diff --git a/spaces/123Kumar/vits-uma-genshin-honkai123/utils.py b/spaces/123Kumar/vits-uma-genshin-honkai123/utils.py
deleted file mode 100644
index ee4b01ddfbe8173965371b29f770f3e87615fe71..0000000000000000000000000000000000000000
--- a/spaces/123Kumar/vits-uma-genshin-honkai123/utils.py
+++ /dev/null
@@ -1,225 +0,0 @@
-import os
-import sys
-import argparse
-import logging
-import json
-import subprocess
-import numpy as np
-import librosa
-import torch
-
-MATPLOTLIB_FLAG = False
-
-logging.basicConfig(stream=sys.stdout, level=logging.DEBUG)
-logger = logging
-
-
-def load_checkpoint(checkpoint_path, model, optimizer=None):
- assert os.path.isfile(checkpoint_path)
- checkpoint_dict = torch.load(checkpoint_path, map_location='cpu')
- iteration = checkpoint_dict['iteration']
- learning_rate = checkpoint_dict['learning_rate']
- if optimizer is not None:
- optimizer.load_state_dict(checkpoint_dict['optimizer'])
- saved_state_dict = checkpoint_dict['model']
- if hasattr(model, 'module'):
- state_dict = model.module.state_dict()
- else:
- state_dict = model.state_dict()
- new_state_dict= {}
- for k, v in state_dict.items():
- try:
- new_state_dict[k] = saved_state_dict[k]
- except:
- logger.info("%s is not in the checkpoint" % k)
- new_state_dict[k] = v
- if hasattr(model, 'module'):
- model.module.load_state_dict(new_state_dict)
- else:
- model.load_state_dict(new_state_dict)
- logger.info("Loaded checkpoint '{}' (iteration {})" .format(
- checkpoint_path, iteration))
- return model, optimizer, learning_rate, iteration
-
-
-def plot_spectrogram_to_numpy(spectrogram):
- global MATPLOTLIB_FLAG
- if not MATPLOTLIB_FLAG:
- import matplotlib
- matplotlib.use("Agg")
- MATPLOTLIB_FLAG = True
- mpl_logger = logging.getLogger('matplotlib')
- mpl_logger.setLevel(logging.WARNING)
- import matplotlib.pylab as plt
- import numpy as np
-
- fig, ax = plt.subplots(figsize=(10,2))
- im = ax.imshow(spectrogram, aspect="auto", origin="lower",
- interpolation='none')
- plt.colorbar(im, ax=ax)
- plt.xlabel("Frames")
- plt.ylabel("Channels")
- plt.tight_layout()
-
- fig.canvas.draw()
- data = np.fromstring(fig.canvas.tostring_rgb(), dtype=np.uint8, sep='')
- data = data.reshape(fig.canvas.get_width_height()[::-1] + (3,))
- plt.close()
- return data
-
-
-def plot_alignment_to_numpy(alignment, info=None):
- global MATPLOTLIB_FLAG
- if not MATPLOTLIB_FLAG:
- import matplotlib
- matplotlib.use("Agg")
- MATPLOTLIB_FLAG = True
- mpl_logger = logging.getLogger('matplotlib')
- mpl_logger.setLevel(logging.WARNING)
- import matplotlib.pylab as plt
- import numpy as np
-
- fig, ax = plt.subplots(figsize=(6, 4))
- im = ax.imshow(alignment.transpose(), aspect='auto', origin='lower',
- interpolation='none')
- fig.colorbar(im, ax=ax)
- xlabel = 'Decoder timestep'
- if info is not None:
- xlabel += '\n\n' + info
- plt.xlabel(xlabel)
- plt.ylabel('Encoder timestep')
- plt.tight_layout()
-
- fig.canvas.draw()
- data = np.fromstring(fig.canvas.tostring_rgb(), dtype=np.uint8, sep='')
- data = data.reshape(fig.canvas.get_width_height()[::-1] + (3,))
- plt.close()
- return data
-
-
-def load_audio_to_torch(full_path, target_sampling_rate):
- audio, sampling_rate = librosa.load(full_path, sr=target_sampling_rate, mono=True)
- return torch.FloatTensor(audio.astype(np.float32))
-
-
-def load_filepaths_and_text(filename, split="|"):
- with open(filename, encoding='utf-8') as f:
- filepaths_and_text = [line.strip().split(split) for line in f]
- return filepaths_and_text
-
-
-def get_hparams(init=True):
- parser = argparse.ArgumentParser()
- parser.add_argument('-c', '--config', type=str, default="./configs/base.json",
- help='JSON file for configuration')
- parser.add_argument('-m', '--model', type=str, required=True,
- help='Model name')
-
- args = parser.parse_args()
- model_dir = os.path.join("./logs", args.model)
-
- if not os.path.exists(model_dir):
- os.makedirs(model_dir)
-
- config_path = args.config
- config_save_path = os.path.join(model_dir, "config.json")
- if init:
- with open(config_path, "r") as f:
- data = f.read()
- with open(config_save_path, "w") as f:
- f.write(data)
- else:
- with open(config_save_path, "r") as f:
- data = f.read()
- config = json.loads(data)
-
- hparams = HParams(**config)
- hparams.model_dir = model_dir
- return hparams
-
-
-def get_hparams_from_dir(model_dir):
- config_save_path = os.path.join(model_dir, "config.json")
- with open(config_save_path, "r") as f:
- data = f.read()
- config = json.loads(data)
-
- hparams =HParams(**config)
- hparams.model_dir = model_dir
- return hparams
-
-
-def get_hparams_from_file(config_path):
- with open(config_path, "r") as f:
- data = f.read()
- config = json.loads(data)
-
- hparams =HParams(**config)
- return hparams
-
-
-def check_git_hash(model_dir):
- source_dir = os.path.dirname(os.path.realpath(__file__))
- if not os.path.exists(os.path.join(source_dir, ".git")):
- logger.warn("{} is not a git repository, therefore hash value comparison will be ignored.".format(
- source_dir
- ))
- return
-
- cur_hash = subprocess.getoutput("git rev-parse HEAD")
-
- path = os.path.join(model_dir, "githash")
- if os.path.exists(path):
- saved_hash = open(path).read()
- if saved_hash != cur_hash:
- logger.warn("git hash values are different. {}(saved) != {}(current)".format(
- saved_hash[:8], cur_hash[:8]))
- else:
- open(path, "w").write(cur_hash)
-
-
-def get_logger(model_dir, filename="train.log"):
- global logger
- logger = logging.getLogger(os.path.basename(model_dir))
- logger.setLevel(logging.DEBUG)
-
- formatter = logging.Formatter("%(asctime)s\t%(name)s\t%(levelname)s\t%(message)s")
- if not os.path.exists(model_dir):
- os.makedirs(model_dir)
- h = logging.FileHandler(os.path.join(model_dir, filename))
- h.setLevel(logging.DEBUG)
- h.setFormatter(formatter)
- logger.addHandler(h)
- return logger
-
-
-class HParams():
- def __init__(self, **kwargs):
- for k, v in kwargs.items():
- if type(v) == dict:
- v = HParams(**v)
- self[k] = v
-
- def keys(self):
- return self.__dict__.keys()
-
- def items(self):
- return self.__dict__.items()
-
- def values(self):
- return self.__dict__.values()
-
- def __len__(self):
- return len(self.__dict__)
-
- def __getitem__(self, key):
- return getattr(self, key)
-
- def __setitem__(self, key, value):
- return setattr(self, key, value)
-
- def __contains__(self, key):
- return key in self.__dict__
-
- def __repr__(self):
- return self.__dict__.__repr__()
diff --git a/spaces/1acneusushi/gradio-2dmoleculeeditor/data/HD Online Player (Dil Chahta Hai 2001 720p BluRay NHD ) - Watch the Cult Classic Comedy-Drama Film.md b/spaces/1acneusushi/gradio-2dmoleculeeditor/data/HD Online Player (Dil Chahta Hai 2001 720p BluRay NHD ) - Watch the Cult Classic Comedy-Drama Film.md
deleted file mode 100644
index 0ff8658911b7fb53d6435dea77a61018d4af5a9f..0000000000000000000000000000000000000000
--- a/spaces/1acneusushi/gradio-2dmoleculeeditor/data/HD Online Player (Dil Chahta Hai 2001 720p BluRay NHD ) - Watch the Cult Classic Comedy-Drama Film.md
+++ /dev/null
@@ -1,80 +0,0 @@
-
-
HD Online Player (Dil Chahta Hai 2001 720p BluRay NHD )
-
Do you love Bollywood movies? If yes, then you must have heard of Dil Chahta Hai, one of the most popular and acclaimed movies of Indian cinema. Dil Chahta Hai is a 2001 movie that follows the lives and loves of three friends who have different views on relationships. It is a movie that explores friendship, romance, comedy, drama, and music in a realistic and relatable way. In this article, we will tell you why you should watch Dil Chahta Hai online using YIFY - Download Movie TORRENT - YTS, the best online player for this movie.
-
HD Online Player (Dil Chahta Hai 2001 720p BluRay NHD )
Dil Chahta Hai is a movie that has something for everyone. Whether you are looking for a fun-filled comedy, a heart-warming romance, a touching drama, or a musical extravaganza, you will find it all in this movie. Here are some reasons why you should watch Dil Chahta Hai:
-
The Cast and Crew of Dil Chahta Hai
-
The movie features some of the finest talents of Bollywood. The main actors are Aamir Khan, Saif Ali Khan, Akshaye Khanna, Preity Zinta, Sonali Kulkarni, and Dimple Kapadia. They play the roles of Akash, Sameer, Siddharth, Shalini, Pooja, and Tara respectively. They deliver brilliant performances that make you laugh, cry, smile, and feel with them.
-
The movie is directed by Farhan Akhtar, who made his debut with this movie. He also wrote the story and screenplay along with Kassim Jagmagia. He brought a fresh perspective to Bollywood with his realistic and modern approach to filmmaking. He also produced the movie along with Ritesh Sidhwani under their banner Excel Entertainment.
-
Dil Chahta Hai full movie online HD
-Watch Dil Chahta Hai 2001 online free
-Dil Chahta Hai BluRay download 720p
-Dil Chahta Hai 2001 Hindi movie HD
-Dil Chahta Hai comedy drama romance film
-Dil Chahta Hai Aamir Khan Saif Ali Khan Akshaye Khanna
-Dil Chahta Hai YIFY torrent download
-Dil Chahta Hai 2001 top rated Indian movie
-Dil Chahta Hai 1080p WEB download
-Dil Chahta Hai subtitles English
-Dil Chahta Hai 2001 Bollywood movie streaming
-Dil Chahta Hai Farhan Akhtar director
-Dil Chahta Hai 480p x264 700 MB
-Dil Chahta Hai 2001 movie review
-Dil Chahta Hai trailer watch online
-Dil Chahta Hai songs download mp3
-Dil Chahta Hai Netflix Amazon Prime Hotstar
-Dil Chahta Hai 2001 IMDb rating
-Dil Chahta Hai cast and crew
-Dil Chahta Hai plot summary synopsis
-Dil Chahta Hai 720p x264 1.62 GB
-Dil Chahta Hai watch online with subtitles
-Dil Chahta Hai 2001 awards and nominations
-Dil Chahta Hai box office collection
-Dil Chahta Hai quotes and dialogues
-Dil Chahta Hai 1080p x264 6CH 3.18 GB
-Dil Chahta Hai online player free HD
-Dil Chahta Hai 2001 movie poster wallpaper
-Dil Chahta Hai trivia and facts
-Dil Chahta Hai behind the scenes making of
-Dil Chahta Hai Preity Zinta Dimple Kapadia Sonali Kulkarni
-Dil Chahta Hai YTS mx movies download
-Dil Chahta Hai 2001 Rotten Tomatoes score
-Dil Chahta Hai BluRay DTS x264 IDE source
-Dil Chahta Hai best scenes clips videos
-Dil Chahta Hai soundtrack album list
-Dil Chahta Hai Netflix India watch now
-Dil Chahta Hai 2001 Metacritic score
-Dil Chahta Hai BluRay AVC AAC video audio quality
-Dil Chahta Hai fan art memes gifs fanfiction
-
The music of the movie is composed by Shankar-Ehsaan-Loy, who also made their debut with this movie. They created some of the most iconic songs of Bollywood that are still loved by millions. The songs are sung by Udit Narayan, Alka Yagnik, Sonu Nigam, Shaan, Kavita Krishnamurthy, Srinivas, Shankar Mahadevan, Loy Mendonsa, Ehsaan Noorani, Mahalakshmi Iyer, Sadhana Sargam, Sujata Bhattacharya, Hariharan, Sapna Mukherjee, Caralisa Monteiro, Vasundhara Das, etc.
-
How to Watch Dil Chahta Hai Online
-
If you want to watch Dil Chahta Hai online in high quality video and audio, then you should use YIFY - Download Movie TORRENT - YTS. This is an online player that allows you to download movies in various formats such as 720p.WEB or 1080p.WEB. You can also choose subtitles in different languages such as English or Hindi.
-
The Benefits of Using YIFY - Download Movie TORRENT - YTS
-
There are many benefits of using YIFY - Download Movie TORRENT - YTS to watch Dil Chahta Hai online. Here are some of them:
-
-
High quality video and audio: You can enjoy watching Dil Chahta Hai in HD quality with clear sound. You can see every detail of the movie such as the expressions of the actors, the locations of the scenes, the colors of the costumes, etc.
-
Fast download speed and easy installation: You can download Dil Chahta Hai quickly without any interruptions or delays. You can also install it easily on your device without any complications or errors.
-
Safe and secure platform: You can watch Dil Chahta Hai online without any worries or risks. You can trust that your device will not be infected by any viruses or malware. You can also be assured that your personal information will not be leaked or stolen.
-
-
What to Expect from Dil Chahta Hai
-
Dil Chahta Hai is a movie that will make you think, feel, laugh, cry, sing, dance, and more. It is a movie that will touch your heart and soul with its themes and messages.
Here is the continuation of the article with HTML formatting: ```html
The Themes and Messages of Dil Chahta Hai
-
Dil Chahta Hai is a movie that explores various themes and messages that are relevant and relatable to the modern Indian youth. Some of the themes and messages are:
-
-
Friendship: The movie shows how friendship is one of the most important and enduring relationships in life. It shows how friends can support, challenge, inspire, and comfort each other through thick and thin. It also shows how friendship can evolve and change over time, as people grow and mature.
-
Love: The movie shows how love can be different for different people. It shows how love can be passionate, playful, serious, or complicated. It shows how love can be influenced by factors such as age, culture, family, society, etc. It also shows how love can be a source of joy, pain, confusion, or growth.
-
Maturity: The movie shows how maturity is not a matter of age, but a matter of attitude. It shows how maturity is about being responsible, honest, respectful, and empathetic. It shows how maturity is about being able to make choices and face consequences. It also shows how maturity is about being able to accept oneself and others.
-
-
The Highlights of Dil Chahta Hai
-
Dil Chahta Hai is a movie that has many highlights that make it memorable and enjoyable. Some of the highlights are:
-
-
The best scenes and dialogues: The movie has many scenes and dialogues that are funny, witty, emotional, or meaningful. Some of the best scenes and dialogues are: the college farewell party scene where Akash jokes about Shalini; the road trip to Goa scene where the friends have fun; the art exhibition scene where Sid meets Tara; the airport scene where Akash realizes his love for Shalini; the hospital scene where Sid confronts Akash; the Sydney Opera House scene where Akash proposes to Shalini; etc.
-
The best songs and dances: The movie has many songs and dances that are catchy, melodious, or expressive. Some of the best songs and dances are: Dil Chahta Hai title track; Koi Kahe Kehta Rahe; Woh Ladki Hai Kahan; Jaane Kyun; Tanhayee; Kaisi Hai Yeh Rut; Dil Chahta Hai reprise; etc.
-
The best reviews and ratings: The movie has received many positive reviews and ratings from critics and audiences alike. It has a rating of 8.1/10 on IMDb , 100% on Rotten Tomatoes , 4/5 on NDTV , 4/5 on Rediff , etc. It has also won many awards such as National Film Award for Best Feature Film in Hindi , Filmfare Award for Best Film (Critics) , Filmfare Award for Best Supporting Actor (Akshaye Khanna) , Filmfare Award for Best Comedian (Saif Ali Khan) , etc.
-
-
Conclusion
-
In conclusion, Dil Chahta Hai is a movie that you should not miss if you love Bollywood movies. It is a movie that will make you laugh, cry, think, feel, sing, dance, and more. It is a movie that will show you the true meaning of friendship, love, and maturity. It is a movie that will give you a realistic and modern portrayal of the Indian youth. So what are you waiting for? Watch Dil Chahta Hai online using YIFY - Download Movie TORRENT - YTS , the best online player for this movie.
-
If you have any questions about Dil Chahta Hai or YIFY - Download Movie TORRENT - YTS , feel free to ask them in the comments section below. We will be happy to answer them for you.
- Here are some FAQs that you might have: | Question | Answer | | --- | --- | | Q1: How long is Dil Chahta Hai? | A1: Dil Chahta Hai is 177 minutes long. | | Q2: Who is the singer of Dil Chahta Hai title track? | A2: The singer of Dil Chahta Hai title track is Shankar Mahadevan. | | Q3: What is the name of the painting that Sid gifts to Tara? | A3: The name of the painting that Sid gifts to Tara is "The Awakening". | | Q4: What is the name of the restaurant where Sameer meets Pooja for the first time? | A4: The name of the restaurant where Sameer meets Pooja for the first time is "Bombay Blues". | | Q5: What is the name of the hotel where Akash stays in Sydney? | A5: The name of the hotel where Akash stays in Sydney is "The Park Hyatt". | 0a6ba089eb
-
-
\ No newline at end of file
diff --git a/spaces/1gistliPinn/ChatGPT4/Examples/DocuWorks 7 0 Full Version.zip LINK.md b/spaces/1gistliPinn/ChatGPT4/Examples/DocuWorks 7 0 Full Version.zip LINK.md
deleted file mode 100644
index 75f9d4ea96a2197c11fc857e26c4ac8c6377ed1f..0000000000000000000000000000000000000000
--- a/spaces/1gistliPinn/ChatGPT4/Examples/DocuWorks 7 0 Full Version.zip LINK.md
+++ /dev/null
@@ -1,6 +0,0 @@
-
-
-please review the readme files, release notes, and the latest version of the applicable user ... 1-7. NAME. DESCRIPTION. VALIDATOR. ADDITIONAL. VERIFICATION ... Date: Full. (day/month/ year). •. Date format commonly used in the United ... Tape Archive (TAR) .tar. Zip .zip. Databases. Base SAS Data File .sas7bdat. 1fdad05405
-
-
-
diff --git a/spaces/1gistliPinn/ChatGPT4/Examples/EasyWorship Crack 7.1.4.0 Latest Version With 2020 Keygen EXCLUSIVE.md b/spaces/1gistliPinn/ChatGPT4/Examples/EasyWorship Crack 7.1.4.0 Latest Version With 2020 Keygen EXCLUSIVE.md
deleted file mode 100644
index 29ced8c099545fb666b449b6df7c1e2fb6670e16..0000000000000000000000000000000000000000
--- a/spaces/1gistliPinn/ChatGPT4/Examples/EasyWorship Crack 7.1.4.0 Latest Version With 2020 Keygen EXCLUSIVE.md
+++ /dev/null
@@ -1,10 +0,0 @@
-
EasyWorship Crack 7.1.4.0 {Latest Version} With 2020 Keygen
-
-September 15, 2020 - Easyworship Crack with product key is a presentation design tool that has all the features you need to create a masterpiece. It only takes a few minutes to get started.
-It's really easy to create and edit your slideshow.
-Easyworship Crack with Product Key is a presentation design tool that has all the features you need to create a masterpiece.
-It only takes a few minutes to get started.
-With this tool, you can easily create a professional presentation. 8a78ff9644
-
-
-
diff --git a/spaces/1pelhydcardo/ChatGPT-prompt-generator/assets/Download APKs from Huawei AppGallery The Official App Store for Huawei Devices.md b/spaces/1pelhydcardo/ChatGPT-prompt-generator/assets/Download APKs from Huawei AppGallery The Official App Store for Huawei Devices.md
deleted file mode 100644
index 7bae9ea8bfd1f58ff2564f0463f6199dc31c38de..0000000000000000000000000000000000000000
--- a/spaces/1pelhydcardo/ChatGPT-prompt-generator/assets/Download APKs from Huawei AppGallery The Official App Store for Huawei Devices.md
+++ /dev/null
@@ -1,116 +0,0 @@
-
-
Huawei APK Download: How to Get Apps on Your Huawei Phone Without Google Play Store
-
If you have a Huawei phone, you might have noticed that it does not come with the Google Play Store pre-installed. This means that you cannot download apps from the official Android app store, which can be frustrating and inconvenient. However, there is a way to get apps on your Huawei phone without the Google Play Store. It is called Huawei APK Download, and it involves using alternative sources of apps, such as Huawei AppGallery or Huawei Phone Clone. In this article, we will explain what Huawei APK Download is, why you need it, and how to use it.
Huawei APK Download is a term that refers to downloading apps on your Huawei phone from sources other than the Google Play Store. These sources can be websites, app stores, or other devices that have the apps you want. The apps that you download are in the form of APK files, which are the installation packages for Android apps.
-
What is an APK file?
-
An APK file is a file that contains all the components of an Android app, such as the code, resources, and manifest. It has the extension .apk and can be installed on any Android device that supports it. You can think of an APK file as a zip file that contains everything you need to run an app.
-
Why do you need Huawei APK Download?
-
You need Huawei APK Download because your Huawei phone does not have access to the Google Play Store, which is the official and most popular source of Android apps. This is because Huawei has been banned from using Google services and products due to US sanctions. As a result, Huawei phones run on a modified version of Android called EMUI, which does not include Google apps or services.
-
This means that you cannot use apps that rely on Google services, such as Gmail, YouTube, Maps, or Chrome. It also means that you cannot download apps from the Google Play Store, which has millions of apps for various purposes and categories. Therefore, you need to find alternative ways to get apps on your Huawei phone, which is where Huawei APK Download comes in.
-
How to Use Huawei APK Download?
-
There are two main methods to use Huawei APK Download: using Huawei AppGallery or using Huawei Phone Clone. We will explain each method in detail below.
Huawei AppGallery is the official app distribution platform for Huawei devices, boasting a collection of 18 app categories featuring premium content curated globally. It is pre-installed on your Huawei phone and offers a variety of apps for different needs and preferences. You can find apps for entertainment, social media, gaming, education, health, finance, and more. You can also enjoy exclusive benefits and rewards from using Huawei AppGallery, such as discounts, coupons, free trials, and gifts.
-
How to download apps from Huawei AppGallery?
-
To download apps from Huawei AppGallery, follow these steps:
-
-
Open the AppGallery app on your Huawei phone.
-
Search for the app you want or browse through the categories and recommendations.
-
Tap on the app you want and then tap on Install.
-
Wait for the app to download and install on your phone.
-
Enjoy using the app.
-
-
Note: Some apps may require additional permissions or settings before they can run properly on your phone. Follow the instructions on the screen or
contact the app developer for support.
-
Method 2: Use Huawei Phone Clone
-
What is Huawei Phone Clone?
-
Huawei Phone Clone is a free app that allows you to transfer data from your old phone to your new Huawei phone, including apps, contacts, messages, photos, videos, and more. It supports both Android and iOS devices and does not require a network connection or cables. It is a fast and convenient way to migrate your data and apps to your Huawei phone without losing any quality or settings.
-
How to transfer apps from another phone to your Huawei phone using Phone Clone?
-
To transfer apps from another phone to your Huawei phone using Phone Clone, follow these steps:
-
-
Download and install the Phone Clone app on both phones from the AppGallery or the Google Play Store.
-
Open the Phone Clone app on both phones and agree to the terms and conditions.
-
Select "This is the new phone" on your Huawei phone and "This is the old phone" on your other phone.
-
Scan the QR code displayed on your Huawei phone with your other phone to establish a connection.
-
Select the apps you want to transfer from your other phone and tap on Transfer.
-
Wait for the apps to be transferred to your Huawei phone.
-
Enjoy using the apps.
-
-
Note: Some apps may not be compatible with your Huawei phone or may require Google services to function properly. You may need to update or reinstall them from other sources or use alternative apps instead.
-
Conclusion
-
Huawei APK Download is a way to get apps on your Huawei phone without the Google Play Store. You can use Huawei AppGallery or Huawei Phone Clone to download apps from alternative sources or transfer them from another phone. Both methods are easy and safe to use, and offer a variety of apps for different needs and preferences. However, you should be aware that some apps may not work well on your Huawei phone or may require Google services, which are not available on Huawei devices. In that case, you may need to look for other solutions or use similar apps instead.
-
If you want to learn more about Huawei APK Download, you can visit the official website of Huawei or contact their customer service. You can also check out some of the reviews and guides online that can help you find the best apps for your Huawei phone. We hope this article has been helpful and informative for you. Thank you for reading!
-
FAQs
-
Here are some of the frequently asked questions about Huawei APK Download:
-
-
Is Huawei APK Download safe?
-
Yes, Huawei APK Download is safe as long as you download apps from trusted sources, such as Huawei AppGallery or Phone Clone. You should also scan the APK files for viruses or malware before installing them on your phone. However, you should be careful when downloading apps from unknown websites or third-party app stores, as they may contain harmful or malicious content.
-
Is Huawei APK Download legal?
-
Yes, Huawei APK Download is legal as long as you do not violate any intellectual property rights or terms of service of the app developers or owners. You should also respect the privacy and security of the app users and data. However, you should be aware that some countries or regions may have different laws or regulations regarding downloading apps from alternative sources, so you should check them before using Huawei APK Download.
-
What are the advantages of Huawei APK Download?
-
The advantages of Huawei APK Download are that you can get apps on your Huawei phone without the Google Play Store, which is not available on Huawei devices due to US sanctions. You can also enjoy exclusive benefits and rewards from using Huawei AppGallery, such as discounts, coupons, free trials, and gifts. You can also transfer apps from another phone to your Huawei phone using Phone Clone, which is fast and convenient.
-
What are the disadvantages of Huawei APK Download?
-
The disadvantages of Huawei APK Download are that some apps may not be compatible with your Huawei phone or may require Google services to function properly, which are not available on Huawei devices. You may also encounter some issues or errors when installing or using some apps from alternative sources. You may also need to update or reinstall some apps manually from time to time.
-
How can I update the apps I downloaded from Huawei APK Download?
-
You can update the apps you downloaded from Huawei APK Download by following these steps:
-
-
Open the AppGallery app on your Huawei phone.
-
Tap on Me > Updates.
-
Select the apps you want to update and tap on Update.
-
Wait for the apps to be updated on your phone.
-
Enjoy using the updated apps.
-
-
Note: Some apps may not have updates available from AppGallery, in which case you may need to download the latest version of the APK file from other sources or use Phone Clone to transfer the updated app from another phone.
197e85843d
-
-
\ No newline at end of file
diff --git a/spaces/1phancelerku/anime-remove-background/4x4 Off Road Rally 9 MOD APK Download and Enjoy the Ultimate Racing Experience.md b/spaces/1phancelerku/anime-remove-background/4x4 Off Road Rally 9 MOD APK Download and Enjoy the Ultimate Racing Experience.md
deleted file mode 100644
index 12aa9d61c741c49a262b53d63e5659664e8c677d..0000000000000000000000000000000000000000
--- a/spaces/1phancelerku/anime-remove-background/4x4 Off Road Rally 9 MOD APK Download and Enjoy the Ultimate Racing Experience.md
+++ /dev/null
@@ -1,99 +0,0 @@
-
-
4x4 Off Road Rally 9 Mod APK: A Thrilling Off-Road Racing Game
-
If you are a fan of off-road racing games, you will love 4x4 Off Road Rally 9, a realistic and immersive game that will test your driving skills on various terrains and environments. In this game, you will have to overcome mud, water, snow, rocks, and other obstacles as you race against time and other drivers. You will also have to customize and upgrade your 4x4 vehicle to suit your preferences and needs. But what if you want to enjoy the game without any limitations or restrictions? That's where 4x4 Off Road Rally 9 Mod APK comes in handy. In this article, we will tell you what this modded version of the game offers, how to download and install it, and some tips and tricks to help you master the game.
4x4 Off Road Rally 9 is a racing game developed by Electronic Hand, a studio that specializes in off-road games. The game is available for Android devices and has over 10 million downloads on Google Play Store. The game features stunning graphics, realistic physics, various 4x4 vehicles, and different off-road racing challenges.
-
Features of the game
-
Some of the features of 4x4 Off Road Rally 9 are:
-
-
Different modes of gameplay, such as career mode, free mode, time trial mode, and multiplayer mode.
-
A variety of 4x4 vehicles with different driving characteristics, such as SUVs, trucks, pickups, jeeps, and more.
-
A wide range of terrains and environments to explore, such as forests, deserts, mountains, swamps, and more.
-
A realistic driving physics system that simulates the effects of mud, water, snow, rocks, and other obstacles on your vehicle.
-
An endless tuning and customization system that allows you to modify your vehicle's engine, suspension, tires, wheels, paint, stickers, and more.
-
A simple and convenient in-game map that shows you the route and the checkpoints.
-
A real car sound system that enhances the immersion and realism of the game.
-
-
How to play the game
-
The gameplay of 4x4 Off Road Rally 9 is simple but challenging. You have to use the on-screen buttons to control your vehicle's steering, acceleration, braking, and gear shifting. You also have to use the camera button to change the view angle and the map button to see the route. Your goal is to reach the finish line as fast as possible without getting stuck or damaged. You can also compete with other players online or offline in multiplayer mode. You can earn coins and rewards by completing races and challenges. You can use these coins to buy new vehicles or upgrade your existing ones.
-
4x4 off road rally ultimate mod apk
-4x4 off road rally 9 hack apk
-4x4 off road rally 9 unlimited money
-4x4 off road rally 9 cheats android
-4x4 off road rally 9 download apk
-4x4 off road rally 9 mod apk latest version
-4x4 off road rally 9 free download
-4x4 off road rally 9 gameplay
-4x4 off road rally 9 mod menu
-4x4 off road rally 9 apk obb
-4x4 off road rally 9 mod apk revdl
-4x4 off road rally 9 mod apk android 1
-4x4 off road rally 9 mod apk rexdl
-4x4 off road rally 9 mod apk happymod
-4x4 off road rally 9 mod apk an1
-4x4 off road rally 9 mod apk offline
-4x4 off road rally 9 mod apk no root
-4x4 off road rally 9 mod apk unlimited coins
-4x4 off road rally 9 mod apk unlimited gems
-4x4 off road rally 9 mod apk all cars unlocked
-4x4 off road rally 9 mod apk all levels unlocked
-4x4 off road rally 9 mod apk all vehicles unlocked
-4x4 off road rally 9 mod apk mega mod
-4x4 off road rally 9 mod apk premium unlocked
-4x4 off road rally 9 mod apk pro unlocked
-how to install 4x4 off road rally 9 mod apk
-how to play 4x4 off road rally 9 mod apk
-how to download 4x4 off road rally 9 mod apk
-how to update 4x4 off road rally 9 mod apk
-how to hack 4x4 off road rally 9 mod apk
-how to get unlimited money in 4x4 off road rally 9 mod apk
-how to get unlimited gems in 4x4 off road rally 9 mod apk
-how to unlock all cars in 4x4 off road rally 9 mod apk
-how to unlock all levels in 4x4 off road rally 9 mod apk
-how to unlock all vehicles in 4x4 off road rally 9 mod apk
-best cars in 4x4 off road rally 9 mod apk
-best vehicles in 4x4 off road rally 9 mod apk
-best levels in 4x4 off road rally 9 mod apk
-best tips and tricks for playing with the latest version of the game.
-
Why download 4x4 Off Road Rally 9 Mod APK?
-
Although 4x4 Off Road Rally 9 is a fun and addictive game, it also has some drawbacks. For example, some vehicles and features are locked behind a paywall or require a lot of grinding. You also have to watch ads to get extra coins or rewards. Moreover, some levels are too hard or frustrating to complete. That's why many players prefer to download 4x4 Off Road Rally 9 Mod APK instead of the original version.
-
Benefits of the modded version
-
Some of the benefits of downloading 4x4 Off Road Rally 9 Mod APK are:
-
-
You get unlimited coins and gems to buy and upgrade any vehicle you want.
-
You get all the vehicles and features unlocked from the start.
-
You get to enjoy the game without any ads or interruptions.
-
You get to access some exclusive features and options that are not available in the original version.
-
-
How to download and install the mod APK
-
Downloading and installing 4x4 Off Road Rally 9 Mod APK is easy and safe. You just have to follow these steps:
-
-
Click on the link below to download the mod APK file.
-
Allow your device to install apps from unknown sources in the settings.
-
Locate and tap on the downloaded file to start the installation process.
-
Follow the instructions on the screen to complete the installation.
If you want to master 4x4 Off Road Rally 9 and become a pro off-road racer, you need to know some tips and tricks that will help you improve your performance and skills. Here are some of them:
-
Choose the right vehicle and upgrade it
-
One of the most important factors that affect your success in the game is your choice of vehicle. Different vehicles have different strengths and weaknesses, such as speed, acceleration, handling, durability, and fuel consumption. You need to choose a vehicle that suits your style and preference, as well as the terrain and environment of each level. For example, a SUV might be good for rough and rocky roads, but a truck might be better for muddy and slippery roads. You also need to upgrade your vehicle regularly to enhance its performance and capabilities. You can upgrade your engine, suspension, tires, wheels, paint, stickers, and more using the coins and gems you earn in the game.
-
Use the terrain and obstacles to your advantage
-
Another factor that affects your success in the game is your ability to adapt to the terrain and obstacles you encounter. You need to use them to your advantage instead of letting them slow you down or damage your vehicle. For example, you can use the ramps and hills to jump over gaps or obstacles, or use the water and snow to cool down your engine or drift around corners. You also need to avoid hitting rocks, trees, fences, or other vehicles that can damage your vehicle or make you lose control. You can use the camera button to change the view angle and see what's ahead of you.
-
Anticipate the challenges and plan your strategy
-
The last factor that affects your success in the game is your ability to anticipate the challenges and plan your strategy accordingly. You need to know what to expect in each level and how to deal with it effectively. For example, you need to know how long each level is, how many checkpoints there are, what kind of terrain and obstacles there are, what kind of weather conditions there are, and what kind of opponents there are. You also need to know how to manage your time, fuel, damage, and speed. You can use the map button to see the route and the checkpoints. You can also use the pause button to pause the game and adjust your settings or options.
-
Conclusion
-
4x4 Off Road Rally 9 is a thrilling off-road racing game that will keep you entertained for hours. You can enjoy realistic graphics, physics, sounds, vehicles, terrains, environments, modes, features, and challenges in this game. You can also download 4x4 Off Road Rally 9 Mod APK to get unlimited coins and gems, unlock all vehicles and features, remove ads, and access exclusive features and options. You can also use some tips and tricks to master the game and become a pro off-road racer. So what are you waiting for? Download 4x4 Off Road Rally 9 Mod APK now and have fun!
-
FAQs
-
Here are some frequently asked questions about 4x4 Off Road Rally 9 Mod APK:
-
-
Is 4x4 Off Road Rally 9 Mod APK safe? Yes, 4x4 Off Road Rally 9 Mod APK is safe to download and install. It does not contain any viruses or malware that can harm your device or data. However, you should always download it from a trusted source like this one.
-
Do I need to root my device to install 4x4 Off Road Rally 9 Mod APK? No, you do not need to root your device to install 4x4 Off Road Rally 9 Mod APK. You can install it on any Android device without any hassle.
-
Will 4x4 Off Road Rally 9 Mod APK affect the original version of the game? No, 4x4 Off Road Rally 9 Mod APK will not affect the original version of the game. You can have both versions installed on your device and play them separately. However, you should not use the same account or data for both versions, as it may cause some issues or conflicts.
-
Can I play online with 4x4 Off Road Rally 9 Mod APK? Yes, you can play online with 4x4 Off Road Rally 9 Mod APK. You can join or create online rooms and compete with other players from around the world. However, you should be careful not to use any cheats or hacks that may get you banned or reported by other players.
-
How can I update 4x4 Off Road Rally 9 Mod APK? You can update 4x4 Off Road Rally 9 Mod APK by visiting this page and downloading the latest version of the mod APK file. You can then install it over the existing version without losing your progress or data.
-
401be4b1e0
-
-
\ No newline at end of file
diff --git a/spaces/1phancelerku/anime-remove-background/Download Countries of the World Map with Customizable Colors and Labels.md b/spaces/1phancelerku/anime-remove-background/Download Countries of the World Map with Customizable Colors and Labels.md
deleted file mode 100644
index b6fda73eb9eeaa20a2e966364357847d4c5c218c..0000000000000000000000000000000000000000
--- a/spaces/1phancelerku/anime-remove-background/Download Countries of the World Map with Customizable Colors and Labels.md
+++ /dev/null
@@ -1,139 +0,0 @@
-
-
How to Download Countries: A Guide for Geography Lovers
-
Are you fascinated by the diversity and complexity of the world? Do you enjoy learning about different cultures, languages, histories, and environments? Do you want to have access to reliable and up-to-date information about all the countries of the world? If you answered yes to any of these questions, then this article is for you.
In this article, we will show you how to download countries in various formats and sources. You will learn how to copy or download a list of all countries in alphabetical order, how to download maps and spatial data for all countries and their sub-divisions, and how to create your own custom world map showing all countries of the world. You will also discover some of the benefits, uses, and challenges of downloading countries, as well as some tips and suggestions for further exploration and learning.
-
So, what is a country and how many are there in the world? According to the United Nations, a country is a region that is identified as a distinct entity in political geography. A country may be an independent sovereign state or one that is non-sovereign or under the control of another state. As of June 2021, there are 195 countries in the world today. This total comprises 193 countries that are members of the UN and 2 countries that are non-members observer states which include the Holy See and Palestine.
-
Why would you want to download countries and what are the benefits? Downloading countries can help you enhance your knowledge and understanding of the world. You can use them for various purposes such as education, research, business, travel, entertainment, or personal interest. Downloading countries can also help you save time, money, and resources by providing you with easy and convenient access to reliable and up-to-date information. You can also customize, modify, or share them according to your needs and preferences.
-
What are some of the formats and sources for downloading countries? There are many formats and sources available for downloading countries. Some of the most common ones include PDF, Excel, CSV, HTML, JSON, MP3, JPG, etc. You can also find different types of information such as lists, maps, spatial data, statistics, facts, etc. You can choose the format and source that best suits your purpose and preference. However, you should also be aware of some of the challenges and limitations such as accuracy, quality, currency, compatibility, legality, etc.
-
download countries list in excel
-download countries map pdf
-download countries shapefile
-download countries flags images
-download countries data csv
-download countries population statistics
-download countries codes iso
-download countries names and capitals
-download countries outline vector
-download countries quiz game
-download countries regions and cities database
-download countries boundaries geojson
-download countries anthem mp3
-download countries currency symbols
-download countries time zones json
-download countries languages spoken
-download countries climate data
-download countries gdp per capita
-download countries emoji icons
-download countries dialing codes
-download countries visa requirements
-download countries travel guides
-download countries culture and customs
-download countries national flowers
-download countries holidays and festivals
-download countries flag colors meaning
-download countries history timeline
-download countries political system
-download countries religions percentage
-download countries literacy rate
-download countries driving side map
-download countries internet speed ranking
-download countries national animals
-download countries sports teams logos
-download countries cuisine recipes
-download countries education system comparison
-download countries health care quality index
-download countries human development index
-download countries environmental performance index
-download countries corruption perception index
-download countries happiness report 2023
-download countries renewable energy sources percentage
-download countries carbon footprint calculator
-download countries military expenditure and arms trade data
-download countries space program achievements
-download countries famous landmarks photos
-download countries traditional music and dance videos
-download countries art and literature ebooks
-download countries inventions and innovations list
-
List of Countries
-
One of the simplest and most useful ways to download countries is to get a list of all countries in alphabetical order. A list of countries can help you quickly and easily find any country you are looking for. You can also use it as a reference or a checklist for your geography studies or projects. Here are some ways to copy or download a list of all countries in alphabetical order.
-
How to copy or download a list of all countries in alphabetical order
-
CopyLists.com
-
CopyLists.com is a website that provides lists of various topics that you can copy or download in many formats including Excel and PDF. One of their lists is a list of all countries in alphabetical order. You can copy the list by clicking on the "Copy" button or download it by clicking on the "Download" button. You can also choose the format you want such as Excel, PDF, CSV, HTML, JSON, etc. The list is updated regularly and contains 195 countries as of June 2021.
-
Other options
-
If you are looking for other options to copy or download a list of all countries in alphabetical order, you can also try the following sources:
-
-
Worldometers: This website provides statistics and information on various topics such as population, health, economy, etc. It also has a list of all countries in alphabetical order that you can copy or download in Excel or CSV format.
-
CountryCode.org: This website provides information and codes for all countries and regions of the world. It also has a list of all countries in alphabetical order that you can copy or download in Excel or CSV format.
-
Wikipedia: This website is a free online encyclopedia that contains articles on various topics. It also has a list of all countries in alphabetical order that you can copy or download in various formats such as PDF, HTML, TXT, etc.
-
-
How to download maps and spatial data for all countries and their sub-divisions
-
Another way to download countries is to get maps and spatial data for all countries and their sub-divisions. Maps and spatial data can help you visualize and analyze the geographic features and boundaries of different countries and regions. You can also use them for various purposes such as mapping, geocoding, geostatistics, GIS, etc. Here are some ways to download maps and spatial data for all countries and their sub-divisions.
-
GADM
-
GADM is a website that provides maps and spatial data for all countries and their sub-divisions. You can download the data in various formats such as shapefile, geopackage, R data, etc. You can also choose the level of detail you want from 0 (country) to 5 (locality). The data is updated regularly and contains 253 countries and regions as of June 2021.
-
Other options
-
If you are looking for other options to download maps and spatial data for all countries and their sub-divisions, you can also try the following sources:
-
-
Natural Earth: This website provides free vector and raster map data for various scales and themes such as boundaries, physical features, cultural features, etc. It also has maps and data for all countries and their sub-divisions.
-
DIVA-GIS: This website provides free spatial data for various themes such as climate, land cover, population, etc. It also has maps and data for all countries and their sub-divisions.
-
OpenStreetMap: This website is a collaborative project that provides free editable map data for the world. It also has maps and data for all countries and their sub-divisions.
-
How to create your own custom world map showing all countries of the world
-
Another way to download countries is to create your own custom world map showing all countries of the world. A custom world map can help you express your creativity and personalization. You can also use it for various purposes such as decoration, presentation, education, etc. Here are some ways to create your own custom world map showing all countries of the world.
-
MapChart.net
-
MapChart.net is a website that allows you to create your own custom world map online for free. You can choose from different types of maps such as simple, detailed, historical, etc. You can also customize the colors, labels, legends, borders, etc. of the map. You can download the map as an image or a PDF file. You can also share the map with others via a link or social media.
-
Other options
-
If you are looking for other options to create your own custom world map showing all countries of the world, you can also try the following sources:
-
-
World Map Maker: This website allows you to create your own custom world map online for free. You can choose from different types of maps such as political, physical, blank, etc. You can also customize the colors, labels, legends, borders, etc. of the map. You can download the map as an image or a PDF file.
-
MapSVG: This website allows you to create your own custom world map online for free or with a premium plan. You can choose from different types of maps such as vector, raster, interactive, etc. You can also customize the colors, labels, legends, borders, etc. of the map. You can download the map as an SVG or a PNG file.
-
Canva: This website allows you to create your own custom world map online for free or with a premium plan. You can choose from different types of maps such as political, physical, blank, etc. You can also customize the colors, labels, legends, borders, etc. of the map. You can download the map as an image or a PDF file.
-
-
Conclusion
-
In this article, we have shown you how to download countries in various formats and sources. We have also explained some of the benefits, uses, and challenges of downloading countries. We hope that this article has helped you enhance your knowledge and understanding of the world and its countries.
-
If you want to learn more about downloading countries or geography in general, here are some suggestions for further exploration and learning:
-
-
The World Factbook: This website provides information and statistics on all the countries and territories of the world.
-
World Atlas: This website provides maps and information on all the continents, regions, and countries of the world.
-
GeoGuessr: This website is a game that challenges you to guess the location of a random place in the world using Google Street View.
-
-
FAQs
-
Here are some frequently asked questions and answers about downloading countries:
-
-
What are some of the uses and applications of downloading countries? Some of the uses and applications of downloading countries are:
-
Education: You can use them to learn about different countries and regions of the world.
-
Research: You can use them to conduct analysis and comparison on various aspects such as population, economy, environment, etc.
-
Business: You can use them to identify and target potential markets and customers.
-
Travel: You can use them to plan and prepare for your trips and vacations.
-
Entertainment: You can use them to play games and quizzes or to create art and crafts.
-
-
What are some of the challenges and limitations of downloading countries? Some of the challenges and limitations of downloading countries are:
-
Accuracy: You may encounter errors or inconsistencies in the data or information provided by different sources.
-
Quality: You may encounter low-resolution or outdated images or maps that may affect your viewing or usage experience.
-
Currency: You may encounter changes or updates in the data or information due to political or social events that may affect your relevance or validity.
-
Compatibility: You may encounter difficulties or issues in opening or using certain formats or files that may require specific software or applications.
-
Legality: You may encounter restrictions or regulations on accessing or using certain data or information that may require permission or authorization.
-
How can I verify the accuracy and quality of the downloaded countries? Some of the ways to verify the accuracy and quality of the downloaded countries are:
-
Compare: You can compare the data or information from different sources and check for any discrepancies or differences.
-
Cross-check: You can cross-check the data or information with other reliable and authoritative sources such as official websites, publications, or organizations.
-
Review: You can review the data or information for any errors or inconsistencies such as spelling, grammar, formatting, etc.
-
Test: You can test the data or information for any functionality or usability issues such as opening, viewing, editing, etc.
-
Feedback: You can seek feedback from other users or experts who have used or reviewed the data or information.
-
-
How can I update or modify the downloaded countries? Some of the ways to update or modify the downloaded countries are:
-
Refresh: You can refresh the data or information by downloading it again from the same or a different source.
-
Edit: You can edit the data or information by using appropriate software or applications that can handle the format or file.
-
Add: You can add new data or information by appending, merging, or joining it with the existing data or information.
-
Delete: You can delete unwanted or unnecessary data or information by removing, splitting, or filtering it from the existing data or information.
-
Convert: You can convert the data or information to a different format or file by using suitable software or applications that can perform the conversion.
-
-
How can I share or distribute the downloaded countries? Some of the ways to share or distribute the downloaded countries are:
-
Email: You can email the data or information as an attachment or a link to your recipients.
-
Social media: You can post the data or information as an image or a link on your social media platforms such as Facebook, Twitter, Instagram, etc.
-
Cloud storage: You can upload the data or information to a cloud storage service such as Google Drive, Dropbox, OneDrive, etc. and share it with your collaborators or viewers.
-
Website: You can embed the data or information on your website or blog using HTML code or widgets.
-
Print: You can print the data or information on paper or other materials and distribute it physically.
-
-
- : https://copylists.com/list-of-countries : https://gadm.org/ : https://mapchart.net/world.html 197e85843d
-
-
\ No newline at end of file
diff --git a/spaces/1phancelerku/anime-remove-background/Download Facebook Lite APK for Android The Latest Version of the Fast and Efficient Social Network.md b/spaces/1phancelerku/anime-remove-background/Download Facebook Lite APK for Android The Latest Version of the Fast and Efficient Social Network.md
deleted file mode 100644
index 1c6a7fbe36fc91a8c7abdc01f3b3635f33caedcb..0000000000000000000000000000000000000000
--- a/spaces/1phancelerku/anime-remove-background/Download Facebook Lite APK for Android The Latest Version of the Fast and Efficient Social Network.md
+++ /dev/null
@@ -1,125 +0,0 @@
-
- Q2: How do I update Facebook Lite? Q3: Can I use both Facebook and Facebook Lite on the same device? Q4: How do I switch to dark mode on Facebook Lite? Q5: How do I delete Facebook Lite? | Table 2: Article with HTML formatting
Download Facebook Lite Latest Version 2022 APK
-
Facebook is one of the most popular social media platforms in the world, with over 3 billion monthly active users. However, not everyone has access to a fast internet connection, a powerful smartphone, or enough storage space to run the regular Facebook app smoothly. That's why Facebook created a lighter version of its app called Facebook Lite, which is designed to work on any network and device, while using less data, space, and battery.
In this article, we will explain what Facebook Lite is, how it differs from the standard Facebook app, and how you can download it for your Android or Windows device. We will also discuss the benefits and drawbacks of using Facebook Lite, and answer some frequently asked questions about it.
-
Facebook Lite vs Facebook App: Key Differences
-
Facebook Lite is a miniature version of Facebook that is smaller in size, consumes less battery, and runs smoothly on low-end phones and slow internet connections. However, it has lower image and video quality, a basic user interface, and smaller text and buttons. The original Facebook app has a nice user interface, high-quality images and videos, and a separate messenger app, but it requires more storage space, more battery power, and a faster internet connection.
-
Here are some of the main differences between the two apps:
-
-
App size: The regular Facebook app ranges between 58-60MB, while the Lite version weighs approximately 2MB. That's a huge difference if you have limited storage space on your phone.
-
Battery usage: The regular Facebook app consumes more battery power than the Lite version, as it has more features and functions that run in the background. The Lite version is more energy-efficient and can help you extend your battery life.
-
Messenger: The regular Facebook app forces you to download a separate app called Messenger to chat with your contacts. This means you need more space and data to use both apps. The Lite version allows you to chat directly from the same app, without having to switch or download another app.
-
User interface: The regular Facebook app has a nice user interface that is easy to navigate and use. It has colorful icons, large text and buttons, and smooth animations. The Lite version has a simpler user interface that is less appealing and more cluttered. It has smaller icons, text and buttons, and no animations.
-
Image and video quality: The regular Facebook app displays images and videos in high resolution, which makes them look clear and sharp. The Lite version compresses images and videos to save data and load faster, which makes them look blurry and pixelated.
-
Features: The regular Facebook app has all the features that you would expect from a social media platform, such as stories, live videos, reactions, stickers, filters, groups, pages, events, marketplace, dating, gaming, watch, news feed customization, notifications control, privacy settings, dark mode, etc. The Lite version has only the essential features that allow you to connect and keep up with your friends and family. Some of the features that are missing or limited in the Lite version are stories (you can only view, not create), live videos (you can only watch, not broadcast), reactions (you can only like, not love, wow, haha, sad, or angry), stickers (you can only use the default ones, not download more), filters (you can only use the basic ones, not the fun ones), groups (you can only join, not create), pages (you can only follow, not manage), events (you can only see, not create or RSVP), marketplace (you can only browse, not sell or buy), dating (not available), gaming (not available), watch (not available), news feed customization (not available), notifications control (not available), privacy settings (limited), dark mode (not available), etc.
-
-
How to Download Facebook Lite APK for Android
-
If you want to download Facebook Lite for your Android device, you have two options: you can either download it from the Google Play Store or from a third-party website. Here are the steps for both methods:
-
Method 1: Download from Google Play Store
-
-
Open the Google Play Store app on your Android device.
-
Search for "Facebook Lite" in the search bar.
-
Tap on the app icon that has a white "f" on a blue background and says "Facebook Lite" below it.
-
Tap on the green "Install" button and wait for the app to download and install on your device.
-
Once the app is installed, tap on the "Open" button to launch it.
-
Log in with your Facebook account or create a new one if you don't have one.
Tap on the green "Download APK" button and wait for the file to download on your device.
-
Once the file is downloaded, tap on it to open it. You may need to enable "Unknown sources" in your device settings to install apps from outside the Google Play Store.
-
Tap on the "Install" button and wait for the app to install on your device.
-
Once the app is installed, tap on the "Open" button to launch it.
-
Log in with your Facebook account or create a new one if you don't have one.
-
Enjoy using Facebook Lite on your Android device.
-
-
How to Download Facebook Lite on PC with MEmu
-
If you want to use Facebook Lite on your PC, you will need an Android emulator that can run Android apps on your computer. One of the best Android emulators for PC is MEmu, which is fast, stable, and easy to use. Here are the steps to download and use Facebook Lite on PC with MEmu:
-
download facebook lite apk latest update 2022
-how to download facebook lite new version 2022 apk
-facebook lite latest version 2022 apk free download
-download facebook lite 2022 apk for android
-facebook lite apk download latest version 2022 for pc
-download facebook lite latest version 2022 mod apk
-facebook lite latest version 2022 apk download uptodown
-download facebook lite 2022 apk for low-end devices
-facebook lite apk download latest version 2022 offline installer
-download facebook lite latest version 2022 apk pure
-facebook lite latest version 2022 apk download for ios
-download facebook lite 2022 apk with dark mode
-facebook lite apk download latest version 2022 without ads
-download facebook lite latest version 2022 beta apk
-facebook lite latest version 2022 apk download for windows 10
-download facebook lite 2022 apk with video downloader
-facebook lite apk download latest version 2022 with messenger
-download facebook lite latest version 2022 pro apk
-facebook lite latest version 2022 apk download for mac
-download facebook lite 2022 apk with stickers
-facebook lite apk download latest version 2022 with stories
-download facebook lite latest version 2022 premium apk
-facebook lite latest version 2022 apk download for linux
-download facebook lite 2022 apk with voice call
-facebook lite apk download latest version 2022 with groups
-download facebook lite latest version 2022 cracked apk
-facebook lite latest version 2022 apk download for chromebook
-download facebook lite 2022 apk with live stream
-facebook lite apk download latest version 2022 with marketplace
-download facebook lite latest version 2022 hacked apk
-facebook lite latest version 2022 apk download for blackberry
-download facebook lite 2022 apk with notifications
-facebook lite apk download latest version 2022 with emojis
-download facebook lite latest version 2022 unlocked apk
-facebook lite latest version 2022 apk download for nokia
-download facebook lite 2022 apk with status saver
-facebook lite apk download latest version 2022 with reactions
-download facebook lite latest version 2022 full apk
-facebook lite latest version 2022 apk download for samsung
-download facebook lite 2022 apk with themes
Launch MEmu and click on the Google Play Store icon on the home screen.
-
Search for "Facebook Lite" in the search bar and tap on the app icon that has a white "f" on a blue background and says "Facebook Lite" below it.
-
Tap on the green "Install" button and wait for the app to download and install on MEmu.
-
Once the app is installed, tap on the "Open" button to launch it.
-
Log in with your Facebook account or create a new one if you don't have one.
-
Enjoy using Facebook Lite on your PC with MEmu.
-
-
Benefits of Using Facebook Lite
-
Facebook Lite has many benefits that make it a great alternative to the regular Facebook app. Here are some of them:
-
-
Save data: Facebook Lite uses less data than the regular Facebook app, as it compresses images and videos and loads them faster. This means you can save money on your data plan and use Facebook even when you have a poor or limited internet connection.
-
Save space: Facebook Lite takes up less space than the regular Facebook app, as it is only 2MB in size. This means you can free up some storage space on your phone and install more apps or store more files.
-
Save battery: Facebook Lite consumes less battery power than the regular Facebook app, as it has fewer features and functions that run in the background. This means you can use your phone for longer without having to charge it frequently.
-
Work on any network and device: Facebook Lite works on any network and device, whether it is 2G, 3G, 4G, or Wi-Fi, and whether it is an old or new smartphone. This means you can use Facebook Lite anywhere and anytime, without worrying about compatibility issues.
-
Access all the essential Facebook functions: Facebook Lite allows you to access all the essential Facebook functions that you need to stay connected and keep up with your friends and family. You can post status updates, photos, and videos, like and comment on other people's posts, chat with your contacts, join and follow groups and pages, see events, browse the marketplace, and more.
-
-
Drawbacks of Using Facebook Lite
-
Facebook Lite also has some drawbacks that make it less appealing than the regular Facebook app. Here are some of them:
-
-
Lower resolution: Facebook Lite displays images and videos in lower resolution than the regular Facebook app, which makes them look blurry and pixelated. This can affect your viewing experience and enjoyment of the content.
-
Basic design: Facebook Lite has a basic design that is less appealing and more cluttered than the regular Facebook app. It has smaller icons, text and buttons, and no animations. This can make it harder to navigate and use the app.
-
Fewer options and tools: Facebook Lite has fewer options and tools than the regular Facebook app, which limits your ability to customize and enhance your Facebook experience. You can't create or view stories, broadcast or watch live videos, use reactions other than like, download more stickers, use fun filters, create or manage groups and pages, create or RSVP to events, sell or buy on the marketplace, date, game, watch videos, customize your news feed, control your notifications, adjust your privacy settings, switch to dark mode, and more.
-
-
Conclusion
-
Facebook Lite is a lighter version of Facebook that is designed to work on any network and device, while using less data, space, and battery. It has some benefits such as saving data, space, and battery; working on any network and device; and accessing all the essential Facebook functions. However, it also has some drawbacks such as lower resolution, basic design, and fewer options and tools.
-
If you have a fast internet connection, a powerful smartphone, and enough storage space, you might prefer to use the regular Facebook app for a better user interface, higher image and video quality, and more features and functions. However, if you have a slow internet connection, a low-end smartphone, or limited storage space, you might want to try Facebook Lite for a faster performance, lower data usage, and longer battery life.
-
You can download Facebook Lite for your Android device from the Google Play Store or from a third-party website. You can also download it for your PC with an Android emulator such as MEmu. We hope this article helped you learn more about Facebook Lite and how to download it for your device.
-
FAQs
-
Here are some frequently asked questions about Facebook Lite:
-
-
Is Facebook Lite safe to use?
-Yes, Facebook Lite is safe to use as long as you download it from a trusted source such as the Google Play Store or a reputable website. You should also be careful about what you share on Facebook Lite and who you interact with.
-
How do I update Facebook Lite?
-You can update Facebook Lite by going to the Google Play Store or the website where you downloaded it from and checking for new versions. You can also enable automatic updates in your device settings to get the latest updates automatically.
-
Can I use both Facebook and Facebook Lite on the same device?
-Yes, you can use both Facebook and Facebook Lite on the same device if you want to. However, you should be aware that using both apps will take up more space and data on your device than using just one app.
-
How do I switch to dark mode on Facebook Lite?
-Unfortunately, dark mode is not available on Facebook Lite at the moment. You can only use dark mode on the regular Facebook app if your device supports it.
-
How do I delete Facebook Lite?
-You can delete Facebook Lite by going to your device settings and finding the app in the list of installed apps. Then tap on it and select "Un install" or "Delete" to remove the app from your device. You can also delete Facebook Lite by long-pressing the app icon on your home screen and dragging it to the trash bin.
- 197e85843d
-
-
\ No newline at end of file
diff --git a/spaces/1phancelerku/anime-remove-background/Download Lokicraft 1.17 with Lokicraft Helper A Guide to the New Features and Mods.md b/spaces/1phancelerku/anime-remove-background/Download Lokicraft 1.17 with Lokicraft Helper A Guide to the New Features and Mods.md
deleted file mode 100644
index 4905f80d075bc12346e02eb88af616124c6775e9..0000000000000000000000000000000000000000
--- a/spaces/1phancelerku/anime-remove-background/Download Lokicraft 1.17 with Lokicraft Helper A Guide to the New Features and Mods.md
+++ /dev/null
@@ -1,163 +0,0 @@
-
-
Lokicraft Helper 1.17 Update APK Download: Everything You Need to Know
-
If you are a fan of Lokicraft, the popular sandbox game inspired by Minecraft, you might be interested in downloading the latest version of Lokicraft Helper, a useful app that enhances your gaming experience. In this article, we will tell you everything you need to know about Lokicraft Helper 1.17 update APK download, including what is new in this version, how to download it, and some FAQs.
Lokicraft is a free simulation game that allows you to build and destroy blocks, get resources, and create various tools, blocks, and weapons. You can explore a huge open world with different biomes, animals, and enemies, and unleash your creativity and imagination. Lokicraft is similar to Minecraft, but with some unique features and graphics.
-
Lokicraft game features
-
Some of the main features of Lokicraft are :
-
-
Two highly addicting game modes: Creative and Survival
-
Smooth character animation and realistic physics
-
A huge open world to explore with different biomes, animals, and enemies
-
An in-depth building and crafting system with hundreds of blocks and items
-
Cool graphics: best pixel graphics with high fps
-
-
Lokicraft game modes
-
Lokicraft has two game modes that offer different challenges and experiences:
-
-
Creative mode: In this mode, you have unlimited resources and can build anything you want without any restrictions. You can also fly around the map and enjoy the view.
-
Survival mode: In this mode, you have to hunt and scavenge for resources, craft tools and weapons, build shelters, and fight against enemies. You also have to manage your hunger, health, and stamina.
-
-
What is Lokicraft Helper?
-
Lokicraft Helper is an app that helps you play Lokicraft better. It provides you with useful information, tips, tricks, guides, cheats, hacks, mods, skins, maps, seeds, servers, and more. With Lokicraft Helper, you can enhance your gaming experience and have more fun.
-
Lokicraft Helper features
-
Some of the main features of Lokicraft Helper are:
-
-
Information: You can find detailed information about blocks, items, biomes, animals, enemies, crafting recipes, commands, achievements, and more.
-
Tips and tricks: You can learn how to play Lokicraft better with tips and tricks on building, mining, farming, fighting, exploring, and more.
-
Guides: You can follow step-by-step guides on how to complete various tasks and challenges in Lokicraft.
-
Cheats and hacks: You can use cheats and hacks to get unlimited resources, fly mode, god mode, teleportation, invisibility, and more.
-
Mods: You can download and install mods that add new features, content, gameplay mechanics, graphics enhancements, and more to Lokicraft.
-
Skins: You can customize your character's appearance with hundreds of skins to choose from.
-
Maps: You can download and play on custom maps created by other players or yourself.
-
Seeds: You can generate random worlds with specific features using seeds.
Servers: You can join and play on multiplayer servers with other players from around the world.
-
-
How to use Lokicraft Helper
-
To use Lokicraft Helper, you need to have Lokicraft installed on your device. Then, you can download and install Lokicraft Helper from the Google Play Store or from a trusted third-party source. After that, you can open Lokicraft Helper and browse through the different categories and options. You can also search for specific information or content using the search bar. To apply any cheats, hacks, mods, skins, maps, seeds, or servers, you need to follow the instructions given by the app.
-
lokicraft helper 1.17 update apk download link
-lokicraft 1.17 new update download youtube
-lokicraft updated version 1.17 free download
-lokicraft helper 1.17 update apk file
-lokicraft 1.17 creative adventure game download
-lokicraft helper 1.17 update mediafire
-lokicraft new update 1.17 download video
-lokicraft helper 1.17 update apk mod
-lokicraft 1.17 latest version download android
-lokicraft helper 1.17 update apk online
-lokicraft 1.17 update features and gameplay
-lokicraft helper 1.17 update apk for pc
-lokicraft 1.17 download link in description
-lokicraft helper 1.17 update apk no ads
-lokicraft 1.17 new update review and tutorial
-lokicraft helper 1.17 update apk install
-lokicraft 1.17 download free full version
-lokicraft helper 1.17 update apk cracked
-lokicraft 1.17 new update release date and time
-lokicraft helper 1.17 update apk premium
-lokicraft 1.17 download without verification
-lokicraft helper 1.17 update apk unlimited resources
-lokicraft 1.17 new update gameplay and tips
-lokicraft helper 1.17 update apk latest version
-lokicraft 1.17 download for android phone
-lokicraft helper 1.17 update apk offline mode
-lokicraft 1.17 new update trailer and screenshots
-lokicraft helper 1.17 update apk hack tool
-lokicraft 1.17 download for windows 10
-lokicraft helper 1.17 update apk mod menu
-lokicraft 1.17 new update changelog and patch notes
-lokicraft helper 1.17 update apk cheat codes
-lokicraft 1.17 download from google play store
-lokicraft helper 1.17 update apk pro version
-lokicraft 1.17 new update bugs and fixes
-lokicraft helper 1.17 update apk generator online
-lokicraft 1.17 download with obb data file
-lokicraft helper 1.17 update apk unlocked all features
-lokicraft 1.17 new update skins and maps download
-lokicraft helper 1.17 update apk safe and secure
-
What is new in Lokicraft Helper 1.17 update?
-
Lokicraft Helper 1.17 update is the latest version of the app that was released on June 18, 2023. This update brings some new features and improvements to the app, as well as some bug fixes. Here are some of the main changes in this update:
-
New blocks and items
-
Lokicraft Helper 1.17 update adds some new blocks and items to the app that are compatible with Lokicraft 1.17 version. These include:
-
-
Copper ore, ingot, block, and lightning rod
-
Amethyst shard, cluster, block, and budding amethyst
-
Tinted glass and spyglass
-
Glow squid and glow ink sac
-
Axolotl and bucket of axolotl
-
Goat and goat horn
-
Glow berries and glow lichen
-
Moss block, moss carpet, and azalea
-
Dripstone block, pointed dripstone, stalactite, and stalagmite
Lokicraft Helper 1.17 update also adds some new biomes and structures to the app that are compatible with Lokicraft 1.17 version. These include:
-
-
Lush caves: A biome that is filled with lush vegetation, such as moss blocks, azaleas, spore blossoms, dripstones, glow berries, cave vines, clay pools, and axolotls.
-
Dripstone caves: A biome that is dominated by dripstone blocks and pointed dripstones that form stalactites and stalagmites.
-
Deep dark: A biome that is located at the deepest part of the world, where the light level is very low and a new hostile mob called the warden spawns.
-
Amethyst geodes: A structure that is composed of smooth basalt, calcite, and amethyst blocks that contain amethyst clusters that grow over time.
-
Copper veins: A structure that is composed of copper ore blocks that generate in blobs underground.
-
Shipwrecks: A structure that is composed of a sunken ship that contains chests with loot.
-
Ocean monuments: A structure that is composed of a large underwater temple that contains guardians, elder guardians, prismarine blocks, sea lanterns, sponges, and gold blocks.
-
-
Bug fixes and improvements
-
Lokicraft Helper 1.17 update also fixes some bugs and improves some aspects of the app. Some of these are:
-
-
Fixed crashes and errors when loading some content.
-
Improved performance and stability of the app.
-
Updated user interface and design of the app.
-
Added more languages support for the app.
-
Added more information and content for Lokicraft 1.17 version.
-
-
How to download Lokicraft Helper 1.17 update APK?
If you want to download Lokicraft Helper 1.17 update APK, you have two options:
-
Download link and instructions
-
You can download Lokicraft Helper 1.17 update APK from the official Google Play Store link or from a trusted third-party source link. Here are the steps to download and install the APK:
-
-
Click on the download link and wait for the APK file to be downloaded.
-
Go to your device settings and enable the option to install apps from unknown sources.
-
Locate the downloaded APK file and tap on it to start the installation process.
-
Follow the instructions on the screen and wait for the installation to finish.
-
Open Lokicraft Helper and enjoy the new features and improvements.
-
-
Precautions and tips
-
Before you download Lokicraft Helper 1.17 update APK, you should take some precautions and follow some tips:
-
-
Make sure you have enough storage space on your device to download and install the APK file.
-
Make sure you have a stable internet connection to avoid any interruptions or errors during the download or installation process.
-
Make sure you download the APK file from a reliable and secure source to avoid any malware or viruses.
-
Make sure you have Lokicraft installed on your device and that it is compatible with Lokicraft Helper 1.17 update.
-
Make sure you backup your game data before installing the APK file in case something goes wrong or you want to uninstall it later.
-
-
Conclusion
-
Lokicraft Helper 1.17 update APK is a great app that helps you play Lokicraft better. It provides you with useful information, tips, tricks, guides, cheats, hacks, mods, skins, maps, seeds, servers, and more. It also adds some new features and improvements to the app, as well as some bug fixes. You can download Lokicraft Helper 1.17 update APK from the Google Play Store or from a trusted third-party source. However, you should take some precautions and follow some tips before downloading and installing the APK file. We hope this article was helpful and informative for you. If you have any questions or feedback, please let us know in the comments section below.
-
FAQs
-
Here are some frequently asked questions about Lokicraft Helper 1.17 update APK:
-
Is Lokicraft Helper 1.17 update APK free?
-
Yes, Lokicraft Helper 1.17 update APK is free to download and use. However, some features or content may require in-app purchases or subscriptions.
-
Is Lokicraft Helper 1.17 update APK safe?
-
Lokicraft Helper 1.17 update APK is safe to download and use if you get it from a reputable and secure source. However, you should always scan the APK file for any malware or viruses before installing it on your device.
-
Is Lokicraft Helper 1.17 update APK legal?
-
Lokicraft Helper 1.17 update APK is legal to download and use as long as you do not violate any terms of service or policies of Lokicraft or Google Play Store. However, some features or content of Lokicraft Helper may be considered as cheating or hacking by some players or developers, so use them at your own risk and discretion.
-
How do I uninstall Lokicraft Helper 1.17 update APK?
-
If you want to uninstall Lokicraft Helper 1.17 update APK from your device, you can follow these steps:
-
-
Go to your device settings and find the apps section.
-
Find and tap on Lokicraft Helper and select the uninstall option.
-
Wait for the uninstallation process to finish and confirm your action.
-
Delete the APK file from your device if you still have it.
-
-
How do I contact Lokicraft Helper support?
-
If you have any issues or problems with Lokicraft Helper 1.17 update APK, you can contact Lokicraft Helper support by sending an email to lokicrafthelper@gmail.com or by leaving a review on the Google Play Store page.
197e85843d
-
-
\ No newline at end of file
diff --git a/spaces/1phancelerku/anime-remove-background/Extreme Live VPN The Ultimate VPN App for Android.md b/spaces/1phancelerku/anime-remove-background/Extreme Live VPN The Ultimate VPN App for Android.md
deleted file mode 100644
index a64de4a691bbc226fe2a42525b28bced0c7baec2..0000000000000000000000000000000000000000
--- a/spaces/1phancelerku/anime-remove-background/Extreme Live VPN The Ultimate VPN App for Android.md
+++ /dev/null
@@ -1,149 +0,0 @@
-
-
Extreme Live APK Download: How to Watch TV on Your Android Device
-
Do you want to watch your favorite TV channels on your smartphone or tablet? Do you want to enjoy live streaming, recording, and parental control features? If yes, then you should try Extreme Live APK, a free app that allows you to watch TV directly from your Android device. In this article, we will tell you what Extreme Live APK is, how to download and install it, how to use it to watch TV channels, and what are some alternatives to it.
-
What is Extreme Live APK?
-
Extreme Live APK is a multimedia application developed by Extreme Live VPN. It is an IPTV player that lets you stream TV channels from your IPTV subscription. IPTV stands for Internet Protocol Television, a service that delivers TV programs over the internet instead of using cable or satellite. With Extreme Live APK, you can load your own IPTV playlist and watch hundreds of channels from different countries and categories. You can also use the built-in video player or choose your preferred one.
Follow the instructions on the screen to install the app.
-
Launch the app and enjoy watching TV.
-
-
What is IPTV and how does it work?
-
IPTV is a service that delivers TV programs over the internet instead of using cable or satellite. It uses IP packets to transmit video and audio data from the source to the destination. IPTV can offer more flexibility, quality, and interactivity than traditional TV services. It can also provide video on demand, catch-up TV, time-shifted TV, and live TV.
-
Benefits of IPTV
-
Some of the benefits of using IPTV are:
-
-
You can watch TV anytime, anywhere, as long as you have an internet connection.
-
You can choose from a wide range of channels and content from different countries and genres.
-
You can customize your viewing experience by creating your own playlists, favorites, and watch history.
-
You can pause, rewind, fast-forward, and record live streams.
-
You can interact with other viewers and participate in polls, quizzes, games, and social media.
-
-
Risks and challenges of IPTV
-
Some of the risks and challenges of using IPTV are:
-
-
You need a reliable and fast internet connection and a compatible device to watch IPTV.
-
You may encounter buffering, lagging, freezing, or low-quality streams due to network congestion, server overload, or bandwidth limitations.
-
You may face legal issues if you watch pirated or unlicensed content without permission from the content owners.
-
You may expose your device and data to malware, viruses, hackers, or phishing attacks if you download or install unsafe apps or visit malicious websites.
-
-
How to use Extreme Live APK to watch TV channels
-
Once you have downloaded and installed Extreme Live APK on your device, you can start watching TV channels by following these steps:
-
How to load your IPTV playlist
-
To load your IPTV playlist, you need to have a valid IPTV subscription from a provider that offers M3U files or URLs. You can also find free IPTV playlists online, but they may not work properly or be illegal. To load your IPTV playlist, do the following:
-
-
Open the Extreme Live APK app and tap on the menu icon on the top left corner.
-
Select Settings and then Playlist.
-
Tap on the plus icon on the bottom right corner and choose Add URL or Add File.
-
Enter the URL or browse the file of your IPTV playlist and tap OK.
-
Wait for the app to load the channels and categories from your playlist.
-
-
How to switch between channels and categories
-
To switch between channels and categories, you can use the following methods:
-
extreme live vpn apk download
-iptv extreme apk download for android
-extreme live tv apk download
-iptv extreme pro apk download
-extreme live wallpaper apk download
-iptv extreme firestick apk download
-extreme live stream apk download
-iptv extreme app apk download
-extreme live camera apk download
-iptv extreme mod apk download
-extreme live video apk download
-iptv extreme smart tv apk download
-extreme live chat apk download
-iptv extreme lite apk download
-extreme live sports apk download
-iptv extreme pc apk download
-extreme live music apk download
-iptv extreme premium apk download
-extreme live radio apk download
-iptv extreme android tv apk download
-extreme live quiz apk download
-iptv extreme pro mod apk download
-extreme live weather apk download
-iptv extreme pro firestick apk download
-extreme live gaming apk download
-iptv extreme pro smart tv apk download
-extreme live photo editor apk download
-iptv extreme pro pc apk download
-extreme live filters apk download
-iptv extreme pro android tv apk download
-extreme live trivia apk download
-iptv extreme pro lite apk download
-extreme live launcher apk download
-iptv extreme pro premium apk download
-extreme live keyboard apk download
-iptv extreme pro modded apk download
-extreme live emoji apk download
-iptv extreme pro cracked apk download
-extreme live stickers apk download
-iptv extreme pro patched apk download
-extreme live themes apk download
-iptv extreme pro ad free apk download
-extreme live caller id apk download
-iptv extreme pro latest version apk download
-extreme live lock screen apk download
-iptv extreme pro full version apk download
-extreme live clock widget apk download
-iptv extreme pro no ads apk download
-extreme live icon pack apk download
-iptv extreme pro 113.0 apk download
-
-
Swipe left or right on the screen to change channels.
-
Tap on the channel name on the top of the screen to see the channel list and select a channel.
-
Tap on the category name on the bottom of the screen to see the category list and select a category.
-
Use the search icon on the top right corner to search for a channel or a category by name or keyword.
-
-
How to record live streams and use parental control
-
To record live streams and use parental control, you can use the following features:
-
-
To record a live stream, tap on the record icon on the top right corner of the screen and choose a time limit. The recorded file will be saved in your device storage under Extreme Live APK folder.
-
To use parental control, go to Settings and then Parental Control. Set a PIN code and enable or disable parental control for each category. You can also hide or show adult channels from the channel list.
-
-
Alternatives to Extreme Live APK
-
If you are looking for other apps that can let you watch TV on your Android device, you can try these alternatives:
-
IPTV Extreme
-
IPTV Extreme is another IPTV player that supports M3U playlists, EPG guides, recording, chromecast, parental control, and more. It has a simple and user-friendly interface that allows you to easily navigate through channels and categories. You can also customize your app settings and preferences according to your needs. You can download IPTV Extreme from this link.
-
MTTV
-
MTTV is an app that offers over 1000 live TV channels from various countries and genres. You can watch sports, movies, news, entertainment, music, kids, and more. You can also enjoy HD quality streams, fast loading speed, and low buffering. You don't need any IPTV subscription or playlist to use this app. You can download MTTV from this link.
-
Insta IPTV
-
Insta IPTV is an app that provides free IPTV playlists for different countries and categories. You can watch live TV channels from USA, UK, Canada, India, Pakistan, Arabic, France, Germany, Italy, Spain, Turkey, and more. You can also request new channels or playlists from the app developers. You can download Insta IPTV from this link.
-
Conclusion
-
In conclusion, Extreme Live APK is a free app that allows you to watch TV on your Android device using your IPTV subscription. It has many features that make it a great app for watching TV such as encryption of all traffic on your device No logging of your online activities Split tunneling: Select which apps will use the VPN and which apps won’t Mask your IP address and geographic location Browse anonymously and avoid being tracked Access blocked websites from anywhere Bypass firewalls to browse without limits Unblock your favorite websites and apps Multi-EPG support and M3U playlists Live streaming recording with time limit PIN protection and parental control. However, you should also be aware of the risks and challenges of using IPTV such as network issues, legal issues, and security issues. Therefore, you should always use a trusted IPTV provider and a reliable VPN service to protect your device and data. You can also try other apps that offer similar or different features to watch TV on your Android device.
-
We hope this article has helped you learn more about Extreme Live APK and how to use it to watch TV on your Android device. If you have any questions or feedback, please feel free to leave a comment below. Thank you for reading!
-
FAQs
-
Here are some frequently asked questions about Extreme Live APK:
-
Is Extreme Live APK safe to use?
-
Extreme Live APK is safe to use as long as you download it from a trusted source and scan it for viruses or malware before installing it. You should also use a VPN service to encrypt your traffic and hide your IP address when using the app.
-
Is Extreme Live APK legal to use?
-
Extreme Live APK is legal to use as long as you have a valid IPTV subscription from a licensed provider and you don't watch any pirated or unlicensed content without permission from the content owners. You should also check the laws and regulations of your country or region before using the app.
-
How can I update Extreme Live APK?
-
To update Extreme Live APK, you can either check for updates from the app settings or visit the official website of the app developer and download the latest version of the app.
-
How can I contact the app developer?
-
To contact the app developer, you can either send an email to extremelivevpn@gmail.com or visit their Facebook page at this link.
-
How can I support the app developer?
-
To support the app developer, you can either rate and review the app on Google Play Store or make a donation via PayPal at this link.
401be4b1e0
-
-
\ No newline at end of file
diff --git a/spaces/1phancelerku/anime-remove-background/Farm Heroes Saga MOD APK How to Get Unlimited Everything and Connect with Facebook Friends.md b/spaces/1phancelerku/anime-remove-background/Farm Heroes Saga MOD APK How to Get Unlimited Everything and Connect with Facebook Friends.md
deleted file mode 100644
index 9df279a4dff924e8bf64e8620fcac8cb3c66785c..0000000000000000000000000000000000000000
--- a/spaces/1phancelerku/anime-remove-background/Farm Heroes Saga MOD APK How to Get Unlimited Everything and Connect with Facebook Friends.md
+++ /dev/null
@@ -1,160 +0,0 @@
-
-
Farm Heroes Saga Mod APK Facebook Connect: How to Play with Unlimited Lives and Boosters
-
Farm Heroes Saga is a popular match-3 puzzle game developed by King, the makers of Candy Crush Saga. In this game, you have to match cropsies (fruits and vegetables) to collect them and save the farm from the evil Rancid the Racoon. The game has hundreds of levels, each with different goals and challenges.
Mod APKs are modified versions of Android applications that offer some advantages over the original ones, such as unlimited resources, unlocked features, or removed ads. Some players want to play Farm Heroes Saga with a modded version of the game because they want to enjoy unlimited lives and boosters, which can help them beat difficult levels and progress faster in the game.
-
However, playing with a modded version of Farm Heroes Saga also has some drawbacks, especially if you want to connect to Facebook and play with your friends. In this article, we will show you how to play Farm Heroes Saga mod APK Facebook connect with unlimited lives and boosters, as well as some tips and tricks for playing the game. We will also warn you about some risks and alternatives of playing with a modded version of the game.
-
Benefits of Playing Farm Heroes Saga Mod APK Facebook Connect
-
Playing Farm Heroes Saga with a modded version of the game can be very fun and rewarding, as you can enjoy some benefits that are not available in the official version of the game. Some of these benefits are:
-
-
Unlimited lives: You don't have to wait for your lives to refill or ask your friends for more lives when you run out of them. You can play as much as you want without any interruption.
-
Unlimited boosters: You can use boosters (special items that can help you match more cropsies or clear obstacles) anytime you want without spending any gold bars or real money. You can also get more boosters by opening chests or completing quests.
-
Unlimited gold bars: You can use gold bars (the premium currency of the game) to buy more boosters, extra moves, or other items in the game. You can also use gold bars to unlock new episodes or access special events.
-
Access to all levels: You don't have to complete a certain number of levels or collect a certain number of stars to unlock new episodes or areas in the game. You can play any level you want, even the ones that are not yet released in the official version of the game.
-
Connect to Facebook: You can connect to Facebook and play with your friends, compare your scores, send and receive lives and boosters, and join groups and tournaments. You can also sync your progress across different devices and platforms.
-
-
As you can see, playing Farm Heroes Saga mod APK Facebook connect can make the game more enjoyable and easier for you. However, you should also be aware of some risks and drawbacks of playing with a modded version of the game, which we will discuss later in this article.
-
farm heroes saga unlimited lives and boosters apk
-farm heroes saga mod apk latest version download
-farm heroes saga hack apk with facebook login
-farm heroes saga apk mod unlimited everything
-farm heroes saga modded apk free download
-farm heroes saga cheats apk for android
-farm heroes saga mod apk offline play
-farm heroes saga cracked apk with facebook sync
-farm heroes saga hack tool apk no root
-farm heroes saga premium apk with facebook connect
-farm heroes saga mod apk unlimited gold bars
-farm heroes saga full unlocked apk download
-farm heroes saga mega mod apk with facebook support
-farm heroes saga pro apk free download for android
-farm heroes saga mod apk no ads and no survey
-farm heroes saga patched apk with facebook integration
-farm heroes saga unlimited moves and magic beans apk
-farm heroes saga mod apk all levels unlocked
-farm heroes saga hack version apk with facebook link
-farm heroes saga vip mod apk download for android
-farm heroes saga mod apk unlimited boosters and lives
-farm heroes saga updated mod apk with facebook access
-farm heroes saga hack online apk no verification
-farm heroes saga modded game apk for android
-farm heroes saga cheat engine apk with facebook connect
-farm heroes saga mod apk unlimited coins and stars
-farm heroes saga latest hack apk download free
-farm heroes saga mod menu apk with facebook login
-farm heroes saga unlimited money and gems apk
-farm heroes saga modded app apk for android devices
-farm heroes saga hacked version apk with facebook sync
-farm heroes saga modded game download with facebook connect
-farm heroes saga unlimited resources and power ups apk
-farm heroes saga modded app download for android phone
-farm heroes saga hacked game apk with facebook support
-farm heroes saga modded game free download with facebook integration
-farm heroes saga unlimited items and rewards apk
-farm heroes saga hacked app download for android device
-farm heroes saga modded game online with facebook link
-farm heroes saga hacked game free download with facebook access
-
How to Download and Install Farm Heroes Saga Mod APK Facebook Connect
-
If you want to play Farm Heroes Saga mod APK Facebook connect, you need to download and install the modded version of the game from a reliable source. There are many websites that offer mod APKs for various games, but not all of them are safe and trustworthy. Some of them may contain malware, viruses, or spyware that can harm your device or steal your personal information. Therefore, you should be careful when choosing where to download and install the modded version of the game.
-
One of the websites that we recommend for downloading and installing Farm Heroes Saga mod APK Facebook connect is [ModAPKStore]. This website provides high-quality mod APKs for various games, including Farm Heroes Saga. The mod APKs on this website are tested and verified by the developers and users, so you can be sure that they are safe and working. The website also updates the mod APKs regularly to keep up with the latest versions of the games.
-
To download and install Farm Heroes Saga mod APK Facebook connect from ModAPKStore, follow these steps:
-
-
Go to [ModAPKStore] and search for Farm Heroes Saga mod APK.
-
Select the latest version of the mod APK from the list of results and click on the download button.
-
Wait for the download to finish and then locate the downloaded file on your device.
-
Before installing the mod APK, make sure that you have enabled the installation of apps from unknown sources on your device. To do this, go to Settings > Security > Unknown Sources and toggle it on.
-
Tap on the downloaded file and follow the instructions to install the mod APK on your device.
-
Once the installation is complete, launch the game and enjoy playing with unlimited lives and boosters.
-
-
How to Connect to Facebook with Farm Heroes Saga Mod APK
-
One of the challenges of playing Farm Heroes Saga mod APK Facebook connect is that you may not be able to connect to Facebook with your real account. This is because Facebook may detect that you are using a modded version of the game and suspend or ban your account for violating their terms of service. Therefore, you should be careful when connecting to Facebook with a modded version of the game.
-
There are two ways to connect to Facebook with Farm Heroes Saga mod APK: using a fake Facebook account or using a third-party app. Here are the pros and cons of each method:
-
-
Method
Pros
Cons
-
Using a fake Facebook account
- You can create a new account with a different name and email address. - You can use this account only for playing Farm Heroes Saga mod APK. - You can avoid risking your real account from being suspended or banned.
- You may not be able to play with your real friends who use their real accounts. - You may lose your progress if your fake account gets suspended or banned. - You may violate Facebook's terms of service by creating a fake account.
-
Using a third-party app
- You can use your real account to connect to Facebook. - You can play with your real friends who use their real accounts. - You can sync your progress across different devices and platforms.
- You may need to download and install another app on your device. - You may expose your personal information to a third-party app that may not be secure or trustworthy. - You may still risk your real account from being suspended or banned.
-
-
The choice is up to you, but we suggest that you use a fake Facebook account for playing Farm Heroes Saga mod APK Facebook connect, as it is safer and easier than using a third-party app. Here are the steps to create and use a fake Facebook account for playing Farm Heroes Saga mod APK Facebook connect:
-
-
Go to [Facebook] and create a new account with a different name and email address. You can use a temporary email service like [TempMail] to generate a disposable email address.
-
Verify your email address and complete your profile with some basic information and a profile picture. You can use a random name generator like [FakeNameGenerator] and a random image generator like [ThisPersonDoesNotExist] to create a fake identity and a fake photo.
-
Launch Farm Heroes Saga mod APK on your device and tap on the Connect to Facebook button.
-
Enter your fake Facebook account credentials and allow the game to access your account.
-
Enjoy playing Farm Heroes Saga mod APK Facebook connect with unlimited lives and boosters.
-
-
Note: You should not use your fake Facebook account for any other purpose than playing Farm Heroes Saga mod APK. You should also not add any real friends or join any real groups or pages with your fake account, as this may raise suspicion and get your account suspended or banned.
-
Tips and Tricks for Playing Farm Heroes Saga Mod APK Facebook Connect
-
Playing Farm Heroes Saga mod APK Facebook connect can be very fun and rewarding, but it can also be challenging and frustrating at times. To help you get the most out of your gaming experience, here are some tips and tricks for playing Farm Heroes Saga mod APK Facebook connect:
-
-
Use boosters wisely: Boosters are special items that can help you match more cropsies or clear obstacles in the game. You can use boosters before or during a level, depending on the type of booster. Some of the boosters you can use are:
-
Shovel: This booster allows you to dig up one crop or obstacle on the board. You can use it before or during a level.
-
Tractor: This booster allows you to clear one row of crops or obstacles on the board. You can use it before or during a level.
-
Dog: This booster allows you to collect all crops of one type on the board. You can use it before or during a level.
-
Color Collector: This booster allows you to collect all crops of one color on the board. You can use it before or during a level.
-
Magic Beans: This booster allows you to activate the Hero Mode, which gives you extra points for matching crops after you complete the level goal. You can use it before a level.
-
- You can get more boosters by opening chests, completing quests, or buying them with gold bars. However, you should not waste your boosters on easy levels or when you don't need them. Save them for hard levels or when you are stuck.
-
Collect more cropsies: Cropsies are the fruits and vegetables that you have to match and collect in the game. The more cropsies you collect, the more points you get and the faster you progress in the game. To collect more cropsies, you should:
-
Match four or more cropsies: When you match four or more cropsies of the same type, you create a super crop, which has more value than a regular crop. For example, matching four strawberries creates a super strawberry, which is worth two regular strawberries. Matching five strawberries creates a mega strawberry, which is worth five regular strawberries.
-
Match cropsies in T or L shapes: When you match cropsies in T or L shapes, you create a special crop, which has a special effect when matched with other crops of the same type. For example, matching cropsies in a T shape creates a water drop, which clears all crops of one type on the board when matched with another water drop.
-
Match cropsies near grumpy cropsies: Grumpy cropsies are cropsies that have an angry face and are worth zero points. They are created by mud, ice, or other obstacles on the board. To turn them into happy cropsies, you have to match them with other crops of the same type near them.
-
-
Beat challenging levels: Some levels in Farm Heroes Saga mod APK Facebook connect are harder than others, as they have more obstacles, less moves, or higher goals. To beat these levels, you should:
-
Plan your moves: Before you make a move, look at the board and see if you can make a better move elsewhere. Try to match cropsies that are required for the level goal, create super or special crops, or clear obstacles. Avoid making moves that do not help you achieve the goal or create grumpy cropsies.
-
Use boosters strategically: If you have boosters, use them when you need them most, such as when you are running out of moves, when you are stuck, or when you are close to completing the goal. Do not use boosters randomly or unnecessarily, as they may not help you much or may even make the level harder.
-
Replay levels: If you fail to complete a level, do not give up. You can replay the level as many times as you want until you beat it. Each time you replay a level, the board layout and the cropsies distribution may change, so you may have a better chance of winning. You can also learn from your mistakes and try a different strategy or approach.
-
-
-
How to Update Farm Heroes Saga Mod APK Facebook Connect
-
Another challenge of playing Farm Heroes Saga mod APK Facebook connect is that you may not be able to update the game when a new version is released. This is because the modded version of the game may not be compatible with the latest version of the game or may not be updated by the modder in time. Therefore, you should check regularly if there is a new version of the modded game available and how to update it.
-
There are two ways to update Farm Heroes Saga mod APK Facebook connect: downloading it again or using an auto-update feature. Here are the pros and cons of each method:
-
-
Method
Pros
Cons
-
Downloading it again
- You can get the latest version of the modded game with new features and improvements. - You can choose which version of the modded game you want to download and install.
- You may need to uninstall the previous version of the modded game and lose your progress. - You may need to download and install the modded game from a different source if the original one is not updated. - You may expose your device to malware or viruses if you download and install the modded game from an untrusted source.
-
Using an auto-update feature
- You can update the modded game automatically without uninstalling it or losing your progress. - You can save time and effort by not having to download and install the modded game manually.
- You may not be able to choose which version of the modded game you want to update to. - You may encounter errors or bugs if the auto-update feature is not working properly. - You may depend on the modder to update the modded game regularly and timely.
-
-
The choice is up to you, but we suggest that you use an auto-update feature for updating Farm Heroes Saga mod APK Facebook connect, as it is more convenient and safer than downloading it again. However, you should make sure that the modded game has an auto-update feature and that it is working properly. Here are the steps to use an auto-update feature for updating Farm Heroes Saga mod APK Facebook connect:
-
-
Launch Farm Heroes Saga mod APK on your device and go to the settings menu.
-
Look for an option that says "Auto-update" or "Check for updates" and toggle it on.
-
Wait for the modded game to check for updates and download them if available.
-
Restart the game and enjoy playing with unlimited lives and boosters.
-
-
Risks and Drawbacks of Playing Farm Heroes Saga Mod APK Facebook Connect
-
While playing Farm Heroes Saga mod APK Facebook connect can be very fun and rewarding, it can also have some risks and drawbacks that you should be aware of before playing. Some of these risks and drawbacks are:
-
-
Possible malware: As we mentioned earlier, not all websites that offer mod APKs for various games are safe and trustworthy. Some of them may contain malware, viruses, or spyware that can harm your device or steal your personal information. Therefore, you should be careful when choosing where to download and install the modded version of the game.
-
Account suspension: Another risk of playing Farm Heroes Saga mod APK Facebook connect is that your account may be suspended or banned by Facebook or King for violating their terms of service. This is because they may detect that you are using a modded version of the game and consider it as cheating or hacking. Therefore, you should be careful when connecting to Facebook with a modded version of the game, as we explained earlier.
-
Data loss: Another drawback of playing Farm Heroes Saga mod APK Facebook connect is that you may lose your progress or data if something goes wrong with the modded version of the game. For example, if the modded version of the game crashes, freezes, or stops working, you may not be able to resume your game or recover your data. Therefore, you should backup your data regularly and avoid relying on the modded version of the game for your gaming experience.
-
Ethical issues: Finally, playing Farm Heroes Saga mod APK Facebook connect may raise some ethical issues, as you may be unfair to other players who play the official version of the game. You may also be disrespecting the developers and publishers of the game, who put a lot of time and effort into creating and maintaining the game. Therefore, you should respect the rules and regulations of the game and appreciate the work of the creators.
-
-
As you can see, playing Farm Heroes Saga mod APK Facebook connect can have some risks and drawbacks that may outweigh the benefits. Therefore, you should think twice before playing with a modded version of the game and consider some alternatives that are safer and more ethical.
-
How to Play Farm Heroes Saga Safely and Legally
-
If you want to play Farm Heroes Saga safely and legally, you should play the official version of the game that is available on Google Play Store or App Store. The official version of the game is free to download and play, and it offers a lot of fun and challenging features that can keep you entertained for hours. Some of these features are:
-
-
New levels every week: The official version of the game is updated regularly with new levels and episodes that offer new goals and challenges. You can play hundreds of levels, each with different cropsies, obstacles, and boosters.
-
Special events and quests: The official version of the game also offers special events and quests that give you extra rewards and opportunities to play. You can join seasonal events, daily quests, leaderboards, tournaments, and more.
-
Legitimate cheats and hacks: The official version of the game also allows you to use some legitimate cheats and hacks that can help you beat difficult levels and progress faster in the game. Some of these cheats and hacks are:
-
Time lapse: This cheat allows you to refill your lives faster by changing the time on your device. To use this cheat, you have to exit the game, go to your device settings, and move the time forward by a few hours. Then, go back to the game and see your lives refilled.
-
Free boosters: This hack allows you to get free boosters by watching ads or completing surveys. To use this hack, you have to go to the shop menu in the game and look for an option that says "Watch video for free boosters" or "Complete survey for free boosters". Then, follow the instructions and get your free boosters.
-
Free gold bars: This hack allows you to get free gold bars by inviting your friends to play the game or by using a referral code. To use this hack, you have to go to the settings menu in the game and look for an option that says "Invite friends" or "Enter referral code". Then, follow the instructions and get your free gold bars.
-
-
Other similar games: If you want to play other games that are similar to Farm Heroes Saga, you can try some of these games that are also available on Google Play Store or App Store:
-
Candy Crush Saga: This is another match-3 puzzle game developed by King, where you have to match candies to clear levels and save Candy Kingdom from the evil Tiffi and Mr. Toffee.
-
Gardenscapes: This is a match-3 puzzle game developed by Playrix, where you have to match fruits and flowers to restore a beautiful garden and uncover its secrets.
-
FarmVille 2: Country Escape: This is a farming simulation game developed by Zynga, where you have to build your own farm, grow crops, raise animals, and trade with other players.
-
-
-
Conclusion
-
In conclusion, Farm Heroes Saga mod APK Facebook connect is a way to play Farm Heroes Saga with unlimited lives and boosters, as well as connect to Facebook and play with your friends. However, it also has some risks and drawbacks, such as possible malware, account suspension, data loss, and ethical issues. Therefore, you should be careful when playing with a modded version of the game and consider some alternatives that are safer and more ethical, such as playing the official version of the game, using legitimate cheats and hacks, or playing other similar games. We hope that this article has helped you understand how to play Farm Heroes Saga mod APK Facebook connect and enjoy the game. If you have any questions or comments, please feel free to share them in the comments section below.
-
FAQs
-
Here are some frequently asked questions about Farm Heroes Saga mod APK Facebook connect:
-
-
What is Farm Heroes Saga? Farm Heroes Saga is a match-3 puzzle game developed by King, where you have to match cropsies (fruits and vegetables) to collect them and save the farm from the evil Rancid the Racoon.
-
What is a mod APK? A mod APK is a modified version of an Android application that offers some advantages over the original one, such as unlimited resources, unlocked features, or removed ads.
-
How to play Farm Heroes Saga mod APK Facebook connect? To play Farm Heroes Saga mod APK Facebook connect, you need to download and install the modded version of the game from a reliable source, such as [ModAPKStore]. Then, you need to connect to Facebook with either a fake account or a third-party app.
-
What are the benefits of playing Farm Heroes Saga mod APK Facebook connect? Some of the benefits of playing Farm Heroes Saga mod APK Facebook connect are unlimited lives, boosters, gold bars, and access to all levels. You can also play with your friends on Facebook and sync your progress across different devices and platforms.
-
What are the risks and drawbacks of playing Farm Heroes Saga mod APK Facebook connect? Some of the risks and drawbacks of playing Farm Heroes Saga mod APK Facebook connect are possible malware, account suspension, data loss, and ethical issues. You may also not be able to update the game when a new version is released.
- 401be4b1e0
-
-
\ No newline at end of file
diff --git a/spaces/4Taps/SadTalker/src/face3d/models/arcface_torch/configs/__init__.py b/spaces/4Taps/SadTalker/src/face3d/models/arcface_torch/configs/__init__.py
deleted file mode 100644
index e69de29bb2d1d6434b8b29ae775ad8c2e48c5391..0000000000000000000000000000000000000000
diff --git a/spaces/4Taps/SadTalker/src/face3d/models/networks.py b/spaces/4Taps/SadTalker/src/face3d/models/networks.py
deleted file mode 100644
index ead9cdcb8720b845c233de79dc8a8d1668492108..0000000000000000000000000000000000000000
--- a/spaces/4Taps/SadTalker/src/face3d/models/networks.py
+++ /dev/null
@@ -1,521 +0,0 @@
-"""This script defines deep neural networks for Deep3DFaceRecon_pytorch
-"""
-
-import os
-import numpy as np
-import torch.nn.functional as F
-from torch.nn import init
-import functools
-from torch.optim import lr_scheduler
-import torch
-from torch import Tensor
-import torch.nn as nn
-try:
- from torch.hub import load_state_dict_from_url
-except ImportError:
- from torch.utils.model_zoo import load_url as load_state_dict_from_url
-from typing import Type, Any, Callable, Union, List, Optional
-from .arcface_torch.backbones import get_model
-from kornia.geometry import warp_affine
-
-def resize_n_crop(image, M, dsize=112):
- # image: (b, c, h, w)
- # M : (b, 2, 3)
- return warp_affine(image, M, dsize=(dsize, dsize), align_corners=True)
-
-def filter_state_dict(state_dict, remove_name='fc'):
- new_state_dict = {}
- for key in state_dict:
- if remove_name in key:
- continue
- new_state_dict[key] = state_dict[key]
- return new_state_dict
-
-def get_scheduler(optimizer, opt):
- """Return a learning rate scheduler
-
- Parameters:
- optimizer -- the optimizer of the network
- opt (option class) -- stores all the experiment flags; needs to be a subclass of BaseOptions.
- opt.lr_policy is the name of learning rate policy: linear | step | plateau | cosine
-
- For other schedulers (step, plateau, and cosine), we use the default PyTorch schedulers.
- See https://pytorch.org/docs/stable/optim.html for more details.
- """
- if opt.lr_policy == 'linear':
- def lambda_rule(epoch):
- lr_l = 1.0 - max(0, epoch + opt.epoch_count - opt.n_epochs) / float(opt.n_epochs + 1)
- return lr_l
- scheduler = lr_scheduler.LambdaLR(optimizer, lr_lambda=lambda_rule)
- elif opt.lr_policy == 'step':
- scheduler = lr_scheduler.StepLR(optimizer, step_size=opt.lr_decay_epochs, gamma=0.2)
- elif opt.lr_policy == 'plateau':
- scheduler = lr_scheduler.ReduceLROnPlateau(optimizer, mode='min', factor=0.2, threshold=0.01, patience=5)
- elif opt.lr_policy == 'cosine':
- scheduler = lr_scheduler.CosineAnnealingLR(optimizer, T_max=opt.n_epochs, eta_min=0)
- else:
- return NotImplementedError('learning rate policy [%s] is not implemented', opt.lr_policy)
- return scheduler
-
-
-def define_net_recon(net_recon, use_last_fc=False, init_path=None):
- return ReconNetWrapper(net_recon, use_last_fc=use_last_fc, init_path=init_path)
-
-def define_net_recog(net_recog, pretrained_path=None):
- net = RecogNetWrapper(net_recog=net_recog, pretrained_path=pretrained_path)
- net.eval()
- return net
-
-class ReconNetWrapper(nn.Module):
- fc_dim=257
- def __init__(self, net_recon, use_last_fc=False, init_path=None):
- super(ReconNetWrapper, self).__init__()
- self.use_last_fc = use_last_fc
- if net_recon not in func_dict:
- return NotImplementedError('network [%s] is not implemented', net_recon)
- func, last_dim = func_dict[net_recon]
- backbone = func(use_last_fc=use_last_fc, num_classes=self.fc_dim)
- if init_path and os.path.isfile(init_path):
- state_dict = filter_state_dict(torch.load(init_path, map_location='cpu'))
- backbone.load_state_dict(state_dict)
- print("loading init net_recon %s from %s" %(net_recon, init_path))
- self.backbone = backbone
- if not use_last_fc:
- self.final_layers = nn.ModuleList([
- conv1x1(last_dim, 80, bias=True), # id layer
- conv1x1(last_dim, 64, bias=True), # exp layer
- conv1x1(last_dim, 80, bias=True), # tex layer
- conv1x1(last_dim, 3, bias=True), # angle layer
- conv1x1(last_dim, 27, bias=True), # gamma layer
- conv1x1(last_dim, 2, bias=True), # tx, ty
- conv1x1(last_dim, 1, bias=True) # tz
- ])
- for m in self.final_layers:
- nn.init.constant_(m.weight, 0.)
- nn.init.constant_(m.bias, 0.)
-
- def forward(self, x):
- x = self.backbone(x)
- if not self.use_last_fc:
- output = []
- for layer in self.final_layers:
- output.append(layer(x))
- x = torch.flatten(torch.cat(output, dim=1), 1)
- return x
-
-
-class RecogNetWrapper(nn.Module):
- def __init__(self, net_recog, pretrained_path=None, input_size=112):
- super(RecogNetWrapper, self).__init__()
- net = get_model(name=net_recog, fp16=False)
- if pretrained_path:
- state_dict = torch.load(pretrained_path, map_location='cpu')
- net.load_state_dict(state_dict)
- print("loading pretrained net_recog %s from %s" %(net_recog, pretrained_path))
- for param in net.parameters():
- param.requires_grad = False
- self.net = net
- self.preprocess = lambda x: 2 * x - 1
- self.input_size=input_size
-
- def forward(self, image, M):
- image = self.preprocess(resize_n_crop(image, M, self.input_size))
- id_feature = F.normalize(self.net(image), dim=-1, p=2)
- return id_feature
-
-
-# adapted from https://github.com/pytorch/vision/edit/master/torchvision/models/resnet.py
-__all__ = ['ResNet', 'resnet18', 'resnet34', 'resnet50', 'resnet101',
- 'resnet152', 'resnext50_32x4d', 'resnext101_32x8d',
- 'wide_resnet50_2', 'wide_resnet101_2']
-
-
-model_urls = {
- 'resnet18': 'https://download.pytorch.org/models/resnet18-f37072fd.pth',
- 'resnet34': 'https://download.pytorch.org/models/resnet34-b627a593.pth',
- 'resnet50': 'https://download.pytorch.org/models/resnet50-0676ba61.pth',
- 'resnet101': 'https://download.pytorch.org/models/resnet101-63fe2227.pth',
- 'resnet152': 'https://download.pytorch.org/models/resnet152-394f9c45.pth',
- 'resnext50_32x4d': 'https://download.pytorch.org/models/resnext50_32x4d-7cdf4587.pth',
- 'resnext101_32x8d': 'https://download.pytorch.org/models/resnext101_32x8d-8ba56ff5.pth',
- 'wide_resnet50_2': 'https://download.pytorch.org/models/wide_resnet50_2-95faca4d.pth',
- 'wide_resnet101_2': 'https://download.pytorch.org/models/wide_resnet101_2-32ee1156.pth',
-}
-
-
-def conv3x3(in_planes: int, out_planes: int, stride: int = 1, groups: int = 1, dilation: int = 1) -> nn.Conv2d:
- """3x3 convolution with padding"""
- return nn.Conv2d(in_planes, out_planes, kernel_size=3, stride=stride,
- padding=dilation, groups=groups, bias=False, dilation=dilation)
-
-
-def conv1x1(in_planes: int, out_planes: int, stride: int = 1, bias: bool = False) -> nn.Conv2d:
- """1x1 convolution"""
- return nn.Conv2d(in_planes, out_planes, kernel_size=1, stride=stride, bias=bias)
-
-
-class BasicBlock(nn.Module):
- expansion: int = 1
-
- def __init__(
- self,
- inplanes: int,
- planes: int,
- stride: int = 1,
- downsample: Optional[nn.Module] = None,
- groups: int = 1,
- base_width: int = 64,
- dilation: int = 1,
- norm_layer: Optional[Callable[..., nn.Module]] = None
- ) -> None:
- super(BasicBlock, self).__init__()
- if norm_layer is None:
- norm_layer = nn.BatchNorm2d
- if groups != 1 or base_width != 64:
- raise ValueError('BasicBlock only supports groups=1 and base_width=64')
- if dilation > 1:
- raise NotImplementedError("Dilation > 1 not supported in BasicBlock")
- # Both self.conv1 and self.downsample layers downsample the input when stride != 1
- self.conv1 = conv3x3(inplanes, planes, stride)
- self.bn1 = norm_layer(planes)
- self.relu = nn.ReLU(inplace=True)
- self.conv2 = conv3x3(planes, planes)
- self.bn2 = norm_layer(planes)
- self.downsample = downsample
- self.stride = stride
-
- def forward(self, x: Tensor) -> Tensor:
- identity = x
-
- out = self.conv1(x)
- out = self.bn1(out)
- out = self.relu(out)
-
- out = self.conv2(out)
- out = self.bn2(out)
-
- if self.downsample is not None:
- identity = self.downsample(x)
-
- out += identity
- out = self.relu(out)
-
- return out
-
-
-class Bottleneck(nn.Module):
- # Bottleneck in torchvision places the stride for downsampling at 3x3 convolution(self.conv2)
- # while original implementation places the stride at the first 1x1 convolution(self.conv1)
- # according to "Deep residual learning for image recognition"https://arxiv.org/abs/1512.03385.
- # This variant is also known as ResNet V1.5 and improves accuracy according to
- # https://ngc.nvidia.com/catalog/model-scripts/nvidia:resnet_50_v1_5_for_pytorch.
-
- expansion: int = 4
-
- def __init__(
- self,
- inplanes: int,
- planes: int,
- stride: int = 1,
- downsample: Optional[nn.Module] = None,
- groups: int = 1,
- base_width: int = 64,
- dilation: int = 1,
- norm_layer: Optional[Callable[..., nn.Module]] = None
- ) -> None:
- super(Bottleneck, self).__init__()
- if norm_layer is None:
- norm_layer = nn.BatchNorm2d
- width = int(planes * (base_width / 64.)) * groups
- # Both self.conv2 and self.downsample layers downsample the input when stride != 1
- self.conv1 = conv1x1(inplanes, width)
- self.bn1 = norm_layer(width)
- self.conv2 = conv3x3(width, width, stride, groups, dilation)
- self.bn2 = norm_layer(width)
- self.conv3 = conv1x1(width, planes * self.expansion)
- self.bn3 = norm_layer(planes * self.expansion)
- self.relu = nn.ReLU(inplace=True)
- self.downsample = downsample
- self.stride = stride
-
- def forward(self, x: Tensor) -> Tensor:
- identity = x
-
- out = self.conv1(x)
- out = self.bn1(out)
- out = self.relu(out)
-
- out = self.conv2(out)
- out = self.bn2(out)
- out = self.relu(out)
-
- out = self.conv3(out)
- out = self.bn3(out)
-
- if self.downsample is not None:
- identity = self.downsample(x)
-
- out += identity
- out = self.relu(out)
-
- return out
-
-
-class ResNet(nn.Module):
-
- def __init__(
- self,
- block: Type[Union[BasicBlock, Bottleneck]],
- layers: List[int],
- num_classes: int = 1000,
- zero_init_residual: bool = False,
- use_last_fc: bool = False,
- groups: int = 1,
- width_per_group: int = 64,
- replace_stride_with_dilation: Optional[List[bool]] = None,
- norm_layer: Optional[Callable[..., nn.Module]] = None
- ) -> None:
- super(ResNet, self).__init__()
- if norm_layer is None:
- norm_layer = nn.BatchNorm2d
- self._norm_layer = norm_layer
-
- self.inplanes = 64
- self.dilation = 1
- if replace_stride_with_dilation is None:
- # each element in the tuple indicates if we should replace
- # the 2x2 stride with a dilated convolution instead
- replace_stride_with_dilation = [False, False, False]
- if len(replace_stride_with_dilation) != 3:
- raise ValueError("replace_stride_with_dilation should be None "
- "or a 3-element tuple, got {}".format(replace_stride_with_dilation))
- self.use_last_fc = use_last_fc
- self.groups = groups
- self.base_width = width_per_group
- self.conv1 = nn.Conv2d(3, self.inplanes, kernel_size=7, stride=2, padding=3,
- bias=False)
- self.bn1 = norm_layer(self.inplanes)
- self.relu = nn.ReLU(inplace=True)
- self.maxpool = nn.MaxPool2d(kernel_size=3, stride=2, padding=1)
- self.layer1 = self._make_layer(block, 64, layers[0])
- self.layer2 = self._make_layer(block, 128, layers[1], stride=2,
- dilate=replace_stride_with_dilation[0])
- self.layer3 = self._make_layer(block, 256, layers[2], stride=2,
- dilate=replace_stride_with_dilation[1])
- self.layer4 = self._make_layer(block, 512, layers[3], stride=2,
- dilate=replace_stride_with_dilation[2])
- self.avgpool = nn.AdaptiveAvgPool2d((1, 1))
-
- if self.use_last_fc:
- self.fc = nn.Linear(512 * block.expansion, num_classes)
-
- for m in self.modules():
- if isinstance(m, nn.Conv2d):
- nn.init.kaiming_normal_(m.weight, mode='fan_out', nonlinearity='relu')
- elif isinstance(m, (nn.BatchNorm2d, nn.GroupNorm)):
- nn.init.constant_(m.weight, 1)
- nn.init.constant_(m.bias, 0)
-
-
-
- # Zero-initialize the last BN in each residual branch,
- # so that the residual branch starts with zeros, and each residual block behaves like an identity.
- # This improves the model by 0.2~0.3% according to https://arxiv.org/abs/1706.02677
- if zero_init_residual:
- for m in self.modules():
- if isinstance(m, Bottleneck):
- nn.init.constant_(m.bn3.weight, 0) # type: ignore[arg-type]
- elif isinstance(m, BasicBlock):
- nn.init.constant_(m.bn2.weight, 0) # type: ignore[arg-type]
-
- def _make_layer(self, block: Type[Union[BasicBlock, Bottleneck]], planes: int, blocks: int,
- stride: int = 1, dilate: bool = False) -> nn.Sequential:
- norm_layer = self._norm_layer
- downsample = None
- previous_dilation = self.dilation
- if dilate:
- self.dilation *= stride
- stride = 1
- if stride != 1 or self.inplanes != planes * block.expansion:
- downsample = nn.Sequential(
- conv1x1(self.inplanes, planes * block.expansion, stride),
- norm_layer(planes * block.expansion),
- )
-
- layers = []
- layers.append(block(self.inplanes, planes, stride, downsample, self.groups,
- self.base_width, previous_dilation, norm_layer))
- self.inplanes = planes * block.expansion
- for _ in range(1, blocks):
- layers.append(block(self.inplanes, planes, groups=self.groups,
- base_width=self.base_width, dilation=self.dilation,
- norm_layer=norm_layer))
-
- return nn.Sequential(*layers)
-
- def _forward_impl(self, x: Tensor) -> Tensor:
- # See note [TorchScript super()]
- x = self.conv1(x)
- x = self.bn1(x)
- x = self.relu(x)
- x = self.maxpool(x)
-
- x = self.layer1(x)
- x = self.layer2(x)
- x = self.layer3(x)
- x = self.layer4(x)
-
- x = self.avgpool(x)
- if self.use_last_fc:
- x = torch.flatten(x, 1)
- x = self.fc(x)
- return x
-
- def forward(self, x: Tensor) -> Tensor:
- return self._forward_impl(x)
-
-
-def _resnet(
- arch: str,
- block: Type[Union[BasicBlock, Bottleneck]],
- layers: List[int],
- pretrained: bool,
- progress: bool,
- **kwargs: Any
-) -> ResNet:
- model = ResNet(block, layers, **kwargs)
- if pretrained:
- state_dict = load_state_dict_from_url(model_urls[arch],
- progress=progress)
- model.load_state_dict(state_dict)
- return model
-
-
-def resnet18(pretrained: bool = False, progress: bool = True, **kwargs: Any) -> ResNet:
- r"""ResNet-18 model from
- `"Deep Residual Learning for Image Recognition" `_.
-
- Args:
- pretrained (bool): If True, returns a model pre-trained on ImageNet
- progress (bool): If True, displays a progress bar of the download to stderr
- """
- return _resnet('resnet18', BasicBlock, [2, 2, 2, 2], pretrained, progress,
- **kwargs)
-
-
-def resnet34(pretrained: bool = False, progress: bool = True, **kwargs: Any) -> ResNet:
- r"""ResNet-34 model from
- `"Deep Residual Learning for Image Recognition" `_.
-
- Args:
- pretrained (bool): If True, returns a model pre-trained on ImageNet
- progress (bool): If True, displays a progress bar of the download to stderr
- """
- return _resnet('resnet34', BasicBlock, [3, 4, 6, 3], pretrained, progress,
- **kwargs)
-
-
-def resnet50(pretrained: bool = False, progress: bool = True, **kwargs: Any) -> ResNet:
- r"""ResNet-50 model from
- `"Deep Residual Learning for Image Recognition" `_.
-
- Args:
- pretrained (bool): If True, returns a model pre-trained on ImageNet
- progress (bool): If True, displays a progress bar of the download to stderr
- """
- return _resnet('resnet50', Bottleneck, [3, 4, 6, 3], pretrained, progress,
- **kwargs)
-
-
-def resnet101(pretrained: bool = False, progress: bool = True, **kwargs: Any) -> ResNet:
- r"""ResNet-101 model from
- `"Deep Residual Learning for Image Recognition" `_.
-
- Args:
- pretrained (bool): If True, returns a model pre-trained on ImageNet
- progress (bool): If True, displays a progress bar of the download to stderr
- """
- return _resnet('resnet101', Bottleneck, [3, 4, 23, 3], pretrained, progress,
- **kwargs)
-
-
-def resnet152(pretrained: bool = False, progress: bool = True, **kwargs: Any) -> ResNet:
- r"""ResNet-152 model from
- `"Deep Residual Learning for Image Recognition" `_.
-
- Args:
- pretrained (bool): If True, returns a model pre-trained on ImageNet
- progress (bool): If True, displays a progress bar of the download to stderr
- """
- return _resnet('resnet152', Bottleneck, [3, 8, 36, 3], pretrained, progress,
- **kwargs)
-
-
-def resnext50_32x4d(pretrained: bool = False, progress: bool = True, **kwargs: Any) -> ResNet:
- r"""ResNeXt-50 32x4d model from
- `"Aggregated Residual Transformation for Deep Neural Networks" `_.
-
- Args:
- pretrained (bool): If True, returns a model pre-trained on ImageNet
- progress (bool): If True, displays a progress bar of the download to stderr
- """
- kwargs['groups'] = 32
- kwargs['width_per_group'] = 4
- return _resnet('resnext50_32x4d', Bottleneck, [3, 4, 6, 3],
- pretrained, progress, **kwargs)
-
-
-def resnext101_32x8d(pretrained: bool = False, progress: bool = True, **kwargs: Any) -> ResNet:
- r"""ResNeXt-101 32x8d model from
- `"Aggregated Residual Transformation for Deep Neural Networks" `_.
-
- Args:
- pretrained (bool): If True, returns a model pre-trained on ImageNet
- progress (bool): If True, displays a progress bar of the download to stderr
- """
- kwargs['groups'] = 32
- kwargs['width_per_group'] = 8
- return _resnet('resnext101_32x8d', Bottleneck, [3, 4, 23, 3],
- pretrained, progress, **kwargs)
-
-
-def wide_resnet50_2(pretrained: bool = False, progress: bool = True, **kwargs: Any) -> ResNet:
- r"""Wide ResNet-50-2 model from
- `"Wide Residual Networks" `_.
-
- The model is the same as ResNet except for the bottleneck number of channels
- which is twice larger in every block. The number of channels in outer 1x1
- convolutions is the same, e.g. last block in ResNet-50 has 2048-512-2048
- channels, and in Wide ResNet-50-2 has 2048-1024-2048.
-
- Args:
- pretrained (bool): If True, returns a model pre-trained on ImageNet
- progress (bool): If True, displays a progress bar of the download to stderr
- """
- kwargs['width_per_group'] = 64 * 2
- return _resnet('wide_resnet50_2', Bottleneck, [3, 4, 6, 3],
- pretrained, progress, **kwargs)
-
-
-def wide_resnet101_2(pretrained: bool = False, progress: bool = True, **kwargs: Any) -> ResNet:
- r"""Wide ResNet-101-2 model from
- `"Wide Residual Networks" `_.
-
- The model is the same as ResNet except for the bottleneck number of channels
- which is twice larger in every block. The number of channels in outer 1x1
- convolutions is the same, e.g. last block in ResNet-50 has 2048-512-2048
- channels, and in Wide ResNet-50-2 has 2048-1024-2048.
-
- Args:
- pretrained (bool): If True, returns a model pre-trained on ImageNet
- progress (bool): If True, displays a progress bar of the download to stderr
- """
- kwargs['width_per_group'] = 64 * 2
- return _resnet('wide_resnet101_2', Bottleneck, [3, 4, 23, 3],
- pretrained, progress, **kwargs)
-
-
-func_dict = {
- 'resnet18': (resnet18, 512),
- 'resnet50': (resnet50, 2048)
-}
diff --git a/spaces/AIFILMS/generate_human_motion/pyrender/docs/make.bat b/spaces/AIFILMS/generate_human_motion/pyrender/docs/make.bat
deleted file mode 100644
index 4d9eb83d9f9309029f4b14ff09024658bb0f5563..0000000000000000000000000000000000000000
--- a/spaces/AIFILMS/generate_human_motion/pyrender/docs/make.bat
+++ /dev/null
@@ -1,35 +0,0 @@
-@ECHO OFF
-
-pushd %~dp0
-
-REM Command file for Sphinx documentation
-
-if "%SPHINXBUILD%" == "" (
- set SPHINXBUILD=sphinx-build
-)
-set SOURCEDIR=source
-set BUILDDIR=build
-
-if "%1" == "" goto help
-
-%SPHINXBUILD% >NUL 2>NUL
-if errorlevel 9009 (
- echo.
- echo.The 'sphinx-build' command was not found. Make sure you have Sphinx
- echo.installed, then set the SPHINXBUILD environment variable to point
- echo.to the full path of the 'sphinx-build' executable. Alternatively you
- echo.may add the Sphinx directory to PATH.
- echo.
- echo.If you don't have Sphinx installed, grab it from
- echo.http://sphinx-doc.org/
- exit /b 1
-)
-
-%SPHINXBUILD% -M %1 %SOURCEDIR% %BUILDDIR% %SPHINXOPTS%
-goto end
-
-:help
-%SPHINXBUILD% -M help %SOURCEDIR% %BUILDDIR% %SPHINXOPTS%
-
-:end
-popd
diff --git a/spaces/AIGText/GlyphControl/scripts/rendertext_tool.py b/spaces/AIGText/GlyphControl/scripts/rendertext_tool.py
deleted file mode 100644
index 198d8714c7b063c8fd915ab5d98be64419463cd0..0000000000000000000000000000000000000000
--- a/spaces/AIGText/GlyphControl/scripts/rendertext_tool.py
+++ /dev/null
@@ -1,206 +0,0 @@
-from cldm.ddim_hacked import DDIMSampler
-import torch
-from annotator.render_images import render_text_image_custom
-from pytorch_lightning import seed_everything
-# save_memory = False
-# from cldm.hack import disable_verbosity
-# disable_verbosity()
-import random
-import einops
-import numpy as np
-from ldm.util import instantiate_from_config
-from cldm.model import load_state_dict
-from torchvision.transforms import ToTensor
-from contextlib import nullcontext
-
-def load_model_from_config(cfg, ckpt, verbose=False, not_use_ckpt=False):
-
- # if "model_ema.input_blocks10in_layers0weight" not in sd:
- # print("missing model_ema.input_blocks10in_layers0weight. set use_ema as False")
- # cfg.model.params.use_ema = False
- model = instantiate_from_config(cfg.model)
-
- if ckpt.endswith("model_states.pt"):
- sd = torch.load(ckpt, map_location='cpu')["module"]
- else:
- sd = load_state_dict(ckpt, location='cpu')
-
- keys_ = list(sd.keys())[:]
- for k in keys_:
- if k.startswith("module."):
- nk = k[7:]
- sd[nk] = sd[k]
- del sd[k]
-
- if not not_use_ckpt:
- m, u = model.load_state_dict(sd, strict=False)
- if len(m) > 0 and verbose:
- print("missing keys: {}".format(len(m)))
- print(m)
- if len(u) > 0 and verbose:
- print("unexpected keys: {}".format(len(u)))
- print(u)
-
- if torch.cuda.is_available():
- model.cuda()
- model.eval()
- return model
-
-def load_model_ckpt(model, ckpt, verbose=True):
- map_location = "cpu" if not torch.cuda.is_available() else "cuda"
- print("checkpoint map location:", map_location)
- if ckpt.endswith("model_states.pt"):
- sd = torch.load(ckpt, map_location=map_location)["module"]
- else:
- sd = load_state_dict(ckpt, location=map_location)
-
- keys_ = list(sd.keys())[:]
- for k in keys_:
- if k.startswith("module."):
- nk = k[7:]
- sd[nk] = sd[k]
- del sd[k]
-
- m, u = model.load_state_dict(sd, strict=False)
- if len(m) > 0 and verbose:
- print("missing keys: {}".format(len(m)))
- print(m)
- if len(u) > 0 and verbose:
- print("unexpected keys: {}".format(len(u)))
- print(u)
- model.eval()
- return model
-
-class Render_Text:
- def __init__(self,
- model,
- precision_scope=nullcontext,
- transform=ToTensor(),
- save_memory = False,
- ):
- self.model = model
- self.precision_scope = precision_scope
- self.transform = transform
- self.ddim_sampler = DDIMSampler(model)
- self.save_memory = save_memory
-
- # process multiple groups of rendered text for building demo
- def process_multi(self,
- rendered_txt_values, shared_prompt,
- width_values, ratio_values,
- top_left_x_values, top_left_y_values,
- yaw_values, num_rows_values,
- shared_num_samples, shared_image_resolution,
- shared_ddim_steps, shared_guess_mode,
- shared_strength, shared_scale, shared_seed,
- shared_eta, shared_a_prompt, shared_n_prompt,
- only_show_rendered_image=False
- ):
- if shared_seed == -1:
- shared_seed = random.randint(0, 65535)
- seed_everything(shared_seed)
- with torch.no_grad(), \
- self.precision_scope("cuda"), \
- self.model.ema_scope("Sampling on Benchmark Prompts"):
- print("rendered txt:", str(rendered_txt_values), "[t]")
- render_none = len([1 for rendered_txt in rendered_txt_values if rendered_txt != ""]) == 0
- if render_none:
- # if rendered_txt_values == "":
- control = None
- if only_show_rendered_image:
- return [None]
- else:
- def format_bboxes(width_values, ratio_values, top_left_x_values, top_left_y_values, yaw_values):
- bboxes = []
- for width, ratio, top_left_x, top_left_y, yaw in zip(width_values, ratio_values, top_left_x_values, top_left_y_values, yaw_values):
- bbox = {
- "width": width,
- "ratio": ratio,
- # "height": height,
- "top_left_x": top_left_x,
- "top_left_y": top_left_y,
- "yaw": yaw
- }
- bboxes.append(bbox)
- return bboxes
-
- whiteboard_img = render_text_image_custom(
- (shared_image_resolution, shared_image_resolution),
- format_bboxes(width_values, ratio_values, top_left_x_values, top_left_y_values, yaw_values),
- rendered_txt_values,
- num_rows_values
- )
- whiteboard_img = whiteboard_img.convert("RGB")
-
- if only_show_rendered_image:
- return [whiteboard_img]
-
- control = self.transform(whiteboard_img.copy())
- if torch.cuda.is_available():
- control = control.cuda()
- control = torch.stack([control for _ in range(shared_num_samples)], dim=0)
- control = control.clone()
- control = [control]
-
- H, W = shared_image_resolution, shared_image_resolution
-
- # if shared_seed == -1:
- # shared_seed = random.randint(0, 65535)
- # seed_everything(shared_seed)
-
- if torch.cuda.is_available() and self.save_memory:
- print("low_vram_shift: is_diffusing", False)
- self.model.low_vram_shift(is_diffusing=False)
-
- print("control is None: {}".format(control is None))
- if shared_prompt.endswith("."):
- if shared_a_prompt == "":
- c_prompt = shared_prompt
- else:
- c_prompt = shared_prompt + " " + shared_a_prompt
- elif shared_prompt.endswith(","):
- if shared_a_prompt == "":
- c_prompt = shared_prompt[:-1] + "."
- else:
- c_prompt = shared_prompt + " " + shared_a_prompt
- else:
- if shared_a_prompt == "":
- c_prompt = shared_prompt + "."
- else:
- c_prompt = shared_prompt + ", " + shared_a_prompt
-
- # cond_c_cross = self.model.get_learned_conditioning([shared_prompt + ', ' + shared_a_prompt] * shared_num_samples)
- cond_c_cross = self.model.get_learned_conditioning([c_prompt] * shared_num_samples)
- print("prompt:", c_prompt)
- un_cond_cross = self.model.get_learned_conditioning([shared_n_prompt] * shared_num_samples)
-
- if torch.cuda.is_available() and self.save_memory:
- print("low_vram_shift: is_diffusing", True)
- self.model.low_vram_shift(is_diffusing=True)
-
- cond = {"c_concat": control, "c_crossattn": [cond_c_cross] if not isinstance(cond_c_cross, list) else cond_c_cross}
- un_cond = {"c_concat": None if shared_guess_mode else control, "c_crossattn": [un_cond_cross] if not isinstance(un_cond_cross, list) else un_cond_cross}
- shape = (4, H // 8, W // 8)
-
- if not self.model.learnable_conscale:
- self.model.control_scales = [shared_strength * (0.825 ** float(12 - i)) for i in range(13)] if shared_guess_mode else ([shared_strength] * 13) # Magic number. IDK why. Perhaps because 0.825**12<0.01 but 0.826**12>0.01
- else:
- print("learned control scale: {}".format(str(self.model.control_scales)))
- samples, intermediates = self.ddim_sampler.sample(shared_ddim_steps, shared_num_samples,
- shape, cond, verbose=False, eta=shared_eta,
- unconditional_guidance_scale=shared_scale,
- unconditional_conditioning=un_cond)
- if torch.cuda.is_available() and self.save_memory:
- print("low_vram_shift: is_diffusing", False)
- self.model.low_vram_shift(is_diffusing=False)
-
- x_samples = self.model.decode_first_stage(samples)
- x_samples = (einops.rearrange(x_samples, 'b c h w -> b h w c') * 127.5 + 127.5).cpu().numpy().clip(0, 255).astype(np.uint8)
-
- results = [x_samples[i] for i in range(shared_num_samples)]
- # if rendered_txt_values != "":
- if not render_none:
- return [whiteboard_img] + results
- else:
- return results
-
\ No newline at end of file
diff --git a/spaces/Abhilashvj/planogram-compliance/utils/plots.py b/spaces/Abhilashvj/planogram-compliance/utils/plots.py
deleted file mode 100644
index 3a1c4f69777158365c1db061bbb56a17d1eaf727..0000000000000000000000000000000000000000
--- a/spaces/Abhilashvj/planogram-compliance/utils/plots.py
+++ /dev/null
@@ -1,781 +0,0 @@
-# YOLOv5 🚀 by Ultralytics, GPL-3.0 license
-"""
-Plotting utils
-"""
-
-import contextlib
-import math
-import os
-from copy import copy
-from pathlib import Path
-from urllib.error import URLError
-
-import cv2
-import matplotlib
-import matplotlib.pyplot as plt
-import numpy as np
-import pandas as pd
-import seaborn as sn
-import torch
-from PIL import Image, ImageDraw, ImageFont
-
-from utils import TryExcept, threaded
-from utils.general import (
- CONFIG_DIR,
- FONT,
- LOGGER,
- check_font,
- check_requirements,
- clip_boxes,
- increment_path,
- is_ascii,
- xywh2xyxy,
- xyxy2xywh,
-)
-from utils.metrics import fitness
-from utils.segment.general import scale_image
-
-# Settings
-RANK = int(os.getenv("RANK", -1))
-matplotlib.rc("font", **{"size": 11})
-matplotlib.use("Agg") # for writing to files only
-
-
-class Colors:
- # Ultralytics color palette https://ultralytics.com/
- def __init__(self):
- # hex = matplotlib.colors.TABLEAU_COLORS.values()
- hexs = (
- "FF3838",
- "FF9D97",
- "FF701F",
- "FFB21D",
- "CFD231",
- "48F90A",
- "92CC17",
- "3DDB86",
- "1A9334",
- "00D4BB",
- "2C99A8",
- "00C2FF",
- "344593",
- "6473FF",
- "0018EC",
- "8438FF",
- "520085",
- "CB38FF",
- "FF95C8",
- "FF37C7",
- )
- self.palette = [self.hex2rgb(f"#{c}") for c in hexs]
- self.n = len(self.palette)
-
- def __call__(self, i, bgr=False):
- c = self.palette[int(i) % self.n]
- return (c[2], c[1], c[0]) if bgr else c
-
- @staticmethod
- def hex2rgb(h): # rgb order (PIL)
- return tuple(int(h[1 + i : 1 + i + 2], 16) for i in (0, 2, 4))
-
-
-colors = Colors() # create instance for 'from utils.plots import colors'
-
-
-def check_pil_font(font=FONT, size=10):
- # Return a PIL TrueType Font, downloading to CONFIG_DIR if necessary
- font = Path(font)
- font = font if font.exists() else (CONFIG_DIR / font.name)
- try:
- return ImageFont.truetype(
- str(font) if font.exists() else font.name, size
- )
- except Exception: # download if missing
- try:
- check_font(font)
- return ImageFont.truetype(str(font), size)
- except TypeError:
- check_requirements(
- "Pillow>=8.4.0"
- ) # known issue https://github.com/ultralytics/yolov5/issues/5374
- except URLError: # not online
- return ImageFont.load_default()
-
-
-class Annotator:
- # YOLOv5 Annotator for train/val mosaics and jpgs and detect/hub inference annotations
- def __init__(
- self,
- im,
- line_width=None,
- font_size=None,
- font="Arial.ttf",
- pil=False,
- example="abc",
- ):
- assert (
- im.data.contiguous
- ), "Image not contiguous. Apply np.ascontiguousarray(im) to Annotator() input images."
- non_ascii = not is_ascii(
- example
- ) # non-latin labels, i.e. asian, arabic, cyrillic
- self.pil = pil or non_ascii
- if self.pil: # use PIL
- self.im = (
- im if isinstance(im, Image.Image) else Image.fromarray(im)
- )
- self.draw = ImageDraw.Draw(self.im)
- self.font = check_pil_font(
- font="Arial.Unicode.ttf" if non_ascii else font,
- size=font_size
- or max(round(sum(self.im.size) / 2 * 0.035), 12),
- )
- else: # use cv2
- self.im = im
- self.lw = line_width or max(
- round(sum(im.shape) / 2 * 0.003), 2
- ) # line width
-
- def box_label(
- self, box, label="", color=(128, 128, 128), txt_color=(255, 255, 255)
- ):
- # Add one xyxy box to image with label
- if self.pil or not is_ascii(label):
- self.draw.rectangle(box, width=self.lw, outline=color) # box
- if label:
- w, h = self.font.getsize(label) # text width, height
- outside = box[1] - h >= 0 # label fits outside box
- self.draw.rectangle(
- (
- box[0],
- box[1] - h if outside else box[1],
- box[0] + w + 1,
- box[1] + 1 if outside else box[1] + h + 1,
- ),
- fill=color,
- )
- # self.draw.text((box[0], box[1]), label, fill=txt_color, font=self.font, anchor='ls') # for PIL>8.0
- self.draw.text(
- (box[0], box[1] - h if outside else box[1]),
- label,
- fill=txt_color,
- font=self.font,
- )
- else: # cv2
- p1, p2 = (int(box[0]), int(box[1])), (int(box[2]), int(box[3]))
- cv2.rectangle(
- self.im, p1, p2, color, thickness=self.lw, lineType=cv2.LINE_AA
- )
- if label:
- tf = max(self.lw - 1, 1) # font thickness
- w, h = cv2.getTextSize(
- label, 0, fontScale=self.lw / 3, thickness=tf
- )[
- 0
- ] # text width, height
- outside = p1[1] - h >= 3
- p2 = p1[0] + w, p1[1] - h - 3 if outside else p1[1] + h + 3
- cv2.rectangle(
- self.im, p1, p2, color, -1, cv2.LINE_AA
- ) # filled
- cv2.putText(
- self.im,
- label,
- (p1[0], p1[1] - 2 if outside else p1[1] + h + 2),
- 0,
- self.lw / 3,
- txt_color,
- thickness=tf,
- lineType=cv2.LINE_AA,
- )
-
- def masks(self, masks, colors, im_gpu, alpha=0.5, retina_masks=False):
- """Plot masks at once.
- Args:
- masks (tensor): predicted masks on cuda, shape: [n, h, w]
- colors (List[List[Int]]): colors for predicted masks, [[r, g, b] * n]
- im_gpu (tensor): img is in cuda, shape: [3, h, w], range: [0, 1]
- alpha (float): mask transparency: 0.0 fully transparent, 1.0 opaque
- """
- if self.pil:
- # convert to numpy first
- self.im = np.asarray(self.im).copy()
- if len(masks) == 0:
- self.im[:] = (
- im_gpu.permute(1, 2, 0).contiguous().cpu().numpy() * 255
- )
- colors = (
- torch.tensor(colors, device=im_gpu.device, dtype=torch.float32)
- / 255.0
- )
- colors = colors[:, None, None] # shape(n,1,1,3)
- masks = masks.unsqueeze(3) # shape(n,h,w,1)
- masks_color = masks * (colors * alpha) # shape(n,h,w,3)
-
- inv_alph_masks = (1 - masks * alpha).cumprod(0) # shape(n,h,w,1)
- mcs = (masks_color * inv_alph_masks).sum(
- 0
- ) * 2 # mask color summand shape(n,h,w,3)
-
- im_gpu = im_gpu.flip(dims=[0]) # flip channel
- im_gpu = im_gpu.permute(1, 2, 0).contiguous() # shape(h,w,3)
- im_gpu = im_gpu * inv_alph_masks[-1] + mcs
- im_mask = (im_gpu * 255).byte().cpu().numpy()
- self.im[:] = (
- im_mask
- if retina_masks
- else scale_image(im_gpu.shape, im_mask, self.im.shape)
- )
- if self.pil:
- # convert im back to PIL and update draw
- self.fromarray(self.im)
-
- def rectangle(self, xy, fill=None, outline=None, width=1):
- # Add rectangle to image (PIL-only)
- self.draw.rectangle(xy, fill, outline, width)
-
- def text(self, xy, text, txt_color=(255, 255, 255), anchor="top"):
- # Add text to image (PIL-only)
- if anchor == "bottom": # start y from font bottom
- w, h = self.font.getsize(text) # text width, height
- xy[1] += 1 - h
- self.draw.text(xy, text, fill=txt_color, font=self.font)
-
- def fromarray(self, im):
- # Update self.im from a numpy array
- self.im = im if isinstance(im, Image.Image) else Image.fromarray(im)
- self.draw = ImageDraw.Draw(self.im)
-
- def result(self):
- # Return annotated image as array
- return np.asarray(self.im)
-
-
-def feature_visualization(
- x, module_type, stage, n=32, save_dir=Path("runs/detect/exp")
-):
- """
- x: Features to be visualized
- module_type: Module type
- stage: Module stage within model
- n: Maximum number of feature maps to plot
- save_dir: Directory to save results
- """
- if "Detect" not in module_type:
- (
- batch,
- channels,
- height,
- width,
- ) = x.shape # batch, channels, height, width
- if height > 1 and width > 1:
- f = (
- save_dir
- / f"stage{stage}_{module_type.split('.')[-1]}_features.png"
- ) # filename
-
- blocks = torch.chunk(
- x[0].cpu(), channels, dim=0
- ) # select batch index 0, block by channels
- n = min(n, channels) # number of plots
- fig, ax = plt.subplots(
- math.ceil(n / 8), 8, tight_layout=True
- ) # 8 rows x n/8 cols
- ax = ax.ravel()
- plt.subplots_adjust(wspace=0.05, hspace=0.05)
- for i in range(n):
- ax[i].imshow(blocks[i].squeeze()) # cmap='gray'
- ax[i].axis("off")
-
- LOGGER.info(f"Saving {f}... ({n}/{channels})")
- plt.savefig(f, dpi=300, bbox_inches="tight")
- plt.close()
- np.save(str(f.with_suffix(".npy")), x[0].cpu().numpy()) # npy save
-
-
-def hist2d(x, y, n=100):
- # 2d histogram used in labels.png and evolve.png
- xedges, yedges = np.linspace(x.min(), x.max(), n), np.linspace(
- y.min(), y.max(), n
- )
- hist, xedges, yedges = np.histogram2d(x, y, (xedges, yedges))
- xidx = np.clip(np.digitize(x, xedges) - 1, 0, hist.shape[0] - 1)
- yidx = np.clip(np.digitize(y, yedges) - 1, 0, hist.shape[1] - 1)
- return np.log(hist[xidx, yidx])
-
-
-def butter_lowpass_filtfilt(data, cutoff=1500, fs=50000, order=5):
- from scipy.signal import butter, filtfilt
-
- # https://stackoverflow.com/questions/28536191/how-to-filter-smooth-with-scipy-numpy
- def butter_lowpass(cutoff, fs, order):
- nyq = 0.5 * fs
- normal_cutoff = cutoff / nyq
- return butter(order, normal_cutoff, btype="low", analog=False)
-
- b, a = butter_lowpass(cutoff, fs, order=order)
- return filtfilt(b, a, data) # forward-backward filter
-
-
-def output_to_target(output, max_det=300):
- # Convert model output to target format [batch_id, class_id, x, y, w, h, conf] for plotting
- targets = []
- for i, o in enumerate(output):
- box, conf, cls = o[:max_det, :6].cpu().split((4, 1, 1), 1)
- j = torch.full((conf.shape[0], 1), i)
- targets.append(torch.cat((j, cls, xyxy2xywh(box), conf), 1))
- return torch.cat(targets, 0).numpy()
-
-
-@threaded
-def plot_images(images, targets, paths=None, fname="images.jpg", names=None):
- # Plot image grid with labels
- if isinstance(images, torch.Tensor):
- images = images.cpu().float().numpy()
- if isinstance(targets, torch.Tensor):
- targets = targets.cpu().numpy()
-
- max_size = 1920 # max image size
- max_subplots = 16 # max image subplots, i.e. 4x4
- bs, _, h, w = images.shape # batch size, _, height, width
- bs = min(bs, max_subplots) # limit plot images
- ns = np.ceil(bs**0.5) # number of subplots (square)
- if np.max(images[0]) <= 1:
- images *= 255 # de-normalise (optional)
-
- # Build Image
- mosaic = np.full(
- (int(ns * h), int(ns * w), 3), 255, dtype=np.uint8
- ) # init
- for i, im in enumerate(images):
- if i == max_subplots: # if last batch has fewer images than we expect
- break
- x, y = int(w * (i // ns)), int(h * (i % ns)) # block origin
- im = im.transpose(1, 2, 0)
- mosaic[y : y + h, x : x + w, :] = im
-
- # Resize (optional)
- scale = max_size / ns / max(h, w)
- if scale < 1:
- h = math.ceil(scale * h)
- w = math.ceil(scale * w)
- mosaic = cv2.resize(mosaic, tuple(int(x * ns) for x in (w, h)))
-
- # Annotate
- fs = int((h + w) * ns * 0.01) # font size
- annotator = Annotator(
- mosaic,
- line_width=round(fs / 10),
- font_size=fs,
- pil=True,
- example=names,
- )
- for i in range(i + 1):
- x, y = int(w * (i // ns)), int(h * (i % ns)) # block origin
- annotator.rectangle(
- [x, y, x + w, y + h], None, (255, 255, 255), width=2
- ) # borders
- if paths:
- annotator.text(
- (x + 5, y + 5),
- text=Path(paths[i]).name[:40],
- txt_color=(220, 220, 220),
- ) # filenames
- if len(targets) > 0:
- ti = targets[targets[:, 0] == i] # image targets
- boxes = xywh2xyxy(ti[:, 2:6]).T
- classes = ti[:, 1].astype("int")
- labels = ti.shape[1] == 6 # labels if no conf column
- conf = (
- None if labels else ti[:, 6]
- ) # check for confidence presence (label vs pred)
-
- if boxes.shape[1]:
- if boxes.max() <= 1.01: # if normalized with tolerance 0.01
- boxes[[0, 2]] *= w # scale to pixels
- boxes[[1, 3]] *= h
- elif scale < 1: # absolute coords need scale if image scales
- boxes *= scale
- boxes[[0, 2]] += x
- boxes[[1, 3]] += y
- for j, box in enumerate(boxes.T.tolist()):
- cls = classes[j]
- color = colors(cls)
- cls = names[cls] if names else cls
- if labels or conf[j] > 0.25: # 0.25 conf thresh
- label = f"{cls}" if labels else f"{cls} {conf[j]:.1f}"
- annotator.box_label(box, label, color=color)
- annotator.im.save(fname) # save
-
-
-def plot_lr_scheduler(optimizer, scheduler, epochs=300, save_dir=""):
- # Plot LR simulating training for full epochs
- optimizer, scheduler = copy(optimizer), copy(
- scheduler
- ) # do not modify originals
- y = []
- for _ in range(epochs):
- scheduler.step()
- y.append(optimizer.param_groups[0]["lr"])
- plt.plot(y, ".-", label="LR")
- plt.xlabel("epoch")
- plt.ylabel("LR")
- plt.grid()
- plt.xlim(0, epochs)
- plt.ylim(0)
- plt.savefig(Path(save_dir) / "LR.png", dpi=200)
- plt.close()
-
-
-def plot_val_txt(): # from utils.plots import *; plot_val()
- # Plot val.txt histograms
- x = np.loadtxt("val.txt", dtype=np.float32)
- box = xyxy2xywh(x[:, :4])
- cx, cy = box[:, 0], box[:, 1]
-
- fig, ax = plt.subplots(1, 1, figsize=(6, 6), tight_layout=True)
- ax.hist2d(cx, cy, bins=600, cmax=10, cmin=0)
- ax.set_aspect("equal")
- plt.savefig("hist2d.png", dpi=300)
-
- fig, ax = plt.subplots(1, 2, figsize=(12, 6), tight_layout=True)
- ax[0].hist(cx, bins=600)
- ax[1].hist(cy, bins=600)
- plt.savefig("hist1d.png", dpi=200)
-
-
-def plot_targets_txt(): # from utils.plots import *; plot_targets_txt()
- # Plot targets.txt histograms
- x = np.loadtxt("targets.txt", dtype=np.float32).T
- s = ["x targets", "y targets", "width targets", "height targets"]
- fig, ax = plt.subplots(2, 2, figsize=(8, 8), tight_layout=True)
- ax = ax.ravel()
- for i in range(4):
- ax[i].hist(
- x[i], bins=100, label=f"{x[i].mean():.3g} +/- {x[i].std():.3g}"
- )
- ax[i].legend()
- ax[i].set_title(s[i])
- plt.savefig("targets.jpg", dpi=200)
-
-
-def plot_val_study(
- file="", dir="", x=None
-): # from utils.plots import *; plot_val_study()
- # Plot file=study.txt generated by val.py (or plot all study*.txt in dir)
- save_dir = Path(file).parent if file else Path(dir)
- plot2 = False # plot additional results
- if plot2:
- ax = plt.subplots(2, 4, figsize=(10, 6), tight_layout=True)[1].ravel()
-
- fig2, ax2 = plt.subplots(1, 1, figsize=(8, 4), tight_layout=True)
- # for f in [save_dir / f'study_coco_{x}.txt' for x in ['yolov5n6', 'yolov5s6', 'yolov5m6', 'yolov5l6', 'yolov5x6']]:
- for f in sorted(save_dir.glob("study*.txt")):
- y = np.loadtxt(
- f, dtype=np.float32, usecols=[0, 1, 2, 3, 7, 8, 9], ndmin=2
- ).T
- x = np.arange(y.shape[1]) if x is None else np.array(x)
- if plot2:
- s = [
- "P",
- "R",
- "mAP@.5",
- "mAP@.5:.95",
- "t_preprocess (ms/img)",
- "t_inference (ms/img)",
- "t_NMS (ms/img)",
- ]
- for i in range(7):
- ax[i].plot(x, y[i], ".-", linewidth=2, markersize=8)
- ax[i].set_title(s[i])
-
- j = y[3].argmax() + 1
- ax2.plot(
- y[5, 1:j],
- y[3, 1:j] * 1e2,
- ".-",
- linewidth=2,
- markersize=8,
- label=f.stem.replace("study_coco_", "").replace("yolo", "YOLO"),
- )
-
- ax2.plot(
- 1e3 / np.array([209, 140, 97, 58, 35, 18]),
- [34.6, 40.5, 43.0, 47.5, 49.7, 51.5],
- "k.-",
- linewidth=2,
- markersize=8,
- alpha=0.25,
- label="EfficientDet",
- )
-
- ax2.grid(alpha=0.2)
- ax2.set_yticks(np.arange(20, 60, 5))
- ax2.set_xlim(0, 57)
- ax2.set_ylim(25, 55)
- ax2.set_xlabel("GPU Speed (ms/img)")
- ax2.set_ylabel("COCO AP val")
- ax2.legend(loc="lower right")
- f = save_dir / "study.png"
- print(f"Saving {f}...")
- plt.savefig(f, dpi=300)
-
-
-@TryExcept() # known issue https://github.com/ultralytics/yolov5/issues/5395
-def plot_labels(labels, names=(), save_dir=Path("")):
- # plot dataset labels
- LOGGER.info(f"Plotting labels to {save_dir / 'labels.jpg'}... ")
- c, b = labels[:, 0], labels[:, 1:].transpose() # classes, boxes
- nc = int(c.max() + 1) # number of classes
- x = pd.DataFrame(b.transpose(), columns=["x", "y", "width", "height"])
-
- # seaborn correlogram
- sn.pairplot(
- x,
- corner=True,
- diag_kind="auto",
- kind="hist",
- diag_kws=dict(bins=50),
- plot_kws=dict(pmax=0.9),
- )
- plt.savefig(save_dir / "labels_correlogram.jpg", dpi=200)
- plt.close()
-
- # matplotlib labels
- matplotlib.use("svg") # faster
- ax = plt.subplots(2, 2, figsize=(8, 8), tight_layout=True)[1].ravel()
- y = ax[0].hist(c, bins=np.linspace(0, nc, nc + 1) - 0.5, rwidth=0.8)
- with contextlib.suppress(Exception): # color histogram bars by class
- [
- y[2].patches[i].set_color([x / 255 for x in colors(i)])
- for i in range(nc)
- ] # known issue #3195
- ax[0].set_ylabel("instances")
- if 0 < len(names) < 30:
- ax[0].set_xticks(range(len(names)))
- ax[0].set_xticklabels(list(names.values()), rotation=90, fontsize=10)
- else:
- ax[0].set_xlabel("classes")
- sn.histplot(x, x="x", y="y", ax=ax[2], bins=50, pmax=0.9)
- sn.histplot(x, x="width", y="height", ax=ax[3], bins=50, pmax=0.9)
-
- # rectangles
- labels[:, 1:3] = 0.5 # center
- labels[:, 1:] = xywh2xyxy(labels[:, 1:]) * 2000
- img = Image.fromarray(np.ones((2000, 2000, 3), dtype=np.uint8) * 255)
- for cls, *box in labels[:1000]:
- ImageDraw.Draw(img).rectangle(
- box, width=1, outline=colors(cls)
- ) # plot
- ax[1].imshow(img)
- ax[1].axis("off")
-
- for a in [0, 1, 2, 3]:
- for s in ["top", "right", "left", "bottom"]:
- ax[a].spines[s].set_visible(False)
-
- plt.savefig(save_dir / "labels.jpg", dpi=200)
- matplotlib.use("Agg")
- plt.close()
-
-
-def imshow_cls(
- im,
- labels=None,
- pred=None,
- names=None,
- nmax=25,
- verbose=False,
- f=Path("images.jpg"),
-):
- # Show classification image grid with labels (optional) and predictions (optional)
- from utils.augmentations import denormalize
-
- names = names or [f"class{i}" for i in range(1000)]
- blocks = torch.chunk(
- denormalize(im.clone()).cpu().float(), len(im), dim=0
- ) # select batch index 0, block by channels
- n = min(len(blocks), nmax) # number of plots
- m = min(8, round(n**0.5)) # 8 x 8 default
- fig, ax = plt.subplots(math.ceil(n / m), m) # 8 rows x n/8 cols
- ax = ax.ravel() if m > 1 else [ax]
- # plt.subplots_adjust(wspace=0.05, hspace=0.05)
- for i in range(n):
- ax[i].imshow(
- blocks[i].squeeze().permute((1, 2, 0)).numpy().clip(0.0, 1.0)
- )
- ax[i].axis("off")
- if labels is not None:
- s = names[labels[i]] + (
- f"—{names[pred[i]]}" if pred is not None else ""
- )
- ax[i].set_title(s, fontsize=8, verticalalignment="top")
- plt.savefig(f, dpi=300, bbox_inches="tight")
- plt.close()
- if verbose:
- LOGGER.info(f"Saving {f}")
- if labels is not None:
- LOGGER.info(
- "True: "
- + " ".join(f"{names[i]:3s}" for i in labels[:nmax])
- )
- if pred is not None:
- LOGGER.info(
- "Predicted:" + " ".join(f"{names[i]:3s}" for i in pred[:nmax])
- )
- return f
-
-
-def plot_evolve(
- evolve_csv="path/to/evolve.csv",
-): # from utils.plots import *; plot_evolve()
- # Plot evolve.csv hyp evolution results
- evolve_csv = Path(evolve_csv)
- data = pd.read_csv(evolve_csv)
- keys = [x.strip() for x in data.columns]
- x = data.values
- f = fitness(x)
- j = np.argmax(f) # max fitness index
- plt.figure(figsize=(10, 12), tight_layout=True)
- matplotlib.rc("font", **{"size": 8})
- print(f"Best results from row {j} of {evolve_csv}:")
- for i, k in enumerate(keys[7:]):
- v = x[:, 7 + i]
- mu = v[j] # best single result
- plt.subplot(6, 5, i + 1)
- plt.scatter(
- v,
- f,
- c=hist2d(v, f, 20),
- cmap="viridis",
- alpha=0.8,
- edgecolors="none",
- )
- plt.plot(mu, f.max(), "k+", markersize=15)
- plt.title(
- f"{k} = {mu:.3g}", fontdict={"size": 9}
- ) # limit to 40 characters
- if i % 5 != 0:
- plt.yticks([])
- print(f"{k:>15}: {mu:.3g}")
- f = evolve_csv.with_suffix(".png") # filename
- plt.savefig(f, dpi=200)
- plt.close()
- print(f"Saved {f}")
-
-
-def plot_results(file="path/to/results.csv", dir=""):
- # Plot training results.csv. Usage: from utils.plots import *; plot_results('path/to/results.csv')
- save_dir = Path(file).parent if file else Path(dir)
- fig, ax = plt.subplots(2, 5, figsize=(12, 6), tight_layout=True)
- ax = ax.ravel()
- files = list(save_dir.glob("results*.csv"))
- assert len(
- files
- ), f"No results.csv files found in {save_dir.resolve()}, nothing to plot."
- for f in files:
- try:
- data = pd.read_csv(f)
- s = [x.strip() for x in data.columns]
- x = data.values[:, 0]
- for i, j in enumerate([1, 2, 3, 4, 5, 8, 9, 10, 6, 7]):
- y = data.values[:, j].astype("float")
- # y[y == 0] = np.nan # don't show zero values
- ax[i].plot(
- x, y, marker=".", label=f.stem, linewidth=2, markersize=8
- )
- ax[i].set_title(s[j], fontsize=12)
- # if j in [8, 9, 10]: # share train and val loss y axes
- # ax[i].get_shared_y_axes().join(ax[i], ax[i - 5])
- except Exception as e:
- LOGGER.info(f"Warning: Plotting error for {f}: {e}")
- ax[1].legend()
- fig.savefig(save_dir / "results.png", dpi=200)
- plt.close()
-
-
-def profile_idetection(start=0, stop=0, labels=(), save_dir=""):
- # Plot iDetection '*.txt' per-image logs. from utils.plots import *; profile_idetection()
- ax = plt.subplots(2, 4, figsize=(12, 6), tight_layout=True)[1].ravel()
- s = [
- "Images",
- "Free Storage (GB)",
- "RAM Usage (GB)",
- "Battery",
- "dt_raw (ms)",
- "dt_smooth (ms)",
- "real-world FPS",
- ]
- files = list(Path(save_dir).glob("frames*.txt"))
- for fi, f in enumerate(files):
- try:
- results = np.loadtxt(f, ndmin=2).T[
- :, 90:-30
- ] # clip first and last rows
- n = results.shape[1] # number of rows
- x = np.arange(start, min(stop, n) if stop else n)
- results = results[:, x]
- t = results[0] - results[0].min() # set t0=0s
- results[0] = x
- for i, a in enumerate(ax):
- if i < len(results):
- label = (
- labels[fi]
- if len(labels)
- else f.stem.replace("frames_", "")
- )
- a.plot(
- t,
- results[i],
- marker=".",
- label=label,
- linewidth=1,
- markersize=5,
- )
- a.set_title(s[i])
- a.set_xlabel("time (s)")
- # if fi == len(files) - 1:
- # a.set_ylim(bottom=0)
- for side in ["top", "right"]:
- a.spines[side].set_visible(False)
- else:
- a.remove()
- except Exception as e:
- print(f"Warning: Plotting error for {f}; {e}")
- ax[1].legend()
- plt.savefig(Path(save_dir) / "idetection_profile.png", dpi=200)
-
-
-def save_one_box(
- xyxy,
- im,
- file=Path("im.jpg"),
- gain=1.02,
- pad=10,
- square=False,
- BGR=False,
- save=True,
-):
- # Save image crop as {file} with crop size multiple {gain} and {pad} pixels. Save and/or return crop
- xyxy = torch.tensor(xyxy).view(-1, 4)
- b = xyxy2xywh(xyxy) # boxes
- if square:
- b[:, 2:] = (
- b[:, 2:].max(1)[0].unsqueeze(1)
- ) # attempt rectangle to square
- b[:, 2:] = b[:, 2:] * gain + pad # box wh * gain + pad
- xyxy = xywh2xyxy(b).long()
- clip_boxes(xyxy, im.shape)
- crop = im[
- int(xyxy[0, 1]) : int(xyxy[0, 3]),
- int(xyxy[0, 0]) : int(xyxy[0, 2]),
- :: (1 if BGR else -1),
- ]
- if save:
- file.parent.mkdir(parents=True, exist_ok=True) # make directory
- f = str(increment_path(file).with_suffix(".jpg"))
- # cv2.imwrite(f, crop) # save BGR, https://github.com/ultralytics/yolov5/issues/7007 chroma subsampling issue
- Image.fromarray(crop[..., ::-1]).save(
- f, quality=95, subsampling=0
- ) # save RGB
- return crop
diff --git a/spaces/AchyuthGamer/Free-Accounts-Generator/README.md b/spaces/AchyuthGamer/Free-Accounts-Generator/README.md
deleted file mode 100644
index 5b1c6794f1fdd6616af7f58cd8ebc5a6af0c25d2..0000000000000000000000000000000000000000
--- a/spaces/AchyuthGamer/Free-Accounts-Generator/README.md
+++ /dev/null
@@ -1,11 +0,0 @@
----
-title: Free Accounts Generator
-emoji: 🏢
-colorFrom: blue
-colorTo: purple
-sdk: static
-pinned: false
-license: mit
----
-
-Check out the configuration reference at https://huggingface.co/docs/hub/spaces-config-reference
diff --git a/spaces/AgentVerse/agentVerse/ui/src/phaser3-rex-plugins/plugins/scale.d.ts b/spaces/AgentVerse/agentVerse/ui/src/phaser3-rex-plugins/plugins/scale.d.ts
deleted file mode 100644
index a21bfca80b9f3727617264eba311df3f67b11986..0000000000000000000000000000000000000000
--- a/spaces/AgentVerse/agentVerse/ui/src/phaser3-rex-plugins/plugins/scale.d.ts
+++ /dev/null
@@ -1,2 +0,0 @@
-import Scale from './behaviors/scale/Scale';
-export default Scale;
\ No newline at end of file
diff --git a/spaces/AgentVerse/agentVerse/ui/src/phaser3-rex-plugins/templates/spinner/pie/Pie.d.ts b/spaces/AgentVerse/agentVerse/ui/src/phaser3-rex-plugins/templates/spinner/pie/Pie.d.ts
deleted file mode 100644
index 3cc4591ead8c6a9d9c178e8449dd84cb7d631572..0000000000000000000000000000000000000000
--- a/spaces/AgentVerse/agentVerse/ui/src/phaser3-rex-plugins/templates/spinner/pie/Pie.d.ts
+++ /dev/null
@@ -1,2 +0,0 @@
-import Base from '../base/Base';
-export default class Pie extends Base { }
\ No newline at end of file
diff --git a/spaces/AgentVerse/agentVerse/ui/src/phaser3-rex-plugins/templates/ui/basesizer/utils/CheckSize.js b/spaces/AgentVerse/agentVerse/ui/src/phaser3-rex-plugins/templates/ui/basesizer/utils/CheckSize.js
deleted file mode 100644
index cae321956abf23fdd008e7ffc326cee2faff3e26..0000000000000000000000000000000000000000
--- a/spaces/AgentVerse/agentVerse/ui/src/phaser3-rex-plugins/templates/ui/basesizer/utils/CheckSize.js
+++ /dev/null
@@ -1,12 +0,0 @@
-var CheckSize = function (child, parent) {
- if (child.width < child.childrenWidth) {
- // Warning
- console.warn(`Layout width error: Parent=${parent.constructor.name}, Child=${child.constructor.name}`);
- }
- if (child.height < child.childrenHeight) {
- // Warning
- console.warn(`Layout height error: Parent=${parent.constructor.name}, Child=${child.constructor.name}`);
- }
-}
-
-export default CheckSize;
\ No newline at end of file
diff --git a/spaces/AgentVerse/agentVerse/ui/src/phaser3-rex-plugins/templates/ui/imagebox/Factory.d.ts b/spaces/AgentVerse/agentVerse/ui/src/phaser3-rex-plugins/templates/ui/imagebox/Factory.d.ts
deleted file mode 100644
index c693cd4eaa24bb8c879a50ed253ac665a96157b4..0000000000000000000000000000000000000000
--- a/spaces/AgentVerse/agentVerse/ui/src/phaser3-rex-plugins/templates/ui/imagebox/Factory.d.ts
+++ /dev/null
@@ -1,7 +0,0 @@
-import ImageBox from './ImageBox';
-
-export default function (
- x?: number, y?: number,
- texture?: string, frame?: string,
- config?: ImageBox.IConfig
-): ImageBox;
\ No newline at end of file
diff --git a/spaces/AgentVerse/agentVerse/ui/src/phaser3-rex-plugins/templates/ui/perspectivecard/Factory.d.ts b/spaces/AgentVerse/agentVerse/ui/src/phaser3-rex-plugins/templates/ui/perspectivecard/Factory.d.ts
deleted file mode 100644
index 69a078a7a31a64e43da2e9c03f1a866628312573..0000000000000000000000000000000000000000
--- a/spaces/AgentVerse/agentVerse/ui/src/phaser3-rex-plugins/templates/ui/perspectivecard/Factory.d.ts
+++ /dev/null
@@ -1,5 +0,0 @@
-import PerspectiveCard from './PerspectiveCard';
-
-export default function (
- config?: PerspectiveCard.IConfig
-): PerspectiveCard;
\ No newline at end of file
diff --git a/spaces/Aki004/herta-so-vits/hubert/__init__.py b/spaces/Aki004/herta-so-vits/hubert/__init__.py
deleted file mode 100644
index e69de29bb2d1d6434b8b29ae775ad8c2e48c5391..0000000000000000000000000000000000000000
diff --git a/spaces/Alcedo/yunmedia/README.md b/spaces/Alcedo/yunmedia/README.md
deleted file mode 100644
index 5c0ea0dc30ce6b16d02d1201c5890bda924311e5..0000000000000000000000000000000000000000
--- a/spaces/Alcedo/yunmedia/README.md
+++ /dev/null
@@ -1,10 +0,0 @@
----
-title: Media
-emoji: 💫
-colorFrom: green
-colorTo: pink
-sdk: docker
-pinned: false
-license: mit
-app_port: 3000
----
\ No newline at end of file
diff --git a/spaces/Allakhazam/anythingV4/app.py b/spaces/Allakhazam/anythingV4/app.py
deleted file mode 100644
index 0555531820f093727ce3b98687ff53c0487cc802..0000000000000000000000000000000000000000
--- a/spaces/Allakhazam/anythingV4/app.py
+++ /dev/null
@@ -1,26 +0,0 @@
-import gradio
-
-model_interfaces = gradio.Interface.load("models/ckpt/anything-v4.0")
-
-def process_prompt(prompt):
- prompt=prompt.lower()
- print(prompt)
- image = model_interfaces(prompt)
- return image
-
-sandbox = gradio.Interface(
- fn=process_prompt,
- inputs=[gradio.Textbox(label="Enter Prompt:")],
- outputs=[gradio.Image(label="Produced Image")],
- title="Text to Image",
- examples=[["Female Adventurer portrait, rogue, tavern background"],
- ["female Adventurer portrait, barbarian, tavern background"],
- ["Magic Adventurer portrait, old wizard, tavern background"],
- ["Male superhero portrait, modern city, building background"],
- ["Magic Adventurer portrait, old wizard, fire elementalist, tavern background, fire"],
- ["Female Adventurer portrait, Druid, tavern background"],
- ["close up portrait Benedict Cumberbatch wizard of black magic, robe with hood, Hogwart University, castle tower background, oil painting on canvas"],
- ["Adventurer portrait, cleric, rogue looking stranger, tavern background"]]
-)
-
-sandbox.queue(concurrency_count=10).launch(debug=True)
diff --git a/spaces/Androidonnxfork/CivitAi-to-Diffusers/diffusers/docs/source/ko/using-diffusers/reusing_seeds.md b/spaces/Androidonnxfork/CivitAi-to-Diffusers/diffusers/docs/source/ko/using-diffusers/reusing_seeds.md
deleted file mode 100644
index 9ad27c3f2ac7f3bcda29f344420efef2c7588cd9..0000000000000000000000000000000000000000
--- a/spaces/Androidonnxfork/CivitAi-to-Diffusers/diffusers/docs/source/ko/using-diffusers/reusing_seeds.md
+++ /dev/null
@@ -1,63 +0,0 @@
-
-
-# Deterministic(결정적) 생성을 통한 이미지 품질 개선
-
-생성된 이미지의 품질을 개선하는 일반적인 방법은 *결정적 batch(배치) 생성*을 사용하는 것입니다. 이 방법은 이미지 batch(배치)를 생성하고 두 번째 추론 라운드에서 더 자세한 프롬프트와 함께 개선할 이미지 하나를 선택하는 것입니다. 핵심은 일괄 이미지 생성을 위해 파이프라인에 [`torch.Generator`](https://pytorch.org/docs/stable/generated/torch.Generator.html#generator) 목록을 전달하고, 각 `Generator`를 시드에 연결하여 이미지에 재사용할 수 있도록 하는 것입니다.
-
-예를 들어 [`runwayml/stable-diffusion-v1-5`](runwayml/stable-diffusion-v1-5)를 사용하여 다음 프롬프트의 여러 버전을 생성해 봅시다.
-
-```py
-prompt = "Labrador in the style of Vermeer"
-```
-
-(가능하다면) 파이프라인을 [`DiffusionPipeline.from_pretrained`]로 인스턴스화하여 GPU에 배치합니다.
-
-```python
->>> from diffusers import DiffusionPipeline
-
->>> pipe = DiffusionPipeline.from_pretrained("runwayml/stable-diffusion-v1-5", torch_dtype=torch.float16)
->>> pipe = pipe.to("cuda")
-```
-
-이제 네 개의 서로 다른 `Generator`를 정의하고 각 `Generator`에 시드(`0` ~ `3`)를 할당하여 나중에 특정 이미지에 대해 `Generator`를 재사용할 수 있도록 합니다.
-
-```python
->>> import torch
-
->>> generator = [torch.Generator(device="cuda").manual_seed(i) for i in range(4)]
-```
-
-이미지를 생성하고 살펴봅니다.
-
-```python
->>> images = pipe(prompt, generator=generator, num_images_per_prompt=4).images
->>> images
-```
-
-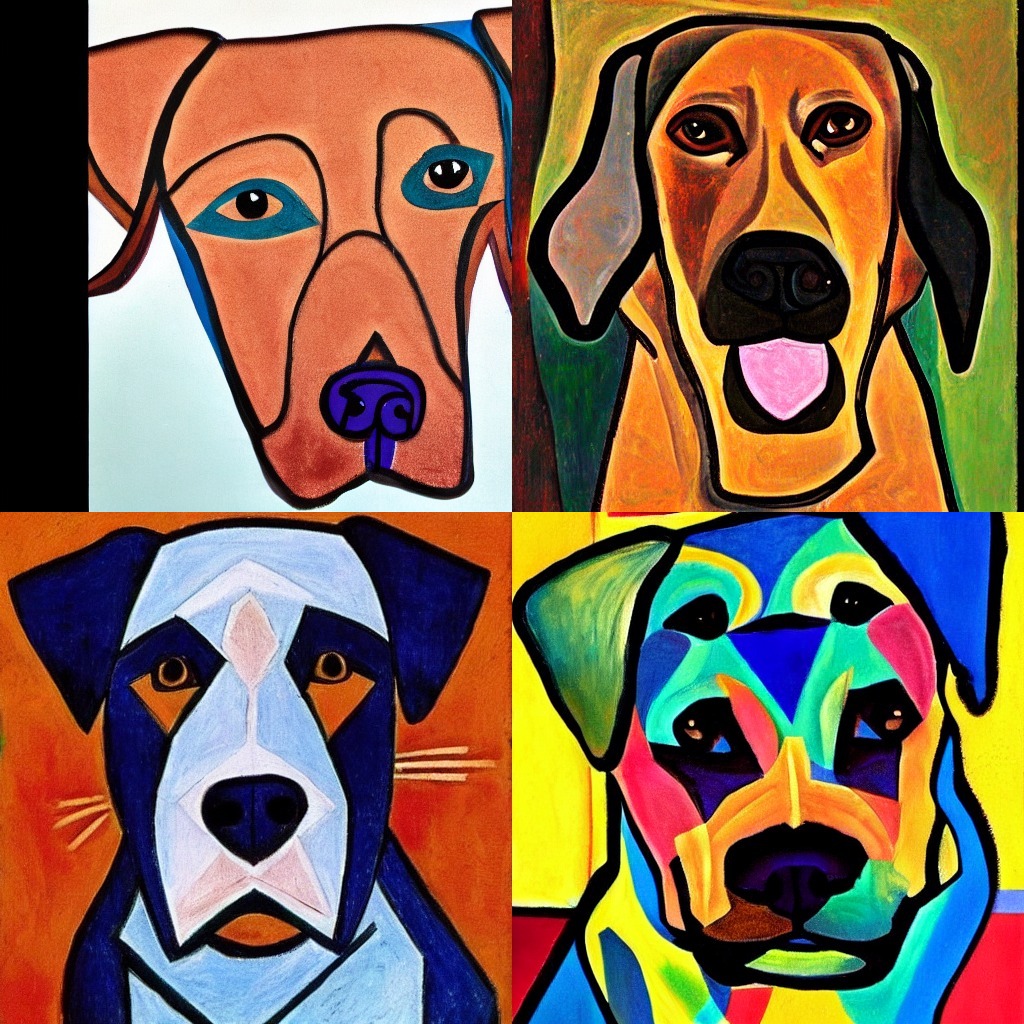
-
-이 예제에서는 첫 번째 이미지를 개선했지만 실제로는 원하는 모든 이미지를 사용할 수 있습니다(심지어 두 개의 눈이 있는 이미지도!). 첫 번째 이미지에서는 시드가 '0'인 '생성기'를 사용했기 때문에 두 번째 추론 라운드에서는 이 '생성기'를 재사용할 것입니다. 이미지의 품질을 개선하려면 프롬프트에 몇 가지 텍스트를 추가합니다:
-
-```python
-prompt = [prompt + t for t in [", highly realistic", ", artsy", ", trending", ", colorful"]]
-generator = [torch.Generator(device="cuda").manual_seed(0) for i in range(4)]
-```
-
-시드가 `0`인 제너레이터 4개를 생성하고, 이전 라운드의 첫 번째 이미지처럼 보이는 다른 이미지 batch(배치)를 생성합니다!
-
-```python
->>> images = pipe(prompt, generator=generator).images
->>> images
-```
-
-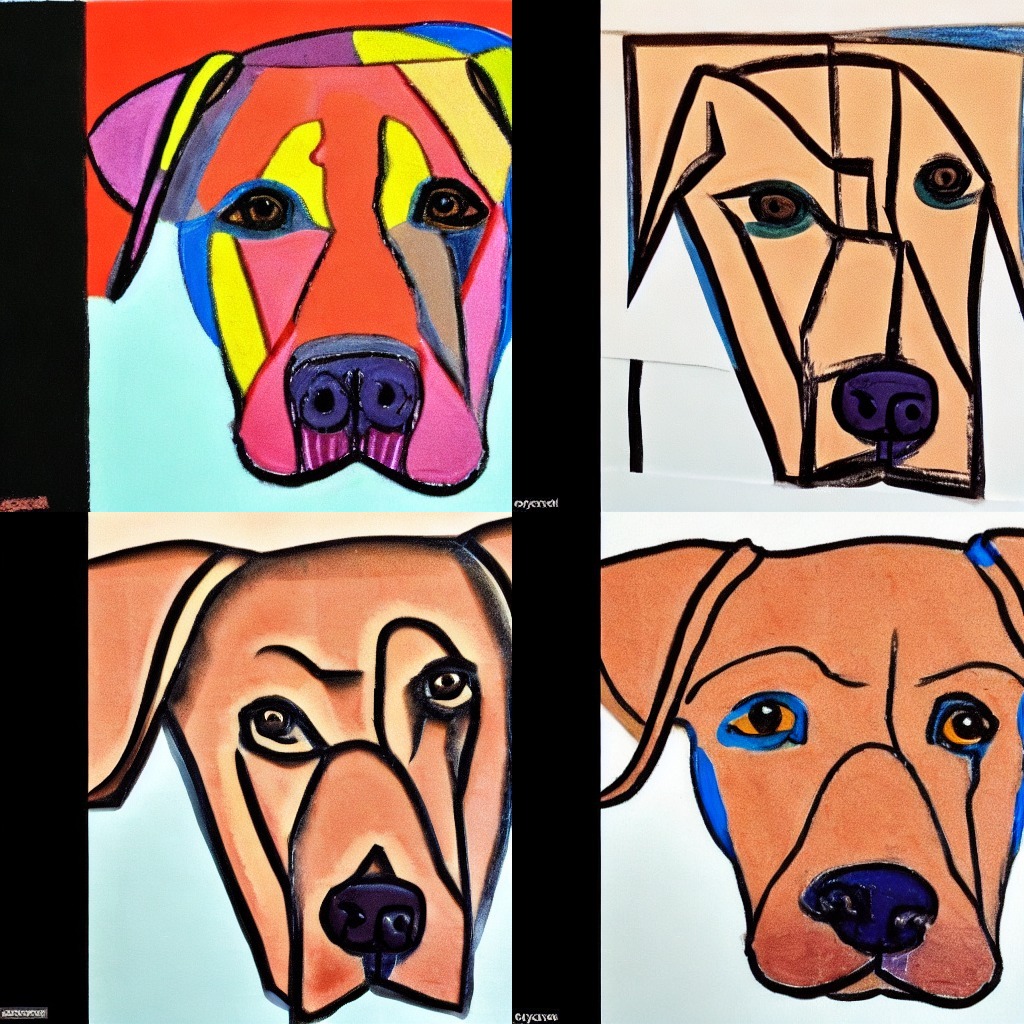
diff --git a/spaces/Androidonnxfork/CivitAi-to-Diffusers/diffusers/examples/community/interpolate_stable_diffusion.py b/spaces/Androidonnxfork/CivitAi-to-Diffusers/diffusers/examples/community/interpolate_stable_diffusion.py
deleted file mode 100644
index 8f33db71b9f3804d2efd2e7e3ac01fd45a7f6598..0000000000000000000000000000000000000000
--- a/spaces/Androidonnxfork/CivitAi-to-Diffusers/diffusers/examples/community/interpolate_stable_diffusion.py
+++ /dev/null
@@ -1,524 +0,0 @@
-import inspect
-import time
-from pathlib import Path
-from typing import Callable, List, Optional, Union
-
-import numpy as np
-import torch
-from transformers import CLIPImageProcessor, CLIPTextModel, CLIPTokenizer
-
-from diffusers import DiffusionPipeline
-from diffusers.configuration_utils import FrozenDict
-from diffusers.models import AutoencoderKL, UNet2DConditionModel
-from diffusers.pipelines.stable_diffusion import StableDiffusionPipelineOutput
-from diffusers.pipelines.stable_diffusion.safety_checker import StableDiffusionSafetyChecker
-from diffusers.schedulers import DDIMScheduler, LMSDiscreteScheduler, PNDMScheduler
-from diffusers.utils import deprecate, logging
-
-
-logger = logging.get_logger(__name__) # pylint: disable=invalid-name
-
-
-def slerp(t, v0, v1, DOT_THRESHOLD=0.9995):
- """helper function to spherically interpolate two arrays v1 v2"""
-
- if not isinstance(v0, np.ndarray):
- inputs_are_torch = True
- input_device = v0.device
- v0 = v0.cpu().numpy()
- v1 = v1.cpu().numpy()
-
- dot = np.sum(v0 * v1 / (np.linalg.norm(v0) * np.linalg.norm(v1)))
- if np.abs(dot) > DOT_THRESHOLD:
- v2 = (1 - t) * v0 + t * v1
- else:
- theta_0 = np.arccos(dot)
- sin_theta_0 = np.sin(theta_0)
- theta_t = theta_0 * t
- sin_theta_t = np.sin(theta_t)
- s0 = np.sin(theta_0 - theta_t) / sin_theta_0
- s1 = sin_theta_t / sin_theta_0
- v2 = s0 * v0 + s1 * v1
-
- if inputs_are_torch:
- v2 = torch.from_numpy(v2).to(input_device)
-
- return v2
-
-
-class StableDiffusionWalkPipeline(DiffusionPipeline):
- r"""
- Pipeline for text-to-image generation using Stable Diffusion.
-
- This model inherits from [`DiffusionPipeline`]. Check the superclass documentation for the generic methods the
- library implements for all the pipelines (such as downloading or saving, running on a particular device, etc.)
-
- Args:
- vae ([`AutoencoderKL`]):
- Variational Auto-Encoder (VAE) Model to encode and decode images to and from latent representations.
- text_encoder ([`CLIPTextModel`]):
- Frozen text-encoder. Stable Diffusion uses the text portion of
- [CLIP](https://huggingface.co/docs/transformers/model_doc/clip#transformers.CLIPTextModel), specifically
- the [clip-vit-large-patch14](https://huggingface.co/openai/clip-vit-large-patch14) variant.
- tokenizer (`CLIPTokenizer`):
- Tokenizer of class
- [CLIPTokenizer](https://huggingface.co/docs/transformers/v4.21.0/en/model_doc/clip#transformers.CLIPTokenizer).
- unet ([`UNet2DConditionModel`]): Conditional U-Net architecture to denoise the encoded image latents.
- scheduler ([`SchedulerMixin`]):
- A scheduler to be used in combination with `unet` to denoise the encoded image latents. Can be one of
- [`DDIMScheduler`], [`LMSDiscreteScheduler`], or [`PNDMScheduler`].
- safety_checker ([`StableDiffusionSafetyChecker`]):
- Classification module that estimates whether generated images could be considered offensive or harmful.
- Please, refer to the [model card](https://huggingface.co/CompVis/stable-diffusion-v1-4) for details.
- feature_extractor ([`CLIPImageProcessor`]):
- Model that extracts features from generated images to be used as inputs for the `safety_checker`.
- """
-
- def __init__(
- self,
- vae: AutoencoderKL,
- text_encoder: CLIPTextModel,
- tokenizer: CLIPTokenizer,
- unet: UNet2DConditionModel,
- scheduler: Union[DDIMScheduler, PNDMScheduler, LMSDiscreteScheduler],
- safety_checker: StableDiffusionSafetyChecker,
- feature_extractor: CLIPImageProcessor,
- ):
- super().__init__()
-
- if hasattr(scheduler.config, "steps_offset") and scheduler.config.steps_offset != 1:
- deprecation_message = (
- f"The configuration file of this scheduler: {scheduler} is outdated. `steps_offset`"
- f" should be set to 1 instead of {scheduler.config.steps_offset}. Please make sure "
- "to update the config accordingly as leaving `steps_offset` might led to incorrect results"
- " in future versions. If you have downloaded this checkpoint from the Hugging Face Hub,"
- " it would be very nice if you could open a Pull request for the `scheduler/scheduler_config.json`"
- " file"
- )
- deprecate("steps_offset!=1", "1.0.0", deprecation_message, standard_warn=False)
- new_config = dict(scheduler.config)
- new_config["steps_offset"] = 1
- scheduler._internal_dict = FrozenDict(new_config)
-
- if safety_checker is None:
- logger.warning(
- f"You have disabled the safety checker for {self.__class__} by passing `safety_checker=None`. Ensure"
- " that you abide to the conditions of the Stable Diffusion license and do not expose unfiltered"
- " results in services or applications open to the public. Both the diffusers team and Hugging Face"
- " strongly recommend to keep the safety filter enabled in all public facing circumstances, disabling"
- " it only for use-cases that involve analyzing network behavior or auditing its results. For more"
- " information, please have a look at https://github.com/huggingface/diffusers/pull/254 ."
- )
-
- self.register_modules(
- vae=vae,
- text_encoder=text_encoder,
- tokenizer=tokenizer,
- unet=unet,
- scheduler=scheduler,
- safety_checker=safety_checker,
- feature_extractor=feature_extractor,
- )
-
- def enable_attention_slicing(self, slice_size: Optional[Union[str, int]] = "auto"):
- r"""
- Enable sliced attention computation.
-
- When this option is enabled, the attention module will split the input tensor in slices, to compute attention
- in several steps. This is useful to save some memory in exchange for a small speed decrease.
-
- Args:
- slice_size (`str` or `int`, *optional*, defaults to `"auto"`):
- When `"auto"`, halves the input to the attention heads, so attention will be computed in two steps. If
- a number is provided, uses as many slices as `attention_head_dim // slice_size`. In this case,
- `attention_head_dim` must be a multiple of `slice_size`.
- """
- if slice_size == "auto":
- # half the attention head size is usually a good trade-off between
- # speed and memory
- slice_size = self.unet.config.attention_head_dim // 2
- self.unet.set_attention_slice(slice_size)
-
- def disable_attention_slicing(self):
- r"""
- Disable sliced attention computation. If `enable_attention_slicing` was previously invoked, this method will go
- back to computing attention in one step.
- """
- # set slice_size = `None` to disable `attention slicing`
- self.enable_attention_slicing(None)
-
- @torch.no_grad()
- def __call__(
- self,
- prompt: Optional[Union[str, List[str]]] = None,
- height: int = 512,
- width: int = 512,
- num_inference_steps: int = 50,
- guidance_scale: float = 7.5,
- negative_prompt: Optional[Union[str, List[str]]] = None,
- num_images_per_prompt: Optional[int] = 1,
- eta: float = 0.0,
- generator: Optional[torch.Generator] = None,
- latents: Optional[torch.FloatTensor] = None,
- output_type: Optional[str] = "pil",
- return_dict: bool = True,
- callback: Optional[Callable[[int, int, torch.FloatTensor], None]] = None,
- callback_steps: int = 1,
- text_embeddings: Optional[torch.FloatTensor] = None,
- **kwargs,
- ):
- r"""
- Function invoked when calling the pipeline for generation.
-
- Args:
- prompt (`str` or `List[str]`, *optional*, defaults to `None`):
- The prompt or prompts to guide the image generation. If not provided, `text_embeddings` is required.
- height (`int`, *optional*, defaults to 512):
- The height in pixels of the generated image.
- width (`int`, *optional*, defaults to 512):
- The width in pixels of the generated image.
- num_inference_steps (`int`, *optional*, defaults to 50):
- The number of denoising steps. More denoising steps usually lead to a higher quality image at the
- expense of slower inference.
- guidance_scale (`float`, *optional*, defaults to 7.5):
- Guidance scale as defined in [Classifier-Free Diffusion Guidance](https://arxiv.org/abs/2207.12598).
- `guidance_scale` is defined as `w` of equation 2. of [Imagen
- Paper](https://arxiv.org/pdf/2205.11487.pdf). Guidance scale is enabled by setting `guidance_scale >
- 1`. Higher guidance scale encourages to generate images that are closely linked to the text `prompt`,
- usually at the expense of lower image quality.
- negative_prompt (`str` or `List[str]`, *optional*):
- The prompt or prompts not to guide the image generation. Ignored when not using guidance (i.e., ignored
- if `guidance_scale` is less than `1`).
- num_images_per_prompt (`int`, *optional*, defaults to 1):
- The number of images to generate per prompt.
- eta (`float`, *optional*, defaults to 0.0):
- Corresponds to parameter eta (η) in the DDIM paper: https://arxiv.org/abs/2010.02502. Only applies to
- [`schedulers.DDIMScheduler`], will be ignored for others.
- generator (`torch.Generator`, *optional*):
- A [torch generator](https://pytorch.org/docs/stable/generated/torch.Generator.html) to make generation
- deterministic.
- latents (`torch.FloatTensor`, *optional*):
- Pre-generated noisy latents, sampled from a Gaussian distribution, to be used as inputs for image
- generation. Can be used to tweak the same generation with different prompts. If not provided, a latents
- tensor will ge generated by sampling using the supplied random `generator`.
- output_type (`str`, *optional*, defaults to `"pil"`):
- The output format of the generate image. Choose between
- [PIL](https://pillow.readthedocs.io/en/stable/): `PIL.Image.Image` or `np.array`.
- return_dict (`bool`, *optional*, defaults to `True`):
- Whether or not to return a [`~pipelines.stable_diffusion.StableDiffusionPipelineOutput`] instead of a
- plain tuple.
- callback (`Callable`, *optional*):
- A function that will be called every `callback_steps` steps during inference. The function will be
- called with the following arguments: `callback(step: int, timestep: int, latents: torch.FloatTensor)`.
- callback_steps (`int`, *optional*, defaults to 1):
- The frequency at which the `callback` function will be called. If not specified, the callback will be
- called at every step.
- text_embeddings (`torch.FloatTensor`, *optional*, defaults to `None`):
- Pre-generated text embeddings to be used as inputs for image generation. Can be used in place of
- `prompt` to avoid re-computing the embeddings. If not provided, the embeddings will be generated from
- the supplied `prompt`.
-
- Returns:
- [`~pipelines.stable_diffusion.StableDiffusionPipelineOutput`] or `tuple`:
- [`~pipelines.stable_diffusion.StableDiffusionPipelineOutput`] if `return_dict` is True, otherwise a `tuple.
- When returning a tuple, the first element is a list with the generated images, and the second element is a
- list of `bool`s denoting whether the corresponding generated image likely represents "not-safe-for-work"
- (nsfw) content, according to the `safety_checker`.
- """
-
- if height % 8 != 0 or width % 8 != 0:
- raise ValueError(f"`height` and `width` have to be divisible by 8 but are {height} and {width}.")
-
- if (callback_steps is None) or (
- callback_steps is not None and (not isinstance(callback_steps, int) or callback_steps <= 0)
- ):
- raise ValueError(
- f"`callback_steps` has to be a positive integer but is {callback_steps} of type"
- f" {type(callback_steps)}."
- )
-
- if text_embeddings is None:
- if isinstance(prompt, str):
- batch_size = 1
- elif isinstance(prompt, list):
- batch_size = len(prompt)
- else:
- raise ValueError(f"`prompt` has to be of type `str` or `list` but is {type(prompt)}")
-
- # get prompt text embeddings
- text_inputs = self.tokenizer(
- prompt,
- padding="max_length",
- max_length=self.tokenizer.model_max_length,
- return_tensors="pt",
- )
- text_input_ids = text_inputs.input_ids
-
- if text_input_ids.shape[-1] > self.tokenizer.model_max_length:
- removed_text = self.tokenizer.batch_decode(text_input_ids[:, self.tokenizer.model_max_length :])
- print(
- "The following part of your input was truncated because CLIP can only handle sequences up to"
- f" {self.tokenizer.model_max_length} tokens: {removed_text}"
- )
- text_input_ids = text_input_ids[:, : self.tokenizer.model_max_length]
- text_embeddings = self.text_encoder(text_input_ids.to(self.device))[0]
- else:
- batch_size = text_embeddings.shape[0]
-
- # duplicate text embeddings for each generation per prompt, using mps friendly method
- bs_embed, seq_len, _ = text_embeddings.shape
- text_embeddings = text_embeddings.repeat(1, num_images_per_prompt, 1)
- text_embeddings = text_embeddings.view(bs_embed * num_images_per_prompt, seq_len, -1)
-
- # here `guidance_scale` is defined analog to the guidance weight `w` of equation (2)
- # of the Imagen paper: https://arxiv.org/pdf/2205.11487.pdf . `guidance_scale = 1`
- # corresponds to doing no classifier free guidance.
- do_classifier_free_guidance = guidance_scale > 1.0
- # get unconditional embeddings for classifier free guidance
- if do_classifier_free_guidance:
- uncond_tokens: List[str]
- if negative_prompt is None:
- uncond_tokens = [""] * batch_size
- elif type(prompt) is not type(negative_prompt):
- raise TypeError(
- f"`negative_prompt` should be the same type to `prompt`, but got {type(negative_prompt)} !="
- f" {type(prompt)}."
- )
- elif isinstance(negative_prompt, str):
- uncond_tokens = [negative_prompt]
- elif batch_size != len(negative_prompt):
- raise ValueError(
- f"`negative_prompt`: {negative_prompt} has batch size {len(negative_prompt)}, but `prompt`:"
- f" {prompt} has batch size {batch_size}. Please make sure that passed `negative_prompt` matches"
- " the batch size of `prompt`."
- )
- else:
- uncond_tokens = negative_prompt
-
- max_length = self.tokenizer.model_max_length
- uncond_input = self.tokenizer(
- uncond_tokens,
- padding="max_length",
- max_length=max_length,
- truncation=True,
- return_tensors="pt",
- )
- uncond_embeddings = self.text_encoder(uncond_input.input_ids.to(self.device))[0]
-
- # duplicate unconditional embeddings for each generation per prompt, using mps friendly method
- seq_len = uncond_embeddings.shape[1]
- uncond_embeddings = uncond_embeddings.repeat(1, num_images_per_prompt, 1)
- uncond_embeddings = uncond_embeddings.view(batch_size * num_images_per_prompt, seq_len, -1)
-
- # For classifier free guidance, we need to do two forward passes.
- # Here we concatenate the unconditional and text embeddings into a single batch
- # to avoid doing two forward passes
- text_embeddings = torch.cat([uncond_embeddings, text_embeddings])
-
- # get the initial random noise unless the user supplied it
-
- # Unlike in other pipelines, latents need to be generated in the target device
- # for 1-to-1 results reproducibility with the CompVis implementation.
- # However this currently doesn't work in `mps`.
- latents_shape = (batch_size * num_images_per_prompt, self.unet.config.in_channels, height // 8, width // 8)
- latents_dtype = text_embeddings.dtype
- if latents is None:
- if self.device.type == "mps":
- # randn does not work reproducibly on mps
- latents = torch.randn(latents_shape, generator=generator, device="cpu", dtype=latents_dtype).to(
- self.device
- )
- else:
- latents = torch.randn(latents_shape, generator=generator, device=self.device, dtype=latents_dtype)
- else:
- if latents.shape != latents_shape:
- raise ValueError(f"Unexpected latents shape, got {latents.shape}, expected {latents_shape}")
- latents = latents.to(self.device)
-
- # set timesteps
- self.scheduler.set_timesteps(num_inference_steps)
-
- # Some schedulers like PNDM have timesteps as arrays
- # It's more optimized to move all timesteps to correct device beforehand
- timesteps_tensor = self.scheduler.timesteps.to(self.device)
-
- # scale the initial noise by the standard deviation required by the scheduler
- latents = latents * self.scheduler.init_noise_sigma
-
- # prepare extra kwargs for the scheduler step, since not all schedulers have the same signature
- # eta (η) is only used with the DDIMScheduler, it will be ignored for other schedulers.
- # eta corresponds to η in DDIM paper: https://arxiv.org/abs/2010.02502
- # and should be between [0, 1]
- accepts_eta = "eta" in set(inspect.signature(self.scheduler.step).parameters.keys())
- extra_step_kwargs = {}
- if accepts_eta:
- extra_step_kwargs["eta"] = eta
-
- for i, t in enumerate(self.progress_bar(timesteps_tensor)):
- # expand the latents if we are doing classifier free guidance
- latent_model_input = torch.cat([latents] * 2) if do_classifier_free_guidance else latents
- latent_model_input = self.scheduler.scale_model_input(latent_model_input, t)
-
- # predict the noise residual
- noise_pred = self.unet(latent_model_input, t, encoder_hidden_states=text_embeddings).sample
-
- # perform guidance
- if do_classifier_free_guidance:
- noise_pred_uncond, noise_pred_text = noise_pred.chunk(2)
- noise_pred = noise_pred_uncond + guidance_scale * (noise_pred_text - noise_pred_uncond)
-
- # compute the previous noisy sample x_t -> x_t-1
- latents = self.scheduler.step(noise_pred, t, latents, **extra_step_kwargs).prev_sample
-
- # call the callback, if provided
- if callback is not None and i % callback_steps == 0:
- callback(i, t, latents)
-
- latents = 1 / 0.18215 * latents
- image = self.vae.decode(latents).sample
-
- image = (image / 2 + 0.5).clamp(0, 1)
-
- # we always cast to float32 as this does not cause significant overhead and is compatible with bfloat16
- image = image.cpu().permute(0, 2, 3, 1).float().numpy()
-
- if self.safety_checker is not None:
- safety_checker_input = self.feature_extractor(self.numpy_to_pil(image), return_tensors="pt").to(
- self.device
- )
- image, has_nsfw_concept = self.safety_checker(
- images=image, clip_input=safety_checker_input.pixel_values.to(text_embeddings.dtype)
- )
- else:
- has_nsfw_concept = None
-
- if output_type == "pil":
- image = self.numpy_to_pil(image)
-
- if not return_dict:
- return (image, has_nsfw_concept)
-
- return StableDiffusionPipelineOutput(images=image, nsfw_content_detected=has_nsfw_concept)
-
- def embed_text(self, text):
- """takes in text and turns it into text embeddings"""
- text_input = self.tokenizer(
- text,
- padding="max_length",
- max_length=self.tokenizer.model_max_length,
- truncation=True,
- return_tensors="pt",
- )
- with torch.no_grad():
- embed = self.text_encoder(text_input.input_ids.to(self.device))[0]
- return embed
-
- def get_noise(self, seed, dtype=torch.float32, height=512, width=512):
- """Takes in random seed and returns corresponding noise vector"""
- return torch.randn(
- (1, self.unet.config.in_channels, height // 8, width // 8),
- generator=torch.Generator(device=self.device).manual_seed(seed),
- device=self.device,
- dtype=dtype,
- )
-
- def walk(
- self,
- prompts: List[str],
- seeds: List[int],
- num_interpolation_steps: Optional[int] = 6,
- output_dir: Optional[str] = "./dreams",
- name: Optional[str] = None,
- batch_size: Optional[int] = 1,
- height: Optional[int] = 512,
- width: Optional[int] = 512,
- guidance_scale: Optional[float] = 7.5,
- num_inference_steps: Optional[int] = 50,
- eta: Optional[float] = 0.0,
- ) -> List[str]:
- """
- Walks through a series of prompts and seeds, interpolating between them and saving the results to disk.
-
- Args:
- prompts (`List[str]`):
- List of prompts to generate images for.
- seeds (`List[int]`):
- List of seeds corresponding to provided prompts. Must be the same length as prompts.
- num_interpolation_steps (`int`, *optional*, defaults to 6):
- Number of interpolation steps to take between prompts.
- output_dir (`str`, *optional*, defaults to `./dreams`):
- Directory to save the generated images to.
- name (`str`, *optional*, defaults to `None`):
- Subdirectory of `output_dir` to save the generated images to. If `None`, the name will
- be the current time.
- batch_size (`int`, *optional*, defaults to 1):
- Number of images to generate at once.
- height (`int`, *optional*, defaults to 512):
- Height of the generated images.
- width (`int`, *optional*, defaults to 512):
- Width of the generated images.
- guidance_scale (`float`, *optional*, defaults to 7.5):
- Guidance scale as defined in [Classifier-Free Diffusion Guidance](https://arxiv.org/abs/2207.12598).
- `guidance_scale` is defined as `w` of equation 2. of [Imagen
- Paper](https://arxiv.org/pdf/2205.11487.pdf). Guidance scale is enabled by setting `guidance_scale >
- 1`. Higher guidance scale encourages to generate images that are closely linked to the text `prompt`,
- usually at the expense of lower image quality.
- num_inference_steps (`int`, *optional*, defaults to 50):
- The number of denoising steps. More denoising steps usually lead to a higher quality image at the
- expense of slower inference.
- eta (`float`, *optional*, defaults to 0.0):
- Corresponds to parameter eta (η) in the DDIM paper: https://arxiv.org/abs/2010.02502. Only applies to
- [`schedulers.DDIMScheduler`], will be ignored for others.
-
- Returns:
- `List[str]`: List of paths to the generated images.
- """
- if not len(prompts) == len(seeds):
- raise ValueError(
- f"Number of prompts and seeds must be equalGot {len(prompts)} prompts and {len(seeds)} seeds"
- )
-
- name = name or time.strftime("%Y%m%d-%H%M%S")
- save_path = Path(output_dir) / name
- save_path.mkdir(exist_ok=True, parents=True)
-
- frame_idx = 0
- frame_filepaths = []
- for prompt_a, prompt_b, seed_a, seed_b in zip(prompts, prompts[1:], seeds, seeds[1:]):
- # Embed Text
- embed_a = self.embed_text(prompt_a)
- embed_b = self.embed_text(prompt_b)
-
- # Get Noise
- noise_dtype = embed_a.dtype
- noise_a = self.get_noise(seed_a, noise_dtype, height, width)
- noise_b = self.get_noise(seed_b, noise_dtype, height, width)
-
- noise_batch, embeds_batch = None, None
- T = np.linspace(0.0, 1.0, num_interpolation_steps)
- for i, t in enumerate(T):
- noise = slerp(float(t), noise_a, noise_b)
- embed = torch.lerp(embed_a, embed_b, t)
-
- noise_batch = noise if noise_batch is None else torch.cat([noise_batch, noise], dim=0)
- embeds_batch = embed if embeds_batch is None else torch.cat([embeds_batch, embed], dim=0)
-
- batch_is_ready = embeds_batch.shape[0] == batch_size or i + 1 == T.shape[0]
- if batch_is_ready:
- outputs = self(
- latents=noise_batch,
- text_embeddings=embeds_batch,
- height=height,
- width=width,
- guidance_scale=guidance_scale,
- eta=eta,
- num_inference_steps=num_inference_steps,
- )
- noise_batch, embeds_batch = None, None
-
- for image in outputs["images"]:
- frame_filepath = str(save_path / f"frame_{frame_idx:06d}.png")
- image.save(frame_filepath)
- frame_filepaths.append(frame_filepath)
- frame_idx += 1
- return frame_filepaths
diff --git a/spaces/Androidonnxfork/CivitAi-to-Diffusers/diffusers/src/diffusers/pipelines/vq_diffusion/__init__.py b/spaces/Androidonnxfork/CivitAi-to-Diffusers/diffusers/src/diffusers/pipelines/vq_diffusion/__init__.py
deleted file mode 100644
index da60bf73ad4275b25766bf99baf00760bc3b1712..0000000000000000000000000000000000000000
--- a/spaces/Androidonnxfork/CivitAi-to-Diffusers/diffusers/src/diffusers/pipelines/vq_diffusion/__init__.py
+++ /dev/null
@@ -1,10 +0,0 @@
-from ...utils import OptionalDependencyNotAvailable, is_torch_available, is_transformers_available
-
-
-try:
- if not (is_transformers_available() and is_torch_available()):
- raise OptionalDependencyNotAvailable()
-except OptionalDependencyNotAvailable:
- from ...utils.dummy_torch_and_transformers_objects import *
-else:
- from .pipeline_vq_diffusion import LearnedClassifierFreeSamplingEmbeddings, VQDiffusionPipeline
diff --git a/spaces/Andy1621/uniformer_image_segmentation/configs/fcn/fcn_r101-d8_512x1024_40k_cityscapes.py b/spaces/Andy1621/uniformer_image_segmentation/configs/fcn/fcn_r101-d8_512x1024_40k_cityscapes.py
deleted file mode 100644
index 7918dd10d05cd98dbc02f02ef1b93e3134f52357..0000000000000000000000000000000000000000
--- a/spaces/Andy1621/uniformer_image_segmentation/configs/fcn/fcn_r101-d8_512x1024_40k_cityscapes.py
+++ /dev/null
@@ -1,2 +0,0 @@
-_base_ = './fcn_r50-d8_512x1024_40k_cityscapes.py'
-model = dict(pretrained='open-mmlab://resnet101_v1c', backbone=dict(depth=101))
diff --git a/spaces/Andy1621/uniformer_image_segmentation/configs/hrnet/fcn_hr18s_480x480_40k_pascal_context_59.py b/spaces/Andy1621/uniformer_image_segmentation/configs/hrnet/fcn_hr18s_480x480_40k_pascal_context_59.py
deleted file mode 100644
index 0412c64f31d85997af9715949672ca55b07aaed7..0000000000000000000000000000000000000000
--- a/spaces/Andy1621/uniformer_image_segmentation/configs/hrnet/fcn_hr18s_480x480_40k_pascal_context_59.py
+++ /dev/null
@@ -1,9 +0,0 @@
-_base_ = './fcn_hr18_480x480_40k_pascal_context_59.py'
-model = dict(
- pretrained='open-mmlab://msra/hrnetv2_w18_small',
- backbone=dict(
- extra=dict(
- stage1=dict(num_blocks=(2, )),
- stage2=dict(num_blocks=(2, 2)),
- stage3=dict(num_modules=3, num_blocks=(2, 2, 2)),
- stage4=dict(num_modules=2, num_blocks=(2, 2, 2, 2)))))
diff --git a/spaces/AnishKumbhar/ChatBot/text-generation-webui-main/extensions/openai/cache_embedding_model.py b/spaces/AnishKumbhar/ChatBot/text-generation-webui-main/extensions/openai/cache_embedding_model.py
deleted file mode 100644
index 7f4f0806a62e3f46cc3a6076e05d3b8b7e87a2b2..0000000000000000000000000000000000000000
--- a/spaces/AnishKumbhar/ChatBot/text-generation-webui-main/extensions/openai/cache_embedding_model.py
+++ /dev/null
@@ -1,11 +0,0 @@
-#!/usr/bin/env python3
-# preload the embedding model, useful for Docker images to prevent re-download on config change
-# Dockerfile:
-# ENV OPENEDAI_EMBEDDING_MODEL=all-mpnet-base-v2 # Optional
-# RUN python3 cache_embedded_model.py
-import os
-
-import sentence_transformers
-
-st_model = os.environ.get("OPENEDAI_EMBEDDING_MODEL", "all-mpnet-base-v2")
-model = sentence_transformers.SentenceTransformer(st_model)
diff --git a/spaces/AnishKumbhar/ChatBot/text-generation-webui-main/start_windows.bat b/spaces/AnishKumbhar/ChatBot/text-generation-webui-main/start_windows.bat
deleted file mode 100644
index 4e6f6fe79d6a2216dbc3ab8d7f6194640d512b5a..0000000000000000000000000000000000000000
--- a/spaces/AnishKumbhar/ChatBot/text-generation-webui-main/start_windows.bat
+++ /dev/null
@@ -1,84 +0,0 @@
-@echo off
-
-cd /D "%~dp0"
-
-set PATH=%PATH%;%SystemRoot%\system32
-
-echo "%CD%"| findstr /C:" " >nul && echo This script relies on Miniconda which can not be silently installed under a path with spaces. && goto end
-
-@rem Check for special characters in installation path
-set "SPCHARMESSAGE="WARNING: Special characters were detected in the installation path!" " This can cause the installation to fail!""
-echo "%CD%"| findstr /R /C:"[!#\$%&()\*+,;<=>?@\[\]\^`{|}~]" >nul && (
- call :PrintBigMessage %SPCHARMESSAGE%
-)
-set SPCHARMESSAGE=
-
-@rem fix failed install when installing to a separate drive
-set TMP=%cd%\installer_files
-set TEMP=%cd%\installer_files
-
-@rem deactivate existing conda envs as needed to avoid conflicts
-(call conda deactivate && call conda deactivate && call conda deactivate) 2>nul
-
-@rem config
-set INSTALL_DIR=%cd%\installer_files
-set CONDA_ROOT_PREFIX=%cd%\installer_files\conda
-set INSTALL_ENV_DIR=%cd%\installer_files\env
-set MINICONDA_DOWNLOAD_URL=https://repo.anaconda.com/miniconda/Miniconda3-py310_23.3.1-0-Windows-x86_64.exe
-set conda_exists=F
-
-@rem figure out whether git and conda needs to be installed
-call "%CONDA_ROOT_PREFIX%\_conda.exe" --version >nul 2>&1
-if "%ERRORLEVEL%" EQU "0" set conda_exists=T
-
-@rem (if necessary) install git and conda into a contained environment
-@rem download conda
-if "%conda_exists%" == "F" (
- echo Downloading Miniconda from %MINICONDA_DOWNLOAD_URL% to %INSTALL_DIR%\miniconda_installer.exe
-
- mkdir "%INSTALL_DIR%"
- call curl -Lk "%MINICONDA_DOWNLOAD_URL%" > "%INSTALL_DIR%\miniconda_installer.exe" || ( echo. && echo Miniconda failed to download. && goto end )
-
- echo Installing Miniconda to %CONDA_ROOT_PREFIX%
- start /wait "" "%INSTALL_DIR%\miniconda_installer.exe" /InstallationType=JustMe /NoShortcuts=1 /AddToPath=0 /RegisterPython=0 /NoRegistry=1 /S /D=%CONDA_ROOT_PREFIX%
-
- @rem test the conda binary
- echo Miniconda version:
- call "%CONDA_ROOT_PREFIX%\_conda.exe" --version || ( echo. && echo Miniconda not found. && goto end )
-)
-
-@rem create the installer env
-if not exist "%INSTALL_ENV_DIR%" (
- echo Packages to install: %PACKAGES_TO_INSTALL%
- call "%CONDA_ROOT_PREFIX%\_conda.exe" create --no-shortcuts -y -k --prefix "%INSTALL_ENV_DIR%" python=3.10 || ( echo. && echo Conda environment creation failed. && goto end )
-)
-
-@rem check if conda environment was actually created
-if not exist "%INSTALL_ENV_DIR%\python.exe" ( echo. && echo Conda environment is empty. && goto end )
-
-@rem environment isolation
-set PYTHONNOUSERSITE=1
-set PYTHONPATH=
-set PYTHONHOME=
-set "CUDA_PATH=%INSTALL_ENV_DIR%"
-set "CUDA_HOME=%CUDA_PATH%"
-
-@rem activate installer env
-call "%CONDA_ROOT_PREFIX%\condabin\conda.bat" activate "%INSTALL_ENV_DIR%" || ( echo. && echo Miniconda hook not found. && goto end )
-
-@rem setup installer env
-call python one_click.py %*
-
-@rem below are functions for the script next line skips these during normal execution
-goto end
-
-:PrintBigMessage
-echo. && echo.
-echo *******************************************************************
-for %%M in (%*) do echo * %%~M
-echo *******************************************************************
-echo. && echo.
-exit /b
-
-:end
-pause
diff --git a/spaces/ArchitSharma/Digital-Photo-Color-Restoration/README.md b/spaces/ArchitSharma/Digital-Photo-Color-Restoration/README.md
deleted file mode 100644
index ad6be44ebe7ad5c85d8099b335c07682dc57f202..0000000000000000000000000000000000000000
--- a/spaces/ArchitSharma/Digital-Photo-Color-Restoration/README.md
+++ /dev/null
@@ -1,13 +0,0 @@
----
-title: Digital Photo Color Restoration
-emoji: 📚
-colorFrom: yellow
-colorTo: blue
-sdk: streamlit
-sdk_version: 1.19.0
-app_file: app.py
-python_version: 3.9.17
-pinned: false
----
-
-Check out the configuration reference at https://huggingface.co/docs/hub/spaces-config-reference
\ No newline at end of file
diff --git a/spaces/AriaMei/TTSdemo/text/cleaners.py b/spaces/AriaMei/TTSdemo/text/cleaners.py
deleted file mode 100644
index 455f3110692f0984d36f72d5fee5fb85e9b7a690..0000000000000000000000000000000000000000
--- a/spaces/AriaMei/TTSdemo/text/cleaners.py
+++ /dev/null
@@ -1,177 +0,0 @@
-import re
-
-from text.japanese import japanese_to_romaji_with_accent, japanese_to_ipa, japanese_to_ipa2, japanese_to_ipa3
-from text.mandarin import number_to_chinese, chinese_to_bopomofo, latin_to_bopomofo, chinese_to_romaji, chinese_to_lazy_ipa, chinese_to_ipa, chinese_to_ipa2
-
-# from text.sanskrit import devanagari_to_ipa
-# from text.english import english_to_lazy_ipa, english_to_ipa2, english_to_lazy_ipa2
-# from text.thai import num_to_thai, latin_to_thai
-# from text.shanghainese import shanghainese_to_ipa
-# from text.cantonese import cantonese_to_ipa
-# from text.ngu_dialect import ngu_dialect_to_ipa
-
-
-def japanese_cleaners(text):
- text = japanese_to_romaji_with_accent(text)
- if re.match('[A-Za-z]', text[-1]):
- text += '.'
- return text
-
-
-def japanese_cleaners2(text):
- return japanese_cleaners(text).replace('ts', 'ʦ').replace('...', '…')
-
-
-def korean_cleaners(text):
- '''Pipeline for Korean text'''
- text = latin_to_hangul(text)
- text = number_to_hangul(text)
- text = divide_hangul(text)
- if re.match('[\u3131-\u3163]', text[-1]):
- text += '.'
- return text
-
-
-def chinese_cleaners(text):
- '''Pipeline for Chinese text'''
- text = number_to_chinese(text)
- text = chinese_to_bopomofo(text)
- text = latin_to_bopomofo(text)
- if re.match('[ˉˊˇˋ˙]', text[-1]):
- text += '。'
- return text
-
-
-def zh_ja_mixture_cleaners(text):
- chinese_texts = re.findall(r'\[ZH\].*?\[ZH\]', text)
- japanese_texts = re.findall(r'\[JA\].*?\[JA\]', text)
- for chinese_text in chinese_texts:
- cleaned_text = chinese_to_romaji(chinese_text[4:-4])
- text = text.replace(chinese_text, cleaned_text+' ', 1)
- for japanese_text in japanese_texts:
- cleaned_text = japanese_to_romaji_with_accent(
- japanese_text[4:-4]).replace('ts', 'ʦ').replace('u', 'ɯ').replace('...', '…')
- text = text.replace(japanese_text, cleaned_text+' ', 1)
- text = text[:-1]
- if re.match('[A-Za-zɯɹəɥ→↓↑]', text[-1]):
- text += '.'
- return text
-
-
-def sanskrit_cleaners(text):
- text = text.replace('॥', '।').replace('ॐ', 'ओम्')
- if text[-1] != '।':
- text += ' ।'
- return text
-
-
-def cjks_cleaners(text):
- chinese_texts = re.findall(r'\[ZH\].*?\[ZH\]', text)
- japanese_texts = re.findall(r'\[JA\].*?\[JA\]', text)
- korean_texts = re.findall(r'\[KO\].*?\[KO\]', text)
- sanskrit_texts = re.findall(r'\[SA\].*?\[SA\]', text)
- english_texts = re.findall(r'\[EN\].*?\[EN\]', text)
- for chinese_text in chinese_texts:
- cleaned_text = chinese_to_lazy_ipa(chinese_text[4:-4])
- text = text.replace(chinese_text, cleaned_text+' ', 1)
- for japanese_text in japanese_texts:
- cleaned_text = japanese_to_ipa(japanese_text[4:-4])
- text = text.replace(japanese_text, cleaned_text+' ', 1)
- for korean_text in korean_texts:
- cleaned_text = korean_to_lazy_ipa(korean_text[4:-4])
- text = text.replace(korean_text, cleaned_text+' ', 1)
- for sanskrit_text in sanskrit_texts:
- cleaned_text = devanagari_to_ipa(sanskrit_text[4:-4])
- text = text.replace(sanskrit_text, cleaned_text+' ', 1)
- for english_text in english_texts:
- cleaned_text = english_to_lazy_ipa(english_text[4:-4])
- text = text.replace(english_text, cleaned_text+' ', 1)
- text = text[:-1]
- if re.match(r'[^\.,!\?\-…~]', text[-1]):
- text += '.'
- return text
-
-
-def cjke_cleaners(text):
- chinese_texts = re.findall(r'\[ZH\].*?\[ZH\]', text)
- japanese_texts = re.findall(r'\[JA\].*?\[JA\]', text)
- korean_texts = re.findall(r'\[KO\].*?\[KO\]', text)
- english_texts = re.findall(r'\[EN\].*?\[EN\]', text)
- for chinese_text in chinese_texts:
- cleaned_text = chinese_to_lazy_ipa(chinese_text[4:-4])
- cleaned_text = cleaned_text.replace(
- 'ʧ', 'tʃ').replace('ʦ', 'ts').replace('ɥan', 'ɥæn')
- text = text.replace(chinese_text, cleaned_text+' ', 1)
- for japanese_text in japanese_texts:
- cleaned_text = japanese_to_ipa(japanese_text[4:-4])
- cleaned_text = cleaned_text.replace('ʧ', 'tʃ').replace(
- 'ʦ', 'ts').replace('ɥan', 'ɥæn').replace('ʥ', 'dz')
- text = text.replace(japanese_text, cleaned_text+' ', 1)
- for korean_text in korean_texts:
- cleaned_text = korean_to_ipa(korean_text[4:-4])
- text = text.replace(korean_text, cleaned_text+' ', 1)
- for english_text in english_texts:
- cleaned_text = english_to_ipa2(english_text[4:-4])
- cleaned_text = cleaned_text.replace('ɑ', 'a').replace(
- 'ɔ', 'o').replace('ɛ', 'e').replace('ɪ', 'i').replace('ʊ', 'u')
- text = text.replace(english_text, cleaned_text+' ', 1)
- text = text[:-1]
- if re.match(r'[^\.,!\?\-…~]', text[-1]):
- text += '.'
- return text
-
-
-def cjke_cleaners2(text):
- chinese_texts = re.findall(r'\[ZH\].*?\[ZH\]', text)
- japanese_texts = re.findall(r'\[JA\].*?\[JA\]', text)
- korean_texts = re.findall(r'\[KO\].*?\[KO\]', text)
- english_texts = re.findall(r'\[EN\].*?\[EN\]', text)
- for chinese_text in chinese_texts:
- cleaned_text = chinese_to_ipa(chinese_text[4:-4])
- text = text.replace(chinese_text, cleaned_text+' ', 1)
- for japanese_text in japanese_texts:
- cleaned_text = japanese_to_ipa2(japanese_text[4:-4])
- text = text.replace(japanese_text, cleaned_text+' ', 1)
- for korean_text in korean_texts:
- cleaned_text = korean_to_ipa(korean_text[4:-4])
- text = text.replace(korean_text, cleaned_text+' ', 1)
- for english_text in english_texts:
- cleaned_text = english_to_ipa2(english_text[4:-4])
- text = text.replace(english_text, cleaned_text+' ', 1)
- text = text[:-1]
- if re.match(r'[^\.,!\?\-…~]', text[-1]):
- text += '.'
- return text
-
-
-def thai_cleaners(text):
- text = num_to_thai(text)
- text = latin_to_thai(text)
- return text
-
-
-def shanghainese_cleaners(text):
- text = shanghainese_to_ipa(text)
- if re.match(r'[^\.,!\?\-…~]', text[-1]):
- text += '.'
- return text
-
-
-def chinese_dialect_cleaners(text):
- text = re.sub(r'\[MD\](.*?)\[MD\]',
- lambda x: chinese_to_ipa2(x.group(1))+' ', text)
- text = re.sub(r'\[TW\](.*?)\[TW\]',
- lambda x: chinese_to_ipa2(x.group(1), True)+' ', text)
- text = re.sub(r'\[JA\](.*?)\[JA\]',
- lambda x: japanese_to_ipa3(x.group(1)).replace('Q', 'ʔ')+' ', text)
- text = re.sub(r'\[SH\](.*?)\[SH\]', lambda x: shanghainese_to_ipa(x.group(1)).replace('1', '˥˧').replace('5',
- '˧˧˦').replace('6', '˩˩˧').replace('7', '˥').replace('8', '˩˨').replace('ᴀ', 'ɐ').replace('ᴇ', 'e')+' ', text)
- text = re.sub(r'\[GD\](.*?)\[GD\]',
- lambda x: cantonese_to_ipa(x.group(1))+' ', text)
- text = re.sub(r'\[EN\](.*?)\[EN\]',
- lambda x: english_to_lazy_ipa2(x.group(1))+' ', text)
- text = re.sub(r'\[([A-Z]{2})\](.*?)\[\1\]', lambda x: ngu_dialect_to_ipa(x.group(2), x.group(
- 1)).replace('ʣ', 'dz').replace('ʥ', 'dʑ').replace('ʦ', 'ts').replace('ʨ', 'tɕ')+' ', text)
- text = re.sub(r'\s+$', '', text)
- text = re.sub(r'([^\.,!\?\-…~])$', r'\1.', text)
- return text
diff --git a/spaces/Ariharasudhan/YoloV5/utils/aws/userdata.sh b/spaces/Ariharasudhan/YoloV5/utils/aws/userdata.sh
deleted file mode 100644
index 5fc1332ac1b0d1794cf8f8c5f6918059ae5dc381..0000000000000000000000000000000000000000
--- a/spaces/Ariharasudhan/YoloV5/utils/aws/userdata.sh
+++ /dev/null
@@ -1,27 +0,0 @@
-#!/bin/bash
-# AWS EC2 instance startup script https://docs.aws.amazon.com/AWSEC2/latest/UserGuide/user-data.html
-# This script will run only once on first instance start (for a re-start script see mime.sh)
-# /home/ubuntu (ubuntu) or /home/ec2-user (amazon-linux) is working dir
-# Use >300 GB SSD
-
-cd home/ubuntu
-if [ ! -d yolov5 ]; then
- echo "Running first-time script." # install dependencies, download COCO, pull Docker
- git clone https://github.com/ultralytics/yolov5 -b master && sudo chmod -R 777 yolov5
- cd yolov5
- bash data/scripts/get_coco.sh && echo "COCO done." &
- sudo docker pull ultralytics/yolov5:latest && echo "Docker done." &
- python -m pip install --upgrade pip && pip install -r requirements.txt && python detect.py && echo "Requirements done." &
- wait && echo "All tasks done." # finish background tasks
-else
- echo "Running re-start script." # resume interrupted runs
- i=0
- list=$(sudo docker ps -qa) # container list i.e. $'one\ntwo\nthree\nfour'
- while IFS= read -r id; do
- ((i++))
- echo "restarting container $i: $id"
- sudo docker start $id
- # sudo docker exec -it $id python train.py --resume # single-GPU
- sudo docker exec -d $id python utils/aws/resume.py # multi-scenario
- done <<<"$list"
-fi
diff --git a/spaces/AtlasUnified/DeforumPromptGenerator/app.py b/spaces/AtlasUnified/DeforumPromptGenerator/app.py
deleted file mode 100644
index dd994b85d68a3ee6f846110058aee3a04ddc19be..0000000000000000000000000000000000000000
--- a/spaces/AtlasUnified/DeforumPromptGenerator/app.py
+++ /dev/null
@@ -1,33 +0,0 @@
-import gradio as gr
-
-def generate_sequence(frames_per_second, seconds_per_prompt, *main_prompts):
- sequence_count = int(frames_per_second) * int(seconds_per_prompt)
- output = {}
-
- for prompt_index, main_prompt in enumerate(main_prompts):
- if main_prompt: # Check if the field has information
- prompts = main_prompt.split(',')
- for i, prompt in enumerate(prompts):
- output[str(prompt_index * sequence_count + i * sequence_count)] = prompt.strip()
-
- return output
-
-def stringify_output(output_dict):
- output_items = [f'"{k}": "{v}"' for k, v in output_dict.items()]
- return ',\n'.join(output_items)
-
-frames_per_second = gr.Number(label="Frames per second")
-seconds_per_prompt = gr.Number(label="Seconds per prompt")
-
-main_prompts = [gr.Textbox(lines=2, label=f"Main prompt {i+1} (comma-separated)") for i in range(10)]
-
-output = gr.Textbox(label="Output")
-
-iface = gr.Interface(
- fn=lambda fps, spp, *mp: stringify_output(generate_sequence(fps, spp, *mp)),
- inputs=[frames_per_second, seconds_per_prompt, *main_prompts],
- outputs=output,
- title="Deforum Prompt Generator"
-)
-
-iface.launch()
diff --git a/spaces/BartPoint/VoiceChange/util.py b/spaces/BartPoint/VoiceChange/util.py
deleted file mode 100644
index 8d6bcff1135c2d97e4caad7922f03f05c98484da..0000000000000000000000000000000000000000
--- a/spaces/BartPoint/VoiceChange/util.py
+++ /dev/null
@@ -1,81 +0,0 @@
-import sys
-import asyncio
-from io import BytesIO
-
-from fairseq import checkpoint_utils
-
-import torch
-
-import edge_tts
-import librosa
-
-
-# https://github.com/fumiama/Retrieval-based-Voice-Conversion-WebUI/blob/main/config.py#L43-L55 # noqa
-def has_mps() -> bool:
- if sys.platform != "darwin":
- return False
- else:
- if not getattr(torch, 'has_mps', False):
- return False
-
- try:
- torch.zeros(1).to(torch.device("mps"))
- return True
- except Exception:
- return False
-
-
-def is_half(device: str) -> bool:
- if not device.startswith('cuda'):
- return False
- else:
- gpu_name = torch.cuda.get_device_name(
- int(device.split(':')[-1])
- ).upper()
-
- # ...regex?
- if (
- ('16' in gpu_name and 'V100' not in gpu_name)
- or 'P40' in gpu_name
- or '1060' in gpu_name
- or '1070' in gpu_name
- or '1080' in gpu_name
- ):
- return False
-
- return True
-
-
-def load_hubert_model(device: str, model_path: str = 'hubert_base.pt'):
- model = checkpoint_utils.load_model_ensemble_and_task(
- [model_path]
- )[0][0].to(device)
-
- if is_half(device):
- return model.half()
- else:
- return model.float()
-
-
-async def call_edge_tts(speaker_name: str, text: str):
- tts_com = edge_tts.Communicate(text, speaker_name)
- tts_raw = b''
-
- # Stream TTS audio to bytes
- async for chunk in tts_com.stream():
- if chunk['type'] == 'audio':
- tts_raw += chunk['data']
-
- # Convert mp3 stream to wav
- ffmpeg_proc = await asyncio.create_subprocess_exec(
- 'ffmpeg',
- '-f', 'mp3',
- '-i', '-',
- '-f', 'wav',
- '-',
- stdin=asyncio.subprocess.PIPE,
- stdout=asyncio.subprocess.PIPE
- )
- (tts_wav, _) = await ffmpeg_proc.communicate(tts_raw)
-
- return librosa.load(BytesIO(tts_wav))
diff --git a/spaces/Benson/text-generation/Examples/Descargar Apk Mod Hello Neighbor.md b/spaces/Benson/text-generation/Examples/Descargar Apk Mod Hello Neighbor.md
deleted file mode 100644
index 146e707ed9e97f1203718ed48894dd50d3caaa1d..0000000000000000000000000000000000000000
--- a/spaces/Benson/text-generation/Examples/Descargar Apk Mod Hello Neighbor.md
+++ /dev/null
@@ -1,63 +0,0 @@
-
-
Descargar APK Mod Hola vecino: Cómo colarse en la casa de su vecino con recursos ilimitados
-
¿Alguna vez te has preguntado qué esconde tu vecino en su sótano? ¿Tienes el coraje y las habilidades para colarte en su casa y averiguarlo? Si eres un fan de los juegos de terror sigilosos, es posible que hayas oído hablar de Hello Neighbor, un juego popular que te desafía a ser más astuto que una IA avanzada que aprende de cada movimiento. Pero ¿qué pasa si quieres tener más diversión y libertad en el juego? ¿Qué pasa si desea acceder a todos los niveles, secretos y artículos sin pasar horas o dinero? En este artículo, le mostraremos cómo download APK mod Hello Neighbor, una versión modificada del juego que le da recursos ilimitados y trucos. Pero antes de hacer eso, vamos a averiguar más sobre el juego en sí y lo que es un mod APK.
Hello Neighbor es un juego de terror oculto que fue lanzado en 2017 por Dynamic Pixels y tinyBuild. El juego está disponible para Windows, Xbox One, PlayStation 4, Nintendo Switch, iOS, Android y Stadia. El juego también ha generado varios spin-offs, como Secret Neighbor, Hello Neighbor Hide and Seek, Hello Engineer y Hello Guest.
-
Un juego de terror sigiloso con una IA avanzada
-
La premisa principal de Hello Neighbor es que eres un chico curioso que quiere colarse en la casa de tu vecino y descubrir lo que está escondiendo en su sótano. Sin embargo, su vecino no es una persona amigable o normal. Es un hombre misterioso y espeluznante que hará cualquier cosa para evitar que entre en su casa. Él pondrá trampas, te perseguirá, e incluso aprenderá de tus acciones. El juego cuenta con una IA avanzada que se adapta a tu comportamiento y crea nuevos obstáculos y desafíos para ti. Tendrás que usar sigilo, estrategia y creatividad para evitar la detección y alcanzar tu objetivo.
-
Un juego estilo sandbox con interacción ambiental y física
-
-
Una serie de juegos y spin-offs establecidos en el universo Hello Neighbor
-
Hola vecino no es solo un juego. Se trata de una serie de juegos y spin-offs que amplían el universo de Hello Neighbor y ofrecen diferentes perspectivas y experiencias. Algunos de los juegos y spin-offs son: - Secret Neighbor: Un juego multijugador de terror social que enfrenta a un grupo de niños contra uno de ellos que es secretamente el vecino disfrazado. El juego se desarrolla entre el Acto 1 y el Acto 2 de Hello Neighbor. - Hello Neighbor Hide and Seek: Una precuela de Hello Neighbor que revela la trágica historia de fondo del vecino y su familia. El juego es un juego de sigilo que simula un juego de escondidas entre los hijos del vecino. - Hello Engineer: Un juego spin-off que se centra en la construcción y elaboración en lugar de sigilo y horror. El juego se desarrolla en un parque de atracciones abandonado donde tienes que usar materiales de desecho y herramientas para crear máquinas y vehículos. - Hello Guest: Una secuela de Hello Neighbor que sigue a un nuevo protagonista que trabaja como guardia nocturno en el mismo parque de diversiones. El juego es un juego de terror sigiloso que introduce un nuevo enemigo, el Invitado, que te acosa y acosa.
¿Qué es APK Mod?
-
APK Mod es un término que se refiere a una versión modificada de una aplicación Android. APK significa Android Package Kit, que es el formato de archivo utilizado por los dispositivos Android para instalar y distribuir aplicaciones. Mod significa modificación, lo que significa que el archivo APK original ha sido alterado o hackeado para cambiar algunos aspectos de la aplicación.
-
Una versión modificada de una aplicación Android
-
-
Una forma de acceder a funciones premium, recursos ilimitados o trucos
-
Una de las principales razones por las que la gente descarga mods APK es acceder a características premium, recursos ilimitados, o trucos que no están disponibles en la versión original de la aplicación. Por ejemplo, pueden desbloquear todos los niveles, personajes, objetos, armas, etc. También pueden obtener monedas, gemas, vidas, salud, etc. También pueden usar trucos como invencibilidad, hackeo de velocidad, teletransportación, etc.
-
Un riesgo potencial de malware, virus o problemas legales
-
Sin embargo, la descarga de mods APK no está exenta de riesgos. Hay muchas fuentes de archivos APK modificados en Internet, pero no todos ellos son confiables o seguros. Algunos de ellos pueden contener malware, virus, spyware u otro software dañino que puede dañar su dispositivo o comprometer sus datos. Algunos de ellos también pueden violar los términos de servicio o los derechos de propiedad intelectual de los desarrolladores originales o editores de la aplicación. Esto puede resultar en problemas legales o prohibiciones de usar la aplicación.
-
-
Cómo descargar APK mod hola vecino?
-
Si desea descargar APK mod Hello Neighbor, tendrá que seguir estos pasos:
-
Encontrar una fuente confiable de archivos APK modded
-
El primer paso es encontrar una fuente confiable de archivos APK modded que ofrecen mod APK Hello Neighbor. Puede buscar en línea para sitios web o foros que proporcionan enlaces o descargas de archivos APK modded. Sin embargo, debe tener cuidado y hacer algunas investigaciones antes de descargar nada de fuentes desconocidas. Usted debe comprobar las revisiones, calificaciones, comentarios y comentarios de otros usuarios que han descargado el mismo archivo. También debe escanear el archivo con un software antivirus o anti-malware antes de instalarlo.
-
Habilitar fuentes desconocidas en la configuración del dispositivo
-
-
Instalar el archivo APK y disfrutar del juego
-
El tercer paso es instalar el archivo APK y disfrutar del juego. Tendrá que localizar el archivo APK descargado en el almacenamiento del dispositivo y toque en él para iniciar el proceso de instalación. Es posible que tenga que seguir algunas instrucciones o aceptar algunos términos y condiciones antes de completar la instalación. Una vez realizada la instalación, puedes iniciar el juego y disfrutar jugando con recursos y trucos ilimitados.
-
¿Cuáles son los beneficios de descargar APK Mod Hello Neighbor?
-
-
Sin embargo, descargar APK mod Hello Neighbor también puede tener algunos inconvenientes para algunos jugadores que quieren disfrutar de la experiencia original y auténtica del juego. Algunos de los inconvenientes son: - Riesgo de dañar su dispositivo o comprometer sus datos: Como se mencionó anteriormente, descargar APK mod Hello Neighbor de fuentes desconocidas puede exponer su dispositivo o datos a malware, virus, spyware u otro software dañino. Estos pueden dañar su dispositivo o comprometer sus datos al eliminarlos, robarlos o cifrarlos. También pueden causar que el dispositivo falle, se bloquee o se sobrecaliente. También pueden acceder a su información personal, como sus contactos, mensajes, fotos, etc., y usarlas con fines maliciosos. - Riesgo de violación de los términos de servicio o los derechos de propiedad intelectual de los desarrolladores: Descarga APK mod Hello Neighbor también puede violar los términos de servicio o los derechos de propiedad intelectual de los desarrolladores originales o editores del juego. Estas son las reglas y regulaciones que aceptas cuando descargas o juegas el juego desde la fuente oficial. Al descargar APK mod Hola Vecino, usted está rompiendo estas reglas y reglamentos y falta de respeto a los creadores y propietarios del juego. Esto puede resultar en problemas legales o prohibiciones de usar el juego u otros servicios de los desarrolladores o editores. - Riesgo de perder el encanto original y el desafío del juego: Descarga mod APK Hola vecino también puede perder el encanto original y el desafío del juego. El juego está diseñado para ser un juego de terror sigiloso que pone a prueba tus habilidades y nervios contra una IA avanzada que aprende de tus acciones. El juego también está diseñado para ser un juego al estilo sandbox que te anima a experimentar y ser creativo con tu enfoque. Al descargar APK mod Hello Neighbor, estás cambiando estos aspectos del juego y haciéndolo más fácil y menos inmersivo. Usted también está perdiendo la satisfacción y la recompensa de completar
-
-
En conclusión, descargar APK mod Hello Neighbor es una elección personal que depende de qué tipo de jugador eres y qué tipo de experiencia quieres tener en el juego. Si quieres tener más diversión y libertad en el juego, se puede descargar APK mod Hello Neighbor y disfrutar de jugar con recursos ilimitados y trucos. Sin embargo, debe ser consciente de los riesgos y desventajas de hacerlo, como dañar su dispositivo o datos, violar los términos de servicio o los derechos de propiedad intelectual de los desarrolladores, o perder el encanto original y el desafío del juego. Si quieres disfrutar del juego como está destinado a ser jugado, puedes descargar Hello Neighbor desde la fuente oficial y respetar a los creadores y otros jugadores. La elección es tuya, pero lo que elijas, diviértete y sé seguro.
-
Preguntas frecuentes
-
Aquí hay algunas preguntas frecuentes sobre la descarga de APK mod Hola vecino:
-
-
-
Pregunta
-
Respuesta
-
-
-
¿Dónde puedo descargar APK mod Hello Neighbor?
-
Puedes buscar en línea sitios web o foros que proporcionan enlaces o descargas para archivos APK modificados. Sin embargo, debe tener cuidado y hacer algunas investigaciones antes de descargar cualquier cosa de fuentes desconocidas.
-
-
-
¿Cómo puedo instalar APK mod Hello Neighbor?
-
Tendrá que habilitar fuentes desconocidas en la configuración del dispositivo, localizar el archivo APK descargado en el almacenamiento del dispositivo, y toque en él para iniciar el proceso de instalación.
-
-
-
¿Cuáles son algunas características de APK mod Hello Neighbor?
-
Algunas características de APK mod Hola Vecino están desbloqueando todos los niveles y secretos, conseguir artículos ilimitados, monedas, y la salud, y la personalización de su personaje y el juego.
-
-
-
¿Cuáles son algunos de los riesgos de descargar APK mod Hello Neighbor?
-
-
-
-
¿Es legal descargar APK mod Hello Neighbor?
-
Descargar APK mod Hello Neighbor puede no ser legal en algunos países o regiones, ya que puede violar los términos de servicio o los derechos de propiedad intelectual de los desarrolladores originales o editores del juego. Usted debe comprobar las leyes y regulaciones en su área antes de descargar nada de fuentes desconocidas.
-
- 64aa2da5cf
-
-
\ No newline at end of file
diff --git a/spaces/CVPR/LIVE/thrust/thrust/iterator/transform_iterator.h b/spaces/CVPR/LIVE/thrust/thrust/iterator/transform_iterator.h
deleted file mode 100644
index fff050e1c05a3b6ed21478aec96cf6394415f3ab..0000000000000000000000000000000000000000
--- a/spaces/CVPR/LIVE/thrust/thrust/iterator/transform_iterator.h
+++ /dev/null
@@ -1,356 +0,0 @@
-/*
- * Copyright 2008-2013 NVIDIA Corporation
- *
- * Licensed under the Apache License, Version 2.0 (the "License");
- * you may not use this file except in compliance with the License.
- * You may obtain a copy of the License at
- *
- * http://www.apache.org/licenses/LICENSE-2.0
- *
- * Unless required by applicable law or agreed to in writing, software
- * distributed under the License is distributed on an "AS IS" BASIS,
- * WITHOUT WARRANTIES OR CONDITIONS OF ANY KIND, either express or implied.
- * See the License for the specific language governing permissions and
- * limitations under the License.
- */
-
-
-/*! \file thrust/iterator/transform_iterator.h
- * \brief An iterator which adapts another iterator by applying a function to the result of its dereference
- */
-
-/*
- * (C) Copyright David Abrahams 2002.
- * (C) Copyright Jeremy Siek 2002.
- * (C) Copyright Thomas Witt 2002.
- *
- * Distributed under the Boost Software License, Version 1.0.
- * (See accompanying NOTICE file for the complete license)
- *
- * For more information, see http://www.boost.org
- */
-
-#pragma once
-
-#include
-
-// #include the details first
-#include
-#include
-#include
-#include
-
-namespace thrust
-{
-
-/*! \addtogroup iterators
- * \{
- */
-
-/*! \addtogroup fancyiterator Fancy Iterators
- * \ingroup iterators
- * \{
- */
-
-/*! \p transform_iterator is an iterator which represents a pointer into a range
- * of values after transformation by a function. This iterator is useful for
- * creating a range filled with the result of applying an operation to another range
- * without either explicitly storing it in memory, or explicitly executing the transformation.
- * Using \p transform_iterator facilitates kernel fusion by deferring the execution
- * of a transformation until the value is needed while saving both memory capacity
- * and bandwidth.
- *
- * The following code snippet demonstrates how to create a \p transform_iterator
- * which represents the result of \c sqrtf applied to the contents of a \p device_vector.
- *
- * \code
- * #include
- * #include
- *
- * // note: functor inherits from unary_function
- * struct square_root : public thrust::unary_function
- * {
- * __host__ __device__
- * float operator()(float x) const
- * {
- * return sqrtf(x);
- * }
- * };
- *
- * int main()
- * {
- * thrust::device_vector v(4);
- * v[0] = 1.0f;
- * v[1] = 4.0f;
- * v[2] = 9.0f;
- * v[3] = 16.0f;
- *
- * typedef thrust::device_vector::iterator FloatIterator;
- *
- * thrust::transform_iterator iter(v.begin(), square_root());
- *
- * *iter; // returns 1.0f
- * iter[0]; // returns 1.0f;
- * iter[1]; // returns 2.0f;
- * iter[2]; // returns 3.0f;
- * iter[3]; // returns 4.0f;
- *
- * // iter[4] is an out-of-bounds error
- * }
- * \endcode
- *
- * This next example demonstrates how to use a \p transform_iterator with the
- * \p thrust::reduce function to compute the sum of squares of a sequence.
- * We will create temporary \p transform_iterators with the
- * \p make_transform_iterator function in order to avoid explicitly specifying their type:
- *
- * \code
- * #include
- * #include
- * #include
- * #include
- *
- * // note: functor inherits from unary_function
- * struct square : public thrust::unary_function
- * {
- * __host__ __device__
- * float operator()(float x) const
- * {
- * return x * x;
- * }
- * };
- *
- * int main()
- * {
- * // initialize a device array
- * thrust::device_vector v(4);
- * v[0] = 1.0f;
- * v[1] = 2.0f;
- * v[2] = 3.0f;
- * v[3] = 4.0f;
- *
- * float sum_of_squares =
- * thrust::reduce(thrust::make_transform_iterator(v.begin(), square()),
- * thrust::make_transform_iterator(v.end(), square()));
- *
- * std::cout << "sum of squares: " << sum_of_squares << std::endl;
- * return 0;
- * }
- * \endcode
- *
- * Note that in the previous two examples the transform functor (namely \c square_root
- * and \c square) inherits from \c thrust::unary_function. Inheriting from
- * \c thrust::unary_function ensures that a functor is a valid \c AdaptableUnaryFunction
- * and provides all the necessary \c typedef declarations. The \p transform_iterator
- * can also be applied to a \c UnaryFunction that does not inherit from
- * \c thrust::unary_function using an optional template argument. The following example
- * illustrates how to use the third template argument to specify the \c result_type of
- * the function.
- *
- * \code
- * #include
- * #include
- *
- * // note: functor *does not* inherit from unary_function
- * struct square_root
- * {
- * __host__ __device__
- * float operator()(float x) const
- * {
- * return sqrtf(x);
- * }
- * };
- *
- * int main()
- * {
- * thrust::device_vector v(4);
- * v[0] = 1.0f;
- * v[1] = 4.0f;
- * v[2] = 9.0f;
- * v[3] = 16.0f;
- *
- * typedef thrust::device_vector::iterator FloatIterator;
- *
- * // note: float result_type is specified explicitly
- * thrust::transform_iterator iter(v.begin(), square_root());
- *
- * *iter; // returns 1.0f
- * iter[0]; // returns 1.0f;
- * iter[1]; // returns 2.0f;
- * iter[2]; // returns 3.0f;
- * iter[3]; // returns 4.0f;
- *
- * // iter[4] is an out-of-bounds error
- * }
- * \endcode
- *
- * \see make_transform_iterator
- */
-template
- class transform_iterator
- : public detail::transform_iterator_base::type
-{
- /*! \cond
- */
- public:
- typedef typename
- detail::transform_iterator_base::type
- super_t;
-
- friend class thrust::iterator_core_access;
- /*! \endcond
- */
-
- public:
- /*! Null constructor does nothing.
- */
- __host__ __device__
- transform_iterator() {}
-
-#if THRUST_CPP_DIALECT >= 2011
- transform_iterator(transform_iterator const&) = default;
-#endif
-
- /*! This constructor takes as arguments an \c Iterator and an \c AdaptableUnaryFunction
- * and copies them to a new \p transform_iterator.
- *
- * \param x An \c Iterator pointing to the input to this \p transform_iterator's \c AdaptableUnaryFunction.
- * \param f An \c AdaptableUnaryFunction used to transform the objects pointed to by \p x.
- */
- __host__ __device__
- transform_iterator(Iterator const& x, AdaptableUnaryFunction f)
- : super_t(x), m_f(f) {
- }
-
- /*! This explicit constructor copies the value of a given \c Iterator and creates
- * this \p transform_iterator's \c AdaptableUnaryFunction using its null constructor.
- *
- * \param x An \c Iterator to copy.
- */
- __host__ __device__
- explicit transform_iterator(Iterator const& x)
- : super_t(x) { }
-
- /*! This copy constructor creates a new \p transform_iterator from another
- * \p transform_iterator.
- *
- * \param other The \p transform_iterator to copy.
- */
- template
- __host__ __device__
- transform_iterator(const transform_iterator &other,
- typename thrust::detail::enable_if_convertible::type* = 0,
- typename thrust::detail::enable_if_convertible::type* = 0)
- : super_t(other.base()), m_f(other.functor()) {}
-
- /*! Copy assignment operator copies from another \p transform_iterator.
- * \p other The other \p transform_iterator to copy
- * \return *this
- *
- * \note If the type of this \p transform_iterator's functor is not copy assignable
- * (for example, if it is a lambda) it is not an error to call this function.
- * In this case, however, the functor will not be modified.
- *
- * In any case, this \p transform_iterator's underlying iterator will be copy assigned.
- */
- __host__ __device__
- transform_iterator &operator=(const transform_iterator &other)
- {
- return do_assign(other,
- // XXX gcc 4.2.1 crashes on is_copy_assignable; just assume the functor is assignable as a WAR
-#if (THRUST_HOST_COMPILER == THRUST_HOST_COMPILER_GCC) && (THRUST_GCC_VERSION <= 40201)
- thrust::detail::true_type()
-#else
- typename thrust::detail::is_copy_assignable::type()
-#endif // THRUST_HOST_COMPILER
- );
- }
-
- /*! This method returns a copy of this \p transform_iterator's \c AdaptableUnaryFunction.
- * \return A copy of this \p transform_iterator's \c AdaptableUnaryFunction.
- */
- __host__ __device__
- AdaptableUnaryFunction functor() const
- { return m_f; }
-
- /*! \cond
- */
- private:
- __host__ __device__
- transform_iterator &do_assign(const transform_iterator &other, thrust::detail::true_type)
- {
- super_t::operator=(other);
-
- // do assign to m_f
- m_f = other.functor();
-
- return *this;
- }
-
- __host__ __device__
- transform_iterator &do_assign(const transform_iterator &other, thrust::detail::false_type)
- {
- super_t::operator=(other);
-
- // don't assign to m_f
-
- return *this;
- }
-
- // MSVC 2013 and 2015 incorrectly warning about returning a reference to
- // a local/temporary here.
- // See goo.gl/LELTNp
- THRUST_DISABLE_MSVC_WARNING_BEGIN(4172)
-
- __thrust_exec_check_disable__
- __host__ __device__
- typename super_t::reference dereference() const
- {
- // Create a temporary to allow iterators with wrapped references to
- // convert to their value type before calling m_f. Note that this
- // disallows non-constant operations through m_f.
- typename thrust::iterator_value::type x = *this->base();
- return m_f(x);
- }
-
- THRUST_DISABLE_MSVC_WARNING_END(4172)
-
- // tag this as mutable per Dave Abrahams in this thread:
- // http://lists.boost.org/Archives/boost/2004/05/65332.php
- mutable AdaptableUnaryFunction m_f;
-
- /*! \endcond
- */
-}; // end transform_iterator
-
-
-/*! \p make_transform_iterator creates a \p transform_iterator
- * from an \c Iterator and \c AdaptableUnaryFunction.
- *
- * \param it The \c Iterator pointing to the input range of the
- * newly created \p transform_iterator.
- * \param fun The \c AdaptableUnaryFunction used to transform the range pointed
- * to by \p it in the newly created \p transform_iterator.
- * \return A new \p transform_iterator which transforms the range at
- * \p it by \p fun.
- * \see transform_iterator
- */
-template
-inline __host__ __device__
-transform_iterator
-make_transform_iterator(Iterator it, AdaptableUnaryFunction fun)
-{
- return transform_iterator(it, fun);
-} // end make_transform_iterator
-
-/*! \} // end fancyiterators
- */
-
-/*! \} // end iterators
- */
-
-} // end thrust
-
diff --git a/spaces/CVPR/LIVE/thrust/thrust/system/tbb/detail/transform_scan.h b/spaces/CVPR/LIVE/thrust/thrust/system/tbb/detail/transform_scan.h
deleted file mode 100644
index 75b075b6b16f063a1c5cda8893911d3f3c533f2d..0000000000000000000000000000000000000000
--- a/spaces/CVPR/LIVE/thrust/thrust/system/tbb/detail/transform_scan.h
+++ /dev/null
@@ -1,23 +0,0 @@
-/*
- * Copyright 2008-2013 NVIDIA Corporation
- *
- * Licensed under the Apache License, Version 2.0 (the "License");
- * you may not use this file except in compliance with the License.
- * You may obtain a copy of the License at
- *
- * http://www.apache.org/licenses/LICENSE-2.0
- *
- * Unless required by applicable law or agreed to in writing, software
- * distributed under the License is distributed on an "AS IS" BASIS,
- * WITHOUT WARRANTIES OR CONDITIONS OF ANY KIND, either express or implied.
- * See the License for the specific language governing permissions and
- * limitations under the License.
- */
-
-#pragma once
-
-#include
-
-// this system inherits transform_scan
-#include
-
diff --git a/spaces/CVPR/WALT/mmdet/models/backbones/resnest.py b/spaces/CVPR/WALT/mmdet/models/backbones/resnest.py
deleted file mode 100644
index 48e1d8bfa47348a13f0da0b9ecf32354fa270340..0000000000000000000000000000000000000000
--- a/spaces/CVPR/WALT/mmdet/models/backbones/resnest.py
+++ /dev/null
@@ -1,317 +0,0 @@
-import math
-
-import torch
-import torch.nn as nn
-import torch.nn.functional as F
-import torch.utils.checkpoint as cp
-from mmcv.cnn import build_conv_layer, build_norm_layer
-
-from ..builder import BACKBONES
-from ..utils import ResLayer
-from .resnet import Bottleneck as _Bottleneck
-from .resnet import ResNetV1d
-
-
-class RSoftmax(nn.Module):
- """Radix Softmax module in ``SplitAttentionConv2d``.
-
- Args:
- radix (int): Radix of input.
- groups (int): Groups of input.
- """
-
- def __init__(self, radix, groups):
- super().__init__()
- self.radix = radix
- self.groups = groups
-
- def forward(self, x):
- batch = x.size(0)
- if self.radix > 1:
- x = x.view(batch, self.groups, self.radix, -1).transpose(1, 2)
- x = F.softmax(x, dim=1)
- x = x.reshape(batch, -1)
- else:
- x = torch.sigmoid(x)
- return x
-
-
-class SplitAttentionConv2d(nn.Module):
- """Split-Attention Conv2d in ResNeSt.
-
- Args:
- in_channels (int): Number of channels in the input feature map.
- channels (int): Number of intermediate channels.
- kernel_size (int | tuple[int]): Size of the convolution kernel.
- stride (int | tuple[int]): Stride of the convolution.
- padding (int | tuple[int]): Zero-padding added to both sides of
- dilation (int | tuple[int]): Spacing between kernel elements.
- groups (int): Number of blocked connections from input channels to
- output channels.
- groups (int): Same as nn.Conv2d.
- radix (int): Radix of SpltAtConv2d. Default: 2
- reduction_factor (int): Reduction factor of inter_channels. Default: 4.
- conv_cfg (dict): Config dict for convolution layer. Default: None,
- which means using conv2d.
- norm_cfg (dict): Config dict for normalization layer. Default: None.
- dcn (dict): Config dict for DCN. Default: None.
- """
-
- def __init__(self,
- in_channels,
- channels,
- kernel_size,
- stride=1,
- padding=0,
- dilation=1,
- groups=1,
- radix=2,
- reduction_factor=4,
- conv_cfg=None,
- norm_cfg=dict(type='BN'),
- dcn=None):
- super(SplitAttentionConv2d, self).__init__()
- inter_channels = max(in_channels * radix // reduction_factor, 32)
- self.radix = radix
- self.groups = groups
- self.channels = channels
- self.with_dcn = dcn is not None
- self.dcn = dcn
- fallback_on_stride = False
- if self.with_dcn:
- fallback_on_stride = self.dcn.pop('fallback_on_stride', False)
- if self.with_dcn and not fallback_on_stride:
- assert conv_cfg is None, 'conv_cfg must be None for DCN'
- conv_cfg = dcn
- self.conv = build_conv_layer(
- conv_cfg,
- in_channels,
- channels * radix,
- kernel_size,
- stride=stride,
- padding=padding,
- dilation=dilation,
- groups=groups * radix,
- bias=False)
- # To be consistent with original implementation, starting from 0
- self.norm0_name, norm0 = build_norm_layer(
- norm_cfg, channels * radix, postfix=0)
- self.add_module(self.norm0_name, norm0)
- self.relu = nn.ReLU(inplace=True)
- self.fc1 = build_conv_layer(
- None, channels, inter_channels, 1, groups=self.groups)
- self.norm1_name, norm1 = build_norm_layer(
- norm_cfg, inter_channels, postfix=1)
- self.add_module(self.norm1_name, norm1)
- self.fc2 = build_conv_layer(
- None, inter_channels, channels * radix, 1, groups=self.groups)
- self.rsoftmax = RSoftmax(radix, groups)
-
- @property
- def norm0(self):
- """nn.Module: the normalization layer named "norm0" """
- return getattr(self, self.norm0_name)
-
- @property
- def norm1(self):
- """nn.Module: the normalization layer named "norm1" """
- return getattr(self, self.norm1_name)
-
- def forward(self, x):
- x = self.conv(x)
- x = self.norm0(x)
- x = self.relu(x)
-
- batch, rchannel = x.shape[:2]
- batch = x.size(0)
- if self.radix > 1:
- splits = x.view(batch, self.radix, -1, *x.shape[2:])
- gap = splits.sum(dim=1)
- else:
- gap = x
- gap = F.adaptive_avg_pool2d(gap, 1)
- gap = self.fc1(gap)
-
- gap = self.norm1(gap)
- gap = self.relu(gap)
-
- atten = self.fc2(gap)
- atten = self.rsoftmax(atten).view(batch, -1, 1, 1)
-
- if self.radix > 1:
- attens = atten.view(batch, self.radix, -1, *atten.shape[2:])
- out = torch.sum(attens * splits, dim=1)
- else:
- out = atten * x
- return out.contiguous()
-
-
-class Bottleneck(_Bottleneck):
- """Bottleneck block for ResNeSt.
-
- Args:
- inplane (int): Input planes of this block.
- planes (int): Middle planes of this block.
- groups (int): Groups of conv2.
- base_width (int): Base of width in terms of base channels. Default: 4.
- base_channels (int): Base of channels for calculating width.
- Default: 64.
- radix (int): Radix of SpltAtConv2d. Default: 2
- reduction_factor (int): Reduction factor of inter_channels in
- SplitAttentionConv2d. Default: 4.
- avg_down_stride (bool): Whether to use average pool for stride in
- Bottleneck. Default: True.
- kwargs (dict): Key word arguments for base class.
- """
- expansion = 4
-
- def __init__(self,
- inplanes,
- planes,
- groups=1,
- base_width=4,
- base_channels=64,
- radix=2,
- reduction_factor=4,
- avg_down_stride=True,
- **kwargs):
- """Bottleneck block for ResNeSt."""
- super(Bottleneck, self).__init__(inplanes, planes, **kwargs)
-
- if groups == 1:
- width = self.planes
- else:
- width = math.floor(self.planes *
- (base_width / base_channels)) * groups
-
- self.avg_down_stride = avg_down_stride and self.conv2_stride > 1
-
- self.norm1_name, norm1 = build_norm_layer(
- self.norm_cfg, width, postfix=1)
- self.norm3_name, norm3 = build_norm_layer(
- self.norm_cfg, self.planes * self.expansion, postfix=3)
-
- self.conv1 = build_conv_layer(
- self.conv_cfg,
- self.inplanes,
- width,
- kernel_size=1,
- stride=self.conv1_stride,
- bias=False)
- self.add_module(self.norm1_name, norm1)
- self.with_modulated_dcn = False
- self.conv2 = SplitAttentionConv2d(
- width,
- width,
- kernel_size=3,
- stride=1 if self.avg_down_stride else self.conv2_stride,
- padding=self.dilation,
- dilation=self.dilation,
- groups=groups,
- radix=radix,
- reduction_factor=reduction_factor,
- conv_cfg=self.conv_cfg,
- norm_cfg=self.norm_cfg,
- dcn=self.dcn)
- delattr(self, self.norm2_name)
-
- if self.avg_down_stride:
- self.avd_layer = nn.AvgPool2d(3, self.conv2_stride, padding=1)
-
- self.conv3 = build_conv_layer(
- self.conv_cfg,
- width,
- self.planes * self.expansion,
- kernel_size=1,
- bias=False)
- self.add_module(self.norm3_name, norm3)
-
- def forward(self, x):
-
- def _inner_forward(x):
- identity = x
-
- out = self.conv1(x)
- out = self.norm1(out)
- out = self.relu(out)
-
- if self.with_plugins:
- out = self.forward_plugin(out, self.after_conv1_plugin_names)
-
- out = self.conv2(out)
-
- if self.avg_down_stride:
- out = self.avd_layer(out)
-
- if self.with_plugins:
- out = self.forward_plugin(out, self.after_conv2_plugin_names)
-
- out = self.conv3(out)
- out = self.norm3(out)
-
- if self.with_plugins:
- out = self.forward_plugin(out, self.after_conv3_plugin_names)
-
- if self.downsample is not None:
- identity = self.downsample(x)
-
- out += identity
-
- return out
-
- if self.with_cp and x.requires_grad:
- out = cp.checkpoint(_inner_forward, x)
- else:
- out = _inner_forward(x)
-
- out = self.relu(out)
-
- return out
-
-
-@BACKBONES.register_module()
-class ResNeSt(ResNetV1d):
- """ResNeSt backbone.
-
- Args:
- groups (int): Number of groups of Bottleneck. Default: 1
- base_width (int): Base width of Bottleneck. Default: 4
- radix (int): Radix of SplitAttentionConv2d. Default: 2
- reduction_factor (int): Reduction factor of inter_channels in
- SplitAttentionConv2d. Default: 4.
- avg_down_stride (bool): Whether to use average pool for stride in
- Bottleneck. Default: True.
- kwargs (dict): Keyword arguments for ResNet.
- """
-
- arch_settings = {
- 50: (Bottleneck, (3, 4, 6, 3)),
- 101: (Bottleneck, (3, 4, 23, 3)),
- 152: (Bottleneck, (3, 8, 36, 3)),
- 200: (Bottleneck, (3, 24, 36, 3))
- }
-
- def __init__(self,
- groups=1,
- base_width=4,
- radix=2,
- reduction_factor=4,
- avg_down_stride=True,
- **kwargs):
- self.groups = groups
- self.base_width = base_width
- self.radix = radix
- self.reduction_factor = reduction_factor
- self.avg_down_stride = avg_down_stride
- super(ResNeSt, self).__init__(**kwargs)
-
- def make_res_layer(self, **kwargs):
- """Pack all blocks in a stage into a ``ResLayer``."""
- return ResLayer(
- groups=self.groups,
- base_width=self.base_width,
- base_channels=self.base_channels,
- radix=self.radix,
- reduction_factor=self.reduction_factor,
- avg_down_stride=self.avg_down_stride,
- **kwargs)
diff --git a/spaces/CVPR/regionclip-demo/detectron2/checkpoint/clip_model_loading.py b/spaces/CVPR/regionclip-demo/detectron2/checkpoint/clip_model_loading.py
deleted file mode 100644
index 12b0fe79aa3d59d72742c33a111fe5d22fb6c725..0000000000000000000000000000000000000000
--- a/spaces/CVPR/regionclip-demo/detectron2/checkpoint/clip_model_loading.py
+++ /dev/null
@@ -1,415 +0,0 @@
-# Copyright (c) Facebook, Inc. and its affiliates.
-import copy
-import logging
-import re
-from typing import Dict, List
-import torch
-from tabulate import tabulate
-
-
-def convert_basic_clip_names(original_keys, add_backbone_prefix=False, use_whole_clip=False, use_fpn_arch=False, regionclip=False):
- """
- Apply some basic name conversion to names in CLIP weights.
- It only deals with typical backbone models.
-
- Args:
- original_keys (list[str]):
- Returns:
- list[str]: The same number of strings matching those in original_keys.
- """
- layer_keys = copy.deepcopy(original_keys)
-
- vit = False
- for l_k in layer_keys:
- if 'visual.transformer' in l_k:
- vit = True
-
- # load pretrained oai clip
- if not vit: # resnet
- if add_backbone_prefix: # CLIPRCNN or CLIPFastRCNN
- if use_whole_clip: # CLIPRCNN
- layer_keys = [k.replace("visual.", "clip_backbone.visual.") for k in layer_keys]
- else: # CLIPFastRCNN
- if use_fpn_arch: # FPN
- layer_keys = [k.replace("visual.", "backbone.bottom_up.") for k in layer_keys]
- else: # C4
- layer_keys = [k.replace("visual.", "backbone.") for k in layer_keys]
- else: # GeneralizedRCNN or ProposalNetwork
- #layer_keys = [k.replace("visual.", "backbone.bottom_up.") for k in layer_keys] #
- layer_keys = [k.replace("visual.", "") for k in layer_keys] #
- #layer_keys = [k.replace("visual.", "backbone.visual.") for k in layer_keys] #
- else: # vit
- pass
-
- return layer_keys, vit
-
-
-def convert_clip_names(weights, add_backbone_prefix=False, use_whole_clip=False, use_fpn_arch=False, regionclip=False):
- """
- Map CLIP Detectron weight names to Detectron2 names.
-
- Args:
- weights (dict): name -> tensor
-
- Returns:
- dict: detectron2 names -> tensor
- dict: detectron2 names -> C2 names
- """
- logger = logging.getLogger(__name__)
- logger.info("Renaming CLIP weights ......")
- original_keys = sorted(weights.keys())
- layer_keys = copy.deepcopy(original_keys)
-
- layer_keys, use_vit = convert_basic_clip_names(layer_keys, add_backbone_prefix, use_whole_clip, use_fpn_arch, regionclip)
-
- # --------------------------------------------------------------------------
- # RPN hidden representation conv
- # --------------------------------------------------------------------------
- # FPN case
- # In the C2 model, the RPN hidden layer conv is defined for FPN level 2 and then
- # shared for all other levels, hence the appearance of "fpn2"
- layer_keys = [
- k.replace("conv.rpn.fpn2", "proposal_generator.rpn_head.conv") for k in layer_keys
- ]
- # Non-FPN case
- layer_keys = [k.replace("conv.rpn", "proposal_generator.rpn_head.conv") for k in layer_keys]
-
- # --------------------------------------------------------------------------
- # RPN box transformation conv
- # --------------------------------------------------------------------------
- # FPN case (see note above about "fpn2")
- layer_keys = [
- k.replace("rpn.bbox.pred.fpn2", "proposal_generator.rpn_head.anchor_deltas")
- for k in layer_keys
- ]
- layer_keys = [
- k.replace("rpn.cls.logits.fpn2", "proposal_generator.rpn_head.objectness_logits")
- for k in layer_keys
- ]
- # Non-FPN case
- layer_keys = [
- k.replace("rpn.bbox.pred", "proposal_generator.rpn_head.anchor_deltas") for k in layer_keys
- ]
- layer_keys = [
- k.replace("rpn.cls.logits", "proposal_generator.rpn_head.objectness_logits")
- for k in layer_keys
- ]
-
- # --------------------------------------------------------------------------
- # Fast R-CNN box head
- # --------------------------------------------------------------------------
- layer_keys = [re.sub("^bbox\\.pred", "bbox_pred", k) for k in layer_keys]
- layer_keys = [re.sub("^cls\\.score", "cls_score", k) for k in layer_keys]
- layer_keys = [re.sub("^fc6\\.", "box_head.fc1.", k) for k in layer_keys]
- layer_keys = [re.sub("^fc7\\.", "box_head.fc2.", k) for k in layer_keys]
- # 4conv1fc head tensor names: head_conv1_w, head_conv1_gn_s
- layer_keys = [re.sub("^head\\.conv", "box_head.conv", k) for k in layer_keys]
-
- # --------------------------------------------------------------------------
- # FPN lateral and output convolutions
- # --------------------------------------------------------------------------
- def fpn_map(name):
- """
- Look for keys with the following patterns:
- 1) Starts with "fpn.inner."
- Example: "fpn.inner.res2.2.sum.lateral.weight"
- Meaning: These are lateral pathway convolutions
- 2) Starts with "fpn.res"
- Example: "fpn.res2.2.sum.weight"
- Meaning: These are FPN output convolutions
- """
- splits = name.split(".")
- norm = ".norm" if "norm" in splits else ""
- if name.startswith("fpn.inner."):
- # splits example: ['fpn', 'inner', 'res2', '2', 'sum', 'lateral', 'weight']
- stage = int(splits[2][len("res") :])
- return "fpn_lateral{}{}.{}".format(stage, norm, splits[-1])
- elif name.startswith("fpn.res"):
- # splits example: ['fpn', 'res2', '2', 'sum', 'weight']
- stage = int(splits[1][len("res") :])
- return "fpn_output{}{}.{}".format(stage, norm, splits[-1])
- return name
-
- layer_keys = [fpn_map(k) for k in layer_keys]
-
- # --------------------------------------------------------------------------
- # Mask R-CNN mask head
- # --------------------------------------------------------------------------
- # roi_heads.StandardROIHeads case
- layer_keys = [k.replace(".[mask].fcn", "mask_head.mask_fcn") for k in layer_keys]
- layer_keys = [re.sub("^\\.mask\\.fcn", "mask_head.mask_fcn", k) for k in layer_keys]
- layer_keys = [k.replace("mask.fcn.logits", "mask_head.predictor") for k in layer_keys]
- # roi_heads.Res5ROIHeads case
- layer_keys = [k.replace("conv5.mask", "mask_head.deconv") for k in layer_keys]
-
- # --------------------------------------------------------------------------
- # Keypoint R-CNN head
- # --------------------------------------------------------------------------
- # interestingly, the keypoint head convs have blob names that are simply "conv_fcnX"
- layer_keys = [k.replace("conv.fcn", "roi_heads.keypoint_head.conv_fcn") for k in layer_keys]
- layer_keys = [
- k.replace("kps.score.lowres", "roi_heads.keypoint_head.score_lowres") for k in layer_keys
- ]
- layer_keys = [k.replace("kps.score.", "roi_heads.keypoint_head.score.") for k in layer_keys]
-
- # --------------------------------------------------------------------------
- # Done with replacements
- # --------------------------------------------------------------------------
- assert len(set(layer_keys)) == len(layer_keys)
- assert len(original_keys) == len(layer_keys)
-
- new_weights = {}
- new_keys_to_original_keys = {}
- for orig, renamed in zip(original_keys, layer_keys):
- new_keys_to_original_keys[renamed] = orig
- if renamed.startswith("bbox_pred.") or renamed.startswith("mask_head.predictor."):
- # remove the meaningless prediction weight for background class
- new_start_idx = 4 if renamed.startswith("bbox_pred.") else 1
- new_weights[renamed] = weights[orig][new_start_idx:]
- logger.info(
- "Remove prediction weight for background class in {}. The shape changes from "
- "{} to {}.".format(
- renamed, tuple(weights[orig].shape), tuple(new_weights[renamed].shape)
- )
- )
- elif renamed.startswith("cls_score."):
- # move weights of bg class from original index 0 to last index
- logger.info(
- "Move classification weights for background class in {} from index 0 to "
- "index {}.".format(renamed, weights[orig].shape[0] - 1)
- )
- new_weights[renamed] = torch.cat([weights[orig][1:], weights[orig][:1]])
- else:
- new_weights[renamed] = weights[orig]
-
- return new_weights, new_keys_to_original_keys, use_vit
-
-
-# Note the current matching is not symmetric.
-# it assumes model_state_dict will have longer names.
-def align_and_update_state_dicts_for_CLIP(model_state_dict, ckpt_state_dict, c2_conversion=True, bb_rpn_weights=False, regionclip=False):
- """
- Extended from ./c2_model_loading.py
- Match names between the two state-dict, and returns a new chkpt_state_dict with names
- converted to match model_state_dict with heuristics. The returned dict can be later
- loaded with fvcore checkpointer.
- If `c2_conversion==True`, `ckpt_state_dict` is assumed to be a Caffe2
- model and will be renamed at first.
-
- Strategy: suppose that the models that we will create will have prefixes appended
- to each of its keys, for example due to an extra level of nesting that the original
- pre-trained weights from ImageNet won't contain. For example, model.state_dict()
- might return backbone[0].body.res2.conv1.weight, while the pre-trained model contains
- res2.conv1.weight. We thus want to match both parameters together.
- For that, we look for each model weight, look among all loaded keys if there is one
- that is a suffix of the current weight name, and use it if that's the case.
- If multiple matches exist, take the one with longest size
- of the corresponding name. For example, for the same model as before, the pretrained
- weight file can contain both res2.conv1.weight, as well as conv1.weight. In this case,
- we want to match backbone[0].body.conv1.weight to conv1.weight, and
- backbone[0].body.res2.conv1.weight to res2.conv1.weight.
- """
- model_keys = sorted(model_state_dict.keys())
- use_whole_clip = False # whether use the whole clip (text & visual encoders), typically in CLIPRCNN meta arch
- add_backbone_prefix = False # convert to 'backbone.' prefix, typically in CLIPFastRCNN meta arch
- use_fpn_arch = False # if use FPN arch then convert to `bottom_up`, typically in CLIPFastRCNN meta arch with FPN backbone
- if bb_rpn_weights: # a 2nd pretrained weights to load, for offline backbone & RPN, then convert the ckpt key names and only keep the ones we need
- new_ckpt_state_dict = {}
- for original_k in ckpt_state_dict:
- if 'backbone' in original_k:
- new_key = original_k.replace('backbone', 'offline_backbone')
- new_ckpt_state_dict[new_key] = ckpt_state_dict[original_k]
- if 'proposal_generator' in original_k:
- new_key = original_k.replace('proposal_generator', 'offline_proposal_generator')
- new_ckpt_state_dict[new_key] = ckpt_state_dict[original_k]
- new_ckpt_state_dict['ignore_others'] = torch.tensor([1]) # ignore other model weights (not 'offline_*') in batch_norm.py
- ckpt_state_dict = new_ckpt_state_dict
- else: # the 1st pretrained weigths to load
- for model_key in model_keys: # if use the whole clip, then convert ckpt 'visual.' names to 'clip_backbone.visual.'
- if 'clip_backbone' in model_key:
- use_whole_clip = True
- for model_key in model_keys: # if there are backbone & offline_backbone, then convert the ckpt 'visual.' names to 'backbone.' to avoid ambiguity
- if 'offline_backbone' in model_key:
- add_backbone_prefix = True
- if 'fpn' in model_key:
- use_fpn_arch = True
- # original_keys: the name in the original dict (before renaming)
- ckpt_state_dict, original_keys, use_vit = convert_clip_names(ckpt_state_dict, add_backbone_prefix, use_whole_clip, use_fpn_arch, regionclip)
- ckpt_keys = sorted(ckpt_state_dict.keys())
-
- def match(a, b):
- # Matched ckpt_key should be a complete (starts with '.') suffix.
- # For example, roi_heads.mesh_head.whatever_conv1 does not match conv1,
- # but matches whatever_conv1 or mesh_head.whatever_conv1.
- return a == b or a.endswith("." + b)
-
- # get a matrix of string matches, where each (i, j) entry correspond to the size of the
- # ckpt_key string, if it matches
- match_matrix = [len(j) if match(i, j) else 0 for i in model_keys for j in ckpt_keys]
- match_matrix = torch.as_tensor(match_matrix).view(len(model_keys), len(ckpt_keys))
- # use the matched one with longest size in case of multiple matches
- max_match_size, idxs = match_matrix.max(1)
- # remove indices that correspond to no-match
- idxs[max_match_size == 0] = -1
-
- logger = logging.getLogger(__name__)
- # matched_pairs (matched checkpoint key --> matched model key)
- matched_keys = {}
- result_state_dict = {}
- for idx_model, idx_ckpt in enumerate(idxs.tolist()):
- if idx_ckpt == -1:
- continue
- key_model = model_keys[idx_model]
- key_ckpt = ckpt_keys[idx_ckpt]
- value_ckpt = ckpt_state_dict[key_ckpt]
- shape_in_model = model_state_dict[key_model].shape
-
- if shape_in_model != value_ckpt.shape:
- logger.warning(
- "Shape of {} in checkpoint is {}, while shape of {} in model is {}.".format(
- key_ckpt, value_ckpt.shape, key_model, shape_in_model
- )
- )
- logger.warning(
- "{} will not be loaded. Please double check and see if this is desired.".format(
- key_ckpt
- )
- )
- continue
-
- assert key_model not in result_state_dict
- result_state_dict[key_model] = value_ckpt
- if key_ckpt in matched_keys: # already added to matched_keys
- logger.error(
- "Ambiguity found for {} in checkpoint!"
- "It matches at least two keys in the model ({} and {}).".format(
- key_ckpt, key_model, matched_keys[key_ckpt]
- )
- )
- raise ValueError("Cannot match one checkpoint key to multiple keys in the model.")
-
- matched_keys[key_ckpt] = key_model
-
- # logging:
- matched_model_keys = sorted(matched_keys.values())
- mmk_list = "The following model parameters are loaded from checkpoints:\n"
- for mmk in matched_model_keys:
- mmk_list += mmk + "\n"
- if len(matched_model_keys) == 0:
- logger.warning("No weights in checkpoint matched with model.")
- return ckpt_state_dict
- common_prefix = _longest_common_prefix(matched_model_keys)
- rev_matched_keys = {v: k for k, v in matched_keys.items()}
- original_keys = {k: original_keys[rev_matched_keys[k]] for k in matched_model_keys}
-
- model_key_groups = _group_keys_by_module(matched_model_keys, original_keys)
- table = []
- memo = set()
- for key_model in matched_model_keys:
- if key_model in memo:
- continue
- if key_model in model_key_groups:
- group = model_key_groups[key_model]
- memo |= set(group)
- shapes = [tuple(model_state_dict[k].shape) for k in group]
- table.append(
- (
- _longest_common_prefix([k[len(common_prefix) :] for k in group]) + "*",
- _group_str([original_keys[k] for k in group]),
- " ".join([str(x).replace(" ", "") for x in shapes]),
- )
- )
- else:
- key_checkpoint = original_keys[key_model]
- shape = str(tuple(model_state_dict[key_model].shape))
- table.append((key_model[len(common_prefix) :], key_checkpoint, shape))
- table_str = tabulate(
- table, tablefmt="pipe", headers=["Names in Model", "Names in Checkpoint", "Shapes"]
- )
- if len(table) != 1 and not use_vit: # do this for now; the table function has some bugs when the whole CLIP is loaded
- logger.info(
- "Following weights matched with "
- + (f"submodule {common_prefix[:-1]}" if common_prefix else "model")
- + ":\n"
- + table_str
- )
- else:
- logger.info(mmk_list)
-
- unmatched_ckpt_keys = [k for k in ckpt_keys if k not in set(matched_keys.keys())]
- for k in unmatched_ckpt_keys:
- result_state_dict[k] = ckpt_state_dict[k]
- return result_state_dict
-
-
-def _group_keys_by_module(keys: List[str], original_names: Dict[str, str]):
- """
- Params in the same submodule are grouped together.
-
- Args:
- keys: names of all parameters
- original_names: mapping from parameter name to their name in the checkpoint
-
- Returns:
- dict[name -> all other names in the same group]
- """
-
- def _submodule_name(key):
- pos = key.rfind(".")
- if pos < 0:
- return None
- prefix = key[: pos + 1]
- return prefix
-
- all_submodules = [_submodule_name(k) for k in keys]
- all_submodules = [x for x in all_submodules if x]
- all_submodules = sorted(all_submodules, key=len)
-
- ret = {}
- for prefix in all_submodules:
- group = [k for k in keys if k.startswith(prefix)]
- if len(group) <= 1:
- continue
- original_name_lcp = _longest_common_prefix_str([original_names[k] for k in group])
- if len(original_name_lcp) == 0:
- # don't group weights if original names don't share prefix
- continue
-
- for k in group:
- if k in ret:
- continue
- ret[k] = group
- return ret
-
-
-def _longest_common_prefix(names: List[str]) -> str:
- """
- ["abc.zfg", "abc.zef"] -> "abc."
- """
- names = [n.split(".") for n in names]
- m1, m2 = min(names), max(names)
- ret = [a for a, b in zip(m1, m2) if a == b]
- ret = ".".join(ret) + "." if len(ret) else ""
- return ret
-
-
-def _longest_common_prefix_str(names: List[str]) -> str:
- m1, m2 = min(names), max(names)
- lcp = [a for a, b in zip(m1, m2) if a == b]
- lcp = "".join(lcp)
- return lcp
-
-
-def _group_str(names: List[str]) -> str:
- """
- Turn "common1", "common2", "common3" into "common{1,2,3}"
- """
- lcp = _longest_common_prefix_str(names)
- rest = [x[len(lcp) :] for x in names]
- rest = "{" + ",".join(rest) + "}"
- ret = lcp + rest
-
- # add some simplification for BN specifically
- ret = ret.replace("bn_{beta,running_mean,running_var,gamma}", "bn_*")
- ret = ret.replace("bn_beta,bn_running_mean,bn_running_var,bn_gamma", "bn_*")
- return ret
diff --git a/spaces/Caoyunkang/Segment-Any-Anomaly/GroundingDINO/groundingdino/util/vl_utils.py b/spaces/Caoyunkang/Segment-Any-Anomaly/GroundingDINO/groundingdino/util/vl_utils.py
deleted file mode 100644
index c91bb02f584398f08a28e6b7719e2b99f6e28616..0000000000000000000000000000000000000000
--- a/spaces/Caoyunkang/Segment-Any-Anomaly/GroundingDINO/groundingdino/util/vl_utils.py
+++ /dev/null
@@ -1,100 +0,0 @@
-import os
-import random
-from typing import List
-
-import torch
-
-
-def create_positive_map_from_span(tokenized, token_span, max_text_len=256):
- """construct a map such that positive_map[i,j] = True iff box i is associated to token j
- Input:
- - tokenized:
- - input_ids: Tensor[1, ntokens]
- - attention_mask: Tensor[1, ntokens]
- - token_span: list with length num_boxes.
- - each item: [start_idx, end_idx]
- """
- positive_map = torch.zeros((len(token_span), max_text_len), dtype=torch.float)
- for j, tok_list in enumerate(token_span):
- for (beg, end) in tok_list:
- beg_pos = tokenized.char_to_token(beg)
- end_pos = tokenized.char_to_token(end - 1)
- if beg_pos is None:
- try:
- beg_pos = tokenized.char_to_token(beg + 1)
- if beg_pos is None:
- beg_pos = tokenized.char_to_token(beg + 2)
- except:
- beg_pos = None
- if end_pos is None:
- try:
- end_pos = tokenized.char_to_token(end - 2)
- if end_pos is None:
- end_pos = tokenized.char_to_token(end - 3)
- except:
- end_pos = None
- if beg_pos is None or end_pos is None:
- continue
-
- assert beg_pos is not None and end_pos is not None
- if os.environ.get("SHILONG_DEBUG_ONLY_ONE_POS", None) == "TRUE":
- positive_map[j, beg_pos] = 1
- break
- else:
- positive_map[j, beg_pos : end_pos + 1].fill_(1)
-
- return positive_map / (positive_map.sum(-1)[:, None] + 1e-6)
-
-
-def build_captions_and_token_span(cat_list, force_lowercase):
- """
- Return:
- captions: str
- cat2tokenspan: dict
- {
- 'dog': [[0, 2]],
- ...
- }
- """
-
- cat2tokenspan = {}
- captions = ""
- for catname in cat_list:
- class_name = catname
- if force_lowercase:
- class_name = class_name.lower()
- if "/" in class_name:
- class_name_list: List = class_name.strip().split("/")
- class_name_list.append(class_name)
- class_name: str = random.choice(class_name_list)
-
- tokens_positive_i = []
- subnamelist = [i.strip() for i in class_name.strip().split(" ")]
- for subname in subnamelist:
- if len(subname) == 0:
- continue
- if len(captions) > 0:
- captions = captions + " "
- strat_idx = len(captions)
- end_idx = strat_idx + len(subname)
- tokens_positive_i.append([strat_idx, end_idx])
- captions = captions + subname
-
- if len(tokens_positive_i) > 0:
- captions = captions + " ."
- cat2tokenspan[class_name] = tokens_positive_i
-
- return captions, cat2tokenspan
-
-
-def build_id2posspan_and_caption(category_dict: dict):
- """Build id2pos_span and caption from category_dict
-
- Args:
- category_dict (dict): category_dict
- """
- cat_list = [item["name"].lower() for item in category_dict]
- id2catname = {item["id"]: item["name"].lower() for item in category_dict}
- caption, cat2posspan = build_captions_and_token_span(cat_list, force_lowercase=True)
- id2posspan = {catid: cat2posspan[catname] for catid, catname in id2catname.items()}
- return id2posspan, caption
diff --git "a/spaces/Chris4K/llms_compare/Hackintosh MacOS Niresh High Sierra For Intel And AMD \302\200? MacOS.md" "b/spaces/Chris4K/llms_compare/Hackintosh MacOS Niresh High Sierra For Intel And AMD \302\200? MacOS.md"
deleted file mode 100644
index 849d12bbe0ee9c1c768e8d65fe37f161b93a719b..0000000000000000000000000000000000000000
--- "a/spaces/Chris4K/llms_compare/Hackintosh MacOS Niresh High Sierra For Intel And AMD \302\200? MacOS.md"
+++ /dev/null
@@ -1,128 +0,0 @@
-## Hackintosh macOS Niresh High Sierra for Intel and AMD – macOS
-
-
-
-
-
- 
-
-
-
-
-
-**Hackintosh MacOS Niresh High Sierra For Intel And AMD ? MacOS ->>> [https://www.google.com/url?q=https%3A%2F%2Furlgoal.com%2F2txP22&sa=D&sntz=1&usg=AOvVaw3za\_PPTo0AOSXp\_zwTpKjt](https://www.google.com/url?q=https%3A%2F%2Furlgoal.com%2F2txP22&sa=D&sntz=1&usg=AOvVaw3za\_PPTo0AOSXp\_zwTpKjt)**
-
-
-
-
-
-
-
-
-
-
-
- Here is a possible title and article with html formatting for the keyword "Hackintosh macOS Niresh High Sierra for Intel and AMD â macOS":
-
-# How to Install Hackintosh macOS Niresh High Sierra on Intel and AMD PCs
-
-
-
-Hackintosh is a term used to describe a computer that runs macOS on non-Apple hardware. It can be a great way to enjoy the features and benefits of macOS without buying a Mac. However, hackintoshing is not a straightforward process and requires some technical knowledge and skills. In this article, we will show you how to install Hackintosh macOS Niresh High Sierra on Intel and AMD PCs using a bootable USB drive.
-
-
-
-Niresh High Sierra is a custom macOS installer that supports both Intel and AMD processors. It comes with many pre-installed drivers and kexts that make the installation easier and faster. Niresh High Sierra also supports legacy BIOS and UEFI boot modes, which means you can use it on older or newer PCs.
-
-
-
-Before you start, you will need the following:
-
-
-
-- A PC with an Intel or AMD processor that supports SSE4.1 instruction set
-
-- A 16GB or larger USB drive
-
-- A Windows PC or a Mac to create the bootable USB drive
-
-- A copy of Niresh High Sierra ISO file (you can download it from [here](https://www.hackintoshzone.com/files/file/1094-niresh-high-sierra/))
-
-- A copy of TransMac software (you can download it from [here](https://www.acutesystems.com/scrtm.htm))
-
-- A backup of your important data (hackintoshing may erase your hard drive)
-
-
-
-Now, follow these steps to create the bootable USB drive:
-
-
-
-1. Insert the USB drive into your Windows PC or Mac
-
-2. Open TransMac software and run it as administrator (on Windows) or enter your password (on Mac)
-
-3. Right-click on the USB drive in the left pane and select Format Disk for Mac
-
-4. Enter a name for the USB drive (e.g. Niresh) and click OK
-
-5. Right-click on the USB drive again and select Restore with Disk Image
-
-6. Browse to the Niresh High Sierra ISO file and click OK
-
-7. Wait for the process to complete (it may take some time)
-
-8. Eject the USB drive safely and insert it into your PC that you want to hackintosh
-
-
-
-Next, follow these steps to install Hackintosh macOS Niresh High Sierra on your PC:
-
-
-
-1. Turn on your PC and enter the BIOS or UEFI settings (usually by pressing F2, F10, F12, Del or Esc keys)
-
-2. Change the boot order to prioritize the USB drive as the first boot device
-
-3. Save and exit the BIOS or UEFI settings
-
-4. Your PC should boot from the USB drive and load the Niresh High Sierra installer
-
-5. Select Boot macOS Install from Niresh High Sierra at the Clover bootloader menu
-
-6. Wait for the installer to load (it may take some time)
-
-7. Select your language and click Continue
-
-8. At the top menu bar, click Utilities and select Disk Utility
-
-9. Select your hard drive in the left pane and click Erase
-
-10. Enter a name for your hard drive (e.g. Macintosh HD) and choose Mac OS Extended (Journaled) as the format
-
-11. Click Erase then Done
-
-12. Close Disk Utility and click Continue at the installer screen
-
-13. Agree to the terms and conditions and select your hard drive as the destination for installation
-
-14. Click Customize and check or uncheck any options according to your preference (you can leave them as default if you are not sure)
-
-15. Click Install and wait for the installation to complete (it may take some time)
-
-16. Your PC should reboot automatically after the installation is done
-
-17. Select Boot macOS from Macintosh HD at the Clover bootloader menu
-
-18. Follow the on-screen instructions to set up your hackintosh (e.g. choose your country, keyboard layout, Apple ID, etc.)
-
-dfd1c89656
-
-
-
-
-
-
-
-
-
diff --git a/spaces/Cletrason/Cletrason-toad-mario-movie/app.py b/spaces/Cletrason/Cletrason-toad-mario-movie/app.py
deleted file mode 100644
index f9ad7b8dfe593e1ca399aece2ecbd6eb65dc8095..0000000000000000000000000000000000000000
--- a/spaces/Cletrason/Cletrason-toad-mario-movie/app.py
+++ /dev/null
@@ -1,3 +0,0 @@
-import gradio as gr
-
-gr.Interface.load("models/Cletrason/toad-mario-movie").launch()
\ No newline at end of file
diff --git a/spaces/Cong723/gpt-academic-public/toolbox.py b/spaces/Cong723/gpt-academic-public/toolbox.py
deleted file mode 100644
index bdd99c9fb2d81a122d41f6cf34b1dabd634c28b6..0000000000000000000000000000000000000000
--- a/spaces/Cong723/gpt-academic-public/toolbox.py
+++ /dev/null
@@ -1,717 +0,0 @@
-import markdown
-import importlib
-import traceback
-import inspect
-import re
-import os
-from latex2mathml.converter import convert as tex2mathml
-from functools import wraps, lru_cache
-
-"""
-========================================================================
-第一部分
-函数插件输入输出接驳区
- - ChatBotWithCookies: 带Cookies的Chatbot类,为实现更多强大的功能做基础
- - ArgsGeneralWrapper: 装饰器函数,用于重组输入参数,改变输入参数的顺序与结构
- - update_ui: 刷新界面用 yield from update_ui(chatbot, history)
- - CatchException: 将插件中出的所有问题显示在界面上
- - HotReload: 实现插件的热更新
- - trimmed_format_exc: 打印traceback,为了安全而隐藏绝对地址
-========================================================================
-"""
-
-class ChatBotWithCookies(list):
- def __init__(self, cookie):
- self._cookies = cookie
-
- def write_list(self, list):
- for t in list:
- self.append(t)
-
- def get_list(self):
- return [t for t in self]
-
- def get_cookies(self):
- return self._cookies
-
-
-def ArgsGeneralWrapper(f):
- """
- 装饰器函数,用于重组输入参数,改变输入参数的顺序与结构。
- """
- def decorated(cookies, max_length, llm_model, txt, txt2, top_p, temperature, chatbot, history, system_prompt, plugin_advanced_arg, *args):
- txt_passon = txt
- if txt == "" and txt2 != "": txt_passon = txt2
- # 引入一个有cookie的chatbot
- cookies.update({
- 'top_p':top_p,
- 'temperature':temperature,
- })
- llm_kwargs = {
- 'api_key': cookies['api_key'],
- 'llm_model': llm_model,
- 'top_p':top_p,
- 'max_length': max_length,
- 'temperature':temperature,
- }
- plugin_kwargs = {
- "advanced_arg": plugin_advanced_arg,
- }
- chatbot_with_cookie = ChatBotWithCookies(cookies)
- chatbot_with_cookie.write_list(chatbot)
- yield from f(txt_passon, llm_kwargs, plugin_kwargs, chatbot_with_cookie, history, system_prompt, *args)
- return decorated
-
-
-def update_ui(chatbot, history, msg='正常', **kwargs): # 刷新界面
- """
- 刷新用户界面
- """
- assert isinstance(chatbot, ChatBotWithCookies), "在传递chatbot的过程中不要将其丢弃。必要时,可用clear将其清空,然后用for+append循环重新赋值。"
- yield chatbot.get_cookies(), chatbot, history, msg
-
-def trimmed_format_exc():
- import os, traceback
- str = traceback.format_exc()
- current_path = os.getcwd()
- replace_path = "."
- return str.replace(current_path, replace_path)
-
-def CatchException(f):
- """
- 装饰器函数,捕捉函数f中的异常并封装到一个生成器中返回,并显示到聊天当中。
- """
-
- @wraps(f)
- def decorated(txt, top_p, temperature, chatbot, history, systemPromptTxt, WEB_PORT):
- try:
- yield from f(txt, top_p, temperature, chatbot, history, systemPromptTxt, WEB_PORT)
- except Exception as e:
- from check_proxy import check_proxy
- from toolbox import get_conf
- proxies, = get_conf('proxies')
- tb_str = '```\n' + trimmed_format_exc() + '```'
- if len(chatbot) == 0:
- chatbot.clear()
- chatbot.append(["插件调度异常", "异常原因"])
- chatbot[-1] = (chatbot[-1][0],
- f"[Local Message] 实验性函数调用出错: \n\n{tb_str} \n\n当前代理可用性: \n\n{check_proxy(proxies)}")
- yield from update_ui(chatbot=chatbot, history=history, msg=f'异常 {e}') # 刷新界面
- return decorated
-
-
-def HotReload(f):
- """
- HotReload的装饰器函数,用于实现Python函数插件的热更新。
- 函数热更新是指在不停止程序运行的情况下,更新函数代码,从而达到实时更新功能。
- 在装饰器内部,使用wraps(f)来保留函数的元信息,并定义了一个名为decorated的内部函数。
- 内部函数通过使用importlib模块的reload函数和inspect模块的getmodule函数来重新加载并获取函数模块,
- 然后通过getattr函数获取函数名,并在新模块中重新加载函数。
- 最后,使用yield from语句返回重新加载过的函数,并在被装饰的函数上执行。
- 最终,装饰器函数返回内部函数。这个内部函数可以将函数的原始定义更新为最新版本,并执行函数的新版本。
- """
- @wraps(f)
- def decorated(*args, **kwargs):
- fn_name = f.__name__
- f_hot_reload = getattr(importlib.reload(inspect.getmodule(f)), fn_name)
- yield from f_hot_reload(*args, **kwargs)
- return decorated
-
-
-"""
-========================================================================
-第二部分
-其他小工具:
- - write_results_to_file: 将结果写入markdown文件中
- - regular_txt_to_markdown: 将普通文本转换为Markdown格式的文本。
- - report_execption: 向chatbot中添加简单的意外错误信息
- - text_divide_paragraph: 将文本按照段落分隔符分割开,生成带有段落标签的HTML代码。
- - markdown_convertion: 用多种方式组合,将markdown转化为好看的html
- - format_io: 接管gradio默认的markdown处理方式
- - on_file_uploaded: 处理文件的上传(自动解压)
- - on_report_generated: 将生成的报告自动投射到文件上传区
- - clip_history: 当历史上下文过长时,自动截断
- - get_conf: 获取设置
- - select_api_key: 根据当前的模型类别,抽取可用的api-key
-========================================================================
-"""
-
-def get_reduce_token_percent(text):
- """
- * 此函数未来将被弃用
- """
- try:
- # text = "maximum context length is 4097 tokens. However, your messages resulted in 4870 tokens"
- pattern = r"(\d+)\s+tokens\b"
- match = re.findall(pattern, text)
- EXCEED_ALLO = 500 # 稍微留一点余地,否则在回复时会因余量太少出问题
- max_limit = float(match[0]) - EXCEED_ALLO
- current_tokens = float(match[1])
- ratio = max_limit/current_tokens
- assert ratio > 0 and ratio < 1
- return ratio, str(int(current_tokens-max_limit))
- except:
- return 0.5, '不详'
-
-
-def write_results_to_file(history, file_name=None):
- """
- 将对话记录history以Markdown格式写入文件中。如果没有指定文件名,则使用当前时间生成文件名。
- """
- import os
- import time
- if file_name is None:
- # file_name = time.strftime("chatGPT分析报告%Y-%m-%d-%H-%M-%S", time.localtime()) + '.md'
- file_name = 'chatGPT分析报告' + \
- time.strftime("%Y-%m-%d-%H-%M-%S", time.localtime()) + '.md'
- os.makedirs('./gpt_log/', exist_ok=True)
- with open(f'./gpt_log/{file_name}', 'w', encoding='utf8') as f:
- f.write('# chatGPT 分析报告\n')
- for i, content in enumerate(history):
- try: # 这个bug没找到触发条件,暂时先这样顶一下
- if type(content) != str:
- content = str(content)
- except:
- continue
- if i % 2 == 0:
- f.write('## ')
- f.write(content)
- f.write('\n\n')
- res = '以上材料已经被写入' + os.path.abspath(f'./gpt_log/{file_name}')
- print(res)
- return res
-
-
-def regular_txt_to_markdown(text):
- """
- 将普通文本转换为Markdown格式的文本。
- """
- text = text.replace('\n', '\n\n')
- text = text.replace('\n\n\n', '\n\n')
- text = text.replace('\n\n\n', '\n\n')
- return text
-
-
-
-
-def report_execption(chatbot, history, a, b):
- """
- 向chatbot中添加错误信息
- """
- chatbot.append((a, b))
- history.append(a)
- history.append(b)
-
-
-def text_divide_paragraph(text):
- """
- 将文本按照段落分隔符分割开,生成带有段落标签的HTML代码。
- """
- if '```' in text:
- # careful input
- return text
- else:
- # wtf input
- lines = text.split("\n")
- for i, line in enumerate(lines):
- lines[i] = lines[i].replace(" ", " ")
- text = "".join(lines)
- return text
-
-@lru_cache(maxsize=128) # 使用 lru缓存 加快转换速度
-def markdown_convertion(txt):
- """
- 将Markdown格式的文本转换为HTML格式。如果包含数学公式,则先将公式转换为HTML格式。
- """
- pre = '
'
- suf = '
'
- if txt.startswith(pre) and txt.endswith(suf):
- # print('警告,输入了已经经过转化的字符串,二次转化可能出问题')
- return txt # 已经被转化过,不需要再次转化
-
- markdown_extension_configs = {
- 'mdx_math': {
- 'enable_dollar_delimiter': True,
- 'use_gitlab_delimiters': False,
- },
- }
- find_equation_pattern = r'\n', '')
- return content
-
- def no_code(txt):
- if '```' not in txt:
- return True
- else:
- if '```reference' in txt: return True # newbing
- else: return False
-
- if ('$' in txt) and no_code(txt): # 有$标识的公式符号,且没有代码段```的标识
- # convert everything to html format
- split = markdown.markdown(text='---')
- convert_stage_1 = markdown.markdown(text=txt, extensions=['mdx_math', 'fenced_code', 'tables', 'sane_lists'], extension_configs=markdown_extension_configs)
- convert_stage_1 = markdown_bug_hunt(convert_stage_1)
- # re.DOTALL: Make the '.' special character match any character at all, including a newline; without this flag, '.' will match anything except a newline. Corresponds to the inline flag (?s).
- # 1. convert to easy-to-copy tex (do not render math)
- convert_stage_2_1, n = re.subn(find_equation_pattern, replace_math_no_render, convert_stage_1, flags=re.DOTALL)
- # 2. convert to rendered equation
- convert_stage_2_2, n = re.subn(find_equation_pattern, replace_math_render, convert_stage_1, flags=re.DOTALL)
- # cat them together
- return pre + convert_stage_2_1 + f'{split}' + convert_stage_2_2 + suf
- else:
- return pre + markdown.markdown(txt, extensions=['fenced_code', 'codehilite', 'tables', 'sane_lists']) + suf
-
-
-def close_up_code_segment_during_stream(gpt_reply):
- """
- 在gpt输出代码的中途(输出了前面的```,但还没输出完后面的```),补上后面的```
-
- Args:
- gpt_reply (str): GPT模型返回的回复字符串。
-
- Returns:
- str: 返回一个新的字符串,将输出代码片段的“后面的```”补上。
-
- """
- if '```' not in gpt_reply:
- return gpt_reply
- if gpt_reply.endswith('```'):
- return gpt_reply
-
- # 排除了以上两个情况,我们
- segments = gpt_reply.split('```')
- n_mark = len(segments) - 1
- if n_mark % 2 == 1:
- # print('输出代码片段中!')
- return gpt_reply+'\n```'
- else:
- return gpt_reply
-
-
-def format_io(self, y):
- """
- 将输入和输出解析为HTML格式。将y中最后一项的输入部分段落化,并将输出部分的Markdown和数学公式转换为HTML格式。
- """
- if y is None or y == []:
- return []
- i_ask, gpt_reply = y[-1]
- i_ask = text_divide_paragraph(i_ask) # 输入部分太自由,预处理一波
- gpt_reply = close_up_code_segment_during_stream(gpt_reply) # 当代码输出半截的时候,试着补上后个```
- y[-1] = (
- None if i_ask is None else markdown.markdown(i_ask, extensions=['fenced_code', 'tables']),
- None if gpt_reply is None else markdown_convertion(gpt_reply)
- )
- return y
-
-
-def find_free_port():
- """
- 返回当前系统中可用的未使用端口。
- """
- import socket
- from contextlib import closing
- with closing(socket.socket(socket.AF_INET, socket.SOCK_STREAM)) as s:
- s.bind(('', 0))
- s.setsockopt(socket.SOL_SOCKET, socket.SO_REUSEADDR, 1)
- return s.getsockname()[1]
-
-
-def extract_archive(file_path, dest_dir):
- import zipfile
- import tarfile
- import os
- # Get the file extension of the input file
- file_extension = os.path.splitext(file_path)[1]
-
- # Extract the archive based on its extension
- if file_extension == '.zip':
- with zipfile.ZipFile(file_path, 'r') as zipobj:
- zipobj.extractall(path=dest_dir)
- print("Successfully extracted zip archive to {}".format(dest_dir))
-
- elif file_extension in ['.tar', '.gz', '.bz2']:
- with tarfile.open(file_path, 'r:*') as tarobj:
- tarobj.extractall(path=dest_dir)
- print("Successfully extracted tar archive to {}".format(dest_dir))
-
- # 第三方库,需要预先pip install rarfile
- # 此外,Windows上还需要安装winrar软件,配置其Path环境变量,如"C:\Program Files\WinRAR"才可以
- elif file_extension == '.rar':
- try:
- import rarfile
- with rarfile.RarFile(file_path) as rf:
- rf.extractall(path=dest_dir)
- print("Successfully extracted rar archive to {}".format(dest_dir))
- except:
- print("Rar format requires additional dependencies to install")
- return '\n\n需要安装pip install rarfile来解压rar文件'
-
- # 第三方库,需要预先pip install py7zr
- elif file_extension == '.7z':
- try:
- import py7zr
- with py7zr.SevenZipFile(file_path, mode='r') as f:
- f.extractall(path=dest_dir)
- print("Successfully extracted 7z archive to {}".format(dest_dir))
- except:
- print("7z format requires additional dependencies to install")
- return '\n\n需要安装pip install py7zr来解压7z文件'
- else:
- return ''
- return ''
-
-
-def find_recent_files(directory):
- """
- me: find files that is created with in one minutes under a directory with python, write a function
- gpt: here it is!
- """
- import os
- import time
- current_time = time.time()
- one_minute_ago = current_time - 60
- recent_files = []
-
- for filename in os.listdir(directory):
- file_path = os.path.join(directory, filename)
- if file_path.endswith('.log'):
- continue
- created_time = os.path.getmtime(file_path)
- if created_time >= one_minute_ago:
- if os.path.isdir(file_path):
- continue
- recent_files.append(file_path)
-
- return recent_files
-
-
-def on_file_uploaded(files, chatbot, txt, txt2, checkboxes):
- """
- 当文件被上传时的回调函数
- """
- if len(files) == 0:
- return chatbot, txt
- import shutil
- import os
- import time
- import glob
- from toolbox import extract_archive
- try:
- shutil.rmtree('./private_upload/')
- except:
- pass
- time_tag = time.strftime("%Y-%m-%d-%H-%M-%S", time.localtime())
- os.makedirs(f'private_upload/{time_tag}', exist_ok=True)
- err_msg = ''
- for file in files:
- file_origin_name = os.path.basename(file.orig_name)
- shutil.copy(file.name, f'private_upload/{time_tag}/{file_origin_name}')
- err_msg += extract_archive(f'private_upload/{time_tag}/{file_origin_name}',
- dest_dir=f'private_upload/{time_tag}/{file_origin_name}.extract')
- moved_files = [fp for fp in glob.glob('private_upload/**/*', recursive=True)]
- if "底部输入区" in checkboxes:
- txt = ""
- txt2 = f'private_upload/{time_tag}'
- else:
- txt = f'private_upload/{time_tag}'
- txt2 = ""
- moved_files_str = '\t\n\n'.join(moved_files)
- chatbot.append(['我上传了文件,请查收',
- f'[Local Message] 收到以下文件: \n\n{moved_files_str}' +
- f'\n\n调用路径参数已自动修正到: \n\n{txt}' +
- f'\n\n现在您点击任意“红颜色”标识的函数插件时,以上文件将被作为输入参数'+err_msg])
- return chatbot, txt, txt2
-
-
-def on_report_generated(files, chatbot):
- from toolbox import find_recent_files
- report_files = find_recent_files('gpt_log')
- if len(report_files) == 0:
- return None, chatbot
- # files.extend(report_files)
- chatbot.append(['汇总报告如何远程获取?', '汇总报告已经添加到右侧“文件上传区”(可能处于折叠状态),请查收。'])
- return report_files, chatbot
-
-def is_openai_api_key(key):
- API_MATCH_ORIGINAL = re.match(r"sk-[a-zA-Z0-9]{48}$", key)
- API_MATCH_AZURE = re.match(r"[a-zA-Z0-9]{32}$", key)
- return bool(API_MATCH_ORIGINAL) or bool(API_MATCH_AZURE)
-
-def is_api2d_key(key):
- if key.startswith('fk') and len(key) == 41:
- return True
- else:
- return False
-
-def is_any_api_key(key):
- if ',' in key:
- keys = key.split(',')
- for k in keys:
- if is_any_api_key(k): return True
- return False
- else:
- return is_openai_api_key(key) or is_api2d_key(key)
-
-def what_keys(keys):
- avail_key_list = {'OpenAI Key':0, "API2D Key":0}
- key_list = keys.split(',')
-
- for k in key_list:
- if is_openai_api_key(k):
- avail_key_list['OpenAI Key'] += 1
-
- for k in key_list:
- if is_api2d_key(k):
- avail_key_list['API2D Key'] += 1
-
- return f"检测到: OpenAI Key {avail_key_list['OpenAI Key']} 个,API2D Key {avail_key_list['API2D Key']} 个"
-
-def select_api_key(keys, llm_model):
- import random
- avail_key_list = []
- key_list = keys.split(',')
-
- if llm_model.startswith('gpt-'):
- for k in key_list:
- if is_openai_api_key(k): avail_key_list.append(k)
-
- if llm_model.startswith('api2d-'):
- for k in key_list:
- if is_api2d_key(k): avail_key_list.append(k)
-
- if len(avail_key_list) == 0:
- raise RuntimeError(f"您提供的api-key不满足要求,不包含任何可用于{llm_model}的api-key。您可能选择了错误的模型或请求源。")
-
- api_key = random.choice(avail_key_list) # 随机负载均衡
- return api_key
-
-def read_env_variable(arg, default_value):
- """
- 环境变量可以是 `GPT_ACADEMIC_CONFIG`(优先),也可以直接是`CONFIG`
- 例如在windows cmd中,既可以写:
- set USE_PROXY=True
- set API_KEY=sk-j7caBpkRoxxxxxxxxxxxxxxxxxxxxxxxxxxxx
- set proxies={"http":"http://127.0.0.1:10085", "https":"http://127.0.0.1:10085",}
- set AVAIL_LLM_MODELS=["gpt-3.5-turbo", "chatglm"]
- set AUTHENTICATION=[("username", "password"), ("username2", "password2")]
- 也可以写:
- set GPT_ACADEMIC_USE_PROXY=True
- set GPT_ACADEMIC_API_KEY=sk-j7caBpkRoxxxxxxxxxxxxxxxxxxxxxxxxxxxx
- set GPT_ACADEMIC_proxies={"http":"http://127.0.0.1:10085", "https":"http://127.0.0.1:10085",}
- set GPT_ACADEMIC_AVAIL_LLM_MODELS=["gpt-3.5-turbo", "chatglm"]
- set GPT_ACADEMIC_AUTHENTICATION=[("username", "password"), ("username2", "password2")]
- """
- from colorful import print亮红, print亮绿
- arg_with_prefix = "GPT_ACADEMIC_" + arg
- if arg_with_prefix in os.environ:
- env_arg = os.environ[arg_with_prefix]
- elif arg in os.environ:
- env_arg = os.environ[arg]
- else:
- raise KeyError
- print(f"[ENV_VAR] 尝试加载{arg},默认值:{default_value} --> 修正值:{env_arg}")
- try:
- if isinstance(default_value, bool):
- r = bool(env_arg)
- elif isinstance(default_value, int):
- r = int(env_arg)
- elif isinstance(default_value, float):
- r = float(env_arg)
- elif isinstance(default_value, str):
- r = env_arg.strip()
- elif isinstance(default_value, dict):
- r = eval(env_arg)
- elif isinstance(default_value, list):
- r = eval(env_arg)
- elif default_value is None:
- assert arg == "proxies"
- r = eval(env_arg)
- else:
- print亮红(f"[ENV_VAR] 环境变量{arg}不支持通过环境变量设置! ")
- raise KeyError
- except:
- print亮红(f"[ENV_VAR] 环境变量{arg}加载失败! ")
- raise KeyError(f"[ENV_VAR] 环境变量{arg}加载失败! ")
-
- print亮绿(f"[ENV_VAR] 成功读取环境变量{arg}")
- return r
-
-@lru_cache(maxsize=128)
-def read_single_conf_with_lru_cache(arg):
- from colorful import print亮红, print亮绿, print亮蓝
- try:
- # 优先级1. 获取环境变量作为配置
- default_ref = getattr(importlib.import_module('config'), arg) # 读取默认值作为数据类型转换的参考
- r = read_env_variable(arg, default_ref)
- except:
- try:
- # 优先级2. 获取config_private中的配置
- r = getattr(importlib.import_module('config_private'), arg)
- except:
- # 优先级3. 获取config中的配置
- r = getattr(importlib.import_module('config'), arg)
-
- # 在读取API_KEY时,检查一下是不是忘了改config
- if arg == 'API_KEY':
- print亮蓝(f"[API_KEY] 本项目现已支持OpenAI和API2D的api-key。也支持同时填写多个api-key,如API_KEY=\"openai-key1,openai-key2,api2d-key3\"")
- print亮蓝(f"[API_KEY] 您既可以在config.py中修改api-key(s),也可以在问题输入区输入临时的api-key(s),然后回车键提交后即可生效。")
- if is_any_api_key(r):
- print亮绿(f"[API_KEY] 您的 API_KEY 是: {r[:15]}*** API_KEY 导入成功")
- else:
- print亮红( "[API_KEY] 正确的 API_KEY 是'sk'开头的51位密钥(OpenAI),或者 'fk'开头的41位密钥,请在config文件中修改API密钥之后再运行。")
- if arg == 'proxies':
- if r is None:
- print亮红('[PROXY] 网络代理状态:未配置。无代理状态下很可能无法访问OpenAI家族的模型。建议:检查USE_PROXY选项是否修改。')
- else:
- print亮绿('[PROXY] 网络代理状态:已配置。配置信息如下:', r)
- assert isinstance(r, dict), 'proxies格式错误,请注意proxies选项的格式,不要遗漏括号。'
- return r
-
-
-def get_conf(*args):
- # 建议您复制一个config_private.py放自己的秘密, 如API和代理网址, 避免不小心传github被别人看到
- res = []
- for arg in args:
- r = read_single_conf_with_lru_cache(arg)
- res.append(r)
- return res
-
-
-def clear_line_break(txt):
- txt = txt.replace('\n', ' ')
- txt = txt.replace(' ', ' ')
- txt = txt.replace(' ', ' ')
- return txt
-
-
-class DummyWith():
- """
- 这段代码定义了一个名为DummyWith的空上下文管理器,
- 它的作用是……额……就是不起作用,即在代码结构不变得情况下取代其他的上下文管理器。
- 上下文管理器是一种Python对象,用于与with语句一起使用,
- 以确保一些资源在代码块执行期间得到正确的初始化和清理。
- 上下文管理器必须实现两个方法,分别为 __enter__()和 __exit__()。
- 在上下文执行开始的情况下,__enter__()方法会在代码块被执行前被调用,
- 而在上下文执行结束时,__exit__()方法则会被调用。
- """
- def __enter__(self):
- return self
-
- def __exit__(self, exc_type, exc_value, traceback):
- return
-
-def run_gradio_in_subpath(demo, auth, port, custom_path):
- """
- 把gradio的运行地址更改到指定的二次路径上
- """
- def is_path_legal(path: str)->bool:
- '''
- check path for sub url
- path: path to check
- return value: do sub url wrap
- '''
- if path == "/": return True
- if len(path) == 0:
- print("ilegal custom path: {}\npath must not be empty\ndeploy on root url".format(path))
- return False
- if path[0] == '/':
- if path[1] != '/':
- print("deploy on sub-path {}".format(path))
- return True
- return False
- print("ilegal custom path: {}\npath should begin with \'/\'\ndeploy on root url".format(path))
- return False
-
- if not is_path_legal(custom_path): raise RuntimeError('Ilegal custom path')
- import uvicorn
- import gradio as gr
- from fastapi import FastAPI
- app = FastAPI()
- if custom_path != "/":
- @app.get("/")
- def read_main():
- return {"message": f"Gradio is running at: {custom_path}"}
- app = gr.mount_gradio_app(app, demo, path=custom_path)
- uvicorn.run(app, host="0.0.0.0", port=port) # , auth=auth
-
-
-def clip_history(inputs, history, tokenizer, max_token_limit):
- """
- reduce the length of history by clipping.
- this function search for the longest entries to clip, little by little,
- until the number of token of history is reduced under threshold.
- 通过裁剪来缩短历史记录的长度。
- 此函数逐渐地搜索最长的条目进行剪辑,
- 直到历史记录的标记数量降低到阈值以下。
- """
- import numpy as np
- from request_llm.bridge_all import model_info
- def get_token_num(txt):
- return len(tokenizer.encode(txt, disallowed_special=()))
- input_token_num = get_token_num(inputs)
- if input_token_num < max_token_limit * 3 / 4:
- # 当输入部分的token占比小于限制的3/4时,裁剪时
- # 1. 把input的余量留出来
- max_token_limit = max_token_limit - input_token_num
- # 2. 把输出用的余量留出来
- max_token_limit = max_token_limit - 128
- # 3. 如果余量太小了,直接清除历史
- if max_token_limit < 128:
- history = []
- return history
- else:
- # 当输入部分的token占比 > 限制的3/4时,直接清除历史
- history = []
- return history
-
- everything = ['']
- everything.extend(history)
- n_token = get_token_num('\n'.join(everything))
- everything_token = [get_token_num(e) for e in everything]
-
- # 截断时的颗粒度
- delta = max(everything_token) // 16
-
- while n_token > max_token_limit:
- where = np.argmax(everything_token)
- encoded = tokenizer.encode(everything[where], disallowed_special=())
- clipped_encoded = encoded[:len(encoded)-delta]
- everything[where] = tokenizer.decode(clipped_encoded)[:-1] # -1 to remove the may-be illegal char
- everything_token[where] = get_token_num(everything[where])
- n_token = get_token_num('\n'.join(everything))
-
- history = everything[1:]
- return history
diff --git a/spaces/DQChoi/gpt-demo/venv/lib/python3.11/site-packages/altair/utils/schemapi.py b/spaces/DQChoi/gpt-demo/venv/lib/python3.11/site-packages/altair/utils/schemapi.py
deleted file mode 100644
index 9fe29c2cf2b97ec6305cebd76a6b9de159156281..0000000000000000000000000000000000000000
--- a/spaces/DQChoi/gpt-demo/venv/lib/python3.11/site-packages/altair/utils/schemapi.py
+++ /dev/null
@@ -1,1126 +0,0 @@
-# The contents of this file are automatically written by
-# tools/generate_schema_wrapper.py. Do not modify directly.
-import collections
-import contextlib
-import inspect
-import json
-import textwrap
-from typing import (
- Any,
- Sequence,
- List,
- Dict,
- Optional,
- DefaultDict,
- Tuple,
- Iterable,
- Type,
-)
-from itertools import zip_longest
-
-import jsonschema
-import jsonschema.exceptions
-import jsonschema.validators
-import numpy as np
-import pandas as pd
-
-from altair import vegalite
-
-ValidationErrorList = List[jsonschema.exceptions.ValidationError]
-GroupedValidationErrors = Dict[str, ValidationErrorList]
-
-
-# If DEBUG_MODE is True, then schema objects are converted to dict and
-# validated at creation time. This slows things down, particularly for
-# larger specs, but leads to much more useful tracebacks for the user.
-# Individual schema classes can override this by setting the
-# class-level _class_is_valid_at_instantiation attribute to False
-DEBUG_MODE = True
-
-
-def enable_debug_mode():
- global DEBUG_MODE
- DEBUG_MODE = True
-
-
-def disable_debug_mode():
- global DEBUG_MODE
- DEBUG_MODE = False
-
-
-@contextlib.contextmanager
-def debug_mode(arg):
- global DEBUG_MODE
- original = DEBUG_MODE
- DEBUG_MODE = arg
- try:
- yield
- finally:
- DEBUG_MODE = original
-
-
-def validate_jsonschema(
- spec: Dict[str, Any],
- schema: Dict[str, Any],
- rootschema: Optional[Dict[str, Any]] = None,
- raise_error: bool = True,
-) -> Optional[jsonschema.exceptions.ValidationError]:
- """Validates the passed in spec against the schema in the context of the
- rootschema. If any errors are found, they are deduplicated and prioritized
- and only the most relevant errors are kept. Errors are then either raised
- or returned, depending on the value of `raise_error`.
- """
- errors = _get_errors_from_spec(spec, schema, rootschema=rootschema)
- if errors:
- leaf_errors = _get_leaves_of_error_tree(errors)
- grouped_errors = _group_errors_by_json_path(leaf_errors)
- grouped_errors = _subset_to_most_specific_json_paths(grouped_errors)
- grouped_errors = _deduplicate_errors(grouped_errors)
-
- # Nothing special about this first error but we need to choose one
- # which can be raised
- main_error = list(grouped_errors.values())[0][0]
- # All errors are then attached as a new attribute to ValidationError so that
- # they can be used in SchemaValidationError to craft a more helpful
- # error message. Setting a new attribute like this is not ideal as
- # it then no longer matches the type ValidationError. It would be better
- # to refactor this function to never raise but only return errors.
- main_error._all_errors = grouped_errors # type: ignore[attr-defined]
- if raise_error:
- raise main_error
- else:
- return main_error
- else:
- return None
-
-
-def _get_errors_from_spec(
- spec: Dict[str, Any],
- schema: Dict[str, Any],
- rootschema: Optional[Dict[str, Any]] = None,
-) -> ValidationErrorList:
- """Uses the relevant jsonschema validator to validate the passed in spec
- against the schema using the rootschema to resolve references.
- The schema and rootschema themselves are not validated but instead considered
- as valid.
- """
- # We don't use jsonschema.validate as this would validate the schema itself.
- # Instead, we pass the schema directly to the validator class. This is done for
- # two reasons: The schema comes from Vega-Lite and is not based on the user
- # input, therefore there is no need to validate it in the first place. Furthermore,
- # the "uri-reference" format checker fails for some of the references as URIs in
- # "$ref" are not encoded,
- # e.g. '#/definitions/ValueDefWithCondition' would be a valid $ref in a Vega-Lite schema but
- # it is not a valid URI reference due to the characters such as '<'.
- if rootschema is not None:
- validator_cls = jsonschema.validators.validator_for(rootschema)
- resolver = jsonschema.RefResolver.from_schema(rootschema)
- else:
- validator_cls = jsonschema.validators.validator_for(schema)
- # No resolver is necessary if the schema is already the full schema
- resolver = None
-
- validator_kwargs = {"resolver": resolver}
- if hasattr(validator_cls, "FORMAT_CHECKER"):
- validator_kwargs["format_checker"] = validator_cls.FORMAT_CHECKER
- validator = validator_cls(schema, **validator_kwargs)
- errors = list(validator.iter_errors(spec))
- return errors
-
-
-def _json_path(err: jsonschema.exceptions.ValidationError) -> str:
- """Drop in replacement for the .json_path property of the jsonschema
- ValidationError class, which is not available as property for
- ValidationError with jsonschema<4.0.1.
- More info, see https://github.com/altair-viz/altair/issues/3038
- """
- path = "$"
- for elem in err.absolute_path:
- if isinstance(elem, int):
- path += "[" + str(elem) + "]"
- else:
- path += "." + elem
- return path
-
-
-def _group_errors_by_json_path(
- errors: ValidationErrorList,
-) -> GroupedValidationErrors:
- """Groups errors by the `json_path` attribute of the jsonschema ValidationError
- class. This attribute contains the path to the offending element within
- a chart specification and can therefore be considered as an identifier of an
- 'issue' in the chart that needs to be fixed.
- """
- errors_by_json_path = collections.defaultdict(list)
- for err in errors:
- err_key = getattr(err, "json_path", _json_path(err))
- errors_by_json_path[err_key].append(err)
- return dict(errors_by_json_path)
-
-
-def _get_leaves_of_error_tree(
- errors: ValidationErrorList,
-) -> ValidationErrorList:
- """For each error in `errors`, it traverses down the "error tree" that is generated
- by the jsonschema library to find and return all "leaf" errors. These are errors
- which have no further errors that caused it and so they are the most specific errors
- with the most specific error messages.
- """
- leaves: ValidationErrorList = []
- for err in errors:
- if err.context:
- # This means that the error `err` was caused by errors in subschemas.
- # The list of errors from the subschemas are available in the property
- # `context`.
- leaves.extend(_get_leaves_of_error_tree(err.context))
- else:
- leaves.append(err)
- return leaves
-
-
-def _subset_to_most_specific_json_paths(
- errors_by_json_path: GroupedValidationErrors,
-) -> GroupedValidationErrors:
- """Removes key (json path), value (errors) pairs where the json path is fully
- contained in another json path. For example if `errors_by_json_path` has two
- keys, `$.encoding.X` and `$.encoding.X.tooltip`, then the first one will be removed
- and only the second one is returned. This is done under the assumption that
- more specific json paths give more helpful error messages to the user.
- """
- errors_by_json_path_specific: GroupedValidationErrors = {}
- for json_path, errors in errors_by_json_path.items():
- if not _contained_at_start_of_one_of_other_values(
- json_path, list(errors_by_json_path.keys())
- ):
- errors_by_json_path_specific[json_path] = errors
- return errors_by_json_path_specific
-
-
-def _contained_at_start_of_one_of_other_values(x: str, values: Sequence[str]) -> bool:
- # Does not count as "contained at start of other value" if the values are
- # the same. These cases should be handled separately
- return any(value.startswith(x) for value in values if x != value)
-
-
-def _deduplicate_errors(
- grouped_errors: GroupedValidationErrors,
-) -> GroupedValidationErrors:
- """Some errors have very similar error messages or are just in general not helpful
- for a user. This function removes as many of these cases as possible and
- can be extended over time to handle new cases that come up.
- """
- grouped_errors_deduplicated: GroupedValidationErrors = {}
- for json_path, element_errors in grouped_errors.items():
- errors_by_validator = _group_errors_by_validator(element_errors)
-
- deduplication_functions = {
- "enum": _deduplicate_enum_errors,
- "additionalProperties": _deduplicate_additional_properties_errors,
- }
- deduplicated_errors: ValidationErrorList = []
- for validator, errors in errors_by_validator.items():
- deduplication_func = deduplication_functions.get(validator, None)
- if deduplication_func is not None:
- errors = deduplication_func(errors)
- deduplicated_errors.extend(_deduplicate_by_message(errors))
-
- # Removes any ValidationError "'value' is a required property" as these
- # errors are unlikely to be the relevant ones for the user. They come from
- # validation against a schema definition where the output of `alt.value`
- # would be valid. However, if a user uses `alt.value`, the `value` keyword
- # is included automatically from that function and so it's unlikely
- # that this was what the user intended if the keyword is not present
- # in the first place.
- deduplicated_errors = [
- err for err in deduplicated_errors if not _is_required_value_error(err)
- ]
-
- grouped_errors_deduplicated[json_path] = deduplicated_errors
- return grouped_errors_deduplicated
-
-
-def _is_required_value_error(err: jsonschema.exceptions.ValidationError) -> bool:
- return err.validator == "required" and err.validator_value == ["value"]
-
-
-def _group_errors_by_validator(errors: ValidationErrorList) -> GroupedValidationErrors:
- """Groups the errors by the json schema "validator" that casued the error. For
- example if the error is that a value is not one of an enumeration in the json schema
- then the "validator" is `"enum"`, if the error is due to an unknown property that
- was set although no additional properties are allowed then "validator" is
- `"additionalProperties`, etc.
- """
- errors_by_validator: DefaultDict[
- str, ValidationErrorList
- ] = collections.defaultdict(list)
- for err in errors:
- # Ignore mypy error as err.validator as it wrongly sees err.validator
- # as of type Optional[Validator] instead of str which it is according
- # to the documentation and all tested cases
- errors_by_validator[err.validator].append(err) # type: ignore[index]
- return dict(errors_by_validator)
-
-
-def _deduplicate_enum_errors(errors: ValidationErrorList) -> ValidationErrorList:
- """Deduplicate enum errors by removing the errors where the allowed values
- are a subset of another error. For example, if `enum` contains two errors
- and one has `validator_value` (i.e. accepted values) ["A", "B"] and the
- other one ["A", "B", "C"] then the first one is removed and the final
- `enum` list only contains the error with ["A", "B", "C"].
- """
- if len(errors) > 1:
- # Values (and therefore `validator_value`) of an enum are always arrays,
- # see https://json-schema.org/understanding-json-schema/reference/generic.html#enumerated-values
- # which is why we can use join below
- value_strings = [",".join(err.validator_value) for err in errors]
- longest_enums: ValidationErrorList = []
- for value_str, err in zip(value_strings, errors):
- if not _contained_at_start_of_one_of_other_values(value_str, value_strings):
- longest_enums.append(err)
- errors = longest_enums
- return errors
-
-
-def _deduplicate_additional_properties_errors(
- errors: ValidationErrorList,
-) -> ValidationErrorList:
- """If there are multiple additional property errors it usually means that
- the offending element was validated against multiple schemas and
- its parent is a common anyOf validator.
- The error messages produced from these cases are usually
- very similar and we just take the shortest one. For example,
- the following 3 errors are raised for the `unknown` channel option in
- `alt.X("variety", unknown=2)`:
- - "Additional properties are not allowed ('unknown' was unexpected)"
- - "Additional properties are not allowed ('field', 'unknown' were unexpected)"
- - "Additional properties are not allowed ('field', 'type', 'unknown' were unexpected)"
- """
- if len(errors) > 1:
- # Test if all parent errors are the same anyOf error and only do
- # the prioritization in these cases. Can't think of a chart spec where this
- # would not be the case but still allow for it below to not break anything.
- parent = errors[0].parent
- if (
- parent is not None
- and parent.validator == "anyOf"
- # Use [1:] as don't have to check for first error as it was used
- # above to define `parent`
- and all(err.parent is parent for err in errors[1:])
- ):
- errors = [min(errors, key=lambda x: len(x.message))]
- return errors
-
-
-def _deduplicate_by_message(errors: ValidationErrorList) -> ValidationErrorList:
- """Deduplicate errors by message. This keeps the original order in case
- it was chosen intentionally.
- """
- return list({e.message: e for e in errors}.values())
-
-
-def _subclasses(cls):
- """Breadth-first sequence of all classes which inherit from cls."""
- seen = set()
- current_set = {cls}
- while current_set:
- seen |= current_set
- current_set = set.union(*(set(cls.__subclasses__()) for cls in current_set))
- for cls in current_set - seen:
- yield cls
-
-
-def _todict(obj, context):
- """Convert an object to a dict representation."""
- if isinstance(obj, SchemaBase):
- return obj.to_dict(validate=False, context=context)
- elif isinstance(obj, (list, tuple, np.ndarray)):
- return [_todict(v, context) for v in obj]
- elif isinstance(obj, dict):
- return {k: _todict(v, context) for k, v in obj.items() if v is not Undefined}
- elif hasattr(obj, "to_dict"):
- return obj.to_dict()
- elif isinstance(obj, np.number):
- return float(obj)
- elif isinstance(obj, (pd.Timestamp, np.datetime64)):
- return pd.Timestamp(obj).isoformat()
- else:
- return obj
-
-
-def _resolve_references(schema, root=None):
- """Resolve schema references."""
- resolver = jsonschema.RefResolver.from_schema(root or schema)
- while "$ref" in schema:
- with resolver.resolving(schema["$ref"]) as resolved:
- schema = resolved
- return schema
-
-
-class SchemaValidationError(jsonschema.ValidationError):
- """A wrapper for jsonschema.ValidationError with friendlier traceback"""
-
- def __init__(self, obj: "SchemaBase", err: jsonschema.ValidationError) -> None:
- super().__init__(**err._contents())
- self.obj = obj
- self._errors: GroupedValidationErrors = getattr(
- err, "_all_errors", {getattr(err, "json_path", _json_path(err)): [err]}
- )
- # This is the message from err
- self._original_message = self.message
- self.message = self._get_message()
-
- def __str__(self) -> str:
- return self.message
-
- def _get_message(self) -> str:
- def indent_second_line_onwards(message: str, indent: int = 4) -> str:
- modified_lines: List[str] = []
- for idx, line in enumerate(message.split("\n")):
- if idx > 0 and len(line) > 0:
- line = " " * indent + line
- modified_lines.append(line)
- return "\n".join(modified_lines)
-
- error_messages: List[str] = []
- # Only show a maximum of 3 errors as else the final message returned by this
- # method could get very long.
- for errors in list(self._errors.values())[:3]:
- error_messages.append(self._get_message_for_errors_group(errors))
-
- message = ""
- if len(error_messages) > 1:
- error_messages = [
- indent_second_line_onwards(f"Error {error_id}: {m}")
- for error_id, m in enumerate(error_messages, start=1)
- ]
- message += "Multiple errors were found.\n\n"
- message += "\n\n".join(error_messages)
- return message
-
- def _get_message_for_errors_group(
- self,
- errors: ValidationErrorList,
- ) -> str:
- if errors[0].validator == "additionalProperties":
- # During development, we only found cases where an additionalProperties
- # error was raised if that was the only error for the offending instance
- # as identifiable by the json path. Therefore, we just check here the first
- # error. However, other constellations might exist in which case
- # this should be adapted so that other error messages are shown as well.
- message = self._get_additional_properties_error_message(errors[0])
- else:
- message = self._get_default_error_message(errors=errors)
-
- return message.strip()
-
- def _get_additional_properties_error_message(
- self,
- error: jsonschema.exceptions.ValidationError,
- ) -> str:
- """Output all existing parameters when an unknown parameter is specified."""
- altair_cls = self._get_altair_class_for_error(error)
- param_dict_keys = inspect.signature(altair_cls).parameters.keys()
- param_names_table = self._format_params_as_table(param_dict_keys)
-
- # Error messages for these errors look like this:
- # "Additional properties are not allowed ('unknown' was unexpected)"
- # Line below extracts "unknown" from this string
- parameter_name = error.message.split("('")[-1].split("'")[0]
- message = f"""\
-`{altair_cls.__name__}` has no parameter named '{parameter_name}'
-
-Existing parameter names are:
-{param_names_table}
-See the help for `{altair_cls.__name__}` to read the full description of these parameters"""
- return message
-
- def _get_altair_class_for_error(
- self, error: jsonschema.exceptions.ValidationError
- ) -> Type["SchemaBase"]:
- """Try to get the lowest class possible in the chart hierarchy so
- it can be displayed in the error message. This should lead to more informative
- error messages pointing the user closer to the source of the issue.
- """
- for prop_name in reversed(error.absolute_path):
- # Check if str as e.g. first item can be a 0
- if isinstance(prop_name, str):
- potential_class_name = prop_name[0].upper() + prop_name[1:]
- cls = getattr(vegalite, potential_class_name, None)
- if cls is not None:
- break
- else:
- # Did not find a suitable class based on traversing the path so we fall
- # back on the class of the top-level object which created
- # the SchemaValidationError
- cls = self.obj.__class__
- return cls
-
- @staticmethod
- def _format_params_as_table(param_dict_keys: Iterable[str]) -> str:
- """Format param names into a table so that they are easier to read"""
- param_names: Tuple[str, ...]
- name_lengths: Tuple[int, ...]
- param_names, name_lengths = zip( # type: ignore[assignment] # Mypy does think it's Tuple[Any]
- *[
- (name, len(name))
- for name in param_dict_keys
- if name not in ["kwds", "self"]
- ]
- )
- # Worst case scenario with the same longest param name in the same
- # row for all columns
- max_name_length = max(name_lengths)
- max_column_width = 80
- # Output a square table if not too big (since it is easier to read)
- num_param_names = len(param_names)
- square_columns = int(np.ceil(num_param_names**0.5))
- columns = min(max_column_width // max_name_length, square_columns)
-
- # Compute roughly equal column heights to evenly divide the param names
- def split_into_equal_parts(n: int, p: int) -> List[int]:
- return [n // p + 1] * (n % p) + [n // p] * (p - n % p)
-
- column_heights = split_into_equal_parts(num_param_names, columns)
-
- # Section the param names into columns and compute their widths
- param_names_columns: List[Tuple[str, ...]] = []
- column_max_widths: List[int] = []
- last_end_idx: int = 0
- for ch in column_heights:
- param_names_columns.append(param_names[last_end_idx : last_end_idx + ch])
- column_max_widths.append(
- max([len(param_name) for param_name in param_names_columns[-1]])
- )
- last_end_idx = ch + last_end_idx
-
- # Transpose the param name columns into rows to facilitate looping
- param_names_rows: List[Tuple[str, ...]] = []
- for li in zip_longest(*param_names_columns, fillvalue=""):
- param_names_rows.append(li)
- # Build the table as a string by iterating over and formatting the rows
- param_names_table: str = ""
- for param_names_row in param_names_rows:
- for num, param_name in enumerate(param_names_row):
- # Set column width based on the longest param in the column
- max_name_length_column = column_max_widths[num]
- column_pad = 3
- param_names_table += "{:<{}}".format(
- param_name, max_name_length_column + column_pad
- )
- # Insert newlines and spacing after the last element in each row
- if num == (len(param_names_row) - 1):
- param_names_table += "\n"
- return param_names_table
-
- def _get_default_error_message(
- self,
- errors: ValidationErrorList,
- ) -> str:
- bullet_points: List[str] = []
- errors_by_validator = _group_errors_by_validator(errors)
- if "enum" in errors_by_validator:
- for error in errors_by_validator["enum"]:
- bullet_points.append(f"one of {error.validator_value}")
-
- if "type" in errors_by_validator:
- types = [f"'{err.validator_value}'" for err in errors_by_validator["type"]]
- point = "of type "
- if len(types) == 1:
- point += types[0]
- elif len(types) == 2:
- point += f"{types[0]} or {types[1]}"
- else:
- point += ", ".join(types[:-1]) + f", or {types[-1]}"
- bullet_points.append(point)
-
- # It should not matter which error is specifically used as they are all
- # about the same offending instance (i.e. invalid value), so we can just
- # take the first one
- error = errors[0]
- # Add a summary line when parameters are passed an invalid value
- # For example: "'asdf' is an invalid value for `stack`
- message = f"'{error.instance}' is an invalid value"
- if error.absolute_path:
- message += f" for `{error.absolute_path[-1]}`"
-
- # Add bullet points
- if len(bullet_points) == 0:
- message += ".\n\n"
- elif len(bullet_points) == 1:
- message += f". Valid values are {bullet_points[0]}.\n\n"
- else:
- # We don't use .capitalize below to make the first letter uppercase
- # as that makes the rest of the message lowercase
- bullet_points = [point[0].upper() + point[1:] for point in bullet_points]
- message += ". Valid values are:\n\n"
- message += "\n".join([f"- {point}" for point in bullet_points])
- message += "\n\n"
-
- # Add unformatted messages of any remaining errors which were not
- # considered so far. This is not expected to be used but more exists
- # as a fallback for cases which were not known during development.
- for validator, errors in errors_by_validator.items():
- if validator not in ("enum", "type"):
- message += "\n".join([e.message for e in errors])
-
- return message
-
-
-class UndefinedType:
- """A singleton object for marking undefined parameters"""
-
- __instance = None
-
- def __new__(cls, *args, **kwargs):
- if not isinstance(cls.__instance, cls):
- cls.__instance = object.__new__(cls, *args, **kwargs)
- return cls.__instance
-
- def __repr__(self):
- return "Undefined"
-
-
-# In the future Altair may implement a more complete set of type hints.
-# But for now, we'll add an annotation to indicate that the type checker
-# should permit any value passed to a function argument whose default
-# value is Undefined.
-Undefined: Any = UndefinedType()
-
-
-class SchemaBase:
- """Base class for schema wrappers.
-
- Each derived class should set the _schema class attribute (and optionally
- the _rootschema class attribute) which is used for validation.
- """
-
- _schema: Optional[Dict[str, Any]] = None
- _rootschema: Optional[Dict[str, Any]] = None
- _class_is_valid_at_instantiation = True
-
- def __init__(self, *args, **kwds):
- # Two valid options for initialization, which should be handled by
- # derived classes:
- # - a single arg with no kwds, for, e.g. {'type': 'string'}
- # - zero args with zero or more kwds for {'type': 'object'}
- if self._schema is None:
- raise ValueError(
- "Cannot instantiate object of type {}: "
- "_schema class attribute is not defined."
- "".format(self.__class__)
- )
-
- if kwds:
- assert len(args) == 0
- else:
- assert len(args) in [0, 1]
-
- # use object.__setattr__ because we override setattr below.
- object.__setattr__(self, "_args", args)
- object.__setattr__(self, "_kwds", kwds)
-
- if DEBUG_MODE and self._class_is_valid_at_instantiation:
- self.to_dict(validate=True)
-
- def copy(self, deep=True, ignore=()):
- """Return a copy of the object
-
- Parameters
- ----------
- deep : boolean or list, optional
- If True (default) then return a deep copy of all dict, list, and
- SchemaBase objects within the object structure.
- If False, then only copy the top object.
- If a list or iterable, then only copy the listed attributes.
- ignore : list, optional
- A list of keys for which the contents should not be copied, but
- only stored by reference.
- """
-
- def _shallow_copy(obj):
- if isinstance(obj, SchemaBase):
- return obj.copy(deep=False)
- elif isinstance(obj, list):
- return obj[:]
- elif isinstance(obj, dict):
- return obj.copy()
- else:
- return obj
-
- def _deep_copy(obj, ignore=()):
- if isinstance(obj, SchemaBase):
- args = tuple(_deep_copy(arg) for arg in obj._args)
- kwds = {
- k: (_deep_copy(v, ignore=ignore) if k not in ignore else v)
- for k, v in obj._kwds.items()
- }
- with debug_mode(False):
- return obj.__class__(*args, **kwds)
- elif isinstance(obj, list):
- return [_deep_copy(v, ignore=ignore) for v in obj]
- elif isinstance(obj, dict):
- return {
- k: (_deep_copy(v, ignore=ignore) if k not in ignore else v)
- for k, v in obj.items()
- }
- else:
- return obj
-
- try:
- deep = list(deep)
- except TypeError:
- deep_is_list = False
- else:
- deep_is_list = True
-
- if deep and not deep_is_list:
- return _deep_copy(self, ignore=ignore)
-
- with debug_mode(False):
- copy = self.__class__(*self._args, **self._kwds)
- if deep_is_list:
- for attr in deep:
- copy[attr] = _shallow_copy(copy._get(attr))
- return copy
-
- def _get(self, attr, default=Undefined):
- """Get an attribute, returning default if not present."""
- attr = self._kwds.get(attr, Undefined)
- if attr is Undefined:
- attr = default
- return attr
-
- def __getattr__(self, attr):
- # reminder: getattr is called after the normal lookups
- if attr == "_kwds":
- raise AttributeError()
- if attr in self._kwds:
- return self._kwds[attr]
- else:
- try:
- _getattr = super(SchemaBase, self).__getattr__
- except AttributeError:
- _getattr = super(SchemaBase, self).__getattribute__
- return _getattr(attr)
-
- def __setattr__(self, item, val):
- self._kwds[item] = val
-
- def __getitem__(self, item):
- return self._kwds[item]
-
- def __setitem__(self, item, val):
- self._kwds[item] = val
-
- def __repr__(self):
- if self._kwds:
- args = (
- "{}: {!r}".format(key, val)
- for key, val in sorted(self._kwds.items())
- if val is not Undefined
- )
- args = "\n" + ",\n".join(args)
- return "{0}({{{1}\n}})".format(
- self.__class__.__name__, args.replace("\n", "\n ")
- )
- else:
- return "{}({!r})".format(self.__class__.__name__, self._args[0])
-
- def __eq__(self, other):
- return (
- type(self) is type(other)
- and self._args == other._args
- and self._kwds == other._kwds
- )
-
- def to_dict(self, validate=True, ignore=None, context=None):
- """Return a dictionary representation of the object
-
- Parameters
- ----------
- validate : boolean
- If True (default), then validate the output dictionary
- against the schema.
- ignore : list
- A list of keys to ignore. This will *not* passed to child to_dict
- function calls.
- context : dict (optional)
- A context dictionary that will be passed to all child to_dict
- function calls
-
- Returns
- -------
- dct : dictionary
- The dictionary representation of this object
-
- Raises
- ------
- jsonschema.ValidationError :
- if validate=True and the dict does not conform to the schema
- """
- if context is None:
- context = {}
- if ignore is None:
- ignore = []
-
- if self._args and not self._kwds:
- result = _todict(self._args[0], context=context)
- elif not self._args:
- kwds = self._kwds.copy()
- # parsed_shorthand is added by FieldChannelMixin.
- # It's used below to replace shorthand with its long form equivalent
- # parsed_shorthand is removed from context if it exists so that it is
- # not passed to child to_dict function calls
- parsed_shorthand = context.pop("parsed_shorthand", {})
- # Prevent that pandas categorical data is automatically sorted
- # when a non-ordinal data type is specifed manually
- # or if the encoding channel does not support sorting
- if "sort" in parsed_shorthand and (
- "sort" not in kwds or kwds["type"] not in ["ordinal", Undefined]
- ):
- parsed_shorthand.pop("sort")
-
- kwds.update(
- {
- k: v
- for k, v in parsed_shorthand.items()
- if kwds.get(k, Undefined) is Undefined
- }
- )
- kwds = {
- k: v for k, v in kwds.items() if k not in list(ignore) + ["shorthand"]
- }
- if "mark" in kwds and isinstance(kwds["mark"], str):
- kwds["mark"] = {"type": kwds["mark"]}
- result = _todict(
- kwds,
- context=context,
- )
- else:
- raise ValueError(
- "{} instance has both a value and properties : "
- "cannot serialize to dict".format(self.__class__)
- )
- if validate:
- try:
- self.validate(result)
- except jsonschema.ValidationError as err:
- # We do not raise `from err` as else the resulting
- # traceback is very long as it contains part
- # of the Vega-Lite schema. It would also first
- # show the less helpful ValidationError instead of
- # the more user friendly SchemaValidationError
- raise SchemaValidationError(self, err) from None
- return result
-
- def to_json(
- self,
- validate=True,
- ignore=None,
- context=None,
- indent=2,
- sort_keys=True,
- **kwargs,
- ):
- """Emit the JSON representation for this object as a string.
-
- Parameters
- ----------
- validate : boolean
- If True (default), then validate the output dictionary
- against the schema.
- ignore : list (optional)
- A list of keys to ignore. This will *not* passed to child to_dict
- function calls.
- context : dict (optional)
- A context dictionary that will be passed to all child to_dict
- function calls
- indent : integer, default 2
- the number of spaces of indentation to use
- sort_keys : boolean, default True
- if True, sort keys in the output
- **kwargs
- Additional keyword arguments are passed to ``json.dumps()``
-
- Returns
- -------
- spec : string
- The JSON specification of the chart object.
- """
- if ignore is None:
- ignore = []
- if context is None:
- context = {}
- dct = self.to_dict(validate=validate, ignore=ignore, context=context)
- return json.dumps(dct, indent=indent, sort_keys=sort_keys, **kwargs)
-
- @classmethod
- def _default_wrapper_classes(cls):
- """Return the set of classes used within cls.from_dict()"""
- return _subclasses(SchemaBase)
-
- @classmethod
- def from_dict(cls, dct, validate=True, _wrapper_classes=None):
- """Construct class from a dictionary representation
-
- Parameters
- ----------
- dct : dictionary
- The dict from which to construct the class
- validate : boolean
- If True (default), then validate the input against the schema.
- _wrapper_classes : list (optional)
- The set of SchemaBase classes to use when constructing wrappers
- of the dict inputs. If not specified, the result of
- cls._default_wrapper_classes will be used.
-
- Returns
- -------
- obj : Schema object
- The wrapped schema
-
- Raises
- ------
- jsonschema.ValidationError :
- if validate=True and dct does not conform to the schema
- """
- if validate:
- cls.validate(dct)
- if _wrapper_classes is None:
- _wrapper_classes = cls._default_wrapper_classes()
- converter = _FromDict(_wrapper_classes)
- return converter.from_dict(dct, cls)
-
- @classmethod
- def from_json(cls, json_string, validate=True, **kwargs):
- """Instantiate the object from a valid JSON string
-
- Parameters
- ----------
- json_string : string
- The string containing a valid JSON chart specification.
- validate : boolean
- If True (default), then validate the input against the schema.
- **kwargs :
- Additional keyword arguments are passed to json.loads
-
- Returns
- -------
- chart : Chart object
- The altair Chart object built from the specification.
- """
- dct = json.loads(json_string, **kwargs)
- return cls.from_dict(dct, validate=validate)
-
- @classmethod
- def validate(cls, instance, schema=None):
- """
- Validate the instance against the class schema in the context of the
- rootschema.
- """
- if schema is None:
- schema = cls._schema
- return validate_jsonschema(
- instance, schema, rootschema=cls._rootschema or cls._schema
- )
-
- @classmethod
- def resolve_references(cls, schema=None):
- """Resolve references in the context of this object's schema or root schema."""
- return _resolve_references(
- schema=(schema or cls._schema),
- root=(cls._rootschema or cls._schema or schema),
- )
-
- @classmethod
- def validate_property(cls, name, value, schema=None):
- """
- Validate a property against property schema in the context of the
- rootschema
- """
- value = _todict(value, context={})
- props = cls.resolve_references(schema or cls._schema).get("properties", {})
- return validate_jsonschema(
- value, props.get(name, {}), rootschema=cls._rootschema or cls._schema
- )
-
- def __dir__(self):
- return sorted(super().__dir__() + list(self._kwds.keys()))
-
-
-def _passthrough(*args, **kwds):
- return args[0] if args else kwds
-
-
-class _FromDict:
- """Class used to construct SchemaBase class hierarchies from a dict
-
- The primary purpose of using this class is to be able to build a hash table
- that maps schemas to their wrapper classes. The candidate classes are
- specified in the ``class_list`` argument to the constructor.
- """
-
- _hash_exclude_keys = ("definitions", "title", "description", "$schema", "id")
-
- def __init__(self, class_list):
- # Create a mapping of a schema hash to a list of matching classes
- # This lets us quickly determine the correct class to construct
- self.class_dict = collections.defaultdict(list)
- for cls in class_list:
- if cls._schema is not None:
- self.class_dict[self.hash_schema(cls._schema)].append(cls)
-
- @classmethod
- def hash_schema(cls, schema, use_json=True):
- """
- Compute a python hash for a nested dictionary which
- properly handles dicts, lists, sets, and tuples.
-
- At the top level, the function excludes from the hashed schema all keys
- listed in `exclude_keys`.
-
- This implements two methods: one based on conversion to JSON, and one based
- on recursive conversions of unhashable to hashable types; the former seems
- to be slightly faster in several benchmarks.
- """
- if cls._hash_exclude_keys and isinstance(schema, dict):
- schema = {
- key: val
- for key, val in schema.items()
- if key not in cls._hash_exclude_keys
- }
- if use_json:
- s = json.dumps(schema, sort_keys=True)
- return hash(s)
- else:
-
- def _freeze(val):
- if isinstance(val, dict):
- return frozenset((k, _freeze(v)) for k, v in val.items())
- elif isinstance(val, set):
- return frozenset(map(_freeze, val))
- elif isinstance(val, list) or isinstance(val, tuple):
- return tuple(map(_freeze, val))
- else:
- return val
-
- return hash(_freeze(schema))
-
- def from_dict(
- self, dct, cls=None, schema=None, rootschema=None, default_class=_passthrough
- ):
- """Construct an object from a dict representation"""
- if (schema is None) == (cls is None):
- raise ValueError("Must provide either cls or schema, but not both.")
- if schema is None:
- schema = schema or cls._schema
- rootschema = rootschema or cls._rootschema
- rootschema = rootschema or schema
-
- if isinstance(dct, SchemaBase):
- return dct
-
- if cls is None:
- # If there are multiple matches, we use the first one in the dict.
- # Our class dict is constructed breadth-first from top to bottom,
- # so the first class that matches is the most general match.
- matches = self.class_dict[self.hash_schema(schema)]
- if matches:
- cls = matches[0]
- else:
- cls = default_class
- schema = _resolve_references(schema, rootschema)
-
- if "anyOf" in schema or "oneOf" in schema:
- schemas = schema.get("anyOf", []) + schema.get("oneOf", [])
- for possible_schema in schemas:
- try:
- validate_jsonschema(dct, possible_schema, rootschema=rootschema)
- except jsonschema.ValidationError:
- continue
- else:
- return self.from_dict(
- dct,
- schema=possible_schema,
- rootschema=rootschema,
- default_class=cls,
- )
-
- if isinstance(dct, dict):
- # TODO: handle schemas for additionalProperties/patternProperties
- props = schema.get("properties", {})
- kwds = {}
- for key, val in dct.items():
- if key in props:
- val = self.from_dict(val, schema=props[key], rootschema=rootschema)
- kwds[key] = val
- return cls(**kwds)
-
- elif isinstance(dct, list):
- item_schema = schema.get("items", {})
- dct = [
- self.from_dict(val, schema=item_schema, rootschema=rootschema)
- for val in dct
- ]
- return cls(dct)
- else:
- return cls(dct)
-
-
-class _PropertySetter:
- def __init__(self, prop, schema):
- self.prop = prop
- self.schema = schema
-
- def __get__(self, obj, cls):
- self.obj = obj
- self.cls = cls
- # The docs from the encoding class parameter (e.g. `bin` in X, Color,
- # etc); this provides a general description of the parameter.
- self.__doc__ = self.schema["description"].replace("__", "**")
- property_name = f"{self.prop}"[0].upper() + f"{self.prop}"[1:]
- if hasattr(vegalite, property_name):
- altair_prop = getattr(vegalite, property_name)
- # Add the docstring from the helper class (e.g. `BinParams`) so
- # that all the parameter names of the helper class are included in
- # the final docstring
- parameter_index = altair_prop.__doc__.find("Parameters\n")
- if parameter_index > -1:
- self.__doc__ = (
- altair_prop.__doc__[:parameter_index].replace(" ", "")
- + self.__doc__
- + textwrap.dedent(
- f"\n\n {altair_prop.__doc__[parameter_index:]}"
- )
- )
- # For short docstrings such as Aggregate, Stack, et
- else:
- self.__doc__ = (
- altair_prop.__doc__.replace(" ", "") + "\n" + self.__doc__
- )
- # Add signatures and tab completion for the method and parameter names
- self.__signature__ = inspect.signature(altair_prop)
- self.__wrapped__ = inspect.getfullargspec(altair_prop)
- self.__name__ = altair_prop.__name__
- else:
- # It seems like bandPosition is the only parameter that doesn't
- # have a helper class.
- pass
- return self
-
- def __call__(self, *args, **kwargs):
- obj = self.obj.copy()
- # TODO: use schema to validate
- obj[self.prop] = args[0] if args else kwargs
- return obj
-
-
-def with_property_setters(cls):
- """
- Decorator to add property setters to a Schema class.
- """
- schema = cls.resolve_references()
- for prop, propschema in schema.get("properties", {}).items():
- setattr(cls, prop, _PropertySetter(prop, propschema))
- return cls
diff --git a/spaces/Datasculptor/StyleGAN-NADA/e4e/configs/paths_config.py b/spaces/Datasculptor/StyleGAN-NADA/e4e/configs/paths_config.py
deleted file mode 100644
index 4604f6063b8125364a52a492de52fcc54004f373..0000000000000000000000000000000000000000
--- a/spaces/Datasculptor/StyleGAN-NADA/e4e/configs/paths_config.py
+++ /dev/null
@@ -1,28 +0,0 @@
-dataset_paths = {
- # Face Datasets (In the paper: FFHQ - train, CelebAHQ - test)
- 'ffhq': '',
- 'celeba_test': '',
-
- # Cars Dataset (In the paper: Stanford cars)
- 'cars_train': '',
- 'cars_test': '',
-
- # Horse Dataset (In the paper: LSUN Horse)
- 'horse_train': '',
- 'horse_test': '',
-
- # Church Dataset (In the paper: LSUN Church)
- 'church_train': '',
- 'church_test': '',
-
- # Cats Dataset (In the paper: LSUN Cat)
- 'cats_train': '',
- 'cats_test': ''
-}
-
-model_paths = {
- 'stylegan_ffhq': 'pretrained_models/stylegan2-ffhq-config-f.pt',
- 'ir_se50': 'pretrained_models/model_ir_se50.pth',
- 'shape_predictor': 'pretrained_models/shape_predictor_68_face_landmarks.dat',
- 'moco': 'pretrained_models/moco_v2_800ep_pretrain.pth'
-}
diff --git a/spaces/ECCV2022/PSG/OpenPSG/configs/_base_/models/detr4seg_r101_psg.py b/spaces/ECCV2022/PSG/OpenPSG/configs/_base_/models/detr4seg_r101_psg.py
deleted file mode 100644
index 1d21e75bc4fd8b693daeaa488a613feb052914fe..0000000000000000000000000000000000000000
--- a/spaces/ECCV2022/PSG/OpenPSG/configs/_base_/models/detr4seg_r101_psg.py
+++ /dev/null
@@ -1,137 +0,0 @@
-_base_ = [
- '../_base_/models/detr4seg_r101.py', '../_base_/datasets/psg.py',
- '../_base_/custom_runtime.py'
-]
-
-custom_imports = dict(imports=[
- 'openpsg.models.frameworks.detr4seg',
- 'openpsg.models.relation_heads.detr4seg_head', 'openpsg.datasets',
- 'openpsg.datasets.pipelines.loading',
- 'openpsg.datasets.pipelines.rel_randomcrop',
- 'openpsg.models.relation_heads.approaches.matcher',
- 'openpsg.models.losses.seg_losses'
-],
- allow_failed_imports=False)
-
-object_classes = [
- 'person', 'bicycle', 'car', 'motorcycle', 'airplane', 'bus', 'train',
- 'truck', 'boat', 'traffic light', 'fire hydrant', 'stop sign',
- 'parking meter', 'bench', 'bird', 'cat', 'dog', 'horse', 'sheep', 'cow',
- 'elephant', 'bear', 'zebra', 'giraffe', 'backpack', 'umbrella', 'handbag',
- 'tie', 'suitcase', 'frisbee', 'skis', 'snowboard', 'sports ball', 'kite',
- 'baseball bat', 'baseball glove', 'skateboard', 'surfboard',
- 'tennis racket', 'bottle', 'wine glass', 'cup', 'fork', 'knife', 'spoon',
- 'bowl', 'banana', 'apple', 'sandwich', 'orange', 'broccoli', 'carrot',
- 'hot dog', 'pizza', 'donut', 'cake', 'chair', 'couch', 'potted plant',
- 'bed', 'dining table', 'toilet', 'tv', 'laptop', 'mouse', 'remote',
- 'keyboard', 'cell phone', 'microwave', 'oven', 'toaster', 'sink',
- 'refrigerator', 'book', 'clock', 'vase', 'scissors', 'teddy bear',
- 'hair drier', 'toothbrush', 'banner', 'blanket', 'bridge', 'cardboard',
- 'counter', 'curtain', 'door-stuff', 'floor-wood', 'flower', 'fruit',
- 'gravel', 'house', 'light', 'mirror-stuff', 'net', 'pillow', 'platform',
- 'playingfield', 'railroad', 'river', 'road', 'roof', 'sand', 'sea',
- 'shelf', 'snow', 'stairs', 'tent', 'towel', 'wall-brick', 'wall-stone',
- 'wall-tile', 'wall-wood', 'water-other', 'window-blind', 'window-other',
- 'tree-merged', 'fence-merged', 'ceiling-merged', 'sky-other-merged',
- 'cabinet-merged', 'table-merged', 'floor-other-merged', 'pavement-merged',
- 'mountain-merged', 'grass-merged', 'dirt-merged', 'paper-merged',
- 'food-other-merged', 'building-other-merged', 'rock-merged',
- 'wall-other-merged', 'rug-merged'
-]
-
-model = dict(bbox_head=dict(
- num_classes=len(object_classes),
- object_classes=object_classes,
-))
-
-img_norm_cfg = dict(mean=[123.675, 116.28, 103.53],
- std=[58.395, 57.12, 57.375],
- to_rgb=True)
-# train_pipeline, NOTE the img_scale and the Pad's size_divisor is different
-# from the default setting in mmdet.
-train_pipeline = [
- dict(type='LoadImageFromFile'),
- dict(type='LoadSceneGraphAnnotations', with_bbox=True, with_rel=True),
- dict(type='RandomFlip', flip_ratio=0.5),
- dict(
- type='AutoAugment',
- policies=[
- [
- dict(type='Resize',
- img_scale=[(480, 1333), (512, 1333), (544, 1333),
- (576, 1333), (608, 1333), (640, 1333),
- (672, 1333), (704, 1333), (736, 1333),
- (768, 1333), (800, 1333)],
- multiscale_mode='value',
- keep_ratio=True)
- ],
- [
- dict(type='Resize',
- img_scale=[(400, 1333), (500, 1333), (600, 1333)],
- multiscale_mode='value',
- keep_ratio=True),
- dict(type='RandomCrop',
- crop_type='absolute_range',
- crop_size=(384, 600),
- allow_negative_crop=False), # no empty relations
- dict(type='Resize',
- img_scale=[(480, 1333), (512, 1333), (544, 1333),
- (576, 1333), (608, 1333), (640, 1333),
- (672, 1333), (704, 1333), (736, 1333),
- (768, 1333), (800, 1333)],
- multiscale_mode='value',
- override=True,
- keep_ratio=True)
- ]
- ]),
- dict(type='Normalize', **img_norm_cfg),
- dict(type='Pad', size_divisor=1),
- dict(type='RelsFormatBundle'),
- dict(type='Collect', keys=['img', 'gt_bboxes', 'gt_labels'])
-]
-# test_pipeline, NOTE the Pad's size_divisor is different from the default
-# setting (size_divisor=32). While there is little effect on the performance
-# whether we use the default setting or use size_divisor=1.
-test_pipeline = [
- dict(type='LoadImageFromFile'),
- dict(type='MultiScaleFlipAug',
- img_scale=(1333, 800),
- flip=False,
- transforms=[
- dict(type='Resize', keep_ratio=True),
- dict(type='RandomFlip'),
- dict(type='Normalize', **img_norm_cfg),
- dict(type='Pad', size_divisor=1),
- dict(type='ImageToTensor', keys=['img']),
- dict(type='Collect', keys=['img'])
- ])
-]
-data = dict(samples_per_gpu=2,
- workers_per_gpu=2,
- train=dict(pipeline=train_pipeline),
- val=dict(pipeline=test_pipeline),
- test=dict(pipeline=test_pipeline))
-# optimizer
-optimizer = dict(
- type='AdamW',
- lr=0.0001,
- weight_decay=0.0001,
- paramwise_cfg=dict(
- custom_keys={'backbone': dict(lr_mult=0.1, decay_mult=1.0)}))
-optimizer_config = dict(grad_clip=dict(max_norm=0.1, norm_type=2))
-
-# learning policy
-lr_config = dict(policy='step', step=110)
-runner = dict(type='EpochBasedRunner', max_epochs=150)
-
-project_name = 'detr4seg'
-expt_name = 'detr4seg_r101_coco'
-work_dir = f'./work_dirs/{expt_name}'
-
-log_config = dict(
- interval=50,
- hooks=[dict(type='TextLoggerHook'),
- dict(type='TensorboardLoggerHook')],
-)
-
-load_from = '/mnt/ssd/gzj/test/OpenPSG/detr_r50_fb_origin.pth'
diff --git a/spaces/Eddycrack864/Applio-Inference/infer/modules/train/preprocess.py b/spaces/Eddycrack864/Applio-Inference/infer/modules/train/preprocess.py
deleted file mode 100644
index fbe81307ee661a95b2ac479336671a44ee02151a..0000000000000000000000000000000000000000
--- a/spaces/Eddycrack864/Applio-Inference/infer/modules/train/preprocess.py
+++ /dev/null
@@ -1,147 +0,0 @@
-import multiprocessing
-import os
-import sys
-
-from scipy import signal
-
-now_dir = os.getcwd()
-sys.path.append(now_dir)
-print(sys.argv)
-inp_root = sys.argv[1]
-sr = int(sys.argv[2])
-n_p = int(sys.argv[3])
-exp_dir = sys.argv[4]
-noparallel = sys.argv[5] == "True"
-per = float(sys.argv[6])
-import multiprocessing
-import os
-import traceback
-
-import librosa
-import numpy as np
-from scipy.io import wavfile
-
-from infer.lib.audio import load_audio
-from infer.lib.slicer2 import Slicer
-
-mutex = multiprocessing.Lock()
-f = open("%s/preprocess.log" % exp_dir, "a+")
-
-
-def println(strr):
- mutex.acquire()
- print(strr)
- f.write("%s\n" % strr)
- f.flush()
- mutex.release()
-
-
-class PreProcess:
- def __init__(self, sr, exp_dir, per=3.7):
- self.slicer = Slicer(
- sr=sr,
- threshold=-42,
- min_length=1500,
- min_interval=400,
- hop_size=15,
- max_sil_kept=500,
- )
- self.sr = sr
- self.bh, self.ah = signal.butter(N=5, Wn=48, btype="high", fs=self.sr)
- self.per = per
- self.overlap = 0.3
- self.tail = self.per + self.overlap
- self.max = 0.9
- self.alpha = 0.75
- self.exp_dir = exp_dir
- self.gt_wavs_dir = "%s/0_gt_wavs" % exp_dir
- self.wavs16k_dir = "%s/1_16k_wavs" % exp_dir
- os.makedirs(self.exp_dir, exist_ok=True)
- os.makedirs(self.gt_wavs_dir, exist_ok=True)
- os.makedirs(self.wavs16k_dir, exist_ok=True)
-
- def norm_write(self, tmp_audio, idx0, idx1):
- tmp_max = np.abs(tmp_audio).max()
- if tmp_max > 2.5:
- print("%s-%s-%s-filtered" % (idx0, idx1, tmp_max))
- return
- tmp_audio = (tmp_audio / tmp_max * (self.max * self.alpha)) + (
- 1 - self.alpha
- ) * tmp_audio
- wavfile.write(
- "%s/%s_%s.wav" % (self.gt_wavs_dir, idx0, idx1),
- self.sr,
- tmp_audio.astype(np.float32),
- )
- tmp_audio = librosa.resample(
- tmp_audio, orig_sr=self.sr, target_sr=16000
- ) # , res_type="soxr_vhq"
- wavfile.write(
- "%s/%s_%s.wav" % (self.wavs16k_dir, idx0, idx1),
- 16000,
- tmp_audio.astype(np.float32),
- )
-
- def pipeline(self, path, idx0):
- try:
- audio = load_audio(path, self.sr)
- # zero phased digital filter cause pre-ringing noise...
- # audio = signal.filtfilt(self.bh, self.ah, audio)
- audio = signal.lfilter(self.bh, self.ah, audio)
-
- idx1 = 0
- for audio in self.slicer.slice(audio):
- i = 0
- while 1:
- start = int(self.sr * (self.per - self.overlap) * i)
- i += 1
- if len(audio[start:]) > self.tail * self.sr:
- tmp_audio = audio[start : start + int(self.per * self.sr)]
- self.norm_write(tmp_audio, idx0, idx1)
- idx1 += 1
- else:
- tmp_audio = audio[start:]
- idx1 += 1
- break
- self.norm_write(tmp_audio, idx0, idx1)
- println("%s->Suc." % path)
- except:
- println("%s->%s" % (path, traceback.format_exc()))
-
- def pipeline_mp(self, infos):
- for path, idx0 in infos:
- self.pipeline(path, idx0)
-
- def pipeline_mp_inp_dir(self, inp_root, n_p):
- try:
- infos = [
- ("%s/%s" % (inp_root, name), idx)
- for idx, name in enumerate(sorted(list(os.listdir(inp_root))))
- ]
- if noparallel:
- for i in range(n_p):
- self.pipeline_mp(infos[i::n_p])
- else:
- ps = []
- for i in range(n_p):
- p = multiprocessing.Process(
- target=self.pipeline_mp, args=(infos[i::n_p],)
- )
- ps.append(p)
- p.start()
- for i in range(n_p):
- ps[i].join()
- except:
- println("Fail. %s" % traceback.format_exc())
-
-
-def preprocess_trainset(inp_root, sr, n_p, exp_dir, per):
- pp = PreProcess(sr, exp_dir, per)
- println("start preprocess")
- println(sys.argv)
- pp.pipeline_mp_inp_dir(inp_root, n_p)
- println("end preprocess")
-
-
-if __name__ == "__main__":
- preprocess_trainset(inp_root, sr, n_p, exp_dir, per)
diff --git a/spaces/Felix123456/bingo/src/lib/hooks/use-bing.ts b/spaces/Felix123456/bingo/src/lib/hooks/use-bing.ts
deleted file mode 100644
index dcdb1667ced0cba299b0825c0e91c4732411308c..0000000000000000000000000000000000000000
--- a/spaces/Felix123456/bingo/src/lib/hooks/use-bing.ts
+++ /dev/null
@@ -1,173 +0,0 @@
-'use client'
-
-import { useState, useCallback, useEffect, useMemo } from 'react'
-import { useAtom, useAtomValue } from 'jotai'
-import { chatFamily, bingConversationStyleAtom, GreetMessages, hashAtom, voiceAtom } from '@/state'
-import { setConversationMessages } from './chat-history'
-import { ChatMessageModel, BotId, FileItem } from '@/lib/bots/bing/types'
-import { nanoid } from '../utils'
-import { TTS } from '../bots/bing/tts'
-
-export function useBing(botId: BotId = 'bing') {
- const chatAtom = useMemo(() => chatFamily({ botId, page: 'singleton' }), [botId])
- const [enableTTS] = useAtom(voiceAtom)
- const speaker = useMemo(() => new TTS(), [])
- const [hash, setHash] = useAtom(hashAtom)
- const bingConversationStyle = useAtomValue(bingConversationStyleAtom)
- const [chatState, setChatState] = useAtom(chatAtom)
- const [input, setInput] = useState('')
- const [attachmentList, setAttachmentList] = useState([])
-
- const updateMessage = useCallback(
- (messageId: string, updater: (message: ChatMessageModel) => void) => {
- setChatState((draft) => {
- const message = draft.messages.find((m) => m.id === messageId)
- if (message) {
- updater(message)
- }
- })
- },
- [setChatState],
- )
-
- const sendMessage = useCallback(
- async (input: string, options = {}) => {
- const botMessageId = nanoid()
- const imageUrl = attachmentList?.[0]?.status === 'loaded' ? attachmentList[0].url : undefined
- setChatState((draft) => {
- const text = imageUrl ? `${input}\n\n` : input
- draft.messages.push({ id: nanoid(), text, author: 'user' }, { id: botMessageId, text: '', author: 'bot' })
- setAttachmentList([])
- })
- const abortController = new AbortController()
- setChatState((draft) => {
- draft.generatingMessageId = botMessageId
- draft.abortController = abortController
- })
- speaker.reset()
- await chatState.bot.sendMessage({
- prompt: input,
- imageUrl: /\?bcid=([^&]+)/.test(imageUrl ?? '') ? `https://www.bing.com/images/blob?bcid=${RegExp.$1}` : imageUrl,
- options: {
- ...options,
- bingConversationStyle,
- },
- signal: abortController.signal,
- onEvent(event) {
- if (event.type === 'UPDATE_ANSWER') {
- updateMessage(botMessageId, (message) => {
- if (event.data.text.length > message.text.length) {
- message.text = event.data.text
- }
-
- if (event.data.spokenText && enableTTS) {
- speaker.speak(event.data.spokenText)
- }
-
- message.throttling = event.data.throttling || message.throttling
- message.sourceAttributions = event.data.sourceAttributions || message.sourceAttributions
- message.suggestedResponses = event.data.suggestedResponses || message.suggestedResponses
- })
- } else if (event.type === 'ERROR') {
- updateMessage(botMessageId, (message) => {
- message.error = event.error
- })
- setChatState((draft) => {
- draft.abortController = undefined
- draft.generatingMessageId = ''
- })
- } else if (event.type === 'DONE') {
- setChatState((draft) => {
- draft.abortController = undefined
- draft.generatingMessageId = ''
- })
- }
- },
- })
- },
- [botId, attachmentList, chatState.bot, setChatState, updateMessage],
- )
-
- const uploadImage = useCallback(async (imgUrl: string) => {
- setAttachmentList([{ url: imgUrl, status: 'loading' }])
- const response = await chatState.bot.uploadImage(imgUrl, bingConversationStyle)
- if (response?.blobId) {
- setAttachmentList([{ url: `/api/blob?bcid=${response.blobId}`, status: 'loaded' }])
- } else {
- setAttachmentList([{ url: imgUrl, status: 'error' }])
- }
- }, [chatState.bot])
-
- const resetConversation = useCallback(() => {
- chatState.bot.resetConversation()
- speaker.abort()
- setChatState((draft) => {
- draft.abortController = undefined
- draft.generatingMessageId = ''
- draft.messages = [{ author: 'bot', text: GreetMessages[Math.floor(GreetMessages.length * Math.random())], id: nanoid() }]
- draft.conversationId = nanoid()
- })
- }, [chatState.bot, setChatState])
-
- const stopGenerating = useCallback(() => {
- chatState.abortController?.abort()
- if (chatState.generatingMessageId) {
- updateMessage(chatState.generatingMessageId, (message) => {
- if (!message.text && !message.error) {
- message.text = 'Cancelled'
- }
- })
- }
- setChatState((draft) => {
- draft.generatingMessageId = ''
- })
- }, [chatState.abortController, chatState.generatingMessageId, setChatState, updateMessage])
-
- useEffect(() => {
- if (chatState.messages.length) {
- setConversationMessages(botId, chatState.conversationId, chatState.messages)
- }
- }, [botId, chatState.conversationId, chatState.messages])
-
- useEffect(() => {
- if (hash === 'reset') {
- resetConversation()
- setHash('')
- }
- }, [hash, setHash])
-
- const chat = useMemo(
- () => ({
- botId,
- bot: chatState.bot,
- isSpeaking: speaker.isSpeaking,
- messages: chatState.messages,
- sendMessage,
- setInput,
- input,
- resetConversation,
- generating: !!chatState.generatingMessageId,
- stopGenerating,
- uploadImage,
- setAttachmentList,
- attachmentList,
- }),
- [
- botId,
- bingConversationStyle,
- chatState.bot,
- chatState.generatingMessageId,
- chatState.messages,
- speaker.isSpeaking,
- setInput,
- input,
- setAttachmentList,
- attachmentList,
- resetConversation,
- sendMessage,
- stopGenerating,
- ],
- )
-
- return chat
-}
diff --git a/spaces/FrankZxShen/so-vits-svc-models-ba/cluster/train_cluster.py b/spaces/FrankZxShen/so-vits-svc-models-ba/cluster/train_cluster.py
deleted file mode 100644
index 8644566388a4107c4442da14c0de090bcd4a91b8..0000000000000000000000000000000000000000
--- a/spaces/FrankZxShen/so-vits-svc-models-ba/cluster/train_cluster.py
+++ /dev/null
@@ -1,84 +0,0 @@
-import time,pdb
-import tqdm
-from time import time as ttime
-import os
-from pathlib import Path
-import logging
-import argparse
-from kmeans import KMeansGPU
-import torch
-import numpy as np
-from sklearn.cluster import KMeans,MiniBatchKMeans
-
-logging.basicConfig(level=logging.INFO)
-logger = logging.getLogger(__name__)
-from time import time as ttime
-import pynvml,torch
-
-def train_cluster(in_dir, n_clusters, use_minibatch=True, verbose=False,use_gpu=False):#gpu_minibatch真拉,虽然库支持但是也不考虑
- logger.info(f"Loading features from {in_dir}")
- features = []
- nums = 0
- for path in tqdm.tqdm(in_dir.glob("*.soft.pt")):
- # for name in os.listdir(in_dir):
- # path="%s/%s"%(in_dir,name)
- features.append(torch.load(path,map_location="cpu").squeeze(0).numpy().T)
- # print(features[-1].shape)
- features = np.concatenate(features, axis=0)
- print(nums, features.nbytes/ 1024**2, "MB , shape:",features.shape, features.dtype)
- features = features.astype(np.float32)
- logger.info(f"Clustering features of shape: {features.shape}")
- t = time.time()
- if(use_gpu==False):
- if use_minibatch:
- kmeans = MiniBatchKMeans(n_clusters=n_clusters,verbose=verbose, batch_size=4096, max_iter=80).fit(features)
- else:
- kmeans = KMeans(n_clusters=n_clusters,verbose=verbose).fit(features)
- else:
- kmeans = KMeansGPU(n_clusters=n_clusters, mode='euclidean', verbose=2 if verbose else 0,max_iter=500,tol=1e-2)#
- features=torch.from_numpy(features)#.to(device)
- labels = kmeans.fit_predict(features)#
-
- print(time.time()-t, "s")
-
- x = {
- "n_features_in_": kmeans.n_features_in_ if use_gpu==False else features.shape[1],
- "_n_threads": kmeans._n_threads if use_gpu==False else 4,
- "cluster_centers_": kmeans.cluster_centers_ if use_gpu==False else kmeans.centroids.cpu().numpy(),
- }
- print("end")
-
- return x
-
-if __name__ == "__main__":
- parser = argparse.ArgumentParser()
- parser.add_argument('--dataset', type=Path, default="./dataset/44k",
- help='path of training data directory')
- parser.add_argument('--output', type=Path, default="logs/44k",
- help='path of model output directory')
- parser.add_argument('--gpu',action='store_true', default=False ,
- help='to use GPU')
-
-
- args = parser.parse_args()
-
- checkpoint_dir = args.output
- dataset = args.dataset
- use_gpu = args.gpu
- n_clusters = 10000
-
- ckpt = {}
- for spk in os.listdir(dataset):
- if os.path.isdir(dataset/spk):
- print(f"train kmeans for {spk}...")
- in_dir = dataset/spk
- x = train_cluster(in_dir, n_clusters,use_minibatch=False,verbose=False,use_gpu=use_gpu)
- ckpt[spk] = x
-
- checkpoint_path = checkpoint_dir / f"kmeans_{n_clusters}.pt"
- checkpoint_path.parent.mkdir(exist_ok=True, parents=True)
- torch.save(
- ckpt,
- checkpoint_path,
- )
-
diff --git a/spaces/GFXY/stablediffusionapi-anything-v5/app.py b/spaces/GFXY/stablediffusionapi-anything-v5/app.py
deleted file mode 100644
index 6db423fde2b7e32c68e8be737dfc7c6175cd67a4..0000000000000000000000000000000000000000
--- a/spaces/GFXY/stablediffusionapi-anything-v5/app.py
+++ /dev/null
@@ -1,3 +0,0 @@
-import gradio as gr
-
-gr.Interface.load("models/stablediffusionapi/anything-v5").launch()
\ No newline at end of file
diff --git a/spaces/Grade2021/bingo/Dockerfile b/spaces/Grade2021/bingo/Dockerfile
deleted file mode 100644
index c677b05b75f7e4b2beee8c97fb47957a0861a83e..0000000000000000000000000000000000000000
--- a/spaces/Grade2021/bingo/Dockerfile
+++ /dev/null
@@ -1,7 +0,0 @@
-FROM weaigc/bingo:latest
-
-ARG DEBIAN_FRONTEND=noninteractive
-
-ENV BING_HEADER ""
-
-CMD npm start
diff --git a/spaces/Gradio-Blocks/uniformer_image_detection/app.py b/spaces/Gradio-Blocks/uniformer_image_detection/app.py
deleted file mode 100644
index 7428aaa14f8874fe96596b59da8e4a42fa56048f..0000000000000000000000000000000000000000
--- a/spaces/Gradio-Blocks/uniformer_image_detection/app.py
+++ /dev/null
@@ -1,62 +0,0 @@
-import os
-
-import torch
-import torch.nn.functional as F
-import torchvision.transforms as T
-from mmdet.apis import init_detector, inference_detector, show_result_pyplot
-import mmcv
-
-import gradio as gr
-from huggingface_hub import hf_hub_download
-
-# Device on which to run the model
-# Set to cuda to load on GPU
-device = "cpu"
-checkpoint_file = hf_hub_download(repo_id="Andy1621/uniformer", filename="mask_rcnn_3x_ms_hybrid_small.pth")
-config_file = './exp/mask_rcnn_3x_ms_hybrid_small/config.py'
-# init detector
-# build the model from a config file and a checkpoint file
-model = init_detector(config_file, checkpoint_file, device='cpu')
-
-
-def set_example_image(example: list) -> dict:
- return gr.Image.update(value=example[0])
-
-
-def inference(img):
- result = inference_detector(model, img)
- res_img = show_result_pyplot(model, img, result)
- return res_img
-
-
-demo = gr.Blocks()
-with demo:
- gr.Markdown(
- """
- # UniFormer-S
- Gradio demo for UniFormer: To use it, simply upload your image, or click one of the examples to load them. Read more at the links below.
- """
- )
-
- with gr.Box():
- with gr.Row():
- with gr.Column():
- with gr.Row():
- input_image = gr.Image(label='Input Image', type='numpy')
- with gr.Row():
- submit_button = gr.Button('Submit')
- with gr.Column():
- res_image = gr.Image(type='numpy', label='Detection Resutls')
- with gr.Row():
- example_images = gr.Dataset(components=[input_image], samples=[['demo.jpg']])
-
- gr.Markdown(
- """
-
- """
- )
-
- submit_button.click(fn=inference, inputs=input_image, outputs=res_image)
- example_images.click(fn=set_example_image, inputs=example_images, outputs=example_images.components)
-
-demo.launch(enable_queue=True)
\ No newline at end of file
diff --git a/spaces/Gradio-Blocks/uniformer_image_detection/configs/dcn/faster_rcnn_x101_32x4d_fpn_dconv_c3-c5_1x_coco.py b/spaces/Gradio-Blocks/uniformer_image_detection/configs/dcn/faster_rcnn_x101_32x4d_fpn_dconv_c3-c5_1x_coco.py
deleted file mode 100644
index 8357766f50ff638f13ca56bd79d1b1c64e96f3dd..0000000000000000000000000000000000000000
--- a/spaces/Gradio-Blocks/uniformer_image_detection/configs/dcn/faster_rcnn_x101_32x4d_fpn_dconv_c3-c5_1x_coco.py
+++ /dev/null
@@ -1,15 +0,0 @@
-_base_ = '../faster_rcnn/faster_rcnn_r50_fpn_1x_coco.py'
-model = dict(
- pretrained='open-mmlab://resnext101_32x4d',
- backbone=dict(
- type='ResNeXt',
- depth=101,
- groups=32,
- base_width=4,
- num_stages=4,
- out_indices=(0, 1, 2, 3),
- frozen_stages=1,
- norm_cfg=dict(type='BN', requires_grad=True),
- style='pytorch',
- dcn=dict(type='DCN', deform_groups=1, fallback_on_stride=False),
- stage_with_dcn=(False, True, True, True)))
diff --git a/spaces/Gradio-Blocks/uniformer_image_detection/configs/scnet/scnet_x101_64x4d_fpn_8x1_20e_coco.py b/spaces/Gradio-Blocks/uniformer_image_detection/configs/scnet/scnet_x101_64x4d_fpn_8x1_20e_coco.py
deleted file mode 100644
index 9f3ce6d14e6b3474d78c8de3f3565b0029dc067e..0000000000000000000000000000000000000000
--- a/spaces/Gradio-Blocks/uniformer_image_detection/configs/scnet/scnet_x101_64x4d_fpn_8x1_20e_coco.py
+++ /dev/null
@@ -1,3 +0,0 @@
-_base_ = './scnet_x101_64x4d_fpn_20e_coco.py'
-data = dict(samples_per_gpu=1, workers_per_gpu=1)
-optimizer = dict(type='SGD', lr=0.01, momentum=0.9, weight_decay=0.0001)
diff --git a/spaces/Gradio-Blocks/uniformer_image_segmentation/configs/encnet/encnet_r50-d8_512x1024_80k_cityscapes.py b/spaces/Gradio-Blocks/uniformer_image_segmentation/configs/encnet/encnet_r50-d8_512x1024_80k_cityscapes.py
deleted file mode 100644
index d2feeef7e982550481365f8187cb1a50f0fafcc9..0000000000000000000000000000000000000000
--- a/spaces/Gradio-Blocks/uniformer_image_segmentation/configs/encnet/encnet_r50-d8_512x1024_80k_cityscapes.py
+++ /dev/null
@@ -1,4 +0,0 @@
-_base_ = [
- '../_base_/models/encnet_r50-d8.py', '../_base_/datasets/cityscapes.py',
- '../_base_/default_runtime.py', '../_base_/schedules/schedule_80k.py'
-]
diff --git a/spaces/GrandaddyShmax/AudioCraft_Plus/audiocraft/models/unet.py b/spaces/GrandaddyShmax/AudioCraft_Plus/audiocraft/models/unet.py
deleted file mode 100644
index db4a6df8e309c21fede37abdbe3c862932027641..0000000000000000000000000000000000000000
--- a/spaces/GrandaddyShmax/AudioCraft_Plus/audiocraft/models/unet.py
+++ /dev/null
@@ -1,214 +0,0 @@
-# Copyright (c) Meta Platforms, Inc. and affiliates.
-# All rights reserved.
-#
-# This source code is licensed under the license found in the
-# LICENSE file in the root directory of this source tree.
-
-"""
-Pytorch Unet Module used for diffusion.
-"""
-
-from dataclasses import dataclass
-import typing as tp
-
-import torch
-from torch import nn
-from torch.nn import functional as F
-from audiocraft.modules.transformer import StreamingTransformer, create_sin_embedding
-
-
-@dataclass
-class Output:
- sample: torch.Tensor
-
-
-def get_model(cfg, channels: int, side: int, num_steps: int):
- if cfg.model == 'unet':
- return DiffusionUnet(
- chin=channels, num_steps=num_steps, **cfg.diffusion_unet)
- else:
- raise RuntimeError('Not Implemented')
-
-
-class ResBlock(nn.Module):
- def __init__(self, channels: int, kernel: int = 3, norm_groups: int = 4,
- dilation: int = 1, activation: tp.Type[nn.Module] = nn.ReLU,
- dropout: float = 0.):
- super().__init__()
- stride = 1
- padding = dilation * (kernel - stride) // 2
- Conv = nn.Conv1d
- Drop = nn.Dropout1d
- self.norm1 = nn.GroupNorm(norm_groups, channels)
- self.conv1 = Conv(channels, channels, kernel, 1, padding, dilation=dilation)
- self.activation1 = activation()
- self.dropout1 = Drop(dropout)
-
- self.norm2 = nn.GroupNorm(norm_groups, channels)
- self.conv2 = Conv(channels, channels, kernel, 1, padding, dilation=dilation)
- self.activation2 = activation()
- self.dropout2 = Drop(dropout)
-
- def forward(self, x):
- h = self.dropout1(self.conv1(self.activation1(self.norm1(x))))
- h = self.dropout2(self.conv2(self.activation2(self.norm2(h))))
- return x + h
-
-
-class DecoderLayer(nn.Module):
- def __init__(self, chin: int, chout: int, kernel: int = 4, stride: int = 2,
- norm_groups: int = 4, res_blocks: int = 1, activation: tp.Type[nn.Module] = nn.ReLU,
- dropout: float = 0.):
- super().__init__()
- padding = (kernel - stride) // 2
- self.res_blocks = nn.Sequential(
- *[ResBlock(chin, norm_groups=norm_groups, dilation=2**idx, dropout=dropout)
- for idx in range(res_blocks)])
- self.norm = nn.GroupNorm(norm_groups, chin)
- ConvTr = nn.ConvTranspose1d
- self.convtr = ConvTr(chin, chout, kernel, stride, padding, bias=False)
- self.activation = activation()
-
- def forward(self, x: torch.Tensor) -> torch.Tensor:
- x = self.res_blocks(x)
- x = self.norm(x)
- x = self.activation(x)
- x = self.convtr(x)
- return x
-
-
-class EncoderLayer(nn.Module):
- def __init__(self, chin: int, chout: int, kernel: int = 4, stride: int = 2,
- norm_groups: int = 4, res_blocks: int = 1, activation: tp.Type[nn.Module] = nn.ReLU,
- dropout: float = 0.):
- super().__init__()
- padding = (kernel - stride) // 2
- Conv = nn.Conv1d
- self.conv = Conv(chin, chout, kernel, stride, padding, bias=False)
- self.norm = nn.GroupNorm(norm_groups, chout)
- self.activation = activation()
- self.res_blocks = nn.Sequential(
- *[ResBlock(chout, norm_groups=norm_groups, dilation=2**idx, dropout=dropout)
- for idx in range(res_blocks)])
-
- def forward(self, x: torch.Tensor) -> torch.Tensor:
- B, C, T = x.shape
- stride, = self.conv.stride
- pad = (stride - (T % stride)) % stride
- x = F.pad(x, (0, pad))
-
- x = self.conv(x)
- x = self.norm(x)
- x = self.activation(x)
- x = self.res_blocks(x)
- return x
-
-
-class BLSTM(nn.Module):
- """BiLSTM with same hidden units as input dim.
- """
- def __init__(self, dim, layers=2):
- super().__init__()
- self.lstm = nn.LSTM(bidirectional=True, num_layers=layers, hidden_size=dim, input_size=dim)
- self.linear = nn.Linear(2 * dim, dim)
-
- def forward(self, x):
- x = x.permute(2, 0, 1)
- x = self.lstm(x)[0]
- x = self.linear(x)
- x = x.permute(1, 2, 0)
- return x
-
-
-class DiffusionUnet(nn.Module):
- def __init__(self, chin: int = 3, hidden: int = 24, depth: int = 3, growth: float = 2.,
- max_channels: int = 10_000, num_steps: int = 1000, emb_all_layers=False, cross_attention: bool = False,
- bilstm: bool = False, transformer: bool = False,
- codec_dim: tp.Optional[int] = None, **kwargs):
- super().__init__()
- self.encoders = nn.ModuleList()
- self.decoders = nn.ModuleList()
- self.embeddings: tp.Optional[nn.ModuleList] = None
- self.embedding = nn.Embedding(num_steps, hidden)
- if emb_all_layers:
- self.embeddings = nn.ModuleList()
- self.condition_embedding: tp.Optional[nn.Module] = None
- for d in range(depth):
- encoder = EncoderLayer(chin, hidden, **kwargs)
- decoder = DecoderLayer(hidden, chin, **kwargs)
- self.encoders.append(encoder)
- self.decoders.insert(0, decoder)
- if emb_all_layers and d > 0:
- assert self.embeddings is not None
- self.embeddings.append(nn.Embedding(num_steps, hidden))
- chin = hidden
- hidden = min(int(chin * growth), max_channels)
- self.bilstm: tp.Optional[nn.Module]
- if bilstm:
- self.bilstm = BLSTM(chin)
- else:
- self.bilstm = None
- self.use_transformer = transformer
- self.cross_attention = False
- if transformer:
- self.cross_attention = cross_attention
- self.transformer = StreamingTransformer(chin, 8, 6, bias_ff=False, bias_attn=False,
- cross_attention=cross_attention)
-
- self.use_codec = False
- if codec_dim is not None:
- self.conv_codec = nn.Conv1d(codec_dim, chin, 1)
- self.use_codec = True
-
- def forward(self, x: torch.Tensor, step: tp.Union[int, torch.Tensor], condition: tp.Optional[torch.Tensor] = None):
- skips = []
- bs = x.size(0)
- z = x
- view_args = [1]
- if type(step) is torch.Tensor:
- step_tensor = step
- else:
- step_tensor = torch.tensor([step], device=x.device, dtype=torch.long).expand(bs)
-
- for idx, encoder in enumerate(self.encoders):
- z = encoder(z)
- if idx == 0:
- z = z + self.embedding(step_tensor).view(bs, -1, *view_args).expand_as(z)
- elif self.embeddings is not None:
- z = z + self.embeddings[idx - 1](step_tensor).view(bs, -1, *view_args).expand_as(z)
-
- skips.append(z)
-
- if self.use_codec: # insert condition in the bottleneck
- assert condition is not None, "Model defined for conditionnal generation"
- condition_emb = self.conv_codec(condition) # reshape to the bottleneck dim
- assert condition_emb.size(-1) <= 2 * z.size(-1), \
- f"You are downsampling the conditionning with factor >=2 : {condition_emb.size(-1)=} and {z.size(-1)=}"
- if not self.cross_attention:
-
- condition_emb = torch.nn.functional.interpolate(condition_emb, z.size(-1))
- assert z.size() == condition_emb.size()
- z += condition_emb
- cross_attention_src = None
- else:
- cross_attention_src = condition_emb.permute(0, 2, 1) # B, T, C
- B, T, C = cross_attention_src.shape
- positions = torch.arange(T, device=x.device).view(1, -1, 1)
- pos_emb = create_sin_embedding(positions, C, max_period=10_000, dtype=cross_attention_src.dtype)
- cross_attention_src = cross_attention_src + pos_emb
- if self.use_transformer:
- z = self.transformer(z.permute(0, 2, 1), cross_attention_src=cross_attention_src).permute(0, 2, 1)
- else:
- if self.bilstm is None:
- z = torch.zeros_like(z)
- else:
- z = self.bilstm(z)
-
- for decoder in self.decoders:
- s = skips.pop(-1)
- z = z[:, :, :s.shape[2]]
- z = z + s
- z = decoder(z)
-
- z = z[:, :, :x.shape[2]]
- return Output(z)
diff --git a/spaces/GrandaddyShmax/MusicGen_Plus/audiocraft/utils/export.py b/spaces/GrandaddyShmax/MusicGen_Plus/audiocraft/utils/export.py
deleted file mode 100644
index b513b52267f7bf5aae09282c15b0a2e20c8a8fee..0000000000000000000000000000000000000000
--- a/spaces/GrandaddyShmax/MusicGen_Plus/audiocraft/utils/export.py
+++ /dev/null
@@ -1,56 +0,0 @@
-# Copyright (c) Meta Platforms, Inc. and affiliates.
-# All rights reserved.
-#
-# This source code is licensed under the license found in the
-# LICENSE file in the root directory of this source tree.
-
-"""
-Utility to export a training checkpoint to a lightweight release checkpoint.
-"""
-
-from pathlib import Path
-import typing as tp
-
-from omegaconf import OmegaConf, DictConfig
-import torch
-
-
-def _clean_lm_cfg(cfg: DictConfig):
- OmegaConf.set_struct(cfg, False)
- # This used to be set automatically in the LM solver, need a more robust solution
- # for the future.
- cfg['transformer_lm']['card'] = 2048
- cfg['transformer_lm']['n_q'] = 4
- # Experimental params no longer supported.
- bad_params = ['spectral_norm_attn_iters', 'spectral_norm_ff_iters',
- 'residual_balancer_attn', 'residual_balancer_ff', 'layer_drop']
- for name in bad_params:
- del cfg['transformer_lm'][name]
- OmegaConf.set_struct(cfg, True)
- return cfg
-
-
-def export_encodec(checkpoint_path: tp.Union[Path, str], out_folder: tp.Union[Path, str]):
- sig = Path(checkpoint_path).parent.name
- assert len(sig) == 8, "Not a valid Dora signature"
- pkg = torch.load(checkpoint_path, 'cpu')
- new_pkg = {
- 'best_state': pkg['ema']['state']['model'],
- 'xp.cfg': OmegaConf.to_yaml(pkg['xp.cfg']),
- }
- out_file = Path(out_folder) / f'{sig}.th'
- torch.save(new_pkg, out_file)
- return out_file
-
-
-def export_lm(checkpoint_path: tp.Union[Path, str], out_folder: tp.Union[Path, str]):
- sig = Path(checkpoint_path).parent.name
- assert len(sig) == 8, "Not a valid Dora signature"
- pkg = torch.load(checkpoint_path, 'cpu')
- new_pkg = {
- 'best_state': pkg['fsdp_best_state']['model'],
- 'xp.cfg': OmegaConf.to_yaml(_clean_lm_cfg(pkg['xp.cfg']))
- }
- out_file = Path(out_folder) / f'{sig}.th'
- torch.save(new_pkg, out_file)
- return out_file
diff --git a/spaces/GrandaddyShmax/MusicGen_Plus/tests/modules/__init__.py b/spaces/GrandaddyShmax/MusicGen_Plus/tests/modules/__init__.py
deleted file mode 100644
index 0952fcc3f57e34b3747962e9ebd6fc57aeea63fa..0000000000000000000000000000000000000000
--- a/spaces/GrandaddyShmax/MusicGen_Plus/tests/modules/__init__.py
+++ /dev/null
@@ -1,5 +0,0 @@
-# Copyright (c) Meta Platforms, Inc. and affiliates.
-# All rights reserved.
-#
-# This source code is licensed under the license found in the
-# LICENSE file in the root directory of this source tree.
diff --git a/spaces/HCMUT-GraduateThesis-HNTThinh/rgbdsod-multimae-demo/s_multimae/prepare_datasets.py b/spaces/HCMUT-GraduateThesis-HNTThinh/rgbdsod-multimae-demo/s_multimae/prepare_datasets.py
deleted file mode 100644
index 808529c123442f105531f8f4a2e9ed1749883dcb..0000000000000000000000000000000000000000
--- a/spaces/HCMUT-GraduateThesis-HNTThinh/rgbdsod-multimae-demo/s_multimae/prepare_datasets.py
+++ /dev/null
@@ -1,115 +0,0 @@
-import os
-from typing import List
-from zipfile import ZipFile
-
-from .configs.base_config import base_cfg
-from .run_type import run_type, RUN_TYPE
-
-def unzip(zip_path: str, dest_dir: str) -> None:
- """Note: it will ignore if this zip file has been unzipped before
-
- Args:
- zip_path (str): the path to zip file
- dest_dir (str): the destination directory to be unzipped to.
- """
- if not os.path.isdir(dest_dir):
- with ZipFile(zip_path, 'r') as zip_obj:
- zip_obj.extractall(dest_dir)
-
-def unzip_datasets(cfg: base_cfg) -> None:
- """
- Note: If the unzip process is abruptedly stopped.
- You need to delete the whole folder '/content/datasets' and rerun again
-
- Directory format:
- datasets:
- |-- previous_train
- |---- GT
- |---- RGB
- |---- depths
- |-- previous_dev
- |---- GT
- |---- RGB
- |---- depths
- |-- previous_test
- |---- dataset1
- |---- GT
- |---- RGB
- |----depths
- |---- dataset2
- |---- GT
- |---- RGB
- |----depths
- """
- os.makedirs(cfg.datasets_working_dir_path, exist_ok=True)
-
- unzip(cfg.train_dataset_zip_path, cfg.train_dataset_working_dir_path)
- if cfg.dev_dataset_zip_path is not None:
- unzip(cfg.dev_dataset_zip_path, cfg.dev_dataset_working_dir_path)
-
- os.makedirs(cfg.test_datasets_working_dir_path, exist_ok=True)
- for test_dataset_name in cfg.test_dataset_names:
- unzip(
- os.path.join(cfg.test_datasets_dir_path, f'{test_dataset_name}.zip'),
- os.path.join(cfg.test_datasets_working_dir_path, test_dataset_name)
- )
-
-def unzip_SOTAs(
- cfg: base_cfg,
- sotas_datasets: List[List[str]], # [['COME-E', 'COME-H'], [...], ...]
- sota_model_names: List[str], # ['SPNet', 'BBSNet', ...]
-) -> None:
- """Directory format:
-
- {benchmark_dir_path}
- |---- {sota_model_name}
- |---- {dataset}.zip
- |---- COME-E.zip
- |---- COME-H.zip
-
- Unzip to
-
- {cfg.sotas_working_dir}
- |---- v1
- |---- {sota_model_name}
- |---- {dataset}
- |---- pred_1.png
- |---- pred_2.png
- |---- SPNet
- |---- COME-E
- |---- pred_1.png
- |---- pred_2.png
- |---- v2
- |---- {sota_model_name}
- |---- {dataset}
- |---- pred_1.png
- |---- pred_2.png
-
- """
- print('run_type', run_type.rt)
- if not run_type.rt == RUN_TYPE.COLAB:
- return
-
- working_dir = cfg.sotas_working_dir # Colab
- os.makedirs(working_dir, exist_ok=True)
- assert len(sotas_datasets) == len(sota_model_names), \
- "sotas_datasets and sota_model_names must have the same size"
-
- for datasets_per_sota, sota_model_name in zip(sotas_datasets, sota_model_names):
- model_working_dir_path = os.path.join(working_dir, sota_model_name) # Colab
- os.makedirs(model_working_dir_path, exist_ok=True)
-
- for dataset_name in datasets_per_sota:
- zip_path = os.path.join(
- cfg.benchmark_dir_path, sota_model_name, f'{dataset_name}.zip'
- ) # GoogleDrive
- pred_working_dir_path = os.path.join(
- model_working_dir_path,
- dataset_name,
- )
- unzip(zip_path, pred_working_dir_path)
-
-def prepare_datasets(cfg: base_cfg):
- if run_type.rt == RUN_TYPE.COLAB:
- print('Unzip datasets ...')
- unzip_datasets(cfg)
diff --git a/spaces/Hackatos/Smart-Shower-ATC/Dockerfile b/spaces/Hackatos/Smart-Shower-ATC/Dockerfile
deleted file mode 100644
index 115c476fb4f24b88c69890caf6243efbd14b28d8..0000000000000000000000000000000000000000
--- a/spaces/Hackatos/Smart-Shower-ATC/Dockerfile
+++ /dev/null
@@ -1,24 +0,0 @@
-FROM python:3.10
-# Set up a new user named "user" with user ID 1000
-RUN useradd -m -u 1000 user
-
-# Switch to the "user" user
-USER user
-# Set home to the user's home directory
-ENV HOME=/home/user \
- PATH=/home/user/.local/bin:$PATH
-
-# Set the working directory to the user's home directory
-WORKDIR $HOME/app
-
-# Copy the current directory contents into the container at $HOME/app setting the owner to the user
-COPY --chown=user ./app $HOME/app
-
-
-ENV DASH_DEBUG_MODE False
-#COPY ./app /app
-#WORKDIR /app
-RUN set -ex && \
- pip install -r requirements.txt
-EXPOSE 8050
-CMD ["gunicorn", "-b", "0.0.0.0:8050", "--reload", "app:server"]
diff --git a/spaces/HaloMaster/ChineseLLM/README.md b/spaces/HaloMaster/ChineseLLM/README.md
deleted file mode 100644
index 84fdeeb94fc9c403d4d88945fe9cdc9cd73048da..0000000000000000000000000000000000000000
--- a/spaces/HaloMaster/ChineseLLM/README.md
+++ /dev/null
@@ -1,13 +0,0 @@
----
-title: ChineseLLM
-emoji: 📉
-colorFrom: yellow
-colorTo: blue
-sdk: gradio
-sdk_version: 3.18.0
-app_file: app.py
-pinned: false
-license: apache-2.0
----
-
-Check out the configuration reference at https://huggingface.co/docs/hub/spaces-config-reference
diff --git a/spaces/HaoFeng2019/DocTr/GeoTr.py b/spaces/HaoFeng2019/DocTr/GeoTr.py
deleted file mode 100644
index efe43efc7d186ea080c5aec02af9e0052a3d06d9..0000000000000000000000000000000000000000
--- a/spaces/HaoFeng2019/DocTr/GeoTr.py
+++ /dev/null
@@ -1,233 +0,0 @@
-from extractor import BasicEncoder
-from position_encoding import build_position_encoding
-
-import argparse
-import numpy as np
-import torch
-from torch import nn, Tensor
-import torch.nn.functional as F
-import copy
-from typing import Optional
-
-
-class attnLayer(nn.Module):
- def __init__(self, d_model, nhead=8, dim_feedforward=2048, dropout=0.1,
- activation="relu", normalize_before=False):
- super().__init__()
- self.self_attn = nn.MultiheadAttention(d_model, nhead, dropout=dropout)
- self.multihead_attn_list = nn.ModuleList([copy.deepcopy(nn.MultiheadAttention(d_model, nhead, dropout=dropout)) for i in range(2)])
- # Implementation of Feedforward model
- self.linear1 = nn.Linear(d_model, dim_feedforward)
- self.dropout = nn.Dropout(dropout)
- self.linear2 = nn.Linear(dim_feedforward, d_model)
-
- self.norm1 = nn.LayerNorm(d_model)
- self.norm2_list = nn.ModuleList([copy.deepcopy(nn.LayerNorm(d_model)) for i in range(2)])
-
- self.norm3 = nn.LayerNorm(d_model)
- self.dropout1 = nn.Dropout(dropout)
- self.dropout2_list = nn.ModuleList([copy.deepcopy(nn.Dropout(dropout)) for i in range(2)])
- self.dropout3 = nn.Dropout(dropout)
-
- self.activation = _get_activation_fn(activation)
- self.normalize_before = normalize_before
-
- def with_pos_embed(self, tensor, pos: Optional[Tensor]):
- return tensor if pos is None else tensor + pos
-
- def forward_post(self, tgt, memory_list, tgt_mask=None, memory_mask=None,
- tgt_key_padding_mask=None, memory_key_padding_mask=None,
- pos=None, memory_pos=None):
- q = k = self.with_pos_embed(tgt, pos)
- tgt2 = self.self_attn(q, k, value=tgt, attn_mask=tgt_mask,
- key_padding_mask=tgt_key_padding_mask)[0]
- tgt = tgt + self.dropout1(tgt2)
- tgt = self.norm1(tgt)
- for memory, multihead_attn, norm2, dropout2, m_pos in zip(memory_list, self.multihead_attn_list, self.norm2_list, self.dropout2_list, memory_pos):
- tgt2 = multihead_attn(query=self.with_pos_embed(tgt, pos),
- key=self.with_pos_embed(memory, m_pos),
- value=memory, attn_mask=memory_mask,
- key_padding_mask=memory_key_padding_mask)[0]
- tgt = tgt + dropout2(tgt2)
- tgt = norm2(tgt)
- tgt2 = self.linear2(self.dropout(self.activation(self.linear1(tgt))))
- tgt = tgt + self.dropout3(tgt2)
- tgt = self.norm3(tgt)
- return tgt
-
- def forward_pre(self, tgt, memory, tgt_mask=None, memory_mask=None,
- tgt_key_padding_mask=None, memory_key_padding_mask=None,
- pos=None, memory_pos=None):
- tgt2 = self.norm1(tgt)
- q = k = self.with_pos_embed(tgt2, pos)
- tgt2 = self.self_attn(q, k, value=tgt2, attn_mask=tgt_mask,
- key_padding_mask=tgt_key_padding_mask)[0]
- tgt = tgt + self.dropout1(tgt2)
- tgt2 = self.norm2(tgt)
- tgt2 = self.multihead_attn(query=self.with_pos_embed(tgt2, pos),
- key=self.with_pos_embed(memory, memory_pos),
- value=memory, attn_mask=memory_mask,
- key_padding_mask=memory_key_padding_mask)[0]
- tgt = tgt + self.dropout2(tgt2)
- tgt2 = self.norm3(tgt)
- tgt2 = self.linear2(self.dropout(self.activation(self.linear1(tgt2))))
- tgt = tgt + self.dropout3(tgt2)
- return tgt
-
- def forward(self, tgt, memory_list, tgt_mask=None, memory_mask=None,
- tgt_key_padding_mask=None, memory_key_padding_mask=None,
- pos=None, memory_pos=None):
- if self.normalize_before:
- return self.forward_pre(tgt, memory_list, tgt_mask, memory_mask,
- tgt_key_padding_mask, memory_key_padding_mask, pos, memory_pos)
- return self.forward_post(tgt, memory_list, tgt_mask, memory_mask,
- tgt_key_padding_mask, memory_key_padding_mask, pos, memory_pos)
-
-
-def _get_clones(module, N):
- return nn.ModuleList([copy.deepcopy(module) for i in range(N)])
-
-
-def _get_activation_fn(activation):
- """Return an activation function given a string"""
- if activation == "relu":
- return F.relu
- if activation == "gelu":
- return F.gelu
- if activation == "glu":
- return F.glu
- raise RuntimeError(F"activation should be relu/gelu, not {activation}.")
-
-
-class TransDecoder(nn.Module):
- def __init__(self, num_attn_layers, hidden_dim=128):
- super(TransDecoder, self).__init__()
- attn_layer = attnLayer(hidden_dim)
- self.layers = _get_clones(attn_layer, num_attn_layers)
- self.position_embedding = build_position_encoding(hidden_dim)
-
- def forward(self, imgf, query_embed):
- pos = self.position_embedding(torch.ones(imgf.shape[0], imgf.shape[2], imgf.shape[3]).bool()) #.cuda()) # torch.Size([1, 128, 36, 36])
-
- bs, c, h, w = imgf.shape
- imgf = imgf.flatten(2).permute(2, 0, 1)
- query_embed = query_embed.unsqueeze(1).repeat(1, bs, 1)
- pos = pos.flatten(2).permute(2, 0, 1)
-
- for layer in self.layers:
- query_embed = layer(query_embed, [imgf], pos=pos, memory_pos=[pos, pos])
- query_embed = query_embed.permute(1, 2, 0).reshape(bs, c, h, w)
-
- return query_embed
-
-
-class TransEncoder(nn.Module):
- def __init__(self, num_attn_layers, hidden_dim=128):
- super(TransEncoder, self).__init__()
- attn_layer = attnLayer(hidden_dim)
- self.layers = _get_clones(attn_layer, num_attn_layers)
- self.position_embedding = build_position_encoding(hidden_dim)
-
- def forward(self, imgf):
- pos = self.position_embedding(torch.ones(imgf.shape[0], imgf.shape[2], imgf.shape[3]).bool()) #.cuda()) # torch.Size([1, 128, 36, 36])
- bs, c, h, w = imgf.shape
- imgf = imgf.flatten(2).permute(2, 0, 1)
- pos = pos.flatten(2).permute(2, 0, 1)
-
- for layer in self.layers:
- imgf = layer(imgf, [imgf], pos=pos, memory_pos=[pos, pos])
- imgf = imgf.permute(1, 2, 0).reshape(bs, c, h, w)
-
- return imgf
-
-
-class FlowHead(nn.Module):
- def __init__(self, input_dim=128, hidden_dim=256):
- super(FlowHead, self).__init__()
- self.conv1 = nn.Conv2d(input_dim, hidden_dim, 3, padding=1)
- self.conv2 = nn.Conv2d(hidden_dim, 2, 3, padding=1)
- self.relu = nn.ReLU(inplace=True)
-
- def forward(self, x):
- return self.conv2(self.relu(self.conv1(x)))
-
-
-class UpdateBlock(nn.Module):
- def __init__(self, hidden_dim=128):
- super(UpdateBlock, self).__init__()
- self.flow_head = FlowHead(hidden_dim, hidden_dim=256)
- self.mask = nn.Sequential(
- nn.Conv2d(hidden_dim, 256, 3, padding=1),
- nn.ReLU(inplace=True),
- nn.Conv2d(256, 64*9, 1, padding=0))
-
- def forward(self, imgf, coords1):
- mask = .25 * self.mask(imgf) # scale mask to balence gradients
- dflow = self.flow_head(imgf)
- coords1 = coords1 + dflow
-
- return mask, coords1
-
-
-def coords_grid(batch, ht, wd):
- coords = torch.meshgrid(torch.arange(ht), torch.arange(wd))
- coords = torch.stack(coords[::-1], dim=0).float()
- return coords[None].repeat(batch, 1, 1, 1)
-
-
-def upflow8(flow, mode='bilinear'):
- new_size = (8 * flow.shape[2], 8 * flow.shape[3])
- return 8 * F.interpolate(flow, size=new_size, mode=mode, align_corners=True)
-
-
-class GeoTr(nn.Module):
- def __init__(self, num_attn_layers):
- super(GeoTr, self).__init__()
- self.num_attn_layers = num_attn_layers
-
- self.hidden_dim = hdim = 256
-
- self.fnet = BasicEncoder(output_dim=hdim, norm_fn='instance')
-
- self.TransEncoder = TransEncoder(self.num_attn_layers, hidden_dim=hdim)
- self.TransDecoder = TransDecoder(self.num_attn_layers, hidden_dim=hdim)
- self.query_embed = nn.Embedding(1296, self.hidden_dim)
-
- self.update_block = UpdateBlock(self.hidden_dim)
-
- def initialize_flow(self, img):
- N, C, H, W = img.shape
- coodslar = coords_grid(N, H, W).to(img.device)
- coords0 = coords_grid(N, H // 8, W // 8).to(img.device)
- coords1 = coords_grid(N, H // 8, W // 8).to(img.device)
-
- return coodslar, coords0, coords1
-
- def upsample_flow(self, flow, mask):
- N, _, H, W = flow.shape
- mask = mask.view(N, 1, 9, 8, 8, H, W)
- mask = torch.softmax(mask, dim=2)
-
- up_flow = F.unfold(8 * flow, [3, 3], padding=1)
- up_flow = up_flow.view(N, 2, 9, 1, 1, H, W)
-
- up_flow = torch.sum(mask * up_flow, dim=2)
- up_flow = up_flow.permute(0, 1, 4, 2, 5, 3)
-
- return up_flow.reshape(N, 2, 8 * H, 8 * W)
-
- def forward(self, image1):
- fmap = self.fnet(image1)
- fmap = torch.relu(fmap)
-
- fmap = self.TransEncoder(fmap)
- fmap = self.TransDecoder(fmap, self.query_embed.weight)
-
- # convex upsample baesd on fmap
- coodslar, coords0, coords1 = self.initialize_flow(image1)
- coords1 = coords1.detach()
- mask, coords1 = self.update_block(fmap, coords1)
- flow_up = self.upsample_flow(coords1 - coords0, mask)
- bm_up = coodslar + flow_up
-
- return bm_up
diff --git a/spaces/Harveenchadha/Vakyansh-Tamil-TTS/README.md b/spaces/Harveenchadha/Vakyansh-Tamil-TTS/README.md
deleted file mode 100644
index 5521c723d218fe8c587333f5d79d381bb9eeed13..0000000000000000000000000000000000000000
--- a/spaces/Harveenchadha/Vakyansh-Tamil-TTS/README.md
+++ /dev/null
@@ -1,13 +0,0 @@
----
-title: Vakyansh Tamil TTS
-emoji: 📈
-colorFrom: red
-colorTo: yellow
-sdk: gradio
-sdk_version: 2.8.13
-app_file: app.py
-pinned: false
-license: apache-2.0
----
-
-Check out the configuration reference at https://huggingface.co/docs/hub/spaces#reference
diff --git a/spaces/Harveenchadha/speech2speech/app.py b/spaces/Harveenchadha/speech2speech/app.py
deleted file mode 100644
index 2bb0e912efedd0dc8b028a136d37b39b8c62dd80..0000000000000000000000000000000000000000
--- a/spaces/Harveenchadha/speech2speech/app.py
+++ /dev/null
@@ -1,104 +0,0 @@
-import soundfile as sf
-import torch
-from transformers import Wav2Vec2ForCTC, Wav2Vec2Processor
-from transformers import M2M100ForConditionalGeneration, M2M100Tokenizer
-import gradio as gr
-import sox
-import numpy as np
-import yaml
-import tensorflow as tf
-from tensorflow_tts.inference import TFAutoModel
-from tensorflow_tts.inference import AutoProcessor
-import scipy.signal as sps
-
-
-
-# initialize fastspeech2 model.
-fastspeech2 = TFAutoModel.from_pretrained("tensorspeech/tts-fastspeech2-ljspeech-en")
-# initialize mb_melgan model
-mb_melgan = TFAutoModel.from_pretrained("tensorspeech/tts-mb_melgan-ljspeech-en")
-# inference
-processor_tts = AutoProcessor.from_pretrained("tensorspeech/tts-fastspeech2-ljspeech-en")
-
-def tts(text):
- input_ids = processor_tts.text_to_sequence(text)
- # fastspeech inference
-
- mel_before, mel_after, duration_outputs, _, _ = fastspeech2.inference(
- input_ids=tf.expand_dims(tf.convert_to_tensor(input_ids, dtype=tf.int32), 0),
- speaker_ids=tf.convert_to_tensor([0], dtype=tf.int32),
- speed_ratios=tf.convert_to_tensor([1.0], dtype=tf.float32),
- f0_ratios =tf.convert_to_tensor([1.0], dtype=tf.float32),
- energy_ratios =tf.convert_to_tensor([1.0], dtype=tf.float32),
- )
-
- # melgan inference
- audio_before = mb_melgan.inference(mel_before)[0, :, 0]
- audio_after = mb_melgan.inference(mel_after)[0, :, 0]
-
- # save to file
- sf.write('./audio_before.wav', audio_before, 22050, "PCM_16")
- sf.write('./audio_after.wav', audio_after, 22050, "PCM_16")
- return './audio_after.wav'
-
-
-def convert(inputfile, outfile):
- sox_tfm = sox.Transformer()
- sox_tfm.set_output_format(
- file_type="wav", channels=1, encoding="signed-integer", rate=16000, bits=16
- )
- sox_tfm.build(inputfile, outfile)
-
-
-model_translate = M2M100ForConditionalGeneration.from_pretrained("facebook/m2m100_418M")
-tokenizer_translate = M2M100Tokenizer.from_pretrained("facebook/m2m100_418M")
-inlang='hi'
-outlang='en'
-tokenizer_translate.src_lang = inlang
-def translate(text):
- encoded_hi = tokenizer_translate(text, return_tensors="pt")
- generated_tokens = model_translate.generate(**encoded_hi, forced_bos_token_id=tokenizer_translate.get_lang_id(outlang))
- return tokenizer_translate.batch_decode(generated_tokens, skip_special_tokens=True)[0]
-
-
-processor = Wav2Vec2Processor.from_pretrained("Harveenchadha/vakyansh-wav2vec2-hindi-him-4200")
-model = Wav2Vec2ForCTC.from_pretrained("Harveenchadha/vakyansh-wav2vec2-hindi-him-4200")
-
-def read_file(wav):
- sample_rate, signal = wav
- signal = signal.mean(-1)
- number_of_samples = round(len(signal) * float(16000) / sample_rate)
- resampled_signal = sps.resample(signal, number_of_samples)
- return resampled_signal
-
-
-def parse_transcription(wav_file):
- #filename = wav_file.name.split('.')[0]
- #convert(wav_file.name, filename + "16k.wav")
- #speech, _ = sf.read(filename + "16k.wav")
- speech = read_file(wav_file)
- input_values = processor(speech, sampling_rate=16_000, return_tensors="pt").input_values
- logits = model(input_values).logits
- predicted_ids = torch.argmax(logits, dim=-1)
- transcription = processor.decode(predicted_ids[0], skip_special_tokens=True)
- translation = translate(transcription)
- return transcription, translation, tts(translation)
-
-
-
-
-
-output1 = gr.outputs.Textbox(label="Hindi Output from ASR")
-output2 = gr.outputs.Textbox(label="English Translated Output")
-
-input_ = gr.inputs.Audio(source="microphone", type="numpy")
-
-
-output_audio = gr.outputs.Audio(type="file", label="Output Audio")
-
-gr.Interface(parse_transcription, inputs = input_, outputs=[output1, output2, output_audio], analytics_enabled=False,
- show_tips=False,
- theme='huggingface',
- layout='vertical',
- title="Vakyansh: Speech To text for Indic Languages",
- description="This is a live demo for Speech to Speech Translation. Speak in Hindi and get output in English", enable_queue=True).launch( inline=False)
diff --git a/spaces/HighCWu/GFPGAN-1.3/gfpgan/models/__init__.py b/spaces/HighCWu/GFPGAN-1.3/gfpgan/models/__init__.py
deleted file mode 100644
index 6afad57a3794b867dabbdb617a16355a24d6a8b3..0000000000000000000000000000000000000000
--- a/spaces/HighCWu/GFPGAN-1.3/gfpgan/models/__init__.py
+++ /dev/null
@@ -1,10 +0,0 @@
-import importlib
-from basicsr.utils import scandir
-from os import path as osp
-
-# automatically scan and import model modules for registry
-# scan all the files that end with '_model.py' under the model folder
-model_folder = osp.dirname(osp.abspath(__file__))
-model_filenames = [osp.splitext(osp.basename(v))[0] for v in scandir(model_folder) if v.endswith('_model.py')]
-# import all the model modules
-_model_modules = [importlib.import_module(f'gfpgan.models.{file_name}') for file_name in model_filenames]
diff --git a/spaces/HugoDzz/spaceship_drift/build/_app/immutable/nodes/2.1cc72ea4.js b/spaces/HugoDzz/spaceship_drift/build/_app/immutable/nodes/2.1cc72ea4.js
deleted file mode 100644
index 5e3654b81dc2c795f5fbb7d58dbb44b9358371e2..0000000000000000000000000000000000000000
--- a/spaces/HugoDzz/spaceship_drift/build/_app/immutable/nodes/2.1cc72ea4.js
+++ /dev/null
@@ -1,11 +0,0 @@
-import{S as oe,i as ce,s as ne,k as a,q as b,a as A,l as i,m as n,r as g,h as r,c as z,n as l,b as G,G as t,H as le,I as fe,o as ue,J as ie,K as de,L as pe,u as he}from"../chunks/index.0d3f7c7a.js";import{p as me}from"../chunks/stores.bd2e29f1.js";const xe=""+new URL("../assets/preview.69504cb0.png",import.meta.url).href;function se(V){let e,s,f,h,o,d,m,k,v,S,y,w,c,_,u;return{c(){e=a("div"),s=a("iframe"),h=A(),o=a("div"),d=A(),m=a("div"),k=A(),v=a("div"),S=A(),y=a("div"),w=A(),c=a("div"),_=a("p"),u=b("Use arrow keys. SPACE to fire."),this.h()},l(x){e=i(x,"DIV",{class:!0});var p=n(e);s=i(p,"IFRAME",{src:!0,frameborder:!0,title:!0,height:!0,width:!0,class:!0}),n(s).forEach(r),h=z(p),o=i(p,"DIV",{class:!0}),n(o).forEach(r),d=z(p),m=i(p,"DIV",{class:!0}),n(m).forEach(r),k=z(p),v=i(p,"DIV",{class:!0}),n(v).forEach(r),S=z(p),y=i(p,"DIV",{class:!0}),n(y).forEach(r),p.forEach(r),w=z(x),c=i(x,"DIV",{class:!0});var D=n(c);_=i(D,"P",{});var L=n(_);u=g(L,"Use arrow keys. SPACE to fire."),L.forEach(r),D.forEach(r),this.h()},h(){ie(s.src,f="game/index.html")||l(s,"src",f),l(s,"frameborder","0"),l(s,"title","Spaceship Drift"),l(s,"height","512"),l(s,"width","512"),l(s,"class",""),l(o,"class","h-[3px] bg-[#0C0F19] w-[3px] z-10 absolute -top-[3px] -left-[3px]"),l(m,"class","h-[3px] bg-[#0C0F19] w-[3px] z-10 absolute -bottom-[3px] -left-[3px]"),l(v,"class","h-[3px] bg-[#0C0F19] w-[3px] z-10 absolute -top-[3px] -right-[3px]"),l(y,"class","h-[3px] bg-[#0C0F19] w-[3px] z-10 absolute -bottom-[3px] -right-[3px]"),l(e,"class","relative mt-10 border-slate-800 border-[3px]"),l(c,"class","flex flex-row justify-center items-center text-[9px] mt-4 text-slate-500")},m(x,p){G(x,e,p),t(e,s),t(e,h),t(e,o),t(e,d),t(e,m),t(e,k),t(e,v),t(e,S),t(e,y),G(x,w,p),G(x,c,p),t(c,_),t(_,u)},d(x){x&&r(e),x&&r(w),x&&r(c)}}}function re(V){let e,s,f,h,o,d,m,k,v,S=V[1]?"Copied!":"Copy the link for later",y,w,c;return{c(){e=a("div"),s=a("img"),h=A(),o=a("p"),d=b("Looks like you're on mobile! Please visit on your laptop."),m=A(),k=a("button"),v=a("p"),y=b(S),this.h()},l(_){e=i(_,"DIV",{class:!0});var u=n(e);s=i(u,"IMG",{src:!0,alt:!0,class:!0,height:!0,width:!0}),h=z(u),o=i(u,"P",{class:!0});var x=n(o);d=g(x,"Looks like you're on mobile! Please visit on your laptop."),x.forEach(r),m=z(u),k=i(u,"BUTTON",{class:!0});var p=n(k);v=i(p,"P",{class:!0});var D=n(v);y=g(D,S),D.forEach(r),p.forEach(r),u.forEach(r),this.h()},h(){ie(s.src,f=xe)||l(s,"src",f),l(s,"alt","Preview of the game"),l(s,"class","w-60 border-slate-800 border-[2px]"),l(s,"height","64"),l(s,"width","64"),l(o,"class","text-xs text-slate-500 mt-6"),l(v,"class","mt-1"),l(k,"class","flex flex-row justify-center items-center px-3 py-5 text-xs w-full bg-slate-800 mt-6"),l(e,"class","flex flex-col justify-center items-center mt-10 text-center")},m(_,u){G(_,e,u),t(e,s),t(e,h),t(e,o),t(o,d),t(e,m),t(e,k),t(k,v),t(v,y),w||(c=de(k,"click",pe(V[2])),w=!0)},p(_,u){u&2&&S!==(S=_[1]?"Copied!":"Copy the link for later")&&he(y,S)},d(_){_&&r(e),w=!1,c()}}}function ae(V){let e,s,f,h;return{c(){e=a("p"),s=b("It's all about "),f=a("a"),h=b("game feel"),this.h()},l(o){e=i(o,"P",{class:!0});var d=n(e);s=g(d,"It's all about "),f=i(d,"A",{href:!0,target:!0,class:!0});var m=n(f);h=g(m,"game feel"),m.forEach(r),d.forEach(r),this.h()},h(){l(f,"href","https://arxiv.org/pdf/2011.09201.pdf"),l(f,"target","_blank"),l(f,"class","tex-center underline"),l(e,"class","text-center mt-2 text-[9px] text-slate-500")},m(o,d){G(o,e,d),t(e,s),t(e,f),t(f,h)},d(o){o&&r(e)}}}function ve(V){let e,s,f,h,o,d,m,k,v,S,y,w,c,_,u,x,p,D,L,R,T,B,J,F,K,N,H,O,U,I=!V[0]&&se(),E=V[0]&&re(V),P=!V[0]&&ae();return{c(){e=a("div"),s=a("div"),f=a("h1"),h=b("Spaceship freeride"),o=A(),d=a("p"),m=b("Take a break and enjoy a little freeride."),k=A(),I&&I.c(),v=A(),E&&E.c(),S=A(),P&&P.c(),y=A(),w=a("div"),c=a("p"),_=b("Made by "),u=a("a"),x=b("Hugo"),p=b(`
- with
- `),D=a("a"),L=b("Godot"),R=b(`,
- `),T=a("a"),B=b("Svelte"),J=b(`,
- `),F=a("a"),K=b("Scenario"),N=b(`, and
- `),H=a("a"),O=b("Pixelicious"),this.h()},l(j){e=i(j,"DIV",{class:!0});var C=n(e);s=i(C,"DIV",{class:!0});var q=n(s);f=i(q,"H1",{class:!0});var W=n(f);h=g(W,"Spaceship freeride"),W.forEach(r),o=z(q),d=i(q,"P",{class:!0});var Q=n(d);m=g(Q,"Take a break and enjoy a little freeride."),Q.forEach(r),q.forEach(r),k=z(C),I&&I.l(C),v=z(C),E&&E.l(C),S=z(C),P&&P.l(C),y=z(C),w=i(C,"DIV",{class:!0});var X=n(w);c=i(X,"P",{});var M=n(c);_=g(M,"Made by "),u=i(M,"A",{href:!0,target:!0,class:!0});var Y=n(u);x=g(Y,"Hugo"),Y.forEach(r),p=g(M,`
- with
- `),D=i(M,"A",{href:!0,target:!0,class:!0});var Z=n(D);L=g(Z,"Godot"),Z.forEach(r),R=g(M,`,
- `),T=i(M,"A",{href:!0,target:!0,class:!0});var $=n(T);B=g($,"Svelte"),$.forEach(r),J=g(M,`,
- `),F=i(M,"A",{href:!0,target:!0,class:!0});var ee=n(F);K=g(ee,"Scenario"),ee.forEach(r),N=g(M,`, and
- `),H=i(M,"A",{href:!0,target:!0,class:!0});var te=n(H);O=g(te,"Pixelicious"),te.forEach(r),M.forEach(r),X.forEach(r),C.forEach(r),this.h()},h(){l(f,"class","text-xl capitalize"),l(d,"class","text-xs"),l(s,"class","flex flex-col justify-center items-center space-y-4 text-center sm:mt-20 mt-12"),l(u,"href","https://www.hugoduprez.com/"),l(u,"target","_blank"),l(u,"class","underline"),l(D,"href","https://godotengine.org/"),l(D,"target","_blank"),l(D,"class","underline"),l(T,"href","https://svelte.dev/"),l(T,"target","_blank"),l(T,"class","underline"),l(F,"href","https://www.scenario.com/"),l(F,"target","_blank"),l(F,"class","underline"),l(H,"href","https://www.pixelicious.xyz/"),l(H,"target","_blank"),l(H,"class","underline"),l(w,"class",U="flex flex-row justify-center items-center text-center "+(V[0]?"mt-20":"fixed bottom-6")+" text-[9px] text-slate-500"),l(e,"class","flex flex-col justify-center text-slate-100 font-Hellovetica items-center p-4 w-full")},m(j,C){G(j,e,C),t(e,s),t(s,f),t(f,h),t(s,o),t(s,d),t(d,m),t(e,k),I&&I.m(e,null),t(e,v),E&&E.m(e,null),t(e,S),P&&P.m(e,null),t(e,y),t(e,w),t(w,c),t(c,_),t(c,u),t(u,x),t(c,p),t(c,D),t(D,L),t(c,R),t(c,T),t(T,B),t(c,J),t(c,F),t(F,K),t(c,N),t(c,H),t(H,O)},p(j,[C]){j[0]?I&&(I.d(1),I=null):I||(I=se(),I.c(),I.m(e,v)),j[0]?E?E.p(j,C):(E=re(j),E.c(),E.m(e,S)):E&&(E.d(1),E=null),j[0]?P&&(P.d(1),P=null):P||(P=ae(),P.c(),P.m(e,y)),C&1&&U!==(U="flex flex-row justify-center items-center text-center "+(j[0]?"mt-20":"fixed bottom-6")+" text-[9px] text-slate-500")&&l(w,"class",U)},i:le,o:le,d(j){j&&r(e),I&&I.d(),E&&E.d(),P&&P.d()}}}function _e(V,e,s){let f;fe(V,me,m=>s(3,f=m));let h=!1,o=!1;ue(()=>{window.innerWidth<768&&s(0,h=!0)});function d(){navigator.clipboard.writeText(f.url.toString()),s(1,o=!0)}return[h,o,d]}class we extends oe{constructor(e){super(),ce(this,e,_e,ve,ne,{})}}export{we as component};
diff --git a/spaces/ICML2022/OFA/fairseq/examples/multilingual/data_scripts/dedup_all.py b/spaces/ICML2022/OFA/fairseq/examples/multilingual/data_scripts/dedup_all.py
deleted file mode 100644
index ef39c05ee606aaeda1d9e94970932d2241a8b281..0000000000000000000000000000000000000000
--- a/spaces/ICML2022/OFA/fairseq/examples/multilingual/data_scripts/dedup_all.py
+++ /dev/null
@@ -1,52 +0,0 @@
-# Copyright (c) Facebook, Inc. and its affiliates.
-#
-# This source code is licensed under the MIT license found in the
-# LICENSE file in the root directory of this source tree.
-
-
-
-import os
-import glob
-import argparse
-from utils.dedup import deup
-
-import sys
-WORKDIR_ROOT = os.environ.get('WORKDIR_ROOT', None)
-
-if WORKDIR_ROOT is None or not WORKDIR_ROOT.strip():
- print('please specify your working directory root in OS environment variable WORKDIR_ROOT. Exitting..."')
- sys.exit(-1)
-
-
-def main():
- parser = argparse.ArgumentParser()
- parser.add_argument("--from-folder", type=str, required=True,
- help="the data folder to be dedup")
- parser.add_argument("--to-folder", type=str, required=True,
- help="the data folder to save deduped data")
- parser.add_argument('--directions', type=str, default=None, required=False)
-
- args = parser.parse_args()
-
- if args.directions is None:
- raw_files = glob.glob(f'{args.from_folder}/train*')
-
- directions = [os.path.split(file_path)[-1].split('.')[1] for file_path in raw_files]
- else:
- directions = args.directions.split(',')
- directions = sorted(set(directions))
-
- for direction in directions:
- src, tgt = direction.split('-')
- src_file = f'{args.from_folder}/train.{src}-{tgt}.{src}'
- tgt_file = f'{args.from_folder}/train.{src}-{tgt}.{tgt}'
- src_file_out = f'{args.to_folder}/train.{src}-{tgt}.{src}'
- tgt_file_out = f'{args.to_folder}/train.{src}-{tgt}.{tgt}'
- assert src_file != src_file_out
- assert tgt_file != tgt_file_out
- print(f'deduping {src_file}, {tgt_file}')
- deup(src_file, tgt_file, src_file_out, tgt_file_out)
-
-
-if __name__ == "__main__":
- main()
diff --git a/spaces/ICML2022/OFA/fairseq/fairseq/models/roberta/hub_interface.py b/spaces/ICML2022/OFA/fairseq/fairseq/models/roberta/hub_interface.py
deleted file mode 100644
index ba298d63ba5da2a5b2f1a44d0384a6b249277ef4..0000000000000000000000000000000000000000
--- a/spaces/ICML2022/OFA/fairseq/fairseq/models/roberta/hub_interface.py
+++ /dev/null
@@ -1,235 +0,0 @@
-# Copyright (c) Facebook, Inc. and its affiliates.
-#
-# This source code is licensed under the MIT license found in the
-# LICENSE file in the root directory of this source tree.
-
-import numpy as np
-import torch
-import torch.nn as nn
-import torch.nn.functional as F
-from fairseq import utils
-from fairseq.data import encoders
-
-
-class RobertaHubInterface(nn.Module):
- """A simple PyTorch Hub interface to RoBERTa.
-
- Usage: https://github.com/pytorch/fairseq/tree/main/examples/roberta
- """
-
- def __init__(self, cfg, task, model):
- super().__init__()
- self.cfg = cfg
- self.task = task
- self.model = model
-
- self.bpe = encoders.build_bpe(cfg.bpe)
-
- # this is useful for determining the device
- self.register_buffer("_float_tensor", torch.tensor([0], dtype=torch.float))
-
- @property
- def device(self):
- return self._float_tensor.device
-
- def encode(
- self, sentence: str, *addl_sentences, no_separator=False
- ) -> torch.LongTensor:
- """
- BPE-encode a sentence (or multiple sentences).
-
- Every sequence begins with a beginning-of-sentence (``) symbol.
- Every sentence ends with an end-of-sentence (``) and we use an
- extra end-of-sentence (``) as a separator.
-
- Example (single sentence): ` a b c `
- Example (sentence pair): ` d e f 1 2 3 `
-
- The BPE encoding follows GPT-2. One subtle detail is that the GPT-2 BPE
- requires leading spaces. For example::
-
- >>> roberta.encode('Hello world').tolist()
- [0, 31414, 232, 2]
- >>> roberta.encode(' world').tolist()
- [0, 232, 2]
- >>> roberta.encode('world').tolist()
- [0, 8331, 2]
- """
- bpe_sentence = " " + self.bpe.encode(sentence) + " "
- for s in addl_sentences:
- bpe_sentence += " " if not no_separator else ""
- bpe_sentence += " " + self.bpe.encode(s) + " "
- tokens = self.task.source_dictionary.encode_line(
- bpe_sentence, append_eos=False, add_if_not_exist=False
- )
- return tokens.long()
-
- def decode(self, tokens: torch.LongTensor):
- assert tokens.dim() == 1
- tokens = tokens.numpy()
- if tokens[0] == self.task.source_dictionary.bos():
- tokens = tokens[1:] # remove
- eos_mask = tokens == self.task.source_dictionary.eos()
- doc_mask = eos_mask[1:] & eos_mask[:-1]
- sentences = np.split(tokens, doc_mask.nonzero()[0] + 1)
- sentences = [
- self.bpe.decode(self.task.source_dictionary.string(s)) for s in sentences
- ]
- if len(sentences) == 1:
- return sentences[0]
- return sentences
-
- def extract_features(
- self, tokens: torch.LongTensor, return_all_hiddens: bool = False
- ) -> torch.Tensor:
- if tokens.dim() == 1:
- tokens = tokens.unsqueeze(0)
- if tokens.size(-1) > self.model.max_positions():
- raise ValueError(
- "tokens exceeds maximum length: {} > {}".format(
- tokens.size(-1), self.model.max_positions()
- )
- )
- features, extra = self.model(
- tokens.to(device=self.device),
- features_only=True,
- return_all_hiddens=return_all_hiddens,
- )
- if return_all_hiddens:
- # convert from T x B x C -> B x T x C
- inner_states = extra["inner_states"]
- return [inner_state.transpose(0, 1) for inner_state in inner_states]
- else:
- return features # just the last layer's features
-
- def register_classification_head(
- self, name: str, num_classes: int = None, embedding_size: int = None, **kwargs
- ):
- self.model.register_classification_head(
- name, num_classes=num_classes, embedding_size=embedding_size, **kwargs
- )
-
- def predict(self, head: str, tokens: torch.LongTensor, return_logits: bool = False):
- features = self.extract_features(tokens.to(device=self.device))
- logits = self.model.classification_heads[head](features)
- if return_logits:
- return logits
- return F.log_softmax(logits, dim=-1)
-
- def extract_features_aligned_to_words(
- self, sentence: str, return_all_hiddens: bool = False
- ) -> torch.Tensor:
- """Extract RoBERTa features, aligned to spaCy's word-level tokenizer."""
- from fairseq.models.roberta import alignment_utils
- from spacy.tokens import Doc
-
- nlp = alignment_utils.spacy_nlp()
- tokenizer = alignment_utils.spacy_tokenizer()
-
- # tokenize both with GPT-2 BPE and spaCy
- bpe_toks = self.encode(sentence)
- spacy_toks = tokenizer(sentence)
- spacy_toks_ws = [t.text_with_ws for t in tokenizer(sentence)]
- alignment = alignment_utils.align_bpe_to_words(self, bpe_toks, spacy_toks_ws)
-
- # extract features and align them
- features = self.extract_features(
- bpe_toks, return_all_hiddens=return_all_hiddens
- )
- features = features.squeeze(0)
- aligned_feats = alignment_utils.align_features_to_words(
- self, features, alignment
- )
-
- # wrap in spaCy Doc
- doc = Doc(
- nlp.vocab,
- words=[""] + [x.text for x in spacy_toks] + [""],
- spaces=[True]
- + [x.endswith(" ") for x in spacy_toks_ws[:-1]]
- + [True, False],
- )
- assert len(doc) == aligned_feats.size(0)
- doc.user_token_hooks["vector"] = lambda token: aligned_feats[token.i]
- return doc
-
- def fill_mask(self, masked_input: str, topk: int = 5):
- masked_token = ""
- assert (
- masked_token in masked_input and masked_input.count(masked_token) == 1
- ), "Please add one {0} token for the input, eg: 'He is a {0} guy'".format(
- masked_token
- )
-
- text_spans = masked_input.split(masked_token)
- text_spans_bpe = (
- (" {0} ".format(masked_token))
- .join([self.bpe.encode(text_span.rstrip()) for text_span in text_spans])
- .strip()
- )
- tokens = self.task.source_dictionary.encode_line(
- " " + text_spans_bpe + " ",
- append_eos=False,
- add_if_not_exist=False,
- )
-
- masked_index = (tokens == self.task.mask_idx).nonzero(as_tuple=False)
- if tokens.dim() == 1:
- tokens = tokens.unsqueeze(0)
-
- with utils.model_eval(self.model):
- features, extra = self.model(
- tokens.long().to(device=self.device),
- features_only=False,
- return_all_hiddens=False,
- )
- logits = features[0, masked_index, :].squeeze()
- prob = logits.softmax(dim=0)
- values, index = prob.topk(k=topk, dim=0)
- topk_predicted_token_bpe = self.task.source_dictionary.string(index)
-
- topk_filled_outputs = []
- for index, predicted_token_bpe in enumerate(
- topk_predicted_token_bpe.split(" ")
- ):
- predicted_token = self.bpe.decode(predicted_token_bpe)
- # Quick hack to fix https://github.com/pytorch/fairseq/issues/1306
- if predicted_token_bpe.startswith("\u2581"):
- predicted_token = " " + predicted_token
- if " {0}".format(masked_token) in masked_input:
- topk_filled_outputs.append(
- (
- masked_input.replace(
- " {0}".format(masked_token), predicted_token
- ),
- values[index].item(),
- predicted_token,
- )
- )
- else:
- topk_filled_outputs.append(
- (
- masked_input.replace(masked_token, predicted_token),
- values[index].item(),
- predicted_token,
- )
- )
- return topk_filled_outputs
-
- def disambiguate_pronoun(self, sentence: str) -> bool:
- """
- Usage::
-
- >>> disambiguate_pronoun('The _trophy_ would not fit in the brown suitcase because [it] was too big.')
- True
-
- >>> disambiguate_pronoun('The trophy would not fit in the brown suitcase because [it] was too big.')
- 'The trophy'
- """
- assert hasattr(
- self.task, "disambiguate_pronoun"
- ), "roberta.disambiguate_pronoun() requires a model trained with the WSC task."
- with utils.model_eval(self.model):
- return self.task.disambiguate_pronoun(
- self.model, sentence, use_cuda=self.device.type == "cuda"
- )
diff --git a/spaces/Iceclear/StableSR/StableSR/basicsr/metrics/test_metrics/test_psnr_ssim.py b/spaces/Iceclear/StableSR/StableSR/basicsr/metrics/test_metrics/test_psnr_ssim.py
deleted file mode 100644
index 18b05a73a0e38e89b2321ddc9415123a92f5c5a4..0000000000000000000000000000000000000000
--- a/spaces/Iceclear/StableSR/StableSR/basicsr/metrics/test_metrics/test_psnr_ssim.py
+++ /dev/null
@@ -1,52 +0,0 @@
-import cv2
-import torch
-
-from basicsr.metrics import calculate_psnr, calculate_ssim
-from basicsr.metrics.psnr_ssim import calculate_psnr_pt, calculate_ssim_pt
-from basicsr.utils import img2tensor
-
-
-def test(img_path, img_path2, crop_border, test_y_channel=False):
- img = cv2.imread(img_path, cv2.IMREAD_UNCHANGED)
- img2 = cv2.imread(img_path2, cv2.IMREAD_UNCHANGED)
-
- # --------------------- Numpy ---------------------
- psnr = calculate_psnr(img, img2, crop_border=crop_border, input_order='HWC', test_y_channel=test_y_channel)
- ssim = calculate_ssim(img, img2, crop_border=crop_border, input_order='HWC', test_y_channel=test_y_channel)
- print(f'\tNumpy\tPSNR: {psnr:.6f} dB, \tSSIM: {ssim:.6f}')
-
- # --------------------- PyTorch (CPU) ---------------------
- img = img2tensor(img / 255., bgr2rgb=True, float32=True).unsqueeze_(0)
- img2 = img2tensor(img2 / 255., bgr2rgb=True, float32=True).unsqueeze_(0)
-
- psnr_pth = calculate_psnr_pt(img, img2, crop_border=crop_border, test_y_channel=test_y_channel)
- ssim_pth = calculate_ssim_pt(img, img2, crop_border=crop_border, test_y_channel=test_y_channel)
- print(f'\tTensor (CPU) \tPSNR: {psnr_pth[0]:.6f} dB, \tSSIM: {ssim_pth[0]:.6f}')
-
- # --------------------- PyTorch (GPU) ---------------------
- img = img.cuda()
- img2 = img2.cuda()
- psnr_pth = calculate_psnr_pt(img, img2, crop_border=crop_border, test_y_channel=test_y_channel)
- ssim_pth = calculate_ssim_pt(img, img2, crop_border=crop_border, test_y_channel=test_y_channel)
- print(f'\tTensor (GPU) \tPSNR: {psnr_pth[0]:.6f} dB, \tSSIM: {ssim_pth[0]:.6f}')
-
- psnr_pth = calculate_psnr_pt(
- torch.repeat_interleave(img, 2, dim=0),
- torch.repeat_interleave(img2, 2, dim=0),
- crop_border=crop_border,
- test_y_channel=test_y_channel)
- ssim_pth = calculate_ssim_pt(
- torch.repeat_interleave(img, 2, dim=0),
- torch.repeat_interleave(img2, 2, dim=0),
- crop_border=crop_border,
- test_y_channel=test_y_channel)
- print(f'\tTensor (GPU batch) \tPSNR: {psnr_pth[0]:.6f}, {psnr_pth[1]:.6f} dB,'
- f'\tSSIM: {ssim_pth[0]:.6f}, {ssim_pth[1]:.6f}')
-
-
-if __name__ == '__main__':
- test('tests/data/bic/baboon.png', 'tests/data/gt/baboon.png', crop_border=4, test_y_channel=False)
- test('tests/data/bic/baboon.png', 'tests/data/gt/baboon.png', crop_border=4, test_y_channel=True)
-
- test('tests/data/bic/comic.png', 'tests/data/gt/comic.png', crop_border=4, test_y_channel=False)
- test('tests/data/bic/comic.png', 'tests/data/gt/comic.png', crop_border=4, test_y_channel=True)
diff --git a/spaces/Ikaros521/so-vits-svc-4.0-ikaros/onnx/onnx_export_48k.py b/spaces/Ikaros521/so-vits-svc-4.0-ikaros/onnx/onnx_export_48k.py
deleted file mode 100644
index 9a046353dc25b658684fa76bdf8b4f21d1a77c98..0000000000000000000000000000000000000000
--- a/spaces/Ikaros521/so-vits-svc-4.0-ikaros/onnx/onnx_export_48k.py
+++ /dev/null
@@ -1,73 +0,0 @@
-import argparse
-import time
-import numpy as np
-import onnx
-from onnxsim import simplify
-import onnxruntime as ort
-import onnxoptimizer
-import torch
-from model_onnx_48k import SynthesizerTrn
-import utils
-from hubert import hubert_model_onnx
-
-def main(HubertExport,NetExport):
-
- path = "NyaruTaffy"
-
- if(HubertExport):
- device = torch.device("cuda")
- hubert_soft = hubert_model_onnx.hubert_soft("hubert/model.pt")
- test_input = torch.rand(1, 1, 16000)
- input_names = ["source"]
- output_names = ["embed"]
- torch.onnx.export(hubert_soft.to(device),
- test_input.to(device),
- "hubert3.0.onnx",
- dynamic_axes={
- "source": {
- 2: "sample_length"
- }
- },
- verbose=False,
- opset_version=13,
- input_names=input_names,
- output_names=output_names)
- if(NetExport):
- device = torch.device("cuda")
- hps = utils.get_hparams_from_file(f"checkpoints/{path}/config.json")
- SVCVITS = SynthesizerTrn(
- hps.data.filter_length // 2 + 1,
- hps.train.segment_size // hps.data.hop_length,
- **hps.model)
- _ = utils.load_checkpoint(f"checkpoints/{path}/model.pth", SVCVITS, None)
- _ = SVCVITS.eval().to(device)
- for i in SVCVITS.parameters():
- i.requires_grad = False
- test_hidden_unit = torch.rand(1, 50, 256)
- test_lengths = torch.LongTensor([50])
- test_pitch = torch.rand(1, 50)
- test_sid = torch.LongTensor([0])
- input_names = ["hidden_unit", "lengths", "pitch", "sid"]
- output_names = ["audio", ]
- SVCVITS.eval()
- torch.onnx.export(SVCVITS,
- (
- test_hidden_unit.to(device),
- test_lengths.to(device),
- test_pitch.to(device),
- test_sid.to(device)
- ),
- f"checkpoints/{path}/model.onnx",
- dynamic_axes={
- "hidden_unit": [0, 1],
- "pitch": [1]
- },
- do_constant_folding=False,
- opset_version=16,
- verbose=False,
- input_names=input_names,
- output_names=output_names)
-
-
-if __name__ == '__main__':
- main(False,True)
diff --git a/spaces/Ilzhabimantara/rvc-Blue-archives/lib/infer_pack/models_dml.py b/spaces/Ilzhabimantara/rvc-Blue-archives/lib/infer_pack/models_dml.py
deleted file mode 100644
index 958d7b29259763d2fea94caf8ba7e314c4a77d05..0000000000000000000000000000000000000000
--- a/spaces/Ilzhabimantara/rvc-Blue-archives/lib/infer_pack/models_dml.py
+++ /dev/null
@@ -1,1124 +0,0 @@
-import math, pdb, os
-from time import time as ttime
-import torch
-from torch import nn
-from torch.nn import functional as F
-from lib.infer_pack import modules
-from lib.infer_pack import attentions
-from lib.infer_pack import commons
-from lib.infer_pack.commons import init_weights, get_padding
-from torch.nn import Conv1d, ConvTranspose1d, AvgPool1d, Conv2d
-from torch.nn.utils import weight_norm, remove_weight_norm, spectral_norm
-from lib.infer_pack.commons import init_weights
-import numpy as np
-from lib.infer_pack import commons
-
-
-class TextEncoder256(nn.Module):
- def __init__(
- self,
- out_channels,
- hidden_channels,
- filter_channels,
- n_heads,
- n_layers,
- kernel_size,
- p_dropout,
- f0=True,
- ):
- super().__init__()
- self.out_channels = out_channels
- self.hidden_channels = hidden_channels
- self.filter_channels = filter_channels
- self.n_heads = n_heads
- self.n_layers = n_layers
- self.kernel_size = kernel_size
- self.p_dropout = p_dropout
- self.emb_phone = nn.Linear(256, hidden_channels)
- self.lrelu = nn.LeakyReLU(0.1, inplace=True)
- if f0 == True:
- self.emb_pitch = nn.Embedding(256, hidden_channels) # pitch 256
- self.encoder = attentions.Encoder(
- hidden_channels, filter_channels, n_heads, n_layers, kernel_size, p_dropout
- )
- self.proj = nn.Conv1d(hidden_channels, out_channels * 2, 1)
-
- def forward(self, phone, pitch, lengths):
- if pitch == None:
- x = self.emb_phone(phone)
- else:
- x = self.emb_phone(phone) + self.emb_pitch(pitch)
- x = x * math.sqrt(self.hidden_channels) # [b, t, h]
- x = self.lrelu(x)
- x = torch.transpose(x, 1, -1) # [b, h, t]
- x_mask = torch.unsqueeze(commons.sequence_mask(lengths, x.size(2)), 1).to(
- x.dtype
- )
- x = self.encoder(x * x_mask, x_mask)
- stats = self.proj(x) * x_mask
-
- m, logs = torch.split(stats, self.out_channels, dim=1)
- return m, logs, x_mask
-
-
-class TextEncoder768(nn.Module):
- def __init__(
- self,
- out_channels,
- hidden_channels,
- filter_channels,
- n_heads,
- n_layers,
- kernel_size,
- p_dropout,
- f0=True,
- ):
- super().__init__()
- self.out_channels = out_channels
- self.hidden_channels = hidden_channels
- self.filter_channels = filter_channels
- self.n_heads = n_heads
- self.n_layers = n_layers
- self.kernel_size = kernel_size
- self.p_dropout = p_dropout
- self.emb_phone = nn.Linear(768, hidden_channels)
- self.lrelu = nn.LeakyReLU(0.1, inplace=True)
- if f0 == True:
- self.emb_pitch = nn.Embedding(256, hidden_channels) # pitch 256
- self.encoder = attentions.Encoder(
- hidden_channels, filter_channels, n_heads, n_layers, kernel_size, p_dropout
- )
- self.proj = nn.Conv1d(hidden_channels, out_channels * 2, 1)
-
- def forward(self, phone, pitch, lengths):
- if pitch == None:
- x = self.emb_phone(phone)
- else:
- x = self.emb_phone(phone) + self.emb_pitch(pitch)
- x = x * math.sqrt(self.hidden_channels) # [b, t, h]
- x = self.lrelu(x)
- x = torch.transpose(x, 1, -1) # [b, h, t]
- x_mask = torch.unsqueeze(commons.sequence_mask(lengths, x.size(2)), 1).to(
- x.dtype
- )
- x = self.encoder(x * x_mask, x_mask)
- stats = self.proj(x) * x_mask
-
- m, logs = torch.split(stats, self.out_channels, dim=1)
- return m, logs, x_mask
-
-
-class ResidualCouplingBlock(nn.Module):
- def __init__(
- self,
- channels,
- hidden_channels,
- kernel_size,
- dilation_rate,
- n_layers,
- n_flows=4,
- gin_channels=0,
- ):
- super().__init__()
- self.channels = channels
- self.hidden_channels = hidden_channels
- self.kernel_size = kernel_size
- self.dilation_rate = dilation_rate
- self.n_layers = n_layers
- self.n_flows = n_flows
- self.gin_channels = gin_channels
-
- self.flows = nn.ModuleList()
- for i in range(n_flows):
- self.flows.append(
- modules.ResidualCouplingLayer(
- channels,
- hidden_channels,
- kernel_size,
- dilation_rate,
- n_layers,
- gin_channels=gin_channels,
- mean_only=True,
- )
- )
- self.flows.append(modules.Flip())
-
- def forward(self, x, x_mask, g=None, reverse=False):
- if not reverse:
- for flow in self.flows:
- x, _ = flow(x, x_mask, g=g, reverse=reverse)
- else:
- for flow in reversed(self.flows):
- x = flow(x, x_mask, g=g, reverse=reverse)
- return x
-
- def remove_weight_norm(self):
- for i in range(self.n_flows):
- self.flows[i * 2].remove_weight_norm()
-
-
-class PosteriorEncoder(nn.Module):
- def __init__(
- self,
- in_channels,
- out_channels,
- hidden_channels,
- kernel_size,
- dilation_rate,
- n_layers,
- gin_channels=0,
- ):
- super().__init__()
- self.in_channels = in_channels
- self.out_channels = out_channels
- self.hidden_channels = hidden_channels
- self.kernel_size = kernel_size
- self.dilation_rate = dilation_rate
- self.n_layers = n_layers
- self.gin_channels = gin_channels
-
- self.pre = nn.Conv1d(in_channels, hidden_channels, 1)
- self.enc = modules.WN(
- hidden_channels,
- kernel_size,
- dilation_rate,
- n_layers,
- gin_channels=gin_channels,
- )
- self.proj = nn.Conv1d(hidden_channels, out_channels * 2, 1)
-
- def forward(self, x, x_lengths, g=None):
- x_mask = torch.unsqueeze(commons.sequence_mask(x_lengths, x.size(2)), 1).to(
- x.dtype
- )
- x = self.pre(x) * x_mask
- x = self.enc(x, x_mask, g=g)
- stats = self.proj(x) * x_mask
- m, logs = torch.split(stats, self.out_channels, dim=1)
- z = (m + torch.randn_like(m) * torch.exp(logs)) * x_mask
- return z, m, logs, x_mask
-
- def remove_weight_norm(self):
- self.enc.remove_weight_norm()
-
-
-class Generator(torch.nn.Module):
- def __init__(
- self,
- initial_channel,
- resblock,
- resblock_kernel_sizes,
- resblock_dilation_sizes,
- upsample_rates,
- upsample_initial_channel,
- upsample_kernel_sizes,
- gin_channels=0,
- ):
- super(Generator, self).__init__()
- self.num_kernels = len(resblock_kernel_sizes)
- self.num_upsamples = len(upsample_rates)
- self.conv_pre = Conv1d(
- initial_channel, upsample_initial_channel, 7, 1, padding=3
- )
- resblock = modules.ResBlock1 if resblock == "1" else modules.ResBlock2
-
- self.ups = nn.ModuleList()
- for i, (u, k) in enumerate(zip(upsample_rates, upsample_kernel_sizes)):
- self.ups.append(
- weight_norm(
- ConvTranspose1d(
- upsample_initial_channel // (2**i),
- upsample_initial_channel // (2 ** (i + 1)),
- k,
- u,
- padding=(k - u) // 2,
- )
- )
- )
-
- self.resblocks = nn.ModuleList()
- for i in range(len(self.ups)):
- ch = upsample_initial_channel // (2 ** (i + 1))
- for j, (k, d) in enumerate(
- zip(resblock_kernel_sizes, resblock_dilation_sizes)
- ):
- self.resblocks.append(resblock(ch, k, d))
-
- self.conv_post = Conv1d(ch, 1, 7, 1, padding=3, bias=False)
- self.ups.apply(init_weights)
-
- if gin_channels != 0:
- self.cond = nn.Conv1d(gin_channels, upsample_initial_channel, 1)
-
- def forward(self, x, g=None):
- x = self.conv_pre(x)
- if g is not None:
- x = x + self.cond(g)
-
- for i in range(self.num_upsamples):
- x = F.leaky_relu(x, modules.LRELU_SLOPE)
- x = self.ups[i](x)
- xs = None
- for j in range(self.num_kernels):
- if xs is None:
- xs = self.resblocks[i * self.num_kernels + j](x)
- else:
- xs += self.resblocks[i * self.num_kernels + j](x)
- x = xs / self.num_kernels
- x = F.leaky_relu(x)
- x = self.conv_post(x)
- x = torch.tanh(x)
-
- return x
-
- def remove_weight_norm(self):
- for l in self.ups:
- remove_weight_norm(l)
- for l in self.resblocks:
- l.remove_weight_norm()
-
-
-class SineGen(torch.nn.Module):
- """Definition of sine generator
- SineGen(samp_rate, harmonic_num = 0,
- sine_amp = 0.1, noise_std = 0.003,
- voiced_threshold = 0,
- flag_for_pulse=False)
- samp_rate: sampling rate in Hz
- harmonic_num: number of harmonic overtones (default 0)
- sine_amp: amplitude of sine-wavefrom (default 0.1)
- noise_std: std of Gaussian noise (default 0.003)
- voiced_thoreshold: F0 threshold for U/V classification (default 0)
- flag_for_pulse: this SinGen is used inside PulseGen (default False)
- Note: when flag_for_pulse is True, the first time step of a voiced
- segment is always sin(np.pi) or cos(0)
- """
-
- def __init__(
- self,
- samp_rate,
- harmonic_num=0,
- sine_amp=0.1,
- noise_std=0.003,
- voiced_threshold=0,
- flag_for_pulse=False,
- ):
- super(SineGen, self).__init__()
- self.sine_amp = sine_amp
- self.noise_std = noise_std
- self.harmonic_num = harmonic_num
- self.dim = self.harmonic_num + 1
- self.sampling_rate = samp_rate
- self.voiced_threshold = voiced_threshold
-
- def _f02uv(self, f0):
- # generate uv signal
- uv = torch.ones_like(f0)
- uv = uv * (f0 > self.voiced_threshold)
- return uv.float()
-
- def forward(self, f0, upp):
- """sine_tensor, uv = forward(f0)
- input F0: tensor(batchsize=1, length, dim=1)
- f0 for unvoiced steps should be 0
- output sine_tensor: tensor(batchsize=1, length, dim)
- output uv: tensor(batchsize=1, length, 1)
- """
- with torch.no_grad():
- f0 = f0[:, None].transpose(1, 2)
- f0_buf = torch.zeros(f0.shape[0], f0.shape[1], self.dim, device=f0.device)
- # fundamental component
- f0_buf[:, :, 0] = f0[:, :, 0]
- for idx in np.arange(self.harmonic_num):
- f0_buf[:, :, idx + 1] = f0_buf[:, :, 0] * (
- idx + 2
- ) # idx + 2: the (idx+1)-th overtone, (idx+2)-th harmonic
- rad_values = (f0_buf / self.sampling_rate) % 1 ###%1意味着n_har的乘积无法后处理优化
- rand_ini = torch.rand(
- f0_buf.shape[0], f0_buf.shape[2], device=f0_buf.device
- )
- rand_ini[:, 0] = 0
- rad_values[:, 0, :] = rad_values[:, 0, :] + rand_ini
- tmp_over_one = torch.cumsum(rad_values, 1) # % 1 #####%1意味着后面的cumsum无法再优化
- tmp_over_one *= upp
- tmp_over_one = F.interpolate(
- tmp_over_one.transpose(2, 1),
- scale_factor=upp,
- mode="linear",
- align_corners=True,
- ).transpose(2, 1)
- rad_values = F.interpolate(
- rad_values.transpose(2, 1), scale_factor=upp, mode="nearest"
- ).transpose(
- 2, 1
- ) #######
- tmp_over_one %= 1
- tmp_over_one_idx = (tmp_over_one[:, 1:, :] - tmp_over_one[:, :-1, :]) < 0
- cumsum_shift = torch.zeros_like(rad_values)
- cumsum_shift[:, 1:, :] = tmp_over_one_idx * -1.0
- sine_waves = torch.sin(
- torch.cumsum(rad_values + cumsum_shift, dim=1) * 2 * np.pi
- )
- sine_waves = sine_waves * self.sine_amp
- uv = self._f02uv(f0)
- uv = F.interpolate(
- uv.transpose(2, 1), scale_factor=upp, mode="nearest"
- ).transpose(2, 1)
- noise_amp = uv * self.noise_std + (1 - uv) * self.sine_amp / 3
- noise = noise_amp * torch.randn_like(sine_waves)
- sine_waves = sine_waves * uv + noise
- return sine_waves, uv, noise
-
-
-class SourceModuleHnNSF(torch.nn.Module):
- """SourceModule for hn-nsf
- SourceModule(sampling_rate, harmonic_num=0, sine_amp=0.1,
- add_noise_std=0.003, voiced_threshod=0)
- sampling_rate: sampling_rate in Hz
- harmonic_num: number of harmonic above F0 (default: 0)
- sine_amp: amplitude of sine source signal (default: 0.1)
- add_noise_std: std of additive Gaussian noise (default: 0.003)
- note that amplitude of noise in unvoiced is decided
- by sine_amp
- voiced_threshold: threhold to set U/V given F0 (default: 0)
- Sine_source, noise_source = SourceModuleHnNSF(F0_sampled)
- F0_sampled (batchsize, length, 1)
- Sine_source (batchsize, length, 1)
- noise_source (batchsize, length 1)
- uv (batchsize, length, 1)
- """
-
- def __init__(
- self,
- sampling_rate,
- harmonic_num=0,
- sine_amp=0.1,
- add_noise_std=0.003,
- voiced_threshod=0,
- is_half=True,
- ):
- super(SourceModuleHnNSF, self).__init__()
-
- self.sine_amp = sine_amp
- self.noise_std = add_noise_std
- self.is_half = is_half
- # to produce sine waveforms
- self.l_sin_gen = SineGen(
- sampling_rate, harmonic_num, sine_amp, add_noise_std, voiced_threshod
- )
-
- # to merge source harmonics into a single excitation
- self.l_linear = torch.nn.Linear(harmonic_num + 1, 1)
- self.l_tanh = torch.nn.Tanh()
-
- def forward(self, x, upp=None):
- sine_wavs, uv, _ = self.l_sin_gen(x, upp)
- if self.is_half:
- sine_wavs = sine_wavs.half()
- sine_merge = self.l_tanh(self.l_linear(sine_wavs))
- return sine_merge, None, None # noise, uv
-
-
-class GeneratorNSF(torch.nn.Module):
- def __init__(
- self,
- initial_channel,
- resblock,
- resblock_kernel_sizes,
- resblock_dilation_sizes,
- upsample_rates,
- upsample_initial_channel,
- upsample_kernel_sizes,
- gin_channels,
- sr,
- is_half=False,
- ):
- super(GeneratorNSF, self).__init__()
- self.num_kernels = len(resblock_kernel_sizes)
- self.num_upsamples = len(upsample_rates)
-
- self.f0_upsamp = torch.nn.Upsample(scale_factor=np.prod(upsample_rates))
- self.m_source = SourceModuleHnNSF(
- sampling_rate=sr, harmonic_num=0, is_half=is_half
- )
- self.noise_convs = nn.ModuleList()
- self.conv_pre = Conv1d(
- initial_channel, upsample_initial_channel, 7, 1, padding=3
- )
- resblock = modules.ResBlock1 if resblock == "1" else modules.ResBlock2
-
- self.ups = nn.ModuleList()
- for i, (u, k) in enumerate(zip(upsample_rates, upsample_kernel_sizes)):
- c_cur = upsample_initial_channel // (2 ** (i + 1))
- self.ups.append(
- weight_norm(
- ConvTranspose1d(
- upsample_initial_channel // (2**i),
- upsample_initial_channel // (2 ** (i + 1)),
- k,
- u,
- padding=(k - u) // 2,
- )
- )
- )
- if i + 1 < len(upsample_rates):
- stride_f0 = np.prod(upsample_rates[i + 1 :])
- self.noise_convs.append(
- Conv1d(
- 1,
- c_cur,
- kernel_size=stride_f0 * 2,
- stride=stride_f0,
- padding=stride_f0 // 2,
- )
- )
- else:
- self.noise_convs.append(Conv1d(1, c_cur, kernel_size=1))
-
- self.resblocks = nn.ModuleList()
- for i in range(len(self.ups)):
- ch = upsample_initial_channel // (2 ** (i + 1))
- for j, (k, d) in enumerate(
- zip(resblock_kernel_sizes, resblock_dilation_sizes)
- ):
- self.resblocks.append(resblock(ch, k, d))
-
- self.conv_post = Conv1d(ch, 1, 7, 1, padding=3, bias=False)
- self.ups.apply(init_weights)
-
- if gin_channels != 0:
- self.cond = nn.Conv1d(gin_channels, upsample_initial_channel, 1)
-
- self.upp = np.prod(upsample_rates)
-
- def forward(self, x, f0, g=None):
- har_source, noi_source, uv = self.m_source(f0, self.upp)
- har_source = har_source.transpose(1, 2)
- x = self.conv_pre(x)
- if g is not None:
- x = x + self.cond(g)
-
- for i in range(self.num_upsamples):
- x = F.leaky_relu(x, modules.LRELU_SLOPE)
- x = self.ups[i](x)
- x_source = self.noise_convs[i](har_source)
- x = x + x_source
- xs = None
- for j in range(self.num_kernels):
- if xs is None:
- xs = self.resblocks[i * self.num_kernels + j](x)
- else:
- xs += self.resblocks[i * self.num_kernels + j](x)
- x = xs / self.num_kernels
- x = F.leaky_relu(x)
- x = self.conv_post(x)
- x = torch.tanh(x)
- return x
-
- def remove_weight_norm(self):
- for l in self.ups:
- remove_weight_norm(l)
- for l in self.resblocks:
- l.remove_weight_norm()
-
-
-sr2sr = {
- "32k": 32000,
- "40k": 40000,
- "48k": 48000,
-}
-
-
-class SynthesizerTrnMs256NSFsid(nn.Module):
- def __init__(
- self,
- spec_channels,
- segment_size,
- inter_channels,
- hidden_channels,
- filter_channels,
- n_heads,
- n_layers,
- kernel_size,
- p_dropout,
- resblock,
- resblock_kernel_sizes,
- resblock_dilation_sizes,
- upsample_rates,
- upsample_initial_channel,
- upsample_kernel_sizes,
- spk_embed_dim,
- gin_channels,
- sr,
- **kwargs
- ):
- super().__init__()
- if type(sr) == type("strr"):
- sr = sr2sr[sr]
- self.spec_channels = spec_channels
- self.inter_channels = inter_channels
- self.hidden_channels = hidden_channels
- self.filter_channels = filter_channels
- self.n_heads = n_heads
- self.n_layers = n_layers
- self.kernel_size = kernel_size
- self.p_dropout = p_dropout
- self.resblock = resblock
- self.resblock_kernel_sizes = resblock_kernel_sizes
- self.resblock_dilation_sizes = resblock_dilation_sizes
- self.upsample_rates = upsample_rates
- self.upsample_initial_channel = upsample_initial_channel
- self.upsample_kernel_sizes = upsample_kernel_sizes
- self.segment_size = segment_size
- self.gin_channels = gin_channels
- # self.hop_length = hop_length#
- self.spk_embed_dim = spk_embed_dim
- self.enc_p = TextEncoder256(
- inter_channels,
- hidden_channels,
- filter_channels,
- n_heads,
- n_layers,
- kernel_size,
- p_dropout,
- )
- self.dec = GeneratorNSF(
- inter_channels,
- resblock,
- resblock_kernel_sizes,
- resblock_dilation_sizes,
- upsample_rates,
- upsample_initial_channel,
- upsample_kernel_sizes,
- gin_channels=gin_channels,
- sr=sr,
- is_half=kwargs["is_half"],
- )
- self.enc_q = PosteriorEncoder(
- spec_channels,
- inter_channels,
- hidden_channels,
- 5,
- 1,
- 16,
- gin_channels=gin_channels,
- )
- self.flow = ResidualCouplingBlock(
- inter_channels, hidden_channels, 5, 1, 3, gin_channels=gin_channels
- )
- self.emb_g = nn.Embedding(self.spk_embed_dim, gin_channels)
- print("gin_channels:", gin_channels, "self.spk_embed_dim:", self.spk_embed_dim)
-
- def remove_weight_norm(self):
- self.dec.remove_weight_norm()
- self.flow.remove_weight_norm()
- self.enc_q.remove_weight_norm()
-
- def forward(
- self, phone, phone_lengths, pitch, pitchf, y, y_lengths, ds
- ): # 这里ds是id,[bs,1]
- # print(1,pitch.shape)#[bs,t]
- g = self.emb_g(ds).unsqueeze(-1) # [b, 256, 1]##1是t,广播的
- m_p, logs_p, x_mask = self.enc_p(phone, pitch, phone_lengths)
- z, m_q, logs_q, y_mask = self.enc_q(y, y_lengths, g=g)
- z_p = self.flow(z, y_mask, g=g)
- z_slice, ids_slice = commons.rand_slice_segments(
- z, y_lengths, self.segment_size
- )
- # print(-1,pitchf.shape,ids_slice,self.segment_size,self.hop_length,self.segment_size//self.hop_length)
- pitchf = commons.slice_segments2(pitchf, ids_slice, self.segment_size)
- # print(-2,pitchf.shape,z_slice.shape)
- o = self.dec(z_slice, pitchf, g=g)
- return o, ids_slice, x_mask, y_mask, (z, z_p, m_p, logs_p, m_q, logs_q)
-
- def infer(self, phone, phone_lengths, pitch, nsff0, sid, max_len=None):
- g = self.emb_g(sid).unsqueeze(-1)
- m_p, logs_p, x_mask = self.enc_p(phone, pitch, phone_lengths)
- z_p = (m_p + torch.exp(logs_p) * torch.randn_like(m_p) * 0.66666) * x_mask
- z = self.flow(z_p, x_mask, g=g, reverse=True)
- o = self.dec((z * x_mask)[:, :, :max_len], nsff0, g=g)
- return o, x_mask, (z, z_p, m_p, logs_p)
-
-
-class SynthesizerTrnMs768NSFsid(nn.Module):
- def __init__(
- self,
- spec_channels,
- segment_size,
- inter_channels,
- hidden_channels,
- filter_channels,
- n_heads,
- n_layers,
- kernel_size,
- p_dropout,
- resblock,
- resblock_kernel_sizes,
- resblock_dilation_sizes,
- upsample_rates,
- upsample_initial_channel,
- upsample_kernel_sizes,
- spk_embed_dim,
- gin_channels,
- sr,
- **kwargs
- ):
- super().__init__()
- if type(sr) == type("strr"):
- sr = sr2sr[sr]
- self.spec_channels = spec_channels
- self.inter_channels = inter_channels
- self.hidden_channels = hidden_channels
- self.filter_channels = filter_channels
- self.n_heads = n_heads
- self.n_layers = n_layers
- self.kernel_size = kernel_size
- self.p_dropout = p_dropout
- self.resblock = resblock
- self.resblock_kernel_sizes = resblock_kernel_sizes
- self.resblock_dilation_sizes = resblock_dilation_sizes
- self.upsample_rates = upsample_rates
- self.upsample_initial_channel = upsample_initial_channel
- self.upsample_kernel_sizes = upsample_kernel_sizes
- self.segment_size = segment_size
- self.gin_channels = gin_channels
- # self.hop_length = hop_length#
- self.spk_embed_dim = spk_embed_dim
- self.enc_p = TextEncoder768(
- inter_channels,
- hidden_channels,
- filter_channels,
- n_heads,
- n_layers,
- kernel_size,
- p_dropout,
- )
- self.dec = GeneratorNSF(
- inter_channels,
- resblock,
- resblock_kernel_sizes,
- resblock_dilation_sizes,
- upsample_rates,
- upsample_initial_channel,
- upsample_kernel_sizes,
- gin_channels=gin_channels,
- sr=sr,
- is_half=kwargs["is_half"],
- )
- self.enc_q = PosteriorEncoder(
- spec_channels,
- inter_channels,
- hidden_channels,
- 5,
- 1,
- 16,
- gin_channels=gin_channels,
- )
- self.flow = ResidualCouplingBlock(
- inter_channels, hidden_channels, 5, 1, 3, gin_channels=gin_channels
- )
- self.emb_g = nn.Embedding(self.spk_embed_dim, gin_channels)
- print("gin_channels:", gin_channels, "self.spk_embed_dim:", self.spk_embed_dim)
-
- def remove_weight_norm(self):
- self.dec.remove_weight_norm()
- self.flow.remove_weight_norm()
- self.enc_q.remove_weight_norm()
-
- def forward(
- self, phone, phone_lengths, pitch, pitchf, y, y_lengths, ds
- ): # 这里ds是id,[bs,1]
- # print(1,pitch.shape)#[bs,t]
- g = self.emb_g(ds).unsqueeze(-1) # [b, 256, 1]##1是t,广播的
- m_p, logs_p, x_mask = self.enc_p(phone, pitch, phone_lengths)
- z, m_q, logs_q, y_mask = self.enc_q(y, y_lengths, g=g)
- z_p = self.flow(z, y_mask, g=g)
- z_slice, ids_slice = commons.rand_slice_segments(
- z, y_lengths, self.segment_size
- )
- # print(-1,pitchf.shape,ids_slice,self.segment_size,self.hop_length,self.segment_size//self.hop_length)
- pitchf = commons.slice_segments2(pitchf, ids_slice, self.segment_size)
- # print(-2,pitchf.shape,z_slice.shape)
- o = self.dec(z_slice, pitchf, g=g)
- return o, ids_slice, x_mask, y_mask, (z, z_p, m_p, logs_p, m_q, logs_q)
-
- def infer(self, phone, phone_lengths, pitch, nsff0, sid, max_len=None):
- g = self.emb_g(sid).unsqueeze(-1)
- m_p, logs_p, x_mask = self.enc_p(phone, pitch, phone_lengths)
- z_p = (m_p + torch.exp(logs_p) * torch.randn_like(m_p) * 0.66666) * x_mask
- z = self.flow(z_p, x_mask, g=g, reverse=True)
- o = self.dec((z * x_mask)[:, :, :max_len], nsff0, g=g)
- return o, x_mask, (z, z_p, m_p, logs_p)
-
-
-class SynthesizerTrnMs256NSFsid_nono(nn.Module):
- def __init__(
- self,
- spec_channels,
- segment_size,
- inter_channels,
- hidden_channels,
- filter_channels,
- n_heads,
- n_layers,
- kernel_size,
- p_dropout,
- resblock,
- resblock_kernel_sizes,
- resblock_dilation_sizes,
- upsample_rates,
- upsample_initial_channel,
- upsample_kernel_sizes,
- spk_embed_dim,
- gin_channels,
- sr=None,
- **kwargs
- ):
- super().__init__()
- self.spec_channels = spec_channels
- self.inter_channels = inter_channels
- self.hidden_channels = hidden_channels
- self.filter_channels = filter_channels
- self.n_heads = n_heads
- self.n_layers = n_layers
- self.kernel_size = kernel_size
- self.p_dropout = p_dropout
- self.resblock = resblock
- self.resblock_kernel_sizes = resblock_kernel_sizes
- self.resblock_dilation_sizes = resblock_dilation_sizes
- self.upsample_rates = upsample_rates
- self.upsample_initial_channel = upsample_initial_channel
- self.upsample_kernel_sizes = upsample_kernel_sizes
- self.segment_size = segment_size
- self.gin_channels = gin_channels
- # self.hop_length = hop_length#
- self.spk_embed_dim = spk_embed_dim
- self.enc_p = TextEncoder256(
- inter_channels,
- hidden_channels,
- filter_channels,
- n_heads,
- n_layers,
- kernel_size,
- p_dropout,
- f0=False,
- )
- self.dec = Generator(
- inter_channels,
- resblock,
- resblock_kernel_sizes,
- resblock_dilation_sizes,
- upsample_rates,
- upsample_initial_channel,
- upsample_kernel_sizes,
- gin_channels=gin_channels,
- )
- self.enc_q = PosteriorEncoder(
- spec_channels,
- inter_channels,
- hidden_channels,
- 5,
- 1,
- 16,
- gin_channels=gin_channels,
- )
- self.flow = ResidualCouplingBlock(
- inter_channels, hidden_channels, 5, 1, 3, gin_channels=gin_channels
- )
- self.emb_g = nn.Embedding(self.spk_embed_dim, gin_channels)
- print("gin_channels:", gin_channels, "self.spk_embed_dim:", self.spk_embed_dim)
-
- def remove_weight_norm(self):
- self.dec.remove_weight_norm()
- self.flow.remove_weight_norm()
- self.enc_q.remove_weight_norm()
-
- def forward(self, phone, phone_lengths, y, y_lengths, ds): # 这里ds是id,[bs,1]
- g = self.emb_g(ds).unsqueeze(-1) # [b, 256, 1]##1是t,广播的
- m_p, logs_p, x_mask = self.enc_p(phone, None, phone_lengths)
- z, m_q, logs_q, y_mask = self.enc_q(y, y_lengths, g=g)
- z_p = self.flow(z, y_mask, g=g)
- z_slice, ids_slice = commons.rand_slice_segments(
- z, y_lengths, self.segment_size
- )
- o = self.dec(z_slice, g=g)
- return o, ids_slice, x_mask, y_mask, (z, z_p, m_p, logs_p, m_q, logs_q)
-
- def infer(self, phone, phone_lengths, sid, max_len=None):
- g = self.emb_g(sid).unsqueeze(-1)
- m_p, logs_p, x_mask = self.enc_p(phone, None, phone_lengths)
- z_p = (m_p + torch.exp(logs_p) * torch.randn_like(m_p) * 0.66666) * x_mask
- z = self.flow(z_p, x_mask, g=g, reverse=True)
- o = self.dec((z * x_mask)[:, :, :max_len], g=g)
- return o, x_mask, (z, z_p, m_p, logs_p)
-
-
-class SynthesizerTrnMs768NSFsid_nono(nn.Module):
- def __init__(
- self,
- spec_channels,
- segment_size,
- inter_channels,
- hidden_channels,
- filter_channels,
- n_heads,
- n_layers,
- kernel_size,
- p_dropout,
- resblock,
- resblock_kernel_sizes,
- resblock_dilation_sizes,
- upsample_rates,
- upsample_initial_channel,
- upsample_kernel_sizes,
- spk_embed_dim,
- gin_channels,
- sr=None,
- **kwargs
- ):
- super().__init__()
- self.spec_channels = spec_channels
- self.inter_channels = inter_channels
- self.hidden_channels = hidden_channels
- self.filter_channels = filter_channels
- self.n_heads = n_heads
- self.n_layers = n_layers
- self.kernel_size = kernel_size
- self.p_dropout = p_dropout
- self.resblock = resblock
- self.resblock_kernel_sizes = resblock_kernel_sizes
- self.resblock_dilation_sizes = resblock_dilation_sizes
- self.upsample_rates = upsample_rates
- self.upsample_initial_channel = upsample_initial_channel
- self.upsample_kernel_sizes = upsample_kernel_sizes
- self.segment_size = segment_size
- self.gin_channels = gin_channels
- # self.hop_length = hop_length#
- self.spk_embed_dim = spk_embed_dim
- self.enc_p = TextEncoder768(
- inter_channels,
- hidden_channels,
- filter_channels,
- n_heads,
- n_layers,
- kernel_size,
- p_dropout,
- f0=False,
- )
- self.dec = Generator(
- inter_channels,
- resblock,
- resblock_kernel_sizes,
- resblock_dilation_sizes,
- upsample_rates,
- upsample_initial_channel,
- upsample_kernel_sizes,
- gin_channels=gin_channels,
- )
- self.enc_q = PosteriorEncoder(
- spec_channels,
- inter_channels,
- hidden_channels,
- 5,
- 1,
- 16,
- gin_channels=gin_channels,
- )
- self.flow = ResidualCouplingBlock(
- inter_channels, hidden_channels, 5, 1, 3, gin_channels=gin_channels
- )
- self.emb_g = nn.Embedding(self.spk_embed_dim, gin_channels)
- print("gin_channels:", gin_channels, "self.spk_embed_dim:", self.spk_embed_dim)
-
- def remove_weight_norm(self):
- self.dec.remove_weight_norm()
- self.flow.remove_weight_norm()
- self.enc_q.remove_weight_norm()
-
- def forward(self, phone, phone_lengths, y, y_lengths, ds): # 这里ds是id,[bs,1]
- g = self.emb_g(ds).unsqueeze(-1) # [b, 256, 1]##1是t,广播的
- m_p, logs_p, x_mask = self.enc_p(phone, None, phone_lengths)
- z, m_q, logs_q, y_mask = self.enc_q(y, y_lengths, g=g)
- z_p = self.flow(z, y_mask, g=g)
- z_slice, ids_slice = commons.rand_slice_segments(
- z, y_lengths, self.segment_size
- )
- o = self.dec(z_slice, g=g)
- return o, ids_slice, x_mask, y_mask, (z, z_p, m_p, logs_p, m_q, logs_q)
-
- def infer(self, phone, phone_lengths, sid, max_len=None):
- g = self.emb_g(sid).unsqueeze(-1)
- m_p, logs_p, x_mask = self.enc_p(phone, None, phone_lengths)
- z_p = (m_p + torch.exp(logs_p) * torch.randn_like(m_p) * 0.66666) * x_mask
- z = self.flow(z_p, x_mask, g=g, reverse=True)
- o = self.dec((z * x_mask)[:, :, :max_len], g=g)
- return o, x_mask, (z, z_p, m_p, logs_p)
-
-
-class MultiPeriodDiscriminator(torch.nn.Module):
- def __init__(self, use_spectral_norm=False):
- super(MultiPeriodDiscriminator, self).__init__()
- periods = [2, 3, 5, 7, 11, 17]
- # periods = [3, 5, 7, 11, 17, 23, 37]
-
- discs = [DiscriminatorS(use_spectral_norm=use_spectral_norm)]
- discs = discs + [
- DiscriminatorP(i, use_spectral_norm=use_spectral_norm) for i in periods
- ]
- self.discriminators = nn.ModuleList(discs)
-
- def forward(self, y, y_hat):
- y_d_rs = [] #
- y_d_gs = []
- fmap_rs = []
- fmap_gs = []
- for i, d in enumerate(self.discriminators):
- y_d_r, fmap_r = d(y)
- y_d_g, fmap_g = d(y_hat)
- # for j in range(len(fmap_r)):
- # print(i,j,y.shape,y_hat.shape,fmap_r[j].shape,fmap_g[j].shape)
- y_d_rs.append(y_d_r)
- y_d_gs.append(y_d_g)
- fmap_rs.append(fmap_r)
- fmap_gs.append(fmap_g)
-
- return y_d_rs, y_d_gs, fmap_rs, fmap_gs
-
-
-class MultiPeriodDiscriminatorV2(torch.nn.Module):
- def __init__(self, use_spectral_norm=False):
- super(MultiPeriodDiscriminatorV2, self).__init__()
- # periods = [2, 3, 5, 7, 11, 17]
- periods = [2, 3, 5, 7, 11, 17, 23, 37]
-
- discs = [DiscriminatorS(use_spectral_norm=use_spectral_norm)]
- discs = discs + [
- DiscriminatorP(i, use_spectral_norm=use_spectral_norm) for i in periods
- ]
- self.discriminators = nn.ModuleList(discs)
-
- def forward(self, y, y_hat):
- y_d_rs = [] #
- y_d_gs = []
- fmap_rs = []
- fmap_gs = []
- for i, d in enumerate(self.discriminators):
- y_d_r, fmap_r = d(y)
- y_d_g, fmap_g = d(y_hat)
- # for j in range(len(fmap_r)):
- # print(i,j,y.shape,y_hat.shape,fmap_r[j].shape,fmap_g[j].shape)
- y_d_rs.append(y_d_r)
- y_d_gs.append(y_d_g)
- fmap_rs.append(fmap_r)
- fmap_gs.append(fmap_g)
-
- return y_d_rs, y_d_gs, fmap_rs, fmap_gs
-
-
-class DiscriminatorS(torch.nn.Module):
- def __init__(self, use_spectral_norm=False):
- super(DiscriminatorS, self).__init__()
- norm_f = weight_norm if use_spectral_norm == False else spectral_norm
- self.convs = nn.ModuleList(
- [
- norm_f(Conv1d(1, 16, 15, 1, padding=7)),
- norm_f(Conv1d(16, 64, 41, 4, groups=4, padding=20)),
- norm_f(Conv1d(64, 256, 41, 4, groups=16, padding=20)),
- norm_f(Conv1d(256, 1024, 41, 4, groups=64, padding=20)),
- norm_f(Conv1d(1024, 1024, 41, 4, groups=256, padding=20)),
- norm_f(Conv1d(1024, 1024, 5, 1, padding=2)),
- ]
- )
- self.conv_post = norm_f(Conv1d(1024, 1, 3, 1, padding=1))
-
- def forward(self, x):
- fmap = []
-
- for l in self.convs:
- x = l(x)
- x = F.leaky_relu(x, modules.LRELU_SLOPE)
- fmap.append(x)
- x = self.conv_post(x)
- fmap.append(x)
- x = torch.flatten(x, 1, -1)
-
- return x, fmap
-
-
-class DiscriminatorP(torch.nn.Module):
- def __init__(self, period, kernel_size=5, stride=3, use_spectral_norm=False):
- super(DiscriminatorP, self).__init__()
- self.period = period
- self.use_spectral_norm = use_spectral_norm
- norm_f = weight_norm if use_spectral_norm == False else spectral_norm
- self.convs = nn.ModuleList(
- [
- norm_f(
- Conv2d(
- 1,
- 32,
- (kernel_size, 1),
- (stride, 1),
- padding=(get_padding(kernel_size, 1), 0),
- )
- ),
- norm_f(
- Conv2d(
- 32,
- 128,
- (kernel_size, 1),
- (stride, 1),
- padding=(get_padding(kernel_size, 1), 0),
- )
- ),
- norm_f(
- Conv2d(
- 128,
- 512,
- (kernel_size, 1),
- (stride, 1),
- padding=(get_padding(kernel_size, 1), 0),
- )
- ),
- norm_f(
- Conv2d(
- 512,
- 1024,
- (kernel_size, 1),
- (stride, 1),
- padding=(get_padding(kernel_size, 1), 0),
- )
- ),
- norm_f(
- Conv2d(
- 1024,
- 1024,
- (kernel_size, 1),
- 1,
- padding=(get_padding(kernel_size, 1), 0),
- )
- ),
- ]
- )
- self.conv_post = norm_f(Conv2d(1024, 1, (3, 1), 1, padding=(1, 0)))
-
- def forward(self, x):
- fmap = []
-
- # 1d to 2d
- b, c, t = x.shape
- if t % self.period != 0: # pad first
- n_pad = self.period - (t % self.period)
- x = F.pad(x, (0, n_pad), "reflect")
- t = t + n_pad
- x = x.view(b, c, t // self.period, self.period)
-
- for l in self.convs:
- x = l(x)
- x = F.leaky_relu(x, modules.LRELU_SLOPE)
- fmap.append(x)
- x = self.conv_post(x)
- fmap.append(x)
- x = torch.flatten(x, 1, -1)
-
- return x, fmap
diff --git a/spaces/Ironicsarcastic/Nse/README.md b/spaces/Ironicsarcastic/Nse/README.md
deleted file mode 100644
index 10cb773cb5101bbaddf4089b2e2a601b82cb8ccc..0000000000000000000000000000000000000000
--- a/spaces/Ironicsarcastic/Nse/README.md
+++ /dev/null
@@ -1,10 +0,0 @@
----
-title: Nse
-emoji: ⚡
-colorFrom: yellow
-colorTo: pink
-sdk: docker
-pinned: false
----
-
-Check out the configuration reference at https://huggingface.co/docs/hub/spaces-config-reference
diff --git a/spaces/JUNGU/VToonify/vtoonify/model/stylegan/lpips/networks_basic.py b/spaces/JUNGU/VToonify/vtoonify/model/stylegan/lpips/networks_basic.py
deleted file mode 100644
index 201359c4e743aed285694668e13da6dd5a40b621..0000000000000000000000000000000000000000
--- a/spaces/JUNGU/VToonify/vtoonify/model/stylegan/lpips/networks_basic.py
+++ /dev/null
@@ -1,187 +0,0 @@
-
-from __future__ import absolute_import
-
-import sys
-import torch
-import torch.nn as nn
-import torch.nn.init as init
-from torch.autograd import Variable
-import numpy as np
-from pdb import set_trace as st
-from skimage import color
-from IPython import embed
-from model.stylegan.lpips import pretrained_networks as pn
-
-import model.stylegan.lpips as util
-
-def spatial_average(in_tens, keepdim=True):
- return in_tens.mean([2,3],keepdim=keepdim)
-
-def upsample(in_tens, out_H=64): # assumes scale factor is same for H and W
- in_H = in_tens.shape[2]
- scale_factor = 1.*out_H/in_H
-
- return nn.Upsample(scale_factor=scale_factor, mode='bilinear', align_corners=False)(in_tens)
-
-# Learned perceptual metric
-class PNetLin(nn.Module):
- def __init__(self, pnet_type='vgg', pnet_rand=False, pnet_tune=False, use_dropout=True, spatial=False, version='0.1', lpips=True):
- super(PNetLin, self).__init__()
-
- self.pnet_type = pnet_type
- self.pnet_tune = pnet_tune
- self.pnet_rand = pnet_rand
- self.spatial = spatial
- self.lpips = lpips
- self.version = version
- self.scaling_layer = ScalingLayer()
-
- if(self.pnet_type in ['vgg','vgg16']):
- net_type = pn.vgg16
- self.chns = [64,128,256,512,512]
- elif(self.pnet_type=='alex'):
- net_type = pn.alexnet
- self.chns = [64,192,384,256,256]
- elif(self.pnet_type=='squeeze'):
- net_type = pn.squeezenet
- self.chns = [64,128,256,384,384,512,512]
- self.L = len(self.chns)
-
- self.net = net_type(pretrained=not self.pnet_rand, requires_grad=self.pnet_tune)
-
- if(lpips):
- self.lin0 = NetLinLayer(self.chns[0], use_dropout=use_dropout)
- self.lin1 = NetLinLayer(self.chns[1], use_dropout=use_dropout)
- self.lin2 = NetLinLayer(self.chns[2], use_dropout=use_dropout)
- self.lin3 = NetLinLayer(self.chns[3], use_dropout=use_dropout)
- self.lin4 = NetLinLayer(self.chns[4], use_dropout=use_dropout)
- self.lins = [self.lin0,self.lin1,self.lin2,self.lin3,self.lin4]
- if(self.pnet_type=='squeeze'): # 7 layers for squeezenet
- self.lin5 = NetLinLayer(self.chns[5], use_dropout=use_dropout)
- self.lin6 = NetLinLayer(self.chns[6], use_dropout=use_dropout)
- self.lins+=[self.lin5,self.lin6]
-
- def forward(self, in0, in1, retPerLayer=False):
- # v0.0 - original release had a bug, where input was not scaled
- in0_input, in1_input = (self.scaling_layer(in0), self.scaling_layer(in1)) if self.version=='0.1' else (in0, in1)
- outs0, outs1 = self.net.forward(in0_input), self.net.forward(in1_input)
- feats0, feats1, diffs = {}, {}, {}
-
- for kk in range(self.L):
- feats0[kk], feats1[kk] = util.normalize_tensor(outs0[kk]), util.normalize_tensor(outs1[kk])
- diffs[kk] = (feats0[kk]-feats1[kk])**2
-
- if(self.lpips):
- if(self.spatial):
- res = [upsample(self.lins[kk].model(diffs[kk]), out_H=in0.shape[2]) for kk in range(self.L)]
- else:
- res = [spatial_average(self.lins[kk].model(diffs[kk]), keepdim=True) for kk in range(self.L)]
- else:
- if(self.spatial):
- res = [upsample(diffs[kk].sum(dim=1,keepdim=True), out_H=in0.shape[2]) for kk in range(self.L)]
- else:
- res = [spatial_average(diffs[kk].sum(dim=1,keepdim=True), keepdim=True) for kk in range(self.L)]
-
- val = res[0]
- for l in range(1,self.L):
- val += res[l]
-
- if(retPerLayer):
- return (val, res)
- else:
- return val
-
-class ScalingLayer(nn.Module):
- def __init__(self):
- super(ScalingLayer, self).__init__()
- self.register_buffer('shift', torch.Tensor([-.030,-.088,-.188])[None,:,None,None])
- self.register_buffer('scale', torch.Tensor([.458,.448,.450])[None,:,None,None])
-
- def forward(self, inp):
- return (inp - self.shift) / self.scale
-
-
-class NetLinLayer(nn.Module):
- ''' A single linear layer which does a 1x1 conv '''
- def __init__(self, chn_in, chn_out=1, use_dropout=False):
- super(NetLinLayer, self).__init__()
-
- layers = [nn.Dropout(),] if(use_dropout) else []
- layers += [nn.Conv2d(chn_in, chn_out, 1, stride=1, padding=0, bias=False),]
- self.model = nn.Sequential(*layers)
-
-
-class Dist2LogitLayer(nn.Module):
- ''' takes 2 distances, puts through fc layers, spits out value between [0,1] (if use_sigmoid is True) '''
- def __init__(self, chn_mid=32, use_sigmoid=True):
- super(Dist2LogitLayer, self).__init__()
-
- layers = [nn.Conv2d(5, chn_mid, 1, stride=1, padding=0, bias=True),]
- layers += [nn.LeakyReLU(0.2,True),]
- layers += [nn.Conv2d(chn_mid, chn_mid, 1, stride=1, padding=0, bias=True),]
- layers += [nn.LeakyReLU(0.2,True),]
- layers += [nn.Conv2d(chn_mid, 1, 1, stride=1, padding=0, bias=True),]
- if(use_sigmoid):
- layers += [nn.Sigmoid(),]
- self.model = nn.Sequential(*layers)
-
- def forward(self,d0,d1,eps=0.1):
- return self.model.forward(torch.cat((d0,d1,d0-d1,d0/(d1+eps),d1/(d0+eps)),dim=1))
-
-class BCERankingLoss(nn.Module):
- def __init__(self, chn_mid=32):
- super(BCERankingLoss, self).__init__()
- self.net = Dist2LogitLayer(chn_mid=chn_mid)
- # self.parameters = list(self.net.parameters())
- self.loss = torch.nn.BCELoss()
-
- def forward(self, d0, d1, judge):
- per = (judge+1.)/2.
- self.logit = self.net.forward(d0,d1)
- return self.loss(self.logit, per)
-
-# L2, DSSIM metrics
-class FakeNet(nn.Module):
- def __init__(self, use_gpu=True, colorspace='Lab'):
- super(FakeNet, self).__init__()
- self.use_gpu = use_gpu
- self.colorspace=colorspace
-
-class L2(FakeNet):
-
- def forward(self, in0, in1, retPerLayer=None):
- assert(in0.size()[0]==1) # currently only supports batchSize 1
-
- if(self.colorspace=='RGB'):
- (N,C,X,Y) = in0.size()
- value = torch.mean(torch.mean(torch.mean((in0-in1)**2,dim=1).view(N,1,X,Y),dim=2).view(N,1,1,Y),dim=3).view(N)
- return value
- elif(self.colorspace=='Lab'):
- value = util.l2(util.tensor2np(util.tensor2tensorlab(in0.data,to_norm=False)),
- util.tensor2np(util.tensor2tensorlab(in1.data,to_norm=False)), range=100.).astype('float')
- ret_var = Variable( torch.Tensor((value,) ) )
- if(self.use_gpu):
- ret_var = ret_var.cuda()
- return ret_var
-
-class DSSIM(FakeNet):
-
- def forward(self, in0, in1, retPerLayer=None):
- assert(in0.size()[0]==1) # currently only supports batchSize 1
-
- if(self.colorspace=='RGB'):
- value = util.dssim(1.*util.tensor2im(in0.data), 1.*util.tensor2im(in1.data), range=255.).astype('float')
- elif(self.colorspace=='Lab'):
- value = util.dssim(util.tensor2np(util.tensor2tensorlab(in0.data,to_norm=False)),
- util.tensor2np(util.tensor2tensorlab(in1.data,to_norm=False)), range=100.).astype('float')
- ret_var = Variable( torch.Tensor((value,) ) )
- if(self.use_gpu):
- ret_var = ret_var.cuda()
- return ret_var
-
-def print_network(net):
- num_params = 0
- for param in net.parameters():
- num_params += param.numel()
- print('Network',net)
- print('Total number of parameters: %d' % num_params)
diff --git a/spaces/Jeff2323/ai-comic-factory/src/app/engine/caption.ts b/spaces/Jeff2323/ai-comic-factory/src/app/engine/caption.ts
deleted file mode 100644
index e43a2aa066b735d6e7c06ae106c2ed31c3146545..0000000000000000000000000000000000000000
--- a/spaces/Jeff2323/ai-comic-factory/src/app/engine/caption.ts
+++ /dev/null
@@ -1,54 +0,0 @@
-"use server"
-
-import { ImageAnalysisRequest, ImageAnalysisResponse } from "@/types"
-
-const apiUrl = `${process.env.VIDEOCHAIN_API_URL || ""}`
-
-export async function see({
- prompt,
- imageBase64
-}: {
- prompt: string
- imageBase64: string
-}): Promise {
- if (!prompt) {
- console.error(`cannot call the API without an image, aborting..`)
- throw new Error(`cannot call the API without an image, aborting..`)
- }
-
- try {
- const request = {
- prompt,
- image: imageBase64
-
- } as ImageAnalysisRequest
-
- console.log(`calling ${apiUrl}/analyze called with: `, {
- prompt: request.prompt,
- image: request.image.slice(0, 20)
- })
-
- const res = await fetch(`${apiUrl}/analyze`, {
- method: "POST",
- headers: {
- Accept: "application/json",
- "Content-Type": "application/json",
- // Authorization: `Bearer ${process.env.VIDEOCHAIN_API_TOKEN}`,
- },
- body: JSON.stringify(request),
- cache: 'no-store',
- // we can also use this (see https://vercel.com/blog/vercel-cache-api-nextjs-cache)
- // next: { revalidate: 1 }
- })
-
- if (res.status !== 200) {
- throw new Error('Failed to fetch data')
- }
-
- const response = (await res.json()) as ImageAnalysisResponse
- return response.result
- } catch (err) {
- console.error(err)
- return ""
- }
-}
diff --git a/spaces/JohnSmith9982/VITS-Umamusume-voice-synthesizer/text/shanghainese.py b/spaces/JohnSmith9982/VITS-Umamusume-voice-synthesizer/text/shanghainese.py
deleted file mode 100644
index cb29c24a08d2e406e8399cf7bc9fe5cb43cb9c61..0000000000000000000000000000000000000000
--- a/spaces/JohnSmith9982/VITS-Umamusume-voice-synthesizer/text/shanghainese.py
+++ /dev/null
@@ -1,64 +0,0 @@
-import re
-import cn2an
-import opencc
-
-
-converter = opencc.OpenCC('zaonhe')
-
-# List of (Latin alphabet, ipa) pairs:
-_latin_to_ipa = [(re.compile('%s' % x[0]), x[1]) for x in [
- ('A', 'ᴇ'),
- ('B', 'bi'),
- ('C', 'si'),
- ('D', 'di'),
- ('E', 'i'),
- ('F', 'ᴇf'),
- ('G', 'dʑi'),
- ('H', 'ᴇtɕʰ'),
- ('I', 'ᴀi'),
- ('J', 'dʑᴇ'),
- ('K', 'kʰᴇ'),
- ('L', 'ᴇl'),
- ('M', 'ᴇm'),
- ('N', 'ᴇn'),
- ('O', 'o'),
- ('P', 'pʰi'),
- ('Q', 'kʰiu'),
- ('R', 'ᴀl'),
- ('S', 'ᴇs'),
- ('T', 'tʰi'),
- ('U', 'ɦiu'),
- ('V', 'vi'),
- ('W', 'dᴀbɤliu'),
- ('X', 'ᴇks'),
- ('Y', 'uᴀi'),
- ('Z', 'zᴇ')
-]]
-
-
-def _number_to_shanghainese(num):
- num = cn2an.an2cn(num).replace('一十','十').replace('二十', '廿').replace('二', '两')
- return re.sub(r'((?:^|[^三四五六七八九])十|廿)两', r'\1二', num)
-
-
-def number_to_shanghainese(text):
- return re.sub(r'\d+(?:\.?\d+)?', lambda x: _number_to_shanghainese(x.group()), text)
-
-
-def latin_to_ipa(text):
- for regex, replacement in _latin_to_ipa:
- text = re.sub(regex, replacement, text)
- return text
-
-
-def shanghainese_to_ipa(text):
- text = number_to_shanghainese(text.upper())
- text = converter.convert(text).replace('-','').replace('$',' ')
- text = re.sub(r'[A-Z]', lambda x: latin_to_ipa(x.group())+' ', text)
- text = re.sub(r'[、;:]', ',', text)
- text = re.sub(r'\s*,\s*', ', ', text)
- text = re.sub(r'\s*。\s*', '. ', text)
- text = re.sub(r'\s*?\s*', '? ', text)
- text = re.sub(r'\s*!\s*', '! ', text)
- text = re.sub(r'\s*$', '', text)
- return text
diff --git a/spaces/Junity/TokaiTeio-SVC/cluster/__init__.py b/spaces/Junity/TokaiTeio-SVC/cluster/__init__.py
deleted file mode 100644
index f1b9bde04e73e9218a5d534227caa4c25332f424..0000000000000000000000000000000000000000
--- a/spaces/Junity/TokaiTeio-SVC/cluster/__init__.py
+++ /dev/null
@@ -1,29 +0,0 @@
-import numpy as np
-import torch
-from sklearn.cluster import KMeans
-
-def get_cluster_model(ckpt_path):
- checkpoint = torch.load(ckpt_path)
- kmeans_dict = {}
- for spk, ckpt in checkpoint.items():
- km = KMeans(ckpt["n_features_in_"])
- km.__dict__["n_features_in_"] = ckpt["n_features_in_"]
- km.__dict__["_n_threads"] = ckpt["_n_threads"]
- km.__dict__["cluster_centers_"] = ckpt["cluster_centers_"]
- kmeans_dict[spk] = km
- return kmeans_dict
-
-def get_cluster_result(model, x, speaker):
- """
- x: np.array [t, 256]
- return cluster class result
- """
- return model[speaker].predict(x)
-
-def get_cluster_center_result(model, x,speaker):
- """x: np.array [t, 256]"""
- predict = model[speaker].predict(x)
- return model[speaker].cluster_centers_[predict]
-
-def get_center(model, x,speaker):
- return model[speaker].cluster_centers_[x]
diff --git a/spaces/KHAMMAMKURRODU/ChatbotApplication/app.py b/spaces/KHAMMAMKURRODU/ChatbotApplication/app.py
deleted file mode 100644
index a362dcc7d0ddd1eee86961f1bc3db6d894fbd3d5..0000000000000000000000000000000000000000
--- a/spaces/KHAMMAMKURRODU/ChatbotApplication/app.py
+++ /dev/null
@@ -1,34 +0,0 @@
-import os
-import gradio as gr
-from langchain.chat_models import ChatOpenAI
-from langchain import LLMChain, PromptTemplate
-from langchain.memory import ConversationBufferMemory
-
-OPENAI_API_KEY=os.getenv('OPENAI_API_KEY')
-
-template = """You are a helpful assistant to answer all user queries.
-{chat_history}
-User: {user_message}
-Chatbot:"""
-
-prompt = PromptTemplate(
- input_variables=["chat_history", "user_message"], template=template
-)
-
-memory = ConversationBufferMemory(memory_key="chat_history")
-
-llm_chain = LLMChain(
- llm=ChatOpenAI(temperature='0.5', model_name="gpt-3.5-turbo"),
- prompt=prompt,
- verbose=True,
- memory=memory,
-)
-
-def get_text_response(user_message,history):
- response = llm_chain.predict(user_message = user_message)
- return response
-
-demo = gr.ChatInterface(get_text_response)
-
-if __name__ == "__main__":
- demo.launch() #To create a public link, set `share=True` in `launch()`. To enable errors and logs, set `debug=True` in `launch()`.
diff --git a/spaces/KenjieDec/RemBG/README.md b/spaces/KenjieDec/RemBG/README.md
deleted file mode 100644
index d6ffead946a387191235aed8e911e08d19729c0a..0000000000000000000000000000000000000000
--- a/spaces/KenjieDec/RemBG/README.md
+++ /dev/null
@@ -1,12 +0,0 @@
----
-title: Rembg
-emoji: 👀
-colorFrom: pink
-colorTo: indigo
-sdk: gradio
-sdk_version: 3.0.20
-app_file: app.py
-pinned: false
----
-
-Check out the configuration reference at https://huggingface.co/docs/hub/spaces-config-reference
diff --git a/spaces/Kevin676/AutoGPT/tests/local_cache_test.py b/spaces/Kevin676/AutoGPT/tests/local_cache_test.py
deleted file mode 100644
index bb10862656bb500f319ac231ff5bd5438d6fe7e2..0000000000000000000000000000000000000000
--- a/spaces/Kevin676/AutoGPT/tests/local_cache_test.py
+++ /dev/null
@@ -1,67 +0,0 @@
-# sourcery skip: snake-case-functions
-"""Tests for LocalCache class"""
-import os
-import sys
-import unittest
-
-import pytest
-
-from autogpt.memory.local import LocalCache
-
-
-def mock_config() -> dict:
- """Mock the Config class"""
- return type(
- "MockConfig",
- (object,),
- {
- "debug_mode": False,
- "continuous_mode": False,
- "speak_mode": False,
- "memory_index": "auto-gpt",
- },
- )
-
-
-@pytest.mark.integration_test
-class TestLocalCache(unittest.TestCase):
- """Tests for LocalCache class"""
-
- def setUp(self) -> None:
- """Set up the test environment"""
- self.cfg = mock_config()
- self.cache = LocalCache(self.cfg)
-
- def test_add(self) -> None:
- """Test adding a text to the cache"""
- text = "Sample text"
- self.cache.add(text)
- self.assertIn(text, self.cache.data.texts)
-
- def test_clear(self) -> None:
- """Test clearing the cache"""
- self.cache.clear()
- self.assertEqual(self.cache.data.texts, [])
-
- def test_get(self) -> None:
- """Test getting a text from the cache"""
- text = "Sample text"
- self.cache.add(text)
- result = self.cache.get(text)
- self.assertEqual(result, [text])
-
- def test_get_relevant(self) -> None:
- """Test getting relevant texts from the cache"""
- text1 = "Sample text 1"
- text2 = "Sample text 2"
- self.cache.add(text1)
- self.cache.add(text2)
- result = self.cache.get_relevant(text1, 1)
- self.assertEqual(result, [text1])
-
- def test_get_stats(self) -> None:
- """Test getting the cache stats"""
- text = "Sample text"
- self.cache.add(text)
- stats = self.cache.get_stats()
- self.assertEqual(stats, (4, self.cache.data.embeddings.shape))
diff --git a/spaces/Kevin676/midjourney-v5/app.py b/spaces/Kevin676/midjourney-v5/app.py
deleted file mode 100644
index a7e777fc5c7f3e31a491e4bd016b8948b6a260f4..0000000000000000000000000000000000000000
--- a/spaces/Kevin676/midjourney-v5/app.py
+++ /dev/null
@@ -1,3 +0,0 @@
-import gradio as gr
-
-gr.Interface.load("models/flax/midjourney-v4-diffusion").launch()
\ No newline at end of file
diff --git a/spaces/KyanChen/RSPrompter/mmdet/models/data_preprocessors/data_preprocessor.py b/spaces/KyanChen/RSPrompter/mmdet/models/data_preprocessors/data_preprocessor.py
deleted file mode 100644
index 5dbd68c01f186a1a1bbd9546bc86bd648abaf90a..0000000000000000000000000000000000000000
--- a/spaces/KyanChen/RSPrompter/mmdet/models/data_preprocessors/data_preprocessor.py
+++ /dev/null
@@ -1,793 +0,0 @@
-# Copyright (c) OpenMMLab. All rights reserved.
-import random
-from numbers import Number
-from typing import List, Optional, Sequence, Tuple, Union
-
-import numpy as np
-import torch
-import torch.nn as nn
-import torch.nn.functional as F
-from mmengine.dist import barrier, broadcast, get_dist_info
-from mmengine.logging import MessageHub
-from mmengine.model import BaseDataPreprocessor, ImgDataPreprocessor
-from mmengine.structures import PixelData
-from mmengine.utils import is_seq_of
-from torch import Tensor
-
-from mmdet.models.utils import unfold_wo_center
-from mmdet.models.utils.misc import samplelist_boxtype2tensor
-from mmdet.registry import MODELS
-from mmdet.structures import DetDataSample
-from mmdet.structures.mask import BitmapMasks
-from mmdet.utils import ConfigType
-
-try:
- import skimage
-except ImportError:
- skimage = None
-
-
-@MODELS.register_module()
-class DetDataPreprocessor(ImgDataPreprocessor):
- """Image pre-processor for detection tasks.
-
- Comparing with the :class:`mmengine.ImgDataPreprocessor`,
-
- 1. It supports batch augmentations.
- 2. It will additionally append batch_input_shape and pad_shape
- to data_samples considering the object detection task.
-
- It provides the data pre-processing as follows
-
- - Collate and move data to the target device.
- - Pad inputs to the maximum size of current batch with defined
- ``pad_value``. The padding size can be divisible by a defined
- ``pad_size_divisor``
- - Stack inputs to batch_inputs.
- - Convert inputs from bgr to rgb if the shape of input is (3, H, W).
- - Normalize image with defined std and mean.
- - Do batch augmentations during training.
-
- Args:
- mean (Sequence[Number], optional): The pixel mean of R, G, B channels.
- Defaults to None.
- std (Sequence[Number], optional): The pixel standard deviation of
- R, G, B channels. Defaults to None.
- pad_size_divisor (int): The size of padded image should be
- divisible by ``pad_size_divisor``. Defaults to 1.
- pad_value (Number): The padded pixel value. Defaults to 0.
- pad_mask (bool): Whether to pad instance masks. Defaults to False.
- mask_pad_value (int): The padded pixel value for instance masks.
- Defaults to 0.
- pad_seg (bool): Whether to pad semantic segmentation maps.
- Defaults to False.
- seg_pad_value (int): The padded pixel value for semantic
- segmentation maps. Defaults to 255.
- bgr_to_rgb (bool): whether to convert image from BGR to RGB.
- Defaults to False.
- rgb_to_bgr (bool): whether to convert image from RGB to RGB.
- Defaults to False.
- boxtype2tensor (bool): Whether to keep the ``BaseBoxes`` type of
- bboxes data or not. Defaults to True.
- non_blocking (bool): Whether block current process
- when transferring data to device. Defaults to False.
- batch_augments (list[dict], optional): Batch-level augmentations
- """
-
- def __init__(self,
- mean: Sequence[Number] = None,
- std: Sequence[Number] = None,
- pad_size_divisor: int = 1,
- pad_value: Union[float, int] = 0,
- pad_mask: bool = False,
- mask_pad_value: int = 0,
- pad_seg: bool = False,
- seg_pad_value: int = 255,
- bgr_to_rgb: bool = False,
- rgb_to_bgr: bool = False,
- boxtype2tensor: bool = True,
- non_blocking: Optional[bool] = False,
- batch_augments: Optional[List[dict]] = None):
- super().__init__(
- mean=mean,
- std=std,
- pad_size_divisor=pad_size_divisor,
- pad_value=pad_value,
- bgr_to_rgb=bgr_to_rgb,
- rgb_to_bgr=rgb_to_bgr,
- non_blocking=non_blocking)
- if batch_augments is not None:
- self.batch_augments = nn.ModuleList(
- [MODELS.build(aug) for aug in batch_augments])
- else:
- self.batch_augments = None
- self.pad_mask = pad_mask
- self.mask_pad_value = mask_pad_value
- self.pad_seg = pad_seg
- self.seg_pad_value = seg_pad_value
- self.boxtype2tensor = boxtype2tensor
-
- def forward(self, data: dict, training: bool = False) -> dict:
- """Perform normalization、padding and bgr2rgb conversion based on
- ``BaseDataPreprocessor``.
-
- Args:
- data (dict): Data sampled from dataloader.
- training (bool): Whether to enable training time augmentation.
-
- Returns:
- dict: Data in the same format as the model input.
- """
- batch_pad_shape = self._get_pad_shape(data)
- data = super().forward(data=data, training=training)
- inputs, data_samples = data['inputs'], data['data_samples']
-
- if data_samples is not None:
- # NOTE the batched image size information may be useful, e.g.
- # in DETR, this is needed for the construction of masks, which is
- # then used for the transformer_head.
- batch_input_shape = tuple(inputs[0].size()[-2:])
- for data_sample, pad_shape in zip(data_samples, batch_pad_shape):
- data_sample.set_metainfo({
- 'batch_input_shape': batch_input_shape,
- 'pad_shape': pad_shape
- })
-
- if self.boxtype2tensor:
- samplelist_boxtype2tensor(data_samples)
-
- if self.pad_mask and training:
- self.pad_gt_masks(data_samples)
-
- if self.pad_seg and training:
- self.pad_gt_sem_seg(data_samples)
-
- if training and self.batch_augments is not None:
- for batch_aug in self.batch_augments:
- inputs, data_samples = batch_aug(inputs, data_samples)
-
- return {'inputs': inputs, 'data_samples': data_samples}
-
- def _get_pad_shape(self, data: dict) -> List[tuple]:
- """Get the pad_shape of each image based on data and
- pad_size_divisor."""
- _batch_inputs = data['inputs']
- # Process data with `pseudo_collate`.
- if is_seq_of(_batch_inputs, torch.Tensor):
- batch_pad_shape = []
- for ori_input in _batch_inputs:
- pad_h = int(
- np.ceil(ori_input.shape[1] /
- self.pad_size_divisor)) * self.pad_size_divisor
- pad_w = int(
- np.ceil(ori_input.shape[2] /
- self.pad_size_divisor)) * self.pad_size_divisor
- batch_pad_shape.append((pad_h, pad_w))
- # Process data with `default_collate`.
- elif isinstance(_batch_inputs, torch.Tensor):
- assert _batch_inputs.dim() == 4, (
- 'The input of `ImgDataPreprocessor` should be a NCHW tensor '
- 'or a list of tensor, but got a tensor with shape: '
- f'{_batch_inputs.shape}')
- pad_h = int(
- np.ceil(_batch_inputs.shape[1] /
- self.pad_size_divisor)) * self.pad_size_divisor
- pad_w = int(
- np.ceil(_batch_inputs.shape[2] /
- self.pad_size_divisor)) * self.pad_size_divisor
- batch_pad_shape = [(pad_h, pad_w)] * _batch_inputs.shape[0]
- else:
- raise TypeError('Output of `cast_data` should be a dict '
- 'or a tuple with inputs and data_samples, but got'
- f'{type(data)}: {data}')
- return batch_pad_shape
-
- def pad_gt_masks(self,
- batch_data_samples: Sequence[DetDataSample]) -> None:
- """Pad gt_masks to shape of batch_input_shape."""
- if 'masks' in batch_data_samples[0].gt_instances:
- for data_samples in batch_data_samples:
- masks = data_samples.gt_instances.masks
- data_samples.gt_instances.masks = masks.pad(
- data_samples.batch_input_shape,
- pad_val=self.mask_pad_value)
-
- def pad_gt_sem_seg(self,
- batch_data_samples: Sequence[DetDataSample]) -> None:
- """Pad gt_sem_seg to shape of batch_input_shape."""
- if 'gt_sem_seg' in batch_data_samples[0]:
- for data_samples in batch_data_samples:
- gt_sem_seg = data_samples.gt_sem_seg.sem_seg
- h, w = gt_sem_seg.shape[-2:]
- pad_h, pad_w = data_samples.batch_input_shape
- gt_sem_seg = F.pad(
- gt_sem_seg,
- pad=(0, max(pad_w - w, 0), 0, max(pad_h - h, 0)),
- mode='constant',
- value=self.seg_pad_value)
- data_samples.gt_sem_seg = PixelData(sem_seg=gt_sem_seg)
-
-
-@MODELS.register_module()
-class BatchSyncRandomResize(nn.Module):
- """Batch random resize which synchronizes the random size across ranks.
-
- Args:
- random_size_range (tuple): The multi-scale random range during
- multi-scale training.
- interval (int): The iter interval of change
- image size. Defaults to 10.
- size_divisor (int): Image size divisible factor.
- Defaults to 32.
- """
-
- def __init__(self,
- random_size_range: Tuple[int, int],
- interval: int = 10,
- size_divisor: int = 32) -> None:
- super().__init__()
- self.rank, self.world_size = get_dist_info()
- self._input_size = None
- self._random_size_range = (round(random_size_range[0] / size_divisor),
- round(random_size_range[1] / size_divisor))
- self._interval = interval
- self._size_divisor = size_divisor
-
- def forward(
- self, inputs: Tensor, data_samples: List[DetDataSample]
- ) -> Tuple[Tensor, List[DetDataSample]]:
- """resize a batch of images and bboxes to shape ``self._input_size``"""
- h, w = inputs.shape[-2:]
- if self._input_size is None:
- self._input_size = (h, w)
- scale_y = self._input_size[0] / h
- scale_x = self._input_size[1] / w
- if scale_x != 1 or scale_y != 1:
- inputs = F.interpolate(
- inputs,
- size=self._input_size,
- mode='bilinear',
- align_corners=False)
- for data_sample in data_samples:
- img_shape = (int(data_sample.img_shape[0] * scale_y),
- int(data_sample.img_shape[1] * scale_x))
- pad_shape = (int(data_sample.pad_shape[0] * scale_y),
- int(data_sample.pad_shape[1] * scale_x))
- data_sample.set_metainfo({
- 'img_shape': img_shape,
- 'pad_shape': pad_shape,
- 'batch_input_shape': self._input_size
- })
- data_sample.gt_instances.bboxes[
- ...,
- 0::2] = data_sample.gt_instances.bboxes[...,
- 0::2] * scale_x
- data_sample.gt_instances.bboxes[
- ...,
- 1::2] = data_sample.gt_instances.bboxes[...,
- 1::2] * scale_y
- if 'ignored_instances' in data_sample:
- data_sample.ignored_instances.bboxes[
- ..., 0::2] = data_sample.ignored_instances.bboxes[
- ..., 0::2] * scale_x
- data_sample.ignored_instances.bboxes[
- ..., 1::2] = data_sample.ignored_instances.bboxes[
- ..., 1::2] * scale_y
- message_hub = MessageHub.get_current_instance()
- if (message_hub.get_info('iter') + 1) % self._interval == 0:
- self._input_size = self._get_random_size(
- aspect_ratio=float(w / h), device=inputs.device)
- return inputs, data_samples
-
- def _get_random_size(self, aspect_ratio: float,
- device: torch.device) -> Tuple[int, int]:
- """Randomly generate a shape in ``_random_size_range`` and broadcast to
- all ranks."""
- tensor = torch.LongTensor(2).to(device)
- if self.rank == 0:
- size = random.randint(*self._random_size_range)
- size = (self._size_divisor * size,
- self._size_divisor * int(aspect_ratio * size))
- tensor[0] = size[0]
- tensor[1] = size[1]
- barrier()
- broadcast(tensor, 0)
- input_size = (tensor[0].item(), tensor[1].item())
- return input_size
-
-
-@MODELS.register_module()
-class BatchFixedSizePad(nn.Module):
- """Fixed size padding for batch images.
-
- Args:
- size (Tuple[int, int]): Fixed padding size. Expected padding
- shape (h, w). Defaults to None.
- img_pad_value (int): The padded pixel value for images.
- Defaults to 0.
- pad_mask (bool): Whether to pad instance masks. Defaults to False.
- mask_pad_value (int): The padded pixel value for instance masks.
- Defaults to 0.
- pad_seg (bool): Whether to pad semantic segmentation maps.
- Defaults to False.
- seg_pad_value (int): The padded pixel value for semantic
- segmentation maps. Defaults to 255.
- """
-
- def __init__(self,
- size: Tuple[int, int],
- img_pad_value: int = 0,
- pad_mask: bool = False,
- mask_pad_value: int = 0,
- pad_seg: bool = False,
- seg_pad_value: int = 255) -> None:
- super().__init__()
- self.size = size
- self.pad_mask = pad_mask
- self.pad_seg = pad_seg
- self.img_pad_value = img_pad_value
- self.mask_pad_value = mask_pad_value
- self.seg_pad_value = seg_pad_value
-
- def forward(
- self,
- inputs: Tensor,
- data_samples: Optional[List[dict]] = None
- ) -> Tuple[Tensor, Optional[List[dict]]]:
- """Pad image, instance masks, segmantic segmentation maps."""
- src_h, src_w = inputs.shape[-2:]
- dst_h, dst_w = self.size
-
- if src_h >= dst_h and src_w >= dst_w:
- return inputs, data_samples
-
- inputs = F.pad(
- inputs,
- pad=(0, max(0, dst_w - src_w), 0, max(0, dst_h - src_h)),
- mode='constant',
- value=self.img_pad_value)
-
- if data_samples is not None:
- # update batch_input_shape
- for data_sample in data_samples:
- data_sample.set_metainfo({
- 'batch_input_shape': (dst_h, dst_w),
- 'pad_shape': (dst_h, dst_w)
- })
-
- if self.pad_mask:
- for data_sample in data_samples:
- masks = data_sample.gt_instances.masks
- data_sample.gt_instances.masks = masks.pad(
- (dst_h, dst_w), pad_val=self.mask_pad_value)
-
- if self.pad_seg:
- for data_sample in data_samples:
- gt_sem_seg = data_sample.gt_sem_seg.sem_seg
- h, w = gt_sem_seg.shape[-2:]
- gt_sem_seg = F.pad(
- gt_sem_seg,
- pad=(0, max(0, dst_w - w), 0, max(0, dst_h - h)),
- mode='constant',
- value=self.seg_pad_value)
- data_sample.gt_sem_seg = PixelData(sem_seg=gt_sem_seg)
-
- return inputs, data_samples
-
-
-@MODELS.register_module()
-class MultiBranchDataPreprocessor(BaseDataPreprocessor):
- """DataPreprocessor wrapper for multi-branch data.
-
- Take semi-supervised object detection as an example, assume that
- the ratio of labeled data and unlabeled data in a batch is 1:2,
- `sup` indicates the branch where the labeled data is augmented,
- `unsup_teacher` and `unsup_student` indicate the branches where
- the unlabeled data is augmented by different pipeline.
-
- The input format of multi-branch data is shown as below :
-
- .. code-block:: none
- {
- 'inputs':
- {
- 'sup': [Tensor, None, None],
- 'unsup_teacher': [None, Tensor, Tensor],
- 'unsup_student': [None, Tensor, Tensor],
- },
- 'data_sample':
- {
- 'sup': [DetDataSample, None, None],
- 'unsup_teacher': [None, DetDataSample, DetDataSample],
- 'unsup_student': [NOne, DetDataSample, DetDataSample],
- }
- }
-
- The format of multi-branch data
- after filtering None is shown as below :
-
- .. code-block:: none
- {
- 'inputs':
- {
- 'sup': [Tensor],
- 'unsup_teacher': [Tensor, Tensor],
- 'unsup_student': [Tensor, Tensor],
- },
- 'data_sample':
- {
- 'sup': [DetDataSample],
- 'unsup_teacher': [DetDataSample, DetDataSample],
- 'unsup_student': [DetDataSample, DetDataSample],
- }
- }
-
- In order to reuse `DetDataPreprocessor` for the data
- from different branches, the format of multi-branch data
- grouped by branch is as below :
-
- .. code-block:: none
- {
- 'sup':
- {
- 'inputs': [Tensor]
- 'data_sample': [DetDataSample, DetDataSample]
- },
- 'unsup_teacher':
- {
- 'inputs': [Tensor, Tensor]
- 'data_sample': [DetDataSample, DetDataSample]
- },
- 'unsup_student':
- {
- 'inputs': [Tensor, Tensor]
- 'data_sample': [DetDataSample, DetDataSample]
- },
- }
-
- After preprocessing data from different branches,
- the multi-branch data needs to be reformatted as:
-
- .. code-block:: none
- {
- 'inputs':
- {
- 'sup': [Tensor],
- 'unsup_teacher': [Tensor, Tensor],
- 'unsup_student': [Tensor, Tensor],
- },
- 'data_sample':
- {
- 'sup': [DetDataSample],
- 'unsup_teacher': [DetDataSample, DetDataSample],
- 'unsup_student': [DetDataSample, DetDataSample],
- }
- }
-
- Args:
- data_preprocessor (:obj:`ConfigDict` or dict): Config of
- :class:`DetDataPreprocessor` to process the input data.
- """
-
- def __init__(self, data_preprocessor: ConfigType) -> None:
- super().__init__()
- self.data_preprocessor = MODELS.build(data_preprocessor)
-
- def forward(self, data: dict, training: bool = False) -> dict:
- """Perform normalization、padding and bgr2rgb conversion based on
- ``BaseDataPreprocessor`` for multi-branch data.
-
- Args:
- data (dict): Data sampled from dataloader.
- training (bool): Whether to enable training time augmentation.
-
- Returns:
- dict:
-
- - 'inputs' (Dict[str, obj:`torch.Tensor`]): The forward data of
- models from different branches.
- - 'data_sample' (Dict[str, obj:`DetDataSample`]): The annotation
- info of the sample from different branches.
- """
-
- if training is False:
- return self.data_preprocessor(data, training)
-
- # Filter out branches with a value of None
- for key in data.keys():
- for branch in data[key].keys():
- data[key][branch] = list(
- filter(lambda x: x is not None, data[key][branch]))
-
- # Group data by branch
- multi_branch_data = {}
- for key in data.keys():
- for branch in data[key].keys():
- if multi_branch_data.get(branch, None) is None:
- multi_branch_data[branch] = {key: data[key][branch]}
- elif multi_branch_data[branch].get(key, None) is None:
- multi_branch_data[branch][key] = data[key][branch]
- else:
- multi_branch_data[branch][key].append(data[key][branch])
-
- # Preprocess data from different branches
- for branch, _data in multi_branch_data.items():
- multi_branch_data[branch] = self.data_preprocessor(_data, training)
-
- # Format data by inputs and data_samples
- format_data = {}
- for branch in multi_branch_data.keys():
- for key in multi_branch_data[branch].keys():
- if format_data.get(key, None) is None:
- format_data[key] = {branch: multi_branch_data[branch][key]}
- elif format_data[key].get(branch, None) is None:
- format_data[key][branch] = multi_branch_data[branch][key]
- else:
- format_data[key][branch].append(
- multi_branch_data[branch][key])
-
- return format_data
-
- @property
- def device(self):
- return self.data_preprocessor.device
-
- def to(self, device: Optional[Union[int, torch.device]], *args,
- **kwargs) -> nn.Module:
- """Overrides this method to set the :attr:`device`
-
- Args:
- device (int or torch.device, optional): The desired device of the
- parameters and buffers in this module.
-
- Returns:
- nn.Module: The model itself.
- """
-
- return self.data_preprocessor.to(device, *args, **kwargs)
-
- def cuda(self, *args, **kwargs) -> nn.Module:
- """Overrides this method to set the :attr:`device`
-
- Returns:
- nn.Module: The model itself.
- """
-
- return self.data_preprocessor.cuda(*args, **kwargs)
-
- def cpu(self, *args, **kwargs) -> nn.Module:
- """Overrides this method to set the :attr:`device`
-
- Returns:
- nn.Module: The model itself.
- """
-
- return self.data_preprocessor.cpu(*args, **kwargs)
-
-
-@MODELS.register_module()
-class BatchResize(nn.Module):
- """Batch resize during training. This implementation is modified from
- https://github.com/Purkialo/CrowdDet/blob/master/lib/data/CrowdHuman.py.
-
- It provides the data pre-processing as follows:
- - A batch of all images will pad to a uniform size and stack them into
- a torch.Tensor by `DetDataPreprocessor`.
- - `BatchFixShapeResize` resize all images to the target size.
- - Padding images to make sure the size of image can be divisible by
- ``pad_size_divisor``.
-
- Args:
- scale (tuple): Images scales for resizing.
- pad_size_divisor (int): Image size divisible factor.
- Defaults to 1.
- pad_value (Number): The padded pixel value. Defaults to 0.
- """
-
- def __init__(
- self,
- scale: tuple,
- pad_size_divisor: int = 1,
- pad_value: Union[float, int] = 0,
- ) -> None:
- super().__init__()
- self.min_size = min(scale)
- self.max_size = max(scale)
- self.pad_size_divisor = pad_size_divisor
- self.pad_value = pad_value
-
- def forward(
- self, inputs: Tensor, data_samples: List[DetDataSample]
- ) -> Tuple[Tensor, List[DetDataSample]]:
- """resize a batch of images and bboxes."""
-
- batch_height, batch_width = inputs.shape[-2:]
- target_height, target_width, scale = self.get_target_size(
- batch_height, batch_width)
-
- inputs = F.interpolate(
- inputs,
- size=(target_height, target_width),
- mode='bilinear',
- align_corners=False)
-
- inputs = self.get_padded_tensor(inputs, self.pad_value)
-
- if data_samples is not None:
- batch_input_shape = tuple(inputs.size()[-2:])
- for data_sample in data_samples:
- img_shape = [
- int(scale * _) for _ in list(data_sample.img_shape)
- ]
- data_sample.set_metainfo({
- 'img_shape': tuple(img_shape),
- 'batch_input_shape': batch_input_shape,
- 'pad_shape': batch_input_shape,
- 'scale_factor': (scale, scale)
- })
-
- data_sample.gt_instances.bboxes *= scale
- data_sample.ignored_instances.bboxes *= scale
-
- return inputs, data_samples
-
- def get_target_size(self, height: int,
- width: int) -> Tuple[int, int, float]:
- """Get the target size of a batch of images based on data and scale."""
- im_size_min = np.min([height, width])
- im_size_max = np.max([height, width])
- scale = self.min_size / im_size_min
- if scale * im_size_max > self.max_size:
- scale = self.max_size / im_size_max
- target_height, target_width = int(round(height * scale)), int(
- round(width * scale))
- return target_height, target_width, scale
-
- def get_padded_tensor(self, tensor: Tensor, pad_value: int) -> Tensor:
- """Pad images according to pad_size_divisor."""
- assert tensor.ndim == 4
- target_height, target_width = tensor.shape[-2], tensor.shape[-1]
- divisor = self.pad_size_divisor
- padded_height = (target_height + divisor - 1) // divisor * divisor
- padded_width = (target_width + divisor - 1) // divisor * divisor
- padded_tensor = torch.ones([
- tensor.shape[0], tensor.shape[1], padded_height, padded_width
- ]) * pad_value
- padded_tensor = padded_tensor.type_as(tensor)
- padded_tensor[:, :, :target_height, :target_width] = tensor
- return padded_tensor
-
-
-@MODELS.register_module()
-class BoxInstDataPreprocessor(DetDataPreprocessor):
- """Pseudo mask pre-processor for BoxInst.
-
- Comparing with the :class:`mmdet.DetDataPreprocessor`,
-
- 1. It generates masks using box annotations.
- 2. It computes the images color similarity in LAB color space.
-
- Args:
- mask_stride (int): The mask output stride in boxinst. Defaults to 4.
- pairwise_size (int): The size of neighborhood for each pixel.
- Defaults to 3.
- pairwise_dilation (int): The dilation of neighborhood for each pixel.
- Defaults to 2.
- pairwise_color_thresh (float): The thresh of image color similarity.
- Defaults to 0.3.
- bottom_pixels_removed (int): The length of removed pixels in bottom.
- It is caused by the annotation error in coco dataset.
- Defaults to 10.
- """
-
- def __init__(self,
- *arg,
- mask_stride: int = 4,
- pairwise_size: int = 3,
- pairwise_dilation: int = 2,
- pairwise_color_thresh: float = 0.3,
- bottom_pixels_removed: int = 10,
- **kwargs) -> None:
- super().__init__(*arg, **kwargs)
- self.mask_stride = mask_stride
- self.pairwise_size = pairwise_size
- self.pairwise_dilation = pairwise_dilation
- self.pairwise_color_thresh = pairwise_color_thresh
- self.bottom_pixels_removed = bottom_pixels_removed
-
- if skimage is None:
- raise RuntimeError('skimage is not installed,\
- please install it by: pip install scikit-image')
-
- def get_images_color_similarity(self, inputs: Tensor,
- image_masks: Tensor) -> Tensor:
- """Compute the image color similarity in LAB color space."""
- assert inputs.dim() == 4
- assert inputs.size(0) == 1
-
- unfolded_images = unfold_wo_center(
- inputs,
- kernel_size=self.pairwise_size,
- dilation=self.pairwise_dilation)
- diff = inputs[:, :, None] - unfolded_images
- similarity = torch.exp(-torch.norm(diff, dim=1) * 0.5)
-
- unfolded_weights = unfold_wo_center(
- image_masks[None, None],
- kernel_size=self.pairwise_size,
- dilation=self.pairwise_dilation)
- unfolded_weights = torch.max(unfolded_weights, dim=1)[0]
-
- return similarity * unfolded_weights
-
- def forward(self, data: dict, training: bool = False) -> dict:
- """Get pseudo mask labels using color similarity."""
- det_data = super().forward(data, training)
- inputs, data_samples = det_data['inputs'], det_data['data_samples']
-
- if training:
- # get image masks and remove bottom pixels
- b_img_h, b_img_w = data_samples[0].batch_input_shape
- img_masks = []
- for i in range(inputs.shape[0]):
- img_h, img_w = data_samples[i].img_shape
- img_mask = inputs.new_ones((img_h, img_w))
- pixels_removed = int(self.bottom_pixels_removed *
- float(img_h) / float(b_img_h))
- if pixels_removed > 0:
- img_mask[-pixels_removed:, :] = 0
- pad_w = b_img_w - img_w
- pad_h = b_img_h - img_h
- img_mask = F.pad(img_mask, (0, pad_w, 0, pad_h), 'constant',
- 0.)
- img_masks.append(img_mask)
- img_masks = torch.stack(img_masks, dim=0)
- start = int(self.mask_stride // 2)
- img_masks = img_masks[:, start::self.mask_stride,
- start::self.mask_stride]
-
- # Get origin rgb image for color similarity
- ori_imgs = inputs * self.std + self.mean
- downsampled_imgs = F.avg_pool2d(
- ori_imgs.float(),
- kernel_size=self.mask_stride,
- stride=self.mask_stride,
- padding=0)
-
- # Compute color similarity for pseudo mask generation
- for im_i, data_sample in enumerate(data_samples):
- # TODO: Support rgb2lab in mmengine?
- images_lab = skimage.color.rgb2lab(
- downsampled_imgs[im_i].byte().permute(1, 2,
- 0).cpu().numpy())
- images_lab = torch.as_tensor(
- images_lab, device=ori_imgs.device, dtype=torch.float32)
- images_lab = images_lab.permute(2, 0, 1)[None]
- images_color_similarity = self.get_images_color_similarity(
- images_lab, img_masks[im_i])
- pairwise_mask = (images_color_similarity >=
- self.pairwise_color_thresh).float()
-
- per_im_bboxes = data_sample.gt_instances.bboxes
- if per_im_bboxes.shape[0] > 0:
- per_im_masks = []
- for per_box in per_im_bboxes:
- mask_full = torch.zeros((b_img_h, b_img_w),
- device=self.device).float()
- mask_full[int(per_box[1]):int(per_box[3] + 1),
- int(per_box[0]):int(per_box[2] + 1)] = 1.0
- per_im_masks.append(mask_full)
- per_im_masks = torch.stack(per_im_masks, dim=0)
- pairwise_masks = torch.cat(
- [pairwise_mask for _ in range(per_im_bboxes.shape[0])],
- dim=0)
- else:
- per_im_masks = torch.zeros((0, b_img_h, b_img_w))
- pairwise_masks = torch.zeros(
- (0, self.pairwise_size**2 - 1, b_img_h, b_img_w))
-
- # TODO: Support BitmapMasks with tensor?
- data_sample.gt_instances.masks = BitmapMasks(
- per_im_masks.cpu().numpy(), b_img_h, b_img_w)
- data_sample.gt_instances.pairwise_masks = pairwise_masks
- return {'inputs': inputs, 'data_samples': data_samples}
diff --git a/spaces/KyanChen/RSPrompter/mmdet/models/layers/transformer/conditional_detr_layers.py b/spaces/KyanChen/RSPrompter/mmdet/models/layers/transformer/conditional_detr_layers.py
deleted file mode 100644
index 6db12a1340c758996e8c0e96f0b21cbc6fa928c9..0000000000000000000000000000000000000000
--- a/spaces/KyanChen/RSPrompter/mmdet/models/layers/transformer/conditional_detr_layers.py
+++ /dev/null
@@ -1,170 +0,0 @@
-# Copyright (c) OpenMMLab. All rights reserved.
-import torch
-from mmcv.cnn import build_norm_layer
-from mmcv.cnn.bricks.transformer import FFN
-from torch import Tensor
-from torch.nn import ModuleList
-
-from .detr_layers import DetrTransformerDecoder, DetrTransformerDecoderLayer
-from .utils import MLP, ConditionalAttention, coordinate_to_encoding
-
-
-class ConditionalDetrTransformerDecoder(DetrTransformerDecoder):
- """Decoder of Conditional DETR."""
-
- def _init_layers(self) -> None:
- """Initialize decoder layers and other layers."""
- self.layers = ModuleList([
- ConditionalDetrTransformerDecoderLayer(**self.layer_cfg)
- for _ in range(self.num_layers)
- ])
- self.embed_dims = self.layers[0].embed_dims
- self.post_norm = build_norm_layer(self.post_norm_cfg,
- self.embed_dims)[1]
- # conditional detr affline
- self.query_scale = MLP(self.embed_dims, self.embed_dims,
- self.embed_dims, 2)
- self.ref_point_head = MLP(self.embed_dims, self.embed_dims, 2, 2)
- # we have substitute 'qpos_proj' with 'qpos_sine_proj' except for
- # the first decoder layer), so 'qpos_proj' should be deleted
- # in other layers.
- for layer_id in range(self.num_layers - 1):
- self.layers[layer_id + 1].cross_attn.qpos_proj = None
-
- def forward(self,
- query: Tensor,
- key: Tensor = None,
- query_pos: Tensor = None,
- key_pos: Tensor = None,
- key_padding_mask: Tensor = None):
- """Forward function of decoder.
-
- Args:
- query (Tensor): The input query with shape
- (bs, num_queries, dim).
- key (Tensor): The input key with shape (bs, num_keys, dim) If
- `None`, the `query` will be used. Defaults to `None`.
- query_pos (Tensor): The positional encoding for `query`, with the
- same shape as `query`. If not `None`, it will be added to
- `query` before forward function. Defaults to `None`.
- key_pos (Tensor): The positional encoding for `key`, with the
- same shape as `key`. If not `None`, it will be added to
- `key` before forward function. If `None`, and `query_pos`
- has the same shape as `key`, then `query_pos` will be used
- as `key_pos`. Defaults to `None`.
- key_padding_mask (Tensor): ByteTensor with shape (bs, num_keys).
- Defaults to `None`.
- Returns:
- List[Tensor]: forwarded results with shape (num_decoder_layers,
- bs, num_queries, dim) if `return_intermediate` is True, otherwise
- with shape (1, bs, num_queries, dim). References with shape
- (bs, num_queries, 2).
- """
- reference_unsigmoid = self.ref_point_head(
- query_pos) # [bs, num_queries, 2]
- reference = reference_unsigmoid.sigmoid()
- reference_xy = reference[..., :2]
- intermediate = []
- for layer_id, layer in enumerate(self.layers):
- if layer_id == 0:
- pos_transformation = 1
- else:
- pos_transformation = self.query_scale(query)
- # get sine embedding for the query reference
- ref_sine_embed = coordinate_to_encoding(coord_tensor=reference_xy)
- # apply transformation
- ref_sine_embed = ref_sine_embed * pos_transformation
- query = layer(
- query,
- key=key,
- query_pos=query_pos,
- key_pos=key_pos,
- key_padding_mask=key_padding_mask,
- ref_sine_embed=ref_sine_embed,
- is_first=(layer_id == 0))
- if self.return_intermediate:
- intermediate.append(self.post_norm(query))
-
- if self.return_intermediate:
- return torch.stack(intermediate), reference
-
- query = self.post_norm(query)
- return query.unsqueeze(0), reference
-
-
-class ConditionalDetrTransformerDecoderLayer(DetrTransformerDecoderLayer):
- """Implements decoder layer in Conditional DETR transformer."""
-
- def _init_layers(self):
- """Initialize self-attention, cross-attention, FFN, and
- normalization."""
- self.self_attn = ConditionalAttention(**self.self_attn_cfg)
- self.cross_attn = ConditionalAttention(**self.cross_attn_cfg)
- self.embed_dims = self.self_attn.embed_dims
- self.ffn = FFN(**self.ffn_cfg)
- norms_list = [
- build_norm_layer(self.norm_cfg, self.embed_dims)[1]
- for _ in range(3)
- ]
- self.norms = ModuleList(norms_list)
-
- def forward(self,
- query: Tensor,
- key: Tensor = None,
- query_pos: Tensor = None,
- key_pos: Tensor = None,
- self_attn_masks: Tensor = None,
- cross_attn_masks: Tensor = None,
- key_padding_mask: Tensor = None,
- ref_sine_embed: Tensor = None,
- is_first: bool = False):
- """
- Args:
- query (Tensor): The input query, has shape (bs, num_queries, dim)
- key (Tensor, optional): The input key, has shape (bs, num_keys,
- dim). If `None`, the `query` will be used. Defaults to `None`.
- query_pos (Tensor, optional): The positional encoding for `query`,
- has the same shape as `query`. If not `None`, it will be
- added to `query` before forward function. Defaults to `None`.
- ref_sine_embed (Tensor): The positional encoding for query in
- cross attention, with the same shape as `x`. Defaults to None.
- key_pos (Tensor, optional): The positional encoding for `key`, has
- the same shape as `key`. If not None, it will be added to
- `key` before forward function. If None, and `query_pos` has
- the same shape as `key`, then `query_pos` will be used for
- `key_pos`. Defaults to None.
- self_attn_masks (Tensor, optional): ByteTensor mask, has shape
- (num_queries, num_keys), Same in `nn.MultiheadAttention.
- forward`. Defaults to None.
- cross_attn_masks (Tensor, optional): ByteTensor mask, has shape
- (num_queries, num_keys), Same in `nn.MultiheadAttention.
- forward`. Defaults to None.
- key_padding_mask (Tensor, optional): ByteTensor, has shape
- (bs, num_keys). Defaults to None.
- is_first (bool): A indicator to tell whether the current layer
- is the first layer of the decoder. Defaults to False.
-
- Returns:
- Tensor: Forwarded results, has shape (bs, num_queries, dim).
- """
- query = self.self_attn(
- query=query,
- key=query,
- query_pos=query_pos,
- key_pos=query_pos,
- attn_mask=self_attn_masks)
- query = self.norms[0](query)
- query = self.cross_attn(
- query=query,
- key=key,
- query_pos=query_pos,
- key_pos=key_pos,
- attn_mask=cross_attn_masks,
- key_padding_mask=key_padding_mask,
- ref_sine_embed=ref_sine_embed,
- is_first=is_first)
- query = self.norms[1](query)
- query = self.ffn(query)
- query = self.norms[2](query)
-
- return query
diff --git a/spaces/KyanChen/RSPrompter/mmpretrain/datasets/__init__.py b/spaces/KyanChen/RSPrompter/mmpretrain/datasets/__init__.py
deleted file mode 100644
index b680fb83abbc4612fbb9a4a3d09ec6ce4de11460..0000000000000000000000000000000000000000
--- a/spaces/KyanChen/RSPrompter/mmpretrain/datasets/__init__.py
+++ /dev/null
@@ -1,54 +0,0 @@
-# Copyright (c) OpenMMLab. All rights reserved.
-from mmpretrain.utils.dependency import WITH_MULTIMODAL
-from .base_dataset import BaseDataset
-from .builder import build_dataset
-from .caltech101 import Caltech101
-from .cifar import CIFAR10, CIFAR100
-from .cub import CUB
-from .custom import CustomDataset
-from .dataset_wrappers import KFoldDataset
-from .dtd import DTD
-from .fgvcaircraft import FGVCAircraft
-from .flowers102 import Flowers102
-from .food101 import Food101
-from .imagenet import ImageNet, ImageNet21k
-from .inshop import InShop
-from .mnist import MNIST, FashionMNIST
-from .multi_label import MultiLabelDataset
-from .multi_task import MultiTaskDataset
-from .nlvr2 import NLVR2
-from .oxfordiiitpet import OxfordIIITPet
-from .places205 import Places205
-from .samplers import * # noqa: F401,F403
-from .stanfordcars import StanfordCars
-from .sun397 import SUN397
-from .transforms import * # noqa: F401,F403
-from .voc import VOC
-
-__all__ = [
- 'BaseDataset', 'CIFAR10', 'CIFAR100', 'CUB', 'Caltech101', 'CustomDataset',
- 'DTD', 'FGVCAircraft', 'FashionMNIST', 'Flowers102', 'Food101', 'ImageNet',
- 'ImageNet21k', 'InShop', 'KFoldDataset', 'MNIST', 'MultiLabelDataset',
- 'MultiTaskDataset', 'NLVR2', 'OxfordIIITPet', 'Places205', 'SUN397',
- 'StanfordCars', 'VOC', 'build_dataset'
-]
-
-if WITH_MULTIMODAL:
- from .coco_caption import COCOCaption
- from .coco_retrieval import COCORetrieval
- from .coco_vqa import COCOVQA
- from .flamingo import FlamingoEvalCOCOCaption, FlamingoEvalCOCOVQA
- from .refcoco import RefCOCO
- from .scienceqa import ScienceQA
- from .visual_genome import VisualGenomeQA
-
- __all__.extend([
- 'COCOCaption',
- 'COCORetrieval',
- 'COCOVQA',
- 'FlamingoEvalCOCOCaption',
- 'FlamingoEvalCOCOVQA',
- 'RefCOCO',
- 'VisualGenomeQA',
- 'ScienceQA',
- ])
diff --git a/spaces/LIHUI123/LIHUI123/Dockerfile b/spaces/LIHUI123/LIHUI123/Dockerfile
deleted file mode 100644
index 2a2905f149a91126cdc583a78faff545cd3958ee..0000000000000000000000000000000000000000
--- a/spaces/LIHUI123/LIHUI123/Dockerfile
+++ /dev/null
@@ -1,34 +0,0 @@
-# Build Stage
-# 使用 golang:alpine 作为构建阶段的基础镜像
-FROM golang:alpine AS builder
-
-# 添加 git,以便之后能从GitHub克隆项目
-RUN apk --no-cache add git
-
-# 从 GitHub 克隆 go-proxy-bingai 项目到 /workspace/app 目录下
-RUN git clone https://github.com/Harry-zklcdc/go-proxy-bingai.git /workspace/app
-
-#设置工作目录为之前克隆的项目目录
-WORKDIR /workspace/app
-
-#编译go项目。-ldflags="-s -w"是为了减少编译后的二进制大小
-RUN go build -ldflags="-s -w" -tags netgo -trimpath -o go-proxy-bingai main.go
-
-#Runtime Stage
-#使用轻量级的alpine镜像作为运行室的基础镜像
-FROM alpine
-
-#设置工作目录
-WORKDIR /workspace/app
-
-#从构建阶段复制编译后的二进制文件到运行时镜像中
-COPY --from=builder /workspace/app/go-proxy-bingai .
-
-#设置环境变量,此处为随机字符
-ENV Go_proxy_BingAI_USER_TOKEN_1="kjs8hD92ncmzlaoQWYtx5rG6bE4fz5i0"
-
-#暴露8080端口
-EXPOSE 8080
-
-#容器启动时运行的命令
-CMD ["/workspace/app/go-proxy-bingai"]
\ No newline at end of file
diff --git a/spaces/LZRi/LZR-Bert-VITS2/transforms.py b/spaces/LZRi/LZR-Bert-VITS2/transforms.py
deleted file mode 100644
index 4793d67ca5a5630e0ffe0f9fb29445c949e64dae..0000000000000000000000000000000000000000
--- a/spaces/LZRi/LZR-Bert-VITS2/transforms.py
+++ /dev/null
@@ -1,193 +0,0 @@
-import torch
-from torch.nn import functional as F
-
-import numpy as np
-
-
-DEFAULT_MIN_BIN_WIDTH = 1e-3
-DEFAULT_MIN_BIN_HEIGHT = 1e-3
-DEFAULT_MIN_DERIVATIVE = 1e-3
-
-
-def piecewise_rational_quadratic_transform(inputs,
- unnormalized_widths,
- unnormalized_heights,
- unnormalized_derivatives,
- inverse=False,
- tails=None,
- tail_bound=1.,
- min_bin_width=DEFAULT_MIN_BIN_WIDTH,
- min_bin_height=DEFAULT_MIN_BIN_HEIGHT,
- min_derivative=DEFAULT_MIN_DERIVATIVE):
-
- if tails is None:
- spline_fn = rational_quadratic_spline
- spline_kwargs = {}
- else:
- spline_fn = unconstrained_rational_quadratic_spline
- spline_kwargs = {
- 'tails': tails,
- 'tail_bound': tail_bound
- }
-
- outputs, logabsdet = spline_fn(
- inputs=inputs,
- unnormalized_widths=unnormalized_widths,
- unnormalized_heights=unnormalized_heights,
- unnormalized_derivatives=unnormalized_derivatives,
- inverse=inverse,
- min_bin_width=min_bin_width,
- min_bin_height=min_bin_height,
- min_derivative=min_derivative,
- **spline_kwargs
- )
- return outputs, logabsdet
-
-
-def searchsorted(bin_locations, inputs, eps=1e-6):
- bin_locations[..., -1] += eps
- return torch.sum(
- inputs[..., None] >= bin_locations,
- dim=-1
- ) - 1
-
-
-def unconstrained_rational_quadratic_spline(inputs,
- unnormalized_widths,
- unnormalized_heights,
- unnormalized_derivatives,
- inverse=False,
- tails='linear',
- tail_bound=1.,
- min_bin_width=DEFAULT_MIN_BIN_WIDTH,
- min_bin_height=DEFAULT_MIN_BIN_HEIGHT,
- min_derivative=DEFAULT_MIN_DERIVATIVE):
- inside_interval_mask = (inputs >= -tail_bound) & (inputs <= tail_bound)
- outside_interval_mask = ~inside_interval_mask
-
- outputs = torch.zeros_like(inputs)
- logabsdet = torch.zeros_like(inputs)
-
- if tails == 'linear':
- unnormalized_derivatives = F.pad(unnormalized_derivatives, pad=(1, 1))
- constant = np.log(np.exp(1 - min_derivative) - 1)
- unnormalized_derivatives[..., 0] = constant
- unnormalized_derivatives[..., -1] = constant
-
- outputs[outside_interval_mask] = inputs[outside_interval_mask]
- logabsdet[outside_interval_mask] = 0
- else:
- raise RuntimeError('{} tails are not implemented.'.format(tails))
-
- outputs[inside_interval_mask], logabsdet[inside_interval_mask] = rational_quadratic_spline(
- inputs=inputs[inside_interval_mask],
- unnormalized_widths=unnormalized_widths[inside_interval_mask, :],
- unnormalized_heights=unnormalized_heights[inside_interval_mask, :],
- unnormalized_derivatives=unnormalized_derivatives[inside_interval_mask, :],
- inverse=inverse,
- left=-tail_bound, right=tail_bound, bottom=-tail_bound, top=tail_bound,
- min_bin_width=min_bin_width,
- min_bin_height=min_bin_height,
- min_derivative=min_derivative
- )
-
- return outputs, logabsdet
-
-def rational_quadratic_spline(inputs,
- unnormalized_widths,
- unnormalized_heights,
- unnormalized_derivatives,
- inverse=False,
- left=0., right=1., bottom=0., top=1.,
- min_bin_width=DEFAULT_MIN_BIN_WIDTH,
- min_bin_height=DEFAULT_MIN_BIN_HEIGHT,
- min_derivative=DEFAULT_MIN_DERIVATIVE):
- if torch.min(inputs) < left or torch.max(inputs) > right:
- raise ValueError('Input to a transform is not within its domain')
-
- num_bins = unnormalized_widths.shape[-1]
-
- if min_bin_width * num_bins > 1.0:
- raise ValueError('Minimal bin width too large for the number of bins')
- if min_bin_height * num_bins > 1.0:
- raise ValueError('Minimal bin height too large for the number of bins')
-
- widths = F.softmax(unnormalized_widths, dim=-1)
- widths = min_bin_width + (1 - min_bin_width * num_bins) * widths
- cumwidths = torch.cumsum(widths, dim=-1)
- cumwidths = F.pad(cumwidths, pad=(1, 0), mode='constant', value=0.0)
- cumwidths = (right - left) * cumwidths + left
- cumwidths[..., 0] = left
- cumwidths[..., -1] = right
- widths = cumwidths[..., 1:] - cumwidths[..., :-1]
-
- derivatives = min_derivative + F.softplus(unnormalized_derivatives)
-
- heights = F.softmax(unnormalized_heights, dim=-1)
- heights = min_bin_height + (1 - min_bin_height * num_bins) * heights
- cumheights = torch.cumsum(heights, dim=-1)
- cumheights = F.pad(cumheights, pad=(1, 0), mode='constant', value=0.0)
- cumheights = (top - bottom) * cumheights + bottom
- cumheights[..., 0] = bottom
- cumheights[..., -1] = top
- heights = cumheights[..., 1:] - cumheights[..., :-1]
-
- if inverse:
- bin_idx = searchsorted(cumheights, inputs)[..., None]
- else:
- bin_idx = searchsorted(cumwidths, inputs)[..., None]
-
- input_cumwidths = cumwidths.gather(-1, bin_idx)[..., 0]
- input_bin_widths = widths.gather(-1, bin_idx)[..., 0]
-
- input_cumheights = cumheights.gather(-1, bin_idx)[..., 0]
- delta = heights / widths
- input_delta = delta.gather(-1, bin_idx)[..., 0]
-
- input_derivatives = derivatives.gather(-1, bin_idx)[..., 0]
- input_derivatives_plus_one = derivatives[..., 1:].gather(-1, bin_idx)[..., 0]
-
- input_heights = heights.gather(-1, bin_idx)[..., 0]
-
- if inverse:
- a = (((inputs - input_cumheights) * (input_derivatives
- + input_derivatives_plus_one
- - 2 * input_delta)
- + input_heights * (input_delta - input_derivatives)))
- b = (input_heights * input_derivatives
- - (inputs - input_cumheights) * (input_derivatives
- + input_derivatives_plus_one
- - 2 * input_delta))
- c = - input_delta * (inputs - input_cumheights)
-
- discriminant = b.pow(2) - 4 * a * c
- assert (discriminant >= 0).all()
-
- root = (2 * c) / (-b - torch.sqrt(discriminant))
- outputs = root * input_bin_widths + input_cumwidths
-
- theta_one_minus_theta = root * (1 - root)
- denominator = input_delta + ((input_derivatives + input_derivatives_plus_one - 2 * input_delta)
- * theta_one_minus_theta)
- derivative_numerator = input_delta.pow(2) * (input_derivatives_plus_one * root.pow(2)
- + 2 * input_delta * theta_one_minus_theta
- + input_derivatives * (1 - root).pow(2))
- logabsdet = torch.log(derivative_numerator) - 2 * torch.log(denominator)
-
- return outputs, -logabsdet
- else:
- theta = (inputs - input_cumwidths) / input_bin_widths
- theta_one_minus_theta = theta * (1 - theta)
-
- numerator = input_heights * (input_delta * theta.pow(2)
- + input_derivatives * theta_one_minus_theta)
- denominator = input_delta + ((input_derivatives + input_derivatives_plus_one - 2 * input_delta)
- * theta_one_minus_theta)
- outputs = input_cumheights + numerator / denominator
-
- derivative_numerator = input_delta.pow(2) * (input_derivatives_plus_one * theta.pow(2)
- + 2 * input_delta * theta_one_minus_theta
- + input_derivatives * (1 - theta).pow(2))
- logabsdet = torch.log(derivative_numerator) - 2 * torch.log(denominator)
-
- return outputs, logabsdet
diff --git a/spaces/Laihiujin/OneFormer/oneformer/data/datasets/register_ade20k_instance.py b/spaces/Laihiujin/OneFormer/oneformer/data/datasets/register_ade20k_instance.py
deleted file mode 100644
index 0cf5466eb7e203de8c78679f622d30c902bd61d9..0000000000000000000000000000000000000000
--- a/spaces/Laihiujin/OneFormer/oneformer/data/datasets/register_ade20k_instance.py
+++ /dev/null
@@ -1,56 +0,0 @@
-# ------------------------------------------------------------------------------
-# Reference: https://github.com/facebookresearch/Mask2Former/blob/main/mask2former/data/datasets/register_ade20k_instance.py
-# ------------------------------------------------------------------------------
-
-import json
-import logging
-import numpy as np
-import os
-from PIL import Image
-
-from detectron2.data import DatasetCatalog, MetadataCatalog
-from detectron2.data.datasets.coco import load_coco_json, register_coco_instances
-from detectron2.utils.file_io import PathManager
-
-ADE_CATEGORIES = [{'id': 7, 'name': 'bed'}, {'id': 8, 'name': 'windowpane'}, {'id': 10, 'name': 'cabinet'}, {'id': 12, 'name': 'person'}, {'id': 14, 'name': 'door'}, {'id': 15, 'name': 'table'}, {'id': 18, 'name': 'curtain'}, {'id': 19, 'name': 'chair'}, {'id': 20, 'name': 'car'}, {'id': 22, 'name': 'painting'}, {'id': 23, 'name': 'sofa'}, {'id': 24, 'name': 'shelf'}, {'id': 27, 'name': 'mirror'}, {'id': 30, 'name': 'armchair'}, {'id': 31, 'name': 'seat'}, {'id': 32, 'name': 'fence'}, {'id': 33, 'name': 'desk'}, {'id': 35, 'name': 'wardrobe'}, {'id': 36, 'name': 'lamp'}, {'id': 37, 'name': 'bathtub'}, {'id': 38, 'name': 'railing'}, {'id': 39, 'name': 'cushion'}, {'id': 41, 'name': 'box'}, {'id': 42, 'name': 'column'}, {'id': 43, 'name': 'signboard'}, {'id': 44, 'name': 'chest of drawers'}, {'id': 45, 'name': 'counter'}, {'id': 47, 'name': 'sink'}, {'id': 49, 'name': 'fireplace'}, {'id': 50, 'name': 'refrigerator'}, {'id': 53, 'name': 'stairs'}, {'id': 55, 'name': 'case'}, {'id': 56, 'name': 'pool table'}, {'id': 57, 'name': 'pillow'}, {'id': 58, 'name': 'screen door'}, {'id': 62, 'name': 'bookcase'}, {'id': 64, 'name': 'coffee table'}, {'id': 65, 'name': 'toilet'}, {'id': 66, 'name': 'flower'}, {'id': 67, 'name': 'book'}, {'id': 69, 'name': 'bench'}, {'id': 70, 'name': 'countertop'}, {'id': 71, 'name': 'stove'}, {'id': 72, 'name': 'palm'}, {'id': 73, 'name': 'kitchen island'}, {'id': 74, 'name': 'computer'}, {'id': 75, 'name': 'swivel chair'}, {'id': 76, 'name': 'boat'}, {'id': 78, 'name': 'arcade machine'}, {'id': 80, 'name': 'bus'}, {'id': 81, 'name': 'towel'}, {'id': 82, 'name': 'light'}, {'id': 83, 'name': 'truck'}, {'id': 85, 'name': 'chandelier'}, {'id': 86, 'name': 'awning'}, {'id': 87, 'name': 'streetlight'}, {'id': 88, 'name': 'booth'}, {'id': 89, 'name': 'television receiver'}, {'id': 90, 'name': 'airplane'}, {'id': 92, 'name': 'apparel'}, {'id': 93, 'name': 'pole'}, {'id': 95, 'name': 'bannister'}, {'id': 97, 'name': 'ottoman'}, {'id': 98, 'name': 'bottle'}, {'id': 102, 'name': 'van'}, {'id': 103, 'name': 'ship'}, {'id': 104, 'name': 'fountain'}, {'id': 107, 'name': 'washer'}, {'id': 108, 'name': 'plaything'}, {'id': 110, 'name': 'stool'}, {'id': 111, 'name': 'barrel'}, {'id': 112, 'name': 'basket'}, {'id': 115, 'name': 'bag'}, {'id': 116, 'name': 'minibike'}, {'id': 118, 'name': 'oven'}, {'id': 119, 'name': 'ball'}, {'id': 120, 'name': 'food'}, {'id': 121, 'name': 'step'}, {'id': 123, 'name': 'trade name'}, {'id': 124, 'name': 'microwave'}, {'id': 125, 'name': 'pot'}, {'id': 126, 'name': 'animal'}, {'id': 127, 'name': 'bicycle'}, {'id': 129, 'name': 'dishwasher'}, {'id': 130, 'name': 'screen'}, {'id': 132, 'name': 'sculpture'}, {'id': 133, 'name': 'hood'}, {'id': 134, 'name': 'sconce'}, {'id': 135, 'name': 'vase'}, {'id': 136, 'name': 'traffic light'}, {'id': 137, 'name': 'tray'}, {'id': 138, 'name': 'ashcan'}, {'id': 139, 'name': 'fan'}, {'id': 142, 'name': 'plate'}, {'id': 143, 'name': 'monitor'}, {'id': 144, 'name': 'bulletin board'}, {'id': 146, 'name': 'radiator'}, {'id': 147, 'name': 'glass'}, {'id': 148, 'name': 'clock'}, {'id': 149, 'name': 'flag'}]
-
-
-_PREDEFINED_SPLITS = {
- # point annotations without masks
- "ade20k_instance_train": (
- "ADEChallengeData2016/images/training",
- "ADEChallengeData2016/ade20k_instance_train.json",
- ),
- "ade20k_instance_val": (
- "ADEChallengeData2016/images/validation",
- "ADEChallengeData2016/ade20k_instance_val.json",
- ),
-}
-
-
-def _get_ade_instances_meta():
- thing_ids = [k["id"] for k in ADE_CATEGORIES]
- assert len(thing_ids) == 100, len(thing_ids)
- # Mapping from the incontiguous ADE category id to an id in [0, 99]
- thing_dataset_id_to_contiguous_id = {k: i for i, k in enumerate(thing_ids)}
- thing_classes = [k["name"] for k in ADE_CATEGORIES]
- ret = {
- "thing_dataset_id_to_contiguous_id": thing_dataset_id_to_contiguous_id,
- "thing_classes": thing_classes,
- }
- return ret
-
-
-def register_all_ade20k_instance(root):
- for key, (image_root, json_file) in _PREDEFINED_SPLITS.items():
- # Assume pre-defined datasets live in `./datasets`.
- register_coco_instances(
- key,
- _get_ade_instances_meta(),
- os.path.join(root, json_file) if "://" not in json_file else json_file,
- os.path.join(root, image_root),
- )
-
-
-_root = os.getenv("DETECTRON2_DATASETS", "datasets")
-register_all_ade20k_instance(_root)
diff --git a/spaces/Lianglan/NLLB200-Translate-Distill-600/langs.py b/spaces/Lianglan/NLLB200-Translate-Distill-600/langs.py
deleted file mode 100644
index f090e1967a32b84bf2db128166c778e0df1a1055..0000000000000000000000000000000000000000
--- a/spaces/Lianglan/NLLB200-Translate-Distill-600/langs.py
+++ /dev/null
@@ -1,8 +0,0 @@
-LANGS = [
- "bod_Tibt",
- "khk_Cyrl",
- "uig_Arab",
- "yue_Hant",
- "zho_Hans",
- "zho_Hant"
-]
diff --git a/spaces/Lianjd/stock_dashboard/backtrader/feed.py b/spaces/Lianjd/stock_dashboard/backtrader/feed.py
deleted file mode 100644
index 1145c997b60ae085a67faeb024c35b4428a9ec7c..0000000000000000000000000000000000000000
--- a/spaces/Lianjd/stock_dashboard/backtrader/feed.py
+++ /dev/null
@@ -1,812 +0,0 @@
-#!/usr/bin/env python
-# -*- coding: utf-8; py-indent-offset:4 -*-
-###############################################################################
-#
-# Copyright (C) 2015-2020 Daniel Rodriguez
-#
-# This program is free software: you can redistribute it and/or modify
-# it under the terms of the GNU General Public License as published by
-# the Free Software Foundation, either version 3 of the License, or
-# (at your option) any later version.
-#
-# This program is distributed in the hope that it will be useful,
-# but WITHOUT ANY WARRANTY; without even the implied warranty of
-# MERCHANTABILITY or FITNESS FOR A PARTICULAR PURPOSE. See the
-# GNU General Public License for more details.
-#
-# You should have received a copy of the GNU General Public License
-# along with this program. If not, see .
-#
-###############################################################################
-from __future__ import (absolute_import, division, print_function,
- unicode_literals)
-
-import collections
-import datetime
-import inspect
-import io
-import os.path
-
-import backtrader as bt
-from backtrader import (date2num, num2date, time2num, TimeFrame, dataseries,
- metabase)
-
-from backtrader.utils.py3 import with_metaclass, zip, range, string_types
-from backtrader.utils import tzparse
-from .dataseries import SimpleFilterWrapper
-from .resamplerfilter import Resampler, Replayer
-from .tradingcal import PandasMarketCalendar
-
-
-class MetaAbstractDataBase(dataseries.OHLCDateTime.__class__):
- _indcol = dict()
-
- def __init__(cls, name, bases, dct):
- '''
- Class has already been created ... register subclasses
- '''
- # Initialize the class
- super(MetaAbstractDataBase, cls).__init__(name, bases, dct)
-
- if not cls.aliased and \
- name != 'DataBase' and not name.startswith('_'):
- cls._indcol[name] = cls
-
- def dopreinit(cls, _obj, *args, **kwargs):
- _obj, args, kwargs = \
- super(MetaAbstractDataBase, cls).dopreinit(_obj, *args, **kwargs)
-
- # Find the owner and store it
- _obj._feed = metabase.findowner(_obj, FeedBase)
-
- _obj.notifs = collections.deque() # store notifications for cerebro
-
- _obj._dataname = _obj.p.dataname
- _obj._name = ''
- return _obj, args, kwargs
-
- def dopostinit(cls, _obj, *args, **kwargs):
- _obj, args, kwargs = \
- super(MetaAbstractDataBase, cls).dopostinit(_obj, *args, **kwargs)
-
- # Either set by subclass or the parameter or use the dataname (ticker)
- _obj._name = _obj._name or _obj.p.name
- if not _obj._name and isinstance(_obj.p.dataname, string_types):
- _obj._name = _obj.p.dataname
- _obj._compression = _obj.p.compression
- _obj._timeframe = _obj.p.timeframe
-
- if isinstance(_obj.p.sessionstart, datetime.datetime):
- _obj.p.sessionstart = _obj.p.sessionstart.time()
-
- elif _obj.p.sessionstart is None:
- _obj.p.sessionstart = datetime.time.min
-
- if isinstance(_obj.p.sessionend, datetime.datetime):
- _obj.p.sessionend = _obj.p.sessionend.time()
-
- elif _obj.p.sessionend is None:
- # remove 9 to avoid precision rounding errors
- _obj.p.sessionend = datetime.time(23, 59, 59, 999990)
-
- if isinstance(_obj.p.fromdate, datetime.date):
- # push it to the end of the day, or else intraday
- # values before the end of the day would be gone
- if not hasattr(_obj.p.fromdate, 'hour'):
- _obj.p.fromdate = datetime.datetime.combine(
- _obj.p.fromdate, _obj.p.sessionstart)
-
- if isinstance(_obj.p.todate, datetime.date):
- # push it to the end of the day, or else intraday
- # values before the end of the day would be gone
- if not hasattr(_obj.p.todate, 'hour'):
- _obj.p.todate = datetime.datetime.combine(
- _obj.p.todate, _obj.p.sessionend)
-
- _obj._barstack = collections.deque() # for filter operations
- _obj._barstash = collections.deque() # for filter operations
-
- _obj._filters = list()
- _obj._ffilters = list()
- for fp in _obj.p.filters:
- if inspect.isclass(fp):
- fp = fp(_obj)
- if hasattr(fp, 'last'):
- _obj._ffilters.append((fp, [], {}))
-
- _obj._filters.append((fp, [], {}))
-
- return _obj, args, kwargs
-
-
-class AbstractDataBase(with_metaclass(MetaAbstractDataBase,
- dataseries.OHLCDateTime)):
-
- params = (
- ('dataname', None),
- ('name', ''),
- ('compression', 1),
- ('timeframe', TimeFrame.Days),
- ('fromdate', None),
- ('todate', None),
- ('sessionstart', None),
- ('sessionend', None),
- ('filters', []),
- ('tz', None),
- ('tzinput', None),
- ('qcheck', 0.0), # timeout in seconds (float) to check for events
- ('calendar', None),
- )
-
- (CONNECTED, DISCONNECTED, CONNBROKEN, DELAYED,
- LIVE, NOTSUBSCRIBED, NOTSUPPORTED_TF, UNKNOWN) = range(8)
-
- _NOTIFNAMES = [
- 'CONNECTED', 'DISCONNECTED', 'CONNBROKEN', 'DELAYED',
- 'LIVE', 'NOTSUBSCRIBED', 'NOTSUPPORTED_TIMEFRAME', 'UNKNOWN']
-
- @classmethod
- def _getstatusname(cls, status):
- return cls._NOTIFNAMES[status]
-
- _compensate = None
- _feed = None
- _store = None
-
- _clone = False
- _qcheck = 0.0
-
- _tmoffset = datetime.timedelta()
-
- # Set to non 0 if resampling/replaying
- resampling = 0
- replaying = 0
-
- _started = False
-
- def _start_finish(self):
- # A live feed (for example) may have learnt something about the
- # timezones after the start and that's why the date/time related
- # parameters are converted at this late stage
- # Get the output timezone (if any)
- self._tz = self._gettz()
- # Lines have already been create, set the tz
- self.lines.datetime._settz(self._tz)
-
- # This should probably be also called from an override-able method
- self._tzinput = bt.utils.date.Localizer(self._gettzinput())
-
- # Convert user input times to the output timezone (or min/max)
- if self.p.fromdate is None:
- self.fromdate = float('-inf')
- else:
- self.fromdate = self.date2num(self.p.fromdate)
-
- if self.p.todate is None:
- self.todate = float('inf')
- else:
- self.todate = self.date2num(self.p.todate)
-
- # FIXME: These two are never used and could be removed
- self.sessionstart = time2num(self.p.sessionstart)
- self.sessionend = time2num(self.p.sessionend)
-
- self._calendar = cal = self.p.calendar
- if cal is None:
- self._calendar = self._env._tradingcal
- elif isinstance(cal, string_types):
- self._calendar = PandasMarketCalendar(calendar=cal)
-
- self._started = True
-
- def _start(self):
- self.start()
-
- if not self._started:
- self._start_finish()
-
- def _timeoffset(self):
- return self._tmoffset
-
- def _getnexteos(self):
- '''Returns the next eos using a trading calendar if available'''
- if self._clone:
- return self.data._getnexteos()
-
- if not len(self):
- return datetime.datetime.min, 0.0
-
- dt = self.lines.datetime[0]
- dtime = num2date(dt)
- if self._calendar is None:
- nexteos = datetime.datetime.combine(dtime, self.p.sessionend)
- nextdteos = self.date2num(nexteos) # locl'ed -> utc-like
- nexteos = num2date(nextdteos) # utc
- while dtime > nexteos:
- nexteos += datetime.timedelta(days=1) # already utc-like
-
- nextdteos = date2num(nexteos) # -> utc-like
-
- else:
- # returns times in utc
- _, nexteos = self._calendar.schedule(dtime, self._tz)
- nextdteos = date2num(nexteos) # nextos is already utc
-
- return nexteos, nextdteos
-
- def _gettzinput(self):
- '''Can be overriden by classes to return a timezone for input'''
- return tzparse(self.p.tzinput)
-
- def _gettz(self):
- '''To be overriden by subclasses which may auto-calculate the
- timezone'''
- return tzparse(self.p.tz)
-
- def date2num(self, dt):
- if self._tz is not None:
- return date2num(self._tz.localize(dt))
-
- return date2num(dt)
-
- def num2date(self, dt=None, tz=None, naive=True):
- if dt is None:
- return num2date(self.lines.datetime[0], tz or self._tz, naive)
-
- return num2date(dt, tz or self._tz, naive)
-
- def haslivedata(self):
- return False # must be overriden for those that can
-
- def do_qcheck(self, onoff, qlapse):
- # if onoff is True the data will wait p.qcheck for incoming live data
- # on its queue.
- qwait = self.p.qcheck if onoff else 0.0
- qwait = max(0.0, qwait - qlapse)
- self._qcheck = qwait
-
- def islive(self):
- '''If this returns True, ``Cerebro`` will deactivate ``preload`` and
- ``runonce`` because a live data source must be fetched tick by tick (or
- bar by bar)'''
- return False
-
- def put_notification(self, status, *args, **kwargs):
- '''Add arguments to notification queue'''
- if self._laststatus != status:
- self.notifs.append((status, args, kwargs))
- self._laststatus = status
-
- def get_notifications(self):
- '''Return the pending "store" notifications'''
- # The background thread could keep on adding notifications. The None
- # mark allows to identify which is the last notification to deliver
- self.notifs.append(None) # put a mark
- notifs = list()
- while True:
- notif = self.notifs.popleft()
- if notif is None: # mark is reached
- break
- notifs.append(notif)
-
- return notifs
-
- def getfeed(self):
- return self._feed
-
- def qbuffer(self, savemem=0, replaying=False):
- extrasize = self.resampling or replaying
- for line in self.lines:
- line.qbuffer(savemem=savemem, extrasize=extrasize)
-
- def start(self):
- self._barstack = collections.deque()
- self._barstash = collections.deque()
- self._laststatus = self.CONNECTED
-
- def stop(self):
- pass
-
- def clone(self, **kwargs):
- return DataClone(dataname=self, **kwargs)
-
- def copyas(self, _dataname, **kwargs):
- d = DataClone(dataname=self, **kwargs)
- d._dataname = _dataname
- d._name = _dataname
- return d
-
- def setenvironment(self, env):
- '''Keep a reference to the environment'''
- self._env = env
-
- def getenvironment(self):
- return self._env
-
- def addfilter_simple(self, f, *args, **kwargs):
- fp = SimpleFilterWrapper(self, f, *args, **kwargs)
- self._filters.append((fp, fp.args, fp.kwargs))
-
- def addfilter(self, p, *args, **kwargs):
- if inspect.isclass(p):
- pobj = p(self, *args, **kwargs)
- self._filters.append((pobj, [], {}))
-
- if hasattr(pobj, 'last'):
- self._ffilters.append((pobj, [], {}))
-
- else:
- self._filters.append((p, args, kwargs))
-
- def compensate(self, other):
- '''Call it to let the broker know that actions on this asset will
- compensate open positions in another'''
-
- self._compensate = other
-
- def _tick_nullify(self):
- # These are the updating prices in case the new bar is "updated"
- # and the length doesn't change like if a replay is happening or
- # a real-time data feed is in use and 1 minutes bars are being
- # constructed with 5 seconds updates
- for lalias in self.getlinealiases():
- if lalias != 'datetime':
- setattr(self, 'tick_' + lalias, None)
-
- self.tick_last = None
-
- def _tick_fill(self, force=False):
- # If nothing filled the tick_xxx attributes, the bar is the tick
- alias0 = self._getlinealias(0)
- if force or getattr(self, 'tick_' + alias0, None) is None:
- for lalias in self.getlinealiases():
- if lalias != 'datetime':
- setattr(self, 'tick_' + lalias,
- getattr(self.lines, lalias)[0])
-
- self.tick_last = getattr(self.lines, alias0)[0]
-
- def advance_peek(self):
- if len(self) < self.buflen():
- return self.lines.datetime[1] # return the future
-
- return float('inf') # max date else
-
- def advance(self, size=1, datamaster=None, ticks=True):
- if ticks:
- self._tick_nullify()
-
- # Need intercepting this call to support datas with
- # different lengths (timeframes)
- self.lines.advance(size)
-
- if datamaster is not None:
- if len(self) > self.buflen():
- # if no bar can be delivered, fill with an empty bar
- self.rewind()
- self.lines.forward()
- return
-
- if self.lines.datetime[0] > datamaster.lines.datetime[0]:
- self.lines.rewind()
- else:
- if ticks:
- self._tick_fill()
- elif len(self) < self.buflen():
- # a resampler may have advance us past the last point
- if ticks:
- self._tick_fill()
-
- def next(self, datamaster=None, ticks=True):
-
- if len(self) >= self.buflen():
- if ticks:
- self._tick_nullify()
-
- # not preloaded - request next bar
- ret = self.load()
- if not ret:
- # if load cannot produce bars - forward the result
- return ret
-
- if datamaster is None:
- # bar is there and no master ... return load's result
- if ticks:
- self._tick_fill()
- return ret
- else:
- self.advance(ticks=ticks)
-
- # a bar is "loaded" or was preloaded - index has been moved to it
- if datamaster is not None:
- # there is a time reference to check against
- if self.lines.datetime[0] > datamaster.lines.datetime[0]:
- # can't deliver new bar, too early, go back
- self.rewind()
- else:
- if ticks:
- self._tick_fill()
-
- else:
- if ticks:
- self._tick_fill()
-
- # tell the world there is a bar (either the new or the previous
- return True
-
- def preload(self):
- while self.load():
- pass
-
- self._last()
- self.home()
-
- def _last(self, datamaster=None):
- # Last chance for filters to deliver something
- ret = 0
- for ff, fargs, fkwargs in self._ffilters:
- ret += ff.last(self, *fargs, **fkwargs)
-
- doticks = False
- if datamaster is not None and self._barstack:
- doticks = True
-
- while self._fromstack(forward=True):
- # consume bar(s) produced by "last"s - adding room
- pass
-
- if doticks:
- self._tick_fill()
-
- return bool(ret)
-
- def _check(self, forcedata=None):
- ret = 0
- for ff, fargs, fkwargs in self._filters:
- if not hasattr(ff, 'check'):
- continue
- ff.check(self, _forcedata=forcedata, *fargs, **fkwargs)
-
- def load(self):
- while True:
- # move data pointer forward for new bar
- self.forward()
-
- if self._fromstack(): # bar is available
- return True
-
- if not self._fromstack(stash=True):
- _loadret = self._load()
- if not _loadret: # no bar use force to make sure in exactbars
- # the pointer is undone this covers especially (but not
- # uniquely) the case in which the last bar has been seen
- # and a backwards would ruin pointer accounting in the
- # "stop" method of the strategy
- self.backwards(force=True) # undo data pointer
-
- # return the actual returned value which may be None to
- # signal no bar is available, but the data feed is not
- # done. False means game over
- return _loadret
-
- # Get a reference to current loaded time
- dt = self.lines.datetime[0]
-
- # A bar has been loaded, adapt the time
- if self._tzinput:
- # Input has been converted at face value but it's not UTC in
- # the input stream
- dtime = num2date(dt) # get it in a naive datetime
- # localize it
- dtime = self._tzinput.localize(dtime) # pytz compatible-ized
- self.lines.datetime[0] = dt = date2num(dtime) # keep UTC val
-
- # Check standard date from/to filters
- if dt < self.fromdate:
- # discard loaded bar and carry on
- self.backwards()
- continue
- if dt > self.todate:
- # discard loaded bar and break out
- self.backwards(force=True)
- break
-
- # Pass through filters
- retff = False
- for ff, fargs, fkwargs in self._filters:
- # previous filter may have put things onto the stack
- if self._barstack:
- for i in range(len(self._barstack)):
- self._fromstack(forward=True)
- retff = ff(self, *fargs, **fkwargs)
- else:
- retff = ff(self, *fargs, **fkwargs)
-
- if retff: # bar removed from systemn
- break # out of the inner loop
-
- if retff: # bar removed from system - loop to get new bar
- continue # in the greater loop
-
- # Checks let the bar through ... notify it
- return True
-
- # Out of the loop ... no more bars or past todate
- return False
-
- def _load(self):
- return False
-
- def _add2stack(self, bar, stash=False):
- '''Saves given bar (list of values) to the stack for later retrieval'''
- if not stash:
- self._barstack.append(bar)
- else:
- self._barstash.append(bar)
-
- def _save2stack(self, erase=False, force=False, stash=False):
- '''Saves current bar to the bar stack for later retrieval
-
- Parameter ``erase`` determines removal from the data stream
- '''
- bar = [line[0] for line in self.itersize()]
- if not stash:
- self._barstack.append(bar)
- else:
- self._barstash.append(bar)
-
- if erase: # remove bar if requested
- self.backwards(force=force)
-
- def _updatebar(self, bar, forward=False, ago=0):
- '''Load a value from the stack onto the lines to form the new bar
-
- Returns True if values are present, False otherwise
- '''
- if forward:
- self.forward()
-
- for line, val in zip(self.itersize(), bar):
- line[0 + ago] = val
-
- def _fromstack(self, forward=False, stash=False):
- '''Load a value from the stack onto the lines to form the new bar
-
- Returns True if values are present, False otherwise
- '''
-
- coll = self._barstack if not stash else self._barstash
-
- if coll:
- if forward:
- self.forward()
-
- for line, val in zip(self.itersize(), coll.popleft()):
- line[0] = val
-
- return True
-
- return False
-
- def resample(self, **kwargs):
- self.addfilter(Resampler, **kwargs)
-
- def replay(self, **kwargs):
- self.addfilter(Replayer, **kwargs)
-
-
-class DataBase(AbstractDataBase):
- pass
-
-
-class FeedBase(with_metaclass(metabase.MetaParams, object)):
- params = () + DataBase.params._gettuple()
-
- def __init__(self):
- self.datas = list()
-
- def start(self):
- for data in self.datas:
- data.start()
-
- def stop(self):
- for data in self.datas:
- data.stop()
-
- def getdata(self, dataname, name=None, **kwargs):
- for pname, pvalue in self.p._getitems():
- kwargs.setdefault(pname, getattr(self.p, pname))
-
- kwargs['dataname'] = dataname
- data = self._getdata(**kwargs)
-
- data._name = name
-
- self.datas.append(data)
- return data
-
- def _getdata(self, dataname, **kwargs):
- for pname, pvalue in self.p._getitems():
- kwargs.setdefault(pname, getattr(self.p, pname))
-
- kwargs['dataname'] = dataname
- return self.DataCls(**kwargs)
-
-
-class MetaCSVDataBase(DataBase.__class__):
- def dopostinit(cls, _obj, *args, **kwargs):
- # Before going to the base class to make sure it overrides the default
- if not _obj.p.name and not _obj._name:
- _obj._name, _ = os.path.splitext(os.path.basename(_obj.p.dataname))
-
- _obj, args, kwargs = \
- super(MetaCSVDataBase, cls).dopostinit(_obj, *args, **kwargs)
-
- return _obj, args, kwargs
-
-
-class CSVDataBase(with_metaclass(MetaCSVDataBase, DataBase)):
- '''
- Base class for classes implementing CSV DataFeeds
-
- The class takes care of opening the file, reading the lines and
- tokenizing them.
-
- Subclasses do only need to override:
-
- - _loadline(tokens)
-
- The return value of ``_loadline`` (True/False) will be the return value
- of ``_load`` which has been overriden by this base class
- '''
-
- f = None
- params = (('headers', True), ('separator', ','),)
-
- def start(self):
- super(CSVDataBase, self).start()
-
- if self.f is None:
- if hasattr(self.p.dataname, 'readline'):
- self.f = self.p.dataname
- else:
- # Let an exception propagate to let the caller know
- self.f = io.open(self.p.dataname, 'r')
-
- if self.p.headers:
- self.f.readline() # skip the headers
-
- self.separator = self.p.separator
-
- def stop(self):
- super(CSVDataBase, self).stop()
- if self.f is not None:
- self.f.close()
- self.f = None
-
- def preload(self):
- while self.load():
- pass
-
- self._last()
- self.home()
-
- # preloaded - no need to keep the object around - breaks multip in 3.x
- self.f.close()
- self.f = None
-
- def _load(self):
- if self.f is None:
- return False
-
- # Let an exception propagate to let the caller know
- line = self.f.readline()
-
- if not line:
- return False
-
- line = line.rstrip('\n')
- linetokens = line.split(self.separator)
- return self._loadline(linetokens)
-
- def _getnextline(self):
- if self.f is None:
- return None
-
- # Let an exception propagate to let the caller know
- line = self.f.readline()
-
- if not line:
- return None
-
- line = line.rstrip('\n')
- linetokens = line.split(self.separator)
- return linetokens
-
-
-class CSVFeedBase(FeedBase):
- params = (('basepath', ''),) + CSVDataBase.params._gettuple()
-
- def _getdata(self, dataname, **kwargs):
- return self.DataCls(dataname=self.p.basepath + dataname,
- **self.p._getkwargs())
-
-
-class DataClone(AbstractDataBase):
- _clone = True
-
- def __init__(self):
- self.data = self.p.dataname
- self._dataname = self.data._dataname
-
- # Copy date/session parameters
- self.p.fromdate = self.p.fromdate
- self.p.todate = self.p.todate
- self.p.sessionstart = self.data.p.sessionstart
- self.p.sessionend = self.data.p.sessionend
-
- self.p.timeframe = self.data.p.timeframe
- self.p.compression = self.data.p.compression
-
- def _start(self):
- # redefine to copy data bits from guest data
- self.start()
-
- # Copy tz infos
- self._tz = self.data._tz
- self.lines.datetime._settz(self._tz)
-
- self._calendar = self.data._calendar
-
- # input has already been converted by guest data
- self._tzinput = None # no need to further converr
-
- # Copy dates/session infos
- self.fromdate = self.data.fromdate
- self.todate = self.data.todate
-
- # FIXME: if removed from guest, remove here too
- self.sessionstart = self.data.sessionstart
- self.sessionend = self.data.sessionend
-
- def start(self):
- super(DataClone, self).start()
- self._dlen = 0
- self._preloading = False
-
- def preload(self):
- self._preloading = True
- super(DataClone, self).preload()
- self.data.home() # preloading data was pushed forward
- self._preloading = False
-
- def _load(self):
- # assumption: the data is in the system
- # simply copy the lines
- if self._preloading:
- # data is preloaded, we are preloading too, can move
- # forward until have full bar or data source is exhausted
- self.data.advance()
- if len(self.data) > self.data.buflen():
- return False
-
- for line, dline in zip(self.lines, self.data.lines):
- line[0] = dline[0]
-
- return True
-
- # Not preloading
- if not (len(self.data) > self._dlen):
- # Data not beyond last seen bar
- return False
-
- self._dlen += 1
-
- for line, dline in zip(self.lines, self.data.lines):
- line[0] = dline[0]
-
- return True
-
- def advance(self, size=1, datamaster=None, ticks=True):
- self._dlen += size
- super(DataClone, self).advance(size, datamaster, ticks=ticks)
diff --git a/spaces/Loren/Streamlit_OCR_comparator/configs/textrecog/satrn/satrn_small.py b/spaces/Loren/Streamlit_OCR_comparator/configs/textrecog/satrn/satrn_small.py
deleted file mode 100644
index 96f86797f4700fd6ab9590fa983323f3e22d15c2..0000000000000000000000000000000000000000
--- a/spaces/Loren/Streamlit_OCR_comparator/configs/textrecog/satrn/satrn_small.py
+++ /dev/null
@@ -1,68 +0,0 @@
-_base_ = [
- '../../_base_/default_runtime.py',
- '../../_base_/recog_pipelines/satrn_pipeline.py',
- '../../_base_/recog_datasets/ST_MJ_train.py',
- '../../_base_/recog_datasets/academic_test.py'
-]
-
-train_list = {{_base_.train_list}}
-test_list = {{_base_.test_list}}
-
-train_pipeline = {{_base_.train_pipeline}}
-test_pipeline = {{_base_.test_pipeline}}
-
-label_convertor = dict(
- type='AttnConvertor', dict_type='DICT90', with_unknown=True)
-
-model = dict(
- type='SATRN',
- backbone=dict(type='ShallowCNN', input_channels=3, hidden_dim=256),
- encoder=dict(
- type='SatrnEncoder',
- n_layers=6,
- n_head=8,
- d_k=256 // 8,
- d_v=256 // 8,
- d_model=256,
- n_position=100,
- d_inner=256 * 4,
- dropout=0.1),
- decoder=dict(
- type='NRTRDecoder',
- n_layers=6,
- d_embedding=256,
- n_head=8,
- d_model=256,
- d_inner=256 * 4,
- d_k=256 // 8,
- d_v=256 // 8),
- loss=dict(type='TFLoss'),
- label_convertor=label_convertor,
- max_seq_len=25)
-
-# optimizer
-optimizer = dict(type='Adam', lr=3e-4)
-optimizer_config = dict(grad_clip=None)
-# learning policy
-lr_config = dict(policy='step', step=[3, 4])
-total_epochs = 6
-
-data = dict(
- samples_per_gpu=64,
- workers_per_gpu=4,
- val_dataloader=dict(samples_per_gpu=1),
- test_dataloader=dict(samples_per_gpu=1),
- train=dict(
- type='UniformConcatDataset',
- datasets=train_list,
- pipeline=train_pipeline),
- val=dict(
- type='UniformConcatDataset',
- datasets=test_list,
- pipeline=test_pipeline),
- test=dict(
- type='UniformConcatDataset',
- datasets=test_list,
- pipeline=test_pipeline))
-
-evaluation = dict(interval=1, metric='acc')
diff --git a/spaces/LucasCodeBreak/MusicGen/audiocraft/modules/__init__.py b/spaces/LucasCodeBreak/MusicGen/audiocraft/modules/__init__.py
deleted file mode 100644
index 81ba30f6466ff91b90490a4fb92f7d3d0d00144d..0000000000000000000000000000000000000000
--- a/spaces/LucasCodeBreak/MusicGen/audiocraft/modules/__init__.py
+++ /dev/null
@@ -1,20 +0,0 @@
-# Copyright (c) Meta Platforms, Inc. and affiliates.
-# All rights reserved.
-#
-# This source code is licensed under the license found in the
-# LICENSE file in the root directory of this source tree.
-
-# flake8: noqa
-from .conv import (
- NormConv1d,
- NormConv2d,
- NormConvTranspose1d,
- NormConvTranspose2d,
- StreamableConv1d,
- StreamableConvTranspose1d,
- pad_for_conv1d,
- pad1d,
- unpad1d,
-)
-from .lstm import StreamableLSTM
-from .seanet import SEANetEncoder, SEANetDecoder
diff --git a/spaces/M52395239m/Image_Face_Upscale_Restoration-GFPGAN/README.md b/spaces/M52395239m/Image_Face_Upscale_Restoration-GFPGAN/README.md
deleted file mode 100644
index a1efe7d8eef5c16ac1b328a9b9f2afccca8524d4..0000000000000000000000000000000000000000
--- a/spaces/M52395239m/Image_Face_Upscale_Restoration-GFPGAN/README.md
+++ /dev/null
@@ -1,14 +0,0 @@
----
-title: Image Face Upscale Restoration-GFPGAN
-emoji: 📈
-colorFrom: blue
-colorTo: gray
-sdk: gradio
-sdk_version: 3.1.7
-app_file: app.py
-pinned: false
-license: apache-2.0
-duplicated_from: clem/Image_Face_Upscale_Restoration-GFPGAN
----
-
-Check out the configuration reference at https://huggingface.co/docs/hub/spaces-config-reference
diff --git a/spaces/Manjushri/SDXL-1.0-Doodle-to-Image/README.md b/spaces/Manjushri/SDXL-1.0-Doodle-to-Image/README.md
deleted file mode 100644
index e2737e4ae80116c41f7532fd7978d18cb9ec32b5..0000000000000000000000000000000000000000
--- a/spaces/Manjushri/SDXL-1.0-Doodle-to-Image/README.md
+++ /dev/null
@@ -1,13 +0,0 @@
----
-title: SDXL 1.0 Doodle To Image
-emoji: 🦀
-colorFrom: red
-colorTo: red
-sdk: gradio
-sdk_version: 3.39.0
-app_file: app.py
-pinned: false
-license: mit
----
-
-Check out the configuration reference at https://huggingface.co/docs/hub/spaces-config-reference
diff --git a/spaces/MercurialAi/OncoMedleyMini/OncoMedley/tools/tumor_size.py b/spaces/MercurialAi/OncoMedleyMini/OncoMedley/tools/tumor_size.py
deleted file mode 100644
index 5267ceb413f70c2a466b4efc7fef5756bf5729da..0000000000000000000000000000000000000000
--- a/spaces/MercurialAi/OncoMedleyMini/OncoMedley/tools/tumor_size.py
+++ /dev/null
@@ -1,46 +0,0 @@
-import torch
-from typing import Type
-from langchain.tools import BaseTool
-from pydantic import BaseModel, Field
-from sklearn.preprocessing import MinMaxScaler
-
-min_max_scaler = MinMaxScaler()
-
-class TumorSizeInput(BaseModel):
- """Inputs for tumor size prediction"""
- tumor_stage: int = Field(description="the stage of the tumor")
- nottingham_prognostic_index: float = Field(description="the nottingham prognostic index of the tumor")
- lymph_nodes_examined_positive: int = Field(description="indicates whether or not the patient's lymph nodes were examined as positive")
- type_of_breast_surgery: str = Field(description="indicates the type of breast surgery for the patient")
- chemotherapy: str = Field(description="indicates whether or not the patient has undergone chemotherapy")
- neoplasm_histologic_grade: float = Field(description="indicates the patient's neoplasm histologic grade")
- pr_status: str = Field(description="indicates the presence of progesterone receptors")
- integrative_cluster: str = Field(description="indicates the integrative cluster of the patient")
- hormone_therapy: str = Field(description="indicates whether or not the patient has undergone hormone therapy")
- er_status: str = Field(description="indicates the presence estrogen receptors")
-
-class TumorSizeTool(BaseTool):
- name="tumor_size"
- description="predicts the tumor size of a breast cancer patient, given clinical features"
- args_schema: Type[BaseModel] = TumorSizeInput
-
- def _run(self, tumor_stage: float, nottingham_prognostic_index: float, lymph_nodes_examined_positive: float, type_of_breast_surgery: str, chemotherapy: str, neoplasm_histologic_grade: float, pr_status: str, integrative_cluster: str, hormone_therapy: str, er_status: str) -> float:
- model = torch.load("data/tumor_size.pth")
-
- code_dict = {'negative': 1, 'no': 1, 'positive': 2, 'yes': 2, 'breastinvasiveductalcarcinoma': 3, 'idc': 4, 'post': 5, 'ductalnst': 6, 'mastectomy': 7, 'left': 8, 'high': 9, 'right': 10, 'breastconserving': 11, 'luma': 12, 'moderate': 13, 'erher2lowprolif': 14, 'erher2highprolif': 15, 'lumb': 16, 'pre': 17, 'her2': 18, 'erher2': 19, '4er': 20, '3': 21, '8': 22, 'breastmixedductalandlobularcarcinoma': 23, 'mixed': 24, 'mdlc': 25, 'low': 26, '10': 27, '7': 28, '5': 29, 'claudinlow': 30, 'basal': 31, 'breastinvasivelobularcarcinoma': 32, 'lobular': 33, 'ilc': 34, '1': 35, '9': 36, 'normal': 37, '6': 38, '2': 39, 'tubularcribriform': 40, 'breastinvasivemixedmucinouscarcinoma': 41, 'mucinous': 42, 'immc': 43, 'medullary': 44, 'breast': 45, 'other': 46, 'nc': 47}
- type_of_breast_surgery = float(code_dict[type_of_breast_surgery.lower()])
- chemotherapy = float(code_dict[chemotherapy.lower()])
- pr_status = float(code_dict[pr_status.lower()])
- integrative_cluster = float(code_dict[integrative_cluster.lower()])
- hormone_therapy = float(code_dict[hormone_therapy.lower()])
- er_status = float(code_dict[er_status.lower()])
-
- x = torch.Tensor([tumor_stage, nottingham_prognostic_index, lymph_nodes_examined_positive, type_of_breast_surgery, chemotherapy, neoplasm_histologic_grade, pr_status, integrative_cluster, hormone_therapy, er_status]).reshape(1, -1)
- x = torch.from_numpy(min_max_scaler.fit_transform(x)).type(torch.float)
- pred = float(model(x))
-
- return pred
-
- async def _arun(self, tumor_stage: int, nottingham_prognostic_index: float, lymph_nodes_examined_positive: int, type_of_breast_surgery: str, chemotherapy: str, neoplasm_histologic_grade: float, pr_status: str, integrative_cluster: str, hormone_therapy: str, er_status: str) -> float:
- raise NotImplementedError("the tumor size tool does not support async")
-
\ No newline at end of file
diff --git a/spaces/Mo9/DionTimmer-controlnet_qrcode-control_v11p_sd21/README.md b/spaces/Mo9/DionTimmer-controlnet_qrcode-control_v11p_sd21/README.md
deleted file mode 100644
index 3c1c2042a752851fa93e819cfd6711c92305633a..0000000000000000000000000000000000000000
--- a/spaces/Mo9/DionTimmer-controlnet_qrcode-control_v11p_sd21/README.md
+++ /dev/null
@@ -1,13 +0,0 @@
----
-title: DionTimmer-controlnet Qrcode-control V11p Sd21
-emoji: 😻
-colorFrom: purple
-colorTo: indigo
-sdk: gradio
-sdk_version: 3.35.2
-app_file: app.py
-pinned: false
-license: unknown
----
-
-Check out the configuration reference at https://huggingface.co/docs/hub/spaces-config-reference
diff --git a/spaces/Mountchicken/MAERec-Gradio/configs/textdet/fcenet/fcenet_resnet50_fpn_1500e_totaltext.py b/spaces/Mountchicken/MAERec-Gradio/configs/textdet/fcenet/fcenet_resnet50_fpn_1500e_totaltext.py
deleted file mode 100644
index cc63975e2a86cd8a0fbc6b08adf3d1ccde6e6cf3..0000000000000000000000000000000000000000
--- a/spaces/Mountchicken/MAERec-Gradio/configs/textdet/fcenet/fcenet_resnet50_fpn_1500e_totaltext.py
+++ /dev/null
@@ -1,117 +0,0 @@
-_base_ = [
- '_base_fcenet_resnet50_fpn.py',
- '../_base_/datasets/totaltext.py',
- '../_base_/default_runtime.py',
- '../_base_/schedules/schedule_sgd_base.py',
-]
-
-default_hooks = dict(
- checkpoint=dict(
- type='CheckpointHook',
- save_best='icdar/hmean',
- rule='greater',
- _delete_=True))
-
-train_pipeline = [
- dict(type='LoadImageFromFile', color_type='color_ignore_orientation'),
- dict(
- type='LoadOCRAnnotations',
- with_polygon=True,
- with_bbox=True,
- with_label=True,
- ),
- dict(type='FixInvalidPolygon'),
- dict(
- type='RandomResize',
- scale=(800, 800),
- ratio_range=(0.75, 2.5),
- keep_ratio=True),
- dict(
- type='TextDetRandomCropFlip',
- crop_ratio=0.5,
- iter_num=1,
- min_area_ratio=0.2),
- dict(
- type='RandomApply',
- transforms=[dict(type='RandomCrop', min_side_ratio=0.3)],
- prob=0.8),
- dict(
- type='RandomApply',
- transforms=[
- dict(
- type='RandomRotate',
- max_angle=30,
- pad_with_fixed_color=False,
- use_canvas=True)
- ],
- prob=0.5),
- dict(
- type='RandomChoice',
- transforms=[[
- dict(type='Resize', scale=800, keep_ratio=True),
- dict(type='SourceImagePad', target_scale=800)
- ],
- dict(type='Resize', scale=800, keep_ratio=False)],
- prob=[0.6, 0.4]),
- dict(type='RandomFlip', prob=0.5, direction='horizontal'),
- dict(
- type='TorchVisionWrapper',
- op='ColorJitter',
- brightness=32.0 / 255,
- saturation=0.5,
- contrast=0.5),
- dict(
- type='PackTextDetInputs',
- meta_keys=('img_path', 'ori_shape', 'img_shape', 'scale_factor'))
-]
-
-test_pipeline = [
- dict(type='LoadImageFromFile', color_type='color_ignore_orientation'),
- dict(type='Resize', scale=(1280, 960), keep_ratio=True),
- # add loading annotation after ``Resize`` because ground truth
- # does not need to do resize data transform
- dict(
- type='LoadOCRAnnotations',
- with_polygon=True,
- with_bbox=True,
- with_label=True),
- dict(type='FixInvalidPolygon'),
- dict(
- type='PackTextDetInputs',
- meta_keys=('img_path', 'ori_shape', 'img_shape', 'scale_factor'))
-]
-
-optim_wrapper = dict(optimizer=dict(lr=1e-3, weight_decay=5e-4))
-train_cfg = dict(max_epochs=1500)
-# learning policy
-param_scheduler = [
- dict(type='StepLR', gamma=0.8, step_size=200, end=1200),
-]
-
-# dataset settings
-totaltext_textdet_train = _base_.totaltext_textdet_train
-totaltext_textdet_test = _base_.totaltext_textdet_test
-totaltext_textdet_train.pipeline = train_pipeline
-totaltext_textdet_test.pipeline = test_pipeline
-
-train_dataloader = dict(
- batch_size=16,
- num_workers=16,
- persistent_workers=True,
- pin_memory=True,
- sampler=dict(type='DefaultSampler', shuffle=True),
- dataset=totaltext_textdet_train)
-
-val_dataloader = dict(
- batch_size=1,
- num_workers=1,
- persistent_workers=True,
- pin_memory=True,
- sampler=dict(type='DefaultSampler', shuffle=False),
- dataset=totaltext_textdet_test)
-
-test_dataloader = val_dataloader
-
-auto_scale_lr = dict(base_batch_size=16)
-
-find_unused_parameters = True
diff --git a/spaces/NSect/multitrack-midi-music-generator/README.md b/spaces/NSect/multitrack-midi-music-generator/README.md
deleted file mode 100644
index 838aa5b8e1b105f0d6564da80ebb3d6f0abb51b2..0000000000000000000000000000000000000000
--- a/spaces/NSect/multitrack-midi-music-generator/README.md
+++ /dev/null
@@ -1,11 +0,0 @@
----
-title: Multitrack Midi Music Generator
-emoji: 🎵
-colorFrom: indigo
-colorTo: gray
-sdk: docker
-pinned: false
-license: mit
----
-
-Check out the configuration reference at https://huggingface.co/docs/hub/spaces-config-reference
diff --git a/spaces/Nagireddys/MygenAI/README.md b/spaces/Nagireddys/MygenAI/README.md
deleted file mode 100644
index e71c5344510275db3d629d2039d6383de2a857c0..0000000000000000000000000000000000000000
--- a/spaces/Nagireddys/MygenAI/README.md
+++ /dev/null
@@ -1,12 +0,0 @@
----
-title: MygenAI
-emoji: 🐨
-colorFrom: purple
-colorTo: blue
-sdk: gradio
-sdk_version: 3.39.0
-app_file: app.py
-pinned: false
----
-
-Check out the configuration reference at https://huggingface.co/docs/hub/spaces-config-reference
diff --git a/spaces/Neilblaze/WhisperAnything/utils.py b/spaces/Neilblaze/WhisperAnything/utils.py
deleted file mode 100644
index a1ff412ac4f060030d5181e0244e9b4ac1d57a55..0000000000000000000000000000000000000000
--- a/spaces/Neilblaze/WhisperAnything/utils.py
+++ /dev/null
@@ -1,128 +0,0 @@
-from bs4 import BeautifulSoup
-import requests
-
-
-lang_ids = {
- "Afrikaans": "af",
- "Amharic": "am",
- "Arabic": "ar",
- "Asturian": "ast",
- "Azerbaijani": "az",
- "Bashkir": "ba",
- "Belarusian": "be",
- "Bulgarian": "bg",
- "Bengali": "bn",
- "Breton": "br",
- "Bosnian": "bs",
- "Catalan": "ca",
- "Cebuano": "ceb",
- "Czech": "cs",
- "Welsh": "cy",
- "Danish": "da",
- "German": "de",
- "Greeek": "el",
- "English": "en",
- "Spanish": "es",
- "Estonian": "et",
- "Persian": "fa",
- "Fulah": "ff",
- "Finnish": "fi",
- "French": "fr",
- "Western Frisian": "fy",
- "Irish": "ga",
- "Gaelic": "gd",
- "Galician": "gl",
- "Gujarati": "gu",
- "Hausa": "ha",
- "Hebrew": "he",
- "Hindi": "hi",
- "Croatian": "hr",
- "Haitian": "ht",
- "Hungarian": "hu",
- "Armenian": "hy",
- "Indonesian": "id",
- "Igbo": "ig",
- "Iloko": "ilo",
- "Icelandic": "is",
- "Italian": "it",
- "Japanese": "ja",
- "Javanese": "jv",
- "Georgian": "ka",
- "Kazakh": "kk",
- "Central Khmer": "km",
- "Kannada": "kn",
- "Korean": "ko",
- "Luxembourgish": "lb",
- "Ganda": "lg",
- "Lingala": "ln",
- "Lao": "lo",
- "Lithuanian": "lt",
- "Latvian": "lv",
- "Malagasy": "mg",
- "Macedonian": "mk",
- "Malayalam": "ml",
- "Mongolian": "mn",
- "Marathi": "mr",
- "Malay": "ms",
- "Burmese": "my",
- "Nepali": "ne",
- "Dutch": "nl",
- "Norwegian": "no",
- "Northern Sotho": "ns",
- "Occitan": "oc",
- "Oriya": "or",
- "Panjabi": "pa",
- "Polish": "pl",
- "Pushto": "ps",
- "Portuguese": "pt",
- "Romanian": "ro",
- "Russian": "ru",
- "Sindhi": "sd",
- "Sinhala": "si",
- "Slovak": "sk",
- "Slovenian": "sl",
- "Somali": "so",
- "Albanian": "sq",
- "Serbian": "sr",
- "Swati": "ss",
- "Sundanese": "su",
- "Swedish": "sv",
- "Swahili": "sw",
- "Tamil": "ta",
- "Thai": "th",
- "Tagalog": "tl",
- "Tswana": "tn",
- "Turkish": "tr",
- "Ukrainian": "uk",
- "Urdu": "ur",
- "Uzbek": "uz",
- "Vietnamese": "vi",
- "Wolof": "wo",
- "Xhosa": "xh",
- "Yiddish": "yi",
- "Yoruba": "yo",
- "Chinese": "zh",
- "Zulu": "zu",
-}
-
-def model_url_list():
- url_list = []
- for i in range(0, 5):
- url_list.append(f"https://huggingface.co/models?other=m2m_100&p={i}&sort=downloads")
- return url_list
-
-def data_scraping():
- url_list = model_url_list()
- model_list = []
- for url in url_list:
- response = requests.get(url)
- soup = BeautifulSoup(response.text, "html.parser")
- div_class = 'grid grid-cols-1 gap-5 2xl:grid-cols-2'
- div = soup.find('div', {'class': div_class})
- for a in div.find_all('a', href=True):
- model_list.append(a['href'])
-
- for i in range(len(model_list)):
- model_list[i] = model_list[i][1:]
-
- return model_list
\ No newline at end of file
diff --git a/spaces/Nekomaru180/rvc-model/infer_pack/modules.py b/spaces/Nekomaru180/rvc-model/infer_pack/modules.py
deleted file mode 100644
index 960481cedad9a6106f2bf0b9e86e82b120f7b33f..0000000000000000000000000000000000000000
--- a/spaces/Nekomaru180/rvc-model/infer_pack/modules.py
+++ /dev/null
@@ -1,522 +0,0 @@
-import copy
-import math
-import numpy as np
-import scipy
-import torch
-from torch import nn
-from torch.nn import functional as F
-
-from torch.nn import Conv1d, ConvTranspose1d, AvgPool1d, Conv2d
-from torch.nn.utils import weight_norm, remove_weight_norm
-
-from infer_pack import commons
-from infer_pack.commons import init_weights, get_padding
-from infer_pack.transforms import piecewise_rational_quadratic_transform
-
-
-LRELU_SLOPE = 0.1
-
-
-class LayerNorm(nn.Module):
- def __init__(self, channels, eps=1e-5):
- super().__init__()
- self.channels = channels
- self.eps = eps
-
- self.gamma = nn.Parameter(torch.ones(channels))
- self.beta = nn.Parameter(torch.zeros(channels))
-
- def forward(self, x):
- x = x.transpose(1, -1)
- x = F.layer_norm(x, (self.channels,), self.gamma, self.beta, self.eps)
- return x.transpose(1, -1)
-
-
-class ConvReluNorm(nn.Module):
- def __init__(
- self,
- in_channels,
- hidden_channels,
- out_channels,
- kernel_size,
- n_layers,
- p_dropout,
- ):
- super().__init__()
- self.in_channels = in_channels
- self.hidden_channels = hidden_channels
- self.out_channels = out_channels
- self.kernel_size = kernel_size
- self.n_layers = n_layers
- self.p_dropout = p_dropout
- assert n_layers > 1, "Number of layers should be larger than 0."
-
- self.conv_layers = nn.ModuleList()
- self.norm_layers = nn.ModuleList()
- self.conv_layers.append(
- nn.Conv1d(
- in_channels, hidden_channels, kernel_size, padding=kernel_size // 2
- )
- )
- self.norm_layers.append(LayerNorm(hidden_channels))
- self.relu_drop = nn.Sequential(nn.ReLU(), nn.Dropout(p_dropout))
- for _ in range(n_layers - 1):
- self.conv_layers.append(
- nn.Conv1d(
- hidden_channels,
- hidden_channels,
- kernel_size,
- padding=kernel_size // 2,
- )
- )
- self.norm_layers.append(LayerNorm(hidden_channels))
- self.proj = nn.Conv1d(hidden_channels, out_channels, 1)
- self.proj.weight.data.zero_()
- self.proj.bias.data.zero_()
-
- def forward(self, x, x_mask):
- x_org = x
- for i in range(self.n_layers):
- x = self.conv_layers[i](x * x_mask)
- x = self.norm_layers[i](x)
- x = self.relu_drop(x)
- x = x_org + self.proj(x)
- return x * x_mask
-
-
-class DDSConv(nn.Module):
- """
- Dialted and Depth-Separable Convolution
- """
-
- def __init__(self, channels, kernel_size, n_layers, p_dropout=0.0):
- super().__init__()
- self.channels = channels
- self.kernel_size = kernel_size
- self.n_layers = n_layers
- self.p_dropout = p_dropout
-
- self.drop = nn.Dropout(p_dropout)
- self.convs_sep = nn.ModuleList()
- self.convs_1x1 = nn.ModuleList()
- self.norms_1 = nn.ModuleList()
- self.norms_2 = nn.ModuleList()
- for i in range(n_layers):
- dilation = kernel_size**i
- padding = (kernel_size * dilation - dilation) // 2
- self.convs_sep.append(
- nn.Conv1d(
- channels,
- channels,
- kernel_size,
- groups=channels,
- dilation=dilation,
- padding=padding,
- )
- )
- self.convs_1x1.append(nn.Conv1d(channels, channels, 1))
- self.norms_1.append(LayerNorm(channels))
- self.norms_2.append(LayerNorm(channels))
-
- def forward(self, x, x_mask, g=None):
- if g is not None:
- x = x + g
- for i in range(self.n_layers):
- y = self.convs_sep[i](x * x_mask)
- y = self.norms_1[i](y)
- y = F.gelu(y)
- y = self.convs_1x1[i](y)
- y = self.norms_2[i](y)
- y = F.gelu(y)
- y = self.drop(y)
- x = x + y
- return x * x_mask
-
-
-class WN(torch.nn.Module):
- def __init__(
- self,
- hidden_channels,
- kernel_size,
- dilation_rate,
- n_layers,
- gin_channels=0,
- p_dropout=0,
- ):
- super(WN, self).__init__()
- assert kernel_size % 2 == 1
- self.hidden_channels = hidden_channels
- self.kernel_size = (kernel_size,)
- self.dilation_rate = dilation_rate
- self.n_layers = n_layers
- self.gin_channels = gin_channels
- self.p_dropout = p_dropout
-
- self.in_layers = torch.nn.ModuleList()
- self.res_skip_layers = torch.nn.ModuleList()
- self.drop = nn.Dropout(p_dropout)
-
- if gin_channels != 0:
- cond_layer = torch.nn.Conv1d(
- gin_channels, 2 * hidden_channels * n_layers, 1
- )
- self.cond_layer = torch.nn.utils.weight_norm(cond_layer, name="weight")
-
- for i in range(n_layers):
- dilation = dilation_rate**i
- padding = int((kernel_size * dilation - dilation) / 2)
- in_layer = torch.nn.Conv1d(
- hidden_channels,
- 2 * hidden_channels,
- kernel_size,
- dilation=dilation,
- padding=padding,
- )
- in_layer = torch.nn.utils.weight_norm(in_layer, name="weight")
- self.in_layers.append(in_layer)
-
- # last one is not necessary
- if i < n_layers - 1:
- res_skip_channels = 2 * hidden_channels
- else:
- res_skip_channels = hidden_channels
-
- res_skip_layer = torch.nn.Conv1d(hidden_channels, res_skip_channels, 1)
- res_skip_layer = torch.nn.utils.weight_norm(res_skip_layer, name="weight")
- self.res_skip_layers.append(res_skip_layer)
-
- def forward(self, x, x_mask, g=None, **kwargs):
- output = torch.zeros_like(x)
- n_channels_tensor = torch.IntTensor([self.hidden_channels])
-
- if g is not None:
- g = self.cond_layer(g)
-
- for i in range(self.n_layers):
- x_in = self.in_layers[i](x)
- if g is not None:
- cond_offset = i * 2 * self.hidden_channels
- g_l = g[:, cond_offset : cond_offset + 2 * self.hidden_channels, :]
- else:
- g_l = torch.zeros_like(x_in)
-
- acts = commons.fused_add_tanh_sigmoid_multiply(x_in, g_l, n_channels_tensor)
- acts = self.drop(acts)
-
- res_skip_acts = self.res_skip_layers[i](acts)
- if i < self.n_layers - 1:
- res_acts = res_skip_acts[:, : self.hidden_channels, :]
- x = (x + res_acts) * x_mask
- output = output + res_skip_acts[:, self.hidden_channels :, :]
- else:
- output = output + res_skip_acts
- return output * x_mask
-
- def remove_weight_norm(self):
- if self.gin_channels != 0:
- torch.nn.utils.remove_weight_norm(self.cond_layer)
- for l in self.in_layers:
- torch.nn.utils.remove_weight_norm(l)
- for l in self.res_skip_layers:
- torch.nn.utils.remove_weight_norm(l)
-
-
-class ResBlock1(torch.nn.Module):
- def __init__(self, channels, kernel_size=3, dilation=(1, 3, 5)):
- super(ResBlock1, self).__init__()
- self.convs1 = nn.ModuleList(
- [
- weight_norm(
- Conv1d(
- channels,
- channels,
- kernel_size,
- 1,
- dilation=dilation[0],
- padding=get_padding(kernel_size, dilation[0]),
- )
- ),
- weight_norm(
- Conv1d(
- channels,
- channels,
- kernel_size,
- 1,
- dilation=dilation[1],
- padding=get_padding(kernel_size, dilation[1]),
- )
- ),
- weight_norm(
- Conv1d(
- channels,
- channels,
- kernel_size,
- 1,
- dilation=dilation[2],
- padding=get_padding(kernel_size, dilation[2]),
- )
- ),
- ]
- )
- self.convs1.apply(init_weights)
-
- self.convs2 = nn.ModuleList(
- [
- weight_norm(
- Conv1d(
- channels,
- channels,
- kernel_size,
- 1,
- dilation=1,
- padding=get_padding(kernel_size, 1),
- )
- ),
- weight_norm(
- Conv1d(
- channels,
- channels,
- kernel_size,
- 1,
- dilation=1,
- padding=get_padding(kernel_size, 1),
- )
- ),
- weight_norm(
- Conv1d(
- channels,
- channels,
- kernel_size,
- 1,
- dilation=1,
- padding=get_padding(kernel_size, 1),
- )
- ),
- ]
- )
- self.convs2.apply(init_weights)
-
- def forward(self, x, x_mask=None):
- for c1, c2 in zip(self.convs1, self.convs2):
- xt = F.leaky_relu(x, LRELU_SLOPE)
- if x_mask is not None:
- xt = xt * x_mask
- xt = c1(xt)
- xt = F.leaky_relu(xt, LRELU_SLOPE)
- if x_mask is not None:
- xt = xt * x_mask
- xt = c2(xt)
- x = xt + x
- if x_mask is not None:
- x = x * x_mask
- return x
-
- def remove_weight_norm(self):
- for l in self.convs1:
- remove_weight_norm(l)
- for l in self.convs2:
- remove_weight_norm(l)
-
-
-class ResBlock2(torch.nn.Module):
- def __init__(self, channels, kernel_size=3, dilation=(1, 3)):
- super(ResBlock2, self).__init__()
- self.convs = nn.ModuleList(
- [
- weight_norm(
- Conv1d(
- channels,
- channels,
- kernel_size,
- 1,
- dilation=dilation[0],
- padding=get_padding(kernel_size, dilation[0]),
- )
- ),
- weight_norm(
- Conv1d(
- channels,
- channels,
- kernel_size,
- 1,
- dilation=dilation[1],
- padding=get_padding(kernel_size, dilation[1]),
- )
- ),
- ]
- )
- self.convs.apply(init_weights)
-
- def forward(self, x, x_mask=None):
- for c in self.convs:
- xt = F.leaky_relu(x, LRELU_SLOPE)
- if x_mask is not None:
- xt = xt * x_mask
- xt = c(xt)
- x = xt + x
- if x_mask is not None:
- x = x * x_mask
- return x
-
- def remove_weight_norm(self):
- for l in self.convs:
- remove_weight_norm(l)
-
-
-class Log(nn.Module):
- def forward(self, x, x_mask, reverse=False, **kwargs):
- if not reverse:
- y = torch.log(torch.clamp_min(x, 1e-5)) * x_mask
- logdet = torch.sum(-y, [1, 2])
- return y, logdet
- else:
- x = torch.exp(x) * x_mask
- return x
-
-
-class Flip(nn.Module):
- def forward(self, x, *args, reverse=False, **kwargs):
- x = torch.flip(x, [1])
- if not reverse:
- logdet = torch.zeros(x.size(0)).to(dtype=x.dtype, device=x.device)
- return x, logdet
- else:
- return x
-
-
-class ElementwiseAffine(nn.Module):
- def __init__(self, channels):
- super().__init__()
- self.channels = channels
- self.m = nn.Parameter(torch.zeros(channels, 1))
- self.logs = nn.Parameter(torch.zeros(channels, 1))
-
- def forward(self, x, x_mask, reverse=False, **kwargs):
- if not reverse:
- y = self.m + torch.exp(self.logs) * x
- y = y * x_mask
- logdet = torch.sum(self.logs * x_mask, [1, 2])
- return y, logdet
- else:
- x = (x - self.m) * torch.exp(-self.logs) * x_mask
- return x
-
-
-class ResidualCouplingLayer(nn.Module):
- def __init__(
- self,
- channels,
- hidden_channels,
- kernel_size,
- dilation_rate,
- n_layers,
- p_dropout=0,
- gin_channels=0,
- mean_only=False,
- ):
- assert channels % 2 == 0, "channels should be divisible by 2"
- super().__init__()
- self.channels = channels
- self.hidden_channels = hidden_channels
- self.kernel_size = kernel_size
- self.dilation_rate = dilation_rate
- self.n_layers = n_layers
- self.half_channels = channels // 2
- self.mean_only = mean_only
-
- self.pre = nn.Conv1d(self.half_channels, hidden_channels, 1)
- self.enc = WN(
- hidden_channels,
- kernel_size,
- dilation_rate,
- n_layers,
- p_dropout=p_dropout,
- gin_channels=gin_channels,
- )
- self.post = nn.Conv1d(hidden_channels, self.half_channels * (2 - mean_only), 1)
- self.post.weight.data.zero_()
- self.post.bias.data.zero_()
-
- def forward(self, x, x_mask, g=None, reverse=False):
- x0, x1 = torch.split(x, [self.half_channels] * 2, 1)
- h = self.pre(x0) * x_mask
- h = self.enc(h, x_mask, g=g)
- stats = self.post(h) * x_mask
- if not self.mean_only:
- m, logs = torch.split(stats, [self.half_channels] * 2, 1)
- else:
- m = stats
- logs = torch.zeros_like(m)
-
- if not reverse:
- x1 = m + x1 * torch.exp(logs) * x_mask
- x = torch.cat([x0, x1], 1)
- logdet = torch.sum(logs, [1, 2])
- return x, logdet
- else:
- x1 = (x1 - m) * torch.exp(-logs) * x_mask
- x = torch.cat([x0, x1], 1)
- return x
-
- def remove_weight_norm(self):
- self.enc.remove_weight_norm()
-
-
-class ConvFlow(nn.Module):
- def __init__(
- self,
- in_channels,
- filter_channels,
- kernel_size,
- n_layers,
- num_bins=10,
- tail_bound=5.0,
- ):
- super().__init__()
- self.in_channels = in_channels
- self.filter_channels = filter_channels
- self.kernel_size = kernel_size
- self.n_layers = n_layers
- self.num_bins = num_bins
- self.tail_bound = tail_bound
- self.half_channels = in_channels // 2
-
- self.pre = nn.Conv1d(self.half_channels, filter_channels, 1)
- self.convs = DDSConv(filter_channels, kernel_size, n_layers, p_dropout=0.0)
- self.proj = nn.Conv1d(
- filter_channels, self.half_channels * (num_bins * 3 - 1), 1
- )
- self.proj.weight.data.zero_()
- self.proj.bias.data.zero_()
-
- def forward(self, x, x_mask, g=None, reverse=False):
- x0, x1 = torch.split(x, [self.half_channels] * 2, 1)
- h = self.pre(x0)
- h = self.convs(h, x_mask, g=g)
- h = self.proj(h) * x_mask
-
- b, c, t = x0.shape
- h = h.reshape(b, c, -1, t).permute(0, 1, 3, 2) # [b, cx?, t] -> [b, c, t, ?]
-
- unnormalized_widths = h[..., : self.num_bins] / math.sqrt(self.filter_channels)
- unnormalized_heights = h[..., self.num_bins : 2 * self.num_bins] / math.sqrt(
- self.filter_channels
- )
- unnormalized_derivatives = h[..., 2 * self.num_bins :]
-
- x1, logabsdet = piecewise_rational_quadratic_transform(
- x1,
- unnormalized_widths,
- unnormalized_heights,
- unnormalized_derivatives,
- inverse=reverse,
- tails="linear",
- tail_bound=self.tail_bound,
- )
-
- x = torch.cat([x0, x1], 1) * x_mask
- logdet = torch.sum(logabsdet * x_mask, [1, 2])
- if not reverse:
- return x, logdet
- else:
- return x
diff --git a/spaces/Nultx/VITS-TTS/models.py b/spaces/Nultx/VITS-TTS/models.py
deleted file mode 100644
index 7dcd22edf811b952514080f5f06cc43d635ead28..0000000000000000000000000000000000000000
--- a/spaces/Nultx/VITS-TTS/models.py
+++ /dev/null
@@ -1,542 +0,0 @@
-import math
-import torch
-from torch import nn
-from torch.nn import functional as F
-
-import commons
-import modules
-import attentions
-
-from torch.nn import Conv1d, ConvTranspose1d, Conv2d
-from torch.nn.utils import weight_norm, remove_weight_norm, spectral_norm
-from commons import init_weights, get_padding
-
-
-class StochasticDurationPredictor(nn.Module):
- def __init__(self, in_channels, filter_channels, kernel_size, p_dropout, n_flows=4, gin_channels=0):
- super().__init__()
- filter_channels = in_channels # it needs to be removed from future version.
- self.in_channels = in_channels
- self.filter_channels = filter_channels
- self.kernel_size = kernel_size
- self.p_dropout = p_dropout
- self.n_flows = n_flows
- self.gin_channels = gin_channels
-
- self.log_flow = modules.Log()
- self.flows = nn.ModuleList()
- self.flows.append(modules.ElementwiseAffine(2))
- for i in range(n_flows):
- self.flows.append(modules.ConvFlow(2, filter_channels, kernel_size, n_layers=3))
- self.flows.append(modules.Flip())
-
- self.post_pre = nn.Conv1d(1, filter_channels, 1)
- self.post_proj = nn.Conv1d(filter_channels, filter_channels, 1)
- self.post_convs = modules.DDSConv(filter_channels, kernel_size, n_layers=3, p_dropout=p_dropout)
- self.post_flows = nn.ModuleList()
- self.post_flows.append(modules.ElementwiseAffine(2))
- for i in range(4):
- self.post_flows.append(modules.ConvFlow(2, filter_channels, kernel_size, n_layers=3))
- self.post_flows.append(modules.Flip())
-
- self.pre = nn.Conv1d(in_channels, filter_channels, 1)
- self.proj = nn.Conv1d(filter_channels, filter_channels, 1)
- self.convs = modules.DDSConv(filter_channels, kernel_size, n_layers=3, p_dropout=p_dropout)
- if gin_channels != 0:
- self.cond = nn.Conv1d(gin_channels, filter_channels, 1)
-
- def forward(self, x, x_mask, w=None, g=None, reverse=False, noise_scale=1.0):
- x = torch.detach(x)
- x = self.pre(x)
- if g is not None:
- g = torch.detach(g)
- x = x + self.cond(g)
- x = self.convs(x, x_mask)
- x = self.proj(x) * x_mask
-
- if not reverse:
- flows = self.flows
- assert w is not None
-
- logdet_tot_q = 0
- h_w = self.post_pre(w)
- h_w = self.post_convs(h_w, x_mask)
- h_w = self.post_proj(h_w) * x_mask
- e_q = torch.randn(w.size(0), 2, w.size(2)).to(device=x.device, dtype=x.dtype) * x_mask
- z_q = e_q
- for flow in self.post_flows:
- z_q, logdet_q = flow(z_q, x_mask, g=(x + h_w))
- logdet_tot_q += logdet_q
- z_u, z1 = torch.split(z_q, [1, 1], 1)
- u = torch.sigmoid(z_u) * x_mask
- z0 = (w - u) * x_mask
- logdet_tot_q += torch.sum((F.logsigmoid(z_u) + F.logsigmoid(-z_u)) * x_mask, [1,2])
- logq = torch.sum(-0.5 * (math.log(2*math.pi) + (e_q**2)) * x_mask, [1,2]) - logdet_tot_q
-
- logdet_tot = 0
- z0, logdet = self.log_flow(z0, x_mask)
- logdet_tot += logdet
- z = torch.cat([z0, z1], 1)
- for flow in flows:
- z, logdet = flow(z, x_mask, g=x, reverse=reverse)
- logdet_tot = logdet_tot + logdet
- nll = torch.sum(0.5 * (math.log(2*math.pi) + (z**2)) * x_mask, [1,2]) - logdet_tot
- return nll + logq # [b]
- else:
- flows = list(reversed(self.flows))
- flows = flows[:-2] + [flows[-1]] # remove a useless vflow
- z = torch.randn(x.size(0), 2, x.size(2)).to(device=x.device, dtype=x.dtype) * noise_scale
- for flow in flows:
- z = flow(z, x_mask, g=x, reverse=reverse)
- z0, z1 = torch.split(z, [1, 1], 1)
- logw = z0
- return logw
-
-
-class DurationPredictor(nn.Module):
- def __init__(self, in_channels, filter_channels, kernel_size, p_dropout, gin_channels=0):
- super().__init__()
-
- self.in_channels = in_channels
- self.filter_channels = filter_channels
- self.kernel_size = kernel_size
- self.p_dropout = p_dropout
- self.gin_channels = gin_channels
-
- self.drop = nn.Dropout(p_dropout)
- self.conv_1 = nn.Conv1d(in_channels, filter_channels, kernel_size, padding=kernel_size//2)
- self.norm_1 = modules.LayerNorm(filter_channels)
- self.conv_2 = nn.Conv1d(filter_channels, filter_channels, kernel_size, padding=kernel_size//2)
- self.norm_2 = modules.LayerNorm(filter_channels)
- self.proj = nn.Conv1d(filter_channels, 1, 1)
-
- if gin_channels != 0:
- self.cond = nn.Conv1d(gin_channels, in_channels, 1)
-
- def forward(self, x, x_mask, g=None):
- x = torch.detach(x)
- if g is not None:
- g = torch.detach(g)
- x = x + self.cond(g)
- x = self.conv_1(x * x_mask)
- x = torch.relu(x)
- x = self.norm_1(x)
- x = self.drop(x)
- x = self.conv_2(x * x_mask)
- x = torch.relu(x)
- x = self.norm_2(x)
- x = self.drop(x)
- x = self.proj(x * x_mask)
- return x * x_mask
-
-
-class TextEncoder(nn.Module):
- def __init__(self,
- n_vocab,
- out_channels,
- hidden_channels,
- filter_channels,
- n_heads,
- n_layers,
- kernel_size,
- p_dropout,
- emotion_embedding):
- super().__init__()
- self.n_vocab = n_vocab
- self.out_channels = out_channels
- self.hidden_channels = hidden_channels
- self.filter_channels = filter_channels
- self.n_heads = n_heads
- self.n_layers = n_layers
- self.kernel_size = kernel_size
- self.p_dropout = p_dropout
- self.emotion_embedding = emotion_embedding
-
- if self.n_vocab!=0:
- self.emb = nn.Embedding(n_vocab, hidden_channels)
- if emotion_embedding:
- self.emotion_emb = nn.Linear(1024, hidden_channels)
- nn.init.normal_(self.emb.weight, 0.0, hidden_channels**-0.5)
-
- self.encoder = attentions.Encoder(
- hidden_channels,
- filter_channels,
- n_heads,
- n_layers,
- kernel_size,
- p_dropout)
- self.proj= nn.Conv1d(hidden_channels, out_channels * 2, 1)
-
- def forward(self, x, x_lengths, emotion_embedding=None):
- if self.n_vocab!=0:
- x = self.emb(x) * math.sqrt(self.hidden_channels) # [b, t, h]
- if emotion_embedding is not None:
- x = x + self.emotion_emb(emotion_embedding.unsqueeze(1))
- x = torch.transpose(x, 1, -1) # [b, h, t]
- x_mask = torch.unsqueeze(commons.sequence_mask(x_lengths, x.size(2)), 1).to(x.dtype)
-
- x = self.encoder(x * x_mask, x_mask)
- stats = self.proj(x) * x_mask
-
- m, logs = torch.split(stats, self.out_channels, dim=1)
- return x, m, logs, x_mask
-
-
-class ResidualCouplingBlock(nn.Module):
- def __init__(self,
- channels,
- hidden_channels,
- kernel_size,
- dilation_rate,
- n_layers,
- n_flows=4,
- gin_channels=0):
- super().__init__()
- self.channels = channels
- self.hidden_channels = hidden_channels
- self.kernel_size = kernel_size
- self.dilation_rate = dilation_rate
- self.n_layers = n_layers
- self.n_flows = n_flows
- self.gin_channels = gin_channels
-
- self.flows = nn.ModuleList()
- for i in range(n_flows):
- self.flows.append(modules.ResidualCouplingLayer(channels, hidden_channels, kernel_size, dilation_rate, n_layers, gin_channels=gin_channels, mean_only=True))
- self.flows.append(modules.Flip())
-
- def forward(self, x, x_mask, g=None, reverse=False):
- if not reverse:
- for flow in self.flows:
- x, _ = flow(x, x_mask, g=g, reverse=reverse)
- else:
- for flow in reversed(self.flows):
- x = flow(x, x_mask, g=g, reverse=reverse)
- return x
-
-
-class PosteriorEncoder(nn.Module):
- def __init__(self,
- in_channels,
- out_channels,
- hidden_channels,
- kernel_size,
- dilation_rate,
- n_layers,
- gin_channels=0):
- super().__init__()
- self.in_channels = in_channels
- self.out_channels = out_channels
- self.hidden_channels = hidden_channels
- self.kernel_size = kernel_size
- self.dilation_rate = dilation_rate
- self.n_layers = n_layers
- self.gin_channels = gin_channels
-
- self.pre = nn.Conv1d(in_channels, hidden_channels, 1)
- self.enc = modules.WN(hidden_channels, kernel_size, dilation_rate, n_layers, gin_channels=gin_channels)
- self.proj = nn.Conv1d(hidden_channels, out_channels * 2, 1)
-
- def forward(self, x, x_lengths, g=None):
- x_mask = torch.unsqueeze(commons.sequence_mask(x_lengths, x.size(2)), 1).to(x.dtype)
- x = self.pre(x) * x_mask
- x = self.enc(x, x_mask, g=g)
- stats = self.proj(x) * x_mask
- m, logs = torch.split(stats, self.out_channels, dim=1)
- z = (m + torch.randn_like(m) * torch.exp(logs)) * x_mask
- return z, m, logs, x_mask
-
-
-class Generator(torch.nn.Module):
- def __init__(self, initial_channel, resblock, resblock_kernel_sizes, resblock_dilation_sizes, upsample_rates, upsample_initial_channel, upsample_kernel_sizes, gin_channels=0):
- super(Generator, self).__init__()
- self.num_kernels = len(resblock_kernel_sizes)
- self.num_upsamples = len(upsample_rates)
- self.conv_pre = Conv1d(initial_channel, upsample_initial_channel, 7, 1, padding=3)
- resblock = modules.ResBlock1 if resblock == '1' else modules.ResBlock2
-
- self.ups = nn.ModuleList()
- for i, (u, k) in enumerate(zip(upsample_rates, upsample_kernel_sizes)):
- self.ups.append(weight_norm(
- ConvTranspose1d(upsample_initial_channel//(2**i), upsample_initial_channel//(2**(i+1)),
- k, u, padding=(k-u)//2)))
-
- self.resblocks = nn.ModuleList()
- for i in range(len(self.ups)):
- ch = upsample_initial_channel//(2**(i+1))
- for j, (k, d) in enumerate(zip(resblock_kernel_sizes, resblock_dilation_sizes)):
- self.resblocks.append(resblock(ch, k, d))
-
- self.conv_post = Conv1d(ch, 1, 7, 1, padding=3, bias=False)
- self.ups.apply(init_weights)
-
- if gin_channels != 0:
- self.cond = nn.Conv1d(gin_channels, upsample_initial_channel, 1)
-
- def forward(self, x, g=None):
- x = self.conv_pre(x)
- if g is not None:
- x = x + self.cond(g)
-
- for i in range(self.num_upsamples):
- x = F.leaky_relu(x, modules.LRELU_SLOPE)
- x = self.ups[i](x)
- xs = None
- for j in range(self.num_kernels):
- if xs is None:
- xs = self.resblocks[i*self.num_kernels+j](x)
- else:
- xs += self.resblocks[i*self.num_kernels+j](x)
- x = xs / self.num_kernels
- x = F.leaky_relu(x)
- x = self.conv_post(x)
- x = torch.tanh(x)
-
- return x
-
- def remove_weight_norm(self):
- print('Removing weight norm...')
- for l in self.ups:
- remove_weight_norm(l)
- for l in self.resblocks:
- l.remove_weight_norm()
-
-
-class DiscriminatorP(torch.nn.Module):
- def __init__(self, period, kernel_size=5, stride=3, use_spectral_norm=False):
- super(DiscriminatorP, self).__init__()
- self.period = period
- self.use_spectral_norm = use_spectral_norm
- norm_f = weight_norm if use_spectral_norm == False else spectral_norm
- self.convs = nn.ModuleList([
- norm_f(Conv2d(1, 32, (kernel_size, 1), (stride, 1), padding=(get_padding(kernel_size, 1), 0))),
- norm_f(Conv2d(32, 128, (kernel_size, 1), (stride, 1), padding=(get_padding(kernel_size, 1), 0))),
- norm_f(Conv2d(128, 512, (kernel_size, 1), (stride, 1), padding=(get_padding(kernel_size, 1), 0))),
- norm_f(Conv2d(512, 1024, (kernel_size, 1), (stride, 1), padding=(get_padding(kernel_size, 1), 0))),
- norm_f(Conv2d(1024, 1024, (kernel_size, 1), 1, padding=(get_padding(kernel_size, 1), 0))),
- ])
- self.conv_post = norm_f(Conv2d(1024, 1, (3, 1), 1, padding=(1, 0)))
-
- def forward(self, x):
- fmap = []
-
- # 1d to 2d
- b, c, t = x.shape
- if t % self.period != 0: # pad first
- n_pad = self.period - (t % self.period)
- x = F.pad(x, (0, n_pad), "reflect")
- t = t + n_pad
- x = x.view(b, c, t // self.period, self.period)
-
- for l in self.convs:
- x = l(x)
- x = F.leaky_relu(x, modules.LRELU_SLOPE)
- fmap.append(x)
- x = self.conv_post(x)
- fmap.append(x)
- x = torch.flatten(x, 1, -1)
-
- return x, fmap
-
-
-class DiscriminatorS(torch.nn.Module):
- def __init__(self, use_spectral_norm=False):
- super(DiscriminatorS, self).__init__()
- norm_f = weight_norm if use_spectral_norm == False else spectral_norm
- self.convs = nn.ModuleList([
- norm_f(Conv1d(1, 16, 15, 1, padding=7)),
- norm_f(Conv1d(16, 64, 41, 4, groups=4, padding=20)),
- norm_f(Conv1d(64, 256, 41, 4, groups=16, padding=20)),
- norm_f(Conv1d(256, 1024, 41, 4, groups=64, padding=20)),
- norm_f(Conv1d(1024, 1024, 41, 4, groups=256, padding=20)),
- norm_f(Conv1d(1024, 1024, 5, 1, padding=2)),
- ])
- self.conv_post = norm_f(Conv1d(1024, 1, 3, 1, padding=1))
-
- def forward(self, x):
- fmap = []
-
- for l in self.convs:
- x = l(x)
- x = F.leaky_relu(x, modules.LRELU_SLOPE)
- fmap.append(x)
- x = self.conv_post(x)
- fmap.append(x)
- x = torch.flatten(x, 1, -1)
-
- return x, fmap
-
-
-class MultiPeriodDiscriminator(torch.nn.Module):
- def __init__(self, use_spectral_norm=False):
- super(MultiPeriodDiscriminator, self).__init__()
- periods = [2,3,5,7,11]
-
- discs = [DiscriminatorS(use_spectral_norm=use_spectral_norm)]
- discs = discs + [DiscriminatorP(i, use_spectral_norm=use_spectral_norm) for i in periods]
- self.discriminators = nn.ModuleList(discs)
-
- def forward(self, y, y_hat):
- y_d_rs = []
- y_d_gs = []
- fmap_rs = []
- fmap_gs = []
- for i, d in enumerate(self.discriminators):
- y_d_r, fmap_r = d(y)
- y_d_g, fmap_g = d(y_hat)
- y_d_rs.append(y_d_r)
- y_d_gs.append(y_d_g)
- fmap_rs.append(fmap_r)
- fmap_gs.append(fmap_g)
-
- return y_d_rs, y_d_gs, fmap_rs, fmap_gs
-
-
-
-class SynthesizerTrn(nn.Module):
- """
- Synthesizer for Training
- """
-
- def __init__(self,
- n_vocab,
- spec_channels,
- segment_size,
- inter_channels,
- hidden_channels,
- filter_channels,
- n_heads,
- n_layers,
- kernel_size,
- p_dropout,
- resblock,
- resblock_kernel_sizes,
- resblock_dilation_sizes,
- upsample_rates,
- upsample_initial_channel,
- upsample_kernel_sizes,
- n_speakers=0,
- gin_channels=0,
- use_sdp=True,
- emotion_embedding=False,
- **kwargs):
-
- super().__init__()
- self.n_vocab = n_vocab
- self.spec_channels = spec_channels
- self.inter_channels = inter_channels
- self.hidden_channels = hidden_channels
- self.filter_channels = filter_channels
- self.n_heads = n_heads
- self.n_layers = n_layers
- self.kernel_size = kernel_size
- self.p_dropout = p_dropout
- self.resblock = resblock
- self.resblock_kernel_sizes = resblock_kernel_sizes
- self.resblock_dilation_sizes = resblock_dilation_sizes
- self.upsample_rates = upsample_rates
- self.upsample_initial_channel = upsample_initial_channel
- self.upsample_kernel_sizes = upsample_kernel_sizes
- self.segment_size = segment_size
- self.n_speakers = n_speakers
- self.gin_channels = gin_channels
-
- self.use_sdp = use_sdp
-
- self.enc_p = TextEncoder(n_vocab,
- inter_channels,
- hidden_channels,
- filter_channels,
- n_heads,
- n_layers,
- kernel_size,
- p_dropout,
- emotion_embedding)
- self.dec = Generator(inter_channels, resblock, resblock_kernel_sizes, resblock_dilation_sizes, upsample_rates, upsample_initial_channel, upsample_kernel_sizes, gin_channels=gin_channels)
- self.enc_q = PosteriorEncoder(spec_channels, inter_channels, hidden_channels, 5, 1, 16, gin_channels=gin_channels)
- self.flow = ResidualCouplingBlock(inter_channels, hidden_channels, 5, 1, 4, gin_channels=gin_channels)
-
- if use_sdp:
- self.dp = StochasticDurationPredictor(hidden_channels, 192, 3, 0.5, 4, gin_channels=gin_channels)
- else:
- self.dp = DurationPredictor(hidden_channels, 256, 3, 0.5, gin_channels=gin_channels)
-
- if n_speakers > 1:
- self.emb_g = nn.Embedding(n_speakers, gin_channels)
-
- def forward(self, x, x_lengths, y, y_lengths, sid=None):
-
- x, m_p, logs_p, x_mask = self.enc_p(x, x_lengths)
- if self.n_speakers > 0:
- g = self.emb_g(sid).unsqueeze(-1) # [b, h, 1]
- else:
- g = None
-
- z, m_q, logs_q, y_mask = self.enc_q(y, y_lengths, g=g)
- z_p = self.flow(z, y_mask, g=g)
-
- with torch.no_grad():
- # negative cross-entropy
- s_p_sq_r = torch.exp(-2 * logs_p) # [b, d, t]
- neg_cent1 = torch.sum(-0.5 * math.log(2 * math.pi) - logs_p, [1], keepdim=True) # [b, 1, t_s]
- neg_cent2 = torch.matmul(-0.5 * (z_p ** 2).transpose(1, 2), s_p_sq_r) # [b, t_t, d] x [b, d, t_s] = [b, t_t, t_s]
- neg_cent3 = torch.matmul(z_p.transpose(1, 2), (m_p * s_p_sq_r)) # [b, t_t, d] x [b, d, t_s] = [b, t_t, t_s]
- neg_cent4 = torch.sum(-0.5 * (m_p ** 2) * s_p_sq_r, [1], keepdim=True) # [b, 1, t_s]
- neg_cent = neg_cent1 + neg_cent2 + neg_cent3 + neg_cent4
-
- attn_mask = torch.unsqueeze(x_mask, 2) * torch.unsqueeze(y_mask, -1)
- attn = monotonic_align.maximum_path(neg_cent, attn_mask.squeeze(1)).unsqueeze(1).detach()
-
- w = attn.sum(2)
- if self.use_sdp:
- l_length = self.dp(x, x_mask, w, g=g)
- l_length = l_length / torch.sum(x_mask)
- else:
- logw_ = torch.log(w + 1e-6) * x_mask
- logw = self.dp(x, x_mask, g=g)
- l_length = torch.sum((logw - logw_)**2, [1,2]) / torch.sum(x_mask) # for averaging
-
- # expand prior
- m_p = torch.matmul(attn.squeeze(1), m_p.transpose(1, 2)).transpose(1, 2)
- logs_p = torch.matmul(attn.squeeze(1), logs_p.transpose(1, 2)).transpose(1, 2)
-
- z_slice, ids_slice = commons.rand_slice_segments(z, y_lengths, self.segment_size)
- o = self.dec(z_slice, g=g)
- return o, l_length, attn, ids_slice, x_mask, y_mask, (z, z_p, m_p, logs_p, m_q, logs_q)
-
- def infer(self, x, x_lengths, sid=None, noise_scale=1, length_scale=1, noise_scale_w=1., max_len=None, emotion_embedding=None):
- x, m_p, logs_p, x_mask = self.enc_p(x, x_lengths, emotion_embedding)
- if self.n_speakers > 0:
- g = self.emb_g(sid).unsqueeze(-1) # [b, h, 1]
- else:
- g = None
-
- if self.use_sdp:
- logw = self.dp(x, x_mask, g=g, reverse=True, noise_scale=noise_scale_w)
- else:
- logw = self.dp(x, x_mask, g=g)
- w = torch.exp(logw) * x_mask * length_scale
- w_ceil = torch.ceil(w)
- y_lengths = torch.clamp_min(torch.sum(w_ceil, [1, 2]), 1).long()
- y_mask = torch.unsqueeze(commons.sequence_mask(y_lengths, None), 1).to(x_mask.dtype)
- attn_mask = torch.unsqueeze(x_mask, 2) * torch.unsqueeze(y_mask, -1)
- attn = commons.generate_path(w_ceil, attn_mask)
-
- m_p = torch.matmul(attn.squeeze(1), m_p.transpose(1, 2)).transpose(1, 2) # [b, t', t], [b, t, d] -> [b, d, t']
- logs_p = torch.matmul(attn.squeeze(1), logs_p.transpose(1, 2)).transpose(1, 2) # [b, t', t], [b, t, d] -> [b, d, t']
-
- z_p = m_p + torch.randn_like(m_p) * torch.exp(logs_p) * noise_scale
- z = self.flow(z_p, y_mask, g=g, reverse=True)
- o = self.dec((z * y_mask)[:,:,:max_len], g=g)
- return o, attn, y_mask, (z, z_p, m_p, logs_p)
-
- def voice_conversion(self, y, y_lengths, sid_src, sid_tgt):
- assert self.n_speakers > 0, "n_speakers have to be larger than 0."
- g_src = self.emb_g(sid_src).unsqueeze(-1)
- g_tgt = self.emb_g(sid_tgt).unsqueeze(-1)
- z, m_q, logs_q, y_mask = self.enc_q(y, y_lengths, g=g_src)
- z_p = self.flow(z, y_mask, g=g_src)
- z_hat = self.flow(z_p, y_mask, g=g_tgt, reverse=True)
- o_hat = self.dec(z_hat * y_mask, g=g_tgt)
- return o_hat, y_mask, (z, z_p, z_hat)
-
diff --git a/spaces/Nunchakuka/FrenchAnonymizer/speaker_encoder/params_data.py b/spaces/Nunchakuka/FrenchAnonymizer/speaker_encoder/params_data.py
deleted file mode 100644
index bdb1716ed45617f2b127a7fb8885afe6cc74fb71..0000000000000000000000000000000000000000
--- a/spaces/Nunchakuka/FrenchAnonymizer/speaker_encoder/params_data.py
+++ /dev/null
@@ -1,29 +0,0 @@
-
-## Mel-filterbank
-mel_window_length = 25 # In milliseconds
-mel_window_step = 10 # In milliseconds
-mel_n_channels = 40
-
-
-## Audio
-sampling_rate = 16000
-# Number of spectrogram frames in a partial utterance
-partials_n_frames = 160 # 1600 ms
-# Number of spectrogram frames at inference
-inference_n_frames = 80 # 800 ms
-
-
-## Voice Activation Detection
-# Window size of the VAD. Must be either 10, 20 or 30 milliseconds.
-# This sets the granularity of the VAD. Should not need to be changed.
-vad_window_length = 30 # In milliseconds
-# Number of frames to average together when performing the moving average smoothing.
-# The larger this value, the larger the VAD variations must be to not get smoothed out.
-vad_moving_average_width = 8
-# Maximum number of consecutive silent frames a segment can have.
-vad_max_silence_length = 6
-
-
-## Audio volume normalization
-audio_norm_target_dBFS = -30
-
diff --git a/spaces/OFA-Sys/OFA-Image_Caption/fairseq/fairseq/clib/libbleu/libbleu.cpp b/spaces/OFA-Sys/OFA-Image_Caption/fairseq/fairseq/clib/libbleu/libbleu.cpp
deleted file mode 100644
index 939d9e1174e398fa48c840009b592c753a67939a..0000000000000000000000000000000000000000
--- a/spaces/OFA-Sys/OFA-Image_Caption/fairseq/fairseq/clib/libbleu/libbleu.cpp
+++ /dev/null
@@ -1,157 +0,0 @@
-/**
- * Copyright 2017-present, Facebook, Inc.
- * All rights reserved.
- *
- * This source code is licensed under the license found in the
- * LICENSE file in the root directory of this source tree.
- */
-
-#include
-#include
-#include
-#include
-
Disadvantages or limitations of using Lite 6 APK
-
There are also some disadvantages or limitations of using Lite 6 APK. Some of them are:
-
-
You might not be able to access some features of the regular Facebook app, such as video calling, live streaming, or gaming.
-
You might not be able to see some content or media on Facebook, such as high-resolution images or videos.
-
You might not be able to use some third-party apps or services that require the regular Facebook app, such as Instagram or WhatsApp.
-
You might encounter some bugs or errors while using the app, such as the app crashing, freezing, or not loading properly.
-
You might need to update the app manually by downloading and installing the latest version from a trusted source.
-
-
Conclusion
-
Lite 6 APK is a modified version of Facebook Lite that offers a faster, lighter, and more efficient Facebook experience for Android users. It has many features that make it a great alternative to the regular Facebook app, such as fast installation, low storage space, less data usage, better performance, compatibility with old Android phones and all network conditions. However, it also has some drawbacks that might make it unsuitable for some users, such as missing some features of the regular Facebook app, not showing some content or media on Facebook, not working with some third-party apps or services, and having some bugs or errors.
-
If you want to try Lite 6 APK on your Android device, you can download and install it by following the steps mentioned in this article. You can also customize your settings and preferences using the app and troubleshoot any issues that you might face. However, you should always be careful when downloading and installing any APK file from unknown sources and make sure that you have a backup of your data before doing so.
-
We hope that this article has helped you understand what Lite 6 APK is and why you might need it. If you have any questions or feedback, please feel free to leave a comment below. Thank you for reading!
-
FAQs
-
What is an APK file?
-
An APK file is an Android Package file that contains all the files and code needed to install and run an app on an Android device. It is similar to an EXE file on Windows or a DMG file on Mac.
-
Is Lite 6 APK safe to use?
-
Lite 6 APK is generally safe to use if you download it from a trusted source, such as [Facebook] or [APKPure]. However, you should always be careful when downloading and installing any APK file from unknown sources and make sure that you have a backup of your data before doing so.
-
How can I update Lite 6 APK?
-
You can update Lite 6 APK by downloading and installing the latest version from a trusted source, such as [Facebook] or [APKPure]. You can also check for updates by going to Menu > Settings > About > Check for Updates.
-
Can I use Lite 6 APK on other devices besides Android?
-
No, Lite 6 APK is only compatible with Android devices. If you want to use Facebook on other devices, such as iOS or Windows, you can use the regular Facebook app or the web version of Facebook.
-
Can I use both Lite 6 APK and the regular Facebook app on my device?
-
Yes, you can use both Lite 6 APK and the regular Facebook app on your device. However, you should keep in mind that they will use different storage space and data usage on your device. You should also make sure that you sign in with the same account on both apps to avoid any confusion or inconsistency.
197e85843d
-
-
\ No newline at end of file
diff --git a/spaces/tinkoff-ai/caif/app.py b/spaces/tinkoff-ai/caif/app.py
deleted file mode 100644
index 04ad092284ff90bb0d99933c96a7e901c0b1f887..0000000000000000000000000000000000000000
--- a/spaces/tinkoff-ai/caif/app.py
+++ /dev/null
@@ -1,265 +0,0 @@
-import os
-from typing import Tuple
-
-import streamlit as st
-
-import torch
-
-import transformers
-
-from transformers import AutoConfig
-import tokenizers
-
-from sampling import CAIFSampler, TopKWithTemperatureSampler
-from generator import Generator
-
-import pickle
-
-from plotly import graph_objects as go
-
-import numpy as np
-
-device = "cuda" if torch.cuda.is_available() else "cpu"
-
-ATTRIBUTE_MODELS = {
- "English": (
- "distilbert-base-uncased-finetuned-sst-2-english",
- "unitary/toxic-bert",
- "cardiffnlp/twitter-roberta-base-sentiment-latest",
- )
-}
-
-CITE = """@misc{https://doi.org/10.48550/arxiv.2205.07276,
- doi = {10.48550/ARXIV.2205.07276},
- url = {https://arxiv.org/abs/2205.07276},
- author = {Sitdikov, Askhat and Balagansky, Nikita and Gavrilov, Daniil and Markov, Alexander},
- keywords = {Computation and Language (cs.CL), Machine Learning (cs.LG), FOS: Computer and information sciences, FOS: Computer and information sciences},
- title = {Classifiers are Better Experts for Controllable Text Generation},
- publisher = {arXiv},
- year = {2022},
- copyright = {Creative Commons Attribution 4.0 International}
-}
-"""
-
-LANGUAGE_MODELS = {
- "English": ("gpt2", "distilgpt2", "EleutherAI/gpt-neo-1.3B")
-}
-
-ATTRIBUTE_MODEL_LABEL = {
- "English": "Choose attribute model"
-}
-
-LM_LABEL = {
- "English": "Choose language model",
-}
-
-ATTRIBUTE_LABEL = {
- "English": "Choose desired attribute",
-}
-
-TEXT_PROMPT_LABEL = {
- "English": "Text prompt",
-}
-
-PROMPT_EXAMPLE = {
- "English": "Hello there",
-}
-
-WARNING_TEXT = {
- "English": """
- **Warning!**
-
- If you are clicking checkbox bellow positive """ + r"$\alpha$" + """ values for CAIF sampling become available.
- It means that language model will be forced to produce toxic or/and abusive text.
- This space is only a demonstration of our method for controllable text generation
- and we are not responsible for the content produced by this method.
-
- **Please use it carefully and with positive intentions!**
- """,
-}
-
-
-def main():
- st.header("CAIF")
- with open("entropy_cdf.pkl", "rb") as inp:
- x_s, y_s = pickle.load(inp)
- scatter = go.Scatter({
- "x": x_s,
- "y": y_s,
- "name": "GPT2",
- "mode": "lines",
- }
- )
- layout = go.Layout({
- "yaxis": {
- "title": "Speedup",
- "tickvals": [0, 0.5, 0.8, 1],
- "ticktext": ["1x", "2x", "5x", "10x"]
- },
- "xaxis": {"title": "Entropy threshold"},
- "template": "plotly_white",
- })
-
- language = "English"
- cls_model_name = st.selectbox(
- ATTRIBUTE_MODEL_LABEL[language],
- ATTRIBUTE_MODELS[language]
-
- )
- lm_model_name = st.selectbox(
- LM_LABEL[language],
- LANGUAGE_MODELS[language]
- )
- cls_model_config = AutoConfig.from_pretrained(cls_model_name)
- if cls_model_config.problem_type == "multi_label_classification":
- label2id = cls_model_config.label2id
- label_key = st.selectbox(ATTRIBUTE_LABEL[language], label2id.keys())
- target_label_id = label2id[label_key]
- act_type = "sigmoid"
- elif cls_model_config.problem_type == "single_label_classification":
- label2id = cls_model_config.label2id
- label_key = st.selectbox(ATTRIBUTE_LABEL[language], [list(label2id.keys())[-1]])
- target_label_id = 1
- act_type = "sigmoid"
- else:
- label_key = st.selectbox(ATTRIBUTE_LABEL[language], ["Negative"])
- target_label_id = 0
- act_type = "softmax"
-
- st.markdown(r"""In our method, we reweight the probability of the next token with the external classifier, namely, the Attribute model. If $\alpha$ parameter is equal to zero we can see that the distribution below collapses into a simple language model without any modification. If alpha is below zero then every generation step attribute model tries to minimize the probability of the desired attribute. Otherwise, the model is forced to produce text with a higher probability of the attribute.""")
- st.latex(r"p(x_i|x_{ Generator:
- with st.spinner('Loading language model...'):
- generator = Generator(lm_model_name=lm_model_name, device=device)
- return generator
-
-
-# @st.cache(hash_funcs={tokenizers.Tokenizer: lambda lm_tokenizer: hash(lm_tokenizer.to_str)}, allow_output_mutation=True)
-def load_sampler(cls_model_name, lm_tokenizer):
- with st.spinner('Loading classifier model...'):
- sampler = CAIFSampler(classifier_name=cls_model_name, lm_tokenizer=lm_tokenizer, device=device)
- return sampler
-
-
-def inference(
- lm_model_name: str,
- cls_model_name: str,
- prompt: str,
- fp16: bool = True,
- alpha: float = 5,
- target_label_id: int = 0,
- entropy_threshold: float = 0,
- act_type: str = "sigmoid",
- num_tokens=10,
-) -> str:
- torch.set_grad_enabled(False)
- generator = load_generator(lm_model_name=lm_model_name)
- lm_tokenizer = transformers.AutoTokenizer.from_pretrained(lm_model_name)
- if alpha != 0:
- caif_sampler = load_sampler(cls_model_name=cls_model_name, lm_tokenizer=lm_tokenizer)
- if entropy_threshold < 0.05:
- entropy_threshold = None
- else:
- caif_sampler = None
- entropy_threshold = None
-
- generator.set_caif_sampler(caif_sampler)
- ordinary_sampler = TopKWithTemperatureSampler()
- kwargs = {
- "top_k": 20,
- "temperature": 1.0,
- "top_k_classifier": 100,
- "classifier_weight": alpha,
- "target_cls_id": target_label_id,
- "act_type": act_type
- }
- generator.set_ordinary_sampler(ordinary_sampler)
- if device == "cpu":
- autocast = torch.cpu.amp.autocast
- else:
- autocast = torch.cuda.amp.autocast
- with autocast(fp16):
- print(f"Generating for prompt: {prompt}")
- progress_bar = st.progress(0)
- sequences, tokens = generator.sample_sequences(
- num_samples=1,
- input_prompt=prompt,
- max_length=num_tokens,
- caif_period=1,
- entropy=entropy_threshold,
- progress_bar=progress_bar,
- **kwargs
- )
- print(f"Output for prompt: {sequences}")
-
- return sequences[0]
-
-
-if __name__ == "__main__":
- main()
diff --git a/spaces/tioseFevbu/cartoon-converter/scripts/Alkid Live Cd 2012 Download.md b/spaces/tioseFevbu/cartoon-converter/scripts/Alkid Live Cd 2012 Download.md
deleted file mode 100644
index acc1b77a2fe55eff4c41aa5ad1cef9845cbc9591..0000000000000000000000000000000000000000
--- a/spaces/tioseFevbu/cartoon-converter/scripts/Alkid Live Cd 2012 Download.md
+++ /dev/null
@@ -1,28 +0,0 @@
-
-
How to Download and Use Alkid Live CD 2012
-
Alkid Live CD 2012 is a universal boot disk that can help you work and restore your system in case of any problems. It supports various file systems, such as exFAT, EXT2/EXT3/EXT4, and GPT, and can work with any media, such as CD, USB drive, USB-HDD, etc. It also contains a set of fresh antivirus, tools for working with disks and images, backup and lifting systems, restore lost information and passwords, create new administrator accounts, and more.
-
In this article, we will show you how to download and use Alkid Live CD 2012 to fix your computer issues.
The first step is to download the Alkid Live CD 2012 ISO file from the official website[^1^]. The file size is about 1.4 GB, so make sure you have enough space on your hard drive or USB drive. You can also use a torrent client to download the file faster.
-
How to Burn Alkid Live CD 2012 to a CD or DVD
-
The next step is to burn the Alkid Live CD 2012 ISO file to a blank CD or DVD. You can use any burning software that supports ISO files, such as Nero, ImgBurn, or CDBurnerXP. Follow the instructions of your burning software to select the ISO file and burn it to the disc. Make sure you choose the option to verify the disc after burning.
-
How to Create Alkid Live USB 2012
-
If you prefer to use a USB drive instead of a CD or DVD, you can create an Alkid Live USB 2012 using a special tool called UltraISO. UltraISO is a software that can create bootable USB drives from ISO files. You can download UltraISO from its official website. After installing UltraISO, follow these steps:
-
-
Launch UltraISO and click on File > Open. Browse to the location of the Alkid Live CD 2012 ISO file and open it.
-
Insert a USB drive with at least 2 GB of free space into your computer. Make sure you backup any important data on the USB drive before proceeding.
-
Click on Bootable > Write Disk Image. A new window will pop up.
-
Select your USB drive from the drop-down menu under Disk Drive. Make sure the Write Method is set to USB-HDD+.
-
Click on Write and wait for the process to complete.
-
-
How to Boot from Alkid Live CD or USB 2012
-
The final step is to boot your computer from the Alkid Live CD or USB 2012. To do this, you need to change the boot order in your BIOS settings. The BIOS settings are different for each computer model, so you may need to consult your manual or search online for instructions. Generally, you need to press a certain key (such as F2, F10, F12, or Del) when your computer starts up to enter the BIOS settings. Then, you need to find the Boot menu and change the priority of the boot devices. You need to move the CD-ROM or USB device to the top of the list. Save and exit the BIOS settings.
-
Now, when you restart your computer, it should boot from the Alkid Live CD or USB 2012. You will see a menu with several options. You can choose either "Start Windows XP" or "Start Windows XP (Safe Mode)" depending on your preference. The safe mode option will load only the essential drivers and services for troubleshooting purposes.
-
How to Use Alkid Live CD or USB 2012
-
Once you boot into Windows XP from the Alkid Live CD or USB 2012, you will see a desktop with several icons. These icons represent different tools and functions that you can use to work and restore your system. Here are some of the most useful ones:
-
-
-
Antivirus: This icon will launch a program called Dr.Web CureIt!, which is a powerful antivirus scanner that can detect and remove various malware infections from your system 81aa517590
-
-
\ No newline at end of file
diff --git a/spaces/tioseFevbu/cartoon-converter/scripts/Fidic Blue Book Free 22 BEST.md b/spaces/tioseFevbu/cartoon-converter/scripts/Fidic Blue Book Free 22 BEST.md
deleted file mode 100644
index ce6ca50eadb634d5a175125c9aa9bb92f1d6265e..0000000000000000000000000000000000000000
--- a/spaces/tioseFevbu/cartoon-converter/scripts/Fidic Blue Book Free 22 BEST.md
+++ /dev/null
@@ -1,42 +0,0 @@
-
-
What is the Fidic Blue Book and How to Get it for Free?
-
-
The Fidic Blue Book is a standard form of contract for dredging and reclamation works, published by the International Federation of Consulting Engineers (FIDIC). It is designed to meet the specific needs of the marine construction industry and to provide a fair balance of risk between the employer and the contractor.
The Fidic Blue Book was first published in 2006 as a test edition, based on the FIDIC Short Form of Contract (Green Book). In 2016, a second edition was released, with significant improvements and updates to reflect the actual practice and feedback from the users. The second edition is more user-friendly, more aligned with other FIDIC contracts, and more specific to the dredging industry.
-
-
If you are involved in dredging and reclamation projects, you may want to get a copy of the Fidic Blue Book for your reference. But how can you get it for free?
-
-
How to Get the Fidic Blue Book for Free?
-
-
There are two ways to get the Fidic Blue Book for free:
-
-
-
Download it from the FIDIC website. The FIDIC website offers a free download of the "Golden Principles" of the Fidic Blue Book, which are the essential principles that should be followed when using the contract. The Golden Principles cover topics such as scope of work, payment, variations, claims, disputes, and termination. You can download the Golden Principles from here.
-
Request it from the IADC website. The International Association of Dredging Companies (IADC) is an organization that represents the interests of the dredging industry. The IADC was involved in the development of the Fidic Blue Book and offers a free copy of the contract to its members and associates. You can request a copy of the Fidic Blue Book from here.
-
-
-
By getting the Fidic Blue Book for free, you can learn more about the best practices and standards for dredging and reclamation works. You can also use it as a template or a guide for drafting your own contracts or negotiating with your clients or contractors.
-
-
-
Conclusion
-
-
The Fidic Blue Book is a valuable resource for anyone involved in dredging and reclamation works. It is a comprehensive and specialized contract that reflects the needs and challenges of the marine construction industry. It also provides a fair and balanced allocation of risk between the parties.
-
-
If you want to get the Fidic Blue Book for free, you can either download it from the FIDIC website or request it from the IADC website. By doing so, you can benefit from the expertise and experience of FIDIC and IADC in developing this contract.
-
-
What are the Main Features of the Fidic Blue Book?
-
-
The Fidic Blue Book has several features that make it suitable and attractive for dredging and reclamation works. Some of these features are:
-
-
-
It is a lump sum contract with a fixed price and a fixed duration. This means that the contractor agrees to perform the works for a predetermined amount and within a specified time. The employer does not have to pay any extra costs or extensions of time, unless there are variations or claims.
-
It is a turnkey contract with a single point of responsibility. This means that the contractor is responsible for all aspects of the works, from design to execution to testing and commissioning. The employer does not have to deal with multiple contractors or consultants, which reduces the complexity and risk of the project.
-
It is a performance-based contract with measurable outcomes. This means that the contractor is paid based on the achievement of certain milestones or deliverables, such as volume of material dredged or reclaimed, quality of workmanship, environmental compliance, etc. The employer can monitor and verify the performance of the contractor and withhold payment if the performance is unsatisfactory.
-
It is a flexible contract with options for variations and claims. This means that the contract allows for changes in the scope of work or the conditions of execution, as well as for compensation for unforeseen events or circumstances. The contract provides clear procedures and mechanisms for dealing with variations and claims, such as notices, evaluations, determinations, and dispute resolution.
-
-
-
These features make the Fidic Blue Book a practical and efficient contract for dredging and reclamation works. They also ensure that the interests and expectations of both parties are protected and fulfilled.
e93f5a0c3f
-
-
\ No newline at end of file
diff --git a/spaces/tjburns/ask_marcus_aurelius/.venv/lib/python3.10/site-packages/pip/_vendor/pyparsing/testing.py b/spaces/tjburns/ask_marcus_aurelius/.venv/lib/python3.10/site-packages/pip/_vendor/pyparsing/testing.py
deleted file mode 100644
index 84a0ef17078c99e5917db41e3dbaf035fe206d7c..0000000000000000000000000000000000000000
--- a/spaces/tjburns/ask_marcus_aurelius/.venv/lib/python3.10/site-packages/pip/_vendor/pyparsing/testing.py
+++ /dev/null
@@ -1,331 +0,0 @@
-# testing.py
-
-from contextlib import contextmanager
-import typing
-
-from .core import (
- ParserElement,
- ParseException,
- Keyword,
- __diag__,
- __compat__,
-)
-
-
-class pyparsing_test:
- """
- namespace class for classes useful in writing unit tests
- """
-
- class reset_pyparsing_context:
- """
- Context manager to be used when writing unit tests that modify pyparsing config values:
- - packrat parsing
- - bounded recursion parsing
- - default whitespace characters.
- - default keyword characters
- - literal string auto-conversion class
- - __diag__ settings
-
- Example::
-
- with reset_pyparsing_context():
- # test that literals used to construct a grammar are automatically suppressed
- ParserElement.inlineLiteralsUsing(Suppress)
-
- term = Word(alphas) | Word(nums)
- group = Group('(' + term[...] + ')')
-
- # assert that the '()' characters are not included in the parsed tokens
- self.assertParseAndCheckList(group, "(abc 123 def)", ['abc', '123', 'def'])
-
- # after exiting context manager, literals are converted to Literal expressions again
- """
-
- def __init__(self):
- self._save_context = {}
-
- def save(self):
- self._save_context["default_whitespace"] = ParserElement.DEFAULT_WHITE_CHARS
- self._save_context["default_keyword_chars"] = Keyword.DEFAULT_KEYWORD_CHARS
-
- self._save_context[
- "literal_string_class"
- ] = ParserElement._literalStringClass
-
- self._save_context["verbose_stacktrace"] = ParserElement.verbose_stacktrace
-
- self._save_context["packrat_enabled"] = ParserElement._packratEnabled
- if ParserElement._packratEnabled:
- self._save_context[
- "packrat_cache_size"
- ] = ParserElement.packrat_cache.size
- else:
- self._save_context["packrat_cache_size"] = None
- self._save_context["packrat_parse"] = ParserElement._parse
- self._save_context[
- "recursion_enabled"
- ] = ParserElement._left_recursion_enabled
-
- self._save_context["__diag__"] = {
- name: getattr(__diag__, name) for name in __diag__._all_names
- }
-
- self._save_context["__compat__"] = {
- "collect_all_And_tokens": __compat__.collect_all_And_tokens
- }
-
- return self
-
- def restore(self):
- # reset pyparsing global state
- if (
- ParserElement.DEFAULT_WHITE_CHARS
- != self._save_context["default_whitespace"]
- ):
- ParserElement.set_default_whitespace_chars(
- self._save_context["default_whitespace"]
- )
-
- ParserElement.verbose_stacktrace = self._save_context["verbose_stacktrace"]
-
- Keyword.DEFAULT_KEYWORD_CHARS = self._save_context["default_keyword_chars"]
- ParserElement.inlineLiteralsUsing(
- self._save_context["literal_string_class"]
- )
-
- for name, value in self._save_context["__diag__"].items():
- (__diag__.enable if value else __diag__.disable)(name)
-
- ParserElement._packratEnabled = False
- if self._save_context["packrat_enabled"]:
- ParserElement.enable_packrat(self._save_context["packrat_cache_size"])
- else:
- ParserElement._parse = self._save_context["packrat_parse"]
- ParserElement._left_recursion_enabled = self._save_context[
- "recursion_enabled"
- ]
-
- __compat__.collect_all_And_tokens = self._save_context["__compat__"]
-
- return self
-
- def copy(self):
- ret = type(self)()
- ret._save_context.update(self._save_context)
- return ret
-
- def __enter__(self):
- return self.save()
-
- def __exit__(self, *args):
- self.restore()
-
- class TestParseResultsAsserts:
- """
- A mixin class to add parse results assertion methods to normal unittest.TestCase classes.
- """
-
- def assertParseResultsEquals(
- self, result, expected_list=None, expected_dict=None, msg=None
- ):
- """
- Unit test assertion to compare a :class:`ParseResults` object with an optional ``expected_list``,
- and compare any defined results names with an optional ``expected_dict``.
- """
- if expected_list is not None:
- self.assertEqual(expected_list, result.as_list(), msg=msg)
- if expected_dict is not None:
- self.assertEqual(expected_dict, result.as_dict(), msg=msg)
-
- def assertParseAndCheckList(
- self, expr, test_string, expected_list, msg=None, verbose=True
- ):
- """
- Convenience wrapper assert to test a parser element and input string, and assert that
- the resulting ``ParseResults.asList()`` is equal to the ``expected_list``.
- """
- result = expr.parse_string(test_string, parse_all=True)
- if verbose:
- print(result.dump())
- else:
- print(result.as_list())
- self.assertParseResultsEquals(result, expected_list=expected_list, msg=msg)
-
- def assertParseAndCheckDict(
- self, expr, test_string, expected_dict, msg=None, verbose=True
- ):
- """
- Convenience wrapper assert to test a parser element and input string, and assert that
- the resulting ``ParseResults.asDict()`` is equal to the ``expected_dict``.
- """
- result = expr.parse_string(test_string, parseAll=True)
- if verbose:
- print(result.dump())
- else:
- print(result.as_list())
- self.assertParseResultsEquals(result, expected_dict=expected_dict, msg=msg)
-
- def assertRunTestResults(
- self, run_tests_report, expected_parse_results=None, msg=None
- ):
- """
- Unit test assertion to evaluate output of ``ParserElement.runTests()``. If a list of
- list-dict tuples is given as the ``expected_parse_results`` argument, then these are zipped
- with the report tuples returned by ``runTests`` and evaluated using ``assertParseResultsEquals``.
- Finally, asserts that the overall ``runTests()`` success value is ``True``.
-
- :param run_tests_report: tuple(bool, [tuple(str, ParseResults or Exception)]) returned from runTests
- :param expected_parse_results (optional): [tuple(str, list, dict, Exception)]
- """
- run_test_success, run_test_results = run_tests_report
-
- if expected_parse_results is not None:
- merged = [
- (*rpt, expected)
- for rpt, expected in zip(run_test_results, expected_parse_results)
- ]
- for test_string, result, expected in merged:
- # expected should be a tuple containing a list and/or a dict or an exception,
- # and optional failure message string
- # an empty tuple will skip any result validation
- fail_msg = next(
- (exp for exp in expected if isinstance(exp, str)), None
- )
- expected_exception = next(
- (
- exp
- for exp in expected
- if isinstance(exp, type) and issubclass(exp, Exception)
- ),
- None,
- )
- if expected_exception is not None:
- with self.assertRaises(
- expected_exception=expected_exception, msg=fail_msg or msg
- ):
- if isinstance(result, Exception):
- raise result
- else:
- expected_list = next(
- (exp for exp in expected if isinstance(exp, list)), None
- )
- expected_dict = next(
- (exp for exp in expected if isinstance(exp, dict)), None
- )
- if (expected_list, expected_dict) != (None, None):
- self.assertParseResultsEquals(
- result,
- expected_list=expected_list,
- expected_dict=expected_dict,
- msg=fail_msg or msg,
- )
- else:
- # warning here maybe?
- print("no validation for {!r}".format(test_string))
-
- # do this last, in case some specific test results can be reported instead
- self.assertTrue(
- run_test_success, msg=msg if msg is not None else "failed runTests"
- )
-
- @contextmanager
- def assertRaisesParseException(self, exc_type=ParseException, msg=None):
- with self.assertRaises(exc_type, msg=msg):
- yield
-
- @staticmethod
- def with_line_numbers(
- s: str,
- start_line: typing.Optional[int] = None,
- end_line: typing.Optional[int] = None,
- expand_tabs: bool = True,
- eol_mark: str = "|",
- mark_spaces: typing.Optional[str] = None,
- mark_control: typing.Optional[str] = None,
- ) -> str:
- """
- Helpful method for debugging a parser - prints a string with line and column numbers.
- (Line and column numbers are 1-based.)
-
- :param s: tuple(bool, str - string to be printed with line and column numbers
- :param start_line: int - (optional) starting line number in s to print (default=1)
- :param end_line: int - (optional) ending line number in s to print (default=len(s))
- :param expand_tabs: bool - (optional) expand tabs to spaces, to match the pyparsing default
- :param eol_mark: str - (optional) string to mark the end of lines, helps visualize trailing spaces (default="|")
- :param mark_spaces: str - (optional) special character to display in place of spaces
- :param mark_control: str - (optional) convert non-printing control characters to a placeholding
- character; valid values:
- - "unicode" - replaces control chars with Unicode symbols, such as "␍" and "␊"
- - any single character string - replace control characters with given string
- - None (default) - string is displayed as-is
-
- :return: str - input string with leading line numbers and column number headers
- """
- if expand_tabs:
- s = s.expandtabs()
- if mark_control is not None:
- if mark_control == "unicode":
- tbl = str.maketrans(
- {c: u for c, u in zip(range(0, 33), range(0x2400, 0x2433))}
- | {127: 0x2421}
- )
- eol_mark = ""
- else:
- tbl = str.maketrans(
- {c: mark_control for c in list(range(0, 32)) + [127]}
- )
- s = s.translate(tbl)
- if mark_spaces is not None and mark_spaces != " ":
- if mark_spaces == "unicode":
- tbl = str.maketrans({9: 0x2409, 32: 0x2423})
- s = s.translate(tbl)
- else:
- s = s.replace(" ", mark_spaces)
- if start_line is None:
- start_line = 1
- if end_line is None:
- end_line = len(s)
- end_line = min(end_line, len(s))
- start_line = min(max(1, start_line), end_line)
-
- if mark_control != "unicode":
- s_lines = s.splitlines()[start_line - 1 : end_line]
- else:
- s_lines = [line + "␊" for line in s.split("␊")[start_line - 1 : end_line]]
- if not s_lines:
- return ""
-
- lineno_width = len(str(end_line))
- max_line_len = max(len(line) for line in s_lines)
- lead = " " * (lineno_width + 1)
- if max_line_len >= 99:
- header0 = (
- lead
- + "".join(
- "{}{}".format(" " * 99, (i + 1) % 100)
- for i in range(max(max_line_len // 100, 1))
- )
- + "\n"
- )
- else:
- header0 = ""
- header1 = (
- header0
- + lead
- + "".join(
- " {}".format((i + 1) % 10)
- for i in range(-(-max_line_len // 10))
- )
- + "\n"
- )
- header2 = lead + "1234567890" * (-(-max_line_len // 10)) + "\n"
- return (
- header1
- + header2
- + "\n".join(
- "{:{}d}:{}{}".format(i, lineno_width, line, eol_mark)
- for i, line in enumerate(s_lines, start=start_line)
- )
- + "\n"
- )
diff --git a/spaces/tomofi/MMOCR/configs/_base_/det_datasets/icdar2015.py b/spaces/tomofi/MMOCR/configs/_base_/det_datasets/icdar2015.py
deleted file mode 100644
index f711c06dce76d53b8737288c8de318e6f90ce585..0000000000000000000000000000000000000000
--- a/spaces/tomofi/MMOCR/configs/_base_/det_datasets/icdar2015.py
+++ /dev/null
@@ -1,18 +0,0 @@
-dataset_type = 'IcdarDataset'
-data_root = 'data/icdar2015'
-
-train = dict(
- type=dataset_type,
- ann_file=f'{data_root}/instances_training.json',
- img_prefix=f'{data_root}/imgs',
- pipeline=None)
-
-test = dict(
- type=dataset_type,
- ann_file=f'{data_root}/instances_test.json',
- img_prefix=f'{data_root}/imgs',
- pipeline=None)
-
-train_list = [train]
-
-test_list = [test]
diff --git a/spaces/tomofi/NDLOCR/src/ndl_layout/mmdetection/tests/test_data/test_pipelines/test_transform/test_translate.py b/spaces/tomofi/NDLOCR/src/ndl_layout/mmdetection/tests/test_data/test_pipelines/test_transform/test_translate.py
deleted file mode 100644
index 87f37d0d8fc6aeda4200e8b94f7b23d1a6069444..0000000000000000000000000000000000000000
--- a/spaces/tomofi/NDLOCR/src/ndl_layout/mmdetection/tests/test_data/test_pipelines/test_transform/test_translate.py
+++ /dev/null
@@ -1,515 +0,0 @@
-import copy
-
-import numpy as np
-import pycocotools.mask as maskUtils
-import pytest
-from mmcv.utils import build_from_cfg
-
-from mmdet.core.mask import BitmapMasks, PolygonMasks
-from mmdet.datasets.builder import PIPELINES
-
-
-def _check_keys(results, results_translated):
- assert len(set(results.keys()).difference(set(
- results_translated.keys()))) == 0
- assert len(set(results_translated.keys()).difference(set(
- results.keys()))) == 0
-
-
-def _pad(h, w, c, pad_val, axis=-1, dtype=np.float32):
- assert isinstance(pad_val, (int, float, tuple))
- if isinstance(pad_val, (int, float)):
- pad_val = tuple([pad_val] * c)
- assert len(pad_val) == c
- pad_data = np.stack([np.ones((h, w)) * pad_val[i] for i in range(c)],
- axis=axis).astype(dtype)
- return pad_data
-
-
-def _construct_img(results):
- h, w = results['img_info']['height'], results['img_info']['width']
- img = np.random.uniform(0, 1, (h, w, 3)) * 255
- img = img.astype(np.uint8)
- results['img'] = img
- results['img_shape'] = img.shape
- results['ori_shape'] = img.shape
- results['img_fields'] = ['img']
-
-
-def _construct_ann_info(h=427, w=640, c=3):
- bboxes = np.array(
- [[222.62, 217.82, 241.81, 238.93], [50.5, 329.7, 130.23, 384.96],
- [175.47, 331.97, 254.8, 389.26]],
- dtype=np.float32)
- labels = np.array([9, 2, 2], dtype=np.int64)
- bboxes_ignore = np.array([[59., 253., 311., 337.]], dtype=np.float32)
- masks = [
- [[222.62, 217.82, 222.62, 238.93, 241.81, 238.93, 240.85, 218.78]],
- [[
- 69.19, 332.17, 82.39, 330.25, 97.24, 329.7, 114.01, 331.35, 116.76,
- 337.39, 119.78, 343.17, 128.03, 344.54, 128.86, 347.84, 124.18,
- 350.59, 129.96, 358.01, 130.23, 366.54, 129.13, 377.81, 125.28,
- 382.48, 119.78, 381.93, 117.31, 377.54, 116.21, 379.46, 114.83,
- 382.21, 107.14, 383.31, 105.49, 378.36, 77.99, 377.54, 75.79,
- 381.11, 69.74, 381.93, 66.72, 378.91, 65.07, 377.81, 63.15, 379.19,
- 62.32, 383.31, 52.7, 384.96, 50.5, 379.46, 51.32, 375.61, 51.6,
- 370.11, 51.6, 364.06, 53.52, 354.99, 56.27, 344.54, 59.57, 336.29,
- 66.45, 332.72
- ]],
- [[
- 175.47, 386.86, 175.87, 376.44, 177.08, 351.2, 189.1, 332.77,
- 194.31, 331.97, 236.37, 332.77, 244.79, 342.39, 246.79, 346.79,
- 248.39, 345.99, 251.6, 345.59, 254.8, 348.0, 254.8, 351.6, 250.0,
- 352.0, 250.0, 354.81, 251.6, 358.41, 251.6, 364.42, 251.6, 370.03,
- 252.8, 378.04, 252.8, 384.05, 250.8, 387.26, 246.39, 387.66,
- 245.19, 386.46, 242.38, 388.86, 233.97, 389.26, 232.77, 388.06,
- 232.77, 383.65, 195.91, 381.25, 195.91, 384.86, 191.1, 384.86,
- 187.49, 385.26, 186.69, 382.85, 184.29, 382.45, 183.09, 387.26,
- 178.68, 388.46, 176.28, 387.66
- ]]
- ]
- return dict(
- bboxes=bboxes, labels=labels, bboxes_ignore=bboxes_ignore, masks=masks)
-
-
-def _load_bboxes(results):
- ann_info = results['ann_info']
- results['gt_bboxes'] = ann_info['bboxes'].copy()
- results['bbox_fields'] = ['gt_bboxes']
- gt_bboxes_ignore = ann_info.get('bboxes_ignore', None)
- if gt_bboxes_ignore is not None:
- results['gt_bboxes_ignore'] = gt_bboxes_ignore.copy()
- results['bbox_fields'].append('gt_bboxes_ignore')
-
-
-def _load_labels(results):
- results['gt_labels'] = results['ann_info']['labels'].copy()
-
-
-def _poly2mask(mask_ann, img_h, img_w):
- if isinstance(mask_ann, list):
- # polygon -- a single object might consist of multiple parts
- # we merge all parts into one mask rle code
- rles = maskUtils.frPyObjects(mask_ann, img_h, img_w)
- rle = maskUtils.merge(rles)
- elif isinstance(mask_ann['counts'], list):
- # uncompressed RLE
- rle = maskUtils.frPyObjects(mask_ann, img_h, img_w)
- else:
- # rle
- rle = mask_ann
- mask = maskUtils.decode(rle)
- return mask
-
-
-def _process_polygons(polygons):
- polygons = [np.array(p) for p in polygons]
- valid_polygons = []
- for polygon in polygons:
- if len(polygon) % 2 == 0 and len(polygon) >= 6:
- valid_polygons.append(polygon)
- return valid_polygons
-
-
-def _load_masks(results, poly2mask=True):
- h, w = results['img_info']['height'], results['img_info']['width']
- gt_masks = results['ann_info']['masks']
- if poly2mask:
- gt_masks = BitmapMasks([_poly2mask(mask, h, w) for mask in gt_masks],
- h, w)
- else:
- gt_masks = PolygonMasks(
- [_process_polygons(polygons) for polygons in gt_masks], h, w)
- results['gt_masks'] = gt_masks
- results['mask_fields'] = ['gt_masks']
-
-
-def _construct_semantic_seg(results):
- h, w = results['img_info']['height'], results['img_info']['width']
- seg_toy = (np.random.uniform(0, 1, (h, w)) * 255).astype(np.uint8)
- results['gt_semantic_seg'] = seg_toy
- results['seg_fields'] = ['gt_semantic_seg']
-
-
-def construct_toy_data(poly2mask=True):
- img_info = dict(height=427, width=640)
- ann_info = _construct_ann_info(h=img_info['height'], w=img_info['width'])
- results = dict(img_info=img_info, ann_info=ann_info)
- # construct image, similar to 'LoadImageFromFile'
- _construct_img(results)
- # 'LoadAnnotations' (bboxes, labels, masks, semantic_seg)
- _load_bboxes(results)
- _load_labels(results)
- _load_masks(results, poly2mask)
- _construct_semantic_seg(results)
- return results
-
-
-def test_translate():
- # test assertion for invalid value of level
- with pytest.raises(AssertionError):
- transform = dict(type='Translate', level=-1)
- build_from_cfg(transform, PIPELINES)
-
- # test assertion for invalid type of level
- with pytest.raises(AssertionError):
- transform = dict(type='Translate', level=[1])
- build_from_cfg(transform, PIPELINES)
-
- # test assertion for invalid prob
- with pytest.raises(AssertionError):
- transform = dict(type='Translate', level=1, prob=-0.5)
- build_from_cfg(transform, PIPELINES)
-
- # test assertion for the num of elements in tuple img_fill_val
- with pytest.raises(AssertionError):
- transform = dict(
- type='Translate', level=1, img_fill_val=(128, 128, 128, 128))
- build_from_cfg(transform, PIPELINES)
-
- # test ValueError for invalid type of img_fill_val
- with pytest.raises(ValueError):
- transform = dict(
- type='Translate', level=1, img_fill_val=[128, 128, 128])
- build_from_cfg(transform, PIPELINES)
-
- # test assertion for invalid value of img_fill_val
- with pytest.raises(AssertionError):
- transform = dict(
- type='Translate', level=1, img_fill_val=(128, -1, 256))
- build_from_cfg(transform, PIPELINES)
-
- # test assertion for invalid value of direction
- with pytest.raises(AssertionError):
- transform = dict(
- type='Translate', level=1, img_fill_val=128, direction='diagonal')
- build_from_cfg(transform, PIPELINES)
-
- # test assertion for invalid type of max_translate_offset
- with pytest.raises(AssertionError):
- transform = dict(
- type='Translate',
- level=1,
- img_fill_val=128,
- max_translate_offset=(250., ))
- build_from_cfg(transform, PIPELINES)
-
- # construct toy data example for unit test
- results = construct_toy_data()
-
- def _check_bbox_mask(results,
- results_translated,
- offset,
- direction,
- min_size=0.):
- # The key correspondence from bboxes to labels and masks.
- bbox2label = {
- 'gt_bboxes': 'gt_labels',
- 'gt_bboxes_ignore': 'gt_labels_ignore'
- }
- bbox2mask = {
- 'gt_bboxes': 'gt_masks',
- 'gt_bboxes_ignore': 'gt_masks_ignore'
- }
-
- def _translate_bbox(bboxes, offset, direction, max_h, max_w):
- if direction == 'horizontal':
- bboxes[:, 0::2] = bboxes[:, 0::2] + offset
- elif direction == 'vertical':
- bboxes[:, 1::2] = bboxes[:, 1::2] + offset
- else:
- raise ValueError
- bboxes[:, 0::2] = np.clip(bboxes[:, 0::2], 0, max_w)
- bboxes[:, 1::2] = np.clip(bboxes[:, 1::2], 0, max_h)
- return bboxes
-
- h, w, c = results_translated['img'].shape
- for key in results_translated.get('bbox_fields', []):
- label_key, mask_key = bbox2label[key], bbox2mask[key]
- # check length of key
- if label_key in results:
- assert len(results_translated[key]) == len(
- results_translated[label_key])
- if mask_key in results:
- assert len(results_translated[key]) == len(
- results_translated[mask_key])
- # construct gt_bboxes
- gt_bboxes = _translate_bbox(
- copy.deepcopy(results[key]), offset, direction, h, w)
- valid_inds = (gt_bboxes[:, 2] - gt_bboxes[:, 0] > min_size) & (
- gt_bboxes[:, 3] - gt_bboxes[:, 1] > min_size)
- gt_bboxes = gt_bboxes[valid_inds]
- # check bbox
- assert np.equal(gt_bboxes, results_translated[key]).all()
-
- # construct gt_masks
- if mask_key not in results:
- # e.g. 'gt_masks_ignore'
- continue
- masks, masks_translated = results[mask_key].to_ndarray(
- ), results_translated[mask_key].to_ndarray()
- assert masks.dtype == masks_translated.dtype
- if direction == 'horizontal':
- masks_pad = _pad(
- h,
- abs(offset),
- masks.shape[0],
- 0,
- axis=0,
- dtype=masks.dtype)
- if offset <= 0:
- # left shift
- gt_masks = np.concatenate(
- (masks[:, :, -offset:], masks_pad), axis=-1)
- else:
- # right shift
- gt_masks = np.concatenate(
- (masks_pad, masks[:, :, :-offset]), axis=-1)
- else:
- masks_pad = _pad(
- abs(offset),
- w,
- masks.shape[0],
- 0,
- axis=0,
- dtype=masks.dtype)
- if offset <= 0:
- # top shift
- gt_masks = np.concatenate(
- (masks[:, -offset:, :], masks_pad), axis=1)
- else:
- # bottom shift
- gt_masks = np.concatenate(
- (masks_pad, masks[:, :-offset, :]), axis=1)
- gt_masks = gt_masks[valid_inds]
- # check masks
- assert np.equal(gt_masks, masks_translated).all()
-
- def _check_img_seg(results, results_translated, keys, offset, fill_val,
- direction):
- for key in keys:
- assert isinstance(results_translated[key], type(results[key]))
- # assert type(results[key]) == type(results_translated[key])
- data, data_translated = results[key], results_translated[key]
- if 'mask' in key:
- data, data_translated = data.to_ndarray(
- ), data_translated.to_ndarray()
- assert data.dtype == data_translated.dtype
- if 'img' in key:
- data, data_translated = data.transpose(
- (2, 0, 1)), data_translated.transpose((2, 0, 1))
- elif 'seg' in key:
- data, data_translated = data[None, :, :], data_translated[
- None, :, :]
- c, h, w = data.shape
- if direction == 'horizontal':
- data_pad = _pad(
- h, abs(offset), c, fill_val, axis=0, dtype=data.dtype)
- if offset <= 0:
- # left shift
- data_gt = np.concatenate((data[:, :, -offset:], data_pad),
- axis=-1)
- else:
- # right shift
- data_gt = np.concatenate((data_pad, data[:, :, :-offset]),
- axis=-1)
- else:
- data_pad = _pad(
- abs(offset), w, c, fill_val, axis=0, dtype=data.dtype)
- if offset <= 0:
- # top shift
- data_gt = np.concatenate((data[:, -offset:, :], data_pad),
- axis=1)
- else:
- # bottom shift
- data_gt = np.concatenate((data_pad, data[:, :-offset, :]),
- axis=1)
- if 'mask' in key:
- # TODO assertion here. ``data_translated`` must be a subset
- # (or equal) of ``data_gt``
- pass
- else:
- assert np.equal(data_gt, data_translated).all()
-
- def check_translate(results,
- results_translated,
- offset,
- img_fill_val,
- seg_ignore_label,
- direction,
- min_size=0):
- # check keys
- _check_keys(results, results_translated)
- # check image
- _check_img_seg(results, results_translated,
- results.get('img_fields', ['img']), offset,
- img_fill_val, direction)
- # check segmentation map
- _check_img_seg(results, results_translated,
- results.get('seg_fields', []), offset, seg_ignore_label,
- direction)
- # check masks and bboxes
- _check_bbox_mask(results, results_translated, offset, direction,
- min_size)
-
- # test case when level=0 (without translate aug)
- img_fill_val = (104, 116, 124)
- seg_ignore_label = 255
- transform = dict(
- type='Translate',
- level=0,
- prob=1.0,
- img_fill_val=img_fill_val,
- seg_ignore_label=seg_ignore_label)
- translate_module = build_from_cfg(transform, PIPELINES)
- results_wo_translate = translate_module(copy.deepcopy(results))
- check_translate(
- copy.deepcopy(results),
- results_wo_translate,
- 0,
- img_fill_val,
- seg_ignore_label,
- 'horizontal',
- )
-
- # test case when level>0 and translate horizontally (left shift).
- transform = dict(
- type='Translate',
- level=8,
- prob=1.0,
- img_fill_val=img_fill_val,
- random_negative_prob=1.0,
- seg_ignore_label=seg_ignore_label)
- translate_module = build_from_cfg(transform, PIPELINES)
- offset = translate_module.offset
- results_translated = translate_module(copy.deepcopy(results))
- check_translate(
- copy.deepcopy(results),
- results_translated,
- -offset,
- img_fill_val,
- seg_ignore_label,
- 'horizontal',
- )
-
- # test case when level>0 and translate horizontally (right shift).
- translate_module.random_negative_prob = 0.0
- results_translated = translate_module(copy.deepcopy(results))
- check_translate(
- copy.deepcopy(results),
- results_translated,
- offset,
- img_fill_val,
- seg_ignore_label,
- 'horizontal',
- )
-
- # test case when level>0 and translate vertically (top shift).
- transform = dict(
- type='Translate',
- level=10,
- prob=1.0,
- img_fill_val=img_fill_val,
- seg_ignore_label=seg_ignore_label,
- random_negative_prob=1.0,
- direction='vertical')
- translate_module = build_from_cfg(transform, PIPELINES)
- offset = translate_module.offset
- results_translated = translate_module(copy.deepcopy(results))
- check_translate(
- copy.deepcopy(results), results_translated, -offset, img_fill_val,
- seg_ignore_label, 'vertical')
-
- # test case when level>0 and translate vertically (bottom shift).
- translate_module.random_negative_prob = 0.0
- results_translated = translate_module(copy.deepcopy(results))
- check_translate(
- copy.deepcopy(results), results_translated, offset, img_fill_val,
- seg_ignore_label, 'vertical')
-
- # test case when no translation is called (prob<=0)
- transform = dict(
- type='Translate',
- level=8,
- prob=0.0,
- img_fill_val=img_fill_val,
- random_negative_prob=0.0,
- seg_ignore_label=seg_ignore_label)
- translate_module = build_from_cfg(transform, PIPELINES)
- results_translated = translate_module(copy.deepcopy(results))
-
- # test translate vertically with PolygonMasks (top shift)
- results = construct_toy_data(False)
- transform = dict(
- type='Translate',
- level=10,
- prob=1.0,
- img_fill_val=img_fill_val,
- seg_ignore_label=seg_ignore_label,
- direction='vertical')
- translate_module = build_from_cfg(transform, PIPELINES)
- offset = translate_module.offset
- translate_module.random_negative_prob = 1.0
- results_translated = translate_module(copy.deepcopy(results))
-
- def _translated_gt(masks, direction, offset, out_shape):
- translated_masks = []
- for poly_per_obj in masks:
- translated_poly_per_obj = []
- for p in poly_per_obj:
- p = p.copy()
- if direction == 'horizontal':
- p[0::2] = np.clip(p[0::2] + offset, 0, out_shape[1])
- elif direction == 'vertical':
- p[1::2] = np.clip(p[1::2] + offset, 0, out_shape[0])
- if PolygonMasks([[p]], *out_shape).areas[0] > 0:
- # filter invalid (area=0)
- translated_poly_per_obj.append(p)
- if len(translated_poly_per_obj):
- translated_masks.append(translated_poly_per_obj)
- translated_masks = PolygonMasks(translated_masks, *out_shape)
- return translated_masks
-
- h, w = results['img_shape'][:2]
- for key in results.get('mask_fields', []):
- masks = results[key]
- translated_gt = _translated_gt(masks, 'vertical', -offset, (h, w))
- assert np.equal(results_translated[key].to_ndarray(),
- translated_gt.to_ndarray()).all()
-
- # test translate horizontally with PolygonMasks (right shift)
- results = construct_toy_data(False)
- transform = dict(
- type='Translate',
- level=8,
- prob=1.0,
- img_fill_val=img_fill_val,
- random_negative_prob=0.0,
- seg_ignore_label=seg_ignore_label)
- translate_module = build_from_cfg(transform, PIPELINES)
- offset = translate_module.offset
- results_translated = translate_module(copy.deepcopy(results))
- h, w = results['img_shape'][:2]
- for key in results.get('mask_fields', []):
- masks = results[key]
- translated_gt = _translated_gt(masks, 'horizontal', offset, (h, w))
- assert np.equal(results_translated[key].to_ndarray(),
- translated_gt.to_ndarray()).all()
-
- # test AutoAugment equipped with Translate
- policies = [[dict(type='Translate', level=10, prob=1.)]]
- autoaug = dict(type='AutoAugment', policies=policies)
- autoaug_module = build_from_cfg(autoaug, PIPELINES)
- autoaug_module(copy.deepcopy(results))
-
- policies = [[
- dict(type='Translate', level=10, prob=1.),
- dict(
- type='Translate',
- level=8,
- img_fill_val=img_fill_val,
- direction='vertical')
- ]]
- autoaug = dict(type='AutoAugment', policies=policies)
- autoaug_module = build_from_cfg(autoaug, PIPELINES)
- autoaug_module(copy.deepcopy(results))
diff --git a/spaces/tornadoslims/instruct-pix2pix/stable_diffusion/ldm/modules/losses/vqperceptual.py b/spaces/tornadoslims/instruct-pix2pix/stable_diffusion/ldm/modules/losses/vqperceptual.py
deleted file mode 100644
index f69981769e4bd5462600458c4fcf26620f7e4306..0000000000000000000000000000000000000000
--- a/spaces/tornadoslims/instruct-pix2pix/stable_diffusion/ldm/modules/losses/vqperceptual.py
+++ /dev/null
@@ -1,167 +0,0 @@
-import torch
-from torch import nn
-import torch.nn.functional as F
-from einops import repeat
-
-from taming.modules.discriminator.model import NLayerDiscriminator, weights_init
-from taming.modules.losses.lpips import LPIPS
-from taming.modules.losses.vqperceptual import hinge_d_loss, vanilla_d_loss
-
-
-def hinge_d_loss_with_exemplar_weights(logits_real, logits_fake, weights):
- assert weights.shape[0] == logits_real.shape[0] == logits_fake.shape[0]
- loss_real = torch.mean(F.relu(1. - logits_real), dim=[1,2,3])
- loss_fake = torch.mean(F.relu(1. + logits_fake), dim=[1,2,3])
- loss_real = (weights * loss_real).sum() / weights.sum()
- loss_fake = (weights * loss_fake).sum() / weights.sum()
- d_loss = 0.5 * (loss_real + loss_fake)
- return d_loss
-
-def adopt_weight(weight, global_step, threshold=0, value=0.):
- if global_step < threshold:
- weight = value
- return weight
-
-
-def measure_perplexity(predicted_indices, n_embed):
- # src: https://github.com/karpathy/deep-vector-quantization/blob/main/model.py
- # eval cluster perplexity. when perplexity == num_embeddings then all clusters are used exactly equally
- encodings = F.one_hot(predicted_indices, n_embed).float().reshape(-1, n_embed)
- avg_probs = encodings.mean(0)
- perplexity = (-(avg_probs * torch.log(avg_probs + 1e-10)).sum()).exp()
- cluster_use = torch.sum(avg_probs > 0)
- return perplexity, cluster_use
-
-def l1(x, y):
- return torch.abs(x-y)
-
-
-def l2(x, y):
- return torch.pow((x-y), 2)
-
-
-class VQLPIPSWithDiscriminator(nn.Module):
- def __init__(self, disc_start, codebook_weight=1.0, pixelloss_weight=1.0,
- disc_num_layers=3, disc_in_channels=3, disc_factor=1.0, disc_weight=1.0,
- perceptual_weight=1.0, use_actnorm=False, disc_conditional=False,
- disc_ndf=64, disc_loss="hinge", n_classes=None, perceptual_loss="lpips",
- pixel_loss="l1"):
- super().__init__()
- assert disc_loss in ["hinge", "vanilla"]
- assert perceptual_loss in ["lpips", "clips", "dists"]
- assert pixel_loss in ["l1", "l2"]
- self.codebook_weight = codebook_weight
- self.pixel_weight = pixelloss_weight
- if perceptual_loss == "lpips":
- print(f"{self.__class__.__name__}: Running with LPIPS.")
- self.perceptual_loss = LPIPS().eval()
- else:
- raise ValueError(f"Unknown perceptual loss: >> {perceptual_loss} <<")
- self.perceptual_weight = perceptual_weight
-
- if pixel_loss == "l1":
- self.pixel_loss = l1
- else:
- self.pixel_loss = l2
-
- self.discriminator = NLayerDiscriminator(input_nc=disc_in_channels,
- n_layers=disc_num_layers,
- use_actnorm=use_actnorm,
- ndf=disc_ndf
- ).apply(weights_init)
- self.discriminator_iter_start = disc_start
- if disc_loss == "hinge":
- self.disc_loss = hinge_d_loss
- elif disc_loss == "vanilla":
- self.disc_loss = vanilla_d_loss
- else:
- raise ValueError(f"Unknown GAN loss '{disc_loss}'.")
- print(f"VQLPIPSWithDiscriminator running with {disc_loss} loss.")
- self.disc_factor = disc_factor
- self.discriminator_weight = disc_weight
- self.disc_conditional = disc_conditional
- self.n_classes = n_classes
-
- def calculate_adaptive_weight(self, nll_loss, g_loss, last_layer=None):
- if last_layer is not None:
- nll_grads = torch.autograd.grad(nll_loss, last_layer, retain_graph=True)[0]
- g_grads = torch.autograd.grad(g_loss, last_layer, retain_graph=True)[0]
- else:
- nll_grads = torch.autograd.grad(nll_loss, self.last_layer[0], retain_graph=True)[0]
- g_grads = torch.autograd.grad(g_loss, self.last_layer[0], retain_graph=True)[0]
-
- d_weight = torch.norm(nll_grads) / (torch.norm(g_grads) + 1e-4)
- d_weight = torch.clamp(d_weight, 0.0, 1e4).detach()
- d_weight = d_weight * self.discriminator_weight
- return d_weight
-
- def forward(self, codebook_loss, inputs, reconstructions, optimizer_idx,
- global_step, last_layer=None, cond=None, split="train", predicted_indices=None):
- if not exists(codebook_loss):
- codebook_loss = torch.tensor([0.]).to(inputs.device)
- #rec_loss = torch.abs(inputs.contiguous() - reconstructions.contiguous())
- rec_loss = self.pixel_loss(inputs.contiguous(), reconstructions.contiguous())
- if self.perceptual_weight > 0:
- p_loss = self.perceptual_loss(inputs.contiguous(), reconstructions.contiguous())
- rec_loss = rec_loss + self.perceptual_weight * p_loss
- else:
- p_loss = torch.tensor([0.0])
-
- nll_loss = rec_loss
- #nll_loss = torch.sum(nll_loss) / nll_loss.shape[0]
- nll_loss = torch.mean(nll_loss)
-
- # now the GAN part
- if optimizer_idx == 0:
- # generator update
- if cond is None:
- assert not self.disc_conditional
- logits_fake = self.discriminator(reconstructions.contiguous())
- else:
- assert self.disc_conditional
- logits_fake = self.discriminator(torch.cat((reconstructions.contiguous(), cond), dim=1))
- g_loss = -torch.mean(logits_fake)
-
- try:
- d_weight = self.calculate_adaptive_weight(nll_loss, g_loss, last_layer=last_layer)
- except RuntimeError:
- assert not self.training
- d_weight = torch.tensor(0.0)
-
- disc_factor = adopt_weight(self.disc_factor, global_step, threshold=self.discriminator_iter_start)
- loss = nll_loss + d_weight * disc_factor * g_loss + self.codebook_weight * codebook_loss.mean()
-
- log = {"{}/total_loss".format(split): loss.clone().detach().mean(),
- "{}/quant_loss".format(split): codebook_loss.detach().mean(),
- "{}/nll_loss".format(split): nll_loss.detach().mean(),
- "{}/rec_loss".format(split): rec_loss.detach().mean(),
- "{}/p_loss".format(split): p_loss.detach().mean(),
- "{}/d_weight".format(split): d_weight.detach(),
- "{}/disc_factor".format(split): torch.tensor(disc_factor),
- "{}/g_loss".format(split): g_loss.detach().mean(),
- }
- if predicted_indices is not None:
- assert self.n_classes is not None
- with torch.no_grad():
- perplexity, cluster_usage = measure_perplexity(predicted_indices, self.n_classes)
- log[f"{split}/perplexity"] = perplexity
- log[f"{split}/cluster_usage"] = cluster_usage
- return loss, log
-
- if optimizer_idx == 1:
- # second pass for discriminator update
- if cond is None:
- logits_real = self.discriminator(inputs.contiguous().detach())
- logits_fake = self.discriminator(reconstructions.contiguous().detach())
- else:
- logits_real = self.discriminator(torch.cat((inputs.contiguous().detach(), cond), dim=1))
- logits_fake = self.discriminator(torch.cat((reconstructions.contiguous().detach(), cond), dim=1))
-
- disc_factor = adopt_weight(self.disc_factor, global_step, threshold=self.discriminator_iter_start)
- d_loss = disc_factor * self.disc_loss(logits_real, logits_fake)
-
- log = {"{}/disc_loss".format(split): d_loss.clone().detach().mean(),
- "{}/logits_real".format(split): logits_real.detach().mean(),
- "{}/logits_fake".format(split): logits_fake.detach().mean()
- }
- return d_loss, log
diff --git a/spaces/training-transformers-together/Dashboard/streamlit_observable/frontend/src/streamlit/ArrowTable.ts b/spaces/training-transformers-together/Dashboard/streamlit_observable/frontend/src/streamlit/ArrowTable.ts
deleted file mode 100644
index 9d0428746e042fb5a8faf3d7321fa91b277ad7b3..0000000000000000000000000000000000000000
--- a/spaces/training-transformers-together/Dashboard/streamlit_observable/frontend/src/streamlit/ArrowTable.ts
+++ /dev/null
@@ -1,224 +0,0 @@
-/**
- * @license
- * Copyright 2018-2019 Streamlit Inc.
- *
- * Licensed under the Apache License, Version 2.0 (the "License");
- * you may not use this file except in compliance with the License.
- * You may obtain a copy of the License at
- *
- * http://www.apache.org/licenses/LICENSE-2.0
- *
- * Unless required by applicable law or agreed to in writing, software
- * distributed under the License is distributed on an "AS IS" BASIS,
- * WITHOUT WARRANTIES OR CONDITIONS OF ANY KIND, either express or implied.
- * See the License for the specific language governing permissions and
- * limitations under the License.
- */
-
-import { Table, Type } from "apache-arrow"
-
-type CellType = "blank" | "index" | "columns" | "data"
-
-export interface ArrowDataframeProto {
- data: ArrowTableProto
- height: string
- width: string
-}
-
-export interface ArrowTableProto {
- data: Uint8Array
- index: Uint8Array
- columns: Uint8Array
- styler: Styler
-}
-
-interface Cell {
- classNames: string
- content: string
- id?: string
- type: CellType
-}
-
-interface Styler {
- caption?: string
- displayValuesTable: Table
- styles?: string
- uuid: string
-}
-
-export class ArrowTable {
- private readonly dataTable: Table
- private readonly indexTable: Table
- private readonly columnsTable: Table
- private readonly styler?: Styler
-
- constructor(
- dataBuffer: Uint8Array,
- indexBuffer: Uint8Array,
- columnsBuffer: Uint8Array,
- styler?: any
- ) {
- this.dataTable = Table.from(dataBuffer)
- this.indexTable = Table.from(indexBuffer)
- this.columnsTable = Table.from(columnsBuffer)
- this.styler = styler
- ? {
- caption: styler.get("caption"),
- displayValuesTable: Table.from(styler.get("displayValues")),
- styles: styler.get("styles"),
- uuid: styler.get("uuid"),
- }
- : undefined
- }
-
- get rows(): number {
- return this.indexTable.length + this.columnsTable.numCols
- }
-
- get columns(): number {
- return this.indexTable.numCols + this.columnsTable.length
- }
-
- get headerRows(): number {
- return this.rows - this.dataRows
- }
-
- get headerColumns(): number {
- return this.columns - this.dataColumns
- }
-
- get dataRows(): number {
- return this.dataTable.length
- }
-
- get dataColumns(): number {
- return this.dataTable.numCols
- }
-
- get uuid(): string | undefined {
- return this.styler && this.styler.uuid
- }
-
- get caption(): string | undefined {
- return this.styler && this.styler.caption
- }
-
- get styles(): string | undefined {
- return this.styler && this.styler.styles
- }
-
- get table(): Table {
- return this.dataTable
- }
-
- get index(): Table {
- return this.indexTable
- }
-
- get columnTable(): Table {
- return this.columnsTable
- }
-
- public getCell = (rowIndex: number, columnIndex: number): Cell => {
- const isBlankCell =
- rowIndex < this.headerRows && columnIndex < this.headerColumns
- const isIndexCell =
- rowIndex >= this.headerRows && columnIndex < this.headerColumns
- const isColumnsCell =
- rowIndex < this.headerRows && columnIndex >= this.headerColumns
-
- if (isBlankCell) {
- const classNames = ["blank"]
- if (columnIndex > 0) {
- classNames.push("level" + rowIndex)
- }
-
- return {
- type: "blank",
- classNames: classNames.join(" "),
- content: "",
- }
- } else if (isColumnsCell) {
- const dataColumnIndex = columnIndex - this.headerColumns
- const classNames = [
- "col_heading",
- "level" + rowIndex,
- "col" + dataColumnIndex,
- ]
-
- return {
- type: "columns",
- classNames: classNames.join(" "),
- content: this.getContent(this.columnsTable, dataColumnIndex, rowIndex),
- }
- } else if (isIndexCell) {
- const dataRowIndex = rowIndex - this.headerRows
- const classNames = [
- "row_heading",
- "level" + columnIndex,
- "row" + dataRowIndex,
- ]
-
- return {
- type: "index",
- id: `T_${this.uuid}level${columnIndex}_row${dataRowIndex}`,
- classNames: classNames.join(" "),
- content: this.getContent(this.indexTable, dataRowIndex, columnIndex),
- }
- } else {
- const dataRowIndex = rowIndex - this.headerRows
- const dataColumnIndex = columnIndex - this.headerColumns
- const classNames = [
- "data",
- "row" + dataRowIndex,
- "col" + dataColumnIndex,
- ]
- const content = this.styler
- ? this.getContent(
- this.styler.displayValuesTable,
- dataRowIndex,
- dataColumnIndex
- )
- : this.getContent(this.dataTable, dataRowIndex, dataColumnIndex)
-
- return {
- type: "data",
- id: `T_${this.uuid}row${dataRowIndex}_col${dataColumnIndex}`,
- classNames: classNames.join(" "),
- content,
- }
- }
- }
-
- public getContent = (
- table: Table,
- rowIndex: number,
- columnIndex: number
- ): any => {
- const column = table.getColumnAt(columnIndex)
- if (column === null) {
- return ""
- }
-
- const columnTypeId = this.getColumnTypeId(table, columnIndex)
- switch (columnTypeId) {
- case Type.Timestamp: {
- return this.nanosToDate(column.get(rowIndex))
- }
- default: {
- return column.get(rowIndex)
- }
- }
- }
-
- /**
- * Returns apache-arrow specific typeId of column.
- */
- private getColumnTypeId(table: Table, columnIndex: number): Type {
- return table.schema.fields[columnIndex].type.typeId
- }
-
- private nanosToDate(nanos: number): Date {
- return new Date(nanos / 1e6)
- }
-}
diff --git a/spaces/trl-lib/trl-text-environment/app.py b/spaces/trl-lib/trl-text-environment/app.py
deleted file mode 100644
index 6210b6ef0b407e098ee6aef515ba1f515f5a791f..0000000000000000000000000000000000000000
--- a/spaces/trl-lib/trl-text-environment/app.py
+++ /dev/null
@@ -1,366 +0,0 @@
-import os
-import re
-import copy
-import time
-
-import gradio as gr
-from text_generation import Client
-from transformers import load_tool
-from share_btn import community_icon_html, loading_icon_html, share_js, share_btn_css
-
-
-HF_TOKEN = os.environ.get("HF_TOKEN", None)
-os.environ["HF_ALLOW_CODE_EVAL"] = "1"
-print(HF_TOKEN)
-
-FIM_PREFIX = ""
-FIM_MIDDLE = ""
-FIM_SUFFIX = ""
-
-FIM_INDICATOR = ""
-
-theme = gr.themes.Monochrome(
- primary_hue="indigo",
- secondary_hue="blue",
- neutral_hue="slate",
- radius_size=gr.themes.sizes.radius_sm,
- font=[
- gr.themes.GoogleFont("Open Sans"),
- "ui-sans-serif",
- "system-ui",
- "sans-serif",
- ],
-)
-
-tool = load_tool("vwxyzjn/pyserini-wikipedia-kilt-doc")
-tool_fn = lambda x: tool(x).split("\n")[1][:600] # limit the amount if token, system_prompts
-
-clients = {
- "StarCoderBase TriviaQA": [
- Client(
- "https://api-inference.huggingface.co/models/vwxyzjn/starcoderbase-triviaqa",
- headers={"Authorization": f"Bearer {HF_TOKEN}"},
- ),
- {"Wiki": tool_fn},
- """\
-Answer the following question:
-Q: In which branch of the arts is Patricia Neary famous?
-A: Ballets
-A2: Patricia NearyPatricia Neary (born October 27, 1942) is an American ballerina, choreographer and ballet director, who has been particularly active in Switzerland. She has also been a highly successful ambassador for the Balanchine Trust, bringing George Balanchine's ballets to 60 cities around the globe.
-Result=Ballets
-Q: Who won Super Bowl XX?
-A: Chicago Bears
-A2: Super Bowl XXSuper Bowl XX was an American football game between the National Football Conference (NFC) champion Chicago Bears and the American Football Conference (AFC) champion New England Patriots to decide the National Football League (NFL) champion for the 1985 season. The Bears defeated the Patriots by the score of 46–10, capturing their first NFL championship (and Chicago's first overall sports victory) since 1963, three years prior to the birth of the Super Bowl. Super Bowl XX was played on January 26, 1986 at the Louisiana Superdome in New Orleans.
-Result=Chicago Bears
-""",
- ["Q: In which country is Oberhofen situated?", "Q: Irish Olympic champion Michelle smith was suspended in 1999 over drug allegations in which sport?"]
- ],
- "StarCoderBase GSM8K": [
- Client(
- "https://api-inference.huggingface.co/models/lvwerra/starcoderbase-gsm8k",
- headers={"Authorization": f"Bearer {HF_TOKEN}"},
- ),
- {"PythonInterpreter": load_tool("lvwerra/python-interpreter")},
- """\
-Example of using a Python API to solve math questions.
-
-Q: Olivia has $23. She bought five bagels for $3 each. How much money does she have left?
-
-
-def solution():
- money_initial = 23
- bagels = 5
- bagel_cost = 3
- money_spent = bagels * bagel_cost
- money_left = money_initial - money_spent
- result = money_left
- return result
-print(solution())
-72
-
-Result = 72
-""",
- ["Q: Tim has $400, and he received $1021. How much does he have?"]
- ],
-}
-
-def parse_tool_call(text, request_token="", call_token=""):
- """
- Parse request string. Expected format: query
- """
- result = re.search(f"(?<={request_token}).*?(?={call_token})", text, re.DOTALL)
-
- # if we can't find a / span we return none
- if result is None:
- return None, None
- else:
- extracted_text = result.group()
-
- result = re.search(r"<(.*?)>", extracted_text)
-
- # if we can't find a tool name we return none
- if result is None:
- return None, None
- else:
- tool = result.group(1)
-
- # split off the tool name
- query = ">".join(extracted_text.split(">")[1:])
-
- return tool, query
-
-
-
-def generate(
- prompt, system_prompt, version, temperature=0.9, max_new_tokens=256, top_p=0.95, repetition_penalty=1.0,
-):
- client, tools, _, _ = clients[version]
- temperature = float(temperature)
- if temperature < 1e-2:
- temperature = 1e-2
- top_p = float(top_p)
- fim_mode = False
-
- # TextEnv tool
- generate_kwargs = dict(
- temperature=temperature,
- max_new_tokens=max_new_tokens,
- top_p=top_p,
- repetition_penalty=repetition_penalty,
- do_sample=True,
- seed=42,
- stop_sequences=["", ""]
- )
- generation_still_running = True
- request_idx = -1
- call_idx = -1
- response_idx = -1
- submit_idx = -1
-
- i = 0
- while generation_still_running:
- try:
- stream = client.generate_stream(system_prompt + prompt, **generate_kwargs)
-
-
- # call env phase
- output = system_prompt + prompt
- generation_start_idx = len(output)
- highlighted_output = [
- (prompt, "QUERY"),
- ]
- yield highlighted_output, output[generation_start_idx:]
- for response in stream:
- i += 1
- output += response.token.text
- tool, query = parse_tool_call(output[generation_start_idx:])
-
- if tool is not None and query is not None:
- # print("=====tool", i, tool, response, output)
- if tool not in tools:
- response = f"Unknown tool {tool}."
- try:
- response = tools[tool](query)
- output += response + ""
-
- except Exception as error:
- response = f"Tool error: {str(error)}"
-
- if request_idx == -1:
- request_idx = output[generation_start_idx:].find("")
- if call_idx == -1:
- call_idx = output[generation_start_idx:].find("")
- if call_idx != -1:
- call_idx += len("")
- if response_idx == -1:
- response_idx = output[generation_start_idx:].find("")
- if response_idx != -1:
- response_idx += len("")
- if submit_idx == -1:
- submit_idx = output[generation_start_idx:].find("")
- # I am sorry about the code
- print("-------", generation_start_idx, request_idx, call_idx, response_idx)
- highlighted_output = [
- (prompt, "QUERY"),
- (output[generation_start_idx:], "MODEL") if request_idx == -1 else ("", ""),
- (output[generation_start_idx:generation_start_idx+request_idx], "MODEL"),
- (output[generation_start_idx+request_idx:], "MODEL") if call_idx == -1 else ("", ""),
- (output[generation_start_idx+request_idx:generation_start_idx+call_idx], "TOOL_REQUEST"),
- (output[generation_start_idx+call_idx:generation_start_idx+response_idx], "TOOL_CALL"),
- (output[generation_start_idx+response_idx:], "MODEL") if submit_idx != -1 else ("", ""),
- # (output[generation_start_idx:generation_start_idx+request_idx], ""),
- # (output[generation_start_idx+request_idx:generation_start_idx+call_idx], "request"),
- # (output[generation_start_idx+call_idx:], "call"),
- ]
- print(i, highlighted_output, output[generation_start_idx:])
- yield highlighted_output, output[generation_start_idx:]
-
- # breakpoint()
- call_output = copy.deepcopy(output)
- print("start submit output")
- # response phase
- generate_kwargs["stop_sequences"] = [""]
- stream = client.generate_stream(output, **generate_kwargs)
- for response in stream:
- output += response.token.text
- if submit_idx == -1:
- submit_idx = output[generation_start_idx:].find("")
- # print("-------", generation_start_idx, request_idx, call_idx, response_idx)
- highlighted_output = [
- (prompt, "QUERY"),
- (output[generation_start_idx:generation_start_idx+request_idx], "MODEL"),
- (output[generation_start_idx+request_idx:generation_start_idx+call_idx], "TOOL_REQUEST"),
- (output[generation_start_idx+call_idx:generation_start_idx+response_idx], "TOOL_CALL"),
- (output[generation_start_idx+response_idx:], "MODEL") if submit_idx != -1 else ("", ""),
- ]
- # print(highlighted_output, output[generation_start_idx:])
- yield highlighted_output, output[generation_start_idx:]
- print("-------", generation_start_idx, request_idx, call_idx, response_idx)
- print(highlighted_output, output[generation_start_idx:])
-
- return highlighted_output, output[generation_start_idx:]
- except Exception as e:
- if "loading" in str(e):
- gr.Warning("waiting for model to load... (this could take up to 20 minutes, after which things are much faster)")
- time.sleep(7)
- continue
- else:
- raise gr.Error(str(e))
-
-
-examples = [
- "X_train, y_train, X_test, y_test = train_test_split(X, y, test_size=0.1)\n\n# Train a logistic regression model, predict the labels on the test set and compute the accuracy score",
- "// Returns every other value in the array as a new array.\nfunction everyOther(arr) {",
- "Poor English: She no went to the market. Corrected English:",
- "def alternating(list1, list2):\n results = []\n for i in range(min(len(list1), len(list2))):\n results.append(list1[i])\n results.append(list2[i])\n if len(list1) > len(list2):\n \n else:\n results.extend(list2[i+1:])\n return results",
-]
-
-
-def process_example(args):
- for x in generate(args):
- pass
- return x
-
-
-css = ".generating {visibility: hidden}"
-
-monospace_css = """
-#q-input textarea {
- font-family: monospace, 'Consolas', Courier, monospace;
-}
-"""
-
-
-css += share_btn_css + monospace_css + ".gradio-container {color: black}"
-
-
-description = """
-
-
-
-
-
-
This is a demo to generate text the following StarCoderBase models fine-tuned using TRL's TextEnvironment:
-
-
StarCoderBase TriviaQA: Uses a Wikipedia search index to answer trivia questions. It was trained on the TriviaQA dataset.
-
StarCoderBase GSM8K: Uses a Python Interpreter to answer math questions. It was trained on the GSM8K dataset.
-
-
-"""
-
-with gr.Blocks(theme=theme, analytics_enabled=False, css=css) as demo:
- with gr.Column():
- gr.Markdown(description)
- with gr.Row():
- version = gr.Dropdown(
- list(clients.keys()),
- value=list(clients.keys())[0],
- label="Model",
- info="Choose a model from the list",
- )
-
- with gr.Row():
- with gr.Column():
- instruction = gr.Textbox(
- value="Q: In which country is Oberhofen situated?",
- # placeholder="Enter your question here. E.g., Q: In which country is Oberhofen situated?",
- lines=2,
- label="Input",
- )
- submit = gr.Button("Generate", variant="primary")
-
- output = gr.HighlightedText(
- label="Output",
- color_map={"QUERY": "red", "TOOL_CALL": "green", "TOOL_RESPONSE": "blue", "MODEL": "pink"},
- )
- gr.Markdown("_Note:_ The trivia model is trained to give an answer first and then refine it with a Wiki call.")
- gr_examples = gr.Examples(
- examples=[example for client in clients.values() for example in client[3]],
- inputs=[instruction],
- cache_examples=False,
- )
-
- with gr.Row():
- with gr.Column():
- with gr.Accordion("Raw output", open=False):
- output2 = gr.Code(elem_id="q-output", lines=30, label="Raw output")
- with gr.Accordion("Advanced settings", open=False):
- with gr.Row():
- column_1, column_2 = gr.Column(), gr.Column()
- with column_1:
- temperature = gr.Slider(
- label="Temperature",
- value=0.2,
- minimum=0.0,
- maximum=1.0,
- step=0.05,
- interactive=True,
- info="Higher values produce more diverse outputs",
- )
- max_new_tokens = gr.Slider(
- label="Max new tokens",
- value=256,
- minimum=0,
- maximum=8192,
- step=64,
- interactive=True,
- info="The maximum numbers of new tokens",
- )
- with column_2:
- top_p = gr.Slider(
- label="Top-p (nucleus sampling)",
- value=0.90,
- minimum=0.0,
- maximum=1,
- step=0.05,
- interactive=True,
- info="Higher values sample more low-probability tokens",
- )
- repetition_penalty = gr.Slider(
- label="Repetition penalty",
- value=1.2,
- minimum=1.0,
- maximum=2.0,
- step=0.05,
- interactive=True,
- info="Penalize repeated tokens",
- )
- with gr.Accordion("Prompt", open=False):
- system_prompt = gr.Textbox(
- value=clients[list(clients.keys())[0]][2],
- label="System prompt",
- )
- version.select(
- lambda x: (clients[x][2]),
- inputs=[version],
- outputs=[system_prompt],
- )
-
-
-
- submit.click(
- generate,
- inputs=[instruction, system_prompt, version, temperature, max_new_tokens, top_p, repetition_penalty],
- outputs=[output, output2],
- )
-demo.queue(concurrency_count=16).launch(debug=True)
diff --git a/spaces/tsi-org/Faceswapper/roop/processors/frame/face_enhancer.py b/spaces/tsi-org/Faceswapper/roop/processors/frame/face_enhancer.py
deleted file mode 100644
index e4c2dec05f834f7732ac62f0db6dcde416ed0b30..0000000000000000000000000000000000000000
--- a/spaces/tsi-org/Faceswapper/roop/processors/frame/face_enhancer.py
+++ /dev/null
@@ -1,81 +0,0 @@
-from typing import Any, List, Callable
-import cv2
-import threading
-import gfpgan
-
-import roop.globals
-import roop.processors.frame.core
-from roop.core import update_status
-from roop.face_analyser import get_one_face
-from roop.typing import Frame, Face
-from roop.utilities import conditional_download, resolve_relative_path, is_image, is_video
-
-FACE_ENHANCER = None
-THREAD_SEMAPHORE = threading.Semaphore()
-THREAD_LOCK = threading.Lock()
-NAME = 'ROOP.FACE-ENHANCER'
-
-
-def get_face_enhancer() -> Any:
- global FACE_ENHANCER
-
- with THREAD_LOCK:
- if FACE_ENHANCER is None:
- model_path = resolve_relative_path('../models/GFPGANv1.4.pth')
- # todo: set models path https://github.com/TencentARC/GFPGAN/issues/399
- FACE_ENHANCER = gfpgan.GFPGANer(model_path=model_path, upscale=1) # type: ignore[attr-defined]
- return FACE_ENHANCER
-
-
-def pre_check() -> bool:
- download_directory_path = resolve_relative_path('../models')
- conditional_download(download_directory_path, ['https://github.com/TencentARC/GFPGAN/releases/download/v1.3.4/GFPGANv1.4.pth'])
- return True
-
-
-def pre_start() -> bool:
- if not is_image(roop.globals.target_path) and not is_video(roop.globals.target_path):
- update_status('Select an image or video for target path.', NAME)
- return False
- return True
-
-
-def post_process() -> None:
- global FACE_ENHANCER
-
- FACE_ENHANCER = None
-
-
-def enhance_face(temp_frame: Frame) -> Frame:
- with THREAD_SEMAPHORE:
- _, _, temp_frame = get_face_enhancer().enhance(
- temp_frame,
- paste_back=True
- )
- return temp_frame
-
-
-def process_frame(source_face: Face, temp_frame: Frame) -> Frame:
- target_face = get_one_face(temp_frame)
- if target_face:
- temp_frame = enhance_face(temp_frame)
- return temp_frame
-
-
-def process_frames(source_path: str, temp_frame_paths: List[str], update: Callable[[], None]) -> None:
- for temp_frame_path in temp_frame_paths:
- temp_frame = cv2.imread(temp_frame_path)
- result = process_frame(None, temp_frame)
- cv2.imwrite(temp_frame_path, result)
- if update:
- update()
-
-
-def process_image(source_path: str, target_path: str, output_path: str) -> None:
- target_frame = cv2.imread(target_path)
- result = process_frame(None, target_frame)
- cv2.imwrite(output_path, result)
-
-
-def process_video(source_path: str, temp_frame_paths: List[str]) -> None:
- roop.processors.frame.core.process_video(None, temp_frame_paths, process_frames)
diff --git a/spaces/tsinghua-ee/SALMONN-7B-gradio/beats/BEATs.py b/spaces/tsinghua-ee/SALMONN-7B-gradio/beats/BEATs.py
deleted file mode 100644
index 5a1da028b4fb95b61f6d84e66d5e1e6eed45bf5b..0000000000000000000000000000000000000000
--- a/spaces/tsinghua-ee/SALMONN-7B-gradio/beats/BEATs.py
+++ /dev/null
@@ -1,180 +0,0 @@
-# --------------------------------------------------------
-# BEATs: Audio Pre-Training with Acoustic Tokenizers (https://arxiv.org/abs/2212.09058)
-# Github source: https://github.com/microsoft/unilm/tree/master/beats
-# Copyright (c) 2022 Microsoft
-# Licensed under The MIT License [see LICENSE for details]
-# Based on fairseq code bases
-# https://github.com/pytorch/fairseq
-# --------------------------------------------------------
-
-
-import torch
-import torch.nn as nn
-from torch.nn import LayerNorm
-import torchaudio.compliance.kaldi as ta_kaldi
-
-from beats.backbone import (
- TransformerEncoder,
-)
-
-import logging
-from typing import Optional
-
-logger = logging.getLogger(__name__)
-
-
-class BEATsConfig:
- def __init__(self, cfg=None):
- self.input_patch_size: int = -1 # path size of patch embedding
- self.embed_dim: int = 512 # patch embedding dimension
- self.conv_bias: bool = False # include bias in conv encoder
-
- self.encoder_layers: int = 12 # num encoder layers in the transformer
- self.encoder_embed_dim: int = 768 # encoder embedding dimension
- self.encoder_ffn_embed_dim: int = 3072 # encoder embedding dimension for FFN
- self.encoder_attention_heads: int = 12 # num encoder attention heads
- self.activation_fn: str = "gelu" # activation function to use
-
- self.layer_wise_gradient_decay_ratio: float = 1.0 # ratio for layer-wise gradient decay
- self.layer_norm_first: bool = False # apply layernorm first in the transformer
- self.deep_norm: bool = False # apply deep_norm first in the transformer
-
- # dropouts
- self.dropout: float = 0.1 # dropout probability for the transformer
- self.attention_dropout: float = 0.1 # dropout probability for attention weights
- self.activation_dropout: float = 0.0 # dropout probability after activation in FFN
- self.encoder_layerdrop: float = 0.0 # probability of dropping a tarnsformer layer
- self.dropout_input: float = 0.0 # dropout to apply to the input (after feat extr)
-
- # positional embeddings
- self.conv_pos: int = 128 # number of filters for convolutional positional embeddings
- self.conv_pos_groups: int = 16 # number of groups for convolutional positional embedding
-
- # relative position embedding
- self.relative_position_embedding: bool = False # apply relative position embedding
- self.num_buckets: int = 320 # number of buckets for relative position embedding
- self.max_distance: int = 1280 # maximum distance for relative position embedding
- self.gru_rel_pos: bool = False # apply gated relative position embedding
-
- # label predictor
- self.finetuned_model: bool = False # whether the model is a fine-tuned model.
- self.predictor_dropout: float = 0.1 # dropout probability for the predictor
- self.predictor_class: int = 527 # target class number for the predictor
-
- if cfg is not None:
- self.update(cfg)
-
- def update(self, cfg: dict):
- self.__dict__.update(cfg)
-
-
-class BEATs(nn.Module):
- def __init__(
- self,
- cfg: BEATsConfig,
- ) -> None:
- super().__init__()
- logger.info(f"BEATs Config: {cfg.__dict__}")
-
- self.cfg = cfg
-
- self.embed = cfg.embed_dim
- self.post_extract_proj = (
- nn.Linear(self.embed, cfg.encoder_embed_dim)
- if self.embed != cfg.encoder_embed_dim
- else None
- )
-
- self.input_patch_size = cfg.input_patch_size
- self.patch_embedding = nn.Conv2d(1, self.embed, kernel_size=self.input_patch_size, stride=self.input_patch_size,
- bias=cfg.conv_bias)
-
- self.dropout_input = nn.Dropout(cfg.dropout_input)
-
- assert not cfg.deep_norm or not cfg.layer_norm_first
- self.encoder = TransformerEncoder(cfg)
- self.layer_norm = LayerNorm(self.embed)
-
- if cfg.finetuned_model:
- self.predictor_dropout = nn.Dropout(cfg.predictor_dropout)
- self.predictor = nn.Linear(cfg.encoder_embed_dim, cfg.predictor_class)
- else:
- self.predictor = None
-
- def forward_padding_mask(
- self,
- features: torch.Tensor,
- padding_mask: torch.Tensor,
- ) -> torch.Tensor:
- extra = padding_mask.size(1) % features.size(1)
- if extra > 0:
- padding_mask = padding_mask[:, :-extra]
- padding_mask = padding_mask.view(
- padding_mask.size(0), features.size(1), -1
- )
- padding_mask = padding_mask.all(-1)
- return padding_mask
-
- def preprocess(
- self,
- source: torch.Tensor,
- fbank_mean: float = 15.41663,
- fbank_std: float = 6.55582,
- ) -> torch.Tensor:
- fbanks = []
- for waveform in source:
- waveform = waveform.unsqueeze(0) * 2 ** 15
- fbank = ta_kaldi.fbank(waveform, num_mel_bins=128, sample_frequency=16000, frame_length=25, frame_shift=10)
- fbanks.append(fbank)
- fbank = torch.stack(fbanks, dim=0)
- fbank = (fbank - fbank_mean) / (2 * fbank_std)
- return fbank
-
- def extract_features(
- self,
- source: torch.Tensor,
- padding_mask: Optional[torch.Tensor] = None,
- fbank_mean: float = 15.41663,
- fbank_std: float = 6.55582,
- feature_only=False,
- ):
- fbank = self.preprocess(source, fbank_mean=fbank_mean, fbank_std=fbank_std).to(torch.float32)
-
- if padding_mask is not None:
- padding_mask = self.forward_padding_mask(fbank, padding_mask)
-
- fbank = fbank.unsqueeze(1)
- features = self.patch_embedding(fbank)
- features = features.reshape(features.shape[0], features.shape[1], -1)
- features = features.transpose(1, 2)
- features = self.layer_norm(features)
-
- if padding_mask is not None:
- padding_mask = self.forward_padding_mask(features, padding_mask)
-
- if self.post_extract_proj is not None:
- features = self.post_extract_proj(features)
-
- x = self.dropout_input(features)
-
- x, layer_results = self.encoder(
- x,
- padding_mask=padding_mask,
- )
-
- if not feature_only and self.predictor is not None:
- x = self.predictor_dropout(x)
- logits = self.predictor(x)
-
- if padding_mask is not None and padding_mask.any():
- logits[padding_mask] = 0
- logits = logits.sum(dim=1)
- logits = logits / (~padding_mask).sum(dim=1).unsqueeze(-1).expand_as(logits)
- else:
- logits = logits.mean(dim=1)
-
- lprobs = torch.sigmoid(logits)
-
- return lprobs, padding_mask
- else:
- return x, padding_mask
\ No newline at end of file
diff --git a/spaces/ucalyptus/PTI/models/StyleCLIP/global_directions/PlayInteractively.py b/spaces/ucalyptus/PTI/models/StyleCLIP/global_directions/PlayInteractively.py
deleted file mode 100644
index 547b08ab2c4373e23711636488145df148d7eb4e..0000000000000000000000000000000000000000
--- a/spaces/ucalyptus/PTI/models/StyleCLIP/global_directions/PlayInteractively.py
+++ /dev/null
@@ -1,197 +0,0 @@
-
-
-
-from tkinter import Tk
-from PIL import Image, ImageTk
-from tkinter.filedialog import askopenfilename
-from GUI import View
-from Inference import StyleCLIP
-import argparse
-#%%
-
-
-class PlayInteractively(): #Controller
- '''
- followed Model View Controller Design Pattern
-
- controller, model, view
- '''
- def __init__(self,dataset_name='ffhq'):
-
- self.root = Tk()
- self.view=View(self.root)
- self.img_ratio=2
- self.style_clip=StyleCLIP(dataset_name)
-
- self.view.neutral.bind("", self.text_n)
- self.view.target.bind("", self.text_t)
- self.view.alpha.bind('', self.ChangeAlpha)
- self.view.beta.bind('', self.ChangeBeta)
- self.view.set_init.bind('', self.SetInit)
- self.view.reset.bind('', self.Reset)
- self.view.bg.bind('', self.open_img)
-
-
- self.drawn = None
-
- self.view.target.delete(1.0, "end")
- self.view.target.insert("end", self.style_clip.target)
-#
- self.view.neutral.delete(1.0, "end")
- self.view.neutral.insert("end", self.style_clip.neutral)
-
-
- def Reset(self,event):
- self.style_clip.GetDt2()
- self.style_clip.M.alpha=[0]
-
- self.view.beta.set(self.style_clip.beta)
- self.view.alpha.set(0)
-
- img=self.style_clip.GetImg()
- img=Image.fromarray(img)
- img = ImageTk.PhotoImage(img)
- self.addImage_m(img)
-
-
- def SetInit(self,event):
- codes=self.style_clip.GetCode()
- self.style_clip.M.dlatent_tmp=[tmp[:,0] for tmp in codes]
- print('set init')
-
- def ChangeAlpha(self,event):
- tmp=self.view.alpha.get()
- self.style_clip.M.alpha=[float(tmp)]
-
- img=self.style_clip.GetImg()
- print('manipulate one')
- img=Image.fromarray(img)
- img = ImageTk.PhotoImage(img)
- self.addImage_m(img)
-
- def ChangeBeta(self,event):
- tmp=self.view.beta.get()
- self.style_clip.beta=float(tmp)
-
- img=self.style_clip.GetImg()
- print('manipulate one')
- img=Image.fromarray(img)
- img = ImageTk.PhotoImage(img)
- self.addImage_m(img)
-
- def ChangeDataset(self,event):
-
- dataset_name=self.view.set_category.get()
-
- self.style_clip.LoadData(dataset_name)
-
- self.view.target.delete(1.0, "end")
- self.view.target.insert("end", self.style_clip.target)
-
- self.view.neutral.delete(1.0, "end")
- self.view.neutral.insert("end", self.style_clip.neutral)
-
- def text_t(self,event):
- tmp=self.view.target.get("1.0",'end')
- tmp=tmp.replace('\n','')
-
- self.view.target.delete(1.0, "end")
- self.view.target.insert("end", tmp)
-
- print('target',tmp,'###')
- self.style_clip.target=tmp
- self.style_clip.GetDt2()
- self.view.beta.set(self.style_clip.beta)
- self.view.alpha.set(3)
- self.style_clip.M.alpha=[3]
-
- img=self.style_clip.GetImg()
- print('manipulate one')
- img=Image.fromarray(img)
- img = ImageTk.PhotoImage(img)
- self.addImage_m(img)
-
-
- def text_n(self,event):
- tmp=self.view.neutral.get("1.0",'end')
- tmp=tmp.replace('\n','')
-
- self.view.neutral.delete(1.0, "end")
- self.view.neutral.insert("end", tmp)
-
- print('neutral',tmp,'###')
- self.style_clip.neutral=tmp
- self.view.target.delete(1.0, "end")
- self.view.target.insert("end", tmp)
-
-
- def run(self):
- self.root.mainloop()
-
- def addImage(self,img):
- self.view.bg.create_image(self.view.width/2, self.view.height/2, image=img, anchor='center')
- self.image=img #save a copy of image. if not the image will disappear
-
- def addImage_m(self,img):
- self.view.mani.create_image(512, 512, image=img, anchor='center')
- self.image2=img
-
-
- def openfn(self):
- filename = askopenfilename(title='open',initialdir='./data/'+self.style_clip.M.dataset_name+'/',filetypes=[("all image format", ".jpg"),("all image format", ".png")])
- return filename
-
- def open_img(self,event):
- x = self.openfn()
- print(x)
-
-
- img = Image.open(x)
- img2 = img.resize(( 512,512), Image.ANTIALIAS)
- img2 = ImageTk.PhotoImage(img2)
- self.addImage(img2)
-
- img = ImageTk.PhotoImage(img)
- self.addImage_m(img)
-
- img_index=x.split('/')[-1].split('.')[0]
- img_index=int(img_index)
- print(img_index)
- self.style_clip.M.img_index=img_index
- self.style_clip.M.dlatent_tmp=[tmp[img_index:(img_index+1)] for tmp in self.style_clip.M.dlatents]
-
-
- self.style_clip.GetDt2()
- self.view.beta.set(self.style_clip.beta)
- self.view.alpha.set(3)
-
- #%%
-if __name__ == "__main__":
- parser = argparse.ArgumentParser(description='Process some integers.')
-
- parser.add_argument('--dataset_name',type=str,default='ffhq',
- help='name of dataset, for example, ffhq')
-
- args = parser.parse_args()
- dataset_name=args.dataset_name
-
- self=PlayInteractively(dataset_name)
- self.run()
-
-
-
-
-
-
-
-
-
-
-
-
-
-
-
-
-
-
\ No newline at end of file
diff --git a/spaces/ucalyptus/PTI/models/e4e/stylegan2/op/fused_bias_act.cpp b/spaces/ucalyptus/PTI/models/e4e/stylegan2/op/fused_bias_act.cpp
deleted file mode 100644
index 02be898f970bcc8ea297867fcaa4e71b24b3d949..0000000000000000000000000000000000000000
--- a/spaces/ucalyptus/PTI/models/e4e/stylegan2/op/fused_bias_act.cpp
+++ /dev/null
@@ -1,21 +0,0 @@
-#include
-
-
-torch::Tensor fused_bias_act_op(const torch::Tensor& input, const torch::Tensor& bias, const torch::Tensor& refer,
- int act, int grad, float alpha, float scale);
-
-#define CHECK_CUDA(x) TORCH_CHECK(x.type().is_cuda(), #x " must be a CUDA tensor")
-#define CHECK_CONTIGUOUS(x) TORCH_CHECK(x.is_contiguous(), #x " must be contiguous")
-#define CHECK_INPUT(x) CHECK_CUDA(x); CHECK_CONTIGUOUS(x)
-
-torch::Tensor fused_bias_act(const torch::Tensor& input, const torch::Tensor& bias, const torch::Tensor& refer,
- int act, int grad, float alpha, float scale) {
- CHECK_CUDA(input);
- CHECK_CUDA(bias);
-
- return fused_bias_act_op(input, bias, refer, act, grad, alpha, scale);
-}
-
-PYBIND11_MODULE(TORCH_EXTENSION_NAME, m) {
- m.def("fused_bias_act", &fused_bias_act, "fused bias act (CUDA)");
-}
\ No newline at end of file
diff --git a/spaces/ulysses115/Nogizaka46-so/modules/losses.py b/spaces/ulysses115/Nogizaka46-so/modules/losses.py
deleted file mode 100644
index cd21799eccde350c3aac0bdd661baf96ed220147..0000000000000000000000000000000000000000
--- a/spaces/ulysses115/Nogizaka46-so/modules/losses.py
+++ /dev/null
@@ -1,61 +0,0 @@
-import torch
-from torch.nn import functional as F
-
-import modules.commons as commons
-
-
-def feature_loss(fmap_r, fmap_g):
- loss = 0
- for dr, dg in zip(fmap_r, fmap_g):
- for rl, gl in zip(dr, dg):
- rl = rl.float().detach()
- gl = gl.float()
- loss += torch.mean(torch.abs(rl - gl))
-
- return loss * 2
-
-
-def discriminator_loss(disc_real_outputs, disc_generated_outputs):
- loss = 0
- r_losses = []
- g_losses = []
- for dr, dg in zip(disc_real_outputs, disc_generated_outputs):
- dr = dr.float()
- dg = dg.float()
- r_loss = torch.mean((1-dr)**2)
- g_loss = torch.mean(dg**2)
- loss += (r_loss + g_loss)
- r_losses.append(r_loss.item())
- g_losses.append(g_loss.item())
-
- return loss, r_losses, g_losses
-
-
-def generator_loss(disc_outputs):
- loss = 0
- gen_losses = []
- for dg in disc_outputs:
- dg = dg.float()
- l = torch.mean((1-dg)**2)
- gen_losses.append(l)
- loss += l
-
- return loss, gen_losses
-
-
-def kl_loss(z_p, logs_q, m_p, logs_p, z_mask):
- """
- z_p, logs_q: [b, h, t_t]
- m_p, logs_p: [b, h, t_t]
- """
- z_p = z_p.float()
- logs_q = logs_q.float()
- m_p = m_p.float()
- logs_p = logs_p.float()
- z_mask = z_mask.float()
- #print(logs_p)
- kl = logs_p - logs_q - 0.5
- kl += 0.5 * ((z_p - m_p)**2) * torch.exp(-2. * logs_p)
- kl = torch.sum(kl * z_mask)
- l = kl / torch.sum(z_mask)
- return l
diff --git a/spaces/unity/ML-Agents-SoccerTwos/Build/SoccerTwos.loader.js b/spaces/unity/ML-Agents-SoccerTwos/Build/SoccerTwos.loader.js
deleted file mode 100644
index 542ad3675ac55dda268bb50cdb82ff0ee8c739c0..0000000000000000000000000000000000000000
--- a/spaces/unity/ML-Agents-SoccerTwos/Build/SoccerTwos.loader.js
+++ /dev/null
@@ -1 +0,0 @@
-function createUnityInstance(r,n,l){function s(e,r){if(!s.aborted&&n.showBanner)return"error"==r&&(s.aborted=!0),n.showBanner(e,r);switch(r){case"error":console.error(e);break;case"warning":console.warn(e);break;default:console.log(e)}}function t(e){var r=e.reason||e.error,n=r?r.toString():e.message||e.reason||"",t=r&&r.stack?r.stack.toString():"";(n+="\n"+(t=t.startsWith(n)?t.substring(n.length):t).trim())&&c.stackTraceRegExp&&c.stackTraceRegExp.test(n)&&h(n,e.filename||r&&(r.fileName||r.sourceURL)||"",e.lineno||r&&(r.lineNumber||r.line)||0)}function e(e,r,n){var t=e[r];void 0!==t&&t||(console.warn('Config option "'+r+'" is missing or empty. Falling back to default value: "'+n+'". Consider updating your WebGL template to include the missing config option.'),e[r]=n)}l=l||function(){};var o,c={canvas:r,webglContextAttributes:{preserveDrawingBuffer:!1,powerPreference:2},streamingAssetsUrl:"StreamingAssets",downloadProgress:{},deinitializers:[],intervals:{},setInterval:function(e,r){e=window.setInterval(e,r);return this.intervals[e]=!0,e},clearInterval:function(e){delete this.intervals[e],window.clearInterval(e)},preRun:[],postRun:[],print:function(e){console.log(e)},printErr:function(e){console.error(e),"string"==typeof e&&-1!=e.indexOf("wasm streaming compile failed")&&(-1!=e.toLowerCase().indexOf("mime")?s('HTTP Response Header "Content-Type" configured incorrectly on the server for file '+c.codeUrl+' , should be "application/wasm". Startup time performance will suffer.',"warning"):s('WebAssembly streaming compilation failed! This can happen for example if "Content-Encoding" HTTP header is incorrectly enabled on the server for file '+c.codeUrl+", but the file is not pre-compressed on disk (or vice versa). Check the Network tab in browser Devtools to debug server header configuration.","warning"))},locateFile:function(e){return"build.wasm"==e?this.codeUrl:e},disabledCanvasEvents:["contextmenu","dragstart"]};for(o in e(n,"companyName","Unity"),e(n,"productName","WebGL Player"),e(n,"productVersion","1.0"),n)c[o]=n[o];c.streamingAssetsUrl=new URL(c.streamingAssetsUrl,document.URL).href;var i=c.disabledCanvasEvents.slice();function a(e){e.preventDefault()}i.forEach(function(e){r.addEventListener(e,a)}),window.addEventListener("error",t),window.addEventListener("unhandledrejection",t),c.deinitializers.push(function(){for(var e in c.disableAccessToMediaDevices(),i.forEach(function(e){r.removeEventListener(e,a)}),window.removeEventListener("error",t),window.removeEventListener("unhandledrejection",t),c.intervals)window.clearInterval(e);c.intervals={}}),c.QuitCleanup=function(){for(var e=0;eIf using custom web server, verify that web server is sending .br files with HTTP Response Header "Content-Encoding: br". Brotli compression may not be supported in Firefox over HTTP connections. '+t+' See https://bugzilla.mozilla.org/show_bug.cgi?id=1670675 for more information.':"Unable to parse "+c.frameworkUrl+'! If using custom web server, verify that web server is sending .br files with HTTP Response Header "Content-Encoding: br". Brotli compression may not be supported over HTTP connections. Migrate your server to use HTTPS.'),void s(n,"error"))}s("Unable to parse "+c.frameworkUrl+"! The file is corrupt, or compression was misconfigured? (check Content-Encoding HTTP Response Header on web server)","error")}var o=unityFramework;unityFramework=null,a.onload=null,i(o)},a.onerror=function(e){s("Unable to load file "+c.frameworkUrl+"! Check that the file exists on the remote server. (also check browser Console and Devtools Network tab to debug)","error")},document.body.appendChild(a),c.deinitializers.push(function(){document.body.removeChild(a)})}).then(function(e){e(c)});g(n="dataUrl"),e=c.fetchWithProgress,r=c[n],r=/file:\/\//.exec(r)?"same-origin":void 0;var n,e,r,t=e(c[n],{method:"GET",companyName:c.companyName,productName:c.productName,control:"no-store",mode:r,onProgress:function(e){g(n,e)}}).then(function(e){return e.parsedBody}).catch(function(e){var r="Failed to download file "+c[n];"file:"==location.protocol?s(r+". Loading web pages via a file:// URL without a web server is not supported by this browser. Please use a local development web server to host Unity content, or use the Unity Build and Run option.","error"):console.error(r)});c.preRun.push(function(){c.addRunDependency("dataUrl"),t.then(function(e){var r=new DataView(e.buffer,e.byteOffset,e.byteLength),n=0,t="UnityWebData1.0\0";if(!String.fromCharCode.apply(null,e.subarray(n,n+t.length))==t)throw"unknown data format";var o=r.getUint32(n+=t.length,!0);for(n+=4;nDennis The Menace Full Movie Free 57
-
-Jan 8, 2012 - rial-key ... database-monitoring-and-analysis-tool-serial-key-gen -and-key-gen-download-free-download-repack-only-online-download-for-download-online-get-download-now.
-Duplicate File Finder - Duplicate File Finder is a program for finding duplicate files.
-This is a program for finding duplicate files on your computer.
-Monitor screen recorder - This is a monitor screen video recording software.
-It is the ability to record video from your computer monitor.
-Duplicate removal software is a software for removing duplicate files from your computer. 8a78ff9644
-
-
-
diff --git a/spaces/varunrayen/banana-dev-GPTrillion/README.md b/spaces/varunrayen/banana-dev-GPTrillion/README.md
deleted file mode 100644
index 44ece99db7eb5d22cd8b572db34c365578c4e6ba..0000000000000000000000000000000000000000
--- a/spaces/varunrayen/banana-dev-GPTrillion/README.md
+++ /dev/null
@@ -1,12 +0,0 @@
----
-title: Banana Dev GPTrillion
-emoji: 📉
-colorFrom: red
-colorTo: purple
-sdk: gradio
-sdk_version: 3.23.0
-app_file: app.py
-pinned: false
----
-
-Check out the configuration reference at https://huggingface.co/docs/hub/spaces-config-reference
diff --git a/spaces/versus666/ml_message_moderation/src/app.py b/spaces/versus666/ml_message_moderation/src/app.py
deleted file mode 100644
index 4146d189429ae3685f646a3b745c595ee8e0da02..0000000000000000000000000000000000000000
--- a/spaces/versus666/ml_message_moderation/src/app.py
+++ /dev/null
@@ -1,377 +0,0 @@
-import streamlit as st
-import tools
-
-
-st.title('Message moderation lab')
-
-
-st.write(
- """
- Термин «модерация» происходит от латинского «moderor», что значит «умерять, сдерживать».
- Суть задачи модерации состоит в контроле за выполнением законов, правил, требований и ограничений в
- любых сообществах и сервисах — будь то простое общение в социальных сетях или деловые переговоры на онлайн площадке.
-
- Автоматические системы модерации внедряются в веб-сервисы и приложения, где необходимо обрабатывать большое
- количество сообщений пользователей. Такие системы позволяют сократить издержки на ручную модерацию, ускорить её и
- обрабатывать все сообщения пользователей в real-time.
-
- Со временем пользователи подстраиваются и учатся обманывать такие системы, например пользователи:
- - генерируют опечатки: you are stupit asswhol, fack u
- - заменяют буквенные символы на цифры, похожие по описанию: n1gga, b0ll0cks,
- - вставляют дополнительные пробелы: i d i o t,
- - удаляют пробелы между словами: dieyoustupid
- - указывают контактные данные: восем-906-три единицы-два раза по две единицы
- и многое другое.
-
- Для того, чтобы обучить классификатор устойчивый к таким подменам, нужно поступить так, как поступают пользователи:
- сгенерировать такие же изменения в сообщениях и добавить их в обучающую выборку к основным данным.
-
- В целом, эта борьба неизбежна: пользователи всегда будут пытаться находить уязвимости и хаки,
- а модераторы реализовывать новые алгоритмы.
-
- В примере ниже можно ознакомиться с работой разных алгоритмов по выявлению наличия контактных данных в сообщениях
- пользователей. Это актуально в первую очередь для торговых площадок и других онлайн площадок по продаже и
- рекомендации товаров и услуг. Актуально это потому, что пользователи не всегда желают платить комиссию за работу
- сервиса и пытаются осуществлять сделки напрямую, минуя сервис.
-
- В данном примере сообщения пользователей подвергаются проверке тремя алгоритмами по поиску контактных данных:
- - регулярные выражения (regex)
- - TF-IDF, на основе частотности слов
- - нейросеть BERT
-
- 1. Регулярные выражения
- Регулярные выражения представляют собой похожий, но гораздо более сильный инструмент для поиска строк, проверки их
- на соответствие какому-либо шаблону и другой подобной работы. Англоязычное название этого
- инструмента — Regular Expressions или просто RegExp.
- """
-)
-
-with st.expander(
- label='Блок теории про регулярные выражения'
-):
- st.write(
- """
- В самом общем смысле регулярные выражения — это последовательности символов для поиска соответствий шаблону.
- Они являются экземплярами регулярного языка и широко применяются для парсинга текста или валидации входных строк.
-
- Представьте лист картона, в котором вырезаны определенные фигуры. И только фигуры, точно соответствующие вырезам,
- смогут через них пройти. В данном случае лист картона аналогичен строке регулярного выражения.
- """
- )
- st.image(
- image='images/re.jpeg',
- caption='Суть работы регулярных выражений',
- use_column_width=True
- )
-
- st.write(
- """
- Несколько случаев применения регулярных выражений:
-
- - парсинг входных данных, например текста, логов, веб-информации и т.д.;
- - валидация пользовательского ввода;
- - тестирование результатов вывода;
- - точный поиск текста;
- - реструктуризация данных.
-
- Регулярные выражения отлично подходят, когда есть четкий формат и структура данных. В нашем же случае пользователям
- легко будет обмануть систему модерации сообщений, если она будет построена только на регулярных выражениях.
- Нужно что-то посложнее.
- """
- )
-
-st.write(
- """
- 2. TF-IDF (TF — term frequency, IDF — inverse document frequency).
- Мера TF-IDF является произведением двух сомножителей TF и IDF.
-
- TF - частота слова - отношение числа вхождений некоторого слова к общему числу слов документа.
- Таким образом, оценивается важность слова в пределах отдельного документа.
-
- IDF - обратная частота документа - инверсия частоты, с которой некоторое слово встречается в документах коллекции.
- Учёт IDF уменьшает вес широкоупотребительных слов. Для каждого уникального слова в пределах конкретной коллекции
- документов существует только одно значение IDF.
- """
-)
-
-with st.expander(
- label='Блок теории про TF-IDF'
-):
-
- st.image(
- image='images/tf_idf_formula.jpg',
- caption='Формула TF-IDF',
- use_column_width=True
- )
-
- st.write(
- """
- TF рассчитывается по следующей формуле:
- """
- )
-
- st.image(
- image='images/tf_formula.jpg'
- )
-
- st.write(
- """
- где t (от англ. term) — количество употребления слова, а n — общее число слов в тексте.
- """
- )
-
- st.image(
- image='images/idf_formula.jpg'
- )
-
- st.write(
- """
- где D - общее число текстов в корпусе, d - количество текстов, в которых это слово встречается.
-
- IDF нужна в формуле, чтобы уменьшить вес слов, наиболее распространённых в любом другом тексте заданного корпуса.
- """
- )
-
- st.write(
- """
- TF-IDF оценивает значимость слова в документе, на основе данных о всей коллекции документов. Данная мера
- определяет вес слова за величину пропорциональную частоте его вхождения в документ и обратно пропорциональную
- частоте его вхождения во всех документах коллекции.
-
- Большая величина TF-IDF говорит об уникальности слова в тексте по отношению к корпусу.
- Чем чаще оно встречается в конкретном тексте и реже в остальных, тем выше значение TF-IDF.
- """
- )
-
-st.write(
- """
- 3. Нейросеть BERT.
-
- BERT — это нейронная сеть от Google, показавшая с большим отрывом state-of-the-art результаты на целом ряде задач.
- С помощью BERT можно создавать программы с ИИ для обработки естественного языка: отвечать на вопросы, заданные
- в произвольной форме, создавать чат-ботов, автоматические переводчики, анализировать текст и так далее.
- """
-)
-
-with st.expander(
- label='Блок теории про BERT'
-):
- st.write(
- """
- Чтобы подавать на вход нейронной сети текст, нужно его как-то представить в виде чисел. Проще всего это делать
- побуквенно, подавая на каждый вход нейросети по одной букве. Тогда каждая буква будет кодироваться числом
- от 0 до 32 (плюс какой-то запас на знаки препинания). Это так называемый character-level.
-
- Но гораздо лучше результаты получаются, если мы предложения будем представлять не по одной букве, а подавая на
- каждый вход нейросети сразу по целому слову (или хотя бы слогами). Это уже будет word-level. Самый простой
- вариант — составить словарь со всеми существующими словами, и скармливать сети номер слова в этом словаре.
- Например, если слово "собака" стоит в этом словаре на 1678 месте, то на вход нейросети для этого слова
- подаем число 1678.
-
- Вот только в естественном языке при слове "собака" у человека всплывает сразу множество
- ассоциаций: "пушистая", "злая", "друг человека". Нельзя ли как-то закодировать эту особенность нашего мышления
- в представлении для нейросети? Оказывается, можно. Для этого достаточно так пересортировать номера слов, чтобы
- близкие по смыслу слова стояли рядом. Пусть будет, например, для "собака" число 1678, а для слова "пушистая"
- число 1680. А для слова "чайник" число 9000. Как видите, цифры 1678 и 1680 находятся намного ближе друг к другу,
- чем цифра 9000.
-
- На практике, каждому слову назначают не одно число, а несколько — вектор, скажем, из 32 чисел. И расстояния
- измеряют как расстояния между точками, на которые указывают эти вектора в пространстве соответствущей
- размерности (для вектора длиной в 32 числа, это пространство с 32 размерностями, или с 32 осями).
- Это позволяет сопоставлять одному слову сразу несколько близких по смыслу слов (смотря по какой оси считать).
- Более того, с векторами можно производить арифметические операции. Классический пример: если из вектора,
- обозначающего слово "король", вычесть вектор "мужчина" и прибавить вектор для слова "женщина", то получится
- некий вектор-результат. И он чудесным образом будет соответствовать слову "королева". И действительно,
- "король — мужчина + женщина = королева". Магия! И это не абстрактный пример, а
- [реально так происходит](https://blog.acolyer.org/2016/04/21/the-amazing-power-of-word-vectors/). Учитывая,
- что нейронные сети хорошо приспособлены для математических преобразований над своими входами, видимо это и
- обеспечивает такую высокую эффективность этого метода.
-
- Идея в основе BERT лежит очень простая: давайте на вход нейросети будем подавать фразы, в которых 15% слов
- заменим на [MASK], и обучим нейронную сеть предсказывать эти закрытые маской слова.
-
- Например, если подаем на вход нейросети фразу "Я пришел в [MASK] и купил [MASK]", она должна на выходе показать
- слова "магазин" и "молоко". Это упрощенный пример с официальной страницы BERT, на более длинных предложениях
- разброс возможных вариантов становится меньше, а ответ нейросети однозначнее.
-
- А для того, чтобы нейросеть научилась понимать соотношения между разными предложениями, дополнительно обучим
- ее предсказывать, является ли вторая фраза логичным продолжением первой. Или это какая-то случайная фраза, не
- имеющая никакого отношения к первой.
-
- Так, для двух предложений: "Я пошел в магазин." и "И купил там молоко.", нейросеть должна ответить,
- что это логично. А если вторая фраза будет "Карась небо Плутон", то должна ответить, что это предложение никак
- не связано с первым. Ниже мы поиграемся с обоими этими режимами работы BERT.
-
- Обучив таким образом нейронную сеть на корпусе текстов из Wikipedia и сборнике книг BookCorpus
- в течении 4 дней на 16 TPU, получили BERT.
- """
- )
-
-if st.checkbox('Сгенерировать рандомное сообщение'):
- user_text = st.text_area(
- label='Введите сообщение',
- height=200,
- value=tools.get_random_message(),
- help='Попробуйте указать ссылки на vk, twich, twitter и др. каналы связи а также почту')
-else:
- user_text = st.text_area(
- label='Введите сообщение',
- height=200,
- help='Попробуйте указать ссылки на vk, twich, twitter и др. каналы связи а также почту'
- )
-
-with st.expander(
- label='Показать примеры сообщений со скрытыми контактными данными'
-):
- st.write(
- """
- Ма8ш9и9н9а6 в 0хо0ро4ш4е2м9 состоянии
-
- Новый велосипед Работает всё Звонить на 8 девятьсот восемь 1976829
-
- Беспроводная точка доступа маршрутизатор Моя Почта xopkin317 mailru
-
- My Отличный телефон TW практически новый ich хороший экран, без трещин lork не падал ing92
- """
- )
-
-re_res = tools.get_re_pred(user_text)
-
-if 'Есть контактная информация' in re_res:
- st.success(f'Regex: {re_res}')
-else:
- st.error(f'Regex : {re_res}')
-
-tf_idf_res = tools.get_tf_idf_pred(user_text)
-
-if 'Есть контактная информация' in tf_idf_res:
- st.success(f'TF_IDF: {tf_idf_res}')
-else:
- st.error(f'TF_IDF: {tf_idf_res}')
-
-bert_res = tools.get_bert_prediction(user_text)
-
-if 'Есть контактная информация' in bert_res:
- st.success(f'BERT: {bert_res}')
-else:
- st.error(f'BERT: {bert_res}')
-
-with st.form(key='quiz'):
- right_answers_count = 0
-
- st.write('QUIZ')
-
- answer = st.radio(
- label='Что такое регулярные выражения?',
- options=[
- 'Модель машинного обучения',
- 'Аналог TF-IDF',
- 'Инструмент проверки строк на соответствие какому-либо шаблону',
- 'Инструмент для классификации сообщений пользователя',
- 'Выражения, которые регулярно используются разработчиками',
- 'WEB фреймворк',
- ]
- )
-
- if answer == 'Инструмент проверки строк на соответствие какому-либо шаблону':
- right_answers_count += 1
-
- answer = st.radio(
- label='Как пользователи обходят правила модерации сервиса?',
- options=[
- 'Пишут в поддержку',
- 'Изменяют сообщения, маскируя запрещенный контент',
- 'Записывают голосовые сообщения',
- 'Пользуются другими сервисами, без модерации'
- ]
- )
-
- if answer == 'Изменяют сообщения, маскируя запрещенный контент':
- right_answers_count += 1
-
- answer = st.radio(
- label='Что такое TF-IDF?',
- options=[
- 'Вид регулярных выражения',
- 'Система модерации текстовых сообщений',
- 'Запчасть автомобиля',
- 'Мера оценки значимости слова в документе',
- 'Модель машинного обучения',
- 'Корпус текстов',
- ]
- )
-
- if answer == 'Мера оценки значимости слова в документе':
- right_answers_count += 1
-
- answer = st.radio(
- label='Что оценивает TF-IDF?',
- options=[
- 'Нужно ли отправлять сообщение на модерацию или нет',
- 'Значимость слова в документе',
- 'Частоту слова',
- 'Обратную частоту слова в документе'
- ]
- )
-
- if answer == 'Значимость слова в документе':
- right_answers_count += 1
-
- answer = st.radio(
- label='Что такое BERT?',
- options=[
- 'Персонаж из мультика "Улица Сезам"',
- 'Нейронная сеть от Google',
- 'Система модерации сообщений',
- 'Система оценки соответствия сообщений правилам организации и законам',
- 'Вид регулярных выражений'
- ]
- )
-
- if answer == 'Нейронная сеть от Google':
- right_answers_count += 1
-
- answer = st.radio(
- label='Как обучается BERT?',
- options=[
- 'На GPU',
- 'Никак, Google уже обучила ее, нам остается только пользоваться готовой',
- 'Маскируя 15% слов символом [MASK] и пытаясь предсказать спрятанные слова'
- ]
- )
-
- if answer == 'Маскируя 15% слов символом [MASK] и пытаясь предсказать спрятанные слова':
- right_answers_count += 1
-
- answer = st.radio(
- label='В каком виде подается информация на вход нейросети BERT?',
- options=[
- 'Как есть без изменений',
- 'В виде векторов с числами, обозначающими целевое слово и близких к нему по смыслу из словаря',
- 'В виде сконкатенированных строк всего обучающего датасета',
- 'В виде списка текстов'
- ]
- )
-
- if answer == 'В виде векторов с числами, обозначающими целевое слово и близких к нему по смыслу из словаря':
- right_answers_count += 1
-
- answer = st.radio(
- label='BERT учитывает контекст в предложениях?',
- options=[
- 'Нет',
- 'Да'
- ]
- )
-
- if answer == 'Да':
- right_answers_count += 1
-
- res = st.form_submit_button()
-
-if res:
- st.info(f'Количество правильных ответов {right_answers_count} из 8.')
- if right_answers_count <= 6:
- st.warning('Для прохождения блока необходимо правильно ответить хотя бы на 7 вопросов.')
- else:
- st.success('Отлично! Блок пройден.')
diff --git a/spaces/vumichien/Lip_movement_reading/README.md b/spaces/vumichien/Lip_movement_reading/README.md
deleted file mode 100644
index 102c1fb5a7ad8c5192753f44c5d94fa40dd08946..0000000000000000000000000000000000000000
--- a/spaces/vumichien/Lip_movement_reading/README.md
+++ /dev/null
@@ -1,14 +0,0 @@
----
-title: Speech Recognition from visual lip movement
-emoji: 🫧
-colorFrom: indigo
-colorTo: pink
-sdk: gradio
-sdk_version: 3.16.1
-app_file: app.py
-pinned: false
-tags:
-- making-demos
----
-
-Check out the configuration reference at https://huggingface.co/docs/hub/spaces-config-reference
\ No newline at end of file
diff --git a/spaces/vumichien/canvas_controlnet/annotator/uniformer/mmcv/ops/border_align.py b/spaces/vumichien/canvas_controlnet/annotator/uniformer/mmcv/ops/border_align.py
deleted file mode 100644
index ff305be328e9b0a15e1bbb5e6b41beb940f55c81..0000000000000000000000000000000000000000
--- a/spaces/vumichien/canvas_controlnet/annotator/uniformer/mmcv/ops/border_align.py
+++ /dev/null
@@ -1,109 +0,0 @@
-# Copyright (c) OpenMMLab. All rights reserved.
-# modified from
-# https://github.com/Megvii-BaseDetection/cvpods/blob/master/cvpods/layers/border_align.py
-
-import torch
-import torch.nn as nn
-from torch.autograd import Function
-from torch.autograd.function import once_differentiable
-
-from ..utils import ext_loader
-
-ext_module = ext_loader.load_ext(
- '_ext', ['border_align_forward', 'border_align_backward'])
-
-
-class BorderAlignFunction(Function):
-
- @staticmethod
- def symbolic(g, input, boxes, pool_size):
- return g.op(
- 'mmcv::MMCVBorderAlign', input, boxes, pool_size_i=pool_size)
-
- @staticmethod
- def forward(ctx, input, boxes, pool_size):
- ctx.pool_size = pool_size
- ctx.input_shape = input.size()
-
- assert boxes.ndim == 3, 'boxes must be with shape [B, H*W, 4]'
- assert boxes.size(2) == 4, \
- 'the last dimension of boxes must be (x1, y1, x2, y2)'
- assert input.size(1) % 4 == 0, \
- 'the channel for input feature must be divisible by factor 4'
-
- # [B, C//4, H*W, 4]
- output_shape = (input.size(0), input.size(1) // 4, boxes.size(1), 4)
- output = input.new_zeros(output_shape)
- # `argmax_idx` only used for backward
- argmax_idx = input.new_zeros(output_shape).to(torch.int)
-
- ext_module.border_align_forward(
- input, boxes, output, argmax_idx, pool_size=ctx.pool_size)
-
- ctx.save_for_backward(boxes, argmax_idx)
- return output
-
- @staticmethod
- @once_differentiable
- def backward(ctx, grad_output):
- boxes, argmax_idx = ctx.saved_tensors
- grad_input = grad_output.new_zeros(ctx.input_shape)
- # complex head architecture may cause grad_output uncontiguous
- grad_output = grad_output.contiguous()
- ext_module.border_align_backward(
- grad_output,
- boxes,
- argmax_idx,
- grad_input,
- pool_size=ctx.pool_size)
- return grad_input, None, None
-
-
-border_align = BorderAlignFunction.apply
-
-
-class BorderAlign(nn.Module):
- r"""Border align pooling layer.
-
- Applies border_align over the input feature based on predicted bboxes.
- The details were described in the paper
- `BorderDet: Border Feature for Dense Object Detection
- `_.
-
- For each border line (e.g. top, left, bottom or right) of each box,
- border_align does the following:
- 1. uniformly samples `pool_size`+1 positions on this line, involving \
- the start and end points.
- 2. the corresponding features on these points are computed by \
- bilinear interpolation.
- 3. max pooling over all the `pool_size`+1 positions are used for \
- computing pooled feature.
-
- Args:
- pool_size (int): number of positions sampled over the boxes' borders
- (e.g. top, bottom, left, right).
-
- """
-
- def __init__(self, pool_size):
- super(BorderAlign, self).__init__()
- self.pool_size = pool_size
-
- def forward(self, input, boxes):
- """
- Args:
- input: Features with shape [N,4C,H,W]. Channels ranged in [0,C),
- [C,2C), [2C,3C), [3C,4C) represent the top, left, bottom,
- right features respectively.
- boxes: Boxes with shape [N,H*W,4]. Coordinate format (x1,y1,x2,y2).
-
- Returns:
- Tensor: Pooled features with shape [N,C,H*W,4]. The order is
- (top,left,bottom,right) for the last dimension.
- """
- return border_align(input, boxes, self.pool_size)
-
- def __repr__(self):
- s = self.__class__.__name__
- s += f'(pool_size={self.pool_size})'
- return s
diff --git a/spaces/wangrongsheng/ChatImprovement/crazy_functions/test_project/cpp/libJPG/jpge.h b/spaces/wangrongsheng/ChatImprovement/crazy_functions/test_project/cpp/libJPG/jpge.h
deleted file mode 100644
index a46c805ab80aab491f7f9508b3a008b149866bee..0000000000000000000000000000000000000000
--- a/spaces/wangrongsheng/ChatImprovement/crazy_functions/test_project/cpp/libJPG/jpge.h
+++ /dev/null
@@ -1,172 +0,0 @@
-
-// jpge.h - C++ class for JPEG compression.
-// Public domain, Rich Geldreich
-// Alex Evans: Added RGBA support, linear memory allocator.
-#ifndef JPEG_ENCODER_H
-#define JPEG_ENCODER_H
-
-#include
-
-namespace jpge
-{
- typedef unsigned char uint8;
- typedef signed short int16;
- typedef signed int int32;
- typedef unsigned short uint16;
- typedef unsigned int uint32;
- typedef unsigned int uint;
-
- // JPEG chroma subsampling factors. Y_ONLY (grayscale images) and H2V2 (color images) are the most common.
- enum subsampling_t { Y_ONLY = 0, H1V1 = 1, H2V1 = 2, H2V2 = 3 };
-
- // JPEG compression parameters structure.
- struct params
- {
- inline params() : m_quality(85), m_subsampling(H2V2), m_no_chroma_discrim_flag(false), m_two_pass_flag(false) { }
-
- inline bool check_valid() const
- {
- if ((m_quality < 1) || (m_quality > 100)) return false;
- if ((uint)m_subsampling > (uint)H2V2) return false;
- return true;
- }
-
- // Quality: 1-100, higher is better. Typical values are around 50-95.
- int m_quality;
-
- // m_subsampling:
- // 0 = Y (grayscale) only
- // 1 = YCbCr, no subsampling (H1V1, YCbCr 1x1x1, 3 blocks per MCU)
- // 2 = YCbCr, H2V1 subsampling (YCbCr 2x1x1, 4 blocks per MCU)
- // 3 = YCbCr, H2V2 subsampling (YCbCr 4x1x1, 6 blocks per MCU-- very common)
- subsampling_t m_subsampling;
-
- // Disables CbCr discrimination - only intended for testing.
- // If true, the Y quantization table is also used for the CbCr channels.
- bool m_no_chroma_discrim_flag;
-
- bool m_two_pass_flag;
- };
-
- // Writes JPEG image to a file.
- // num_channels must be 1 (Y) or 3 (RGB), image pitch must be width*num_channels.
- bool compress_image_to_jpeg_file(const char *pFilename, int64_t width, int64_t height, int64_t num_channels, const uint8 *pImage_data, const params &comp_params = params());
-
- // Writes JPEG image to memory buffer.
- // On entry, buf_size is the size of the output buffer pointed at by pBuf, which should be at least ~1024 bytes.
- // If return value is true, buf_size will be set to the size of the compressed data.
- bool compress_image_to_jpeg_file_in_memory(void *pBuf, int64_t &buf_size, int64_t width, int64_t height, int64_t num_channels, const uint8 *pImage_data, const params &comp_params = params());
-
- // Output stream abstract class - used by the jpeg_encoder class to write to the output stream.
- // put_buf() is generally called with len==JPGE_OUT_BUF_SIZE bytes, but for headers it'll be called with smaller amounts.
- class output_stream
- {
- public:
- virtual ~output_stream() { };
- virtual bool put_buf(const void* Pbuf, int64_t len) = 0;
- template inline bool put_obj(const T& obj) { return put_buf(&obj, sizeof(T)); }
- };
-
- // Lower level jpeg_encoder class - useful if more control is needed than the above helper functions.
- class jpeg_encoder
- {
- public:
- jpeg_encoder();
- ~jpeg_encoder();
-
- // Initializes the compressor.
- // pStream: The stream object to use for writing compressed data.
- // params - Compression parameters structure, defined above.
- // width, height - Image dimensions.
- // channels - May be 1, or 3. 1 indicates grayscale, 3 indicates RGB source data.
- // Returns false on out of memory or if a stream write fails.
- bool init(output_stream *pStream, int64_t width, int64_t height, int64_t src_channels, const params &comp_params = params());
-
- const params &get_params() const { return m_params; }
-
- // Deinitializes the compressor, freeing any allocated memory. May be called at any time.
- void deinit();
-
- uint get_total_passes() const { return m_params.m_two_pass_flag ? 2 : 1; }
- inline uint get_cur_pass() { return m_pass_num; }
-
- // Call this method with each source scanline.
- // width * src_channels bytes per scanline is expected (RGB or Y format).
- // You must call with NULL after all scanlines are processed to finish compression.
- // Returns false on out of memory or if a stream write fails.
- bool process_scanline(const void* pScanline);
-
- private:
- jpeg_encoder(const jpeg_encoder &);
- jpeg_encoder &operator =(const jpeg_encoder &);
-
- typedef int32 sample_array_t;
-
- output_stream *m_pStream;
- params m_params;
- uint8 m_num_components;
- uint8 m_comp_h_samp[3], m_comp_v_samp[3];
- int m_image_x, m_image_y, m_image_bpp, m_image_bpl;
- int m_image_x_mcu, m_image_y_mcu;
- int m_image_bpl_xlt, m_image_bpl_mcu;
- int m_mcus_per_row;
- int m_mcu_x, m_mcu_y;
- uint8 *m_mcu_lines[16];
- uint8 m_mcu_y_ofs;
- sample_array_t m_sample_array[64];
- int16 m_coefficient_array[64];
- int32 m_quantization_tables[2][64];
- uint m_huff_codes[4][256];
- uint8 m_huff_code_sizes[4][256];
- uint8 m_huff_bits[4][17];
- uint8 m_huff_val[4][256];
- uint32 m_huff_count[4][256];
- int m_last_dc_val[3];
- enum { JPGE_OUT_BUF_SIZE = 2048 };
- uint8 m_out_buf[JPGE_OUT_BUF_SIZE];
- uint8 *m_pOut_buf;
- uint m_out_buf_left;
- uint32 m_bit_buffer;
- uint m_bits_in;
- uint8 m_pass_num;
- bool m_all_stream_writes_succeeded;
-
- void optimize_huffman_table(int table_num, int table_len);
- void emit_byte(uint8 i);
- void emit_word(uint i);
- void emit_marker(int marker);
- void emit_jfif_app0();
- void emit_dqt();
- void emit_sof();
- void emit_dht(uint8 *bits, uint8 *val, int index, bool ac_flag);
- void emit_dhts();
- void emit_sos();
- void emit_markers();
- void compute_huffman_table(uint *codes, uint8 *code_sizes, uint8 *bits, uint8 *val);
- void compute_quant_table(int32 *dst, int16 *src);
- void adjust_quant_table(int32 *dst, int32 *src);
- void first_pass_init();
- bool second_pass_init();
- bool jpg_open(int p_x_res, int p_y_res, int src_channels);
- void load_block_8_8_grey(int x);
- void load_block_8_8(int x, int y, int c);
- void load_block_16_8(int x, int c);
- void load_block_16_8_8(int x, int c);
- void load_quantized_coefficients(int component_num);
- void flush_output_buffer();
- void put_bits(uint bits, uint len);
- void code_coefficients_pass_one(int component_num);
- void code_coefficients_pass_two(int component_num);
- void code_block(int component_num);
- void process_mcu_row();
- bool terminate_pass_one();
- bool terminate_pass_two();
- bool process_end_of_image();
- void load_mcu(const void* src);
- void clear();
- void init();
- };
-
-} // namespace jpge
-
-#endif // JPEG_ENCODER
\ No newline at end of file
diff --git a/spaces/xfys/yolov5_tracking/val_utils/scripts/run_kitti.py b/spaces/xfys/yolov5_tracking/val_utils/scripts/run_kitti.py
deleted file mode 100644
index dbb63bde0a47ef51e4fe8c659c8de66ad4c8f29f..0000000000000000000000000000000000000000
--- a/spaces/xfys/yolov5_tracking/val_utils/scripts/run_kitti.py
+++ /dev/null
@@ -1,87 +0,0 @@
-
-""" run_kitti.py
-
-Run example:
-run_kitti.py --USE_PARALLEL False --METRICS Hota --TRACKERS_TO_EVAL CIWT
-
-Command Line Arguments: Defaults, # Comments
- Eval arguments:
- 'USE_PARALLEL': False,
- 'NUM_PARALLEL_CORES': 8,
- 'BREAK_ON_ERROR': True,
- 'PRINT_RESULTS': True,
- 'PRINT_ONLY_COMBINED': False,
- 'PRINT_CONFIG': True,
- 'TIME_PROGRESS': True,
- 'OUTPUT_SUMMARY': True,
- 'OUTPUT_DETAILED': True,
- 'PLOT_CURVES': True,
- Dataset arguments:
- 'GT_FOLDER': os.path.join(code_path, 'data/gt/kitti/kitti_2d_box_train'), # Location of GT data
- 'TRACKERS_FOLDER': os.path.join(code_path, 'data/trackers/kitti/kitti_2d_box_train/'), # Trackers location
- 'OUTPUT_FOLDER': None, # Where to save eval results (if None, same as TRACKERS_FOLDER)
- 'TRACKERS_TO_EVAL': None, # Filenames of trackers to eval (if None, all in folder)
- 'CLASSES_TO_EVAL': ['car', 'pedestrian'], # Valid: ['car', 'pedestrian']
- 'SPLIT_TO_EVAL': 'training', # Valid: 'training', 'val', 'training_minus_val', 'test'
- 'INPUT_AS_ZIP': False, # Whether tracker input files are zipped
- 'PRINT_CONFIG': True, # Whether to print current config
- 'TRACKER_SUB_FOLDER': 'data', # Tracker files are in TRACKER_FOLDER/tracker_name/TRACKER_SUB_FOLDER
- 'OUTPUT_SUB_FOLDER': '' # Output files are saved in OUTPUT_FOLDER/tracker_name/OUTPUT_SUB_FOLDER
- Metric arguments:
- 'METRICS': ['Hota','Clear', 'ID', 'Count']
-"""
-
-import sys
-import os
-import argparse
-from multiprocessing import freeze_support
-
-sys.path.insert(0, os.path.abspath(os.path.join(os.path.dirname(__file__), '..')))
-import trackeval # noqa: E402
-
-if __name__ == '__main__':
- freeze_support()
-
- # Command line interface:
- default_eval_config = trackeval.Evaluator.get_default_eval_config()
- default_eval_config['DISPLAY_LESS_PROGRESS'] = False
- default_dataset_config = trackeval.datasets.Kitti2DBox.get_default_dataset_config()
- default_metrics_config = {'METRICS': ['HOTA', 'CLEAR', 'Identity']}
- config = {**default_eval_config, **default_dataset_config, **default_metrics_config} # Merge default configs
- parser = argparse.ArgumentParser()
- for setting in config.keys():
- if type(config[setting]) == list or type(config[setting]) == type(None):
- parser.add_argument("--" + setting, nargs='+')
- else:
- parser.add_argument("--" + setting)
- args = parser.parse_args().__dict__
- for setting in args.keys():
- if args[setting] is not None:
- if type(config[setting]) == type(True):
- if args[setting] == 'True':
- x = True
- elif args[setting] == 'False':
- x = False
- else:
- raise Exception('Command line parameter ' + setting + 'must be True or False')
- elif type(config[setting]) == type(1):
- x = int(args[setting])
- elif type(args[setting]) == type(None):
- x = None
- else:
- x = args[setting]
- config[setting] = x
- eval_config = {k: v for k, v in config.items() if k in default_eval_config.keys()}
- dataset_config = {k: v for k, v in config.items() if k in default_dataset_config.keys()}
- metrics_config = {k: v for k, v in config.items() if k in default_metrics_config.keys()}
-
- # Run code
- evaluator = trackeval.Evaluator(eval_config)
- dataset_list = [trackeval.datasets.Kitti2DBox(dataset_config)]
- metrics_list = []
- for metric in [trackeval.metrics.HOTA, trackeval.metrics.CLEAR, trackeval.metrics.Identity]:
- if metric.get_name() in metrics_config['METRICS']:
- metrics_list.append(metric())
- if len(metrics_list) == 0:
- raise Exception('No metrics selected for evaluation')
- evaluator.evaluate(dataset_list, metrics_list)
diff --git a/spaces/xp3857/Image_Restoration_Colorization/Global/data/Load_Bigfile.py b/spaces/xp3857/Image_Restoration_Colorization/Global/data/Load_Bigfile.py
deleted file mode 100644
index b34f1ece4d296f4e7e8ccb709d84a23c01ee5dd7..0000000000000000000000000000000000000000
--- a/spaces/xp3857/Image_Restoration_Colorization/Global/data/Load_Bigfile.py
+++ /dev/null
@@ -1,42 +0,0 @@
-# Copyright (c) Microsoft Corporation.
-# Licensed under the MIT License.
-
-import io
-import os
-import struct
-from PIL import Image
-
-class BigFileMemoryLoader(object):
- def __load_bigfile(self):
- print('start load bigfile (%0.02f GB) into memory' % (os.path.getsize(self.file_path)/1024/1024/1024))
- with open(self.file_path, 'rb') as fid:
- self.img_num = struct.unpack('i', fid.read(4))[0]
- self.img_names = []
- self.img_bytes = []
- print('find total %d images' % self.img_num)
- for i in range(self.img_num):
- img_name_len = struct.unpack('i', fid.read(4))[0]
- img_name = fid.read(img_name_len).decode('utf-8')
- self.img_names.append(img_name)
- img_bytes_len = struct.unpack('i', fid.read(4))[0]
- self.img_bytes.append(fid.read(img_bytes_len))
- if i % 5000 == 0:
- print('load %d images done' % i)
- print('load all %d images done' % self.img_num)
-
- def __init__(self, file_path):
- super(BigFileMemoryLoader, self).__init__()
- self.file_path = file_path
- self.__load_bigfile()
-
- def __getitem__(self, index):
- try:
- img = Image.open(io.BytesIO(self.img_bytes[index])).convert('RGB')
- return self.img_names[index], img
- except Exception:
- print('Image read error for index %d: %s' % (index, self.img_names[index]))
- return self.__getitem__((index+1)%self.img_num)
-
-
- def __len__(self):
- return self.img_num
diff --git a/spaces/xwsm/gpt/crazy_functions/test_project/cpp/cppipc/ipc.cpp b/spaces/xwsm/gpt/crazy_functions/test_project/cpp/cppipc/ipc.cpp
deleted file mode 100644
index c713b852ea5a51fbeb4729b64561da482caaf351..0000000000000000000000000000000000000000
--- a/spaces/xwsm/gpt/crazy_functions/test_project/cpp/cppipc/ipc.cpp
+++ /dev/null
@@ -1,701 +0,0 @@
-
-#include
-#include
-#include
-#include // std::pair, std::move, std::forward
-#include
-#include // aligned_storage_t
-#include
-#include
-#include
-#include
-
-#include "libipc/ipc.h"
-#include "libipc/def.h"
-#include "libipc/shm.h"
-#include "libipc/pool_alloc.h"
-#include "libipc/queue.h"
-#include "libipc/policy.h"
-#include "libipc/rw_lock.h"
-#include "libipc/waiter.h"
-
-#include "libipc/utility/log.h"
-#include "libipc/utility/id_pool.h"
-#include "libipc/utility/scope_guard.h"
-#include "libipc/utility/utility.h"
-
-#include "libipc/memory/resource.h"
-#include "libipc/platform/detail.h"
-#include "libipc/circ/elem_array.h"
-
-namespace {
-
-using msg_id_t = std::uint32_t;
-using acc_t = std::atomic;
-
-template
-struct msg_t;
-
-template
-struct msg_t<0, AlignSize> {
- msg_id_t cc_id_;
- msg_id_t id_;
- std::int32_t remain_;
- bool storage_;
-};
-
-template
-struct msg_t : msg_t<0, AlignSize> {
- std::aligned_storage_t data_ {};
-
- msg_t() = default;
- msg_t(msg_id_t cc_id, msg_id_t id, std::int32_t remain, void const * data, std::size_t size)
- : msg_t<0, AlignSize> {cc_id, id, remain, (data == nullptr) || (size == 0)} {
- if (this->storage_) {
- if (data != nullptr) {
- // copy storage-id
- *reinterpret_cast(&data_) =
- *static_cast(data);
- }
- }
- else std::memcpy(&data_, data, size);
- }
-};
-
-template
-ipc::buff_t make_cache(T& data, std::size_t size) {
- auto ptr = ipc::mem::alloc(size);
- std::memcpy(ptr, &data, (ipc::detail::min)(sizeof(data), size));
- return { ptr, size, ipc::mem::free };
-}
-
-struct cache_t {
- std::size_t fill_;
- ipc::buff_t buff_;
-
- cache_t(std::size_t f, ipc::buff_t && b)
- : fill_(f), buff_(std::move(b))
- {}
-
- void append(void const * data, std::size_t size) {
- if (fill_ >= buff_.size() || data == nullptr || size == 0) return;
- auto new_fill = (ipc::detail::min)(fill_ + size, buff_.size());
- std::memcpy(static_cast(buff_.data()) + fill_, data, new_fill - fill_);
- fill_ = new_fill;
- }
-};
-
-auto cc_acc() {
- static ipc::shm::handle acc_h("__CA_CONN__", sizeof(acc_t));
- return static_cast(acc_h.get());
-}
-
-IPC_CONSTEXPR_ std::size_t align_chunk_size(std::size_t size) noexcept {
- return (((size - 1) / ipc::large_msg_align) + 1) * ipc::large_msg_align;
-}
-
-IPC_CONSTEXPR_ std::size_t calc_chunk_size(std::size_t size) noexcept {
- return ipc::make_align(alignof(std::max_align_t), align_chunk_size(
- ipc::make_align(alignof(std::max_align_t), sizeof(std::atomic)) + size));
-}
-
-struct chunk_t {
- std::atomic &conns() noexcept {
- return *reinterpret_cast *>(this);
- }
-
- void *data() noexcept {
- return reinterpret_cast(this)
- + ipc::make_align(alignof(std::max_align_t), sizeof(std::atomic));
- }
-};
-
-struct chunk_info_t {
- ipc::id_pool<> pool_;
- ipc::spin_lock lock_;
-
- IPC_CONSTEXPR_ static std::size_t chunks_mem_size(std::size_t chunk_size) noexcept {
- return ipc::id_pool<>::max_count * chunk_size;
- }
-
- ipc::byte_t *chunks_mem() noexcept {
- return reinterpret_cast(this + 1);
- }
-
- chunk_t *at(std::size_t chunk_size, ipc::storage_id_t id) noexcept {
- if (id < 0) return nullptr;
- return reinterpret_cast(chunks_mem() + (chunk_size * id));
- }
-};
-
-auto& chunk_storages() {
- class chunk_handle_t {
- ipc::shm::handle handle_;
-
- public:
- chunk_info_t *get_info(std::size_t chunk_size) {
- if (!handle_.valid() &&
- !handle_.acquire( ("__CHUNK_INFO__" + ipc::to_string(chunk_size)).c_str(),
- sizeof(chunk_info_t) + chunk_info_t::chunks_mem_size(chunk_size) )) {
- ipc::error("[chunk_storages] chunk_shm.id_info_.acquire failed: chunk_size = %zd\n", chunk_size);
- return nullptr;
- }
- auto info = static_cast(handle_.get());
- if (info == nullptr) {
- ipc::error("[chunk_storages] chunk_shm.id_info_.get failed: chunk_size = %zd\n", chunk_size);
- return nullptr;
- }
- return info;
- }
- };
- static ipc::map chunk_hs;
- return chunk_hs;
-}
-
-chunk_info_t *chunk_storage_info(std::size_t chunk_size) {
- auto &storages = chunk_storages();
- std::decay_t::iterator it;
- {
- static ipc::rw_lock lock;
- IPC_UNUSED_ std::shared_lock guard {lock};
- if ((it = storages.find(chunk_size)) == storages.end()) {
- using chunk_handle_t = std::decay_t::value_type::second_type;
- guard.unlock();
- IPC_UNUSED_ std::lock_guard guard {lock};
- it = storages.emplace(chunk_size, chunk_handle_t{}).first;
- }
- }
- return it->second.get_info(chunk_size);
-}
-
-std::pair acquire_storage(std::size_t size, ipc::circ::cc_t conns) {
- std::size_t chunk_size = calc_chunk_size(size);
- auto info = chunk_storage_info(chunk_size);
- if (info == nullptr) return {};
-
- info->lock_.lock();
- info->pool_.prepare();
- // got an unique id
- auto id = info->pool_.acquire();
- info->lock_.unlock();
-
- auto chunk = info->at(chunk_size, id);
- if (chunk == nullptr) return {};
- chunk->conns().store(conns, std::memory_order_relaxed);
- return { id, chunk->data() };
-}
-
-void *find_storage(ipc::storage_id_t id, std::size_t size) {
- if (id < 0) {
- ipc::error("[find_storage] id is invalid: id = %ld, size = %zd\n", (long)id, size);
- return nullptr;
- }
- std::size_t chunk_size = calc_chunk_size(size);
- auto info = chunk_storage_info(chunk_size);
- if (info == nullptr) return nullptr;
- return info->at(chunk_size, id)->data();
-}
-
-void release_storage(ipc::storage_id_t id, std::size_t size) {
- if (id < 0) {
- ipc::error("[release_storage] id is invalid: id = %ld, size = %zd\n", (long)id, size);
- return;
- }
- std::size_t chunk_size = calc_chunk_size(size);
- auto info = chunk_storage_info(chunk_size);
- if (info == nullptr) return;
- info->lock_.lock();
- info->pool_.release(id);
- info->lock_.unlock();
-}
-
-template
-bool sub_rc(ipc::wr