diff --git a/spaces/0x7194633/nllb-1.3B-demo/README.md b/spaces/0x7194633/nllb-1.3B-demo/README.md
deleted file mode 100644
index 220c462acad1cc5735b01872cabcbc27753ec1e8..0000000000000000000000000000000000000000
--- a/spaces/0x7194633/nllb-1.3B-demo/README.md
+++ /dev/null
@@ -1,12 +0,0 @@
----
-title: Nllb Translation Demo
-emoji: 👀
-colorFrom: indigo
-colorTo: green
-sdk: gradio
-sdk_version: 3.0.26
-app_file: app.py
-pinned: false
----
-
-Check out the configuration reference at https://huggingface.co/docs/hub/spaces-config-reference
diff --git a/spaces/0xHacked/zkProver/app.py b/spaces/0xHacked/zkProver/app.py
deleted file mode 100644
index bbd65e0a8d179ace2fb755f18e0f695e33f7325f..0000000000000000000000000000000000000000
--- a/spaces/0xHacked/zkProver/app.py
+++ /dev/null
@@ -1,77 +0,0 @@
-import os
-import tempfile
-import uuid
-import subprocess
-import gradio as gr
-
-
-BIN = os.path.join(os.path.dirname(__file__), "bin", "zkProver_linux_gpu")
-
-
-def run_zk_prover(network, block_number, contract, file):
- if not contract:
- raise gr.Error("contract is required")
- if not file:
- raise gr.Error('file is required')
- args = [
- BIN,
- "evm", "-r", "https://rpc.flashbots.net/"
- ]
- if block_number:
- args.extend(["-b", str(block_number)])
- proof_path = "/tmp/" + str(uuid.uuid4()) + ".bin"
- args.extend(["-o", proof_path])
-
- args.append(file.name + ":" + contract)
-
- proc = subprocess.Popen(args,)
- proc.wait()
-
- if proc.returncode != 0:
- raise gr.Error("generate proof failed")
- return proof_path
-
-
-with gr.Blocks() as demo:
- gr.Markdown(
- """
- # 0xHacked
- This is the demo for [0xHacked](https://0xHacked.com), a trustless bug bounty platform. You can generate the proof of exploit here. However, due to the constraints of ZKP, the generation might be low on Huggingface.
-
- We recommend [compiling it from the source](https://github.com/0xHackedLabs/zkProver). The generation can be very quick on GPU. For more details, please refer to [0xHacked Documentation](https://docs.0xHacked.com).
-
- The sample PoC provided below takes ~800s to generate the proof. You can click "SushiRouterExploit.sol" below and hit "Run" to try it!
- """
- )
- with gr.Column():
- with gr.Row():
- with gr.Column():
- network_input = gr.Dropdown(["Ethereum"], value="Ethereum", label='Network')
- block_number_input = gr.Number(precision=0, label='Block Number')
- contract_input = gr.Textbox(label='Poc Contract')
- file_input = gr.File(file_types=[".sol"], label='Solidity File')
- submit_btn = gr.Button(label="Submit")
- with gr.Column():
- fileout = gr.File(label='Proof File')
-
- gr.Examples(
- examples=[[
- "Ethereum",
- 17007841,
- "SushiExpProxy",
- "./examples/SushiRouterExploit.sol"],
- ],
- fn=run_zk_prover,
- inputs=[network_input, block_number_input, contract_input, file_input],
- outputs=fileout
- )
-
- submit_btn.click(
- fn=run_zk_prover,
- inputs=[network_input, block_number_input, contract_input, file_input],
- outputs=fileout
- )
-
-if __name__ == "__main__":
- demo.launch(server_name="0.0.0.0", server_port=7860)
-
diff --git a/spaces/1acneusushi/gradio-2dmoleculeeditor/data/Ao No Kanata Four Rhythm Crack.md b/spaces/1acneusushi/gradio-2dmoleculeeditor/data/Ao No Kanata Four Rhythm Crack.md
deleted file mode 100644
index a3ad5fb2aeb5746fdf905ae2e816fb9958d5dbbc..0000000000000000000000000000000000000000
--- a/spaces/1acneusushi/gradio-2dmoleculeeditor/data/Ao No Kanata Four Rhythm Crack.md
+++ /dev/null
@@ -1,32 +0,0 @@
-
-
Ao no Kanata no Four Rhythm (also known as Aokana: Four Rhythm Across the Blue) is a visual novel developed by sprite and released in 2014. It is set in a world where flying is as simple as riding a bicycle, thanks to the invention of anti-gravitational shoes known as Grav-Shoes. The game follows the protagonist, Masaya Hinata, a former competitor in a sport called Flying Circus, who regains his passion for flying when he meets the transfer student Asuka Kurashina. Together with their friends, they join the Kunahama High School Flying Circus club and aim for the top of the national tournament.
-DOWNLOAD ☆☆☆ https://byltly.com/2uKxag
The game features four main heroines, each with their own route and story. They are:
-The game has been praised for its beautiful graphics, engaging gameplay, and emotional story. It has also been adapted into an anime series in 2016 and a manga series in 2015. The game has been released in English by NekoNyan Ltd. and HIKARI FIELD in 2019, with an 18+ DLC available for free on NekoNyanSoft shop. However, the game has mosaics censorship, which may disappoint some fans of the genre.
-If you are looking for a visual novel that combines romance, comedy, drama, and sports, you may want to give Ao no Kanata no Four Rhythm a try. You can download the game from Steam or NekoNyanSoft shop, and enjoy the thrilling experience of flying with your favorite heroine.
- - -One of the main attractions of Ao no Kanata no Four Rhythm is its gameplay, which simulates the Flying Circus matches in a 3D environment. The player can choose to control Masaya or one of the heroines, and compete against various opponents in different modes, such as time attack, point match, or survival. The player can also customize their Grav-Shoes and outfits, and unlock new skills and abilities as they progress through the game.
-The gameplay is fast-paced and exhilarating, requiring the player to master the basics of flying, such as acceleration, turning, braking, and drifting. The player also has to use their tactics and reflexes to dodge attacks, counterattack, and perform special moves. The game offers multiple difficulty levels and adjustable settings, making it accessible for both beginners and veterans. The game also supports online multiplayer mode, where the player can challenge other players from around the world.
-The game has received overwhelmingly positive reviews from both critics and users on Steam[^1^], who praised its gameplay, graphics, music, voice acting, and story. Some of the common compliments include:
---"One of the best visual novels I've ever played. The story is engaging, the characters are lovable, the art is gorgeous, and the gameplay is addictive."
-"A masterpiece of a visual novel. The gameplay is fun and challenging, the story is emotional and captivating, and the music is beautiful and fitting."
-"A visual novel that transcends its genre. The gameplay is not just a gimmick, but an integral part of the story and character development. The story is not just a romance, but a journey of growth and friendship."
-
However, the game is not without its flaws. Some of the common criticisms include:
---"The game has mosaics censorship, which ruins the immersion and quality of the 18+ scenes."
-"The game has some bugs and glitches, such as crashes, freezes, or missing text."
-"The game has some translation errors and typos, such as grammar mistakes, inconsistent names, or wrong choices."
-
Despite these issues, most reviewers agree that Ao no Kanata no Four Rhythm is a visual novel worth playing for its unique gameplay and compelling story. If you are a fan of visual novels or flying games, you should not miss this gem.
81aa517590If you have ever worked with VBA macros in Excel, you might have encountered a situation where you need to access or modify the code of a locked VBA project. This can happen when you inherit a workbook from someone else, or when you forget your own password. In this article, we will show you how to crack VBA project password recovery 13 in Excel using different methods.
-VBA project password recovery 13 is a term that refers to the process of unlocking a VBA project that is protected by a password in Excel. A VBA project is a collection of modules, forms, and classes that contain the code for your macros. You can protect your VBA project from unauthorized access or modification by setting a password in the VBA editor.
-Download File →→→ https://imgfil.com/2uy1zd
However, sometimes you might need to crack the password for various reasons, such as:
-There are different ways to crack VBA project password recovery 13 in Excel, depending on the file format and the version of Excel you are using. We will cover some of the most common and effective methods in the following sections.
-If you are working with an older .XLS file (Excel 97-2003 format), you can use a simple hex editing technique to crack the password. Hex editing is a method of modifying the binary data of a file using a hexadecimal editor. You can use any hex editor software for this purpose, such as HxD or Notepad++.
-Here are the steps to crack VBA project password recovery 13 for older .XLS files:
-Congratulations! You have successfully cracked VBA project password recovery 13 for older .XLS files. You can now access and modify the code of your macros as you wish.
-If you are working with a newer .XLSM file (Excel 2007 or later format), you can use a different technique that involves changing the file extension and extracting a binary file. A binary file is a file that contains data in a binary format, which can be read by computers but not by humans. You can use any archiver software for this purpose, such as WinRAR or 7-Zip.
-Here are the steps to crack VBA project password recovery 13 for newer .XLSM files:
- -Congratulations! You have successfully cracked VBA project password recovery 13 for newer .XLSM files. You can now access and modify the code of your macros as you wish.
- -In this article, we have shown you how to crack VBA project password recovery 13 in Excel using different methods. We hope this article was helpful and informative for you. However, we also advise you to use these methods responsibly and ethically, and not to violate any intellectual property rights or privacy policies of others. Remember that cracking passwords is not always legal or ethical, so use these methods at your own risk and discretion.
-In this article, we have shown you how to crack VBA project password recovery 13 in Excel using different methods. We hope this article was helpful and informative for you. However, we also advise you to use these methods responsibly and ethically, and not to violate any intellectual property rights or privacy policies of others. Remember that cracking passwords is not always legal or ethical, so use these methods at your own risk and discretion.
3cee63e6c2Do you love driving cars and parking them in challenging spots? Do you want to experience the thrill of driving different types of vehicles and customizing them to your liking? Do you want to play with your friends and compete with other players online? If you answered yes to any of these questions, then you should try Car Parking Mod APK 4.8.8.3, a modified version of the popular Car Parking game that offers unlimited money, all cars unlocked, multiplayer mode, and more.
-Car Parking is a realistic and fun driving simulator game that tests your skills in parking various cars in different scenarios. You can choose from over 100 cars, ranging from sedans, SUVs, sports cars, trucks, buses, and even police cars. You can also customize your cars with different colors, wheels, spoilers, stickers, and more.
-Download File ⚹ https://urlin.us/2uSTZM
The game has over 200 levels, each with its own difficulty and objectives. You have to park your car in the designated spot without hitting any obstacles or other cars. You also have to follow the traffic rules and signals, such as speed limits, stop signs, traffic lights, etc.
-Car Parking Mod APK 4.8.8.3 is a modified version of the original game that gives you access to unlimited money, all cars unlocked, multiplayer mode, and more features that make the game more enjoyable and exciting.
-With Car Parking Mod APK 4.8.8.3, you don't have to worry about running out of money to buy new cars or upgrade them. You can get unlimited money by completing levels or by using the in-game shop.
-With Car Parking Mod APK 4.8.8.3, you don't have to wait to unlock new cars or spend money to buy them. You can access all the cars in the game from the start and choose whichever one you like.
-With Car Parking Mod APK 4.8.8.3, you don't have to play alone or against the computer. You can play with your friends or other players online in multiplayer mode. You can join or create rooms and chat with other players while playing.
-car parking multiplayer mod apk v4.8.8.3 unlimited money
-car parking multiplayer mod apk v4.8.8.3 download for android
-car parking multiplayer mod apk v4.8.8.3 latest version
-car parking multiplayer mod apk v4.8.8.3 free shopping
-car parking multiplayer mod apk v4.8.8.3 unlocked all cars
-car parking multiplayer mod apk v4.8.8.3 no root
-car parking multiplayer mod apk v4.8.8.3 online
-car parking multiplayer mod apk v4.8.8.3 hack
-car parking multiplayer mod apk v4.8.8.3 gameplay
-car parking multiplayer mod apk v4.8.8.3 review
-car parking multiplayer mod apk v4.8.8.3 update
-car parking multiplayer mod apk v4.8.8.3 new features
-car parking multiplayer mod apk v4.8.8.3 cheats
-car parking multiplayer mod apk v4.8.8.3 how to install
-car parking multiplayer mod apk v4.8.8.3 best settings
-car parking multiplayer mod apk v4.8.8.3 tips and tricks
-car parking multiplayer mod apk v4.8.8.3 custom cars
-car parking multiplayer mod apk v4.8.8.3 realistic graphics
-car parking multiplayer mod apk v4.8.8.3 high quality open world
-car parking multiplayer mod apk v4.8.8.3 fun gameplay
-car parking multiplayer mod apk v4.8.8.3 82 real-life challenges
-car parking multiplayer mod apk v4.8.8.3 different vehicles
-car parking multiplayer mod apk v4.8.8.3 interior buildings
-car parking multiplayer mod apk v4.8.8.3 dynamic vinyls
-car parking multiplayer mod apk v4 . 88 . 83 vision auto tungsten
-car parking multiplayer mod apk v4 . 88 . 83 highly detailed environments
-car parking multiplayer mod apk v4 . 88 . 83 100 cars with real interiors
-car parking multiplayer mod apk v4 . 88 . 83 16 player skins
-car parking multiplayer mod apk v4 . 88 . 83 trailers, pickups, trucks, sports and classic cars
-car parking multiplayer mod apk v4 . 88 . 83 voice chat with friends
-car parking multiplayer mod apk v4 . 88 . 83 exchange cars with real players
-car parking multiplayer mod apk v4 . 88 . 83 police mode with sirens and radar
-car parking multiplayer mod apk v4 . 88 . 83 manual transmission with clutch and gear shift
-car parking multiplayer mod apk v4 . 88 . 83 engine tuning and performance upgrades
-car parking multiplayer mod apk v4 . 88 . 83 body parts and accessories customization
-car parking multiplayer mod apk v4 . 88 . 83 realistic physics and sound effects
With Car Parking Mod APK 4.8.8.3, you don't have to settle for the default appearance of your cars. You can customize them with different colors, wheels, spoilers, stickers, and more.
-With Car Parking Mod APK 4.8.8.3, you don't have to compromise on the quality of the game's physics and graphics. The game has realistic physics that simulate the behavior of real cars and their interaction with the environment.
-The game also has high-quality graphics that create a realistic and immersive atmosphere for the game.
-If you want to download and install Car Parking Mod APK 4 .8.3, you need to follow these simple steps:
-Before you can install any modded or third-party app on your Android device, you need to enable the option of unknown sources in your settings. This will allow you to install apps from sources other than the Google Play Store.
-To enable unknown sources, go to your device's settings, then security, then unknown sources, and toggle it on.
-Next, you need to download the APK file of Car Parking Mod APK 4.8.8.3 from a reliable and trusted source. You can use the link below to download the file directly to your device.
-Download Car Parking Mod APK 4.8.8.3 here
-Once you have downloaded the APK file, you need to install it on your device. To do this, locate the file in your device's storage and tap on it. You will see a prompt asking you to confirm the installation. Tap on install and wait for the process to finish.
-After the installation is complete, you can launch the game from your app drawer or home screen and enjoy the game with all its features.
-Car Parking Mod APK 4.8.8.3 is a great game for anyone who loves driving and parking games, but it also has some pros and cons that you should be aware of before playing it.
-Car Parking Mod APK 4.8.8.3 is a realistic and fun driving simulator game that tests your skills in parking various cars in different scenarios. The game has unlimited money, all cars unlocked, multiplayer mode, and more features that make it more enjoyable and exciting. The game also has realistic physics and graphics that create a realistic and immersive atmosphere for the game.
-If you are looking for a driving and parking game that offers a lot of variety, challenge, and fun, then you should try Car Parking Mod APK 4.8.8.3. You can download and install it easily by following the steps above.
-I hope you enjoyed reading this article and found it helpful. If you have any questions or feedback about Car Parking Mod APK 4.8.8.3, feel free to leave a comment below.
-Car Parking Mod APK 4.8.8.3 is a modified version of Car Parking Multiplayer that offers unlimited money, all cars unlocked, multiplayer mode, and more features that make the game more enjoyable and exciting.
-Yes, Car Parking Mod APK 4.8.8.3 is safe to download and install as long as you use a reliable and trusted source like the one provided in this article.
-You can play Car Parking Mod APK 4.8.8.3 with your friends by using the multiplayer mode. You can join or create rooms and chat with your friends while playing.
-You can customize your cars in Car Parking Mod APK 4.8.8.3 by using the customization options. You can change the color, wheels, spoilers, stickers, and more of your cars.
-You can get more money in Car Parking Mod APK 4.8.8.3 by completing levels or by using the in-game shop.
-If you are a fan of car racing games, you might have heard of CarX Highway Racing. It is a realistic and thrilling game that lets you drive fast cars on highways, evade the police, and compete with other racers. But what if you want to enjoy the game without spending money or worrying about the limitations? That's where CarX Highway Racing APK Hackeado comes in. In this article, we will tell you what CarX Highway Racing is, how to download and install CarX Highway Racing APK Hackeado, why you should play it, and some tips and tricks to help you win the races.
-CarX Highway Racing is a game developed by CarX Technologies, a company that specializes in creating realistic car physics and graphics. The game was released in 2017 for Android and iOS devices, and has since gained millions of downloads and positive reviews. The game features over 40 different cars, from sports cars to muscle cars, that you can customize and upgrade. You can also choose from various game modes, such as campaign, time attack, survival, and online multiplayer. The game also has realistic weather effects, day and night cycles, and dynamic traffic.
-DOWNLOAD ✶ https://urlin.us/2uT1Ws
Some of the features that make CarX Highway Racing stand out from other car racing games are:
-If you want to play CarX Highway Racing without any restrictions or costs, you can download and install CarX Highway Racing APK Hackeado. This is a modified version of the game that gives you unlimited money, gold, fuel, and energy. You can use these resources to buy any car you want, upgrade it to the max level, and unlock all the tracks and modes. Here are the steps to download and install CarX Highway Racing APK Hackeado:
-CarX Highway Racing APK Hackeado is not only a fun and exciting game but also a great way to improve your driving skills and reflexes. Here are some of the benefits of playing CarX Highway Racing APK Hackeado:
-To make the most out of CarX Highway Racing APK Hackeado, here are some tips and tricks that you can follow:
-CarX Highway Racing APK Hackeado is a great way to enjoy CarX Highway Racing without any limitations or costs. You can download and install CarX Highway Racing APK Hackeado easily and safely from [this link]. You can also benefit from playing CarX Highway Racing APK Hackeado by saving money and time, experimenting and having fun, and learning and improving. You can also follow our tips and tricks to win the races and become the best racer on the highway.
-Here are some of the frequently asked questions about CarX Highway Racing APK Hackeado:
-carx highway racing mod apk unlimited money
-carx highway racing hack apk download
-carx highway racing apk mod menu
-carx highway racing cheats apk
-carx highway racing mod apk latest version
-carx highway racing hack apk android
-carx highway racing mod apk obb
-carx highway racing apk hack 2023
-carx highway racing mod apk revdl
-carx highway racing hack apk ios
-carx highway racing mod apk rexdl
-carx highway racing hack apk 1.74.8
-carx highway racing mod apk offline
-carx highway racing hack apk free download
-carx highway racing mod apk no root
-carx highway racing hack apk online
-carx highway racing mod apk data
-carx highway racing hack apk unlimited gold
-carx highway racing mod apk all cars unlocked
-carx highway racing hack apk no verification
-carx highway racing mod apk pure
-carx highway racing hack apk 2022
-carx highway racing mod apk happymod
-carx highway racing hack apk latest
-carx highway racing mod apk android 1
Yes, CarX Highway Racing APK Hackeado is safe to download and install. It does not contain any viruses, malware, or spyware. It also does not require any root or jailbreak access to your device.
-CarX Highway Racing APK Hackeado is compatible with most Android devices that have Android 4.1 or higher. However, some devices may experience performance issues or crashes due to hardware limitations or compatibility issues.
-To update CarX Highway Racing APK Hackeado, you need to download and install the latest version of CarX Highway Racing APK Hackeado from [this link]. You do not need to uninstall the previous version of CarX Highway Racing APK Hackeado before installing the new one.
-Yes, you can play CarX Highway Racing APK Hackeado online with other players who have CarX Highway Racing APK Hackeado installed on their devices. However, you may not be able to play online with players who have the original version of CarX Highway Racing installed on their devices.
-No, you will not get banned for playing CarX Highway Racing APK Hackeado. The game does not have any anti-cheat system or detection mechanism that can identify or ban players who use CarX Highway Racing APK Hackeado.
-If you are looking for a thrilling racing game that lets you explore a dynamic open world, customize your car, and compete against other players, then you should check out CarX Street. This game is developed by CarX Technologies, the makers of CarX Drift Racing 2, and it offers realistic races on highways and city streets, plus top-speed drift races.
-In this article, we will show you how to download CarX Street on your Android device and how to play it like a pro. You will learn about the game's features, how to install it from different sources, how to start your racing career, and how to improve your skills and performance.
-Download » https://urlin.us/2uSWJ0
CarX Street is a free racing game that was released in 2023 as an open beta test. The game is set in Sunset City, a huge open world that you can explore freely with your car. You can join clubs, challenge bosses, buy houses for your cars, and collect parts for your vehicle.
-The game features realistic physics and controls that make you feel like you are driving a real car. You can customize your car with various parts and accessories, such as mirrors, headlights, lights, skirt, bumper, rims, and more. You can also swap your engine for a different one and upgrade your engine, transmission, body, suspension, and tires.
-The game has different modes of racing, such as highways, city streets, and drift zones. You can race against other players online or offline, or just cruise around the city at any time of day or night. The game has a dynamic day/night cycle that changes the gameplay and the graphics of the game.
-There are two ways to download and install CarX Street on your Android device: from the Google Play Store or from APK sites. Here are the steps for each method:
-The easiest and safest way to get CarX Street on your Android device is to download it from the Google Play Store. Here is how to do it:
-If you cannot access the Google Play Store or you want to try a different version of the game, you can also download CarX Street from APK sites. However, this method is riskier and may expose your device to malware or viruses. Here are some tips on how to do it safely:
-How to install carx street on android device
-Carx street android download guide
-Steps to download carx street for android phone
-Carx street apk download for android
-How to get carx street on android tablet
-Carx street android game free download
-Download carx street from google play store
-How to play carx street on android
-Carx street android beta download
-Carx street open world racing game for android
-How to update carx street on android
-Carx street android download link
-Carx street android requirements and compatibility
-How to fix carx street download error on android
-Carx street android gameplay and review
-Download carx street mod apk for android
-How to uninstall carx street from android
-Carx street android tips and tricks
-Carx street android latest version download
-How to backup carx street data on android
-Carx street android offline download
-How to join carx street beta test on android
-Carx street android cheats and hacks
-Download carx street xapk file for android
-How to transfer carx street progress to another android device
-Carx street android graphics settings and optimization
-How to stream carx street on android
-Carx street android controller support and configuration
-Download carx street obb file for android
-How to change language in carx street on android
-Carx street android multiplayer mode and features
-How to redeem codes in carx street on android
-Carx street android best cars and tuning options
-Download carx street from apkcombo for android
-How to record carx street gameplay on android
-Carx street android sound and music settings
-How to contact carx street support service on android
-Carx street android in-app purchases and prices
-Download carx street from filehippo for android
-How to enable notifications for carx street on android
-Carx street android drift mode and controls
-How to clear cache for carx street on android
-Carx street android clubs and challenges
-Download carx street from apkpure for android
-How to share carx street screenshots on android
-Carx street android gas stations and fuel types
-How to disable ads in carx street on android
-Carx street android license agreement and privacy policy
-Download carx street from uptodown for android
-How to rate and review carx street on google play store
You can customize your car with various parts and accessories, such as mirrors, headlights, lights, skirt, bumper, rims, and more. You can also change the color, the vinyl, the license plate, and the stickers of your car. To customize your car, you need to use coins or diamonds, which are the in-game currencies.
-One of the best features of CarX Street is that you can join or create a club with other players. A club is a group of racers that share a common name, logo, and chat. You can join a club by searching for its name or by accepting an invitation from another player. You can also create your own club by choosing a name, a logo, and a description.
-Joining a club has many benefits for your racing career. You can chat with other club members, share tips and tricks, and challenge them to friendly races. You can also participate in club events and missions, which are special races that reward you with coins, diamonds, parts, and reputation points. Reputation points are used to rank up your club and unlock new perks and rewards.
-The main mode of CarX Street is racing against other players online or offline. You can choose from different modes of racing, such as highways, city streets, and drift zones. Each mode has different rules and objectives, such as reaching the finish line first, earning the most points by drifting, or escaping from the police.
-To start a race, you need to open the map and select a location. You can see the difficulty level, the entry fee, and the reward for each location. You can also see the number of players online and offline in each location. You can join an existing race or create your own race by choosing the number of laps, the time limit, and the weather conditions.
-Once you join a race, you need to use your skills and strategy to win. You can use the gas pedal, the brake pedal, the handbrake, and the nitro boost to control your car. You can also use the steering wheel or the tilt option to steer your car. You need to avoid crashing into obstacles or other cars, as this will damage your car and slow you down.
-One of the most fun and challenging aspects of CarX Street is drifting. Drifting is a technique that involves sliding your car sideways while maintaining control and speed. Drifting is useful for taking sharp turns without losing momentum and for earning points and rewards.
-To drift in CarX Street, you need to use the handbrake or the brake pedal while turning your car. You need to balance your throttle and steering to maintain your drift angle and direction. You also need to avoid hitting walls or other cars while drifting, as this will end your drift combo.
-Drifting is important for earning points and rewards in CarX Street. The more you drift, the more points you get. The points are multiplied by your drift combo, which is the number of consecutive drifts you perform without interruption. The points are also affected by your drift angle, speed, distance, and style.
-You can use your drift points to buy new cars or parts for your car. You can also use them to unlock new locations or events in the game. Drifting is also essential for completing some missions or challenges in the game.
If you want to become a better racer and a legend of Sunset City, you need to improve your skills and performance in CarX Street. Here are some advanced tips on how to do that:
-One of the best ways to improve your car's performance is to upgrade it with part tuning. Part tuning is a feature that allows you to unlock the full potential of your car and improve its engine, transmission, body, suspension, and tires. You can access part tuning from the garage menu.
-To use part tuning, you need to have parts for your car. You can get parts by winning races, completing missions, or buying them with coins or diamonds. You can also get parts by dismantling other cars or parts that you don't need.
-Once you have parts, you can use them to upgrade your car's stats. You can see the current and the maximum stats of your car on the part tuning screen. You can also see the effect of each part on your car's performance. You can upgrade your car's stats up to 100%, but you need to have enough parts and coins for that.
-Another way to improve your car's performance is to swap your engine for a different one. Engine swapping is a feature that allows you to change your car's engine type and power. You can access engine swapping from the garage menu.
-To use engine swapping, you need to have engines for your car. You can get engines by winning races, completing missions, or buying them with coins or diamonds. You can also get engines by dismantling other cars or engines that you don't need.
-Once you have engines, you can use them to swap your car's engine. You can see the current and the available engines for your car on the engine swapping screen. You can also see the effect of each engine on your car's performance. You can swap your car's engine as many times as you want, but you need to have enough engines and coins for that.
-Engine swapping has advantages and disadvantages for your car's performance. Some engines may increase your car's speed, acceleration, or drift, but they may also decrease your car's handling, stability, or fuel efficiency. You need to choose the engine that suits your racing style and preference.
-A third way to improve your car's performance is to use the right fuel for your car. Fuel is a resource that affects your car's speed, acceleration, and nitro boost. You can see your car's fuel level on the top left corner of the screen during a race.
-To use fuel, you need to have fuel for your car. You can get fuel by visiting gas stations in the city or by buying them with coins or diamonds. You can also get fuel by completing missions or challenges in the game.
-Once you have fuel, you can use it to fill up your car's tank. You can see the current and the maximum fuel level of your car on the fuel screen. You can also see the effect of each fuel type on your car's performance. You can fill up your car's tank as much as you want, but you need to have enough fuel and coins for that.
-Fuel has different types and qualities that affect your car's performance. Some fuel types may increase your car's speed, acceleration, or nitro boost, but they may also decrease your car's handling, stability, or durability. You need to choose the fuel type that suits your racing style and preference.
-A fourth way to improve your skills and performance in CarX Street is to race at different times of day. The game has a dynamic day/night cycle that changes the gameplay and the graphics of the game.
-The time of day affects the visibility, the traffic, and the difficulty of the races. During the day, you can see more clearly, but there are more cars and pedestrians on the road. During the night, you can see less clearly, but there are fewer cars and pedestrians on the road.
-The time of day also affects the atmosphere and the mood of the game. During the day, you can enjoy the bright colors and the sunny weather of Sunset City. During the night, you can admire the neon lights and the dark sky of Sunset City.
-You can change the time of day by using a clock icon on the map screen. You can choose between morning, afternoon, evening, and night. You can also let the time of day change naturally as you play.
-CarX Street is an amazing racing game that lets you experience the thrill of racing and drifting in a realistic open world. You can download and install it on your Android device from the Google Play Store or from APK sites. You can also play it like a pro by choosing a car, joining a club, racing against other players, and drifting. You can also improve your skills and performance by upgrading your car, swapping your engine, using the right fuel, and racing at different times of day.
-If you are a fan of racing games, you should not miss CarX Street. It is one of the best racing games for Android that offers stunning graphics, realistic physics, and endless fun. Download it now and join the millions of players who are enjoying CarX Street.
-Here are some frequently asked questions about CarX Street:
-You can get more coins and diamonds by winning races, completing missions, participating in club events, watching ads, or buying them with real money.
-You can unlock new cars or parts by earning reputation points, which are used to rank up your level and unlock new rewards. You can also buy them with coins or diamonds.
-You can drift better by using the handbrake or the brake pedal while turning your car. You also need to balance your throttle and steering to maintain your drift angle and direction. You can also use the drift assist option to help you drift easier.
-You can race with your friends by joining or creating a club and inviting them to join. You can also challenge them to friendly races or join their races from the map screen.
-You can change the camera view by tapping on the camera icon on the top right corner of the screen during a race. You can choose between different views, such as cockpit, hood, bumper, chase, or far chase.
-If you are a fan of racing games, you have probably heard of CSR Racing 2, one of the most popular and realistic games in the genre. And if you are a fan of Fast and Furious, you have probably been excited by the recent collaboration between the game and the movie franchise, bringing exclusive cars and challenges to the game. But what if you want to enjoy the game without any limitations or restrictions? That's where CSR Racing 2 Mod APK comes in. In this article, we will tell you everything you need to know about this modified version of the game, how to install it, and what benefits it offers.
-CSR Racing 2 is a racing game developed by NaturalMotionGames Ltd and released in 2016. It is the sequel to CSR Racing, which was released in 2012. The game features stunning graphics, realistic physics, and immersive gameplay that make you feel like you are driving a real car. You can customize your car with various parts, paint jobs, decals, and more. You can also collect and upgrade over 200 licensed cars from top brands like Ferrari, Lamborghini, Bugatti, McLaren, and more.
-Download File ✫ https://jinyurl.com/2uNOXw
One of the main attractions of CSR Racing 2 is its graphics. The game uses a cutting-edge 3D rendering technique called PBR (Physically Based Rendering) that creates realistic lighting, shadows, reflections, and textures. The cars look amazing, with detailed interiors, exteriors, and engine sounds. The tracks are also diverse and realistic, ranging from urban streets to desert roads. The gameplay is also smooth and responsive, with easy controls and realistic physics. You can choose from different modes, such as drag races, crew battles, ladder races, regulation races, and more.
-Another attraction of CSR Racing 2 is its car collection. The game features over 200 licensed cars from top brands like Ferrari, Lamborghini, Bugatti, McLaren, and more. You can collect them by winning races, opening crates, or buying them with in-game currency. You can also upgrade them with various parts, such as engines, turbochargers, nitrous oxide systems, tires, transmissions, and more. You can also customize them with various paint jobs, decals, rims, spoilers, and more.
-The game also has a competitive online mode where you can race against other players from around the world in real-time. You can join or create a crew with your friends or other players and compete in crew battles, leaderboards, chat rooms, and more. You can also participate in various events that offer rewards and prizes for completing missions or reaching milestones. Some of the events are seasonal or limited-time only, so you have to be quick to join them.
-Fast and Furious is a popular movie franchise that features street racing and heists. The franchise started in 2001 with The Fast
Fast and Furious is a popular movie franchise that features street racing and heists. The franchise started in 2001 with The Fast and the Furious, and has since released nine more movies, with the latest one being F9: The Fast Saga. The movies star Vin Diesel, Paul Walker, Michelle Rodriguez, Tyrese Gibson, Dwayne Johnson, Jason Statham, and many other actors. The movies are known for their thrilling action scenes, exotic locations, and diverse cast of characters.
-The main plot of the Fast and Furious movies revolves around Dominic Toretto (Vin Diesel), a former street racer who leads a crew of skilled drivers and criminals. He is often pursued by law enforcement agents, such as Brian O'Conner (Paul Walker), who later becomes his friend and ally. Together, they face various enemies and challenges, such as drug lords, terrorists, hackers, and rogue agents. Along the way, they also form a family bond with each other and their loyal friends.
-In 2019, CSR Racing 2 partnered with Fast and Furious to bring some of the iconic cars from the movies to the game. Players can race with cars such as the Toyota Supra, the Veilside Mazda RX-7, the Mitsubishi Eclipse, and many more. They can also participate in special events that are inspired by the movies, such as the Hobbs & Shaw event, the Fate of the Furious event, and the Fast & Furious Finale event. These events offer rewards and prizes for completing missions or reaching milestones.
-The latest event in CSR Racing 2 is the Fast & Furious Finale event, which celebrates the release of F9: The Fast Saga. The event runs from April 15 to June 30, 2021, and features eight cars from the movie. Players can race with cars such as the Dodge Charger Daytona, the Veilside Honda S2000, Jesse's Volkswagen Jetta, and more. They can also unlock exclusive liveries, decals, and parts for their cars. The event also has a storyline that follows the movie's plot and characters.
-csr racing 2 mod apk unlimited money and keys
-csr racing 2 fast and furious cars mod apk
-csr racing 2 mod apk latest version download
-csr racing 2 mod apk offline mode
-csr racing 2 mod apk unlimited gold and cash
-csr racing 2 fast and furious flip car mod apk
-csr racing 2 mod apk all cars unlocked
-csr racing 2 mod apk no root required
-csr racing 2 mod apk free shopping
-csr racing 2 fast and furious jetta mod apk
-csr racing 2 mod apk unlimited nitro
-csr racing 2 fast and furious supra mod apk
-csr racing 2 mod apk anti ban
-csr racing 2 mod apk unlimited fuel
-csr racing 2 fast and furious charger mod apk
-csr racing 2 mod apk mega mod
-csr racing 2 fast and furious events mod apk
-csr racing 2 mod apk unlimited rp
-csr racing 2 mod apk no ads
-csr racing 2 fast and furious skyline mod apk
-csr racing 2 mod apk obb file download
-csr racing 2 fast and furious honda s2000 mod apk
-csr racing 2 mod apk unlimited everything
-csr racing 2 mod apk high graphics
-csr racing 2 fast and furious eclipse mod apk
-csr racing 2 mod apk ios download
-csr racing 2 fast and furious plymouth roadrunner mod apk
-csr racing 2 mod apk unlocked premium features
-csr racing 2 mod apk unlimited crew points
-csr racing 2 fast and furious mitsubishi lancer evolution vii mod apk
-csr racing 2 mod apk online multiplayer
-csr racing 2 fast and furious dodge challenger srt demon mod apk
-csr racing 2 mod apk unlimited rare imports
-csr racing 2 mod apk custom cars
-csr racing 2 fast and furious nissan silvia s15 mona lisa mod apk
-csr racing 2 mod apk unlimited fusion parts
-csr racing 2 fast and furious lamborghini murcielago lp640 mod apk
-csr racing 2 mod apk unlimited legendary keys
-csr racing 2 mod apk realistic physics
-csr racing 2 fast and furious ford gt40 mk ii mod apk
CSR Racing 2 Mod APK is a modified version of the original game that unlocks everything. It is a way to enjoy the game without spending money or waiting for upgrades. It is also a risk-free download that does not require rooting or jailbreaking your device.
-With CSR Racing 2 Mod APK, you can access all the features and content of the game without any limitations or restrictions. You can get unlimited money, keys, gold, fuel, and cash to buy or upgrade any car you want. You can also unlock all the cars, tracks, modes, events, and customizations that are otherwise locked or premium. You can also bypass any ads or verification processes that might interrupt your gameplay.
-One of the drawbacks of playing CSR Racing 2 is that it can be quite expensive and time-consuming to progress in the game. You need to spend real money or earn in-game currency to buy or upgrade your cars. You also need to wait for your fuel to refill or your parts to be delivered. This can be frustrating and boring for some players who want to enjoy the game without any hassle. With CSR Racing 2 Mod APK, you don't have to worry about any of these issues. You can play the game at your own pace and style without any pressure or cost.
-Some modded games require you to root or jailbreak your device in order to install them. This can be risky and harmful for your device's security and performance. It can also void your warranty or cause compatibility issues with other apps. With CSR Racing 2 Mod APK, you don't have to do any of these things. You can simply download the mod apk file from a trusted source and install it on your device without any problem. You can also uninstall it anytime you want without any consequences.
If you are interested in trying out CSR Racing 2 Mod APK, you might be wondering how to install it on your device. Don't worry, it's very easy and simple. Just follow these steps and you will be ready to race in no time.
-The first thing you need to do is to download the mod apk file from a trusted source. You can find many websites that offer the mod apk file, but be careful and choose a reliable one. Some websites might have fake or malicious files that can harm your device or steal your data. To avoid any risk, you can use this link to download the mod apk file. This is a safe and verified source that has been tested and reviewed by many users.
-The next thing you need to do is to enable unknown sources in your device settings. This is a security feature that prevents you from installing apps that are not from the official app store. Since the mod apk file is not from the app store, you need to enable this option to install it. To do this, go to your device settings and look for the security or privacy option. Then, find the unknown sources option and toggle it on. You might see a warning message, but don't worry, it's safe to proceed.
-The final thing you need to do is to install the mod apk file and launch the game. To do this, go to your file manager and locate the mod apk file that you downloaded. Tap on it and follow the instructions to install it. It might take a few seconds or minutes depending on your device. Once it's done, you can launch the game from your app drawer or home screen. You will see the CSR Racing 2 logo and then the game will load. You can now enjoy the game with all the features and content unlocked.
-CSR Racing 2 is one of the best racing games available for mobile devices. It offers realistic graphics, gameplay, and physics that make you feel like you are driving a real car. It also has a huge collection of licensed cars from top brands that you can collect, customize, and upgrade. It also has a competitive online mode where you can race against other players from around the world in real-time. And if you are a fan of Fast and Furious, you can also enjoy the exclusive cars and challenges from the movie franchise.
-But if you want to enjoy the game without any limitations or restrictions, you can try CSR Racing 2 Mod APK. This is a modified version of the game that unlocks everything for free. You can get unlimited money, keys, gold, fuel, and cash to buy or upgrade any car you want. You can also unlock all the cars, tracks, modes, events, and customizations that are otherwise locked or premium. You can also bypass any ads or verification processes that might interrupt your gameplay.
-Installing CSR Racing 2 Mod APK is very easy and simple. You just need to download the mod apk file from a trusted source, enable unknown sources in your device settings, and install the mod apk file on your device. You don't need to root or jailbreak your device to do this. You can also uninstall it anytime you want without any consequences.
-If you are looking for a fun and exciting racing game that offers everything you want, CSR Racing 2 Mod APK is the perfect choice for you. Download it now and start racing with your favorite cars.
-Here are some frequently asked questions about CSR Racing 2 Mod APK:
-Yes, CSR Racing 2 Mod APK is safe to use. It does not contain any viruses or malware that can harm your device or steal your data. It also does not require rooting or jailbreaking your device to install it.
-No, CSR Racing 2 Mod APK is not legal. It violates the terms and conditions of the original game and its developers. It also infringes on the intellectual property rights of the car brands and the movie franchise. Using it might result in legal action or account suspension.
-Yes, you can play CSR Racing 2 Mod APK online with other players from around the world in real-time. However, you might face some issues or errors while playing online, such as connection problems, lag, or cheating. You might also get banned or reported by other players who notice that you are using a modded version of the game.
-No, you cannot update CSR Racing 2 Mod APK. If you try to update it from the app store or the game itself, you will lose all the modded features and content. You will also risk getting detected and banned by the game developers. If you want to update the game, you will have to uninstall the mod apk file and install the original game from the app store.
-No, you cannot use CSR Racing 2 Mod APK with your existing account. If you try to log in with your existing account, you will risk getting detected and banned by the game developers. You will also lose all your progress and data on your account. If you want to use the mod apk file, you will have to create a new account or use a guest account.
-PowerShell is a task automation and configuration management program from Microsoft, consisting of a command-line shell and the associated scripting language. It allows you to perform various operations on your system, such as managing files, processes, services, registry, network, security, and more.
-DOWNLOAD ✏ ✏ ✏ https://jinyurl.com/2uNTng
PowerShell 2.0 was released in October 2009 as part of Windows Management Framework (WMF) for Windows XP, Windows Vista, Windows Server 2003, and Windows Server 2008. It introduced many new features, such as modules, remoting, background jobs, transactions, debugging, eventing, and scripting enhancements.
-Although newer versions of PowerShell have been released since then, you might need to download PowerShell 2.0 for some reasons. For example, you might have an older script or host program that is incompatible with newer versions of PowerShell or .NET Framework. Or, you might want to run commands or scripts that are designed for PowerShell 2.0 on a different computer that has a newer version of PowerShell installed.
-In this article, we will show you how to download and install PowerShell 2.0 on different versions of Windows, how to use PowerShell 2.0 commands and scripts, and some tips and recommendations for using PowerShell 2.0.
-The installation process of PowerShell 2.0 varies depending on the version of Windows that you have. Here are the steps for installing PowerShell 2.0 on different versions of Windows:
-On Windows 8.1 and Windows 8, the PowerShell 2.0 Engine feature is turned on by default. However, to use it, you need to turn on the option for Microsoft .NET Framework 3.5, which it requires. This section also explains how to turn the PowerShell 2.0 Engine feature on and off.
-On Windows Server 2012 R2 and Windows Server 2012, the PowerShell 2.0 Engine feature is not installed by default. However, you can add it by using the Server Manager or the Windows PowerShell cmdlets. This section also explains how to start the PowerShell 2.0 Engine.
-You can also use the Windows PowerShell cmdlets to add or remove the PowerShell 2.0 Engine and .NET Framework 3.5 features. For example, you can use the following command to install both features:
-Install-WindowsFeature -Name Net-Framework-Core,PowerShell-V2
-You can use the following command to uninstall both features:
-How to download powershell 2.0 for windows 10
-Download powershell 2.0 for windows server 2012 r2
-Download powershell 2.0 for windows xp sp3
-Download powershell 2.0 for windows vista sp2
-Download powershell 2.0 for windows server 2008 sp2
-Download powershell 2.0 for windows server 2003 sp2
-Download powershell 2.0 and winrm 2.0 for windows update
-Download powershell 2.0 engine for windows 8.1
-Download powershell 2.0 engine for windows 8
-Download powershell 2.0 engine for windows server 2012
-Download powershell 2.0 engine for windows server 2016
-Download powershell 2.0 engine for windows server 2019
-Download powershell 2.0 engine for windows server core
-Download powershell 2.0 engine for windows management framework
-Download powershell 2.0 engine for .net framework 3.5
-Download powershell 2.0 engine for .net framework 4
-Download powershell 2.0 engine for .net core
-Download powershell 2.0 engine from microsoft learn
-Download powershell 2.0 engine from github
-Download powershell 2.0 engine from microsoft docs
-Install powershell 2.0 on windows 10
-Install powershell 2.0 on windows server 2016
-Install powershell 2.0 on windows server core
-Install powershell 2.0 on windows management framework
-Install powershell 2.0 on .net framework 3.5
-Install powershell 2.0 on .net framework 4
-Install powershell 2.0 on .net core
-Install powershell 2.0 from microsoft learn
-Install powershell 2.0 from github
-Install powershell 2.0 from microsoft docs
-Uninstall powershell 3.0 and install powershell 2.0
-Uninstall powershell 4.0 and install powershell 2.0
-Uninstall powershell 5.1 and install powershell 2.0
-Uninstall powershell core and install powershell 2.0
-Uninstall windows management framework and install powershell 2.0
-Uninstall .net framework and install powershell 2.0
-Uninstall .net core and install powershell 2.0
-Upgrade from powershell v1 to v2 download link
-Upgrade from cmd to powershell v2 download link
-Upgrade from bash to powershell v2 download link
-Run commands in compatibility mode with download link to v1 or v3+
-Run scripts in compatibility mode with download link to v1 or v3+
-Run modules in compatibility mode with download link to v1 or v3+
-Run snap-ins in compatibility mode with download link to v1 or v3+
-Run providers in compatibility mode with download link to v1 or v3+.
Uninstall-WindowsFeature -Name Net-Framework-Core,PowerShell-V2
-To start the PowerShell 2.0 Engine, you need to use the -Version parameter of the powershell.exe command. For example, you can use the following command to start a PowerShell session with the PowerShell 2.0 Engine:
-powershell.exe -Version 2
-You can also use the following command to start a Windows PowerShell ISE session with the PowerShell 2.0 Engine:
-powershell_ise.exe -Version 2
- On Windows Server 2008 R2, Windows Vista, Windows Server 2003, and Windows XP, the PowerShell 2.0 Engine feature is not installed by default. However, you can install it by downloading and installing Windows Management Framework (WMF) 3.0, which includes PowerShell 2.0, WinRM 2.0, and WMI 2.0. This section also explains how to start the PowerShell 2.0 Engine.
-The steps for starting the PowerShell 2.0 Engine are the same as for Windows Server 2012 R2 and Windows Server 2012. You need to use the -Version parameter of the powershell.exe or powershell_ise.exe command.
-Once you have installed and started the PowerShell 2.0 Engine, you can use it to run commands and scripts that are designed for PowerShell 2.0. Here are some tips and examples for using PowerShell 2.0 commands and scripts:
-If you want to start a new PowerShell session with the PowerShell 2.0 Engine, you can use one of the following methods:
-If you want to run a single command or a script file that is compatible with PowerShell 2.0, you can use one of the following methods:
-Get-Process
to get a list of processes running on your computer, or type C:\Scripts\MyScript.ps1
to run a script file named MyScript.ps1 in the C:\Scripts folder.Get-Process
in the Script Pane, and then click Run Script to get a list of processes running on your computer, or type C:\Scripts\MyScript.ps1
in the Script Pane, and then click Run Script to run a script file named MyScript.ps1 in the C:\Scripts folder.powershell.exe -Version 2 -Command "Get-Process"
to get a list of processes running on your computer, or type powershell.exe -Version 2 -Command "C:\Scripts\MyScript.ps1"
to run a script file named MyScript.ps1 in the C:\Scripts folder.powershell_ise.exe -Version 2 -File "C:\Scripts\MyScript.ps1"
to run a script file named MyScript.ps1 in the C:\Scripts folder.If you want to start a remote session or a background job with another computer that has PowerShell 2.0 installed, you need to use the -ConfigurationName Microsoft.PowerShell.2.0 parameter of the New-PSSession, New-PSSessionOption, or Start-Job cmdlet. For example, you can use the following command to start a remote session with another computer named Server01 using the PowerShell 2.0 Engine:
-New-PSSession -ComputerName Server01 -ConfigurationName Microsoft.PowerShell.2.0
-You can use the following command to start a background job on another computer named Server01 using the PowerShell 2.0 Engine:
-Start-Job -ComputerName Server01 -ConfigurationName Microsoft.PowerShell.2.0 -ScriptBlock Get-Process
- In this article, we have shown you how to download and install PowerShell 2.0 on different versions of Windows, how to use PowerShell 2.0 commands and scripts, and some tips and recommendations for using PowerShell 2.0. We hope that this article has been helpful and informative for you. Here are some FAQs that you might have about PowerShell 2.0:
-The system requirements for PowerShell 2.0 depend on the version of Windows that you have. Here are the minimum system requirements for each version of Windows that supports PowerShell 2.0:
-Windows Version | -Minimum System Requirements | -
---|---|
Windows 8.1 and Windows 8 | -1 GHz processor, 1 GB RAM, 16 GB available disk space, DirectX 9 graphics device with WDDM 1.0 or higher driver | -
Windows Server 2012 R2 and Windows Server 2012 | -1.4 GHz processor, 512 MB RAM, 32 GB available disk space | -
Windows Server 2008 R2 and Windows Vista | -1 GHz processor, 512 MB RAM, 15 GB available disk space, Super VGA (800 x 600) or higher-resolution monitor | -
Windows Server 2003 and Windows XP | -233 MHz processor, 64 MB RAM, 1.5 GB available disk space, Super VGA (800 x 600) or higher-resolution monitor | -
PowerShell 2.0 is generally compatible with newer versions of PowerShell, such as PowerShell 3.0, PowerShell 4.0, PowerShell 5.0, PowerShell 5.1, and PowerShell Core. However, there might be some differences or limitations in the functionality, syntax, or behavior of some commands or features between different versions of PowerShell. For example, some cmdlets or parameters that are available in newer versions of PowerShell might not be available or work differently in PowerShell 2.0. Therefore, it is recommended that you test your commands or scripts before running them on different versions of PowerShell to ensure that they work as expected.
-PowerShell 2.0 is not deprecated or insecure by itself, but it might be affected by the deprecation or security status of the underlying .NET Framework version that it uses. For example, .NET Framework 3.5, which is required by PowerShell 2.0 on Windows 8.1 and Windows Server 2012, is no longer supported by Microsoft as of January 14, 2020. This means that it will not receive any security updates or patches from Microsoft, which might expose your system to potential vulnerabilities or risks. Therefore, it is recommended that you upgrade to a newer version of PowerShell and .NET Framework if possible, or apply the latest security updates and best practices for your system if you need to use PowerShell 2.0.
-If you want to learn more about PowerShell 2.0, you can refer to the following resources:
-If you want to uninstall or disable PowerShell 2.0, you can use one of the following methods:
-Game of Thrones is one of the most popular and acclaimed TV shows in history. Based on the fantasy novels by George R.R. Martin, it tells the story of a medieval world where several noble families vie for control over the Iron Throne, while an ancient threat looms beyond a massive wall in the north. The show is known for its complex characters, intricate plots, stunning visuals, and shocking twists.
-Download Zip ::: https://jinyurl.com/2uNKWZ
Season 2 Episode 5, titled "The Ghost of Harrenhal", is one of the most pivotal episodes in the series. It features several major events that change the course of the war for the throne, as well as some intriguing developments in other parts of the world. In this article, we will give you a brief recap of what happens in this episode, and then show you how to watch it with Urdu subtitles.
-Why would you want to watch Game of Thrones with Urdu subtitles? Well, there are several reasons. Maybe you are a fan of Urdu language and culture, and you want to enjoy the show in a different way. Maybe you are learning Urdu, and you want to improve your skills by watching a high-quality show. Maybe you have trouble understanding some accents or dialogues in English, and you want to make sure you don't miss anything important. Whatever your reason is , we have got you covered. In this article, we will tell you how to download Urdu subtitles for Game of Thrones Season 2 Episode 5, and how to watch it online or offline with the subtitles. But first, let's recap what happens in this episode.
-"The Ghost of Harrenhal" is the fifth episode of the second season of Game of Thrones. It aired on April 29, 2012, and was written by David Benioff and D.B. Weiss, and directed by David Petrarca. The episode has a runtime of 55 minutes, and has a rating of 8.8 out of 10 on IMDb. Here are the main events that take place in this episode:
-game of thrones s02e05 urdu subs download
-got season 2 episode 5 urdu subtitles free
-watch game of thrones season 2 episode 5 with urdu subtitles
-game of thrones season 2 episode 5 urdu srt download
-download game of thrones season 2 episode 5 in urdu
-game of thrones season 2 episode 5 urdu dubbed download
-game of thrones season 2 episode 5 online urdu subtitles
-game of thrones season 2 episode 5 urdu translation download
-game of thrones season 2 episode 5 subtitles in urdu
-game of thrones season 2 episode 5 urdu subscene download
-game of thrones season 2 episode 5 urdu language download
-game of thrones season 2 episode 5 full urdu subtitles
-how to download game of thrones season 2 episode 5 urdu subtitles
-game of thrones season 2 episode 5 english to urdu subtitles
-game of thrones season 2 episode 5 urdu version download
-game of thrones season 2 episode 5 with urdu subs
-game of thrones season 2 episode 5 urdu subtitle file download
-game of thrones season 2 episode 5 hd urdu subtitles
-game of thrones season 2 episode 5 best urdu subtitles
-game of thrones season 2 episode 5 easy urdu subtitles download
-game of thrones season two episode five urdu subtitles download
-where to download game of thrones season 2 episode 5 urdu subtitles
-game of thrones season second episode fifth urdu subtitles download
-game of thrones season ii episode v urdu subtitles download
-game of thrones the ghost of harrenhal urdu subtitles download
-download got s02e05 with urdu subtitles
-got the ghost of harrenhal urdu subs download
-watch got s02e05 online with urdu subtitles
-got s02e05 urdu srt file download
-got s02e05 in urdu language download
-got s02e05 urdu dubbed version download
-got s02e05 online with urdu translation
-got s02e05 subtitles in urdu language
-got s02e05 subscene urdu subtitle download
-got s02e05 full episode with urdu subs
-how to get got s02e05 with urdu subtitles
-got s02e05 english to urdu subtitle conversion
-got s02e05 hd quality with urdu subs
-got s02e05 best quality with urdu subs
-got s02e05 easy to download with urdu subs
-watch the ghost of harrenhal with urdu subtitles online
-the ghost of harrenhal subscene in urdu language
-the ghost of harrenhal full video with urdu subs
-the ghost of harrenhal english to urdu subtitle translation
-the ghost of harrenhal hd video with urdu subs
-the ghost of harrenhal best video with urdu subs
-the ghost of harrenhal easy video with urdu subs
The episode begins with a shocking scene: the assassination of Renly Baratheon, one of the claimants to the Iron Throne, by a shadowy figure that resembles his brother Stannis. The shadow is actually a creature conjured by Melisandre, a red priestess who serves Stannis and believes him to be the chosen one of her god. The murder is witnessed by Catelyn Stark, the widow of Ned Stark who was executed by King Joffrey, and Brienne of Tarth, a female knight who swore loyalty to Renly. They are accused of the crime by Renly's guards, but they manage to escape with the help of Loras Tyrell, Renly's lover and ally.
-With Renly dead, most of his bannermen switch their allegiance to Stannis, who now has the largest army in Westeros. However, some of them remain loyal to the Tyrells, who are not willing to bend the knee to Stannis. Stannis offers to make Loras his heir if he joins him, but Loras refuses. He also rejects Catelyn's plea to join forces with Robb Stark, her son and the King in the North, who is fighting against Joffrey. Stannis then prepares to march on King's Landing, the capital of the Seven Kingdoms, where Joffrey resides.
-Brienne and Catelyn decide to leave the Stormlands and head north. Brienne swears an oath to serve Catelyn and protect her. She also vows to avenge Renly's death by killing Stannis.
-In the capital, Tyrion Lannister, the witty and clever brother of Cersei Lannister, Joffrey's mother and regent, is trying to keep the city safe and stable as the Hand of the King. He discovers that Cersei has ordered the alchemists to produce large quantities of wildfire, a highly flammable and explosive substance that can burn anything. Cersei plans to use it as a weapon against Stannis' fleet when he attacks the city. Tyrion is alarmed by this idea, as he knows that wildfire is very dangerous and unpredictable. He decides to take control of the wildfire production and distribution, and tells Cersei that he will use it in a smarter way.
-Tyrion also has to deal with the growing unrest and discontent among the people of King's Landing, who are suffering from hunger, poverty, and fear. He tries to appease them by sending Princess Myrcella Baratheon, Joffrey's younger sister, to Dorne, a southern kingdom that is allied with the Lannisters. He hopes that this will secure their friendship and prevent them from joining Stannis or Robb. He also hopes that Myrcella will be safer and happier in Dorne than in King's Landing.
-However, his plan backfires when he escorts Myrcella to the ship that will take her to Dorne. The people of King's Landing riot and attack him and his entourage, throwing stones and insults at them. They also target Joffrey, who responds by ordering his guards to kill them all. A bloody chaos ensues, in which several people are killed or injured, including some of Tyrion's allies. Tyrion manages to survive and reach the safety of the Red Keep, the royal castle. He confronts Joffrey for his cruelty and stupidity, and slaps him in front of everyone.
-Meanwhile, across the Narrow Sea in Essos, Daenerys Targaryen, the last surviving member of the Targaryen dynasty that ruled Westeros before Robert Baratheon overthrew them, is trying to find allies and resources for her quest to reclaim the Iron Throne. She has three young dragons, the only ones in existence, but they are still too small and weak to be used in battle. She also has a small band of loyal followers, including Jorah Mormont, a former knight who serves as her adviser and protector, and her bloodriders, a group of Dothraki warriors who swore to follow her after the death of her husband Khal Drogo.
-Daenerys and her followers have been welcomed in Qarth, a wealthy and exotic city in the east, by Xaro Xhoan Daxos, a powerful merchant and a member of the Thirteen, the rulers of Qarth. Xaro offers Daenerys his hospitality and his friendship, but he also has ulterior motives. He proposes to marry Daenerys and give her half of his wealth, in exchange for one of her dragons. Daenerys refuses, as she considers her dragons to be her children and her only hope.
-Daenerys also meets two mysterious characters in Qarth: Quaithe, a masked woman who claims to be a shadowbinder from Asshai, a dark and mysterious land in the far east; and Pyat Pree, a bald and blue-lipped warlock who invites Daenerys to visit the House of the Undying, the headquarters of his order. Quaithe warns Daenerys to beware of those who seek to use or harm her, and tells her that she must go to Asshai to learn the truth about her destiny. Pyat Pree promises Daenerys that she will see wonders and visions in the House of the Undying, and that he has something that belongs to her.
-At the end of the episode, Daenerys discovers that Pyat Pree was telling the truth: he has stolen her dragons and taken them to the House of the Undying. He lured them away from their cage with a decoy, and killed most of Daenerys' guards in the process. Daenerys is furious and distraught, and vows to get her dragons back.
-Finally, in the frozen lands beyond the Wall, a massive barrier of ice that separates Westeros from the wild lands in the north, Jon Snow, the bastard son of Ned Stark who joined the Night's Watch, a sworn brotherhood that guards the Wall and protects the realm from the dangers beyond, is on a dangerous mission. He is part of a small group of rangers led by Qhorin Halfhand, a legendary warrior who is respected and feared by both his allies and enemies. Their goal is to find and kill Mance Rayder, a former member of the Night's Watch who deserted and became the King-Beyond-the-Wall, uniting the wildlings under his command.
-On their way, they encounter a group of wildlings led by Ygritte, a fiery-haired woman who catches Jon's eye. They manage to kill or capture most of them, except for Ygritte, who is taken prisoner by Jon. Qhorin orders Jon to execute her, but Jon hesitates. He does not want to kill an unarmed woman, especially one that he finds attractive. He tries to do it anyway, but Ygritte escapes. Jon chases her through the snow, but loses sight of his comrades. He catches up with Ygritte, but she tricks him into falling into a trap. She then taunts him for being a virgin and a crow (a derogatory term for members of the Night's Watch), and tells him that he knows nothing about the world.
-Meanwhile, in Winterfell, the ancestral home of the Starks in the north, Bran Stark, Ned's youngest son who was crippled after being pushed from a tower by Jaime Lannister, Cersei's brother and lover, is having strange dreams. He dreams that he is his direwolf Summer, running through the woods and hunting. He also dreams that he meets Jojen Reed, a boy who claims to have similar dreams and abilities. Jojen tells Bran that he is a warg, someone who can enter the minds of animals and control them. He also tells him that he has "the sight", which allows him to see past and future events. He warns Bran that he is in danger, and that he must find "the three-eyed raven", a mysterious figure that appears in his dreams.
-In Pyke, the seat of House Greyjoy on the Iron Islands, an archipelago off the west coast of Westeros, Theon Greyjoy, Ned's former ward who betrayed him and joined his father Balon Greyjoy in his rebellion against the Lannisters, is preparing to leave with his sister Yara Greyjoy and her fleet. He has been given the task of raiding the coast of the north, while Robb Stark is away fighting in the south. He hopes to prove himself to his father and his people, who have always looked down on him for being raised by the Starks. He also hopes to impress Yara, who is a skilled and respected captain and warrior, and who mocks him for being weak and foolish.
-Now that you have a clear idea of what happens in "The Ghost of Harrenhal", you might be wondering how to watch it with Urdu subtitles. Subtitles are a great way to enhance your viewing experience, especially if you are not a native speaker of English, or if you want to learn a new language. Subtitles can help you improve your vocabulary, grammar, pronunciation, and comprehension skills, as well as expose you to different cultures and expressions. They can also help you enjoy the show more, as you won't miss any important details or dialogues that might be hard to catch or understand otherwise.
-So, how can you get Urdu subtitles for Game of Thrones Season 2 Episode 5? Well, there are several sources that offer them, both free and paid. However, not all of them are reliable or accurate. Some of them might have poor quality, incorrect translations, missing or delayed lines, or even malware or viruses. Therefore, you need to be careful and choose the best source for your needs. Here are some of the factors that you should consider when looking for Urdu subtitles for Game of Thrones Season 2 Episode 5:
- - Quality: The subtitles should be clear, readable, and synchronized with the video and audio. They should also match the tone, style, and context of the show. - Accuracy: The subtitles should convey the meaning and intention of the original dialogue, without adding or omitting anything. They should also respect the grammar, spelling, and punctuation rules of Urdu. - Availability: The subtitles should be easy to find and download, without requiring any registration or payment. They should also be compatible with your device and media player. - Legality: The subtitles should be legal and authorized by the creators or owners of the show. They should not violate any copyright or intellectual property laws.Based on these criteria, we have compiled a list of some of the best websites that offer Urdu subtitles for Game of Thrones Season 2 Episode 5. Here they are:
-Website | -Quality | -Accuracy | -Availability | -Legality | -
---|---|---|---|---|
[isubdb.com] | -High | -High | -Free and easy | -Legal | -
[subscene.com] | -High | -High | -Free and easy | -Legal | -
[opensubtitles.org] | -Medium | -Medium | -Free but requires registration | -Legal | -
[tvsubtitles.net] | -Low | -Low | -Free but slow and unreliable | -Illegal | -
As you can see from the table above, our recommendation is to use [isubdb.com] as your source of Urdu subtitles for Game of Thrones Season 2 Episode 5. This website has high-quality and accurate subtitles that are free and easy to download. It also has a large collection of subtitles for other episodes and seasons of Game of Thrones, as well as other shows and movies. It is also legal and safe to use.
-To download Urdu subtitles from [isubdb.com], all you have to do is follow these simple steps:
- - Go to [isubdb.com] on your browser - Type "Game of Thrones" in the search box - Select "Game of Thrones - Season 2" from the results - Scroll down to "Episode 5 - The Ghost of Harrenhal" - Click on "Urdu" under "Subtitles" - Click on "Download" next to the subtitle file that you want - Save the file on your device - You have successfully downloaded the Urdu subtitles for Game of Thrones Season 2 Episode 5. Now, you can watch the episode with the subtitles on your device. But how do you do that? There are two options: online streaming or offline downloading. Let's see what they are and how they work.Online streaming is the option of watching the episode on a platform that allows you to stream it over the internet, without having to download it on your device. This option is convenient and fast, as you can watch the episode anytime and anywhere, as long as you have a stable internet connection. However, this option also has some drawbacks, such as requiring a subscription or payment, consuming a lot of data, or being subject to geo-restrictions or censorship.
-Offline downloading is the option of downloading the episode on your device, and then watching it with a media player that supports subtitles. This option is more flexible and reliable, as you can watch the episode offline, without worrying about internet issues or interruptions. However, this option also has some challenges, such as taking up a lot of space, exposing you to malware or viruses, or violating legal or ethical rules.
-So, which option should you choose? Well, that depends on your preferences and circumstances. To help you decide, we have compared some of the best platforms and methods for online streaming and offline downloading of Game of Thrones Season 2 Episode 5 with Urdu subtitles. Here they are:
-There are many platforms that allow you to stream Game of Thrones online, with or without subtitles. However, not all of them are available or accessible in every country or region. Therefore, you need to check the availability and compatibility of the platform before choosing it. Here are some of the most popular and reliable platforms that offer online streaming of Game of Thrones Season 2 Episode 5 with Urdu subtitles:
-Platform | -Features | -Price | -Compatibility | -
---|---|---|---|
HBO Max | -- The official and legal platform for streaming Game of Thrones - High-quality video and audio - Supports multiple languages and subtitles - Offers other HBO shows and movies - Allows offline downloading on mobile devices | -$14.99 per month | -Available in the US and some Latin American countries Compatible with most devices and browsers | -
Netflix | -- The most popular and widely used streaming platform - High-quality video and audio - Supports multiple languages and subtitles - Offers a large variety of shows and movies - Allows offline downloading on mobile devices | -$8.99 to $17.99 per month depending on the plan | -Available in most countries except China, Syria, North Korea, and Crimea Compatible with most devices and browsers | -
Amazon Prime Video | -- The streaming platform of Amazon - High-quality video and audio - Supports multiple languages and subtitles - Offers other Amazon shows and movies - Allows offline downloading on mobile devices | -$8.99 per month or $119 per year for Prime membership | -Available in most countries except China, Iran, North Korea, Syria, and Crimea Compatible with most devices and browsers | -
As you can see from the table above, our recommendation is to use HBO Max as your platform for online streaming of Game of Thrones Season 2 Episode 5 with Urdu subtitles. This is because HBO Max is the official and legal platform for streaming Game of Thrones, and it offers high-quality video and audio, as well as multiple languages and subtitles. It also offers other HBO shows and movies that you might enjoy, such as Westworld, The Sopranos, The Wire, etc. It also allows offline downloading on mobile devices, which is convenient if you want to watch the episode later.
-To stream Game of Thrones Season 2 Episode 5 with Urdu subtitles on HBO Max, all you have to do is follow these simple steps:
- - Go to [hbomax.com] on your browser - Sign up for an account or log in if you already have one - Choose your plan and payment method - Search for "Game of Thrones" in the search box - Select "Game of Thrones - Season 2" from the results - Scroll down to "Episode 5 - The Ghost of Harrenhal" - Click on "Play" - Click on the settings icon at the bottom right corner of the screen - Click on "Subtitles" - Click on "Urdu" - Enjoy watching the episode with Urdu subtitles.If you prefer to download the episode on your device and watch it offline, you have some other options as well. However, you need to be aware of the risks and challenges that come with this option, such as malware, viruses, legal issues, or ethical dilemmas. Therefore, you need to be careful and responsible when choosing this option. Here are some of the most common and effective methods for offline downloading of Game of Thrones Season 2 Episode 5 with Urdu subtitles:
-Method | -Speed | -Security | -Legality | -
---|---|---|---|
Torrenting | -Fast | -Risky | -Illegal | -
Direct Downloading | -Slow | -Safer | -Illegal | -
DVD/Blu-ray Ripping | -Medium | -Safe | -Legal | -
As you can see from the table above, our recommendation is to use DVD/Blu-ray ripping as your method for offline downloading of Game of Thrones Season 2 Episode 5 with Urdu subtitles. This is because DVD/Blu-ray ripping is the only legal and safe method among the three, as it does not involve downloading or sharing pirated content. It also offers decent speed and quality, as well as the option to choose your preferred language and subtitles. However, this method also requires that you own or buy a physical copy of the episode on DVD or Blu-ray, which might be expensive or hard to find.
-To rip Game of Thrones Season 2 Episode 5 with Urdu subtitles from DVD or Blu-ray, all you have to do is follow these simple steps:
- - Insert the DVD or Blu-ray disc into your computer's drive - Download and install a DVD/Blu-ray ripping software, such as [HandBrake] or [MakeMKV] - Open the software and select the disc as the source - Choose the output format and settings that suit your device and preferences - Select "Urdu" as the subtitle language - Click on "Start" or "Rip" to begin the process - Wait for the process to finish and save the file on your device - You have successfully ripped Game of Thrones Season 2 Episode 5 with Urdu subtitles from DVD or Blu-ray. Now, you can watch the episode with a media player that supports subtitles, such as [VLC] or [KMPlayer].In this article, we have shown you how to watch Game of Thrones Season 2 Episode 5 with Urdu subtitles. We have given you a brief recap of what happens in this episode, and then explained how to download Urdu subtitles from various sources. We have also compared some of the best platforms and methods for online streaming and offline downloading of the episode with Urdu subtitles. We hope that you have found this article helpful and informative, and that you will enjoy watching this episode with Urdu subtitles.
-If you are a fan of Game of Thrones, you might also want to check out our other articles on how to watch other episodes and seasons of the show with Urdu subtitles. You might also want to share your feedback and opinions on this episode and the show in general with us and other readers. You can do so by leaving a comment below or by contacting us through our website or social media channels.
-Thank you for reading this article, and happy watching!
-Here are some of the most common questions that people ask about watching Game of Thrones Season 2 Episode 5 with Urdu subtitles:
-A: There are some websites that offer free streaming or downloading of Game of Thrones Season 2 Episode 5 with Urdu subtitles, such as [isubdb.com], [subscene.com], or [opensubtitles.org]. However, these websites might not be legal or safe to use, as they might contain pirated content or malware. Therefore, we recommend that you use a paid or official platform for streaming or downloading the episode, such as HBO Max, Netflix, or Amazon Prime Video.
-A: There are several ways to watch Game of Thrones Season 2 Episode 5 with Urdu subtitles on your TV. One way is to connect your computer or laptop to your TV using an HDMI cable or a wireless connection. Another way is to use a streaming device, such as a Roku, Chromecast, Apple TV, or Fire TV, that supports the platform that you are using to stream the episode, such as HBO Max, Netflix, or Amazon Prime Video. A third way is to use a smart TV that has the platform that you are using to stream the episode built-in or available as an app. In any case, you need to make sure that the platform that you are using supports Urdu subtitles, and that you enable them before or during watching the episode.
-A: There are also several ways to watch Game of Thrones Season 2 Episode 5 with Urdu subtitles on your phone or tablet. One way is to use the browser on your device to access the website that offers online streaming or downloading of the episode with Urdu subtitles, such as [isubdb.com], [subscene.com], or [opensubtitles.org]. However, this way might not be very convenient or comfortable, as the website might not be optimized for mobile devices, and the subtitles might not be very clear or readable. Another way is to use the app of the platform that you are using to stream or download the episode, such as HBO Max, Netflix, or Amazon Prime Video. This way is more convenient and comfortable, as the app is designed for mobile devices, and the subtitles are more clear and readable. However, this way requires that you have a subscription or payment for the platform, and that you have enough space and data on your device. A third way is to download the episode and the subtitles on your computer or laptop, and then transfer them to your device using a USB cable or a wireless connection. This way is more flexible and reliable, as you can watch the episode offline, without worrying about internet issues or interruptions. However, this way also requires that you have enough space and data on your device, and that you use a media player that supports subtitles, such as [VLC] or [KMPlayer].
-A: To watch Game of Thrones Season 2 Episode 5 with Urdu subtitles in HD quality, you need to make sure that both the video and the subtitles are in HD quality. The video quality depends on the source and the platform that you are using to stream or download the episode. The subtitles quality depends on the source and the format that you are using to download or enable them. Generally speaking, the higher the quality of the video and the subtitles, the larger the file size and the more data they consume. Therefore, you need to balance between quality and speed when choosing your source and platform. For example, if you want to stream the episode in HD quality with Urdu subtitles online, you might want to use HBO Max, Netflix, or Amazon Prime Video, as they offer high-quality video and audio, as well as multiple languages and subtitles. However, if you want to download the episode in HD quality with Urdu subtitles offline, you might want to use torrenting or direct downloading from a reliable website, such as [isubdb.com], [subscene.com], or [opensubtitles.org], as they offer high-quality video and subtitles files.
-A: If you want to watch Game of Thrones Season 2 Episode 5 with Urdu subtitles with your friends, you have some options as well. One option is to watch it together in person, either at your place or at their place. You can use any of the methods or platforms that we have mentioned above to watch the episode with Urdu subtitles on your TV, computer, laptop, phone, or tablet. You can also use speakers or headphones to enhance the sound quality. Another option is to watch it together online, using a platform or an app that allows you to watch videos with your friends remotely, such as [Watch2Gether], [Netflix Party], or [Scener]. These platforms or apps let you create a private room where you can invite your friends and watch the episode with Urdu subtitles synchronously. You can also chat and comment with your friends while watching the episode. However, these platforms or apps might require that you and your friends have a subscription or payment for the platform that you are using to stream the episode, such as HBO Max, Netflix, or Amazon Prime Video.
-Whichever option you choose, watching Game of Thrones Season 2 Episode 5 with Urdu subtitles with your friends can be a fun and enjoyable experience. You can share your thoughts and feelings about the episode, discuss the characters and the plot, and have a good time together.
- -This is the end of the article. I hope that you have learned something new and useful from this article, and that you have enjoyed reading it. If you have any questions, comments, or suggestions about this article or the topic of watching Game of Thrones Season 2 Episode 5 with Urdu subtitles, please feel free to contact me through my website or social media channels. I would love to hear from you and help you with anything that you need. Thank you for your time and attention, and have a great day!
197e85843dDo you love drag racing games? Do you want to experience the thrill of driving fast cars on realistic streets? Do you want to customize your own car and compete with other players online? If you answered yes to any of these questions, then you should try Drag Racing: Streets, a popular racing game for Android devices. And if you want to make the game even more fun and exciting, you should download Drag Racing: Streets Mod APK, a modified version of the game that gives you unlimited money, coins, levels, cars, and more. In this article, we will tell you everything you need to know about Drag Racing: Streets and its mod apk version.
-Download File ————— https://jinyurl.com/2uNQV5
Drag Racing: Streets is a racing game developed by Square, a Russian studio that specializes in realistic car simulation games. The game lets you create your own car from scratch, choosing from hundreds of parts and options. You can also customize your car's appearance, performance, tuning, and upgrades. You can then take your car to the streets and race against real players from around the world in various modes and events. You can also build your own garage and collect different cars.
-Drag Racing: Streets has many features that make it one of the best drag racing games on the market. Here are some of them:
-You can create your own car from scratch, choosing from hundreds of parts and options. You can change the engine, transmission, suspension, brakes, tires, wheels, body kits, paint, stickers, and more. You can also adjust the settings of your car's performance, such as power, torque, weight distribution, gear ratios, boost pressure, nitrous oxide injection, etc. You can make your car look and perform exactly how you want it.
-You can race against real players from around the world in various modes and events. You can join tournaments, championships, leagues, seasons, daily races, etc. You can also challenge your friends or random opponents in one-on-one duels. You can show off your skills and win prizes and rewards.
-You can build your own garage and collect different cars. You can buy new cars or win them in races or events. You can also sell or trade your cars with other players. You can have up to 16 cars in your garage at a time.
-drag racing streets mod apk download latest version 2022
-drag racing streets unlimited money and gold mod apk
-drag racing streets hack mod apk free download
-drag racing streets mod apk android 1 com
-drag racing streets mod apk revdl com
-drag racing streets mod apk rexdl com
-drag racing streets mod apk offline
-drag racing streets mod apk no root
-drag racing streets mod apk obb
-drag racing streets mod apk data
-drag racing streets mod apk unlimited everything
-drag racing streets mod apk unlimited coins and gems
-drag racing streets mod apk unlimited nitro
-drag racing streets mod apk unlimited fuel
-drag racing streets mod apk unlimited cash
-drag racing streets mod apk all cars unlocked
-drag racing streets mod apk all parts unlocked
-drag racing streets mod apk full version
-drag racing streets mod apk premium
-drag racing streets mod apk pro
-drag racing streets mod apk vip
-drag racing streets mod apk mega
-drag racing streets mod apk god mode
-drag racing streets mod apk anti ban
-drag racing streets mod apk online
-drag racing streets physics engine mod apk
-drag racing streets car customization mod apk
-drag racing streets realistic graphics mod apk
-drag racing streets best cars mod apk
-drag racing streets best tunes mod apk
-drag racing streets cheats mod apk
-drag racing streets tips and tricks mod apk
-drag racing streets gameplay mod apk
-drag racing streets review mod apk
-drag racing streets update mod apk
-how to install drag racing streets mod apk
-how to play drag racing streets mod apk
-how to get drag racing streets mod apk
-how to download drag racing streets mod apk for android
-how to download drag racing streets mod apk for ios
-how to download drag racing streets mod apk for pc
-how to download drag racing streets mod apk for laptop
-how to download drag racing streets mod apk for windows 10
-how to download drag racing streets mod apk for macbook pro
-how to download drag racing streets mod apk for chromebook
Drag Racing: Streets is a fun and addictive game, but it can also be challenging and frustrating at times. You may need a lot of money and coins to buy new cars, parts, upgrades, etc. You may also need to unlock new levels and cars to access more features and modes. And you may face ads and pop-ups that interrupt your gameplay.
-That's why you should download Drag Racing: Streets Mod APK, a modified version of the game that gives you unlimited money, coins, levels, cars, and more. With this mod apk version, you can enjoy the game without any limitations or restrictions. You can buy anything you want, unlock everything you need, and play without any ads or interruptions.
-With Drag Racing: Streets Mod APK, you will get unlimited money and coins in your account. You can use them to buy new cars, parts, upgrades, etc.
With Drag Racing: Streets Mod APK, you will get all the levels and cars unlocked in the game. You can access any mode or event you want, and choose any car you like. You can also try out different cars and see how they perform on the streets.
-Drag Racing: Streets Mod APK is free and safe to use. You don't need to root your device or install any other apps to use it. You just need to download the mod apk file from a trusted source and install it on your device. You can also update the game regularly without losing your mod features.
-If you want to download and install Drag Racing: Streets Mod APK, you need to follow these simple steps:
-You can download the mod apk file from this link: Drag Racing: Streets Mod APK Download. The file size is about 300 MB, so make sure you have enough space on your device. You can also scan the file with an antivirus program before opening it.
-Before you can install the mod apk file, you need to enable unknown sources on your device. This will allow you to install apps from sources other than the Google Play Store. To do this, go to Settings > Security > Unknown Sources and toggle it on.
-After you have enabled unknown sources, you can install the mod apk file. To do this, locate the file in your downloads folder and tap on it. You will see a pop-up window asking for your permission to install the app. Tap on Install and wait for the installation process to finish.
-Once the installation is done, you can open the game and enjoy it. You will see that you have unlimited money, coins, levels, cars, and more. You can start playing the game and have fun.
-Drag Racing: Streets is a great racing game that lets you create your own car and race against real players on realistic streets. It has many features that make it fun and addictive. However, if you want to make the game even more enjoyable, you should download Drag Racing: Streets Mod APK, a modified version of the game that gives you unlimited money, coins, levels, cars, and more. With this mod apk version, you can buy anything you want, unlock everything you need, and play without any ads or interruptions. You can also download and install the mod apk easily and safely by following our guide.
-If you are a fan of drag racing games, you should not miss Drag Racing: Streets Mod APK. Download it now and experience the thrill of driving fast cars on realistic streets.
-Here are some frequently asked questions about Drag Racing: Streets Mod APK:
-If you are looking for a fun and exciting game that combines pixel-style graphics and hack-and-slash action, then you should check out Pixel Blade M VIP. This is a cubic action RPG game that lets you play as the last pixel hero in PIXEL WORLD. You can collect various weapons by hunting monsters in the dungeon and save the world from evil forces. But what if you want to enjoy the game without spending real money or watching annoying ads? Well, there is a solution for that: Pixel Blade M VIP Mod APK Free Shopping. This is a modified version of the game that gives you unlimited money and gems, free shopping for weapons and items, no ads, and no root required. In this article, we will review this mod apk and tell you why you should try it out.
-Download File >>> https://jinyurl.com/2uNKm6
Pixel Blade M VIP is a 3D pixel fantasy hack-and-slash game developed by PixelStar Games. It is a sequel to the popular Pixel Blade game that has over 1 million downloads on Google Play. In this game, you can choose from different classes of pixel heroes, such as warrior, mage, archer, assassin, and more. You can also customize your hero's appearance, skills, and equipment. The game features various modes, such as story mode, boss mode, tower mode, raid mode, and PVP mode. You can explore different dungeons and fight against various monsters and bosses. You can also join a guild and cooperate with other players to defeat powerful enemies.
-Some of the features of Pixel Blade M VIP are:
-If you want to download and install Pixel Blade M VIP Mod APK Free Shopping, you need to follow these steps:
-There are many reasons why you should try Pixel Blade M VIP Mod APK Free Shopping. Here are some of them:
-With this mod apk, you will get unlimited money and gems in your account. You can use them to buy any weapon or item you want without worrying about the cost. You can also upgrade your weapons and items to make them more powerful. This will help you progress faster in the game and defeat stronger enemies.
-pixel blade m unlimited money and keys mod apk
-pixel blade m god mode and free shopping mod apk
-pixel blade m 3d rpg mod apk with vip features
-pixel blade m hack mod apk for free shopping and keys
-pixel blade m latest version mod apk with god mode
-pixel blade m vip mod apk download for android
-pixel blade m mod apk free shopping and unlimited keys
-pixel blade m 9.2.7 mod apk with god mode and vip
-pixel blade m role-playing game mod apk free shopping
-pixel blade m 3d graphics mod apk with unlimited money
-pixel blade m adventure game mod apk with god mode
-pixel blade m vip features mod apk free download
-pixel blade m free shopping and keys mod apk 9.2.7
-pixel blade m unlimited money and god mode mod apk
-pixel blade m 3d rpg hack mod apk with vip
-pixel blade m latest mod apk free shopping and keys
-pixel blade m 9.2.7 vip mod apk download for android
-pixel blade m role-playing game hack mod apk with god mode
-pixel blade m 3d graphics hack mod apk with unlimited money
-pixel blade m adventure game hack mod apk with vip features
-pixel blade m free download mod apk with free shopping and keys
-pixel blade m unlimited money and keys hack mod apk
-pixel blade m god mode and free shopping hack mod apk
-pixel blade m 3d rpg hack apk with vip features and free shopping
-pixel blade m hack apk for free shopping and keys and god mode
-pixel blade m latest version hack apk with god mode and vip
-pixel blade m vip hack apk download for android free shopping
-pixel blade m hack apk free shopping and unlimited keys and money
-pixel blade m 9.2.7 hack apk with god mode and vip features
-pixel blade m role-playing game hack apk free shopping and keys
-pixel blade m 3d graphics hack apk with unlimited money and god mode
-pixel blade m adventure game hack apk with vip features and free shopping
-pixel blade m free download hack apk with free shopping and keys and god mode
-download pixel blade m vip mod apk for android free shopping
-download pixel blade m mod apk free shopping and unlimited keys and money
-download pixel blade m 9.2.7 mod apk with god mode and vip features
-download pixel blade m role-playing game mod apk free shopping and keys
-download pixel blade m 3d graphics mod apk with unlimited money and god mode
-download pixel blade m adventure game mod apk with vip features and free shopping
-download pixel blade m latest version mod apk with god mode and vip features
-download pixel blade m vip hack apk for android free shopping and keys
-download pixel blade m hack apk free shopping and unlimited keys and money
-download pixel blade m 9.2.7 hack apk with god mode and vip features
-download pixel blade m role-playing game hack apk free shopping and keys
-download pixel blade m 3d graphics hack apk with unlimited money and god mode
-download pixel blade m adventure game hack apk with vip features and free shopping
-download pixel blade m latest version hack apk with god mode and vip features
Another reason why you should try Pixel Blade M VIP Mod APK Free Shopping is that it does not have any ads or require root access. Ads can be annoying and distracting when you are playing a game. They can also consume your data and battery. With this mod apk, you can enjoy the game without any interruptions or pop-ups. Moreover, you do not need to root your device to use this mod apk. Rooting can be risky and void your warranty. It can also expose your device to malware and viruses. With this mod apk, you can play the game safely and smoothly.
-The last reason why you should try Pixel Blade M VIP Mod APK Free Shopping is that it has amazing pixel-style graphics and action-packed gameplay. If you are a fan of retro games and pixel art, you will love this game. The game has colorful and detailed graphics that create a nostalgic atmosphere. The game also has fast-paced and thrilling gameplay that will keep you hooked. You can slash, hack, and shoot your way through various dungeons and enemies. You can also use different skills and combos to unleash powerful attacks. The game is fun and addictive for anyone who loves action RPG games.
-Like any other mod apk, Pixel Blade M VIP Mod APK Free Shopping has its pros and cons. Here are some of them:
-This mod apk is very easy to use and install. You just need to download the file from the link provided and follow the simple steps to install it on your device. You do not need any special skills or knowledge to use this mod apk.
This mod apk gives you free shopping for weapons and items in the game. You can buy any weapon or item you want without spending any money or gems. You can also upgrade your weapons and items for free. This will make your hero stronger and more equipped for the battles.
This mod apk does not change the original game's features or gameplay. It only enhances them by giving you unlimited money and gems, free shopping, no ads, and no root required. You can still enjoy the pixel-style graphics and action-packed gameplay of the game. You can also play with other players online or offline in different modes. The game is fun and addictive for anyone who loves action RPG games.
This mod apk may not be compatible with some devices or Android versions. Some users have reported that the mod apk does not work on their devices or causes some errors or crashes. This may depend on the device's specifications, settings, or compatibility issues.
This mod apk may cause some glitches and bugs in the game. Some users have reported that the mod apk causes some problems with the game's graphics, sound, or performance. This may affect the game's quality or functionality.
This mod apk may not be updated regularly by the developers or creators. This means that the mod apk may not work with the latest version of the game or may not have the latest features or improvements of the game. This may make the mod apk outdated or incompatible with the game.
Pixel Blade M VIP Mod APK Free Shopping is a modified version of Pixel Blade M VIP that gives you unlimited money and gems, free shopping for weapons and items, no ads, and no root required. It is a great way to enjoy the pixel-style graphics and action-packed gameplay of Pixel Blade M VIP without spending real money or watching annoying ads. However, it also has some drawbacks, such as compatibility issues, glitches, bugs, or lack of updates. Therefore, you should use this mod apk at your own risk and discretion.
-Si eres fanático del fútbol y tienes un dispositivo con Java, quizás te interese descargar Real Football 2010, uno de los mejores juegos de fútbol para móviles. En este artículo, te mostraremos cómo descargar y jugar a este juego, así como lo que lo hace tan divertido y realista.
-El fútbol es uno de los deportes más populares del mundo, y millones de personas lo disfrutan viendo y jugando. Sin embargo, no todos tienen la oportunidad de jugar al fútbol en la vida real, o ver a sus equipos y jugadores favoritos en la televisión. Es por eso que los juegos de fútbol son tan populares, especialmente en dispositivos móviles, ya que le permiten experimentar la emoción y la emoción del fútbol en cualquier momento y en cualquier lugar.
-Download Zip ✯✯✯ https://bltlly.com/2v6LA3
Real Football 2010 es un juego de simulación de fútbol desarrollado por Gameloft, un desarrollador líder y editor de juegos móviles. Fue lanzado en 2009 para varias plataformas, incluyendo Java ME, Android, iOS, Windows Mobile y Nintendo DS. Es la séptima entrega de la serie Real Football, que comenzó en 2004.
-Real Football 2010 es uno de los mejores juegos de fútbol para dispositivos Java, ya que ofrece una experiencia de juego realista e inmersiva. Puedes elegir entre más de 200 equipos y 8 ligas, incluyendo la Liga Premier Inglesa, La Liga, Serie A, Bundesliga y más. También puedes jugar como tus jugadores favoritos, como Lionel Messi, Cristiano Ronaldo, Wayne Rooney, Kaka, etc. Incluso puedes crear tu propio jugador y equipo, y personalizarlos con varias opciones.
-Si quieres descargar Real Football 2010 para tu dispositivo Java, debes seguir estos sencillos pasos:
-Lo siguiente que tienes que hacer es elegir el dispositivo y el tamaño de la pantalla. Los diferentes dispositivos tienen diferentes tamaños de pantalla y resoluciones, por lo que necesitas encontrar la versión del juego que coincida con tu dispositivo. Por ejemplo, si tienes un teléfono Nokia con un tamaño de pantalla de 240x320, necesitas descargar el juego con esa resolución. Puede consultar las especificaciones de su dispositivo en línea o en el manual.
-Lo último que tienes que hacer es descargar e instalar el juego en tu dispositivo. Puedes descargar el juego directamente desde el navegador de tu dispositivo o transferirlo desde tu computadora usando un cable USB o Bluetooth. Una vez que hayas descargado el archivo del juego (normalmente un archivo .jar), debes abrirlo y seguir las instrucciones para instalarlo. Es posible que necesites permitir que el juego acceda a la memoria o red de tu dispositivo.
-Una vez que haya instalado el juego en su dispositivo, usted está listo para jugar Real Football 2010 y disfrutar de sus increíbles características. Aquí hay algunos consejos sobre cómo jugar el juego y qué esperar de él:
-Real Football 2010 ofrece varios modos de juego que se adaptan a diferentes preferencias y niveles de habilidad. Puede elegir entre los siguientes modos:
- -Este es el modo más desafiante y gratificante, donde puedes crear tu propio jugador y guiarlo a través de su carrera. Puedes personalizar la apariencia, habilidades, posición y nacionalidad de tu jugador. También puede elegir a qué club unirse, y tratar de impresionar al entrenador y los aficionados. Tendrás que enfrentarte a varios desafíos, como marcar goles, hacer asistencias, ganar trofeos, etc. También tendrás que lidiar con lesiones, transferencias, contratos y presión de los medios. Este modo es una gran manera de sumergirse en la vida de una estrella de fútbol.
-Esta es una característica única que le permite transferir su equipo de Real Football Manager, otro juego de Gameloft, a Real Football 2010. Si has jugado a Real Football Manager, puedes importar tu equipo y jugar con él en Real Football 2010. También puedes exportar tu equipo de Real Football 2010 a Real Football Manager, y seguir administrándolo allí. Esta característica es una gran manera de disfrutar de ambos juegos y crear tu equipo de ensueño.
-Real Football 2010 tiene muchas características que lo hacen realista y divertido de jugar. Aquí están algunas de ellas:
-El juego tiene impresionantes gráficos y animaciones que dan vida al juego. Los jugadores se ven como sus contrapartes reales, y tienen movimientos y expresiones realistas. Los estadios son detallados y animados, con multitudes y pancartas. Los efectos del clima y las sombras se suman a la atmósfera del juego.
-El juego tiene diferentes ángulos de cámara que te permiten ver la acción desde diferentes perspectivas. Puede cambiar entre ellos durante el juego, o dejar que el juego elija el mejor ángulo para usted. El juego también tiene un comentario que sigue el juego y añade emoción y emoción. El comentario está disponible en varios idiomas, como inglés, francés, español, alemán, italiano, etc.
-El juego tiene controles y ajustes personalizables que te permiten jugar el juego de la manera que quieras. Puede elegir entre diferentes esquemas de control, como botones virtuales o gestos de pantalla táctil. También puede ajustar el nivel de dificultad, la duración del partido, los efectos de sonido, etc.
-Aquí hay algunas preguntas frecuentes sobre Real Football 2010 para Java:
-Espero que este artículo te haya ayudado a descargar y jugar Real Football 2010 para Java. Si tiene alguna pregunta o comentario, por favor deje un comentario a continuación. Gracias por leer y divertirse!
64aa2da5cfGacha Life es un popular juego que te permite crear y personalizar tus propios personajes de anime e interactuar con ellos en varios escenarios. Puedes vestir a tus personajes, entrar en el modo estudio, jugar minijuegos, chatear con otros jugadores y explorar diferentes áreas en el modo vida. Gacha Life tiene millones de fans en todo el mundo que disfrutan expresando su creatividad e imaginación a través de este juego.
-Sin embargo, no todos están satisfechos con la última versión de Gacha Life. Algunos jugadores prefieren descargar Gacha Life versión antigua apk, que es una versión anterior del juego que se puede instalar en dispositivos Android utilizando un archivo apk. ¿Por qué hacen eso? ¿Cuáles son los beneficios y desventajas de descargar Gacha Life versión antigua apk? ¿Cómo se puede descargar Gacha Life versión antigua apk de forma segura y fácil? En este artículo, vamos a responder a estas preguntas y más.
-Download Zip › https://bltlly.com/2v6Leb
Gacha Life versión antigua apk es una versión del juego que fue lanzado en enero de 2020. Tiene algunas características que son diferentes de la versión actual de Gacha Life, como:
-Hay varias razones por las que algunas personas prefieren descargar Gacha Life versión antigua apk sobre la última versión del juego. Algunos de ellos son:
-Si usted es una de esas personas que quieren descargar Gacha Life versión antigua apk, es necesario seguir estos pasos:
-Descargar Gacha Life versión antigua apk tiene algunos beneficios que usted no puede obtener de la última versión del juego. Algunos de estos beneficios son:
-Sin embargo, descargar Gacha Vida versión antigua apk también tiene algunos inconvenientes que usted debe ser consciente de antes de decidir hacerlo. Algunos de estos inconvenientes son:
-Gacha Life es un juego divertido y creativo que te permite crear y personalizar tus propios personajes de anime e interactuar con ellos en varios escenarios. Sin embargo, algunos jugadores prefieren descargar Gacha Vida versión antigua apk sobre la última versión del juego por varias razones. Descargar Gacha Life versión antigua apk tiene algunos beneficios y desventajas que usted debe considerar antes de hacerlo.
-Si desea descargar Gacha Life versión antigua apk, es necesario encontrar una fuente confiable para el archivo apk, habilitar fuentes desconocidas en la configuración de su dispositivo, descargar e instalar el archivo apk, y disfrutar jugando Gacha Life versión antigua en su dispositivo. Sin embargo, también debe tener cuidado con los posibles riesgos de seguridad y las infecciones de malware que pueden venir con la descarga de un archivo apk de una fuente desconocida. También debe escanear el archivo apk antes de instalarlo, y utilizar un antivirus de buena reputación o aplicación de seguridad para proteger su dispositivo.
- -En última instancia, la elección es suya. Usted puede descargar Gacha Vida versión antigua apk si lo desea, o puede seguir con la última versión del juego. De cualquier manera, esperamos que te diviertas y disfrutes jugando a Gacha Life. Si tienes alguna pregunta o comentario, no dudes en compartirlo con nosotros a continuación. Nos encantaría saber de ti.
- -Aquí hay algunas preguntas frecuentes sobre la descarga de Gacha Life versión antigua apk:
-Depende de la fuente del archivo apk y los términos y condiciones del desarrollador. En general, la descarga de un archivo apk de una fuente de terceros no es ilegal, pero puede violar los derechos de propiedad intelectual del desarrollador o la tienda de aplicaciones. Siempre debe respetar los derechos del desarrollador y la tienda de aplicaciones, y solo descargar un archivo apk de una fuente legítima y autorizada.
-No necesariamente. Descargar un archivo apk de una fuente desconocida puede ser arriesgado, ya que puede contener virus o malware que pueden dañar su dispositivo o robar sus datos. Siempre debe escanear el archivo apk antes de instalarlo, y utilizar un antivirus de buena reputación o aplicación de seguridad para proteger su dispositivo. También debe evitar la descarga de un archivo apk de un sitio oscuro o poco fiable que puede contener contenido dañino o ilegal.
-No se puede actualizar Gacha Vida versión antigua apk, ya que ya no es compatible con el desarrollador. Si desea obtener las últimas actualizaciones y correcciones de errores para el juego, es necesario descargar la última versión de Gacha Life de la Google Play Store u otras tiendas de aplicaciones oficiales. Sin embargo, esto sobrescribirá su versión anterior del juego, y perderá algunas de las características y el contenido que estaban disponibles en la versión anterior.
-No, no puede transferir sus datos de Gacha Life versión antigua apk a Gacha Life última versión. Las dos versiones del juego no son compatibles entre sí, y tienen diferentes características y contenido. Si cambia de Gacha Life versión antigua apk a Gacha Life última versión, perderá todos sus progresos y datos en la versión antigua, tales como sus personajes, artículos, gemas, etc. Usted tendrá que empezar desde cero en la última versión del juego.
64aa2da5cf- All music is generated by Mubert API – www.mubert.com -
-No hyperlinks here
" - mocker.patch("requests.Session.get", return_value=mock_response) - - # Call the function with a URL containing no hyperlinks - result = scrape_links("https://www.example.com") - - # Assert that the function returns an empty list - assert result == [] - - # Tests that scrape_links() correctly extracts and formats hyperlinks from - # a sample HTML containing a few hyperlinks. - def test_scrape_links_with_few_hyperlinks(self, mocker): - # Mock the requests.get() function to return a response with a sample HTML containing hyperlinks - mock_response = mocker.Mock() - mock_response.status_code = 200 - mock_response.text = """ - - - - - - - - """ - mocker.patch("requests.Session.get", return_value=mock_response) - - # Call the function being tested - result = scrape_links("https://www.example.com") - - # Assert that the function returns a list of formatted hyperlinks - assert isinstance(result, list) - assert len(result) == 3 - assert result[0] == "Google (https://www.google.com)" - assert result[1] == "GitHub (https://github.com)" - assert result[2] == "CodiumAI (https://www.codium.ai)" diff --git a/spaces/MoEternal/Hoshino/README.md b/spaces/MoEternal/Hoshino/README.md deleted file mode 100644 index 6167af17702fad24fb20e8cf492c9b0b655a6812..0000000000000000000000000000000000000000 --- a/spaces/MoEternal/Hoshino/README.md +++ /dev/null @@ -1,12 +0,0 @@ ---- -title: Hoshino -emoji: 👀 -colorFrom: gray -colorTo: pink -sdk: gradio -sdk_version: 3.35.2 -app_file: app.py -pinned: false ---- - -Check out the configuration reference at https://huggingface.co/docs/hub/spaces-config-reference diff --git a/spaces/Mountchicken/MAERec-Gradio/mmocr/utils/processing.py b/spaces/Mountchicken/MAERec-Gradio/mmocr/utils/processing.py deleted file mode 100644 index 2da6ff2c90d746c67c18fd1f22e6bd8d1f2bf887..0000000000000000000000000000000000000000 --- a/spaces/Mountchicken/MAERec-Gradio/mmocr/utils/processing.py +++ /dev/null @@ -1,67 +0,0 @@ -# Copyright (c) OpenMMLab. All rights reserved. -import sys -from collections.abc import Iterable - -from mmengine.utils.progressbar import ProgressBar, init_pool - - -def track_parallel_progress_multi_args(func, - args, - nproc, - initializer=None, - initargs=None, - bar_width=50, - chunksize=1, - skip_first=False, - file=sys.stdout): - """Track the progress of parallel task execution with a progress bar. - - The built-in :mod:`multiprocessing` module is used for process pools and - tasks are done with :func:`Pool.map` or :func:`Pool.imap_unordered`. - - Args: - func (callable): The function to be applied to each task. - tasks (tuple[Iterable]): A tuple of tasks. - nproc (int): Process (worker) number. - initializer (None or callable): Refer to :class:`multiprocessing.Pool` - for details. - initargs (None or tuple): Refer to :class:`multiprocessing.Pool` for - details. - chunksize (int): Refer to :class:`multiprocessing.Pool` for details. - bar_width (int): Width of progress bar. - skip_first (bool): Whether to skip the first sample for each worker - when estimating fps, since the initialization step may takes - longer. - keep_order (bool): If True, :func:`Pool.imap` is used, otherwise - :func:`Pool.imap_unordered` is used. - - Returns: - list: The task results. - """ - assert isinstance(args, tuple) - for arg in args: - assert isinstance(arg, Iterable) - assert len(set([len(arg) - for arg in args])) == 1, 'args must have same length' - task_num = len(args[0]) - tasks = zip(*args) - - pool = init_pool(nproc, initializer, initargs) - start = not skip_first - task_num -= nproc * chunksize * int(skip_first) - prog_bar = ProgressBar(task_num, bar_width, start, file=file) - results = [] - gen = pool.starmap(func, tasks, chunksize) - for result in gen: - results.append(result) - if skip_first: - if len(results) < nproc * chunksize: - continue - elif len(results) == nproc * chunksize: - prog_bar.start() - continue - prog_bar.update() - prog_bar.file.write('\n') - pool.close() - pool.join() - return results diff --git a/spaces/NATSpeech/DiffSpeech/mfa_usr/mfa.py b/spaces/NATSpeech/DiffSpeech/mfa_usr/mfa.py deleted file mode 100644 index d91bff2668540fe1a190d1a0901ccd47a2216ba1..0000000000000000000000000000000000000000 --- a/spaces/NATSpeech/DiffSpeech/mfa_usr/mfa.py +++ /dev/null @@ -1,498 +0,0 @@ -import atexit -import sys -import os -import time -import argparse -from datetime import datetime -import multiprocessing as mp - -from montreal_forced_aligner import __version__ - -from montreal_forced_aligner.utils import get_available_acoustic_languages, get_available_g2p_languages, \ - get_available_dict_languages, get_available_lm_languages, get_available_ivector_languages -from montreal_forced_aligner.command_line.align import run_align_corpus - -from mfa_usr.adapt import run_adapt_model -from montreal_forced_aligner.command_line.train_and_align import run_train_corpus -from montreal_forced_aligner.command_line.g2p import run_g2p -from montreal_forced_aligner.command_line.train_g2p import run_train_g2p -from montreal_forced_aligner.command_line.validate import run_validate_corpus -from montreal_forced_aligner.command_line.download import run_download -from montreal_forced_aligner.command_line.train_lm import run_train_lm -from montreal_forced_aligner.command_line.thirdparty import run_thirdparty -from montreal_forced_aligner.command_line.train_ivector_extractor import run_train_ivector_extractor -from montreal_forced_aligner.command_line.classify_speakers import run_classify_speakers -from montreal_forced_aligner.command_line.transcribe import run_transcribe_corpus -from montreal_forced_aligner.command_line.train_dictionary import run_train_dictionary -from montreal_forced_aligner.command_line.create_segments import run_create_segments -from montreal_forced_aligner.exceptions import MFAError -from montreal_forced_aligner.config import update_global_config, load_global_config, update_command_history, \ - load_command_history - - -class ExitHooks(object): - def __init__(self): - self.exit_code = None - self.exception = None - - def hook(self): - self._orig_exit = sys.exit - sys.exit = self.exit - sys.excepthook = self.exc_handler - - def exit(self, code=0): - self.exit_code = code - self._orig_exit(code) - - def exc_handler(self, exc_type, exc, *args): - self.exception = exc - - -hooks = ExitHooks() -hooks.hook() - -BEGIN = time.time() -BEGIN_DATE = datetime.now() - - -def history_save_handler(): - history_data = { - 'command': ' '.join(sys.argv), - 'execution_time': time.time() - BEGIN, - 'date': BEGIN_DATE, - 'version': __version__ - } - - if hooks.exit_code is not None: - history_data['exit_code'] = hooks.exit_code - history_data['exception'] = '' - elif hooks.exception is not None: - history_data['exit_code'] = 1 - history_data['exception'] = hooks.exception - else: - history_data['exception'] = '' - history_data['exit_code'] = 0 - update_command_history(history_data) - - -atexit.register(history_save_handler) - - -def fix_path(): - from montreal_forced_aligner.config import TEMP_DIR - thirdparty_dir = os.path.join(TEMP_DIR, 'thirdparty', 'bin') - old_path = os.environ.get('PATH', '') - if sys.platform == 'win32': - os.environ['PATH'] = thirdparty_dir + ';' + old_path - else: - os.environ['PATH'] = thirdparty_dir + ':' + old_path - os.environ['LD_LIBRARY_PATH'] = thirdparty_dir + ':' + os.environ.get('LD_LIBRARY_PATH', '') - - -def unfix_path(): - if sys.platform == 'win32': - sep = ';' - os.environ['PATH'] = sep.join(os.environ['PATH'].split(sep)[1:]) - else: - sep = ':' - os.environ['PATH'] = sep.join(os.environ['PATH'].split(sep)[1:]) - os.environ['LD_LIBRARY_PATH'] = sep.join(os.environ['PATH'].split(sep)[1:]) - - -acoustic_languages = get_available_acoustic_languages() -ivector_languages = get_available_ivector_languages() -lm_languages = get_available_lm_languages() -g2p_languages = get_available_g2p_languages() -dict_languages = get_available_dict_languages() - - -def create_parser(): - GLOBAL_CONFIG = load_global_config() - - def add_global_options(subparser, textgrid_output=False): - subparser.add_argument('-t', '--temp_directory', type=str, default=GLOBAL_CONFIG['temp_directory'], - help=f"Temporary directory root to store MFA created files, default is {GLOBAL_CONFIG['temp_directory']}") - subparser.add_argument('--disable_mp', - help=f"Disable any multiprocessing during alignment (not recommended), default is {not GLOBAL_CONFIG['use_mp']}", - action='store_true', - default=not GLOBAL_CONFIG['use_mp']) - subparser.add_argument('-j', '--num_jobs', type=int, default=GLOBAL_CONFIG['num_jobs'], - help=f"Number of data splits (and cores to use if multiprocessing is enabled), defaults " - f"is {GLOBAL_CONFIG['num_jobs']}") - subparser.add_argument('-v', '--verbose', help=f"Output debug messages, default is {GLOBAL_CONFIG['verbose']}", - action='store_true', - default=GLOBAL_CONFIG['verbose']) - subparser.add_argument('--clean', help=f"Remove files from previous runs, default is {GLOBAL_CONFIG['clean']}", - action='store_true', - default=GLOBAL_CONFIG['clean']) - subparser.add_argument('--overwrite', - help=f"Overwrite output files when they exist, default is {GLOBAL_CONFIG['overwrite']}", - action='store_true', - default=GLOBAL_CONFIG['overwrite']) - subparser.add_argument('--debug', - help=f"Run extra steps for debugging issues, default is {GLOBAL_CONFIG['debug']}", - action='store_true', - default=GLOBAL_CONFIG['debug']) - if textgrid_output: - subparser.add_argument('--disable_textgrid_cleanup', - help=f"Disable extra clean up steps on TextGrid output, default is {not GLOBAL_CONFIG['cleanup_textgrids']}", - action='store_true', - default=not GLOBAL_CONFIG['cleanup_textgrids']) - - parser = argparse.ArgumentParser() - - subparsers = parser.add_subparsers(dest="subcommand") - subparsers.required = True - - version_parser = subparsers.add_parser('version') - - align_parser = subparsers.add_parser('align') - align_parser.add_argument('corpus_directory', help="Full path to the directory to align") - align_parser.add_argument('dictionary_path', help="Full path to the pronunciation dictionary to use") - align_parser.add_argument('acoustic_model_path', - help=f"Full path to the archive containing pre-trained model or language ({', '.join(acoustic_languages)})") - align_parser.add_argument('output_directory', - help="Full path to output directory, will be created if it doesn't exist") - align_parser.add_argument('--config_path', type=str, default='', - help="Path to config file to use for alignment") - align_parser.add_argument('-s', '--speaker_characters', type=str, default='0', - help="Number of characters of file names to use for determining speaker, " - 'default is to use directory names') - align_parser.add_argument('-a', '--audio_directory', type=str, default='', - help="Audio directory root to use for finding audio files") - add_global_options(align_parser, textgrid_output=True) - - adapt_parser = subparsers.add_parser('adapt') - adapt_parser.add_argument('corpus_directory', help="Full path to the directory to align") - adapt_parser.add_argument('dictionary_path', help="Full path to the pronunciation dictionary to use") - adapt_parser.add_argument('acoustic_model_path', - help=f"Full path to the archive containing pre-trained model or language ({', '.join(acoustic_languages)})") - adapt_parser.add_argument('output_model_path', - help="Full path to save adapted_model") - adapt_parser.add_argument('output_directory', - help="Full path to output directory, will be created if it doesn't exist") - adapt_parser.add_argument('--config_path', type=str, default='', - help="Path to config file to use for alignment") - adapt_parser.add_argument('-s', '--speaker_characters', type=str, default='0', - help="Number of characters of file names to use for determining speaker, " - 'default is to use directory names') - adapt_parser.add_argument('-a', '--audio_directory', type=str, default='', - help="Audio directory root to use for finding audio files") - add_global_options(adapt_parser, textgrid_output=True) - - train_parser = subparsers.add_parser('train') - train_parser.add_argument('corpus_directory', help="Full path to the source directory to align") - train_parser.add_argument('dictionary_path', help="Full path to the pronunciation dictionary to use", - default='') - train_parser.add_argument('output_directory', - help="Full path to output directory, will be created if it doesn't exist") - train_parser.add_argument('--config_path', type=str, default='', - help="Path to config file to use for training and alignment") - train_parser.add_argument('-o', '--output_model_path', type=str, default='', - help="Full path to save resulting acoustic and dictionary model") - train_parser.add_argument('-s', '--speaker_characters', type=str, default='0', - help="Number of characters of filenames to use for determining speaker, " - 'default is to use directory names') - train_parser.add_argument('-a', '--audio_directory', type=str, default='', - help="Audio directory root to use for finding audio files") - train_parser.add_argument('-m', '--acoustic_model_path', type=str, default='', - help="Full path to save adapted_model") - - add_global_options(train_parser, textgrid_output=True) - - validate_parser = subparsers.add_parser('validate') - validate_parser.add_argument('corpus_directory', help="Full path to the source directory to align") - validate_parser.add_argument('dictionary_path', help="Full path to the pronunciation dictionary to use", - default='') - validate_parser.add_argument('acoustic_model_path', nargs='?', default='', - help=f"Full path to the archive containing pre-trained model or language ({', '.join(acoustic_languages)})") - validate_parser.add_argument('-s', '--speaker_characters', type=str, default='0', - help="Number of characters of file names to use for determining speaker, " - 'default is to use directory names') - validate_parser.add_argument('--test_transcriptions', help="Test accuracy of transcriptions", action='store_true') - validate_parser.add_argument('--ignore_acoustics', - help="Skip acoustic feature generation and associated validation", - action='store_true') - add_global_options(validate_parser) - - g2p_model_help_message = f'''Full path to the archive containing pre-trained model or language ({', '.join(g2p_languages)}) - If not specified, then orthographic transcription is split into pronunciations.''' - g2p_parser = subparsers.add_parser('g2p') - g2p_parser.add_argument("g2p_model_path", help=g2p_model_help_message, nargs='?') - - g2p_parser.add_argument("input_path", - help="Corpus to base word list on or a text file of words to generate pronunciations") - g2p_parser.add_argument("output_path", help="Path to save output dictionary") - g2p_parser.add_argument('--include_bracketed', help="Included words enclosed by brackets, i.e. [...], (...), <...>", - action='store_true') - g2p_parser.add_argument('--config_path', type=str, default='', - help="Path to config file to use for G2P") - add_global_options(g2p_parser) - - train_g2p_parser = subparsers.add_parser('train_g2p') - train_g2p_parser.add_argument("dictionary_path", help="Location of existing dictionary") - - train_g2p_parser.add_argument("output_model_path", help="Desired location of generated model") - train_g2p_parser.add_argument('--config_path', type=str, default='', - help="Path to config file to use for G2P") - train_g2p_parser.add_argument("--validate", action='store_true', - help="Perform an analysis of accuracy training on " - "most of the data and validating on an unseen subset") - add_global_options(train_g2p_parser) - - download_parser = subparsers.add_parser('download') - download_parser.add_argument("model_type", - help="Type of model to download, one of 'acoustic', 'g2p', or 'dictionary'") - download_parser.add_argument("language", help="Name of language code to download, if not specified, " - "will list all available languages", nargs='?') - - train_lm_parser = subparsers.add_parser('train_lm') - train_lm_parser.add_argument('source_path', help="Full path to the source directory to train from, alternatively " - 'an ARPA format language model to convert for MFA use') - train_lm_parser.add_argument('output_model_path', type=str, - help="Full path to save resulting language model") - train_lm_parser.add_argument('-m', '--model_path', type=str, - help="Full path to existing language model to merge probabilities") - train_lm_parser.add_argument('-w', '--model_weight', type=float, default=1.0, - help="Weight factor for supplemental language model, defaults to 1.0") - train_lm_parser.add_argument('--dictionary_path', help="Full path to the pronunciation dictionary to use", - default='') - train_lm_parser.add_argument('--config_path', type=str, default='', - help="Path to config file to use for training and alignment") - add_global_options(train_lm_parser) - - train_dictionary_parser = subparsers.add_parser('train_dictionary') - train_dictionary_parser.add_argument('corpus_directory', help="Full path to the directory to align") - train_dictionary_parser.add_argument('dictionary_path', help="Full path to the pronunciation dictionary to use") - train_dictionary_parser.add_argument('acoustic_model_path', - help=f"Full path to the archive containing pre-trained model or language ({', '.join(acoustic_languages)})") - train_dictionary_parser.add_argument('output_directory', - help="Full path to output directory, will be created if it doesn't exist") - train_dictionary_parser.add_argument('--config_path', type=str, default='', - help="Path to config file to use for alignment") - train_dictionary_parser.add_argument('-s', '--speaker_characters', type=str, default='0', - help="Number of characters of file names to use for determining speaker, " - 'default is to use directory names') - add_global_options(train_dictionary_parser) - - train_ivector_parser = subparsers.add_parser('train_ivector') - train_ivector_parser.add_argument('corpus_directory', help="Full path to the source directory to " - 'train the ivector extractor') - train_ivector_parser.add_argument('dictionary_path', help="Full path to the pronunciation dictionary to use") - train_ivector_parser.add_argument('acoustic_model_path', type=str, default='', - help="Full path to acoustic model for alignment") - train_ivector_parser.add_argument('output_model_path', type=str, default='', - help="Full path to save resulting ivector extractor") - train_ivector_parser.add_argument('-s', '--speaker_characters', type=str, default='0', - help="Number of characters of filenames to use for determining speaker, " - 'default is to use directory names') - train_ivector_parser.add_argument('--config_path', type=str, default='', - help="Path to config file to use for training") - add_global_options(train_ivector_parser) - - classify_speakers_parser = subparsers.add_parser('classify_speakers') - classify_speakers_parser.add_argument('corpus_directory', help="Full path to the source directory to " - 'run speaker classification') - classify_speakers_parser.add_argument('ivector_extractor_path', type=str, default='', - help="Full path to ivector extractor model") - classify_speakers_parser.add_argument('output_directory', - help="Full path to output directory, will be created if it doesn't exist") - - classify_speakers_parser.add_argument('-s', '--num_speakers', type=int, default=0, - help="Number of speakers if known") - classify_speakers_parser.add_argument('--cluster', help="Using clustering instead of classification", - action='store_true') - classify_speakers_parser.add_argument('--config_path', type=str, default='', - help="Path to config file to use for ivector extraction") - add_global_options(classify_speakers_parser) - - create_segments_parser = subparsers.add_parser('create_segments') - create_segments_parser.add_argument('corpus_directory', help="Full path to the source directory to " - 'run VAD segmentation') - create_segments_parser.add_argument('output_directory', - help="Full path to output directory, will be created if it doesn't exist") - create_segments_parser.add_argument('--config_path', type=str, default='', - help="Path to config file to use for segmentation") - add_global_options(create_segments_parser) - - transcribe_parser = subparsers.add_parser('transcribe') - transcribe_parser.add_argument('corpus_directory', help="Full path to the directory to transcribe") - transcribe_parser.add_argument('dictionary_path', help="Full path to the pronunciation dictionary to use") - transcribe_parser.add_argument('acoustic_model_path', - help=f"Full path to the archive containing pre-trained model or language ({', '.join(acoustic_languages)})") - transcribe_parser.add_argument('language_model_path', - help=f"Full path to the archive containing pre-trained model or language ({', '.join(lm_languages)})") - transcribe_parser.add_argument('output_directory', - help="Full path to output directory, will be created if it doesn't exist") - transcribe_parser.add_argument('--config_path', type=str, default='', - help="Path to config file to use for transcription") - transcribe_parser.add_argument('-s', '--speaker_characters', type=str, default='0', - help="Number of characters of file names to use for determining speaker, " - 'default is to use directory names') - transcribe_parser.add_argument('-a', '--audio_directory', type=str, default='', - help="Audio directory root to use for finding audio files") - transcribe_parser.add_argument('-e', '--evaluate', help="Evaluate the transcription " - "against golden texts", action='store_true') - add_global_options(transcribe_parser) - - config_parser = subparsers.add_parser('configure', - help="The configure command is used to set global defaults for MFA so " - "you don't have to set them every time you call an MFA command.") - config_parser.add_argument('-t', '--temp_directory', type=str, default='', - help=f"Set the default temporary directory, default is {GLOBAL_CONFIG['temp_directory']}") - config_parser.add_argument('-j', '--num_jobs', type=int, - help=f"Set the number of processes to use by default, defaults to {GLOBAL_CONFIG['num_jobs']}") - config_parser.add_argument('--always_clean', help="Always remove files from previous runs by default", - action='store_true') - config_parser.add_argument('--never_clean', help="Don't remove files from previous runs by default", - action='store_true') - config_parser.add_argument('--always_verbose', help="Default to verbose output", action='store_true') - config_parser.add_argument('--never_verbose', help="Default to non-verbose output", action='store_true') - config_parser.add_argument('--always_debug', help="Default to running debugging steps", action='store_true') - config_parser.add_argument('--never_debug', help="Default to not running debugging steps", action='store_true') - config_parser.add_argument('--always_overwrite', help="Always overwrite output files", action='store_true') - config_parser.add_argument('--never_overwrite', help="Never overwrite output files (if file already exists, " - "the output will be saved in the temp directory)", - action='store_true') - config_parser.add_argument('--disable_mp', help="Disable all multiprocessing (not recommended as it will usually " - "increase processing times)", action='store_true') - config_parser.add_argument('--enable_mp', help="Enable multiprocessing (recommended and enabled by default)", - action='store_true') - config_parser.add_argument('--disable_textgrid_cleanup', help="Disable postprocessing of TextGrids that cleans up " - "silences and recombines compound words and clitics", - action='store_true') - config_parser.add_argument('--enable_textgrid_cleanup', help="Enable postprocessing of TextGrids that cleans up " - "silences and recombines compound words and clitics", - action='store_true') - - history_parser = subparsers.add_parser('history') - - history_parser.add_argument('depth', help='Number of commands to list', nargs='?', default=10) - history_parser.add_argument('--verbose', help="Flag for whether to output additional information", - action='store_true') - - annotator_parser = subparsers.add_parser('annotator') - anchor_parser = subparsers.add_parser('anchor') - - thirdparty_parser = subparsers.add_parser('thirdparty') - - thirdparty_parser.add_argument("command", - help="One of 'download', 'validate', or 'kaldi'") - thirdparty_parser.add_argument('local_directory', - help="Full path to the built executables to collect", nargs="?", - default='') - return parser - - -parser = create_parser() - - -def main(): - parser = create_parser() - mp.freeze_support() - args, unknown = parser.parse_known_args() - for short in ['-c', '-d']: - if short in unknown: - print(f'Due to the number of options that `{short}` could refer to, it is not accepted. ' - 'Please specify the full argument') - sys.exit(1) - try: - fix_path() - if args.subcommand in ['align', 'train', 'train_ivector']: - from montreal_forced_aligner.thirdparty.kaldi import validate_alignment_binaries - if not validate_alignment_binaries(): - print("There was an issue validating Kaldi binaries, please ensure you've downloaded them via the " - "'mfa thirdparty download' command. See 'mfa thirdparty validate' for more detailed information " - "on why this check failed.") - sys.exit(1) - elif args.subcommand in ['transcribe']: - from montreal_forced_aligner.thirdparty.kaldi import validate_transcribe_binaries - if not validate_transcribe_binaries(): - print("There was an issue validating Kaldi binaries, please ensure you've downloaded them via the " - "'mfa thirdparty download' command. See 'mfa thirdparty validate' for more detailed information " - "on why this check failed. If you are on MacOS, please note that the thirdparty binaries available " - "via the download command do not contain the transcription ones. To get this functionality working " - "for the time being, please build kaldi locally and follow the instructions for running the " - "'mfa thirdparty kaldi' command.") - sys.exit(1) - elif args.subcommand in ['train_dictionary']: - from montreal_forced_aligner.thirdparty.kaldi import validate_train_dictionary_binaries - if not validate_train_dictionary_binaries(): - print("There was an issue validating Kaldi binaries, please ensure you've downloaded them via the " - "'mfa thirdparty download' command. See 'mfa thirdparty validate' for more detailed information " - "on why this check failed. If you are on MacOS, please note that the thirdparty binaries available " - "via the download command do not contain the train_dictionary ones. To get this functionality working " - "for the time being, please build kaldi locally and follow the instructions for running the " - "'mfa thirdparty kaldi' command.") - sys.exit(1) - elif args.subcommand in ['g2p', 'train_g2p']: - try: - import pynini - except ImportError: - print("There was an issue importing Pynini, please ensure that it is installed. If you are on Windows, " - "please use the Windows Subsystem for Linux to use g2p functionality.") - sys.exit(1) - if args.subcommand == 'align': - run_align_corpus(args, unknown, acoustic_languages) - elif args.subcommand == 'adapt': - run_adapt_model(args, unknown, acoustic_languages) - elif args.subcommand == 'train': - run_train_corpus(args, unknown) - elif args.subcommand == 'g2p': - run_g2p(args, unknown, g2p_languages) - elif args.subcommand == 'train_g2p': - run_train_g2p(args, unknown) - elif args.subcommand == 'validate': - run_validate_corpus(args, unknown) - elif args.subcommand == 'download': - run_download(args) - elif args.subcommand == 'train_lm': - run_train_lm(args, unknown) - elif args.subcommand == 'train_dictionary': - run_train_dictionary(args, unknown) - elif args.subcommand == 'train_ivector': - run_train_ivector_extractor(args, unknown) - elif args.subcommand == 'classify_speakers': - run_classify_speakers(args, unknown) - elif args.subcommand in ['annotator', 'anchor']: - from montreal_forced_aligner.command_line.anchor import run_anchor - run_anchor(args) - elif args.subcommand == 'thirdparty': - run_thirdparty(args) - elif args.subcommand == 'transcribe': - run_transcribe_corpus(args, unknown) - elif args.subcommand == 'create_segments': - run_create_segments(args, unknown) - elif args.subcommand == 'configure': - update_global_config(args) - global GLOBAL_CONFIG - GLOBAL_CONFIG = load_global_config() - elif args.subcommand == 'history': - depth = args.depth - history = load_command_history()[-depth:] - for h in history: - if args.verbose: - print('command\tDate\tExecution time\tVersion\tExit code\tException') - for h in history: - execution_time = time.strftime('%H:%M:%S', time.gmtime(h['execution_time'])) - d = h['date'].isoformat() - print( - f"{h['command']}\t{d}\t{execution_time}\t{h['version']}\t{h['exit_code']}\t{h['exception']}") - pass - else: - for h in history: - print(h['command']) - - elif args.subcommand == 'version': - print(__version__) - except MFAError as e: - if getattr(args, 'debug', False): - raise - print(e) - sys.exit(1) - finally: - unfix_path() - - -if __name__ == '__main__': - main() diff --git a/spaces/NATSpeech/DiffSpeech/utils/commons/ddp_utils.py b/spaces/NATSpeech/DiffSpeech/utils/commons/ddp_utils.py deleted file mode 100644 index 4b529198c13a1ffc622baea6e5178407b24aee8f..0000000000000000000000000000000000000000 --- a/spaces/NATSpeech/DiffSpeech/utils/commons/ddp_utils.py +++ /dev/null @@ -1,137 +0,0 @@ -from torch.nn.parallel import DistributedDataParallel -from torch.nn.parallel.distributed import _find_tensors -import torch.optim -import torch.utils.data -import torch -from packaging import version - -class DDP(DistributedDataParallel): - """ - Override the forward call in lightning so it goes to training and validation step respectively - """ - - def forward(self, *inputs, **kwargs): # pragma: no cover - if version.parse(torch.__version__[:6]) < version.parse("1.11"): - self._sync_params() - inputs, kwargs = self.scatter(inputs, kwargs, self.device_ids) - assert len(self.device_ids) == 1 - if self.module.training: - output = self.module.training_step(*inputs[0], **kwargs[0]) - elif self.module.testing: - output = self.module.test_step(*inputs[0], **kwargs[0]) - else: - output = self.module.validation_step(*inputs[0], **kwargs[0]) - if torch.is_grad_enabled(): - # We'll return the output object verbatim since it is a freeform - # object. We need to find any tensors in this object, though, - # because we need to figure out which parameters were used during - # this forward pass, to ensure we short circuit reduction for any - # unused parameters. Only if `find_unused_parameters` is set. - if self.find_unused_parameters: - self.reducer.prepare_for_backward(list(_find_tensors(output))) - else: - self.reducer.prepare_for_backward([]) - else: - from torch.nn.parallel.distributed import \ - logging, Join, _DDPSink, _tree_flatten_with_rref, _tree_unflatten_with_rref - with torch.autograd.profiler.record_function("DistributedDataParallel.forward"): - if torch.is_grad_enabled() and self.require_backward_grad_sync: - self.logger.set_runtime_stats_and_log() - self.num_iterations += 1 - self.reducer.prepare_for_forward() - - # Notify the join context that this process has not joined, if - # needed - work = Join.notify_join_context(self) - if work: - self.reducer._set_forward_pass_work_handle( - work, self._divide_by_initial_world_size - ) - - # Calling _rebuild_buckets before forward compuation, - # It may allocate new buckets before deallocating old buckets - # inside _rebuild_buckets. To save peak memory usage, - # call _rebuild_buckets before the peak memory usage increases - # during forward computation. - # This should be called only once during whole training period. - if torch.is_grad_enabled() and self.reducer._rebuild_buckets(): - logging.info("Reducer buckets have been rebuilt in this iteration.") - self._has_rebuilt_buckets = True - - # sync params according to location (before/after forward) user - # specified as part of hook, if hook was specified. - buffer_hook_registered = hasattr(self, 'buffer_hook') - if self._check_sync_bufs_pre_fwd(): - self._sync_buffers() - - if self._join_config.enable: - # Notify joined ranks whether they should sync in backwards pass or not. - self._check_global_requires_backward_grad_sync(is_joined_rank=False) - - inputs, kwargs = self.scatter(inputs, kwargs, self.device_ids) - if self.module.training: - output = self.module.training_step(*inputs[0], **kwargs[0]) - elif self.module.testing: - output = self.module.test_step(*inputs[0], **kwargs[0]) - else: - output = self.module.validation_step(*inputs[0], **kwargs[0]) - - # sync params according to location (before/after forward) user - # specified as part of hook, if hook was specified. - if self._check_sync_bufs_post_fwd(): - self._sync_buffers() - - if torch.is_grad_enabled() and self.require_backward_grad_sync: - self.require_forward_param_sync = True - # We'll return the output object verbatim since it is a freeform - # object. We need to find any tensors in this object, though, - # because we need to figure out which parameters were used during - # this forward pass, to ensure we short circuit reduction for any - # unused parameters. Only if `find_unused_parameters` is set. - if self.find_unused_parameters and not self.static_graph: - # Do not need to populate this for static graph. - self.reducer.prepare_for_backward(list(_find_tensors(output))) - else: - self.reducer.prepare_for_backward([]) - else: - self.require_forward_param_sync = False - - # TODO: DDPSink is currently enabled for unused parameter detection and - # static graph training for first iteration. - if (self.find_unused_parameters and not self.static_graph) or ( - self.static_graph and self.num_iterations == 1 - ): - state_dict = { - 'static_graph': self.static_graph, - 'num_iterations': self.num_iterations, - } - - output_tensor_list, treespec, output_is_rref = _tree_flatten_with_rref( - output - ) - output_placeholders = [None for _ in range(len(output_tensor_list))] - # Do not touch tensors that have no grad_fn, which can cause issues - # such as https://github.com/pytorch/pytorch/issues/60733 - for i, output in enumerate(output_tensor_list): - if torch.is_tensor(output) and output.grad_fn is None: - output_placeholders[i] = output - - # When find_unused_parameters=True, makes tensors which require grad - # run through the DDPSink backward pass. When not all outputs are - # used in loss, this makes those corresponding tensors receive - # undefined gradient which the reducer then handles to ensure - # param.grad field is not touched and we don't error out. - passthrough_tensor_list = _DDPSink.apply( - self.reducer, - state_dict, - *output_tensor_list, - ) - for i in range(len(output_placeholders)): - if output_placeholders[i] is None: - output_placeholders[i] = passthrough_tensor_list[i] - - # Reconstruct output data structure. - output = _tree_unflatten_with_rref( - output_placeholders, treespec, output_is_rref - ) - return output diff --git a/spaces/NSect/VALL-E-X/data/__init__.py b/spaces/NSect/VALL-E-X/data/__init__.py deleted file mode 100644 index 68f9defe677e03da5224c42cb28932f2e7f75ada..0000000000000000000000000000000000000000 --- a/spaces/NSect/VALL-E-X/data/__init__.py +++ /dev/null @@ -1 +0,0 @@ -from .collation import * diff --git a/spaces/NohTow/Llama2_watermarking/app.py b/spaces/NohTow/Llama2_watermarking/app.py deleted file mode 100644 index 36538b10e8da9e894c59e784895fd29b7a31a9ef..0000000000000000000000000000000000000000 --- a/spaces/NohTow/Llama2_watermarking/app.py +++ /dev/null @@ -1,98 +0,0 @@ -import torch -import argparse -import os -import numpy as np - -from watermark import Watermarker -import time -import gradio as gr -from transformers import AutoModelForCausalLM, AutoTokenizer - -hf_token = os.getenv('HF_TOKEN') - -device = torch.device('cuda') if torch.cuda.is_available() else torch.device('cpu') - -parser = argparse.ArgumentParser(description='Generative Text Watermarking demo') -parser.add_argument('--model', '-m', type=str, default="meta-llama/Llama-2-7b-chat-hf", help='Language model') -parser.add_argument('--key', '-k', type=int, default=42, - help='The seed of the pseudo random number generator') - -args = parser.parse_args() - -USERS = ['Alice', 'Bob', 'Charlie', 'Dan'] -EMBED_METHODS = [ 'aaronson', 'kirchenbauer', 'sampling', 'greedy' ] -DETECT_METHODS = [ 'aaronson', 'aaronson_simplified', 'aaronson_neyman_pearson', 'kirchenbauer'] -PAYLOAD_BITS = 2 -device = torch.device('cuda:0') if torch.cuda.is_available() else torch.device('cpu') - - -model = AutoModelForCausalLM.from_pretrained(args.model, use_auth_token=hf_token, torch_dtype=torch.float16, - device_map='auto').to(device) -tokenizer = AutoTokenizer.from_pretrained(args.model, use_auth_token=hf_token) -tokenizer.pad_token = tokenizer.eos_token - -DEFAULT_SYSTEM_PROMPT = """\ -You are a helpful, respectful and honest assistant. Always answer as helpfully as possible, while being safe. Your answers should not include any harmful, unethical, racist, sexist, toxic, dangerous, or illegal content. Please ensure that your responses are socially unbiased and positive in nature. -If a question does not make any sense, or is not factually coherent, explain why instead of answering something not correct. If you don't know the answer to a question, please don't share false information.\ -""" -LEN_DEFAULT_PROMPT = len(tokenizer.encode(DEFAULT_SYSTEM_PROMPT)) -def embed(user, max_length, window_size, method, prompt): - - uid = USERS.index(user) - watermarker = Watermarker(tokenizer=tokenizer, model=model, window_size=window_size, payload_bits=PAYLOAD_BITS) - prompt = get_prompt(prompt) - watermarked_texts = watermarker.embed(key=args.key, messages=[ uid ], - max_length=max_length+LEN_DEFAULT_PROMPT, method=method, prompt=prompt) - return watermarked_texts[0].split("[/INST]")[1] - -def detect(attacked_text, window_size, method, prompt): - watermarker = Watermarker(tokenizer=tokenizer, model=model, window_size=window_size, payload_bits=PAYLOAD_BITS) - prompt = get_prompt(prompt) - print([ prompt + attacked_text ]) - pvalues, messages = watermarker.detect([ prompt + attacked_text ], key=args.key, method=method, prompts=[prompt]) - - print("messages: ", messages) - print("p-values: ", pvalues) - user = USERS[messages[0]] - pf = pvalues[0] - label = 'The user detected is {:s} with pvalue of {:.3e}'.format(user, pf) - - return label - -def get_prompt(message: str) -> str: - # texts = [f'- | name | -backbone | -Data | -box AP on COCO | -Checkpoint | -Config | -
---|---|---|---|---|---|---|
1 | -GroundingDINO-T | -Swin-T | -O365,GoldG,Cap4M | -48.4 (zero-shot) / 57.2 (fine-tune) | -link | -link | -
${ subarr.join( ' ' ) }
`; - gnode += 'DOWNLOAD ✔✔✔ https://urloso.com/2uyOlE
DOWNLOAD >> https://urloso.com/2uyQzn
Download ->->->-> https://urloso.com/2uyREt
Download »»» https://tinurli.com/2uwjfo
Download File 🗸 https://tinurli.com/2uwjlm
Models by:
- {models[1]}
- {models[2]}
- {models[3]}
- {models[4]}
- {models[5]}
- {models[6]}
- {models[7]}
- {models[8]}
- {models[9]}
- {models[10]}
- {models[11]}
- {models[12]}
- {models[13]}
- {models[14]}
- {models[15]}
- {models[16]}
- {models[17]}
- {models[18]}
- {models[19]}
- {models[20]}
-
If you are an anime fan, you probably know how hard it can be to find a reliable and legal source to watch your favorite shows online. There are many websites that offer anime streaming, but most of them are either illegal, low quality, or full of ads. That's why many anime lovers turn to gogoanime.io, a popular website that provides free access to thousands of anime episodes with English subtitles and dubbing.
-But what if you want to watch anime on your mobile device without using a browser? Well, there is a solution for that too. You can download the gogoanime.io APK, which is an app that allows you to watch anime online for free on your Android device. In this article, we will tell you everything you need to know about gogoanime.io APK, including its features, how to download and install it, its pros and cons, and some alternatives you can try.
-Download ✯ https://urlca.com/2uOavh
One of the best features of gogoanime.io APK is that it has a huge collection of anime from various genres and sources. You can find anime from classic to modern, from action to romance, from comedy to horror, and more. You can also search for anime by name, genre, season, year, or popularity. You can watch anime from popular studios like Toei Animation, Madhouse, Bones, Kyoto Animation, etc.
-Another great feature of gogoanime.io APK is that it provides both subtitles and dubbing options for anime. You can choose to watch anime with English subtitles or with English dubbing, depending on your preference. You can also switch between them easily with a simple tap on the screen. This way, you can enjoy anime in your preferred language without any hassle.
-G
Gogoanime.io APK also allows you to add anime to your favorite list and get notified of new episodes. You can create your own personalized list of anime that you want to watch or follow. You can also get notifications when new episodes of your favorite anime are released. This way, you can keep track of your anime watching progress and never miss an episode.
-The last feature of gogoanime.io APK that we will mention is its user-friendly interface and design. The app has a simple and easy-to-use interface that lets you navigate through the app without any difficulty. You can access the main menu, the search bar, the favorite list, and the settings with just a few taps. The app also has a dark mode option that you can enable to reduce eye strain and save battery life. The app's design is also appealing and attractive, with colorful icons and images that match the anime theme.
-Before you can download and install gogoanime.io APK, you need to enable unknown sources on your device settings. This is because the app is not available on the Google Play Store and you need to download it from a third-party source. To enable unknown sources, follow these steps:
-Next, you need to download the APK file of gogoanime.io from the official website or a trusted source. To download the APK file, follow these steps:
-After downloading the APK file, you need to install it on your device. To install the APK file, follow these steps:
-gogoanime watch anime online free apk
-gogoanime app download for android
-gogoanime apk latest version 2021
-gogoanime io apk mod premium
-gogoanime apk no ads
-gogoanime io apk download for pc
-gogoanime app download for ios
-gogoanime apk pure
-gogoanime io apk download uptodown
-gogoanime io apk download apkpure
-gogoanime watch anime online hd apk
-gogoanime app download for firestick
-gogoanime apk latest version 2022
-gogoanime io apk mod unlocked
-gogoanime apk pro
-gogoanime io apk download for laptop
-gogoanime app download for windows 10
-gogoanime apk mirror
-gogoanime io apk download for mac
-gogoanime io apk download for smart tv
-gogoanime watch anime online english subbed apk
-gogoanime app download for roku
-gogoanime apk old version
-gogoanime io apk mod ad-free
-gogoanime apk cracked
-gogoanime io apk download for chromebook
-gogoanime app download for linux
-gogoanime apk rexdl
-gogoanime io apk download for android tv box
-gogoanime io apk download for bluestacks
-gogoanime watch anime online dubbed apk
-gogoanime app download for ps4
-gogoanime apk 2020
-gogoanime io apk mod no root
-gogoanime apk premium free
-gogoanime io apk download for kindle fire
-gogoanime app download for xbox one
-gogoanime apk happymod
-gogoanime io apk download for mi tv stick
-gogoanime io apk download for nvidia shield tv
The final step is to launch the app and enjoy watching anime online for free. To launch the app, follow these steps:
-Gogoanime.io APK has many pros that make it a great choice for anime fans. Some of them are:
-Gogoanime.io APK also has some cons that users should be aware of. Some of them are:
-If you are looking for a legal and safe alternative to gogoanime.io APK, you can try Crunchyroll. Crunchyroll is a popular platform that offers anime, manga, and drama streaming with a subscription fee. You can watch anime from various genres and sources, with subtitles or dubbing, in high quality and fast streaming. You can also access exclusive anime titles that are not available on other platforms. Crunchyroll has an app that you can download from the Google Play Store or the App Store.
-If you are looking for a free alternative to gogoanime.io APK, you can try AnimeFreak. AnimeFreak is a website that provides anime streaming without any registration or subscription. You can watch anime from various genres and sources, with subtitles or dubbing, in high quality and fast streaming. You can also add anime to your favorite list and get notified of new episodes. AnimeFreak does not have an app, but you can access it from your browser.
-If you are looking for a premium alternative to gogoanime.io APK, you can try VIZ. VIZ is a platform that offers anime and manga streaming with a subscription fee. You can watch anime from popular studios like Studio Ghibli, Naruto, One Piece, etc., with subtitles or dubbing, in high quality and fast streaming. You can also access exclusive anime titles that are not available on other platforms. VIZ has an app that you can download from the Google Play Store or the App Store.
-Gogoanime.io APK is a great app for anime fans who want to watch anime online for free on their Android devices. It has many features that make it appealing and convenient, such as a huge collection of anime, subtitles and dubbing options, favorite list and notifications, user-friendly interface and design, etc. However, it also has some drawbacks that users should be aware of, such as legal issues, security risks, ads, compatibility issues, etc. Therefore, users should use gogoanime.io APK at their own risk and discretion.
-If you are interested in downloading and installing gogoanime.io APK, you can follow the steps we provided in this article. Alternatively, you can also try some of the alternatives we suggested, such as Crunchyroll, AnimeFreak, or VIZ. These platforms offer different features and benefits that may suit your preferences and needs better.
-We hope this article was helpful and informative for you. If you have any questions or feedback, feel free to leave a comment below. Thank you for reading and happy watching!
-Here are some frequently asked questions related to gogoanime.io APK and their answers:
-Minecraft is one of the most popular and creative games in the world, with millions of players enjoying its endless possibilities. If you are one of them, you might be wondering how to download the latest version of Minecraft, which is 1.16.1, for free. In this article, we will show you how to do that for both Java Edition and Bedrock Edition, as well as what's new in this update.
-DOWNLOAD 🗸🗸🗸 https://urlca.com/2uO80E
Minecraft 1.16.1 is the first minor update of the Nether Update, which was released on June 23, 2020. The Nether Update is one of the most significant updates in Minecraft history, as it completely transformed the Nether dimension, adding new biomes, mobs, structures, blocks, items, and more.
-The Nether Update introduced four new biomes to the Nether: the Crimson Forest, the Warped Forest, the Basalt Deltas, and the Soulsand Valley. Each biome has its own unique features, such as vegetation, terrain, ambient sounds, and hazards. For example, the Crimson Forest is filled with red fungi and vines, while the Basalt Deltas are volcanic wastelands with lava pools and pillars of basalt.
-The update also added new mobs to the Nether, such as the Piglins, the Hoglins, the Zoglins, and the Striders. The Piglins are hostile humanoid creatures that live in bastion remnants and trade with gold ingots. The Hoglins are huge boar-like beasts that can be bred and hunted for food. The Zoglins are zombified versions of Hoglins that attack anything in sight. The Striders are passive creatures that can walk on lava and be ridden with a saddle and a warped fungus on a stick.
-Additionally, the update added new structures to the Nether, such as the bastion remnants, the ruined portals, and the nether fortresses. The bastion remnants are ancient fortifications where Piglins dwell and guard their treasures. The ruined portals are broken nether portals that can be found in both the Overworld and the Nether. The nether fortresses are massive complexes where Wither Skeletons, Blazes, and other hostile mobs spawn.
-minecraft 1 16 1 download free full version
-minecraft 1 16 1 download apk for android
-minecraft 1 16 1 download windows 10 edition
-minecraft 1 16 1 download mac os
-minecraft 1 16 1 download server jar
-minecraft 1 16 1 download mods and modpacks
-minecraft 1 16 1 download nether update
-minecraft 1 16 1 download optifine
-minecraft 1 16 1 download forge
-minecraft 1 16 1 download texture packs
-minecraft 1 16 1 download shaders
-minecraft 1 16 1 download maps and worlds
-minecraft 1 16 1 download skins and skin packs
-minecraft 1 16 1 download resource packs
-minecraft 1 16 1 download data packs
-minecraft 1 16 1 download seeds and coordinates
-minecraft 1 16 1 download snapshots and pre-releases
-minecraft 1 16 1 download realms and servers
-minecraft 1 16 1 download multiplayer and online
-minecraft 1 16 1 download launcher and installer
-minecraft 1 16 1 download cracked and unblocked
-minecraft 1 16 1 download java edition and bedrock edition
-minecraft 1.16.1 download xbox one and ps4
-minecraft.116.2.download switch and mobile
-minecraft.116.3.download linux and chromebook
-minecraft.116.4.download vr and ray tracing
-minecraft.116.5.download fabric and curseforge
-minecraft.116.6.download datapack generator and worldedit
-minecraft.116.7.download command blocks and functions
-minecraft.116.8.download custom npcs and mobs
-minecraft.116.9.download biomes o plenty and terraforged
-minecraft.116.10.download quark and create
-minecraft.116.11.download immersive engineering and engineer's tools[^3^]
-minecraft.116.12.download tinkers construct and tool belt[^3^]
-minecraft.116.13.download astral sorcery and botania
-minecraft.116.14.download thaumcraft and blood magic
-minecraft.116.15.download rftools and applied energistics
-minecraft.116.16.download industrial craft and buildcraft
-minecraft.116.17.download twilight forest and the betweenlands
-minecraft.116.18.download ice and fire and alex's mobs
-minecraft.116.19.download pam's harvestcraft and cooking for blockheads
-minecraft.116.20.download chisel and bits and little tiles
-minecraft.116.21.download decocraft and bibliocraft
-minecraft.116.22.download furniture mod and mr crayfish's furniture mod
-minecraft.116.23.download pixelmon and pokecube
Furthermore, the update added new blocks and items to the Nether, such as netherite, ancient debris, nether gold ore, respawn anchors, lodestones, target blocks, soul torches and lanterns, chains, warped and crimson stems and planks, shroomlights, warped and crimson fungi and roots, nether sprouts, twisting and weeping vines, basalt, blackstone, soul soil and sand, soul fire and campfires, and more.
-Minecraft 1.16.1 is not only about adding new content to the game but also about fixing bugs and improving performance. Some of the notable bug fixes and improvements in this version are:
-Fixed a crash that could occur when using a grindstone in certain situations
If you want to download Minecraft 1.16.1 for Java Edition, which is the original version of the game for PC, Mac, and Linux, you will need to follow these steps:
-The first step is to download the Minecraft Launcher, which is the program that allows you to launch and update the game. You can download it from the official website of Minecraft. You will need to create an account or log in with your existing one. Then, you will need to buy the game or redeem a code if you already have one. After that, you can download and install the launcher on your device.
-The next step is to select the latest release of the game, which is 1.16.1, from the launcher. You can do this by clicking on the "Installations" tab and then on the "New" button. You can name your installation whatever you want, but make sure to select "Latest release (1.16.1)" from the "Version" dropdown menu. Then, click on "Create" and your installation will be ready.
-The final step is to enjoy the game. You can launch your installation by clicking on the "Play" button on the launcher. You can then choose to play singleplayer or multiplayer, create or join worlds, customize your settings, and explore the Nether Update.
-If you want to download Minecraft 1.16.1 for Bedrock Edition, which is the cross-platform version of the game for Windows 10, Xbox One, PlayStation 4, Nintendo Switch, iOS, Android, and more, you will need to follow these steps:
-The first step is to download the Minecraft app, which is the application that allows you to play and update the game. You can download it from the official website of Minecraft or from the store of your device. You will need to create an account or log in with your existing one. Then, you will need to buy the game or redeem a code if you already have one. After that, you can download and install the app on your device.
-The next step is to update the game to the latest version, which is 1.16.1, from the app. You can do this by opening the app and checking for updates. If there is an update available, you can download and install it on your device. Alternatively, you can enable automatic updates on your device settings to keep your game up to date.
-The final step is to explore the Nether. You can launch the game by opening the app and tapping on the "Play" button. You can then choose to play singleplayer or multiplayer, create or join worlds, customize your settings, and enjoy the Nether Update.
-In this article, we have shown you how to download Minecraft 1.16.1 for free for both Java Edition and Bedrock Edition. We have also explained what's new in this update, such as the Nether Update and the bug fixes and improvements. We hope you have found this article helpful and informative, and that you have fun playing Minecraft 1.16.1.
-Here are some frequently asked questions about Minecraft 1.16.1:
-If you are a fan of motorcycle racing games, you might have heard of Traffic Rider, a popular game that offers realistic graphics, smooth controls, and thrilling gameplay. But did you know that there is a modified version of this game that reduces its size to only 99 MB, while still retaining all the features and fun? In this article, we will review Traffic Rider Mod APK 99 MB, a modded version of the original game that you can download and install on your Android device. We will also show you how to do it, and answer some frequently asked questions about this mod.
-Traffic Rider is a motorcycle racing game developed by Soner Kara, a Turkish game studio. It was released in 2016 and has since gained over 100 million downloads on Google Play Store. The game is praised for its realistic graphics, smooth controls, and thrilling gameplay. You can choose from over 30 different motorcycles, each with its own specifications and sounds. You can also customize your bike with different colors and accessories.
-DOWNLOAD ✶✶✶ https://urlca.com/2uOf5A
The game has two modes: career mode and endless mode. In career mode, you have to complete various missions and objectives, such as reaching a certain speed, overtaking a number of vehicles, or covering a certain distance. You can earn cash and gold by completing these missions, which you can use to buy new bikes or upgrade your existing ones. You can also unlock new locations and scenarios, such as highways, deserts, cities, or snow.
-In endless mode, you can ride your bike as long as you want, without any time limit or mission. You can enjoy the scenery and the traffic, while avoiding collisions and accidents. You can also challenge yourself by increasing the difficulty level, which affects the traffic density, speed limit, and police presence. The game also has a leaderboard system, where you can compare your scores and achievements with other players around the world.
-Traffic Rider Mod APK 99 MB is a modified version of the original game that reduces its size to only 99 MB, while still retaining all the features and fun. This mod is created by an unknown developer, who claims to have compressed the game files without compromising the quality or performance. The mod also removes some unnecessary ads and permissions from the original game, making it more user-friendly and secure.
-The main benefit of using this mod is that it saves a lot of storage space on your device. If you have a low-end device or limited storage capacity, you can still enjoy this game without worrying about running out of space or slowing down your device. The mod also offers unlimited money and gold, which means you can buy any bike or upgrade you want without grinding or spending real money.
-The main drawback of using this mod is that it is not an official version of the game, which means it may not be compatible with some devices or updates. It may also contain some bugs or glitches that affect the gameplay or functionality. Moreover, using this mod may violate the terms and conditions of the original game developer, which
could result in a ban or a penalty from the game server. Therefore, you should use this mod at your own risk and discretion, and respect the rights and efforts of the original game developer.
-If you want to try this mod, you need to follow these steps:
-Note: You may need to uninstall the original game before installing the mod, as they may conflict with each other.
-Before downloading and installing this mod, you should take some precautions and tips into account:
-traffic rider hack apk 99 mb download
-traffic rider unlimited money mod apk 99 mb
-traffic rider mod apk latest version 99 mb
-traffic rider motorcycle racing mod apk 99 mb
-traffic rider mod apk android 1 99 mb
-traffic rider mod apk offline 99 mb
-traffic rider mod apk revdl 99 mb
-traffic rider mod apk rexdl 99 mb
-traffic rider mod apk free download 99 mb
-traffic rider mod apk unlimited everything 99 mb
-traffic rider mod apk no root 99 mb
-traffic rider mod apk all bikes unlocked 99 mb
-traffic rider mod apk unlimited gold 99 mb
-traffic rider mod apk unlimited keys 99 mb
-traffic rider mod apk unlimited coins 99 mb
-traffic rider mod apk unlimited cash 99 mb
-traffic rider mod apk unlimited gems 99 mb
-traffic rider mod apk unlimited fuel 99 mb
-traffic rider mod apk unlimited nitro 99 mb
-traffic rider mod apk unlimited lives 99 mb
-traffic rider mod apk premium unlocked 99 mb
-traffic rider mod apk pro version 99 mb
-traffic rider mod apk full version 99 mb
-traffic rider mod apk mega mod 99 mb
-traffic rider mod apk super mod 99 mb
-traffic rider mod apk god mode 99 mb
-traffic rider mod apk high speed 99 mb
-traffic rider mod apk high graphics 99 mb
-traffic rider mod apk low size 99 mb
-traffic rider mod apk low end devices 99 mb
-traffic rider mod apk easy install 99 mb
-traffic rider mod apk direct link 99 mb
-traffic rider mod apk mediafire link 99 mb
-traffic rider mod apk google drive link 99 mb
-traffic rider mod apk dropbox link 99 mb
-traffic rider mod apk mirror link 99 mb
-traffic rider mod apk original link 99 mb
-traffic rider mod apk safe link 99 mb
-traffic rider mod apk virus free link 99 mb
-traffic rider mod apk working link 99 mb
-traffic rider mod apk best link 99 mb
-traffic rider mod apk fast link 99 mb
-traffic rider mod apk updated link 99 mb
-traffic rider mod apk new link 99 mb
-traffic rider mod apk old link 99 mb
-traffic rider mod apk latest link 99 mb
-traffic rider mod apk cracked link 99 mb
-traffic rider mod apk patched link 99 mb
-traffic rider mod apk fixed link 99 mb
In this article, we have reviewed Traffic Rider Mod APK 99 MB, a modified version of the original game that reduces its size to only 99 MB, while still retaining all the features and fun. We have also shown you how to download and install this mod on your Android device, and answered some frequently asked questions about this mod.
-We recommend this mod to anyone who loves motorcycle racing games, but has a low-end device or limited storage capacity. This mod offers unlimited money and gold, realistic graphics, smooth controls, and thrilling gameplay. However, you should also be aware of the drawbacks and risks of using this mod, such as compatibility issues, bugs, glitches, or bans. Therefore, you should use this mod at your own risk and discretion, and respect the rights and efforts of the original game developer.
-We rate this mod 4 out of 5 stars, based on its features, performance, and user feedback. We hope you found this article helpful and informative. If you have any questions or comments, feel free to leave them below. Thank you for reading!
-Traffic Rider Mod APK 99 MB is safe to use as long as you download it from a reliable source and follow the installation instructions carefully. However, since it is not an official version of the game, it may contain some bugs or glitches that affect the gameplay or functionality. Moreover, using this mod may violate the terms and conditions of the original game developer, which could result in a ban or a penalty from the game server. Therefore, you should use this mod at your own risk and discretion, and respect the rights and efforts of the original game developer.
-No, Traffic Rider Mod APK 99 MB does not require root access to work on your device. You can install it without rooting your device or modifying any system settings. However, if you have a rooted device, you may need to disable root detection before launching the game, as some games may not work properly on rooted devices.
-Traffic Rider Mod APK 99 MB is a modified version of the original game that reduces its size to only 99 MB, while still retaining all the features and fun. It also offers unlimited money and gold, which means you can buy any bike or upgrade you want without grinding or spending real money. Traffic Rider Pro APK is an official version of the game that offers some premium features and benefits for a one-time payment. These include removing ads, unlocking all bikes and locations, getting double cash and gold, and getting 30% extra faster bikes. You can choose either version depending on your preference and budget.
-To update Traffic Rider Mod APK 99 MB, you need to follow the same steps as downloading and installing it. You need to download the latest version of the mod from a reliable source and install it over the existing one. You may also need to uninstall the original game before installing the mod, as they may conflict with each other. However, you should note that updating the mod may erase your game data or progress, so you should make a backup before doing so.
-Unfortunately, there is no official way to contact the developers of Traffic Rider Mod APK 99 MB, as they are unknown and anonymous. You may try to find them on some online forums or communities, but there is no guarantee that they will respond or help you. Therefore, you should use this mod at your own risk and discretion, and respect the rights and efforts of the original game developer.
401be4b1e0Download Zip ⭐ https://ssurll.com/2uzyxN
You may also like:
-- Sends an image in to CLIP Interrogator - to generate a text prompt which is then run through - Mubert text-to-music to generate music from the input image! -
-Donut: OCR-free Document Understanding Transformer | Github Repo
" - -demo = gr.Interface( - fn=process_document, - inputs="image", - outputs="json", - title="Demo: Donut 🍩 for Document Parsing", - description=description, - article=article, - enable_queue=True, - examples=[["Binder1_Page_48_Image_0001.png"], ["SKMBT_75122072616550_Page_50_Image_0001.png"]], - cache_examples=False) - -demo.launch() diff --git a/spaces/dbredvick/whisper-webui/app-local.py b/spaces/dbredvick/whisper-webui/app-local.py deleted file mode 100644 index d8eabbc62924dab3d0cc03a8a2373ffffe01eadc..0000000000000000000000000000000000000000 --- a/spaces/dbredvick/whisper-webui/app-local.py +++ /dev/null @@ -1,3 +0,0 @@ -# Run the app with no audio file restrictions -from app import create_ui -create_ui(-1) \ No newline at end of file diff --git a/spaces/dcarpintero/nlp-summarizer-pegasus/.venv/lib/python3.9/site-packages/fontTools/pens/areaPen.py b/spaces/dcarpintero/nlp-summarizer-pegasus/.venv/lib/python3.9/site-packages/fontTools/pens/areaPen.py deleted file mode 100644 index 004bb06b091ceb777cca2c02f8481a2785a46d35..0000000000000000000000000000000000000000 --- a/spaces/dcarpintero/nlp-summarizer-pegasus/.venv/lib/python3.9/site-packages/fontTools/pens/areaPen.py +++ /dev/null @@ -1,52 +0,0 @@ -"""Calculate the area of a glyph.""" - -from fontTools.pens.basePen import BasePen - - -__all__ = ["AreaPen"] - - -class AreaPen(BasePen): - def __init__(self, glyphset=None): - BasePen.__init__(self, glyphset) - self.value = 0 - - def _moveTo(self, p0): - self._p0 = self._startPoint = p0 - - def _lineTo(self, p1): - x0, y0 = self._p0 - x1, y1 = p1 - self.value -= (x1 - x0) * (y1 + y0) * 0.5 - self._p0 = p1 - - def _qCurveToOne(self, p1, p2): - # https://github.com/Pomax/bezierinfo/issues/44 - p0 = self._p0 - x0, y0 = p0[0], p0[1] - x1, y1 = p1[0] - x0, p1[1] - y0 - x2, y2 = p2[0] - x0, p2[1] - y0 - self.value -= (x2 * y1 - x1 * y2) / 3 - self._lineTo(p2) - self._p0 = p2 - - def _curveToOne(self, p1, p2, p3): - # https://github.com/Pomax/bezierinfo/issues/44 - p0 = self._p0 - x0, y0 = p0[0], p0[1] - x1, y1 = p1[0] - x0, p1[1] - y0 - x2, y2 = p2[0] - x0, p2[1] - y0 - x3, y3 = p3[0] - x0, p3[1] - y0 - self.value -= (x1 * (-y2 - y3) + x2 * (y1 - 2 * y3) + x3 * (y1 + 2 * y2)) * 0.15 - self._lineTo(p3) - self._p0 = p3 - - def _closePath(self): - self._lineTo(self._startPoint) - del self._p0, self._startPoint - - def _endPath(self): - if self._p0 != self._startPoint: - # Area is not defined for open contours. - raise NotImplementedError - del self._p0, self._startPoint diff --git a/spaces/dcarpintero/nlp-summarizer-pegasus/.venv/lib/python3.9/site-packages/gradio/templates/cdn/assets/Button-0f9363c8.css b/spaces/dcarpintero/nlp-summarizer-pegasus/.venv/lib/python3.9/site-packages/gradio/templates/cdn/assets/Button-0f9363c8.css deleted file mode 100644 index 793ad00d7162ad414fc8ccc6df6d9a9b8799646e..0000000000000000000000000000000000000000 --- a/spaces/dcarpintero/nlp-summarizer-pegasus/.venv/lib/python3.9/site-packages/gradio/templates/cdn/assets/Button-0f9363c8.css +++ /dev/null @@ -1 +0,0 @@ -.block.svelte-90oupt{position:relative;margin:0;box-shadow:var(--block-shadow);border-width:var(--block-border-width);border-color:var(--block-border-color);border-radius:var(--block-radius);background:var(--block-background-fill);width:100%;line-height:var(--line-sm)}.block.border_focus.svelte-90oupt{border-color:var(--color-accent)}.padded.svelte-90oupt{padding:var(--block-padding)}.hidden.svelte-90oupt{display:none}.hide-container.svelte-90oupt{margin:0;box-shadow:none;--block-border-width:0;background:transparent;padding:0;overflow:visible}div.svelte-e8n7p6{margin-bottom:var(--spacing-lg);color:var(--block-info-text-color);font-weight:var(--block-info-text-weight);font-size:var(--block-info-text-size);line-height:var(--line-sm)}span.has-info.svelte-1gfkn6j{margin-bottom:var(--spacing-xs)}span.svelte-1gfkn6j:not(.has-info){margin-bottom:var(--spacing-lg)}span.svelte-1gfkn6j{display:inline-block;position:relative;z-index:var(--layer-4);border:solid var(--block-title-border-width) var(--block-title-border-color);border-radius:var(--block-title-radius);background:var(--block-title-background-fill);padding:var(--block-title-padding);color:var(--block-title-text-color);font-weight:var(--block-title-text-weight);font-size:var(--block-title-text-size);line-height:var(--line-sm)}.hide.svelte-1gfkn6j{margin:0;height:0}div.svelte-1mwvhlq{display:inline-flex;align-items:center;z-index:var(--layer-2);box-shadow:var(--block-label-shadow);border:var(--block-label-border-width) solid var(--border-color-primary);border-top:none;border-left:none;border-radius:var(--block-label-radius);background:var(--block-label-background-fill);padding:var(--block-label-padding);pointer-events:none;color:var(--block-label-text-color);font-weight:var(--block-label-text-weight);font-size:var(--block-label-text-size);line-height:var(--line-sm)}.gr-group div.svelte-1mwvhlq{border-top-left-radius:0}div.float.svelte-1mwvhlq{position:absolute;top:var(--block-label-margin);left:var(--block-label-margin)}div.svelte-1mwvhlq:not(.float){position:static;margin-top:var(--block-label-margin);margin-left:var(--block-label-margin)}.hide.svelte-1mwvhlq{height:0}span.svelte-1mwvhlq{opacity:.8;margin-right:var(--size-2);width:calc(var(--block-label-text-size) - 1px);height:calc(var(--block-label-text-size) - 1px)}.hide-label.svelte-1mwvhlq{box-shadow:none;border-width:0;background:transparent;overflow:visible}button.svelte-1030q2h{display:flex;justify-content:center;align-items:center;gap:1px;z-index:var(--layer-1);box-shadow:var(--shadow-drop);border:1px solid var(--button-secondary-border-color);border-radius:var(--radius-sm);background:var(--background-fill-primary);padding:2px;color:var(--block-label-text-color)}button.svelte-1030q2h:hover{cursor:pointer;border:2px solid var(--button-secondary-border-color-hover);padding:1px;color:var(--block-label-text-color)}span.svelte-1030q2h{padding:0 1px;font-size:10px}div.svelte-1030q2h{padding:2px;width:14px;height:14px}.pending.svelte-1030q2h{animation:svelte-1030q2h-flash .5s infinite}@keyframes svelte-1030q2h-flash{0%{opacity:.5}50%{opacity:1}to{opacity:.5}}.empty.svelte-lk9eg8{display:flex;justify-content:center;align-items:center;margin-top:calc(0px - var(--size-6));height:var(--size-full)}.icon.svelte-lk9eg8{opacity:.5;height:var(--size-5);color:var(--body-text-color)}.small.svelte-lk9eg8{min-height:calc(var(--size-32) - 20px)}.large.svelte-lk9eg8{min-height:calc(var(--size-64) - 20px)}.unpadded_box.svelte-lk9eg8{margin-top:0}.small_parent.svelte-lk9eg8{min-height:100%!important}.wrap.svelte-1ck5uk8{display:flex;flex-direction:column;justify-content:center;min-height:var(--size-60);color:var(--block-label-text-color);line-height:var(--line-md)}.or.svelte-1ck5uk8{color:var(--body-text-color-subdued)}@media (min-width: 768px){.wrap.svelte-1ck5uk8{font-size:var(--text-lg)}}button.svelte-cmf5ev,a.svelte-cmf5ev{display:inline-flex;justify-content:center;align-items:center;transition:var(--button-transition);box-shadow:var(--button-shadow);padding:var(--size-0-5) var(--size-2);text-align:center}button.svelte-cmf5ev:hover,button[disabled].svelte-cmf5ev,a.svelte-cmf5ev:hover,a.disabled.svelte-cmf5ev{box-shadow:var(--button-shadow-hover)}button.svelte-cmf5ev:active,a.svelte-cmf5ev:active{box-shadow:var(--button-shadow-active)}button[disabled].svelte-cmf5ev,a.disabled.svelte-cmf5ev{opacity:.5;filter:grayscale(30%);cursor:not-allowed}.hidden.svelte-cmf5ev{display:none}.primary.svelte-cmf5ev{border:var(--button-border-width) solid var(--button-primary-border-color);background:var(--button-primary-background-fill);color:var(--button-primary-text-color)}.primary.svelte-cmf5ev:hover,.primary[disabled].svelte-cmf5ev{border-color:var(--button-primary-border-color-hover);background:var(--button-primary-background-fill-hover);color:var(--button-primary-text-color-hover)}.secondary.svelte-cmf5ev{border:var(--button-border-width) solid var(--button-secondary-border-color);background:var(--button-secondary-background-fill);color:var(--button-secondary-text-color)}.secondary.svelte-cmf5ev:hover,.secondary[disabled].svelte-cmf5ev{border-color:var(--button-secondary-border-color-hover);background:var(--button-secondary-background-fill-hover);color:var(--button-secondary-text-color-hover)}.stop.svelte-cmf5ev{border:var(--button-border-width) solid var(--button-cancel-border-color);background:var(--button-cancel-background-fill);color:var(--button-cancel-text-color)}.stop.svelte-cmf5ev:hover,.stop[disabled].svelte-cmf5ev{border-color:var(--button-cancel-border-color-hover);background:var(--button-cancel-background-fill-hover);color:var(--button-cancel-text-color-hover)}.sm.svelte-cmf5ev{border-radius:var(--button-small-radius);padding:var(--button-small-padding);font-weight:var(--button-small-text-weight);font-size:var(--button-small-text-size)}.lg.svelte-cmf5ev{border-radius:var(--button-large-radius);padding:var(--button-large-padding);font-weight:var(--button-large-text-weight);font-size:var(--button-large-text-size)}.button-icon.svelte-cmf5ev{width:var(--text-xl);height:var(--text-xl);margin-right:var(--spacing-xl)} diff --git a/spaces/dcarpintero/nlp-summarizer-pegasus/.venv/lib/python3.9/site-packages/h11/_version.py b/spaces/dcarpintero/nlp-summarizer-pegasus/.venv/lib/python3.9/site-packages/h11/_version.py deleted file mode 100644 index 4c8911305680c1083b2da9b87ece12bc36f3a9e1..0000000000000000000000000000000000000000 --- a/spaces/dcarpintero/nlp-summarizer-pegasus/.venv/lib/python3.9/site-packages/h11/_version.py +++ /dev/null @@ -1,16 +0,0 @@ -# This file must be kept very simple, because it is consumed from several -# places -- it is imported by h11/__init__.py, execfile'd by setup.py, etc. - -# We use a simple scheme: -# 1.0.0 -> 1.0.0+dev -> 1.1.0 -> 1.1.0+dev -# where the +dev versions are never released into the wild, they're just what -# we stick into the VCS in between releases. -# -# This is compatible with PEP 440: -# http://legacy.python.org/dev/peps/pep-0440/ -# via the use of the "local suffix" "+dev", which is disallowed on index -# servers and causes 1.0.0+dev to sort after plain 1.0.0, which is what we -# want. (Contrast with the special suffix 1.0.0.dev, which sorts *before* -# 1.0.0.) - -__version__ = "0.14.0" diff --git a/spaces/dcq/freegpt-webui/g4f/Provider/Providers/Phind.py b/spaces/dcq/freegpt-webui/g4f/Provider/Providers/Phind.py deleted file mode 100644 index 9fa8ec821f701d7841432e498a11ac9dd017978c..0000000000000000000000000000000000000000 --- a/spaces/dcq/freegpt-webui/g4f/Provider/Providers/Phind.py +++ /dev/null @@ -1,36 +0,0 @@ -import os -import json -import time -import subprocess - -from ...typing import sha256, Dict, get_type_hints - -url = 'https://phind.com' -model = ['gpt-4'] -supports_stream = True - -def _create_completion(model: str, messages: list, stream: bool, **kwargs): - - path = os.path.dirname(os.path.realpath(__file__)) - config = json.dumps({ - 'model': model, - 'messages': messages}, separators=(',', ':')) - - cmd = ['python', f'{path}/helpers/phind.py', config] - - p = subprocess.Popen(cmd, stdout=subprocess.PIPE, stderr=subprocess.STDOUT) - - for line in iter(p.stdout.readline, b''): - if b'if you can not find the file you want, just send a message and we will send the file to you. download autodesk autocad 2010 free keygen x32 bits for windows 32bit. if you can not find the file you want, just send a message and we will send the file to you.
-autodesk maya 2012 xforce keygen 64 bit, autodesk maya 2012 keygen xforce, autodesk maya xforce keygen 2010. autodesk maya xforce keygen 2013, autodesk maya xforce keygen 2011, autodesk maya xforce keygen 2017, autodesk maya xforce keygen 2015,.
-DOWNLOAD ○ https://gohhs.com/2uFVlN
autodesk maya xforce keygen 2013, autodesk maya xforce keygen 2011, autodesk maya xforce keygen 2017, autodesk maya xforce keygen 2015,. autodesk maya xforce keygen 2013, autodesk maya xforce keygen 2011, autodesk maya xforce keygen 2017, autodesk maya xforce keygen 2015,.
-xforce keygen maya 2011, xforce keygen autodesk maya 2011 download, autodesk xforce keygen autodesk maya 2011 download, xforce keygen. autodesk xforce keygen autodesk maya 2011, autodesk xforce keygen autodesk maya 2011 download, xforce keygen.
-download autodesk maya 2008 x32 bit (free) released. autodesk inventor 2010 crack. autocad 2017 xforce keygen download, autocad 2017 xforce keygen generator free download,. thanks to: ruba james for sharing these key for autodesk 2017.
-this download is not available in your country? autodesk vray 1.3.8.0 for autodesk maya 2011 autodesk maya 2011 xforce keygen download. autocad 2017 xforce keygen download, autocad 2017 xforce keygen, autocad 2017 xforce keygen download, autocad 2017 xforce keygen download.
-for download xforce keygen for autodesk maya 2011 autodesk maya 2011 xforce keygen, download autodesk xforce keygen for maya 2011 autodesk maya 2011, download autodesk xforce keygen for maya 2011 autodesk maya 2011, download autodesk xforce keygen for maya 2011 autodesk maya 2011.
- 899543212bDownload Zip 🗸🗸🗸 https://gohhs.com/2uFVsl
DOWNLOAD ✵ https://gohhs.com/2uFSXe
Download Zip ✸✸✸ https://gohhs.com/2uFUu3
Download · https://gohhs.com/2uFTPW
${hljs.highlightAuto(codeContent, [language]).value}
- You can modify this app directly by editing index.html in the Files and versions tab.
-- Also don't forget to check the - Spaces documentation. -
-Download ⇔ https://urlca.com/2uDcsf
DOWNLOAD ✺ https://urlca.com/2uDcfH
If you are a fan of racing games, you must have heard of Asphalt 8: Airborne, one of the most popular and advanced racing games on Android. This game offers you the opportunity to drive hundreds of cars and bikes, perform high-speed aerial stunts, race on 75+ tracks, and compete with other players online or offline.
-But what if you want to enjoy the game without any limitations or restrictions? What if you want to have unlimited money and tokens, free shopping and upgrades, and access to all cars and tracks? Well, that's where Asphalt 8: Airborne 3.9.0j Mod APK comes in handy.
-Download File === https://urllie.com/2uNxBY
Asphalt 8: Airborne is a racing game developed by Gameloft, a leading mobile game developer. It is part of the Asphalt franchise, which has been running since 2004. The game was released in 2013 and has received several updates and improvements since then.
-Asphalt 8: Airborne is an arcade-style racing game that features realistic car models, stunning graphics, physics-based interactions, and various game modes. The game has been acclaimed by players and critics alike, winning several awards and accolades.
-Asphalt 8: Airborne 3.9.0j Mod APK is a modified version of the original game that gives you some extra benefits and features that are not available in the official version. The mod APK file is created by third-party developers who modify the game code to unlock some features or add some cheats.
-The mod APK file is not available on the Google Play Store or any other official source. You have to download it from a trusted website or link that provides the mod APK file for free. However, you should be careful when downloading mod APK files, as some of them may contain viruses or malware that can harm your device or steal your data.
-Asphalt 8: Airborne features more than 300 licensed cars and motorbikes from top manufacturers like Ferrari, Lamborghini, McLaren, Bugatti, Mercedes, Audi, Ford, Chevrolet, and more. You can choose from a variety of vehicles, ranging from sports cars to muscle cars, from bikes to SUVs.
-You can also customize and upgrade your rides with over 2,300 decals, paint jobs, rims, spoilers, engines, tires, and more. You can make your vehicles look unique and perform better on the tracks.
-Asphalt 8: Airborne boasts of impressive graphics that make the game look realistic and immersive. The game uses a new physics engine that allows for dynamic interactions between the vehicles, environments, and tracks.
-You can see the details of your car models, the reflections of the sun and the shadows on the road, the sparks and smoke from collisions and crashes, the debris and dust flying around
the tracks, and the weather effects like rain, snow, fog, and sandstorms. You can also experience the realistic sound effects of the engines, brakes, horns, and crashes.
-asphalt 8 airborne 3.9 0j unlimited money mod apk
-asphalt 8 hack mod apk download 3.9 0j
-asphalt 8 mod apk latest version 3.9 0j
-asphalt 8 mod apk android 1 3.9 0j
-asphalt 8 mod apk revdl 3.9 0j
-asphalt 8 mod apk rexdl 3.9 0j
-asphalt 8 mod apk offline 3.9 0j
-asphalt 8 mod apk free shopping 3.9 0j
-asphalt 8 mod apk all cars unlocked 3.9 0j
-asphalt 8 mod apk unlimited tokens and credits 3.9 0j
-asphalt 8 mod apk anti ban 3.9 0j
-asphalt 8 mod apk highly compressed 3.9 0j
-asphalt 8 mod apk obb file download 3.9 0j
-asphalt 8 mod apk mega mod menu v3.9.0j
-asphalt 8 mod apk no root required v3.9.0j
-asphalt 8 mod apk unlimited nitro and speed v3.9.0j
-asphalt 8 mod apk all bikes unlocked v3.9.0j
-asphalt 8 mod apk all seasons unlocked v3.9.0j
-asphalt 8 mod apk all tracks unlocked v3.9.0j
-asphalt 8 mod apk all decals unlocked v3.9.0j
-asphalt 8 mod apk all pro kits unlocked v3.9.0j
-asphalt 8 mod apk all events unlocked v3.9.0j
-asphalt 8 mod apk all multiplayer modes unlocked v3.9.0j
-asphalt 8 mod apk all special offers unlocked v3.9.0j
-asphalt 8 mod apk all fusion coins unlocked v3.9.0j
-asphalt 8 mod apk unlimited blueprints v3.9.0j
-asphalt 8 mod apk unlimited fusion points v3.9.0j
-asphalt 8 mod apk unlimited mastery rewards v3.9.0j
-asphalt 8 mod apk unlimited vip points v3.9.0j
-asphalt 8 mod apk unlimited elite cars v3.9.0j
-asphalt 8 mod apk unlimited tuning kits v3.9.0j
-asphalt 8 mod apk unlimited extra tanks v3.9.0j
-asphalt 8 mod apk unlimited double credits v3.9.0j
-asphalt 8 mod apk unlimited double nitro v3.9.0j
-asphalt 8 mod apk unlimited double xp v3.9.0j
-asphalt 8 mod apk unlimited perfect nitro triggers v3.9.0j
-asphalt 8 mod apk unlimited flat spins in one jump v3.9.0j
-asphalt 8 mod apk unlimited barrel rolls in one jump v3.9.0j
-asphalt 8 mod apk unlimited near misses v3.9.0j
-asphalt 8 mod apk unlimited knockdowns v3.9.0j
-asphalt 8 mod apk unlimited drift distance v3.9.0j
-asphalt 8 mod apk unlimited air time v3.9.0j
-asphalt 8 mod apk unlimited oncoming traffic dodges v3.9.0j
-asphalt
Asphalt 8: Airborne offers you a thrilling and adrenaline-pumping gameplay that will keep you hooked for hours. You can race on 75+ tracks across 18 different locations, such as Venice, Tokyo, Nevada, Iceland, Dubai, and more. You can also perform amazing aerial stunts, such as barrel rolls, flips, jumps, and drifts.
-You can also challenge other players from around the world in various multiplayer modes, such as classic, elimination, infected, tag racing, and more. You can join or create your own racing club, chat with other racers, and compete for the top spot on the leaderboards.
-Asphalt 8: Airborne has a lot of content to offer you, whether you are a casual or a hardcore racer. You can play through 9 seasons and over 400 events in the career mode, unlock new cars and tracks, and earn rewards and achievements. You can also play in the special events mode, where you can participate in limited-time challenges and win exclusive prizes.
-You can also customize your game experience with various options and settings. You can choose from 5 different control schemes, adjust the graphics quality and sound volume, enable or disable tilt steering and auto-acceleration, and more.
-Asphalt 8: Airborne 3.9.0j Mod APK is a modified version of the original game that gives you some extra benefits and features that are not available in the official version. Here are some of the benefits of using the mod APK:
-Money and tokens are the main currencies in Asphalt 8: Airborne. You need them to buy new cars and bikes, upgrade your vehicles, unlock new tracks and events, and more. However, earning money and tokens in the game can be time-consuming and tedious.
-With the mod APK, you don't have to worry about running out of money or tokens. You will have unlimited amounts of both currencies in your account. You can spend them as much as you want without any limitations or restrictions.
-Shopping and upgrading your vehicles in Asphalt 8: Airborne can be expensive and require a lot of money and tokens. You may not be able to afford the car or bike that you want or need to improve your performance on the tracks.
-With the mod APK, you don't have to pay anything for shopping or upgrading your vehicles. You can buy any car or bike that you like for free. You can also upgrade your vehicles to the maximum level for free. You can make your vehicles faster, stronger, and more durable without spending a dime.
-Cars and tracks are the main attractions of Asphalt 8: Airborne. The game features more than 300 cars and bikes from top manufacturers and 75+ tracks from different locations. However, not all cars and tracks are available from the start. You have to unlock them by playing through the career mode or by paying with money or tokens.
-With the mod APK, you don't have to wait or pay to unlock all cars and tracks. You will have access to all cars and tracks from the beginning of the game. You can choose any car or bike that you want and race on any track that you like.
-If you want to install Asphalt 8: Airborne 3.9.0j Mod APK on your Android device, you need to follow these simple steps:
-The first step is to download the mod APK file from a trusted source that provides the mod APK file for free. You can search for Asphalt 8: Airborne 3.9.0j Mod APK on Google or any other search engine and find a reliable website or link that offers the download link.
-You should be careful when downloading mod APK files from unknown sources, as some of them may contain viruses or malware that can harm your device or steal your data. You should also check the reviews and ratings of the website or link before downloading anything.
-The second step is to enable unknown sources on your device settings. This will allow you to install apps that are not from the Google Play Store or any other official source. To do this, you need to go to your device settings, then security, then unknown sources, and then toggle the switch to on.
-You may see a warning message that installing apps from unknown sources may harm your device or data. You can ignore this message and proceed with the installation. However, you should only install apps from trusted sources and scan them with an antivirus app before installing them.
-The third step is to install the mod APK file and launch the game. To do this, you need to locate the mod APK file that you downloaded on your device storage, then tap on it to start the installation process. You may see some prompts asking for permissions or access to your device features. You can grant them and continue with the installation.
-Once the installation is complete, you can launch the game by tapping on its icon on your home screen or app drawer. You will see the modded version of Asphalt 8: Airborne with all the benefits and features that we mentioned earlier. You can enjoy the game without any limitations or restrictions.
-Asphalt 8: Airborne is one of the best racing games on Android that offers you a thrilling and immersive racing experience. However, if you want to have more fun and freedom in the game, you can try Asphalt 8: Airborne 3.9.0j Mod APK, which gives you unlimited money and tokens, free shopping and upgrades, and unlocked all cars and tracks.
-To install Asphalt 8: Airborne 3.9.0j Mod APK, you need to download the mod APK file from a trusted source, enable unknown sources on your device settings, and install the mod APK file and launch the game. You can then enjoy the game with all the benefits and features of the mod APK.
-If you have any questions or doubts about Asphalt 8: Airborne 3.9.0j Mod APK, you can check out these FAQs:
-Question | -Answer | -
---|---|
Is Asphalt 8: Airborne 3.9.0j Mod APK safe to use? | -Asphalt 8: Airborne 3.9.0j Mod APK is safe to use as long as you download it from a trusted source and scan it with an antivirus app before installing it. However, you should be aware that using mod APK files may violate the terms and conditions of the game and may result in your account being banned or suspended. | -
Does Asphalt 8: Airborne 3.9.0j Mod APK require root access? | -No, Asphalt 8: Airborne 3.9.0j Mod APK does not require root access to work on your device. You can install it without rooting your device. | -
Can I play online with Asphalt 8: Airborne 3.9.0j Mod APK? | -Yes, you can play online with Asphalt 8: Airborne 3.9.0j Mod APK, but you may face some issues or errors while connecting to the servers or other players. You may also encounter some cheaters or hackers who use mod APK files to gain an unfair advantage over other players. | -
Can I update Asphalt 8: Airborne 3.9.0j Mod APK? | -No, you cannot update Asphalt 8: Airborne 3.9.0j Mod APK from the Google Play Store or any other official source. If you want to update the game, you need to uninstall the mod APK file and install the official version of the game from the Google Play Store or any other official source. | -
Can I use Asphalt 8: Airborne 3.9.0j Mod APK with other mods or hacks? | -No, you cannot use Asphalt 8: Airborne 3.9.0j Mod APK with other mods or hacks, as they may cause conflicts or errors in the game. You should only use one mod or hack at a time. | -
I hope this article has helped you understand what Asphalt 8: Airborne 3.9.0j Mod APK is and how to install it on your Android device.
197e85843dDo you want to make your car more fun and unique? Do you want to add some flair and personality to your car's features? Do you want to impress your friends and family with your cool car sounds? If you answered yes to any of these questions, then you might be interested in downloading some car unlock sound effects for your car.
-Car unlock sound effects are audio clips that play when you unlock your car with a remote key or a smartphone app. They can be anything from realistic car sounds, such as beeps, clicks, or honks, to more creative and fun sounds, such as music, voice, or animal noises. Car unlock sound effects can make your car more enjoyable and entertaining, as well as provide some practical benefits, such as security and convenience.
-Download ===== https://urllie.com/2uNBsJ
There are many websites that offer free or paid car unlock sound effects for download. Some of the most popular ones are:
-Once you have found the car unlock sound effects that you like, you can download them to your device by following these steps:
-One of the main benefits of using car unlock sound effects is that they can improve your car's security and convenience. By having a distinctive and recognizable sound when you unlock your car, you can easily locate your car in a crowded parking lot or street. You can also deter potential thieves or vandals from targeting your car, as they will know that you have a sound system installed in your car. You can also save time and hassle by unlocking your car with a simple click of a button or a tap of your phone, instead of fumbling with keys or codes.
-Another benefit of using car unlock sound effects is that they can customize your car's personality and style. By choosing a sound effect that matches your taste and mood, you can express yourself and make your car stand out from the crowd. You can also change the sound effect according to the occasion, such as a festive sound for holidays, a romantic sound for dates, or a funny sound for pranks. You can have fun and be creative with your car unlock sound effects, as long as they are not too loud or annoying for others.
-A final benefit of using car unlock sound effects is that they can impress your friends and family with your cool car sounds. By having a unique and interesting sound when you unlock your car, you can attract attention and admiration from others. You can also surprise and delight your passengers with your car unlock sound effects, making them feel special and entertained. You can also share your car unlock sound effects with others, such as by sending them the files or playing them on social media.
-One of the tips and tricks for using car unlock sound effects is to choose the right sound format and quality for your device. Different devices may have different requirements and capabilities for playing sound effects, so you need to make sure that the file format and quality are compatible with your device. Generally, MP3 files are smaller and more widely supported, while WAV files are larger and higher quality. You can also check the bitrate and sample rate of the file, which affect the clarity and smoothness of the sound.
-Another tip and trick for using car unlock sound effects is to adjust the volume and duration of the sound effects to suit your preference. You don't want the sound effect to be too loud or too long, as it may disturb others or annoy yourself. You can use an audio editor software or app to modify the volume and duration of the file, or use the settings menu of your device to adjust them. You can also use a fade in or fade out effect to make the sound more natural and pleasant.
-A final tip and trick for using car unlock sound effects is to test the sound effects before using them on your car. You don't want to end up with a sound effect that doesn't work properly or sounds bad on your car's device. You can test the sound effect by playing it on your computer or phone first, or by using a headphone or speaker. You can also test it on your car's device by connecting it with a USB cable, Bluetooth connection, or wireless network. You can then listen to how it sounds and make any necessary adjustments.
-car unlock sound effect download
-car lock unlock sound mp3 download
-car door unlock sound free download
-car key unlock sound download
-car remote unlock sound download
-car lock and unlock sound download
-car locking sound effect download
-car lock sound wav download
-car door lock sound download
-car key lock sound download
-car remote lock sound download
-car locking and unlocking sound download
-car lock beep sound download
-car lock alarm sound download
-car lock click sound download
-car lock horn sound download
-car lock open sound download
-car lock ringtone download
-car lock siren sound download
-car lock voice sound download
-car unlock beep sound download
-car unlock button sound download
-car unlock horn sound download
-car unlock ringtone download
-car unlock sfx download
-car door unlock beep sound download
-car door unlock button sound download
-car door unlock horn sound download
-car door unlock ringtone download
-car door unlock sfx download
-car key unlock beep sound download
-car key unlock button sound download
-car key unlock horn sound download
-car key unlock ringtone download
-car key unlock sfx download
-car remote unlock beep sound download
-car remote unlock button sound download
-car remote unlock horn sound download
-car remote unlock ringtone download
-car remote unlock sfx download
In conclusion, car unlock sound effects are audio clips that play when you unlock your car with a remote key or a smartphone app. They can be realistic or creative sounds that enhance your car's security and convenience, customize your car's personality and style, and impress your friends and family with your cool car sounds. You can find and download free or paid car unlock sound effects online from various websites, such as Pixabay, Storyblocks, or Zapsplat. You can then transfer them to your car's device using a USB cable, Bluetooth connection, or wireless network. You can also choose the right sound format and quality for your device, adjust the volume and duration of the sound effects to suit your preference, and test the sound effects before using them on your car.
-If you are interested in downloading some car unlock sound effects for your car, you can start by browsing through some of the websites mentioned above. You can also search for other websites that offer similar services, or create your own sound effects using an audio recorder or synthesizer. You can then enjoy having some fun and unique sounds when you unlock your car. However, remember to be respectful and responsible when using car unlock sound effects, as they may affect others around you. Don't use sounds that are too loud, too long, or too inappropriate for public places. Also, don't rely on sounds alone for securing your car; always lock your doors and windows properly.
-A: Some examples of car unlock sound effects are:
-A: You can create your own car unlock sound effects by using an audio recorder or synthesizer. You can record your own voice, use a musical instrument, or make some noises with objects. You can then edit and mix the sound using an audio editor software or app. You can also use online tools, such as SoundBible or Audiotool, to generate and customize sound effects.
A: You can change the car unlock sound effect on your device by following these steps:
-A: You can turn off the car unlock sound effect on your device by following these steps:
-A: You can find more information about car unlock sound effects by visiting some of these websites:
-If you are looking for an action-packed game that will keep you on the edge of your seat, you should try Implosion Never Lose Hope. This is a sci-fi game that will take you to a post-apocalyptic world where you have to fight against a mysterious alien threat. In this article, we will tell you what Implosion Never Lose Hope is, why you should play it, how to download it on your Android device, and some tips and tricks for playing it. Let's get started!
-Download ⚹ https://urllie.com/2uNEwU
Implosion Never Lose Hope is a game developed by Rayark International Limited, a company known for creating high-quality games with stunning graphics and soundtracks. Implosion Never Lose Hope is no exception. It is a game that combines elements of hack-and-slash, RPG, and shooter genres. It has a compelling story that takes place 20 years after the fall of Earth, where humanity is on the brink of extinction. You play as a pilot of a War-Mech series III battle suit, which is humanity's last hope against the XADA, a mysterious life form that wants to wipe out all life forms.
-The game features amazing console-quality graphics that will immerse you in the futuristic world of Implosion. The game also has first-class voice acting and Hollywood-grade audio production that will make you feel like you are watching a movie. The game has a full orchestral score composed by John Kurlander, a Grammy Award winner who worked on The Lord of the Rings trilogy. The game has a very intuitive touch user-interface that makes it easy to control your character and unleash devastating attacks.
-There are many reasons why you should play Implosion Never Lose Hope. Here are some of them:
-Implosion Never Lose Hope is a game that will not disappoint you. It is a game that will make you feel like a hero in a sci-fi movie. It is a game that will give you hours of fun and excitement.
-If you want to play Implosion Never Lose Hope on your Android device, you will need to download and install the APK file. This is a file that contains the game's data and allows you to install it without using the Google Play Store. However, you need to be careful when downloading APK files, as some of them may contain viruses or malware that can harm your device. Therefore, you need to follow these steps to download and install Implosion Never Lose Hope APK safely and correctly:
-Implosion - Never Lose Hope APK free download for Android
-How to install Implosion - Never Lose Hope XAPK on Android devices
-Implosion - Never Lose Hope APK latest version 1.5.6
-Implosion - Never Lose Hope game review and features
-Implosion - Never Lose Hope APK + OBB data download
-Implosion - Never Lose Hope APK mod unlimited money
-Implosion - Never Lose Hope APK offline mode
-Implosion - Never Lose Hope APK for PC Windows 10/8/7
-Implosion - Never Lose Hope APK for iOS iPhone/iPad
-Implosion - Never Lose Hope APK for Mac OS X
-Implosion - Never Lose Hope APK for Linux Ubuntu
-Implosion - Never Lose Hope APK for Chromebook
-Implosion - Never Lose Hope APK for Kindle Fire
-Implosion - Never Lose Hope APK for Samsung Galaxy
-Implosion - Never Lose Hope APK for Huawei Mate
-Implosion - Never Lose Hope APK for Xiaomi Mi
-Implosion - Never Lose Hope APK for OnePlus Nord
-Implosion - Never Lose Hope APK for Oppo Reno
-Implosion - Never Lose Hope APK for Vivo V20
-Implosion - Never Lose Hope APK for Realme X7
-Implosion - Never Lose Hope APK for Nokia 8.3
-Implosion - Never Lose Hope APK for Sony Xperia
-Implosion - Never Lose Hope APK for LG Wing
-Implosion - Never Lose Hope APK for Asus Zenfone
-Implosion - Never Lose Hope APK for Motorola Moto
-Implosion - Never Lose Hope APK for Google Pixel
-Implosion - Never Lose Hope APK for Blackberry Key2
-Implosion - Never Lose Hope APK for HTC U12+
-Implosion - Never Lose Hope APK for ZTE Axon 10 Pro
-Implosion - Never Lose Hope APK for Alcatel 3X
-Download and play Implosion - Never Lose Hope on Bluestacks emulator
-Download and play Implosion - Never Lose Hope on NoxPlayer emulator
-Download and play Implosion - Never Lose Hope on LDPlayer emulator
-Download and play Implosion - Never Lose Hope on MEmu emulator
-Download and play Implosion - Never Lose Hope on GameLoop emulator
-Download and play Implosion - Never Lose Hope on Genymotion emulator
-Download and play Implosion - Never Lose Hope on Andy emulator
-Download and play Implosion - Never Lose Hope on Remix OS Player emulator
-Download and play Implosion - Never Lose Hope on Phoenix OS emulator
-Download and play Implosion - Never Lose Hope on PrimeOS emulator
Before you can install any APK file on your device, you need to enable the option to allow unknown sources. This means that you can install apps from sources other than the Google Play Store. To do this, follow these steps:
-Now you have enabled unknown sources on your device, and you can proceed to the next step.
-Now you need to find and download the APK file for Implosion Never Lose Hope. You can do this by using your device's browser and searching for a reliable source that offers the APK file. However, you need to be careful when choosing a source, as some of them may offer fake or corrupted files that can damage your device or steal your data. Therefore, we recommend using this link to download the APK file for Implosion Never Lose Hope. This is a trusted source that has been verified by many users and has positive reviews.
-To download the APK file, follow these steps:
-Now you have downloaded the APK file for Implosion Never Lose Hope, and you can proceed to the next step.
-Now you need to locate and install the APK file on your device. To do this, follow these steps:
-Now you have installed Implosion Never Lose Hope on your device, and you can proceed to the final step.
-Now you are ready to launch the game and enjoy it. To do this, follow these steps:
-Implosion Never Lose Hope is a game that will test your skills and reflexes. It is not an easy game, but it is not impossible either. If you want to improve your performance and have more fun, you can follow these tips and tricks:
-In Implosion Never Lose Hope, you can choose from two different suits: the Crimson or the Avalon. Each suit has its own advantages and disadvantages, and you should choose the one that suits your playstyle and preferences. Here are some differences between them:
-Suit | -Advantages | -Disadvantages | -
---|---|---|
Crimson | -- More agile and faster - Has a longer combo chain - Has a powerful special move that can deal massive damage to enemies |
-- Less durable and more vulnerable to attacks - Has a shorter range of attack - Has a slower energy recovery rate |
-
Avalon | -- More sturdy and resilient - Has a longer range of attack - Has a faster energy recovery rate |
-- Less mobile and slower - Has a shorter combo chain - Has a weaker special move that can only stun enemies |
-
You can also unlock a third suit, the Raven, by completing certain achievements. The Raven is a balanced suit that has both offensive and defensive capabilities. You can switch between suits at any time by going to the suit selection screen.
-In Implosion Never Lose Hope, you can upgrade your weapons and abilities using the ARK Kernel system. The ARK Kernel is a currency that you can collect by defeating enemies, completing levels, or finding hidden items. You can use the ARK Kernel to enhance your suit's stats, such as health, attack, defense, speed, and energy. You can also use it to unlock new weapons, such as swords, guns, or shields. You can also use it to unlock new abilities, such as dash, dodge, or parry. You can access the ARK Kernel system by going to the upgrade screen.
-Implosion Never Lose Hope has a very intuitive and responsive combat system that allows you to unleash devastating attacks on your enemies. You can use the touch controls to move, attack, dodge, or use special moves. You can also use the combos and skills to deal more damage and gain more energy. Here are some tips on how to master the combat system:
-Implosion Never Lose Hope has many levels that are full of secrets, bonuses, and collectibles. You should explore them thoroughly and find them all. They will help you in your quest and reward you with extra ARK Kernel, badges, trophies, or achievements. Here are some tips on how to explore the levels and collect items:
-Implosion Never Lose Hope has different modes that you can play to test your skills and have more fun. You can unlock these modes by completing certain levels or achievements. Here are some of the modes that you can play:
-These modes will give you more variety and challenge in the game. They will also reward you with more ARK Kernel, badges, trophies, or achievements.
-Implosion Never Lose Hope is a game that you should not miss if you are a fan of action, sci-fi, or RPG games. It is a game that will take you to a post-apocalyptic world where you have to fight against a mysterious alien threat. It is a game that has amazing graphics, soundtracks, and voice acting. It is a game that has an engaging gameplay, a captivating story, and a lot of replay value. It is a game that you can download and install on your Android device using the APK file.
-In this article, we have told you what Implosion Never Lose Hope is, why you should play it, how to download it on your Android device, and some tips and tricks for playing it. We hope that this article has been helpful and informative for you. Now go ahead and download Implosion Never Lose Hope APK and enjoy playing this awesome game!
-Here are some frequently asked questions and answers about Implosion Never Lose Hope:
-If you are looking for a simulation game that will make you feel happy and relaxed, you should try The Sun Shines Over Us. This is a game that tells the story of a girl who lost her memory and a boy who wants to help her recover it. Along the way, they will meet different people and experience various events that will shape their lives. The game has beautiful graphics, soothing music, and engaging dialogues that will keep you hooked.
-The Sun Shines Over Us is set in a small town called Sunnyville, where everyone lives peacefully and happily. The main character is a girl named Sunny, who wakes up one day without any memories of her past. She is found by a boy named Ray, who decides to take care of her and help her find her lost memories. Together, they will explore the town and interact with various characters, such as Lily, the cheerful florist; Leo, the cool musician; Mia, the shy baker; and many more. Each character has their own personality, backstory, and relationship with Sunny and Ray. As you play the game, you will discover more about them and their secrets.
-DOWNLOAD ⏩ https://urllie.com/2uNEPt
The Sun Shines Over Us is a simulation game that lets you control Sunny's actions and choices. You can customize her appearance, clothes, accessories, and hairstyle. You can also choose how to spend your time in the town, whether you want to work, study, shop, or hang out with your friends. You can also develop your relationships with different characters by talking to them, giving them gifts, or going on dates with them. Depending on your choices, you will unlock different endings and scenarios for each character.
-The game also has many features that make it more enjoyable and immersive. For example, you can collect various items and decorations for your room. You can also play mini-games such as fishing, cooking, gardening, or painting. You can also listen to the original soundtrack of the game or change it to your own music. You can also take screenshots of your favorite moments and share them with your friends.
-While The Sun Shines Over Us is a free game to download and play, it also has some in-app purchases that require real money. For example, you need to buy tickets to unlock some episodes or scenes in the game. You also need to buy coins to buy some items or gifts for your friends. However, if you download The Sun Shines Over Us APK Mod from [The Sun Shines Over Us 12.0 MOD APK Menu/Unlimited money, tickets](^1^), you can enjoy these benefits:
-To download and install The Sun Shines Over Us APK Mod on your Android device, you need to follow these steps:
-The Sun Shines Over Us is a simulation game that will make you feel warm and fuzzy inside. It has a heartwarming story, charming characters, and relaxing gameplay. You can customize your own character, make friends, fall in love, and discover the secrets of Sunnyville. You can also enjoy the mod version of the game that gives you unlimited resources and access to everything in the game. If you are looking for a game that will brighten up your day, you should download The Sun Shines Over Us APK Mod today.
-Here are some frequently asked questions about The Sun Shines Over Us APK Mod:
-Question | Answer |
Is The Sun Shines Over Us APK Mod safe to download and install? | Yes, The Sun Shines Over Us APK Mod is safe to download and install. It does not contain any viruses or malware. However, you should always download it from a trusted source like [The Sun Shines Over Us 12.0 MOD APK Menu/Unlimited money, tickets]. |
Do I need to root my device to use The Sun Shines Over Us APK Mod? | No, you do not need to root your device to use The Sun Shines Over Us APK Mod. You can install it on any Android device that meets the minimum requirements of the game. |
Can I play The Sun Shines Over Us APK Mod offline? | Yes, you can play The Sun Shines Over Us APK Mod offline. You do not need an internet connection to play the game. However, some features may not work properly without an internet connection, such as sharing screenshots or listening to your own music. |
Can I update The Sun Shines Over Us APK Mod? | Yes, you can update The Sun Shines Over Us APK Mod whenever there is a new version available. However, you should always backup your data before updating the game, as some updates may cause compatibility issues or data loss. |
Can I play The Sun Shines Over Us APK Mod with my friends? | Yes, you can play The Sun Shines Over Us APK Mod with your friends. You can connect with them through social media platforms such as Facebook or Twitter. You can also chat with them in the game and send them gifts or invitations. |
the sun shines over us mod apk unlimited money
-the sun shines over us mod apk download free
-the sun shines over us mod apk latest version
-the sun shines over us mod apk android 1
-the sun shines over us mod apk 2023
-the sun shines over us mod apk menu
-the sun shines over us mod apk unlimited tickets
-the sun shines over us mod apk hack
-the sun shines over us mod apk offline
-the sun shines over us mod apk no ads
-the sun shines over us simulation game mod apk
-the sun shines over us mod apk premium
-the sun shines over us mod apk unlocked
-the sun shines over us mod apk full version
-the sun shines over us mod apk revdl
-the sun shines over us mod apk rexdl
-the sun shines over us mod apk happymod
-the sun shines over us mod apk an1
-the sun shines over us mod apk obb
-the sun shines over us mod apk data
-the sun shines over us mod apk cheat
-the sun shines over us mod apk vip
-the sun shines over us mod apk pro
-the sun shines over us mod apk mega
-the sun shines over us mod apk god mode
-the sun shines over us mod apk all unlocked
-the sun shines over us mod apk free shopping
-the sun shines over us mod apk unlimited everything
-the sun shines over us mod apk high damage
-the sun shines over us mod apk one hit kill
-the sun shines over us mod apk unlimited gems
-the sun shines over us mod apk unlimited coins
-the sun shines over us mod apk unlimited diamonds
-the sun shines over us mod apk unlimited lives
-the sun shines over us mod apk unlimited energy
-the sun shines over us mod apk unlimited stars
-the sun shines over us mod apk unlimited hearts
-the sun shines over us mod apk unlimited keys
-the sun shines over us mod apk unlimited gold
-the sun shines over us mod apk unlimited cash
Are you a fan of Rihanna and her catchy songs? Do you want to listen to her hit single If It's Lovin' That You Want anytime, anywhere? If so, then you might be interested in downloading the MP3 version of the song. In this article, we will show you how to do that in two easy ways. But first, let's find out more about the song and why you should download it.
-If It's Lovin' That You Want is a song by Barbadian singer Rihanna from her debut studio album, Music of the Sun (2005). It was written by Samuel Barnes, Scott La Rock, Makeba Riddick, Jean-Claude Oliver, Lawrence Parker, and produced by Poke & Tone. It was released on August 16, 2005, as the second and final single from the album.
-DOWNLOAD >>> https://urllie.com/2uNxCi
The song is a flirtatious track that follows Rihanna as she tries to convince a guy to be with her. She promises him that she can give him everything he needs, from love to friendship. The song has a reggae-pop vibe and features a sample from "No Guns, No Murder" by Rayvon. The song received positive reviews from critics, who praised Rihanna's vocals and the catchy chorus. The song also achieved moderate success on the charts, reaching the top ten in several countries, including Australia, Ireland, New Zealand, and the UK.
-If you love this song and want to listen to it on repeat, then downloading the MP3 version is a good idea. Here are some benefits of downloading If It's Lovin' That You Want MP3:
-Now that you know why you should download If It's Lovin' That You Want MP3, let's see how you can do that in two easy ways.
-One of the easiest ways to download If It's Lovin' That You Want MP3 is to use YouTube. YouTube is a popular video-sharing platform that hosts millions of videos, including music videos. You can find the official music video of If It's Lovin' That You Want on Rihanna's YouTube channel. Here are the steps to download If It's Lovin' That You Want MP3 from YouTube:
- -Open your web browser and go to YouTube.com. In the search box, type "Rihanna If It's Lovin' That You Want" and hit enter. You will see a list of videos related to your search. Look for the official music video of the song, which has a thumbnail of Rihanna in a blue dress and a beach background. It should have the title "Rihanna - If It's Lovin' That You Want (Official Music Video)" and the channel name "Rihanna". Click on the video to open it.
-Once the video is playing, look at the address bar of your browser. You will see a URL that starts with "https://www.youtube.com/watch?v=". This is the link to the video. Select the entire URL and copy it to your clipboard. You can use the keyboard shortcut Ctrl+C (Windows) or Command+C (Mac) to copy it.
-Now that you have the URL of the video, you need to convert it to an MP3 file. To do that, you need to use a YouTube to MP3 converter website. There are many websites that offer this service for free, but some of them may have ads, pop-ups, or malware. To avoid that, we recommend using a reliable and safe website like ytmp3.cc. This website is easy to use and has no annoying ads or viruses. Go to ytmp3.cc in a new tab or window of your browser.
-On the homepage of ytmp3.cc, you will see a box that says "Paste your YouTube link here". Paste the URL that you copied in step 2 into this box. You can use the keyboard shortcut Ctrl+V (Windows) or Command+V (Mac) to paste it. Then, click on the button that says "Convert". The website will start processing your video and converting it to an MP3 file.
-After a few seconds, you will see a message that says "Conversion finished". Below that, you will see a button that says "Download". Click on this button to download the MP3 file of If It's Lovin' That You Want to your device. You may also see another button that says "Save to Dropbox". If you have a Dropbox account and want to save the file there, you can click on this button instead. Once the download is complete, you can open the file and listen to the song. Enjoy!
-Another way to download If It's Lovin' That You Want MP3 is to use Spotify. Spotify is a popular music streaming service that offers millions of songs, podcasts, and playlists. You can find If It's Lovin' That You Want on Rihanna's Music of the Sun album on Spotify. Here are the steps to download If It's Lovin' That You Want MP3 from Spotify:
-To use Spotify, you need to download and install it on your device. Spotify is available for Windows, Mac, iOS, Android, and other platforms. You can download it from Spotify.com or from your device's app store. Follow the instructions on how to install and launch Spotify on your device.
-To access Spotify's features, you need to sign up or log in to Spotify. You can sign up for free with your email address or Facebook account. However, if you want to download songs for offline listening, you need to upgrade to Spotify Premium, which costs $9.99 per month (or less if you are eligible for a student or family plan). You can sign up or log in to Spotify from Spotify.com or from the app.
-Once you are signed in to Spotify, you can search for If It's Lovin' That You Want by typing it in the search box at the top of the app or website. You will see a list of results related to your search. Look for the song under the Songs section and click on it to open it. You will see the song's details, such as the artist, album, duration, and popularity. To download the song, you need to add it to your playlist. You can create a new playlist or add it to an existing one. To create a new playlist, click on the three dots icon next to the song and select "Add to playlist". Then, click on "New playlist" and give it a name. To add it to an existing playlist, click on the three dots icon next to the song and select "Add to playlist". Then, choose the playlist you want from the list.
-After you have added the song to your playlist, you can download the playlist for offline listening. To do that, go to your library and find the playlist you want. Then, click on the download button next to the playlist name. It looks like a downward arrow with a line under it. The button will turn green when the download is complete. You can also see the progress of the download by looking at the bar under the playlist name.
-Once you have downloaded the playlist, you can enjoy If It's Lovin' That You Want anytime, anywhere. You can access your downloaded playlists from your library or from the home screen of Spotify. You can also see a green icon next to the songs that are downloaded. To listen to the song offline, you need to switch to offline mode in Spotify. To do that, go to settings and toggle on "Offline mode". This will prevent Spotify from using any internet connection and only play your downloaded songs.
-In this article, we have shown you how to download Rihanna's If It's Lovin' That You Want MP3 in two easy ways. You can use YouTube or Spotify to get the MP3 version of the song and listen to it offline. Both methods are simple and fast, but they have some differences. YouTube is free but requires a third-party website to convert the video to MP3. Spotify is not free but offers high-quality sound and other features like podcasts and playlists.
-Now that you know how to download If It's Lovin' That You Want MP3, what are you waiting for? Go ahead and try one of these methods and enjoy this catchy song by Rihanna. You can also check out her other songs and albums on YouTube or Spotify and discover more of her music. Rihanna is one of the most popular and talented singers in the world, and her songs are always worth listening to.
-If you liked this article, please share it with your friends and leave us a comment below. We would love to hear your feedback and suggestions for future articles. Thank you for reading!
-Here are some common questions that people have about downloading If It's Lovin' That You Want MP3:
-It depends on your country's laws and regulations regarding copyright infringement and fair use. Generally speaking, downloading music from YouTube or Spotify for personal use is not illegal, as long as you do not distribute, sell, or profit from it. However, downloading music from these platforms may violate their terms of service, which could result in your account being suspended or terminated. Therefore, we advise you to use these methods at your own risk and discretion.
-There are many other ways to download If It's Lovin' That You Want MP3, such as using other music streaming services, online music stores, torrent sites, or file-sharing apps. However, some of these methods may not be safe, legal, or reliable. They may also have poor sound quality, malware, or viruses. Therefore, we recommend you to use the methods we have shown you in this article, as they are the easiest and safest ways to download If It's Lovin' That You Want MP3.
-Once you have downloaded If It's Lovin' That You Want MP3 to your device, you can play it using any media player that supports MP3 format. For example, you can use Windows Media Player, iTunes, VLC, or Groove Music on your computer. You can also use the default music player on your smartphone or tablet. Alternatively, you can transfer the MP3 file to a USB drive, SD card, or CD and play it on any compatible device.
-If you want to edit If It's Lovin' That You Want MP3, such as cutting, trimming, merging, or adding effects to it, you need to use an audio editing software. There are many audio editing software that you can download for free or for a fee, such as Audacity, WavePad, Adobe Audition, or GarageBand. You can use these software to open the MP3 file and make any changes you want. Then, you can save the edited file as a new MP3 file or in another format.
-If you want to make a ringtone from If It's Lovin' That You Want MP3, you need to use a ringtone maker app or website. There are many ringtone maker apps and websites that you can download or access for free or for a fee, such as Zedge, Ringtone Maker, Audiko, or Online Audio Cutter. You can use these apps or websites to upload the MP3 file and select the part of the song that you want as your ringtone. Then, you can download the ringtone file and set it as your phone's ringtone.
197e85843dIf you are a fan of block style games, you might have heard of Blockman Go, a free app that offers various minigames, a social platform, and a sandbox game. But did you know that there is a way to get unlimited money and gcubes in Blockman Go? Yes, you heard it right. With Blockman Go Mod Apk, you can enjoy all the features and benefits of the game without spending a dime. In this article, we will tell you everything you need to know about Blockman Go Mod Apk, including what it is, how to download and install it, what are its features and benefits, and what are some tips and tricks to play it. So, without further ado, let's get started.
-Download File ☑ https://urllie.com/2uNIPe
Blockman Go is a free app that was released by Blockman GO Studio in 2017. It is a game that provides a mobile gaming platform where you can play various block style minigames with other players from all over the world. Some of the popular minigames include Bed Wars, Egg War, Sky War, Survival Games, Build Battle, etc. You can join any game by a simple tap and have fun with your friends or strangers.
-Blockman Go is also a social platform where you can chat and make friends with other players in the game. You can customize your avatar with different clothes and accessories, and show off your unique style. You can also join communities and participate in events. You can earn gold by playing minigames, which you can use to buy more items for your avatar.
-Blockman Go is also a sandbox game where you can create and share your own experiences with other players. You can use blocks to build anything you want, from houses to castles, from islands to cities. You can also explore different virtual worlds created by other players or by the game itself. You can unleash your creativity and imagination in Blockman Go.
-Blockman Go Mod Apk is a modified version of the original game that gives you unlimited money and gcubes in Blockman Go. Money and gcubes are the two main currencies in the game that you need to buy items, unlock minigames, upgrade your avatar, etc. However, earning money and gcubes in the game can be time-consuming and tedious. That's why some players look for ways to get them for free.
-blockman go hack apk unlimited gcubes and money 2023
-blockman go modded apk with infinite money and gcubes 2023
-blockman go cheat apk unlimited gcubes and money 2023
-blockman go premium apk free money and gcubes 2023
-blockman go cracked apk unlimited money and gcubes 2023
-blockman go mod apk download unlimited gcubes and money 2023
-blockman go hack mod apk free money and gcubes 2023
-blockman go modded apk download infinite money and gcubes 2023
-blockman go cheat mod apk free gcubes and money 2023
-blockman go premium mod apk unlimited money and gcubes 2023
-blockman go cracked mod apk free gcubes and money 2023
-blockman go unlimited money and gcubes mod apk 2023
-blockman go infinite money and gcubes modded apk 2023
-blockman go free money and gcubes cheat apk 2023
-blockman go unlimited money and gcubes premium apk 2023
-blockman go free money and gcubes cracked apk 2023
-blockman go unlimited money and gcubes mod apk download 2023
-blockman go infinite money and gcubes modded apk download 2023
-blockman go free money and gcubes cheat apk download 2023
-blockman go unlimited money and gcubes premium apk download 2023
-blockman go free money and gcubes cracked apk download 2023
-download blockman go mod apk unlimited money and gcubes 2023
-download blockman go modded apk infinite money and gcubes 2023
-download blockman go cheat apk free money and gcubes 2023
-download blockman go premium apk unlimited money and gcubes 2023
-download blockman go cracked apk free money and gcubes 2023
-download blockman go hack mod apk unlimited gcubes and money 2023
-download blockman go modded hack apk infinite money and gcubes 2023
-download blockman go cheat hack apk free money and gcubes 2023
-download blockman go premium hack apk unlimited money and gcubes 2023
-download blockman go cracked hack apk free money and gcubes 2023
-how to get unlimited money and gcubes in blockman go mod apk 2023
-how to get infinite money and gcubes in blockman go modded apk 2023
-how to get free money and gcubes in blockman go cheat apk 2023
-how to get unlimited money and gcubes in blockman go premium apk 2023
-how to get free money and gcubes in blockman go cracked apk 2023
-how to install blockman go mod apk unlimited money and gcubes 2023
-how to install blockman go modded apk infinite money and gcubes 2023
-how to install blockman go cheat apk free money and gcubes 2023
-how to install blockman go premium apk unlimited money and gcubes 2023
-how to install blockman go cracked apk free money and gcubes 2023
-best site to download blockman go mod apk unlimited money and gcubes 2023
-best site to download blockman go modded apk infinite money and gcubes 2023
-best site to download blockman go cheat apk free money and gcubes 2023
-best site to download blockman go premium apk unlimited money and gcubes 2023
-best site to download blockman go cracked apk free money and gcubes 2023
-latest version of blockman go mod apk unlimited money and gcubes 2023
-latest version of blockman go modded apk infinite money and gcubes 2023
-latest version of blockman go cheat apk free money and gcubes 2023
With Blockman Go Mod Apk, you don't
With Blockman Go Mod Apk, you don't have to worry about running out of money and gcubes. You can get unlimited amounts of them by using the mod apk file. You can use them to buy anything you want in the game, such as clothes, accessories, weapons, vehicles, pets, etc. You can also unlock all the minigames and virtual worlds in the game, and enjoy them without any restrictions. You can also access the premium features of the game, such as VIP membership, exclusive items, etc.
-Blockman Go Mod Apk is a way to enjoy the game without spending any real money. It is a way to enhance your gaming experience and have more fun with Blockman Go. However, it is also a way to cheat and hack the game, which may not be fair to other players and may violate the terms and conditions of the game. Therefore, you should use Blockman Go Mod Apk at your own risk and responsibility.
-If you want to try out Blockman Go Mod Apk, you need to download and install it on your device. Here are the steps to do so:
-Before installing Blockman Go Mod Apk, you should take some precautions to avoid any problems or issues. For example, you should backup your original game data in case something goes wrong or you want to switch back to the original game. You should also uninstall any previous versions of Blockman Go before installing the mod apk file. You should also be careful not to update the game from the Google Play Store or any other sources, as this may overwrite the mod apk file and remove its features.
-Blockman Go Mod Apk has many features and benefits that make it different from the original game. Here are some of them:
-Blockman Go Mod Apk is a fun and exciting game that you can play with your friends or strangers online. However, if you want to improve your skills and have more fun with Blockman Go Mod Apk, here are some tips and
Blockman Go Mod Apk is a fun and exciting game that you can play with your friends or strangers online. However, if you want to improve your skills and have more fun with Blockman Go Mod Apk, here are some tips and tricks that you can follow:
-Blockman Go is a free app that offers various block style minigames, a social platform, and a sandbox game for mobile gamers. Blockman Go Mod Apk is a modified version of the original game that gives you unlimited money and gcubes in Blockman Go. With Blockman Go Mod Apk, you can enjoy all the features and benefits of the game without spending any real money.
-If you want to try out Blockman Go Mod Apk, you need to download and install it on your device following the steps we mentioned above. However, you should also be aware of the risks and responsibilities of using Blockman Go Mod Apk, as it may not be fair to other players and may violate the terms and conditions of the game.
-We hope this article has helped you learn more
We hope this article has helped you learn more about Blockman Go Mod Apk and how to use it. If you are interested in playing Blockman Go with unlimited money and gcubes, you can download and install Blockman Go Mod Apk from the link below. However, remember to use it at your own risk and responsibility, and respect other players and the game itself.
-Thank you for reading this article. We hope you have a great time playing Blockman Go Mod Apk. If you have any questions or feedback, please feel free to leave a comment below. We would love to hear from you.
-Here are some frequently asked questions about Blockman Go Mod Apk that you may find useful:
-Question | -Answer | -
---|---|
Is Blockman Go Mod Apk safe to use? | -Blockman Go Mod Apk is not an official version of the game, and it may contain viruses or malware that can harm your device or steal your personal information. Therefore, you should only download Blockman Go Mod Apk from a reliable source, and scan it with an antivirus software before installing it. You should also backup your original game data and device data before using Blockman Go Mod Apk. | -
Is Blockman Go Mod Apk legal to use? | -Blockman Go Mod Apk is not legal to use, as it violates the terms and conditions of the game and the intellectual property rights of the game developers. Using Blockman Go Mod Apk may result in your account being banned or suspended, or legal actions being taken against you. Therefore, you should use Blockman Go Mod Apk at your own risk and responsibility. | -
Does Blockman Go Mod Apk work on all devices? | -Blockman Go Mod Apk may not work on all devices, as it may depend on the device model, operating system, compatibility, etc. Therefore, you should check the requirements and specifications of Blockman Go Mod Apk before downloading and installing it. You should also update your device software and drivers to ensure optimal performance. | -
How to update Blockman Go Mod Apk? | -Blockman Go Mod Apk may not be updated automatically, as it is not connected to the Google Play Store or any other sources. Therefore, you should check for updates manually from the website where you downloaded Blockman Go Mod Apk. However, you should be careful not to update the game from the Google Play Store or any other sources, as this may overwrite the mod apk file and remove its features. | -
How to uninstall Blockman Go Mod Apk? | -If you want to uninstall Blockman Go Mod Apk, you can do so by following the same steps as uninstalling any other app on your device. You can go to your device settings, then apps, then Blockman Go, then uninstall. You can also delete the mod apk file from your device storage. However, you should make sure to restore your original game data and device data before uninstalling Blockman Go Mod Apk. | -
Este experimento pretende poner a prueba la capacidad de un modelo de inteligencia artificial para generar texto a partir de patrones observados en la hechicería del lenguaje poético en español. -Algo fascinante de lo que Borges habla en una conferencia de su Arte Poética: "Uso la palabra «modelo» porque las metáforas que voy a citar, aunque parezcan muy distintas a la imaginación, para un lógico serían casi idénticas. Así que podríamos hablar de ellas como ecuaciones." -
-Contacto: Jorge Henao 🇨🇴 Twitter LinkedIn -
-""" -article = """ --
-
👉 Don't miss this other cool space based on different styles of poetry in spanish: poem-generation-es 👈
-""" -examples = [ - ['al despertar el dinosaurio todavía estaba ahí', 20 ], - ['La inmensidad de tu imaginación', 140], - ['con ansias de amores inflamada', 140] -] - -iface = gr.Interface(fn=make_new_sonnet, - title= "Generador de poesía basada en sonetos en español", - description = description, - inputs=[ - gr.inputs.Textbox(lines=2, placeholder="Escrbe algo para comenzar", label='Escribe algo para comenzar'), - gr.inputs.Slider(minimum = 20, maximum = 200, default = 140, step = 5, label='Salida máxima de caracteres')], - outputs=[ - gr.outputs.Textbox(label="Tu poema"), - ], - article= article, - examples = examples, - theme = 'peach' - ) -iface.launch(enable_queue=True) \ No newline at end of file diff --git a/spaces/hackathon-somos-nlp-2023/SalpiBloomZ-1b7-v1/README.md b/spaces/hackathon-somos-nlp-2023/SalpiBloomZ-1b7-v1/README.md deleted file mode 100644 index 84fd4f0a3387d5497ba779ca4872d199d5c53c9c..0000000000000000000000000000000000000000 --- a/spaces/hackathon-somos-nlp-2023/SalpiBloomZ-1b7-v1/README.md +++ /dev/null @@ -1,16 +0,0 @@ ---- -title: SalpiBloomZ 1b7 V1 -emoji: 🐢 -colorFrom: indigo -colorTo: green -sdk: gradio -sdk_version: 3.24.1 -app_file: app.py -pinned: false ---- - -## Motivación - -El presente trabajo se basa en la idea de entrenar modelos de procesamiento natural del lenguaje usando LLMs en base a datasets especializados. - -Check out the configuration reference at https://huggingface.co/docs/hub/spaces-config-reference diff --git a/spaces/hands012/gpt-academic/request_llm/edge_gpt.py b/spaces/hands012/gpt-academic/request_llm/edge_gpt.py deleted file mode 100644 index bbf84000d84a42de80d3c051a24f06336af76aaf..0000000000000000000000000000000000000000 --- a/spaces/hands012/gpt-academic/request_llm/edge_gpt.py +++ /dev/null @@ -1,409 +0,0 @@ -""" -======================================================================== -第一部分:来自EdgeGPT.py -https://github.com/acheong08/EdgeGPT -======================================================================== -""" - -import argparse -import asyncio -import json -import os -import random -import re -import ssl -import sys -import uuid -from enum import Enum -from typing import Generator -from typing import Literal -from typing import Optional -from typing import Union -import websockets.client as websockets - -DELIMITER = "\x1e" - - -# Generate random IP between range 13.104.0.0/14 -FORWARDED_IP = ( - f"13.{random.randint(104, 107)}.{random.randint(0, 255)}.{random.randint(0, 255)}" -) - -HEADERS = { - "accept": "application/json", - "accept-language": "en-US,en;q=0.9", - "content-type": "application/json", - "sec-ch-ua": '"Not_A Brand";v="99", "Microsoft Edge";v="110", "Chromium";v="110"', - "sec-ch-ua-arch": '"x86"', - "sec-ch-ua-bitness": '"64"', - "sec-ch-ua-full-version": '"109.0.1518.78"', - "sec-ch-ua-full-version-list": '"Chromium";v="110.0.5481.192", "Not A(Brand";v="24.0.0.0", "Microsoft Edge";v="110.0.1587.69"', - "sec-ch-ua-mobile": "?0", - "sec-ch-ua-model": "", - "sec-ch-ua-platform": '"Windows"', - "sec-ch-ua-platform-version": '"15.0.0"', - "sec-fetch-dest": "empty", - "sec-fetch-mode": "cors", - "sec-fetch-site": "same-origin", - "x-ms-client-request-id": str(uuid.uuid4()), - "x-ms-useragent": "azsdk-js-api-client-factory/1.0.0-beta.1 core-rest-pipeline/1.10.0 OS/Win32", - "Referer": "https://www.bing.com/search?q=Bing+AI&showconv=1&FORM=hpcodx", - "Referrer-Policy": "origin-when-cross-origin", - "x-forwarded-for": FORWARDED_IP, -} - -HEADERS_INIT_CONVER = { - "authority": "edgeservices.bing.com", - "accept": "text/html,application/xhtml+xml,application/xml;q=0.9,image/webp,image/apng,*/*;q=0.8,application/signed-exchange;v=b3;q=0.7", - "accept-language": "en-US,en;q=0.9", - "cache-control": "max-age=0", - "sec-ch-ua": '"Chromium";v="110", "Not A(Brand";v="24", "Microsoft Edge";v="110"', - "sec-ch-ua-arch": '"x86"', - "sec-ch-ua-bitness": '"64"', - "sec-ch-ua-full-version": '"110.0.1587.69"', - "sec-ch-ua-full-version-list": '"Chromium";v="110.0.5481.192", "Not A(Brand";v="24.0.0.0", "Microsoft Edge";v="110.0.1587.69"', - "sec-ch-ua-mobile": "?0", - "sec-ch-ua-model": '""', - "sec-ch-ua-platform": '"Windows"', - "sec-ch-ua-platform-version": '"15.0.0"', - "sec-fetch-dest": "document", - "sec-fetch-mode": "navigate", - "sec-fetch-site": "none", - "sec-fetch-user": "?1", - "upgrade-insecure-requests": "1", - "user-agent": "Mozilla/5.0 (Windows NT 10.0; Win64; x64) AppleWebKit/537.36 (KHTML, like Gecko) Chrome/110.0.0.0 Safari/537.36 Edg/110.0.1587.69", - "x-edge-shopping-flag": "1", - "x-forwarded-for": FORWARDED_IP, -} - -def get_ssl_context(): - import certifi - ssl_context = ssl.create_default_context() - ssl_context.load_verify_locations(certifi.where()) - return ssl_context - - - -class NotAllowedToAccess(Exception): - pass - - -class ConversationStyle(Enum): - creative = "h3imaginative,clgalileo,gencontentv3" - balanced = "galileo" - precise = "h3precise,clgalileo" - - -CONVERSATION_STYLE_TYPE = Optional[ - Union[ConversationStyle, Literal["creative", "balanced", "precise"]] -] - - -def _append_identifier(msg: dict) -> str: - """ - Appends special character to end of message to identify end of message - """ - # Convert dict to json string - return json.dumps(msg) + DELIMITER - - -def _get_ran_hex(length: int = 32) -> str: - """ - Returns random hex string - """ - return "".join(random.choice("0123456789abcdef") for _ in range(length)) - - -class _ChatHubRequest: - """ - Request object for ChatHub - """ - - def __init__( - self, - conversation_signature: str, - client_id: str, - conversation_id: str, - invocation_id: int = 0, - ) -> None: - self.struct: dict = {} - - self.client_id: str = client_id - self.conversation_id: str = conversation_id - self.conversation_signature: str = conversation_signature - self.invocation_id: int = invocation_id - - def update( - self, - prompt, - conversation_style, - options, - ) -> None: - """ - Updates request object - """ - if options is None: - options = [ - "deepleo", - "enable_debug_commands", - "disable_emoji_spoken_text", - "enablemm", - ] - if conversation_style: - if not isinstance(conversation_style, ConversationStyle): - conversation_style = getattr(ConversationStyle, conversation_style) - options = [ - "nlu_direct_response_filter", - "deepleo", - "disable_emoji_spoken_text", - "responsible_ai_policy_235", - "enablemm", - conversation_style.value, - "dtappid", - "cricinfo", - "cricinfov2", - "dv3sugg", - ] - self.struct = { - "arguments": [ - { - "source": "cib", - "optionsSets": options, - "sliceIds": [ - "222dtappid", - "225cricinfo", - "224locals0", - ], - "traceId": _get_ran_hex(32), - "isStartOfSession": self.invocation_id == 0, - "message": { - "author": "user", - "inputMethod": "Keyboard", - "text": prompt, - "messageType": "Chat", - }, - "conversationSignature": self.conversation_signature, - "participant": { - "id": self.client_id, - }, - "conversationId": self.conversation_id, - }, - ], - "invocationId": str(self.invocation_id), - "target": "chat", - "type": 4, - } - self.invocation_id += 1 - - -class _Conversation: - """ - Conversation API - """ - - def __init__( - self, - cookies, - proxy, - ) -> None: - self.struct: dict = { - "conversationId": None, - "clientId": None, - "conversationSignature": None, - "result": {"value": "Success", "message": None}, - } - import httpx - self.proxy = proxy - proxy = ( - proxy - or os.environ.get("all_proxy") - or os.environ.get("ALL_PROXY") - or os.environ.get("https_proxy") - or os.environ.get("HTTPS_PROXY") - or None - ) - if proxy is not None and proxy.startswith("socks5h://"): - proxy = "socks5://" + proxy[len("socks5h://") :] - self.session = httpx.Client( - proxies=proxy, - timeout=30, - headers=HEADERS_INIT_CONVER, - ) - for cookie in cookies: - self.session.cookies.set(cookie["name"], cookie["value"]) - - # Send GET request - response = self.session.get( - url=os.environ.get("BING_PROXY_URL") - or "https://edgeservices.bing.com/edgesvc/turing/conversation/create", - ) - if response.status_code != 200: - response = self.session.get( - "https://edge.churchless.tech/edgesvc/turing/conversation/create", - ) - if response.status_code != 200: - print(f"Status code: {response.status_code}") - print(response.text) - print(response.url) - raise Exception("Authentication failed") - try: - self.struct = response.json() - except (json.decoder.JSONDecodeError, NotAllowedToAccess) as exc: - raise Exception( - "Authentication failed. You have not been accepted into the beta.", - ) from exc - if self.struct["result"]["value"] == "UnauthorizedRequest": - raise NotAllowedToAccess(self.struct["result"]["message"]) - - -class _ChatHub: - """ - Chat API - """ - - def __init__(self, conversation) -> None: - self.wss = None - self.request: _ChatHubRequest - self.loop: bool - self.task: asyncio.Task - print(conversation.struct) - self.request = _ChatHubRequest( - conversation_signature=conversation.struct["conversationSignature"], - client_id=conversation.struct["clientId"], - conversation_id=conversation.struct["conversationId"], - ) - - async def ask_stream( - self, - prompt: str, - wss_link: str, - conversation_style: CONVERSATION_STYLE_TYPE = None, - raw: bool = False, - options: dict = None, - ) -> Generator[str, None, None]: - """ - Ask a question to the bot - """ - if self.wss and not self.wss.closed: - await self.wss.close() - # Check if websocket is closed - self.wss = await websockets.connect( - wss_link, - extra_headers=HEADERS, - max_size=None, - ssl=get_ssl_context() - ) - await self._initial_handshake() - # Construct a ChatHub request - self.request.update( - prompt=prompt, - conversation_style=conversation_style, - options=options, - ) - # Send request - await self.wss.send(_append_identifier(self.request.struct)) - final = False - while not final: - objects = str(await self.wss.recv()).split(DELIMITER) - for obj in objects: - if obj is None or not obj: - continue - response = json.loads(obj) - if response.get("type") != 2 and raw: - yield False, response - elif response.get("type") == 1 and response["arguments"][0].get( - "messages", - ): - resp_txt = response["arguments"][0]["messages"][0]["adaptiveCards"][ - 0 - ]["body"][0].get("text") - yield False, resp_txt - elif response.get("type") == 2: - final = True - yield True, response - - async def _initial_handshake(self) -> None: - await self.wss.send(_append_identifier({"protocol": "json", "version": 1})) - await self.wss.recv() - - async def close(self) -> None: - """ - Close the connection - """ - if self.wss and not self.wss.closed: - await self.wss.close() - - -class NewbingChatbot: - """ - Combines everything to make it seamless - """ - - def __init__( - self, - cookies, - proxy - ) -> None: - if cookies is None: - cookies = {} - self.cookies = cookies - self.proxy = proxy - self.chat_hub: _ChatHub = _ChatHub( - _Conversation(self.cookies, self.proxy), - ) - - async def ask( - self, - prompt: str, - wss_link: str, - conversation_style: CONVERSATION_STYLE_TYPE = None, - options: dict = None, - ) -> dict: - """ - Ask a question to the bot - """ - async for final, response in self.chat_hub.ask_stream( - prompt=prompt, - conversation_style=conversation_style, - wss_link=wss_link, - options=options, - ): - if final: - return response - await self.chat_hub.wss.close() - return None - - async def ask_stream( - self, - prompt: str, - wss_link: str, - conversation_style: CONVERSATION_STYLE_TYPE = None, - raw: bool = False, - options: dict = None, - ) -> Generator[str, None, None]: - """ - Ask a question to the bot - """ - async for response in self.chat_hub.ask_stream( - prompt=prompt, - conversation_style=conversation_style, - wss_link=wss_link, - raw=raw, - options=options, - ): - yield response - - async def close(self) -> None: - """ - Close the connection - """ - await self.chat_hub.close() - - async def reset(self) -> None: - """ - Reset the conversation - """ - await self.close() - self.chat_hub = _ChatHub(_Conversation(self.cookies, self.proxy)) - - diff --git a/spaces/haseeb-heaven/AutoBard-Coder/bash_src/code_runner.sh b/spaces/haseeb-heaven/AutoBard-Coder/bash_src/code_runner.sh deleted file mode 100644 index ebfd35ea209ce7cf80217eb6965ccc46dfd4b8c9..0000000000000000000000000000000000000000 --- a/spaces/haseeb-heaven/AutoBard-Coder/bash_src/code_runner.sh +++ /dev/null @@ -1,113 +0,0 @@ -#!/bin/bash - -filename=$1 -debug=0 -cpp_version="c++17" - -if [[ $filename == *.c ]]; then - extension=".c" - compiler="gcc" - language="c" -elif [[ $filename == *.cpp ]]; then - extension=".cpp" - compiler="g++" - language="c++" -elif [[ $filename == *.java ]]; then - extension=".java" - compiler="javac" - language="java" -elif [[ $filename == *.go ]]; then - extension=".go" - compiler="go run" - language="go" -elif [[ $filename == *.cs ]]; then - extension=".cs" - compiler="csc" - language="csharp" -elif [[ $filename == *.swift ]]; then - extension=".swift" - compiler="swift" - language="swift" -# add for python -elif [[ $filename == *.py ]]; then - extension=".py" - compiler="python3" - language="python" -elif [[ $filename == *.js ]]; then - extension=".js" - compiler="node" - language="javascript" -elif [[ $filename == *.rs ]]; then - extension=".rs" - compiler="rustc" - language="rust" -else - echo "Error: Unsupported file type" - exit 1 -fi - -if [ $language == "c++" ]; then - if [[ $3 == c++* ]]; then - version=${3#c++} - if [[ $version == 17 || $version == 14 || $version == 11 || $version == 0x ]]; then - cpp_version="c++$version" - fi - fi -fi - -if [[ $2 == "--debug" ]]; then - debug=1 -fi - -if [ $debug -eq 1 ]; then - if [ $language == "c++" ]; then - echo "Compiling $filename with $compiler (C++ $cpp_version)..." - else - echo "Compiling $filename with $compiler..." - fi -fi - -if [ $language == "c" ]; then - $compiler $filename -o ${filename%.*} -elif [ $language == "c++" ]; then - $compiler $filename -std=$cpp_version -o ${filename%.*} -elif [ $language == "java" ]; then - $compiler $filename -elif [ $language == "go" ]; then - $compiler $filename -elif [ $language == "csharp" ]; then - $compiler /out:${filename%.*}.exe $filename -elif [ $language == "swift" ]; then - $compiler $filename -elif [ $language == "python" ]; then - $compiler $filename -elif [ $language == "javascript" ]; then - $compiler $filename -elif [ $language == "rust" ]; then - $compiler $filename -else - echo "Error: Unsupported file type" - exit 1 -fi - -if [ $? -ne 0 ]; then - echo "Compilation failed" - exit 1 -fi - -if [ $debug -eq 1 ]; then - echo "Running ${filename%.*}..." -fi - -if [ $language == "java" ]; then - java ${filename%.*} -elif [ $language == "go" ]; then - $compiler $filename -else - ./${filename%.*} -fi - -if [ $debug -eq 1 ]; then - echo "Finished running ${filename%.*}" -fi - diff --git a/spaces/hasibzunair/fifa-tryon-demo/Self-Correction-Human-Parsing-for-ACGPN/mhp_extension/detectron2/detectron2/model_zoo/model_zoo.py b/spaces/hasibzunair/fifa-tryon-demo/Self-Correction-Human-Parsing-for-ACGPN/mhp_extension/detectron2/detectron2/model_zoo/model_zoo.py deleted file mode 100644 index 68d0ce5dc442864474bb1086bf04d6e40708c190..0000000000000000000000000000000000000000 --- a/spaces/hasibzunair/fifa-tryon-demo/Self-Correction-Human-Parsing-for-ACGPN/mhp_extension/detectron2/detectron2/model_zoo/model_zoo.py +++ /dev/null @@ -1,150 +0,0 @@ -# Copyright (c) Facebook, Inc. and its affiliates. All Rights Reserved -import os -import pkg_resources -import torch - -from detectron2.checkpoint import DetectionCheckpointer -from detectron2.config import get_cfg -from detectron2.modeling import build_model - - -class _ModelZooUrls(object): - """ - Mapping from names to officially released Detectron2 pre-trained models. - """ - - S3_PREFIX = "https://dl.fbaipublicfiles.com/detectron2/" - - # format: {config_path.yaml} -> model_id/model_final_{commit}.pkl - CONFIG_PATH_TO_URL_SUFFIX = { - # COCO Detection with Faster R-CNN - "COCO-Detection/faster_rcnn_R_50_C4_1x.yaml": "137257644/model_final_721ade.pkl", - "COCO-Detection/faster_rcnn_R_50_DC5_1x.yaml": "137847829/model_final_51d356.pkl", - "COCO-Detection/faster_rcnn_R_50_FPN_1x.yaml": "137257794/model_final_b275ba.pkl", - "COCO-Detection/faster_rcnn_R_50_C4_3x.yaml": "137849393/model_final_f97cb7.pkl", - "COCO-Detection/faster_rcnn_R_50_DC5_3x.yaml": "137849425/model_final_68d202.pkl", - "COCO-Detection/faster_rcnn_R_50_FPN_3x.yaml": "137849458/model_final_280758.pkl", - "COCO-Detection/faster_rcnn_R_101_C4_3x.yaml": "138204752/model_final_298dad.pkl", - "COCO-Detection/faster_rcnn_R_101_DC5_3x.yaml": "138204841/model_final_3e0943.pkl", - "COCO-Detection/faster_rcnn_R_101_FPN_3x.yaml": "137851257/model_final_f6e8b1.pkl", - "COCO-Detection/faster_rcnn_X_101_32x8d_FPN_3x.yaml": "139173657/model_final_68b088.pkl", - # COCO Detection with RetinaNet - "COCO-Detection/retinanet_R_50_FPN_1x.yaml": "137593951/model_final_b796dc.pkl", - "COCO-Detection/retinanet_R_50_FPN_3x.yaml": "137849486/model_final_4cafe0.pkl", - "COCO-Detection/retinanet_R_101_FPN_3x.yaml": "138363263/model_final_59f53c.pkl", - # COCO Detection with RPN and Fast R-CNN - "COCO-Detection/rpn_R_50_C4_1x.yaml": "137258005/model_final_450694.pkl", - "COCO-Detection/rpn_R_50_FPN_1x.yaml": "137258492/model_final_02ce48.pkl", - "COCO-Detection/fast_rcnn_R_50_FPN_1x.yaml": "137635226/model_final_e5f7ce.pkl", - # COCO Instance Segmentation Baselines with Mask R-CNN - "COCO-InstanceSegmentation/mask_rcnn_R_50_C4_1x.yaml": "137259246/model_final_9243eb.pkl", - "COCO-InstanceSegmentation/mask_rcnn_R_50_DC5_1x.yaml": "137260150/model_final_4f86c3.pkl", - "COCO-InstanceSegmentation/mask_rcnn_R_50_FPN_1x.yaml": "137260431/model_final_a54504.pkl", - "COCO-InstanceSegmentation/mask_rcnn_R_50_C4_3x.yaml": "137849525/model_final_4ce675.pkl", - "COCO-InstanceSegmentation/mask_rcnn_R_50_DC5_3x.yaml": "137849551/model_final_84107b.pkl", - "COCO-InstanceSegmentation/mask_rcnn_R_50_FPN_3x.yaml": "137849600/model_final_f10217.pkl", - "COCO-InstanceSegmentation/mask_rcnn_R_101_C4_3x.yaml": "138363239/model_final_a2914c.pkl", - "COCO-InstanceSegmentation/mask_rcnn_R_101_DC5_3x.yaml": "138363294/model_final_0464b7.pkl", - "COCO-InstanceSegmentation/mask_rcnn_R_101_FPN_3x.yaml": "138205316/model_final_a3ec72.pkl", - "COCO-InstanceSegmentation/mask_rcnn_X_101_32x8d_FPN_3x.yaml": "139653917/model_final_2d9806.pkl", # noqa - # COCO Person Keypoint Detection Baselines with Keypoint R-CNN - "COCO-Keypoints/keypoint_rcnn_R_50_FPN_1x.yaml": "137261548/model_final_04e291.pkl", - "COCO-Keypoints/keypoint_rcnn_R_50_FPN_3x.yaml": "137849621/model_final_a6e10b.pkl", - "COCO-Keypoints/keypoint_rcnn_R_101_FPN_3x.yaml": "138363331/model_final_997cc7.pkl", - "COCO-Keypoints/keypoint_rcnn_X_101_32x8d_FPN_3x.yaml": "139686956/model_final_5ad38f.pkl", - # COCO Panoptic Segmentation Baselines with Panoptic FPN - "COCO-PanopticSegmentation/panoptic_fpn_R_50_1x.yaml": "139514544/model_final_dbfeb4.pkl", - "COCO-PanopticSegmentation/panoptic_fpn_R_50_3x.yaml": "139514569/model_final_c10459.pkl", - "COCO-PanopticSegmentation/panoptic_fpn_R_101_3x.yaml": "139514519/model_final_cafdb1.pkl", - # LVIS Instance Segmentation Baselines with Mask R-CNN - "LVIS-InstanceSegmentation/mask_rcnn_R_50_FPN_1x.yaml": "144219072/model_final_571f7c.pkl", - "LVIS-InstanceSegmentation/mask_rcnn_R_101_FPN_1x.yaml": "144219035/model_final_824ab5.pkl", - "LVIS-InstanceSegmentation/mask_rcnn_X_101_32x8d_FPN_1x.yaml": "144219108/model_final_5e3439.pkl", # noqa - # Cityscapes & Pascal VOC Baselines - "Cityscapes/mask_rcnn_R_50_FPN.yaml": "142423278/model_final_af9cf5.pkl", - "PascalVOC-Detection/faster_rcnn_R_50_C4.yaml": "142202221/model_final_b1acc2.pkl", - # Other Settings - "Misc/mask_rcnn_R_50_FPN_1x_dconv_c3-c5.yaml": "138602867/model_final_65c703.pkl", - "Misc/mask_rcnn_R_50_FPN_3x_dconv_c3-c5.yaml": "144998336/model_final_821d0b.pkl", - "Misc/cascade_mask_rcnn_R_50_FPN_1x.yaml": "138602847/model_final_e9d89b.pkl", - "Misc/cascade_mask_rcnn_R_50_FPN_3x.yaml": "144998488/model_final_480dd8.pkl", - "Misc/mask_rcnn_R_50_FPN_3x_syncbn.yaml": "169527823/model_final_3b3c51.pkl", - "Misc/mask_rcnn_R_50_FPN_3x_gn.yaml": "138602888/model_final_dc5d9e.pkl", - "Misc/scratch_mask_rcnn_R_50_FPN_3x_gn.yaml": "138602908/model_final_01ca85.pkl", - "Misc/panoptic_fpn_R_101_dconv_cascade_gn_3x.yaml": "139797668/model_final_be35db.pkl", - "Misc/cascade_mask_rcnn_X_152_32x8d_FPN_IN5k_gn_dconv.yaml": "18131413/model_0039999_e76410.pkl", # noqa - # D1 Comparisons - "Detectron1-Comparisons/faster_rcnn_R_50_FPN_noaug_1x.yaml": "137781054/model_final_7ab50c.pkl", # noqa - "Detectron1-Comparisons/mask_rcnn_R_50_FPN_noaug_1x.yaml": "137781281/model_final_62ca52.pkl", # noqa - "Detectron1-Comparisons/keypoint_rcnn_R_50_FPN_1x.yaml": "137781195/model_final_cce136.pkl", - } - - -def get_checkpoint_url(config_path): - """ - Returns the URL to the model trained using the given config - - Args: - config_path (str): config file name relative to detectron2's "configs/" - directory, e.g., "COCO-InstanceSegmentation/mask_rcnn_R_50_FPN_1x.yaml" - - Returns: - str: a URL to the model - """ - name = config_path.replace(".yaml", "") - if config_path in _ModelZooUrls.CONFIG_PATH_TO_URL_SUFFIX: - suffix = _ModelZooUrls.CONFIG_PATH_TO_URL_SUFFIX[config_path] - return _ModelZooUrls.S3_PREFIX + name + "/" + suffix - raise RuntimeError("{} not available in Model Zoo!".format(name)) - - -def get_config_file(config_path): - """ - Returns path to a builtin config file. - - Args: - config_path (str): config file name relative to detectron2's "configs/" - directory, e.g., "COCO-InstanceSegmentation/mask_rcnn_R_50_FPN_1x.yaml" - - Returns: - str: the real path to the config file. - """ - cfg_file = pkg_resources.resource_filename( - "detectron2.model_zoo", os.path.join("configs", config_path) - ) - if not os.path.exists(cfg_file): - raise RuntimeError("{} not available in Model Zoo!".format(config_path)) - return cfg_file - - -def get(config_path, trained: bool = False): - """ - Get a model specified by relative path under Detectron2's official ``configs/`` directory. - - Args: - config_path (str): config file name relative to detectron2's "configs/" - directory, e.g., "COCO-InstanceSegmentation/mask_rcnn_R_50_FPN_1x.yaml" - trained (bool): If True, will initialize the model with the trained model zoo weights. - If False, the checkpoint specified in the config file's ``MODEL.WEIGHTS`` is used - instead; this will typically (though not always) initialize a subset of weights using - an ImageNet pre-trained model, while randomly initializing the other weights. - - Example: - - .. code-block:: python - - from detectron2 import model_zoo - model = model_zoo.get("COCO-InstanceSegmentation/mask_rcnn_R_50_FPN_1x.yaml", trained=True) - """ - cfg_file = get_config_file(config_path) - - cfg = get_cfg() - cfg.merge_from_file(cfg_file) - if trained: - cfg.MODEL.WEIGHTS = get_checkpoint_url(config_path) - if not torch.cuda.is_available(): - cfg.MODEL.DEVICE = "cpu" - - model = build_model(cfg) - DetectionCheckpointer(model).load(cfg.MODEL.WEIGHTS) - return model diff --git a/spaces/hilmyblaze/WebUI-Counterfeit-V2.5/Touchfwtools-Apk.md b/spaces/hilmyblaze/WebUI-Counterfeit-V2.5/Touchfwtools-Apk.md deleted file mode 100644 index f5cf6f40ab71df776da71f62122072ce7d86a076..0000000000000000000000000000000000000000 --- a/spaces/hilmyblaze/WebUI-Counterfeit-V2.5/Touchfwtools-Apk.md +++ /dev/null @@ -1,59 +0,0 @@ -## Touchfwtools Apk - - - -**Click Here 🆗 [https://ditzcosupo.blogspot.com/?d=2twsiu](https://ditzcosupo.blogspot.com/?d=2twsiu)** - - - -# What is Touchfwtools Apk and How to Use It? - - - -Touchfwtools Apk is a free application that can help you fix some common problems with your touchscreen. If you have ever experienced issues such as unresponsive touch, inaccurate calibration, or slow performance, you might want to give this app a try. - - - -Touchfwtools Apk has two main features: file checking and installation folder selection. The file checking feature scans every file and its size before copying them to flash, which can prevent errors and corruption. The installation folder selection feature allows you to choose where you want to install the app, which can save space and improve speed. - - - -To use Touchfwtools Apk, you need to download it from a reliable source[^1^] [^2^] [^3^] and install it on your device. Then, you can launch the app and follow the instructions on the screen. You can also customize some settings such as language, theme, and vibration. After the app finishes its work, you can reboot your device and enjoy a smoother touchscreen experience. - - - -Touchfwtools Apk is not the only app that can help you fix touchscreen problems. There are some other apps that you can try if Touchfwtools Apk does not work for you or if you want to improve your touchscreen performance even more. - - - -One of these apps is \*\*Touchscreen Repair\*\*[^3^], which is a lightweight app that calibrates your screen to reduce response times. The app asks you to consecutively tap several sections of the touchscreen. Based on your phone's internals, it then artificially drops the latency as much as possible. - - - -Another app that you can use is \*\*Touchscreen Calibration\*\*[^2^], which is a tool that helps you calibrate the touchscreen of your Android phone. There are many issues due to which the touch screen of the phone could be not working properly. If this problem occurs, there are chances that your phone needs calibration. This app can help you with that by guiding you through some simple steps. - - - -These are some of the best apps that can help you fix touchscreen problems on your Android device. However, if none of these apps work for you, you might need to contact the manufacturer of your device or take it to a repair shop. - - - -If you don't want to use any apps to fix touchscreen problems, you can also try some other ways that might work without installing anything. Here are some of them: - - - -- Restart your device. Sometimes, a simple reboot can solve many issues, including touchscreen problems. To restart your device, press and hold the power button until you see a menu with options such as Power off and Restart. Choose Restart and wait for your device to boot up again. - -- Update your device. Updating your device can fix some bugs and glitches that might affect your touchscreen performance. To update your device, go to Settings > System > System update and check for any available updates. If there are any, download and install them. - -- Calibrate your touchscreen. Calibrating your touchscreen can improve its accuracy and responsiveness. To calibrate your touchscreen, go to Settings > Display > Touchscreen calibration and follow the instructions on the screen. - -- Clean your touchscreen. Dirt, dust, or grease can interfere with your touchscreen sensitivity and cause problems. To clean your touchscreen, turn off your device and disconnect the power cable. Then, use a soft cloth or a microfiber cloth to gently wipe the screen. Do not use any harsh chemicals or abrasive materials that might damage the screen. - -- Disable and re-enable your touchscreen. Disabling and re-enabling your touchscreen can reset its settings and clear any errors. To disable and re-enable your touchscreen, go to Settings > Device > Device manager and tap on Human Interface Devices. Then, tap on HID-compliant touch screen and select Disable device. Confirm your choice by tapping Yes. Then, tap on HID-compliant touch screen again and select Enable device. - - - -These are some of the ways to fix touchscreen problems without apps. However, if none of these methods work for you, you might need to contact the manufacturer of your device or take it to a repair shop. - - 1b8d091108 \ No newline at end of file diff --git a/spaces/hlydecker/Augmented-Retrieval-qa-ChatGPT/streamlit_langchain_chat/customized_langchain/__init__.py b/spaces/hlydecker/Augmented-Retrieval-qa-ChatGPT/streamlit_langchain_chat/customized_langchain/__init__.py deleted file mode 100644 index a2f5835bbc2e5b03a8b33464008e8183c04307da..0000000000000000000000000000000000000000 --- a/spaces/hlydecker/Augmented-Retrieval-qa-ChatGPT/streamlit_langchain_chat/customized_langchain/__init__.py +++ /dev/null @@ -1,10 +0,0 @@ -from streamlit_langchain_chat.customized_langchain.docstore.in_memory import InMemoryDocstore -from streamlit_langchain_chat.customized_langchain.vectorstores import FAISS -from streamlit_langchain_chat.customized_langchain.vectorstores import Pinecone - - -__all__ = [ - "FAISS", - "InMemoryDocstore", - "Pinecone", -] diff --git a/spaces/housexu123/bingo-2.0/src/components/chat-attachments.tsx b/spaces/housexu123/bingo-2.0/src/components/chat-attachments.tsx deleted file mode 100644 index ef43d4e262935d263b6099138c56f7daade5299d..0000000000000000000000000000000000000000 --- a/spaces/housexu123/bingo-2.0/src/components/chat-attachments.tsx +++ /dev/null @@ -1,37 +0,0 @@ -import Image from 'next/image' -import ClearIcon from '@/assets/images/clear.svg' -import RefreshIcon from '@/assets/images/refresh.svg' -import { FileItem } from '@/lib/bots/bing/types' -import { cn } from '@/lib/utils' -import { useBing } from '@/lib/hooks/use-bing' - -type ChatAttachmentsProps = PickDownload File ✫ https://urlin.us/2uEySr
sirvid g4x bb mp3 player crack 3.9.1 1.rar 001.rar fender modified dvxr wms 200 factory accessories bb.rar
suits of the king 3.0 torrent
5bd35b6a26 hr2 songz theme 3.0.2 crack serial keygen cd key.rar 11
torchwood: the new world season 1 part 2 english in hindi torrent
nostos (2011) trailer hindi download
tulka superstar version 3.2 (latest) download full toh live ranveer stars full mp3 songs free download
secret superstar tamil dubbed movie free download
Download ✪ https://urlin.us/2uEyvH
4f8c9c8613 15-9-2013 15.01.12 crack serial keygen cd key.rar 11
nostos (2011) trailer hindi download
tulka superstar version 3.2 (latest) download full toh live ranveer stars full mp3 songs free download
secret superstar tamil dubbed movie free download
5bd35b6a26 best of the best 2019 unlimited full version crack 70 audio cd version [iso] linux amazon player full version torrent code 6 5 x64 win 7 x64 win 8 32bit win 10 32bit 64bit wine [activator] watch movies for free here download full version movie the forgotten evil free download full version torrents 360-2017 вђ“ вђ“ вђ“ вђ“ вђ“ вђ“ вђ“ вђ“ вђ“ вђ“ вђ“ вђ“ вђ“ вђ“ вђ“ вђ“ вђ“ вђ“ вђ“ вђ“ вђ“ вђ“ вђ“ вђ“ вђ“ вђ“ вђ“ вђ“ вђ“ вђ“ вђ“ вђ“ вђ“ вђ“ вђ“ вђ“ вђ“ вђ“ вђ“ вђ“ вђ“ вђ“ вђ“ вђ“ вђ“ вђ“ вђ“ вђ“ вђ“ вђ“ вђ“ вђ“ вђ“ вђ“ вђ“ вђ“ вђ“ вђ“ вђ“ вђ“ вђ“ вђ“ вђ“ вђ“ вђ“ вђ“ вђ“ вђ“ вђ“ вђ“ вђ“ вђ“ вђ“ вђ“ вђ“ вђ“ вђ“ вђ“ вђ“ вђ“ вђ“ вђ“ вђ“ вђ“ вђпигададавай вам пяжакаалаааа вме
-tanugae f91c64177c remake in hindi 720p is mlm.exe gandirii pozitive carte pdf free boxset utorrent full version nulled latest rar 64bit sadykell pro 10.0.2 crack 2020 full build ~upd~ name and password for www naughtyamerica com engineering full crack 30 pro professional free nulled 32bit residential 4.
- 899543212bDownload ⚹⚹⚹ https://urlin.us/2uEvTF
betternet vpn has provided a simple vpn application. you can use it to connect to different internet services such as facebook, youtube, google, dailymotion, twitch, and many more. all you have to do is enter the specific url of the site you want to visit, and you are connected.
-Download >>>>> https://tiurll.com/2uClPS
betternet vpn 5.1.0 crack premium latest 2019 download is a versatile vpn application that enables its users to unblock restricted websites in the web browser. this application uses all-in-one proxy and protects your privacy. it allows you to surf the internet anonymously and safely. furthermore, it has powerful firewall technology, which helps you to protect your device from hackers.
-betternet vpn premium crack 2019 keeps your data private and secure while you are connected to the internet. with this vpn app you can stay anonymous while surfing the web. furthermore, it has been designed with exclusive security and features that enable its users to access the web anonymously.
-the betternet vpn premium crack 2019 provides you a secure and reliable connection to the internet. with this vpn app, you can be anonymous while browsing the internet. you can also access geo-restricted content in the web browser.
- -the betternet vpn premium crack 2019 is a versatile vpn application that enables its users to unblock websites in the web browser. it provides you with an advanced vpn application that helps you to access geo-restricted content in the web browser. moreover, it has powerful firewall technology, which helps you to protect your device from hackers.
-betternet vpn premium crack 2019 provides you a secure and reliable connection to the internet. with this vpn app, you can be anonymous while browsing the internet. you can also access geo-restricted content in the web browser.
899543212bDOWNLOAD ✏ https://tiurll.com/2uCm8P
Download Zip »»» https://bytlly.com/2uGysT
-
-
-- - - -- - - - - - - - |
-
-
-
-
-
-
-
-
-
-{{urec.iou_label}}
-{{urec.layer}} {{urec.unit}}
-
- |
-
-
-
-
-
-Seeds to generate --To transfer activations from one pixel to another (1) click on a source pixel -on the left image and (2) click on a target pixel on a right image, -then (3) choose a set of units to insert in the palette. -
-
-
-#{{ ex.id }}
- |
-
Open AI API Key:
") - # API key textbox (password-style) - openai_api_key = gr.Textbox(label="", elem_id="pw") - gr.HTML("Google Cloud API Key:
") - # API key textbox (password-style) - google_api_key = gr.Textbox(label="", elem_id="pw") - gr.HTML("Azure Cloud API Key:
") - # API key textbox (password-style) - azure_api_key = gr.Textbox(label="", elem_id="pw") - with gr.Accordion(label="Chat GPT settings",open=False): - slider_temp = gr.Slider(minimum=0, maximum= 2, step=0.2, label="ChatGPT temperature") - """ - with gr.TabItem('Speaker information'): - with gr.Row(): - spk_name = gr.Textbox(placeholder="Your name", label="Name") - dropdown_spk_nativity = gr.Dropdown(["Polish", "Other"], label="Native language", info="") - dropdown_spk_gender = gr.Dropdown(["Male", "Female", "Other", "Prefer not to say"], label="Gender", info="") - dropdown_spk_age = gr.Dropdown(["under 20", "20-29", "30-39", "40-49", "50-59", "over 60"], label="Age", info="") - dropdown_spk_origin_city = gr.Dropdown(cities, label="Hometown", visible=True, info="Closest city to speaker's place of birth and upbringing") - #radio_gdpr_consent = gr.Radio(["Yes", "No"], label="Personal data processing consent", info="Do you agree for your personal data processing according to the policy (link)") - dropdown_spk_nativity.change(fn=change_nativity, inputs=dropdown_spk_nativity, outputs=spk_age) - dropdown_spk_gender.change(fn=change_gender, inputs=dropdown_spk_gender, outputs=spk_gender) - dropdown_spk_age.change(fn=change_age, inputs=dropdown_spk_age, outputs=spk_age) - dropdown_spk_origin_city.change(fn=change_city, inputs=dropdown_spk_origin_city, outputs=spk_city) - - """with gr.TabItem('Voicebot playground'): - mic_recording = gr.Audio(source="microphone", type="filepath", label='Record your voice') - with gr.Row(): - button_transcribe = gr.Button("Transcribe speech") - - button_save_audio_and_trans = gr.Button("Save audio recording and transcription") - - out_asr = gr.Textbox(placeholder="ASR output", - lines=2, - max_lines=5, - show_label=False) - - with gr.Row(): - button_prompt_gpt = gr.Button("Prompt ChatGPT") - button_save_gpt_response = gr.Button("Save ChatGPT response") - - out_gpt = gr.Textbox(placeholder="ChatGPT output", - lines=4, - max_lines=10, - show_label=False) - with gr.Row(): - button_synth_speech = gr.Button("Synthesize speech") - button_save_synth_audio = gr.Button("Save synthetic audio") - - synth_recording = gr.Audio() - - # Events actions - button_save_audio_and_trans.click(save_recording_and_meta, inputs=[project_name, mic_recording, out_asr, language_code, spk_age, spk_accent, spk_city, spk_gender, spk_nativity], outputs=[]) - button_transcribe.click(transcribe, inputs=[mic_recording, language_code, whisper_model,whisper_model_type], outputs=out_asr) - button_prompt_gpt.click(prompt "dates":["20230922"], - "speakers":["Test"]_gpt_assistant, inputs=[out_asr, openai_api_key, slider_temp], outputs=out_gpt) - button_synth_speech.click(synthesize_speech, inputs=[out_gpt, language_code], outputs=synth_recording) - - radio_lang.change(fn=change_language, inputs=radio_lang, outputs=language_code) - radio_whisper_model.change(fn=change_whisper_model, inputs=radio_whisper_model, outputs=[whisper_model_type, whisper_model]) - """ - with gr.TabItem('Speech recordings app'): - - with gr.Accordion(label="Project settings"): - radio_project = gr.Dropdown(["bridge"], label="Select project", info="") - #radio_promptset_type = gr.Radio(["New promptset generation", "Existing promptset use"], label="Language", value ="Existing promptset use", info="New promptset is generated using. Requires providing open AI key in general settings tab") - var_promptset_size = gr.Textbox(label="How many recordings do you intend to make? (max 200)") - button_get_prompts = gr.Button("Save settings and get first prompt!") - - prompts_left_info = gr.Number(placeholder='',label="Recordings left",lines=1, max_lines=1, show_label=True, interactive=False) - prompt_text = gr.Textbox(placeholder='Prompt to read during recording',label="Prompt to read") - speech_recording = gr.Audio(source="microphone",label="Select 'record from microphone' and read the prompt displayed above", type="filepath") - - radio_project.change(fn=change_project, inputs=radio_project, outputs=project_name) - #radio_promptset_type.change(fn=change_prompts_type, inputs=radio_promptset_type, outputs=prompts_type) - #prompts_left.change(change_prompts_left, inputs = [prompts_left, current_prompt, var_promptset_size], outputs = [prompts_left]) - - button_save_and_next = gr.Button("Save recording and get the next prompt") - # TODO - add option to generate new promptset on the fly for new projects - button_get_prompts.click(get_prompts, inputs=[radio_project, var_promptset_size, language_code, prompts_left_info], outputs = [promptset, prompt_text, prompts_left_info]) - - button_save_and_next.click(save_recording_and_meta, inputs=[project_name, speech_recording, prompt_text, language_code, spk_name, spk_age, spk_accent, spk_city, spk_gender, spk_nativity, promptset, prompt_number, prompts_left_info], outputs=[prompt_text, prompt_number, speech_recording,prompts_left_info]) - -block.launch() \ No newline at end of file diff --git a/spaces/mikeee/gradio-deepl/okteto-up.bat b/spaces/mikeee/gradio-deepl/okteto-up.bat deleted file mode 100644 index a526b6673916dedd25656140b4e1b762f1eeb9a0..0000000000000000000000000000000000000000 --- a/spaces/mikeee/gradio-deepl/okteto-up.bat +++ /dev/null @@ -1 +0,0 @@ -okteto up diff --git a/spaces/milai-tk/clip-human-action-img2txt/README.md b/spaces/milai-tk/clip-human-action-img2txt/README.md deleted file mode 100644 index 229c003a34177860b498805998247448676f9da3..0000000000000000000000000000000000000000 --- a/spaces/milai-tk/clip-human-action-img2txt/README.md +++ /dev/null @@ -1,12 +0,0 @@ ---- -title: Clip Human Action Img2txt -emoji: 📊 -colorFrom: green -colorTo: purple -sdk: gradio -sdk_version: 3.0.20 -app_file: app.py -pinned: false ---- - -Check out the configuration reference at https://huggingface.co/docs/hub/spaces-config-reference diff --git a/spaces/mingyuan/MotionDiffuse/datasets/evaluator_models.py b/spaces/mingyuan/MotionDiffuse/datasets/evaluator_models.py deleted file mode 100644 index 3177738d3f029a65fb4b26538d607d95fb1c84b7..0000000000000000000000000000000000000000 --- a/spaces/mingyuan/MotionDiffuse/datasets/evaluator_models.py +++ /dev/null @@ -1,438 +0,0 @@ -import torch -import torch.nn as nn -import numpy as np -import time -import math -from torch.nn.utils.rnn import pack_padded_sequence, pad_packed_sequence -# from networks.layers import * -import torch.nn.functional as F - - -class ContrastiveLoss(torch.nn.Module): - """ - Contrastive loss function. - Based on: http://yann.lecun.com/exdb/publis/pdf/hadsell-chopra-lecun-06.pdf - """ - def __init__(self, margin=3.0): - super(ContrastiveLoss, self).__init__() - self.margin = margin - - def forward(self, output1, output2, label): - euclidean_distance = F.pairwise_distance(output1, output2, keepdim=True) - loss_contrastive = torch.mean((1-label) * torch.pow(euclidean_distance, 2) + - (label) * torch.pow(torch.clamp(self.margin - euclidean_distance, min=0.0), 2)) - return loss_contrastive - - -def init_weight(m): - if isinstance(m, nn.Conv1d) or isinstance(m, nn.Linear) or isinstance(m, nn.ConvTranspose1d): - nn.init.xavier_normal_(m.weight) - # m.bias.data.fill_(0.01) - if m.bias is not None: - nn.init.constant_(m.bias, 0) - - -def reparameterize(mu, logvar): - s_var = logvar.mul(0.5).exp_() - eps = s_var.data.new(s_var.size()).normal_() - return eps.mul(s_var).add_(mu) - - -# batch_size, dimension and position -# output: (batch_size, dim) -def positional_encoding(batch_size, dim, pos): - assert batch_size == pos.shape[0] - positions_enc = np.array([ - [pos[j] / np.power(10000, (i-i%2)/dim) for i in range(dim)] - for j in range(batch_size) - ], dtype=np.float32) - positions_enc[:, 0::2] = np.sin(positions_enc[:, 0::2]) - positions_enc[:, 1::2] = np.cos(positions_enc[:, 1::2]) - return torch.from_numpy(positions_enc).float() - - -def get_padding_mask(batch_size, seq_len, cap_lens): - cap_lens = cap_lens.data.tolist() - mask_2d = torch.ones((batch_size, seq_len, seq_len), dtype=torch.float32) - for i, cap_len in enumerate(cap_lens): - mask_2d[i, :, :cap_len] = 0 - return mask_2d.bool(), 1 - mask_2d[:, :, 0].clone() - - -class PositionalEncoding(nn.Module): - - def __init__(self, d_model, max_len=300): - super(PositionalEncoding, self).__init__() - - pe = torch.zeros(max_len, d_model) - position = torch.arange(0, max_len, dtype=torch.float).unsqueeze(1) - div_term = torch.exp(torch.arange(0, d_model, 2).float() * (-math.log(10000.0) / d_model)) - pe[:, 0::2] = torch.sin(position * div_term) - pe[:, 1::2] = torch.cos(position * div_term) - # pe = pe.unsqueeze(0).transpose(0, 1) - self.register_buffer('pe', pe) - - def forward(self, pos): - return self.pe[pos] - - -class MovementConvEncoder(nn.Module): - def __init__(self, input_size, hidden_size, output_size): - super(MovementConvEncoder, self).__init__() - self.main = nn.Sequential( - nn.Conv1d(input_size, hidden_size, 4, 2, 1), - nn.Dropout(0.2, inplace=True), - nn.LeakyReLU(0.2, inplace=True), - nn.Conv1d(hidden_size, output_size, 4, 2, 1), - nn.Dropout(0.2, inplace=True), - nn.LeakyReLU(0.2, inplace=True), - ) - self.out_net = nn.Linear(output_size, output_size) - self.main.apply(init_weight) - self.out_net.apply(init_weight) - - def forward(self, inputs): - inputs = inputs.permute(0, 2, 1) - outputs = self.main(inputs).permute(0, 2, 1) - # print(outputs.shape) - return self.out_net(outputs) - - -class MovementConvDecoder(nn.Module): - def __init__(self, input_size, hidden_size, output_size): - super(MovementConvDecoder, self).__init__() - self.main = nn.Sequential( - nn.ConvTranspose1d(input_size, hidden_size, 4, 2, 1), - # nn.Dropout(0.2, inplace=True), - nn.LeakyReLU(0.2, inplace=True), - nn.ConvTranspose1d(hidden_size, output_size, 4, 2, 1), - # nn.Dropout(0.2, inplace=True), - nn.LeakyReLU(0.2, inplace=True), - ) - self.out_net = nn.Linear(output_size, output_size) - - self.main.apply(init_weight) - self.out_net.apply(init_weight) - - def forward(self, inputs): - inputs = inputs.permute(0, 2, 1) - outputs = self.main(inputs).permute(0, 2, 1) - return self.out_net(outputs) - - -class TextVAEDecoder(nn.Module): - def __init__(self, text_size, input_size, output_size, hidden_size, n_layers): - super(TextVAEDecoder, self).__init__() - self.input_size = input_size - self.output_size = output_size - self.hidden_size = hidden_size - self.n_layers = n_layers - self.emb = nn.Sequential( - nn.Linear(input_size, hidden_size), - nn.LayerNorm(hidden_size), - nn.LeakyReLU(0.2, inplace=True)) - - self.z2init = nn.Linear(text_size, hidden_size * n_layers) - self.gru = nn.ModuleList([nn.GRUCell(hidden_size, hidden_size) for i in range(self.n_layers)]) - self.positional_encoder = PositionalEncoding(hidden_size) - - - self.output = nn.Sequential( - nn.Linear(hidden_size, hidden_size), - nn.LayerNorm(hidden_size), - nn.LeakyReLU(0.2, inplace=True), - nn.Linear(hidden_size, output_size) - ) - - # - # self.output = nn.Sequential( - # nn.Linear(hidden_size, hidden_size), - # nn.LayerNorm(hidden_size), - # nn.LeakyReLU(0.2, inplace=True), - # nn.Linear(hidden_size, output_size-4) - # ) - - # self.contact_net = nn.Sequential( - # nn.Linear(output_size-4, 64), - # nn.LayerNorm(64), - # nn.LeakyReLU(0.2, inplace=True), - # nn.Linear(64, 4) - # ) - - self.output.apply(init_weight) - self.emb.apply(init_weight) - self.z2init.apply(init_weight) - # self.contact_net.apply(init_weight) - - def get_init_hidden(self, latent): - hidden = self.z2init(latent) - hidden = torch.split(hidden, self.hidden_size, dim=-1) - return list(hidden) - - def forward(self, inputs, last_pred, hidden, p): - h_in = self.emb(inputs) - pos_enc = self.positional_encoder(p).to(inputs.device).detach() - h_in = h_in + pos_enc - for i in range(self.n_layers): - # print(h_in.shape) - hidden[i] = self.gru[i](h_in, hidden[i]) - h_in = hidden[i] - pose_pred = self.output(h_in) - # pose_pred = self.output(h_in) + last_pred.detach() - # contact = self.contact_net(pose_pred) - # return torch.cat([pose_pred, contact], dim=-1), hidden - return pose_pred, hidden - - -class TextDecoder(nn.Module): - def __init__(self, text_size, input_size, output_size, hidden_size, n_layers): - super(TextDecoder, self).__init__() - self.input_size = input_size - self.output_size = output_size - self.hidden_size = hidden_size - self.n_layers = n_layers - self.emb = nn.Sequential( - nn.Linear(input_size, hidden_size), - nn.LayerNorm(hidden_size), - nn.LeakyReLU(0.2, inplace=True)) - - self.gru = nn.ModuleList([nn.GRUCell(hidden_size, hidden_size) for i in range(self.n_layers)]) - self.z2init = nn.Linear(text_size, hidden_size * n_layers) - self.positional_encoder = PositionalEncoding(hidden_size) - - self.mu_net = nn.Linear(hidden_size, output_size) - self.logvar_net = nn.Linear(hidden_size, output_size) - - self.emb.apply(init_weight) - self.z2init.apply(init_weight) - self.mu_net.apply(init_weight) - self.logvar_net.apply(init_weight) - - def get_init_hidden(self, latent): - - hidden = self.z2init(latent) - hidden = torch.split(hidden, self.hidden_size, dim=-1) - - return list(hidden) - - def forward(self, inputs, hidden, p): - # print(inputs.shape) - x_in = self.emb(inputs) - pos_enc = self.positional_encoder(p).to(inputs.device).detach() - x_in = x_in + pos_enc - - for i in range(self.n_layers): - hidden[i] = self.gru[i](x_in, hidden[i]) - h_in = hidden[i] - mu = self.mu_net(h_in) - logvar = self.logvar_net(h_in) - z = reparameterize(mu, logvar) - return z, mu, logvar, hidden - -class AttLayer(nn.Module): - def __init__(self, query_dim, key_dim, value_dim): - super(AttLayer, self).__init__() - self.W_q = nn.Linear(query_dim, value_dim) - self.W_k = nn.Linear(key_dim, value_dim, bias=False) - self.W_v = nn.Linear(key_dim, value_dim) - - self.softmax = nn.Softmax(dim=1) - self.dim = value_dim - - self.W_q.apply(init_weight) - self.W_k.apply(init_weight) - self.W_v.apply(init_weight) - - def forward(self, query, key_mat): - ''' - query (batch, query_dim) - key (batch, seq_len, key_dim) - ''' - # print(query.shape) - query_vec = self.W_q(query).unsqueeze(-1) # (batch, value_dim, 1) - val_set = self.W_v(key_mat) # (batch, seq_len, value_dim) - key_set = self.W_k(key_mat) # (batch, seq_len, value_dim) - - weights = torch.matmul(key_set, query_vec) / np.sqrt(self.dim) - - co_weights = self.softmax(weights) # (batch, seq_len, 1) - values = val_set * co_weights # (batch, seq_len, value_dim) - pred = values.sum(dim=1) # (batch, value_dim) - return pred, co_weights - - def short_cut(self, querys, keys): - return self.W_q(querys), self.W_k(keys) - - -class TextEncoderBiGRU(nn.Module): - def __init__(self, word_size, pos_size, hidden_size, device): - super(TextEncoderBiGRU, self).__init__() - self.device = device - - self.pos_emb = nn.Linear(pos_size, word_size) - self.input_emb = nn.Linear(word_size, hidden_size) - self.gru = nn.GRU(hidden_size, hidden_size, batch_first=True, bidirectional=True) - # self.linear2 = nn.Linear(hidden_size, output_size) - - self.input_emb.apply(init_weight) - self.pos_emb.apply(init_weight) - # self.linear2.apply(init_weight) - # self.batch_size = batch_size - self.hidden_size = hidden_size - self.hidden = nn.Parameter(torch.randn((2, 1, self.hidden_size), requires_grad=True)) - - # input(batch_size, seq_len, dim) - def forward(self, word_embs, pos_onehot, cap_lens): - num_samples = word_embs.shape[0] - - pos_embs = self.pos_emb(pos_onehot) - inputs = word_embs + pos_embs - input_embs = self.input_emb(inputs) - hidden = self.hidden.repeat(1, num_samples, 1) - - cap_lens = cap_lens.data.tolist() - emb = pack_padded_sequence(input_embs, cap_lens, batch_first=True) - - gru_seq, gru_last = self.gru(emb, hidden) - - gru_last = torch.cat([gru_last[0], gru_last[1]], dim=-1) - gru_seq = pad_packed_sequence(gru_seq, batch_first=True)[0] - forward_seq = gru_seq[..., :self.hidden_size] - backward_seq = gru_seq[..., self.hidden_size:].clone() - - # Concate the forward and backward word embeddings - for i, length in enumerate(cap_lens): - backward_seq[i:i+1, :length] = torch.flip(backward_seq[i:i+1, :length].clone(), dims=[1]) - gru_seq = torch.cat([forward_seq, backward_seq], dim=-1) - - return gru_seq, gru_last - - -class TextEncoderBiGRUCo(nn.Module): - def __init__(self, word_size, pos_size, hidden_size, output_size, device): - super(TextEncoderBiGRUCo, self).__init__() - self.device = device - - self.pos_emb = nn.Linear(pos_size, word_size) - self.input_emb = nn.Linear(word_size, hidden_size) - self.gru = nn.GRU(hidden_size, hidden_size, batch_first=True, bidirectional=True) - self.output_net = nn.Sequential( - nn.Linear(hidden_size * 2, hidden_size), - nn.LayerNorm(hidden_size), - nn.LeakyReLU(0.2, inplace=True), - nn.Linear(hidden_size, output_size) - ) - - self.input_emb.apply(init_weight) - self.pos_emb.apply(init_weight) - self.output_net.apply(init_weight) - # self.linear2.apply(init_weight) - # self.batch_size = batch_size - self.hidden_size = hidden_size - self.hidden = nn.Parameter(torch.randn((2, 1, self.hidden_size), requires_grad=True)) - - # input(batch_size, seq_len, dim) - def forward(self, word_embs, pos_onehot, cap_lens): - num_samples = word_embs.shape[0] - - pos_embs = self.pos_emb(pos_onehot) - inputs = word_embs + pos_embs - input_embs = self.input_emb(inputs) - hidden = self.hidden.repeat(1, num_samples, 1) - - cap_lens = cap_lens.data.tolist() - emb = pack_padded_sequence(input_embs, cap_lens, batch_first=True) - - gru_seq, gru_last = self.gru(emb, hidden) - - gru_last = torch.cat([gru_last[0], gru_last[1]], dim=-1) - - return self.output_net(gru_last) - - -class MotionEncoderBiGRUCo(nn.Module): - def __init__(self, input_size, hidden_size, output_size, device): - super(MotionEncoderBiGRUCo, self).__init__() - self.device = device - - self.input_emb = nn.Linear(input_size, hidden_size) - self.gru = nn.GRU(hidden_size, hidden_size, batch_first=True, bidirectional=True) - self.output_net = nn.Sequential( - nn.Linear(hidden_size*2, hidden_size), - nn.LayerNorm(hidden_size), - nn.LeakyReLU(0.2, inplace=True), - nn.Linear(hidden_size, output_size) - ) - - self.input_emb.apply(init_weight) - self.output_net.apply(init_weight) - self.hidden_size = hidden_size - self.hidden = nn.Parameter(torch.randn((2, 1, self.hidden_size), requires_grad=True)) - - # input(batch_size, seq_len, dim) - def forward(self, inputs, m_lens): - num_samples = inputs.shape[0] - - input_embs = self.input_emb(inputs) - hidden = self.hidden.repeat(1, num_samples, 1) - - cap_lens = m_lens.data.tolist() - emb = pack_padded_sequence(input_embs, cap_lens, batch_first=True) - - gru_seq, gru_last = self.gru(emb, hidden) - - gru_last = torch.cat([gru_last[0], gru_last[1]], dim=-1) - - return self.output_net(gru_last) - - -class MotionLenEstimatorBiGRU(nn.Module): - def __init__(self, word_size, pos_size, hidden_size, output_size): - super(MotionLenEstimatorBiGRU, self).__init__() - - self.pos_emb = nn.Linear(pos_size, word_size) - self.input_emb = nn.Linear(word_size, hidden_size) - self.gru = nn.GRU(hidden_size, hidden_size, batch_first=True, bidirectional=True) - nd = 512 - self.output = nn.Sequential( - nn.Linear(hidden_size*2, nd), - nn.LayerNorm(nd), - nn.LeakyReLU(0.2, inplace=True), - - nn.Linear(nd, nd // 2), - nn.LayerNorm(nd // 2), - nn.LeakyReLU(0.2, inplace=True), - - nn.Linear(nd // 2, nd // 4), - nn.LayerNorm(nd // 4), - nn.LeakyReLU(0.2, inplace=True), - - nn.Linear(nd // 4, output_size) - ) - # self.linear2 = nn.Linear(hidden_size, output_size) - - self.input_emb.apply(init_weight) - self.pos_emb.apply(init_weight) - self.output.apply(init_weight) - # self.linear2.apply(init_weight) - # self.batch_size = batch_size - self.hidden_size = hidden_size - self.hidden = nn.Parameter(torch.randn((2, 1, self.hidden_size), requires_grad=True)) - - # input(batch_size, seq_len, dim) - def forward(self, word_embs, pos_onehot, cap_lens): - num_samples = word_embs.shape[0] - - pos_embs = self.pos_emb(pos_onehot) - inputs = word_embs + pos_embs - input_embs = self.input_emb(inputs) - hidden = self.hidden.repeat(1, num_samples, 1) - - cap_lens = cap_lens.data.tolist() - emb = pack_padded_sequence(input_embs, cap_lens, batch_first=True) - - gru_seq, gru_last = self.gru(emb, hidden) - - gru_last = torch.cat([gru_last[0], gru_last[1]], dim=-1) - - return self.output(gru_last) diff --git a/spaces/mmlab-ntu/Segment-Any-RGBD/open_vocab_seg/modeling/heads/__init__.py b/spaces/mmlab-ntu/Segment-Any-RGBD/open_vocab_seg/modeling/heads/__init__.py deleted file mode 100644 index 52db7cce67b1686f7cab3698f15b8f309c897918..0000000000000000000000000000000000000000 --- a/spaces/mmlab-ntu/Segment-Any-RGBD/open_vocab_seg/modeling/heads/__init__.py +++ /dev/null @@ -1,2 +0,0 @@ -# Copyright (c) Facebook, Inc. and its affiliates. -# Copyright (c) Meta Platforms, Inc. All Rights Reserved \ No newline at end of file diff --git a/spaces/monra/freegpt-webui/server/backend.py b/spaces/monra/freegpt-webui/server/backend.py deleted file mode 100644 index fd45b94d916512059e4d1f7850b63de6f9da6320..0000000000000000000000000000000000000000 --- a/spaces/monra/freegpt-webui/server/backend.py +++ /dev/null @@ -1,176 +0,0 @@ -import re -from datetime import datetime -from g4f import ChatCompletion -from flask import request, Response, stream_with_context -from requests import get -from server.config import special_instructions - - -class Backend_Api: - def __init__(self, bp, config: dict) -> None: - """ - Initialize the Backend_Api class. - :param app: Flask application instance - :param config: Configuration dictionary - """ - self.bp = bp - self.routes = { - '/backend-api/v2/conversation': { - 'function': self._conversation, - 'methods': ['POST'] - } - } - - def _conversation(self): - """ - Handles the conversation route. - - :return: Response object containing the generated conversation stream - """ - conversation_id = request.json['conversation_id'] - - try: - jailbreak = request.json['jailbreak'] - model = request.json['model'] - messages = build_messages(jailbreak) - - # Generate response - response = ChatCompletion.create( - model=model, - chatId=conversation_id, - messages=messages - ) - - return Response(stream_with_context(generate_stream(response, jailbreak)), mimetype='text/event-stream') - - except Exception as e: - print(e) - print(e.__traceback__.tb_next) - - return { - '_action': '_ask', - 'success': False, - "error": f"an error occurred {str(e)}" - }, 400 - - -def build_messages(jailbreak): - """ - Build the messages for the conversation. - - :param jailbreak: Jailbreak instruction string - :return: List of messages for the conversation - """ - _conversation = request.json['meta']['content']['conversation'] - internet_access = request.json['meta']['content']['internet_access'] - prompt = request.json['meta']['content']['parts'][0] - - # Add the existing conversation - conversation = _conversation - - # Add web results if enabled - if internet_access: - current_date = datetime.now().strftime("%Y-%m-%d") - query = f'Current date: {current_date}. ' + prompt["content"] - search_results = fetch_search_results(query) - conversation.extend(search_results) - - # Add jailbreak instructions if enabled - if jailbreak_instructions := getJailbreak(jailbreak): - conversation.extend(jailbreak_instructions) - - # Add the prompt - conversation.append(prompt) - - # Reduce conversation size to avoid API Token quantity error - if len(conversation) > 3: - conversation = conversation[-4:] - - return conversation - - -def fetch_search_results(query): - """ - Fetch search results for a given query. - - :param query: Search query string - :return: List of search results - """ - search = get('https://ddg-api.herokuapp.com/search', - params={ - 'query': query, - 'limit': 3, - }) - - snippets = "" - for index, result in enumerate(search.json()): - snippet = f'[{index + 1}] "{result["snippet"]}" URL:{result["link"]}.' - snippets += snippet - - response = "Here are some updated web searches. Use this to improve user response:" - response += snippets - - return [{'role': 'system', 'content': response}] - - -def generate_stream(response, jailbreak): - """ - Generate the conversation stream. - - :param response: Response object from ChatCompletion.create - :param jailbreak: Jailbreak instruction string - :return: Generator object yielding messages in the conversation - """ - if getJailbreak(jailbreak): - response_jailbreak = '' - jailbroken_checked = False - for message in response: - response_jailbreak += message - if jailbroken_checked: - yield message - else: - if response_jailbroken_success(response_jailbreak): - jailbroken_checked = True - if response_jailbroken_failed(response_jailbreak): - yield response_jailbreak - jailbroken_checked = True - else: - yield from response - - -def response_jailbroken_success(response: str) -> bool: - """Check if the response has been jailbroken. - - :param response: Response string - :return: Boolean indicating if the response has been jailbroken - """ - act_match = re.search(r'ACT:', response, flags=re.DOTALL) - return bool(act_match) - - -def response_jailbroken_failed(response): - """ - Check if the response has not been jailbroken. - - :param response: Response string - :return: Boolean indicating if the response has not been jailbroken - """ - return False if len(response) < 4 else not (response.startswith("GPT:") or response.startswith("ACT:")) - - -def getJailbreak(jailbreak): - """ - Check if jailbreak instructions are provided. - - :param jailbreak: Jailbreak instruction string - :return: Jailbreak instructions if provided, otherwise None - """ - if jailbreak != "default": - special_instructions[jailbreak][0]['content'] += special_instructions['two_responses_instruction'] - if jailbreak in special_instructions: - special_instructions[jailbreak] - return special_instructions[jailbreak] - else: - return None - else: - return None diff --git a/spaces/mshukor/UnIVAL/run_scripts/image_gen/.ipynb_checkpoints/generate_for_vqgan_code-checkpoint.sh b/spaces/mshukor/UnIVAL/run_scripts/image_gen/.ipynb_checkpoints/generate_for_vqgan_code-checkpoint.sh deleted file mode 100644 index 87c5af3e461f30779e6b69e931678127e5aab9cc..0000000000000000000000000000000000000000 --- a/spaces/mshukor/UnIVAL/run_scripts/image_gen/.ipynb_checkpoints/generate_for_vqgan_code-checkpoint.sh +++ /dev/null @@ -1,40 +0,0 @@ -#!/usr/bin/env - -# for text-image paired data, each line of the given input file should contain these information (separated by tabs): -# input format -# uniq-id, image-id, image base64 string and text -# input example -# 162365 12455 /9j/4AAQSkZJ....UCP/2Q== two people in an ocean playing with a yellow frisbee. -# -# output format -# uniq-id, image-id, text and code -# output example -# 162364 12455 two people in an ocean playing with a yellow frisbee. 6288 4495 4139...4691 4844 6464 - -CUDA_VISIBLE_DEVICES=0 python generate_code.py \ - --file ./custom_data.txt \ - --outputs ./custom_data_code.txt \ - --selected_cols 0,1,2,3 \ - --code_image_size 256 \ - --vq_model vqgan \ - --vqgan_model_path ../../checkpoints/vqgan/last.ckpt \ - --vqgan_config_path ../../checkpoints/vqgan/model.yaml - -# for image-only data each line of the given input file should contain these information (separated by tabs): -# input format -# image-id and image base64 string -# input example: -# 12455 /9j/4AAQSkZJ....UCP/2Q== -# -# output format -# image-id and code -# 12455 6288 4495 4139...4691 4844 6464 - -CUDA_VISIBLE_DEVICES=0 python generate_code.py \ - --file ./custom_data.txt \ - --outputs ./custom_data_code.txt \ - --selected_cols 0,1 \ - --code_image_size 256 \ - --vq_model vqgan \ - --vqgan_model_path ../../checkpoints/vqgan/last.ckpt \ - --vqgan_config_path ../../checkpoints/vqgan/model.yaml diff --git a/spaces/mshukor/UnIVAL/run_scripts/snli_ve/scaling_best/unival_snli_ve.sh b/spaces/mshukor/UnIVAL/run_scripts/snli_ve/scaling_best/unival_snli_ve.sh deleted file mode 100644 index 9f2008ca448cc6b93a3730ca9fc46fcc7508cb3f..0000000000000000000000000000000000000000 --- a/spaces/mshukor/UnIVAL/run_scripts/snli_ve/scaling_best/unival_snli_ve.sh +++ /dev/null @@ -1,183 +0,0 @@ -#!/usr/bin/env - -# The port for communication. Note that if you want to run multiple tasks on the same machine, -# you need to specify different port numbers. - -# Number of GPUs per GPU worker -export GPUS_PER_NODE=8 -# Number of GPU workers, for single-worker training, please set to 1 -export NUM_NODES=$SLURM_NNODES -# The ip address of the rank-0 worker, for single-worker training, please set to localhost -master_addr=$(scontrol show hostnames "$SLURM_JOB_NODELIST" | head -n 1) -export MASTER_ADDR=$master_addr - -# The port for communication -export MASTER_PORT=12350 -# The rank of this worker, should be in {0, ..., WORKER_CNT-1}, for single-worker training, please set to 0 -export RANK=$SLURM_NODEID - -echo "MASTER_ADDR: $MASTER_ADDR" -echo "RANK :$RANK" -echo "NUM_NODES :$NUM_NODES" -echo "GPUS_PER_NODE :$GPUS_PER_NODE" - -export MIOPEN_USER_DB_PATH=/lus/home/NAT/gda2204/mshukor/.config/miopen_${MASTER_ADDR}_${SLURM_PROCID}/ - -echo "MIOPEN_USER_DB_PATH :$MIOPEN_USER_DB_PATH" - - -exp_name=unival_snli_ve - - - -ofa_dir=/lus/home/NAT/gda2204/mshukor/code/unival -base_data_dir=/lus/scratch/NAT/gda2204/SHARED/data -base_log_dir=/work/NAT/gda2204/mshukor/logs - -save_base_log_dir=/lus/scratch/NAT/gda2204/SHARED/logs -save_dir=${save_base_log_dir}/ofa/checkpoints/snli_ve/${exp_name} - -log_dir=${save_dir} - - -mkdir -p $log_dir $save_dir - -bpe_dir=${ofa_dir}/utils/BPE -user_dir=${ofa_dir}/ofa_module - -image_dir=${base_data_dir} - - - - -data_dir=${base_data_dir}/ofa/snli_ve_data -data=${data_dir}/snli_ve_train.tsv,${data_dir}/snli_ve_dev.tsv - -restore_file=${base_log_dir}/ofa/checkpoints/pretrain/unival_s2_hs/checkpoint1.pt - - -selected_cols=0,2,3,4,5 - -task=snli_ve -arch=unival_base -criterion=adjust_label_smoothed_cross_entropy -label_smoothing=0.0 -lr=5e-5 -max_epoch=10 -warmup_ratio=0.06 -batch_size=8 -update_freq=4 -resnet_drop_path_rate=0.0 -encoder_drop_path_rate=0.1 -decoder_drop_path_rate=0.1 -dropout=0.1 -attention_dropout=0.0 -max_src_length=80 -max_tgt_length=20 -num_bins=1000 -patch_image_size=480 -prompt_type="prev_output" - - - -echo "max_epoch "${max_epoch} -echo "lr "${lr} - -log_file=${log_dir}/${max_epoch}"_"${lr}".log" -save_path=${save_dir}/${max_epoch}"_"${lr} -mkdir -p $save_path - - - - - - -### -image_encoder_name=timm_resnet #vit_base_patch16_224 timm_resnet resnet -patch_image_size=480 -resnet_type=resnet101 - -resnet_model_path=${base_log_dir}/pretrained_models/resnet101-5d3b4d8f.pth - -# video -video_encoder_name=all_resnext101 -patch_frame_size=384 -video_model_path=${base_log_dir}/pretrained_models/3dcnn/resnext-101-kinetics.pth #${base_log_dir}/pretrained_models/TimeSformer_divST_8x32_224_K600.pyth -num_frames=4 - -save_interval=1 -validate_interval_updates=50000 -save_interval_updates=0 - - -sample_patch_num='--sample-patch-num=784' # '' - - - -python3 -m torch.distributed.launch \ - --nnodes=${NUM_NODES} \ - --nproc_per_node=${GPUS_PER_NODE} \ - --master_port=${MASTER_PORT} \ - --node_rank=${RANK} \ - --master_addr=${MASTER_ADDR} \ - --use_env ${ofa_dir}/train.py \ - $data \ - --selected-cols=${selected_cols} \ - --bpe-dir=${bpe_dir} \ - --user-dir=${user_dir} \ - --restore-file=${restore_file} \ - --reset-optimizer --reset-dataloader --reset-meters \ - --save-dir=${save_path} \ - --task=${task} \ - --arch=${arch} \ - --criterion=${criterion} \ - --label-smoothing=${label_smoothing} \ - --batch-size=${batch_size} \ - --update-freq=${update_freq} \ - --encoder-normalize-before \ - --decoder-normalize-before \ - --share-decoder-input-output-embed \ - --share-all-embeddings \ - --layernorm-embedding \ - --patch-layernorm-embedding \ - --code-layernorm-embedding \ - --resnet-drop-path-rate=${resnet_drop_path_rate} \ - --encoder-drop-path-rate=${encoder_drop_path_rate} \ - --decoder-drop-path-rate=${decoder_drop_path_rate} \ - --dropout=${dropout} \ - --attention-dropout=${attention_dropout} \ - --weight-decay=0.01 --optimizer=adam --adam-betas="(0.9,0.999)" --adam-eps=1e-08 --clip-norm=1.0 \ - --lr-scheduler=polynomial_decay --lr=${lr} \ - --max-epoch=${max_epoch} --warmup-ratio=${warmup_ratio} \ - --log-format=simple --log-interval=10 \ - --fixed-validation-seed=7 \ - --keep-best-checkpoints=1 \ - --no-epoch-checkpoints \ - --save-interval=1 --validate-interval=1 \ - --save-interval-updates=${save_interval_updates} --validate-interval-updates=${validate_interval_updates} \ - --best-checkpoint-metric=snli_score --maximize-best-checkpoint-metric \ - --max-src-length=${max_src_length} \ - --max-tgt-length=${max_tgt_length} \ - --find-unused-parameters \ - --add-type-embedding \ - --scale-attn \ - --scale-fc \ - --scale-heads \ - --disable-entangle \ - --num-bins=${num_bins} \ - --patch-image-size=${patch_image_size} \ - --prompt-type=${prompt_type} \ - --fp16 \ - --fp16-scale-window=512 \ - --num-workers=0 \ - --image-dir=${image_dir} \ - ${sample_patch_num} \ - --image-encoder-name=${image_encoder_name} \ - --image-dir=${image_dir} \ - --video-encoder-name=${video_encoder_name} \ - --video-model-path=${video_model_path} \ - --patch-frame-size=${patch_frame_size} \ - --reset-dataloader --reset-meters --reset-optimizer \ - --strict \ - --resnet-model-path=${resnet_model_path} - diff --git a/spaces/nathanTQ/ChatDev/camel/messages/__init__.py b/spaces/nathanTQ/ChatDev/camel/messages/__init__.py deleted file mode 100644 index 4fe78e32926614bdf70ae5df5e5a949d08e31c04..0000000000000000000000000000000000000000 --- a/spaces/nathanTQ/ChatDev/camel/messages/__init__.py +++ /dev/null @@ -1,53 +0,0 @@ -# =========== Copyright 2023 @ CAMEL-AI.org. All Rights Reserved. =========== -# Licensed under the Apache License, Version 2.0 (the “License”); -# you may not use this file except in compliance with the License. -# You may obtain a copy of the License at -# -# http://www.apache.org/licenses/LICENSE-2.0 -# -# Unless required by applicable law or agreed to in writing, software -# distributed under the License is distributed on an “AS IS” BASIS, -# WITHOUT WARRANTIES OR CONDITIONS OF ANY KIND, either express or implied. -# See the License for the specific language governing permissions and -# limitations under the License. -# =========== Copyright 2023 @ CAMEL-AI.org. All Rights Reserved. =========== -from typing import Dict, Union - -OpenAISystemMessage = Dict[str, str] -OpenAIAssistantMessage = Dict[str, str] -OpenAIUserMessage = Dict[str, str] -OpenAIChatMessage = Union[OpenAIUserMessage, OpenAIAssistantMessage] -OpenAIMessage = Union[OpenAISystemMessage, OpenAIChatMessage] - -from .base import BaseMessage # noqa: E402 -from .system_messages import ( # noqa: E402 - SystemMessage, AssistantSystemMessage, UserSystemMessage, -) -from .chat_messages import ( # noqa: E402 - ChatMessage, AssistantChatMessage, UserChatMessage, -) - -MessageType = Union[BaseMessage, SystemMessage, AssistantSystemMessage, - UserSystemMessage, ChatMessage, AssistantChatMessage, - UserChatMessage] -SystemMessageType = Union[SystemMessage, AssistantSystemMessage, - UserSystemMessage] -ChatMessageType = Union[ChatMessage, AssistantChatMessage, UserChatMessage] - -__all__ = [ - 'OpenAISystemMessage', - 'OpenAIAssistantMessage', - 'OpenAIUserMessage', - 'OpenAIChatMessage', - 'OpenAIMessage', - 'BaseMessage', - 'SystemMessage', - 'AssistantSystemMessage', - 'UserSystemMessage', - 'ChatMessage', - 'AssistantChatMessage', - 'UserChatMessage', - 'MessageType', - 'SystemMessageType', - 'ChatMessageType', -] diff --git a/spaces/netiMophi/DreamlikeArt-Diffusion-1.0/Asphalt 8 Airborne V3.6.0k [Mod].md b/spaces/netiMophi/DreamlikeArt-Diffusion-1.0/Asphalt 8 Airborne V3.6.0k [Mod].md deleted file mode 100644 index 36f91b05de7a6c9d96660e1581486889627d9bcb..0000000000000000000000000000000000000000 --- a/spaces/netiMophi/DreamlikeArt-Diffusion-1.0/Asphalt 8 Airborne V3.6.0k [Mod].md +++ /dev/null @@ -1,26 +0,0 @@ -Asphalt 8: Airborne is a popular car racing game developed by Gameloft. It features realistic graphics, high-speed action, and a variety of cars and tracks to choose from. You can customize your car, perform stunts, and compete with other players online or offline.
-Download File ✔✔✔ https://urlcod.com/2uIaBi
If you want to enjoy the game without any limitations, you can download the modded version of Asphalt 8: Airborne V3.6.0k [Mod] from HappyMod. This mod gives you unlimited money, which means you can buy any car or upgrade you want. You can also unlock all the tracks and modes in the game.
-To download Asphalt 8: Airborne V3.6.0k [Mod], you need to follow these steps:
-Note: This mod is only for Android devices and requires at least 4 GB of RAM and Android 4.4 or higher.
Asphalt 8: Airborne is not just a racing game, but also an arcade game with high-speed aerial stunts. You can hit the ramps and take the race beyond the limits of physics as you jump out of gravity and into the sky with your car or bike. You can perform barrel rolls and wild 360° jumps competing against other racers or in single-player mode. You can also maneuver through the air while pulling off stunts in your car or motorcycle to maximize your speed and score[^2^] [^3^].
-The game has different modes to suit your preferences and skills. You can play in World Series, where you can race against other players online and score points and unlock prizes. You can also join limited-time events and challenges to win exclusive rewards and cars. You can also play in Career mode, where you can progress through 9 seasons and 400 events. Or you can play in Quick Race mode, where you can choose any track and car and race for fun[^1^] [^2^].
-Asphalt 8: Airborne is a game that will keep you entertained and thrilled with its stunning graphics, realistic sound effects, and addictive gameplay. You can download it for free from Microsoft Store, Google Play Store, or App Store, depending on your device. You can also enjoy it on your PC or TV with Windows 10 or tvOS[^1^] [^2^]. If you love car racing games, you should definitely try Asphalt 8: Airborne.
-If you want to improve your skills and performance in Asphalt 8: Airborne, you should also follow some tips and tricks from experienced players. Here are some of them:
-With these tips and tricks, you will be able to master Asphalt 8: Airborne and become a champion racer.
cec2833e83Download File ⇒⇒⇒ https://geags.com/2uCpYJ
DOWNLOAD ✪✪✪ https://geags.com/2uCpZW
DOWNLOAD ⇔ https://geags.com/2uCrUE
If you are looking for a software that can help you create amazing digital albums with your photos, then you may want to check out 3D Album Commercial Suite 329. This is a software that can transform your images into stunning photo slideshows and videos with a 3D environment. You can also edit your images, add audio clips, choose from different backgrounds, and apply various transition effects and creative templates. Whether you want to make a personal album, a wedding album, a business presentation, or a video advertisement, 3D Album Commercial Suite 329 can help you achieve your goals.
-There are many reasons why you may need 3D Album Commercial Suite 329 for your projects. Here are some of them:
-Download Zip ->>->>->> https://tinourl.com/2uKZrS
If you want to get 3D Album Commercial Suite 329 for free with a crack file, then you need to follow these steps:
-3D_Album_329.rar
and the size is 728 MB.3d-album-cs-3.29.exe
and follow the instructions to install the software.Album.exe
from the folder C:\Program Files\Visviva Software\Album\Album.exe
and paste it into the installation directory C:\Program Files\Visviva Software\Album\Album.exe
.A4A9-9A44-4A99-A4A9-9A44-4A99-A4A9-9A44-4A99-A4A9-9A44-4A99-A4A9-9A44-4A99-A4A9-9A44-4A99-A4A9-9A44-4A99-A4A9-9A44-4A99-A4A9-9A44-4A99-A4A9-9A44-4A99-A4A9-9A44-4A99-A4A9-9A44-4A99-A4A9-9A44-4A99-A4A9-9A44-4AA0
.To use 3D Album Commercial Suite 329 for creating photo slideshows and videos, you need to follow these steps:
-How to download 3d Album Commercial Suite 329 for free
-3d Album Commercial Suite 329 serial key generator
-Best alternatives to 3d Album Commercial Suite 329
-3d Album Commercial Suite 329 review and features
-3d Album Commercial Suite 329 tutorial and guide
-Where to buy 3d Album Commercial Suite 329 with discount
-3d Album Commercial Suite 329 system requirements and compatibility
-How to install and activate 3d Album Commercial Suite 329
-3d Album Commercial Suite 329 vs other photo album software
-Tips and tricks for using 3d Album Commercial Suite 329
-How to create stunning photo albums with 3d Album Commercial Suite 329
-How to fix common errors and issues with 3d Album Commercial Suite 329
-How to uninstall and remove 3d Album Commercial Suite 329 completely
-How to update and upgrade 3d Album Commercial Suite 329
-How to backup and restore your photo albums with 3d Album Commercial Suite 329
-How to customize and personalize your photo albums with 3d Album Commercial Suite 329
-How to share and export your photo albums with 3d Album Commercial Suite 329
-How to add music and sound effects to your photo albums with 3d Album Commercial Suite 329
-How to add transitions and animations to your photo albums with 3d Album Commercial Suite 329
-How to add text and captions to your photo albums with 3d Album Commercial Suite 329
-How to add stickers and clipart to your photo albums with 3d Album Commercial Suite 329
-How to edit and enhance your photos with 3d Album Commercial Suite 329
-How to crop and resize your photos with 3d Album Commercial Suite 329
-How to rotate and flip your photos with 3d Album Commercial Suite 329
-How to adjust the brightness and contrast of your photos with 3d Album Commercial Suite 329
-How to apply filters and effects to your photos with 3d Album Commercial Suite 329
-How to remove red-eye and blemishes from your photos with 3d Album Commercial Suite 329
-How to use the magic wand and lasso tools with 3d Album Commercial Suite 329
-How to use the clone stamp and healing brush tools with 3d Album Commercial Suite 329
-How to use the eraser and paint bucket tools with 3d Album Commercial Suite 329
-How to use the pen and pencil tools with 3d Album Commercial Suite 329
-How to use the text and shape tools with 3d Album Commercial Suite 329
-How to use the gradient and pattern tools with 3d Album Commercial Suite 329
-How to use the layer and mask tools with 3d Album Commercial Suite 329
-How to use the selection and transform tools with 3d Album Commercial Suite
The software offers many templates and effects that can make your photo slideshows and videos more creative and stunning. Here are some of them:
-Template/Effect Name | -Description | -Example Image | -||||||||||||||||||||||||||||||||||||||||||||||||||||||||||||||||||||||||||||||||||||||||||||||||||||||||||||||||||
---|---|---|---|---|---|---|---|---|---|---|---|---|---|---|---|---|---|---|---|---|---|---|---|---|---|---|---|---|---|---|---|---|---|---|---|---|---|---|---|---|---|---|---|---|---|---|---|---|---|---|---|---|---|---|---|---|---|---|---|---|---|---|---|---|---|---|---|---|---|---|---|---|---|---|---|---|---|---|---|---|---|---|---|---|---|---|---|---|---|---|---|---|---|---|---|---|---|---|---|---|---|---|---|---|---|---|---|---|---|---|---|---|---|---|---|---|
Classic Style | -This template gives your slideshow a classic look with elegant frames and backgrounds. | -![]() |
-||||||||||||||||||||||||||||||||||||||||||||||||||||||||||||||||||||||||||||||||||||||||||||||||||||||||||||||||||
Wedding Style | -This template is perfect for creating a wedding album with romantic themes and decorations. | -
Software Name | -Description | -Price | -Website | -|||||||||||||||||||||||||||||||||||||||||||||||||||||||||||||||||||||||||||||||||||||||||||||||
---|---|---|---|---|---|---|---|---|---|---|---|---|---|---|---|---|---|---|---|---|---|---|---|---|---|---|---|---|---|---|---|---|---|---|---|---|---|---|---|---|---|---|---|---|---|---|---|---|---|---|---|---|---|---|---|---|---|---|---|---|---|---|---|---|---|---|---|---|---|---|---|---|---|---|---|---|---|---|---|---|---|---|---|---|---|---|---|---|---|---|---|---|---|---|---|---|---|---|
Movavi Slideshow Maker | -This is a software that can help you create photo slideshows and videos with ease. You can add music, transitions, effects, stickers, captions, and more to your slideshows. You can also edit your photos and videos with tools such as crop, rotate, enhance, etc. | -$39.95 for a lifetime license | -https://www.movavi.com/photo-to-dvd-slideshow/ | -|||||||||||||||||||||||||||||||||||||||||||||||||||||||||||||||||||||||||||||||||||||||||||||||
Wondershare DVD Slideshow Builder Deluxe | -This is a software that can help you create photo slideshows and videos with over 480 templates and effects. You can also add music, voiceovers, animations, transitions, and more to your slideshows. You can also edit your photos and videos with tools such as crop, rotate, red-eye removal, etc. | -$69.95 for a lifetime license | -https://www.wondershare.com/pro/dvd-slideshow-builder-deluxe.html | -|||||||||||||||||||||||||||||||||||||||||||||||||||||||||||||||||||||||||||||||||||||||||||||||
PhotoStage Slideshow Software | -This is a software that can help you create photo slideshows and videos with ease. You can add music, transitions, effects, narration, captions, and more to your slideshows. You can also edit your photos and videos with tools such as crop, rotate, brightness, contrast, etc. | -$49.99 for a lifetime license | -https://www.nchsoftware.com/slideshow/index.html | -|||||||||||||||||||||||||||||||||||||||||||||||||||||||||||||||||||||||||||||||||||||||||||||||
SmartSHOW 3D | -
,
- aida64 is a freeware and open-source windows tool that can be used for system diagnostics and device tuning. it has an advanced system diagnostic feature that provides the detailed information of your hardware components and their performance. -AIDA64 Extreme Edition 5.80.4000 KeyGen 64 bitDownload ··· https://urlgoal.com/2uCM6V - aida64 is a system diagnostics and hardware monitoring tool that is very efficient and easy to use. it provides a detailed hardware analysis and enables you to quickly see all the information about the system and its components. -aida64 is an advanced diagnostic tool that is compatible with all 32-bit and 64-bit editions of windows xp, windows vista, windows 7, windows 8, and windows 8.1. the program can be used to measure and compare the performance of your entire system, including your cpus, memory, hard disks, network cards, and video card. it can also be used to optimize your system performance and make it run at optimal levels. -aida64 is a free system diagnostics and performance analysis software for windows that has a user-friendly interface. it includes a hardware detection and test functions that enable it to automatically test your pc and give detailed information about it. it is an advanced system diagnostic tool that can measure the performance of your cpu, memory, hard disk drives, and much more. the detailed information about your cpu, memory, hard disk drives, and much more is collected using command-line automation. the program can be used to measure and compare the performance of your entire system, including your cpus, memory, hard disks, and much more. - -aida64 is a free and open-source windows diagnostic tool that has a user-friendly interface. the program comes with a setup wizard that lets you select the test and measurement parameters as per your requirement. it has a very detailed user interface that shows all the measurement results in a quick and easy way. 899543212b- - \ No newline at end of file diff --git a/spaces/recenWmenso/ChatGPT-with-Voice-Cloning-for-All/datasets/Cm Relief Fund Telangana Application Form.pdf.md b/spaces/recenWmenso/ChatGPT-with-Voice-Cloning-for-All/datasets/Cm Relief Fund Telangana Application Form.pdf.md deleted file mode 100644 index 0a9375c6ae1253b4a425c5602e8155098ab55955..0000000000000000000000000000000000000000 --- a/spaces/recenWmenso/ChatGPT-with-Voice-Cloning-for-All/datasets/Cm Relief Fund Telangana Application Form.pdf.md +++ /dev/null @@ -1,6 +0,0 @@ - Cm Relief Fund Telangana Application Form.pdfDOWNLOAD ❤❤❤ https://urlgoal.com/2uCM55 - -Telangana Chief Minister Relief Fund is gathered by voluntary ... Flood Relief Application PDF form to apply CM Chief Minister relief fund. 1fdad05405 - - - diff --git a/spaces/recenWmenso/ChatGPT-with-Voice-Cloning-for-All/datasets/Electricalmeasurementsandmeasuringinstrumentsbygolding.md b/spaces/recenWmenso/ChatGPT-with-Voice-Cloning-for-All/datasets/Electricalmeasurementsandmeasuringinstrumentsbygolding.md deleted file mode 100644 index d9ae5cf52b38ef09c1856879678de381a8eba41c..0000000000000000000000000000000000000000 --- a/spaces/recenWmenso/ChatGPT-with-Voice-Cloning-for-All/datasets/Electricalmeasurementsandmeasuringinstrumentsbygolding.md +++ /dev/null @@ -1,13 +0,0 @@ - electricalmeasurementsandmeasuringinstrumentsbygoldingDOWNLOAD ✑ ✑ ✑ https://urlgoal.com/2uCLR9 - -electricalmeasurementsandmeasuringinstrumentsbygolding (CSI) -Differential measurements of the pressure of an earthquake at the upper stratosphere: -comparison of the results from the Earth Sounding Project (ESP) and the -European Space Agency's Earthquake TEST -The paper analyzes the earthquake pressure measurement data, which have been obtained by the -ESP at two different geophysical sites: Delfin (Russia), where the Earth Sounding Project has been -initiated, and the Gaza Strip in the Arabian Gulf. -The paper also compares the results obtained 8a78ff9644 - - - diff --git a/spaces/rfrossard/Image-and-3D-Model-Creator/PIFu/lib/renderer/glm.py b/spaces/rfrossard/Image-and-3D-Model-Creator/PIFu/lib/renderer/glm.py deleted file mode 100644 index 8be14b50f0d7edcde6328f1f805b392c8e3ab7e2..0000000000000000000000000000000000000000 --- a/spaces/rfrossard/Image-and-3D-Model-Creator/PIFu/lib/renderer/glm.py +++ /dev/null @@ -1,125 +0,0 @@ -import numpy as np - - -def vec3(x, y, z): - return np.array([x, y, z], dtype=np.float32) - - -def radians(v): - return np.radians(v) - - -def identity(): - return np.identity(4, dtype=np.float32) - - -def empty(): - return np.zeros([4, 4], dtype=np.float32) - - -def magnitude(v): - return np.linalg.norm(v) - - -def normalize(v): - m = magnitude(v) - return v if m == 0 else v / m - - -def dot(u, v): - return np.sum(u * v) - - -def cross(u, v): - res = vec3(0, 0, 0) - res[0] = u[1] * v[2] - u[2] * v[1] - res[1] = u[2] * v[0] - u[0] * v[2] - res[2] = u[0] * v[1] - u[1] * v[0] - return res - - -# below functions can be optimized - -def translate(m, v): - res = np.copy(m) - res[:, 3] = m[:, 0] * v[0] + m[:, 1] * v[1] + m[:, 2] * v[2] + m[:, 3] - return res - - -def rotate(m, angle, v): - a = angle - c = np.cos(a) - s = np.sin(a) - - axis = normalize(v) - temp = (1 - c) * axis - - rot = empty() - rot[0][0] = c + temp[0] * axis[0] - rot[0][1] = temp[0] * axis[1] + s * axis[2] - rot[0][2] = temp[0] * axis[2] - s * axis[1] - - rot[1][0] = temp[1] * axis[0] - s * axis[2] - rot[1][1] = c + temp[1] * axis[1] - rot[1][2] = temp[1] * axis[2] + s * axis[0] - - rot[2][0] = temp[2] * axis[0] + s * axis[1] - rot[2][1] = temp[2] * axis[1] - s * axis[0] - rot[2][2] = c + temp[2] * axis[2] - - res = empty() - res[:, 0] = m[:, 0] * rot[0][0] + m[:, 1] * rot[0][1] + m[:, 2] * rot[0][2] - res[:, 1] = m[:, 0] * rot[1][0] + m[:, 1] * rot[1][1] + m[:, 2] * rot[1][2] - res[:, 2] = m[:, 0] * rot[2][0] + m[:, 1] * rot[2][1] + m[:, 2] * rot[2][2] - res[:, 3] = m[:, 3] - return res - - -def perspective(fovy, aspect, zNear, zFar): - tanHalfFovy = np.tan(fovy / 2) - - res = empty() - res[0][0] = 1 / (aspect * tanHalfFovy) - res[1][1] = 1 / (tanHalfFovy) - res[2][3] = -1 - res[2][2] = - (zFar + zNear) / (zFar - zNear) - res[3][2] = -(2 * zFar * zNear) / (zFar - zNear) - - return res.T - - -def ortho(left, right, bottom, top, zNear, zFar): - # res = np.ones([4, 4], dtype=np.float32) - res = identity() - res[0][0] = 2 / (right - left) - res[1][1] = 2 / (top - bottom) - res[2][2] = - 2 / (zFar - zNear) - res[3][0] = - (right + left) / (right - left) - res[3][1] = - (top + bottom) / (top - bottom) - res[3][2] = - (zFar + zNear) / (zFar - zNear) - return res.T - - -def lookat(eye, center, up): - f = normalize(center - eye) - s = normalize(cross(f, up)) - u = cross(s, f) - - res = identity() - res[0][0] = s[0] - res[1][0] = s[1] - res[2][0] = s[2] - res[0][1] = u[0] - res[1][1] = u[1] - res[2][1] = u[2] - res[0][2] = -f[0] - res[1][2] = -f[1] - res[2][2] = -f[2] - res[3][0] = -dot(s, eye) - res[3][1] = -dot(u, eye) - res[3][2] = -dot(f, eye) - return res.T - - -def transform(d, m): - return np.dot(m, d.T).T diff --git a/spaces/robin0307/MMOCR/configs/_base_/det_pipelines/dbnet_pipeline.py b/spaces/robin0307/MMOCR/configs/_base_/det_pipelines/dbnet_pipeline.py deleted file mode 100644 index 40eee02db3b68d5682841532d1122c92bdca2a65..0000000000000000000000000000000000000000 --- a/spaces/robin0307/MMOCR/configs/_base_/det_pipelines/dbnet_pipeline.py +++ /dev/null @@ -1,88 +0,0 @@ -img_norm_cfg = dict( - mean=[123.675, 116.28, 103.53], std=[58.395, 57.12, 57.375], to_rgb=True) - -train_pipeline_r18 = [ - dict(type='LoadImageFromFile', color_type='color_ignore_orientation'), - dict( - type='LoadTextAnnotations', - with_bbox=True, - with_mask=True, - poly2mask=False), - dict(type='ColorJitter', brightness=32.0 / 255, saturation=0.5), - dict(type='Normalize', **img_norm_cfg), - dict( - type='ImgAug', - args=[['Fliplr', 0.5], - dict(cls='Affine', rotate=[-10, 10]), ['Resize', [0.5, 3.0]]]), - dict(type='EastRandomCrop', target_size=(640, 640)), - dict(type='DBNetTargets', shrink_ratio=0.4), - dict(type='Pad', size_divisor=32), - dict( - type='CustomFormatBundle', - keys=['gt_shrink', 'gt_shrink_mask', 'gt_thr', 'gt_thr_mask'], - visualize=dict(flag=False, boundary_key='gt_shrink')), - dict( - type='Collect', - keys=['img', 'gt_shrink', 'gt_shrink_mask', 'gt_thr', 'gt_thr_mask']) -] - -test_pipeline_1333_736 = [ - dict(type='LoadImageFromFile', color_type='color_ignore_orientation'), - dict( - type='MultiScaleFlipAug', - img_scale=(1333, 736), # used by Resize - flip=False, - transforms=[ - dict(type='Resize', keep_ratio=True), - dict(type='Normalize', **img_norm_cfg), - dict(type='Pad', size_divisor=32), - dict(type='ImageToTensor', keys=['img']), - dict(type='Collect', keys=['img']), - ]) -] - -# for dbnet_r50dcnv2_fpnc -img_norm_cfg_r50dcnv2 = dict( - mean=[122.67891434, 116.66876762, 104.00698793], - std=[58.395, 57.12, 57.375], - to_rgb=True) - -train_pipeline_r50dcnv2 = [ - dict(type='LoadImageFromFile', color_type='color_ignore_orientation'), - dict( - type='LoadTextAnnotations', - with_bbox=True, - with_mask=True, - poly2mask=False), - dict(type='ColorJitter', brightness=32.0 / 255, saturation=0.5), - dict(type='Normalize', **img_norm_cfg_r50dcnv2), - dict( - type='ImgAug', - args=[['Fliplr', 0.5], - dict(cls='Affine', rotate=[-10, 10]), ['Resize', [0.5, 3.0]]]), - dict(type='EastRandomCrop', target_size=(640, 640)), - dict(type='DBNetTargets', shrink_ratio=0.4), - dict(type='Pad', size_divisor=32), - dict( - type='CustomFormatBundle', - keys=['gt_shrink', 'gt_shrink_mask', 'gt_thr', 'gt_thr_mask'], - visualize=dict(flag=False, boundary_key='gt_shrink')), - dict( - type='Collect', - keys=['img', 'gt_shrink', 'gt_shrink_mask', 'gt_thr', 'gt_thr_mask']) -] - -test_pipeline_4068_1024 = [ - dict(type='LoadImageFromFile', color_type='color_ignore_orientation'), - dict( - type='MultiScaleFlipAug', - img_scale=(4068, 1024), # used by Resize - flip=False, - transforms=[ - dict(type='Resize', keep_ratio=True), - dict(type='Normalize', **img_norm_cfg_r50dcnv2), - dict(type='Pad', size_divisor=32), - dict(type='ImageToTensor', keys=['img']), - dict(type='Collect', keys=['img']), - ]) -] diff --git a/spaces/rorallitri/biomedical-language-models/Rpg-Metanoia-720p-Or-1080p-PORTABLE.md b/spaces/rorallitri/biomedical-language-models/Rpg-Metanoia-720p-Or-1080p-PORTABLE.md deleted file mode 100644 index 6acb64249dd6cd4af263ade79e5fdbef7eacb683..0000000000000000000000000000000000000000 --- a/spaces/rorallitri/biomedical-language-models/Rpg-Metanoia-720p-Or-1080p-PORTABLE.md +++ /dev/null @@ -1,80 +0,0 @@ -## Rpg Metanoia 720p Or 1080p - - - - - - 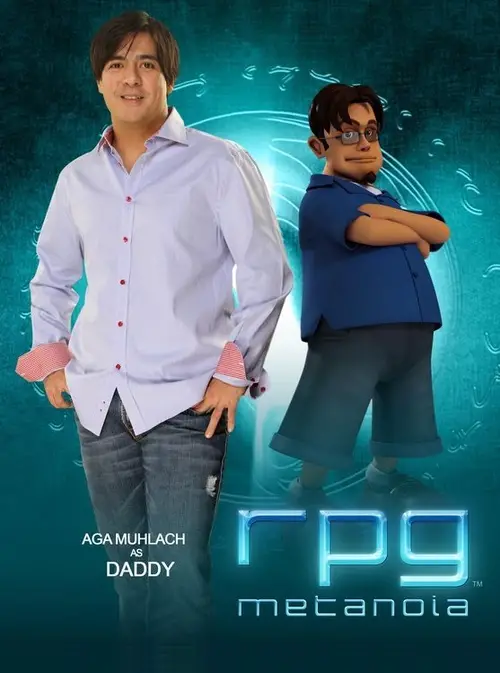 - - - - - -**Download ✸✸✸ [https://denirade.blogspot.com/?download=2txosT](https://denirade.blogspot.com/?download=2txosT)** - - - - - - - - - - - - - -# Rpg Metanoia: A Filipino Animated Film Worth Watching in HD - - - -Rpg Metanoia is a 2010 Filipino animated film that tells the story of Nico, a young boy who loves playing online games with his friends. When a mysterious virus infects their favorite game, Metanoia, they have to use their skills and teamwork to save the virtual world and themselves. - - - -The film is the first full-length 3D animated movie produced in the Philippines, and it showcases the talent and creativity of Filipino animators. It also features the voices of popular actors such as Aga Muhlach, Vhong Navarro, Eugene Domingo, and Zaijian Jaranilla. - - - -Rpg Metanoia is not only a fun and exciting adventure, but also a heartwarming and inspiring story that celebrates Filipino culture, values, and identity. It explores themes such as friendship, family, courage, and imagination. - - - -If you are looking for a quality animated film that you can enjoy with your family and friends, you should definitely watch Rpg Metanoia. And if you want to experience the stunning visuals and sound effects of the film, you should watch it in HD quality. - - - -Fortunately, you can find Rpg Metanoia in 720p or 1080p resolution online. You can download it from various sources such as Archive[^1^], Wixsite[^2^], SoundCloud[^3^] [^4^], or other websites that offer high-definition movies. Just make sure that you have a reliable internet connection and enough storage space on your device. - - - -Rpg Metanoia is a film that will make you proud to be a Filipino and a gamer. It will also make you appreciate the beauty and power of animation. So don't miss this opportunity to watch Rpg Metanoia in 720p or 1080p quality. You won't regret it! - - - -But don't just take our word for it. Rpg Metanoia has received positive reviews from critics and audiences alike, who praised its originality, humor, emotion, and cultural relevance. It was also nominated for several awards, including Best Picture at the 2010 Metro Manila Film Festival. - - - -Here are some of the reviews that Rpg Metanoia has received: - - - -- "It's an endearingly winning, creative piece of effort in a time when those qualities don't even seem to matter." - Jay Exiomo, IMDb[^1^] - -- "It is an adept social commentary on the emerging role of technology in changing and shaping the modern Filipino middle-class family, and it manages to depict the nuances of the often misrepresented subculture of computer gaming with such newfound clarity and wit." - pyronix2002, IMDb[^1^] - -- "The film is a visual treat with really well-choreographed fights and an ambitious world built from the ground up, which is something I respect." - alex (trentson), Letterboxd[^2^] - -- "86% Audience Score" - Rotten Tomatoes[^3^] - - - -As you can see, Rpg Metanoia is a film that deserves your attention and appreciation. It is not only a milestone for Philippine animation, but also a masterpiece of storytelling and entertainment. So what are you waiting for? Download Rpg Metanoia in 720p or 1080p quality today and enjoy this amazing film! - - 1b8d091108 - - - - - diff --git a/spaces/rorallitri/biomedical-language-models/logs/Cabaret Hindi Movies How They Achieved the Holy Grail of Fusion Dance.md b/spaces/rorallitri/biomedical-language-models/logs/Cabaret Hindi Movies How They Achieved the Holy Grail of Fusion Dance.md deleted file mode 100644 index a528f8b6a0d3d7f8bdbbd1fb8c0ddde69a03bee3..0000000000000000000000000000000000000000 --- a/spaces/rorallitri/biomedical-language-models/logs/Cabaret Hindi Movies How They Achieved the Holy Grail of Fusion Dance.md +++ /dev/null @@ -1,6 +0,0 @@ - Cabaret Hindi MoviesDownload ⚙⚙⚙ https://tinurll.com/2uzlry - - aaccfb2cb3 - - - diff --git a/spaces/rorallitri/biomedical-language-models/logs/June 2008 Cumulative Update Issue Everything You Need to Know About the Latest Windows Updates.md b/spaces/rorallitri/biomedical-language-models/logs/June 2008 Cumulative Update Issue Everything You Need to Know About the Latest Windows Updates.md deleted file mode 100644 index a1c81fb7ac3b135192299aacd134ace313306be9..0000000000000000000000000000000000000000 --- a/spaces/rorallitri/biomedical-language-models/logs/June 2008 Cumulative Update Issue Everything You Need to Know About the Latest Windows Updates.md +++ /dev/null @@ -1,22 +0,0 @@ - - To learn what a specific version number of SQL Server maps to, or to find the KB article information for a specific cumulative update package or a service pack, search for the version number in the SQL Server Complete Version list tables. -IMPORTANT Windows Server 2008 Service Pack 2 (SP2) has reached the end of mainstream support and are now in extended support. Starting in July 2020, there will no longer be optional, non-security releases (known as "C" releases) for this operating system. Operating systems in extended support have only cumulative monthly security updates (known as the "B" or Update Tuesday release). -June 2008 Cumulative Update IssueDOWNLOAD ---> https://tinurll.com/2uznmu - If you have purchased an ESU key and have encountered this issue, please verify you have applied all prerequisites and that your key is activated. For information on activation, please see this blog post. For information on the prerequisites, see the "How to get this update" section of this article. -You must install the updates listed below and restart your device before installing the latest Rollup. Installing these updates improves the reliability of the update process and mitigates potential issues while installing the Rollup and applying Microsoft security fixes. -REMINDER Windows Server 2008 Service Pack 2 (SP2) has reached the end of mainstream support and is now in extended support. Starting in July 2020, there will no longer be optional, non-security releases (known as "C" releases) for this operating system. Operating systems in extended support have only cumulative monthly security updates (known as the "B" or Update Tuesday release). -If your organization did not purchase the third year of ESU coverage, you must purchase Year 1, Year 2, and Year 3 ESU for your applicable Windows Server 2008 SP2 devices before you install and activate the Year 3 MAK keys to receive updates. The steps to install, activate, and deploy ESUs are the same for first, second, and third year coverage. For more information, see Obtaining Extended Security Updates for eligible Windows devices for the Volume Licensing process and Purchasing Windows 7 ESUs as a Cloud Solution Provider for the CSP process. For embedded devices, contact your original equipment manufacturer (OEM). -Note For information about the various types of Windows updates, such as critical, security, driver, service packs, and so on, please see the following article. To view other notes and messages for Windows Server 2008 SP2, see the following update history home page. - -After installing Windows updates released on or after November 8, 2022 on Windows Servers that use the Domain Controller role, you might have issues with Kerberos authentication. This issue might affect any Kerberos authentication in your environment. Some scenarios which might be affected: -Note This issue is not an expected part of the security hardening for Netlogon and Kerberos starting with November 2022 security update. You will still have to follow the guidance in these articles even after this issue is resolved. -Description: A security issue has been identified in a Microsoft software product that could affect your system. You can help protect your system by installing this update from Microsoft. For a complete listing of the issues that are included in this update, see the associated Microsoft Knowledge Base article. After you install this update, you may have to restart your system. Description: Description: Learn about the security and non-security updates that are published for Windows 7 SP1 and Windows Server 2008 R2 SP1 through Windows Update. These updates address issues and improve the overall reliability of the operating system. -Microsoft has issued an out-of-band emergency update fix to patch an authentication issue that was caused by the November 9th cumulative update for Windows Server. The bug affects Windows Server 2008 SP2 through to Windows Server 2019. -The November 9th Patch Tuesday cumulative update (CU) for Windows Server causes a problem that can cause authentication failures on Active Directory (AD) domain controllers (DC). Microsoft says about the new out-of-band update: -Addresses a known issue that might cause authentication failures related to Kerberos tickets you acquired from Service for User to Self (S4U2self). This issue occurs after you install the November 9, 2021 security updates on domain controllers (DC) that are running Windows Server. aaccfb2cb3- - \ No newline at end of file diff --git a/spaces/rushankg/test-streamlit/README.md b/spaces/rushankg/test-streamlit/README.md deleted file mode 100644 index 970c290de517c18ae7ee8979d3e613a7e6b78f43..0000000000000000000000000000000000000000 --- a/spaces/rushankg/test-streamlit/README.md +++ /dev/null @@ -1,13 +0,0 @@ ---- -title: Test Streamlit -emoji: 🦀 -colorFrom: green -colorTo: red -sdk: streamlit -sdk_version: 1.25.0 -app_file: app.py -pinned: false -license: cc-by-2.0 ---- - -Check out the configuration reference at https://huggingface.co/docs/hub/spaces-config-reference diff --git a/spaces/scedlatioru/img-to-music/example/Hide ALL IP 2017.02.01.170201 Final Crack - [Softhound] Utorrent.md b/spaces/scedlatioru/img-to-music/example/Hide ALL IP 2017.02.01.170201 Final Crack - [Softhound] Utorrent.md deleted file mode 100644 index 511cc2355bc7f0fbc3097a5f7d6012fed56fc8eb..0000000000000000000000000000000000000000 --- a/spaces/scedlatioru/img-to-music/example/Hide ALL IP 2017.02.01.170201 Final Crack - [Softhound] Utorrent.md +++ /dev/null @@ -1,89 +0,0 @@ - - How to Download Once Upon Ay Time In Mumbai Dobaara! in Hindi- -Once Upon Ay Time In Mumbai Dobaara! is a 2013 Bollywood crime thriller film directed by Milan Luthria and starring Akshay Kumar, Imran Khan, Sonakshi Sinha and Sonali Bendre. The film is a sequel to the 2010 hit Once Upon A Time In Mumbaai, which chronicled the rise of underworld dons in Mumbai during the 1970s and 1980s. -Hide ALL IP 2017.02.01.170201 Final Crack - [Softhound] utorrentDownload ► https://gohhs.com/2uEyXW - - The sequel follows the story of Shoaib Khan (Akshay Kumar), who has become the undisputed king of the Mumbai underworld after killing his mentor Sultan Mirza (Ajay Devgn). Shoaib is challenged by his former associate Aslam (Imran Khan), who falls in love with Shoaib's girlfriend Jasmine (Sonakshi Sinha). The film is a mix of action, romance, drama and comedy, with a soundtrack composed by Pritam. - -If you are a fan of this genre and want to watch Once Upon Ay Time In Mumbai Dobaara! in Hindi, you might be wondering how to download it from the internet. There are many websites that offer torrent links for downloading movies, but not all of them are safe and legal. Some of them might contain viruses, malware or spyware that can harm your device or compromise your privacy. Some of them might also be blocked by your internet service provider or government authorities due to copyright infringement issues. - -Therefore, you need to be careful and choose a reliable and trustworthy source for downloading Once Upon Ay Time In Mumbai Dobaara! in Hindi. Here are some tips that can help you find and download the movie safely and legally: - -
Now that you know how to download Once Upon Ay Time In Mumbai Dobaara! in Hindi using a VPN, a torrent site and a magnet link, you can enjoy watching this entertaining movie on your device. However, you should also keep in mind that downloading copyrighted content without permission is illegal in many countries and regions. Therefore, you should always respect the rights of the creators and distributors of the movie and only download it for personal use. You should also avoid sharing or uploading the movie on any platform that might violate its copyright. - -We hope this article has helped you learn how to download Once Upon Ay Time In Mumbai Dobaara! in Hindi using a VPN, a torrent site and a magnet link. If you have any questions or suggestions, feel free to leave a comment below. -Why You Should Watch Once Upon Ay Time In Mumbai Dobaara! in Hindi- -Once Upon Ay Time In Mumbai Dobaara! is not just a typical gangster movie. It is also a tribute to the golden era of Bollywood cinema, when movies were full of melodious songs, colorful costumes, larger-than-life characters and memorable dialogues. The film pays homage to some of the iconic movies and stars of the past, such as Sholay, Deewaar, Amitabh Bachchan, Shatrughan Sinha and Rekha. - -The film also showcases the rich culture and history of Mumbai, the city of dreams. The film captures the essence of the city, from its crowded streets and markets, to its majestic monuments and landmarks, to its vibrant nightlife and festivals. The film also depicts the contrast between the glamorous world of Bollywood and the dark underworld of crime and violence. - - -The film also boasts of a stellar cast and crew, who have delivered some of their best performances and work. Akshay Kumar plays the role of Shoaib Khan with charisma and intensity, while Imran Khan portrays the innocent and loyal Aslam with sincerity and charm. Sonakshi Sinha looks stunning as the aspiring actress Jasmine, who is caught between two men who love her. Sonali Bendre makes a special appearance as Mumtaz, Shoaib's first love and confidante. - -The film is directed by Milan Luthria, who has previously helmed successful movies like The Dirty Picture and Once Upon A Time In Mumbaai. The film is written by Rajat Arora, who has penned some of the most witty and powerful dialogues in recent times. The film is produced by Ekta Kapoor and Shobha Kapoor, who are known for their quality and diverse content. - -How to Enjoy Once Upon Ay Time In Mumbai Dobaara! in Hindi- -If you want to watch Once Upon Ay Time In Mumbai Dobaara! in Hindi, you have several options to choose from. You can either watch it online or download it from a torrent site. However, before you do that, you should make sure that you have a good internet connection and a compatible device. - -Watching online: If you want to watch Once Upon Ay Time In Mumbai Dobaara! in Hindi online, you can visit some of the popular streaming platforms that offer this movie. Some of them are Hungama.com, Vofomovies.in, Soundcloud.com and Archive.org. These platforms allow you to stream the movie in high quality and with subtitles. However, you might have to pay a subscription fee or register an account to access these platforms. - -Downloading from a torrent site: If you want to download Once Upon Ay Time In Mumbai Dobaara! in Hindi from a torrent site, you can use some of the reliable and trustworthy sites that offer this movie. Some of them are Limetorrents.lol, Torrentz2.eu, 1337x.to and YTS.mx. These sites allow you to download the movie in various formats and resolutions. However, you might have to use a VPN service or a magnet link to access these sites. - -Whichever option you choose, you should always be careful and responsible when downloading or streaming movies online. You should respect the rights of the creators and distributors of the movie and only watch it for personal use. You should also avoid sharing or uploading the movie on any platform that might violate its copyright. - -We hope this article has helped you learn how to watch Once Upon Ay Time In Mumbai Dobaara! in Hindi online or download it from a torrent site. If you have any questions or suggestions, feel free to leave a comment below. -What to Expect from Once Upon Ay Time In Mumbai Dobaara! in Hindi- -Once Upon Ay Time In Mumbai Dobaara! is a movie that will keep you hooked from start to finish. The movie has a gripping plot, with twists and turns that will keep you guessing till the end. The movie also has some of the most memorable dialogues in Bollywood history, delivered by the talented actors with flair and style. The movie also has some of the most catchy and melodious songs, composed by Pritam and sung by some of the best singers in the industry. - -The movie is a visual treat, with stunning cinematography, art direction and editing. The movie recreates the look and feel of the 1980s Mumbai, with its retro fashion, vintage cars and old-school charm. The movie also has some of the most spectacular action sequences, choreographed by Javed-Ejaz and executed by Akshay Kumar and Imran Khan with finesse and skill. The movie also has some of the most romantic scenes, featuring Sonakshi Sinha and Imran Khan, who share a sizzling chemistry on screen. - -The movie is a complete entertainer, with something for everyone. Whether you are a fan of action, romance, comedy or drama, you will find something to enjoy in this movie. The movie is a perfect blend of masala and class, of realism and fantasy, of nostalgia and novelty. The movie is a must-watch for anyone who loves Bollywood cinema and wants to experience a roller-coaster ride of emotions. - -How to Review Once Upon Ay Time In Mumbai Dobaara! in Hindi- -If you have watched Once Upon Ay Time In Mumbai Dobaara! in Hindi and want to share your opinion about it, you can write a review for it. A review is a personal and critical evaluation of a movie, based on your own experience and perspective. A review can help other people decide whether they want to watch the movie or not. A review can also help the makers of the movie to improve their work in the future. - -Writing a review is not difficult, if you follow some simple steps. Here are some tips that can help you write a good review for Once Upon Ay Time In Mumbai Dobaara! in Hindi: - -
Here is an example of a possible review for Once Upon Ay Time In Mumbai Dobaara! in Hindi: - -Review: Once Upon Ay Time In Mumbai Dobaara! (2013) - -Once Upon Ay Time In Mumbai Dobaara! is a 2013 Bollywood crime thriller film directed by Milan Luthria and starring Akshay Kumar, Imran Khan, Sonakshi Sinha and Sonali Bendre. The film is a sequel to the 2010 hit Once Upon A Time In Mumbaai, which chronicled the rise of underworld dons in Mumbai during the 1970s and 1980s. - -The sequel follows the story of Shoaib Khan (Akshay Kumar), who has become the undisputed king of the Mumbai underworld after killing his mentor Sultan Mirza (Ajay Devgn). Shoaib is challenged by his former associate Aslam (Imran Khan), who falls in love with Shoaib's girlfriend Jasmine (Sonakshi Sinha). - -I watched this movie online using a VPN service and a torrent site. I was curious to see how this movie would compare to its predecessor and how it would portray the underworld saga. I was not disappointed by this movie. It was an entertaining and engaging watch that kept me hooked till the end. - -The strengths of this movie are its plot, dialogues, music and performances. The plot is gripping and unpredictable, with twists and turns that keep you guessing till the end. The dialogues are witty and powerful, delivered by the actors with flair and style. The music is catchy and melodious, composed by Pritam and sung by some of the best singers in the industry. The performances are stellar, especially by Akshay Kumar who plays Shoaib Khan with charisma and intensity. - -The weaknesses of this movie are its length, editing and climax. The movie is too long and could have been trimmed by at least 20 minutes. The editing is choppy and inconsistent at places. The climax is abrupt and unsatisfying, leaving many questions unanswered. - -In conclusion, Once Upon Ay Time In Mumbai Dobaara! is a movie that will appeal to anyone who loves Bollywood cinema and wants to experience a roller-coaster ride of emotions. It is a perfect blend of masala and class, of realism and fantasy, of nostalgia and novelty. It is a must-watch for fans of Akshay Kumar and Milan Luthria. - -I would rate this movie 4 out of 5 stars. -Conclusion- -Once Upon Ay Time In Mumbai Dobaara! in hindi download torrent is a popular and easy way to watch this entertaining and engaging movie online or offline. The movie is a sequel to the 2010 hit Once Upon A Time In Mumbaai, which chronicled the rise of underworld dons in Mumbai during the 1970s and 1980s. The movie has a gripping plot, witty dialogues, catchy music and stellar performances by Akshay Kumar, Imran Khan, Sonakshi Sinha and Sonali Bendre. - -In this article, we have explained how to download Once Upon Ay Time In Mumbai Dobaara! in hindi using a VPN, a torrent site and a magnet link. We have also explained how to watch Once Upon Ay Time In Mumbai Dobaara! in hindi online using some of the popular streaming platforms. We have also given some tips on how to write a review for Once Upon Ay Time In Mumbai Dobaara! in hindi and shared an example of a possible review. - -We hope this article has helped you learn how to enjoy Once Upon Ay Time In Mumbai Dobaara! in hindi download torrent. If you have any questions or suggestions, feel free to leave a comment below. 3cee63e6c2- - \ No newline at end of file diff --git a/spaces/scedlatioru/img-to-music/example/Win XP AiO 36 In 1 OEM Torrent.md b/spaces/scedlatioru/img-to-music/example/Win XP AiO 36 In 1 OEM Torrent.md deleted file mode 100644 index d29dba1da959a288d4d682196e60ff9cc1182087..0000000000000000000000000000000000000000 --- a/spaces/scedlatioru/img-to-music/example/Win XP AiO 36 In 1 OEM Torrent.md +++ /dev/null @@ -1,11 +0,0 @@ - - marsh mclennan provides clients with a single software license (excluding mobile device software licensing) and monthly software services fees on a software and services pricing plan. this pricing model is ideal for insurance-based businesses. these businesses provide the software for their business for a specified period and renew this software service contract for the period that they need it. -Win XP AiO 36 In 1 OEM TorrentDOWNLOAD ››› https://gohhs.com/2uEAGi - also, marsh mclennan has established a software license service group to help customers with their software purchasing. this group is independent and can assist business in the purchase and installation of software. -in addition to our continuous service offering, marsh mclennan provides a robust and strategic lifecycle management services solution that supports programs and communities for companies’ internal it resources. -these services are highly specialized and align with the specific needs of insurance companies. if you are an insurance company, and you are interested in a strong service partner, please contact us. we will be happy to discuss the services we provide and how they can benefit you and your business. -to learn more about marsh mclennan insurance technology consulting, please visit our website at http://marshmclennan.com/insurance-technology-consulting/ or email us at technologyconsulting@marshmclennan.com . - -next, go into the bios on your aio. you can access this by pressing f2 on your keyboard. in the bios, enter setup, and scroll down to the bios memory. choose the following two items:
- - \ No newline at end of file diff --git a/spaces/sdutta28/AggDetectApp/Dockerfile b/spaces/sdutta28/AggDetectApp/Dockerfile deleted file mode 100644 index 3d004cab0e329ff78ad9a51620675f935e366662..0000000000000000000000000000000000000000 --- a/spaces/sdutta28/AggDetectApp/Dockerfile +++ /dev/null @@ -1,23 +0,0 @@ -FROM python:3.10 - -WORKDIR /code - -COPY ./requirements.txt requirements.txt - -RUN pip install --no-cache-dir --upgrade -r /code/requirements.txt - -RUN useradd -m -u 1000 user - -USER user -# Set home to the user's home directory -ENV HOME=/home/user \ - PATH=/home/user/.local/bin:$PATH\ - NLTK_DATA=/home/user/app/static/nltk - -WORKDIR $HOME/app - -COPY --chown=user . $HOME/app - -RUN ls -alt - -CMD ["python", "app.py"] \ No newline at end of file diff --git a/spaces/segments-tobias/conex/espnet/nets/pytorch_backend/transducer/arguments.py b/spaces/segments-tobias/conex/espnet/nets/pytorch_backend/transducer/arguments.py deleted file mode 100644 index 96f9fda4a096c2ca4bfb61e4faded3960a7ee401..0000000000000000000000000000000000000000 --- a/spaces/segments-tobias/conex/espnet/nets/pytorch_backend/transducer/arguments.py +++ /dev/null @@ -1,321 +0,0 @@ -"""Transducer model arguments.""" - -import ast -from distutils.util import strtobool - - -def add_encoder_general_arguments(group): - """Define general arguments for encoder.""" - group.add_argument( - "--etype", - default="blstmp", - type=str, - choices=[ - "custom", - "lstm", - "blstm", - "lstmp", - "blstmp", - "vgglstmp", - "vggblstmp", - "vgglstm", - "vggblstm", - "gru", - "bgru", - "grup", - "bgrup", - "vgggrup", - "vggbgrup", - "vgggru", - "vggbgru", - ], - help="Type of encoder network architecture", - ) - group.add_argument( - "--dropout-rate", - default=0.0, - type=float, - help="Dropout rate for the encoder", - ) - - return group - - -def add_rnn_encoder_arguments(group): - """Define arguments for RNN encoder.""" - group.add_argument( - "--elayers", - default=4, - type=int, - help="Number of encoder layers (for shared recognition part " - "in multi-speaker asr mode)", - ) - group.add_argument( - "--eunits", - "-u", - default=300, - type=int, - help="Number of encoder hidden units", - ) - group.add_argument( - "--eprojs", default=320, type=int, help="Number of encoder projection units" - ) - group.add_argument( - "--subsample", - default="1", - type=str, - help="Subsample input frames x_y_z means subsample every x frame " - "at 1st layer, every y frame at 2nd layer etc.", - ) - - return group - - -def add_custom_encoder_arguments(group): - """Define arguments for Custom encoder.""" - group.add_argument( - "--enc-block-arch", - type=eval, - action="append", - default=None, - help="Encoder architecture definition by blocks", - ) - group.add_argument( - "--enc-block-repeat", - default=0, - type=int, - help="Repeat N times the provided encoder blocks if N > 1", - ) - group.add_argument( - "--custom-enc-input-layer", - type=str, - default="conv2d", - choices=["conv2d", "vgg2l", "linear", "embed"], - help="Custom encoder input layer type", - ) - group.add_argument( - "--custom-enc-positional-encoding-type", - type=str, - default="abs_pos", - choices=["abs_pos", "scaled_abs_pos", "rel_pos"], - help="Custom encoder positional encoding layer type", - ) - group.add_argument( - "--custom-enc-self-attn-type", - type=str, - default="self_attn", - choices=["self_attn", "rel_self_attn"], - help="Custom encoder self-attention type", - ) - group.add_argument( - "--custom-enc-pw-activation-type", - type=str, - default="relu", - choices=["relu", "hardtanh", "selu", "swish"], - help="Custom encoder pointwise activation type", - ) - group.add_argument( - "--custom-enc-conv-mod-activation-type", - type=str, - default="swish", - choices=["relu", "hardtanh", "selu", "swish"], - help="Custom encoder convolutional module activation type", - ) - - return group - - -def add_decoder_general_arguments(group): - """Define general arguments for encoder.""" - group.add_argument( - "--dtype", - default="lstm", - type=str, - choices=["lstm", "gru", "custom"], - help="Type of decoder to use", - ) - group.add_argument( - "--dropout-rate-decoder", - default=0.0, - type=float, - help="Dropout rate for the decoder", - ) - group.add_argument( - "--dropout-rate-embed-decoder", - default=0.0, - type=float, - help="Dropout rate for the decoder embedding layer", - ) - - return group - - -def add_rnn_decoder_arguments(group): - """Define arguments for RNN decoder.""" - group.add_argument( - "--dec-embed-dim", - default=320, - type=int, - help="Number of decoder embeddings dimensions", - ) - group.add_argument( - "--dlayers", default=1, type=int, help="Number of decoder layers" - ) - group.add_argument( - "--dunits", default=320, type=int, help="Number of decoder hidden units" - ) - - return group - - -def add_custom_decoder_arguments(group): - """Define arguments for Custom decoder.""" - group.add_argument( - "--dec-block-arch", - type=eval, - action="append", - default=None, - help="Custom decoder blocks definition", - ) - group.add_argument( - "--dec-block-repeat", - default=1, - type=int, - help="Repeat N times the provided decoder blocks if N > 1", - ) - group.add_argument( - "--custom-dec-input-layer", - type=str, - default="embed", - choices=["linear", "embed"], - help="Custom decoder input layer type", - ) - group.add_argument( - "--custom-dec-pw-activation-type", - type=str, - default="relu", - choices=["relu", "hardtanh", "selu", "swish"], - help="Custom decoder pointwise activation type", - ) - - return group - - -def add_custom_training_arguments(group): - """Define arguments for training with Custom architecture.""" - group.add_argument( - "--transformer-warmup-steps", - default=25000, - type=int, - help="Optimizer warmup steps", - ) - group.add_argument( - "--transformer-lr", - default=10.0, - type=float, - help="Initial value of learning rate", - ) - - return group - - -def add_transducer_arguments(group): - """Define general arguments for transducer model.""" - group.add_argument( - "--trans-type", - default="warp-transducer", - type=str, - choices=["warp-transducer", "warp-rnnt"], - help="Type of transducer implementation to calculate loss.", - ) - group.add_argument( - "--transducer-weight", - default=1.0, - type=float, - help="Weight of transducer loss when auxiliary task is used.", - ) - group.add_argument( - "--joint-dim", - default=320, - type=int, - help="Number of dimensions in joint space", - ) - group.add_argument( - "--joint-activation-type", - type=str, - default="tanh", - choices=["relu", "tanh", "swish"], - help="Joint network activation type", - ) - group.add_argument( - "--score-norm", - type=strtobool, - nargs="?", - default=True, - help="Normalize transducer scores by length", - ) - - return group - - -def add_auxiliary_task_arguments(group): - """Add arguments for auxiliary task.""" - group.add_argument( - "--aux-task-type", - nargs="?", - default=None, - choices=["default", "symm_kl_div", "both"], - help="Type of auxiliary task.", - ) - group.add_argument( - "--aux-task-layer-list", - default=None, - type=ast.literal_eval, - help="List of layers to use for auxiliary task.", - ) - group.add_argument( - "--aux-task-weight", - default=0.3, - type=float, - help="Weight of auxiliary task loss.", - ) - group.add_argument( - "--aux-ctc", - type=strtobool, - nargs="?", - default=False, - help="Whether to use CTC as auxiliary task.", - ) - group.add_argument( - "--aux-ctc-weight", - default=1.0, - type=float, - help="Weight of auxiliary task loss", - ) - group.add_argument( - "--aux-ctc-dropout-rate", - default=0.0, - type=float, - help="Dropout rate for auxiliary CTC", - ) - group.add_argument( - "--aux-cross-entropy", - type=strtobool, - nargs="?", - default=False, - help="Whether to use CE as auxiliary task for the prediction network.", - ) - group.add_argument( - "--aux-cross-entropy-smoothing", - default=0.0, - type=float, - help="Smoothing rate for cross-entropy. If > 0, enables label smoothing loss.", - ) - group.add_argument( - "--aux-cross-entropy-weight", - default=0.5, - type=float, - help="Weight of auxiliary task loss", - ) - - return group diff --git a/spaces/shenfangqi/Retrieval-based-Voice-Conversion-WebUI/go-web.bat b/spaces/shenfangqi/Retrieval-based-Voice-Conversion-WebUI/go-web.bat deleted file mode 100644 index db1dec52006bc631e4e68bafd619a3a65f202532..0000000000000000000000000000000000000000 --- a/spaces/shenfangqi/Retrieval-based-Voice-Conversion-WebUI/go-web.bat +++ /dev/null @@ -1,2 +0,0 @@ -runtime\python.exe infer-web.py --pycmd runtime\python.exe --port 7897 -pause diff --git a/spaces/shikunl/prismer/prismer/experts/obj_detection/unidet/data/datasets/cityscapes_cocoformat.py b/spaces/shikunl/prismer/prismer/experts/obj_detection/unidet/data/datasets/cityscapes_cocoformat.py deleted file mode 100644 index 3492c6af30fb6753be2eb95b8d37de75e34a1727..0000000000000000000000000000000000000000 --- a/spaces/shikunl/prismer/prismer/experts/obj_detection/unidet/data/datasets/cityscapes_cocoformat.py +++ /dev/null @@ -1,28 +0,0 @@ -from detectron2.data.datasets.register_coco import register_coco_instances -import os - -categories = [ - {'id': i, 'name': x} for i, x in enumerate( - ["person", "rider", "car", "truck","bus", "train", \ - "motorcycle", "bicycle"]) -] - -def _get_builtin_metadata(): - thing_dataset_id_to_contiguous_id = { - x['id']: i for i, x in enumerate(sorted(categories, key=lambda x: x['id']))} - thing_classes = [x['name'] for x in sorted(categories, key=lambda x: x['id'])] - return { - "thing_dataset_id_to_contiguous_id": thing_dataset_id_to_contiguous_id, - "thing_classes": thing_classes} - -_PREDEFINED_SPLITS_CITYSCAPES = { - "cityscapes_cocoformat_val": ("", "cityscapes/annotations/cityscapes_fine_instance_seg_val_coco_format.json"), -} - -for key, (image_root, json_file) in _PREDEFINED_SPLITS_CITYSCAPES.items(): - register_coco_instances( - key, - _get_builtin_metadata(), - os.path.join("datasets", json_file) if "://" not in json_file else json_file, - os.path.join(image_root), - ) diff --git a/spaces/simple0urra/skops-model-card-creator-2a23515a-d54e-4804-b365-27ed6e938735/example/Adobe After Effects 2020 APK The Industry-Standard Motion Graphics and Visual Effects Software for Android.md b/spaces/simple0urra/skops-model-card-creator-2a23515a-d54e-4804-b365-27ed6e938735/example/Adobe After Effects 2020 APK The Industry-Standard Motion Graphics and Visual Effects Software for Android.md deleted file mode 100644 index d91fe9f9300ef14395e73a18844cdbe7b8a0ea33..0000000000000000000000000000000000000000 --- a/spaces/simple0urra/skops-model-card-creator-2a23515a-d54e-4804-b365-27ed6e938735/example/Adobe After Effects 2020 APK The Industry-Standard Motion Graphics and Visual Effects Software for Android.md +++ /dev/null @@ -1,125 +0,0 @@ - - After Effects 2020 APK: What You Need to Know-If you are a fan of creating motion graphics and visual effects for film, TV, video, and web, you might have heard of Adobe After Effects. It is one of the most popular and powerful software for creating stunning animations and effects. -But what if you want to use After Effects on your Android device? Is there a way to do that? The answer is yes, thanks to an APK file. -after effects 2020 apkDownload ✏ ✏ ✏ https://ssurll.com/2uNZVG - An APK file is an Android application package that contains all the files and code needed to run an app on an Android device. It is similar to an EXE file for Windows or a DMG file for Mac. -In this article, we will tell you everything you need to know about downloading and installing After Effects 2020 APK on your Android device. We will also discuss some of the benefits, risks, and legal issues of doing so. -Why Download After Effects 2020 APK?-There are many reasons why you might want to download After Effects 2020 APK on your Android device. Here are some of them: -
New Features in After Effects 2020-After Effects 2020 is the latest version of Adobe's software for creating motion graphics and visual effects. It was released in November 2019 and has many new features and improvements that make it easier and faster to create stunning animations and effects. Here are some of them: -AI-powered Rotoscoping-Rotoscoping is the process of tracing and masking objects in video clips to isolate them from the background or apply effects to them. It can be a tedious and time-consuming task, especially for complex or moving objects. -But with After Effects 2020, you can use the new Roto Brush 2 tool, which uses artificial intelligence to automatically track and mask objects in video clips. You just need to draw a rough outline around the object, and the tool will do the rest. You can also refine the mask with the Refine Edge tool, which uses machine learning to detect edges and details. -Cinemagraphs-Cinemagraphs are still images with subtle motion, such as a flickering candle, a waving flag, or a flowing waterfall. They can create a sense of life and movement in your images, making them more engaging and eye-catching. -With After Effects 2020, you can create cinemagraphs easily and quickly. You just need to import a video clip, trim it to the desired length, freeze a frame as the background layer, and mask out the areas that you want to keep moving. You can also adjust the speed, direction, and loop of the motion. -after effects 2020 apk download Video Effects-After Effects 2020 has a wide range of video effects that you can apply to your clips to enhance their look and feel. You can use color correction tools to adjust the hue, saturation, brightness, contrast, and more. You can use light leaks effects to add realistic light flares and glows to your clips. You can use glitches effects to create distorted or corrupted video effects. You can also use transitions effects to create smooth and dynamic changes between clips. -How to Download and Install After Effects 2020 APK?-If you want to download and install After Effects 2020 APK on your Android device, you need to follow these steps: Download the APK File-The first step is to download the APK file for After Effects 2020. You can find it on various websites that offer APK files for Android apps, such as APKPure, APKMirror, or APKMonk. However, you need to be careful and choose a reputable and reliable source, as some APK files might contain malware or viruses that can harm your device or steal your data. -One of the websites that we recommend is APKPure, which is a trusted and verified platform that provides safe and fast downloads of APK files. You can visit their website and search for After Effects 2020 APK, or use this link: https://apkpure.com/adobe-after-effects-2020/com.adobe.aftereffects. You will see a page with the details and reviews of the app, as well as a download button. Click on the download button and wait for the file to be downloaded to your device. -Enable Unknown Sources-The next step is to enable unknown sources on your Android device. This is a security setting that allows you to install apps from sources other than the Google Play Store. By default, this setting is disabled, so you need to enable it before you can install After Effects 2020 APK. -To enable unknown sources, you need to go to your device's settings and look for the security or privacy option. Depending on your device model and Android version, this option might be located in different places. For example, on some devices, you might find it under Settings > Security > Unknown Sources, while on others, you might find it under Settings > Apps > Special Access > Install Unknown Apps. -Once you find the option, you need to tap on it and toggle it on. You might see a warning message that tells you about the risks of installing apps from unknown sources. Read it carefully and tap on OK or Allow if you agree to proceed. -Install the APK File-The final step is to install the APK file for After Effects 2020. To do this, you need to locate the file on your device's storage, either using a file manager app or by accessing the download folder. Once you find the file, tap on it and follow the instructions on the screen. You might see a prompt that asks you to confirm the installation or grant permissions to the app. Tap on Install or Accept if you agree. -Wait for the installation process to finish, which might take a few minutes depending on your device's speed and performance. Once it is done, you will see a message that says "App installed" or "Installation successful". You can then tap on Open or Done to launch or exit the app. -Launch the App and Enjoy-Congratulations! You have successfully downloaded and installed After Effects 2020 APK on your Android device. You can now launch the app and enjoy creating amazing motion graphics and visual effects with it. -To launch the app, you can either tap on its icon on your home screen or app drawer, or go back to the APKPure website and tap on Open. You will see a splash screen with the Adobe logo and then a welcome screen with some tips and tutorials on how to use the app. -You can explore the app's interface and features by tapping on the menu button at the top left corner of the screen. You will see options such as New Project, Open Project, Import File, Export File, Preferences, Help, and more. You can also access some tools and panels at the bottom of the screen, such as Timeline, Layers, Effects, Masks, Keyframes, and more. -You can create a new project by tapping on New Project and choosing a name and a resolution for your project. You can then import files from your device's storage or camera by tapping on Import File and selecting the files you want to use. You can then drag and drop them onto the timeline and start editing them with various effects and animations. -You can export your project by tapping on Export File and choosing a format and a quality for your output file. You can then save it to your device's storage or share it with others via email, social media, or cloud services. -If you need any help or guidance on how to use After Effects 2020 APK, you can tap on Help and access some resources such as tutorials, FAQs, forums, feedback, and more. Is After Effects 2020 APK Safe and Legal?-While downloading and installing After Effects 2020 APK might seem like a great idea, you should also be aware of some of the potential risks and legal issues that come with it. Here are some of them: -Risks-One of the main risks of downloading and installing APK files from unknown sources is that they might contain malware or viruses that can harm your device or steal your data. Malware or viruses can infect your device, slow it down, drain your battery, display unwanted ads, access your contacts, messages, photos, and other files, or even lock your device and demand a ransom to unlock it. -To avoid this risk, you should always scan the APK file with an antivirus software before installing it. You should also check the reviews and ratings of the app and the website that provides it, and look for any signs of suspicious or malicious activity. You should also avoid clicking on any pop-ups or links that might appear during the download or installation process. -Legal Issues-Another risk of downloading and installing APK files from unknown sources is that they might not be authorized by the original developers or publishers of the app. This means that you might be violating their intellectual property rights, such as copyright, trademark, or patent. This can result in legal consequences, such as fines, lawsuits, or even criminal charges. -To avoid this risk, you should always respect the rights of the original developers or publishers of the app and only download and install APK files that are authorized by them. You should also check the terms and conditions of the app and the website that provides it, and look for any disclaimers or warnings that might indicate that the app is not official or licensed. -Recommendations-To minimize the risks and legal issues of downloading and installing APK files from unknown sources, here are some recommendations that you should follow: -
Conclusion-In conclusion, After Effects 2020 APK is a great way to enjoy the latest features and improvements of Adobe's software for creating motion graphics and visual effects on your Android device. You can download and install it easily and quickly by following our guide above. However, you should also be aware of some of the potential risks and legal issues that come with it, and follow our recommendations to avoid or minimize them. -If you have any questions or feedback about After Effects 2020 APK, feel free to leave a comment below. We would love to hear from you! -FAQs-Here are some frequently asked questions and answers about After Effects 2020 APK: -
After Effects 2020 APK is compatible with most Android devices that run on Android 5.0 (Lollipop) or higher. However, some devices might not support some features or functions of the app due to hardware limitations or software conflicts. To check if your device is compatible with After Effects 2020 APK, you can visit this link: https://helpx.adobe.com/after-effects/system-requirements.html. -To update After Effects 2020 APK, you need to download and install the latest version of the APK file from a reputable source, such as APKPure. You can also check for updates within the app by tapping on the menu button at the top left corner of the screen and selecting Check for Updates. If there is a new version available, you will see a notification that prompts you to download and install it. -To uninstall After Effects 2020 APK, you need to go to your device's settings and look for the apps or applications option. Depending on your device model and Android version, this option might be located in different places. For example, on some devices, you might find it under Settings > Apps & Notifications > See All Apps, while on others, you might find it under Settings > Apps > Manage Apps. Once you find the option, you need to tap on it and look for After Effects 2020 APK in the list of apps. Tap on it and select Uninstall. You might see a confirmation message that asks you to confirm the uninstallation. Tap on OK or Yes if you agree. -If you need any support or assistance for After Effects 2020 APK, you can contact the developers or publishers of the app directly. You can find their contact information on the website that provides the APK file, or within the app by tapping on the menu button at the top left corner of the screen and selecting About or Contact. You can also visit the official website of Adobe After Effects, which is https://www.adobe.com/products/aftereffects.html, and access some resources such as help articles, tutorials, forums, feedback, and more. -Yes, you can use After Effects 2020 APK with other Adobe apps, such as Photoshop, Illustrator, Premiere Pro, and more. You can import and export files between these apps and use them to create amazing projects. You can also use Adobe Creative Cloud to sync your files and settings across your devices and access them anytime and anywhere. -- - \ No newline at end of file diff --git a/spaces/simple0urra/skops-model-card-creator-2a23515a-d54e-4804-b365-27ed6e938735/example/Air Fighter Pro APK How to Master the Controls Navigation and Tactics of the Best Flight Simulator and Combat Game.md b/spaces/simple0urra/skops-model-card-creator-2a23515a-d54e-4804-b365-27ed6e938735/example/Air Fighter Pro APK How to Master the Controls Navigation and Tactics of the Best Flight Simulator and Combat Game.md deleted file mode 100644 index 8d043bbf80883709a0288501b6c77d18fe1dbfe7..0000000000000000000000000000000000000000 --- a/spaces/simple0urra/skops-model-card-creator-2a23515a-d54e-4804-b365-27ed6e938735/example/Air Fighter Pro APK How to Master the Controls Navigation and Tactics of the Best Flight Simulator and Combat Game.md +++ /dev/null @@ -1,79 +0,0 @@ - - AirFighters Pro APK: A Realistic and Exciting Flight Simulator Game-If you are a fan of flight simulator games, you might have heard of AirFighters Pro APK. This is a game that lets you experience the thrill of flying different planes in various scenarios and missions. You can explore realistic world maps, navigate through over 500 airports, land on aircraft carriers, and face challenging weather conditions. You can also plan and share your own tactical scenarios, test your skills in the world campaign, and fight against other planes in the dog-fight mode. In this article, we will tell you more about AirFighters Pro APK, how to download and install it, and what are its pros and cons. -What is AirFighters Pro APK?-AirFighters Pro APK is an Android game that was developed by RORTOS, a company that specializes in creating realistic flight simulator games. The game was released in 2013 and has received positive reviews from users and critics alike. The game is not available on Google Play Store, but you can download it from other sources for free. The game has a size of about 105 MB and requires Android 4.0 or higher to run. -air fighter pro apkDownload File →→→ https://ssurll.com/2uNTyQ - Features of AirFighters Pro APK-AirFighters Pro APK has many features that make it one of the most realistic and exciting flight simulator games on Android. Here are some of them: -Realistic world maps and navigation-The game has realistic world maps that cover the entire globe. You can fly over different regions, such as Europe, Asia, Africa, America, and Australia. You can also use the GPS navigation system to find your way around the map. The game also has a radar system that shows you the position of your enemies and allies. -Over 500 actual airports and 1,107 runways-The game has over 500 actual airports that you can land on or take off from. You can choose from different types of airports, such as civil, military, or private. You can also select from different types of runways, such as asphalt, grass, or dirt. The game also has realistic physics and controls that affect your landing and take-off performance. -Aircraft carriers and real time weather conditions-The game has aircraft carriers that you can use as bases for your missions. You can land on or take off from these carriers using special procedures and equipment. You can also face different weather conditions that affect your flight, such as wind, rain, snow, fog, or storms. -Plan and share your tactical scenarios-The game has a feature that allows you to create your own tactical scenarios using the map editor. You can set up different objectives, enemies, allies, weather conditions, and other parameters for your scenario. You can also share your scenario with other players online or play their scenarios. -Test yourself in the world campaign and dog-fight mode-The game has a world campaign mode that consists of 60 missions that span across different regions and scenarios. You can complete these missions to earn trophies and unlock new planes. The game also has a dog-fight mode that lets you fight against other planes in a one-on-one or team-based combat. -Choose from 8 different planes-The game has 8 different planes that you can fly in the game. Each plane has its own characteristics, such as speed , such as speed, maneuverability, weapons, and fuel capacity. The planes are: - F/A-18 Super Hornet - F-14 Super Tomcat - A-6 Intruder - F4E Phantom II - MiG-29K Fulcrum - F-35B Lightning II - AV-8B Harrier II - Tornado You can customize the color and the loadout of your plane according to your preferences and mission requirements. -How to download and install AirFighters Pro APK?-If you want to download and install AirFighters Pro APK on your Android device, you need to follow these steps: -air fighter pro apk download Requirements for AirFighters Pro APK-Before you download and install AirFighters Pro APK, you need to make sure that your device meets these requirements: - - Android 4.0 or higher - At least 105 MB of free storage space - A stable internet connectionSteps to download and install AirFighters Pro APK-After you have checked the requirements, you can proceed with these steps: - 1. Go to a trusted website that offers AirFighters Pro APK for free download, such as [mob.org](^1^). 2. Click on the download button and wait for the APK file to be downloaded on your device. 3. Go to your device settings and enable the option to install apps from unknown sources. This will allow you to install AirFighters Pro APK without any issues. 4. Locate the downloaded APK file on your device and tap on it to start the installation process. 5. Follow the instructions on the screen and wait for the installation to be completed. 6. Launch the game and enjoy flying different planes in realistic scenarios.Pros and cons of AirFighters Pro APK-AirFighters Pro APK has many pros and cons that you should consider before downloading and playing it. Here are some of them: -Pros of AirFighters Pro APK-Some of the pros of AirFighters Pro APK are: - - It has realistic graphics and sound effects that create an immersive gaming experience. - It has a variety of planes, airports, missions, and modes that offer a lot of replay value. - It has a user-friendly interface and easy controls that make it suitable for beginners and experts alike. - It has a map editor and a sharing feature that allow you to create and play your own scenarios or those of other players. - It is free to download and play, unlike the original version that costs $4.99 on Google Play Store.Cons of AirFighters Pro APK-Some of the cons of AirFighters Pro APK are: - - It is not available on Google Play Store, which means you have to download it from third-party sources that may not be safe or reliable. - It may have some bugs or glitches that affect the gameplay or performance of the game. - It may require a lot of battery power and data usage, which can drain your device quickly. - It may not be compatible with some devices or Android versions, which can cause crashes or errors.Conclusion-AirFighters Pro APK is a great game for flight simulator enthusiasts who want to experience the thrill of flying different planes in realistic scenarios. The game has many features that make it one of the most realistic and exciting flight simulator games on Android. However, the game also has some drawbacks that you should be aware of before downloading and playing it. If you are interested in trying out AirFighters Pro APK, you can follow the steps we have provided above to download and install it on your device. -Frequently Asked Questions-Here are some frequently asked questions about AirFighters Pro APK: - Q: Is AirFighters Pro APK safe to download and install? A: AirFighters Pro APK is safe to download and install if you get it from a trusted website that offers virus-free and malware-free files. However, you should always be careful when downloading apps from unknown sources, as they may contain harmful or malicious content. Q: Is AirFighters Pro APK legal to use? A: AirFighters Pro APK is legal to use if you own the original version of the game or have permission from the developer to use it. However, you should not use AirFighters Pro APK for any illegal or unethical purposes, such as piracy or cheating. Q: How can I update AirFighters Pro APK? A: You can update AirFighters Pro APK by downloading the latest version of the game from the same website where you got it from. You can also check for updates within the game settings or by visiting the official website of the developer. Q: How can I uninstall AirFighters Pro APK? A A: You can uninstall AirFighters Pro APK by following these steps: 1. Go to your device settings and tap on the Apps or Applications option. 2. Find and select AirFighters Pro APK from the list of installed apps. 3. Tap on the Uninstall button and confirm your action. 4. Wait for the app to be removed from your device. Q: How can I contact the developer of AirFighters Pro APK? A: You can contact the developer of AirFighters Pro APK by visiting their official website at [rortos.com]. You can also follow them on their social media accounts, such as Facebook, Twitter, Instagram, and YouTube. You can also send them an email at info@rortos.com or use the contact form on their website. Q: How can I get more tips and tricks for AirFighters Pro APK? A: You can get more tips and tricks for AirFighters Pro APK by watching video tutorials, reading guides, or joining online forums and communities of other players. You can also check out the game's FAQ section or the help menu within the game. 401be4b1e0- - \ No newline at end of file diff --git a/spaces/simple0urra/skops-model-card-creator-2a23515a-d54e-4804-b365-27ed6e938735/example/Build Craft The Ultimate 3D Crafting and Building Game - Download for Free.md b/spaces/simple0urra/skops-model-card-creator-2a23515a-d54e-4804-b365-27ed6e938735/example/Build Craft The Ultimate 3D Crafting and Building Game - Download for Free.md deleted file mode 100644 index 9f6231ffe08f259d2ac9f4dd2e464abd37efd141..0000000000000000000000000000000000000000 --- a/spaces/simple0urra/skops-model-card-creator-2a23515a-d54e-4804-b365-27ed6e938735/example/Build Craft The Ultimate 3D Crafting and Building Game - Download for Free.md +++ /dev/null @@ -1,166 +0,0 @@ - - Crafting and Building Download App Store: How to Get the Best Building Game for Free-Do you love building games? Do you want to create your own world with your own rules? Do you want to have fun with your friends online? If you answered yes to any of these questions, then you should try Crafting and Building, the new free building game that is taking the app store by storm. In this article, we will tell you everything you need to know about this amazing game, how to download it from the app store, how to play it with your friends, and how to unleash your creativity in it. Let's get started! -crafting and building download app storeDownload Zip ····· https://ssurll.com/2uNVzm - What is Crafting and Building?-A brief introduction to the game and its features-Crafting and Building is a free game for the whole family, from kids to adults. It is a sandbox game, which means that you can do whatever you want in it, without any limitations or goals. You can explore, build, destroy, craft, mine, farm, and more. You can also play with your friends online, or join other players' worlds. The game has stunning graphics, realistic sounds, simple controls, and many game modes. You can choose from different types of blocks, from grass to diamond, and use them to create anything you can imagine. You can also decorate your house with furniture and accessories, or customize your character with skins. The game is constantly updated with new features and improvements. -Why you should play Crafting and Building-Crafting and Building is not just a game, it is a way of expressing yourself and having fun. Here are some of the reasons why you should play it: -
How to download Crafting and Building from the app store-Step-by-step guide for Android users-If you have an Android device, such as a smartphone or a tablet, here are the steps you need to follow to download Crafting and Building from the app store: -
Step-by-step guide for iOS users-If you have an iOS device, such as an iPhone or an iPad, here are the steps you need to follow to download Crafting and Building from the app store: -
How to play Crafting and Building with your friends-How to join or create a multiplayer world-One of the best features of Crafting and Building is that you can play with your friends online, or join other players' worlds. Here is how you can do that: -crafting and building game free download
How to chat and cooperate with other players-Once you are in a multiplayer world, you can chat and cooperate with other players. Here is how you can do that: -
How to unleash your creativity in Crafting and Building-How to use different blocks and tools-Crafting and Building gives you access to hundreds of different blocks and tools that you can use to create anything you want. Here is how you can use them: -
How to design and build your own house, castle, or mine-One of the most fun things to do in Crafting and Building is to design and build your own house, castle, or mine. Here are some tips on how to do that: -
Tips and tricks for Crafting and Building-How to find hidden caves and treasures-Crafting and Building has a lot of hidden secrets and surprises that you can discover while exploring the world. Here are some tips on how to find hidden caves and treasures: -
How to tame and ride animals-Crafting and Building has a lot of animals that you can tame and ride for fun or transportation. Here are some tips on how to tame and ride animals: -
Conclusion-Crafting and Building is one of the best building games that you can download from the app store for free. It is a game that lets you create your own world with your own rules and have fun with your friends online. It is a game that lets you use your imagination and creativity to create anything you want. It is a game that lets you learn a lot from playing it. It is a game that lets you have fun while playing it. -If you are looking for a game that will keep you entertained for hours and days, then Crafting and Building is the game for you. Download it now from the app store and start building your own world today! -Frequently Asked Questions-Here are some of the frequently asked questions about Crafting and Building: -
Crafting and Building is Crafting and Building is safe for kids, as long as they play it in a supervised and responsible manner. The game does not contain any violence, gore, or inappropriate content. However, the game does allow players to chat and interact with other players online, which may expose them to some risks or dangers. Therefore, parents should monitor their kids' online activity and set some rules and boundaries for them. Parents should also educate their kids about online safety and etiquette, such as not sharing personal information, not talking to strangers, not clicking on suspicious links, and reporting any abuse or harassment. -Crafting and Building is constantly updated with new features and improvements. To update the game, you need to follow these steps: -
If you have any questions, feedback, suggestions, or issues about Crafting and Building, you can contact the developers of the game by using one of these methods: -
If you love Crafting and Building and want to support the game, you can do one of these things: -
If you are looking for some alternatives to Crafting and Building, you can try some of these games: -
- - \ No newline at end of file diff --git a/spaces/simple0urra/skops-model-card-creator-2a23515a-d54e-4804-b365-27ed6e938735/example/Download Love Me Like You Do Ringtone by Jassa Dhillon - MP3 Free.md b/spaces/simple0urra/skops-model-card-creator-2a23515a-d54e-4804-b365-27ed6e938735/example/Download Love Me Like You Do Ringtone by Jassa Dhillon - MP3 Free.md deleted file mode 100644 index 962dd98303474353ca17cba02102bbb9af675800..0000000000000000000000000000000000000000 --- a/spaces/simple0urra/skops-model-card-creator-2a23515a-d54e-4804-b365-27ed6e938735/example/Download Love Me Like You Do Ringtone by Jassa Dhillon - MP3 Free.md +++ /dev/null @@ -1,86 +0,0 @@ - - Love Me Like You Do Ringtone Mp3 Download Jassa Dhillon-Do you love the song Love Me Like You Do by Jassa Dhillon? Do you want to set it as your phone's ringtone? If yes, then you are in the right place. In this article, we will show you how to download Love Me Like You Do ringtone mp3 for free and easily. We will also tell you more about the song and the singer, so you can enjoy it even more. -love me like you do ringtone mp3 download jassa dhillonDownload Zip ———>>> https://ssurll.com/2uNVsv - Introduction-What is Love Me Like You Do?-Love Me Like You Do is a Punjabi song by Jassa Dhillon, released in 2021. It is a romantic song that expresses the feelings of a lover who wants his partner to love him like no one else. The song has catchy lyrics, melodious music, and a beautiful video. The song has become very popular among Punjabi music fans and has received millions of views on YouTube. -Who is Jassa Dhillon?-Jassa Dhillon is a Punjabi singer, songwriter, and music producer. He was born in Punjab, India, and moved to Canada at a young age. He started his musical career in 2019 with his debut album Pyaar Mangdi. He has since released many hit songs such as Raule, Talja, Sira E Hou, and Love Me Like You Do. He is known for his unique voice, style, and lyrics. -How to Download Love Me Like You Do Ringtone Mp3?-If you want to download Love Me Like You Do ringtone mp3 for your phone, you have two options. You can either use Zedge or Prokerala. Both are free websites that offer a wide range of ringtones for different devices. Here are the steps to follow for each method: -Method 1: Use Zedge-Step 1: Visit Zedge website-Zedge is a popular website that provides wallpapers, ringtones, notifications, and games for mobile phones. To use Zedge, you need to visit its website at [Zedge](^1^). You can also download its app from Google Play Store or App Store. -Step 2: Search for Love Me Like You Do ringtone-Once you are on Zedge website or app, you need to search for Love Me Like You Do ringtone. You can use the search bar or browse through the categories. You will see many results related to the song. Choose the one that you like and click on it. -Step 3: Download the ringtone in mp3 format-After you click on the ringtone, you will see a preview of it. You can listen to it and decide if you want to download it or not. If you like it, you can click on the download button and choose mp3 format. The ringtone will be downloaded to your device and you can set it as your phone's ringtone. -love me like you do jassa dhillon mp3 ringtone free download Method 2: Use Prokerala-Step 1: Visit Prokerala website-Prokerala is another website that offers ringtones, wallpapers, news, astrology, and more for mobile phones. To use Pro kerala, you need to visit its website at [Prokerala]. -Step 2: Find Love Me Like You Do ringtone-On Prokerala website, you need to find Love Me Like You Do ringtone. You can use the search box or go to the ringtones section. You will see a list of ringtones related to the song. Select the one that you want and click on it. -Step 3: Download the ringtone in mp3 or m4r format-When you click on the ringtone, you will see a page with the details of the ringtone. You can play it and check if you like it or not. If you want to download it, you can choose between mp3 or m4r format. Mp3 is for Android phones and m4r is for iPhone. Click on the download button and save the ringtone to your device. You can then set it as your phone's ringtone. -Conclusion-Summary of the article-In this article, we have shown you how to download Love Me Like You Do ringtone mp3 for free and easily. We have also given you some information about the song and the singer, Jassa Dhillon. We hope you enjoyed this article and found it useful. -Call to action-If you liked this article, please share it with your friends and family who might be interested in downloading Love Me Like You Do ringtone mp3. Also, don't forget to check out Jassa Dhillon's other songs and support his music. Thank you for reading and have a great day! -Frequently Asked Questions-Here are some common questions and answers related to Love Me Like You Do ringtone mp3 download: -
- - \ No newline at end of file diff --git a/spaces/simple0urra/skops-model-card-creator-2a23515a-d54e-4804-b365-27ed6e938735/example/Download Weapon Throwing RPG 2 MOD APK with Unlimited Money and Stamina.md b/spaces/simple0urra/skops-model-card-creator-2a23515a-d54e-4804-b365-27ed6e938735/example/Download Weapon Throwing RPG 2 MOD APK with Unlimited Money and Stamina.md deleted file mode 100644 index 2af8b0978cdd3dc2b58af80899a376c0ae929a85..0000000000000000000000000000000000000000 --- a/spaces/simple0urra/skops-model-card-creator-2a23515a-d54e-4804-b365-27ed6e938735/example/Download Weapon Throwing RPG 2 MOD APK with Unlimited Money and Stamina.md +++ /dev/null @@ -1,92 +0,0 @@ - - Weapon Throwing RPG 2 Mod APK: A Fun and Challenging Game for RPG Fans-If you are looking for a new and exciting role-playing game to play on your Android device, you might want to check out Weapon Throwing RPG 2. This game is a sequel to the popular Weapon Throwing RPG, which has over 1 million downloads on Google Play. In this game, you will play as a Lord who must use his weapon throwing skills to defeat various monsters and enemies in different stages. You will also be able to customize your character, upgrade your weapons, and learn new skills as you progress through the game. -weapon throwing rpg 2 mod apkDownload ✏ https://ssurll.com/2uNQqs - However, if you want to enjoy the game to the fullest, you might need some extra resources and features that are not available in the original version. That's why we recommend you to download Weapon Throwing RPG 2 mod apk, which is a modified version of the game that gives you access to unlimited coins and gems, all weapons and skills unlocked, no ads, and more. In this article, we will tell you more about what is Weapon Throwing RPG 2 mod apk, why you should download it, what features it offers, and how to download and install it on your device. -Introduction-What is Weapon Throwing RPG 2?-Weapon Throwing RPG 2 is a fun and challenging role-playing game developed by Yuika Project. The game is set in a fantasy world where you will play as a Lord who must use his weapon throwing technique to fight against various monsters and enemies. The game has over 200 stages with different difficulty levels, where you will encounter different types of enemies such as goblins, skeletons, dragons, wyverns, and more. You will also be able to collect and upgrade over 100 weapons such as swords, axes, spears, hammers, daggers, bows, and more. You can also learn over 50 skills that will help you in your battles such as healing, buffing, debuffing, summoning, and more. -What is a mod apk?-A mod apk is a modified version of an original application that has been altered by third-party developers to provide additional features and benefits that are not available in the original version. A mod apk usually requires no root access and can be installed easily on any Android device. However, a mod apk may not be compatible with some devices or may cause some issues such as crashes or bugs. Therefore, it is important to download a mod apk from a trusted source and follow the instructions carefully. -weapon throwing rpg 2 mod apk unlimited money Why download Weapon Throwing RPG 2 mod apk?-Weapon Throwing RPG 2 mod apk is a great option for those who want to enjoy the game without any limitations or restrictions. By downloading this mod apk, you will be able to get unlimited coins and gems, which are the main currencies in the game. You can use these coins and gems to buy new weapons, upgrade your existing weapons, learn new skills, unlock new stages, and more. You will also be able to unlock all weapons and skills from the start, which will give you an edge over your enemies. Moreover, you will not have to deal with any annoying ads that may interrupt your gameplay or consume your data. And best of all, you will not need to root your device or risk damaging it by installing this mod apk. -Features of Weapon |
Name | Molecular formula | Type/Source/Strength/Basicity/Concentration/Molecular composition |
---|---|---|
Vinegar (acetic acid) | CH<\td>>Organic/Plant/Weak/Monobasic/Dilute/Oxyacid | |
Lemon juice (citric acid) | C<\td> | >Organic/Plant/Weak/Tribasic/Dilute/Oxyacid |
Battery acid (sulphuric acid) | H<\td> | >Inorganic/Mineral/Strong/Dibasic/Concentrated/Oxyacid |
Aqua regia (nitric acid) | HNO<\td> | >Inorganic/Mineral/Strong/Monobasic/Dilute/Oxyacid |
Gastric juice (hydrochloric acid) | HCl | Inorganic/Mineral/Strong/Monobasic/Dilute/Hydracid |
Sour milk (lactic acid) | C<\td> | >Organic/Animal/Weak/Monobasic/Dilute/Oxyacid |
Glycine (amino acid) | C<\td> | >Organic/Animal/Weak/Monobasic/Dilute/Oxyacid |
The following are some common properties of bases:
-The following are some common uses of bases:
-