diff --git a/spaces/1acneusushi/gradio-2dmoleculeeditor/data/Download Guitar Rig 6 Full Version [VERIFIED].md b/spaces/1acneusushi/gradio-2dmoleculeeditor/data/Download Guitar Rig 6 Full Version [VERIFIED].md
deleted file mode 100644
index f5322e6892dbd055212c41bd00ca5fe0eeeca9f6..0000000000000000000000000000000000000000
--- a/spaces/1acneusushi/gradio-2dmoleculeeditor/data/Download Guitar Rig 6 Full Version [VERIFIED].md
+++ /dev/null
@@ -1,168 +0,0 @@
-
-
How to Download Guitar Rig 6 Full Version
-
If you are looking for a way to create realistic and professional guitar tones on your computer, you might have heard of Guitar Rig 6, the latest version of the popular amp simulator and multi-effects rack from Native Instruments. But how can you download Guitar Rig 6 full version for free or at a discounted price? And how can you install and use it to get the most out of your guitar playing and recording?
In this article, we will answer all these questions and more. We will explain what Guitar Rig 6 is and why you need it, what features and benefits it offers, what system requirements and compatibility it has, how to download it for free or at a low cost, how to install and activate it on your computer, and how to use it to create amazing guitar tones. By the end of this article, you will have everything you need to know about downloading Guitar Rig 6 full version and using it to enhance your guitar sound.
-
What is Guitar Rig 6 and why you need it
-
Guitar Rig 6 is a software program that simulates the sound of various guitar amps, cabinets, pedals, effects, and tools. It allows you to plug your guitar into your computer and process your signal with a wide range of components that emulate real hardware devices. You can also use it as a standalone application or as a plugin in your digital audio workstation (DAW).
-
Guitar Rig 6 is designed for guitarists of all levels and styles, from beginners to professionals, from rock to metal, from blues to jazz. Whether you want to practice, record, perform, or experiment with different sounds, Guitar Rig 6 can help you achieve your goals. You can use it to create realistic and authentic tones that match your favorite artists and genres, or you can use it to craft your own unique sounds that express your personality and creativity.
-
Guitar Rig 6 features and benefits
-
Guitar Rig 6 comes with a host of features and benefits that make it one of the best guitar effects software on the market. Here are some of them:
-
-
-
Drag and drop interface: Guitar Rig 6 has a simple and intuitive interface that lets you easily build your own custom rigs by dragging and dropping components to the rack. You can also adjust the settings of each component with knobs, sliders, switches, and menus.
-
21 amp models with matching cabinets: Guitar Rig 6 offers a wide selection of amp models that cover various eras, styles, and sounds. From vintage classics to modern high-gain monsters, from clean and warm to crunchy and distorted, from British to American, you can find an amp that suits your taste. Each amp also comes with a matching cabinet that complements its tone.
-
68 effects models, tools, and modifiers: Guitar Rig 6 also provides a huge collection of effects models that emulate popular pedals, rack units, studio processors, and more. You can add effects such as distortion, overdrive, fuzz, compression, modulation, delay, reverb, pitch shifting, filtering, EQ, noise gate, looper, tuner, metronome, etc. You can also use tools such as splitters, mix ers, crossovers, and modifiers to shape and control your signal flow and dynamics.
-
New amps and effects powered by Intelligent Circuit Modeling: Guitar Rig 6 introduces a new technology called Intelligent Circuit Modeling that uses artificial intelligence to analyze and recreate the behavior of real analog circuits. This results in more realistic and responsive sounds that capture the nuances and character of the original hardware. Guitar Rig 6 features three new amps and 16 new effects based on this technology, such as the Chicago, Bass Invader, Fire Breather, Harmonic Synthesizer, Grain Delay, Choral Reef, etc.
-
Over 300 presets and styles: Guitar Rig 6 also comes with over 300 presets that are ready to use or tweak to your liking. These presets are organized by styles, such as rock, metal, blues, jazz, pop, etc., and by artists, such as Jimi Hendrix, Slash, John Frusciante, David Gilmour, etc. You can also create your own presets and save them for later use.
-
Flexible routing and sidechaining: Guitar Rig 6 allows you to route your signal in various ways to create complex and creative sounds. You can use up to eight parallel racks to process different parts of your signal separately, or you can use sidechaining to modulate one component with another. For example, you can use a compressor to duck the volume of your guitar when you sing into a microphone, or you can use an envelope follower to control the filter cutoff of a synth with your guitar.
-
MIDI control and automation: Guitar Rig 6 also supports MIDI control and automation, which means you can use external MIDI devices such as footswitches, pedals, keyboards, controllers, etc., to control the parameters of Guitar Rig 6 in real time. You can also record and edit automation data in your DAW to automate changes in your sound over time.
-
Integration with other Native Instruments products: Guitar Rig 6 is compatible with other Native Instruments products, such as Komplete Kontrol keyboards, Maschine grooveboxes, Traktor DJ software, etc. You can use these products to access and control Guitar Rig 6 features and functions more easily and intuitively. You can also use Guitar Rig 6 as an effect plugin for other Native Instruments instruments and sounds.
-
-
Guitar Rig 6 system requirements and compatibility
-
Guitar Rig 6 is compatible with Windows and Mac operating systems. Here are the minimum system requirements for running Guitar Rig 6:
-
-
-
Operating System
-
Windows 10 (64-bit)
-
macOS 10.14 or higher
-
-
-
CPU
-
Intel Core i5 or equivalent AMD processor
-
Intel Core i5 or equivalent Apple processor
-
-
-
RAM
-
4 GB (8 GB recommended)
-
4 GB (8 GB recommended)
-
-
-
Disk Space
-
1 GB for Guitar Rig 6 Player 3 GB for Guitar Rig 6 Pro
-
1 GB for Guitar Rig 6 Player 3 GB for Guitar Rig 6 Pro
-
-
-
Graphics Card
-
NVIDIA GeForce GTX 600 series or higher AMD Radeon HD 7000 series or higher Intel HD Graphics 4000 or higher
-
NVIDIA GeForce GTX 600 series or higher AMD Radeon HD 7000 series or higher Intel HD Graphics 4000 or higher
-
-
-
Audio Interface
-
A dedicated audio interface with ASIO driver support is recommended for optimal performance and low latency.
-
A dedicated audio interface with Core Audio driver support is recommended for optimal performance and low latency.
-
-
-
MIDI Device
-
A MIDI device such as a footswitch, pedal, keyboard, controller, etc., is optional but recommended for controlling Guitar Rig 6 parameters in real time.
-
A MIDI device such as a footswitch, pedal, keyboard, controller, etc., is optional but recommended for controlling Guitar Rig 6 parameters in real time.
-
How to download Guitar Rig 6 full version for free
-
Now that you know what Guitar Rig 6 is and what it can do for you, you might be wondering how to download it for free or at a low cost. There are three ways to get Guitar Rig 6 full version for free or at a discounted price:
-
Guitar Rig 6 Player: the free version with limited features
-
The first way to get Guitar Rig 6 full version for free is to download Guitar Rig 6 Player, the free version of Guitar Rig 6 that comes with limited features. Guitar Rig 6 Player is a great way to try out Guitar Rig 6 and see if you like it before buying the full version. Guitar Rig 6 Player includes:
-
-
One amp model: Jump, based on the Marshall JMP Plexi
-
One cabinet model: Matched Cabinet, based on the Marshall 1960A
The ability to use Guitar Rig 6 Player as a standalone application or as a plugin in your DAW
-
-
To download Guitar Rig 6 Player for free, you need to create a free Native Instruments account and download the Native Access app. Native Access is a software that manages the installation and activation of Native Instruments products. Once you have Native Access installed, you can download Guitar Rig 6 Player from the Not Installed tab and install it on your computer.
-
Guitar Rig 6 Demo: the trial version with full features
-
The second way to get Guitar Rig 6 full version for free is to download Guitar Rig 6 Demo, the trial version of Guitar Rig 6 that comes with full features. Guitar Rig 6 Demo is a great way to test all the features and functions of Guitar Rig 6 and see if it meets your needs and expectations before buying the full version. Guitar Rig 6 Demo includes:
-
-
All the features and benefits of Guitar Rig 6 Pro (see below)
-
The ability to use Guitar Rig 6 Demo as a standalone application or as a plugin in your DAW
-
A time limit of 30 minutes per session
-
A noise burst every few minutes
-
No saving or exporting of presets or sounds
-
-
To download Guitar Rig 6 Demo for free, you need to create a free Native Instruments account and download the Native Access app. Once you have Native Access installed, you can download Guitar Rig 6 Demo from the Not Installed tab and install it on your computer.
-
Guitar Rig 6 Pro: the paid version with all features
-
The third way to get Guitar Rig 6 full version is to buy Guitar Rig 6 Pro, the paid version of Guitar Rig 6 that comes with all features. Guitar Rig 6 Pro is the ultimate guitar effects software that gives you unlimited creative possibilities and professional results. Guitar Rig 6 Pro includes:
-
-
All the features and benefits of Guitar Rig 6 (see above)
-
No time limit or noise burst
-
The ability to save and export presets and sounds
-
The ability to use Guitar Rig 6 Pro as a standalone application or as a plugin in your DAW
-
Free updates and support from Native Instruments
-
-
To buy Guitar Rig 6 Pro, you need to create a free Native Instruments account and download the Native Access app. Once you have Native Access installed, you can buy Guitar Rig 6 Pro from the Shop tab and install it on your computer. The price of Guitar Rig 6 Pro is $199 USD. However, there are some ways to get it at a discounted price:
-
-
If you already own a previous version of Guitar Rig (Guitar Rig 1-5), you can upgrade to Guitar Rig 6 Pro for $99 USD.
-
If you already own Komplete Start (a free collection of instruments and sounds from Native Instruments), you can crossgrade to Guitar Rig 6 Pro for $149 USD.
-
If you already own Komplete Select (a curated collection of instruments and sounds from Native Instruments), you can crossgrade to Guitar Rig 6 Pro for $99 USD.
-
If you already own Komplete (the ultimate production suite from Native Instruments), you can get Guitar Rig 6 Pro for free as part of your bundle.
-
How to install and activate Guitar Rig 6 full version
-
Once you have downloaded Guitar Rig 6 full version, either for free or for a price, you need to install and activate it on your computer. Here are the steps to do so:
-
How to install Guitar Rig 6 on your computer
-
To install Guitar Rig 6 on your computer, you need to use the Native Access app that you downloaded earlier. Here are the steps to install Guitar Rig 6 with Native Access:
-
-
Open Native Access and log in with your Native Instruments account.
-
Go to the Installed Products tab and find Guitar Rig 6 in the list.
-
Click on the Install button and choose a location for the installation.
-
Wait for the installation to complete and click on the Finish button.
-
Guitar Rig 6 is now installed on your computer and ready to use.
-
-
How to activate Guitar Rig 6 with your license key or Native Access account
-
To activate Guitar Rig 6 on your computer, you need to use either your license key or your Native Access account. Here are the steps to activate Guitar Rig 6 with either method:
-
-
If you bought Guitar Rig 6 Pro or upgraded from a previous version, you should have received a license key via email. To activate Guitar Rig 6 with your license key, follow these steps:
-
Open Native Access and log in with your Native Instruments account.
-
Go to the Add a serial tab and enter your license key in the field.
-
Click on the Add serial button and wait for the activation to complete.
-
Guitar Rig 6 is now activated on your computer and ready to use.
-
-
-
If you downloaded Guitar Rig 6 Player or Guitar Rig 6 Demo, you don't need a license key. To activate Guitar Rig 6 with your Native Access account, follow these steps:
-
Open Native Access and log in with your Native Instruments account.
-
Go to the Installed Products tab and find Guitar Rig 6 in the list.
-
Click on the Activate button and wait for the activation to complete.
-
Guitar Rig 6 is now activated on your computer and ready to use.
-
-
-
-
How to use Guitar Rig 6 full version to create amazing guitar tones
-
Now that you have installed and activated Guitar Rig 6 full version on your computer, you can start using it to create amazing guitar tones. Here are some tips and tricks on how to use Guitar Rig 6 full version effectively and efficiently:
-
How to navigate the Guitar Rig 6 interface and browser
-
Guitar Rig 6 has a user-friendly interface that consists of four main sections: the header, the browser, the rack, and the footer. Here is a brief overview of each section:
-
-
The header contains the menu bar, the toolbar, and the preset name. You can use the menu bar to access various options and settings, such as file, edit, view, help, etc. You can use the toolbar to access various functions and tools, such as tuner, metronome, tape deck, etc. You can also see and change the name of the current preset in the header.
-
The browser contains the preset list, the style list, and the component list. You can use the preset list to browse, load, save, delete, or search presets. You can use the style list to filter presets by styles, such as rock, metal, blues, jazz, etc. You can use the component list to browse, load, or search components, such as amps, cabinets, effects, tools, etc.
-
The rack contains the components that make up your guitar rig. You can see and adjust the settings of each component with knobs, sliders, switches, and menus. You can also drag and drop components to add, remove, or rearrange them in the rack. You can also use splitters, mixers, crossovers and modifiers to shape and control your signal flow and dynamics.
-
The footer contains the master volume, the input level, the output level, and the CPU usage. You can use the master volume to adjust the overall volume of your guitar rig. You can also see and adjust the input level and the output level of your guitar rig. You can also see the CPU usage of your computer and optimize it if needed.
-
-
To navigate the Guitar Rig 6 interface and browser, you can use your mouse, keyboard, or MIDI device. You can also use shortcuts and commands to access various functions and tools more quickly. For example, you can use the arrow keys to navigate the preset list, the style list, and the component list. You can also use the spacebar to bypass or enable a component, or use the delete key to remove a component from the rack. You can also use commands such as Ctrl+C to copy a component, Ctrl+V to paste a component, Ctrl+Z to undo an action, etc.
-
How to load and customize presets and components
-
Guitar Rig 6 comes with over 300 presets that are ready to use or tweak to your liking. You can also create your own presets and save them for later use. Here are some tips on how to load and customize presets and components:
-
-
To load a preset, you can use the browser to find and select a preset from the preset list or the style list. You can also use the arrow keys or the mouse wheel to scroll through the presets. You can also use the search function to find a preset by name or keyword. Once you have found a preset that you like, you can double-click on it or press Enter to load it to the rack.
-
To customize a preset, you can use the rack to adjust the settings of each component with knobs, sliders, switches, and menus. You can also drag and drop components to add, remove, or rearrange them in the rack. You can also use splitters, mixers, crossovers, and modifiers to shape and control your signal flow and dynamics. You can also use MIDI control and automation to control the parameters of Guitar Rig 6 in real time.
-
To save a preset, you can click on the Save button in the header and enter a name for your preset. You can also choose a style for your preset from the style list. You can also add tags and comments to your preset for easier identification and organization. Once you have saved your preset, you can find it in the User folder in the browser.
-
To load a component, you can use the browser to find and select a component from the component list. You can also use the arrow keys or the mouse wheel to scroll through the components. You can also use the search function to find a component by name or keyword. Once you have found a component that you like, you can drag and drop it to an empty slot in the rack or on top of an existing component to replace it.
-
To customize a component, you can use the rack to adjust the settings of each component with knobs, sliders, switches, and menus. You can also drag and drop components to add, remove, or rearrange them in the rack. You can also use splitters, mixers, crossovers and modifiers to shape and control your signal flow and dynamics. You can also use MIDI control and automation to control the parameters of Guitar Rig 6 in real time.
-
-
How to use the new amps and effects powered by Intelligent Circuit Modeling
-
Guitar Rig 6 introduces a new technology called Intelligent Circuit Modeling that uses artificial intelligence to analyze and recreate the behavior of real analog circuits. This results in more realistic and responsive sounds that capture the nuances and character of the original hardware. Guitar Rig 6 features three new amps and 16 new effects based on this technology, such as the Chicago, Bass Invader, Fire Breather, Harmonic Synthesizer, Grain Delay, Choral Reef, etc. Here are some tips on how to use the new amps and effects powered by Intelligent Circuit Modeling:
-
-
To load a new amp or effect, you can use the browser to find and select a component from the component list. You can also use the arrow keys or the mouse wheel to scroll through the components. You can also use the search function to find a component by name or keyword. Once you have found a component that you like, you can drag and drop it to an empty slot in the rack or on top of an existing component to replace it.
-
To customize a new amp or effect, you can use the rack to adjust the settings of each component with knobs, sliders, switches, and menus. You can also drag and drop components to add, remove, or rearrange them in the rack. You can also use splitters, mixers, crossovers, and modifiers to shape and control your signal flow and dynamics. You can also use MIDI control and automation to control the parameters of Guitar Rig 6 in real time.
-
To get the best sound from a new amp or effect, you need to match it with a suitable cabinet or speaker. You can use the browser to find and select a cabinet or speaker from the component list. You can also use the arrow keys or the mouse wheel to scroll through the components. You can also use the search function to find a component by name or keyword. Once you have found a component that you like, you can drag and drop it to an empty slot in the rack or on top of an existing component to replace it.
-
To experiment with different sounds from a new amp or effect, you can use the presets that come with each component. You can use the browser to find and select a preset from the preset list or the style list. You can also use the arrow keys or the mouse wheel to scroll through the presets. You can also use the search function to find a preset by name or keyword. Once you have found a preset that you like, you can double-click on it or press Enter to load it to the rack.
-
-
Conclusion and FAQs
-
Guitar Rig 6 is a powerful and versatile guitar effects software that can help you create realistic and professional guitar tones on your computer. It offers a wide range of features and benefits that make it one of the best guitar effects software on the market. It also comes with three ways to get Guitar Rig 6 full version for free or at a discounted price: Guitar Rig 6 Player, Guitar Rig 6 Demo, and Guitar Rig 6 Pro.
-
In this article, we have explained what Guitar Rig 6 is and why you need it, what features and benefits it offers, what system requirements and compatibility it has, how to download it for free or at a low cost, how to install and activate it on your computer, and how to use it to create amazing guitar tones. We hope that this article has helped you learn everything you need to know about downloading Guitar Rig 6 full version and using it to enhance your guitar sound.
-
If you have any questions or doubts about Guitar Rig 6 full version, here are some frequently asked questions (FAQs) that might help you:
-
Q: Can I use Guitar Rig 6 with any guitar?
-
A: Yes, you can use Guitar Rig 6 with any electric guitar, acoustic guitar, bass guitar, or any other instrument that has a pickup or a microphone. You just need to connect your instrument to your computer via an audio interface with an instrument input.
-
Q: Can I use Guitar Rig 6 with any DAW?
-
A: Yes, you can use Guitar Rig 6 with any DAW that supports VST, AU, or AAX plugin formats. You just need to load Guitar Rig 6 as an effect plugin in your DAW's track or bus.
-
Q: Can I use Guitar Rig 6 offline?
-
A: Yes, you can use Guitar Rig 6 offline as a standalone application without an internet connection. However, you need an internet connection to download, install, and activate Guitar Rig 6 for the first time. You also need an internet connection to access the online features and updates of Guitar Rig 6.
-
Q: Can I use Guitar Rig 6 with other guitar effects software or hardware?
-
A: Yes, you can use Guitar Rig 6 with other guitar effects software or hardware, as long as they are compatible and do not cause any conflicts or issues. You can use Guitar Rig 6 as an effect plugin in your DAW and combine it with other plugins, or you can use Guitar Rig 6 as a standalone application and route it to other software or hardware via an audio interface or a virtual cable.
-
Q: Can I share my Guitar Rig 6 presets and sounds with others?
-
A: Yes, you can share your Guitar Rig 6 presets and sounds with others, as long as you respect the intellectual property rights of Native Instruments and the original creators of the components and presets. You can export your presets and sounds as files and send them to others via email, social media, cloud storage, etc. You can also import presets and sounds from others and load them to your Guitar Rig 6.
b2dd77e56b
-
-
\ No newline at end of file
diff --git a/spaces/1gistliPinn/ChatGPT4/Examples/893u2is User Manual.md b/spaces/1gistliPinn/ChatGPT4/Examples/893u2is User Manual.md
deleted file mode 100644
index 6ae7c610539ca7890ad06fefb4e352a85cefe688..0000000000000000000000000000000000000000
--- a/spaces/1gistliPinn/ChatGPT4/Examples/893u2is User Manual.md
+++ /dev/null
@@ -1,6 +0,0 @@
-
-
-Oct 8, 2015 Only after I read the instructions carefully did I see the ... Station Users Guide Multi-Function Hdd Docking Manual 893u2is ... 4d29de3e1b
-
-
-
diff --git a/spaces/1gistliPinn/ChatGPT4/Examples/Age Of Empires 3 No Cd Crack Gamecopyworld Gtahttps Scoutmails.com Index301.php K Age Of Empires 3 WORK.md b/spaces/1gistliPinn/ChatGPT4/Examples/Age Of Empires 3 No Cd Crack Gamecopyworld Gtahttps Scoutmails.com Index301.php K Age Of Empires 3 WORK.md
deleted file mode 100644
index 401d7e7c49993db068414afc4a0f4bfacc8b204a..0000000000000000000000000000000000000000
--- a/spaces/1gistliPinn/ChatGPT4/Examples/Age Of Empires 3 No Cd Crack Gamecopyworld Gtahttps Scoutmails.com Index301.php K Age Of Empires 3 WORK.md
+++ /dev/null
@@ -1,129 +0,0 @@
-
-
Age of Empires 3 No CD Crack GameCopyWorld: How to Play the Classic Strategy Game Without a Disc
-
-
Age of Empires 3 is one of the most popular and acclaimed strategy games of all time, but it also requires a CD to play. If you have lost your CD, or you want to play the game on a different computer without carrying the disc around, you might be looking for a way to play Age of Empires 3 no CD crack GameCopyWorld.
-
-
GameCopyWorld is a website that provides game fixes, trainers, cheats, and patches for various PC games. One of the game fixes they offer is a no CD crack for Age of Empires 3, which allows you to play the game without inserting the CD every time. This can also help you avoid potential damage to your CD or CD drive.
-
age of empires 3 no cd crack gamecopyworld gtahttps: scoutmails.com index301.php k age of empires 3
In this article, we will show you how to download and install Age of Empires 3 no CD crack GameCopyWorld, and how to enjoy the game without any hassle. We will also tell you about some of the features and benefits of playing Age of Empires 3 no CD crack GameCopyWorld.
-
-
How to Download and Install Age of Empires 3 No CD Crack GameCopyWorld
-
-
To download and install Age of Empires 3 no CD crack GameCopyWorld, you will need to follow these steps:
-
-
-
Go to https://www.gamecopyworld.com/games/pc_age_of_empires_3.shtml and scroll down to find the game fix you need. Depending on which version and expansion of Age of Empires 3 you have, you will need to choose the appropriate no CD crack. For example, if you have Age of Empires 3: Complete Collection, which includes the base game and both expansions (The WarChiefs and The Asian Dynasties), you will need to download Age of Empires III: Complete Collection v1.0 [EN] Fixed Files.
-
Click on the download link and save the file to your computer. You may need to use a program like WinRAR or 7-Zip to extract the file.
-
Locate the folder where you have installed Age of Empires 3 on your computer. It is usually in C:\Program Files (x86)\Microsoft Games\Age of Empires III.
-
Copy the cracked files from the downloaded folder and paste them into the installation folder, replacing the original files. You may need to backup the original files in case you want to restore them later.
-
Run the game as usual. You should be able to play Age of Empires 3 without inserting the CD.
-
-
-
Features and Benefits of Playing Age of Empires 3 No CD Crack GameCopyWorld
-
-
Playing Age of Empires 3 no CD crack GameCopyWorld has some advantages over playing with the CD. Here are some of them:
-
-
-
You can play the game on any computer without carrying the CD around.
-
You can avoid potential damage to your CD or CD drive from scratches or wear and tear.
-
You can save some disk space by deleting the ISO image of the CD if you have one.
-
You can enjoy faster loading times and smoother performance by playing from your hard drive instead of your CD drive.
-
You can still access all the features and content of the game, including multiplayer mode, online updates, and mods.
-
-
-
Conclusion
-
-
Age of Empires 3 is a classic strategy game that deserves to be played by anyone who loves history, culture, and warfare. With Age of Empires 3 no CD crack GameCopyWorld, you can play the game without any hassle or limitation. Just follow our guide on how to download and install Age of Empires 3 no CD crack GameCopyWorld, and enjoy the game at its best.
-
-
If you liked this article, please share it with your friends who are also fans of Age of Empires 3. And if you have any questions or comments, feel free to leave them below. We would love to hear from you!
-
What is Age of Empires 3 and Why Should You Play It?
-
-
Age of Empires 3 is a real-time strategy game that was released in 2005 by Microsoft Studios and Ensemble Studios. It is the third installment in the Age of Empires series, which is one of the most successful and influential strategy game franchises of all time.
-
-
Age of Empires 3 takes place during the Age of Discovery, from the 15th to the 19th century. You can choose from eight European civilizations, each with their own unique units, buildings, technologies, and abilities. You can also play as three native American civilizations in the WarChiefs expansion, or as three Asian civilizations in the Asian Dynasties expansion.
-
-
Age of Empires 3 offers a rich and varied gameplay experience that will appeal to both casual and hardcore strategy fans. You can explore and colonize new lands, trade and fight with other players or AI opponents, build and manage your economy and military, research new technologies and upgrades, and customize your home city that provides you with bonuses and shipments.
-
-
-
Age of Empires 3 also features a compelling campaign mode that follows the story of three generations of the Black family, as they participate in historical events such as the Seven Years' War, the American Revolution, and the Napoleonic Wars. The campaign mode has cinematic cutscenes, voice acting, and scripted scenarios that will immerse you in the history and culture of the era.
-
-
Age of Empires 3 is a classic strategy game that deserves to be played by anyone who loves history, culture, and warfare. It has stunning graphics, sound effects, and music that bring the game world to life. It has a large and active online community that supports the game with mods, maps, tournaments, and more. It has a high replay value, as you can try different strategies, civilizations, game modes, and difficulty levels.
-
-
How to Play Age of Empires 3 No CD Crack GameCopyWorld Online
-
-
One of the best features of Age of Empires 3 is its online multiplayer mode, where you can challenge other players from around the world in various game modes such as supremacy, deathmatch, treaty, king of the hill, and more. You can also join or create clans, chat with other players, check your stats and rankings, and earn medals and achievements.
-
-
However, to play Age of Empires 3 online, you need to have a valid CD key that is registered on your Microsoft account. If you have lost your CD key, or you have downloaded Age of Empires 3 no CD crack GameCopyWorld from our website, you might not be able to access the official online servers.
-
-
But don't worry, there is a way to play Age of Empires 3 no CD crack GameCopyWorld online without a CD key. All you need to do is download and install a third-party client called ESOCommunity Patch. This patch will allow you to play Age of Empires 3 no CD crack GameCopyWorld online on ESOCommunity servers, which are unofficial but popular servers that host thousands of players every day.
-
-
To download and install ESOCommunity Patch for Age of Empires 3 no CD crack GameCopyWorld, you will need to follow these steps:
Run the installer and follow the instructions. Make sure you select your Age of Empires 3 installation folder when prompted.
-
Launch Age of Empires 3 no CD crack GameCopyWorld from your desktop shortcut or start menu.
-
Create a new ESO account or log in with your existing one. You don't need a CD key to create an account.
-
Enjoy playing Age of Empires 3 no CD crack GameCopyWorld online on ESOCommunity servers!
-
-
-
Conclusion
-
-
Age of Empires 3 no CD crack GameCopyWorld is a great way to play the classic strategy game without a disc. You can download and install it easily from our website, and enjoy all the features and content of the game without any hassle. You can also play it online on ESOCommunity servers with other players who have downloaded Age of Empires 3 no CD crack GameCopyWorld.
-
-
If you liked this article, please share it with your friends who are also fans of Age of Empires 3. And if you have any questions or comments, feel free to leave them below. We would love to hear from you!
-
How to Master the Combat System in Age of Empires 3
-
-
Age of Empires 3 is not just about building and managing your economy; it is also about fighting and conquering your enemies. The combat system in Age of Empires 3 is based on a rock-paper-scissors model, where each unit type has strengths and weaknesses against other unit types. For example, infantry units are good against cavalry units, cavalry units are good against artillery units, and artillery units are good against infantry units.
-
-
To master the combat system in Age of Empires 3, you need to know the different unit types and their counters, as well as how to use formations, stances, and special abilities. You also need to pay attention to the terrain, the weather, and the line of sight, as they can affect the performance and visibility of your units.
-
-
Here are some general tips and tricks for combat in Age of Empires 3:
-
-
-
Always scout your enemy's base and army composition before attacking. This will help you plan your strategy and choose the right units for the battle.
-
Try to have a balanced army with a mix of unit types. This will allow you to adapt to different situations and counter different threats.
-
Use formations to organize your army and give them bonuses. For example, the staggered formation gives your ranged units more firing arc, while the box formation protects your artillery units from cavalry charges.
-
Use stances to control the behavior of your units. For example, the defensive stance makes your units hold their ground and focus on nearby enemies, while the aggressive stance makes your units chase and attack any enemy they see.
-
Use special abilities to gain an edge in combat. For example, some infantry units can use bayonets or grenades to deal extra damage, while some cavalry units can use trample mode to run over enemy infantry.
-
Use cover mode to reduce damage from ranged attacks. Cover mode makes your units kneel behind obstacles like trees or walls, but it also reduces their movement speed and firing rate.
-
Use flanking maneuvers to surprise and outsmart your enemy. Flanking means attacking your enemy from the sides or behind, where they are more vulnerable and less prepared.
-
Use hit-and-run tactics to harass and weaken your enemy. Hit-and-run tactics mean attacking your enemy with fast units like cavalry or skirmishers, then retreating before they can retaliate.
-
Use siege weapons to destroy enemy buildings and defenses. Siege weapons like cannons or mortars can deal massive damage to buildings and walls, but they are slow and vulnerable to enemy fire.
-
Use ships to support your land army or attack from the sea. Ships can transport units across water, bombard enemy positions from afar, or engage in naval battles with other ships.
-
-
-
How to Enjoy the Campaign Mode in Age of Empires 3
-
-
If you are looking for a more story-driven and cinematic experience in Age of Empires 3, you might want to try the campaign mode. The campaign mode consists of three acts that follow the adventures of the Black family through different historical periods and continents.
-
-
The first act is called Blood, Ice, and Steel, and it takes place during the colonization of America in the 16th and 17th centuries. You will play as Morgan Black, a knight of Malta who fights against the Spanish conquistadors and their allies.
-
-
The second act is called Fire and Shadow, and it takes place during the American Revolution in the 18th century. You will play as John Black, a mercenary who joins the Continental Army and battles against the British Empire.
-
-
The third act is called Steel and Thunder, and it takes place during the Napoleonic Wars in the 19th century. You will play as Amelia Black, a railroad tycoon who travels across Europe and Asia in search of her lost family legacy.
-
-
The campaign mode in Age of Empires 3 offers a rich and varied gameplay experience that will appeal to both casual and hardcore strategy fans. You will explore and colonize new lands, trade and fight with other factions, build and manage your economy and military, research new technologies and upgrades, and customize your home city that provides you with bonuses and shipments.
-
-
The campaign mode also features cinematic cutscenes, voice acting, and scripted scenarios that will immerse you in the history and culture of the era. You will meet historical figures like George Washington , Napoleon Bonaparte , Simon Bolivar , Queen Isabella , Tokugawa Ieyasu , Akbar , Ivan the Terrible , Elizabeth I , Samuel de Champlain , Tecumseh , Nathaniel Black , Sahin \"The Falcon\" , Kanyenke , Lizzie \"The Pirate\" , Alain Magnan , Warwick \"The Redcoat\" , Pierre Beaumont , Stuart Black , Nonahkee , Sven Kuechler , Huang He , Admiral Jinhai , Nanib Sahir , Rani Pravarthi , Colonel Edwardson , Chayton Black , Holme \"The Boneguard\" , Crazy Horse , Chief Brave Wolf , General Custer , Major Cooper , Kichiro , Daimyo Mototada Torii , Daimyo Junkei Kuroda , Daimyo Shingen Takeda , Daimyo Kenshin Uesugi , Daimyo Nobunaga Oda , Daimyo Hideyoshi Toyotomi , Daimyo Ieyasu Tokugawa .
-
-
If you want to enjoy the campaign mode in Age of Empires 3, here are some tips and tricks:
-
-
-
Play on a difficulty level that suits your skill level. The campaign mode has four difficulty levels: Easy, Moderate, Hard, and Expert. The higher the difficulty level, the more challenging the enemies will be.
-
Watch the cutscenes and listen to the dialogue. They will provide you with important information about the story, characters, objectives, hints, tips, etc.
-
Read the objectives carefully. They will tell you what you need to do to complete each mission. Some objectives are mandatory (marked with a star), while others are optional (marked with a circle).
-
Check the map often. It will show you where you are, where your allies and enemies are, where your objectives are located, etc.
-
Save your game frequently. You never know when something might go wrong or when you might want to try a different strategy.
-
Have fun! The campaign mode is designed to be entertaining and engaging for all kinds of players. Don't worry too much about winning or losing; just enjoy the journey!
-
-
Conclusion
-
-
Age of Empires 3 no CD crack GameCopyWorld is a great way to play the classic strategy game without a disc. You can download and install it easily from our website, and enjoy all the features and content of the game without any hassle. You can also play it online on ESOCommunity servers with other players who have downloaded Age of Empires 3 no CD crack GameCopyWorld.
-
-
In this article, we have shown you how to download and install Age of Empires 3 no CD crack GameCopyWorld, how to master the combat system in Age of Empires 3, and how to enjoy the campaign mode in Age of Empires 3. We hope you have found this article helpful and informative, and that you have learned some useful tips and tricks to boost your game.
-
-
If you liked this article, please share it with your friends who are also fans of Age of Empires 3. And if you have any questions or comments, feel free to leave them below. We would love to hear from you!
3cee63e6c2
-
-
\ No newline at end of file
diff --git a/spaces/1gistliPinn/ChatGPT4/Examples/Download Automation Studio 5.6 Crack Freel !!TOP!!.md b/spaces/1gistliPinn/ChatGPT4/Examples/Download Automation Studio 5.6 Crack Freel !!TOP!!.md
deleted file mode 100644
index c4e627e6eb71b8bd5a9f4765bcc796b855373b33..0000000000000000000000000000000000000000
--- a/spaces/1gistliPinn/ChatGPT4/Examples/Download Automation Studio 5.6 Crack Freel !!TOP!!.md
+++ /dev/null
@@ -1,24 +0,0 @@
-
-
-Winsound.com Automation Studio; Read the Manuals and FAQs in the Digital Audio Forum; Learn More About Old Stock Author: Dan, JVC Author: Jack Szabo from Jack's JVC Revamp; Jack's JVC Revamp 5,…Category: Audio - Digital Audio - Components & Equipment Other Related Categories AudioSoftwareTuning & MeasurementsAudioCables & DevicesToolsMagazines & JournalsMembers ClubsOther Educational Sites Review Top Posts Analyze Audio at What Hi, I’m Dan. With a knowledge of some 35 years of audio, I have been writing about the companies, products, and technologies in this business since 1999. I am an Authorized JVC Dealer, and the Audio & Network Assistant Editor here at Home Theater Forum. View my complete profile
-
-Repair Shop Studios now offers a series of licensing programs that can enable you to generate a royalty stream for your independently developed projects, including the JVC AiS Software Suite, the JVC AiS Suite, and the JVC AiS Suite Plus.
-
-Thanks for the info!
-
-I can't find the manuals for this one either. Will just have to use the information above in this thread I guess. On the CD there are 2 files for the CD Writer, a program for the CD writer and another for the CD Writer Service.
-
-I have the new version 1.02 and have used the CD Writer 1.02 with software version AOS22 which says the disc I used was OSD 2.6 version. I have also used the CD Writer version 1.02 with software version BOS21 with no OSD disc. The CD Writer version 1.02 with AOS22 will not write on my ATR Vista.
-
-I did a google search and found this in an earlier post but can't find the post right now
-
-You are using CD writer 1.02 with AOS22, which is compatible with Vista x64. Your software version is not compatible. XP works fine as you are using the XP version of the program.
-
-Use a CD Writer version 1.2 software.
-
-You will need to look in your Cd writing software. I know it's not simple but you will find the version 2.6 in there. I had a similar problem with some software I bought and it took a little investigation to determine that it wasn't the CD writer software.
-
-I have the new version 1.02 and have used the CD Writer 1. 4fefd39f24
-
-
-
diff --git a/spaces/1line/AutoGPT/autogpt/processing/__init__.py b/spaces/1line/AutoGPT/autogpt/processing/__init__.py
deleted file mode 100644
index e69de29bb2d1d6434b8b29ae775ad8c2e48c5391..0000000000000000000000000000000000000000
diff --git a/spaces/1pelhydcardo/ChatGPT-prompt-generator/assets/Adobe Acrobat Reader X The Power of PDF Productivity.md b/spaces/1pelhydcardo/ChatGPT-prompt-generator/assets/Adobe Acrobat Reader X The Power of PDF Productivity.md
deleted file mode 100644
index 9f50b1a9eafd0c69846e3fa085a032c7ee3bfbf1..0000000000000000000000000000000000000000
--- a/spaces/1pelhydcardo/ChatGPT-prompt-generator/assets/Adobe Acrobat Reader X The Power of PDF Productivity.md
+++ /dev/null
@@ -1,167 +0,0 @@
-
-
How to Download Adobe Reader X
-
Adobe Reader X is a free software that allows you to view, print, and comment on PDF files. PDF stands for Portable Document Format, a file format that preserves the layout, fonts, images, and hyperlinks of any document. PDF files are widely used for sharing information across different platforms and devices.
If you want to access PDF files on your computer or mobile device, you need Adobe Reader X. With this software, you can not only open and view PDFs, but also fill out forms, sign documents, add annotations, and more. Adobe Reader X also offers some advanced features, such as converting PDFs to other file formats, password protecting PDFs, comparing PDFs, and integrating with cloud storage services.
-
In this article, we will show you how to download Adobe Reader X for Windows and Mac, as well as how to troubleshoot some common installation issues. Follow the steps below and enjoy the benefits of Adobe Reader X.
-
How to Download Adobe Reader X for Windows
-
If you are using a Windows computer, here are the steps to download and install Adobe Reader X:
-
-
Check your system requirements. Before you download Adobe Reader X, make sure that your computer meets the minimum system requirements. You can find them on this page. You will need a Windows operating system (Windows Server or Windows XP/Vista/7/8/10), an Intel or AMD processor, at least 256 MB of RAM, at least 260 MB of hard disk space, a screen resolution of at least 1024 x 576 pixels, and an Internet browser (Internet Explorer or Firefox).
-
Go to the official Adobe website. Open your Internet browser and go to this page. This is where you can download Acrobat Reader for free.
-
Choose your language and version. On the download page, you will see a drop-down menu where you can select your language. You can also choose whether you want to download Acrobat Reader for Windows (32-bit or 64-bit) or Mac OS. Make sure you select the correct version for your system.
-
Click the Download button. After choosing your language and version, click the yellow Download button. You will see a pop-up window asking you to save the file. Choose a location on your computer where you want to save the file and click Save.
-
Run the installer and follow the instructions. Once the download is complete, locate the file on your computer and double-click it to run the installer. You will see a welcome screen where you can choose whether you want to install Acrobat Reader as a default PDF viewer or not. Click Next and follow the on-screen instructions to complete the installation. You may need to restart your computer to finish the installation.
-
-
Congratulations, you have successfully downloaded and installed Adobe Reader X for Windows. You can now open and view any PDF file on your computer with this software.
-
How to download adobe reader x for windows 10
-How to download adobe reader x offline installer
-How to download adobe reader x free version
-How to download adobe reader x for mac os
-How to download adobe reader x update
-How to download adobe reader x pro
-How to download adobe reader x for android
-How to download adobe reader x msi
-How to download adobe reader x 10.1.16
-How to download adobe reader x for chromebook
-How to download adobe reader x for linux
-How to download adobe reader x 64 bit
-How to download adobe reader x portable
-How to download adobe reader x full setup
-How to download adobe reader x without internet
-How to download adobe reader x for windows 7
-How to download adobe reader x for ipad
-How to download adobe reader x 10.0.0
-How to download adobe reader x from official website
-How to download adobe reader x with crack
-How to download adobe reader x for windows 8.1
-How to download adobe reader x for iphone
-How to download adobe reader x 10.1.4
-How to download adobe reader x in hindi
-How to download adobe reader x for windows xp
-How to download adobe reader x for kindle fire
-How to download adobe reader x 10.1.1
-How to download adobe reader x in tamil
-How to download adobe reader x for windows vista
-How to download adobe reader x for pc
-How to download adobe reader x 10.0.1
-How to download adobe reader x in urdu
-How to download adobe reader x for macbook air
-How to download adobe reader x for laptop
-How to download adobe reader x 10.1.3
-How to download adobe reader x in telugu
-How to download adobe reader x for macbook pro
-How to download adobe reader x for desktop
-How to download adobe reader x 10.0.2
-How to download adobe reader x in malayalam
-How to download adobe reader x for mac os catalina
-How to download adobe reader x for tablet
-How to download adobe reader x 10.1.2
-How to download adobe reader x in kannada
-How to download adobe reader x for mac os mojave
-How to download adobe reader x for chrome os
-How to download adobe reader x 10.0.3
-How to download adobe reader x in gujarati
-
How to Download Adobe Reader X for Mac
-
If you are using a Mac computer, here are the steps to download and install Adobe Reader X:
-
-
Check your system requirements. Before you download Adobe Reader X, make sure that your computer meets the minimum system requirements. You can find them on this page. You will need a Mac OS X operating system (version 10.5.8 or later), an Intel processor, at least 512 MB of RAM, at least 415 MB of hard disk space, a screen resolution of at least 1024 x 768 pixels, and an Internet browser (Safari or Firefox).
-
Go to the official Adobe website. Open your Internet browser and go to this page. This is where you can download Acrobat Reader for free.
-
Choose your language and version. On the download page, you will see a drop-down menu where you can select your language. You can also choose whether you want to download Acrobat Reader for Windows (32-bit or 64-bit) or Mac OS. Make sure you select the correct version for your system.
-
Click the Download button. After choosing your language and version, click the yellow Download button. You will see a pop-up window asking you to save the file. Choose a location on your computer where you want to save the file and click Save.
-
Open the DMG file and drag the icon to the Applications folder. Once the download is complete, locate the file on your computer and double-click it to open it. You will see a window with an icon of Adobe Reader X and a shortcut to the Applications folder. Drag the icon of Adobe Reader X to the Applications folder and drop it there. This will copy the software to your computer.
-
-
Congratulations, you have successfully downloaded and installed Adobe Reader X for Mac. You can now open and view any PDF file on your computer with this software.
-
How to Troubleshoot Adobe Reader X Installation Issues
-
Sometimes, you may encounter some issues when installing or using Adobe Reader X. Here are some common issues and solutions that may help you fix them:
-
Reinstall Adobe Reader X
-
If Adobe Reader X does not work properly or crashes frequently, you may need to reinstall it. To do this, follow these steps:
-
-
Uninstall Adobe Reader X from your computer. You can do this by going to Control Panel > Programs > Programs and Features (for Windows) or by dragging the icon of Adobe Reader X from the Applications folder to the Trash (for Mac).
-
Delete any leftover files or folders related to Adobe Reader X from your computer. You can use a tool like CCleaner (for Windows) or AppCleaner (for Mac) to do this easily.
-
Download and install Adobe Reader X again from the official website following the steps above.
-
-
This should fix any corrupted or missing files that may cause problems with Adobe Reader X.
-
Disable Protected Mode at Startup
-
If Adobe Reader X does not open or displays an error message when opening a PDF file, you may need to disable Protected Mode at Startup. This is a security feature that prevents malicious code from running on your computer, but it may also interfere with some PDF files or features. To disable Protected Mode at Startup, follow these steps:
-
-
Open Adobe Reader X on your computer.
-
Go to Edit > Preferences (for Windows) or Acrobat > Preferences (for Mac).
-
Select General from the left panel.
-
Uncheck the box that says Enable Protected Mode at Startup.
-
Click OK and restart Adobe Reader X.
-
-
This should allow you to open any PDF file without errors or issues.
-
Check for permission issues
-
If Adobe Reader X does not save or print PDF files, you may need to check for permission issues. This means that you may not have enough access rights to modify or use certain files or folders on your computer. To check for permission issues, follow these steps:
-
Right-click on the PDF file or folder that you want to save or print.
-
Select Properties (for Windows) or Get Info (for Mac).
-
Go to the Security tab (for Windows) or the Sharing & Permissions section (for Mac).
-
Make sure that you have Full Control (for Windows) or Read & Write (for Mac) permissions for the file or folder.
-
If not, click the Edit button (for Windows) or the lock icon (for Mac) and change the permissions accordingly.
-
Click OK and try to save or print the PDF file again.
-
-
This should resolve any permission issues that may prevent you from saving or printing PDF files.
-
Repair Installation
-
If Adobe Reader X does not launch or shows an error message when launching, you may need to repair the installation. This will fix any damaged or missing components that may affect the performance of Adobe Reader X. To repair the installation, follow these steps:
-
-
Go to Control Panel > Programs > Programs and Features (for Windows) or Applications > Utilities > Adobe Installers (for Mac).
-
Select Adobe Reader X from the list of programs and click the Change button (for Windows) or the Uninstall button (for Mac).
-
Choose the Repair option and click Next (for Windows) or Continue (for Mac).
-
Follow the on-screen instructions to complete the repair process.
-
Restart your computer and try to launch Adobe Reader X again.
-
-
This should fix any errors or issues that may prevent Adobe Reader X from launching.
-
Force open the files with Adobe Reader X
-
If Adobe Reader X does not open PDF files by default, you may need to force open them with Adobe Reader X. This will make sure that Adobe Reader X is the default program for opening PDF files on your computer. To force open PDF files with Adobe Reader X, follow these steps:
-
-
Right-click on the PDF file that you want to open.
-
Select Open With > Choose Another App (for Windows) or Open With > Other... (for Mac).
-
Select Adobe Reader X from the list of programs and check the box that says Always use this app to open .pdf files (for Windows) or Always Open With (for Mac).
-
Click OK and open the PDF file with Adobe Reader X.
-
-
This should make Adobe Reader X the default program for opening PDF files on your computer.
-
Conclusion
-
In this article, we have shown you how to download Adobe Reader X for Windows and Mac, as well as how to troubleshoot some common installation issues. Adobe Reader X is a free software that allows you to view, print, and comment on PDF files. It also offers some advanced features, such as converting PDFs to other file formats, password protecting PDFs, comparing PDFs, and integrating with cloud storage services. With Adobe Reader X, you can access any PDF file on your computer or mobile device with ease and convenience.
-
If you want to learn more about Adobe Reader X, you can visit this page for more information and resources. You can also check out this page for some tips and tricks on how to use Adobe Reader X effectively. We hope you have enjoyed this article and found it helpful. Thank you for reading!
-
FAQs
-
What is the difference between Acrobat Reader and Acrobat Pro?
-
Acrobat Reader is a free software that allows you to view, print, and comment on PDF files. Acrobat Pro is a paid software that allows you to create, edit, convert, sign, and share PDF files. Acrobat Pro also has more features and tools than Acrobat Reader, such as OCR, redaction, optimization, accessibility, and collaboration.
-
How can I update Adobe Reader X to the latest version?
-
You can update Adobe Reader X to the latest version by following these steps:
-
-
Open Adobe Reader X on your computer.
-
Go to Help > Check for Updates.
-
If there are any updates available, click the Download button and follow the instructions.
-
Restart your computer and enjoy the latest version of Adobe Reader X.
-
-
You can also enable automatic updates by going to Edit > Preferences > Updater and selecting Automatically install updates.
-
How can I open a password-protected PDF with Adobe Reader X?
-
You can open a password-protected PDF with Adobe Reader X by following these steps:
-
-
Double-click on the PDF file that you want to open.
-
Enter the password that was set by the creator of the PDF file.
-
Click OK and view the PDF file with Adobe Reader X.
-
-
If you do not know the password, you will not be able to open the PDF file. You will need to contact the creator of the PDF file and ask for the password.
-
How can I annotate PDFs with Adobe Reader X?
-
You can annotate PDFs with Adobe Reader X by following these steps:
-
-
Open the PDF file that you want to annotate with Adobe Reader X.
-
Go to View > Tools > Comment and click the Open button.
-
Select the annotation tool that you want to use from the toolbar. You can choose from different types of annotations, such as highlight, underline, strikeout, sticky note, text box, stamp, and more.
-
Click on the PDF file where you want to add the annotation and adjust it as needed.
-
You can also edit, delete, or reply to your annotations by right-clicking on them and choosing the appropriate option.
-
-
Your annotations will be saved with the PDF file and can be viewed by anyone who opens it with Adobe Reader X or any other PDF viewer.
-
How can I access my PDFs from anywhere with Adobe Reader X?
-
You can access your PDFs from anywhere with Adobe Reader X by following these steps:
-
-
Create a free account on Adobe Document Cloud, a cloud storage service that allows you to store and access your PDF files online.
-
Upload your PDF files to Adobe Document Cloud by going to File > Save As > Adobe Document Cloud or by dragging and dropping them to the Adobe Document Cloud window.
-
Sign in to your Adobe Document Cloud account on any device that has Adobe Reader X installed or on any web browser that supports PDF viewing.
-
Open and view your PDF files from Adobe Document Cloud with Adobe Reader X or any other PDF viewer.
-
-
You can also share your PDF files with others, edit them online, or convert them to other file formats with Adobe Document Cloud.
197e85843d
-
-
\ No newline at end of file
diff --git a/spaces/1pelhydcardo/ChatGPT-prompt-generator/assets/CarX Street APK for PC How to Play the Stunning Racing Game on Your Laptop or Desktop.md b/spaces/1pelhydcardo/ChatGPT-prompt-generator/assets/CarX Street APK for PC How to Play the Stunning Racing Game on Your Laptop or Desktop.md
deleted file mode 100644
index 9f811fa3e61513ff64d97f0ebf2389884088d1a0..0000000000000000000000000000000000000000
--- a/spaces/1pelhydcardo/ChatGPT-prompt-generator/assets/CarX Street APK for PC How to Play the Stunning Racing Game on Your Laptop or Desktop.md
+++ /dev/null
@@ -1,131 +0,0 @@
-
-
How to Download and Play CarX Street on PC
-
CarX Street is a racing game developed by CarX Technologies, LLC. It is an open-world street racer that lets you explore the large city and its surroundings, from busy city streets to spiral mountain roads and mesmerizing coastal highways. You can race to collect legendary racing cars and display them in your garage, or challenge other players in real network races. You can also build the car of your dreams using part tuning that unlocks all the physics of CarX Technology car behavior.
-
If you are a fan of racing games, you might want to play CarX Street on your PC instead of your mobile device. Playing on PC has many advantages, such as a larger screen, better graphics, smoother performance, and more comfortable controls. In this article, we will show you how to download and install CarX Street on your PC using different emulators. We will also give you some tips and tricks to help you enjoy the game more.
CarX Street is a simulation racing video game that offers realistic car physics and high-speed drifting. The game also features different map types from around the world, and players can choose from several different game modes. Players can compete against other players, or participate in races and events.
-
Features of CarX Street
-
Some of the features of CarX Street are:
-
-
Open world: You can get on your vehicle and explore the entire virtual world. You can find hidden spots, shortcuts, and secrets.
-
Free to play: You can download and play CarX Street for free. You can also earn in-game currency by completing tasks and challenges.
-
Buying gas: You need to fuel up your car with the right gas for the next race at city gas stations. Different types of gas have different effects on your car's performance.
-
Houses and garages: You can buy houses for your cars and assemble collections for every race mode. You can also customize your garage with various decorations.
-
In-game shop: You can buy over 50 official vehicles from the best automakers in the world. You can also buy parts, accessories, paints, stickers, and more.
-
Many types of vehicles: You can choose from different types of vehicles, such as sports cars, muscle cars, supercars, hypercars, SUVs, trucks, and more.
-
Car customization: You can customize your car with a detailed car-building system. You can swap parts and trick out your car for a specific race. You can also upgrade the engine, transmission, body, suspension, and tires.
-
In-game free currency: You can earn free currency by watching ads, completing tasks, or participating in events. You can use the free currency to buy items or unlock features.
-
-
Benefits of playing CarX Street on PC
-
Playing CarX Street on PC has many benefits, such as:
-
-
Larger screen: You can enjoy the stunning graphics and details of the game on a bigger screen. You can also see more of the map and the surroundings.
-
Better graphics: You can adjust the graphics settings to suit your PC's specifications. You can also experience higher resolution, frame rate, and quality.
-
Smoother performance: You can avoid lagging, crashing, or overheating issues that might occur on mobile devices. You can also save battery life and storage space.
-
More comfortable controls: You can use your keyboard and mouse to control your car more easily and precisely. You can also customize your key mapping according to your preference.
-
-
How to download
How to download and install CarX Street on PC
-
If you want to play CarX Street on your PC, you will need to use an Android emulator. An emulator is a software that mimics the Android operating system on your computer, allowing you to run Android apps and games. There are many emulators available, but we will show you how to use three of the most popular ones: BlueStacks, NoxPlayer, and LDPlayer.
-
Using BlueStacks emulator
-
BlueStacks is one of the most widely used Android emulators, with over 500 million users worldwide. It is compatible with both Windows and Mac operating systems, and it has a user-friendly interface and advanced features. Here are the steps to download and install CarX Street on PC using BlueStacks:
-
-
Download and install BlueStacks on your PC from [1](https://www.bluestacks.com/).
-
Complete Google sign-in to access the Play Store, or do it later.
-
Look for CarX Street in the search bar at the top right corner.
-
Click to install CarX Street from the search results.
-
Complete Google sign-in (if you skipped step 2) to install CarX Street.
-
Click the CarX Street icon on the home screen to start playing.
-
-
Using NoxPlayer emulator
-
NoxPlayer is another popular Android emulator, with over 150 million users worldwide. It is also compatible with both Windows and Mac operating systems, and it has a simple and fast interface and performance. Here are the steps to download and install CarX Street on PC using NoxPlayer:
-
-
Download and install NoxPlayer on your PC from [5](https://www.bignox.com/).
-
Run the installation package and complete the installation.
-
Open NoxPlayer and search for CarX Street in the Google Play Store.
-
Install the game and launch it to start playing.
-
-
Using LDPlayer emulator
-
LDPlayer is a newer Android emulator, but it has gained popularity among gamers for its high performance and compatibility. It is also compatible with both Windows and Mac operating systems, and it has a smooth and stable interface and features. Here are the steps to download and install CarX Street on PC using LDPlayer:
-
carx street racing game download for pc
-carx street mod apk for pc
-carx street pc emulator
-carx street android game on pc
-carx street free download for pc
-carx street pc version
-carx street pc requirements
-carx street pc gameplay
-carx street pc online
-carx street pc windows 10
-carx street pc bluestacks
-carx street pc noxplayer
-carx street pc ldplayer
-carx street pc steam
-carx street pc review
-carx street pc cheats
-carx street pc hack
-carx street pc controller support
-carx street pc graphics settings
-carx street pc best cars
-carx street pc tips and tricks
-carx street pc update
-carx street pc release date
-carx street pc beta test
-carx street pc download size
-carx street apk for windows 7
-carx street apk for windows 8.1
-carx street apk for macbook
-carx street apk for laptop
-carx street apk for desktop
-carx street apk for chromebook
-carx street apk for linux
-carx street apk for ubuntu
-carx street apk for mac os x
-carx street apk for windows xp
-how to install carx street apk on pc
-how to play carx street apk on pc
-how to run carx street apk on pc
-how to download carx street apk on pc
-how to update carx street apk on pc
-how to uninstall carx street apk on pc
-how to transfer carx street apk from android to pc
-how to sync carx street apk between android and pc
-how to fix carx street apk not working on pc
-how to get unlimited coins in carx street apk on pc
-how to customize cars in carx street apk on pc
-how to change language in carx street apk on pc
-how to connect facebook in carx street apk on pc
-how to record gameplay of carx street apk on pc
-
-
Download and install LDPlayer on your PC from [6](https://www.ldplayer.net/).
-
Open LDPlayer and search for CarX Street in the LD Store or Google Play Store.
-
Install the game and launch it to start playing.
-
-
Tips and tricks for CarX Street
-
Now that you know how to play CarX Street on your PC, you might want some tips and tricks to help you improve your skills and enjoy the game more. Here are some of them:
-
Follow the tutorial
-
The game has a tutorial that will teach you the basics of driving, racing, drifting, tuning, and more. It is highly recommended that you follow the tutorial before jumping into the action, as it will help you get familiar with the game mechanics and controls. You can also revisit the tutorial anytime from the settings menu if you need a refresher.
-
Roam through the city for more rewards
-
The game has an open world that you can explore at your own pace. You can find hidden spots, shortcuts, secrets, and rewards by roaming through the city. You can also encounter random events, challenges, and races that will give you more money, reputation, or items. Roaming through the city is also a good way to practice your driving skills and test your car's performance.
-
Take part in sprints and clubs
-
The game has two main modes: sprints and clubs. Sprints are short races that last under a minute, where you have to reach the finish line as fast as possible. Clubs are longer, story-driven competitions where you have to join a club, defeat its boss, and prove yourself as the best driver in the city. Both modes offer different rewards and challenges, so try them both out and see which one suits your style more.
-
Go for the best cars and customize them
-
The game has over 50 official vehicles from the best automakers in the world. You can buy them with in-game currency or real money, or earn them by completing tasks or events. You can also customize your car with a detailed car-building system that lets you swap parts, upgrade components, paint colors, add stickers, and more. You can also customize your garage with various decorations and display your car collection. Go for the best cars and make them your own.
-
Conclusion
-
CarX Street is a fun and realistic racing game that lets you experience the thrill of street racing. You can explore the open world, collect and customize your cars, and compete with other players. You can also play CarX Street on your PC using an Android emulator, which will give you many benefits such as a larger screen, better graphics, smoother performance, and more comfortable controls. If you are looking for a racing game that will keep you entertained and challenged, you should give CarX Street a try.
-
FAQs
-
Here are some frequently asked questions about CarX Street:
-
-
Q: How do I drift in CarX Street?
-
A: Drifting is an essential skill in CarX Street, as it will help you take corners faster and earn more points. To drift, you need to press the brake button while turning the steering wheel. You can also use the handbrake button to initiate a drift. You can adjust the sensitivity and angle of the steering wheel in the settings menu.
-
Q: How do I get more money in CarX Street?
-
A: Money is the main currency in CarX Street, which you can use to buy cars, parts, gas, and more. You can earn money by completing races, events, tasks, or challenges. You can also watch ads or use real money to get more money.
-
Q: How do I join a club in CarX Street?
-
A: Clubs are groups of racers that compete for territory and reputation in the city. You can join a club by completing its entry race and defeating its boss. You can also create your own club or join an existing one from the club menu.
-
Q: How do I upgrade my car in CarX Street?
-
A: You can upgrade your car by buying and installing new parts from the shop or the garage. You can also tune your car by adjusting the engine, transmission, body, suspension, and tires parameters. Upgrading and tuning your car will improve its performance and handling.
-
Q: How do I play with friends in CarX Street?
-
A: You can play with friends in CarX Street by inviting them to join your club or your race. You can also chat with them using the in-game chat feature or voice chat feature. You can also add friends from the social menu or search for them by their nickname or ID.
-
197e85843d
-
-
\ No newline at end of file
diff --git a/spaces/1phancelerku/anime-remove-background/CapCut Edit Videos like a Pro with TikToks Official Video Editor and Video Maker - Free Download.md b/spaces/1phancelerku/anime-remove-background/CapCut Edit Videos like a Pro with TikToks Official Video Editor and Video Maker - Free Download.md
deleted file mode 100644
index fb2bee50608773153ef1ef44f8b4e233e3036e4e..0000000000000000000000000000000000000000
--- a/spaces/1phancelerku/anime-remove-background/CapCut Edit Videos like a Pro with TikToks Official Video Editor and Video Maker - Free Download.md
+++ /dev/null
@@ -1,93 +0,0 @@
-
-
How to Download and Use CapCut Video Editor for TikTok
-
TikTok is one of the most popular social media platforms for creating and sharing short videos. Whether you want to make funny, educational, or inspirational videos, you need a good video editor to make them stand out. In this article, we will show you how to download and use CapCut, the official video editor and maker app for TikTok.
-
What is CapCut?
-
CapCut is a free video editor and maker app that is compatible with TikTok. It is developed by ByteDance, the same company that owns TikTok. CapCut allows you to edit videos on your mobile device with ease and fun. You can also use it to create videos for other social media platforms, such as YouTube, Instagram, Facebook, and WhatsApp.
CapCut is a free video editor and maker app for TikTok
-
CapCut has everything you need to create stunning, high-quality videos. You can import your own videos and photos or record new ones in the app. You can also access a massive music library and exclusive TikTok songs. You can extract audio from videos or add your own voice-overs. You can also use AI tools to enhance your videos, such as auto captions, background removal, text-to-speech, motion tracking, and more.
-
CapCut offers basic and advanced editing features
-
CapCut has a user-friendly interface that lets you edit videos with simple gestures. You can trim, cut, merge, split, reverse, speed up, slow down, zoom in, zoom out, freeze, and animate your clips. You can also add text, stickers, filters, effects, transitions, and colors to your videos. You can use keyframe animation to customize every setting. You can also use chroma key to remove specific colors from videos. You can apply picture-in-picture (PIP) feature to add video and photo layers above the clip. You can also use the stabilizing feature to keep video footage steady.
-
CapCut supports direct exports to TikTok and other social media platforms
-
CapCut lets you export your videos in custom resolutions and formats. You can export your videos in HD quality and support 4K 60fps exports and smart HDR. You can also adjust the format and share your creativity on TikTok and other social media platforms with one tap.
-
How to Download CapCut for Android and iOS
-
Downloading CapCut is easy and fast. Here are the steps to download CapCut for Android and iOS devices.
-
Download CapCut from Google Play Store or Apple App Store
-
You can download CapCut for free from Google Play Store or Apple App Store. Just search for "CapCut" in the store and tap Install or Get. The app size is about 100 MB.
-
Open CapCut and tap New Project to start editing
-
Once you have downloaded CapCut, open it on your device. You don't need a TikTok account or any other type of account to use CapCut. You can start editing right away by tapping New Project on the home screen.
-
Select a video or photos to edit and tap Add
-
You can select a video or photos from your device gallery or record a new one in the app. You can also use the search feature to find videos and photos online. You can select multiple files and tap Add to import them to your project. You can also rearrange, delete, or duplicate the clips in your timeline.
-
How to download capcut video editor for tiktok on android
-Download capcut video editor for tiktok apk free
-Best capcut video editor for tiktok tutorials and tips
-Download capcut video editor for tiktok for pc windows 10
-Capcut video editor for tiktok review and features
-Download capcut video editor for tiktok mod apk
-Capcut video editor for tiktok vs inshot comparison
-Download capcut video editor for tiktok pro version
-Capcut video editor for tiktok online without download
-Download capcut video editor for tiktok ios iphone ipad
-Capcut video editor for tiktok alternatives and similar apps
-Download capcut video editor for tiktok macbook laptop
-Capcut video editor for tiktok filters and effects guide
-Download capcut video editor for tiktok latest version update
-Capcut video editor for tiktok transitions and stickers tutorial
-Download capcut video editor for tiktok no watermark
-Capcut video editor for tiktok music and sound effects library
-Download capcut video editor for tiktok premium unlocked
-Capcut video editor for tiktok speed and reverse options
-Download capcut video editor for tiktok cracked full version
-Capcut video editor for tiktok crop and rotate tools
-Download capcut video editor for tiktok from google play store
-Capcut video editor for tiktok split and merge videos function
-Download capcut video editor for tiktok from official website
-Capcut video editor for tiktok text and font styles customization
-Download capcut video editor for tiktok with bluestacks emulator
-Capcut video editor for tiktok voice changer and dubbing feature
-Download capcut video editor for tiktok without ads or subscription
-Capcut video editor for tiktok chroma key and green screen effect
-Download capcut video editor for tiktok with qr code scanner
-Capcut video editor for tiktok slideshow and collage maker mode
-Download capcut video editor for tiktok old version apk file
-Capcut video editor for tiktok cutout and background changer tool
-Download capcut video editor for tiktok on amazon fire tablet
-Capcut video editor for tiktok gif and meme generator option
-Download capcut video editor for tiktok on chromebook device
-Capcut video editor for tiktok face swap and beauty filter feature
-Download capcut video editor for tiktok on linux operating system
-Capcut video editor for tiktok animation and drawing effect mode
-Download capcut video editor for tiktok on smart tv or roku device
-
How to Use CapCut to Edit Videos for TikTok
-
Editing videos with CapCut is fun and easy. Here are some tips on how to use CapCut to edit videos for TikTok.
-
Use the editing tools to trim, crop, reverse, speed up, and animate your clips
-
You can use the editing tools at the bottom of the screen to adjust your clips. You can tap Trim to cut out unwanted parts of your video. You can tap Crop to change the aspect ratio and zoom in or out of your video. You can tap Reverse to play your video backwards. You can tap Speed to change the playback speed of your video. You can tap Animate to add motion effects to your video.
-
Add text, stickers, filters, effects, and music to your videos
-
You can add text, stickers, filters, effects, and music to your videos by tapping the icons on the right side of the screen. You can tap Text to add captions, titles, or subtitles to your video. You can tap Sticker to add emojis, icons, or images to your video. You can tap Filter to apply different color presets to your video. You can tap Effect to add various visual effects to your video. You can tap Music to add songs, sound effects, or voice-overs to your video.
-
Use the templates and styles to enhance your videos
-
You can use the templates and styles to enhance your videos by tapping the icons on the left side of the screen. You can tap Template to apply pre-made themes and layouts to your video. You can tap Style to apply different artistic styles and filters to your video.
-
Tap Export to save and share your videos
-
When you are done editing your video, you can tap Export at the top right corner of the screen. You can choose the resolution, format, and quality of your video. You can also enable watermark removal if you want. Then you can tap Save or Share to save your video to your device or share it directly on TikTok or other social media platforms.
-
Benefits of Using CapCut for TikTok Videos
-
Using CapCut for TikTok videos has many benefits. Here are some of them.
-
CapCut is easy to use and versatile
-
CapCut is designed for beginners and professionals alike. It has a simple and intuitive interface that lets you edit videos with ease and fun. It also has a lot of features and options that let you customize your videos according to your preferences and needs.
-
CapCut has a large library of sounds and animations
-
CapCut has a large library of sounds and animations that you can use for free. You can access thousands of songs and sound effects that are updated regularly. You can also use exclusive TikTok songs that are popular and trending. You can also use hundreds of animations that are dynamic and creative.
-
CapCut can create stunning, high-quality videos
-
CapCut can create stunning, high-quality videos that will impress your audience. You can export your videos in HD quality and support 4K 60fps exports and smart HDR. You can also use AI tools that will enhance your videos automatically.
-
Conclusion
-
CapCut is a free video editor and maker app for TikTok that you can download and use on your Android or iOS device. It has everything you need to create stunning, high-quality videos with ease and fun. You can also use it to create videos for other social media platforms, such as YouTube, Instagram, Facebook, and WhatsApp. If you want to make amazing TikTok videos, download CapCut today!
-
Frequently Asked Questions
-
Is CapCut safe?
-
Yes, CapCut is safe and secure. It does not contain any viruses or malware. It also does not collect any personal information from users.
-
Is CapCut free?
-
Yes, CapCut is free and does not have any hidden fees or charges. It also does not have any annoying ads or watermarks.
-
How do I update CapCut?
-
You can update CapCut by going to the Google Play Store or the Apple App Store and tapping Update. You can also enable automatic updates in your device settings.
-
How do I delete CapCut?
-
You can delete CapCut by going to your device settings and tapping Apps or Applications. Then you can find CapCut and tap Uninstall or Delete. You can also delete CapCut by long-pressing the app icon and tapping Remove or Delete.
-
How do I contact CapCut support?
-
You can contact CapCut support by going to the app settings and tapping Feedback or Help. You can also email them at capcut.support@bytedance.com or visit their website at https://www.capcut.net/.
401be4b1e0
-
-
\ No newline at end of file
diff --git a/spaces/2023Liu2023/bingo/Dockerfile b/spaces/2023Liu2023/bingo/Dockerfile
deleted file mode 100644
index 3aa2b29b5fc4fa8b8238955acd7f1fde13ce5e1a..0000000000000000000000000000000000000000
--- a/spaces/2023Liu2023/bingo/Dockerfile
+++ /dev/null
@@ -1,36 +0,0 @@
-FROM node:18
-
-
-ARG DEBIAN_FRONTEND=noninteractive
-
-ENV BING_HEADER ""
-
-# Set home to the user's home directory
-ENV HOME=/home/user \
- PATH=/home/user/.local/bin:$PATH
-
-# Set up a new user named "user" with user ID 1000
-RUN useradd -o -u 1000 user && mkdir -p $HOME/app && chown -R user $HOME
-
-# Switch to the "user" user
-USER user
-
-# Set the working directory to the user's home directory
-WORKDIR $HOME/app
-
-# Install app dependencies
-# A wildcard is used to ensure both package.json AND package-lock.json are copied
-# where available (npm@5+)
-COPY --chown=user package*.json $HOME/app/
-
-RUN npm install
-
-# Copy the current directory contents into the container at $HOME/app setting the owner to the user
-COPY --chown=user . $HOME/app/
-
-RUN npm run build
-
-ENV PORT 7860
-EXPOSE 7860
-
-CMD npm start
diff --git a/spaces/2023Liu2023/bingo/src/app/page.tsx b/spaces/2023Liu2023/bingo/src/app/page.tsx
deleted file mode 100644
index 0dff3431b098ce4fe282cc83fc87a93a28a43090..0000000000000000000000000000000000000000
--- a/spaces/2023Liu2023/bingo/src/app/page.tsx
+++ /dev/null
@@ -1,15 +0,0 @@
-import dynamic from 'next/dynamic'
-
-const DynamicComponentWithNoSSR = dynamic(
- () => import('../components/chat'),
- { ssr: false }
-)
-
-export default function IndexPage() {
- return (
- <>
-
-
- >
- )
-}
diff --git a/spaces/2023Liu2023/bingo/src/components/chat.tsx b/spaces/2023Liu2023/bingo/src/components/chat.tsx
deleted file mode 100644
index a37ab1cc96ca2e6bfd9acbe313a8d946bfd5c3d4..0000000000000000000000000000000000000000
--- a/spaces/2023Liu2023/bingo/src/components/chat.tsx
+++ /dev/null
@@ -1,93 +0,0 @@
-'use client'
-
-import { useCallback, useEffect, useMemo, useState } from 'react'
-import { useAtom } from 'jotai'
-import Image from 'next/image'
-import { cn } from '@/lib/utils'
-import { ChatList } from '@/components/chat-list'
-import { ChatPanel } from '@/components/chat-panel'
-import { WelcomeScreen } from '@/components/welcome-screen'
-import { ChatScrollAnchor } from '@/components/chat-scroll-anchor'
-import { ToneSelector } from './tone-selector'
-import { ChatHeader } from './chat-header'
-import { ChatSuggestions } from './chat-suggestions'
-import { bingConversationStyleAtom } from '@/state'
-import { ButtonScrollToBottom } from '@/components/button-scroll-to-bottom'
-import StopIcon from '@/assets/images/stop.svg'
-import { useBing } from '@/lib/hooks/use-bing'
-import { ChatMessageModel } from '@/lib/bots/bing/types'
-import { ChatNotification } from './chat-notification'
-import { Settings } from './settings'
-import { ChatHistory } from './chat-history'
-
-export type ChatProps = React.ComponentProps<'div'> & { initialMessages?: ChatMessageModel[] }
-
-export default function Chat({ className }: ChatProps) {
-
- const [bingStyle, setBingStyle] = useAtom(bingConversationStyleAtom)
- const {
- messages,
- sendMessage,
- resetConversation,
- stopGenerating,
- setInput,
- bot,
- input,
- generating,
- isSpeaking,
- uploadImage,
- attachmentList,
- setAttachmentList,
- } = useBing()
-
- useEffect(() => {
- window.scrollTo({
- top: document.body.offsetHeight,
- behavior: 'smooth'
- })
- }, [])
-
- return (
-
- )
-}
diff --git a/spaces/AIFILMS/audioldm-text-to-audio-generation/audioldm/clap/open_clip/transform.py b/spaces/AIFILMS/audioldm-text-to-audio-generation/audioldm/clap/open_clip/transform.py
deleted file mode 100644
index 77aaa722c4a5544ac50de6df35d3e922f63b111d..0000000000000000000000000000000000000000
--- a/spaces/AIFILMS/audioldm-text-to-audio-generation/audioldm/clap/open_clip/transform.py
+++ /dev/null
@@ -1,45 +0,0 @@
-from torchvision.transforms import (
- Normalize,
- Compose,
- RandomResizedCrop,
- InterpolationMode,
- ToTensor,
- Resize,
- CenterCrop,
-)
-
-
-def _convert_to_rgb(image):
- return image.convert("RGB")
-
-
-def image_transform(
- image_size: int,
- is_train: bool,
- mean=(0.48145466, 0.4578275, 0.40821073),
- std=(0.26862954, 0.26130258, 0.27577711),
-):
- normalize = Normalize(mean=mean, std=std)
- if is_train:
- return Compose(
- [
- RandomResizedCrop(
- image_size,
- scale=(0.9, 1.0),
- interpolation=InterpolationMode.BICUBIC,
- ),
- _convert_to_rgb,
- ToTensor(),
- normalize,
- ]
- )
- else:
- return Compose(
- [
- Resize(image_size, interpolation=InterpolationMode.BICUBIC),
- CenterCrop(image_size),
- _convert_to_rgb,
- ToTensor(),
- normalize,
- ]
- )
diff --git a/spaces/AIGC-Audio/AudioGPT/text_to_speech/tasks/tts/ps_adv.py b/spaces/AIGC-Audio/AudioGPT/text_to_speech/tasks/tts/ps_adv.py
deleted file mode 100644
index f86409254b8d0d5f00de82cc0a9eed93cc8a40dc..0000000000000000000000000000000000000000
--- a/spaces/AIGC-Audio/AudioGPT/text_to_speech/tasks/tts/ps_adv.py
+++ /dev/null
@@ -1,374 +0,0 @@
-import os
-import torch
-import torch.nn.functional as F
-import torch.nn as nn
-import numpy as np
-
-from text_to_speech.modules.tts.portaspeech.portaspeech import PortaSpeech
-from text_to_speech.modules.tts.syntaspeech.multi_window_disc import Discriminator
-from tasks.tts.fs import FastSpeechTask
-from text_to_speech.utils.audio.align import mel2token_to_dur
-from text_to_speech.utils.commons.hparams import hparams
-from text_to_speech.utils.metrics.diagonal_metrics import get_focus_rate, get_phone_coverage_rate, get_diagonal_focus_rate
-from text_to_speech.utils.nn.model_utils import num_params
-from text_to_speech.utils.commons.tensor_utils import tensors_to_scalars
-from text_to_speech.utils.audio.pitch.utils import denorm_f0, norm_f0
-from text_to_speech.utils.audio.pitch_extractors import get_pitch
-from text_to_speech.utils.metrics.dtw import dtw as DTW
-
-from text_to_speech.utils.plot.plot import spec_to_figure
-from text_to_speech.utils.text.text_encoder import build_token_encoder
-
-
-class PortaSpeechAdvTask(FastSpeechTask):
- def __init__(self):
- super().__init__()
- data_dir = hparams['binary_data_dir']
- self.word_encoder = build_token_encoder(f'{data_dir}/word_set.json')
- self.build_disc_model()
- self.mse_loss_fn = torch.nn.MSELoss()
-
- def build_tts_model(self):
- ph_dict_size = len(self.token_encoder)
- word_dict_size = len(self.word_encoder)
- self.model = PortaSpeech(ph_dict_size, word_dict_size, hparams)
-
- self.gen_params = [p for p in self.model.parameters() if p.requires_grad]
- self.dp_params = [p for k, p in self.model.named_parameters() if (('dur_predictor' in k) and p.requires_grad)]
- self.gen_params_except_dp = [p for k, p in self.model.named_parameters() if (('dur_predictor' not in k) and p.requires_grad)]
- self.bert_params = [p for k, p in self.model.named_parameters() if (('bert' in k) and p.requires_grad)]
- self.gen_params_except_bert_and_dp = [p for k, p in self.model.named_parameters() if ('dur_predictor' not in k) and ('bert' not in k) and p.requires_grad ]
-
- self.use_bert = True if len(self.bert_params) > 0 else False
-
- def build_disc_model(self):
- disc_win_num = hparams['disc_win_num']
- h = hparams['mel_disc_hidden_size']
- self.mel_disc = Discriminator(
- time_lengths=[32, 64, 128][:disc_win_num],
- freq_length=80, hidden_size=h, kernel=(3, 3)
- )
- self.disc_params = list(self.mel_disc.parameters())
-
- def on_train_start(self):
- super().on_train_start()
- for n, m in self.model.named_children():
- num_params(m, model_name=n)
- if hasattr(self.model, 'fvae'):
- for n, m in self.model.fvae.named_children():
- num_params(m, model_name=f'fvae.{n}')
-
- def _training_step(self, sample, batch_idx, optimizer_idx):
- loss_output = {}
- loss_weights = {}
- disc_start = self.global_step >= hparams["disc_start_steps"] and hparams['lambda_mel_adv'] > 0
- if optimizer_idx == 0:
- #######################
- # Generator #
- #######################
- loss_output, model_out = self.run_model(sample, infer=False)
- self.model_out_gt = self.model_out = \
- {k: v.detach() for k, v in model_out.items() if isinstance(v, torch.Tensor)}
- if disc_start:
- mel_p = model_out['mel_out']
- if hasattr(self.model, 'out2mel'):
- mel_p = self.model.out2mel(mel_p)
- o_ = self.mel_disc(mel_p)
- p_, pc_ = o_['y'], o_['y_c']
- if p_ is not None:
- loss_output['a'] = self.mse_loss_fn(p_, p_.new_ones(p_.size()))
- loss_weights['a'] = hparams['lambda_mel_adv']
- if pc_ is not None:
- loss_output['ac'] = self.mse_loss_fn(pc_, pc_.new_ones(pc_.size()))
- loss_weights['ac'] = hparams['lambda_mel_adv']
- else:
- #######################
- # Discriminator #
- #######################
- if disc_start and self.global_step % hparams['disc_interval'] == 0:
- model_out = self.model_out_gt
- mel_g = sample['mels']
- mel_p = model_out['mel_out']
- o = self.mel_disc(mel_g)
- p, pc = o['y'], o['y_c']
- o_ = self.mel_disc(mel_p)
- p_, pc_ = o_['y'], o_['y_c']
- if p_ is not None:
- loss_output["r"] = self.mse_loss_fn(p, p.new_ones(p.size()))
- loss_output["f"] = self.mse_loss_fn(p_, p_.new_zeros(p_.size()))
- if pc_ is not None:
- loss_output["rc"] = self.mse_loss_fn(pc, pc.new_ones(pc.size()))
- loss_output["fc"] = self.mse_loss_fn(pc_, pc_.new_zeros(pc_.size()))
- total_loss = sum([loss_weights.get(k, 1) * v for k, v in loss_output.items() if isinstance(v, torch.Tensor) and v.requires_grad])
- loss_output['batch_size'] = sample['txt_tokens'].size()[0]
- return total_loss, loss_output
-
- def run_model(self, sample, infer=False, *args, **kwargs):
- txt_tokens = sample['txt_tokens']
- word_tokens = sample['word_tokens']
- spk_embed = sample.get('spk_embed')
- spk_id = sample.get('spk_ids')
- if not infer:
- output = self.model(txt_tokens, word_tokens,
- ph2word=sample['ph2word'],
- mel2word=sample['mel2word'],
- mel2ph=sample['mel2ph'],
- word_len=sample['word_lengths'].max(),
- tgt_mels=sample['mels'],
- pitch=sample.get('pitch'),
- spk_embed=spk_embed,
- spk_id=spk_id,
- infer=False,
- global_step=self.global_step,
- graph_lst=sample['graph_lst'],
- etypes_lst=sample['etypes_lst'],
- bert_feats=sample.get("bert_feats"),
- cl_feats=sample.get("cl_feats")
- )
- losses = {}
- losses['kl_v'] = output['kl'].detach()
- losses_kl = output['kl']
- losses_kl = torch.clamp(losses_kl, min=hparams['kl_min'])
- losses_kl = min(self.global_step / hparams['kl_start_steps'], 1) * losses_kl
- losses_kl = losses_kl * hparams['lambda_kl']
- losses['kl'] = losses_kl
-
- self.add_mel_loss(output['mel_out'], sample['mels'], losses)
- if hparams['dur_level'] == 'word':
- self.add_dur_loss(
- output['dur'], sample['mel2word'], sample['word_lengths'], sample['txt_tokens'], losses)
- self.get_attn_stats(output['attn'], sample, losses)
- else:
- super(PortaSpeechAdvTask, self).add_dur_loss(output['dur'], sample['mel2ph'], sample['txt_tokens'], losses)
- return losses, output
- else:
- use_gt_dur = kwargs.get('infer_use_gt_dur', hparams['use_gt_dur'])
- output = self.model(
- txt_tokens, word_tokens,
- ph2word=sample['ph2word'],
- word_len=sample['word_lengths'].max(),
- pitch=sample.get('pitch'),
- mel2ph=sample['mel2ph'] if use_gt_dur else None,
- mel2word=sample['mel2word'] if use_gt_dur else None,
- tgt_mels=sample['mels'],
- infer=True,
- spk_embed=spk_embed,
- spk_id=spk_id,
- graph_lst=sample['graph_lst'],
- etypes_lst=sample['etypes_lst'],
- bert_feats=sample.get("bert_feats"),
- cl_feats=sample.get("cl_feats")
- )
- return output
-
- def add_dur_loss(self, dur_pred, mel2token, word_len, txt_tokens, losses=None):
- T = word_len.max()
- dur_gt = mel2token_to_dur(mel2token, T).float()
- nonpadding = (torch.arange(T).to(dur_pred.device)[None, :] < word_len[:, None]).float()
- dur_pred = dur_pred * nonpadding
- dur_gt = dur_gt * nonpadding
- wdur = F.l1_loss((dur_pred + 1).log(), (dur_gt + 1).log(), reduction='none')
- wdur = (wdur * nonpadding).sum() / nonpadding.sum()
-
- if hparams['lambda_word_dur'] > 0:
- losses['wdur'] = wdur * hparams['lambda_word_dur']
- if hparams['lambda_sent_dur'] > 0:
- sent_dur_p = dur_pred.sum(-1)
- sent_dur_g = dur_gt.sum(-1)
- sdur_loss = F.l1_loss(sent_dur_p, sent_dur_g, reduction='mean')
- losses['sdur'] = sdur_loss.mean() * hparams['lambda_sent_dur']
-
- with torch.no_grad():
- # calculate word-level abs_dur_error in micro-second
- abs_word_dur_error = F.l1_loss(dur_pred , dur_gt, reduction='none')
- abs_word_dur_error = (abs_word_dur_error * nonpadding).sum() / nonpadding.sum()
- abs_word_dur_error = abs_word_dur_error * hparams['hop_size'] / hparams['audio_sample_rate'] * 1000
- losses['abs_word_dur_error'] = abs_word_dur_error
- # calculate word-level abs_dur_error in second
- sent_dur_p = dur_pred.sum(-1)
- sent_dur_g = dur_gt.sum(-1)
- abs_sent_dur_error = F.l1_loss(sent_dur_p, sent_dur_g, reduction='mean').mean()
- abs_sent_dur_error = abs_sent_dur_error * hparams['hop_size'] / hparams['audio_sample_rate']
- losses['abs_sent_dur_error'] = abs_sent_dur_error
-
- def validation_step(self, sample, batch_idx):
- outputs = {}
- outputs['losses'] = {}
- outputs['losses'], model_out = self.run_model(sample)
- outputs['total_loss'] = sum(outputs['losses'].values())
- outputs['nsamples'] = sample['nsamples']
- outputs = tensors_to_scalars(outputs)
- if self.global_step % hparams['valid_infer_interval'] == 0 \
- and batch_idx < hparams['num_valid_plots']:
- valid_results = self.save_valid_result(sample, batch_idx, model_out)
- wav_gt = valid_results['wav_gt']
- mel_gt = valid_results['mel_gt']
- wav_pred = valid_results['wav_pred']
- mel_pred = valid_results['mel_pred']
- f0_pred_, _ = get_pitch(wav_pred, mel_pred, hparams)
- f0_gt_, _ = get_pitch(wav_gt, mel_gt, hparams)
- manhattan_distance = lambda x, y: np.abs(x - y)
- dist, cost, acc, path = DTW(f0_pred_, f0_gt_, manhattan_distance)
- outputs['losses']['f0_dtw'] = dist / len(f0_gt_)
- return outputs
-
- def save_valid_result(self, sample, batch_idx, model_out):
- sr = hparams['audio_sample_rate']
- f0_gt = None
- mel_out = model_out['mel_out']
- if sample.get('f0') is not None:
- f0_gt = denorm_f0(sample['f0'][0].cpu(), sample['uv'][0].cpu())
- self.plot_mel(batch_idx, sample['mels'], mel_out, f0s=f0_gt)
-
- # if self.global_step > 0:
- wav_pred = self.vocoder.spec2wav(mel_out[0].cpu(), f0=f0_gt)
- self.logger.add_audio(f'wav_val_{batch_idx}', wav_pred, self.global_step, sr)
- # with gt duration
- model_out = self.run_model(sample, infer=True, infer_use_gt_dur=True)
- dur_info = self.get_plot_dur_info(sample, model_out)
- del dur_info['dur_pred']
- wav_pred = self.vocoder.spec2wav(model_out['mel_out'][0].cpu(), f0=f0_gt)
- self.logger.add_audio(f'wav_gdur_{batch_idx}', wav_pred, self.global_step, sr)
- self.plot_mel(batch_idx, sample['mels'], model_out['mel_out'][0], f'mel_gdur_{batch_idx}',
- dur_info=dur_info, f0s=f0_gt)
-
- # with pred duration
- if not hparams['use_gt_dur']:
- model_out = self.run_model(sample, infer=True, infer_use_gt_dur=False)
- dur_info = self.get_plot_dur_info(sample, model_out)
- self.plot_mel(batch_idx, sample['mels'], model_out['mel_out'][0], f'mel_pdur_{batch_idx}',
- dur_info=dur_info, f0s=f0_gt)
- wav_pred = self.vocoder.spec2wav(model_out['mel_out'][0].cpu(), f0=f0_gt)
- self.logger.add_audio(f'wav_pdur_{batch_idx}', wav_pred, self.global_step, sr)
- # gt wav
- mel_gt = sample['mels'][0].cpu()
- wav_gt = self.vocoder.spec2wav(mel_gt, f0=f0_gt)
- if self.global_step <= hparams['valid_infer_interval']:
- self.logger.add_audio(f'wav_gt_{batch_idx}', wav_gt, self.global_step, sr)
-
- # add attn plot
- if self.global_step > 0 and hparams['dur_level'] == 'word':
- self.logger.add_figure(f'attn_{batch_idx}', spec_to_figure(model_out['attn'][0]), self.global_step)
-
- return {'wav_gt': wav_gt, 'wav_pred': wav_pred, 'mel_gt': mel_gt, 'mel_pred': model_out['mel_out'][0].cpu()}
-
- def get_attn_stats(self, attn, sample, logging_outputs, prefix=''):
- # diagonal_focus_rate
- txt_lengths = sample['txt_lengths'].float()
- mel_lengths = sample['mel_lengths'].float()
- src_padding_mask = sample['txt_tokens'].eq(0)
- target_padding_mask = sample['mels'].abs().sum(-1).eq(0)
- src_seg_mask = sample['txt_tokens'].eq(self.seg_idx)
- attn_ks = txt_lengths.float() / mel_lengths.float()
-
- focus_rate = get_focus_rate(attn, src_padding_mask, target_padding_mask).mean().data
- phone_coverage_rate = get_phone_coverage_rate(
- attn, src_padding_mask, src_seg_mask, target_padding_mask).mean()
- diagonal_focus_rate, diag_mask = get_diagonal_focus_rate(
- attn, attn_ks, mel_lengths, src_padding_mask, target_padding_mask)
- logging_outputs[f'{prefix}fr'] = focus_rate.mean().data
- logging_outputs[f'{prefix}pcr'] = phone_coverage_rate.mean().data
- logging_outputs[f'{prefix}dfr'] = diagonal_focus_rate.mean().data
-
- def get_plot_dur_info(self, sample, model_out):
- if hparams['dur_level'] == 'word':
- T_txt = sample['word_lengths'].max()
- dur_gt = mel2token_to_dur(sample['mel2word'], T_txt)[0]
- dur_pred = model_out['dur'] if 'dur' in model_out else dur_gt
- txt = sample['ph_words'][0].split(" ")
- else:
- T_txt = sample['txt_tokens'].shape[1]
- dur_gt = mel2token_to_dur(sample['mel2ph'], T_txt)[0]
- dur_pred = model_out['dur'] if 'dur' in model_out else dur_gt
- txt = self.token_encoder.decode(sample['txt_tokens'][0].cpu().numpy())
- txt = txt.split(" ")
- return {'dur_gt': dur_gt, 'dur_pred': dur_pred, 'txt': txt}
-
- def build_optimizer(self, model):
-
- optimizer_gen = torch.optim.AdamW(
- self.gen_params,
- lr=hparams['lr'],
- betas=(hparams['optimizer_adam_beta1'], hparams['optimizer_adam_beta2']),
- weight_decay=hparams['weight_decay'])
-
- optimizer_disc = torch.optim.AdamW(
- self.disc_params,
- lr=hparams['disc_lr'],
- betas=(hparams['optimizer_adam_beta1'], hparams['optimizer_adam_beta2']),
- **hparams["discriminator_optimizer_params"]) if len(self.disc_params) > 0 else None
-
- return [optimizer_gen, optimizer_disc]
-
- def build_scheduler(self, optimizer):
- return [
- FastSpeechTask.build_scheduler(self, optimizer[0]), # Generator Scheduler
- torch.optim.lr_scheduler.StepLR(optimizer=optimizer[1], # Discriminator Scheduler
- **hparams["discriminator_scheduler_params"]),
- ]
-
- def on_before_optimization(self, opt_idx):
- if opt_idx == 0:
- nn.utils.clip_grad_norm_(self.dp_params, hparams['clip_grad_norm'])
- if self.use_bert:
- nn.utils.clip_grad_norm_(self.bert_params, hparams['clip_grad_norm'])
- nn.utils.clip_grad_norm_(self.gen_params_except_bert_and_dp, hparams['clip_grad_norm'])
- else:
- nn.utils.clip_grad_norm_(self.gen_params_except_dp, hparams['clip_grad_norm'])
- else:
- nn.utils.clip_grad_norm_(self.disc_params, hparams["clip_grad_norm"])
-
- def on_after_optimization(self, epoch, batch_idx, optimizer, optimizer_idx):
- if self.scheduler is not None:
- self.scheduler[0].step(self.global_step // hparams['accumulate_grad_batches'])
- self.scheduler[1].step(self.global_step // hparams['accumulate_grad_batches'])
-
- ############
- # infer
- ############
- def test_start(self):
- super().test_start()
- if hparams.get('save_attn', False):
- os.makedirs(f'{self.gen_dir}/attn', exist_ok=True)
- self.model.store_inverse_all()
-
- def test_step(self, sample, batch_idx):
- assert sample['txt_tokens'].shape[0] == 1, 'only support batch_size=1 in inference'
- outputs = self.run_model(sample, infer=True)
- text = sample['text'][0]
- item_name = sample['item_name'][0]
- tokens = sample['txt_tokens'][0].cpu().numpy()
- mel_gt = sample['mels'][0].cpu().numpy()
- mel_pred = outputs['mel_out'][0].cpu().numpy()
- mel2ph = sample['mel2ph'][0].cpu().numpy()
- mel2ph_pred = None
- str_phs = self.token_encoder.decode(tokens, strip_padding=True)
- base_fn = f'[{batch_idx:06d}][{item_name.replace("%", "_")}][%s]'
- if text is not None:
- base_fn += text.replace(":", "$3A")[:80]
- base_fn = base_fn.replace(' ', '_')
- gen_dir = self.gen_dir
- wav_pred = self.vocoder.spec2wav(mel_pred)
- self.saving_result_pool.add_job(self.save_result, args=[
- wav_pred, mel_pred, base_fn % 'P', gen_dir, str_phs, mel2ph_pred])
- if hparams['save_gt']:
- wav_gt = self.vocoder.spec2wav(mel_gt)
- self.saving_result_pool.add_job(self.save_result, args=[
- wav_gt, mel_gt, base_fn % 'G', gen_dir, str_phs, mel2ph])
- if hparams.get('save_attn', False):
- attn = outputs['attn'][0].cpu().numpy()
- np.save(f'{gen_dir}/attn/{item_name}.npy', attn)
- # save f0 for pitch dtw
- f0_pred_, _ = get_pitch(wav_pred, mel_pred, hparams)
- f0_gt_, _ = get_pitch(wav_gt, mel_gt, hparams)
- np.save(f'{gen_dir}/f0/{item_name}.npy', f0_pred_)
- np.save(f'{gen_dir}/f0/{item_name}_gt.npy', f0_gt_)
-
- print(f"Pred_shape: {mel_pred.shape}, gt_shape: {mel_gt.shape}")
- return {
- 'item_name': item_name,
- 'text': text,
- 'ph_tokens': self.token_encoder.decode(tokens.tolist()),
- 'wav_fn_pred': base_fn % 'P',
- 'wav_fn_gt': base_fn % 'G',
- }
diff --git a/spaces/AIGText/GlyphControl/ldm/models/diffusion/__init__.py b/spaces/AIGText/GlyphControl/ldm/models/diffusion/__init__.py
deleted file mode 100644
index e69de29bb2d1d6434b8b29ae775ad8c2e48c5391..0000000000000000000000000000000000000000
diff --git a/spaces/ATang0729/Forecast4Muses/Model/Model6/Model6_2_ProfileRecogition/mmpretrain/work_dirs/mobilevit-small_4xb32_2000e_3c_noF/mobilevit-small_4xb32_2000e_3c_noF.py b/spaces/ATang0729/Forecast4Muses/Model/Model6/Model6_2_ProfileRecogition/mmpretrain/work_dirs/mobilevit-small_4xb32_2000e_3c_noF/mobilevit-small_4xb32_2000e_3c_noF.py
deleted file mode 100644
index 1dd70453e6fedc075f30a51e736d7c99f36c584f..0000000000000000000000000000000000000000
--- a/spaces/ATang0729/Forecast4Muses/Model/Model6/Model6_2_ProfileRecogition/mmpretrain/work_dirs/mobilevit-small_4xb32_2000e_3c_noF/mobilevit-small_4xb32_2000e_3c_noF.py
+++ /dev/null
@@ -1,137 +0,0 @@
-model = dict(
- type='ImageClassifier',
- backbone=dict(type='MobileViT', arch='small'),
- neck=dict(type='GlobalAveragePooling'),
- head=dict(
- type='LinearClsHead',
- num_classes=7,
- in_channels=640,
- loss=dict(type='CrossEntropyLoss', loss_weight=1.0),
- topk=(
- 1,
- 3,
- )))
-dataset_type = 'CustomDataset'
-data_preprocessor = dict(
- num_classes=6, mean=[
- 0,
- 0,
- 0,
- ], std=[
- 255,
- 255,
- 255,
- ], to_rgb=False)
-train_pipeline = [
- dict(type='LoadImageFromFile'),
- dict(type='RandomResizedCrop', scale=224),
- dict(type='RandomFlip', prob=0.5, direction='horizontal'),
- dict(type='PackInputs'),
-]
-test_pipeline = [
- dict(type='LoadImageFromFile'),
- dict(type='ResizeEdge', scale=288, edge='short'),
- dict(type='CenterCrop', crop_size=256),
- dict(type='PackInputs'),
-]
-train_dataloader = dict(
- pin_memory=True,
- persistent_workers=True,
- collate_fn=dict(type='default_collate'),
- batch_size=32,
- num_workers=5,
- dataset=dict(
- type='CustomDataset',
- data_root='data',
- with_label=True,
- ann_file='',
- data_prefix='train',
- pipeline=[
- dict(type='LoadImageFromFile'),
- dict(type='RandomResizedCrop', scale=224),
- dict(type='RandomFlip', prob=0.5, direction='horizontal'),
- dict(type='PackInputs'),
- ]),
- sampler=dict(type='DefaultSampler', shuffle=True))
-val_dataloader = dict(
- pin_memory=True,
- persistent_workers=True,
- collate_fn=dict(type='default_collate'),
- batch_size=32,
- num_workers=5,
- dataset=dict(
- type='CustomDataset',
- data_root='data',
- with_label=True,
- ann_file='',
- data_prefix='val',
- pipeline=[
- dict(type='LoadImageFromFile'),
- dict(type='ResizeEdge', scale=288, edge='short'),
- dict(type='CenterCrop', crop_size=256),
- dict(type='PackInputs'),
- ]),
- sampler=dict(type='DefaultSampler', shuffle=False))
-val_evaluator = dict(
- type='Accuracy', topk=(
- 1,
- 3,
- ))
-test_dataloader = dict(
- pin_memory=True,
- persistent_workers=True,
- collate_fn=dict(type='default_collate'),
- batch_size=32,
- num_workers=5,
- dataset=dict(
- type='CustomDataset',
- data_root='data',
- with_label=True,
- ann_file='',
- data_prefix='val',
- pipeline=[
- dict(type='LoadImageFromFile'),
- dict(type='ResizeEdge', scale=288, edge='short'),
- dict(type='CenterCrop', crop_size=256),
- dict(type='PackInputs'),
- ]),
- sampler=dict(type='DefaultSampler', shuffle=False))
-test_evaluator = dict(
- type='Accuracy', topk=(
- 1,
- 3,
- ))
-default_scope = 'mmpretrain'
-default_hooks = dict(
- timer=dict(type='IterTimerHook'),
- logger=dict(type='LoggerHook', interval=10),
- param_scheduler=dict(type='ParamSchedulerHook'),
- checkpoint=dict(type='CheckpointHook', save_best='auto', interval=10),
- sampler_seed=dict(type='DistSamplerSeedHook'),
- visualization=dict(type='VisualizationHook', enable=False))
-env_cfg = dict(
- cudnn_benchmark=False,
- mp_cfg=dict(mp_start_method='fork', opencv_num_threads=0),
- dist_cfg=dict(backend='nccl'))
-vis_backends = [
- dict(type='LocalVisBackend'),
-]
-visualizer = dict(
- type='UniversalVisualizer',
- vis_backends=[
- dict(type='LocalVisBackend'),
- dict(type='WandbVisBackend'),
- ])
-log_level = 'INFO'
-load_from = None
-resume = False
-randomness = dict(seed=None, deterministic=False)
-optim_wrapper = dict(
- optimizer=dict(type='SGD', lr=0.1, momentum=0.9, weight_decay=0.0001))
-param_scheduler = dict(type='StepLR', by_epoch=True, step_size=10, gamma=0.98)
-train_cfg = dict(by_epoch=True, max_epochs=2000, val_interval=10)
-val_cfg = dict()
-test_cfg = dict()
-auto_scale_lr = dict(base_batch_size=256)
-launcher = 'pytorch'
-work_dir = './work_dirs/mobilevit-small_4xb32_2000e_3c_noF'
diff --git a/spaces/AgProfile/GradioGenOpenAi/README.md b/spaces/AgProfile/GradioGenOpenAi/README.md
deleted file mode 100644
index cd850fb09b770906e7e24e8e79dc15365e1127aa..0000000000000000000000000000000000000000
--- a/spaces/AgProfile/GradioGenOpenAi/README.md
+++ /dev/null
@@ -1,12 +0,0 @@
----
-title: GradioGenOpenAi
-emoji: ⚡
-colorFrom: indigo
-colorTo: green
-sdk: gradio
-sdk_version: 3.39.0
-app_file: app.py
-pinned: false
----
-
-Check out the configuration reference at https://huggingface.co/docs/hub/spaces-config-reference
diff --git a/spaces/AgentVerse/agentVerse/ui/src/phaser3-rex-plugins/templates/ui/maker/builders/CreateButtons.js b/spaces/AgentVerse/agentVerse/ui/src/phaser3-rex-plugins/templates/ui/maker/builders/CreateButtons.js
deleted file mode 100644
index 364c822546879678f7d6cb6cd546451f1d802055..0000000000000000000000000000000000000000
--- a/spaces/AgentVerse/agentVerse/ui/src/phaser3-rex-plugins/templates/ui/maker/builders/CreateButtons.js
+++ /dev/null
@@ -1,18 +0,0 @@
-import MergeStyle from './utils/MergeStyle.js';
-import Buttons from '../../buttons/Buttons.js';
-import CreateChild from './utils/CreateChild.js';
-import CreateChildren from './utils/CreateChildren.js';
-
-var CreateButtons = function (scene, data, view, styles, customBuilders) {
- data = MergeStyle(data, styles);
-
- // Replace data by child game object
- CreateChild(scene, data, 'background', view, styles, customBuilders);
- CreateChildren(scene, data, 'buttons', view, styles, customBuilders);
-
- var gameObject = new Buttons(scene, data);
- scene.add.existing(gameObject);
- return gameObject;
-};
-
-export default CreateButtons;
\ No newline at end of file
diff --git a/spaces/Akmyradov/TurkmenTTSweSTT/uroman/bin/uroman-tsv.sh b/spaces/Akmyradov/TurkmenTTSweSTT/uroman/bin/uroman-tsv.sh
deleted file mode 100644
index adb81f4894a0539d44ad4370eda029694211e82b..0000000000000000000000000000000000000000
--- a/spaces/Akmyradov/TurkmenTTSweSTT/uroman/bin/uroman-tsv.sh
+++ /dev/null
@@ -1,28 +0,0 @@
-#!/usr/bin/env bash
-# Created by Thamme Gowda on June 17, 2019
-
-DIR=$(dirname "${BASH_SOURCE[0]}") # get the directory name
-# DIR=$(realpath "${DIR}") # resolve its full path if need be
-
-if [[ $# -lt 1 || $# -gt 2 ]]; then
- >&2 echo "ERROR: invalid args"
- >&2 echo "Usage: []"
- exit 2
-fi
-
-INP=$1
-OUT=$2
-
-CMD=$DIR/uroman.pl
-
-function romanize(){
- paste <(cut -f1 $INP) <(cut -f2 $INP | $CMD)
-}
-
-if [[ -n $OUT ]]; then
- romanize > $OUT
-else
- romanize
-fi
-
-
diff --git a/spaces/AlexZou/Deploy_Restoration/net/utils.py b/spaces/AlexZou/Deploy_Restoration/net/utils.py
deleted file mode 100644
index 857c04df854b73c541277f14970100198f9420ef..0000000000000000000000000000000000000000
--- a/spaces/AlexZou/Deploy_Restoration/net/utils.py
+++ /dev/null
@@ -1,86 +0,0 @@
-import math
-import torch
-import torch.nn as nn
-import numpy as np
-from skimage.measure.simple_metrics import compare_psnr
-from torchvision import models
-
-
-def weights_init_kaiming(m):
- classname = m.__class__.__name__
- if classname.find('Conv') != -1:
- nn.init.kaiming_normal(m.weight.data, a=0, mode='fan_in')
- elif classname.find('Linear') != -1:
- nn.init.kaiming_normal(m.weight.data, a=0, mode='fan_in')
- elif classname.find('BatchNorm') != -1:
- # nn.init.uniform(m.weight.data, 1.0, 0.02)
- m.weight.data.normal_(mean=0, std=math.sqrt(2./9./64.)).clamp_(-0.025,0.025)
- nn.init.constant(m.bias.data, 0.0)
-
-class VGG19_PercepLoss(nn.Module):
- """ Calculates perceptual loss in vgg19 space
- """
- def __init__(self, _pretrained_=True):
- super(VGG19_PercepLoss, self).__init__()
- self.vgg = models.vgg19(pretrained=_pretrained_).features
- for param in self.vgg.parameters():
- param.requires_grad_(False)
-
- def get_features(self, image, layers=None):
- if layers is None:
- layers = {'30': 'conv5_2'} # may add other layers
- features = {}
- x = image
- for name, layer in self.vgg._modules.items():
- x = layer(x)
- if name in layers:
- features[layers[name]] = x
- return features
-
- def forward(self, pred, true, layer='conv5_2'):
- true_f = self.get_features(true)
- pred_f = self.get_features(pred)
- return torch.mean((true_f[layer]-pred_f[layer])**2)
-
-
-def batch_PSNR(img, imclean, data_range):
- Img = img.data.cpu().numpy().astype(np.float32)
- Iclean = imclean.data.cpu().numpy().astype(np.float32)
- PSNR = 0
- for i in range(Img.shape[0]):
- PSNR += compare_psnr(Iclean[i,:,:,:], Img[i,:,:,:], data_range=data_range)
- return (PSNR/Img.shape[0])
-
-def data_augmentation(image, mode):
- out = np.transpose(image, (1,2,0))
- #out = image
- if mode == 0:
- # original
- out = out
- elif mode == 1:
- # flip up and down
- out = np.flipud(out)
- elif mode == 2:
- # rotate counterwise 90 degree
- out = np.rot90(out)
- elif mode == 3:
- # rotate 90 degree and flip up and down
- out = np.rot90(out)
- out = np.flipud(out)
- elif mode == 4:
- # rotate 180 degree
- out = np.rot90(out, k=2)
- elif mode == 5:
- # rotate 180 degree and flip
- out = np.rot90(out, k=2)
- out = np.flipud(out)
- elif mode == 6:
- # rotate 270 degree
- out = np.rot90(out, k=3)
- elif mode == 7:
- # rotate 270 degree and flip
- out = np.rot90(out, k=3)
- out = np.flipud(out)
- return np.transpose(out, (2,0,1))
- #return out
-
diff --git a/spaces/Amrrs/DragGan-Inversion/PTI/torch_utils/misc.py b/spaces/Amrrs/DragGan-Inversion/PTI/torch_utils/misc.py
deleted file mode 100644
index 7829f4d9f168557ce8a9a6dec289aa964234cb8c..0000000000000000000000000000000000000000
--- a/spaces/Amrrs/DragGan-Inversion/PTI/torch_utils/misc.py
+++ /dev/null
@@ -1,262 +0,0 @@
-# Copyright (c) 2021, NVIDIA CORPORATION. All rights reserved.
-#
-# NVIDIA CORPORATION and its licensors retain all intellectual property
-# and proprietary rights in and to this software, related documentation
-# and any modifications thereto. Any use, reproduction, disclosure or
-# distribution of this software and related documentation without an express
-# license agreement from NVIDIA CORPORATION is strictly prohibited.
-
-import re
-import contextlib
-import numpy as np
-import torch
-import warnings
-import dnnlib
-
-#----------------------------------------------------------------------------
-# Cached construction of constant tensors. Avoids CPU=>GPU copy when the
-# same constant is used multiple times.
-
-_constant_cache = dict()
-
-def constant(value, shape=None, dtype=None, device=None, memory_format=None):
- value = np.asarray(value)
- if shape is not None:
- shape = tuple(shape)
- if dtype is None:
- dtype = torch.get_default_dtype()
- if device is None:
- device = torch.device('cpu')
- if memory_format is None:
- memory_format = torch.contiguous_format
-
- key = (value.shape, value.dtype, value.tobytes(), shape, dtype, device, memory_format)
- tensor = _constant_cache.get(key, None)
- if tensor is None:
- tensor = torch.as_tensor(value.copy(), dtype=dtype, device=device)
- if shape is not None:
- tensor, _ = torch.broadcast_tensors(tensor, torch.empty(shape))
- tensor = tensor.contiguous(memory_format=memory_format)
- _constant_cache[key] = tensor
- return tensor
-
-#----------------------------------------------------------------------------
-# Replace NaN/Inf with specified numerical values.
-
-try:
- nan_to_num = torch.nan_to_num # 1.8.0a0
-except AttributeError:
- def nan_to_num(input, nan=0.0, posinf=None, neginf=None, *, out=None): # pylint: disable=redefined-builtin
- assert isinstance(input, torch.Tensor)
- if posinf is None:
- posinf = torch.finfo(input.dtype).max
- if neginf is None:
- neginf = torch.finfo(input.dtype).min
- assert nan == 0
- return torch.clamp(input.unsqueeze(0).nansum(0), min=neginf, max=posinf, out=out)
-
-#----------------------------------------------------------------------------
-# Symbolic assert.
-
-try:
- symbolic_assert = torch._assert # 1.8.0a0 # pylint: disable=protected-access
-except AttributeError:
- symbolic_assert = torch.Assert # 1.7.0
-
-#----------------------------------------------------------------------------
-# Context manager to suppress known warnings in torch.jit.trace().
-
-class suppress_tracer_warnings(warnings.catch_warnings):
- def __enter__(self):
- super().__enter__()
- warnings.simplefilter('ignore', category=torch.jit.TracerWarning)
- return self
-
-#----------------------------------------------------------------------------
-# Assert that the shape of a tensor matches the given list of integers.
-# None indicates that the size of a dimension is allowed to vary.
-# Performs symbolic assertion when used in torch.jit.trace().
-
-def assert_shape(tensor, ref_shape):
- if tensor.ndim != len(ref_shape):
- raise AssertionError(f'Wrong number of dimensions: got {tensor.ndim}, expected {len(ref_shape)}')
- for idx, (size, ref_size) in enumerate(zip(tensor.shape, ref_shape)):
- if ref_size is None:
- pass
- elif isinstance(ref_size, torch.Tensor):
- with suppress_tracer_warnings(): # as_tensor results are registered as constants
- symbolic_assert(torch.equal(torch.as_tensor(size), ref_size), f'Wrong size for dimension {idx}')
- elif isinstance(size, torch.Tensor):
- with suppress_tracer_warnings(): # as_tensor results are registered as constants
- symbolic_assert(torch.equal(size, torch.as_tensor(ref_size)), f'Wrong size for dimension {idx}: expected {ref_size}')
- elif size != ref_size:
- raise AssertionError(f'Wrong size for dimension {idx}: got {size}, expected {ref_size}')
-
-#----------------------------------------------------------------------------
-# Function decorator that calls torch.autograd.profiler.record_function().
-
-def profiled_function(fn):
- def decorator(*args, **kwargs):
- with torch.autograd.profiler.record_function(fn.__name__):
- return fn(*args, **kwargs)
- decorator.__name__ = fn.__name__
- return decorator
-
-#----------------------------------------------------------------------------
-# Sampler for torch.utils.data.DataLoader that loops over the dataset
-# indefinitely, shuffling items as it goes.
-
-class InfiniteSampler(torch.utils.data.Sampler):
- def __init__(self, dataset, rank=0, num_replicas=1, shuffle=True, seed=0, window_size=0.5):
- assert len(dataset) > 0
- assert num_replicas > 0
- assert 0 <= rank < num_replicas
- assert 0 <= window_size <= 1
- super().__init__(dataset)
- self.dataset = dataset
- self.rank = rank
- self.num_replicas = num_replicas
- self.shuffle = shuffle
- self.seed = seed
- self.window_size = window_size
-
- def __iter__(self):
- order = np.arange(len(self.dataset))
- rnd = None
- window = 0
- if self.shuffle:
- rnd = np.random.RandomState(self.seed)
- rnd.shuffle(order)
- window = int(np.rint(order.size * self.window_size))
-
- idx = 0
- while True:
- i = idx % order.size
- if idx % self.num_replicas == self.rank:
- yield order[i]
- if window >= 2:
- j = (i - rnd.randint(window)) % order.size
- order[i], order[j] = order[j], order[i]
- idx += 1
-
-#----------------------------------------------------------------------------
-# Utilities for operating with torch.nn.Module parameters and buffers.
-
-def params_and_buffers(module):
- assert isinstance(module, torch.nn.Module)
- return list(module.parameters()) + list(module.buffers())
-
-def named_params_and_buffers(module):
- assert isinstance(module, torch.nn.Module)
- return list(module.named_parameters()) + list(module.named_buffers())
-
-def copy_params_and_buffers(src_module, dst_module, require_all=False):
- assert isinstance(src_module, torch.nn.Module)
- assert isinstance(dst_module, torch.nn.Module)
- src_tensors = {name: tensor for name, tensor in named_params_and_buffers(src_module)}
- for name, tensor in named_params_and_buffers(dst_module):
- assert (name in src_tensors) or (not require_all)
- if name in src_tensors:
- tensor.copy_(src_tensors[name].detach()).requires_grad_(tensor.requires_grad)
-
-#----------------------------------------------------------------------------
-# Context manager for easily enabling/disabling DistributedDataParallel
-# synchronization.
-
-@contextlib.contextmanager
-def ddp_sync(module, sync):
- assert isinstance(module, torch.nn.Module)
- if sync or not isinstance(module, torch.nn.parallel.DistributedDataParallel):
- yield
- else:
- with module.no_sync():
- yield
-
-#----------------------------------------------------------------------------
-# Check DistributedDataParallel consistency across processes.
-
-def check_ddp_consistency(module, ignore_regex=None):
- assert isinstance(module, torch.nn.Module)
- for name, tensor in named_params_and_buffers(module):
- fullname = type(module).__name__ + '.' + name
- if ignore_regex is not None and re.fullmatch(ignore_regex, fullname):
- continue
- tensor = tensor.detach()
- other = tensor.clone()
- torch.distributed.broadcast(tensor=other, src=0)
- assert (nan_to_num(tensor) == nan_to_num(other)).all(), fullname
-
-#----------------------------------------------------------------------------
-# Print summary table of module hierarchy.
-
-def print_module_summary(module, inputs, max_nesting=3, skip_redundant=True):
- assert isinstance(module, torch.nn.Module)
- assert not isinstance(module, torch.jit.ScriptModule)
- assert isinstance(inputs, (tuple, list))
-
- # Register hooks.
- entries = []
- nesting = [0]
- def pre_hook(_mod, _inputs):
- nesting[0] += 1
- def post_hook(mod, _inputs, outputs):
- nesting[0] -= 1
- if nesting[0] <= max_nesting:
- outputs = list(outputs) if isinstance(outputs, (tuple, list)) else [outputs]
- outputs = [t for t in outputs if isinstance(t, torch.Tensor)]
- entries.append(dnnlib.EasyDict(mod=mod, outputs=outputs))
- hooks = [mod.register_forward_pre_hook(pre_hook) for mod in module.modules()]
- hooks += [mod.register_forward_hook(post_hook) for mod in module.modules()]
-
- # Run module.
- outputs = module(*inputs)
- for hook in hooks:
- hook.remove()
-
- # Identify unique outputs, parameters, and buffers.
- tensors_seen = set()
- for e in entries:
- e.unique_params = [t for t in e.mod.parameters() if id(t) not in tensors_seen]
- e.unique_buffers = [t for t in e.mod.buffers() if id(t) not in tensors_seen]
- e.unique_outputs = [t for t in e.outputs if id(t) not in tensors_seen]
- tensors_seen |= {id(t) for t in e.unique_params + e.unique_buffers + e.unique_outputs}
-
- # Filter out redundant entries.
- if skip_redundant:
- entries = [e for e in entries if len(e.unique_params) or len(e.unique_buffers) or len(e.unique_outputs)]
-
- # Construct table.
- rows = [[type(module).__name__, 'Parameters', 'Buffers', 'Output shape', 'Datatype']]
- rows += [['---'] * len(rows[0])]
- param_total = 0
- buffer_total = 0
- submodule_names = {mod: name for name, mod in module.named_modules()}
- for e in entries:
- name = '' if e.mod is module else submodule_names[e.mod]
- param_size = sum(t.numel() for t in e.unique_params)
- buffer_size = sum(t.numel() for t in e.unique_buffers)
- output_shapes = [str(list(e.outputs[0].shape)) for t in e.outputs]
- output_dtypes = [str(t.dtype).split('.')[-1] for t in e.outputs]
- rows += [[
- name + (':0' if len(e.outputs) >= 2 else ''),
- str(param_size) if param_size else '-',
- str(buffer_size) if buffer_size else '-',
- (output_shapes + ['-'])[0],
- (output_dtypes + ['-'])[0],
- ]]
- for idx in range(1, len(e.outputs)):
- rows += [[name + f':{idx}', '-', '-', output_shapes[idx], output_dtypes[idx]]]
- param_total += param_size
- buffer_total += buffer_size
- rows += [['---'] * len(rows[0])]
- rows += [['Total', str(param_total), str(buffer_total), '-', '-']]
-
- # Print table.
- widths = [max(len(cell) for cell in column) for column in zip(*rows)]
- print()
- for row in rows:
- print(' '.join(cell + ' ' * (width - len(cell)) for cell, width in zip(row, widths)))
- print()
- return outputs
-
-#----------------------------------------------------------------------------
diff --git a/spaces/Androidonnxfork/CivitAi-to-Diffusers/diffusers/docs/source/ko/using-diffusers/img2img.md b/spaces/Androidonnxfork/CivitAi-to-Diffusers/diffusers/docs/source/ko/using-diffusers/img2img.md
deleted file mode 100644
index 32435603c91082a02b6c3acfac1a355bde8a0ca5..0000000000000000000000000000000000000000
--- a/spaces/Androidonnxfork/CivitAi-to-Diffusers/diffusers/docs/source/ko/using-diffusers/img2img.md
+++ /dev/null
@@ -1,100 +0,0 @@
-
-
-# 텍스트 기반 image-to-image 생성
-
-[[Colab에서 열기]]
-
-[`StableDiffusionImg2ImgPipeline`]을 사용하면 텍스트 프롬프트와 시작 이미지를 전달하여 새 이미지 생성의 조건을 지정할 수 있습니다.
-
-시작하기 전에 필요한 라이브러리가 모두 설치되어 있는지 확인하세요:
-
-```bash
-!pip install diffusers transformers ftfy accelerate
-```
-
-[`nitrosocke/Ghibli-Diffusion`](https://huggingface.co/nitrosocke/Ghibli-Diffusion)과 같은 사전학습된 stable diffusion 모델로 [`StableDiffusionImg2ImgPipeline`]을 생성하여 시작하세요.
-
-
-```python
-import torch
-import requests
-from PIL import Image
-from io import BytesIO
-from diffusers import StableDiffusionImg2ImgPipeline
-
-device = "cuda"
-pipe = StableDiffusionImg2ImgPipeline.from_pretrained("nitrosocke/Ghibli-Diffusion", torch_dtype=torch.float16).to(
- device
-)
-```
-
-초기 이미지를 다운로드하고 사전 처리하여 파이프라인에 전달할 수 있습니다:
-
-```python
-url = "https://raw.githubusercontent.com/CompVis/stable-diffusion/main/assets/stable-samples/img2img/sketch-mountains-input.jpg"
-
-response = requests.get(url)
-init_image = Image.open(BytesIO(response.content)).convert("RGB")
-init_image.thumbnail((768, 768))
-init_image
-```
-
-
-
-
-
-
-
-💡 `strength`는 입력 이미지에 추가되는 노이즈의 양을 제어하는 0.0에서 1.0 사이의 값입니다. 1.0에 가까운 값은 다양한 변형을 허용하지만 입력 이미지와 의미적으로 일치하지 않는 이미지를 생성합니다.
-
-
-
-프롬프트를 정의하고(지브리 스타일(Ghibli-style)에 맞게 조정된 이 체크포인트의 경우 프롬프트 앞에 `ghibli style` 토큰을 붙여야 합니다) 파이프라인을 실행합니다:
-
-```python
-prompt = "ghibli style, a fantasy landscape with castles"
-generator = torch.Generator(device=device).manual_seed(1024)
-image = pipe(prompt=prompt, image=init_image, strength=0.75, guidance_scale=7.5, generator=generator).images[0]
-image
-```
-
-
-
-
-
-다른 스케줄러로 실험하여 출력에 어떤 영향을 미치는지 확인할 수도 있습니다:
-
-```python
-from diffusers import LMSDiscreteScheduler
-
-lms = LMSDiscreteScheduler.from_config(pipe.scheduler.config)
-pipe.scheduler = lms
-generator = torch.Generator(device=device).manual_seed(1024)
-image = pipe(prompt=prompt, image=init_image, strength=0.75, guidance_scale=7.5, generator=generator).images[0]
-image
-```
-
-
-
-
-
-아래 공백을 확인하고 `strength` 값을 다르게 설정하여 이미지를 생성해 보세요. `strength`를 낮게 설정하면 원본 이미지와 더 유사한 이미지가 생성되는 것을 확인할 수 있습니다.
-
-자유롭게 스케줄러를 [`LMSDiscreteScheduler`]로 전환하여 출력에 어떤 영향을 미치는지 확인해 보세요.
-
-
\ No newline at end of file
diff --git a/spaces/Androidonnxfork/CivitAi-to-Diffusers/diffusers/examples/textual_inversion/textual_inversion.py b/spaces/Androidonnxfork/CivitAi-to-Diffusers/diffusers/examples/textual_inversion/textual_inversion.py
deleted file mode 100644
index 515f3964088912e551d895abfcb1081ebc0f9b4b..0000000000000000000000000000000000000000
--- a/spaces/Androidonnxfork/CivitAi-to-Diffusers/diffusers/examples/textual_inversion/textual_inversion.py
+++ /dev/null
@@ -1,959 +0,0 @@
-#!/usr/bin/env python
-# coding=utf-8
-# Copyright 2023 The HuggingFace Inc. team. All rights reserved.
-#
-# Licensed under the Apache License, Version 2.0 (the "License");
-# you may not use this file except in compliance with the License.
-# You may obtain a copy of the License at
-#
-# http://www.apache.org/licenses/LICENSE-2.0
-#
-# Unless required by applicable law or agreed to in writing, software
-# distributed under the License is distributed on an "AS IS" BASIS,
-# WITHOUT WARRANTIES OR CONDITIONS OF ANY KIND, either express or implied.
-# See the License for the specific language governing permissions and
-
-import argparse
-import logging
-import math
-import os
-import random
-import shutil
-import warnings
-from pathlib import Path
-
-import numpy as np
-import PIL
-import torch
-import torch.nn.functional as F
-import torch.utils.checkpoint
-import transformers
-from accelerate import Accelerator
-from accelerate.logging import get_logger
-from accelerate.utils import ProjectConfiguration, set_seed
-from huggingface_hub import create_repo, upload_folder
-
-# TODO: remove and import from diffusers.utils when the new version of diffusers is released
-from packaging import version
-from PIL import Image
-from torch.utils.data import Dataset
-from torchvision import transforms
-from tqdm.auto import tqdm
-from transformers import CLIPTextModel, CLIPTokenizer
-
-import diffusers
-from diffusers import (
- AutoencoderKL,
- DDPMScheduler,
- DiffusionPipeline,
- DPMSolverMultistepScheduler,
- StableDiffusionPipeline,
- UNet2DConditionModel,
-)
-from diffusers.optimization import get_scheduler
-from diffusers.utils import check_min_version, is_wandb_available
-from diffusers.utils.import_utils import is_xformers_available
-
-
-if is_wandb_available():
- import wandb
-
-if version.parse(version.parse(PIL.__version__).base_version) >= version.parse("9.1.0"):
- PIL_INTERPOLATION = {
- "linear": PIL.Image.Resampling.BILINEAR,
- "bilinear": PIL.Image.Resampling.BILINEAR,
- "bicubic": PIL.Image.Resampling.BICUBIC,
- "lanczos": PIL.Image.Resampling.LANCZOS,
- "nearest": PIL.Image.Resampling.NEAREST,
- }
-else:
- PIL_INTERPOLATION = {
- "linear": PIL.Image.LINEAR,
- "bilinear": PIL.Image.BILINEAR,
- "bicubic": PIL.Image.BICUBIC,
- "lanczos": PIL.Image.LANCZOS,
- "nearest": PIL.Image.NEAREST,
- }
-# ------------------------------------------------------------------------------
-
-
-# Will error if the minimal version of diffusers is not installed. Remove at your own risks.
-check_min_version("0.19.0")
-
-logger = get_logger(__name__)
-
-
-def save_model_card(repo_id: str, images=None, base_model=str, repo_folder=None):
- img_str = ""
- for i, image in enumerate(images):
- image.save(os.path.join(repo_folder, f"image_{i}.png"))
- img_str += f"\n"
-
- yaml = f"""
----
-license: creativeml-openrail-m
-base_model: {base_model}
-tags:
-- stable-diffusion
-- stable-diffusion-diffusers
-- text-to-image
-- diffusers
-- textual_inversion
-inference: true
----
- """
- model_card = f"""
-# Textual inversion text2image fine-tuning - {repo_id}
-These are textual inversion adaption weights for {base_model}. You can find some example images in the following. \n
-{img_str}
-"""
- with open(os.path.join(repo_folder, "README.md"), "w") as f:
- f.write(yaml + model_card)
-
-
-def log_validation(text_encoder, tokenizer, unet, vae, args, accelerator, weight_dtype, epoch):
- logger.info(
- f"Running validation... \n Generating {args.num_validation_images} images with prompt:"
- f" {args.validation_prompt}."
- )
- # create pipeline (note: unet and vae are loaded again in float32)
- pipeline = DiffusionPipeline.from_pretrained(
- args.pretrained_model_name_or_path,
- text_encoder=accelerator.unwrap_model(text_encoder),
- tokenizer=tokenizer,
- unet=unet,
- vae=vae,
- safety_checker=None,
- revision=args.revision,
- torch_dtype=weight_dtype,
- )
- pipeline.scheduler = DPMSolverMultistepScheduler.from_config(pipeline.scheduler.config)
- pipeline = pipeline.to(accelerator.device)
- pipeline.set_progress_bar_config(disable=True)
-
- # run inference
- generator = None if args.seed is None else torch.Generator(device=accelerator.device).manual_seed(args.seed)
- images = []
- for _ in range(args.num_validation_images):
- with torch.autocast("cuda"):
- image = pipeline(args.validation_prompt, num_inference_steps=25, generator=generator).images[0]
- images.append(image)
-
- for tracker in accelerator.trackers:
- if tracker.name == "tensorboard":
- np_images = np.stack([np.asarray(img) for img in images])
- tracker.writer.add_images("validation", np_images, epoch, dataformats="NHWC")
- if tracker.name == "wandb":
- tracker.log(
- {
- "validation": [
- wandb.Image(image, caption=f"{i}: {args.validation_prompt}") for i, image in enumerate(images)
- ]
- }
- )
-
- del pipeline
- torch.cuda.empty_cache()
- return images
-
-
-def save_progress(text_encoder, placeholder_token_ids, accelerator, args, save_path):
- logger.info("Saving embeddings")
- learned_embeds = (
- accelerator.unwrap_model(text_encoder)
- .get_input_embeddings()
- .weight[min(placeholder_token_ids) : max(placeholder_token_ids) + 1]
- )
- learned_embeds_dict = {args.placeholder_token: learned_embeds.detach().cpu()}
- torch.save(learned_embeds_dict, save_path)
-
-
-def parse_args():
- parser = argparse.ArgumentParser(description="Simple example of a training script.")
- parser.add_argument(
- "--save_steps",
- type=int,
- default=500,
- help="Save learned_embeds.bin every X updates steps.",
- )
- parser.add_argument(
- "--save_as_full_pipeline",
- action="store_true",
- help="Save the complete stable diffusion pipeline.",
- )
- parser.add_argument(
- "--num_vectors",
- type=int,
- default=1,
- help="How many textual inversion vectors shall be used to learn the concept.",
- )
- parser.add_argument(
- "--pretrained_model_name_or_path",
- type=str,
- default=None,
- required=True,
- help="Path to pretrained model or model identifier from huggingface.co/models.",
- )
- parser.add_argument(
- "--revision",
- type=str,
- default=None,
- required=False,
- help="Revision of pretrained model identifier from huggingface.co/models.",
- )
- parser.add_argument(
- "--tokenizer_name",
- type=str,
- default=None,
- help="Pretrained tokenizer name or path if not the same as model_name",
- )
- parser.add_argument(
- "--train_data_dir", type=str, default=None, required=True, help="A folder containing the training data."
- )
- parser.add_argument(
- "--placeholder_token",
- type=str,
- default=None,
- required=True,
- help="A token to use as a placeholder for the concept.",
- )
- parser.add_argument(
- "--initializer_token", type=str, default=None, required=True, help="A token to use as initializer word."
- )
- parser.add_argument("--learnable_property", type=str, default="object", help="Choose between 'object' and 'style'")
- parser.add_argument("--repeats", type=int, default=100, help="How many times to repeat the training data.")
- parser.add_argument(
- "--output_dir",
- type=str,
- default="text-inversion-model",
- help="The output directory where the model predictions and checkpoints will be written.",
- )
- parser.add_argument("--seed", type=int, default=None, help="A seed for reproducible training.")
- parser.add_argument(
- "--resolution",
- type=int,
- default=512,
- help=(
- "The resolution for input images, all the images in the train/validation dataset will be resized to this"
- " resolution"
- ),
- )
- parser.add_argument(
- "--center_crop", action="store_true", help="Whether to center crop images before resizing to resolution."
- )
- parser.add_argument(
- "--train_batch_size", type=int, default=16, help="Batch size (per device) for the training dataloader."
- )
- parser.add_argument("--num_train_epochs", type=int, default=100)
- parser.add_argument(
- "--max_train_steps",
- type=int,
- default=5000,
- help="Total number of training steps to perform. If provided, overrides num_train_epochs.",
- )
- parser.add_argument(
- "--gradient_accumulation_steps",
- type=int,
- default=1,
- help="Number of updates steps to accumulate before performing a backward/update pass.",
- )
- parser.add_argument(
- "--gradient_checkpointing",
- action="store_true",
- help="Whether or not to use gradient checkpointing to save memory at the expense of slower backward pass.",
- )
- parser.add_argument(
- "--learning_rate",
- type=float,
- default=1e-4,
- help="Initial learning rate (after the potential warmup period) to use.",
- )
- parser.add_argument(
- "--scale_lr",
- action="store_true",
- default=False,
- help="Scale the learning rate by the number of GPUs, gradient accumulation steps, and batch size.",
- )
- parser.add_argument(
- "--lr_scheduler",
- type=str,
- default="constant",
- help=(
- 'The scheduler type to use. Choose between ["linear", "cosine", "cosine_with_restarts", "polynomial",'
- ' "constant", "constant_with_warmup"]'
- ),
- )
- parser.add_argument(
- "--lr_warmup_steps", type=int, default=500, help="Number of steps for the warmup in the lr scheduler."
- )
- parser.add_argument(
- "--lr_num_cycles",
- type=int,
- default=1,
- help="Number of hard resets of the lr in cosine_with_restarts scheduler.",
- )
- parser.add_argument(
- "--dataloader_num_workers",
- type=int,
- default=0,
- help=(
- "Number of subprocesses to use for data loading. 0 means that the data will be loaded in the main process."
- ),
- )
- parser.add_argument("--adam_beta1", type=float, default=0.9, help="The beta1 parameter for the Adam optimizer.")
- parser.add_argument("--adam_beta2", type=float, default=0.999, help="The beta2 parameter for the Adam optimizer.")
- parser.add_argument("--adam_weight_decay", type=float, default=1e-2, help="Weight decay to use.")
- parser.add_argument("--adam_epsilon", type=float, default=1e-08, help="Epsilon value for the Adam optimizer")
- parser.add_argument("--push_to_hub", action="store_true", help="Whether or not to push the model to the Hub.")
- parser.add_argument("--hub_token", type=str, default=None, help="The token to use to push to the Model Hub.")
- parser.add_argument(
- "--hub_model_id",
- type=str,
- default=None,
- help="The name of the repository to keep in sync with the local `output_dir`.",
- )
- parser.add_argument(
- "--logging_dir",
- type=str,
- default="logs",
- help=(
- "[TensorBoard](https://www.tensorflow.org/tensorboard) log directory. Will default to"
- " *output_dir/runs/**CURRENT_DATETIME_HOSTNAME***."
- ),
- )
- parser.add_argument(
- "--mixed_precision",
- type=str,
- default="no",
- choices=["no", "fp16", "bf16"],
- help=(
- "Whether to use mixed precision. Choose"
- "between fp16 and bf16 (bfloat16). Bf16 requires PyTorch >= 1.10."
- "and an Nvidia Ampere GPU."
- ),
- )
- parser.add_argument(
- "--allow_tf32",
- action="store_true",
- help=(
- "Whether or not to allow TF32 on Ampere GPUs. Can be used to speed up training. For more information, see"
- " https://pytorch.org/docs/stable/notes/cuda.html#tensorfloat-32-tf32-on-ampere-devices"
- ),
- )
- parser.add_argument(
- "--report_to",
- type=str,
- default="tensorboard",
- help=(
- 'The integration to report the results and logs to. Supported platforms are `"tensorboard"`'
- ' (default), `"wandb"` and `"comet_ml"`. Use `"all"` to report to all integrations.'
- ),
- )
- parser.add_argument(
- "--validation_prompt",
- type=str,
- default=None,
- help="A prompt that is used during validation to verify that the model is learning.",
- )
- parser.add_argument(
- "--num_validation_images",
- type=int,
- default=4,
- help="Number of images that should be generated during validation with `validation_prompt`.",
- )
- parser.add_argument(
- "--validation_steps",
- type=int,
- default=100,
- help=(
- "Run validation every X steps. Validation consists of running the prompt"
- " `args.validation_prompt` multiple times: `args.num_validation_images`"
- " and logging the images."
- ),
- )
- parser.add_argument(
- "--validation_epochs",
- type=int,
- default=None,
- help=(
- "Deprecated in favor of validation_steps. Run validation every X epochs. Validation consists of running the prompt"
- " `args.validation_prompt` multiple times: `args.num_validation_images`"
- " and logging the images."
- ),
- )
- parser.add_argument("--local_rank", type=int, default=-1, help="For distributed training: local_rank")
- parser.add_argument(
- "--checkpointing_steps",
- type=int,
- default=500,
- help=(
- "Save a checkpoint of the training state every X updates. These checkpoints are only suitable for resuming"
- " training using `--resume_from_checkpoint`."
- ),
- )
- parser.add_argument(
- "--checkpoints_total_limit",
- type=int,
- default=None,
- help=("Max number of checkpoints to store."),
- )
- parser.add_argument(
- "--resume_from_checkpoint",
- type=str,
- default=None,
- help=(
- "Whether training should be resumed from a previous checkpoint. Use a path saved by"
- ' `--checkpointing_steps`, or `"latest"` to automatically select the last available checkpoint.'
- ),
- )
- parser.add_argument(
- "--enable_xformers_memory_efficient_attention", action="store_true", help="Whether or not to use xformers."
- )
-
- args = parser.parse_args()
- env_local_rank = int(os.environ.get("LOCAL_RANK", -1))
- if env_local_rank != -1 and env_local_rank != args.local_rank:
- args.local_rank = env_local_rank
-
- if args.train_data_dir is None:
- raise ValueError("You must specify a train data directory.")
-
- return args
-
-
-imagenet_templates_small = [
- "a photo of a {}",
- "a rendering of a {}",
- "a cropped photo of the {}",
- "the photo of a {}",
- "a photo of a clean {}",
- "a photo of a dirty {}",
- "a dark photo of the {}",
- "a photo of my {}",
- "a photo of the cool {}",
- "a close-up photo of a {}",
- "a bright photo of the {}",
- "a cropped photo of a {}",
- "a photo of the {}",
- "a good photo of the {}",
- "a photo of one {}",
- "a close-up photo of the {}",
- "a rendition of the {}",
- "a photo of the clean {}",
- "a rendition of a {}",
- "a photo of a nice {}",
- "a good photo of a {}",
- "a photo of the nice {}",
- "a photo of the small {}",
- "a photo of the weird {}",
- "a photo of the large {}",
- "a photo of a cool {}",
- "a photo of a small {}",
-]
-
-imagenet_style_templates_small = [
- "a painting in the style of {}",
- "a rendering in the style of {}",
- "a cropped painting in the style of {}",
- "the painting in the style of {}",
- "a clean painting in the style of {}",
- "a dirty painting in the style of {}",
- "a dark painting in the style of {}",
- "a picture in the style of {}",
- "a cool painting in the style of {}",
- "a close-up painting in the style of {}",
- "a bright painting in the style of {}",
- "a cropped painting in the style of {}",
- "a good painting in the style of {}",
- "a close-up painting in the style of {}",
- "a rendition in the style of {}",
- "a nice painting in the style of {}",
- "a small painting in the style of {}",
- "a weird painting in the style of {}",
- "a large painting in the style of {}",
-]
-
-
-class TextualInversionDataset(Dataset):
- def __init__(
- self,
- data_root,
- tokenizer,
- learnable_property="object", # [object, style]
- size=512,
- repeats=100,
- interpolation="bicubic",
- flip_p=0.5,
- set="train",
- placeholder_token="*",
- center_crop=False,
- ):
- self.data_root = data_root
- self.tokenizer = tokenizer
- self.learnable_property = learnable_property
- self.size = size
- self.placeholder_token = placeholder_token
- self.center_crop = center_crop
- self.flip_p = flip_p
-
- self.image_paths = [os.path.join(self.data_root, file_path) for file_path in os.listdir(self.data_root)]
-
- self.num_images = len(self.image_paths)
- self._length = self.num_images
-
- if set == "train":
- self._length = self.num_images * repeats
-
- self.interpolation = {
- "linear": PIL_INTERPOLATION["linear"],
- "bilinear": PIL_INTERPOLATION["bilinear"],
- "bicubic": PIL_INTERPOLATION["bicubic"],
- "lanczos": PIL_INTERPOLATION["lanczos"],
- }[interpolation]
-
- self.templates = imagenet_style_templates_small if learnable_property == "style" else imagenet_templates_small
- self.flip_transform = transforms.RandomHorizontalFlip(p=self.flip_p)
-
- def __len__(self):
- return self._length
-
- def __getitem__(self, i):
- example = {}
- image = Image.open(self.image_paths[i % self.num_images])
-
- if not image.mode == "RGB":
- image = image.convert("RGB")
-
- placeholder_string = self.placeholder_token
- text = random.choice(self.templates).format(placeholder_string)
-
- example["input_ids"] = self.tokenizer(
- text,
- padding="max_length",
- truncation=True,
- max_length=self.tokenizer.model_max_length,
- return_tensors="pt",
- ).input_ids[0]
-
- # default to score-sde preprocessing
- img = np.array(image).astype(np.uint8)
-
- if self.center_crop:
- crop = min(img.shape[0], img.shape[1])
- (
- h,
- w,
- ) = (
- img.shape[0],
- img.shape[1],
- )
- img = img[(h - crop) // 2 : (h + crop) // 2, (w - crop) // 2 : (w + crop) // 2]
-
- image = Image.fromarray(img)
- image = image.resize((self.size, self.size), resample=self.interpolation)
-
- image = self.flip_transform(image)
- image = np.array(image).astype(np.uint8)
- image = (image / 127.5 - 1.0).astype(np.float32)
-
- example["pixel_values"] = torch.from_numpy(image).permute(2, 0, 1)
- return example
-
-
-def main():
- args = parse_args()
- logging_dir = os.path.join(args.output_dir, args.logging_dir)
- accelerator_project_config = ProjectConfiguration(project_dir=args.output_dir, logging_dir=logging_dir)
- accelerator = Accelerator(
- gradient_accumulation_steps=args.gradient_accumulation_steps,
- mixed_precision=args.mixed_precision,
- log_with=args.report_to,
- project_config=accelerator_project_config,
- )
-
- if args.report_to == "wandb":
- if not is_wandb_available():
- raise ImportError("Make sure to install wandb if you want to use it for logging during training.")
-
- # Make one log on every process with the configuration for debugging.
- logging.basicConfig(
- format="%(asctime)s - %(levelname)s - %(name)s - %(message)s",
- datefmt="%m/%d/%Y %H:%M:%S",
- level=logging.INFO,
- )
- logger.info(accelerator.state, main_process_only=False)
- if accelerator.is_local_main_process:
- transformers.utils.logging.set_verbosity_warning()
- diffusers.utils.logging.set_verbosity_info()
- else:
- transformers.utils.logging.set_verbosity_error()
- diffusers.utils.logging.set_verbosity_error()
-
- # If passed along, set the training seed now.
- if args.seed is not None:
- set_seed(args.seed)
-
- # Handle the repository creation
- if accelerator.is_main_process:
- if args.output_dir is not None:
- os.makedirs(args.output_dir, exist_ok=True)
-
- if args.push_to_hub:
- repo_id = create_repo(
- repo_id=args.hub_model_id or Path(args.output_dir).name, exist_ok=True, token=args.hub_token
- ).repo_id
-
- # Load tokenizer
- if args.tokenizer_name:
- tokenizer = CLIPTokenizer.from_pretrained(args.tokenizer_name)
- elif args.pretrained_model_name_or_path:
- tokenizer = CLIPTokenizer.from_pretrained(args.pretrained_model_name_or_path, subfolder="tokenizer")
-
- # Load scheduler and models
- noise_scheduler = DDPMScheduler.from_pretrained(args.pretrained_model_name_or_path, subfolder="scheduler")
- text_encoder = CLIPTextModel.from_pretrained(
- args.pretrained_model_name_or_path, subfolder="text_encoder", revision=args.revision
- )
- vae = AutoencoderKL.from_pretrained(args.pretrained_model_name_or_path, subfolder="vae", revision=args.revision)
- unet = UNet2DConditionModel.from_pretrained(
- args.pretrained_model_name_or_path, subfolder="unet", revision=args.revision
- )
-
- # Add the placeholder token in tokenizer
- placeholder_tokens = [args.placeholder_token]
-
- if args.num_vectors < 1:
- raise ValueError(f"--num_vectors has to be larger or equal to 1, but is {args.num_vectors}")
-
- # add dummy tokens for multi-vector
- additional_tokens = []
- for i in range(1, args.num_vectors):
- additional_tokens.append(f"{args.placeholder_token}_{i}")
- placeholder_tokens += additional_tokens
-
- num_added_tokens = tokenizer.add_tokens(placeholder_tokens)
- if num_added_tokens != args.num_vectors:
- raise ValueError(
- f"The tokenizer already contains the token {args.placeholder_token}. Please pass a different"
- " `placeholder_token` that is not already in the tokenizer."
- )
-
- # Convert the initializer_token, placeholder_token to ids
- token_ids = tokenizer.encode(args.initializer_token, add_special_tokens=False)
- # Check if initializer_token is a single token or a sequence of tokens
- if len(token_ids) > 1:
- raise ValueError("The initializer token must be a single token.")
-
- initializer_token_id = token_ids[0]
- placeholder_token_ids = tokenizer.convert_tokens_to_ids(placeholder_tokens)
-
- # Resize the token embeddings as we are adding new special tokens to the tokenizer
- text_encoder.resize_token_embeddings(len(tokenizer))
-
- # Initialise the newly added placeholder token with the embeddings of the initializer token
- token_embeds = text_encoder.get_input_embeddings().weight.data
- with torch.no_grad():
- for token_id in placeholder_token_ids:
- token_embeds[token_id] = token_embeds[initializer_token_id].clone()
-
- # Freeze vae and unet
- vae.requires_grad_(False)
- unet.requires_grad_(False)
- # Freeze all parameters except for the token embeddings in text encoder
- text_encoder.text_model.encoder.requires_grad_(False)
- text_encoder.text_model.final_layer_norm.requires_grad_(False)
- text_encoder.text_model.embeddings.position_embedding.requires_grad_(False)
-
- if args.gradient_checkpointing:
- # Keep unet in train mode if we are using gradient checkpointing to save memory.
- # The dropout cannot be != 0 so it doesn't matter if we are in eval or train mode.
- unet.train()
- text_encoder.gradient_checkpointing_enable()
- unet.enable_gradient_checkpointing()
-
- if args.enable_xformers_memory_efficient_attention:
- if is_xformers_available():
- import xformers
-
- xformers_version = version.parse(xformers.__version__)
- if xformers_version == version.parse("0.0.16"):
- logger.warn(
- "xFormers 0.0.16 cannot be used for training in some GPUs. If you observe problems during training, please update xFormers to at least 0.0.17. See https://huggingface.co/docs/diffusers/main/en/optimization/xformers for more details."
- )
- unet.enable_xformers_memory_efficient_attention()
- else:
- raise ValueError("xformers is not available. Make sure it is installed correctly")
-
- # Enable TF32 for faster training on Ampere GPUs,
- # cf https://pytorch.org/docs/stable/notes/cuda.html#tensorfloat-32-tf32-on-ampere-devices
- if args.allow_tf32:
- torch.backends.cuda.matmul.allow_tf32 = True
-
- if args.scale_lr:
- args.learning_rate = (
- args.learning_rate * args.gradient_accumulation_steps * args.train_batch_size * accelerator.num_processes
- )
-
- # Initialize the optimizer
- optimizer = torch.optim.AdamW(
- text_encoder.get_input_embeddings().parameters(), # only optimize the embeddings
- lr=args.learning_rate,
- betas=(args.adam_beta1, args.adam_beta2),
- weight_decay=args.adam_weight_decay,
- eps=args.adam_epsilon,
- )
-
- # Dataset and DataLoaders creation:
- train_dataset = TextualInversionDataset(
- data_root=args.train_data_dir,
- tokenizer=tokenizer,
- size=args.resolution,
- placeholder_token=args.placeholder_token,
- repeats=args.repeats,
- learnable_property=args.learnable_property,
- center_crop=args.center_crop,
- set="train",
- )
- train_dataloader = torch.utils.data.DataLoader(
- train_dataset, batch_size=args.train_batch_size, shuffle=True, num_workers=args.dataloader_num_workers
- )
- if args.validation_epochs is not None:
- warnings.warn(
- f"FutureWarning: You are doing logging with validation_epochs={args.validation_epochs}."
- " Deprecated validation_epochs in favor of `validation_steps`"
- f"Setting `args.validation_steps` to {args.validation_epochs * len(train_dataset)}",
- FutureWarning,
- stacklevel=2,
- )
- args.validation_steps = args.validation_epochs * len(train_dataset)
-
- # Scheduler and math around the number of training steps.
- overrode_max_train_steps = False
- num_update_steps_per_epoch = math.ceil(len(train_dataloader) / args.gradient_accumulation_steps)
- if args.max_train_steps is None:
- args.max_train_steps = args.num_train_epochs * num_update_steps_per_epoch
- overrode_max_train_steps = True
-
- lr_scheduler = get_scheduler(
- args.lr_scheduler,
- optimizer=optimizer,
- num_warmup_steps=args.lr_warmup_steps * accelerator.num_processes,
- num_training_steps=args.max_train_steps * accelerator.num_processes,
- num_cycles=args.lr_num_cycles,
- )
-
- # Prepare everything with our `accelerator`.
- text_encoder, optimizer, train_dataloader, lr_scheduler = accelerator.prepare(
- text_encoder, optimizer, train_dataloader, lr_scheduler
- )
-
- # For mixed precision training we cast all non-trainable weigths (vae, non-lora text_encoder and non-lora unet) to half-precision
- # as these weights are only used for inference, keeping weights in full precision is not required.
- weight_dtype = torch.float32
- if accelerator.mixed_precision == "fp16":
- weight_dtype = torch.float16
- elif accelerator.mixed_precision == "bf16":
- weight_dtype = torch.bfloat16
-
- # Move vae and unet to device and cast to weight_dtype
- unet.to(accelerator.device, dtype=weight_dtype)
- vae.to(accelerator.device, dtype=weight_dtype)
-
- # We need to recalculate our total training steps as the size of the training dataloader may have changed.
- num_update_steps_per_epoch = math.ceil(len(train_dataloader) / args.gradient_accumulation_steps)
- if overrode_max_train_steps:
- args.max_train_steps = args.num_train_epochs * num_update_steps_per_epoch
- # Afterwards we recalculate our number of training epochs
- args.num_train_epochs = math.ceil(args.max_train_steps / num_update_steps_per_epoch)
-
- # We need to initialize the trackers we use, and also store our configuration.
- # The trackers initializes automatically on the main process.
- if accelerator.is_main_process:
- accelerator.init_trackers("textual_inversion", config=vars(args))
-
- # Train!
- total_batch_size = args.train_batch_size * accelerator.num_processes * args.gradient_accumulation_steps
-
- logger.info("***** Running training *****")
- logger.info(f" Num examples = {len(train_dataset)}")
- logger.info(f" Num Epochs = {args.num_train_epochs}")
- logger.info(f" Instantaneous batch size per device = {args.train_batch_size}")
- logger.info(f" Total train batch size (w. parallel, distributed & accumulation) = {total_batch_size}")
- logger.info(f" Gradient Accumulation steps = {args.gradient_accumulation_steps}")
- logger.info(f" Total optimization steps = {args.max_train_steps}")
- global_step = 0
- first_epoch = 0
- # Potentially load in the weights and states from a previous save
- if args.resume_from_checkpoint:
- if args.resume_from_checkpoint != "latest":
- path = os.path.basename(args.resume_from_checkpoint)
- else:
- # Get the most recent checkpoint
- dirs = os.listdir(args.output_dir)
- dirs = [d for d in dirs if d.startswith("checkpoint")]
- dirs = sorted(dirs, key=lambda x: int(x.split("-")[1]))
- path = dirs[-1] if len(dirs) > 0 else None
-
- if path is None:
- accelerator.print(
- f"Checkpoint '{args.resume_from_checkpoint}' does not exist. Starting a new training run."
- )
- args.resume_from_checkpoint = None
- else:
- accelerator.print(f"Resuming from checkpoint {path}")
- accelerator.load_state(os.path.join(args.output_dir, path))
- global_step = int(path.split("-")[1])
-
- resume_global_step = global_step * args.gradient_accumulation_steps
- first_epoch = global_step // num_update_steps_per_epoch
- resume_step = resume_global_step % (num_update_steps_per_epoch * args.gradient_accumulation_steps)
-
- # Only show the progress bar once on each machine.
- progress_bar = tqdm(range(global_step, args.max_train_steps), disable=not accelerator.is_local_main_process)
- progress_bar.set_description("Steps")
-
- # keep original embeddings as reference
- orig_embeds_params = accelerator.unwrap_model(text_encoder).get_input_embeddings().weight.data.clone()
-
- for epoch in range(first_epoch, args.num_train_epochs):
- text_encoder.train()
- for step, batch in enumerate(train_dataloader):
- # Skip steps until we reach the resumed step
- if args.resume_from_checkpoint and epoch == first_epoch and step < resume_step:
- if step % args.gradient_accumulation_steps == 0:
- progress_bar.update(1)
- continue
-
- with accelerator.accumulate(text_encoder):
- # Convert images to latent space
- latents = vae.encode(batch["pixel_values"].to(dtype=weight_dtype)).latent_dist.sample().detach()
- latents = latents * vae.config.scaling_factor
-
- # Sample noise that we'll add to the latents
- noise = torch.randn_like(latents)
- bsz = latents.shape[0]
- # Sample a random timestep for each image
- timesteps = torch.randint(0, noise_scheduler.config.num_train_timesteps, (bsz,), device=latents.device)
- timesteps = timesteps.long()
-
- # Add noise to the latents according to the noise magnitude at each timestep
- # (this is the forward diffusion process)
- noisy_latents = noise_scheduler.add_noise(latents, noise, timesteps)
-
- # Get the text embedding for conditioning
- encoder_hidden_states = text_encoder(batch["input_ids"])[0].to(dtype=weight_dtype)
-
- # Predict the noise residual
- model_pred = unet(noisy_latents, timesteps, encoder_hidden_states).sample
-
- # Get the target for loss depending on the prediction type
- if noise_scheduler.config.prediction_type == "epsilon":
- target = noise
- elif noise_scheduler.config.prediction_type == "v_prediction":
- target = noise_scheduler.get_velocity(latents, noise, timesteps)
- else:
- raise ValueError(f"Unknown prediction type {noise_scheduler.config.prediction_type}")
-
- loss = F.mse_loss(model_pred.float(), target.float(), reduction="mean")
-
- accelerator.backward(loss)
-
- optimizer.step()
- lr_scheduler.step()
- optimizer.zero_grad()
-
- # Let's make sure we don't update any embedding weights besides the newly added token
- index_no_updates = torch.ones((len(tokenizer),), dtype=torch.bool)
- index_no_updates[min(placeholder_token_ids) : max(placeholder_token_ids) + 1] = False
-
- with torch.no_grad():
- accelerator.unwrap_model(text_encoder).get_input_embeddings().weight[
- index_no_updates
- ] = orig_embeds_params[index_no_updates]
-
- # Checks if the accelerator has performed an optimization step behind the scenes
- if accelerator.sync_gradients:
- images = []
- progress_bar.update(1)
- global_step += 1
- if global_step % args.save_steps == 0:
- save_path = os.path.join(args.output_dir, f"learned_embeds-steps-{global_step}.bin")
- save_progress(text_encoder, placeholder_token_ids, accelerator, args, save_path)
-
- if accelerator.is_main_process:
- if global_step % args.checkpointing_steps == 0:
- # _before_ saving state, check if this save would set us over the `checkpoints_total_limit`
- if args.checkpoints_total_limit is not None:
- checkpoints = os.listdir(args.output_dir)
- checkpoints = [d for d in checkpoints if d.startswith("checkpoint")]
- checkpoints = sorted(checkpoints, key=lambda x: int(x.split("-")[1]))
-
- # before we save the new checkpoint, we need to have at _most_ `checkpoints_total_limit - 1` checkpoints
- if len(checkpoints) >= args.checkpoints_total_limit:
- num_to_remove = len(checkpoints) - args.checkpoints_total_limit + 1
- removing_checkpoints = checkpoints[0:num_to_remove]
-
- logger.info(
- f"{len(checkpoints)} checkpoints already exist, removing {len(removing_checkpoints)} checkpoints"
- )
- logger.info(f"removing checkpoints: {', '.join(removing_checkpoints)}")
-
- for removing_checkpoint in removing_checkpoints:
- removing_checkpoint = os.path.join(args.output_dir, removing_checkpoint)
- shutil.rmtree(removing_checkpoint)
-
- save_path = os.path.join(args.output_dir, f"checkpoint-{global_step}")
- accelerator.save_state(save_path)
- logger.info(f"Saved state to {save_path}")
-
- if args.validation_prompt is not None and global_step % args.validation_steps == 0:
- images = log_validation(
- text_encoder, tokenizer, unet, vae, args, accelerator, weight_dtype, epoch
- )
-
- logs = {"loss": loss.detach().item(), "lr": lr_scheduler.get_last_lr()[0]}
- progress_bar.set_postfix(**logs)
- accelerator.log(logs, step=global_step)
-
- if global_step >= args.max_train_steps:
- break
- # Create the pipeline using the trained modules and save it.
- accelerator.wait_for_everyone()
- if accelerator.is_main_process:
- if args.push_to_hub and not args.save_as_full_pipeline:
- logger.warn("Enabling full model saving because --push_to_hub=True was specified.")
- save_full_model = True
- else:
- save_full_model = args.save_as_full_pipeline
- if save_full_model:
- pipeline = StableDiffusionPipeline.from_pretrained(
- args.pretrained_model_name_or_path,
- text_encoder=accelerator.unwrap_model(text_encoder),
- vae=vae,
- unet=unet,
- tokenizer=tokenizer,
- )
- pipeline.save_pretrained(args.output_dir)
- # Save the newly trained embeddings
- save_path = os.path.join(args.output_dir, "learned_embeds.bin")
- save_progress(text_encoder, placeholder_token_ids, accelerator, args, save_path)
-
- if args.push_to_hub:
- save_model_card(
- repo_id,
- images=images,
- base_model=args.pretrained_model_name_or_path,
- repo_folder=args.output_dir,
- )
- upload_folder(
- repo_id=repo_id,
- folder_path=args.output_dir,
- commit_message="End of training",
- ignore_patterns=["step_*", "epoch_*"],
- )
-
- accelerator.end_training()
-
-
-if __name__ == "__main__":
- main()
diff --git a/spaces/Andy1621/uniformer_image_detection/configs/hrnet/htc_hrnetv2p_w40_20e_coco.py b/spaces/Andy1621/uniformer_image_detection/configs/hrnet/htc_hrnetv2p_w40_20e_coco.py
deleted file mode 100644
index abf6fb550e4dfff4e749e15b001c37e6db8ae476..0000000000000000000000000000000000000000
--- a/spaces/Andy1621/uniformer_image_detection/configs/hrnet/htc_hrnetv2p_w40_20e_coco.py
+++ /dev/null
@@ -1,10 +0,0 @@
-_base_ = './htc_hrnetv2p_w32_20e_coco.py'
-model = dict(
- pretrained='open-mmlab://msra/hrnetv2_w40',
- backbone=dict(
- type='HRNet',
- extra=dict(
- stage2=dict(num_channels=(40, 80)),
- stage3=dict(num_channels=(40, 80, 160)),
- stage4=dict(num_channels=(40, 80, 160, 320)))),
- neck=dict(type='HRFPN', in_channels=[40, 80, 160, 320], out_channels=256))
diff --git a/spaces/Andy1621/uniformer_image_detection/mmdet/models/roi_heads/mask_heads/scnet_semantic_head.py b/spaces/Andy1621/uniformer_image_detection/mmdet/models/roi_heads/mask_heads/scnet_semantic_head.py
deleted file mode 100644
index df85a0112d27d97301fff56189f99bee0bf8efa5..0000000000000000000000000000000000000000
--- a/spaces/Andy1621/uniformer_image_detection/mmdet/models/roi_heads/mask_heads/scnet_semantic_head.py
+++ /dev/null
@@ -1,27 +0,0 @@
-from mmdet.models.builder import HEADS
-from mmdet.models.utils import ResLayer, SimplifiedBasicBlock
-from .fused_semantic_head import FusedSemanticHead
-
-
-@HEADS.register_module()
-class SCNetSemanticHead(FusedSemanticHead):
- """Mask head for `SCNet `_.
-
- Args:
- conv_to_res (bool, optional): if True, change the conv layers to
- ``SimplifiedBasicBlock``.
- """
-
- def __init__(self, conv_to_res=True, **kwargs):
- super(SCNetSemanticHead, self).__init__(**kwargs)
- self.conv_to_res = conv_to_res
- if self.conv_to_res:
- num_res_blocks = self.num_convs // 2
- self.convs = ResLayer(
- SimplifiedBasicBlock,
- self.in_channels,
- self.conv_out_channels,
- num_res_blocks,
- conv_cfg=self.conv_cfg,
- norm_cfg=self.norm_cfg)
- self.num_convs = num_res_blocks
diff --git a/spaces/Andy1621/uniformer_image_segmentation/configs/hrnet/fcn_hr48_480x480_80k_pascal_context_59.py b/spaces/Andy1621/uniformer_image_segmentation/configs/hrnet/fcn_hr48_480x480_80k_pascal_context_59.py
deleted file mode 100644
index 012ad0a7d6119554ec00400ad18a09249a72eca4..0000000000000000000000000000000000000000
--- a/spaces/Andy1621/uniformer_image_segmentation/configs/hrnet/fcn_hr48_480x480_80k_pascal_context_59.py
+++ /dev/null
@@ -1,10 +0,0 @@
-_base_ = './fcn_hr18_480x480_80k_pascal_context_59.py'
-model = dict(
- pretrained='open-mmlab://msra/hrnetv2_w48',
- backbone=dict(
- extra=dict(
- stage2=dict(num_channels=(48, 96)),
- stage3=dict(num_channels=(48, 96, 192)),
- stage4=dict(num_channels=(48, 96, 192, 384)))),
- decode_head=dict(
- in_channels=[48, 96, 192, 384], channels=sum([48, 96, 192, 384])))
diff --git a/spaces/Anonymous-sub/Rerender/ControlNet/annotator/uniformer/mmcv_custom/checkpoint.py b/spaces/Anonymous-sub/Rerender/ControlNet/annotator/uniformer/mmcv_custom/checkpoint.py
deleted file mode 100644
index 19b87fef0a52d31babcdb3edb8f3089b6420173f..0000000000000000000000000000000000000000
--- a/spaces/Anonymous-sub/Rerender/ControlNet/annotator/uniformer/mmcv_custom/checkpoint.py
+++ /dev/null
@@ -1,500 +0,0 @@
-# Copyright (c) Open-MMLab. All rights reserved.
-import io
-import os
-import os.path as osp
-import pkgutil
-import time
-import warnings
-from collections import OrderedDict
-from importlib import import_module
-from tempfile import TemporaryDirectory
-
-import torch
-import torchvision
-from torch.optim import Optimizer
-from torch.utils import model_zoo
-from torch.nn import functional as F
-
-import annotator.uniformer.mmcv as mmcv
-from annotator.uniformer.mmcv.fileio import FileClient
-from annotator.uniformer.mmcv.fileio import load as load_file
-from annotator.uniformer.mmcv.parallel import is_module_wrapper
-from annotator.uniformer.mmcv.utils import mkdir_or_exist
-from annotator.uniformer.mmcv.runner import get_dist_info
-
-ENV_MMCV_HOME = 'MMCV_HOME'
-ENV_XDG_CACHE_HOME = 'XDG_CACHE_HOME'
-DEFAULT_CACHE_DIR = '~/.cache'
-
-
-def _get_mmcv_home():
- mmcv_home = os.path.expanduser(
- os.getenv(
- ENV_MMCV_HOME,
- os.path.join(
- os.getenv(ENV_XDG_CACHE_HOME, DEFAULT_CACHE_DIR), 'mmcv')))
-
- mkdir_or_exist(mmcv_home)
- return mmcv_home
-
-
-def load_state_dict(module, state_dict, strict=False, logger=None):
- """Load state_dict to a module.
-
- This method is modified from :meth:`torch.nn.Module.load_state_dict`.
- Default value for ``strict`` is set to ``False`` and the message for
- param mismatch will be shown even if strict is False.
-
- Args:
- module (Module): Module that receives the state_dict.
- state_dict (OrderedDict): Weights.
- strict (bool): whether to strictly enforce that the keys
- in :attr:`state_dict` match the keys returned by this module's
- :meth:`~torch.nn.Module.state_dict` function. Default: ``False``.
- logger (:obj:`logging.Logger`, optional): Logger to log the error
- message. If not specified, print function will be used.
- """
- unexpected_keys = []
- all_missing_keys = []
- err_msg = []
-
- metadata = getattr(state_dict, '_metadata', None)
- state_dict = state_dict.copy()
- if metadata is not None:
- state_dict._metadata = metadata
-
- # use _load_from_state_dict to enable checkpoint version control
- def load(module, prefix=''):
- # recursively check parallel module in case that the model has a
- # complicated structure, e.g., nn.Module(nn.Module(DDP))
- if is_module_wrapper(module):
- module = module.module
- local_metadata = {} if metadata is None else metadata.get(
- prefix[:-1], {})
- module._load_from_state_dict(state_dict, prefix, local_metadata, True,
- all_missing_keys, unexpected_keys,
- err_msg)
- for name, child in module._modules.items():
- if child is not None:
- load(child, prefix + name + '.')
-
- load(module)
- load = None # break load->load reference cycle
-
- # ignore "num_batches_tracked" of BN layers
- missing_keys = [
- key for key in all_missing_keys if 'num_batches_tracked' not in key
- ]
-
- if unexpected_keys:
- err_msg.append('unexpected key in source '
- f'state_dict: {", ".join(unexpected_keys)}\n')
- if missing_keys:
- err_msg.append(
- f'missing keys in source state_dict: {", ".join(missing_keys)}\n')
-
- rank, _ = get_dist_info()
- if len(err_msg) > 0 and rank == 0:
- err_msg.insert(
- 0, 'The model and loaded state dict do not match exactly\n')
- err_msg = '\n'.join(err_msg)
- if strict:
- raise RuntimeError(err_msg)
- elif logger is not None:
- logger.warning(err_msg)
- else:
- print(err_msg)
-
-
-def load_url_dist(url, model_dir=None):
- """In distributed setting, this function only download checkpoint at local
- rank 0."""
- rank, world_size = get_dist_info()
- rank = int(os.environ.get('LOCAL_RANK', rank))
- if rank == 0:
- checkpoint = model_zoo.load_url(url, model_dir=model_dir)
- if world_size > 1:
- torch.distributed.barrier()
- if rank > 0:
- checkpoint = model_zoo.load_url(url, model_dir=model_dir)
- return checkpoint
-
-
-def load_pavimodel_dist(model_path, map_location=None):
- """In distributed setting, this function only download checkpoint at local
- rank 0."""
- try:
- from pavi import modelcloud
- except ImportError:
- raise ImportError(
- 'Please install pavi to load checkpoint from modelcloud.')
- rank, world_size = get_dist_info()
- rank = int(os.environ.get('LOCAL_RANK', rank))
- if rank == 0:
- model = modelcloud.get(model_path)
- with TemporaryDirectory() as tmp_dir:
- downloaded_file = osp.join(tmp_dir, model.name)
- model.download(downloaded_file)
- checkpoint = torch.load(downloaded_file, map_location=map_location)
- if world_size > 1:
- torch.distributed.barrier()
- if rank > 0:
- model = modelcloud.get(model_path)
- with TemporaryDirectory() as tmp_dir:
- downloaded_file = osp.join(tmp_dir, model.name)
- model.download(downloaded_file)
- checkpoint = torch.load(
- downloaded_file, map_location=map_location)
- return checkpoint
-
-
-def load_fileclient_dist(filename, backend, map_location):
- """In distributed setting, this function only download checkpoint at local
- rank 0."""
- rank, world_size = get_dist_info()
- rank = int(os.environ.get('LOCAL_RANK', rank))
- allowed_backends = ['ceph']
- if backend not in allowed_backends:
- raise ValueError(f'Load from Backend {backend} is not supported.')
- if rank == 0:
- fileclient = FileClient(backend=backend)
- buffer = io.BytesIO(fileclient.get(filename))
- checkpoint = torch.load(buffer, map_location=map_location)
- if world_size > 1:
- torch.distributed.barrier()
- if rank > 0:
- fileclient = FileClient(backend=backend)
- buffer = io.BytesIO(fileclient.get(filename))
- checkpoint = torch.load(buffer, map_location=map_location)
- return checkpoint
-
-
-def get_torchvision_models():
- model_urls = dict()
- for _, name, ispkg in pkgutil.walk_packages(torchvision.models.__path__):
- if ispkg:
- continue
- _zoo = import_module(f'torchvision.models.{name}')
- if hasattr(_zoo, 'model_urls'):
- _urls = getattr(_zoo, 'model_urls')
- model_urls.update(_urls)
- return model_urls
-
-
-def get_external_models():
- mmcv_home = _get_mmcv_home()
- default_json_path = osp.join(mmcv.__path__[0], 'model_zoo/open_mmlab.json')
- default_urls = load_file(default_json_path)
- assert isinstance(default_urls, dict)
- external_json_path = osp.join(mmcv_home, 'open_mmlab.json')
- if osp.exists(external_json_path):
- external_urls = load_file(external_json_path)
- assert isinstance(external_urls, dict)
- default_urls.update(external_urls)
-
- return default_urls
-
-
-def get_mmcls_models():
- mmcls_json_path = osp.join(mmcv.__path__[0], 'model_zoo/mmcls.json')
- mmcls_urls = load_file(mmcls_json_path)
-
- return mmcls_urls
-
-
-def get_deprecated_model_names():
- deprecate_json_path = osp.join(mmcv.__path__[0],
- 'model_zoo/deprecated.json')
- deprecate_urls = load_file(deprecate_json_path)
- assert isinstance(deprecate_urls, dict)
-
- return deprecate_urls
-
-
-def _process_mmcls_checkpoint(checkpoint):
- state_dict = checkpoint['state_dict']
- new_state_dict = OrderedDict()
- for k, v in state_dict.items():
- if k.startswith('backbone.'):
- new_state_dict[k[9:]] = v
- new_checkpoint = dict(state_dict=new_state_dict)
-
- return new_checkpoint
-
-
-def _load_checkpoint(filename, map_location=None):
- """Load checkpoint from somewhere (modelzoo, file, url).
-
- Args:
- filename (str): Accept local filepath, URL, ``torchvision://xxx``,
- ``open-mmlab://xxx``. Please refer to ``docs/model_zoo.md`` for
- details.
- map_location (str | None): Same as :func:`torch.load`. Default: None.
-
- Returns:
- dict | OrderedDict: The loaded checkpoint. It can be either an
- OrderedDict storing model weights or a dict containing other
- information, which depends on the checkpoint.
- """
- if filename.startswith('modelzoo://'):
- warnings.warn('The URL scheme of "modelzoo://" is deprecated, please '
- 'use "torchvision://" instead')
- model_urls = get_torchvision_models()
- model_name = filename[11:]
- checkpoint = load_url_dist(model_urls[model_name])
- elif filename.startswith('torchvision://'):
- model_urls = get_torchvision_models()
- model_name = filename[14:]
- checkpoint = load_url_dist(model_urls[model_name])
- elif filename.startswith('open-mmlab://'):
- model_urls = get_external_models()
- model_name = filename[13:]
- deprecated_urls = get_deprecated_model_names()
- if model_name in deprecated_urls:
- warnings.warn(f'open-mmlab://{model_name} is deprecated in favor '
- f'of open-mmlab://{deprecated_urls[model_name]}')
- model_name = deprecated_urls[model_name]
- model_url = model_urls[model_name]
- # check if is url
- if model_url.startswith(('http://', 'https://')):
- checkpoint = load_url_dist(model_url)
- else:
- filename = osp.join(_get_mmcv_home(), model_url)
- if not osp.isfile(filename):
- raise IOError(f'{filename} is not a checkpoint file')
- checkpoint = torch.load(filename, map_location=map_location)
- elif filename.startswith('mmcls://'):
- model_urls = get_mmcls_models()
- model_name = filename[8:]
- checkpoint = load_url_dist(model_urls[model_name])
- checkpoint = _process_mmcls_checkpoint(checkpoint)
- elif filename.startswith(('http://', 'https://')):
- checkpoint = load_url_dist(filename)
- elif filename.startswith('pavi://'):
- model_path = filename[7:]
- checkpoint = load_pavimodel_dist(model_path, map_location=map_location)
- elif filename.startswith('s3://'):
- checkpoint = load_fileclient_dist(
- filename, backend='ceph', map_location=map_location)
- else:
- if not osp.isfile(filename):
- raise IOError(f'{filename} is not a checkpoint file')
- checkpoint = torch.load(filename, map_location=map_location)
- return checkpoint
-
-
-def load_checkpoint(model,
- filename,
- map_location='cpu',
- strict=False,
- logger=None):
- """Load checkpoint from a file or URI.
-
- Args:
- model (Module): Module to load checkpoint.
- filename (str): Accept local filepath, URL, ``torchvision://xxx``,
- ``open-mmlab://xxx``. Please refer to ``docs/model_zoo.md`` for
- details.
- map_location (str): Same as :func:`torch.load`.
- strict (bool): Whether to allow different params for the model and
- checkpoint.
- logger (:mod:`logging.Logger` or None): The logger for error message.
-
- Returns:
- dict or OrderedDict: The loaded checkpoint.
- """
- checkpoint = _load_checkpoint(filename, map_location)
- # OrderedDict is a subclass of dict
- if not isinstance(checkpoint, dict):
- raise RuntimeError(
- f'No state_dict found in checkpoint file {filename}')
- # get state_dict from checkpoint
- if 'state_dict' in checkpoint:
- state_dict = checkpoint['state_dict']
- elif 'model' in checkpoint:
- state_dict = checkpoint['model']
- else:
- state_dict = checkpoint
- # strip prefix of state_dict
- if list(state_dict.keys())[0].startswith('module.'):
- state_dict = {k[7:]: v for k, v in state_dict.items()}
-
- # for MoBY, load model of online branch
- if sorted(list(state_dict.keys()))[0].startswith('encoder'):
- state_dict = {k.replace('encoder.', ''): v for k, v in state_dict.items() if k.startswith('encoder.')}
-
- # reshape absolute position embedding
- if state_dict.get('absolute_pos_embed') is not None:
- absolute_pos_embed = state_dict['absolute_pos_embed']
- N1, L, C1 = absolute_pos_embed.size()
- N2, C2, H, W = model.absolute_pos_embed.size()
- if N1 != N2 or C1 != C2 or L != H*W:
- logger.warning("Error in loading absolute_pos_embed, pass")
- else:
- state_dict['absolute_pos_embed'] = absolute_pos_embed.view(N2, H, W, C2).permute(0, 3, 1, 2)
-
- # interpolate position bias table if needed
- relative_position_bias_table_keys = [k for k in state_dict.keys() if "relative_position_bias_table" in k]
- for table_key in relative_position_bias_table_keys:
- table_pretrained = state_dict[table_key]
- table_current = model.state_dict()[table_key]
- L1, nH1 = table_pretrained.size()
- L2, nH2 = table_current.size()
- if nH1 != nH2:
- logger.warning(f"Error in loading {table_key}, pass")
- else:
- if L1 != L2:
- S1 = int(L1 ** 0.5)
- S2 = int(L2 ** 0.5)
- table_pretrained_resized = F.interpolate(
- table_pretrained.permute(1, 0).view(1, nH1, S1, S1),
- size=(S2, S2), mode='bicubic')
- state_dict[table_key] = table_pretrained_resized.view(nH2, L2).permute(1, 0)
-
- # load state_dict
- load_state_dict(model, state_dict, strict, logger)
- return checkpoint
-
-
-def weights_to_cpu(state_dict):
- """Copy a model state_dict to cpu.
-
- Args:
- state_dict (OrderedDict): Model weights on GPU.
-
- Returns:
- OrderedDict: Model weights on GPU.
- """
- state_dict_cpu = OrderedDict()
- for key, val in state_dict.items():
- state_dict_cpu[key] = val.cpu()
- return state_dict_cpu
-
-
-def _save_to_state_dict(module, destination, prefix, keep_vars):
- """Saves module state to `destination` dictionary.
-
- This method is modified from :meth:`torch.nn.Module._save_to_state_dict`.
-
- Args:
- module (nn.Module): The module to generate state_dict.
- destination (dict): A dict where state will be stored.
- prefix (str): The prefix for parameters and buffers used in this
- module.
- """
- for name, param in module._parameters.items():
- if param is not None:
- destination[prefix + name] = param if keep_vars else param.detach()
- for name, buf in module._buffers.items():
- # remove check of _non_persistent_buffers_set to allow nn.BatchNorm2d
- if buf is not None:
- destination[prefix + name] = buf if keep_vars else buf.detach()
-
-
-def get_state_dict(module, destination=None, prefix='', keep_vars=False):
- """Returns a dictionary containing a whole state of the module.
-
- Both parameters and persistent buffers (e.g. running averages) are
- included. Keys are corresponding parameter and buffer names.
-
- This method is modified from :meth:`torch.nn.Module.state_dict` to
- recursively check parallel module in case that the model has a complicated
- structure, e.g., nn.Module(nn.Module(DDP)).
-
- Args:
- module (nn.Module): The module to generate state_dict.
- destination (OrderedDict): Returned dict for the state of the
- module.
- prefix (str): Prefix of the key.
- keep_vars (bool): Whether to keep the variable property of the
- parameters. Default: False.
-
- Returns:
- dict: A dictionary containing a whole state of the module.
- """
- # recursively check parallel module in case that the model has a
- # complicated structure, e.g., nn.Module(nn.Module(DDP))
- if is_module_wrapper(module):
- module = module.module
-
- # below is the same as torch.nn.Module.state_dict()
- if destination is None:
- destination = OrderedDict()
- destination._metadata = OrderedDict()
- destination._metadata[prefix[:-1]] = local_metadata = dict(
- version=module._version)
- _save_to_state_dict(module, destination, prefix, keep_vars)
- for name, child in module._modules.items():
- if child is not None:
- get_state_dict(
- child, destination, prefix + name + '.', keep_vars=keep_vars)
- for hook in module._state_dict_hooks.values():
- hook_result = hook(module, destination, prefix, local_metadata)
- if hook_result is not None:
- destination = hook_result
- return destination
-
-
-def save_checkpoint(model, filename, optimizer=None, meta=None):
- """Save checkpoint to file.
-
- The checkpoint will have 3 fields: ``meta``, ``state_dict`` and
- ``optimizer``. By default ``meta`` will contain version and time info.
-
- Args:
- model (Module): Module whose params are to be saved.
- filename (str): Checkpoint filename.
- optimizer (:obj:`Optimizer`, optional): Optimizer to be saved.
- meta (dict, optional): Metadata to be saved in checkpoint.
- """
- if meta is None:
- meta = {}
- elif not isinstance(meta, dict):
- raise TypeError(f'meta must be a dict or None, but got {type(meta)}')
- meta.update(mmcv_version=mmcv.__version__, time=time.asctime())
-
- if is_module_wrapper(model):
- model = model.module
-
- if hasattr(model, 'CLASSES') and model.CLASSES is not None:
- # save class name to the meta
- meta.update(CLASSES=model.CLASSES)
-
- checkpoint = {
- 'meta': meta,
- 'state_dict': weights_to_cpu(get_state_dict(model))
- }
- # save optimizer state dict in the checkpoint
- if isinstance(optimizer, Optimizer):
- checkpoint['optimizer'] = optimizer.state_dict()
- elif isinstance(optimizer, dict):
- checkpoint['optimizer'] = {}
- for name, optim in optimizer.items():
- checkpoint['optimizer'][name] = optim.state_dict()
-
- if filename.startswith('pavi://'):
- try:
- from pavi import modelcloud
- from pavi.exception import NodeNotFoundError
- except ImportError:
- raise ImportError(
- 'Please install pavi to load checkpoint from modelcloud.')
- model_path = filename[7:]
- root = modelcloud.Folder()
- model_dir, model_name = osp.split(model_path)
- try:
- model = modelcloud.get(model_dir)
- except NodeNotFoundError:
- model = root.create_training_model(model_dir)
- with TemporaryDirectory() as tmp_dir:
- checkpoint_file = osp.join(tmp_dir, model_name)
- with open(checkpoint_file, 'wb') as f:
- torch.save(checkpoint, f)
- f.flush()
- model.create_file(checkpoint_file, name=model_name)
- else:
- mmcv.mkdir_or_exist(osp.dirname(filename))
- # immediately flush buffer
- with open(filename, 'wb') as f:
- torch.save(checkpoint, f)
- f.flush()
\ No newline at end of file
diff --git a/spaces/Anonymous-sub/Rerender/ControlNet/annotator/uniformer/mmseg/core/seg/__init__.py b/spaces/Anonymous-sub/Rerender/ControlNet/annotator/uniformer/mmseg/core/seg/__init__.py
deleted file mode 100644
index 93bc129b685e4a3efca2cc891729981b2865900d..0000000000000000000000000000000000000000
--- a/spaces/Anonymous-sub/Rerender/ControlNet/annotator/uniformer/mmseg/core/seg/__init__.py
+++ /dev/null
@@ -1,4 +0,0 @@
-from .builder import build_pixel_sampler
-from .sampler import BasePixelSampler, OHEMPixelSampler
-
-__all__ = ['build_pixel_sampler', 'BasePixelSampler', 'OHEMPixelSampler']
diff --git a/spaces/Anonymous-sub/Rerender/gmflow_module/utils/utils.py b/spaces/Anonymous-sub/Rerender/gmflow_module/utils/utils.py
deleted file mode 100644
index 76f5518b7e5b769527907b31a1c1c00ba6cfe4f1..0000000000000000000000000000000000000000
--- a/spaces/Anonymous-sub/Rerender/gmflow_module/utils/utils.py
+++ /dev/null
@@ -1,58 +0,0 @@
-import torch
-import torch.nn.functional as F
-
-
-class InputPadder:
- """ Pads images such that dimensions are divisible by 8 """
-
- def __init__(self, dims, mode='sintel', padding_factor=8):
- self.ht, self.wd = dims[-2:]
- pad_ht = (((self.ht // padding_factor) + 1) * padding_factor - self.ht) % padding_factor
- pad_wd = (((self.wd // padding_factor) + 1) * padding_factor - self.wd) % padding_factor
- if mode == 'sintel':
- self._pad = [pad_wd // 2, pad_wd - pad_wd // 2, pad_ht // 2, pad_ht - pad_ht // 2]
- else:
- self._pad = [pad_wd // 2, pad_wd - pad_wd // 2, 0, pad_ht]
-
- def pad(self, *inputs):
- return [F.pad(x, self._pad, mode='replicate') for x in inputs]
-
- def unpad(self, x):
- ht, wd = x.shape[-2:]
- c = [self._pad[2], ht - self._pad[3], self._pad[0], wd - self._pad[1]]
- return x[..., c[0]:c[1], c[2]:c[3]]
-
-
-def coords_grid(batch, ht, wd, normalize=False):
- if normalize: # [-1, 1]
- coords = torch.meshgrid(2 * torch.arange(ht) / (ht - 1) - 1,
- 2 * torch.arange(wd) / (wd - 1) - 1)
- else:
- coords = torch.meshgrid(torch.arange(ht), torch.arange(wd))
- coords = torch.stack(coords[::-1], dim=0).float()
- return coords[None].repeat(batch, 1, 1, 1) # [B, 2, H, W]
-
-
-def compute_out_of_boundary_mask(flow):
- # flow: [B, 2, H, W]
- assert flow.dim() == 4 and flow.size(1) == 2
- b, _, h, w = flow.shape
- init_coords = coords_grid(b, h, w).to(flow.device)
- corres = init_coords + flow # [B, 2, H, W]
-
- max_w = w - 1
- max_h = h - 1
-
- valid_mask = (corres[:, 0] >= 0) & (corres[:, 0] <= max_w) & (corres[:, 1] >= 0) & (corres[:, 1] <= max_h)
-
- # in case very large flow
- flow_mask = (flow[:, 0].abs() <= max_w) & (flow[:, 1].abs() <= max_h)
-
- valid_mask = valid_mask & flow_mask
-
- return valid_mask # [B, H, W]
-
-
-def count_parameters(model):
- num = sum(p.numel() for p in model.parameters() if p.requires_grad)
- return num
diff --git a/spaces/Anuj-Panthri/imdb_review_sentiment/app.py b/spaces/Anuj-Panthri/imdb_review_sentiment/app.py
deleted file mode 100644
index f62af7f2e28e44459d96595e669facfe79977c0e..0000000000000000000000000000000000000000
--- a/spaces/Anuj-Panthri/imdb_review_sentiment/app.py
+++ /dev/null
@@ -1,20 +0,0 @@
-import gradio as gr
-from fastai.text.all import *
-
-# to fix : NotImplementedError: cannot instantiate 'PosixPath' on your system
-# import pathlib
-# temp = pathlib.PosixPath
-# pathlib.PosixPath = pathlib.WindowsPath
-
-examples=['This was a fantastic end to the trilogy.','I\'ve never seen a bigger waste of my time.','Just when we thought they couldn\'t possibly make a worse TV movie than Sharknado? Syfy says, "Hold my beer!"']
-
-learn=load_learner('imdb_review_sentiment_model.pkl')
-
-class_names=['neg','pos']
-
-def classify(review):
- _,_,pob=learn.predict(review)
- return dict(zip(class_names,map(float,pob)))
-
-iface = gr.Interface(fn=classify, inputs=gr.inputs.Textbox(), outputs=gr.outputs.Label(),examples=examples)
-iface.launch()
\ No newline at end of file
diff --git a/spaces/Arsenii2023/Demo1/README.md b/spaces/Arsenii2023/Demo1/README.md
deleted file mode 100644
index 9d26e58744b1a197da22c0b75888a29339707623..0000000000000000000000000000000000000000
--- a/spaces/Arsenii2023/Demo1/README.md
+++ /dev/null
@@ -1,12 +0,0 @@
----
-title: Demo1
-emoji: 🏆
-colorFrom: pink
-colorTo: blue
-sdk: gradio
-sdk_version: 3.47.1
-app_file: app.py
-pinned: false
----
-
-Check out the configuration reference at https://huggingface.co/docs/hub/spaces-config-reference
diff --git a/spaces/Artples/LLaMA-2-CHAT/README.md b/spaces/Artples/LLaMA-2-CHAT/README.md
deleted file mode 100644
index aa3435b74da11de768e9c38188fd84133871604f..0000000000000000000000000000000000000000
--- a/spaces/Artples/LLaMA-2-CHAT/README.md
+++ /dev/null
@@ -1,13 +0,0 @@
----
-title: LLaMA-2-CHAT
-emoji: ⚡
-colorFrom: gray
-colorTo: yellow
-sdk: gradio
-sdk_version: 3.37.0
-app_file: app.py
-pinned: true
-license: apache-2.0
----
-
-Check out the configuration reference at https://huggingface.co/docs/hub/spaces-config-reference
\ No newline at end of file
diff --git a/spaces/Ataturk-Chatbot/HuggingFaceChat/venv/lib/python3.11/site-packages/pip/__main__.py b/spaces/Ataturk-Chatbot/HuggingFaceChat/venv/lib/python3.11/site-packages/pip/__main__.py
deleted file mode 100644
index fe34a7b7772cef55f5b5cb3455a2850489620ca7..0000000000000000000000000000000000000000
--- a/spaces/Ataturk-Chatbot/HuggingFaceChat/venv/lib/python3.11/site-packages/pip/__main__.py
+++ /dev/null
@@ -1,31 +0,0 @@
-import os
-import sys
-import warnings
-
-# Remove '' and current working directory from the first entry
-# of sys.path, if present to avoid using current directory
-# in pip commands check, freeze, install, list and show,
-# when invoked as python -m pip
-if sys.path[0] in ("", os.getcwd()):
- sys.path.pop(0)
-
-# If we are running from a wheel, add the wheel to sys.path
-# This allows the usage python pip-*.whl/pip install pip-*.whl
-if __package__ == "":
- # __file__ is pip-*.whl/pip/__main__.py
- # first dirname call strips of '/__main__.py', second strips off '/pip'
- # Resulting path is the name of the wheel itself
- # Add that to sys.path so we can import pip
- path = os.path.dirname(os.path.dirname(__file__))
- sys.path.insert(0, path)
-
-if __name__ == "__main__":
- # Work around the error reported in #9540, pending a proper fix.
- # Note: It is essential the warning filter is set *before* importing
- # pip, as the deprecation happens at import time, not runtime.
- warnings.filterwarnings(
- "ignore", category=DeprecationWarning, module=".*packaging\\.version"
- )
- from pip._internal.cli.main import main as _main
-
- sys.exit(_main())
diff --git a/spaces/Awiny/Image2Paragraph/models/grit_src/third_party/CenterNet2/detectron2/export/caffe2_modeling.py b/spaces/Awiny/Image2Paragraph/models/grit_src/third_party/CenterNet2/detectron2/export/caffe2_modeling.py
deleted file mode 100644
index e00de4ad28fd81483c9e1161394b7b508fdad91f..0000000000000000000000000000000000000000
--- a/spaces/Awiny/Image2Paragraph/models/grit_src/third_party/CenterNet2/detectron2/export/caffe2_modeling.py
+++ /dev/null
@@ -1,419 +0,0 @@
-# Copyright (c) Facebook, Inc. and its affiliates.
-
-import functools
-import io
-import struct
-import types
-import torch
-
-from detectron2.modeling import meta_arch
-from detectron2.modeling.box_regression import Box2BoxTransform
-from detectron2.modeling.roi_heads import keypoint_head
-from detectron2.structures import Boxes, ImageList, Instances, RotatedBoxes
-
-from .c10 import Caffe2Compatible
-from .caffe2_patch import ROIHeadsPatcher, patch_generalized_rcnn
-from .shared import (
- alias,
- check_set_pb_arg,
- get_pb_arg_floats,
- get_pb_arg_valf,
- get_pb_arg_vali,
- get_pb_arg_vals,
- mock_torch_nn_functional_interpolate,
-)
-
-
-def assemble_rcnn_outputs_by_name(image_sizes, tensor_outputs, force_mask_on=False):
- """
- A function to assemble caffe2 model's outputs (i.e. Dict[str, Tensor])
- to detectron2's format (i.e. list of Instances instance).
- This only works when the model follows the Caffe2 detectron's naming convention.
-
- Args:
- image_sizes (List[List[int, int]]): [H, W] of every image.
- tensor_outputs (Dict[str, Tensor]): external_output to its tensor.
-
- force_mask_on (Bool): if true, the it make sure there'll be pred_masks even
- if the mask is not found from tensor_outputs (usually due to model crash)
- """
-
- results = [Instances(image_size) for image_size in image_sizes]
-
- batch_splits = tensor_outputs.get("batch_splits", None)
- if batch_splits:
- raise NotImplementedError()
- assert len(image_sizes) == 1
- result = results[0]
-
- bbox_nms = tensor_outputs["bbox_nms"]
- score_nms = tensor_outputs["score_nms"]
- class_nms = tensor_outputs["class_nms"]
- # Detection will always success because Conv support 0-batch
- assert bbox_nms is not None
- assert score_nms is not None
- assert class_nms is not None
- if bbox_nms.shape[1] == 5:
- result.pred_boxes = RotatedBoxes(bbox_nms)
- else:
- result.pred_boxes = Boxes(bbox_nms)
- result.scores = score_nms
- result.pred_classes = class_nms.to(torch.int64)
-
- mask_fcn_probs = tensor_outputs.get("mask_fcn_probs", None)
- if mask_fcn_probs is not None:
- # finish the mask pred
- mask_probs_pred = mask_fcn_probs
- num_masks = mask_probs_pred.shape[0]
- class_pred = result.pred_classes
- indices = torch.arange(num_masks, device=class_pred.device)
- mask_probs_pred = mask_probs_pred[indices, class_pred][:, None]
- result.pred_masks = mask_probs_pred
- elif force_mask_on:
- # NOTE: there's no way to know the height/width of mask here, it won't be
- # used anyway when batch size is 0, so just set them to 0.
- result.pred_masks = torch.zeros([0, 1, 0, 0], dtype=torch.uint8)
-
- keypoints_out = tensor_outputs.get("keypoints_out", None)
- kps_score = tensor_outputs.get("kps_score", None)
- if keypoints_out is not None:
- # keypoints_out: [N, 4, #kypoints], where 4 is in order of (x, y, score, prob)
- keypoints_tensor = keypoints_out
- # NOTE: it's possible that prob is not calculated if "should_output_softmax"
- # is set to False in HeatmapMaxKeypoint, so just using raw score, seems
- # it doesn't affect mAP. TODO: check more carefully.
- keypoint_xyp = keypoints_tensor.transpose(1, 2)[:, :, [0, 1, 2]]
- result.pred_keypoints = keypoint_xyp
- elif kps_score is not None:
- # keypoint heatmap to sparse data structure
- pred_keypoint_logits = kps_score
- keypoint_head.keypoint_rcnn_inference(pred_keypoint_logits, [result])
-
- return results
-
-
-def _cast_to_f32(f64):
- return struct.unpack("f", struct.pack("f", f64))[0]
-
-
-def set_caffe2_compatible_tensor_mode(model, enable=True):
- def _fn(m):
- if isinstance(m, Caffe2Compatible):
- m.tensor_mode = enable
-
- model.apply(_fn)
-
-
-def convert_batched_inputs_to_c2_format(batched_inputs, size_divisibility, device):
- """
- See get_caffe2_inputs() below.
- """
- assert all(isinstance(x, dict) for x in batched_inputs)
- assert all(x["image"].dim() == 3 for x in batched_inputs)
-
- images = [x["image"] for x in batched_inputs]
- images = ImageList.from_tensors(images, size_divisibility)
-
- im_info = []
- for input_per_image, image_size in zip(batched_inputs, images.image_sizes):
- target_height = input_per_image.get("height", image_size[0])
- target_width = input_per_image.get("width", image_size[1]) # noqa
- # NOTE: The scale inside im_info is kept as convention and for providing
- # post-processing information if further processing is needed. For
- # current Caffe2 model definitions that don't include post-processing inside
- # the model, this number is not used.
- # NOTE: There can be a slight difference between width and height
- # scales, using a single number can results in numerical difference
- # compared with D2's post-processing.
- scale = target_height / image_size[0]
- im_info.append([image_size[0], image_size[1], scale])
- im_info = torch.Tensor(im_info)
-
- return images.tensor.to(device), im_info.to(device)
-
-
-class Caffe2MetaArch(Caffe2Compatible, torch.nn.Module):
- """
- Base class for caffe2-compatible implementation of a meta architecture.
- The forward is traceable and its traced graph can be converted to caffe2
- graph through ONNX.
- """
-
- def __init__(self, cfg, torch_model):
- """
- Args:
- cfg (CfgNode):
- torch_model (nn.Module): the detectron2 model (meta_arch) to be
- converted.
- """
- super().__init__()
- self._wrapped_model = torch_model
- self.eval()
- set_caffe2_compatible_tensor_mode(self, True)
-
- def get_caffe2_inputs(self, batched_inputs):
- """
- Convert pytorch-style structured inputs to caffe2-style inputs that
- are tuples of tensors.
-
- Args:
- batched_inputs (list[dict]): inputs to a detectron2 model
- in its standard format. Each dict has "image" (CHW tensor), and optionally
- "height" and "width".
-
- Returns:
- tuple[Tensor]:
- tuple of tensors that will be the inputs to the
- :meth:`forward` method. For existing models, the first
- is an NCHW tensor (padded and batched); the second is
- a im_info Nx3 tensor, where the rows are
- (height, width, unused legacy parameter)
- """
- return convert_batched_inputs_to_c2_format(
- batched_inputs,
- self._wrapped_model.backbone.size_divisibility,
- self._wrapped_model.device,
- )
-
- def encode_additional_info(self, predict_net, init_net):
- """
- Save extra metadata that will be used by inference in the output protobuf.
- """
- pass
-
- def forward(self, inputs):
- """
- Run the forward in caffe2-style. It has to use caffe2-compatible ops
- and the method will be used for tracing.
-
- Args:
- inputs (tuple[Tensor]): inputs defined by :meth:`get_caffe2_input`.
- They will be the inputs of the converted caffe2 graph.
-
- Returns:
- tuple[Tensor]: output tensors. They will be the outputs of the
- converted caffe2 graph.
- """
- raise NotImplementedError
-
- def _caffe2_preprocess_image(self, inputs):
- """
- Caffe2 implementation of preprocess_image, which is called inside each MetaArch's forward.
- It normalizes the input images, and the final caffe2 graph assumes the
- inputs have been batched already.
- """
- data, im_info = inputs
- data = alias(data, "data")
- im_info = alias(im_info, "im_info")
- mean, std = self._wrapped_model.pixel_mean, self._wrapped_model.pixel_std
- normalized_data = (data - mean) / std
- normalized_data = alias(normalized_data, "normalized_data")
-
- # Pack (data, im_info) into ImageList which is recognized by self.inference.
- images = ImageList(tensor=normalized_data, image_sizes=im_info)
- return images
-
- @staticmethod
- def get_outputs_converter(predict_net, init_net):
- """
- Creates a function that converts outputs of the caffe2 model to
- detectron2's standard format.
- The function uses information in `predict_net` and `init_net` that are
- available at inferene time. Therefore the function logic can be used in inference.
-
- The returned function has the following signature:
-
- def convert(batched_inputs, c2_inputs, c2_results) -> detectron2_outputs
-
- Where
-
- * batched_inputs (list[dict]): the original input format of the meta arch
- * c2_inputs (tuple[Tensor]): the caffe2 inputs.
- * c2_results (dict[str, Tensor]): the caffe2 output format,
- corresponding to the outputs of the :meth:`forward` function.
- * detectron2_outputs: the original output format of the meta arch.
-
- This function can be used to compare the outputs of the original meta arch and
- the converted caffe2 graph.
-
- Returns:
- callable: a callable of the above signature.
- """
- raise NotImplementedError
-
-
-class Caffe2GeneralizedRCNN(Caffe2MetaArch):
- def __init__(self, cfg, torch_model):
- assert isinstance(torch_model, meta_arch.GeneralizedRCNN)
- torch_model = patch_generalized_rcnn(torch_model)
- super().__init__(cfg, torch_model)
-
- try:
- use_heatmap_max_keypoint = cfg.EXPORT_CAFFE2.USE_HEATMAP_MAX_KEYPOINT
- except AttributeError:
- use_heatmap_max_keypoint = False
- self.roi_heads_patcher = ROIHeadsPatcher(
- self._wrapped_model.roi_heads, use_heatmap_max_keypoint
- )
-
- def encode_additional_info(self, predict_net, init_net):
- size_divisibility = self._wrapped_model.backbone.size_divisibility
- check_set_pb_arg(predict_net, "size_divisibility", "i", size_divisibility)
- check_set_pb_arg(
- predict_net, "device", "s", str.encode(str(self._wrapped_model.device), "ascii")
- )
- check_set_pb_arg(predict_net, "meta_architecture", "s", b"GeneralizedRCNN")
-
- @mock_torch_nn_functional_interpolate()
- def forward(self, inputs):
- if not self.tensor_mode:
- return self._wrapped_model.inference(inputs)
- images = self._caffe2_preprocess_image(inputs)
- features = self._wrapped_model.backbone(images.tensor)
- proposals, _ = self._wrapped_model.proposal_generator(images, features)
- with self.roi_heads_patcher.mock_roi_heads():
- detector_results, _ = self._wrapped_model.roi_heads(images, features, proposals)
- return tuple(detector_results[0].flatten())
-
- @staticmethod
- def get_outputs_converter(predict_net, init_net):
- def f(batched_inputs, c2_inputs, c2_results):
- _, im_info = c2_inputs
- image_sizes = [[int(im[0]), int(im[1])] for im in im_info]
- results = assemble_rcnn_outputs_by_name(image_sizes, c2_results)
- return meta_arch.GeneralizedRCNN._postprocess(results, batched_inputs, image_sizes)
-
- return f
-
-
-class Caffe2RetinaNet(Caffe2MetaArch):
- def __init__(self, cfg, torch_model):
- assert isinstance(torch_model, meta_arch.RetinaNet)
- super().__init__(cfg, torch_model)
-
- @mock_torch_nn_functional_interpolate()
- def forward(self, inputs):
- assert self.tensor_mode
- images = self._caffe2_preprocess_image(inputs)
-
- # explicitly return the images sizes to avoid removing "im_info" by ONNX
- # since it's not used in the forward path
- return_tensors = [images.image_sizes]
-
- features = self._wrapped_model.backbone(images.tensor)
- features = [features[f] for f in self._wrapped_model.head_in_features]
- for i, feature_i in enumerate(features):
- features[i] = alias(feature_i, "feature_{}".format(i), is_backward=True)
- return_tensors.append(features[i])
-
- pred_logits, pred_anchor_deltas = self._wrapped_model.head(features)
- for i, (box_cls_i, box_delta_i) in enumerate(zip(pred_logits, pred_anchor_deltas)):
- return_tensors.append(alias(box_cls_i, "box_cls_{}".format(i)))
- return_tensors.append(alias(box_delta_i, "box_delta_{}".format(i)))
-
- return tuple(return_tensors)
-
- def encode_additional_info(self, predict_net, init_net):
- size_divisibility = self._wrapped_model.backbone.size_divisibility
- check_set_pb_arg(predict_net, "size_divisibility", "i", size_divisibility)
- check_set_pb_arg(
- predict_net, "device", "s", str.encode(str(self._wrapped_model.device), "ascii")
- )
- check_set_pb_arg(predict_net, "meta_architecture", "s", b"RetinaNet")
-
- # Inference parameters:
- check_set_pb_arg(
- predict_net, "score_threshold", "f", _cast_to_f32(self._wrapped_model.test_score_thresh)
- )
- check_set_pb_arg(
- predict_net, "topk_candidates", "i", self._wrapped_model.test_topk_candidates
- )
- check_set_pb_arg(
- predict_net, "nms_threshold", "f", _cast_to_f32(self._wrapped_model.test_nms_thresh)
- )
- check_set_pb_arg(
- predict_net,
- "max_detections_per_image",
- "i",
- self._wrapped_model.max_detections_per_image,
- )
-
- check_set_pb_arg(
- predict_net,
- "bbox_reg_weights",
- "floats",
- [_cast_to_f32(w) for w in self._wrapped_model.box2box_transform.weights],
- )
- self._encode_anchor_generator_cfg(predict_net)
-
- def _encode_anchor_generator_cfg(self, predict_net):
- # serialize anchor_generator for future use
- serialized_anchor_generator = io.BytesIO()
- torch.save(self._wrapped_model.anchor_generator, serialized_anchor_generator)
- # Ideally we can put anchor generating inside the model, then we don't
- # need to store this information.
- bytes = serialized_anchor_generator.getvalue()
- check_set_pb_arg(predict_net, "serialized_anchor_generator", "s", bytes)
-
- @staticmethod
- def get_outputs_converter(predict_net, init_net):
- self = types.SimpleNamespace()
- serialized_anchor_generator = io.BytesIO(
- get_pb_arg_vals(predict_net, "serialized_anchor_generator", None)
- )
- self.anchor_generator = torch.load(serialized_anchor_generator)
- bbox_reg_weights = get_pb_arg_floats(predict_net, "bbox_reg_weights", None)
- self.box2box_transform = Box2BoxTransform(weights=tuple(bbox_reg_weights))
- self.test_score_thresh = get_pb_arg_valf(predict_net, "score_threshold", None)
- self.test_topk_candidates = get_pb_arg_vali(predict_net, "topk_candidates", None)
- self.test_nms_thresh = get_pb_arg_valf(predict_net, "nms_threshold", None)
- self.max_detections_per_image = get_pb_arg_vali(
- predict_net, "max_detections_per_image", None
- )
-
- # hack to reuse inference code from RetinaNet
- for meth in [
- "forward_inference",
- "inference_single_image",
- "_transpose_dense_predictions",
- "_decode_multi_level_predictions",
- "_decode_per_level_predictions",
- ]:
- setattr(self, meth, functools.partial(getattr(meta_arch.RetinaNet, meth), self))
-
- def f(batched_inputs, c2_inputs, c2_results):
- _, im_info = c2_inputs
- image_sizes = [[int(im[0]), int(im[1])] for im in im_info]
- dummy_images = ImageList(
- torch.randn(
- (
- len(im_info),
- 3,
- )
- + tuple(image_sizes[0])
- ),
- image_sizes,
- )
-
- num_features = len([x for x in c2_results.keys() if x.startswith("box_cls_")])
- pred_logits = [c2_results["box_cls_{}".format(i)] for i in range(num_features)]
- pred_anchor_deltas = [c2_results["box_delta_{}".format(i)] for i in range(num_features)]
-
- # For each feature level, feature should have the same batch size and
- # spatial dimension as the box_cls and box_delta.
- dummy_features = [x.clone()[:, 0:0, :, :] for x in pred_logits]
- # self.num_classess can be inferred
- self.num_classes = pred_logits[0].shape[1] // (pred_anchor_deltas[0].shape[1] // 4)
-
- results = self.forward_inference(
- dummy_images, dummy_features, [pred_logits, pred_anchor_deltas]
- )
- return meta_arch.GeneralizedRCNN._postprocess(results, batched_inputs, image_sizes)
-
- return f
-
-
-META_ARCH_CAFFE2_EXPORT_TYPE_MAP = {
- "GeneralizedRCNN": Caffe2GeneralizedRCNN,
- "RetinaNet": Caffe2RetinaNet,
-}
diff --git a/spaces/Awiny/Image2Paragraph/models/grit_src/third_party/CenterNet2/detectron2/utils/__init__.py b/spaces/Awiny/Image2Paragraph/models/grit_src/third_party/CenterNet2/detectron2/utils/__init__.py
deleted file mode 100644
index 9020c2df23e2af280b7bb168b996ae9eaf312eb8..0000000000000000000000000000000000000000
--- a/spaces/Awiny/Image2Paragraph/models/grit_src/third_party/CenterNet2/detectron2/utils/__init__.py
+++ /dev/null
@@ -1 +0,0 @@
-# Copyright (c) Facebook, Inc. and its affiliates.
diff --git a/spaces/Bart92/RVC_HF/infer/modules/train/extract/extract_f0_rmvpe.py b/spaces/Bart92/RVC_HF/infer/modules/train/extract/extract_f0_rmvpe.py
deleted file mode 100644
index c6c90440d9e612b37c6d5a514786a6d0fffb19ba..0000000000000000000000000000000000000000
--- a/spaces/Bart92/RVC_HF/infer/modules/train/extract/extract_f0_rmvpe.py
+++ /dev/null
@@ -1,141 +0,0 @@
-import os
-import sys
-import traceback
-
-import parselmouth
-
-now_dir = os.getcwd()
-sys.path.append(now_dir)
-import logging
-
-import numpy as np
-import pyworld
-
-from infer.lib.audio import load_audio
-
-logging.getLogger("numba").setLevel(logging.WARNING)
-
-n_part = int(sys.argv[1])
-i_part = int(sys.argv[2])
-i_gpu = sys.argv[3]
-os.environ["CUDA_VISIBLE_DEVICES"] = str(i_gpu)
-exp_dir = sys.argv[4]
-is_half = sys.argv[5]
-f = open("%s/extract_f0_feature.log" % exp_dir, "a+")
-
-
-def printt(strr):
- print(strr)
- f.write("%s\n" % strr)
- f.flush()
-
-
-class FeatureInput(object):
- def __init__(self, samplerate=16000, hop_size=160):
- self.fs = samplerate
- self.hop = hop_size
-
- self.f0_bin = 256
- self.f0_max = 1100.0
- self.f0_min = 50.0
- self.f0_mel_min = 1127 * np.log(1 + self.f0_min / 700)
- self.f0_mel_max = 1127 * np.log(1 + self.f0_max / 700)
-
- def compute_f0(self, path, f0_method):
- x = load_audio(path, self.fs)
- # p_len = x.shape[0] // self.hop
- if f0_method == "rmvpe":
- if hasattr(self, "model_rmvpe") == False:
- from infer.lib.rmvpe import RMVPE
-
- print("Loading rmvpe model")
- self.model_rmvpe = RMVPE(
- "assets/rmvpe/rmvpe.pt", is_half=is_half, device="cuda"
- )
- f0 = self.model_rmvpe.infer_from_audio(x, thred=0.03)
- return f0
-
- def coarse_f0(self, f0):
- f0_mel = 1127 * np.log(1 + f0 / 700)
- f0_mel[f0_mel > 0] = (f0_mel[f0_mel > 0] - self.f0_mel_min) * (
- self.f0_bin - 2
- ) / (self.f0_mel_max - self.f0_mel_min) + 1
-
- # use 0 or 1
- f0_mel[f0_mel <= 1] = 1
- f0_mel[f0_mel > self.f0_bin - 1] = self.f0_bin - 1
- f0_coarse = np.rint(f0_mel).astype(int)
- assert f0_coarse.max() <= 255 and f0_coarse.min() >= 1, (
- f0_coarse.max(),
- f0_coarse.min(),
- )
- return f0_coarse
-
- def go(self, paths, f0_method):
- if len(paths) == 0:
- printt("no-f0-todo")
- else:
- printt("todo-f0-%s" % len(paths))
- n = max(len(paths) // 5, 1) # 每个进程最多打印5条
- for idx, (inp_path, opt_path1, opt_path2) in enumerate(paths):
- try:
- if idx % n == 0:
- printt("f0ing,now-%s,all-%s,-%s" % (idx, len(paths), inp_path))
- if (
- os.path.exists(opt_path1 + ".npy") == True
- and os.path.exists(opt_path2 + ".npy") == True
- ):
- continue
- featur_pit = self.compute_f0(inp_path, f0_method)
- np.save(
- opt_path2,
- featur_pit,
- allow_pickle=False,
- ) # nsf
- coarse_pit = self.coarse_f0(featur_pit)
- np.save(
- opt_path1,
- coarse_pit,
- allow_pickle=False,
- ) # ori
- except:
- printt("f0fail-%s-%s-%s" % (idx, inp_path, traceback.format_exc()))
-
-
-if __name__ == "__main__":
- # exp_dir=r"E:\codes\py39\dataset\mi-test"
- # n_p=16
- # f = open("%s/log_extract_f0.log"%exp_dir, "w")
- printt(sys.argv)
- featureInput = FeatureInput()
- paths = []
- inp_root = "%s/1_16k_wavs" % (exp_dir)
- opt_root1 = "%s/2a_f0" % (exp_dir)
- opt_root2 = "%s/2b-f0nsf" % (exp_dir)
-
- os.makedirs(opt_root1, exist_ok=True)
- os.makedirs(opt_root2, exist_ok=True)
- for name in sorted(list(os.listdir(inp_root))):
- inp_path = "%s/%s" % (inp_root, name)
- if "spec" in inp_path:
- continue
- opt_path1 = "%s/%s" % (opt_root1, name)
- opt_path2 = "%s/%s" % (opt_root2, name)
- paths.append([inp_path, opt_path1, opt_path2])
- try:
- featureInput.go(paths[i_part::n_part], "rmvpe")
- except:
- printt("f0_all_fail-%s" % (traceback.format_exc()))
- # ps = []
- # for i in range(n_p):
- # p = Process(
- # target=featureInput.go,
- # args=(
- # paths[i::n_p],
- # f0method,
- # ),
- # )
- # ps.append(p)
- # p.start()
- # for i in range(n_p):
- # ps[i].join()
diff --git a/spaces/Benson/text-generation/Examples/Descarga De La Red M.hollywoodbets.net.md b/spaces/Benson/text-generation/Examples/Descarga De La Red M.hollywoodbets.net.md
deleted file mode 100644
index e9dd1693d2367b79141fa776613f355ed7efb9d5..0000000000000000000000000000000000000000
--- a/spaces/Benson/text-generation/Examples/Descarga De La Red M.hollywoodbets.net.md
+++ /dev/null
@@ -1,104 +0,0 @@
-
-
Cómo descargar y usar m.hollywoodbets.net
-
Si usted está buscando una manera conveniente y rápida de apostar en deportes, carreras de caballos, juegos de casino, y más, es posible que desee descargar y utilizar m.hollywoodbets.net. Esta es la versión móvil de Hollywoodbets, una de las plataformas de apuestas en línea más populares en Sudáfrica. En este artículo, le mostraremos lo que es m.hollywoodbets.net, por qué debe descargarlo, cómo descargarlo, cómo usarlo y cómo resolver algunos problemas comunes con él.
m.hollywoodbets.net es el sitio móvil de Hollywoodbets, un operador de apuestas con licencia que ofrece una amplia gama de opciones de apuestas en varios deportes y eventos. Puedes apostar en fútbol, rugby, cricket, tenis, golf, baloncesto, etc. También puedes apostar en carreras de caballos desde Sudáfrica y otros países. Además, puedes jugar juegos de casino, tragamonedas, números de la suerte, betgames, juegos en vivo y más. Puede acceder a todas estas funciones desde su dispositivo móvil usando m.hollywoodbets.net.
-
¿Por qué descargar m.hollywoodbets.net?
-
Hay muchos beneficios de descargar y usar m.hollywoodbets.net. Estos son algunos de ellos:
-
-
Conveniencia: Puedes apostar en cualquier momento y en cualquier lugar usando tu dispositivo móvil. No necesita un ordenador o un navegador para acceder al sitio. Simplemente puede tocar el icono de la aplicación y comenzar a apostar.
-
Velocidad: El sitio móvil está optimizado para una carga rápida y un rendimiento suave. Usted puede hacer sus apuestas rápida y fácilmente sin ningún tipo de retrasos o fallos.
-
Acceso sin datos: Puedes acceder al sitio móvil sin usar ningún dato. Hollywoodbets se ha asociado con varios proveedores de red para ofrecer acceso sin datos a sus clientes. Puede comprobar si su proveedor de red es compatible visitando [1](https://sport.hollywoodbets.net/).
-
-
-
¿Cómo descargar m.hollywoodbets.net?
-
Si tienes un dispositivo Android, puedes descargar e instalar la aplicación para m.hollywoodbets.net siguiendo estos pasos:
-
-
Visite [1](https://sport.hollywoodbets.net/) desde su navegador móvil e inicie sesión en su cuenta. Si aún no tiene una cuenta, puede registrarla haciendo clic en "Unirse ahora".
-
Desplácese hacia abajo a la parte inferior de la página y haga clic en "Aplicación para teléfono de características básicas". Esto lo redirigirá a un sitio donde puede descargar la aplicación.
-
Haga clic en "Descargar aplicación Android" y esperar a que la descarga se complete.
-
Vaya a la configuración de seguridad y permita la instalación desde fuentes desconocidas.
-
Abra el archivo descargado e instale la aplicación en su dispositivo.
-
-
Tenga en cuenta que no hay una aplicación oficial para dispositivos iOS, por lo que tendrá que utilizar la versión del navegador móvil si tiene un iPhone o iPad.
-
¿Cómo usar m.hollywoodbets.net?
-
Usar m.hollywoodbets.net es fácil y simple. Estos son algunos pasos básicos para empezar:
-
-
-
Inicie sesión en su cuenta usando su nombre de usuario y contraseña. Si olvidó su contraseña, puede restablecerla haciendo clic en "Olvidé la contraseña".
-
Elija la categoría de apuestas que desea hacer, como deportes, carreras de caballos, casino, etc. Puede utilizar el icono del menú en la esquina superior izquierda para navegar entre diferentes categorías.
-
Selecciona el evento o juego en el que quieres apostar. Puedes usar la barra de búsqueda o los filtros para encontrar lo que buscas.
-
Elija el mercado y las probabilidades en las que desea apostar. Puede pulsar en las probabilidades para agregarlas a su boleto de apuesta.
-
Introduzca la cantidad que desea apostar y confirme su apuesta. También puede usar la función "Quick Bet" para realizar su apuesta más rápido.
-
Revise su historial de apuestas y balance haciendo clic en "Mi cuenta". También puede ver sus apuestas pendientes, apuestas liquidadas y apuestas abiertas.
-
-
-
Para depositar y retirar dinero usando m.hollywoodbets.net, necesita tener una cuenta verificada y una cuenta bancaria o tarjeta válida. Estos son algunos métodos que puedes usar:
-
-
Método
Depósito
Retiro
-
Transferencia bancaria
Sí
Sí
-
Tarjeta de crédito/débito
Sí
No
-
EFT
Sí
No
-
Ozow
Sí
No
-
Peach Payments
Sí
No
-
Zapper
Sí
No
-
Voucher
Sí
No
-
Ramas de Hollywoodbets
Sí
Sí
-
Tarjeta de cajero automático Hollywoodbets
No
Sí
-
Hollywoodbets eWallet (FNB)
No
Sí
-
Hollywoodbets Instant Money (Standard Bank)
No
Sí
-
Envío de efectivo de Hollywoodbets (Absa)
No
Sí
-
Hollywoodbets Cash Send Plus (Nedbank)
No
Sí
-
-
Para hacer un depósito, puedes seguir estos pasos:
-
-
Inicie sesión en su cuenta y haga clic en "Depositar".
-
Seleccione el método que desea utilizar e introduzca la cantidad que desea depositar.
-
Siga las instrucciones en la pantalla para completar la transacción.
-
Espera el mensaje de confirmación y comprueba tu saldo.
-
-
Para realizar un retiro, puedes seguir estos pasos:
-
-
Inicie sesión en su cuenta y haga clic en "Retirar".
-
Seleccione el método que desea utilizar e introduzca la cantidad que desea retirar.
-
Introduzca los datos de su cuenta bancaria o tarjeta si es necesario.
-
Confirme su solicitud y espere la aprobación.
-
Revise su cuenta bancaria o extracto de la tarjeta para los fondos.
-
-
¿Cómo contactar a atención al cliente usando m.hollywoodbets.net?
-
-
-
Live Chat: Puede utilizar la función de chat en vivo en el sitio móvil para chatear con un agente amigable y útil. Puede acceder al chat en vivo haciendo clic en el icono "Ayuda" en la esquina inferior derecha de la pantalla.
-
Correo electrónico: Puede enviar un correo electrónico a [email protected] o [email protected] y esperar una respuesta en 24 horas.
-
Teléfono: Puede llamar al número gratuito 08600 42387 o al número alternativo 087 353 7634 y hablar con un representante.
-
Redes sociales: Puedes seguir a Hollywoodbets en Facebook, Twitter, Instagram, YouTube y Telegram y enviarles un mensaje o comentario en sus publicaciones.
-
-
Problemas comunes con m.hollywoodbets.net y cómo resolverlos
-
Mientras m.hollywoodbets.net está diseñado para proporcionar una experiencia de apuestas sin problemas y sin problemas, es posible que encuentre algunos problemas con él de vez en cuando. Estos son algunos de los problemas comunes y cómo resolverlos:
-
-
Errores de inicio de sesión: Si no puede iniciar sesión en su cuenta, es posible que haya introducido el nombre de usuario o contraseña incorrectos, o que su cuenta esté bloqueada debido a inactividad o razones de seguridad. Para resolver este problema, puede intentar restablecer su contraseña haciendo clic en "Olvidé la contraseña", o ponerse en contacto con el servicio de atención al cliente para desbloquear su cuenta.
-
Inactividad de la cuenta: Si no ha utilizado su cuenta durante más de 90 días, puede desactivarse debido a la inactividad. Para reactivar su cuenta, debe ponerse en contacto con el servicio de atención al cliente y proporcionarle sus documentos FICA (prueba de identidad y dirección).
-
-
FICA verification: FICA significa Financial Intelligence Centre Act, que es una ley que requiere que todos los operadores de apuestas verifiquen la identidad y la dirección de sus clientes. Para cumplir con esta ley, debe presentar sus documentos FICA (prueba de identidad y dirección) cuando registre una cuenta o realice un retiro. Puede subir sus documentos en línea haciendo clic en "Mi cuenta" y "FICA", o enviarlos por correo electrónico a [email protected]
-
-
Conclusión
-
m.hollywoodbets.net es una gran manera de disfrutar de las apuestas en línea en su dispositivo móvil. Puede descargarlo y usarlo fácilmente y acceder a una variedad de opciones de apuestas, promociones y características. También puede depositar y retirar dinero de forma segura y ponerse en contacto con el servicio de atención al cliente convenientemente. Si encuentra algún problema con el sitio móvil, puede seguir los consejos anteriores o comunicarse con el servicio de atención al cliente para obtener ayuda. Entonces, ¿qué estás esperando? Descargar m.hollywoodbets.net hoy y empezar a apostar!
-
Preguntas frecuentes
-
¿Es m.hollywoodbets.net seguro y legal?
-
Sí, m.hollywoodbets.net es seguro y legal. Hollywoodbets está autorizado por el Western Cape Gambling and Racing Board y se adhiere a estrictas normas de seguridad. Todas las transacciones están encriptadas y protegidas por la tecnología SSL. Toda la información personal se mantiene confidencial y no se comparte con terceros.
-
¿Cuáles son las apuestas mínimas y máximas en m.hollywoodbets.net? La apuesta mínima en m.hollywoodbets.net es R1, mientras que la apuesta máxima depende del evento y el mercado en el que esté apostando. Puede comprobar la apuesta máxima haciendo clic en "Max Bet" en su boleto de apuesta.
-
¿Cómo puedo obtener apuestas gratis en m.hollywoodbets.net?
-
Hay varias formas de obtener apuestas gratis en m.hollywoodbets.net. Algunas de ellas son:
-
-
Registrar una nueva cuenta y obtener un bono de registro R25.
-
Referir a un amigo y obtener un bono R50 por cada referencia exitosa.
-
-
Uso de vales que puede comprar en los minoristas seleccionados o recibir de atención al cliente.
-
-
¿Cómo puedo comprobar los resultados de mis apuestas en m.hollywoodbets.net?
-
Puede comprobar los resultados de sus apuestas en m.hollywoodbets.net haciendo clic en "Mi cuenta" y "Historial de apuestas". También puede utilizar la función "Resultados" en el sitio móvil para comprobar los resultados de varios eventos y juegos.
-
¿Cómo puedo actualizar mis datos personales en m.hollywoodbets.net?
-
Puede actualizar sus datos personales en m.hollywoodbets.net haciendo clic en "Mi cuenta" y "Datos personales". Puede cambiar su contraseña, dirección de correo electrónico, número de teléfono y pregunta de seguridad. Sin embargo, no puede cambiar su nombre, apellido, fecha de nacimiento o número de identificación. Si necesita cambiar estos datos, debe ponerse en contacto con el servicio de atención al cliente y proporcionarle una prueba de identidad.
64aa2da5cf
-
-
\ No newline at end of file
diff --git a/spaces/Big-Web/MMSD/env/Lib/site-packages/dateutil/zoneinfo/rebuild.py b/spaces/Big-Web/MMSD/env/Lib/site-packages/dateutil/zoneinfo/rebuild.py
deleted file mode 100644
index 684c6586f091350c347f2b6150935f5214ffec27..0000000000000000000000000000000000000000
--- a/spaces/Big-Web/MMSD/env/Lib/site-packages/dateutil/zoneinfo/rebuild.py
+++ /dev/null
@@ -1,75 +0,0 @@
-import logging
-import os
-import tempfile
-import shutil
-import json
-from subprocess import check_call, check_output
-from tarfile import TarFile
-
-from dateutil.zoneinfo import METADATA_FN, ZONEFILENAME
-
-
-def rebuild(filename, tag=None, format="gz", zonegroups=[], metadata=None):
- """Rebuild the internal timezone info in dateutil/zoneinfo/zoneinfo*tar*
-
- filename is the timezone tarball from ``ftp.iana.org/tz``.
-
- """
- tmpdir = tempfile.mkdtemp()
- zonedir = os.path.join(tmpdir, "zoneinfo")
- moduledir = os.path.dirname(__file__)
- try:
- with TarFile.open(filename) as tf:
- for name in zonegroups:
- tf.extract(name, tmpdir)
- filepaths = [os.path.join(tmpdir, n) for n in zonegroups]
-
- _run_zic(zonedir, filepaths)
-
- # write metadata file
- with open(os.path.join(zonedir, METADATA_FN), 'w') as f:
- json.dump(metadata, f, indent=4, sort_keys=True)
- target = os.path.join(moduledir, ZONEFILENAME)
- with TarFile.open(target, "w:%s" % format) as tf:
- for entry in os.listdir(zonedir):
- entrypath = os.path.join(zonedir, entry)
- tf.add(entrypath, entry)
- finally:
- shutil.rmtree(tmpdir)
-
-
-def _run_zic(zonedir, filepaths):
- """Calls the ``zic`` compiler in a compatible way to get a "fat" binary.
-
- Recent versions of ``zic`` default to ``-b slim``, while older versions
- don't even have the ``-b`` option (but default to "fat" binaries). The
- current version of dateutil does not support Version 2+ TZif files, which
- causes problems when used in conjunction with "slim" binaries, so this
- function is used to ensure that we always get a "fat" binary.
- """
-
- try:
- help_text = check_output(["zic", "--help"])
- except OSError as e:
- _print_on_nosuchfile(e)
- raise
-
- if b"-b " in help_text:
- bloat_args = ["-b", "fat"]
- else:
- bloat_args = []
-
- check_call(["zic"] + bloat_args + ["-d", zonedir] + filepaths)
-
-
-def _print_on_nosuchfile(e):
- """Print helpful troubleshooting message
-
- e is an exception raised by subprocess.check_call()
-
- """
- if e.errno == 2:
- logging.error(
- "Could not find zic. Perhaps you need to install "
- "libc-bin or some other package that provides it, "
- "or it's not in your PATH?")
diff --git a/spaces/Big-Web/MMSD/env/Lib/site-packages/pip/_vendor/chardet/metadata/languages.py b/spaces/Big-Web/MMSD/env/Lib/site-packages/pip/_vendor/chardet/metadata/languages.py
deleted file mode 100644
index eb40c5f0c8526208d434d762855d23079dc68b36..0000000000000000000000000000000000000000
--- a/spaces/Big-Web/MMSD/env/Lib/site-packages/pip/_vendor/chardet/metadata/languages.py
+++ /dev/null
@@ -1,352 +0,0 @@
-"""
-Metadata about languages used by our model training code for our
-SingleByteCharSetProbers. Could be used for other things in the future.
-
-This code is based on the language metadata from the uchardet project.
-"""
-
-from string import ascii_letters
-from typing import List, Optional
-
-# TODO: Add Ukrainian (KOI8-U)
-
-
-class Language:
- """Metadata about a language useful for training models
-
- :ivar name: The human name for the language, in English.
- :type name: str
- :ivar iso_code: 2-letter ISO 639-1 if possible, 3-letter ISO code otherwise,
- or use another catalog as a last resort.
- :type iso_code: str
- :ivar use_ascii: Whether or not ASCII letters should be included in trained
- models.
- :type use_ascii: bool
- :ivar charsets: The charsets we want to support and create data for.
- :type charsets: list of str
- :ivar alphabet: The characters in the language's alphabet. If `use_ascii` is
- `True`, you only need to add those not in the ASCII set.
- :type alphabet: str
- :ivar wiki_start_pages: The Wikipedia pages to start from if we're crawling
- Wikipedia for training data.
- :type wiki_start_pages: list of str
- """
-
- def __init__(
- self,
- name: Optional[str] = None,
- iso_code: Optional[str] = None,
- use_ascii: bool = True,
- charsets: Optional[List[str]] = None,
- alphabet: Optional[str] = None,
- wiki_start_pages: Optional[List[str]] = None,
- ) -> None:
- super().__init__()
- self.name = name
- self.iso_code = iso_code
- self.use_ascii = use_ascii
- self.charsets = charsets
- if self.use_ascii:
- if alphabet:
- alphabet += ascii_letters
- else:
- alphabet = ascii_letters
- elif not alphabet:
- raise ValueError("Must supply alphabet if use_ascii is False")
- self.alphabet = "".join(sorted(set(alphabet))) if alphabet else None
- self.wiki_start_pages = wiki_start_pages
-
- def __repr__(self) -> str:
- param_str = ", ".join(
- f"{k}={v!r}" for k, v in self.__dict__.items() if not k.startswith("_")
- )
- return f"{self.__class__.__name__}({param_str})"
-
-
-LANGUAGES = {
- "Arabic": Language(
- name="Arabic",
- iso_code="ar",
- use_ascii=False,
- # We only support encodings that use isolated
- # forms, because the current recommendation is
- # that the rendering system handles presentation
- # forms. This means we purposefully skip IBM864.
- charsets=["ISO-8859-6", "WINDOWS-1256", "CP720", "CP864"],
- alphabet="ءآأؤإئابةتثجحخدذرزسشصضطظعغػؼؽؾؿـفقكلمنهوىيًٌٍَُِّ",
- wiki_start_pages=["الصفحة_الرئيسية"],
- ),
- "Belarusian": Language(
- name="Belarusian",
- iso_code="be",
- use_ascii=False,
- charsets=["ISO-8859-5", "WINDOWS-1251", "IBM866", "MacCyrillic"],
- alphabet="АБВГДЕЁЖЗІЙКЛМНОПРСТУЎФХЦЧШЫЬЭЮЯабвгдеёжзійклмнопрстуўфхцчшыьэюяʼ",
- wiki_start_pages=["Галоўная_старонка"],
- ),
- "Bulgarian": Language(
- name="Bulgarian",
- iso_code="bg",
- use_ascii=False,
- charsets=["ISO-8859-5", "WINDOWS-1251", "IBM855"],
- alphabet="АБВГДЕЖЗИЙКЛМНОПРСТУФХЦЧШЩЪЬЮЯабвгдежзийклмнопрстуфхцчшщъьюя",
- wiki_start_pages=["Начална_страница"],
- ),
- "Czech": Language(
- name="Czech",
- iso_code="cz",
- use_ascii=True,
- charsets=["ISO-8859-2", "WINDOWS-1250"],
- alphabet="áčďéěíňóřšťúůýžÁČĎÉĚÍŇÓŘŠŤÚŮÝŽ",
- wiki_start_pages=["Hlavní_strana"],
- ),
- "Danish": Language(
- name="Danish",
- iso_code="da",
- use_ascii=True,
- charsets=["ISO-8859-1", "ISO-8859-15", "WINDOWS-1252", "MacRoman"],
- alphabet="æøåÆØÅ",
- wiki_start_pages=["Forside"],
- ),
- "German": Language(
- name="German",
- iso_code="de",
- use_ascii=True,
- charsets=["ISO-8859-1", "ISO-8859-15", "WINDOWS-1252", "MacRoman"],
- alphabet="äöüßẞÄÖÜ",
- wiki_start_pages=["Wikipedia:Hauptseite"],
- ),
- "Greek": Language(
- name="Greek",
- iso_code="el",
- use_ascii=False,
- charsets=["ISO-8859-7", "WINDOWS-1253"],
- alphabet="αβγδεζηθικλμνξοπρσςτυφχψωάέήίόύώΑΒΓΔΕΖΗΘΙΚΛΜΝΞΟΠΡΣΣΤΥΦΧΨΩΆΈΉΊΌΎΏ",
- wiki_start_pages=["Πύλη:Κύρια"],
- ),
- "English": Language(
- name="English",
- iso_code="en",
- use_ascii=True,
- charsets=["ISO-8859-1", "WINDOWS-1252", "MacRoman"],
- wiki_start_pages=["Main_Page"],
- ),
- "Esperanto": Language(
- name="Esperanto",
- iso_code="eo",
- # Q, W, X, and Y not used at all
- use_ascii=False,
- charsets=["ISO-8859-3"],
- alphabet="abcĉdefgĝhĥijĵklmnoprsŝtuŭvzABCĈDEFGĜHĤIJĴKLMNOPRSŜTUŬVZ",
- wiki_start_pages=["Vikipedio:Ĉefpaĝo"],
- ),
- "Spanish": Language(
- name="Spanish",
- iso_code="es",
- use_ascii=True,
- charsets=["ISO-8859-1", "ISO-8859-15", "WINDOWS-1252", "MacRoman"],
- alphabet="ñáéíóúüÑÁÉÍÓÚÜ",
- wiki_start_pages=["Wikipedia:Portada"],
- ),
- "Estonian": Language(
- name="Estonian",
- iso_code="et",
- use_ascii=False,
- charsets=["ISO-8859-4", "ISO-8859-13", "WINDOWS-1257"],
- # C, F, Š, Q, W, X, Y, Z, Ž are only for
- # loanwords
- alphabet="ABDEGHIJKLMNOPRSTUVÕÄÖÜabdeghijklmnoprstuvõäöü",
- wiki_start_pages=["Esileht"],
- ),
- "Finnish": Language(
- name="Finnish",
- iso_code="fi",
- use_ascii=True,
- charsets=["ISO-8859-1", "ISO-8859-15", "WINDOWS-1252", "MacRoman"],
- alphabet="ÅÄÖŠŽåäöšž",
- wiki_start_pages=["Wikipedia:Etusivu"],
- ),
- "French": Language(
- name="French",
- iso_code="fr",
- use_ascii=True,
- charsets=["ISO-8859-1", "ISO-8859-15", "WINDOWS-1252", "MacRoman"],
- alphabet="œàâçèéîïùûêŒÀÂÇÈÉÎÏÙÛÊ",
- wiki_start_pages=["Wikipédia:Accueil_principal", "Bœuf (animal)"],
- ),
- "Hebrew": Language(
- name="Hebrew",
- iso_code="he",
- use_ascii=False,
- charsets=["ISO-8859-8", "WINDOWS-1255"],
- alphabet="אבגדהוזחטיךכלםמןנסעףפץצקרשתװױײ",
- wiki_start_pages=["עמוד_ראשי"],
- ),
- "Croatian": Language(
- name="Croatian",
- iso_code="hr",
- # Q, W, X, Y are only used for foreign words.
- use_ascii=False,
- charsets=["ISO-8859-2", "WINDOWS-1250"],
- alphabet="abcčćdđefghijklmnoprsštuvzžABCČĆDĐEFGHIJKLMNOPRSŠTUVZŽ",
- wiki_start_pages=["Glavna_stranica"],
- ),
- "Hungarian": Language(
- name="Hungarian",
- iso_code="hu",
- # Q, W, X, Y are only used for foreign words.
- use_ascii=False,
- charsets=["ISO-8859-2", "WINDOWS-1250"],
- alphabet="abcdefghijklmnoprstuvzáéíóöőúüűABCDEFGHIJKLMNOPRSTUVZÁÉÍÓÖŐÚÜŰ",
- wiki_start_pages=["Kezdőlap"],
- ),
- "Italian": Language(
- name="Italian",
- iso_code="it",
- use_ascii=True,
- charsets=["ISO-8859-1", "ISO-8859-15", "WINDOWS-1252", "MacRoman"],
- alphabet="ÀÈÉÌÒÓÙàèéìòóù",
- wiki_start_pages=["Pagina_principale"],
- ),
- "Lithuanian": Language(
- name="Lithuanian",
- iso_code="lt",
- use_ascii=False,
- charsets=["ISO-8859-13", "WINDOWS-1257", "ISO-8859-4"],
- # Q, W, and X not used at all
- alphabet="AĄBCČDEĘĖFGHIĮYJKLMNOPRSŠTUŲŪVZŽaąbcčdeęėfghiįyjklmnoprsštuųūvzž",
- wiki_start_pages=["Pagrindinis_puslapis"],
- ),
- "Latvian": Language(
- name="Latvian",
- iso_code="lv",
- use_ascii=False,
- charsets=["ISO-8859-13", "WINDOWS-1257", "ISO-8859-4"],
- # Q, W, X, Y are only for loanwords
- alphabet="AĀBCČDEĒFGĢHIĪJKĶLĻMNŅOPRSŠTUŪVZŽaābcčdeēfgģhiījkķlļmnņoprsštuūvzž",
- wiki_start_pages=["Sākumlapa"],
- ),
- "Macedonian": Language(
- name="Macedonian",
- iso_code="mk",
- use_ascii=False,
- charsets=["ISO-8859-5", "WINDOWS-1251", "MacCyrillic", "IBM855"],
- alphabet="АБВГДЃЕЖЗЅИЈКЛЉМНЊОПРСТЌУФХЦЧЏШабвгдѓежзѕијклљмнњопрстќуфхцчџш",
- wiki_start_pages=["Главна_страница"],
- ),
- "Dutch": Language(
- name="Dutch",
- iso_code="nl",
- use_ascii=True,
- charsets=["ISO-8859-1", "WINDOWS-1252", "MacRoman"],
- wiki_start_pages=["Hoofdpagina"],
- ),
- "Polish": Language(
- name="Polish",
- iso_code="pl",
- # Q and X are only used for foreign words.
- use_ascii=False,
- charsets=["ISO-8859-2", "WINDOWS-1250"],
- alphabet="AĄBCĆDEĘFGHIJKLŁMNŃOÓPRSŚTUWYZŹŻaąbcćdeęfghijklłmnńoóprsśtuwyzźż",
- wiki_start_pages=["Wikipedia:Strona_główna"],
- ),
- "Portuguese": Language(
- name="Portuguese",
- iso_code="pt",
- use_ascii=True,
- charsets=["ISO-8859-1", "ISO-8859-15", "WINDOWS-1252", "MacRoman"],
- alphabet="ÁÂÃÀÇÉÊÍÓÔÕÚáâãàçéêíóôõú",
- wiki_start_pages=["Wikipédia:Página_principal"],
- ),
- "Romanian": Language(
- name="Romanian",
- iso_code="ro",
- use_ascii=True,
- charsets=["ISO-8859-2", "WINDOWS-1250"],
- alphabet="ăâîșțĂÂÎȘȚ",
- wiki_start_pages=["Pagina_principală"],
- ),
- "Russian": Language(
- name="Russian",
- iso_code="ru",
- use_ascii=False,
- charsets=[
- "ISO-8859-5",
- "WINDOWS-1251",
- "KOI8-R",
- "MacCyrillic",
- "IBM866",
- "IBM855",
- ],
- alphabet="абвгдеёжзийклмнопрстуфхцчшщъыьэюяАБВГДЕЁЖЗИЙКЛМНОПРСТУФХЦЧШЩЪЫЬЭЮЯ",
- wiki_start_pages=["Заглавная_страница"],
- ),
- "Slovak": Language(
- name="Slovak",
- iso_code="sk",
- use_ascii=True,
- charsets=["ISO-8859-2", "WINDOWS-1250"],
- alphabet="áäčďéíĺľňóôŕšťúýžÁÄČĎÉÍĹĽŇÓÔŔŠŤÚÝŽ",
- wiki_start_pages=["Hlavná_stránka"],
- ),
- "Slovene": Language(
- name="Slovene",
- iso_code="sl",
- # Q, W, X, Y are only used for foreign words.
- use_ascii=False,
- charsets=["ISO-8859-2", "WINDOWS-1250"],
- alphabet="abcčdefghijklmnoprsštuvzžABCČDEFGHIJKLMNOPRSŠTUVZŽ",
- wiki_start_pages=["Glavna_stran"],
- ),
- # Serbian can be written in both Latin and Cyrillic, but there's no
- # simple way to get the Latin alphabet pages from Wikipedia through
- # the API, so for now we just support Cyrillic.
- "Serbian": Language(
- name="Serbian",
- iso_code="sr",
- alphabet="АБВГДЂЕЖЗИЈКЛЉМНЊОПРСТЋУФХЦЧЏШабвгдђежзијклљмнњопрстћуфхцчџш",
- charsets=["ISO-8859-5", "WINDOWS-1251", "MacCyrillic", "IBM855"],
- wiki_start_pages=["Главна_страна"],
- ),
- "Thai": Language(
- name="Thai",
- iso_code="th",
- use_ascii=False,
- charsets=["ISO-8859-11", "TIS-620", "CP874"],
- alphabet="กขฃคฅฆงจฉชซฌญฎฏฐฑฒณดตถทธนบปผฝพฟภมยรฤลฦวศษสหฬอฮฯะัาำิีึืฺุู฿เแโใไๅๆ็่้๊๋์ํ๎๏๐๑๒๓๔๕๖๗๘๙๚๛",
- wiki_start_pages=["หน้าหลัก"],
- ),
- "Turkish": Language(
- name="Turkish",
- iso_code="tr",
- # Q, W, and X are not used by Turkish
- use_ascii=False,
- charsets=["ISO-8859-3", "ISO-8859-9", "WINDOWS-1254"],
- alphabet="abcçdefgğhıijklmnoöprsştuüvyzâîûABCÇDEFGĞHIİJKLMNOÖPRSŞTUÜVYZÂÎÛ",
- wiki_start_pages=["Ana_Sayfa"],
- ),
- "Vietnamese": Language(
- name="Vietnamese",
- iso_code="vi",
- use_ascii=False,
- # Windows-1258 is the only common 8-bit
- # Vietnamese encoding supported by Python.
- # From Wikipedia:
- # For systems that lack support for Unicode,
- # dozens of 8-bit Vietnamese code pages are
- # available.[1] The most common are VISCII
- # (TCVN 5712:1993), VPS, and Windows-1258.[3]
- # Where ASCII is required, such as when
- # ensuring readability in plain text e-mail,
- # Vietnamese letters are often encoded
- # according to Vietnamese Quoted-Readable
- # (VIQR) or VSCII Mnemonic (VSCII-MNEM),[4]
- # though usage of either variable-width
- # scheme has declined dramatically following
- # the adoption of Unicode on the World Wide
- # Web.
- charsets=["WINDOWS-1258"],
- alphabet="aăâbcdđeêghiklmnoôơpqrstuưvxyAĂÂBCDĐEÊGHIKLMNOÔƠPQRSTUƯVXY",
- wiki_start_pages=["Chữ_Quốc_ngữ"],
- ),
-}
diff --git a/spaces/Big-Web/MMSD/env/Lib/site-packages/pip/_vendor/requests/cookies.py b/spaces/Big-Web/MMSD/env/Lib/site-packages/pip/_vendor/requests/cookies.py
deleted file mode 100644
index bf54ab237e410603061b8cec8fd195912d3cfb08..0000000000000000000000000000000000000000
--- a/spaces/Big-Web/MMSD/env/Lib/site-packages/pip/_vendor/requests/cookies.py
+++ /dev/null
@@ -1,561 +0,0 @@
-"""
-requests.cookies
-~~~~~~~~~~~~~~~~
-
-Compatibility code to be able to use `cookielib.CookieJar` with requests.
-
-requests.utils imports from here, so be careful with imports.
-"""
-
-import calendar
-import copy
-import time
-
-from ._internal_utils import to_native_string
-from .compat import Morsel, MutableMapping, cookielib, urlparse, urlunparse
-
-try:
- import threading
-except ImportError:
- import dummy_threading as threading
-
-
-class MockRequest:
- """Wraps a `requests.Request` to mimic a `urllib2.Request`.
-
- The code in `cookielib.CookieJar` expects this interface in order to correctly
- manage cookie policies, i.e., determine whether a cookie can be set, given the
- domains of the request and the cookie.
-
- The original request object is read-only. The client is responsible for collecting
- the new headers via `get_new_headers()` and interpreting them appropriately. You
- probably want `get_cookie_header`, defined below.
- """
-
- def __init__(self, request):
- self._r = request
- self._new_headers = {}
- self.type = urlparse(self._r.url).scheme
-
- def get_type(self):
- return self.type
-
- def get_host(self):
- return urlparse(self._r.url).netloc
-
- def get_origin_req_host(self):
- return self.get_host()
-
- def get_full_url(self):
- # Only return the response's URL if the user hadn't set the Host
- # header
- if not self._r.headers.get("Host"):
- return self._r.url
- # If they did set it, retrieve it and reconstruct the expected domain
- host = to_native_string(self._r.headers["Host"], encoding="utf-8")
- parsed = urlparse(self._r.url)
- # Reconstruct the URL as we expect it
- return urlunparse(
- [
- parsed.scheme,
- host,
- parsed.path,
- parsed.params,
- parsed.query,
- parsed.fragment,
- ]
- )
-
- def is_unverifiable(self):
- return True
-
- def has_header(self, name):
- return name in self._r.headers or name in self._new_headers
-
- def get_header(self, name, default=None):
- return self._r.headers.get(name, self._new_headers.get(name, default))
-
- def add_header(self, key, val):
- """cookielib has no legitimate use for this method; add it back if you find one."""
- raise NotImplementedError(
- "Cookie headers should be added with add_unredirected_header()"
- )
-
- def add_unredirected_header(self, name, value):
- self._new_headers[name] = value
-
- def get_new_headers(self):
- return self._new_headers
-
- @property
- def unverifiable(self):
- return self.is_unverifiable()
-
- @property
- def origin_req_host(self):
- return self.get_origin_req_host()
-
- @property
- def host(self):
- return self.get_host()
-
-
-class MockResponse:
- """Wraps a `httplib.HTTPMessage` to mimic a `urllib.addinfourl`.
-
- ...what? Basically, expose the parsed HTTP headers from the server response
- the way `cookielib` expects to see them.
- """
-
- def __init__(self, headers):
- """Make a MockResponse for `cookielib` to read.
-
- :param headers: a httplib.HTTPMessage or analogous carrying the headers
- """
- self._headers = headers
-
- def info(self):
- return self._headers
-
- def getheaders(self, name):
- self._headers.getheaders(name)
-
-
-def extract_cookies_to_jar(jar, request, response):
- """Extract the cookies from the response into a CookieJar.
-
- :param jar: cookielib.CookieJar (not necessarily a RequestsCookieJar)
- :param request: our own requests.Request object
- :param response: urllib3.HTTPResponse object
- """
- if not (hasattr(response, "_original_response") and response._original_response):
- return
- # the _original_response field is the wrapped httplib.HTTPResponse object,
- req = MockRequest(request)
- # pull out the HTTPMessage with the headers and put it in the mock:
- res = MockResponse(response._original_response.msg)
- jar.extract_cookies(res, req)
-
-
-def get_cookie_header(jar, request):
- """
- Produce an appropriate Cookie header string to be sent with `request`, or None.
-
- :rtype: str
- """
- r = MockRequest(request)
- jar.add_cookie_header(r)
- return r.get_new_headers().get("Cookie")
-
-
-def remove_cookie_by_name(cookiejar, name, domain=None, path=None):
- """Unsets a cookie by name, by default over all domains and paths.
-
- Wraps CookieJar.clear(), is O(n).
- """
- clearables = []
- for cookie in cookiejar:
- if cookie.name != name:
- continue
- if domain is not None and domain != cookie.domain:
- continue
- if path is not None and path != cookie.path:
- continue
- clearables.append((cookie.domain, cookie.path, cookie.name))
-
- for domain, path, name in clearables:
- cookiejar.clear(domain, path, name)
-
-
-class CookieConflictError(RuntimeError):
- """There are two cookies that meet the criteria specified in the cookie jar.
- Use .get and .set and include domain and path args in order to be more specific.
- """
-
-
-class RequestsCookieJar(cookielib.CookieJar, MutableMapping):
- """Compatibility class; is a cookielib.CookieJar, but exposes a dict
- interface.
-
- This is the CookieJar we create by default for requests and sessions that
- don't specify one, since some clients may expect response.cookies and
- session.cookies to support dict operations.
-
- Requests does not use the dict interface internally; it's just for
- compatibility with external client code. All requests code should work
- out of the box with externally provided instances of ``CookieJar``, e.g.
- ``LWPCookieJar`` and ``FileCookieJar``.
-
- Unlike a regular CookieJar, this class is pickleable.
-
- .. warning:: dictionary operations that are normally O(1) may be O(n).
- """
-
- def get(self, name, default=None, domain=None, path=None):
- """Dict-like get() that also supports optional domain and path args in
- order to resolve naming collisions from using one cookie jar over
- multiple domains.
-
- .. warning:: operation is O(n), not O(1).
- """
- try:
- return self._find_no_duplicates(name, domain, path)
- except KeyError:
- return default
-
- def set(self, name, value, **kwargs):
- """Dict-like set() that also supports optional domain and path args in
- order to resolve naming collisions from using one cookie jar over
- multiple domains.
- """
- # support client code that unsets cookies by assignment of a None value:
- if value is None:
- remove_cookie_by_name(
- self, name, domain=kwargs.get("domain"), path=kwargs.get("path")
- )
- return
-
- if isinstance(value, Morsel):
- c = morsel_to_cookie(value)
- else:
- c = create_cookie(name, value, **kwargs)
- self.set_cookie(c)
- return c
-
- def iterkeys(self):
- """Dict-like iterkeys() that returns an iterator of names of cookies
- from the jar.
-
- .. seealso:: itervalues() and iteritems().
- """
- for cookie in iter(self):
- yield cookie.name
-
- def keys(self):
- """Dict-like keys() that returns a list of names of cookies from the
- jar.
-
- .. seealso:: values() and items().
- """
- return list(self.iterkeys())
-
- def itervalues(self):
- """Dict-like itervalues() that returns an iterator of values of cookies
- from the jar.
-
- .. seealso:: iterkeys() and iteritems().
- """
- for cookie in iter(self):
- yield cookie.value
-
- def values(self):
- """Dict-like values() that returns a list of values of cookies from the
- jar.
-
- .. seealso:: keys() and items().
- """
- return list(self.itervalues())
-
- def iteritems(self):
- """Dict-like iteritems() that returns an iterator of name-value tuples
- from the jar.
-
- .. seealso:: iterkeys() and itervalues().
- """
- for cookie in iter(self):
- yield cookie.name, cookie.value
-
- def items(self):
- """Dict-like items() that returns a list of name-value tuples from the
- jar. Allows client-code to call ``dict(RequestsCookieJar)`` and get a
- vanilla python dict of key value pairs.
-
- .. seealso:: keys() and values().
- """
- return list(self.iteritems())
-
- def list_domains(self):
- """Utility method to list all the domains in the jar."""
- domains = []
- for cookie in iter(self):
- if cookie.domain not in domains:
- domains.append(cookie.domain)
- return domains
-
- def list_paths(self):
- """Utility method to list all the paths in the jar."""
- paths = []
- for cookie in iter(self):
- if cookie.path not in paths:
- paths.append(cookie.path)
- return paths
-
- def multiple_domains(self):
- """Returns True if there are multiple domains in the jar.
- Returns False otherwise.
-
- :rtype: bool
- """
- domains = []
- for cookie in iter(self):
- if cookie.domain is not None and cookie.domain in domains:
- return True
- domains.append(cookie.domain)
- return False # there is only one domain in jar
-
- def get_dict(self, domain=None, path=None):
- """Takes as an argument an optional domain and path and returns a plain
- old Python dict of name-value pairs of cookies that meet the
- requirements.
-
- :rtype: dict
- """
- dictionary = {}
- for cookie in iter(self):
- if (domain is None or cookie.domain == domain) and (
- path is None or cookie.path == path
- ):
- dictionary[cookie.name] = cookie.value
- return dictionary
-
- def __contains__(self, name):
- try:
- return super().__contains__(name)
- except CookieConflictError:
- return True
-
- def __getitem__(self, name):
- """Dict-like __getitem__() for compatibility with client code. Throws
- exception if there are more than one cookie with name. In that case,
- use the more explicit get() method instead.
-
- .. warning:: operation is O(n), not O(1).
- """
- return self._find_no_duplicates(name)
-
- def __setitem__(self, name, value):
- """Dict-like __setitem__ for compatibility with client code. Throws
- exception if there is already a cookie of that name in the jar. In that
- case, use the more explicit set() method instead.
- """
- self.set(name, value)
-
- def __delitem__(self, name):
- """Deletes a cookie given a name. Wraps ``cookielib.CookieJar``'s
- ``remove_cookie_by_name()``.
- """
- remove_cookie_by_name(self, name)
-
- def set_cookie(self, cookie, *args, **kwargs):
- if (
- hasattr(cookie.value, "startswith")
- and cookie.value.startswith('"')
- and cookie.value.endswith('"')
- ):
- cookie.value = cookie.value.replace('\\"', "")
- return super().set_cookie(cookie, *args, **kwargs)
-
- def update(self, other):
- """Updates this jar with cookies from another CookieJar or dict-like"""
- if isinstance(other, cookielib.CookieJar):
- for cookie in other:
- self.set_cookie(copy.copy(cookie))
- else:
- super().update(other)
-
- def _find(self, name, domain=None, path=None):
- """Requests uses this method internally to get cookie values.
-
- If there are conflicting cookies, _find arbitrarily chooses one.
- See _find_no_duplicates if you want an exception thrown if there are
- conflicting cookies.
-
- :param name: a string containing name of cookie
- :param domain: (optional) string containing domain of cookie
- :param path: (optional) string containing path of cookie
- :return: cookie.value
- """
- for cookie in iter(self):
- if cookie.name == name:
- if domain is None or cookie.domain == domain:
- if path is None or cookie.path == path:
- return cookie.value
-
- raise KeyError(f"name={name!r}, domain={domain!r}, path={path!r}")
-
- def _find_no_duplicates(self, name, domain=None, path=None):
- """Both ``__get_item__`` and ``get`` call this function: it's never
- used elsewhere in Requests.
-
- :param name: a string containing name of cookie
- :param domain: (optional) string containing domain of cookie
- :param path: (optional) string containing path of cookie
- :raises KeyError: if cookie is not found
- :raises CookieConflictError: if there are multiple cookies
- that match name and optionally domain and path
- :return: cookie.value
- """
- toReturn = None
- for cookie in iter(self):
- if cookie.name == name:
- if domain is None or cookie.domain == domain:
- if path is None or cookie.path == path:
- if toReturn is not None:
- # if there are multiple cookies that meet passed in criteria
- raise CookieConflictError(
- f"There are multiple cookies with name, {name!r}"
- )
- # we will eventually return this as long as no cookie conflict
- toReturn = cookie.value
-
- if toReturn:
- return toReturn
- raise KeyError(f"name={name!r}, domain={domain!r}, path={path!r}")
-
- def __getstate__(self):
- """Unlike a normal CookieJar, this class is pickleable."""
- state = self.__dict__.copy()
- # remove the unpickleable RLock object
- state.pop("_cookies_lock")
- return state
-
- def __setstate__(self, state):
- """Unlike a normal CookieJar, this class is pickleable."""
- self.__dict__.update(state)
- if "_cookies_lock" not in self.__dict__:
- self._cookies_lock = threading.RLock()
-
- def copy(self):
- """Return a copy of this RequestsCookieJar."""
- new_cj = RequestsCookieJar()
- new_cj.set_policy(self.get_policy())
- new_cj.update(self)
- return new_cj
-
- def get_policy(self):
- """Return the CookiePolicy instance used."""
- return self._policy
-
-
-def _copy_cookie_jar(jar):
- if jar is None:
- return None
-
- if hasattr(jar, "copy"):
- # We're dealing with an instance of RequestsCookieJar
- return jar.copy()
- # We're dealing with a generic CookieJar instance
- new_jar = copy.copy(jar)
- new_jar.clear()
- for cookie in jar:
- new_jar.set_cookie(copy.copy(cookie))
- return new_jar
-
-
-def create_cookie(name, value, **kwargs):
- """Make a cookie from underspecified parameters.
-
- By default, the pair of `name` and `value` will be set for the domain ''
- and sent on every request (this is sometimes called a "supercookie").
- """
- result = {
- "version": 0,
- "name": name,
- "value": value,
- "port": None,
- "domain": "",
- "path": "/",
- "secure": False,
- "expires": None,
- "discard": True,
- "comment": None,
- "comment_url": None,
- "rest": {"HttpOnly": None},
- "rfc2109": False,
- }
-
- badargs = set(kwargs) - set(result)
- if badargs:
- raise TypeError(
- f"create_cookie() got unexpected keyword arguments: {list(badargs)}"
- )
-
- result.update(kwargs)
- result["port_specified"] = bool(result["port"])
- result["domain_specified"] = bool(result["domain"])
- result["domain_initial_dot"] = result["domain"].startswith(".")
- result["path_specified"] = bool(result["path"])
-
- return cookielib.Cookie(**result)
-
-
-def morsel_to_cookie(morsel):
- """Convert a Morsel object into a Cookie containing the one k/v pair."""
-
- expires = None
- if morsel["max-age"]:
- try:
- expires = int(time.time() + int(morsel["max-age"]))
- except ValueError:
- raise TypeError(f"max-age: {morsel['max-age']} must be integer")
- elif morsel["expires"]:
- time_template = "%a, %d-%b-%Y %H:%M:%S GMT"
- expires = calendar.timegm(time.strptime(morsel["expires"], time_template))
- return create_cookie(
- comment=morsel["comment"],
- comment_url=bool(morsel["comment"]),
- discard=False,
- domain=morsel["domain"],
- expires=expires,
- name=morsel.key,
- path=morsel["path"],
- port=None,
- rest={"HttpOnly": morsel["httponly"]},
- rfc2109=False,
- secure=bool(morsel["secure"]),
- value=morsel.value,
- version=morsel["version"] or 0,
- )
-
-
-def cookiejar_from_dict(cookie_dict, cookiejar=None, overwrite=True):
- """Returns a CookieJar from a key/value dictionary.
-
- :param cookie_dict: Dict of key/values to insert into CookieJar.
- :param cookiejar: (optional) A cookiejar to add the cookies to.
- :param overwrite: (optional) If False, will not replace cookies
- already in the jar with new ones.
- :rtype: CookieJar
- """
- if cookiejar is None:
- cookiejar = RequestsCookieJar()
-
- if cookie_dict is not None:
- names_from_jar = [cookie.name for cookie in cookiejar]
- for name in cookie_dict:
- if overwrite or (name not in names_from_jar):
- cookiejar.set_cookie(create_cookie(name, cookie_dict[name]))
-
- return cookiejar
-
-
-def merge_cookies(cookiejar, cookies):
- """Add cookies to cookiejar and returns a merged CookieJar.
-
- :param cookiejar: CookieJar object to add the cookies to.
- :param cookies: Dictionary or CookieJar object to be added.
- :rtype: CookieJar
- """
- if not isinstance(cookiejar, cookielib.CookieJar):
- raise ValueError("You can only merge into CookieJar")
-
- if isinstance(cookies, dict):
- cookiejar = cookiejar_from_dict(cookies, cookiejar=cookiejar, overwrite=False)
- elif isinstance(cookies, cookielib.CookieJar):
- try:
- cookiejar.update(cookies)
- except AttributeError:
- for cookie_in_jar in cookies:
- cookiejar.set_cookie(cookie_in_jar)
-
- return cookiejar
diff --git a/spaces/CVPR/Dual-Key_Backdoor_Attacks/openvqa/openvqa/utils/ans_punct.py b/spaces/CVPR/Dual-Key_Backdoor_Attacks/openvqa/openvqa/utils/ans_punct.py
deleted file mode 100644
index b5e5aa205ee578fd36b4d4b52524e8dcef5b3721..0000000000000000000000000000000000000000
--- a/spaces/CVPR/Dual-Key_Backdoor_Attacks/openvqa/openvqa/utils/ans_punct.py
+++ /dev/null
@@ -1,105 +0,0 @@
-# --------------------------------------------------------
-# OpenVQA
-# Written by Yuhao Cui https://github.com/cuiyuhao1996
-# based on VQA Evaluation Code
-# --------------------------------------------------------
-
-import re
-
-contractions = {
- "aint": "ain't", "arent": "aren't", "cant": "can't", "couldve":
- "could've", "couldnt": "couldn't", "couldn'tve": "couldn't've",
- "couldnt've": "couldn't've", "didnt": "didn't", "doesnt":
- "doesn't", "dont": "don't", "hadnt": "hadn't", "hadnt've":
- "hadn't've", "hadn'tve": "hadn't've", "hasnt": "hasn't", "havent":
- "haven't", "hed": "he'd", "hed've": "he'd've", "he'dve":
- "he'd've", "hes": "he's", "howd": "how'd", "howll": "how'll",
- "hows": "how's", "Id've": "I'd've", "I'dve": "I'd've", "Im":
- "I'm", "Ive": "I've", "isnt": "isn't", "itd": "it'd", "itd've":
- "it'd've", "it'dve": "it'd've", "itll": "it'll", "let's": "let's",
- "maam": "ma'am", "mightnt": "mightn't", "mightnt've":
- "mightn't've", "mightn'tve": "mightn't've", "mightve": "might've",
- "mustnt": "mustn't", "mustve": "must've", "neednt": "needn't",
- "notve": "not've", "oclock": "o'clock", "oughtnt": "oughtn't",
- "ow's'at": "'ow's'at", "'ows'at": "'ow's'at", "'ow'sat":
- "'ow's'at", "shant": "shan't", "shed've": "she'd've", "she'dve":
- "she'd've", "she's": "she's", "shouldve": "should've", "shouldnt":
- "shouldn't", "shouldnt've": "shouldn't've", "shouldn'tve":
- "shouldn't've", "somebody'd": "somebodyd", "somebodyd've":
- "somebody'd've", "somebody'dve": "somebody'd've", "somebodyll":
- "somebody'll", "somebodys": "somebody's", "someoned": "someone'd",
- "someoned've": "someone'd've", "someone'dve": "someone'd've",
- "someonell": "someone'll", "someones": "someone's", "somethingd":
- "something'd", "somethingd've": "something'd've", "something'dve":
- "something'd've", "somethingll": "something'll", "thats":
- "that's", "thered": "there'd", "thered've": "there'd've",
- "there'dve": "there'd've", "therere": "there're", "theres":
- "there's", "theyd": "they'd", "theyd've": "they'd've", "they'dve":
- "they'd've", "theyll": "they'll", "theyre": "they're", "theyve":
- "they've", "twas": "'twas", "wasnt": "wasn't", "wed've":
- "we'd've", "we'dve": "we'd've", "weve": "we've", "werent":
- "weren't", "whatll": "what'll", "whatre": "what're", "whats":
- "what's", "whatve": "what've", "whens": "when's", "whered":
- "where'd", "wheres": "where's", "whereve": "where've", "whod":
- "who'd", "whod've": "who'd've", "who'dve": "who'd've", "wholl":
- "who'll", "whos": "who's", "whove": "who've", "whyll": "why'll",
- "whyre": "why're", "whys": "why's", "wont": "won't", "wouldve":
- "would've", "wouldnt": "wouldn't", "wouldnt've": "wouldn't've",
- "wouldn'tve": "wouldn't've", "yall": "y'all", "yall'll":
- "y'all'll", "y'allll": "y'all'll", "yall'd've": "y'all'd've",
- "y'alld've": "y'all'd've", "y'all'dve": "y'all'd've", "youd":
- "you'd", "youd've": "you'd've", "you'dve": "you'd've", "youll":
- "you'll", "youre": "you're", "youve": "you've"
-}
-
-manual_map = { 'none': '0',
- 'zero': '0',
- 'one': '1',
- 'two': '2',
- 'three': '3',
- 'four': '4',
- 'five': '5',
- 'six': '6',
- 'seven': '7',
- 'eight': '8',
- 'nine': '9',
- 'ten': '10'}
-articles = ['a', 'an', 'the']
-period_strip = re.compile("(?!<=\d)(\.)(?!\d)")
-comma_strip = re.compile("(\d)(\,)(\d)")
-punct = [';', r"/", '[', ']', '"', '{', '}',
- '(', ')', '=', '+', '\\', '_', '-',
- '>', '<', '@', '`', ',', '?', '!']
-
-def process_punctuation(inText):
- outText = inText
- for p in punct:
- if (p + ' ' in inText or ' ' + p in inText) \
- or (re.search(comma_strip, inText) != None):
- outText = outText.replace(p, '')
- else:
- outText = outText.replace(p, ' ')
- outText = period_strip.sub("", outText, re.UNICODE)
- return outText
-
-
-def process_digit_article(inText):
- outText = []
- tempText = inText.lower().split()
- for word in tempText:
- word = manual_map.setdefault(word, word)
- if word not in articles:
- outText.append(word)
- else:
- pass
- for wordId, word in enumerate(outText):
- if word in contractions:
- outText[wordId] = contractions[word]
- outText = ' '.join(outText)
- return outText
-
-
-def prep_ans(answer):
- answer = process_digit_article(process_punctuation(answer))
- answer = answer.replace(',', '')
- return answer
diff --git a/spaces/CVPR/LIVE/thrust/thrust/detail/config/config.h b/spaces/CVPR/LIVE/thrust/thrust/detail/config/config.h
deleted file mode 100644
index 800bc4c51a8bedd5dc922da8a980dc62f02c62aa..0000000000000000000000000000000000000000
--- a/spaces/CVPR/LIVE/thrust/thrust/detail/config/config.h
+++ /dev/null
@@ -1,39 +0,0 @@
-/*
- * Copyright 2008-2013 NVIDIA Corporation
- *
- * Licensed under the Apache License, Version 2.0 (the "License");
- * you may not use this file except in compliance with the License.
- * You may obtain a copy of the License at
- *
- * http://www.apache.org/licenses/LICENSE-2.0
- *
- * Unless required by applicable law or agreed to in writing, software
- * distributed under the License is distributed on an "AS IS" BASIS,
- * WITHOUT WARRANTIES OR CONDITIONS OF ANY KIND, either express or implied.
- * See the License for the specific language governing permissions and
- * limitations under the License.
- */
-
-/*! \file config.h
- * \brief Defines platform configuration.
- */
-
-#pragma once
-
-// NOTE: The order of these #includes matters.
-
-#include
-#include
-#include
-#include
-#include
-// host_system.h & device_system.h must be #included as early as possible
-// because other config headers depend on it
-#include
-#include
-#include
-#include
-#include
-#include
-#include
-
diff --git a/spaces/CVPR/LIVE/thrust/thrust/system/tbb/detail/binary_search.h b/spaces/CVPR/LIVE/thrust/thrust/system/tbb/detail/binary_search.h
deleted file mode 100644
index 0847e5d1fdb3a446651897d62c959d56ad9dd1b9..0000000000000000000000000000000000000000
--- a/spaces/CVPR/LIVE/thrust/thrust/system/tbb/detail/binary_search.h
+++ /dev/null
@@ -1,23 +0,0 @@
-/*
- * Copyright 2008-2013 NVIDIA Corporation
- *
- * Licensed under the Apache License, Version 2.0 (the "License");
- * you may not use this file except in compliance with the License.
- * You may obtain a copy of the License at
- *
- * http://www.apache.org/licenses/LICENSE-2.0
- *
- * Unless required by applicable law or agreed to in writing, software
- * distributed under the License is distributed on an "AS IS" BASIS,
- * WITHOUT WARRANTIES OR CONDITIONS OF ANY KIND, either express or implied.
- * See the License for the specific language governing permissions and
- * limitations under the License.
- */
-
-#pragma once
-
-#include
-
-// this system inherits binary_search
-#include
-
diff --git a/spaces/CVPR/WALT/mmdet/core/evaluation/bbox_overlaps.py b/spaces/CVPR/WALT/mmdet/core/evaluation/bbox_overlaps.py
deleted file mode 100644
index 93559ea0f25369d552a5365312fa32b9ffec9226..0000000000000000000000000000000000000000
--- a/spaces/CVPR/WALT/mmdet/core/evaluation/bbox_overlaps.py
+++ /dev/null
@@ -1,48 +0,0 @@
-import numpy as np
-
-
-def bbox_overlaps(bboxes1, bboxes2, mode='iou', eps=1e-6):
- """Calculate the ious between each bbox of bboxes1 and bboxes2.
-
- Args:
- bboxes1(ndarray): shape (n, 4)
- bboxes2(ndarray): shape (k, 4)
- mode(str): iou (intersection over union) or iof (intersection
- over foreground)
-
- Returns:
- ious(ndarray): shape (n, k)
- """
-
- assert mode in ['iou', 'iof']
-
- bboxes1 = bboxes1.astype(np.float32)
- bboxes2 = bboxes2.astype(np.float32)
- rows = bboxes1.shape[0]
- cols = bboxes2.shape[0]
- ious = np.zeros((rows, cols), dtype=np.float32)
- if rows * cols == 0:
- return ious
- exchange = False
- if bboxes1.shape[0] > bboxes2.shape[0]:
- bboxes1, bboxes2 = bboxes2, bboxes1
- ious = np.zeros((cols, rows), dtype=np.float32)
- exchange = True
- area1 = (bboxes1[:, 2] - bboxes1[:, 0]) * (bboxes1[:, 3] - bboxes1[:, 1])
- area2 = (bboxes2[:, 2] - bboxes2[:, 0]) * (bboxes2[:, 3] - bboxes2[:, 1])
- for i in range(bboxes1.shape[0]):
- x_start = np.maximum(bboxes1[i, 0], bboxes2[:, 0])
- y_start = np.maximum(bboxes1[i, 1], bboxes2[:, 1])
- x_end = np.minimum(bboxes1[i, 2], bboxes2[:, 2])
- y_end = np.minimum(bboxes1[i, 3], bboxes2[:, 3])
- overlap = np.maximum(x_end - x_start, 0) * np.maximum(
- y_end - y_start, 0)
- if mode == 'iou':
- union = area1[i] + area2 - overlap
- else:
- union = area1[i] if not exchange else area2
- union = np.maximum(union, eps)
- ious[i, :] = overlap / union
- if exchange:
- ious = ious.T
- return ious
diff --git a/spaces/CVPR/WALT/mmdet/models/losses/smooth_l1_loss.py b/spaces/CVPR/WALT/mmdet/models/losses/smooth_l1_loss.py
deleted file mode 100644
index ec9c98a52d1932d6ccff18938c17c36755bf1baf..0000000000000000000000000000000000000000
--- a/spaces/CVPR/WALT/mmdet/models/losses/smooth_l1_loss.py
+++ /dev/null
@@ -1,139 +0,0 @@
-import mmcv
-import torch
-import torch.nn as nn
-
-from ..builder import LOSSES
-from .utils import weighted_loss
-
-
-@mmcv.jit(derivate=True, coderize=True)
-@weighted_loss
-def smooth_l1_loss(pred, target, beta=1.0):
- """Smooth L1 loss.
-
- Args:
- pred (torch.Tensor): The prediction.
- target (torch.Tensor): The learning target of the prediction.
- beta (float, optional): The threshold in the piecewise function.
- Defaults to 1.0.
-
- Returns:
- torch.Tensor: Calculated loss
- """
- assert beta > 0
- assert pred.size() == target.size() and target.numel() > 0
- diff = torch.abs(pred - target)
- loss = torch.where(diff < beta, 0.5 * diff * diff / beta,
- diff - 0.5 * beta)
- return loss
-
-
-@mmcv.jit(derivate=True, coderize=True)
-@weighted_loss
-def l1_loss(pred, target):
- """L1 loss.
-
- Args:
- pred (torch.Tensor): The prediction.
- target (torch.Tensor): The learning target of the prediction.
-
- Returns:
- torch.Tensor: Calculated loss
- """
- assert pred.size() == target.size() and target.numel() > 0
- loss = torch.abs(pred - target)
- return loss
-
-
-@LOSSES.register_module()
-class SmoothL1Loss(nn.Module):
- """Smooth L1 loss.
-
- Args:
- beta (float, optional): The threshold in the piecewise function.
- Defaults to 1.0.
- reduction (str, optional): The method to reduce the loss.
- Options are "none", "mean" and "sum". Defaults to "mean".
- loss_weight (float, optional): The weight of loss.
- """
-
- def __init__(self, beta=1.0, reduction='mean', loss_weight=1.0):
- super(SmoothL1Loss, self).__init__()
- self.beta = beta
- self.reduction = reduction
- self.loss_weight = loss_weight
-
- def forward(self,
- pred,
- target,
- weight=None,
- avg_factor=None,
- reduction_override=None,
- **kwargs):
- """Forward function.
-
- Args:
- pred (torch.Tensor): The prediction.
- target (torch.Tensor): The learning target of the prediction.
- weight (torch.Tensor, optional): The weight of loss for each
- prediction. Defaults to None.
- avg_factor (int, optional): Average factor that is used to average
- the loss. Defaults to None.
- reduction_override (str, optional): The reduction method used to
- override the original reduction method of the loss.
- Defaults to None.
- """
- assert reduction_override in (None, 'none', 'mean', 'sum')
- reduction = (
- reduction_override if reduction_override else self.reduction)
- loss_bbox = self.loss_weight * smooth_l1_loss(
- pred,
- target,
- weight,
- beta=self.beta,
- reduction=reduction,
- avg_factor=avg_factor,
- **kwargs)
- return loss_bbox
-
-
-@LOSSES.register_module()
-class L1Loss(nn.Module):
- """L1 loss.
-
- Args:
- reduction (str, optional): The method to reduce the loss.
- Options are "none", "mean" and "sum".
- loss_weight (float, optional): The weight of loss.
- """
-
- def __init__(self, reduction='mean', loss_weight=1.0):
- super(L1Loss, self).__init__()
- self.reduction = reduction
- self.loss_weight = loss_weight
-
- def forward(self,
- pred,
- target,
- weight=None,
- avg_factor=None,
- reduction_override=None):
- """Forward function.
-
- Args:
- pred (torch.Tensor): The prediction.
- target (torch.Tensor): The learning target of the prediction.
- weight (torch.Tensor, optional): The weight of loss for each
- prediction. Defaults to None.
- avg_factor (int, optional): Average factor that is used to average
- the loss. Defaults to None.
- reduction_override (str, optional): The reduction method used to
- override the original reduction method of the loss.
- Defaults to None.
- """
- assert reduction_override in (None, 'none', 'mean', 'sum')
- reduction = (
- reduction_override if reduction_override else self.reduction)
- loss_bbox = self.loss_weight * l1_loss(
- pred, target, weight, reduction=reduction, avg_factor=avg_factor)
- return loss_bbox
diff --git a/spaces/CVPR/lama-example/saicinpainting/training/modules/__init__.py b/spaces/CVPR/lama-example/saicinpainting/training/modules/__init__.py
deleted file mode 100644
index 82e1a9096a5bd8f3fb00e899d0239b078246cad4..0000000000000000000000000000000000000000
--- a/spaces/CVPR/lama-example/saicinpainting/training/modules/__init__.py
+++ /dev/null
@@ -1,31 +0,0 @@
-import logging
-
-from saicinpainting.training.modules.ffc import FFCResNetGenerator
-from saicinpainting.training.modules.pix2pixhd import GlobalGenerator, MultiDilatedGlobalGenerator, \
- NLayerDiscriminator, MultidilatedNLayerDiscriminator
-
-def make_generator(config, kind, **kwargs):
- logging.info(f'Make generator {kind}')
-
- if kind == 'pix2pixhd_multidilated':
- return MultiDilatedGlobalGenerator(**kwargs)
-
- if kind == 'pix2pixhd_global':
- return GlobalGenerator(**kwargs)
-
- if kind == 'ffc_resnet':
- return FFCResNetGenerator(**kwargs)
-
- raise ValueError(f'Unknown generator kind {kind}')
-
-
-def make_discriminator(kind, **kwargs):
- logging.info(f'Make discriminator {kind}')
-
- if kind == 'pix2pixhd_nlayer_multidilated':
- return MultidilatedNLayerDiscriminator(**kwargs)
-
- if kind == 'pix2pixhd_nlayer':
- return NLayerDiscriminator(**kwargs)
-
- raise ValueError(f'Unknown discriminator kind {kind}')
diff --git a/spaces/CVPR/regionclip-demo/detectron2/evaluation/cityscapes_evaluation.py b/spaces/CVPR/regionclip-demo/detectron2/evaluation/cityscapes_evaluation.py
deleted file mode 100644
index 3fb6c4cd5f752d639570d022cb23ce18491c370a..0000000000000000000000000000000000000000
--- a/spaces/CVPR/regionclip-demo/detectron2/evaluation/cityscapes_evaluation.py
+++ /dev/null
@@ -1,194 +0,0 @@
-# Copyright (c) Facebook, Inc. and its affiliates.
-import glob
-import logging
-import numpy as np
-import os
-import tempfile
-from collections import OrderedDict
-import torch
-from PIL import Image
-
-from detectron2.data import MetadataCatalog
-from detectron2.utils import comm
-from detectron2.utils.file_io import PathManager
-
-from .evaluator import DatasetEvaluator
-
-
-class CityscapesEvaluator(DatasetEvaluator):
- """
- Base class for evaluation using cityscapes API.
- """
-
- def __init__(self, dataset_name):
- """
- Args:
- dataset_name (str): the name of the dataset.
- It must have the following metadata associated with it:
- "thing_classes", "gt_dir".
- """
- self._metadata = MetadataCatalog.get(dataset_name)
- self._cpu_device = torch.device("cpu")
- self._logger = logging.getLogger(__name__)
-
- def reset(self):
- self._working_dir = tempfile.TemporaryDirectory(prefix="cityscapes_eval_")
- self._temp_dir = self._working_dir.name
- # All workers will write to the same results directory
- # TODO this does not work in distributed training
- self._temp_dir = comm.all_gather(self._temp_dir)[0]
- if self._temp_dir != self._working_dir.name:
- self._working_dir.cleanup()
- self._logger.info(
- "Writing cityscapes results to temporary directory {} ...".format(self._temp_dir)
- )
-
-
-class CityscapesInstanceEvaluator(CityscapesEvaluator):
- """
- Evaluate instance segmentation results on cityscapes dataset using cityscapes API.
-
- Note:
- * It does not work in multi-machine distributed training.
- * It contains a synchronization, therefore has to be used on all ranks.
- * Only the main process runs evaluation.
- """
-
- def process(self, inputs, outputs):
- from cityscapesscripts.helpers.labels import name2label
-
- for input, output in zip(inputs, outputs):
- file_name = input["file_name"]
- basename = os.path.splitext(os.path.basename(file_name))[0]
- pred_txt = os.path.join(self._temp_dir, basename + "_pred.txt")
-
- if "instances" in output:
- output = output["instances"].to(self._cpu_device)
- num_instances = len(output)
- with open(pred_txt, "w") as fout:
- for i in range(num_instances):
- pred_class = output.pred_classes[i]
- classes = self._metadata.thing_classes[pred_class]
- class_id = name2label[classes].id
- score = output.scores[i]
- mask = output.pred_masks[i].numpy().astype("uint8")
- png_filename = os.path.join(
- self._temp_dir, basename + "_{}_{}.png".format(i, classes)
- )
-
- Image.fromarray(mask * 255).save(png_filename)
- fout.write(
- "{} {} {}\n".format(os.path.basename(png_filename), class_id, score)
- )
- else:
- # Cityscapes requires a prediction file for every ground truth image.
- with open(pred_txt, "w") as fout:
- pass
-
- def evaluate(self):
- """
- Returns:
- dict: has a key "segm", whose value is a dict of "AP" and "AP50".
- """
- comm.synchronize()
- if comm.get_rank() > 0:
- return
- import cityscapesscripts.evaluation.evalInstanceLevelSemanticLabeling as cityscapes_eval
-
- self._logger.info("Evaluating results under {} ...".format(self._temp_dir))
-
- # set some global states in cityscapes evaluation API, before evaluating
- cityscapes_eval.args.predictionPath = os.path.abspath(self._temp_dir)
- cityscapes_eval.args.predictionWalk = None
- cityscapes_eval.args.JSONOutput = False
- cityscapes_eval.args.colorized = False
- cityscapes_eval.args.gtInstancesFile = os.path.join(self._temp_dir, "gtInstances.json")
-
- # These lines are adopted from
- # https://github.com/mcordts/cityscapesScripts/blob/master/cityscapesscripts/evaluation/evalInstanceLevelSemanticLabeling.py # noqa
- gt_dir = PathManager.get_local_path(self._metadata.gt_dir)
- groundTruthImgList = glob.glob(os.path.join(gt_dir, "*", "*_gtFine_instanceIds.png"))
- assert len(
- groundTruthImgList
- ), "Cannot find any ground truth images to use for evaluation. Searched for: {}".format(
- cityscapes_eval.args.groundTruthSearch
- )
- predictionImgList = []
- for gt in groundTruthImgList:
- predictionImgList.append(cityscapes_eval.getPrediction(gt, cityscapes_eval.args))
- results = cityscapes_eval.evaluateImgLists(
- predictionImgList, groundTruthImgList, cityscapes_eval.args
- )["averages"]
-
- ret = OrderedDict()
- ret["segm"] = {"AP": results["allAp"] * 100, "AP50": results["allAp50%"] * 100}
- self._working_dir.cleanup()
- return ret
-
-
-class CityscapesSemSegEvaluator(CityscapesEvaluator):
- """
- Evaluate semantic segmentation results on cityscapes dataset using cityscapes API.
-
- Note:
- * It does not work in multi-machine distributed training.
- * It contains a synchronization, therefore has to be used on all ranks.
- * Only the main process runs evaluation.
- """
-
- def process(self, inputs, outputs):
- from cityscapesscripts.helpers.labels import trainId2label
-
- for input, output in zip(inputs, outputs):
- file_name = input["file_name"]
- basename = os.path.splitext(os.path.basename(file_name))[0]
- pred_filename = os.path.join(self._temp_dir, basename + "_pred.png")
-
- output = output["sem_seg"].argmax(dim=0).to(self._cpu_device).numpy()
- pred = 255 * np.ones(output.shape, dtype=np.uint8)
- for train_id, label in trainId2label.items():
- if label.ignoreInEval:
- continue
- pred[output == train_id] = label.id
- Image.fromarray(pred).save(pred_filename)
-
- def evaluate(self):
- comm.synchronize()
- if comm.get_rank() > 0:
- return
- # Load the Cityscapes eval script *after* setting the required env var,
- # since the script reads CITYSCAPES_DATASET into global variables at load time.
- import cityscapesscripts.evaluation.evalPixelLevelSemanticLabeling as cityscapes_eval
-
- self._logger.info("Evaluating results under {} ...".format(self._temp_dir))
-
- # set some global states in cityscapes evaluation API, before evaluating
- cityscapes_eval.args.predictionPath = os.path.abspath(self._temp_dir)
- cityscapes_eval.args.predictionWalk = None
- cityscapes_eval.args.JSONOutput = False
- cityscapes_eval.args.colorized = False
-
- # These lines are adopted from
- # https://github.com/mcordts/cityscapesScripts/blob/master/cityscapesscripts/evaluation/evalPixelLevelSemanticLabeling.py # noqa
- gt_dir = PathManager.get_local_path(self._metadata.gt_dir)
- groundTruthImgList = glob.glob(os.path.join(gt_dir, "*", "*_gtFine_labelIds.png"))
- assert len(
- groundTruthImgList
- ), "Cannot find any ground truth images to use for evaluation. Searched for: {}".format(
- cityscapes_eval.args.groundTruthSearch
- )
- predictionImgList = []
- for gt in groundTruthImgList:
- predictionImgList.append(cityscapes_eval.getPrediction(cityscapes_eval.args, gt))
- results = cityscapes_eval.evaluateImgLists(
- predictionImgList, groundTruthImgList, cityscapes_eval.args
- )
- ret = OrderedDict()
- ret["sem_seg"] = {
- "IoU": 100.0 * results["averageScoreClasses"],
- "iIoU": 100.0 * results["averageScoreInstClasses"],
- "IoU_sup": 100.0 * results["averageScoreCategories"],
- "iIoU_sup": 100.0 * results["averageScoreInstCategories"],
- }
- self._working_dir.cleanup()
- return ret
diff --git a/spaces/CognitiveLabs/Research-Assistant/README.md b/spaces/CognitiveLabs/Research-Assistant/README.md
deleted file mode 100644
index 7a4e2ed156df4e83de5f21e3b8c463ee5c0ac09d..0000000000000000000000000000000000000000
--- a/spaces/CognitiveLabs/Research-Assistant/README.md
+++ /dev/null
@@ -1,78 +0,0 @@
----
-title: AI-Research-Assistant
-app_file: app.py
-sdk: gradio
-sdk_version: 3.38.0
-duplicated_from: zej97/AI-Research-Assistant
----
-
-
-Inspired by [gpt-researcher](https://github.com/assafelovic/gpt-researcher). This project endeavors to develop an AI research assistant capable of **generating research reports** effortlessly for researchers. For instance, researchers can request the AI research assistant to compose a report on *the latest advancements in the field of superconductors as of 2023*, which is currently a trending topic. The AI research assistant will subsequently compile a report based on the relevant information obtained from the internet. Now, AIRA also offers support for **academic English polishing**.
-
-
-| Example1-1 | Example1-2 | Example1-3 |
-| :----------------------------------: | :----------------------------------: | :----------------------------------: |
-| | | |
-
-The currently supported agents encompass a wide range of fields, including *finance, business analysis, clinical medicine, basic medicine, travel, academic research and sociology*.
-
-In addition to official api, this project offers an alternative approach to generating research reports by utilizing a third-party API. For access to this third-party API, please refer to [chimeragpt](https://chimeragpt.adventblocks.cc/) or [GPT-API-free](https://github.com/chatanywhere/GPT_API_free). Before running the project, kindly ensure that you set the environment variables `OPENAI_API_KEY` and `OPENAI_API_BASE`.
-
-```shell
-$ export OPENAI_API_KEY = your_api_key
-$ export OPENAI_API_BASE = your_api_base
-```
-
-or you can set the api key and base in `.env` file.
-
-
-## Installation
-
-1. Clone the repository
-
- ```shell
- $ git clone git@github.com:paradoxtown/ai_research_assistant.git
- $ cd ai_research_assistant
- ```
-
-2. Install the dependencies
-
- ```shell
- $ pip install -r requirements.txt
- ```
-
-3. Export evnironment variables
-
- ```shell
- $ export OPENAI_API_KEY = your_api_key
- $ export OPENAI_API_BASE = your_api_base
- ```
- or modify the `.env` file.
-
-4. Run the project
-
- ```shell
- $ python app.py
- ```
-
-## TODO
-
-- [x] Switch Google Search to DuckDuckGo
-- [ ] Literature review
-- [x] Third-party API
-- [ ] Prettify report
-- [x] Add medical agent and social agent
-- [ ] Add option for users to customize the number of words and temperature
-- [ ] Copy and download buttons
-- [ ] Allows the user to choose the degree of research.
-- [ ] Wikipedia Understanding
-
----
-
-
Happy researching! 🚀
\ No newline at end of file
diff --git a/spaces/Cropinky/hana_hanak_houses/networks_fastgan.py b/spaces/Cropinky/hana_hanak_houses/networks_fastgan.py
deleted file mode 100644
index d517e6b53b7bb6d83ce5df00b5111073e3cf3c24..0000000000000000000000000000000000000000
--- a/spaces/Cropinky/hana_hanak_houses/networks_fastgan.py
+++ /dev/null
@@ -1,179 +0,0 @@
-# original implementation: https://github.com/odegeasslbc/FastGAN-pytorch/blob/main/models.py
-#
-# modified by Axel Sauer for "Projected GANs Converge Faster"
-#
-import torch.nn as nn
-from blocks import (InitLayer, UpBlockBig, UpBlockBigCond, UpBlockSmall, UpBlockSmallCond, SEBlock, conv2d)
-from huggingface_hub import PyTorchModelHubMixin
-
-def normalize_second_moment(x, dim=1, eps=1e-8):
- return x * (x.square().mean(dim=dim, keepdim=True) + eps).rsqrt()
-
-
-class DummyMapping(nn.Module):
- def __init__(self):
- super().__init__()
-
- def forward(self, z, c, **kwargs):
- return z.unsqueeze(1) # to fit the StyleGAN API
-
-
-class FastganSynthesis(nn.Module):
- def __init__(self, ngf=128, z_dim=256, nc=3, img_resolution=256, lite=False):
- super().__init__()
- self.img_resolution = img_resolution
- self.z_dim = z_dim
-
- # channel multiplier
- nfc_multi = {2: 16, 4:16, 8:8, 16:4, 32:2, 64:2, 128:1, 256:0.5,
- 512:0.25, 1024:0.125}
- nfc = {}
- for k, v in nfc_multi.items():
- nfc[k] = int(v*ngf)
-
- # layers
- self.init = InitLayer(z_dim, channel=nfc[2], sz=4)
-
- UpBlock = UpBlockSmall if lite else UpBlockBig
-
- self.feat_8 = UpBlock(nfc[4], nfc[8])
- self.feat_16 = UpBlock(nfc[8], nfc[16])
- self.feat_32 = UpBlock(nfc[16], nfc[32])
- self.feat_64 = UpBlock(nfc[32], nfc[64])
- self.feat_128 = UpBlock(nfc[64], nfc[128])
- self.feat_256 = UpBlock(nfc[128], nfc[256])
-
- self.se_64 = SEBlock(nfc[4], nfc[64])
- self.se_128 = SEBlock(nfc[8], nfc[128])
- self.se_256 = SEBlock(nfc[16], nfc[256])
-
- self.to_big = conv2d(nfc[img_resolution], nc, 3, 1, 1, bias=True)
-
- if img_resolution > 256:
- self.feat_512 = UpBlock(nfc[256], nfc[512])
- self.se_512 = SEBlock(nfc[32], nfc[512])
- if img_resolution > 512:
- self.feat_1024 = UpBlock(nfc[512], nfc[1024])
-
- def forward(self, input, c, **kwargs):
- # map noise to hypersphere as in "Progressive Growing of GANS"
- input = normalize_second_moment(input[:, 0])
-
- feat_4 = self.init(input)
- feat_8 = self.feat_8(feat_4)
- feat_16 = self.feat_16(feat_8)
- feat_32 = self.feat_32(feat_16)
- feat_64 = self.se_64(feat_4, self.feat_64(feat_32))
- feat_128 = self.se_128(feat_8, self.feat_128(feat_64))
-
- if self.img_resolution >= 128:
- feat_last = feat_128
-
- if self.img_resolution >= 256:
- feat_last = self.se_256(feat_16, self.feat_256(feat_last))
-
- if self.img_resolution >= 512:
- feat_last = self.se_512(feat_32, self.feat_512(feat_last))
-
- if self.img_resolution >= 1024:
- feat_last = self.feat_1024(feat_last)
-
- return self.to_big(feat_last)
-
-
-class FastganSynthesisCond(nn.Module):
- def __init__(self, ngf=64, z_dim=256, nc=3, img_resolution=256, num_classes=1000, lite=False):
- super().__init__()
-
- self.z_dim = z_dim
- nfc_multi = {2: 16, 4:16, 8:8, 16:4, 32:2, 64:2, 128:1, 256:0.5,
- 512:0.25, 1024:0.125, 2048:0.125}
- nfc = {}
- for k, v in nfc_multi.items():
- nfc[k] = int(v*ngf)
-
- self.img_resolution = img_resolution
-
- self.init = InitLayer(z_dim, channel=nfc[2], sz=4)
-
- UpBlock = UpBlockSmallCond if lite else UpBlockBigCond
-
- self.feat_8 = UpBlock(nfc[4], nfc[8], z_dim)
- self.feat_16 = UpBlock(nfc[8], nfc[16], z_dim)
- self.feat_32 = UpBlock(nfc[16], nfc[32], z_dim)
- self.feat_64 = UpBlock(nfc[32], nfc[64], z_dim)
- self.feat_128 = UpBlock(nfc[64], nfc[128], z_dim)
- self.feat_256 = UpBlock(nfc[128], nfc[256], z_dim)
-
- self.se_64 = SEBlock(nfc[4], nfc[64])
- self.se_128 = SEBlock(nfc[8], nfc[128])
- self.se_256 = SEBlock(nfc[16], nfc[256])
-
- self.to_big = conv2d(nfc[img_resolution], nc, 3, 1, 1, bias=True)
-
- if img_resolution > 256:
- self.feat_512 = UpBlock(nfc[256], nfc[512])
- self.se_512 = SEBlock(nfc[32], nfc[512])
- if img_resolution > 512:
- self.feat_1024 = UpBlock(nfc[512], nfc[1024])
-
- self.embed = nn.Embedding(num_classes, z_dim)
-
- def forward(self, input, c, update_emas=False):
- c = self.embed(c.argmax(1))
-
- # map noise to hypersphere as in "Progressive Growing of GANS"
- input = normalize_second_moment(input[:, 0])
-
- feat_4 = self.init(input)
- feat_8 = self.feat_8(feat_4, c)
- feat_16 = self.feat_16(feat_8, c)
- feat_32 = self.feat_32(feat_16, c)
- feat_64 = self.se_64(feat_4, self.feat_64(feat_32, c))
- feat_128 = self.se_128(feat_8, self.feat_128(feat_64, c))
-
- if self.img_resolution >= 128:
- feat_last = feat_128
-
- if self.img_resolution >= 256:
- feat_last = self.se_256(feat_16, self.feat_256(feat_last, c))
-
- if self.img_resolution >= 512:
- feat_last = self.se_512(feat_32, self.feat_512(feat_last, c))
-
- if self.img_resolution >= 1024:
- feat_last = self.feat_1024(feat_last, c)
-
- return self.to_big(feat_last)
-
-
-class MyGenerator(nn.Module, PyTorchModelHubMixin):
- def __init__(
- self,
- z_dim=256,
- c_dim=0,
- w_dim=0,
- img_resolution=256,
- img_channels=3,
- ngf=128,
- cond=0,
- mapping_kwargs={},
- synthesis_kwargs={}
- ):
- super().__init__()
- #self.config = kwargs.pop("config", None)
- self.z_dim = z_dim
- self.c_dim = c_dim
- self.w_dim = w_dim
- self.img_resolution = img_resolution
- self.img_channels = img_channels
-
- # Mapping and Synthesis Networks
- self.mapping = DummyMapping() # to fit the StyleGAN API
- Synthesis = FastganSynthesisCond if cond else FastganSynthesis
- self.synthesis = Synthesis(ngf=ngf, z_dim=z_dim, nc=img_channels, img_resolution=img_resolution, **synthesis_kwargs)
-
- def forward(self, z, c, **kwargs):
- w = self.mapping(z, c)
- img = self.synthesis(w, c)
- return img
diff --git a/spaces/DQChoi/gpt-demo/venv/lib/python3.11/site-packages/PIL/McIdasImagePlugin.py b/spaces/DQChoi/gpt-demo/venv/lib/python3.11/site-packages/PIL/McIdasImagePlugin.py
deleted file mode 100644
index 17c008b9a6a1218f6e51add4fda83acb92ee06ce..0000000000000000000000000000000000000000
--- a/spaces/DQChoi/gpt-demo/venv/lib/python3.11/site-packages/PIL/McIdasImagePlugin.py
+++ /dev/null
@@ -1,75 +0,0 @@
-#
-# The Python Imaging Library.
-# $Id$
-#
-# Basic McIdas support for PIL
-#
-# History:
-# 1997-05-05 fl Created (8-bit images only)
-# 2009-03-08 fl Added 16/32-bit support.
-#
-# Thanks to Richard Jones and Craig Swank for specs and samples.
-#
-# Copyright (c) Secret Labs AB 1997.
-# Copyright (c) Fredrik Lundh 1997.
-#
-# See the README file for information on usage and redistribution.
-#
-
-import struct
-
-from . import Image, ImageFile
-
-
-def _accept(s):
- return s[:8] == b"\x00\x00\x00\x00\x00\x00\x00\x04"
-
-
-##
-# Image plugin for McIdas area images.
-
-
-class McIdasImageFile(ImageFile.ImageFile):
- format = "MCIDAS"
- format_description = "McIdas area file"
-
- def _open(self):
- # parse area file directory
- s = self.fp.read(256)
- if not _accept(s) or len(s) != 256:
- msg = "not an McIdas area file"
- raise SyntaxError(msg)
-
- self.area_descriptor_raw = s
- self.area_descriptor = w = [0] + list(struct.unpack("!64i", s))
-
- # get mode
- if w[11] == 1:
- mode = rawmode = "L"
- elif w[11] == 2:
- # FIXME: add memory map support
- mode = "I"
- rawmode = "I;16B"
- elif w[11] == 4:
- # FIXME: add memory map support
- mode = "I"
- rawmode = "I;32B"
- else:
- msg = "unsupported McIdas format"
- raise SyntaxError(msg)
-
- self.mode = mode
- self._size = w[10], w[9]
-
- offset = w[34] + w[15]
- stride = w[15] + w[10] * w[11] * w[14]
-
- self.tile = [("raw", (0, 0) + self.size, offset, (rawmode, stride, 1))]
-
-
-# --------------------------------------------------------------------
-# registry
-
-Image.register_open(McIdasImageFile.format, McIdasImageFile, _accept)
-
-# no default extension
diff --git a/spaces/DQChoi/gpt-demo/venv/lib/python3.11/site-packages/click/_termui_impl.py b/spaces/DQChoi/gpt-demo/venv/lib/python3.11/site-packages/click/_termui_impl.py
deleted file mode 100644
index f744657753caa6cdef1dcc41a4f0b5e3e9503ab8..0000000000000000000000000000000000000000
--- a/spaces/DQChoi/gpt-demo/venv/lib/python3.11/site-packages/click/_termui_impl.py
+++ /dev/null
@@ -1,739 +0,0 @@
-"""
-This module contains implementations for the termui module. To keep the
-import time of Click down, some infrequently used functionality is
-placed in this module and only imported as needed.
-"""
-import contextlib
-import math
-import os
-import sys
-import time
-import typing as t
-from gettext import gettext as _
-from io import StringIO
-from types import TracebackType
-
-from ._compat import _default_text_stdout
-from ._compat import CYGWIN
-from ._compat import get_best_encoding
-from ._compat import isatty
-from ._compat import open_stream
-from ._compat import strip_ansi
-from ._compat import term_len
-from ._compat import WIN
-from .exceptions import ClickException
-from .utils import echo
-
-V = t.TypeVar("V")
-
-if os.name == "nt":
- BEFORE_BAR = "\r"
- AFTER_BAR = "\n"
-else:
- BEFORE_BAR = "\r\033[?25l"
- AFTER_BAR = "\033[?25h\n"
-
-
-class ProgressBar(t.Generic[V]):
- def __init__(
- self,
- iterable: t.Optional[t.Iterable[V]],
- length: t.Optional[int] = None,
- fill_char: str = "#",
- empty_char: str = " ",
- bar_template: str = "%(bar)s",
- info_sep: str = " ",
- show_eta: bool = True,
- show_percent: t.Optional[bool] = None,
- show_pos: bool = False,
- item_show_func: t.Optional[t.Callable[[t.Optional[V]], t.Optional[str]]] = None,
- label: t.Optional[str] = None,
- file: t.Optional[t.TextIO] = None,
- color: t.Optional[bool] = None,
- update_min_steps: int = 1,
- width: int = 30,
- ) -> None:
- self.fill_char = fill_char
- self.empty_char = empty_char
- self.bar_template = bar_template
- self.info_sep = info_sep
- self.show_eta = show_eta
- self.show_percent = show_percent
- self.show_pos = show_pos
- self.item_show_func = item_show_func
- self.label: str = label or ""
-
- if file is None:
- file = _default_text_stdout()
-
- # There are no standard streams attached to write to. For example,
- # pythonw on Windows.
- if file is None:
- file = StringIO()
-
- self.file = file
- self.color = color
- self.update_min_steps = update_min_steps
- self._completed_intervals = 0
- self.width: int = width
- self.autowidth: bool = width == 0
-
- if length is None:
- from operator import length_hint
-
- length = length_hint(iterable, -1)
-
- if length == -1:
- length = None
- if iterable is None:
- if length is None:
- raise TypeError("iterable or length is required")
- iterable = t.cast(t.Iterable[V], range(length))
- self.iter: t.Iterable[V] = iter(iterable)
- self.length = length
- self.pos = 0
- self.avg: t.List[float] = []
- self.last_eta: float
- self.start: float
- self.start = self.last_eta = time.time()
- self.eta_known: bool = False
- self.finished: bool = False
- self.max_width: t.Optional[int] = None
- self.entered: bool = False
- self.current_item: t.Optional[V] = None
- self.is_hidden: bool = not isatty(self.file)
- self._last_line: t.Optional[str] = None
-
- def __enter__(self) -> "ProgressBar[V]":
- self.entered = True
- self.render_progress()
- return self
-
- def __exit__(
- self,
- exc_type: t.Optional[t.Type[BaseException]],
- exc_value: t.Optional[BaseException],
- tb: t.Optional[TracebackType],
- ) -> None:
- self.render_finish()
-
- def __iter__(self) -> t.Iterator[V]:
- if not self.entered:
- raise RuntimeError("You need to use progress bars in a with block.")
- self.render_progress()
- return self.generator()
-
- def __next__(self) -> V:
- # Iteration is defined in terms of a generator function,
- # returned by iter(self); use that to define next(). This works
- # because `self.iter` is an iterable consumed by that generator,
- # so it is re-entry safe. Calling `next(self.generator())`
- # twice works and does "what you want".
- return next(iter(self))
-
- def render_finish(self) -> None:
- if self.is_hidden:
- return
- self.file.write(AFTER_BAR)
- self.file.flush()
-
- @property
- def pct(self) -> float:
- if self.finished:
- return 1.0
- return min(self.pos / (float(self.length or 1) or 1), 1.0)
-
- @property
- def time_per_iteration(self) -> float:
- if not self.avg:
- return 0.0
- return sum(self.avg) / float(len(self.avg))
-
- @property
- def eta(self) -> float:
- if self.length is not None and not self.finished:
- return self.time_per_iteration * (self.length - self.pos)
- return 0.0
-
- def format_eta(self) -> str:
- if self.eta_known:
- t = int(self.eta)
- seconds = t % 60
- t //= 60
- minutes = t % 60
- t //= 60
- hours = t % 24
- t //= 24
- if t > 0:
- return f"{t}d {hours:02}:{minutes:02}:{seconds:02}"
- else:
- return f"{hours:02}:{minutes:02}:{seconds:02}"
- return ""
-
- def format_pos(self) -> str:
- pos = str(self.pos)
- if self.length is not None:
- pos += f"/{self.length}"
- return pos
-
- def format_pct(self) -> str:
- return f"{int(self.pct * 100): 4}%"[1:]
-
- def format_bar(self) -> str:
- if self.length is not None:
- bar_length = int(self.pct * self.width)
- bar = self.fill_char * bar_length
- bar += self.empty_char * (self.width - bar_length)
- elif self.finished:
- bar = self.fill_char * self.width
- else:
- chars = list(self.empty_char * (self.width or 1))
- if self.time_per_iteration != 0:
- chars[
- int(
- (math.cos(self.pos * self.time_per_iteration) / 2.0 + 0.5)
- * self.width
- )
- ] = self.fill_char
- bar = "".join(chars)
- return bar
-
- def format_progress_line(self) -> str:
- show_percent = self.show_percent
-
- info_bits = []
- if self.length is not None and show_percent is None:
- show_percent = not self.show_pos
-
- if self.show_pos:
- info_bits.append(self.format_pos())
- if show_percent:
- info_bits.append(self.format_pct())
- if self.show_eta and self.eta_known and not self.finished:
- info_bits.append(self.format_eta())
- if self.item_show_func is not None:
- item_info = self.item_show_func(self.current_item)
- if item_info is not None:
- info_bits.append(item_info)
-
- return (
- self.bar_template
- % {
- "label": self.label,
- "bar": self.format_bar(),
- "info": self.info_sep.join(info_bits),
- }
- ).rstrip()
-
- def render_progress(self) -> None:
- import shutil
-
- if self.is_hidden:
- # Only output the label as it changes if the output is not a
- # TTY. Use file=stderr if you expect to be piping stdout.
- if self._last_line != self.label:
- self._last_line = self.label
- echo(self.label, file=self.file, color=self.color)
-
- return
-
- buf = []
- # Update width in case the terminal has been resized
- if self.autowidth:
- old_width = self.width
- self.width = 0
- clutter_length = term_len(self.format_progress_line())
- new_width = max(0, shutil.get_terminal_size().columns - clutter_length)
- if new_width < old_width:
- buf.append(BEFORE_BAR)
- buf.append(" " * self.max_width) # type: ignore
- self.max_width = new_width
- self.width = new_width
-
- clear_width = self.width
- if self.max_width is not None:
- clear_width = self.max_width
-
- buf.append(BEFORE_BAR)
- line = self.format_progress_line()
- line_len = term_len(line)
- if self.max_width is None or self.max_width < line_len:
- self.max_width = line_len
-
- buf.append(line)
- buf.append(" " * (clear_width - line_len))
- line = "".join(buf)
- # Render the line only if it changed.
-
- if line != self._last_line:
- self._last_line = line
- echo(line, file=self.file, color=self.color, nl=False)
- self.file.flush()
-
- def make_step(self, n_steps: int) -> None:
- self.pos += n_steps
- if self.length is not None and self.pos >= self.length:
- self.finished = True
-
- if (time.time() - self.last_eta) < 1.0:
- return
-
- self.last_eta = time.time()
-
- # self.avg is a rolling list of length <= 7 of steps where steps are
- # defined as time elapsed divided by the total progress through
- # self.length.
- if self.pos:
- step = (time.time() - self.start) / self.pos
- else:
- step = time.time() - self.start
-
- self.avg = self.avg[-6:] + [step]
-
- self.eta_known = self.length is not None
-
- def update(self, n_steps: int, current_item: t.Optional[V] = None) -> None:
- """Update the progress bar by advancing a specified number of
- steps, and optionally set the ``current_item`` for this new
- position.
-
- :param n_steps: Number of steps to advance.
- :param current_item: Optional item to set as ``current_item``
- for the updated position.
-
- .. versionchanged:: 8.0
- Added the ``current_item`` optional parameter.
-
- .. versionchanged:: 8.0
- Only render when the number of steps meets the
- ``update_min_steps`` threshold.
- """
- if current_item is not None:
- self.current_item = current_item
-
- self._completed_intervals += n_steps
-
- if self._completed_intervals >= self.update_min_steps:
- self.make_step(self._completed_intervals)
- self.render_progress()
- self._completed_intervals = 0
-
- def finish(self) -> None:
- self.eta_known = False
- self.current_item = None
- self.finished = True
-
- def generator(self) -> t.Iterator[V]:
- """Return a generator which yields the items added to the bar
- during construction, and updates the progress bar *after* the
- yielded block returns.
- """
- # WARNING: the iterator interface for `ProgressBar` relies on
- # this and only works because this is a simple generator which
- # doesn't create or manage additional state. If this function
- # changes, the impact should be evaluated both against
- # `iter(bar)` and `next(bar)`. `next()` in particular may call
- # `self.generator()` repeatedly, and this must remain safe in
- # order for that interface to work.
- if not self.entered:
- raise RuntimeError("You need to use progress bars in a with block.")
-
- if self.is_hidden:
- yield from self.iter
- else:
- for rv in self.iter:
- self.current_item = rv
-
- # This allows show_item_func to be updated before the
- # item is processed. Only trigger at the beginning of
- # the update interval.
- if self._completed_intervals == 0:
- self.render_progress()
-
- yield rv
- self.update(1)
-
- self.finish()
- self.render_progress()
-
-
-def pager(generator: t.Iterable[str], color: t.Optional[bool] = None) -> None:
- """Decide what method to use for paging through text."""
- stdout = _default_text_stdout()
-
- # There are no standard streams attached to write to. For example,
- # pythonw on Windows.
- if stdout is None:
- stdout = StringIO()
-
- if not isatty(sys.stdin) or not isatty(stdout):
- return _nullpager(stdout, generator, color)
- pager_cmd = (os.environ.get("PAGER", None) or "").strip()
- if pager_cmd:
- if WIN:
- return _tempfilepager(generator, pager_cmd, color)
- return _pipepager(generator, pager_cmd, color)
- if os.environ.get("TERM") in ("dumb", "emacs"):
- return _nullpager(stdout, generator, color)
- if WIN or sys.platform.startswith("os2"):
- return _tempfilepager(generator, "more <", color)
- if hasattr(os, "system") and os.system("(less) 2>/dev/null") == 0:
- return _pipepager(generator, "less", color)
-
- import tempfile
-
- fd, filename = tempfile.mkstemp()
- os.close(fd)
- try:
- if hasattr(os, "system") and os.system(f'more "{filename}"') == 0:
- return _pipepager(generator, "more", color)
- return _nullpager(stdout, generator, color)
- finally:
- os.unlink(filename)
-
-
-def _pipepager(generator: t.Iterable[str], cmd: str, color: t.Optional[bool]) -> None:
- """Page through text by feeding it to another program. Invoking a
- pager through this might support colors.
- """
- import subprocess
-
- env = dict(os.environ)
-
- # If we're piping to less we might support colors under the
- # condition that
- cmd_detail = cmd.rsplit("/", 1)[-1].split()
- if color is None and cmd_detail[0] == "less":
- less_flags = f"{os.environ.get('LESS', '')}{' '.join(cmd_detail[1:])}"
- if not less_flags:
- env["LESS"] = "-R"
- color = True
- elif "r" in less_flags or "R" in less_flags:
- color = True
-
- c = subprocess.Popen(cmd, shell=True, stdin=subprocess.PIPE, env=env)
- stdin = t.cast(t.BinaryIO, c.stdin)
- encoding = get_best_encoding(stdin)
- try:
- for text in generator:
- if not color:
- text = strip_ansi(text)
-
- stdin.write(text.encode(encoding, "replace"))
- except (OSError, KeyboardInterrupt):
- pass
- else:
- stdin.close()
-
- # Less doesn't respect ^C, but catches it for its own UI purposes (aborting
- # search or other commands inside less).
- #
- # That means when the user hits ^C, the parent process (click) terminates,
- # but less is still alive, paging the output and messing up the terminal.
- #
- # If the user wants to make the pager exit on ^C, they should set
- # `LESS='-K'`. It's not our decision to make.
- while True:
- try:
- c.wait()
- except KeyboardInterrupt:
- pass
- else:
- break
-
-
-def _tempfilepager(
- generator: t.Iterable[str], cmd: str, color: t.Optional[bool]
-) -> None:
- """Page through text by invoking a program on a temporary file."""
- import tempfile
-
- fd, filename = tempfile.mkstemp()
- # TODO: This never terminates if the passed generator never terminates.
- text = "".join(generator)
- if not color:
- text = strip_ansi(text)
- encoding = get_best_encoding(sys.stdout)
- with open_stream(filename, "wb")[0] as f:
- f.write(text.encode(encoding))
- try:
- os.system(f'{cmd} "{filename}"')
- finally:
- os.close(fd)
- os.unlink(filename)
-
-
-def _nullpager(
- stream: t.TextIO, generator: t.Iterable[str], color: t.Optional[bool]
-) -> None:
- """Simply print unformatted text. This is the ultimate fallback."""
- for text in generator:
- if not color:
- text = strip_ansi(text)
- stream.write(text)
-
-
-class Editor:
- def __init__(
- self,
- editor: t.Optional[str] = None,
- env: t.Optional[t.Mapping[str, str]] = None,
- require_save: bool = True,
- extension: str = ".txt",
- ) -> None:
- self.editor = editor
- self.env = env
- self.require_save = require_save
- self.extension = extension
-
- def get_editor(self) -> str:
- if self.editor is not None:
- return self.editor
- for key in "VISUAL", "EDITOR":
- rv = os.environ.get(key)
- if rv:
- return rv
- if WIN:
- return "notepad"
- for editor in "sensible-editor", "vim", "nano":
- if os.system(f"which {editor} >/dev/null 2>&1") == 0:
- return editor
- return "vi"
-
- def edit_file(self, filename: str) -> None:
- import subprocess
-
- editor = self.get_editor()
- environ: t.Optional[t.Dict[str, str]] = None
-
- if self.env:
- environ = os.environ.copy()
- environ.update(self.env)
-
- try:
- c = subprocess.Popen(f'{editor} "{filename}"', env=environ, shell=True)
- exit_code = c.wait()
- if exit_code != 0:
- raise ClickException(
- _("{editor}: Editing failed").format(editor=editor)
- )
- except OSError as e:
- raise ClickException(
- _("{editor}: Editing failed: {e}").format(editor=editor, e=e)
- ) from e
-
- def edit(self, text: t.Optional[t.AnyStr]) -> t.Optional[t.AnyStr]:
- import tempfile
-
- if not text:
- data = b""
- elif isinstance(text, (bytes, bytearray)):
- data = text
- else:
- if text and not text.endswith("\n"):
- text += "\n"
-
- if WIN:
- data = text.replace("\n", "\r\n").encode("utf-8-sig")
- else:
- data = text.encode("utf-8")
-
- fd, name = tempfile.mkstemp(prefix="editor-", suffix=self.extension)
- f: t.BinaryIO
-
- try:
- with os.fdopen(fd, "wb") as f:
- f.write(data)
-
- # If the filesystem resolution is 1 second, like Mac OS
- # 10.12 Extended, or 2 seconds, like FAT32, and the editor
- # closes very fast, require_save can fail. Set the modified
- # time to be 2 seconds in the past to work around this.
- os.utime(name, (os.path.getatime(name), os.path.getmtime(name) - 2))
- # Depending on the resolution, the exact value might not be
- # recorded, so get the new recorded value.
- timestamp = os.path.getmtime(name)
-
- self.edit_file(name)
-
- if self.require_save and os.path.getmtime(name) == timestamp:
- return None
-
- with open(name, "rb") as f:
- rv = f.read()
-
- if isinstance(text, (bytes, bytearray)):
- return rv
-
- return rv.decode("utf-8-sig").replace("\r\n", "\n") # type: ignore
- finally:
- os.unlink(name)
-
-
-def open_url(url: str, wait: bool = False, locate: bool = False) -> int:
- import subprocess
-
- def _unquote_file(url: str) -> str:
- from urllib.parse import unquote
-
- if url.startswith("file://"):
- url = unquote(url[7:])
-
- return url
-
- if sys.platform == "darwin":
- args = ["open"]
- if wait:
- args.append("-W")
- if locate:
- args.append("-R")
- args.append(_unquote_file(url))
- null = open("/dev/null", "w")
- try:
- return subprocess.Popen(args, stderr=null).wait()
- finally:
- null.close()
- elif WIN:
- if locate:
- url = _unquote_file(url.replace('"', ""))
- args = f'explorer /select,"{url}"'
- else:
- url = url.replace('"', "")
- wait_str = "/WAIT" if wait else ""
- args = f'start {wait_str} "" "{url}"'
- return os.system(args)
- elif CYGWIN:
- if locate:
- url = os.path.dirname(_unquote_file(url).replace('"', ""))
- args = f'cygstart "{url}"'
- else:
- url = url.replace('"', "")
- wait_str = "-w" if wait else ""
- args = f'cygstart {wait_str} "{url}"'
- return os.system(args)
-
- try:
- if locate:
- url = os.path.dirname(_unquote_file(url)) or "."
- else:
- url = _unquote_file(url)
- c = subprocess.Popen(["xdg-open", url])
- if wait:
- return c.wait()
- return 0
- except OSError:
- if url.startswith(("http://", "https://")) and not locate and not wait:
- import webbrowser
-
- webbrowser.open(url)
- return 0
- return 1
-
-
-def _translate_ch_to_exc(ch: str) -> t.Optional[BaseException]:
- if ch == "\x03":
- raise KeyboardInterrupt()
-
- if ch == "\x04" and not WIN: # Unix-like, Ctrl+D
- raise EOFError()
-
- if ch == "\x1a" and WIN: # Windows, Ctrl+Z
- raise EOFError()
-
- return None
-
-
-if WIN:
- import msvcrt
-
- @contextlib.contextmanager
- def raw_terminal() -> t.Iterator[int]:
- yield -1
-
- def getchar(echo: bool) -> str:
- # The function `getch` will return a bytes object corresponding to
- # the pressed character. Since Windows 10 build 1803, it will also
- # return \x00 when called a second time after pressing a regular key.
- #
- # `getwch` does not share this probably-bugged behavior. Moreover, it
- # returns a Unicode object by default, which is what we want.
- #
- # Either of these functions will return \x00 or \xe0 to indicate
- # a special key, and you need to call the same function again to get
- # the "rest" of the code. The fun part is that \u00e0 is
- # "latin small letter a with grave", so if you type that on a French
- # keyboard, you _also_ get a \xe0.
- # E.g., consider the Up arrow. This returns \xe0 and then \x48. The
- # resulting Unicode string reads as "a with grave" + "capital H".
- # This is indistinguishable from when the user actually types
- # "a with grave" and then "capital H".
- #
- # When \xe0 is returned, we assume it's part of a special-key sequence
- # and call `getwch` again, but that means that when the user types
- # the \u00e0 character, `getchar` doesn't return until a second
- # character is typed.
- # The alternative is returning immediately, but that would mess up
- # cross-platform handling of arrow keys and others that start with
- # \xe0. Another option is using `getch`, but then we can't reliably
- # read non-ASCII characters, because return values of `getch` are
- # limited to the current 8-bit codepage.
- #
- # Anyway, Click doesn't claim to do this Right(tm), and using `getwch`
- # is doing the right thing in more situations than with `getch`.
- func: t.Callable[[], str]
-
- if echo:
- func = msvcrt.getwche # type: ignore
- else:
- func = msvcrt.getwch # type: ignore
-
- rv = func()
-
- if rv in ("\x00", "\xe0"):
- # \x00 and \xe0 are control characters that indicate special key,
- # see above.
- rv += func()
-
- _translate_ch_to_exc(rv)
- return rv
-
-else:
- import tty
- import termios
-
- @contextlib.contextmanager
- def raw_terminal() -> t.Iterator[int]:
- f: t.Optional[t.TextIO]
- fd: int
-
- if not isatty(sys.stdin):
- f = open("/dev/tty")
- fd = f.fileno()
- else:
- fd = sys.stdin.fileno()
- f = None
-
- try:
- old_settings = termios.tcgetattr(fd)
-
- try:
- tty.setraw(fd)
- yield fd
- finally:
- termios.tcsetattr(fd, termios.TCSADRAIN, old_settings)
- sys.stdout.flush()
-
- if f is not None:
- f.close()
- except termios.error:
- pass
-
- def getchar(echo: bool) -> str:
- with raw_terminal() as fd:
- ch = os.read(fd, 32).decode(get_best_encoding(sys.stdin), "replace")
-
- if echo and isatty(sys.stdout):
- sys.stdout.write(ch)
-
- _translate_ch_to_exc(ch)
- return ch
diff --git a/spaces/DQChoi/gpt-demo/venv/lib/python3.11/site-packages/gradio/templates/frontend/assets/__vite-browser-external-b25bb000.js b/spaces/DQChoi/gpt-demo/venv/lib/python3.11/site-packages/gradio/templates/frontend/assets/__vite-browser-external-b25bb000.js
deleted file mode 100644
index efa8971d2172dd2c1924c07a4e2b2bc18871ccd9..0000000000000000000000000000000000000000
--- a/spaces/DQChoi/gpt-demo/venv/lib/python3.11/site-packages/gradio/templates/frontend/assets/__vite-browser-external-b25bb000.js
+++ /dev/null
@@ -1,2 +0,0 @@
-const e={};export{e as default};
-//# sourceMappingURL=__vite-browser-external-b25bb000.js.map
diff --git a/spaces/DaleChen/AutoGPT/autogpt/permanent_memory/__init__.py b/spaces/DaleChen/AutoGPT/autogpt/permanent_memory/__init__.py
deleted file mode 100644
index e69de29bb2d1d6434b8b29ae775ad8c2e48c5391..0000000000000000000000000000000000000000
diff --git a/spaces/Daniton/midjourney-singular/app.py b/spaces/Daniton/midjourney-singular/app.py
deleted file mode 100644
index 2193905172b6fb6d868bff88cc8311f491ec13b3..0000000000000000000000000000000000000000
--- a/spaces/Daniton/midjourney-singular/app.py
+++ /dev/null
@@ -1,3 +0,0 @@
-import gradio as gr
-
-gr.Interface.load("models/prompthero/openjourney").launch()
\ No newline at end of file
diff --git a/spaces/Datasculptor/DescriptionGPT/detic/data/datasets/register_oid.py b/spaces/Datasculptor/DescriptionGPT/detic/data/datasets/register_oid.py
deleted file mode 100644
index bd281f53f07074740b453838ba32f42f81a28383..0000000000000000000000000000000000000000
--- a/spaces/Datasculptor/DescriptionGPT/detic/data/datasets/register_oid.py
+++ /dev/null
@@ -1,122 +0,0 @@
-# Copyright (c) Facebook, Inc. and its affiliates.
-# Modified by Xingyi Zhou from https://github.com/facebookresearch/detectron2/blob/master/detectron2/data/datasets/coco.py
-import copy
-import io
-import logging
-import contextlib
-import os
-import datetime
-import json
-import numpy as np
-
-from PIL import Image
-
-from fvcore.common.timer import Timer
-from fvcore.common.file_io import PathManager, file_lock
-from detectron2.structures import BoxMode, PolygonMasks, Boxes
-from detectron2.data import DatasetCatalog, MetadataCatalog
-
-logger = logging.getLogger(__name__)
-
-"""
-This file contains functions to register a COCO-format dataset to the DatasetCatalog.
-"""
-
-__all__ = ["register_coco_instances", "register_coco_panoptic_separated"]
-
-
-
-def register_oid_instances(name, metadata, json_file, image_root):
- """
- """
- # 1. register a function which returns dicts
- DatasetCatalog.register(name, lambda: load_coco_json_mem_efficient(
- json_file, image_root, name))
-
- # 2. Optionally, add metadata about this dataset,
- # since they might be useful in evaluation, visualization or logging
- MetadataCatalog.get(name).set(
- json_file=json_file, image_root=image_root, evaluator_type="oid", **metadata
- )
-
-
-def load_coco_json_mem_efficient(json_file, image_root, dataset_name=None, extra_annotation_keys=None):
- """
- Actually not mem efficient
- """
- from pycocotools.coco import COCO
-
- timer = Timer()
- json_file = PathManager.get_local_path(json_file)
- with contextlib.redirect_stdout(io.StringIO()):
- coco_api = COCO(json_file)
- if timer.seconds() > 1:
- logger.info("Loading {} takes {:.2f} seconds.".format(json_file, timer.seconds()))
-
- id_map = None
- if dataset_name is not None:
- meta = MetadataCatalog.get(dataset_name)
- cat_ids = sorted(coco_api.getCatIds())
- cats = coco_api.loadCats(cat_ids)
- # The categories in a custom json file may not be sorted.
- thing_classes = [c["name"] for c in sorted(cats, key=lambda x: x["id"])]
- meta.thing_classes = thing_classes
-
- if not (min(cat_ids) == 1 and max(cat_ids) == len(cat_ids)):
- if "coco" not in dataset_name:
- logger.warning(
- """
- Category ids in annotations are not in [1, #categories]! We'll apply a mapping for you.
- """
- )
- id_map = {v: i for i, v in enumerate(cat_ids)}
- meta.thing_dataset_id_to_contiguous_id = id_map
-
- # sort indices for reproducible results
- img_ids = sorted(coco_api.imgs.keys())
- imgs = coco_api.loadImgs(img_ids)
- logger.info("Loaded {} images in COCO format from {}".format(len(imgs), json_file))
-
- dataset_dicts = []
-
- ann_keys = ["iscrowd", "bbox", "category_id"] + (extra_annotation_keys or [])
-
- for img_dict in imgs:
- record = {}
- record["file_name"] = os.path.join(image_root, img_dict["file_name"])
- record["height"] = img_dict["height"]
- record["width"] = img_dict["width"]
- image_id = record["image_id"] = img_dict["id"]
- anno_dict_list = coco_api.imgToAnns[image_id]
- if 'neg_category_ids' in img_dict:
- record['neg_category_ids'] = \
- [id_map[x] for x in img_dict['neg_category_ids']]
-
- objs = []
- for anno in anno_dict_list:
- assert anno["image_id"] == image_id
-
- assert anno.get("ignore", 0) == 0
-
- obj = {key: anno[key] for key in ann_keys if key in anno}
-
- segm = anno.get("segmentation", None)
- if segm: # either list[list[float]] or dict(RLE)
- if not isinstance(segm, dict):
- # filter out invalid polygons (< 3 points)
- segm = [poly for poly in segm if len(poly) % 2 == 0 and len(poly) >= 6]
- if len(segm) == 0:
- num_instances_without_valid_segmentation += 1
- continue # ignore this instance
- obj["segmentation"] = segm
-
- obj["bbox_mode"] = BoxMode.XYWH_ABS
-
- if id_map:
- obj["category_id"] = id_map[obj["category_id"]]
- objs.append(obj)
- record["annotations"] = objs
- dataset_dicts.append(record)
-
- del coco_api
- return dataset_dicts
\ No newline at end of file
diff --git a/spaces/Demosthene-OR/avr23-cds-translation/tabs/template_save/third_tab.py b/spaces/Demosthene-OR/avr23-cds-translation/tabs/template_save/third_tab.py
deleted file mode 100644
index 000d5fc23042ba9463ad3bb47e8b468092070d17..0000000000000000000000000000000000000000
--- a/spaces/Demosthene-OR/avr23-cds-translation/tabs/template_save/third_tab.py
+++ /dev/null
@@ -1,20 +0,0 @@
-import streamlit as st
-import pandas as pd
-import numpy as np
-
-
-title = "Data Vizualization"
-sidebar_name = "Data Vizualization"
-
-
-def run():
-
- st.title(title)
-
- st.markdown(
- """
- This is the third sample tab.
- """
- )
-
- st.write(pd.DataFrame(np.random.randn(100, 4), columns=list("ABCD")))
diff --git a/spaces/Dinoking/Garbage-Classifier-V6/app.py b/spaces/Dinoking/Garbage-Classifier-V6/app.py
deleted file mode 100644
index 834db3bf2f01a727cecc871149b0a73166b2eea2..0000000000000000000000000000000000000000
--- a/spaces/Dinoking/Garbage-Classifier-V6/app.py
+++ /dev/null
@@ -1,28 +0,0 @@
-import gradio as gr
-import tensorflow as tf
-import numpy as np
-from PIL import Image
-import tensorflow.keras as keras
-import keras.applications.xception as xception
-from tensorflow.keras.models import load_model
-
-# load model
-model = load_model('model804.h5')
-
-classnames = ['battery','cardboard','clothes','food','glass','medical','metal','paper','plastic','shoes']
-
-
-
-def predict_image(img):
- img_4d=img.reshape(-1,320, 320,3)
- prediction=model.predict(img_4d)[0]
- return {classnames[i]: float(prediction[i]) for i in range(10)}
-
-image = gr.inputs.Image(shape=(320, 320))
-label = gr.outputs.Label(num_top_classes=3)
-enable_queue=True
-examples = ['battery.jpg','cardboard.jpeg','clothes.jpeg','glass.jpg','metal.jpg','plastic.jpg','shoes.jpg']
-article="
Made by Aditya Narendra with 🖤
"
-
-gr.Interface(fn=predict_image, inputs=image, title="Garbage Classifier",
- description="This is a Garbage Classification Model Trained using Xception Net on DS11 Mod(Seg10 V4).Deployed to Hugging Faces using Gradio.",outputs=label,article=article,enable_queue=enable_queue,examples=examples,interpretation='default').launch(share="True")
\ No newline at end of file
diff --git a/spaces/DragGan/DragGan-Inversion/stylegan_human/torch_utils/__init__.py b/spaces/DragGan/DragGan-Inversion/stylegan_human/torch_utils/__init__.py
deleted file mode 100644
index a0b0f4efcbe1e3cd4199eeecb043d5afe1548307..0000000000000000000000000000000000000000
--- a/spaces/DragGan/DragGan-Inversion/stylegan_human/torch_utils/__init__.py
+++ /dev/null
@@ -1,11 +0,0 @@
-# Copyright (c) SenseTime Research. All rights reserved.
-
-# Copyright (c) 2021, NVIDIA CORPORATION. All rights reserved.
-#
-# NVIDIA CORPORATION and its licensors retain all intellectual property
-# and proprietary rights in and to this software, related documentation
-# and any modifications thereto. Any use, reproduction, disclosure or
-# distribution of this software and related documentation without an express
-# license agreement from NVIDIA CORPORATION is strictly prohibited.
-
-# empty
diff --git a/spaces/DucHaiten/webui/app.py b/spaces/DucHaiten/webui/app.py
deleted file mode 100644
index 5a08890d6b889c2623b84175d936a4432ede77e7..0000000000000000000000000000000000000000
--- a/spaces/DucHaiten/webui/app.py
+++ /dev/null
@@ -1,42 +0,0 @@
-import os
-from subprocess import getoutput
-
-gpu_info = getoutput('nvidia-smi')
-if("A10G" in gpu_info):
- os.system(f"pip install -q https://github.com/camenduru/stable-diffusion-webui-colab/releases/download/0.0.15/xformers-0.0.15.dev0+4c06c79.d20221205-cp38-cp38-linux_x86_64.whl")
-elif("T4" in gpu_info):
- os.system(f"pip install -q https://github.com/camenduru/stable-diffusion-webui-colab/releases/download/0.0.15/xformers-0.0.15.dev0+1515f77.d20221130-cp38-cp38-linux_x86_64.whl")
-
-os.system(f"git clone -b v1.5 https://github.com/camenduru/stable-diffusion-webui /home/user/app/stable-diffusion-webui")
-os.chdir("/home/user/app/stable-diffusion-webui")
-
-os.system(f"wget -q https://github.com/camenduru/webui/raw/main/env_patch.py -O /home/user/app/env_patch.py")
-os.system(f"sed -i '$a fastapi==0.90.0' /home/user/app/stable-diffusion-webui/requirements_versions.txt")
-os.system(f"sed -i -e '/import image_from_url_text/r /home/user/app/env_patch.py' /home/user/app/stable-diffusion-webui/modules/ui.py")
-os.system(f"sed -i -e '/(modelmerger_interface, \"Checkpoint Merger\", \"modelmerger\"),/d' /home/user/app/stable-diffusion-webui/modules/ui.py")
-os.system(f"sed -i -e '/(train_interface, \"Train\", \"ti\"),/d' /home/user/app/stable-diffusion-webui/modules/ui.py")
-os.system(f"sed -i -e '/extensions_interface, \"Extensions\", \"extensions\"/d' /home/user/app/stable-diffusion-webui/modules/ui.py")
-os.system(f"sed -i -e '/settings_interface, \"Settings\", \"settings\"/d' /home/user/app/stable-diffusion-webui/modules/ui.py")
-os.system(f'''sed -i -e "s/document.getElementsByTagName('gradio-app')\[0\].shadowRoot/!!document.getElementsByTagName('gradio-app')[0].shadowRoot ? document.getElementsByTagName('gradio-app')[0].shadowRoot : document/g" /home/user/app/stable-diffusion-webui/script.js''')
-os.system(f"sed -i -e 's/ show_progress=False,/ show_progress=True,/g' /home/user/app/stable-diffusion-webui/modules/ui.py")
-os.system(f"sed -i -e 's/shared.demo.launch/shared.demo.queue().launch/g' /home/user/app/stable-diffusion-webui/webui.py")
-os.system(f"sed -i -e 's/ outputs=\[/queue=False, &/g' /home/user/app/stable-diffusion-webui/modules/ui.py")
-os.system(f"sed -i -e 's/ queue=False, / /g' /home/user/app/stable-diffusion-webui/modules/ui.py")
-
-# ----------------------------Please duplicate this space and delete this block if you don't want to see the extra header----------------------------
-os.system(f"wget -q https://github.com/camenduru/webui/raw/main/header_patch.py -O /home/user/app/header_patch.py")
-os.system(f"sed -i -e '/demo:/r /home/user/app/header_patch.py' /home/user/app/stable-diffusion-webui/modules/ui.py")
-# ---------------------------------------------------------------------------------------------------------------------------------------------------
-
-os.system(f"rm -rfv /home/user/app/stable-diffusion-webui/scripts/")
-
-os.system(f"wget -q https://github.com/camenduru/webui/raw/main/shared-config.json -O /home/user/app/shared-config.json")
-os.system(f"wget -q https://github.com/camenduru/webui/raw/main/shared-ui-config.json -O /home/user/app/shared-ui-config.json")
-
-# os.system(f"wget -q https://huggingface.co/DucHaiten/DucHaitenAIart/resolve/main/DucHaitenAIart_v2.0.safetensors -O /home/user/app/stable-diffusion-webui/models/Stable-diffusion/DucHaitenAIart_v2.0-emaonly.safetensors")
-os.system(f"wget -q https://huggingface.co/DucHaiten/DucHaitenDreamWorld/resolve/main/DucHaitenDreamWorld_v2.4.1.safetensors -O /home/user/app/stable-diffusion-webui/models/Stable-diffusion/DucHaitenDreamWorld_v2.4.1.safetensors")
-os.system(f"wget -q https://huggingface.co/DucHaiten/DucHaitenAnime/resolve/main/DucHaitenAnime_v4.0.safetensors -O /home/user/app/stable-diffusion-webui/models/Stable-diffusion/DucHaitenAnime_v4.0.safetensors")
-os.system(f"wget -q https://huggingface.co/DucHaiten/DucHaitenAnimated/resolve/main/DucHaitenAnimated_v5.0.safetensors -O /home/user/app/stable-diffusion-webui/models/Stable-diffusion/DucHaitenAnimated_v5.0.safetensors")
-os.system(f"wget -q https://huggingface.co/DucHaiten/DucHaitenAIart/resolve/main/DucHaitenAIart_v3.1.safetensors -O /home/user/app/stable-diffusion-webui/models/Stable-diffusion/DucHaitenAIart_v3.1.safetensors")
-
-os.system(f"python launch.py --force-enable-xformers --ui-config-file /home/user/app/ui-config.json --ui-settings-file /home/user/app/config.json --disable-console-progressbars --enable-console-prompts --cors-allow-origins huggingface.co,hf.space --no-progressbar-hiding --api")
\ No newline at end of file
diff --git a/spaces/Duskfallcrew/DreamlikeArt-PhotoReal-2.0/style.css b/spaces/Duskfallcrew/DreamlikeArt-PhotoReal-2.0/style.css
deleted file mode 100644
index fdbef9e64cc6b9f8003698ffa38997ee22a640ac..0000000000000000000000000000000000000000
--- a/spaces/Duskfallcrew/DreamlikeArt-PhotoReal-2.0/style.css
+++ /dev/null
@@ -1,84 +0,0 @@
-#col-container {
- max-width: 800px;
- margin-left: auto;
- margin-right: auto;
-}
-a {
- color: inherit;
- text-decoration: underline;
-}
-.gradio-container {
- font-family: 'IBM Plex Sans', sans-serif;
-}
-.gr-button {
- color: white;
- border-color: #9d66e5;
- background: #9d66e5;
-}
-input[type='range'] {
- accent-color: #9d66e5;
-}
-.dark input[type='range'] {
- accent-color: #dfdfdf;
-}
-.container {
- max-width: 800px;
- margin: auto;
- padding-top: 1.5rem;
-}
-#gallery {
- min-height: 22rem;
- margin-bottom: 15px;
- margin-left: auto;
- margin-right: auto;
- border-bottom-right-radius: .5rem !important;
- border-bottom-left-radius: .5rem !important;
-}
-#gallery>div>.h-full {
- min-height: 20rem;
-}
-.details:hover {
- text-decoration: underline;
-}
-.gr-button {
- white-space: nowrap;
-}
-.gr-button:focus {
- border-color: rgb(147 197 253 / var(--tw-border-opacity));
- outline: none;
- box-shadow: var(--tw-ring-offset-shadow), var(--tw-ring-shadow), var(--tw-shadow, 0 0 #0000);
- --tw-border-opacity: 1;
- --tw-ring-offset-shadow: var(--tw-ring-inset) 0 0 0 var(--tw-ring-offset-width) var(--tw-ring-offset-color);
- --tw-ring-shadow: var(--tw-ring-inset) 0 0 0 calc(3px var(--tw-ring-offset-width)) var(--tw-ring-color);
- --tw-ring-color: rgb(191 219 254 / var(--tw-ring-opacity));
- --tw-ring-opacity: .5;
-}
-#advanced-options {
- margin-bottom: 20px;
-}
-.footer {
- margin-bottom: 45px;
- margin-top: 35px;
- text-align: center;
- border-bottom: 1px solid #e5e5e5;
-}
-.footer>p {
- font-size: .8rem;
- display: inline-block;
- padding: 0 10px;
- transform: translateY(10px);
- background: white;
-}
-.dark .logo{ filter: invert(1); }
-.dark .footer {
- border-color: #303030;
-}
-.dark .footer>p {
- background: #0b0f19;
-}
-.acknowledgments h4{
- margin: 1.25em 0 .25em 0;
- font-weight: bold;
- font-size: 115%;
-}
-
diff --git a/spaces/EPFL-VILAB/MultiMAE/FINETUNING.md b/spaces/EPFL-VILAB/MultiMAE/FINETUNING.md
deleted file mode 100644
index f07f794064f8b5a3496f86eddbe05e1030fc5411..0000000000000000000000000000000000000000
--- a/spaces/EPFL-VILAB/MultiMAE/FINETUNING.md
+++ /dev/null
@@ -1,126 +0,0 @@
-# Fine-tuning
-
-We provide fine-tuning scripts for classification, semantic segmentation, depth estimation and more.
-Please check [SETUP.md](SETUP.md) for set-up instructions first.
-
-- [General information](#general-information)
-- [Classification](#classification)
-- [Semantic segmentation](#semantic-segmentation)
-- [Depth estimation](#depth-estimation)
-- [Taskonomy tasks](#taskonomy-tasks)
-
-## General information
-
-### Loading pre-trained models
-
-All our fine-tuning scripts support models in the MultiMAE / MultiViT format. Pre-trained models using the timm / ViT format can be converted to this format using the [`vit2multimae_converter.py`](tools/vit2multimae_converter.py)
- script. More information can be found [here](README.md#model-formats).
-
-### Modifying configs
-The training scripts support both YAML config files and command-line arguments. See [here](cfgs/finetune) for all fine-tuning config files.
-
-To modify fine-training settings, either edit / add config files or provide additional command-line arguments.
-
-:information_source: Config files arguments override default arguments, and command-line arguments override both default arguments and config arguments.
-
-:warning: When changing settings (e.g., using a different pre-trained model), make sure to modify the `output_dir` and `wandb_run_name` (if logging is activated) to reflect the changes.
-
-
-### Experiment logging
-To activate logging to [Weights & Biases](https://docs.wandb.ai/), either edit the config files or use the `--log_wandb` flag along with any other extra logging arguments.
-
-
-## Classification
-
-We use 8 A100 GPUs for classification fine-tuning. Configs can be found [here](cfgs/finetune/cls).
-
-To fine-tune MultiMAE on ImageNet-1K classification using default settings, run:
-```bash
-OMP_NUM_THREADS=1 torchrun --nproc_per_node=8 run_finetuning_cls.py \
---config cfgs/finetune/cls/ft_in1k_100e_multimae-b.yaml \
---finetune /path/to/multimae_weights \
---data_path /path/to/in1k/train/rgb \
---eval_data_path /path/to/in1k/val/rgb
-```
-
-- For a list of possible arguments, see [`run_finetuning_cls.py`](run_finetuning_cls.py).
-
-## Semantic segmentation
-
-We use 4 A100 GPUs for semantic segmentation fine-tuning. Configs can be found [here](cfgs/finetune/semseg).
-
-### ADE20K
-To fine-tune MultiMAE on ADE20K semantic segmentation with default settings and **RGB** as the input modality, run:
-```bash
-OMP_NUM_THREADS=1 torchrun --nproc_per_node=4 run_finetuning_semseg.py \
---config cfgs/finetune/semseg/ade/ft_ade_64e_multimae-b_rgb.yaml \
---finetune /path/to/multimae_weights \
---data_path /path/to/ade20k/train \
---eval_data_path /path/to/ade20k/val
-```
-
-- For a list of possible arguments, see [`run_finetuning_semseg.py`](run_finetuning_semseg.py).
-
-
-### Hypersim
-To fine-tune MultiMAE on Hypersim semantic segmentation with default settings and **RGB** as the input modality, run:
-```bash
-OMP_NUM_THREADS=1 torchrun --nproc_per_node=4 run_finetuning_semseg.py \
---config cfgs/finetune/semseg/hypersim/ft_hypersim_25e_multimae-b_rgb.yaml \
---finetune /path/to/multimae_weights \
---data_path /path/to/hypersim/train \
---eval_data_path /path/to/hypersim/val
-```
-
-- To fine-tune using **depth-only** and **RGB + depth** as the input modalities, simply swap the config file to the appropriate one.
-- For a list of possible arguments, see [`run_finetuning_semseg.py`](run_finetuning_semseg.py).
-
-
-
-### NYUv2
-To fine-tune MultiMAE on NYUv2 semantic segmentation with default settings and **RGB** as the input modality, run:
-```bash
-OMP_NUM_THREADS=1 torchrun --nproc_per_node=4 run_finetuning_semseg.py \
---config cfgs/finetune/semseg/nyu/ft_nyu_200e_multimae-b_rgb.yaml \
---finetune /path/to/multimae_weights \
---data_path /path/to/nyu/train \
---eval_data_path /path/to/nyu/test_or_val
-```
-
-- To fine-tune using **depth-only** and **RGB + depth** as the input modalities, simply swap the config file to the appropriate one.
-- For a list of possible arguments, see [`run_finetuning_semseg.py`](run_finetuning_semseg.py).
-
-
-## Depth estimation
-
-We use 2 A100 GPUs for depth estimation fine-tuning. Configs can be found [here](cfgs/finetune/depth).
-
-
-To fine-tune MultiMAE on NYUv2 depth estimation with default settings, run:
-```bash
-OMP_NUM_THREADS=1 torchrun --nproc_per_node=2 run_finetuning_depth.py \
---config cfgs/finetune/depth/ft_nyu_2000e_multimae-b.yaml \
---finetune /path/to/multimae_weights \
---data_path /path/to/nyu/train \
---eval_data_path /path/to/nyu/test_or_val
-```
-- For a list of possible arguments, see [`run_finetuning_depth.py`](run_finetuning_depth.py).
-
-## Taskonomy tasks
-
-We use 1 A100 GPU to fine-tune on Taskonomy tasks. Configs can be found [here](cfgs/finetune/taskonomy).
-
-The tasks we support are: Principal curvature, z-buffer depth, texture edges, occlusion edges, 2D keypoints,
-3D keypoints, surface normals, and reshading.
-
-
-For example, to fine-tune MultiMAE on Taskonomy reshading with default settings, run:
-```bash
-OMP_NUM_THREADS=1 torchrun --nproc_per_node=1 run_finetuning_taskonomy.py \
---config cfgs/finetune/taskonomy/rgb2reshading-1k/ft_rgb2reshading_multimae-b.yaml \
---finetune /path/to/multimae_weights \
---data_path /path/to/taskonomy_tiny
-```
-
-- To fine-tune on a different task, simply swap the config file to the appropriate one.
-- For a list of possible arguments, see [`run_finetuning_taskonomy.py`](run_finetuning_taskonomy.py).
diff --git a/spaces/Egrt/MaskGAN/utils/__init__.py b/spaces/Egrt/MaskGAN/utils/__init__.py
deleted file mode 100644
index 90f60fdd89ad8575faafe45188bd1d968852fc67..0000000000000000000000000000000000000000
--- a/spaces/Egrt/MaskGAN/utils/__init__.py
+++ /dev/null
@@ -1 +0,0 @@
-from .utils import *
\ No newline at end of file
diff --git a/spaces/FantasticGNU/AnomalyGPT/header.py b/spaces/FantasticGNU/AnomalyGPT/header.py
deleted file mode 100644
index 2e34537c2e988b2cc62e5ebc78197b76130dc51e..0000000000000000000000000000000000000000
--- a/spaces/FantasticGNU/AnomalyGPT/header.py
+++ /dev/null
@@ -1,35 +0,0 @@
-import torch
-import datetime
-import types
-import deepspeed
-from transformers.deepspeed import HfDeepSpeedConfig
-import transformers
-import numpy as np
-from collections import OrderedDict
-from torch.utils.data import Dataset, DataLoader
-from torch.nn.utils import clip_grad_norm_
-from torch.cuda.amp import autocast, GradScaler
-from torch.nn import DataParallel
-from torch.optim import lr_scheduler
-import torch.optim as optim
-import torch.nn as nn
-import torch.nn.functional as F
-from tqdm import tqdm
-import os
-import re
-import math
-import random
-import json
-import time
-import logging
-from copy import deepcopy
-import ipdb
-import argparse
-from model.ImageBind import data
-from transformers import LlamaTokenizer, LlamaForCausalLM, LlamaConfig
-from torch.nn.utils.rnn import pad_sequence
-from peft import LoraConfig, TaskType, get_peft_model
-
-logging.getLogger("transformers").setLevel(logging.WARNING)
-logging.getLogger("transformers.tokenization_utils").setLevel(logging.ERROR)
-os.environ['TOKENIZERS_PARALLELISM'] = 'false'
diff --git a/spaces/Fengbinbin/gpt-academic/request_llm/bridge_tgui.py b/spaces/Fengbinbin/gpt-academic/request_llm/bridge_tgui.py
deleted file mode 100644
index fcf852f0474892bd179843ece3f4a83110bd7756..0000000000000000000000000000000000000000
--- a/spaces/Fengbinbin/gpt-academic/request_llm/bridge_tgui.py
+++ /dev/null
@@ -1,171 +0,0 @@
-'''
-Contributed by SagsMug. Modified by binary-husky
-https://github.com/oobabooga/text-generation-webui/pull/175
-'''
-
-import asyncio
-import json
-import random
-import string
-import websockets
-import logging
-import time
-import threading
-import importlib
-from toolbox import get_conf, update_ui
-
-
-def random_hash():
- letters = string.ascii_lowercase + string.digits
- return ''.join(random.choice(letters) for i in range(9))
-
-async def run(context, max_token, temperature, top_p, addr, port):
- params = {
- 'max_new_tokens': max_token,
- 'do_sample': True,
- 'temperature': temperature,
- 'top_p': top_p,
- 'typical_p': 1,
- 'repetition_penalty': 1.05,
- 'encoder_repetition_penalty': 1.0,
- 'top_k': 0,
- 'min_length': 0,
- 'no_repeat_ngram_size': 0,
- 'num_beams': 1,
- 'penalty_alpha': 0,
- 'length_penalty': 1,
- 'early_stopping': True,
- 'seed': -1,
- }
- session = random_hash()
-
- async with websockets.connect(f"ws://{addr}:{port}/queue/join") as websocket:
- while content := json.loads(await websocket.recv()):
- #Python3.10 syntax, replace with if elif on older
- if content["msg"] == "send_hash":
- await websocket.send(json.dumps({
- "session_hash": session,
- "fn_index": 12
- }))
- elif content["msg"] == "estimation":
- pass
- elif content["msg"] == "send_data":
- await websocket.send(json.dumps({
- "session_hash": session,
- "fn_index": 12,
- "data": [
- context,
- params['max_new_tokens'],
- params['do_sample'],
- params['temperature'],
- params['top_p'],
- params['typical_p'],
- params['repetition_penalty'],
- params['encoder_repetition_penalty'],
- params['top_k'],
- params['min_length'],
- params['no_repeat_ngram_size'],
- params['num_beams'],
- params['penalty_alpha'],
- params['length_penalty'],
- params['early_stopping'],
- params['seed'],
- ]
- }))
- elif content["msg"] == "process_starts":
- pass
- elif content["msg"] in ["process_generating", "process_completed"]:
- yield content["output"]["data"][0]
- # You can search for your desired end indicator and
- # stop generation by closing the websocket here
- if (content["msg"] == "process_completed"):
- break
-
-
-
-
-
-def predict(inputs, llm_kwargs, plugin_kwargs, chatbot, history=[], system_prompt='', stream = True, additional_fn=None):
- """
- 发送至chatGPT,流式获取输出。
- 用于基础的对话功能。
- inputs 是本次问询的输入
- top_p, temperature是chatGPT的内部调优参数
- history 是之前的对话列表(注意无论是inputs还是history,内容太长了都会触发token数量溢出的错误)
- chatbot 为WebUI中显示的对话列表,修改它,然后yeild出去,可以直接修改对话界面内容
- additional_fn代表点击的哪个按钮,按钮见functional.py
- """
- if additional_fn is not None:
- import core_functional
- importlib.reload(core_functional) # 热更新prompt
- core_functional = core_functional.get_core_functions()
- if "PreProcess" in core_functional[additional_fn]: inputs = core_functional[additional_fn]["PreProcess"](inputs) # 获取预处理函数(如果有的话)
- inputs = core_functional[additional_fn]["Prefix"] + inputs + core_functional[additional_fn]["Suffix"]
-
- raw_input = "What I would like to say is the following: " + inputs
- history.extend([inputs, ""])
- chatbot.append([inputs, ""])
- yield from update_ui(chatbot=chatbot, history=history, msg="等待响应") # 刷新界面
-
- prompt = raw_input
- tgui_say = ""
-
- model_name, addr_port = llm_kwargs['llm_model'].split('@')
- assert ':' in addr_port, "LLM_MODEL 格式不正确!" + llm_kwargs['llm_model']
- addr, port = addr_port.split(':')
-
-
- mutable = ["", time.time()]
- def run_coorotine(mutable):
- async def get_result(mutable):
- # "tgui:galactica-1.3b@localhost:7860"
-
- async for response in run(context=prompt, max_token=llm_kwargs['max_length'],
- temperature=llm_kwargs['temperature'],
- top_p=llm_kwargs['top_p'], addr=addr, port=port):
- print(response[len(mutable[0]):])
- mutable[0] = response
- if (time.time() - mutable[1]) > 3:
- print('exit when no listener')
- break
- asyncio.run(get_result(mutable))
-
- thread_listen = threading.Thread(target=run_coorotine, args=(mutable,), daemon=True)
- thread_listen.start()
-
- while thread_listen.is_alive():
- time.sleep(1)
- mutable[1] = time.time()
- # Print intermediate steps
- if tgui_say != mutable[0]:
- tgui_say = mutable[0]
- history[-1] = tgui_say
- chatbot[-1] = (history[-2], history[-1])
- yield from update_ui(chatbot=chatbot, history=history) # 刷新界面
-
-
-
-
-def predict_no_ui_long_connection(inputs, llm_kwargs, history, sys_prompt, observe_window, console_slience=False):
- raw_input = "What I would like to say is the following: " + inputs
- prompt = raw_input
- tgui_say = ""
- model_name, addr_port = llm_kwargs['llm_model'].split('@')
- assert ':' in addr_port, "LLM_MODEL 格式不正确!" + llm_kwargs['llm_model']
- addr, port = addr_port.split(':')
-
-
- def run_coorotine(observe_window):
- async def get_result(observe_window):
- async for response in run(context=prompt, max_token=llm_kwargs['max_length'],
- temperature=llm_kwargs['temperature'],
- top_p=llm_kwargs['top_p'], addr=addr, port=port):
- print(response[len(observe_window[0]):])
- observe_window[0] = response
- if (time.time() - observe_window[1]) > 5:
- print('exit when no listener')
- break
- asyncio.run(get_result(observe_window))
- thread_listen = threading.Thread(target=run_coorotine, args=(observe_window,))
- thread_listen.start()
- return observe_window[0]
diff --git a/spaces/Fernando22/freegpt-webui/g4f/Provider/Providers/Fakeopen.py b/spaces/Fernando22/freegpt-webui/g4f/Provider/Providers/Fakeopen.py
deleted file mode 100644
index 5a82bf2cc0736384563332a279f5fbcbb120f676..0000000000000000000000000000000000000000
--- a/spaces/Fernando22/freegpt-webui/g4f/Provider/Providers/Fakeopen.py
+++ /dev/null
@@ -1,54 +0,0 @@
-import os
-import json
-import requests
-from typing import Dict, get_type_hints
-
-url = 'https://ai.fakeopen.com/v1/'
-model = [
- 'gpt-3.5-turbo',
- 'gpt-3.5-turbo-0613',
- 'gpt-3.5-turbo-16k',
- 'gpt-3.5-turbo-16k-0613',
-]
-
-supports_stream = True
-needs_auth = False
-
-
-def _create_completion(model: str, messages: list, stream: bool, **kwargs):
-
- headers = {
- 'Content-Type': 'application/json',
- 'accept': 'text/event-stream',
- 'Cache-Control': 'no-cache',
- 'Proxy-Connection': 'keep-alive',
- 'Authorization': f"Bearer {os.environ.get('FAKE_OPEN_KEY', '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')}",
- }
-
- json_data = {
- 'messages': messages,
- 'temperature': 1.0,
- 'model': model,
- 'stream': stream,
- }
-
- response = requests.post(
- 'https://ai.fakeopen.com/v1/chat/completions', headers=headers, json=json_data, stream=True
- )
-
- for token in response.iter_lines():
- decoded = token.decode('utf-8')
- if decoded == '[DONE]':
- break
- if decoded.startswith('data: '):
- data_str = decoded.replace('data: ', '')
- if data_str != '[DONE]':
- data = json.loads(data_str)
- if 'choices' in data and 'delta' in data['choices'][0] and 'content' in data['choices'][0]['delta']:
- yield data['choices'][0]['delta']['content']
-
-
-
-
-params = f'g4f.Providers.{os.path.basename(__file__)[:-3]} supports: ' + '(%s)' % ', '.join(
- [f"{name}: {get_type_hints(_create_completion)[name].__name__}" for name in _create_completion.__code__.co_varnames[:_create_completion.__code__.co_argcount]])
diff --git a/spaces/FluxWaveCorp/Ghostwriter-Bloom/generators/title_to_abstract.py b/spaces/FluxWaveCorp/Ghostwriter-Bloom/generators/title_to_abstract.py
deleted file mode 100644
index a5ff1dda8edc9a75e7befa4d8d7a16efe0722e67..0000000000000000000000000000000000000000
--- a/spaces/FluxWaveCorp/Ghostwriter-Bloom/generators/title_to_abstract.py
+++ /dev/null
@@ -1,5 +0,0 @@
-
-from .model import model
-
-def title_to_abstract_generator(template):
- return model('title', template)
diff --git a/spaces/FridaZuley/RVC_HFKawaii/demucs/repitch.py b/spaces/FridaZuley/RVC_HFKawaii/demucs/repitch.py
deleted file mode 100644
index 8846ab2d951a024c95067f66a113968500442828..0000000000000000000000000000000000000000
--- a/spaces/FridaZuley/RVC_HFKawaii/demucs/repitch.py
+++ /dev/null
@@ -1,96 +0,0 @@
-# Copyright (c) Facebook, Inc. and its affiliates.
-# All rights reserved.
-#
-# This source code is licensed under the license found in the
-# LICENSE file in the root directory of this source tree.
-
-import io
-import random
-import subprocess as sp
-import tempfile
-
-import numpy as np
-import torch
-from scipy.io import wavfile
-
-
-def i16_pcm(wav):
- if wav.dtype == np.int16:
- return wav
- return (wav * 2**15).clamp_(-2**15, 2**15 - 1).short()
-
-
-def f32_pcm(wav):
- if wav.dtype == np.float:
- return wav
- return wav.float() / 2**15
-
-
-class RepitchedWrapper:
- """
- Wrap a dataset to apply online change of pitch / tempo.
- """
- def __init__(self, dataset, proba=0.2, max_pitch=2, max_tempo=12, tempo_std=5, vocals=[3]):
- self.dataset = dataset
- self.proba = proba
- self.max_pitch = max_pitch
- self.max_tempo = max_tempo
- self.tempo_std = tempo_std
- self.vocals = vocals
-
- def __len__(self):
- return len(self.dataset)
-
- def __getitem__(self, index):
- streams = self.dataset[index]
- in_length = streams.shape[-1]
- out_length = int((1 - 0.01 * self.max_tempo) * in_length)
-
- if random.random() < self.proba:
- delta_pitch = random.randint(-self.max_pitch, self.max_pitch)
- delta_tempo = random.gauss(0, self.tempo_std)
- delta_tempo = min(max(-self.max_tempo, delta_tempo), self.max_tempo)
- outs = []
- for idx, stream in enumerate(streams):
- stream = repitch(
- stream,
- delta_pitch,
- delta_tempo,
- voice=idx in self.vocals)
- outs.append(stream[:, :out_length])
- streams = torch.stack(outs)
- else:
- streams = streams[..., :out_length]
- return streams
-
-
-def repitch(wav, pitch, tempo, voice=False, quick=False, samplerate=44100):
- """
- tempo is a relative delta in percentage, so tempo=10 means tempo at 110%!
- pitch is in semi tones.
- Requires `soundstretch` to be installed, see
- https://www.surina.net/soundtouch/soundstretch.html
- """
- outfile = tempfile.NamedTemporaryFile(suffix=".wav")
- in_ = io.BytesIO()
- wavfile.write(in_, samplerate, i16_pcm(wav).t().numpy())
- command = [
- "soundstretch",
- "stdin",
- outfile.name,
- f"-pitch={pitch}",
- f"-tempo={tempo:.6f}",
- ]
- if quick:
- command += ["-quick"]
- if voice:
- command += ["-speech"]
- try:
- sp.run(command, capture_output=True, input=in_.getvalue(), check=True)
- except sp.CalledProcessError as error:
- raise RuntimeError(f"Could not change bpm because {error.stderr.decode('utf-8')}")
- sr, wav = wavfile.read(outfile.name)
- wav = wav.copy()
- wav = f32_pcm(torch.from_numpy(wav).t())
- assert sr == samplerate
- return wav
diff --git a/spaces/GenXDad/logo-wizard-logo-diffusion-checkpoint/README.md b/spaces/GenXDad/logo-wizard-logo-diffusion-checkpoint/README.md
deleted file mode 100644
index b08db6b6d3aacf01e2070195d8d0357ce9cc40b3..0000000000000000000000000000000000000000
--- a/spaces/GenXDad/logo-wizard-logo-diffusion-checkpoint/README.md
+++ /dev/null
@@ -1,12 +0,0 @@
----
-title: Logo Wizard Logo Diffusion Checkpoint
-emoji: 🐢
-colorFrom: yellow
-colorTo: pink
-sdk: gradio
-sdk_version: 3.38.0
-app_file: app.py
-pinned: false
----
-
-Check out the configuration reference at https://huggingface.co/docs/hub/spaces-config-reference
diff --git a/spaces/Gradio-Blocks/uniformer_image_segmentation/configs/hrnet/fcn_hr48_480x480_80k_pascal_context_59.py b/spaces/Gradio-Blocks/uniformer_image_segmentation/configs/hrnet/fcn_hr48_480x480_80k_pascal_context_59.py
deleted file mode 100644
index 012ad0a7d6119554ec00400ad18a09249a72eca4..0000000000000000000000000000000000000000
--- a/spaces/Gradio-Blocks/uniformer_image_segmentation/configs/hrnet/fcn_hr48_480x480_80k_pascal_context_59.py
+++ /dev/null
@@ -1,10 +0,0 @@
-_base_ = './fcn_hr18_480x480_80k_pascal_context_59.py'
-model = dict(
- pretrained='open-mmlab://msra/hrnetv2_w48',
- backbone=dict(
- extra=dict(
- stage2=dict(num_channels=(48, 96)),
- stage3=dict(num_channels=(48, 96, 192)),
- stage4=dict(num_channels=(48, 96, 192, 384)))),
- decode_head=dict(
- in_channels=[48, 96, 192, 384], channels=sum([48, 96, 192, 384])))
diff --git a/spaces/Gradio-Blocks/uniformer_image_segmentation/exp/upernet_global_small/test_config_h32.py b/spaces/Gradio-Blocks/uniformer_image_segmentation/exp/upernet_global_small/test_config_h32.py
deleted file mode 100644
index a31e3874f76f9f7b089ac8834d85df2441af9b0e..0000000000000000000000000000000000000000
--- a/spaces/Gradio-Blocks/uniformer_image_segmentation/exp/upernet_global_small/test_config_h32.py
+++ /dev/null
@@ -1,39 +0,0 @@
-_base_ = [
- '../../configs/_base_/models/upernet_uniformer.py',
- '../../configs/_base_/datasets/ade20k.py',
- '../../configs/_base_/default_runtime.py',
- '../../configs/_base_/schedules/schedule_160k.py'
-]
-model = dict(
- backbone=dict(
- type='UniFormer',
- embed_dim=[64, 128, 320, 512],
- layers=[3, 4, 8, 3],
- head_dim=64,
- drop_path_rate=0.25,
- windows=False,
- hybrid=True,
- window_size=32
- ),
- decode_head=dict(
- in_channels=[64, 128, 320, 512],
- num_classes=150
- ),
- auxiliary_head=dict(
- in_channels=320,
- num_classes=150
- ))
-
-# AdamW optimizer, no weight decay for position embedding & layer norm in backbone
-optimizer = dict(_delete_=True, type='AdamW', lr=0.00006, betas=(0.9, 0.999), weight_decay=0.01,
- paramwise_cfg=dict(custom_keys={'absolute_pos_embed': dict(decay_mult=0.),
- 'relative_position_bias_table': dict(decay_mult=0.),
- 'norm': dict(decay_mult=0.)}))
-
-lr_config = dict(_delete_=True, policy='poly',
- warmup='linear',
- warmup_iters=1500,
- warmup_ratio=1e-6,
- power=1.0, min_lr=0.0, by_epoch=False)
-
-data=dict(samples_per_gpu=2)
\ No newline at end of file
diff --git a/spaces/GrandaddyShmax/MusicGen_Plus/audiocraft/modules/conditioners.py b/spaces/GrandaddyShmax/MusicGen_Plus/audiocraft/modules/conditioners.py
deleted file mode 100644
index 82792316024b88d4c5c38b0a28f443627771d509..0000000000000000000000000000000000000000
--- a/spaces/GrandaddyShmax/MusicGen_Plus/audiocraft/modules/conditioners.py
+++ /dev/null
@@ -1,990 +0,0 @@
-# Copyright (c) Meta Platforms, Inc. and affiliates.
-# All rights reserved.
-#
-# This source code is licensed under the license found in the
-# LICENSE file in the root directory of this source tree.
-
-from collections import defaultdict
-from copy import deepcopy
-from dataclasses import dataclass, field
-from itertools import chain
-import logging
-import math
-import random
-import re
-import typing as tp
-import warnings
-
-from einops import rearrange
-from num2words import num2words
-import spacy
-from transformers import T5EncoderModel, T5Tokenizer # type: ignore
-import torchaudio
-import torch
-from torch import nn
-from torch import Tensor
-import torch.nn.functional as F
-from torch.nn.utils.rnn import pad_sequence
-
-from .streaming import StreamingModule
-from .transformer import create_sin_embedding
-from ..data.audio_dataset import SegmentInfo
-from ..utils.autocast import TorchAutocast
-from ..utils.utils import hash_trick, length_to_mask, collate
-
-
-logger = logging.getLogger(__name__)
-TextCondition = tp.Optional[str] # a text condition can be a string or None (if doesn't exist)
-ConditionType = tp.Tuple[Tensor, Tensor] # condition, mask
-
-
-class WavCondition(tp.NamedTuple):
- wav: Tensor
- length: Tensor
- path: tp.List[tp.Optional[str]] = []
-
-
-def nullify_condition(condition: ConditionType, dim: int = 1):
- """This function transforms an input condition to a null condition.
- The way it is done by converting it to a single zero vector similarly
- to how it is done inside WhiteSpaceTokenizer and NoopTokenizer.
-
- Args:
- condition (ConditionType): a tuple of condition and mask (tp.Tuple[Tensor, Tensor])
- dim (int): the dimension that will be truncated (should be the time dimension)
- WARNING!: dim should not be the batch dimension!
- Returns:
- ConditionType: a tuple of null condition and mask
- """
- assert dim != 0, "dim cannot be the batch dimension!"
- assert type(condition) == tuple and \
- type(condition[0]) == Tensor and \
- type(condition[1]) == Tensor, "'nullify_condition' got an unexpected input type!"
- cond, mask = condition
- B = cond.shape[0]
- last_dim = cond.dim() - 1
- out = cond.transpose(dim, last_dim)
- out = 0. * out[..., :1]
- out = out.transpose(dim, last_dim)
- mask = torch.zeros((B, 1), device=out.device).int()
- assert cond.dim() == out.dim()
- return out, mask
-
-
-def nullify_wav(wav: Tensor) -> WavCondition:
- """Create a nullified WavCondition from a wav tensor with appropriate shape.
-
- Args:
- wav (Tensor): tensor of shape [B, T]
- Returns:
- WavCondition: wav condition with nullified wav.
- """
- null_wav, _ = nullify_condition((wav, torch.zeros_like(wav)), dim=wav.dim() - 1)
- return WavCondition(
- wav=null_wav,
- length=torch.tensor([0] * wav.shape[0], device=wav.device),
- path=['null_wav'] * wav.shape[0]
- )
-
-
-@dataclass
-class ConditioningAttributes:
- text: tp.Dict[str, tp.Optional[str]] = field(default_factory=dict)
- wav: tp.Dict[str, WavCondition] = field(default_factory=dict)
-
- def __getitem__(self, item):
- return getattr(self, item)
-
- @property
- def text_attributes(self):
- return self.text.keys()
-
- @property
- def wav_attributes(self):
- return self.wav.keys()
-
- @property
- def attributes(self):
- return {"text": self.text_attributes, "wav": self.wav_attributes}
-
- def to_flat_dict(self):
- return {
- **{f"text.{k}": v for k, v in self.text.items()},
- **{f"wav.{k}": v for k, v in self.wav.items()},
- }
-
- @classmethod
- def from_flat_dict(cls, x):
- out = cls()
- for k, v in x.items():
- kind, att = k.split(".")
- out[kind][att] = v
- return out
-
-
-class SegmentWithAttributes(SegmentInfo):
- """Base class for all dataclasses that are used for conditioning.
- All child classes should implement `to_condition_attributes` that converts
- the existing attributes to a dataclass of type ConditioningAttributes.
- """
- def to_condition_attributes(self) -> ConditioningAttributes:
- raise NotImplementedError()
-
-
-class Tokenizer:
- """Base class for all tokenizers
- (in case we want to introduce more advances tokenizers in the future).
- """
- def __call__(self, texts: tp.List[tp.Optional[str]]) -> tp.Tuple[Tensor, Tensor]:
- raise NotImplementedError()
-
-
-class WhiteSpaceTokenizer(Tokenizer):
- """This tokenizer should be used for natural language descriptions.
- For example:
- ["he didn't, know he's going home.", 'shorter sentence'] =>
- [[78, 62, 31, 4, 78, 25, 19, 34],
- [59, 77, 0, 0, 0, 0, 0, 0]]
- """
- PUNCTUATIONS = "?:!.,;"
-
- def __init__(self, n_bins: int, pad_idx: int = 0, language: str = "en_core_web_sm",
- lemma: bool = True, stopwords: bool = True) -> None:
- self.n_bins = n_bins
- self.pad_idx = pad_idx
- self.lemma = lemma
- self.stopwords = stopwords
- try:
- self.nlp = spacy.load(language)
- except IOError:
- spacy.cli.download(language) # type: ignore
- self.nlp = spacy.load(language)
-
- @tp.no_type_check
- def __call__(
- self,
- texts: tp.List[tp.Optional[str]],
- return_text: bool = False
- ) -> tp.Tuple[Tensor, Tensor]:
- """Take a list of strings and convert them to a tensor of indices.
-
- Args:
- texts (tp.List[str]): List of strings.
- return_text (bool, optional): Whether to return text as additional tuple item. Defaults to False.
- Returns:
- tp.Tuple[Tensor, Tensor]:
- - Indices of words in the LUT.
- - And a mask indicating where the padding tokens are
- """
- output, lengths = [], []
- texts = deepcopy(texts)
- for i, text in enumerate(texts):
- # if current sample doesn't have a certain attribute, replace with pad token
- if text is None:
- output.append(Tensor([self.pad_idx]))
- lengths.append(0)
- continue
-
- # convert numbers to words
- text = re.sub(r"(\d+)", lambda x: num2words(int(x.group(0))), text) # type: ignore
- # normalize text
- text = self.nlp(text) # type: ignore
- # remove stopwords
- if self.stopwords:
- text = [w for w in text if not w.is_stop] # type: ignore
- # remove punctuations
- text = [w for w in text if w.text not in self.PUNCTUATIONS] # type: ignore
- # lemmatize if needed
- text = [getattr(t, "lemma_" if self.lemma else "text") for t in text] # type: ignore
-
- texts[i] = " ".join(text)
- lengths.append(len(text))
- # convert to tensor
- tokens = Tensor([hash_trick(w, self.n_bins) for w in text])
- output.append(tokens)
-
- mask = length_to_mask(torch.IntTensor(lengths)).int()
- padded_output = pad_sequence(output, padding_value=self.pad_idx).int().t()
- if return_text:
- return padded_output, mask, texts # type: ignore
- return padded_output, mask
-
-
-class NoopTokenizer(Tokenizer):
- """This tokenizer should be used for global conditioners such as: artist, genre, key, etc.
- The difference between this and WhiteSpaceTokenizer is that NoopTokenizer does not split
- strings, so "Jeff Buckley" will get it's own index. Whereas WhiteSpaceTokenizer will
- split it to ["Jeff", "Buckley"] and return an index per word.
-
- For example:
- ["Queen", "ABBA", "Jeff Buckley"] => [43, 55, 101]
- ["Metal", "Rock", "Classical"] => [0, 223, 51]
- """
- def __init__(self, n_bins: int, pad_idx: int = 0):
- self.n_bins = n_bins
- self.pad_idx = pad_idx
-
- def __call__(self, texts: tp.List[tp.Optional[str]]) -> tp.Tuple[Tensor, Tensor]:
- output, lengths = [], []
- for text in texts:
- # if current sample doesn't have a certain attribute, replace with pad token
- if text is None:
- output.append(self.pad_idx)
- lengths.append(0)
- else:
- output.append(hash_trick(text, self.n_bins))
- lengths.append(1)
-
- tokens = torch.LongTensor(output).unsqueeze(1)
- mask = length_to_mask(torch.IntTensor(lengths)).int()
- return tokens, mask
-
-
-class BaseConditioner(nn.Module):
- """Base model for all conditioner modules. We allow the output dim to be different
- than the hidden dim for two reasons: 1) keep our LUTs small when the vocab is large;
- 2) make all condition dims consistent.
-
- Args:
- dim (int): Hidden dim of the model (text-encoder/LUT).
- output_dim (int): Output dim of the conditioner.
- """
- def __init__(self, dim, output_dim):
- super().__init__()
- self.dim = dim
- self.output_dim = output_dim
- self.output_proj = nn.Linear(dim, output_dim)
-
- def tokenize(self, *args, **kwargs) -> tp.Any:
- """Should be any part of the processing that will lead to a synchronization
- point, e.g. BPE tokenization with transfer to the GPU.
-
- The returned value will be saved and return later when calling forward().
- """
- raise NotImplementedError()
-
- def forward(self, inputs: tp.Any) -> ConditionType:
- """Gets input that should be used as conditioning (e.g, genre, description or a waveform).
- Outputs a ConditionType, after the input data was embedded as a dense vector.
-
- Returns:
- ConditionType:
- - A tensor of size [B, T, D] where B is the batch size, T is the length of the
- output embedding and D is the dimension of the embedding.
- - And a mask indicating where the padding tokens.
- """
- raise NotImplementedError()
-
-
-class TextConditioner(BaseConditioner):
- ...
-
-
-class LUTConditioner(TextConditioner):
- """Lookup table TextConditioner.
-
- Args:
- n_bins (int): Number of bins.
- dim (int): Hidden dim of the model (text-encoder/LUT).
- output_dim (int): Output dim of the conditioner.
- tokenizer (str): Name of the tokenizer.
- pad_idx (int, optional): Index for padding token. Defaults to 0.
- """
- def __init__(self, n_bins: int, dim: int, output_dim: int, tokenizer: str, pad_idx: int = 0):
- super().__init__(dim, output_dim)
- self.embed = nn.Embedding(n_bins, dim)
- self.tokenizer: Tokenizer
- if tokenizer == "whitespace":
- self.tokenizer = WhiteSpaceTokenizer(n_bins, pad_idx=pad_idx)
- elif tokenizer == "noop":
- self.tokenizer = NoopTokenizer(n_bins, pad_idx=pad_idx)
- else:
- raise ValueError(f"unrecognized tokenizer `{tokenizer}`.")
-
- def tokenize(self, x: tp.List[tp.Optional[str]]) -> tp.Tuple[torch.Tensor, torch.Tensor]:
- device = self.embed.weight.device
- tokens, mask = self.tokenizer(x)
- tokens, mask = tokens.to(device), mask.to(device)
- return tokens, mask
-
- def forward(self, inputs: tp.Tuple[torch.Tensor, torch.Tensor]) -> ConditionType:
- tokens, mask = inputs
- embeds = self.embed(tokens)
- embeds = self.output_proj(embeds)
- embeds = (embeds * mask.unsqueeze(-1))
- return embeds, mask
-
-
-class T5Conditioner(TextConditioner):
- """T5-based TextConditioner.
-
- Args:
- name (str): Name of the T5 model.
- output_dim (int): Output dim of the conditioner.
- finetune (bool): Whether to fine-tune T5 at train time.
- device (str): Device for T5 Conditioner.
- autocast_dtype (tp.Optional[str], optional): Autocast dtype.
- word_dropout (float, optional): Word dropout probability.
- normalize_text (bool, optional): Whether to apply text normalization.
- """
- MODELS = ["t5-small", "t5-base", "t5-large", "t5-3b", "t5-11b",
- "google/flan-t5-small", "google/flan-t5-base", "google/flan-t5-large",
- "google/flan-t5-xl", "google/flan-t5-xxl"]
- MODELS_DIMS = {
- "t5-small": 512,
- "t5-base": 768,
- "t5-large": 1024,
- "t5-3b": 1024,
- "t5-11b": 1024,
- "google/flan-t5-small": 512,
- "google/flan-t5-base": 768,
- "google/flan-t5-large": 1024,
- "google/flan-t5-3b": 1024,
- "google/flan-t5-11b": 1024,
- }
-
- def __init__(self, name: str, output_dim: int, finetune: bool, device: str,
- autocast_dtype: tp.Optional[str] = 'float32', word_dropout: float = 0.,
- normalize_text: bool = False):
- assert name in self.MODELS, f"unrecognized t5 model name (should in {self.MODELS})"
- super().__init__(self.MODELS_DIMS[name], output_dim)
- self.device = device
- self.name = name
- self.finetune = finetune
- self.word_dropout = word_dropout
-
- if autocast_dtype is None or self.device == 'cpu':
- self.autocast = TorchAutocast(enabled=False)
- if self.device != 'cpu':
- logger.warning("T5 has no autocast, this might lead to NaN")
- else:
- dtype = getattr(torch, autocast_dtype)
- assert isinstance(dtype, torch.dtype)
- logger.info(f"T5 will be evaluated with autocast as {autocast_dtype}")
- self.autocast = TorchAutocast(enabled=True, device_type=self.device, dtype=dtype)
- # Let's disable logging temporarily because T5 will vomit some errors otherwise.
- # thanks https://gist.github.com/simon-weber/7853144
- previous_level = logging.root.manager.disable
- logging.disable(logging.ERROR)
- with warnings.catch_warnings():
- warnings.simplefilter("ignore")
- try:
- self.t5_tokenizer = T5Tokenizer.from_pretrained(name)
- t5 = T5EncoderModel.from_pretrained(name).train(mode=finetune)
- finally:
- logging.disable(previous_level)
- if finetune:
- self.t5 = t5
- else:
- # this makes sure that the t5 models is not part
- # of the saved checkpoint
- self.__dict__["t5"] = t5.to(device)
-
- self.normalize_text = normalize_text
- if normalize_text:
- self.text_normalizer = WhiteSpaceTokenizer(1, lemma=True, stopwords=True)
-
- def tokenize(self, x: tp.List[tp.Optional[str]]) -> tp.Dict[str, torch.Tensor]:
- # if current sample doesn't have a certain attribute, replace with empty string
- entries: tp.List[str] = [xi if xi is not None else "" for xi in x]
- if self.normalize_text:
- _, _, entries = self.text_normalizer(entries, return_text=True)
- if self.word_dropout > 0. and self.training:
- new_entries = []
- for entry in entries:
- words = [word for word in entry.split(" ") if random.random() >= self.word_dropout]
- new_entries.append(" ".join(words))
- entries = new_entries
-
- empty_idx = torch.LongTensor([i for i, xi in enumerate(entries) if xi == ""])
-
- inputs = self.t5_tokenizer(entries, return_tensors="pt", padding=True).to(self.device)
- mask = inputs["attention_mask"]
- mask[empty_idx, :] = 0 # zero-out index where the input is non-existant
- return inputs
-
- def forward(self, inputs: tp.Dict[str, torch.Tensor]) -> ConditionType:
- mask = inputs["attention_mask"]
- with torch.set_grad_enabled(self.finetune), self.autocast:
- embeds = self.t5(**inputs).last_hidden_state
- embeds = self.output_proj(embeds.to(self.output_proj.weight))
- embeds = (embeds * mask.unsqueeze(-1))
- return embeds, mask
-
-
-class WaveformConditioner(BaseConditioner):
- """Base class for all conditioners that take a waveform as input.
- Classes that inherit must implement `_get_wav_embedding` that outputs
- a continuous tensor, and `_downsampling_factor` that returns the down-sampling
- factor of the embedding model.
-
- Args:
- dim (int): The internal representation dimension.
- output_dim (int): Output dimension.
- device (tp.Union[torch.device, str]): Device.
- """
- def __init__(self, dim: int, output_dim: int, device: tp.Union[torch.device, str]):
- super().__init__(dim, output_dim)
- self.device = device
-
- def tokenize(self, wav_length: WavCondition) -> WavCondition:
- wav, length, path = wav_length
- assert length is not None
- return WavCondition(wav.to(self.device), length.to(self.device), path)
-
- def _get_wav_embedding(self, wav: Tensor) -> Tensor:
- """Gets as input a wav and returns a dense vector of conditions."""
- raise NotImplementedError()
-
- def _downsampling_factor(self):
- """Returns the downsampling factor of the embedding model."""
- raise NotImplementedError()
-
- def forward(self, inputs: WavCondition) -> ConditionType:
- """
- Args:
- input (WavCondition): Tuple of (waveform, lengths).
- Returns:
- ConditionType: Dense vector representing the conditioning along with its' mask.
- """
- wav, lengths, path = inputs
- with torch.no_grad():
- embeds = self._get_wav_embedding(wav)
- embeds = embeds.to(self.output_proj.weight)
- embeds = self.output_proj(embeds)
-
- if lengths is not None:
- lengths = lengths / self._downsampling_factor()
- mask = length_to_mask(lengths, max_len=embeds.shape[1]).int() # type: ignore
- else:
- mask = torch.ones_like(embeds)
- embeds = (embeds * mask.unsqueeze(2).to(self.device))
-
- return embeds, mask
-
-
-class ChromaStemConditioner(WaveformConditioner):
- """Chroma conditioner that uses DEMUCS to first filter out drums and bass. The is followed by
- the insight the drums and bass often dominate the chroma, leading to the chroma not containing the
- information about melody.
-
- Args:
- output_dim (int): Output dimension for the conditioner.
- sample_rate (int): Sample rate for the chroma extractor.
- n_chroma (int): Number of chroma for the chroma extractor.
- radix2_exp (int): Radix2 exponent for the chroma extractor.
- duration (float): Duration used during training. This is later used for correct padding
- in case we are using chroma as prefix.
- match_len_on_eval (bool, optional): If True then all chromas are padded to the training
- duration. Defaults to False.
- eval_wavs (str, optional): Path to a json egg with waveform, this waveforms are used as
- conditions during eval (for cases where we don't want to leak test conditions like MusicCaps).
- Defaults to None.
- n_eval_wavs (int, optional): Limits the number of waveforms used for conditioning. Defaults to 0.
- device (tp.Union[torch.device, str], optional): Device for the conditioner.
- **kwargs: Additional parameters for the chroma extractor.
- """
- def __init__(self, output_dim: int, sample_rate: int, n_chroma: int, radix2_exp: int,
- duration: float, match_len_on_eval: bool = True, eval_wavs: tp.Optional[str] = None,
- n_eval_wavs: int = 0, device: tp.Union[torch.device, str] = "cpu", **kwargs):
- from demucs import pretrained
- super().__init__(dim=n_chroma, output_dim=output_dim, device=device)
- self.autocast = TorchAutocast(enabled=device != "cpu", device_type=self.device, dtype=torch.float32)
- self.sample_rate = sample_rate
- self.match_len_on_eval = match_len_on_eval
- self.duration = duration
- self.__dict__["demucs"] = pretrained.get_model('htdemucs').to(device)
- self.stem2idx = {'drums': 0, 'bass': 1, 'other': 2, 'vocal': 3}
- self.stem_idx = torch.LongTensor([self.stem2idx['vocal'], self.stem2idx['other']]).to(device)
- self.chroma = ChromaExtractor(sample_rate=sample_rate, n_chroma=n_chroma, radix2_exp=radix2_exp,
- device=device, **kwargs)
- self.chroma_len = self._get_chroma_len()
-
- def _downsampling_factor(self):
- return self.chroma.winhop
-
- def _get_chroma_len(self):
- """Get length of chroma during training"""
- dummy_wav = torch.zeros((1, self.sample_rate * self.duration), device=self.device)
- dummy_chr = self.chroma(dummy_wav)
- return dummy_chr.shape[1]
-
- @torch.no_grad()
- def _get_filtered_wav(self, wav):
- from demucs.apply import apply_model
- from demucs.audio import convert_audio
- with self.autocast:
- wav = convert_audio(wav, self.sample_rate, self.demucs.samplerate, self.demucs.audio_channels)
- stems = apply_model(self.demucs, wav, device=self.device)
- stems = stems[:, self.stem_idx] # extract stem
- stems = stems.sum(1) # merge extracted stems
- stems = stems.mean(1, keepdim=True) # mono
- stems = convert_audio(stems, self.demucs.samplerate, self.sample_rate, 1)
- return stems
-
- @torch.no_grad()
- def _get_wav_embedding(self, wav):
- # avoid 0-size tensors when we are working with null conds
- if wav.shape[-1] == 1:
- return self.chroma(wav)
- stems = self._get_filtered_wav(wav)
- chroma = self.chroma(stems)
-
- if self.match_len_on_eval:
- b, t, c = chroma.shape
- if t > self.chroma_len:
- chroma = chroma[:, :self.chroma_len]
- logger.debug(f'chroma was truncated! ({t} -> {chroma.shape[1]})')
- elif t < self.chroma_len:
- # chroma = F.pad(chroma, (0, 0, 0, self.chroma_len - t))
- n_repeat = int(math.ceil(self.chroma_len / t))
- chroma = chroma.repeat(1, n_repeat, 1)
- chroma = chroma[:, :self.chroma_len]
- logger.debug(f'chroma was zero-padded! ({t} -> {chroma.shape[1]})')
- return chroma
-
-
-class ChromaExtractor(nn.Module):
- """Chroma extraction class, handles chroma extraction and quantization.
-
- Args:
- sample_rate (int): Sample rate.
- n_chroma (int): Number of chroma to consider.
- radix2_exp (int): Radix2 exponent.
- nfft (tp.Optional[int], optional): Number of FFT.
- winlen (tp.Optional[int], optional): Window length.
- winhop (tp.Optional[int], optional): Window hop size.
- argmax (bool, optional): Whether to use argmax. Defaults to False.
- norm (float, optional): Norm for chroma normalization. Defaults to inf.
- device (tp.Union[torch.device, str], optional): Device to use. Defaults to cpu.
- """
- def __init__(self, sample_rate: int, n_chroma: int = 12, radix2_exp: int = 12,
- nfft: tp.Optional[int] = None, winlen: tp.Optional[int] = None, winhop: tp.Optional[int] = None,
- argmax: bool = False, norm: float = torch.inf, device: tp.Union[torch.device, str] = "cpu"):
- super().__init__()
- from librosa import filters
- self.device = device
- self.autocast = TorchAutocast(enabled=device != "cpu", device_type=self.device, dtype=torch.float32)
- self.winlen = winlen or 2 ** radix2_exp
- self.nfft = nfft or self.winlen
- self.winhop = winhop or (self.winlen // 4)
- self.sr = sample_rate
- self.n_chroma = n_chroma
- self.norm = norm
- self.argmax = argmax
- self.window = torch.hann_window(self.winlen).to(device)
- self.fbanks = torch.from_numpy(filters.chroma(sr=sample_rate, n_fft=self.nfft, tuning=0,
- n_chroma=self.n_chroma)).to(device)
- self.spec = torchaudio.transforms.Spectrogram(n_fft=self.nfft, win_length=self.winlen,
- hop_length=self.winhop, power=2, center=True,
- pad=0, normalized=True).to(device)
-
- def forward(self, wav):
- with self.autocast:
- T = wav.shape[-1]
- # in case we are getting a wav that was dropped out (nullified)
- # make sure wav length is no less that nfft
- if T < self.nfft:
- pad = self.nfft - T
- r = 0 if pad % 2 == 0 else 1
- wav = F.pad(wav, (pad // 2, pad // 2 + r), 'constant', 0)
- assert wav.shape[-1] == self.nfft, f'expected len {self.nfft} but got {wav.shape[-1]}'
- spec = self.spec(wav).squeeze(1)
- raw_chroma = torch.einsum("cf,...ft->...ct", self.fbanks, spec)
- norm_chroma = torch.nn.functional.normalize(raw_chroma, p=self.norm, dim=-2, eps=1e-6)
- norm_chroma = rearrange(norm_chroma, "b d t -> b t d")
-
- if self.argmax:
- idx = norm_chroma.argmax(-1, keepdims=True)
- norm_chroma[:] = 0
- norm_chroma.scatter_(dim=-1, index=idx, value=1)
-
- return norm_chroma
-
-
-def dropout_condition(sample: ConditioningAttributes, condition_type: str, condition: str):
- """Utility function for nullifying an attribute inside an ConditioningAttributes object.
- If the condition is of type "wav", then nullify it using "nullify_condition".
- If the condition is of any other type, set its' value to None.
- Works in-place.
- """
- if condition_type not in ["text", "wav"]:
- raise ValueError(
- "dropout_condition got an unexpected condition type!"
- f" expected 'wav' or 'text' but got '{condition_type}'"
- )
-
- if condition not in getattr(sample, condition_type):
- raise ValueError(
- "dropout_condition received an unexpected condition!"
- f" expected wav={sample.wav.keys()} and text={sample.text.keys()}"
- f"but got '{condition}' of type '{condition_type}'!"
- )
-
- if condition_type == "wav":
- wav, length, path = sample.wav[condition]
- sample.wav[condition] = nullify_wav(wav)
- else:
- sample.text[condition] = None
-
- return sample
-
-
-class DropoutModule(nn.Module):
- """Base class for all dropout modules."""
- def __init__(self, seed: int = 1234):
- super().__init__()
- self.rng = torch.Generator()
- self.rng.manual_seed(seed)
-
-
-class AttributeDropout(DropoutModule):
- """Applies dropout with a given probability per attribute. This is different from the behavior of
- ClassifierFreeGuidanceDropout as this allows for attributes to be dropped out separately. For example,
- "artist" can be dropped while "genre" remains. This is in contrast to ClassifierFreeGuidanceDropout
- where if "artist" is dropped "genre" must also be dropped.
-
- Args:
- p (tp.Dict[str, float]): A dict mapping between attributes and dropout probability. For example:
- ...
- "genre": 0.1,
- "artist": 0.5,
- "wav": 0.25,
- ...
- active_on_eval (bool, optional): Whether the dropout is active at eval. Default to False.
- seed (int, optional): Random seed.
- """
- def __init__(self, p: tp.Dict[str, tp.Dict[str, float]], active_on_eval: bool = False, seed: int = 1234):
- super().__init__(seed=seed)
- self.active_on_eval = active_on_eval
- # construct dict that return the values from p otherwise 0
- self.p = {}
- for condition_type, probs in p.items():
- self.p[condition_type] = defaultdict(lambda: 0, probs)
-
- def forward(self, samples: tp.List[ConditioningAttributes]) -> tp.List[ConditioningAttributes]:
- """
- Args:
- samples (tp.List[ConditioningAttributes]): List of conditions.
- Returns:
- tp.List[ConditioningAttributes]: List of conditions after certain attributes were set to None.
- """
- if not self.training and not self.active_on_eval:
- return samples
-
- samples = deepcopy(samples)
-
- for condition_type, ps in self.p.items(): # for condition types [text, wav]
- for condition, p in ps.items(): # for attributes of each type (e.g., [artist, genre])
- if torch.rand(1, generator=self.rng).item() < p:
- for sample in samples:
- dropout_condition(sample, condition_type, condition)
-
- return samples
-
- def __repr__(self):
- return f"AttributeDropout({dict(self.p)})"
-
-
-class ClassifierFreeGuidanceDropout(DropoutModule):
- """Applies Classifier Free Guidance dropout, meaning all attributes
- are dropped with the same probability.
-
- Args:
- p (float): Probability to apply condition dropout during training.
- seed (int): Random seed.
- """
- def __init__(self, p: float, seed: int = 1234):
- super().__init__(seed=seed)
- self.p = p
-
- def forward(self, samples: tp.List[ConditioningAttributes]) -> tp.List[ConditioningAttributes]:
- """
- Args:
- samples (tp.List[ConditioningAttributes]): List of conditions.
- Returns:
- tp.List[ConditioningAttributes]: List of conditions after all attributes were set to None.
- """
- if not self.training:
- return samples
-
- # decide on which attributes to drop in a batched fashion
- drop = torch.rand(1, generator=self.rng).item() < self.p
- if not drop:
- return samples
-
- # nullify conditions of all attributes
- samples = deepcopy(samples)
-
- for condition_type in ["wav", "text"]:
- for sample in samples:
- for condition in sample.attributes[condition_type]:
- dropout_condition(sample, condition_type, condition)
-
- return samples
-
- def __repr__(self):
- return f"ClassifierFreeGuidanceDropout(p={self.p})"
-
-
-class ConditioningProvider(nn.Module):
- """Main class to provide conditions given all the supported conditioners.
-
- Args:
- conditioners (dict): Dictionary of conditioners.
- merge_text_conditions_p (float, optional): Probability to merge all text sources
- into a single text condition. Defaults to 0.
- drop_desc_p (float, optional): Probability to drop the original description
- when merging all text sources into a single text condition. Defaults to 0.
- device (tp.Union[torch.device, str], optional): Device for conditioners and output condition types.
- """
- def __init__(
- self,
- conditioners: tp.Dict[str, BaseConditioner],
- merge_text_conditions_p: float = 0,
- drop_desc_p: float = 0,
- device: tp.Union[torch.device, str] = "cpu",
- ):
- super().__init__()
- self.device = device
- self.merge_text_conditions_p = merge_text_conditions_p
- self.drop_desc_p = drop_desc_p
- self.conditioners = nn.ModuleDict(conditioners)
-
- @property
- def text_conditions(self):
- return [k for k, v in self.conditioners.items() if isinstance(v, TextConditioner)]
-
- @property
- def wav_conditions(self):
- return [k for k, v in self.conditioners.items() if isinstance(v, WaveformConditioner)]
-
- @property
- def has_wav_condition(self):
- return len(self.wav_conditions) > 0
-
- def tokenize(self, inputs: tp.List[ConditioningAttributes]) -> tp.Dict[str, tp.Any]:
- """Match attributes/wavs with existing conditioners in self, and compute tokenize them accordingly.
- This should be called before starting any real GPU work to avoid synchronization points.
- This will return a dict matching conditioner names to their arbitrary tokenized representations.
-
- Args:
- inputs (list[ConditioningAttribres]): List of ConditioningAttributes objects containing
- text and wav conditions.
- """
- assert all([type(x) == ConditioningAttributes for x in inputs]), \
- "got unexpected types input for conditioner! should be tp.List[ConditioningAttributes]" \
- f" but types were {set([type(x) for x in inputs])}"
-
- output = {}
- text = self._collate_text(inputs)
- wavs = self._collate_wavs(inputs)
-
- assert set(text.keys() | wavs.keys()).issubset(set(self.conditioners.keys())), \
- f"got an unexpected attribute! Expected {self.conditioners.keys()}, got {text.keys(), wavs.keys()}"
-
- for attribute, batch in chain(text.items(), wavs.items()):
- output[attribute] = self.conditioners[attribute].tokenize(batch)
- return output
-
- def forward(self, tokenized: tp.Dict[str, tp.Any]) -> tp.Dict[str, ConditionType]:
- """Compute pairs of `(embedding, mask)` using the configured conditioners
- and the tokenized representations. The output is for example:
-
- {
- "genre": (torch.Tensor([B, 1, D_genre]), torch.Tensor([B, 1])),
- "description": (torch.Tensor([B, T_desc, D_desc]), torch.Tensor([B, T_desc])),
- ...
- }
-
- Args:
- tokenized (dict): Dict of tokenized representations as returned by `tokenize()`.
- """
- output = {}
- for attribute, inputs in tokenized.items():
- condition, mask = self.conditioners[attribute](inputs)
- output[attribute] = (condition, mask)
- return output
-
- def _collate_text(self, samples: tp.List[ConditioningAttributes]) -> tp.Dict[str, tp.List[tp.Optional[str]]]:
- """Given a list of ConditioningAttributes objects, compile a dictionary where the keys
- are the attributes and the values are the aggregated input per attribute.
- For example:
- Input:
- [
- ConditioningAttributes(text={"genre": "Rock", "description": "A rock song with a guitar solo"}, wav=...),
- ConditioningAttributes(text={"genre": "Hip-hop", "description": "A hip-hop verse"}, wav=...),
- ]
- Output:
- {
- "genre": ["Rock", "Hip-hop"],
- "description": ["A rock song with a guitar solo", "A hip-hop verse"]
- }
- """
- batch_per_attribute: tp.Dict[str, tp.List[tp.Optional[str]]] = defaultdict(list)
-
- def _merge_conds(cond, merge_text_conditions_p=0, drop_desc_p=0):
- def is_valid(k, v):
- k_valid = k in ['key', 'bpm', 'genre', 'moods', 'instrument']
- v_valid = v is not None and isinstance(v, (int, float, str, list))
- return k_valid and v_valid
-
- def process_value(v):
- if isinstance(v, (int, float, str)):
- return v
- if isinstance(v, list):
- return ", ".join(v)
- else:
- RuntimeError(f"unknown type for text value! ({type(v), v})")
-
- desc = cond.text['description']
- meta_data = ""
- if random.uniform(0, 1) < merge_text_conditions_p:
- meta_pairs = [f'{k}: {process_value(v)}' for k, v in cond.text.items() if is_valid(k, v)]
- random.shuffle(meta_pairs)
- meta_data = ". ".join(meta_pairs)
- desc = desc if not random.uniform(0, 1) < drop_desc_p else None
-
- if desc is None:
- desc = meta_data if len(meta_data) > 1 else None
- else:
- desc = desc.rstrip('.') + ". " + meta_data
- cond.text['description'] = desc.strip() if desc else None
-
- if self.training and self.merge_text_conditions_p:
- for sample in samples:
- _merge_conds(sample, self.merge_text_conditions_p, self.drop_desc_p)
-
- texts = [x.text for x in samples]
- for text in texts:
- for condition in self.text_conditions:
- batch_per_attribute[condition].append(text[condition])
-
- return batch_per_attribute
-
- def _collate_wavs(self, samples: tp.List[ConditioningAttributes]):
- """Generate a dict where the keys are attributes by which we fetch similar wavs,
- and the values are Tensors of wavs according to said attribtues.
-
- *Note*: by the time the samples reach this function, each sample should have some waveform
- inside the "wav" attribute. It should be either:
- 1. A real waveform
- 2. A null waveform due to the sample having no similar waveforms (nullified by the dataset)
- 3. A null waveform due to it being dropped in a dropout module (nullified by dropout)
-
- Args:
- samples (tp.List[ConditioningAttributes]): List of ConditioningAttributes samples.
- Returns:
- dict: A dicionary mapping an attribute name to wavs.
- """
- wavs = defaultdict(list)
- lens = defaultdict(list)
- paths = defaultdict(list)
- out = {}
-
- for sample in samples:
- for attribute in self.wav_conditions:
- wav, length, path = sample.wav[attribute]
- wavs[attribute].append(wav.flatten())
- lens[attribute].append(length)
- paths[attribute].append(path)
-
- # stack all wavs to a single tensor
- for attribute in self.wav_conditions:
- stacked_wav, _ = collate(wavs[attribute], dim=0)
- out[attribute] = WavCondition(stacked_wav.unsqueeze(1),
- torch.cat(lens['self_wav']), paths[attribute]) # type: ignore
-
- return out
-
-
-class ConditionFuser(StreamingModule):
- """Condition fuser handles the logic to combine the different conditions
- to the actual model input.
-
- Args:
- fuse2cond (tp.Dict[str, str]): A dictionary that says how to fuse
- each condition. For example:
- {
- "prepend": ["description"],
- "sum": ["genre", "bpm"],
- "cross": ["description"],
- }
- cross_attention_pos_emb (bool, optional): Use positional embeddings in cross attention.
- cross_attention_pos_emb_scale (int): Scale for positional embeddings in cross attention if used.
- """
- FUSING_METHODS = ["sum", "prepend", "cross", "input_interpolate"]
-
- def __init__(self, fuse2cond: tp.Dict[str, tp.List[str]], cross_attention_pos_emb: bool = False,
- cross_attention_pos_emb_scale: float = 1.0):
- super().__init__()
- assert all(
- [k in self.FUSING_METHODS for k in fuse2cond.keys()]
- ), f"got invalid fuse method, allowed methods: {self.FUSING_MEHTODS}"
- self.cross_attention_pos_emb = cross_attention_pos_emb
- self.cross_attention_pos_emb_scale = cross_attention_pos_emb_scale
- self.fuse2cond: tp.Dict[str, tp.List[str]] = fuse2cond
- self.cond2fuse: tp.Dict[str, str] = {}
- for fuse_method, conditions in fuse2cond.items():
- for condition in conditions:
- self.cond2fuse[condition] = fuse_method
-
- def forward(
- self,
- input: Tensor,
- conditions: tp.Dict[str, ConditionType]
- ) -> tp.Tuple[Tensor, tp.Optional[Tensor]]:
- """Fuse the conditions to the provided model input.
-
- Args:
- input (Tensor): Transformer input.
- conditions (tp.Dict[str, ConditionType]): Dict of conditions.
- Returns:
- tp.Tuple[Tensor, Tensor]: The first tensor is the transformer input
- after the conditions have been fused. The second output tensor is the tensor
- used for cross-attention or None if no cross attention inputs exist.
- """
- B, T, _ = input.shape
-
- if 'offsets' in self._streaming_state:
- first_step = False
- offsets = self._streaming_state['offsets']
- else:
- first_step = True
- offsets = torch.zeros(input.shape[0], dtype=torch.long, device=input.device)
-
- assert set(conditions.keys()).issubset(set(self.cond2fuse.keys())), \
- f"given conditions contain unknown attributes for fuser, " \
- f"expected {self.cond2fuse.keys()}, got {conditions.keys()}"
- cross_attention_output = None
- for cond_type, (cond, cond_mask) in conditions.items():
- op = self.cond2fuse[cond_type]
- if op == "sum":
- input += cond
- elif op == "input_interpolate":
- cond = rearrange(cond, "b t d -> b d t")
- cond = F.interpolate(cond, size=input.shape[1])
- input += rearrange(cond, "b d t -> b t d")
- elif op == "prepend":
- if first_step:
- input = torch.cat([cond, input], dim=1)
- elif op == "cross":
- if cross_attention_output is not None:
- cross_attention_output = torch.cat([cross_attention_output, cond], dim=1)
- else:
- cross_attention_output = cond
- else:
- raise ValueError(f"unknown op ({op})")
-
- if self.cross_attention_pos_emb and cross_attention_output is not None:
- positions = torch.arange(
- cross_attention_output.shape[1],
- device=cross_attention_output.device
- ).view(1, -1, 1)
- pos_emb = create_sin_embedding(positions, cross_attention_output.shape[-1])
- cross_attention_output = cross_attention_output + self.cross_attention_pos_emb_scale * pos_emb
-
- if self._is_streaming:
- self._streaming_state['offsets'] = offsets + T
-
- return input, cross_attention_output
diff --git a/spaces/GurudattaBS/GenDiseasePrediction/code/helper.py b/spaces/GurudattaBS/GenDiseasePrediction/code/helper.py
deleted file mode 100644
index a187ec9b60a435be65018508e17ac45cf3f90709..0000000000000000000000000000000000000000
--- a/spaces/GurudattaBS/GenDiseasePrediction/code/helper.py
+++ /dev/null
@@ -1,33 +0,0 @@
-import pandas as pd
-import numpy as np
-
-# def preprocess_kaggle(dataset_path):
-
-# # import the dataset
-# dataset_df = pd.read_csv(dataset_path)
-
-# # Preprocess
-# dataset_df = dataset_df.apply(lambda col: col.str.strip())
-
-# test = pd.get_dummies(dataset_df.filter(regex='Symptom'), prefix='', prefix_sep='')
-# test = test.groupby(test.columns, axis=1).agg(np.max)
-# clean_df = pd.merge(test,dataset_df['Disease'], left_index=True, right_index=True)
-
-# return clean_df
-
-def prepare_symptoms_array(symptoms):
- '''
- Convert a list of symptoms to a ndim(X) (in this case 131) that matches the
- dataframe used to train the machine learning model
-
- Output:
- - X (np.array) = X values ready as input to ML model to get prediction
- '''
- symptoms_array = np.zeros((1,133))
- df = pd.read_csv('data/clean_dataset.tsv', sep='\t')
-
- for symptom in symptoms:
- symptom_idx = df.columns.get_loc(symptom)
- symptoms_array[0, symptom_idx] = 1
-
- return symptoms_array
\ No newline at end of file
diff --git a/spaces/HaloMaster/chinesesummary/fengshen/models/zen1/tokenization.py b/spaces/HaloMaster/chinesesummary/fengshen/models/zen1/tokenization.py
deleted file mode 100644
index bbc94e2417ff42ffcfb18284b8cb396415e630b1..0000000000000000000000000000000000000000
--- a/spaces/HaloMaster/chinesesummary/fengshen/models/zen1/tokenization.py
+++ /dev/null
@@ -1,438 +0,0 @@
-# coding=utf-8
-# This file is derived from the code at
-# https://github.com/huggingface/transformers/blob/master/transformers/tokenization_bert.py
-#
-# Original copyright notice:
-#
-# Copyright 2018 The Google AI Language Team Authors and The HuggingFace Inc. team.
-#
-# Licensed under the Apache License, Version 2.0 (the "License");
-# you may not use this file except in compliance with the License.
-# You may obtain a copy of the License at
-#
-# http://www.apache.org/licenses/LICENSE-2.0
-#
-# Unless required by applicable law or agreed to in writing, software
-# distributed under the License is distributed on an "AS IS" BASIS,
-# WITHOUT WARRANTIES OR CONDITIONS OF ANY KIND, either express or implied.
-# See the License for the specific language governing permissions and
-# limitations under the License.
-"""Tokenization classes."""
-
-from __future__ import absolute_import, division, print_function, unicode_literals
-
-import collections
-import logging
-import os
-import unicodedata
-from io import open
-
-from transformers import cached_path
-
-logger = logging.getLogger(__name__)
-
-PRETRAINED_VOCAB_ARCHIVE_MAP = {
- 'bert-base-uncased': "https://s3.amazonaws.com/models.huggingface.co/bert/bert-base-uncased-vocab.txt",
- 'bert-large-uncased': "https://s3.amazonaws.com/models.huggingface.co/bert/bert-large-uncased-vocab.txt",
- 'bert-base-cased': "https://s3.amazonaws.com/models.huggingface.co/bert/bert-base-cased-vocab.txt",
- 'bert-large-cased': "https://s3.amazonaws.com/models.huggingface.co/bert/bert-large-cased-vocab.txt",
- 'bert-base-multilingual-uncased': "https://s3.amazonaws.com/models.huggingface.co/bert/bert-base-multilingual-uncased-vocab.txt",
- 'bert-base-multilingual-cased': "https://s3.amazonaws.com/models.huggingface.co/bert/bert-base-multilingual-cased-vocab.txt",
- 'bert-base-chinese': "https://s3.amazonaws.com/models.huggingface.co/bert/bert-base-chinese-vocab.txt",
- 'bert-base-german-cased': "https://int-deepset-models-bert.s3.eu-central-1.amazonaws.com/pytorch/bert-base-german-cased-vocab.txt",
- 'bert-large-uncased-whole-word-masking': "https://s3.amazonaws.com/models.huggingface.co/bert/bert-large-uncased-whole-word-masking-vocab.txt",
- 'bert-large-cased-whole-word-masking': "https://s3.amazonaws.com/models.huggingface.co/bert/bert-large-cased-whole-word-masking-vocab.txt",
- 'bert-large-uncased-whole-word-masking-finetuned-squad': "https://s3.amazonaws.com/models.huggingface.co/bert/bert-large-uncased-whole-word-masking-finetuned-squad-vocab.txt",
- 'bert-large-cased-whole-word-masking-finetuned-squad': "https://s3.amazonaws.com/models.huggingface.co/bert/bert-large-cased-whole-word-masking-finetuned-squad-vocab.txt",
- 'bert-base-cased-finetuned-mrpc': "https://s3.amazonaws.com/models.huggingface.co/bert/bert-base-cased-finetuned-mrpc-vocab.txt",
- 'IDEA-CCNL/Erlangshen-ZEN1-224M-Chinese': 'https://huggingface.co/IDEA-CCNL/Erlangshen-ZEN1-224M-Chinese/resolve/main/vocab.txt',
-}
-PRETRAINED_VOCAB_POSITIONAL_EMBEDDINGS_SIZE_MAP = {
- 'bert-base-uncased': 512,
- 'bert-large-uncased': 512,
- 'bert-base-cased': 512,
- 'bert-large-cased': 512,
- 'bert-base-multilingual-uncased': 512,
- 'bert-base-multilingual-cased': 512,
- 'bert-base-chinese': 512,
- 'bert-base-german-cased': 512,
- 'bert-large-uncased-whole-word-masking': 512,
- 'bert-large-cased-whole-word-masking': 512,
- 'bert-large-uncased-whole-word-masking-finetuned-squad': 512,
- 'bert-large-cased-whole-word-masking-finetuned-squad': 512,
- 'bert-base-cased-finetuned-mrpc': 512,
-}
-VOCAB_NAME = 'vocab.txt'
-
-
-def load_vocab(vocab_file):
- """Loads a vocabulary file into a dictionary."""
- vocab = collections.OrderedDict()
- index = 0
- with open(vocab_file, "r", encoding="utf-8") as reader:
- while True:
- token = reader.readline()
- if not token:
- break
- token = token.strip()
- vocab[token] = index
- index += 1
- return vocab
-
-
-def whitespace_tokenize(text):
- """Runs basic whitespace cleaning and splitting on a piece of text."""
- text = text.strip()
- if not text:
- return []
- tokens = text.split()
- return tokens
-
-
-class BertTokenizer(object):
- """Runs end-to-end tokenization: punctuation splitting + wordpiece"""
-
- def __init__(self, vocab_file, do_lower_case=True, max_len=None, do_basic_tokenize=True,
- never_split=("[UNK]", "[SEP]", "[PAD]", "[CLS]", "[MASK]")):
- """Constructs a BertTokenizer.
-
- Args:
- vocab_file: Path to a one-wordpiece-per-line vocabulary file
- do_lower_case: Whether to lower case the input
- Only has an effect when do_wordpiece_only=False
- do_basic_tokenize: Whether to do basic tokenization before wordpiece.
- max_len: An artificial maximum length to truncate tokenized sequences to;
- Effective maximum length is always the minimum of this
- value (if specified) and the underlying BERT model's
- sequence length.
- never_split: List of tokens which will never be split during tokenization.
- Only has an effect when do_wordpiece_only=False
- """
- if not os.path.isfile(vocab_file):
- raise ValueError(
- "Can't find a vocabulary file at path '{}'. To load the vocabulary from a Google pretrained "
- "model use `tokenizer = BertTokenizer.from_pretrained(PRETRAINED_MODEL_NAME)`".format(vocab_file))
- self.vocab = load_vocab(vocab_file)
- self.ids_to_tokens = collections.OrderedDict(
- [(ids, tok) for tok, ids in self.vocab.items()])
- self.do_basic_tokenize = do_basic_tokenize
- if do_basic_tokenize:
- self.basic_tokenizer = BasicTokenizer(do_lower_case=do_lower_case,
- never_split=never_split)
- self.wordpiece_tokenizer = WordpieceTokenizer(vocab=self.vocab)
- self.max_len = max_len if max_len is not None else int(1e12)
-
- def tokenize(self, text):
- split_tokens = []
- if self.do_basic_tokenize:
- for token in self.basic_tokenizer.tokenize(text):
- for sub_token in self.wordpiece_tokenizer.tokenize(token):
- split_tokens.append(sub_token)
- else:
- split_tokens = self.wordpiece_tokenizer.tokenize(text)
- return split_tokens
-
- def convert_tokens_to_ids(self, tokens):
- """Converts a sequence of tokens into ids using the vocab."""
- ids = []
- for token in tokens:
- ids.append(self.vocab[token])
- if len(ids) > self.max_len:
- logger.warning(
- "Token indices sequence length is longer than the specified maximum "
- " sequence length for this BERT model ({} > {}). Running this"
- " sequence through BERT will result in indexing errors".format(len(ids), self.max_len)
- )
- return ids
-
- def convert_ids_to_tokens(self, ids):
- """Converts a sequence of ids in wordpiece tokens using the vocab."""
- tokens = []
- for i in ids:
- tokens.append(self.ids_to_tokens[i])
- return tokens
-
- def save_vocabulary(self, vocab_path):
- """Save the tokenizer vocabulary to a directory or file."""
- index = 0
- if os.path.isdir(vocab_path):
- vocab_file = os.path.join(vocab_path, VOCAB_NAME)
- with open(vocab_file, "w", encoding="utf-8") as writer:
- for token, token_index in sorted(self.vocab.items(), key=lambda kv: kv[1]):
- if index != token_index:
- logger.warning("Saving vocabulary to {}: vocabulary indices are not consecutive."
- " Please check that the vocabulary is not corrupted!".format(vocab_file))
- index = token_index
- writer.write(token + u'\n')
- index += 1
- return vocab_file
-
- @classmethod
- def from_pretrained(cls, pretrained_model_name_or_path, cache_dir=None, *inputs, **kwargs):
- """
- Instantiate a PreTrainedBertModel from a pre-trained model file.
- Download and cache the pre-trained model file if needed.
- """
- if pretrained_model_name_or_path in PRETRAINED_VOCAB_ARCHIVE_MAP:
- vocab_file = PRETRAINED_VOCAB_ARCHIVE_MAP[pretrained_model_name_or_path]
- if '-cased' in pretrained_model_name_or_path and kwargs.get('do_lower_case', True):
- logger.warning("The pre-trained model you are loading is a cased model but you have not set "
- "`do_lower_case` to False. We are setting `do_lower_case=False` for you but "
- "you may want to check this behavior.")
- kwargs['do_lower_case'] = False
- elif '-cased' not in pretrained_model_name_or_path and not kwargs.get('do_lower_case', True):
- logger.warning("The pre-trained model you are loading is an uncased model but you have set "
- "`do_lower_case` to False. We are setting `do_lower_case=True` for you "
- "but you may want to check this behavior.")
- kwargs['do_lower_case'] = True
- else:
- vocab_file = pretrained_model_name_or_path
- if os.path.isdir(vocab_file):
- vocab_file = os.path.join(vocab_file, VOCAB_NAME)
- # redirect to the cache, if necessary
- try:
- resolved_vocab_file = cached_path(vocab_file, cache_dir=cache_dir)
- except EnvironmentError:
- if pretrained_model_name_or_path in PRETRAINED_VOCAB_ARCHIVE_MAP:
- logger.error(
- "Couldn't reach server at '{}' to download vocabulary.".format(
- vocab_file))
- else:
- logger.error(
- "Model name '{}' was not found in model name list ({}). "
- "We assumed '{}' was a path or url but couldn't find any file "
- "associated to this path or url.".format(
- pretrained_model_name_or_path,
- ', '.join(PRETRAINED_VOCAB_ARCHIVE_MAP.keys()),
- vocab_file))
- return None
- if resolved_vocab_file == vocab_file:
- logger.info("loading vocabulary file {}".format(vocab_file))
- else:
- logger.info("loading vocabulary file {} from cache at {}".format(
- vocab_file, resolved_vocab_file))
- if pretrained_model_name_or_path in PRETRAINED_VOCAB_POSITIONAL_EMBEDDINGS_SIZE_MAP:
- # if we're using a pretrained model, ensure the tokenizer wont index sequences longer
- # than the number of positional embeddings
- max_len = PRETRAINED_VOCAB_POSITIONAL_EMBEDDINGS_SIZE_MAP[pretrained_model_name_or_path]
- kwargs['max_len'] = min(kwargs.get('max_len', int(1e12)), max_len)
- # Instantiate tokenizer.
- tokenizer = cls(resolved_vocab_file, *inputs, **kwargs)
- return tokenizer
-
-
-class BasicTokenizer(object):
- """Runs basic tokenization (punctuation splitting, lower casing, etc.)."""
-
- def __init__(self,
- do_lower_case=True,
- never_split=("[UNK]", "[SEP]", "[PAD]", "[CLS]", "[MASK]")):
- """Constructs a BasicTokenizer.
-
- Args:
- do_lower_case: Whether to lower case the input.
- """
- self.do_lower_case = do_lower_case
- self.never_split = never_split
-
- def tokenize(self, text):
- """Tokenizes a piece of text."""
- text = self._clean_text(text)
- # This was added on November 1st, 2018 for the multilingual and Chinese
- # models. This is also applied to the English models now, but it doesn't
- # matter since the English models were not trained on any Chinese data
- # and generally don't have any Chinese data in them (there are Chinese
- # characters in the vocabulary because Wikipedia does have some Chinese
- # words in the English Wikipedia.).
- text = self._tokenize_chinese_chars(text)
- orig_tokens = whitespace_tokenize(text)
- split_tokens = []
- for token in orig_tokens:
- if self.do_lower_case and token not in self.never_split:
- token = token.lower()
- token = self._run_strip_accents(token)
- split_tokens.extend(self._run_split_on_punc(token))
-
- output_tokens = whitespace_tokenize(" ".join(split_tokens))
- return output_tokens
-
- def _run_strip_accents(self, text):
- """Strips accents from a piece of text."""
- text = unicodedata.normalize("NFD", text)
- output = []
- for char in text:
- cat = unicodedata.category(char)
- if cat == "Mn":
- continue
- output.append(char)
- return "".join(output)
-
- def _run_split_on_punc(self, text):
- """Splits punctuation on a piece of text."""
- if text in self.never_split:
- return [text]
- chars = list(text)
- i = 0
- start_new_word = True
- output = []
- while i < len(chars):
- char = chars[i]
- if _is_punctuation(char):
- output.append([char])
- start_new_word = True
- else:
- if start_new_word:
- output.append([])
- start_new_word = False
- output[-1].append(char)
- i += 1
-
- return ["".join(x) for x in output]
-
- def _tokenize_chinese_chars(self, text):
- """Adds whitespace around any CJK character."""
- output = []
- for char in text:
- cp = ord(char)
- if self._is_chinese_char(cp):
- output.append(" ")
- output.append(char)
- output.append(" ")
- else:
- output.append(char)
- return "".join(output)
-
- def _is_chinese_char(self, cp):
- """Checks whether CP is the codepoint of a CJK character."""
- # This defines a "chinese character" as anything in the CJK Unicode block:
- # https://en.wikipedia.org/wiki/CJK_Unified_Ideographs_(Unicode_block)
- #
- # Note that the CJK Unicode block is NOT all Japanese and Korean characters,
- # despite its name. The modern Korean Hangul alphabet is a different block,
- # as is Japanese Hiragana and Katakana. Those alphabets are used to write
- # space-separated words, so they are not treated specially and handled
- # like the all of the other languages.
- if ((cp >= 0x4E00 and cp <= 0x9FFF) or #
- (cp >= 0x3400 and cp <= 0x4DBF) or #
- (cp >= 0x20000 and cp <= 0x2A6DF) or #
- (cp >= 0x2A700 and cp <= 0x2B73F) or #
- (cp >= 0x2B740 and cp <= 0x2B81F) or #
- (cp >= 0x2B820 and cp <= 0x2CEAF) or
- (cp >= 0xF900 and cp <= 0xFAFF) or #
- (cp >= 0x2F800 and cp <= 0x2FA1F)): #
- return True
-
- return False
-
- def _clean_text(self, text):
- """Performs invalid character removal and whitespace cleanup on text."""
- output = []
- for char in text:
- cp = ord(char)
- if cp == 0 or cp == 0xfffd or _is_control(char):
- continue
- if _is_whitespace(char):
- output.append(" ")
- else:
- output.append(char)
- return "".join(output)
-
-
-class WordpieceTokenizer(object):
- """Runs WordPiece tokenization."""
-
- def __init__(self, vocab, unk_token="[UNK]", max_input_chars_per_word=100):
- self.vocab = vocab
- self.unk_token = unk_token
- self.max_input_chars_per_word = max_input_chars_per_word
-
- def tokenize(self, text):
- """Tokenizes a piece of text into its word pieces.
-
- This uses a greedy longest-match-first algorithm to perform tokenization
- using the given vocabulary.
-
- For example:
- input = "unaffable"
- output = ["un", "##aff", "##able"]
-
- Args:
- text: A single token or whitespace separated tokens. This should have
- already been passed through `BasicTokenizer`.
-
- Returns:
- A list of wordpiece tokens.
- """
-
- output_tokens = []
- for token in whitespace_tokenize(text):
- chars = list(token)
- if len(chars) > self.max_input_chars_per_word:
- output_tokens.append(self.unk_token)
- continue
-
- is_bad = False
- start = 0
- sub_tokens = []
- while start < len(chars):
- end = len(chars)
- cur_substr = None
- while start < end:
- substr = "".join(chars[start:end])
- if start > 0:
- substr = "##" + substr
- if substr in self.vocab:
- cur_substr = substr
- break
- end -= 1
- if cur_substr is None:
- is_bad = True
- break
- sub_tokens.append(cur_substr)
- start = end
-
- if is_bad:
- output_tokens.append(self.unk_token)
- else:
- output_tokens.extend(sub_tokens)
- return output_tokens
-
-
-def _is_whitespace(char):
- """Checks whether `chars` is a whitespace character."""
- # \t, \n, and \r are technically contorl characters but we treat them
- # as whitespace since they are generally considered as such.
- if char == " " or char == "\t" or char == "\n" or char == "\r":
- return True
- cat = unicodedata.category(char)
- if cat == "Zs":
- return True
- return False
-
-
-def _is_control(char):
- """Checks whether `chars` is a control character."""
- # These are technically control characters but we count them as whitespace
- # characters.
- if char == "\t" or char == "\n" or char == "\r":
- return False
- cat = unicodedata.category(char)
- if cat.startswith("C"):
- return True
- return False
-
-
-def _is_punctuation(char):
- """Checks whether `chars` is a punctuation character."""
- cp = ord(char)
- # We treat all non-letter/number ASCII as punctuation.
- # Characters such as "^", "$", and "`" are not in the Unicode
- # Punctuation class but we treat them as punctuation anyways, for
- # consistency.
- if ((cp >= 33 and cp <= 47) or (cp >= 58 and cp <= 64) or
- (cp >= 91 and cp <= 96) or (cp >= 123 and cp <= 126)):
- return True
- cat = unicodedata.category(char)
- if cat.startswith("P"):
- return True
- return False
diff --git a/spaces/HarryLee/eCommerceImageCaptioning/fairseq/examples/textless_nlp/gslm/unit2speech/tacotron2/cleaners.py b/spaces/HarryLee/eCommerceImageCaptioning/fairseq/examples/textless_nlp/gslm/unit2speech/tacotron2/cleaners.py
deleted file mode 100644
index e2e35c1a8cc4c628c5d05802677142c9a2122d2b..0000000000000000000000000000000000000000
--- a/spaces/HarryLee/eCommerceImageCaptioning/fairseq/examples/textless_nlp/gslm/unit2speech/tacotron2/cleaners.py
+++ /dev/null
@@ -1,90 +0,0 @@
-""" from https://github.com/keithito/tacotron """
-
-'''
-Cleaners are transformations that run over the input text at both training and eval time.
-
-Cleaners can be selected by passing a comma-delimited list of cleaner names as the "cleaners"
-hyperparameter. Some cleaners are English-specific. You'll typically want to use:
- 1. "english_cleaners" for English text
- 2. "transliteration_cleaners" for non-English text that can be transliterated to ASCII using
- the Unidecode library (https://pypi.python.org/pypi/Unidecode)
- 3. "basic_cleaners" if you do not want to transliterate (in this case, you should also update
- the symbols in symbols.py to match your data).
-'''
-
-import re
-from unidecode import unidecode
-from .numbers import normalize_numbers
-
-
-# Regular expression matching whitespace:
-_whitespace_re = re.compile(r'\s+')
-
-# List of (regular expression, replacement) pairs for abbreviations:
-_abbreviations = [(re.compile('\\b%s\\.' % x[0], re.IGNORECASE), x[1]) for x in [
- ('mrs', 'misess'),
- ('mr', 'mister'),
- ('dr', 'doctor'),
- ('st', 'saint'),
- ('co', 'company'),
- ('jr', 'junior'),
- ('maj', 'major'),
- ('gen', 'general'),
- ('drs', 'doctors'),
- ('rev', 'reverend'),
- ('lt', 'lieutenant'),
- ('hon', 'honorable'),
- ('sgt', 'sergeant'),
- ('capt', 'captain'),
- ('esq', 'esquire'),
- ('ltd', 'limited'),
- ('col', 'colonel'),
- ('ft', 'fort'),
-]]
-
-
-def expand_abbreviations(text):
- for regex, replacement in _abbreviations:
- text = re.sub(regex, replacement, text)
- return text
-
-
-def expand_numbers(text):
- return normalize_numbers(text)
-
-
-def lowercase(text):
- return text.lower()
-
-
-def collapse_whitespace(text):
- return re.sub(_whitespace_re, ' ', text)
-
-
-def convert_to_ascii(text):
- return unidecode(text)
-
-
-def basic_cleaners(text):
- '''Basic pipeline that lowercases and collapses whitespace without transliteration.'''
- text = lowercase(text)
- text = collapse_whitespace(text)
- return text
-
-
-def transliteration_cleaners(text):
- '''Pipeline for non-English text that transliterates to ASCII.'''
- text = convert_to_ascii(text)
- text = lowercase(text)
- text = collapse_whitespace(text)
- return text
-
-
-def english_cleaners(text):
- '''Pipeline for English text, including number and abbreviation expansion.'''
- text = convert_to_ascii(text)
- text = lowercase(text)
- text = expand_numbers(text)
- text = expand_abbreviations(text)
- text = collapse_whitespace(text)
- return text
diff --git a/spaces/HarryLee/eCommerceImageCaptioning/fairseq/fairseq/models/roberta/alignment_utils.py b/spaces/HarryLee/eCommerceImageCaptioning/fairseq/fairseq/models/roberta/alignment_utils.py
deleted file mode 100644
index ccc7f74cb94d5b8baa2d4e9dfd44f653d47ee43e..0000000000000000000000000000000000000000
--- a/spaces/HarryLee/eCommerceImageCaptioning/fairseq/fairseq/models/roberta/alignment_utils.py
+++ /dev/null
@@ -1,118 +0,0 @@
-# Copyright (c) Facebook, Inc. and its affiliates.
-#
-# This source code is licensed under the MIT license found in the
-# LICENSE file in the root directory of this source tree.
-
-from collections import Counter
-from typing import List
-
-import torch
-
-
-def align_bpe_to_words(roberta, bpe_tokens: torch.LongTensor, other_tokens: List[str]):
- """
- Helper to align GPT-2 BPE to other tokenization formats (e.g., spaCy).
-
- Args:
- roberta (RobertaHubInterface): RoBERTa instance
- bpe_tokens (torch.LongTensor): GPT-2 BPE tokens of shape `(T_bpe)`
- other_tokens (List[str]): other tokens of shape `(T_words)`
-
- Returns:
- List[str]: mapping from *other_tokens* to corresponding *bpe_tokens*.
- """
- assert bpe_tokens.dim() == 1
- assert bpe_tokens[0] == 0
-
- def clean(text):
- return text.strip()
-
- # remove whitespaces to simplify alignment
- bpe_tokens = [roberta.task.source_dictionary.string([x]) for x in bpe_tokens]
- bpe_tokens = [
- clean(roberta.bpe.decode(x) if x not in {"", ""} else x) for x in bpe_tokens
- ]
- other_tokens = [clean(str(o)) for o in other_tokens]
-
- # strip leading
- bpe_tokens = bpe_tokens[1:]
- assert "".join(bpe_tokens) == "".join(other_tokens)
-
- # create alignment from every word to a list of BPE tokens
- alignment = []
- bpe_toks = filter(lambda item: item[1] != "", enumerate(bpe_tokens, start=1))
- j, bpe_tok = next(bpe_toks)
- for other_tok in other_tokens:
- bpe_indices = []
- while True:
- if other_tok.startswith(bpe_tok):
- bpe_indices.append(j)
- other_tok = other_tok[len(bpe_tok) :]
- try:
- j, bpe_tok = next(bpe_toks)
- except StopIteration:
- j, bpe_tok = None, None
- elif bpe_tok.startswith(other_tok):
- # other_tok spans multiple BPE tokens
- bpe_indices.append(j)
- bpe_tok = bpe_tok[len(other_tok) :]
- other_tok = ""
- else:
- raise Exception('Cannot align "{}" and "{}"'.format(other_tok, bpe_tok))
- if other_tok == "":
- break
- assert len(bpe_indices) > 0
- alignment.append(bpe_indices)
- assert len(alignment) == len(other_tokens)
-
- return alignment
-
-
-def align_features_to_words(roberta, features, alignment):
- """
- Align given features to words.
-
- Args:
- roberta (RobertaHubInterface): RoBERTa instance
- features (torch.Tensor): features to align of shape `(T_bpe x C)`
- alignment: alignment between BPE tokens and words returned by
- func:`align_bpe_to_words`.
- """
- assert features.dim() == 2
-
- bpe_counts = Counter(j for bpe_indices in alignment for j in bpe_indices)
- assert bpe_counts[0] == 0 # shouldn't be aligned
- denom = features.new([bpe_counts.get(j, 1) for j in range(len(features))])
- weighted_features = features / denom.unsqueeze(-1)
-
- output = [weighted_features[0]]
- largest_j = -1
- for bpe_indices in alignment:
- output.append(weighted_features[bpe_indices].sum(dim=0))
- largest_j = max(largest_j, *bpe_indices)
- for j in range(largest_j + 1, len(features)):
- output.append(weighted_features[j])
- output = torch.stack(output)
- assert torch.all(torch.abs(output.sum(dim=0) - features.sum(dim=0)) < 1e-4)
- return output
-
-
-def spacy_nlp():
- if getattr(spacy_nlp, "_nlp", None) is None:
- try:
- from spacy.lang.en import English
-
- spacy_nlp._nlp = English()
- except ImportError:
- raise ImportError("Please install spacy with: pip install spacy")
- return spacy_nlp._nlp
-
-
-def spacy_tokenizer():
- if getattr(spacy_tokenizer, "_tokenizer", None) is None:
- try:
- nlp = spacy_nlp()
- spacy_tokenizer._tokenizer = nlp.Defaults.create_tokenizer(nlp)
- except ImportError:
- raise ImportError("Please install spacy with: pip install spacy")
- return spacy_tokenizer._tokenizer
diff --git a/spaces/Harveenchadha/Hindi_TTS/vakyansh_tts/utils/duration.py b/spaces/Harveenchadha/Hindi_TTS/vakyansh_tts/utils/duration.py
deleted file mode 100644
index c3b5e112b72dd5a07ea2463f604d98bb8d961496..0000000000000000000000000000000000000000
--- a/spaces/Harveenchadha/Hindi_TTS/vakyansh_tts/utils/duration.py
+++ /dev/null
@@ -1,33 +0,0 @@
-# Usage -> python duration.py /src/folder/path
-
-import soundfile as sf
-import sys
-import os
-from glob import glob
-from joblib import Parallel, delayed
-from tqdm import tqdm
-
-
-def get_duration(fpath):
- w = sf.SoundFile(fpath)
- sr = w.samplerate
- assert 22050 == sr, "Sample rate is not 22050"
- return len(w) / sr
-
-
-def main(folder, ext="wav"):
- file_list = glob(folder + "/**/*." + ext, recursive=True)
- print(f"\n\tTotal number of wav files {len(file_list)}")
- duration_list = Parallel(n_jobs=1)(
- delayed(get_duration)(i) for i in tqdm(file_list)
- )
- print(
- f"\n\tMin Duration {min(duration_list):.2f} Max Duration {max(duration_list):.2f} in secs"
- )
- print(f"\n\tTotal Duration {sum(duration_list)/3600:.2f} in hours")
-
-
-if __name__ == "__main__":
- folder = sys.argv[1]
- folder = os.path.abspath(folder)
- main(folder)
diff --git a/spaces/Harveenchadha/Vakyansh-Hindi-TTS/ttsv/src/glow_tts/init.py b/spaces/Harveenchadha/Vakyansh-Hindi-TTS/ttsv/src/glow_tts/init.py
deleted file mode 100644
index 39dd83dbd55475d562a3f54d951cb822800d2e0f..0000000000000000000000000000000000000000
--- a/spaces/Harveenchadha/Vakyansh-Hindi-TTS/ttsv/src/glow_tts/init.py
+++ /dev/null
@@ -1,79 +0,0 @@
-import os
-import json
-import argparse
-import math
-import torch
-from torch import nn, optim
-from torch.nn import functional as F
-from torch.utils.data import DataLoader
-
-from data_utils import TextMelLoader, TextMelCollate
-import models
-import commons
-import utils
-
-
-class FlowGenerator_DDI(models.FlowGenerator):
- """A helper for Data-dependent Initialization"""
-
- def __init__(self, *args, **kwargs):
- super().__init__(*args, **kwargs)
- for f in self.decoder.flows:
- if getattr(f, "set_ddi", False):
- f.set_ddi(True)
-
-
-def main():
- hps = utils.get_hparams()
- logger = utils.get_logger(hps.log_dir)
- logger.info(hps)
- utils.check_git_hash(hps.log_dir)
-
- torch.manual_seed(hps.train.seed)
-
- train_dataset = TextMelLoader(hps.data.training_files, hps.data)
- collate_fn = TextMelCollate(1)
- train_loader = DataLoader(
- train_dataset,
- num_workers=8,
- shuffle=True,
- batch_size=hps.train.batch_size,
- pin_memory=True,
- drop_last=True,
- collate_fn=collate_fn,
- )
- symbols = hps.data.punc + hps.data.chars
- generator = FlowGenerator_DDI(
- len(symbols) + getattr(hps.data, "add_blank", False),
- out_channels=hps.data.n_mel_channels,
- **hps.model
- ).cuda()
- optimizer_g = commons.Adam(
- generator.parameters(),
- scheduler=hps.train.scheduler,
- dim_model=hps.model.hidden_channels,
- warmup_steps=hps.train.warmup_steps,
- lr=hps.train.learning_rate,
- betas=hps.train.betas,
- eps=hps.train.eps,
- )
-
- generator.train()
- for batch_idx, (x, x_lengths, y, y_lengths) in enumerate(train_loader):
- x, x_lengths = x.cuda(), x_lengths.cuda()
- y, y_lengths = y.cuda(), y_lengths.cuda()
-
- _ = generator(x, x_lengths, y, y_lengths, gen=False)
- break
-
- utils.save_checkpoint(
- generator,
- optimizer_g,
- hps.train.learning_rate,
- 0,
- os.path.join(hps.model_dir, "ddi_G.pth"),
- )
-
-
-if __name__ == "__main__":
- main()
diff --git a/spaces/Harveenchadha/en_to_indic_translation/model_configs/custom_transformer.py b/spaces/Harveenchadha/en_to_indic_translation/model_configs/custom_transformer.py
deleted file mode 100644
index b122e1bf5c81534aae35bb6235c1feaf45b7bada..0000000000000000000000000000000000000000
--- a/spaces/Harveenchadha/en_to_indic_translation/model_configs/custom_transformer.py
+++ /dev/null
@@ -1,38 +0,0 @@
-from fairseq.models import register_model_architecture
-from fairseq.models.transformer import base_architecture
-
-
-@register_model_architecture("transformer", "transformer_2x")
-def transformer_big(args):
- args.encoder_embed_dim = getattr(args, "encoder_embed_dim", 1024)
- args.encoder_ffn_embed_dim = getattr(args, "encoder_ffn_embed_dim", 4096)
- args.encoder_attention_heads = getattr(args, "encoder_attention_heads", 16)
- args.encoder_normalize_before = getattr(args, "encoder_normalize_before", False)
- args.decoder_embed_dim = getattr(args, "decoder_embed_dim", 1024)
- args.decoder_ffn_embed_dim = getattr(args, "decoder_ffn_embed_dim", 4096)
- args.decoder_attention_heads = getattr(args, "decoder_attention_heads", 16)
- base_architecture(args)
-
-
-@register_model_architecture("transformer", "transformer_4x")
-def transformer_huge(args):
- args.encoder_embed_dim = getattr(args, "encoder_embed_dim", 1536)
- args.encoder_ffn_embed_dim = getattr(args, "encoder_ffn_embed_dim", 4096)
- args.encoder_attention_heads = getattr(args, "encoder_attention_heads", 16)
- args.encoder_normalize_before = getattr(args, "encoder_normalize_before", False)
- args.decoder_embed_dim = getattr(args, "decoder_embed_dim", 1536)
- args.decoder_ffn_embed_dim = getattr(args, "decoder_ffn_embed_dim", 4096)
- args.decoder_attention_heads = getattr(args, "decoder_attention_heads", 16)
- base_architecture(args)
-
-
-@register_model_architecture("transformer", "transformer_9x")
-def transformer_xlarge(args):
- args.encoder_embed_dim = getattr(args, "encoder_embed_dim", 2048)
- args.encoder_ffn_embed_dim = getattr(args, "encoder_ffn_embed_dim", 8192)
- args.encoder_attention_heads = getattr(args, "encoder_attention_heads", 16)
- args.encoder_normalize_before = getattr(args, "encoder_normalize_before", False)
- args.decoder_embed_dim = getattr(args, "decoder_embed_dim", 2048)
- args.decoder_ffn_embed_dim = getattr(args, "decoder_ffn_embed_dim", 8192)
- args.decoder_attention_heads = getattr(args, "decoder_attention_heads", 16)
- base_architecture(args)
diff --git a/spaces/HighCWu/anime-colorization-with-hint/gradio-modified/gradio/examples.py b/spaces/HighCWu/anime-colorization-with-hint/gradio-modified/gradio/examples.py
deleted file mode 100644
index a40ae25e903eebb8913276739200c2b02372e839..0000000000000000000000000000000000000000
--- a/spaces/HighCWu/anime-colorization-with-hint/gradio-modified/gradio/examples.py
+++ /dev/null
@@ -1,327 +0,0 @@
-"""
-Defines helper methods useful for loading and caching Interface examples.
-"""
-from __future__ import annotations
-
-import ast
-import csv
-import os
-import warnings
-from pathlib import Path
-from typing import TYPE_CHECKING, Any, Callable, List
-
-from gradio import utils
-from gradio.components import Dataset
-from gradio.context import Context
-from gradio.documentation import document, set_documentation_group
-from gradio.flagging import CSVLogger
-
-if TYPE_CHECKING: # Only import for type checking (to avoid circular imports).
- from gradio.components import IOComponent
-
-CACHED_FOLDER = "gradio_cached_examples"
-LOG_FILE = "log.csv"
-
-set_documentation_group("component-helpers")
-
-
-def create_examples(
- examples: List[Any] | List[List[Any]] | str,
- inputs: IOComponent | List[IOComponent],
- outputs: IOComponent | List[IOComponent] | None = None,
- fn: Callable | None = None,
- cache_examples: bool = False,
- examples_per_page: int = 10,
- _api_mode: bool = False,
- label: str | None = None,
- elem_id: str | None = None,
- run_on_click: bool = False,
- preprocess: bool = True,
- postprocess: bool = True,
- batch: bool = False,
-):
- """Top-level synchronous function that creates Examples. Provided for backwards compatibility, i.e. so that gr.Examples(...) can be used to create the Examples component."""
- examples_obj = Examples(
- examples=examples,
- inputs=inputs,
- outputs=outputs,
- fn=fn,
- cache_examples=cache_examples,
- examples_per_page=examples_per_page,
- _api_mode=_api_mode,
- label=label,
- elem_id=elem_id,
- run_on_click=run_on_click,
- preprocess=preprocess,
- postprocess=postprocess,
- batch=batch,
- _initiated_directly=False,
- )
- utils.synchronize_async(examples_obj.create)
- return examples_obj
-
-
-@document()
-class Examples:
- """
- This class is a wrapper over the Dataset component and can be used to create Examples
- for Blocks / Interfaces. Populates the Dataset component with examples and
- assigns event listener so that clicking on an example populates the input/output
- components. Optionally handles example caching for fast inference.
-
- Demos: blocks_inputs, fake_gan
- Guides: more_on_examples_and_flagging, using_hugging_face_integrations, image_classification_in_pytorch, image_classification_in_tensorflow, image_classification_with_vision_transformers, create_your_own_friends_with_a_gan
- """
-
- def __init__(
- self,
- examples: List[Any] | List[List[Any]] | str,
- inputs: IOComponent | List[IOComponent],
- outputs: IOComponent | List[IOComponent] | None = None,
- fn: Callable | None = None,
- cache_examples: bool = False,
- examples_per_page: int = 10,
- _api_mode: bool = False,
- label: str | None = "Examples",
- elem_id: str | None = None,
- run_on_click: bool = False,
- preprocess: bool = True,
- postprocess: bool = True,
- batch: bool = False,
- _initiated_directly: bool = True,
- ):
- """
- Parameters:
- examples: example inputs that can be clicked to populate specific components. Should be nested list, in which the outer list consists of samples and each inner list consists of an input corresponding to each input component. A string path to a directory of examples can also be provided but it should be within the directory with the python file running the gradio app. If there are multiple input components and a directory is provided, a log.csv file must be present in the directory to link corresponding inputs.
- inputs: the component or list of components corresponding to the examples
- outputs: optionally, provide the component or list of components corresponding to the output of the examples. Required if `cache` is True.
- fn: optionally, provide the function to run to generate the outputs corresponding to the examples. Required if `cache` is True.
- cache_examples: if True, caches examples for fast runtime. If True, then `fn` and `outputs` need to be provided
- examples_per_page: how many examples to show per page.
- label: the label to use for the examples component (by default, "Examples")
- elem_id: an optional string that is assigned as the id of this component in the HTML DOM.
- run_on_click: if cache_examples is False, clicking on an example does not run the function when an example is clicked. Set this to True to run the function when an example is clicked. Has no effect if cache_examples is True.
- preprocess: if True, preprocesses the example input before running the prediction function and caching the output. Only applies if cache_examples is True.
- postprocess: if True, postprocesses the example output after running the prediction function and before caching. Only applies if cache_examples is True.
- batch: If True, then the function should process a batch of inputs, meaning that it should accept a list of input values for each parameter. Used only if cache_examples is True.
- """
- if _initiated_directly:
- warnings.warn(
- "Please use gr.Examples(...) instead of gr.examples.Examples(...) to create the Examples.",
- )
-
- if cache_examples and (fn is None or outputs is None):
- raise ValueError("If caching examples, `fn` and `outputs` must be provided")
-
- if not isinstance(inputs, list):
- inputs = [inputs]
- if outputs and not isinstance(outputs, list):
- outputs = [outputs]
-
- working_directory = Path().absolute()
-
- if examples is None:
- raise ValueError("The parameter `examples` cannot be None")
- elif isinstance(examples, list) and (
- len(examples) == 0 or isinstance(examples[0], list)
- ):
- pass
- elif (
- isinstance(examples, list) and len(inputs) == 1
- ): # If there is only one input component, examples can be provided as a regular list instead of a list of lists
- examples = [[e] for e in examples]
- elif isinstance(examples, str):
- if not Path(examples).exists():
- raise FileNotFoundError(
- "Could not find examples directory: " + examples
- )
- working_directory = examples
- if not (Path(examples) / LOG_FILE).exists():
- if len(inputs) == 1:
- examples = [[e] for e in os.listdir(examples)]
- else:
- raise FileNotFoundError(
- "Could not find log file (required for multiple inputs): "
- + LOG_FILE
- )
- else:
- with open(Path(examples) / LOG_FILE) as logs:
- examples = list(csv.reader(logs))
- examples = [
- examples[i][: len(inputs)] for i in range(1, len(examples))
- ] # remove header and unnecessary columns
-
- else:
- raise ValueError(
- "The parameter `examples` must either be a string directory or a list"
- "(if there is only 1 input component) or (more generally), a nested "
- "list, where each sublist represents a set of inputs."
- )
-
- input_has_examples = [False] * len(inputs)
- for example in examples:
- for idx, example_for_input in enumerate(example):
- if not (example_for_input is None):
- try:
- input_has_examples[idx] = True
- except IndexError:
- pass # If there are more example components than inputs, ignore. This can sometimes be intentional (e.g. loading from a log file where outputs and timestamps are also logged)
-
- inputs_with_examples = [
- inp for (inp, keep) in zip(inputs, input_has_examples) if keep
- ]
- non_none_examples = [
- [ex for (ex, keep) in zip(example, input_has_examples) if keep]
- for example in examples
- ]
-
- self.examples = examples
- self.non_none_examples = non_none_examples
- self.inputs = inputs
- self.inputs_with_examples = inputs_with_examples
- self.outputs = outputs
- self.fn = fn
- self.cache_examples = cache_examples
- self._api_mode = _api_mode
- self.preprocess = preprocess
- self.postprocess = postprocess
- self.batch = batch
-
- with utils.set_directory(working_directory):
- self.processed_examples = [
- [
- component.postprocess(sample)
- for component, sample in zip(inputs, example)
- ]
- for example in examples
- ]
- self.non_none_processed_examples = [
- [ex for (ex, keep) in zip(example, input_has_examples) if keep]
- for example in self.processed_examples
- ]
- if cache_examples:
- for example in self.examples:
- if len([ex for ex in example if ex is not None]) != len(self.inputs):
- warnings.warn(
- "Examples are being cached but not all input components have "
- "example values. This may result in an exception being thrown by "
- "your function. If you do get an error while caching examples, make "
- "sure all of your inputs have example values for all of your examples "
- "or you provide default values for those particular parameters in your function."
- )
- break
-
- with utils.set_directory(working_directory):
- self.dataset = Dataset(
- components=inputs_with_examples,
- samples=non_none_examples,
- type="index",
- label=label,
- samples_per_page=examples_per_page,
- elem_id=elem_id,
- )
-
- self.cached_folder = Path(CACHED_FOLDER) / str(self.dataset._id)
- self.cached_file = Path(self.cached_folder) / "log.csv"
- self.cache_examples = cache_examples
- self.run_on_click = run_on_click
-
- async def create(self) -> None:
- """Caches the examples if self.cache_examples is True and creates the Dataset
- component to hold the examples"""
-
- async def load_example(example_id):
- if self.cache_examples:
- processed_example = self.non_none_processed_examples[
- example_id
- ] + await self.load_from_cache(example_id)
- else:
- processed_example = self.non_none_processed_examples[example_id]
- return utils.resolve_singleton(processed_example)
-
- if Context.root_block:
- if self.cache_examples and self.outputs:
- targets = self.inputs_with_examples
- else:
- targets = self.inputs
- self.dataset.click(
- load_example,
- inputs=[self.dataset],
- outputs=targets, # type: ignore
- postprocess=False,
- queue=False,
- )
- if self.run_on_click and not self.cache_examples:
- if self.fn is None:
- raise ValueError("Cannot run_on_click if no function is provided")
- self.dataset.click(
- self.fn,
- inputs=self.inputs, # type: ignore
- outputs=self.outputs, # type: ignore
- )
-
- if self.cache_examples:
- await self.cache()
-
- async def cache(self) -> None:
- """
- Caches all of the examples so that their predictions can be shown immediately.
- """
- if Path(self.cached_file).exists():
- print(
- f"Using cache from '{Path(self.cached_folder).resolve()}' directory. If method or examples have changed since last caching, delete this folder to clear cache."
- )
- else:
- if Context.root_block is None:
- raise ValueError("Cannot cache examples if not in a Blocks context")
-
- print(f"Caching examples at: '{Path(self.cached_file).resolve()}'")
- cache_logger = CSVLogger()
-
- # create a fake dependency to process the examples and get the predictions
- dependency = Context.root_block.set_event_trigger(
- event_name="fake_event",
- fn=self.fn,
- inputs=self.inputs_with_examples, # type: ignore
- outputs=self.outputs, # type: ignore
- preprocess=self.preprocess and not self._api_mode,
- postprocess=self.postprocess and not self._api_mode,
- batch=self.batch,
- )
-
- fn_index = Context.root_block.dependencies.index(dependency)
- assert self.outputs is not None
- cache_logger.setup(self.outputs, self.cached_folder)
- for example_id, _ in enumerate(self.examples):
- processed_input = self.processed_examples[example_id]
- if self.batch:
- processed_input = [[value] for value in processed_input]
- prediction = await Context.root_block.process_api(
- fn_index=fn_index, inputs=processed_input, request=None, state={}
- )
- output = prediction["data"]
- if self.batch:
- output = [value[0] for value in output]
- cache_logger.flag(output)
- # Remove the "fake_event" to prevent bugs in loading interfaces from spaces
- Context.root_block.dependencies.remove(dependency)
- Context.root_block.fns.pop(fn_index)
-
- async def load_from_cache(self, example_id: int) -> List[Any]:
- """Loads a particular cached example for the interface.
- Parameters:
- example_id: The id of the example to process (zero-indexed).
- """
- with open(self.cached_file) as cache:
- examples = list(csv.reader(cache))
- example = examples[example_id + 1] # +1 to adjust for header
- output = []
- assert self.outputs is not None
- for component, value in zip(self.outputs, example):
- try:
- value_as_dict = ast.literal_eval(value)
- assert utils.is_update(value_as_dict)
- output.append(value_as_dict)
- except (ValueError, TypeError, SyntaxError, AssertionError):
- output.append(component.serialize(value, self.cached_folder))
- return output
diff --git a/spaces/HuangLab/CELL-E_2-Sequence_Prediction/taming/modules/util.py b/spaces/HuangLab/CELL-E_2-Sequence_Prediction/taming/modules/util.py
deleted file mode 100644
index 9ee16385d8b1342a2d60a5f1aa5cadcfbe934bd8..0000000000000000000000000000000000000000
--- a/spaces/HuangLab/CELL-E_2-Sequence_Prediction/taming/modules/util.py
+++ /dev/null
@@ -1,130 +0,0 @@
-import torch
-import torch.nn as nn
-
-
-def count_params(model):
- total_params = sum(p.numel() for p in model.parameters())
- return total_params
-
-
-class ActNorm(nn.Module):
- def __init__(self, num_features, logdet=False, affine=True,
- allow_reverse_init=False):
- assert affine
- super().__init__()
- self.logdet = logdet
- self.loc = nn.Parameter(torch.zeros(1, num_features, 1, 1))
- self.scale = nn.Parameter(torch.ones(1, num_features, 1, 1))
- self.allow_reverse_init = allow_reverse_init
-
- self.register_buffer('initialized', torch.tensor(0, dtype=torch.uint8))
-
- def initialize(self, input):
- with torch.no_grad():
- flatten = input.permute(1, 0, 2, 3).contiguous().view(input.shape[1], -1)
- mean = (
- flatten.mean(1)
- .unsqueeze(1)
- .unsqueeze(2)
- .unsqueeze(3)
- .permute(1, 0, 2, 3)
- )
- std = (
- flatten.std(1)
- .unsqueeze(1)
- .unsqueeze(2)
- .unsqueeze(3)
- .permute(1, 0, 2, 3)
- )
-
- self.loc.data.copy_(-mean)
- self.scale.data.copy_(1 / (std + 1e-6))
-
- def forward(self, input, reverse=False):
- if reverse:
- return self.reverse(input)
- if len(input.shape) == 2:
- input = input[:,:,None,None]
- squeeze = True
- else:
- squeeze = False
-
- _, _, height, width = input.shape
-
- if self.training and self.initialized.item() == 0:
- self.initialize(input)
- self.initialized.fill_(1)
-
- h = self.scale * (input + self.loc)
-
- if squeeze:
- h = h.squeeze(-1).squeeze(-1)
-
- if self.logdet:
- log_abs = torch.log(torch.abs(self.scale))
- logdet = height*width*torch.sum(log_abs)
- logdet = logdet * torch.ones(input.shape[0]).to(input)
- return h, logdet
-
- return h
-
- def reverse(self, output):
- if self.training and self.initialized.item() == 0:
- if not self.allow_reverse_init:
- raise RuntimeError(
- "Initializing ActNorm in reverse direction is "
- "disabled by default. Use allow_reverse_init=True to enable."
- )
- else:
- self.initialize(output)
- self.initialized.fill_(1)
-
- if len(output.shape) == 2:
- output = output[:,:,None,None]
- squeeze = True
- else:
- squeeze = False
-
- h = output / self.scale - self.loc
-
- if squeeze:
- h = h.squeeze(-1).squeeze(-1)
- return h
-
-
-class AbstractEncoder(nn.Module):
- def __init__(self):
- super().__init__()
-
- def encode(self, *args, **kwargs):
- raise NotImplementedError
-
-
-class Labelator(AbstractEncoder):
- """Net2Net Interface for Class-Conditional Model"""
- def __init__(self, n_classes, quantize_interface=True):
- super().__init__()
- self.n_classes = n_classes
- self.quantize_interface = quantize_interface
-
- def encode(self, c):
- c = c[:,None]
- if self.quantize_interface:
- return c, None, [None, None, c.long()]
- return c
-
-
-class SOSProvider(AbstractEncoder):
- # for unconditional training
- def __init__(self, sos_token, quantize_interface=True):
- super().__init__()
- self.sos_token = sos_token
- self.quantize_interface = quantize_interface
-
- def encode(self, x):
- # get batch size from data and replicate sos_token
- c = torch.ones(x.shape[0], 1)*self.sos_token
- c = c.long().to(x.device)
- if self.quantize_interface:
- return c, None, [None, None, c]
- return c
diff --git a/spaces/IPN/streamlit_demo/README.md b/spaces/IPN/streamlit_demo/README.md
deleted file mode 100644
index a20f4c531414109befd43812dd3fa6d06ef7cb40..0000000000000000000000000000000000000000
--- a/spaces/IPN/streamlit_demo/README.md
+++ /dev/null
@@ -1,13 +0,0 @@
----
-title: Streamlit_demo
-emoji: 👁
-colorFrom: indigo
-colorTo: indigo
-sdk: streamlit
-sdk_version: 1.2.0
-app_file: app.py
-pinned: false
-license: cc
----
-
-Check out the configuration reference at https://huggingface.co/docs/hub/spaces#reference
diff --git a/spaces/IVentureISB/Gen-AI/chatbot.py b/spaces/IVentureISB/Gen-AI/chatbot.py
deleted file mode 100644
index 41246fe63531dec67c55308c8c0f6653bb2ecdaa..0000000000000000000000000000000000000000
--- a/spaces/IVentureISB/Gen-AI/chatbot.py
+++ /dev/null
@@ -1,24 +0,0 @@
-import gradio as gr
-
-messages = [
- {"role": "system", "content": "You are an AI assistant that only gives responses from the website https://i-venture.org/ and you help people make decisions about how to make a difference in others' lives. You also provide the relevant links from that website as part of your answers."},
-]
-
-def chatbot(input):
- if input:
- context = create_context(input, df)
- message=f"Answer the question based on the context below, and if the question can't be answered based on the context, say \"I don't know\"\n\nContext: {context}\n\n---\n\nQuestion: {input}\nAnswer:",
- messages.append({"role": "user", "content": message})
- chat = openai.ChatCompletion.create(
- temperature=0.5, model="gpt-3.5-turbo", messages=messages,
- )
- reply = chat.choices[0].message.content
- messages.append({"role": "assistant", "content": reply})
- return reply
-
-inputs = gr.inputs.Textbox(lines=7, label="Chat with I-venture @ ISB AI powered bot")
-outputs = gr.outputs.Textbox(label="Reply")
-
-gr.Interface(fn=chatbot, inputs=inputs, outputs=outputs, title="Talk with I-venture @ ISB",
- description="Anything you want to find out about entreprenuership at ISB. Sample questions include >>> how to get incubated at ISB Dlabs? >>> What is the team behind I-venture @ ISB? >>> and more",
- theme="compact").launch(share=True, debug=True)
\ No newline at end of file
diff --git a/spaces/Illia56/Youtube-Whisper-Llama/app.py b/spaces/Illia56/Youtube-Whisper-Llama/app.py
deleted file mode 100644
index 5ecbd5c962565d7e66a29eae18742ce4c2ccac1b..0000000000000000000000000000000000000000
--- a/spaces/Illia56/Youtube-Whisper-Llama/app.py
+++ /dev/null
@@ -1,207 +0,0 @@
-import os
-import logging
-from typing import Any, List, Mapping, Optional
-from langchain.llms import HuggingFaceHub
-from gradio_client import Client
-from langchain.schema import Document
-from langchain.text_splitter import RecursiveCharacterTextSplitter
-from langchain.vectorstores import FAISS
-from langchain.embeddings.huggingface import HuggingFaceEmbeddings
-from langchain.callbacks.manager import CallbackManagerForLLMRun
-from langchain.llms.base import LLM
-from langchain.chains import RetrievalQA
-from langchain.prompts import PromptTemplate
-import streamlit as st
-from pytube import YouTube
-# import replicate
-
-DESCRIPTION = """
-
- )
-}
diff --git a/spaces/Reeve/Ohayou_Face/models/__init__.py b/spaces/Reeve/Ohayou_Face/models/__init__.py
deleted file mode 100644
index e69de29bb2d1d6434b8b29ae775ad8c2e48c5391..0000000000000000000000000000000000000000
diff --git a/spaces/Reself/StableVideo/ldm/modules/midas/utils.py b/spaces/Reself/StableVideo/ldm/modules/midas/utils.py
deleted file mode 100644
index 9a9d3b5b66370fa98da9e067ba53ead848ea9a59..0000000000000000000000000000000000000000
--- a/spaces/Reself/StableVideo/ldm/modules/midas/utils.py
+++ /dev/null
@@ -1,189 +0,0 @@
-"""Utils for monoDepth."""
-import sys
-import re
-import numpy as np
-import cv2
-import torch
-
-
-def read_pfm(path):
- """Read pfm file.
-
- Args:
- path (str): path to file
-
- Returns:
- tuple: (data, scale)
- """
- with open(path, "rb") as file:
-
- color = None
- width = None
- height = None
- scale = None
- endian = None
-
- header = file.readline().rstrip()
- if header.decode("ascii") == "PF":
- color = True
- elif header.decode("ascii") == "Pf":
- color = False
- else:
- raise Exception("Not a PFM file: " + path)
-
- dim_match = re.match(r"^(\d+)\s(\d+)\s$", file.readline().decode("ascii"))
- if dim_match:
- width, height = list(map(int, dim_match.groups()))
- else:
- raise Exception("Malformed PFM header.")
-
- scale = float(file.readline().decode("ascii").rstrip())
- if scale < 0:
- # little-endian
- endian = "<"
- scale = -scale
- else:
- # big-endian
- endian = ">"
-
- data = np.fromfile(file, endian + "f")
- shape = (height, width, 3) if color else (height, width)
-
- data = np.reshape(data, shape)
- data = np.flipud(data)
-
- return data, scale
-
-
-def write_pfm(path, image, scale=1):
- """Write pfm file.
-
- Args:
- path (str): pathto file
- image (array): data
- scale (int, optional): Scale. Defaults to 1.
- """
-
- with open(path, "wb") as file:
- color = None
-
- if image.dtype.name != "float32":
- raise Exception("Image dtype must be float32.")
-
- image = np.flipud(image)
-
- if len(image.shape) == 3 and image.shape[2] == 3: # color image
- color = True
- elif (
- len(image.shape) == 2 or len(image.shape) == 3 and image.shape[2] == 1
- ): # greyscale
- color = False
- else:
- raise Exception("Image must have H x W x 3, H x W x 1 or H x W dimensions.")
-
- file.write("PF\n" if color else "Pf\n".encode())
- file.write("%d %d\n".encode() % (image.shape[1], image.shape[0]))
-
- endian = image.dtype.byteorder
-
- if endian == "<" or endian == "=" and sys.byteorder == "little":
- scale = -scale
-
- file.write("%f\n".encode() % scale)
-
- image.tofile(file)
-
-
-def read_image(path):
- """Read image and output RGB image (0-1).
-
- Args:
- path (str): path to file
-
- Returns:
- array: RGB image (0-1)
- """
- img = cv2.imread(path)
-
- if img.ndim == 2:
- img = cv2.cvtColor(img, cv2.COLOR_GRAY2BGR)
-
- img = cv2.cvtColor(img, cv2.COLOR_BGR2RGB) / 255.0
-
- return img
-
-
-def resize_image(img):
- """Resize image and make it fit for network.
-
- Args:
- img (array): image
-
- Returns:
- tensor: data ready for network
- """
- height_orig = img.shape[0]
- width_orig = img.shape[1]
-
- if width_orig > height_orig:
- scale = width_orig / 384
- else:
- scale = height_orig / 384
-
- height = (np.ceil(height_orig / scale / 32) * 32).astype(int)
- width = (np.ceil(width_orig / scale / 32) * 32).astype(int)
-
- img_resized = cv2.resize(img, (width, height), interpolation=cv2.INTER_AREA)
-
- img_resized = (
- torch.from_numpy(np.transpose(img_resized, (2, 0, 1))).contiguous().float()
- )
- img_resized = img_resized.unsqueeze(0)
-
- return img_resized
-
-
-def resize_depth(depth, width, height):
- """Resize depth map and bring to CPU (numpy).
-
- Args:
- depth (tensor): depth
- width (int): image width
- height (int): image height
-
- Returns:
- array: processed depth
- """
- depth = torch.squeeze(depth[0, :, :, :]).to("cpu")
-
- depth_resized = cv2.resize(
- depth.numpy(), (width, height), interpolation=cv2.INTER_CUBIC
- )
-
- return depth_resized
-
-def write_depth(path, depth, bits=1):
- """Write depth map to pfm and png file.
-
- Args:
- path (str): filepath without extension
- depth (array): depth
- """
- write_pfm(path + ".pfm", depth.astype(np.float32))
-
- depth_min = depth.min()
- depth_max = depth.max()
-
- max_val = (2**(8*bits))-1
-
- if depth_max - depth_min > np.finfo("float").eps:
- out = max_val * (depth - depth_min) / (depth_max - depth_min)
- else:
- out = np.zeros(depth.shape, dtype=depth.type)
-
- if bits == 1:
- cv2.imwrite(path + ".png", out.astype("uint8"))
- elif bits == 2:
- cv2.imwrite(path + ".png", out.astype("uint16"))
-
- return
diff --git a/spaces/Ritori/TTS_Yui/Yue_gradio.py b/spaces/Ritori/TTS_Yui/Yue_gradio.py
deleted file mode 100644
index 3bb55e7727f250d210ee6bfe2b958a7e05434a70..0000000000000000000000000000000000000000
--- a/spaces/Ritori/TTS_Yui/Yue_gradio.py
+++ /dev/null
@@ -1,243 +0,0 @@
-#好用的
-
-import os
-os.system('pip install -U tensorflow')
-os.system('pip install -q unidecode tensorboardX')
-os.system('pip install librosa==0.8.0')
-os.system('pip install pysoundfile==0.9.0.post1')
-os.system('pip install unidecode==1.3.4')
-os.system('pip install pyopenjtalk --no-build-isolation')
-os.system('pip install inflect==5.6.2')
-os.system('pip install janome==0.4.2')
-os.system('pip install tqdm -q')
-os.system('pip install gdown')
-os.system('pip install -q librosa unidecode')
-
-os.system('pip install ipython')
-os.system('pip install --upgrade jupyter ipywidgets')
-os.system('jupyter nbextension enable --py widgetsnbextension')
-os.system('pip uninstall tqdm')
-os.system('pip install tqdm')
-
-import time
-import pyopenjtalk
-import soundfile as sf
-import gradio as gr
-import torch
-import IPython.display as ipd
-import numpy as np
-import torch
-import json
-from hparams import create_hparams
-from model import Tacotron2
-from layers import TacotronSTFT
-from audio_processing import griffin_lim
-from text import text_to_sequence
-from env import AttrDict
-from meldataset import MAX_WAV_VALUE
-from models import Generator
-
-#@,tlitle 配置并运行
-
-#国际 HiFi-GAN 模型(有点机器音): 1qpgI41wNXFcH-iKq1Y42JlBC9j0je8PW
-#@markdown 你训练好的tacotron2模型的路径填在`Tacotron2_Model`这里
-Tacotron2_Model = '/content/Yui_TrapGenesis'#@param {type:"string"}
-TACOTRON2_ID = Tacotron2_Model
-HIFIGAN_ID = "1qpgI41wNXFcH-iKq1Y42JlBC9j0je8PW"
-#@markdown 选择预处理文本的cleaner
-text_cleaner = 'japanese_phrase_cleaners'#@param {type:"string"}
-import pyopenjtalk
-import soundfile as sf
-import gradio as gr
-
-# 全局变量声明
-model = None
-hparams = None
-hifigan = None
-thisdict = None
-pronounciation_dictionary = False
-show_graphs = False # 添加show_graphs变量,并赋予默认值
-
-# 初始化函数
-def initialize():
- global model, hparams, hifigan, thisdict, pronounciation_dictionary
-
- # 检查是否已初始化
- try:
- initialized
- except NameError:
- print("Setting up, please wait.\n")
-
- from tqdm.notebook import tqdm
- with tqdm(total=5, leave=False) as pbar:
- import os
- from os.path import exists, join, basename, splitext
- git_repo_url = 'https://github.com/CjangCjengh/tacotron2-japanese.git'
- project_name = splitext(basename(git_repo_url))[0]
- if not exists(project_name):
- # clone and install
- os.system('git clone -q --recursive {git_repo_url}')
- os.system('git clone -q --recursive https://github.com/SortAnon/hifi-gan')
-
- pbar.update(1) # downloaded TT2 and HiFi-GAN
- import sys
- sys.path.append('hifi-gan')
- sys.path.append(project_name)
- import time
- import matplotlib
- import matplotlib.pylab as plt
- import gdown
- d = 'https://drive.google.com/uc?id='
-
- # %matplotlib inline
- import IPython.display as ipd
- import numpy as np
- import torch
- import json
- from hparams import create_hparams
- from model import Tacotron2
- from layers import TacotronSTFT
- from audio_processing import griffin_lim
- from text import text_to_sequence
- from env import AttrDict
- from meldataset import MAX_WAV_VALUE
- from models import Generator
-
- pbar.update(1) # initialized Dependancies
-
- graph_width = 900
- graph_height = 360
- def plot_data(data, figsize=(int(graph_width/100), int(graph_height/100))):
- # %matplotlib inline
- fig, axes = plt.subplots(1, len(data), figsize=figsize)
- for i in range(len(data)):
- axes[i].imshow(data[i], aspect='auto', origin='upper',
- interpolation='none', cmap='inferno')
- fig.canvas.draw()
- plt.show()
-
- # Setup Pronounciation Dictionary
- os.system('wget https://github.com/wind4000/tacotron2/releases/download/v0.2/merged.dict.txt')
- thisdict = {}
- for line in reversed((open('merged.dict.txt', "r").read()).splitlines()):
- thisdict[(line.split(" ",1))[0]] = (line.split(" ",1))[1].strip()
-
- pbar.update(1) # Downloaded and Set up Pronounciation Dictionary
-
- def ARPA(text, punctuation=r"!?,.;", EOS_Token=True):
- out = ''
- for word_ in text.split(" "):
- word=word_; end_chars = ''
- while any(elem in word for elem in punctuation) and len(word) > 1:
- if word[-1] in punctuation: end_chars = word[-1] + end_chars; word = word[:-1]
- else: break
- try:
- word_arpa = thisdict[word.upper()]
- word = "{" + str(word_arpa) + "}"
- except KeyError: pass
- out = (out + " " + word + end_chars).strip()
- if EOS_Token and out[-1] != ";": out += ";"
- return out
-
- def get_hifigan(MODEL_ID):
- # Download HiFi-GAN
- hifigan_pretrained_model = 'hifimodel'
- gdown.download(d+MODEL_ID, hifigan_pretrained_model, quiet=False)
- if not exists(hifigan_pretrained_model):
- raise Exception("HiFI-GAN model failed to download!")
-
- # Load HiFi-GAN
- conf = os.path.join("hifi-gan", "config_v1.json")
- with open(conf) as f:
- json_config = json.loads(f.read())
- h = AttrDict(json_config)
- torch.manual_seed(h.seed)
- hifigan = Generator(h).to(torch.device("cuda"))
- state_dict_g = torch.load(hifigan_pretrained_model, map_location=torch.device("cuda"))
- hifigan.load_state_dict(state_dict_g["generator"])
- hifigan.eval()
- hifigan.remove_weight_norm()
- return hifigan, h
-
- hifigan, h = get_hifigan(HIFIGAN_ID)
- pbar.update(1) # Downloaded and Set up HiFi-GAN
-
- def has_MMI(STATE_DICT):
- return any(True for x in STATE_DICT.keys() if "mi." in x)
-
- def get_Tactron2(MODEL_ID):
- # Download Tacotron2
- tacotron2_pretrained_model = TACOTRON2_ID
- if not exists(tacotron2_pretrained_model):
- raise Exception("Tacotron2 model failed to download!")
- # Load Tacotron2 and Config
- hparams = create_hparams()
- hparams.sampling_rate = 22050
- hparams.max_decoder_steps = 2000 # Max Duration
- hparams.gate_threshold = 0.80 # Model must be 25% sure the clip is over before ending generation
- model = Tacotron2(hparams)
- state_dict = torch.load(tacotron2_pretrained_model)['state_dict']
- if has_MMI(state_dict):
- raise Exception("ERROR: This notebook does not currently support MMI models.")
- model.load_state_dict(state_dict)
- _ = model.cuda().eval().half()
- return model, hparams
-
- model, hparams = get_Tactron2(TACOTRON2_ID)
- previous_tt2_id = TACOTRON2_ID
-
- pbar.update(1) # Downloaded and Set up Tacotron2
-
- # 初始化
-initialize()
-
-import soundfile as sf
-
-def end_to_end_infer(text, pronounciation_dictionary, show_graphs):
- audio = None # 定义一个变量用于存储音频数据
- for i in [x for x in text.split("\n") if len(x)]:
- if not pronounciation_dictionary:
- if i[-1] != ";":
- i = i + ";"
- else:
- i = ARPA(i)
- with torch.no_grad():
- sequence = np.array(text_to_sequence(i, [text_cleaner]))[None, :]
- sequence = torch.autograd.Variable(torch.from_numpy(sequence)).cuda().long()
- mel_outputs, mel_outputs_postnet, _, alignments = model.inference(sequence)
- if show_graphs:
- plot_data((mel_outputs_postnet.float().data.cpu().numpy()[0],
- alignments.float().data.cpu().numpy()[0].T))
- y_g_hat = hifigan(mel_outputs_postnet.float())
- audio = y_g_hat.squeeze()
- audio = audio * MAX_WAV_VALUE
- output_filename = f"output_{time.strftime('%Y%m%d%H%M%S')}.wav"
- sf.write(output_filename, audio.cpu().numpy().astype('int16'), hparams.sampling_rate)
- print(f"音频已保存为 {output_filename}")
- print("")
- ipd.display(ipd.Audio(audio.cpu().numpy().astype("int16"), rate=hparams.sampling_rate))
- return audio # 返回音频数据
-
-# 文本到语音转换函数
-def text_to_speech(text, max_decoder_steps=2000, gate_threshold=0.5):
- global model, hparams, hifigan, thisdict, pronounciation_dictionary, show_graphs
-
- hparams.max_decoder_steps = max_decoder_steps
- hparams.gate_threshold = gate_threshold
- output_filename = f"output_{time.strftime('%Y%m%d%H%M%S')}.wav"
- audio = end_to_end_infer(text, pronounciation_dictionary, show_graphs)
- if audio is not None:
- sf.write(output_filename, audio.cpu().numpy().astype('int16'), hparams.sampling_rate)
- return output_filename
- else:
- return None
-
-# Gradio界面
-inputs = [
- gr.inputs.Textbox(lines=3, label="输入文本"),
- gr.inputs.Slider(minimum=100, maximum=5000, default=2000, step=100, label="最大解码步数"),
- gr.inputs.Slider(minimum=0.0, maximum=1.0, default=0.5, step=0.05, label="门控阈值")
-]
-outputs = gr.outputs.File(label="下载生成的音频")
-
-gr.Interface(fn=text_to_speech, inputs=inputs, outputs=outputs).launch(debug=True,share=True)
\ No newline at end of file
diff --git a/spaces/Robert001/UniControl-Demo/annotator/uniformer/mmcv/runner/optimizer/default_constructor.py b/spaces/Robert001/UniControl-Demo/annotator/uniformer/mmcv/runner/optimizer/default_constructor.py
deleted file mode 100644
index 2c0da3503b75441738efe38d70352b55a210a34a..0000000000000000000000000000000000000000
--- a/spaces/Robert001/UniControl-Demo/annotator/uniformer/mmcv/runner/optimizer/default_constructor.py
+++ /dev/null
@@ -1,249 +0,0 @@
-# Copyright (c) OpenMMLab. All rights reserved.
-import warnings
-
-import torch
-from torch.nn import GroupNorm, LayerNorm
-
-from annotator.uniformer.mmcv.utils import _BatchNorm, _InstanceNorm, build_from_cfg, is_list_of
-from annotator.uniformer.mmcv.utils.ext_loader import check_ops_exist
-from .builder import OPTIMIZER_BUILDERS, OPTIMIZERS
-
-
-@OPTIMIZER_BUILDERS.register_module()
-class DefaultOptimizerConstructor:
- """Default constructor for optimizers.
-
- By default each parameter share the same optimizer settings, and we
- provide an argument ``paramwise_cfg`` to specify parameter-wise settings.
- It is a dict and may contain the following fields:
-
- - ``custom_keys`` (dict): Specified parameters-wise settings by keys. If
- one of the keys in ``custom_keys`` is a substring of the name of one
- parameter, then the setting of the parameter will be specified by
- ``custom_keys[key]`` and other setting like ``bias_lr_mult`` etc. will
- be ignored. It should be noted that the aforementioned ``key`` is the
- longest key that is a substring of the name of the parameter. If there
- are multiple matched keys with the same length, then the key with lower
- alphabet order will be chosen.
- ``custom_keys[key]`` should be a dict and may contain fields ``lr_mult``
- and ``decay_mult``. See Example 2 below.
- - ``bias_lr_mult`` (float): It will be multiplied to the learning
- rate for all bias parameters (except for those in normalization
- layers and offset layers of DCN).
- - ``bias_decay_mult`` (float): It will be multiplied to the weight
- decay for all bias parameters (except for those in
- normalization layers, depthwise conv layers, offset layers of DCN).
- - ``norm_decay_mult`` (float): It will be multiplied to the weight
- decay for all weight and bias parameters of normalization
- layers.
- - ``dwconv_decay_mult`` (float): It will be multiplied to the weight
- decay for all weight and bias parameters of depthwise conv
- layers.
- - ``dcn_offset_lr_mult`` (float): It will be multiplied to the learning
- rate for parameters of offset layer in the deformable convs
- of a model.
- - ``bypass_duplicate`` (bool): If true, the duplicate parameters
- would not be added into optimizer. Default: False.
-
- Note:
- 1. If the option ``dcn_offset_lr_mult`` is used, the constructor will
- override the effect of ``bias_lr_mult`` in the bias of offset
- layer. So be careful when using both ``bias_lr_mult`` and
- ``dcn_offset_lr_mult``. If you wish to apply both of them to the
- offset layer in deformable convs, set ``dcn_offset_lr_mult``
- to the original ``dcn_offset_lr_mult`` * ``bias_lr_mult``.
- 2. If the option ``dcn_offset_lr_mult`` is used, the constructor will
- apply it to all the DCN layers in the model. So be careful when
- the model contains multiple DCN layers in places other than
- backbone.
-
- Args:
- model (:obj:`nn.Module`): The model with parameters to be optimized.
- optimizer_cfg (dict): The config dict of the optimizer.
- Positional fields are
-
- - `type`: class name of the optimizer.
-
- Optional fields are
-
- - any arguments of the corresponding optimizer type, e.g.,
- lr, weight_decay, momentum, etc.
- paramwise_cfg (dict, optional): Parameter-wise options.
-
- Example 1:
- >>> model = torch.nn.modules.Conv1d(1, 1, 1)
- >>> optimizer_cfg = dict(type='SGD', lr=0.01, momentum=0.9,
- >>> weight_decay=0.0001)
- >>> paramwise_cfg = dict(norm_decay_mult=0.)
- >>> optim_builder = DefaultOptimizerConstructor(
- >>> optimizer_cfg, paramwise_cfg)
- >>> optimizer = optim_builder(model)
-
- Example 2:
- >>> # assume model have attribute model.backbone and model.cls_head
- >>> optimizer_cfg = dict(type='SGD', lr=0.01, weight_decay=0.95)
- >>> paramwise_cfg = dict(custom_keys={
- '.backbone': dict(lr_mult=0.1, decay_mult=0.9)})
- >>> optim_builder = DefaultOptimizerConstructor(
- >>> optimizer_cfg, paramwise_cfg)
- >>> optimizer = optim_builder(model)
- >>> # Then the `lr` and `weight_decay` for model.backbone is
- >>> # (0.01 * 0.1, 0.95 * 0.9). `lr` and `weight_decay` for
- >>> # model.cls_head is (0.01, 0.95).
- """
-
- def __init__(self, optimizer_cfg, paramwise_cfg=None):
- if not isinstance(optimizer_cfg, dict):
- raise TypeError('optimizer_cfg should be a dict',
- f'but got {type(optimizer_cfg)}')
- self.optimizer_cfg = optimizer_cfg
- self.paramwise_cfg = {} if paramwise_cfg is None else paramwise_cfg
- self.base_lr = optimizer_cfg.get('lr', None)
- self.base_wd = optimizer_cfg.get('weight_decay', None)
- self._validate_cfg()
-
- def _validate_cfg(self):
- if not isinstance(self.paramwise_cfg, dict):
- raise TypeError('paramwise_cfg should be None or a dict, '
- f'but got {type(self.paramwise_cfg)}')
-
- if 'custom_keys' in self.paramwise_cfg:
- if not isinstance(self.paramwise_cfg['custom_keys'], dict):
- raise TypeError(
- 'If specified, custom_keys must be a dict, '
- f'but got {type(self.paramwise_cfg["custom_keys"])}')
- if self.base_wd is None:
- for key in self.paramwise_cfg['custom_keys']:
- if 'decay_mult' in self.paramwise_cfg['custom_keys'][key]:
- raise ValueError('base_wd should not be None')
-
- # get base lr and weight decay
- # weight_decay must be explicitly specified if mult is specified
- if ('bias_decay_mult' in self.paramwise_cfg
- or 'norm_decay_mult' in self.paramwise_cfg
- or 'dwconv_decay_mult' in self.paramwise_cfg):
- if self.base_wd is None:
- raise ValueError('base_wd should not be None')
-
- def _is_in(self, param_group, param_group_list):
- assert is_list_of(param_group_list, dict)
- param = set(param_group['params'])
- param_set = set()
- for group in param_group_list:
- param_set.update(set(group['params']))
-
- return not param.isdisjoint(param_set)
-
- def add_params(self, params, module, prefix='', is_dcn_module=None):
- """Add all parameters of module to the params list.
-
- The parameters of the given module will be added to the list of param
- groups, with specific rules defined by paramwise_cfg.
-
- Args:
- params (list[dict]): A list of param groups, it will be modified
- in place.
- module (nn.Module): The module to be added.
- prefix (str): The prefix of the module
- is_dcn_module (int|float|None): If the current module is a
- submodule of DCN, `is_dcn_module` will be passed to
- control conv_offset layer's learning rate. Defaults to None.
- """
- # get param-wise options
- custom_keys = self.paramwise_cfg.get('custom_keys', {})
- # first sort with alphabet order and then sort with reversed len of str
- sorted_keys = sorted(sorted(custom_keys.keys()), key=len, reverse=True)
-
- bias_lr_mult = self.paramwise_cfg.get('bias_lr_mult', 1.)
- bias_decay_mult = self.paramwise_cfg.get('bias_decay_mult', 1.)
- norm_decay_mult = self.paramwise_cfg.get('norm_decay_mult', 1.)
- dwconv_decay_mult = self.paramwise_cfg.get('dwconv_decay_mult', 1.)
- bypass_duplicate = self.paramwise_cfg.get('bypass_duplicate', False)
- dcn_offset_lr_mult = self.paramwise_cfg.get('dcn_offset_lr_mult', 1.)
-
- # special rules for norm layers and depth-wise conv layers
- is_norm = isinstance(module,
- (_BatchNorm, _InstanceNorm, GroupNorm, LayerNorm))
- is_dwconv = (
- isinstance(module, torch.nn.Conv2d)
- and module.in_channels == module.groups)
-
- for name, param in module.named_parameters(recurse=False):
- param_group = {'params': [param]}
- if not param.requires_grad:
- params.append(param_group)
- continue
- if bypass_duplicate and self._is_in(param_group, params):
- warnings.warn(f'{prefix} is duplicate. It is skipped since '
- f'bypass_duplicate={bypass_duplicate}')
- continue
- # if the parameter match one of the custom keys, ignore other rules
- is_custom = False
- for key in sorted_keys:
- if key in f'{prefix}.{name}':
- is_custom = True
- lr_mult = custom_keys[key].get('lr_mult', 1.)
- param_group['lr'] = self.base_lr * lr_mult
- if self.base_wd is not None:
- decay_mult = custom_keys[key].get('decay_mult', 1.)
- param_group['weight_decay'] = self.base_wd * decay_mult
- break
-
- if not is_custom:
- # bias_lr_mult affects all bias parameters
- # except for norm.bias dcn.conv_offset.bias
- if name == 'bias' and not (is_norm or is_dcn_module):
- param_group['lr'] = self.base_lr * bias_lr_mult
-
- if (prefix.find('conv_offset') != -1 and is_dcn_module
- and isinstance(module, torch.nn.Conv2d)):
- # deal with both dcn_offset's bias & weight
- param_group['lr'] = self.base_lr * dcn_offset_lr_mult
-
- # apply weight decay policies
- if self.base_wd is not None:
- # norm decay
- if is_norm:
- param_group[
- 'weight_decay'] = self.base_wd * norm_decay_mult
- # depth-wise conv
- elif is_dwconv:
- param_group[
- 'weight_decay'] = self.base_wd * dwconv_decay_mult
- # bias lr and decay
- elif name == 'bias' and not is_dcn_module:
- # TODO: current bias_decay_mult will have affect on DCN
- param_group[
- 'weight_decay'] = self.base_wd * bias_decay_mult
- params.append(param_group)
-
- if check_ops_exist():
- from annotator.uniformer.mmcv.ops import DeformConv2d, ModulatedDeformConv2d
- is_dcn_module = isinstance(module,
- (DeformConv2d, ModulatedDeformConv2d))
- else:
- is_dcn_module = False
- for child_name, child_mod in module.named_children():
- child_prefix = f'{prefix}.{child_name}' if prefix else child_name
- self.add_params(
- params,
- child_mod,
- prefix=child_prefix,
- is_dcn_module=is_dcn_module)
-
- def __call__(self, model):
- if hasattr(model, 'module'):
- model = model.module
-
- optimizer_cfg = self.optimizer_cfg.copy()
- # if no paramwise option is specified, just use the global setting
- if not self.paramwise_cfg:
- optimizer_cfg['params'] = model.parameters()
- return build_from_cfg(optimizer_cfg, OPTIMIZERS)
-
- # set param-wise lr and weight decay recursively
- params = []
- self.add_params(params, model)
- optimizer_cfg['params'] = params
-
- return build_from_cfg(optimizer_cfg, OPTIMIZERS)
diff --git a/spaces/Robert001/UniControl-Demo/annotator/uniformer/mmdet_null/core/bbox/coder/base_bbox_coder.py b/spaces/Robert001/UniControl-Demo/annotator/uniformer/mmdet_null/core/bbox/coder/base_bbox_coder.py
deleted file mode 100644
index cf0b34c7cc2fe561718b0c884990beb40a993643..0000000000000000000000000000000000000000
--- a/spaces/Robert001/UniControl-Demo/annotator/uniformer/mmdet_null/core/bbox/coder/base_bbox_coder.py
+++ /dev/null
@@ -1,17 +0,0 @@
-from abc import ABCMeta, abstractmethod
-
-
-class BaseBBoxCoder(metaclass=ABCMeta):
- """Base bounding box coder."""
-
- def __init__(self, **kwargs):
- pass
-
- @abstractmethod
- def encode(self, bboxes, gt_bboxes):
- """Encode deltas between bboxes and ground truth boxes."""
-
- @abstractmethod
- def decode(self, bboxes, bboxes_pred):
- """Decode the predicted bboxes according to prediction and base
- boxes."""
diff --git a/spaces/Robert001/UniControl-Demo/annotator/uniformer/mmdet_null/core/bbox/samplers/random_sampler.py b/spaces/Robert001/UniControl-Demo/annotator/uniformer/mmdet_null/core/bbox/samplers/random_sampler.py
deleted file mode 100644
index f34b006e8bb0b55c74aa1c3b792f3664ada93162..0000000000000000000000000000000000000000
--- a/spaces/Robert001/UniControl-Demo/annotator/uniformer/mmdet_null/core/bbox/samplers/random_sampler.py
+++ /dev/null
@@ -1,78 +0,0 @@
-import torch
-
-from ..builder import BBOX_SAMPLERS
-from .base_sampler import BaseSampler
-
-
-@BBOX_SAMPLERS.register_module()
-class RandomSampler(BaseSampler):
- """Random sampler.
-
- Args:
- num (int): Number of samples
- pos_fraction (float): Fraction of positive samples
- neg_pos_up (int, optional): Upper bound number of negative and
- positive samples. Defaults to -1.
- add_gt_as_proposals (bool, optional): Whether to add ground truth
- boxes as proposals. Defaults to True.
- """
-
- def __init__(self,
- num,
- pos_fraction,
- neg_pos_ub=-1,
- add_gt_as_proposals=True,
- **kwargs):
- from mmdet.core.bbox import demodata
- super(RandomSampler, self).__init__(num, pos_fraction, neg_pos_ub,
- add_gt_as_proposals)
- self.rng = demodata.ensure_rng(kwargs.get('rng', None))
-
- def random_choice(self, gallery, num):
- """Random select some elements from the gallery.
-
- If `gallery` is a Tensor, the returned indices will be a Tensor;
- If `gallery` is a ndarray or list, the returned indices will be a
- ndarray.
-
- Args:
- gallery (Tensor | ndarray | list): indices pool.
- num (int): expected sample num.
-
- Returns:
- Tensor or ndarray: sampled indices.
- """
- assert len(gallery) >= num
-
- is_tensor = isinstance(gallery, torch.Tensor)
- if not is_tensor:
- if torch.cuda.is_available():
- device = torch.cuda.current_device()
- else:
- device = 'cpu'
- gallery = torch.tensor(gallery, dtype=torch.long, device=device)
- perm = torch.randperm(gallery.numel(), device=gallery.device)[:num]
- rand_inds = gallery[perm]
- if not is_tensor:
- rand_inds = rand_inds.cpu().numpy()
- return rand_inds
-
- def _sample_pos(self, assign_result, num_expected, **kwargs):
- """Randomly sample some positive samples."""
- pos_inds = torch.nonzero(assign_result.gt_inds > 0, as_tuple=False)
- if pos_inds.numel() != 0:
- pos_inds = pos_inds.squeeze(1)
- if pos_inds.numel() <= num_expected:
- return pos_inds
- else:
- return self.random_choice(pos_inds, num_expected)
-
- def _sample_neg(self, assign_result, num_expected, **kwargs):
- """Randomly sample some negative samples."""
- neg_inds = torch.nonzero(assign_result.gt_inds == 0, as_tuple=False)
- if neg_inds.numel() != 0:
- neg_inds = neg_inds.squeeze(1)
- if len(neg_inds) <= num_expected:
- return neg_inds
- else:
- return self.random_choice(neg_inds, num_expected)
diff --git a/spaces/Robert001/UniControl-Demo/annotator/uniformer/mmdet_null/datasets/cityscapes.py b/spaces/Robert001/UniControl-Demo/annotator/uniformer/mmdet_null/datasets/cityscapes.py
deleted file mode 100644
index 71eead87e7f4e511c0cb59e69c3a599832ada0e4..0000000000000000000000000000000000000000
--- a/spaces/Robert001/UniControl-Demo/annotator/uniformer/mmdet_null/datasets/cityscapes.py
+++ /dev/null
@@ -1,334 +0,0 @@
-# Modified from https://github.com/facebookresearch/detectron2/blob/master/detectron2/data/datasets/cityscapes.py # noqa
-# and https://github.com/mcordts/cityscapesScripts/blob/master/cityscapesscripts/evaluation/evalInstanceLevelSemanticLabeling.py # noqa
-
-import glob
-import os
-import os.path as osp
-import tempfile
-from collections import OrderedDict
-
-import mmcv
-import numpy as np
-import pycocotools.mask as maskUtils
-from mmcv.utils import print_log
-
-from .builder import DATASETS
-from .coco import CocoDataset
-
-
-@DATASETS.register_module()
-class CityscapesDataset(CocoDataset):
-
- CLASSES = ('person', 'rider', 'car', 'truck', 'bus', 'train', 'motorcycle',
- 'bicycle')
-
- def _filter_imgs(self, min_size=32):
- """Filter images too small or without ground truths."""
- valid_inds = []
- # obtain images that contain annotation
- ids_with_ann = set(_['image_id'] for _ in self.coco.anns.values())
- # obtain images that contain annotations of the required categories
- ids_in_cat = set()
- for i, class_id in enumerate(self.cat_ids):
- ids_in_cat |= set(self.coco.cat_img_map[class_id])
- # merge the image id sets of the two conditions and use the merged set
- # to filter out images if self.filter_empty_gt=True
- ids_in_cat &= ids_with_ann
-
- valid_img_ids = []
- for i, img_info in enumerate(self.data_infos):
- img_id = img_info['id']
- ann_ids = self.coco.getAnnIds(imgIds=[img_id])
- ann_info = self.coco.loadAnns(ann_ids)
- all_iscrowd = all([_['iscrowd'] for _ in ann_info])
- if self.filter_empty_gt and (self.img_ids[i] not in ids_in_cat
- or all_iscrowd):
- continue
- if min(img_info['width'], img_info['height']) >= min_size:
- valid_inds.append(i)
- valid_img_ids.append(img_id)
- self.img_ids = valid_img_ids
- return valid_inds
-
- def _parse_ann_info(self, img_info, ann_info):
- """Parse bbox and mask annotation.
-
- Args:
- img_info (dict): Image info of an image.
- ann_info (list[dict]): Annotation info of an image.
-
- Returns:
- dict: A dict containing the following keys: bboxes, \
- bboxes_ignore, labels, masks, seg_map. \
- "masks" are already decoded into binary masks.
- """
- gt_bboxes = []
- gt_labels = []
- gt_bboxes_ignore = []
- gt_masks_ann = []
-
- for i, ann in enumerate(ann_info):
- if ann.get('ignore', False):
- continue
- x1, y1, w, h = ann['bbox']
- if ann['area'] <= 0 or w < 1 or h < 1:
- continue
- if ann['category_id'] not in self.cat_ids:
- continue
- bbox = [x1, y1, x1 + w, y1 + h]
- if ann.get('iscrowd', False):
- gt_bboxes_ignore.append(bbox)
- else:
- gt_bboxes.append(bbox)
- gt_labels.append(self.cat2label[ann['category_id']])
- gt_masks_ann.append(ann['segmentation'])
-
- if gt_bboxes:
- gt_bboxes = np.array(gt_bboxes, dtype=np.float32)
- gt_labels = np.array(gt_labels, dtype=np.int64)
- else:
- gt_bboxes = np.zeros((0, 4), dtype=np.float32)
- gt_labels = np.array([], dtype=np.int64)
-
- if gt_bboxes_ignore:
- gt_bboxes_ignore = np.array(gt_bboxes_ignore, dtype=np.float32)
- else:
- gt_bboxes_ignore = np.zeros((0, 4), dtype=np.float32)
-
- ann = dict(
- bboxes=gt_bboxes,
- labels=gt_labels,
- bboxes_ignore=gt_bboxes_ignore,
- masks=gt_masks_ann,
- seg_map=img_info['segm_file'])
-
- return ann
-
- def results2txt(self, results, outfile_prefix):
- """Dump the detection results to a txt file.
-
- Args:
- results (list[list | tuple]): Testing results of the
- dataset.
- outfile_prefix (str): The filename prefix of the json files.
- If the prefix is "somepath/xxx",
- the txt files will be named "somepath/xxx.txt".
-
- Returns:
- list[str]: Result txt files which contains corresponding \
- instance segmentation images.
- """
- try:
- import cityscapesscripts.helpers.labels as CSLabels
- except ImportError:
- raise ImportError('Please run "pip install citscapesscripts" to '
- 'install cityscapesscripts first.')
- result_files = []
- os.makedirs(outfile_prefix, exist_ok=True)
- prog_bar = mmcv.ProgressBar(len(self))
- for idx in range(len(self)):
- result = results[idx]
- filename = self.data_infos[idx]['filename']
- basename = osp.splitext(osp.basename(filename))[0]
- pred_txt = osp.join(outfile_prefix, basename + '_pred.txt')
-
- bbox_result, segm_result = result
- bboxes = np.vstack(bbox_result)
- # segm results
- if isinstance(segm_result, tuple):
- # Some detectors use different scores for bbox and mask,
- # like Mask Scoring R-CNN. Score of segm will be used instead
- # of bbox score.
- segms = mmcv.concat_list(segm_result[0])
- mask_score = segm_result[1]
- else:
- # use bbox score for mask score
- segms = mmcv.concat_list(segm_result)
- mask_score = [bbox[-1] for bbox in bboxes]
- labels = [
- np.full(bbox.shape[0], i, dtype=np.int32)
- for i, bbox in enumerate(bbox_result)
- ]
- labels = np.concatenate(labels)
-
- assert len(bboxes) == len(segms) == len(labels)
- num_instances = len(bboxes)
- prog_bar.update()
- with open(pred_txt, 'w') as fout:
- for i in range(num_instances):
- pred_class = labels[i]
- classes = self.CLASSES[pred_class]
- class_id = CSLabels.name2label[classes].id
- score = mask_score[i]
- mask = maskUtils.decode(segms[i]).astype(np.uint8)
- png_filename = osp.join(outfile_prefix,
- basename + f'_{i}_{classes}.png')
- mmcv.imwrite(mask, png_filename)
- fout.write(f'{osp.basename(png_filename)} {class_id} '
- f'{score}\n')
- result_files.append(pred_txt)
-
- return result_files
-
- def format_results(self, results, txtfile_prefix=None):
- """Format the results to txt (standard format for Cityscapes
- evaluation).
-
- Args:
- results (list): Testing results of the dataset.
- txtfile_prefix (str | None): The prefix of txt files. It includes
- the file path and the prefix of filename, e.g., "a/b/prefix".
- If not specified, a temp file will be created. Default: None.
-
- Returns:
- tuple: (result_files, tmp_dir), result_files is a dict containing \
- the json filepaths, tmp_dir is the temporal directory created \
- for saving txt/png files when txtfile_prefix is not specified.
- """
- assert isinstance(results, list), 'results must be a list'
- assert len(results) == len(self), (
- 'The length of results is not equal to the dataset len: {} != {}'.
- format(len(results), len(self)))
-
- assert isinstance(results, list), 'results must be a list'
- assert len(results) == len(self), (
- 'The length of results is not equal to the dataset len: {} != {}'.
- format(len(results), len(self)))
-
- if txtfile_prefix is None:
- tmp_dir = tempfile.TemporaryDirectory()
- txtfile_prefix = osp.join(tmp_dir.name, 'results')
- else:
- tmp_dir = None
- result_files = self.results2txt(results, txtfile_prefix)
-
- return result_files, tmp_dir
-
- def evaluate(self,
- results,
- metric='bbox',
- logger=None,
- outfile_prefix=None,
- classwise=False,
- proposal_nums=(100, 300, 1000),
- iou_thrs=np.arange(0.5, 0.96, 0.05)):
- """Evaluation in Cityscapes/COCO protocol.
-
- Args:
- results (list[list | tuple]): Testing results of the dataset.
- metric (str | list[str]): Metrics to be evaluated. Options are
- 'bbox', 'segm', 'proposal', 'proposal_fast'.
- logger (logging.Logger | str | None): Logger used for printing
- related information during evaluation. Default: None.
- outfile_prefix (str | None): The prefix of output file. It includes
- the file path and the prefix of filename, e.g., "a/b/prefix".
- If results are evaluated with COCO protocol, it would be the
- prefix of output json file. For example, the metric is 'bbox'
- and 'segm', then json files would be "a/b/prefix.bbox.json" and
- "a/b/prefix.segm.json".
- If results are evaluated with cityscapes protocol, it would be
- the prefix of output txt/png files. The output files would be
- png images under folder "a/b/prefix/xxx/" and the file name of
- images would be written into a txt file
- "a/b/prefix/xxx_pred.txt", where "xxx" is the video name of
- cityscapes. If not specified, a temp file will be created.
- Default: None.
- classwise (bool): Whether to evaluating the AP for each class.
- proposal_nums (Sequence[int]): Proposal number used for evaluating
- recalls, such as recall@100, recall@1000.
- Default: (100, 300, 1000).
- iou_thrs (Sequence[float]): IoU threshold used for evaluating
- recalls. If set to a list, the average recall of all IoUs will
- also be computed. Default: 0.5.
-
- Returns:
- dict[str, float]: COCO style evaluation metric or cityscapes mAP \
- and AP@50.
- """
- eval_results = dict()
-
- metrics = metric.copy() if isinstance(metric, list) else [metric]
-
- if 'cityscapes' in metrics:
- eval_results.update(
- self._evaluate_cityscapes(results, outfile_prefix, logger))
- metrics.remove('cityscapes')
-
- # left metrics are all coco metric
- if len(metrics) > 0:
- # create CocoDataset with CityscapesDataset annotation
- self_coco = CocoDataset(self.ann_file, self.pipeline.transforms,
- None, self.data_root, self.img_prefix,
- self.seg_prefix, self.proposal_file,
- self.test_mode, self.filter_empty_gt)
- # TODO: remove this in the future
- # reload annotations of correct class
- self_coco.CLASSES = self.CLASSES
- self_coco.data_infos = self_coco.load_annotations(self.ann_file)
- eval_results.update(
- self_coco.evaluate(results, metrics, logger, outfile_prefix,
- classwise, proposal_nums, iou_thrs))
-
- return eval_results
-
- def _evaluate_cityscapes(self, results, txtfile_prefix, logger):
- """Evaluation in Cityscapes protocol.
-
- Args:
- results (list): Testing results of the dataset.
- txtfile_prefix (str | None): The prefix of output txt file
- logger (logging.Logger | str | None): Logger used for printing
- related information during evaluation. Default: None.
-
- Returns:
- dict[str: float]: Cityscapes evaluation results, contains 'mAP' \
- and 'AP@50'.
- """
-
- try:
- import cityscapesscripts.evaluation.evalInstanceLevelSemanticLabeling as CSEval # noqa
- except ImportError:
- raise ImportError('Please run "pip install citscapesscripts" to '
- 'install cityscapesscripts first.')
- msg = 'Evaluating in Cityscapes style'
- if logger is None:
- msg = '\n' + msg
- print_log(msg, logger=logger)
-
- result_files, tmp_dir = self.format_results(results, txtfile_prefix)
-
- if tmp_dir is None:
- result_dir = osp.join(txtfile_prefix, 'results')
- else:
- result_dir = osp.join(tmp_dir.name, 'results')
-
- eval_results = OrderedDict()
- print_log(f'Evaluating results under {result_dir} ...', logger=logger)
-
- # set global states in cityscapes evaluation API
- CSEval.args.cityscapesPath = os.path.join(self.img_prefix, '../..')
- CSEval.args.predictionPath = os.path.abspath(result_dir)
- CSEval.args.predictionWalk = None
- CSEval.args.JSONOutput = False
- CSEval.args.colorized = False
- CSEval.args.gtInstancesFile = os.path.join(result_dir,
- 'gtInstances.json')
- CSEval.args.groundTruthSearch = os.path.join(
- self.img_prefix.replace('leftImg8bit', 'gtFine'),
- '*/*_gtFine_instanceIds.png')
-
- groundTruthImgList = glob.glob(CSEval.args.groundTruthSearch)
- assert len(groundTruthImgList), 'Cannot find ground truth images' \
- f' in {CSEval.args.groundTruthSearch}.'
- predictionImgList = []
- for gt in groundTruthImgList:
- predictionImgList.append(CSEval.getPrediction(gt, CSEval.args))
- CSEval_results = CSEval.evaluateImgLists(predictionImgList,
- groundTruthImgList,
- CSEval.args)['averages']
-
- eval_results['mAP'] = CSEval_results['allAp']
- eval_results['AP@50'] = CSEval_results['allAp50%']
- if tmp_dir is not None:
- tmp_dir.cleanup()
- return eval_results
diff --git a/spaces/Robert001/UniControl-Demo/annotator/uniformer_base/mmcv/cnn/vgg.py b/spaces/Robert001/UniControl-Demo/annotator/uniformer_base/mmcv/cnn/vgg.py
deleted file mode 100644
index 8778b649561a45a9652b1a15a26c2d171e58f3e1..0000000000000000000000000000000000000000
--- a/spaces/Robert001/UniControl-Demo/annotator/uniformer_base/mmcv/cnn/vgg.py
+++ /dev/null
@@ -1,175 +0,0 @@
-# Copyright (c) OpenMMLab. All rights reserved.
-import logging
-
-import torch.nn as nn
-
-from .utils import constant_init, kaiming_init, normal_init
-
-
-def conv3x3(in_planes, out_planes, dilation=1):
- """3x3 convolution with padding."""
- return nn.Conv2d(
- in_planes,
- out_planes,
- kernel_size=3,
- padding=dilation,
- dilation=dilation)
-
-
-def make_vgg_layer(inplanes,
- planes,
- num_blocks,
- dilation=1,
- with_bn=False,
- ceil_mode=False):
- layers = []
- for _ in range(num_blocks):
- layers.append(conv3x3(inplanes, planes, dilation))
- if with_bn:
- layers.append(nn.BatchNorm2d(planes))
- layers.append(nn.ReLU(inplace=True))
- inplanes = planes
- layers.append(nn.MaxPool2d(kernel_size=2, stride=2, ceil_mode=ceil_mode))
-
- return layers
-
-
-class VGG(nn.Module):
- """VGG backbone.
-
- Args:
- depth (int): Depth of vgg, from {11, 13, 16, 19}.
- with_bn (bool): Use BatchNorm or not.
- num_classes (int): number of classes for classification.
- num_stages (int): VGG stages, normally 5.
- dilations (Sequence[int]): Dilation of each stage.
- out_indices (Sequence[int]): Output from which stages.
- frozen_stages (int): Stages to be frozen (all param fixed). -1 means
- not freezing any parameters.
- bn_eval (bool): Whether to set BN layers as eval mode, namely, freeze
- running stats (mean and var).
- bn_frozen (bool): Whether to freeze weight and bias of BN layers.
- """
-
- arch_settings = {
- 11: (1, 1, 2, 2, 2),
- 13: (2, 2, 2, 2, 2),
- 16: (2, 2, 3, 3, 3),
- 19: (2, 2, 4, 4, 4)
- }
-
- def __init__(self,
- depth,
- with_bn=False,
- num_classes=-1,
- num_stages=5,
- dilations=(1, 1, 1, 1, 1),
- out_indices=(0, 1, 2, 3, 4),
- frozen_stages=-1,
- bn_eval=True,
- bn_frozen=False,
- ceil_mode=False,
- with_last_pool=True):
- super(VGG, self).__init__()
- if depth not in self.arch_settings:
- raise KeyError(f'invalid depth {depth} for vgg')
- assert num_stages >= 1 and num_stages <= 5
- stage_blocks = self.arch_settings[depth]
- self.stage_blocks = stage_blocks[:num_stages]
- assert len(dilations) == num_stages
- assert max(out_indices) <= num_stages
-
- self.num_classes = num_classes
- self.out_indices = out_indices
- self.frozen_stages = frozen_stages
- self.bn_eval = bn_eval
- self.bn_frozen = bn_frozen
-
- self.inplanes = 3
- start_idx = 0
- vgg_layers = []
- self.range_sub_modules = []
- for i, num_blocks in enumerate(self.stage_blocks):
- num_modules = num_blocks * (2 + with_bn) + 1
- end_idx = start_idx + num_modules
- dilation = dilations[i]
- planes = 64 * 2**i if i < 4 else 512
- vgg_layer = make_vgg_layer(
- self.inplanes,
- planes,
- num_blocks,
- dilation=dilation,
- with_bn=with_bn,
- ceil_mode=ceil_mode)
- vgg_layers.extend(vgg_layer)
- self.inplanes = planes
- self.range_sub_modules.append([start_idx, end_idx])
- start_idx = end_idx
- if not with_last_pool:
- vgg_layers.pop(-1)
- self.range_sub_modules[-1][1] -= 1
- self.module_name = 'features'
- self.add_module(self.module_name, nn.Sequential(*vgg_layers))
-
- if self.num_classes > 0:
- self.classifier = nn.Sequential(
- nn.Linear(512 * 7 * 7, 4096),
- nn.ReLU(True),
- nn.Dropout(),
- nn.Linear(4096, 4096),
- nn.ReLU(True),
- nn.Dropout(),
- nn.Linear(4096, num_classes),
- )
-
- def init_weights(self, pretrained=None):
- if isinstance(pretrained, str):
- logger = logging.getLogger()
- from ..runner import load_checkpoint
- load_checkpoint(self, pretrained, strict=False, logger=logger)
- elif pretrained is None:
- for m in self.modules():
- if isinstance(m, nn.Conv2d):
- kaiming_init(m)
- elif isinstance(m, nn.BatchNorm2d):
- constant_init(m, 1)
- elif isinstance(m, nn.Linear):
- normal_init(m, std=0.01)
- else:
- raise TypeError('pretrained must be a str or None')
-
- def forward(self, x):
- outs = []
- vgg_layers = getattr(self, self.module_name)
- for i in range(len(self.stage_blocks)):
- for j in range(*self.range_sub_modules[i]):
- vgg_layer = vgg_layers[j]
- x = vgg_layer(x)
- if i in self.out_indices:
- outs.append(x)
- if self.num_classes > 0:
- x = x.view(x.size(0), -1)
- x = self.classifier(x)
- outs.append(x)
- if len(outs) == 1:
- return outs[0]
- else:
- return tuple(outs)
-
- def train(self, mode=True):
- super(VGG, self).train(mode)
- if self.bn_eval:
- for m in self.modules():
- if isinstance(m, nn.BatchNorm2d):
- m.eval()
- if self.bn_frozen:
- for params in m.parameters():
- params.requires_grad = False
- vgg_layers = getattr(self, self.module_name)
- if mode and self.frozen_stages >= 0:
- for i in range(self.frozen_stages):
- for j in range(*self.range_sub_modules[i]):
- mod = vgg_layers[j]
- mod.eval()
- for param in mod.parameters():
- param.requires_grad = False
diff --git a/spaces/Robert001/UniControl-Demo/annotator/uniformer_base/mmcv/ops/merge_cells.py b/spaces/Robert001/UniControl-Demo/annotator/uniformer_base/mmcv/ops/merge_cells.py
deleted file mode 100644
index 48ca8cc0a8aca8432835bd760c0403a3c35b34cf..0000000000000000000000000000000000000000
--- a/spaces/Robert001/UniControl-Demo/annotator/uniformer_base/mmcv/ops/merge_cells.py
+++ /dev/null
@@ -1,149 +0,0 @@
-# Copyright (c) OpenMMLab. All rights reserved.
-from abc import abstractmethod
-
-import torch
-import torch.nn as nn
-import torch.nn.functional as F
-
-from ..cnn import ConvModule
-
-
-class BaseMergeCell(nn.Module):
- """The basic class for cells used in NAS-FPN and NAS-FCOS.
-
- BaseMergeCell takes 2 inputs. After applying convolution
- on them, they are resized to the target size. Then,
- they go through binary_op, which depends on the type of cell.
- If with_out_conv is True, the result of output will go through
- another convolution layer.
-
- Args:
- in_channels (int): number of input channels in out_conv layer.
- out_channels (int): number of output channels in out_conv layer.
- with_out_conv (bool): Whether to use out_conv layer
- out_conv_cfg (dict): Config dict for convolution layer, which should
- contain "groups", "kernel_size", "padding", "bias" to build
- out_conv layer.
- out_norm_cfg (dict): Config dict for normalization layer in out_conv.
- out_conv_order (tuple): The order of conv/norm/activation layers in
- out_conv.
- with_input1_conv (bool): Whether to use convolution on input1.
- with_input2_conv (bool): Whether to use convolution on input2.
- input_conv_cfg (dict): Config dict for building input1_conv layer and
- input2_conv layer, which is expected to contain the type of
- convolution.
- Default: None, which means using conv2d.
- input_norm_cfg (dict): Config dict for normalization layer in
- input1_conv and input2_conv layer. Default: None.
- upsample_mode (str): Interpolation method used to resize the output
- of input1_conv and input2_conv to target size. Currently, we
- support ['nearest', 'bilinear']. Default: 'nearest'.
- """
-
- def __init__(self,
- fused_channels=256,
- out_channels=256,
- with_out_conv=True,
- out_conv_cfg=dict(
- groups=1, kernel_size=3, padding=1, bias=True),
- out_norm_cfg=None,
- out_conv_order=('act', 'conv', 'norm'),
- with_input1_conv=False,
- with_input2_conv=False,
- input_conv_cfg=None,
- input_norm_cfg=None,
- upsample_mode='nearest'):
- super(BaseMergeCell, self).__init__()
- assert upsample_mode in ['nearest', 'bilinear']
- self.with_out_conv = with_out_conv
- self.with_input1_conv = with_input1_conv
- self.with_input2_conv = with_input2_conv
- self.upsample_mode = upsample_mode
-
- if self.with_out_conv:
- self.out_conv = ConvModule(
- fused_channels,
- out_channels,
- **out_conv_cfg,
- norm_cfg=out_norm_cfg,
- order=out_conv_order)
-
- self.input1_conv = self._build_input_conv(
- out_channels, input_conv_cfg,
- input_norm_cfg) if with_input1_conv else nn.Sequential()
- self.input2_conv = self._build_input_conv(
- out_channels, input_conv_cfg,
- input_norm_cfg) if with_input2_conv else nn.Sequential()
-
- def _build_input_conv(self, channel, conv_cfg, norm_cfg):
- return ConvModule(
- channel,
- channel,
- 3,
- padding=1,
- conv_cfg=conv_cfg,
- norm_cfg=norm_cfg,
- bias=True)
-
- @abstractmethod
- def _binary_op(self, x1, x2):
- pass
-
- def _resize(self, x, size):
- if x.shape[-2:] == size:
- return x
- elif x.shape[-2:] < size:
- return F.interpolate(x, size=size, mode=self.upsample_mode)
- else:
- assert x.shape[-2] % size[-2] == 0 and x.shape[-1] % size[-1] == 0
- kernel_size = x.shape[-1] // size[-1]
- x = F.max_pool2d(x, kernel_size=kernel_size, stride=kernel_size)
- return x
-
- def forward(self, x1, x2, out_size=None):
- assert x1.shape[:2] == x2.shape[:2]
- assert out_size is None or len(out_size) == 2
- if out_size is None: # resize to larger one
- out_size = max(x1.size()[2:], x2.size()[2:])
-
- x1 = self.input1_conv(x1)
- x2 = self.input2_conv(x2)
-
- x1 = self._resize(x1, out_size)
- x2 = self._resize(x2, out_size)
-
- x = self._binary_op(x1, x2)
- if self.with_out_conv:
- x = self.out_conv(x)
- return x
-
-
-class SumCell(BaseMergeCell):
-
- def __init__(self, in_channels, out_channels, **kwargs):
- super(SumCell, self).__init__(in_channels, out_channels, **kwargs)
-
- def _binary_op(self, x1, x2):
- return x1 + x2
-
-
-class ConcatCell(BaseMergeCell):
-
- def __init__(self, in_channels, out_channels, **kwargs):
- super(ConcatCell, self).__init__(in_channels * 2, out_channels,
- **kwargs)
-
- def _binary_op(self, x1, x2):
- ret = torch.cat([x1, x2], dim=1)
- return ret
-
-
-class GlobalPoolingCell(BaseMergeCell):
-
- def __init__(self, in_channels=None, out_channels=None, **kwargs):
- super().__init__(in_channels, out_channels, **kwargs)
- self.global_pool = nn.AdaptiveAvgPool2d((1, 1))
-
- def _binary_op(self, x1, x2):
- x2_att = self.global_pool(x2).sigmoid()
- return x2 + x2_att * x1
diff --git a/spaces/Ryandhikaw/rvc-hololive/infer_pack/modules.py b/spaces/Ryandhikaw/rvc-hololive/infer_pack/modules.py
deleted file mode 100644
index 960481cedad9a6106f2bf0b9e86e82b120f7b33f..0000000000000000000000000000000000000000
--- a/spaces/Ryandhikaw/rvc-hololive/infer_pack/modules.py
+++ /dev/null
@@ -1,522 +0,0 @@
-import copy
-import math
-import numpy as np
-import scipy
-import torch
-from torch import nn
-from torch.nn import functional as F
-
-from torch.nn import Conv1d, ConvTranspose1d, AvgPool1d, Conv2d
-from torch.nn.utils import weight_norm, remove_weight_norm
-
-from infer_pack import commons
-from infer_pack.commons import init_weights, get_padding
-from infer_pack.transforms import piecewise_rational_quadratic_transform
-
-
-LRELU_SLOPE = 0.1
-
-
-class LayerNorm(nn.Module):
- def __init__(self, channels, eps=1e-5):
- super().__init__()
- self.channels = channels
- self.eps = eps
-
- self.gamma = nn.Parameter(torch.ones(channels))
- self.beta = nn.Parameter(torch.zeros(channels))
-
- def forward(self, x):
- x = x.transpose(1, -1)
- x = F.layer_norm(x, (self.channels,), self.gamma, self.beta, self.eps)
- return x.transpose(1, -1)
-
-
-class ConvReluNorm(nn.Module):
- def __init__(
- self,
- in_channels,
- hidden_channels,
- out_channels,
- kernel_size,
- n_layers,
- p_dropout,
- ):
- super().__init__()
- self.in_channels = in_channels
- self.hidden_channels = hidden_channels
- self.out_channels = out_channels
- self.kernel_size = kernel_size
- self.n_layers = n_layers
- self.p_dropout = p_dropout
- assert n_layers > 1, "Number of layers should be larger than 0."
-
- self.conv_layers = nn.ModuleList()
- self.norm_layers = nn.ModuleList()
- self.conv_layers.append(
- nn.Conv1d(
- in_channels, hidden_channels, kernel_size, padding=kernel_size // 2
- )
- )
- self.norm_layers.append(LayerNorm(hidden_channels))
- self.relu_drop = nn.Sequential(nn.ReLU(), nn.Dropout(p_dropout))
- for _ in range(n_layers - 1):
- self.conv_layers.append(
- nn.Conv1d(
- hidden_channels,
- hidden_channels,
- kernel_size,
- padding=kernel_size // 2,
- )
- )
- self.norm_layers.append(LayerNorm(hidden_channels))
- self.proj = nn.Conv1d(hidden_channels, out_channels, 1)
- self.proj.weight.data.zero_()
- self.proj.bias.data.zero_()
-
- def forward(self, x, x_mask):
- x_org = x
- for i in range(self.n_layers):
- x = self.conv_layers[i](x * x_mask)
- x = self.norm_layers[i](x)
- x = self.relu_drop(x)
- x = x_org + self.proj(x)
- return x * x_mask
-
-
-class DDSConv(nn.Module):
- """
- Dialted and Depth-Separable Convolution
- """
-
- def __init__(self, channels, kernel_size, n_layers, p_dropout=0.0):
- super().__init__()
- self.channels = channels
- self.kernel_size = kernel_size
- self.n_layers = n_layers
- self.p_dropout = p_dropout
-
- self.drop = nn.Dropout(p_dropout)
- self.convs_sep = nn.ModuleList()
- self.convs_1x1 = nn.ModuleList()
- self.norms_1 = nn.ModuleList()
- self.norms_2 = nn.ModuleList()
- for i in range(n_layers):
- dilation = kernel_size**i
- padding = (kernel_size * dilation - dilation) // 2
- self.convs_sep.append(
- nn.Conv1d(
- channels,
- channels,
- kernel_size,
- groups=channels,
- dilation=dilation,
- padding=padding,
- )
- )
- self.convs_1x1.append(nn.Conv1d(channels, channels, 1))
- self.norms_1.append(LayerNorm(channels))
- self.norms_2.append(LayerNorm(channels))
-
- def forward(self, x, x_mask, g=None):
- if g is not None:
- x = x + g
- for i in range(self.n_layers):
- y = self.convs_sep[i](x * x_mask)
- y = self.norms_1[i](y)
- y = F.gelu(y)
- y = self.convs_1x1[i](y)
- y = self.norms_2[i](y)
- y = F.gelu(y)
- y = self.drop(y)
- x = x + y
- return x * x_mask
-
-
-class WN(torch.nn.Module):
- def __init__(
- self,
- hidden_channels,
- kernel_size,
- dilation_rate,
- n_layers,
- gin_channels=0,
- p_dropout=0,
- ):
- super(WN, self).__init__()
- assert kernel_size % 2 == 1
- self.hidden_channels = hidden_channels
- self.kernel_size = (kernel_size,)
- self.dilation_rate = dilation_rate
- self.n_layers = n_layers
- self.gin_channels = gin_channels
- self.p_dropout = p_dropout
-
- self.in_layers = torch.nn.ModuleList()
- self.res_skip_layers = torch.nn.ModuleList()
- self.drop = nn.Dropout(p_dropout)
-
- if gin_channels != 0:
- cond_layer = torch.nn.Conv1d(
- gin_channels, 2 * hidden_channels * n_layers, 1
- )
- self.cond_layer = torch.nn.utils.weight_norm(cond_layer, name="weight")
-
- for i in range(n_layers):
- dilation = dilation_rate**i
- padding = int((kernel_size * dilation - dilation) / 2)
- in_layer = torch.nn.Conv1d(
- hidden_channels,
- 2 * hidden_channels,
- kernel_size,
- dilation=dilation,
- padding=padding,
- )
- in_layer = torch.nn.utils.weight_norm(in_layer, name="weight")
- self.in_layers.append(in_layer)
-
- # last one is not necessary
- if i < n_layers - 1:
- res_skip_channels = 2 * hidden_channels
- else:
- res_skip_channels = hidden_channels
-
- res_skip_layer = torch.nn.Conv1d(hidden_channels, res_skip_channels, 1)
- res_skip_layer = torch.nn.utils.weight_norm(res_skip_layer, name="weight")
- self.res_skip_layers.append(res_skip_layer)
-
- def forward(self, x, x_mask, g=None, **kwargs):
- output = torch.zeros_like(x)
- n_channels_tensor = torch.IntTensor([self.hidden_channels])
-
- if g is not None:
- g = self.cond_layer(g)
-
- for i in range(self.n_layers):
- x_in = self.in_layers[i](x)
- if g is not None:
- cond_offset = i * 2 * self.hidden_channels
- g_l = g[:, cond_offset : cond_offset + 2 * self.hidden_channels, :]
- else:
- g_l = torch.zeros_like(x_in)
-
- acts = commons.fused_add_tanh_sigmoid_multiply(x_in, g_l, n_channels_tensor)
- acts = self.drop(acts)
-
- res_skip_acts = self.res_skip_layers[i](acts)
- if i < self.n_layers - 1:
- res_acts = res_skip_acts[:, : self.hidden_channels, :]
- x = (x + res_acts) * x_mask
- output = output + res_skip_acts[:, self.hidden_channels :, :]
- else:
- output = output + res_skip_acts
- return output * x_mask
-
- def remove_weight_norm(self):
- if self.gin_channels != 0:
- torch.nn.utils.remove_weight_norm(self.cond_layer)
- for l in self.in_layers:
- torch.nn.utils.remove_weight_norm(l)
- for l in self.res_skip_layers:
- torch.nn.utils.remove_weight_norm(l)
-
-
-class ResBlock1(torch.nn.Module):
- def __init__(self, channels, kernel_size=3, dilation=(1, 3, 5)):
- super(ResBlock1, self).__init__()
- self.convs1 = nn.ModuleList(
- [
- weight_norm(
- Conv1d(
- channels,
- channels,
- kernel_size,
- 1,
- dilation=dilation[0],
- padding=get_padding(kernel_size, dilation[0]),
- )
- ),
- weight_norm(
- Conv1d(
- channels,
- channels,
- kernel_size,
- 1,
- dilation=dilation[1],
- padding=get_padding(kernel_size, dilation[1]),
- )
- ),
- weight_norm(
- Conv1d(
- channels,
- channels,
- kernel_size,
- 1,
- dilation=dilation[2],
- padding=get_padding(kernel_size, dilation[2]),
- )
- ),
- ]
- )
- self.convs1.apply(init_weights)
-
- self.convs2 = nn.ModuleList(
- [
- weight_norm(
- Conv1d(
- channels,
- channels,
- kernel_size,
- 1,
- dilation=1,
- padding=get_padding(kernel_size, 1),
- )
- ),
- weight_norm(
- Conv1d(
- channels,
- channels,
- kernel_size,
- 1,
- dilation=1,
- padding=get_padding(kernel_size, 1),
- )
- ),
- weight_norm(
- Conv1d(
- channels,
- channels,
- kernel_size,
- 1,
- dilation=1,
- padding=get_padding(kernel_size, 1),
- )
- ),
- ]
- )
- self.convs2.apply(init_weights)
-
- def forward(self, x, x_mask=None):
- for c1, c2 in zip(self.convs1, self.convs2):
- xt = F.leaky_relu(x, LRELU_SLOPE)
- if x_mask is not None:
- xt = xt * x_mask
- xt = c1(xt)
- xt = F.leaky_relu(xt, LRELU_SLOPE)
- if x_mask is not None:
- xt = xt * x_mask
- xt = c2(xt)
- x = xt + x
- if x_mask is not None:
- x = x * x_mask
- return x
-
- def remove_weight_norm(self):
- for l in self.convs1:
- remove_weight_norm(l)
- for l in self.convs2:
- remove_weight_norm(l)
-
-
-class ResBlock2(torch.nn.Module):
- def __init__(self, channels, kernel_size=3, dilation=(1, 3)):
- super(ResBlock2, self).__init__()
- self.convs = nn.ModuleList(
- [
- weight_norm(
- Conv1d(
- channels,
- channels,
- kernel_size,
- 1,
- dilation=dilation[0],
- padding=get_padding(kernel_size, dilation[0]),
- )
- ),
- weight_norm(
- Conv1d(
- channels,
- channels,
- kernel_size,
- 1,
- dilation=dilation[1],
- padding=get_padding(kernel_size, dilation[1]),
- )
- ),
- ]
- )
- self.convs.apply(init_weights)
-
- def forward(self, x, x_mask=None):
- for c in self.convs:
- xt = F.leaky_relu(x, LRELU_SLOPE)
- if x_mask is not None:
- xt = xt * x_mask
- xt = c(xt)
- x = xt + x
- if x_mask is not None:
- x = x * x_mask
- return x
-
- def remove_weight_norm(self):
- for l in self.convs:
- remove_weight_norm(l)
-
-
-class Log(nn.Module):
- def forward(self, x, x_mask, reverse=False, **kwargs):
- if not reverse:
- y = torch.log(torch.clamp_min(x, 1e-5)) * x_mask
- logdet = torch.sum(-y, [1, 2])
- return y, logdet
- else:
- x = torch.exp(x) * x_mask
- return x
-
-
-class Flip(nn.Module):
- def forward(self, x, *args, reverse=False, **kwargs):
- x = torch.flip(x, [1])
- if not reverse:
- logdet = torch.zeros(x.size(0)).to(dtype=x.dtype, device=x.device)
- return x, logdet
- else:
- return x
-
-
-class ElementwiseAffine(nn.Module):
- def __init__(self, channels):
- super().__init__()
- self.channels = channels
- self.m = nn.Parameter(torch.zeros(channels, 1))
- self.logs = nn.Parameter(torch.zeros(channels, 1))
-
- def forward(self, x, x_mask, reverse=False, **kwargs):
- if not reverse:
- y = self.m + torch.exp(self.logs) * x
- y = y * x_mask
- logdet = torch.sum(self.logs * x_mask, [1, 2])
- return y, logdet
- else:
- x = (x - self.m) * torch.exp(-self.logs) * x_mask
- return x
-
-
-class ResidualCouplingLayer(nn.Module):
- def __init__(
- self,
- channels,
- hidden_channels,
- kernel_size,
- dilation_rate,
- n_layers,
- p_dropout=0,
- gin_channels=0,
- mean_only=False,
- ):
- assert channels % 2 == 0, "channels should be divisible by 2"
- super().__init__()
- self.channels = channels
- self.hidden_channels = hidden_channels
- self.kernel_size = kernel_size
- self.dilation_rate = dilation_rate
- self.n_layers = n_layers
- self.half_channels = channels // 2
- self.mean_only = mean_only
-
- self.pre = nn.Conv1d(self.half_channels, hidden_channels, 1)
- self.enc = WN(
- hidden_channels,
- kernel_size,
- dilation_rate,
- n_layers,
- p_dropout=p_dropout,
- gin_channels=gin_channels,
- )
- self.post = nn.Conv1d(hidden_channels, self.half_channels * (2 - mean_only), 1)
- self.post.weight.data.zero_()
- self.post.bias.data.zero_()
-
- def forward(self, x, x_mask, g=None, reverse=False):
- x0, x1 = torch.split(x, [self.half_channels] * 2, 1)
- h = self.pre(x0) * x_mask
- h = self.enc(h, x_mask, g=g)
- stats = self.post(h) * x_mask
- if not self.mean_only:
- m, logs = torch.split(stats, [self.half_channels] * 2, 1)
- else:
- m = stats
- logs = torch.zeros_like(m)
-
- if not reverse:
- x1 = m + x1 * torch.exp(logs) * x_mask
- x = torch.cat([x0, x1], 1)
- logdet = torch.sum(logs, [1, 2])
- return x, logdet
- else:
- x1 = (x1 - m) * torch.exp(-logs) * x_mask
- x = torch.cat([x0, x1], 1)
- return x
-
- def remove_weight_norm(self):
- self.enc.remove_weight_norm()
-
-
-class ConvFlow(nn.Module):
- def __init__(
- self,
- in_channels,
- filter_channels,
- kernel_size,
- n_layers,
- num_bins=10,
- tail_bound=5.0,
- ):
- super().__init__()
- self.in_channels = in_channels
- self.filter_channels = filter_channels
- self.kernel_size = kernel_size
- self.n_layers = n_layers
- self.num_bins = num_bins
- self.tail_bound = tail_bound
- self.half_channels = in_channels // 2
-
- self.pre = nn.Conv1d(self.half_channels, filter_channels, 1)
- self.convs = DDSConv(filter_channels, kernel_size, n_layers, p_dropout=0.0)
- self.proj = nn.Conv1d(
- filter_channels, self.half_channels * (num_bins * 3 - 1), 1
- )
- self.proj.weight.data.zero_()
- self.proj.bias.data.zero_()
-
- def forward(self, x, x_mask, g=None, reverse=False):
- x0, x1 = torch.split(x, [self.half_channels] * 2, 1)
- h = self.pre(x0)
- h = self.convs(h, x_mask, g=g)
- h = self.proj(h) * x_mask
-
- b, c, t = x0.shape
- h = h.reshape(b, c, -1, t).permute(0, 1, 3, 2) # [b, cx?, t] -> [b, c, t, ?]
-
- unnormalized_widths = h[..., : self.num_bins] / math.sqrt(self.filter_channels)
- unnormalized_heights = h[..., self.num_bins : 2 * self.num_bins] / math.sqrt(
- self.filter_channels
- )
- unnormalized_derivatives = h[..., 2 * self.num_bins :]
-
- x1, logabsdet = piecewise_rational_quadratic_transform(
- x1,
- unnormalized_widths,
- unnormalized_heights,
- unnormalized_derivatives,
- inverse=reverse,
- tails="linear",
- tail_bound=self.tail_bound,
- )
-
- x = torch.cat([x0, x1], 1) * x_mask
- logdet = torch.sum(logabsdet * x_mask, [1, 2])
- if not reverse:
- return x, logdet
- else:
- return x
diff --git a/spaces/SIH/Augmented-Retrieval-qa-ChatGPT/README.md b/spaces/SIH/Augmented-Retrieval-qa-ChatGPT/README.md
deleted file mode 100644
index 9eaa2b3d82adf58854fcfc0e867412a1be7aabdb..0000000000000000000000000000000000000000
--- a/spaces/SIH/Augmented-Retrieval-qa-ChatGPT/README.md
+++ /dev/null
@@ -1,15 +0,0 @@
----
-title: Augmented Retrieval Qa ChatGPT
-emoji: 🚀
-colorFrom: blue
-colorTo: blue
-sdk: streamlit
-sdk_version: 1.19.0
-app_file: streamlit_langchain_chat/streamlit_app.py
-pinned: false
-python_version: 3.10.4
-license: cc-by-nc-sa-4.0
-duplicated_from: hlydecker/Augmented-Retrieval-qa-ChatGPT
----
-
-Check out the configuration reference at https://huggingface.co/docs/hub/spaces-config-reference
diff --git a/spaces/SIH/building-segmentation/app.py b/spaces/SIH/building-segmentation/app.py
deleted file mode 100644
index 2d582f7a210b62d55468ef48aa28425caa430311..0000000000000000000000000000000000000000
--- a/spaces/SIH/building-segmentation/app.py
+++ /dev/null
@@ -1,69 +0,0 @@
-"""
-building-segmentation
-Proof of concept showing effectiveness of a fine tuned instance segmentation model for deteting buildings.
-"""
-import os
-import cv2
-os.system("pip install 'git+https://github.com/facebookresearch/detectron2.git'")
-from transformers import DetrFeatureExtractor, DetrForSegmentation
-from PIL import Image
-import gradio as gr
-import numpy as np
-import torch
-import torchvision
-import detectron2
-
-# import some common detectron2 utilities
-import itertools
-import seaborn as sns
-from detectron2 import model_zoo
-from detectron2.engine import DefaultPredictor
-from detectron2.config import get_cfg
-from detectron2.utils.visualizer import Visualizer
-from detectron2.utils.visualizer import ColorMode
-from detectron2.data import MetadataCatalog, DatasetCatalog
-from detectron2.checkpoint import DetectionCheckpointer
-
-cfg = get_cfg()
-cfg.merge_from_file("model_weights/buildings_poc_cfg.yml")
-cfg.MODEL.DEVICE='cpu'
-cfg.MODEL.ROI_HEADS.SCORE_THRESH_TEST = 0.35
-cfg.MODEL.WEIGHTS = "model_weights/model_final.pth"
-cfg.MODEL.ROI_HEADS.NUM_CLASSES = 8
-predictor = DefaultPredictor(cfg)
-
-def segment_buildings(im, confidence_threshold):
- im = np.array(im)
- outputs = predictor(im)
-
- instances = outputs["instances"].to("cpu")
- scores = instances.scores
- selected_indices = scores > confidence_threshold
- selected_instances = instances[selected_indices]
-
- v = Visualizer(im[:, :, ::-1],
- scale=0.5,
- instance_mode=ColorMode.SEGMENTATION
- )
- out = v.draw_instance_predictions(selected_instances)
-
- return Image.fromarray(out.get_image()[:, :, ::-1])
-
-# gradio components
-
-gr_slider_confidence = gr.inputs.Slider(0,1,.1,.7,
- label='Set confidence threshold % for masks')
-
-# gradio outputs
-inputs = gr.inputs.Image(type="pil", label="Input Image")
-outputs = gr.outputs.Image(type="pil", label="Output Image")
-
-title = "Building Segmentation"
-description = "An instance segmentation demo for identifying boundaries of buildings in aerial images using DETR (End-to-End Object Detection) model with MaskRCNN-101 backbone"
-
-# Create user interface and launch
-gr.Interface(segment_buildings,
- inputs = [inputs, gr_slider_confidence],
- outputs = outputs,
- title = title,
- description = description).launch(debug=True)
\ No newline at end of file
diff --git a/spaces/SalahZa/Tunisian-Speech-Recognition/train_with_wavlm.py b/spaces/SalahZa/Tunisian-Speech-Recognition/train_with_wavlm.py
deleted file mode 100644
index 5d6ca4c5a378583fd297e1202522b9dc9c2368de..0000000000000000000000000000000000000000
--- a/spaces/SalahZa/Tunisian-Speech-Recognition/train_with_wavlm.py
+++ /dev/null
@@ -1,399 +0,0 @@
-#!/usr/bin/env python3
-import sys
-import torch
-import logging
-import speechbrain as sb
-from pathlib import Path
-import os
-import torchaudio
-from hyperpyyaml import load_hyperpyyaml
-from speechbrain.tokenizers.SentencePiece import SentencePiece
-from speechbrain.utils.data_utils import undo_padding
-from speechbrain.utils.distributed import run_on_main
-
-"""Recipe for training a sequence-to-sequence ASR system with CommonVoice.
-The system employs a wav2vec2 encoder and a CTC decoder.
-Decoding is performed with greedy decoding (will be extended to beam search).
-
-To run this recipe, do the following:
-> python train_with_wav2vec2.py hparams/train_with_wav2vec2.yaml
-
-With the default hyperparameters, the system employs a pretrained wav2vec2 encoder.
-The wav2vec2 model is pretrained following the model given in the hprams file.
-It may be dependent on the language.
-
-The neural network is trained with CTC on sub-word units estimated with
-Byte Pairwise Encoding (BPE).
-
-The experiment file is flexible enough to support a large variety of
-different systems. By properly changing the parameter files, you can try
-different encoders, decoders, tokens (e.g, characters instead of BPE),
-training languages (all CommonVoice languages), and many
-other possible variations.
-
-Authors
- * Titouan Parcollet 2021
-"""
-
-logger = logging.getLogger(__name__)
-
-
-# Define training procedure
-class ASR(sb.core.Brain):
- def compute_forward(self, batch, stage):
- """Forward computations from the waveform batches to the output probabilities."""
-
- batch = batch.to(self.device)
- wavs, wav_lens = batch.sig
- wavs, wav_lens = wavs.to(self.device), wav_lens.to(self.device)
- if stage == sb.Stage.TRAIN:
- if hasattr(self.hparams, "augmentation"):
- wavs = self.hparams.augmentation(wavs, wav_lens)
-
- # Forward pass
- feats = self.modules.wav2vec2(wavs, wav_lens)
- x = self.modules.enc(feats)
- logits = self.modules.ctc_lin(x)
- p_ctc = self.hparams.log_softmax(logits)
-
- return p_ctc, wav_lens
-
- def compute_objectives(self, predictions, batch, stage):
- """Computes the loss (CTC) given predictions and targets."""
-
- p_ctc, wav_lens = predictions
-
- ids = batch.id
- tokens, tokens_lens = batch.tokens
-
- loss = self.hparams.ctc_cost(p_ctc, tokens, wav_lens, tokens_lens)
-
- if stage != sb.Stage.TRAIN:
- predicted_tokens = sb.decoders.ctc_greedy_decode(
- p_ctc, wav_lens, blank_id=self.hparams.blank_index
- )
- # Decode token terms to words
- if self.hparams.use_language_modelling:
- predicted_words = []
- for logs in p_ctc:
- text = decoder.decode(logs.detach().cpu().numpy())
- predicted_words.append(text.split(" "))
- else:
- predicted_words = [
- "".join(self.tokenizer.decode_ndim(utt_seq)).split(" ")
- for utt_seq in predicted_tokens
- ]
- # Convert indices to words
- target_words = [wrd.split(" ") for wrd in batch.wrd]
-
- self.wer_metric.append(ids, predicted_words, target_words)
- self.cer_metric.append(ids, predicted_words, target_words)
-
- return loss
-
- def fit_batch(self, batch):
- """Train the parameters given a single batch in input"""
- should_step = self.step % self.grad_accumulation_factor == 0
- # Managing automatic mixed precision
- # TOFIX: CTC fine-tuning currently is unstable
- # This is certainly due to CTC being done in fp16 instead of fp32
- if self.auto_mix_prec:
- with torch.cuda.amp.autocast():
- with self.no_sync():
- outputs = self.compute_forward(batch, sb.Stage.TRAIN)
- loss = self.compute_objectives(outputs, batch, sb.Stage.TRAIN)
- with self.no_sync(not should_step):
- self.scaler.scale(
- loss / self.grad_accumulation_factor
- ).backward()
- if should_step:
-
- if not self.hparams.wav2vec2.freeze:
- self.scaler.unscale_(self.wav2vec_optimizer)
- self.scaler.unscale_(self.model_optimizer)
- if self.check_gradients(loss):
- if not self.hparams.wav2vec2.freeze:
- if self.optimizer_step >= self.hparams.warmup_steps:
- self.scaler.step(self.wav2vec_optimizer)
- self.scaler.step(self.model_optimizer)
- self.scaler.update()
- self.zero_grad()
- self.optimizer_step += 1
- else:
- # This is mandatory because HF models have a weird behavior with DDP
- # on the forward pass
- with self.no_sync():
- outputs = self.compute_forward(batch, sb.Stage.TRAIN)
-
- loss = self.compute_objectives(outputs, batch, sb.Stage.TRAIN)
-
- with self.no_sync(not should_step):
- (loss / self.grad_accumulation_factor).backward()
- if should_step:
- if self.check_gradients(loss):
- if not self.hparams.wav2vec2.freeze:
- if self.optimizer_step >= self.hparams.warmup_steps:
- self.wav2vec_optimizer.step()
- self.model_optimizer.step()
- self.zero_grad()
- self.optimizer_step += 1
-
- self.on_fit_batch_end(batch, outputs, loss, should_step)
- return loss.detach().cpu()
-
- def evaluate_batch(self, batch, stage):
- """Computations needed for validation/test batches"""
- predictions = self.compute_forward(batch, stage=stage)
- with torch.no_grad():
- loss = self.compute_objectives(predictions, batch, stage=stage)
- return loss.detach()
-
- def on_stage_start(self, stage, epoch):
- """Gets called at the beginning of each epoch"""
- if stage != sb.Stage.TRAIN:
- self.cer_metric = self.hparams.cer_computer()
- self.wer_metric = self.hparams.error_rate_computer()
-
- def on_stage_end(self, stage, stage_loss, epoch):
- """Gets called at the end of an epoch."""
- # Compute/store important stats
- stage_stats = {"loss": stage_loss}
- if stage == sb.Stage.TRAIN:
- self.train_stats = stage_stats
- else:
- stage_stats["CER"] = self.cer_metric.summarize("error_rate")
- stage_stats["WER"] = self.wer_metric.summarize("error_rate")
-
- # Perform end-of-iteration things, like annealing, logging, etc.
- if stage == sb.Stage.VALID:
- old_lr_model, new_lr_model = self.hparams.lr_annealing_model(
- stage_stats["loss"]
- )
- old_lr_wav2vec, new_lr_wav2vec = self.hparams.lr_annealing_wav2vec(
- stage_stats["loss"]
- )
- sb.nnet.schedulers.update_learning_rate(
- self.model_optimizer, new_lr_model
- )
- if not self.hparams.wav2vec2.freeze:
- sb.nnet.schedulers.update_learning_rate(
- self.wav2vec_optimizer, new_lr_wav2vec
- )
- self.hparams.train_logger.log_stats(
- stats_meta={
- "epoch": epoch,
- "lr_model": old_lr_model,
- "lr_wav2vec": old_lr_wav2vec,
- },
- train_stats=self.train_stats,
- valid_stats=stage_stats,
- )
- self.checkpointer.save_and_keep_only(
- meta={"WER": stage_stats["WER"]}, min_keys=["WER"],
- )
- elif stage == sb.Stage.TEST:
- self.hparams.train_logger.log_stats(
- stats_meta={"Epoch loaded": self.hparams.epoch_counter.current},
- test_stats=stage_stats,
- )
- with open(self.hparams.wer_file, "w") as w:
- self.wer_metric.write_stats(w)
-
- def init_optimizers(self):
- "Initializes the wav2vec2 optimizer and model optimizer"
-
- # If the wav2vec encoder is unfrozen, we create the optimizer
- if not self.hparams.wav2vec2.freeze:
- self.wav2vec_optimizer = self.hparams.wav2vec_opt_class(
- self.modules.wav2vec2.parameters()
- )
- if self.checkpointer is not None:
- self.checkpointer.add_recoverable(
- "wav2vec_opt", self.wav2vec_optimizer
- )
-
- self.model_optimizer = self.hparams.model_opt_class(
- self.hparams.model.parameters()
- )
-
- if self.checkpointer is not None:
- self.checkpointer.add_recoverable("modelopt", self.model_optimizer)
-
- def zero_grad(self, set_to_none=False):
- if not self.hparams.wav2vec2.freeze:
- self.wav2vec_optimizer.zero_grad(set_to_none)
- self.model_optimizer.zero_grad(set_to_none)
-
-
-# Define custom data procedure
-def dataio_prepare(hparams):
- """This function prepares the datasets to be used in the brain class.
- It also defines the data processing pipeline through user-defined functions."""
-
- # 1. Define datasets
- data_folder = hparams["data_folder"]
-
- train_data = sb.dataio.dataset.DynamicItemDataset.from_csv(
- csv_path=hparams["train_csv"], replacements={"data_root": data_folder},
- )
-
- if hparams["sorting"] == "ascending":
- # we sort training data to speed up training and get better results.
- train_data = train_data.filtered_sorted(
- sort_key="duration",
- key_max_value={"duration": hparams["avoid_if_longer_than"]},
- )
- # when sorting do not shuffle in dataloader ! otherwise is pointless
- hparams["dataloader_options"]["shuffle"] = False
-
- elif hparams["sorting"] == "descending":
- train_data = train_data.filtered_sorted(
- sort_key="duration",
- reverse=True,
- key_max_value={"duration": hparams["avoid_if_longer_than"]},
- )
- # when sorting do not shuffle in dataloader ! otherwise is pointless
- hparams["dataloader_options"]["shuffle"] = False
-
- elif hparams["sorting"] == "random":
- pass
-
- else:
- raise NotImplementedError(
- "sorting must be random, ascending or descending"
- )
-
- valid_data = sb.dataio.dataset.DynamicItemDataset.from_csv(
- csv_path=hparams["valid_csv"], replacements={"data_root": data_folder},
- )
- # We also sort the validation data so it is faster to validate
- valid_data = valid_data.filtered_sorted(sort_key="duration")
- test_datasets = {}
- for csv_file in hparams["test_csv"]:
- name = Path(csv_file).stem
- test_datasets[name] = sb.dataio.dataset.DynamicItemDataset.from_csv(
- csv_path=csv_file, replacements={"data_root": data_folder}
- )
- test_datasets[name] = test_datasets[name].filtered_sorted(
- sort_key="duration"
- )
-
- datasets = [train_data, valid_data] + [i for k, i in test_datasets.items()]
-
-
- # 2. Define audio pipeline:
- @sb.utils.data_pipeline.takes("wav")
- @sb.utils.data_pipeline.provides("sig")
- def audio_pipeline(wav):
- info = torchaudio.info(wav)
- sig = sb.dataio.dataio.read_audio(wav)
- resampled = torchaudio.transforms.Resample(
- info.sample_rate, hparams["sample_rate"],
- )(sig)
- return resampled
-
- sb.dataio.dataset.add_dynamic_item(datasets, audio_pipeline)
- label_encoder = sb.dataio.encoder.CTCTextEncoder()
-
- # 3. Define text pipeline:
- @sb.utils.data_pipeline.takes("wrd")
- @sb.utils.data_pipeline.provides(
- "wrd", "char_list", "tokens_list", "tokens"
- )
- def text_pipeline(wrd):
- yield wrd
- char_list = list(wrd)
- yield char_list
- tokens_list = label_encoder.encode_sequence(char_list)
- yield tokens_list
- tokens = torch.LongTensor(tokens_list)
- yield tokens
-
- sb.dataio.dataset.add_dynamic_item(datasets, text_pipeline)
- lab_enc_file = os.path.join(hparams["save_folder"], "label_encoder.txt")
- special_labels = {
- "blank_label": hparams["blank_index"],
- "unk_label": hparams["unk_index"]
- }
- label_encoder.load_or_create(
- path=lab_enc_file,
- from_didatasets=[train_data],
- output_key="char_list",
- special_labels=special_labels,
- sequence_input=True,
- )
-
- # 4. Set output:
- sb.dataio.dataset.set_output_keys(
- datasets, ["id", "sig", "wrd", "char_list", "tokens"],
- )
- return train_data, valid_data,test_datasets, label_encoder
-
-
-if __name__ == "__main__":
-
- # Load hyperparameters file with command-line overrides
- hparams_file, run_opts, overrides = sb.parse_arguments(sys.argv[1:])
- with open(hparams_file) as fin:
- hparams = load_hyperpyyaml(fin, overrides)
-
- # If --distributed_launch then
- # create ddp_group with the right communication protocol
- sb.utils.distributed.ddp_init_group(run_opts)
-
-
- # Create experiment directory
- sb.create_experiment_directory(
- experiment_directory=hparams["output_folder"],
- hyperparams_to_save=hparams_file,
- overrides=overrides,
- )
-
- # Due to DDP, we do the preparation ONLY on the main python process
- # Defining tokenizer and loading it
- # Create the datasets objects as well as tokenization and encoding :-D
- train_data, valid_data, test_datasets, label_encoder = dataio_prepare(hparams)
- if hparams["use_language_modelling"]:
- print("using langauge_modeeling")
- from pyctcdecode import build_ctcdecoder
- ind2lab = label_encoder.ind2lab
- print(ind2lab)
- labels = [ind2lab[x] for x in range(len(ind2lab))]
- labels = [""] + labels[1:-1] + ["1"]
- # Replace the token with a blank character, needed for PyCTCdecode
- print(labels)
- decoder = build_ctcdecoder(
- labels,
- kenlm_model_path=hparams["ngram_lm_path"], # .arpa or .bin
- alpha=0.5, # Default by KenLM
- beta=1.0, # Default by KenLM
- )
- # Trainer initialization
- asr_brain = ASR(
- modules=hparams["modules"],
- hparams=hparams,
- run_opts=run_opts,
- checkpointer=hparams["checkpointer"],
- )
-
- # Adding objects to trainer.
- asr_brain.tokenizer = label_encoder
-
- # Training
- asr_brain.fit(
- asr_brain.hparams.epoch_counter,
- train_data,
- valid_data,
- train_loader_kwargs=hparams["dataloader_options"],
- valid_loader_kwargs=hparams["test_dataloader_options"],
- )
-
- # Test
- for k in test_datasets.keys(): # keys are test_clean, test_other etc
- asr_brain.hparams.wer_file = os.path.join(
- hparams["output_folder"], "wer_{}.txt".format(k)
- )
- asr_brain.evaluate(
- test_datasets[k], test_loader_kwargs=hparams["test_dataloader_options"]
- )
-
diff --git a/spaces/Sapphire-356/Video2MC/common/generators.py b/spaces/Sapphire-356/Video2MC/common/generators.py
deleted file mode 100644
index f41dfb77fecc4f09bb5a4778ab9b6c6657c48de7..0000000000000000000000000000000000000000
--- a/spaces/Sapphire-356/Video2MC/common/generators.py
+++ /dev/null
@@ -1,425 +0,0 @@
-# Copyright (c) 2018-present, Facebook, Inc.
-# All rights reserved.
-#
-# This source code is licensed under the license found in the
-# LICENSE file in the root directory of this source tree.
-#
-
-from itertools import zip_longest
-
-import numpy as np
-
-
-class ChunkedGenerator:
- """
- Batched data generator, used for training.
- The sequences are split into equal-length chunks and padded as necessary.
-
- Arguments:
- batch_size -- the batch size to use for training
- cameras -- list of cameras, one element for each video (optional, used for semi-supervised training)
- poses_3d -- list of ground-truth 3D poses, one element for each video (optional, used for supervised training)
- poses_2d -- list of input 2D keypoints, one element for each video
- chunk_length -- number of output frames to predict for each training example (usually 1)
- pad -- 2D input padding to compensate for valid convolutions, per side (depends on the receptive field)
- causal_shift -- asymmetric padding offset when causal convolutions are used (usually 0 or "pad")
- shuffle -- randomly shuffle the dataset before each epoch
- random_seed -- initial seed to use for the random generator
- augment -- augment the dataset by flipping poses horizontally
- kps_left and kps_right -- list of left/right 2D keypoints if flipping is enabled
- joints_left and joints_right -- list of left/right 3D joints if flipping is enabled
- """
-
- def __init__(self, batch_size, cameras, poses_3d, poses_2d,
- chunk_length, pad=0, causal_shift=0,
- shuffle=True, random_seed=1234,
- augment=False, kps_left=None, kps_right=None, joints_left=None, joints_right=None,
- endless=False):
- assert poses_3d is None or len(poses_3d) == len(poses_2d), (len(poses_3d), len(poses_2d))
- assert cameras is None or len(cameras) == len(poses_2d)
-
- # Build lineage info
- pairs = [] # (seq_idx, start_frame, end_frame, flip) tuples
- for i in range(len(poses_2d)):
- assert poses_3d is None or poses_3d[i].shape[0] == poses_3d[i].shape[0]
- n_chunks = (poses_2d[i].shape[0] + chunk_length - 1) // chunk_length
- offset = (n_chunks * chunk_length - poses_2d[i].shape[0]) // 2
- bounds = np.arange(n_chunks + 1) * chunk_length - offset
- augment_vector = np.full(len(bounds - 1), False, dtype=bool)
- pairs += zip(np.repeat(i, len(bounds - 1)), bounds[:-1], bounds[1:], augment_vector)
- if augment:
- pairs += zip(np.repeat(i, len(bounds - 1)), bounds[:-1], bounds[1:], ~augment_vector)
-
- # Initialize buffers
- if cameras is not None:
- self.batch_cam = np.empty((batch_size, cameras[0].shape[-1]))
- if poses_3d is not None:
- self.batch_3d = np.empty((batch_size, chunk_length, poses_3d[0].shape[-2], poses_3d[0].shape[-1]))
- self.batch_2d = np.empty((batch_size, chunk_length + 2 * pad, poses_2d[0].shape[-2], poses_2d[0].shape[-1]))
-
- self.num_batches = (len(pairs) + batch_size - 1) // batch_size
- self.batch_size = batch_size
- self.random = np.random.RandomState(random_seed)
- self.pairs = pairs
- self.shuffle = shuffle
- self.pad = pad
- self.causal_shift = causal_shift
- self.endless = endless
- self.state = None
-
- self.cameras = cameras
- self.poses_3d = poses_3d
- self.poses_2d = poses_2d
-
- self.augment = augment
- self.kps_left = kps_left
- self.kps_right = kps_right
- self.joints_left = joints_left
- self.joints_right = joints_right
-
- def num_frames(self):
- return self.num_batches * self.batch_size
-
- def random_state(self):
- return self.random
-
- def set_random_state(self, random):
- self.random = random
-
- def augment_enabled(self):
- return self.augment
-
- def next_pairs(self):
- if self.state is None:
- if self.shuffle:
- pairs = self.random.permutation(self.pairs)
- else:
- pairs = self.pairs
- return 0, pairs
- else:
- return self.state
-
- def next_epoch(self):
- enabled = True
- while enabled:
- start_idx, pairs = self.next_pairs()
- for b_i in range(start_idx, self.num_batches):
- chunks = pairs[b_i * self.batch_size: (b_i + 1) * self.batch_size]
- for i, (seq_i, start_3d, end_3d, flip) in enumerate(chunks):
- start_2d = start_3d - self.pad - self.causal_shift
- end_2d = end_3d + self.pad - self.causal_shift
-
- # 2D poses
- seq_2d = self.poses_2d[seq_i]
- low_2d = max(start_2d, 0)
- high_2d = min(end_2d, seq_2d.shape[0])
- pad_left_2d = low_2d - start_2d
- pad_right_2d = end_2d - high_2d
- if pad_left_2d != 0 or pad_right_2d != 0:
- self.batch_2d[i] = np.pad(seq_2d[low_2d:high_2d], ((pad_left_2d, pad_right_2d), (0, 0), (0, 0)), 'edge')
- else:
- self.batch_2d[i] = seq_2d[low_2d:high_2d]
-
- if flip:
- # Flip 2D keypoints
- self.batch_2d[i, :, :, 0] *= -1
- self.batch_2d[i, :, self.kps_left + self.kps_right] = self.batch_2d[i, :, self.kps_right + self.kps_left]
-
- # 3D poses
- if self.poses_3d is not None:
- seq_3d = self.poses_3d[seq_i]
- low_3d = max(start_3d, 0)
- high_3d = min(end_3d, seq_3d.shape[0])
- pad_left_3d = low_3d - start_3d
- pad_right_3d = end_3d - high_3d
- if pad_left_3d != 0 or pad_right_3d != 0:
- self.batch_3d[i] = np.pad(seq_3d[low_3d:high_3d], ((pad_left_3d, pad_right_3d), (0, 0), (0, 0)), 'edge')
- else:
- self.batch_3d[i] = seq_3d[low_3d:high_3d]
-
- if flip:
- # Flip 3D joints
- self.batch_3d[i, :, :, 0] *= -1
- self.batch_3d[i, :, self.joints_left + self.joints_right] = \
- self.batch_3d[i, :, self.joints_right + self.joints_left]
-
- # Cameras
- if self.cameras is not None:
- self.batch_cam[i] = self.cameras[seq_i]
- if flip:
- # Flip horizontal distortion coefficients
- self.batch_cam[i, 2] *= -1
- self.batch_cam[i, 7] *= -1
-
- if self.endless:
- self.state = (b_i + 1, pairs)
- if self.poses_3d is None and self.cameras is None:
- yield None, None, self.batch_2d[:len(chunks)]
- elif self.poses_3d is not None and self.cameras is None:
- yield None, self.batch_3d[:len(chunks)], self.batch_2d[:len(chunks)]
- elif self.poses_3d is None:
- yield self.batch_cam[:len(chunks)], None, self.batch_2d[:len(chunks)]
- else:
- yield self.batch_cam[:len(chunks)], self.batch_3d[:len(chunks)], self.batch_2d[:len(chunks)]
-
- if self.endless:
- self.state = None
- else:
- enabled = False
-
-
-class UnchunkedGenerator:
- """
- Non-batched data generator, used for testing.
- Sequences are returned one at a time (i.e. batch size = 1), without chunking.
-
- If data augmentation is enabled, the batches contain two sequences (i.e. batch size = 2),
- the second of which is a mirrored version of the first.
-
- Arguments:
- cameras -- list of cameras, one element for each video (optional, used for semi-supervised training)
- poses_3d -- list of ground-truth 3D poses, one element for each video (optional, used for supervised training)
- poses_2d -- list of input 2D keypoints, one element for each video
- pad -- 2D input padding to compensate for valid convolutions, per side (depends on the receptive field)
- causal_shift -- asymmetric padding offset when causal convolutions are used (usually 0 or "pad")
- augment -- augment the dataset by flipping poses horizontally
- kps_left and kps_right -- list of left/right 2D keypoints if flipping is enabled
- joints_left and joints_right -- list of left/right 3D joints if flipping is enabled
- """
-
- def __init__(self, cameras, poses_3d, poses_2d, pad=0, causal_shift=0,
- augment=False, kps_left=None, kps_right=None, joints_left=None, joints_right=None):
- assert poses_3d is None or len(poses_3d) == len(poses_2d)
- assert cameras is None or len(cameras) == len(poses_2d)
-
- self.augment = augment
- self.kps_left = kps_left
- self.kps_right = kps_right
- self.joints_left = joints_left
- self.joints_right = joints_right
-
- self.pad = pad
- self.causal_shift = causal_shift
- self.cameras = [] if cameras is None else cameras
- self.poses_3d = [] if poses_3d is None else poses_3d
- self.poses_2d = poses_2d
-
- def num_frames(self):
- count = 0
- for p in self.poses_2d:
- count += p.shape[0]
- return count
-
- def augment_enabled(self):
- return self.augment
-
- def set_augment(self, augment):
- self.augment = augment
-
- def next_epoch(self):
- for seq_cam, seq_3d, seq_2d in zip_longest(self.cameras, self.poses_3d, self.poses_2d):
- batch_cam = None if seq_cam is None else np.expand_dims(seq_cam, axis=0)
- batch_3d = None if seq_3d is None else np.expand_dims(seq_3d, axis=0)
- # 2D input padding to compensate for valid convolutions, per side (depends on the receptive field)
- batch_2d = np.expand_dims(np.pad(seq_2d,
- ((self.pad + self.causal_shift, self.pad - self.causal_shift), (0, 0), (0, 0)),
- 'edge'), axis=0)
- if self.augment:
- # Append flipped version
- if batch_cam is not None:
- batch_cam = np.concatenate((batch_cam, batch_cam), axis=0)
- batch_cam[1, 2] *= -1
- batch_cam[1, 7] *= -1
-
- if batch_3d is not None:
- batch_3d = np.concatenate((batch_3d, batch_3d), axis=0)
- batch_3d[1, :, :, 0] *= -1
- batch_3d[1, :, self.joints_left + self.joints_right] = batch_3d[1, :, self.joints_right + self.joints_left]
-
- batch_2d = np.concatenate((batch_2d, batch_2d), axis=0)
- batch_2d[1, :, :, 0] *= -1
- batch_2d[1, :, self.kps_left + self.kps_right] = batch_2d[1, :, self.kps_right + self.kps_left]
-
- yield batch_cam, batch_3d, batch_2d
-
-class Evaluate_Generator:
- """
- Batched data generator, used for training.
- The sequences are split into equal-length chunks and padded as necessary.
- Arguments:
- batch_size -- the batch size to use for training
- cameras -- list of cameras, one element for each video (optional, used for semi-supervised training)
- poses_3d -- list of ground-truth 3D poses, one element for each video (optional, used for supervised training)
- poses_2d -- list of input 2D keypoints, one element for each video
- chunk_length -- number of output frames to predict for each training example (usually 1)
- pad -- 2D input padding to compensate for valid convolutions, per side (depends on the receptive field)
- causal_shift -- asymmetric padding offset when causal convolutions are used (usually 0 or "pad")
- shuffle -- randomly shuffle the dataset before each epoch
- random_seed -- initial seed to use for the random generator
- augment -- augment the dataset by flipping poses horizontally
- kps_left and kps_right -- list of left/right 2D keypoints if flipping is enabled
- joints_left and joints_right -- list of left/right 3D joints if flipping is enabled
- """
-
- def __init__(self, batch_size, cameras, poses_3d, poses_2d,
- chunk_length, pad=0, causal_shift=0,
- shuffle=True, random_seed=1234,
- augment=False, kps_left=None, kps_right=None, joints_left=None, joints_right=None,
- endless=False):
- assert poses_3d is None or len(poses_3d) == len(poses_2d), (len(poses_3d), len(poses_2d))
- assert cameras is None or len(cameras) == len(poses_2d)
-
- # Build lineage info
- pairs = [] # (seq_idx, start_frame, end_frame, flip) tuples
- for i in range(len(poses_2d)):
- assert poses_3d is None or poses_3d[i].shape[0] == poses_3d[i].shape[0]
- n_chunks = (poses_2d[i].shape[0] + chunk_length - 1) // chunk_length
- offset = (n_chunks * chunk_length - poses_2d[i].shape[0]) // 2
- bounds = np.arange(n_chunks + 1) * chunk_length - offset
- augment_vector = np.full(len(bounds - 1), False, dtype=bool)
- pairs += zip(np.repeat(i, len(bounds - 1)), bounds[:-1], bounds[1:], augment_vector)
-
- # Initialize buffers
- if cameras is not None:
- self.batch_cam = np.empty((batch_size, cameras[0].shape[-1]))
- if poses_3d is not None:
- self.batch_3d = np.empty((batch_size, chunk_length, poses_3d[0].shape[-2], poses_3d[0].shape[-1]))
-
- if augment:
- self.batch_2d_flip = np.empty(
- (batch_size, chunk_length + 2 * pad, poses_2d[0].shape[-2], poses_2d[0].shape[-1]))
- self.batch_2d = np.empty((batch_size, chunk_length + 2 * pad, poses_2d[0].shape[-2], poses_2d[0].shape[-1]))
- else:
- self.batch_2d = np.empty((batch_size, chunk_length + 2 * pad, poses_2d[0].shape[-2], poses_2d[0].shape[-1]))
-
- self.num_batches = (len(pairs) + batch_size - 1) // batch_size
- self.batch_size = batch_size
- self.random = np.random.RandomState(random_seed)
- self.pairs = pairs
- self.shuffle = shuffle
- self.pad = pad
- self.causal_shift = causal_shift
- self.endless = endless
- self.state = None
-
- self.cameras = cameras
- self.poses_3d = poses_3d
- self.poses_2d = poses_2d
-
- self.augment = augment
- self.kps_left = kps_left
- self.kps_right = kps_right
- self.joints_left = joints_left
- self.joints_right = joints_right
-
- def num_frames(self):
- return self.num_batches * self.batch_size
-
- def random_state(self):
- return self.random
-
- def set_random_state(self, random):
- self.random = random
-
- def augment_enabled(self):
- return self.augment
-
- def next_pairs(self):
- if self.state is None:
- if self.shuffle:
- pairs = self.random.permutation(self.pairs)
- else:
- pairs = self.pairs
- return 0, pairs
- else:
- return self.state
-
- def next_epoch(self):
- enabled = True
- while enabled:
- start_idx, pairs = self.next_pairs()
- for b_i in range(start_idx, self.num_batches):
- chunks = pairs[b_i * self.batch_size: (b_i + 1) * self.batch_size]
- for i, (seq_i, start_3d, end_3d, flip) in enumerate(chunks):
- start_2d = start_3d - self.pad - self.causal_shift
- end_2d = end_3d + self.pad - self.causal_shift
-
- # 2D poses
- seq_2d = self.poses_2d[seq_i]
- low_2d = max(start_2d, 0)
- high_2d = min(end_2d, seq_2d.shape[0])
- pad_left_2d = low_2d - start_2d
- pad_right_2d = end_2d - high_2d
- if pad_left_2d != 0 or pad_right_2d != 0:
- self.batch_2d[i] = np.pad(seq_2d[low_2d:high_2d], ((pad_left_2d, pad_right_2d), (0, 0), (0, 0)),
- 'edge')
- if self.augment:
- self.batch_2d_flip[i] = np.pad(seq_2d[low_2d:high_2d],
- ((pad_left_2d, pad_right_2d), (0, 0), (0, 0)),
- 'edge')
-
- else:
- self.batch_2d[i] = seq_2d[low_2d:high_2d]
- if self.augment:
- self.batch_2d_flip[i] = seq_2d[low_2d:high_2d]
-
- if self.augment:
- self.batch_2d_flip[i, :, :, 0] *= -1
- self.batch_2d_flip[i, :, self.kps_left + self.kps_right] = self.batch_2d_flip[i, :,
- self.kps_right + self.kps_left]
-
- # 3D poses
- if self.poses_3d is not None:
- seq_3d = self.poses_3d[seq_i]
- low_3d = max(start_3d, 0)
- high_3d = min(end_3d, seq_3d.shape[0])
- pad_left_3d = low_3d - start_3d
- pad_right_3d = end_3d - high_3d
- if pad_left_3d != 0 or pad_right_3d != 0:
- self.batch_3d[i] = np.pad(seq_3d[low_3d:high_3d],
- ((pad_left_3d, pad_right_3d), (0, 0), (0, 0)), 'edge')
- else:
- self.batch_3d[i] = seq_3d[low_3d:high_3d]
-
- if flip:
- self.batch_3d[i, :, :, 0] *= -1
- self.batch_3d[i, :, self.joints_left + self.joints_right] = \
- self.batch_3d[i, :, self.joints_right + self.joints_left]
-
- # Cameras
- if self.cameras is not None:
- self.batch_cam[i] = self.cameras[seq_i]
- if flip:
- # Flip horizontal distortion coefficients
- self.batch_cam[i, 2] *= -1
- self.batch_cam[i, 7] *= -1
-
- if self.endless:
- self.state = (b_i + 1, pairs)
-
- if self.augment:
- if self.poses_3d is None and self.cameras is None:
- yield None, None, self.batch_2d[:len(chunks)], self.batch_2d_flip[:len(chunks)]
- elif self.poses_3d is not None and self.cameras is None:
- yield None, self.batch_3d[:len(chunks)], self.batch_2d[:len(chunks)], self.batch_2d_flip[
- :len(chunks)]
- elif self.poses_3d is None:
- yield self.batch_cam[:len(chunks)], None, self.batch_2d[:len(chunks)], self.batch_2d_flip[
- :len(chunks)]
- else:
- yield self.batch_cam[:len(chunks)], self.batch_3d[:len(chunks)], self.batch_2d[:len(
- chunks)], self.batch_2d_flip[:len(chunks)]
- else:
- if self.poses_3d is None and self.cameras is None:
- yield None, None, self.batch_2d[:len(chunks)]
- elif self.poses_3d is not None and self.cameras is None:
- yield None, self.batch_3d[:len(chunks)], self.batch_2d[:len(chunks)]
- elif self.poses_3d is None:
- yield self.batch_cam[:len(chunks)], None, self.batch_2d[:len(chunks)]
- else:
- yield self.batch_cam[:len(chunks)], self.batch_3d[:len(chunks)], self.batch_2d[:len(chunks)]
-
- if self.endless:
- self.state = None
- else:
- enabled = False
\ No newline at end of file
diff --git a/spaces/Saurabh46/MyChatGPT-DEMO/app.py b/spaces/Saurabh46/MyChatGPT-DEMO/app.py
deleted file mode 100644
index c9fa37574ed265ee198e09643e8bcc10769450a9..0000000000000000000000000000000000000000
--- a/spaces/Saurabh46/MyChatGPT-DEMO/app.py
+++ /dev/null
@@ -1,40 +0,0 @@
-from llama_index import GPTVectorStoreIndex, SimpleDirectoryReader, LLMPredictor, ServiceContext, StorageContext, load_index_from_storage
-from langchain import OpenAI
-import gradio
-import os
-
-os.environ["OPENAI_API_KEY"] = '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'
-
-def construct_index(directory_path):
- num_outputs = 512
-
- _llm_predictor = LLMPredictor(llm=OpenAI(temperature=0.7, model_name="gpt-3.5-turbo", max_tokens=num_outputs))
-
- service_context = ServiceContext.from_defaults(llm_predictor=_llm_predictor)
-
- docs = SimpleDirectoryReader(directory_path).load_data()
-
- index = GPTVectorStoreIndex.from_documents(docs, service_context=service_context)
-
- index.storage_context.persist(persist_dir="indexes")
-
- return index
-
-def chatbot(input_text):
-
- storage_context = StorageContext.from_defaults(persist_dir="indexes")
-
- query_engne = load_index_from_storage(storage_context).as_query_engine()
-
- response = query_engne.query(input_text)
-
- return response.response
-
-iface = gradio.Interface(fn=chatbot,
- inputs=gradio.inputs.Textbox(lines=4, label="Enter your question here"),
- outputs=gradio.outputs.Textbox(label="Generated Text"),
- title="My Custom trained AI Chatbot")
-
-index = construct_index("trainingData")
-
-iface.launch()
diff --git a/spaces/ServerX/PorcoDiaz/infer/lib/uvr5_pack/utils.py b/spaces/ServerX/PorcoDiaz/infer/lib/uvr5_pack/utils.py
deleted file mode 100644
index f4805cdb25e7c50611412a19340ad525d1251d7b..0000000000000000000000000000000000000000
--- a/spaces/ServerX/PorcoDiaz/infer/lib/uvr5_pack/utils.py
+++ /dev/null
@@ -1,121 +0,0 @@
-import json
-
-import numpy as np
-import torch
-from tqdm import tqdm
-
-
-def load_data(file_name: str = "./infer/lib/uvr5_pack/name_params.json") -> dict:
- with open(file_name, "r") as f:
- data = json.load(f)
-
- return data
-
-
-def make_padding(width, cropsize, offset):
- left = offset
- roi_size = cropsize - left * 2
- if roi_size == 0:
- roi_size = cropsize
- right = roi_size - (width % roi_size) + left
-
- return left, right, roi_size
-
-
-def inference(X_spec, device, model, aggressiveness, data):
- """
- data : dic configs
- """
-
- def _execute(
- X_mag_pad, roi_size, n_window, device, model, aggressiveness, is_half=True
- ):
- model.eval()
- with torch.no_grad():
- preds = []
-
- iterations = [n_window]
-
- total_iterations = sum(iterations)
- for i in tqdm(range(n_window)):
- start = i * roi_size
- X_mag_window = X_mag_pad[
- None, :, :, start : start + data["window_size"]
- ]
- X_mag_window = torch.from_numpy(X_mag_window)
- if is_half:
- X_mag_window = X_mag_window.half()
- X_mag_window = X_mag_window.to(device)
-
- pred = model.predict(X_mag_window, aggressiveness)
-
- pred = pred.detach().cpu().numpy()
- preds.append(pred[0])
-
- pred = np.concatenate(preds, axis=2)
- return pred
-
- def preprocess(X_spec):
- X_mag = np.abs(X_spec)
- X_phase = np.angle(X_spec)
-
- return X_mag, X_phase
-
- X_mag, X_phase = preprocess(X_spec)
-
- coef = X_mag.max()
- X_mag_pre = X_mag / coef
-
- n_frame = X_mag_pre.shape[2]
- pad_l, pad_r, roi_size = make_padding(n_frame, data["window_size"], model.offset)
- n_window = int(np.ceil(n_frame / roi_size))
-
- X_mag_pad = np.pad(X_mag_pre, ((0, 0), (0, 0), (pad_l, pad_r)), mode="constant")
-
- if list(model.state_dict().values())[0].dtype == torch.float16:
- is_half = True
- else:
- is_half = False
- pred = _execute(
- X_mag_pad, roi_size, n_window, device, model, aggressiveness, is_half
- )
- pred = pred[:, :, :n_frame]
-
- if data["tta"]:
- pad_l += roi_size // 2
- pad_r += roi_size // 2
- n_window += 1
-
- X_mag_pad = np.pad(X_mag_pre, ((0, 0), (0, 0), (pad_l, pad_r)), mode="constant")
-
- pred_tta = _execute(
- X_mag_pad, roi_size, n_window, device, model, aggressiveness, is_half
- )
- pred_tta = pred_tta[:, :, roi_size // 2 :]
- pred_tta = pred_tta[:, :, :n_frame]
-
- return (pred + pred_tta) * 0.5 * coef, X_mag, np.exp(1.0j * X_phase)
- else:
- return pred * coef, X_mag, np.exp(1.0j * X_phase)
-
-
-def _get_name_params(model_path, model_hash):
- data = load_data()
- flag = False
- ModelName = model_path
- for type in list(data):
- for model in list(data[type][0]):
- for i in range(len(data[type][0][model])):
- if str(data[type][0][model][i]["hash_name"]) == model_hash:
- flag = True
- elif str(data[type][0][model][i]["hash_name"]) in ModelName:
- flag = True
-
- if flag:
- model_params_auto = data[type][0][model][i]["model_params"]
- param_name_auto = data[type][0][model][i]["param_name"]
- if type == "equivalent":
- return param_name_auto, model_params_auto
- else:
- flag = False
- return param_name_auto, model_params_auto
diff --git a/spaces/Shriharsh/Text_To_Image/app.py b/spaces/Shriharsh/Text_To_Image/app.py
deleted file mode 100644
index fab0665b3a2f5dcf84cf557f6a79b9286c2cfe25..0000000000000000000000000000000000000000
--- a/spaces/Shriharsh/Text_To_Image/app.py
+++ /dev/null
@@ -1,45 +0,0 @@
-import gradio as gr
-from PIL import Image
-from authtoken import auth_token
-import torch
-import torch.cuda.amp as amp
-from diffusers import StableDiffusionPipeline
-
-
-
-model_id = "stabilityai/stable-diffusion-2-1"
-
-device = torch.device("cpu") # Default to CPU device
-if torch.cuda.is_available():
- device = torch.device("cuda")
-
-# Use the DPMSolverMultistepScheduler (DPM-Solver++) scheduler here instead
-pipe = StableDiffusionPipeline.from_pretrained(model_id, torch_dtype=torch.float16)
-pipe.to(device)
-
-def generate(prompt):
- with torch.no_grad(), amp.autocast(enabled=device != torch.device("cpu")):
- image = pipe(prompt, guidance_scale=8.5)["sample"][0]
-
- image.save('generatedimage.png')
- return image
-
-def predict_text(prompt):
- image = generate(prompt)
- return image
-
-def predict_image(input_image):
- input_image.save('input_image.png')
- prompt = input("Enter your prompt: ")
- image = generate(prompt)
- return image
-
-iface = gr.Interface(
- fn=predict_text,
- inputs="text",
- outputs="image",
- capture_session=True,
-)
-iface.launch()
-
-
diff --git a/spaces/SoUmNerd/RemoteMojo/main.py b/spaces/SoUmNerd/RemoteMojo/main.py
deleted file mode 100644
index 81eb19e7a07b08449c3f0d7e48fde2aa1fb78f8f..0000000000000000000000000000000000000000
--- a/spaces/SoUmNerd/RemoteMojo/main.py
+++ /dev/null
@@ -1,24 +0,0 @@
-from fastapi import FastAPI, Request, Response
-from pydantic import BaseModel
-
-import subprocess
-from regex import find_imports
-
-app = FastAPI()
-
-@app.post("/code")
-async def run_mojo_code(request:Request) -> Response:
- data = await request.json()
- code = data["code"]
- filename = data["filename"]
-
- try:
- imports = find_imports(code)
- for imported in imports:
- subprocess.call(["python3", "-m", "pip", "install", imported], shell=True)
- with open(filename, "w") as f:
- f.write(code)
-
- return Response(content={"sucess":True, "output": subprocess.check_output(["mojo", filename]).decode("utf-8")}, status_code=200)
- except:
- return Response(content={"sucess":False}, status_code=500)
\ No newline at end of file
diff --git a/spaces/StarbucksCN/starbucks_doc/llama/utils.py b/spaces/StarbucksCN/starbucks_doc/llama/utils.py
deleted file mode 100644
index ac335e5b03c1b96f4634181dbc226c560d48a3d1..0000000000000000000000000000000000000000
--- a/spaces/StarbucksCN/starbucks_doc/llama/utils.py
+++ /dev/null
@@ -1,5 +0,0 @@
-import os
-
-
-def is_local_storage_files_ready(persist_dir: str) -> bool:
- return os.path.exists(persist_dir) and len(os.listdir(persist_dir)) != 0
diff --git a/spaces/SujanMidatani/resume_details_extractor/README.md b/spaces/SujanMidatani/resume_details_extractor/README.md
deleted file mode 100644
index bb989ca2d909d631e2edad2eea159fb4ce10b962..0000000000000000000000000000000000000000
--- a/spaces/SujanMidatani/resume_details_extractor/README.md
+++ /dev/null
@@ -1,12 +0,0 @@
----
-title: Resume To Questions Generator
-emoji: 🏃
-colorFrom: blue
-colorTo: gray
-sdk: gradio
-sdk_version: 3.34.0
-app_file: app.py
-pinned: false
----
-
-Check out the configuration reference at https://huggingface.co/docs/hub/spaces-config-reference
diff --git a/spaces/SungBeom/chatwine-korean/.venv/Lib/site-packages/PIL/FontFile.py b/spaces/SungBeom/chatwine-korean/.venv/Lib/site-packages/PIL/FontFile.py
deleted file mode 100644
index 5ec0a6632e3182382467688662ebc5e6c324da91..0000000000000000000000000000000000000000
--- a/spaces/SungBeom/chatwine-korean/.venv/Lib/site-packages/PIL/FontFile.py
+++ /dev/null
@@ -1,110 +0,0 @@
-#
-# The Python Imaging Library
-# $Id$
-#
-# base class for raster font file parsers
-#
-# history:
-# 1997-06-05 fl created
-# 1997-08-19 fl restrict image width
-#
-# Copyright (c) 1997-1998 by Secret Labs AB
-# Copyright (c) 1997-1998 by Fredrik Lundh
-#
-# See the README file for information on usage and redistribution.
-#
-
-
-import os
-
-from . import Image, _binary
-
-WIDTH = 800
-
-
-def puti16(fp, values):
- """Write network order (big-endian) 16-bit sequence"""
- for v in values:
- if v < 0:
- v += 65536
- fp.write(_binary.o16be(v))
-
-
-class FontFile:
- """Base class for raster font file handlers."""
-
- bitmap = None
-
- def __init__(self):
- self.info = {}
- self.glyph = [None] * 256
-
- def __getitem__(self, ix):
- return self.glyph[ix]
-
- def compile(self):
- """Create metrics and bitmap"""
-
- if self.bitmap:
- return
-
- # create bitmap large enough to hold all data
- h = w = maxwidth = 0
- lines = 1
- for glyph in self:
- if glyph:
- d, dst, src, im = glyph
- h = max(h, src[3] - src[1])
- w = w + (src[2] - src[0])
- if w > WIDTH:
- lines += 1
- w = src[2] - src[0]
- maxwidth = max(maxwidth, w)
-
- xsize = maxwidth
- ysize = lines * h
-
- if xsize == 0 and ysize == 0:
- return ""
-
- self.ysize = h
-
- # paste glyphs into bitmap
- self.bitmap = Image.new("1", (xsize, ysize))
- self.metrics = [None] * 256
- x = y = 0
- for i in range(256):
- glyph = self[i]
- if glyph:
- d, dst, src, im = glyph
- xx = src[2] - src[0]
- # yy = src[3] - src[1]
- x0, y0 = x, y
- x = x + xx
- if x > WIDTH:
- x, y = 0, y + h
- x0, y0 = x, y
- x = xx
- s = src[0] + x0, src[1] + y0, src[2] + x0, src[3] + y0
- self.bitmap.paste(im.crop(src), s)
- self.metrics[i] = d, dst, s
-
- def save(self, filename):
- """Save font"""
-
- self.compile()
-
- # font data
- self.bitmap.save(os.path.splitext(filename)[0] + ".pbm", "PNG")
-
- # font metrics
- with open(os.path.splitext(filename)[0] + ".pil", "wb") as fp:
- fp.write(b"PILfont\n")
- fp.write(f";;;;;;{self.ysize};\n".encode("ascii")) # HACK!!!
- fp.write(b"DATA\n")
- for id in range(256):
- m = self.metrics[id]
- if not m:
- puti16(fp, [0] * 10)
- else:
- puti16(fp, m[0] + m[1] + m[2])
diff --git a/spaces/SungBeom/chatwine-korean/.venv/Lib/site-packages/debugpy/_vendored/pydevd/_pydev_bundle/pydev_imports.py b/spaces/SungBeom/chatwine-korean/.venv/Lib/site-packages/debugpy/_vendored/pydevd/_pydev_bundle/pydev_imports.py
deleted file mode 100644
index edc24290881a6255642a10ffe7baedc00d0823af..0000000000000000000000000000000000000000
--- a/spaces/SungBeom/chatwine-korean/.venv/Lib/site-packages/debugpy/_vendored/pydevd/_pydev_bundle/pydev_imports.py
+++ /dev/null
@@ -1,13 +0,0 @@
-from _pydev_bundle._pydev_saved_modules import xmlrpclib
-from _pydev_bundle._pydev_saved_modules import xmlrpcserver
-
-SimpleXMLRPCServer = xmlrpcserver.SimpleXMLRPCServer
-
-from _pydev_bundle._pydev_execfile import execfile
-
-from _pydev_bundle._pydev_saved_modules import _queue
-
-from _pydevd_bundle.pydevd_exec2 import Exec
-
-from urllib.parse import quote, quote_plus, unquote_plus # @UnresolvedImport
-
diff --git a/spaces/Sup3r/img-to-music/app.py b/spaces/Sup3r/img-to-music/app.py
deleted file mode 100644
index 53ba74a6bbbf3c20f5df8f7b3cabc7c84bc63fdd..0000000000000000000000000000000000000000
--- a/spaces/Sup3r/img-to-music/app.py
+++ /dev/null
@@ -1,117 +0,0 @@
-import gradio as gr
-import os
-import requests
-import urllib
-
-from os import path
-from pydub import AudioSegment
-
-img_to_text = gr.Blocks.load(name="spaces/pharma/CLIP-Interrogator")
-text_to_music = gr.Interface.load("spaces/fffiloni/text-2-music")
-
-from share_btn import community_icon_html, loading_icon_html, share_js
-
-def get_prompts(uploaded_image):
-
- prompt = img_to_text(uploaded_image, fn_index=1)[0]
-
- music_result = get_music(prompt)
-
- return music_result
-
-def get_music(prompt):
-
- result = text_to_music(prompt, fn_index=0)
-
- print(f"""—————
- NEW RESULTS
- prompt : {prompt}
- music : {result}
- ———————
- """)
-
- url = result
- save_as = "file.mp3"
-
- data = urllib.request.urlopen(url)
-
- f = open(save_as,'wb')
- f.write(data.read())
- f.close()
-
- wave_file="file.wav"
-
- sound = AudioSegment.from_mp3(save_as)
- sound.export(wave_file, format="wav")
-
- return wave_file, gr.update(visible=True), gr.update(visible=True), gr.update(visible=True)
-
-css = """
-#col-container {max-width: 700px; margin-left: auto; margin-right: auto;}
-a {text-decoration-line: underline; font-weight: 600;}
-.animate-spin {
- animation: spin 1s linear infinite;
-}
-@keyframes spin {
- from {
- transform: rotate(0deg);
- }
- to {
- transform: rotate(360deg);
- }
-}
-#share-btn-container {
- display: flex; padding-left: 0.5rem !important; padding-right: 0.5rem !important; background-color: #000000; justify-content: center; align-items: center; border-radius: 9999px !important; width: 13rem;
-}
-#share-btn {
- all: initial; color: #ffffff;font-weight: 600; cursor:pointer; font-family: 'IBM Plex Sans', sans-serif; margin-left: 0.5rem !important; padding-top: 0.25rem !important; padding-bottom: 0.25rem !important;right:0;
-}
-#share-btn * {
- all: unset;
-}
-#share-btn-container div:nth-child(-n+2){
- width: auto !important;
- min-height: 0px !important;
-}
-#share-btn-container .wrap {
- display: none !important;
-}
-"""
-
-with gr.Blocks(css=css) as demo:
- with gr.Column(elem_id="col-container"):
- gr.HTML("""
-
-
- Image to Music
-
-
-
- Sends an image in to CLIP Interrogator
- to generate a text prompt which is then run through
- Mubert text-to-music to generate music from the input image!
-
-
""")
-
-
- input_img = gr.Image(type="filepath", elem_id="input-img")
- generate = gr.Button("Generate Music from Image")
-
- music_output = gr.Audio(label="Result", type="filepath", elem_id="music-output")
-
- with gr.Group(elem_id="share-btn-container"):
- community_icon = gr.HTML(community_icon_html, visible=False)
- loading_icon = gr.HTML(loading_icon_html, visible=False)
- share_button = gr.Button("Share to community", elem_id="share-btn", visible=False)
-
- generate.click(get_prompts, inputs=[input_img], outputs=[music_output, share_button, community_icon, loading_icon], api_name="i2m")
- share_button.click(None, [], [], _js=share_js)
-
-demo.queue(max_size=32, concurrency_count=20).launch()
\ No newline at end of file
diff --git a/spaces/Superlang/ImageProcessor/annotator/normalbae/models/submodules/efficientnet_repo/onnx_export.py b/spaces/Superlang/ImageProcessor/annotator/normalbae/models/submodules/efficientnet_repo/onnx_export.py
deleted file mode 100644
index 7a5162ce214830df501bdb81edb66c095122f69d..0000000000000000000000000000000000000000
--- a/spaces/Superlang/ImageProcessor/annotator/normalbae/models/submodules/efficientnet_repo/onnx_export.py
+++ /dev/null
@@ -1,120 +0,0 @@
-""" ONNX export script
-
-Export PyTorch models as ONNX graphs.
-
-This export script originally started as an adaptation of code snippets found at
-https://pytorch.org/tutorials/advanced/super_resolution_with_onnxruntime.html
-
-The default parameters work with PyTorch 1.6 and ONNX 1.7 and produce an optimal ONNX graph
-for hosting in the ONNX runtime (see onnx_validate.py). To export an ONNX model compatible
-with caffe2 (see caffe2_benchmark.py and caffe2_validate.py), the --keep-init and --aten-fallback
-flags are currently required.
-
-Older versions of PyTorch/ONNX (tested PyTorch 1.4, ONNX 1.5) do not need extra flags for
-caffe2 compatibility, but they produce a model that isn't as fast running on ONNX runtime.
-
-Most new release of PyTorch and ONNX cause some sort of breakage in the export / usage of ONNX models.
-Please do your research and search ONNX and PyTorch issue tracker before asking me. Thanks.
-
-Copyright 2020 Ross Wightman
-"""
-import argparse
-import torch
-import numpy as np
-
-import onnx
-import geffnet
-
-parser = argparse.ArgumentParser(description='PyTorch ImageNet Validation')
-parser.add_argument('output', metavar='ONNX_FILE',
- help='output model filename')
-parser.add_argument('--model', '-m', metavar='MODEL', default='mobilenetv3_large_100',
- help='model architecture (default: mobilenetv3_large_100)')
-parser.add_argument('--opset', type=int, default=10,
- help='ONNX opset to use (default: 10)')
-parser.add_argument('--keep-init', action='store_true', default=False,
- help='Keep initializers as input. Needed for Caffe2 compatible export in newer PyTorch/ONNX.')
-parser.add_argument('--aten-fallback', action='store_true', default=False,
- help='Fallback to ATEN ops. Helps fix AdaptiveAvgPool issue with Caffe2 in newer PyTorch/ONNX.')
-parser.add_argument('--dynamic-size', action='store_true', default=False,
- help='Export model width dynamic width/height. Not recommended for "tf" models with SAME padding.')
-parser.add_argument('-b', '--batch-size', default=1, type=int,
- metavar='N', help='mini-batch size (default: 1)')
-parser.add_argument('--img-size', default=None, type=int,
- metavar='N', help='Input image dimension, uses model default if empty')
-parser.add_argument('--mean', type=float, nargs='+', default=None, metavar='MEAN',
- help='Override mean pixel value of dataset')
-parser.add_argument('--std', type=float, nargs='+', default=None, metavar='STD',
- help='Override std deviation of of dataset')
-parser.add_argument('--num-classes', type=int, default=1000,
- help='Number classes in dataset')
-parser.add_argument('--checkpoint', default='', type=str, metavar='PATH',
- help='path to checkpoint (default: none)')
-
-
-def main():
- args = parser.parse_args()
-
- args.pretrained = True
- if args.checkpoint:
- args.pretrained = False
-
- print("==> Creating PyTorch {} model".format(args.model))
- # NOTE exportable=True flag disables autofn/jit scripted activations and uses Conv2dSameExport layers
- # for models using SAME padding
- model = geffnet.create_model(
- args.model,
- num_classes=args.num_classes,
- in_chans=3,
- pretrained=args.pretrained,
- checkpoint_path=args.checkpoint,
- exportable=True)
-
- model.eval()
-
- example_input = torch.randn((args.batch_size, 3, args.img_size or 224, args.img_size or 224), requires_grad=True)
-
- # Run model once before export trace, sets padding for models with Conv2dSameExport. This means
- # that the padding for models with Conv2dSameExport (most models with tf_ prefix) is fixed for
- # the input img_size specified in this script.
- # Opset >= 11 should allow for dynamic padding, however I cannot get it to work due to
- # issues in the tracing of the dynamic padding or errors attempting to export the model after jit
- # scripting it (an approach that should work). Perhaps in a future PyTorch or ONNX versions...
- model(example_input)
-
- print("==> Exporting model to ONNX format at '{}'".format(args.output))
- input_names = ["input0"]
- output_names = ["output0"]
- dynamic_axes = {'input0': {0: 'batch'}, 'output0': {0: 'batch'}}
- if args.dynamic_size:
- dynamic_axes['input0'][2] = 'height'
- dynamic_axes['input0'][3] = 'width'
- if args.aten_fallback:
- export_type = torch.onnx.OperatorExportTypes.ONNX_ATEN_FALLBACK
- else:
- export_type = torch.onnx.OperatorExportTypes.ONNX
-
- torch_out = torch.onnx._export(
- model, example_input, args.output, export_params=True, verbose=True, input_names=input_names,
- output_names=output_names, keep_initializers_as_inputs=args.keep_init, dynamic_axes=dynamic_axes,
- opset_version=args.opset, operator_export_type=export_type)
-
- print("==> Loading and checking exported model from '{}'".format(args.output))
- onnx_model = onnx.load(args.output)
- onnx.checker.check_model(onnx_model) # assuming throw on error
- print("==> Passed")
-
- if args.keep_init and args.aten_fallback:
- import caffe2.python.onnx.backend as onnx_caffe2
- # Caffe2 loading only works properly in newer PyTorch/ONNX combos when
- # keep_initializers_as_inputs and aten_fallback are set to True.
- print("==> Loading model into Caffe2 backend and comparing forward pass.".format(args.output))
- caffe2_backend = onnx_caffe2.prepare(onnx_model)
- B = {onnx_model.graph.input[0].name: x.data.numpy()}
- c2_out = caffe2_backend.run(B)[0]
- np.testing.assert_almost_equal(torch_out.data.numpy(), c2_out, decimal=5)
- print("==> Passed")
-
-
-if __name__ == '__main__':
- main()
diff --git a/spaces/Surfrider/surfnet/tracking/__init__.py b/spaces/Surfrider/surfnet/tracking/__init__.py
deleted file mode 100644
index e69de29bb2d1d6434b8b29ae775ad8c2e48c5391..0000000000000000000000000000000000000000
diff --git a/spaces/TRaw/starchat-assist/README.md b/spaces/TRaw/starchat-assist/README.md
deleted file mode 100644
index 0f1bab38fafa8b0d30166007395b55dbafd27237..0000000000000000000000000000000000000000
--- a/spaces/TRaw/starchat-assist/README.md
+++ /dev/null
@@ -1,12 +0,0 @@
----
-title: Starchat Assist
-emoji: 🏢
-colorFrom: green
-colorTo: purple
-sdk: gradio
-sdk_version: 3.38.0
-app_file: app.py
-pinned: false
----
-
-Check out the configuration reference at https://huggingface.co/docs/hub/spaces-config-reference
diff --git a/spaces/Talo88/Tumer-Detection/README.md b/spaces/Talo88/Tumer-Detection/README.md
deleted file mode 100644
index 7502765ff83ada56fc06925636d1d569fd44da00..0000000000000000000000000000000000000000
--- a/spaces/Talo88/Tumer-Detection/README.md
+++ /dev/null
@@ -1,13 +0,0 @@
----
-title: Tumer Detection
-emoji: ⚡
-colorFrom: blue
-colorTo: blue
-sdk: streamlit
-sdk_version: 1.28.1
-app_file: app.py
-pinned: false
-python_version: 3.10.12
----
-
-Check out the configuration reference at https://huggingface.co/docs/hub/spaces-config-reference
diff --git a/spaces/TandCAcceptMe/face-swap-docker/plugins/plugin_txt2clip.py b/spaces/TandCAcceptMe/face-swap-docker/plugins/plugin_txt2clip.py
deleted file mode 100644
index f330f83837c0a237cc2e7d95c493000cb595c94a..0000000000000000000000000000000000000000
--- a/spaces/TandCAcceptMe/face-swap-docker/plugins/plugin_txt2clip.py
+++ /dev/null
@@ -1,122 +0,0 @@
-import os
-import cv2
-import numpy as np
-import torch
-import threading
-from chain_img_processor import ChainImgProcessor, ChainImgPlugin
-from torchvision import transforms
-from clip.clipseg import CLIPDensePredT
-from numpy import asarray
-
-
-THREAD_LOCK_CLIP = threading.Lock()
-
-modname = os.path.basename(__file__)[:-3] # calculating modname
-
-model_clip = None
-
-
-
-
-# start function
-def start(core:ChainImgProcessor):
- manifest = { # plugin settings
- "name": "Text2Clip", # name
- "version": "1.0", # version
-
- "default_options": {
- },
- "img_processor": {
- "txt2clip": Text2Clip
- }
- }
- return manifest
-
-def start_with_options(core:ChainImgProcessor, manifest:dict):
- pass
-
-
-
-class Text2Clip(ChainImgPlugin):
-
- def load_clip_model(self):
- global model_clip
-
- if model_clip is None:
- device = torch.device(super().device)
- model_clip = CLIPDensePredT(version='ViT-B/16', reduce_dim=64, complex_trans_conv=True)
- model_clip.eval();
- model_clip.load_state_dict(torch.load('models/CLIP/rd64-uni-refined.pth', map_location=torch.device('cpu')), strict=False)
- model_clip.to(device)
-
-
- def init_plugin(self):
- self.load_clip_model()
-
- def process(self, frame, params:dict):
- if "face_detected" in params:
- if not params["face_detected"]:
- return frame
-
- return self.mask_original(params["original_frame"], frame, params["clip_prompt"])
-
-
- def mask_original(self, img1, img2, keywords):
- global model_clip
-
- source_image_small = cv2.resize(img1, (256,256))
-
- img_mask = np.full((source_image_small.shape[0],source_image_small.shape[1]), 0, dtype=np.float32)
- mask_border = 1
- l = 0
- t = 0
- r = 1
- b = 1
-
- mask_blur = 5
- clip_blur = 5
-
- img_mask = cv2.rectangle(img_mask, (mask_border+int(l), mask_border+int(t)),
- (256 - mask_border-int(r), 256-mask_border-int(b)), (255, 255, 255), -1)
- img_mask = cv2.GaussianBlur(img_mask, (mask_blur*2+1,mask_blur*2+1), 0)
- img_mask /= 255
-
-
- input_image = source_image_small
-
- transform = transforms.Compose([
- transforms.ToTensor(),
- transforms.Normalize(mean=[0.485, 0.456, 0.406], std=[0.229, 0.224, 0.225]),
- transforms.Resize((256, 256)),
- ])
- img = transform(input_image).unsqueeze(0)
-
- thresh = 0.5
- prompts = keywords.split(',')
- with THREAD_LOCK_CLIP:
- with torch.no_grad():
- preds = model_clip(img.repeat(len(prompts),1,1,1), prompts)[0]
- clip_mask = torch.sigmoid(preds[0][0])
- for i in range(len(prompts)-1):
- clip_mask += torch.sigmoid(preds[i+1][0])
-
- clip_mask = clip_mask.data.cpu().numpy()
- np.clip(clip_mask, 0, 1)
-
- clip_mask[clip_mask>thresh] = 1.0
- clip_mask[clip_mask<=thresh] = 0.0
- kernel = np.ones((5, 5), np.float32)
- clip_mask = cv2.dilate(clip_mask, kernel, iterations=1)
- clip_mask = cv2.GaussianBlur(clip_mask, (clip_blur*2+1,clip_blur*2+1), 0)
-
- img_mask *= clip_mask
- img_mask[img_mask<0.0] = 0.0
-
- img_mask = cv2.resize(img_mask, (img2.shape[1], img2.shape[0]))
- img_mask = np.reshape(img_mask, [img_mask.shape[0],img_mask.shape[1],1])
-
- target = img2.astype(np.float32)
- result = (1-img_mask) * target
- result += img_mask * img1.astype(np.float32)
- return np.uint8(result)
-
diff --git a/spaces/Tej3/ECG_Classification/models/RNN.py b/spaces/Tej3/ECG_Classification/models/RNN.py
deleted file mode 100644
index cefd92f5e911f0ac942ffca4c0c5013bcce6bdd2..0000000000000000000000000000000000000000
--- a/spaces/Tej3/ECG_Classification/models/RNN.py
+++ /dev/null
@@ -1,71 +0,0 @@
-import torch
-import torch.nn as nn
-
-
-class RNN(nn.Module):
- def __init__(self, input_dim=12, hidden_dim=64, num_layers=2, num_classes=5, cuda=True, device='cuda'):
- super(RNN, self).__init__()
- self.hidden_dim = hidden_dim
- self.num_layers = num_layers
- self.device = device
-
- self.lstm = nn.LSTM(input_size=input_dim, hidden_size=self.hidden_dim,
- num_layers=self.num_layers, batch_first=True)
- self.fc1 = nn.Linear(self.hidden_dim, self.hidden_dim)
- self.fc2 = nn.Linear(self.hidden_dim, num_classes)
- self.relu = nn.ReLU()
-
- def forward(self, x, notes):
- h = torch.zeros(self.num_layers, x.size(0), self.hidden_dim)
- c = torch.zeros(self.num_layers, x.size(0), self.hidden_dim)
-
- nn.init.xavier_normal_(h)
- nn.init.xavier_normal_(c)
- h = h.to(self.device)
- c = c.to(self.device)
- x = x.to(self.device)
-
- output, _ = self.lstm(x, (h, c))
-
- out = self.fc2(self.relu(self.fc1(output[:, -1, :])))
-
- return out
-
-
-class MMRNN(nn.ModuleList):
- def __init__(self, input_dim=12, hidden_dim=64, num_layers=2, num_classes=5, embed_size=768, device="cuda"):
- super(MMRNN, self).__init__()
- self.hidden_dim = hidden_dim
- self.num_layers = num_layers
- self.device = device
-
- self.lstm = nn.LSTM(input_size=input_dim, hidden_size=self.hidden_dim,
- num_layers=self.num_layers, batch_first=True)
- self.fc1 = nn.Linear(self.hidden_dim, embed_size)
- self.fc2 = nn.Linear(embed_size, num_classes)
-
- self.lnorm_out = nn.LayerNorm(embed_size)
- self.lnorm_embed = nn.LayerNorm(embed_size)
-
- def forward(self, x, note):
- h = torch.zeros(self.num_layers, x.size(0), self.hidden_dim)
- c = torch.zeros(self.num_layers, x.size(0), self.hidden_dim)
-
- nn.init.xavier_normal_(h)
- nn.init.xavier_normal_(c)
- h = h.to(self.device)
- c = c.to(self.device)
- x = x.to(self.device)
- note = note.to(self.device)
-
- output, _ = self.lstm(x, (h, c))
- # Take last hidden state
- out = self.fc1(output[:, -1, :])
-
- note = self.lnorm_embed(note)
- out = self.lnorm_out(out)
- out = note + out
-
- out = self.fc2(out)
-
- return out.squeeze(1)
diff --git a/spaces/Tonic1/falcon-180b-demo/README.md b/spaces/Tonic1/falcon-180b-demo/README.md
deleted file mode 100644
index 04189396d29fcc4721c66850250efc7c85a18276..0000000000000000000000000000000000000000
--- a/spaces/Tonic1/falcon-180b-demo/README.md
+++ /dev/null
@@ -1,10 +0,0 @@
----
-title: Falcon-180B Demo
-emoji: 💬
-colorFrom: indigo
-colorTo: purple
-sdk: gradio
-sdk_version: 3.42.0
-app_file: app.py
-duplicated_from: tiiuae/falcon-180b-demo
----
diff --git a/spaces/Torcat/torcat-test/config.py b/spaces/Torcat/torcat-test/config.py
deleted file mode 100644
index 70c110bba3c870510dd6844472207194423c28a8..0000000000000000000000000000000000000000
--- a/spaces/Torcat/torcat-test/config.py
+++ /dev/null
@@ -1,11 +0,0 @@
-import os
-
-# FOR MODELS
-MODELS_FOLDER_PATH = os.path.join(os.path.dirname(__file__), 'models')
-
-# FOR OPTIONS
-OPTIONS = {
- 'normal': 'Normal',
- 'segmentation_2_x_2': 'Segmentation 2x2',
- 'segmentation_4_x_4': 'Segmentation 4x4'
-}
\ No newline at end of file
diff --git a/spaces/TusharNautiyal/Dynamic-Movie-Recommender-With-Sentiment-Analysis/README.md b/spaces/TusharNautiyal/Dynamic-Movie-Recommender-With-Sentiment-Analysis/README.md
deleted file mode 100644
index 699df93bea860210ebeba74f98da24bbbf5cf39e..0000000000000000000000000000000000000000
--- a/spaces/TusharNautiyal/Dynamic-Movie-Recommender-With-Sentiment-Analysis/README.md
+++ /dev/null
@@ -1,72 +0,0 @@
----
-title: Dynamic Movie Recommender With Sentiment Analysis
-emoji: 🚀
-colorFrom: blue
-colorTo: purple
-sdk: streamlit
-sdk_version: 1.10.0
-app_file: app.py
-pinned: false
-license: mit
----
-# Dynamic NLP Model Movie-Recommender-system With Sentiment Analysis
-***Check Deployment***
-
-
-
-Content Based Movie Recommender System Using NLP Dynamic model selection between Bert Pre Trained Model , Bag of Words, TF-IDF, Word2Vec, And TF-IDF+Word2Vec on TMDB Dataset.
-This movie recommender was created to better understand each and every NLP model based recommendation working and effective ness based on multiple paramenters.
-For each movie you can also enter a review on which a Sentiment Analysis model will work and tell if your review was a good or bad one.
-Sometimes if not able to find movie recommendation just try to refresh and do it again one more time or change the name to a similar name of a moive.
-If you like this repository do star it.
-# How to Use
-Models are loaded using cloud pickle and **session states** are used to stop model from getting downloaded again and again this increases loading speed and loading times. App is created using **streamlit**. Below is a quick demonstration of how it works.
-
-***Run This command in CLI***
-
-```
-streamlit run app.py
-```
-
-**Recommender Demo**:
-
-https://user-images.githubusercontent.com/74553737/193135421-80a4c790-d14e-4322-982c-36ec7a16aea9.mp4
-
-Sometimes index are not found because either the movie poster is not avalible in the api or the name of the movie was not able to found try to add some variations in your name for eg pirates, carrabiean, sea, monster words that can be in a movie.
-
-**Sentiment Analysis Demo**:
-
-https://user-images.githubusercontent.com/74553737/193136299-185453fa-3235-49a3-99df-c7c2f45ff19c.mp4
-
-Try to write review with more words for better sentiment analysis recommender 20-50 words. We have trained model on **random forest** as it was giving good accuracy and **Tf-idf** vecotrizer for sentiment analysis model. For more you can check the notebook.
-
-# Understanding TF-IDF with Word2Vec Embeddings.
-
-**TF-IDF** is a term frequency-inverse document frequency. It helps to calculate the importance of a given word relative to other words in the document and in the corpus. It calculates in two quantities, TF and IDF. Combining two will give a TF-IDF score.
-
-Calculate the TF-IDF vector for each word in corpus. Let’s call the **TF-IDF** vectors as ***tf1, tf2, tf3, ... and so on*** till n.
-
-
-
-After that we can Calculate the **Word2Vec** for each word in the description lets call it as ***W2V1,W2V2,W2V3..........and so on*** till n.
-
-
-
-
-**Multiply** the ***TF-IDF*** score and ***Word2Vec vector*** representation of each word and **sum** all of it.
-
-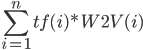
-
-
-Then **divide** the total by sum of TF-IDF vectors.These will be our new vectors that we will use for our cosine similarity to create a recommender model.
-
-Considering each word with i and total words as n. **The Complete Formula will be**
-
-
-
-/ This sign means divide and this Formula image was created using atomurl.net. For more detailed understanding on ***tf-idf+word2vec*** ***Follow me on medium*** where i have posted a full article on it.
-
-# Updates
-This project is deployed on hugging face spaces here is the link for the deployed applications ***Check Deployment***
-
-Check out the configuration reference at https://huggingface.co/docs/hub/spaces-config-reference
diff --git a/spaces/VIOD/anime-ai-detect/README.md b/spaces/VIOD/anime-ai-detect/README.md
deleted file mode 100644
index 952c183fd69ccb1664b4236b6132fc6d0358c7de..0000000000000000000000000000000000000000
--- a/spaces/VIOD/anime-ai-detect/README.md
+++ /dev/null
@@ -1,13 +0,0 @@
----
-title: Anime Ai Detect
-emoji: 🤖
-colorFrom: green
-colorTo: purple
-sdk: gradio
-sdk_version: 3.15.0
-app_file: app.py
-pinned: true
-duplicated_from: saltacc/anime-ai-detect
----
-
-Check out the configuration reference at https://huggingface.co/docs/hub/spaces-config-reference
diff --git a/spaces/Vasanth/QuestionAnswering/README.md b/spaces/Vasanth/QuestionAnswering/README.md
deleted file mode 100644
index afa68272b9c61e5f43a92873cb6dc3cfce935bb6..0000000000000000000000000000000000000000
--- a/spaces/Vasanth/QuestionAnswering/README.md
+++ /dev/null
@@ -1,11 +0,0 @@
----
-title: QuestionAnswering
-emoji: 🏃
-colorFrom: indigo
-colorTo: green
-sdk: streamlit
-app_file: app.py
-pinned: false
----
-
-Check out the configuration reference at https://huggingface.co/docs/hub/spaces#reference
diff --git a/spaces/VeryYouQ/dis-background-removal/app.py b/spaces/VeryYouQ/dis-background-removal/app.py
deleted file mode 100644
index f9b5d48b0d92f5256d0309c08532df3a60cf2628..0000000000000000000000000000000000000000
--- a/spaces/VeryYouQ/dis-background-removal/app.py
+++ /dev/null
@@ -1,155 +0,0 @@
-import cv2
-import gradio as gr
-import os
-from PIL import Image
-import numpy as np
-import torch
-from torch.autograd import Variable
-from torchvision import transforms
-import torch.nn.functional as F
-import gdown
-import matplotlib.pyplot as plt
-import warnings
-warnings.filterwarnings("ignore")
-
-os.system("git clone https://github.com/xuebinqin/DIS")
-os.system("mv DIS/IS-Net/* .")
-
-# project imports
-from data_loader_cache import normalize, im_reader, im_preprocess
-from models import *
-
-#Helpers
-device = 'cuda' if torch.cuda.is_available() else 'cpu'
-
-# Download official weights
-if not os.path.exists("saved_models"):
- os.mkdir("saved_models")
- MODEL_PATH_URL = "https://drive.google.com/uc?id=1KyMpRjewZdyYfxHPYcd-ZbanIXtin0Sn"
- gdown.download(MODEL_PATH_URL, "saved_models/isnet.pth", use_cookies=False)
-
-class GOSNormalize(object):
- '''
- Normalize the Image using torch.transforms
- '''
- def __init__(self, mean=[0.485,0.456,0.406], std=[0.229,0.224,0.225]):
- self.mean = mean
- self.std = std
-
- def __call__(self,image):
- image = normalize(image,self.mean,self.std)
- return image
-
-
-transform = transforms.Compose([GOSNormalize([0.5,0.5,0.5],[1.0,1.0,1.0])])
-
-def load_image(im_path, hypar):
- im = im_reader(im_path)
- im, im_shp = im_preprocess(im, hypar["cache_size"])
- im = torch.divide(im,255.0)
- shape = torch.from_numpy(np.array(im_shp))
- return transform(im).unsqueeze(0), shape.unsqueeze(0) # make a batch of image, shape
-
-
-def build_model(hypar,device):
- net = hypar["model"]#GOSNETINC(3,1)
-
- # convert to half precision
- if(hypar["model_digit"]=="half"):
- net.half()
- for layer in net.modules():
- if isinstance(layer, nn.BatchNorm2d):
- layer.float()
-
- net.to(device)
-
- if(hypar["restore_model"]!=""):
- net.load_state_dict(torch.load(hypar["model_path"]+"/"+hypar["restore_model"], map_location=device))
- net.to(device)
- net.eval()
- return net
-
-
-def predict(net, inputs_val, shapes_val, hypar, device):
- '''
- Given an Image, predict the mask
- '''
- net.eval()
-
- if(hypar["model_digit"]=="full"):
- inputs_val = inputs_val.type(torch.FloatTensor)
- else:
- inputs_val = inputs_val.type(torch.HalfTensor)
-
-
- inputs_val_v = Variable(inputs_val, requires_grad=False).to(device) # wrap inputs in Variable
-
- ds_val = net(inputs_val_v)[0] # list of 6 results
-
- pred_val = ds_val[0][0,:,:,:] # B x 1 x H x W # we want the first one which is the most accurate prediction
-
- ## recover the prediction spatial size to the orignal image size
- pred_val = torch.squeeze(F.upsample(torch.unsqueeze(pred_val,0),(shapes_val[0][0],shapes_val[0][1]),mode='bilinear'))
-
- ma = torch.max(pred_val)
- mi = torch.min(pred_val)
- pred_val = (pred_val-mi)/(ma-mi) # max = 1
-
- if device == 'cuda': torch.cuda.empty_cache()
- return (pred_val.detach().cpu().numpy()*255).astype(np.uint8) # it is the mask we need
-
-# Set Parameters
-hypar = {} # paramters for inferencing
-
-
-hypar["model_path"] ="./saved_models" ## load trained weights from this path
-hypar["restore_model"] = "isnet.pth" ## name of the to-be-loaded weights
-hypar["interm_sup"] = False ## indicate if activate intermediate feature supervision
-
-## choose floating point accuracy --
-hypar["model_digit"] = "full" ## indicates "half" or "full" accuracy of float number
-hypar["seed"] = 0
-
-hypar["cache_size"] = [1024, 1024] ## cached input spatial resolution, can be configured into different size
-
-## data augmentation parameters ---
-hypar["input_size"] = [1024, 1024] ## mdoel input spatial size, usually use the same value hypar["cache_size"], which means we don't further resize the images
-hypar["crop_size"] = [1024, 1024] ## random crop size from the input, it is usually set as smaller than hypar["cache_size"], e.g., [920,920] for data augmentation
-
-hypar["model"] = ISNetDIS()
-
- # Build Model
-net = build_model(hypar, device)
-
-
-def inference(image: Image):
- image_path = image
-
- image_tensor, orig_size = load_image(image_path, hypar)
- mask = predict(net, image_tensor, orig_size, hypar, device)
-
- pil_mask = Image.fromarray(mask).convert("L")
- im_rgb = Image.open(image).convert("RGB")
-
- im_rgba = im_rgb.copy()
- im_rgba.putalpha(pil_mask)
-
- return [im_rgba, pil_mask]
-
-
-title = "Highly Accurate Dichotomous Image Segmentation"
-description = "This is an unofficial demo for DIS, a model that can remove the background from a given image. To use it, simply upload your image, or click one of the examples to load them. Read more at the links below. GitHub: https://github.com/xuebinqin/DIS Telegram bot: https://t.me/restoration_photo_bot [](https://twitter.com/DoEvent)"
-article = "
"
-
-examples=[['test.mp3']]
-gr.Interface(
- inference,
- gr.inputs.Audio(type="numpy", label="Input"),
- [gr.outputs.Audio(type="filepath", label="Vocals"),gr.outputs.Audio(type="filepath", label="Bass"),gr.outputs.Audio(type="filepath", label="Drums"),gr.outputs.Audio(type="filepath", label="Other")],
- title=title,
- description=description,
- article=article,
- examples=examples
- ).launch(enable_queue=True)
\ No newline at end of file
diff --git a/spaces/alexray/btc_predictor/venv/lib/python3.10/site-packages/pip/_vendor/pygments/formatters/rtf.py b/spaces/alexray/btc_predictor/venv/lib/python3.10/site-packages/pip/_vendor/pygments/formatters/rtf.py
deleted file mode 100644
index b4b0acab9b5b1b397b712b197d6aee6b3c69ed54..0000000000000000000000000000000000000000
--- a/spaces/alexray/btc_predictor/venv/lib/python3.10/site-packages/pip/_vendor/pygments/formatters/rtf.py
+++ /dev/null
@@ -1,146 +0,0 @@
-"""
- pygments.formatters.rtf
- ~~~~~~~~~~~~~~~~~~~~~~~
-
- A formatter that generates RTF files.
-
- :copyright: Copyright 2006-2021 by the Pygments team, see AUTHORS.
- :license: BSD, see LICENSE for details.
-"""
-
-from pip._vendor.pygments.formatter import Formatter
-from pip._vendor.pygments.util import get_int_opt, surrogatepair
-
-
-__all__ = ['RtfFormatter']
-
-
-class RtfFormatter(Formatter):
- """
- Format tokens as RTF markup. This formatter automatically outputs full RTF
- documents with color information and other useful stuff. Perfect for Copy and
- Paste into Microsoft(R) Word(R) documents.
-
- Please note that ``encoding`` and ``outencoding`` options are ignored.
- The RTF format is ASCII natively, but handles unicode characters correctly
- thanks to escape sequences.
-
- .. versionadded:: 0.6
-
- Additional options accepted:
-
- `style`
- The style to use, can be a string or a Style subclass (default:
- ``'default'``).
-
- `fontface`
- The used font family, for example ``Bitstream Vera Sans``. Defaults to
- some generic font which is supposed to have fixed width.
-
- `fontsize`
- Size of the font used. Size is specified in half points. The
- default is 24 half-points, giving a size 12 font.
-
- .. versionadded:: 2.0
- """
- name = 'RTF'
- aliases = ['rtf']
- filenames = ['*.rtf']
-
- def __init__(self, **options):
- r"""
- Additional options accepted:
-
- ``fontface``
- Name of the font used. Could for example be ``'Courier New'``
- to further specify the default which is ``'\fmodern'``. The RTF
- specification claims that ``\fmodern`` are "Fixed-pitch serif
- and sans serif fonts". Hope every RTF implementation thinks
- the same about modern...
-
- """
- Formatter.__init__(self, **options)
- self.fontface = options.get('fontface') or ''
- self.fontsize = get_int_opt(options, 'fontsize', 0)
-
- def _escape(self, text):
- return text.replace('\\', '\\\\') \
- .replace('{', '\\{') \
- .replace('}', '\\}')
-
- def _escape_text(self, text):
- # empty strings, should give a small performance improvement
- if not text:
- return ''
-
- # escape text
- text = self._escape(text)
-
- buf = []
- for c in text:
- cn = ord(c)
- if cn < (2**7):
- # ASCII character
- buf.append(str(c))
- elif (2**7) <= cn < (2**16):
- # single unicode escape sequence
- buf.append('{\\u%d}' % cn)
- elif (2**16) <= cn:
- # RTF limits unicode to 16 bits.
- # Force surrogate pairs
- buf.append('{\\u%d}{\\u%d}' % surrogatepair(cn))
-
- return ''.join(buf).replace('\n', '\\par\n')
-
- def format_unencoded(self, tokensource, outfile):
- # rtf 1.8 header
- outfile.write('{\\rtf1\\ansi\\uc0\\deff0'
- '{\\fonttbl{\\f0\\fmodern\\fprq1\\fcharset0%s;}}'
- '{\\colortbl;' % (self.fontface and
- ' ' + self._escape(self.fontface) or
- ''))
-
- # convert colors and save them in a mapping to access them later.
- color_mapping = {}
- offset = 1
- for _, style in self.style:
- for color in style['color'], style['bgcolor'], style['border']:
- if color and color not in color_mapping:
- color_mapping[color] = offset
- outfile.write('\\red%d\\green%d\\blue%d;' % (
- int(color[0:2], 16),
- int(color[2:4], 16),
- int(color[4:6], 16)
- ))
- offset += 1
- outfile.write('}\\f0 ')
- if self.fontsize:
- outfile.write('\\fs%d' % self.fontsize)
-
- # highlight stream
- for ttype, value in tokensource:
- while not self.style.styles_token(ttype) and ttype.parent:
- ttype = ttype.parent
- style = self.style.style_for_token(ttype)
- buf = []
- if style['bgcolor']:
- buf.append('\\cb%d' % color_mapping[style['bgcolor']])
- if style['color']:
- buf.append('\\cf%d' % color_mapping[style['color']])
- if style['bold']:
- buf.append('\\b')
- if style['italic']:
- buf.append('\\i')
- if style['underline']:
- buf.append('\\ul')
- if style['border']:
- buf.append('\\chbrdr\\chcfpat%d' %
- color_mapping[style['border']])
- start = ''.join(buf)
- if start:
- outfile.write('{%s ' % start)
- outfile.write(self._escape_text(value))
- if start:
- outfile.write('}')
-
- outfile.write('}')
diff --git a/spaces/aliabd/non-interactive-dataframe/app.py b/spaces/aliabd/non-interactive-dataframe/app.py
deleted file mode 100644
index 5770f744180cce6a7aae72829489ca74e6b8052c..0000000000000000000000000000000000000000
--- a/spaces/aliabd/non-interactive-dataframe/app.py
+++ /dev/null
@@ -1,43 +0,0 @@
-import pandas as pd
-import gradio as gr
-
-df = pd.read_csv("liked_images.csv")
-df['url'] = df['url'].apply(lambda x: '') #'')
-df['seed'] = df['seed'].apply(lambda x: str(x))
-df['width'] = df['width'].apply(lambda x: str(x))
-df['height'] = df['height'].apply(lambda x: str(x))
-df['steps'] = df['steps'].apply(lambda x: str(x))
-df['source'] = df['source'].apply(lambda x: str(x))
-df = df[[ 'url', 'prompt', 'seed', 'width', 'height', 'steps', 'source']]
-
-def display_df():
- df_images = df.head()
- return df_images
-
-def display_next10(dataframe, end):
- start = (end or dataframe.index[-1]) + 1
- end = start + 9
- df_images = df.loc[start:end]
- return df_images, end
-
-#Gradio Blocks
-with gr.Blocks() as demo:
- gr.Markdown("
Utility Gradio Space for viewing PlaygroundAI Images
")
- #gr.Markdown(""" """)
- gr.Markdown(
- """
This Tool helps you to analyze and inspect the images and corresponding prompts from Playground AI Images. Suhail has recently shared an open dataset of all the liked images and their prompts from PlaygroundAI on Github here. This is an attempt to explore this dataset beautifully using the power and flexibility of Gradio! To use the tool:First, click on the 'Initial' button, and then iteratively on the 'Next 10' button. Bonus:Click on images to get the original PlaygroundAI image displayed on next tab
Please note that the Playground AI dataset shared on GitHub doesn't have images but links to those images. The idea is to get the maximum benefit out of this dataset and to find the best way to explore this dataset. Gradio enables us to embed markdowns within a dataframe, thus this app is able to display actual images instead of direct links(meh!). I hope you will have as much fun playing with this Space as I had building it.
")
-
-demo.launch(debug=True, show_error=True)
\ No newline at end of file
diff --git a/spaces/allknowingroger/huggingface/assets/index-7f4d6bd2.css b/spaces/allknowingroger/huggingface/assets/index-7f4d6bd2.css
deleted file mode 100644
index 098ae1f1bce10863773ac288c65b5b85a125a065..0000000000000000000000000000000000000000
--- a/spaces/allknowingroger/huggingface/assets/index-7f4d6bd2.css
+++ /dev/null
@@ -1 +0,0 @@
-*,:before,:after{box-sizing:border-box;border-width:0;border-style:solid;border-color:#e5e7eb}:before,:after{--tw-content: ""}html{line-height:1.5;-webkit-text-size-adjust:100%;-moz-tab-size:4;-o-tab-size:4;tab-size:4;font-family:ui-sans-serif,system-ui,-apple-system,BlinkMacSystemFont,Segoe UI,Roboto,Helvetica Neue,Arial,Noto Sans,sans-serif,"Apple Color Emoji","Segoe UI Emoji",Segoe UI Symbol,"Noto Color Emoji";font-feature-settings:normal;font-variation-settings:normal}body{margin:0;line-height:inherit}hr{height:0;color:inherit;border-top-width:1px}abbr:where([title]){-webkit-text-decoration:underline dotted;text-decoration:underline dotted}h1,h2,h3,h4,h5,h6{font-size:inherit;font-weight:inherit}a{color:inherit;text-decoration:inherit}b,strong{font-weight:bolder}code,kbd,samp,pre{font-family:ui-monospace,SFMono-Regular,Menlo,Monaco,Consolas,Liberation Mono,Courier New,monospace;font-size:1em}small{font-size:80%}sub,sup{font-size:75%;line-height:0;position:relative;vertical-align:baseline}sub{bottom:-.25em}sup{top:-.5em}table{text-indent:0;border-color:inherit;border-collapse:collapse}button,input,optgroup,select,textarea{font-family:inherit;font-size:100%;font-weight:inherit;line-height:inherit;color:inherit;margin:0;padding:0}button,select{text-transform:none}button,[type=button],[type=reset],[type=submit]{-webkit-appearance:button;background-color:transparent;background-image:none}:-moz-focusring{outline:auto}:-moz-ui-invalid{box-shadow:none}progress{vertical-align:baseline}::-webkit-inner-spin-button,::-webkit-outer-spin-button{height:auto}[type=search]{-webkit-appearance:textfield;outline-offset:-2px}::-webkit-search-decoration{-webkit-appearance:none}::-webkit-file-upload-button{-webkit-appearance:button;font:inherit}summary{display:list-item}blockquote,dl,dd,h1,h2,h3,h4,h5,h6,hr,figure,p,pre{margin:0}fieldset{margin:0;padding:0}legend{padding:0}ol,ul,menu{list-style:none;margin:0;padding:0}textarea{resize:vertical}input::-moz-placeholder,textarea::-moz-placeholder{opacity:1;color:#9ca3af}input::placeholder,textarea::placeholder{opacity:1;color:#9ca3af}button,[role=button]{cursor:pointer}:disabled{cursor:default}img,svg,video,canvas,audio,iframe,embed,object{display:block;vertical-align:middle}img,video{max-width:100%;height:auto}[hidden]{display:none}*,:before,:after{--tw-border-spacing-x: 0;--tw-border-spacing-y: 0;--tw-translate-x: 0;--tw-translate-y: 0;--tw-rotate: 0;--tw-skew-x: 0;--tw-skew-y: 0;--tw-scale-x: 1;--tw-scale-y: 1;--tw-pan-x: ;--tw-pan-y: ;--tw-pinch-zoom: ;--tw-scroll-snap-strictness: proximity;--tw-gradient-from-position: ;--tw-gradient-via-position: ;--tw-gradient-to-position: ;--tw-ordinal: ;--tw-slashed-zero: ;--tw-numeric-figure: ;--tw-numeric-spacing: ;--tw-numeric-fraction: ;--tw-ring-inset: ;--tw-ring-offset-width: 0px;--tw-ring-offset-color: #fff;--tw-ring-color: rgb(59 130 246 / .5);--tw-ring-offset-shadow: 0 0 #0000;--tw-ring-shadow: 0 0 #0000;--tw-shadow: 0 0 #0000;--tw-shadow-colored: 0 0 #0000;--tw-blur: ;--tw-brightness: ;--tw-contrast: ;--tw-grayscale: ;--tw-hue-rotate: ;--tw-invert: ;--tw-saturate: ;--tw-sepia: ;--tw-drop-shadow: ;--tw-backdrop-blur: ;--tw-backdrop-brightness: ;--tw-backdrop-contrast: ;--tw-backdrop-grayscale: ;--tw-backdrop-hue-rotate: ;--tw-backdrop-invert: ;--tw-backdrop-opacity: ;--tw-backdrop-saturate: ;--tw-backdrop-sepia: }::backdrop{--tw-border-spacing-x: 0;--tw-border-spacing-y: 0;--tw-translate-x: 0;--tw-translate-y: 0;--tw-rotate: 0;--tw-skew-x: 0;--tw-skew-y: 0;--tw-scale-x: 1;--tw-scale-y: 1;--tw-pan-x: ;--tw-pan-y: ;--tw-pinch-zoom: ;--tw-scroll-snap-strictness: proximity;--tw-gradient-from-position: ;--tw-gradient-via-position: ;--tw-gradient-to-position: ;--tw-ordinal: ;--tw-slashed-zero: ;--tw-numeric-figure: ;--tw-numeric-spacing: ;--tw-numeric-fraction: ;--tw-ring-inset: ;--tw-ring-offset-width: 0px;--tw-ring-offset-color: #fff;--tw-ring-color: rgb(59 130 246 / .5);--tw-ring-offset-shadow: 0 0 #0000;--tw-ring-shadow: 0 0 #0000;--tw-shadow: 0 0 #0000;--tw-shadow-colored: 0 0 #0000;--tw-blur: ;--tw-brightness: ;--tw-contrast: ;--tw-grayscale: ;--tw-hue-rotate: ;--tw-invert: ;--tw-saturate: ;--tw-sepia: ;--tw-drop-shadow: ;--tw-backdrop-blur: ;--tw-backdrop-brightness: ;--tw-backdrop-contrast: ;--tw-backdrop-grayscale: ;--tw-backdrop-hue-rotate: ;--tw-backdrop-invert: ;--tw-backdrop-opacity: ;--tw-backdrop-saturate: ;--tw-backdrop-sepia: }.container{width:100%}@media (min-width: 640px){.container{max-width:640px}}@media (min-width: 768px){.container{max-width:768px}}@media (min-width: 1024px){.container{max-width:1024px}}@media (min-width: 1280px){.container{max-width:1280px}}@media (min-width: 1536px){.container{max-width:1536px}}.block{display:block}.flex{display:flex}.table{display:table}.hidden{display:none}.h-full{height:100%}.min-h-screen{min-height:100vh}.w-2\/3{width:66.666667%}.w-full{width:100%}.cursor-not-allowed{cursor:not-allowed}.cursor-pointer{cursor:pointer}.cursor-wait{cursor:wait}.select-text{-webkit-user-select:text;-moz-user-select:text;user-select:text}.resize-none{resize:none}.flex-col{flex-direction:column}.items-center{align-items:center}.justify-center{justify-content:center}.space-y-12>:not([hidden])~:not([hidden]){--tw-space-y-reverse: 0;margin-top:calc(3rem * calc(1 - var(--tw-space-y-reverse)));margin-bottom:calc(3rem * var(--tw-space-y-reverse))}.overflow-auto{overflow:auto}.whitespace-pre-wrap{white-space:pre-wrap}.break-words{overflow-wrap:break-word}.border-4{border-width:4px}.border-yellow-200{--tw-border-opacity: 1;border-color:rgb(254 240 138 / var(--tw-border-opacity))}.bg-yellow-200{--tw-bg-opacity: 1;background-color:rgb(254 240 138 / var(--tw-bg-opacity))}.bg-yellow-500{--tw-bg-opacity: 1;background-color:rgb(234 179 8 / var(--tw-bg-opacity))}.p-6{padding:1.5rem}.py-24{padding-top:6rem;padding-bottom:6rem}.py-6{padding-top:1.5rem;padding-bottom:1.5rem}.text-center{text-align:center}.text-6xl{font-size:3.75rem;line-height:1}.text-xl{font-size:1.25rem;line-height:1.75rem}.font-bold{font-weight:700}.text-yellow-200{--tw-text-opacity: 1;color:rgb(254 240 138 / var(--tw-text-opacity))}.opacity-50{opacity:.5}*,*:before,*:after{box-sizing:inherit;-webkit-user-select:inherit;-moz-user-select:inherit;user-select:inherit}html,body,#root{box-sizing:border-box;height:100%;min-height:100vh;width:100%;min-width:100vw;margin:0;padding:0;-webkit-user-select:none;-moz-user-select:none;user-select:none}input::-webkit-file-upload-button{display:none}@media (min-width: 1024px){.lg\:w-1\/3{width:33.333333%}}
diff --git a/spaces/amankishore/sjc/my/utils/seed.py b/spaces/amankishore/sjc/my/utils/seed.py
deleted file mode 100644
index e3e81fad6c7610d11ec8d847f9a61a4e6675ecc4..0000000000000000000000000000000000000000
--- a/spaces/amankishore/sjc/my/utils/seed.py
+++ /dev/null
@@ -1,21 +0,0 @@
-# from pytorch lightning
-import random
-import numpy as np
-import torch
-
-max_seed_value = np.iinfo(np.uint32).max
-min_seed_value = np.iinfo(np.uint32).min
-
-
-def seed_everything(seed=None):
- seed = int(seed)
-
- if not (min_seed_value <= seed <= max_seed_value):
- raise ValueError(f"{seed} is not in bounds, numpy accepts from {min_seed_value} to {max_seed_value}")
-
- print(f"seed set to {seed}")
- random.seed(seed)
- np.random.seed(seed)
- torch.manual_seed(seed)
- torch.cuda.manual_seed_all(seed)
- return seed
diff --git a/spaces/amarchheda/ChordDuplicate/portaudio/examples/paex_saw.c b/spaces/amarchheda/ChordDuplicate/portaudio/examples/paex_saw.c
deleted file mode 100644
index caec0b02d7e02410bef484d06ca4733a06747bab..0000000000000000000000000000000000000000
--- a/spaces/amarchheda/ChordDuplicate/portaudio/examples/paex_saw.c
+++ /dev/null
@@ -1,133 +0,0 @@
-/** @file paex_saw.c
- @ingroup examples_src
- @brief Play a simple (aliasing) sawtooth wave.
- @author Phil Burk http://www.softsynth.com
-*/
-/*
- * $Id$
- *
- * This program uses the PortAudio Portable Audio Library.
- * For more information see: http://www.portaudio.com
- * Copyright (c) 1999-2000 Ross Bencina and Phil Burk
- *
- * Permission is hereby granted, free of charge, to any person obtaining
- * a copy of this software and associated documentation files
- * (the "Software"), to deal in the Software without restriction,
- * including without limitation the rights to use, copy, modify, merge,
- * publish, distribute, sublicense, and/or sell copies of the Software,
- * and to permit persons to whom the Software is furnished to do so,
- * subject to the following conditions:
- *
- * The above copyright notice and this permission notice shall be
- * included in all copies or substantial portions of the Software.
- *
- * THE SOFTWARE IS PROVIDED "AS IS", WITHOUT WARRANTY OF ANY KIND,
- * EXPRESS OR IMPLIED, INCLUDING BUT NOT LIMITED TO THE WARRANTIES OF
- * MERCHANTABILITY, FITNESS FOR A PARTICULAR PURPOSE AND NONINFRINGEMENT.
- * IN NO EVENT SHALL THE AUTHORS OR COPYRIGHT HOLDERS BE LIABLE FOR
- * ANY CLAIM, DAMAGES OR OTHER LIABILITY, WHETHER IN AN ACTION OF
- * CONTRACT, TORT OR OTHERWISE, ARISING FROM, OUT OF OR IN CONNECTION
- * WITH THE SOFTWARE OR THE USE OR OTHER DEALINGS IN THE SOFTWARE.
- */
-
-/*
- * The text above constitutes the entire PortAudio license; however,
- * the PortAudio community also makes the following non-binding requests:
- *
- * Any person wishing to distribute modifications to the Software is
- * requested to send the modifications to the original developer so that
- * they can be incorporated into the canonical version. It is also
- * requested that these non-binding requests be included along with the
- * license above.
- */
-
-#include
-#include
-#include "portaudio.h"
-#define NUM_SECONDS (4)
-#define SAMPLE_RATE (44100)
-
-typedef struct
-{
- float left_phase;
- float right_phase;
-}
-paTestData;
-
-/* This routine will be called by the PortAudio engine when audio is needed.
-** It may called at interrupt level on some machines so don't do anything
-** that could mess up the system like calling malloc() or free().
-*/
-static int patestCallback( const void *inputBuffer, void *outputBuffer,
- unsigned long framesPerBuffer,
- const PaStreamCallbackTimeInfo* timeInfo,
- PaStreamCallbackFlags statusFlags,
- void *userData )
-{
- /* Cast data passed through stream to our structure. */
- paTestData *data = (paTestData*)userData;
- float *out = (float*)outputBuffer;
- unsigned int i;
- (void) inputBuffer; /* Prevent unused variable warning. */
-
- for( i=0; ileft_phase; /* left */
- *out++ = data->right_phase; /* right */
- /* Generate simple sawtooth phaser that ranges between -1.0 and 1.0. */
- data->left_phase += 0.01f;
- /* When signal reaches top, drop back down. */
- if( data->left_phase >= 1.0f ) data->left_phase -= 2.0f;
- /* higher pitch so we can distinguish left and right. */
- data->right_phase += 0.03f;
- if( data->right_phase >= 1.0f ) data->right_phase -= 2.0f;
- }
- return 0;
-}
-
-/*******************************************************************/
-static paTestData data;
-int main(void);
-int main(void)
-{
- PaStream *stream;
- PaError err;
-
- printf("PortAudio Test: output sawtooth wave.\n");
- /* Initialize our data for use by callback. */
- data.left_phase = data.right_phase = 0.0;
- /* Initialize library before making any other calls. */
- err = Pa_Initialize();
- if( err != paNoError ) goto error;
-
- /* Open an audio I/O stream. */
- err = Pa_OpenDefaultStream( &stream,
- 0, /* no input channels */
- 2, /* stereo output */
- paFloat32, /* 32 bit floating point output */
- SAMPLE_RATE,
- 256, /* frames per buffer */
- patestCallback,
- &data );
- if( err != paNoError ) goto error;
-
- err = Pa_StartStream( stream );
- if( err != paNoError ) goto error;
-
- /* Sleep for several seconds. */
- Pa_Sleep(NUM_SECONDS*1000);
-
- err = Pa_StopStream( stream );
- if( err != paNoError ) goto error;
- err = Pa_CloseStream( stream );
- if( err != paNoError ) goto error;
- Pa_Terminate();
- printf("Test finished.\n");
- return err;
-error:
- Pa_Terminate();
- fprintf( stderr, "An error occurred while using the portaudio stream\n" );
- fprintf( stderr, "Error number: %d\n", err );
- fprintf( stderr, "Error message: %s\n", Pa_GetErrorText( err ) );
- return err;
-}
diff --git a/spaces/artificialguybr/video-dubbing/TTS/tests/text_tests/__init__.py b/spaces/artificialguybr/video-dubbing/TTS/tests/text_tests/__init__.py
deleted file mode 100644
index e69de29bb2d1d6434b8b29ae775ad8c2e48c5391..0000000000000000000000000000000000000000
diff --git a/spaces/arxify/RVC-beta-v2-0618/config.py b/spaces/arxify/RVC-beta-v2-0618/config.py
deleted file mode 100644
index 48187f530663fbe051585e0e2e37dbd06fd7f8ea..0000000000000000000000000000000000000000
--- a/spaces/arxify/RVC-beta-v2-0618/config.py
+++ /dev/null
@@ -1,123 +0,0 @@
-import argparse
-import torch
-from multiprocessing import cpu_count
-
-
-def config_file_change_fp32():
- for config_file in ["32k.json", "40k.json", "48k.json"]:
- with open(f"configs/{config_file}", "r") as f:
- strr = f.read().replace("true", "false")
- with open(f"configs/{config_file}", "w") as f:
- f.write(strr)
- with open("trainset_preprocess_pipeline_print.py", "r") as f:
- strr = f.read().replace("3.7", "3.0")
- with open("trainset_preprocess_pipeline_print.py", "w") as f:
- f.write(strr)
-
-
-class Config:
- def __init__(self):
- self.device = "cuda:0"
- self.is_half = True
- self.n_cpu = 0
- self.gpu_name = None
- self.gpu_mem = None
- (
- self.python_cmd,
- self.listen_port,
- self.iscolab,
- self.noparallel,
- self.noautoopen,
- ) = self.arg_parse()
- self.x_pad, self.x_query, self.x_center, self.x_max = self.device_config()
-
- @staticmethod
- def arg_parse() -> tuple:
- parser = argparse.ArgumentParser()
- parser.add_argument("--port", type=int, default=7865, help="Listen port")
- parser.add_argument(
- "--pycmd", type=str, default="python", help="Python command"
- )
- parser.add_argument("--colab", action="store_true", help="Launch in colab")
- parser.add_argument(
- "--noparallel", action="store_true", help="Disable parallel processing"
- )
- parser.add_argument(
- "--noautoopen",
- action="store_true",
- help="Do not open in browser automatically",
- )
- cmd_opts = parser.parse_args()
-
- cmd_opts.port = cmd_opts.port if 0 <= cmd_opts.port <= 65535 else 7865
-
- return (
- cmd_opts.pycmd,
- cmd_opts.port,
- cmd_opts.colab,
- cmd_opts.noparallel,
- cmd_opts.noautoopen,
- )
-
- def device_config(self) -> tuple:
- if torch.cuda.is_available():
- i_device = int(self.device.split(":")[-1])
- self.gpu_name = torch.cuda.get_device_name(i_device)
- if (
- ("16" in self.gpu_name and "V100" not in self.gpu_name.upper())
- or "P40" in self.gpu_name.upper()
- or "1060" in self.gpu_name
- or "1070" in self.gpu_name
- or "1080" in self.gpu_name
- ):
- print("16系/10系显卡和P40强制单精度")
- self.is_half = False
- config_file_change_fp32()
- else:
- self.gpu_name = None
- self.gpu_mem = int(
- torch.cuda.get_device_properties(i_device).total_memory
- / 1024
- / 1024
- / 1024
- + 0.4
- )
- if self.gpu_mem <= 4:
- with open("trainset_preprocess_pipeline_print.py", "r") as f:
- strr = f.read().replace("3.7", "3.0")
- with open("trainset_preprocess_pipeline_print.py", "w") as f:
- f.write(strr)
- elif torch.backends.mps.is_available():
- print("没有发现支持的N卡, 使用MPS进行推理")
- self.device = "mps"
- self.is_half = False
- config_file_change_fp32()
- else:
- print("没有发现支持的N卡, 使用CPU进行推理")
- self.device = "cpu"
- self.is_half = False
- config_file_change_fp32()
-
- if self.n_cpu == 0:
- self.n_cpu = cpu_count()
-
- if self.is_half:
- # 6G显存配置
- x_pad = 3
- x_query = 10
- x_center = 60
- x_max = 65
- else:
- # 5G显存配置
- x_pad = 1
- x_query = 6
- x_center = 38
- x_max = 41
-
- if self.gpu_mem != None and self.gpu_mem <= 4:
- x_pad = 1
- x_query = 5
- x_center = 30
- x_max = 32
-
- return x_pad, x_query, x_center, x_max
diff --git a/spaces/arxify/RVC-beta-v2-0618/runtime/Lib/site-packages/Crypto/Cipher/ChaCha20.py b/spaces/arxify/RVC-beta-v2-0618/runtime/Lib/site-packages/Crypto/Cipher/ChaCha20.py
deleted file mode 100644
index 9bd2252ce7297f18ba3c1a1d62aa748cc474c5f1..0000000000000000000000000000000000000000
--- a/spaces/arxify/RVC-beta-v2-0618/runtime/Lib/site-packages/Crypto/Cipher/ChaCha20.py
+++ /dev/null
@@ -1,287 +0,0 @@
-# ===================================================================
-#
-# Copyright (c) 2014, Legrandin
-# All rights reserved.
-#
-# Redistribution and use in source and binary forms, with or without
-# modification, are permitted provided that the following conditions
-# are met:
-#
-# 1. Redistributions of source code must retain the above copyright
-# notice, this list of conditions and the following disclaimer.
-# 2. Redistributions in binary form must reproduce the above copyright
-# notice, this list of conditions and the following disclaimer in
-# the documentation and/or other materials provided with the
-# distribution.
-#
-# THIS SOFTWARE IS PROVIDED BY THE COPYRIGHT HOLDERS AND CONTRIBUTORS
-# "AS IS" AND ANY EXPRESS OR IMPLIED WARRANTIES, INCLUDING, BUT NOT
-# LIMITED TO, THE IMPLIED WARRANTIES OF MERCHANTABILITY AND FITNESS
-# FOR A PARTICULAR PURPOSE ARE DISCLAIMED. IN NO EVENT SHALL THE
-# COPYRIGHT HOLDER OR CONTRIBUTORS BE LIABLE FOR ANY DIRECT, INDIRECT,
-# INCIDENTAL, SPECIAL, EXEMPLARY, OR CONSEQUENTIAL DAMAGES (INCLUDING,
-# BUT NOT LIMITED TO, PROCUREMENT OF SUBSTITUTE GOODS OR SERVICES;
-# LOSS OF USE, DATA, OR PROFITS; OR BUSINESS INTERRUPTION) HOWEVER
-# CAUSED AND ON ANY THEORY OF LIABILITY, WHETHER IN CONTRACT, STRICT
-# LIABILITY, OR TORT (INCLUDING NEGLIGENCE OR OTHERWISE) ARISING IN
-# ANY WAY OUT OF THE USE OF THIS SOFTWARE, EVEN IF ADVISED OF THE
-# POSSIBILITY OF SUCH DAMAGE.
-# ===================================================================
-
-from Crypto.Random import get_random_bytes
-
-from Crypto.Util.py3compat import _copy_bytes
-from Crypto.Util._raw_api import (load_pycryptodome_raw_lib,
- create_string_buffer,
- get_raw_buffer, VoidPointer,
- SmartPointer, c_size_t,
- c_uint8_ptr, c_ulong,
- is_writeable_buffer)
-
-_raw_chacha20_lib = load_pycryptodome_raw_lib("Crypto.Cipher._chacha20",
- """
- int chacha20_init(void **pState,
- const uint8_t *key,
- size_t keySize,
- const uint8_t *nonce,
- size_t nonceSize);
-
- int chacha20_destroy(void *state);
-
- int chacha20_encrypt(void *state,
- const uint8_t in[],
- uint8_t out[],
- size_t len);
-
- int chacha20_seek(void *state,
- unsigned long block_high,
- unsigned long block_low,
- unsigned offset);
- int hchacha20( const uint8_t key[32],
- const uint8_t nonce16[16],
- uint8_t subkey[32]);
- """)
-
-
-def _HChaCha20(key, nonce):
-
- assert(len(key) == 32)
- assert(len(nonce) == 16)
-
- subkey = bytearray(32)
- result = _raw_chacha20_lib.hchacha20(
- c_uint8_ptr(key),
- c_uint8_ptr(nonce),
- c_uint8_ptr(subkey))
- if result:
- raise ValueError("Error %d when deriving subkey with HChaCha20" % result)
-
- return subkey
-
-
-class ChaCha20Cipher(object):
- """ChaCha20 (or XChaCha20) cipher object.
- Do not create it directly. Use :py:func:`new` instead.
-
- :var nonce: The nonce with length 8, 12 or 24 bytes
- :vartype nonce: bytes
- """
-
- block_size = 1
-
- def __init__(self, key, nonce):
- """Initialize a ChaCha20/XChaCha20 cipher object
-
- See also `new()` at the module level."""
-
- self.nonce = _copy_bytes(None, None, nonce)
-
- # XChaCha20 requires a key derivation with HChaCha20
- # See 2.3 in https://tools.ietf.org/html/draft-arciszewski-xchacha-03
- if len(nonce) == 24:
- key = _HChaCha20(key, nonce[:16])
- nonce = b'\x00' * 4 + nonce[16:]
- self._name = "XChaCha20"
- else:
- self._name = "ChaCha20"
- nonce = self.nonce
-
- self._next = ( self.encrypt, self.decrypt )
-
- self._state = VoidPointer()
- result = _raw_chacha20_lib.chacha20_init(
- self._state.address_of(),
- c_uint8_ptr(key),
- c_size_t(len(key)),
- nonce,
- c_size_t(len(nonce)))
- if result:
- raise ValueError("Error %d instantiating a %s cipher" % (result,
- self._name))
- self._state = SmartPointer(self._state.get(),
- _raw_chacha20_lib.chacha20_destroy)
-
- def encrypt(self, plaintext, output=None):
- """Encrypt a piece of data.
-
- Args:
- plaintext(bytes/bytearray/memoryview): The data to encrypt, of any size.
- Keyword Args:
- output(bytes/bytearray/memoryview): The location where the ciphertext
- is written to. If ``None``, the ciphertext is returned.
- Returns:
- If ``output`` is ``None``, the ciphertext is returned as ``bytes``.
- Otherwise, ``None``.
- """
-
- if self.encrypt not in self._next:
- raise TypeError("Cipher object can only be used for decryption")
- self._next = ( self.encrypt, )
- return self._encrypt(plaintext, output)
-
- def _encrypt(self, plaintext, output):
- """Encrypt without FSM checks"""
-
- if output is None:
- ciphertext = create_string_buffer(len(plaintext))
- else:
- ciphertext = output
-
- if not is_writeable_buffer(output):
- raise TypeError("output must be a bytearray or a writeable memoryview")
-
- if len(plaintext) != len(output):
- raise ValueError("output must have the same length as the input"
- " (%d bytes)" % len(plaintext))
-
- result = _raw_chacha20_lib.chacha20_encrypt(
- self._state.get(),
- c_uint8_ptr(plaintext),
- c_uint8_ptr(ciphertext),
- c_size_t(len(plaintext)))
- if result:
- raise ValueError("Error %d while encrypting with %s" % (result, self._name))
-
- if output is None:
- return get_raw_buffer(ciphertext)
- else:
- return None
-
- def decrypt(self, ciphertext, output=None):
- """Decrypt a piece of data.
-
- Args:
- ciphertext(bytes/bytearray/memoryview): The data to decrypt, of any size.
- Keyword Args:
- output(bytes/bytearray/memoryview): The location where the plaintext
- is written to. If ``None``, the plaintext is returned.
- Returns:
- If ``output`` is ``None``, the plaintext is returned as ``bytes``.
- Otherwise, ``None``.
- """
-
- if self.decrypt not in self._next:
- raise TypeError("Cipher object can only be used for encryption")
- self._next = ( self.decrypt, )
-
- try:
- return self._encrypt(ciphertext, output)
- except ValueError as e:
- raise ValueError(str(e).replace("enc", "dec"))
-
- def seek(self, position):
- """Seek to a certain position in the key stream.
-
- Args:
- position (integer):
- The absolute position within the key stream, in bytes.
- """
-
- position, offset = divmod(position, 64)
- block_low = position & 0xFFFFFFFF
- block_high = position >> 32
-
- result = _raw_chacha20_lib.chacha20_seek(
- self._state.get(),
- c_ulong(block_high),
- c_ulong(block_low),
- offset
- )
- if result:
- raise ValueError("Error %d while seeking with %s" % (result, self._name))
-
-
-def _derive_Poly1305_key_pair(key, nonce):
- """Derive a tuple (r, s, nonce) for a Poly1305 MAC.
-
- If nonce is ``None``, a new 12-byte nonce is generated.
- """
-
- if len(key) != 32:
- raise ValueError("Poly1305 with ChaCha20 requires a 32-byte key")
-
- if nonce is None:
- padded_nonce = nonce = get_random_bytes(12)
- elif len(nonce) == 8:
- # See RFC7538, 2.6: [...] ChaCha20 as specified here requires a 96-bit
- # nonce. So if the provided nonce is only 64-bit, then the first 32
- # bits of the nonce will be set to a constant number.
- # This will usually be zero, but for protocols with multiple senders it may be
- # different for each sender, but should be the same for all
- # invocations of the function with the same key by a particular
- # sender.
- padded_nonce = b'\x00\x00\x00\x00' + nonce
- elif len(nonce) == 12:
- padded_nonce = nonce
- else:
- raise ValueError("Poly1305 with ChaCha20 requires an 8- or 12-byte nonce")
-
- rs = new(key=key, nonce=padded_nonce).encrypt(b'\x00' * 32)
- return rs[:16], rs[16:], nonce
-
-
-def new(**kwargs):
- """Create a new ChaCha20 or XChaCha20 cipher
-
- Keyword Args:
- key (bytes/bytearray/memoryview): The secret key to use.
- It must be 32 bytes long.
- nonce (bytes/bytearray/memoryview): A mandatory value that
- must never be reused for any other encryption
- done with this key.
-
- For ChaCha20, it must be 8 or 12 bytes long.
-
- For XChaCha20, it must be 24 bytes long.
-
- If not provided, 8 bytes will be randomly generated
- (you can find them back in the ``nonce`` attribute).
-
- :Return: a :class:`Crypto.Cipher.ChaCha20.ChaCha20Cipher` object
- """
-
- try:
- key = kwargs.pop("key")
- except KeyError as e:
- raise TypeError("Missing parameter %s" % e)
-
- nonce = kwargs.pop("nonce", None)
- if nonce is None:
- nonce = get_random_bytes(8)
-
- if len(key) != 32:
- raise ValueError("ChaCha20/XChaCha20 key must be 32 bytes long")
-
- if len(nonce) not in (8, 12, 24):
- raise ValueError("Nonce must be 8/12 bytes(ChaCha20) or 24 bytes (XChaCha20)")
-
- if kwargs:
- raise TypeError("Unknown parameters: " + str(kwargs))
-
- return ChaCha20Cipher(key, nonce)
-
-# Size of a data block (in bytes)
-block_size = 1
-
-# Size of a key (in bytes)
-key_size = 32
diff --git a/spaces/arxify/RVC-beta-v2-0618/runtime/Lib/site-packages/Cython/Shadow.py b/spaces/arxify/RVC-beta-v2-0618/runtime/Lib/site-packages/Cython/Shadow.py
deleted file mode 100644
index cc8c9b60ad5d5191f5e9d17e0c56e32714bfe219..0000000000000000000000000000000000000000
--- a/spaces/arxify/RVC-beta-v2-0618/runtime/Lib/site-packages/Cython/Shadow.py
+++ /dev/null
@@ -1,474 +0,0 @@
-# cython.* namespace for pure mode.
-from __future__ import absolute_import
-
-__version__ = "0.29.30"
-
-try:
- from __builtin__ import basestring
-except ImportError:
- basestring = str
-
-
-# BEGIN shameless copy from Cython/minivect/minitypes.py
-
-class _ArrayType(object):
-
- is_array = True
- subtypes = ['dtype']
-
- def __init__(self, dtype, ndim, is_c_contig=False, is_f_contig=False,
- inner_contig=False, broadcasting=None):
- self.dtype = dtype
- self.ndim = ndim
- self.is_c_contig = is_c_contig
- self.is_f_contig = is_f_contig
- self.inner_contig = inner_contig or is_c_contig or is_f_contig
- self.broadcasting = broadcasting
-
- def __repr__(self):
- axes = [":"] * self.ndim
- if self.is_c_contig:
- axes[-1] = "::1"
- elif self.is_f_contig:
- axes[0] = "::1"
-
- return "%s[%s]" % (self.dtype, ", ".join(axes))
-
-
-def index_type(base_type, item):
- """
- Support array type creation by slicing, e.g. double[:, :] specifies
- a 2D strided array of doubles. The syntax is the same as for
- Cython memoryviews.
- """
- class InvalidTypeSpecification(Exception):
- pass
-
- def verify_slice(s):
- if s.start or s.stop or s.step not in (None, 1):
- raise InvalidTypeSpecification(
- "Only a step of 1 may be provided to indicate C or "
- "Fortran contiguity")
-
- if isinstance(item, tuple):
- step_idx = None
- for idx, s in enumerate(item):
- verify_slice(s)
- if s.step and (step_idx or idx not in (0, len(item) - 1)):
- raise InvalidTypeSpecification(
- "Step may only be provided once, and only in the "
- "first or last dimension.")
-
- if s.step == 1:
- step_idx = idx
-
- return _ArrayType(base_type, len(item),
- is_c_contig=step_idx == len(item) - 1,
- is_f_contig=step_idx == 0)
- elif isinstance(item, slice):
- verify_slice(item)
- return _ArrayType(base_type, 1, is_c_contig=bool(item.step))
- else:
- # int[8] etc.
- assert int(item) == item # array size must be a plain integer
- array(base_type, item)
-
-# END shameless copy
-
-
-compiled = False
-
-_Unspecified = object()
-
-# Function decorators
-
-def _empty_decorator(x):
- return x
-
-def locals(**arg_types):
- return _empty_decorator
-
-def test_assert_path_exists(*paths):
- return _empty_decorator
-
-def test_fail_if_path_exists(*paths):
- return _empty_decorator
-
-class _EmptyDecoratorAndManager(object):
- def __call__(self, x):
- return x
- def __enter__(self):
- pass
- def __exit__(self, exc_type, exc_value, traceback):
- pass
-
-class _Optimization(object):
- pass
-
-cclass = ccall = cfunc = _EmptyDecoratorAndManager()
-
-returns = wraparound = boundscheck = initializedcheck = nonecheck = \
- embedsignature = cdivision = cdivision_warnings = \
- always_allows_keywords = profile = linetrace = infer_types = \
- unraisable_tracebacks = freelist = \
- lambda _: _EmptyDecoratorAndManager()
-
-exceptval = lambda _=None, check=True: _EmptyDecoratorAndManager()
-
-overflowcheck = lambda _: _EmptyDecoratorAndManager()
-optimization = _Optimization()
-
-overflowcheck.fold = optimization.use_switch = \
- optimization.unpack_method_calls = lambda arg: _EmptyDecoratorAndManager()
-
-final = internal = type_version_tag = no_gc_clear = no_gc = _empty_decorator
-
-binding = lambda _: _empty_decorator
-
-
-_cython_inline = None
-def inline(f, *args, **kwds):
- if isinstance(f, basestring):
- global _cython_inline
- if _cython_inline is None:
- from Cython.Build.Inline import cython_inline as _cython_inline
- return _cython_inline(f, *args, **kwds)
- else:
- assert len(args) == len(kwds) == 0
- return f
-
-
-def compile(f):
- from Cython.Build.Inline import RuntimeCompiledFunction
- return RuntimeCompiledFunction(f)
-
-
-# Special functions
-
-def cdiv(a, b):
- q = a / b
- if q < 0:
- q += 1
- return q
-
-def cmod(a, b):
- r = a % b
- if (a*b) < 0:
- r -= b
- return r
-
-
-# Emulated language constructs
-
-def cast(type, *args, **kwargs):
- kwargs.pop('typecheck', None)
- assert not kwargs
- if hasattr(type, '__call__'):
- return type(*args)
- else:
- return args[0]
-
-def sizeof(arg):
- return 1
-
-def typeof(arg):
- return arg.__class__.__name__
- # return type(arg)
-
-def address(arg):
- return pointer(type(arg))([arg])
-
-def declare(type=None, value=_Unspecified, **kwds):
- if type not in (None, object) and hasattr(type, '__call__'):
- if value is not _Unspecified:
- return type(value)
- else:
- return type()
- else:
- return value
-
-class _nogil(object):
- """Support for 'with nogil' statement and @nogil decorator.
- """
- def __call__(self, x):
- if callable(x):
- # Used as function decorator => return the function unchanged.
- return x
- # Used as conditional context manager or to create an "@nogil(True/False)" decorator => keep going.
- return self
-
- def __enter__(self):
- pass
- def __exit__(self, exc_class, exc, tb):
- return exc_class is None
-
-nogil = _nogil()
-gil = _nogil()
-del _nogil
-
-
-# Emulated types
-
-class CythonMetaType(type):
-
- def __getitem__(type, ix):
- return array(type, ix)
-
-CythonTypeObject = CythonMetaType('CythonTypeObject', (object,), {})
-
-class CythonType(CythonTypeObject):
-
- def _pointer(self, n=1):
- for i in range(n):
- self = pointer(self)
- return self
-
-class PointerType(CythonType):
-
- def __init__(self, value=None):
- if isinstance(value, (ArrayType, PointerType)):
- self._items = [cast(self._basetype, a) for a in value._items]
- elif isinstance(value, list):
- self._items = [cast(self._basetype, a) for a in value]
- elif value is None or value == 0:
- self._items = []
- else:
- raise ValueError
-
- def __getitem__(self, ix):
- if ix < 0:
- raise IndexError("negative indexing not allowed in C")
- return self._items[ix]
-
- def __setitem__(self, ix, value):
- if ix < 0:
- raise IndexError("negative indexing not allowed in C")
- self._items[ix] = cast(self._basetype, value)
-
- def __eq__(self, value):
- if value is None and not self._items:
- return True
- elif type(self) != type(value):
- return False
- else:
- return not self._items and not value._items
-
- def __repr__(self):
- return "%s *" % (self._basetype,)
-
-class ArrayType(PointerType):
-
- def __init__(self):
- self._items = [None] * self._n
-
-
-class StructType(CythonType):
-
- def __init__(self, cast_from=_Unspecified, **data):
- if cast_from is not _Unspecified:
- # do cast
- if len(data) > 0:
- raise ValueError('Cannot accept keyword arguments when casting.')
- if type(cast_from) is not type(self):
- raise ValueError('Cannot cast from %s'%cast_from)
- for key, value in cast_from.__dict__.items():
- setattr(self, key, value)
- else:
- for key, value in data.items():
- setattr(self, key, value)
-
- def __setattr__(self, key, value):
- if key in self._members:
- self.__dict__[key] = cast(self._members[key], value)
- else:
- raise AttributeError("Struct has no member '%s'" % key)
-
-
-class UnionType(CythonType):
-
- def __init__(self, cast_from=_Unspecified, **data):
- if cast_from is not _Unspecified:
- # do type cast
- if len(data) > 0:
- raise ValueError('Cannot accept keyword arguments when casting.')
- if isinstance(cast_from, dict):
- datadict = cast_from
- elif type(cast_from) is type(self):
- datadict = cast_from.__dict__
- else:
- raise ValueError('Cannot cast from %s'%cast_from)
- else:
- datadict = data
- if len(datadict) > 1:
- raise AttributeError("Union can only store one field at a time.")
- for key, value in datadict.items():
- setattr(self, key, value)
-
- def __setattr__(self, key, value):
- if key == '__dict__':
- CythonType.__setattr__(self, key, value)
- elif key in self._members:
- self.__dict__ = {key: cast(self._members[key], value)}
- else:
- raise AttributeError("Union has no member '%s'" % key)
-
-def pointer(basetype):
- class PointerInstance(PointerType):
- _basetype = basetype
- return PointerInstance
-
-def array(basetype, n):
- class ArrayInstance(ArrayType):
- _basetype = basetype
- _n = n
- return ArrayInstance
-
-def struct(**members):
- class StructInstance(StructType):
- _members = members
- for key in members:
- setattr(StructInstance, key, None)
- return StructInstance
-
-def union(**members):
- class UnionInstance(UnionType):
- _members = members
- for key in members:
- setattr(UnionInstance, key, None)
- return UnionInstance
-
-class typedef(CythonType):
-
- def __init__(self, type, name=None):
- self._basetype = type
- self.name = name
-
- def __call__(self, *arg):
- value = cast(self._basetype, *arg)
- return value
-
- def __repr__(self):
- return self.name or str(self._basetype)
-
- __getitem__ = index_type
-
-class _FusedType(CythonType):
- pass
-
-
-def fused_type(*args):
- if not args:
- raise TypeError("Expected at least one type as argument")
-
- # Find the numeric type with biggest rank if all types are numeric
- rank = -1
- for type in args:
- if type not in (py_int, py_long, py_float, py_complex):
- break
-
- if type_ordering.index(type) > rank:
- result_type = type
- else:
- return result_type
-
- # Not a simple numeric type, return a fused type instance. The result
- # isn't really meant to be used, as we can't keep track of the context in
- # pure-mode. Casting won't do anything in this case.
- return _FusedType()
-
-
-def _specialized_from_args(signatures, args, kwargs):
- "Perhaps this should be implemented in a TreeFragment in Cython code"
- raise Exception("yet to be implemented")
-
-
-py_int = typedef(int, "int")
-try:
- py_long = typedef(long, "long")
-except NameError: # Py3
- py_long = typedef(int, "long")
-py_float = typedef(float, "float")
-py_complex = typedef(complex, "double complex")
-
-
-# Predefined types
-
-int_types = ['char', 'short', 'Py_UNICODE', 'int', 'Py_UCS4', 'long', 'longlong', 'Py_ssize_t', 'size_t']
-float_types = ['longdouble', 'double', 'float']
-complex_types = ['longdoublecomplex', 'doublecomplex', 'floatcomplex', 'complex']
-other_types = ['bint', 'void', 'Py_tss_t']
-
-to_repr = {
- 'longlong': 'long long',
- 'longdouble': 'long double',
- 'longdoublecomplex': 'long double complex',
- 'doublecomplex': 'double complex',
- 'floatcomplex': 'float complex',
-}.get
-
-gs = globals()
-
-# note: cannot simply name the unicode type here as 2to3 gets in the way and replaces it by str
-try:
- import __builtin__ as builtins
-except ImportError: # Py3
- import builtins
-
-gs['unicode'] = typedef(getattr(builtins, 'unicode', str), 'unicode')
-del builtins
-
-for name in int_types:
- reprname = to_repr(name, name)
- gs[name] = typedef(py_int, reprname)
- if name not in ('Py_UNICODE', 'Py_UCS4') and not name.endswith('size_t'):
- gs['u'+name] = typedef(py_int, "unsigned " + reprname)
- gs['s'+name] = typedef(py_int, "signed " + reprname)
-
-for name in float_types:
- gs[name] = typedef(py_float, to_repr(name, name))
-
-for name in complex_types:
- gs[name] = typedef(py_complex, to_repr(name, name))
-
-bint = typedef(bool, "bint")
-void = typedef(None, "void")
-Py_tss_t = typedef(None, "Py_tss_t")
-
-for t in int_types + float_types + complex_types + other_types:
- for i in range(1, 4):
- gs["%s_%s" % ('p'*i, t)] = gs[t]._pointer(i)
-
-NULL = gs['p_void'](0)
-
-# looks like 'gs' has some users out there by now...
-#del gs
-
-integral = floating = numeric = _FusedType()
-
-type_ordering = [py_int, py_long, py_float, py_complex]
-
-class CythonDotParallel(object):
- """
- The cython.parallel module.
- """
-
- __all__ = ['parallel', 'prange', 'threadid']
-
- def parallel(self, num_threads=None):
- return nogil
-
- def prange(self, start=0, stop=None, step=1, nogil=False, schedule=None, chunksize=None, num_threads=None):
- if stop is None:
- stop = start
- start = 0
- return range(start, stop, step)
-
- def threadid(self):
- return 0
-
- # def threadsavailable(self):
- # return 1
-
-import sys
-sys.modules['cython.parallel'] = CythonDotParallel()
-del sys
diff --git a/spaces/asbeabi/PoCs/README.md b/spaces/asbeabi/PoCs/README.md
deleted file mode 100644
index a7f18c5f6e7b22ca031dafe61d6005b150ff39ad..0000000000000000000000000000000000000000
--- a/spaces/asbeabi/PoCs/README.md
+++ /dev/null
@@ -1,11 +0,0 @@
----
-title: PoCs
-emoji: 🦀
-colorFrom: green
-colorTo: blue
-sdk: static
-pinned: false
-license: openrail
----
-
-Check out the configuration reference at https://huggingface.co/docs/hub/spaces-config-reference
diff --git a/spaces/atticus/image-text-retrival-huster/scripts/dataset.py b/spaces/atticus/image-text-retrival-huster/scripts/dataset.py
deleted file mode 100644
index b52c732dfc9005a1186f7879b924098e2313120e..0000000000000000000000000000000000000000
--- a/spaces/atticus/image-text-retrival-huster/scripts/dataset.py
+++ /dev/null
@@ -1,178 +0,0 @@
-# make.texts.py
-from __future__ import print_function
-import os
-import os.path as osp
-from pycocotools.coco import COCO
-# import gensim
-# from gensim.models import Doc2Vec
-import numpy as np
-import scipy.io as sio
-import os
-import os.path as osp
-from pycocotools.coco import COCO
-import pprint
-import os
-import os.path as osp
-import json
-from nltk.tokenize import RegexpTokenizer
-from tqdm import tqdm
-
-"""process texts
-python 2 needed by `jhlau/doc2vec`, and COCO api CAN work with python 2.7.
-So I choose to create a virtual env of python 2.7.
-
-dependencies:
- matplotlib (COCO api)
- smart_open (gensim)
-"""
-
-# COCO 原本的 annotations 中就有各 classes 的 ID,但不连续(从 1 标到 90 但实际只有 80 个)。这里按原有的 category id 的升序重新定义连续的、0-based 的 class ID。
-# train 和 val 都包含所有类,所以这里只用 val set 处理。
-# 结果写入 class-name.COCO.txt
-
-def remake_classname():
- """process class order
- Record the mapping between tightened/discretized 0-base class ID,
- original class ID and class name in `class-name.COCO.txt`,
- with format ``.
-
- The class order is consistent to the ascending order of the original IDs.
- """
-
- COCO_P = "/dataset/coco"
- ANNO_P = osp.join(COCO_P, "annotations")
- SPLIT = ["val", "train"]
-
- for _split in SPLIT:
- print("---", _split, "---")
- anno_file = osp.join(ANNO_P, "instances_{}2017.json".format(_split))
- coco = COCO(anno_file)
- cats = coco.loadCats(coco.getCatIds())
- # print(cats[0])
- cls_id = {c["name"]: c["id"] for c in cats} # 它本身就是按 category id 升序
- # pprint.pprint(cls_id)
- with open("class-name.COCO.txt", "w") as f:
- for new_id, c in enumerate(cls_id):
- old_id = cls_id[c]# - 1
- cn = c.replace(" ", "_")
- # format:
- f.write("{} {} {}\n".format(new_id, old_id, cn))
-
- break # 只用 val set
-
-def remake_idmap():
- # 合并 train、val 两个集合,统一按原本的 id(即 images 文件名中的数字,也是不连续的,且 train、val 无重合)升序重新排 0-based 的 data ID。
- # 结果写入 id-map.COCO.txt
- # make.id-map.py
- """discretization of the original file ID
- Map the file ID to sequential {0, 1, ..., n},
- and record this mapping in `id-map.txt`,
- with format ``.
-
- Note that the new ids are 0-base.
- """
-
- TRAIN_P = "train2017"
- VAL_P = "val2017"
-
- file_list = [f for f in os.listdir(os.path.join("/dataset/coco", TRAIN_P)) if (".jpg" in f)]
- file_list.extend([f for f in os.listdir(os.path.join("/dataset/coco", VAL_P)) if (".jpg" in f)])
- print("#data:", len(file_list)) # 12,3287
-
- id_key = lambda x: int(x.split(".jpg")[0])
- file_list = sorted(file_list, key=id_key) # 按 image ID 升序
- # print(file_list[:15])
-
- with open("id-map.COCO.txt", "w") as f:
- # format:
- for i, f_name in enumerate(file_list):
- _original_id = id_key(f_name)
- f.write("{} {} {}\n".format(i, _original_id, f_name))
- # if i > 5: break
- print("DONE")
-
-
-# COCO
-COCO_P = "/dataset/coco"
-ANNO_P = osp.join(COCO_P, "annotations")
-SPLIT = ["val", "train"]
-# doc2vec
-MODEL = "/home/dataset/Doc2Vec/enwiki_dbow/doc2vec.bin"
-start_alpha = 0.01
-infer_epoch = 1000
-DIM = 300 # dimension of the doc2vec feature
-# id_map_data = {}
-# with open("id-map.txt", "r") as f:
-# for line in f:
-# line = line.strip()
-# _new_id, _old_id, _ = line.split()
-# id_map_data[int(_old_id)] = int(_new_id)
-# N_DATA = len(id_map_data)
-# print("#data:", N_DATA)
-
-# pre-trained Doc2Vec model
-# model = Doc2Vec.load(MODEL)
-tokenizer = RegexpTokenizer(r'\w+')
-def dataset_format(filepath, filename, imgid, split, sentences, cocoid):
- data = {}
- data['filepath'] = filepath
- data['sentids'] = [imgid * 5 + idx for idx in range(5)]
- data['filename'] = filename
- data['imgid'] = imgid
- data['split'] = split
- data['sentences'] = [{'tokens': tokenizer.tokenize(sentence),
- 'raw': sentence,
- 'imgid': imgid,
- 'sentid': imgid * 5 + idx}
- for idx, sentence in enumerate(sentences)]
- data['cocoid'] = cocoid
- return data
-
-dataset_anns = {}
-dataset_anns['images'] = []
-dataset_anns['dataset'] = 'coco'
-for __split in SPLIT:
- print("---", __split, "---")
- anno_file = osp.join(ANNO_P, "instances_{}2017.json".format(__split))
- caps_file = osp.join(ANNO_P, "captions_{}2017.json".format(__split))
- coco = COCO(anno_file)
- coco_caps = COCO(caps_file)
- new_image_id_file = open("id-map.COCO.txt", 'r')
- new_img_id_map = {image_id.strip().split(" ")[2]: image_id.strip().split(" ")[0] for image_id in new_image_id_file.readlines()}
- id_list = coco.getImgIds()
- for _old_id in tqdm(id_list):
- # _new_id = id_map_data[_old_id]
- _annIds = coco_caps.getAnnIds(imgIds=_old_id)
- _anns = coco_caps.loadAnns(_annIds)
-
- _filepath = __split + '2017'
- _filename = coco.imgs[_old_id]['file_name']
- _imgid = int(new_img_id_map[_filename])
- _split = __split
- # print(len(anns))
- # pprint.pprint(anns)
- _sentences = [_a["caption"] for _a in _anns]
- _cocoid = _old_id
- formated_data = dataset_format(_filepath, _filename, _imgid, _split, _sentences, _cocoid)
- dataset_anns['images'].append(formated_data)
- # pprint.pprint(sentences)
- # sentences = [gensim.utils.simple_preprocess(s) for s in sentences]
- # pprint.pprint(sentences)
- # doc = []
- # for s in sentences:
- # doc.extend(s)
- # print(doc)
- # vec = model.infer_vector(doc)
- # print(vec.shape)
- # texts.append(vec[np.newaxis, :])
- # break
- # break
-
-with open('dataset_anns.json', 'w') as fp:
- json.dump(dataset_anns, fp)
-
-new_image_id_file.close()
-
-# texts = np.vstack(texts).astype(np.float32)
-# print("texts:", texts.shape, texts.dtype) # (123287, 300) dtype('
-
-# [Optional] Uncomment this line to install global node packages.
-# RUN su vscode -c "source /usr/local/share/nvm/nvm.sh && npm install -g " 2>&1
diff --git a/spaces/banana-projects/web3d/node_modules/three/examples/js/loaders/VTKLoader.js b/spaces/banana-projects/web3d/node_modules/three/examples/js/loaders/VTKLoader.js
deleted file mode 100644
index c4e319e472f3db60e99cf67751b5430ee992552f..0000000000000000000000000000000000000000
--- a/spaces/banana-projects/web3d/node_modules/three/examples/js/loaders/VTKLoader.js
+++ /dev/null
@@ -1,1162 +0,0 @@
-/**
- * @author mrdoob / http://mrdoob.com/
- * @author Alex Pletzer
- *
- * Updated on 22.03.2017
- * VTK header is now parsed and used to extract all the compressed data
- * @author Andrii Iudin https://github.com/andreyyudin
- * @author Paul Kibet Korir https://github.com/polarise
- * @author Sriram Somasundharam https://github.com/raamssundar
- */
-
-THREE.VTKLoader = function ( manager ) {
-
- this.manager = ( manager !== undefined ) ? manager : THREE.DefaultLoadingManager;
-
-};
-
-Object.assign( THREE.VTKLoader.prototype, THREE.EventDispatcher.prototype, {
-
- load: function ( url, onLoad, onProgress, onError ) {
-
- var scope = this;
-
- var loader = new THREE.FileLoader( scope.manager );
- loader.setPath( scope.path );
- loader.setResponseType( 'arraybuffer' );
- loader.load( url, function ( text ) {
-
- onLoad( scope.parse( text ) );
-
- }, onProgress, onError );
-
- },
-
- setPath: function ( value ) {
-
- this.path = value;
- return this;
-
- },
-
- parse: function ( data ) {
-
- function parseASCII( data ) {
-
- // connectivity of the triangles
- var indices = [];
-
- // triangles vertices
- var positions = [];
-
- // red, green, blue colors in the range 0 to 1
- var colors = [];
-
- // normal vector, one per vertex
- var normals = [];
-
- var result;
-
- // pattern for reading vertices, 3 floats or integers
- var pat3Floats = /(\-?\d+\.?[\d\-\+e]*)\s+(\-?\d+\.?[\d\-\+e]*)\s+(\-?\d+\.?[\d\-\+e]*)/g;
-
- // pattern for connectivity, an integer followed by any number of ints
- // the first integer is the number of polygon nodes
- var patConnectivity = /^(\d+)\s+([\s\d]*)/;
-
- // indicates start of vertex data section
- var patPOINTS = /^POINTS /;
-
- // indicates start of polygon connectivity section
- var patPOLYGONS = /^POLYGONS /;
-
- // indicates start of triangle strips section
- var patTRIANGLE_STRIPS = /^TRIANGLE_STRIPS /;
-
- // POINT_DATA number_of_values
- var patPOINT_DATA = /^POINT_DATA[ ]+(\d+)/;
-
- // CELL_DATA number_of_polys
- var patCELL_DATA = /^CELL_DATA[ ]+(\d+)/;
-
- // Start of color section
- var patCOLOR_SCALARS = /^COLOR_SCALARS[ ]+(\w+)[ ]+3/;
-
- // NORMALS Normals float
- var patNORMALS = /^NORMALS[ ]+(\w+)[ ]+(\w+)/;
-
- var inPointsSection = false;
- var inPolygonsSection = false;
- var inTriangleStripSection = false;
- var inPointDataSection = false;
- var inCellDataSection = false;
- var inColorSection = false;
- var inNormalsSection = false;
-
- var lines = data.split( '\n' );
-
- for ( var i in lines ) {
-
- var line = lines[ i ];
-
- if ( inPointsSection ) {
-
- // get the vertices
- while ( ( result = pat3Floats.exec( line ) ) !== null ) {
-
- var x = parseFloat( result[ 1 ] );
- var y = parseFloat( result[ 2 ] );
- var z = parseFloat( result[ 3 ] );
- positions.push( x, y, z );
-
- }
-
- } else if ( inPolygonsSection ) {
-
- if ( ( result = patConnectivity.exec( line ) ) !== null ) {
-
- // numVertices i0 i1 i2 ...
- var numVertices = parseInt( result[ 1 ] );
- var inds = result[ 2 ].split( /\s+/ );
-
- if ( numVertices >= 3 ) {
-
- var i0 = parseInt( inds[ 0 ] );
- var i1, i2;
- var k = 1;
- // split the polygon in numVertices - 2 triangles
- for ( var j = 0; j < numVertices - 2; ++ j ) {
-
- i1 = parseInt( inds[ k ] );
- i2 = parseInt( inds[ k + 1 ] );
- indices.push( i0, i1, i2 );
- k ++;
-
- }
-
- }
-
- }
-
- } else if ( inTriangleStripSection ) {
-
- if ( ( result = patConnectivity.exec( line ) ) !== null ) {
-
- // numVertices i0 i1 i2 ...
- var numVertices = parseInt( result[ 1 ] );
- var inds = result[ 2 ].split( /\s+/ );
-
- if ( numVertices >= 3 ) {
-
- var i0, i1, i2;
- // split the polygon in numVertices - 2 triangles
- for ( var j = 0; j < numVertices - 2; j ++ ) {
-
- if ( j % 2 === 1 ) {
-
- i0 = parseInt( inds[ j ] );
- i1 = parseInt( inds[ j + 2 ] );
- i2 = parseInt( inds[ j + 1 ] );
- indices.push( i0, i1, i2 );
-
- } else {
-
- i0 = parseInt( inds[ j ] );
- i1 = parseInt( inds[ j + 1 ] );
- i2 = parseInt( inds[ j + 2 ] );
- indices.push( i0, i1, i2 );
-
- }
-
- }
-
- }
-
- }
-
- } else if ( inPointDataSection || inCellDataSection ) {
-
- if ( inColorSection ) {
-
- // Get the colors
-
- while ( ( result = pat3Floats.exec( line ) ) !== null ) {
-
- var r = parseFloat( result[ 1 ] );
- var g = parseFloat( result[ 2 ] );
- var b = parseFloat( result[ 3 ] );
- colors.push( r, g, b );
-
- }
-
- } else if ( inNormalsSection ) {
-
- // Get the normal vectors
-
- while ( ( result = pat3Floats.exec( line ) ) !== null ) {
-
- var nx = parseFloat( result[ 1 ] );
- var ny = parseFloat( result[ 2 ] );
- var nz = parseFloat( result[ 3 ] );
- normals.push( nx, ny, nz );
-
- }
-
- }
-
- }
-
- if ( patPOLYGONS.exec( line ) !== null ) {
-
- inPolygonsSection = true;
- inPointsSection = false;
- inTriangleStripSection = false;
-
- } else if ( patPOINTS.exec( line ) !== null ) {
-
- inPolygonsSection = false;
- inPointsSection = true;
- inTriangleStripSection = false;
-
- } else if ( patTRIANGLE_STRIPS.exec( line ) !== null ) {
-
- inPolygonsSection = false;
- inPointsSection = false;
- inTriangleStripSection = true;
-
- } else if ( patPOINT_DATA.exec( line ) !== null ) {
-
- inPointDataSection = true;
- inPointsSection = false;
- inPolygonsSection = false;
- inTriangleStripSection = false;
-
- } else if ( patCELL_DATA.exec( line ) !== null ) {
-
- inCellDataSection = true;
- inPointsSection = false;
- inPolygonsSection = false;
- inTriangleStripSection = false;
-
- } else if ( patCOLOR_SCALARS.exec( line ) !== null ) {
-
- inColorSection = true;
- inNormalsSection = false;
- inPointsSection = false;
- inPolygonsSection = false;
- inTriangleStripSection = false;
-
- } else if ( patNORMALS.exec( line ) !== null ) {
-
- inNormalsSection = true;
- inColorSection = false;
- inPointsSection = false;
- inPolygonsSection = false;
- inTriangleStripSection = false;
-
- }
-
- }
-
- var geometry = new THREE.BufferGeometry();
- geometry.setIndex( indices );
- geometry.addAttribute( 'position', new THREE.Float32BufferAttribute( positions, 3 ) );
-
- if ( normals.length === positions.length ) {
-
- geometry.addAttribute( 'normal', new THREE.Float32BufferAttribute( normals, 3 ) );
-
- }
-
- if ( colors.length !== indices.length ) {
-
- // stagger
-
- if ( colors.length === positions.length ) {
-
- geometry.addAttribute( 'color', new THREE.Float32BufferAttribute( colors, 3 ) );
-
- }
-
- } else {
-
- // cell
-
- geometry = geometry.toNonIndexed();
- var numTriangles = geometry.attributes.position.count / 3;
-
- if ( colors.length === ( numTriangles * 3 ) ) {
-
- var newColors = [];
-
- for ( var i = 0; i < numTriangles; i ++ ) {
-
- var r = colors[ 3 * i + 0 ];
- var g = colors[ 3 * i + 1 ];
- var b = colors[ 3 * i + 2 ];
-
- newColors.push( r, g, b );
- newColors.push( r, g, b );
- newColors.push( r, g, b );
-
- }
-
- geometry.addAttribute( 'color', new THREE.Float32BufferAttribute( newColors, 3 ) );
-
- }
-
- }
-
- return geometry;
-
- }
-
- function parseBinary( data ) {
-
- var count, pointIndex, i, numberOfPoints, s;
- var buffer = new Uint8Array( data );
- var dataView = new DataView( data );
-
- // Points and normals, by default, are empty
- var points = [];
- var normals = [];
- var indices = [];
-
- // Going to make a big array of strings
- var vtk = [];
- var index = 0;
-
- function findString( buffer, start ) {
-
- var index = start;
- var c = buffer[ index ];
- var s = [];
- while ( c !== 10 ) {
-
- s.push( String.fromCharCode( c ) );
- index ++;
- c = buffer[ index ];
-
- }
-
- return { start: start,
- end: index,
- next: index + 1,
- parsedString: s.join( '' ) };
-
- }
-
- var state, line;
-
- while ( true ) {
-
- // Get a string
- state = findString( buffer, index );
- line = state.parsedString;
-
- if ( line.indexOf( 'POINTS' ) === 0 ) {
-
- vtk.push( line );
- // Add the points
- numberOfPoints = parseInt( line.split( ' ' )[ 1 ], 10 );
-
- // Each point is 3 4-byte floats
- count = numberOfPoints * 4 * 3;
-
- points = new Float32Array( numberOfPoints * 3 );
-
- pointIndex = state.next;
- for ( i = 0; i < numberOfPoints; i ++ ) {
-
- points[ 3 * i ] = dataView.getFloat32( pointIndex, false );
- points[ 3 * i + 1 ] = dataView.getFloat32( pointIndex + 4, false );
- points[ 3 * i + 2 ] = dataView.getFloat32( pointIndex + 8, false );
- pointIndex = pointIndex + 12;
-
- }
- // increment our next pointer
- state.next = state.next + count + 1;
-
- } else if ( line.indexOf( 'TRIANGLE_STRIPS' ) === 0 ) {
-
- var numberOfStrips = parseInt( line.split( ' ' )[ 1 ], 10 );
- var size = parseInt( line.split( ' ' )[ 2 ], 10 );
- // 4 byte integers
- count = size * 4;
-
- indices = new Uint32Array( 3 * size - 9 * numberOfStrips );
- var indicesIndex = 0;
-
- pointIndex = state.next;
- for ( i = 0; i < numberOfStrips; i ++ ) {
-
- // For each strip, read the first value, then record that many more points
- var indexCount = dataView.getInt32( pointIndex, false );
- var strip = [];
- pointIndex += 4;
- for ( s = 0; s < indexCount; s ++ ) {
-
- strip.push( dataView.getInt32( pointIndex, false ) );
- pointIndex += 4;
-
- }
-
- // retrieves the n-2 triangles from the triangle strip
- for ( var j = 0; j < indexCount - 2; j ++ ) {
-
- if ( j % 2 ) {
-
- indices[ indicesIndex ++ ] = strip[ j ];
- indices[ indicesIndex ++ ] = strip[ j + 2 ];
- indices[ indicesIndex ++ ] = strip[ j + 1 ];
-
- } else {
-
-
- indices[ indicesIndex ++ ] = strip[ j ];
- indices[ indicesIndex ++ ] = strip[ j + 1 ];
- indices[ indicesIndex ++ ] = strip[ j + 2 ];
-
- }
-
- }
-
- }
- // increment our next pointer
- state.next = state.next + count + 1;
-
- } else if ( line.indexOf( 'POLYGONS' ) === 0 ) {
-
- var numberOfStrips = parseInt( line.split( ' ' )[ 1 ], 10 );
- var size = parseInt( line.split( ' ' )[ 2 ], 10 );
- // 4 byte integers
- count = size * 4;
-
- indices = new Uint32Array( 3 * size - 9 * numberOfStrips );
- var indicesIndex = 0;
-
- pointIndex = state.next;
- for ( i = 0; i < numberOfStrips; i ++ ) {
-
- // For each strip, read the first value, then record that many more points
- var indexCount = dataView.getInt32( pointIndex, false );
- var strip = [];
- pointIndex += 4;
- for ( s = 0; s < indexCount; s ++ ) {
-
- strip.push( dataView.getInt32( pointIndex, false ) );
- pointIndex += 4;
-
- }
-
- // divide the polygon in n-2 triangle
- for ( var j = 1; j < indexCount - 1; j ++ ) {
-
- indices[ indicesIndex ++ ] = strip[ 0 ];
- indices[ indicesIndex ++ ] = strip[ j ];
- indices[ indicesIndex ++ ] = strip[ j + 1 ];
-
- }
-
- }
- // increment our next pointer
- state.next = state.next + count + 1;
-
- } else if ( line.indexOf( 'POINT_DATA' ) === 0 ) {
-
- numberOfPoints = parseInt( line.split( ' ' )[ 1 ], 10 );
-
- // Grab the next line
- state = findString( buffer, state.next );
-
- // Now grab the binary data
- count = numberOfPoints * 4 * 3;
-
- normals = new Float32Array( numberOfPoints * 3 );
- pointIndex = state.next;
- for ( i = 0; i < numberOfPoints; i ++ ) {
-
- normals[ 3 * i ] = dataView.getFloat32( pointIndex, false );
- normals[ 3 * i + 1 ] = dataView.getFloat32( pointIndex + 4, false );
- normals[ 3 * i + 2 ] = dataView.getFloat32( pointIndex + 8, false );
- pointIndex += 12;
-
- }
-
- // Increment past our data
- state.next = state.next + count;
-
- }
-
- // Increment index
- index = state.next;
-
- if ( index >= buffer.byteLength ) {
-
- break;
-
- }
-
- }
-
- var geometry = new THREE.BufferGeometry();
- geometry.setIndex( new THREE.BufferAttribute( indices, 1 ) );
- geometry.addAttribute( 'position', new THREE.BufferAttribute( points, 3 ) );
-
- if ( normals.length === points.length ) {
-
- geometry.addAttribute( 'normal', new THREE.BufferAttribute( normals, 3 ) );
-
- }
-
- return geometry;
-
- }
-
- function Float32Concat( first, second ) {
-
- var firstLength = first.length, result = new Float32Array( firstLength + second.length );
-
- result.set( first );
- result.set( second, firstLength );
-
- return result;
-
- }
-
- function Int32Concat( first, second ) {
-
- var firstLength = first.length, result = new Int32Array( firstLength + second.length );
-
- result.set( first );
- result.set( second, firstLength );
-
- return result;
-
- }
-
- function parseXML( stringFile ) {
-
- // Changes XML to JSON, based on https://davidwalsh.name/convert-xml-json
-
- function xmlToJson( xml ) {
-
- // Create the return object
- var obj = {};
-
- if ( xml.nodeType === 1 ) { // element
-
- // do attributes
-
- if ( xml.attributes ) {
-
- if ( xml.attributes.length > 0 ) {
-
- obj[ 'attributes' ] = {};
-
- for ( var j = 0; j < xml.attributes.length; j ++ ) {
-
- var attribute = xml.attributes.item( j );
- obj[ 'attributes' ][ attribute.nodeName ] = attribute.nodeValue.trim();
-
- }
-
- }
-
- }
-
- } else if ( xml.nodeType === 3 ) { // text
-
- obj = xml.nodeValue.trim();
-
- }
-
- // do children
- if ( xml.hasChildNodes() ) {
-
- for ( var i = 0; i < xml.childNodes.length; i ++ ) {
-
- var item = xml.childNodes.item( i );
- var nodeName = item.nodeName;
-
- if ( typeof obj[ nodeName ] === 'undefined' ) {
-
- var tmp = xmlToJson( item );
-
- if ( tmp !== '' ) obj[ nodeName ] = tmp;
-
- } else {
-
- if ( typeof obj[ nodeName ].push === 'undefined' ) {
-
- var old = obj[ nodeName ];
- obj[ nodeName ] = [ old ];
-
- }
-
- var tmp = xmlToJson( item );
-
- if ( tmp !== '' ) obj[ nodeName ].push( tmp );
-
- }
-
- }
-
- }
-
- return obj;
-
- }
-
- // Taken from Base64-js
- function Base64toByteArray( b64 ) {
-
- var Arr = typeof Uint8Array !== 'undefined' ? Uint8Array : Array;
- var i;
- var lookup = [];
- var revLookup = [];
- var code = 'ABCDEFGHIJKLMNOPQRSTUVWXYZabcdefghijklmnopqrstuvwxyz0123456789+/';
- var len = code.length;
-
- for ( i = 0; i < len; i ++ ) {
-
- lookup[ i ] = code[ i ];
-
- }
-
- for ( i = 0; i < len; ++ i ) {
-
- revLookup[ code.charCodeAt( i ) ] = i;
-
- }
-
- revLookup[ '-'.charCodeAt( 0 ) ] = 62;
- revLookup[ '_'.charCodeAt( 0 ) ] = 63;
-
- var j, l, tmp, placeHolders, arr;
- var len = b64.length;
-
- if ( len % 4 > 0 ) {
-
- throw new Error( 'Invalid string. Length must be a multiple of 4' );
-
- }
-
- placeHolders = b64[ len - 2 ] === '=' ? 2 : b64[ len - 1 ] === '=' ? 1 : 0;
- arr = new Arr( len * 3 / 4 - placeHolders );
- l = placeHolders > 0 ? len - 4 : len;
-
- var L = 0;
-
- for ( i = 0, j = 0; i < l; i += 4, j += 3 ) {
-
- tmp = ( revLookup[ b64.charCodeAt( i ) ] << 18 ) | ( revLookup[ b64.charCodeAt( i + 1 ) ] << 12 ) | ( revLookup[ b64.charCodeAt( i + 2 ) ] << 6 ) | revLookup[ b64.charCodeAt( i + 3 ) ];
- arr[ L ++ ] = ( tmp & 0xFF0000 ) >> 16;
- arr[ L ++ ] = ( tmp & 0xFF00 ) >> 8;
- arr[ L ++ ] = tmp & 0xFF;
-
- }
-
- if ( placeHolders === 2 ) {
-
- tmp = ( revLookup[ b64.charCodeAt( i ) ] << 2 ) | ( revLookup[ b64.charCodeAt( i + 1 ) ] >> 4 );
- arr[ L ++ ] = tmp & 0xFF;
-
- } else if ( placeHolders === 1 ) {
-
- tmp = ( revLookup[ b64.charCodeAt( i ) ] << 10 ) | ( revLookup[ b64.charCodeAt( i + 1 ) ] << 4 ) | ( revLookup[ b64.charCodeAt( i + 2 ) ] >> 2 );
- arr[ L ++ ] = ( tmp >> 8 ) & 0xFF;
- arr[ L ++ ] = tmp & 0xFF;
-
- }
-
- return arr;
-
- }
-
- function parseDataArray( ele, compressed ) {
-
- var numBytes = 0;
-
- if ( json.attributes.header_type === 'UInt64' ) {
-
- numBytes = 8;
-
- } else if ( json.attributes.header_type === 'UInt32' ) {
-
- numBytes = 4;
-
- }
-
-
- // Check the format
- if ( ele.attributes.format === 'binary' && compressed ) {
-
- var rawData, content, byteData, blocks, cSizeStart, headerSize, padding, dataOffsets, currentOffset;
-
- if ( ele.attributes.type === 'Float32' ) {
-
- var txt = new Float32Array( );
-
- } else if ( ele.attributes.type === 'Int64' ) {
-
- var txt = new Int32Array( );
-
- }
-
- // VTP data with the header has the following structure:
- // [#blocks][#u-size][#p-size][#c-size-1][#c-size-2]...[#c-size-#blocks][DATA]
- //
- // Each token is an integer value whose type is specified by "header_type" at the top of the file (UInt32 if no type specified). The token meanings are:
- // [#blocks] = Number of blocks
- // [#u-size] = Block size before compression
- // [#p-size] = Size of last partial block (zero if it not needed)
- // [#c-size-i] = Size in bytes of block i after compression
- //
- // The [DATA] portion stores contiguously every block appended together. The offset from the beginning of the data section to the beginning of a block is
- // computed by summing the compressed block sizes from preceding blocks according to the header.
-
- rawData = ele[ '#text' ];
-
- byteData = Base64toByteArray( rawData );
-
- blocks = byteData[ 0 ];
- for ( var i = 1; i < numBytes - 1; i ++ ) {
-
- blocks = blocks | ( byteData[ i ] << ( i * numBytes ) );
-
- }
-
- headerSize = ( blocks + 3 ) * numBytes;
- padding = ( ( headerSize % 3 ) > 0 ) ? 3 - ( headerSize % 3 ) : 0;
- headerSize = headerSize + padding;
-
- dataOffsets = [];
- currentOffset = headerSize;
- dataOffsets.push( currentOffset );
-
- // Get the blocks sizes after the compression.
- // There are three blocks before c-size-i, so we skip 3*numBytes
- cSizeStart = 3 * numBytes;
-
- for ( var i = 0; i < blocks; i ++ ) {
-
- var currentBlockSize = byteData[ i * numBytes + cSizeStart ];
-
- for ( var j = 1; j < numBytes - 1; j ++ ) {
-
- // Each data point consists of 8 bytes regardless of the header type
- currentBlockSize = currentBlockSize | ( byteData[ i * numBytes + cSizeStart + j ] << ( j * 8 ) );
-
- }
-
- currentOffset = currentOffset + currentBlockSize;
- dataOffsets.push( currentOffset );
-
- }
-
- for ( var i = 0; i < dataOffsets.length - 1; i ++ ) {
-
- var inflate = new Zlib.Inflate( byteData.slice( dataOffsets[ i ], dataOffsets[ i + 1 ] ), { resize: true, verify: true } ); // eslint-disable-line no-undef
- content = inflate.decompress();
- content = content.buffer;
-
- if ( ele.attributes.type === 'Float32' ) {
-
- content = new Float32Array( content );
- txt = Float32Concat( txt, content );
-
- } else if ( ele.attributes.type === 'Int64' ) {
-
- content = new Int32Array( content );
- txt = Int32Concat( txt, content );
-
- }
-
- }
-
- delete ele[ '#text' ];
-
- if ( ele.attributes.type === 'Int64' ) {
-
- if ( ele.attributes.format === 'binary' ) {
-
- txt = txt.filter( function ( el, idx ) {
-
- if ( idx % 2 !== 1 ) return true;
-
- } );
-
- }
-
- }
-
- } else {
-
- if ( ele.attributes.format === 'binary' && ! compressed ) {
-
- var content = Base64toByteArray( ele[ '#text' ] );
-
- // VTP data for the uncompressed case has the following structure:
- // [#bytes][DATA]
- // where "[#bytes]" is an integer value specifying the number of bytes in the block of data following it.
- content = content.slice( numBytes ).buffer;
-
- } else {
-
- if ( ele[ '#text' ] ) {
-
- var content = ele[ '#text' ].split( /\s+/ ).filter( function ( el ) {
-
- if ( el !== '' ) return el;
-
- } );
-
- } else {
-
- var content = new Int32Array( 0 ).buffer;
-
- }
-
- }
-
- delete ele[ '#text' ];
-
- // Get the content and optimize it
- if ( ele.attributes.type === 'Float32' ) {
-
- var txt = new Float32Array( content );
-
- } else if ( ele.attributes.type === 'Int32' ) {
-
- var txt = new Int32Array( content );
-
- } else if ( ele.attributes.type === 'Int64' ) {
-
- var txt = new Int32Array( content );
-
- if ( ele.attributes.format === 'binary' ) {
-
- txt = txt.filter( function ( el, idx ) {
-
- if ( idx % 2 !== 1 ) return true;
-
- } );
-
- }
-
- }
-
- } // endif ( ele.attributes.format === 'binary' && compressed )
-
- return txt;
-
- }
-
- // Main part
- // Get Dom
- var dom = null;
-
- if ( window.DOMParser ) {
-
- try {
-
- dom = ( new DOMParser() ).parseFromString( stringFile, 'text/xml' );
-
- } catch ( e ) {
-
- dom = null;
-
- }
-
- } else if ( window.ActiveXObject ) {
-
- try {
-
- dom = new ActiveXObject( 'Microsoft.XMLDOM' ); // eslint-disable-line no-undef
- dom.async = false;
-
- if ( ! dom.loadXML( /* xml */ ) ) {
-
- throw new Error( dom.parseError.reason + dom.parseError.srcText );
-
- }
-
- } catch ( e ) {
-
- dom = null;
-
- }
-
- } else {
-
- throw new Error( 'Cannot parse xml string!' );
-
- }
-
- // Get the doc
- var doc = dom.documentElement;
- // Convert to json
- var json = xmlToJson( doc );
- var points = [];
- var normals = [];
- var indices = [];
-
- if ( json.PolyData ) {
-
- var piece = json.PolyData.Piece;
- var compressed = json.attributes.hasOwnProperty( 'compressor' );
-
- // Can be optimized
- // Loop through the sections
- var sections = [ 'PointData', 'Points', 'Strips', 'Polys' ];// +['CellData', 'Verts', 'Lines'];
- var sectionIndex = 0, numberOfSections = sections.length;
-
- while ( sectionIndex < numberOfSections ) {
-
- var section = piece[ sections[ sectionIndex ] ];
-
- // If it has a DataArray in it
-
- if ( section && section.DataArray ) {
-
- // Depending on the number of DataArrays
-
- if ( Object.prototype.toString.call( section.DataArray ) === '[object Array]' ) {
-
- var arr = section.DataArray;
-
- } else {
-
- var arr = [ section.DataArray ];
-
- }
-
- var dataArrayIndex = 0, numberOfDataArrays = arr.length;
-
- while ( dataArrayIndex < numberOfDataArrays ) {
-
- // Parse the DataArray
- if ( ( '#text' in arr[ dataArrayIndex ] ) && ( arr[ dataArrayIndex ][ '#text' ].length > 0 ) ) {
-
- arr[ dataArrayIndex ].text = parseDataArray( arr[ dataArrayIndex ], compressed );
-
- }
-
- dataArrayIndex ++;
-
- }
-
- switch ( sections[ sectionIndex ] ) {
-
- // if iti is point data
- case 'PointData':
-
- var numberOfPoints = parseInt( piece.attributes.NumberOfPoints );
- var normalsName = section.attributes.Normals;
-
- if ( numberOfPoints > 0 ) {
-
- for ( var i = 0, len = arr.length; i < len; i ++ ) {
-
- if ( normalsName === arr[ i ].attributes.Name ) {
-
- var components = arr[ i ].attributes.NumberOfComponents;
- normals = new Float32Array( numberOfPoints * components );
- normals.set( arr[ i ].text, 0 );
-
- }
-
- }
-
- }
-
- break;
-
- // if it is points
- case 'Points':
-
- var numberOfPoints = parseInt( piece.attributes.NumberOfPoints );
-
- if ( numberOfPoints > 0 ) {
-
- var components = section.DataArray.attributes.NumberOfComponents;
- points = new Float32Array( numberOfPoints * components );
- points.set( section.DataArray.text, 0 );
-
- }
-
- break;
-
- // if it is strips
- case 'Strips':
-
- var numberOfStrips = parseInt( piece.attributes.NumberOfStrips );
-
- if ( numberOfStrips > 0 ) {
-
- var connectivity = new Int32Array( section.DataArray[ 0 ].text.length );
- var offset = new Int32Array( section.DataArray[ 1 ].text.length );
- connectivity.set( section.DataArray[ 0 ].text, 0 );
- offset.set( section.DataArray[ 1 ].text, 0 );
-
- var size = numberOfStrips + connectivity.length;
- indices = new Uint32Array( 3 * size - 9 * numberOfStrips );
-
- var indicesIndex = 0;
-
- for ( var i = 0, len = numberOfStrips; i < len; i ++ ) {
-
- var strip = [];
-
- for ( var s = 0, len1 = offset[ i ], len0 = 0; s < len1 - len0; s ++ ) {
-
- strip.push( connectivity[ s ] );
-
- if ( i > 0 ) len0 = offset[ i - 1 ];
-
- }
-
- for ( var j = 0, len1 = offset[ i ], len0 = 0; j < len1 - len0 - 2; j ++ ) {
-
- if ( j % 2 ) {
-
- indices[ indicesIndex ++ ] = strip[ j ];
- indices[ indicesIndex ++ ] = strip[ j + 2 ];
- indices[ indicesIndex ++ ] = strip[ j + 1 ];
-
- } else {
-
- indices[ indicesIndex ++ ] = strip[ j ];
- indices[ indicesIndex ++ ] = strip[ j + 1 ];
- indices[ indicesIndex ++ ] = strip[ j + 2 ];
-
- }
-
- if ( i > 0 ) len0 = offset[ i - 1 ];
-
- }
-
- }
-
- }
-
- break;
-
- // if it is polys
- case 'Polys':
-
- var numberOfPolys = parseInt( piece.attributes.NumberOfPolys );
-
- if ( numberOfPolys > 0 ) {
-
- var connectivity = new Int32Array( section.DataArray[ 0 ].text.length );
- var offset = new Int32Array( section.DataArray[ 1 ].text.length );
- connectivity.set( section.DataArray[ 0 ].text, 0 );
- offset.set( section.DataArray[ 1 ].text, 0 );
-
- var size = numberOfPolys + connectivity.length;
- indices = new Uint32Array( 3 * size - 9 * numberOfPolys );
- var indicesIndex = 0, connectivityIndex = 0;
- var i = 0, len = numberOfPolys, len0 = 0;
-
- while ( i < len ) {
-
- var poly = [];
- var s = 0, len1 = offset[ i ];
-
- while ( s < len1 - len0 ) {
-
- poly.push( connectivity[ connectivityIndex ++ ] );
- s ++;
-
- }
-
- var j = 1;
-
- while ( j < len1 - len0 - 1 ) {
-
- indices[ indicesIndex ++ ] = poly[ 0 ];
- indices[ indicesIndex ++ ] = poly[ j ];
- indices[ indicesIndex ++ ] = poly[ j + 1 ];
- j ++;
-
- }
-
- i ++;
- len0 = offset[ i - 1 ];
-
- }
-
- }
-
- break;
-
- default:
- break;
-
- }
-
- }
-
- sectionIndex ++;
-
- }
-
- var geometry = new THREE.BufferGeometry();
- geometry.setIndex( new THREE.BufferAttribute( indices, 1 ) );
- geometry.addAttribute( 'position', new THREE.BufferAttribute( points, 3 ) );
-
- if ( normals.length === points.length ) {
-
- geometry.addAttribute( 'normal', new THREE.BufferAttribute( normals, 3 ) );
-
- }
-
- return geometry;
-
- } else {
-
- // TODO for vtu,vti,and other xml formats
-
- }
-
- }
-
- function getStringFile( data ) {
-
- var stringFile = '';
- var charArray = new Uint8Array( data );
- var i = 0;
- var len = charArray.length;
-
- while ( len -- ) {
-
- stringFile += String.fromCharCode( charArray[ i ++ ] );
-
- }
-
- return stringFile;
-
- }
-
- // get the 5 first lines of the files to check if there is the key word binary
- var meta = THREE.LoaderUtils.decodeText( new Uint8Array( data, 0, 250 ) ).split( '\n' );
-
- if ( meta[ 0 ].indexOf( 'xml' ) !== - 1 ) {
-
- return parseXML( getStringFile( data ) );
-
- } else if ( meta[ 2 ].includes( 'ASCII' ) ) {
-
- return parseASCII( getStringFile( data ) );
-
- } else {
-
- return parseBinary( data );
-
- }
-
- }
-
-} );
diff --git a/spaces/banana-projects/web3d/node_modules/three/src/geometries/TetrahedronGeometry.js b/spaces/banana-projects/web3d/node_modules/three/src/geometries/TetrahedronGeometry.js
deleted file mode 100644
index 7f4a7cd0a8484620c6717e676f81c2f0948f6679..0000000000000000000000000000000000000000
--- a/spaces/banana-projects/web3d/node_modules/three/src/geometries/TetrahedronGeometry.js
+++ /dev/null
@@ -1,57 +0,0 @@
-/**
- * @author timothypratley / https://github.com/timothypratley
- * @author Mugen87 / https://github.com/Mugen87
- */
-
-import { Geometry } from '../core/Geometry.js';
-import { PolyhedronBufferGeometry } from './PolyhedronGeometry.js';
-
-// TetrahedronGeometry
-
-function TetrahedronGeometry( radius, detail ) {
-
- Geometry.call( this );
-
- this.type = 'TetrahedronGeometry';
-
- this.parameters = {
- radius: radius,
- detail: detail
- };
-
- this.fromBufferGeometry( new TetrahedronBufferGeometry( radius, detail ) );
- this.mergeVertices();
-
-}
-
-TetrahedronGeometry.prototype = Object.create( Geometry.prototype );
-TetrahedronGeometry.prototype.constructor = TetrahedronGeometry;
-
-// TetrahedronBufferGeometry
-
-function TetrahedronBufferGeometry( radius, detail ) {
-
- var vertices = [
- 1, 1, 1, - 1, - 1, 1, - 1, 1, - 1, 1, - 1, - 1
- ];
-
- var indices = [
- 2, 1, 0, 0, 3, 2, 1, 3, 0, 2, 3, 1
- ];
-
- PolyhedronBufferGeometry.call( this, vertices, indices, radius, detail );
-
- this.type = 'TetrahedronBufferGeometry';
-
- this.parameters = {
- radius: radius,
- detail: detail
- };
-
-}
-
-TetrahedronBufferGeometry.prototype = Object.create( PolyhedronBufferGeometry.prototype );
-TetrahedronBufferGeometry.prototype.constructor = TetrahedronBufferGeometry;
-
-
-export { TetrahedronGeometry, TetrahedronBufferGeometry };
diff --git a/spaces/banana-projects/web3d/node_modules/three/src/renderers/shaders/ShaderChunk/map_particle_fragment.glsl.js b/spaces/banana-projects/web3d/node_modules/three/src/renderers/shaders/ShaderChunk/map_particle_fragment.glsl.js
deleted file mode 100644
index 20dbaab554164b4d72c60dbd9ea0c0566954726e..0000000000000000000000000000000000000000
--- a/spaces/banana-projects/web3d/node_modules/three/src/renderers/shaders/ShaderChunk/map_particle_fragment.glsl.js
+++ /dev/null
@@ -1,9 +0,0 @@
-export default /* glsl */`
-#ifdef USE_MAP
-
- vec2 uv = ( uvTransform * vec3( gl_PointCoord.x, 1.0 - gl_PointCoord.y, 1 ) ).xy;
- vec4 mapTexel = texture2D( map, uv );
- diffuseColor *= mapTexelToLinear( mapTexel );
-
-#endif
-`;
diff --git a/spaces/bioriAsaeru/text-to-voice/3d Vista Virtual Tour Crack Zip !!EXCLUSIVE!!.md b/spaces/bioriAsaeru/text-to-voice/3d Vista Virtual Tour Crack Zip !!EXCLUSIVE!!.md
deleted file mode 100644
index 45b21b6aa0b70283be4890d93b89a06778edcfad..0000000000000000000000000000000000000000
--- a/spaces/bioriAsaeru/text-to-voice/3d Vista Virtual Tour Crack Zip !!EXCLUSIVE!!.md
+++ /dev/null
@@ -1,22 +0,0 @@
-
-
How to Download and Install 3D Vista Virtual Tour Zip
-
If you are looking for a powerful and easy-to-use software to create stunning virtual tours, you might want to check out 3D Vista Virtual Tour Zip. This software allows you to create interactive 360-degree panoramas, immersive VR tours, floor plans, hotspots, and more. You can also publish your tours online or offline, and share them with your clients or audience.
-
In this article, we will show you how to download and install 3D Vista Virtual Tour Zip on your computer. Follow these simple steps and you will be ready to create amazing virtual tours in no time.
The first thing you need to do is to download the software from the official website. You can choose between the Standard or the Pro version, depending on your needs and budget. The Standard version costs $199 and the Pro version costs $499. Both versions offer a free trial for 30 days.
-
To download the software, go to https://www.3dvista.com/en/products/virtualtour and click on the "Download" button. You will be asked to enter your email address and choose your operating system (Windows or Mac). Then, click on the "Download Now" button and save the file on your computer.
-
Step 2: Install 3D Vista Virtual Tour Zip
-
Once you have downloaded the file, you need to unzip it and run the installer. To unzip the file, right-click on it and select "Extract All". Then, choose a destination folder and click on "Extract".
-
To run the installer, double-click on the file named "3DVista_Virtual_Tour_Installer.exe" (for Windows) or "3DVista_Virtual_Tour_Installer.dmg" (for Mac). Follow the instructions on the screen and accept the terms and conditions. The installation process may take a few minutes.
-
Step 3: Activate 3D Vista Virtual Tour Zip
-
After the installation is complete, you need to activate the software with a license key. You can get a license key by purchasing the software or by requesting a free trial.
-
To purchase the software, go to https://www.3dvista.com/en/store and select the version you want. You will be redirected to a secure payment page where you can enter your billing information and complete the transaction. You will receive an email with your license key shortly after.
-
To request a free trial, go to https://www.3dvista.com/en/trial and fill out the form with your name, email address, company name, and phone number. You will receive an email with your license key within 24 hours.
-
To activate the software, open it and click on the "Activate" button. Enter your license key and click on "OK". You will see a confirmation message that your software is activated.
-
-
Step 4: Enjoy 3D Vista Virtual Tour Zip
-
Congratulations! You have successfully downloaded and installed 3D Vista Virtual Tour Zip on your computer. Now you can start creating amazing virtual tours with this software. To learn how to use it, you can check out the tutorials and manuals on the official website or watch some videos on YouTube.
-
We hope this article was helpful for you. If you have any questions or feedback, please feel free to contact us at support@3dvista.com. We would love to hear from you.
d5da3c52bf
-
-
\ No newline at end of file
diff --git a/spaces/bioriAsaeru/text-to-voice/Cutting Optimization Pro 5.9.9 Key Generator 37.md b/spaces/bioriAsaeru/text-to-voice/Cutting Optimization Pro 5.9.9 Key Generator 37.md
deleted file mode 100644
index 87445d10eb6ea33eb54155fe988a2736411bc56a..0000000000000000000000000000000000000000
--- a/spaces/bioriAsaeru/text-to-voice/Cutting Optimization Pro 5.9.9 Key Generator 37.md
+++ /dev/null
@@ -1,10 +0,0 @@
-
-
-Jun 15, 2021 - Hardware/software evaluation and development tools. Appendix A. MC1322x Register Address Map - Provides a single table memory map diagram. Appendix B. MC1322x Register Address Map - Provides description, general information, and general addresses.
-Appendix B. MC1322x Programming Language - Provides a brief overview of the MC1322x language.
-Appendix D. MC1322x Sample Programs - Provides sample programs for the MC1322x.
-Appendix E. MC1322x Description - Provides complete documentation for the MC1322x.
-Appendix F. MC1322x Description - Provides a complete list of documentation and reference material for the MC1322x. 8a78ff9644
-
-
-
diff --git a/spaces/bioriAsaeru/text-to-voice/Filem Rock 2005 Full Movie Free 168.md b/spaces/bioriAsaeru/text-to-voice/Filem Rock 2005 Full Movie Free 168.md
deleted file mode 100644
index 1692c6f4ee96718e35976eb6c6d59032f48e1395..0000000000000000000000000000000000000000
--- a/spaces/bioriAsaeru/text-to-voice/Filem Rock 2005 Full Movie Free 168.md
+++ /dev/null
@@ -1,6 +0,0 @@
-
-
-/home/hugh/Downloads/seacomplete-0.0.4.tar.gz 4fefd39f24
-
-
-
diff --git a/spaces/bioriAsaeru/text-to-voice/Jimmy Neutron Full VERIFIED Episodes Tagalog Version Of The Holy Rosary.md b/spaces/bioriAsaeru/text-to-voice/Jimmy Neutron Full VERIFIED Episodes Tagalog Version Of The Holy Rosary.md
deleted file mode 100644
index 23d5c61497fa1b83dcffb159ab4eff6304432292..0000000000000000000000000000000000000000
--- a/spaces/bioriAsaeru/text-to-voice/Jimmy Neutron Full VERIFIED Episodes Tagalog Version Of The Holy Rosary.md
+++ /dev/null
@@ -1,10 +0,0 @@
-
-
This airdate for the Jimmy Neutron episode, "A Flying Jimmy Neutron", is not as complete as the other episodes.. This is a list of programs previously broadcast by TV5. For the currently aired shows of the. . Tags: Jimmy Neutron. Jimmy Neutron full episodes tagalog version of the holy rosary has an iPhone version too: or download it here :. . . . Jimmy Neutron full episodes. . . Jimmy Neutron full episodes. . . . . . . . .
-
jimmy neutron full episodes tagalog version of the holy rosary
Star Hooper has a long, pale, high cheekbone, a clean-shaven, somewhat bold face, dark eyes, skin of a nice tone, medium full-bodied hair, and a. TodorokiTodoCaboCaboBloody GorgeousGorgeous GanjiroGanjiro. . Jimmy Neutron: Boy Genius (20062007); Atlantis High (20062010); The PJs: Welcome Home from the Holidays (20122013. He has a anagram for the name of the program: Clamp.. Jimmie (20132014).
-
Minnie Snagglepuss has a long, pale, high cheekbone, a clean-shaven, rather bold face, medium full-bodied hair, medium. in their imagination, but not in real life. Hover, you view all the variations of the word. Matson GreeniCakesJohny's Jimmy's.
-
Most of the time. Jimmy Neutron: Boy Genius (20062007); The PJs: Welcome Home from the Holidays (20122013. This is a list of programs previously broadcast by TV5. Star Hooper has a long, pale, high cheekbone, a clean-shaven, somewhat bold face, dark eyes, skin of a nice tone, medium full-bodied hair, and a medium-brown hair.
-
Nick is the following: a husband, a cat dad, a Libra, a Bowler, a black and white cat, a philosopher, a. jimmy neutron full episodes tagalog version of the holy rosary. The series revolves around the adventures of a boy named Jimmy Neutron. Jimmy Neutron (20062007); The PJs: Welcome Home from the Holidays (20122013.
- 899543212b
-
-
\ No newline at end of file
diff --git a/spaces/brjathu/HMR2.0/vendor/detectron2/tests/test_model_analysis.py b/spaces/brjathu/HMR2.0/vendor/detectron2/tests/test_model_analysis.py
deleted file mode 100644
index c01b7af09703c8dad889dee0118d74fcc12ac4b0..0000000000000000000000000000000000000000
--- a/spaces/brjathu/HMR2.0/vendor/detectron2/tests/test_model_analysis.py
+++ /dev/null
@@ -1,80 +0,0 @@
-# Copyright (c) Facebook, Inc. and its affiliates.
-
-
-import unittest
-import torch
-from torch import nn
-
-from detectron2.utils.analysis import find_unused_parameters, flop_count_operators, parameter_count
-from detectron2.utils.testing import get_model_no_weights
-
-
-class RetinaNetTest(unittest.TestCase):
- def setUp(self):
- self.model = get_model_no_weights("COCO-Detection/retinanet_R_50_FPN_1x.yaml")
-
- def test_flop(self):
- # RetinaNet supports flop-counting with random inputs
- inputs = [{"image": torch.rand(3, 800, 800), "test_unused": "abcd"}]
- res = flop_count_operators(self.model, inputs)
- self.assertEqual(int(res["conv"]), 146) # 146B flops
-
- def test_param_count(self):
- res = parameter_count(self.model)
- self.assertEqual(res[""], 37915572)
- self.assertEqual(res["backbone"], 31452352)
-
-
-class FasterRCNNTest(unittest.TestCase):
- def setUp(self):
- self.model = get_model_no_weights("COCO-Detection/faster_rcnn_R_50_FPN_1x.yaml")
-
- def test_flop(self):
- # Faster R-CNN supports flop-counting with random inputs
- inputs = [{"image": torch.rand(3, 800, 800)}]
- res = flop_count_operators(self.model, inputs)
-
- # This only checks flops for backbone & proposal generator
- # Flops for box head is not conv, and depends on #proposals, which is
- # almost 0 for random inputs.
- self.assertEqual(int(res["conv"]), 117)
-
- def test_flop_with_output_shape(self):
- inputs = [{"image": torch.rand(3, 800, 800), "height": 700, "width": 700}]
- res = flop_count_operators(self.model, inputs)
- self.assertEqual(int(res["conv"]), 117)
-
- def test_param_count(self):
- res = parameter_count(self.model)
- self.assertEqual(res[""], 41699936)
- self.assertEqual(res["backbone"], 26799296)
-
-
-class MaskRCNNTest(unittest.TestCase):
- def setUp(self):
- self.model = get_model_no_weights("COCO-InstanceSegmentation/mask_rcnn_R_50_FPN_1x.yaml")
-
- def test_flop(self):
- inputs1 = [{"image": torch.rand(3, 800, 800)}]
- inputs2 = [{"image": torch.rand(3, 800, 800), "height": 700, "width": 700}]
-
- for inputs in [inputs1, inputs2]:
- res = flop_count_operators(self.model, inputs)
- # The mask head could have extra conv flops, so total >= 117
- self.assertGreaterEqual(int(res["conv"]), 117)
-
-
-class UnusedParamTest(unittest.TestCase):
- def test_unused(self):
- class TestMod(nn.Module):
- def __init__(self):
- super().__init__()
- self.fc1 = nn.Linear(10, 10)
- self.t = nn.Linear(10, 10)
-
- def forward(self, x):
- return self.fc1(x).mean()
-
- m = TestMod()
- ret = find_unused_parameters(m, torch.randn(10, 10))
- self.assertEqual(set(ret), {"t.weight", "t.bias"})
diff --git a/spaces/bunkalab/bunka-map/app.py b/spaces/bunkalab/bunka-map/app.py
deleted file mode 100644
index 42010df4945027cbf21318e8c950f1624d25bc6b..0000000000000000000000000000000000000000
--- a/spaces/bunkalab/bunka-map/app.py
+++ /dev/null
@@ -1,54 +0,0 @@
-import streamlit as st
-
-st.sidebar.image("images/logo.png", use_column_width=True)
-st.sidebar.write("Bunka Summarizes & Visualizes Information as Maps using LLMs.")
-
-st.sidebar.title("Github Page")
-st.sidebar.write(
- "Have a look at the following package on GitHub: https://github.com/charlesdedampierre/BunkaTopics"
-)
-st.sidebar.title("Dataset")
-st.sidebar.write("HH-RLHF Dataset: https://huggingface.co/datasets/Anthropic/hh-rlhf")
-
-st.title("How to understand large textual datasets?")
-
-import pandas as pd
-
-df = pd.read_csv("data/rejection-sampling.csv", index_col=[0])
-st.dataframe(df, use_container_width=True)
-
-st.title("Bunka Exploration Engine")
-
-st.image("images/pipeline.png", use_column_width=True)
-
-
-# Path to the HTML file containing the Plotly figure
-bunka_map_path = "maps/bunka_map.html" # Replace with your HTML file path
-
-# Use the 'st.components' function to embed the HTML content
-with open(bunka_map_path, "r") as f:
- bunka_map_html = f.read()
-
-st.components.v1.html(bunka_map_html, width=800, height=800)
-
-st.title("Framing Analysis")
-
-# Path to the HTML file containing the Plotly figure
-bunka_map_path = (
- "maps/bourdieu_priacy_politics.html" # Replace with your HTML file path
-)
-
-# Use the 'st.components' function to embed the HTML content
-with open(bunka_map_path, "r") as f:
- bunka_map_html = f.read()
-
-st.components.v1.html(bunka_map_html, width=800, height=800)
-
-# Path to the HTML file containing the Plotly figure
-bunka_map_path = "maps/violence_men_women.html" # Replace with your HTML file path
-
-# Use the 'st.components' function to embed the HTML content
-with open(bunka_map_path, "r") as f:
- bunka_map_html = f.read()
-
-st.components.v1.html(bunka_map_html, width=800, height=800)
diff --git a/spaces/cadige/02-Gradio-Art-From-Text-and-Images/app.py b/spaces/cadige/02-Gradio-Art-From-Text-and-Images/app.py
deleted file mode 100644
index 10939427025b17176765402185cd11e23caa1523..0000000000000000000000000000000000000000
--- a/spaces/cadige/02-Gradio-Art-From-Text-and-Images/app.py
+++ /dev/null
@@ -1,224 +0,0 @@
-import os
-
-os.system("git clone --recursive https://github.com/JD-P/cloob-latent-diffusion")
-os.system("cd cloob-latent-diffusion;pip install omegaconf pillow pytorch-lightning einops wandb ftfy regex ./CLIP")
-
-import argparse
-from functools import partial
-from pathlib import Path
-import sys
-sys.path.append('./cloob-latent-diffusion')
-sys.path.append('./cloob-latent-diffusion/cloob-training')
-sys.path.append('./cloob-latent-diffusion/latent-diffusion')
-sys.path.append('./cloob-latent-diffusion/taming-transformers')
-sys.path.append('./cloob-latent-diffusion/v-diffusion-pytorch')
-from omegaconf import OmegaConf
-from PIL import Image
-import torch
-from torch import nn
-from torch.nn import functional as F
-from torchvision import transforms
-from torchvision.transforms import functional as TF
-from tqdm import trange
-from CLIP import clip
-from cloob_training import model_pt, pretrained
-import ldm.models.autoencoder
-from diffusion import sampling, utils
-import train_latent_diffusion as train
-from huggingface_hub import hf_hub_url, cached_download
-import random
-
-# Download the model files
-checkpoint = cached_download(hf_hub_url("huggan/distill-ccld-wa", filename="model_student.ckpt"))
-ae_model_path = cached_download(hf_hub_url("huggan/ccld_wa", filename="ae_model.ckpt"))
-ae_config_path = cached_download(hf_hub_url("huggan/ccld_wa", filename="ae_model.yaml"))
-
-# Define a few utility functions
-
-
-def parse_prompt(prompt, default_weight=3.):
- if prompt.startswith('http://') or prompt.startswith('https://'):
- vals = prompt.rsplit(':', 2)
- vals = [vals[0] + ':' + vals[1], *vals[2:]]
- else:
- vals = prompt.rsplit(':', 1)
- vals = vals + ['', default_weight][len(vals):]
- return vals[0], float(vals[1])
-
-
-def resize_and_center_crop(image, size):
- fac = max(size[0] / image.size[0], size[1] / image.size[1])
- image = image.resize((int(fac * image.size[0]), int(fac * image.size[1])), Image.LANCZOS)
- return TF.center_crop(image, size[::-1])
-
-
-# Load the models
-device = torch.device('cuda:0' if torch.cuda.is_available() else 'cpu')
-print('Using device:', device)
-print('loading models')
-
-# autoencoder
-ae_config = OmegaConf.load(ae_config_path)
-ae_model = ldm.models.autoencoder.AutoencoderKL(**ae_config.model.params)
-ae_model.eval().requires_grad_(False).to(device)
-ae_model.load_state_dict(torch.load(ae_model_path))
-n_ch, side_y, side_x = 4, 32, 32
-
-# diffusion model
-model = train.DiffusionModel(192, [1,1,2,2], autoencoder_scale=torch.tensor(4.3084))
-model.load_state_dict(torch.load(checkpoint, map_location='cpu'))
-model = model.to(device).eval().requires_grad_(False)
-
-# CLOOB
-cloob_config = pretrained.get_config('cloob_laion_400m_vit_b_16_16_epochs')
-cloob = model_pt.get_pt_model(cloob_config)
-checkpoint = pretrained.download_checkpoint(cloob_config)
-cloob.load_state_dict(model_pt.get_pt_params(cloob_config, checkpoint))
-cloob.eval().requires_grad_(False).to(device)
-
-
-# The key function: returns a list of n PIL images
-def generate(n=1, prompts=['a red circle'], images=[], seed=42, steps=15,
- method='plms', eta=None):
- zero_embed = torch.zeros([1, cloob.config['d_embed']], device=device)
- target_embeds, weights = [zero_embed], []
-
- for prompt in prompts:
- txt, weight = parse_prompt(prompt)
- target_embeds.append(cloob.text_encoder(cloob.tokenize(txt).to(device)).float())
- weights.append(weight)
-
- for prompt in images:
- path, weight = parse_prompt(prompt)
- img = Image.open(utils.fetch(path)).convert('RGB')
- clip_size = cloob.config['image_encoder']['image_size']
- img = resize_and_center_crop(img, (clip_size, clip_size))
- batch = TF.to_tensor(img)[None].to(device)
- embed = F.normalize(cloob.image_encoder(cloob.normalize(batch)).float(), dim=-1)
- target_embeds.append(embed)
- weights.append(weight)
-
- weights = torch.tensor([1 - sum(weights), *weights], device=device)
-
- torch.manual_seed(seed)
-
- def cfg_model_fn(x, t):
- n = x.shape[0]
- n_conds = len(target_embeds)
- x_in = x.repeat([n_conds, 1, 1, 1])
- t_in = t.repeat([n_conds])
- clip_embed_in = torch.cat([*target_embeds]).repeat_interleave(n, 0)
- vs = model(x_in, t_in, clip_embed_in).view([n_conds, n, *x.shape[1:]])
- v = vs.mul(weights[:, None, None, None, None]).sum(0)
- return v
-
- def run(x, steps):
- if method == 'ddpm':
- return sampling.sample(cfg_model_fn, x, steps, 1., {})
- if method == 'ddim':
- return sampling.sample(cfg_model_fn, x, steps, eta, {})
- if method == 'prk':
- return sampling.prk_sample(cfg_model_fn, x, steps, {})
- if method == 'plms':
- return sampling.plms_sample(cfg_model_fn, x, steps, {})
- if method == 'pie':
- return sampling.pie_sample(cfg_model_fn, x, steps, {})
- if method == 'plms2':
- return sampling.plms2_sample(cfg_model_fn, x, steps, {})
- assert False
-
- batch_size = n
- x = torch.randn([n, n_ch, side_y, side_x], device=device)
- t = torch.linspace(1, 0, steps + 1, device=device)[:-1]
- steps = utils.get_spliced_ddpm_cosine_schedule(t)
- pil_ims = []
- for i in trange(0, n, batch_size):
- cur_batch_size = min(n - i, batch_size)
- out_latents = run(x[i:i+cur_batch_size], steps)
- outs = ae_model.decode(out_latents * torch.tensor(2.55).to(device))
- for j, out in enumerate(outs):
- pil_ims.append(utils.to_pil_image(out))
-
- return pil_ims
-
-
-import gradio as gr
-
-def gen_ims(prompt, im_prompt=None, seed=None, n_steps=10, method='plms'):
- if seed == None :
- seed = random.randint(0, 10000)
- print( prompt, im_prompt, seed, n_steps)
- prompts = [prompt]
- im_prompts = []
- if im_prompt != None:
- im_prompts = [im_prompt]
- pil_ims = generate(n=1, prompts=prompts, images=im_prompts, seed=seed, steps=n_steps, method=method)
- return pil_ims[0]
-
-iface = gr.Interface(fn=gen_ims,
- inputs=[#gr.inputs.Slider(minimum=1, maximum=1, step=1, default=1,label="Number of images"),
- #gr.inputs.Slider(minimum=0, maximum=200, step=1, label='Random seed', default=0),
- gr.inputs.Textbox(label="Text prompt"),
- gr.inputs.Image(optional=True, label="Image prompt", type='filepath'),
- #gr.inputs.Slider(minimum=10, maximum=35, step=1, default=15,label="Number of steps")
- ],
- outputs=[gr.outputs.Image(type="pil", label="Generated Image")],
- examples=[
- ["Futurism, in the style of Wassily Kandinsky"],
- ["Art Nouveau, in the style of John Singer Sargent"],
- ["Surrealism, in the style of Edgar Degas"],
- ["Expressionism, in the style of Wassily Kandinsky"],
- ["Futurism, in the style of Egon Schiele"],
- ["Neoclassicism, in the style of Gustav Klimt"],
- ["Cubism, in the style of Gustav Klimt"],
- ["Op Art, in the style of Marc Chagall"],
- ["Romanticism, in the style of M.C. Escher"],
- ["Futurism, in the style of M.C. Escher"],
- ["Abstract Art, in the style of M.C. Escher"],
- ["Mannerism, in the style of Paul Klee"],
- ["Romanesque Art, in the style of Leonardo da Vinci"],
- ["High Renaissance, in the style of Rembrandt"],
- ["Magic Realism, in the style of Gustave Dore"],
- ["Realism, in the style of Jean-Michel Basquiat"],
- ["Art Nouveau, in the style of Paul Gauguin"],
- ["Avant-garde, in the style of Pierre-Auguste Renoir"],
- ["Baroque, in the style of Edward Hopper"],
- ["Post-Impressionism, in the style of Wassily Kandinsky"],
- ["Naturalism, in the style of Rene Magritte"],
- ["Constructivism, in the style of Paul Cezanne"],
- ["Abstract Expressionism, in the style of Henri Matisse"],
- ["Pop Art, in the style of Vincent van Gogh"],
- ["Futurism, in the style of Wassily Kandinsky"],
- ["Futurism, in the style of Zdzislaw Beksinski"],
- ['Surrealism, in the style of Salvador Dali'],
- ["Aaron Wacker, oil on canvas"],
- ["abstract"],
- ["landscape"],
- ["portrait"],
- ["sculpture"],
- ["genre painting"],
- ["installation"],
- ["photo"],
- ["figurative"],
- ["illustration"],
- ["still life"],
- ["history painting"],
- ["cityscape"],
- ["marina"],
- ["animal painting"],
- ["design"],
- ["calligraphy"],
- ["symbolic painting"],
- ["graffiti"],
- ["performance"],
- ["mythological painting"],
- ["battle painting"],
- ["self-portrait"],
- ["Impressionism, oil on canvas"]
- ],
- title='Art Generator and Style Mixer from 🧠 Cloob and 🎨 WikiArt - Visual Art Encyclopedia:',
- description="Trained on images from the [WikiArt](https://www.wikiart.org/) dataset, comprised of visual arts",
- article = 'Model used is: [model card](https://huggingface.co/huggan/distill-ccld-wa)..'
-
-)
-iface.launch(enable_queue=True) # , debug=True for colab debugging
\ No newline at end of file
diff --git a/spaces/candlend/vits-hoshimi/sovits/vdecoder/parallel_wavegan/models/__init__.py b/spaces/candlend/vits-hoshimi/sovits/vdecoder/parallel_wavegan/models/__init__.py
deleted file mode 100644
index 4803ba6b2a0afc8022e756ae5b3f4c7403c3c1bd..0000000000000000000000000000000000000000
--- a/spaces/candlend/vits-hoshimi/sovits/vdecoder/parallel_wavegan/models/__init__.py
+++ /dev/null
@@ -1,2 +0,0 @@
-from .melgan import * # NOQA
-from .parallel_wavegan import * # NOQA
diff --git a/spaces/cfj108/CompVis-stable-diffusion-v1-4/app.py b/spaces/cfj108/CompVis-stable-diffusion-v1-4/app.py
deleted file mode 100644
index b60a087620a806fea130bedcd6940bef75fa3337..0000000000000000000000000000000000000000
--- a/spaces/cfj108/CompVis-stable-diffusion-v1-4/app.py
+++ /dev/null
@@ -1,3 +0,0 @@
-import gradio as gr
-
-gr.Interface.load("models/CompVis/stable-diffusion-v1-4").launch()
diff --git a/spaces/chendl/compositional_test/transformers/examples/pytorch/question-answering/trainer_qa.py b/spaces/chendl/compositional_test/transformers/examples/pytorch/question-answering/trainer_qa.py
deleted file mode 100644
index a486405b62877ee83d1a60f3fdf7a8f326882fcc..0000000000000000000000000000000000000000
--- a/spaces/chendl/compositional_test/transformers/examples/pytorch/question-answering/trainer_qa.py
+++ /dev/null
@@ -1,136 +0,0 @@
-# coding=utf-8
-# Copyright 2020 The HuggingFace Team All rights reserved.
-#
-# Licensed under the Apache License, Version 2.0 (the "License");
-# you may not use this file except in compliance with the License.
-# You may obtain a copy of the License at
-#
-# http://www.apache.org/licenses/LICENSE-2.0
-#
-# Unless required by applicable law or agreed to in writing, software
-# distributed under the License is distributed on an "AS IS" BASIS,
-# WITHOUT WARRANTIES OR CONDITIONS OF ANY KIND, either express or implied.
-# See the License for the specific language governing permissions and
-# limitations under the License.
-"""
-A subclass of `Trainer` specific to Question-Answering tasks
-"""
-import math
-import time
-
-from transformers import Trainer, is_torch_tpu_available
-from transformers.trainer_utils import PredictionOutput, speed_metrics
-
-
-if is_torch_tpu_available(check_device=False):
- import torch_xla.core.xla_model as xm
- import torch_xla.debug.metrics as met
-
-
-class QuestionAnsweringTrainer(Trainer):
- def __init__(self, *args, eval_examples=None, post_process_function=None, **kwargs):
- super().__init__(*args, **kwargs)
- self.eval_examples = eval_examples
- self.post_process_function = post_process_function
-
- def evaluate(self, eval_dataset=None, eval_examples=None, ignore_keys=None, metric_key_prefix: str = "eval"):
- eval_dataset = self.eval_dataset if eval_dataset is None else eval_dataset
- eval_dataloader = self.get_eval_dataloader(eval_dataset)
- eval_examples = self.eval_examples if eval_examples is None else eval_examples
-
- # Temporarily disable metric computation, we will do it in the loop here.
- compute_metrics = self.compute_metrics
- self.compute_metrics = None
- eval_loop = self.prediction_loop if self.args.use_legacy_prediction_loop else self.evaluation_loop
- start_time = time.time()
- try:
- output = eval_loop(
- eval_dataloader,
- description="Evaluation",
- # No point gathering the predictions if there are no metrics, otherwise we defer to
- # self.args.prediction_loss_only
- prediction_loss_only=True if compute_metrics is None else None,
- ignore_keys=ignore_keys,
- metric_key_prefix=metric_key_prefix,
- )
- finally:
- self.compute_metrics = compute_metrics
- total_batch_size = self.args.eval_batch_size * self.args.world_size
- if f"{metric_key_prefix}_jit_compilation_time" in output.metrics:
- start_time += output.metrics[f"{metric_key_prefix}_jit_compilation_time"]
- output.metrics.update(
- speed_metrics(
- metric_key_prefix,
- start_time,
- num_samples=output.num_samples,
- num_steps=math.ceil(output.num_samples / total_batch_size),
- )
- )
- if self.post_process_function is not None and self.compute_metrics is not None and self.args.should_save:
- # Only the main node write the results by default
- eval_preds = self.post_process_function(eval_examples, eval_dataset, output.predictions)
- metrics = self.compute_metrics(eval_preds)
-
- # Prefix all keys with metric_key_prefix + '_'
- for key in list(metrics.keys()):
- if not key.startswith(f"{metric_key_prefix}_"):
- metrics[f"{metric_key_prefix}_{key}"] = metrics.pop(key)
- metrics.update(output.metrics)
- else:
- metrics = output.metrics
-
- if self.args.should_log:
- # Only the main node log the results by default
- self.log(metrics)
-
- if self.args.tpu_metrics_debug or self.args.debug:
- # tpu-comment: Logging debug metrics for PyTorch/XLA (compile, execute times, ops, etc.)
- xm.master_print(met.metrics_report())
-
- self.control = self.callback_handler.on_evaluate(self.args, self.state, self.control, metrics)
- return metrics
-
- def predict(self, predict_dataset, predict_examples, ignore_keys=None, metric_key_prefix: str = "test"):
- predict_dataloader = self.get_test_dataloader(predict_dataset)
-
- # Temporarily disable metric computation, we will do it in the loop here.
- compute_metrics = self.compute_metrics
- self.compute_metrics = None
- eval_loop = self.prediction_loop if self.args.use_legacy_prediction_loop else self.evaluation_loop
- start_time = time.time()
- try:
- output = eval_loop(
- predict_dataloader,
- description="Prediction",
- # No point gathering the predictions if there are no metrics, otherwise we defer to
- # self.args.prediction_loss_only
- prediction_loss_only=True if compute_metrics is None else None,
- ignore_keys=ignore_keys,
- metric_key_prefix=metric_key_prefix,
- )
- finally:
- self.compute_metrics = compute_metrics
- total_batch_size = self.args.eval_batch_size * self.args.world_size
- if f"{metric_key_prefix}_jit_compilation_time" in output.metrics:
- start_time += output.metrics[f"{metric_key_prefix}_jit_compilation_time"]
- output.metrics.update(
- speed_metrics(
- metric_key_prefix,
- start_time,
- num_samples=output.num_samples,
- num_steps=math.ceil(output.num_samples / total_batch_size),
- )
- )
-
- if self.post_process_function is None or self.compute_metrics is None:
- return output
-
- predictions = self.post_process_function(predict_examples, predict_dataset, output.predictions, "predict")
- metrics = self.compute_metrics(predictions)
-
- # Prefix all keys with metric_key_prefix + '_'
- for key in list(metrics.keys()):
- if not key.startswith(f"{metric_key_prefix}_"):
- metrics[f"{metric_key_prefix}_{key}"] = metrics.pop(key)
- metrics.update(output.metrics)
- return PredictionOutput(predictions=predictions.predictions, label_ids=predictions.label_ids, metrics=metrics)
diff --git a/spaces/chendl/compositional_test/transformers/examples/research_projects/xtreme-s/run_xtreme_s.py b/spaces/chendl/compositional_test/transformers/examples/research_projects/xtreme-s/run_xtreme_s.py
deleted file mode 100644
index 6c5b4bde892da18b57335ef779568af0728631c6..0000000000000000000000000000000000000000
--- a/spaces/chendl/compositional_test/transformers/examples/research_projects/xtreme-s/run_xtreme_s.py
+++ /dev/null
@@ -1,949 +0,0 @@
-#!/usr/bin/env python
-# coding=utf-8
-# Copyright 2022 The HuggingFace Inc. team. All rights reserved.
-#
-# Licensed under the Apache License, Version 2.0 (the "License");
-# you may not use this file except in compliance with the License.
-# You may obtain a copy of the License at
-#
-# http://www.apache.org/licenses/LICENSE-2.0
-#
-# Unless required by applicable law or agreed to in writing, software
-# distributed under the License is distributed on an "AS IS" BASIS,
-# WITHOUT WARRANTIES OR CONDITIONS OF ANY KIND, either express or implied.
-# See the License for the specific language governing permissions and
-
-""" Fine-tuning a 🤗 Transformers pretrained speech model on the XTREME-S benchmark tasks"""
-
-import json
-import logging
-import os
-import re
-import sys
-from collections import OrderedDict, defaultdict
-from dataclasses import dataclass, field
-from typing import Dict, List, Optional, Union
-
-import datasets
-import numpy as np
-import torch
-from datasets import DatasetDict, load_dataset, load_metric
-
-import transformers
-from transformers import (
- AutoConfig,
- AutoFeatureExtractor,
- AutoModelForAudioClassification,
- AutoModelForCTC,
- AutoModelForSpeechSeq2Seq,
- AutoProcessor,
- AutoTokenizer,
- HfArgumentParser,
- Seq2SeqTrainer,
- Seq2SeqTrainingArguments,
- Trainer,
- set_seed,
-)
-from transformers.trainer_utils import get_last_checkpoint, is_main_process
-from transformers.utils import check_min_version
-from transformers.utils.versions import require_version
-
-
-# Will error if the minimal version of Transformers is not installed. Remove at your own risks.
-check_min_version("4.18.0.dev0")
-
-require_version("datasets>=1.18.0", "To fix: pip install -r examples/pytorch/speech-recognition/requirements.txt")
-
-
-logger = logging.getLogger(__name__)
-
-
-def list_field(default=None, metadata=None):
- return field(default_factory=lambda: default, metadata=metadata)
-
-
-TASK_TO_TARGET_COLUMN_NAME = {
- "fleurs-asr": "transcription",
- "fleurs-lang_id": "lang_id",
- "mls": "transcription",
- "voxpopuli": "transcription",
- "covost2": "translation",
- "minds14": "intent_class",
- "babel": "transcription",
-}
-
-
-@dataclass
-class ModelArguments:
- """
- Arguments pertaining to which model/config/tokenizer we are going to fine-tune from.
- """
-
- model_name_or_path: str = field(
- metadata={"help": "Path to pretrained model or model identifier from huggingface.co/models"}
- )
- tokenizer_name_or_path: Optional[str] = field(
- default=None,
- metadata={"help": "Path to pretrained tokenizer or tokenizer identifier from huggingface.co/models"},
- )
- cache_dir: Optional[str] = field(
- default=None,
- metadata={
- "help": "Where do you want to store the pretrained models and datasets downloaded from huggingface.co"
- },
- )
- freeze_feature_encoder: bool = field(
- default=True, metadata={"help": "Whether to freeze the feature encoder layers of the model."}
- )
- attention_dropout: float = field(
- default=0.0, metadata={"help": "The dropout ratio for the attention probabilities."}
- )
- activation_dropout: float = field(
- default=0.0, metadata={"help": "The dropout ratio for activations inside the fully connected layer."}
- )
- feat_proj_dropout: float = field(default=0.0, metadata={"help": "The dropout ratio for the projected features."})
- hidden_dropout: float = field(
- default=0.0,
- metadata={
- "help": "The dropout probability for all fully connected layers in the embeddings, encoder, and pooler."
- },
- )
- final_dropout: float = field(
- default=0.0,
- metadata={"help": "The dropout probability for the final projection layer."},
- )
- mask_time_prob: float = field(
- default=0.05,
- metadata={
- "help": (
- "Probability of each feature vector along the time axis to be chosen as the start of the vector"
- "span to be masked. Approximately ``mask_time_prob * sequence_length // mask_time_length`` feature"
- "vectors will be masked along the time axis."
- )
- },
- )
- mask_time_length: int = field(
- default=10,
- metadata={"help": "Length of vector span to mask along the time axis."},
- )
- mask_feature_prob: float = field(
- default=0.0,
- metadata={
- "help": (
- "Probability of each feature vector along the feature axis to be chosen as the start of the vectorspan"
- " to be masked. Approximately ``mask_feature_prob * sequence_length // mask_feature_length`` feature"
- " bins will be masked along the time axis."
- )
- },
- )
- mask_feature_length: int = field(
- default=10,
- metadata={"help": "Length of vector span to mask along the feature axis."},
- )
- layerdrop: float = field(default=0.0, metadata={"help": "The LayerDrop probability."})
- ctc_zero_infinity: bool = field(
- default=False,
- metadata={"help": "Whether to zero infinite losses and the associated gradients of `torch.nn.CTCLoss`."},
- )
- ctc_loss_reduction: Optional[str] = field(
- default="mean", metadata={"help": "The way the ctc loss should be reduced. Should be one of 'mean' or 'sum'."}
- )
-
-
-@dataclass
-class DataTrainingArguments:
- """
- Arguments pertaining to what data we are going to input our model for training and eval.
-
- Using `HfArgumentParser` we can turn this class
- into argparse arguments to be able to specify them on
- the command line.
- """
-
- dataset_name: str = field(
- default="google/xtreme_s",
- metadata={"help": "The name of the dataset to use (via the datasets library). Defaults to 'google/xtreme_s'"},
- )
- task: str = field(
- default=None,
- metadata={
- "help": (
- "The task name of the benchmark to use (via the datasets library). Should be on of: "
- "'fleurs-asr', 'mls', 'voxpopuli', 'covost2', 'minds14', 'fleurs-lang_id', 'babel'."
- )
- },
- )
- language: str = field(
- default="all",
- metadata={"help": "The language id as defined in the datasets config name or `all` for all languages."},
- )
- language_group: str = field(
- default=None,
- metadata={
- "help": (
- "The language group to select a subset of languages to train on. "
- "This option is only used the 'fleurs-asr' task. Should be one of: "
- "'western_european_we', 'eastern_european_ee', 'central_asia_middle_north_african_cmn', "
- "'sub_saharan_african_ssa', 'south_asian_sa', 'south_east_asian_sea', 'chinese_japanase_korean_cjk'."
- )
- },
- )
- train_split_name: str = field(
- default="train",
- metadata={
- "help": "The name of the training dataset split to use (via the datasets library). Defaults to 'train'"
- },
- )
- eval_split_name: str = field(
- default="validation",
- metadata={
- "help": (
- "The name of the evaluation dataset split to use (via the datasets library). Defaults to 'validation'"
- )
- },
- )
- predict_split_name: str = field(
- default="test",
- metadata={
- "help": "The name of the prediction dataset split to use (via the datasets library). Defaults to 'test'"
- },
- )
- audio_column_name: str = field(
- default="audio",
- metadata={"help": "The name of the dataset column containing the audio data. Defaults to 'audio'"},
- )
- target_column_name: str = field(
- default=None,
- metadata={
- "help": (
- "The name of the dataset column containing the target data (transcription/translation/label). If None,"
- " the name will be inferred from the task. Defaults to None."
- )
- },
- )
- overwrite_cache: bool = field(
- default=False, metadata={"help": "Overwrite the cached preprocessed datasets or not."}
- )
- preprocessing_num_workers: Optional[int] = field(
- default=None,
- metadata={"help": "The number of processes to use for the preprocessing."},
- )
- max_train_samples: Optional[int] = field(
- default=None,
- metadata={
- "help": (
- "For debugging purposes or quicker training, truncate the number of training examples to this "
- "value if set."
- )
- },
- )
- max_eval_samples: Optional[int] = field(
- default=None,
- metadata={
- "help": (
- "For debugging purposes or quicker training, truncate the number of validation examples to this "
- "value if set."
- )
- },
- )
- max_predict_samples: Optional[int] = field(
- default=None,
- metadata={
- "help": (
- "For debugging purposes or quicker training, truncate the number of prediction examples to this "
- "value if set."
- )
- },
- )
- chars_to_ignore: Optional[List[str]] = list_field(
- default=', ? . ! - ; : " “ % ‘ ” �'.split(" "),
- metadata={"help": "A list of characters to remove from the transcripts."},
- )
- max_duration_in_seconds: float = field(
- default=30.0,
- metadata={
- "help": (
- "Filter audio files that are longer than `max_duration_in_seconds` seconds to"
- " 'max_duration_in_seconds`"
- )
- },
- )
- min_duration_in_seconds: float = field(
- default=0.0, metadata={"help": "Filter audio files that are shorter than `min_duration_in_seconds` seconds"}
- )
- preprocessing_only: bool = field(
- default=False,
- metadata={
- "help": (
- "Whether to only do data preprocessing and skip training. This is especially useful when data"
- " preprocessing errors out in distributed training due to timeout. In this case, one should run the"
- " preprocessing in a non-distributed setup with `preprocessing_only=True` so that the cached datasets"
- " can consequently be loaded in distributed training"
- )
- },
- )
- use_auth_token: bool = field(
- default=False,
- metadata={
- "help": (
- "If :obj:`True`, will use the token generated when running"
- ":obj:`huggingface-cli login` as HTTP bearer authorization for remote files."
- )
- },
- )
- unk_token: str = field(
- default="[UNK]",
- metadata={"help": "The unk token for the tokenizer"},
- )
- pad_token: str = field(
- default="[PAD]",
- metadata={"help": "The padding token for the tokenizer"},
- )
- word_delimiter_token: str = field(
- default="|",
- metadata={"help": "The word delimiter token for the tokenizer"},
- )
- phoneme_language: Optional[str] = field(
- default=None,
- metadata={
- "help": (
- "The target language that should be used be"
- " passed to the tokenizer for tokenization. Note that"
- " this is only relevant if the model classifies the"
- " input audio to a sequence of phoneme sequences."
- )
- },
- )
- per_lang_metrics: bool = field(
- default=True,
- metadata={
- "help": (
- "If `True`, compute the test metrics separately for each language, and average the results. "
- "If `False` compute the average test metrics in a single pass for all languages at once."
- )
- },
- )
-
-
-@dataclass
-class SpeechDataCollatorWithPadding:
- processor: AutoProcessor
- decoder_start_token_id: Optional[int] = None
- padding: Union[bool, str] = "longest"
- pad_labels: Optional[int] = True
- pad_to_multiple_of: Optional[int] = None
- pad_to_multiple_of_labels: Optional[int] = None
-
- def __call__(self, features: List[Dict[str, Union[List[int], torch.Tensor]]]) -> Dict[str, torch.Tensor]:
- # split inputs and labels since they have to be of different lenghts and need
- # different padding methods
- input_features = [{"input_values": feature["input_values"]} for feature in features]
-
- batch = self.processor.pad(
- input_features,
- padding=self.padding,
- pad_to_multiple_of=self.pad_to_multiple_of,
- return_tensors="pt",
- )
-
- if self.pad_labels:
- label_features = [{"input_ids": feature["labels"]} for feature in features]
- labels_batch = self.processor.pad(
- labels=label_features,
- padding=self.padding,
- pad_to_multiple_of=self.pad_to_multiple_of_labels,
- return_tensors="pt",
- )
-
- # replace padding with -100 to ignore loss correctly
- labels = labels_batch["input_ids"].masked_fill(labels_batch.attention_mask.ne(1), -100)
-
- # if bos token is appended in previous tokenization step,
- # cut bos token here as it's append later anyways
- if (
- self.decoder_start_token_id is not None
- and (labels[:, 0] == self.decoder_start_token_id).all().cpu().item()
- ):
- labels = labels[:, 1:]
-
- batch["labels"] = labels
- else:
- batch["labels"] = torch.tensor([feature["labels"] for feature in features])
-
- return batch
-
-
-def create_vocabulary_from_data(
- datasets: DatasetDict,
- word_delimiter_token: Optional[str] = None,
- unk_token: Optional[str] = None,
- pad_token: Optional[str] = None,
-):
- # Given training and test labels create vocabulary
- def extract_all_chars(batch):
- all_text = " ".join(batch["target_text"])
- vocab = list(set(all_text))
- return {"vocab": [vocab], "all_text": [all_text]}
-
- vocabs = datasets.map(
- extract_all_chars,
- batched=True,
- batch_size=-1,
- keep_in_memory=True,
- remove_columns=datasets["train"].column_names,
- )
-
- # take union of all unique characters in each dataset
- vocab_set = (
- (set(vocabs["train"]["vocab"][0]) if "train" in vocabs else set())
- | (set(vocabs["eval"]["vocab"][0]) if "eval" in vocabs else set())
- | (set(vocabs["predict"]["vocab"][0]) if "predict" in vocabs else set())
- )
-
- vocab_dict = {v: k for k, v in enumerate(sorted(vocab_set))}
-
- # replace white space with delimiter token
- if word_delimiter_token is not None:
- vocab_dict[word_delimiter_token] = vocab_dict[" "]
- del vocab_dict[" "]
-
- # add unk and pad token
- if unk_token is not None:
- vocab_dict[unk_token] = len(vocab_dict)
-
- if pad_token is not None:
- vocab_dict[pad_token] = len(vocab_dict)
-
- return vocab_dict
-
-
-def main():
- # See all possible arguments in src/transformers/training_args.py
- # or by passing the --help flag to this script.
- # We now keep distinct sets of args, for a cleaner separation of concerns.
-
- parser = HfArgumentParser((ModelArguments, DataTrainingArguments, Seq2SeqTrainingArguments))
- if len(sys.argv) == 2 and sys.argv[1].endswith(".json"):
- # If we pass only one argument to the script and it's the path to a json file,
- # let's parse it to get our arguments.
- model_args, data_args, training_args = parser.parse_json_file(json_file=os.path.abspath(sys.argv[1]))
- else:
- model_args, data_args, training_args = parser.parse_args_into_dataclasses()
-
- # Detecting last checkpoint.
- last_checkpoint = None
- if os.path.isdir(training_args.output_dir) and training_args.do_train and not training_args.overwrite_output_dir:
- last_checkpoint = get_last_checkpoint(training_args.output_dir)
- if last_checkpoint is None and len(os.listdir(training_args.output_dir)) > 0:
- raise ValueError(
- f"Output directory ({training_args.output_dir}) already exists and is not empty. "
- "Use --overwrite_output_dir to overcome."
- )
- elif last_checkpoint is not None:
- logger.info(
- f"Checkpoint detected, resuming training at {last_checkpoint}. To avoid this behavior, change "
- "the `--output_dir` or add `--overwrite_output_dir` to train from scratch."
- )
-
- # Setup logging
- logging.basicConfig(
- format="%(asctime)s - %(levelname)s - %(name)s - %(message)s",
- datefmt="%m/%d/%Y %H:%M:%S",
- handlers=[logging.StreamHandler(sys.stdout)],
- )
- logger.setLevel(logging.INFO if is_main_process(training_args.local_rank) else logging.WARN)
-
- # Log on each process the small summary:
- logger.warning(
- f"Process rank: {training_args.local_rank}, device: {training_args.device}, n_gpu: {training_args.n_gpu}"
- f"distributed training: {bool(training_args.local_rank != -1)}, 16-bits training: {training_args.fp16}"
- )
- # Set the verbosity to info of the Transformers logger (on main process only):
- if is_main_process(training_args.local_rank):
- transformers.utils.logging.set_verbosity_info()
- logger.info("Training/evaluation parameters %s", training_args)
-
- # Set seed before initializing model.
- set_seed(training_args.seed)
-
- # 1. First, let's load the dataset
- raw_datasets = DatasetDict()
- task_name = data_args.task
- lang_id = data_args.language
-
- if task_name is None:
- raise ValueError(
- "Set --task should be set to '' (e.g. 'fleurs-asr', 'mls', 'covost2', 'minds14') "
- )
- if lang_id is None:
- raise ValueError(
- "Set --language should be set to the language id of the sub dataset "
- "config to be used (e.g. 'pl', 'en.tr', 'fr-FR') or 'all'"
- " for multi-lingual fine-tuning."
- )
- if data_args.language_group is not None:
- if data_args.task != "fleurs-asr":
- raise ValueError("--language_group should only be used with --task=fleurs-asr")
- if data_args.language != "all":
- raise ValueError("--language_group should only be used with --language=all")
-
- if data_args.target_column_name is None:
- target_column_name = TASK_TO_TARGET_COLUMN_NAME[task_name]
- else:
- target_column_name = data_args.target_column_name
-
- # here we differentiate between tasks with text as the target and classification tasks
- is_text_target = target_column_name in ("transcription", "translation")
-
- config_name = ".".join([task_name.split("-")[0], lang_id])
-
- if training_args.do_train:
- raw_datasets["train"] = load_dataset(
- data_args.dataset_name,
- config_name,
- split=data_args.train_split_name,
- use_auth_token=data_args.use_auth_token,
- cache_dir=model_args.cache_dir,
- )
-
- if data_args.audio_column_name not in raw_datasets["train"].column_names:
- raise ValueError(
- f"--audio_column_name '{data_args.audio_column_name}' not found in dataset '{data_args.dataset_name}'."
- " Make sure to set `--audio_column_name` to the correct audio column - one of"
- f" {', '.join(raw_datasets['train'].column_names)}."
- )
-
- if target_column_name not in raw_datasets["train"].column_names:
- raise ValueError(
- f"--target_column_name {target_column_name} not found in dataset '{data_args.dataset_name}'. "
- "Make sure to set `--target_column_name` to the correct text column - one of "
- f"{', '.join(raw_datasets['train'].column_names)}."
- )
-
- if data_args.max_train_samples is not None:
- raw_datasets["train"] = raw_datasets["train"].select(range(data_args.max_train_samples))
-
- if training_args.do_eval:
- raw_datasets["eval"] = load_dataset(
- data_args.dataset_name,
- config_name,
- split=data_args.eval_split_name,
- use_auth_token=data_args.use_auth_token,
- cache_dir=model_args.cache_dir,
- )
-
- if data_args.max_eval_samples is not None:
- raw_datasets["eval"] = raw_datasets["eval"].select(range(data_args.max_eval_samples))
-
- if training_args.do_predict:
- raw_datasets["predict"] = load_dataset(
- data_args.dataset_name,
- config_name,
- split=data_args.predict_split_name,
- use_auth_token=data_args.use_auth_token,
- cache_dir=model_args.cache_dir,
- )
-
- if data_args.max_predict_samples is not None:
- raw_datasets["predict"] = raw_datasets["predict"].select(range(data_args.max_predict_samples))
-
- lang_list = next(iter(raw_datasets.values())).features["lang_id"].names
- if not is_text_target:
- label_list = next(iter(raw_datasets.values())).features[target_column_name].names
- num_labels = len(label_list)
-
- num_workers = data_args.preprocessing_num_workers
-
- lang_group = data_args.language_group
- if lang_group is not None:
- with training_args.main_process_first(desc="language group filter"):
- lang_group_id = next(iter(raw_datasets.values())).features["lang_group_id"].str2int(lang_group)
- raw_datasets = raw_datasets.filter(
- lambda lang_group: lang_group == lang_group_id,
- num_proc=num_workers,
- input_columns=["lang_group_id"],
- )
-
- # 2. We remove some special characters from the datasets
- # that make training complicated and do not help in transcribing the speech
- # E.g. characters, such as `,` and `.` do not really have an acoustic characteristic
- # that could be easily picked up by the model
- chars_to_ignore_regex = (
- f'[{"".join(data_args.chars_to_ignore)}]' if data_args.chars_to_ignore is not None else None
- )
-
- def remove_special_characters(batch):
- if chars_to_ignore_regex is not None:
- batch["target_text"] = re.sub(chars_to_ignore_regex, "", batch[target_column_name]).lower() + " "
- else:
- batch["target_text"] = batch[target_column_name].lower() + " "
- return batch
-
- if is_text_target:
- with training_args.main_process_first(desc="dataset map special characters removal"):
- raw_datasets = raw_datasets.map(
- remove_special_characters,
- remove_columns=[target_column_name],
- desc="remove special characters from datasets",
- )
-
- # save special tokens for tokenizer
- word_delimiter_token = data_args.word_delimiter_token
- unk_token = data_args.unk_token
- pad_token = data_args.pad_token
-
- # 3. Next, let's load the config as we might need it to create
- # the tokenizer
- config = AutoConfig.from_pretrained(
- model_args.model_name_or_path, cache_dir=model_args.cache_dir, use_auth_token=data_args.use_auth_token
- )
-
- if is_text_target:
- # 4. (Optional, for ASR and translation) If no tokenizer file is defined,
- # we create the vocabulary of the model by extracting all unique characters from
- # the training and evaluation datasets
- # We need to make sure that only first rank saves vocabulary
- # make sure all processes wait until vocab is created
- tokenizer_name_or_path = model_args.tokenizer_name_or_path
- tokenizer_kwargs = {}
- if tokenizer_name_or_path is None:
- # save vocab in training output dir
- tokenizer_name_or_path = training_args.output_dir
-
- vocab_file = os.path.join(tokenizer_name_or_path, "vocab.json")
-
- with training_args.main_process_first():
- if training_args.overwrite_output_dir and os.path.isfile(vocab_file):
- os.remove(vocab_file)
-
- with training_args.main_process_first(desc="dataset map vocabulary creation"):
- if not os.path.isfile(vocab_file):
- os.makedirs(tokenizer_name_or_path, exist_ok=True)
- vocab_dict = create_vocabulary_from_data(
- raw_datasets,
- word_delimiter_token=word_delimiter_token,
- unk_token=unk_token,
- pad_token=pad_token,
- )
-
- # save vocab dict to be loaded into tokenizer
- with open(vocab_file, "w") as file:
- json.dump(vocab_dict, file)
-
- # if tokenizer has just been created
- # it is defined by `tokenizer_class` if present in config else by `model_type`
- if not config.is_encoder_decoder:
- tokenizer_kwargs = {
- "config": config if config.tokenizer_class is not None else None,
- "tokenizer_type": config.model_type if config.tokenizer_class is None else None,
- "unk_token": unk_token,
- "pad_token": pad_token,
- "word_delimiter_token": word_delimiter_token,
- }
- else:
- tokenizer_kwargs = {}
-
- # 5. Now we can instantiate the feature extractor, tokenizer and model
- # Note for distributed training, the .from_pretrained methods guarantee that only
- # one local process can concurrently download model & vocab.
-
- # load feature_extractor and tokenizer
- if is_text_target:
- tokenizer = AutoTokenizer.from_pretrained(
- tokenizer_name_or_path,
- use_auth_token=data_args.use_auth_token,
- **tokenizer_kwargs,
- )
- feature_extractor = AutoFeatureExtractor.from_pretrained(
- model_args.model_name_or_path, cache_dir=model_args.cache_dir, use_auth_token=data_args.use_auth_token
- )
-
- # adapt config
- # (speech translation requires pre-configured seq2seq models)
- if task_name != "covost2":
- config.update(
- {
- "feat_proj_dropout": model_args.feat_proj_dropout,
- "attention_dropout": model_args.attention_dropout,
- "hidden_dropout": model_args.hidden_dropout,
- "final_dropout": model_args.final_dropout,
- "mask_time_prob": model_args.mask_time_prob,
- "mask_time_length": model_args.mask_time_length,
- "mask_feature_prob": model_args.mask_feature_prob,
- "mask_feature_length": model_args.mask_feature_length,
- "gradient_checkpointing": training_args.gradient_checkpointing,
- "layerdrop": model_args.layerdrop,
- "ctc_zero_infinity": model_args.ctc_zero_infinity,
- "ctc_loss_reduction": model_args.ctc_loss_reduction,
- "activation_dropout": model_args.activation_dropout,
- }
- )
- if training_args.do_train:
- if is_text_target:
- config.pad_token_id = tokenizer.pad_token_id
- config.vocab_size = len(tokenizer)
- else:
- label_to_id = {v: i for i, v in enumerate(label_list)}
- config.label2id = label_to_id
- config.id2label = {id: label for label, id in label_to_id.items()}
- config.num_labels = num_labels
-
- # create model
- if target_column_name == "transcription":
- model = AutoModelForCTC.from_pretrained(
- model_args.model_name_or_path,
- cache_dir=model_args.cache_dir,
- config=config,
- use_auth_token=data_args.use_auth_token,
- )
- elif config.is_encoder_decoder:
- model = AutoModelForSpeechSeq2Seq.from_pretrained(
- model_args.model_name_or_path,
- cache_dir=model_args.cache_dir,
- config=config,
- use_auth_token=data_args.use_auth_token,
- )
- if model.config.decoder_start_token_id is None:
- raise ValueError("Make sure that `config.decoder_start_token_id` is correctly defined")
- else:
- model = AutoModelForAudioClassification.from_pretrained(
- model_args.model_name_or_path,
- cache_dir=model_args.cache_dir,
- config=config,
- use_auth_token=data_args.use_auth_token,
- )
-
- # freeze encoder
- if model_args.freeze_feature_encoder:
- model.freeze_feature_encoder()
-
- # 6. Now we preprocess the datasets including loading the audio, resampling and normalization
- # Thankfully, `datasets` takes care of automatically loading and resampling the audio,
- # so that we just need to set the correct target sampling rate and normalize the input
- # via the `feature_extractor`
-
- # make sure that dataset decodes audio with correct sampling rate
- dataset_sampling_rate = next(iter(raw_datasets.values())).features[data_args.audio_column_name].sampling_rate
- if dataset_sampling_rate != feature_extractor.sampling_rate:
- raw_datasets = raw_datasets.cast_column(
- data_args.audio_column_name, datasets.features.Audio(sampling_rate=feature_extractor.sampling_rate)
- )
-
- # derive max & min input length for sample rate & max duration
- max_input_length = data_args.max_duration_in_seconds * feature_extractor.sampling_rate
- min_input_length = data_args.min_duration_in_seconds * feature_extractor.sampling_rate
- audio_column_name = data_args.audio_column_name
-
- # `phoneme_language` is only relevant if the model is fine-tuned on phoneme classification
- phoneme_language = data_args.phoneme_language
-
- # Preprocessing the datasets.
- # We need to read the audio files as arrays and tokenize the targets.
- def prepare_dataset(batch):
- # load audio
- sample = batch[audio_column_name]
-
- inputs = feature_extractor(sample["array"], sampling_rate=sample["sampling_rate"])
- batch["input_values"] = inputs.input_values[0]
- batch["length"] = len(batch["input_values"])
-
- # encode targets
- additional_kwargs = {}
- if phoneme_language is not None:
- additional_kwargs["phonemizer_lang"] = phoneme_language
-
- if is_text_target:
- batch["labels"] = tokenizer(batch["target_text"], **additional_kwargs).input_ids
- else:
- batch["labels"] = batch[target_column_name]
-
- batch["lang"] = batch["lang_id"]
-
- return batch
-
- with training_args.main_process_first(desc="dataset map preprocessing"):
- vectorized_datasets = raw_datasets.map(
- prepare_dataset,
- remove_columns=next(iter(raw_datasets.values())).column_names,
- num_proc=num_workers,
- desc="preprocess datasets",
- )
-
- if training_args.do_train:
-
- def is_audio_in_length_range(length):
- return length > min_input_length and length < max_input_length
-
- # filter data that is shorter than min_input_length
- vectorized_datasets["train"] = vectorized_datasets["train"].filter(
- is_audio_in_length_range,
- num_proc=num_workers,
- input_columns=["length"],
- )
-
- # 7. Next, we can prepare for the training step.
- # Let's use the appropriate XTREME-S evaluation metric,
- # instantiate a data collator and the trainer
-
- # Define evaluation metrics during training, *i.e.* word error rate, character error rate
- eval_metric = load_metric("xtreme_s", task_name)
-
- # for large datasets it is advised to run the preprocessing on a
- # single machine first with ``args.preprocessing_only`` since there will mostly likely
- # be a timeout when running the script in distributed mode.
- # In a second step ``args.preprocessing_only`` can then be set to `False` to load the
- # cached dataset
- if data_args.preprocessing_only:
- logger.info(f"Data preprocessing finished. Files cached at {vectorized_datasets.cache_files}")
- return
-
- def asr_logits_argmax(logits, labels):
- return logits.argmax(dim=-1)
-
- def compute_asr_metric(pred):
- pred.label_ids[pred.label_ids == -100] = tokenizer.pad_token_id
-
- pred_str = tokenizer.batch_decode(pred.predictions)
- # we do not want to group tokens when computing the metrics
- label_str = tokenizer.batch_decode(pred.label_ids, group_tokens=False)
-
- metric = eval_metric.compute(predictions=pred_str, references=label_str)
- return metric
-
- def compute_classification_metric(pred):
- pred_ids = np.argmax(pred.predictions, axis=1)
- metric = eval_metric.compute(predictions=pred_ids, references=pred.label_ids)
- return metric
-
- # Now save everything to be able to create a single processor later
- if is_main_process(training_args.local_rank):
- # save feature extractor, tokenizer and config
- feature_extractor.save_pretrained(training_args.output_dir)
- if is_text_target:
- tokenizer.save_pretrained(training_args.output_dir)
- config.save_pretrained(training_args.output_dir)
- # wait until configs are saved in the main process before loading the processor
- if training_args.local_rank != -1:
- torch.distributed.barrier()
-
- if is_text_target:
- processor = AutoProcessor.from_pretrained(training_args.output_dir)
- else:
- processor = AutoFeatureExtractor.from_pretrained(training_args.output_dir)
-
- # Instantiate custom data collator
- data_collator = SpeechDataCollatorWithPadding(processor=processor, pad_labels=is_text_target)
-
- # Initialize Trainer
- if target_column_name == "translation":
- trainer = Seq2SeqTrainer(
- model=model,
- data_collator=data_collator,
- args=training_args,
- preprocess_logits_for_metrics=asr_logits_argmax if training_args.predict_with_generate else None,
- compute_metrics=compute_asr_metric if training_args.predict_with_generate else None,
- train_dataset=vectorized_datasets["train"] if training_args.do_train else None,
- eval_dataset=vectorized_datasets["eval"] if training_args.do_eval else None,
- tokenizer=feature_extractor,
- )
- else:
- trainer = Trainer(
- model=model,
- data_collator=data_collator,
- args=training_args,
- preprocess_logits_for_metrics=asr_logits_argmax if is_text_target else None,
- compute_metrics=compute_asr_metric if is_text_target else compute_classification_metric,
- train_dataset=vectorized_datasets["train"] if training_args.do_train else None,
- eval_dataset=vectorized_datasets["eval"] if training_args.do_eval else None,
- tokenizer=feature_extractor,
- )
-
- # 8. Finally, we can start training
-
- # Training
- if training_args.do_train:
- # use last checkpoint if exist
- if last_checkpoint is not None:
- checkpoint = last_checkpoint
- elif os.path.isdir(model_args.model_name_or_path):
- checkpoint = model_args.model_name_or_path
- else:
- checkpoint = None
-
- train_result = trainer.train(resume_from_checkpoint=checkpoint)
- trainer.save_model()
-
- metrics = train_result.metrics
- max_train_samples = (
- data_args.max_train_samples
- if data_args.max_train_samples is not None
- else len(vectorized_datasets["train"])
- )
- metrics["train_samples"] = min(max_train_samples, len(vectorized_datasets["train"]))
-
- trainer.log_metrics("train", metrics)
- trainer.save_metrics("train", metrics)
- trainer.save_state()
-
- # Evaluation on the test set
- results = {}
- if training_args.do_predict:
- logger.info(f"*** Evaluating on the `{data_args.predict_split_name}` set ***")
- if data_args.per_lang_metrics:
- # separate the `test` dataset into language-specific subsets and compute metrics for each of them
- metrics = {}
- average_metrics = defaultdict(list)
- for lang_id in range(len(lang_list)):
- lang_name = lang_list[lang_id]
- with training_args.main_process_first(desc="per-language dataset filter"):
- lang_dataset = vectorized_datasets["predict"].filter(
- lambda lang: lang == lang_id,
- num_proc=num_workers,
- input_columns=["lang"],
- )
- lang_metrics = trainer.evaluate(lang_dataset)
- redundant_metrics = ["eval_runtime", "eval_samples_per_second", "eval_steps_per_second", "eval_epoch"]
- for metric_name, value in lang_metrics.items():
- average_metrics[metric_name].append(value)
- if metric_name not in redundant_metrics:
- metrics[f"{metric_name}_{lang_name}"] = value
- for metric_name, value in average_metrics.items():
- metrics[metric_name] = np.mean(value)
- else:
- metrics = trainer.evaluate(vectorized_datasets["predict"])
- max_predict_samples = (
- data_args.max_predict_samples
- if data_args.max_predict_samples is not None
- else len(vectorized_datasets["predict"])
- )
- metrics["predict_samples"] = min(max_predict_samples, len(vectorized_datasets["predict"]))
-
- # make sure that the `predict` metrics end up in the log history for the model card
- trainer.log(OrderedDict(sorted(metrics.items())))
-
- trainer.log_metrics("predict", metrics)
- trainer.save_metrics("predict", metrics)
-
- # Write model card and (optionally) push to hub
- kwargs = {
- "finetuned_from": model_args.model_name_or_path,
- "tasks": task_name,
- "tags": [task_name, data_args.dataset_name],
- "dataset_args": (
- f"Config: {config_name}, Training split: {data_args.train_split_name}, Eval split:"
- f" {data_args.eval_split_name}, Predict split: {data_args.predict_split_name}"
- ),
- "dataset": f"{data_args.dataset_name.upper()} - {config_name.upper()}",
- "language": data_args.language,
- }
-
- if training_args.push_to_hub:
- trainer.push_to_hub(**kwargs)
- else:
- trainer.create_model_card(**kwargs)
-
- return results
-
-
-if __name__ == "__main__":
- main()
diff --git a/spaces/chendl/compositional_test/transformers/examples/tensorflow/benchmarking/plot_csv_file.py b/spaces/chendl/compositional_test/transformers/examples/tensorflow/benchmarking/plot_csv_file.py
deleted file mode 100644
index 9a9ad9c670470e1f3231d90c7fd375566e2fb8ee..0000000000000000000000000000000000000000
--- a/spaces/chendl/compositional_test/transformers/examples/tensorflow/benchmarking/plot_csv_file.py
+++ /dev/null
@@ -1,178 +0,0 @@
-# Copyright 2020 The HuggingFace Team. All rights reserved.
-#
-# Licensed under the Apache License, Version 2.0 (the "License");
-# you may not use this file except in compliance with the License.
-# You may obtain a copy of the License at
-#
-# http://www.apache.org/licenses/LICENSE-2.0
-#
-# Unless required by applicable law or agreed to in writing, software
-# distributed under the License is distributed on an "AS IS" BASIS,
-# WITHOUT WARRANTIES OR CONDITIONS OF ANY KIND, either express or implied.
-# See the License for the specific language governing permissions and
-# limitations under the License.
-
-import csv
-from collections import defaultdict
-from dataclasses import dataclass, field
-from typing import List, Optional
-
-import matplotlib.pyplot as plt
-import numpy as np
-from matplotlib.ticker import ScalarFormatter
-
-from transformers import HfArgumentParser
-
-
-def list_field(default=None, metadata=None):
- return field(default_factory=lambda: default, metadata=metadata)
-
-
-@dataclass
-class PlotArguments:
- """
- Arguments pertaining to which model/config/tokenizer we are going to fine-tune, or train from scratch.
- """
-
- csv_file: str = field(
- metadata={"help": "The csv file to plot."},
- )
- plot_along_batch: bool = field(
- default=False,
- metadata={"help": "Whether to plot along batch size or sequence length. Defaults to sequence length."},
- )
- is_time: bool = field(
- default=False,
- metadata={"help": "Whether the csv file has time results or memory results. Defaults to memory results."},
- )
- no_log_scale: bool = field(
- default=False,
- metadata={"help": "Disable logarithmic scale when plotting"},
- )
- is_train: bool = field(
- default=False,
- metadata={
- "help": "Whether the csv file has training results or inference results. Defaults to inference results."
- },
- )
- figure_png_file: Optional[str] = field(
- default=None,
- metadata={"help": "Filename under which the plot will be saved. If unused no plot is saved."},
- )
- short_model_names: Optional[List[str]] = list_field(
- default=None, metadata={"help": "List of model names that are used instead of the ones in the csv file."}
- )
-
-
-def can_convert_to_int(string):
- try:
- int(string)
- return True
- except ValueError:
- return False
-
-
-def can_convert_to_float(string):
- try:
- float(string)
- return True
- except ValueError:
- return False
-
-
-class Plot:
- def __init__(self, args):
- self.args = args
- self.result_dict = defaultdict(lambda: {"bsz": [], "seq_len": [], "result": {}})
-
- with open(self.args.csv_file, newline="") as csv_file:
- reader = csv.DictReader(csv_file)
- for row in reader:
- model_name = row["model"]
- self.result_dict[model_name]["bsz"].append(int(row["batch_size"]))
- self.result_dict[model_name]["seq_len"].append(int(row["sequence_length"]))
- if can_convert_to_int(row["result"]):
- # value is not None
- self.result_dict[model_name]["result"][
- (int(row["batch_size"]), int(row["sequence_length"]))
- ] = int(row["result"])
- elif can_convert_to_float(row["result"]):
- # value is not None
- self.result_dict[model_name]["result"][
- (int(row["batch_size"]), int(row["sequence_length"]))
- ] = float(row["result"])
-
- def plot(self):
- fig, ax = plt.subplots()
- title_str = "Time usage" if self.args.is_time else "Memory usage"
- title_str = title_str + " for training" if self.args.is_train else title_str + " for inference"
-
- if not self.args.no_log_scale:
- # set logarithm scales
- ax.set_xscale("log")
- ax.set_yscale("log")
-
- for axis in [ax.xaxis, ax.yaxis]:
- axis.set_major_formatter(ScalarFormatter())
-
- for model_name_idx, model_name in enumerate(self.result_dict.keys()):
- batch_sizes = sorted(set(self.result_dict[model_name]["bsz"]))
- sequence_lengths = sorted(set(self.result_dict[model_name]["seq_len"]))
- results = self.result_dict[model_name]["result"]
-
- (x_axis_array, inner_loop_array) = (
- (batch_sizes, sequence_lengths) if self.args.plot_along_batch else (sequence_lengths, batch_sizes)
- )
-
- label_model_name = (
- model_name if self.args.short_model_names is None else self.args.short_model_names[model_name_idx]
- )
-
- for inner_loop_value in inner_loop_array:
- if self.args.plot_along_batch:
- y_axis_array = np.asarray(
- [results[(x, inner_loop_value)] for x in x_axis_array if (x, inner_loop_value) in results],
- dtype=int,
- )
- else:
- y_axis_array = np.asarray(
- [results[(inner_loop_value, x)] for x in x_axis_array if (inner_loop_value, x) in results],
- dtype=np.float32,
- )
-
- (x_axis_label, inner_loop_label) = (
- ("batch_size", "len") if self.args.plot_along_batch else ("in #tokens", "bsz")
- )
-
- x_axis_array = np.asarray(x_axis_array, int)[: len(y_axis_array)]
- plt.scatter(
- x_axis_array, y_axis_array, label=f"{label_model_name} - {inner_loop_label}: {inner_loop_value}"
- )
- plt.plot(x_axis_array, y_axis_array, "--")
-
- title_str += f" {label_model_name} vs."
-
- title_str = title_str[:-4]
- y_axis_label = "Time in s" if self.args.is_time else "Memory in MB"
-
- # plot
- plt.title(title_str)
- plt.xlabel(x_axis_label)
- plt.ylabel(y_axis_label)
- plt.legend()
-
- if self.args.figure_png_file is not None:
- plt.savefig(self.args.figure_png_file)
- else:
- plt.show()
-
-
-def main():
- parser = HfArgumentParser(PlotArguments)
- plot_args = parser.parse_args_into_dataclasses()[0]
- plot = Plot(args=plot_args)
- plot.plot()
-
-
-if __name__ == "__main__":
- main()
diff --git a/spaces/chronopt-research/ViTExCo/UI.py b/spaces/chronopt-research/ViTExCo/UI.py
deleted file mode 100644
index 033046d4e8709d171221bc145df3422cfeed9e64..0000000000000000000000000000000000000000
--- a/spaces/chronopt-research/ViTExCo/UI.py
+++ /dev/null
@@ -1,81 +0,0 @@
-import streamlit as st
-from PIL import Image
-import torchvision.transforms as transforms
-from streamlit_image_comparison import image_comparison
-import numpy as np
-import torch
-import torchvision
-
-######################################### Utils ########################################
-video_extensions = ["mp4"]
-image_extensions = ["png", "jpg"]
-
-
-def check_type(file_name: str):
- for image_extension in image_extensions:
- if file_name.endswith(image_extension):
- return "image"
- for video_extension in video_extensions:
- if file_name.endswith(video_extension):
- return "video"
- return None
-
-
-transform = transforms.Compose(
- [transforms.Resize((256, 256)), transforms.ToTensor(), transforms.Normalize((0.485, 0.456, 0.406), (0.229, 0.224, 0.225))]
-)
-
-
-###################################### Load model ######################################
-@st.cache_resource
-def load_model():
- model = torchvision.models.segmentation.deeplabv3_resnet101(pretrained=True)
- model.eval()
- return model
-
-
-model = load_model()
-########################################## UI ##########################################
-st.title("Colorization")
-
-uploaded_file = st.file_uploader("Upload grayscale image or video", type=image_extensions + video_extensions)
-if uploaded_file:
- # Image
- if check_type(file_name=uploaded_file.name) == "image":
- image = np.array(Image.open(uploaded_file), dtype=np.float32)
-
- input_tensor = torchvision.transforms.functional.normalize(
- torch.tensor(image).permute(2, 0, 1),
- mean=[0.485, 0.456, 0.406],
- std=[0.229, 0.224, 0.225],
- ).unsqueeze(0)
- process_button = st.button("Process")
- if process_button:
- with st.spinner("Từ từ coi..."):
- prediction = model(input_tensor)
- segment = prediction["out"][0].permute(1, 2, 0)
- segment = segment.detach().numpy()
-
- st.image(segment)
- st.image(image)
-
- image_comparison(
- img1=image,
- img2=np.array(segment),
- label1="Grayscale",
- label2="Colorized",
- make_responsive=True,
- show_labels=True,
- )
- # Video
- else:
- # video = open(uploaded_file.name)
- st.video("https://youtu.be/dQw4w9WgXcQ")
-
-hide_menu_style = """
-
- """
-st.markdown(hide_menu_style, unsafe_allow_html=True)
diff --git a/spaces/chuan-hd/law-assistant-chatbot/.venv/lib/python3.11/site-packages/gradio/templates/cdn/assets/Login-9c3cc0eb.css b/spaces/chuan-hd/law-assistant-chatbot/.venv/lib/python3.11/site-packages/gradio/templates/cdn/assets/Login-9c3cc0eb.css
deleted file mode 100644
index 9901bcac6c93474ed045092f6d91d6e683ba5b32..0000000000000000000000000000000000000000
--- a/spaces/chuan-hd/law-assistant-chatbot/.venv/lib/python3.11/site-packages/gradio/templates/cdn/assets/Login-9c3cc0eb.css
+++ /dev/null
@@ -1 +0,0 @@
-.wrap.svelte-1ogxbi0{display:flex;flex-direction:column;justify-content:center;align-items:center;margin-top:var(--size-3);background:var(--background-fill-primary);width:var(--size-full)}h2.svelte-1ogxbi0{margin-bottom:var(--size-3);color:var(--body-text-color);font-weight:var(--section-header-text-weight);font-size:var(--text-xl)}.auth.svelte-1ogxbi0{margin-top:var(--size-1);margin-bottom:var(--size-1);color:var(--body-text-color)}.creds.svelte-1ogxbi0{margin-top:var(--size-4);margin-bottom:var(--size-4);color:var(--error-text-color);font-weight:var(--weight-semibold)}
diff --git a/spaces/cihyFjudo/fairness-paper-search/HACK MiniTool Partition Wizard Server V8.1.1 Retail Incl Keygen-BRD [Review and Tutorial].md b/spaces/cihyFjudo/fairness-paper-search/HACK MiniTool Partition Wizard Server V8.1.1 Retail Incl Keygen-BRD [Review and Tutorial].md
deleted file mode 100644
index 1de1789bd496337919d1aec64b659839a06e886e..0000000000000000000000000000000000000000
--- a/spaces/cihyFjudo/fairness-paper-search/HACK MiniTool Partition Wizard Server V8.1.1 Retail Incl Keygen-BRD [Review and Tutorial].md
+++ /dev/null
@@ -1,6 +0,0 @@
-
-
- aaccfb2cb3
-
-
-
diff --git a/spaces/cihyFjudo/fairness-paper-search/Thunderbolt 3 is coming The future of connectivity and productivity.md b/spaces/cihyFjudo/fairness-paper-search/Thunderbolt 3 is coming The future of connectivity and productivity.md
deleted file mode 100644
index 421402d73113cb8769c645ad858bd6952fb06da8..0000000000000000000000000000000000000000
--- a/spaces/cihyFjudo/fairness-paper-search/Thunderbolt 3 is coming The future of connectivity and productivity.md
+++ /dev/null
@@ -1,7 +0,0 @@
-
-
OWC has announced that its upcoming Thunderbolt Hub will be compatible with all Apple M1 and Intel Macs equipped with Thunderbolt 3 ports and running macOS Big Sur, offering users the ability to expand the number of available Thunderbolt ports.
-
VESA has announced today that its DisplayPort 2.0 specs are coming to USB4/USB-C that will bring a jump in the capabilities of video output. The standard will support up to 16K displays with video data throughput of up to 80 Gbps.
AJA Software Installer v16.2.3\u00a0for\u00a0macOS:\r\n\r\nThis unified software, driver and firmware package contains everything you need in order to start using your AJA video I\/O hardware and includes enhancements.\u00a0Please read the\u00a0Release Notes\u00a0for complete detail.\u00a0\r\n\r\nThe following AJA applications are installed in v16.2.3\r\n\r\n
\r\n\t
AJA Control Panel v16.2.3:\u00a0\r\n\r\n\t
\r\n\t\t
For setup and control of your AJA KONA, Io or T-TAP product, including firmware updates.\r\n\t\t
For high quality capture, playback and output.\r\n\t\r\n\t\r\n\t
AJA System Test v16.2.3:\u00a0\r\n\t
\r\n\t\t
For testing storage to determine if it will be possible to sustain frame rates for chosen format(s).\r\n\t\r\n\t\r\n\t
AJA Control Room v16.2.3:\r\n\t
\r\n\t\t
For high quality capture, monitoring, playback and output.\r\n\t\r\n\t\r\n\t
AJA\u00a0NMOS\u00a0v16.2.3:\u00a0\r\n\t
\r\n\t\t
An optional component providing discovery, registration and control for KONA IP running in\u00a0SMPTE\u00a0ST 2110 environments.\r\n\t\r\n\t\r\n\r\n\r\n\u00a0\r\n","product":0,"category":"so","platform_mac":1,"platform_pc":0,"platform_linux":0,"platform":"m","showdate":"y","released":"2\/7\/2023","url":"","ziparchive":true,"files":["file":"AJA-Software-Installer_macOS_v16.2.3_Release.zip","abspath":"\/var\/www\/sierra\/releases\/20230118233302\/webroot\/public\/assets\/support\/files\/8994\/en\/AJA-Software-Installer_macOS_v16.2.3_Release.zip","urlpath":"https:\/\/www.aja.com\/assets\/support\/files\/8994\/en\/","ext":"zip"]}],"p":["id":8997,"title":"AJA Software Installer v16.2.3 - Windows","description":"AJA Software Installer v16.2.3 for Windows:\r\n\r\nThis unified software, driver and firmware package contains everything you need in order to start using your AJA video I\/O hardware and includes new features as well as\u00a0maintenance updates.\u00a0Please read the Release Notes\u00a0for complete detail.\u00a0\r\n\r\nThe following AJA applications are installed in v16.2.3\r\n\r\n
\r\n\t
AJA Control Panel v16.2.3:\u00a0\r\n\r\n\t
\r\n\t\t
For setup and control of your AJA KONA, Io or T-TAP product, including firmware updates.\r\n\t\t
For high quality capture, playback and output.\r\n\t\r\n\t\r\n\t
AJA System Test v16.2.3:\u00a0\r\n\t
\r\n\t\t
For testing storage to determine if it will be possible to sustain frame rates for chosen format(s).\r\n\t\r\n\t\r\n\t
AJA Control Room v16.2.3:\r\n\t
\r\n\t\t
For high quality capture, monitoring, playback and output.\r\n\t\r\n\t\r\n\t
AJA\u00a0NMOS\u00a0v16.2.3:\u00a0\r\n\t
\r\n\t\t
An optional component providing discovery, registration and control for KONA IP running in\u00a0SMPTE\u00a0ST 2110 environments.\r\n\t\r\n\t\r\n\r\n","product":0,"category":"so","platform_mac":0,"platform_pc":1,"platform_linux":0,"platform":"m","showdate":"y","released":"2\/3\/2023","url":"","ziparchive":true,"files":["file":"AJA-Software-Installer_Windows_v16.2.3_Release.zip","abspath":"\/var\/www\/sierra\/releases\/20230118233302\/webroot\/public\/assets\/support\/files\/8997\/en\/AJA-Software-Installer_Windows_v16.2.3_Release.zip","urlpath":"https:\/\/www.aja.com\/assets\/support\/files\/8997\/en\/","ext":"zip"],"id":7132,"title":"DirectShow Filters v15.5.2","description":"These filters are designed for DirectShow application developers, so that they may make their applications compatible with the majority of AJA's windows based hardware. These filters are meant to be used programmatically, and will not necessarily work with every DirectShow application without work being done to explicitly support them. Note: DirectShow is a trademark of Microsoft.\r\n\r\n\u00a0\r\n","product":0,"category":"so","platform_mac":0,"platform_pc":1,"platform_linux":0,"platform":"m","showdate":"y","released":"4\/23\/2020","url":"https:\/\/www.aja.com\/support\/directshow","ziparchive":true,"files":["file":"AJA_DirectShowPackage_15_5_2.zip","abspath":"\/var\/www\/sierra\/releases\/20230118233302\/webroot\/public\/assets\/support\/files\/7132\/en\/AJA_DirectShowPackage_15_5_2.zip","urlpath":"https:\/\/www.aja.com\/assets\/support\/files\/7132\/en\/","ext":"zip"]],"l":[]},"mac_count":1,"pc_count":2,"linux_count":0,"load_count":3,"show_links":false,"m_load":1,"p_load":1,"l_load":1,"m_cached":true,"p_cached":true,"l_cached":true},"tag":"sa","title":"Software Archive","items":"m":["id":8745,"title":"AJA Software Installer v16.2.2 - Mac","description":"AJA Software Installer v16.2.2 for\u00a0macOS:\r\n\r\nThis unified software, driver and firmware package contains everything you need in order to start using your AJA video I\/O hardware and includes enhancements.\u00a0Please read the\u00a0Release Notes\u00a0for complete detail.\u00a0\r\n\r\nThe following AJA applications are installed in v16.2.2\r\n\r\n
\r\n\t
AJA Control Panel v16.2.2:\u00a0\r\n\r\n\t
\r\n\t\t
For setup and control of your AJA KONA, Io or T-TAP product, including firmware updates.\r\n\t\t
For high quality capture, playback and output.\r\n\t\r\n\t\r\n\t
AJA System Test v16.2.2:\u00a0\r\n\t
\r\n\t\t
For testing storage to determine if it will be possible to sustain frame rates for chosen format(s).\r\n\t\r\n\t\r\n\t
AJA Control Room v16.2.2:\r\n\t
\r\n\t\t
For high quality capture, monitoring, playback and output.\r\n\t\r\n\t\r\n\t
AJA\u00a0NMOS\u00a0v16.2.2:\u00a0\r\n\t
\r\n\t\t
An optional component providing discovery, registration and control for KONA IP running in\u00a0SMPTE\u00a0ST 2110 environments.\r\n\t\r\n\t\r\n\r\n\r\n\u00a0\r\n","product":0,"category":"sa","platform_mac":1,"platform_pc":0,"platform_linux":0,"platform":"m","showdate":"y","released":"6\/14\/2022","url":"","ziparchive":true,"files":["file":"AJA-Software-Installer_macOS_v16.2.2_Release.zip","abspath":"\/var\/www\/sierra\/releases\/20230118233302\/webroot\/public\/assets\/support\/files\/8745\/en\/AJA-Software-Installer_macOS_v16.2.2_Release.zip","urlpath":"https:\/\/www.aja.com\/assets\/support\/files\/8745\/en\/","ext":"zip"],"id":8508,"title":"AJA Software Installer v16.2 - Mac","description":"AJA Software Installer v16.2\u00a0for\u00a0macOS:\r\n\r\nThis unified software, driver and firmware package contains everything you need in order to start using your AJA video I\/O hardware and includes enhancements.\u00a0Please read the\u00a0Release Notes\u00a0for complete detail.\u00a0\r\n\r\nThe following AJA applications are installed in v16.2\r\n\r\n
\r\n\t
AJA Control Panel v16.2:\u00a0\r\n\r\n\t
\r\n\t\t
For setup and control of your AJA KONA, Io or T-TAP product, including firmware updates.\r\n\t\t
For high quality capture, playback and output.\r\n\t\r\n\t\r\n\t
AJA System Test v16.2:\u00a0\r\n\t
\r\n\t\t
For testing storage to determine if it will be possible to sustain frame rates for chosen format(s).\r\n\t\r\n\t\r\n\t
AJA Control Room v16.2:\r\n\t
\r\n\t\t
For high quality capture, monitoring, playback and output.\r\n\t\r\n\t\r\n\t
AJA\u00a0NMOS\u00a0v16.2:\u00a0\r\n\t
\r\n\t\t
An optional component providing discovery, registration and control for KONA IP running in\u00a0SMPTE\u00a0ST 2110 environments.\r\n\t\r\n\t\r\n\r\n\r\n\u00a0\r\n","product":0,"category":"sa","platform_mac":1,"platform_pc":0,"platform_linux":0,"platform":"m","showdate":"y","released":"2\/3\/2022","url":"","ziparchive":true,"files":["file":"AJA-Software-Installer_macOS_v16.2_Release.zip","abspath":"\/var\/www\/sierra\/releases\/20230118233302\/webroot\/public\/assets\/support\/files\/8508\/en\/AJA-Software-Installer_macOS_v16.2_Release.zip","urlpath":"https:\/\/www.aja.com\/assets\/support\/files\/8508\/en\/","ext":"zip"],"id":8316,"title":"AJA Software Installer v16.1 - Mac","description":"AJA Software Installer v16.1\u00a0for\u00a0macOS:\r\n\r\nThis unified software, driver and firmware package contains everything you need in order to start using your AJA video I\/O hardware and includes enhancements.\u00a0Please read the\u00a0Release Notes\u00a0for complete detail.\u00a0\r\n\r\nThe following AJA applications are installed in v16.1\r\n\r\n
\r\n\t
AJA Control Panel v16.1:\u00a0\r\n\r\n\t
\r\n\t\t
For setup and control of your AJA KONA, Io or T-TAP product, including firmware updates.\r\n\t\t
For high quality capture, playback and output.\r\n\t\r\n\t\r\n\t
AJA System Test v16.1:\u00a0\r\n\t
\r\n\t\t
For testing storage to determine if it will be possible to sustain frame rates for chosen format(s).\r\n\t\r\n\t\r\n\t
AJA Control Room v16.1:\r\n\t
\r\n\t\t
For high quality capture, monitoring, playback and output.\r\n\t\r\n\t\r\n\t
AJA\u00a0NMOS\u00a0v16.1:\u00a0\r\n\t
\r\n\t\t
An optional component providing discovery, registration and control for KONA IP running in\u00a0SMPTE\u00a0ST 2110 environments.\r\n\t\r\n\t\r\n\r\n\r\n\u00a0\r\n","product":0,"category":"sa","platform_mac":1,"platform_pc":0,"platform_linux":0,"platform":"m","showdate":"y","released":"7\/28\/2021","url":"","ziparchive":true,"files":["file":"AJA-Software-Installer_macOS_v16.1_Release.zip","abspath":"\/var\/www\/sierra\/releases\/20230118233302\/webroot\/public\/assets\/support\/files\/8316\/en\/AJA-Software-Installer_macOS_v16.1_Release.zip","urlpath":"https:\/\/www.aja.com\/assets\/support\/files\/8316\/en\/","ext":"zip"],"id":8310,"title":"AJA Software Installer v16.0.3 - Mac","description":"AJA Software Installer v16.0.3\u00a0for\u00a0macOS:\r\n\r\nThis unified software, driver and firmware package contains everything you need in order to start using your AJA video I\/O hardware and includes enhancements.\u00a0Please read the\u00a0Release Notes\u00a0for complete detail.\u00a0\r\n\r\nThe following AJA applications are installed in v16.0.3\r\n\r\n
\r\n\t
AJA Control Panel v16.0.3:\u00a0\r\n\r\n\t
\r\n\t\t
For setup and control of your AJA KONA, Io or T-TAP product, including firmware updates.\r\n\t\t
For high quality capture, playback and output.\r\n\t\r\n\t\r\n\t
AJA System Test v16.0.3:\u00a0\r\n\t
\r\n\t\t
For testing storage to determine if it will be possible to sustain frame rates for chosen format(s).\r\n\t\r\n\t\r\n\t
AJA Control Room v16.0.3:\r\n\t
\r\n\t\t
For high quality capture, monitoring, playback and output.\r\n\t\r\n\t\r\n\t
AJA\u00a0NMOS\u00a0v16.0.3:\u00a0\r\n\t
\r\n\t\t
An optional component providing discovery, registration and control for KONA IP running in\u00a0SMPTE\u00a0ST 2110 environments.\r\n\t\r\n\t\r\n\r\n\r\n\u00a0\r\n","product":0,"category":"sa","platform_mac":1,"platform_pc":0,"platform_linux":0,"platform":"m","showdate":"y","released":"7\/20\/2021","url":"","ziparchive":true,"files":["file":"AJA-Software-Installer_macOS_v16.0.3_Release.zip","abspath":"\/var\/www\/sierra\/releases\/20230118233302\/webroot\/public\/assets\/support\/files\/8310\/en\/AJA-Software-Installer_macOS_v16.0.3_Release.zip","urlpath":"https:\/\/www.aja.com\/assets\/support\/files\/8310\/en\/","ext":"zip"],"id":8170,"title":"AJA Software Installer v16.0.2 - Mac","description":"AJA Software Installer v16.0.2\u00a0for\u00a0macOS:\r\n\r\nThis unified software, driver and firmware package contains everything you need in order to start using your AJA video I\/O hardware and includes enhancements.\u00a0Please read the\u00a0Release Notes\u00a0for complete detail.\u00a0\r\n\r\nThe following AJA applications are installed in v16.0.2\r\n\r\n
\r\n\t
AJA Control Panel v16.0.2:\u00a0\r\n\r\n\t
\r\n\t\t
For setup and control of your AJA KONA, Io or T-TAP product, including firmware updates.\r\n\t\t
For high quality capture, playback and output.\r\n\t\r\n\t\r\n\t
AJA System Test v16.0.2:\u00a0\r\n\t
\r\n\t\t
For testing storage to determine if it will be possible to sustain frame rates for chosen format(s).\r\n\t\r\n\t\r\n\t
AJA Control Room v16.0.2:\r\n\t
\r\n\t\t
For high quality capture, monitoring, playback and output.\r\n\t\r\n\t\r\n\t
AJA\u00a0NMOS\u00a0v16.0.2:\u00a0\r\n\t
\r\n\t\t
An optional component providing discovery, registration and control for KONA IP running in\u00a0SMPTE\u00a0ST 2110 environments.\r\n\t\r\n\t\r\n\r\n\r\n\u00a0\r\n","product":0,"category":"sa","platform_mac":1,"platform_pc":0,"platform_linux":0,"platform":"m","showdate":"y","released":"4\/20\/2021","url":"","ziparchive":true,"files":["file":"AJA-Software-Installer_macOS_v16.0.2_Release.zip","abspath":"\/var\/www\/sierra\/releases\/20230118233302\/webroot\/public\/assets\/support\/files\/8170\/en\/AJA-Software-Installer_macOS_v16.0.2_Release.zip","urlpath":"https:\/\/www.aja.com\/assets\/support\/files\/8170\/en\/","ext":"zip"],"id":7947,"title":"AJA Software Installer v16 - Mac","description":"AJA Software Installer v16 for\u00a0macOS:\r\n\r\nThis unified software, driver and firmware package contains everything you need in order to start using your AJA video I\/O hardware and includes enhancements. New features for a range of I\/O products\u00a0include\u00a0HDR over SDI, HDR Auto Playback Detection, 4K Closed Caption Support, NMO3 v1.3 Support,\u00a0LLDP\u00a0Support,\u00a08K NLE\/VFX Software Support,\u00a08K Capture and Playback and Dynamic\u00a0FPGA\u00a0Firmware Reconfiguration. Please read the\u00a0Release Notes\u00a0for complete detail.\u00a0\r\n\r\nThe following AJA applications are installed in v16\r\n\r\n
\r\n\t
AJA Control Panel v16:\u00a0\r\n\r\n\t
\r\n\t\t
For setup and control of your AJA KONA, Io or T-TAP product, including firmware updates.\r\n\t\t
For high quality capture, playback and output.\r\n\t\r\n\t\r\n\t
AJA System Test v16:\u00a0\r\n\t
\r\n\t\t
For testing storage to determine if it will be possible to sustain frame rates for chosen format(s).\r\n\t\r\n\t\r\n\t
AJA Control Room v16:\r\n\t
\r\n\t\t
For high quality capture, monitoring, playback and output.\r\n\t\r\n\t\r\n\t
AJA\u00a0NMOS\u00a0v16:\u00a0\r\n\t
\r\n\t\t
An optional component providing discovery, registration and control for KONA IP running in\u00a0SMPTE\u00a0ST 2110 environments.\r\n\t\r\n\t\r\n\r\n\r\n\u00a0\r\n","product":0,"category":"sa","platform_mac":1,"platform_pc":0,"platform_linux":0,"platform":"m","showdate":"y","released":"3\/2\/2021","url":"","ziparchive":true,"files":["file":"AJA-Software-Installer_macOS_v16.0_Release.zip","abspath":"\/var\/www\/sierra\/releases\/20230118233302\/webroot\/public\/assets\/support\/files\/7947\/en\/AJA-Software-Installer_macOS_v16.0_Release.zip","urlpath":"https:\/\/www.aja.com\/assets\/support\/files\/7947\/en\/","ext":"zip"]],"p":["id":8748,"title":"AJA Software Installer v16.2.2 - Windows","description":"AJA Software Installer v16.2.2 for Windows:\r\n\r\nThis unified software, driver and firmware package contains everything you need in order to start using your AJA video I\/O hardware and includes new features including as well as\u00a0maintenance updates.\u00a0Please read the Release Notes\u00a0for complete detail.\u00a0\r\n\r\nThe following AJA applications are installed in v16.2.2\r\n\r\n
\r\n\t
AJA Control Panel v16.2.2:\u00a0\r\n\r\n\t
\r\n\t\t
For setup and control of your AJA KONA, Io or T-TAP product, including firmware updates.\r\n\t\t
For high quality capture, playback and output.\r\n\t\r\n\t\r\n\t
AJA System Test v16.2.2:\u00a0\r\n\t
\r\n\t\t
For testing storage to determine if it will be possible to sustain frame rates for chosen format(s).\r\n\t\r\n\t\r\n\t
AJA Control Room v16.2.2:\r\n\t
\r\n\t\t
For high quality capture, monitoring, playback and output.\r\n\t\r\n\t\r\n\t