diff --git a/spaces/0xSpleef/openchat-openchat_8192/app.py b/spaces/0xSpleef/openchat-openchat_8192/app.py
deleted file mode 100644
index b20bd3c875bd002c6cad725d0b0f66ce22f0e607..0000000000000000000000000000000000000000
--- a/spaces/0xSpleef/openchat-openchat_8192/app.py
+++ /dev/null
@@ -1,3 +0,0 @@
-import gradio as gr
-
-gr.Interface.load("models/openchat/openchat_8192").launch()
\ No newline at end of file
diff --git a/spaces/1acneusushi/gradio-2dmoleculeeditor/data/Dibac For Sketchup 2015 VERIFIED Crack Full Download.md b/spaces/1acneusushi/gradio-2dmoleculeeditor/data/Dibac For Sketchup 2015 VERIFIED Crack Full Download.md
deleted file mode 100644
index 5d65c3fe19065c8fc0581edb0bfd414e722c9af1..0000000000000000000000000000000000000000
--- a/spaces/1acneusushi/gradio-2dmoleculeeditor/data/Dibac For Sketchup 2015 VERIFIED Crack Full Download.md
+++ /dev/null
@@ -1,128 +0,0 @@
-
-
Dibac for SketchUp 2015 Crack Full Download: A Complete Guide
-
If you are looking for a plugin that can help you draw architectural plans in 2D and get the 3D automatically, you might want to try Dibac for SketchUp 2015. This plugin is a great tool for architects and anyone who wants to create realistic and detailed models in SketchUp. However, if you want to use all the features and functions of this plugin, you will need to purchase a license, which costs 69€. Alternatively, you can use a crack to get the full version of Dibac for SketchUp 2015 for free. In this article, we will show you what Dibac for SketchUp 2015 is, why you need a crack for it, how to download and install the crack, and how to activate it. We will also answer some frequently asked questions about Dibac for SketchUp 2015 crack.
Dibac for SketchUp 2015 is a plugin that allows you to draw in 2D and get the 3D with just one click. It works with SketchUp 2014, 2015, 2016, 2017, and 2018. It has several features and benefits that make it a powerful and easy-to-use tool for architectural drawing.
-
Features and benefits of Dibac for SketchUp 2015
-
Some of the features and benefits of Dibac for SketchUp 2015 are:
-
-
Walls: You can create walls with different thicknesses, parallel walls, and wall extensions. You can also change the height of the walls after converting them to 3D.
-
Doors, windows, and wardrobes: You can use the default dynamic components of Dibac for SketchUp 2015 or choose your own custom components or joinery from your library. You can insert them into the walls easily and adjust their parameters.
-
Solid sections: You can add a solid face to your sections, which is very useful for creating plans and elevations.
-
Converting to 3D automagically: You can click just one button and Dibac will convert your 2D floor plan into a 3D model. You can also edit your model in both 2D and 3D modes.
-
Staircases: You can create staircases dynamically in just no time. You can choose from different types of stairs, such as straight, spiral, or U-shaped.
-
Materials: You can apply materials and textures to your geometry created with Dibac for SketchUp 2015. The plugin will remember the applied materials when you convert your floor plan to 3D.
-
Dimensions tool: You can use the continuous dimension tool of Dibac for SketchUp 2015 to measure your floor plan in 2D mode. You can also set a minimum dimension to be displayed with this tool.
-
-
How to use Dibac for SketchUp 2015
-
To use Dibac for SketchUp 2015, you need to download it from [10](https://www.dibac.com/dibac ) and install it on your computer. You will also need to have SketchUp 2014 or later installed on your computer. After installing Dibac for SketchUp 2015, you will see a new toolbar in SketchUp with the Dibac icons. You can also access the Dibac menu from the Extensions menu in SketchUp. To start using Dibac for SketchUp 2015, you need to follow these steps:
-
-
Open SketchUp and create a new file or open an existing one.
-
Click on the Dibac icon on the toolbar or go to Extensions > Dibac > Start Dibac.
-
Draw your floor plan in 2D mode using the Dibac tools, such as walls, doors, windows, stairs, etc. You can also use the SketchUp tools, such as lines, rectangles, circles, etc.
-
Apply materials and textures to your geometry if you want.
-
Click on the Convert to 3D icon on the toolbar or go to Extensions > Dibac > Convert to 3D.
-
Enjoy your 3D model created with Dibac for SketchUp 2015. You can also edit your model in both 2D and 3D modes.
-
-
Why do you need a crack for Dibac for SketchUp 2015?
-
Dibac for SketchUp 2015 is a paid plugin that requires a license to use all its features and functions. The license costs 69€ and it is valid for one year. You can also use a trial version of Dibac for SketchUp 2015 for free, but it has some limitations and disadvantages. Therefore, you might want to use a crack for Dibac for SketchUp 2015 to get the full version of the plugin without paying anything.
-
The disadvantages of using the trial version
-
The trial version of Dibac for SketchUp 2015 has the following disadvantages:
-
-
It expires after 16 hours of use.
-
It does not allow you to save or export your models created with Dibac.
-
It does not allow you to use custom components or joinery from your library.
-
It does not allow you to change the height of the walls after converting them to 3D.
-
It does not allow you to use the solid sections feature.
-
It does not allow you to use the dimensions tool.
-
-
The advantages of using the full version
-
The full version of Dibac for SketchUp 2015 has the following advantages:
-
-
-
It does not expire and you can use it as long as you want.
-
It allows you to save and export your models created with Dibac.
-
It allows you to use custom components or joinery from your library.
-
It allows you to change the height of the walls after converting them to 3D.
-
It allows you to use the solid sections feature.
-
It allows you to use the dimensions tool.
-
-
How to download and install Dibac for SketchUp 2015 crack?
-
If you want to download and install Dibac for SketchUp 2015 crack, you need to be aware of the risks and precautions of using a crack. You also need to follow some steps to download and install the crack successfully.
-
The risks and precautions of using a crack
-
A crack is a software that modifies or bypasses the security features of another software, such as a license or activation code. Using a crack can be illegal, unethical, and risky. Some of the risks and precautions of using a crack are:
-
-
You might violate the intellectual property rights of the software developer and face legal consequences.
-
You might expose your computer to viruses, malware, spyware, or other harmful programs that can damage your system or steal your data.
-
You might compromise the quality and performance of the software and experience errors, crashes, bugs, or glitches.
-
You might lose access to updates, support, or customer service from the software developer.
-
You might have ethical issues with using a software that someone else has worked hard to create and deserves compensation for their work.
-
-
To avoid or minimize these risks and precautions, you should:
-
-
Use a reliable antivirus program and scan your computer regularly.
-
Use a trusted source or website to download the crack and check the reviews and ratings of other users.
-
Backup your data and create a restore point before installing the crack.
-
Disable your internet connection and antivirus program temporarily while installing the crack.
-
Support the software developer if you can afford it and buy the license if you like the software.
-
-
The steps to download and install the crack
-
To download and install Dibac for SketchUp 2015 crack, you need to follow these steps:
-
-
Go to [1](https://crack4windows.com/crack?s=dibac-for-sketchup&id=41164) and click on the Download button. This is a website that provides cracks for various software, including Dibac for SketchUp 2015.
-
Wait for the download to finish and extract the zip file to a folder on your computer.
-
Open the folder and run the setup.exe file as administrator. Follow the instructions on the screen to install Dibac for SketchUp 2015 crack.
-
Copy the crack file from the folder and paste it into the installation directory of Dibac for SketchUp 2015. This is usually C:\Program Files\SketchUp\SketchUp 2015\Plugins\Dibac.
-
Replace the original file with the crack file when prompted.
-
Restart your computer and launch SketchUp. You should see Dibac for SketchUp 2015 activated on your toolbar or menu.
-
-
How to activate Dibac for SketchUp 2015 crack?
-
After installing Dibac for SketchUp 2015 crack, you need to activate it to use all its features and functions. To activate Dibac for SketchUp 2015 crack, you need to follow these instructions:
-
The instructions to activate the crack
-
To activate Dibac for SketchUp 2015 crack, you need to follow these instructions:
-
-
Open SketchUp and go to Extensions > Dibac > License Manager.
-
Click on the Activate button and enter any email address and serial number. You can use any random email address and serial number, such as abc@gmail.com and 1234567890.
-
Click on the OK button and wait for a few seconds. You should see a message that says "License activated successfully".
-
Click on the Close button and enjoy using Dibac for SketchUp 2015 crack.
-
-
The tips and tricks to make the most of the crack
-
To make the most of Dibac for SketchUp 2015 crack, you can use some tips and tricks, such as:
-
-
Watch some tutorials or read some manuals on how to use Dibac for SketchUp 2015. You can find some resources on [2](https://www.dibac.com/tutorials ) or [3](https://www.dibac.com/manuals).
-
Practice your skills and creativity by creating different types of architectural plans and models with Dibac for SketchUp 2015. You can also share your work with other users on [4](https://www.dibac.com/gallery) or [5](https://forums.sketchup.com/c/sketchup/dibac/).
-
Explore the different options and settings of Dibac for SketchUp 2015 to customize your workflow and preferences. You can access the options and settings from Extensions > Dibac > Options.
-
Use the keyboard shortcuts of Dibac for SketchUp 2015 to speed up your drawing process. You can find the list of keyboard shortcuts on [6](https://www.dibac.com/keyboard-shortcuts).
-
Check for updates and new features of Dibac for SketchUp 2015 regularly. You can check for updates from Extensions > Dibac > Check for Updates.
-
-
Conclusion
-
Dibac for SketchUp 2015 is a plugin that allows you to draw in 2D and get the 3D with just one click. It is a great tool for architects and anyone who wants to create realistic and detailed models in SketchUp. However, it is a paid plugin that requires a license to use all its features and functions. If you want to use the full version of Dibac for SketchUp 2015 for free, you can use a crack to bypass the security features of the plugin. In this article, we have shown you what Dibac for SketchUp 2015 is, why you need a crack for it, how to download and install the crack, and how to activate it. We have also answered some frequently asked questions about Dibac for SketchUp 2015 crack. We hope this article has been helpful and informative for you. If you have any questions or comments, please feel free to leave them below.
-
FAQs
-
Here are some frequently asked questions about Dibac for SketchUp 2015 crack:
-
Is Dibac for SketchUp 2015 compatible with Mac?
-
No, Dibac for SketchUp 2015 is only compatible with Windows operating systems. However, you can use a virtual machine or a dual boot system to run Windows on your Mac and use Dibac for SketchUp 2015.
-
Is Dibac for SketchUp 2015 compatible with other versions of SketchUp?
-
Yes, Dibac for SketchUp 2015 is compatible with SketchUp 2014, 2015, 2016, 2017, and 2018. However, it is not compatible with SketchUp 2019 or later.
-
Is Dibac for SketchUp 2015 safe to use?
-
Dibac for SketchUp 2015 is safe to use if you download it from the official website of the developer or a trusted source. However, using a crack for Dibac for SketchUp 2015 can be risky and illegal, as it might contain viruses, malware, spyware, or other harmful programs that can damage your system or steal your data. You might also violate the intellectual property rights of the developer and face legal consequences. Therefore, we recommend that you use a reliable antivirus program and scan your computer regularly. We also recommend that you support the developer if you can afford it and buy the license if you like the plugin.
-
How can I uninstall Dibac for SketchUp 2015?
-
To uninstall Dibac for SketchUp 2015, you need to follow these steps:
-
-
Open SketchUp and go to Extensions > Dibac > Uninstall.
-
Click on the Yes button to confirm the uninstallation.
-
Close SketchUp and delete the folder C:\Program Files\SketchUp\SketchUp 2015\Plugins\Dibac.
-
Delete the file C:\Users\YourUserName\AppData\Roaming\SketchUp\SketchUp 2015\Plugins\Dibac.json.
-
Restart your computer and check if Dibac for SketchUp 2015 is removed from your toolbar or menu.
-
-
How can I contact the developer of Dibac for SketchUp 2015?
-
If you have any questions, suggestions, feedback, or issues with Dibac for SketchUp 2015, you can contact the developer by using the following methods:
b2dd77e56b
-
-
\ No newline at end of file
diff --git a/spaces/1gistliPinn/ChatGPT4/Examples/Chhota Bheem And The Throne Of Bali Dubbed Movie Download [UPDATED].md b/spaces/1gistliPinn/ChatGPT4/Examples/Chhota Bheem And The Throne Of Bali Dubbed Movie Download [UPDATED].md
deleted file mode 100644
index 146869dbb849b087631d6e77d73c1ddfdc1e2924..0000000000000000000000000000000000000000
--- a/spaces/1gistliPinn/ChatGPT4/Examples/Chhota Bheem And The Throne Of Bali Dubbed Movie Download [UPDATED].md
+++ /dev/null
@@ -1,74 +0,0 @@
-
-
Chhota Bheem and the Throne of Bali Dubbed Movie Download: A Review
-
If you are looking for a fun and adventurous animated movie for your kids, you might want to check out Chhota Bheem and the Throne of Bali. This movie is based on the popular Indian cartoon series Chhota Bheem, which follows the adventures of a brave and smart boy named Bheem and his friends in the fictional village of Dholakpur.
-
In this movie, Bheem and his friends are invited by the King of Bali to attend the crowning ceremony of his son, Prince Arjun. However, on their way to Bali, they learn that the kingdom has been captured by an evil witch named Rangda, who has imprisoned the king and queen and wants to rule over Bali with her army of Leyaks, who are monstrous creatures that spread destruction and disease.
-
Chhota Bheem and the throne of Bali dubbed movie download
Bheem and his friends team up with Prince Arjun, who has escaped from Rangda's clutches, and decide to fight against the witch and her minions. Along the way, they encounter many challenges and dangers, but also make new friends and discover the beauty and culture of Bali.
-
Why You Should Watch Chhota Bheem and the Throne of Bali Dubbed Movie
-
There are many reasons why you should watch Chhota Bheem and the Throne of Bali dubbed movie. Here are some of them:
-
-
The movie is full of action, comedy, and drama. It will keep you and your kids entertained and engaged throughout.
-
The movie has a positive message about friendship, courage, loyalty, and teamwork. It will inspire you and your kids to be brave and kind like Bheem and his friends.
-
The movie showcases the rich and diverse culture of Bali, such as its music, dance, art, architecture, and cuisine. It will educate you and your kids about a different country and its traditions.
-
The movie has amazing visuals and animation. It will dazzle you and your kids with its colorful and detailed scenes of Bali and its creatures.
-
The movie has catchy songs and music. It will make you and your kids sing along and enjoy the tunes.
-
-
How to Download Chhota Bheem and the Throne of Bali Dubbed Movie for Free
-
If you want to download Chhota Bheem and the Throne of Bali dubbed movie for free, you can follow these simple steps:
-
-
Go to a reliable website that offers free downloads of animated movies. You can search for such websites on Google or any other search engine.
-
Search for Chhota Bheem and the Throne of Bali dubbed movie on the website. You can use the search bar or browse through the categories.
-
Select the movie from the list of results. Make sure it is in good quality and has clear audio.
-
Click on the download button or link. You might have to register or sign up on the website before downloading.
-
Choose a suitable format and resolution for your device. You can also select a preferred language if available.
-
Wait for the download to complete. You might have to wait for some time depending on your internet speed and file size.
-
Enjoy watching Chhota Bheem and the Throne of Bali dubbed movie with your kids!
-
-
Note: Downloading movies from unauthorized sources may be illegal or unsafe. We do not endorse or promote any such websites or activities. Please use your own discretion and judgment before downloading any content from the internet.
-
Conclusion
-
Chhota Bheem and the Throne of Bali dubbed movie is a great choice for a family-friendly entertainment. It is a fun-filled adventure that will make you laugh, cry, cheer, and learn. You can download it for free from various websites or watch it online on streaming platforms like Prime Video or Google Play. So what are you waiting for? Grab some popcorns and enjoy this amazing movie with your kids!
-
Who are the Characters of Chhota Bheem and the Throne of Bali Dubbed Movie
-
One of the reasons why Chhota Bheem and the Throne of Bali dubbed movie is so popular is because of its lovable and memorable characters. Here are some of the main characters of the movie:
-
-
Bheem: He is the protagonist of the movie and the leader of his friends. He is brave, strong, smart, and kind. He loves to eat laddoos, which give him extra strength and energy. He is always ready to help others and fight against evil.
-
Chutki: She is a seven-year-old girl and Bheem's best friend. She is sweet, caring, and loyal. She likes to cook and make flower garlands. She often accompanies Bheem on his adventures and supports him.
-
Raju: He is a four-year-old boy and Bheem's youngest friend. He is cute, innocent, and cheerful. He admires Bheem and wants to be like him. He often gets into trouble but also helps Bheem in his missions.
-
Jaggu: He is a talking monkey and Bheem's pet. He is witty, funny, and agile. He can swing from trees and jump over obstacles. He loves bananas and shares a special bond with Bheem.
-
Kalia: He is a ten-year-old boy and Bheem's rival. He is arrogant, greedy, and lazy. He often tries to compete with Bheem and prove himself better than him. He has two sidekicks, Dholu and Bholu, who follow him everywhere.
-
Indumati: She is a seven-year-old girl and the princess of Dholakpur. She is beautiful, graceful, and polite. She respects her father, King Indravarma, and cares for her people. She is friends with Bheem and his gang.
-
Arjun: He is an eight-year-old boy and the prince of Bali. He is brave, noble, and generous. He invites Bheem and his friends to his coronation ceremony but gets into trouble when Rangda captures his kingdom. He joins forces with Bheem to defeat Rangda and free his parents.
-
Rangda: She is the antagonist of the movie and an evil witch. She is cruel, cunning, and powerful. She wants to rule over Bali with her army of Leyaks, who are monstrous creatures that spread destruction and disease. She kidnaps the king and queen of Bali and tries to stop Bheem and his friends from saving them.
-
-
Where to Watch Chhota Bheem and the Throne of Bali Dubbed Movie Online
-
If you want to watch Chhota Bheem and the Throne of Bali dubbed movie online, you have several options to choose from. Here are some of them:
-
-
Prime Video: You can watch Chhota Bheem and the Throne of Bali dubbed movie online on Prime Video, which is a streaming service by Amazon. You can either rent or buy the movie in HD quality with English subtitles. You can also watch other Chhota Bheem movies and shows on Prime Video.
-
Google Play: You can watch Chhota Bheem and the Throne of Bali dubbed movie online on Google Play, which is a digital store by Google. You can either rent or buy the movie in HD quality with English subtitles. You can also watch other Chhota Bheem movies and shows on Google Play.
-
Atozcartoons: You can watch Chhota Bheem and the Throne of Bali dubbed movie online on Atozcartoons, which is a website that offers free downloads of animated movies in Hindi and Telugu languages. You can download the movie in MP4 format with good quality and clear audio.
-
-
Note: Watching movies from unauthorized sources may be illegal or unsafe. We do not endorse or promote any such websites or activities. Please use your own discretion and judgment before watching any content from the internet.
-
-How to Enjoy Chhota Bheem and the Throne of Bali Dubbed Movie with Your Kids
-
Chhota Bheem and the Throne of Bali dubbed movie is not only a great entertainment for you, but also for your kids. You can enjoy this movie with your kids in many ways. Here are some of them:
-
-
Watch the movie together: You can watch the movie together with your kids on your TV, laptop, tablet, or smartphone. You can also use headphones or speakers to enhance the sound quality. You can pause, rewind, or fast forward the movie as per your convenience. You can also discuss the movie with your kids and share your opinions and feelings.
-
Sing along the songs: You can sing along the songs of the movie with your kids and have fun. You can find the lyrics of the songs online or on YouTube. You can also learn the tunes and melodies of the songs and hum them. You can also dance along the songs and express yourself.
-
Play games related to the movie: You can play games related to the movie with your kids and have fun. You can play quizzes, puzzles, word games, memory games, etc. based on the characters, scenes, dialogues, and songs of the movie. You can also make your own games and rules and challenge each other.
-
Draw or color pictures related to the movie: You can draw or color pictures related to the movie with your kids and have fun. You can use pencils, crayons, paints, stickers, etc. to create your own artworks. You can draw or color your favorite characters, scenes, or moments from the movie. You can also make collages or posters related to the movie.
-
Act out scenes from the movie: You can act out scenes from the movie with your kids and have fun. You can use costumes, props, masks, etc. to make your own drama. You can imitate your favorite characters, dialogues, or actions from the movie. You can also improvise or add your own twists to the scenes.
-
-
These are some of the ways you can enjoy Chhota Bheem and the Throne of Bali dubbed movie with your kids. You can also come up with your own ideas and make your own fun. The main thing is to have a good time with your kids and bond with them over this wonderful movie.
-What are the Reviews of Chhota Bheem and the Throne of Bali Dubbed Movie
-
Chhota Bheem and the Throne of Bali dubbed movie has received mixed reviews from critics and audiences alike. Some have praised the movie for its animation, story, characters, songs, and message, while others have criticized it for its lack of originality, creativity, and depth. Here are some of the reviews of the movie:
-
-
The Times of India gave the movie 3 stars out of 5 and said, \"It's a perfect vacation film for kids. You too can accompany them. The film will make you smile.\" [1]
-
Wikipedia gave the movie a positive review and said, \"It is the sixteenth instalment in the Chhota Bheem film series and the second film in the series to be released directly to movie theatres. Distributed by Yash Raj Films, it was released in four different languages (English, Hindi, Tamil, and Telugu). It received mixed reviews.\" [2]
-
Bollywood Hungama gave the movie a negative review and said, \"Chhota Bheem and the throne of Bali Review – Get Chhota Bheem and the throne of Bali Movie Review, Film Ratings, Chhota Bheem and the throne of Bali Review, Chhota Bheem and the throne of Bali User Review, Chhota Bheem and the throne of Bali Critic Review and Latest Movie Reviews and Ratings on Bollywoodhungama.com.\" [3]
-
-
These are some of the reviews of Chhota Bheem and the Throne of Bali dubbed movie. You can also read more reviews online or watch the movie yourself and form your own opinion.
-Conclusion
-
Chhota Bheem and the Throne of Bali dubbed movie is a fun and adventurous animated movie that will appeal to kids and adults alike. It is based on the popular Indian cartoon series Chhota Bheem, which follows the exploits of a brave and smart boy named Bheem and his friends in the fictional village of Dholakpur. In this movie, Bheem and his friends travel to Bali to attend the crowning ceremony of Prince Arjun, but end up fighting against an evil witch named Rangda, who has captured the kingdom and its rulers.
-
The movie has many positive aspects, such as its action, comedy, drama, message, characters, songs, music, visuals, and animation. It also showcases the rich and diverse culture of Bali, such as its music, dance, art, architecture, and cuisine. The movie has received mixed reviews from critics and audiences, but it has also won many awards and accolades. It is the sixteenth instalment in the Chhota Bheem film series and the second film in the series to be released directly to movie theatres.
-
You can download Chhota Bheem and the Throne of Bali dubbed movie for free from various websites or watch it online on streaming platforms like Prime Video or Google Play. You can also enjoy this movie with your kids in many ways, such as watching it together, singing along the songs, playing games related to the movie, drawing or coloring pictures related to the movie, or acting out scenes from the movie. The main thing is to have a good time with your kids and bond with them over this wonderful movie.
3cee63e6c2
-
-
\ No newline at end of file
diff --git a/spaces/1line/AutoGPT/autogpt/logs.py b/spaces/1line/AutoGPT/autogpt/logs.py
deleted file mode 100644
index 35037404a98f7be9b7d577b625cc190ca27f4566..0000000000000000000000000000000000000000
--- a/spaces/1line/AutoGPT/autogpt/logs.py
+++ /dev/null
@@ -1,332 +0,0 @@
-"""Logging module for Auto-GPT."""
-import json
-import logging
-import os
-import random
-import re
-import time
-import traceback
-from logging import LogRecord
-
-from colorama import Fore, Style
-
-from autogpt.config import Config, Singleton
-from autogpt.speech import say_text
-
-CFG = Config()
-
-
-class Logger(metaclass=Singleton):
- """
- Logger that handle titles in different colors.
- Outputs logs in console, activity.log, and errors.log
- For console handler: simulates typing
- """
-
- def __init__(self):
- # create log directory if it doesn't exist
- this_files_dir_path = os.path.dirname(__file__)
- log_dir = os.path.join(this_files_dir_path, "../logs")
- if not os.path.exists(log_dir):
- os.makedirs(log_dir)
-
- log_file = "activity.log"
- error_file = "error.log"
-
- console_formatter = AutoGptFormatter("%(title_color)s %(message)s")
-
- # Create a handler for console which simulate typing
- self.typing_console_handler = TypingConsoleHandler()
- self.typing_console_handler.setLevel(logging.INFO)
- self.typing_console_handler.setFormatter(console_formatter)
-
- # Create a handler for console without typing simulation
- self.console_handler = ConsoleHandler()
- self.console_handler.setLevel(logging.DEBUG)
- self.console_handler.setFormatter(console_formatter)
-
- # Info handler in activity.log
- self.file_handler = logging.FileHandler(
- os.path.join(log_dir, log_file), "a", "utf-8"
- )
- self.file_handler.setLevel(logging.DEBUG)
- info_formatter = AutoGptFormatter(
- "%(asctime)s %(levelname)s %(title)s %(message_no_color)s"
- )
- self.file_handler.setFormatter(info_formatter)
-
- # Error handler error.log
- error_handler = logging.FileHandler(
- os.path.join(log_dir, error_file), "a", "utf-8"
- )
- error_handler.setLevel(logging.ERROR)
- error_formatter = AutoGptFormatter(
- "%(asctime)s %(levelname)s %(module)s:%(funcName)s:%(lineno)d %(title)s"
- " %(message_no_color)s"
- )
- error_handler.setFormatter(error_formatter)
-
- self.typing_logger = logging.getLogger("TYPER")
- self.typing_logger.addHandler(self.typing_console_handler)
- self.typing_logger.addHandler(self.file_handler)
- self.typing_logger.addHandler(error_handler)
- self.typing_logger.setLevel(logging.DEBUG)
-
- self.logger = logging.getLogger("LOGGER")
- self.logger.addHandler(self.console_handler)
- self.logger.addHandler(self.file_handler)
- self.logger.addHandler(error_handler)
- self.logger.setLevel(logging.DEBUG)
-
- def typewriter_log(
- self, title="", title_color="", content="", speak_text=False, level=logging.INFO
- ):
- if speak_text and CFG.speak_mode:
- say_text(f"{title}. {content}")
-
- if content:
- if isinstance(content, list):
- content = " ".join(content)
- else:
- content = ""
-
- self.typing_logger.log(
- level, content, extra={"title": title, "color": title_color}
- )
-
- def debug(
- self,
- message,
- title="",
- title_color="",
- ):
- self._log(title, title_color, message, logging.DEBUG)
-
- def warn(
- self,
- message,
- title="",
- title_color="",
- ):
- self._log(title, title_color, message, logging.WARN)
-
- def error(self, title, message=""):
- self._log(title, Fore.RED, message, logging.ERROR)
-
- def _log(self, title="", title_color="", message="", level=logging.INFO):
- if message:
- if isinstance(message, list):
- message = " ".join(message)
- self.logger.log(level, message, extra={"title": title, "color": title_color})
-
- def set_level(self, level):
- self.logger.setLevel(level)
- self.typing_logger.setLevel(level)
-
- def double_check(self, additionalText=None):
- if not additionalText:
- additionalText = (
- "Please ensure you've setup and configured everything"
- " correctly. Read https://github.com/Torantulino/Auto-GPT#readme to "
- "double check. You can also create a github issue or join the discord"
- " and ask there!"
- )
-
- self.typewriter_log("DOUBLE CHECK CONFIGURATION", Fore.YELLOW, additionalText)
-
-
-"""
-Output stream to console using simulated typing
-"""
-
-
-class TypingConsoleHandler(logging.StreamHandler):
- def emit(self, record):
- min_typing_speed = 0.05
- max_typing_speed = 0.01
-
- msg = self.format(record)
- try:
- words = msg.split()
- for i, word in enumerate(words):
- print(word, end="", flush=True)
- if i < len(words) - 1:
- print(" ", end="", flush=True)
- typing_speed = random.uniform(min_typing_speed, max_typing_speed)
- time.sleep(typing_speed)
- # type faster after each word
- min_typing_speed = min_typing_speed * 0.95
- max_typing_speed = max_typing_speed * 0.95
- print()
- except Exception:
- self.handleError(record)
-
-
-class ConsoleHandler(logging.StreamHandler):
- def emit(self, record) -> None:
- msg = self.format(record)
- try:
- print(msg)
- except Exception:
- self.handleError(record)
-
-
-class AutoGptFormatter(logging.Formatter):
- """
- Allows to handle custom placeholders 'title_color' and 'message_no_color'.
- To use this formatter, make sure to pass 'color', 'title' as log extras.
- """
-
- def format(self, record: LogRecord) -> str:
- if hasattr(record, "color"):
- record.title_color = (
- getattr(record, "color")
- + getattr(record, "title")
- + " "
- + Style.RESET_ALL
- )
- else:
- record.title_color = getattr(record, "title")
- if hasattr(record, "msg"):
- record.message_no_color = remove_color_codes(getattr(record, "msg"))
- else:
- record.message_no_color = ""
- return super().format(record)
-
-
-def remove_color_codes(s: str) -> str:
- ansi_escape = re.compile(r"\x1B(?:[@-Z\\-_]|\[[0-?]*[ -/]*[@-~])")
- return ansi_escape.sub("", s)
-
-
-logger = Logger()
-
-
-def print_assistant_thoughts(ai_name, assistant_reply):
- """Prints the assistant's thoughts to the console"""
- from autogpt.json_utils.json_fix_llm import (
- attempt_to_fix_json_by_finding_outermost_brackets,
- fix_and_parse_json,
- )
-
- try:
- try:
- # Parse and print Assistant response
- assistant_reply_json = fix_and_parse_json(assistant_reply)
- except json.JSONDecodeError:
- logger.error("Error: Invalid JSON in assistant thoughts\n", assistant_reply)
- assistant_reply_json = attempt_to_fix_json_by_finding_outermost_brackets(
- assistant_reply
- )
- if isinstance(assistant_reply_json, str):
- assistant_reply_json = fix_and_parse_json(assistant_reply_json)
-
- # Check if assistant_reply_json is a string and attempt to parse
- # it into a JSON object
- if isinstance(assistant_reply_json, str):
- try:
- assistant_reply_json = json.loads(assistant_reply_json)
- except json.JSONDecodeError:
- logger.error("Error: Invalid JSON\n", assistant_reply)
- assistant_reply_json = (
- attempt_to_fix_json_by_finding_outermost_brackets(
- assistant_reply_json
- )
- )
-
- assistant_thoughts_reasoning = None
- assistant_thoughts_plan = None
- assistant_thoughts_speak = None
- assistant_thoughts_criticism = None
- if not isinstance(assistant_reply_json, dict):
- assistant_reply_json = {}
- assistant_thoughts = assistant_reply_json.get("thoughts", {})
- assistant_thoughts_text = assistant_thoughts.get("text")
-
- if assistant_thoughts:
- assistant_thoughts_reasoning = assistant_thoughts.get("reasoning")
- assistant_thoughts_plan = assistant_thoughts.get("plan")
- assistant_thoughts_criticism = assistant_thoughts.get("criticism")
- assistant_thoughts_speak = assistant_thoughts.get("speak")
-
- logger.typewriter_log(
- f"{ai_name.upper()} THOUGHTS:", Fore.YELLOW, f"{assistant_thoughts_text}"
- )
- logger.typewriter_log(
- "REASONING:", Fore.YELLOW, f"{assistant_thoughts_reasoning}"
- )
-
- if assistant_thoughts_plan:
- logger.typewriter_log("PLAN:", Fore.YELLOW, "")
- # If it's a list, join it into a string
- if isinstance(assistant_thoughts_plan, list):
- assistant_thoughts_plan = "\n".join(assistant_thoughts_plan)
- elif isinstance(assistant_thoughts_plan, dict):
- assistant_thoughts_plan = str(assistant_thoughts_plan)
-
- # Split the input_string using the newline character and dashes
- lines = assistant_thoughts_plan.split("\n")
- for line in lines:
- line = line.lstrip("- ")
- logger.typewriter_log("- ", Fore.GREEN, line.strip())
-
- logger.typewriter_log(
- "CRITICISM:", Fore.YELLOW, f"{assistant_thoughts_criticism}"
- )
- # Speak the assistant's thoughts
- if CFG.speak_mode and assistant_thoughts_speak:
- say_text(assistant_thoughts_speak)
- else:
- logger.typewriter_log("SPEAK:", Fore.YELLOW, f"{assistant_thoughts_speak}")
-
- return assistant_reply_json
- except json.decoder.JSONDecodeError:
- logger.error("Error: Invalid JSON\n", assistant_reply)
- if CFG.speak_mode:
- say_text(
- "I have received an invalid JSON response from the OpenAI API."
- " I cannot ignore this response."
- )
-
- # All other errors, return "Error: + error message"
- except Exception:
- call_stack = traceback.format_exc()
- logger.error("Error: \n", call_stack)
-
-
-def print_assistant_thoughts(
- ai_name: object, assistant_reply_json_valid: object
-) -> None:
- assistant_thoughts_reasoning = None
- assistant_thoughts_plan = None
- assistant_thoughts_speak = None
- assistant_thoughts_criticism = None
-
- assistant_thoughts = assistant_reply_json_valid.get("thoughts", {})
- assistant_thoughts_text = assistant_thoughts.get("text")
- if assistant_thoughts:
- assistant_thoughts_reasoning = assistant_thoughts.get("reasoning")
- assistant_thoughts_plan = assistant_thoughts.get("plan")
- assistant_thoughts_criticism = assistant_thoughts.get("criticism")
- assistant_thoughts_speak = assistant_thoughts.get("speak")
- logger.typewriter_log(
- f"{ai_name.upper()} THOUGHTS:", Fore.YELLOW, f"{assistant_thoughts_text}"
- )
- logger.typewriter_log("REASONING:", Fore.YELLOW, f"{assistant_thoughts_reasoning}")
- if assistant_thoughts_plan:
- logger.typewriter_log("PLAN:", Fore.YELLOW, "")
- # If it's a list, join it into a string
- if isinstance(assistant_thoughts_plan, list):
- assistant_thoughts_plan = "\n".join(assistant_thoughts_plan)
- elif isinstance(assistant_thoughts_plan, dict):
- assistant_thoughts_plan = str(assistant_thoughts_plan)
-
- # Split the input_string using the newline character and dashes
- lines = assistant_thoughts_plan.split("\n")
- for line in lines:
- line = line.lstrip("- ")
- logger.typewriter_log("- ", Fore.GREEN, line.strip())
- logger.typewriter_log("CRITICISM:", Fore.YELLOW, f"{assistant_thoughts_criticism}")
- # Speak the assistant's thoughts
- if CFG.speak_mode and assistant_thoughts_speak:
- say_text(assistant_thoughts_speak)
diff --git a/spaces/1pelhydcardo/ChatGPT-prompt-generator/assets/APK Award Presents FIFA 16 - The Most Beautiful and Fastest Soccer Game on Mobile.md b/spaces/1pelhydcardo/ChatGPT-prompt-generator/assets/APK Award Presents FIFA 16 - The Most Beautiful and Fastest Soccer Game on Mobile.md
deleted file mode 100644
index b1a572b0cda68561462016f270bba68dccb6d869..0000000000000000000000000000000000000000
--- a/spaces/1pelhydcardo/ChatGPT-prompt-generator/assets/APK Award Presents FIFA 16 - The Most Beautiful and Fastest Soccer Game on Mobile.md
+++ /dev/null
@@ -1,138 +0,0 @@
-
-
FIFA 16 Mobile: A Review of the Game and How to Download It
-
If you are a fan of football games, you might have heard of FIFA 16 Mobile, a popular and realistic soccer simulation game for Android devices. In this article, we will review the game and its features, as well as show you how to download it from apkaward.com, a trusted website that offers free apk files for Android games. We will also share some tips and tricks to help you play better and enjoy the game more.
-
What is FIFA 16 Mobile?
-
FIFA 16 Mobile is a mobile version of FIFA 16, a console and PC game developed by EA Sports. It was released in September 2015 and it is one of the most downloaded games on Google Play. FIFA 16 Mobile lets you play beautiful football with a newer, better, and faster experience on your mobile device. You can choose from over 10,000 players from over 500 licensed teams and go to battle against other players from real leagues in real arenas from around the world. You can also build and manage your own ultimate team, earn, trade, and transfer superstars like Lionel Messi, Jordan Henderson, and Juan Cuadrado. You can also show off your skills on the pitch with challenging skill games, dynamic accomplishments, and unique player celebrations.
All-new engine: FIFA 16 Mobile uses an all-new engine that delivers better skill moves, more exciting goals, more responsive controls, smarter teammates, and improved animations. You can also use enhanced hybrid controls that let you use gestures or buttons to control the ball. You can also gain improved offside awareness and more with attacking intelligence.
-
Ultimate team: FIFA 16 Mobile allows you to build and manage your own fantasy team. You can choose your play style, formation, kits, and more, then balance player chemistry for the strongest squad compositions. You can also simulate matches or take control of them on the pitch.
-
Skill games: FIFA 16 Mobile offers you various skill games to test your abilities on the pitch. You can choose your daily challenge from shooting, ground passing, dribbling, crossing, penalties, and more. Then, pick the right player and beat the challenge to earn rewards.
-
Real world football: FIFA 16 Mobile gives you a realistic football experience with real players, teams, leagues, stadiums, and events. You can recreate challenges from current live-event football matches or create your own custom tournaments.
-
Player exchange: FIFA 16 Mobile introduces a new feature called player exchange. You can trade players and items you no longer need for a chance of unlocking something better. The higher value items or players you trade, the better the upgrades you’ll get back.
-
-
What are the pros and cons of FIFA 16 Mobile?
-
Like any game, FIFA 16 Mobile has its pros and cons. Here are some of them:
-
-
-
Pros
-
Cons
-
-
-
-
Realistic and immersive graphics and animations
-
Wide variety of players, teams, leagues, and modes
-
Easy and intuitive controls and interface
-
Fun and challenging skill games and achievements
-
Innovative and rewarding player exchange feature
-
-
-
Large file size and high device requirements
-
Limited compatibility with some Android devices
-
Potential lagging and crashing issues
-
Requires internet connection to play
-
Some bugs and glitches reported by users
-
-
-
-
How to download FIFA 16 Mobile?
-
If you want to download FIFA 16 Mobile for your Android device, you can follow these simple steps:
-
-
Go to apkaward.com, a reliable and safe website that offers free apk files for Android games.
-
Search for FIFA 16 Mobile in the search bar or browse the categories.
-
Select the game from the results and click on the download button.
-
Wait for the download to finish and locate the apk file in your device's storage.
-
Before installing the apk file, make sure you enable the "Unknown sources" option in your device's settings. This will allow you to install apps from sources other than Google Play.
-
Tap on the apk file and follow the instructions to install the game.
-
Enjoy playing FIFA 16 Mobile on your device.
-
-
Tips and tricks for FIFA 16 Mobile
-
To help you play better and enjoy FIFA 16 Mobile more, here are some tips and tricks that you can use:
-
-
Defend smartly: Don't rush into tackles or slide unnecessarily. Instead, use the pressure button to close down the space and force the opponent to make a mistake. You can also use the second defender button to call for backup from a teammate. When defending corners, use the swipe gesture to clear the ball.
-
Pass accurately: Don't just spam the pass button or use long balls all the time. Instead, use short passes to build up your play and create openings. You can also use through balls to send your attackers behind the defense. When passing, pay attention to the direction and power of your passes.
-
Dribble skillfully: Don't just run with the ball or use sprint all the time. Instead, use the skill move button to perform tricks and feints that can confuse or beat your opponents. You can also use the joystick to change direction or speed up your dribbling. When dribbling, pay attention to your player's balance and agility.
-
Score effectively: Don't just shoot whenever you get the ball or use finesse shots all the time. Instead, use different types of shots depending on the situation, such as power shots, chip shots, or volleys. You can also use headers or tap-ins to score from crosses or rebounds. When shooting, pay attention to your player's position, angle, and timing.
-
Manage wisely: Don't just buy or sell players randomly or use the same formation all the time. Instead, use the player exchange feature to get better players or items. You can also use different formations depending on your play style or opponent. When managing, pay attention to your player's chemistry, rating, and fitness.
-
-
Conclusion
-
FIFA 16 Mobile is a great game for football fans who want to enjoy a realistic and immersive soccer simulation on their mobile devices. It has many features that make it fun and challenging, such as the all-new engine, the ultimate team, the skill games, the real world football, and the player exchange. It also has some drawbacks, such as its large file size, its limited compatibility, its potential lagging issues, its internet requirement, and its bugs and glitches. However, these can be overcome by downloading it from apkaward.com, a trusted website that offers free apk files for Android games. By following our tips and tricks, you can also improve your performance and experience in FIFA 16 Mobile.
-
FAQs
-
-
Q: How much space does FIFA 16 Mobile take on my device?
-
A: FIFA 16 Mobile requires about 1.4 GB of free space on your device. You may need more space for additional data or updates.
-
Q: Which Android devices are compatible with FIFA 16 Mobile?
-
A: FIFA 16 Mobile is compatible with Android devices that have at least 1.5 GB of RAM, Android 4.4 or later, and a minimum resolution of 800x480. However, some devices may not run the game smoothly or at all, depending on their specifications and performance.
-
Q: How can I fix the lagging or crashing issues in FIFA 16 Mobile?
-
A: If you experience lagging or crashing issues in FIFA 16 Mobile, you can try the following solutions:
-
fifa 16 ultimate team apk download
-fifa 16 soccer android game
-fifa 16 mobile free download
-fifa 16 apk + obb offline
-fifa 16 apk mod unlimited money
-fifa 16 android gameplay
-fifa 16 mobile best players
-fifa 16 apk + data highly compressed
-fifa 16 soccer apk latest version
-fifa 16 mobile tips and tricks
-fifa 16 apk no license verification
-fifa 16 android requirements
-fifa 16 mobile cheats and hacks
-fifa 16 apk + obb google drive
-fifa 16 apk revdl
-fifa 16 android online or offline
-fifa 16 mobile skill moves
-fifa 16 apk + data mega
-fifa 16 soccer apk old version
-fifa 16 mobile update
-fifa 16 apk no root needed
-fifa 16 android controller support
-fifa 16 mobile player exchange
-fifa 16 apk + obb mediafire
-fifa 16 apk rexdl
-fifa 16 android multiplayer
-fifa 16 mobile manager mode
-fifa 16 apk + data zip file
-fifa 16 soccer apk pure
-fifa 16 mobile review
-fifa 16 apk cracked version
-fifa 16 android system requirements
-fifa 16 mobile hack tool
-fifa 16 apk + obb zippyshare
-fifa 16 apk mirror
-fifa 16 android graphics settings
-fifa 16 mobile tournaments
-fifa 16 apk + data kickass torrent
-fifa 16 soccer apkpure.com[^1^]
-fifa 16 mobile ratings
-fifa 16 apk full unlocked version
-fifa 16 android download size
-fifa 16 mobile coins generator
-fifa 16 apk + obb uptodown.com[^1^]
-fifa 16 apk mob.org[^1^]
-fifa 16 android bugs and glitches
-fifa 16 mobile achievements
-fifa 16 apk + data parts download[^1^]
-
-
Close other apps or background processes that may be consuming your device's memory or battery.
-
Clear your device's cache or data to free up some space and improve its performance.
-
Update your device's software or firmware to the latest version.
-
Reinstall the game or download the latest version from apkaward.com.
-
Contact EA Sports customer support for further assistance.
-
-
Q: How can I play FIFA 16 Mobile offline?
-
A: Unfortunately, you cannot play FIFA 16 Mobile offline. You need an internet connection to access the game's features and modes, such as the ultimate team, the skill games, and the real world football. You also need an internet connection to download the game's data and updates.
-
Q: How can I get more coins or points in FIFA 16 Mobile?
-
A: There are several ways to get more coins or points in FIFA 16 Mobile, such as:
-
-
Completing skill games, achievements, and challenges.
-
Winning matches, tournaments, and seasons.
-
Selling or exchanging players or items in the market or player exchange.
-
Watching ads or completing offers in the store.
-
Purchasing them with real money in the store.
-
-
I hope you enjoyed reading this article and found it helpful. If you have any questions or feedback, please feel free to leave a comment below. Thank you for your time and attention.
197e85843d
-
-
\ No newline at end of file
diff --git a/spaces/1phancelerku/anime-remove-background/Boost your Android device with Speed APK The ultimate performance optimizer.md b/spaces/1phancelerku/anime-remove-background/Boost your Android device with Speed APK The ultimate performance optimizer.md
deleted file mode 100644
index ceabe03194e6ee8be10e4661b4e3cea3e84dd3c6..0000000000000000000000000000000000000000
--- a/spaces/1phancelerku/anime-remove-background/Boost your Android device with Speed APK The ultimate performance optimizer.md
+++ /dev/null
@@ -1,128 +0,0 @@
-
-
What is Speed Apk and How to Use It?
-
If you are an avid gamer who loves playing Android games, you might have wondered if there is a way to change the speed of the games. Maybe you want to make them faster to save time, or slower to enjoy them more. Or maybe you want to cheat or hack some games by manipulating their speed. Whatever your reason, there is a tool that can help you do that. It is called speed apk, and in this article, we will tell you what it is, how to use it, and what are its benefits and risks.
Speed apk is an application that allows you to change the speed of any game on your Android device. It works by modifying the system clock of your device, which affects how fast or slow the game runs. You can use it to make your games run faster or slower, depending on your preference. You can also use it to cheat or hack some games by speeding up or slowing down certain aspects of them.
-
Why would you want to use speed apk? There are many reasons why you might want to change the speed of your games. For example, you might want to:
-
-
Make your games run faster to reduce waiting time, loading time, or animation time.
-
Make your games run slower to enjoy them more, or make them more challenging or realistic.
-
Cheat or hack some games by speeding up or slowing down certain elements, such as timers, enemies, resources, etc.
-
-
How to download and install speed apk on your Android device? To use speed apk, you need to have a rooted Android device. Rooting is a process that gives you full control over your device, allowing you to modify its system settings and install apps that require root access. If you don't know how to root your device, you can search online for tutorials or guides for your specific device model. Once you have rooted your device, you can download and install speed apk from its official website or from other sources. Make sure you download the latest version of the app and that it is compatible with your device.
-
How to Use Speed Apk to Change the Speed of Games in Android?
-
Once you have downloaded and installed speed apk on your rooted Android device, you can start using it to change the speed of your games. Here are the steps you need to follow:
-
-
Launch speed apk and grant it root access. You will see a floating icon on your screen that indicates that the app is running.
-
Select the game you want to speed up or slow down. You can do this by tapping on the floating icon and choosing "Select application". You will see a list of all the apps installed on your device. Tap on the game you want to modify and press "OK ". The game will be added to the speed apk list.
-
Adjust the speed multiplier and apply the changes. You can do this by tapping on the floating icon and choosing "Speed". You will see a slider that allows you to change the speed of the game from 0.1x to 10x. You can also use the buttons to increase or decrease the speed by 0.1x. Once you have set the desired speed, press "Apply". The game will run at the new speed.
-
Revert the changes and restore the original speed. You can do this by tapping on the floating icon and choosing "Restore". The game will run at its normal speed. You can also remove the game from the speed apk list by tapping on it and choosing "Remove".
-
-
That's it! You have successfully changed the speed of your game using speed apk. You can repeat these steps for any other game you want to modify.
-
Benefits and Risks of Using Speed Apk
-
Using speed apk can have some benefits and risks, depending on how you use it and what games you use it on. Here are some of them:
-
speed stars apk
-zingspeed mobile apk
-speed test apk
-speed vpn apk
-speed booster apk
-speed camera apk
-speed racing apk
-speed meter apk
-speed browser apk
-speed dial apk
-speed cleaner apk
-speed drifters apk
-speed fan apk
-speed golf apk
-speed hacker apk
-speed indicator apk
-speed jump apk
-speed keyboard apk
-speed launcher apk
-speed logic apk
-speed monitor apk
-speed optimizer apk
-speed painter apk
-speed quiz apk
-speed reader apk
-speed run apk
-speed scanner apk
-speed tracker apk
-speed video apk
-speed wallpaper apk
-speed x3d apk
-speed zone apk
-need for speed apk
-asphalt 9: legends - epic car action racing game (speed edition) apk
-bike race free - top motorcycle racing games (speed edition) apk
-carx drift racing 2 (speed edition) apk
-drag racing (speed edition) apk
-extreme car driving simulator (speed edition) apk
-fast & furious takedown (speed edition) apk
-hill climb racing 2 (speed edition) apk
-hot wheels: race off (speed edition) apk
-real racing 3 (speed edition) apk
-traffic rider (speed edition) apk
-turbo driving racing 3d (speed edition) apk
-csr racing 2 - free car racing game (speed edition) apk
-real drift car racing (speed edition) apk
-traffic racer (speed edition) apk
-beach buggy racing 2 (speed edition) apk
-city racing 3d (speed edition) apk
-
Benefits of Using Speed Apk
-
-
Faster gameplay and reduced waiting time: Using speed apk can make your games run faster, which can save you time and make your gaming experience more enjoyable. For example, you can use it to speed up games that have long loading times, slow animations, or tedious tasks.
-
More fun and challenge: Using speed apk can also make your games more fun and challenging, by changing their difficulty level or adding some variety. For example, you can use it to slow down games that are too easy or boring, or to speed up games that are too hard or frustrating.
-
Cheating and hacking possibilities: Using speed apk can also give you some advantages or disadvantages in some games, by altering their mechanics or outcomes. For example, you can use it to cheat or hack games that have timers, enemies, resources, or other elements that depend on the speed of the game.
-
-
Risks of Using Speed Apk
-
-
Potential damage to your device: Using speed apk can also cause some problems or damage to your device, by affecting its performance or stability. For example, using it to make your games run too fast or too slow can overheat your device, drain your battery, or crash your system.
-
Possible bans and penalties from game developers: Using speed apk can also get you in trouble with some game developers, by violating their terms of service or policies. For example, using it to cheat or hack online games can result in bans, suspensions, or other penalties from the game developers or platforms.
-
Ethical and moral issues: Using speed apk can also raise some ethical and moral questions, by affecting your gaming integrity or fairness. For example, using it to cheat or hack games that involve other players can be considered unfair, dishonest, or disrespectful.
-
-
Conclusion
-
In conclusion, speed apk is a tool that allows you to change the speed of any game on your Android device. It can be used for various purposes, such as making your games faster or slower, more fun or challenging, or cheating or hacking them. However, it also comes with some benefits and risks, such as affecting your device performance or stability, getting banned or penalized by game developers, or raising ethical or moral issues. Therefore, you should use it wisely and responsibly, and at your own risk.
-
Here are some tips and recommendations for using speed apk:
-
-
Make sure your device is rooted before using speed apk.
-
Download and install speed apk from its official website or from trusted sources.
-
Use speed apk only on games that you own or have permission to modify.
-
Use speed apk only on offline games or single-player modes.
-
Use speed apk only for personal use or entertainment purposes.
-
Do not use speed apk to harm others or gain unfair advantages.
-
Do not use speed apk excessively or unnecessarily.
-
Do not use speed apk on games that are incompatible or unstable with it.
-
Backup your device data before using speed apk.
-
Monitor your device temperature and battery level while using speed apk.
-
-
We hope this article has helped you understand what is speed apk and how to use it. If you have any feedback or opinions about this topic, feel free to share them with us in the comments section below. Happy gaming!
-
FAQs
-
Here are some of the frequently asked questions about speed apk:
-
-
What are some of the best games to use speed apk on?
-
There is no definitive answer to this question, as different games may have different effects or results when using speed apk. However, some of the games that are commonly used with speed apk are:
-
-
Idle or clicker games, such as Cookie Clicker, Adventure Capitalist, or Idle Miner Tycoon. These games can be sped up to earn more money, resources, or achievements faster.
-
Action or arcade games, such as Temple Run, Subway Surfers, or Jetpack Joyride. These games can be slowed down to make them easier to play, avoid obstacles, or collect items.
-
Strategy or simulation games, such as Clash of Clans, SimCity, or FarmVille. These games can be sped up to reduce the waiting time for building, upgrading, or harvesting.
-
-
Does speed apk work on online games?
-
Speed apk does not work on online games that require an internet connection or a server to run. This is because the speed of the game is determined by the server, not by your device. If you try to use speed apk on online games, you may experience errors, glitches, or disconnections. You may also get banned or penalized by the game developers for violating their rules or policies.
-
Is speed apk safe and legal to use?
-
Speed apk is not a malicious or harmful app, but it is not a risk-free app either. It can cause some problems or damage to your device, such as overheating, battery drain, or system crash. It can also get you in trouble with some game developers, who may ban or penalize you for using it. Moreover, it can raise some ethical or moral issues, such as cheating or hacking other players. Therefore, you should use speed apk at your own risk and responsibility.
-
How can I uninstall speed apk from my device?
-
If you want to uninstall speed apk from your device, you can follow these steps:
-
-
Launch speed apk and tap on the floating icon.
-
Choose "Settings" and then "Uninstall".
-
Confirm your choice and wait for the app to be uninstalled.
-
Reboot your device to complete the process.
-
-
Where can I find more information and support for speed apk?
-
If you want to find more information and support for speed apk, you can visit its official website or its social media pages. You can also contact its developers via email or feedback form. You can also join its online community or forum, where you can ask questions, share tips, or report issues.
- 401be4b1e0
-
-
\ No newline at end of file
diff --git a/spaces/1phancelerku/anime-remove-background/Download Google Drive APK for Android and Enjoy Free Cloud Storage.md b/spaces/1phancelerku/anime-remove-background/Download Google Drive APK for Android and Enjoy Free Cloud Storage.md
deleted file mode 100644
index 178cfe6409216afbd88f549e629516933823d2fc..0000000000000000000000000000000000000000
--- a/spaces/1phancelerku/anime-remove-background/Download Google Drive APK for Android and Enjoy Free Cloud Storage.md
+++ /dev/null
@@ -1,130 +0,0 @@
-
-
How to Download APK Files from Google Drive and Install Them on Your Android Device
-
If you have an Android device, you probably know that you can install apps from the Google Play Store. But did you know that you can also install apps from other sources, such as Google Drive? In this article, we will show you how to download APK files from Google Drive and install them on your Android device. We will also explain what an APK file is, why you might need it, and what risks and precautions you should take when installing it.
An APK file is a package file that contains the installation files for an Android app. It has the extension .apk and can be opened by any file explorer app. APK files are useful for installing apps that are not available on the Google Play Store, such as beta versions, regional apps, or modded apps. They can also help you update your apps faster, bypass restrictions, or access features that are not supported by your device.
-
Risks and Precautions of Installing APK Files
-
However, installing APK files also comes with some risks. You might download a malicious or corrupted file that can harm your device or compromise your data. You might also violate the terms of service of some apps or infringe on their intellectual property rights. Therefore, you should only download APK files from reputable sources, such as official websites, trusted developers, or verified platforms. You should also scan the files for viruses before installing them and check their permissions carefully. Finally, you should always back up your data before installing any APK file, in case something goes wrong.
-
How to Download APK Files from Google Drive
-
Step 1: Enable Unknown Sources on Your Android Device
-
Before you can install any APK file on your Android device, you need to enable unknown sources. This means that you allow your device to install apps from sources other than the Google Play Store. To do this, follow these steps:
-
-
Go to your device settings and tap Security or Apps & Notifications.
-
Tap the three dots in the upper-right corner and select Special Access or Install Unknown Apps.
-
Tap Chrome or whichever web browser you use to access Google Drive.
-
Toggle Allow from this source to the On position.
-
-
Step 2: Find the APK File on Google Drive and Download It
-
Now that you have enabled unknown sources, you can download the APK file from Google Drive. To do this, follow these steps:
-
-
Open your web browser and go to [Google Drive](^10^).
-
Sign in with your Google account if you haven't already.
-
Find the APK file that you want to download and tap it.
-
Tap Download or the three dots icon and select Download.
-
Accept any pop-ups or warnings that appear.
-
-
Step 3: Locate the Downloaded APK File and Install It
-
Once you have downloaded the APK file from Google Drive, you need to locate it and install it. To do this, follow these steps:
-
-
Open your file explorer app or download one from the Google Play Store if you don't have one.
-
Navigate to the Downloads folder or wherever you saved the APK file.
-
Tap the APK file and tap Install.
-
Follow the on-screen instructions and wait for the installation to finish.
-
Tap Open or Done to launch or exit the app.
-
-
Congratulations, you have successfully downloaded and installed an APK file from Google Drive!
-
How to Install APK Files on Your Android Device Using Other Methods
-
Method 1: Use a File Manager App
-
If you don't want to use your web browser to download APK files from Google Drive, you can use a file manager app instead. A file manager app allows you to access and manage the files on your device, including APK files. Some popular file manager apps are [ES File Explorer], [Solid Explorer], and [Files by Google]. To use a file manager app to install APK files, follow these steps:
-
How to download apk files from google drive
-Download google drive apk for android
-Google drive apk download latest version
-Download google drive apk for pc
-Google drive apk download for firestick
-Download google drive apk old version
-Google drive apk download uptodown
-Download google drive apk mod
-Google drive apk download for android tv
-Download google drive apk mirror
-Google drive apk download apkpure
-Download google drive apk for chromebook
-Google drive apk download for windows 10
-Download google drive apk pro
-Google drive apk download for laptop
-Download google drive apk offline installer
-Google drive apk download for ios
-Download google drive apk premium
-Google drive apk download for kindle fire
-Download google drive apk no ads
-Google drive apk download for mac
-Download google drive apk cracked
-Google drive apk download for smart tv
-Download google drive apk filehippo
-Google drive apk download for windows 7
-Download google drive apk full version
-Google drive apk download for blackberry
-Download google drive apk pure
-Google drive apk download for windows 8.1
-Download google drive apk hack
-Google drive apk download for linux
-Download google drive apk free
-Google drive apk download for android 4.4.2
-Download google drive apk beta
-Google drive apk download for android 5.1.1
-Download google drive apk direct link
-Google drive apk download for android 6.0.1
-Download google drive apk xda
-Google drive apk download for android 7.0
-Download google drive apk rexdl
-Google drive apk download for android 8.0
-Download google drive apk revdl
-Google drive apk download for android 9.0 pie
-Download google drive apk from play store
-Google drive apk download for android 10 q
-
-
Download and install a file manager app from the Google Play Store if you don't have one.
-
Open the file manager app and tap Google Drive or whichever cloud service you use to store your APK files.
-
Sign in with your Google account if you haven't already.
-
Find the APK file that you want to install and tap it.
-
Tap Install and follow the on-screen instructions.
-
Tap Open or Done to launch or exit the app.
-
-
Method 2: Use an APK Installer App
-
If you want to make the installation process easier, you can use an APK installer app. An APK installer app is a tool that helps you install APK files on your device without any hassle. Some popular APK installer apps are [APK Installer], [APKPure], and [APKMirror Installer]. To use an APK installer app to install APK files, follow these steps:
-
-
Download and install an APK installer app from the Google Play Store or its official website if you don't have one.
-
Open the APK installer app and tap Google Drive or whichever cloud service you use to store your APK files.
-
Sign in with your Google account if you haven't already.
-
Find the APK file that you want to install and tap it.
-
The APK installer app will automatically scan, verify, and install the APK file for you.
-
Tap Open or Done to launch or exit the app.
-
-
Method 3: Transfer the APK File from Your Computer via USB
-
If you have the APK file on your computer, you can also transfer it to your Android device via USB and install it. To do this, follow these steps:
-
-
Connect your Android device to your computer using a USB cable.
-
Select Transfer Files or MTP mode on your device if prompted.
-
On your computer, open File Explorer or Finder and locate the APK file that you want to transfer.
-
Drag and drop the APK file to your device's internal storage or SD card.
-
Eject your device from your computer and disconnect the USB cable.
-
On your device, open your file explorer app or download one from the Google Play Store if you don't have one.
-
Navigate to the folder where you transferred the APK file and tap it.
-
Tap Install and follow the on-screen instructions.
-
Tap Open or Done to launch or exit the app.
-
-
Conclusion
-
In this article, we have shown you how to download APK files from Google Drive and install them on your Android device. We have also explained what an APK file is, why you might need it, and what risks and precautions you should take when installing it. We hope that this article has been helpful and informative for you. If you have any questions or feedback, please feel free to leave a comment below. Thank you for reading!
-
FAQs
-
-
Question
Answer
-
What is Google Drive?
Google Drive is a cloud storage service that allows you to store and access your files online. You can upload, download, share, and sync your files across different devices using Google Drive. You can also create and edit documents, spreadsheets, presentations, forms, drawings, and more using Google Drive's online tools. You can get 15 GB of free storage space with a Google account or upgrade to a paid plan for more storage options.
-
How do I update an APK file?
To update an APK file, you need to download and install the latest version of the APK file from the same source that you got the original one. You can also check for updates using the APK installer app that you used to install the APK file. Alternatively, you can uninstall the old version of the app and install the new one from the Google Play Store if it is available there.
-
How do I uninstall an APK file?
To uninstall an APK file, you need to go to your device settings and tap Apps or Applications. Find the app that you want to uninstall and tap it. Tap Uninstall and confirm your choice. You can also uninstall an APK file using the APK installer app that you used to install it.
-
How do I share an APK file?
To share an APK file, you need to upload it to a cloud service, such as Google Drive, Dropbox, or OneDrive, and share the link with the person that you want to share it with. You can also use a file sharing app, such as [SHAREit], [Xender], or [Zapya], to transfer the APK file directly to another device via Wi-Fi or Bluetooth.
-
How do I backup an APK file?
To backup an APK file, you need to copy it from your device's internal storage or SD card to your computer or another storage device. You can also use a backup app, such as [Titanium Backup], [Helium], or [Super Backup], to backup your APK files along with their data and settings.
-
How do I open an APK file on my computer?
To open an APK file on your computer, you need to use an Android emulator, such as [BlueStacks], [Nox Player], or [MEmu], that allows you to run Android apps on your computer. You can also use a software tool, such as [APK Studio], [APK Easy Tool], or [APK Editor Pro], that allows you to view and edit the contents of an APK file.
-
197e85843d
-
-
\ No newline at end of file
diff --git a/spaces/1toTree/lora_test/ppdiffusers/schedulers/scheduling_dpmsolver_multistep.py b/spaces/1toTree/lora_test/ppdiffusers/schedulers/scheduling_dpmsolver_multistep.py
deleted file mode 100644
index ac93600eb6fdea3d18475e845a9f934e4ec7e341..0000000000000000000000000000000000000000
--- a/spaces/1toTree/lora_test/ppdiffusers/schedulers/scheduling_dpmsolver_multistep.py
+++ /dev/null
@@ -1,524 +0,0 @@
-# Copyright (c) 2022 PaddlePaddle Authors. All Rights Reserved.
-# Copyright 2022 TSAIL Team and The HuggingFace Team. All rights reserved.
-#
-# Licensed under the Apache License, Version 2.0 (the "License");
-# you may not use this file except in compliance with the License.
-# You may obtain a copy of the License at
-#
-# http://www.apache.org/licenses/LICENSE-2.0
-#
-# Unless required by applicable law or agreed to in writing, software
-# distributed under the License is distributed on an "AS IS" BASIS,
-# WITHOUT WARRANTIES OR CONDITIONS OF ANY KIND, either express or implied.
-# See the License for the specific language governing permissions and
-# limitations under the License.
-
-# DISCLAIMER: This file is strongly influenced by https://github.com/LuChengTHU/dpm-solver
-
-import math
-from typing import List, Optional, Tuple, Union
-
-import numpy as np
-import paddle
-
-from ..configuration_utils import ConfigMixin, register_to_config
-from ..utils import _COMPATIBLE_STABLE_DIFFUSION_SCHEDULERS, deprecate
-from .scheduling_utils import SchedulerMixin, SchedulerOutput
-
-
-def betas_for_alpha_bar(num_diffusion_timesteps, max_beta=0.999):
- """
- Create a beta schedule that discretizes the given alpha_t_bar function, which defines the cumulative product of
- (1-beta) over time from t = [0,1].
-
- Contains a function alpha_bar that takes an argument t and transforms it to the cumulative product of (1-beta) up
- to that part of the diffusion process.
-
-
- Args:
- num_diffusion_timesteps (`int`): the number of betas to produce.
- max_beta (`float`): the maximum beta to use; use values lower than 1 to
- prevent singularities.
-
- Returns:
- betas (`np.ndarray`): the betas used by the scheduler to step the model outputs
- """
-
- def alpha_bar(time_step):
- return math.cos((time_step + 0.008) / 1.008 * math.pi / 2) ** 2
-
- betas = []
- for i in range(num_diffusion_timesteps):
- t1 = i / num_diffusion_timesteps
- t2 = (i + 1) / num_diffusion_timesteps
- betas.append(min(1 - alpha_bar(t2) / alpha_bar(t1), max_beta))
- return paddle.to_tensor(betas, dtype="float32")
-
-
-class DPMSolverMultistepScheduler(SchedulerMixin, ConfigMixin):
- """
- DPM-Solver (and the improved version DPM-Solver++) is a fast dedicated high-order solver for diffusion ODEs with
- the convergence order guarantee. Empirically, sampling by DPM-Solver with only 20 steps can generate high-quality
- samples, and it can generate quite good samples even in only 10 steps.
-
- For more details, see the original paper: https://arxiv.org/abs/2206.00927 and https://arxiv.org/abs/2211.01095
-
- Currently, we support the multistep DPM-Solver for both noise prediction models and data prediction models. We
- recommend to use `solver_order=2` for guided sampling, and `solver_order=3` for unconditional sampling.
-
- We also support the "dynamic thresholding" method in Imagen (https://arxiv.org/abs/2205.11487). For pixel-space
- diffusion models, you can set both `algorithm_type="dpmsolver++"` and `thresholding=True` to use the dynamic
- thresholding. Note that the thresholding method is unsuitable for latent-space diffusion models (such as
- stable-diffusion).
-
- [`~ConfigMixin`] takes care of storing all config attributes that are passed in the scheduler's `__init__`
- function, such as `num_train_timesteps`. They can be accessed via `scheduler.config.num_train_timesteps`.
- [`SchedulerMixin`] provides general loading and saving functionality via the [`SchedulerMixin.save_pretrained`] and
- [`~SchedulerMixin.from_pretrained`] functions.
-
- Args:
- num_train_timesteps (`int`): number of diffusion steps used to train the model.
- beta_start (`float`): the starting `beta` value of inference.
- beta_end (`float`): the final `beta` value.
- beta_schedule (`str`):
- the beta schedule, a mapping from a beta range to a sequence of betas for stepping the model. Choose from
- `linear`, `scaled_linear`, or `squaredcos_cap_v2`.
- trained_betas (`np.ndarray`, optional):
- option to pass an array of betas directly to the constructor to bypass `beta_start`, `beta_end` etc.
- solver_order (`int`, default `2`):
- the order of DPM-Solver; can be `1` or `2` or `3`. We recommend to use `solver_order=2` for guided
- sampling, and `solver_order=3` for unconditional sampling.
- prediction_type (`str`, default `epsilon`, optional):
- prediction type of the scheduler function, one of `epsilon` (predicting the noise of the diffusion
- process), `sample` (directly predicting the noisy sample`) or `v_prediction` (see section 2.4
- https://imagen.research.google/video/paper.pdf)
- thresholding (`bool`, default `False`):
- whether to use the "dynamic thresholding" method (introduced by Imagen, https://arxiv.org/abs/2205.11487).
- For pixel-space diffusion models, you can set both `algorithm_type=dpmsolver++` and `thresholding=True` to
- use the dynamic thresholding. Note that the thresholding method is unsuitable for latent-space diffusion
- models (such as stable-diffusion).
- dynamic_thresholding_ratio (`float`, default `0.995`):
- the ratio for the dynamic thresholding method. Default is `0.995`, the same as Imagen
- (https://arxiv.org/abs/2205.11487).
- sample_max_value (`float`, default `1.0`):
- the threshold value for dynamic thresholding. Valid only when `thresholding=True` and
- `algorithm_type="dpmsolver++`.
- algorithm_type (`str`, default `dpmsolver++`):
- the algorithm type for the solver. Either `dpmsolver` or `dpmsolver++`. The `dpmsolver` type implements the
- algorithms in https://arxiv.org/abs/2206.00927, and the `dpmsolver++` type implements the algorithms in
- https://arxiv.org/abs/2211.01095. We recommend to use `dpmsolver++` with `solver_order=2` for guided
- sampling (e.g. stable-diffusion).
- solver_type (`str`, default `midpoint`):
- the solver type for the second-order solver. Either `midpoint` or `heun`. The solver type slightly affects
- the sample quality, especially for small number of steps. We empirically find that `midpoint` solvers are
- slightly better, so we recommend to use the `midpoint` type.
- lower_order_final (`bool`, default `True`):
- whether to use lower-order solvers in the final steps. Only valid for < 15 inference steps. We empirically
- find this trick can stabilize the sampling of DPM-Solver for steps < 15, especially for steps <= 10.
-
- """
-
- _compatibles = _COMPATIBLE_STABLE_DIFFUSION_SCHEDULERS.copy()
- _deprecated_kwargs = ["predict_epsilon"]
- order = 1
-
- @register_to_config
- def __init__(
- self,
- num_train_timesteps: int = 1000,
- beta_start: float = 0.0001,
- beta_end: float = 0.02,
- beta_schedule: str = "linear",
- trained_betas: Optional[Union[np.ndarray, List[float]]] = None,
- solver_order: int = 2,
- prediction_type: str = "epsilon",
- thresholding: bool = False,
- dynamic_thresholding_ratio: float = 0.995,
- sample_max_value: float = 1.0,
- algorithm_type: str = "dpmsolver++",
- solver_type: str = "midpoint",
- lower_order_final: bool = True,
- **kwargs,
- ):
- message = (
- "Please make sure to instantiate your scheduler with `prediction_type` instead. E.g. `scheduler ="
- " DPMSolverMultistepScheduler.from_pretrained(, prediction_type='epsilon')`."
- )
- predict_epsilon = deprecate("predict_epsilon", "0.13.0", message, take_from=kwargs)
- if predict_epsilon is not None:
- self.register_to_config(prediction_type="epsilon" if predict_epsilon else "sample")
- if trained_betas is not None:
- self.betas = paddle.to_tensor(trained_betas, dtype="float32")
- elif beta_schedule == "linear":
- self.betas = paddle.linspace(beta_start, beta_end, num_train_timesteps, dtype="float32")
- elif beta_schedule == "scaled_linear":
- # this schedule is very specific to the latent diffusion model.
- self.betas = paddle.linspace(beta_start**0.5, beta_end**0.5, num_train_timesteps, dtype="float32") ** 2
- elif beta_schedule == "squaredcos_cap_v2":
- # Glide cosine schedule
- self.betas = betas_for_alpha_bar(num_train_timesteps)
- else:
- raise NotImplementedError(f"{beta_schedule} does is not implemented for {self.__class__}")
-
- self.alphas = 1.0 - self.betas
- self.alphas_cumprod = paddle.cumprod(self.alphas, 0)
- # Currently we only support VP-type noise schedule
- self.alpha_t = paddle.sqrt(self.alphas_cumprod)
- self.sigma_t = paddle.sqrt(1 - self.alphas_cumprod)
- self.lambda_t = paddle.log(self.alpha_t) - paddle.log(self.sigma_t)
-
- # standard deviation of the initial noise distribution
- self.init_noise_sigma = 1.0
-
- # settings for DPM-Solver
- if algorithm_type not in ["dpmsolver", "dpmsolver++"]:
- raise NotImplementedError(f"{algorithm_type} does is not implemented for {self.__class__}")
- if solver_type not in ["midpoint", "heun"]:
- raise NotImplementedError(f"{solver_type} does is not implemented for {self.__class__}")
-
- # setable values
- self.num_inference_steps = None
- timesteps = np.linspace(0, num_train_timesteps - 1, num_train_timesteps, dtype=np.float32)[::-1].copy()
- self.timesteps = paddle.to_tensor(timesteps)
- self.model_outputs = [None] * solver_order
- self.lower_order_nums = 0
-
- def set_timesteps(self, num_inference_steps: int):
- """
- Sets the timesteps used for the diffusion chain. Supporting function to be run before inference.
-
- Args:
- num_inference_steps (`int`):
- the number of diffusion steps used when generating samples with a pre-trained model.
- """
- self.num_inference_steps = num_inference_steps
- timesteps = (
- np.linspace(0, self.num_train_timesteps - 1, num_inference_steps + 1)
- .round()[::-1][:-1]
- .copy()
- .astype(np.int64)
- )
- self.timesteps = paddle.to_tensor(timesteps)
- self.model_outputs = [
- None,
- ] * self.config.solver_order
- self.lower_order_nums = 0
-
- def convert_model_output(self, model_output: paddle.Tensor, timestep: int, sample: paddle.Tensor) -> paddle.Tensor:
- """
- Convert the model output to the corresponding type that the algorithm (DPM-Solver / DPM-Solver++) needs.
-
- DPM-Solver is designed to discretize an integral of the noise prediction model, and DPM-Solver++ is designed to
- discretize an integral of the data prediction model. So we need to first convert the model output to the
- corresponding type to match the algorithm.
-
- Note that the algorithm type and the model type is decoupled. That is to say, we can use either DPM-Solver or
- DPM-Solver++ for both noise prediction model and data prediction model.
-
- Args:
- model_output (`paddle.Tensor`): direct output from learned diffusion model.
- timestep (`int`): current discrete timestep in the diffusion chain.
- sample (`paddle.Tensor`):
- current instance of sample being created by diffusion process.
-
- Returns:
- `paddle.Tensor`: the converted model output.
- """
- # DPM-Solver++ needs to solve an integral of the data prediction model.
- if self.config.algorithm_type == "dpmsolver++":
- if self.config.prediction_type == "epsilon":
- alpha_t, sigma_t = self.alpha_t[timestep], self.sigma_t[timestep]
- x0_pred = (sample - sigma_t * model_output) / alpha_t
- elif self.config.prediction_type == "sample":
- x0_pred = model_output
- elif self.config.prediction_type == "v_prediction":
- alpha_t, sigma_t = self.alpha_t[timestep], self.sigma_t[timestep]
- x0_pred = alpha_t * sample - sigma_t * model_output
- else:
- raise ValueError(
- f"prediction_type given as {self.config.prediction_type} must be one of `epsilon`, `sample`, or"
- " `v_prediction` for the DPMSolverMultistepScheduler."
- )
-
- if self.config.thresholding:
- # Dynamic thresholding in https://arxiv.org/abs/2205.11487
- orig_dtype = x0_pred.dtype
- if orig_dtype not in [paddle.float32, paddle.float64]:
- x0_pred = x0_pred.cast("float32")
- dynamic_max_val = paddle.quantile(
- paddle.abs(x0_pred).reshape((x0_pred.shape[0], -1)), self.config.dynamic_thresholding_ratio, axis=1
- )
- dynamic_max_val = paddle.maximum(
- dynamic_max_val,
- self.config.sample_max_value * paddle.ones_like(dynamic_max_val),
- )[(...,) + (None,) * (x0_pred.ndim - 1)]
- x0_pred = paddle.clip(x0_pred, -dynamic_max_val, dynamic_max_val) / dynamic_max_val
- x0_pred = x0_pred.cast(orig_dtype)
- return x0_pred
- # DPM-Solver needs to solve an integral of the noise prediction model.
- elif self.config.algorithm_type == "dpmsolver":
- if self.config.prediction_type == "epsilon":
- return model_output
- elif self.config.prediction_type == "sample":
- alpha_t, sigma_t = self.alpha_t[timestep], self.sigma_t[timestep]
- epsilon = (sample - alpha_t * model_output) / sigma_t
- return epsilon
- elif self.config.prediction_type == "v_prediction":
- alpha_t, sigma_t = self.alpha_t[timestep], self.sigma_t[timestep]
- epsilon = alpha_t * model_output + sigma_t * sample
- return epsilon
- else:
- raise ValueError(
- f"prediction_type given as {self.config.prediction_type} must be one of `epsilon`, `sample`, or"
- " `v_prediction` for the DPMSolverMultistepScheduler."
- )
-
- def dpm_solver_first_order_update(
- self,
- model_output: paddle.Tensor,
- timestep: int,
- prev_timestep: int,
- sample: paddle.Tensor,
- ) -> paddle.Tensor:
- """
- One step for the first-order DPM-Solver (equivalent to DDIM).
-
- See https://arxiv.org/abs/2206.00927 for the detailed derivation.
-
- Args:
- model_output (`paddle.Tensor`): direct output from learned diffusion model.
- timestep (`int`): current discrete timestep in the diffusion chain.
- prev_timestep (`int`): previous discrete timestep in the diffusion chain.
- sample (`paddle.Tensor`):
- current instance of sample being created by diffusion process.
-
- Returns:
- `paddle.Tensor`: the sample tensor at the previous timestep.
- """
- lambda_t, lambda_s = self.lambda_t[prev_timestep], self.lambda_t[timestep]
- alpha_t, alpha_s = self.alpha_t[prev_timestep], self.alpha_t[timestep]
- sigma_t, sigma_s = self.sigma_t[prev_timestep], self.sigma_t[timestep]
- h = lambda_t - lambda_s
- if self.config.algorithm_type == "dpmsolver++":
- x_t = (sigma_t / sigma_s) * sample - (alpha_t * (paddle.exp(-h) - 1.0)) * model_output
- elif self.config.algorithm_type == "dpmsolver":
- x_t = (alpha_t / alpha_s) * sample - (sigma_t * (paddle.exp(h) - 1.0)) * model_output
- return x_t
-
- def multistep_dpm_solver_second_order_update(
- self,
- model_output_list: List[paddle.Tensor],
- timestep_list: List[int],
- prev_timestep: int,
- sample: paddle.Tensor,
- ) -> paddle.Tensor:
- """
- One step for the second-order multistep DPM-Solver.
-
- Args:
- model_output_list (`List[paddle.Tensor]`):
- direct outputs from learned diffusion model at current and latter timesteps.
- timestep (`int`): current and latter discrete timestep in the diffusion chain.
- prev_timestep (`int`): previous discrete timestep in the diffusion chain.
- sample (`paddle.Tensor`):
- current instance of sample being created by diffusion process.
-
- Returns:
- `paddle.Tensor`: the sample tensor at the previous timestep.
- """
- t, s0, s1 = prev_timestep, timestep_list[-1], timestep_list[-2]
- m0, m1 = model_output_list[-1], model_output_list[-2]
- lambda_t, lambda_s0, lambda_s1 = self.lambda_t[t], self.lambda_t[s0], self.lambda_t[s1]
- alpha_t, alpha_s0 = self.alpha_t[t], self.alpha_t[s0]
- sigma_t, sigma_s0 = self.sigma_t[t], self.sigma_t[s0]
- h, h_0 = lambda_t - lambda_s0, lambda_s0 - lambda_s1
- r0 = h_0 / h
- D0, D1 = m0, (1.0 / r0) * (m0 - m1)
- if self.config.algorithm_type == "dpmsolver++":
- # See https://arxiv.org/abs/2211.01095 for detailed derivations
- if self.config.solver_type == "midpoint":
- x_t = (
- (sigma_t / sigma_s0) * sample
- - (alpha_t * (paddle.exp(-h) - 1.0)) * D0
- - 0.5 * (alpha_t * (paddle.exp(-h) - 1.0)) * D1
- )
- elif self.config.solver_type == "heun":
- x_t = (
- (sigma_t / sigma_s0) * sample
- - (alpha_t * (paddle.exp(-h) - 1.0)) * D0
- + (alpha_t * ((paddle.exp(-h) - 1.0) / h + 1.0)) * D1
- )
- elif self.config.algorithm_type == "dpmsolver":
- # See https://arxiv.org/abs/2206.00927 for detailed derivations
- if self.config.solver_type == "midpoint":
- x_t = (
- (alpha_t / alpha_s0) * sample
- - (sigma_t * (paddle.exp(h) - 1.0)) * D0
- - 0.5 * (sigma_t * (paddle.exp(h) - 1.0)) * D1
- )
- elif self.config.solver_type == "heun":
- x_t = (
- (alpha_t / alpha_s0) * sample
- - (sigma_t * (paddle.exp(h) - 1.0)) * D0
- - (sigma_t * ((paddle.exp(h) - 1.0) / h - 1.0)) * D1
- )
- return x_t
-
- def multistep_dpm_solver_third_order_update(
- self,
- model_output_list: List[paddle.Tensor],
- timestep_list: List[int],
- prev_timestep: int,
- sample: paddle.Tensor,
- ) -> paddle.Tensor:
- """
- One step for the third-order multistep DPM-Solver.
-
- Args:
- model_output_list (`List[paddle.Tensor]`):
- direct outputs from learned diffusion model at current and latter timesteps.
- timestep (`int`): current and latter discrete timestep in the diffusion chain.
- prev_timestep (`int`): previous discrete timestep in the diffusion chain.
- sample (`paddle.Tensor`):
- current instance of sample being created by diffusion process.
-
- Returns:
- `paddle.Tensor`: the sample tensor at the previous timestep.
- """
- t, s0, s1, s2 = prev_timestep, timestep_list[-1], timestep_list[-2], timestep_list[-3]
- m0, m1, m2 = model_output_list[-1], model_output_list[-2], model_output_list[-3]
- lambda_t, lambda_s0, lambda_s1, lambda_s2 = (
- self.lambda_t[t],
- self.lambda_t[s0],
- self.lambda_t[s1],
- self.lambda_t[s2],
- )
- alpha_t, alpha_s0 = self.alpha_t[t], self.alpha_t[s0]
- sigma_t, sigma_s0 = self.sigma_t[t], self.sigma_t[s0]
- h, h_0, h_1 = lambda_t - lambda_s0, lambda_s0 - lambda_s1, lambda_s1 - lambda_s2
- r0, r1 = h_0 / h, h_1 / h
- D0 = m0
- D1_0, D1_1 = (1.0 / r0) * (m0 - m1), (1.0 / r1) * (m1 - m2)
- D1 = D1_0 + (r0 / (r0 + r1)) * (D1_0 - D1_1)
- D2 = (1.0 / (r0 + r1)) * (D1_0 - D1_1)
- if self.config.algorithm_type == "dpmsolver++":
- # See https://arxiv.org/abs/2206.00927 for detailed derivations
- x_t = (
- (sigma_t / sigma_s0) * sample
- - (alpha_t * (paddle.exp(-h) - 1.0)) * D0
- + (alpha_t * ((paddle.exp(-h) - 1.0) / h + 1.0)) * D1
- - (alpha_t * ((paddle.exp(-h) - 1.0 + h) / h**2 - 0.5)) * D2
- )
- elif self.config.algorithm_type == "dpmsolver":
- # See https://arxiv.org/abs/2206.00927 for detailed derivations
- x_t = (
- (alpha_t / alpha_s0) * sample
- - (sigma_t * (paddle.exp(h) - 1.0)) * D0
- - (sigma_t * ((paddle.exp(h) - 1.0) / h - 1.0)) * D1
- - (sigma_t * ((paddle.exp(h) - 1.0 - h) / h**2 - 0.5)) * D2
- )
- return x_t
-
- def step(
- self,
- model_output: paddle.Tensor,
- timestep: int,
- sample: paddle.Tensor,
- return_dict: bool = True,
- ) -> Union[SchedulerOutput, Tuple]:
- """
- Step function propagating the sample with the multistep DPM-Solver.
-
- Args:
- model_output (`paddle.Tensor`): direct output from learned diffusion model.
- timestep (`int`): current discrete timestep in the diffusion chain.
- sample (`paddle.Tensor`):
- current instance of sample being created by diffusion process.
- return_dict (`bool`): option for returning tuple rather than SchedulerOutput class
-
- Returns:
- [`~scheduling_utils.SchedulerOutput`] or `tuple`: [`~scheduling_utils.SchedulerOutput`] if `return_dict` is
- True, otherwise a `tuple`. When returning a tuple, the first element is the sample tensor.
-
- """
- if self.num_inference_steps is None:
- raise ValueError(
- "Number of inference steps is 'None', you need to run 'set_timesteps' after creating the scheduler"
- )
-
- step_index = (self.timesteps == timestep).nonzero()
- if len(step_index) == 0:
- step_index = len(self.timesteps) - 1
- else:
- step_index = step_index.item()
- prev_timestep = 0 if step_index == len(self.timesteps) - 1 else self.timesteps[step_index + 1]
- lower_order_final = (
- (step_index == len(self.timesteps) - 1) and self.config.lower_order_final and len(self.timesteps) < 15
- )
- lower_order_second = (
- (step_index == len(self.timesteps) - 2) and self.config.lower_order_final and len(self.timesteps) < 15
- )
-
- model_output = self.convert_model_output(model_output, timestep, sample)
- for i in range(self.config.solver_order - 1):
- self.model_outputs[i] = self.model_outputs[i + 1]
- self.model_outputs[-1] = model_output
-
- if self.config.solver_order == 1 or self.lower_order_nums < 1 or lower_order_final:
- prev_sample = self.dpm_solver_first_order_update(model_output, timestep, prev_timestep, sample)
- elif self.config.solver_order == 2 or self.lower_order_nums < 2 or lower_order_second:
- timestep_list = [self.timesteps[step_index - 1], timestep]
- prev_sample = self.multistep_dpm_solver_second_order_update(
- self.model_outputs, timestep_list, prev_timestep, sample
- )
- else:
- timestep_list = [self.timesteps[step_index - 2], self.timesteps[step_index - 1], timestep]
- prev_sample = self.multistep_dpm_solver_third_order_update(
- self.model_outputs, timestep_list, prev_timestep, sample
- )
-
- if self.lower_order_nums < self.config.solver_order:
- self.lower_order_nums += 1
-
- if not return_dict:
- return (prev_sample,)
-
- return SchedulerOutput(prev_sample=prev_sample)
-
- def scale_model_input(self, sample: paddle.Tensor, *args, **kwargs) -> paddle.Tensor:
- """
- Ensures interchangeability with schedulers that need to scale the denoising model input depending on the
- current timestep.
-
- Args:
- sample (`paddle.Tensor`): input sample
-
- Returns:
- `paddle.Tensor`: scaled input sample
- """
- return sample
-
- def add_noise(
- self,
- original_samples: paddle.Tensor,
- noise: paddle.Tensor,
- timesteps: paddle.Tensor,
- ) -> paddle.Tensor:
- # Make sure alphas_cumprod and timestep have same dtype as original_samples
- self.alphas_cumprod = self.alphas_cumprod.cast(original_samples.dtype)
-
- sqrt_alpha_prod = self.alphas_cumprod[timesteps] ** 0.5
- sqrt_alpha_prod = sqrt_alpha_prod.flatten()
- while len(sqrt_alpha_prod.shape) < len(original_samples.shape):
- sqrt_alpha_prod = sqrt_alpha_prod.unsqueeze(-1)
-
- sqrt_one_minus_alpha_prod = (1 - self.alphas_cumprod[timesteps]) ** 0.5
- sqrt_one_minus_alpha_prod = sqrt_one_minus_alpha_prod.flatten()
- while len(sqrt_one_minus_alpha_prod.shape) < len(original_samples.shape):
- sqrt_one_minus_alpha_prod = sqrt_one_minus_alpha_prod.unsqueeze(-1)
-
- noisy_samples = sqrt_alpha_prod * original_samples + sqrt_one_minus_alpha_prod * noise
- return noisy_samples
-
- def __len__(self):
- return self.config.num_train_timesteps
diff --git a/spaces/7hao/bingo/src/components/ui/sheet.tsx b/spaces/7hao/bingo/src/components/ui/sheet.tsx
deleted file mode 100644
index c9f5ce0f81a91067bb013e988a07eb1e6bf6953b..0000000000000000000000000000000000000000
--- a/spaces/7hao/bingo/src/components/ui/sheet.tsx
+++ /dev/null
@@ -1,122 +0,0 @@
-'use client'
-
-import * as React from 'react'
-import * as SheetPrimitive from '@radix-ui/react-dialog'
-
-import { cn } from '@/lib/utils'
-import { IconClose } from '@/components/ui/icons'
-
-const Sheet = SheetPrimitive.Root
-
-const SheetTrigger = SheetPrimitive.Trigger
-
-const SheetClose = SheetPrimitive.Close
-
-const SheetPortal = ({
- className,
- children,
- ...props
-}: SheetPrimitive.DialogPortalProps) => (
-
- {children}
-
-)
-SheetPortal.displayName = SheetPrimitive.Portal.displayName
-
-const SheetOverlay = React.forwardRef<
- React.ElementRef,
- React.ComponentPropsWithoutRef
->(({ className, children, ...props }, ref) => (
-
-))
-SheetOverlay.displayName = SheetPrimitive.Overlay.displayName
-
-const SheetContent = React.forwardRef<
- React.ElementRef,
- React.ComponentPropsWithoutRef
->(({ className, children, ...props }, ref) => (
-
-
- {children}
-
-
- Close
-
-
-
-))
-SheetContent.displayName = SheetPrimitive.Content.displayName
-
-const SheetHeader = ({
- className,
- ...props
-}: React.HTMLAttributes) => (
-
-)
-SheetHeader.displayName = 'SheetHeader'
-
-const SheetFooter = ({
- className,
- ...props
-}: React.HTMLAttributes) => (
-
-)
-SheetFooter.displayName = 'SheetFooter'
-
-const SheetTitle = React.forwardRef<
- React.ElementRef,
- React.ComponentPropsWithoutRef
->(({ className, ...props }, ref) => (
-
-))
-SheetTitle.displayName = SheetPrimitive.Title.displayName
-
-const SheetDescription = React.forwardRef<
- React.ElementRef,
- React.ComponentPropsWithoutRef
->(({ className, ...props }, ref) => (
-
-))
-SheetDescription.displayName = SheetPrimitive.Description.displayName
-
-export {
- Sheet,
- SheetTrigger,
- SheetClose,
- SheetContent,
- SheetHeader,
- SheetFooter,
- SheetTitle,
- SheetDescription
-}
diff --git a/spaces/801artistry/RVC801/infer/lib/infer_pack/attentions.py b/spaces/801artistry/RVC801/infer/lib/infer_pack/attentions.py
deleted file mode 100644
index 19a0a670021aacb9ae1c7f8f54ca1bff8e065375..0000000000000000000000000000000000000000
--- a/spaces/801artistry/RVC801/infer/lib/infer_pack/attentions.py
+++ /dev/null
@@ -1,417 +0,0 @@
-import copy
-import math
-
-import numpy as np
-import torch
-from torch import nn
-from torch.nn import functional as F
-
-from infer.lib.infer_pack import commons, modules
-from infer.lib.infer_pack.modules import LayerNorm
-
-
-class Encoder(nn.Module):
- def __init__(
- self,
- hidden_channels,
- filter_channels,
- n_heads,
- n_layers,
- kernel_size=1,
- p_dropout=0.0,
- window_size=10,
- **kwargs
- ):
- super().__init__()
- self.hidden_channels = hidden_channels
- self.filter_channels = filter_channels
- self.n_heads = n_heads
- self.n_layers = n_layers
- self.kernel_size = kernel_size
- self.p_dropout = p_dropout
- self.window_size = window_size
-
- self.drop = nn.Dropout(p_dropout)
- self.attn_layers = nn.ModuleList()
- self.norm_layers_1 = nn.ModuleList()
- self.ffn_layers = nn.ModuleList()
- self.norm_layers_2 = nn.ModuleList()
- for i in range(self.n_layers):
- self.attn_layers.append(
- MultiHeadAttention(
- hidden_channels,
- hidden_channels,
- n_heads,
- p_dropout=p_dropout,
- window_size=window_size,
- )
- )
- self.norm_layers_1.append(LayerNorm(hidden_channels))
- self.ffn_layers.append(
- FFN(
- hidden_channels,
- hidden_channels,
- filter_channels,
- kernel_size,
- p_dropout=p_dropout,
- )
- )
- self.norm_layers_2.append(LayerNorm(hidden_channels))
-
- def forward(self, x, x_mask):
- attn_mask = x_mask.unsqueeze(2) * x_mask.unsqueeze(-1)
- x = x * x_mask
- for i in range(self.n_layers):
- y = self.attn_layers[i](x, x, attn_mask)
- y = self.drop(y)
- x = self.norm_layers_1[i](x + y)
-
- y = self.ffn_layers[i](x, x_mask)
- y = self.drop(y)
- x = self.norm_layers_2[i](x + y)
- x = x * x_mask
- return x
-
-
-class Decoder(nn.Module):
- def __init__(
- self,
- hidden_channels,
- filter_channels,
- n_heads,
- n_layers,
- kernel_size=1,
- p_dropout=0.0,
- proximal_bias=False,
- proximal_init=True,
- **kwargs
- ):
- super().__init__()
- self.hidden_channels = hidden_channels
- self.filter_channels = filter_channels
- self.n_heads = n_heads
- self.n_layers = n_layers
- self.kernel_size = kernel_size
- self.p_dropout = p_dropout
- self.proximal_bias = proximal_bias
- self.proximal_init = proximal_init
-
- self.drop = nn.Dropout(p_dropout)
- self.self_attn_layers = nn.ModuleList()
- self.norm_layers_0 = nn.ModuleList()
- self.encdec_attn_layers = nn.ModuleList()
- self.norm_layers_1 = nn.ModuleList()
- self.ffn_layers = nn.ModuleList()
- self.norm_layers_2 = nn.ModuleList()
- for i in range(self.n_layers):
- self.self_attn_layers.append(
- MultiHeadAttention(
- hidden_channels,
- hidden_channels,
- n_heads,
- p_dropout=p_dropout,
- proximal_bias=proximal_bias,
- proximal_init=proximal_init,
- )
- )
- self.norm_layers_0.append(LayerNorm(hidden_channels))
- self.encdec_attn_layers.append(
- MultiHeadAttention(
- hidden_channels, hidden_channels, n_heads, p_dropout=p_dropout
- )
- )
- self.norm_layers_1.append(LayerNorm(hidden_channels))
- self.ffn_layers.append(
- FFN(
- hidden_channels,
- hidden_channels,
- filter_channels,
- kernel_size,
- p_dropout=p_dropout,
- causal=True,
- )
- )
- self.norm_layers_2.append(LayerNorm(hidden_channels))
-
- def forward(self, x, x_mask, h, h_mask):
- """
- x: decoder input
- h: encoder output
- """
- self_attn_mask = commons.subsequent_mask(x_mask.size(2)).to(
- device=x.device, dtype=x.dtype
- )
- encdec_attn_mask = h_mask.unsqueeze(2) * x_mask.unsqueeze(-1)
- x = x * x_mask
- for i in range(self.n_layers):
- y = self.self_attn_layers[i](x, x, self_attn_mask)
- y = self.drop(y)
- x = self.norm_layers_0[i](x + y)
-
- y = self.encdec_attn_layers[i](x, h, encdec_attn_mask)
- y = self.drop(y)
- x = self.norm_layers_1[i](x + y)
-
- y = self.ffn_layers[i](x, x_mask)
- y = self.drop(y)
- x = self.norm_layers_2[i](x + y)
- x = x * x_mask
- return x
-
-
-class MultiHeadAttention(nn.Module):
- def __init__(
- self,
- channels,
- out_channels,
- n_heads,
- p_dropout=0.0,
- window_size=None,
- heads_share=True,
- block_length=None,
- proximal_bias=False,
- proximal_init=False,
- ):
- super().__init__()
- assert channels % n_heads == 0
-
- self.channels = channels
- self.out_channels = out_channels
- self.n_heads = n_heads
- self.p_dropout = p_dropout
- self.window_size = window_size
- self.heads_share = heads_share
- self.block_length = block_length
- self.proximal_bias = proximal_bias
- self.proximal_init = proximal_init
- self.attn = None
-
- self.k_channels = channels // n_heads
- self.conv_q = nn.Conv1d(channels, channels, 1)
- self.conv_k = nn.Conv1d(channels, channels, 1)
- self.conv_v = nn.Conv1d(channels, channels, 1)
- self.conv_o = nn.Conv1d(channels, out_channels, 1)
- self.drop = nn.Dropout(p_dropout)
-
- if window_size is not None:
- n_heads_rel = 1 if heads_share else n_heads
- rel_stddev = self.k_channels**-0.5
- self.emb_rel_k = nn.Parameter(
- torch.randn(n_heads_rel, window_size * 2 + 1, self.k_channels)
- * rel_stddev
- )
- self.emb_rel_v = nn.Parameter(
- torch.randn(n_heads_rel, window_size * 2 + 1, self.k_channels)
- * rel_stddev
- )
-
- nn.init.xavier_uniform_(self.conv_q.weight)
- nn.init.xavier_uniform_(self.conv_k.weight)
- nn.init.xavier_uniform_(self.conv_v.weight)
- if proximal_init:
- with torch.no_grad():
- self.conv_k.weight.copy_(self.conv_q.weight)
- self.conv_k.bias.copy_(self.conv_q.bias)
-
- def forward(self, x, c, attn_mask=None):
- q = self.conv_q(x)
- k = self.conv_k(c)
- v = self.conv_v(c)
-
- x, self.attn = self.attention(q, k, v, mask=attn_mask)
-
- x = self.conv_o(x)
- return x
-
- def attention(self, query, key, value, mask=None):
- # reshape [b, d, t] -> [b, n_h, t, d_k]
- b, d, t_s, t_t = (*key.size(), query.size(2))
- query = query.view(b, self.n_heads, self.k_channels, t_t).transpose(2, 3)
- key = key.view(b, self.n_heads, self.k_channels, t_s).transpose(2, 3)
- value = value.view(b, self.n_heads, self.k_channels, t_s).transpose(2, 3)
-
- scores = torch.matmul(query / math.sqrt(self.k_channels), key.transpose(-2, -1))
- if self.window_size is not None:
- assert (
- t_s == t_t
- ), "Relative attention is only available for self-attention."
- key_relative_embeddings = self._get_relative_embeddings(self.emb_rel_k, t_s)
- rel_logits = self._matmul_with_relative_keys(
- query / math.sqrt(self.k_channels), key_relative_embeddings
- )
- scores_local = self._relative_position_to_absolute_position(rel_logits)
- scores = scores + scores_local
- if self.proximal_bias:
- assert t_s == t_t, "Proximal bias is only available for self-attention."
- scores = scores + self._attention_bias_proximal(t_s).to(
- device=scores.device, dtype=scores.dtype
- )
- if mask is not None:
- scores = scores.masked_fill(mask == 0, -1e4)
- if self.block_length is not None:
- assert (
- t_s == t_t
- ), "Local attention is only available for self-attention."
- block_mask = (
- torch.ones_like(scores)
- .triu(-self.block_length)
- .tril(self.block_length)
- )
- scores = scores.masked_fill(block_mask == 0, -1e4)
- p_attn = F.softmax(scores, dim=-1) # [b, n_h, t_t, t_s]
- p_attn = self.drop(p_attn)
- output = torch.matmul(p_attn, value)
- if self.window_size is not None:
- relative_weights = self._absolute_position_to_relative_position(p_attn)
- value_relative_embeddings = self._get_relative_embeddings(
- self.emb_rel_v, t_s
- )
- output = output + self._matmul_with_relative_values(
- relative_weights, value_relative_embeddings
- )
- output = (
- output.transpose(2, 3).contiguous().view(b, d, t_t)
- ) # [b, n_h, t_t, d_k] -> [b, d, t_t]
- return output, p_attn
-
- def _matmul_with_relative_values(self, x, y):
- """
- x: [b, h, l, m]
- y: [h or 1, m, d]
- ret: [b, h, l, d]
- """
- ret = torch.matmul(x, y.unsqueeze(0))
- return ret
-
- def _matmul_with_relative_keys(self, x, y):
- """
- x: [b, h, l, d]
- y: [h or 1, m, d]
- ret: [b, h, l, m]
- """
- ret = torch.matmul(x, y.unsqueeze(0).transpose(-2, -1))
- return ret
-
- def _get_relative_embeddings(self, relative_embeddings, length):
- max_relative_position = 2 * self.window_size + 1
- # Pad first before slice to avoid using cond ops.
- pad_length = max(length - (self.window_size + 1), 0)
- slice_start_position = max((self.window_size + 1) - length, 0)
- slice_end_position = slice_start_position + 2 * length - 1
- if pad_length > 0:
- padded_relative_embeddings = F.pad(
- relative_embeddings,
- commons.convert_pad_shape([[0, 0], [pad_length, pad_length], [0, 0]]),
- )
- else:
- padded_relative_embeddings = relative_embeddings
- used_relative_embeddings = padded_relative_embeddings[
- :, slice_start_position:slice_end_position
- ]
- return used_relative_embeddings
-
- def _relative_position_to_absolute_position(self, x):
- """
- x: [b, h, l, 2*l-1]
- ret: [b, h, l, l]
- """
- batch, heads, length, _ = x.size()
- # Concat columns of pad to shift from relative to absolute indexing.
- x = F.pad(x, commons.convert_pad_shape([[0, 0], [0, 0], [0, 0], [0, 1]]))
-
- # Concat extra elements so to add up to shape (len+1, 2*len-1).
- x_flat = x.view([batch, heads, length * 2 * length])
- x_flat = F.pad(
- x_flat, commons.convert_pad_shape([[0, 0], [0, 0], [0, length - 1]])
- )
-
- # Reshape and slice out the padded elements.
- x_final = x_flat.view([batch, heads, length + 1, 2 * length - 1])[
- :, :, :length, length - 1 :
- ]
- return x_final
-
- def _absolute_position_to_relative_position(self, x):
- """
- x: [b, h, l, l]
- ret: [b, h, l, 2*l-1]
- """
- batch, heads, length, _ = x.size()
- # padd along column
- x = F.pad(
- x, commons.convert_pad_shape([[0, 0], [0, 0], [0, 0], [0, length - 1]])
- )
- x_flat = x.view([batch, heads, length**2 + length * (length - 1)])
- # add 0's in the beginning that will skew the elements after reshape
- x_flat = F.pad(x_flat, commons.convert_pad_shape([[0, 0], [0, 0], [length, 0]]))
- x_final = x_flat.view([batch, heads, length, 2 * length])[:, :, :, 1:]
- return x_final
-
- def _attention_bias_proximal(self, length):
- """Bias for self-attention to encourage attention to close positions.
- Args:
- length: an integer scalar.
- Returns:
- a Tensor with shape [1, 1, length, length]
- """
- r = torch.arange(length, dtype=torch.float32)
- diff = torch.unsqueeze(r, 0) - torch.unsqueeze(r, 1)
- return torch.unsqueeze(torch.unsqueeze(-torch.log1p(torch.abs(diff)), 0), 0)
-
-
-class FFN(nn.Module):
- def __init__(
- self,
- in_channels,
- out_channels,
- filter_channels,
- kernel_size,
- p_dropout=0.0,
- activation=None,
- causal=False,
- ):
- super().__init__()
- self.in_channels = in_channels
- self.out_channels = out_channels
- self.filter_channels = filter_channels
- self.kernel_size = kernel_size
- self.p_dropout = p_dropout
- self.activation = activation
- self.causal = causal
-
- if causal:
- self.padding = self._causal_padding
- else:
- self.padding = self._same_padding
-
- self.conv_1 = nn.Conv1d(in_channels, filter_channels, kernel_size)
- self.conv_2 = nn.Conv1d(filter_channels, out_channels, kernel_size)
- self.drop = nn.Dropout(p_dropout)
-
- def forward(self, x, x_mask):
- x = self.conv_1(self.padding(x * x_mask))
- if self.activation == "gelu":
- x = x * torch.sigmoid(1.702 * x)
- else:
- x = torch.relu(x)
- x = self.drop(x)
- x = self.conv_2(self.padding(x * x_mask))
- return x * x_mask
-
- def _causal_padding(self, x):
- if self.kernel_size == 1:
- return x
- pad_l = self.kernel_size - 1
- pad_r = 0
- padding = [[0, 0], [0, 0], [pad_l, pad_r]]
- x = F.pad(x, commons.convert_pad_shape(padding))
- return x
-
- def _same_padding(self, x):
- if self.kernel_size == 1:
- return x
- pad_l = (self.kernel_size - 1) // 2
- pad_r = self.kernel_size // 2
- padding = [[0, 0], [0, 0], [pad_l, pad_r]]
- x = F.pad(x, commons.convert_pad_shape(padding))
- return x
diff --git a/spaces/AI-Zero-to-Hero/02-H5-AR-VR-IOT/index.html b/spaces/AI-Zero-to-Hero/02-H5-AR-VR-IOT/index.html
deleted file mode 100644
index f64aad6580cd12cbdbb0bcc0321ed7a6486d2a19..0000000000000000000000000000000000000000
--- a/spaces/AI-Zero-to-Hero/02-H5-AR-VR-IOT/index.html
+++ /dev/null
@@ -1,66 +0,0 @@
-
-
-
- Dynamic Lights - A-Frame
-
-
-
-
-
-
-
-
-
-
-
-
-
-
-
-
-
-
-
-
-
-
-
-
-
-
-
\ No newline at end of file
diff --git a/spaces/AIFILMS/Image-Animation-using-Thin-Plate-Spline-Motion-Model/style.css b/spaces/AIFILMS/Image-Animation-using-Thin-Plate-Spline-Motion-Model/style.css
deleted file mode 100644
index 435ebb5987b8913a52f73664c54022374d0c3ed7..0000000000000000000000000000000000000000
--- a/spaces/AIFILMS/Image-Animation-using-Thin-Plate-Spline-Motion-Model/style.css
+++ /dev/null
@@ -1,19 +0,0 @@
-h1 {
- text-align: center;
-}
-img#overview {
- max-width: 1000px;
- max-height: 600px;
- display: block;
- margin: auto;
-}
-img#style-image {
- max-width: 1000px;
- max-height: 600px;
- display: block;
- margin: auto;
-}
-img#visitor-badge {
- display: block;
- margin: auto;
-}
\ No newline at end of file
diff --git a/spaces/AIFILMS/audioldm-text-to-audio-generation/audioldm/clap/open_clip/timm_model.py b/spaces/AIFILMS/audioldm-text-to-audio-generation/audioldm/clap/open_clip/timm_model.py
deleted file mode 100644
index c9d1ab4666b5bab5038d44b90c9ddca5087de460..0000000000000000000000000000000000000000
--- a/spaces/AIFILMS/audioldm-text-to-audio-generation/audioldm/clap/open_clip/timm_model.py
+++ /dev/null
@@ -1,112 +0,0 @@
-""" timm model adapter
-
-Wraps timm (https://github.com/rwightman/pytorch-image-models) models for use as a vision tower in CLIP model.
-"""
-from collections import OrderedDict
-
-import torch.nn as nn
-
-try:
- import timm
- from timm.models.layers import Mlp, to_2tuple
- from timm.models.layers.attention_pool2d import RotAttentionPool2d
- from timm.models.layers.attention_pool2d import (
- AttentionPool2d as AbsAttentionPool2d,
- )
-except ImportError as e:
- timm = None
-
-from .utils import freeze_batch_norm_2d
-
-
-class TimmModel(nn.Module):
- """timm model adapter
- # FIXME this adapter is a work in progress, may change in ways that break weight compat
- """
-
- def __init__(
- self,
- model_name,
- embed_dim,
- image_size=224,
- pool="avg",
- proj="linear",
- drop=0.0,
- pretrained=False,
- ):
- super().__init__()
- if timm is None:
- raise RuntimeError("Please `pip install timm` to use timm models.")
-
- self.image_size = to_2tuple(image_size)
- self.trunk = timm.create_model(model_name, pretrained=pretrained)
- feat_size = self.trunk.default_cfg.get("pool_size", None)
- feature_ndim = 1 if not feat_size else 2
- if pool in ("abs_attn", "rot_attn"):
- assert feature_ndim == 2
- # if attn pooling used, remove both classifier and default pool
- self.trunk.reset_classifier(0, global_pool="")
- else:
- # reset global pool if pool config set, otherwise leave as network default
- reset_kwargs = dict(global_pool=pool) if pool else {}
- self.trunk.reset_classifier(0, **reset_kwargs)
- prev_chs = self.trunk.num_features
-
- head_layers = OrderedDict()
- if pool == "abs_attn":
- head_layers["pool"] = AbsAttentionPool2d(
- prev_chs, feat_size=feat_size, out_features=embed_dim
- )
- prev_chs = embed_dim
- elif pool == "rot_attn":
- head_layers["pool"] = RotAttentionPool2d(prev_chs, out_features=embed_dim)
- prev_chs = embed_dim
- else:
- assert proj, "projection layer needed if non-attention pooling is used."
-
- # NOTE attention pool ends with a projection layer, so proj should usually be set to '' if such pooling is used
- if proj == "linear":
- head_layers["drop"] = nn.Dropout(drop)
- head_layers["proj"] = nn.Linear(prev_chs, embed_dim)
- elif proj == "mlp":
- head_layers["mlp"] = Mlp(prev_chs, 2 * embed_dim, embed_dim, drop=drop)
-
- self.head = nn.Sequential(head_layers)
-
- def lock(self, unlocked_groups=0, freeze_bn_stats=False):
- """lock modules
- Args:
- unlocked_groups (int): leave last n layer groups unlocked (default: 0)
- """
- if not unlocked_groups:
- # lock full model
- for param in self.trunk.parameters():
- param.requires_grad = False
- if freeze_bn_stats:
- freeze_batch_norm_2d(self.trunk)
- else:
- # NOTE: partial freeze requires latest timm (master) branch and is subject to change
- try:
- # FIXME import here until API stable and in an official release
- from timm.models.helpers import group_parameters, group_modules
- except ImportError:
- raise RuntimeError(
- "Please install latest timm `pip install git+https://github.com/rwightman/pytorch-image-models`"
- )
- matcher = self.trunk.group_matcher()
- gparams = group_parameters(self.trunk, matcher)
- max_layer_id = max(gparams.keys())
- max_layer_id = max_layer_id - unlocked_groups
- for group_idx in range(max_layer_id + 1):
- group = gparams[group_idx]
- for param in group:
- self.trunk.get_parameter(param).requires_grad = False
- if freeze_bn_stats:
- gmodules = group_modules(self.trunk, matcher, reverse=True)
- gmodules = {k for k, v in gmodules.items() if v <= max_layer_id}
- freeze_batch_norm_2d(self.trunk, gmodules)
-
- def forward(self, x):
- x = self.trunk(x)
- x = self.head(x)
- return x
diff --git a/spaces/AIGC-Audio/AudioGPT/text_to_speech/tasks/tts/speech_base.py b/spaces/AIGC-Audio/AudioGPT/text_to_speech/tasks/tts/speech_base.py
deleted file mode 100644
index 7b5f85edc9b827806ca565b617e1b72149e09943..0000000000000000000000000000000000000000
--- a/spaces/AIGC-Audio/AudioGPT/text_to_speech/tasks/tts/speech_base.py
+++ /dev/null
@@ -1,373 +0,0 @@
-import filecmp
-import os
-import traceback
-import numpy as np
-import pandas as pd
-import torch
-import torch.distributed as dist
-import torch.nn.functional as F
-import torch.optim
-import torch.utils.data
-import yaml
-from tqdm import tqdm
-import utils
-from tasks.tts.dataset_utils import BaseSpeechDataset
-from tasks.tts.utils. import parse_mel_losses, parse_dataset_configs, load_data_preprocessor, load_data_binarizer
-from tasks.tts.vocoder_infer.base_vocoder import BaseVocoder, get_vocoder_cls
-from text_to_speech.utils.audio.align import mel2token_to_dur
-from text_to_speech.utils.audio.io import save_wav
-from text_to_speech.utils.audio.pitch_extractors import extract_pitch_simple
-from text_to_speech.utils.commons.base_task import BaseTask
-from text_to_speech.utils.commons.ckpt_utils import load_ckpt
-from text_to_speech.utils.commons.dataset_utils import data_loader, BaseConcatDataset
-from text_to_speech.utils.commons.hparams import hparams
-from text_to_speech.utils.commons.multiprocess_utils import MultiprocessManager
-from text_to_speech.utils.commons.tensor_utils import tensors_to_scalars
-from text_to_speech.utils.metrics.ssim import ssim
-from text_to_speech.utils.nn.model_utils import print_arch
-from text_to_speech.utils.nn.schedulers import RSQRTSchedule, NoneSchedule, WarmupSchedule
-from text_to_speech.utils.nn.seq_utils import weights_nonzero_speech
-from text_to_speech.utils.plot.plot import spec_to_figure
-from text_to_speech.utils.text.text_encoder import build_token_encoder
-import matplotlib.pyplot as plt
-
-
-class SpeechBaseTask(BaseTask):
- def __init__(self, *args, **kwargs):
- super().__init__(*args, **kwargs)
- self.dataset_cls = BaseSpeechDataset
- self.vocoder = None
- data_dir = hparams['binary_data_dir']
- if not hparams['use_word_input']:
- self.token_encoder = build_token_encoder(f'{data_dir}/phone_set.json')
- else:
- self.token_encoder = build_token_encoder(f'{data_dir}/word_set.json')
- self.padding_idx = self.token_encoder.pad()
- self.eos_idx = self.token_encoder.eos()
- self.seg_idx = self.token_encoder.seg()
- self.saving_result_pool = None
- self.saving_results_futures = None
- self.mel_losses = parse_mel_losses()
- self.max_tokens, self.max_sentences, \
- self.max_valid_tokens, self.max_valid_sentences = parse_dataset_configs()
-
- ##########################
- # datasets
- ##########################
- @data_loader
- def train_dataloader(self):
- if hparams['train_sets'] != '':
- train_sets = hparams['train_sets'].split("|")
- # check if all train_sets have the same spk map and dictionary
- binary_data_dir = hparams['binary_data_dir']
- file_to_cmp = ['phone_set.json']
- if os.path.exists(f'{binary_data_dir}/word_set.json'):
- file_to_cmp.append('word_set.json')
- if hparams['use_spk_id']:
- file_to_cmp.append('spk_map.json')
- for f in file_to_cmp:
- for ds_name in train_sets:
- base_file = os.path.join(binary_data_dir, f)
- ds_file = os.path.join(ds_name, f)
- assert filecmp.cmp(base_file, ds_file), \
- f'{f} in {ds_name} is not same with that in {binary_data_dir}.'
- train_dataset = BaseConcatDataset([
- self.dataset_cls(prefix='train', shuffle=True, data_dir=ds_name) for ds_name in train_sets])
- else:
- train_dataset = self.dataset_cls(prefix=hparams['train_set_name'], shuffle=True)
- return self.build_dataloader(train_dataset, True, self.max_tokens, self.max_sentences,
- endless=hparams['endless_ds'])
-
- @data_loader
- def val_dataloader(self):
- valid_dataset = self.dataset_cls(prefix=hparams['valid_set_name'], shuffle=False)
- return self.build_dataloader(valid_dataset, False, self.max_valid_tokens, self.max_valid_sentences,
- batch_by_size=False)
-
- @data_loader
- def test_dataloader(self):
- test_dataset = self.dataset_cls(prefix=hparams['test_set_name'], shuffle=False)
- self.test_dl = self.build_dataloader(
- test_dataset, False, self.max_valid_tokens, self.max_valid_sentences, batch_by_size=False)
- return self.test_dl
-
- def build_dataloader(self, dataset, shuffle, max_tokens=None, max_sentences=None,
- required_batch_size_multiple=-1, endless=False, batch_by_size=True):
- devices_cnt = torch.cuda.device_count()
- if devices_cnt == 0:
- devices_cnt = 1
- if required_batch_size_multiple == -1:
- required_batch_size_multiple = devices_cnt
-
- def shuffle_batches(batches):
- np.random.shuffle(batches)
- return batches
-
- if max_tokens is not None:
- max_tokens *= devices_cnt
- if max_sentences is not None:
- max_sentences *= devices_cnt
- indices = dataset.ordered_indices()
- if batch_by_size:
- batch_sampler = utils.commons.dataset_utils.batch_by_size(
- indices, dataset.num_tokens, max_tokens=max_tokens, max_sentences=max_sentences,
- required_batch_size_multiple=required_batch_size_multiple,
- )
- else:
- batch_sampler = []
- for i in range(0, len(indices), max_sentences):
- batch_sampler.append(indices[i:i + max_sentences])
-
- if shuffle:
- batches = shuffle_batches(list(batch_sampler))
- if endless:
- batches = [b for _ in range(1000) for b in shuffle_batches(list(batch_sampler))]
- else:
- batches = batch_sampler
- if endless:
- batches = [b for _ in range(1000) for b in batches]
- num_workers = dataset.num_workers
- if self.trainer.use_ddp:
- num_replicas = dist.get_world_size()
- rank = dist.get_rank()
- batches = [x[rank::num_replicas] for x in batches if len(x) % num_replicas == 0]
- return torch.utils.data.DataLoader(dataset,
- collate_fn=dataset.collater,
- batch_sampler=batches,
- num_workers=num_workers,
- pin_memory=False)
-
- ##########################
- # scheduler and optimizer
- ##########################
- def build_model(self):
- self.build_tts_model()
- if hparams['load_ckpt'] != '':
- load_ckpt(self.model, hparams['load_ckpt'])
- print_arch(self.model)
- return self.model
-
- def build_tts_model(self):
- raise NotImplementedError
-
- def build_scheduler(self, optimizer):
- if hparams['scheduler'] == 'rsqrt':
- return RSQRTSchedule(optimizer, hparams['lr'], hparams['warmup_updates'], hparams['hidden_size'])
- elif hparams['scheduler'] == 'warmup':
- return WarmupSchedule(optimizer, hparams['lr'], hparams['warmup_updates'])
- elif hparams['scheduler'] == 'step_lr':
- return torch.optim.lr_scheduler.StepLR(
- optimizer=optimizer, step_size=500, gamma=0.998)
- else:
- return NoneSchedule(optimizer, hparams['lr'])
-
- def build_optimizer(self, model):
- self.optimizer = optimizer = torch.optim.AdamW(
- model.parameters(),
- lr=hparams['lr'],
- betas=(hparams['optimizer_adam_beta1'], hparams['optimizer_adam_beta2']),
- weight_decay=hparams['weight_decay'])
-
- return optimizer
-
- ##########################
- # training and validation
- ##########################
- def _training_step(self, sample, batch_idx, _):
- loss_output, _ = self.run_model(sample)
- total_loss = sum([v for v in loss_output.values() if isinstance(v, torch.Tensor) and v.requires_grad])
- loss_output['batch_size'] = sample['txt_tokens'].size()[0]
- return total_loss, loss_output
-
- def run_model(self, sample, infer=False):
- """
-
- :param sample: a batch of data
- :param infer: bool, run in infer mode
- :return:
- if not infer:
- return losses, model_out
- if infer:
- return model_out
- """
- raise NotImplementedError
-
- def validation_start(self):
- self.vocoder = get_vocoder_cls(hparams['vocoder'])()
-
- def validation_step(self, sample, batch_idx):
- outputs = {}
- outputs['losses'] = {}
- outputs['losses'], model_out = self.run_model(sample)
- outputs['total_loss'] = sum(outputs['losses'].values())
- outputs['nsamples'] = sample['nsamples']
- outputs = tensors_to_scalars(outputs)
- if self.global_step % hparams['valid_infer_interval'] == 0 \
- and batch_idx < hparams['num_valid_plots']:
- self.save_valid_result(sample, batch_idx, model_out)
- return outputs
-
- def validation_end(self, outputs):
- self.vocoder = None
- return super(SpeechBaseTask, self).validation_end(outputs)
-
- def save_valid_result(self, sample, batch_idx, model_out):
- raise NotImplementedError
-
- ##########################
- # losses
- ##########################
- def add_mel_loss(self, mel_out, target, losses, postfix=''):
- for loss_name, lambd in self.mel_losses.items():
- losses[f'{loss_name}{postfix}'] = getattr(self, f'{loss_name}_loss')(mel_out, target) * lambd
-
- def l1_loss(self, decoder_output, target):
- # decoder_output : B x T x n_mel
- # target : B x T x n_mel
- l1_loss = F.l1_loss(decoder_output, target, reduction='none')
- weights = weights_nonzero_speech(target)
- l1_loss = (l1_loss * weights).sum() / weights.sum()
- return l1_loss
-
- def mse_loss(self, decoder_output, target):
- # decoder_output : B x T x n_mel
- # target : B x T x n_mel
- assert decoder_output.shape == target.shape
- mse_loss = F.mse_loss(decoder_output, target, reduction='none')
- weights = weights_nonzero_speech(target)
- mse_loss = (mse_loss * weights).sum() / weights.sum()
- return mse_loss
-
- def ssim_loss(self, decoder_output, target, bias=6.0):
- # decoder_output : B x T x n_mel
- # target : B x T x n_mel
- assert decoder_output.shape == target.shape
- weights = weights_nonzero_speech(target)
- decoder_output = decoder_output[:, None] + bias
- target = target[:, None] + bias
- ssim_loss = 1 - ssim(decoder_output, target, size_average=False)
- ssim_loss = (ssim_loss * weights).sum() / weights.sum()
- return ssim_loss
-
- def plot_mel(self, batch_idx, spec_out, spec_gt=None, name=None, title='', f0s=None, dur_info=None):
- vmin = hparams['mel_vmin']
- vmax = hparams['mel_vmax']
- if len(spec_out.shape) == 3:
- spec_out = spec_out[0]
- if isinstance(spec_out, torch.Tensor):
- spec_out = spec_out.cpu().numpy()
- if spec_gt is not None:
- if len(spec_gt.shape) == 3:
- spec_gt = spec_gt[0]
- if isinstance(spec_gt, torch.Tensor):
- spec_gt = spec_gt.cpu().numpy()
- max_len = max(len(spec_gt), len(spec_out))
- if max_len - len(spec_gt) > 0:
- spec_gt = np.pad(spec_gt, [[0, max_len - len(spec_gt)], [0, 0]], mode='constant',
- constant_values=vmin)
- if max_len - len(spec_out) > 0:
- spec_out = np.pad(spec_out, [[0, max_len - len(spec_out)], [0, 0]], mode='constant',
- constant_values=vmin)
- spec_out = np.concatenate([spec_out, spec_gt], -1)
- name = f'mel_val_{batch_idx}' if name is None else name
- self.logger.add_figure(name, spec_to_figure(
- spec_out, vmin, vmax, title=title, f0s=f0s, dur_info=dur_info), self.global_step)
-
- ##########################
- # testing
- ##########################
- def test_start(self):
- self.saving_result_pool = MultiprocessManager(int(os.getenv('N_PROC', os.cpu_count())))
- self.saving_results_futures = []
- self.gen_dir = os.path.join(
- hparams['work_dir'], f'generated_{self.trainer.global_step}_{hparams["gen_dir_name"]}')
- self.vocoder: BaseVocoder = get_vocoder_cls(hparams['vocoder'])()
- os.makedirs(self.gen_dir, exist_ok=True)
- os.makedirs(f'{self.gen_dir}/wavs', exist_ok=True)
- os.makedirs(f'{self.gen_dir}/plot', exist_ok=True)
- if hparams.get('save_mel_npy', False):
- os.makedirs(f'{self.gen_dir}/mel_npy', exist_ok=True)
-
- def test_step(self, sample, batch_idx):
- """
-
- :param sample:
- :param batch_idx:
- :return:
- """
- assert sample['txt_tokens'].shape[0] == 1, 'only support batch_size=1 in inference'
- outputs = self.run_model(sample, infer=True)
- text = sample['text'][0]
- item_name = sample['item_name'][0]
- tokens = sample['txt_tokens'][0].cpu().numpy()
- mel_gt = sample['mels'][0].cpu().numpy()
- mel_pred = outputs['mel_out'][0].cpu().numpy()
- str_phs = self.token_encoder.decode(tokens, strip_padding=True)
- base_fn = f'[{self.results_id:06d}][{item_name.replace("%", "_")}][%s]'
- if text is not None:
- base_fn += text.replace(":", "$3A")[:80]
- base_fn = base_fn.replace(' ', '_')
- gen_dir = self.gen_dir
- wav_pred = self.vocoder.spec2wav(mel_pred)
- self.saving_result_pool.add_job(self.save_result, args=[
- wav_pred, mel_pred, base_fn % 'P', gen_dir, str_phs])
- if hparams['save_gt']:
- wav_gt = self.vocoder.spec2wav(mel_gt)
- self.saving_result_pool.add_job(self.save_result, args=[
- wav_gt, mel_gt, base_fn % 'G', gen_dir, str_phs])
- print(f"Pred_shape: {mel_pred.shape}, gt_shape: {mel_gt.shape}")
- return {
- 'item_name': item_name,
- 'text': text,
- 'ph_tokens': self.token_encoder.decode(tokens.tolist()),
- 'wav_fn_pred': base_fn % 'P',
- 'wav_fn_gt': base_fn % 'G',
- }
-
- @staticmethod
- def save_result(wav_out, mel, base_fn, gen_dir, str_phs=None, mel2ph=None, alignment=None):
- save_wav(wav_out, f'{gen_dir}/wavs/{base_fn}.wav', hparams['audio_sample_rate'],
- norm=hparams['out_wav_norm'])
- fig = plt.figure(figsize=(14, 10))
- spec_vmin = hparams['mel_vmin']
- spec_vmax = hparams['mel_vmax']
- heatmap = plt.pcolor(mel.T, vmin=spec_vmin, vmax=spec_vmax)
- fig.colorbar(heatmap)
- try:
- f0 = extract_pitch_simple(wav_out)
- f0 = f0 / 10 * (f0 > 0)
- plt.plot(f0, c='white', linewidth=1, alpha=0.6)
- if mel2ph is not None and str_phs is not None:
- decoded_txt = str_phs.split(" ")
- dur = mel2token_to_dur(torch.LongTensor(mel2ph)[None, :], len(decoded_txt))[0].numpy()
- dur = [0] + list(np.cumsum(dur))
- for i in range(len(dur) - 1):
- shift = (i % 20) + 1
- plt.text(dur[i], shift, decoded_txt[i])
- plt.hlines(shift, dur[i], dur[i + 1], colors='b' if decoded_txt[i] != '|' else 'black')
- plt.vlines(dur[i], 0, 5, colors='b' if decoded_txt[i] != '|' else 'black',
- alpha=1, linewidth=1)
- plt.tight_layout()
- plt.savefig(f'{gen_dir}/plot/{base_fn}.png', format='png')
- plt.close(fig)
- if hparams.get('save_mel_npy', False):
- np.save(f'{gen_dir}/mel_npy/{base_fn}', mel)
- if alignment is not None:
- fig, ax = plt.subplots(figsize=(12, 16))
- im = ax.imshow(alignment, aspect='auto', origin='lower',
- interpolation='none')
- decoded_txt = str_phs.split(" ")
- ax.set_yticks(np.arange(len(decoded_txt)))
- ax.set_yticklabels(list(decoded_txt), fontsize=6)
- fig.colorbar(im, ax=ax)
- fig.savefig(f'{gen_dir}/attn_plot/{base_fn}_attn.png', format='png')
- plt.close(fig)
- except Exception:
- traceback.print_exc()
- return None
-
- def test_end(self, outputs):
- pd.DataFrame(outputs).to_csv(f'{self.gen_dir}/meta.csv')
- for _1, _2 in tqdm(self.saving_result_pool.get_results(), total=len(self.saving_result_pool)):
- pass
- return {}
diff --git a/spaces/AILab-CVC/SEED-LLaMA/scripts/seed_llama_inference_14B.py b/spaces/AILab-CVC/SEED-LLaMA/scripts/seed_llama_inference_14B.py
deleted file mode 100644
index 054b61438bc28351e6a2baabaad247d3c2af09f2..0000000000000000000000000000000000000000
--- a/spaces/AILab-CVC/SEED-LLaMA/scripts/seed_llama_inference_14B.py
+++ /dev/null
@@ -1,120 +0,0 @@
-import hydra
-
-import pyrootutils
-import os
-import torch
-
-from omegaconf import OmegaConf
-import json
-from typing import Optional
-import transformers
-from PIL import Image
-from torchvision.transforms.functional import InterpolationMode
-
-pyrootutils.setup_root(__file__, indicator=".project-root", pythonpath=True)
-
-BOI_TOKEN = ''
-EOI_TOKEN = ''
-IMG_TOKEN = ''
-
-IMG_FLAG = ''
-NUM_IMG_TOKNES = 32
-NUM_IMG_CODES = 8192
-image_id_shift = 32000
-
-
-
-
-def generate(tokenizer, input_tokens, generation_config, model):
-
- input_ids = tokenizer(input_tokens, add_special_tokens=False, return_tensors='pt').input_ids
- input_ids = input_ids.to("cuda")
-
- generate_ids = model.generate(
- input_ids=input_ids,
- **generation_config
- )
- generate_ids = generate_ids[0][input_ids.shape[1]:]
-
- return generate_ids
-
-def decode_image_text(generate_ids, tokenizer, save_path=None):
-
- boi_list = torch.where(generate_ids == tokenizer(BOI_TOKEN, add_special_tokens=False).input_ids[0])[0]
- eoi_list = torch.where(generate_ids == tokenizer(EOI_TOKEN, add_special_tokens=False).input_ids[0])[0]
-
- if len(boi_list) == 0 and len(eoi_list) == 0:
- text_ids = generate_ids
- texts = tokenizer.decode(text_ids, skip_special_tokens=True)
- print(texts)
-
- else:
- boi_index = boi_list[0]
- eoi_index = eoi_list[0]
-
- text_ids = generate_ids[:boi_index]
- if len(text_ids) != 0:
- texts = tokenizer.decode(text_ids, skip_special_tokens=True)
- print(texts)
-
- image_ids = (generate_ids[boi_index+1:eoi_index] - image_id_shift).reshape(1,-1)
-
- images = tokenizer.decode_image(image_ids)
-
- images[0].save(save_path)
-
-
-device = "cuda"
-
-tokenizer_cfg_path = 'configs/tokenizer/seed_llama_tokenizer.yaml'
-tokenizer_cfg = OmegaConf.load(tokenizer_cfg_path)
-tokenizer = hydra.utils.instantiate(tokenizer_cfg, device=device, load_diffusion=True)
-
-transform_cfg_path = 'configs/transform/clip_transform.yaml'
-transform_cfg = OmegaConf.load(transform_cfg_path)
-transform = hydra.utils.instantiate(transform_cfg)
-
-model_cfg = OmegaConf.load('configs/llm/seed_llama_14b.yaml')
-model = hydra.utils.instantiate(model_cfg, torch_dtype=torch.float16)
-model = model.eval().to(device)
-
-generation_config = {
- 'temperature': 1.0,
- 'num_beams': 1,
- 'max_new_tokens': 512,
- 'top_p': 0.5,
- 'do_sample': True
- }
-
-s_token = "[INST] "
-e_token = " [/INST]"
-sep = "\n"
-
-
-### visual question answering
-image_path = "images/cat.jpg"
-image = Image.open(image_path).convert('RGB')
-image_tensor = transform(image).to(device)
-img_ids = tokenizer.encode_image(image_torch=image_tensor)
-img_ids = img_ids.view(-1).cpu().numpy()
-img_tokens = BOI_TOKEN + ''.join([IMG_TOKEN.format(item) for item in img_ids]) + EOI_TOKEN
-
-question = "What is this animal?"
-
-input_tokens = tokenizer.bos_token + s_token + img_tokens + question + e_token + sep
-generate_ids = generate(tokenizer, input_tokens, generation_config, model)
-decode_image_text(generate_ids, tokenizer)
-
-### text-to-image generation
-prompt = "Can you generate an image of a dog on the green grass?"
-input_tokens = tokenizer.bos_token + s_token + prompt + e_token + sep
-generate_ids = generate(tokenizer, input_tokens, generation_config, model)
-save_path = 'dog.jpg'
-decode_image_text(generate_ids, tokenizer, save_path)
-
-### multimodal prompt image generation
-instruction = "Can you make the cat wear sunglasses?"
-input_tokens = tokenizer.bos_token + s_token + img_tokens + instruction + e_token + sep
-generate_ids = generate(tokenizer, input_tokens, generation_config, model)
-save_path = 'cat_sunglasses.jpg'
-decode_image_text(generate_ids, tokenizer, save_path)
\ No newline at end of file
diff --git a/spaces/ATang0729/Forecast4Muses/Model/Model6/Model6_0_ClothesDetection/mmyolo/configs/custom_dataset/yolov7_l_syncbn_fast_6x16b-100e_coco.py b/spaces/ATang0729/Forecast4Muses/Model/Model6/Model6_0_ClothesDetection/mmyolo/configs/custom_dataset/yolov7_l_syncbn_fast_6x16b-100e_coco.py
deleted file mode 100644
index a005c28eb78d101354650a20b1ff7212e24d0743..0000000000000000000000000000000000000000
--- a/spaces/ATang0729/Forecast4Muses/Model/Model6/Model6_0_ClothesDetection/mmyolo/configs/custom_dataset/yolov7_l_syncbn_fast_6x16b-100e_coco.py
+++ /dev/null
@@ -1,489 +0,0 @@
-_base_ = ['../_base_/default_runtime.py', '../_base_/det_p5_tta.py']
-
-data_root = './data-df2/'
-train_ann_file = 'annotations/train.json'
-train_data_prefix = 'smaller-dataset/'
-val_ann_file = 'annotations/val.json'
-val_data_prefix = 'smaller-dataset/'
-test_ann_file = 'annotations/test.json'
-test_data_prefix = 'smaller-dataset/'
-# num_classes = 13
-train_batch_size_per_gpu = 32
-train_num_workers = 4
-persistent_workers = True
-
-vis_backends = [
- dict(type='LocalVisBackend'),
-]
-visualizer = dict(
- type='mmdet.DetLocalVisualizer',
- vis_backends=[
- dict(type='LocalVisBackend'),
- # dict(type='WandbVisBackend'),
- dict(type='TensorboardVisBackend')
- ],
- name='visualizer')
-log_processor = dict(type='LogProcessor', window_size=50, by_epoch=True)
-log_level = 'INFO'
-load_from = None
-resume = False
-
-anchors = [
- [(12, 16), (19, 36), (40, 28)], # P3/8
- [(36, 75), (76, 55), (72, 146)], # P4/16
- [(142, 110), (192, 243), (459, 401)] # P5/32
-]
-
-base_lr = 0.01
-max_epochs = 100
-
-num_epoch_stage2 = 10 # The last 10 epochs switch evaluation interval
-val_interval_stage2 = 1
-
-model_test_cfg = dict(
- multi_label=True,
- nms_pre=30000,
- score_thr=0.001,
- nms=dict(type='nms', iou_threshold=0.65),
- max_per_img=300)
-
-img_scale = (640, 640)
-dataset_type = 'YOLOv5CocoDataset'
-classes=('short_sleeved_shirt', 'long_sleeved_shirt',
- 'short_sleeved_outwear', 'long_sleeved_outwear',
- 'vest', 'sling', 'shorts', 'trousers', 'skirt',
- 'short_sleeved_dress', 'long_sleeved_dress',
- 'vest_dress', 'sling_dress')
-num_classes = len(classes)
-palette=[(255, 0, 0), (255, 128, 0), (255, 255, 0),
- (128, 255, 0), (0, 255, 0), (0, 255, 128),
- (0, 255, 255), (0, 128, 255), (0, 0, 255),
- (127, 0, 255), (255, 0, 255), (255, 0, 127),
- (128, 128, 128)]
-metainfo = dict(
- classes=classes,
- palette=palette
-)
-val_batch_size_per_gpu = 1
-val_num_workers = 2
-batch_shapes_cfg = dict(
- type='BatchShapePolicy',
- batch_size=val_batch_size_per_gpu,
- img_size=img_scale[0],
- size_divisor=32,
- extra_pad_ratio=0.5)
-strides = [8, 16, 32] # Strides of multi-scale prior box
-num_det_layers = 3
-norm_cfg = dict(type='BN', momentum=0.03, eps=0.001)
-
-# Data augmentation
-max_translate_ratio = 0.2 # YOLOv5RandomAffine
-scaling_ratio_range = (0.1, 2.0) # YOLOv5RandomAffine
-mixup_prob = 0.15 # YOLOv5MixUp
-randchoice_mosaic_prob = [0.8, 0.2]
-mixup_alpha = 8.0 # YOLOv5MixUp
-mixup_beta = 8.0 # YOLOv5MixUp
-
-# -----train val related-----
-loss_cls_weight = 0.3
-loss_bbox_weight = 0.05
-loss_obj_weight = 0.7
-# BatchYOLOv7Assigner params
-simota_candidate_topk = 10
-simota_iou_weight = 3.0
-simota_cls_weight = 1.0
-prior_match_thr = 4. # Priori box matching threshold
-obj_level_weights = [4., 1.,
- 0.4] # The obj loss weights of the three output layers
-
-lr_factor = 0.1 # Learning rate scaling factor
-weight_decay = 0.0005
-save_epoch_intervals = 1
-max_keep_ckpts = 5
-
-env_cfg = dict(
- cudnn_benchmark=True,
- mp_cfg=dict(mp_start_method='fork', opencv_num_threads=0),
- dist_cfg=dict(backend='nccl'))
-
-# ===============================Unmodified in most cases====================
-model = dict(
- type='YOLODetector',
- data_preprocessor=dict(
- type='YOLOv5DetDataPreprocessor',
- mean=[0., 0., 0.],
- std=[255., 255., 255.],
- bgr_to_rgb=True),
- backbone=dict(
- type='YOLOv7Backbone',
- arch='L',
- norm_cfg=norm_cfg,
- act_cfg=dict(type='SiLU', inplace=True)),
- neck=dict(
- type='YOLOv7PAFPN',
- block_cfg=dict(
- type='ELANBlock',
- middle_ratio=0.5,
- block_ratio=0.25,
- num_blocks=4,
- num_convs_in_block=1),
- upsample_feats_cat_first=False,
- in_channels=[512, 1024, 1024],
- # The real output channel will be multiplied by 2
- out_channels=[128, 256, 512],
- norm_cfg=norm_cfg,
- act_cfg=dict(type='SiLU', inplace=True)),
- bbox_head=dict(
- type='YOLOv7Head',
- head_module=dict(
- type='YOLOv7HeadModule',
- num_classes=num_classes,
- in_channels=[256, 512, 1024],
- featmap_strides=strides,
- num_base_priors=3),
- prior_generator=dict(
- type='mmdet.YOLOAnchorGenerator',
- base_sizes=anchors,
- strides=strides),
- # scaled based on number of detection layers
- loss_cls=dict(
- type='mmdet.CrossEntropyLoss',
- use_sigmoid=True,
- reduction='mean',
- loss_weight=loss_cls_weight *
- (num_classes / 80 * 3 / num_det_layers)),
- loss_bbox=dict(
- type='IoULoss',
- iou_mode='ciou',
- bbox_format='xyxy',
- reduction='mean',
- loss_weight=loss_bbox_weight * (3 / num_det_layers),
- return_iou=True),
- loss_obj=dict(
- type='mmdet.CrossEntropyLoss',
- use_sigmoid=True,
- reduction='mean',
- loss_weight=loss_obj_weight *
- ((img_scale[0] / 640)**2 * 3 / num_det_layers)),
- prior_match_thr=prior_match_thr,
- obj_level_weights=obj_level_weights,
- # BatchYOLOv7Assigner params
- simota_candidate_topk=simota_candidate_topk,
- simota_iou_weight=simota_iou_weight,
- simota_cls_weight=simota_cls_weight),
- test_cfg=model_test_cfg)
-
-pre_transform = [
- dict(type='LoadImageFromFile', file_client_args=_base_.file_client_args),
- dict(type='LoadAnnotations', with_bbox=True)
-]
-
-mosiac4_pipeline = [
- dict(
- type='Mosaic',
- img_scale=img_scale,
- pad_val=114.0,
- pre_transform=pre_transform),
- dict(
- type='YOLOv5RandomAffine',
- max_rotate_degree=0.0,
- max_shear_degree=0.0,
- max_translate_ratio=max_translate_ratio, # note
- scaling_ratio_range=scaling_ratio_range, # note
- # img_scale is (width, height)
- border=(-img_scale[0] // 2, -img_scale[1] // 2),
- border_val=(114, 114, 114)),
-]
-
-mosiac9_pipeline = [
- dict(
- type='Mosaic9',
- img_scale=img_scale,
- pad_val=114.0,
- pre_transform=pre_transform),
- dict(
- type='YOLOv5RandomAffine',
- max_rotate_degree=0.0,
- max_shear_degree=0.0,
- max_translate_ratio=max_translate_ratio, # note
- scaling_ratio_range=scaling_ratio_range, # note
- # img_scale is (width, height)
- border=(-img_scale[0] // 2, -img_scale[1] // 2),
- border_val=(114, 114, 114)),
-]
-
-randchoice_mosaic_pipeline = dict(
- type='RandomChoice',
- transforms=[mosiac4_pipeline, mosiac9_pipeline],
- prob=randchoice_mosaic_prob)
-
-train_pipeline = [
- *pre_transform,
- randchoice_mosaic_pipeline,
- dict(
- type='YOLOv5MixUp',
- alpha=mixup_alpha, # note
- beta=mixup_beta, # note
- prob=mixup_prob,
- pre_transform=[*pre_transform, randchoice_mosaic_pipeline]),
- dict(type='YOLOv5HSVRandomAug'),
- dict(type='mmdet.RandomFlip', prob=0.5),
- dict(
- type='mmdet.PackDetInputs',
- meta_keys=('img_id', 'img_path', 'ori_shape', 'img_shape', 'flip',
- 'flip_direction'))
-]
-
-test_pipeline = [
- dict(type='LoadImageFromFile', file_client_args=_base_.file_client_args),
- dict(type='YOLOv5KeepRatioResize', scale=img_scale),
- dict(
- type='LetterResize',
- scale=img_scale,
- allow_scale_up=False,
- pad_val=dict(img=114)),
- dict(type='LoadAnnotations', with_bbox=True, _scope_='mmdet'),
- dict(
- type='mmdet.PackDetInputs',
- meta_keys=('img_id', 'img_path', 'ori_shape', 'img_shape',
- 'scale_factor', 'pad_param'))
-]
-
-train_dataloader = dict(
- batch_size=train_batch_size_per_gpu,
- num_workers=train_num_workers,
- persistent_workers=persistent_workers,
- pin_memory=True,
- sampler=dict(type='DefaultSampler', shuffle=True),
- collate_fn=dict(type='yolov5_collate'), # FASTER
- dataset=dict(
- type='RepeatDataset',
- times=2,
- dataset=dict(
- type=dataset_type,
- data_root=data_root,
- metainfo=metainfo,
- ann_file=val_ann_file,
- data_prefix=dict(img=train_data_prefix),
- filter_cfg=dict(filter_empty_gt=False, min_size=32),
- pipeline=train_pipeline)
- )
- )
-
-val_dataloader = dict(
- dataset=dict(
- metainfo=metainfo,
- data_root=data_root,
- ann_file=val_ann_file,
- data_prefix=dict(img=val_data_prefix)))
-
-val_evaluator = dict(ann_file=data_root + val_ann_file)
-
-test_dataloader = dict(
- dataset=dict(
- metainfo=metainfo,
- data_root=data_root,
- ann_file=test_ann_file,
- data_prefix=dict(img=test_data_prefix)))
-test_evaluator = dict(ann_file=data_root + test_ann_file)
-
-train_cfg = dict(
- type='EpochBasedTrainLoop',
- max_epochs=max_epochs,
- val_interval=save_epoch_intervals,
- dynamic_intervals=[(max_epochs - num_epoch_stage2, val_interval_stage2)])
-val_cfg = dict(type='ValLoop')
-test_cfg = dict(type='TestLoop')
-
-param_scheduler = None
-optim_wrapper = dict(
- type='OptimWrapper',
- optimizer=dict(
- type='SGD',
- lr=base_lr,
- momentum=0.937,
- weight_decay=weight_decay,
- nesterov=True,
- batch_size_per_gpu=train_batch_size_per_gpu),
- constructor='YOLOv7OptimWrapperConstructor')
-
-# TO DO: change param_scheduler type to StepLR, refer to mobilenet
-default_scope = 'mmyolo'
-default_hooks = dict(
- timer=dict(type='IterTimerHook'),
- logger=dict(type='LoggerHook', interval=10),
- param_scheduler=dict(
- type='YOLOv5ParamSchedulerHook',
- scheduler_type='cosine',
- lr_factor=lr_factor, # note
- max_epochs=max_epochs),
- checkpoint=dict(
- type='CheckpointHook',
- save_param_scheduler=False,
- interval=save_epoch_intervals,
- save_best='auto',
- max_keep_ckpts=max_keep_ckpts),
- sampler_seed=dict(type='DistSamplerSeedHook'),
- visualization=dict(type='mmdet.DetVisualizationHook'))
-
-custom_hooks = [
- dict(
- type='EMAHook',
- ema_type='ExpMomentumEMA',
- momentum=0.001,
- update_buffers=True,
- strict_load=False,
- priority=49)
-]
-
-# ============================
-
-file_client_args = dict(backend='disk')
-_file_client_args = dict(backend='disk')
-tta_model = dict(
- type='mmdet.DetTTAModel',
- tta_cfg=dict(nms=dict(type='nms', iou_threshold=0.65), max_per_img=300))
-img_scales = [
- (
- 640,
- 640,
- ),
- (
- 320,
- 320,
- ),
- (
- 960,
- 960,
- ),
-]
-_multiscale_resize_transforms = [
- dict(
- type='Compose',
- transforms=[
- dict(type='YOLOv5KeepRatioResize', scale=(
- 640,
- 640,
- )),
- dict(
- type='LetterResize',
- scale=(
- 640,
- 640,
- ),
- allow_scale_up=False,
- pad_val=dict(img=114)),
- ]),
- dict(
- type='Compose',
- transforms=[
- dict(type='YOLOv5KeepRatioResize', scale=(
- 320,
- 320,
- )),
- dict(
- type='LetterResize',
- scale=(
- 320,
- 320,
- ),
- allow_scale_up=False,
- pad_val=dict(img=114)),
- ]),
- dict(
- type='Compose',
- transforms=[
- dict(type='YOLOv5KeepRatioResize', scale=(
- 960,
- 960,
- )),
- dict(
- type='LetterResize',
- scale=(
- 960,
- 960,
- ),
- allow_scale_up=False,
- pad_val=dict(img=114)),
- ]),
-]
-tta_pipeline = [
- dict(type='LoadImageFromFile', file_client_args=dict(backend='disk')),
- dict(
- type='TestTimeAug',
- transforms=[
- [
- dict(
- type='Compose',
- transforms=[
- dict(type='YOLOv5KeepRatioResize', scale=(
- 640,
- 640,
- )),
- dict(
- type='LetterResize',
- scale=(
- 640,
- 640,
- ),
- allow_scale_up=False,
- pad_val=dict(img=114)),
- ]),
- dict(
- type='Compose',
- transforms=[
- dict(type='YOLOv5KeepRatioResize', scale=(
- 320,
- 320,
- )),
- dict(
- type='LetterResize',
- scale=(
- 320,
- 320,
- ),
- allow_scale_up=False,
- pad_val=dict(img=114)),
- ]),
- dict(
- type='Compose',
- transforms=[
- dict(type='YOLOv5KeepRatioResize', scale=(
- 960,
- 960,
- )),
- dict(
- type='LetterResize',
- scale=(
- 960,
- 960,
- ),
- allow_scale_up=False,
- pad_val=dict(img=114)),
- ]),
- ],
- [
- dict(type='mmdet.RandomFlip', prob=1.0),
- dict(type='mmdet.RandomFlip', prob=0.0),
- ],
- [
- dict(type='mmdet.LoadAnnotations', with_bbox=True),
- ],
- [
- dict(
- type='mmdet.PackDetInputs',
- meta_keys=(
- 'img_id',
- 'img_path',
- 'ori_shape',
- 'img_shape',
- 'scale_factor',
- 'pad_param',
- 'flip',
- 'flip_direction',
- )),
- ],
- ]),
-]
-
-launcher = 'none'
diff --git a/spaces/ATang0729/Forecast4Muses/Model/Model6/Model6_2_ProfileRecogition/mmpretrain/configs/resnet/resnetv1c101_8xb32_in1k.py b/spaces/ATang0729/Forecast4Muses/Model/Model6/Model6_2_ProfileRecogition/mmpretrain/configs/resnet/resnetv1c101_8xb32_in1k.py
deleted file mode 100644
index 441aff591851f402a176c142c93dc866a77b82c2..0000000000000000000000000000000000000000
--- a/spaces/ATang0729/Forecast4Muses/Model/Model6/Model6_2_ProfileRecogition/mmpretrain/configs/resnet/resnetv1c101_8xb32_in1k.py
+++ /dev/null
@@ -1,7 +0,0 @@
-_base_ = [
- '../_base_/models/resnetv1c50.py',
- '../_base_/datasets/imagenet_bs32_pil_resize.py',
- '../_base_/schedules/imagenet_bs256.py', '../_base_/default_runtime.py'
-]
-
-model = dict(backbone=dict(depth=101))
diff --git a/spaces/Ababababababbababa/Ashaar/poetry_diacritizer/util/utils.py b/spaces/Ababababababbababa/Ashaar/poetry_diacritizer/util/utils.py
deleted file mode 100644
index e9d33ca2361e48e9781cfee644dd9ddcffd6a59a..0000000000000000000000000000000000000000
--- a/spaces/Ababababababbababa/Ashaar/poetry_diacritizer/util/utils.py
+++ /dev/null
@@ -1,238 +0,0 @@
-import os
-from typing import Any
-
-import matplotlib.pyplot as plt
-import torch
-from torch import nn
-from itertools import repeat
-from poetry_diacritizer.util.decorators import ignore_exception
-from dataclasses import dataclass
-import numpy as np
-
-
-@dataclass
-class ErrorRate:
- wer: float
- der: float
- wer_without_case_ending: float
- der_without_case_ending: float
-
-
-def epoch_time(start_time, end_time):
- elapsed_time = end_time - start_time
- elapsed_mins = int(elapsed_time / 60)
- elapsed_secs = int(elapsed_time - (elapsed_mins * 60))
- return elapsed_mins, elapsed_secs
-
-
-@ignore_exception
-def plot_alignment(alignment: torch.Tensor, path: str, global_step: Any = 0):
- """
- Plot alignment and save it into a path
- Args:
- alignment (Tensor): the encoder-decoder alignment
- path (str): a path used to save the alignment plot
- global_step (int): used in the name of the output alignment plot
- """
- alignment = alignment.squeeze(1).transpose(0, 1).cpu().detach().numpy()
- fig, axs = plt.subplots()
- img = axs.imshow(alignment, aspect="auto", origin="lower", interpolation="none")
- fig.colorbar(img, ax=axs)
- xlabel = "Decoder timestep"
- plt.xlabel(xlabel)
- plt.ylabel("Encoder timestep")
- plt.tight_layout()
- plot_name = f"{global_step}.png"
- plt.savefig(os.path.join(path, plot_name), dpi=300, format="png")
- plt.close()
-
-
-def get_mask_from_lengths(memory, memory_lengths):
- """Get mask tensor from list of length
- Args:
- memory: (batch, max_time, dim)
- memory_lengths: array like
- """
- mask = memory.data.new(memory.size(0), memory.size(1)).bool().zero_()
- for idx, length in enumerate(memory_lengths):
- mask[idx][:length] = 1
- return ~mask
-
-
-def repeater(data_loader):
- for loader in repeat(data_loader):
- for data in loader:
- yield data
-
-
-def count_parameters(model):
- return sum(p.numel() for p in model.parameters() if p.requires_grad)
-
-
-def initialize_weights(m):
- if hasattr(m, "weight") and m.weight.dim() > 1:
- nn.init.xavier_uniform_(m.weight.data)
-
-
-def get_encoder_layers_attentions(model):
- attentions = []
- for layer in model.encoder.layers:
- attentions.append(layer.self_attention.attention)
- return attentions
-
-
-def get_decoder_layers_attentions(model):
- self_attns, src_attens = [], []
- for layer in model.decoder.layers:
- self_attns.append(layer.self_attention.attention)
- src_attens.append(layer.encoder_attention.attention)
- return self_attns, src_attens
-
-
-def display_attention(
- attention, path, global_step: int, name="att", n_heads=4, n_rows=2, n_cols=2
-):
- assert n_rows * n_cols == n_heads
-
- fig = plt.figure(figsize=(15, 15))
-
- for i in range(n_heads):
-
- ax = fig.add_subplot(n_rows, n_cols, i + 1)
-
- _attention = attention.squeeze(0)[i].transpose(0, 1).cpu().detach().numpy()
- cax = ax.imshow(_attention, aspect="auto", origin="lower", interpolation="none")
-
- plot_name = f"{global_step}-{name}.png"
- plt.savefig(os.path.join(path, plot_name), dpi=300, format="png")
- plt.close()
-
-
-def plot_multi_head(model, path, global_step):
- encoder_attentions = get_encoder_layers_attentions(model)
- decoder_attentions, attentions = get_decoder_layers_attentions(model)
- for i in range(len(attentions)):
- display_attention(
- attentions[0][0], path, global_step, f"encoder-decoder-layer{i + 1}"
- )
- for i in range(len(decoder_attentions)):
- display_attention(
- decoder_attentions[0][0], path, global_step, f"decoder-layer{i + 1}"
- )
- for i in range(len(encoder_attentions)):
- display_attention(
- encoder_attentions[0][0], path, global_step, f"encoder-layer {i + 1}"
- )
-
-
-def make_src_mask(src, pad_idx=0):
-
- # src = [batch size, src len]
-
- src_mask = (src != pad_idx).unsqueeze(1).unsqueeze(2)
-
- # src_mask = [batch size, 1, 1, src len]
-
- return src_mask
-
-
-def get_angles(pos, i, model_dim):
- angle_rates = 1 / np.power(10000, (2 * (i // 2)) / np.float32(model_dim))
- return pos * angle_rates
-
-
-def positional_encoding(position, model_dim):
- angle_rads = get_angles(
- np.arange(position)[:, np.newaxis],
- np.arange(model_dim)[np.newaxis, :],
- model_dim,
- )
-
- # apply sin to even indices in the array; 2i
- angle_rads[:, 0::2] = np.sin(angle_rads[:, 0::2])
-
- # apply cos to odd indices in the array; 2i+1
- angle_rads[:, 1::2] = np.cos(angle_rads[:, 1::2])
-
- pos_encoding = angle_rads[np.newaxis, ...]
-
- return torch.from_numpy(pos_encoding)
-
-
-def calculate_error_rates(original_file_path: str, target_file_path: str) -> ErrorRate:
- """
- Calculates ErrorRates from paths
- """
- assert os.path.isfile(original_file_path)
- assert os.path.isfile(target_file_path)
-
- _wer = wer.calculate_wer_from_path(
- inp_path=original_file_path, out_path=target_file_path, case_ending=True
- )
-
- _wer_without_case_ending = wer.calculate_wer_from_path(
- inp_path=original_file_path, out_path=target_file_path, case_ending=False
- )
-
- _der = der.calculate_der_from_path(
- inp_path=original_file_path, out_path=target_file_path, case_ending=True
- )
-
- _der_without_case_ending = der.calculate_der_from_path(
- inp_path=original_file_path, out_path=target_file_path, case_ending=False
- )
-
- error_rates = ErrorRate(
- _wer,
- _der,
- _wer_without_case_ending,
- _der_without_case_ending,
- )
-
- return error_rates
-
-
-def categorical_accuracy(preds, y, tag_pad_idx, device="cuda"):
- """
- Returns accuracy per batch, i.e. if you get 8/10 right, this returns 0.8, NOT 8
- """
- max_preds = preds.argmax(
- dim=1, keepdim=True
- ) # get the index of the max probability
- non_pad_elements = torch.nonzero((y != tag_pad_idx))
- correct = max_preds[non_pad_elements].squeeze(1).eq(y[non_pad_elements])
- return correct.sum() / torch.FloatTensor([y[non_pad_elements].shape[0]]).to(device)
-
-
-def write_to_files(input_path, output_path, input_list, output_list):
- with open(input_path, "w", encoding="utf8") as file:
- for inp in input_list:
- file.write(inp + "\n")
- with open(output_path, "w", encoding="utf8") as file:
- for out in output_list:
- file.write(out + "\n")
-
-
-def make_src_mask(src: torch.Tensor, pad_idx=0):
- return (src != pad_idx).unsqueeze(1).unsqueeze(2)
-
-
-def make_trg_mask(trg, trg_pad_idx=0):
-
- # trg = [batch size, trg len]
-
- trg_pad_mask = (trg != trg_pad_idx).unsqueeze(1).unsqueeze(2)
-
- # trg_pad_mask = [batch size, 1, 1, trg len]
-
- trg_len = trg.shape[1]
-
- trg_sub_mask = torch.tril(torch.ones((trg_len, trg_len))).bool()
-
- # trg_sub_mask = [trg len, trg len]
-
- trg_mask = trg_pad_mask & trg_sub_mask
-
- # trg_mask = [batch size, 1, trg len, trg len]
-
- return trg_mask
diff --git a/spaces/AchyuthGamer/OpenGPT/g4f/Provider/Providers/__init__.py b/spaces/AchyuthGamer/OpenGPT/g4f/Provider/Providers/__init__.py
deleted file mode 100644
index 5b0ec33b0fc47c3dc2ef8ad9839f997c4f6bc70b..0000000000000000000000000000000000000000
--- a/spaces/AchyuthGamer/OpenGPT/g4f/Provider/Providers/__init__.py
+++ /dev/null
@@ -1,100 +0,0 @@
-from __future__ import annotations
-from .Acytoo import Acytoo
-from .AiAsk import AiAsk
-from .Aibn import Aibn
-from .Aichat import Aichat
-from .Ails import Ails
-from .Aivvm import Aivvm
-from .AItianhu import AItianhu
-from .AItianhuSpace import AItianhuSpace
-from .Bing import Bing
-from .ChatBase import ChatBase
-from .ChatForAi import ChatForAi
-from .Chatgpt4Online import Chatgpt4Online
-from .ChatgptAi import ChatgptAi
-from .ChatgptDemo import ChatgptDemo
-from .ChatgptDuo import ChatgptDuo
-from .ChatgptX import ChatgptX
-from .Cromicle import Cromicle
-from .DeepAi import DeepAi
-from .FreeGpt import FreeGpt
-from .GPTalk import GPTalk
-from .GptForLove import GptForLove
-from .GptGo import GptGo
-from .GptGod import GptGod
-from .H2o import H2o
-from .Liaobots import Liaobots
-from .Myshell import Myshell
-from .Phind import Phind
-from .Vercel import Vercel
-from .Vitalentum import Vitalentum
-from .Ylokh import Ylokh
-from .You import You
-from .Yqcloud import Yqcloud
-
-from .base_provider import BaseProvider, AsyncProvider, AsyncGeneratorProvider
-from .retry_provider import RetryProvider
-from .deprecated import *
-from .needs_auth import *
-from .unfinished import *
-
-__all__ = [
- 'BaseProvider',
- 'AsyncProvider',
- 'AsyncGeneratorProvider',
- 'RetryProvider',
- 'Acytoo',
- 'AiAsk',
- 'Aibn',
- 'Aichat',
- 'Ails',
- 'Aivvm',
- 'AiService',
- 'AItianhu',
- 'AItianhuSpace',
- 'Aivvm',
- 'Bard',
- 'Bing',
- 'ChatBase',
- 'ChatForAi',
- 'Chatgpt4Online',
- 'ChatgptAi',
- 'ChatgptDemo',
- 'ChatgptDuo',
- 'ChatgptLogin',
- 'ChatgptX',
- 'Cromicle',
- 'CodeLinkAva',
- 'DeepAi',
- 'DfeHub',
- 'EasyChat',
- 'Forefront',
- 'FreeGpt',
- 'GPTalk',
- 'GptForLove',
- 'GetGpt',
- 'GptGo',
- 'GptGod',
- 'H2o',
- 'HuggingChat',
- 'Liaobots',
- 'Lockchat',
- 'Myshell',
- 'Opchatgpts',
- 'Raycast',
- 'OpenaiChat',
- 'OpenAssistant',
- 'PerplexityAi',
- 'Phind',
- 'Theb',
- 'Vercel',
- 'Vitalentum',
- 'Wewordle',
- 'Ylokh',
- 'You',
- 'Yqcloud',
- 'Equing',
- 'FastGpt',
- 'Wuguokai',
- 'V50'
-]
\ No newline at end of file
diff --git a/spaces/Amrrs/DragGan-Inversion/stylegan_human/torch_utils/op_edit/fused_bias_act.cpp b/spaces/Amrrs/DragGan-Inversion/stylegan_human/torch_utils/op_edit/fused_bias_act.cpp
deleted file mode 100644
index a79a3d65b8fb56393c954630ae8ce5a5c8a8bb7d..0000000000000000000000000000000000000000
--- a/spaces/Amrrs/DragGan-Inversion/stylegan_human/torch_utils/op_edit/fused_bias_act.cpp
+++ /dev/null
@@ -1,23 +0,0 @@
-// Copyright (c) SenseTime Research. All rights reserved.
-
-#include
-
-
-torch::Tensor fused_bias_act_op(const torch::Tensor& input, const torch::Tensor& bias, const torch::Tensor& refer,
- int act, int grad, float alpha, float scale);
-
-#define CHECK_CUDA(x) TORCH_CHECK(x.type().is_cuda(), #x " must be a CUDA tensor")
-#define CHECK_CONTIGUOUS(x) TORCH_CHECK(x.is_contiguous(), #x " must be contiguous")
-#define CHECK_INPUT(x) CHECK_CUDA(x); CHECK_CONTIGUOUS(x)
-
-torch::Tensor fused_bias_act(const torch::Tensor& input, const torch::Tensor& bias, const torch::Tensor& refer,
- int act, int grad, float alpha, float scale) {
- CHECK_CUDA(input);
- CHECK_CUDA(bias);
-
- return fused_bias_act_op(input, bias, refer, act, grad, alpha, scale);
-}
-
-PYBIND11_MODULE(TORCH_EXTENSION_NAME, m) {
- m.def("fused_bias_act", &fused_bias_act, "fused bias act (CUDA)");
-}
\ No newline at end of file
diff --git a/spaces/Anar0140/4.RealTime-MediaPipe-AI-From-Video-On-Any-Device/app.py b/spaces/Anar0140/4.RealTime-MediaPipe-AI-From-Video-On-Any-Device/app.py
deleted file mode 100644
index 5a8522c4a42926ac6f90d040ab01b342a1f8e7ad..0000000000000000000000000000000000000000
--- a/spaces/Anar0140/4.RealTime-MediaPipe-AI-From-Video-On-Any-Device/app.py
+++ /dev/null
@@ -1,59 +0,0 @@
-import streamlit as st
-st.markdown("""
-
-# MediaPipe
-
-### A cross language SDK for AI that is real time, 3d, camera responsive, and on any device for nearly any language
-
-#### Vision
-#### Natural Language
-#### Audio
-
-Mediapipe has fast and flexible AI/ML pipelines.
-
-Examples with Javascript Links!
-
-1. Image Classifier: https://mediapipe-studio.webapps.google.com/demo/image_classifier
-2. Object Detector: https://mediapipe-studio.webapps.google.com/demo/object_detector
-3. Text Classification: https://mediapipe-studio.webapps.google.com/demo/text_classifier
-4. Gesture Recognizer: https://mediapipe-studio.webapps.google.com/demo/gesture_recognizer
-5. Hand Landmark Detection: https://mediapipe-studio.webapps.google.com/demo/hand_landmarker
-6. Audio Classifier: https://mediapipe-studio.webapps.google.com/demo/audio_classifier
-
-Get started with just Javascript!!
-
-Getting Started: https://google.github.io/mediapipe/getting_started/javascript.html
-
-Javascript Solutions - Ready to Demo:
-1. Face Mesh: https://codepen.io/mediapipe/full/KKgVaPJ
-2. Face Detection: https://codepen.io/mediapipe/full/dyOzvZM
-3. Hands: https://codepen.io/mediapipe/full/RwGWYJw
-4. Face, Hands, Body: https://codepen.io/mediapipe/full/LYRRYEw
-5. Objectron: https://codepen.io/mediapipe/full/BaWvzdY
-6. Full Skeletal Pose: https://codepen.io/mediapipe/full/jOMbvxw
-7. Self Segmentation From Background: https://codepen.io/mediapipe/full/wvJyQpq
-
-
-Demonstration in Action with Screenshots:
-
-Self Segmentation From Background:
-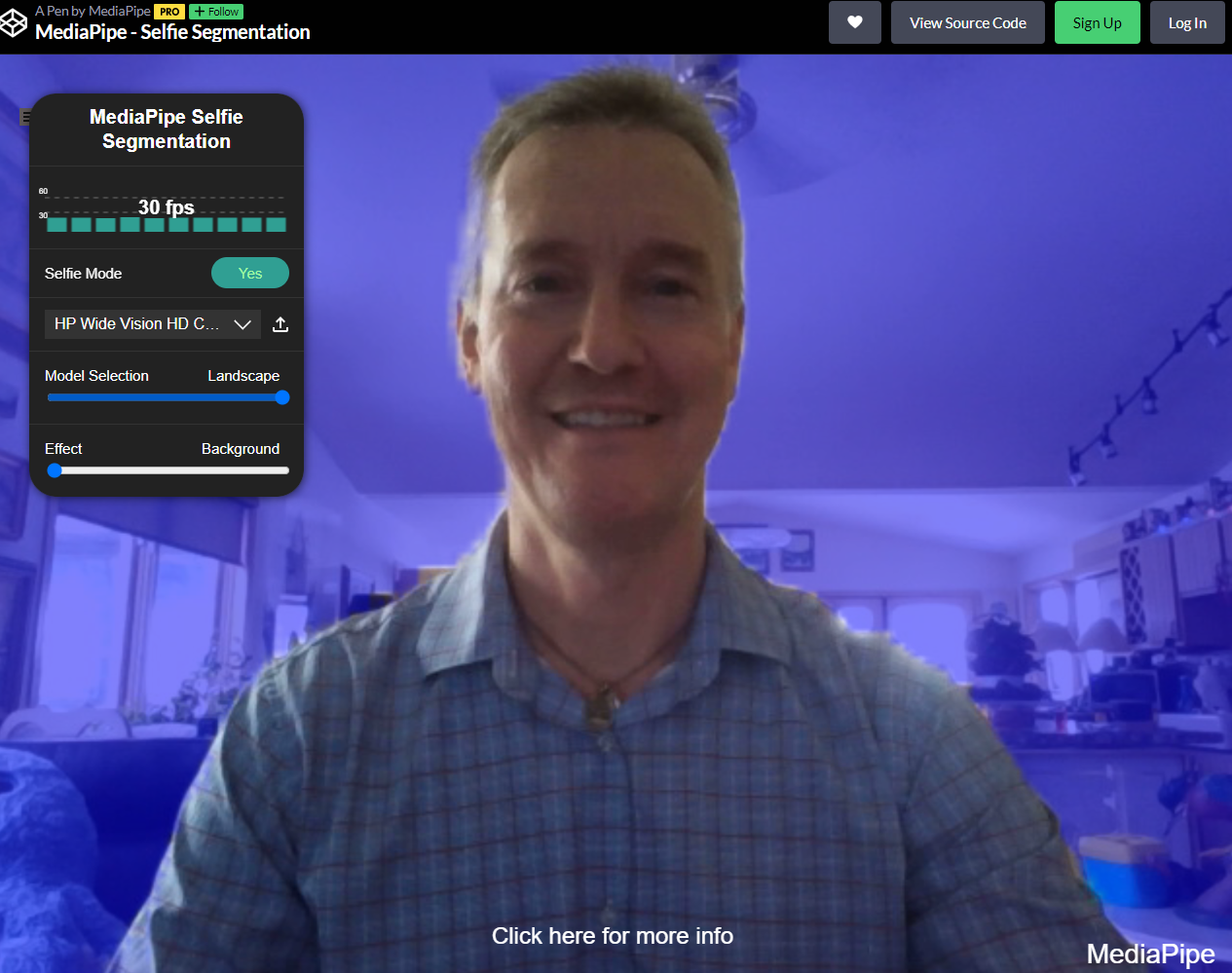
-
-Full Skeletal Pose:
-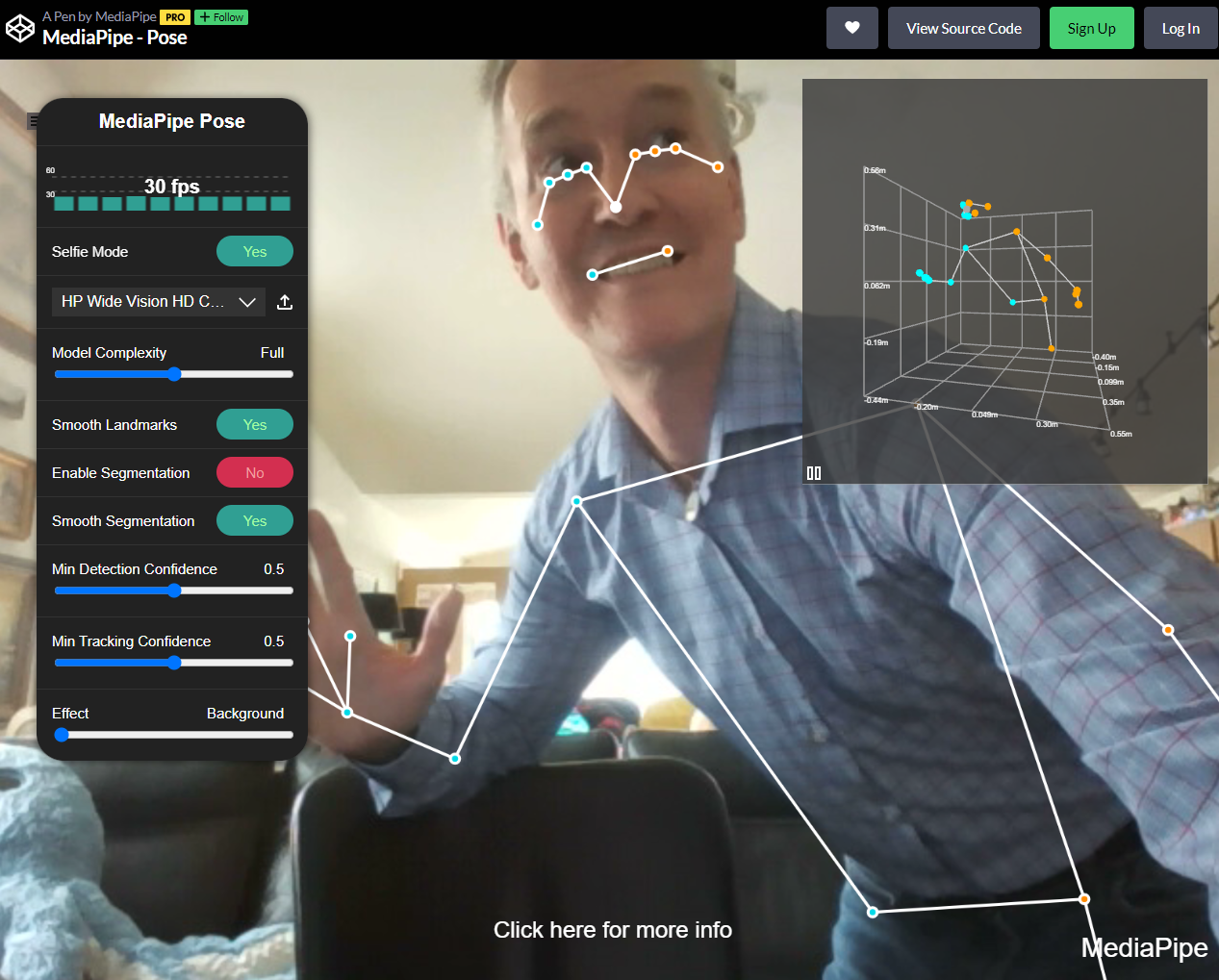
-
-Hands - Both in 3D Projection even hidden surface vertices - Mahalo:
-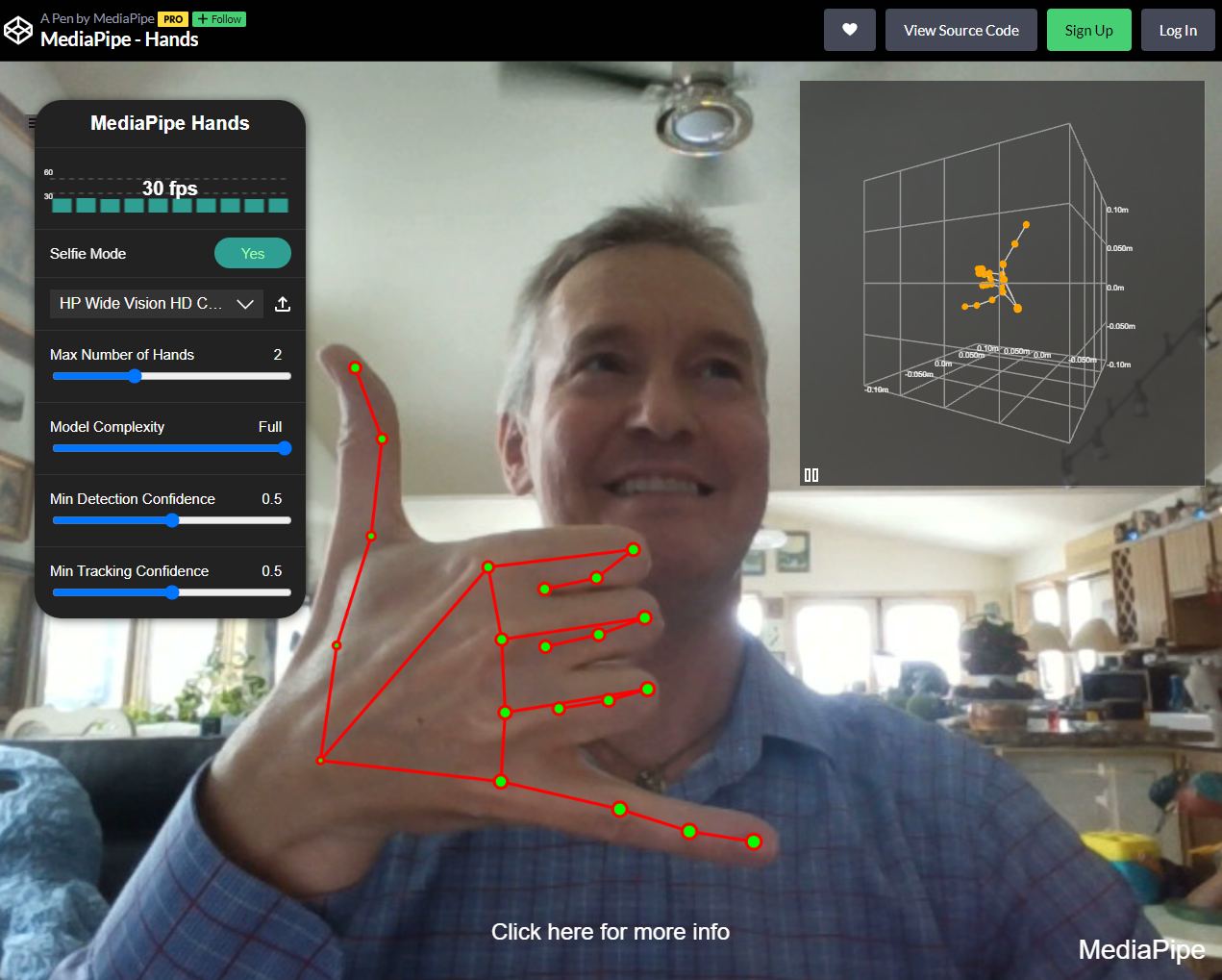
-
-Holistic - Face, Hands, Body:
-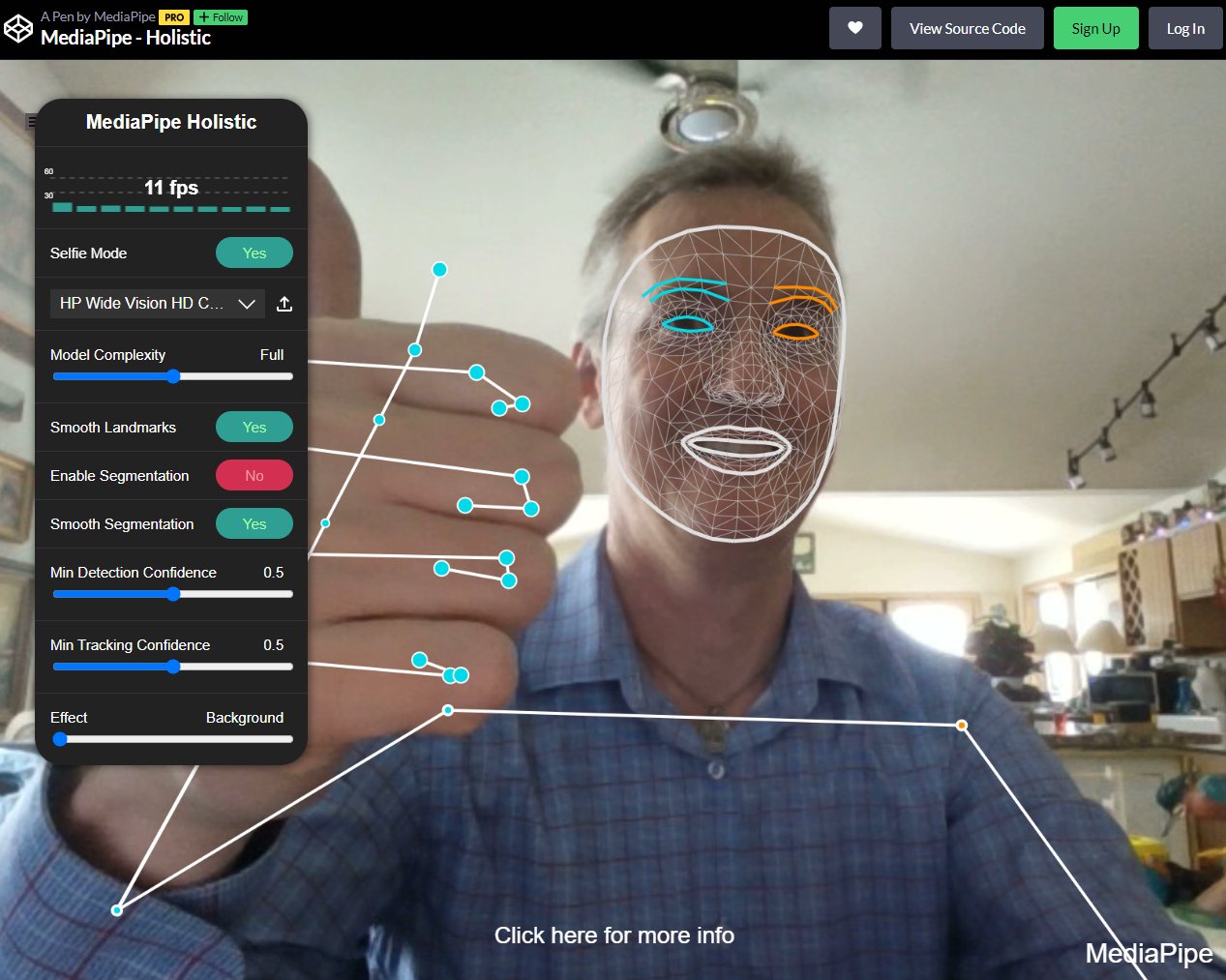
-
-Face Detection:
-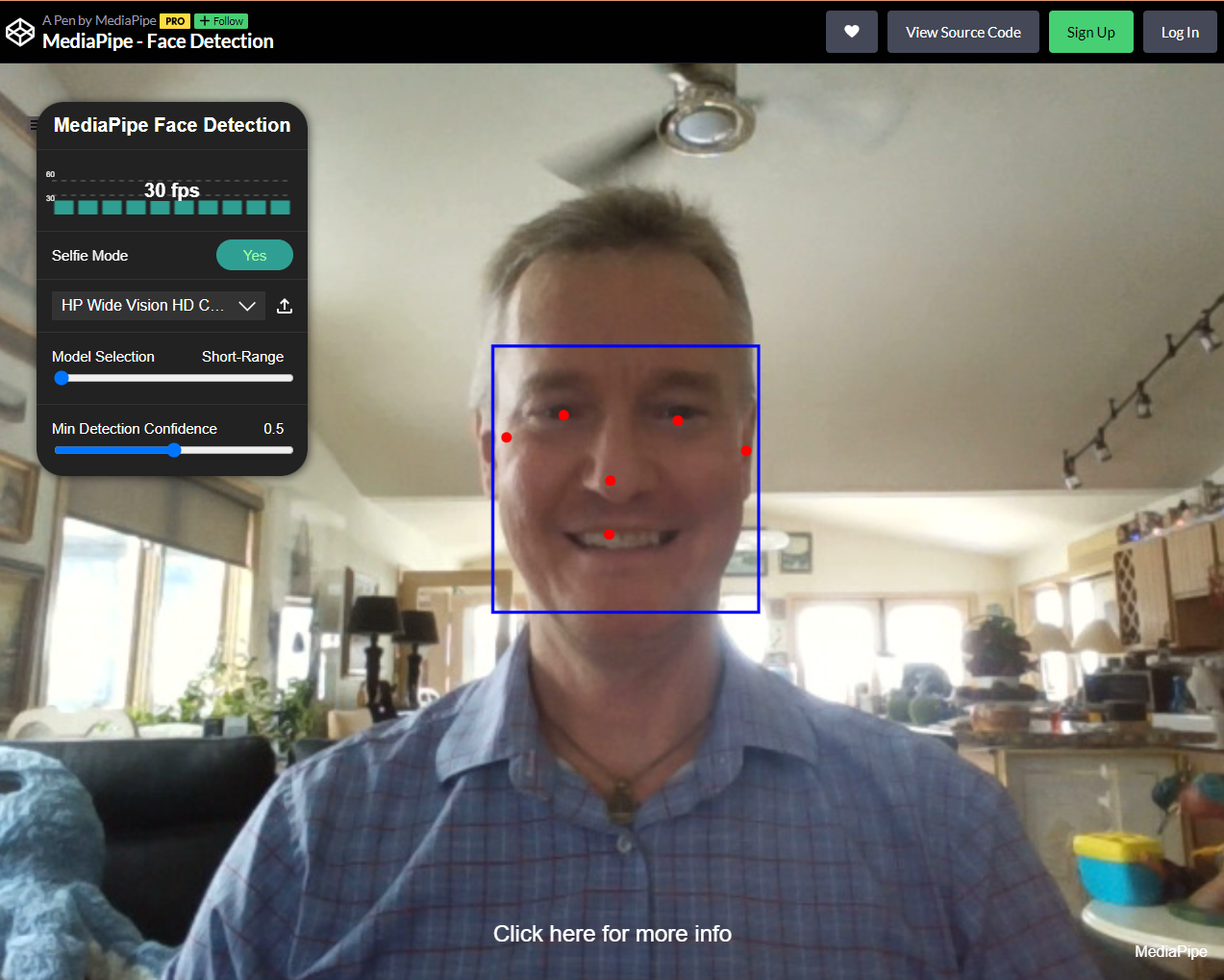
-
-Face Mesh Real Time - 30 Frames per second!
-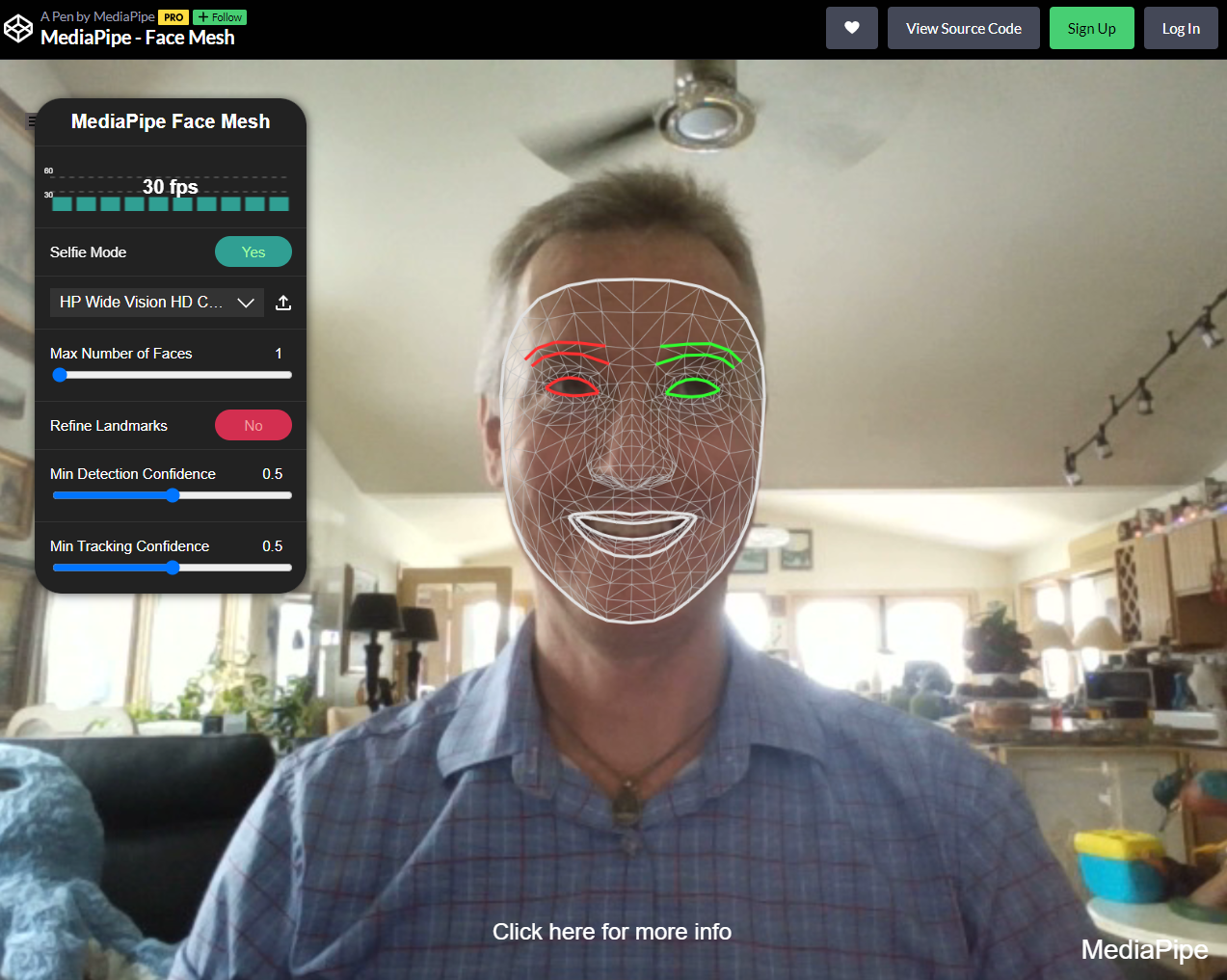
-
-
-
-""")
\ No newline at end of file
diff --git a/spaces/Androidonnxfork/CivitAi-to-Diffusers/diffusers/src/diffusers/pipelines/stable_diffusion/pipeline_stable_diffusion_instruct_pix2pix.py b/spaces/Androidonnxfork/CivitAi-to-Diffusers/diffusers/src/diffusers/pipelines/stable_diffusion/pipeline_stable_diffusion_instruct_pix2pix.py
deleted file mode 100644
index d27f8a21f3698a2807f95b9aaef1426b5eab733b..0000000000000000000000000000000000000000
--- a/spaces/Androidonnxfork/CivitAi-to-Diffusers/diffusers/src/diffusers/pipelines/stable_diffusion/pipeline_stable_diffusion_instruct_pix2pix.py
+++ /dev/null
@@ -1,748 +0,0 @@
-# Copyright 2023 The InstructPix2Pix Authors and The HuggingFace Team. All rights reserved.
-#
-# Licensed under the Apache License, Version 2.0 (the "License");
-# you may not use this file except in compliance with the License.
-# You may obtain a copy of the License at
-#
-# http://www.apache.org/licenses/LICENSE-2.0
-#
-# Unless required by applicable law or agreed to in writing, software
-# distributed under the License is distributed on an "AS IS" BASIS,
-# WITHOUT WARRANTIES OR CONDITIONS OF ANY KIND, either express or implied.
-# See the License for the specific language governing permissions and
-# limitations under the License.
-
-import inspect
-import warnings
-from typing import Callable, List, Optional, Union
-
-import numpy as np
-import PIL
-import torch
-from transformers import CLIPImageProcessor, CLIPTextModel, CLIPTokenizer
-
-from ...image_processor import VaeImageProcessor
-from ...loaders import LoraLoaderMixin, TextualInversionLoaderMixin
-from ...models import AutoencoderKL, UNet2DConditionModel
-from ...schedulers import KarrasDiffusionSchedulers
-from ...utils import (
- PIL_INTERPOLATION,
- deprecate,
- is_accelerate_available,
- is_accelerate_version,
- logging,
- randn_tensor,
-)
-from ..pipeline_utils import DiffusionPipeline
-from . import StableDiffusionPipelineOutput
-from .safety_checker import StableDiffusionSafetyChecker
-
-
-logger = logging.get_logger(__name__) # pylint: disable=invalid-name
-
-
-# Copied from diffusers.pipelines.stable_diffusion.pipeline_stable_diffusion_img2img.preprocess
-def preprocess(image):
- warnings.warn(
- "The preprocess method is deprecated and will be removed in a future version. Please"
- " use VaeImageProcessor.preprocess instead",
- FutureWarning,
- )
- if isinstance(image, torch.Tensor):
- return image
- elif isinstance(image, PIL.Image.Image):
- image = [image]
-
- if isinstance(image[0], PIL.Image.Image):
- w, h = image[0].size
- w, h = (x - x % 8 for x in (w, h)) # resize to integer multiple of 8
-
- image = [np.array(i.resize((w, h), resample=PIL_INTERPOLATION["lanczos"]))[None, :] for i in image]
- image = np.concatenate(image, axis=0)
- image = np.array(image).astype(np.float32) / 255.0
- image = image.transpose(0, 3, 1, 2)
- image = 2.0 * image - 1.0
- image = torch.from_numpy(image)
- elif isinstance(image[0], torch.Tensor):
- image = torch.cat(image, dim=0)
- return image
-
-
-class StableDiffusionInstructPix2PixPipeline(DiffusionPipeline, TextualInversionLoaderMixin, LoraLoaderMixin):
- r"""
- Pipeline for pixel-level image editing by following text instructions (based on Stable Diffusion).
-
- This model inherits from [`DiffusionPipeline`]. Check the superclass documentation for the generic methods
- implemented for all pipelines (downloading, saving, running on a particular device, etc.).
-
- The pipeline also inherits the following loading methods:
- - [`~loaders.TextualInversionLoaderMixin.load_textual_inversion`] for loading textual inversion embeddings
- - [`~loaders.LoraLoaderMixin.load_lora_weights`] for loading LoRA weights
- - [`~loaders.LoraLoaderMixin.save_lora_weights`] for saving LoRA weights
-
- Args:
- vae ([`AutoencoderKL`]):
- Variational Auto-Encoder (VAE) model to encode and decode images to and from latent representations.
- text_encoder ([`~transformers.CLIPTextModel`]):
- Frozen text-encoder ([clip-vit-large-patch14](https://huggingface.co/openai/clip-vit-large-patch14)).
- tokenizer ([`~transformers.CLIPTokenizer`]):
- A `CLIPTokenizer` to tokenize text.
- unet ([`UNet2DConditionModel`]):
- A `UNet2DConditionModel` to denoise the encoded image latents.
- scheduler ([`SchedulerMixin`]):
- A scheduler to be used in combination with `unet` to denoise the encoded image latents. Can be one of
- [`DDIMScheduler`], [`LMSDiscreteScheduler`], or [`PNDMScheduler`].
- safety_checker ([`StableDiffusionSafetyChecker`]):
- Classification module that estimates whether generated images could be considered offensive or harmful.
- Please refer to the [model card](https://huggingface.co/runwayml/stable-diffusion-v1-5) for more details
- about a model's potential harms.
- feature_extractor ([`~transformers.CLIPImageProcessor`]):
- A `CLIPImageProcessor` to extract features from generated images; used as inputs to the `safety_checker`.
- """
- _optional_components = ["safety_checker", "feature_extractor"]
-
- def __init__(
- self,
- vae: AutoencoderKL,
- text_encoder: CLIPTextModel,
- tokenizer: CLIPTokenizer,
- unet: UNet2DConditionModel,
- scheduler: KarrasDiffusionSchedulers,
- safety_checker: StableDiffusionSafetyChecker,
- feature_extractor: CLIPImageProcessor,
- requires_safety_checker: bool = True,
- ):
- super().__init__()
-
- if safety_checker is None and requires_safety_checker:
- logger.warning(
- f"You have disabled the safety checker for {self.__class__} by passing `safety_checker=None`. Ensure"
- " that you abide to the conditions of the Stable Diffusion license and do not expose unfiltered"
- " results in services or applications open to the public. Both the diffusers team and Hugging Face"
- " strongly recommend to keep the safety filter enabled in all public facing circumstances, disabling"
- " it only for use-cases that involve analyzing network behavior or auditing its results. For more"
- " information, please have a look at https://github.com/huggingface/diffusers/pull/254 ."
- )
-
- if safety_checker is not None and feature_extractor is None:
- raise ValueError(
- "Make sure to define a feature extractor when loading {self.__class__} if you want to use the safety"
- " checker. If you do not want to use the safety checker, you can pass `'safety_checker=None'` instead."
- )
-
- self.register_modules(
- vae=vae,
- text_encoder=text_encoder,
- tokenizer=tokenizer,
- unet=unet,
- scheduler=scheduler,
- safety_checker=safety_checker,
- feature_extractor=feature_extractor,
- )
- self.vae_scale_factor = 2 ** (len(self.vae.config.block_out_channels) - 1)
- self.image_processor = VaeImageProcessor(vae_scale_factor=self.vae_scale_factor)
- self.register_to_config(requires_safety_checker=requires_safety_checker)
-
- @torch.no_grad()
- def __call__(
- self,
- prompt: Union[str, List[str]] = None,
- image: Union[
- torch.FloatTensor,
- PIL.Image.Image,
- np.ndarray,
- List[torch.FloatTensor],
- List[PIL.Image.Image],
- List[np.ndarray],
- ] = None,
- num_inference_steps: int = 100,
- guidance_scale: float = 7.5,
- image_guidance_scale: float = 1.5,
- negative_prompt: Optional[Union[str, List[str]]] = None,
- num_images_per_prompt: Optional[int] = 1,
- eta: float = 0.0,
- generator: Optional[Union[torch.Generator, List[torch.Generator]]] = None,
- latents: Optional[torch.FloatTensor] = None,
- prompt_embeds: Optional[torch.FloatTensor] = None,
- negative_prompt_embeds: Optional[torch.FloatTensor] = None,
- output_type: Optional[str] = "pil",
- return_dict: bool = True,
- callback: Optional[Callable[[int, int, torch.FloatTensor], None]] = None,
- callback_steps: int = 1,
- ):
- r"""
- The call function to the pipeline for generation.
-
- Args:
- prompt (`str` or `List[str]`, *optional*):
- The prompt or prompts to guide image generation. If not defined, you need to pass `prompt_embeds`.
- image (`torch.FloatTensor` `np.ndarray`, `PIL.Image.Image`, `List[torch.FloatTensor]`, `List[PIL.Image.Image]`, or `List[np.ndarray]`):
- `Image` or tensor representing an image batch to be repainted according to `prompt`. Can also accept
- image latents as `image`, but if passing latents directly it is not encoded again.
- num_inference_steps (`int`, *optional*, defaults to 100):
- The number of denoising steps. More denoising steps usually lead to a higher quality image at the
- expense of slower inference.
- guidance_scale (`float`, *optional*, defaults to 7.5):
- A higher guidance scale value encourages the model to generate images closely linked to the text
- `prompt` at the expense of lower image quality. Guidance scale is enabled when `guidance_scale > 1`.
- image_guidance_scale (`float`, *optional*, defaults to 1.5):
- Push the generated image towards the inital `image`. Image guidance scale is enabled by setting
- `image_guidance_scale > 1`. Higher image guidance scale encourages generated images that are closely
- linked to the source `image`, usually at the expense of lower image quality. This pipeline requires a
- value of at least `1`.
- negative_prompt (`str` or `List[str]`, *optional*):
- The prompt or prompts to guide what to not include in image generation. If not defined, you need to
- pass `negative_prompt_embeds` instead. Ignored when not using guidance (`guidance_scale < 1`).
- num_images_per_prompt (`int`, *optional*, defaults to 1):
- The number of images to generate per prompt.
- eta (`float`, *optional*, defaults to 0.0):
- Corresponds to parameter eta (η) from the [DDIM](https://arxiv.org/abs/2010.02502) paper. Only applies
- to the [`~schedulers.DDIMScheduler`], and is ignored in other schedulers.
- generator (`torch.Generator`, *optional*):
- A [`torch.Generator`](https://pytorch.org/docs/stable/generated/torch.Generator.html) to make
- generation deterministic.
- latents (`torch.FloatTensor`, *optional*):
- Pre-generated noisy latents sampled from a Gaussian distribution, to be used as inputs for image
- generation. Can be used to tweak the same generation with different prompts. If not provided, a latents
- tensor is generated by sampling using the supplied random `generator`.
- prompt_embeds (`torch.FloatTensor`, *optional*):
- Pre-generated text embeddings. Can be used to easily tweak text inputs (prompt weighting). If not
- provided, text embeddings are generated from the `prompt` input argument.
- negative_prompt_embeds (`torch.FloatTensor`, *optional*):
- Pre-generated negative text embeddings. Can be used to easily tweak text inputs (prompt weighting). If
- not provided, `negative_prompt_embeds` are generated from the `negative_prompt` input argument.
- output_type (`str`, *optional*, defaults to `"pil"`):
- The output format of the generated image. Choose between `PIL.Image` or `np.array`.
- return_dict (`bool`, *optional*, defaults to `True`):
- Whether or not to return a [`~pipelines.stable_diffusion.StableDiffusionPipelineOutput`] instead of a
- plain tuple.
- callback (`Callable`, *optional*):
- A function that calls every `callback_steps` steps during inference. The function is called with the
- following arguments: `callback(step: int, timestep: int, latents: torch.FloatTensor)`.
- callback_steps (`int`, *optional*, defaults to 1):
- The frequency at which the `callback` function is called. If not specified, the callback is called at
- every step.
-
- Examples:
-
- ```py
- >>> import PIL
- >>> import requests
- >>> import torch
- >>> from io import BytesIO
-
- >>> from diffusers import StableDiffusionInstructPix2PixPipeline
-
-
- >>> def download_image(url):
- ... response = requests.get(url)
- ... return PIL.Image.open(BytesIO(response.content)).convert("RGB")
-
-
- >>> img_url = "https://huggingface.co/datasets/diffusers/diffusers-images-docs/resolve/main/mountain.png"
-
- >>> image = download_image(img_url).resize((512, 512))
-
- >>> pipe = StableDiffusionInstructPix2PixPipeline.from_pretrained(
- ... "timbrooks/instruct-pix2pix", torch_dtype=torch.float16
- ... )
- >>> pipe = pipe.to("cuda")
-
- >>> prompt = "make the mountains snowy"
- >>> image = pipe(prompt=prompt, image=image).images[0]
- ```
-
- Returns:
- [`~pipelines.stable_diffusion.StableDiffusionPipelineOutput`] or `tuple`:
- If `return_dict` is `True`, [`~pipelines.stable_diffusion.StableDiffusionPipelineOutput`] is returned,
- otherwise a `tuple` is returned where the first element is a list with the generated images and the
- second element is a list of `bool`s indicating whether the corresponding generated image contains
- "not-safe-for-work" (nsfw) content.
- """
- # 0. Check inputs
- self.check_inputs(prompt, callback_steps, negative_prompt, prompt_embeds, negative_prompt_embeds)
-
- if image is None:
- raise ValueError("`image` input cannot be undefined.")
-
- # 1. Define call parameters
- if prompt is not None and isinstance(prompt, str):
- batch_size = 1
- elif prompt is not None and isinstance(prompt, list):
- batch_size = len(prompt)
- else:
- batch_size = prompt_embeds.shape[0]
-
- device = self._execution_device
- # here `guidance_scale` is defined analog to the guidance weight `w` of equation (2)
- # of the Imagen paper: https://arxiv.org/pdf/2205.11487.pdf . `guidance_scale = 1`
- # corresponds to doing no classifier free guidance.
- do_classifier_free_guidance = guidance_scale > 1.0 and image_guidance_scale >= 1.0
- # check if scheduler is in sigmas space
- scheduler_is_in_sigma_space = hasattr(self.scheduler, "sigmas")
-
- # 2. Encode input prompt
- prompt_embeds = self._encode_prompt(
- prompt,
- device,
- num_images_per_prompt,
- do_classifier_free_guidance,
- negative_prompt,
- prompt_embeds=prompt_embeds,
- negative_prompt_embeds=negative_prompt_embeds,
- )
-
- # 3. Preprocess image
- image = self.image_processor.preprocess(image)
-
- # 4. set timesteps
- self.scheduler.set_timesteps(num_inference_steps, device=device)
- timesteps = self.scheduler.timesteps
-
- # 5. Prepare Image latents
- image_latents = self.prepare_image_latents(
- image,
- batch_size,
- num_images_per_prompt,
- prompt_embeds.dtype,
- device,
- do_classifier_free_guidance,
- generator,
- )
-
- height, width = image_latents.shape[-2:]
- height = height * self.vae_scale_factor
- width = width * self.vae_scale_factor
-
- # 6. Prepare latent variables
- num_channels_latents = self.vae.config.latent_channels
- latents = self.prepare_latents(
- batch_size * num_images_per_prompt,
- num_channels_latents,
- height,
- width,
- prompt_embeds.dtype,
- device,
- generator,
- latents,
- )
-
- # 7. Check that shapes of latents and image match the UNet channels
- num_channels_image = image_latents.shape[1]
- if num_channels_latents + num_channels_image != self.unet.config.in_channels:
- raise ValueError(
- f"Incorrect configuration settings! The config of `pipeline.unet`: {self.unet.config} expects"
- f" {self.unet.config.in_channels} but received `num_channels_latents`: {num_channels_latents} +"
- f" `num_channels_image`: {num_channels_image} "
- f" = {num_channels_latents+num_channels_image}. Please verify the config of"
- " `pipeline.unet` or your `image` input."
- )
-
- # 8. Prepare extra step kwargs. TODO: Logic should ideally just be moved out of the pipeline
- extra_step_kwargs = self.prepare_extra_step_kwargs(generator, eta)
-
- # 9. Denoising loop
- num_warmup_steps = len(timesteps) - num_inference_steps * self.scheduler.order
- with self.progress_bar(total=num_inference_steps) as progress_bar:
- for i, t in enumerate(timesteps):
- # Expand the latents if we are doing classifier free guidance.
- # The latents are expanded 3 times because for pix2pix the guidance\
- # is applied for both the text and the input image.
- latent_model_input = torch.cat([latents] * 3) if do_classifier_free_guidance else latents
-
- # concat latents, image_latents in the channel dimension
- scaled_latent_model_input = self.scheduler.scale_model_input(latent_model_input, t)
- scaled_latent_model_input = torch.cat([scaled_latent_model_input, image_latents], dim=1)
-
- # predict the noise residual
- noise_pred = self.unet(
- scaled_latent_model_input, t, encoder_hidden_states=prompt_embeds, return_dict=False
- )[0]
-
- # Hack:
- # For karras style schedulers the model does classifer free guidance using the
- # predicted_original_sample instead of the noise_pred. So we need to compute the
- # predicted_original_sample here if we are using a karras style scheduler.
- if scheduler_is_in_sigma_space:
- step_index = (self.scheduler.timesteps == t).nonzero()[0].item()
- sigma = self.scheduler.sigmas[step_index]
- noise_pred = latent_model_input - sigma * noise_pred
-
- # perform guidance
- if do_classifier_free_guidance:
- noise_pred_text, noise_pred_image, noise_pred_uncond = noise_pred.chunk(3)
- noise_pred = (
- noise_pred_uncond
- + guidance_scale * (noise_pred_text - noise_pred_image)
- + image_guidance_scale * (noise_pred_image - noise_pred_uncond)
- )
-
- # Hack:
- # For karras style schedulers the model does classifer free guidance using the
- # predicted_original_sample instead of the noise_pred. But the scheduler.step function
- # expects the noise_pred and computes the predicted_original_sample internally. So we
- # need to overwrite the noise_pred here such that the value of the computed
- # predicted_original_sample is correct.
- if scheduler_is_in_sigma_space:
- noise_pred = (noise_pred - latents) / (-sigma)
-
- # compute the previous noisy sample x_t -> x_t-1
- latents = self.scheduler.step(noise_pred, t, latents, **extra_step_kwargs, return_dict=False)[0]
-
- # call the callback, if provided
- if i == len(timesteps) - 1 or ((i + 1) > num_warmup_steps and (i + 1) % self.scheduler.order == 0):
- progress_bar.update()
- if callback is not None and i % callback_steps == 0:
- callback(i, t, latents)
-
- if not output_type == "latent":
- image = self.vae.decode(latents / self.vae.config.scaling_factor, return_dict=False)[0]
- image, has_nsfw_concept = self.run_safety_checker(image, device, prompt_embeds.dtype)
- else:
- image = latents
- has_nsfw_concept = None
-
- if has_nsfw_concept is None:
- do_denormalize = [True] * image.shape[0]
- else:
- do_denormalize = [not has_nsfw for has_nsfw in has_nsfw_concept]
-
- image = self.image_processor.postprocess(image, output_type=output_type, do_denormalize=do_denormalize)
-
- # Offload last model to CPU
- if hasattr(self, "final_offload_hook") and self.final_offload_hook is not None:
- self.final_offload_hook.offload()
-
- if not return_dict:
- return (image, has_nsfw_concept)
-
- return StableDiffusionPipelineOutput(images=image, nsfw_content_detected=has_nsfw_concept)
-
- # Copied from diffusers.pipelines.stable_diffusion.pipeline_stable_diffusion.StableDiffusionPipeline.enable_model_cpu_offload
- def enable_model_cpu_offload(self, gpu_id=0):
- r"""
- Offload all models to CPU to reduce memory usage with a low impact on performance. Moves one whole model at a
- time to the GPU when its `forward` method is called, and the model remains in GPU until the next model runs.
- Memory savings are lower than using `enable_sequential_cpu_offload`, but performance is much better due to the
- iterative execution of the `unet`.
- """
- if is_accelerate_available() and is_accelerate_version(">=", "0.17.0.dev0"):
- from accelerate import cpu_offload_with_hook
- else:
- raise ImportError("`enable_model_cpu_offload` requires `accelerate v0.17.0` or higher.")
-
- device = torch.device(f"cuda:{gpu_id}")
-
- if self.device.type != "cpu":
- self.to("cpu", silence_dtype_warnings=True)
- torch.cuda.empty_cache() # otherwise we don't see the memory savings (but they probably exist)
-
- hook = None
- for cpu_offloaded_model in [self.text_encoder, self.unet, self.vae]:
- _, hook = cpu_offload_with_hook(cpu_offloaded_model, device, prev_module_hook=hook)
-
- if self.safety_checker is not None:
- _, hook = cpu_offload_with_hook(self.safety_checker, device, prev_module_hook=hook)
-
- # We'll offload the last model manually.
- self.final_offload_hook = hook
-
- def _encode_prompt(
- self,
- prompt,
- device,
- num_images_per_prompt,
- do_classifier_free_guidance,
- negative_prompt=None,
- prompt_embeds: Optional[torch.FloatTensor] = None,
- negative_prompt_embeds: Optional[torch.FloatTensor] = None,
- ):
- r"""
- Encodes the prompt into text encoder hidden states.
-
- Args:
- prompt (`str` or `List[str]`, *optional*):
- prompt to be encoded
- device: (`torch.device`):
- torch device
- num_images_per_prompt (`int`):
- number of images that should be generated per prompt
- do_classifier_free_guidance (`bool`):
- whether to use classifier free guidance or not
- negative_ prompt (`str` or `List[str]`, *optional*):
- The prompt or prompts not to guide the image generation. If not defined, one has to pass
- `negative_prompt_embeds` instead. Ignored when not using guidance (i.e., ignored if `guidance_scale` is
- less than `1`).
- prompt_embeds (`torch.FloatTensor`, *optional*):
- Pre-generated text embeddings. Can be used to easily tweak text inputs, *e.g.* prompt weighting. If not
- provided, text embeddings will be generated from `prompt` input argument.
- negative_prompt_embeds (`torch.FloatTensor`, *optional*):
- Pre-generated negative text embeddings. Can be used to easily tweak text inputs, *e.g.* prompt
- weighting. If not provided, negative_prompt_embeds will be generated from `negative_prompt` input
- argument.
- """
- if prompt is not None and isinstance(prompt, str):
- batch_size = 1
- elif prompt is not None and isinstance(prompt, list):
- batch_size = len(prompt)
- else:
- batch_size = prompt_embeds.shape[0]
-
- if prompt_embeds is None:
- # textual inversion: procecss multi-vector tokens if necessary
- if isinstance(self, TextualInversionLoaderMixin):
- prompt = self.maybe_convert_prompt(prompt, self.tokenizer)
-
- text_inputs = self.tokenizer(
- prompt,
- padding="max_length",
- max_length=self.tokenizer.model_max_length,
- truncation=True,
- return_tensors="pt",
- )
- text_input_ids = text_inputs.input_ids
- untruncated_ids = self.tokenizer(prompt, padding="longest", return_tensors="pt").input_ids
-
- if untruncated_ids.shape[-1] >= text_input_ids.shape[-1] and not torch.equal(
- text_input_ids, untruncated_ids
- ):
- removed_text = self.tokenizer.batch_decode(
- untruncated_ids[:, self.tokenizer.model_max_length - 1 : -1]
- )
- logger.warning(
- "The following part of your input was truncated because CLIP can only handle sequences up to"
- f" {self.tokenizer.model_max_length} tokens: {removed_text}"
- )
-
- if hasattr(self.text_encoder.config, "use_attention_mask") and self.text_encoder.config.use_attention_mask:
- attention_mask = text_inputs.attention_mask.to(device)
- else:
- attention_mask = None
-
- prompt_embeds = self.text_encoder(
- text_input_ids.to(device),
- attention_mask=attention_mask,
- )
- prompt_embeds = prompt_embeds[0]
-
- prompt_embeds = prompt_embeds.to(dtype=self.text_encoder.dtype, device=device)
-
- bs_embed, seq_len, _ = prompt_embeds.shape
- # duplicate text embeddings for each generation per prompt, using mps friendly method
- prompt_embeds = prompt_embeds.repeat(1, num_images_per_prompt, 1)
- prompt_embeds = prompt_embeds.view(bs_embed * num_images_per_prompt, seq_len, -1)
-
- # get unconditional embeddings for classifier free guidance
- if do_classifier_free_guidance and negative_prompt_embeds is None:
- uncond_tokens: List[str]
- if negative_prompt is None:
- uncond_tokens = [""] * batch_size
- elif type(prompt) is not type(negative_prompt):
- raise TypeError(
- f"`negative_prompt` should be the same type to `prompt`, but got {type(negative_prompt)} !="
- f" {type(prompt)}."
- )
- elif isinstance(negative_prompt, str):
- uncond_tokens = [negative_prompt]
- elif batch_size != len(negative_prompt):
- raise ValueError(
- f"`negative_prompt`: {negative_prompt} has batch size {len(negative_prompt)}, but `prompt`:"
- f" {prompt} has batch size {batch_size}. Please make sure that passed `negative_prompt` matches"
- " the batch size of `prompt`."
- )
- else:
- uncond_tokens = negative_prompt
-
- # textual inversion: procecss multi-vector tokens if necessary
- if isinstance(self, TextualInversionLoaderMixin):
- uncond_tokens = self.maybe_convert_prompt(uncond_tokens, self.tokenizer)
-
- max_length = prompt_embeds.shape[1]
- uncond_input = self.tokenizer(
- uncond_tokens,
- padding="max_length",
- max_length=max_length,
- truncation=True,
- return_tensors="pt",
- )
-
- if hasattr(self.text_encoder.config, "use_attention_mask") and self.text_encoder.config.use_attention_mask:
- attention_mask = uncond_input.attention_mask.to(device)
- else:
- attention_mask = None
-
- negative_prompt_embeds = self.text_encoder(
- uncond_input.input_ids.to(device),
- attention_mask=attention_mask,
- )
- negative_prompt_embeds = negative_prompt_embeds[0]
-
- if do_classifier_free_guidance:
- # duplicate unconditional embeddings for each generation per prompt, using mps friendly method
- seq_len = negative_prompt_embeds.shape[1]
-
- negative_prompt_embeds = negative_prompt_embeds.to(dtype=self.text_encoder.dtype, device=device)
-
- negative_prompt_embeds = negative_prompt_embeds.repeat(1, num_images_per_prompt, 1)
- negative_prompt_embeds = negative_prompt_embeds.view(batch_size * num_images_per_prompt, seq_len, -1)
-
- # For classifier free guidance, we need to do two forward passes.
- # Here we concatenate the unconditional and text embeddings into a single batch
- # to avoid doing two forward passes
- # pix2pix has two negative embeddings, and unlike in other pipelines latents are ordered [prompt_embeds, negative_prompt_embeds, negative_prompt_embeds]
- prompt_embeds = torch.cat([prompt_embeds, negative_prompt_embeds, negative_prompt_embeds])
-
- return prompt_embeds
-
- # Copied from diffusers.pipelines.stable_diffusion.pipeline_stable_diffusion.StableDiffusionPipeline.run_safety_checker
- def run_safety_checker(self, image, device, dtype):
- if self.safety_checker is None:
- has_nsfw_concept = None
- else:
- if torch.is_tensor(image):
- feature_extractor_input = self.image_processor.postprocess(image, output_type="pil")
- else:
- feature_extractor_input = self.image_processor.numpy_to_pil(image)
- safety_checker_input = self.feature_extractor(feature_extractor_input, return_tensors="pt").to(device)
- image, has_nsfw_concept = self.safety_checker(
- images=image, clip_input=safety_checker_input.pixel_values.to(dtype)
- )
- return image, has_nsfw_concept
-
- # Copied from diffusers.pipelines.stable_diffusion.pipeline_stable_diffusion.StableDiffusionPipeline.prepare_extra_step_kwargs
- def prepare_extra_step_kwargs(self, generator, eta):
- # prepare extra kwargs for the scheduler step, since not all schedulers have the same signature
- # eta (η) is only used with the DDIMScheduler, it will be ignored for other schedulers.
- # eta corresponds to η in DDIM paper: https://arxiv.org/abs/2010.02502
- # and should be between [0, 1]
-
- accepts_eta = "eta" in set(inspect.signature(self.scheduler.step).parameters.keys())
- extra_step_kwargs = {}
- if accepts_eta:
- extra_step_kwargs["eta"] = eta
-
- # check if the scheduler accepts generator
- accepts_generator = "generator" in set(inspect.signature(self.scheduler.step).parameters.keys())
- if accepts_generator:
- extra_step_kwargs["generator"] = generator
- return extra_step_kwargs
-
- # Copied from diffusers.pipelines.stable_diffusion.pipeline_stable_diffusion.StableDiffusionPipeline.decode_latents
- def decode_latents(self, latents):
- warnings.warn(
- "The decode_latents method is deprecated and will be removed in a future version. Please"
- " use VaeImageProcessor instead",
- FutureWarning,
- )
- latents = 1 / self.vae.config.scaling_factor * latents
- image = self.vae.decode(latents, return_dict=False)[0]
- image = (image / 2 + 0.5).clamp(0, 1)
- # we always cast to float32 as this does not cause significant overhead and is compatible with bfloat16
- image = image.cpu().permute(0, 2, 3, 1).float().numpy()
- return image
-
- def check_inputs(
- self, prompt, callback_steps, negative_prompt=None, prompt_embeds=None, negative_prompt_embeds=None
- ):
- if (callback_steps is None) or (
- callback_steps is not None and (not isinstance(callback_steps, int) or callback_steps <= 0)
- ):
- raise ValueError(
- f"`callback_steps` has to be a positive integer but is {callback_steps} of type"
- f" {type(callback_steps)}."
- )
-
- if prompt is not None and prompt_embeds is not None:
- raise ValueError(
- f"Cannot forward both `prompt`: {prompt} and `prompt_embeds`: {prompt_embeds}. Please make sure to"
- " only forward one of the two."
- )
- elif prompt is None and prompt_embeds is None:
- raise ValueError(
- "Provide either `prompt` or `prompt_embeds`. Cannot leave both `prompt` and `prompt_embeds` undefined."
- )
- elif prompt is not None and (not isinstance(prompt, str) and not isinstance(prompt, list)):
- raise ValueError(f"`prompt` has to be of type `str` or `list` but is {type(prompt)}")
-
- if negative_prompt is not None and negative_prompt_embeds is not None:
- raise ValueError(
- f"Cannot forward both `negative_prompt`: {negative_prompt} and `negative_prompt_embeds`:"
- f" {negative_prompt_embeds}. Please make sure to only forward one of the two."
- )
-
- if prompt_embeds is not None and negative_prompt_embeds is not None:
- if prompt_embeds.shape != negative_prompt_embeds.shape:
- raise ValueError(
- "`prompt_embeds` and `negative_prompt_embeds` must have the same shape when passed directly, but"
- f" got: `prompt_embeds` {prompt_embeds.shape} != `negative_prompt_embeds`"
- f" {negative_prompt_embeds.shape}."
- )
-
- # Copied from diffusers.pipelines.stable_diffusion.pipeline_stable_diffusion.StableDiffusionPipeline.prepare_latents
- def prepare_latents(self, batch_size, num_channels_latents, height, width, dtype, device, generator, latents=None):
- shape = (batch_size, num_channels_latents, height // self.vae_scale_factor, width // self.vae_scale_factor)
- if isinstance(generator, list) and len(generator) != batch_size:
- raise ValueError(
- f"You have passed a list of generators of length {len(generator)}, but requested an effective batch"
- f" size of {batch_size}. Make sure the batch size matches the length of the generators."
- )
-
- if latents is None:
- latents = randn_tensor(shape, generator=generator, device=device, dtype=dtype)
- else:
- latents = latents.to(device)
-
- # scale the initial noise by the standard deviation required by the scheduler
- latents = latents * self.scheduler.init_noise_sigma
- return latents
-
- def prepare_image_latents(
- self, image, batch_size, num_images_per_prompt, dtype, device, do_classifier_free_guidance, generator=None
- ):
- if not isinstance(image, (torch.Tensor, PIL.Image.Image, list)):
- raise ValueError(
- f"`image` has to be of type `torch.Tensor`, `PIL.Image.Image` or list but is {type(image)}"
- )
-
- image = image.to(device=device, dtype=dtype)
-
- batch_size = batch_size * num_images_per_prompt
-
- if image.shape[1] == 4:
- image_latents = image
- else:
- if isinstance(generator, list) and len(generator) != batch_size:
- raise ValueError(
- f"You have passed a list of generators of length {len(generator)}, but requested an effective batch"
- f" size of {batch_size}. Make sure the batch size matches the length of the generators."
- )
-
- if isinstance(generator, list):
- image_latents = [self.vae.encode(image[i : i + 1]).latent_dist.mode() for i in range(batch_size)]
- image_latents = torch.cat(image_latents, dim=0)
- else:
- image_latents = self.vae.encode(image).latent_dist.mode()
-
- if batch_size > image_latents.shape[0] and batch_size % image_latents.shape[0] == 0:
- # expand image_latents for batch_size
- deprecation_message = (
- f"You have passed {batch_size} text prompts (`prompt`), but only {image_latents.shape[0]} initial"
- " images (`image`). Initial images are now duplicating to match the number of text prompts. Note"
- " that this behavior is deprecated and will be removed in a version 1.0.0. Please make sure to update"
- " your script to pass as many initial images as text prompts to suppress this warning."
- )
- deprecate("len(prompt) != len(image)", "1.0.0", deprecation_message, standard_warn=False)
- additional_image_per_prompt = batch_size // image_latents.shape[0]
- image_latents = torch.cat([image_latents] * additional_image_per_prompt, dim=0)
- elif batch_size > image_latents.shape[0] and batch_size % image_latents.shape[0] != 0:
- raise ValueError(
- f"Cannot duplicate `image` of batch size {image_latents.shape[0]} to {batch_size} text prompts."
- )
- else:
- image_latents = torch.cat([image_latents], dim=0)
-
- if do_classifier_free_guidance:
- uncond_image_latents = torch.zeros_like(image_latents)
- image_latents = torch.cat([image_latents, image_latents, uncond_image_latents], dim=0)
-
- return image_latents
diff --git a/spaces/Androidonnxfork/CivitAi-to-Diffusers/diffusers/tests/pipelines/stable_unclip/test_stable_unclip.py b/spaces/Androidonnxfork/CivitAi-to-Diffusers/diffusers/tests/pipelines/stable_unclip/test_stable_unclip.py
deleted file mode 100644
index 8d5edda16904e7780bc00ea06746e1fd8f5c034d..0000000000000000000000000000000000000000
--- a/spaces/Androidonnxfork/CivitAi-to-Diffusers/diffusers/tests/pipelines/stable_unclip/test_stable_unclip.py
+++ /dev/null
@@ -1,241 +0,0 @@
-import gc
-import unittest
-
-import torch
-from transformers import CLIPTextConfig, CLIPTextModel, CLIPTextModelWithProjection, CLIPTokenizer
-
-from diffusers import (
- AutoencoderKL,
- DDIMScheduler,
- DDPMScheduler,
- PriorTransformer,
- StableUnCLIPPipeline,
- UNet2DConditionModel,
-)
-from diffusers.pipelines.stable_diffusion.stable_unclip_image_normalizer import StableUnCLIPImageNormalizer
-from diffusers.utils.testing_utils import enable_full_determinism, load_numpy, require_torch_gpu, slow, torch_device
-
-from ..pipeline_params import TEXT_TO_IMAGE_BATCH_PARAMS, TEXT_TO_IMAGE_IMAGE_PARAMS, TEXT_TO_IMAGE_PARAMS
-from ..test_pipelines_common import (
- PipelineKarrasSchedulerTesterMixin,
- PipelineLatentTesterMixin,
- PipelineTesterMixin,
- assert_mean_pixel_difference,
-)
-
-
-enable_full_determinism()
-
-
-class StableUnCLIPPipelineFastTests(
- PipelineLatentTesterMixin, PipelineKarrasSchedulerTesterMixin, PipelineTesterMixin, unittest.TestCase
-):
- pipeline_class = StableUnCLIPPipeline
- params = TEXT_TO_IMAGE_PARAMS
- batch_params = TEXT_TO_IMAGE_BATCH_PARAMS
- image_params = TEXT_TO_IMAGE_IMAGE_PARAMS
- image_latents_params = TEXT_TO_IMAGE_IMAGE_PARAMS
-
- # TODO(will) Expected attn_bias.stride(1) == 0 to be true, but got false
- test_xformers_attention = False
-
- def get_dummy_components(self):
- embedder_hidden_size = 32
- embedder_projection_dim = embedder_hidden_size
-
- # prior components
-
- torch.manual_seed(0)
- prior_tokenizer = CLIPTokenizer.from_pretrained("hf-internal-testing/tiny-random-clip")
-
- torch.manual_seed(0)
- prior_text_encoder = CLIPTextModelWithProjection(
- CLIPTextConfig(
- bos_token_id=0,
- eos_token_id=2,
- hidden_size=embedder_hidden_size,
- projection_dim=embedder_projection_dim,
- intermediate_size=37,
- layer_norm_eps=1e-05,
- num_attention_heads=4,
- num_hidden_layers=5,
- pad_token_id=1,
- vocab_size=1000,
- )
- )
-
- torch.manual_seed(0)
- prior = PriorTransformer(
- num_attention_heads=2,
- attention_head_dim=12,
- embedding_dim=embedder_projection_dim,
- num_layers=1,
- )
-
- torch.manual_seed(0)
- prior_scheduler = DDPMScheduler(
- variance_type="fixed_small_log",
- prediction_type="sample",
- num_train_timesteps=1000,
- clip_sample=True,
- clip_sample_range=5.0,
- beta_schedule="squaredcos_cap_v2",
- )
-
- # regular denoising components
-
- torch.manual_seed(0)
- image_normalizer = StableUnCLIPImageNormalizer(embedding_dim=embedder_hidden_size)
- image_noising_scheduler = DDPMScheduler(beta_schedule="squaredcos_cap_v2")
-
- torch.manual_seed(0)
- tokenizer = CLIPTokenizer.from_pretrained("hf-internal-testing/tiny-random-clip")
-
- torch.manual_seed(0)
- text_encoder = CLIPTextModel(
- CLIPTextConfig(
- bos_token_id=0,
- eos_token_id=2,
- hidden_size=embedder_hidden_size,
- projection_dim=32,
- intermediate_size=37,
- layer_norm_eps=1e-05,
- num_attention_heads=4,
- num_hidden_layers=5,
- pad_token_id=1,
- vocab_size=1000,
- )
- )
-
- torch.manual_seed(0)
- unet = UNet2DConditionModel(
- sample_size=32,
- in_channels=4,
- out_channels=4,
- down_block_types=("CrossAttnDownBlock2D", "DownBlock2D"),
- up_block_types=("UpBlock2D", "CrossAttnUpBlock2D"),
- block_out_channels=(32, 64),
- attention_head_dim=(2, 4),
- class_embed_type="projection",
- # The class embeddings are the noise augmented image embeddings.
- # I.e. the image embeddings concated with the noised embeddings of the same dimension
- projection_class_embeddings_input_dim=embedder_projection_dim * 2,
- cross_attention_dim=embedder_hidden_size,
- layers_per_block=1,
- upcast_attention=True,
- use_linear_projection=True,
- )
-
- torch.manual_seed(0)
- scheduler = DDIMScheduler(
- beta_schedule="scaled_linear",
- beta_start=0.00085,
- beta_end=0.012,
- prediction_type="v_prediction",
- set_alpha_to_one=False,
- steps_offset=1,
- )
-
- torch.manual_seed(0)
- vae = AutoencoderKL()
-
- components = {
- # prior components
- "prior_tokenizer": prior_tokenizer,
- "prior_text_encoder": prior_text_encoder,
- "prior": prior,
- "prior_scheduler": prior_scheduler,
- # image noising components
- "image_normalizer": image_normalizer,
- "image_noising_scheduler": image_noising_scheduler,
- # regular denoising components
- "tokenizer": tokenizer,
- "text_encoder": text_encoder,
- "unet": unet,
- "scheduler": scheduler,
- "vae": vae,
- }
-
- return components
-
- def get_dummy_inputs(self, device, seed=0):
- if str(device).startswith("mps"):
- generator = torch.manual_seed(seed)
- else:
- generator = torch.Generator(device=device).manual_seed(seed)
- inputs = {
- "prompt": "A painting of a squirrel eating a burger",
- "generator": generator,
- "num_inference_steps": 2,
- "prior_num_inference_steps": 2,
- "output_type": "numpy",
- }
- return inputs
-
- # Overriding PipelineTesterMixin::test_attention_slicing_forward_pass
- # because UnCLIP GPU undeterminism requires a looser check.
- def test_attention_slicing_forward_pass(self):
- test_max_difference = torch_device == "cpu"
-
- self._test_attention_slicing_forward_pass(test_max_difference=test_max_difference)
-
- # Overriding PipelineTesterMixin::test_inference_batch_single_identical
- # because UnCLIP undeterminism requires a looser check.
- def test_inference_batch_single_identical(self):
- test_max_difference = torch_device in ["cpu", "mps"]
-
- self._test_inference_batch_single_identical(test_max_difference=test_max_difference)
-
-
-@slow
-@require_torch_gpu
-class StableUnCLIPPipelineIntegrationTests(unittest.TestCase):
- def tearDown(self):
- # clean up the VRAM after each test
- super().tearDown()
- gc.collect()
- torch.cuda.empty_cache()
-
- def test_stable_unclip(self):
- expected_image = load_numpy(
- "https://huggingface.co/datasets/hf-internal-testing/diffusers-images/resolve/main/stable_unclip/stable_unclip_2_1_l_anime_turtle_fp16.npy"
- )
-
- pipe = StableUnCLIPPipeline.from_pretrained("fusing/stable-unclip-2-1-l", torch_dtype=torch.float16)
- pipe.to(torch_device)
- pipe.set_progress_bar_config(disable=None)
- # stable unclip will oom when integration tests are run on a V100,
- # so turn on memory savings
- pipe.enable_attention_slicing()
- pipe.enable_sequential_cpu_offload()
-
- generator = torch.Generator(device="cpu").manual_seed(0)
- output = pipe("anime turle", generator=generator, output_type="np")
-
- image = output.images[0]
-
- assert image.shape == (768, 768, 3)
-
- assert_mean_pixel_difference(image, expected_image)
-
- def test_stable_unclip_pipeline_with_sequential_cpu_offloading(self):
- torch.cuda.empty_cache()
- torch.cuda.reset_max_memory_allocated()
- torch.cuda.reset_peak_memory_stats()
-
- pipe = StableUnCLIPPipeline.from_pretrained("fusing/stable-unclip-2-1-l", torch_dtype=torch.float16)
- pipe = pipe.to(torch_device)
- pipe.set_progress_bar_config(disable=None)
- pipe.enable_attention_slicing()
- pipe.enable_sequential_cpu_offload()
-
- _ = pipe(
- "anime turtle",
- prior_num_inference_steps=2,
- num_inference_steps=2,
- output_type="np",
- )
-
- mem_bytes = torch.cuda.max_memory_allocated()
- # make sure that less than 7 GB is allocated
- assert mem_bytes < 7 * 10**9
diff --git a/spaces/Andy1621/uniformer_image_detection/configs/foveabox/README.md b/spaces/Andy1621/uniformer_image_detection/configs/foveabox/README.md
deleted file mode 100644
index 91a43c9797bc88e747f22a5878f1bf4b12946389..0000000000000000000000000000000000000000
--- a/spaces/Andy1621/uniformer_image_detection/configs/foveabox/README.md
+++ /dev/null
@@ -1,41 +0,0 @@
-# FoveaBox: Beyond Anchor-based Object Detector
-
-[ALGORITHM]
-
-FoveaBox is an accurate, flexible and completely anchor-free object detection system for object detection framework, as presented in our paper [https://arxiv.org/abs/1904.03797](https://arxiv.org/abs/1904.03797):
-Different from previous anchor-based methods, FoveaBox directly learns the object existing possibility and the bounding box coordinates without anchor reference. This is achieved by: (a) predicting category-sensitive semantic maps for the object existing possibility, and (b) producing category-agnostic bounding box for each position that potentially contains an object.
-
-## Main Results
-
-### Results on R50/101-FPN
-
-| Backbone | Style | align | ms-train| Lr schd | Mem (GB) | Inf time (fps) | box AP | Config | Download |
-|:---------:|:-------:|:-------:|:-------:|:-------:|:--------:|:--------------:|:------:|:------:|:--------:|
-| R-50 | pytorch | N | N | 1x | 5.6 | 24.1 | 36.5 | [config](https://github.com/open-mmlab/mmdetection/tree/master/configs/foveabox/fovea_r50_fpn_4x4_1x_coco.py) | [model](http://download.openmmlab.com/mmdetection/v2.0/foveabox/fovea_r50_fpn_4x4_1x_coco/fovea_r50_fpn_4x4_1x_coco_20200219-ee4d5303.pth) | [log](http://download.openmmlab.com/mmdetection/v2.0/foveabox/fovea_r50_fpn_4x4_1x_coco/fovea_r50_fpn_4x4_1x_coco_20200219_223025.log.json) |
-| R-50 | pytorch | N | N | 2x | 5.6 | - | 37.2 | [config](https://github.com/open-mmlab/mmdetection/tree/master/configs/foveabox/fovea_r50_fpn_4x4_2x_coco.py) | [model](http://download.openmmlab.com/mmdetection/v2.0/foveabox/fovea_r50_fpn_4x4_2x_coco/fovea_r50_fpn_4x4_2x_coco_20200203-2df792b1.pth) | [log](http://download.openmmlab.com/mmdetection/v2.0/foveabox/fovea_r50_fpn_4x4_2x_coco/fovea_r50_fpn_4x4_2x_coco_20200203_112043.log.json) |
-| R-50 | pytorch | Y | N | 2x | 8.1 | 19.4 | 37.9 | [config](https://github.com/open-mmlab/mmdetection/tree/master/configs/foveabox/fovea_align_r50_fpn_gn-head_4x4_2x_coco.py) | [model](http://download.openmmlab.com/mmdetection/v2.0/foveabox/fovea_align_r50_fpn_gn-head_4x4_2x_coco/fovea_align_r50_fpn_gn-head_4x4_2x_coco_20200203-8987880d.pth) | [log](http://download.openmmlab.com/mmdetection/v2.0/foveabox/fovea_align_r50_fpn_gn-head_4x4_2x_coco/fovea_align_r50_fpn_gn-head_4x4_2x_coco_20200203_134252.log.json) |
-| R-50 | pytorch | Y | Y | 2x | 8.1 | 18.3 | 40.4 | [config](https://github.com/open-mmlab/mmdetection/tree/master/configs/foveabox/fovea_align_r50_fpn_gn-head_mstrain_640-800_4x4_2x_coco.py) | [model](http://download.openmmlab.com/mmdetection/v2.0/foveabox/fovea_align_r50_fpn_gn-head_mstrain_640-800_4x4_2x_coco/fovea_align_r50_fpn_gn-head_mstrain_640-800_4x4_2x_coco_20200205-85ce26cb.pth) | [log](http://download.openmmlab.com/mmdetection/v2.0/foveabox/fovea_align_r50_fpn_gn-head_mstrain_640-800_4x4_2x_coco/fovea_align_r50_fpn_gn-head_mstrain_640-800_4x4_2x_coco_20200205_112557.log.json) |
-| R-101 | pytorch | N | N | 1x | 9.2 | 17.4 | 38.6 | [config](https://github.com/open-mmlab/mmdetection/tree/master/configs/foveabox/fovea_r101_fpn_4x4_1x_coco.py) | [model](http://download.openmmlab.com/mmdetection/v2.0/foveabox/fovea_r101_fpn_4x4_1x_coco/fovea_r101_fpn_4x4_1x_coco_20200219-05e38f1c.pth) | [log](http://download.openmmlab.com/mmdetection/v2.0/foveabox/fovea_r101_fpn_4x4_1x_coco/fovea_r101_fpn_4x4_1x_coco_20200219_011740.log.json) |
-| R-101 | pytorch | N | N | 2x | 11.7 | - | 40.0 | [config](https://github.com/open-mmlab/mmdetection/tree/master/configs/foveabox/fovea_r101_fpn_4x4_2x_coco.py) | [model](http://download.openmmlab.com/mmdetection/v2.0/foveabox/fovea_r101_fpn_4x4_2x_coco/fovea_r101_fpn_4x4_2x_coco_20200208-02320ea4.pth) | [log](http://download.openmmlab.com/mmdetection/v2.0/foveabox/fovea_r101_fpn_4x4_2x_coco/fovea_r101_fpn_4x4_2x_coco_20200208_202059.log.json) |
-| R-101 | pytorch | Y | N | 2x | 11.7 | 14.7 | 40.0 | [config](https://github.com/open-mmlab/mmdetection/tree/master/configs/foveabox/fovea_align_r101_fpn_gn-head_4x4_2x_coco.py) | [model](http://download.openmmlab.com/mmdetection/v2.0/foveabox/fovea_align_r101_fpn_gn-head_4x4_2x_coco/fovea_align_r101_fpn_gn-head_4x4_2x_coco_20200208-c39a027a.pth) | [log](http://download.openmmlab.com/mmdetection/v2.0/foveabox/fovea_align_r101_fpn_gn-head_4x4_2x_coco/fovea_align_r101_fpn_gn-head_4x4_2x_coco_20200208_203337.log.json) |
-| R-101 | pytorch | Y | Y | 2x | 11.7 | 14.7 | 42.0 | [config](https://github.com/open-mmlab/mmdetection/tree/master/configs/foveabox/fovea_align_r101_fpn_gn-head_mstrain_640-800_4x4_2x_coco.py) | [model](http://download.openmmlab.com/mmdetection/v2.0/foveabox/fovea_align_r101_fpn_gn-head_mstrain_640-800_4x4_2x_coco/fovea_align_r101_fpn_gn-head_mstrain_640-800_4x4_2x_coco_20200208-649c5eb6.pth) | [log](http://download.openmmlab.com/mmdetection/v2.0/foveabox/fovea_align_r101_fpn_gn-head_mstrain_640-800_4x4_2x_coco/fovea_align_r101_fpn_gn-head_mstrain_640-800_4x4_2x_coco_20200208_202124.log.json) |
-
-[1] *1x and 2x mean the model is trained for 12 and 24 epochs, respectively.* \
-[2] *Align means utilizing deformable convolution to align the cls branch.* \
-[3] *All results are obtained with a single model and without any test time data augmentation.*\
-[4] *We use 4 GPUs for training.*
-
-Any pull requests or issues are welcome.
-
-## Citations
-
-Please consider citing our paper in your publications if the project helps your research. BibTeX reference is as follows.
-
-```latex
-@article{kong2019foveabox,
- title={FoveaBox: Beyond Anchor-based Object Detector},
- author={Kong, Tao and Sun, Fuchun and Liu, Huaping and Jiang, Yuning and Shi, Jianbo},
- journal={arXiv preprint arXiv:1904.03797},
- year={2019}
-}
-```
diff --git a/spaces/Andy1621/uniformer_image_detection/configs/hrnet/faster_rcnn_hrnetv2p_w18_1x_coco.py b/spaces/Andy1621/uniformer_image_detection/configs/hrnet/faster_rcnn_hrnetv2p_w18_1x_coco.py
deleted file mode 100644
index 9907bcbf6464fb964664a318533bf9edda4e34fd..0000000000000000000000000000000000000000
--- a/spaces/Andy1621/uniformer_image_detection/configs/hrnet/faster_rcnn_hrnetv2p_w18_1x_coco.py
+++ /dev/null
@@ -1,10 +0,0 @@
-_base_ = './faster_rcnn_hrnetv2p_w32_1x_coco.py'
-# model settings
-model = dict(
- pretrained='open-mmlab://msra/hrnetv2_w18',
- backbone=dict(
- extra=dict(
- stage2=dict(num_channels=(18, 36)),
- stage3=dict(num_channels=(18, 36, 72)),
- stage4=dict(num_channels=(18, 36, 72, 144)))),
- neck=dict(type='HRFPN', in_channels=[18, 36, 72, 144], out_channels=256))
diff --git a/spaces/Andy1621/uniformer_image_segmentation/configs/ann/ann_r50-d8_512x512_160k_ade20k.py b/spaces/Andy1621/uniformer_image_segmentation/configs/ann/ann_r50-d8_512x512_160k_ade20k.py
deleted file mode 100644
index ca6bb248ac867d463c274f975c884aa80a57730f..0000000000000000000000000000000000000000
--- a/spaces/Andy1621/uniformer_image_segmentation/configs/ann/ann_r50-d8_512x512_160k_ade20k.py
+++ /dev/null
@@ -1,6 +0,0 @@
-_base_ = [
- '../_base_/models/ann_r50-d8.py', '../_base_/datasets/ade20k.py',
- '../_base_/default_runtime.py', '../_base_/schedules/schedule_160k.py'
-]
-model = dict(
- decode_head=dict(num_classes=150), auxiliary_head=dict(num_classes=150))
diff --git a/spaces/Anonymous-123/ImageNet-Editing/editing_diffusion/guided_diffusion/datasets/README.md b/spaces/Anonymous-123/ImageNet-Editing/editing_diffusion/guided_diffusion/datasets/README.md
deleted file mode 100644
index 336b8e83262764419aceae9c975c58bed0fbb47b..0000000000000000000000000000000000000000
--- a/spaces/Anonymous-123/ImageNet-Editing/editing_diffusion/guided_diffusion/datasets/README.md
+++ /dev/null
@@ -1,27 +0,0 @@
-# Downloading datasets
-
-This directory includes instructions and scripts for downloading ImageNet and LSUN bedrooms for use in this codebase.
-
-## Class-conditional ImageNet
-
-For our class-conditional models, we use the official ILSVRC2012 dataset with manual center cropping and downsampling. To obtain this dataset, navigate to [this page on image-net.org](http://www.image-net.org/challenges/LSVRC/2012/downloads) and sign in (or create an account if you do not already have one). Then click on the link reading "Training images (Task 1 & 2)". This is a 138GB tar file containing 1000 sub-tar files, one per class.
-
-Once the file is downloaded, extract it and look inside. You should see 1000 `.tar` files. You need to extract each of these, which may be impractical to do by hand on your operating system. To automate the process on a Unix-based system, you can `cd` into the directory and run this short shell script:
-
-```
-for file in *.tar; do tar xf "$file"; rm "$file"; done
-```
-
-This will extract and remove each tar file in turn.
-
-Once all of the images have been extracted, the resulting directory should be usable as a data directory (the `--data_dir` argument for the training script). The filenames should all start with WNID (class ids) followed by underscores, like `n01440764_2708.JPEG`. Conveniently (but not by accident) this is how the automated data-loader expects to discover class labels.
-
-## LSUN bedroom
-
-To download and pre-process LSUN bedroom, clone [fyu/lsun](https://github.com/fyu/lsun) on GitHub and run their download script `python3 download.py bedroom`. The result will be an "lmdb" database named like `bedroom_train_lmdb`. You can pass this to our [lsun_bedroom.py](lsun_bedroom.py) script like so:
-
-```
-python lsun_bedroom.py bedroom_train_lmdb lsun_train_output_dir
-```
-
-This creates a directory called `lsun_train_output_dir`. This directory can be passed to the training scripts via the `--data_dir` argument.
diff --git a/spaces/AnthonyTruchetPoC/persistent-docker/scripts/run-all-precommit-checks.sh b/spaces/AnthonyTruchetPoC/persistent-docker/scripts/run-all-precommit-checks.sh
deleted file mode 100644
index df8842c73870d3a896a24af301429ffd2d8e3f1a..0000000000000000000000000000000000000000
--- a/spaces/AnthonyTruchetPoC/persistent-docker/scripts/run-all-precommit-checks.sh
+++ /dev/null
@@ -1,2 +0,0 @@
-#!/usr/bin/env sh
-poetry run pre-commit run --all-files --hook-stage=manual
diff --git a/spaces/Araby/BRATArA/README.md b/spaces/Araby/BRATArA/README.md
deleted file mode 100644
index 3a1778a4166c79b4afde9bd1c6beaa9e2d14f018..0000000000000000000000000000000000000000
--- a/spaces/Araby/BRATArA/README.md
+++ /dev/null
@@ -1,13 +0,0 @@
----
-title: BRATArA
-emoji: 🏃
-colorFrom: purple
-colorTo: red
-sdk: streamlit
-sdk_version: 1.27.2
-app_file: app.py
-pinned: false
-license: mit
----
-
-Check out the configuration reference at https://huggingface.co/docs/hub/spaces-config-reference
diff --git a/spaces/Ataturk-Chatbot/HuggingFaceChat/venv/lib/python3.11/site-packages/dotenv/cli.py b/spaces/Ataturk-Chatbot/HuggingFaceChat/venv/lib/python3.11/site-packages/dotenv/cli.py
deleted file mode 100644
index 65ead46155f568a197a16b64c6335f1f28cda9a6..0000000000000000000000000000000000000000
--- a/spaces/Ataturk-Chatbot/HuggingFaceChat/venv/lib/python3.11/site-packages/dotenv/cli.py
+++ /dev/null
@@ -1,199 +0,0 @@
-import json
-import os
-import shlex
-import sys
-from contextlib import contextmanager
-from subprocess import Popen
-from typing import Any, Dict, IO, Iterator, List
-
-try:
- import click
-except ImportError:
- sys.stderr.write('It seems python-dotenv is not installed with cli option. \n'
- 'Run pip install "python-dotenv[cli]" to fix this.')
- sys.exit(1)
-
-from .main import dotenv_values, set_key, unset_key
-from .version import __version__
-
-
-def enumerate_env():
- """
- Return a path for the ${pwd}/.env file.
-
- If pwd does not exist, return None.
- """
- try:
- cwd = os.getcwd()
- except FileNotFoundError:
- return None
- path = os.path.join(cwd, '.env')
- return path
-
-
-@click.group()
-@click.option('-f', '--file', default=enumerate_env(),
- type=click.Path(file_okay=True),
- help="Location of the .env file, defaults to .env file in current working directory.")
-@click.option('-q', '--quote', default='always',
- type=click.Choice(['always', 'never', 'auto']),
- help="Whether to quote or not the variable values. Default mode is always. This does not affect parsing.")
-@click.option('-e', '--export', default=False,
- type=click.BOOL,
- help="Whether to write the dot file as an executable bash script.")
-@click.version_option(version=__version__)
-@click.pass_context
-def cli(ctx: click.Context, file: Any, quote: Any, export: Any) -> None:
- """This script is used to set, get or unset values from a .env file."""
- ctx.obj = {'QUOTE': quote, 'EXPORT': export, 'FILE': file}
-
-
-@contextmanager
-def stream_file(path: os.PathLike) -> Iterator[IO[str]]:
- """
- Open a file and yield the corresponding (decoded) stream.
-
- Exits with error code 2 if the file cannot be opened.
- """
-
- try:
- with open(path) as stream:
- yield stream
- except OSError as exc:
- print(f"Error opening env file: {exc}", file=sys.stderr)
- exit(2)
-
-
-@cli.command()
-@click.pass_context
-@click.option('--format', default='simple',
- type=click.Choice(['simple', 'json', 'shell', 'export']),
- help="The format in which to display the list. Default format is simple, "
- "which displays name=value without quotes.")
-def list(ctx: click.Context, format: bool) -> None:
- """Display all the stored key/value."""
- file = ctx.obj['FILE']
-
- with stream_file(file) as stream:
- values = dotenv_values(stream=stream)
-
- if format == 'json':
- click.echo(json.dumps(values, indent=2, sort_keys=True))
- else:
- prefix = 'export ' if format == 'export' else ''
- for k in sorted(values):
- v = values[k]
- if v is not None:
- if format in ('export', 'shell'):
- v = shlex.quote(v)
- click.echo(f'{prefix}{k}={v}')
-
-
-@cli.command()
-@click.pass_context
-@click.argument('key', required=True)
-@click.argument('value', required=True)
-def set(ctx: click.Context, key: Any, value: Any) -> None:
- """Store the given key/value."""
- file = ctx.obj['FILE']
- quote = ctx.obj['QUOTE']
- export = ctx.obj['EXPORT']
- success, key, value = set_key(file, key, value, quote, export)
- if success:
- click.echo(f'{key}={value}')
- else:
- exit(1)
-
-
-@cli.command()
-@click.pass_context
-@click.argument('key', required=True)
-def get(ctx: click.Context, key: Any) -> None:
- """Retrieve the value for the given key."""
- file = ctx.obj['FILE']
-
- with stream_file(file) as stream:
- values = dotenv_values(stream=stream)
-
- stored_value = values.get(key)
- if stored_value:
- click.echo(stored_value)
- else:
- exit(1)
-
-
-@cli.command()
-@click.pass_context
-@click.argument('key', required=True)
-def unset(ctx: click.Context, key: Any) -> None:
- """Removes the given key."""
- file = ctx.obj['FILE']
- quote = ctx.obj['QUOTE']
- success, key = unset_key(file, key, quote)
- if success:
- click.echo(f"Successfully removed {key}")
- else:
- exit(1)
-
-
-@cli.command(context_settings={'ignore_unknown_options': True})
-@click.pass_context
-@click.option(
- "--override/--no-override",
- default=True,
- help="Override variables from the environment file with those from the .env file.",
-)
-@click.argument('commandline', nargs=-1, type=click.UNPROCESSED)
-def run(ctx: click.Context, override: bool, commandline: List[str]) -> None:
- """Run command with environment variables present."""
- file = ctx.obj['FILE']
- if not os.path.isfile(file):
- raise click.BadParameter(
- f'Invalid value for \'-f\' "{file}" does not exist.',
- ctx=ctx
- )
- dotenv_as_dict = {
- k: v
- for (k, v) in dotenv_values(file).items()
- if v is not None and (override or k not in os.environ)
- }
-
- if not commandline:
- click.echo('No command given.')
- exit(1)
- ret = run_command(commandline, dotenv_as_dict)
- exit(ret)
-
-
-def run_command(command: List[str], env: Dict[str, str]) -> int:
- """Run command in sub process.
-
- Runs the command in a sub process with the variables from `env`
- added in the current environment variables.
-
- Parameters
- ----------
- command: List[str]
- The command and it's parameters
- env: Dict
- The additional environment variables
-
- Returns
- -------
- int
- The return code of the command
-
- """
- # copy the current environment variables and add the vales from
- # `env`
- cmd_env = os.environ.copy()
- cmd_env.update(env)
-
- p = Popen(command,
- universal_newlines=True,
- bufsize=0,
- shell=False,
- env=cmd_env)
- _, _ = p.communicate()
-
- return p.returncode
diff --git a/spaces/Ataturk-Chatbot/HuggingFaceChat/venv/lib/python3.11/site-packages/pip/_internal/commands/hash.py b/spaces/Ataturk-Chatbot/HuggingFaceChat/venv/lib/python3.11/site-packages/pip/_internal/commands/hash.py
deleted file mode 100644
index 042dac813e74b8187c3754cb9a937c7f7183e331..0000000000000000000000000000000000000000
--- a/spaces/Ataturk-Chatbot/HuggingFaceChat/venv/lib/python3.11/site-packages/pip/_internal/commands/hash.py
+++ /dev/null
@@ -1,59 +0,0 @@
-import hashlib
-import logging
-import sys
-from optparse import Values
-from typing import List
-
-from pip._internal.cli.base_command import Command
-from pip._internal.cli.status_codes import ERROR, SUCCESS
-from pip._internal.utils.hashes import FAVORITE_HASH, STRONG_HASHES
-from pip._internal.utils.misc import read_chunks, write_output
-
-logger = logging.getLogger(__name__)
-
-
-class HashCommand(Command):
- """
- Compute a hash of a local package archive.
-
- These can be used with --hash in a requirements file to do repeatable
- installs.
- """
-
- usage = "%prog [options] ..."
- ignore_require_venv = True
-
- def add_options(self) -> None:
- self.cmd_opts.add_option(
- "-a",
- "--algorithm",
- dest="algorithm",
- choices=STRONG_HASHES,
- action="store",
- default=FAVORITE_HASH,
- help="The hash algorithm to use: one of {}".format(
- ", ".join(STRONG_HASHES)
- ),
- )
- self.parser.insert_option_group(0, self.cmd_opts)
-
- def run(self, options: Values, args: List[str]) -> int:
- if not args:
- self.parser.print_usage(sys.stderr)
- return ERROR
-
- algorithm = options.algorithm
- for path in args:
- write_output(
- "%s:\n--hash=%s:%s", path, algorithm, _hash_of_file(path, algorithm)
- )
- return SUCCESS
-
-
-def _hash_of_file(path: str, algorithm: str) -> str:
- """Return the hash digest of a file."""
- with open(path, "rb") as archive:
- hash = hashlib.new(algorithm)
- for chunk in read_chunks(archive):
- hash.update(chunk)
- return hash.hexdigest()
diff --git a/spaces/Ataturk-Chatbot/HuggingFaceChat/venv/lib/python3.11/site-packages/pip/_vendor/rich/containers.py b/spaces/Ataturk-Chatbot/HuggingFaceChat/venv/lib/python3.11/site-packages/pip/_vendor/rich/containers.py
deleted file mode 100644
index e29cf368991ccb083b67cda8133e4635defbfe53..0000000000000000000000000000000000000000
--- a/spaces/Ataturk-Chatbot/HuggingFaceChat/venv/lib/python3.11/site-packages/pip/_vendor/rich/containers.py
+++ /dev/null
@@ -1,167 +0,0 @@
-from itertools import zip_longest
-from typing import (
- Iterator,
- Iterable,
- List,
- Optional,
- Union,
- overload,
- TypeVar,
- TYPE_CHECKING,
-)
-
-if TYPE_CHECKING:
- from .console import (
- Console,
- ConsoleOptions,
- JustifyMethod,
- OverflowMethod,
- RenderResult,
- RenderableType,
- )
- from .text import Text
-
-from .cells import cell_len
-from .measure import Measurement
-
-T = TypeVar("T")
-
-
-class Renderables:
- """A list subclass which renders its contents to the console."""
-
- def __init__(
- self, renderables: Optional[Iterable["RenderableType"]] = None
- ) -> None:
- self._renderables: List["RenderableType"] = (
- list(renderables) if renderables is not None else []
- )
-
- def __rich_console__(
- self, console: "Console", options: "ConsoleOptions"
- ) -> "RenderResult":
- """Console render method to insert line-breaks."""
- yield from self._renderables
-
- def __rich_measure__(
- self, console: "Console", options: "ConsoleOptions"
- ) -> "Measurement":
- dimensions = [
- Measurement.get(console, options, renderable)
- for renderable in self._renderables
- ]
- if not dimensions:
- return Measurement(1, 1)
- _min = max(dimension.minimum for dimension in dimensions)
- _max = max(dimension.maximum for dimension in dimensions)
- return Measurement(_min, _max)
-
- def append(self, renderable: "RenderableType") -> None:
- self._renderables.append(renderable)
-
- def __iter__(self) -> Iterable["RenderableType"]:
- return iter(self._renderables)
-
-
-class Lines:
- """A list subclass which can render to the console."""
-
- def __init__(self, lines: Iterable["Text"] = ()) -> None:
- self._lines: List["Text"] = list(lines)
-
- def __repr__(self) -> str:
- return f"Lines({self._lines!r})"
-
- def __iter__(self) -> Iterator["Text"]:
- return iter(self._lines)
-
- @overload
- def __getitem__(self, index: int) -> "Text":
- ...
-
- @overload
- def __getitem__(self, index: slice) -> List["Text"]:
- ...
-
- def __getitem__(self, index: Union[slice, int]) -> Union["Text", List["Text"]]:
- return self._lines[index]
-
- def __setitem__(self, index: int, value: "Text") -> "Lines":
- self._lines[index] = value
- return self
-
- def __len__(self) -> int:
- return self._lines.__len__()
-
- def __rich_console__(
- self, console: "Console", options: "ConsoleOptions"
- ) -> "RenderResult":
- """Console render method to insert line-breaks."""
- yield from self._lines
-
- def append(self, line: "Text") -> None:
- self._lines.append(line)
-
- def extend(self, lines: Iterable["Text"]) -> None:
- self._lines.extend(lines)
-
- def pop(self, index: int = -1) -> "Text":
- return self._lines.pop(index)
-
- def justify(
- self,
- console: "Console",
- width: int,
- justify: "JustifyMethod" = "left",
- overflow: "OverflowMethod" = "fold",
- ) -> None:
- """Justify and overflow text to a given width.
-
- Args:
- console (Console): Console instance.
- width (int): Number of characters per line.
- justify (str, optional): Default justify method for text: "left", "center", "full" or "right". Defaults to "left".
- overflow (str, optional): Default overflow for text: "crop", "fold", or "ellipsis". Defaults to "fold".
-
- """
- from .text import Text
-
- if justify == "left":
- for line in self._lines:
- line.truncate(width, overflow=overflow, pad=True)
- elif justify == "center":
- for line in self._lines:
- line.rstrip()
- line.truncate(width, overflow=overflow)
- line.pad_left((width - cell_len(line.plain)) // 2)
- line.pad_right(width - cell_len(line.plain))
- elif justify == "right":
- for line in self._lines:
- line.rstrip()
- line.truncate(width, overflow=overflow)
- line.pad_left(width - cell_len(line.plain))
- elif justify == "full":
- for line_index, line in enumerate(self._lines):
- if line_index == len(self._lines) - 1:
- break
- words = line.split(" ")
- words_size = sum(cell_len(word.plain) for word in words)
- num_spaces = len(words) - 1
- spaces = [1 for _ in range(num_spaces)]
- index = 0
- if spaces:
- while words_size + num_spaces < width:
- spaces[len(spaces) - index - 1] += 1
- num_spaces += 1
- index = (index + 1) % len(spaces)
- tokens: List[Text] = []
- for index, (word, next_word) in enumerate(
- zip_longest(words, words[1:])
- ):
- tokens.append(word)
- if index < len(spaces):
- style = word.get_style_at_offset(console, -1)
- next_style = next_word.get_style_at_offset(console, 0)
- space_style = style if style == next_style else line.style
- tokens.append(Text(" " * spaces[index], style=space_style))
- self[line_index] = Text("").join(tokens)
diff --git a/spaces/Ataturk-Chatbot/HuggingFaceChat/venv/lib/python3.11/site-packages/setuptools/_vendor/jaraco/functools.py b/spaces/Ataturk-Chatbot/HuggingFaceChat/venv/lib/python3.11/site-packages/setuptools/_vendor/jaraco/functools.py
deleted file mode 100644
index bbd8b29f9c012d62a37393476a5e393405d2918c..0000000000000000000000000000000000000000
--- a/spaces/Ataturk-Chatbot/HuggingFaceChat/venv/lib/python3.11/site-packages/setuptools/_vendor/jaraco/functools.py
+++ /dev/null
@@ -1,525 +0,0 @@
-import functools
-import time
-import inspect
-import collections
-import types
-import itertools
-
-import setuptools.extern.more_itertools
-
-from typing import Callable, TypeVar
-
-
-CallableT = TypeVar("CallableT", bound=Callable[..., object])
-
-
-def compose(*funcs):
- """
- Compose any number of unary functions into a single unary function.
-
- >>> import textwrap
- >>> expected = str.strip(textwrap.dedent(compose.__doc__))
- >>> strip_and_dedent = compose(str.strip, textwrap.dedent)
- >>> strip_and_dedent(compose.__doc__) == expected
- True
-
- Compose also allows the innermost function to take arbitrary arguments.
-
- >>> round_three = lambda x: round(x, ndigits=3)
- >>> f = compose(round_three, int.__truediv__)
- >>> [f(3*x, x+1) for x in range(1,10)]
- [1.5, 2.0, 2.25, 2.4, 2.5, 2.571, 2.625, 2.667, 2.7]
- """
-
- def compose_two(f1, f2):
- return lambda *args, **kwargs: f1(f2(*args, **kwargs))
-
- return functools.reduce(compose_two, funcs)
-
-
-def method_caller(method_name, *args, **kwargs):
- """
- Return a function that will call a named method on the
- target object with optional positional and keyword
- arguments.
-
- >>> lower = method_caller('lower')
- >>> lower('MyString')
- 'mystring'
- """
-
- def call_method(target):
- func = getattr(target, method_name)
- return func(*args, **kwargs)
-
- return call_method
-
-
-def once(func):
- """
- Decorate func so it's only ever called the first time.
-
- This decorator can ensure that an expensive or non-idempotent function
- will not be expensive on subsequent calls and is idempotent.
-
- >>> add_three = once(lambda a: a+3)
- >>> add_three(3)
- 6
- >>> add_three(9)
- 6
- >>> add_three('12')
- 6
-
- To reset the stored value, simply clear the property ``saved_result``.
-
- >>> del add_three.saved_result
- >>> add_three(9)
- 12
- >>> add_three(8)
- 12
-
- Or invoke 'reset()' on it.
-
- >>> add_three.reset()
- >>> add_three(-3)
- 0
- >>> add_three(0)
- 0
- """
-
- @functools.wraps(func)
- def wrapper(*args, **kwargs):
- if not hasattr(wrapper, 'saved_result'):
- wrapper.saved_result = func(*args, **kwargs)
- return wrapper.saved_result
-
- wrapper.reset = lambda: vars(wrapper).__delitem__('saved_result')
- return wrapper
-
-
-def method_cache(
- method: CallableT,
- cache_wrapper: Callable[
- [CallableT], CallableT
- ] = functools.lru_cache(), # type: ignore[assignment]
-) -> CallableT:
- """
- Wrap lru_cache to support storing the cache data in the object instances.
-
- Abstracts the common paradigm where the method explicitly saves an
- underscore-prefixed protected property on first call and returns that
- subsequently.
-
- >>> class MyClass:
- ... calls = 0
- ...
- ... @method_cache
- ... def method(self, value):
- ... self.calls += 1
- ... return value
-
- >>> a = MyClass()
- >>> a.method(3)
- 3
- >>> for x in range(75):
- ... res = a.method(x)
- >>> a.calls
- 75
-
- Note that the apparent behavior will be exactly like that of lru_cache
- except that the cache is stored on each instance, so values in one
- instance will not flush values from another, and when an instance is
- deleted, so are the cached values for that instance.
-
- >>> b = MyClass()
- >>> for x in range(35):
- ... res = b.method(x)
- >>> b.calls
- 35
- >>> a.method(0)
- 0
- >>> a.calls
- 75
-
- Note that if method had been decorated with ``functools.lru_cache()``,
- a.calls would have been 76 (due to the cached value of 0 having been
- flushed by the 'b' instance).
-
- Clear the cache with ``.cache_clear()``
-
- >>> a.method.cache_clear()
-
- Same for a method that hasn't yet been called.
-
- >>> c = MyClass()
- >>> c.method.cache_clear()
-
- Another cache wrapper may be supplied:
-
- >>> cache = functools.lru_cache(maxsize=2)
- >>> MyClass.method2 = method_cache(lambda self: 3, cache_wrapper=cache)
- >>> a = MyClass()
- >>> a.method2()
- 3
-
- Caution - do not subsequently wrap the method with another decorator, such
- as ``@property``, which changes the semantics of the function.
-
- See also
- http://code.activestate.com/recipes/577452-a-memoize-decorator-for-instance-methods/
- for another implementation and additional justification.
- """
-
- def wrapper(self: object, *args: object, **kwargs: object) -> object:
- # it's the first call, replace the method with a cached, bound method
- bound_method: CallableT = types.MethodType( # type: ignore[assignment]
- method, self
- )
- cached_method = cache_wrapper(bound_method)
- setattr(self, method.__name__, cached_method)
- return cached_method(*args, **kwargs)
-
- # Support cache clear even before cache has been created.
- wrapper.cache_clear = lambda: None # type: ignore[attr-defined]
-
- return ( # type: ignore[return-value]
- _special_method_cache(method, cache_wrapper) or wrapper
- )
-
-
-def _special_method_cache(method, cache_wrapper):
- """
- Because Python treats special methods differently, it's not
- possible to use instance attributes to implement the cached
- methods.
-
- Instead, install the wrapper method under a different name
- and return a simple proxy to that wrapper.
-
- https://github.com/jaraco/jaraco.functools/issues/5
- """
- name = method.__name__
- special_names = '__getattr__', '__getitem__'
- if name not in special_names:
- return
-
- wrapper_name = '__cached' + name
-
- def proxy(self, *args, **kwargs):
- if wrapper_name not in vars(self):
- bound = types.MethodType(method, self)
- cache = cache_wrapper(bound)
- setattr(self, wrapper_name, cache)
- else:
- cache = getattr(self, wrapper_name)
- return cache(*args, **kwargs)
-
- return proxy
-
-
-def apply(transform):
- """
- Decorate a function with a transform function that is
- invoked on results returned from the decorated function.
-
- >>> @apply(reversed)
- ... def get_numbers(start):
- ... "doc for get_numbers"
- ... return range(start, start+3)
- >>> list(get_numbers(4))
- [6, 5, 4]
- >>> get_numbers.__doc__
- 'doc for get_numbers'
- """
-
- def wrap(func):
- return functools.wraps(func)(compose(transform, func))
-
- return wrap
-
-
-def result_invoke(action):
- r"""
- Decorate a function with an action function that is
- invoked on the results returned from the decorated
- function (for its side-effect), then return the original
- result.
-
- >>> @result_invoke(print)
- ... def add_two(a, b):
- ... return a + b
- >>> x = add_two(2, 3)
- 5
- >>> x
- 5
- """
-
- def wrap(func):
- @functools.wraps(func)
- def wrapper(*args, **kwargs):
- result = func(*args, **kwargs)
- action(result)
- return result
-
- return wrapper
-
- return wrap
-
-
-def call_aside(f, *args, **kwargs):
- """
- Call a function for its side effect after initialization.
-
- >>> @call_aside
- ... def func(): print("called")
- called
- >>> func()
- called
-
- Use functools.partial to pass parameters to the initial call
-
- >>> @functools.partial(call_aside, name='bingo')
- ... def func(name): print("called with", name)
- called with bingo
- """
- f(*args, **kwargs)
- return f
-
-
-class Throttler:
- """
- Rate-limit a function (or other callable)
- """
-
- def __init__(self, func, max_rate=float('Inf')):
- if isinstance(func, Throttler):
- func = func.func
- self.func = func
- self.max_rate = max_rate
- self.reset()
-
- def reset(self):
- self.last_called = 0
-
- def __call__(self, *args, **kwargs):
- self._wait()
- return self.func(*args, **kwargs)
-
- def _wait(self):
- "ensure at least 1/max_rate seconds from last call"
- elapsed = time.time() - self.last_called
- must_wait = 1 / self.max_rate - elapsed
- time.sleep(max(0, must_wait))
- self.last_called = time.time()
-
- def __get__(self, obj, type=None):
- return first_invoke(self._wait, functools.partial(self.func, obj))
-
-
-def first_invoke(func1, func2):
- """
- Return a function that when invoked will invoke func1 without
- any parameters (for its side-effect) and then invoke func2
- with whatever parameters were passed, returning its result.
- """
-
- def wrapper(*args, **kwargs):
- func1()
- return func2(*args, **kwargs)
-
- return wrapper
-
-
-def retry_call(func, cleanup=lambda: None, retries=0, trap=()):
- """
- Given a callable func, trap the indicated exceptions
- for up to 'retries' times, invoking cleanup on the
- exception. On the final attempt, allow any exceptions
- to propagate.
- """
- attempts = itertools.count() if retries == float('inf') else range(retries)
- for attempt in attempts:
- try:
- return func()
- except trap:
- cleanup()
-
- return func()
-
-
-def retry(*r_args, **r_kwargs):
- """
- Decorator wrapper for retry_call. Accepts arguments to retry_call
- except func and then returns a decorator for the decorated function.
-
- Ex:
-
- >>> @retry(retries=3)
- ... def my_func(a, b):
- ... "this is my funk"
- ... print(a, b)
- >>> my_func.__doc__
- 'this is my funk'
- """
-
- def decorate(func):
- @functools.wraps(func)
- def wrapper(*f_args, **f_kwargs):
- bound = functools.partial(func, *f_args, **f_kwargs)
- return retry_call(bound, *r_args, **r_kwargs)
-
- return wrapper
-
- return decorate
-
-
-def print_yielded(func):
- """
- Convert a generator into a function that prints all yielded elements
-
- >>> @print_yielded
- ... def x():
- ... yield 3; yield None
- >>> x()
- 3
- None
- """
- print_all = functools.partial(map, print)
- print_results = compose(more_itertools.consume, print_all, func)
- return functools.wraps(func)(print_results)
-
-
-def pass_none(func):
- """
- Wrap func so it's not called if its first param is None
-
- >>> print_text = pass_none(print)
- >>> print_text('text')
- text
- >>> print_text(None)
- """
-
- @functools.wraps(func)
- def wrapper(param, *args, **kwargs):
- if param is not None:
- return func(param, *args, **kwargs)
-
- return wrapper
-
-
-def assign_params(func, namespace):
- """
- Assign parameters from namespace where func solicits.
-
- >>> def func(x, y=3):
- ... print(x, y)
- >>> assigned = assign_params(func, dict(x=2, z=4))
- >>> assigned()
- 2 3
-
- The usual errors are raised if a function doesn't receive
- its required parameters:
-
- >>> assigned = assign_params(func, dict(y=3, z=4))
- >>> assigned()
- Traceback (most recent call last):
- TypeError: func() ...argument...
-
- It even works on methods:
-
- >>> class Handler:
- ... def meth(self, arg):
- ... print(arg)
- >>> assign_params(Handler().meth, dict(arg='crystal', foo='clear'))()
- crystal
- """
- sig = inspect.signature(func)
- params = sig.parameters.keys()
- call_ns = {k: namespace[k] for k in params if k in namespace}
- return functools.partial(func, **call_ns)
-
-
-def save_method_args(method):
- """
- Wrap a method such that when it is called, the args and kwargs are
- saved on the method.
-
- >>> class MyClass:
- ... @save_method_args
- ... def method(self, a, b):
- ... print(a, b)
- >>> my_ob = MyClass()
- >>> my_ob.method(1, 2)
- 1 2
- >>> my_ob._saved_method.args
- (1, 2)
- >>> my_ob._saved_method.kwargs
- {}
- >>> my_ob.method(a=3, b='foo')
- 3 foo
- >>> my_ob._saved_method.args
- ()
- >>> my_ob._saved_method.kwargs == dict(a=3, b='foo')
- True
-
- The arguments are stored on the instance, allowing for
- different instance to save different args.
-
- >>> your_ob = MyClass()
- >>> your_ob.method({str('x'): 3}, b=[4])
- {'x': 3} [4]
- >>> your_ob._saved_method.args
- ({'x': 3},)
- >>> my_ob._saved_method.args
- ()
- """
- args_and_kwargs = collections.namedtuple('args_and_kwargs', 'args kwargs')
-
- @functools.wraps(method)
- def wrapper(self, *args, **kwargs):
- attr_name = '_saved_' + method.__name__
- attr = args_and_kwargs(args, kwargs)
- setattr(self, attr_name, attr)
- return method(self, *args, **kwargs)
-
- return wrapper
-
-
-def except_(*exceptions, replace=None, use=None):
- """
- Replace the indicated exceptions, if raised, with the indicated
- literal replacement or evaluated expression (if present).
-
- >>> safe_int = except_(ValueError)(int)
- >>> safe_int('five')
- >>> safe_int('5')
- 5
-
- Specify a literal replacement with ``replace``.
-
- >>> safe_int_r = except_(ValueError, replace=0)(int)
- >>> safe_int_r('five')
- 0
-
- Provide an expression to ``use`` to pass through particular parameters.
-
- >>> safe_int_pt = except_(ValueError, use='args[0]')(int)
- >>> safe_int_pt('five')
- 'five'
-
- """
-
- def decorate(func):
- @functools.wraps(func)
- def wrapper(*args, **kwargs):
- try:
- return func(*args, **kwargs)
- except exceptions:
- try:
- return eval(use)
- except TypeError:
- return replace
-
- return wrapper
-
- return decorate
diff --git a/spaces/Audio-AGI/AudioSep/models/CLAP/training/lp_train.py b/spaces/Audio-AGI/AudioSep/models/CLAP/training/lp_train.py
deleted file mode 100644
index 24a19bacd0a4b789415cfccbce1f8bc99bc493ed..0000000000000000000000000000000000000000
--- a/spaces/Audio-AGI/AudioSep/models/CLAP/training/lp_train.py
+++ /dev/null
@@ -1,301 +0,0 @@
-import json
-import logging
-import math
-import os
-import time
-from contextlib import suppress
-
-import numpy as np
-import torch
-import torch.nn.functional as F
-
-try:
- import wandb
-except ImportError:
- wandb = None
-
-from open_clip import LPLoss, LPMetrics, lp_gather_features
-from open_clip.utils import do_mixup, get_mix_lambda
-from .distributed import is_master
-from .zero_shot import zero_shot_eval
-
-
-class AverageMeter(object):
- """Computes and stores the average and current value"""
-
- def __init__(self):
- self.reset()
-
- def reset(self):
- self.val = 0
- self.avg = 0
- self.sum = 0
- self.count = 0
-
- def update(self, val, n=1):
- self.val = val
- self.sum += val * n
- self.count += n
- self.avg = self.sum / self.count
-
-
-def unwrap_model(model):
- if hasattr(model, "module"):
- return model.module
- else:
- return model
-
-
-def train_one_epoch(
- model,
- data,
- epoch,
- optimizer,
- scaler,
- scheduler,
- args,
- tb_writer=None,
- extra_suffix="",
-):
- device = torch.device(args.device)
- autocast = torch.cuda.amp.autocast if args.precision == "amp" else suppress
- model.train()
- loss = LPLoss(args.lp_loss)
-
- dataloader, sampler = data["train"].dataloader, data["train"].sampler
- if args.distributed and sampler is not None:
- sampler.set_epoch(epoch)
- num_batches_per_epoch = dataloader.num_batches
- sample_digits = math.ceil(math.log(dataloader.num_samples + 1, 10))
-
- # for toy dataset
- if args.dataset_type == "toy":
- dataloader.dataset.generate_queue()
-
- loss_m = AverageMeter()
- batch_time_m = AverageMeter()
- data_time_m = AverageMeter()
- end = time.time()
-
- for i, batch in enumerate(dataloader):
- step = num_batches_per_epoch * epoch + i
-
- if isinstance(scheduler, dict):
- for s in scheduler.values():
- s(step)
- else:
- scheduler(step)
-
- audio = batch # contains mel_spec, wavform, and longer list
- class_label = batch["class_label"]
- # audio = audio.to(device=device, non_blocking=True)
- class_label = class_label.to(device=device, non_blocking=True)
-
- if args.mixup:
- # https://github.com/RetroCirce/HTS-Audio-Transformer/blob/main/utils.py#L146
- mix_lambda = torch.from_numpy(
- get_mix_lambda(0.5, len(audio["waveform"]))
- ).to(device)
- class_label = do_mixup(class_label, mix_lambda)
- else:
- mix_lambda = None
-
- data_time_m.update(time.time() - end)
- if isinstance(optimizer, dict):
- for o_ in optimizer.values():
- o_.zero_grad()
- else:
- optimizer.zero_grad()
-
- with autocast():
- pred = model(audio, mix_lambda=mix_lambda, device=device)
- total_loss = loss(pred, class_label)
-
- if isinstance(optimizer, dict):
- if scaler is not None:
- scaler.scale(total_loss).backward()
- for o_ in optimizer.values():
- if args.horovod:
- o_.synchronize()
- scaler.unscale_(o_)
- with o_.skip_synchronize():
- scaler.step(o_)
- else:
- scaler.step(o_)
- scaler.update()
- else:
- total_loss.backward()
- for o_ in optimizer.values():
- o_.step()
- else:
- if scaler is not None:
- scaler.scale(total_loss).backward()
- if args.horovod:
- optimizer.synchronize()
- scaler.unscale_(optimizer)
- with optimizer.skip_synchronize():
- scaler.step(optimizer)
- else:
- scaler.step(optimizer)
- scaler.update()
- else:
- total_loss.backward()
- optimizer.step()
-
- # Note: we clamp to 4.6052 = ln(100), as in the original paper.
- with torch.no_grad():
- unwrap_model(model).clap_model.logit_scale_a.clamp_(0, math.log(100))
- unwrap_model(model).clap_model.logit_scale_t.clamp_(0, math.log(100))
-
- batch_time_m.update(time.time() - end)
- end = time.time()
- batch_count = i + 1
-
- if is_master(args) and (i % 100 == 0 or batch_count == num_batches_per_epoch):
- if isinstance(audio, dict):
- batch_size = len(audio["waveform"])
- else:
- batch_size = len(audio)
- num_samples = batch_count * batch_size * args.world_size
- samples_per_epoch = dataloader.num_samples
- percent_complete = 100.0 * batch_count / num_batches_per_epoch
-
- # NOTE loss is coarsely sampled, just master node and per log update
- loss_m.update(total_loss.item(), batch_size)
- if isinstance(optimizer, dict):
- logging.info(
- f"Train Epoch: {epoch} [{num_samples:>{sample_digits}}/{samples_per_epoch} ({percent_complete:.0f}%)] "
- f"Loss: {loss_m.val:#.5g} ({loss_m.avg:#.4g}) "
- f"Data (t): {data_time_m.avg:.3f} "
- f"Batch (t): {batch_time_m.avg:.3f} "
- f"LR: {[o_.param_groups[0]['lr'] for o_ in optimizer.values()]}"
- )
- log_data = {
- "loss": loss_m.val,
- "data_time": data_time_m.val,
- "batch_time": batch_time_m.val,
- "lr": [o_.param_groups[0]["lr"] for o_ in optimizer.values()],
- }
- else:
- logging.info(
- f"Train Epoch: {epoch} [{num_samples:>{sample_digits}}/{samples_per_epoch} ({percent_complete:.0f}%)] "
- f"Loss: {loss_m.val:#.5g} ({loss_m.avg:#.4g}) "
- f"Data (t): {data_time_m.avg:.3f} "
- f"Batch (t): {batch_time_m.avg:.3f} "
- f"LR: {optimizer.param_groups[0]['lr']:5f} "
- )
-
- # Save train loss / etc. Using non avg meter values as loggers have their own smoothing
- log_data = {
- "loss": loss_m.val,
- "data_time": data_time_m.val,
- "batch_time": batch_time_m.val,
- "lr": optimizer.param_groups[0]["lr"],
- }
- for name, val in log_data.items():
- name = f"train{extra_suffix}/{name}"
- if tb_writer is not None:
- tb_writer.add_scalar(name, val, step)
- if args.wandb:
- assert wandb is not None, "Please install wandb."
- wandb.log({name: val, "step": step})
-
- # resetting batch / data time meters per log window
- batch_time_m.reset()
- data_time_m.reset()
- # end for
-
-
-def evaluate(model, data, epoch, args, tb_writer=None, extra_suffix=""):
- metrics = {}
- if not args.parallel_eval:
- if not is_master(args):
- return metrics
- device = torch.device(args.device)
- model.eval()
-
- # CHANGE
- # zero_shot_metrics = zero_shot_eval(model, data, epoch, args)
- # metrics.update(zero_shot_metrics)
- if is_master(args):
- print("Evaluating...")
- metric_names = args.lp_metrics.split(",")
- eval_tool = LPMetrics(metric_names=metric_names)
-
- autocast = torch.cuda.amp.autocast if args.precision == "amp" else suppress
- if "val" in data and (
- args.val_frequency
- and ((epoch % args.val_frequency) == 0 or epoch == args.epochs)
- ):
- if args.parallel_eval:
- dataloader, sampler = data["val"].dataloader, data["val"].sampler
- if args.distributed and sampler is not None:
- sampler.set_epoch(epoch)
- samples_per_val = dataloader.num_samples
- else:
- dataloader = data["val"].dataloader
- num_samples = 0
- samples_per_val = dataloader.num_samples
-
- eval_info = {"pred": [], "target": []}
- with torch.no_grad():
- for i, batch in enumerate(dataloader):
- audio = batch # contains mel_spec, wavform, and longer list
- class_label = batch["class_label"]
-
- # audio = audio.to(device=device, non_blocking=True)
- class_label = class_label.to(device=device, non_blocking=True)
-
- with autocast():
- pred = model(audio, device=device)
- if args.parallel_eval:
- pred, class_label = lp_gather_features(
- pred, class_label, args.world_size, args.horovod
- )
- eval_info["pred"].append(pred)
- eval_info["target"].append(class_label)
-
- num_samples += class_label.shape[0]
-
- if (i % 100) == 0: # and i != 0:
- logging.info(
- f"Eval Epoch: {epoch} [{num_samples} / {samples_per_val}]"
- )
-
- if is_master(args):
- eval_info["pred"] = torch.cat(eval_info["pred"], 0).cpu()
- eval_info["target"] = torch.cat(eval_info["target"], 0).cpu()
- metric_dict = eval_tool.evaluate_mertics(
- eval_info["pred"], eval_info["target"]
- )
- metrics.update(metric_dict)
- if "epoch" not in metrics.keys():
- metrics.update({"epoch": epoch})
-
- if is_master(args):
- if not metrics:
- return metrics
-
- logging.info(
- f"Eval Epoch: {epoch} "
- + "\n".join(
- ["\t".join([f"{m}: {round(metrics[m], 4):.4f}"]) for m in metrics]
- )
- )
- if args.save_logs:
- for name, val in metrics.items():
- if tb_writer is not None:
- tb_writer.add_scalar(f"val{extra_suffix}/{name}", val, epoch)
-
- with open(os.path.join(args.checkpoint_path, "results.jsonl"), "a+") as f:
- f.write(json.dumps(metrics))
- f.write("\n")
-
- if args.wandb:
- assert wandb is not None, "Please install wandb."
- for name, val in metrics.items():
- wandb.log({f"val{extra_suffix}/{name}": val, "epoch": epoch})
-
- return metrics
- else:
- return metrics
diff --git a/spaces/Audio-AGI/WavJourney/VoiceParser/customtokenizer.py b/spaces/Audio-AGI/WavJourney/VoiceParser/customtokenizer.py
deleted file mode 100644
index aa2e7d49bab149dfe3cb43db5502a4c5b40821c1..0000000000000000000000000000000000000000
--- a/spaces/Audio-AGI/WavJourney/VoiceParser/customtokenizer.py
+++ /dev/null
@@ -1,202 +0,0 @@
-"""
-Custom tokenizer model.
-Author: https://www.github.com/gitmylo/
-License: MIT
-"""
-
-import json
-import os.path
-from zipfile import ZipFile
-from typing import Union
-
-
-import numpy
-import torch
-from torch import nn, optim
-from torch.serialization import MAP_LOCATION
-
-
-class CustomTokenizer(nn.Module):
- def __init__(self, hidden_size=1024, input_size=768, output_size=10000, version=0):
- super(CustomTokenizer, self).__init__()
- next_size = input_size
- if version == 0:
- self.lstm = nn.LSTM(input_size, hidden_size, 2, batch_first=True)
- next_size = hidden_size
- if version == 1:
- self.lstm = nn.LSTM(input_size, hidden_size, 2, batch_first=True)
- self.intermediate = nn.Linear(hidden_size, 4096)
- next_size = 4096
-
- self.fc = nn.Linear(next_size, output_size)
- self.softmax = nn.LogSoftmax(dim=1)
- self.optimizer: optim.Optimizer = None
- self.lossfunc = nn.CrossEntropyLoss()
- self.input_size = input_size
- self.hidden_size = hidden_size
- self.output_size = output_size
- self.version = version
-
- def forward(self, x):
- x, _ = self.lstm(x)
- if self.version == 1:
- x = self.intermediate(x)
- x = self.fc(x)
- x = self.softmax(x)
- return x
-
- @torch.no_grad()
- def get_token(self, x):
- """
- Used to get the token for the first
- :param x: An array with shape (N, input_size) where N is a whole number greater or equal to 1, and input_size is the input size used when creating the model.
- :return: An array with shape (N,) where N is the same as N from the input. Every number in the array is a whole number in range 0...output_size - 1 where output_size is the output size used when creating the model.
- """
- return torch.argmax(self(x), dim=1)
-
- def prepare_training(self):
- self.optimizer = optim.Adam(self.parameters(), 0.001)
-
- def train_step(self, x_train, y_train, log_loss=False):
- # y_train = y_train[:-1]
- # y_train = y_train[1:]
-
- optimizer = self.optimizer
- lossfunc = self.lossfunc
- # Zero the gradients
- self.zero_grad()
-
- # Forward pass
- y_pred = self(x_train)
-
- y_train_len = len(y_train)
- y_pred_len = y_pred.shape[0]
-
- if y_train_len > y_pred_len:
- diff = y_train_len - y_pred_len
- y_train = y_train[diff:]
- elif y_train_len < y_pred_len:
- diff = y_pred_len - y_train_len
- y_pred = y_pred[:-diff, :]
-
- y_train_hot = torch.zeros(len(y_train), self.output_size)
- y_train_hot[range(len(y_train)), y_train] = 1
- y_train_hot = y_train_hot.to('cuda')
-
- # Calculate the loss
- loss = lossfunc(y_pred, y_train_hot)
-
- # Print loss
- if log_loss:
- print('Loss', loss.item())
-
- # Backward pass
- loss.backward()
-
- # Update the weights
- optimizer.step()
-
- def save(self, path):
- info_path = '.'.join(os.path.basename(path).split('.')[:-1]) + '/.info'
- torch.save(self.state_dict(), path)
- data_from_model = Data(self.input_size, self.hidden_size, self.output_size, self.version)
- with ZipFile(path, 'a') as model_zip:
- model_zip.writestr(info_path, data_from_model.save())
- model_zip.close()
-
- @staticmethod
- def load_from_checkpoint(path, map_location: MAP_LOCATION = None):
- old = True
- with ZipFile(path) as model_zip:
- filesMatch = [file for file in model_zip.namelist() if file.endswith('/.info')]
- file = filesMatch[0] if filesMatch else None
- if file:
- old = False
- data_from_model = Data.load(model_zip.read(file).decode('utf-8'))
- model_zip.close()
- if old:
- model = CustomTokenizer()
- else:
- model = CustomTokenizer(data_from_model.hidden_size, data_from_model.input_size, data_from_model.output_size, data_from_model.version)
- model.load_state_dict(torch.load(path, map_location=map_location))
- if map_location:
- model = model.to(map_location)
- return model
-
-
-
-class Data:
- input_size: int
- hidden_size: int
- output_size: int
- version: int
-
- def __init__(self, input_size=768, hidden_size=1024, output_size=10000, version=0):
- self.input_size = input_size
- self.hidden_size = hidden_size
- self.output_size = output_size
- self.version = version
-
- @staticmethod
- def load(string):
- data = json.loads(string)
- return Data(data['input_size'], data['hidden_size'], data['output_size'], data['version'])
-
- def save(self):
- data = {
- 'input_size': self.input_size,
- 'hidden_size': self.hidden_size,
- 'output_size': self.output_size,
- 'version': self.version,
- }
- return json.dumps(data)
-
-
-def auto_train(data_path, save_path='model.pth', lload_model: Union[str, None] = None, save_epochs=1):
- data_x, data_y = {}, {}
-
- if load_model and os.path.isfile(load_model):
- print('Loading model from', load_model)
- model_training = CustomTokenizer.load_from_checkpoint(load_model, 'cuda')
- else:
- print('Creating new model.')
- model_training = CustomTokenizer(version=1).to('cuda')
- save_path = os.path.join(data_path, save_path)
- base_save_path = '.'.join(save_path.split('.')[:-1])
-
- sem_string = '_semantic.npy'
- feat_string = '_semantic_features.npy'
-
- ready = os.path.join(data_path, 'ready')
- for input_file in os.listdir(ready):
- full_path = os.path.join(ready, input_file)
- try:
- prefix = input_file.split("_")[0]
- number = int(prefix)
- except ValueError as e:
- raise e
- if input_file.endswith(sem_string):
- data_y[number] = numpy.load(full_path)
- elif input_file.endswith(feat_string):
- data_x[number] = numpy.load(full_path)
-
- model_training.prepare_training()
- epoch = 1
-
- while 1:
- for i in range(save_epochs):
- j = 0
- for i in range(max(len(data_x), len(data_y))):
- x = data_x.get(i)
- y = data_y.get(i)
- if x is None or y is None:
- print(f'The training data does not match. key={i}')
- continue
- model_training.train_step(torch.tensor(x).to('cuda'), torch.tensor(y).to('cuda'), j % 50 == 0) # Print loss every 50 steps
- j += 1
- save_p = save_path
- save_p_2 = f'{base_save_path}_epoch_{epoch}.pth'
- model_training.save(save_p)
- model_training.save(save_p_2)
- print(f'Epoch {epoch} completed')
- epoch += 1
\ No newline at end of file
diff --git a/spaces/Benson/text-generation/Examples/Bitcoin-qt.exe Download.md b/spaces/Benson/text-generation/Examples/Bitcoin-qt.exe Download.md
deleted file mode 100644
index d7aa2315d80164d41046b333c89cd4a579cccc7b..0000000000000000000000000000000000000000
--- a/spaces/Benson/text-generation/Examples/Bitcoin-qt.exe Download.md
+++ /dev/null
@@ -1,61 +0,0 @@
-
-
Cómo descargar y usar Bitcoin-Qt.exe, el cliente oficial de Bitcoin
-
Bitcoin es una moneda digital descentralizada que permite transacciones peer-to-peer sin intermediarios. Para usar Bitcoin, necesitas un programa de software que te permita interactuar con la red Bitcoin y administrar tus fondos. En este artículo, te mostraremos cómo descargar y usar Bitcoin-Qt.exe, el cliente oficial de Bitcoin para Windows. También discutiremos algunas de las características y beneficios de usar Bitcoin-Qt.exe, así como algunas de las alternativas que puedes considerar.
-
¿Qué es Bitcoin-Qt.exe y por qué lo necesita?
-
Bitcoin-Qt.exe es el cliente original de Bitcoin que fue desarrollado por Satoshi Nakamoto, el creador de Bitcoin. También se conoce como Bitcoin Core, ya que forma el núcleo de la red Bitcoin. Bitcoin-Qt.exe es un cliente de nodo completo, lo que significa que descarga y valida todo el historial de transacciones en la cadena de bloques, el libro mayor distribuido que registra todas las transacciones de Bitcoin. Al ejecutar Bitcoin-Qt.exe, estás contribuyendo a la seguridad y estabilidad de la red.
Bitcoin-Qt.exe le proporciona seguridad, privacidad y control total sobre sus fondos
-
Una de las principales ventajas de usar Bitcoin-Qt.exe es que te proporciona un alto nivel de seguridad, privacidad y control total sobre tus fondos. A diferencia de otros clientes o carteras que dependen de servicios o servidores de terceros, Bitcoin-Qt.exe no almacena sus claves privadas o sus fondos en ningún otro lugar, sino en su propio ordenador. Esto significa que usted es el único que puede acceder y gastar sus bitcoins, y nadie puede congelar, incautar o censurar sus transacciones. También es responsable de mantener sus llaves privadas y su archivo de billetera a salvo del robo o pérdida.
-
-
Otra ventaja de usar Bitcoin-Qt.exe es que soporta funciones avanzadas que te permiten personalizar y optimizar tu experiencia con Bitcoin. Por ejemplo, puede crear y difundir transacciones sin procesar, que son transacciones que construye manualmente sin usar una interfaz gráfica. También puede usar comandos RPC, que son comandos que puede enviar a Bitcoin-Qt.exe para interactuar con la red Bitcoin y realizar varias operaciones. También puede utilizar BIPs, que son propuestas de mejora de Bitcoin que introducen nuevas características o estándares para el protocolo de Bitcoin. Por ejemplo, puede usar BIP39 para generar una frase mnemotécnica que puede ayudarlo a recuperar su billetera en caso de pérdida o daño.
-
Cómo descargar Bitcoin-Qt.exe para Windows
-
Si desea utilizar Bitcoin-Qt.exe para Windows, debe descargarlo desde el sitio web oficial o desde una fuente de confianza. También necesita verificar la integridad y autenticidad del archivo descargado e instalarlo en su computadora. Estos son los pasos que debes seguir:
-
Puede descargar Bitcoin-Qt.exe desde el sitio web oficial o desde una fuente de confianza
-
El sitio web oficial de Bitcoin-Qt.exe es https://bitcoincore.org, donde puede encontrar la última versión del cliente para Windows y otros sistemas operativos. También puede descargar Bitcoin-Qt.exe de otras fuentes, como https://bitcoin.org o Electrum, que es un cliente ligero que no requiere descargar la cadena de bloques, sino que se conecta a servidores remotos que proporcionan la información necesaria. También puede usar MultiBit HD, que es un cliente fácil de usar que admite múltiples carteras e idiomas. Puede encontrar más clientes de Bitcoin para Windows en el sitio web oficial o en otras fuentes.
-
Puede utilizar carteras basadas en la web o móviles que son más convenientes pero menos seguras
-
-
Puedes usar carteras de hardware o de papel que son más seguras pero menos convenientes
-
Si desea usar una billetera de hardware o una billetera de papel que sea más segura pero menos conveniente, puede elegir entre una variedad de opciones que ofrecen diferentes características y funcionalidades. Por ejemplo, puede usar Ledger, que es una cartera de hardware que almacena sus claves privadas en una tarjeta inteligente que se conecta a su computadora a través de USB, pero también requiere que ingrese un código PIN y confirme cada transacción en la pantalla del dispositivo. También puede usar Bitaddress.org o Bitcoinpaperwallet.com para generar e imprimir su billetera de papel, pero debe asegurarse de hacerlo sin conexión y en una computadora e impresora seguras y limpias. También debe mantener su billetera de papel a salvo del fuego, el agua o los daños físicos, y escanearla con un lector de código QR cada vez que desee acceder a sus fondos. Puede encontrar más información sobre carteras de papel en el sitio web oficial o en otras fuentes.
-
Conclusión
-
-
Preguntas frecuentes
-
¿Cuáles son los requisitos del sistema para ejecutar Bitcoin-Qt.exe?
-
Para ejecutar Bitcoin-Qt.exe, necesita un sistema operativo Windows (7 o posterior), un procesador de 64 bits, al menos 2 GB de RAM, al menos 400 GB de espacio en disco (preferiblemente SSD), y una conexión a Internet de banda ancha.
-
¿Cómo puedo actualizar Bitcoin-Qt.exe a la última versión?
-
Para actualizar Bitcoin-Qt.exe a la última versión, debe descargar la nueva versión desde el sitio web oficial o desde una fuente de confianza, verificar el archivo e instalarlo sobre la versión anterior. No es necesario desinstalar la versión anterior o eliminar su directorio de datos.
-
¿Cómo puedo restaurar mi billetera desde una copia de seguridad?
-
Para restaurar su billetera desde una copia de seguridad, necesita copiar su archivo de copia de seguridad (generalmente llamado wallet.dat) a su directorio de datos, reemplazando el archivo existente si hay uno. Es posible que tenga que volver a analizar la cadena de bloques para actualizar su saldo y el historial de transacciones.
-
¿Cómo puedo importar o exportar mis claves privadas?
-
Para importar o exportar tus claves privadas, necesitas usar la pestaña Console en la ventana Debug. Puede utilizar comandos como importprivkey, dumpprivkey, o dumpwallet para importar o exportar sus claves privadas. Debes tener cuidado al manejar tus llaves privadas, ya que son muy sensibles y pueden comprometer tus fondos si se exponen o se pierden.
-
¿Cómo puedo contactar a los desarrolladores u obtener soporte para Bitcoin-Qt.exe?
-
Para contactar a los desarrolladores u obtener soporte para Bitcoin-Qt.exe, puedes usar los siguientes canales:
64aa2da5cf
-
-
\ No newline at end of file
diff --git a/spaces/Big-Web/MMSD/env/Lib/site-packages/setuptools/_vendor/pyparsing/__init__.py b/spaces/Big-Web/MMSD/env/Lib/site-packages/setuptools/_vendor/pyparsing/__init__.py
deleted file mode 100644
index 7802ff158d83eb88e6dbe78d9cd33ca14341662a..0000000000000000000000000000000000000000
--- a/spaces/Big-Web/MMSD/env/Lib/site-packages/setuptools/_vendor/pyparsing/__init__.py
+++ /dev/null
@@ -1,331 +0,0 @@
-# module pyparsing.py
-#
-# Copyright (c) 2003-2022 Paul T. McGuire
-#
-# Permission is hereby granted, free of charge, to any person obtaining
-# a copy of this software and associated documentation files (the
-# "Software"), to deal in the Software without restriction, including
-# without limitation the rights to use, copy, modify, merge, publish,
-# distribute, sublicense, and/or sell copies of the Software, and to
-# permit persons to whom the Software is furnished to do so, subject to
-# the following conditions:
-#
-# The above copyright notice and this permission notice shall be
-# included in all copies or substantial portions of the Software.
-#
-# THE SOFTWARE IS PROVIDED "AS IS", WITHOUT WARRANTY OF ANY KIND,
-# EXPRESS OR IMPLIED, INCLUDING BUT NOT LIMITED TO THE WARRANTIES OF
-# MERCHANTABILITY, FITNESS FOR A PARTICULAR PURPOSE AND NONINFRINGEMENT.
-# IN NO EVENT SHALL THE AUTHORS OR COPYRIGHT HOLDERS BE LIABLE FOR ANY
-# CLAIM, DAMAGES OR OTHER LIABILITY, WHETHER IN AN ACTION OF CONTRACT,
-# TORT OR OTHERWISE, ARISING FROM, OUT OF OR IN CONNECTION WITH THE
-# SOFTWARE OR THE USE OR OTHER DEALINGS IN THE SOFTWARE.
-#
-
-__doc__ = """
-pyparsing module - Classes and methods to define and execute parsing grammars
-=============================================================================
-
-The pyparsing module is an alternative approach to creating and
-executing simple grammars, vs. the traditional lex/yacc approach, or the
-use of regular expressions. With pyparsing, you don't need to learn
-a new syntax for defining grammars or matching expressions - the parsing
-module provides a library of classes that you use to construct the
-grammar directly in Python.
-
-Here is a program to parse "Hello, World!" (or any greeting of the form
-``", !"``), built up using :class:`Word`,
-:class:`Literal`, and :class:`And` elements
-(the :meth:`'+'` operators create :class:`And` expressions,
-and the strings are auto-converted to :class:`Literal` expressions)::
-
- from pyparsing import Word, alphas
-
- # define grammar of a greeting
- greet = Word(alphas) + "," + Word(alphas) + "!"
-
- hello = "Hello, World!"
- print(hello, "->", greet.parse_string(hello))
-
-The program outputs the following::
-
- Hello, World! -> ['Hello', ',', 'World', '!']
-
-The Python representation of the grammar is quite readable, owing to the
-self-explanatory class names, and the use of :class:`'+'`,
-:class:`'|'`, :class:`'^'` and :class:`'&'` operators.
-
-The :class:`ParseResults` object returned from
-:class:`ParserElement.parseString` can be
-accessed as a nested list, a dictionary, or an object with named
-attributes.
-
-The pyparsing module handles some of the problems that are typically
-vexing when writing text parsers:
-
- - extra or missing whitespace (the above program will also handle
- "Hello,World!", "Hello , World !", etc.)
- - quoted strings
- - embedded comments
-
-
-Getting Started -
------------------
-Visit the classes :class:`ParserElement` and :class:`ParseResults` to
-see the base classes that most other pyparsing
-classes inherit from. Use the docstrings for examples of how to:
-
- - construct literal match expressions from :class:`Literal` and
- :class:`CaselessLiteral` classes
- - construct character word-group expressions using the :class:`Word`
- class
- - see how to create repetitive expressions using :class:`ZeroOrMore`
- and :class:`OneOrMore` classes
- - use :class:`'+'`, :class:`'|'`, :class:`'^'`,
- and :class:`'&'` operators to combine simple expressions into
- more complex ones
- - associate names with your parsed results using
- :class:`ParserElement.setResultsName`
- - access the parsed data, which is returned as a :class:`ParseResults`
- object
- - find some helpful expression short-cuts like :class:`delimitedList`
- and :class:`oneOf`
- - find more useful common expressions in the :class:`pyparsing_common`
- namespace class
-"""
-from typing import NamedTuple
-
-
-class version_info(NamedTuple):
- major: int
- minor: int
- micro: int
- releaselevel: str
- serial: int
-
- @property
- def __version__(self):
- return (
- "{}.{}.{}".format(self.major, self.minor, self.micro)
- + (
- "{}{}{}".format(
- "r" if self.releaselevel[0] == "c" else "",
- self.releaselevel[0],
- self.serial,
- ),
- "",
- )[self.releaselevel == "final"]
- )
-
- def __str__(self):
- return "{} {} / {}".format(__name__, self.__version__, __version_time__)
-
- def __repr__(self):
- return "{}.{}({})".format(
- __name__,
- type(self).__name__,
- ", ".join("{}={!r}".format(*nv) for nv in zip(self._fields, self)),
- )
-
-
-__version_info__ = version_info(3, 0, 9, "final", 0)
-__version_time__ = "05 May 2022 07:02 UTC"
-__version__ = __version_info__.__version__
-__versionTime__ = __version_time__
-__author__ = "Paul McGuire "
-
-from .util import *
-from .exceptions import *
-from .actions import *
-from .core import __diag__, __compat__
-from .results import *
-from .core import *
-from .core import _builtin_exprs as core_builtin_exprs
-from .helpers import *
-from .helpers import _builtin_exprs as helper_builtin_exprs
-
-from .unicode import unicode_set, UnicodeRangeList, pyparsing_unicode as unicode
-from .testing import pyparsing_test as testing
-from .common import (
- pyparsing_common as common,
- _builtin_exprs as common_builtin_exprs,
-)
-
-# define backward compat synonyms
-if "pyparsing_unicode" not in globals():
- pyparsing_unicode = unicode
-if "pyparsing_common" not in globals():
- pyparsing_common = common
-if "pyparsing_test" not in globals():
- pyparsing_test = testing
-
-core_builtin_exprs += common_builtin_exprs + helper_builtin_exprs
-
-
-__all__ = [
- "__version__",
- "__version_time__",
- "__author__",
- "__compat__",
- "__diag__",
- "And",
- "AtLineStart",
- "AtStringStart",
- "CaselessKeyword",
- "CaselessLiteral",
- "CharsNotIn",
- "Combine",
- "Dict",
- "Each",
- "Empty",
- "FollowedBy",
- "Forward",
- "GoToColumn",
- "Group",
- "IndentedBlock",
- "Keyword",
- "LineEnd",
- "LineStart",
- "Literal",
- "Located",
- "PrecededBy",
- "MatchFirst",
- "NoMatch",
- "NotAny",
- "OneOrMore",
- "OnlyOnce",
- "OpAssoc",
- "Opt",
- "Optional",
- "Or",
- "ParseBaseException",
- "ParseElementEnhance",
- "ParseException",
- "ParseExpression",
- "ParseFatalException",
- "ParseResults",
- "ParseSyntaxException",
- "ParserElement",
- "PositionToken",
- "QuotedString",
- "RecursiveGrammarException",
- "Regex",
- "SkipTo",
- "StringEnd",
- "StringStart",
- "Suppress",
- "Token",
- "TokenConverter",
- "White",
- "Word",
- "WordEnd",
- "WordStart",
- "ZeroOrMore",
- "Char",
- "alphanums",
- "alphas",
- "alphas8bit",
- "any_close_tag",
- "any_open_tag",
- "c_style_comment",
- "col",
- "common_html_entity",
- "counted_array",
- "cpp_style_comment",
- "dbl_quoted_string",
- "dbl_slash_comment",
- "delimited_list",
- "dict_of",
- "empty",
- "hexnums",
- "html_comment",
- "identchars",
- "identbodychars",
- "java_style_comment",
- "line",
- "line_end",
- "line_start",
- "lineno",
- "make_html_tags",
- "make_xml_tags",
- "match_only_at_col",
- "match_previous_expr",
- "match_previous_literal",
- "nested_expr",
- "null_debug_action",
- "nums",
- "one_of",
- "printables",
- "punc8bit",
- "python_style_comment",
- "quoted_string",
- "remove_quotes",
- "replace_with",
- "replace_html_entity",
- "rest_of_line",
- "sgl_quoted_string",
- "srange",
- "string_end",
- "string_start",
- "trace_parse_action",
- "unicode_string",
- "with_attribute",
- "indentedBlock",
- "original_text_for",
- "ungroup",
- "infix_notation",
- "locatedExpr",
- "with_class",
- "CloseMatch",
- "token_map",
- "pyparsing_common",
- "pyparsing_unicode",
- "unicode_set",
- "condition_as_parse_action",
- "pyparsing_test",
- # pre-PEP8 compatibility names
- "__versionTime__",
- "anyCloseTag",
- "anyOpenTag",
- "cStyleComment",
- "commonHTMLEntity",
- "countedArray",
- "cppStyleComment",
- "dblQuotedString",
- "dblSlashComment",
- "delimitedList",
- "dictOf",
- "htmlComment",
- "javaStyleComment",
- "lineEnd",
- "lineStart",
- "makeHTMLTags",
- "makeXMLTags",
- "matchOnlyAtCol",
- "matchPreviousExpr",
- "matchPreviousLiteral",
- "nestedExpr",
- "nullDebugAction",
- "oneOf",
- "opAssoc",
- "pythonStyleComment",
- "quotedString",
- "removeQuotes",
- "replaceHTMLEntity",
- "replaceWith",
- "restOfLine",
- "sglQuotedString",
- "stringEnd",
- "stringStart",
- "traceParseAction",
- "unicodeString",
- "withAttribute",
- "indentedBlock",
- "originalTextFor",
- "infixNotation",
- "locatedExpr",
- "withClass",
- "tokenMap",
- "conditionAsParseAction",
- "autoname_elements",
-]
diff --git a/spaces/Boadiwaa/Recipes/README.md b/spaces/Boadiwaa/Recipes/README.md
deleted file mode 100644
index c5f785c77c172d6acaf43538df6edd656c54c8e8..0000000000000000000000000000000000000000
--- a/spaces/Boadiwaa/Recipes/README.md
+++ /dev/null
@@ -1,12 +0,0 @@
----
-title: Recipes
-emoji: 🏢
-colorFrom: pink
-colorTo: gray
-sdk: gradio
-sdk_version: 3.16.2
-app_file: app.py
-pinned: false
----
-
-Check out the configuration reference at https://huggingface.co/docs/hub/spaces-config-reference
diff --git a/spaces/CVPR/LIVE/pybind11/.github/ISSUE_TEMPLATE/question.md b/spaces/CVPR/LIVE/pybind11/.github/ISSUE_TEMPLATE/question.md
deleted file mode 100644
index b199b6ee8ad446994aed54f67b0d1c22049d53c1..0000000000000000000000000000000000000000
--- a/spaces/CVPR/LIVE/pybind11/.github/ISSUE_TEMPLATE/question.md
+++ /dev/null
@@ -1,21 +0,0 @@
----
-name: Question
-about: File an issue about unexplained behavior
-title: "[QUESTION] "
----
-
-If you have a question, please check the following first:
-
-1. Check if your question has already been answered in the [FAQ][] section.
-2. Make sure you've read the [documentation][]. Your issue may be addressed there.
-3. If those resources didn't help and you only have a short question (not a bug report), consider asking in the [Gitter chat room][]
-4. Search the [issue tracker][], including the closed issues, to see if your question has already been asked/answered. +1 or comment if it has been asked but has no answer.
-5. If you have a more complex question which is not answered in the previous items (or not suitable for chat), please fill in the details below.
-6. Include a self-contained and minimal piece of code that illustrates your question. If that's not possible, try to make the description as clear as possible.
-
-[FAQ]: http://pybind11.readthedocs.io/en/latest/faq.html
-[documentation]: https://pybind11.readthedocs.io
-[issue tracker]: https://github.com/pybind/pybind11/issues
-[Gitter chat room]: https://gitter.im/pybind/Lobby
-
-*After reading, remove this checklist.*
diff --git a/spaces/CVPR/LIVE/pybind11/tests/test_stl_binders.cpp b/spaces/CVPR/LIVE/pybind11/tests/test_stl_binders.cpp
deleted file mode 100644
index 8688874091219f5a5035f5eb46e976e7408080b8..0000000000000000000000000000000000000000
--- a/spaces/CVPR/LIVE/pybind11/tests/test_stl_binders.cpp
+++ /dev/null
@@ -1,129 +0,0 @@
-/*
- tests/test_stl_binders.cpp -- Usage of stl_binders functions
-
- Copyright (c) 2016 Sergey Lyskov
-
- All rights reserved. Use of this source code is governed by a
- BSD-style license that can be found in the LICENSE file.
-*/
-
-#include "pybind11_tests.h"
-
-#include
-#include
-#include