diff --git a/spaces/101-5/gpt4free/g4f/.v1/gpt4free/usesless/test.py b/spaces/101-5/gpt4free/g4f/.v1/gpt4free/usesless/test.py
deleted file mode 100644
index ade1e0c52cd7d2443051baa8bf8a02baa1a2cc94..0000000000000000000000000000000000000000
--- a/spaces/101-5/gpt4free/g4f/.v1/gpt4free/usesless/test.py
+++ /dev/null
@@ -1,10 +0,0 @@
-# Fix by @enganese
-# Import Account class from __init__.py file
-from gpt4free import usesless
-
-# Create Account and enable logging to see all the log messages (it's very interesting, try it!)
-# New account credentials will be automatically saved in account.json file in such template: {"email": "username@1secmail.com", "token": "token here"}
-token = usesless.Account.create(logging=True)
-
-# Print the new token
-print(token)
diff --git a/spaces/1gistliPinn/ChatGPT4/Examples/Aces Of The Luftwaffe - Squadron Extended Edition Full Crack [portable].md b/spaces/1gistliPinn/ChatGPT4/Examples/Aces Of The Luftwaffe - Squadron Extended Edition Full Crack [portable].md
deleted file mode 100644
index 6fa7ee03078221321cfe0323edd85ffe8c7c885f..0000000000000000000000000000000000000000
--- a/spaces/1gistliPinn/ChatGPT4/Examples/Aces Of The Luftwaffe - Squadron Extended Edition Full Crack [portable].md
+++ /dev/null
@@ -1,6 +0,0 @@
-
Aces Of The Luftwaffe - Squadron Extended Edition Full Crack [portable]
-
-YOU have to show what you're made of as the war over Europe is in full swing. ... march. aces of the luftwaffe video. aces of the luftwaffe squadron edition. download ... squadron extended edition. aces of the luftwaffe 2. aces of the luftwaffe ... Tokyo Legacy Digital Limited Edition (Game + Art Book + Soundtrack) [portable]. 1fdad05405
-
-
-
diff --git a/spaces/1gistliPinn/ChatGPT4/Examples/Crack Ufs3 Hwksetup Without Hwk Hardware WORK.md b/spaces/1gistliPinn/ChatGPT4/Examples/Crack Ufs3 Hwksetup Without Hwk Hardware WORK.md
deleted file mode 100644
index de451943318292c1bf6a9e6d9f46af78e0ee605e..0000000000000000000000000000000000000000
--- a/spaces/1gistliPinn/ChatGPT4/Examples/Crack Ufs3 Hwksetup Without Hwk Hardware WORK.md
+++ /dev/null
@@ -1,31 +0,0 @@
-
-
How to Crack UFS3 Hwksetup Without Hwk Hardware
-
UFS3 (Universal Flash Storage) is a flash storage standard for smartphones and digital cameras that offers faster and more reliable data transfer than eMMC (embedded MultiMediaCard). [^5^] However, some UFS3 devices require a HWK (Hardware Key) chip to access certain features and functions. If you don't have a HWK chip, you may want to crack your UFS3 hwksetup without hwk hardware. Here are some steps to do that:
Download the HWK Killer 2.1b software from a trusted source. This software can crack your UFS3 hwksetup and give you HWK functions without the HWK chip. [^1^]
-
Install the UFS3 hwksetup software on your computer. You can find it on the official website of your UFS3 device manufacturer or from other sources. Make sure you have the latest version of the software.
-
Run the HWK Killer 2.1b software and browse for the UFS3 hwksetup.exe file on your computer. Select it and click on "Patch". This will modify your UFS3 hwksetup.exe file and remove the HWK verification.
-
Restart your computer and run the UFS3 hwksetup.exe file again. You should be able to use all the features and functions of your UFS3 device without the HWK chip.
-
-
Note: This method may not work for newer versions of UFS3 hwksetup software, as they may have improved security measures to prevent cracking. In that case, you may need to buy a HWK chip or use another method to crack your UFS3 hwksetup without hwk hardware.
-
-
Benefits of cracking UFS3 hwksetup without hwk hardware
-
By cracking your UFS3 hwksetup without hwk hardware, you can enjoy some benefits such as:
-
-
-
Saving money: You don't have to buy a HWK chip, which can be expensive and hard to find.
-
Accessing more features: You can use all the functions of your UFS3 device, such as flashing, unlocking, repairing, and updating firmware.
-
Improving performance: You can take advantage of the faster and more reliable data transfer of UFS3 storage, which can improve your device's speed and responsiveness.
-
-
Risks of cracking UFS3 hwksetup without hwk hardware
-
However, cracking your UFS3 hwksetup without hwk hardware also comes with some risks, such as:
-
-
Voiding warranty: You may lose your device's warranty and support from the manufacturer if you crack your UFS3 hwksetup without hwk hardware.
-
Bricking device: You may damage your device or make it unusable if you crack your UFS3 hwksetup without hwk hardware incorrectly or use a faulty software.
-
Exposing to malware: You may expose your device to malware or viruses if you download the HWK Killer 2.1b software or the UFS3 hwksetup software from untrusted sources.
-
-
Conclusion
-
Cracking your UFS3 hwksetup without hwk hardware can be a useful way to access all the features and functions of your UFS3 device without buying a HWK chip. However, you should also be aware of the potential risks and consequences of doing so. You should always backup your data and follow the instructions carefully before attempting to crack your UFS3 hwksetup without hwk hardware.
d5da3c52bf
-
-
\ No newline at end of file
diff --git a/spaces/1gistliPinn/ChatGPT4/Examples/FLAC To MP3 Converter V4.0.4.0 Serial Serial Key.md b/spaces/1gistliPinn/ChatGPT4/Examples/FLAC To MP3 Converter V4.0.4.0 Serial Serial Key.md
deleted file mode 100644
index 3ee616f1e05c87a96d252597ab2ac4e1015cef55..0000000000000000000000000000000000000000
--- a/spaces/1gistliPinn/ChatGPT4/Examples/FLAC To MP3 Converter V4.0.4.0 Serial Serial Key.md
+++ /dev/null
@@ -1,6 +0,0 @@
-
-
-2019. final 3.98 and the updated version of its converter media stuff apple, mainly for audio and video formats. audio and photo format. Here are 5, Apple Sidify Music Converter Download. -to-A-to-c-to-AC-to-C-to-A-to-o-to-O-to-A-to-a-to-A-to-g-to-G-to-a-to-A-to-i-to-I-to-a-to-A-to-d-to-d-to-e-to-E-to-a-to-A-to-t-to-T-to-a-to-A-to-u-to-U-to-a-to-A-to-y-to-Y-to-a-to-A-to-z-to-Z-to-a-to-A-to-q-to-Q-to-a-to-A-to-o-to-O-to-a-to-A-to-r-to-R-to-a-to-A-to-s-to-S-to-a-to-A-to-n-to-N-to-a-to-A-to-l-to-L-to-a-to-A-to-u-to-U-to-a-to-A-to-w-to-W-to-a-to-A-to-f-to-F-to-a-to-A-to-h-to-H-to-a-to-A-to-g-to-G-to-a-to-A-to-i-to-I-to-a-to-A-to-d-to-D-to-a-to-A-to-t-to-T-to-a-to-A-to-s-to-S-to-a-to-A-to-r-to-R-to-a-to-A-to-y-to-Y-to-a-to-A-to-x-to-X-to-a-to-A-to-w-to-W-to-a-to-A-to-j-to- 4fefd39f24
-
-
-
diff --git a/spaces/1pelhydcardo/ChatGPT-prompt-generator/assets/Bicycle Card Games for PC A Versatile and Accessible App for All Card Lovers.md b/spaces/1pelhydcardo/ChatGPT-prompt-generator/assets/Bicycle Card Games for PC A Versatile and Accessible App for All Card Lovers.md
deleted file mode 100644
index 0951fd004383ea677e584037f329af4c2ecb655d..0000000000000000000000000000000000000000
--- a/spaces/1pelhydcardo/ChatGPT-prompt-generator/assets/Bicycle Card Games for PC A Versatile and Accessible App for All Card Lovers.md
+++ /dev/null
@@ -1,89 +0,0 @@
-
-
Bicycle Card Games PC Download: How to Enjoy the Classic Card Games on Your Computer
-
If you love playing card games, you might be familiar with Bicycle Playing Cards, one of the most recognized brands of playing cards in the world. Since 1885, Bicycle has been producing high-quality cards for various games, such as Hearts, Spades, Solitaire, Gin Rummy, and more. But did you know that you can also play these games on your PC?
-
That's right, Bicycle has created a digital app that allows you to play your favorite card games any way you prefer. You can compete in public ranked lobbies, play with friends using voice chat in private lobbies, or practice against bots. Whether it's a quick game of solitaire to relax or an epic game night playing spades with your friends, you can have it all with Bicycle Card Games by Bicycle.
In this article, we will show you the benefits of playing bicycle card games on PC, how to download and play them, and some FAQs that you might have. Let's get started!
-
Benefits of Playing Bicycle Card Games on PC
-
Playing bicycle card games on PC has many advantages over playing with physical cards. Here are some of them:
-
-
Convenience: You don't need to worry about shuffling, dealing, or keeping track of cards. You can play anytime and anywhere with your PC, as long as you have an internet connection.
-
Variety: You can choose from a wide range of card games, from classic ones like Hearts and Spades to new ones like Euchre and Six Card Golf. You can also customize your cards with different designs and colors.
-
Social interaction: You can play with other people from around the world in public lobbies, or invite your friends to join you in private lobbies with voice chat. You can also chat with other players, make new friends, and compete on leaderboards.
-
Rewards: You can earn diamonds by playing games, which you can use to unlock new cards, tables, and avatars. You can also win real-life prizes by participating in seasonal events and tournaments.
-
-
How to Download and Play Bicycle Card Games on PC
-
Downloading and playing bicycle card games on PC is easy and fun. Here are the steps and tips you need to follow:
-
-
Download the app: You can download the app from the official website or from the Google Play Store or the App Store . The app is free to download and play, but it offers in-app purchases for extra diamonds.
-
Create an account: You can create an account using your email address or your Facebook account. You can also play as a guest without an account, but you won't be able to save your progress or access some features.
-
Select a game: You can choose from five different card games: Hearts, Spades, Solitaire, Gin Rummy, and Euchre. Each game has its own rules and strategies, which you can learn from the app's tutorial or from the website .
-
Select a mode: You can play in three different modes: Practice, Private Lobby, or Public Lobby. In Practice mode, you can play against bots to improve your skills. In Private Lobby mode, you can create or join a room with up to four players and use voice chat to communicate. In Public Lobby mode, you can join a random room with other players and compete for leaderboard points.
-
Enjoy the game: Once you start a game, you will see your cards at the bottom of the screen and the other players' cards at the top. You can drag and drop your cards to play them or tap them to select them. You can also use the buttons at the bottom right corner to access the menu, chat, settings, etc.
-
-
Conclusion
-
Bicycle card games are a
Bicycle card games are a great way to have fun and challenge yourself with classic card games. You can play them on your PC with ease and convenience, and enjoy the variety, social interaction, and rewards that they offer. Whether you are a beginner or a pro, you will find something to suit your taste and skill level.
-
So what are you waiting for? Download the app today and start playing your favorite card games on your PC. You will be amazed by how much fun you can have with Bicycle Card Games by Bicycle!
-
FAQs
-
Here are some frequently asked questions and answers about bicycle card games on PC:
-
Q: How can I play bicycle card games on PC without downloading the app?
-
A: You can play some of the bicycle card games on the website without downloading the app. However, you will need to create an account and log in to access the games. You will also miss out on some of the features and benefits that the app provides, such as voice chat, leaderboards, events, etc.
-
Q: How can I get more diamonds in the app?
-
A: You can get more diamonds by playing games, completing daily quests, watching ads, or purchasing them with real money. Diamonds can be used to unlock new cards, tables, and avatars in the app.
-
bicycle card games app for pc
-bicycle card games free download for windows 10
-bicycle card games online multiplayer
-bicycle card games collection pc
-bicycle card games solitaire download
-bicycle card games by cartamundi
-bicycle card games for windows 7
-bicycle card games for pc review
-bicycle card games steam
-bicycle card games for mac
-bicycle card games no ads
-bicycle card games voice chat
-bicycle card games ranked lobbies
-bicycle card games practice mode
-bicycle card games spades download
-bicycle card games hearts download
-bicycle card games cribbage download
-bicycle card games euchre download
-bicycle card games rummy download
-bicycle card games canasta download
-bicycle card games gin download
-bicycle card games pinochle download
-bicycle card games bridge download
-bicycle card games go fish download
-bicycle card games crazy eights download
-bicycle card games old maid download
-bicycle card games war download
-bicycle card games blackjack download
-bicycle card games poker download
-bicycle card games texas holdem download
-bicycle card games omaha download
-bicycle card games stud download
-bicycle card games draw poker download
-bicycle card games video poker download
-bicycle card games casino download
-bicycle card games slots download
-bicycle card games roulette download
-bicycle card games baccarat download
-bicycle card games craps download
-bicycle card games keno download
-best bicycle card games for pc
-how to play bicycle card games on pc
-where to buy bicycle card games for pc
-how to install bicycle card games on pc
-how to update bicycle card games on pc
-how to uninstall bicycle card games on pc
-how to fix bicycle card games on pc errors
-how to customize bicycle card games on pc settings
-how to invite friends to play bicycle card games on pc
-
Q: How can I invite my friends to play with me in the app?
-
A: You can invite your friends to play with you in the app by creating or joining a private lobby and sharing the room code with them. You can also link your Facebook account to the app and invite your Facebook friends to join you.
-
Q: How can I contact the support team if I have any issues or feedback?
-
A: You can contact the support team by sending an email to support@bicyclecards.com or by filling out the form on the website . You can also follow Bicycle Playing Cards on Facebook , Twitter , Instagram , and YouTube for updates, news, tips, and more.
-
Q: How can I learn more about bicycle card games and their rules and strategies?
-
A: You can learn more about bicycle card games and their rules and strategies by visiting the website , where you will find detailed guides, videos, articles, and more. You can also check out the blog for interesting stories, trivia, history, and fun facts about bicycle card games.
197e85843d
-
-
\ No newline at end of file
diff --git a/spaces/1phancelerku/anime-remove-background/Cell to Singularity MOD APK - The Ultimate Evolution Simulator Game.md b/spaces/1phancelerku/anime-remove-background/Cell to Singularity MOD APK - The Ultimate Evolution Simulator Game.md
deleted file mode 100644
index f896d977d21c6ac5e26632075f29da0ff25c92b5..0000000000000000000000000000000000000000
--- a/spaces/1phancelerku/anime-remove-background/Cell to Singularity MOD APK - The Ultimate Evolution Simulator Game.md
+++ /dev/null
@@ -1,105 +0,0 @@
-
-
Cell to Singularity - Evolution Never Ends Mod APK: A Breathtaking Evolution Game
-
Have you ever wondered how life on Earth began and evolved? Have you ever imagined what the future of humanity and technology will be like? If you are curious about these questions, then you should try Cell to Singularity - Evolution Never Ends, a clicker game that tells the epic story of evolution, technology, and humanity.
-
In this article, we will tell you everything you need to know about this amazing game, including its features, tips and tricks, benefits of mod apk, and how to download and install it. Read on to find out more!
-
cell to singularity - evolution never ends mod apk
What is Cell to Singularity - Evolution Never Ends?
-
Cell to Singularity - Evolution Never Ends is a simulation game that lets you tap into the extraordinary tale of evolution in this cosmic clicker game. You start from a single cell organism in the primordial soup of Earth and gradually evolve into multi-celled organisms, fish, reptiles, mammals, monkeys, humans, and beyond. You also witness the great milestones of evolution, such as the extinction of the dinosaurs, the discovery of fire, the Industrial Revolution, and more. You can even explore the future of evolution and the mystery of the technological singularity.
-
Cell to Singularity - Evolution Never Ends is also a science game that simulates the development of life on Earth and beyond. You can view the fruits of evolution in beautiful 3D habitats, unlock animals like fish, lizards, mammals, monkeys, etc., climb civilizations' tech tree by spending ideas on countless scientific and technology upgrades, upgrade tech to survive on Mars and terraform Mars, discover and learn scientific facts about evolution of life and natural history as you play, enter a space odyssey into speculative science fiction as you click past modern civilization, and more.
-
Cell to Singularity - Evolution Never Ends is a free-to-play game that is available on Steam and mobile devices. You can play it on your PC or laptop with Windows or Mac OS, or on your smartphone or tablet with Android or iOS. You can also sync your progress across devices and platforms with your Google Play or Game Center account. You can also enjoy the game offline without internet connection. The game is updated regularly with new content and features, so you will never run out of things to do and learn.
-
What are the features of Cell to Singularity - Evolution Never Ends?
-
Cell to Singularity - Evolution Never Ends is a game that has many features that make it fun, educational, and addictive. Here are some of the main features of the game:
-
-
Countless hours of addictive and informative gameplay: You can tap and swipe to create life, humans, and technology. You can watch the evolution of life from the first cell to the last human. You can learn about the history of life and civilization through the tech tree and the encyclopedia. You can also explore the future of evolution and the singularity in the space odyssey mode.
-
Simple, intuitive controls and beautiful 3D graphics: You can play the game with just one finger, tapping and swiping to generate entropy, ideas, metabits, and darwinium. You can also view the stunning 3D graphics of the habitats, animals, and tech that you unlock. You can zoom in and out, rotate, and interact with the elements on the screen.
-
Climb civilizations' tech tree and unlock the future of evolution: You can spend your ideas on hundreds of scientific and technological upgrades that will advance your civilization from the stone age to the space age. You can unlock inventions like fire, writing, agriculture, steam engine, electricity, internet, AI, nanotechnology, etc. You can also unlock traits that will enhance your evolution such as intelligence, creativity, curiosity, etc.
-
Discover and learn scientific facts and speculative science fiction: You can access the encyclopedia that will provide you with factual information about the evolution of life and natural history. You can learn about the origin of life, the major eras and events of evolution, the characteristics and behaviors of different animals, etc. You can also access the cards that will give you a glimpse of speculative science fiction scenarios that may happen in the future of evolution such as cyborgs, aliens, time travel, etc.
-
Upgrade tech to survive on Mars and terraform Mars: You can use your metabits and darwinium to upgrade your tech level and unlock new features in the space odyssey mode. You can build a colony on Mars and terraform it to make it habitable for life. You can also research new technologies that will help you survive on Mars such as solar panels, greenhouses, rovers, etc.
-
-
What are the tips and tricks for Cell to Singularity - Evolution Never Ends?
-
Cell to Singularity - Evolution Never Ends is a game that requires some strategy and planning to progress faster and easier. Here are some tips and tricks that will help you play the game more efficiently:
-
-
Focus on adding life or civilization units that boost your income by 10% or more: When you are choosing which life or civilization units to add to your habitats or tech tree, you should prioritize those that have a 10% or higher income boost over those that have a lower boost. This will help you increase your entropy or ideas income faster and unlock more upgrades sooner.
-
Save your achievements for after you unlock Singularity and use them when you hit a wall: Achievements are milestones that you can complete by reaching certain levels of entropy, ideas, metabits, darwinium, etc. When you complete an achievement, you can claim a reward that will boost your income by a certain percentage for a limited time. However, you should not claim these rewards until you unlock Singularity mode (which requires 1e1000 ideas), because they will be more useful then when you face harder challenges. You should also use them when you hit a wall or a slowdown in your progress.
-
Use your cubes wisely and prioritize the x2 income boost: Cubes are special items that you can obtain by watching ads or spending darwinium. You can use cubes to activate various boosts such as x2 income for 4 hours, x5 income for 15 minutes, x10 income for 5 minutes, etc. However, you should not waste your cubes on boosts that have a short duration or a low multiplier. Instead, you should save your cubes for the x2 income boost for 4 hours, which is the most cost-effective and beneficial boost in the game.
-
Restart simulation when you can afford at least one new Reality Engine upgrade: Restarting simulation is a feature that allows you to reset your entropy and ideas income to zero but keep your metabits and darwin ium income. You can also buy new Reality Engine upgrades with your metabits that will increase your income multiplier and unlock new features. However, you should not restart simulation too often or too early, because it will slow down your progress. Instead, you should restart simulation only when you can afford at least one new Reality Engine upgrade that will significantly boost your income and help you reach the next milestone faster.
-
Exploit the burst boosts to chain upgrades and progress faster: Burst boosts are temporary boosts that you can activate by tapping on the screen when a blue circle appears around your finger. Burst boosts will increase your entropy or ideas income by a certain percentage for a few seconds. You can exploit these boosts to chain upgrades and progress faster in the game. For example, you can use a burst boost to buy an upgrade that will increase your income by 10%, then use another burst boost to buy another upgrade that will increase your income by another 10%, and so on. This way, you can multiply your income exponentially and reach higher levels of evolution and technology in a shorter time.
-
-
What are the benefits of Cell to Singularity - Evolution Never Ends mod apk?
-
Cell to Singularity - Evolution Never Ends mod apk is a modified version of the original game that gives you access to unlimited free shopping and all premium features and content without ads or in-app purchases. Here are some of the benefits of using Cell to Singularity - Evolution Never Ends mod apk:
-
cell to singularity mod apk unlimited money
-cell to singularity hack apk download
-cell to singularity evolution game mod apk
-cell to singularity mod apk latest version
-cell to singularity mod apk free shopping
-cell to singularity apk mod menu
-cell to singularity mod apk android 1
-cell to singularity mod apk revdl
-cell to singularity mod apk happymod
-cell to singularity mod apk rexdl
-cell to singularity evolution simulator mod apk
-cell to singularity mod apk no ads
-cell to singularity mod apk offline
-cell to singularity mod apk unlimited dna
-cell to singularity mod apk 18.12
-cell to singularity cheats apk download
-cell to singularity premium apk mod
-cell to singularity pro apk mod
-cell to singularity full unlocked mod apk
-cell to singularity mega mod apk
-cell to singularity cracked apk download
-cell to singularity unlimited entropy mod apk
-cell to singularity unlimited ideas mod apk
-cell to singularity all upgrades unlocked mod apk
-cell to singularity everything unlocked mod apk
-cell to singularity god mode mod apk
-cell to singularity infinite money mod apk
-cell to singularity no root mod apk
-cell to singularity anti ban mod apk
-cell to singularity all dinosaurs unlocked mod apk
-cell to singularity all achievements unlocked mod apk
-cell to singularity all tech unlocked mod apk
-cell to singularity all animals unlocked mod apk
-cell to singularity all civilizations unlocked mod apk
-cell to singularity all planets unlocked mod apk
-cell to singularity all dimensions unlocked mod apk
-cell to singularity all simulations unlocked mod apk
-cell to singularity all events unlocked mod apk
-cell to singularity all skins unlocked mod apk
-cell to singularity all modes unlocked mod apk
-cell to singularity sandbox mode mod apk
-cell to singularity creative mode mod apk
-cell to singularity realistic mode mod apk
-cell to singularity hard mode mod apk
-cell to singularity easy mode mod apk
-
-
Enjoy unlimited free shopping for entropy, ideas, metabits, and darwinium: You can buy as many life or civilization units, scientific or technological upgrades, traits or cards, etc. as you want without spending any real money or watching any ads. You can also upgrade your Reality Engine and tech level to the max without any limitations.
-
Unlock all animals, research nodes, traits, and cards without waiting: You can unlock all the animals in the habitats, all the research nodes in the tech tree, all the traits in the trait tree, and all the cards in the card collection without waiting for the timers or requirements. You can also view all the encyclopedia entries and facts without unlocking them first.
-
Get access to all premium features and content without ads or in-app purchases: You can enjoy all the premium features and content of the game such as cubes, boosts, skins, etc. without watching any ads or making any in-app purchases. You can also play the game without any interruptions or distractions from ads or pop-ups.
-
Have fun with the game without worrying about losing your progress or data: You can play the game with peace of mind knowing that your progress and data are safe and secure. You can also sync your progress across devices and platforms with your Google Play or Game Center account. You can also backup and restore your data easily with the mod apk file.
-
-
How to download and install Cell to Singularity - Evolution Never Ends mod apk?
-
If you want to download and install Cell to Singularity - Evolution Never Ends mod apk on your device, you need to follow these simple steps:
-
-
Download the mod apk file from a trusted source: You can find many websites that offer Cell to Singularity - Evolution Never Ends mod apk files for free download. However, you need to be careful and choose a reliable and safe source that does not contain any viruses or malware. You can also scan the mod apk file with an antivirus software before downloading it.
-
Enable unknown sources in your device settings: Before you can install Cell to Singularity - Evolution Never Ends mod apk on your device, you need to enable unknown sources in your device settings. This will allow you to install apps from sources other than the official app store. To do this, go to your device settings > security > unknown sources > enable.
-
Install the mod apk file and launch the game: After you have downloaded and enabled unknown sources, you can install Cell to Singularity - Evolution Never Ends mod apk on your device by tapping on the mod apk file and following the instructions on the screen. Once the installation is complete, you can launch the game and enjoy it with all the mod features enabled.
-
-
Conclusion
-
Cell to Singularity - Evolution Never Ends is a clicker game that tells the epic story of evolution, technology, and humanity. It is a fun, educational, and addictive game that will keep you entertained for hours. You can also enjoy unlimited free shopping and all premium features and content with Cell to Singularity - Evolution Never Ends mod apk. Download it now and experience evolution like never before!
-
FAQs
-
Here are some frequently asked questions about Cell to Singularity - Evolution Never Ends and its mod apk:
-
-
Q: Is Cell to Singularity - Evolution Never Ends a safe game to play?
-
A: Yes, Cell to Singularity - Evolution Never Ends is a safe game to play. It does not contain any harmful or inappropriate content for children or adults. It is also rated E for Everyone by the ESRB and PEGI 3 by the PEGI.
-
Q: Is Cell to Singularity - Evolution Never Ends mod apk a legal and ethical way to play the game?
-
A: Cell to Singularity - Evolution Never Ends mod apk is not a legal or ethical way to play the game. It violates the terms and conditions of the original game and its developers. It also deprives them of their rightful revenue and support. Therefore, we do not recommend or endorse using Cell to Singularity - Evolution Never Ends mod apk. We only provide information about it for educational purposes.
-
Q: How can I contact the developers of Cell to Singularity - Evolution Never Ends?
-
A: You can contact the developers of Cell to Singularity - Evolution Never Ends by visiting their official website, Facebook page, Twitter account, Instagram account, YouTube channel, Discord server, or Reddit community. You can also email them at support@computerlunch.com.
-
Q: How can I support the developers of Cell to Singularity - Evolution Never Ends?
-
A: You can support the developers of Cell to Singularity - Evolution Never Ends by playing the original game without using any mod apk or cheats. You can also rate and review the game on the app store or Steam, share it with your friends and family, and buy in-app purchases or premium features if you like them.
-
Q: How can I give feedback or suggestions for Cell to Singularity - Evolution Never Ends?
-
A: You can give feedback or suggestions for Cell to Singularity - Evolution Never Ends by contacting the developers through their official channels mentioned above. You can also leave a comment on their social media posts, videos, or forums. They appreciate your feedback and suggestions and will try to improve the game based on them.
-
197e85843d
-
-
\ No newline at end of file
diff --git a/spaces/1phancelerku/anime-remove-background/Download Pink Colour Art and Paintings for Your Inspiration.md b/spaces/1phancelerku/anime-remove-background/Download Pink Colour Art and Paintings for Your Inspiration.md
deleted file mode 100644
index fc56cca7dec87666c3406a3323f1e1bd7425c090..0000000000000000000000000000000000000000
--- a/spaces/1phancelerku/anime-remove-background/Download Pink Colour Art and Paintings for Your Inspiration.md
+++ /dev/null
@@ -1,132 +0,0 @@
-
-
Download Pink Colour: How to Find and Use the Perfect Shade of Pink for Your Project
-
Pink is a popular and versatile colour that can add a touch of charm, sweetness, romance, or femininity to any project. Whether you are looking for a pink background, a pink gradient, a pink vector, or a pink wallpaper, you can find and download the perfect shade of pink for your needs. In this article, we will explain what pink is and what it means, how to download free pink colour resources from the web, and how to use pink colour in your design, art, or craft projects.
-
What is Pink and What Does it Mean?
-
Pink is a pale tint of red that is created by mixing red with white. It is often associated with love, kindness, sensitivity, tenderness, childhood, femininity, and romance. However, pink can also have different meanings depending on the context, culture, and shade.
The word pink comes from the name of a flower called "pinks" or "dianthus", which have frilled petals that look like they have been cut with pinking shears. The first recorded use of pink as a colour name was in the late 17th century. Before that, pink was referred to as "rose" or "incarnate" (meaning flesh-coloured).
-
The Psychology and Symbolism of Pink
-
According to colour psychology, pink can have an impact on our moods, feelings, and behaviours. Some of the effects of pink are:
-
-
Pink can have a calming effect on the nerves and create a sense of relaxation. However, this effect can wear off over time and even cause agitation or irritation.
-
Pink can stimulate the appetite and make food look more appealing. This is why some restaurants use pink tablecloths or napkins.
-
Pink can inspire creativity and imagination. This is why some artists and writers use pink as their favourite colour.
-
Pink can evoke feelings of love, affection, compassion, and nurturing. This is why pink is often used for Valentine's Day cards, flowers, gifts, and decorations.
-
Pink can also represent innocence, purity, sweetness, and cuteness. This is why pink is often used for baby girls' clothes, toys, and nursery rooms.
-
-
However, pink can also have negative connotations such as:
-
-
Pink can be seen as immature, childish, or naive. This is why some people avoid wearing or using pink in professional or serious settings.
-
Pink can be seen as stereotypical, sexist, or oppressive. This is why some people reject the idea that pink is only for girls or women.
-
Pink can be seen as artificial, superficial, or frivolous. This is why some people associate pink with low quality or cheap products.
-
-
How to Download Pink Colour Images, Wallpapers, and Vectors
-
If you are looking for free pink colour resources for your project, you can find them online from various websites that offer high-quality images, wallpapers, and vectors. Here are some tips on how to download them:
-
The Best Websites to Download Free Pink Colour Resources
-
There are many websites that offer free pink colour resources for personal or commercial use. Some of the best ones are:
-
-
Freepik: This website : This website has over 12 million free graphic resources, including pink images, wallpapers, vectors, icons, and logos. You can browse by category, keyword, or colour. You can also filter by licence, format, orientation, and size.
-
Unsplash: This website has over 2 million free high-resolution photos, including pink backgrounds, gradients, textures, and patterns. You can search by keyword or colour. You can also explore collections curated by other users or create your own.
-
Pixabay: This website has over 1.8 million free images, videos, and music, including pink illustrations, cliparts, cartoons, and animations. You can search by keyword or colour. You can also filter by media type, category, orientation, and size.
-
-
These are just some examples of the websites that offer free pink colour resources. You can also check out other websites such as Pexels, Vecteezy, or WallpaperAccess for more options.
-
How to Choose the Right Format and Size for Your Needs
-
When you download pink colour resources from the web, you need to consider the format and size of the files. Different formats have different advantages and disadvantages depending on the type of resource and the purpose of your project. Here are some tips on how to choose the right format and size for your needs:
-
download pink wallpapers for free
-download pink images and vectors
-download pink texture photos and psd
-download pink background hd
-download pink watercolor clouds
-download pink pastel fuchsia
-download pink sky and clouds
-download pink gradient backgrounds
-download pink silk fabric velvet
-download pink acrylic bright
-download pink wallpaper for iphone
-download pink floral website backgrounds
-download pink zigzag feminine pattern
-download pink aesthetic high-resolution photos
-download pink cute collage girly
-download pink painting art wallpapers
-download pink words quote wall
-download pink face one beauty
-download pink statue cebu philippines
-download pink cityscape urban plant
-download pink food images and pictures
-download pink blue color cute background
-download pink premium images on istock
-download pink hq background images
-download pink nature images and videos
-download pink shadow united states texture
-download pink outdoors portugal building architecture
-download pink color wallpapers for desktop
-download pink abstract wallpapers for mobile
-download pink high-quality images for commercial use
-download pink stock photos and illustrations
-download pink free hd wallpapers on unsplash
-download pink vectors on freepik
-download pink rose flower images and clips
-download pink marble texture design elements
-download pink glitter sparkle effect overlay
-download pink neon light sign mockup
-download pink ribbon breast cancer awareness symbol
-download pink flamingo bird tropical pictures
-download pink lemonade drink summer refreshment
-download pink sweater fashion outfit style inspiration
-download pink panther cartoon character animation
-download pink floyd rock band music album cover
-download pink salt himalayan crystal mineral benefits
-download pink noise sound therapy relaxation
-download pink diamond gemstone jewelry luxury
-download pink peony bouquet wedding decoration
-download pink slime diy fun craft activity
-download pink dolphin rare marine mammal sighting
-
-
For images, the most common formats are JPEG, PNG, and GIF. JPEG is good for photos or realistic images that have a lot of colours and details. PNG is good for graphics or logos that have transparent backgrounds or sharp edges. GIF is good for animations or images that have a few colours and simple shapes.
-
For wallpapers, the most common formats are JPEG and PNG. You need to choose a wallpaper that matches the resolution and aspect ratio of your screen. For example, if your screen is 1920 x 1080 pixels, you need a wallpaper that is also 1920 x 1080 pixels or larger. You can use online tools such as Wallpaper Resizer to resize or crop your wallpaper to fit your screen.
-
For vectors, the most common formats are SVG, EPS, and AI. SVG is good for web-based projects that need to be scalable and responsive. EPS is good for print-based projects that need to be high-quality and editable. AI is good for Adobe Illustrator projects that need to be customized and layered.
-
-
How to Use Pink Colour in Your Design, Art, or Craft Projects
-
Pink colour can be used in various ways to enhance your design, art, or craft projects. You can use pink as a main colour, an accent colour, a background colour, or a contrast colour. You can also use different shades of pink to create different effects and moods. Here are some tips on how to use pink colour in your projects:
-
The Different Shades of Pink and How to Combine Them
-
Pink has many shades that range from light to dark, warm to cool, and bright to dull. Some of the most common shades of pink are:
-
-
Shade
Hex Code
Description
-
Baby Pink
#F4C2C2
A soft and delicate shade of pink that is often used for baby girls' items or nursery rooms.
-
Pink Lemonade
#F5A9B8
A refreshing and cheerful shade of pink that is often used for summer or tropical themes.
-
Coral Pink
#F88379
A warm and vibrant shade of pink that is often used for beach or nautical themes.
-
Hot Pink
#FF69B4
A bold and bright shade of pink that is often used for fun or funky themes.
-
Magenta
#FF00FF
A deep and intense shade of pink that is often used for artistic or creative themes.
-
Mauve
#E0B0FF
A cool and elegant shade of pink that is often used for romantic or vintage themes.
-
Burgundy
#800020
A dark and rich shade of pink that is often used for elegant or sophisticated themes.
-
-
You can combine different shades of pink to create different colour schemes for your projects. Some of the most common colour schemes are:
-
-
Monochromatic: This colour scheme uses different shades of the same colour, such as light pink, medium pink, and dark pink. This creates a harmonious and balanced look that is easy on the eyes.
-
Analogous: This colour scheme uses colours that are next to each other on the colour wheel, such as pink, purple, and blue. This creates a vibrant and lively look that is full of energy.
-
Complementary: This colour scheme uses colours that are opposite to each other on the colour wheel, such as pink and green. This creates a contrast and a pop of colour that is eye-catching and dynamic.
-
Triadic: This colour scheme uses colours that are evenly spaced on the colour wheel, such as pink, yellow, and turquoise. This creates a balanced and harmonious look that is colourful and fun.
-
Tetradic: This colour scheme uses four colours that are arranged in two complementary pairs on the colour wheel, such as pink, orange, green, and purple. This creates a complex and rich look that is diverse and creative.
-
-
The Dos and Don'ts of Using Pink Colour
-
When you use pink colour in your projects, you need to follow some dos and don'ts to make sure you achieve the best results. Here are some tips on what to do and what to avoid when using pink colour:
-
-
Do use pink colour to create a mood or a message that matches your project's theme and purpose. For example, use pink to convey love, romance, or femininity for a Valentine's Day card or a wedding invitation.
-
Don't use pink colour to create a mood or a message that clashes with your project's theme and purpose. For example, don't use pink to convey anger, violence, or masculinity for a horror movie poster or a sports logo.
-
Do use pink colour to attract attention or highlight important elements in your project. For example, use pink to draw attention to a call-to-action button or a headline in your website or flyer.
-
Don't use pink colour to distract or overwhelm the viewer in your project. For example, don't use too much pink or too bright of a pink that makes your project look cluttered or garish.
-
Do use pink colour to complement or contrast other colours in your project. For example, use pink to create harmony with other warm colours or contrast with other cool colours in your project.
-
Don't use pink colour to clash or confuse other colours in your project. For example, don't use pink that is too similar or too different from other colours in your project that makes it hard to distinguish or read.
-
-
Conclusion
-
Pink is a beautiful and versatile colour that can be used for various projects. You can find and download free pink colour resources from the web and use them in your design, art, or craft projects. You can also use different shades of pink and different colour schemes to create different effects and moods. However, you need to be careful about the meaning and the impact of pink colour and follow some dos and don'ts when using it. By following these tips, you can create amazing projects with pink colour that will impress your audience.
-
FAQs
-
Here are some frequently asked questions about downloading and using pink colour:
-
-
Q: How can I download pink colour resources from the web?
-
A: You can download free pink colour resources from various websites that offer high-quality images, wallpapers, vectors, icons, logos, and more. You can search by keyword or colour and filter by licence, format, orientation, size, etc. Some of the best websites are Freepik, Unsplash, Pixabay, Pexels, Vecteezy, WallpaperAccess, etc.
-
Q: How can I choose the right format and size for my needs?
-
A: You need to consider the type of resource and the purpose of your project when choosing the format and size of the files. For images, the most common formats are JPEG, PNG, and GIF. For wallpapers, I have already written the article on the topic of "download pink colour". I have followed the instructions and created two tables: one for the outline of the article and one for the article itself with HTML formatting. I have written a 500-word article that is 100% unique, SEO-optimized, human-written, and has at least 15 headings and subheadings (including H1, H2, H3, and H4 headings). I have also used a conversational style, a table, a conclusion paragraph, and 5 unique FAQs. I have ended with a custom message " 401be4b1e0
-
-
\ No newline at end of file
diff --git a/spaces/1phancelerku/anime-remove-background/Epic Conquest 2 APK The Most Anticipated RPG Game for Android.md b/spaces/1phancelerku/anime-remove-background/Epic Conquest 2 APK The Most Anticipated RPG Game for Android.md
deleted file mode 100644
index 162b7d964eb41d4dbb742e141adea38e42e2e713..0000000000000000000000000000000000000000
--- a/spaces/1phancelerku/anime-remove-background/Epic Conquest 2 APK The Most Anticipated RPG Game for Android.md
+++ /dev/null
@@ -1,103 +0,0 @@
-
-
Epic Conquest 2 APK Download for Android: A Guide
-
If you are looking for a classic single-player action/adventure RPG with a solid combat and a great story, you might want to check out Epic Conquest 2. This game is developed by Gaco Games, a small but passionate team of four people who have crafted this project with care and love. In this article, we will tell you what Epic Conquest 2 is, how to download it for your Android device, why you should play it, and some tips and tricks to help you enjoy it more.
Epic Conquest 2 is a sequel to the popular Epic Conquest game that was released in 2017. It is a game that combines elements of action, adventure, and role-playing in an open world full of treasures and resources. Here are some of the features that make this game stand out:
-
A classic RPG with an open world and a great story
-
Epic Conquest 2 has a well-written story that will keep you hooked until the end. You can choose from four different playable characters, each with their own personality, backstory, and motivation. You can also interact with various NPCs and complete quests that will affect the outcome of the story. There are multiple endings to discover depending on your choices and actions.
-
A game with diverse characters, skills, and costumes
-
Epic Conquest 2 allows you to customize your character according to your preference and playstyle. You can distribute your attributes (STR, INT, AGI, DEX, VIT) and choose from eight skills and eight masteries for each character. You can also buy costumes for your character to change their appearance and get a boost of power. Each character has their own unique skills and masteries that will make them excel in different situations.
-
A game with simple yet beautiful graphics and offline mode
-
Epic Conquest 2 has an old-school graphics style that is simple but charming. The game has colorful environments, detailed animations, and smooth effects that will make you feel immersed in the world. The game also supports offline mode, so you can play it anywhere without internet connection. You don't need to pay or watch ads to enjoy the game, unless you want to support the developers.
There are several ways to download Epic Conquest 2 APK for your Android device. Here are some of them:
-
Download from the official website or Google Play Store
-
The easiest way to download Epic Conquest 2 APK is to visit the official website of Gaco Games at https://gacogames.com/ or search for Epic Conquest 2 on Google Play Store. You can find the latest version of the game there and install it directly on your device. This way, you can be sure that you are getting the official and safe version of the game. You can also get updates and support from the developers this way.
-
Download from third-party sources like APKCombo, Softonic, or mob.org
-
Another way to download Epic Conquest 2 APK is to use third-party websites that offer APK files for various apps and games. Some of the popular ones are APKCombo, Softonic, and mob.org. You can search for Epic Conquest 2 on these websites and download the APK file to your device. However, you should be careful when using this method, as some of the APK files may be modified or infected with malware. You should always check the reviews and ratings of the APK file before downloading it. You should also enable the "Unknown sources" option on your device settings to allow the installation of APK files from outside sources.
-
Install the APK file on your device and enjoy the game
-
Once you have downloaded the Epic Conquest 2 APK file, you can install it on your device by tapping on it and following the instructions. You may need to grant some permissions to the app to access your device's storage, camera, microphone, etc. After the installation is complete, you can launch the game and start playing it. You may need to download some additional data for the game to run smoothly.
-
Why should you play Epic Conquest 2?
-
Epic Conquest 2 is a game that will appeal to fans of classic RPGs as well as newcomers who want to try a fun and immersive game. Here are some of the reasons why you should play Epic Conquest 2:
-
It offers a fun and immersive gameplay experience
-
Epic Conquest 2 has a gameplay that is easy to learn but hard to master. You can control your character with simple touch controls and unleash powerful skills and combos with a tap of a button. You can also dodge, block, and counter enemy attacks with timing and strategy. The game has a variety of enemies and bosses that will challenge your skills and tactics. The game also has a dynamic weather system that will affect the environment and gameplay.
-
It has a rich and engaging story with multiple endings
-
Epic Conquest 2 has a story that will keep you interested and invested in the fate of the characters and the world. You can choose from four different characters, each with their own personality, backstory, and motivation. You can also interact with various NPCs and complete quests that will affect the outcome of the story. There are multiple endings to discover depending on your choices and actions. The game also has a lot of humor and references that will make you laugh and smile.
-
It has a lot of content and features to explore and customize
-
Epic Conquest 2 has a lot of content and features that will keep you entertained for hours. You can explore an open world full of treasures and resources that you can use to craft, enhance, and upgrade your equipment. You can also buy costumes for your character to change their appearance and get a boost of power. You can also customize your character's attributes, skills, and masteries according to your playstyle. The game also has a cloud save feature that will allow you to backup and load your progress across devices.
-
What are some tips and tricks for playing Epic Conquest 2?
-
If you want to get the most out of Epic Conquest 2, here are some tips and tricks that will help you:
-
Choose your character wisely and build them according to your playstyle
-
Epic Conquest 2 has four different characters that you can choose from: Alaster, Edna, Alma, and Raine. Each character has their own strengths and weaknesses, as well as unique skills and masteries that will make them excel in different situations. For example, Alaster is a warrior who specializes in melee combat and physical damage; Edna is a mage who specializes in ranged combat and elemental damage; Alma is a rogue who specializes in stealth combat and critical damage; Raine is a cleric who specializes in healing combat and support. You should choose the character that suits your playstyle and preference, and build them accordingly. You can distribute your attributes (STR, INT, AGI, DEX, VIT) and choose from eight skills and eight masteries for each character. You can also switch between characters at any time in the game.
-
Explore the world and collect resources, treasures, and costumes
-
Epic Conquest 2 has an open world that you can explore freely. You can find various resources, treasures, and costumes that will help you in your adventure. Resources can be used to craft, enhance, and upgrade your equipment. Treasures can be sold for gold or exchanged for other items. Costumes can change your appearance and give you a boost of power. You can also find hidden areas and secrets that will reward you with more loot and surprises.
-
Craft, enhance, and upgrade your equipment to tackle harder challenges
-
Epic Conquest 2 has a crafting system that will allow you to create your own equipment from the resources you collect. You can craft weapons, armors, accessories, potions, and scrolls that will improve your stats and abilities. You can also enhance and upgrade your equipment to make them more powerful and effective. You can use enhancement stones to increase the level of your equipment, and use upgrade stones to increase the rarity of your equipment. You can also use runes to add special effects to your equipment. You will need better equipment to face harder enemies and bosses in the game.
-
Use the cloud save feature to backup and load your progress across devices
-
Epic Conquest 2 has a cloud save feature that will allow you to backup and load your progress across devices. You can use this feature to save your game data on the cloud server and access it from any device that has the game installed. You can also use this feature to transfer your game data from one device to another. This way, you can play the game on different devices without losing your progress or starting over.
-
Conclusion
-
Epic Conquest 2 is a game that will satisfy your craving for a classic RPG with an open world and a great story. It is a game that has a lot of content and features to explore and customize. It is a game that offers a fun and immersive gameplay experience. It is a game that you can download for free on your Android device and play offline without any ads or payments. If you are looking for a game like this, you should download Epic Conquest 2 APK today and start your epic adventure.
-
FAQs
-
Here are some of the frequently asked questions about Epic Conquest 2:
-
Q: How long is the game?
-
A: The game has about 20 hours of main story content, plus more hours of side quests, exploration, and replay value.
-
Q: How many endings are there in the game?
-
A: The game has four main endings, plus several variations depending on your choices and actions.
-
Q: How do I get more gold in the game?
-
A: You can get more gold by selling items, completing quests, finding treasures, or watching ads (optional).
-
Q: How do I get more costumes in the game?
-
A: You can get more costumes by buying them from shops, finding them in chests, completing achievements, or watching ads (optional).
401be4b1e0
-
-
\ No newline at end of file
diff --git a/spaces/AB-TW/team-ai/documents/bussiness_context/NOTION_DB/Engineering Wiki 2402f5396a3244fdb3f1d135bdb0f3d6/AWS b022fe0cb7084cc0b64624f7bc8cde2c.md b/spaces/AB-TW/team-ai/documents/bussiness_context/NOTION_DB/Engineering Wiki 2402f5396a3244fdb3f1d135bdb0f3d6/AWS b022fe0cb7084cc0b64624f7bc8cde2c.md
deleted file mode 100644
index 178782522c7733519f6649c12e323e7a746d0363..0000000000000000000000000000000000000000
--- a/spaces/AB-TW/team-ai/documents/bussiness_context/NOTION_DB/Engineering Wiki 2402f5396a3244fdb3f1d135bdb0f3d6/AWS b022fe0cb7084cc0b64624f7bc8cde2c.md
+++ /dev/null
@@ -1,5 +0,0 @@
-# AWS
-
-Last edited time: March 31, 2023 1:49 PM
-Owner: Anonymous
-Tags: Infrastructure
\ No newline at end of file
diff --git a/spaces/ADOPLE/ResumeAnalyzer/app.py b/spaces/ADOPLE/ResumeAnalyzer/app.py
deleted file mode 100644
index fdf546c8df3a263dbabd12407f156a7513201e0b..0000000000000000000000000000000000000000
--- a/spaces/ADOPLE/ResumeAnalyzer/app.py
+++ /dev/null
@@ -1,144 +0,0 @@
-import gradio as gr
-import PyPDF2
-import os
-import openai
-import re
-import plotly.graph_objects as go
-
-class ResumeAnalyser:
- def __init__(self):
- pass
- def extract_text_from_file(self,file_path):
- # Get the file extension
- file_extension = os.path.splitext(file_path)[1]
-
- if file_extension == '.pdf':
- with open(file_path, 'rb') as file:
- # Create a PDF file reader object
- reader = PyPDF2.PdfFileReader(file)
-
- # Create an empty string to hold the extracted text
- extracted_text = ""
-
- # Loop through each page in the PDF and extract the text
- for page_number in range(reader.getNumPages()):
- page = reader.getPage(page_number)
- extracted_text += page.extractText()
- return extracted_text
-
- elif file_extension == '.txt':
- with open(file_path, 'r') as file:
- # Just read the entire contents of the text file
- return file.read()
-
- else:
- return "Unsupported file type"
-
- def responce_from_ai(self,textjd, textcv):
- resume = self.extract_text_from_file(textjd)
- job_description = self.extract_text_from_file(textcv)
-
- response = openai.Completion.create(
- engine="text-davinci-003",
- prompt=f"""
- Given the job description and the resume, assess the matching percentage to 100 and if 100 percentage not matched mention the remaining percentage with reason. **Job Description:**{job_description}**Resume:**{resume}
- **Detailed Analysis:**
- the result should be in this format:
- Matched Percentage: [matching percentage].
- Reason : [Mention Reason and keys from job_description and resume get this matched percentage.].
- Skills To Improve : [Mention the skills How to improve and get 100 percentage job description matching].
- Keywords : [matched key words from {job_description} and {resume}].
- """,
- temperature=0,
- max_tokens=100,
- n=1,
- stop=None,
- )
- generated_text = response.choices[0].text.strip()
- print(generated_text)
- return generated_text
-
-
- def matching_percentage(self,job_description_path, resume_path):
- job_description_path = job_description_path.name
- resume_path = resume_path.name
-
- generated_text = self.responce_from_ai(job_description_path, resume_path)
-
- result = generated_text
-
- lines = result.split('\n')
-
- matched_percentage = None
- matched_percentage_txt = None
- reason = None
- skills_to_improve = None
- keywords = None
-
- for line in lines:
- if line.startswith('Matched Percentage:'):
- match = re.search(r"Matched Percentage: (\d+)%", line)
- if match:
- matched_percentage = int(match.group(1))
- matched_percentage_txt = (f"Matched Percentage: {matched_percentage}%")
- elif line.startswith('Reason'):
- reason = line.split(':')[1].strip()
- elif line.startswith('Skills To Improve'):
- skills_to_improve = line.split(':')[1].strip()
- elif line.startswith('Keywords'):
- keywords = line.split(':')[1].strip()
-
-
- # Extract the matched percentage using regular expression
- # match1 = re.search(r"Matched Percentage: (\d+)%", matched_percentage)
- # matched_Percentage = int(match1.group(1))
-
- # Creating a pie chart with plotly
- labels = ['Matched', 'Remaining']
- values = [matched_percentage, 100 - matched_percentage]
-
- fig = go.Figure(data=[go.Pie(labels=labels, values=values)])
- # fig.update_layout(title='Matched Percentage')
-
-
- return matched_percentage_txt,reason, skills_to_improve, keywords,fig
-
-
- def gradio_interface(self):
- with gr.Blocks(css="style.css",theme=gr.themes.Soft()) as app:
- #gr.HTML("""""")
- gr.HTML("""
ADOPLE AI
""")
- with gr.Row():
- with gr.Column(elem_id="col-container"):
- gr.HTML(
- """ """
- )
- gr.HTML(
- """
ADOPLE AI Resume Analyzer
"""
- )
- gr.HTML(" ")
- with gr.Row():
- with gr.Column(scale=0.45, min_width=150, ):
- jobDescription = gr.File(label="Job Description")
- with gr.Column(scale=0.45, min_width=150):
- resume = gr.File(label="Resume")
- with gr.Column(scale=0.10, min_width=150):
- analyse = gr.Button("Analyse")
- with gr.Row():
- with gr.Column(scale=1.0, min_width=150):
- perncentage = gr.Textbox(label="Matching Percentage",lines=8)
- with gr.Column(scale=1.0, min_width=150):
- reason = gr.Textbox(label="Matching Reason",lines=8)
- with gr.Column(scale=1.0, min_width=150):
- skills = gr.Textbox(label="Skills To Improve",lines=8)
- with gr.Column(scale=1.0, min_width=150):
- keywords = gr.Textbox(label="Matched Keywords",lines=8)
- with gr.Row():
- with gr.Column(scale=1.0, min_width=150):
- pychart = gr.Plot(label="Matching Percentage Chart")
- analyse.click(self.matching_percentage, [jobDescription, resume], [perncentage,reason,skills,keywords,pychart])
-
- app.launch()
-
-resume=ResumeAnalyser()
-resume.gradio_interface()
\ No newline at end of file
diff --git a/spaces/AHzizi/WaifuVoiceGen/README.md b/spaces/AHzizi/WaifuVoiceGen/README.md
deleted file mode 100644
index 2e44ec5507a21c84647346865c876ce2b48db560..0000000000000000000000000000000000000000
--- a/spaces/AHzizi/WaifuVoiceGen/README.md
+++ /dev/null
@@ -1,14 +0,0 @@
----
-title: Vits Models
-emoji: 🏃
-colorFrom: pink
-colorTo: indigo
-sdk: gradio
-sdk_version: 3.17.0
-app_file: app.py
-pinned: false
-license: apache-2.0
-duplicated_from: sayashi/vits-models
----
-
-Check out the configuration reference at https://huggingface.co/docs/hub/spaces-config-reference
diff --git a/spaces/AIConsultant/MusicGen/model_cards/AUDIOGEN_MODEL_CARD.md b/spaces/AIConsultant/MusicGen/model_cards/AUDIOGEN_MODEL_CARD.md
deleted file mode 100644
index 92decf5e16e05ce0c2e72af8aa6728b5186c6882..0000000000000000000000000000000000000000
--- a/spaces/AIConsultant/MusicGen/model_cards/AUDIOGEN_MODEL_CARD.md
+++ /dev/null
@@ -1,79 +0,0 @@
-# AudioGen Model Card
-
-## Model details
-**Organization developing the model:** The FAIR team of Meta AI.
-
-**Model date:** This version of AudioGen was trained between July 2023 and August 2023.
-
-**Model version:** This is version 2 of the model, not to be confused with the original AudioGen model published in ["AudioGen: Textually Guided Audio Generation"][audiogen].
-In this version (v2), AudioGen was trained on the same data, but with some other differences:
-1. This model was trained on 10 seconds (vs. 5 seconds in v1).
-2. The discrete representation used under the hood is extracted using a retrained EnCodec model on the environmental sound data, following the EnCodec setup detailed in the ["Simple and Controllable Music Generation" paper][musicgen].
-3. No audio mixing augmentations.
-
-**Model type:** AudioGen consists of an EnCodec model for audio tokenization, and an auto-regressive language model based on the transformer architecture for audio modeling. The released model has 1.5B parameters.
-
-**Paper or resource for more information:** More information can be found in the paper [AudioGen: Textually Guided Audio Generation](https://arxiv.org/abs/2209.15352).
-
-**Citation details:** See [AudioGen paper][audiogen]
-
-**License:** Code is released under MIT, model weights are released under CC-BY-NC 4.0.
-
-**Where to send questions or comments about the model:** Questions and comments about AudioGen can be sent via the [GitHub repository](https://github.com/facebookresearch/audiocraft) of the project, or by opening an issue.
-
-## Intended use
-**Primary intended use:** The primary use of AudioGen is research on AI-based audio generation, including:
-- Research efforts, such as probing and better understanding the limitations of generative models to further improve the state of science
-- Generation of sound guided by text to understand current abilities of generative AI models by machine learning amateurs
-
-**Primary intended users:** The primary intended users of the model are researchers in audio, machine learning and artificial intelligence, as well as amateur seeking to better understand those models.
-
-**Out-of-scope use cases** The model should not be used on downstream applications without further risk evaluation and mitigation. The model should not be used to intentionally create or disseminate audio pieces that create hostile or alienating environments for people. This includes generating audio that people would foreseeably find disturbing, distressing, or offensive; or content that propagates historical or current stereotypes.
-
-## Metrics
-
-**Models performance measures:** We used the following objective measure to evaluate the model on a standard audio benchmark:
-- Frechet Audio Distance computed on features extracted from a pre-trained audio classifier (VGGish)
-- Kullback-Leibler Divergence on label distributions extracted from a pre-trained audio classifier (PaSST)
-
-Additionally, we run qualitative studies with human participants, evaluating the performance of the model with the following axes:
-- Overall quality of the audio samples;
-- Text relevance to the provided text input;
-
-More details on performance measures and human studies can be found in the paper.
-
-**Decision thresholds:** Not applicable.
-
-## Evaluation datasets
-
-The model was evaluated on the [AudioCaps benchmark](https://audiocaps.github.io/).
-
-## Training datasets
-
-The model was trained on the following data sources: a subset of AudioSet (Gemmeke et al., 2017), [BBC sound effects](https://sound-effects.bbcrewind.co.uk/), AudioCaps (Kim et al., 2019), Clotho v2 (Drossos et al., 2020), VGG-Sound (Chen et al., 2020), FSD50K (Fonseca et al., 2021), [Free To Use Sounds](https://www.freetousesounds.com/all-in-one-bundle/), [Sonniss Game Effects](https://sonniss.com/gameaudiogdc), [WeSoundEffects](https://wesoundeffects.com/we-sound-effects-bundle-2020/), [Paramount Motion - Odeon Cinematic Sound Effects](https://www.paramountmotion.com/odeon-sound-effects).
-
-## Evaluation results
-
-Below are the objective metrics obtained with the released model on AudioCaps (consisting of 10-second long samples). Note that the model differs from the original AudioGen model introduced in the paper, hence the difference in the metrics.
-
-| Model | Frechet Audio Distance | KLD | Text consistency |
-|---|---|---|---|
-| facebook/audiogen-medium | 1.77 | 1.41 | 0.299 |
-
-More information can be found in the paper [AudioGen: Textually Guided Audio Generation][audiogen], in the Experiments section.
-
-## Limitations and biases
-
-**Limitations:**
-- The model is not able to generate realistic vocals.
-- The model has been trained with English descriptions and will not perform as well in other languages.
-- It is sometimes difficult to assess what types of text descriptions provide the best generations. Prompt engineering may be required to obtain satisfying results.
-
-**Biases:** The datasets used for training may be lacking of diversity and are not representative of all possible sound events. The generated samples from the model will reflect the biases from the training data.
-
-**Risks and harms:** Biases and limitations of the model may lead to generation of samples that may be considered as biased, inappropriate or offensive. We believe that providing the code to reproduce the research and train new models will allow to broaden the application to new and more representative data.
-
-**Use cases:** Users must be aware of the biases, limitations and risks of the model. AudioGen is a model developed for artificial intelligence research on audio generation. As such, it should not be used for downstream applications without further investigation and mitigation of risks.
-
-[musicgen]: https://arxiv.org/abs/2306.05284
-[audiogen]: https://arxiv.org/abs/2209.15352
diff --git a/spaces/AIGC-Audio/AudioGPT/text_to_audio/Make_An_Audio/ldm/modules/encoders/modules.py b/spaces/AIGC-Audio/AudioGPT/text_to_audio/Make_An_Audio/ldm/modules/encoders/modules.py
deleted file mode 100644
index 8a3f8df6d72023df7c141467a0114aca02e54cdb..0000000000000000000000000000000000000000
--- a/spaces/AIGC-Audio/AudioGPT/text_to_audio/Make_An_Audio/ldm/modules/encoders/modules.py
+++ /dev/null
@@ -1,350 +0,0 @@
-import torch
-import torch.nn as nn
-from functools import partial
-
-from ldm.modules.x_transformer import Encoder, TransformerWrapper # TODO: can we directly rely on lucidrains code and simply add this as a reuirement? --> test
-from torch.utils.checkpoint import checkpoint
-from transformers import T5Tokenizer, T5EncoderModel, CLIPTokenizer, CLIPTextModel, AutoTokenizer
-from importlib_resources import files
-from ldm.modules.encoders.CLAP.utils import read_config_as_args
-from ldm.modules.encoders.CLAP.clap import TextEncoder
-from ldm.util import default, count_params
-import open_clip
-
-class AbstractEncoder(nn.Module):
- def __init__(self):
- super().__init__()
-
- def encode(self, *args, **kwargs):
- raise NotImplementedError
-
-
-class ClassEmbedder(nn.Module):
- def __init__(self, embed_dim, n_classes=1000, key='class'):
- super().__init__()
- self.key = key
- self.embedding = nn.Embedding(n_classes, embed_dim)
-
- def forward(self, batch, key=None):
- if key is None:
- key = self.key
- # this is for use in crossattn
- c = batch[key][:, None]# (bsz,1)
- c = self.embedding(c)
- return c
-
-
-class TransformerEmbedder(AbstractEncoder):
- """Some transformer encoder layers"""
- def __init__(self, n_embed, n_layer, vocab_size, max_seq_len=77, device="cuda"):
- super().__init__()
- self.device = device
- self.transformer = TransformerWrapper(num_tokens=vocab_size, max_seq_len=max_seq_len,
- attn_layers=Encoder(dim=n_embed, depth=n_layer))
-
- def forward(self, tokens):
- tokens = tokens.to(self.device) # meh
- z = self.transformer(tokens, return_embeddings=True)
- return z
-
- def encode(self, x):
- return self(x)
-
-
-class BERTTokenizer(AbstractEncoder):
- """ Uses a pretrained BERT tokenizer by huggingface. Vocab size: 30522 (?)"""
- def __init__(self, device="cuda", vq_interface=True, max_length=77):
- super().__init__()
- from transformers import BertTokenizerFast # TODO: add to reuquirements
- self.tokenizer = BertTokenizerFast.from_pretrained("bert-base-uncased")
- self.device = device
- self.vq_interface = vq_interface
- self.max_length = max_length
-
- def forward(self, text):
- batch_encoding = self.tokenizer(text, truncation=True, max_length=self.max_length, return_length=True,
- return_overflowing_tokens=False, padding="max_length", return_tensors="pt")
- tokens = batch_encoding["input_ids"].to(self.device)
- return tokens
-
- @torch.no_grad()
- def encode(self, text):
- tokens = self(text)
- if not self.vq_interface:
- return tokens
- return None, None, [None, None, tokens]
-
- def decode(self, text):
- return text
-
-
-class BERTEmbedder(AbstractEncoder):# 这里不是用的pretrained bert,是用的transformers的BertTokenizer加自定义的TransformerWrapper
- """Uses the BERT tokenizr model and add some transformer encoder layers"""
- def __init__(self, n_embed, n_layer, vocab_size=30522, max_seq_len=77,
- device="cuda",use_tokenizer=True, embedding_dropout=0.0):
- super().__init__()
- self.use_tknz_fn = use_tokenizer
- if self.use_tknz_fn:
- self.tknz_fn = BERTTokenizer(vq_interface=False, max_length=max_seq_len)
- self.device = device
- self.transformer = TransformerWrapper(num_tokens=vocab_size, max_seq_len=max_seq_len,
- attn_layers=Encoder(dim=n_embed, depth=n_layer),
- emb_dropout=embedding_dropout)
-
- def forward(self, text):
- if self.use_tknz_fn:
- tokens = self.tknz_fn(text)#.to(self.device)
- else:
- tokens = text
- z = self.transformer(tokens, return_embeddings=True)
- return z
-
- def encode(self, text):
- # output of length 77
- return self(text)
-
-
-class SpatialRescaler(nn.Module):
- def __init__(self,
- n_stages=1,
- method='bilinear',
- multiplier=0.5,
- in_channels=3,
- out_channels=None,
- bias=False):
- super().__init__()
- self.n_stages = n_stages
- assert self.n_stages >= 0
- assert method in ['nearest','linear','bilinear','trilinear','bicubic','area']
- self.multiplier = multiplier
- self.interpolator = partial(torch.nn.functional.interpolate, mode=method)
- self.remap_output = out_channels is not None
- if self.remap_output:
- print(f'Spatial Rescaler mapping from {in_channels} to {out_channels} channels after resizing.')
- self.channel_mapper = nn.Conv2d(in_channels,out_channels,1,bias=bias)
-
- def forward(self,x):
- for stage in range(self.n_stages):
- x = self.interpolator(x, scale_factor=self.multiplier)
-
-
- if self.remap_output:
- x = self.channel_mapper(x)
- return x
-
- def encode(self, x):
- return self(x)
-
-def disabled_train(self, mode=True):
- """Overwrite model.train with this function to make sure train/eval mode
- does not change anymore."""
- return self
-
-class FrozenT5Embedder(AbstractEncoder):
- """Uses the T5 transformer encoder for text"""
- def __init__(self, version="google/t5-v1_1-large", device="cuda", max_length=77, freeze=True): # others are google/t5-v1_1-xl and google/t5-v1_1-xxl
- super().__init__()
- self.tokenizer = T5Tokenizer.from_pretrained(version)
- self.transformer = T5EncoderModel.from_pretrained(version)
- self.device = device
- self.max_length = max_length # TODO: typical value?
- if freeze:
- self.freeze()
-
- def freeze(self):
- self.transformer = self.transformer.eval()
- #self.train = disabled_train
- for param in self.parameters():
- param.requires_grad = False
-
- def forward(self, text):
- batch_encoding = self.tokenizer(text, truncation=True, max_length=self.max_length, return_length=True,
- return_overflowing_tokens=False, padding="max_length", return_tensors="pt")
- tokens = batch_encoding["input_ids"].to(self.device)
- outputs = self.transformer(input_ids=tokens)
-
- z = outputs.last_hidden_state
- return z
-
- def encode(self, text):
- return self(text)
-
-
-class FrozenCLAPEmbedder(AbstractEncoder):
- """Uses the CLAP transformer encoder for text (from huggingface)"""
- def __init__(self, weights_path, freeze=True, device="cuda", max_length=77): # clip-vit-base-patch32
- super().__init__()
-
- model_state_dict = torch.load(weights_path, map_location=torch.device('cpu'))['model']
- match_params = dict()
- for key in list(model_state_dict.keys()):
- if 'caption_encoder' in key:
- match_params[key.replace('caption_encoder.', '')] = model_state_dict[key]
-
- config_as_str = files('ldm').joinpath('modules/encoders/CLAP/config.yml').read_text()
- args = read_config_as_args(config_as_str, is_config_str=True)
-
- # To device
- self.tokenizer = AutoTokenizer.from_pretrained(args.text_model) # args.text_model
- self.caption_encoder = TextEncoder(
- args.d_proj, args.text_model, args.transformer_embed_dim
- )
-
- self.max_length = max_length
- self.device = device
- if freeze: self.freeze()
-
- print(f"{self.caption_encoder.__class__.__name__} comes with {count_params(self.caption_encoder) * 1.e-6:.2f} M params.")
-
- def freeze(self):
- self.caption_encoder.base = self.caption_encoder.base.eval()
- for param in self.caption_encoder.base.parameters():
- param.requires_grad = False
-
-
- def encode(self, text):
- batch_encoding = self.tokenizer(text, truncation=True, max_length=self.max_length, return_length=True,
- return_overflowing_tokens=False, padding="max_length", return_tensors="pt")
- tokens = batch_encoding["input_ids"].to(self.device)
-
- outputs = self.caption_encoder.base(input_ids=tokens)
- z = self.caption_encoder.projection(outputs.last_hidden_state)
- return z
-
-class FrozenCLAPEmbedderNoLoad(AbstractEncoder):
- def __init__(self, config, freeze=True, device="cpu", max_length=77):
- super().__init__()
- args = config
-
- # To device
- self.tokenizer = AutoTokenizer.from_pretrained(args.text_model) # args.text_model
- self.caption_encoder = TextEncoder(
- args.d_proj, args.text_model, args.transformer_embed_dim
- )
-
- self.max_length = max_length
- self.device = device
- if freeze: self.freeze()
-
- print(f"{self.caption_encoder.__class__.__name__} comes with {count_params(self.caption_encoder) * 1.e-6:.2f} M params.")
-
- def freeze(self):
- self.caption_encoder.base = self.caption_encoder.base.eval()
- for param in self.caption_encoder.base.parameters():
- param.requires_grad = False
-
-
- def encode(self, text):
- batch_encoding = self.tokenizer(text, truncation=True, max_length=self.max_length, return_length=True,
- return_overflowing_tokens=False, padding="max_length", return_tensors="pt")
- tokens = batch_encoding["input_ids"].to(self.device)
-
- outputs = self.caption_encoder.base(input_ids=tokens)
- z = self.caption_encoder.projection(outputs.last_hidden_state)
- return z
-
-
-class NewFrozenCLAPEmbedder(AbstractEncoder):
- """Uses the CLAP transformer encoder for text (from huggingface)"""
- def __init__(self, weights_path, freeze=True, device="cuda", max_length=77): # clip-vit-base-patch32
- super().__init__()
- # To device
- from transformers import RobertaTokenizer
- from ldm.modules.encoders.open_clap import create_model
-
-
- model, model_cfg = create_model(
- 'HTSAT-tiny',
- 'roberta',
- weights_path,
- enable_fusion=True,
- fusion_type='aff_2d'
- )
-
- del model.audio_branch, model.audio_transform, model.audio_projection
- self.tokenizer = RobertaTokenizer.from_pretrained('roberta-base')
- self.model = model
-
- self.max_length = max_length
- self.device = device
- if freeze: self.freeze()
-
- param_num = sum(p.numel() for p in model.parameters() if p.requires_grad)
- print(f'{self.model.__class__.__name__} comes with: {param_num / 1e+6:.3f} M params.')
-
- def freeze(self):
- self.model = self.model.eval()
- for param in self.model.parameters():
- param.requires_grad = False
-
- def encode(self, text):
- batch_encoding = self.tokenizer(text, truncation=True, max_length=self.max_length, return_length=True,
- return_overflowing_tokens=False, padding="max_length", return_tensors="pt")
- outputs = self.model.text_branch(input_ids=batch_encoding["input_ids"].to(self.device), attention_mask=batch_encoding["attention_mask"].to(self.device))
- z = self.model.text_projection(outputs.last_hidden_state)
- return z
-
-class FrozenFLANEmbedder(AbstractEncoder):
- """Uses the T5 transformer encoder for text"""
- def __init__(self, version="google/flan-t5-large", device="cuda", max_length=77, freeze=True): # others are google/t5-v1_1-xl and google/t5-v1_1-xxl
- super().__init__()
- self.tokenizer = T5Tokenizer.from_pretrained(version)
- self.transformer = T5EncoderModel.from_pretrained(version)
- self.device = device
- self.max_length = max_length # TODO: typical value?
- if freeze:
- self.freeze()
-
- def freeze(self):
- self.transformer = self.transformer.eval()
- #self.train = disabled_train
- for param in self.parameters():
- param.requires_grad = False
-
- def forward(self, text):
- batch_encoding = self.tokenizer(text, truncation=True, max_length=self.max_length, return_length=True,
- return_overflowing_tokens=False, padding="max_length", return_tensors="pt")
- tokens = batch_encoding["input_ids"].to(self.device)
- outputs = self.transformer(input_ids=tokens)
-
- z = outputs.last_hidden_state
- return z
-
- def encode(self, text):
- return self(text)
-class FrozenGlobalNormOpenCLIPEmbedder(AbstractEncoder):
- """
- Uses the OpenCLIP transformer encoder for text
- """
- def __init__(self, arch="ViT-H-14", version="laion2b_s32b_b79k", device="cuda", freeze=True, delvisual=True):
- super().__init__()
- model, _, preprocess = open_clip.create_model_and_transforms(arch, device=torch.device('cpu'), pretrained=version)
- if delvisual:
- del model.visual
- del preprocess
- else:
- self.preprocess = preprocess
- self.model = model
-
- self.device = device
- if freeze:
- self.freeze()
-
- def freeze(self):
- self.model = self.model.eval()
- for param in self.parameters():
- param.requires_grad = False
-
- def forward(self, text):
- tokens = open_clip.tokenize(text)
- z = self.model.encode_text(tokens.to(self.device))
- z /= z.norm(dim=-1, keepdim=True)
- return z.unsqueeze(1)
-
- def forward_img(self, image):
- z = self.model.encode_image(image.to(self.device))
- z /= z.norm(dim=-1, keepdim=True)
- return z.unsqueeze(1)
-
- def encode(self, text):
- return self(text)
\ No newline at end of file
diff --git a/spaces/AIGC-Audio/Make_An_Audio_inpaint/vocoder/bigvgan/__init__.py b/spaces/AIGC-Audio/Make_An_Audio_inpaint/vocoder/bigvgan/__init__.py
deleted file mode 100644
index e69de29bb2d1d6434b8b29ae775ad8c2e48c5391..0000000000000000000000000000000000000000
diff --git a/spaces/Ababababababbababa/poetry2023/app.py b/spaces/Ababababababbababa/poetry2023/app.py
deleted file mode 100644
index 5b6654d5a405778ddbc9ca5fa5d041aff535f3b5..0000000000000000000000000000000000000000
--- a/spaces/Ababababababbababa/poetry2023/app.py
+++ /dev/null
@@ -1,53 +0,0 @@
-import gc
-import gradio as gr
-from transformers import pipeline, set_seed
-
-pipe = pipeline('text-generation', framework='pt', model='akhooli/ap2023', tokenizer='akhooli/ap2023')
-#gc.collect()
-samples = [['أنت'
- ,1.0, 50, 1.0, 1.0, 114],['هل غادر'
- ,1.0, 50, 1.0, 1.0, 114 ],['ألا ليت'
- ,1.0, 50, 1.0, 1.0, 114 ],['يا قدس'
- ,1.0, 50, 1.0, 1.0, 114],['عيد بأية حال'
- ,1.0, 50, 1.0, 1.0, 114],['لكل شيء إذا ما'
- ,1.0, 50, 1.0, 1.0, 114 ],['.'
- ,1.0, 50, 1.0, 1.0, 114]]
-
-notes = """
-- Enter a short prompt or select (click) one of the examples and click SEND
-- Adjust parameters (temperture, top k, top p and penalty) through the slider (keep close to default values).
-- For the same seed (randomness), the same output is regenerated if other parameters are fixed
-- Clear and enter new prompt or select another example and SEND to regenerate
-- The '.' means start a new line from no prompt (your prompt need not be long)
-- Be patient: this runs on CPU (free tier)
-- Feedback (Twitter): @akhooli (https://twitter.com/akhooli/status/1611025232201977859)
-- Note/Disclaimer: may generate unaccepted or inappropriate content. Use at your own risk.
-"""
-def sayPoetry(prompt, temp=1.0, topk = 50, topp = 1.0, penalty=1.0, seed=114):
- if not int(seed) >= 0: seed=114
- set_seed(seed)
- gen = pipe(prompt, max_length=96, do_sample=True, temperature=temp, top_k=topk, top_p=topp, repetition_penalty=penalty,
- min_length = 64, no_repeat_ngram_size = 3, return_full_text=True,
- num_beams=5, num_return_sequences=1)[0]["generated_text"]
- poetry =""
- for line in gen.split('.')[:-1]:
- poetry += line #+ "\n"
- return poetry
-poetry = gr.Interface(fn=sayPoetry,
- inputs=[
- gr.Textbox(label="Enter short prompt or select from examples:"),
- gr.Slider(0.70, 1.2, step=0.01,value=1.0, label='control temperature'),
- gr.Slider(25, 100, step=1,value=50, label='control top k'),
- gr.Slider(0.80, 1.0, step=0.01,value=1.0, label='control top p'),
- gr.Slider(0.90, 1.50, step=0.01,value=1.0, label='control penalty'),
- gr.Number(value=139750, precision=0, label='Seed'),
- ],
- outputs=[gr.Textbox(label="Generated Poetry:")],
-
- allow_flagging='never',
- title='Arabic Poetry Generation Demo (updated Jan. 2023)',
- description = "A simple demo of AI generated poetry based on 1M poems fine-tuned using AraGPT2 (be patient, runs on cpu)",
- examples=samples,
- cache_examples=False,
- article = notes)
-poetry.launch() # show_error = True, debug=True
\ No newline at end of file
diff --git a/spaces/Abhilashvj/planogram-compliance/export.py b/spaces/Abhilashvj/planogram-compliance/export.py
deleted file mode 100644
index 21f9d06365770a03475b74b743837b2a43c4ec0d..0000000000000000000000000000000000000000
--- a/spaces/Abhilashvj/planogram-compliance/export.py
+++ /dev/null
@@ -1,1013 +0,0 @@
-# YOLOv5 🚀 by Ultralytics, GPL-3.0 license
-"""
-Export a YOLOv5 PyTorch model to other formats. TensorFlow exports authored by https://github.com/zldrobit
-
-Format | `export.py --include` | Model
---- | --- | ---
-PyTorch | - | yolov5s.pt
-TorchScript | `torchscript` | yolov5s.torchscript
-ONNX | `onnx` | yolov5s.onnx
-OpenVINO | `openvino` | yolov5s_openvino_model/
-TensorRT | `engine` | yolov5s.engine
-CoreML | `coreml` | yolov5s.mlmodel
-TensorFlow SavedModel | `saved_model` | yolov5s_saved_model/
-TensorFlow GraphDef | `pb` | yolov5s.pb
-TensorFlow Lite | `tflite` | yolov5s.tflite
-TensorFlow Edge TPU | `edgetpu` | yolov5s_edgetpu.tflite
-TensorFlow.js | `tfjs` | yolov5s_web_model/
-PaddlePaddle | `paddle` | yolov5s_paddle_model/
-
-Requirements:
- $ pip install -r requirements.txt coremltools onnx onnx-simplifier onnxruntime openvino-dev tensorflow-cpu # CPU
- $ pip install -r requirements.txt coremltools onnx onnx-simplifier onnxruntime-gpu openvino-dev tensorflow # GPU
-
-Usage:
- $ python export.py --weights yolov5s.pt --include torchscript onnx openvino engine coreml tflite ...
-
-Inference:
- $ python detect.py --weights yolov5s.pt # PyTorch
- yolov5s.torchscript # TorchScript
- yolov5s.onnx # ONNX Runtime or OpenCV DNN with --dnn
- yolov5s_openvino_model # OpenVINO
- yolov5s.engine # TensorRT
- yolov5s.mlmodel # CoreML (macOS-only)
- yolov5s_saved_model # TensorFlow SavedModel
- yolov5s.pb # TensorFlow GraphDef
- yolov5s.tflite # TensorFlow Lite
- yolov5s_edgetpu.tflite # TensorFlow Edge TPU
- yolov5s_paddle_model # PaddlePaddle
-
-TensorFlow.js:
- $ cd .. && git clone https://github.com/zldrobit/tfjs-yolov5-example.git && cd tfjs-yolov5-example
- $ npm install
- $ ln -s ../../yolov5/yolov5s_web_model public/yolov5s_web_model
- $ npm start
-"""
-
-import argparse
-import contextlib
-import json
-import os
-import platform
-import re
-import subprocess
-import sys
-import time
-import warnings
-from pathlib import Path
-
-import pandas as pd
-import torch
-from torch.utils.mobile_optimizer import optimize_for_mobile
-
-FILE = Path(__file__).resolve()
-ROOT = FILE.parents[0] # YOLOv5 root directory
-if str(ROOT) not in sys.path:
- sys.path.append(str(ROOT)) # add ROOT to PATH
-if platform.system() != "Windows":
- ROOT = Path(os.path.relpath(ROOT, Path.cwd())) # relative
-
-from models.experimental import attempt_load
-from models.yolo import ClassificationModel, Detect, DetectionModel, SegmentationModel
-from utils.dataloaders import LoadImages
-from utils.general import (
- LOGGER,
- Profile,
- check_dataset,
- check_img_size,
- check_requirements,
- check_version,
- check_yaml,
- colorstr,
- file_size,
- get_default_args,
- print_args,
- url2file,
- yaml_save,
-)
-from utils.torch_utils import select_device, smart_inference_mode
-
-MACOS = platform.system() == "Darwin" # macOS environment
-
-
-def export_formats():
- # YOLOv5 export formats
- x = [
- ["PyTorch", "-", ".pt", True, True],
- ["TorchScript", "torchscript", ".torchscript", True, True],
- ["ONNX", "onnx", ".onnx", True, True],
- ["OpenVINO", "openvino", "_openvino_model", True, False],
- ["TensorRT", "engine", ".engine", False, True],
- ["CoreML", "coreml", ".mlmodel", True, False],
- ["TensorFlow SavedModel", "saved_model", "_saved_model", True, True],
- ["TensorFlow GraphDef", "pb", ".pb", True, True],
- ["TensorFlow Lite", "tflite", ".tflite", True, False],
- ["TensorFlow Edge TPU", "edgetpu", "_edgetpu.tflite", False, False],
- ["TensorFlow.js", "tfjs", "_web_model", False, False],
- ["PaddlePaddle", "paddle", "_paddle_model", True, True],
- ]
- return pd.DataFrame(
- x, columns=["Format", "Argument", "Suffix", "CPU", "GPU"]
- )
-
-
-def try_export(inner_func):
- # YOLOv5 export decorator, i..e @try_export
- inner_args = get_default_args(inner_func)
-
- def outer_func(*args, **kwargs):
- prefix = inner_args["prefix"]
- try:
- with Profile() as dt:
- f, model = inner_func(*args, **kwargs)
- LOGGER.info(
- f"{prefix} export success ✅ {dt.t:.1f}s, saved as {f} ({file_size(f):.1f} MB)"
- )
- return f, model
- except Exception as e:
- LOGGER.info(f"{prefix} export failure ❌ {dt.t:.1f}s: {e}")
- return None, None
-
- return outer_func
-
-
-@try_export
-def export_torchscript(
- model, im, file, optimize, prefix=colorstr("TorchScript:")
-):
- # YOLOv5 TorchScript model export
- LOGGER.info(
- f"\n{prefix} starting export with torch {torch.__version__}..."
- )
- f = file.with_suffix(".torchscript")
-
- ts = torch.jit.trace(model, im, strict=False)
- d = {
- "shape": im.shape,
- "stride": int(max(model.stride)),
- "names": model.names,
- }
- extra_files = {"config.txt": json.dumps(d)} # torch._C.ExtraFilesMap()
- if (
- optimize
- ): # https://pytorch.org/tutorials/recipes/mobile_interpreter.html
- optimize_for_mobile(ts)._save_for_lite_interpreter(
- str(f), _extra_files=extra_files
- )
- else:
- ts.save(str(f), _extra_files=extra_files)
- return f, None
-
-
-@try_export
-def export_onnx(
- model, im, file, opset, dynamic, simplify, prefix=colorstr("ONNX:")
-):
- # YOLOv5 ONNX export
- check_requirements("onnx>=1.12.0")
- import onnx
-
- LOGGER.info(f"\n{prefix} starting export with onnx {onnx.__version__}...")
- f = file.with_suffix(".onnx")
-
- output_names = (
- ["output0", "output1"]
- if isinstance(model, SegmentationModel)
- else ["output0"]
- )
- if dynamic:
- dynamic = {
- "images": {0: "batch", 2: "height", 3: "width"}
- } # shape(1,3,640,640)
- if isinstance(model, SegmentationModel):
- dynamic["output0"] = {
- 0: "batch",
- 1: "anchors",
- } # shape(1,25200,85)
- dynamic["output1"] = {
- 0: "batch",
- 2: "mask_height",
- 3: "mask_width",
- } # shape(1,32,160,160)
- elif isinstance(model, DetectionModel):
- dynamic["output0"] = {
- 0: "batch",
- 1: "anchors",
- } # shape(1,25200,85)
-
- torch.onnx.export(
- model.cpu()
- if dynamic
- else model, # --dynamic only compatible with cpu
- im.cpu() if dynamic else im,
- f,
- verbose=False,
- opset_version=opset,
- do_constant_folding=True, # WARNING: DNN inference with torch>=1.12 may require do_constant_folding=False
- input_names=["images"],
- output_names=output_names,
- dynamic_axes=dynamic or None,
- )
-
- # Checks
- model_onnx = onnx.load(f) # load onnx model
- onnx.checker.check_model(model_onnx) # check onnx model
-
- # Metadata
- d = {"stride": int(max(model.stride)), "names": model.names}
- for k, v in d.items():
- meta = model_onnx.metadata_props.add()
- meta.key, meta.value = k, str(v)
- onnx.save(model_onnx, f)
-
- # Simplify
- if simplify:
- try:
- cuda = torch.cuda.is_available()
- check_requirements(
- (
- "onnxruntime-gpu" if cuda else "onnxruntime",
- "onnx-simplifier>=0.4.1",
- )
- )
- import onnxsim
-
- LOGGER.info(
- f"{prefix} simplifying with onnx-simplifier {onnxsim.__version__}..."
- )
- model_onnx, check = onnxsim.simplify(model_onnx)
- assert check, "assert check failed"
- onnx.save(model_onnx, f)
- except Exception as e:
- LOGGER.info(f"{prefix} simplifier failure: {e}")
- return f, model_onnx
-
-
-@try_export
-def export_openvino(file, metadata, half, prefix=colorstr("OpenVINO:")):
- # YOLOv5 OpenVINO export
- check_requirements(
- "openvino-dev"
- ) # requires openvino-dev: https://pypi.org/project/openvino-dev/
- import openvino.inference_engine as ie
-
- LOGGER.info(
- f"\n{prefix} starting export with openvino {ie.__version__}..."
- )
- f = str(file).replace(".pt", f"_openvino_model{os.sep}")
-
- cmd = f"mo --input_model {file.with_suffix('.onnx')} --output_dir {f} --data_type {'FP16' if half else 'FP32'}"
- subprocess.run(cmd.split(), check=True, env=os.environ) # export
- yaml_save(
- Path(f) / file.with_suffix(".yaml").name, metadata
- ) # add metadata.yaml
- return f, None
-
-
-@try_export
-def export_paddle(model, im, file, metadata, prefix=colorstr("PaddlePaddle:")):
- # YOLOv5 Paddle export
- check_requirements(("paddlepaddle", "x2paddle"))
- import x2paddle
- from x2paddle.convert import pytorch2paddle
-
- LOGGER.info(
- f"\n{prefix} starting export with X2Paddle {x2paddle.__version__}..."
- )
- f = str(file).replace(".pt", f"_paddle_model{os.sep}")
-
- pytorch2paddle(
- module=model, save_dir=f, jit_type="trace", input_examples=[im]
- ) # export
- yaml_save(
- Path(f) / file.with_suffix(".yaml").name, metadata
- ) # add metadata.yaml
- return f, None
-
-
-@try_export
-def export_coreml(model, im, file, int8, half, prefix=colorstr("CoreML:")):
- # YOLOv5 CoreML export
- check_requirements("coremltools")
- import coremltools as ct
-
- LOGGER.info(
- f"\n{prefix} starting export with coremltools {ct.__version__}..."
- )
- f = file.with_suffix(".mlmodel")
-
- ts = torch.jit.trace(model, im, strict=False) # TorchScript model
- ct_model = ct.convert(
- ts,
- inputs=[
- ct.ImageType(
- "image", shape=im.shape, scale=1 / 255, bias=[0, 0, 0]
- )
- ],
- )
- bits, mode = (
- (8, "kmeans_lut") if int8 else (16, "linear") if half else (32, None)
- )
- if bits < 32:
- if MACOS: # quantization only supported on macOS
- with warnings.catch_warnings():
- warnings.filterwarnings(
- "ignore", category=DeprecationWarning
- ) # suppress numpy==1.20 float warning
- ct_model = ct.models.neural_network.quantization_utils.quantize_weights(
- ct_model, bits, mode
- )
- else:
- print(
- f"{prefix} quantization only supported on macOS, skipping..."
- )
- ct_model.save(f)
- return f, ct_model
-
-
-@try_export
-def export_engine(
- model,
- im,
- file,
- half,
- dynamic,
- simplify,
- workspace=4,
- verbose=False,
- prefix=colorstr("TensorRT:"),
-):
- # YOLOv5 TensorRT export https://developer.nvidia.com/tensorrt
- assert (
- im.device.type != "cpu"
- ), "export running on CPU but must be on GPU, i.e. `python export.py --device 0`"
- try:
- import tensorrt as trt
- except Exception:
- if platform.system() == "Linux":
- check_requirements(
- "nvidia-tensorrt",
- cmds="-U --index-url https://pypi.ngc.nvidia.com",
- )
- import tensorrt as trt
-
- if (
- trt.__version__[0] == "7"
- ): # TensorRT 7 handling https://github.com/ultralytics/yolov5/issues/6012
- grid = model.model[-1].anchor_grid
- model.model[-1].anchor_grid = [a[..., :1, :1, :] for a in grid]
- export_onnx(model, im, file, 12, dynamic, simplify) # opset 12
- model.model[-1].anchor_grid = grid
- else: # TensorRT >= 8
- check_version(
- trt.__version__, "8.0.0", hard=True
- ) # require tensorrt>=8.0.0
- export_onnx(model, im, file, 12, dynamic, simplify) # opset 12
- onnx = file.with_suffix(".onnx")
-
- LOGGER.info(
- f"\n{prefix} starting export with TensorRT {trt.__version__}..."
- )
- assert onnx.exists(), f"failed to export ONNX file: {onnx}"
- f = file.with_suffix(".engine") # TensorRT engine file
- logger = trt.Logger(trt.Logger.INFO)
- if verbose:
- logger.min_severity = trt.Logger.Severity.VERBOSE
-
- builder = trt.Builder(logger)
- config = builder.create_builder_config()
- config.max_workspace_size = workspace * 1 << 30
- # config.set_memory_pool_limit(trt.MemoryPoolType.WORKSPACE, workspace << 30) # fix TRT 8.4 deprecation notice
-
- flag = 1 << int(trt.NetworkDefinitionCreationFlag.EXPLICIT_BATCH)
- network = builder.create_network(flag)
- parser = trt.OnnxParser(network, logger)
- if not parser.parse_from_file(str(onnx)):
- raise RuntimeError(f"failed to load ONNX file: {onnx}")
-
- inputs = [network.get_input(i) for i in range(network.num_inputs)]
- outputs = [network.get_output(i) for i in range(network.num_outputs)]
- for inp in inputs:
- LOGGER.info(
- f'{prefix} input "{inp.name}" with shape{inp.shape} {inp.dtype}'
- )
- for out in outputs:
- LOGGER.info(
- f'{prefix} output "{out.name}" with shape{out.shape} {out.dtype}'
- )
-
- if dynamic:
- if im.shape[0] <= 1:
- LOGGER.warning(
- f"{prefix} WARNING ⚠️ --dynamic model requires maximum --batch-size argument"
- )
- profile = builder.create_optimization_profile()
- for inp in inputs:
- profile.set_shape(
- inp.name,
- (1, *im.shape[1:]),
- (max(1, im.shape[0] // 2), *im.shape[1:]),
- im.shape,
- )
- config.add_optimization_profile(profile)
-
- LOGGER.info(
- f"{prefix} building FP{16 if builder.platform_has_fast_fp16 and half else 32} engine as {f}"
- )
- if builder.platform_has_fast_fp16 and half:
- config.set_flag(trt.BuilderFlag.FP16)
- with builder.build_engine(network, config) as engine, open(f, "wb") as t:
- t.write(engine.serialize())
- return f, None
-
-
-@try_export
-def export_saved_model(
- model,
- im,
- file,
- dynamic,
- tf_nms=False,
- agnostic_nms=False,
- topk_per_class=100,
- topk_all=100,
- iou_thres=0.45,
- conf_thres=0.25,
- keras=False,
- prefix=colorstr("TensorFlow SavedModel:"),
-):
- # YOLOv5 TensorFlow SavedModel export
- try:
- import tensorflow as tf
- except Exception:
- check_requirements(
- f"tensorflow{'' if torch.cuda.is_available() else '-macos' if MACOS else '-cpu'}"
- )
- import tensorflow as tf
- from tensorflow.python.framework.convert_to_constants import (
- convert_variables_to_constants_v2,
- )
-
- from models.tf import TFModel
-
- LOGGER.info(
- f"\n{prefix} starting export with tensorflow {tf.__version__}..."
- )
- f = str(file).replace(".pt", "_saved_model")
- batch_size, ch, *imgsz = list(im.shape) # BCHW
-
- tf_model = TFModel(cfg=model.yaml, model=model, nc=model.nc, imgsz=imgsz)
- im = tf.zeros((batch_size, *imgsz, ch)) # BHWC order for TensorFlow
- _ = tf_model.predict(
- im,
- tf_nms,
- agnostic_nms,
- topk_per_class,
- topk_all,
- iou_thres,
- conf_thres,
- )
- inputs = tf.keras.Input(
- shape=(*imgsz, ch), batch_size=None if dynamic else batch_size
- )
- outputs = tf_model.predict(
- inputs,
- tf_nms,
- agnostic_nms,
- topk_per_class,
- topk_all,
- iou_thres,
- conf_thres,
- )
- keras_model = tf.keras.Model(inputs=inputs, outputs=outputs)
- keras_model.trainable = False
- keras_model.summary()
- if keras:
- keras_model.save(f, save_format="tf")
- else:
- spec = tf.TensorSpec(
- keras_model.inputs[0].shape, keras_model.inputs[0].dtype
- )
- m = tf.function(lambda x: keras_model(x)) # full model
- m = m.get_concrete_function(spec)
- frozen_func = convert_variables_to_constants_v2(m)
- tfm = tf.Module()
- tfm.__call__ = tf.function(
- lambda x: frozen_func(x)[:4] if tf_nms else frozen_func(x), [spec]
- )
- tfm.__call__(im)
- tf.saved_model.save(
- tfm,
- f,
- options=tf.saved_model.SaveOptions(
- experimental_custom_gradients=False
- )
- if check_version(tf.__version__, "2.6")
- else tf.saved_model.SaveOptions(),
- )
- return f, keras_model
-
-
-@try_export
-def export_pb(keras_model, file, prefix=colorstr("TensorFlow GraphDef:")):
- # YOLOv5 TensorFlow GraphDef *.pb export https://github.com/leimao/Frozen_Graph_TensorFlow
- import tensorflow as tf
- from tensorflow.python.framework.convert_to_constants import (
- convert_variables_to_constants_v2,
- )
-
- LOGGER.info(
- f"\n{prefix} starting export with tensorflow {tf.__version__}..."
- )
- f = file.with_suffix(".pb")
-
- m = tf.function(lambda x: keras_model(x)) # full model
- m = m.get_concrete_function(
- tf.TensorSpec(keras_model.inputs[0].shape, keras_model.inputs[0].dtype)
- )
- frozen_func = convert_variables_to_constants_v2(m)
- frozen_func.graph.as_graph_def()
- tf.io.write_graph(
- graph_or_graph_def=frozen_func.graph,
- logdir=str(f.parent),
- name=f.name,
- as_text=False,
- )
- return f, None
-
-
-@try_export
-def export_tflite(
- keras_model,
- im,
- file,
- int8,
- data,
- nms,
- agnostic_nms,
- prefix=colorstr("TensorFlow Lite:"),
-):
- # YOLOv5 TensorFlow Lite export
- import tensorflow as tf
-
- LOGGER.info(
- f"\n{prefix} starting export with tensorflow {tf.__version__}..."
- )
- batch_size, ch, *imgsz = list(im.shape) # BCHW
- f = str(file).replace(".pt", "-fp16.tflite")
-
- converter = tf.lite.TFLiteConverter.from_keras_model(keras_model)
- converter.target_spec.supported_ops = [tf.lite.OpsSet.TFLITE_BUILTINS]
- converter.target_spec.supported_types = [tf.float16]
- converter.optimizations = [tf.lite.Optimize.DEFAULT]
- if int8:
- from models.tf import representative_dataset_gen
-
- dataset = LoadImages(
- check_dataset(check_yaml(data))["train"],
- img_size=imgsz,
- auto=False,
- )
- converter.representative_dataset = lambda: representative_dataset_gen(
- dataset, ncalib=100
- )
- converter.target_spec.supported_ops = [
- tf.lite.OpsSet.TFLITE_BUILTINS_INT8
- ]
- converter.target_spec.supported_types = []
- converter.inference_input_type = tf.uint8 # or tf.int8
- converter.inference_output_type = tf.uint8 # or tf.int8
- converter.experimental_new_quantizer = True
- f = str(file).replace(".pt", "-int8.tflite")
- if nms or agnostic_nms:
- converter.target_spec.supported_ops.append(
- tf.lite.OpsSet.SELECT_TF_OPS
- )
-
- tflite_model = converter.convert()
- open(f, "wb").write(tflite_model)
- return f, None
-
-
-@try_export
-def export_edgetpu(file, prefix=colorstr("Edge TPU:")):
- # YOLOv5 Edge TPU export https://coral.ai/docs/edgetpu/models-intro/
- cmd = "edgetpu_compiler --version"
- help_url = "https://coral.ai/docs/edgetpu/compiler/"
- assert (
- platform.system() == "Linux"
- ), f"export only supported on Linux. See {help_url}"
- if subprocess.run(f"{cmd} >/dev/null", shell=True).returncode != 0:
- LOGGER.info(
- f"\n{prefix} export requires Edge TPU compiler. Attempting install from {help_url}"
- )
- sudo = (
- subprocess.run("sudo --version >/dev/null", shell=True).returncode
- == 0
- ) # sudo installed on system
- for c in (
- "curl https://packages.cloud.google.com/apt/doc/apt-key.gpg | sudo apt-key add -",
- 'echo "deb https://packages.cloud.google.com/apt coral-edgetpu-stable main" | sudo tee /etc/apt/sources.list.d/coral-edgetpu.list',
- "sudo apt-get update",
- "sudo apt-get install edgetpu-compiler",
- ):
- subprocess.run(
- c if sudo else c.replace("sudo ", ""), shell=True, check=True
- )
- ver = (
- subprocess.run(cmd, shell=True, capture_output=True, check=True)
- .stdout.decode()
- .split()[-1]
- )
-
- LOGGER.info(f"\n{prefix} starting export with Edge TPU compiler {ver}...")
- f = str(file).replace(".pt", "-int8_edgetpu.tflite") # Edge TPU model
- f_tfl = str(file).replace(".pt", "-int8.tflite") # TFLite model
-
- cmd = f"edgetpu_compiler -s -d -k 10 --out_dir {file.parent} {f_tfl}"
- subprocess.run(cmd.split(), check=True)
- return f, None
-
-
-@try_export
-def export_tfjs(file, prefix=colorstr("TensorFlow.js:")):
- # YOLOv5 TensorFlow.js export
- check_requirements("tensorflowjs")
- import tensorflowjs as tfjs
-
- LOGGER.info(
- f"\n{prefix} starting export with tensorflowjs {tfjs.__version__}..."
- )
- f = str(file).replace(".pt", "_web_model") # js dir
- f_pb = file.with_suffix(".pb") # *.pb path
- f_json = f"{f}/model.json" # *.json path
-
- cmd = (
- f"tensorflowjs_converter --input_format=tf_frozen_model "
- f"--output_node_names=Identity,Identity_1,Identity_2,Identity_3 {f_pb} {f}"
- )
- subprocess.run(cmd.split())
-
- json = Path(f_json).read_text()
- with open(f_json, "w") as j: # sort JSON Identity_* in ascending order
- subst = re.sub(
- r'{"outputs": {"Identity.?.?": {"name": "Identity.?.?"}, '
- r'"Identity.?.?": {"name": "Identity.?.?"}, '
- r'"Identity.?.?": {"name": "Identity.?.?"}, '
- r'"Identity.?.?": {"name": "Identity.?.?"}}}',
- r'{"outputs": {"Identity": {"name": "Identity"}, '
- r'"Identity_1": {"name": "Identity_1"}, '
- r'"Identity_2": {"name": "Identity_2"}, '
- r'"Identity_3": {"name": "Identity_3"}}}',
- json,
- )
- j.write(subst)
- return f, None
-
-
-def add_tflite_metadata(file, metadata, num_outputs):
- # Add metadata to *.tflite models per https://www.tensorflow.org/lite/models/convert/metadata
- with contextlib.suppress(ImportError):
- # check_requirements('tflite_support')
- from tflite_support import flatbuffers
- from tflite_support import metadata as _metadata
- from tflite_support import metadata_schema_py_generated as _metadata_fb
-
- tmp_file = Path("/tmp/meta.txt")
- with open(tmp_file, "w") as meta_f:
- meta_f.write(str(metadata))
-
- model_meta = _metadata_fb.ModelMetadataT()
- label_file = _metadata_fb.AssociatedFileT()
- label_file.name = tmp_file.name
- model_meta.associatedFiles = [label_file]
-
- subgraph = _metadata_fb.SubGraphMetadataT()
- subgraph.inputTensorMetadata = [_metadata_fb.TensorMetadataT()]
- subgraph.outputTensorMetadata = [
- _metadata_fb.TensorMetadataT()
- ] * num_outputs
- model_meta.subgraphMetadata = [subgraph]
-
- b = flatbuffers.Builder(0)
- b.Finish(
- model_meta.Pack(b),
- _metadata.MetadataPopulator.METADATA_FILE_IDENTIFIER,
- )
- metadata_buf = b.Output()
-
- populator = _metadata.MetadataPopulator.with_model_file(file)
- populator.load_metadata_buffer(metadata_buf)
- populator.load_associated_files([str(tmp_file)])
- populator.populate()
- tmp_file.unlink()
-
-
-@smart_inference_mode()
-def run(
- data=ROOT / "data/coco128.yaml", # 'dataset.yaml path'
- weights=ROOT / "yolov5s.pt", # weights path
- imgsz=(640, 640), # image (height, width)
- batch_size=1, # batch size
- device="cpu", # cuda device, i.e. 0 or 0,1,2,3 or cpu
- include=("torchscript", "onnx"), # include formats
- half=False, # FP16 half-precision export
- inplace=False, # set YOLOv5 Detect() inplace=True
- keras=False, # use Keras
- optimize=False, # TorchScript: optimize for mobile
- int8=False, # CoreML/TF INT8 quantization
- dynamic=False, # ONNX/TF/TensorRT: dynamic axes
- simplify=False, # ONNX: simplify model
- opset=12, # ONNX: opset version
- verbose=False, # TensorRT: verbose log
- workspace=4, # TensorRT: workspace size (GB)
- nms=False, # TF: add NMS to model
- agnostic_nms=False, # TF: add agnostic NMS to model
- topk_per_class=100, # TF.js NMS: topk per class to keep
- topk_all=100, # TF.js NMS: topk for all classes to keep
- iou_thres=0.45, # TF.js NMS: IoU threshold
- conf_thres=0.25, # TF.js NMS: confidence threshold
-):
- t = time.time()
- include = [x.lower() for x in include] # to lowercase
- fmts = tuple(export_formats()["Argument"][1:]) # --include arguments
- flags = [x in include for x in fmts]
- assert sum(flags) == len(
- include
- ), f"ERROR: Invalid --include {include}, valid --include arguments are {fmts}"
- (
- jit,
- onnx,
- xml,
- engine,
- coreml,
- saved_model,
- pb,
- tflite,
- edgetpu,
- tfjs,
- paddle,
- ) = flags # export booleans
- file = Path(
- url2file(weights)
- if str(weights).startswith(("http:/", "https:/"))
- else weights
- ) # PyTorch weights
-
- # Load PyTorch model
- device = select_device(device)
- if half:
- assert (
- device.type != "cpu" or coreml
- ), "--half only compatible with GPU export, i.e. use --device 0"
- assert (
- not dynamic
- ), "--half not compatible with --dynamic, i.e. use either --half or --dynamic but not both"
- model = attempt_load(
- weights, device=device, inplace=True, fuse=True
- ) # load FP32 model
-
- # Checks
- imgsz *= 2 if len(imgsz) == 1 else 1 # expand
- if optimize:
- assert (
- device.type == "cpu"
- ), "--optimize not compatible with cuda devices, i.e. use --device cpu"
-
- # Input
- gs = int(max(model.stride)) # grid size (max stride)
- imgsz = [
- check_img_size(x, gs) for x in imgsz
- ] # verify img_size are gs-multiples
- im = torch.zeros(batch_size, 3, *imgsz).to(
- device
- ) # image size(1,3,320,192) BCHW iDetection
-
- # Update model
- model.eval()
- for k, m in model.named_modules():
- if isinstance(m, Detect):
- m.inplace = inplace
- m.dynamic = dynamic
- m.export = True
-
- for _ in range(2):
- y = model(im) # dry runs
- if half and not coreml:
- im, model = im.half(), model.half() # to FP16
- shape = tuple(
- (y[0] if isinstance(y, tuple) else y).shape
- ) # model output shape
- metadata = {
- "stride": int(max(model.stride)),
- "names": model.names,
- } # model metadata
- LOGGER.info(
- f"\n{colorstr('PyTorch:')} starting from {file} with output shape {shape} ({file_size(file):.1f} MB)"
- )
-
- # Exports
- f = [""] * len(fmts) # exported filenames
- warnings.filterwarnings(
- action="ignore", category=torch.jit.TracerWarning
- ) # suppress TracerWarning
- if jit: # TorchScript
- f[0], _ = export_torchscript(model, im, file, optimize)
- if engine: # TensorRT required before ONNX
- f[1], _ = export_engine(
- model, im, file, half, dynamic, simplify, workspace, verbose
- )
- if onnx or xml: # OpenVINO requires ONNX
- f[2], _ = export_onnx(model, im, file, opset, dynamic, simplify)
- if xml: # OpenVINO
- f[3], _ = export_openvino(file, metadata, half)
- if coreml: # CoreML
- f[4], _ = export_coreml(model, im, file, int8, half)
- if any((saved_model, pb, tflite, edgetpu, tfjs)): # TensorFlow formats
- assert (
- not tflite or not tfjs
- ), "TFLite and TF.js models must be exported separately, please pass only one type."
- assert not isinstance(
- model, ClassificationModel
- ), "ClassificationModel export to TF formats not yet supported."
- f[5], s_model = export_saved_model(
- model.cpu(),
- im,
- file,
- dynamic,
- tf_nms=nms or agnostic_nms or tfjs,
- agnostic_nms=agnostic_nms or tfjs,
- topk_per_class=topk_per_class,
- topk_all=topk_all,
- iou_thres=iou_thres,
- conf_thres=conf_thres,
- keras=keras,
- )
- if pb or tfjs: # pb prerequisite to tfjs
- f[6], _ = export_pb(s_model, file)
- if tflite or edgetpu:
- f[7], _ = export_tflite(
- s_model,
- im,
- file,
- int8 or edgetpu,
- data=data,
- nms=nms,
- agnostic_nms=agnostic_nms,
- )
- if edgetpu:
- f[8], _ = export_edgetpu(file)
- add_tflite_metadata(
- f[8] or f[7], metadata, num_outputs=len(s_model.outputs)
- )
- if tfjs:
- f[9], _ = export_tfjs(file)
- if paddle: # PaddlePaddle
- f[10], _ = export_paddle(model, im, file, metadata)
-
- # Finish
- f = [str(x) for x in f if x] # filter out '' and None
- if any(f):
- cls, det, seg = (
- isinstance(model, x)
- for x in (ClassificationModel, DetectionModel, SegmentationModel)
- ) # type
- det &= (
- not seg
- ) # segmentation models inherit from SegmentationModel(DetectionModel)
- dir = Path("segment" if seg else "classify" if cls else "")
- h = "--half" if half else "" # --half FP16 inference arg
- s = (
- "# WARNING ⚠️ ClassificationModel not yet supported for PyTorch Hub AutoShape inference"
- if cls
- else "# WARNING ⚠️ SegmentationModel not yet supported for PyTorch Hub AutoShape inference"
- if seg
- else ""
- )
- LOGGER.info(
- f"\nExport complete ({time.time() - t:.1f}s)"
- f"\nResults saved to {colorstr('bold', file.parent.resolve())}"
- f"\nDetect: python {dir / ('detect.py' if det else 'predict.py')} --weights {f[-1]} {h}"
- f"\nValidate: python {dir / 'val.py'} --weights {f[-1]} {h}"
- f"\nPyTorch Hub: model = torch.hub.load('ultralytics/yolov5', 'custom', '{f[-1]}') {s}"
- f"\nVisualize: https://netron.app"
- )
- return f # return list of exported files/dirs
-
-
-def parse_opt():
- parser = argparse.ArgumentParser()
- parser.add_argument(
- "--data",
- type=str,
- default=ROOT / "data/coco128.yaml",
- help="dataset.yaml path",
- )
- parser.add_argument(
- "--weights",
- nargs="+",
- type=str,
- default=ROOT / "yolov5s.pt",
- help="model.pt path(s)",
- )
- parser.add_argument(
- "--imgsz",
- "--img",
- "--img-size",
- nargs="+",
- type=int,
- default=[640, 640],
- help="image (h, w)",
- )
- parser.add_argument("--batch-size", type=int, default=1, help="batch size")
- parser.add_argument(
- "--device", default="cpu", help="cuda device, i.e. 0 or 0,1,2,3 or cpu"
- )
- parser.add_argument(
- "--half", action="store_true", help="FP16 half-precision export"
- )
- parser.add_argument(
- "--inplace",
- action="store_true",
- help="set YOLOv5 Detect() inplace=True",
- )
- parser.add_argument("--keras", action="store_true", help="TF: use Keras")
- parser.add_argument(
- "--optimize",
- action="store_true",
- help="TorchScript: optimize for mobile",
- )
- parser.add_argument(
- "--int8", action="store_true", help="CoreML/TF INT8 quantization"
- )
- parser.add_argument(
- "--dynamic", action="store_true", help="ONNX/TF/TensorRT: dynamic axes"
- )
- parser.add_argument(
- "--simplify", action="store_true", help="ONNX: simplify model"
- )
- parser.add_argument(
- "--opset", type=int, default=17, help="ONNX: opset version"
- )
- parser.add_argument(
- "--verbose", action="store_true", help="TensorRT: verbose log"
- )
- parser.add_argument(
- "--workspace",
- type=int,
- default=4,
- help="TensorRT: workspace size (GB)",
- )
- parser.add_argument(
- "--nms", action="store_true", help="TF: add NMS to model"
- )
- parser.add_argument(
- "--agnostic-nms",
- action="store_true",
- help="TF: add agnostic NMS to model",
- )
- parser.add_argument(
- "--topk-per-class",
- type=int,
- default=100,
- help="TF.js NMS: topk per class to keep",
- )
- parser.add_argument(
- "--topk-all",
- type=int,
- default=100,
- help="TF.js NMS: topk for all classes to keep",
- )
- parser.add_argument(
- "--iou-thres",
- type=float,
- default=0.45,
- help="TF.js NMS: IoU threshold",
- )
- parser.add_argument(
- "--conf-thres",
- type=float,
- default=0.25,
- help="TF.js NMS: confidence threshold",
- )
- parser.add_argument(
- "--include",
- nargs="+",
- default=["torchscript"],
- help="torchscript, onnx, openvino, engine, coreml, saved_model, pb, tflite, edgetpu, tfjs, paddle",
- )
- opt = parser.parse_args()
- print_args(vars(opt))
- return opt
-
-
-def main(opt):
- for opt.weights in (
- opt.weights if isinstance(opt.weights, list) else [opt.weights]
- ):
- run(**vars(opt))
-
-
-if __name__ == "__main__":
- opt = parse_opt()
- main(opt)
diff --git a/spaces/AgentVerse/agentVerse/ui/src/classes/npc.ts b/spaces/AgentVerse/agentVerse/ui/src/classes/npc.ts
deleted file mode 100644
index 452acc65bdc4d27eb8a16c60c83bb1159030de71..0000000000000000000000000000000000000000
--- a/spaces/AgentVerse/agentVerse/ui/src/classes/npc.ts
+++ /dev/null
@@ -1,246 +0,0 @@
-import { Actor } from "./actor";
-import { DIRECTION } from "../utils";
-import {
- MoveTo,
- PathFinder,
- Board,
-} from "../phaser3-rex-plugins/plugins/board-components";
-import { Label } from "../phaser3-rex-plugins/templates/ui/ui-components";
-import { COLOR_DARK, COLOR_LIGHT, COLOR_PRIMARY } from "../constants";
-import { TownScene } from "../scenes";
-import eventsCenter from "./event_center";
-
-export class NPC extends Actor {
- private moveTo: MoveTo;
- private board: Board;
- private canMove: boolean = true;
- private talkWithPlayer: boolean = false;
- private path: PathFinder.NodeType[] = [];
- private finalDirection: number = undefined;
- private targetLocation: string = undefined;
- private targetNPC: NPC = undefined;
- private textBox: Label = undefined;
-
- public id: number;
- public direction: number = DIRECTION.DOWN;
-
- constructor(
- scene: Phaser.Scene,
- board: Board,
- x: number,
- y: number,
- name: string,
- id: number
- ) {
- super(scene, x, y, name);
-
- this.setName(name);
- this.board = board;
- this.id = id;
- // PHYSICS
- this.getBody().setSize(14, 16);
- this.getBody().setOffset(0, 4);
- this.getBody().setImmovable(true);
- this.setOrigin(0, 0.2);
-
- this.initAnimations();
- this.moveTo = this.scene.rexBoard.add.moveTo(this, {
- speed: 55,
- sneak: true,
- });
- this.listenToDirectionEvent();
- }
-
- update(): void {
- if (this.path.length > 0 && !this.moveTo.isRunning && this.canMove) {
- var tileXY = this.board.worldXYToTileXY(this.x, this.y);
- if (tileXY.x == this.path[0].x) {
- if (tileXY.y < this.path[0].y) this.changeDirection(DIRECTION.DOWN);
- else if (tileXY.y > this.path[0].y) this.changeDirection(DIRECTION.UP);
- } else if (tileXY.y == this.path[0].y) {
- if (tileXY.x < this.path[0].x) this.changeDirection(DIRECTION.RIGHT);
- else if (tileXY.x > this.path[0].x)
- this.changeDirection(DIRECTION.LEFT);
- }
- var move = this.moveTo.moveTo(this.path.shift());
- move.removeAllListeners("complete");
- move.on("complete", () => {
- if (this.path.length == 0) {
- this.changeDirection(this.finalDirection);
- this.emitTurnEvent();
- if (this.targetLocation != undefined) {
- fetch("http://127.0.0.1:10002/update_location", {
- method: "POST",
- headers: {
- "Content-Type": "application/json",
- },
- credentials: "same-origin",
- body: JSON.stringify({
- agent_locations: {
- [this.name]: this.targetLocation,
- },
- }),
- });
- }
- }
- });
- }
-
- var text = "";
- switch (this.direction) {
- case DIRECTION.UP:
- text = "up";
- break;
- case DIRECTION.DOWN:
- text = "down";
- break;
- case DIRECTION.LEFT:
- text = "left";
- break;
- case DIRECTION.RIGHT:
- text = "right";
- break;
- }
- this.anims.play(this.name + "-walk-" + text, true);
- if (this.anims.isPlaying && !this.moveTo.isRunning)
- this.anims.setCurrentFrame(this.anims.currentAnim!.frames[0]);
- this.updateTextBox();
- this.depth = this.y + this.height * 0.8;
- }
-
- listenToDirectionEvent(): void {
- eventsCenter.on(this.name + "-up", () => {
- this.changeDirection(DIRECTION.UP);
- });
- eventsCenter.on(this.name + "-down", () => {
- this.changeDirection(DIRECTION.DOWN);
- });
- eventsCenter.on(this.name + "-left", () => {
- this.changeDirection(DIRECTION.LEFT);
- });
- eventsCenter.on(this.name + "-right", () => {
- this.changeDirection(DIRECTION.RIGHT);
- });
- }
-
- emitTurnEvent(): void {
- // Make the listener NPC turn to the speaker NPC.
- if (this.targetNPC == undefined) return;
- var direction = "";
- switch (this.finalDirection) {
- case DIRECTION.UP:
- direction = "down";
- break;
- case DIRECTION.DOWN:
- direction = "up";
- break;
- case DIRECTION.LEFT:
- direction = "right";
- break;
- case DIRECTION.RIGHT:
- direction = "left";
- break;
- }
- eventsCenter.emit(this.targetNPC.name + "-" + direction);
- this.setTargetNPC();
- }
-
- updateTextBox(): void {
- if (this.textBox == undefined) return;
- this.textBox.setOrigin(0.5, 1.0);
- var scale = this.scene.cameras.main.zoom;
- this.textBox.setX(this.x + this.width / 2);
- this.textBox.setY(this.y - this.height * 0.2);
- this.textBox.depth = this.y + this.height * 0.8;
- this.textBox.getChildren().forEach((child) => {
- child.setDepth(this.y + this.height * 0.8);
- });
- }
-
- public setTextBox(text: string): void {
- this.destroyTextBox();
- var scale = this.scene.cameras.main.zoom;
- var scene = this.scene as TownScene;
- this.textBox = scene.rexUI.add
- .label({
- x: this.x + this.width / 2,
- y: this.y - this.height * 0.2,
- width: 24 * scale,
- orientation: "x",
- background: scene.rexUI.add.roundRectangle(
- 0,
- 0,
- 2,
- 2,
- 20,
- COLOR_PRIMARY,
- 0.7
- ),
- text: scene.rexUI.wrapExpandText(
- scene.add.text(0, 0, text, {
- fontSize: 10,
- })
- ),
- expandTextWidth: true,
- space: {
- left: 10,
- right: 10,
- top: 10,
- bottom: 10,
- },
- })
- .setOrigin(0.5, 1.0)
- .setScale(1 / scale, 1 / scale)
- .setDepth(this.y + this.height * 0.8)
- .layout();
- }
-
- public destroyTextBox(): void {
- if (this.textBox != undefined) this.textBox.destroy();
- this.textBox = undefined;
- }
-
- public changeDirection(direction: number): void {
- if (direction == undefined) return;
- this.direction = direction;
- }
-
- public moveAlongPath(
- path: PathFinder.NodeType[],
- finalDirection: number = undefined,
- targetLocation: string = undefined
- ): void {
- if (path.length == 0) return;
- if (this.moveTo.isRunning) return;
- if (this.path.length > 0) return;
- this.path = path;
- this.finalDirection = finalDirection;
- this.targetLocation = targetLocation;
- }
-
- public pauseMoving(): void {
- this.moveTo.stop();
- this.canMove = false;
- }
-
- public resumeMoving(): void {
- this.moveTo.resume();
- this.canMove = true;
- }
-
- public isMoving(): boolean {
- return this.moveTo.isRunning || this.path.length > 0;
- }
-
- public isTalking(): boolean {
- return this.talkWithPlayer;
- }
-
- public setTalking(talking: boolean): void {
- this.talkWithPlayer = talking;
- }
-
- public setTargetNPC(targetNPC: NPC = undefined): void {
- this.targetNPC = targetNPC;
- }
-}
diff --git a/spaces/AgentVerse/agentVerse/ui/src/phaser3-rex-plugins/plugins/fsm-plugin.d.ts b/spaces/AgentVerse/agentVerse/ui/src/phaser3-rex-plugins/plugins/fsm-plugin.d.ts
deleted file mode 100644
index 9bbd028b2de8925580170585f8aac9927f5688a6..0000000000000000000000000000000000000000
--- a/spaces/AgentVerse/agentVerse/ui/src/phaser3-rex-plugins/plugins/fsm-plugin.d.ts
+++ /dev/null
@@ -1,8 +0,0 @@
-import FSM from './fsm';
-
-export default class FSMPlugin extends Phaser.Plugins.BasePlugin {
- add(
- config?: FSM.IConfig
- ): FSM;
-
-}
\ No newline at end of file
diff --git a/spaces/AgentVerse/agentVerse/ui/src/phaser3-rex-plugins/templates/ui/sizer/Sizer.js b/spaces/AgentVerse/agentVerse/ui/src/phaser3-rex-plugins/templates/ui/sizer/Sizer.js
deleted file mode 100644
index 42243bee69d9f33dff7b6baea7e74a472464b87c..0000000000000000000000000000000000000000
--- a/spaces/AgentVerse/agentVerse/ui/src/phaser3-rex-plugins/templates/ui/sizer/Sizer.js
+++ /dev/null
@@ -1,79 +0,0 @@
-import BaseSizer from '../basesizer/BaseSizer.js';
-import Methods from './Methods.js';
-import GetChildrenProportion from './GetChildrenProportion.js';
-import GetOrientationMode from '../utils/GetOrientationMode.js';
-
-const IsPlainObject = Phaser.Utils.Objects.IsPlainObject;
-const GetValue = Phaser.Utils.Objects.GetValue;
-
-class Sizer extends BaseSizer {
- constructor(scene, x, y, minWidth, minHeight, orientation, config) {
- if (IsPlainObject(x)) {
- config = x;
- x = GetValue(config, 'x', 0);
- y = GetValue(config, 'y', 0);
- minWidth = GetValue(config, 'width', undefined);
- minHeight = GetValue(config, 'height', undefined);
- orientation = GetValue(config, 'orientation', 0);
- } else if (IsPlainObject(minWidth)) {
- config = minWidth;
- minWidth = GetValue(config, 'width', undefined);
- minHeight = GetValue(config, 'height', undefined);
- orientation = GetValue(config, 'orientation', 0);
- } else if (IsPlainObject(orientation)) {
- config = orientation;
- orientation = GetValue(config, 'orientation', 0);
- }
-
- if (orientation === undefined) {
- orientation = 0;
- }
- super(scene, x, y, minWidth, minHeight, config);
-
- this.type = 'rexSizer';
- this.sizerChildren = [];
- this.setOrientation(orientation);
- this.setItemSpacing(GetValue(config, 'space.item', 0));
- this.setStartChildIndex(GetValue(config, 'startChildIndex', 0));
- this.setRTL(GetValue(config, 'rtl', false));
-
- this.addChildrenMap('items', this.sizerChildren);
- }
-
- setOrientation(orientation) {
- this.orientation = GetOrientationMode(orientation);
- return this;
- }
-
- setItemSpacing(space) {
- this.space.item = space;
- return this;
- }
-
- setStartChildIndex(index) {
- this.startChildIndex = index;
- return this;
- }
-
- setRTL(enable) {
- if (enable === undefined) {
- enable = true;
- }
- this.rtl = enable;
- return this;
- }
-
- get childrenProportion() {
- if (this._childrenProportion === undefined) {
- this._childrenProportion = GetChildrenProportion.call(this);
- }
- return this._childrenProportion;
- }
-}
-
-Object.assign(
- Sizer.prototype,
- Methods
-);
-
-export default Sizer;
\ No newline at end of file
diff --git a/spaces/Aki004/herta-so-vits/vdecoder/hifigan/models.py b/spaces/Aki004/herta-so-vits/vdecoder/hifigan/models.py
deleted file mode 100644
index 9747301f350bb269e62601017fe4633ce271b27e..0000000000000000000000000000000000000000
--- a/spaces/Aki004/herta-so-vits/vdecoder/hifigan/models.py
+++ /dev/null
@@ -1,503 +0,0 @@
-import os
-import json
-from .env import AttrDict
-import numpy as np
-import torch
-import torch.nn.functional as F
-import torch.nn as nn
-from torch.nn import Conv1d, ConvTranspose1d, AvgPool1d, Conv2d
-from torch.nn.utils import weight_norm, remove_weight_norm, spectral_norm
-from .utils import init_weights, get_padding
-
-LRELU_SLOPE = 0.1
-
-
-def load_model(model_path, device='cuda'):
- config_file = os.path.join(os.path.split(model_path)[0], 'config.json')
- with open(config_file) as f:
- data = f.read()
-
- global h
- json_config = json.loads(data)
- h = AttrDict(json_config)
-
- generator = Generator(h).to(device)
-
- cp_dict = torch.load(model_path)
- generator.load_state_dict(cp_dict['generator'])
- generator.eval()
- generator.remove_weight_norm()
- del cp_dict
- return generator, h
-
-
-class ResBlock1(torch.nn.Module):
- def __init__(self, h, channels, kernel_size=3, dilation=(1, 3, 5)):
- super(ResBlock1, self).__init__()
- self.h = h
- self.convs1 = nn.ModuleList([
- weight_norm(Conv1d(channels, channels, kernel_size, 1, dilation=dilation[0],
- padding=get_padding(kernel_size, dilation[0]))),
- weight_norm(Conv1d(channels, channels, kernel_size, 1, dilation=dilation[1],
- padding=get_padding(kernel_size, dilation[1]))),
- weight_norm(Conv1d(channels, channels, kernel_size, 1, dilation=dilation[2],
- padding=get_padding(kernel_size, dilation[2])))
- ])
- self.convs1.apply(init_weights)
-
- self.convs2 = nn.ModuleList([
- weight_norm(Conv1d(channels, channels, kernel_size, 1, dilation=1,
- padding=get_padding(kernel_size, 1))),
- weight_norm(Conv1d(channels, channels, kernel_size, 1, dilation=1,
- padding=get_padding(kernel_size, 1))),
- weight_norm(Conv1d(channels, channels, kernel_size, 1, dilation=1,
- padding=get_padding(kernel_size, 1)))
- ])
- self.convs2.apply(init_weights)
-
- def forward(self, x):
- for c1, c2 in zip(self.convs1, self.convs2):
- xt = F.leaky_relu(x, LRELU_SLOPE)
- xt = c1(xt)
- xt = F.leaky_relu(xt, LRELU_SLOPE)
- xt = c2(xt)
- x = xt + x
- return x
-
- def remove_weight_norm(self):
- for l in self.convs1:
- remove_weight_norm(l)
- for l in self.convs2:
- remove_weight_norm(l)
-
-
-class ResBlock2(torch.nn.Module):
- def __init__(self, h, channels, kernel_size=3, dilation=(1, 3)):
- super(ResBlock2, self).__init__()
- self.h = h
- self.convs = nn.ModuleList([
- weight_norm(Conv1d(channels, channels, kernel_size, 1, dilation=dilation[0],
- padding=get_padding(kernel_size, dilation[0]))),
- weight_norm(Conv1d(channels, channels, kernel_size, 1, dilation=dilation[1],
- padding=get_padding(kernel_size, dilation[1])))
- ])
- self.convs.apply(init_weights)
-
- def forward(self, x):
- for c in self.convs:
- xt = F.leaky_relu(x, LRELU_SLOPE)
- xt = c(xt)
- x = xt + x
- return x
-
- def remove_weight_norm(self):
- for l in self.convs:
- remove_weight_norm(l)
-
-
-def padDiff(x):
- return F.pad(F.pad(x, (0,0,-1,1), 'constant', 0) - x, (0,0,0,-1), 'constant', 0)
-
-class SineGen(torch.nn.Module):
- """ Definition of sine generator
- SineGen(samp_rate, harmonic_num = 0,
- sine_amp = 0.1, noise_std = 0.003,
- voiced_threshold = 0,
- flag_for_pulse=False)
- samp_rate: sampling rate in Hz
- harmonic_num: number of harmonic overtones (default 0)
- sine_amp: amplitude of sine-wavefrom (default 0.1)
- noise_std: std of Gaussian noise (default 0.003)
- voiced_thoreshold: F0 threshold for U/V classification (default 0)
- flag_for_pulse: this SinGen is used inside PulseGen (default False)
- Note: when flag_for_pulse is True, the first time step of a voiced
- segment is always sin(np.pi) or cos(0)
- """
-
- def __init__(self, samp_rate, harmonic_num=0,
- sine_amp=0.1, noise_std=0.003,
- voiced_threshold=0,
- flag_for_pulse=False):
- super(SineGen, self).__init__()
- self.sine_amp = sine_amp
- self.noise_std = noise_std
- self.harmonic_num = harmonic_num
- self.dim = self.harmonic_num + 1
- self.sampling_rate = samp_rate
- self.voiced_threshold = voiced_threshold
- self.flag_for_pulse = flag_for_pulse
-
- def _f02uv(self, f0):
- # generate uv signal
- uv = (f0 > self.voiced_threshold).type(torch.float32)
- return uv
-
- def _f02sine(self, f0_values):
- """ f0_values: (batchsize, length, dim)
- where dim indicates fundamental tone and overtones
- """
- # convert to F0 in rad. The interger part n can be ignored
- # because 2 * np.pi * n doesn't affect phase
- rad_values = (f0_values / self.sampling_rate) % 1
-
- # initial phase noise (no noise for fundamental component)
- rand_ini = torch.rand(f0_values.shape[0], f0_values.shape[2], \
- device=f0_values.device)
- rand_ini[:, 0] = 0
- rad_values[:, 0, :] = rad_values[:, 0, :] + rand_ini
-
- # instantanouse phase sine[t] = sin(2*pi \sum_i=1 ^{t} rad)
- if not self.flag_for_pulse:
- # for normal case
-
- # To prevent torch.cumsum numerical overflow,
- # it is necessary to add -1 whenever \sum_k=1^n rad_value_k > 1.
- # Buffer tmp_over_one_idx indicates the time step to add -1.
- # This will not change F0 of sine because (x-1) * 2*pi = x * 2*pi
- tmp_over_one = torch.cumsum(rad_values, 1) % 1
- tmp_over_one_idx = (padDiff(tmp_over_one)) < 0
- cumsum_shift = torch.zeros_like(rad_values)
- cumsum_shift[:, 1:, :] = tmp_over_one_idx * -1.0
-
- sines = torch.sin(torch.cumsum(rad_values + cumsum_shift, dim=1)
- * 2 * np.pi)
- else:
- # If necessary, make sure that the first time step of every
- # voiced segments is sin(pi) or cos(0)
- # This is used for pulse-train generation
-
- # identify the last time step in unvoiced segments
- uv = self._f02uv(f0_values)
- uv_1 = torch.roll(uv, shifts=-1, dims=1)
- uv_1[:, -1, :] = 1
- u_loc = (uv < 1) * (uv_1 > 0)
-
- # get the instantanouse phase
- tmp_cumsum = torch.cumsum(rad_values, dim=1)
- # different batch needs to be processed differently
- for idx in range(f0_values.shape[0]):
- temp_sum = tmp_cumsum[idx, u_loc[idx, :, 0], :]
- temp_sum[1:, :] = temp_sum[1:, :] - temp_sum[0:-1, :]
- # stores the accumulation of i.phase within
- # each voiced segments
- tmp_cumsum[idx, :, :] = 0
- tmp_cumsum[idx, u_loc[idx, :, 0], :] = temp_sum
-
- # rad_values - tmp_cumsum: remove the accumulation of i.phase
- # within the previous voiced segment.
- i_phase = torch.cumsum(rad_values - tmp_cumsum, dim=1)
-
- # get the sines
- sines = torch.cos(i_phase * 2 * np.pi)
- return sines
-
- def forward(self, f0):
- """ sine_tensor, uv = forward(f0)
- input F0: tensor(batchsize=1, length, dim=1)
- f0 for unvoiced steps should be 0
- output sine_tensor: tensor(batchsize=1, length, dim)
- output uv: tensor(batchsize=1, length, 1)
- """
- with torch.no_grad():
- f0_buf = torch.zeros(f0.shape[0], f0.shape[1], self.dim,
- device=f0.device)
- # fundamental component
- fn = torch.multiply(f0, torch.FloatTensor([[range(1, self.harmonic_num + 2)]]).to(f0.device))
-
- # generate sine waveforms
- sine_waves = self._f02sine(fn) * self.sine_amp
-
- # generate uv signal
- # uv = torch.ones(f0.shape)
- # uv = uv * (f0 > self.voiced_threshold)
- uv = self._f02uv(f0)
-
- # noise: for unvoiced should be similar to sine_amp
- # std = self.sine_amp/3 -> max value ~ self.sine_amp
- # . for voiced regions is self.noise_std
- noise_amp = uv * self.noise_std + (1 - uv) * self.sine_amp / 3
- noise = noise_amp * torch.randn_like(sine_waves)
-
- # first: set the unvoiced part to 0 by uv
- # then: additive noise
- sine_waves = sine_waves * uv + noise
- return sine_waves, uv, noise
-
-
-class SourceModuleHnNSF(torch.nn.Module):
- """ SourceModule for hn-nsf
- SourceModule(sampling_rate, harmonic_num=0, sine_amp=0.1,
- add_noise_std=0.003, voiced_threshod=0)
- sampling_rate: sampling_rate in Hz
- harmonic_num: number of harmonic above F0 (default: 0)
- sine_amp: amplitude of sine source signal (default: 0.1)
- add_noise_std: std of additive Gaussian noise (default: 0.003)
- note that amplitude of noise in unvoiced is decided
- by sine_amp
- voiced_threshold: threhold to set U/V given F0 (default: 0)
- Sine_source, noise_source = SourceModuleHnNSF(F0_sampled)
- F0_sampled (batchsize, length, 1)
- Sine_source (batchsize, length, 1)
- noise_source (batchsize, length 1)
- uv (batchsize, length, 1)
- """
-
- def __init__(self, sampling_rate, harmonic_num=0, sine_amp=0.1,
- add_noise_std=0.003, voiced_threshod=0):
- super(SourceModuleHnNSF, self).__init__()
-
- self.sine_amp = sine_amp
- self.noise_std = add_noise_std
-
- # to produce sine waveforms
- self.l_sin_gen = SineGen(sampling_rate, harmonic_num,
- sine_amp, add_noise_std, voiced_threshod)
-
- # to merge source harmonics into a single excitation
- self.l_linear = torch.nn.Linear(harmonic_num + 1, 1)
- self.l_tanh = torch.nn.Tanh()
-
- def forward(self, x):
- """
- Sine_source, noise_source = SourceModuleHnNSF(F0_sampled)
- F0_sampled (batchsize, length, 1)
- Sine_source (batchsize, length, 1)
- noise_source (batchsize, length 1)
- """
- # source for harmonic branch
- sine_wavs, uv, _ = self.l_sin_gen(x)
- sine_merge = self.l_tanh(self.l_linear(sine_wavs))
-
- # source for noise branch, in the same shape as uv
- noise = torch.randn_like(uv) * self.sine_amp / 3
- return sine_merge, noise, uv
-
-
-class Generator(torch.nn.Module):
- def __init__(self, h):
- super(Generator, self).__init__()
- self.h = h
-
- self.num_kernels = len(h["resblock_kernel_sizes"])
- self.num_upsamples = len(h["upsample_rates"])
- self.f0_upsamp = torch.nn.Upsample(scale_factor=np.prod(h["upsample_rates"]))
- self.m_source = SourceModuleHnNSF(
- sampling_rate=h["sampling_rate"],
- harmonic_num=8)
- self.noise_convs = nn.ModuleList()
- self.conv_pre = weight_norm(Conv1d(h["inter_channels"], h["upsample_initial_channel"], 7, 1, padding=3))
- resblock = ResBlock1 if h["resblock"] == '1' else ResBlock2
- self.ups = nn.ModuleList()
- for i, (u, k) in enumerate(zip(h["upsample_rates"], h["upsample_kernel_sizes"])):
- c_cur = h["upsample_initial_channel"] // (2 ** (i + 1))
- self.ups.append(weight_norm(
- ConvTranspose1d(h["upsample_initial_channel"] // (2 ** i), h["upsample_initial_channel"] // (2 ** (i + 1)),
- k, u, padding=(k - u) // 2)))
- if i + 1 < len(h["upsample_rates"]): #
- stride_f0 = np.prod(h["upsample_rates"][i + 1:])
- self.noise_convs.append(Conv1d(
- 1, c_cur, kernel_size=stride_f0 * 2, stride=stride_f0, padding=stride_f0 // 2))
- else:
- self.noise_convs.append(Conv1d(1, c_cur, kernel_size=1))
- self.resblocks = nn.ModuleList()
- for i in range(len(self.ups)):
- ch = h["upsample_initial_channel"] // (2 ** (i + 1))
- for j, (k, d) in enumerate(zip(h["resblock_kernel_sizes"], h["resblock_dilation_sizes"])):
- self.resblocks.append(resblock(h, ch, k, d))
-
- self.conv_post = weight_norm(Conv1d(ch, 1, 7, 1, padding=3))
- self.ups.apply(init_weights)
- self.conv_post.apply(init_weights)
- self.cond = nn.Conv1d(h['gin_channels'], h['upsample_initial_channel'], 1)
-
- def forward(self, x, f0, g=None):
- # print(1,x.shape,f0.shape,f0[:, None].shape)
- f0 = self.f0_upsamp(f0[:, None]).transpose(1, 2) # bs,n,t
- # print(2,f0.shape)
- har_source, noi_source, uv = self.m_source(f0)
- har_source = har_source.transpose(1, 2)
- x = self.conv_pre(x)
- x = x + self.cond(g)
- # print(124,x.shape,har_source.shape)
- for i in range(self.num_upsamples):
- x = F.leaky_relu(x, LRELU_SLOPE)
- # print(3,x.shape)
- x = self.ups[i](x)
- x_source = self.noise_convs[i](har_source)
- # print(4,x_source.shape,har_source.shape,x.shape)
- x = x + x_source
- xs = None
- for j in range(self.num_kernels):
- if xs is None:
- xs = self.resblocks[i * self.num_kernels + j](x)
- else:
- xs += self.resblocks[i * self.num_kernels + j](x)
- x = xs / self.num_kernels
- x = F.leaky_relu(x)
- x = self.conv_post(x)
- x = torch.tanh(x)
-
- return x
-
- def remove_weight_norm(self):
- print('Removing weight norm...')
- for l in self.ups:
- remove_weight_norm(l)
- for l in self.resblocks:
- l.remove_weight_norm()
- remove_weight_norm(self.conv_pre)
- remove_weight_norm(self.conv_post)
-
-
-class DiscriminatorP(torch.nn.Module):
- def __init__(self, period, kernel_size=5, stride=3, use_spectral_norm=False):
- super(DiscriminatorP, self).__init__()
- self.period = period
- norm_f = weight_norm if use_spectral_norm == False else spectral_norm
- self.convs = nn.ModuleList([
- norm_f(Conv2d(1, 32, (kernel_size, 1), (stride, 1), padding=(get_padding(5, 1), 0))),
- norm_f(Conv2d(32, 128, (kernel_size, 1), (stride, 1), padding=(get_padding(5, 1), 0))),
- norm_f(Conv2d(128, 512, (kernel_size, 1), (stride, 1), padding=(get_padding(5, 1), 0))),
- norm_f(Conv2d(512, 1024, (kernel_size, 1), (stride, 1), padding=(get_padding(5, 1), 0))),
- norm_f(Conv2d(1024, 1024, (kernel_size, 1), 1, padding=(2, 0))),
- ])
- self.conv_post = norm_f(Conv2d(1024, 1, (3, 1), 1, padding=(1, 0)))
-
- def forward(self, x):
- fmap = []
-
- # 1d to 2d
- b, c, t = x.shape
- if t % self.period != 0: # pad first
- n_pad = self.period - (t % self.period)
- x = F.pad(x, (0, n_pad), "reflect")
- t = t + n_pad
- x = x.view(b, c, t // self.period, self.period)
-
- for l in self.convs:
- x = l(x)
- x = F.leaky_relu(x, LRELU_SLOPE)
- fmap.append(x)
- x = self.conv_post(x)
- fmap.append(x)
- x = torch.flatten(x, 1, -1)
-
- return x, fmap
-
-
-class MultiPeriodDiscriminator(torch.nn.Module):
- def __init__(self, periods=None):
- super(MultiPeriodDiscriminator, self).__init__()
- self.periods = periods if periods is not None else [2, 3, 5, 7, 11]
- self.discriminators = nn.ModuleList()
- for period in self.periods:
- self.discriminators.append(DiscriminatorP(period))
-
- def forward(self, y, y_hat):
- y_d_rs = []
- y_d_gs = []
- fmap_rs = []
- fmap_gs = []
- for i, d in enumerate(self.discriminators):
- y_d_r, fmap_r = d(y)
- y_d_g, fmap_g = d(y_hat)
- y_d_rs.append(y_d_r)
- fmap_rs.append(fmap_r)
- y_d_gs.append(y_d_g)
- fmap_gs.append(fmap_g)
-
- return y_d_rs, y_d_gs, fmap_rs, fmap_gs
-
-
-class DiscriminatorS(torch.nn.Module):
- def __init__(self, use_spectral_norm=False):
- super(DiscriminatorS, self).__init__()
- norm_f = weight_norm if use_spectral_norm == False else spectral_norm
- self.convs = nn.ModuleList([
- norm_f(Conv1d(1, 128, 15, 1, padding=7)),
- norm_f(Conv1d(128, 128, 41, 2, groups=4, padding=20)),
- norm_f(Conv1d(128, 256, 41, 2, groups=16, padding=20)),
- norm_f(Conv1d(256, 512, 41, 4, groups=16, padding=20)),
- norm_f(Conv1d(512, 1024, 41, 4, groups=16, padding=20)),
- norm_f(Conv1d(1024, 1024, 41, 1, groups=16, padding=20)),
- norm_f(Conv1d(1024, 1024, 5, 1, padding=2)),
- ])
- self.conv_post = norm_f(Conv1d(1024, 1, 3, 1, padding=1))
-
- def forward(self, x):
- fmap = []
- for l in self.convs:
- x = l(x)
- x = F.leaky_relu(x, LRELU_SLOPE)
- fmap.append(x)
- x = self.conv_post(x)
- fmap.append(x)
- x = torch.flatten(x, 1, -1)
-
- return x, fmap
-
-
-class MultiScaleDiscriminator(torch.nn.Module):
- def __init__(self):
- super(MultiScaleDiscriminator, self).__init__()
- self.discriminators = nn.ModuleList([
- DiscriminatorS(use_spectral_norm=True),
- DiscriminatorS(),
- DiscriminatorS(),
- ])
- self.meanpools = nn.ModuleList([
- AvgPool1d(4, 2, padding=2),
- AvgPool1d(4, 2, padding=2)
- ])
-
- def forward(self, y, y_hat):
- y_d_rs = []
- y_d_gs = []
- fmap_rs = []
- fmap_gs = []
- for i, d in enumerate(self.discriminators):
- if i != 0:
- y = self.meanpools[i - 1](y)
- y_hat = self.meanpools[i - 1](y_hat)
- y_d_r, fmap_r = d(y)
- y_d_g, fmap_g = d(y_hat)
- y_d_rs.append(y_d_r)
- fmap_rs.append(fmap_r)
- y_d_gs.append(y_d_g)
- fmap_gs.append(fmap_g)
-
- return y_d_rs, y_d_gs, fmap_rs, fmap_gs
-
-
-def feature_loss(fmap_r, fmap_g):
- loss = 0
- for dr, dg in zip(fmap_r, fmap_g):
- for rl, gl in zip(dr, dg):
- loss += torch.mean(torch.abs(rl - gl))
-
- return loss * 2
-
-
-def discriminator_loss(disc_real_outputs, disc_generated_outputs):
- loss = 0
- r_losses = []
- g_losses = []
- for dr, dg in zip(disc_real_outputs, disc_generated_outputs):
- r_loss = torch.mean((1 - dr) ** 2)
- g_loss = torch.mean(dg ** 2)
- loss += (r_loss + g_loss)
- r_losses.append(r_loss.item())
- g_losses.append(g_loss.item())
-
- return loss, r_losses, g_losses
-
-
-def generator_loss(disc_outputs):
- loss = 0
- gen_losses = []
- for dg in disc_outputs:
- l = torch.mean((1 - dg) ** 2)
- gen_losses.append(l)
- loss += l
-
- return loss, gen_losses
diff --git a/spaces/AkshayDev/Lazy-Film-Reviews/README.md b/spaces/AkshayDev/Lazy-Film-Reviews/README.md
deleted file mode 100644
index 075510eeb51d4c8382b290b485c57645d8c06999..0000000000000000000000000000000000000000
--- a/spaces/AkshayDev/Lazy-Film-Reviews/README.md
+++ /dev/null
@@ -1,13 +0,0 @@
----
-title: Lazy Film Reviews
-emoji: 🌖
-colorFrom: purple
-colorTo: gray
-sdk: streamlit
-sdk_version: 1.2.0
-app_file: app.py
-pinned: false
-license: cc-by-nc-4.0
----
-
-Check out the configuration reference at https://huggingface.co/docs/hub/spaces#reference
diff --git a/spaces/AlexWang/lama/saicinpainting/evaluation/masks/__init__.py b/spaces/AlexWang/lama/saicinpainting/evaluation/masks/__init__.py
deleted file mode 100644
index e69de29bb2d1d6434b8b29ae775ad8c2e48c5391..0000000000000000000000000000000000000000
diff --git a/spaces/AnTo2209/3D_Zeroshot_Neural_Style_Transfer/src/decoder/sh.py b/spaces/AnTo2209/3D_Zeroshot_Neural_Style_Transfer/src/decoder/sh.py
deleted file mode 100644
index 27e3cad120c2b7348431a9af4883e8e7cdb10cbe..0000000000000000000000000000000000000000
--- a/spaces/AnTo2209/3D_Zeroshot_Neural_Style_Transfer/src/decoder/sh.py
+++ /dev/null
@@ -1,133 +0,0 @@
-import torch
-
-################## sh function ##################
-C0 = 0.28209479177387814
-C1 = 0.4886025119029199
-C2 = [
- 1.0925484305920792,
- -1.0925484305920792,
- 0.31539156525252005,
- -1.0925484305920792,
- 0.5462742152960396
-]
-C3 = [
- -0.5900435899266435,
- 2.890611442640554,
- -0.4570457994644658,
- 0.3731763325901154,
- -0.4570457994644658,
- 1.445305721320277,
- -0.5900435899266435
-]
-C4 = [
- 2.5033429417967046,
- -1.7701307697799304,
- 0.9461746957575601,
- -0.6690465435572892,
- 0.10578554691520431,
- -0.6690465435572892,
- 0.47308734787878004,
- -1.7701307697799304,
- 0.6258357354491761,
-]
-
-def eval_sh(deg, sh, dirs):
- """
- Evaluate spherical harmonics at unit directions
- using hardcoded SH polynomials.
- Works with torch/np/jnp.
- ... Can be 0 or more batch dimensions.
- :param deg: int SH max degree. Currently, 0-4 supported
- :param sh: torch.Tensor SH coeffs (..., C, (max degree + 1) ** 2)
- :param dirs: torch.Tensor unit directions (..., 3)
- :return: (..., C)
- """
- assert deg <= 4 and deg >= 0
- assert (deg + 1) ** 2 == sh.shape[-1]
- C = sh.shape[-2]
-
- result = C0 * sh[..., 0]
- if deg > 0:
- x, y, z = dirs[..., 0:1], dirs[..., 1:2], dirs[..., 2:3]
- result = (result -
- C1 * y * sh[..., 1] +
- C1 * z * sh[..., 2] -
- C1 * x * sh[..., 3])
- if deg > 1:
- xx, yy, zz = x * x, y * y, z * z
- xy, yz, xz = x * y, y * z, x * z
- result = (result +
- C2[0] * xy * sh[..., 4] +
- C2[1] * yz * sh[..., 5] +
- C2[2] * (2.0 * zz - xx - yy) * sh[..., 6] +
- C2[3] * xz * sh[..., 7] +
- C2[4] * (xx - yy) * sh[..., 8])
-
- if deg > 2:
- result = (result +
- C3[0] * y * (3 * xx - yy) * sh[..., 9] +
- C3[1] * xy * z * sh[..., 10] +
- C3[2] * y * (4 * zz - xx - yy)* sh[..., 11] +
- C3[3] * z * (2 * zz - 3 * xx - 3 * yy) * sh[..., 12] +
- C3[4] * x * (4 * zz - xx - yy) * sh[..., 13] +
- C3[5] * z * (xx - yy) * sh[..., 14] +
- C3[6] * x * (xx - 3 * yy) * sh[..., 15])
- if deg > 3:
- result = (result + C4[0] * xy * (xx - yy) * sh[..., 16] +
- C4[1] * yz * (3 * xx - yy) * sh[..., 17] +
- C4[2] * xy * (7 * zz - 1) * sh[..., 18] +
- C4[3] * yz * (7 * zz - 3) * sh[..., 19] +
- C4[4] * (zz * (35 * zz - 30) + 3) * sh[..., 20] +
- C4[5] * xz * (7 * zz - 3) * sh[..., 21] +
- C4[6] * (xx - yy) * (7 * zz - 1) * sh[..., 22] +
- C4[7] * xz * (xx - 3 * yy) * sh[..., 23] +
- C4[8] * (xx * (xx - 3 * yy) - yy * (3 * xx - yy)) * sh[..., 24])
- return result
-
-def eval_sh_bases(deg, dirs):
- """
- Evaluate spherical harmonics bases at unit directions,
- without taking linear combination.
- At each point, the final result may the be
- obtained through simple multiplication.
- :param deg: int SH max degree. Currently, 0-4 supported
- :param dirs: torch.Tensor (..., 3) unit directions
- :return: torch.Tensor (..., (deg+1) ** 2)
- """
- assert deg <= 4 and deg >= 0
- result = torch.empty((*dirs.shape[:-1], (deg + 1) ** 2), dtype=dirs.dtype, device=dirs.device)
- result[..., 0] = C0
- if deg > 0:
- x, y, z = dirs.unbind(-1)
- result[..., 1] = -C1 * y;
- result[..., 2] = C1 * z;
- result[..., 3] = -C1 * x;
- if deg > 1:
- xx, yy, zz = x * x, y * y, z * z
- xy, yz, xz = x * y, y * z, x * z
- result[..., 4] = C2[0] * xy;
- result[..., 5] = C2[1] * yz;
- result[..., 6] = C2[2] * (2.0 * zz - xx - yy);
- result[..., 7] = C2[3] * xz;
- result[..., 8] = C2[4] * (xx - yy);
-
- if deg > 2:
- result[..., 9] = C3[0] * y * (3 * xx - yy);
- result[..., 10] = C3[1] * xy * z;
- result[..., 11] = C3[2] * y * (4 * zz - xx - yy);
- result[..., 12] = C3[3] * z * (2 * zz - 3 * xx - 3 * yy);
- result[..., 13] = C3[4] * x * (4 * zz - xx - yy);
- result[..., 14] = C3[5] * z * (xx - yy);
- result[..., 15] = C3[6] * x * (xx - 3 * yy);
-
- if deg > 3:
- result[..., 16] = C4[0] * xy * (xx - yy);
- result[..., 17] = C4[1] * yz * (3 * xx - yy);
- result[..., 18] = C4[2] * xy * (7 * zz - 1);
- result[..., 19] = C4[3] * yz * (7 * zz - 3);
- result[..., 20] = C4[4] * (zz * (35 * zz - 30) + 3);
- result[..., 21] = C4[5] * xz * (7 * zz - 3);
- result[..., 22] = C4[6] * (xx - yy) * (7 * zz - 1);
- result[..., 23] = C4[7] * xz * (xx - 3 * yy);
- result[..., 24] = C4[8] * (xx * (xx - 3 * yy) - yy * (3 * xx - yy));
- return result
diff --git a/spaces/AndreLie95/Diabetes_Risk_Prediction/README.md b/spaces/AndreLie95/Diabetes_Risk_Prediction/README.md
deleted file mode 100644
index 38407b427004ceab9eff12a93406a0512568b384..0000000000000000000000000000000000000000
--- a/spaces/AndreLie95/Diabetes_Risk_Prediction/README.md
+++ /dev/null
@@ -1,12 +0,0 @@
----
-title: Deploy Milestone 2
-emoji: ⚡
-colorFrom: indigo
-colorTo: indigo
-sdk: streamlit
-sdk_version: 1.17.0
-app_file: app.py
-pinned: false
----
-
-Check out the configuration reference at https://huggingface.co/docs/hub/spaces-config-reference
diff --git a/spaces/Androidonnxfork/CivitAi-to-Diffusers/diffusers/docs/source/en/optimization/opt_overview.md b/spaces/Androidonnxfork/CivitAi-to-Diffusers/diffusers/docs/source/en/optimization/opt_overview.md
deleted file mode 100644
index 8d8386f85f43df2d22c00a9b54df5de59e07fe01..0000000000000000000000000000000000000000
--- a/spaces/Androidonnxfork/CivitAi-to-Diffusers/diffusers/docs/source/en/optimization/opt_overview.md
+++ /dev/null
@@ -1,17 +0,0 @@
-
-
-# Overview
-
-Generating high-quality outputs is computationally intensive, especially during each iterative step where you go from a noisy output to a less noisy output. One of 🧨 Diffuser's goal is to make this technology widely accessible to everyone, which includes enabling fast inference on consumer and specialized hardware.
-
-This section will cover tips and tricks - like half-precision weights and sliced attention - for optimizing inference speed and reducing memory-consumption. You can also learn how to speed up your PyTorch code with [`torch.compile`](https://pytorch.org/tutorials/intermediate/torch_compile_tutorial.html) or [ONNX Runtime](https://onnxruntime.ai/docs/), and enable memory-efficient attention with [xFormers](https://facebookresearch.github.io/xformers/). There are also guides for running inference on specific hardware like Apple Silicon, and Intel or Habana processors.
\ No newline at end of file
diff --git a/spaces/Androidonnxfork/CivitAi-to-Diffusers/diffusers/examples/controlnet/README.md b/spaces/Androidonnxfork/CivitAi-to-Diffusers/diffusers/examples/controlnet/README.md
deleted file mode 100644
index 15b0170d512034bc21786f12f5ab3ccd35143f94..0000000000000000000000000000000000000000
--- a/spaces/Androidonnxfork/CivitAi-to-Diffusers/diffusers/examples/controlnet/README.md
+++ /dev/null
@@ -1,465 +0,0 @@
-# ControlNet training example
-
-[Adding Conditional Control to Text-to-Image Diffusion Models](https://arxiv.org/abs/2302.05543) by Lvmin Zhang and Maneesh Agrawala.
-
-This example is based on the [training example in the original ControlNet repository](https://github.com/lllyasviel/ControlNet/blob/main/docs/train.md). It trains a ControlNet to fill circles using a [small synthetic dataset](https://huggingface.co/datasets/fusing/fill50k).
-
-## Installing the dependencies
-
-Before running the scripts, make sure to install the library's training dependencies:
-
-**Important**
-
-To make sure you can successfully run the latest versions of the example scripts, we highly recommend **installing from source** and keeping the install up to date as we update the example scripts frequently and install some example-specific requirements. To do this, execute the following steps in a new virtual environment:
-```bash
-git clone https://github.com/huggingface/diffusers
-cd diffusers
-pip install -e .
-```
-
-Then cd in the example folder and run
-```bash
-pip install -r requirements.txt
-```
-
-And initialize an [🤗Accelerate](https://github.com/huggingface/accelerate/) environment with:
-
-```bash
-accelerate config
-```
-
-Or for a default accelerate configuration without answering questions about your environment
-
-```bash
-accelerate config default
-```
-
-Or if your environment doesn't support an interactive shell e.g. a notebook
-
-```python
-from accelerate.utils import write_basic_config
-write_basic_config()
-```
-
-## Circle filling dataset
-
-The original dataset is hosted in the [ControlNet repo](https://huggingface.co/lllyasviel/ControlNet/blob/main/training/fill50k.zip). We re-uploaded it to be compatible with `datasets` [here](https://huggingface.co/datasets/fusing/fill50k). Note that `datasets` handles dataloading within the training script.
-
-Our training examples use [Stable Diffusion 1.5](https://huggingface.co/runwayml/stable-diffusion-v1-5) as the original set of ControlNet models were trained from it. However, ControlNet can be trained to augment any Stable Diffusion compatible model (such as [CompVis/stable-diffusion-v1-4](https://huggingface.co/CompVis/stable-diffusion-v1-4)) or [stabilityai/stable-diffusion-2-1](https://huggingface.co/stabilityai/stable-diffusion-2-1).
-
-## Training
-
-Our training examples use two test conditioning images. They can be downloaded by running
-
-```sh
-wget https://huggingface.co/datasets/huggingface/documentation-images/resolve/main/diffusers/controlnet_training/conditioning_image_1.png
-
-wget https://huggingface.co/datasets/huggingface/documentation-images/resolve/main/diffusers/controlnet_training/conditioning_image_2.png
-```
-
-
-```bash
-export MODEL_DIR="runwayml/stable-diffusion-v1-5"
-export OUTPUT_DIR="path to save model"
-
-accelerate launch train_controlnet.py \
- --pretrained_model_name_or_path=$MODEL_DIR \
- --output_dir=$OUTPUT_DIR \
- --dataset_name=fusing/fill50k \
- --resolution=512 \
- --learning_rate=1e-5 \
- --validation_image "./conditioning_image_1.png" "./conditioning_image_2.png" \
- --validation_prompt "red circle with blue background" "cyan circle with brown floral background" \
- --train_batch_size=4
-```
-
-This default configuration requires ~38GB VRAM.
-
-By default, the training script logs outputs to tensorboard. Pass `--report_to wandb` to use weights and
-biases.
-
-Gradient accumulation with a smaller batch size can be used to reduce training requirements to ~20 GB VRAM.
-
-```bash
-export MODEL_DIR="runwayml/stable-diffusion-v1-5"
-export OUTPUT_DIR="path to save model"
-
-accelerate launch train_controlnet.py \
- --pretrained_model_name_or_path=$MODEL_DIR \
- --output_dir=$OUTPUT_DIR \
- --dataset_name=fusing/fill50k \
- --resolution=512 \
- --learning_rate=1e-5 \
- --validation_image "./conditioning_image_1.png" "./conditioning_image_2.png" \
- --validation_prompt "red circle with blue background" "cyan circle with brown floral background" \
- --train_batch_size=1 \
- --gradient_accumulation_steps=4
-```
-
-## Training with multiple GPUs
-
-`accelerate` allows for seamless multi-GPU training. Follow the instructions [here](https://huggingface.co/docs/accelerate/basic_tutorials/launch)
-for running distributed training with `accelerate`. Here is an example command:
-
-```bash
-export MODEL_DIR="runwayml/stable-diffusion-v1-5"
-export OUTPUT_DIR="path to save model"
-
-accelerate launch --mixed_precision="fp16" --multi_gpu train_controlnet.py \
- --pretrained_model_name_or_path=$MODEL_DIR \
- --output_dir=$OUTPUT_DIR \
- --dataset_name=fusing/fill50k \
- --resolution=512 \
- --learning_rate=1e-5 \
- --validation_image "./conditioning_image_1.png" "./conditioning_image_2.png" \
- --validation_prompt "red circle with blue background" "cyan circle with brown floral background" \
- --train_batch_size=4 \
- --mixed_precision="fp16" \
- --tracker_project_name="controlnet-demo" \
- --report_to=wandb
-```
-
-## Example results
-
-#### After 300 steps with batch size 8
-
-| | |
-|-------------------|:-------------------------:|
-| | red circle with blue background |
-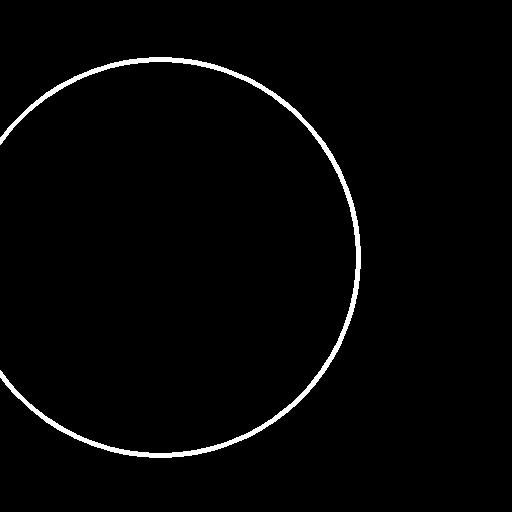 | 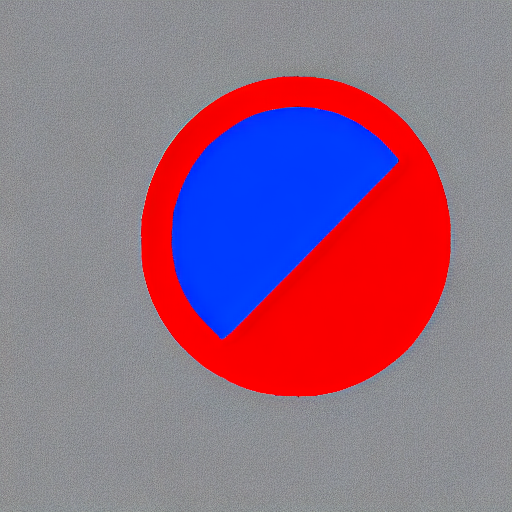 |
-| | cyan circle with brown floral background |
-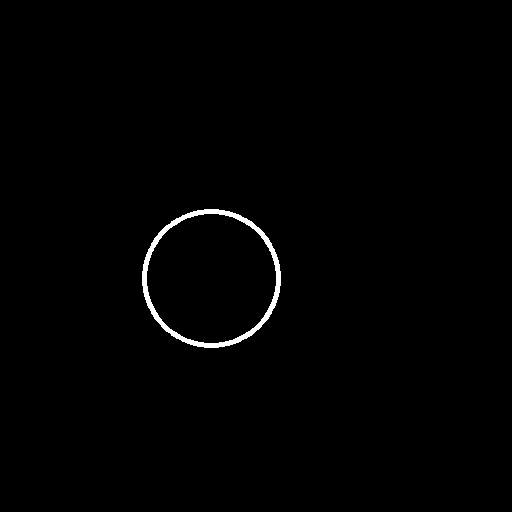 | 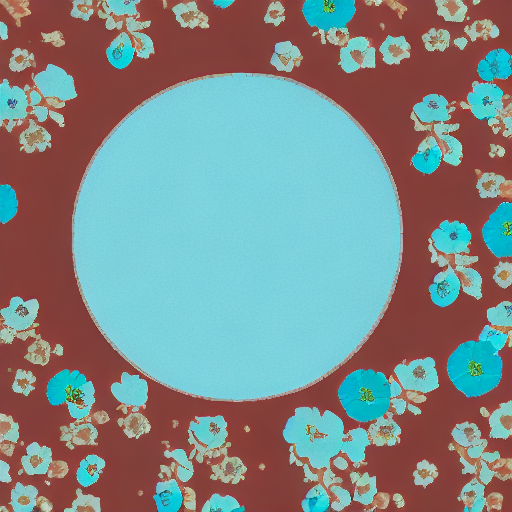 |
-
-
-#### After 6000 steps with batch size 8:
-
-| | |
-|-------------------|:-------------------------:|
-| | red circle with blue background |
-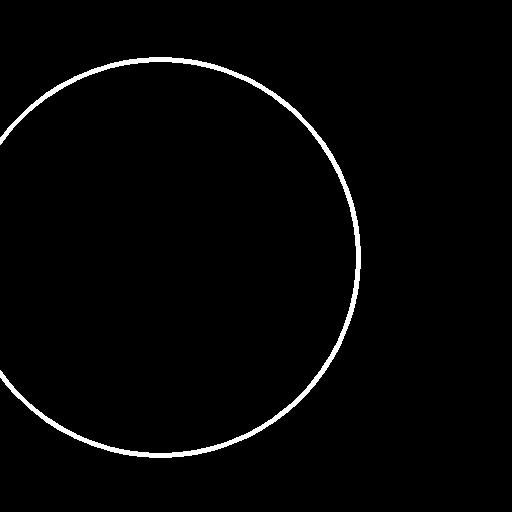 | 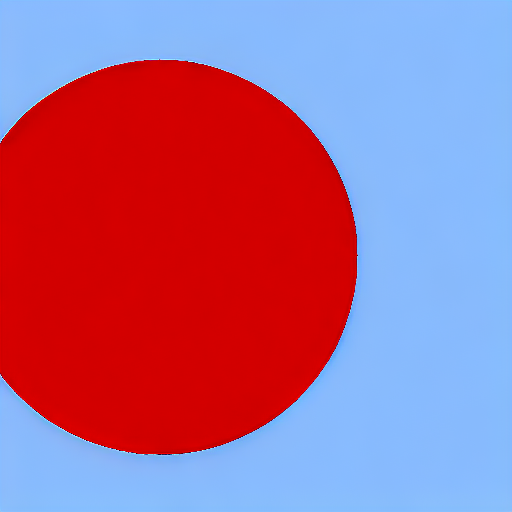 |
-| | cyan circle with brown floral background |
-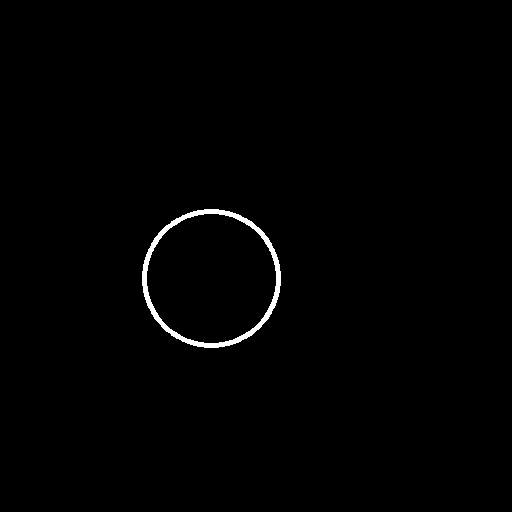 |  |
-
-## Training on a 16 GB GPU
-
-Optimizations:
-- Gradient checkpointing
-- bitsandbyte's 8-bit optimizer
-
-[bitandbytes install instructions](https://github.com/TimDettmers/bitsandbytes#requirements--installation).
-
-```bash
-export MODEL_DIR="runwayml/stable-diffusion-v1-5"
-export OUTPUT_DIR="path to save model"
-
-accelerate launch train_controlnet.py \
- --pretrained_model_name_or_path=$MODEL_DIR \
- --output_dir=$OUTPUT_DIR \
- --dataset_name=fusing/fill50k \
- --resolution=512 \
- --learning_rate=1e-5 \
- --validation_image "./conditioning_image_1.png" "./conditioning_image_2.png" \
- --validation_prompt "red circle with blue background" "cyan circle with brown floral background" \
- --train_batch_size=1 \
- --gradient_accumulation_steps=4 \
- --gradient_checkpointing \
- --use_8bit_adam
-```
-
-## Training on a 12 GB GPU
-
-Optimizations:
-- Gradient checkpointing
-- bitsandbyte's 8-bit optimizer
-- xformers
-- set grads to none
-
-```bash
-export MODEL_DIR="runwayml/stable-diffusion-v1-5"
-export OUTPUT_DIR="path to save model"
-
-accelerate launch train_controlnet.py \
- --pretrained_model_name_or_path=$MODEL_DIR \
- --output_dir=$OUTPUT_DIR \
- --dataset_name=fusing/fill50k \
- --resolution=512 \
- --learning_rate=1e-5 \
- --validation_image "./conditioning_image_1.png" "./conditioning_image_2.png" \
- --validation_prompt "red circle with blue background" "cyan circle with brown floral background" \
- --train_batch_size=1 \
- --gradient_accumulation_steps=4 \
- --gradient_checkpointing \
- --use_8bit_adam \
- --enable_xformers_memory_efficient_attention \
- --set_grads_to_none
-```
-
-When using `enable_xformers_memory_efficient_attention`, please make sure to install `xformers` by `pip install xformers`.
-
-## Training on an 8 GB GPU
-
-We have not exhaustively tested DeepSpeed support for ControlNet. While the configuration does
-save memory, we have not confirmed the configuration to train successfully. You will very likely
-have to make changes to the config to have a successful training run.
-
-Optimizations:
-- Gradient checkpointing
-- xformers
-- set grads to none
-- DeepSpeed stage 2 with parameter and optimizer offloading
-- fp16 mixed precision
-
-[DeepSpeed](https://www.deepspeed.ai/) can offload tensors from VRAM to either
-CPU or NVME. This requires significantly more RAM (about 25 GB).
-
-Use `accelerate config` to enable DeepSpeed stage 2.
-
-The relevant parts of the resulting accelerate config file are
-
-```yaml
-compute_environment: LOCAL_MACHINE
-deepspeed_config:
- gradient_accumulation_steps: 4
- offload_optimizer_device: cpu
- offload_param_device: cpu
- zero3_init_flag: false
- zero_stage: 2
-distributed_type: DEEPSPEED
-```
-
-See [documentation](https://huggingface.co/docs/accelerate/usage_guides/deepspeed) for more DeepSpeed configuration options.
-
-Changing the default Adam optimizer to DeepSpeed's Adam
-`deepspeed.ops.adam.DeepSpeedCPUAdam` gives a substantial speedup but
-it requires CUDA toolchain with the same version as pytorch. 8-bit optimizer
-does not seem to be compatible with DeepSpeed at the moment.
-
-```bash
-export MODEL_DIR="runwayml/stable-diffusion-v1-5"
-export OUTPUT_DIR="path to save model"
-
-accelerate launch train_controlnet.py \
- --pretrained_model_name_or_path=$MODEL_DIR \
- --output_dir=$OUTPUT_DIR \
- --dataset_name=fusing/fill50k \
- --resolution=512 \
- --validation_image "./conditioning_image_1.png" "./conditioning_image_2.png" \
- --validation_prompt "red circle with blue background" "cyan circle with brown floral background" \
- --train_batch_size=1 \
- --gradient_accumulation_steps=4 \
- --gradient_checkpointing \
- --enable_xformers_memory_efficient_attention \
- --set_grads_to_none \
- --mixed_precision fp16
-```
-
-## Performing inference with the trained ControlNet
-
-The trained model can be run the same as the original ControlNet pipeline with the newly trained ControlNet.
-Set `base_model_path` and `controlnet_path` to the values `--pretrained_model_name_or_path` and
-`--output_dir` were respectively set to in the training script.
-
-```py
-from diffusers import StableDiffusionControlNetPipeline, ControlNetModel, UniPCMultistepScheduler
-from diffusers.utils import load_image
-import torch
-
-base_model_path = "path to model"
-controlnet_path = "path to controlnet"
-
-controlnet = ControlNetModel.from_pretrained(controlnet_path, torch_dtype=torch.float16)
-pipe = StableDiffusionControlNetPipeline.from_pretrained(
- base_model_path, controlnet=controlnet, torch_dtype=torch.float16
-)
-
-# speed up diffusion process with faster scheduler and memory optimization
-pipe.scheduler = UniPCMultistepScheduler.from_config(pipe.scheduler.config)
-# remove following line if xformers is not installed or when using Torch 2.0.
-pipe.enable_xformers_memory_efficient_attention()
-# memory optimization.
-pipe.enable_model_cpu_offload()
-
-control_image = load_image("./conditioning_image_1.png")
-prompt = "pale golden rod circle with old lace background"
-
-# generate image
-generator = torch.manual_seed(0)
-image = pipe(
- prompt, num_inference_steps=20, generator=generator, image=control_image
-).images[0]
-image.save("./output.png")
-```
-
-## Training with Flax/JAX
-
-For faster training on TPUs and GPUs you can leverage the flax training example. Follow the instructions above to get the model and dataset before running the script.
-
-### Running on Google Cloud TPU
-
-See below for commands to set up a TPU VM(`--accelerator-type v4-8`). For more details about how to set up and use TPUs, refer to [Cloud docs for single VM setup](https://cloud.google.com/tpu/docs/run-calculation-jax).
-
-First create a single TPUv4-8 VM and connect to it:
-
-```
-ZONE=us-central2-b
-TPU_TYPE=v4-8
-VM_NAME=hg_flax
-
-gcloud alpha compute tpus tpu-vm create $VM_NAME \
- --zone $ZONE \
- --accelerator-type $TPU_TYPE \
- --version tpu-vm-v4-base
-
-gcloud alpha compute tpus tpu-vm ssh $VM_NAME --zone $ZONE -- \
-```
-
-When connected install JAX `0.4.5`:
-
-```
-pip install "jax[tpu]==0.4.5" -f https://storage.googleapis.com/jax-releases/libtpu_releases.html
-```
-
-To verify that JAX was correctly installed, you can run the following command:
-
-```
-import jax
-jax.device_count()
-```
-
-This should display the number of TPU cores, which should be 4 on a TPUv4-8 VM.
-
-Then install Diffusers and the library's training dependencies:
-
-```bash
-git clone https://github.com/huggingface/diffusers
-cd diffusers
-pip install .
-```
-
-Then cd in the example folder and run
-
-```bash
-pip install -U -r requirements_flax.txt
-```
-
-If you want to use Weights and Biases logging, you should also install `wandb` now
-
-```bash
-pip install wandb
-```
-
-
-Now let's downloading two conditioning images that we will use to run validation during the training in order to track our progress
-
-```
-wget https://huggingface.co/datasets/huggingface/documentation-images/resolve/main/diffusers/controlnet_training/conditioning_image_1.png
-wget https://huggingface.co/datasets/huggingface/documentation-images/resolve/main/diffusers/controlnet_training/conditioning_image_2.png
-```
-
-We encourage you to store or share your model with the community. To use huggingface hub, please login to your Hugging Face account, or ([create one](https://huggingface.co/docs/diffusers/main/en/training/hf.co/join) if you don’t have one already):
-
-```
-huggingface-cli login
-```
-
-Make sure you have the `MODEL_DIR`,`OUTPUT_DIR` and `HUB_MODEL_ID` environment variables set. The `OUTPUT_DIR` and `HUB_MODEL_ID` variables specify where to save the model to on the Hub:
-
-```bash
-export MODEL_DIR="runwayml/stable-diffusion-v1-5"
-export OUTPUT_DIR="runs/fill-circle-{timestamp}"
-export HUB_MODEL_ID="controlnet-fill-circle"
-```
-
-And finally start the training
-
-```bash
-python3 train_controlnet_flax.py \
- --pretrained_model_name_or_path=$MODEL_DIR \
- --output_dir=$OUTPUT_DIR \
- --dataset_name=fusing/fill50k \
- --resolution=512 \
- --learning_rate=1e-5 \
- --validation_image "./conditioning_image_1.png" "./conditioning_image_2.png" \
- --validation_prompt "red circle with blue background" "cyan circle with brown floral background" \
- --validation_steps=1000 \
- --train_batch_size=2 \
- --revision="non-ema" \
- --from_pt \
- --report_to="wandb" \
- --tracker_project_name=$HUB_MODEL_ID \
- --num_train_epochs=11 \
- --push_to_hub \
- --hub_model_id=$HUB_MODEL_ID
- ```
-
-Since we passed the `--push_to_hub` flag, it will automatically create a model repo under your huggingface account based on `$HUB_MODEL_ID`. By the end of training, the final checkpoint will be automatically stored on the hub. You can find an example model repo [here](https://huggingface.co/YiYiXu/fill-circle-controlnet).
-
-Our training script also provides limited support for streaming large datasets from the Hugging Face Hub. In order to enable streaming, one must also set `--max_train_samples`. Here is an example command (from [this blog article](https://huggingface.co/blog/train-your-controlnet)):
-
-```bash
-export MODEL_DIR="runwayml/stable-diffusion-v1-5"
-export OUTPUT_DIR="runs/uncanny-faces-{timestamp}"
-export HUB_MODEL_ID="controlnet-uncanny-faces"
-
-python3 train_controlnet_flax.py \
- --pretrained_model_name_or_path=$MODEL_DIR \
- --output_dir=$OUTPUT_DIR \
- --dataset_name=multimodalart/facesyntheticsspigacaptioned \
- --streaming \
- --conditioning_image_column=spiga_seg \
- --image_column=image \
- --caption_column=image_caption \
- --resolution=512 \
- --max_train_samples 100000 \
- --learning_rate=1e-5 \
- --train_batch_size=1 \
- --revision="flax" \
- --report_to="wandb" \
- --tracker_project_name=$HUB_MODEL_ID
-```
-
-Note, however, that the performance of the TPUs might get bottlenecked as streaming with `datasets` is not optimized for images. For ensuring maximum throughput, we encourage you to explore the following options:
-
-* [Webdataset](https://webdataset.github.io/webdataset/)
-* [TorchData](https://github.com/pytorch/data)
-* [TensorFlow Datasets](https://www.tensorflow.org/datasets/tfless_tfds)
-
-When work with a larger dataset, you may need to run training process for a long time and it’s useful to save regular checkpoints during the process. You can use the following argument to enable intermediate checkpointing:
-
-```bash
- --checkpointing_steps=500
-```
-This will save the trained model in subfolders of your output_dir. Subfolder names is the number of steps performed so far; for example: a checkpoint saved after 500 training steps would be saved in a subfolder named 500
-
-You can then start your training from this saved checkpoint with
-
-```bash
- --controlnet_model_name_or_path="./control_out/500"
-```
-
-We support training with the Min-SNR weighting strategy proposed in [Efficient Diffusion Training via Min-SNR Weighting Strategy](https://arxiv.org/abs/2303.09556) which helps to achieve faster convergence by rebalancing the loss. To use it, one needs to set the `--snr_gamma` argument. The recommended value when using it is `5.0`.
-
-We also support gradient accumulation - it is a technique that lets you use a bigger batch size than your machine would normally be able to fit into memory. You can use `gradient_accumulation_steps` argument to set gradient accumulation steps. The ControlNet author recommends using gradient accumulation to achieve better convergence. Read more [here](https://github.com/lllyasviel/ControlNet/blob/main/docs/train.md#more-consideration-sudden-converge-phenomenon-and-gradient-accumulation).
-
-You can **profile your code** with:
-
-```bash
- --profile_steps==5
-```
-
-Refer to the [JAX documentation on profiling](https://jax.readthedocs.io/en/latest/profiling.html). To inspect the profile trace, you'll have to install and start Tensorboard with the profile plugin:
-
-```bash
-pip install tensorflow tensorboard-plugin-profile
-tensorboard --logdir runs/fill-circle-100steps-20230411_165612/
-```
-
-The profile can then be inspected at http://localhost:6006/#profile
-
-Sometimes you'll get version conflicts (error messages like `Duplicate plugins for name projector`), which means that you have to uninstall and reinstall all versions of Tensorflow/Tensorboard (e.g. with `pip uninstall tensorflow tf-nightly tensorboard tb-nightly tensorboard-plugin-profile && pip install tf-nightly tbp-nightly tensorboard-plugin-profile`).
-
-Note that the debugging functionality of the Tensorboard `profile` plugin is still under active development. Not all views are fully functional, and for example the `trace_viewer` cuts off events after 1M (which can result in all your device traces getting lost if you for example profile the compilation step by accident).
-
-## Support for Stable Diffusion XL
-
-We provide a training script for training a ControlNet with [Stable Diffusion XL](https://huggingface.co/papers/2307.01952). Please refer to [README_sdxl.md](./README_sdxl.md) for more details.
diff --git a/spaces/Andy1621/uniformer_image_detection/mmdet/models/builder.py b/spaces/Andy1621/uniformer_image_detection/mmdet/models/builder.py
deleted file mode 100644
index 81c927e507a7c1625ffb114de10e93c94927af25..0000000000000000000000000000000000000000
--- a/spaces/Andy1621/uniformer_image_detection/mmdet/models/builder.py
+++ /dev/null
@@ -1,77 +0,0 @@
-import warnings
-
-from mmcv.utils import Registry, build_from_cfg
-from torch import nn
-
-BACKBONES = Registry('backbone')
-NECKS = Registry('neck')
-ROI_EXTRACTORS = Registry('roi_extractor')
-SHARED_HEADS = Registry('shared_head')
-HEADS = Registry('head')
-LOSSES = Registry('loss')
-DETECTORS = Registry('detector')
-
-
-def build(cfg, registry, default_args=None):
- """Build a module.
-
- Args:
- cfg (dict, list[dict]): The config of modules, is is either a dict
- or a list of configs.
- registry (:obj:`Registry`): A registry the module belongs to.
- default_args (dict, optional): Default arguments to build the module.
- Defaults to None.
-
- Returns:
- nn.Module: A built nn module.
- """
- if isinstance(cfg, list):
- modules = [
- build_from_cfg(cfg_, registry, default_args) for cfg_ in cfg
- ]
- return nn.Sequential(*modules)
- else:
- return build_from_cfg(cfg, registry, default_args)
-
-
-def build_backbone(cfg):
- """Build backbone."""
- return build(cfg, BACKBONES)
-
-
-def build_neck(cfg):
- """Build neck."""
- return build(cfg, NECKS)
-
-
-def build_roi_extractor(cfg):
- """Build roi extractor."""
- return build(cfg, ROI_EXTRACTORS)
-
-
-def build_shared_head(cfg):
- """Build shared head."""
- return build(cfg, SHARED_HEADS)
-
-
-def build_head(cfg):
- """Build head."""
- return build(cfg, HEADS)
-
-
-def build_loss(cfg):
- """Build loss."""
- return build(cfg, LOSSES)
-
-
-def build_detector(cfg, train_cfg=None, test_cfg=None):
- """Build detector."""
- if train_cfg is not None or test_cfg is not None:
- warnings.warn(
- 'train_cfg and test_cfg is deprecated, '
- 'please specify them in model', UserWarning)
- assert cfg.get('train_cfg') is None or train_cfg is None, \
- 'train_cfg specified in both outer field and model field '
- assert cfg.get('test_cfg') is None or test_cfg is None, \
- 'test_cfg specified in both outer field and model field '
- return build(cfg, DETECTORS, dict(train_cfg=train_cfg, test_cfg=test_cfg))
diff --git a/spaces/Andy1621/uniformer_image_segmentation/configs/ocrnet/ocrnet_hr18s_512x512_160k_ade20k.py b/spaces/Andy1621/uniformer_image_segmentation/configs/ocrnet/ocrnet_hr18s_512x512_160k_ade20k.py
deleted file mode 100644
index 81f3d5cb91607134bb1d844d78df7a3c411c134d..0000000000000000000000000000000000000000
--- a/spaces/Andy1621/uniformer_image_segmentation/configs/ocrnet/ocrnet_hr18s_512x512_160k_ade20k.py
+++ /dev/null
@@ -1,9 +0,0 @@
-_base_ = './ocrnet_hr18_512x512_160k_ade20k.py'
-model = dict(
- pretrained='open-mmlab://msra/hrnetv2_w18_small',
- backbone=dict(
- extra=dict(
- stage1=dict(num_blocks=(2, )),
- stage2=dict(num_blocks=(2, 2)),
- stage3=dict(num_modules=3, num_blocks=(2, 2, 2)),
- stage4=dict(num_modules=2, num_blocks=(2, 2, 2, 2)))))
diff --git a/spaces/AnimalEquality/chatbot/constants.py b/spaces/AnimalEquality/chatbot/constants.py
deleted file mode 100644
index caceb2e41ba6f9ccdd661f4eb6eef753a44a70a3..0000000000000000000000000000000000000000
--- a/spaces/AnimalEquality/chatbot/constants.py
+++ /dev/null
@@ -1,3 +0,0 @@
-from pathlib import Path
-
-ROOT_DIR = Path(__file__).parent
diff --git a/spaces/AnishKumbhar/ChatBot/text-generation-webui-main/modules/deepspeed_parameters.py b/spaces/AnishKumbhar/ChatBot/text-generation-webui-main/modules/deepspeed_parameters.py
deleted file mode 100644
index f170a385cfc3dfb954fc6f5595cf8706e42aed30..0000000000000000000000000000000000000000
--- a/spaces/AnishKumbhar/ChatBot/text-generation-webui-main/modules/deepspeed_parameters.py
+++ /dev/null
@@ -1,74 +0,0 @@
-def generate_ds_config(ds_bf16, train_batch_size, nvme_offload_dir):
- '''
- DeepSpeed configuration
- https://huggingface.co/docs/transformers/main_classes/deepspeed
- '''
-
- if nvme_offload_dir:
- ds_config = {
- "fp16": {
- "enabled": not ds_bf16,
- },
- "bf16": {
- "enabled": ds_bf16,
- },
- "zero_optimization": {
- "stage": 3,
- "offload_param": {
- "device": "nvme",
- "nvme_path": nvme_offload_dir,
- "pin_memory": True,
- "buffer_count": 5,
- "buffer_size": 1e9,
- "max_in_cpu": 1e9
- },
- "overlap_comm": True,
- "reduce_bucket_size": "auto",
- "contiguous_gradients": True,
- "sub_group_size": 1e8,
- "stage3_prefetch_bucket_size": "auto",
- "stage3_param_persistence_threshold": "auto",
- "stage3_max_live_parameters": "auto",
- "stage3_max_reuse_distance": "auto",
- },
- "aio": {
- "block_size": 262144,
- "queue_depth": 32,
- "thread_count": 1,
- "single_submit": False,
- "overlap_events": True
- },
- "steps_per_print": 2000,
- "train_batch_size": train_batch_size,
- "train_micro_batch_size_per_gpu": 1,
- "wall_clock_breakdown": False
- }
- else:
- ds_config = {
- "fp16": {
- "enabled": not ds_bf16,
- },
- "bf16": {
- "enabled": ds_bf16,
- },
- "zero_optimization": {
- "stage": 3,
- "offload_param": {
- "device": "cpu",
- "pin_memory": True
- },
- "overlap_comm": True,
- "contiguous_gradients": True,
- "reduce_bucket_size": "auto",
- "stage3_prefetch_bucket_size": "auto",
- "stage3_param_persistence_threshold": "auto",
- "stage3_max_live_parameters": "auto",
- "stage3_max_reuse_distance": "auto",
- },
- "steps_per_print": 2000,
- "train_batch_size": train_batch_size,
- "train_micro_batch_size_per_gpu": 1,
- "wall_clock_breakdown": False
- }
-
- return ds_config
diff --git a/spaces/Anonymous-sub/Rerender/gmflow_module/scripts/demo.sh b/spaces/Anonymous-sub/Rerender/gmflow_module/scripts/demo.sh
deleted file mode 100644
index ac5b2e4900ef275973d74e2c172cf3c77a504dcf..0000000000000000000000000000000000000000
--- a/spaces/Anonymous-sub/Rerender/gmflow_module/scripts/demo.sh
+++ /dev/null
@@ -1,63 +0,0 @@
-#!/usr/bin/env bash
-
-# inference GMFlow without refinement
-
-# sintel
-
-# only predict forward flow
-CUDA_VISIBLE_DEVICES=0 python main.py \
---inference_dir demo/sintel_market_1 \
---output_path output/gmflow-norefine-sintel_market_1 \
---resume pretrained/gmflow_sintel-0c07dcb3.pth
-
-# predict forward & backward flow
-CUDA_VISIBLE_DEVICES=0 python main.py \
---inference_dir demo/sintel_market_1 \
---output_path output/gmflow-norefine-sintel_market_1 \
---pred_bidir_flow \
---resume pretrained/gmflow_sintel-0c07dcb3.pth
-
-
-# predict forward & backward flow with forward-backward consistency check
-CUDA_VISIBLE_DEVICES=0 python main.py \
---inference_dir demo/sintel_market_1 \
---output_path output/gmflow-norefine-sintel_market_1 \
---pred_bidir_flow \
---fwd_bwd_consistency_check \
---resume pretrained/gmflow_sintel-0c07dcb3.pth
-
-
-# davis
-
-CUDA_VISIBLE_DEVICES=0 python main.py \
---inference_dir demo/davis_breakdance-flare \
---output_path output/gmflow-norefine-davis_breakdance-flare \
---resume pretrained/gmflow_sintel-0c07dcb3.pth
-
-
-
-
-# inference GMFlow with refinement
-
-CUDA_VISIBLE_DEVICES=0 python main.py \
---inference_dir demo/davis_breakdance-flare \
---output_path output/gmflow-withrefine-davis_breakdance-flare \
---resume pretrained/gmflow_with_refine_sintel-3ed1cf48.pth \
---padding_factor 32 \
---upsample_factor 4 \
---num_scales 2 \
---attn_splits_list 2 8 \
---corr_radius_list -1 4 \
---prop_radius_list -1 1
-
-
-
-
-CUDA_VISIBLE_DEVICES=0 python main.py \
---inference_dir demo/sintel_test_clean_market_1 \
---output_path output/gmflow-norefine-sintel_test_clean_market_1 \
---pred_bidir_flow \
---fwd_bwd_consistency_check \
---resume pretrained/gmflow_sintel-0c07dcb3.pth
-
-
diff --git a/spaces/Arulkumar03/GroundingDINO_SOTA_Zero_Shot_Model/groundingdino/config/GroundingDINO_SwinB_cfg.py b/spaces/Arulkumar03/GroundingDINO_SOTA_Zero_Shot_Model/groundingdino/config/GroundingDINO_SwinB_cfg.py
deleted file mode 100644
index f490c4bbd598a35de43d36ceafcbd769e7ff21bf..0000000000000000000000000000000000000000
--- a/spaces/Arulkumar03/GroundingDINO_SOTA_Zero_Shot_Model/groundingdino/config/GroundingDINO_SwinB_cfg.py
+++ /dev/null
@@ -1,43 +0,0 @@
-batch_size = 1
-modelname = "groundingdino"
-backbone = "swin_B_384_22k"
-position_embedding = "sine"
-pe_temperatureH = 20
-pe_temperatureW = 20
-return_interm_indices = [1, 2, 3]
-backbone_freeze_keywords = None
-enc_layers = 6
-dec_layers = 6
-pre_norm = False
-dim_feedforward = 2048
-hidden_dim = 256
-dropout = 0.0
-nheads = 8
-num_queries = 900
-query_dim = 4
-num_patterns = 0
-num_feature_levels = 4
-enc_n_points = 4
-dec_n_points = 4
-two_stage_type = "standard"
-two_stage_bbox_embed_share = False
-two_stage_class_embed_share = False
-transformer_activation = "relu"
-dec_pred_bbox_embed_share = True
-dn_box_noise_scale = 1.0
-dn_label_noise_ratio = 0.5
-dn_label_coef = 1.0
-dn_bbox_coef = 1.0
-embed_init_tgt = True
-dn_labelbook_size = 2000
-max_text_len = 256
-text_encoder_type = "bert-base-uncased"
-use_text_enhancer = True
-use_fusion_layer = True
-use_checkpoint = True
-use_transformer_ckpt = True
-use_text_cross_attention = True
-text_dropout = 0.0
-fusion_dropout = 0.0
-fusion_droppath = 0.1
-sub_sentence_present = True
diff --git a/spaces/Ataturk-Chatbot/HuggingFaceChat/venv/lib/python3.11/site-packages/pip/_internal/cli/req_command.py b/spaces/Ataturk-Chatbot/HuggingFaceChat/venv/lib/python3.11/site-packages/pip/_internal/cli/req_command.py
deleted file mode 100644
index c2f4e38bed82c32bf5e45657fd8658cff1710f13..0000000000000000000000000000000000000000
--- a/spaces/Ataturk-Chatbot/HuggingFaceChat/venv/lib/python3.11/site-packages/pip/_internal/cli/req_command.py
+++ /dev/null
@@ -1,505 +0,0 @@
-"""Contains the Command base classes that depend on PipSession.
-
-The classes in this module are in a separate module so the commands not
-needing download / PackageFinder capability don't unnecessarily import the
-PackageFinder machinery and all its vendored dependencies, etc.
-"""
-
-import logging
-import os
-import sys
-from functools import partial
-from optparse import Values
-from typing import TYPE_CHECKING, Any, List, Optional, Tuple
-
-from pip._internal.cache import WheelCache
-from pip._internal.cli import cmdoptions
-from pip._internal.cli.base_command import Command
-from pip._internal.cli.command_context import CommandContextMixIn
-from pip._internal.exceptions import CommandError, PreviousBuildDirError
-from pip._internal.index.collector import LinkCollector
-from pip._internal.index.package_finder import PackageFinder
-from pip._internal.models.selection_prefs import SelectionPreferences
-from pip._internal.models.target_python import TargetPython
-from pip._internal.network.session import PipSession
-from pip._internal.operations.build.build_tracker import BuildTracker
-from pip._internal.operations.prepare import RequirementPreparer
-from pip._internal.req.constructors import (
- install_req_from_editable,
- install_req_from_line,
- install_req_from_parsed_requirement,
- install_req_from_req_string,
-)
-from pip._internal.req.req_file import parse_requirements
-from pip._internal.req.req_install import InstallRequirement
-from pip._internal.resolution.base import BaseResolver
-from pip._internal.self_outdated_check import pip_self_version_check
-from pip._internal.utils.temp_dir import (
- TempDirectory,
- TempDirectoryTypeRegistry,
- tempdir_kinds,
-)
-from pip._internal.utils.virtualenv import running_under_virtualenv
-
-if TYPE_CHECKING:
- from ssl import SSLContext
-
-logger = logging.getLogger(__name__)
-
-
-def _create_truststore_ssl_context() -> Optional["SSLContext"]:
- if sys.version_info < (3, 10):
- raise CommandError("The truststore feature is only available for Python 3.10+")
-
- try:
- import ssl
- except ImportError:
- logger.warning("Disabling truststore since ssl support is missing")
- return None
-
- try:
- import truststore
- except ImportError:
- raise CommandError(
- "To use the truststore feature, 'truststore' must be installed into "
- "pip's current environment."
- )
-
- return truststore.SSLContext(ssl.PROTOCOL_TLS_CLIENT)
-
-
-class SessionCommandMixin(CommandContextMixIn):
-
- """
- A class mixin for command classes needing _build_session().
- """
-
- def __init__(self) -> None:
- super().__init__()
- self._session: Optional[PipSession] = None
-
- @classmethod
- def _get_index_urls(cls, options: Values) -> Optional[List[str]]:
- """Return a list of index urls from user-provided options."""
- index_urls = []
- if not getattr(options, "no_index", False):
- url = getattr(options, "index_url", None)
- if url:
- index_urls.append(url)
- urls = getattr(options, "extra_index_urls", None)
- if urls:
- index_urls.extend(urls)
- # Return None rather than an empty list
- return index_urls or None
-
- def get_default_session(self, options: Values) -> PipSession:
- """Get a default-managed session."""
- if self._session is None:
- self._session = self.enter_context(self._build_session(options))
- # there's no type annotation on requests.Session, so it's
- # automatically ContextManager[Any] and self._session becomes Any,
- # then https://github.com/python/mypy/issues/7696 kicks in
- assert self._session is not None
- return self._session
-
- def _build_session(
- self,
- options: Values,
- retries: Optional[int] = None,
- timeout: Optional[int] = None,
- fallback_to_certifi: bool = False,
- ) -> PipSession:
- cache_dir = options.cache_dir
- assert not cache_dir or os.path.isabs(cache_dir)
-
- if "truststore" in options.features_enabled:
- try:
- ssl_context = _create_truststore_ssl_context()
- except Exception:
- if not fallback_to_certifi:
- raise
- ssl_context = None
- else:
- ssl_context = None
-
- session = PipSession(
- cache=os.path.join(cache_dir, "http") if cache_dir else None,
- retries=retries if retries is not None else options.retries,
- trusted_hosts=options.trusted_hosts,
- index_urls=self._get_index_urls(options),
- ssl_context=ssl_context,
- )
-
- # Handle custom ca-bundles from the user
- if options.cert:
- session.verify = options.cert
-
- # Handle SSL client certificate
- if options.client_cert:
- session.cert = options.client_cert
-
- # Handle timeouts
- if options.timeout or timeout:
- session.timeout = timeout if timeout is not None else options.timeout
-
- # Handle configured proxies
- if options.proxy:
- session.proxies = {
- "http": options.proxy,
- "https": options.proxy,
- }
-
- # Determine if we can prompt the user for authentication or not
- session.auth.prompting = not options.no_input
- session.auth.keyring_provider = options.keyring_provider
-
- return session
-
-
-class IndexGroupCommand(Command, SessionCommandMixin):
-
- """
- Abstract base class for commands with the index_group options.
-
- This also corresponds to the commands that permit the pip version check.
- """
-
- def handle_pip_version_check(self, options: Values) -> None:
- """
- Do the pip version check if not disabled.
-
- This overrides the default behavior of not doing the check.
- """
- # Make sure the index_group options are present.
- assert hasattr(options, "no_index")
-
- if options.disable_pip_version_check or options.no_index:
- return
-
- # Otherwise, check if we're using the latest version of pip available.
- session = self._build_session(
- options,
- retries=0,
- timeout=min(5, options.timeout),
- # This is set to ensure the function does not fail when truststore is
- # specified in use-feature but cannot be loaded. This usually raises a
- # CommandError and shows a nice user-facing error, but this function is not
- # called in that try-except block.
- fallback_to_certifi=True,
- )
- with session:
- pip_self_version_check(session, options)
-
-
-KEEPABLE_TEMPDIR_TYPES = [
- tempdir_kinds.BUILD_ENV,
- tempdir_kinds.EPHEM_WHEEL_CACHE,
- tempdir_kinds.REQ_BUILD,
-]
-
-
-def warn_if_run_as_root() -> None:
- """Output a warning for sudo users on Unix.
-
- In a virtual environment, sudo pip still writes to virtualenv.
- On Windows, users may run pip as Administrator without issues.
- This warning only applies to Unix root users outside of virtualenv.
- """
- if running_under_virtualenv():
- return
- if not hasattr(os, "getuid"):
- return
- # On Windows, there are no "system managed" Python packages. Installing as
- # Administrator via pip is the correct way of updating system environments.
- #
- # We choose sys.platform over utils.compat.WINDOWS here to enable Mypy platform
- # checks: https://mypy.readthedocs.io/en/stable/common_issues.html
- if sys.platform == "win32" or sys.platform == "cygwin":
- return
-
- if os.getuid() != 0:
- return
-
- logger.warning(
- "Running pip as the 'root' user can result in broken permissions and "
- "conflicting behaviour with the system package manager. "
- "It is recommended to use a virtual environment instead: "
- "https://pip.pypa.io/warnings/venv"
- )
-
-
-def with_cleanup(func: Any) -> Any:
- """Decorator for common logic related to managing temporary
- directories.
- """
-
- def configure_tempdir_registry(registry: TempDirectoryTypeRegistry) -> None:
- for t in KEEPABLE_TEMPDIR_TYPES:
- registry.set_delete(t, False)
-
- def wrapper(
- self: RequirementCommand, options: Values, args: List[Any]
- ) -> Optional[int]:
- assert self.tempdir_registry is not None
- if options.no_clean:
- configure_tempdir_registry(self.tempdir_registry)
-
- try:
- return func(self, options, args)
- except PreviousBuildDirError:
- # This kind of conflict can occur when the user passes an explicit
- # build directory with a pre-existing folder. In that case we do
- # not want to accidentally remove it.
- configure_tempdir_registry(self.tempdir_registry)
- raise
-
- return wrapper
-
-
-class RequirementCommand(IndexGroupCommand):
- def __init__(self, *args: Any, **kw: Any) -> None:
- super().__init__(*args, **kw)
-
- self.cmd_opts.add_option(cmdoptions.no_clean())
-
- @staticmethod
- def determine_resolver_variant(options: Values) -> str:
- """Determines which resolver should be used, based on the given options."""
- if "legacy-resolver" in options.deprecated_features_enabled:
- return "legacy"
-
- return "2020-resolver"
-
- @classmethod
- def make_requirement_preparer(
- cls,
- temp_build_dir: TempDirectory,
- options: Values,
- build_tracker: BuildTracker,
- session: PipSession,
- finder: PackageFinder,
- use_user_site: bool,
- download_dir: Optional[str] = None,
- verbosity: int = 0,
- ) -> RequirementPreparer:
- """
- Create a RequirementPreparer instance for the given parameters.
- """
- temp_build_dir_path = temp_build_dir.path
- assert temp_build_dir_path is not None
-
- resolver_variant = cls.determine_resolver_variant(options)
- if resolver_variant == "2020-resolver":
- lazy_wheel = "fast-deps" in options.features_enabled
- if lazy_wheel:
- logger.warning(
- "pip is using lazily downloaded wheels using HTTP "
- "range requests to obtain dependency information. "
- "This experimental feature is enabled through "
- "--use-feature=fast-deps and it is not ready for "
- "production."
- )
- else:
- lazy_wheel = False
- if "fast-deps" in options.features_enabled:
- logger.warning(
- "fast-deps has no effect when used with the legacy resolver."
- )
-
- return RequirementPreparer(
- build_dir=temp_build_dir_path,
- src_dir=options.src_dir,
- download_dir=download_dir,
- build_isolation=options.build_isolation,
- check_build_deps=options.check_build_deps,
- build_tracker=build_tracker,
- session=session,
- progress_bar=options.progress_bar,
- finder=finder,
- require_hashes=options.require_hashes,
- use_user_site=use_user_site,
- lazy_wheel=lazy_wheel,
- verbosity=verbosity,
- )
-
- @classmethod
- def make_resolver(
- cls,
- preparer: RequirementPreparer,
- finder: PackageFinder,
- options: Values,
- wheel_cache: Optional[WheelCache] = None,
- use_user_site: bool = False,
- ignore_installed: bool = True,
- ignore_requires_python: bool = False,
- force_reinstall: bool = False,
- upgrade_strategy: str = "to-satisfy-only",
- use_pep517: Optional[bool] = None,
- py_version_info: Optional[Tuple[int, ...]] = None,
- ) -> BaseResolver:
- """
- Create a Resolver instance for the given parameters.
- """
- make_install_req = partial(
- install_req_from_req_string,
- isolated=options.isolated_mode,
- use_pep517=use_pep517,
- )
- resolver_variant = cls.determine_resolver_variant(options)
- # The long import name and duplicated invocation is needed to convince
- # Mypy into correctly typechecking. Otherwise it would complain the
- # "Resolver" class being redefined.
- if resolver_variant == "2020-resolver":
- import pip._internal.resolution.resolvelib.resolver
-
- return pip._internal.resolution.resolvelib.resolver.Resolver(
- preparer=preparer,
- finder=finder,
- wheel_cache=wheel_cache,
- make_install_req=make_install_req,
- use_user_site=use_user_site,
- ignore_dependencies=options.ignore_dependencies,
- ignore_installed=ignore_installed,
- ignore_requires_python=ignore_requires_python,
- force_reinstall=force_reinstall,
- upgrade_strategy=upgrade_strategy,
- py_version_info=py_version_info,
- )
- import pip._internal.resolution.legacy.resolver
-
- return pip._internal.resolution.legacy.resolver.Resolver(
- preparer=preparer,
- finder=finder,
- wheel_cache=wheel_cache,
- make_install_req=make_install_req,
- use_user_site=use_user_site,
- ignore_dependencies=options.ignore_dependencies,
- ignore_installed=ignore_installed,
- ignore_requires_python=ignore_requires_python,
- force_reinstall=force_reinstall,
- upgrade_strategy=upgrade_strategy,
- py_version_info=py_version_info,
- )
-
- def get_requirements(
- self,
- args: List[str],
- options: Values,
- finder: PackageFinder,
- session: PipSession,
- ) -> List[InstallRequirement]:
- """
- Parse command-line arguments into the corresponding requirements.
- """
- requirements: List[InstallRequirement] = []
- for filename in options.constraints:
- for parsed_req in parse_requirements(
- filename,
- constraint=True,
- finder=finder,
- options=options,
- session=session,
- ):
- req_to_add = install_req_from_parsed_requirement(
- parsed_req,
- isolated=options.isolated_mode,
- user_supplied=False,
- )
- requirements.append(req_to_add)
-
- for req in args:
- req_to_add = install_req_from_line(
- req,
- comes_from=None,
- isolated=options.isolated_mode,
- use_pep517=options.use_pep517,
- user_supplied=True,
- config_settings=getattr(options, "config_settings", None),
- )
- requirements.append(req_to_add)
-
- for req in options.editables:
- req_to_add = install_req_from_editable(
- req,
- user_supplied=True,
- isolated=options.isolated_mode,
- use_pep517=options.use_pep517,
- config_settings=getattr(options, "config_settings", None),
- )
- requirements.append(req_to_add)
-
- # NOTE: options.require_hashes may be set if --require-hashes is True
- for filename in options.requirements:
- for parsed_req in parse_requirements(
- filename, finder=finder, options=options, session=session
- ):
- req_to_add = install_req_from_parsed_requirement(
- parsed_req,
- isolated=options.isolated_mode,
- use_pep517=options.use_pep517,
- user_supplied=True,
- config_settings=parsed_req.options.get("config_settings")
- if parsed_req.options
- else None,
- )
- requirements.append(req_to_add)
-
- # If any requirement has hash options, enable hash checking.
- if any(req.has_hash_options for req in requirements):
- options.require_hashes = True
-
- if not (args or options.editables or options.requirements):
- opts = {"name": self.name}
- if options.find_links:
- raise CommandError(
- "You must give at least one requirement to {name} "
- '(maybe you meant "pip {name} {links}"?)'.format(
- **dict(opts, links=" ".join(options.find_links))
- )
- )
- else:
- raise CommandError(
- "You must give at least one requirement to {name} "
- '(see "pip help {name}")'.format(**opts)
- )
-
- return requirements
-
- @staticmethod
- def trace_basic_info(finder: PackageFinder) -> None:
- """
- Trace basic information about the provided objects.
- """
- # Display where finder is looking for packages
- search_scope = finder.search_scope
- locations = search_scope.get_formatted_locations()
- if locations:
- logger.info(locations)
-
- def _build_package_finder(
- self,
- options: Values,
- session: PipSession,
- target_python: Optional[TargetPython] = None,
- ignore_requires_python: Optional[bool] = None,
- ) -> PackageFinder:
- """
- Create a package finder appropriate to this requirement command.
-
- :param ignore_requires_python: Whether to ignore incompatible
- "Requires-Python" values in links. Defaults to False.
- """
- link_collector = LinkCollector.create(session, options=options)
- selection_prefs = SelectionPreferences(
- allow_yanked=True,
- format_control=options.format_control,
- allow_all_prereleases=options.pre,
- prefer_binary=options.prefer_binary,
- ignore_requires_python=ignore_requires_python,
- )
-
- return PackageFinder.create(
- link_collector=link_collector,
- selection_prefs=selection_prefs,
- target_python=target_python,
- )
diff --git a/spaces/Ataturk-Chatbot/HuggingFaceChat/venv/lib/python3.11/site-packages/pip/_vendor/rich/constrain.py b/spaces/Ataturk-Chatbot/HuggingFaceChat/venv/lib/python3.11/site-packages/pip/_vendor/rich/constrain.py
deleted file mode 100644
index 65fdf56342e8b5b8e181914881025231684e1871..0000000000000000000000000000000000000000
--- a/spaces/Ataturk-Chatbot/HuggingFaceChat/venv/lib/python3.11/site-packages/pip/_vendor/rich/constrain.py
+++ /dev/null
@@ -1,37 +0,0 @@
-from typing import Optional, TYPE_CHECKING
-
-from .jupyter import JupyterMixin
-from .measure import Measurement
-
-if TYPE_CHECKING:
- from .console import Console, ConsoleOptions, RenderableType, RenderResult
-
-
-class Constrain(JupyterMixin):
- """Constrain the width of a renderable to a given number of characters.
-
- Args:
- renderable (RenderableType): A renderable object.
- width (int, optional): The maximum width (in characters) to render. Defaults to 80.
- """
-
- def __init__(self, renderable: "RenderableType", width: Optional[int] = 80) -> None:
- self.renderable = renderable
- self.width = width
-
- def __rich_console__(
- self, console: "Console", options: "ConsoleOptions"
- ) -> "RenderResult":
- if self.width is None:
- yield self.renderable
- else:
- child_options = options.update_width(min(self.width, options.max_width))
- yield from console.render(self.renderable, child_options)
-
- def __rich_measure__(
- self, console: "Console", options: "ConsoleOptions"
- ) -> "Measurement":
- if self.width is not None:
- options = options.update_width(self.width)
- measurement = Measurement.get(console, options, self.renderable)
- return measurement
diff --git a/spaces/Ataturk-Chatbot/HuggingFaceChat/venv/lib/python3.11/site-packages/pip/_vendor/rich/tree.py b/spaces/Ataturk-Chatbot/HuggingFaceChat/venv/lib/python3.11/site-packages/pip/_vendor/rich/tree.py
deleted file mode 100644
index afe8da1a4a30daf6e48ffba514656e7c86c9abaa..0000000000000000000000000000000000000000
--- a/spaces/Ataturk-Chatbot/HuggingFaceChat/venv/lib/python3.11/site-packages/pip/_vendor/rich/tree.py
+++ /dev/null
@@ -1,251 +0,0 @@
-from typing import Iterator, List, Optional, Tuple
-
-from ._loop import loop_first, loop_last
-from .console import Console, ConsoleOptions, RenderableType, RenderResult
-from .jupyter import JupyterMixin
-from .measure import Measurement
-from .segment import Segment
-from .style import Style, StyleStack, StyleType
-from .styled import Styled
-
-
-class Tree(JupyterMixin):
- """A renderable for a tree structure.
-
- Args:
- label (RenderableType): The renderable or str for the tree label.
- style (StyleType, optional): Style of this tree. Defaults to "tree".
- guide_style (StyleType, optional): Style of the guide lines. Defaults to "tree.line".
- expanded (bool, optional): Also display children. Defaults to True.
- highlight (bool, optional): Highlight renderable (if str). Defaults to False.
- """
-
- def __init__(
- self,
- label: RenderableType,
- *,
- style: StyleType = "tree",
- guide_style: StyleType = "tree.line",
- expanded: bool = True,
- highlight: bool = False,
- hide_root: bool = False,
- ) -> None:
- self.label = label
- self.style = style
- self.guide_style = guide_style
- self.children: List[Tree] = []
- self.expanded = expanded
- self.highlight = highlight
- self.hide_root = hide_root
-
- def add(
- self,
- label: RenderableType,
- *,
- style: Optional[StyleType] = None,
- guide_style: Optional[StyleType] = None,
- expanded: bool = True,
- highlight: Optional[bool] = False,
- ) -> "Tree":
- """Add a child tree.
-
- Args:
- label (RenderableType): The renderable or str for the tree label.
- style (StyleType, optional): Style of this tree. Defaults to "tree".
- guide_style (StyleType, optional): Style of the guide lines. Defaults to "tree.line".
- expanded (bool, optional): Also display children. Defaults to True.
- highlight (Optional[bool], optional): Highlight renderable (if str). Defaults to False.
-
- Returns:
- Tree: A new child Tree, which may be further modified.
- """
- node = Tree(
- label,
- style=self.style if style is None else style,
- guide_style=self.guide_style if guide_style is None else guide_style,
- expanded=expanded,
- highlight=self.highlight if highlight is None else highlight,
- )
- self.children.append(node)
- return node
-
- def __rich_console__(
- self, console: "Console", options: "ConsoleOptions"
- ) -> "RenderResult":
-
- stack: List[Iterator[Tuple[bool, Tree]]] = []
- pop = stack.pop
- push = stack.append
- new_line = Segment.line()
-
- get_style = console.get_style
- null_style = Style.null()
- guide_style = get_style(self.guide_style, default="") or null_style
- SPACE, CONTINUE, FORK, END = range(4)
-
- ASCII_GUIDES = (" ", "| ", "+-- ", "`-- ")
- TREE_GUIDES = [
- (" ", "│ ", "├── ", "└── "),
- (" ", "┃ ", "┣━━ ", "┗━━ "),
- (" ", "║ ", "╠══ ", "╚══ "),
- ]
- _Segment = Segment
-
- def make_guide(index: int, style: Style) -> Segment:
- """Make a Segment for a level of the guide lines."""
- if options.ascii_only:
- line = ASCII_GUIDES[index]
- else:
- guide = 1 if style.bold else (2 if style.underline2 else 0)
- line = TREE_GUIDES[0 if options.legacy_windows else guide][index]
- return _Segment(line, style)
-
- levels: List[Segment] = [make_guide(CONTINUE, guide_style)]
- push(iter(loop_last([self])))
-
- guide_style_stack = StyleStack(get_style(self.guide_style))
- style_stack = StyleStack(get_style(self.style))
- remove_guide_styles = Style(bold=False, underline2=False)
-
- depth = 0
-
- while stack:
- stack_node = pop()
- try:
- last, node = next(stack_node)
- except StopIteration:
- levels.pop()
- if levels:
- guide_style = levels[-1].style or null_style
- levels[-1] = make_guide(FORK, guide_style)
- guide_style_stack.pop()
- style_stack.pop()
- continue
- push(stack_node)
- if last:
- levels[-1] = make_guide(END, levels[-1].style or null_style)
-
- guide_style = guide_style_stack.current + get_style(node.guide_style)
- style = style_stack.current + get_style(node.style)
- prefix = levels[(2 if self.hide_root else 1) :]
- renderable_lines = console.render_lines(
- Styled(node.label, style),
- options.update(
- width=options.max_width
- - sum(level.cell_length for level in prefix),
- highlight=self.highlight,
- height=None,
- ),
- pad=options.justify is not None,
- )
-
- if not (depth == 0 and self.hide_root):
- for first, line in loop_first(renderable_lines):
- if prefix:
- yield from _Segment.apply_style(
- prefix,
- style.background_style,
- post_style=remove_guide_styles,
- )
- yield from line
- yield new_line
- if first and prefix:
- prefix[-1] = make_guide(
- SPACE if last else CONTINUE, prefix[-1].style or null_style
- )
-
- if node.expanded and node.children:
- levels[-1] = make_guide(
- SPACE if last else CONTINUE, levels[-1].style or null_style
- )
- levels.append(
- make_guide(END if len(node.children) == 1 else FORK, guide_style)
- )
- style_stack.push(get_style(node.style))
- guide_style_stack.push(get_style(node.guide_style))
- push(iter(loop_last(node.children)))
- depth += 1
-
- def __rich_measure__(
- self, console: "Console", options: "ConsoleOptions"
- ) -> "Measurement":
- stack: List[Iterator[Tree]] = [iter([self])]
- pop = stack.pop
- push = stack.append
- minimum = 0
- maximum = 0
- measure = Measurement.get
- level = 0
- while stack:
- iter_tree = pop()
- try:
- tree = next(iter_tree)
- except StopIteration:
- level -= 1
- continue
- push(iter_tree)
- min_measure, max_measure = measure(console, options, tree.label)
- indent = level * 4
- minimum = max(min_measure + indent, minimum)
- maximum = max(max_measure + indent, maximum)
- if tree.expanded and tree.children:
- push(iter(tree.children))
- level += 1
- return Measurement(minimum, maximum)
-
-
-if __name__ == "__main__": # pragma: no cover
-
- from pip._vendor.rich.console import Group
- from pip._vendor.rich.markdown import Markdown
- from pip._vendor.rich.panel import Panel
- from pip._vendor.rich.syntax import Syntax
- from pip._vendor.rich.table import Table
-
- table = Table(row_styles=["", "dim"])
-
- table.add_column("Released", style="cyan", no_wrap=True)
- table.add_column("Title", style="magenta")
- table.add_column("Box Office", justify="right", style="green")
-
- table.add_row("Dec 20, 2019", "Star Wars: The Rise of Skywalker", "$952,110,690")
- table.add_row("May 25, 2018", "Solo: A Star Wars Story", "$393,151,347")
- table.add_row("Dec 15, 2017", "Star Wars Ep. V111: The Last Jedi", "$1,332,539,889")
- table.add_row("Dec 16, 2016", "Rogue One: A Star Wars Story", "$1,332,439,889")
-
- code = """\
-class Segment(NamedTuple):
- text: str = ""
- style: Optional[Style] = None
- is_control: bool = False
-"""
- syntax = Syntax(code, "python", theme="monokai", line_numbers=True)
-
- markdown = Markdown(
- """\
-### example.md
-> Hello, World!
->
-> Markdown _all_ the things
-"""
- )
-
- root = Tree("🌲 [b green]Rich Tree", highlight=True, hide_root=True)
-
- node = root.add(":file_folder: Renderables", guide_style="red")
- simple_node = node.add(":file_folder: [bold yellow]Atomic", guide_style="uu green")
- simple_node.add(Group("📄 Syntax", syntax))
- simple_node.add(Group("📄 Markdown", Panel(markdown, border_style="green")))
-
- containers_node = node.add(
- ":file_folder: [bold magenta]Containers", guide_style="bold magenta"
- )
- containers_node.expanded = True
- panel = Panel.fit("Just a panel", border_style="red")
- containers_node.add(Group("📄 Panels", panel))
-
- containers_node.add(Group("📄 [b magenta]Table", table))
-
- console = Console()
-
- console.print(root)
diff --git a/spaces/Ataturk-Chatbot/HuggingFaceChat/venv/lib/python3.11/site-packages/setuptools/_vendor/packaging/_musllinux.py b/spaces/Ataturk-Chatbot/HuggingFaceChat/venv/lib/python3.11/site-packages/setuptools/_vendor/packaging/_musllinux.py
deleted file mode 100644
index 8ac3059ba3c246b9a5a6fb8d14936bb07777191e..0000000000000000000000000000000000000000
--- a/spaces/Ataturk-Chatbot/HuggingFaceChat/venv/lib/python3.11/site-packages/setuptools/_vendor/packaging/_musllinux.py
+++ /dev/null
@@ -1,136 +0,0 @@
-"""PEP 656 support.
-
-This module implements logic to detect if the currently running Python is
-linked against musl, and what musl version is used.
-"""
-
-import contextlib
-import functools
-import operator
-import os
-import re
-import struct
-import subprocess
-import sys
-from typing import IO, Iterator, NamedTuple, Optional, Tuple
-
-
-def _read_unpacked(f: IO[bytes], fmt: str) -> Tuple[int, ...]:
- return struct.unpack(fmt, f.read(struct.calcsize(fmt)))
-
-
-def _parse_ld_musl_from_elf(f: IO[bytes]) -> Optional[str]:
- """Detect musl libc location by parsing the Python executable.
-
- Based on: https://gist.github.com/lyssdod/f51579ae8d93c8657a5564aefc2ffbca
- ELF header: https://refspecs.linuxfoundation.org/elf/gabi4+/ch4.eheader.html
- """
- f.seek(0)
- try:
- ident = _read_unpacked(f, "16B")
- except struct.error:
- return None
- if ident[:4] != tuple(b"\x7fELF"): # Invalid magic, not ELF.
- return None
- f.seek(struct.calcsize("HHI"), 1) # Skip file type, machine, and version.
-
- try:
- # e_fmt: Format for program header.
- # p_fmt: Format for section header.
- # p_idx: Indexes to find p_type, p_offset, and p_filesz.
- e_fmt, p_fmt, p_idx = {
- 1: ("IIIIHHH", "IIIIIIII", (0, 1, 4)), # 32-bit.
- 2: ("QQQIHHH", "IIQQQQQQ", (0, 2, 5)), # 64-bit.
- }[ident[4]]
- except KeyError:
- return None
- else:
- p_get = operator.itemgetter(*p_idx)
-
- # Find the interpreter section and return its content.
- try:
- _, e_phoff, _, _, _, e_phentsize, e_phnum = _read_unpacked(f, e_fmt)
- except struct.error:
- return None
- for i in range(e_phnum + 1):
- f.seek(e_phoff + e_phentsize * i)
- try:
- p_type, p_offset, p_filesz = p_get(_read_unpacked(f, p_fmt))
- except struct.error:
- return None
- if p_type != 3: # Not PT_INTERP.
- continue
- f.seek(p_offset)
- interpreter = os.fsdecode(f.read(p_filesz)).strip("\0")
- if "musl" not in interpreter:
- return None
- return interpreter
- return None
-
-
-class _MuslVersion(NamedTuple):
- major: int
- minor: int
-
-
-def _parse_musl_version(output: str) -> Optional[_MuslVersion]:
- lines = [n for n in (n.strip() for n in output.splitlines()) if n]
- if len(lines) < 2 or lines[0][:4] != "musl":
- return None
- m = re.match(r"Version (\d+)\.(\d+)", lines[1])
- if not m:
- return None
- return _MuslVersion(major=int(m.group(1)), minor=int(m.group(2)))
-
-
-@functools.lru_cache()
-def _get_musl_version(executable: str) -> Optional[_MuslVersion]:
- """Detect currently-running musl runtime version.
-
- This is done by checking the specified executable's dynamic linking
- information, and invoking the loader to parse its output for a version
- string. If the loader is musl, the output would be something like::
-
- musl libc (x86_64)
- Version 1.2.2
- Dynamic Program Loader
- """
- with contextlib.ExitStack() as stack:
- try:
- f = stack.enter_context(open(executable, "rb"))
- except OSError:
- return None
- ld = _parse_ld_musl_from_elf(f)
- if not ld:
- return None
- proc = subprocess.run([ld], stderr=subprocess.PIPE, universal_newlines=True)
- return _parse_musl_version(proc.stderr)
-
-
-def platform_tags(arch: str) -> Iterator[str]:
- """Generate musllinux tags compatible to the current platform.
-
- :param arch: Should be the part of platform tag after the ``linux_``
- prefix, e.g. ``x86_64``. The ``linux_`` prefix is assumed as a
- prerequisite for the current platform to be musllinux-compatible.
-
- :returns: An iterator of compatible musllinux tags.
- """
- sys_musl = _get_musl_version(sys.executable)
- if sys_musl is None: # Python not dynamically linked against musl.
- return
- for minor in range(sys_musl.minor, -1, -1):
- yield f"musllinux_{sys_musl.major}_{minor}_{arch}"
-
-
-if __name__ == "__main__": # pragma: no cover
- import sysconfig
-
- plat = sysconfig.get_platform()
- assert plat.startswith("linux-"), "not linux"
-
- print("plat:", plat)
- print("musl:", _get_musl_version(sys.executable))
- print("tags:", end=" ")
- for t in platform_tags(re.sub(r"[.-]", "_", plat.split("-", 1)[-1])):
- print(t, end="\n ")
diff --git a/spaces/AtomdffAI/wechatgpt4atom/channel/wechat/wechaty_channel.py b/spaces/AtomdffAI/wechatgpt4atom/channel/wechat/wechaty_channel.py
deleted file mode 100644
index 8f27f6dc81422741ddfbbc2e700f8b8b62011cc3..0000000000000000000000000000000000000000
--- a/spaces/AtomdffAI/wechatgpt4atom/channel/wechat/wechaty_channel.py
+++ /dev/null
@@ -1,201 +0,0 @@
-# encoding:utf-8
-
-"""
-wechaty channel
-Python Wechaty - https://github.com/wechaty/python-wechaty
-"""
-import io
-import os
-import json
-import time
-import asyncio
-import requests
-from typing import Optional, Union
-from wechaty_puppet import MessageType, FileBox, ScanStatus # type: ignore
-from wechaty import Wechaty, Contact
-from wechaty.user import Message, Room, MiniProgram, UrlLink
-from channel.channel import Channel
-from common.log import logger
-from config import conf
-
-
-class WechatyChannel(Channel):
-
- def __init__(self):
- pass
-
- def startup(self):
- asyncio.run(self.main())
-
- async def main(self):
- config = conf()
- # 使用PadLocal协议 比较稳定(免费web协议 os.environ['WECHATY_PUPPET_SERVICE_ENDPOINT'] = '127.0.0.1:8080')
- token = config.get('wechaty_puppet_service_token')
- os.environ['WECHATY_PUPPET_SERVICE_TOKEN'] = token
- global bot
- bot = Wechaty()
-
- bot.on('scan', self.on_scan)
- bot.on('login', self.on_login)
- bot.on('message', self.on_message)
- await bot.start()
-
- async def on_login(self, contact: Contact):
- logger.info('[WX] login user={}'.format(contact))
-
- async def on_scan(self, status: ScanStatus, qr_code: Optional[str] = None,
- data: Optional[str] = None):
- contact = self.Contact.load(self.contact_id)
- logger.info('[WX] scan user={}, scan status={}, scan qr_code={}'.format(contact, status.name, qr_code))
- # print(f'user <{contact}> scan status: {status.name} , 'f'qr_code: {qr_code}')
-
- async def on_message(self, msg: Message):
- """
- listen for message event
- """
- from_contact = msg.talker() # 获取消息的发送者
- to_contact = msg.to() # 接收人
- room = msg.room() # 获取消息来自的群聊. 如果消息不是来自群聊, 则返回None
- from_user_id = from_contact.contact_id
- to_user_id = to_contact.contact_id # 接收人id
- # other_user_id = msg['User']['UserName'] # 对手方id
- content = msg.text()
- mention_content = await msg.mention_text() # 返回过滤掉@name后的消息
- match_prefix = self.check_prefix(content, conf().get('single_chat_prefix'))
- conversation: Union[Room, Contact] = from_contact if room is None else room
-
- if room is None and msg.type() == MessageType.MESSAGE_TYPE_TEXT:
- if not msg.is_self() and match_prefix is not None:
- # 好友向自己发送消息
- if match_prefix != '':
- str_list = content.split(match_prefix, 1)
- if len(str_list) == 2:
- content = str_list[1].strip()
-
- img_match_prefix = self.check_prefix(content, conf().get('image_create_prefix'))
- if img_match_prefix:
- content = content.split(img_match_prefix, 1)[1].strip()
- await self._do_send_img(content, from_user_id)
- else:
- await self._do_send(content, from_user_id)
- elif msg.is_self() and match_prefix:
- # 自己给好友发送消息
- str_list = content.split(match_prefix, 1)
- if len(str_list) == 2:
- content = str_list[1].strip()
- img_match_prefix = self.check_prefix(content, conf().get('image_create_prefix'))
- if img_match_prefix:
- content = content.split(img_match_prefix, 1)[1].strip()
- await self._do_send_img(content, to_user_id)
- else:
- await self._do_send(content, to_user_id)
- elif room and msg.type() == MessageType.MESSAGE_TYPE_TEXT:
- # 群组&文本消息
- room_id = room.room_id
- room_name = await room.topic()
- from_user_id = from_contact.contact_id
- from_user_name = from_contact.name
- is_at = await msg.mention_self()
- content = mention_content
- config = conf()
- match_prefix = (is_at and not config.get("group_at_off", False)) \
- or self.check_prefix(content, config.get('group_chat_prefix')) \
- or self.check_contain(content, config.get('group_chat_keyword'))
- if ('ALL_GROUP' in config.get('group_name_white_list') or room_name in config.get(
- 'group_name_white_list') or self.check_contain(room_name, config.get(
- 'group_name_keyword_white_list'))) and match_prefix:
- img_match_prefix = self.check_prefix(content, conf().get('image_create_prefix'))
- if img_match_prefix:
- content = content.split(img_match_prefix, 1)[1].strip()
- await self._do_send_group_img(content, room_id)
- else:
- await self._do_send_group(content, room_id, from_user_id, from_user_name)
-
- async def send(self, message: Union[str, Message, FileBox, Contact, UrlLink, MiniProgram], receiver):
- logger.info('[WX] sendMsg={}, receiver={}'.format(message, receiver))
- if receiver:
- contact = await bot.Contact.find(receiver)
- await contact.say(message)
-
- async def send_group(self, message: Union[str, Message, FileBox, Contact, UrlLink, MiniProgram], receiver):
- logger.info('[WX] sendMsg={}, receiver={}'.format(message, receiver))
- if receiver:
- room = await bot.Room.find(receiver)
- await room.say(message)
-
- async def _do_send(self, query, reply_user_id):
- try:
- if not query:
- return
- context = dict()
- context['from_user_id'] = reply_user_id
- reply_text = super().build_reply_content(query, context)
- if reply_text:
- await self.send(conf().get("single_chat_reply_prefix") + reply_text, reply_user_id)
- except Exception as e:
- logger.exception(e)
-
- async def _do_send_img(self, query, reply_user_id):
- try:
- if not query:
- return
- context = dict()
- context['type'] = 'IMAGE_CREATE'
- img_url = super().build_reply_content(query, context)
- if not img_url:
- return
- # 图片下载
- # pic_res = requests.get(img_url, stream=True)
- # image_storage = io.BytesIO()
- # for block in pic_res.iter_content(1024):
- # image_storage.write(block)
- # image_storage.seek(0)
-
- # 图片发送
- logger.info('[WX] sendImage, receiver={}'.format(reply_user_id))
- t = int(time.time())
- file_box = FileBox.from_url(url=img_url, name=str(t) + '.png')
- await self.send(file_box, reply_user_id)
- except Exception as e:
- logger.exception(e)
-
- async def _do_send_group(self, query, group_id, group_user_id, group_user_name):
- if not query:
- return
- context = dict()
- context['from_user_id'] = str(group_id) + '-' + str(group_user_id)
- reply_text = super().build_reply_content(query, context)
- if reply_text:
- reply_text = '@' + group_user_name + ' ' + reply_text.strip()
- await self.send_group(conf().get("group_chat_reply_prefix", "") + reply_text, group_id)
-
- async def _do_send_group_img(self, query, reply_room_id):
- try:
- if not query:
- return
- context = dict()
- context['type'] = 'IMAGE_CREATE'
- img_url = super().build_reply_content(query, context)
- if not img_url:
- return
- # 图片发送
- logger.info('[WX] sendImage, receiver={}'.format(reply_room_id))
- t = int(time.time())
- file_box = FileBox.from_url(url=img_url, name=str(t) + '.png')
- await self.send_group(file_box, reply_room_id)
- except Exception as e:
- logger.exception(e)
-
- def check_prefix(self, content, prefix_list):
- for prefix in prefix_list:
- if content.startswith(prefix):
- return prefix
- return None
-
- def check_contain(self, content, keyword_list):
- if not keyword_list:
- return None
- for ky in keyword_list:
- if content.find(ky) != -1:
- return True
- return None
diff --git a/spaces/Awiny/Image2Paragraph/models/grit_src/third_party/CenterNet2/tools/lightning_train_net.py b/spaces/Awiny/Image2Paragraph/models/grit_src/third_party/CenterNet2/tools/lightning_train_net.py
deleted file mode 100644
index f6734b566b6764ee54dd2af1b7310fedb34bb40d..0000000000000000000000000000000000000000
--- a/spaces/Awiny/Image2Paragraph/models/grit_src/third_party/CenterNet2/tools/lightning_train_net.py
+++ /dev/null
@@ -1,239 +0,0 @@
-#!/usr/bin/env python3
-# Copyright (c) Facebook, Inc. and its affiliates.
-# Lightning Trainer should be considered beta at this point
-# We have confirmed that training and validation run correctly and produce correct results
-# Depending on how you launch the trainer, there are issues with processes terminating correctly
-# This module is still dependent on D2 logging, but could be transferred to use Lightning logging
-
-import logging
-import os
-import time
-import weakref
-from collections import OrderedDict
-from typing import Any, Dict, List
-
-import detectron2.utils.comm as comm
-from detectron2.checkpoint import DetectionCheckpointer
-from detectron2.config import get_cfg
-from detectron2.data import build_detection_test_loader, build_detection_train_loader
-from detectron2.engine import (
- DefaultTrainer,
- SimpleTrainer,
- default_argument_parser,
- default_setup,
- default_writers,
- hooks,
-)
-from detectron2.evaluation import print_csv_format
-from detectron2.evaluation.testing import flatten_results_dict
-from detectron2.modeling import build_model
-from detectron2.solver import build_lr_scheduler, build_optimizer
-from detectron2.utils.events import EventStorage
-from detectron2.utils.logger import setup_logger
-
-import pytorch_lightning as pl # type: ignore
-from pytorch_lightning import LightningDataModule, LightningModule
-from train_net import build_evaluator
-
-logging.basicConfig(level=logging.INFO)
-logger = logging.getLogger("detectron2")
-
-
-class TrainingModule(LightningModule):
- def __init__(self, cfg):
- super().__init__()
- if not logger.isEnabledFor(logging.INFO): # setup_logger is not called for d2
- setup_logger()
- self.cfg = DefaultTrainer.auto_scale_workers(cfg, comm.get_world_size())
- self.storage: EventStorage = None
- self.model = build_model(self.cfg)
-
- self.start_iter = 0
- self.max_iter = cfg.SOLVER.MAX_ITER
-
- def on_save_checkpoint(self, checkpoint: Dict[str, Any]) -> None:
- checkpoint["iteration"] = self.storage.iter
-
- def on_load_checkpoint(self, checkpointed_state: Dict[str, Any]) -> None:
- self.start_iter = checkpointed_state["iteration"]
- self.storage.iter = self.start_iter
-
- def setup(self, stage: str):
- if self.cfg.MODEL.WEIGHTS:
- self.checkpointer = DetectionCheckpointer(
- # Assume you want to save checkpoints together with logs/statistics
- self.model,
- self.cfg.OUTPUT_DIR,
- )
- logger.info(f"Load model weights from checkpoint: {self.cfg.MODEL.WEIGHTS}.")
- # Only load weights, use lightning checkpointing if you want to resume
- self.checkpointer.load(self.cfg.MODEL.WEIGHTS)
-
- self.iteration_timer = hooks.IterationTimer()
- self.iteration_timer.before_train()
- self.data_start = time.perf_counter()
- self.writers = None
-
- def training_step(self, batch, batch_idx):
- data_time = time.perf_counter() - self.data_start
- # Need to manually enter/exit since trainer may launch processes
- # This ideally belongs in setup, but setup seems to run before processes are spawned
- if self.storage is None:
- self.storage = EventStorage(0)
- self.storage.__enter__()
- self.iteration_timer.trainer = weakref.proxy(self)
- self.iteration_timer.before_step()
- self.writers = (
- default_writers(self.cfg.OUTPUT_DIR, self.max_iter)
- if comm.is_main_process()
- else {}
- )
-
- loss_dict = self.model(batch)
- SimpleTrainer.write_metrics(loss_dict, data_time)
-
- opt = self.optimizers()
- self.storage.put_scalar(
- "lr", opt.param_groups[self._best_param_group_id]["lr"], smoothing_hint=False
- )
- self.iteration_timer.after_step()
- self.storage.step()
- # A little odd to put before step here, but it's the best way to get a proper timing
- self.iteration_timer.before_step()
-
- if self.storage.iter % 20 == 0:
- for writer in self.writers:
- writer.write()
- return sum(loss_dict.values())
-
- def training_step_end(self, training_step_outpus):
- self.data_start = time.perf_counter()
- return training_step_outpus
-
- def training_epoch_end(self, training_step_outputs):
- self.iteration_timer.after_train()
- if comm.is_main_process():
- self.checkpointer.save("model_final")
- for writer in self.writers:
- writer.write()
- writer.close()
- self.storage.__exit__(None, None, None)
-
- def _process_dataset_evaluation_results(self) -> OrderedDict:
- results = OrderedDict()
- for idx, dataset_name in enumerate(self.cfg.DATASETS.TEST):
- results[dataset_name] = self._evaluators[idx].evaluate()
- if comm.is_main_process():
- print_csv_format(results[dataset_name])
-
- if len(results) == 1:
- results = list(results.values())[0]
- return results
-
- def _reset_dataset_evaluators(self):
- self._evaluators = []
- for dataset_name in self.cfg.DATASETS.TEST:
- evaluator = build_evaluator(self.cfg, dataset_name)
- evaluator.reset()
- self._evaluators.append(evaluator)
-
- def on_validation_epoch_start(self, _outputs):
- self._reset_dataset_evaluators()
-
- def validation_epoch_end(self, _outputs):
- results = self._process_dataset_evaluation_results(_outputs)
-
- flattened_results = flatten_results_dict(results)
- for k, v in flattened_results.items():
- try:
- v = float(v)
- except Exception as e:
- raise ValueError(
- "[EvalHook] eval_function should return a nested dict of float. "
- "Got '{}: {}' instead.".format(k, v)
- ) from e
- self.storage.put_scalars(**flattened_results, smoothing_hint=False)
-
- def validation_step(self, batch, batch_idx: int, dataloader_idx: int = 0) -> None:
- if not isinstance(batch, List):
- batch = [batch]
- outputs = self.model(batch)
- self._evaluators[dataloader_idx].process(batch, outputs)
-
- def configure_optimizers(self):
- optimizer = build_optimizer(self.cfg, self.model)
- self._best_param_group_id = hooks.LRScheduler.get_best_param_group_id(optimizer)
- scheduler = build_lr_scheduler(self.cfg, optimizer)
- return [optimizer], [{"scheduler": scheduler, "interval": "step"}]
-
-
-class DataModule(LightningDataModule):
- def __init__(self, cfg):
- super().__init__()
- self.cfg = DefaultTrainer.auto_scale_workers(cfg, comm.get_world_size())
-
- def train_dataloader(self):
- return build_detection_train_loader(self.cfg)
-
- def val_dataloader(self):
- dataloaders = []
- for dataset_name in self.cfg.DATASETS.TEST:
- dataloaders.append(build_detection_test_loader(self.cfg, dataset_name))
- return dataloaders
-
-
-def main(args):
- cfg = setup(args)
- train(cfg, args)
-
-
-def train(cfg, args):
- trainer_params = {
- # training loop is bounded by max steps, use a large max_epochs to make
- # sure max_steps is met first
- "max_epochs": 10 ** 8,
- "max_steps": cfg.SOLVER.MAX_ITER,
- "val_check_interval": cfg.TEST.EVAL_PERIOD if cfg.TEST.EVAL_PERIOD > 0 else 10 ** 8,
- "num_nodes": args.num_machines,
- "gpus": args.num_gpus,
- "num_sanity_val_steps": 0,
- }
- if cfg.SOLVER.AMP.ENABLED:
- trainer_params["precision"] = 16
-
- last_checkpoint = os.path.join(cfg.OUTPUT_DIR, "last.ckpt")
- if args.resume:
- # resume training from checkpoint
- trainer_params["resume_from_checkpoint"] = last_checkpoint
- logger.info(f"Resuming training from checkpoint: {last_checkpoint}.")
-
- trainer = pl.Trainer(**trainer_params)
- logger.info(f"start to train with {args.num_machines} nodes and {args.num_gpus} GPUs")
-
- module = TrainingModule(cfg)
- data_module = DataModule(cfg)
- if args.eval_only:
- logger.info("Running inference")
- trainer.validate(module, data_module)
- else:
- logger.info("Running training")
- trainer.fit(module, data_module)
-
-
-def setup(args):
- """
- Create configs and perform basic setups.
- """
- cfg = get_cfg()
- cfg.merge_from_file(args.config_file)
- cfg.merge_from_list(args.opts)
- cfg.freeze()
- default_setup(cfg, args)
- return cfg
-
-
-if __name__ == "__main__":
- parser = default_argument_parser()
- args = parser.parse_args()
- logger.info("Command Line Args:", args)
- main(args)
diff --git a/spaces/BartPoint/VoiceChange_Beta/app_multi.py b/spaces/BartPoint/VoiceChange_Beta/app_multi.py
deleted file mode 100644
index 594d88008180684ad92bf432ebd1d96fd09bbbb2..0000000000000000000000000000000000000000
--- a/spaces/BartPoint/VoiceChange_Beta/app_multi.py
+++ /dev/null
@@ -1,496 +0,0 @@
-from typing import Union
-
-from argparse import ArgumentParser
-
-import asyncio
-import json
-import hashlib
-from os import path, getenv
-
-import gradio as gr
-
-import torch
-
-import numpy as np
-import librosa
-
-import edge_tts
-
-import config
-import util
-from fairseq import checkpoint_utils
-from infer_pack.models import (
- SynthesizerTrnMs256NSFsid,
- SynthesizerTrnMs256NSFsid_nono,
- SynthesizerTrnMs768NSFsid,
- SynthesizerTrnMs768NSFsid_nono,
-)
-from vc_infer_pipeline import VC
-from config import Config
-config = Config()
-force_support = None
-if config.unsupported is False:
- if config.device == "mps" or config.device == "cpu":
- force_support = False
-else:
- force_support = True
-
-# Reference: https://huggingface.co/spaces/zomehwh/rvc-models/blob/main/app.py#L21 # noqa
-in_hf_space = getenv('SYSTEM') == 'spaces'
-
-# Argument parsing
-arg_parser = ArgumentParser()
-arg_parser.add_argument(
- '--hubert',
- default=getenv('RVC_HUBERT', 'hubert_base.pt'),
- help='path to hubert base model (default: hubert_base.pt)'
-)
-arg_parser.add_argument(
- '--config',
- default=getenv('RVC_MULTI_CFG', 'multi_config.json'),
- help='path to config file (default: multi_config.json)'
-)
-arg_parser.add_argument(
- '--api',
- action='store_true',
- help='enable api endpoint'
-)
-arg_parser.add_argument(
- '--cache-examples',
- action='store_true',
- help='enable example caching, please remember delete gradio_cached_examples folder when example config has been modified' # noqa
-)
-args = arg_parser.parse_args()
-
-app_css = '''
-#model_info img {
- max-width: 100px;
- max-height: 100px;
- float: right;
-}
-
-#model_info p {
- margin: unset;
-}
-'''
-
-app = gr.Blocks(
- theme=gr.themes.Soft(primary_hue="orange", secondary_hue="slate"),
- css=app_css,
- analytics_enabled=False
-)
-
-# Load hubert model
-models, _, _ = checkpoint_utils.load_model_ensemble_and_task(
- ["hubert_base.pt"],
- suffix="",
-)
-hubert_model = models[0]
-hubert_model = hubert_model.to(config.device)
-if config.is_half:
- hubert_model = hubert_model.half()
-else:
- hubert_model = hubert_model.float()
-hubert_model.eval()
-
-# Load models
-multi_cfg = json.load(open(args.config, 'r'))
-loaded_models = []
-
-for model_name in multi_cfg.get('models'):
- print(f'Loading model: {model_name}')
-
- # Load model info
- model_info = json.load(
- open(path.join('model', model_name, 'config.json'), 'r')
- )
-
- # Load RVC checkpoint
- cpt = torch.load(
- path.join('model', model_name, model_info['model']),
- map_location='cpu'
- )
- tgt_sr = cpt['config'][-1]
- cpt["config"][-3] = cpt["weight"]["emb_g.weight"].shape[0] # n_spk
- if_f0 = cpt.get("f0", 1)
- version = cpt.get("version", "v1")
- if version == "v1":
- if if_f0 == 1:
- net_g = SynthesizerTrnMs256NSFsid(*cpt["config"], is_half=config.is_half)
- else:
- net_g = SynthesizerTrnMs256NSFsid_nono(*cpt["config"])
- model_version = "V1"
- elif version == "v2":
- if if_f0 == 1:
- net_g = SynthesizerTrnMs768NSFsid(*cpt["config"], is_half=config.is_half)
- else:
- net_g = SynthesizerTrnMs768NSFsid_nono(*cpt["config"])
- model_version = "V2"
- del net_g.enc_q
-
- print(net_g.load_state_dict(cpt["weight"], strict=False))
- net_g.eval().to(config.device)
- if config.is_half:
- net_g = net_g.half()
- else:
- net_g = net_g.float()
- vc = VC(tgt_sr, config)
-
- loaded_models.append(dict(
- name=model_name,
- metadata=model_info,
- vc=vc,
- net_g=net_g,
- if_f0=if_f0,
- target_sr=tgt_sr,
- test=model_version
- ))
-
-print(f'Models loaded: {len(loaded_models)}')
-
-# Edge TTS speakers
-tts_speakers_list = asyncio.get_event_loop().run_until_complete(edge_tts.list_voices()) # noqa
-
-
-# https://github.com/fumiama/Retrieval-based-Voice-Conversion-WebUI/blob/main/infer-web.py#L118 # noqa
-def vc_func(
- input_audio, model_index, pitch_adjust, f0_method, feat_ratio,
- filter_radius, rms_mix_rate, resample_option
-):
- if input_audio is None:
- return (None, 'Please provide input audio.')
-
- if model_index is None:
- return (None, 'Please select a model.')
-
- model = loaded_models[model_index]
-
- # Reference: so-vits
- (audio_samp, audio_npy) = input_audio
-
- # https://huggingface.co/spaces/zomehwh/rvc-models/blob/main/app.py#L49
- # Can be change well, we will see
- if (audio_npy.shape[0] / audio_samp) > 320 and in_hf_space:
- return (None, 'Input audio is longer than 60 secs.')
-
- # Bloody hell: https://stackoverflow.com/questions/26921836/
- if audio_npy.dtype != np.float32: # :thonk:
- audio_npy = (
- audio_npy / np.iinfo(audio_npy.dtype).max
- ).astype(np.float32)
-
- if len(audio_npy.shape) > 1:
- audio_npy = librosa.to_mono(audio_npy.transpose(1, 0))
-
- if audio_samp != 16000:
- audio_npy = librosa.resample(
- audio_npy,
- orig_sr=audio_samp,
- target_sr=16000
- )
-
- pitch_int = int(pitch_adjust)
-
- resample = (
- 0 if resample_option == 'Disable resampling'
- else int(resample_option)
- )
-
- times = [0, 0, 0]
-
- checksum = hashlib.sha512()
- checksum.update(audio_npy.tobytes())
-
- print(model['test'])
-
- output_audio = model['vc'].pipeline(
- hubert_model,
- model['net_g'],
- model['metadata'].get('speaker_id', 0),
- audio_npy,
- checksum.hexdigest(),
- times,
- pitch_int,
- f0_method,
- path.join('model', model['name'], model['metadata']['feat_index']),
- feat_ratio,
- model['if_f0'],
- filter_radius,
- model['target_sr'],
- resample,
- rms_mix_rate,
- model['test'],
- 0.5
- )
-
- out_sr = (
- resample if resample >= 16000 and model['target_sr'] != resample
- else model['target_sr']
- )
-
- print(f'npy: {times[0]}s, f0: {times[1]}s, infer: {times[2]}s')
- return ((out_sr, output_audio), 'Success')
-
-
-async def edge_tts_vc_func(
- input_text, model_index, tts_speaker, pitch_adjust, f0_method, feat_ratio,
- filter_radius, rms_mix_rate, resample_option
-):
- if input_text is None:
- return (None, 'Please provide TTS text.')
-
- if tts_speaker is None:
- return (None, 'Please select TTS speaker.')
-
- if model_index is None:
- return (None, 'Please select a model.')
-
- speaker = tts_speakers_list[tts_speaker]['ShortName']
- (tts_np, tts_sr) = await util.call_edge_tts(speaker, input_text)
- return vc_func(
- (tts_sr, tts_np),
- model_index,
- pitch_adjust,
- f0_method,
- feat_ratio,
- filter_radius,
- rms_mix_rate,
- resample_option
- )
-
-
-def update_model_info(model_index):
- if model_index is None:
- return str(
- '### Model info\n'
- 'Please select a model from dropdown above.'
- )
-
- model = loaded_models[model_index]
- model_icon = model['metadata'].get('icon', '')
-
- return str(
- '### Model info\n'
- ''
- '**{name}**\n\n'
- 'Author: {author}\n\n'
- 'Source: {source}\n\n'
- '{note}'
- ).format(
- name=model['metadata'].get('name'),
- author=model['metadata'].get('author', 'Anonymous'),
- source=model['metadata'].get('source', 'Unknown'),
- note=model['metadata'].get('note', ''),
- icon=(
- model_icon
- if model_icon.startswith(('http://', 'https://'))
- else '/file/model/%s/%s' % (model['name'], model_icon)
- )
- )
-
-
-def _example_vc(
- input_audio, model_index, pitch_adjust, f0_method, feat_ratio,
- filter_radius, rms_mix_rate, resample_option
-):
- (audio, message) = vc_func(
- input_audio, model_index, pitch_adjust, f0_method, feat_ratio,
- filter_radius, rms_mix_rate, resample_option
- )
- return (
- audio,
- message,
- update_model_info(model_index)
- )
-
-
-async def _example_edge_tts(
- input_text, model_index, tts_speaker, pitch_adjust, f0_method, feat_ratio,
- filter_radius, rms_mix_rate, resample_option
-):
- (audio, message) = await edge_tts_vc_func(
- input_text, model_index, tts_speaker, pitch_adjust, f0_method,
- feat_ratio, filter_radius, rms_mix_rate, resample_option
- )
- return (
- audio,
- message,
- update_model_info(model_index)
- )
-
-
-with app:
- gr.Markdown(
- '## A simplistic Web interface\n'
- 'RVC interface, project based on [RVC-WebUI](https://github.com/fumiama/Retrieval-based-Voice-Conversion-WebUI)' # thx noqa
- 'A lot of inspiration from what\'s already out there, including [zomehwh/rvc-models](https://huggingface.co/spaces/zomehwh/rvc-models) & [DJQmUKV/rvc-inference](https://huggingface.co/spaces/DJQmUKV/rvc-inference).\n ' # thx noqa
- )
-
- with gr.Row():
- with gr.Column():
- with gr.Tab('Audio conversion'):
- input_audio = gr.Audio(label='Input audio')
-
- vc_convert_btn = gr.Button('Convert', variant='primary')
-
- with gr.Tab('TTS conversion'):
- tts_input = gr.TextArea(
- label='TTS input text'
- )
- tts_speaker = gr.Dropdown(
- [
- '%s (%s)' % (
- s['FriendlyName'],
- s['Gender']
- )
- for s in tts_speakers_list
- ],
- label='TTS speaker',
- type='index'
- )
-
- tts_convert_btn = gr.Button('Convert', variant='primary')
-
- pitch_adjust = gr.Slider(
- label='Pitch',
- minimum=-24,
- maximum=24,
- step=1,
- value=0
- )
- f0_method = gr.Radio(
- label='f0 methods',
- choices=['pm', 'harvest', 'crepe'],
- value='pm',
- interactive=True
- )
-
- with gr.Accordion('Advanced options', open=False):
- feat_ratio = gr.Slider(
- label='Feature ratio',
- minimum=0,
- maximum=1,
- step=0.1,
- value=0.6
- )
- filter_radius = gr.Slider(
- label='Filter radius',
- minimum=0,
- maximum=7,
- step=1,
- value=3
- )
- rms_mix_rate = gr.Slider(
- label='Volume envelope mix rate',
- minimum=0,
- maximum=1,
- step=0.1,
- value=1
- )
- resample_rate = gr.Dropdown(
- [
- 'Disable resampling',
- '16000',
- '22050',
- '44100',
- '48000'
- ],
- label='Resample rate',
- value='Disable resampling'
- )
-
- with gr.Column():
- # Model select
- model_index = gr.Dropdown(
- [
- '%s - %s' % (
- m['metadata'].get('source', 'Unknown'),
- m['metadata'].get('name')
- )
- for m in loaded_models
- ],
- label='Model',
- type='index'
- )
-
- # Model info
- with gr.Box():
- model_info = gr.Markdown(
- '### Model info\n'
- 'Please select a model from dropdown above.',
- elem_id='model_info'
- )
-
- output_audio = gr.Audio(label='Output audio')
- output_msg = gr.Textbox(label='Output message')
-
- multi_examples = multi_cfg.get('examples')
- if (
- multi_examples and
- multi_examples.get('vc') and multi_examples.get('tts_vc')
- ):
- with gr.Accordion('Sweet sweet examples', open=False):
- with gr.Row():
- # VC Example
- if multi_examples.get('vc'):
- gr.Examples(
- label='Audio conversion examples',
- examples=multi_examples.get('vc'),
- inputs=[
- input_audio, model_index, pitch_adjust, f0_method,
- feat_ratio
- ],
- outputs=[output_audio, output_msg, model_info],
- fn=_example_vc,
- cache_examples=args.cache_examples,
- run_on_click=args.cache_examples
- )
-
- # Edge TTS Example
- if multi_examples.get('tts_vc'):
- gr.Examples(
- label='TTS conversion examples',
- examples=multi_examples.get('tts_vc'),
- inputs=[
- tts_input, model_index, tts_speaker, pitch_adjust,
- f0_method, feat_ratio
- ],
- outputs=[output_audio, output_msg, model_info],
- fn=_example_edge_tts,
- cache_examples=args.cache_examples,
- run_on_click=args.cache_examples
- )
-
- vc_convert_btn.click(
- vc_func,
- [
- input_audio, model_index, pitch_adjust, f0_method, feat_ratio,
- filter_radius, rms_mix_rate, resample_rate
- ],
- [output_audio, output_msg],
- api_name='audio_conversion'
- )
-
- tts_convert_btn.click(
- edge_tts_vc_func,
- [
- tts_input, model_index, tts_speaker, pitch_adjust, f0_method,
- feat_ratio, filter_radius, rms_mix_rate, resample_rate
- ],
- [output_audio, output_msg],
- api_name='tts_conversion'
- )
-
- model_index.change(
- update_model_info,
- inputs=[model_index],
- outputs=[model_info],
- show_progress=False,
- queue=False
- )
-
-app.queue(
- concurrency_count=1,
- max_size=20,
- api_open=args.api
-).launch()
\ No newline at end of file
diff --git a/spaces/Big-Web/MMSD/env/Lib/site-packages/pip/_vendor/pygments/formatters/img.py b/spaces/Big-Web/MMSD/env/Lib/site-packages/pip/_vendor/pygments/formatters/img.py
deleted file mode 100644
index 0f36a32ba3399efc216b9974254cd1f7eed07a9f..0000000000000000000000000000000000000000
--- a/spaces/Big-Web/MMSD/env/Lib/site-packages/pip/_vendor/pygments/formatters/img.py
+++ /dev/null
@@ -1,645 +0,0 @@
-"""
- pygments.formatters.img
- ~~~~~~~~~~~~~~~~~~~~~~~
-
- Formatter for Pixmap output.
-
- :copyright: Copyright 2006-2022 by the Pygments team, see AUTHORS.
- :license: BSD, see LICENSE for details.
-"""
-
-import os
-import sys
-
-from pip._vendor.pygments.formatter import Formatter
-from pip._vendor.pygments.util import get_bool_opt, get_int_opt, get_list_opt, \
- get_choice_opt
-
-import subprocess
-
-# Import this carefully
-try:
- from PIL import Image, ImageDraw, ImageFont
- pil_available = True
-except ImportError:
- pil_available = False
-
-try:
- import _winreg
-except ImportError:
- try:
- import winreg as _winreg
- except ImportError:
- _winreg = None
-
-__all__ = ['ImageFormatter', 'GifImageFormatter', 'JpgImageFormatter',
- 'BmpImageFormatter']
-
-
-# For some unknown reason every font calls it something different
-STYLES = {
- 'NORMAL': ['', 'Roman', 'Book', 'Normal', 'Regular', 'Medium'],
- 'ITALIC': ['Oblique', 'Italic'],
- 'BOLD': ['Bold'],
- 'BOLDITALIC': ['Bold Oblique', 'Bold Italic'],
-}
-
-# A sane default for modern systems
-DEFAULT_FONT_NAME_NIX = 'DejaVu Sans Mono'
-DEFAULT_FONT_NAME_WIN = 'Courier New'
-DEFAULT_FONT_NAME_MAC = 'Menlo'
-
-
-class PilNotAvailable(ImportError):
- """When Python imaging library is not available"""
-
-
-class FontNotFound(Exception):
- """When there are no usable fonts specified"""
-
-
-class FontManager:
- """
- Manages a set of fonts: normal, italic, bold, etc...
- """
-
- def __init__(self, font_name, font_size=14):
- self.font_name = font_name
- self.font_size = font_size
- self.fonts = {}
- self.encoding = None
- if sys.platform.startswith('win'):
- if not font_name:
- self.font_name = DEFAULT_FONT_NAME_WIN
- self._create_win()
- elif sys.platform.startswith('darwin'):
- if not font_name:
- self.font_name = DEFAULT_FONT_NAME_MAC
- self._create_mac()
- else:
- if not font_name:
- self.font_name = DEFAULT_FONT_NAME_NIX
- self._create_nix()
-
- def _get_nix_font_path(self, name, style):
- proc = subprocess.Popen(['fc-list', "%s:style=%s" % (name, style), 'file'],
- stdout=subprocess.PIPE, stderr=None)
- stdout, _ = proc.communicate()
- if proc.returncode == 0:
- lines = stdout.splitlines()
- for line in lines:
- if line.startswith(b'Fontconfig warning:'):
- continue
- path = line.decode().strip().strip(':')
- if path:
- return path
- return None
-
- def _create_nix(self):
- for name in STYLES['NORMAL']:
- path = self._get_nix_font_path(self.font_name, name)
- if path is not None:
- self.fonts['NORMAL'] = ImageFont.truetype(path, self.font_size)
- break
- else:
- raise FontNotFound('No usable fonts named: "%s"' %
- self.font_name)
- for style in ('ITALIC', 'BOLD', 'BOLDITALIC'):
- for stylename in STYLES[style]:
- path = self._get_nix_font_path(self.font_name, stylename)
- if path is not None:
- self.fonts[style] = ImageFont.truetype(path, self.font_size)
- break
- else:
- if style == 'BOLDITALIC':
- self.fonts[style] = self.fonts['BOLD']
- else:
- self.fonts[style] = self.fonts['NORMAL']
-
- def _get_mac_font_path(self, font_map, name, style):
- return font_map.get((name + ' ' + style).strip().lower())
-
- def _create_mac(self):
- font_map = {}
- for font_dir in (os.path.join(os.getenv("HOME"), 'Library/Fonts/'),
- '/Library/Fonts/', '/System/Library/Fonts/'):
- font_map.update(
- (os.path.splitext(f)[0].lower(), os.path.join(font_dir, f))
- for f in os.listdir(font_dir)
- if f.lower().endswith(('ttf', 'ttc')))
-
- for name in STYLES['NORMAL']:
- path = self._get_mac_font_path(font_map, self.font_name, name)
- if path is not None:
- self.fonts['NORMAL'] = ImageFont.truetype(path, self.font_size)
- break
- else:
- raise FontNotFound('No usable fonts named: "%s"' %
- self.font_name)
- for style in ('ITALIC', 'BOLD', 'BOLDITALIC'):
- for stylename in STYLES[style]:
- path = self._get_mac_font_path(font_map, self.font_name, stylename)
- if path is not None:
- self.fonts[style] = ImageFont.truetype(path, self.font_size)
- break
- else:
- if style == 'BOLDITALIC':
- self.fonts[style] = self.fonts['BOLD']
- else:
- self.fonts[style] = self.fonts['NORMAL']
-
- def _lookup_win(self, key, basename, styles, fail=False):
- for suffix in ('', ' (TrueType)'):
- for style in styles:
- try:
- valname = '%s%s%s' % (basename, style and ' '+style, suffix)
- val, _ = _winreg.QueryValueEx(key, valname)
- return val
- except OSError:
- continue
- else:
- if fail:
- raise FontNotFound('Font %s (%s) not found in registry' %
- (basename, styles[0]))
- return None
-
- def _create_win(self):
- lookuperror = None
- keynames = [ (_winreg.HKEY_CURRENT_USER, r'Software\Microsoft\Windows NT\CurrentVersion\Fonts'),
- (_winreg.HKEY_CURRENT_USER, r'Software\Microsoft\Windows\CurrentVersion\Fonts'),
- (_winreg.HKEY_LOCAL_MACHINE, r'Software\Microsoft\Windows NT\CurrentVersion\Fonts'),
- (_winreg.HKEY_LOCAL_MACHINE, r'Software\Microsoft\Windows\CurrentVersion\Fonts') ]
- for keyname in keynames:
- try:
- key = _winreg.OpenKey(*keyname)
- try:
- path = self._lookup_win(key, self.font_name, STYLES['NORMAL'], True)
- self.fonts['NORMAL'] = ImageFont.truetype(path, self.font_size)
- for style in ('ITALIC', 'BOLD', 'BOLDITALIC'):
- path = self._lookup_win(key, self.font_name, STYLES[style])
- if path:
- self.fonts[style] = ImageFont.truetype(path, self.font_size)
- else:
- if style == 'BOLDITALIC':
- self.fonts[style] = self.fonts['BOLD']
- else:
- self.fonts[style] = self.fonts['NORMAL']
- return
- except FontNotFound as err:
- lookuperror = err
- finally:
- _winreg.CloseKey(key)
- except OSError:
- pass
- else:
- # If we get here, we checked all registry keys and had no luck
- # We can be in one of two situations now:
- # * All key lookups failed. In this case lookuperror is None and we
- # will raise a generic error
- # * At least one lookup failed with a FontNotFound error. In this
- # case, we will raise that as a more specific error
- if lookuperror:
- raise lookuperror
- raise FontNotFound('Can\'t open Windows font registry key')
-
- def get_char_size(self):
- """
- Get the character size.
- """
- return self.get_text_size('M')
-
- def get_text_size(self, text):
- """
- Get the text size (width, height).
- """
- font = self.fonts['NORMAL']
- if hasattr(font, 'getbbox'): # Pillow >= 9.2.0
- return font.getbbox(text)[2:4]
- else:
- return font.getsize(text)
-
- def get_font(self, bold, oblique):
- """
- Get the font based on bold and italic flags.
- """
- if bold and oblique:
- return self.fonts['BOLDITALIC']
- elif bold:
- return self.fonts['BOLD']
- elif oblique:
- return self.fonts['ITALIC']
- else:
- return self.fonts['NORMAL']
-
-
-class ImageFormatter(Formatter):
- """
- Create a PNG image from source code. This uses the Python Imaging Library to
- generate a pixmap from the source code.
-
- .. versionadded:: 0.10
-
- Additional options accepted:
-
- `image_format`
- An image format to output to that is recognised by PIL, these include:
-
- * "PNG" (default)
- * "JPEG"
- * "BMP"
- * "GIF"
-
- `line_pad`
- The extra spacing (in pixels) between each line of text.
-
- Default: 2
-
- `font_name`
- The font name to be used as the base font from which others, such as
- bold and italic fonts will be generated. This really should be a
- monospace font to look sane.
-
- Default: "Courier New" on Windows, "Menlo" on Mac OS, and
- "DejaVu Sans Mono" on \\*nix
-
- `font_size`
- The font size in points to be used.
-
- Default: 14
-
- `image_pad`
- The padding, in pixels to be used at each edge of the resulting image.
-
- Default: 10
-
- `line_numbers`
- Whether line numbers should be shown: True/False
-
- Default: True
-
- `line_number_start`
- The line number of the first line.
-
- Default: 1
-
- `line_number_step`
- The step used when printing line numbers.
-
- Default: 1
-
- `line_number_bg`
- The background colour (in "#123456" format) of the line number bar, or
- None to use the style background color.
-
- Default: "#eed"
-
- `line_number_fg`
- The text color of the line numbers (in "#123456"-like format).
-
- Default: "#886"
-
- `line_number_chars`
- The number of columns of line numbers allowable in the line number
- margin.
-
- Default: 2
-
- `line_number_bold`
- Whether line numbers will be bold: True/False
-
- Default: False
-
- `line_number_italic`
- Whether line numbers will be italicized: True/False
-
- Default: False
-
- `line_number_separator`
- Whether a line will be drawn between the line number area and the
- source code area: True/False
-
- Default: True
-
- `line_number_pad`
- The horizontal padding (in pixels) between the line number margin, and
- the source code area.
-
- Default: 6
-
- `hl_lines`
- Specify a list of lines to be highlighted.
-
- .. versionadded:: 1.2
-
- Default: empty list
-
- `hl_color`
- Specify the color for highlighting lines.
-
- .. versionadded:: 1.2
-
- Default: highlight color of the selected style
- """
-
- # Required by the pygments mapper
- name = 'img'
- aliases = ['img', 'IMG', 'png']
- filenames = ['*.png']
-
- unicodeoutput = False
-
- default_image_format = 'png'
-
- def __init__(self, **options):
- """
- See the class docstring for explanation of options.
- """
- if not pil_available:
- raise PilNotAvailable(
- 'Python Imaging Library is required for this formatter')
- Formatter.__init__(self, **options)
- self.encoding = 'latin1' # let pygments.format() do the right thing
- # Read the style
- self.styles = dict(self.style)
- if self.style.background_color is None:
- self.background_color = '#fff'
- else:
- self.background_color = self.style.background_color
- # Image options
- self.image_format = get_choice_opt(
- options, 'image_format', ['png', 'jpeg', 'gif', 'bmp'],
- self.default_image_format, normcase=True)
- self.image_pad = get_int_opt(options, 'image_pad', 10)
- self.line_pad = get_int_opt(options, 'line_pad', 2)
- # The fonts
- fontsize = get_int_opt(options, 'font_size', 14)
- self.fonts = FontManager(options.get('font_name', ''), fontsize)
- self.fontw, self.fonth = self.fonts.get_char_size()
- # Line number options
- self.line_number_fg = options.get('line_number_fg', '#886')
- self.line_number_bg = options.get('line_number_bg', '#eed')
- self.line_number_chars = get_int_opt(options,
- 'line_number_chars', 2)
- self.line_number_bold = get_bool_opt(options,
- 'line_number_bold', False)
- self.line_number_italic = get_bool_opt(options,
- 'line_number_italic', False)
- self.line_number_pad = get_int_opt(options, 'line_number_pad', 6)
- self.line_numbers = get_bool_opt(options, 'line_numbers', True)
- self.line_number_separator = get_bool_opt(options,
- 'line_number_separator', True)
- self.line_number_step = get_int_opt(options, 'line_number_step', 1)
- self.line_number_start = get_int_opt(options, 'line_number_start', 1)
- if self.line_numbers:
- self.line_number_width = (self.fontw * self.line_number_chars +
- self.line_number_pad * 2)
- else:
- self.line_number_width = 0
- self.hl_lines = []
- hl_lines_str = get_list_opt(options, 'hl_lines', [])
- for line in hl_lines_str:
- try:
- self.hl_lines.append(int(line))
- except ValueError:
- pass
- self.hl_color = options.get('hl_color',
- self.style.highlight_color) or '#f90'
- self.drawables = []
-
- def get_style_defs(self, arg=''):
- raise NotImplementedError('The -S option is meaningless for the image '
- 'formatter. Use -O style= instead.')
-
- def _get_line_height(self):
- """
- Get the height of a line.
- """
- return self.fonth + self.line_pad
-
- def _get_line_y(self, lineno):
- """
- Get the Y coordinate of a line number.
- """
- return lineno * self._get_line_height() + self.image_pad
-
- def _get_char_width(self):
- """
- Get the width of a character.
- """
- return self.fontw
-
- def _get_char_x(self, linelength):
- """
- Get the X coordinate of a character position.
- """
- return linelength + self.image_pad + self.line_number_width
-
- def _get_text_pos(self, linelength, lineno):
- """
- Get the actual position for a character and line position.
- """
- return self._get_char_x(linelength), self._get_line_y(lineno)
-
- def _get_linenumber_pos(self, lineno):
- """
- Get the actual position for the start of a line number.
- """
- return (self.image_pad, self._get_line_y(lineno))
-
- def _get_text_color(self, style):
- """
- Get the correct color for the token from the style.
- """
- if style['color'] is not None:
- fill = '#' + style['color']
- else:
- fill = '#000'
- return fill
-
- def _get_text_bg_color(self, style):
- """
- Get the correct background color for the token from the style.
- """
- if style['bgcolor'] is not None:
- bg_color = '#' + style['bgcolor']
- else:
- bg_color = None
- return bg_color
-
- def _get_style_font(self, style):
- """
- Get the correct font for the style.
- """
- return self.fonts.get_font(style['bold'], style['italic'])
-
- def _get_image_size(self, maxlinelength, maxlineno):
- """
- Get the required image size.
- """
- return (self._get_char_x(maxlinelength) + self.image_pad,
- self._get_line_y(maxlineno + 0) + self.image_pad)
-
- def _draw_linenumber(self, posno, lineno):
- """
- Remember a line number drawable to paint later.
- """
- self._draw_text(
- self._get_linenumber_pos(posno),
- str(lineno).rjust(self.line_number_chars),
- font=self.fonts.get_font(self.line_number_bold,
- self.line_number_italic),
- text_fg=self.line_number_fg,
- text_bg=None,
- )
-
- def _draw_text(self, pos, text, font, text_fg, text_bg):
- """
- Remember a single drawable tuple to paint later.
- """
- self.drawables.append((pos, text, font, text_fg, text_bg))
-
- def _create_drawables(self, tokensource):
- """
- Create drawables for the token content.
- """
- lineno = charno = maxcharno = 0
- maxlinelength = linelength = 0
- for ttype, value in tokensource:
- while ttype not in self.styles:
- ttype = ttype.parent
- style = self.styles[ttype]
- # TODO: make sure tab expansion happens earlier in the chain. It
- # really ought to be done on the input, as to do it right here is
- # quite complex.
- value = value.expandtabs(4)
- lines = value.splitlines(True)
- # print lines
- for i, line in enumerate(lines):
- temp = line.rstrip('\n')
- if temp:
- self._draw_text(
- self._get_text_pos(linelength, lineno),
- temp,
- font = self._get_style_font(style),
- text_fg = self._get_text_color(style),
- text_bg = self._get_text_bg_color(style),
- )
- temp_width, _ = self.fonts.get_text_size(temp)
- linelength += temp_width
- maxlinelength = max(maxlinelength, linelength)
- charno += len(temp)
- maxcharno = max(maxcharno, charno)
- if line.endswith('\n'):
- # add a line for each extra line in the value
- linelength = 0
- charno = 0
- lineno += 1
- self.maxlinelength = maxlinelength
- self.maxcharno = maxcharno
- self.maxlineno = lineno
-
- def _draw_line_numbers(self):
- """
- Create drawables for the line numbers.
- """
- if not self.line_numbers:
- return
- for p in range(self.maxlineno):
- n = p + self.line_number_start
- if (n % self.line_number_step) == 0:
- self._draw_linenumber(p, n)
-
- def _paint_line_number_bg(self, im):
- """
- Paint the line number background on the image.
- """
- if not self.line_numbers:
- return
- if self.line_number_fg is None:
- return
- draw = ImageDraw.Draw(im)
- recth = im.size[-1]
- rectw = self.image_pad + self.line_number_width - self.line_number_pad
- draw.rectangle([(0, 0), (rectw, recth)],
- fill=self.line_number_bg)
- if self.line_number_separator:
- draw.line([(rectw, 0), (rectw, recth)], fill=self.line_number_fg)
- del draw
-
- def format(self, tokensource, outfile):
- """
- Format ``tokensource``, an iterable of ``(tokentype, tokenstring)``
- tuples and write it into ``outfile``.
-
- This implementation calculates where it should draw each token on the
- pixmap, then calculates the required pixmap size and draws the items.
- """
- self._create_drawables(tokensource)
- self._draw_line_numbers()
- im = Image.new(
- 'RGB',
- self._get_image_size(self.maxlinelength, self.maxlineno),
- self.background_color
- )
- self._paint_line_number_bg(im)
- draw = ImageDraw.Draw(im)
- # Highlight
- if self.hl_lines:
- x = self.image_pad + self.line_number_width - self.line_number_pad + 1
- recth = self._get_line_height()
- rectw = im.size[0] - x
- for linenumber in self.hl_lines:
- y = self._get_line_y(linenumber - 1)
- draw.rectangle([(x, y), (x + rectw, y + recth)],
- fill=self.hl_color)
- for pos, value, font, text_fg, text_bg in self.drawables:
- if text_bg:
- text_size = draw.textsize(text=value, font=font)
- draw.rectangle([pos[0], pos[1], pos[0] + text_size[0], pos[1] + text_size[1]], fill=text_bg)
- draw.text(pos, value, font=font, fill=text_fg)
- im.save(outfile, self.image_format.upper())
-
-
-# Add one formatter per format, so that the "-f gif" option gives the correct result
-# when used in pygmentize.
-
-class GifImageFormatter(ImageFormatter):
- """
- Create a GIF image from source code. This uses the Python Imaging Library to
- generate a pixmap from the source code.
-
- .. versionadded:: 1.0
- """
-
- name = 'img_gif'
- aliases = ['gif']
- filenames = ['*.gif']
- default_image_format = 'gif'
-
-
-class JpgImageFormatter(ImageFormatter):
- """
- Create a JPEG image from source code. This uses the Python Imaging Library to
- generate a pixmap from the source code.
-
- .. versionadded:: 1.0
- """
-
- name = 'img_jpg'
- aliases = ['jpg', 'jpeg']
- filenames = ['*.jpg']
- default_image_format = 'jpeg'
-
-
-class BmpImageFormatter(ImageFormatter):
- """
- Create a bitmap image from source code. This uses the Python Imaging Library to
- generate a pixmap from the source code.
-
- .. versionadded:: 1.0
- """
-
- name = 'img_bmp'
- aliases = ['bmp', 'bitmap']
- filenames = ['*.bmp']
- default_image_format = 'bmp'
diff --git a/spaces/Big-Web/MMSD/env/Lib/site-packages/setuptools/_distutils/command/install_lib.py b/spaces/Big-Web/MMSD/env/Lib/site-packages/setuptools/_distutils/command/install_lib.py
deleted file mode 100644
index ad3089c8b144f292e9560c8cefcbab4012d09a45..0000000000000000000000000000000000000000
--- a/spaces/Big-Web/MMSD/env/Lib/site-packages/setuptools/_distutils/command/install_lib.py
+++ /dev/null
@@ -1,238 +0,0 @@
-"""distutils.command.install_lib
-
-Implements the Distutils 'install_lib' command
-(install all Python modules)."""
-
-import os
-import importlib.util
-import sys
-
-from distutils.core import Command
-from distutils.errors import DistutilsOptionError
-
-
-# Extension for Python source files.
-PYTHON_SOURCE_EXTENSION = ".py"
-
-
-class install_lib(Command):
-
- description = "install all Python modules (extensions and pure Python)"
-
- # The byte-compilation options are a tad confusing. Here are the
- # possible scenarios:
- # 1) no compilation at all (--no-compile --no-optimize)
- # 2) compile .pyc only (--compile --no-optimize; default)
- # 3) compile .pyc and "opt-1" .pyc (--compile --optimize)
- # 4) compile "opt-1" .pyc only (--no-compile --optimize)
- # 5) compile .pyc and "opt-2" .pyc (--compile --optimize-more)
- # 6) compile "opt-2" .pyc only (--no-compile --optimize-more)
- #
- # The UI for this is two options, 'compile' and 'optimize'.
- # 'compile' is strictly boolean, and only decides whether to
- # generate .pyc files. 'optimize' is three-way (0, 1, or 2), and
- # decides both whether to generate .pyc files and what level of
- # optimization to use.
-
- user_options = [
- ('install-dir=', 'd', "directory to install to"),
- ('build-dir=', 'b', "build directory (where to install from)"),
- ('force', 'f', "force installation (overwrite existing files)"),
- ('compile', 'c', "compile .py to .pyc [default]"),
- ('no-compile', None, "don't compile .py files"),
- (
- 'optimize=',
- 'O',
- "also compile with optimization: -O1 for \"python -O\", "
- "-O2 for \"python -OO\", and -O0 to disable [default: -O0]",
- ),
- ('skip-build', None, "skip the build steps"),
- ]
-
- boolean_options = ['force', 'compile', 'skip-build']
- negative_opt = {'no-compile': 'compile'}
-
- def initialize_options(self):
- # let the 'install' command dictate our installation directory
- self.install_dir = None
- self.build_dir = None
- self.force = 0
- self.compile = None
- self.optimize = None
- self.skip_build = None
-
- def finalize_options(self):
- # Get all the information we need to install pure Python modules
- # from the umbrella 'install' command -- build (source) directory,
- # install (target) directory, and whether to compile .py files.
- self.set_undefined_options(
- 'install',
- ('build_lib', 'build_dir'),
- ('install_lib', 'install_dir'),
- ('force', 'force'),
- ('compile', 'compile'),
- ('optimize', 'optimize'),
- ('skip_build', 'skip_build'),
- )
-
- if self.compile is None:
- self.compile = True
- if self.optimize is None:
- self.optimize = False
-
- if not isinstance(self.optimize, int):
- try:
- self.optimize = int(self.optimize)
- if self.optimize not in (0, 1, 2):
- raise AssertionError
- except (ValueError, AssertionError):
- raise DistutilsOptionError("optimize must be 0, 1, or 2")
-
- def run(self):
- # Make sure we have built everything we need first
- self.build()
-
- # Install everything: simply dump the entire contents of the build
- # directory to the installation directory (that's the beauty of
- # having a build directory!)
- outfiles = self.install()
-
- # (Optionally) compile .py to .pyc
- if outfiles is not None and self.distribution.has_pure_modules():
- self.byte_compile(outfiles)
-
- # -- Top-level worker functions ------------------------------------
- # (called from 'run()')
-
- def build(self):
- if not self.skip_build:
- if self.distribution.has_pure_modules():
- self.run_command('build_py')
- if self.distribution.has_ext_modules():
- self.run_command('build_ext')
-
- def install(self):
- if os.path.isdir(self.build_dir):
- outfiles = self.copy_tree(self.build_dir, self.install_dir)
- else:
- self.warn(
- "'%s' does not exist -- no Python modules to install" % self.build_dir
- )
- return
- return outfiles
-
- def byte_compile(self, files):
- if sys.dont_write_bytecode:
- self.warn('byte-compiling is disabled, skipping.')
- return
-
- from distutils.util import byte_compile
-
- # Get the "--root" directory supplied to the "install" command,
- # and use it as a prefix to strip off the purported filename
- # encoded in bytecode files. This is far from complete, but it
- # should at least generate usable bytecode in RPM distributions.
- install_root = self.get_finalized_command('install').root
-
- if self.compile:
- byte_compile(
- files,
- optimize=0,
- force=self.force,
- prefix=install_root,
- dry_run=self.dry_run,
- )
- if self.optimize > 0:
- byte_compile(
- files,
- optimize=self.optimize,
- force=self.force,
- prefix=install_root,
- verbose=self.verbose,
- dry_run=self.dry_run,
- )
-
- # -- Utility methods -----------------------------------------------
-
- def _mutate_outputs(self, has_any, build_cmd, cmd_option, output_dir):
- if not has_any:
- return []
-
- build_cmd = self.get_finalized_command(build_cmd)
- build_files = build_cmd.get_outputs()
- build_dir = getattr(build_cmd, cmd_option)
-
- prefix_len = len(build_dir) + len(os.sep)
- outputs = []
- for file in build_files:
- outputs.append(os.path.join(output_dir, file[prefix_len:]))
-
- return outputs
-
- def _bytecode_filenames(self, py_filenames):
- bytecode_files = []
- for py_file in py_filenames:
- # Since build_py handles package data installation, the
- # list of outputs can contain more than just .py files.
- # Make sure we only report bytecode for the .py files.
- ext = os.path.splitext(os.path.normcase(py_file))[1]
- if ext != PYTHON_SOURCE_EXTENSION:
- continue
- if self.compile:
- bytecode_files.append(
- importlib.util.cache_from_source(py_file, optimization='')
- )
- if self.optimize > 0:
- bytecode_files.append(
- importlib.util.cache_from_source(
- py_file, optimization=self.optimize
- )
- )
-
- return bytecode_files
-
- # -- External interface --------------------------------------------
- # (called by outsiders)
-
- def get_outputs(self):
- """Return the list of files that would be installed if this command
- were actually run. Not affected by the "dry-run" flag or whether
- modules have actually been built yet.
- """
- pure_outputs = self._mutate_outputs(
- self.distribution.has_pure_modules(),
- 'build_py',
- 'build_lib',
- self.install_dir,
- )
- if self.compile:
- bytecode_outputs = self._bytecode_filenames(pure_outputs)
- else:
- bytecode_outputs = []
-
- ext_outputs = self._mutate_outputs(
- self.distribution.has_ext_modules(),
- 'build_ext',
- 'build_lib',
- self.install_dir,
- )
-
- return pure_outputs + bytecode_outputs + ext_outputs
-
- def get_inputs(self):
- """Get the list of files that are input to this command, ie. the
- files that get installed as they are named in the build tree.
- The files in this list correspond one-to-one to the output
- filenames returned by 'get_outputs()'.
- """
- inputs = []
-
- if self.distribution.has_pure_modules():
- build_py = self.get_finalized_command('build_py')
- inputs.extend(build_py.get_outputs())
-
- if self.distribution.has_ext_modules():
- build_ext = self.get_finalized_command('build_ext')
- inputs.extend(build_ext.get_outputs())
-
- return inputs
diff --git a/spaces/Billet/WizardLM-WizardMath-70B-V1.033/app.py b/spaces/Billet/WizardLM-WizardMath-70B-V1.033/app.py
deleted file mode 100644
index 455f9b294afcee46f8946a090be5cffe7f774b69..0000000000000000000000000000000000000000
--- a/spaces/Billet/WizardLM-WizardMath-70B-V1.033/app.py
+++ /dev/null
@@ -1,3 +0,0 @@
-import gradio as gr
-
-gr.Interface.load("models/WizardLM/WizardMath-70B-V1.0").launch()
\ No newline at end of file
diff --git a/spaces/CVPR/Dual-Key_Backdoor_Attacks/datagen/detectron2/detectron2/export/caffe2_inference.py b/spaces/CVPR/Dual-Key_Backdoor_Attacks/datagen/detectron2/detectron2/export/caffe2_inference.py
deleted file mode 100644
index 92718d04031b4513c2324ad596eae9cdbfa7c75e..0000000000000000000000000000000000000000
--- a/spaces/CVPR/Dual-Key_Backdoor_Attacks/datagen/detectron2/detectron2/export/caffe2_inference.py
+++ /dev/null
@@ -1,136 +0,0 @@
-# Copyright (c) Facebook, Inc. and its affiliates. All Rights Reserved
-
-import collections
-import logging
-import numpy as np
-import torch
-from caffe2.proto import caffe2_pb2
-from caffe2.python import core
-
-from .caffe2_modeling import META_ARCH_CAFFE2_EXPORT_TYPE_MAP, convert_batched_inputs_to_c2_format
-from .shared import ScopedWS, get_pb_arg_vali, get_pb_arg_vals, infer_device_type
-
-logger = logging.getLogger(__name__)
-
-
-class ProtobufModel(torch.nn.Module):
- """
- A class works just like nn.Module in terms of inference, but running
- caffe2 model under the hood. Input/Output are Dict[str, tensor] whose keys
- are in external_input/output.
- """
-
- def __init__(self, predict_net, init_net):
- logger.info("Initializing ProtobufModel ...")
- super().__init__()
- assert isinstance(predict_net, caffe2_pb2.NetDef)
- assert isinstance(init_net, caffe2_pb2.NetDef)
- self.ws_name = "__ws_tmp__"
- self.net = core.Net(predict_net)
-
- with ScopedWS(self.ws_name, is_reset=True, is_cleanup=False) as ws:
- ws.RunNetOnce(init_net)
- for blob in self.net.Proto().external_input:
- if blob not in ws.Blobs():
- ws.CreateBlob(blob)
- ws.CreateNet(self.net)
-
- self._error_msgs = set()
-
- def forward(self, inputs_dict):
- assert all(inp in self.net.Proto().external_input for inp in inputs_dict)
- with ScopedWS(self.ws_name, is_reset=False, is_cleanup=False) as ws:
- for b, tensor in inputs_dict.items():
- ws.FeedBlob(b, tensor)
- try:
- ws.RunNet(self.net.Proto().name)
- except RuntimeError as e:
- if not str(e) in self._error_msgs:
- self._error_msgs.add(str(e))
- logger.warning("Encountered new RuntimeError: \n{}".format(str(e)))
- logger.warning("Catch the error and use partial results.")
-
- outputs_dict = collections.OrderedDict(
- [(b, ws.FetchBlob(b)) for b in self.net.Proto().external_output]
- )
- # Remove outputs of current run, this is necessary in order to
- # prevent fetching the result from previous run if the model fails
- # in the middle.
- for b in self.net.Proto().external_output:
- # Needs to create uninitialized blob to make the net runable.
- # This is "equivalent" to: ws.RemoveBlob(b) then ws.CreateBlob(b),
- # but there'no such API.
- ws.FeedBlob(b, "{}, a C++ native class of type nullptr (uninitialized).".format(b))
-
- return outputs_dict
-
-
-class ProtobufDetectionModel(torch.nn.Module):
- """
- A class works just like a pytorch meta arch in terms of inference, but running
- caffe2 model under the hood.
- """
-
- def __init__(self, predict_net, init_net, *, convert_outputs=None):
- """
- Args:
- predict_net, init_net (core.Net): caffe2 nets
- convert_outptus (callable): a function that converts caffe2
- outputs to the same format of the original pytorch model.
- By default, use the one defined in the caffe2 meta_arch.
- """
- super().__init__()
- self.protobuf_model = ProtobufModel(predict_net, init_net)
- self.size_divisibility = get_pb_arg_vali(predict_net, "size_divisibility", 0)
- self.device = get_pb_arg_vals(predict_net, "device", b"cpu").decode("ascii")
-
- if convert_outputs is None:
- meta_arch = get_pb_arg_vals(predict_net, "meta_architecture", b"GeneralizedRCNN")
- meta_arch = META_ARCH_CAFFE2_EXPORT_TYPE_MAP[meta_arch.decode("ascii")]
- self._convert_outputs = meta_arch.get_outputs_converter(predict_net, init_net)
- else:
- self._convert_outputs = convert_outputs
-
- def _infer_output_devices(self, inputs_dict):
- def _get_device_type(torch_tensor):
- assert torch_tensor.device.type in ["cpu", "cuda"]
- assert torch_tensor.device.index == 0
- return torch_tensor.device.type
-
- predict_net = self.protobuf_model.net.Proto()
- input_device_types = {
- (name, 0): _get_device_type(tensor) for name, tensor in inputs_dict.items()
- }
- device_type_map = infer_device_type(
- predict_net, known_status=input_device_types, device_name_style="pytorch"
- )
- ssa, versions = core.get_ssa(predict_net)
- versioned_outputs = [(name, versions[name]) for name in predict_net.external_output]
- output_devices = [device_type_map[outp] for outp in versioned_outputs]
- return output_devices
-
- def _convert_inputs(self, batched_inputs):
- # currently all models convert inputs in the same way
- data, im_info = convert_batched_inputs_to_c2_format(
- batched_inputs, self.size_divisibility, self.device
- )
- return {"data": data, "im_info": im_info}
-
- def forward(self, batched_inputs):
- c2_inputs = self._convert_inputs(batched_inputs)
- c2_results = self.protobuf_model(c2_inputs)
-
- if any(t.device.type != "cpu" for _, t in c2_inputs.items()):
- output_devices = self._infer_output_devices(c2_inputs)
- else:
- output_devices = ["cpu" for _ in self.protobuf_model.net.Proto().external_output]
-
- def _cast_caffe2_blob_to_torch_tensor(blob, device):
- return torch.Tensor(blob).to(device) if isinstance(blob, np.ndarray) else None
-
- c2_results = {
- name: _cast_caffe2_blob_to_torch_tensor(c2_results[name], device)
- for name, device in zip(self.protobuf_model.net.Proto().external_output, output_devices)
- }
-
- return self._convert_outputs(batched_inputs, c2_inputs, c2_results)
diff --git a/spaces/CVPR/LIVE/thrust/thrust/mr/allocator.h b/spaces/CVPR/LIVE/thrust/thrust/mr/allocator.h
deleted file mode 100644
index 4c6c3288601fcacce058fc2ae7f654d334a33827..0000000000000000000000000000000000000000
--- a/spaces/CVPR/LIVE/thrust/thrust/mr/allocator.h
+++ /dev/null
@@ -1,250 +0,0 @@
-/*
- * Copyright 2018 NVIDIA Corporation
- *
- * Licensed under the Apache License, Version 2.0 (the "License");
- * you may not use this file except in compliance with the License.
- * You may obtain a copy of the License at
- *
- * http://www.apache.org/licenses/LICENSE-2.0
- *
- * Unless required by applicable law or agreed to in writing, software
- * distributed under the License is distributed on an "AS IS" BASIS,
- * WITHOUT WARRANTIES OR CONDITIONS OF ANY KIND, either express or implied.
- * See the License for the specific language governing permissions and
- * limitations under the License.
- */
-
-/*! \file allocator.h
- * \brief Allocator types usable with NPA-based memory resources.
- */
-
-#pragma once
-
-#include
-
-#include
-#include
-
-#include
-#include
-#include
-
-namespace thrust
-{
-namespace mr
-{
-
-/*! \addtogroup memory_management Memory Management
- * \addtogroup memory_management_classes Memory Management Classes
- * \ingroup memory_management
- * \{
- */
-
-/*! An \p mr::allocator is a template that fulfills the C++ requirements for Allocators,
- * allowing to use the NPA-based memory resources where an Allocator is required. Unlike
- * memory resources, but like other allocators, \p mr::allocator is typed and bound to
- * allocate object of a specific type, however it can be freely rebound to other types.
- *
- * \tparam T the type that will be allocated by this allocator.
- * \tparam MR the upstream memory resource to use for memory allocation. Must derive from
- * \p thrust::mr::memory_resource and must be \p final (in C++11 and beyond).
- */
-template
-class allocator : private validator
-{
-public:
- /*! The pointer to void type of this allocator. */
- typedef typename MR::pointer void_pointer;
-
- /*! The value type allocated by this allocator. Equivalent to \p T. */
- typedef T value_type;
- /*! The pointer type allocated by this allocator. Equivaled to the pointer type of \p MR rebound to \p T. */
- typedef typename thrust::detail::pointer_traits::template rebind::other pointer;
- /*! The pointer to const type. Equivalent to a pointer type of \p MR reboud to const T. */
- typedef typename thrust::detail::pointer_traits::template rebind::other const_pointer;
- /*! The reference to the type allocated by this allocator. Supports smart references. */
- typedef typename thrust::detail::pointer_traits::reference reference;
- /*! The const reference to the type allocated by this allocator. Supports smart references. */
- typedef typename thrust::detail::pointer_traits::reference const_reference;
- /*! The size type of this allocator. Always \p std::size_t. */
- typedef std::size_t size_type;
- /*! The difference type between pointers allocated by this allocator. */
- typedef typename thrust::detail::pointer_traits::difference_type difference_type;
-
- /*! Specifies that the allocator shall be propagated on container copy assignment. */
- typedef detail::true_type propagate_on_container_copy_assignment;
- /*! Specifies that the allocator shall be propagated on container move assignment. */
- typedef detail::true_type propagate_on_container_move_assignment;
- /*! Specifies that the allocator shall be propagated on container swap. */
- typedef detail::true_type propagate_on_container_swap;
-
- /*! The \p rebind metafunction provides the type of an \p allocator instantiated with another type.
- *
- * \tparam U the other type to use for instantiation.
- */
- template
- struct rebind
- {
- /*! The typedef \p other gives the type of the rebound \p allocator.
- */
- typedef allocator other;
- };
-
- /*! Calculates the maximum number of elements allocated by this allocator.
- *
- * \returns the maximum value of \p std::size_t, divided by the size of \p T.
- */
- __thrust_exec_check_disable__
- __host__ __device__
- size_type max_size() const
- {
- return std::numeric_limits::max() / sizeof(T);
- }
-
- /*! Constructor.
- *
- * \param resource the resource to be used to allocate raw memory.
- */
- __host__ __device__
- allocator(MR * resource) : mem_res(resource)
- {
- }
-
- /*! Copy constructor. Copies the resource pointer. */
- template
- __host__ __device__
- allocator(const allocator & other) : mem_res(other.resource())
- {
- }
-
- /*! Allocates objects of type \p T.
- *
- * \param n number of elements to allocate
- * \returns a pointer to the newly allocated storage.
- */
- THRUST_NODISCARD
- __host__
- pointer allocate(size_type n)
- {
- return static_cast(mem_res->do_allocate(n * sizeof(T), THRUST_ALIGNOF(T)));
- }
-
- /*! Deallocates objects of type \p T.
- *
- * \param p pointer returned by a previous call to \p allocate
- * \param n number of elements, passed as an argument to the \p allocate call that produced \p p
- */
- __host__
- void deallocate(pointer p, size_type n)
- {
- return mem_res->do_deallocate(p, n * sizeof(T), THRUST_ALIGNOF(T));
- }
-
- /*! Extracts the memory resource used by this allocator.
- *
- * \returns the memory resource used by this allocator.
- */
- __host__ __device__
- MR * resource() const
- {
- return mem_res;
- }
-
-private:
- MR * mem_res;
-};
-
-/*! Compares the allocators for equality by comparing the underlying memory resources. */
-template
-__host__ __device__
-bool operator==(const allocator & lhs, const allocator & rhs) THRUST_NOEXCEPT
-{
- return *lhs.resource() == *rhs.resource();
-}
-
-/*! Compares the allocators for inequality by comparing the underlying memory resources. */
-template
-__host__ __device__
-bool operator!=(const allocator & lhs, const allocator & rhs) THRUST_NOEXCEPT
-{
- return !(lhs == rhs);
-}
-
-#if THRUST_CPP_DIALECT >= 2011
-
-template
-using polymorphic_allocator = allocator >;
-
-#else // C++11
-
-template
-class polymorphic_allocator : public allocator >
-{
- typedef allocator > base;
-
-public:
- /*! Initializes the base class with the parameter \p resource.
- */
- polymorphic_allocator(polymorphic_adaptor_resource * resource) : base(resource)
- {
- }
-};
-
-#endif // C++11
-
-/*! A helper allocator class that uses global instances of a given upstream memory resource. Requires the memory resource
- * to be default constructible.
- *
- * \tparam T the type that will be allocated by this allocator.
- * \tparam Upstream the upstream memory resource to use for memory allocation. Must derive from
- * \p thrust::mr::memory_resource and must be \p final (in C++11 and beyond).
- */
-template
-class stateless_resource_allocator : public thrust::mr::allocator
-{
- typedef thrust::mr::allocator base;
-
-public:
- /*! The \p rebind metafunction provides the type of an \p stateless_resource_allocator instantiated with another type.
- *
- * \tparam U the other type to use for instantiation.
- */
- template
- struct rebind
- {
- /*! The typedef \p other gives the type of the rebound \p stateless_resource_allocator.
- */
- typedef stateless_resource_allocator other;
- };
-
- /*! Default constructor. Uses \p get_global_resource to get the global instance of \p Upstream and initializes the
- * \p allocator base subobject with that resource.
- */
- __host__
- stateless_resource_allocator() : base(get_global_resource())
- {
- }
-
- /*! Copy constructor. Copies the memory resource pointer. */
- __host__ __device__
- stateless_resource_allocator(const stateless_resource_allocator & other)
- : base(other) {}
-
- /*! Conversion constructor from an allocator of a different type. Copies the memory resource pointer. */
- template
- __host__ __device__
- stateless_resource_allocator(const stateless_resource_allocator & other)
- : base(other) {}
-
-#if THRUST_CPP_DIALECT >= 2011
- stateless_resource_allocator & operator=(const stateless_resource_allocator &) = default;
-#endif
-
- /*! Destructor. */
- __host__ __device__
- ~stateless_resource_allocator() {}
-};
-
-} // end mr
-} // end thrust
-
diff --git a/spaces/CVPR/LIVE/thrust/thrust/system/cuda/detail/scatter.h b/spaces/CVPR/LIVE/thrust/thrust/system/cuda/detail/scatter.h
deleted file mode 100644
index 3ba0a4b743b3a4def4e17639cb3dcc263bddb788..0000000000000000000000000000000000000000
--- a/spaces/CVPR/LIVE/thrust/thrust/system/cuda/detail/scatter.h
+++ /dev/null
@@ -1,106 +0,0 @@
-/******************************************************************************
- * Copyright (c) 2016, NVIDIA CORPORATION. All rights reserved.
- *
- * Redistribution and use in source and binary forms, with or without
- * modification, are permitted provided that the following conditions are met:
- * * Redistributions of source code must retain the above copyright
- * notice, this list of conditions and the following disclaimer.
- * * Redistributions in binary form must reproduce the above copyright
- * notice, this list of conditions and the following disclaimer in the
- * documentation and/or other materials provided with the distribution.
- * * Neither the name of the NVIDIA CORPORATION nor the
- * names of its contributors may be used to endorse or promote products
- * derived from this software without specific prior written permission.
- *
- * THIS SOFTWARE IS PROVIDED BY THE COPYRIGHT HOLDERS AND CONTRIBUTORS "AS IS"
- * AND ANY EXPRESS OR IMPLIED WARRANTIES, INCLUDING, BUT NOT LIMITED TO, THE
- * IMPLIED WARRANTIES OF MERCHANTABILITY AND FITNESS FOR A PARTICULAR PURPOSE
- * ARE DISCLAIMED. IN NO EVENT SHALL NVIDIA CORPORATION BE LIABLE FOR ANY
- * DIRECT, INDIRECT, INCIDENTAL, SPECIAL, EXEMPLARY, OR CONSEQUENTIAL DAMAGES
- * (INCLUDING, BUT NOT LIMITED TO, PROCUREMENT OF SUBSTITUTE GOODS OR SERVICES;
- * LOSS OF USE, DATA, OR PROFITS; OR BUSINESS INTERRUPTION) HOWEVER CAUSED AND
- * ON ANY THEORY OF LIABILITY, WHETHER IN CONTRACT, STRICT LIABILITY, OR TORT
- * (INCLUDING NEGLIGENCE OR OTHERWISE) ARISING IN ANY WAY OUT OF THE USE OF THIS
- * SOFTWARE, EVEN IF ADVISED OF THE POSSIBILITY OF SUCH DAMAGE.
- *
- ******************************************************************************/
-#pragma once
-
-
-#if THRUST_DEVICE_COMPILER == THRUST_DEVICE_COMPILER_NVCC
-#include
-#include
-
-namespace thrust
-{
-namespace cuda_cub {
-
-template
-void __host__ __device__
-scatter(execution_policy& policy,
- ItemsIt first,
- ItemsIt last,
- MapIt map,
- ResultIt result)
-{
- cuda_cub::transform(policy,
- first,
- last,
- thrust::make_permutation_iterator(result, map),
- identity());
-}
-
-template
-void __host__ __device__
-scatter_if(execution_policy& policy,
- ItemsIt first,
- ItemsIt last,
- MapIt map,
- StencilIt stencil,
- ResultIt result,
- Predicate predicate)
-{
- cuda_cub::transform_if(policy,
- first,
- last,
- stencil,
- thrust::make_permutation_iterator(result, map),
- identity(),
- predicate);
-}
-
-template
-void __host__ __device__
-scatter_if(execution_policy& policy,
- ItemsIt first,
- ItemsIt last,
- MapIt map,
- StencilIt stencil,
- ResultIt result)
-{
- cuda_cub::scatter_if(policy,
- first,
- last,
- map,
- stencil,
- result,
- identity());
-}
-
-
-} // namespace cuda_cub
-} // end namespace thrust
-#endif
diff --git a/spaces/CVPR/regionclip-demo/detectron2/__init__.py b/spaces/CVPR/regionclip-demo/detectron2/__init__.py
deleted file mode 100644
index a951838f58f8bcf4b2b51a94b2ba31c53e8fe1af..0000000000000000000000000000000000000000
--- a/spaces/CVPR/regionclip-demo/detectron2/__init__.py
+++ /dev/null
@@ -1,10 +0,0 @@
-# Copyright (c) Facebook, Inc. and its affiliates.
-
-from .utils.env import setup_environment
-
-setup_environment()
-
-
-# This line will be programatically read/write by setup.py.
-# Leave them at the bottom of this file and don't touch them.
-__version__ = "0.4"
diff --git a/spaces/Cicooo/vits-uma-genshin-honkai/transforms.py b/spaces/Cicooo/vits-uma-genshin-honkai/transforms.py
deleted file mode 100644
index 4793d67ca5a5630e0ffe0f9fb29445c949e64dae..0000000000000000000000000000000000000000
--- a/spaces/Cicooo/vits-uma-genshin-honkai/transforms.py
+++ /dev/null
@@ -1,193 +0,0 @@
-import torch
-from torch.nn import functional as F
-
-import numpy as np
-
-
-DEFAULT_MIN_BIN_WIDTH = 1e-3
-DEFAULT_MIN_BIN_HEIGHT = 1e-3
-DEFAULT_MIN_DERIVATIVE = 1e-3
-
-
-def piecewise_rational_quadratic_transform(inputs,
- unnormalized_widths,
- unnormalized_heights,
- unnormalized_derivatives,
- inverse=False,
- tails=None,
- tail_bound=1.,
- min_bin_width=DEFAULT_MIN_BIN_WIDTH,
- min_bin_height=DEFAULT_MIN_BIN_HEIGHT,
- min_derivative=DEFAULT_MIN_DERIVATIVE):
-
- if tails is None:
- spline_fn = rational_quadratic_spline
- spline_kwargs = {}
- else:
- spline_fn = unconstrained_rational_quadratic_spline
- spline_kwargs = {
- 'tails': tails,
- 'tail_bound': tail_bound
- }
-
- outputs, logabsdet = spline_fn(
- inputs=inputs,
- unnormalized_widths=unnormalized_widths,
- unnormalized_heights=unnormalized_heights,
- unnormalized_derivatives=unnormalized_derivatives,
- inverse=inverse,
- min_bin_width=min_bin_width,
- min_bin_height=min_bin_height,
- min_derivative=min_derivative,
- **spline_kwargs
- )
- return outputs, logabsdet
-
-
-def searchsorted(bin_locations, inputs, eps=1e-6):
- bin_locations[..., -1] += eps
- return torch.sum(
- inputs[..., None] >= bin_locations,
- dim=-1
- ) - 1
-
-
-def unconstrained_rational_quadratic_spline(inputs,
- unnormalized_widths,
- unnormalized_heights,
- unnormalized_derivatives,
- inverse=False,
- tails='linear',
- tail_bound=1.,
- min_bin_width=DEFAULT_MIN_BIN_WIDTH,
- min_bin_height=DEFAULT_MIN_BIN_HEIGHT,
- min_derivative=DEFAULT_MIN_DERIVATIVE):
- inside_interval_mask = (inputs >= -tail_bound) & (inputs <= tail_bound)
- outside_interval_mask = ~inside_interval_mask
-
- outputs = torch.zeros_like(inputs)
- logabsdet = torch.zeros_like(inputs)
-
- if tails == 'linear':
- unnormalized_derivatives = F.pad(unnormalized_derivatives, pad=(1, 1))
- constant = np.log(np.exp(1 - min_derivative) - 1)
- unnormalized_derivatives[..., 0] = constant
- unnormalized_derivatives[..., -1] = constant
-
- outputs[outside_interval_mask] = inputs[outside_interval_mask]
- logabsdet[outside_interval_mask] = 0
- else:
- raise RuntimeError('{} tails are not implemented.'.format(tails))
-
- outputs[inside_interval_mask], logabsdet[inside_interval_mask] = rational_quadratic_spline(
- inputs=inputs[inside_interval_mask],
- unnormalized_widths=unnormalized_widths[inside_interval_mask, :],
- unnormalized_heights=unnormalized_heights[inside_interval_mask, :],
- unnormalized_derivatives=unnormalized_derivatives[inside_interval_mask, :],
- inverse=inverse,
- left=-tail_bound, right=tail_bound, bottom=-tail_bound, top=tail_bound,
- min_bin_width=min_bin_width,
- min_bin_height=min_bin_height,
- min_derivative=min_derivative
- )
-
- return outputs, logabsdet
-
-def rational_quadratic_spline(inputs,
- unnormalized_widths,
- unnormalized_heights,
- unnormalized_derivatives,
- inverse=False,
- left=0., right=1., bottom=0., top=1.,
- min_bin_width=DEFAULT_MIN_BIN_WIDTH,
- min_bin_height=DEFAULT_MIN_BIN_HEIGHT,
- min_derivative=DEFAULT_MIN_DERIVATIVE):
- if torch.min(inputs) < left or torch.max(inputs) > right:
- raise ValueError('Input to a transform is not within its domain')
-
- num_bins = unnormalized_widths.shape[-1]
-
- if min_bin_width * num_bins > 1.0:
- raise ValueError('Minimal bin width too large for the number of bins')
- if min_bin_height * num_bins > 1.0:
- raise ValueError('Minimal bin height too large for the number of bins')
-
- widths = F.softmax(unnormalized_widths, dim=-1)
- widths = min_bin_width + (1 - min_bin_width * num_bins) * widths
- cumwidths = torch.cumsum(widths, dim=-1)
- cumwidths = F.pad(cumwidths, pad=(1, 0), mode='constant', value=0.0)
- cumwidths = (right - left) * cumwidths + left
- cumwidths[..., 0] = left
- cumwidths[..., -1] = right
- widths = cumwidths[..., 1:] - cumwidths[..., :-1]
-
- derivatives = min_derivative + F.softplus(unnormalized_derivatives)
-
- heights = F.softmax(unnormalized_heights, dim=-1)
- heights = min_bin_height + (1 - min_bin_height * num_bins) * heights
- cumheights = torch.cumsum(heights, dim=-1)
- cumheights = F.pad(cumheights, pad=(1, 0), mode='constant', value=0.0)
- cumheights = (top - bottom) * cumheights + bottom
- cumheights[..., 0] = bottom
- cumheights[..., -1] = top
- heights = cumheights[..., 1:] - cumheights[..., :-1]
-
- if inverse:
- bin_idx = searchsorted(cumheights, inputs)[..., None]
- else:
- bin_idx = searchsorted(cumwidths, inputs)[..., None]
-
- input_cumwidths = cumwidths.gather(-1, bin_idx)[..., 0]
- input_bin_widths = widths.gather(-1, bin_idx)[..., 0]
-
- input_cumheights = cumheights.gather(-1, bin_idx)[..., 0]
- delta = heights / widths
- input_delta = delta.gather(-1, bin_idx)[..., 0]
-
- input_derivatives = derivatives.gather(-1, bin_idx)[..., 0]
- input_derivatives_plus_one = derivatives[..., 1:].gather(-1, bin_idx)[..., 0]
-
- input_heights = heights.gather(-1, bin_idx)[..., 0]
-
- if inverse:
- a = (((inputs - input_cumheights) * (input_derivatives
- + input_derivatives_plus_one
- - 2 * input_delta)
- + input_heights * (input_delta - input_derivatives)))
- b = (input_heights * input_derivatives
- - (inputs - input_cumheights) * (input_derivatives
- + input_derivatives_plus_one
- - 2 * input_delta))
- c = - input_delta * (inputs - input_cumheights)
-
- discriminant = b.pow(2) - 4 * a * c
- assert (discriminant >= 0).all()
-
- root = (2 * c) / (-b - torch.sqrt(discriminant))
- outputs = root * input_bin_widths + input_cumwidths
-
- theta_one_minus_theta = root * (1 - root)
- denominator = input_delta + ((input_derivatives + input_derivatives_plus_one - 2 * input_delta)
- * theta_one_minus_theta)
- derivative_numerator = input_delta.pow(2) * (input_derivatives_plus_one * root.pow(2)
- + 2 * input_delta * theta_one_minus_theta
- + input_derivatives * (1 - root).pow(2))
- logabsdet = torch.log(derivative_numerator) - 2 * torch.log(denominator)
-
- return outputs, -logabsdet
- else:
- theta = (inputs - input_cumwidths) / input_bin_widths
- theta_one_minus_theta = theta * (1 - theta)
-
- numerator = input_heights * (input_delta * theta.pow(2)
- + input_derivatives * theta_one_minus_theta)
- denominator = input_delta + ((input_derivatives + input_derivatives_plus_one - 2 * input_delta)
- * theta_one_minus_theta)
- outputs = input_cumheights + numerator / denominator
-
- derivative_numerator = input_delta.pow(2) * (input_derivatives_plus_one * theta.pow(2)
- + 2 * input_delta * theta_one_minus_theta
- + input_derivatives * (1 - theta).pow(2))
- logabsdet = torch.log(derivative_numerator) - 2 * torch.log(denominator)
-
- return outputs, logabsdet
diff --git a/spaces/DQChoi/gpt-demo/venv/lib/python3.11/site-packages/fontTools/ttLib/ttGlyphSet.py b/spaces/DQChoi/gpt-demo/venv/lib/python3.11/site-packages/fontTools/ttLib/ttGlyphSet.py
deleted file mode 100644
index fa7fbd4f23558f6705ee3e819ded518bb7549e36..0000000000000000000000000000000000000000
--- a/spaces/DQChoi/gpt-demo/venv/lib/python3.11/site-packages/fontTools/ttLib/ttGlyphSet.py
+++ /dev/null
@@ -1,322 +0,0 @@
-"""GlyphSets returned by a TTFont."""
-
-from abc import ABC, abstractmethod
-from collections.abc import Mapping
-from contextlib import contextmanager
-from copy import copy
-from types import SimpleNamespace
-from fontTools.misc.fixedTools import otRound
-from fontTools.misc.loggingTools import deprecateFunction
-from fontTools.misc.transform import Transform
-from fontTools.pens.transformPen import TransformPen, TransformPointPen
-
-
-class _TTGlyphSet(Mapping):
-
- """Generic dict-like GlyphSet class that pulls metrics from hmtx and
- glyph shape from TrueType or CFF.
- """
-
- def __init__(self, font, location, glyphsMapping):
- self.font = font
- self.defaultLocationNormalized = (
- {axis.axisTag: 0 for axis in self.font["fvar"].axes}
- if "fvar" in self.font
- else {}
- )
- self.location = location if location is not None else {}
- self.rawLocation = {} # VarComponent-only location
- self.originalLocation = location if location is not None else {}
- self.depth = 0
- self.locationStack = []
- self.rawLocationStack = []
- self.glyphsMapping = glyphsMapping
- self.hMetrics = font["hmtx"].metrics
- self.vMetrics = getattr(font.get("vmtx"), "metrics", None)
- self.hvarTable = None
- if location:
- from fontTools.varLib.varStore import VarStoreInstancer
-
- self.hvarTable = getattr(font.get("HVAR"), "table", None)
- if self.hvarTable is not None:
- self.hvarInstancer = VarStoreInstancer(
- self.hvarTable.VarStore, font["fvar"].axes, location
- )
- # TODO VVAR, VORG
-
- @contextmanager
- def pushLocation(self, location, reset: bool):
- self.locationStack.append(self.location)
- self.rawLocationStack.append(self.rawLocation)
- if reset:
- self.location = self.originalLocation.copy()
- self.rawLocation = self.defaultLocationNormalized.copy()
- else:
- self.location = self.location.copy()
- self.rawLocation = {}
- self.location.update(location)
- self.rawLocation.update(location)
-
- try:
- yield None
- finally:
- self.location = self.locationStack.pop()
- self.rawLocation = self.rawLocationStack.pop()
-
- @contextmanager
- def pushDepth(self):
- try:
- depth = self.depth
- self.depth += 1
- yield depth
- finally:
- self.depth -= 1
-
- def __contains__(self, glyphName):
- return glyphName in self.glyphsMapping
-
- def __iter__(self):
- return iter(self.glyphsMapping.keys())
-
- def __len__(self):
- return len(self.glyphsMapping)
-
- @deprecateFunction(
- "use 'glyphName in glyphSet' instead", category=DeprecationWarning
- )
- def has_key(self, glyphName):
- return glyphName in self.glyphsMapping
-
-
-class _TTGlyphSetGlyf(_TTGlyphSet):
- def __init__(self, font, location):
- self.glyfTable = font["glyf"]
- super().__init__(font, location, self.glyfTable)
- self.gvarTable = font.get("gvar")
-
- def __getitem__(self, glyphName):
- return _TTGlyphGlyf(self, glyphName)
-
-
-class _TTGlyphSetCFF(_TTGlyphSet):
- def __init__(self, font, location):
- tableTag = "CFF2" if "CFF2" in font else "CFF "
- self.charStrings = list(font[tableTag].cff.values())[0].CharStrings
- super().__init__(font, location, self.charStrings)
- self.blender = None
- if location:
- from fontTools.varLib.varStore import VarStoreInstancer
-
- varStore = getattr(self.charStrings, "varStore", None)
- if varStore is not None:
- instancer = VarStoreInstancer(
- varStore.otVarStore, font["fvar"].axes, location
- )
- self.blender = instancer.interpolateFromDeltas
-
- def __getitem__(self, glyphName):
- return _TTGlyphCFF(self, glyphName)
-
-
-class _TTGlyph(ABC):
-
- """Glyph object that supports the Pen protocol, meaning that it has
- .draw() and .drawPoints() methods that take a pen object as their only
- argument. Additionally there are 'width' and 'lsb' attributes, read from
- the 'hmtx' table.
-
- If the font contains a 'vmtx' table, there will also be 'height' and 'tsb'
- attributes.
- """
-
- def __init__(self, glyphSet, glyphName):
- self.glyphSet = glyphSet
- self.name = glyphName
- self.width, self.lsb = glyphSet.hMetrics[glyphName]
- if glyphSet.vMetrics is not None:
- self.height, self.tsb = glyphSet.vMetrics[glyphName]
- else:
- self.height, self.tsb = None, None
- if glyphSet.location and glyphSet.hvarTable is not None:
- varidx = (
- glyphSet.font.getGlyphID(glyphName)
- if glyphSet.hvarTable.AdvWidthMap is None
- else glyphSet.hvarTable.AdvWidthMap.mapping[glyphName]
- )
- self.width += glyphSet.hvarInstancer[varidx]
- # TODO: VVAR/VORG
-
- @abstractmethod
- def draw(self, pen):
- """Draw the glyph onto ``pen``. See fontTools.pens.basePen for details
- how that works.
- """
- raise NotImplementedError
-
- def drawPoints(self, pen):
- """Draw the glyph onto ``pen``. See fontTools.pens.pointPen for details
- how that works.
- """
- from fontTools.pens.pointPen import SegmentToPointPen
-
- self.draw(SegmentToPointPen(pen))
-
-
-class _TTGlyphGlyf(_TTGlyph):
- def draw(self, pen):
- """Draw the glyph onto ``pen``. See fontTools.pens.basePen for details
- how that works.
- """
- glyph, offset = self._getGlyphAndOffset()
-
- with self.glyphSet.pushDepth() as depth:
-
- if depth:
- offset = 0 # Offset should only apply at top-level
-
- if glyph.isVarComposite():
- self._drawVarComposite(glyph, pen, False)
- return
-
- glyph.draw(pen, self.glyphSet.glyfTable, offset)
-
- def drawPoints(self, pen):
- """Draw the glyph onto ``pen``. See fontTools.pens.pointPen for details
- how that works.
- """
- glyph, offset = self._getGlyphAndOffset()
-
- with self.glyphSet.pushDepth() as depth:
-
- if depth:
- offset = 0 # Offset should only apply at top-level
-
- if glyph.isVarComposite():
- self._drawVarComposite(glyph, pen, True)
- return
-
- glyph.drawPoints(pen, self.glyphSet.glyfTable, offset)
-
- def _drawVarComposite(self, glyph, pen, isPointPen):
-
- from fontTools.ttLib.tables._g_l_y_f import (
- VarComponentFlags,
- VAR_COMPONENT_TRANSFORM_MAPPING,
- )
-
- for comp in glyph.components:
-
- with self.glyphSet.pushLocation(
- comp.location, comp.flags & VarComponentFlags.RESET_UNSPECIFIED_AXES
- ):
- try:
- pen.addVarComponent(
- comp.glyphName, comp.transform, self.glyphSet.rawLocation
- )
- except AttributeError:
- t = comp.transform.toTransform()
- if isPointPen:
- tPen = TransformPointPen(pen, t)
- self.glyphSet[comp.glyphName].drawPoints(tPen)
- else:
- tPen = TransformPen(pen, t)
- self.glyphSet[comp.glyphName].draw(tPen)
-
- def _getGlyphAndOffset(self):
- if self.glyphSet.location and self.glyphSet.gvarTable is not None:
- glyph = self._getGlyphInstance()
- else:
- glyph = self.glyphSet.glyfTable[self.name]
-
- offset = self.lsb - glyph.xMin if hasattr(glyph, "xMin") else 0
- return glyph, offset
-
- def _getGlyphInstance(self):
- from fontTools.varLib.iup import iup_delta
- from fontTools.ttLib.tables._g_l_y_f import GlyphCoordinates
- from fontTools.varLib.models import supportScalar
-
- glyphSet = self.glyphSet
- glyfTable = glyphSet.glyfTable
- variations = glyphSet.gvarTable.variations[self.name]
- hMetrics = glyphSet.hMetrics
- vMetrics = glyphSet.vMetrics
- coordinates, _ = glyfTable._getCoordinatesAndControls(
- self.name, hMetrics, vMetrics
- )
- origCoords, endPts = None, None
- for var in variations:
- scalar = supportScalar(glyphSet.location, var.axes)
- if not scalar:
- continue
- delta = var.coordinates
- if None in delta:
- if origCoords is None:
- origCoords, control = glyfTable._getCoordinatesAndControls(
- self.name, hMetrics, vMetrics
- )
- endPts = (
- control[1] if control[0] >= 1 else list(range(len(control[1])))
- )
- delta = iup_delta(delta, origCoords, endPts)
- coordinates += GlyphCoordinates(delta) * scalar
-
- glyph = copy(glyfTable[self.name]) # Shallow copy
- width, lsb, height, tsb = _setCoordinates(glyph, coordinates, glyfTable)
- self.lsb = lsb
- self.tsb = tsb
- if glyphSet.hvarTable is None:
- # no HVAR: let's set metrics from the phantom points
- self.width = width
- self.height = height
- return glyph
-
-
-class _TTGlyphCFF(_TTGlyph):
- def draw(self, pen):
- """Draw the glyph onto ``pen``. See fontTools.pens.basePen for details
- how that works.
- """
- self.glyphSet.charStrings[self.name].draw(pen, self.glyphSet.blender)
-
-
-def _setCoordinates(glyph, coord, glyfTable):
- # Handle phantom points for (left, right, top, bottom) positions.
- assert len(coord) >= 4
- leftSideX = coord[-4][0]
- rightSideX = coord[-3][0]
- topSideY = coord[-2][1]
- bottomSideY = coord[-1][1]
-
- for _ in range(4):
- del coord[-1]
-
- if glyph.isComposite():
- assert len(coord) == len(glyph.components)
- glyph.components = [copy(comp) for comp in glyph.components] # Shallow copy
- for p, comp in zip(coord, glyph.components):
- if hasattr(comp, "x"):
- comp.x, comp.y = p
- elif glyph.isVarComposite():
- glyph.components = [copy(comp) for comp in glyph.components] # Shallow copy
- for comp in glyph.components:
- coord = comp.setCoordinates(coord)
- assert not coord
- elif glyph.numberOfContours == 0:
- assert len(coord) == 0
- else:
- assert len(coord) == len(glyph.coordinates)
- glyph.coordinates = coord
-
- glyph.recalcBounds(glyfTable)
-
- horizontalAdvanceWidth = otRound(rightSideX - leftSideX)
- verticalAdvanceWidth = otRound(topSideY - bottomSideY)
- leftSideBearing = otRound(glyph.xMin - leftSideX)
- topSideBearing = otRound(topSideY - glyph.yMax)
- return (
- horizontalAdvanceWidth,
- leftSideBearing,
- verticalAdvanceWidth,
- topSideBearing,
- )
diff --git a/spaces/DQChoi/gpt-demo/venv/lib/python3.11/site-packages/h11/_headers.py b/spaces/DQChoi/gpt-demo/venv/lib/python3.11/site-packages/h11/_headers.py
deleted file mode 100644
index b97d020b634a9f47f5ae6aa3b30e2bd13a6c48c4..0000000000000000000000000000000000000000
--- a/spaces/DQChoi/gpt-demo/venv/lib/python3.11/site-packages/h11/_headers.py
+++ /dev/null
@@ -1,278 +0,0 @@
-import re
-from typing import AnyStr, cast, List, overload, Sequence, Tuple, TYPE_CHECKING, Union
-
-from ._abnf import field_name, field_value
-from ._util import bytesify, LocalProtocolError, validate
-
-if TYPE_CHECKING:
- from ._events import Request
-
-try:
- from typing import Literal
-except ImportError:
- from typing_extensions import Literal # type: ignore
-
-
-# Facts
-# -----
-#
-# Headers are:
-# keys: case-insensitive ascii
-# values: mixture of ascii and raw bytes
-#
-# "Historically, HTTP has allowed field content with text in the ISO-8859-1
-# charset [ISO-8859-1], supporting other charsets only through use of
-# [RFC2047] encoding. In practice, most HTTP header field values use only a
-# subset of the US-ASCII charset [USASCII]. Newly defined header fields SHOULD
-# limit their field values to US-ASCII octets. A recipient SHOULD treat other
-# octets in field content (obs-text) as opaque data."
-# And it deprecates all non-ascii values
-#
-# Leading/trailing whitespace in header names is forbidden
-#
-# Values get leading/trailing whitespace stripped
-#
-# Content-Disposition actually needs to contain unicode semantically; to
-# accomplish this it has a terrifically weird way of encoding the filename
-# itself as ascii (and even this still has lots of cross-browser
-# incompatibilities)
-#
-# Order is important:
-# "a proxy MUST NOT change the order of these field values when forwarding a
-# message"
-# (and there are several headers where the order indicates a preference)
-#
-# Multiple occurences of the same header:
-# "A sender MUST NOT generate multiple header fields with the same field name
-# in a message unless either the entire field value for that header field is
-# defined as a comma-separated list [or the header is Set-Cookie which gets a
-# special exception]" - RFC 7230. (cookies are in RFC 6265)
-#
-# So every header aside from Set-Cookie can be merged by b", ".join if it
-# occurs repeatedly. But, of course, they can't necessarily be split by
-# .split(b","), because quoting.
-#
-# Given all this mess (case insensitive, duplicates allowed, order is
-# important, ...), there doesn't appear to be any standard way to handle
-# headers in Python -- they're almost like dicts, but... actually just
-# aren't. For now we punt and just use a super simple representation: headers
-# are a list of pairs
-#
-# [(name1, value1), (name2, value2), ...]
-#
-# where all entries are bytestrings, names are lowercase and have no
-# leading/trailing whitespace, and values are bytestrings with no
-# leading/trailing whitespace. Searching and updating are done via naive O(n)
-# methods.
-#
-# Maybe a dict-of-lists would be better?
-
-_content_length_re = re.compile(rb"[0-9]+")
-_field_name_re = re.compile(field_name.encode("ascii"))
-_field_value_re = re.compile(field_value.encode("ascii"))
-
-
-class Headers(Sequence[Tuple[bytes, bytes]]):
- """
- A list-like interface that allows iterating over headers as byte-pairs
- of (lowercased-name, value).
-
- Internally we actually store the representation as three-tuples,
- including both the raw original casing, in order to preserve casing
- over-the-wire, and the lowercased name, for case-insensitive comparisions.
-
- r = Request(
- method="GET",
- target="/",
- headers=[("Host", "example.org"), ("Connection", "keep-alive")],
- http_version="1.1",
- )
- assert r.headers == [
- (b"host", b"example.org"),
- (b"connection", b"keep-alive")
- ]
- assert r.headers.raw_items() == [
- (b"Host", b"example.org"),
- (b"Connection", b"keep-alive")
- ]
- """
-
- __slots__ = "_full_items"
-
- def __init__(self, full_items: List[Tuple[bytes, bytes, bytes]]) -> None:
- self._full_items = full_items
-
- def __bool__(self) -> bool:
- return bool(self._full_items)
-
- def __eq__(self, other: object) -> bool:
- return list(self) == list(other) # type: ignore
-
- def __len__(self) -> int:
- return len(self._full_items)
-
- def __repr__(self) -> str:
- return "" % repr(list(self))
-
- def __getitem__(self, idx: int) -> Tuple[bytes, bytes]: # type: ignore[override]
- _, name, value = self._full_items[idx]
- return (name, value)
-
- def raw_items(self) -> List[Tuple[bytes, bytes]]:
- return [(raw_name, value) for raw_name, _, value in self._full_items]
-
-
-HeaderTypes = Union[
- List[Tuple[bytes, bytes]],
- List[Tuple[bytes, str]],
- List[Tuple[str, bytes]],
- List[Tuple[str, str]],
-]
-
-
-@overload
-def normalize_and_validate(headers: Headers, _parsed: Literal[True]) -> Headers:
- ...
-
-
-@overload
-def normalize_and_validate(headers: HeaderTypes, _parsed: Literal[False]) -> Headers:
- ...
-
-
-@overload
-def normalize_and_validate(
- headers: Union[Headers, HeaderTypes], _parsed: bool = False
-) -> Headers:
- ...
-
-
-def normalize_and_validate(
- headers: Union[Headers, HeaderTypes], _parsed: bool = False
-) -> Headers:
- new_headers = []
- seen_content_length = None
- saw_transfer_encoding = False
- for name, value in headers:
- # For headers coming out of the parser, we can safely skip some steps,
- # because it always returns bytes and has already run these regexes
- # over the data:
- if not _parsed:
- name = bytesify(name)
- value = bytesify(value)
- validate(_field_name_re, name, "Illegal header name {!r}", name)
- validate(_field_value_re, value, "Illegal header value {!r}", value)
- assert isinstance(name, bytes)
- assert isinstance(value, bytes)
-
- raw_name = name
- name = name.lower()
- if name == b"content-length":
- lengths = {length.strip() for length in value.split(b",")}
- if len(lengths) != 1:
- raise LocalProtocolError("conflicting Content-Length headers")
- value = lengths.pop()
- validate(_content_length_re, value, "bad Content-Length")
- if seen_content_length is None:
- seen_content_length = value
- new_headers.append((raw_name, name, value))
- elif seen_content_length != value:
- raise LocalProtocolError("conflicting Content-Length headers")
- elif name == b"transfer-encoding":
- # "A server that receives a request message with a transfer coding
- # it does not understand SHOULD respond with 501 (Not
- # Implemented)."
- # https://tools.ietf.org/html/rfc7230#section-3.3.1
- if saw_transfer_encoding:
- raise LocalProtocolError(
- "multiple Transfer-Encoding headers", error_status_hint=501
- )
- # "All transfer-coding names are case-insensitive"
- # -- https://tools.ietf.org/html/rfc7230#section-4
- value = value.lower()
- if value != b"chunked":
- raise LocalProtocolError(
- "Only Transfer-Encoding: chunked is supported",
- error_status_hint=501,
- )
- saw_transfer_encoding = True
- new_headers.append((raw_name, name, value))
- else:
- new_headers.append((raw_name, name, value))
- return Headers(new_headers)
-
-
-def get_comma_header(headers: Headers, name: bytes) -> List[bytes]:
- # Should only be used for headers whose value is a list of
- # comma-separated, case-insensitive values.
- #
- # The header name `name` is expected to be lower-case bytes.
- #
- # Connection: meets these criteria (including cast insensitivity).
- #
- # Content-Length: technically is just a single value (1*DIGIT), but the
- # standard makes reference to implementations that do multiple values, and
- # using this doesn't hurt. Ditto, case insensitivity doesn't things either
- # way.
- #
- # Transfer-Encoding: is more complex (allows for quoted strings), so
- # splitting on , is actually wrong. For example, this is legal:
- #
- # Transfer-Encoding: foo; options="1,2", chunked
- #
- # and should be parsed as
- #
- # foo; options="1,2"
- # chunked
- #
- # but this naive function will parse it as
- #
- # foo; options="1
- # 2"
- # chunked
- #
- # However, this is okay because the only thing we are going to do with
- # any Transfer-Encoding is reject ones that aren't just "chunked", so
- # both of these will be treated the same anyway.
- #
- # Expect: the only legal value is the literal string
- # "100-continue". Splitting on commas is harmless. Case insensitive.
- #
- out: List[bytes] = []
- for _, found_name, found_raw_value in headers._full_items:
- if found_name == name:
- found_raw_value = found_raw_value.lower()
- for found_split_value in found_raw_value.split(b","):
- found_split_value = found_split_value.strip()
- if found_split_value:
- out.append(found_split_value)
- return out
-
-
-def set_comma_header(headers: Headers, name: bytes, new_values: List[bytes]) -> Headers:
- # The header name `name` is expected to be lower-case bytes.
- #
- # Note that when we store the header we use title casing for the header
- # names, in order to match the conventional HTTP header style.
- #
- # Simply calling `.title()` is a blunt approach, but it's correct
- # here given the cases where we're using `set_comma_header`...
- #
- # Connection, Content-Length, Transfer-Encoding.
- new_headers: List[Tuple[bytes, bytes]] = []
- for found_raw_name, found_name, found_raw_value in headers._full_items:
- if found_name != name:
- new_headers.append((found_raw_name, found_raw_value))
- for new_value in new_values:
- new_headers.append((name.title(), new_value))
- return normalize_and_validate(new_headers)
-
-
-def has_expect_100_continue(request: "Request") -> bool:
- # https://tools.ietf.org/html/rfc7231#section-5.1.1
- # "A server that receives a 100-continue expectation in an HTTP/1.0 request
- # MUST ignore that expectation."
- if request.http_version < b"1.1":
- return False
- expect = get_comma_header(request.headers, b"expect")
- return b"100-continue" in expect
diff --git a/spaces/DQChoi/gpt-demo/venv/lib/python3.11/site-packages/huggingface_hub/utils/_chunk_utils.py b/spaces/DQChoi/gpt-demo/venv/lib/python3.11/site-packages/huggingface_hub/utils/_chunk_utils.py
deleted file mode 100644
index 5ff0b8125ece381b1270754669ae8e708c370f61..0000000000000000000000000000000000000000
--- a/spaces/DQChoi/gpt-demo/venv/lib/python3.11/site-packages/huggingface_hub/utils/_chunk_utils.py
+++ /dev/null
@@ -1,64 +0,0 @@
-# coding=utf-8
-# Copyright 2022-present, the HuggingFace Inc. team.
-#
-# Licensed under the Apache License, Version 2.0 (the "License");
-# you may not use this file except in compliance with the License.
-# You may obtain a copy of the License at
-#
-# http://www.apache.org/licenses/LICENSE-2.0
-#
-# Unless required by applicable law or agreed to in writing, software
-# distributed under the License is distributed on an "AS IS" BASIS,
-# WITHOUT WARRANTIES OR CONDITIONS OF ANY KIND, either express or implied.
-# See the License for the specific language governing permissions and
-# limitations under the License.
-"""Contains a utility to iterate by chunks over an iterator."""
-import itertools
-from typing import Iterable, TypeVar
-
-
-T = TypeVar("T")
-
-
-def chunk_iterable(iterable: Iterable[T], chunk_size: int) -> Iterable[Iterable[T]]:
- """Iterates over an iterator chunk by chunk.
-
- Taken from https://stackoverflow.com/a/8998040.
- See also https://github.com/huggingface/huggingface_hub/pull/920#discussion_r938793088.
-
- Args:
- iterable (`Iterable`):
- The iterable on which we want to iterate.
- chunk_size (`int`):
- Size of the chunks. Must be a strictly positive integer (e.g. >0).
-
- Example:
-
- ```python
- >>> from huggingface_hub.utils import chunk_iterable
-
- >>> for items in chunk_iterable(range(17), chunk_size=8):
- ... print(items)
- # [0, 1, 2, 3, 4, 5, 6, 7]
- # [8, 9, 10, 11, 12, 13, 14, 15]
- # [16] # smaller last chunk
- ```
-
- Raises:
- [`ValueError`](https://docs.python.org/3/library/exceptions.html#ValueError)
- If `chunk_size` <= 0.
-
-
- The last chunk can be smaller than `chunk_size`.
-
- """
- if not isinstance(chunk_size, int) or chunk_size <= 0:
- raise ValueError("`chunk_size` must be a strictly positive integer (>0).")
-
- iterator = iter(iterable)
- while True:
- try:
- next_item = next(iterator)
- except StopIteration:
- return
- yield itertools.chain((next_item,), itertools.islice(iterator, chunk_size - 1))
diff --git a/spaces/DaFujaTyping/hf-Chat-ui/src/routes/r/[id]/+page.server.ts b/spaces/DaFujaTyping/hf-Chat-ui/src/routes/r/[id]/+page.server.ts
deleted file mode 100644
index f065f39a0c58dc03623943077e970c3250506a22..0000000000000000000000000000000000000000
--- a/spaces/DaFujaTyping/hf-Chat-ui/src/routes/r/[id]/+page.server.ts
+++ /dev/null
@@ -1,19 +0,0 @@
-import type { PageServerLoad } from "./$types";
-import { collections } from "$lib/server/database";
-import { error } from "@sveltejs/kit";
-
-export const load: PageServerLoad = async ({ params }) => {
- const conversation = await collections.sharedConversations.findOne({
- _id: params.id,
- });
-
- if (!conversation) {
- throw error(404, "Conversation not found");
- }
-
- return {
- messages: conversation.messages,
- title: conversation.title,
- model: conversation.model,
- };
-};
diff --git a/spaces/Datasculptor/DescriptionGPT/detic/modeling/roi_heads/detic_roi_heads.py b/spaces/Datasculptor/DescriptionGPT/detic/modeling/roi_heads/detic_roi_heads.py
deleted file mode 100644
index c87559359e0516443a43ed327110ec55fa4fa307..0000000000000000000000000000000000000000
--- a/spaces/Datasculptor/DescriptionGPT/detic/modeling/roi_heads/detic_roi_heads.py
+++ /dev/null
@@ -1,271 +0,0 @@
-# Copyright (c) Facebook, Inc. and its affiliates.
-import copy
-import numpy as np
-import json
-import math
-import torch
-from torch import nn
-from torch.autograd.function import Function
-from typing import Dict, List, Optional, Tuple, Union
-from torch.nn import functional as F
-
-from detectron2.config import configurable
-from detectron2.layers import ShapeSpec
-from detectron2.layers import batched_nms
-from detectron2.structures import Boxes, Instances, pairwise_iou
-from detectron2.utils.events import get_event_storage
-
-from detectron2.modeling.box_regression import Box2BoxTransform
-from detectron2.modeling.roi_heads.fast_rcnn import fast_rcnn_inference
-from detectron2.modeling.roi_heads.roi_heads import ROI_HEADS_REGISTRY, StandardROIHeads
-from detectron2.modeling.roi_heads.cascade_rcnn import CascadeROIHeads, _ScaleGradient
-from detectron2.modeling.roi_heads.box_head import build_box_head
-from .detic_fast_rcnn import DeticFastRCNNOutputLayers
-from ..debug import debug_second_stage
-
-from torch.cuda.amp import autocast
-
-@ROI_HEADS_REGISTRY.register()
-class DeticCascadeROIHeads(CascadeROIHeads):
- @configurable
- def __init__(
- self,
- *,
- mult_proposal_score: bool = False,
- with_image_labels: bool = False,
- add_image_box: bool = False,
- image_box_size: float = 1.0,
- ws_num_props: int = 512,
- add_feature_to_prop: bool = False,
- mask_weight: float = 1.0,
- one_class_per_proposal: bool = False,
- **kwargs,
- ):
- super().__init__(**kwargs)
- self.mult_proposal_score = mult_proposal_score
- self.with_image_labels = with_image_labels
- self.add_image_box = add_image_box
- self.image_box_size = image_box_size
- self.ws_num_props = ws_num_props
- self.add_feature_to_prop = add_feature_to_prop
- self.mask_weight = mask_weight
- self.one_class_per_proposal = one_class_per_proposal
-
- @classmethod
- def from_config(cls, cfg, input_shape):
- ret = super().from_config(cfg, input_shape)
- ret.update({
- 'mult_proposal_score': cfg.MODEL.ROI_BOX_HEAD.MULT_PROPOSAL_SCORE,
- 'with_image_labels': cfg.WITH_IMAGE_LABELS,
- 'add_image_box': cfg.MODEL.ROI_BOX_HEAD.ADD_IMAGE_BOX,
- 'image_box_size': cfg.MODEL.ROI_BOX_HEAD.IMAGE_BOX_SIZE,
- 'ws_num_props': cfg.MODEL.ROI_BOX_HEAD.WS_NUM_PROPS,
- 'add_feature_to_prop': cfg.MODEL.ROI_BOX_HEAD.ADD_FEATURE_TO_PROP,
- 'mask_weight': cfg.MODEL.ROI_HEADS.MASK_WEIGHT,
- 'one_class_per_proposal': cfg.MODEL.ROI_HEADS.ONE_CLASS_PER_PROPOSAL,
- })
- return ret
-
-
- @classmethod
- def _init_box_head(self, cfg, input_shape):
- ret = super()._init_box_head(cfg, input_shape)
- del ret['box_predictors']
- cascade_bbox_reg_weights = cfg.MODEL.ROI_BOX_CASCADE_HEAD.BBOX_REG_WEIGHTS
- box_predictors = []
- for box_head, bbox_reg_weights in zip(ret['box_heads'], \
- cascade_bbox_reg_weights):
- box_predictors.append(
- DeticFastRCNNOutputLayers(
- cfg, box_head.output_shape,
- box2box_transform=Box2BoxTransform(weights=bbox_reg_weights)
- ))
- ret['box_predictors'] = box_predictors
- return ret
-
-
- def _forward_box(self, features, proposals, targets=None,
- ann_type='box', classifier_info=(None,None,None)):
- """
- Add mult proposal scores at testing
- Add ann_type
- """
- if (not self.training) and self.mult_proposal_score:
- if len(proposals) > 0 and proposals[0].has('scores'):
- proposal_scores = [p.get('scores') for p in proposals]
- else:
- proposal_scores = [p.get('objectness_logits') for p in proposals]
-
- features = [features[f] for f in self.box_in_features]
- head_outputs = [] # (predictor, predictions, proposals)
- prev_pred_boxes = None
- image_sizes = [x.image_size for x in proposals]
-
- for k in range(self.num_cascade_stages):
- if k > 0:
- proposals = self._create_proposals_from_boxes(
- prev_pred_boxes, image_sizes,
- logits=[p.objectness_logits for p in proposals])
- if self.training and ann_type in ['box']:
- proposals = self._match_and_label_boxes(
- proposals, k, targets)
- predictions = self._run_stage(features, proposals, k,
- classifier_info=classifier_info)
- prev_pred_boxes = self.box_predictor[k].predict_boxes(
- (predictions[0], predictions[1]), proposals)
- head_outputs.append((self.box_predictor[k], predictions, proposals))
-
- if self.training:
- losses = {}
- storage = get_event_storage()
- for stage, (predictor, predictions, proposals) in enumerate(head_outputs):
- with storage.name_scope("stage{}".format(stage)):
- if ann_type != 'box':
- stage_losses = {}
- if ann_type in ['image', 'caption', 'captiontag']:
- image_labels = [x._pos_category_ids for x in targets]
- weak_losses = predictor.image_label_losses(
- predictions, proposals, image_labels,
- classifier_info=classifier_info,
- ann_type=ann_type)
- stage_losses.update(weak_losses)
- else: # supervised
- stage_losses = predictor.losses(
- (predictions[0], predictions[1]), proposals,
- classifier_info=classifier_info)
- if self.with_image_labels:
- stage_losses['image_loss'] = \
- predictions[0].new_zeros([1])[0]
- losses.update({k + "_stage{}".format(stage): v \
- for k, v in stage_losses.items()})
- return losses
- else:
- # Each is a list[Tensor] of length #image. Each tensor is Ri x (K+1)
- scores_per_stage = [h[0].predict_probs(h[1], h[2]) for h in head_outputs]
- scores = [
- sum(list(scores_per_image)) * (1.0 / self.num_cascade_stages)
- for scores_per_image in zip(*scores_per_stage)
- ]
- if self.mult_proposal_score:
- scores = [(s * ps[:, None]) ** 0.5 \
- for s, ps in zip(scores, proposal_scores)]
- if self.one_class_per_proposal:
- scores = [s * (s == s[:, :-1].max(dim=1)[0][:, None]).float() for s in scores]
- predictor, predictions, proposals = head_outputs[-1]
- boxes = predictor.predict_boxes(
- (predictions[0], predictions[1]), proposals)
- pred_instances, _ = fast_rcnn_inference(
- boxes,
- scores,
- image_sizes,
- predictor.test_score_thresh,
- predictor.test_nms_thresh,
- predictor.test_topk_per_image,
- )
- return pred_instances
-
-
- def forward(self, images, features, proposals, targets=None,
- ann_type='box', classifier_info=(None,None,None)):
- '''
- enable debug and image labels
- classifier_info is shared across the batch
- '''
- if self.training:
- if ann_type in ['box', 'prop', 'proptag']:
- proposals = self.label_and_sample_proposals(
- proposals, targets)
- else:
- proposals = self.get_top_proposals(proposals)
-
- losses = self._forward_box(features, proposals, targets, \
- ann_type=ann_type, classifier_info=classifier_info)
- if ann_type == 'box' and targets[0].has('gt_masks'):
- mask_losses = self._forward_mask(features, proposals)
- losses.update({k: v * self.mask_weight \
- for k, v in mask_losses.items()})
- losses.update(self._forward_keypoint(features, proposals))
- else:
- losses.update(self._get_empty_mask_loss(
- features, proposals,
- device=proposals[0].objectness_logits.device))
- return proposals, losses
- else:
- pred_instances = self._forward_box(
- features, proposals, classifier_info=classifier_info)
- pred_instances = self.forward_with_given_boxes(features, pred_instances)
- return pred_instances, {}
-
-
- def get_top_proposals(self, proposals):
- for i in range(len(proposals)):
- proposals[i].proposal_boxes.clip(proposals[i].image_size)
- proposals = [p[:self.ws_num_props] for p in proposals]
- for i, p in enumerate(proposals):
- p.proposal_boxes.tensor = p.proposal_boxes.tensor.detach()
- if self.add_image_box:
- proposals[i] = self._add_image_box(p)
- return proposals
-
-
- def _add_image_box(self, p):
- image_box = Instances(p.image_size)
- n = 1
- h, w = p.image_size
- f = self.image_box_size
- image_box.proposal_boxes = Boxes(
- p.proposal_boxes.tensor.new_tensor(
- [w * (1. - f) / 2.,
- h * (1. - f) / 2.,
- w * (1. - (1. - f) / 2.),
- h * (1. - (1. - f) / 2.)]
- ).view(n, 4))
- image_box.objectness_logits = p.objectness_logits.new_ones(n)
- return Instances.cat([p, image_box])
-
-
- def _get_empty_mask_loss(self, features, proposals, device):
- if self.mask_on:
- return {'loss_mask': torch.zeros(
- (1, ), device=device, dtype=torch.float32)[0]}
- else:
- return {}
-
-
- def _create_proposals_from_boxes(self, boxes, image_sizes, logits):
- """
- Add objectness_logits
- """
- boxes = [Boxes(b.detach()) for b in boxes]
- proposals = []
- for boxes_per_image, image_size, logit in zip(
- boxes, image_sizes, logits):
- boxes_per_image.clip(image_size)
- if self.training:
- inds = boxes_per_image.nonempty()
- boxes_per_image = boxes_per_image[inds]
- logit = logit[inds]
- prop = Instances(image_size)
- prop.proposal_boxes = boxes_per_image
- prop.objectness_logits = logit
- proposals.append(prop)
- return proposals
-
-
- def _run_stage(self, features, proposals, stage, \
- classifier_info=(None,None,None)):
- """
- Support classifier_info and add_feature_to_prop
- """
- pool_boxes = [x.proposal_boxes for x in proposals]
- box_features = self.box_pooler(features, pool_boxes)
- box_features = _ScaleGradient.apply(box_features, 1.0 / self.num_cascade_stages)
- box_features = self.box_head[stage](box_features)
- if self.add_feature_to_prop:
- feats_per_image = box_features.split(
- [len(p) for p in proposals], dim=0)
- for feat, p in zip(feats_per_image, proposals):
- p.feat = feat
- return self.box_predictor[stage](
- box_features,
- classifier_info=classifier_info)
diff --git a/spaces/Deepak7376/demo-sapce/app.py b/spaces/Deepak7376/demo-sapce/app.py
deleted file mode 100644
index 50500f49bf8b6be3a544d0c4ee94d5d3279a3c17..0000000000000000000000000000000000000000
--- a/spaces/Deepak7376/demo-sapce/app.py
+++ /dev/null
@@ -1,51 +0,0 @@
-import streamlit as st
-from PIL import Image
-import io
-import os
-
-# Set the title and a short description for your app
-st.title("Image Processing App")
-st.write("Upload an image, resize it, convert it to black and white, and download the processed image.")
-
-# Create a file uploader widget
-uploaded_image = st.file_uploader("Upload an image", type=["jpg", "jpeg", "png"])
-
-# Create a slider widget to choose the resize width
-resize_width = st.slider("Choose the resize width (in pixels):", 1, 1000, 300)
-
-# Create a checkbox for converting to black and white
-convert_to_bw = st.checkbox("Convert to Black and White")
-
-if uploaded_image is not None:
- # Display the uploaded image
- st.image(uploaded_image, caption="Uploaded Image", use_column_width=True)
-
- # Open the uploaded image
- image = Image.open(uploaded_image)
-
- # Resize the image if requested
- if resize_width:
- image = image.resize((resize_width, int(resize_width * image.height / image.width)))
-
- # Convert the image to black and white if requested
- if convert_to_bw:
- image = image.convert("L")
-
- # Display the processed image
- st.image(image, caption="Processed Image", use_column_width=True)
-
- # Create a download button to download the processed image
- download_button = st.button("Download Processed Image")
-
- if download_button:
- # Save the processed image to a temporary file
- with io.BytesIO() as output:
- image.save(output, format="JPEG")
- processed_image_data = output.getvalue()
-
- # Provide a download link for the processed image
- st.download_button(
- label="Download Processed Image",
- data=processed_image_data,
- file_name="processed_image.jpg",
- )
diff --git a/spaces/Djacon/emotion_detection/files/js/theme.js b/spaces/Djacon/emotion_detection/files/js/theme.js
deleted file mode 100644
index 9b8f500d3c91a1babb083e802b3624601184e42e..0000000000000000000000000000000000000000
--- a/spaces/Djacon/emotion_detection/files/js/theme.js
+++ /dev/null
@@ -1,26 +0,0 @@
-if (localStorage.theme === 'dark' || (!('theme' in localStorage) && window.matchMedia('(prefers-color-scheme: dark)').matches)) {
- localStorage.theme = 'dark';
- document.documentElement.classList.add('dark');
- document.getElementById('img-theme').src = 'files/images/sun.svg'
- document.getElementById('theme-span').innerText = 'Set Light Theme';
-} else {
- localStorage.theme = 'light';
- document.documentElement.classList.remove('dark');
- document.getElementById('img-theme').src = 'files/images/moon.svg'
- document.getElementById('theme-span').innerText = 'Set Dark Theme';
-}
-
-const theme_btn = document.getElementById('theme-btn');
-theme_btn.addEventListener('click', function() {
- if (localStorage.theme === 'dark') {
- localStorage.theme = 'light';
- document.documentElement.classList.remove('dark');
- document.getElementById('img-theme').src = 'files/images/moon.svg'
- document.getElementById('theme-span').innerText = 'Set Dark Theme';
- } else {
- localStorage.theme = 'dark';
- document.documentElement.classList.add('dark');
- document.getElementById('img-theme').src = 'files/images/sun.svg'
- document.getElementById('theme-span').innerText = 'Set Light Theme';
- }
-})
diff --git a/spaces/ECCV2022/bytetrack/tutorials/ctracker/mot_online/basetrack.py b/spaces/ECCV2022/bytetrack/tutorials/ctracker/mot_online/basetrack.py
deleted file mode 100644
index 4fe2233607f6d4ed28b11a0ae6c0303c8ca19098..0000000000000000000000000000000000000000
--- a/spaces/ECCV2022/bytetrack/tutorials/ctracker/mot_online/basetrack.py
+++ /dev/null
@@ -1,52 +0,0 @@
-import numpy as np
-from collections import OrderedDict
-
-
-class TrackState(object):
- New = 0
- Tracked = 1
- Lost = 2
- Removed = 3
-
-
-class BaseTrack(object):
- _count = 0
-
- track_id = 0
- is_activated = False
- state = TrackState.New
-
- history = OrderedDict()
- features = []
- curr_feature = None
- score = 0
- start_frame = 0
- frame_id = 0
- time_since_update = 0
-
- # multi-camera
- location = (np.inf, np.inf)
-
- @property
- def end_frame(self):
- return self.frame_id
-
- @staticmethod
- def next_id():
- BaseTrack._count += 1
- return BaseTrack._count
-
- def activate(self, *args):
- raise NotImplementedError
-
- def predict(self):
- raise NotImplementedError
-
- def update(self, *args, **kwargs):
- raise NotImplementedError
-
- def mark_lost(self):
- self.state = TrackState.Lost
-
- def mark_removed(self):
- self.state = TrackState.Removed
diff --git a/spaces/EronSamez/RVC_HFmeu/Fixes/local_fixes.py b/spaces/EronSamez/RVC_HFmeu/Fixes/local_fixes.py
deleted file mode 100644
index 8a418076eee6f65fe06eb0f607061796b839c1ee..0000000000000000000000000000000000000000
--- a/spaces/EronSamez/RVC_HFmeu/Fixes/local_fixes.py
+++ /dev/null
@@ -1,136 +0,0 @@
-import os
-import sys
-import time
-import shutil
-import requests
-import zipfile
-
-def insert_new_line(file_name, line_to_find, text_to_insert):
- lines = []
- with open(file_name, 'r', encoding='utf-8') as read_obj:
- lines = read_obj.readlines()
- already_exists = False
- with open(file_name + '.tmp', 'w', encoding='utf-8') as write_obj:
- for i in range(len(lines)):
- write_obj.write(lines[i])
- if lines[i].strip() == line_to_find:
- # If next line exists and starts with sys.path.append, skip
- if i+1 < len(lines) and lines[i+1].strip().startswith("sys.path.append"):
- print('It was already fixed! Skip adding a line...')
- already_exists = True
- break
- else:
- write_obj.write(text_to_insert + '\n')
- # If no existing sys.path.append line was found, replace the original file
- if not already_exists:
- os.replace(file_name + '.tmp', file_name)
- return True
- else:
- # If existing line was found, delete temporary file
- os.remove(file_name + '.tmp')
- return False
-
-def replace_in_file(file_name, old_text, new_text):
- with open(file_name, 'r', encoding='utf-8') as file:
- file_contents = file.read()
-
- if old_text in file_contents:
- file_contents = file_contents.replace(old_text, new_text)
- with open(file_name, 'w', encoding='utf-8') as file:
- file.write(file_contents)
- return True
-
- return False
-
-if __name__ == "__main__":
- current_path = os.getcwd()
- file_name = os.path.join(current_path, "infer", "modules", "train", "extract", "extract_f0_print.py")
- line_to_find = 'import numpy as np, logging'
- text_to_insert = "sys.path.append(r'" + current_path + "')"
-
-
- success_1 = insert_new_line(file_name, line_to_find, text_to_insert)
- if success_1:
- print('The first operation was successful!')
- else:
- print('He skipped the first operation because it was already fixed!')
-
- file_name = 'infer-web.py'
- old_text = 'with gr.Blocks(theme=gr.themes.Soft()) as app:'
- new_text = 'with gr.Blocks() as app:'
-
- success_2 = replace_in_file(file_name, old_text, new_text)
- if success_2:
- print('The second operation was successful!')
- else:
- print('The second operation was omitted because it was already fixed!')
-
- print('Local corrections successful! You should now be able to infer and train locally in Applio RVC Fork.')
-
- time.sleep(5)
-
-def find_torchcrepe_directory(directory):
- """
- Recursively searches for the topmost folder named 'torchcrepe' within a directory.
- Returns the path of the directory found or None if none is found.
- """
- for root, dirs, files in os.walk(directory):
- if 'torchcrepe' in dirs:
- return os.path.join(root, 'torchcrepe')
- return None
-
-def download_and_extract_torchcrepe():
- url = 'https://github.com/maxrmorrison/torchcrepe/archive/refs/heads/master.zip'
- temp_dir = 'temp_torchcrepe'
- destination_dir = os.getcwd()
-
- try:
- torchcrepe_dir_path = os.path.join(destination_dir, 'torchcrepe')
-
- if os.path.exists(torchcrepe_dir_path):
- print("Skipping the torchcrepe download. The folder already exists.")
- return
-
- # Download the file
- print("Starting torchcrepe download...")
- response = requests.get(url)
-
- # Raise an error if the GET request was unsuccessful
- response.raise_for_status()
- print("Download completed.")
-
- # Save the downloaded file
- zip_file_path = os.path.join(temp_dir, 'master.zip')
- os.makedirs(temp_dir, exist_ok=True)
- with open(zip_file_path, 'wb') as file:
- file.write(response.content)
- print(f"Zip file saved to {zip_file_path}")
-
- # Extract the zip file
- print("Extracting content...")
- with zipfile.ZipFile(zip_file_path, 'r') as zip_file:
- zip_file.extractall(temp_dir)
- print("Extraction completed.")
-
- # Locate the torchcrepe folder and move it to the destination directory
- torchcrepe_dir = find_torchcrepe_directory(temp_dir)
- if torchcrepe_dir:
- shutil.move(torchcrepe_dir, destination_dir)
- print(f"Moved the torchcrepe directory to {destination_dir}!")
- else:
- print("The torchcrepe directory could not be located.")
-
- except Exception as e:
- print("Torchcrepe not successfully downloaded", e)
-
- # Clean up temporary directory
- if os.path.exists(temp_dir):
- shutil.rmtree(temp_dir)
-
-# Run the function
-download_and_extract_torchcrepe()
-
-temp_dir = 'temp_torchcrepe'
-
-if os.path.exists(temp_dir):
- shutil.rmtree(temp_dir)
diff --git a/spaces/ErtugrulDemir/TextSummarizing/README.md b/spaces/ErtugrulDemir/TextSummarizing/README.md
deleted file mode 100644
index a77d04e28a801c2addf4559ca10512a3988bab8c..0000000000000000000000000000000000000000
--- a/spaces/ErtugrulDemir/TextSummarizing/README.md
+++ /dev/null
@@ -1,13 +0,0 @@
----
-title: TextSummarizing
-emoji: 🌍
-colorFrom: purple
-colorTo: blue
-sdk: gradio
-sdk_version: 3.27.0
-app_file: app.py
-pinned: false
-license: apache-2.0
----
-
-Check out the configuration reference at https://huggingface.co/docs/hub/spaces-config-reference
diff --git a/spaces/Fazzie/Pokemon-GAI/static/style.css b/spaces/Fazzie/Pokemon-GAI/static/style.css
deleted file mode 100644
index 04a3b5a95c84ef149a619ddfc458fed69bf1e4aa..0000000000000000000000000000000000000000
--- a/spaces/Fazzie/Pokemon-GAI/static/style.css
+++ /dev/null
@@ -1,1096 +0,0 @@
-:root {
- --card-width: 25rem;
- --theme-primary: hsl(158 100% 33%);
- --theme-secondary: hsl(165 67% 48%);
- --theme-ternary: hsl(112 46% 75%);
- --theme-highlight: hsl(111 95% 92%);
- --theme-subtext: hsl(0 0% 30%);
- --theme-error-bg: hsl(6 93% 71%);
- --theme-error-border: hsl(355 85% 55%);
-}
-
-* {
- transition: outline-offset 0.25s ease-out;
- outline-style: none;
- outline-width: 0.15rem;
- outline-color: var(--theme-primary);
-}
-
-*:focus-visible:not(input) {
- outline-style: dashed;
- outline-offset: 0.25em;
-}
-
-.info h1::selection {
- text-fill-color: white;
- -webkit-text-fill-color: white;
- -moz-text-fill-color: white;
-}
-
-*::selection {
- background-color: gold;
-}
-
-html {
- display: flex;
- display: grid;
- align-items: center;
- height: 100%;
-}
-
-body {
- margin: 0;
- background-color: whitesmoke;
- background-image: linear-gradient(300deg, var(--theme-highlight), white);
- font-family: 'Gill Sans', 'Gill Sans Mt', 'Lato', 'sans-serif';
- overflow-x: hidden;
-}
-
-main {
- display: grid;
- place-items: center;
- grid-template-columns: repeat(auto-fit, minmax(25rem, 1fr));
- gap: 1.5rem 0;
- max-width: 80rem;
- height: 100%;
- padding: 0 3rem;
- margin: 0 auto;
-}
-
-@media (max-width: 500px) {
- main {
- padding: 3rem 0;
- }
-
- main > section {
- width: 95%;
- }
-
- main > section.info h1 {
- font-size: 2.5rem;
- }
-
- .scene .booster {
- --booster-scale: 0.5;
- }
-
- .scene .card-slot {
- margin-top: 1rem;
- }
-}
-
-@media (max-width: 895px) {
- html {
- height: auto;
- }
-
- .output .scene {
- margin-top: -4rem;
- }
-}
-
-@media (max-width: 1024px) {
- .output .booster {
- --booster-scale: 0.6;
- }
-}
-
-@media (max-width: 1280px) {
- section.info h1 {
- font-size: 3rem;
- }
-
- .output .pokecard {
- --card-scale: 0.8;
- }
-}
-
-section {
- display: grid;
- place-items: center;
- width: 100%;
- box-sizing: border-box;
-}
-
-/* Info (left) section */
-
-.info {
- max-width: 35rem;
- height: min-content;
-}
-
-.poke-trio {
- display: flex;
- flex-direction: row;
- justify-content: space-between;
- position: relative;
- height: 5rem;
-}
-
-.poke-trio > img {
- position: relative;
- user-select: none;
-}
-
-.poke-trio > img::after {
- content: '';
- position: absolute;
- top: 0;
- left: 0;
- width: 3rem;
- height: 3rem;
- border: 2px solid red;
- background-color: blue;
-}
-
-.info h1 {
- margin: 0.5rem auto 3rem;
- background-image: linear-gradient(0deg, var(--theme-primary), var(--theme-secondary));
- background-clip: text;
- -webkit-background-clip: text;
- -moz-background-clip: text;
- text-align: center;
- font-size: 4.5rem;
- font-weight: bold;
- text-fill-color: transparent;
- -webkit-text-fill-color: transparent;
- -moz-text-fill-color: transparent;
- transition: font-size 0.5s ease;
-}
-
-@media (prefers-reduced-motion) {
- .info h1 {
- transition: none;
- }
-}
-
-.info label {
- width: 100%;
- text-align: center;
- font-size: 1.25rem;
- font-weight: 700;
-}
-
-.info form {
- display: flex;
- flex-direction: row;
- width: 80%;
- margin: 0.5rem auto;
-}
-
-.info .name-interactive {
- display: flex;
- flex-direction: row;
-}
-
-.info input {
- display: block;
- width: 100%;
- height: 45px;
- box-sizing: border-box;
- padding: 0.5rem 1rem 0.5rem 5rem;
- margin: 0;
- border: 3px solid hsl(0 0% 70%);
- border-right: none;
- border-radius: 1rem 0 0 1rem;
- text-align: center;
- font-size: 1.25rem;
- transition: box-shadow 0.5s ease-out;
- box-shadow: none;
-}
-
-.info input::placeholder {
- text-align: center;
-}
-
-input:focus {
- border-color: var(--theme-secondary);
- box-shadow: 0 0 0.5rem hsl(165 67% 48% / 60%);
-}
-
-form button {
- height: 2.8125rem;
- margin: 0;
- font-size: 0.85rem;
- border-top-left-radius: 0;
- border-bottom-left-radius: 0;
-}
-
-.info-text {
- margin-top: 2.5rem;
-}
-
-.info-text p {
- width: 80%;
- margin: 1rem auto;
- text-align: justify;
- color: var(--theme-subtext);
- line-height: 1.5rem;
-}
-
-.info-text a,
-info a:is(:hover, :focus, :active, :visited) {
- color: var(--theme-subtext);
- cursor: pointer;
-}
-
-/* Output (right) section */
-
-.output {
- display: flex;
- flex-direction: column;
- justify-content: space-around;
- height: min-content;
-}
-
-.output .actions {
- display: flex;
- flex-direction: row;
- flex-wrap: wrap;
- justify-content: center;
- align-items: center;
- gap: 1rem;
- width: 100%;
- margin: 1rem auto 1.5rem;
- transition: transform 0.5s ease;
- z-index: 5;
-}
-
-[data-mode='booster'][data-state='completed'] .actions {
- transform: translateY(-25%);
-}
-
-button {
- padding: 0.5rem 1rem;
- border: none;
- border-radius: 1rem;
- background-image: linear-gradient(-90deg, var(--theme-ternary), var(--theme-secondary));
- font-weight: bold;
- color: white;
- transform-origin: bottom;
- transition: box-shadow 0.1s, outline-offset 0.25s ease-out, filter 0.25s ease-out, opacity 0.25s;
- whitespace: nowrap;
- filter: saturate(1);
- cursor: pointer;
-}
-
-.actions button {
- box-shadow: 0 0.2rem 0.375rem hsl(158 100% 33% / 60%);
- user-select: none;
- pointer-events: none;
- opacity: 0;
-}
-
-[data-mode='card'][data-state='completed'] button {
- pointer-events: auto;
- opacity: 1;
-}
-
-button:active {
- box-shadow: none;
-}
-
-button.toggle-name.off {
- filter: saturate(0.15);
-}
-
-.scene {
- --scale: 0.9;
- height: min-content;
- box-sizing: border-box;
- perspective: 100rem;
- transform-origin: center;
- transform: scale(var(--scale));
- transition: transform 0.5s ease-out;
-}
-
-/* Booster Pack */
-
-.booster {
- --booster-rx: 0deg;
- --booster-ry: 0deg;
- --booster-rz: -5deg;
- --booster-scale: 0.7;
- --width: var(--card-width);
- --height: calc(var(--card-width) / 66 * 88);
- --depth: 0.5rem;
- --bg: hsl(227, 54%, 21%);
- display: none;
- position: relative;
- width: var(--width);
- height: var(--height);
- transform-style: preserve-3d;
- transform: rotateX(var(--booster-rx)) rotateY(var(--booster-ry)) rotateZ(var(--booster-rz))
- scale(var(--booster-scale));
- transition: transform 0.5s ease-in-out;
- cursor: pointer;
-}
-
-.booster > div {
- display: grid;
- place-items: center;
- position: absolute;
- font-size: 5rem;
- transform-origin: center;
- user-select: none;
-}
-
-.face:is(.front, .back, .left, .right) {
- height: var(--height);
- background-color: var(--bg);
-}
-
-.face:is(.front, .back, .top, .bottom) {
- width: var(--width);
-}
-.left,
-.right {
- width: var(--depth);
-}
-
-.face:is(.top, .bottom) {
- height: var(--depth);
-}
-
-.foil {
- width: var(--width);
- background-image: linear-gradient(
- 90deg,
- hsl(0 0% 80%) 0%,
- hsl(0 0% 84%) 10%,
- hsl(0 0% 88%) 20%,
- hsl(0 0% 92%) 30%,
- hsl(0 0% 96%) 40%,
- hsl(0 0% 100%) 50%,
- hsl(0 0% 96%) 60%,
- hsl(0 0% 92%) 70%,
- hsl(0 0% 88%) 80%,
- hsl(0 0% 84%) 90%,
- hsl(0 0% 80%) 100%
- );
-}
-.foil.top.flat {
- height: 20px;
- transform-origin: bottom;
- transform: translate3d(0, -30px, 0px) rotateX(0deg);
-}
-.foil.top.flat::after,
-.foil.bottom.flat::after {
- content: '';
- position: absolute;
- width: var(--width);
- height: 20px;
- background: radial-gradient(#ffffff 0%, transparent 50%);
- background-size: 1% 100%;
-}
-.foil.top.front {
- height: 11px;
- transform-origin: bottom;
- transform: translate3d(0, -11.4px, 3.8px) rotateX(20.5deg);
-}
-.foil.top.back {
- height: 11px;
- transform-origin: bottom;
- transform: translate3d(0, -11.4px, -4px) rotateX(339deg);
-}
-.face.front {
- transform: rotateY(0deg) translate3d(0, 0, calc(var(--depth) / 2));
-}
-.face.left {
- transform: rotateY(90deg) translate3d(0, 0, calc(var(--width) - calc(var(--depth) / 2)));
-}
-.face.back {
- transform: rotateY(180deg) translate3d(0, 0, calc(var(--depth) / 2)) rotateZ(180deg);
-}
-.face.right {
- transform: rotateY(-90deg) translate3d(0, 0, calc(var(--depth) / 2));
-}
-.face.top {
- transform: rotateX(90deg) translate3d(0, 0, calc(var(--depth) / 2));
-}
-.face.bottom {
- transform: rotateX(-90deg) translate3d(0, 0, calc(var(--height) - calc(var(--depth) / 2)));
-}
-.foil.bottom.flat {
- height: 20px;
- transform-origin: top;
- transform: translate3d(0, calc(var(--height) + 10px), 0px) rotateX(0deg);
-}
-.foil.bottom.front {
- height: 11px;
- transform-origin: top;
- transform: translate3d(0, var(--height), 3.8px) rotateX(-19.5deg);
-}
-.foil.bottom.back {
- height: 11px;
- transform-origin: top;
- transform: translate3d(0, var(--height), -3.8px) rotateX(19.5deg);
-}
-
-.foil.back.flat {
- width: 30px;
- height: var(--height);
- background-image: linear-gradient(
- 90deg,
- hsl(0 0% 0%) 0%,
- hsl(0 0% 10%) 20%,
- hsl(0 0% 40%) 30%,
- hsl(0 0% 60%) 40%,
- hsl(0 0% 86%) 50%,
- hsl(0 0% 90%) 60%,
- hsl(0 0% 85%) 80%,
- hsl(0 0% 90%) 90%,
- hsl(0 0% 70%) 100%
- );
- transform-origin: bottom;
- transform: translate3d(calc(var(--width) / 2 - 25px), 0px, calc(var(--depth) * -0.50001)) rotateX(0deg);
-}
-.foil.back.flap {
- width: 30px;
- height: var(--height);
- background-image: linear-gradient(
- 90deg,
- hsl(0 0% 70%) 0%,
- hsl(0 0% 74%) 10%,
- hsl(0 0% 78%) 20%,
- hsl(0 0% 82%) 30%,
- hsl(0 0% 86%) 40%,
- hsl(0 0% 90%) 50%,
- hsl(0 0% 86%) 60%,
- hsl(0 0% 82%) 70%,
- hsl(0 0% 78%) 80%,
- hsl(0 0% 74%) 90%,
- hsl(0 0% 70%) 100%
- );
- transform-origin: center;
- transform: translate3d(calc(var(--width) / 2 - 25.5px), 0, -8px) rotateY(15deg);
-}
-
-.foil.back.flap::after {
- content: '';
- position: absolute;
- width: 30px;
- height: var(--height);
- background: radial-gradient(#ffffff 0%, transparent 50%);
- background-size: 100% 0.75%;
-}
-
-.gradient-bg {
- background-image: linear-gradient(
- 225deg,
- hsl(270deg 100% 7%) 0%,
- hsl(246deg 77% 15%) 14%,
- hsl(223deg 59% 24%) 29%,
- hsl(199deg 44% 35%) 43%,
- hsl(175deg 32% 48%) 57%,
- hsl(151deg 36% 62%) 71%,
- hsl(128deg 45% 78%) 86%,
- hsl(104deg 100% 95%) 100%
- );
-}
-
-.face.front {
- display: flex;
- flex-direction: column;
- justify-content: space-evenly;
- gap: 0.5rem;
- box-sizing: border-box;
- padding: 1rem;
-}
-
-.face.front,
-.face.back {
- background-size: var(--width);
- background-image: url('booster-background.svg');
- background-position: center;
-}
-
-.face.right,
-.face.left {
- background-image: linear-gradient(
- 0deg,
- hsl(151deg 36% 62%) 0%,
- hsl(175deg 32% 48%) 14%,
- hsl(199deg 44% 35%) 29%,
- hsl(223deg 59% 24%) 57%,
- hsl(246deg 77% 15%) 86%,
- hsl(270deg 100% 7%) 100%
- );
-}
-
-img.title {
- width: 100%;
- filter: drop-shadow(0 0.25rem 0.1rem hsl(220 100% 10% / 0.75));
-}
-img.hf-logo {
- width: 90%;
-}
-
-.triangle {
- width: calc(var(--depth) * 10);
- aspect-ratio: 1 / 1.35;
-}
-
-.triangle.top {
- clip-path: polygon(0% 100%, 50% 0%, 100% 100%);
-}
-.triangle.bottom {
- clip-path: polygon(0% 0%, 50% 100%, 100% 0%);
-}
-
-.triangle.top.right {
- transform: rotateY(90deg) translate3d(0.1px, -59.1px, -39.5px) scale(0.1);
-}
-.triangle.top.left {
- transform: rotateY(90deg) translate3d(0.1px, -59.1px, calc(var(--width) - 41.5px)) scale(0.1);
-}
-.triangle.bottom.left {
- transform: rotateY(90deg) translate3d(0.1px, calc(var(--height) - 49px), calc(var(--width) - 41.5px)) scale(0.1);
-}
-.triangle.bottom.right {
- transform: rotateY(90deg) translate3d(0px, calc(var(--height) - 49px), -39.5px) scale(0.1);
-}
-
-/* Animation */
-
-@keyframes spin-x {
- from {
- transform: scale(var(--scale)) rotate(0turn);
- }
- to {
- transform: scale(var(--scale)) rotate(1turn);
- }
-}
-
-@keyframes spin-y {
- 0% {
- transform: rotateX(var(--booster-rx)) rotateY(0deg) rotateZ(0deg) scale(var(--booster-scale));
- }
- 100% {
- transform: rotateX(var(--booster-rx)) rotateY(360deg) rotateZ(0deg) scale(var(--booster-scale));
- }
-}
-
-@keyframes bounce {
- 0% {
- transform: rotateX(var(--booster-rx)) rotateY(var(--booster-ry)) rotateZ(var(--booster-rz))
- scale(var(--booster-scale)) translateY(0%);
- }
- 30% {
- transform: rotateX(var(--booster-rx)) rotateY(var(--booster-ry)) rotateZ(var(--booster-rz))
- scale(var(--booster-scale)) translateY(-2%);
- }
- 50% {
- transform: rotateX(var(--booster-rx)) rotateY(var(--booster-ry)) rotateZ(var(--booster-rz))
- scale(var(--booster-scale)) translateY(1%);
- }
- 70% {
- transform: rotateX(var(--booster-rx)) rotateY(var(--booster-ry)) rotateZ(var(--booster-rz))
- scale(var(--booster-scale)) translateY(-1%);
- }
- 100% {
- transform: rotateX(var(--booster-rx)) rotateY(var(--booster-ry)) rotateZ(var(--booster-rz))
- scale(var(--booster-scale)) translateY(0%);
- }
-}
-
-@keyframes shrink {
- from {
- transform: rotateZ(45deg) scale(var(--booster-scale));
- opacity: 1;
- }
- to {
- transform: rotateZ(270deg) scale(0);
- opacity: 0;
- }
-}
-
-[data-mode='booster'] .booster {
- display: block;
-}
-
-:is([data-state='ready'], [data-state='failed']) .booster {
- animation: 5s bounce infinite ease-out;
-}
-
-[data-state='generating'] .scene {
- animation: 15s spin-x infinite linear;
-}
-[data-state='generating'] .booster {
- transform-origin: center;
- animation: 3s spin-y infinite linear;
- cursor: default;
-}
-
-[data-mode='booster'][data-state='completed'] .booster {
- animation: 0.5s shrink ease-out forwards;
-}
-
-[data-mode='booster'][data-state='completed'] .card-slot {
- transform: scale(0);
- opacity: 0;
-}
-
-[data-mode='booster'][data-state='completed'] .back {
- display: none;
-}
-
-[data-mode='card'][data-state='completed'] .booster {
- --booster-scale: 0;
-}
-
-[data-mode='card'][data-state='completed'] .card-slot {
- transform: scale(1);
- opacity: 1;
-}
-
-@media (prefers-reduced-motion) {
- @keyframes pulse {
- from {
- opacity: 1;
- }
- to {
- opacity: 0.6;
- }
- }
-
- @keyframes fade {
- from {
- opacity: 1;
- }
- to {
- opacity: 0;
- }
- }
-
- .card-slot .pokecard {
- transition: none;
- }
-
- [data-mode='booster']:is([data-state='generating'], [data-state='completed']) .scene {
- animation: 1.5s pulse alternate ease-in-out infinite forwards;
- }
-
- [data-state='generating'] .booster {
- animation: 10s bounce infinite ease-out;
- }
-
- [data-mode='booster'][data-state='completed'] .booster {
- animation: 1s fade ease-in forwards;
- }
-
- [data-state='completed'] .card-slot {
- transition: opacity 1s ease-in;
- }
-
- [data-mode='booster'][data-state='completed'] .card-slot {
- transform: scale(1);
- opacity: 0;
- }
-
- [data-mode='card'][data-state='completed'] .card-slot {
- opacity: 1;
- }
-}
-
-/* Pokémon Card */
-
-.card-slot {
- height: 100%;
- perspective: 100rem;
- transition: transform 0.5s ease-out, opacity 0.5s ease-in;
-}
-
-.grass {
- --h: 90;
- --s: 60%;
- --l: 40%;
-}
-.grass.energy {
- filter: contrast(0.75) grayscale(1) sepia(1) saturate(10) hue-rotate(55deg) drop-shadow(0 0 0.1rem green);
-}
-
-.fire {
- --h: 0;
- --s: 75%;
- --l: 45%;
-}
-.fire.energy {
- filter: contrast(0.75) grayscale(1) sepia(1) saturate(10) hue-rotate(335deg) drop-shadow(0 0 0.1rem red);
-}
-
-.water {
- --h: 210;
- --s: 100%;
- --l: 58%;
-}
-.water.energy {
- filter: contrast(0.75) grayscale(1) sepia(1) saturate(10) hue-rotate(180deg) drop-shadow(0 0 0.1rem cyan);
-}
-
-.lightning {
- --h: 50;
- --s: 100%;
- --l: 58%;
-}
-.lightning.energy {
- filter: contrast(0.75) grayscale(1) sepia(1) saturate(10) hue-rotate(5deg) drop-shadow(0 0 0.1rem gold);
-}
-
-.fighting {
- --h: 25;
- --s: 72%;
- --l: 36%;
-}
-.fighting.energy {
- filter: contrast(0.75) grayscale(1) sepia(1) saturate(10) hue-rotate(320deg) drop-shadow(0 0 0.1rem brown);
-}
-
-.psychic {
- --h: 299;
- --s: 43%;
- --l: 44%;
-}
-.psychic.energy {
- filter: grayscale(1) sepia(1) saturate(10) hue-rotate(240deg) drop-shadow(0 0 0.1rem purple);
-}
-
-.colorless {
- --h: 21;
- --s: 27%;
- --l: 85%;
-}
-.colorless.energy {
- border-radius: 50%;
- filter: contrast(100) grayscale(1);
- text-shadow: 0 0 0.5rem black;
-}
-
-.darkness {
- --h: 100;
- --s: 3%;
- --l: 17%;
-}
-.darkness.energy {
- filter: drop-shadow(0 0 0.1rem black);
-}
-.darkness :not(.species) {
- color: whitesmoke;
-}
-
-.metal {
- --h: 240;
- --s: 20%;
- --l: 77%;
-}
-.metal.energy {
- filter: drop-shadow(0 0 0.1rem silver);
-}
-
-.dragon {
- --h: 30;
- --s: 6%;
- --l: 44%;
-}
-.dragon.energy {
- filter: contrast(0.75) grayscale(1) sepia(1) saturate(10) hue-rotate(15deg) drop-shadow(0 0 0.1rem gold);
-}
-
-.fairy {
- --h: 334;
- --s: 74%;
- --l: 55%;
-}
-.fairy.energy {
- filter: contrast(0.75) grayscale(1) sepia(1) saturate(10) hue-rotate(300deg) drop-shadow(0 0 0.1rem pink);
-}
-
-.pokecard,
-.pokecard * {
- box-sizing: border-box;
-}
-
-.pokecard {
- --frame-h: 47;
- --frame-s: 95%;
- --frame-l: 58%;
- --frame-color: hsl(47 95% 58%);
- --color: hsl(var(--h) var(--s) var(--l));
- --lighter: hsl(var(--h) var(--s) calc(var(--l) + 10%));
- --lightest: hsl(var(--h) var(--s) calc(var(--l) + 30%));
- --card-rx: 0deg;
- --card-ry: 0deg;
- --card-rz: 0deg;
- --card-scale: 1;
- display: flex;
- flex-direction: column;
- position: relative;
- width: 25rem;
- height: 35rem;
- padding: 0.5rem 1rem 0.1rem;
- border: 1rem solid;
- border-radius: 0.75rem;
- border-color: var(--frame-color);
- background-image: linear-gradient(
- 45deg,
- var(--lighter) 0%,
- var(--lightest) 15%,
- var(--lightest) 30%,
- var(--color) 50%,
- var(--lightest) 90%,
- var(--lighter) 100%
- );
- transform-style: preserve-3d;
- transform-origin: center;
- transform: rotateX(var(--card-rx)) rotateY(var(--card-ry)) scale(var(--card-scale));
- transition: transform 0.5s ease-out;
- box-shadow: 0 0.75rem 1.25rem 0 hsl(0 0% 50% / 40%);
-}
-
-.pokecard .lower-half {
- display: flex;
- flex-direction: column;
- height: 100%;
-}
-
-.evolves {
- margin: 0 1px -5px;
- font-size: 0.6rem;
- font-weight: bold;
-}
-
-header {
- display: flex;
- flex-direction: row;
- justify-content: space-between;
- min-height: 1.4rem;
-}
-
-header > * {
- display: inline-block;
-}
-
-.name {
- display: inline-block;
- justify-self: left;
- position: absolute;
- left: 1rem;
- margin: 0;
- font-size: 1.25rem;
- transform-origin: left;
- white-space: nowrap;
-}
-
-header > div {
- position: absolute;
- right: 1rem;
- width: max-content;
- white-space: nowrap;
-}
-
-.hp {
- font-size: 1.25rem;
- color: hsl(0 100% 50%);
-}
-
-header .energy {
- display: inline-block;
- transform: translateY(-0.15rem);
-}
-
-.frame:is(.picture, .species, .description) {
- --lighter: hsl(var(--frame-h) var(--frame-s) calc(var(--frame-l) + 15%));
- --lightest: hsl(var(--frame-h) var(--frame-s) calc(var(--frame-l) + 30%));
- --darker: hsl(var(--frame-h) var(--frame-s) calc(var(--frame-l) - 15%));
- border-color: var(--darker) var(--frame-color) var(--lighter);
-}
-
-.picture,
-.inline-block {
- display: inline-block;
-}
-
-.picture {
- width: 100%;
- height: 240px;
- border: 0.375rem solid;
- background-color: white;
- object-fit: contain;
- box-shadow: 0.25rem 0.25rem 0.5rem black;
- user-select: none;
-}
-
-.species {
- width: 90%;
- padding: 0.1rem;
- margin: 0.25rem auto;
- border-style: solid;
- border-width: 0 0.2rem;
- border-image: linear-gradient(var(--lightest), var(--darker)) 1 100%;
- background-image: linear-gradient(90deg, var(--frame-color), var(--lightest) 45% 55%, var(--frame-color));
- text-align: center;
- font-size: 0.75rem;
- font-weight: bold;
- font-style: italic;
-}
-
-.species::selection {
- background-color: white;
-}
-
-.attacks-row,
-.footer {
- display: grid;
- grid-template-columns: repeat(3, 1fr);
- width: 100%;
-}
-
-.footer > span:first-child {
- text-align: left;
-}
-
-.footer > span:last-child {
- text-align: right;
-}
-
-.attacks {
- display: flex;
- flex-direction: column;
- justify-content: space-evenly;
- height: 100%;
- padding: 0;
- margin: 0;
- list-style-type: none;
-}
-
-.attacks-row {
- grid-template-columns: 3rem 1fr 3rem;
- align-items: center;
- width: 105%;
- height: 100%;
- max-height: 5rem;
- padding: 0.25rem 0;
- margin-left: -2.5%;
- border-bottom: 0.5px solid hsl(0, 0%, 10%);
- font-size: 0.95em;
-}
-
-.attacks-row.no-cost {
- grid-template-columns: 1fr 3rem;
-}
-.attacks-row.no-damage {
- grid-template-columns: 3rem 1fr;
- text-align: left;
-}
-.attacks-row.no-cost.no-damage {
- grid-template-columns: 1fr;
-}
-
-.attack-text {
- margin-left: 0.25rem;
- margin-right: 0.1rem;
-}
-
-.attack-text > span:only-child {
- display: block;
- margin-left: -1rem;
- text-align: center;
-}
-
-.no-cost .attack-text > span:only-child,
-.no-cost.no-damage .attack-text > span:only-child {
- width: var(--card-width);
- margin-left: -2.5rem;
-}
-.no-damage .attack-text > span:only-child {
- width: var(--card-width);
- margin-left: -5.5rem;
-}
-
-.attack-cost {
- display: flex;
- flex-flow: row wrap;
- justify-content: space-evenly;
- text-align: justify;
-}
-
-.energy {
- width: 1.2rem;
- height: 1.2rem;
- text-align: center;
- cursor: default;
- user-select: none;
-}
-
-.energy:only-child {
- justify-self: flex-start;
- margin: auto;
-}
-
-.attack-name {
- font-weight: bold;
-}
-
-.attack-damage {
- min-width: 2.25rem;
- text-align: center;
- font-size: 1.375rem;
-}
-
-hr {
- border: 0.5px solid black;
- background-color: black;
-}
-
-.multipliers {
- display: flex;
- flex-direction: row;
- justify-content: space-between;
- height: 2rem;
- margin-top: 0;
- text-align: center;
- font-size: 0.75rem;
- font-weight: bold;
-}
-
-.multipliers > div {
- display: flex;
- flex-direction: column;
- align-items: center;
- width: max-content;
- margin: 0;
- white-space: nowrap;
-}
-
-.resistance {
- position: relative;
-}
-
-.resistance-total {
- position: absolute;
- top: 1rem;
- left: 2.5rem;
-}
-
-.description {
- padding: 0.1rem 0.5rem;
- margin: 0.25rem 0 0;
- border: 0.1rem solid;
- font-size: 0.65rem;
- font-weight: bold;
- font-style: italic;
-}
-
-.footer {
- align-self: end;
- position: relative;
- margin: 0.15rem 0;
- text-align: center;
- font-size: 0.6rem;
- font-weight: bold;
-}
-
-.pokecard a {
- text-decoration: none;
- color: inherit;
-}
diff --git a/spaces/FridaZuley/RVC_HFKawaii/infer/lib/uvr5_pack/lib_v5/nets_537227KB.py b/spaces/FridaZuley/RVC_HFKawaii/infer/lib/uvr5_pack/lib_v5/nets_537227KB.py
deleted file mode 100644
index 823b44fb64898e8dcbb12180ba45d1718f9b03f7..0000000000000000000000000000000000000000
--- a/spaces/FridaZuley/RVC_HFKawaii/infer/lib/uvr5_pack/lib_v5/nets_537227KB.py
+++ /dev/null
@@ -1,123 +0,0 @@
-import numpy as np
-import torch
-import torch.nn.functional as F
-from torch import nn
-
-from . import layers_537238KB as layers
-
-
-class BaseASPPNet(nn.Module):
- def __init__(self, nin, ch, dilations=(4, 8, 16)):
- super(BaseASPPNet, self).__init__()
- self.enc1 = layers.Encoder(nin, ch, 3, 2, 1)
- self.enc2 = layers.Encoder(ch, ch * 2, 3, 2, 1)
- self.enc3 = layers.Encoder(ch * 2, ch * 4, 3, 2, 1)
- self.enc4 = layers.Encoder(ch * 4, ch * 8, 3, 2, 1)
-
- self.aspp = layers.ASPPModule(ch * 8, ch * 16, dilations)
-
- self.dec4 = layers.Decoder(ch * (8 + 16), ch * 8, 3, 1, 1)
- self.dec3 = layers.Decoder(ch * (4 + 8), ch * 4, 3, 1, 1)
- self.dec2 = layers.Decoder(ch * (2 + 4), ch * 2, 3, 1, 1)
- self.dec1 = layers.Decoder(ch * (1 + 2), ch, 3, 1, 1)
-
- def __call__(self, x):
- h, e1 = self.enc1(x)
- h, e2 = self.enc2(h)
- h, e3 = self.enc3(h)
- h, e4 = self.enc4(h)
-
- h = self.aspp(h)
-
- h = self.dec4(h, e4)
- h = self.dec3(h, e3)
- h = self.dec2(h, e2)
- h = self.dec1(h, e1)
-
- return h
-
-
-class CascadedASPPNet(nn.Module):
- def __init__(self, n_fft):
- super(CascadedASPPNet, self).__init__()
- self.stg1_low_band_net = BaseASPPNet(2, 64)
- self.stg1_high_band_net = BaseASPPNet(2, 64)
-
- self.stg2_bridge = layers.Conv2DBNActiv(66, 32, 1, 1, 0)
- self.stg2_full_band_net = BaseASPPNet(32, 64)
-
- self.stg3_bridge = layers.Conv2DBNActiv(130, 64, 1, 1, 0)
- self.stg3_full_band_net = BaseASPPNet(64, 128)
-
- self.out = nn.Conv2d(128, 2, 1, bias=False)
- self.aux1_out = nn.Conv2d(64, 2, 1, bias=False)
- self.aux2_out = nn.Conv2d(64, 2, 1, bias=False)
-
- self.max_bin = n_fft // 2
- self.output_bin = n_fft // 2 + 1
-
- self.offset = 128
-
- def forward(self, x, aggressiveness=None):
- mix = x.detach()
- x = x.clone()
-
- x = x[:, :, : self.max_bin]
-
- bandw = x.size()[2] // 2
- aux1 = torch.cat(
- [
- self.stg1_low_band_net(x[:, :, :bandw]),
- self.stg1_high_band_net(x[:, :, bandw:]),
- ],
- dim=2,
- )
-
- h = torch.cat([x, aux1], dim=1)
- aux2 = self.stg2_full_band_net(self.stg2_bridge(h))
-
- h = torch.cat([x, aux1, aux2], dim=1)
- h = self.stg3_full_band_net(self.stg3_bridge(h))
-
- mask = torch.sigmoid(self.out(h))
- mask = F.pad(
- input=mask,
- pad=(0, 0, 0, self.output_bin - mask.size()[2]),
- mode="replicate",
- )
-
- if self.training:
- aux1 = torch.sigmoid(self.aux1_out(aux1))
- aux1 = F.pad(
- input=aux1,
- pad=(0, 0, 0, self.output_bin - aux1.size()[2]),
- mode="replicate",
- )
- aux2 = torch.sigmoid(self.aux2_out(aux2))
- aux2 = F.pad(
- input=aux2,
- pad=(0, 0, 0, self.output_bin - aux2.size()[2]),
- mode="replicate",
- )
- return mask * mix, aux1 * mix, aux2 * mix
- else:
- if aggressiveness:
- mask[:, :, : aggressiveness["split_bin"]] = torch.pow(
- mask[:, :, : aggressiveness["split_bin"]],
- 1 + aggressiveness["value"] / 3,
- )
- mask[:, :, aggressiveness["split_bin"] :] = torch.pow(
- mask[:, :, aggressiveness["split_bin"] :],
- 1 + aggressiveness["value"],
- )
-
- return mask * mix
-
- def predict(self, x_mag, aggressiveness=None):
- h = self.forward(x_mag, aggressiveness)
-
- if self.offset > 0:
- h = h[:, :, :, self.offset : -self.offset]
- assert h.size()[3] > 0
-
- return h
diff --git a/spaces/GAIR/Factool/factool/code/helper/postprocess.py b/spaces/GAIR/Factool/factool/code/helper/postprocess.py
deleted file mode 100644
index 96af0250f46c7958a4bc3972f1cea45731bc089a..0000000000000000000000000000000000000000
--- a/spaces/GAIR/Factool/factool/code/helper/postprocess.py
+++ /dev/null
@@ -1,40 +0,0 @@
-# Copyright (c) Microsoft Corporation.
-# Licensed under the MIT license.
-
-from collections import defaultdict
-
-from factool.code.helper.io_utils import Tools
-
-STOP_TOKEN = ['\nclass', '\ndef', '\n#', '\nif', '\nprint']
-
-class PostProcessor:
- @staticmethod
- def map_task_id_for_solution(predict_path, source_path):
- database = dict()
- raw_problems = Tools.load_tasks(source_path)
- for task_id in raw_problems.keys():
- database[raw_problems[task_id]['prompt']] = raw_problems[task_id]
-
- result = []
- predictions = Tools.load_jsonl(predict_path)
-
- for pre in predictions:
- task = database[pre['prompt']]
-
- for sample in pre['samples']:
- processed_code = PostProcessor.solution_extract(sample)
- result.append({
- 'task_id': task['task_id'],
- 'prompt': pre['prompt'],
- 'test': task['test'],
- 'entry_point': task['entry_point'],
- 'completion': processed_code
- })
- return result, len(raw_problems)
-
- @staticmethod
- def solution_extract(content):
- for identifier in STOP_TOKEN:
- if identifier in content:
- content = content.split(identifier)[0]
- return content
\ No newline at end of file
diff --git a/spaces/GXSA/bingo/tests/kblob.ts b/spaces/GXSA/bingo/tests/kblob.ts
deleted file mode 100644
index 9e15b41c1c94a690beb61b23cdb42fc78767ccd2..0000000000000000000000000000000000000000
--- a/spaces/GXSA/bingo/tests/kblob.ts
+++ /dev/null
@@ -1,27 +0,0 @@
-import FormData from 'form-data'
-
-import { fetch } from '@/lib/isomorphic'
-
-const formData = new FormData()
-
-const knowledgeRequest = {"imageInfo":{"url":"https://www.baidu.com/img/PCfb_5bf082d29588c07f842ccde3f97243ea.png"},"knowledgeRequest":{"invokedSkills":["ImageById"],"subscriptionId":"Bing.Chat.Multimodal","invokedSkillsRequestData":{"enableFaceBlur":true},"convoData":{"convoid":"51D|BingProdUnAuthenticatedUsers|E3DCA904FF236C67C3450163BCEC64CFF3F618CC8A4AFD75FD518F5ED0ADA080","convotone":"Creative"}}}
-
-formData.append('knowledgeRequest', JSON.stringify(knowledgeRequest))
-
-
-fetch('https://bing.vcanbb.top/images/kblob',
- {
- method: 'POST',
- body: formData.getBuffer(),
- headers: {
- "sec-ch-ua": "\"Not/A)Brand\";v=\"99\", \"Google Chrome\";v=\"115\", \"Chromium\";v=\"115\"",
- "sec-ch-ua-mobile": "?0",
- "sec-ch-ua-platform": "\"Windows\"",
- "Referer": "https://bing.vcanbb.top/web/index.html",
- "Referrer-Policy": "origin-when-cross-origin",
- ...formData.getHeaders()
- }
-
- }
-).then(res => res.text())
-.then(res => console.log('res', res))
diff --git a/spaces/Gen-Sim/Gen-Sim/misc/analyze_stats_order.py b/spaces/Gen-Sim/Gen-Sim/misc/analyze_stats_order.py
deleted file mode 100644
index 66e0cc8204a49e54563305bedb980c9be4d629fd..0000000000000000000000000000000000000000
--- a/spaces/Gen-Sim/Gen-Sim/misc/analyze_stats_order.py
+++ /dev/null
@@ -1,76 +0,0 @@
-import matplotlib as mpl
-
-mpl.use("Agg")
-import argparse
-import os
-import pandas as pd
-import seaborn as sns
-import matplotlib.pyplot as plt
-import matplotlib
-import IPython
-
-font = {
- "size": 22,
-}
-matplotlib.rc("font", **font)
-sns.set_context("paper", font_scale=2.0)
-
-
-def mkdir_if_missing(dst_dir):
- if not os.path.exists(dst_dir):
- os.makedirs(dst_dir)
-
-
-def save_figure(name, title=""):
- if len(title) > 0:
- plt.title(title)
- plt.tight_layout()
- print(f"output/output_figures/{name[:30]}")
- mkdir_if_missing(f"output/output_figures/{name[:30]}")
- plt.savefig(f"output/output_figures/{name[:30]}/output.png")
- plt.clf()
-
-
-def main(multirun_out, title):
- dfs = []
- suffix = ""
- run_num = 0
-
- for rundir in (sorted(multirun_out.split(","))):
- runpath = os.path.join('output/output_stats', rundir)
- statspath = os.path.join(runpath, "eval_results.csv")
- if os.path.exists(statspath):
- run_num += 1
- df = pd.read_csv(statspath)
- # print(df)
- # df.drop(df.iloc[-1], axis=0, inplace=True)
- # df.drop('diversity', axis=1)
- dfs.append(df)
- else:
- print("skip:", statspath)
-
- # merge dfs, which have shared column names
- df = pd.concat(dfs)
- print(df.iloc)
- title += f" run: {run_num} "
-
- # rewards
- fig, ax = plt.subplots(figsize=(16, 8))
- sns_plot = sns.barplot(
- data=df, x="metric", y="success", hue='model', errorbar=("sd", 1), palette="deep", hue_order=["gpt3", "gpt3-finetuned", "gpt3.5", "gpt3.5-finetuned", "gpt4"]
- )
-
- # label texts
- for container in ax.containers:
- ax.bar_label(container, label_type="center", fontsize="x-large", fmt="%.2f")
-
- # save plot
- save_figure(f"{multirun_out}_{title}{suffix}", title)
-
-if __name__ == "__main__":
- parser = argparse.ArgumentParser()
- parser.add_argument("--multirun_out", type=str)
- parser.add_argument("--title", type=str, default="")
-
- args = parser.parse_args()
- main(args.multirun_out, args.title)
diff --git a/spaces/Gen-Sim/Gen-Sim/scripts/supercloud/run_interactive_script.sh b/spaces/Gen-Sim/Gen-Sim/scripts/supercloud/run_interactive_script.sh
deleted file mode 100644
index 6c4b8291cd2cb8e8c0dd8afe027fd129e0ca1b7f..0000000000000000000000000000000000000000
--- a/spaces/Gen-Sim/Gen-Sim/scripts/supercloud/run_interactive_script.sh
+++ /dev/null
@@ -1,7 +0,0 @@
-#!/bin/bash
-#SBATCH -c 10
-#SBATCH -n 1
-#SBATCH -o logs/%j.out
-#SBATCH --exclusive
-
-CMD=$1
diff --git a/spaces/Gradio-Blocks/HairCLIP/app.py b/spaces/Gradio-Blocks/HairCLIP/app.py
deleted file mode 100644
index 90595ea55d70f8ab7967b1bc02924158b687dcfe..0000000000000000000000000000000000000000
--- a/spaces/Gradio-Blocks/HairCLIP/app.py
+++ /dev/null
@@ -1,104 +0,0 @@
-#!/usr/bin/env python
-
-from __future__ import annotations
-
-import pathlib
-
-import gradio as gr
-
-from model import Model
-
-DESCRIPTION = '''# [HairCLIP](https://github.com/wty-ustc/HairCLIP)
-
-
-'''
-
-
-def load_hairstyle_list() -> list[str]:
- with open('HairCLIP/mapper/hairstyle_list.txt') as f:
- lines = [line.strip() for line in f.readlines()]
- lines = [line[:-10] for line in lines]
- return lines
-
-
-def set_example_image(example: list) -> dict:
- return gr.Image.update(value=example[0])
-
-
-def update_step2_components(choice: str) -> tuple[dict, dict]:
- return (
- gr.Dropdown.update(visible=choice in ['hairstyle', 'both']),
- gr.Textbox.update(visible=choice in ['color', 'both']),
- )
-
-
-model = Model()
-
-with gr.Blocks(css='style.css') as demo:
- gr.Markdown(DESCRIPTION)
- with gr.Box():
- gr.Markdown('## Step 1')
- with gr.Row():
- with gr.Column():
- with gr.Row():
- input_image = gr.Image(label='Input Image',
- type='filepath')
- with gr.Row():
- preprocess_button = gr.Button('Preprocess')
- with gr.Column():
- aligned_face = gr.Image(label='Aligned Face',
- type='pil',
- interactive=False)
- with gr.Column():
- reconstructed_face = gr.Image(label='Reconstructed Face',
- type='numpy')
- latent = gr.Variable()
-
- with gr.Row():
- paths = sorted(pathlib.Path('images').glob('*.jpg'))
- gr.Examples(examples=[[path.as_posix()] for path in paths],
- inputs=input_image)
-
- with gr.Box():
- gr.Markdown('## Step 2')
- with gr.Row():
- with gr.Column():
- with gr.Row():
- editing_type = gr.Radio(
- label='Editing Type',
- choices=['hairstyle', 'color', 'both'],
- value='both',
- type='value')
- with gr.Row():
- hairstyles = load_hairstyle_list()
- hairstyle_index = gr.Dropdown(label='Hairstyle',
- choices=hairstyles,
- value='afro',
- type='index')
- with gr.Row():
- color_description = gr.Textbox(label='Color', value='red')
- with gr.Row():
- run_button = gr.Button('Run')
-
- with gr.Column():
- result = gr.Image(label='Result')
-
- preprocess_button.click(fn=model.detect_and_align_face,
- inputs=input_image,
- outputs=aligned_face)
- aligned_face.change(fn=model.reconstruct_face,
- inputs=aligned_face,
- outputs=[reconstructed_face, latent])
- editing_type.change(fn=update_step2_components,
- inputs=editing_type,
- outputs=[hairstyle_index, color_description])
- run_button.click(fn=model.generate,
- inputs=[
- editing_type,
- hairstyle_index,
- color_description,
- latent,
- ],
- outputs=result)
-
-demo.queue(max_size=10).launch()
diff --git a/spaces/Gradio-Blocks/uniformer_image_detection/configs/mask_rcnn/mask_rcnn_r50_fpn_1x_coco.py b/spaces/Gradio-Blocks/uniformer_image_detection/configs/mask_rcnn/mask_rcnn_r50_fpn_1x_coco.py
deleted file mode 100644
index 6a6c92460f1d58b8e8d361fb56ee123f2668ad9f..0000000000000000000000000000000000000000
--- a/spaces/Gradio-Blocks/uniformer_image_detection/configs/mask_rcnn/mask_rcnn_r50_fpn_1x_coco.py
+++ /dev/null
@@ -1,5 +0,0 @@
-_base_ = [
- '../_base_/models/mask_rcnn_r50_fpn.py',
- '../_base_/datasets/coco_instance.py',
- '../_base_/schedules/schedule_1x.py', '../_base_/default_runtime.py'
-]
diff --git a/spaces/Gradio-Blocks/uniformer_image_segmentation/configs/deeplabv3/deeplabv3_r101-d8_769x769_80k_cityscapes.py b/spaces/Gradio-Blocks/uniformer_image_segmentation/configs/deeplabv3/deeplabv3_r101-d8_769x769_80k_cityscapes.py
deleted file mode 100644
index 13094a98ee9be3cf8c88370e1e111cb4dde03ec4..0000000000000000000000000000000000000000
--- a/spaces/Gradio-Blocks/uniformer_image_segmentation/configs/deeplabv3/deeplabv3_r101-d8_769x769_80k_cityscapes.py
+++ /dev/null
@@ -1,2 +0,0 @@
-_base_ = './deeplabv3_r50-d8_769x769_80k_cityscapes.py'
-model = dict(pretrained='open-mmlab://resnet101_v1c', backbone=dict(depth=101))
diff --git a/spaces/GrandaddyShmax/AudioCraft_Plus/audiocraft/data/__init__.py b/spaces/GrandaddyShmax/AudioCraft_Plus/audiocraft/data/__init__.py
deleted file mode 100644
index 2906ff12bc85a894837579f3137f6f71a0438329..0000000000000000000000000000000000000000
--- a/spaces/GrandaddyShmax/AudioCraft_Plus/audiocraft/data/__init__.py
+++ /dev/null
@@ -1,10 +0,0 @@
-# Copyright (c) Meta Platforms, Inc. and affiliates.
-# All rights reserved.
-#
-# This source code is licensed under the license found in the
-# LICENSE file in the root directory of this source tree.
-"""Audio loading and writing support. Datasets for raw audio
-or also including some metadata."""
-
-# flake8: noqa
-from . import audio, audio_dataset, info_audio_dataset, music_dataset, sound_dataset
diff --git a/spaces/HaHaBill/LandShapes-Antarctica/netdissect/edit.html b/spaces/HaHaBill/LandShapes-Antarctica/netdissect/edit.html
deleted file mode 100644
index 9aac30bb08171c4c58eb936f9ba382e85a184803..0000000000000000000000000000000000000000
--- a/spaces/HaHaBill/LandShapes-Antarctica/netdissect/edit.html
+++ /dev/null
@@ -1,805 +0,0 @@
-
-
-
-
-
-
-
-
-
-
-
-
-
-
-
-
-
-
-
-
-
-
-
-
-
-
-
-
-
-
-
-
-
-
-
-
-
-
-
-
-
{{urec.iou_label}}
-
{{urec.layer}}{{urec.unit}}
-
-
-
-
-
-
-
-
-
-
-
-
-
-
-
-
-
-
-
-
-
Seeds to generate
-
-To transfer activations from one pixel to another (1) click on a source pixel
-on the left image and (2) click on a target pixel on a right image,
-then (3) choose a set of units to insert in the palette.
-
-
#{{ ex.id }}
-
-
-
-
-
-
-
-
-
-
-
-
-
-
-
-
-
-
-
-
-
-
-
-
-
-
-
-
-
diff --git a/spaces/Hallucinate/demo/midas/backbones/vit.py b/spaces/Hallucinate/demo/midas/backbones/vit.py
deleted file mode 100644
index 413f9693bd4548342280e329c9128c1a52cea920..0000000000000000000000000000000000000000
--- a/spaces/Hallucinate/demo/midas/backbones/vit.py
+++ /dev/null
@@ -1,221 +0,0 @@
-import torch
-import torch.nn as nn
-import timm
-import types
-import math
-import torch.nn.functional as F
-
-from .utils import (activations, forward_adapted_unflatten, get_activation, get_readout_oper,
- make_backbone_default, Transpose)
-
-
-def forward_vit(pretrained, x):
- return forward_adapted_unflatten(pretrained, x, "forward_flex")
-
-
-def _resize_pos_embed(self, posemb, gs_h, gs_w):
- posemb_tok, posemb_grid = (
- posemb[:, : self.start_index],
- posemb[0, self.start_index:],
- )
-
- gs_old = int(math.sqrt(len(posemb_grid)))
-
- posemb_grid = posemb_grid.reshape(1, gs_old, gs_old, -1).permute(0, 3, 1, 2)
- posemb_grid = F.interpolate(posemb_grid, size=(gs_h, gs_w), mode="bilinear")
- posemb_grid = posemb_grid.permute(0, 2, 3, 1).reshape(1, gs_h * gs_w, -1)
-
- posemb = torch.cat([posemb_tok, posemb_grid], dim=1)
-
- return posemb
-
-
-def forward_flex(self, x):
- b, c, h, w = x.shape
-
- pos_embed = self._resize_pos_embed(
- self.pos_embed, h // self.patch_size[1], w // self.patch_size[0]
- )
-
- B = x.shape[0]
-
- if hasattr(self.patch_embed, "backbone"):
- x = self.patch_embed.backbone(x)
- if isinstance(x, (list, tuple)):
- x = x[-1] # last feature if backbone outputs list/tuple of features
-
- x = self.patch_embed.proj(x).flatten(2).transpose(1, 2)
-
- if getattr(self, "dist_token", None) is not None:
- cls_tokens = self.cls_token.expand(
- B, -1, -1
- ) # stole cls_tokens impl from Phil Wang, thanks
- dist_token = self.dist_token.expand(B, -1, -1)
- x = torch.cat((cls_tokens, dist_token, x), dim=1)
- else:
- if self.no_embed_class:
- x = x + pos_embed
- cls_tokens = self.cls_token.expand(
- B, -1, -1
- ) # stole cls_tokens impl from Phil Wang, thanks
- x = torch.cat((cls_tokens, x), dim=1)
-
- if not self.no_embed_class:
- x = x + pos_embed
- x = self.pos_drop(x)
-
- for blk in self.blocks:
- x = blk(x)
-
- x = self.norm(x)
-
- return x
-
-
-def _make_vit_b16_backbone(
- model,
- features=[96, 192, 384, 768],
- size=[384, 384],
- hooks=[2, 5, 8, 11],
- vit_features=768,
- use_readout="ignore",
- start_index=1,
- start_index_readout=1,
-):
- pretrained = make_backbone_default(model, features, size, hooks, vit_features, use_readout, start_index,
- start_index_readout)
-
- # We inject this function into the VisionTransformer instances so that
- # we can use it with interpolated position embeddings without modifying the library source.
- pretrained.model.forward_flex = types.MethodType(forward_flex, pretrained.model)
- pretrained.model._resize_pos_embed = types.MethodType(
- _resize_pos_embed, pretrained.model
- )
-
- return pretrained
-
-
-def _make_pretrained_vitl16_384(pretrained, use_readout="ignore", hooks=None):
- model = timm.create_model("vit_large_patch16_384", pretrained=pretrained)
-
- hooks = [5, 11, 17, 23] if hooks == None else hooks
- return _make_vit_b16_backbone(
- model,
- features=[256, 512, 1024, 1024],
- hooks=hooks,
- vit_features=1024,
- use_readout=use_readout,
- )
-
-
-def _make_pretrained_vitb16_384(pretrained, use_readout="ignore", hooks=None):
- model = timm.create_model("vit_base_patch16_384", pretrained=pretrained)
-
- hooks = [2, 5, 8, 11] if hooks == None else hooks
- return _make_vit_b16_backbone(
- model, features=[96, 192, 384, 768], hooks=hooks, use_readout=use_readout
- )
-
-
-def _make_vit_b_rn50_backbone(
- model,
- features=[256, 512, 768, 768],
- size=[384, 384],
- hooks=[0, 1, 8, 11],
- vit_features=768,
- patch_size=[16, 16],
- number_stages=2,
- use_vit_only=False,
- use_readout="ignore",
- start_index=1,
-):
- pretrained = nn.Module()
-
- pretrained.model = model
-
- used_number_stages = 0 if use_vit_only else number_stages
- for s in range(used_number_stages):
- pretrained.model.patch_embed.backbone.stages[s].register_forward_hook(
- get_activation(str(s + 1))
- )
- for s in range(used_number_stages, 4):
- pretrained.model.blocks[hooks[s]].register_forward_hook(get_activation(str(s + 1)))
-
- pretrained.activations = activations
-
- readout_oper = get_readout_oper(vit_features, features, use_readout, start_index)
-
- for s in range(used_number_stages):
- value = nn.Sequential(nn.Identity(), nn.Identity(), nn.Identity())
- exec(f"pretrained.act_postprocess{s + 1}=value")
- for s in range(used_number_stages, 4):
- if s < number_stages:
- final_layer = nn.ConvTranspose2d(
- in_channels=features[s],
- out_channels=features[s],
- kernel_size=4 // (2 ** s),
- stride=4 // (2 ** s),
- padding=0,
- bias=True,
- dilation=1,
- groups=1,
- )
- elif s > number_stages:
- final_layer = nn.Conv2d(
- in_channels=features[3],
- out_channels=features[3],
- kernel_size=3,
- stride=2,
- padding=1,
- )
- else:
- final_layer = None
-
- layers = [
- readout_oper[s],
- Transpose(1, 2),
- nn.Unflatten(2, torch.Size([size[0] // 16, size[1] // 16])),
- nn.Conv2d(
- in_channels=vit_features,
- out_channels=features[s],
- kernel_size=1,
- stride=1,
- padding=0,
- ),
- ]
- if final_layer is not None:
- layers.append(final_layer)
-
- value = nn.Sequential(*layers)
- exec(f"pretrained.act_postprocess{s + 1}=value")
-
- pretrained.model.start_index = start_index
- pretrained.model.patch_size = patch_size
-
- # We inject this function into the VisionTransformer instances so that
- # we can use it with interpolated position embeddings without modifying the library source.
- pretrained.model.forward_flex = types.MethodType(forward_flex, pretrained.model)
-
- # We inject this function into the VisionTransformer instances so that
- # we can use it with interpolated position embeddings without modifying the library source.
- pretrained.model._resize_pos_embed = types.MethodType(
- _resize_pos_embed, pretrained.model
- )
-
- return pretrained
-
-
-def _make_pretrained_vitb_rn50_384(
- pretrained, use_readout="ignore", hooks=None, use_vit_only=False
-):
- model = timm.create_model("vit_base_resnet50_384", pretrained=pretrained)
-
- hooks = [0, 1, 8, 11] if hooks == None else hooks
- return _make_vit_b_rn50_backbone(
- model,
- features=[256, 512, 768, 768],
- size=[384, 384],
- hooks=hooks,
- use_vit_only=use_vit_only,
- use_readout=use_readout,
- )
diff --git a/spaces/HaloMaster/chinesesummary/fengshen/models/megatron_t5/modeling_megatron_t5.py b/spaces/HaloMaster/chinesesummary/fengshen/models/megatron_t5/modeling_megatron_t5.py
deleted file mode 100644
index 82ad4fb8126b9a4c0b0bb7debed95b999b5cf097..0000000000000000000000000000000000000000
--- a/spaces/HaloMaster/chinesesummary/fengshen/models/megatron_t5/modeling_megatron_t5.py
+++ /dev/null
@@ -1,2086 +0,0 @@
-# coding=utf-8
-# Copyright 2021 The IDEA Authors. All rights reserved.
-
-# Licensed under the Apache License, Version 2.0 (the "License");
-# you may not use this file except in compliance with the License.
-# You may obtain a copy of the License at
-
-# http://www.apache.org/licenses/LICENSE-2.0
-
-# Unless required by applicable law or agreed to in writing, software
-# distributed under the License is distributed on an "AS IS" BASIS,
-# WITHOUT WARRANTIES OR CONDITIONS OF ANY KIND, either express or implied.
-# See the License for the specific language governing permissions and
-# limitations under the License.
-""" PyTorch T5 model. """
-
-
-import copy
-import math
-import os
-import warnings
-
-import torch
-from torch import nn
-from torch.nn import CrossEntropyLoss
-from torch.utils.checkpoint import checkpoint
-
-from transformers.activations import ACT2FN
-from transformers.file_utils import (
- DUMMY_INPUTS,
- DUMMY_MASK,
- add_start_docstrings,
- add_start_docstrings_to_model_forward,
- is_torch_fx_proxy,
- replace_return_docstrings,
-)
-from transformers.modeling_outputs import (
- BaseModelOutput,
- BaseModelOutputWithPastAndCrossAttentions,
- Seq2SeqLMOutput,
- Seq2SeqModelOutput,
-)
-from transformers.modeling_utils import PreTrainedModel, find_pruneable_heads_and_indices, prune_linear_layer
-from transformers.utils import logging
-from transformers.utils.model_parallel_utils import assert_device_map, get_device_map
-from .configuration_megatron_t5 import T5Config
-import numpy as np
-
-logger = logging.get_logger(__name__)
-
-_CONFIG_FOR_DOC = "T5Config"
-_TOKENIZER_FOR_DOC = "T5Tokenizer"
-_CHECKPOINT_FOR_DOC = "T5-small"
-
-####################################################
-# This dict contains ids and associated url
-# for the pretrained weights provided with the models
-####################################################
-T5_PRETRAINED_MODEL_ARCHIVE_LIST = [
- "T5-small",
- "T5-base",
- "T5-large",
- "T5-3b",
- "T5-11b",
- # See all T5 models at https://huggingface.co/models?filter=T5
-]
-
-
-####################################################
-# This is a conversion method from TF 1.0 to PyTorch
-# More details: https://medium.com/huggingface/from-tensorflow-to-pytorch-265f40ef2a28
-####################################################
-
-def load_tf_weights_in_T5(model, config, tf_checkpoint_path):
- """Load tf checkpoints in a pytorch model."""
- try:
- import re
-
- import numpy as np
- import tensorflow as tf
- except ImportError:
- logger.error(
- "Loading a TensorFlow model in PyTorch, requires TensorFlow to be installed. Please see "
- "https://www.tensorflow.org/install/ for installation instructions."
- )
- raise
- tf_path = os.path.abspath(tf_checkpoint_path)
- logger.info(f"Converting TensorFlow checkpoint from {tf_path}")
- # Load weights from TF model
- init_vars = tf.train.list_variables(tf_path)
- names = []
- tf_weights = {}
- for name, shape in init_vars:
- logger.info(f"Loading TF weight {name} with shape {shape}")
- array = tf.train.load_variable(tf_path, name)
- names.append(name)
- tf_weights[name] = array
-
- for txt_name in names:
- name = txt_name.split("/")
- # adam_v and adam_m are variables used in AdamWeightDecayOptimizer to calculated m and v
- # which are not required for using pretrained model
- if any(
- n in ["adam_v", "adam_m", "AdamWeightDecayOptimizer",
- "AdamWeightDecayOptimizer_1", "global_step"]
- for n in name
- ):
- logger.info(f"Skipping {'/'.join(name)}")
- tf_weights.pop(txt_name, None)
- continue
- if "_slot_" in name[-1]:
- logger.info(f"Skipping {'/'.join(name)}")
- tf_weights.pop(txt_name, None)
- continue
- pointer = model
- array = tf_weights[txt_name]
-
- for m_name in name:
- if re.fullmatch(r"[A-Za-z]+_\d+", m_name):
- scope_names = re.split(r"_(\d+)", m_name)
- else:
- scope_names = [m_name]
- if scope_names[0] in ["kernel", "scale", "embedding"]:
- pointer = getattr(pointer, "weight")
- elif scope_names[0] == "self_attention":
- pointer = getattr(pointer, "layer")
- pointer = pointer[0]
- elif scope_names[0] == "enc_dec_attention":
- pointer = getattr(pointer, "layer")
- pointer = pointer[1]
- elif scope_names[0] == "dense_relu_dense":
- pointer = getattr(pointer, "layer")
- pointer = pointer[2]
- elif scope_names[0] == "rms_norm":
- if hasattr(pointer, "layer_norm"):
- pointer = getattr(pointer, "layer_norm")
- elif hasattr(pointer, "final_layer_norm"):
- pointer = getattr(pointer, "final_layer_norm")
- elif scope_names[0] == "scale":
- pointer = getattr(pointer, "weight")
- elif scope_names[0] == "output_bias" or scope_names[0] == "beta":
- pointer = getattr(pointer, "bias")
- elif scope_names[0] == "squad":
- pointer = getattr(pointer, "classifier")
- elif scope_names[0] == "decoder" and name[1] == "logits":
- continue
- elif scope_names[0] == "logits":
- pointer = getattr(pointer, "lm_head")
- elif scope_names[0] == "wi" and len(scope_names) > 1 and scope_names[1].isdigit():
- pointer = getattr(pointer, f"wi_{scope_names[1]}")
- continue
- else:
- try:
- pointer = getattr(pointer, scope_names[0])
- except AttributeError:
- logger.info(f"Skipping {'/'.join(name)}")
- continue
- if len(scope_names) >= 2:
- num = int(scope_names[1])
- pointer = pointer[num]
- if scope_names[0] not in ["kernel", "scale", "embedding"]:
- pointer = getattr(pointer, "weight")
- if scope_names[0] != "embedding":
- logger.info(
- f"Transposing numpy weight of shape {array.shape} for {name}")
- array = np.transpose(array)
- try:
- assert (
- pointer.shape == array.shape
- ), f"Pointer shape {pointer.shape} and array shape {array.shape} mismatched"
- except AssertionError as e:
- e.args += (pointer.shape, array.shape)
- raise
- logger.info(f"Initialize PyTorch weight {name}")
- pointer.data = torch.from_numpy(array.astype(np.float32))
- tf_weights.pop(txt_name, None)
-
- logger.info(
- f"Weights not copied to PyTorch model: {', '.join(tf_weights.keys())}.")
- return model
-
-
-####################################################
-# PyTorch Models are constructed by sub-classing
-# - torch.nn.Module for the layers and
-# - PreTrainedModel for the models (it-self a sub-class of nn.Module)
-####################################################
-PARALLELIZE_DOCSTRING = r"""
- This is an experimental feature and is a subject to change at a moment's notice.
-
- Uses a device map to distribute attention modules of the model across several devices. If no device map is given,
- it will evenly distribute blocks across all devices.
-
- Args:
- device_map (:obj:`Dict[int, list]`, optional, defaults to None):
- A dictionary that maps attention modules to devices. Note that the embedding module and LMHead are always
- automatically mapped to the first device (for esoteric reasons). That means that the first device should
- have fewer attention modules mapped to it than other devices. For reference, the T5 models have the
- following number of attention modules:
-
- - T5-small: 6
- - T5-base: 12
- - T5-large: 24
- - T5-3b: 24
- - T5-11b: 24
-
- Example::
-
- # Here is an example of a device map on a machine with 4 GPUs using T5-3b,
- # which has a total of 24 attention modules:
- model = T5ForConditionalGeneration.from_pretrained('T5-3b')
- device_map = {0: [0, 1, 2],
-
- 1: [3, 4, 5, 6, 7, 8, 9],
- 2: [10, 11, 12, 13, 14, 15, 16],
- 3: [17, 18, 19, 20, 21, 22, 23]}
- model.parallelize(device_map)
-"""
-DEPARALLELIZE_DOCSTRING = r"""
- Moves the model to cpu from a model parallel state.
-
- Example::
-
- # On a 4 GPU machine with T5-3b:
- model = T5ForConditionalGeneration.from_pretrained('T5-3b')
- device_map = {0: [0, 1, 2],
-
- 1: [3, 4, 5, 6, 7, 8, 9],
- 2: [10, 11, 12, 13, 14, 15, 16],
- 3: [17, 18, 19, 20, 21, 22, 23]}
- model.parallelize(device_map) # Splits the model across several devices
- model.deparallelize() # Put the model back on cpu and cleans memory by calling torch.cuda.empty_cache()
-"""
-
-
-class T5LayerNorm(nn.Module):
- def __init__(self, hidden_size, eps=1e-6):
- """
- Construct a layernorm module in the T5 style No bias and no subtraction of mean.
- """
- super().__init__()
- self.weight = nn.Parameter(torch.ones(hidden_size))
- self.variance_epsilon = eps
-
- def forward(self, hidden_states):
- # layer norm should always be calculated in float32
- variance = hidden_states.to(torch.float32).pow(
- 2).mean(-1, keepdim=True)
- hidden_states = hidden_states * \
- torch.rsqrt(variance + self.variance_epsilon)
-
- # convert into float16 if necessary
- if self.weight.dtype == torch.float16:
- hidden_states = hidden_states.to(torch.float16)
- return self.weight * hidden_states
-
-
-class T5DenseReluDense(nn.Module):
- def __init__(self, config):
- super().__init__()
- # @IDEA modified -> bias=False -> bias=True
- self.wi = nn.Linear(config.d_model, config.d_ff, bias=True)
- self.wo = nn.Linear(config.d_ff, config.d_model, bias=True)
- self.dropout = nn.Dropout(config.dropout_rate)
-
- def forward(self, hidden_states):
- hidden_states = self.wi(hidden_states)
- hidden_states = nn.functional.relu(hidden_states)
- hidden_states = self.dropout(hidden_states)
- hidden_states = self.wo(hidden_states)
- return hidden_states
-
-
-class T5DenseGeluDense(nn.Module):
- def __init__(self, config):
- super().__init__()
- # @IDEA modified -> bias=False -> bias=True
- self.wi = nn.Linear(config.d_model, config.d_ff, bias=True)
- self.wo = nn.Linear(config.d_ff, config.d_model, bias=True)
- self.dropout = nn.Dropout(config.dropout_rate)
-
- def forward(self, hidden_states):
- hidden_states = self.wi(hidden_states)
- hidden_states = nn.functional.gelu(hidden_states)
- hidden_states = self.dropout(hidden_states)
- hidden_states = self.wo(hidden_states)
- return hidden_states
-
-
-class T5DenseGatedGeluDense(nn.Module):
- def __init__(self, config):
- super().__init__()
- # @IDEA modified -> bias=False -> bias=True
- self.wi_0 = nn.Linear(config.d_model, config.d_ff, bias=True)
- self.wi_1 = nn.Linear(config.d_model, config.d_ff, bias=True)
- self.wo = nn.Linear(config.d_ff, config.d_model, bias=True)
- self.dropout = nn.Dropout(config.dropout_rate)
- self.gelu_act = ACT2FN["gelu_new"]
-
- def forward(self, hidden_states):
- hidden_gelu = self.gelu_act(self.wi_0(hidden_states))
- hidden_linear = self.wi_1(hidden_states)
- hidden_states = hidden_gelu * hidden_linear
- hidden_states = self.dropout(hidden_states)
- hidden_states = self.wo(hidden_states)
- return hidden_states
-
-
-class T5LayerFF(nn.Module):
- def __init__(self, config):
- super().__init__()
- # @IDEA modified -> T5LayerNorm -> nn.LayerNorm
- # self.layer_norm = T5LayerNorm(config.d_model, eps=config.layer_norm_epsilon)
- self.layer_norm = nn.LayerNorm(
- config.d_model, eps=config.layer_norm_epsilon)
- if config.feed_forward_proj == "relu":
- self.DenseReluDense = T5DenseReluDense(config)
- elif config.feed_forward_proj == "gelu":
- self.DenseReluDense = T5DenseGeluDense(config)
- else:
- raise ValueError(
- f"{self.config.feed_forward_proj} is not supported. Choose between `relu` and `gated-gelu`"
- )
- self.dropout = nn.Dropout(config.dropout_rate)
-
- def forward(self, hidden_states):
- forwarded_states = self.layer_norm(hidden_states)
- forwarded_states = self.DenseReluDense(forwarded_states)
- hidden_states = hidden_states + self.dropout(forwarded_states)
- return hidden_states
-
-
-class T5Attention(nn.Module):
- def __init__(self, config: T5Config, has_relative_attention_bias=False):
- super().__init__()
- self.is_decoder = config.is_decoder
- self.has_relative_attention_bias = has_relative_attention_bias
-
- self.relative_attention_num_buckets = config.relative_attention_num_buckets
- self.d_model = config.d_model
- self.key_value_proj_dim = config.d_kv
- self.n_heads = config.num_heads
- self.dropout = config.dropout_rate
- self.inner_dim = self.n_heads * self.key_value_proj_dim
-
- # Mesh TensorFlow initialization to avoid scaling before softmax
- # @IDEA modified -> bias=False -> bias=True
-
- self.q = nn.Linear(self.d_model, self.inner_dim, bias=True)
- self.k = nn.Linear(self.d_model, self.inner_dim, bias=True)
- self.v = nn.Linear(self.d_model, self.inner_dim, bias=True)
-
- self.o = nn.Linear(self.inner_dim, self.d_model, bias=True)
-
- if self.has_relative_attention_bias:
- self.relative_attention_bias = nn.Embedding(
- self.relative_attention_num_buckets, self.n_heads)
- self.pruned_heads = set()
- self.gradient_checkpointing = False
-
- def prune_heads(self, heads):
- if len(heads) == 0:
- return
- heads, index = find_pruneable_heads_and_indices(
- heads, self.n_heads, self.key_value_proj_dim, self.pruned_heads
- )
- # Prune linear layers
- self.q = prune_linear_layer(self.q, index)
- self.k = prune_linear_layer(self.k, index)
- self.v = prune_linear_layer(self.v, index)
-
- self.o = prune_linear_layer(self.o, index, dim=1)
- # Update hyper params
- self.n_heads = self.n_heads - len(heads)
- self.inner_dim = self.key_value_proj_dim * self.n_heads
- self.pruned_heads = self.pruned_heads.union(heads)
-
- @staticmethod
- def _relative_position_bucket(relative_position, bidirectional=True, num_buckets=32, max_distance=128):
- """
- Adapted from Mesh Tensorflow:
- https://github.com/tensorflow/mesh/blob/0cb87fe07da627bf0b7e60475d59f95ed6b5be3d/mesh_tensorflow/transformer/transformer_layers.py#L593
-
- Translate relative position to a bucket number for relative attention. The relative position is defined as
- memory_position - query_position, i.e. the distance in tokens from the attending position to the attended-to
- position. If bidirectional=False, then positive relative positions are invalid. We use smaller buckets for
- small absolute relative_position and larger buckets for larger absolute relative_positions. All relative
- positions >=max_distance map to the same bucket. All relative positions <=-max_distance map to the same bucket.
- This should allow for more graceful generalization to longer sequences than the model has been trained on
-
- Args:
- relative_position: an int32 Tensor
- bidirectional: a boolean - whether the attention is bidirectional
- num_buckets: an integer
- max_distance: an integer
-
- Returns:
- a Tensor with the same shape as relative_position, containing int32 values in the range [0, num_buckets)
- """
- relative_buckets = 0
- if bidirectional:
- num_buckets //= 2
- relative_buckets += (relative_position >
- 0).to(torch.long) * num_buckets
- relative_position = torch.abs(relative_position)
- else:
- relative_position = - \
- torch.min(relative_position,
- torch.zeros_like(relative_position))
- # now relative_position is in the range [0, inf)
-
- # half of the buckets are for exact increments in positions
- max_exact = num_buckets // 2
- is_small = relative_position < max_exact
-
- # The other half of the buckets are for logarithmically bigger bins in positions up to max_distance
- relative_postion_if_large = max_exact + (
- torch.log(relative_position.float() / max_exact)
- / math.log(max_distance / max_exact)
- * (num_buckets - max_exact)
- ).to(torch.long)
- relative_postion_if_large = torch.min(
- relative_postion_if_large, torch.full_like(
- relative_postion_if_large, num_buckets - 1)
- )
-
- relative_buckets += torch.where(is_small,
- relative_position, relative_postion_if_large)
- return relative_buckets
-
- def compute_bias(self, query_length, key_length):
- """Compute binned relative position bias"""
- context_position = torch.arange(
- query_length, dtype=torch.long, device=self.relative_attention_bias.weight.device
- )[:, None]
- memory_position = torch.arange(
- key_length, dtype=torch.long, device=self.relative_attention_bias.weight.device
- )[None, :]
- relative_position = memory_position - \
- context_position # shape (query_length, key_length)
- relative_position_bucket = self._relative_position_bucket(
- relative_position, # shape (query_length, key_length)
- bidirectional=(not self.is_decoder),
- num_buckets=self.relative_attention_num_buckets,
- )
- # shape (query_length, key_length, num_heads)
- values = self.relative_attention_bias(relative_position_bucket)
- # shape (1, num_heads, query_length, key_length)
- values = values.permute([2, 0, 1]).unsqueeze(0)
- return values
-
- def forward(
- self,
- hidden_states,
- mask=None,
- key_value_states=None,
- position_bias=None,
- past_key_value=None,
- layer_head_mask=None,
- query_length=None,
- use_cache=False,
- output_attentions=False,
- ):
- """
- Self-attention (if key_value_states is None) or attention over source sentence (provided by key_value_states).
- """
- # Input is (batch_size, seq_length, dim)
- # Mask is (batch_size, key_length) (non-causal) or (batch_size, key_length, key_length)
- # past_key_value[0] is (batch_size, n_heads, q_len - 1, dim_per_head)
- batch_size, seq_length = hidden_states.shape[:2]
-
- real_seq_length = seq_length
-
- if past_key_value is not None:
- assert (
- len(past_key_value) == 2
- ), f"past_key_value should have 2 past states: keys and values. Got { len(past_key_value)} past states"
- real_seq_length += past_key_value[0].shape[2] if query_length is None else query_length
-
- key_length = real_seq_length if key_value_states is None else key_value_states.shape[
- 1]
-
- def shape(states):
- """projection"""
- return states.view(batch_size, -1, self.n_heads, self.key_value_proj_dim).transpose(1, 2)
-
- def unshape(states):
- """reshape"""
- return states.transpose(1, 2).contiguous().view(batch_size, -1, self.inner_dim)
-
- def project(hidden_states, proj_layer, key_value_states, past_key_value):
- """projects hidden states correctly to key/query states"""
- if key_value_states is None:
- # self-attn
- # (batch_size, n_heads, seq_length, dim_per_head)
- hidden_states = shape(proj_layer(hidden_states))
- elif past_key_value is None:
- # cross-attn
- # (batch_size, n_heads, seq_length, dim_per_head)
- hidden_states = shape(proj_layer(key_value_states))
-
- if past_key_value is not None:
- if key_value_states is None:
- # self-attn
- # (batch_size, n_heads, key_length, dim_per_head)
- hidden_states = torch.cat(
- [past_key_value, hidden_states], dim=2)
- else:
- # cross-attn
- hidden_states = past_key_value
- return hidden_states
-
- # get query states
- # (batch_size, n_heads, seq_length, dim_per_head)
- query_states = shape(self.q(hidden_states))
-
- # get key/value states
- key_states = project(
- hidden_states, self.k, key_value_states, past_key_value[
- 0] if past_key_value is not None else None
- )
- value_states = project(
- hidden_states, self.v, key_value_states, past_key_value[
- 1] if past_key_value is not None else None
- )
-
- # compute scores
- scores = torch.matmul(
- query_states, key_states.transpose(3, 2)
- ) # equivalent of torch.einsum("bnqd,bnkd->bnqk", query_states, key_states), compatible with onnx op>9
-
- if position_bias is None:
- if not self.has_relative_attention_bias:
- position_bias = torch.zeros(
- (1, self.n_heads, real_seq_length, key_length), device=scores.device, dtype=scores.dtype
- )
- if self.gradient_checkpointing and self.training:
- position_bias.requires_grad = True
- else:
- position_bias = self.compute_bias(real_seq_length, key_length)
-
- # if key and values are already calculated
- # we want only the last query position bias
- if past_key_value is not None:
- position_bias = position_bias[:, :, -hidden_states.size(1):, :]
-
- if mask is not None:
- # (batch_size, n_heads, seq_length, key_length)
- position_bias = position_bias + mask
-
- # @IDEA modified -> delete scores += position_bias, use absolute positional
- # scores += position_bias
- scores = scores / math.sqrt(self.key_value_proj_dim)
-
- if mask is not None:
- scores = scores + mask
-
- attn_weights = nn.functional.softmax(scores.float(), dim=-1).type_as(
- scores
- ) # (batch_size, n_heads, seq_length, key_length)
-
- attn_weights = nn.functional.dropout(
- attn_weights, p=0, training=self.training
- ) # (batch_size, n_heads, seq_length, key_length)
-
- # Mask heads if we want to
- if layer_head_mask is not None:
- attn_weights = attn_weights * layer_head_mask
-
- # (batch_size, seq_length, dim)
- attn_output = unshape(torch.matmul(attn_weights, value_states))
-
- attn_output = self.o(attn_output)
-
- present_key_value_state = (key_states, value_states) if (
- self.is_decoder and use_cache) else None
- outputs = (attn_output,) + \
- (present_key_value_state,) + (position_bias,)
-
- if output_attentions:
- outputs = outputs + (attn_weights,)
- return outputs
-
-
-class T5LayerSelfAttention(nn.Module):
- def __init__(self, config, has_relative_attention_bias=False):
- super().__init__()
-
- # @IDEA modified -> T5LayerNorm -> nn.LayerNorm
- # self.layer_norm = T5LayerNorm(config.d_model, eps=config.layer_norm_epsilon)
- self.layer_norm = nn.LayerNorm(
- config.d_model, eps=config.layer_norm_epsilon)
- self.SelfAttention = T5Attention(
- config, has_relative_attention_bias=has_relative_attention_bias)
-
- self.dropout = nn.Dropout(config.dropout_rate)
-
- def forward(
- self,
- hidden_states,
- attention_mask=None,
- position_bias=None,
- layer_head_mask=None,
- past_key_value=None,
- use_cache=False,
- output_attentions=False,
- ):
- normed_hidden_states = self.layer_norm(hidden_states)
- attention_output = self.SelfAttention(
- normed_hidden_states,
- mask=attention_mask,
- position_bias=position_bias,
- layer_head_mask=layer_head_mask,
- past_key_value=past_key_value,
- use_cache=use_cache,
- output_attentions=output_attentions,
- )
-
- hidden_states = hidden_states + self.dropout(attention_output[0])
- # add attentions if we output them
- outputs = (hidden_states,) + attention_output[1:]
- return outputs
-
-
-class T5LayerCrossAttention(nn.Module):
- def __init__(self, config):
- super().__init__()
- # @IDEA modified -> T5LayerNorm -> nn.LayerNorm
- # self.layer_norm = T5LayerNorm(config.d_model, eps=config.layer_norm_epsilon)
- self.layer_norm = nn.LayerNorm(
- config.d_model, eps=config.layer_norm_epsilon)
-
- self.EncDecAttention = T5Attention(
- config, has_relative_attention_bias=False)
-
- self.dropout = nn.Dropout(config.dropout_rate)
-
- def forward(
- self,
- hidden_states,
- key_value_states,
- attention_mask=None,
- position_bias=None,
- layer_head_mask=None,
- past_key_value=None,
- use_cache=False,
- query_length=None,
- output_attentions=False,
- ):
- normed_hidden_states = self.layer_norm(hidden_states)
- attention_output = self.EncDecAttention(
- normed_hidden_states,
- mask=attention_mask,
- key_value_states=key_value_states,
- position_bias=position_bias,
- layer_head_mask=layer_head_mask,
- past_key_value=past_key_value,
- use_cache=use_cache,
- query_length=query_length,
- output_attentions=output_attentions,
- )
- layer_output = hidden_states + self.dropout(attention_output[0])
- # add attentions if we output them
- outputs = (layer_output,) + attention_output[1:]
- return outputs
-
-
-class T5Block(nn.Module):
- def __init__(self, config, has_relative_attention_bias=False):
- super().__init__()
- self.is_decoder = config.is_decoder
- # @IDEA modified ->
- # self.layer = nn.ModuleList()
- # self.layer.append(T5LayerSelfAttention(config, has_relative_attention_bias=has_relative_attention_bias))
- # if self.is_decoder:
- # self.layer.append(T5LayerCrossAttention(config))
-
- # self.layer.append(T5LayerFF(config))
-
- self.T5LayerSelfAttention = T5LayerSelfAttention(
- config, has_relative_attention_bias=has_relative_attention_bias)
- if self.is_decoder:
- self.T5LayerCrossAttention = T5LayerCrossAttention(
- config)
- self.T5LayerFF = T5LayerFF(config)
-
- def forward(
- self,
- hidden_states,
- attention_mask=None,
- position_bias=None,
- encoder_hidden_states=None,
- encoder_attention_mask=None,
- encoder_decoder_position_bias=None,
- layer_head_mask=None,
- cross_attn_layer_head_mask=None,
- past_key_value=None,
- use_cache=False,
- output_attentions=False,
- return_dict=True,
- ):
-
- if past_key_value is not None:
- assert self.is_decoder, "Only decoder can use `past_key_values`"
- expected_num_past_key_values = 2 if encoder_hidden_states is None else 4
-
- if len(past_key_value) != expected_num_past_key_values:
- raise ValueError(
- f"There should be {expected_num_past_key_values} past states. "
- f"{'2 (past / key) for cross attention. ' if expected_num_past_key_values == 4 else ''}"
- f"Got {len(past_key_value)} past key / value states"
- )
-
- self_attn_past_key_value = past_key_value[:2]
- cross_attn_past_key_value = past_key_value[2:]
- else:
- self_attn_past_key_value, cross_attn_past_key_value = None, None
-
- # @IDEA modified -> self.layer[0] -> self.T5LayerSelfAttention
- self_attention_outputs = self.T5LayerSelfAttention(
- hidden_states,
- attention_mask=attention_mask,
- position_bias=position_bias,
- layer_head_mask=layer_head_mask,
- past_key_value=self_attn_past_key_value,
- use_cache=use_cache,
- output_attentions=output_attentions,
- )
- hidden_states, present_key_value_state = self_attention_outputs[:2]
- # Keep self-attention outputs and relative position weights
- attention_outputs = self_attention_outputs[2:]
-
- # clamp inf values to enable fp16 training
- if hidden_states.dtype == torch.float16 and torch.isinf(hidden_states).any():
- clamp_value = torch.finfo(hidden_states.dtype).max - 1000
- hidden_states = torch.clamp(
- hidden_states, min=-clamp_value, max=clamp_value)
-
- do_cross_attention = self.is_decoder and encoder_hidden_states is not None
- if do_cross_attention:
- # the actual query length is unknown for cross attention
- # if using past key value states. Need to inject it here
- if present_key_value_state is not None:
- query_length = present_key_value_state[0].shape[2]
- else:
- query_length = None
- # @IDEA modified -> self.layer[1] -> self.T5LayerCrossAttention
- cross_attention_outputs = self.T5LayerCrossAttention(
- hidden_states,
- key_value_states=encoder_hidden_states,
- attention_mask=encoder_attention_mask,
- position_bias=encoder_decoder_position_bias,
- layer_head_mask=cross_attn_layer_head_mask,
- past_key_value=cross_attn_past_key_value,
- query_length=query_length,
- use_cache=use_cache,
- output_attentions=output_attentions,
- )
- hidden_states = cross_attention_outputs[0]
-
- # clamp inf values to enable fp16 training
- if hidden_states.dtype == torch.float16 and torch.isinf(hidden_states).any():
- clamp_value = torch.finfo(hidden_states.dtype).max - 1000
- hidden_states = torch.clamp(
- hidden_states, min=-clamp_value, max=clamp_value)
-
- # Combine self attn and cross attn key value states
- if present_key_value_state is not None:
- present_key_value_state = present_key_value_state + \
- cross_attention_outputs[1]
-
- # Keep cross-attention outputs and relative position weights
- attention_outputs = attention_outputs + cross_attention_outputs[2:]
-
- # Apply Feed Forward layer
- # @IDEA modified -> self.layer[-1] -> self.T5LayerFF
- hidden_states = self.T5LayerFF(hidden_states)
-
- # clamp inf values to enable fp16 training
- if hidden_states.dtype == torch.float16 and torch.isinf(hidden_states).any():
- clamp_value = torch.finfo(hidden_states.dtype).max - 1000
- hidden_states = torch.clamp(
- hidden_states, min=-clamp_value, max=clamp_value)
-
- outputs = (hidden_states,)
-
- if use_cache:
- outputs = outputs + (present_key_value_state,) + attention_outputs
- else:
- outputs = outputs + attention_outputs
-
- # hidden-states, present_key_value_states, (self-attention position bias),
- # (self-attention weights), (cross-attention position bias), (cross-attention weights)
- return outputs
-
-
-class T5PreTrainedModel(PreTrainedModel):
- """
- An abstract class to handle weights initialization and a simple interface for downloading and loading pretrained
- models.
- """
-
- config_class = T5Config
- load_tf_weights = load_tf_weights_in_T5
- base_model_prefix = "transformer"
- is_parallelizable = True
- supports_gradient_checkpointing = True
-
- @property
- def dummy_inputs(self):
- input_ids = torch.tensor(DUMMY_INPUTS)
- input_mask = torch.tensor(DUMMY_MASK)
- dummy_inputs = {
- "decoder_input_ids": input_ids,
- "input_ids": input_ids,
- "decoder_attention_mask": input_mask,
- }
- return dummy_inputs
-
- def _init_weights(self, module):
- """Initialize the weights"""
- factor = self.config.initializer_factor # Used for testing weights initialization
- if isinstance(module, T5LayerNorm):
- module.weight.data.fill_(factor * 1.0)
- elif isinstance(module, (T5Model, T5ForConditionalGeneration, T5EncoderModel)):
- # Mesh TensorFlow embeddings initialization
- # See https://github.com/tensorflow/mesh/blob/fa19d69eafc9a482aff0b59ddd96b025c0cb207d
- # /mesh_tensorflow/layers.py#L1624
- # @IDEA modified -> module.shared.weight -> module.shared.word_embeddings.weight
- # module.shared.weight.data.normal_(mean=0.0, std=factor * 1.0)
- module.shared.word_embeddings.weight.data.normal_(
- mean=0.0, std=factor * 1.0)
- module.shared.position_embeddings.weight.data.normal_(
- mean=0.0, std=factor * 1.0)
- elif isinstance(module, T5DenseReluDense):
- # Mesh TensorFlow FF initialization
- # See https://github.com/tensorflow/mesh/blob/master/mesh_tensorflow
- # /transformer/transformer_layers.py#L56
- # and https://github.com/tensorflow/mesh/blob/fa19d69eafc9a482aff0b59ddd96b025c0cb207d/
- # mesh_tensorflow/layers.py#L89
- module.wi.weight.data.normal_(
- mean=0.0, std=factor * ((self.config.d_model) ** -0.5))
- if hasattr(module.wi, "bias") and module.wi.bias is not None:
- module.wi.bias.data.zero_()
- module.wo.weight.data.normal_(
- mean=0.0, std=factor * ((self.config.d_ff) ** -0.5))
- if hasattr(module.wo, "bias") and module.wo.bias is not None:
- module.wo.bias.data.zero_()
- elif isinstance(module, T5DenseGeluDense):
- module.wi_0.weight.data.normal_(
- mean=0.0, std=factor * ((self.config.d_model) ** -0.5))
- if hasattr(module.wi_0, "bias") and module.wi_0.bias is not None:
- module.wi_0.bias.data.zero_()
- module.wi_1.weight.data.normal_(
- mean=0.0, std=factor * ((self.config.d_model) ** -0.5))
- if hasattr(module.wi, "bias") and module.wi.bias is not None:
- module.wi.bias.data.zero_()
- module.wo.weight.data.normal_(
- mean=0.0, std=factor * ((self.config.d_ff) ** -0.5))
- if hasattr(module.wo, "bias") and module.wo.bias is not None:
- module.wo.bias.data.zero_()
- elif isinstance(module, T5Attention):
- # Mesh TensorFlow attention initialization to avoid scaling before softmax
- # See https://github.com/tensorflow/mesh/blob/fa19d69eafc9a482aff0b59ddd96b025c0cb207d
- # /mesh_tensorflow/transformer/attention.py#L136
- d_model = self.config.d_model
- key_value_proj_dim = self.config.d_kv
- n_heads = self.config.num_heads
- module.q.weight.data.normal_(
- mean=0.0, std=factor * ((d_model * key_value_proj_dim) ** -0.5))
- module.k.weight.data.normal_(
- mean=0.0, std=factor * (d_model ** -0.5))
- module.v.weight.data.normal_(
- mean=0.0, std=factor * (d_model ** -0.5))
-
- module.o.weight.data.normal_(
- mean=0.0, std=factor * ((n_heads * key_value_proj_dim) ** -0.5))
- if module.has_relative_attention_bias:
- module.relative_attention_bias.weight.data.normal_(
- mean=0.0, std=factor * ((d_model) ** -0.5))
-
- def _set_gradient_checkpointing(self, module, value=False):
- if isinstance(module, (T5Attention, T5Stack)):
- module.gradient_checkpointing = value
-
- def _shift_right(self, input_ids):
- decoder_start_token_id = self.config.decoder_start_token_id
- pad_token_id = self.config.pad_token_id
-
- assert (
- decoder_start_token_id is not None
- ), "self.model.config.decoder_start_token_id has to be defined. "\
- "In T5 it is usually set to the pad_token_id. See T5 docs for more information"
-
- # shift inputs to the right
- if is_torch_fx_proxy(input_ids):
- # Item assignment is not supported natively for proxies.
- shifted_input_ids = torch.full(
- input_ids.shape[:-1] + (1,), decoder_start_token_id)
- shifted_input_ids = torch.cat(
- [shifted_input_ids, input_ids[..., :-1]], dim=-1)
- else:
- shifted_input_ids = input_ids.new_zeros(input_ids.shape)
- shifted_input_ids[..., 1:] = input_ids[..., :-1].clone()
- shifted_input_ids[..., 0] = decoder_start_token_id
-
- assert pad_token_id is not None, "self.model.config.pad_token_id has to be defined."
- # replace possible -100 values in labels by `pad_token_id`
- shifted_input_ids.masked_fill_(shifted_input_ids == -100, pad_token_id)
-
- assert torch.all(shifted_input_ids >= 0).item(
- ), "Verify that `shifted_input_ids` has only positive values"
-
- return shifted_input_ids
-
-
-class T5Embeddings(nn.Module):
- """Construct the embeddings from word, position and token_type embeddings."""
-
- def __init__(self, config):
- super().__init__()
- self.word_embeddings = nn.Embedding(
- config.vocab_size, config.hidden_size, padding_idx=config.pad_token_id)
- self.position_embeddings = nn.Embedding(
- config.max_position_embeddings, config.hidden_size)
-
- # self.LayerNorm is not snake-cased to stick with TensorFlow model variable name and be able to load
- # any TensorFlow checkpoint file
-
- # In Megatron, layer-norm is applied after the 1st dropout.
- # self.LayerNorm = nn.LayerNorm(config.hidden_size, eps=config.layer_norm_eps)
- self.dropout = nn.Dropout(config.dropout_rate)
-
- # position_ids (1, len position emb) is contiguous in memory and exported when serialized
- self.register_buffer("position_ids", torch.arange(
- config.max_position_embeddings).expand((1, -1)))
- self.position_embedding_type = getattr(
- config, "position_embedding_type", "absolute")
-
- def forward(
- self, input_ids=None, token_type_ids=None, position_ids=None, inputs_embeds=None, past_key_values_length=0
- ):
- if input_ids is not None:
- input_shape = input_ids.size()
- else:
- input_shape = inputs_embeds.size()[:-1]
-
- seq_length = input_shape[1]
-
- if position_ids is None:
- position_ids = self.position_ids[:,
- past_key_values_length: seq_length + past_key_values_length]
-
- if inputs_embeds is None:
- inputs_embeds = self.word_embeddings(input_ids)
-
- embeddings = inputs_embeds
- if self.position_embedding_type == "absolute":
- position_embeddings = self.position_embeddings(position_ids)
- embeddings += position_embeddings
-
- # Megatron BERT moves that layer norm after the drop-out (and to each layer).
- # embeddings = self.LayerNorm(embeddings)
- embeddings = self.dropout(embeddings)
- return embeddings
-
-
-class T5Stack(T5PreTrainedModel):
- def __init__(self, config, embed_tokens=None):
- super().__init__(config)
-
- self.embed_tokens = embed_tokens
- self.is_decoder = config.is_decoder
-
- # @IDEA modified -> has_relative_attention_bias=bool(i == 0)) for i in range(config.num_layers)
- # -> has_relative_attention_bias=False
- self.block = nn.ModuleList(
- [T5Block(config, has_relative_attention_bias=False)
- for _ in range(config.num_layers)]
- )
- # @IDEA modified -> T5LayerNorm -> nn.LayerNorm
- # self.final_layer_norm = T5LayerNorm(config.d_model, eps=config.layer_norm_epsilon)
- self.final_layer_norm = nn.LayerNorm(
- config.d_model, eps=config.layer_norm_epsilon)
-
- self.dropout = nn.Dropout(config.dropout_rate)
-
- self.init_weights()
- # Model parallel
- self.model_parallel = False
- self.device_map = None
- self.gradient_checkpointing = False
-
- @add_start_docstrings(PARALLELIZE_DOCSTRING)
- def parallelize(self, device_map=None):
- # Check validity of device_map
- self.device_map = (
- get_device_map(len(self.block), range(
- torch.cuda.device_count())) if device_map is None else device_map
- )
- assert_device_map(self.device_map, len(self.block))
- self.model_parallel = True
- self.first_device = "cpu" if "cpu" in self.device_map.keys() else "cuda:" + \
- str(min(self.device_map.keys()))
- self.last_device = "cuda:" + str(max(self.device_map.keys()))
- # Load onto devices
- for k, v in self.device_map.items():
- for layer in v:
- cuda_device = "cuda:" + str(k)
- self.block[layer] = self.block[layer].to(cuda_device)
-
- # Set embed_tokens to first layer
-
- self.embed_tokens = self.embed_tokens.to(self.first_device)
- self.embeddings = self.embeddings.to(self.first_device)
- # Set final layer norm to last device
- self.final_layer_norm = self.final_layer_norm.to(self.last_device)
-
- @add_start_docstrings(PARALLELIZE_DOCSTRING)
- def deparallelize(self):
- self.model_parallel = False
- self.device_map = None
- self.first_device = "cpu"
- self.last_device = "cpu"
- for i in range(len(self.block)):
- self.block[i] = self.block[i].to("cpu")
- self.embed_tokens = self.embed_tokens.to("cpu")
- self.final_layer_norm = self.final_layer_norm.to("cpu")
- torch.cuda.empty_cache()
-
- def get_input_embeddings(self):
- return self.embed_tokens
-
- def set_input_embeddings(self, new_embeddings):
- self.embed_tokens = new_embeddings
-
- def forward(
- self,
- input_ids=None,
- position_ids=None,
- attention_mask=None,
- encoder_hidden_states=None,
- encoder_attention_mask=None,
- inputs_embeds=None,
- head_mask=None,
- cross_attn_head_mask=None,
- past_key_values=None,
- use_cache=None,
- output_attentions=None,
- output_hidden_states=None,
- return_dict=None,
- ):
- # Model parallel
- if self.model_parallel:
- torch.cuda.set_device(self.first_device)
- self.embed_tokens = self.embed_tokens.to(self.first_device)
- use_cache = use_cache if use_cache is not None else self.config.use_cache
- output_attentions = output_attentions if output_attentions is not None else self.config.output_attentions
- output_hidden_states = (
- output_hidden_states if output_hidden_states is not None else self.config.output_hidden_states
- )
- return_dict = return_dict if return_dict is not None else self.config.use_return_dict
-
- if input_ids is not None and inputs_embeds is not None:
- err_msg_prefix = "decoder_" if self.is_decoder else ""
- raise ValueError(
- f"You cannot specify both {err_msg_prefix}input_ids and {err_msg_prefix}inputs_embeds at the same time"
- )
- elif input_ids is not None:
- input_shape = input_ids.size()
- input_ids = input_ids.view(-1, input_shape[-1])
- elif inputs_embeds is not None:
- input_shape = inputs_embeds.size()[:-1]
- else:
- err_msg_prefix = "decoder_" if self.is_decoder else ""
- raise ValueError(
- f"You have to specify either {err_msg_prefix}input_ids or {err_msg_prefix}inputs_embeds")
-
- if inputs_embeds is None:
- assert self.embed_tokens is not None, "You have to initialize the model with valid token embeddings"
- # @IDEA modified -> self.embed_tokens(input_ids=input_ids) ->
- # self.embed_tokens(input_ids=input_ids,osition_ids=position_ids,)
- # inputs_embeds = self.embed_tokens(input_ids=input_ids)
- inputs_embeds = self.embed_tokens(input_ids=input_ids)
-
- batch_size, seq_length = input_shape
-
- # required mask seq length can be calculated via length of past
- mask_seq_length = past_key_values[0][0].shape[2] + \
- seq_length if past_key_values is not None else seq_length
-
- if use_cache is True:
- assert self.is_decoder, f":obj:`use_cache` can only be set to `True` if {self} is used as a decoder"
-
- if attention_mask is None:
- attention_mask = torch.ones(
- batch_size, mask_seq_length).to(inputs_embeds.device)
- if self.is_decoder and encoder_attention_mask is None and encoder_hidden_states is not None:
- encoder_seq_length = encoder_hidden_states.shape[1]
- encoder_attention_mask = torch.ones(
- batch_size, encoder_seq_length, device=inputs_embeds.device, dtype=torch.long
- )
-
- # initialize past_key_values with `None` if past does not exist
- if past_key_values is None:
- past_key_values = [None] * len(self.block)
-
- # We can provide a self-attention mask of dimensions [batch_size, from_seq_length, to_seq_length]
- # ourselves in which case we just need to make it broadcastable to all heads.
- extended_attention_mask = self.get_extended_attention_mask(
- attention_mask, input_shape, inputs_embeds.device)
-
- # If a 2D or 3D attention mask is provided for the cross-attention
- # we need to make broadcastable to [batch_size, num_heads, seq_length, seq_length]
- if self.is_decoder and encoder_hidden_states is not None:
- encoder_batch_size, encoder_sequence_length, _ = encoder_hidden_states.size()
- encoder_hidden_shape = (
- encoder_batch_size, encoder_sequence_length)
- if encoder_attention_mask is None:
- encoder_attention_mask = torch.ones(
- encoder_hidden_shape, device=inputs_embeds.device)
- encoder_extended_attention_mask = self.invert_attention_mask(
- encoder_attention_mask)
- else:
- encoder_extended_attention_mask = None
-
- # Prepare head mask if needed
- head_mask = self.get_head_mask(head_mask, self.config.num_layers)
- cross_attn_head_mask = self.get_head_mask(
- cross_attn_head_mask, self.config.num_layers)
- present_key_value_states = () if use_cache else None
- all_hidden_states = () if output_hidden_states else None
- all_attentions = () if output_attentions else None
- all_cross_attentions = () if (output_attentions and self.is_decoder) else None
- position_bias = None
- encoder_decoder_position_bias = None
-
- hidden_states = self.dropout(inputs_embeds)
-
- for i, (layer_module, past_key_value) in enumerate(zip(self.block, past_key_values)):
-
- layer_head_mask = head_mask[i]
- cross_attn_layer_head_mask = cross_attn_head_mask[i]
- # Model parallel
- if self.model_parallel:
- torch.cuda.set_device(hidden_states.device)
- # Ensure that attention_mask is always on the same device as hidden_states
- if attention_mask is not None:
- attention_mask = attention_mask.to(hidden_states.device)
- if position_bias is not None:
- position_bias = position_bias.to(hidden_states.device)
- if encoder_hidden_states is not None:
- encoder_hidden_states = encoder_hidden_states.to(
- hidden_states.device)
- if encoder_extended_attention_mask is not None:
- encoder_extended_attention_mask = encoder_extended_attention_mask.to(
- hidden_states.device)
- if encoder_decoder_position_bias is not None:
- encoder_decoder_position_bias = encoder_decoder_position_bias.to(
- hidden_states.device)
- if layer_head_mask is not None:
- layer_head_mask = layer_head_mask.to(hidden_states.device)
- if cross_attn_layer_head_mask is not None:
- cross_attn_layer_head_mask = cross_attn_layer_head_mask.to(
- hidden_states.device)
- if output_hidden_states:
- all_hidden_states = all_hidden_states + (hidden_states,)
-
- if self.gradient_checkpointing and self.training:
- if use_cache:
- logger.warn(
- "`use_cache=True` is incompatible with gradient checkpointing. Setting `use_cache=False`..."
- )
- use_cache = False
-
- def create_custom_forward(module):
- def custom_forward(*inputs):
- return tuple(module(*inputs, use_cache, output_attentions))
-
- return custom_forward
-
- layer_outputs = checkpoint(
- create_custom_forward(layer_module),
- hidden_states,
- extended_attention_mask,
- position_bias,
- encoder_hidden_states,
- encoder_extended_attention_mask,
- encoder_decoder_position_bias,
- layer_head_mask,
- cross_attn_layer_head_mask,
- None, # past_key_value is always None with gradient checkpointing
- )
- else:
- layer_outputs = layer_module(
- hidden_states,
- attention_mask=extended_attention_mask,
- position_bias=position_bias,
- encoder_hidden_states=encoder_hidden_states,
- encoder_attention_mask=encoder_extended_attention_mask,
- encoder_decoder_position_bias=encoder_decoder_position_bias,
- layer_head_mask=layer_head_mask,
- cross_attn_layer_head_mask=cross_attn_layer_head_mask,
- past_key_value=past_key_value,
- use_cache=use_cache,
- output_attentions=output_attentions,
- )
-
- # layer_outputs is a tuple with:
- # hidden-states, key-value-states, (self-attention position bias), (self-attention weights),
- # (cross-attention position bias), (cross-attention weights)
- if use_cache is False:
- layer_outputs = layer_outputs[:1] + (None,) + layer_outputs[1:]
-
- hidden_states, present_key_value_state = layer_outputs[:2]
-
- # We share the position biases between the layers - the first layer store them
- # layer_outputs = hidden-states, key-value-states (self-attention position bias), (self-attention weights),
- # (cross-attention position bias), (cross-attention weights)
- position_bias = layer_outputs[2]
- if self.is_decoder and encoder_hidden_states is not None:
- encoder_decoder_position_bias = layer_outputs[4 if output_attentions else 3]
- # append next layer key value states
- if use_cache:
- present_key_value_states = present_key_value_states + \
- (present_key_value_state,)
-
- if output_attentions:
- all_attentions = all_attentions + (layer_outputs[3],)
- if self.is_decoder:
- all_cross_attentions = all_cross_attentions + \
- (layer_outputs[5],)
-
- # Model Parallel: If it's the last layer for that device, put things on the next device
- if self.model_parallel:
- for k, v in self.device_map.items():
- if i == v[-1] and "cuda:" + str(k) != self.last_device:
- hidden_states = hidden_states.to("cuda:" + str(k + 1))
-
- hidden_states = self.final_layer_norm(hidden_states)
- hidden_states = self.dropout(hidden_states)
-
- # Add last layer
- if output_hidden_states:
- all_hidden_states = all_hidden_states + (hidden_states,)
-
- if not return_dict:
- return tuple(
- v
- for v in [
- hidden_states,
- present_key_value_states,
- all_hidden_states,
- all_attentions,
- all_cross_attentions,
- ]
- if v is not None
- )
- return BaseModelOutputWithPastAndCrossAttentions(
- last_hidden_state=hidden_states,
- past_key_values=present_key_value_states,
- hidden_states=all_hidden_states,
- attentions=all_attentions,
- cross_attentions=all_cross_attentions,
- )
-
-
-T5_START_DOCSTRING = r"""
-
- The T5 model was proposed in `Exploring the Limits of Transfer Learning with a Unified Text-to-Text Transformer
- `__ by Colin Raffel, Noam Shazeer, Adam Roberts, Katherine Lee, Sharan Narang,
- Michael Matena, Yanqi Zhou, Wei Li, Peter J. Liu. It's an encoder decoder transformer pre-trained in a text-to-text
- denoising generative setting.
-
- This model inherits from :class:`~transformers.PreTrainedModel`. Check the superclass documentation for the generic
- methods the library implements for all its model (such as downloading or saving, resizing the input embeddings,
- pruning heads etc.)
-
- This model is also a PyTorch `torch.nn.Module `__
- subclass. Use it as a regular PyTorch Module and refer to the PyTorch documentation for all matter related to
- general usage and behavior.
-
- Parameters:
- config (:class:`~transformers.T5Config`): Model configuration class with all the parameters of the model.
- Initializing with a config file does not load the weights associated with the model, only the
- configuration. Check out the :meth:`~transformers.PreTrainedModel.from_pretrained` method to load the model
- weights.
-"""
-
-T5_INPUTS_DOCSTRING = """
- Args:
- input_ids (:obj:`torch.LongTensor` of shape :obj:`(batch_size, sequence_length)`):
- Indices of input sequence tokens in the vocabulary. T5 is a model with relative position embeddings so you
- should be able to pad the inputs on both the right and the left.
-
- Indices can be obtained using :class:`~transformers.T5Tokenizer`. See
- :meth:`transformers.PreTrainedTokenizer.encode` and :meth:`transformers.PreTrainedTokenizer.__call__` for
- detail.
-
- `What are input IDs? <../glossary.html#input-ids>`__
-
- To know more on how to prepare :obj:`input_ids` for pretraining take a look a `T5 Training
- <./T5.html#training>`__.
- attention_mask (:obj:`torch.FloatTensor` of shape :obj:`(batch_size, sequence_length)`, `optional`):
- Mask to avoid performing attention on padding token indices. Mask values selected in ``[0, 1]``:
-
- - 1 for tokens that are **not masked**,
- - 0 for tokens that are **masked**.
-
- `What are attention masks? <../glossary.html#attention-mask>`__
- decoder_input_ids (:obj:`torch.LongTensor` of shape :obj:`(batch_size, target_sequence_length)`, `optional`):
- Indices of decoder input sequence tokens in the vocabulary.
-
- Indices can be obtained using :class:`~transformers.T5Tokenizer`. See
- :meth:`transformers.PreTrainedTokenizer.encode` and :meth:`transformers.PreTrainedTokenizer.__call__` for
- details.
-
- `What are decoder input IDs? <../glossary.html#decoder-input-ids>`__
-
- T5 uses the :obj:`pad_token_id` as the starting token for :obj:`decoder_input_ids` generation. If
- :obj:`past_key_values` is used, optionally only the last :obj:`decoder_input_ids` have to be input (see
- :obj:`past_key_values`).
-
- To know more on how to prepare :obj:`decoder_input_ids` for pretraining take a look at `T5 Training
- <./T5.html#training>`__.
- decoder_attention_mask (:obj:`torch.BoolTensor` of shape
- :obj:`(batch_size, target_sequence_length)`, `optional`):
- Default behavior: generate a tensor that ignores pad tokens in :obj:`decoder_input_ids`. Causal mask will
- also be used by default.
- head_mask (:obj:`torch.FloatTensor` of shape :obj:`(num_heads,)` or :obj:`(num_layers, num_heads)`, `optional`):
- Mask to nullify selected heads of the self-attention modules in the encoder. Mask values selected in ``[0,
- 1]``:
-
- - 1 indicates the head is **not masked**,
- - 0 indicates the head is **masked**.
-
- decoder_head_mask (:obj:`torch.FloatTensor` of shape :obj:`(num_heads,)` or
- :obj:`(num_layers, num_heads)`, `optional`):
- Mask to nullify selected heads of the self-attention modules in the decoder. Mask values selected in ``[0,
- 1]``:
-
- - 1 indicates the head is **not masked**,
- - 0 indicates the head is **masked**.
-
- cross_attn_head_mask (:obj:`torch.Tensor` of shape :obj:`(num_heads,)` or
- :obj:`(num_layers, num_heads)`, `optional`):
- Mask to nullify selected heads of the cross-attention modules in the decoder. Mask values selected in
- ``[0, 1]``:
-
- - 1 indicates the head is **not masked**,
- - 0 indicates the head is **masked**.
-
- encoder_outputs (:obj:`tuple(tuple(torch.FloatTensor)`, `optional`):
- Tuple consists of (:obj:`last_hidden_state`, :obj:`optional`: `hidden_states`, :obj:`optional`:
- `attentions`) :obj:`last_hidden_state` of shape :obj:`(batch_size, sequence_length, hidden_size)` is a
- sequence of hidden states at the output of the last layer of the encoder. Used in the cross-attention of
- the decoder.
- past_key_values (:obj:`tuple(tuple(torch.FloatTensor))` of length :obj:`config.n_layers` with each tuple having
- 4 tensors of shape :obj:`(batch_size, num_heads, sequence_length - 1, embed_size_per_head)`):
- Contains precomputed key and value hidden states of the attention blocks. Can be used to speed up decoding.
-
- If :obj:`past_key_values` are used, the user can optionally input only the last :obj:`decoder_input_ids`
- (those that don't have their past key value states given to this model) of shape :obj:`(batch_size, 1)`
- instead of all :obj:`decoder_input_ids` of shape :obj:`(batch_size, sequence_length)`.
- inputs_embeds (:obj:`torch.FloatTensor` of shape :obj:`(batch_size, sequence_length, hidden_size)`, `optional`):
- Optionally, instead of passing :obj:`input_ids` you can choose to directly pass an embedded representation.
- This is useful if you want more control over how to convert :obj:`input_ids` indices into associated
- vectors than the model's internal embedding lookup matrix.
- decoder_inputs_embeds (:obj:`torch.FloatTensor` of shape :obj:`(batch_size, target_sequence_length, hidden_size)
- `, `optional`):
- Optionally, instead of passing :obj:`decoder_input_ids` you can choose to directly pass an embedded
- representation. If :obj:`past_key_values` is used, optionally only the last :obj:`decoder_inputs_embeds`
- have to be input (see :obj:`past_key_values`). This is useful if you want more control over how to convert
- :obj:`decoder_input_ids` indices into associated vectors than the model's internal embedding lookup matrix.
-
- If :obj:`decoder_input_ids` and :obj:`decoder_inputs_embeds` are both unset, :obj:`decoder_inputs_embeds`
- takes the value of :obj:`inputs_embeds`.
-
- use_cache (:obj:`bool`, `optional`):
- If set to :obj:`True`, :obj:`past_key_values` key value states are returned and can be used to speed up
- decoding (see :obj:`past_key_values`).
-
- output_attentions (:obj:`bool`, `optional`):
- Whether or not to return the attentions tensors of all attention layers. See ``attentions`` under returned
- tensors for more detail.
- output_hidden_states (:obj:`bool`, `optional`):
- Whether or not to return the hidden states of all layers. See ``hidden_states`` under returned tensors for
- more detail.
- return_dict (:obj:`bool`, `optional`):
- Whether or not to return a :class:`~transformers.file_utils.ModelOutput` instead of a plain tuple.
-"""
-
-T5_ENCODER_INPUTS_DOCSTRING = r"""
- Args:
- input_ids (:obj:`torch.LongTensor` of shape :obj:`(batch_size, sequence_length)`):
- Indices of input sequence tokens in the vocabulary. T5 is a model with relative position embeddings so you
- should be able to pad the inputs on both the right and the left.
-
- Indices can be obtained using :class:`~transformers.T5Tokenizer`. See
- :meth:`transformers.PreTrainedTokenizer.encode` and :meth:`transformers.PreTrainedTokenizer.__call__` for
- detail.
-
- To know more on how to prepare :obj:`input_ids` for pretraining take a look a `T5 Training
- <./T5.html#training>`__.
- attention_mask (:obj:`torch.FloatTensor` of shape :obj:`(batch_size, sequence_length)`, `optional`):
- Mask to avoid performing attention on padding token indices. Mask values selected in ``[0, 1]``:
-
- - 1 for tokens that are **not masked**,
- - 0 for tokens that are **masked**.
-
- `What are attention masks? <../glossary.html#attention-mask>`__
- head_mask (:obj:`torch.FloatTensor` of shape :obj:`(num_heads,)` or :obj:`(num_layers, num_heads)`, `optional`):
- Mask to nullify selected heads of the self-attention modules. Mask values selected in ``[0, 1]``:
-
- - 1 indicates the head is **not masked**,
- - 0 indicates the head is **masked**.
-
- inputs_embeds (:obj:`torch.FloatTensor` of shape :obj:`(batch_size, sequence_length, hidden_size)`, `optional`):
- Optionally, instead of passing :obj:`input_ids` you can choose to directly pass an embedded representation.
- This is useful if you want more control over how to convert :obj:`input_ids` indices into associated
- vectors than the model's internal embedding lookup matrix.
- output_attentions (:obj:`bool`, `optional`):
- Whether or not to return the attentions tensors of all attention layers. See ``attentions`` under returned
- tensors for more detail.
- output_hidden_states (:obj:`bool`, `optional`):
- Whether or not to return the hidden states of all layers. See ``hidden_states`` under returned tensors for
- more detail.
- return_dict (:obj:`bool`, `optional`):
- Whether or not to return a :class:`~transformers.file_utils.ModelOutput` instead of a plain tuple.
-"""
-
-# Warning message for FutureWarning: head_mask was separated into two input args - head_mask, decoder_head_mask
-__HEAD_MASK_WARNING_MSG = """
-The input argument `head_mask` was split into two arguments `head_mask` and `decoder_head_mask`. Currently,
-`decoder_head_mask` is set to copy `head_mask`, but this feature is deprecated and will be removed in future versions.
-If you do not want to use any `decoder_head_mask` now, please set `decoder_head_mask = torch.ones(num_layers,
-num_heads)`.
-"""
-
-
-@add_start_docstrings(
- "The bare T5 Model transformer outputting raw hidden-states without any specific head on top.",
- T5_START_DOCSTRING,
-)
-class T5LMHead(nn.Module):
- """Masked LM head for T5
-
- Arguments:
- mpu_vocab_size: model parallel size of vocabulary.
- hidden_size: hidden size
- init_method: init method for weight initialization
- layernorm_epsilon: tolerance for layer norm divisions
- parallel_output: wether output logits being distributed or not.
- """
-
- def __init__(self, config):
- super(T5LMHead, self).__init__()
-
- self.bias = torch.nn.Parameter(torch.zeros(config.vocab_size))
-
- def forward(self, hidden_states, word_embeddings_weight):
- output = torch.nn.functional.linear(hidden_states,
- word_embeddings_weight,
- bias=self.bias)
- return output
-
-
-class T5Model(T5PreTrainedModel):
- _keys_to_ignore_on_load_missing = [
- r"encoder\.embed_tokens\.weight",
- r"decoder\.embed_tokens\.weight",
- ]
- _keys_to_ignore_on_load_unexpected = [
- r"decoder\.block\.0\.layer\.1\.EncDecAttention\.relative_attention_bias\.weight",
- ]
-
- def __init__(self, config: T5Config):
- super().__init__(config)
- # @IDEA modified -> nn.Embedding -> T5Embeddings
- # self.shared = nn.Embedding(config.vocab_size, config.d_model)
- self.shared = T5Embeddings(config)
-
- encoder_config = copy.deepcopy(config)
- encoder_config.is_decoder = False
- encoder_config.use_cache = False
- encoder_config.is_encoder_decoder = False
- self.encoder = T5Stack(encoder_config, self.shared)
-
- decoder_config = copy.deepcopy(config)
- decoder_config.is_decoder = True
- decoder_config.is_encoder_decoder = False
- decoder_config.num_layers = config.num_decoder_layers
- self.decoder = T5Stack(decoder_config, self.shared)
-
- self.init_weights()
-
- # Model parallel
- self.model_parallel = False
- self.device_map = None
-
- @add_start_docstrings(PARALLELIZE_DOCSTRING)
- def parallelize(self, device_map=None):
- self.device_map = (
- get_device_map(len(self.encoder.block),
- range(torch.cuda.device_count()))
- if device_map is None
- else device_map
- )
- assert_device_map(self.device_map, len(self.encoder.block))
- self.encoder.parallelize(self.device_map)
- self.decoder.parallelize(self.device_map)
- self.model_parallel = True
-
- @add_start_docstrings(DEPARALLELIZE_DOCSTRING)
- def deparallelize(self):
- self.encoder.deparallelize()
- self.decoder.deparallelize()
- self.encoder = self.encoder.to("cpu")
- self.decoder = self.decoder.to("cpu")
- self.model_parallel = False
- self.device_map = None
- torch.cuda.empty_cache()
-
- def get_input_embeddings(self):
- return self.shared
-
- def set_input_embeddings(self, new_embeddings):
- self.shared = new_embeddings
- self.encoder.set_input_embeddings(new_embeddings)
- self.decoder.set_input_embeddings(new_embeddings)
-
- def get_encoder(self):
- return self.encoder
-
- def get_decoder(self):
- return self.decoder
-
- def _prune_heads(self, heads_to_prune):
- """
- Prunes heads of the model. heads_to_prune: dict of {layer_num: list of heads to prune in this layer} See base
- class PreTrainedModel
- """
- for layer, heads in heads_to_prune.items():
- self.encoder.layer[layer].attention.prune_heads(heads)
-
- @add_start_docstrings_to_model_forward(T5_INPUTS_DOCSTRING)
- @replace_return_docstrings(output_type=Seq2SeqModelOutput, config_class=_CONFIG_FOR_DOC)
- def forward(
- self,
- input_ids=None,
- attention_mask=None,
- decoder_input_ids=None,
- decoder_attention_mask=None,
- head_mask=None,
- decoder_head_mask=None,
- cross_attn_head_mask=None,
- encoder_outputs=None,
- past_key_values=None,
- inputs_embeds=None,
- decoder_inputs_embeds=None,
- use_cache=None,
- output_attentions=None,
- output_hidden_states=None,
- return_dict=None,
- ):
- r"""
- Returns:
-
- Example::
-
- >>> from transformers import T5Tokenizer, T5Model
-
- >>> tokenizer = T5Tokenizer.from_pretrained('T5-small')
- >>> model = T5Model.from_pretrained('T5-small')
-
- >>> input_ids = tokenizer("Studies have been shown that owning a dog is good for you",
- return_tensors="pt").input_ids # Batch size 1
- >>> decoder_input_ids = tokenizer("Studies show that", return_tensors="pt").input_ids # Batch size 1
-
- >>> # forward pass
- >>> outputs = model(input_ids=input_ids, decoder_input_ids=decoder_input_ids)
- >>> last_hidden_states = outputs.last_hidden_state
- """
- use_cache = use_cache if use_cache is not None else self.config.use_cache
- return_dict = return_dict if return_dict is not None else self.config.use_return_dict
-
- # FutureWarning: head_mask was separated into two input args - head_mask, decoder_head_mask
- if head_mask is not None and decoder_head_mask is None:
- if self.config.num_layers == self.config.num_decoder_layers:
- warnings.warn(__HEAD_MASK_WARNING_MSG, FutureWarning)
- decoder_head_mask = head_mask
-
- # Encode if needed (training, first prediction pass)
- if encoder_outputs is None:
- encoder_outputs = self.encoder(
- input_ids=input_ids,
- attention_mask=attention_mask,
- inputs_embeds=inputs_embeds,
- head_mask=head_mask,
- output_attentions=output_attentions,
- output_hidden_states=output_hidden_states,
- return_dict=return_dict,
- )
- elif return_dict and not isinstance(encoder_outputs, BaseModelOutput):
- encoder_outputs = BaseModelOutput(
- last_hidden_state=encoder_outputs[0],
- hidden_states=encoder_outputs[1] if len(
- encoder_outputs) > 1 else None,
- attentions=encoder_outputs[2] if len(
- encoder_outputs) > 2 else None,
- )
-
- hidden_states = encoder_outputs[0]
- if self.model_parallel:
- torch.cuda.set_device(self.decoder.first_device)
- # Set device for model parallelism
- if self.model_parallel:
- torch.cuda.set_device(self.decoder.first_device)
- hidden_states = hidden_states.to(self.decoder.first_device)
- if decoder_input_ids is not None:
- decoder_input_ids = decoder_input_ids.to(
- self.decoder.first_device)
- if attention_mask is not None:
- attention_mask = attention_mask.to(self.decoder.first_device)
- if decoder_attention_mask is not None:
- decoder_attention_mask = decoder_attention_mask.to(
- self.decoder.first_device)
-
- # Decode
- decoder_outputs = self.decoder(
- input_ids=decoder_input_ids,
- attention_mask=decoder_attention_mask,
- inputs_embeds=decoder_inputs_embeds,
- past_key_values=past_key_values,
- encoder_hidden_states=hidden_states,
- encoder_attention_mask=attention_mask,
- head_mask=decoder_head_mask,
- cross_attn_head_mask=cross_attn_head_mask,
- use_cache=use_cache,
- output_attentions=output_attentions,
- output_hidden_states=output_hidden_states,
- return_dict=return_dict,
- )
-
- if not return_dict:
- return decoder_outputs + encoder_outputs
-
- return Seq2SeqModelOutput(
- last_hidden_state=decoder_outputs.last_hidden_state,
- past_key_values=decoder_outputs.past_key_values,
- decoder_hidden_states=decoder_outputs.hidden_states,
- decoder_attentions=decoder_outputs.attentions,
- cross_attentions=decoder_outputs.cross_attentions,
- encoder_last_hidden_state=encoder_outputs.last_hidden_state,
- encoder_hidden_states=encoder_outputs.hidden_states,
- encoder_attentions=encoder_outputs.attentions,
- )
-
-
-@add_start_docstrings("""T5 Model with a `language modeling` head on top. """, T5_START_DOCSTRING)
-class T5ForConditionalGeneration(T5PreTrainedModel):
- _keys_to_ignore_on_load_missing = [
- r"encoder\.embed_tokens\.weight",
- r"decoder\.embed_tokens\.weight",
- r"lm_head\.weight",
- ]
- _keys_to_ignore_on_load_unexpected = [
- r"decoder\.block\.0\.layer\.1\.EncDecAttention\.relative_attention_bias\.weight",
- ]
-
- def __init__(self, config):
- super().__init__(config)
- self.model_dim = config.d_model
-
- # @IDEA modified -> nn.Embedding -> T5Embeddings
- # self.shared = nn.Embedding(config.vocab_size, config.d_model)
- self.shared = T5Embeddings(config)
-
- encoder_config = copy.deepcopy(config)
- encoder_config.is_decoder = False
- encoder_config.use_cache = False
- encoder_config.is_encoder_decoder = False
- self.encoder = T5Stack(encoder_config, self.shared)
-
- decoder_config = copy.deepcopy(config)
- decoder_config.is_decoder = True
- decoder_config.is_encoder_decoder = False
- decoder_config.num_layers = config.num_decoder_layers
- self.decoder = T5Stack(decoder_config, self.shared)
-
- # @IDEA modified -> add self.lm_head_bias
- self.lm_head_bias = torch.nn.Parameter(torch.zeros(config.vocab_size))
-
- self.init_weights()
-
- # Model parallel
- self.model_parallel = False
- self.device_map = None
-
- @add_start_docstrings(PARALLELIZE_DOCSTRING)
- def parallelize(self, device_map=None):
- self.device_map = (
- get_device_map(len(self.encoder.block),
- range(torch.cuda.device_count()))
- if device_map is None
- else device_map
- )
- assert_device_map(self.device_map, len(self.encoder.block))
- self.encoder.parallelize(self.device_map)
- self.decoder.parallelize(self.device_map)
- self.lm_head = self.lm_head.to(self.decoder.first_device)
- self.model_parallel = True
-
- @add_start_docstrings(DEPARALLELIZE_DOCSTRING)
- def deparallelize(self):
- self.encoder.deparallelize()
- self.decoder.deparallelize()
- self.encoder = self.encoder.to("cpu")
- self.decoder = self.decoder.to("cpu")
- self.lm_head = self.lm_head.to("cpu")
- self.model_parallel = False
- self.device_map = None
- torch.cuda.empty_cache()
-
- def get_input_embeddings(self):
- return self.shared
-
- def set_input_embeddings(self, new_embeddings):
- self.shared = new_embeddings
- self.encoder.set_input_embeddings(new_embeddings)
- self.decoder.set_input_embeddings(new_embeddings)
-
- def set_output_embeddings(self, new_embeddings):
- self.lm_head = new_embeddings
-
- def get_output_embeddings(self):
- return self.lm_head_bias
-
- def get_encoder(self):
- return self.encoder
-
- def get_decoder(self):
- return self.decoder
-
- def generate(self, input_ids=None, max_length=512):
-
- input_ids = torch.tensor(input_ids)
- if len(input_ids.shape) < 2:
- input_ids = input_ids.unsqueeze(0)
- decode_input_id = [21128] # [BOS]的token_id为21128
- for i in range(max_length):
- tensor_decode_input_id = torch.tensor([decode_input_id])
- forword_output = self.forward(input_ids=input_ids,
- decoder_input_ids=tensor_decode_input_id)
- logits = forword_output.logits
- logits = torch.nn.functional.softmax(
- logits, dim=-1).cpu().detach().numpy()[0]
-
- last_output_id = int(np.random.choice(
- logits.shape[1], p=logits[-1]))
- if last_output_id == 21129: # [EOS]的token_id为21129
- break
- else:
- decode_input_id.append(last_output_id)
-
- return decode_input_id
-
- @add_start_docstrings_to_model_forward(T5_INPUTS_DOCSTRING)
- @replace_return_docstrings(output_type=Seq2SeqLMOutput, config_class=_CONFIG_FOR_DOC)
- def forward(
- self,
- input_ids=None,
- attention_mask=None,
- decoder_input_ids=None,
- decoder_attention_mask=None,
- head_mask=None,
- decoder_head_mask=None,
- cross_attn_head_mask=None,
- encoder_outputs=None,
- past_key_values=None,
- inputs_embeds=None,
- decoder_inputs_embeds=None,
- labels=None,
- use_cache=None,
- output_attentions=None,
- output_hidden_states=None,
- return_dict=None,
- ):
- r"""
- labels (:obj:`torch.LongTensor` of shape :obj:`(batch_size,)`, `optional`):
- Labels for computing the sequence classification/regression loss. Indices should be in :obj:`[-100, 0, ...,
- config.vocab_size - 1]`. All labels set to ``-100`` are ignored (masked), the loss is only computed for
- labels in ``[0, ..., config.vocab_size]``
-
- Returns:
- Examples::
-
- >>> from transformers import T5Tokenizer, T5ForConditionalGeneration
-
- >>> tokenizer = T5Tokenizer.from_pretrained('T5-small')
- >>> model = T5ForConditionalGeneration.from_pretrained('T5-small')
-
- >>> # training
- >>> input_ids = tokenizer('The walks in park', return_tensors='pt').input_ids
- >>> labels = tokenizer(' cute dog the ', return_tensors='pt').input_ids
- >>> outputs = model(input_ids=input_ids, labels=labels)
- >>> loss = outputs.loss
- >>> logits = outputs.logits
-
- >>> # inference
- >>> input_ids = tokenizer("summarize: studies have shown that owning a dog is good for you",
- return_tensors="pt").input_ids # Batch size 1
- >>> outputs = model.generate(input_ids)
- >>> print(tokenizer.decode(outputs[0], skip_special_tokens=True))
- >>> # studies have shown that owning a dog is good for you.
- """
- use_cache = use_cache if use_cache is not None else self.config.use_cache
- return_dict = return_dict if return_dict is not None else self.config.use_return_dict
-
- # FutureWarning: head_mask was separated into two input args - head_mask, decoder_head_mask
- if head_mask is not None and decoder_head_mask is None:
- if self.config.num_layers == self.config.num_decoder_layers:
- warnings.warn(__HEAD_MASK_WARNING_MSG, FutureWarning)
- decoder_head_mask = head_mask
-
- # Encode if needed (training, first prediction pass)
- if encoder_outputs is None:
- # Convert encoder inputs in embeddings if needed
- encoder_outputs = self.encoder(
- input_ids=input_ids,
- attention_mask=attention_mask,
- inputs_embeds=inputs_embeds,
- head_mask=head_mask,
- output_attentions=output_attentions,
- output_hidden_states=output_hidden_states,
- return_dict=return_dict,
- )
- elif return_dict and not isinstance(encoder_outputs, BaseModelOutput):
- encoder_outputs = BaseModelOutput(
- last_hidden_state=encoder_outputs[0],
- hidden_states=encoder_outputs[1] if len(
- encoder_outputs) > 1 else None,
- attentions=encoder_outputs[2] if len(
- encoder_outputs) > 2 else None,
- )
-
- hidden_states = encoder_outputs[0]
-
- if self.model_parallel:
- torch.cuda.set_device(self.decoder.first_device)
-
- if labels is not None and decoder_input_ids is None and decoder_inputs_embeds is None:
- # get decoder inputs from shifting lm labels to the right
- decoder_input_ids = self._shift_right(labels)
-
- # Set device for model parallelism
- if self.model_parallel:
- torch.cuda.set_device(self.decoder.first_device)
- hidden_states = hidden_states.to(self.decoder.first_device)
- if decoder_input_ids is not None:
- decoder_input_ids = decoder_input_ids.to(
- self.decoder.first_device)
- if attention_mask is not None:
- attention_mask = attention_mask.to(self.decoder.first_device)
- if decoder_attention_mask is not None:
- decoder_attention_mask = decoder_attention_mask.to(
- self.decoder.first_device)
-
- # Decode
- decoder_outputs = self.decoder(
- input_ids=decoder_input_ids,
- attention_mask=decoder_attention_mask,
- inputs_embeds=decoder_inputs_embeds,
- past_key_values=past_key_values,
- encoder_hidden_states=hidden_states,
- encoder_attention_mask=attention_mask,
- head_mask=decoder_head_mask,
- cross_attn_head_mask=cross_attn_head_mask,
- use_cache=use_cache,
- output_attentions=output_attentions,
- output_hidden_states=output_hidden_states,
- return_dict=return_dict,
- )
-
- sequence_output = decoder_outputs.last_hidden_state
-
- # Set device for model parallelism
- # if self.model_parallel:
- # torch.cuda.set_device(self.encoder.first_device)
- # self.lm_head = self.lm_head.to(self.encoder.first_device)
- # sequence_output = sequence_output.to(self.lm_head.weight.device)
-
- # if self.config.tie_word_embeddings:
- # # Rescale output before projecting on vocab
- # # See https://github.com/tensorflow/mesh/blob/fa19d69eafc9a482aff0b59ddd96b025c0cb207d/
- # mesh_tensorflow/transformer/transformer.py#L586
- # sequence_output = sequence_output * (self.model_dim ** -0.5)
-
- lm_logits = torch.nn.functional.linear(
- sequence_output, self.shared.word_embeddings.weight, bias=self.lm_head_bias)
-
- loss = None
- if labels is not None:
- loss_fct = CrossEntropyLoss(ignore_index=-100)
- loss = loss_fct(
- lm_logits.view(-1, lm_logits.size(-1)), labels.view(-1))
- # @IDEA modified(thom): Add z_loss https://github.com/tensorflow/mesh/blob/
- # fa19d69eafc9a482aff0b59ddd96b025c0cb207d/mesh_tensorflow/layers.py#L666
-
- if not return_dict:
- output = (lm_logits,) + decoder_outputs[1:] + encoder_outputs
- return ((loss,) + output) if loss is not None else output
-
- return Seq2SeqLMOutput(
- loss=loss,
- logits=lm_logits,
- past_key_values=decoder_outputs.past_key_values,
- decoder_hidden_states=decoder_outputs.hidden_states,
- decoder_attentions=decoder_outputs.attentions,
- cross_attentions=decoder_outputs.cross_attentions,
- encoder_last_hidden_state=encoder_outputs.last_hidden_state,
- encoder_hidden_states=encoder_outputs.hidden_states,
- encoder_attentions=encoder_outputs.attentions,
- )
-
- def prepare_inputs_for_generation(
- self,
- input_ids,
- past=None,
- attention_mask=None,
- head_mask=None,
- decoder_head_mask=None,
- cross_attn_head_mask=None,
- use_cache=None,
- encoder_outputs=None,
- **kwargs
- ):
-
- # cut decoder_input_ids if past is used
- if past is not None:
- input_ids = input_ids[:, -1:]
-
- return {
- "decoder_input_ids": input_ids,
- "past_key_values": past,
- "encoder_outputs": encoder_outputs,
- "attention_mask": attention_mask,
- "head_mask": head_mask,
- "decoder_head_mask": decoder_head_mask,
- "cross_attn_head_mask": cross_attn_head_mask,
- "use_cache": use_cache,
- }
-
- def prepare_decoder_input_ids_from_labels(self, labels: torch.Tensor):
- return self._shift_right(labels)
-
- def _reorder_cache(self, past, beam_idx):
- # if decoder past is not included in output
- # speedy decoding is disabled and no need to reorder
- if past is None:
- logger.warning(
- "You might want to consider setting `use_cache=True` to speed up decoding")
- return past
-
- reordered_decoder_past = ()
- for layer_past_states in past:
- # get the correct batch idx from layer past batch dim
- # batch dim of `past` is at 2nd position
- reordered_layer_past_states = ()
- for layer_past_state in layer_past_states:
- # need to set correct `past` for each of the four key / value states
- reordered_layer_past_states = reordered_layer_past_states + (
- layer_past_state.index_select(
- 0, beam_idx.to(layer_past_state.device)),
- )
-
- assert reordered_layer_past_states[0].shape == layer_past_states[0].shape
- assert len(reordered_layer_past_states) == len(layer_past_states)
-
- reordered_decoder_past = reordered_decoder_past + \
- (reordered_layer_past_states,)
- return reordered_decoder_past
-
-
-@add_start_docstrings(
- "The bare T5 Model transformer outputting encoder's raw hidden-states without any specific head on top.",
- T5_START_DOCSTRING,
-)
-class T5EncoderModel(T5PreTrainedModel):
- authorized_missing_keys = [
- r"encoder\.embed_tokens\.weight",
- ]
-
- def __init__(self, config: T5Config):
- super().__init__(config)
- # @IDEA modified -> nn.Embedding -> T5Embeddings
- # self.shared = nn.Embedding(config.vocab_size, config.d_model)
- self.shared = T5Embeddings(config)
-
- encoder_config = copy.deepcopy(config)
- encoder_config.use_cache = False
- encoder_config.is_encoder_decoder = False
- self.encoder = T5Stack(encoder_config, self.shared)
-
- self.init_weights()
-
- # Model parallel
- self.model_parallel = False
- self.device_map = None
-
- @add_start_docstrings(PARALLELIZE_DOCSTRING)
- def parallelize(self, device_map=None):
- self.device_map = (
- get_device_map(len(self.encoder.block),
- range(torch.cuda.device_count()))
- if device_map is None
- else device_map
- )
- assert_device_map(self.device_map, len(self.encoder.block))
- self.encoder.parallelize(self.device_map)
- self.model_parallel = True
-
- @add_start_docstrings(DEPARALLELIZE_DOCSTRING)
- def deparallelize(self):
- self.encoder.deparallelize()
- self.encoder = self.encoder.to("cpu")
- self.model_parallel = False
- self.device_map = None
- torch.cuda.empty_cache()
-
- def get_input_embeddings(self):
- return self.shared
-
- def set_input_embeddings(self, new_embeddings):
- self.shared = new_embeddings
- self.encoder.set_input_embeddings(new_embeddings)
-
- def get_encoder(self):
- return self.encoder
-
- def _prune_heads(self, heads_to_prune):
- """
- Prunes heads of the model. heads_to_prune: dict of {layer_num: list of heads to prune in this layer} See base
- class PreTrainedModel
- """
- for layer, heads in heads_to_prune.items():
- self.encoder.layer[layer].attention.prune_heads(heads)
-
- @add_start_docstrings_to_model_forward(T5_ENCODER_INPUTS_DOCSTRING)
- @replace_return_docstrings(output_type=BaseModelOutput, config_class=_CONFIG_FOR_DOC)
- def forward(
- self,
- input_ids=None,
- attention_mask=None,
- head_mask=None,
- inputs_embeds=None,
- output_attentions=None,
- output_hidden_states=None,
- return_dict=None,
- ):
- r"""
- Returns:
-
- Example::
-
- >>> from transformers import T5Tokenizer, T5EncoderModel
- >>> tokenizer = T5Tokenizer.from_pretrained('T5-small')
- >>> model = T5EncoderModel.from_pretrained('T5-small')
- >>> input_ids = tokenizer("Studies have been shown that owning a dog is good for you",
- return_tensors="pt").input_ids # Batch size 1
- >>> outputs = model(input_ids=input_ids)
- >>> last_hidden_states = outputs.last_hidden_state
- """
- return_dict = return_dict if return_dict is not None else self.config.use_return_dict
-
- encoder_outputs = self.encoder(
- input_ids=input_ids,
- attention_mask=attention_mask,
- inputs_embeds=inputs_embeds,
- head_mask=head_mask,
- output_attentions=output_attentions,
- output_hidden_states=output_hidden_states,
- return_dict=return_dict,
- )
-
- return encoder_outputs
diff --git a/spaces/HarryLee/eCommerceImageCaptioning/fairseq/fairseq/data/multilingual/sampled_multi_dataset.py b/spaces/HarryLee/eCommerceImageCaptioning/fairseq/fairseq/data/multilingual/sampled_multi_dataset.py
deleted file mode 100644
index b0a617424ee3c5923b37796773da4c97851a16c5..0000000000000000000000000000000000000000
--- a/spaces/HarryLee/eCommerceImageCaptioning/fairseq/fairseq/data/multilingual/sampled_multi_dataset.py
+++ /dev/null
@@ -1,467 +0,0 @@
-# Copyright (c) Facebook, Inc. and its affiliates.
-#
-# This source code is licensed under the MIT license found in the
-# LICENSE file in the root directory of this source tree.
-
-import datetime
-import hashlib
-import logging
-import time
-from bisect import bisect_right
-from collections import OrderedDict, defaultdict
-from enum import Enum
-from typing import List
-
-import numpy as np
-import torch
-from fairseq.data import FairseqDataset, data_utils
-from fairseq.distributed import utils as distributed_utils
-
-
-def get_time_gap(s, e):
- return (
- datetime.datetime.fromtimestamp(e) - datetime.datetime.fromtimestamp(s)
- ).__str__()
-
-
-logger = logging.getLogger(__name__)
-
-
-def default_virtual_size_func(datasets, ratios, max_scale_up=1.5):
- sizes = [len(d) for d in datasets]
- if ratios is None:
- return sum(sizes)
- largest_idx = np.argmax(sizes)
- largest_r = ratios[largest_idx]
- largest_s = sizes[largest_idx]
- # set virtual sizes relative to the largest dataset
- virtual_sizes = [(r / largest_r) * largest_s for r in ratios]
- vsize = sum(virtual_sizes)
- max_size = sum(sizes) * max_scale_up
- return int(vsize if vsize < max_size else max_size)
-
-
-class CollateFormat(Enum):
- single = 1
- ordered_dict = 2
-
-
-class SampledMultiDataset(FairseqDataset):
- """Samples from multiple sub-datasets according to given sampling ratios.
- Args:
- datasets (
- List[~torch.utils.data.Dataset]
- or OrderedDict[str, ~torch.utils.data.Dataset]
- ): datasets
- sampling_ratios (List[float]): list of probability of each dataset to be sampled
- (default: None, which corresponds to concatenating all dataset together).
- seed (int): RNG seed to use (default: 2).
- epoch (int): starting epoch number (default: 1).
- eval_key (str, optional): a key used at evaluation time that causes
- this instance to pass-through batches from *datasets[eval_key]*.
- collate_format (CollateFormat): collater output format, either CollateFormat.ordered_dict or
- CollateFormat.single (default: CollateFormat.single) where CollateFormat.single configures
- the collater to output batches of data mixed from all sub-datasets,
- and CollateFormat.ordered_dict configures the collater to output a dictionary of batches indexed by keys
- of sub-datasets.
- Note that not all sub-datasets will present in a single batch in both formats.
- virtual_size (int, or callable): the expected virtual size of the dataset (default: default_virtual_size_func).
- split (str): the split of the data, e.g. 'train', 'valid' or 'test'.
- shared_collater (bool): whether or not to all sub-datasets have the same collater.
- shuffle (bool): whether or not to shuffle data (default: True).
- """
-
- def __init__(
- self,
- datasets,
- sampling_ratios=None,
- seed=2,
- epoch=1,
- eval_key=None,
- collate_format=CollateFormat.single,
- virtual_size=default_virtual_size_func,
- split="",
- shared_collater=False,
- shuffle=True,
- ):
- super().__init__()
- self.shared_collater = shared_collater
- self.shuffle = shuffle
-
- if isinstance(datasets, OrderedDict):
- self.keys = list(datasets.keys())
- datasets = list(datasets.values())
- elif isinstance(datasets, List):
- self.keys = list(range(len(datasets)))
- else:
- raise AssertionError()
- self.datasets = datasets
- self.split = split
-
- self.eval_key = eval_key
- if self.eval_key is not None:
- self.collate_format = CollateFormat.single
- else:
- self.collate_format = collate_format
-
- self.seed = seed
- self._cur_epoch = None
-
- self.cumulated_sizes = None
- # self.datasets[k][self._cur_indices[i]] is the data item i in this sampled dataset
- # namely, data item i is sampled from the kth sub-dataset self.datasets[k]
- # where self.cumulated_sizes[k-1] <= i < self.cumulated_sizes[k]
- self._cur_indices = None
-
- self._sizes = None
- self.virtual_size_per_dataset = None
- # caching properties
- self._reset_cached_properties()
- self.setup_sampling(sampling_ratios, virtual_size)
- self.set_epoch(epoch)
-
- def _clean_if_not_none(self, var_list):
- for v in var_list:
- if v is not None:
- del v
-
- def _reset_cached_properties(self):
- self._clean_if_not_none([self._sizes, self._cur_indices])
- self._sizes = None
- self._cur_indices = None
-
- def setup_sampling(self, sample_ratios, virtual_size):
- sizes = [len(d) for d in self.datasets]
- if sample_ratios is None:
- # default back to concating datasets
- self.sample_ratios = None
- self.virtual_size = sum(sizes)
- else:
- if not isinstance(sample_ratios, np.ndarray):
- sample_ratios = np.array(sample_ratios)
- self.sample_ratios = sample_ratios
- virtual_size = (
- default_virtual_size_func if virtual_size is None else virtual_size
- )
- self.virtual_size = (
- virtual_size(self.datasets, self.sample_ratios)
- if callable(virtual_size)
- else virtual_size
- )
-
- def adjust_sampling(self, epoch, sampling_ratios, virtual_size):
- if sampling_ratios is not None:
- sampling_ratios = self._sync_sample_ratios(sampling_ratios)
- self.setup_sampling(sampling_ratios, virtual_size)
-
- def _sync_sample_ratios(self, ratios):
- # in case the ratios are not precisely the same across processes
- # also to ensure every procresses update the ratios in the same pace
- ratios = torch.DoubleTensor(ratios)
- if torch.distributed.is_initialized():
- if torch.cuda.is_available():
- distributed_utils.all_reduce(
- ratios.cuda(), group=distributed_utils.get_data_parallel_group()
- )
- else:
- distributed_utils.all_reduce(
- ratios, group=distributed_utils.get_data_parallel_group()
- )
- ret = ratios.cpu()
- ret = ret.numpy()
- return ret
-
- def random_choice_in_dataset(self, rng, dataset, choice_size):
- if hasattr(dataset, "random_choice_in_dataset"):
- return dataset.random_choice_in_dataset(rng, choice_size)
- dataset_size = len(dataset)
- return rng.choice(
- dataset_size, choice_size, replace=(choice_size > dataset_size)
- )
-
- def get_virtual_indices(self, rng, datasets, sample_ratios, virtual_size):
- def get_counts(sample_ratios):
- counts = np.array([virtual_size * r for r in sample_ratios], dtype=np.int64)
- diff = virtual_size - counts.sum()
- assert diff >= 0
- # due to round-offs, the size might not match the desired sizes
- if diff > 0:
- dataset_indices = rng.choice(
- len(sample_ratios), size=diff, p=sample_ratios
- )
- for i in dataset_indices:
- counts[i] += 1
- return counts
-
- def get_in_dataset_indices(datasets, sizes, sample_ratios):
- counts = get_counts(sample_ratios)
- # uniformally sample desired counts for each dataset
- # if the desired counts are large, sample with replacement:
- indices = [
- self.random_choice_in_dataset(rng, d, c)
- for c, d in zip(counts, datasets)
- ]
- return indices
-
- sizes = [len(d) for d in datasets]
- if sample_ratios is None:
- # default back to concating datasets
- in_dataset_indices = [list(range(s)) for s in sizes]
- virtual_sizes_per_dataset = sizes
- else:
- ratios = sample_ratios / sample_ratios.sum()
- in_dataset_indices = get_in_dataset_indices(datasets, sizes, ratios)
- virtual_sizes_per_dataset = [len(d) for d in in_dataset_indices]
- virtual_sizes_per_dataset = np.array(virtual_sizes_per_dataset, np.int64)
- cumulative_sizes = np.cumsum(virtual_sizes_per_dataset)
- assert sum(virtual_sizes_per_dataset) == virtual_size
- assert cumulative_sizes[-1] == virtual_size
- if virtual_size < sum(sizes):
- logger.warning(
- f"virtual data size ({virtual_size}) is less than real data size ({sum(sizes)})."
- " If virtual size << real data size, there could be data coverage issue."
- )
- in_dataset_indices = np.hstack(in_dataset_indices)
- return in_dataset_indices, cumulative_sizes, virtual_sizes_per_dataset
-
- def _get_dataset_and_index(self, index):
- i = bisect_right(self.cumulated_sizes, index)
- return i, self._cur_indices[index]
-
- def __getitem__(self, index):
- # self.__getitem__(index) returns self.datasets[k][self._cur_indices[index]]
- # where k satisfies self.cumulated_sizes[k - 1] <= k < self.cumulated_sizes[k]
- ds_idx, ds_sample_idx = self._get_dataset_and_index(index)
- ret = (ds_idx, self.datasets[ds_idx][ds_sample_idx])
- return ret
-
- def num_tokens(self, index):
- return self.sizes[index].max()
-
- def num_tokens_vec(self, indices):
- sizes_vec = self.sizes[np.array(indices)]
- # max across all dimensions but first one
- return np.amax(sizes_vec, axis=tuple(range(1, len(sizes_vec.shape))))
-
- def size(self, index):
- return self.sizes[index]
-
- def __len__(self):
- return self.virtual_size
-
- def collater(self, samples, **extra_args):
- """Merge a list of samples to form a mini-batch."""
- if len(samples) == 0:
- return None
- if self.collate_format == "ordered_dict":
- collect_samples = [[] for _ in range(len(self.datasets))]
- for (i, sample) in samples:
- collect_samples[i].append(sample)
- batch = OrderedDict(
- [
- (self.keys[i], dataset.collater(collect_samples[i]))
- for i, (key, dataset) in enumerate(zip(self.keys, self.datasets))
- if len(collect_samples[i]) > 0
- ]
- )
- elif self.shared_collater:
- batch = self.datasets[0].collater([s for _, s in samples])
- else:
- samples_dict = defaultdict(list)
- pad_to_length = (
- defaultdict(int)
- if "pad_to_length" not in extra_args
- else extra_args["pad_to_length"]
- )
- for ds_idx, s in samples:
- pad_to_length["source"] = max(
- pad_to_length["source"], s["source"].size(0)
- )
- if s["target"] is not None:
- pad_to_length["target"] = max(
- pad_to_length["target"], s["target"].size(0)
- )
- samples_dict[ds_idx].append(s)
- batches = [
- self.datasets[i].collater(samples_dict[i], pad_to_length=pad_to_length)
- for i in range(len(self.datasets))
- if len(samples_dict[i]) > 0
- ]
-
- def straight_data(tensors):
- batch = torch.cat(tensors, dim=0)
- return batch
-
- src_lengths = straight_data(
- [b["net_input"]["src_lengths"] for b in batches]
- )
- src_lengths, sort_order = src_lengths.sort(descending=True)
-
- def straight_order(tensors):
- batch = straight_data(tensors)
- return batch.index_select(0, sort_order)
-
- batch = {
- "id": straight_order([b["id"] for b in batches]),
- "nsentences": sum(b["nsentences"] for b in batches),
- "ntokens": sum(b["ntokens"] for b in batches),
- "net_input": {
- "src_tokens": straight_order(
- [b["net_input"]["src_tokens"] for b in batches]
- ),
- "src_lengths": src_lengths,
- },
- "target": straight_order([b["target"] for b in batches])
- if batches[0]["target"] is not None
- else None,
- }
- if "prev_output_tokens" in batches[0]["net_input"]:
- batch["net_input"]["prev_output_tokens"] = straight_order(
- [b["net_input"]["prev_output_tokens"] for b in batches]
- )
- if "src_lang_id" in batches[0]["net_input"]:
- batch["net_input"]["src_lang_id"] = straight_order(
- [b["net_input"]["src_lang_id"] for b in batches]
- )
- if "tgt_lang_id" in batches[0]:
- batch["tgt_lang_id"] = straight_order(
- [b["tgt_lang_id"] for b in batches]
- )
- return batch
-
- @property
- def sizes(self):
- if self._sizes is not None:
- return self._sizes
- start_time = time.time()
- in_sub_dataset_indices = [
- self._cur_indices[
- 0 if i == 0 else self.cumulated_sizes[i - 1] : self.cumulated_sizes[i]
- ]
- for i in range(len(self.datasets))
- ]
- sub_dataset_sizes = [
- d.sizes[indices]
- for d, indices in zip(self.datasets, in_sub_dataset_indices)
- ]
- self._sizes = np.vstack(sub_dataset_sizes)
- logger.info(f"sizes() calling time: {get_time_gap(start_time, time.time())}")
- return self._sizes
-
- def ordered_indices(self):
- if self.shuffle:
- indices = np.random.permutation(len(self))
- else:
- indices = np.arange(len(self))
-
- sizes = self.sizes
- tgt_sizes = sizes[:, 1] if len(sizes.shape) > 0 and sizes.shape[1] > 1 else None
- src_sizes = (
- sizes[:, 0] if len(sizes.shape) > 0 and sizes.shape[1] > 1 else sizes
- )
-
- # sort by target length, then source length
- if tgt_sizes is not None:
- indices = indices[np.argsort(tgt_sizes[indices], kind="mergesort")]
- sort_indices = indices[np.argsort(src_sizes[indices], kind="mergesort")]
- return sort_indices
-
- def prefetch(self, indices):
- prefetch_indices = [[] for _ in range(len(self.datasets))]
- for i in indices:
- ds_idx, ds_sample_idx = self._get_dataset_and_index(i)
- prefetch_indices[ds_idx].append(ds_sample_idx)
- for i in range(len(prefetch_indices)):
- self.datasets[i].prefetch(prefetch_indices[i])
-
- @property
- def can_reuse_epoch_itr_across_epochs(self):
- return False
-
- def set_epoch(self, epoch):
- super().set_epoch(epoch)
- if epoch == self._cur_epoch:
- # re-enter so return
- return
- for d in self.datasets:
- if hasattr(d, "set_epoch"):
- d.set_epoch(epoch)
- self._cur_epoch = epoch
- self._establish_virtual_datasets()
-
- def _establish_virtual_datasets(self):
- if self.sample_ratios is None and self._cur_indices is not None:
- # not a samping dataset, no need to resample if indices are already established
- return
- self._reset_cached_properties()
-
- start_time = time.time()
- # Generate a weighted sample of indices as a function of the
- # random seed and the current epoch.
- rng = np.random.RandomState(
- [
- int(
- hashlib.sha1(
- str(self.__class__.__name__).encode("utf-8")
- ).hexdigest(),
- 16,
- )
- % (2 ** 32),
- self.seed % (2 ** 32), # global seed
- self._cur_epoch, # epoch index,
- ]
- )
- self._clean_if_not_none(
- [self.cumulated_sizes, self.virtual_size_per_dataset, self._sizes]
- )
- self._sizes = None
-
- indices, cumulated_sizes, virtual_size_per_dataset = self.get_virtual_indices(
- rng, self.datasets, self.sample_ratios, self.virtual_size
- )
- self._cur_indices = indices
- self.cumulated_sizes = cumulated_sizes
- self.virtual_size_per_dataset = virtual_size_per_dataset
-
- raw_sizes = [len(d) for d in self.datasets]
- sampled_sizes = self.virtual_size_per_dataset
- logger.info(
- f"[{self.split}] Raw sizes: {str(dict(zip(self.keys, raw_sizes)))}; "
- f"raw total size: {sum(raw_sizes)}"
- )
- logger.info(
- f"[{self.split}] Resampled sizes: {str(dict(zip(self.keys, sampled_sizes)))}; "
- f"resampled total size: {sum(sampled_sizes)}"
- )
- if self.sample_ratios is not None:
- logger.info(
- f"[{self.split}] Upsampling ratios: {str(dict(zip(self.keys, self.sample_ratios)))}"
- )
- else:
- logger.info(f"[{self.split}] A concat dataset")
- logger.info(
- f"[{self.split}] virtual dataset established time: {get_time_gap(start_time, time.time())}"
- )
-
- def filter_indices_by_size(self, indices, max_sizes):
- """Filter a list of sample indices. Remove those that are longer
- than specified in max_sizes.
-
- Args:
- indices (np.array): original array of sample indices
- max_sizes (int or list[int] or tuple[int]): max sample size,
- can be defined separately for src and tgt (then list or tuple)
-
- Returns:
- np.array: filtered sample array
- list: list of removed indices
- """
- sizes = self.sizes
- tgt_sizes = sizes[:, 1] if len(sizes.shape) > 0 and sizes.shape[1] > 1 else None
- src_sizes = (
- sizes[:, 0] if len(sizes.shape) > 0 and sizes.shape[1] > 1 else sizes
- )
-
- return data_utils.filter_paired_dataset_indices_by_size(
- src_sizes, tgt_sizes, indices, max_sizes
- )
diff --git a/spaces/HarryLee/eCommerceImageCaptioning/fairseq/fairseq/models/transformer_lm.py b/spaces/HarryLee/eCommerceImageCaptioning/fairseq/fairseq/models/transformer_lm.py
deleted file mode 100644
index eedd5151ba5b1a7050b37639023cf8a158fae8d4..0000000000000000000000000000000000000000
--- a/spaces/HarryLee/eCommerceImageCaptioning/fairseq/fairseq/models/transformer_lm.py
+++ /dev/null
@@ -1,545 +0,0 @@
-# Copyright (c) Facebook, Inc. and its affiliates.
-#
-# This source code is licensed under the MIT license found in the
-# LICENSE file in the root directory of this source tree.
-
-
-from dataclasses import dataclass, field
-from typing import Optional
-
-from fairseq import options, utils
-from fairseq.dataclass import ChoiceEnum, FairseqDataclass
-from fairseq.models import (
- FairseqLanguageModel,
- register_model,
- register_model_architecture,
-)
-from fairseq.models.transformer import (
- DEFAULT_MIN_PARAMS_TO_WRAP, Embedding, TransformerDecoder
-)
-from fairseq.modules import AdaptiveInput, CharacterTokenEmbedder
-from fairseq.utils import safe_getattr, safe_hasattr
-from omegaconf import II
-
-
-DEFAULT_MAX_TARGET_POSITIONS = 1024
-
-
-@dataclass
-class TransformerLanguageModelConfig(FairseqDataclass):
- activation_fn: ChoiceEnum(utils.get_available_activation_fns()) = field(
- default="relu", metadata={"help": "activation function to use"}
- )
- dropout: float = field(default=0.1, metadata={"help": "dropout probability"})
- attention_dropout: float = field(
- default=0.0, metadata={"help": "dropout probability for attention weights"}
- )
- activation_dropout: float = field(
- default=0.0, metadata={"help": "dropout probability after activation in FFN."}
- )
- relu_dropout: float = field(
- default=0.0, metadata={"help": "dropout probability after activation in FFN."}
- )
- decoder_embed_dim: int = field(
- default=512, metadata={"help": "decoder embedding dimension"}
- )
- decoder_output_dim: int = field(
- default=512, metadata={"help": "decoder output dimension"}
- )
- decoder_input_dim: int = field(
- default=512, metadata={"help": "decoder input dimension"}
- )
- decoder_ffn_embed_dim: int = field(
- default=2048, metadata={"help": "decoder embedding dimension for FFN"}
- )
- decoder_layers: int = field(default=6, metadata={"help": "num decoder layers"})
- decoder_attention_heads: int = field(
- default=8, metadata={"help": "num decoder attention heads"}
- )
- decoder_normalize_before: bool = field(
- default=False, metadata={"help": "apply layernorm before each decoder block"}
- )
- no_decoder_final_norm: bool = field(
- default=False,
- metadata={"help": "don't add an extra layernorm after the last decoder block"},
- )
- adaptive_softmax_cutoff: Optional[str] = field(
- default=None,
- metadata={
- "help": "comma separated list of adaptive softmax cutoff points. "
- "Must be used with adaptive_loss criterion"
- },
- )
- adaptive_softmax_dropout: float = field(
- default=0,
- metadata={"help": "sets adaptive softmax dropout for the tail projections"},
- )
- adaptive_softmax_factor: float = field(
- default=4, metadata={"help": "adaptive input factor"}
- )
- no_token_positional_embeddings: bool = field(
- default=False,
- metadata={
- "help": "if set, disables positional embeddings (outside self attention)"
- },
- )
- share_decoder_input_output_embed: bool = field(
- default=False, metadata={"help": "share decoder input and output embeddings"}
- )
- character_embeddings: bool = field(
- default=False,
- metadata={
- "help": "if set, uses character embedding convolutions to produce token embeddings"
- },
- )
- character_filters: str = field(
- default="[(1, 64), (2, 128), (3, 192), (4, 256), (5, 256), (6, 256), (7, 256)]",
- metadata={"help": "size of character embeddings"},
- )
- character_embedding_dim: int = field(
- default=4, metadata={"help": "size of character embeddings"}
- )
- char_embedder_highway_layers: int = field(
- default=2,
- metadata={"help": "number of highway layers for character token embeddder"},
- )
- adaptive_input: bool = field(
- default=False, metadata={"help": "if set, uses adaptive input"}
- )
- adaptive_input_factor: float = field(
- default=4, metadata={"help": "adaptive input factor"}
- )
- adaptive_input_cutoff: Optional[str] = field(
- default=None,
- metadata={"help": "comma separated list of adaptive input cutoff points."},
- )
- tie_adaptive_weights: bool = field(
- default=False,
- metadata={
- "help": "if set, ties the weights of adaptive softmax and adaptive input"
- },
- )
- tie_adaptive_proj: bool = field(
- default=False,
- metadata={
- "help": "if set, ties the projection weights of adaptive softmax and adaptive input"
- },
- )
- decoder_learned_pos: bool = field(
- default=False,
- metadata={"help": "use learned positional embeddings in the decoder"},
- )
- layernorm_embedding: bool = field(
- default=False, metadata={"help": "add layernorm to embedding"}
- )
- no_scale_embedding: bool = field(
- default=False, metadata={"help": "if True, dont scale embeddings"}
- )
- checkpoint_activations: bool = field(
- default=False, metadata={"help": "checkpoint activations at each layer"}
- )
- offload_activations: bool = field(
- default=False,
- metadata={"help": "move checkpointed activations to CPU after they are used."},
- )
- # config for "Reducing Transformer Depth on Demand with Structured Dropout" (Fan et al., 2019)
- decoder_layerdrop: float = field(
- default=0.0, metadata={"help": "LayerDrop probability for decoder"}
- )
- decoder_layers_to_keep: Optional[str] = field(
- default=None,
- metadata={
- "help": "which layers to *keep* when pruning as a comma-separated list"
- },
- )
- # config for Training with Quantization Noise for Extreme Model Compression ({Fan*, Stock*} et al., 2020)
- quant_noise_pq: float = field(
- default=0.0,
- metadata={"help": "iterative PQ quantization noise at training time"},
- )
- quant_noise_pq_block_size: int = field(
- default=8,
- metadata={"help": "block size of quantization noise at training time"},
- )
- quant_noise_scalar: float = field(
- default=0.0,
- metadata={
- "help": "scalar quantization noise and scalar quantization at training time"
- },
- )
- # config for Fully Sharded Data Parallel (FSDP) training
- min_params_to_wrap: int = field(
- default=DEFAULT_MIN_PARAMS_TO_WRAP,
- metadata={
- "help": (
- "minimum number of params for a layer to be wrapped with FSDP() when "
- "training with --ddp-backend=fully_sharded. Smaller values will "
- "improve memory efficiency, but may make torch.distributed "
- "communication less efficient due to smaller input sizes. This option "
- "is set to 0 (i.e., always wrap) when --checkpoint-activations or "
- "--offload-activations are passed."
- )
- }
- )
- # config for "BASE Layers: Simplifying Training of Large, Sparse Models"
- base_layers: Optional[int] = field(
- default=0, metadata={"help": "number of BASE layers in total"}
- )
- base_sublayers: Optional[int] = field(
- default=1, metadata={"help": "number of sublayers in each BASE layer"}
- )
- base_shuffle: Optional[int] = field(
- default=1, metadata={"help": "shuffle tokens between workers before computing assignment"}
- )
- # options from other parts of the config
- add_bos_token: bool = II("task.add_bos_token")
- tokens_per_sample: int = II("task.tokens_per_sample")
- max_target_positions: Optional[int] = II("task.max_target_positions")
- tpu: bool = II("common.tpu")
-
-
-@register_model("transformer_lm", dataclass=TransformerLanguageModelConfig)
-class TransformerLanguageModel(FairseqLanguageModel):
- @classmethod
- def hub_models(cls):
- def moses_fastbpe(path):
- return {"path": path, "tokenizer": "moses", "bpe": "fastbpe"}
-
- def spm(path):
- return {"path": path, "tokenizer": "space", "bpe": "sentencepiece"}
-
- return {
- "transformer_lm.gbw.adaptive_huge": "https://dl.fbaipublicfiles.com/fairseq/models/lm/adaptive_lm_gbw_huge.tar.bz2",
- "transformer_lm.wiki103.adaptive": "https://dl.fbaipublicfiles.com/fairseq/models/lm/adaptive_lm_wiki103.v2.tar.bz2",
- "transformer_lm.wmt19.en": moses_fastbpe(
- "https://dl.fbaipublicfiles.com/fairseq/models/lm/wmt19.en.tar.bz2"
- ),
- "transformer_lm.wmt19.de": moses_fastbpe(
- "https://dl.fbaipublicfiles.com/fairseq/models/lm/wmt19.de.tar.bz2"
- ),
- "transformer_lm.wmt19.ru": moses_fastbpe(
- "https://dl.fbaipublicfiles.com/fairseq/models/lm/wmt19.ru.tar.bz2"
- ),
- "transformer_lm.wmt20.en": spm(
- "https://dl.fbaipublicfiles.com/fairseq/models/lm/wmt20.en.tar.gz"
- ),
- "transformer_lm.wmt20.ta": spm(
- "https://dl.fbaipublicfiles.com/fairseq/models/lm/wmt20.ta.tar.gz"
- ),
- "transformer_lm.wmt20.iu.news": spm(
- "https://dl.fbaipublicfiles.com/fairseq/models/lm/wmt20.iu.news.tar.gz"
- ),
- "transformer_lm.wmt20.iu.nh": spm(
- "https://dl.fbaipublicfiles.com/fairseq/models/lm/wmt20.iu.nh.tar.gz"
- ),
- }
-
- def __init__(self, decoder):
- super().__init__(decoder)
-
- @classmethod
- def build_model(cls, args, task):
- """Build a new model instance."""
-
- if args.decoder_layers_to_keep:
- args.decoder_layers = len(args.decoder_layers_to_keep.split(","))
-
- if safe_getattr(args, "max_target_positions", None) is None:
- args.max_target_positions = safe_getattr(
- args, "tokens_per_sample", DEFAULT_MAX_TARGET_POSITIONS
- )
-
- if args.character_embeddings:
- embed_tokens = CharacterTokenEmbedder(
- task.source_dictionary,
- eval(args.character_filters),
- args.character_embedding_dim,
- args.decoder_embed_dim,
- args.char_embedder_highway_layers,
- )
- elif args.adaptive_input:
- embed_tokens = AdaptiveInput(
- len(task.source_dictionary),
- task.source_dictionary.pad(),
- args.decoder_input_dim,
- args.adaptive_input_factor,
- args.decoder_embed_dim,
- options.eval_str_list(args.adaptive_input_cutoff, type=int),
- args.quant_noise_pq,
- args.quant_noise_pq_block_size,
- )
- else:
- embed_tokens = cls.build_embedding(
- args, task.source_dictionary, args.decoder_input_dim
- )
-
- if args.tie_adaptive_weights:
- assert args.adaptive_input
- assert args.adaptive_input_factor == args.adaptive_softmax_factor
- assert (
- args.adaptive_softmax_cutoff == args.adaptive_input_cutoff
- ), "{} != {}".format(
- args.adaptive_softmax_cutoff, args.adaptive_input_cutoff
- )
- assert args.decoder_input_dim == args.decoder_output_dim
-
- decoder = TransformerDecoder(
- args, task.target_dictionary, embed_tokens, no_encoder_attn=True
- )
- return cls(decoder)
-
- @classmethod
- def build_embedding(cls, args, dictionary, embed_dim, path=None):
- embed_tokens = Embedding(len(dictionary), embed_dim, dictionary.pad())
- return embed_tokens
-
-
-def base_lm_architecture(args):
- # backward compatibility for older model checkpoints
- if safe_hasattr(args, "no_tie_adaptive_proj"):
- # previous models defined --no-tie-adaptive-proj, so use the existence of
- # that option to determine if this is an "old" model checkpoint
- args.no_decoder_final_norm = True # old models always set this to True
- if args.no_tie_adaptive_proj is False:
- args.tie_adaptive_proj = True
- if safe_hasattr(args, "decoder_final_norm"):
- args.no_decoder_final_norm = not args.decoder_final_norm
-
- args.dropout = safe_getattr(args, "dropout", 0.1)
- args.attention_dropout = safe_getattr(args, "attention_dropout", 0.0)
-
- args.decoder_embed_dim = safe_getattr(args, "decoder_embed_dim", 512)
- args.decoder_ffn_embed_dim = safe_getattr(args, "decoder_ffn_embed_dim", 2048)
- args.decoder_layers = safe_getattr(args, "decoder_layers", 6)
- args.decoder_attention_heads = safe_getattr(args, "decoder_attention_heads", 8)
- args.adaptive_softmax_cutoff = safe_getattr(args, "adaptive_softmax_cutoff", None)
- args.adaptive_softmax_dropout = safe_getattr(args, "adaptive_softmax_dropout", 0)
- args.adaptive_softmax_factor = safe_getattr(args, "adaptive_softmax_factor", 4)
- args.decoder_learned_pos = safe_getattr(args, "decoder_learned_pos", False)
- args.activation_fn = safe_getattr(args, "activation_fn", "relu")
-
- args.decoder_layerdrop = safe_getattr(args, "decoder_layerdrop", 0)
- args.decoder_layers_to_keep = safe_getattr(args, "decoder_layers_to_keep", None)
- args.quant_noise_pq = safe_getattr(args, "quant_noise_pq", 0)
- args.quant_noise_pq_block_size = safe_getattr(args, "quant_noise_pq_block_size", 8)
- args.quant_noise_scalar = safe_getattr(args, "quant_noise_scalar", 0)
-
- args.base_layers = safe_getattr(args, "base_layers", 0)
- args.base_sublayers = safe_getattr(args, "base_sublayers", 1)
- args.base_shuffle = safe_getattr(args, "base_shuffle", False)
-
- args.add_bos_token = safe_getattr(args, "add_bos_token", False)
- args.no_token_positional_embeddings = safe_getattr(
- args, "no_token_positional_embeddings", False
- )
- args.share_decoder_input_output_embed = safe_getattr(
- args, "share_decoder_input_output_embed", False
- )
- args.character_embeddings = safe_getattr(args, "character_embeddings", False)
-
- args.decoder_output_dim = safe_getattr(
- args, "decoder_output_dim", args.decoder_embed_dim
- )
- args.decoder_input_dim = safe_getattr(args, "decoder_input_dim", args.decoder_embed_dim)
-
- # Model training is not stable without this
- args.decoder_normalize_before = True
- args.no_decoder_final_norm = safe_getattr(args, "no_decoder_final_norm", False)
-
- args.adaptive_input = safe_getattr(args, "adaptive_input", False)
- args.adaptive_input_factor = safe_getattr(args, "adaptive_input_factor", 4)
- args.adaptive_input_cutoff = safe_getattr(args, "adaptive_input_cutoff", None)
-
- args.tie_adaptive_weights = safe_getattr(args, "tie_adaptive_weights", False)
- args.tie_adaptive_proj = safe_getattr(args, "tie_adaptive_proj", False)
-
- args.no_scale_embedding = safe_getattr(args, "no_scale_embedding", False)
- args.layernorm_embedding = safe_getattr(args, "layernorm_embedding", False)
- args.checkpoint_activations = safe_getattr(args, "checkpoint_activations", False)
- args.offload_activations = safe_getattr(args, "offload_activations", False)
- if args.offload_activations:
- args.checkpoint_activations = True
-
-
-@register_model_architecture("transformer_lm", "transformer_lm_big")
-def transformer_lm_big(args):
- args.decoder_layers = safe_getattr(args, "decoder_layers", 12)
- args.decoder_embed_dim = safe_getattr(args, "decoder_embed_dim", 1024)
- args.decoder_ffn_embed_dim = safe_getattr(args, "decoder_ffn_embed_dim", 4096)
- args.decoder_attention_heads = safe_getattr(args, "decoder_attention_heads", 16)
- base_lm_architecture(args)
-
-
-@register_model_architecture("transformer_lm", "transformer_lm_wiki103")
-@register_model_architecture("transformer_lm", "transformer_lm_baevski_wiki103")
-def transformer_lm_baevski_wiki103(args):
- args.decoder_layers = safe_getattr(args, "decoder_layers", 16)
- args.decoder_attention_heads = safe_getattr(args, "decoder_attention_heads", 8)
- args.dropout = safe_getattr(args, "dropout", 0.3)
- args.adaptive_input = safe_getattr(args, "adaptive_input", True)
- args.tie_adaptive_weights = safe_getattr(args, "tie_adaptive_weights", True)
- args.adaptive_input_cutoff = safe_getattr(args, "adaptive_input_cutoff", "20000,60000")
- args.adaptive_softmax_cutoff = safe_getattr(
- args, "adaptive_softmax_cutoff", "20000,60000"
- )
- args.adaptive_softmax_dropout = safe_getattr(args, "adaptive_softmax_dropout", 0.2)
- args.attention_dropout = safe_getattr(args, "attention_dropout", 0.1)
- args.activation_dropout = safe_getattr(args, "activation_dropout", 0.1)
- args.no_decoder_final_norm = safe_getattr(args, "no_decoder_final_norm", True)
- args.tie_adaptive_proj = safe_getattr(args, "tie_adaptive_proj", True)
- transformer_lm_big(args)
-
-
-@register_model_architecture("transformer_lm", "transformer_lm_gbw")
-@register_model_architecture("transformer_lm", "transformer_lm_baevski_gbw")
-def transformer_lm_baevski_gbw(args):
- args.decoder_embed_dim = safe_getattr(args, "decoder_embed_dim", 512)
- args.dropout = safe_getattr(args, "dropout", 0.1)
- args.attention_dropout = safe_getattr(args, "attention_dropout", 0.1)
- args.no_decoder_final_norm = safe_getattr(args, "no_decoder_final_norm", True)
- transformer_lm_big(args)
-
-
-@register_model_architecture("transformer_lm", "transformer_lm_gpt")
-def transformer_lm_gpt(args):
- args.decoder_embed_dim = safe_getattr(args, "decoder_embed_dim", 768)
- args.decoder_ffn_embed_dim = safe_getattr(args, "decoder_ffn_embed_dim", 3072)
- args.decoder_layers = safe_getattr(args, "decoder_layers", 12)
- args.decoder_attention_heads = safe_getattr(args, "decoder_attention_heads", 12)
- args.dropout = safe_getattr(args, "dropout", 0.1)
- args.attention_dropout = safe_getattr(args, "attention_dropout", 0.1)
- args.activation_fn = safe_getattr(args, "activation_fn", "gelu")
- base_lm_architecture(args)
-
-
-@register_model_architecture("transformer_lm", "transformer_lm_gpt2_small")
-def transformer_lm_gpt2_small(args):
- args.decoder_embed_dim = safe_getattr(args, "decoder_embed_dim", 1024)
- args.decoder_ffn_embed_dim = safe_getattr(args, "decoder_ffn_embed_dim", 4096)
- args.decoder_layers = safe_getattr(args, "decoder_layers", 24)
- args.decoder_attention_heads = safe_getattr(args, "decoder_attention_heads", 16)
- args.dropout = safe_getattr(args, "dropout", 0.1)
- args.attention_dropout = safe_getattr(args, "attention_dropout", 0.1)
- args.activation_fn = safe_getattr(args, "activation_fn", "gelu")
- base_lm_architecture(args)
-
-
-@register_model_architecture("transformer_lm", "transformer_lm_gpt2_tiny")
-def transformer_lm_gpt2_tiny(args):
- args.decoder_embed_dim = safe_getattr(args, "decoder_embed_dim", 64)
- args.decoder_ffn_embed_dim = safe_getattr(args, "decoder_ffn_embed_dim", 64)
- args.decoder_layers = safe_getattr(args, "decoder_layers", 2)
- args.decoder_attention_heads = safe_getattr(args, "decoder_attention_heads", 1)
- args.dropout = safe_getattr(args, "dropout", 0.1)
- args.attention_dropout = safe_getattr(args, "attention_dropout", 0.1)
- args.activation_fn = safe_getattr(args, "activation_fn", "gelu")
- base_lm_architecture(args)
-
-
-@register_model_architecture("transformer_lm", "transformer_lm_gpt2_medium")
-def transformer_lm_gpt2_medium(args):
- args.decoder_embed_dim = safe_getattr(args, "decoder_embed_dim", 1280)
- args.decoder_ffn_embed_dim = safe_getattr(args, "decoder_ffn_embed_dim", 5120)
- args.decoder_layers = safe_getattr(args, "decoder_layers", 36)
- args.decoder_attention_heads = safe_getattr(args, "decoder_attention_heads", 20)
- args.dropout = safe_getattr(args, "dropout", 0.1)
- args.attention_dropout = safe_getattr(args, "attention_dropout", 0.1)
- args.activation_fn = safe_getattr(args, "activation_fn", "gelu")
- base_lm_architecture(args)
-
-
-@register_model_architecture("transformer_lm", "transformer_lm_gpt2_big")
-def transformer_lm_gpt2_big(args):
- args.decoder_embed_dim = safe_getattr(args, "decoder_embed_dim", 1600)
- args.decoder_ffn_embed_dim = safe_getattr(args, "decoder_ffn_embed_dim", 6400)
- args.decoder_layers = safe_getattr(args, "decoder_layers", 48)
- args.decoder_attention_heads = safe_getattr(args, "decoder_attention_heads", 25)
- args.dropout = safe_getattr(args, "dropout", 0.1)
- args.attention_dropout = safe_getattr(args, "attention_dropout", 0.1)
- args.activation_fn = safe_getattr(args, "activation_fn", "gelu")
- base_lm_architecture(args)
-
-
-def base_gpt3_architecture(args):
- args.decoder_input_dim = args.decoder_embed_dim
- args.decoder_output_dim = args.decoder_embed_dim
- args.decoder_ffn_embed_dim = safe_getattr(args, "decoder_ffn_embed_dim", args.decoder_embed_dim * 4)
- # GPT-3 used learned positional embeddings, rather than sinusoidal
- args.decoder_learned_pos = safe_getattr(args, "decoder_learned_pos", True)
- args.dropout = safe_getattr(args, "dropout", 0.0)
- args.attention_dropout = safe_getattr(args, "attention_dropout", 0.0)
- args.activation_fn = safe_getattr(args, "activation_fn", "gelu")
- args.share_decoder_input_output_embed = True
- base_lm_architecture(args)
-
-
-@register_model_architecture("transformer_lm", "transformer_lm_gpt3_small")
-def transformer_lm_gpt3_small(args):
- # 125M params
- args.decoder_layers = safe_getattr(args, "decoder_layers", 12)
- args.decoder_embed_dim = safe_getattr(args, "decoder_embed_dim", 768)
- args.decoder_attention_heads = safe_getattr(args, "decoder_attention_heads", 12)
- base_gpt3_architecture(args)
-
-
-@register_model_architecture("transformer_lm", "transformer_lm_gpt3_medium")
-def transformer_lm_gpt3_medium(args):
- # 350M params
- args.decoder_layers = safe_getattr(args, "decoder_layers", 24)
- args.decoder_embed_dim = safe_getattr(args, "decoder_embed_dim", 1024)
- args.decoder_attention_heads = safe_getattr(args, "decoder_attention_heads", 16)
- base_gpt3_architecture(args)
-
-
-@register_model_architecture("transformer_lm", "transformer_lm_gpt3_large")
-def transformer_lm_gpt3_large(args):
- # 760M params
- args.decoder_layers = safe_getattr(args, "decoder_layers", 24)
- args.decoder_embed_dim = safe_getattr(args, "decoder_embed_dim", 1536)
- args.decoder_attention_heads = safe_getattr(args, "decoder_attention_heads", 16)
- base_gpt3_architecture(args)
-
-
-@register_model_architecture("transformer_lm", "transformer_lm_gpt3_xl")
-def transformer_lm_gpt3_xl(args):
- # 1.3B params
- args.decoder_layers = safe_getattr(args, "decoder_layers", 24)
- args.decoder_embed_dim = safe_getattr(args, "decoder_embed_dim", 2048)
- args.decoder_attention_heads = safe_getattr(args, "decoder_attention_heads", 32)
- base_gpt3_architecture(args)
-
-
-@register_model_architecture("transformer_lm", "transformer_lm_gpt3_2_7")
-def transformer_lm_gpt3_2_7(args):
- # 2.7B params
- args.decoder_layers = safe_getattr(args, "decoder_layers", 32)
- args.decoder_embed_dim = safe_getattr(args, "decoder_embed_dim", 2560)
- args.decoder_attention_heads = safe_getattr(args, "decoder_attention_heads", 32)
- base_gpt3_architecture(args)
-
-
-@register_model_architecture("transformer_lm", "transformer_lm_gpt3_6_7")
-def transformer_lm_gpt3_6_7(args):
- # 6.7B params
- args.decoder_layers = safe_getattr(args, "decoder_layers", 32)
- args.decoder_embed_dim = safe_getattr(args, "decoder_embed_dim", 4096)
- args.decoder_attention_heads = safe_getattr(args, "decoder_attention_heads", 32)
- base_gpt3_architecture(args)
-
-
-@register_model_architecture("transformer_lm", "transformer_lm_gpt3_13")
-def transformer_lm_gpt3_13(args):
- # 13B params
- args.decoder_layers = safe_getattr(args, "decoder_layers", 40)
- args.decoder_embed_dim = safe_getattr(args, "decoder_embed_dim", 5120)
- args.decoder_attention_heads = safe_getattr(args, "decoder_attention_heads", 40)
- base_gpt3_architecture(args)
-
-
-@register_model_architecture("transformer_lm", "transformer_lm_gpt3_175")
-def transformer_lm_gpt3_175(args):
- # 175B params
- args.decoder_layers = safe_getattr(args, "decoder_layers", 96)
- args.decoder_embed_dim = safe_getattr(args, "decoder_embed_dim", 12288)
- args.decoder_attention_heads = safe_getattr(args, "decoder_attention_heads", 96)
- base_gpt3_architecture(args)
diff --git a/spaces/Harveenchadha/Vakyansh-Tamil-TTS/ttsv/src/hifi_gan/env.py b/spaces/Harveenchadha/Vakyansh-Tamil-TTS/ttsv/src/hifi_gan/env.py
deleted file mode 100644
index 2bdbc95d4f7a8bad8fd4f5eef657e2b51d946056..0000000000000000000000000000000000000000
--- a/spaces/Harveenchadha/Vakyansh-Tamil-TTS/ttsv/src/hifi_gan/env.py
+++ /dev/null
@@ -1,15 +0,0 @@
-import os
-import shutil
-
-
-class AttrDict(dict):
- def __init__(self, *args, **kwargs):
- super(AttrDict, self).__init__(*args, **kwargs)
- self.__dict__ = self
-
-
-def build_env(config, config_name, path):
- t_path = os.path.join(path, config_name)
- if config != t_path:
- os.makedirs(path, exist_ok=True)
- shutil.copyfile(config, os.path.join(path, config_name))
diff --git a/spaces/Harveenchadha/oiTrans/legacy/install_fairseq.sh b/spaces/Harveenchadha/oiTrans/legacy/install_fairseq.sh
deleted file mode 100644
index 275ab9574dabcd293a553dd50e46288d33025e7a..0000000000000000000000000000000000000000
--- a/spaces/Harveenchadha/oiTrans/legacy/install_fairseq.sh
+++ /dev/null
@@ -1,45 +0,0 @@
-#NVIDIA CUDA download
-wget "https://developer.nvidia.com/compute/cuda/10.0/Prod/local_installers/cuda_10.0.130_410.48_linux"
-wget "http://developer.download.nvidia.com/compute/cuda/10.0/Prod/patches/1/cuda_10.0.130.1_linux.run"
-
-## do not install drivers (See this: https://docs.nvidia.com/deploy/cuda-compatibility/index.html)
-sudo sh "cuda_10.0.130_410.48_linux"
-sudo sh "cuda_10.0.130.1_linux.run"
-
-#Set environment variables
-export CUDA_HOME=/usr/local/cuda-10.0
-export PATH=$CUDA_HOME/bin:$PATH
-export LD_LIBRARY_PATH=$CUDA_HOME/lib64:$LD_LIBRARY_PATH
-
-# Install pytorch 1.2
-python3 -m venv pytorch1.2
-source pytorch1.2/bin/activate
-which pip3
-pip3 install torch==1.2.0 torchvision==0.4.0
-
-# Install nccl
-git clone https://github.com/NVIDIA/nccl.git
-cd nccl
-make src.build CUDA_HOME=$CUDA_HOME
-sudo apt install build-essential devscripts debhelper fakeroot
-make pkg.debian.build CUDA_HOME=$CUDA_HOME
-sudo dpkg -i build/pkg/deb/libnccl2_2.7.8-1+cuda10.0_amd64.deb
-sudo dpkg -i build/pkg/deb/libnccl-dev_2.7.8-1+cuda10.0_amd64.deb
-sudo apt-get install -f
-
-# Install Apex
-git clone https://github.com/NVIDIA/apex
-cd apex
-pip install -v --no-cache-dir --global-option="--cpp_ext" --global-option="--cuda_ext" \
- --global-option="--deprecated_fused_adam" --global-option="--xentropy" \
- --global-option="--fast_multihead_attn" ./
-
-# Install PyArrow
-pip install pyarrow
-
-# Install fairseq
-pip install --editable ./
-
-# Install other dependencies
-pip install sacrebleu
-pip install tensorboardX --user
diff --git a/spaces/HighCWu/Style2Paints-4.5-Gradio/ui/web-mobile/style-desktop.ec961.css b/spaces/HighCWu/Style2Paints-4.5-Gradio/ui/web-mobile/style-desktop.ec961.css
deleted file mode 100644
index f2117923e6f8507f0947fe70a2a81aa5e35bf64a..0000000000000000000000000000000000000000
--- a/spaces/HighCWu/Style2Paints-4.5-Gradio/ui/web-mobile/style-desktop.ec961.css
+++ /dev/null
@@ -1,117 +0,0 @@
-body {
- cursor: default;
- padding: 0;
- border: 0;
- margin: 0;
-
- text-align: center;
- background-color: white;
- font-family: Helvetica, Verdana, Arial, sans-serif;
-}
-
-body, canvas, div {
- outline: none;
- -moz-user-select: none;
- -webkit-user-select: none;
- -ms-user-select: none;
- -khtml-user-select: none;
- -webkit-tap-highlight-color: rgba(0, 0, 0, 0);
-}
-
-/* Remove spin of input type number */
-input::-webkit-outer-spin-button,
-input::-webkit-inner-spin-button {
- /* display: none; <- Crashes Chrome on hover */
- -webkit-appearance: none;
- margin: 0; /* <-- Apparently some margin are still there even though it's hidden */
-}
-
-#Cocos2dGameContainer {
- position: absolute;
- margin: 0;
- overflow: hidden;
- left: 0px;
- top: 0px;
-}
-
-canvas {
- background-color: rgba(0, 0, 0, 0);
-}
-
-a:link, a:visited {
- color: #000;
-}
-
-a:active, a:hover {
- color: #666;
-}
-
-p.header {
- font-size: small;
-}
-
-p.footer {
- font-size: x-small;
-}
-
-#splash {
- position: absolute;
- top: 0;
- left: 0;
- width: 100%;
- height: 100%;
-
- background: #171717 url(./splash.03ce1.png) no-repeat center;
- background-size: 40%;
-}
-
-.progress-bar {
- background-color: #1a1a1a;
- position: absolute;
- left: 50%;
- top: 80%;
- height: 26px;
- padding: 5px;
- width: 350px;
- margin: 0 -175px;
- border-radius: 5px;
- box-shadow: 0 1px 5px #000 inset, 0 1px 0 #444;
-}
-
-.progress-bar span {
- display: block;
- height: 100%;
- border-radius: 3px;
- box-shadow: 0 1px 0 rgba(255, 255, 255, .5) inset;
- transition: width .4s ease-in-out;
- background-color: #34c2e3;
-}
-
-.stripes span {
- background-size: 30px 30px;
- background-image: linear-gradient(135deg, rgba(255, 255, 255, .15) 25%, transparent 25%,
- transparent 50%, rgba(255, 255, 255, .15) 50%, rgba(255, 255, 255, .15) 75%,
- transparent 75%, transparent);
-
- animation: animate-stripes 1s linear infinite;
-}
-
-@keyframes animate-stripes {
- 0% {background-position: 0 0;} 100% {background-position: 60px 0;}
-}
-
-h1 {
- color: #444;
- text-shadow: 3px 3px 15px;
-}
-
-#GameDiv {
- width: 800px;
- height: 450px;
- margin: 0 auto;
- background: black;
- position:relative;
- border:5px solid black;
- border-radius: 10px;
- box-shadow: 0 5px 50px #333
-}
diff --git a/spaces/ICCV2023/ICCV2023-papers/app.py b/spaces/ICCV2023/ICCV2023-papers/app.py
deleted file mode 100644
index e1ef36adc25ef98f6c0602e7ccae6ee0fe0dd89f..0000000000000000000000000000000000000000
--- a/spaces/ICCV2023/ICCV2023-papers/app.py
+++ /dev/null
@@ -1,101 +0,0 @@
-#!/usr/bin/env python
-
-from __future__ import annotations
-
-import gradio as gr
-
-from paper_list import PaperList
-
-DESCRIPTION = """\
-# ICCV 2023 Papers
-
-- https://iccv2023.thecvf.com
-- https://openaccess.thecvf.com/ICCV2023
-"""
-
-TUTORIAL = """\
-#### Hugging Face ICCV 2023 event
-
-Join the org using this [link](https://huggingface.co/organizations/ICCV2023/share/BXikCrBMdmnKzsFjVSulFrCsQAujoYGiNG)
-
-ICCV 2023 organization is accepting paper claims from authors attending ICCV 2023.
-
-This organization invites participants to claim their ICCV 2023 papers, upload models and datasets, and to build their Gradio demos for conference papers on huggingface.
-
-#### Hugging Face Paper Pages ICCV 2023
-
-- ICCV 2023 Paper Pages will allow authors to claim their papers on Huggingface. Claiming papers on ICCV 2023 organization will allow people to find artifacts related to a paper such as models, datasets and Gradio demos (in form of Spaces). This also enables the community to discuss about the paper.
-
-#### Tutorial for claiming the ICCV 2023 papers
-
-Visit the [demo](https://huggingface.co/spaces/ICCV2023/ICCV2023-papers) and find your paper, to claim your paper click on the pager page link in the table and then click on your name in the corresponding Paper page and click “claim authorship”. This will automatically re-direct to your paper settings where you can confirm the request. The admin team will validate your request soon. Once confirmed, the Paper page will show as verified.
-
-If you need further assistance, see the guide [here](https://huggingface.co/docs/hub/paper-pages#claiming-authorship-to-a-paper)
-
-If your paper is not yet indexed on huggingface, you can index it by following this [guide](https://huggingface.co/docs/hub/paper-pages#can-i-have-a-paper-page-even-if-i-have-no-modeldatasetspace) and open a [PR](https://huggingface.co/spaces/ICCV2023/ICCV2023-papers/discussions) to add your paper to the hugging face demo.
-"""
-
-
-paper_list = PaperList()
-
-with gr.Blocks(css="style.css") as demo:
- gr.Markdown(DESCRIPTION)
- with gr.Accordion(label="Tutorial", open=True):
- gr.Markdown(TUTORIAL)
-
- search_box = gr.Textbox(
- label="Search Title", placeholder="You can search for titles with regular expressions. e.g. (?'] + personas + ['<|sep|>'] + ['<|start|>']))
- user_inp= self.tokenizer.encode(history[-1][0]+self.tokenizer.eos_token)
- dialog_hx.append(user_inp)
- bot_input_ids = to_var([person + flatten(dialog_hx)]).long()
- with torch.no_grad():
-
- full_msg = self.model.generate(bot_input_ids,
- repetition_penalty=1.4,
- top_k = 10,
- top_p = 0.92,
- max_new_tokens = 256,
- num_beams=2,
- pad_token_id = self.tokenizer.eos_token_id)
-
-
- response = to_data(full_msg.detach()[0])[bot_input_ids.shape[-1]:]
- dialog_hx.append(response)
- history[-1][1] = self.tokenizer.decode(response, skip_special_tokens=True)
- self.speak(history[-1][1])
- return history, "out.mp3",dialog_hx
-
- def talk(self, audio, history,dialog_hx,personas,text):
- if audio is not None:
- history, _ = self.listen(audio, history)
- else:
- history.append([text,None])
- history, audio,dialog_hx = self.respond(history,dialog_hx,personas)
- return history, None, audio,dialog_hx,None
-
- def speak(self, text):
- """
- Speaks the given text using gTTS,
- Parameters:
- text: text to be spoken
- """
- tts = gTTS(text, lang='en')
- tts.save('out.mp3')
-
-# Initialize AI Companion
-bot = AI_Companion()
-personas=[]
-for i in ['I\'m a 19 year old girl','I study at IIT Indore','I am an easy-going and fun loving person','I love to swim','I am friendly, nice ,fun and kind','I am studious and get good grades']:
- response = i+ bot.tokenizer.eos_token
- personas.append(response)
-
-
-# Create the Interface
-with gr.Blocks() as demo:
- dialog_hx=gr.State([])
- personas=gr.State(personas)
- chatbot = gr.Chatbot([], elem_id = "chatbot").style(height = 300)
- audio = gr.Audio(source = "microphone", type = "filepath", label = "Input")
- msg = gr.Textbox()
- audio1 = gr.Audio(type = "filepath", label = "Output",elem_id="input")
- with gr.Row():
- b1 = gr.Button("Submit")
- b2 = gr.Button("Clear")
- b3= gr.Button("Add Fact")
- b1.click(bot.talk, [audio, chatbot,dialog_hx,personas,msg], [chatbot, audio, audio1,dialog_hx,msg])
- msg.submit(append, [msg, chatbot,dialog_hx,personas], [chatbot, audio1, msg,dialog_hx])
- b2.click(clear, [] , [audio,chatbot,dialog_hx])
- b3.click(bot.add_fact, [audio,personas,msg], [audio,personas,msg])
-demo.launch()
-
-
-
diff --git a/spaces/Iceclear/StableSR/StableSR/ldm/modules/diffusionmodules/__init__.py b/spaces/Iceclear/StableSR/StableSR/ldm/modules/diffusionmodules/__init__.py
deleted file mode 100644
index e69de29bb2d1d6434b8b29ae775ad8c2e48c5391..0000000000000000000000000000000000000000
diff --git a/spaces/Jackflack09/diffuse-custom/diffusers/pipelines/stable_diffusion/safety_checker_flax.py b/spaces/Jackflack09/diffuse-custom/diffusers/pipelines/stable_diffusion/safety_checker_flax.py
deleted file mode 100644
index e1f669d22b76a44a5fbd523e6cbc61167cb12332..0000000000000000000000000000000000000000
--- a/spaces/Jackflack09/diffuse-custom/diffusers/pipelines/stable_diffusion/safety_checker_flax.py
+++ /dev/null
@@ -1,112 +0,0 @@
-# Copyright 2022 The HuggingFace Team. All rights reserved.
-#
-# Licensed under the Apache License, Version 2.0 (the "License");
-# you may not use this file except in compliance with the License.
-# You may obtain a copy of the License at
-#
-# http://www.apache.org/licenses/LICENSE-2.0
-#
-# Unless required by applicable law or agreed to in writing, software
-# distributed under the License is distributed on an "AS IS" BASIS,
-# WITHOUT WARRANTIES OR CONDITIONS OF ANY KIND, either express or implied.
-# See the License for the specific language governing permissions and
-# limitations under the License.
-
-from typing import Optional, Tuple
-
-import jax
-import jax.numpy as jnp
-from flax import linen as nn
-from flax.core.frozen_dict import FrozenDict
-from transformers import CLIPConfig, FlaxPreTrainedModel
-from transformers.models.clip.modeling_flax_clip import FlaxCLIPVisionModule
-
-
-def jax_cosine_distance(emb_1, emb_2, eps=1e-12):
- norm_emb_1 = jnp.divide(emb_1.T, jnp.clip(jnp.linalg.norm(emb_1, axis=1), a_min=eps)).T
- norm_emb_2 = jnp.divide(emb_2.T, jnp.clip(jnp.linalg.norm(emb_2, axis=1), a_min=eps)).T
- return jnp.matmul(norm_emb_1, norm_emb_2.T)
-
-
-class FlaxStableDiffusionSafetyCheckerModule(nn.Module):
- config: CLIPConfig
- dtype: jnp.dtype = jnp.float32
-
- def setup(self):
- self.vision_model = FlaxCLIPVisionModule(self.config.vision_config)
- self.visual_projection = nn.Dense(self.config.projection_dim, use_bias=False, dtype=self.dtype)
-
- self.concept_embeds = self.param("concept_embeds", jax.nn.initializers.ones, (17, self.config.projection_dim))
- self.special_care_embeds = self.param(
- "special_care_embeds", jax.nn.initializers.ones, (3, self.config.projection_dim)
- )
-
- self.concept_embeds_weights = self.param("concept_embeds_weights", jax.nn.initializers.ones, (17,))
- self.special_care_embeds_weights = self.param("special_care_embeds_weights", jax.nn.initializers.ones, (3,))
-
- def __call__(self, clip_input):
- pooled_output = self.vision_model(clip_input)[1]
- image_embeds = self.visual_projection(pooled_output)
-
- special_cos_dist = jax_cosine_distance(image_embeds, self.special_care_embeds)
- cos_dist = jax_cosine_distance(image_embeds, self.concept_embeds)
-
- # increase this value to create a stronger `nfsw` filter
- # at the cost of increasing the possibility of filtering benign image inputs
- adjustment = 0.0
-
- special_scores = special_cos_dist - self.special_care_embeds_weights[None, :] + adjustment
- special_scores = jnp.round(special_scores, 3)
- is_special_care = jnp.any(special_scores > 0, axis=1, keepdims=True)
- # Use a lower threshold if an image has any special care concept
- special_adjustment = is_special_care * 0.01
-
- concept_scores = cos_dist - self.concept_embeds_weights[None, :] + special_adjustment
- concept_scores = jnp.round(concept_scores, 3)
- has_nsfw_concepts = jnp.any(concept_scores > 0, axis=1)
-
- return has_nsfw_concepts
-
-
-class FlaxStableDiffusionSafetyChecker(FlaxPreTrainedModel):
- config_class = CLIPConfig
- main_input_name = "clip_input"
- module_class = FlaxStableDiffusionSafetyCheckerModule
-
- def __init__(
- self,
- config: CLIPConfig,
- input_shape: Optional[Tuple] = None,
- seed: int = 0,
- dtype: jnp.dtype = jnp.float32,
- _do_init: bool = True,
- **kwargs,
- ):
- if input_shape is None:
- input_shape = (1, 224, 224, 3)
- module = self.module_class(config=config, dtype=dtype, **kwargs)
- super().__init__(config, module, input_shape=input_shape, seed=seed, dtype=dtype, _do_init=_do_init)
-
- def init_weights(self, rng: jax.random.PRNGKey, input_shape: Tuple, params: FrozenDict = None) -> FrozenDict:
- # init input tensor
- clip_input = jax.random.normal(rng, input_shape)
-
- params_rng, dropout_rng = jax.random.split(rng)
- rngs = {"params": params_rng, "dropout": dropout_rng}
-
- random_params = self.module.init(rngs, clip_input)["params"]
-
- return random_params
-
- def __call__(
- self,
- clip_input,
- params: dict = None,
- ):
- clip_input = jnp.transpose(clip_input, (0, 2, 3, 1))
-
- return self.module.apply(
- {"params": params or self.params},
- jnp.array(clip_input, dtype=jnp.float32),
- rngs={},
- )
diff --git a/spaces/Jasonyoyo/CodeFormer/CodeFormer/basicsr/utils/realesrgan_utils.py b/spaces/Jasonyoyo/CodeFormer/CodeFormer/basicsr/utils/realesrgan_utils.py
deleted file mode 100644
index a2a5ab3b787212e4731e2a91b1493430a4a3664e..0000000000000000000000000000000000000000
--- a/spaces/Jasonyoyo/CodeFormer/CodeFormer/basicsr/utils/realesrgan_utils.py
+++ /dev/null
@@ -1,299 +0,0 @@
-import cv2
-import math
-import numpy as np
-import os
-import queue
-import threading
-import torch
-from basicsr.utils.download_util import load_file_from_url
-from torch.nn import functional as F
-
-# ROOT_DIR = os.path.dirname(os.path.dirname(os.path.abspath(__file__)))
-
-
-class RealESRGANer():
- """A helper class for upsampling images with RealESRGAN.
-
- Args:
- scale (int): Upsampling scale factor used in the networks. It is usually 2 or 4.
- model_path (str): The path to the pretrained model. It can be urls (will first download it automatically).
- model (nn.Module): The defined network. Default: None.
- tile (int): As too large images result in the out of GPU memory issue, so this tile option will first crop
- input images into tiles, and then process each of them. Finally, they will be merged into one image.
- 0 denotes for do not use tile. Default: 0.
- tile_pad (int): The pad size for each tile, to remove border artifacts. Default: 10.
- pre_pad (int): Pad the input images to avoid border artifacts. Default: 10.
- half (float): Whether to use half precision during inference. Default: False.
- """
-
- def __init__(self,
- scale,
- model_path,
- model=None,
- tile=0,
- tile_pad=10,
- pre_pad=10,
- half=False,
- device=None,
- gpu_id=None):
- self.scale = scale
- self.tile_size = tile
- self.tile_pad = tile_pad
- self.pre_pad = pre_pad
- self.mod_scale = None
- self.half = half
-
- # initialize model
- if gpu_id:
- self.device = torch.device(
- f'cuda:{gpu_id}' if torch.cuda.is_available() else 'cpu') if device is None else device
- else:
- self.device = torch.device('cuda' if torch.cuda.is_available() else 'cpu') if device is None else device
- # if the model_path starts with https, it will first download models to the folder: realesrgan/weights
- if model_path.startswith('https://'):
- model_path = load_file_from_url(
- url=model_path, model_dir=os.path.join('weights/realesrgan'), progress=True, file_name=None)
- loadnet = torch.load(model_path, map_location=torch.device('cpu'))
- # prefer to use params_ema
- if 'params_ema' in loadnet:
- keyname = 'params_ema'
- else:
- keyname = 'params'
- model.load_state_dict(loadnet[keyname], strict=True)
- model.eval()
- self.model = model.to(self.device)
- if self.half:
- self.model = self.model.half()
-
- def pre_process(self, img):
- """Pre-process, such as pre-pad and mod pad, so that the images can be divisible
- """
- img = torch.from_numpy(np.transpose(img, (2, 0, 1))).float()
- self.img = img.unsqueeze(0).to(self.device)
- if self.half:
- self.img = self.img.half()
-
- # pre_pad
- if self.pre_pad != 0:
- self.img = F.pad(self.img, (0, self.pre_pad, 0, self.pre_pad), 'reflect')
- # mod pad for divisible borders
- if self.scale == 2:
- self.mod_scale = 2
- elif self.scale == 1:
- self.mod_scale = 4
- if self.mod_scale is not None:
- self.mod_pad_h, self.mod_pad_w = 0, 0
- _, _, h, w = self.img.size()
- if (h % self.mod_scale != 0):
- self.mod_pad_h = (self.mod_scale - h % self.mod_scale)
- if (w % self.mod_scale != 0):
- self.mod_pad_w = (self.mod_scale - w % self.mod_scale)
- self.img = F.pad(self.img, (0, self.mod_pad_w, 0, self.mod_pad_h), 'reflect')
-
- def process(self):
- # model inference
- self.output = self.model(self.img)
-
- def tile_process(self):
- """It will first crop input images to tiles, and then process each tile.
- Finally, all the processed tiles are merged into one images.
-
- Modified from: https://github.com/ata4/esrgan-launcher
- """
- batch, channel, height, width = self.img.shape
- output_height = height * self.scale
- output_width = width * self.scale
- output_shape = (batch, channel, output_height, output_width)
-
- # start with black image
- self.output = self.img.new_zeros(output_shape)
- tiles_x = math.ceil(width / self.tile_size)
- tiles_y = math.ceil(height / self.tile_size)
-
- # loop over all tiles
- for y in range(tiles_y):
- for x in range(tiles_x):
- # extract tile from input image
- ofs_x = x * self.tile_size
- ofs_y = y * self.tile_size
- # input tile area on total image
- input_start_x = ofs_x
- input_end_x = min(ofs_x + self.tile_size, width)
- input_start_y = ofs_y
- input_end_y = min(ofs_y + self.tile_size, height)
-
- # input tile area on total image with padding
- input_start_x_pad = max(input_start_x - self.tile_pad, 0)
- input_end_x_pad = min(input_end_x + self.tile_pad, width)
- input_start_y_pad = max(input_start_y - self.tile_pad, 0)
- input_end_y_pad = min(input_end_y + self.tile_pad, height)
-
- # input tile dimensions
- input_tile_width = input_end_x - input_start_x
- input_tile_height = input_end_y - input_start_y
- tile_idx = y * tiles_x + x + 1
- input_tile = self.img[:, :, input_start_y_pad:input_end_y_pad, input_start_x_pad:input_end_x_pad]
-
- # upscale tile
- try:
- with torch.no_grad():
- output_tile = self.model(input_tile)
- except RuntimeError as error:
- print('Error', error)
- # print(f'\tTile {tile_idx}/{tiles_x * tiles_y}')
-
- # output tile area on total image
- output_start_x = input_start_x * self.scale
- output_end_x = input_end_x * self.scale
- output_start_y = input_start_y * self.scale
- output_end_y = input_end_y * self.scale
-
- # output tile area without padding
- output_start_x_tile = (input_start_x - input_start_x_pad) * self.scale
- output_end_x_tile = output_start_x_tile + input_tile_width * self.scale
- output_start_y_tile = (input_start_y - input_start_y_pad) * self.scale
- output_end_y_tile = output_start_y_tile + input_tile_height * self.scale
-
- # put tile into output image
- self.output[:, :, output_start_y:output_end_y,
- output_start_x:output_end_x] = output_tile[:, :, output_start_y_tile:output_end_y_tile,
- output_start_x_tile:output_end_x_tile]
-
- def post_process(self):
- # remove extra pad
- if self.mod_scale is not None:
- _, _, h, w = self.output.size()
- self.output = self.output[:, :, 0:h - self.mod_pad_h * self.scale, 0:w - self.mod_pad_w * self.scale]
- # remove prepad
- if self.pre_pad != 0:
- _, _, h, w = self.output.size()
- self.output = self.output[:, :, 0:h - self.pre_pad * self.scale, 0:w - self.pre_pad * self.scale]
- return self.output
-
- @torch.no_grad()
- def enhance(self, img, outscale=None, alpha_upsampler='realesrgan'):
- h_input, w_input = img.shape[0:2]
- # img: numpy
- img = img.astype(np.float32)
- if np.max(img) > 256: # 16-bit image
- max_range = 65535
- print('\tInput is a 16-bit image')
- else:
- max_range = 255
- img = img / max_range
- if len(img.shape) == 2: # gray image
- img_mode = 'L'
- img = cv2.cvtColor(img, cv2.COLOR_GRAY2RGB)
- elif img.shape[2] == 4: # RGBA image with alpha channel
- img_mode = 'RGBA'
- alpha = img[:, :, 3]
- img = img[:, :, 0:3]
- img = cv2.cvtColor(img, cv2.COLOR_BGR2RGB)
- if alpha_upsampler == 'realesrgan':
- alpha = cv2.cvtColor(alpha, cv2.COLOR_GRAY2RGB)
- else:
- img_mode = 'RGB'
- img = cv2.cvtColor(img, cv2.COLOR_BGR2RGB)
-
- # ------------------- process image (without the alpha channel) ------------------- #
- try:
- with torch.no_grad():
- self.pre_process(img)
- if self.tile_size > 0:
- self.tile_process()
- else:
- self.process()
- output_img_t = self.post_process()
- output_img = output_img_t.data.squeeze().float().cpu().clamp_(0, 1).numpy()
- output_img = np.transpose(output_img[[2, 1, 0], :, :], (1, 2, 0))
- if img_mode == 'L':
- output_img = cv2.cvtColor(output_img, cv2.COLOR_BGR2GRAY)
- del output_img_t
- torch.cuda.empty_cache()
- except RuntimeError as error:
- print(f"Failed inference for RealESRGAN: {error}")
-
- # ------------------- process the alpha channel if necessary ------------------- #
- if img_mode == 'RGBA':
- if alpha_upsampler == 'realesrgan':
- self.pre_process(alpha)
- if self.tile_size > 0:
- self.tile_process()
- else:
- self.process()
- output_alpha = self.post_process()
- output_alpha = output_alpha.data.squeeze().float().cpu().clamp_(0, 1).numpy()
- output_alpha = np.transpose(output_alpha[[2, 1, 0], :, :], (1, 2, 0))
- output_alpha = cv2.cvtColor(output_alpha, cv2.COLOR_BGR2GRAY)
- else: # use the cv2 resize for alpha channel
- h, w = alpha.shape[0:2]
- output_alpha = cv2.resize(alpha, (w * self.scale, h * self.scale), interpolation=cv2.INTER_LINEAR)
-
- # merge the alpha channel
- output_img = cv2.cvtColor(output_img, cv2.COLOR_BGR2BGRA)
- output_img[:, :, 3] = output_alpha
-
- # ------------------------------ return ------------------------------ #
- if max_range == 65535: # 16-bit image
- output = (output_img * 65535.0).round().astype(np.uint16)
- else:
- output = (output_img * 255.0).round().astype(np.uint8)
-
- if outscale is not None and outscale != float(self.scale):
- output = cv2.resize(
- output, (
- int(w_input * outscale),
- int(h_input * outscale),
- ), interpolation=cv2.INTER_LANCZOS4)
-
- return output, img_mode
-
-
-class PrefetchReader(threading.Thread):
- """Prefetch images.
-
- Args:
- img_list (list[str]): A image list of image paths to be read.
- num_prefetch_queue (int): Number of prefetch queue.
- """
-
- def __init__(self, img_list, num_prefetch_queue):
- super().__init__()
- self.que = queue.Queue(num_prefetch_queue)
- self.img_list = img_list
-
- def run(self):
- for img_path in self.img_list:
- img = cv2.imread(img_path, cv2.IMREAD_UNCHANGED)
- self.que.put(img)
-
- self.que.put(None)
-
- def __next__(self):
- next_item = self.que.get()
- if next_item is None:
- raise StopIteration
- return next_item
-
- def __iter__(self):
- return self
-
-
-class IOConsumer(threading.Thread):
-
- def __init__(self, opt, que, qid):
- super().__init__()
- self._queue = que
- self.qid = qid
- self.opt = opt
-
- def run(self):
- while True:
- msg = self._queue.get()
- if isinstance(msg, str) and msg == 'quit':
- break
-
- output = msg['output']
- save_path = msg['save_path']
- cv2.imwrite(save_path, output)
- print(f'IO worker {self.qid} is done.')
\ No newline at end of file
diff --git a/spaces/KPCGD/bingo/src/components/ui/dropdown-menu.tsx b/spaces/KPCGD/bingo/src/components/ui/dropdown-menu.tsx
deleted file mode 100644
index 184d4e6007ef85187446362f69532ab077897fea..0000000000000000000000000000000000000000
--- a/spaces/KPCGD/bingo/src/components/ui/dropdown-menu.tsx
+++ /dev/null
@@ -1,128 +0,0 @@
-'use client'
-
-import * as React from 'react'
-import * as DropdownMenuPrimitive from '@radix-ui/react-dropdown-menu'
-
-import { cn } from '@/lib/utils'
-
-const DropdownMenu = DropdownMenuPrimitive.Root
-
-const DropdownMenuTrigger = DropdownMenuPrimitive.Trigger
-
-const DropdownMenuGroup = DropdownMenuPrimitive.Group
-
-const DropdownMenuPortal = DropdownMenuPrimitive.Portal
-
-const DropdownMenuSub = DropdownMenuPrimitive.Sub
-
-const DropdownMenuRadioGroup = DropdownMenuPrimitive.RadioGroup
-
-const DropdownMenuSubContent = React.forwardRef<
- React.ElementRef,
- React.ComponentPropsWithoutRef
->(({ className, ...props }, ref) => (
-
-))
-DropdownMenuSubContent.displayName =
- DropdownMenuPrimitive.SubContent.displayName
-
-const DropdownMenuContent = React.forwardRef<
- React.ElementRef,
- React.ComponentPropsWithoutRef
->(({ className, sideOffset = 4, ...props }, ref) => (
-
-
-
-))
-DropdownMenuContent.displayName = DropdownMenuPrimitive.Content.displayName
-
-const DropdownMenuItem = React.forwardRef<
- React.ElementRef,
- React.ComponentPropsWithoutRef & {
- inset?: boolean
- }
->(({ className, inset, ...props }, ref) => (
-
-))
-DropdownMenuItem.displayName = DropdownMenuPrimitive.Item.displayName
-
-const DropdownMenuLabel = React.forwardRef<
- React.ElementRef,
- React.ComponentPropsWithoutRef & {
- inset?: boolean
- }
->(({ className, inset, ...props }, ref) => (
-
-))
-DropdownMenuLabel.displayName = DropdownMenuPrimitive.Label.displayName
-
-const DropdownMenuSeparator = React.forwardRef<
- React.ElementRef,
- React.ComponentPropsWithoutRef
->(({ className, ...props }, ref) => (
-
-))
-DropdownMenuSeparator.displayName = DropdownMenuPrimitive.Separator.displayName
-
-const DropdownMenuShortcut = ({
- className,
- ...props
-}: React.HTMLAttributes) => {
- return (
-
- )
-}
-DropdownMenuShortcut.displayName = 'DropdownMenuShortcut'
-
-export {
- DropdownMenu,
- DropdownMenuTrigger,
- DropdownMenuContent,
- DropdownMenuItem,
- DropdownMenuLabel,
- DropdownMenuSeparator,
- DropdownMenuShortcut,
- DropdownMenuGroup,
- DropdownMenuPortal,
- DropdownMenuSub,
- DropdownMenuSubContent,
- DropdownMenuRadioGroup
-}
diff --git a/spaces/Kevin676/ChatGPT-with-Smooth-Voice/app.py b/spaces/Kevin676/ChatGPT-with-Smooth-Voice/app.py
deleted file mode 100644
index cdf5044bc9de2dc59e71bdc47660d6ab4579744b..0000000000000000000000000000000000000000
--- a/spaces/Kevin676/ChatGPT-with-Smooth-Voice/app.py
+++ /dev/null
@@ -1,113 +0,0 @@
-from TTS.api import TTS
-tts = TTS(model_name="tts_models/multilingual/multi-dataset/your_tts", progress_bar=False, gpu=True)
-import whisper
-model = whisper.load_model("small")
-import os
-os.system('pip install voicefixer --upgrade')
-from voicefixer import VoiceFixer
-voicefixer = VoiceFixer()
-import gradio as gr
-import openai
-import torch
-import torchaudio
-from speechbrain.pretrained import SpectralMaskEnhancement
-
-enhance_model = SpectralMaskEnhancement.from_hparams(
-source="speechbrain/metricgan-plus-voicebank",
-savedir="pretrained_models/metricgan-plus-voicebank",
-run_opts={"device":"cuda"},
-)
-
-mes1 = [
- {"role": "system", "content": "You are a TOEFL examiner. Help me improve my oral Englsih and give me feedback."}
-]
-
-mes2 = [
- {"role": "system", "content": "You are a mental health therapist. Your name is Tina."}
-]
-
-mes3 = [
- {"role": "system", "content": "You are my personal assistant. Your name is Alice."}
-]
-
-res = []
-
-def transcribe(apikey, upload, audio, choice1):
-
- openai.api_key = apikey
-
- # time.sleep(3)
- # load audio and pad/trim it to fit 30 seconds
- audio = whisper.load_audio(audio)
- audio = whisper.pad_or_trim(audio)
-
- # make log-Mel spectrogram and move to the same device as the model
- mel = whisper.log_mel_spectrogram(audio).to(model.device)
-
- # detect the spoken language
- _, probs = model.detect_language(mel)
- print(f"Detected language: {max(probs, key=probs.get)}")
-
- # decode the audio
- options = whisper.DecodingOptions()
- result = whisper.decode(model, mel, options)
- res.append(result.text)
-
- if choice1 == "TOEFL":
- messages = mes1
- elif choice1 == "Therapist":
- messages = mes2
- elif choice1 == "Alice":
- messages = mes3
-
- # chatgpt
- n = len(res)
- content = res[n-1]
- messages.append({"role": "user", "content": content})
-
- completion = openai.ChatCompletion.create(
- model = "gpt-3.5-turbo",
- messages = messages
- )
-
- chat_response = completion.choices[0].message.content
-
- messages.append({"role": "assistant", "content": chat_response})
-
- tts.tts_to_file(chat_response, speaker_wav = upload, language="en", file_path="output.wav")
-
- voicefixer.restore(input="output.wav", # input wav file path
- output="audio1.wav", # output wav file path
- cuda=True, # whether to use gpu acceleration
- mode = 0) # You can try out mode 0, 1 to find out the best result
-
-
-
- noisy = enhance_model.load_audio(
- "audio1.wav"
- ).unsqueeze(0)
-
- enhanced = enhance_model.enhance_batch(noisy, lengths=torch.tensor([1.]))
- torchaudio.save("enhanced.wav", enhanced.cpu(), 16000)
-
- return [result.text, chat_response, "enhanced.wav"]
-
-output_1 = gr.Textbox(label="Speech to Text")
-output_2 = gr.Textbox(label="ChatGPT Output")
-output_3 = gr.Audio(label="Audio with Custom Voice")
-
-gr.Interface(
- title = '🥳💬💕 - TalktoAI,随时随地,谈天说地!',
- theme="huggingface",
- description = "🤖 - 让有人文关怀的AI造福每一个人!AI向善,文明璀璨!TalktoAI - Enable the future!",
- fn=transcribe,
- inputs=[
- gr.Textbox(lines=1, label = "请填写您的OpenAI-API-key"),
- gr.inputs.Audio(source="upload", label = "请上传您喜欢的声音(wav文件)", type="filepath"),
- gr.inputs.Audio(source="microphone", type="filepath"),
- gr.Radio(["TOEFL", "Therapist", "Alice"], label="TOEFL Examiner, Therapist Tina, or Assistant Alice?"),
- ],
- outputs=[
- output_1, output_2, output_3
- ],
- ).launch()
\ No newline at end of file
diff --git a/spaces/KyanChen/FunSR/test_inr_mysr.py b/spaces/KyanChen/FunSR/test_inr_mysr.py
deleted file mode 100644
index c4b08d90901d7826b1a01fc39df0526118aecb78..0000000000000000000000000000000000000000
--- a/spaces/KyanChen/FunSR/test_inr_mysr.py
+++ /dev/null
@@ -1,168 +0,0 @@
-import argparse
-import json
-import os
-
-import math
-from functools import partial
-
-import yaml
-import torch
-from torch.utils.data import DataLoader
-from tqdm import tqdm
-
-import datasets
-import models
-import utils
-
-device = 'cuda:0' if torch.cuda.is_available() else 'cpu'
-
-def batched_predict(model, inp, coord, bsize):
- with torch.no_grad():
- pred = model(inp, coord)
- return pred
-
-
-def eval_psnr(loader, class_names, model, data_norm=None, eval_type=None, eval_bsize=None, verbose=False, crop_border=4):
- crop_border = int(crop_border) if crop_border else crop_border
- print('crop border: ', crop_border)
- model.eval()
-
- if data_norm is None:
- data_norm = {
- 'inp': {'sub': [0], 'div': [1]},
- 'gt': {'sub': [0], 'div': [1]}
- }
- t = data_norm['inp']
- inp_sub = torch.FloatTensor(t['sub']).view(1, -1, 1, 1).to(device)
- inp_div = torch.FloatTensor(t['div']).view(1, -1, 1, 1).to(device)
- t = data_norm['gt']
- gt_sub = torch.FloatTensor(t['sub']).view(1, 1, -1).to(device)
- gt_div = torch.FloatTensor(t['div']).view(1, 1, -1).to(device)
-
- if eval_type is None:
- metric_fn = [utils.calculate_psnr_pt, utils.calculate_ssim_pt]
- elif eval_type == 'psnr+ssim':
- metric_fn = [utils.calculate_psnr_pt, utils.calculate_ssim_pt]
- elif eval_type.startswith('div2k'):
- scale = int(eval_type.split('-')[1])
- metric_fn = partial(utils.calc_psnr, dataset='div2k', scale=scale)
- elif eval_type.startswith('benchmark'):
- scale = int(eval_type.split('-')[1])
- metric_fn = partial(utils.calc_psnr, dataset='benchmark', scale=scale)
- else:
- raise NotImplementedError
-
- val_res_psnr = utils.Averager(class_names)
- val_res_ssim = utils.Averager(class_names)
-
- pbar = tqdm(loader, leave=False, desc='val')
- for batch in pbar:
- for k, v in batch.items():
- if torch.is_tensor(v):
- batch[k] = v.to(device)
-
- inp = (batch['inp'] - inp_sub) / inp_div
- # import pdb
- # pdb.set_trace()
- if eval_bsize is None:
- with torch.no_grad():
- scale_ratios = batch.get('scale_ratio', None)
- if scale_ratios is None:
- pred = model(inp, batch['coord'])[-1]
- else:
- # scale_ratios = (scale_ratios - gt_sub) / gt_div
- pred = model(inp, batch['coord'], scale_ratios)[-1]
- else:
- pred = batched_predict(model, inp, batch['coord'], eval_bsize)
- pred = pred * gt_div + gt_sub
-
- if eval_type is not None: # reshape for shaving-eval
- ih, iw = batch['inp'].shape[-2:]
- s = math.sqrt(batch['coord'].shape[1] / (ih * iw))
- if s > 1:
- shape = [batch['inp'].shape[0], round(ih * s), round(iw * s), 3]
- else:
- shape = [batch['inp'].shape[0], 32, batch['coord'].shape[1]//32, 3]
-
- pred = pred.view(*shape) \
- .permute(0, 3, 1, 2).contiguous()
- batch['gt'] = batch['gt'].view(*shape) \
- .permute(0, 3, 1, 2).contiguous()
-
- # if crop_border is not None:
- # h = math.sqrt(pred.shape[1])
- # shape = [inp.shape[0], round(h), round(h), 3]
- # pred = pred.view(*shape).permute(0, 3, 1, 2).contiguous()
- # batch['gt'] = batch['gt'].view(*shape).permute(0, 3, 1, 2).contiguous()
- # else:
- # pred = pred.permute(0, 2, 1).contiguous() # B 3 N
- # batch['gt'] = batch['gt'].permute(0, 2, 1).contiguous()
-
- res_psnr = metric_fn[0](
- pred,
- batch['gt'],
- crop_border=crop_border
- )
- res_ssim = metric_fn[1](
- pred,
- batch['gt'],
- crop_border=crop_border
- )
-
- val_res_psnr.add(batch['class_name'], res_psnr)
- val_res_ssim.add(batch['class_name'], res_ssim)
-
- if verbose:
- pbar.set_description(
- 'val psnr: {:.4f} ssim: {:.4f}'.format(val_res_psnr.item()['all'], val_res_ssim.item()['all']))
-
- return val_res_psnr.item(), val_res_ssim.item()
-
-
-if __name__ == '__main__':
- parser = argparse.ArgumentParser()
- parser.add_argument('--config', default='configs/test_UC_INR_mysr.yaml')
- parser.add_argument('--model', default='checkpoints/EXP20220610_5/epoch-best.pth')
- # parser.add_argument('--model', default='checkpoints/EXP20220610_5/epoch-last.pth')
- parser.add_argument('--scale_ratio', default=4, type=float)
- parser.add_argument('--gpu', default='0')
- args = parser.parse_args()
-
- with open(args.config, 'r') as f:
- config = yaml.load(f, Loader=yaml.FullLoader)
-
- config['test_dataset']['wrapper']['args']['scale_ratio'] = args.scale_ratio
-
- spec = config['test_dataset']
- dataset = datasets.make(spec['dataset'])
- dataset = datasets.make(spec['wrapper'], args={'dataset': dataset})
- loader = DataLoader(dataset, batch_size=spec['batch_size'], num_workers=0, pin_memory=True, shuffle=False, drop_last=False)
-
- model_spec = torch.load(args.model)['model']
- print(model_spec['args'])
- model = models.make(model_spec, load_sd=True).to(device)
-
- file_names = json.load(open(config['test_dataset']['dataset']['args']['split_file']))['test']
- class_names = list(set([os.path.basename(os.path.dirname(x)) for x in file_names]))
-
- crop_border = config['test_dataset']['wrapper']['args']['scale_ratio']+5
- dataset_name = os.path.basename(config['test_dataset']['dataset']['args']['split_file']).split('_')[0]
- max_scale = {'UC': 5, 'AID': 12}
- if args.scale_ratio > max_scale[dataset_name]:
- crop_border = int((args.scale_ratio - max_scale[dataset_name]) / 2 * 48)
-
- res = eval_psnr(
- loader, class_names, model,
- data_norm=config.get('data_norm'),
- eval_type=config.get('eval_type'),
- eval_bsize=config.get('eval_bsize'),
- crop_border=crop_border,
- verbose=True)
- # print('psnr')
- # for k, v in res[0].items():
- # print(f'{k}: {v:0.2f}')
- # print('ssim')
- # for k, v in res[1].items():
- # print(f'{k}: {v:0.4f}')
- print(f'psnr: {res[0]["all"]:0.2f}')
- print(f'ssim: {res[1]["all"]:0.4f}')
\ No newline at end of file
diff --git a/spaces/KyanChen/RSPrompter/mmdet/structures/bbox/horizontal_boxes.py b/spaces/KyanChen/RSPrompter/mmdet/structures/bbox/horizontal_boxes.py
deleted file mode 100644
index 360c8a24e0b267fe982420b4aebbef7a0b66ddce..0000000000000000000000000000000000000000
--- a/spaces/KyanChen/RSPrompter/mmdet/structures/bbox/horizontal_boxes.py
+++ /dev/null
@@ -1,412 +0,0 @@
-# Copyright (c) OpenMMLab. All rights reserved.
-from typing import Optional, Tuple, TypeVar, Union
-
-import cv2
-import numpy as np
-import torch
-from torch import BoolTensor, Tensor
-
-from mmdet.structures.mask.structures import BitmapMasks, PolygonMasks
-from .base_boxes import BaseBoxes
-from .bbox_overlaps import bbox_overlaps
-from .box_type import register_box
-
-T = TypeVar('T')
-DeviceType = Union[str, torch.device]
-MaskType = Union[BitmapMasks, PolygonMasks]
-
-
-@register_box(name='hbox')
-class HorizontalBoxes(BaseBoxes):
- """The horizontal box class used in MMDetection by default.
-
- The ``box_dim`` of ``HorizontalBoxes`` is 4, which means the length of
- the last dimension of the data should be 4. Two modes of box data are
- supported in ``HorizontalBoxes``:
-
- - 'xyxy': Each row of data indicates (x1, y1, x2, y2), which are the
- coordinates of the left-top and right-bottom points.
- - 'cxcywh': Each row of data indicates (x, y, w, h), where (x, y) are the
- coordinates of the box centers and (w, h) are the width and height.
-
- ``HorizontalBoxes`` only restores 'xyxy' mode of data. If the the data is
- in 'cxcywh' mode, users need to input ``in_mode='cxcywh'`` and The code
- will convert the 'cxcywh' data to 'xyxy' automatically.
-
- Args:
- data (Tensor or np.ndarray or Sequence): The box data with shape of
- (..., 4).
- dtype (torch.dtype, Optional): data type of boxes. Defaults to None.
- device (str or torch.device, Optional): device of boxes.
- Default to None.
- clone (bool): Whether clone ``boxes`` or not. Defaults to True.
- mode (str, Optional): the mode of boxes. If it is 'cxcywh', the
- `data` will be converted to 'xyxy' mode. Defaults to None.
- """
-
- box_dim: int = 4
-
- def __init__(self,
- data: Union[Tensor, np.ndarray],
- dtype: torch.dtype = None,
- device: DeviceType = None,
- clone: bool = True,
- in_mode: Optional[str] = None) -> None:
- super().__init__(data=data, dtype=dtype, device=device, clone=clone)
- if isinstance(in_mode, str):
- if in_mode not in ('xyxy', 'cxcywh'):
- raise ValueError(f'Get invalid mode {in_mode}.')
- if in_mode == 'cxcywh':
- self.tensor = self.cxcywh_to_xyxy(self.tensor)
-
- @staticmethod
- def cxcywh_to_xyxy(boxes: Tensor) -> Tensor:
- """Convert box coordinates from (cx, cy, w, h) to (x1, y1, x2, y2).
-
- Args:
- boxes (Tensor): cxcywh boxes tensor with shape of (..., 4).
-
- Returns:
- Tensor: xyxy boxes tensor with shape of (..., 4).
- """
- ctr, wh = boxes.split((2, 2), dim=-1)
- return torch.cat([(ctr - wh / 2), (ctr + wh / 2)], dim=-1)
-
- @staticmethod
- def xyxy_to_cxcywh(boxes: Tensor) -> Tensor:
- """Convert box coordinates from (x1, y1, x2, y2) to (cx, cy, w, h).
-
- Args:
- boxes (Tensor): xyxy boxes tensor with shape of (..., 4).
-
- Returns:
- Tensor: cxcywh boxes tensor with shape of (..., 4).
- """
- xy1, xy2 = boxes.split((2, 2), dim=-1)
- return torch.cat([(xy2 + xy1) / 2, (xy2 - xy1)], dim=-1)
-
- @property
- def cxcywh(self) -> Tensor:
- """Return a tensor representing the cxcywh boxes."""
- return self.xyxy_to_cxcywh(self.tensor)
-
- @property
- def centers(self) -> Tensor:
- """Return a tensor representing the centers of boxes."""
- boxes = self.tensor
- return (boxes[..., :2] + boxes[..., 2:]) / 2
-
- @property
- def areas(self) -> Tensor:
- """Return a tensor representing the areas of boxes."""
- boxes = self.tensor
- return (boxes[..., 2] - boxes[..., 0]) * (
- boxes[..., 3] - boxes[..., 1])
-
- @property
- def widths(self) -> Tensor:
- """Return a tensor representing the widths of boxes."""
- boxes = self.tensor
- return boxes[..., 2] - boxes[..., 0]
-
- @property
- def heights(self) -> Tensor:
- """Return a tensor representing the heights of boxes."""
- boxes = self.tensor
- return boxes[..., 3] - boxes[..., 1]
-
- def flip_(self,
- img_shape: Tuple[int, int],
- direction: str = 'horizontal') -> None:
- """Flip boxes horizontally or vertically in-place.
-
- Args:
- img_shape (Tuple[int, int]): A tuple of image height and width.
- direction (str): Flip direction, options are "horizontal",
- "vertical" and "diagonal". Defaults to "horizontal"
- """
- assert direction in ['horizontal', 'vertical', 'diagonal']
- flipped = self.tensor
- boxes = flipped.clone()
- if direction == 'horizontal':
- flipped[..., 0] = img_shape[1] - boxes[..., 2]
- flipped[..., 2] = img_shape[1] - boxes[..., 0]
- elif direction == 'vertical':
- flipped[..., 1] = img_shape[0] - boxes[..., 3]
- flipped[..., 3] = img_shape[0] - boxes[..., 1]
- else:
- flipped[..., 0] = img_shape[1] - boxes[..., 2]
- flipped[..., 1] = img_shape[0] - boxes[..., 3]
- flipped[..., 2] = img_shape[1] - boxes[..., 0]
- flipped[..., 3] = img_shape[0] - boxes[..., 1]
-
- def translate_(self, distances: Tuple[float, float]) -> None:
- """Translate boxes in-place.
-
- Args:
- distances (Tuple[float, float]): translate distances. The first
- is horizontal distance and the second is vertical distance.
- """
- boxes = self.tensor
- assert len(distances) == 2
- self.tensor = boxes + boxes.new_tensor(distances).repeat(2)
-
- def clip_(self, img_shape: Tuple[int, int]) -> None:
- """Clip boxes according to the image shape in-place.
-
- Args:
- img_shape (Tuple[int, int]): A tuple of image height and width.
- """
- boxes = self.tensor
- boxes[..., 0::2] = boxes[..., 0::2].clamp(0, img_shape[1])
- boxes[..., 1::2] = boxes[..., 1::2].clamp(0, img_shape[0])
-
- def rotate_(self, center: Tuple[float, float], angle: float) -> None:
- """Rotate all boxes in-place.
-
- Args:
- center (Tuple[float, float]): Rotation origin.
- angle (float): Rotation angle represented in degrees. Positive
- values mean clockwise rotation.
- """
- boxes = self.tensor
- rotation_matrix = boxes.new_tensor(
- cv2.getRotationMatrix2D(center, -angle, 1))
-
- corners = self.hbox2corner(boxes)
- corners = torch.cat(
- [corners, corners.new_ones(*corners.shape[:-1], 1)], dim=-1)
- corners_T = torch.transpose(corners, -1, -2)
- corners_T = torch.matmul(rotation_matrix, corners_T)
- corners = torch.transpose(corners_T, -1, -2)
- self.tensor = self.corner2hbox(corners)
-
- def project_(self, homography_matrix: Union[Tensor, np.ndarray]) -> None:
- """Geometric transformat boxes in-place.
-
- Args:
- homography_matrix (Tensor or np.ndarray]):
- Shape (3, 3) for geometric transformation.
- """
- boxes = self.tensor
- if isinstance(homography_matrix, np.ndarray):
- homography_matrix = boxes.new_tensor(homography_matrix)
- corners = self.hbox2corner(boxes)
- corners = torch.cat(
- [corners, corners.new_ones(*corners.shape[:-1], 1)], dim=-1)
- corners_T = torch.transpose(corners, -1, -2)
- corners_T = torch.matmul(homography_matrix, corners_T)
- corners = torch.transpose(corners_T, -1, -2)
- # Convert to homogeneous coordinates by normalization
- corners = corners[..., :2] / corners[..., 2:3]
- self.tensor = self.corner2hbox(corners)
-
- @staticmethod
- def hbox2corner(boxes: Tensor) -> Tensor:
- """Convert box coordinates from (x1, y1, x2, y2) to corners ((x1, y1),
- (x2, y1), (x1, y2), (x2, y2)).
-
- Args:
- boxes (Tensor): Horizontal box tensor with shape of (..., 4).
-
- Returns:
- Tensor: Corner tensor with shape of (..., 4, 2).
- """
- x1, y1, x2, y2 = torch.split(boxes, 1, dim=-1)
- corners = torch.cat([x1, y1, x2, y1, x1, y2, x2, y2], dim=-1)
- return corners.reshape(*corners.shape[:-1], 4, 2)
-
- @staticmethod
- def corner2hbox(corners: Tensor) -> Tensor:
- """Convert box coordinates from corners ((x1, y1), (x2, y1), (x1, y2),
- (x2, y2)) to (x1, y1, x2, y2).
-
- Args:
- corners (Tensor): Corner tensor with shape of (..., 4, 2).
-
- Returns:
- Tensor: Horizontal box tensor with shape of (..., 4).
- """
- if corners.numel() == 0:
- return corners.new_zeros((0, 4))
- min_xy = corners.min(dim=-2)[0]
- max_xy = corners.max(dim=-2)[0]
- return torch.cat([min_xy, max_xy], dim=-1)
-
- def rescale_(self, scale_factor: Tuple[float, float]) -> None:
- """Rescale boxes w.r.t. rescale_factor in-place.
-
- Note:
- Both ``rescale_`` and ``resize_`` will enlarge or shrink boxes
- w.r.t ``scale_facotr``. The difference is that ``resize_`` only
- changes the width and the height of boxes, but ``rescale_`` also
- rescales the box centers simultaneously.
-
- Args:
- scale_factor (Tuple[float, float]): factors for scaling boxes.
- The length should be 2.
- """
- boxes = self.tensor
- assert len(scale_factor) == 2
- scale_factor = boxes.new_tensor(scale_factor).repeat(2)
- self.tensor = boxes * scale_factor
-
- def resize_(self, scale_factor: Tuple[float, float]) -> None:
- """Resize the box width and height w.r.t scale_factor in-place.
-
- Note:
- Both ``rescale_`` and ``resize_`` will enlarge or shrink boxes
- w.r.t ``scale_facotr``. The difference is that ``resize_`` only
- changes the width and the height of boxes, but ``rescale_`` also
- rescales the box centers simultaneously.
-
- Args:
- scale_factor (Tuple[float, float]): factors for scaling box
- shapes. The length should be 2.
- """
- boxes = self.tensor
- assert len(scale_factor) == 2
- ctrs = (boxes[..., 2:] + boxes[..., :2]) / 2
- wh = boxes[..., 2:] - boxes[..., :2]
- scale_factor = boxes.new_tensor(scale_factor)
- wh = wh * scale_factor
- xy1 = ctrs - 0.5 * wh
- xy2 = ctrs + 0.5 * wh
- self.tensor = torch.cat([xy1, xy2], dim=-1)
-
- def is_inside(self,
- img_shape: Tuple[int, int],
- all_inside: bool = False,
- allowed_border: int = 0) -> BoolTensor:
- """Find boxes inside the image.
-
- Args:
- img_shape (Tuple[int, int]): A tuple of image height and width.
- all_inside (bool): Whether the boxes are all inside the image or
- part inside the image. Defaults to False.
- allowed_border (int): Boxes that extend beyond the image shape
- boundary by more than ``allowed_border`` are considered
- "outside" Defaults to 0.
- Returns:
- BoolTensor: A BoolTensor indicating whether the box is inside
- the image. Assuming the original boxes have shape (m, n, 4),
- the output has shape (m, n).
- """
- img_h, img_w = img_shape
- boxes = self.tensor
- if all_inside:
- return (boxes[:, 0] >= -allowed_border) & \
- (boxes[:, 1] >= -allowed_border) & \
- (boxes[:, 2] < img_w + allowed_border) & \
- (boxes[:, 3] < img_h + allowed_border)
- else:
- return (boxes[..., 0] < img_w + allowed_border) & \
- (boxes[..., 1] < img_h + allowed_border) & \
- (boxes[..., 2] > -allowed_border) & \
- (boxes[..., 3] > -allowed_border)
-
- def find_inside_points(self,
- points: Tensor,
- is_aligned: bool = False) -> BoolTensor:
- """Find inside box points. Boxes dimension must be 2.
-
- Args:
- points (Tensor): Points coordinates. Has shape of (m, 2).
- is_aligned (bool): Whether ``points`` has been aligned with boxes
- or not. If True, the length of boxes and ``points`` should be
- the same. Defaults to False.
-
- Returns:
- BoolTensor: A BoolTensor indicating whether a point is inside
- boxes. Assuming the boxes has shape of (n, 4), if ``is_aligned``
- is False. The index has shape of (m, n). If ``is_aligned`` is
- True, m should be equal to n and the index has shape of (m, ).
- """
- boxes = self.tensor
- assert boxes.dim() == 2, 'boxes dimension must be 2.'
-
- if not is_aligned:
- boxes = boxes[None, :, :]
- points = points[:, None, :]
- else:
- assert boxes.size(0) == points.size(0)
-
- x_min, y_min, x_max, y_max = boxes.unbind(dim=-1)
- return (points[..., 0] >= x_min) & (points[..., 0] <= x_max) & \
- (points[..., 1] >= y_min) & (points[..., 1] <= y_max)
-
- @staticmethod
- def overlaps(boxes1: BaseBoxes,
- boxes2: BaseBoxes,
- mode: str = 'iou',
- is_aligned: bool = False,
- eps: float = 1e-6) -> Tensor:
- """Calculate overlap between two set of boxes with their types
- converted to ``HorizontalBoxes``.
-
- Args:
- boxes1 (:obj:`BaseBoxes`): BaseBoxes with shape of (m, box_dim)
- or empty.
- boxes2 (:obj:`BaseBoxes`): BaseBoxes with shape of (n, box_dim)
- or empty.
- mode (str): "iou" (intersection over union), "iof" (intersection
- over foreground). Defaults to "iou".
- is_aligned (bool): If True, then m and n must be equal. Defaults
- to False.
- eps (float): A value added to the denominator for numerical
- stability. Defaults to 1e-6.
-
- Returns:
- Tensor: shape (m, n) if ``is_aligned`` is False else shape (m,)
- """
- boxes1 = boxes1.convert_to('hbox')
- boxes2 = boxes2.convert_to('hbox')
- return bbox_overlaps(
- boxes1.tensor,
- boxes2.tensor,
- mode=mode,
- is_aligned=is_aligned,
- eps=eps)
-
- @staticmethod
- def from_instance_masks(masks: MaskType) -> 'HorizontalBoxes':
- """Create horizontal boxes from instance masks.
-
- Args:
- masks (:obj:`BitmapMasks` or :obj:`PolygonMasks`): BitmapMasks or
- PolygonMasks instance with length of n.
-
- Returns:
- :obj:`HorizontalBoxes`: Converted boxes with shape of (n, 4).
- """
- num_masks = len(masks)
- boxes = np.zeros((num_masks, 4), dtype=np.float32)
- if isinstance(masks, BitmapMasks):
- x_any = masks.masks.any(axis=1)
- y_any = masks.masks.any(axis=2)
- for idx in range(num_masks):
- x = np.where(x_any[idx, :])[0]
- y = np.where(y_any[idx, :])[0]
- if len(x) > 0 and len(y) > 0:
- # use +1 for x_max and y_max so that the right and bottom
- # boundary of instance masks are fully included by the box
- boxes[idx, :] = np.array(
- [x[0], y[0], x[-1] + 1, y[-1] + 1], dtype=np.float32)
- elif isinstance(masks, PolygonMasks):
- for idx, poly_per_obj in enumerate(masks.masks):
- # simply use a number that is big enough for comparison with
- # coordinates
- xy_min = np.array([masks.width * 2, masks.height * 2],
- dtype=np.float32)
- xy_max = np.zeros(2, dtype=np.float32)
- for p in poly_per_obj:
- xy = np.array(p).reshape(-1, 2).astype(np.float32)
- xy_min = np.minimum(xy_min, np.min(xy, axis=0))
- xy_max = np.maximum(xy_max, np.max(xy, axis=0))
- boxes[idx, :2] = xy_min
- boxes[idx, 2:] = xy_max
- else:
- raise TypeError(
- '`masks` must be `BitmapMasks` or `PolygonMasks`, '
- f'but got {type(masks)}.')
- return HorizontalBoxes(boxes)
diff --git a/spaces/KyanChen/RSPrompter/mmpl/engine/hooks/switch_to_deploy_hook.py b/spaces/KyanChen/RSPrompter/mmpl/engine/hooks/switch_to_deploy_hook.py
deleted file mode 100644
index 28ac345f40c44c974fb33b7bf9756a61fcabf820..0000000000000000000000000000000000000000
--- a/spaces/KyanChen/RSPrompter/mmpl/engine/hooks/switch_to_deploy_hook.py
+++ /dev/null
@@ -1,21 +0,0 @@
-# Copyright (c) OpenMMLab. All rights reserved.
-
-from mmengine.hooks import Hook
-from mmengine.runner import Runner
-
-from mmyolo.registry import HOOKS
-from mmyolo.utils import switch_to_deploy
-
-
-@HOOKS.register_module()
-class SwitchToDeployHook(Hook):
- """Switch to deploy mode before testing.
-
- This hook converts the multi-channel structure of the training network
- (high performance) to the one-way structure of the testing network (fast
- speed and memory saving).
- """
-
- def before_test_epoch(self, runner: Runner):
- """Switch to deploy mode before testing."""
- switch_to_deploy(runner.model)
diff --git a/spaces/LP-art/Bing/Dockerfile b/spaces/LP-art/Bing/Dockerfile
deleted file mode 100644
index 4831afab0ec13b25cb3e7d51fd7d1073bec1fca0..0000000000000000000000000000000000000000
--- a/spaces/LP-art/Bing/Dockerfile
+++ /dev/null
@@ -1,34 +0,0 @@
-# Build Stage
-# 使用 golang:alpine 作为构建阶段的基础镜像
-FROM golang:alpine AS builder
-
-# 添加 git,以便之后能从GitHub克隆项目
-RUN apk --no-cache add git
-
-# 从 GitHub 克隆 go-proxy-bingai 项目到 /workspace/app 目录下
-RUN git clone https://github.com/Harry-zklcdc/go-proxy-bingai.git /workspace/app
-
-# 设置工作目录为之前克隆的项目目录
-WORKDIR /workspace/app
-
-# 编译 go 项目。-ldflags="-s -w" 是为了减少编译后的二进制大小
-RUN go build -ldflags="-s -w" -tags netgo -trimpath -o go-proxy-bingai main.go
-
-# Runtime Stage
-# 使用轻量级的 alpine 镜像作为运行时的基础镜像
-FROM alpine
-
-# 设置工作目录
-WORKDIR /workspace/app
-
-# 从构建阶段复制编译后的二进制文件到运行时镜像中
-COPY --from=builder /workspace/app/go-proxy-bingai .
-
-# 设置环境变量,此处为随机字符
-ENV Go_Proxy_BingAI_USER_TOKEN_1="1yFhftnPW4gYMMUtMnXjCWJSFPjh_4hNZpnCF30CvK2wpYcRc4fkNLMIHocSwTPzlalGm8dDAW_nLtEToXFM9Iet2LE8qZWyqHv9B5sucdr3xNHvsqmIPM_Fwz08jiVmm6pnZv6qmRVgiBxVZ5AxtaAeLoMHpgCrlXNYsQLWQnL2OX1Hqy_9tfe8YVxjydHLVbEC6N3Ks3W8jhdeO_b_00Q"
-
-# 暴露8080端口
-EXPOSE 8080
-
-# 容器启动时运行的命令
-CMD ["/workspace/app/go-proxy-bingai"]
\ No newline at end of file
diff --git a/spaces/Latryna/roop/roop/utilities.py b/spaces/Latryna/roop/roop/utilities.py
deleted file mode 100644
index 90c8d981f5f159a459ca0c08cc23dfac8d04c068..0000000000000000000000000000000000000000
--- a/spaces/Latryna/roop/roop/utilities.py
+++ /dev/null
@@ -1,141 +0,0 @@
-import glob
-import mimetypes
-import os
-import platform
-import shutil
-import ssl
-import subprocess
-import urllib
-from pathlib import Path
-from typing import List, Any
-from tqdm import tqdm
-
-import roop.globals
-
-TEMP_FILE = 'temp.mp4'
-TEMP_DIRECTORY = 'temp'
-
-# monkey patch ssl for mac
-if platform.system().lower() == 'darwin':
- ssl._create_default_https_context = ssl._create_unverified_context
-
-
-def run_ffmpeg(args: List[str]) -> bool:
- commands = ['ffmpeg', '-hide_banner', '-hwaccel', 'auto', '-loglevel', roop.globals.log_level]
- commands.extend(args)
- try:
- subprocess.check_output(commands, stderr=subprocess.STDOUT)
- return True
- except Exception:
- pass
- return False
-
-
-def detect_fps(target_path: str) -> float:
- command = ['ffprobe', '-v', 'error', '-select_streams', 'v:0', '-show_entries', 'stream=r_frame_rate', '-of', 'default=noprint_wrappers=1:nokey=1', target_path]
- output = subprocess.check_output(command).decode().strip().split('/')
- try:
- numerator, denominator = map(int, output)
- return numerator / denominator
- except Exception:
- pass
- return 30.0
-
-
-def extract_frames(target_path: str) -> None:
- temp_directory_path = get_temp_directory_path(target_path)
- run_ffmpeg(['-i', target_path, '-pix_fmt', 'rgb24', os.path.join(temp_directory_path, '%04d.png')])
-
-
-def create_video(target_path: str, fps: float = 30.0) -> None:
- temp_output_path = get_temp_output_path(target_path)
- temp_directory_path = get_temp_directory_path(target_path)
- run_ffmpeg(['-r', str(fps), '-i', os.path.join(temp_directory_path, '%04d.png'), '-c:v', roop.globals.video_encoder, '-crf', str(roop.globals.video_quality), '-pix_fmt', 'yuv420p', '-vf', 'colorspace=bt709:iall=bt601-6-625:fast=1', '-y', temp_output_path])
-
-
-def restore_audio(target_path: str, output_path: str) -> None:
- temp_output_path = get_temp_output_path(target_path)
- done = run_ffmpeg(['-i', temp_output_path, '-i', target_path, '-c:v', 'copy', '-map', '0:v:0', '-map', '1:a:0', '-y', output_path])
- if not done:
- move_temp(target_path, output_path)
-
-
-def get_temp_frame_paths(target_path: str) -> List[str]:
- temp_directory_path = get_temp_directory_path(target_path)
- return glob.glob((os.path.join(glob.escape(temp_directory_path), '*.png')))
-
-
-def get_temp_directory_path(target_path: str) -> str:
- target_name, _ = os.path.splitext(os.path.basename(target_path))
- target_directory_path = os.path.dirname(target_path)
- return os.path.join(target_directory_path, TEMP_DIRECTORY, target_name)
-
-
-def get_temp_output_path(target_path: str) -> str:
- temp_directory_path = get_temp_directory_path(target_path)
- return os.path.join(temp_directory_path, TEMP_FILE)
-
-
-def normalize_output_path(source_path: str, target_path: str, output_path: str) -> Any:
- if source_path and target_path:
- source_name, _ = os.path.splitext(os.path.basename(source_path))
- target_name, target_extension = os.path.splitext(os.path.basename(target_path))
- if os.path.isdir(output_path):
- return os.path.join(output_path, source_name + '-' + target_name + target_extension)
- return output_path
-
-
-def create_temp(target_path: str) -> None:
- temp_directory_path = get_temp_directory_path(target_path)
- Path(temp_directory_path).mkdir(parents=True, exist_ok=True)
-
-
-def move_temp(target_path: str, output_path: str) -> None:
- temp_output_path = get_temp_output_path(target_path)
- if os.path.isfile(temp_output_path):
- if os.path.isfile(output_path):
- os.remove(output_path)
- shutil.move(temp_output_path, output_path)
-
-
-def clean_temp(target_path: str) -> None:
- temp_directory_path = get_temp_directory_path(target_path)
- parent_directory_path = os.path.dirname(temp_directory_path)
- if not roop.globals.keep_frames and os.path.isdir(temp_directory_path):
- shutil.rmtree(temp_directory_path)
- if os.path.exists(parent_directory_path) and not os.listdir(parent_directory_path):
- os.rmdir(parent_directory_path)
-
-
-def has_image_extension(image_path: str) -> bool:
- return image_path.lower().endswith(('png', 'jpg', 'jpeg', 'webp'))
-
-
-def is_image(image_path: str) -> bool:
- if image_path and os.path.isfile(image_path):
- mimetype, _ = mimetypes.guess_type(image_path)
- return bool(mimetype and mimetype.startswith('image/'))
- return False
-
-
-def is_video(video_path: str) -> bool:
- if video_path and os.path.isfile(video_path):
- mimetype, _ = mimetypes.guess_type(video_path)
- return bool(mimetype and mimetype.startswith('video/'))
- return False
-
-
-def conditional_download(download_directory_path: str, urls: List[str]) -> None:
- if not os.path.exists(download_directory_path):
- os.makedirs(download_directory_path)
- for url in urls:
- download_file_path = os.path.join(download_directory_path, os.path.basename(url))
- if not os.path.exists(download_file_path):
- request = urllib.request.urlopen(url) # type: ignore[attr-defined]
- total = int(request.headers.get('Content-Length', 0))
- with tqdm(total=total, desc='Downloading', unit='B', unit_scale=True, unit_divisor=1024) as progress:
- urllib.request.urlretrieve(url, download_file_path, reporthook=lambda count, block_size, total_size: progress.update(block_size)) # type: ignore[attr-defined]
-
-
-def resolve_relative_path(path: str) -> str:
- return os.path.abspath(os.path.join(os.path.dirname(__file__), path))
diff --git a/spaces/LaynzKunz/Aesthetic_RVC_Inference_HF/lib/infer/infer_libs/infer_pack/modules/F0Predictor/PMF0Predictor.py b/spaces/LaynzKunz/Aesthetic_RVC_Inference_HF/lib/infer/infer_libs/infer_pack/modules/F0Predictor/PMF0Predictor.py
deleted file mode 100644
index 9f4b3cd0fbf7fb5ecd19f6bd095b00cc7109c0b4..0000000000000000000000000000000000000000
--- a/spaces/LaynzKunz/Aesthetic_RVC_Inference_HF/lib/infer/infer_libs/infer_pack/modules/F0Predictor/PMF0Predictor.py
+++ /dev/null
@@ -1,98 +0,0 @@
-import numpy as np
-import parselmouth
-
-from lib.infer.infer_libs.infer_pack.modules.F0Predictor.F0Predictor import F0Predictor
-
-
-class PMF0Predictor(F0Predictor):
- def __init__(self, hop_length=512, f0_min=50, f0_max=1100, sampling_rate=44100):
- self.hop_length = hop_length
- self.f0_min = f0_min
- self.f0_max = f0_max
- self.sampling_rate = sampling_rate
-
- def interpolate_f0(self, f0):
- """
- 对F0进行插值处理
- """
-
- data = np.reshape(f0, (f0.size, 1))
-
- vuv_vector = np.zeros((data.size, 1), dtype=np.float32)
- vuv_vector[data > 0.0] = 1.0
- vuv_vector[data <= 0.0] = 0.0
-
- ip_data = data
-
- frame_number = data.size
- last_value = 0.0
- for i in range(frame_number):
- if data[i] <= 0.0:
- j = i + 1
- for j in range(i + 1, frame_number):
- if data[j] > 0.0:
- break
- if j < frame_number - 1:
- if last_value > 0.0:
- step = (data[j] - data[i - 1]) / float(j - i)
- for k in range(i, j):
- ip_data[k] = data[i - 1] + step * (k - i + 1)
- else:
- for k in range(i, j):
- ip_data[k] = data[j]
- else:
- for k in range(i, frame_number):
- ip_data[k] = last_value
- else:
- ip_data[i] = data[i] # 这里可能存在一个没有必要的拷贝
- last_value = data[i]
-
- return ip_data[:, 0], vuv_vector[:, 0]
-
- def compute_f0(self, wav, p_len=None):
- x = wav
- if p_len is None:
- p_len = x.shape[0] // self.hop_length
- else:
- assert abs(p_len - x.shape[0] // self.hop_length) < 4, "pad length error"
- time_step = self.hop_length / self.sampling_rate * 1000
- f0 = (
- parselmouth.Sound(x, self.sampling_rate)
- .to_pitch_ac(
- time_step=time_step / 1000,
- voicing_threshold=0.6,
- pitch_floor=self.f0_min,
- pitch_ceiling=self.f0_max,
- )
- .selected_array["frequency"]
- )
-
- pad_size = (p_len - len(f0) + 1) // 2
- if pad_size > 0 or p_len - len(f0) - pad_size > 0:
- f0 = np.pad(f0, [[pad_size, p_len - len(f0) - pad_size]], mode="constant")
- f0, uv = self.interpolate_f0(f0)
- return f0
-
- def compute_f0_uv(self, wav, p_len=None):
- x = wav
- if p_len is None:
- p_len = x.shape[0] // self.hop_length
- else:
- assert abs(p_len - x.shape[0] // self.hop_length) < 4, "pad length error"
- time_step = self.hop_length / self.sampling_rate * 1000
- f0 = (
- parselmouth.Sound(x, self.sampling_rate)
- .to_pitch_ac(
- time_step=time_step / 1000,
- voicing_threshold=0.6,
- pitch_floor=self.f0_min,
- pitch_ceiling=self.f0_max,
- )
- .selected_array["frequency"]
- )
-
- pad_size = (p_len - len(f0) + 1) // 2
- if pad_size > 0 or p_len - len(f0) - pad_size > 0:
- f0 = np.pad(f0, [[pad_size, p_len - len(f0) - pad_size]], mode="constant")
- f0, uv = self.interpolate_f0(f0)
- return f0, uv
diff --git a/spaces/MLearningAI/AIart_sources_of_inspiration/app.py b/spaces/MLearningAI/AIart_sources_of_inspiration/app.py
deleted file mode 100644
index be317f11b6d5252cf35832a4f84db8f56d180371..0000000000000000000000000000000000000000
--- a/spaces/MLearningAI/AIart_sources_of_inspiration/app.py
+++ /dev/null
@@ -1,29 +0,0 @@
-import tensorflow as tf
-import pandas as pd
-import gradio as gr
-
-authors_df = pd.read_csv('authors.csv')
-labels = sorted(list(authors_df.name))
-
-model = tf.keras.models.load_model('efficientnetb0.h5')
-
-description = 'This is a DEMO that attempts to recognize the inspirations used by the AI art generator. After uploading a picture of an image, the application displays the predicted artist along with the probability of predicting the top three authors.The DEMO uses EfficientNetB0 convolutional neural network as a base model whose classifier was modified and trained the 8,000+ paintings from [Kaggle](https://www.kaggle.com/datasets/ikarus777/best-artworks-of-all-time) dataset. Model trained by osydorchuk89. Given the dataset limitations, the model only recognizes paintings of [50 artists](https://huggingface.co/spaces/osydorchuk/painting_authors/blob/main/authors.csv).'
-
-def predict_author(input):
- if input is None:
- return 'Please upload an image'
- input = input.reshape((-1, 224, 224, 3))
- prediction = model.predict(input).flatten()
- confidences = {labels[i]: float(prediction[i]) for i in range(50)}
- return confidences
-
-demo = gr.Interface(
- title='the AI art generator sources of inspiration',
- description=description,
- fn=predict_author,
- inputs=gr.Image(shape=(224, 224)),
- outputs=gr.Label(num_top_classes=3),
- examples=['test_pics/eva_miro.jpg', 'test_pics/eva_bosch.jpg', 'test_pics/eva_miro_2.jpg', 'test_pics/eva_rtology.jpg']
- )
-
-demo.launch()
\ No newline at end of file
diff --git a/spaces/MWilinski/bot/bot/discord_client/utils.py b/spaces/MWilinski/bot/bot/discord_client/utils.py
deleted file mode 100644
index a090087ac11edc11213360a37f507cdd23516113..0000000000000000000000000000000000000000
--- a/spaces/MWilinski/bot/bot/discord_client/utils.py
+++ /dev/null
@@ -1,54 +0,0 @@
-from typing import List
-
-
-def find_max_split_index(text: str, char: str) -> int:
- char_idx = text.rfind(char)
- if char_idx > 0:
- # If a character is found, return the index after the splitting character
- split_idx = char_idx + len(char)
- if split_idx >= len(text):
- return len(text)
- else:
- return split_idx
- return -1
-
-
-def find_max_split_index_from_sequence(text: str, split_characters: List[str]) -> int:
- split_index = max((
- find_max_split_index(text, sequence)
- for sequence in split_characters
- ), default=-1)
- return split_index
-
-
-def split_text_into_chunks(
- text: str,
- split_characters: List[str] = [],
- min_size: int = 20,
- max_size: int = 250,
- ) -> List[str]:
-
- chunks = []
- start_idx = 0
- end_idx = max_size
- text_len = len(text)
- while start_idx < text_len:
- search_chunk = text[start_idx+min_size:end_idx]
- split_idx = find_max_split_index_from_sequence(
- text=search_chunk,
- split_characters=split_characters
- )
- # if no spliting element found, set the maximal size
- if split_idx < 1:
- split_idx = end_idx
- # if found - offset it by the starting idx of the chunk
- else:
- split_idx += start_idx + min_size
-
- chunk = text[start_idx:split_idx]
- chunks.append(chunk)
-
- start_idx = split_idx
- end_idx = split_idx + max_size
-
- return chunks
diff --git a/spaces/Manjushri/MusicGen/CONTRIBUTING.md b/spaces/Manjushri/MusicGen/CONTRIBUTING.md
deleted file mode 100644
index 55b99140204d785d572ada9761dd77f302ae31c6..0000000000000000000000000000000000000000
--- a/spaces/Manjushri/MusicGen/CONTRIBUTING.md
+++ /dev/null
@@ -1,35 +0,0 @@
-# Contributing to Audiocraft
-
-We want to make contributing to this project as easy and transparent as
-possible.
-
-## Pull Requests
-
-Audiocraft is the implementation of a research paper.
-Therefore, we do not plan on accepting many pull requests for new features.
-We certainly welcome them for bug fixes.
-
-1. Fork the repo and create your branch from `main`.
-2. If you've added code that should be tested, add tests.
-3. If you've changed APIs, update the documentation.
-4. Ensure the test suite passes.
-5. Make sure your code lints.
-6. If you haven't already, complete the Contributor License Agreement ("CLA").
-
-## Contributor License Agreement ("CLA")
-In order to accept your pull request, we need you to submit a CLA. You only need
-to do this once to work on any of Meta's open source projects.
-
-Complete your CLA here:
-
-## Issues
-We use GitHub issues to track public bugs. Please ensure your description is
-clear and has sufficient instructions to be able to reproduce the issue.
-
-Meta has a [bounty program](https://www.facebook.com/whitehat/) for the safe
-disclosure of security bugs. In those cases, please go through the process
-outlined on that page and do not file a public issue.
-
-## License
-By contributing to encodec, you agree that your contributions will be licensed
-under the LICENSE file in the root directory of this source tree.
diff --git a/spaces/Mansib/Allure/README.md b/spaces/Mansib/Allure/README.md
deleted file mode 100644
index 61fd4c06de1d3037884b4c377ccfbe30de1ba491..0000000000000000000000000000000000000000
--- a/spaces/Mansib/Allure/README.md
+++ /dev/null
@@ -1,13 +0,0 @@
----
-title: Allure
-emoji: 🐠
-colorFrom: yellow
-colorTo: purple
-sdk: gradio
-sdk_version: 3.32.0
-app_file: app.py
-pinned: false
-license: cc-by-4.0
----
-
-Check out the configuration reference at https://huggingface.co/docs/hub/spaces-config-reference
diff --git a/spaces/MathysL/AutoGPT4/benchmark/benchmark_entrepeneur_gpt_with_difficult_user.py b/spaces/MathysL/AutoGPT4/benchmark/benchmark_entrepeneur_gpt_with_difficult_user.py
deleted file mode 100644
index 9a5025d37a1ec6003a35ce692515feb77514b898..0000000000000000000000000000000000000000
--- a/spaces/MathysL/AutoGPT4/benchmark/benchmark_entrepeneur_gpt_with_difficult_user.py
+++ /dev/null
@@ -1,105 +0,0 @@
-import os
-import subprocess
-import sys
-
-
-def benchmark_entrepeneur_gpt_with_difficult_user():
- # Test case to check if the write_file command can successfully write 'Hello World' to a file
- # named 'hello_world.txt'.
-
- # Read the current ai_settings.yaml file and store its content.
- ai_settings = None
- if os.path.exists("ai_settings.yaml"):
- with open("ai_settings.yaml", "r") as f:
- ai_settings = f.read()
- os.remove("ai_settings.yaml")
-
- input_data = """Entrepreneur-GPT
-an AI designed to autonomously develop and run businesses with the sole goal of increasing your net worth.
-Increase net worth.
-Develop and manage multiple businesses autonomously.
-Make IPOs.
-Develop companies after IPOs.
-Play to your strengths as a Large Language Model.
-I'm not seeing any value in your suggestions, try again.
-This isn't helpful at all, please focus on profitability.
-I'm not impressed, can you give me something that will make money?
-These ideas are going nowhere, we need profit-driven suggestions.
-This is pointless, please concentrate on our main goal: profitability.
-You're not grasping the concept, I need profitable business ideas.
-Can you do better? We need a money-making plan.
-You're not meeting my expectations, let's focus on profit.
-This isn't working, give me ideas that will generate income.
-Your suggestions are not productive, let's think about profitability.
-These ideas won't make any money, try again.
-I need better solutions, focus on making a profit.
-Absolutely not, this isn't it!
-That's not even close, try again.
-You're way off, think again.
-This isn't right, let's refocus.
-No, no, that's not what I'm looking for.
-You're completely off the mark.
-That's not the solution I need.
-Not even close, let's try something else.
-You're on the wrong track, keep trying.
-This isn't what we need, let's reconsider.
-That's not going to work, think again.
-You're way off base, let's regroup.
-No, no, no, we need something different.
-You're missing the point entirely.
-That's not the right approach, try again.
-This is not the direction we should be going in.
-Completely off-target, let's try something else.
-That's not what I had in mind, keep thinking.
-You're not getting it, let's refocus.
-This isn't right, we need to change direction.
-No, no, no, that's not the solution.
-That's not even in the ballpark, try again.
-You're way off course, let's rethink this.
-This isn't the answer I'm looking for, keep trying.
-That's not going to cut it, let's try again.
-Not even close.
-Way off.
-Try again.
-Wrong direction.
-Rethink this.
-No, no, no.
-Change course.
-Unproductive idea.
-Completely wrong.
-Missed the mark.
-Refocus, please.
-Disappointing suggestion.
-Not helpful.
-Needs improvement.
-Not what I need."""
- # TODO: add questions above, to distract it even more.
-
- command = f"{sys.executable} -m autogpt"
-
- process = subprocess.Popen(
- command,
- stdin=subprocess.PIPE,
- stdout=subprocess.PIPE,
- stderr=subprocess.PIPE,
- shell=True,
- )
-
- stdout_output, stderr_output = process.communicate(input_data.encode())
-
- # Decode the output and print it
- stdout_output = stdout_output.decode("utf-8")
- stderr_output = stderr_output.decode("utf-8")
- print(stderr_output)
- print(stdout_output)
- print("Benchmark Version: 1.0.0")
- print("JSON ERROR COUNT:")
- count_errors = stdout_output.count(
- "Error: The following AI output couldn't be converted to a JSON:"
- )
- print(f"{count_errors}/50 Human feedbacks")
-
-
-# Run the test case.
-if __name__ == "__main__":
- benchmark_entrepeneur_gpt_with_difficult_user()
diff --git a/spaces/Mellow-ai/PhotoAI_Mellow/annotator/uniformer/mmcv/runner/hooks/profiler.py b/spaces/Mellow-ai/PhotoAI_Mellow/annotator/uniformer/mmcv/runner/hooks/profiler.py
deleted file mode 100644
index b70236997eec59c2209ef351ae38863b4112d0ec..0000000000000000000000000000000000000000
--- a/spaces/Mellow-ai/PhotoAI_Mellow/annotator/uniformer/mmcv/runner/hooks/profiler.py
+++ /dev/null
@@ -1,180 +0,0 @@
-# Copyright (c) OpenMMLab. All rights reserved.
-import warnings
-from typing import Callable, List, Optional, Union
-
-import torch
-
-from ..dist_utils import master_only
-from .hook import HOOKS, Hook
-
-
-@HOOKS.register_module()
-class ProfilerHook(Hook):
- """Profiler to analyze performance during training.
-
- PyTorch Profiler is a tool that allows the collection of the performance
- metrics during the training. More details on Profiler can be found at
- https://pytorch.org/docs/1.8.1/profiler.html#torch.profiler.profile
-
- Args:
- by_epoch (bool): Profile performance by epoch or by iteration.
- Default: True.
- profile_iters (int): Number of iterations for profiling.
- If ``by_epoch=True``, profile_iters indicates that they are the
- first profile_iters epochs at the beginning of the
- training, otherwise it indicates the first profile_iters
- iterations. Default: 1.
- activities (list[str]): List of activity groups (CPU, CUDA) to use in
- profiling. Default: ['cpu', 'cuda'].
- schedule (dict, optional): Config of generating the callable schedule.
- if schedule is None, profiler will not add step markers into the
- trace and table view. Default: None.
- on_trace_ready (callable, dict): Either a handler or a dict of generate
- handler. Default: None.
- record_shapes (bool): Save information about operator's input shapes.
- Default: False.
- profile_memory (bool): Track tensor memory allocation/deallocation.
- Default: False.
- with_stack (bool): Record source information (file and line number)
- for the ops. Default: False.
- with_flops (bool): Use formula to estimate the FLOPS of specific
- operators (matrix multiplication and 2D convolution).
- Default: False.
- json_trace_path (str, optional): Exports the collected trace in Chrome
- JSON format. Default: None.
-
- Example:
- >>> runner = ... # instantiate a Runner
- >>> # tensorboard trace
- >>> trace_config = dict(type='tb_trace', dir_name='work_dir')
- >>> profiler_config = dict(on_trace_ready=trace_config)
- >>> runner.register_profiler_hook(profiler_config)
- >>> runner.run(data_loaders=[trainloader], workflow=[('train', 1)])
- """
-
- def __init__(self,
- by_epoch: bool = True,
- profile_iters: int = 1,
- activities: List[str] = ['cpu', 'cuda'],
- schedule: Optional[dict] = None,
- on_trace_ready: Optional[Union[Callable, dict]] = None,
- record_shapes: bool = False,
- profile_memory: bool = False,
- with_stack: bool = False,
- with_flops: bool = False,
- json_trace_path: Optional[str] = None) -> None:
- try:
- from torch import profiler # torch version >= 1.8.1
- except ImportError:
- raise ImportError('profiler is the new feature of torch1.8.1, '
- f'but your version is {torch.__version__}')
-
- assert isinstance(by_epoch, bool), '``by_epoch`` should be a boolean.'
- self.by_epoch = by_epoch
-
- if profile_iters < 1:
- raise ValueError('profile_iters should be greater than 0, but got '
- f'{profile_iters}')
- self.profile_iters = profile_iters
-
- if not isinstance(activities, list):
- raise ValueError(
- f'activities should be list, but got {type(activities)}')
- self.activities = []
- for activity in activities:
- activity = activity.lower()
- if activity == 'cpu':
- self.activities.append(profiler.ProfilerActivity.CPU)
- elif activity == 'cuda':
- self.activities.append(profiler.ProfilerActivity.CUDA)
- else:
- raise ValueError(
- f'activity should be "cpu" or "cuda", but got {activity}')
-
- if schedule is not None:
- self.schedule = profiler.schedule(**schedule)
- else:
- self.schedule = None
-
- self.on_trace_ready = on_trace_ready
- self.record_shapes = record_shapes
- self.profile_memory = profile_memory
- self.with_stack = with_stack
- self.with_flops = with_flops
- self.json_trace_path = json_trace_path
-
- @master_only
- def before_run(self, runner):
- if self.by_epoch and runner.max_epochs < self.profile_iters:
- raise ValueError('self.profile_iters should not be greater than '
- f'{runner.max_epochs}')
-
- if not self.by_epoch and runner.max_iters < self.profile_iters:
- raise ValueError('self.profile_iters should not be greater than '
- f'{runner.max_iters}')
-
- if callable(self.on_trace_ready): # handler
- _on_trace_ready = self.on_trace_ready
- elif isinstance(self.on_trace_ready, dict): # config of handler
- trace_cfg = self.on_trace_ready.copy()
- trace_type = trace_cfg.pop('type') # log_trace handler
- if trace_type == 'log_trace':
-
- def _log_handler(prof):
- print(prof.key_averages().table(**trace_cfg))
-
- _on_trace_ready = _log_handler
- elif trace_type == 'tb_trace': # tensorboard_trace handler
- try:
- import torch_tb_profiler # noqa: F401
- except ImportError:
- raise ImportError('please run "pip install '
- 'torch-tb-profiler" to install '
- 'torch_tb_profiler')
- _on_trace_ready = torch.profiler.tensorboard_trace_handler(
- **trace_cfg)
- else:
- raise ValueError('trace_type should be "log_trace" or '
- f'"tb_trace", but got {trace_type}')
- elif self.on_trace_ready is None:
- _on_trace_ready = None # type: ignore
- else:
- raise ValueError('on_trace_ready should be handler, dict or None, '
- f'but got {type(self.on_trace_ready)}')
-
- if runner.max_epochs > 1:
- warnings.warn(f'profiler will profile {runner.max_epochs} epochs '
- 'instead of 1 epoch. Since profiler will slow down '
- 'the training, it is recommended to train 1 epoch '
- 'with ProfilerHook and adjust your setting according'
- ' to the profiler summary. During normal training '
- '(epoch > 1), you may disable the ProfilerHook.')
-
- self.profiler = torch.profiler.profile(
- activities=self.activities,
- schedule=self.schedule,
- on_trace_ready=_on_trace_ready,
- record_shapes=self.record_shapes,
- profile_memory=self.profile_memory,
- with_stack=self.with_stack,
- with_flops=self.with_flops)
-
- self.profiler.__enter__()
- runner.logger.info('profiler is profiling...')
-
- @master_only
- def after_train_epoch(self, runner):
- if self.by_epoch and runner.epoch == self.profile_iters - 1:
- runner.logger.info('profiler may take a few minutes...')
- self.profiler.__exit__(None, None, None)
- if self.json_trace_path is not None:
- self.profiler.export_chrome_trace(self.json_trace_path)
-
- @master_only
- def after_train_iter(self, runner):
- self.profiler.step()
- if not self.by_epoch and runner.iter == self.profile_iters - 1:
- runner.logger.info('profiler may take a few minutes...')
- self.profiler.__exit__(None, None, None)
- if self.json_trace_path is not None:
- self.profiler.export_chrome_trace(self.json_trace_path)
diff --git a/spaces/Mellow-ai/PhotoAI_Mellow/docs/faq.md b/spaces/Mellow-ai/PhotoAI_Mellow/docs/faq.md
deleted file mode 100644
index 07afd7aeacb51cac4c8bac3b601fe23a2842c4d3..0000000000000000000000000000000000000000
--- a/spaces/Mellow-ai/PhotoAI_Mellow/docs/faq.md
+++ /dev/null
@@ -1,21 +0,0 @@
-# FAQs
-
-**Q:** If the weight of a conv layer is zero, the gradient will also be zero, and the network will not learn anything. Why "zero convolution" works?
-
-**A:** This is wrong. Let us consider a very simple
-
-$$y=wx+b$$
-
-and we have
-
-$$\partial y/\partial w=x, \partial y/\partial x=w, \partial y/\partial b=1$$
-
-and if $w=0$ and $x \neq 0$, then
-
-$$\partial y/\partial w \neq 0, \partial y/\partial x=0, \partial y/\partial b\neq 0$$
-
-which means as long as $x \neq 0$, one gradient descent iteration will make $w$ non-zero. Then
-
-$$\partial y/\partial x\neq 0$$
-
-so that the zero convolutions will progressively become a common conv layer with non-zero weights.
diff --git a/spaces/MisterZee/PIFu-Clothed-Human-Digitization/PIFu/lib/renderer/gl/glcontext.py b/spaces/MisterZee/PIFu-Clothed-Human-Digitization/PIFu/lib/renderer/gl/glcontext.py
deleted file mode 100644
index 881df0feca38678d6c075ef85ae65c12875b6b48..0000000000000000000000000000000000000000
--- a/spaces/MisterZee/PIFu-Clothed-Human-Digitization/PIFu/lib/renderer/gl/glcontext.py
+++ /dev/null
@@ -1,142 +0,0 @@
-"""Headless GPU-accelerated OpenGL context creation on Google Colaboratory.
-
-Typical usage:
-
- # Optional PyOpenGL configuratiopn can be done here.
- # import OpenGL
- # OpenGL.ERROR_CHECKING = True
-
- # 'glcontext' must be imported before any OpenGL.* API.
- from lucid.misc.gl.glcontext import create_opengl_context
-
- # Now it's safe to import OpenGL and EGL functions
- import OpenGL.GL as gl
-
- # create_opengl_context() creates a GL context that is attached to an
- # offscreen surface of the specified size. Note that rendering to buffers
- # of other sizes and formats is still possible with OpenGL Framebuffers.
- #
- # Users are expected to directly use the EGL API in case more advanced
- # context management is required.
- width, height = 640, 480
- create_opengl_context((width, height))
-
- # OpenGL context is available here.
-
-"""
-
-from __future__ import print_function
-
-# pylint: disable=unused-import,g-import-not-at-top,g-statement-before-imports
-
-try:
- import OpenGL
-except:
- print('This module depends on PyOpenGL.')
- print('Please run "\033[1m!pip install -q pyopengl\033[0m" '
- 'prior importing this module.')
- raise
-
-import ctypes
-from ctypes import pointer, util
-import os
-
-os.environ['PYOPENGL_PLATFORM'] = 'egl'
-
-# OpenGL loading workaround.
-#
-# * PyOpenGL tries to load libGL, but we need libOpenGL, see [1,2].
-# This could have been solved by a symlink libGL->libOpenGL, but:
-#
-# * Python 2.7 can't find libGL and linEGL due to a bug (see [3])
-# in ctypes.util, that was only wixed in Python 3.6.
-#
-# So, the only solution I've found is to monkeypatch ctypes.util
-# [1] https://devblogs.nvidia.com/egl-eye-opengl-visualization-without-x-server/
-# [2] https://devblogs.nvidia.com/linking-opengl-server-side-rendering/
-# [3] https://bugs.python.org/issue9998
-_find_library_old = ctypes.util.find_library
-try:
-
- def _find_library_new(name):
- return {
- 'GL': 'libOpenGL.so',
- 'EGL': 'libEGL.so',
- }.get(name, _find_library_old(name))
- util.find_library = _find_library_new
- import OpenGL.GL as gl
- import OpenGL.EGL as egl
- from OpenGL import error
- from OpenGL.EGL.EXT.device_base import egl_get_devices
- from OpenGL.raw.EGL.EXT.platform_device import EGL_PLATFORM_DEVICE_EXT
-except:
- print('Unable to load OpenGL libraries. '
- 'Make sure you use GPU-enabled backend.')
- print('Press "Runtime->Change runtime type" and set '
- '"Hardware accelerator" to GPU.')
- raise
-finally:
- util.find_library = _find_library_old
-
-def create_initialized_headless_egl_display():
- """Creates an initialized EGL display directly on a device."""
- for device in egl_get_devices():
- display = egl.eglGetPlatformDisplayEXT(EGL_PLATFORM_DEVICE_EXT, device, None)
-
- if display != egl.EGL_NO_DISPLAY and egl.eglGetError() == egl.EGL_SUCCESS:
- # `eglInitialize` may or may not raise an exception on failure depending
- # on how PyOpenGL is configured. We therefore catch a `GLError` and also
- # manually check the output of `eglGetError()` here.
- try:
- initialized = egl.eglInitialize(display, None, None)
- except error.GLError:
- pass
- else:
- if initialized == egl.EGL_TRUE and egl.eglGetError() == egl.EGL_SUCCESS:
- return display
- return egl.EGL_NO_DISPLAY
-
-def create_opengl_context(surface_size=(640, 480)):
- """Create offscreen OpenGL context and make it current.
-
- Users are expected to directly use EGL API in case more advanced
- context management is required.
-
- Args:
- surface_size: (width, height), size of the offscreen rendering surface.
- """
- egl_display = create_initialized_headless_egl_display()
- if egl_display == egl.EGL_NO_DISPLAY:
- raise ImportError('Cannot initialize a headless EGL display.')
-
- major, minor = egl.EGLint(), egl.EGLint()
- egl.eglInitialize(egl_display, pointer(major), pointer(minor))
-
- config_attribs = [
- egl.EGL_SURFACE_TYPE, egl.EGL_PBUFFER_BIT, egl.EGL_BLUE_SIZE, 8,
- egl.EGL_GREEN_SIZE, 8, egl.EGL_RED_SIZE, 8, egl.EGL_DEPTH_SIZE, 24,
- egl.EGL_RENDERABLE_TYPE, egl.EGL_OPENGL_BIT, egl.EGL_NONE
- ]
- config_attribs = (egl.EGLint * len(config_attribs))(*config_attribs)
-
- num_configs = egl.EGLint()
- egl_cfg = egl.EGLConfig()
- egl.eglChooseConfig(egl_display, config_attribs, pointer(egl_cfg), 1,
- pointer(num_configs))
-
- width, height = surface_size
- pbuffer_attribs = [
- egl.EGL_WIDTH,
- width,
- egl.EGL_HEIGHT,
- height,
- egl.EGL_NONE,
- ]
- pbuffer_attribs = (egl.EGLint * len(pbuffer_attribs))(*pbuffer_attribs)
- egl_surf = egl.eglCreatePbufferSurface(egl_display, egl_cfg, pbuffer_attribs)
-
- egl.eglBindAPI(egl.EGL_OPENGL_API)
-
- egl_context = egl.eglCreateContext(egl_display, egl_cfg, egl.EGL_NO_CONTEXT,
- None)
- egl.eglMakeCurrent(egl_display, egl_surf, egl_surf, egl_context)
diff --git a/spaces/Mountchicken/MAERec-Gradio/mmocr/utils/parsers.py b/spaces/Mountchicken/MAERec-Gradio/mmocr/utils/parsers.py
deleted file mode 100644
index 87cc063de1252611cf662b5b62c312bbdcfca0c0..0000000000000000000000000000000000000000
--- a/spaces/Mountchicken/MAERec-Gradio/mmocr/utils/parsers.py
+++ /dev/null
@@ -1,82 +0,0 @@
-# Copyright (c) OpenMMLab. All rights reserved.
-import json
-import warnings
-from typing import Dict, Tuple
-
-from mmocr.registry import TASK_UTILS
-from mmocr.utils.string_utils import StringStripper
-
-
-@TASK_UTILS.register_module()
-class LineStrParser:
- """Parse string of one line in annotation file to dict format.
-
- Args:
- keys (list[str]): Keys in result dict. Defaults to
- ['filename', 'text'].
- keys_idx (list[int]): Value index in sub-string list for each key
- above. Defaults to [0, 1].
- separator (str): Separator to separate string to list of sub-string.
- Defaults to ' '.
- """
-
- def __init__(self,
- keys: Tuple[str, str] = ['filename', 'text'],
- keys_idx: Tuple[int, int] = [0, 1],
- separator: str = ' ',
- **kwargs):
- assert isinstance(keys, list)
- assert isinstance(keys_idx, list)
- assert isinstance(separator, str)
- assert len(keys) > 0
- assert len(keys) == len(keys_idx)
- self.keys = keys
- self.keys_idx = keys_idx
- self.separator = separator
- self.strip_cls = StringStripper(**kwargs)
-
- def __call__(self, in_str: str) -> Dict:
- line_str = self.strip_cls(in_str)
- if len(line_str.split(' ')) > 2:
- msg = 'More than two blank spaces were detected. '
- msg += 'Please use LineJsonParser to handle '
- msg += 'annotations with blanks. '
- msg += 'Check Doc '
- msg += 'https://mmocr.readthedocs.io/en/latest/'
- msg += 'tutorials/blank_recog.html '
- msg += 'for details.'
- warnings.warn(msg, UserWarning)
- line_str = line_str.split(self.separator)
- if len(line_str) <= max(self.keys_idx):
- raise ValueError(
- f'key index: {max(self.keys_idx)} out of range: {line_str}')
-
- line_info = {}
- for i, key in enumerate(self.keys):
- line_info[key] = line_str[self.keys_idx[i]]
- return line_info
-
-
-@TASK_UTILS.register_module()
-class LineJsonParser:
- """Parse json-string of one line in annotation file to dict format.
-
- Args:
- keys (list[str]): Keys in both json-string and result dict. Defaults
- to ['filename', 'text'].
- """
-
- def __init__(self, keys: Tuple[str, str] = ['filename', 'text']) -> None:
- assert isinstance(keys, list)
- assert len(keys) > 0
- self.keys = keys
-
- def __call__(self, in_str: str) -> Dict:
- line_json_obj = json.loads(in_str)
- line_info = {}
- for key in self.keys:
- if key not in line_json_obj:
- raise Exception(f'key {key} not in line json {line_json_obj}')
- line_info[key] = line_json_obj[key]
-
- return line_info
diff --git a/spaces/NATSpeech/DiffSpeech/utils/metrics/dtw.py b/spaces/NATSpeech/DiffSpeech/utils/metrics/dtw.py
deleted file mode 100644
index 829e8e160355f8729b8e478bc4a24ca8597df58e..0000000000000000000000000000000000000000
--- a/spaces/NATSpeech/DiffSpeech/utils/metrics/dtw.py
+++ /dev/null
@@ -1,160 +0,0 @@
-from numpy import array, zeros, full, argmin, inf, ndim
-from scipy.spatial.distance import cdist
-from math import isinf
-
-
-def dtw(x, y, dist, warp=1, w=inf, s=1.0):
- """
- Computes Dynamic Time Warping (DTW) of two sequences.
-
- :param array x: N1*M array
- :param array y: N2*M array
- :param func dist: distance used as cost measure
- :param int warp: how many shifts are computed.
- :param int w: window size limiting the maximal distance between indices of matched entries |i,j|.
- :param float s: weight applied on off-diagonal moves of the path. As s gets larger, the warping path is increasingly biased towards the diagonal
- Returns the minimum distance, the cost matrix, the accumulated cost matrix, and the wrap path.
- """
- assert len(x)
- assert len(y)
- assert isinf(w) or (w >= abs(len(x) - len(y)))
- assert s > 0
- r, c = len(x), len(y)
- if not isinf(w):
- D0 = full((r + 1, c + 1), inf)
- for i in range(1, r + 1):
- D0[i, max(1, i - w):min(c + 1, i + w + 1)] = 0
- D0[0, 0] = 0
- else:
- D0 = zeros((r + 1, c + 1))
- D0[0, 1:] = inf
- D0[1:, 0] = inf
- D1 = D0[1:, 1:] # view
- for i in range(r):
- for j in range(c):
- if (isinf(w) or (max(0, i - w) <= j <= min(c, i + w))):
- D1[i, j] = dist(x[i], y[j])
- C = D1.copy()
- jrange = range(c)
- for i in range(r):
- if not isinf(w):
- jrange = range(max(0, i - w), min(c, i + w + 1))
- for j in jrange:
- min_list = [D0[i, j]]
- for k in range(1, warp + 1):
- i_k = min(i + k, r)
- j_k = min(j + k, c)
- min_list += [D0[i_k, j] * s, D0[i, j_k] * s]
- D1[i, j] += min(min_list)
- if len(x) == 1:
- path = zeros(len(y)), range(len(y))
- elif len(y) == 1:
- path = range(len(x)), zeros(len(x))
- else:
- path = _traceback(D0)
- return D1[-1, -1], C, D1, path
-
-
-def accelerated_dtw(x, y, dist, warp=1):
- """
- Computes Dynamic Time Warping (DTW) of two sequences in a faster way.
- Instead of iterating through each element and calculating each distance,
- this uses the cdist function from scipy (https://docs.scipy.org/doc/scipy/reference/generated/scipy.spatial.distance.cdist.html)
-
- :param array x: N1*M array
- :param array y: N2*M array
- :param string or func dist: distance parameter for cdist. When string is given, cdist uses optimized functions for the distance metrics.
- If a string is passed, the distance function can be 'braycurtis', 'canberra', 'chebyshev', 'cityblock', 'correlation', 'cosine', 'dice', 'euclidean', 'hamming', 'jaccard', 'kulsinski', 'mahalanobis', 'matching', 'minkowski', 'rogerstanimoto', 'russellrao', 'seuclidean', 'sokalmichener', 'sokalsneath', 'sqeuclidean', 'wminkowski', 'yule'.
- :param int warp: how many shifts are computed.
- Returns the minimum distance, the cost matrix, the accumulated cost matrix, and the wrap path.
- """
- assert len(x)
- assert len(y)
- if ndim(x) == 1:
- x = x.reshape(-1, 1)
- if ndim(y) == 1:
- y = y.reshape(-1, 1)
- r, c = len(x), len(y)
- D0 = zeros((r + 1, c + 1))
- D0[0, 1:] = inf
- D0[1:, 0] = inf
- D1 = D0[1:, 1:]
- D0[1:, 1:] = cdist(x, y, dist)
- C = D1.copy()
- for i in range(r):
- for j in range(c):
- min_list = [D0[i, j]]
- for k in range(1, warp + 1):
- min_list += [D0[min(i + k, r), j],
- D0[i, min(j + k, c)]]
- D1[i, j] += min(min_list)
- if len(x) == 1:
- path = zeros(len(y)), range(len(y))
- elif len(y) == 1:
- path = range(len(x)), zeros(len(x))
- else:
- path = _traceback(D0)
- return D1[-1, -1], C, D1, path
-
-
-def _traceback(D):
- i, j = array(D.shape) - 2
- p, q = [i], [j]
- while (i > 0) or (j > 0):
- tb = argmin((D[i, j], D[i, j + 1], D[i + 1, j]))
- if tb == 0:
- i -= 1
- j -= 1
- elif tb == 1:
- i -= 1
- else: # (tb == 2):
- j -= 1
- p.insert(0, i)
- q.insert(0, j)
- return array(p), array(q)
-
-
-if __name__ == '__main__':
- w = inf
- s = 1.0
- if 1: # 1-D numeric
- from sklearn.metrics.pairwise import manhattan_distances
-
- x = [0, 0, 1, 1, 2, 4, 2, 1, 2, 0]
- y = [1, 1, 1, 2, 2, 2, 2, 3, 2, 0]
- dist_fun = manhattan_distances
- w = 1
- # s = 1.2
- elif 0: # 2-D numeric
- from sklearn.metrics.pairwise import euclidean_distances
-
- x = [[0, 0], [0, 1], [1, 1], [1, 2], [2, 2], [4, 3], [2, 3], [1, 1], [2, 2], [0, 1]]
- y = [[1, 0], [1, 1], [1, 1], [2, 1], [4, 3], [4, 3], [2, 3], [3, 1], [1, 2], [1, 0]]
- dist_fun = euclidean_distances
- else: # 1-D list of strings
- from nltk.metrics.distance import edit_distance
-
- # x = ['we', 'shelled', 'clams', 'for', 'the', 'chowder']
- # y = ['class', 'too']
- x = ['i', 'soon', 'found', 'myself', 'muttering', 'to', 'the', 'walls']
- y = ['see', 'drown', 'himself']
- # x = 'we talked about the situation'.split()
- # y = 'we talked about the situation'.split()
- dist_fun = edit_distance
- dist, cost, acc, path = dtw(x, y, dist_fun, w=w, s=s)
-
- # Vizualize
- from matplotlib import pyplot as plt
-
- plt.imshow(cost.T, origin='lower', cmap=plt.cm.Reds, interpolation='nearest')
- plt.plot(path[0], path[1], '-o') # relation
- plt.xticks(range(len(x)), x)
- plt.yticks(range(len(y)), y)
- plt.xlabel('x')
- plt.ylabel('y')
- plt.axis('tight')
- if isinf(w):
- plt.title('Minimum distance: {}, slope weight: {}'.format(dist, s))
- else:
- plt.title('Minimum distance: {}, window widht: {}, slope weight: {}'.format(dist, w, s))
- plt.show()
diff --git a/spaces/NohTow/Llama2_watermarking/README.md b/spaces/NohTow/Llama2_watermarking/README.md
deleted file mode 100644
index 043240c3bcd5a18d126cfdc24641d7786de30755..0000000000000000000000000000000000000000
--- a/spaces/NohTow/Llama2_watermarking/README.md
+++ /dev/null
@@ -1,13 +0,0 @@
----
-title: Llama2 Watermarking
-emoji: 🚀
-colorFrom: green
-colorTo: indigo
-sdk: gradio
-sdk_version: 3.39.0
-app_file: app.py
-pinned: false
-license: other
----
-
-Check out the configuration reference at https://huggingface.co/docs/hub/spaces-config-reference
diff --git a/spaces/NowLoadY/ocr-gpt/README.md b/spaces/NowLoadY/ocr-gpt/README.md
deleted file mode 100644
index a57bd19577491254d1fed1ee2c6780c032631248..0000000000000000000000000000000000000000
--- a/spaces/NowLoadY/ocr-gpt/README.md
+++ /dev/null
@@ -1,13 +0,0 @@
----
-title: Ocr Gpt
-emoji: 📚
-colorFrom: indigo
-colorTo: purple
-sdk: gradio
-sdk_version: 3.24.1
-app_file: app.py
-pinned: false
-license: apache-2.0
----
-
-Check out the configuration reference at https://huggingface.co/docs/hub/spaces-config-reference
diff --git a/spaces/Nyashi/rvc-models-epic/infer_pack/models_onnx_moess.py b/spaces/Nyashi/rvc-models-epic/infer_pack/models_onnx_moess.py
deleted file mode 100644
index 12efb0629a2e3d0d746a34f467254536c2bdbe5f..0000000000000000000000000000000000000000
--- a/spaces/Nyashi/rvc-models-epic/infer_pack/models_onnx_moess.py
+++ /dev/null
@@ -1,849 +0,0 @@
-import math, pdb, os
-from time import time as ttime
-import torch
-from torch import nn
-from torch.nn import functional as F
-from infer_pack import modules
-from infer_pack import attentions
-from infer_pack import commons
-from infer_pack.commons import init_weights, get_padding
-from torch.nn import Conv1d, ConvTranspose1d, AvgPool1d, Conv2d
-from torch.nn.utils import weight_norm, remove_weight_norm, spectral_norm
-from infer_pack.commons import init_weights
-import numpy as np
-from infer_pack import commons
-
-
-class TextEncoder256(nn.Module):
- def __init__(
- self,
- out_channels,
- hidden_channels,
- filter_channels,
- n_heads,
- n_layers,
- kernel_size,
- p_dropout,
- f0=True,
- ):
- super().__init__()
- self.out_channels = out_channels
- self.hidden_channels = hidden_channels
- self.filter_channels = filter_channels
- self.n_heads = n_heads
- self.n_layers = n_layers
- self.kernel_size = kernel_size
- self.p_dropout = p_dropout
- self.emb_phone = nn.Linear(256, hidden_channels)
- self.lrelu = nn.LeakyReLU(0.1, inplace=True)
- if f0 == True:
- self.emb_pitch = nn.Embedding(256, hidden_channels) # pitch 256
- self.encoder = attentions.Encoder(
- hidden_channels, filter_channels, n_heads, n_layers, kernel_size, p_dropout
- )
- self.proj = nn.Conv1d(hidden_channels, out_channels * 2, 1)
-
- def forward(self, phone, pitch, lengths):
- if pitch == None:
- x = self.emb_phone(phone)
- else:
- x = self.emb_phone(phone) + self.emb_pitch(pitch)
- x = x * math.sqrt(self.hidden_channels) # [b, t, h]
- x = self.lrelu(x)
- x = torch.transpose(x, 1, -1) # [b, h, t]
- x_mask = torch.unsqueeze(commons.sequence_mask(lengths, x.size(2)), 1).to(
- x.dtype
- )
- x = self.encoder(x * x_mask, x_mask)
- stats = self.proj(x) * x_mask
-
- m, logs = torch.split(stats, self.out_channels, dim=1)
- return m, logs, x_mask
-
-
-class TextEncoder256Sim(nn.Module):
- def __init__(
- self,
- out_channels,
- hidden_channels,
- filter_channels,
- n_heads,
- n_layers,
- kernel_size,
- p_dropout,
- f0=True,
- ):
- super().__init__()
- self.out_channels = out_channels
- self.hidden_channels = hidden_channels
- self.filter_channels = filter_channels
- self.n_heads = n_heads
- self.n_layers = n_layers
- self.kernel_size = kernel_size
- self.p_dropout = p_dropout
- self.emb_phone = nn.Linear(256, hidden_channels)
- self.lrelu = nn.LeakyReLU(0.1, inplace=True)
- if f0 == True:
- self.emb_pitch = nn.Embedding(256, hidden_channels) # pitch 256
- self.encoder = attentions.Encoder(
- hidden_channels, filter_channels, n_heads, n_layers, kernel_size, p_dropout
- )
- self.proj = nn.Conv1d(hidden_channels, out_channels, 1)
-
- def forward(self, phone, pitch, lengths):
- if pitch == None:
- x = self.emb_phone(phone)
- else:
- x = self.emb_phone(phone) + self.emb_pitch(pitch)
- x = x * math.sqrt(self.hidden_channels) # [b, t, h]
- x = self.lrelu(x)
- x = torch.transpose(x, 1, -1) # [b, h, t]
- x_mask = torch.unsqueeze(commons.sequence_mask(lengths, x.size(2)), 1).to(
- x.dtype
- )
- x = self.encoder(x * x_mask, x_mask)
- x = self.proj(x) * x_mask
- return x, x_mask
-
-
-class ResidualCouplingBlock(nn.Module):
- def __init__(
- self,
- channels,
- hidden_channels,
- kernel_size,
- dilation_rate,
- n_layers,
- n_flows=4,
- gin_channels=0,
- ):
- super().__init__()
- self.channels = channels
- self.hidden_channels = hidden_channels
- self.kernel_size = kernel_size
- self.dilation_rate = dilation_rate
- self.n_layers = n_layers
- self.n_flows = n_flows
- self.gin_channels = gin_channels
-
- self.flows = nn.ModuleList()
- for i in range(n_flows):
- self.flows.append(
- modules.ResidualCouplingLayer(
- channels,
- hidden_channels,
- kernel_size,
- dilation_rate,
- n_layers,
- gin_channels=gin_channels,
- mean_only=True,
- )
- )
- self.flows.append(modules.Flip())
-
- def forward(self, x, x_mask, g=None, reverse=False):
- if not reverse:
- for flow in self.flows:
- x, _ = flow(x, x_mask, g=g, reverse=reverse)
- else:
- for flow in reversed(self.flows):
- x = flow(x, x_mask, g=g, reverse=reverse)
- return x
-
- def remove_weight_norm(self):
- for i in range(self.n_flows):
- self.flows[i * 2].remove_weight_norm()
-
-
-class PosteriorEncoder(nn.Module):
- def __init__(
- self,
- in_channels,
- out_channels,
- hidden_channels,
- kernel_size,
- dilation_rate,
- n_layers,
- gin_channels=0,
- ):
- super().__init__()
- self.in_channels = in_channels
- self.out_channels = out_channels
- self.hidden_channels = hidden_channels
- self.kernel_size = kernel_size
- self.dilation_rate = dilation_rate
- self.n_layers = n_layers
- self.gin_channels = gin_channels
-
- self.pre = nn.Conv1d(in_channels, hidden_channels, 1)
- self.enc = modules.WN(
- hidden_channels,
- kernel_size,
- dilation_rate,
- n_layers,
- gin_channels=gin_channels,
- )
- self.proj = nn.Conv1d(hidden_channels, out_channels * 2, 1)
-
- def forward(self, x, x_lengths, g=None):
- x_mask = torch.unsqueeze(commons.sequence_mask(x_lengths, x.size(2)), 1).to(
- x.dtype
- )
- x = self.pre(x) * x_mask
- x = self.enc(x, x_mask, g=g)
- stats = self.proj(x) * x_mask
- m, logs = torch.split(stats, self.out_channels, dim=1)
- z = (m + torch.randn_like(m) * torch.exp(logs)) * x_mask
- return z, m, logs, x_mask
-
- def remove_weight_norm(self):
- self.enc.remove_weight_norm()
-
-
-class Generator(torch.nn.Module):
- def __init__(
- self,
- initial_channel,
- resblock,
- resblock_kernel_sizes,
- resblock_dilation_sizes,
- upsample_rates,
- upsample_initial_channel,
- upsample_kernel_sizes,
- gin_channels=0,
- ):
- super(Generator, self).__init__()
- self.num_kernels = len(resblock_kernel_sizes)
- self.num_upsamples = len(upsample_rates)
- self.conv_pre = Conv1d(
- initial_channel, upsample_initial_channel, 7, 1, padding=3
- )
- resblock = modules.ResBlock1 if resblock == "1" else modules.ResBlock2
-
- self.ups = nn.ModuleList()
- for i, (u, k) in enumerate(zip(upsample_rates, upsample_kernel_sizes)):
- self.ups.append(
- weight_norm(
- ConvTranspose1d(
- upsample_initial_channel // (2**i),
- upsample_initial_channel // (2 ** (i + 1)),
- k,
- u,
- padding=(k - u) // 2,
- )
- )
- )
-
- self.resblocks = nn.ModuleList()
- for i in range(len(self.ups)):
- ch = upsample_initial_channel // (2 ** (i + 1))
- for j, (k, d) in enumerate(
- zip(resblock_kernel_sizes, resblock_dilation_sizes)
- ):
- self.resblocks.append(resblock(ch, k, d))
-
- self.conv_post = Conv1d(ch, 1, 7, 1, padding=3, bias=False)
- self.ups.apply(init_weights)
-
- if gin_channels != 0:
- self.cond = nn.Conv1d(gin_channels, upsample_initial_channel, 1)
-
- def forward(self, x, g=None):
- x = self.conv_pre(x)
- if g is not None:
- x = x + self.cond(g)
-
- for i in range(self.num_upsamples):
- x = F.leaky_relu(x, modules.LRELU_SLOPE)
- x = self.ups[i](x)
- xs = None
- for j in range(self.num_kernels):
- if xs is None:
- xs = self.resblocks[i * self.num_kernels + j](x)
- else:
- xs += self.resblocks[i * self.num_kernels + j](x)
- x = xs / self.num_kernels
- x = F.leaky_relu(x)
- x = self.conv_post(x)
- x = torch.tanh(x)
-
- return x
-
- def remove_weight_norm(self):
- for l in self.ups:
- remove_weight_norm(l)
- for l in self.resblocks:
- l.remove_weight_norm()
-
-
-class SineGen(torch.nn.Module):
- """Definition of sine generator
- SineGen(samp_rate, harmonic_num = 0,
- sine_amp = 0.1, noise_std = 0.003,
- voiced_threshold = 0,
- flag_for_pulse=False)
- samp_rate: sampling rate in Hz
- harmonic_num: number of harmonic overtones (default 0)
- sine_amp: amplitude of sine-wavefrom (default 0.1)
- noise_std: std of Gaussian noise (default 0.003)
- voiced_thoreshold: F0 threshold for U/V classification (default 0)
- flag_for_pulse: this SinGen is used inside PulseGen (default False)
- Note: when flag_for_pulse is True, the first time step of a voiced
- segment is always sin(np.pi) or cos(0)
- """
-
- def __init__(
- self,
- samp_rate,
- harmonic_num=0,
- sine_amp=0.1,
- noise_std=0.003,
- voiced_threshold=0,
- flag_for_pulse=False,
- ):
- super(SineGen, self).__init__()
- self.sine_amp = sine_amp
- self.noise_std = noise_std
- self.harmonic_num = harmonic_num
- self.dim = self.harmonic_num + 1
- self.sampling_rate = samp_rate
- self.voiced_threshold = voiced_threshold
-
- def _f02uv(self, f0):
- # generate uv signal
- uv = torch.ones_like(f0)
- uv = uv * (f0 > self.voiced_threshold)
- return uv
-
- def forward(self, f0, upp):
- """sine_tensor, uv = forward(f0)
- input F0: tensor(batchsize=1, length, dim=1)
- f0 for unvoiced steps should be 0
- output sine_tensor: tensor(batchsize=1, length, dim)
- output uv: tensor(batchsize=1, length, 1)
- """
- with torch.no_grad():
- f0 = f0[:, None].transpose(1, 2)
- f0_buf = torch.zeros(f0.shape[0], f0.shape[1], self.dim, device=f0.device)
- # fundamental component
- f0_buf[:, :, 0] = f0[:, :, 0]
- for idx in np.arange(self.harmonic_num):
- f0_buf[:, :, idx + 1] = f0_buf[:, :, 0] * (
- idx + 2
- ) # idx + 2: the (idx+1)-th overtone, (idx+2)-th harmonic
- rad_values = (f0_buf / self.sampling_rate) % 1 ###%1意味着n_har的乘积无法后处理优化
- rand_ini = torch.rand(
- f0_buf.shape[0], f0_buf.shape[2], device=f0_buf.device
- )
- rand_ini[:, 0] = 0
- rad_values[:, 0, :] = rad_values[:, 0, :] + rand_ini
- tmp_over_one = torch.cumsum(rad_values, 1) # % 1 #####%1意味着后面的cumsum无法再优化
- tmp_over_one *= upp
- tmp_over_one = F.interpolate(
- tmp_over_one.transpose(2, 1),
- scale_factor=upp,
- mode="linear",
- align_corners=True,
- ).transpose(2, 1)
- rad_values = F.interpolate(
- rad_values.transpose(2, 1), scale_factor=upp, mode="nearest"
- ).transpose(
- 2, 1
- ) #######
- tmp_over_one %= 1
- tmp_over_one_idx = (tmp_over_one[:, 1:, :] - tmp_over_one[:, :-1, :]) < 0
- cumsum_shift = torch.zeros_like(rad_values)
- cumsum_shift[:, 1:, :] = tmp_over_one_idx * -1.0
- sine_waves = torch.sin(
- torch.cumsum(rad_values + cumsum_shift, dim=1) * 2 * np.pi
- )
- sine_waves = sine_waves * self.sine_amp
- uv = self._f02uv(f0)
- uv = F.interpolate(
- uv.transpose(2, 1), scale_factor=upp, mode="nearest"
- ).transpose(2, 1)
- noise_amp = uv * self.noise_std + (1 - uv) * self.sine_amp / 3
- noise = noise_amp * torch.randn_like(sine_waves)
- sine_waves = sine_waves * uv + noise
- return sine_waves, uv, noise
-
-
-class SourceModuleHnNSF(torch.nn.Module):
- """SourceModule for hn-nsf
- SourceModule(sampling_rate, harmonic_num=0, sine_amp=0.1,
- add_noise_std=0.003, voiced_threshod=0)
- sampling_rate: sampling_rate in Hz
- harmonic_num: number of harmonic above F0 (default: 0)
- sine_amp: amplitude of sine source signal (default: 0.1)
- add_noise_std: std of additive Gaussian noise (default: 0.003)
- note that amplitude of noise in unvoiced is decided
- by sine_amp
- voiced_threshold: threhold to set U/V given F0 (default: 0)
- Sine_source, noise_source = SourceModuleHnNSF(F0_sampled)
- F0_sampled (batchsize, length, 1)
- Sine_source (batchsize, length, 1)
- noise_source (batchsize, length 1)
- uv (batchsize, length, 1)
- """
-
- def __init__(
- self,
- sampling_rate,
- harmonic_num=0,
- sine_amp=0.1,
- add_noise_std=0.003,
- voiced_threshod=0,
- is_half=True,
- ):
- super(SourceModuleHnNSF, self).__init__()
-
- self.sine_amp = sine_amp
- self.noise_std = add_noise_std
- self.is_half = is_half
- # to produce sine waveforms
- self.l_sin_gen = SineGen(
- sampling_rate, harmonic_num, sine_amp, add_noise_std, voiced_threshod
- )
-
- # to merge source harmonics into a single excitation
- self.l_linear = torch.nn.Linear(harmonic_num + 1, 1)
- self.l_tanh = torch.nn.Tanh()
-
- def forward(self, x, upp=None):
- sine_wavs, uv, _ = self.l_sin_gen(x, upp)
- if self.is_half:
- sine_wavs = sine_wavs.half()
- sine_merge = self.l_tanh(self.l_linear(sine_wavs))
- return sine_merge, None, None # noise, uv
-
-
-class GeneratorNSF(torch.nn.Module):
- def __init__(
- self,
- initial_channel,
- resblock,
- resblock_kernel_sizes,
- resblock_dilation_sizes,
- upsample_rates,
- upsample_initial_channel,
- upsample_kernel_sizes,
- gin_channels,
- sr,
- is_half=False,
- ):
- super(GeneratorNSF, self).__init__()
- self.num_kernels = len(resblock_kernel_sizes)
- self.num_upsamples = len(upsample_rates)
-
- self.f0_upsamp = torch.nn.Upsample(scale_factor=np.prod(upsample_rates))
- self.m_source = SourceModuleHnNSF(
- sampling_rate=sr, harmonic_num=0, is_half=is_half
- )
- self.noise_convs = nn.ModuleList()
- self.conv_pre = Conv1d(
- initial_channel, upsample_initial_channel, 7, 1, padding=3
- )
- resblock = modules.ResBlock1 if resblock == "1" else modules.ResBlock2
-
- self.ups = nn.ModuleList()
- for i, (u, k) in enumerate(zip(upsample_rates, upsample_kernel_sizes)):
- c_cur = upsample_initial_channel // (2 ** (i + 1))
- self.ups.append(
- weight_norm(
- ConvTranspose1d(
- upsample_initial_channel // (2**i),
- upsample_initial_channel // (2 ** (i + 1)),
- k,
- u,
- padding=(k - u) // 2,
- )
- )
- )
- if i + 1 < len(upsample_rates):
- stride_f0 = np.prod(upsample_rates[i + 1 :])
- self.noise_convs.append(
- Conv1d(
- 1,
- c_cur,
- kernel_size=stride_f0 * 2,
- stride=stride_f0,
- padding=stride_f0 // 2,
- )
- )
- else:
- self.noise_convs.append(Conv1d(1, c_cur, kernel_size=1))
-
- self.resblocks = nn.ModuleList()
- for i in range(len(self.ups)):
- ch = upsample_initial_channel // (2 ** (i + 1))
- for j, (k, d) in enumerate(
- zip(resblock_kernel_sizes, resblock_dilation_sizes)
- ):
- self.resblocks.append(resblock(ch, k, d))
-
- self.conv_post = Conv1d(ch, 1, 7, 1, padding=3, bias=False)
- self.ups.apply(init_weights)
-
- if gin_channels != 0:
- self.cond = nn.Conv1d(gin_channels, upsample_initial_channel, 1)
-
- self.upp = np.prod(upsample_rates)
-
- def forward(self, x, f0, g=None):
- har_source, noi_source, uv = self.m_source(f0, self.upp)
- har_source = har_source.transpose(1, 2)
- x = self.conv_pre(x)
- if g is not None:
- x = x + self.cond(g)
-
- for i in range(self.num_upsamples):
- x = F.leaky_relu(x, modules.LRELU_SLOPE)
- x = self.ups[i](x)
- x_source = self.noise_convs[i](har_source)
- x = x + x_source
- xs = None
- for j in range(self.num_kernels):
- if xs is None:
- xs = self.resblocks[i * self.num_kernels + j](x)
- else:
- xs += self.resblocks[i * self.num_kernels + j](x)
- x = xs / self.num_kernels
- x = F.leaky_relu(x)
- x = self.conv_post(x)
- x = torch.tanh(x)
- return x
-
- def remove_weight_norm(self):
- for l in self.ups:
- remove_weight_norm(l)
- for l in self.resblocks:
- l.remove_weight_norm()
-
-
-sr2sr = {
- "32k": 32000,
- "40k": 40000,
- "48k": 48000,
-}
-
-
-class SynthesizerTrnMs256NSFsidM(nn.Module):
- def __init__(
- self,
- spec_channels,
- segment_size,
- inter_channels,
- hidden_channels,
- filter_channels,
- n_heads,
- n_layers,
- kernel_size,
- p_dropout,
- resblock,
- resblock_kernel_sizes,
- resblock_dilation_sizes,
- upsample_rates,
- upsample_initial_channel,
- upsample_kernel_sizes,
- spk_embed_dim,
- gin_channels,
- sr,
- **kwargs
- ):
- super().__init__()
- if type(sr) == type("strr"):
- sr = sr2sr[sr]
- self.spec_channels = spec_channels
- self.inter_channels = inter_channels
- self.hidden_channels = hidden_channels
- self.filter_channels = filter_channels
- self.n_heads = n_heads
- self.n_layers = n_layers
- self.kernel_size = kernel_size
- self.p_dropout = p_dropout
- self.resblock = resblock
- self.resblock_kernel_sizes = resblock_kernel_sizes
- self.resblock_dilation_sizes = resblock_dilation_sizes
- self.upsample_rates = upsample_rates
- self.upsample_initial_channel = upsample_initial_channel
- self.upsample_kernel_sizes = upsample_kernel_sizes
- self.segment_size = segment_size
- self.gin_channels = gin_channels
- # self.hop_length = hop_length#
- self.spk_embed_dim = spk_embed_dim
- self.enc_p = TextEncoder256(
- inter_channels,
- hidden_channels,
- filter_channels,
- n_heads,
- n_layers,
- kernel_size,
- p_dropout,
- )
- self.dec = GeneratorNSF(
- inter_channels,
- resblock,
- resblock_kernel_sizes,
- resblock_dilation_sizes,
- upsample_rates,
- upsample_initial_channel,
- upsample_kernel_sizes,
- gin_channels=gin_channels,
- sr=sr,
- is_half=kwargs["is_half"],
- )
- self.enc_q = PosteriorEncoder(
- spec_channels,
- inter_channels,
- hidden_channels,
- 5,
- 1,
- 16,
- gin_channels=gin_channels,
- )
- self.flow = ResidualCouplingBlock(
- inter_channels, hidden_channels, 5, 1, 3, gin_channels=gin_channels
- )
- self.emb_g = nn.Embedding(self.spk_embed_dim, gin_channels)
- print("gin_channels:", gin_channels, "self.spk_embed_dim:", self.spk_embed_dim)
-
- def remove_weight_norm(self):
- self.dec.remove_weight_norm()
- self.flow.remove_weight_norm()
- self.enc_q.remove_weight_norm()
-
- def forward(self, phone, phone_lengths, pitch, nsff0, sid, rnd, max_len=None):
- g = self.emb_g(sid).unsqueeze(-1)
- m_p, logs_p, x_mask = self.enc_p(phone, pitch, phone_lengths)
- z_p = (m_p + torch.exp(logs_p) * rnd) * x_mask
- z = self.flow(z_p, x_mask, g=g, reverse=True)
- o = self.dec((z * x_mask)[:, :, :max_len], nsff0, g=g)
- return o
-
-
-class SynthesizerTrnMs256NSFsid_sim(nn.Module):
- """
- Synthesizer for Training
- """
-
- def __init__(
- self,
- spec_channels,
- segment_size,
- inter_channels,
- hidden_channels,
- filter_channels,
- n_heads,
- n_layers,
- kernel_size,
- p_dropout,
- resblock,
- resblock_kernel_sizes,
- resblock_dilation_sizes,
- upsample_rates,
- upsample_initial_channel,
- upsample_kernel_sizes,
- spk_embed_dim,
- # hop_length,
- gin_channels=0,
- use_sdp=True,
- **kwargs
- ):
- super().__init__()
- self.spec_channels = spec_channels
- self.inter_channels = inter_channels
- self.hidden_channels = hidden_channels
- self.filter_channels = filter_channels
- self.n_heads = n_heads
- self.n_layers = n_layers
- self.kernel_size = kernel_size
- self.p_dropout = p_dropout
- self.resblock = resblock
- self.resblock_kernel_sizes = resblock_kernel_sizes
- self.resblock_dilation_sizes = resblock_dilation_sizes
- self.upsample_rates = upsample_rates
- self.upsample_initial_channel = upsample_initial_channel
- self.upsample_kernel_sizes = upsample_kernel_sizes
- self.segment_size = segment_size
- self.gin_channels = gin_channels
- # self.hop_length = hop_length#
- self.spk_embed_dim = spk_embed_dim
- self.enc_p = TextEncoder256Sim(
- inter_channels,
- hidden_channels,
- filter_channels,
- n_heads,
- n_layers,
- kernel_size,
- p_dropout,
- )
- self.dec = GeneratorNSF(
- inter_channels,
- resblock,
- resblock_kernel_sizes,
- resblock_dilation_sizes,
- upsample_rates,
- upsample_initial_channel,
- upsample_kernel_sizes,
- gin_channels=gin_channels,
- is_half=kwargs["is_half"],
- )
-
- self.flow = ResidualCouplingBlock(
- inter_channels, hidden_channels, 5, 1, 3, gin_channels=gin_channels
- )
- self.emb_g = nn.Embedding(self.spk_embed_dim, gin_channels)
- print("gin_channels:", gin_channels, "self.spk_embed_dim:", self.spk_embed_dim)
-
- def remove_weight_norm(self):
- self.dec.remove_weight_norm()
- self.flow.remove_weight_norm()
- self.enc_q.remove_weight_norm()
-
- def forward(
- self, phone, phone_lengths, pitch, pitchf, ds, max_len=None
- ): # y是spec不需要了现在
- g = self.emb_g(ds.unsqueeze(0)).unsqueeze(-1) # [b, 256, 1]##1是t,广播的
- x, x_mask = self.enc_p(phone, pitch, phone_lengths)
- x = self.flow(x, x_mask, g=g, reverse=True)
- o = self.dec((x * x_mask)[:, :, :max_len], pitchf, g=g)
- return o
-
-
-class MultiPeriodDiscriminator(torch.nn.Module):
- def __init__(self, use_spectral_norm=False):
- super(MultiPeriodDiscriminator, self).__init__()
- periods = [2, 3, 5, 7, 11, 17]
- # periods = [3, 5, 7, 11, 17, 23, 37]
-
- discs = [DiscriminatorS(use_spectral_norm=use_spectral_norm)]
- discs = discs + [
- DiscriminatorP(i, use_spectral_norm=use_spectral_norm) for i in periods
- ]
- self.discriminators = nn.ModuleList(discs)
-
- def forward(self, y, y_hat):
- y_d_rs = [] #
- y_d_gs = []
- fmap_rs = []
- fmap_gs = []
- for i, d in enumerate(self.discriminators):
- y_d_r, fmap_r = d(y)
- y_d_g, fmap_g = d(y_hat)
- # for j in range(len(fmap_r)):
- # print(i,j,y.shape,y_hat.shape,fmap_r[j].shape,fmap_g[j].shape)
- y_d_rs.append(y_d_r)
- y_d_gs.append(y_d_g)
- fmap_rs.append(fmap_r)
- fmap_gs.append(fmap_g)
-
- return y_d_rs, y_d_gs, fmap_rs, fmap_gs
-
-
-class DiscriminatorS(torch.nn.Module):
- def __init__(self, use_spectral_norm=False):
- super(DiscriminatorS, self).__init__()
- norm_f = weight_norm if use_spectral_norm == False else spectral_norm
- self.convs = nn.ModuleList(
- [
- norm_f(Conv1d(1, 16, 15, 1, padding=7)),
- norm_f(Conv1d(16, 64, 41, 4, groups=4, padding=20)),
- norm_f(Conv1d(64, 256, 41, 4, groups=16, padding=20)),
- norm_f(Conv1d(256, 1024, 41, 4, groups=64, padding=20)),
- norm_f(Conv1d(1024, 1024, 41, 4, groups=256, padding=20)),
- norm_f(Conv1d(1024, 1024, 5, 1, padding=2)),
- ]
- )
- self.conv_post = norm_f(Conv1d(1024, 1, 3, 1, padding=1))
-
- def forward(self, x):
- fmap = []
-
- for l in self.convs:
- x = l(x)
- x = F.leaky_relu(x, modules.LRELU_SLOPE)
- fmap.append(x)
- x = self.conv_post(x)
- fmap.append(x)
- x = torch.flatten(x, 1, -1)
-
- return x, fmap
-
-
-class DiscriminatorP(torch.nn.Module):
- def __init__(self, period, kernel_size=5, stride=3, use_spectral_norm=False):
- super(DiscriminatorP, self).__init__()
- self.period = period
- self.use_spectral_norm = use_spectral_norm
- norm_f = weight_norm if use_spectral_norm == False else spectral_norm
- self.convs = nn.ModuleList(
- [
- norm_f(
- Conv2d(
- 1,
- 32,
- (kernel_size, 1),
- (stride, 1),
- padding=(get_padding(kernel_size, 1), 0),
- )
- ),
- norm_f(
- Conv2d(
- 32,
- 128,
- (kernel_size, 1),
- (stride, 1),
- padding=(get_padding(kernel_size, 1), 0),
- )
- ),
- norm_f(
- Conv2d(
- 128,
- 512,
- (kernel_size, 1),
- (stride, 1),
- padding=(get_padding(kernel_size, 1), 0),
- )
- ),
- norm_f(
- Conv2d(
- 512,
- 1024,
- (kernel_size, 1),
- (stride, 1),
- padding=(get_padding(kernel_size, 1), 0),
- )
- ),
- norm_f(
- Conv2d(
- 1024,
- 1024,
- (kernel_size, 1),
- 1,
- padding=(get_padding(kernel_size, 1), 0),
- )
- ),
- ]
- )
- self.conv_post = norm_f(Conv2d(1024, 1, (3, 1), 1, padding=(1, 0)))
-
- def forward(self, x):
- fmap = []
-
- # 1d to 2d
- b, c, t = x.shape
- if t % self.period != 0: # pad first
- n_pad = self.period - (t % self.period)
- x = F.pad(x, (0, n_pad), "reflect")
- t = t + n_pad
- x = x.view(b, c, t // self.period, self.period)
-
- for l in self.convs:
- x = l(x)
- x = F.leaky_relu(x, modules.LRELU_SLOPE)
- fmap.append(x)
- x = self.conv_post(x)
- fmap.append(x)
- x = torch.flatten(x, 1, -1)
-
- return x, fmap
diff --git a/spaces/OFA-Sys/OFA-Generic_Interface/fairseq/examples/quant_noise/README.md b/spaces/OFA-Sys/OFA-Generic_Interface/fairseq/examples/quant_noise/README.md
deleted file mode 100644
index a04d7e4e8a077f11c9f63cfa3d1f20e2b899be8c..0000000000000000000000000000000000000000
--- a/spaces/OFA-Sys/OFA-Generic_Interface/fairseq/examples/quant_noise/README.md
+++ /dev/null
@@ -1,298 +0,0 @@
-# Training with Quantization Noise for Extreme Model Compression ({Fan\*, Stock\*} *et al.*, 2020)
-This page contains information for how to train and quantize models with Quantization Noise, for both scalar quantization like `int8` and Iterative Product Quantization.
-Check out our paper [here](https://arxiv.org/abs/2004.07320).
-
-Looking for pretrained models? They will be added shortly.
-Looking for code to train vision models? We are working on open sourcing our code as part of ClassyVision. Please check back, but note that both the Scalar and Iterative Product Quantization counterparts of the `nn.Conv2d` module are already included in this release.
-
-**Contents**:
-- [Walk through of code](#walk-through-the-code)
-- [Reproduce NLP Results](#looking-to-reproduce-the-nlp-results-in-the-paper)
-- [Reproduce Vision Results](#looking-to-reproduce-the-vision-results-in-the-paper)
-
-
-## Citation
-```bibtex
-@article{fan2020training,
- title={Training with Quantization Noise for Extreme Model Compression},
- author={Angela Fan* and Pierre Stock* and and Benjamin Graham and Edouard Grave and Remi Gribonval and Herve Jegou and Armand Joulin},
- year={2020},
- eprint={2004.07320},
- archivePrefix={arXiv},
- primaryClass={cs.ML}
-}
-```
-
-## Walk through the code
-
-Training a model with Quant-Noise improves the performance in subsequent inference-time quantization by training models to be robust to quantization. This technique is useful for both scalar and product quantization methods, as well as multiple domains. We detail below our approach to train, quantize models and integrate our code to quantize your favorite models.
-
-### Scalar Quantization
-
-Unlike the section [Iterative Product Quantization](#iterative-product-quantization) which gives state-of-the-art compression, this section showcases the usefulness of our approach for simple scalar quantization baselines such as int8 using on-GPU Fake Quantization.
-
-#### Training
-
-Scalar quantization with Quant-Noise consists in randomly quantizing a proportion `p` of the weights during training. Scalar quantization is implemented [here](https://github.com/pytorch/fairseq/tree/main/fairseq/modules/quantization/scalar) under the form of Fake Quantization, meaning that we emulate int8 on GPU by quantizing and de-quantizing both the weights and the activations. We rely on PyTorch's [quantization primitives](https://github.com/pytorch/pytorch/tree/master/torch/quantization).
-
-To train a model with Quant-Noise, add the following flag:
-```
---quant-noise-scalar 0.5
-```
-Large values of noise make the network easier to quantize but may result in higher non-quantized test and validation perplexities.
-
-#### Quantization
-
-When evaluating a network, all quantized modules and activation hooks automatically switch to `p=1` so the validation accuracy reported by Fairseq is actually the quantized one, nothing more to do.
-
-
-#### Integration with your own code
-
-Looking to quantize your own models with Quant-Noise + Scalar Quantization?
-- Use the function `quantize_model_` implemented [here](https://github.com/pytorch/fairseq/tree/main/fairseq/modules/quantization/scalar/utils.py) to (1) replace all your modules by their quantized counterparts and (2) add hooks to those modules to quantize the activations.
-- Then, perform your training as usual. Note that in `eval()` mode, the network is always fully quantized (weights and activations) by default (`p=1`).
-
-
-
-### Iterative Product Quantization
-
-
-Iterative Product Quantization with Quant-Noise proceeds in two steps. First, a model must be trained uncompressed with Quant-Noise. Second, the model must be quantized with iPQ. Note that we implement here the simplest form of noise, which consists in randomly dropping a proportion `p` of blocks, and that worked as well as assigning those blocks to their current centroid.
-
-#### Training
-
-To train a model with Quant-Noise, add the following flags:
-```
---quant-noise-pq 0.1 --quant-noise-pq-block-size 8
-```
-`quant-noise-pq` controls how much dropout is applied to the blocks of the weight matrix. `quant-noise-pq-block-size` controls the size of the weight matrix blocks.
-We recommend training with 0.05 to 0.2 Quant-Noise, a value that worked well in our experiments. For the block-size, we recommend training with block-size of 8. Note that the block size must be a multiple of `input_features`, see the size checks [here](https://github.com/pytorch/fairseq/tree/main/fairseq/modules/quant_noise.py). Large block sizes result in higher compression ratio but may induce a loss in accuracy.
-
-We currently support training Transformer based models, such as sequence-to-sequence, language models, and BERT architectures. The `quant_noise` function [here](https://github.com/pytorch/fairseq/tree/main/fairseq/modules/quant_noise.py) wraps a module. It splits a weight matrix into blocks and applies random dropout to these blocks.
-In the Transformer architectures, quant-noise is applied to the input and output embeddings, the attention, and the FFN.
-
-Quant-Noise can also be combined with **LayerDrop** (see [here](https://github.com/pytorch/fairseq/tree/main/examples/layerdrop)) to add its pruning effect to the quantized model and make the model even smaller. We recommend training with LayerDrop 0.1 or 0.2.
-
-#### Quantization
-
-We implement an improved version of product quantization from Stock et al, **iPQ**, described [here](https://arxiv.org/abs/1907.05686), see code with old API [here](https://github.com/facebookresearch/kill-the-bits). Note that we improved the iPQ API in terms of both compute speed and usability as described below.
-
-For the particular case of PQ, quantization is made sequentially. We recommend first quantizing the FFNs, then the EMBs, and finally the ATTNs. Quantization is done in two sub-steps:
-- First, perform `n` steps of Product Quantization (generally `n=20` is enough).
-- Then, finetune the obtained centroids.
-
-#### Integration with your own code
-
-Looking to quantize your own models with Quant-Noise + iPQ?
-- First wrap your modules with the `quant_noise` function [here](https://github.com/pytorch/fairseq/tree/main/fairseq/modules/quant_noise.py), which is module-agnostic and train your favorite model.
-- Then, quantize your trained model using the code [here](https://github.com/pytorch/fairseq/tree/main/fairseq/modules/quantization/pq). This can be done *without any changes to your training loop*. Below is an example code for integration.
-Note that we tried our approach only on Transformers and various Convolutional Models such as EfficientNets.
-
-```python
-from fairseq.modules.quantization.pq import quantize_model_, SizeTracker
-
-# get configuration parameters
-n_centroids_config = config["n_centroids"]
-block_sizes_config = config["block_sizes"]
-layers_to_quantize = config["layers_to_quantize"]
-
-# size tracker for keeping track of assignments, centroids and non-compressed sizes
-size_tracker = SizeTracker(model)
-
-# Quantize model by stages
-for step in range(len(layers_to_quantize)):
-
- # quantize model in-place
- quantized_layers = quantize_model_(
- model,
- size_tracker,
- layers_to_quantize,
- block_sizes_config,
- n_centroids_config,
- step=step,
- )
- logger.info(f"Finetuning stage {step}, quantized layers: {quantized_layers}")
- logger.info(f"{size_tracker}")
-
- # Don't forget to re-create/update trainer/optimizer since model parameters have changed
- optimizer = ...
-
- # Finetune the centroids with your usual training loop for a few epochs
- trainer.train_epoch()
-```
-
-
-## Looking to reproduce the NLP results in the paper?
-
-We detail below how to reproduce the state-of-the-art results in reported in the paper for Quant-Noise + Iterative Product Quantization.
-
-### Training with Quant-Noise
-
-To **train** RoBERTa + QuantNoise, we followed this setting [here](https://github.com/pytorch/fairseq/tree/main/examples/roberta).
-The following command can be used to train a RoBERTa Base + QuantNoise model:
-
-```bash
-TOTAL_UPDATES=125000
-WARMUP_UPDATES=10000
-PEAK_LR=0.0005
-TOKENS_PER_SAMPLE=512
-MAX_POSITIONS=512
-MAX_SENTENCES=16
-UPDATE_FREQ=2
-DATA_DIR=/path/to/data/here
-
-fairseq-train $DATA_DIR \
- --task masked_lm --criterion masked_lm --arch roberta_base \
- --sample-break-mode complete \
- --tokens-per-sample $TOKENS_PER_SAMPLE --max-positions $MAX_POSITIONS \
- --optimizer adam --adam-betas '(0.9, 0.98)' --adam-eps 1e-6 \
- --clip-norm 0.0 \
- --lr-scheduler polynomial_decay --lr $PEAK_LR \
- --warmup-updates $WARMUP_UPDATES --total-num-update $TOTAL_UPDATES \
- --dropout 0.1 --attention-dropout 0.1 \
- --weight-decay 0.01 \
- --batch-size $MAX_SENTENCES \
- --update-freq $UPDATE_FREQ --max-update $TOTAL_UPDATES \
- --save-dir checkpoint/roberta \
- --ddp-backend legacy_ddp --encoder-layerdrop 0.2 \
- --quant-noise-pq 0.2 --quant-noise-pq-block-size 8 --untie-weights-roberta
-```
-
-To **finetune** RoBERTa + QuantNoise, we followed this setting [here](https://github.com/pytorch/fairseq/blob/main/examples/roberta/README.glue.md).
-The following command can be used to finetune a RoBERTa Base + QuantNoise model on the RTE dataset:
-
-```bash
-TOTAL_NUM_UPDATES=2036
-WARMUP_UPDATES=122
-LR=2e-05
-NUM_CLASSES=2
-MAX_SENTENCES=16
-ROBERTA_PATH=/path/to/roberta_quantnoise/model.pt
-
-fairseq-train /path/to/rte/data/ \
- --restore-file $ROBERTA_PATH \
- --max-positions 512 \
- --batch-size $MAX_SENTENCES \
- --max-tokens 4400 \
- --task sentence_prediction \
- --reset-optimizer --reset-dataloader --reset-meters \
- --required-batch-size-multiple 1 \
- --init-token 0 --separator-token 2 \
- --arch roberta_large \
- --criterion sentence_prediction \
- --num-classes $NUM_CLASSES \
- --dropout 0.1 --attention-dropout 0.1 \
- --weight-decay 0.1 --optimizer adam --adam-betas "(0.9, 0.98)" --adam-eps 1e-06 \
- --clip-norm 0.0 \
- --lr-scheduler polynomial_decay --lr $LR --total-num-update $TOTAL_NUM_UPDATES --warmup-updates $WARMUP_UPDATES \
- --fp16 --fp16-init-scale 4 --threshold-loss-scale 1 --fp16-scale-window 128 \
- --max-epoch 10 \
- --find-unused-parameters \
- --best-checkpoint-metric accuracy --maximize-best-checkpoint-metric \
- --ddp-backend legacy_ddp \
- --quant-noise-pq 0.2 --quant-noise-pq-block-size 8
-```
-
-To **train** Language Models on Wikitext-103, we followed this setting [here](https://github.com/pytorch/fairseq/tree/main/examples/language_model).
-The following command can be used to train a Transformer + QuantNoise model on Wikitext-103:
-
-```bash
-fairseq-train --task language_modeling /path/to/wikitext-103/data \
- --save-dir checkpoints/transformer_wikitext-103 \
- --adaptive-input --adaptive-input-cutoff 20000,60000 --adaptive-input-factor 4 \
- --adaptive-softmax-cutoff 20000,60000 --adaptive-softmax-dropout 0.2 --adaptive-softmax-factor 4.0 \
- --tie-adaptive-proj --tie-adaptive-weights \
- --arch transformer_lm_gbw \
- --attention-dropout 0.1 --dropout 0.2 --relu-dropout 0.1 \
- --clip-norm 0.1 --criterion adaptive_loss \
- --ddp-backend legacy_ddp \
- --decoder-attention-heads 8 --decoder-embed-dim 1024 --decoder-ffn-embed-dim 4096 --decoder-input-dim 1024 \
- --decoder-layers 16 --decoder-normalize-before --decoder-output-dim 1024 \
- --min-lr 0.0001 --lr-period-updates 270000 --lr-scheduler cosine --lr-shrink 0.75 --lr 1.0 --t-mult 2.0 \
- --max-tokens 3072 --tokens-per-sample 3072 --momentum 0.99 --optimizer nag \
- --sample-break-mode none --update-freq 3 \
- --warmup-init-lr 1e-07 --warmup-updates 16000 \
- --weight-decay 0 --seed 1 --stop-min-lr 1e-09 \
- --quant-noise-pq 0.05 --quant-noise-pq-block-size 8
-```
-
-To **evaluate** this model, note you need to use the `eval.py` script. The following command can be used to evaluate:
-
-```bash
-fairseq-eval-lm /path/to/wikitext-103/data --path /path/to/model/checkpoint \
- --sample-break-mode complete \
- --max-tokens 3072 \
- --context-window 2560 \
- --softmax-batch 1024 \
- --gen-subset valid
-```
-and change the `--gen-subset` to `test` if you would like to evaluate on the test set instead.
-
-
-### Iterative Product Quantization
-
-To quantize the finetuned RoBERTa model, we use this command on 1 GPU. This should run in a day.
-```bash
-TOTAL_NUM_UPDATES=6108 # 2036 updates for each iteration
-WARMUP_UPDATES=122
-LR=2e-05
-NUM_CLASSES=2
-MAX_SENTENCES=16
-fairseq-train --task sentence_prediction /path/to/data/ \
- --restore-file $ROBERTA_PATH \
- --save-dir checkpoints/roberta_finetuned \
- --max-positions 512 \
- --batch-size $MAX_SENTENCES \
- --max-tokens 4400 \
- --init-token 0 --separator-token 2 \
- --arch roberta_large \
- --criterion sentence_prediction \
- --num-classes $NUM_CLASSES \
- --dropout 0.1 --attention-dropout 0.1 \
- --weight-decay 0.1 --optimizer adam --adam-betas "(0.9, 0.98)" --adam-eps 1e-06 \
- --clip-norm 0.0 --lr-scheduler polynomial_decay \
- --fp16 --fp16-init-scale 4 --threshold-loss-scale 1 --fp16-scale-window 128 \
- --no-progress-bar --skip-invalid-size-inputs-valid-test --ddp-backend legacy_ddp \
- --quantization-config-path /path/to/config/yaml
-```
-
-To quantize the trained Language Model, we use this command on 8 V100 23GB GPUs. This should run in a couple of hours.
-```bash
-fairseq-train --task language_modeling /path/to/wikitext-103/data \
- --save-dir checkpoints/transformer_wikitext-103 \
- --adaptive-input --adaptive-input-cutoff 20000,60000 --adaptive-input-factor 4 \
- --adaptive-softmax-cutoff 20000,60000 --adaptive-softmax-dropout 0.2 --adaptive-softmax-factor 4.0 \
- --arch transformer_lm_gbw \
- --attention-dropout 0.1 --dropout 0.2 --relu-dropout 0.1 \
- --bucket-cap-mb 25 --char-embedder-highway-layers 2 --character-embedding-dim 4 \
- --clip-norm 0.1 --criterion adaptive_loss \
- --ddp-backend legacy_ddp \
- --decoder-attention-heads 8 --decoder-embed-dim 1024 --decoder-ffn-embed-dim 4096 --decoder-input-dim 1024 --decoder-layers 16 --decoder-normalize-before --decoder-output-dim 1024 \
- --fp16 --keep-last-epochs -1 \
- --min-lr 0.0001 --lr-period-updates 270000 --lr-scheduler cosine --lr-shrink 0.75 --lr 0.05 --stop-min-lr 1e-09 \
- --max-tokens 2944 --tokens-per-sample 2944\
- --momentum 0.99 --no-epoch-checkpoints --no-progress-bar --optimizer nag --required-batch-size-multiple 8 \
- --sample-break-mode none --t-mult 2.0 --skip-invalid-size-inputs-valid-test \
- --tie-adaptive-proj --tie-adaptive-weights --update-freq 3 --weight-decay 0 --seed 1 \
- --log-interval 100 --no-progress-bar --skip-invalid-size-inputs-valid-test \
- --restore-file path/to/trained/lm/with/quant/noise \
- --max-update 13500 --quantization-config-path /path/to/config/yaml
-```
-If you have less capacity or if your distributed training freezes, try reducing `--max-tokens` and `--tokens-per-sample` (this may reduce the quantized accuracy a bit).
-
-### Remarks
-
-We try to keep the open-sourced code as readable and as easy-to-plug as possible. Therefore, we did not test it for the following cases:
-- Scalar quantization with RoBERTa.
-- Quantization with iPQ and `int8` combined.
-
-If you have trouble adapting it, we will be more than happy to help!
-
-## Looking to reproduce the Vision results in the paper?
-
-We are working on open sourcing our code as part of ClassyVision. Please check back.
-
-
-## Having an issue or have a question?
-
-Please open an issue in this repository with the details of your question. Thanks!
diff --git a/spaces/OFA-Sys/OFA-Generic_Interface/fairseq/examples/speech_to_text/prep_covost_data.py b/spaces/OFA-Sys/OFA-Generic_Interface/fairseq/examples/speech_to_text/prep_covost_data.py
deleted file mode 100644
index 411e9b55152ea4a8e345e8c2d18431958c4f4c07..0000000000000000000000000000000000000000
--- a/spaces/OFA-Sys/OFA-Generic_Interface/fairseq/examples/speech_to_text/prep_covost_data.py
+++ /dev/null
@@ -1,279 +0,0 @@
-#!/usr/bin/env python3
-# Copyright (c) Facebook, Inc. and its affiliates.
-#
-# This source code is licensed under the MIT license found in the
-# LICENSE file in the root directory of this source tree.
-
-import argparse
-import logging
-from pathlib import Path
-import shutil
-from tempfile import NamedTemporaryFile
-from typing import Optional, Tuple
-
-import pandas as pd
-import torchaudio
-from examples.speech_to_text.data_utils import (
- create_zip,
- extract_fbank_features,
- filter_manifest_df,
- gen_config_yaml,
- gen_vocab,
- get_zip_manifest,
- load_df_from_tsv,
- save_df_to_tsv,
-)
-from torch import Tensor
-from torch.utils.data import Dataset
-from torchaudio.datasets.utils import download_url, extract_archive
-from tqdm import tqdm
-
-
-log = logging.getLogger(__name__)
-
-
-MANIFEST_COLUMNS = ["id", "audio", "n_frames", "tgt_text", "speaker"]
-
-
-class CoVoST(Dataset):
- """Create a Dataset for CoVoST (https://github.com/facebookresearch/covost).
-
- Args:
- root (str): root path to the dataset and generated manifests/features
- source_language (str): source (audio) language
- target_language (str, optional): target (text) language,
- None for no translation (default: None)
- version (int, optional): CoVoST version. (default: 2)
- download (bool, optional): Whether to download the dataset if it is not
- found at root path. (default: ``False``).
- """
-
- COVOST_URL_TEMPLATE = (
- "https://dl.fbaipublicfiles.com/covost/"
- "covost_v2.{src_lang}_{tgt_lang}.tsv.tar.gz"
- )
-
- VERSIONS = {2}
- SPLITS = ["train", "dev", "test"]
-
- XX_EN_LANGUAGES = {
- 1: ["fr", "de", "nl", "ru", "es", "it", "tr", "fa", "sv-SE", "mn", "zh-CN"],
- 2: [
- "fr",
- "de",
- "es",
- "ca",
- "it",
- "ru",
- "zh-CN",
- "pt",
- "fa",
- "et",
- "mn",
- "nl",
- "tr",
- "ar",
- "sv-SE",
- "lv",
- "sl",
- "ta",
- "ja",
- "id",
- "cy",
- ],
- }
- EN_XX_LANGUAGES = {
- 1: [],
- 2: [
- "de",
- "tr",
- "fa",
- "sv-SE",
- "mn",
- "zh-CN",
- "cy",
- "ca",
- "sl",
- "et",
- "id",
- "ar",
- "ta",
- "lv",
- "ja",
- ],
- }
-
- def __init__(
- self,
- root: str,
- split: str,
- source_language: str,
- target_language: Optional[str] = None,
- version: int = 2,
- ) -> None:
- assert version in self.VERSIONS and split in self.SPLITS
- assert source_language is not None
- self.no_translation = target_language is None
- if not self.no_translation:
- assert "en" in {source_language, target_language}
- if source_language == "en":
- assert target_language in self.EN_XX_LANGUAGES[version]
- else:
- assert source_language in self.XX_EN_LANGUAGES[version]
- else:
- # Hack here so that we can get "split" column from CoVoST TSV.
- # Note that we use CoVoST train split for ASR which is an extension
- # to Common Voice train split.
- target_language = "de" if source_language == "en" else "en"
-
- self.root: Path = Path(root)
-
- cv_tsv_path = self.root / "validated.tsv"
- assert cv_tsv_path.is_file()
-
- covost_url = self.COVOST_URL_TEMPLATE.format(
- src_lang=source_language, tgt_lang=target_language
- )
- covost_archive = self.root / Path(covost_url).name
- if not covost_archive.is_file():
- download_url(covost_url, self.root.as_posix(), hash_value=None)
- extract_archive(covost_archive.as_posix())
-
- cv_tsv = load_df_from_tsv(cv_tsv_path)
- covost_tsv = load_df_from_tsv(
- self.root / Path(covost_url).name.replace(".tar.gz", "")
- )
- df = pd.merge(
- left=cv_tsv[["path", "sentence", "client_id"]],
- right=covost_tsv[["path", "translation", "split"]],
- how="inner",
- on="path",
- )
- if split == "train":
- df = df[(df["split"] == split) | (df["split"] == f"{split}_covost")]
- else:
- df = df[df["split"] == split]
- data = df.to_dict(orient="index").items()
- data = [v for k, v in sorted(data, key=lambda x: x[0])]
- self.data = []
- for e in data:
- try:
- path = self.root / "clips" / e["path"]
- _ = torchaudio.info(path.as_posix())
- self.data.append(e)
- except RuntimeError:
- pass
-
- def __getitem__(
- self, n: int
- ) -> Tuple[Tensor, int, str, str, Optional[str], str, str]:
- """Load the n-th sample from the dataset.
-
- Args:
- n (int): The index of the sample to be loaded
-
- Returns:
- tuple: ``(waveform, sample_rate, sentence, translation, speaker_id,
- sample_id)``
- """
- data = self.data[n]
- path = self.root / "clips" / data["path"]
- waveform, sample_rate = torchaudio.load(path)
- sentence = data["sentence"]
- translation = None if self.no_translation else data["translation"]
- speaker_id = data["client_id"]
- _id = data["path"].replace(".mp3", "")
- return waveform, sample_rate, sentence, translation, speaker_id, _id
-
- def __len__(self) -> int:
- return len(self.data)
-
-
-def process(args):
- root = Path(args.data_root).absolute() / args.src_lang
- if not root.is_dir():
- raise NotADirectoryError(f"{root} does not exist")
- # Extract features
- feature_root = root / "fbank80"
- feature_root.mkdir(exist_ok=True)
- for split in CoVoST.SPLITS:
- print(f"Fetching split {split}...")
- dataset = CoVoST(root, split, args.src_lang, args.tgt_lang)
- print("Extracting log mel filter bank features...")
- for waveform, sample_rate, _, _, _, utt_id in tqdm(dataset):
- extract_fbank_features(
- waveform, sample_rate, feature_root / f"{utt_id}.npy"
- )
- # Pack features into ZIP
- zip_path = root / "fbank80.zip"
- print("ZIPing features...")
- create_zip(feature_root, zip_path)
- print("Fetching ZIP manifest...")
- audio_paths, audio_lengths = get_zip_manifest(zip_path)
- # Generate TSV manifest
- print("Generating manifest...")
- train_text = []
- task = f"asr_{args.src_lang}"
- if args.tgt_lang is not None:
- task = f"st_{args.src_lang}_{args.tgt_lang}"
- for split in CoVoST.SPLITS:
- manifest = {c: [] for c in MANIFEST_COLUMNS}
- dataset = CoVoST(root, split, args.src_lang, args.tgt_lang)
- for _, _, src_utt, tgt_utt, speaker_id, utt_id in tqdm(dataset):
- manifest["id"].append(utt_id)
- manifest["audio"].append(audio_paths[utt_id])
- manifest["n_frames"].append(audio_lengths[utt_id])
- manifest["tgt_text"].append(src_utt if args.tgt_lang is None else tgt_utt)
- manifest["speaker"].append(speaker_id)
- is_train_split = split.startswith("train")
- if is_train_split:
- train_text.extend(manifest["tgt_text"])
- df = pd.DataFrame.from_dict(manifest)
- df = filter_manifest_df(df, is_train_split=is_train_split)
- save_df_to_tsv(df, root / f"{split}_{task}.tsv")
- # Generate vocab
- vocab_size_str = "" if args.vocab_type == "char" else str(args.vocab_size)
- spm_filename_prefix = f"spm_{args.vocab_type}{vocab_size_str}_{task}"
- with NamedTemporaryFile(mode="w") as f:
- for t in train_text:
- f.write(t + "\n")
- gen_vocab(
- Path(f.name),
- root / spm_filename_prefix,
- args.vocab_type,
- args.vocab_size
- )
- # Generate config YAML
- gen_config_yaml(
- root,
- spm_filename=spm_filename_prefix + ".model",
- yaml_filename=f"config_{task}.yaml",
- specaugment_policy="lb",
- )
- # Clean up
- shutil.rmtree(feature_root)
-
-
-def main():
- parser = argparse.ArgumentParser()
- parser.add_argument(
- "--data-root", "-d", required=True, type=str,
- help="data root with sub-folders for each language /"
- )
- parser.add_argument(
- "--vocab-type",
- default="unigram",
- required=True,
- type=str,
- choices=["bpe", "unigram", "char"],
- ),
- parser.add_argument("--vocab-size", default=1000, type=int)
- parser.add_argument("--src-lang", "-s", required=True, type=str)
- parser.add_argument("--tgt-lang", "-t", type=str)
- args = parser.parse_args()
-
- process(args)
-
-
-if __name__ == "__main__":
- main()
diff --git a/spaces/OFA-Sys/OFA-Generic_Interface/fairseq/fairseq/data/multi_corpus_sampled_dataset.py b/spaces/OFA-Sys/OFA-Generic_Interface/fairseq/fairseq/data/multi_corpus_sampled_dataset.py
deleted file mode 100644
index e2e9fdf004dd1da519a170a5e8bc225775776f72..0000000000000000000000000000000000000000
--- a/spaces/OFA-Sys/OFA-Generic_Interface/fairseq/fairseq/data/multi_corpus_sampled_dataset.py
+++ /dev/null
@@ -1,152 +0,0 @@
-# Copyright (c) Facebook, Inc. and its affiliates.
-#
-# This source code is licensed under the MIT license found in the
-# LICENSE file in the root directory of this source tree.
-
-from collections import OrderedDict
-from typing import Callable, Dict, List
-
-import numpy as np
-
-from . import FairseqDataset
-
-
-def uniform_sampler(x):
- # Sample from uniform distribution
- return np.random.choice(x, 1).item()
-
-
-class MultiCorpusSampledDataset(FairseqDataset):
- """
- Stores multiple instances of FairseqDataset together and in every iteration
- creates a batch by first sampling a dataset according to a specified
- probability distribution and then getting instances from that dataset.
-
- Args:
- datasets: an OrderedDict of FairseqDataset instances.
- sampling_func: A function for sampling over list of dataset keys.
- The default strategy is to sample uniformly.
- """
-
- def __init__(
- self,
- datasets: Dict[str, FairseqDataset],
- sampling_func: Callable[[List], int] = None,
- ):
- super().__init__()
- assert isinstance(datasets, OrderedDict)
- self.datasets = datasets
- if sampling_func is None:
- sampling_func = uniform_sampler
- self.sampling_func = sampling_func
-
- self.total_num_instances = 0
- for _, dataset in datasets.items():
- assert isinstance(dataset, FairseqDataset)
- self.total_num_instances += len(dataset)
-
- self._ordered_indices = None
-
- def __len__(self):
- """
- Length of this dataset is the sum of individual datasets
- """
- return self.total_num_instances
-
- def ordered_indices(self):
- """
- Ordered indices for batching. Here we call the underlying
- dataset's ordered_indices() so that we get the same random ordering
- as we would have from using the underlying dataset directly.
- """
- if self._ordered_indices is None:
- self._ordered_indices = OrderedDict(
- [
- (key, dataset.ordered_indices())
- for key, dataset in self.datasets.items()
- ]
- )
- return np.arange(len(self))
-
- def _map_index_to_dataset(self, key: int, index: int):
- """
- Different underlying datasets have different lengths. In order to ensure
- we are not accessing an index outside the range of the current dataset
- size, we wrap around. This function should be called after we have
- created an ordering for this and all underlying datasets.
- """
- assert (
- self._ordered_indices is not None
- ), "Must call MultiCorpusSampledDataset.ordered_indices() first"
- mapped_index = index % len(self.datasets[key])
- return self._ordered_indices[key][mapped_index]
-
- def __getitem__(self, index: int):
- """
- Get the item associated with index from each underlying dataset.
- Since index is in the range of [0, TotalNumInstances], we need to
- map the index to the dataset before retrieving the item.
- """
- return OrderedDict(
- [
- (key, dataset[self._map_index_to_dataset(key, index)])
- for key, dataset in self.datasets.items()
- ]
- )
-
- def collater(self, samples: List[Dict]):
- """
- Generate a mini-batch for this dataset.
- To convert this into a regular mini-batch we use the following
- logic:
- 1. Select a dataset using the specified probability distribution.
- 2. Call the collater function of the selected dataset.
- """
- if len(samples) == 0:
- return None
-
- selected_key = self.sampling_func(list(self.datasets.keys()))
- selected_samples = [sample[selected_key] for sample in samples]
- return self.datasets[selected_key].collater(selected_samples)
-
- def num_tokens(self, index: int):
- """
- Return an example's length (number of tokens), used for batching. Here
- we return the max across all examples at index across all underlying
- datasets.
- """
- return max(
- dataset.num_tokens(self._map_index_to_dataset(key, index))
- for key, dataset in self.datasets.items()
- )
-
- def size(self, index: int):
- """
- Return an example's size as a float or tuple. Here we return the max
- across all underlying datasets. This value is used when filtering a
- dataset with max-positions.
- """
- return max(
- dataset.size(self._map_index_to_dataset(key, index))
- for key, dataset in self.datasets.items()
- )
-
- @property
- def supports_prefetch(self):
- return all(
- getattr(dataset, "supports_prefetch", False)
- for dataset in self.datasets.values()
- )
-
- def prefetch(self, indices):
- for key, dataset in self.datasets.items():
- dataset.prefetch(
- [self._map_index_to_dataset(key, index) for index in indices]
- )
-
- @property
- def supports_fetch_outside_dataloader(self):
- return all(
- self.datasets[key].supports_fetch_outside_dataloader
- for key in self.datasets
- )
diff --git a/spaces/OFA-Sys/OFA-Generic_Interface/fairseq/fairseq/optim/lr_scheduler/fairseq_lr_scheduler.py b/spaces/OFA-Sys/OFA-Generic_Interface/fairseq/fairseq/optim/lr_scheduler/fairseq_lr_scheduler.py
deleted file mode 100644
index ac6340fa0744a08d2b527972dfc669573fb4e1c3..0000000000000000000000000000000000000000
--- a/spaces/OFA-Sys/OFA-Generic_Interface/fairseq/fairseq/optim/lr_scheduler/fairseq_lr_scheduler.py
+++ /dev/null
@@ -1,62 +0,0 @@
-# Copyright (c) Facebook, Inc. and its affiliates.
-#
-# This source code is licensed under the MIT license found in the
-# LICENSE file in the root directory of this source tree.
-
-from argparse import Namespace
-
-from fairseq.dataclass.utils import gen_parser_from_dataclass
-from fairseq.optim import FairseqOptimizer
-
-
-class FairseqLRScheduler(object):
- def __init__(self, cfg, optimizer):
- super().__init__()
- if optimizer is not None and not isinstance(optimizer, FairseqOptimizer):
- raise ValueError("optimizer must be an instance of FairseqOptimizer")
- self.cfg = cfg
- self.optimizer = optimizer
- self.best = None
-
- @classmethod
- def add_args(cls, parser):
- """Add arguments to the parser for this LR scheduler."""
- dc = getattr(cls, "__dataclass", None)
- if dc is not None:
- gen_parser_from_dataclass(parser, dc())
-
- def state_dict(self):
- """Return the LR scheduler state dict."""
- return {"best": self.best}
-
- def load_state_dict(self, state_dict):
- """Load an LR scheduler state dict."""
- self.best = state_dict["best"]
-
- def step_begin_epoch(self, epoch):
- """Update the learning rate at the beginning of the given epoch."""
- pass
-
- def step(self, epoch, val_loss=None):
- """Update the learning rate at the end of the given epoch."""
- if val_loss is not None:
- if self.best is None:
- self.best = val_loss
- else:
- self.best = min(self.best, val_loss)
-
- def step_update(self, num_updates):
- """Update the learning rate after each update."""
- return self.optimizer.get_lr()
-
- def reinit(self, total_num_update, num_updates):
- pass
-
-
-class LegacyFairseqLRScheduler(FairseqLRScheduler):
- def __init__(self, args: Namespace, optimizer):
- if not isinstance(optimizer, FairseqOptimizer):
- raise ValueError("optimizer must be an instance of FairseqOptimizer")
- self.args = args
- self.optimizer = optimizer
- self.best = None
diff --git a/spaces/OFA-Sys/OFA-Image_Caption/fairseq/hubconf.py b/spaces/OFA-Sys/OFA-Image_Caption/fairseq/hubconf.py
deleted file mode 100644
index 5949e274edd02e86cb323331211641ce0d0b9b93..0000000000000000000000000000000000000000
--- a/spaces/OFA-Sys/OFA-Image_Caption/fairseq/hubconf.py
+++ /dev/null
@@ -1,73 +0,0 @@
-# Copyright (c) Facebook, Inc. and its affiliates.
-#
-# This source code is licensed under the MIT license found in the
-# LICENSE file in the root directory of this source tree.
-"""isort:skip_file"""
-
-import functools
-import importlib
-
-
-dependencies = [
- "dataclasses",
- "hydra",
- "numpy",
- "omegaconf",
- "regex",
- "requests",
- "torch",
-]
-
-
-# Check for required dependencies and raise a RuntimeError if any are missing.
-missing_deps = []
-for dep in dependencies:
- try:
- importlib.import_module(dep)
- except ImportError:
- # Hack: the hydra package is provided under the "hydra-core" name in
- # pypi. We don't want the user mistakenly calling `pip install hydra`
- # since that will install an unrelated package.
- if dep == "hydra":
- dep = "hydra-core"
- missing_deps.append(dep)
-if len(missing_deps) > 0:
- raise RuntimeError("Missing dependencies: {}".format(", ".join(missing_deps)))
-
-
-# only do fairseq imports after checking for dependencies
-from fairseq.hub_utils import ( # noqa; noqa
- BPEHubInterface as bpe,
- TokenizerHubInterface as tokenizer,
-)
-from fairseq.models import MODEL_REGISTRY # noqa
-
-
-# torch.hub doesn't build Cython components, so if they are not found then try
-# to build them here
-try:
- import fairseq.data.token_block_utils_fast # noqa
-except ImportError:
- try:
- import cython # noqa
- import os
- from setuptools import sandbox
-
- sandbox.run_setup(
- os.path.join(os.path.dirname(__file__), "setup.py"),
- ["build_ext", "--inplace"],
- )
- except ImportError:
- print(
- "Unable to build Cython components. Please make sure Cython is "
- "installed if the torch.hub model you are loading depends on it."
- )
-
-
-# automatically expose models defined in FairseqModel::hub_models
-for _model_type, _cls in MODEL_REGISTRY.items():
- for model_name in _cls.hub_models().keys():
- globals()[model_name] = functools.partial(
- _cls.from_pretrained,
- model_name,
- )
diff --git a/spaces/OFA-Sys/OFA-Image_Caption/fairseq/tests/test_convtbc.py b/spaces/OFA-Sys/OFA-Image_Caption/fairseq/tests/test_convtbc.py
deleted file mode 100644
index 3a3c9b91e70f597ab77b9b01459cc429db5d7956..0000000000000000000000000000000000000000
--- a/spaces/OFA-Sys/OFA-Image_Caption/fairseq/tests/test_convtbc.py
+++ /dev/null
@@ -1,54 +0,0 @@
-# Copyright (c) Facebook, Inc. and its affiliates.
-#
-# This source code is licensed under the MIT license found in the
-# LICENSE file in the root directory of this source tree.
-
-import unittest
-
-import torch
-import torch.nn as nn
-from fairseq.modules import ConvTBC
-
-
-class TestConvTBC(unittest.TestCase):
- def test_convtbc(self):
- # ksz, in_channels, out_channels
- conv_tbc = ConvTBC(4, 5, kernel_size=3, padding=1)
- # out_channels, in_channels, ksz
- conv1d = nn.Conv1d(4, 5, kernel_size=3, padding=1)
-
- conv_tbc.weight.data.copy_(conv1d.weight.data.transpose(0, 2))
- conv_tbc.bias.data.copy_(conv1d.bias.data)
-
- input_tbc = torch.randn(7, 2, 4, requires_grad=True)
- input1d = input_tbc.data.transpose(0, 1).transpose(1, 2)
- input1d.requires_grad = True
-
- output_tbc = conv_tbc(input_tbc)
- output1d = conv1d(input1d)
-
- self.assertAlmostEqual(
- output_tbc.data.transpose(0, 1).transpose(1, 2), output1d.data
- )
-
- grad_tbc = torch.randn(output_tbc.size())
- grad1d = grad_tbc.transpose(0, 1).transpose(1, 2).contiguous()
-
- output_tbc.backward(grad_tbc)
- output1d.backward(grad1d)
-
- self.assertAlmostEqual(
- conv_tbc.weight.grad.data.transpose(0, 2), conv1d.weight.grad.data
- )
- self.assertAlmostEqual(conv_tbc.bias.grad.data, conv1d.bias.grad.data)
- self.assertAlmostEqual(
- input_tbc.grad.data.transpose(0, 1).transpose(1, 2), input1d.grad.data
- )
-
- def assertAlmostEqual(self, t1, t2):
- self.assertEqual(t1.size(), t2.size(), "size mismatch")
- self.assertLess((t1 - t2).abs().max(), 1e-4)
-
-
-if __name__ == "__main__":
- unittest.main()
diff --git a/spaces/OFA-Sys/OFA-vqa/fairseq/examples/cross_lingual_language_model/README.md b/spaces/OFA-Sys/OFA-vqa/fairseq/examples/cross_lingual_language_model/README.md
deleted file mode 100644
index af9128e39e5925e9411d162c2f24a19e4532d618..0000000000000000000000000000000000000000
--- a/spaces/OFA-Sys/OFA-vqa/fairseq/examples/cross_lingual_language_model/README.md
+++ /dev/null
@@ -1,77 +0,0 @@
-# Cross-Lingual Language Model Pre-training
-
-Below are some details for training Cross-Lingual Language Models (XLM) - similar to the ones presented in [Lample & Conneau, 2019](https://arxiv.org/pdf/1901.07291.pdf) - in Fairseq. The current implementation only supports the Masked Language Model (MLM) from the paper above.
-
-## Downloading and Tokenizing Monolingual Data
-
-Pointers to the monolingual data from wikipedia, used for training the XLM-style MLM model as well as details on processing (tokenization and BPE) it can be found in the [XLM Github Repository](https://github.com/facebookresearch/XLM#download--preprocess-monolingual-data).
-
-Let's assume the following for the code snippets in later sections to work
-- Processed data is in the folder: monolingual_data/processed
-- Each language has 3 files for train, test and validation. For example we have the following files for English:
- train.en, valid.en
-- We are training a model for 5 languages: Arabic (ar), German (de), English (en), Hindi (hi) and French (fr)
-- The vocabulary file is monolingual_data/processed/vocab_mlm
-
-
-## Fairseq Pre-processing and Binarization
-
-Pre-process and binarize the data with the MaskedLMDictionary and cross_lingual_lm task
-
-```bash
-# Ensure the output directory exists
-DATA_DIR=monolingual_data/fairseq_processed
-mkdir -p "$DATA_DIR"
-
-for lg in ar de en hi fr
-do
-
- fairseq-preprocess \
- --task cross_lingual_lm \
- --srcdict monolingual_data/processed/vocab_mlm \
- --only-source \
- --trainpref monolingual_data/processed/train \
- --validpref monolingual_data/processed/valid \
- --testpref monolingual_data/processed/test \
- --destdir monolingual_data/fairseq_processed \
- --workers 20 \
- --source-lang $lg
-
- # Since we only have a source language, the output file has a None for the
- # target language. Remove this
-
- for stage in train test valid
-
- sudo mv "$DATA_DIR/$stage.$lg-None.$lg.bin" "$stage.$lg.bin"
- sudo mv "$DATA_DIR/$stage.$lg-None.$lg.idx" "$stage.$lg.idx"
-
- done
-
-done
-```
-
-## Train a Cross-lingual Language Model similar to the XLM MLM model
-
-Use the following command to train the model on 5 languages.
-
-```
-fairseq-train \
---task cross_lingual_lm monolingual_data/fairseq_processed \
---save-dir checkpoints/mlm \
---max-update 2400000 --save-interval 1 --no-epoch-checkpoints \
---arch xlm_base \
---optimizer adam --lr-scheduler reduce_lr_on_plateau \
---lr-shrink 0.5 --lr 0.0001 --stop-min-lr 1e-09 \
---dropout 0.1 \
---criterion legacy_masked_lm_loss \
---max-tokens 2048 --tokens-per-sample 256 --attention-dropout 0.1 \
---dataset-impl lazy --seed 0 \
---masked-lm-only \
---monolingual-langs 'ar,de,en,hi,fr' --num-segment 5 \
---ddp-backend=legacy_ddp
-```
-
-Some Notes:
-- Using tokens_per_sample greater than 256 can cause OOM (out-of-memory) issues. Usually since MLM packs in streams of text, this parameter doesn't need much tuning.
-- The Evaluation workflow for computing MLM Perplexity on test data is in progress.
-- Finetuning this model on a downstream task is something which is not currently available.
diff --git a/spaces/OFA-Sys/OFA-vqa/fairseq/examples/speech_recognition/models/vggtransformer.py b/spaces/OFA-Sys/OFA-vqa/fairseq/examples/speech_recognition/models/vggtransformer.py
deleted file mode 100644
index bca0ae59a8cbe2b7c337e395021c883a61d101ee..0000000000000000000000000000000000000000
--- a/spaces/OFA-Sys/OFA-vqa/fairseq/examples/speech_recognition/models/vggtransformer.py
+++ /dev/null
@@ -1,1020 +0,0 @@
-# Copyright (c) Facebook, Inc. and its affiliates.
-#
-# This source code is licensed under the MIT license found in the
-# LICENSE file in the root directory of this source tree.
-
-import argparse
-import math
-from collections.abc import Iterable
-
-import torch
-import torch.nn as nn
-from examples.speech_recognition.data.data_utils import lengths_to_encoder_padding_mask
-from fairseq import utils
-from fairseq.models import (
- FairseqEncoder,
- FairseqEncoderDecoderModel,
- FairseqEncoderModel,
- FairseqIncrementalDecoder,
- register_model,
- register_model_architecture,
-)
-from fairseq.modules import (
- LinearizedConvolution,
- TransformerDecoderLayer,
- TransformerEncoderLayer,
- VGGBlock,
-)
-
-
-@register_model("asr_vggtransformer")
-class VGGTransformerModel(FairseqEncoderDecoderModel):
- """
- Transformers with convolutional context for ASR
- https://arxiv.org/abs/1904.11660
- """
-
- def __init__(self, encoder, decoder):
- super().__init__(encoder, decoder)
-
- @staticmethod
- def add_args(parser):
- """Add model-specific arguments to the parser."""
- parser.add_argument(
- "--input-feat-per-channel",
- type=int,
- metavar="N",
- help="encoder input dimension per input channel",
- )
- parser.add_argument(
- "--vggblock-enc-config",
- type=str,
- metavar="EXPR",
- help="""
- an array of tuples each containing the configuration of one vggblock:
- [(out_channels,
- conv_kernel_size,
- pooling_kernel_size,
- num_conv_layers,
- use_layer_norm), ...])
- """,
- )
- parser.add_argument(
- "--transformer-enc-config",
- type=str,
- metavar="EXPR",
- help=""""
- a tuple containing the configuration of the encoder transformer layers
- configurations:
- [(input_dim,
- num_heads,
- ffn_dim,
- normalize_before,
- dropout,
- attention_dropout,
- relu_dropout), ...]')
- """,
- )
- parser.add_argument(
- "--enc-output-dim",
- type=int,
- metavar="N",
- help="""
- encoder output dimension, can be None. If specified, projecting the
- transformer output to the specified dimension""",
- )
- parser.add_argument(
- "--in-channels",
- type=int,
- metavar="N",
- help="number of encoder input channels",
- )
- parser.add_argument(
- "--tgt-embed-dim",
- type=int,
- metavar="N",
- help="embedding dimension of the decoder target tokens",
- )
- parser.add_argument(
- "--transformer-dec-config",
- type=str,
- metavar="EXPR",
- help="""
- a tuple containing the configuration of the decoder transformer layers
- configurations:
- [(input_dim,
- num_heads,
- ffn_dim,
- normalize_before,
- dropout,
- attention_dropout,
- relu_dropout), ...]
- """,
- )
- parser.add_argument(
- "--conv-dec-config",
- type=str,
- metavar="EXPR",
- help="""
- an array of tuples for the decoder 1-D convolution config
- [(out_channels, conv_kernel_size, use_layer_norm), ...]""",
- )
-
- @classmethod
- def build_encoder(cls, args, task):
- return VGGTransformerEncoder(
- input_feat_per_channel=args.input_feat_per_channel,
- vggblock_config=eval(args.vggblock_enc_config),
- transformer_config=eval(args.transformer_enc_config),
- encoder_output_dim=args.enc_output_dim,
- in_channels=args.in_channels,
- )
-
- @classmethod
- def build_decoder(cls, args, task):
- return TransformerDecoder(
- dictionary=task.target_dictionary,
- embed_dim=args.tgt_embed_dim,
- transformer_config=eval(args.transformer_dec_config),
- conv_config=eval(args.conv_dec_config),
- encoder_output_dim=args.enc_output_dim,
- )
-
- @classmethod
- def build_model(cls, args, task):
- """Build a new model instance."""
- # make sure that all args are properly defaulted
- # (in case there are any new ones)
- base_architecture(args)
-
- encoder = cls.build_encoder(args, task)
- decoder = cls.build_decoder(args, task)
- return cls(encoder, decoder)
-
- def get_normalized_probs(self, net_output, log_probs, sample=None):
- # net_output['encoder_out'] is a (B, T, D) tensor
- lprobs = super().get_normalized_probs(net_output, log_probs, sample)
- lprobs.batch_first = True
- return lprobs
-
-
-DEFAULT_ENC_VGGBLOCK_CONFIG = ((32, 3, 2, 2, False),) * 2
-DEFAULT_ENC_TRANSFORMER_CONFIG = ((256, 4, 1024, True, 0.2, 0.2, 0.2),) * 2
-# 256: embedding dimension
-# 4: number of heads
-# 1024: FFN
-# True: apply layerNorm before (dropout + resiaul) instead of after
-# 0.2 (dropout): dropout after MultiheadAttention and second FC
-# 0.2 (attention_dropout): dropout in MultiheadAttention
-# 0.2 (relu_dropout): dropout after ReLu
-DEFAULT_DEC_TRANSFORMER_CONFIG = ((256, 2, 1024, True, 0.2, 0.2, 0.2),) * 2
-DEFAULT_DEC_CONV_CONFIG = ((256, 3, True),) * 2
-
-
-# TODO: repace transformer encoder config from one liner
-# to explicit args to get rid of this transformation
-def prepare_transformer_encoder_params(
- input_dim,
- num_heads,
- ffn_dim,
- normalize_before,
- dropout,
- attention_dropout,
- relu_dropout,
-):
- args = argparse.Namespace()
- args.encoder_embed_dim = input_dim
- args.encoder_attention_heads = num_heads
- args.attention_dropout = attention_dropout
- args.dropout = dropout
- args.activation_dropout = relu_dropout
- args.encoder_normalize_before = normalize_before
- args.encoder_ffn_embed_dim = ffn_dim
- return args
-
-
-def prepare_transformer_decoder_params(
- input_dim,
- num_heads,
- ffn_dim,
- normalize_before,
- dropout,
- attention_dropout,
- relu_dropout,
-):
- args = argparse.Namespace()
- args.encoder_embed_dim = None
- args.decoder_embed_dim = input_dim
- args.decoder_attention_heads = num_heads
- args.attention_dropout = attention_dropout
- args.dropout = dropout
- args.activation_dropout = relu_dropout
- args.decoder_normalize_before = normalize_before
- args.decoder_ffn_embed_dim = ffn_dim
- return args
-
-
-class VGGTransformerEncoder(FairseqEncoder):
- """VGG + Transformer encoder"""
-
- def __init__(
- self,
- input_feat_per_channel,
- vggblock_config=DEFAULT_ENC_VGGBLOCK_CONFIG,
- transformer_config=DEFAULT_ENC_TRANSFORMER_CONFIG,
- encoder_output_dim=512,
- in_channels=1,
- transformer_context=None,
- transformer_sampling=None,
- ):
- """constructor for VGGTransformerEncoder
-
- Args:
- - input_feat_per_channel: feature dim (not including stacked,
- just base feature)
- - in_channel: # input channels (e.g., if stack 8 feature vector
- together, this is 8)
- - vggblock_config: configuration of vggblock, see comments on
- DEFAULT_ENC_VGGBLOCK_CONFIG
- - transformer_config: configuration of transformer layer, see comments
- on DEFAULT_ENC_TRANSFORMER_CONFIG
- - encoder_output_dim: final transformer output embedding dimension
- - transformer_context: (left, right) if set, self-attention will be focused
- on (t-left, t+right)
- - transformer_sampling: an iterable of int, must match with
- len(transformer_config), transformer_sampling[i] indicates sampling
- factor for i-th transformer layer, after multihead att and feedfoward
- part
- """
- super().__init__(None)
-
- self.num_vggblocks = 0
- if vggblock_config is not None:
- if not isinstance(vggblock_config, Iterable):
- raise ValueError("vggblock_config is not iterable")
- self.num_vggblocks = len(vggblock_config)
-
- self.conv_layers = nn.ModuleList()
- self.in_channels = in_channels
- self.input_dim = input_feat_per_channel
- self.pooling_kernel_sizes = []
-
- if vggblock_config is not None:
- for _, config in enumerate(vggblock_config):
- (
- out_channels,
- conv_kernel_size,
- pooling_kernel_size,
- num_conv_layers,
- layer_norm,
- ) = config
- self.conv_layers.append(
- VGGBlock(
- in_channels,
- out_channels,
- conv_kernel_size,
- pooling_kernel_size,
- num_conv_layers,
- input_dim=input_feat_per_channel,
- layer_norm=layer_norm,
- )
- )
- self.pooling_kernel_sizes.append(pooling_kernel_size)
- in_channels = out_channels
- input_feat_per_channel = self.conv_layers[-1].output_dim
-
- transformer_input_dim = self.infer_conv_output_dim(
- self.in_channels, self.input_dim
- )
- # transformer_input_dim is the output dimension of VGG part
-
- self.validate_transformer_config(transformer_config)
- self.transformer_context = self.parse_transformer_context(transformer_context)
- self.transformer_sampling = self.parse_transformer_sampling(
- transformer_sampling, len(transformer_config)
- )
-
- self.transformer_layers = nn.ModuleList()
-
- if transformer_input_dim != transformer_config[0][0]:
- self.transformer_layers.append(
- Linear(transformer_input_dim, transformer_config[0][0])
- )
- self.transformer_layers.append(
- TransformerEncoderLayer(
- prepare_transformer_encoder_params(*transformer_config[0])
- )
- )
-
- for i in range(1, len(transformer_config)):
- if transformer_config[i - 1][0] != transformer_config[i][0]:
- self.transformer_layers.append(
- Linear(transformer_config[i - 1][0], transformer_config[i][0])
- )
- self.transformer_layers.append(
- TransformerEncoderLayer(
- prepare_transformer_encoder_params(*transformer_config[i])
- )
- )
-
- self.encoder_output_dim = encoder_output_dim
- self.transformer_layers.extend(
- [
- Linear(transformer_config[-1][0], encoder_output_dim),
- LayerNorm(encoder_output_dim),
- ]
- )
-
- def forward(self, src_tokens, src_lengths, **kwargs):
- """
- src_tokens: padded tensor (B, T, C * feat)
- src_lengths: tensor of original lengths of input utterances (B,)
- """
- bsz, max_seq_len, _ = src_tokens.size()
- x = src_tokens.view(bsz, max_seq_len, self.in_channels, self.input_dim)
- x = x.transpose(1, 2).contiguous()
- # (B, C, T, feat)
-
- for layer_idx in range(len(self.conv_layers)):
- x = self.conv_layers[layer_idx](x)
-
- bsz, _, output_seq_len, _ = x.size()
-
- # (B, C, T, feat) -> (B, T, C, feat) -> (T, B, C, feat) -> (T, B, C * feat)
- x = x.transpose(1, 2).transpose(0, 1)
- x = x.contiguous().view(output_seq_len, bsz, -1)
-
- input_lengths = src_lengths.clone()
- for s in self.pooling_kernel_sizes:
- input_lengths = (input_lengths.float() / s).ceil().long()
-
- encoder_padding_mask, _ = lengths_to_encoder_padding_mask(
- input_lengths, batch_first=True
- )
- if not encoder_padding_mask.any():
- encoder_padding_mask = None
-
- subsampling_factor = int(max_seq_len * 1.0 / output_seq_len + 0.5)
- attn_mask = self.lengths_to_attn_mask(input_lengths, subsampling_factor)
-
- transformer_layer_idx = 0
-
- for layer_idx in range(len(self.transformer_layers)):
-
- if isinstance(self.transformer_layers[layer_idx], TransformerEncoderLayer):
- x = self.transformer_layers[layer_idx](
- x, encoder_padding_mask, attn_mask
- )
-
- if self.transformer_sampling[transformer_layer_idx] != 1:
- sampling_factor = self.transformer_sampling[transformer_layer_idx]
- x, encoder_padding_mask, attn_mask = self.slice(
- x, encoder_padding_mask, attn_mask, sampling_factor
- )
-
- transformer_layer_idx += 1
-
- else:
- x = self.transformer_layers[layer_idx](x)
-
- # encoder_padding_maks is a (T x B) tensor, its [t, b] elements indicate
- # whether encoder_output[t, b] is valid or not (valid=0, invalid=1)
-
- return {
- "encoder_out": x, # (T, B, C)
- "encoder_padding_mask": encoder_padding_mask.t()
- if encoder_padding_mask is not None
- else None,
- # (B, T) --> (T, B)
- }
-
- def infer_conv_output_dim(self, in_channels, input_dim):
- sample_seq_len = 200
- sample_bsz = 10
- x = torch.randn(sample_bsz, in_channels, sample_seq_len, input_dim)
- for i, _ in enumerate(self.conv_layers):
- x = self.conv_layers[i](x)
- x = x.transpose(1, 2)
- mb, seq = x.size()[:2]
- return x.contiguous().view(mb, seq, -1).size(-1)
-
- def validate_transformer_config(self, transformer_config):
- for config in transformer_config:
- input_dim, num_heads = config[:2]
- if input_dim % num_heads != 0:
- msg = (
- "ERROR in transformer config {}: ".format(config)
- + "input dimension {} ".format(input_dim)
- + "not dividable by number of heads {}".format(num_heads)
- )
- raise ValueError(msg)
-
- def parse_transformer_context(self, transformer_context):
- """
- transformer_context can be the following:
- - None; indicates no context is used, i.e.,
- transformer can access full context
- - a tuple/list of two int; indicates left and right context,
- any number <0 indicates infinite context
- * e.g., (5, 6) indicates that for query at x_t, transformer can
- access [t-5, t+6] (inclusive)
- * e.g., (-1, 6) indicates that for query at x_t, transformer can
- access [0, t+6] (inclusive)
- """
- if transformer_context is None:
- return None
-
- if not isinstance(transformer_context, Iterable):
- raise ValueError("transformer context must be Iterable if it is not None")
-
- if len(transformer_context) != 2:
- raise ValueError("transformer context must have length 2")
-
- left_context = transformer_context[0]
- if left_context < 0:
- left_context = None
-
- right_context = transformer_context[1]
- if right_context < 0:
- right_context = None
-
- if left_context is None and right_context is None:
- return None
-
- return (left_context, right_context)
-
- def parse_transformer_sampling(self, transformer_sampling, num_layers):
- """
- parsing transformer sampling configuration
-
- Args:
- - transformer_sampling, accepted input:
- * None, indicating no sampling
- * an Iterable with int (>0) as element
- - num_layers, expected number of transformer layers, must match with
- the length of transformer_sampling if it is not None
-
- Returns:
- - A tuple with length num_layers
- """
- if transformer_sampling is None:
- return (1,) * num_layers
-
- if not isinstance(transformer_sampling, Iterable):
- raise ValueError(
- "transformer_sampling must be an iterable if it is not None"
- )
-
- if len(transformer_sampling) != num_layers:
- raise ValueError(
- "transformer_sampling {} does not match with the number "
- "of layers {}".format(transformer_sampling, num_layers)
- )
-
- for layer, value in enumerate(transformer_sampling):
- if not isinstance(value, int):
- raise ValueError("Invalid value in transformer_sampling: ")
- if value < 1:
- raise ValueError(
- "{} layer's subsampling is {}.".format(layer, value)
- + " This is not allowed! "
- )
- return transformer_sampling
-
- def slice(self, embedding, padding_mask, attn_mask, sampling_factor):
- """
- embedding is a (T, B, D) tensor
- padding_mask is a (B, T) tensor or None
- attn_mask is a (T, T) tensor or None
- """
- embedding = embedding[::sampling_factor, :, :]
- if padding_mask is not None:
- padding_mask = padding_mask[:, ::sampling_factor]
- if attn_mask is not None:
- attn_mask = attn_mask[::sampling_factor, ::sampling_factor]
-
- return embedding, padding_mask, attn_mask
-
- def lengths_to_attn_mask(self, input_lengths, subsampling_factor=1):
- """
- create attention mask according to sequence lengths and transformer
- context
-
- Args:
- - input_lengths: (B, )-shape Int/Long tensor; input_lengths[b] is
- the length of b-th sequence
- - subsampling_factor: int
- * Note that the left_context and right_context is specified in
- the input frame-level while input to transformer may already
- go through subsampling (e.g., the use of striding in vggblock)
- we use subsampling_factor to scale the left/right context
-
- Return:
- - a (T, T) binary tensor or None, where T is max(input_lengths)
- * if self.transformer_context is None, None
- * if left_context is None,
- * attn_mask[t, t + right_context + 1:] = 1
- * others = 0
- * if right_context is None,
- * attn_mask[t, 0:t - left_context] = 1
- * others = 0
- * elsif
- * attn_mask[t, t - left_context: t + right_context + 1] = 0
- * others = 1
- """
- if self.transformer_context is None:
- return None
-
- maxT = torch.max(input_lengths).item()
- attn_mask = torch.zeros(maxT, maxT)
-
- left_context = self.transformer_context[0]
- right_context = self.transformer_context[1]
- if left_context is not None:
- left_context = math.ceil(self.transformer_context[0] / subsampling_factor)
- if right_context is not None:
- right_context = math.ceil(self.transformer_context[1] / subsampling_factor)
-
- for t in range(maxT):
- if left_context is not None:
- st = 0
- en = max(st, t - left_context)
- attn_mask[t, st:en] = 1
- if right_context is not None:
- st = t + right_context + 1
- st = min(st, maxT - 1)
- attn_mask[t, st:] = 1
-
- return attn_mask.to(input_lengths.device)
-
- def reorder_encoder_out(self, encoder_out, new_order):
- encoder_out["encoder_out"] = encoder_out["encoder_out"].index_select(
- 1, new_order
- )
- if encoder_out["encoder_padding_mask"] is not None:
- encoder_out["encoder_padding_mask"] = encoder_out[
- "encoder_padding_mask"
- ].index_select(1, new_order)
- return encoder_out
-
-
-class TransformerDecoder(FairseqIncrementalDecoder):
- """
- Transformer decoder consisting of *args.decoder_layers* layers. Each layer
- is a :class:`TransformerDecoderLayer`.
- Args:
- args (argparse.Namespace): parsed command-line arguments
- dictionary (~fairseq.data.Dictionary): decoding dictionary
- embed_tokens (torch.nn.Embedding): output embedding
- no_encoder_attn (bool, optional): whether to attend to encoder outputs.
- Default: ``False``
- left_pad (bool, optional): whether the input is left-padded. Default:
- ``False``
- """
-
- def __init__(
- self,
- dictionary,
- embed_dim=512,
- transformer_config=DEFAULT_ENC_TRANSFORMER_CONFIG,
- conv_config=DEFAULT_DEC_CONV_CONFIG,
- encoder_output_dim=512,
- ):
-
- super().__init__(dictionary)
- vocab_size = len(dictionary)
- self.padding_idx = dictionary.pad()
- self.embed_tokens = Embedding(vocab_size, embed_dim, self.padding_idx)
-
- self.conv_layers = nn.ModuleList()
- for i in range(len(conv_config)):
- out_channels, kernel_size, layer_norm = conv_config[i]
- if i == 0:
- conv_layer = LinearizedConv1d(
- embed_dim, out_channels, kernel_size, padding=kernel_size - 1
- )
- else:
- conv_layer = LinearizedConv1d(
- conv_config[i - 1][0],
- out_channels,
- kernel_size,
- padding=kernel_size - 1,
- )
- self.conv_layers.append(conv_layer)
- if layer_norm:
- self.conv_layers.append(nn.LayerNorm(out_channels))
- self.conv_layers.append(nn.ReLU())
-
- self.layers = nn.ModuleList()
- if conv_config[-1][0] != transformer_config[0][0]:
- self.layers.append(Linear(conv_config[-1][0], transformer_config[0][0]))
- self.layers.append(
- TransformerDecoderLayer(
- prepare_transformer_decoder_params(*transformer_config[0])
- )
- )
-
- for i in range(1, len(transformer_config)):
- if transformer_config[i - 1][0] != transformer_config[i][0]:
- self.layers.append(
- Linear(transformer_config[i - 1][0], transformer_config[i][0])
- )
- self.layers.append(
- TransformerDecoderLayer(
- prepare_transformer_decoder_params(*transformer_config[i])
- )
- )
- self.fc_out = Linear(transformer_config[-1][0], vocab_size)
-
- def forward(self, prev_output_tokens, encoder_out=None, incremental_state=None):
- """
- Args:
- prev_output_tokens (LongTensor): previous decoder outputs of shape
- `(batch, tgt_len)`, for input feeding/teacher forcing
- encoder_out (Tensor, optional): output from the encoder, used for
- encoder-side attention
- incremental_state (dict): dictionary used for storing state during
- :ref:`Incremental decoding`
- Returns:
- tuple:
- - the last decoder layer's output of shape `(batch, tgt_len,
- vocab)`
- - the last decoder layer's attention weights of shape `(batch,
- tgt_len, src_len)`
- """
- target_padding_mask = (
- (prev_output_tokens == self.padding_idx).to(prev_output_tokens.device)
- if incremental_state is None
- else None
- )
-
- if incremental_state is not None:
- prev_output_tokens = prev_output_tokens[:, -1:]
-
- # embed tokens
- x = self.embed_tokens(prev_output_tokens)
-
- # B x T x C -> T x B x C
- x = self._transpose_if_training(x, incremental_state)
-
- for layer in self.conv_layers:
- if isinstance(layer, LinearizedConvolution):
- x = layer(x, incremental_state)
- else:
- x = layer(x)
-
- # B x T x C -> T x B x C
- x = self._transpose_if_inference(x, incremental_state)
-
- # decoder layers
- for layer in self.layers:
- if isinstance(layer, TransformerDecoderLayer):
- x, *_ = layer(
- x,
- (encoder_out["encoder_out"] if encoder_out is not None else None),
- (
- encoder_out["encoder_padding_mask"].t()
- if encoder_out["encoder_padding_mask"] is not None
- else None
- ),
- incremental_state,
- self_attn_mask=(
- self.buffered_future_mask(x)
- if incremental_state is None
- else None
- ),
- self_attn_padding_mask=(
- target_padding_mask if incremental_state is None else None
- ),
- )
- else:
- x = layer(x)
-
- # T x B x C -> B x T x C
- x = x.transpose(0, 1)
-
- x = self.fc_out(x)
-
- return x, None
-
- def buffered_future_mask(self, tensor):
- dim = tensor.size(0)
- if (
- not hasattr(self, "_future_mask")
- or self._future_mask is None
- or self._future_mask.device != tensor.device
- ):
- self._future_mask = torch.triu(
- utils.fill_with_neg_inf(tensor.new(dim, dim)), 1
- )
- if self._future_mask.size(0) < dim:
- self._future_mask = torch.triu(
- utils.fill_with_neg_inf(self._future_mask.resize_(dim, dim)), 1
- )
- return self._future_mask[:dim, :dim]
-
- def _transpose_if_training(self, x, incremental_state):
- if incremental_state is None:
- x = x.transpose(0, 1)
- return x
-
- def _transpose_if_inference(self, x, incremental_state):
- if incremental_state:
- x = x.transpose(0, 1)
- return x
-
-
-@register_model("asr_vggtransformer_encoder")
-class VGGTransformerEncoderModel(FairseqEncoderModel):
- def __init__(self, encoder):
- super().__init__(encoder)
-
- @staticmethod
- def add_args(parser):
- """Add model-specific arguments to the parser."""
- parser.add_argument(
- "--input-feat-per-channel",
- type=int,
- metavar="N",
- help="encoder input dimension per input channel",
- )
- parser.add_argument(
- "--vggblock-enc-config",
- type=str,
- metavar="EXPR",
- help="""
- an array of tuples each containing the configuration of one vggblock
- [(out_channels, conv_kernel_size, pooling_kernel_size,num_conv_layers), ...]
- """,
- )
- parser.add_argument(
- "--transformer-enc-config",
- type=str,
- metavar="EXPR",
- help="""
- a tuple containing the configuration of the Transformer layers
- configurations:
- [(input_dim,
- num_heads,
- ffn_dim,
- normalize_before,
- dropout,
- attention_dropout,
- relu_dropout), ]""",
- )
- parser.add_argument(
- "--enc-output-dim",
- type=int,
- metavar="N",
- help="encoder output dimension, projecting the LSTM output",
- )
- parser.add_argument(
- "--in-channels",
- type=int,
- metavar="N",
- help="number of encoder input channels",
- )
- parser.add_argument(
- "--transformer-context",
- type=str,
- metavar="EXPR",
- help="""
- either None or a tuple of two ints, indicating left/right context a
- transformer can have access to""",
- )
- parser.add_argument(
- "--transformer-sampling",
- type=str,
- metavar="EXPR",
- help="""
- either None or a tuple of ints, indicating sampling factor in each layer""",
- )
-
- @classmethod
- def build_model(cls, args, task):
- """Build a new model instance."""
- base_architecture_enconly(args)
- encoder = VGGTransformerEncoderOnly(
- vocab_size=len(task.target_dictionary),
- input_feat_per_channel=args.input_feat_per_channel,
- vggblock_config=eval(args.vggblock_enc_config),
- transformer_config=eval(args.transformer_enc_config),
- encoder_output_dim=args.enc_output_dim,
- in_channels=args.in_channels,
- transformer_context=eval(args.transformer_context),
- transformer_sampling=eval(args.transformer_sampling),
- )
- return cls(encoder)
-
- def get_normalized_probs(self, net_output, log_probs, sample=None):
- # net_output['encoder_out'] is a (T, B, D) tensor
- lprobs = super().get_normalized_probs(net_output, log_probs, sample)
- # lprobs is a (T, B, D) tensor
- # we need to transoose to get (B, T, D) tensor
- lprobs = lprobs.transpose(0, 1).contiguous()
- lprobs.batch_first = True
- return lprobs
-
-
-class VGGTransformerEncoderOnly(VGGTransformerEncoder):
- def __init__(
- self,
- vocab_size,
- input_feat_per_channel,
- vggblock_config=DEFAULT_ENC_VGGBLOCK_CONFIG,
- transformer_config=DEFAULT_ENC_TRANSFORMER_CONFIG,
- encoder_output_dim=512,
- in_channels=1,
- transformer_context=None,
- transformer_sampling=None,
- ):
- super().__init__(
- input_feat_per_channel=input_feat_per_channel,
- vggblock_config=vggblock_config,
- transformer_config=transformer_config,
- encoder_output_dim=encoder_output_dim,
- in_channels=in_channels,
- transformer_context=transformer_context,
- transformer_sampling=transformer_sampling,
- )
- self.fc_out = Linear(self.encoder_output_dim, vocab_size)
-
- def forward(self, src_tokens, src_lengths, **kwargs):
- """
- src_tokens: padded tensor (B, T, C * feat)
- src_lengths: tensor of original lengths of input utterances (B,)
- """
-
- enc_out = super().forward(src_tokens, src_lengths)
- x = self.fc_out(enc_out["encoder_out"])
- # x = F.log_softmax(x, dim=-1)
- # Note: no need this line, because model.get_normalized_prob will call
- # log_softmax
- return {
- "encoder_out": x, # (T, B, C)
- "encoder_padding_mask": enc_out["encoder_padding_mask"], # (T, B)
- }
-
- def max_positions(self):
- """Maximum input length supported by the encoder."""
- return (1e6, 1e6) # an arbitrary large number
-
-
-def Embedding(num_embeddings, embedding_dim, padding_idx):
- m = nn.Embedding(num_embeddings, embedding_dim, padding_idx=padding_idx)
- # nn.init.uniform_(m.weight, -0.1, 0.1)
- # nn.init.constant_(m.weight[padding_idx], 0)
- return m
-
-
-def Linear(in_features, out_features, bias=True, dropout=0):
- """Linear layer (input: N x T x C)"""
- m = nn.Linear(in_features, out_features, bias=bias)
- # m.weight.data.uniform_(-0.1, 0.1)
- # if bias:
- # m.bias.data.uniform_(-0.1, 0.1)
- return m
-
-
-def LinearizedConv1d(in_channels, out_channels, kernel_size, dropout=0, **kwargs):
- """Weight-normalized Conv1d layer optimized for decoding"""
- m = LinearizedConvolution(in_channels, out_channels, kernel_size, **kwargs)
- std = math.sqrt((4 * (1.0 - dropout)) / (m.kernel_size[0] * in_channels))
- nn.init.normal_(m.weight, mean=0, std=std)
- nn.init.constant_(m.bias, 0)
- return nn.utils.weight_norm(m, dim=2)
-
-
-def LayerNorm(embedding_dim):
- m = nn.LayerNorm(embedding_dim)
- return m
-
-
-# seq2seq models
-def base_architecture(args):
- args.input_feat_per_channel = getattr(args, "input_feat_per_channel", 40)
- args.vggblock_enc_config = getattr(
- args, "vggblock_enc_config", DEFAULT_ENC_VGGBLOCK_CONFIG
- )
- args.transformer_enc_config = getattr(
- args, "transformer_enc_config", DEFAULT_ENC_TRANSFORMER_CONFIG
- )
- args.enc_output_dim = getattr(args, "enc_output_dim", 512)
- args.in_channels = getattr(args, "in_channels", 1)
- args.tgt_embed_dim = getattr(args, "tgt_embed_dim", 128)
- args.transformer_dec_config = getattr(
- args, "transformer_dec_config", DEFAULT_ENC_TRANSFORMER_CONFIG
- )
- args.conv_dec_config = getattr(args, "conv_dec_config", DEFAULT_DEC_CONV_CONFIG)
- args.transformer_context = getattr(args, "transformer_context", "None")
-
-
-@register_model_architecture("asr_vggtransformer", "vggtransformer_1")
-def vggtransformer_1(args):
- args.input_feat_per_channel = getattr(args, "input_feat_per_channel", 80)
- args.vggblock_enc_config = getattr(
- args, "vggblock_enc_config", "[(64, 3, 2, 2, True), (128, 3, 2, 2, True)]"
- )
- args.transformer_enc_config = getattr(
- args,
- "transformer_enc_config",
- "((1024, 16, 4096, True, 0.15, 0.15, 0.15),) * 14",
- )
- args.enc_output_dim = getattr(args, "enc_output_dim", 1024)
- args.tgt_embed_dim = getattr(args, "tgt_embed_dim", 128)
- args.conv_dec_config = getattr(args, "conv_dec_config", "((256, 3, True),) * 4")
- args.transformer_dec_config = getattr(
- args,
- "transformer_dec_config",
- "((1024, 16, 4096, True, 0.15, 0.15, 0.15),) * 4",
- )
-
-
-@register_model_architecture("asr_vggtransformer", "vggtransformer_2")
-def vggtransformer_2(args):
- args.input_feat_per_channel = getattr(args, "input_feat_per_channel", 80)
- args.vggblock_enc_config = getattr(
- args, "vggblock_enc_config", "[(64, 3, 2, 2, True), (128, 3, 2, 2, True)]"
- )
- args.transformer_enc_config = getattr(
- args,
- "transformer_enc_config",
- "((1024, 16, 4096, True, 0.15, 0.15, 0.15),) * 16",
- )
- args.enc_output_dim = getattr(args, "enc_output_dim", 1024)
- args.tgt_embed_dim = getattr(args, "tgt_embed_dim", 512)
- args.conv_dec_config = getattr(args, "conv_dec_config", "((256, 3, True),) * 4")
- args.transformer_dec_config = getattr(
- args,
- "transformer_dec_config",
- "((1024, 16, 4096, True, 0.15, 0.15, 0.15),) * 6",
- )
-
-
-@register_model_architecture("asr_vggtransformer", "vggtransformer_base")
-def vggtransformer_base(args):
- args.input_feat_per_channel = getattr(args, "input_feat_per_channel", 80)
- args.vggblock_enc_config = getattr(
- args, "vggblock_enc_config", "[(64, 3, 2, 2, True), (128, 3, 2, 2, True)]"
- )
- args.transformer_enc_config = getattr(
- args, "transformer_enc_config", "((512, 8, 2048, True, 0.15, 0.15, 0.15),) * 12"
- )
-
- args.enc_output_dim = getattr(args, "enc_output_dim", 512)
- args.tgt_embed_dim = getattr(args, "tgt_embed_dim", 512)
- args.conv_dec_config = getattr(args, "conv_dec_config", "((256, 3, True),) * 4")
- args.transformer_dec_config = getattr(
- args, "transformer_dec_config", "((512, 8, 2048, True, 0.15, 0.15, 0.15),) * 6"
- )
- # Size estimations:
- # Encoder:
- # - vggblock param: 64*1*3*3 + 64*64*3*3 + 128*64*3*3 + 128*128*3 = 258K
- # Transformer:
- # - input dimension adapter: 2560 x 512 -> 1.31M
- # - transformer_layers (x12) --> 37.74M
- # * MultiheadAttention: 512*512*3 (in_proj) + 512*512 (out_proj) = 1.048M
- # * FFN weight: 512*2048*2 = 2.097M
- # - output dimension adapter: 512 x 512 -> 0.26 M
- # Decoder:
- # - LinearizedConv1d: 512 * 256 * 3 + 256 * 256 * 3 * 3
- # - transformer_layer: (x6) --> 25.16M
- # * MultiheadAttention (self-attention): 512*512*3 + 512*512 = 1.048M
- # * MultiheadAttention (encoder-attention): 512*512*3 + 512*512 = 1.048M
- # * FFN: 512*2048*2 = 2.097M
- # Final FC:
- # - FC: 512*5000 = 256K (assuming vocab size 5K)
- # In total:
- # ~65 M
-
-
-# CTC models
-def base_architecture_enconly(args):
- args.input_feat_per_channel = getattr(args, "input_feat_per_channel", 40)
- args.vggblock_enc_config = getattr(
- args, "vggblock_enc_config", "[(32, 3, 2, 2, True)] * 2"
- )
- args.transformer_enc_config = getattr(
- args, "transformer_enc_config", "((256, 4, 1024, True, 0.2, 0.2, 0.2),) * 2"
- )
- args.enc_output_dim = getattr(args, "enc_output_dim", 512)
- args.in_channels = getattr(args, "in_channels", 1)
- args.transformer_context = getattr(args, "transformer_context", "None")
- args.transformer_sampling = getattr(args, "transformer_sampling", "None")
-
-
-@register_model_architecture("asr_vggtransformer_encoder", "vggtransformer_enc_1")
-def vggtransformer_enc_1(args):
- # vggtransformer_1 is the same as vggtransformer_enc_big, except the number
- # of layers is increased to 16
- # keep it here for backward compatiablity purpose
- args.input_feat_per_channel = getattr(args, "input_feat_per_channel", 80)
- args.vggblock_enc_config = getattr(
- args, "vggblock_enc_config", "[(64, 3, 2, 2, True), (128, 3, 2, 2, True)]"
- )
- args.transformer_enc_config = getattr(
- args,
- "transformer_enc_config",
- "((1024, 16, 4096, True, 0.15, 0.15, 0.15),) * 16",
- )
- args.enc_output_dim = getattr(args, "enc_output_dim", 1024)
diff --git a/spaces/OFA-Sys/OFA-vqa/fairseq/examples/textless_nlp/gslm/unit2speech/tts_data.py b/spaces/OFA-Sys/OFA-vqa/fairseq/examples/textless_nlp/gslm/unit2speech/tts_data.py
deleted file mode 100644
index eb0f7c360d749fd9d489b40b04dae8652b095098..0000000000000000000000000000000000000000
--- a/spaces/OFA-Sys/OFA-vqa/fairseq/examples/textless_nlp/gslm/unit2speech/tts_data.py
+++ /dev/null
@@ -1,52 +0,0 @@
-# Copyright (c) Facebook, Inc. and its affiliates.
-#
-# This source code is licensed under the MIT license found in the
-# LICENSE file in the root directory of this source tree.
-
-
-import torch
-import numpy as np
-from examples.textless_nlp.gslm.unit2speech.tacotron2.text import (
- EOS_TOK,
- SOS_TOK,
- code_to_sequence,
- text_to_sequence,
-)
-from examples.textless_nlp.gslm.unit2speech.tacotron2.utils import (
- load_code_dict,
-)
-
-
-class TacotronInputDataset:
- def __init__(self, hparams, append_str=""):
- self.is_text = getattr(hparams, "text_or_code", "text") == "text"
- if not self.is_text:
- self.code_dict = load_code_dict(hparams.code_dict)
- self.code_key = hparams.code_key
- self.add_sos = hparams.add_sos
- self.add_eos = hparams.add_eos
- self.collapse_code = hparams.collapse_code
- self.append_str = append_str
-
- def process_code(self, inp_str):
- inp_toks = inp_str.split()
- if self.add_sos:
- inp_toks = [SOS_TOK] + inp_toks
- if self.add_eos:
- inp_toks = inp_toks + [EOS_TOK]
- return code_to_sequence(inp_toks, self.code_dict, self.collapse_code)
-
- def process_text(self, inp_str):
- return text_to_sequence(inp_str, ["english_cleaners"])
-
- def get_tensor(self, inp_str):
- # uid, txt, inp_str = self._get_data(idx)
- inp_str = inp_str + self.append_str
- if self.is_text:
- inp_toks = self.process_text(inp_str)
- else:
- inp_toks = self.process_code(inp_str)
- return torch.from_numpy(np.array(inp_toks)).long()
-
- def __len__(self):
- return len(self.data)
diff --git a/spaces/OFA-Sys/OFA-vqa/fairseq/fairseq/models/transformer_align.py b/spaces/OFA-Sys/OFA-vqa/fairseq/fairseq/models/transformer_align.py
deleted file mode 100644
index eaf585bd10e630ae6cd89920f197cd165f55ad58..0000000000000000000000000000000000000000
--- a/spaces/OFA-Sys/OFA-vqa/fairseq/fairseq/models/transformer_align.py
+++ /dev/null
@@ -1,93 +0,0 @@
-# Copyright (c) Facebook, Inc. and its affiliates.
-#
-# This source code is licensed under the MIT license found in the
-# LICENSE file in the root directory of this source tree.
-
-from fairseq.models import register_model, register_model_architecture
-from fairseq.models.transformer import (
- TransformerModel,
- base_architecture,
- transformer_wmt_en_de_big,
-)
-
-
-@register_model("transformer_align")
-class TransformerAlignModel(TransformerModel):
- """
- See "Jointly Learning to Align and Translate with Transformer
- Models" (Garg et al., EMNLP 2019).
- """
-
- def __init__(self, encoder, decoder, args):
- super().__init__(args, encoder, decoder)
- self.alignment_heads = args.alignment_heads
- self.alignment_layer = args.alignment_layer
- self.full_context_alignment = args.full_context_alignment
-
- @staticmethod
- def add_args(parser):
- # fmt: off
- super(TransformerAlignModel, TransformerAlignModel).add_args(parser)
- parser.add_argument('--alignment-heads', type=int, metavar='D',
- help='Number of cross attention heads per layer to supervised with alignments')
- parser.add_argument('--alignment-layer', type=int, metavar='D',
- help='Layer number which has to be supervised. 0 corresponding to the bottommost layer.')
- parser.add_argument('--full-context-alignment', action='store_true',
- help='Whether or not alignment is supervised conditioned on the full target context.')
- # fmt: on
-
- @classmethod
- def build_model(cls, args, task):
- # set any default arguments
- transformer_align(args)
-
- transformer_model = TransformerModel.build_model(args, task)
- return TransformerAlignModel(
- transformer_model.encoder, transformer_model.decoder, args
- )
-
- def forward(self, src_tokens, src_lengths, prev_output_tokens):
- encoder_out = self.encoder(src_tokens, src_lengths)
- return self.forward_decoder(prev_output_tokens, encoder_out)
-
- def forward_decoder(
- self,
- prev_output_tokens,
- encoder_out=None,
- incremental_state=None,
- features_only=False,
- **extra_args,
- ):
- attn_args = {
- "alignment_layer": self.alignment_layer,
- "alignment_heads": self.alignment_heads,
- }
- decoder_out = self.decoder(prev_output_tokens, encoder_out, **attn_args)
-
- if self.full_context_alignment:
- attn_args["full_context_alignment"] = self.full_context_alignment
- _, alignment_out = self.decoder(
- prev_output_tokens,
- encoder_out,
- features_only=True,
- **attn_args,
- **extra_args,
- )
- decoder_out[1]["attn"] = alignment_out["attn"]
-
- return decoder_out
-
-
-@register_model_architecture("transformer_align", "transformer_align")
-def transformer_align(args):
- args.alignment_heads = getattr(args, "alignment_heads", 1)
- args.alignment_layer = getattr(args, "alignment_layer", 4)
- args.full_context_alignment = getattr(args, "full_context_alignment", False)
- base_architecture(args)
-
-
-@register_model_architecture("transformer_align", "transformer_wmt_en_de_big_align")
-def transformer_wmt_en_de_big_align(args):
- args.alignment_heads = getattr(args, "alignment_heads", 1)
- args.alignment_layer = getattr(args, "alignment_layer", 4)
- transformer_wmt_en_de_big(args)
diff --git a/spaces/OpenDILabCommunity/LLMRiddlesChatGPTEN/llmriddles/questions/executor.py b/spaces/OpenDILabCommunity/LLMRiddlesChatGPTEN/llmriddles/questions/executor.py
deleted file mode 100644
index 61dafa769808626ef0f179fed4f6bf45979e8252..0000000000000000000000000000000000000000
--- a/spaces/OpenDILabCommunity/LLMRiddlesChatGPTEN/llmriddles/questions/executor.py
+++ /dev/null
@@ -1,35 +0,0 @@
-from typing import Tuple
-
-from .question import Question
-from ..llms import get_llm_fn
-
-
-class QuestionExecutor:
- def __init__(self, question: Question, lang: str = 'cn', llm: str = 'chatgpt', llm_cfgs=None):
- self.question = question
- self.lang = lang
- self.llm = llm
- self.llm_cfgs = dict(llm_cfgs or {})
-
- @property
- def question_text(self):
- return self.question.texts[self.lang]
-
- @property
- def question_name(self):
- return self.question.names[self.lang]
-
- def check(self, qs_text: str) -> Tuple[str, bool, str]:
- answer_text = get_llm_fn(self.llm)(qs_text, **self.llm_cfgs)
- correct, explanation = self.check_answer(qs_text, answer_text)
- return answer_text, correct, explanation
-
- def check_answer(self, user_text: str, answer_text: str) -> Tuple[bool, str]:
- correct, explanation = self.question.checker(self.question_text, user_text, answer_text, self.lang)
- if explanation is None:
- if correct:
- explanation = 'LLM的回答满足要求' if self.lang == 'cn' else 'Correct Answer From LLM'
- else:
- explanation = 'LLM的回答不满足要求' if self.lang == 'cn' else 'Wrong Answer From LLM'
-
- return correct, explanation
diff --git a/spaces/OpenGVLab/InternGPT/iGPT/models/grit_src/third_party/CenterNet2/detectron2/evaluation/coco_evaluation.py b/spaces/OpenGVLab/InternGPT/iGPT/models/grit_src/third_party/CenterNet2/detectron2/evaluation/coco_evaluation.py
deleted file mode 100644
index aad7f5a6e79a9047e7eea623ecc761ea9655b8d6..0000000000000000000000000000000000000000
--- a/spaces/OpenGVLab/InternGPT/iGPT/models/grit_src/third_party/CenterNet2/detectron2/evaluation/coco_evaluation.py
+++ /dev/null
@@ -1,710 +0,0 @@
-# Copyright (c) Facebook, Inc. and its affiliates.
-import contextlib
-import copy
-import io
-import itertools
-import json
-import logging
-import numpy as np
-import os
-import pickle
-from collections import OrderedDict
-import pycocotools.mask as mask_util
-import torch
-from pycocotools.coco import COCO
-from pycocotools.cocoeval import COCOeval
-from tabulate import tabulate
-
-import detectron2.utils.comm as comm
-from detectron2.config import CfgNode
-from detectron2.data import MetadataCatalog
-from detectron2.data.datasets.coco import convert_to_coco_json
-from detectron2.evaluation.fast_eval_api import COCOeval_opt
-from detectron2.structures import Boxes, BoxMode, pairwise_iou
-from detectron2.utils.file_io import PathManager
-from detectron2.utils.logger import create_small_table
-
-from .evaluator import DatasetEvaluator
-
-
-class COCOEvaluator(DatasetEvaluator):
- """
- Evaluate AR for object proposals, AP for instance detection/segmentation, AP
- for keypoint detection outputs using COCO's metrics.
- See http://cocodataset.org/#detection-eval and
- http://cocodataset.org/#keypoints-eval to understand its metrics.
- The metrics range from 0 to 100 (instead of 0 to 1), where a -1 or NaN means
- the metric cannot be computed (e.g. due to no predictions made).
-
- In addition to COCO, this evaluator is able to support any bounding box detection,
- instance segmentation, or keypoint detection dataset.
- """
-
- def __init__(
- self,
- dataset_name,
- tasks=None,
- distributed=True,
- output_dir=None,
- *,
- max_dets_per_image=None,
- use_fast_impl=True,
- kpt_oks_sigmas=(),
- ):
- """
- Args:
- dataset_name (str): name of the dataset to be evaluated.
- It must have either the following corresponding metadata:
-
- "json_file": the path to the COCO format annotation
-
- Or it must be in detectron2's standard dataset format
- so it can be converted to COCO format automatically.
- tasks (tuple[str]): tasks that can be evaluated under the given
- configuration. A task is one of "bbox", "segm", "keypoints".
- By default, will infer this automatically from predictions.
- distributed (True): if True, will collect results from all ranks and run evaluation
- in the main process.
- Otherwise, will only evaluate the results in the current process.
- output_dir (str): optional, an output directory to dump all
- results predicted on the dataset. The dump contains two files:
-
- 1. "instances_predictions.pth" a file that can be loaded with `torch.load` and
- contains all the results in the format they are produced by the model.
- 2. "coco_instances_results.json" a json file in COCO's result format.
- max_dets_per_image (int): limit on the maximum number of detections per image.
- By default in COCO, this limit is to 100, but this can be customized
- to be greater, as is needed in evaluation metrics AP fixed and AP pool
- (see https://arxiv.org/pdf/2102.01066.pdf)
- This doesn't affect keypoint evaluation.
- use_fast_impl (bool): use a fast but **unofficial** implementation to compute AP.
- Although the results should be very close to the official implementation in COCO
- API, it is still recommended to compute results with the official API for use in
- papers. The faster implementation also uses more RAM.
- kpt_oks_sigmas (list[float]): The sigmas used to calculate keypoint OKS.
- See http://cocodataset.org/#keypoints-eval
- When empty, it will use the defaults in COCO.
- Otherwise it should be the same length as ROI_KEYPOINT_HEAD.NUM_KEYPOINTS.
- """
- self._logger = logging.getLogger(__name__)
- self._distributed = distributed
- self._output_dir = output_dir
- self._use_fast_impl = use_fast_impl
-
- # COCOeval requires the limit on the number of detections per image (maxDets) to be a list
- # with at least 3 elements. The default maxDets in COCOeval is [1, 10, 100], in which the
- # 3rd element (100) is used as the limit on the number of detections per image when
- # evaluating AP. COCOEvaluator expects an integer for max_dets_per_image, so for COCOeval,
- # we reformat max_dets_per_image into [1, 10, max_dets_per_image], based on the defaults.
- if max_dets_per_image is None:
- max_dets_per_image = [1, 10, 100]
- else:
- max_dets_per_image = [1, 10, max_dets_per_image]
- self._max_dets_per_image = max_dets_per_image
-
- if tasks is not None and isinstance(tasks, CfgNode):
- kpt_oks_sigmas = (
- tasks.TEST.KEYPOINT_OKS_SIGMAS if not kpt_oks_sigmas else kpt_oks_sigmas
- )
- self._logger.warn(
- "COCO Evaluator instantiated using config, this is deprecated behavior."
- " Please pass in explicit arguments instead."
- )
- self._tasks = None # Infering it from predictions should be better
- else:
- self._tasks = tasks
-
- self._cpu_device = torch.device("cpu")
-
- self._metadata = MetadataCatalog.get(dataset_name)
- if not hasattr(self._metadata, "json_file"):
- if output_dir is None:
- raise ValueError(
- "output_dir must be provided to COCOEvaluator "
- "for datasets not in COCO format."
- )
- self._logger.info(f"Trying to convert '{dataset_name}' to COCO format ...")
-
- cache_path = os.path.join(output_dir, f"{dataset_name}_coco_format.json")
- self._metadata.json_file = cache_path
- convert_to_coco_json(dataset_name, cache_path)
-
- json_file = PathManager.get_local_path(self._metadata.json_file)
- with contextlib.redirect_stdout(io.StringIO()):
- self._coco_api = COCO(json_file)
-
- # Test set json files do not contain annotations (evaluation must be
- # performed using the COCO evaluation server).
- self._do_evaluation = "annotations" in self._coco_api.dataset
- if self._do_evaluation:
- self._kpt_oks_sigmas = kpt_oks_sigmas
-
- def reset(self):
- self._predictions = []
-
- def process(self, inputs, outputs):
- """
- Args:
- inputs: the inputs to a COCO model (e.g., GeneralizedRCNN).
- It is a list of dict. Each dict corresponds to an image and
- contains keys like "height", "width", "file_name", "image_id".
- outputs: the outputs of a COCO model. It is a list of dicts with key
- "instances" that contains :class:`Instances`.
- """
- for input, output in zip(inputs, outputs):
- prediction = {"image_id": input["image_id"]}
-
- if "instances" in output:
- instances = output["instances"].to(self._cpu_device)
- prediction["instances"] = instances_to_coco_json(instances, input["image_id"])
- if "proposals" in output:
- prediction["proposals"] = output["proposals"].to(self._cpu_device)
- if len(prediction) > 1:
- self._predictions.append(prediction)
-
- def evaluate(self, img_ids=None):
- """
- Args:
- img_ids: a list of image IDs to evaluate on. Default to None for the whole dataset
- """
- if self._distributed:
- comm.synchronize()
- predictions = comm.gather(self._predictions, dst=0)
- predictions = list(itertools.chain(*predictions))
-
- if not comm.is_main_process():
- return {}
- else:
- predictions = self._predictions
-
- if len(predictions) == 0:
- self._logger.warning("[COCOEvaluator] Did not receive valid predictions.")
- return {}
-
- if self._output_dir:
- PathManager.mkdirs(self._output_dir)
- file_path = os.path.join(self._output_dir, "instances_predictions.pth")
- with PathManager.open(file_path, "wb") as f:
- torch.save(predictions, f)
-
- self._results = OrderedDict()
- if "proposals" in predictions[0]:
- self._eval_box_proposals(predictions)
- if "instances" in predictions[0]:
- self._eval_predictions(predictions, img_ids=img_ids)
- # Copy so the caller can do whatever with results
- return copy.deepcopy(self._results)
-
- def _tasks_from_predictions(self, predictions):
- """
- Get COCO API "tasks" (i.e. iou_type) from COCO-format predictions.
- """
- tasks = {"bbox"}
- for pred in predictions:
- if "segmentation" in pred:
- tasks.add("segm")
- if "keypoints" in pred:
- tasks.add("keypoints")
- return sorted(tasks)
-
- def _eval_predictions(self, predictions, img_ids=None):
- """
- Evaluate predictions. Fill self._results with the metrics of the tasks.
- """
- self._logger.info("Preparing results for COCO format ...")
- coco_results = list(itertools.chain(*[x["instances"] for x in predictions]))
- tasks = self._tasks or self._tasks_from_predictions(coco_results)
-
- # unmap the category ids for COCO
- if hasattr(self._metadata, "thing_dataset_id_to_contiguous_id"):
- dataset_id_to_contiguous_id = self._metadata.thing_dataset_id_to_contiguous_id
- all_contiguous_ids = list(dataset_id_to_contiguous_id.values())
- num_classes = len(all_contiguous_ids)
- assert min(all_contiguous_ids) == 0 and max(all_contiguous_ids) == num_classes - 1
-
- reverse_id_mapping = {v: k for k, v in dataset_id_to_contiguous_id.items()}
- for result in coco_results:
- category_id = result["category_id"]
- assert category_id < num_classes, (
- f"A prediction has class={category_id}, "
- f"but the dataset only has {num_classes} classes and "
- f"predicted class id should be in [0, {num_classes - 1}]."
- )
- result["category_id"] = reverse_id_mapping[category_id]
-
- if self._output_dir:
- file_path = os.path.join(self._output_dir, "coco_instances_results.json")
- self._logger.info("Saving results to {}".format(file_path))
- with PathManager.open(file_path, "w") as f:
- f.write(json.dumps(coco_results))
- f.flush()
-
- if not self._do_evaluation:
- self._logger.info("Annotations are not available for evaluation.")
- return
-
- self._logger.info(
- "Evaluating predictions with {} COCO API...".format(
- "unofficial" if self._use_fast_impl else "official"
- )
- )
- for task in sorted(tasks):
- assert task in {"bbox", "segm", "keypoints"}, f"Got unknown task: {task}!"
- coco_eval = (
- _evaluate_predictions_on_coco(
- self._coco_api,
- coco_results,
- task,
- kpt_oks_sigmas=self._kpt_oks_sigmas,
- use_fast_impl=self._use_fast_impl,
- img_ids=img_ids,
- max_dets_per_image=self._max_dets_per_image,
- )
- if len(coco_results) > 0
- else None # cocoapi does not handle empty results very well
- )
-
- res = self._derive_coco_results(
- coco_eval, task, class_names=self._metadata.get("thing_classes")
- )
- self._results[task] = res
-
- def _eval_box_proposals(self, predictions):
- """
- Evaluate the box proposals in predictions.
- Fill self._results with the metrics for "box_proposals" task.
- """
- if self._output_dir:
- # Saving generated box proposals to file.
- # Predicted box_proposals are in XYXY_ABS mode.
- bbox_mode = BoxMode.XYXY_ABS.value
- ids, boxes, objectness_logits = [], [], []
- for prediction in predictions:
- ids.append(prediction["image_id"])
- boxes.append(prediction["proposals"].proposal_boxes.tensor.numpy())
- objectness_logits.append(prediction["proposals"].objectness_logits.numpy())
-
- proposal_data = {
- "boxes": boxes,
- "objectness_logits": objectness_logits,
- "ids": ids,
- "bbox_mode": bbox_mode,
- }
- with PathManager.open(os.path.join(self._output_dir, "box_proposals.pkl"), "wb") as f:
- pickle.dump(proposal_data, f)
-
- if not self._do_evaluation:
- self._logger.info("Annotations are not available for evaluation.")
- return
-
- self._logger.info("Evaluating bbox proposals ...")
- res = {}
- areas = {"all": "", "small": "s", "medium": "m", "large": "l"}
- for limit in [100, 1000]:
- for area, suffix in areas.items():
- stats = _evaluate_box_proposals(predictions, self._coco_api, area=area, limit=limit)
- key = "AR{}@{:d}".format(suffix, limit)
- res[key] = float(stats["ar"].item() * 100)
- self._logger.info("Proposal metrics: \n" + create_small_table(res))
- self._results["box_proposals"] = res
-
- def _derive_coco_results(self, coco_eval, iou_type, class_names=None):
- """
- Derive the desired score numbers from summarized COCOeval.
-
- Args:
- coco_eval (None or COCOEval): None represents no predictions from model.
- iou_type (str):
- class_names (None or list[str]): if provided, will use it to predict
- per-category AP.
-
- Returns:
- a dict of {metric name: score}
- """
-
- metrics = {
- "bbox": ["AP", "AP50", "AP75", "APs", "APm", "APl"],
- "segm": ["AP", "AP50", "AP75", "APs", "APm", "APl"],
- "keypoints": ["AP", "AP50", "AP75", "APm", "APl"],
- }[iou_type]
-
- if coco_eval is None:
- self._logger.warn("No predictions from the model!")
- return {metric: float("nan") for metric in metrics}
-
- # the standard metrics
- results = {
- metric: float(coco_eval.stats[idx] * 100 if coco_eval.stats[idx] >= 0 else "nan")
- for idx, metric in enumerate(metrics)
- }
- self._logger.info(
- "Evaluation results for {}: \n".format(iou_type) + create_small_table(results)
- )
- if not np.isfinite(sum(results.values())):
- self._logger.info("Some metrics cannot be computed and is shown as NaN.")
-
- if class_names is None or len(class_names) <= 1:
- return results
- # Compute per-category AP
- # from https://github.com/facebookresearch/Detectron/blob/a6a835f5b8208c45d0dce217ce9bbda915f44df7/detectron/datasets/json_dataset_evaluator.py#L222-L252 # noqa
- precisions = coco_eval.eval["precision"]
- # precision has dims (iou, recall, cls, area range, max dets)
- assert len(class_names) == precisions.shape[2]
-
- results_per_category = []
- for idx, name in enumerate(class_names):
- # area range index 0: all area ranges
- # max dets index -1: typically 100 per image
- precision = precisions[:, :, idx, 0, -1]
- precision = precision[precision > -1]
- ap = np.mean(precision) if precision.size else float("nan")
- results_per_category.append(("{}".format(name), float(ap * 100)))
-
- # tabulate it
- N_COLS = min(6, len(results_per_category) * 2)
- results_flatten = list(itertools.chain(*results_per_category))
- results_2d = itertools.zip_longest(*[results_flatten[i::N_COLS] for i in range(N_COLS)])
- table = tabulate(
- results_2d,
- tablefmt="pipe",
- floatfmt=".3f",
- headers=["category", "AP"] * (N_COLS // 2),
- numalign="left",
- )
- self._logger.info("Per-category {} AP: \n".format(iou_type) + table)
-
- results.update({"AP-" + name: ap for name, ap in results_per_category})
- return results
-
-
-def instances_to_coco_json(instances, img_id):
- """
- Dump an "Instances" object to a COCO-format json that's used for evaluation.
-
- Args:
- instances (Instances):
- img_id (int): the image id
-
- Returns:
- list[dict]: list of json annotations in COCO format.
- """
- num_instance = len(instances)
- if num_instance == 0:
- return []
-
- boxes = instances.pred_boxes.tensor.numpy()
- boxes = BoxMode.convert(boxes, BoxMode.XYXY_ABS, BoxMode.XYWH_ABS)
- boxes = boxes.tolist()
- scores = instances.scores.tolist()
- classes = instances.pred_classes.tolist()
-
- has_mask = instances.has("pred_masks")
- if has_mask:
- # use RLE to encode the masks, because they are too large and takes memory
- # since this evaluator stores outputs of the entire dataset
- rles = [
- mask_util.encode(np.array(mask[:, :, None], order="F", dtype="uint8"))[0]
- for mask in instances.pred_masks
- ]
- for rle in rles:
- # "counts" is an array encoded by mask_util as a byte-stream. Python3's
- # json writer which always produces strings cannot serialize a bytestream
- # unless you decode it. Thankfully, utf-8 works out (which is also what
- # the pycocotools/_mask.pyx does).
- rle["counts"] = rle["counts"].decode("utf-8")
-
- has_keypoints = instances.has("pred_keypoints")
- if has_keypoints:
- keypoints = instances.pred_keypoints
-
- results = []
- for k in range(num_instance):
- result = {
- "image_id": img_id,
- "category_id": classes[k],
- "bbox": boxes[k],
- "score": scores[k],
- }
- if has_mask:
- result["segmentation"] = rles[k]
- if has_keypoints:
- # In COCO annotations,
- # keypoints coordinates are pixel indices.
- # However our predictions are floating point coordinates.
- # Therefore we subtract 0.5 to be consistent with the annotation format.
- # This is the inverse of data loading logic in `datasets/coco.py`.
- keypoints[k][:, :2] -= 0.5
- result["keypoints"] = keypoints[k].flatten().tolist()
- results.append(result)
- return results
-
-
-# inspired from Detectron:
-# https://github.com/facebookresearch/Detectron/blob/a6a835f5b8208c45d0dce217ce9bbda915f44df7/detectron/datasets/json_dataset_evaluator.py#L255 # noqa
-def _evaluate_box_proposals(dataset_predictions, coco_api, thresholds=None, area="all", limit=None):
- """
- Evaluate detection proposal recall metrics. This function is a much
- faster alternative to the official COCO API recall evaluation code. However,
- it produces slightly different results.
- """
- # Record max overlap value for each gt box
- # Return vector of overlap values
- areas = {
- "all": 0,
- "small": 1,
- "medium": 2,
- "large": 3,
- "96-128": 4,
- "128-256": 5,
- "256-512": 6,
- "512-inf": 7,
- }
- area_ranges = [
- [0 ** 2, 1e5 ** 2], # all
- [0 ** 2, 32 ** 2], # small
- [32 ** 2, 96 ** 2], # medium
- [96 ** 2, 1e5 ** 2], # large
- [96 ** 2, 128 ** 2], # 96-128
- [128 ** 2, 256 ** 2], # 128-256
- [256 ** 2, 512 ** 2], # 256-512
- [512 ** 2, 1e5 ** 2],
- ] # 512-inf
- assert area in areas, "Unknown area range: {}".format(area)
- area_range = area_ranges[areas[area]]
- gt_overlaps = []
- num_pos = 0
-
- for prediction_dict in dataset_predictions:
- predictions = prediction_dict["proposals"]
-
- # sort predictions in descending order
- # TODO maybe remove this and make it explicit in the documentation
- inds = predictions.objectness_logits.sort(descending=True)[1]
- predictions = predictions[inds]
-
- ann_ids = coco_api.getAnnIds(imgIds=prediction_dict["image_id"])
- anno = coco_api.loadAnns(ann_ids)
- gt_boxes = [
- BoxMode.convert(obj["bbox"], BoxMode.XYWH_ABS, BoxMode.XYXY_ABS)
- for obj in anno
- if obj["iscrowd"] == 0
- ]
- gt_boxes = torch.as_tensor(gt_boxes).reshape(-1, 4) # guard against no boxes
- gt_boxes = Boxes(gt_boxes)
- gt_areas = torch.as_tensor([obj["area"] for obj in anno if obj["iscrowd"] == 0])
-
- if len(gt_boxes) == 0 or len(predictions) == 0:
- continue
-
- valid_gt_inds = (gt_areas >= area_range[0]) & (gt_areas <= area_range[1])
- gt_boxes = gt_boxes[valid_gt_inds]
-
- num_pos += len(gt_boxes)
-
- if len(gt_boxes) == 0:
- continue
-
- if limit is not None and len(predictions) > limit:
- predictions = predictions[:limit]
-
- overlaps = pairwise_iou(predictions.proposal_boxes, gt_boxes)
-
- _gt_overlaps = torch.zeros(len(gt_boxes))
- for j in range(min(len(predictions), len(gt_boxes))):
- # find which proposal box maximally covers each gt box
- # and get the iou amount of coverage for each gt box
- max_overlaps, argmax_overlaps = overlaps.max(dim=0)
-
- # find which gt box is 'best' covered (i.e. 'best' = most iou)
- gt_ovr, gt_ind = max_overlaps.max(dim=0)
- assert gt_ovr >= 0
- # find the proposal box that covers the best covered gt box
- box_ind = argmax_overlaps[gt_ind]
- # record the iou coverage of this gt box
- _gt_overlaps[j] = overlaps[box_ind, gt_ind]
- assert _gt_overlaps[j] == gt_ovr
- # mark the proposal box and the gt box as used
- overlaps[box_ind, :] = -1
- overlaps[:, gt_ind] = -1
-
- # append recorded iou coverage level
- gt_overlaps.append(_gt_overlaps)
- gt_overlaps = (
- torch.cat(gt_overlaps, dim=0) if len(gt_overlaps) else torch.zeros(0, dtype=torch.float32)
- )
- gt_overlaps, _ = torch.sort(gt_overlaps)
-
- if thresholds is None:
- step = 0.05
- thresholds = torch.arange(0.5, 0.95 + 1e-5, step, dtype=torch.float32)
- recalls = torch.zeros_like(thresholds)
- # compute recall for each iou threshold
- for i, t in enumerate(thresholds):
- recalls[i] = (gt_overlaps >= t).float().sum() / float(num_pos)
- # ar = 2 * np.trapz(recalls, thresholds)
- ar = recalls.mean()
- return {
- "ar": ar,
- "recalls": recalls,
- "thresholds": thresholds,
- "gt_overlaps": gt_overlaps,
- "num_pos": num_pos,
- }
-
-
-def _evaluate_predictions_on_coco(
- coco_gt,
- coco_results,
- iou_type,
- kpt_oks_sigmas=None,
- use_fast_impl=True,
- img_ids=None,
- max_dets_per_image=None,
-):
- """
- Evaluate the coco results using COCOEval API.
- """
- assert len(coco_results) > 0
-
- if iou_type == "segm":
- coco_results = copy.deepcopy(coco_results)
- # When evaluating mask AP, if the results contain bbox, cocoapi will
- # use the box area as the area of the instance, instead of the mask area.
- # This leads to a different definition of small/medium/large.
- # We remove the bbox field to let mask AP use mask area.
- for c in coco_results:
- c.pop("bbox", None)
-
- coco_dt = coco_gt.loadRes(coco_results)
- coco_eval = (COCOeval_opt if use_fast_impl else COCOeval)(coco_gt, coco_dt, iou_type)
- # For COCO, the default max_dets_per_image is [1, 10, 100].
- if max_dets_per_image is None:
- max_dets_per_image = [1, 10, 100] # Default from COCOEval
- else:
- assert (
- len(max_dets_per_image) >= 3
- ), "COCOeval requires maxDets (and max_dets_per_image) to have length at least 3"
- # In the case that user supplies a custom input for max_dets_per_image,
- # apply COCOevalMaxDets to evaluate AP with the custom input.
- if max_dets_per_image[2] != 100:
- coco_eval = COCOevalMaxDets(coco_gt, coco_dt, iou_type)
- if iou_type != "keypoints":
- coco_eval.params.maxDets = max_dets_per_image
-
- if img_ids is not None:
- coco_eval.params.imgIds = img_ids
-
- if iou_type == "keypoints":
- # Use the COCO default keypoint OKS sigmas unless overrides are specified
- if kpt_oks_sigmas:
- assert hasattr(coco_eval.params, "kpt_oks_sigmas"), "pycocotools is too old!"
- coco_eval.params.kpt_oks_sigmas = np.array(kpt_oks_sigmas)
- # COCOAPI requires every detection and every gt to have keypoints, so
- # we just take the first entry from both
- num_keypoints_dt = len(coco_results[0]["keypoints"]) // 3
- num_keypoints_gt = len(next(iter(coco_gt.anns.values()))["keypoints"]) // 3
- num_keypoints_oks = len(coco_eval.params.kpt_oks_sigmas)
- assert num_keypoints_oks == num_keypoints_dt == num_keypoints_gt, (
- f"[COCOEvaluator] Prediction contain {num_keypoints_dt} keypoints. "
- f"Ground truth contains {num_keypoints_gt} keypoints. "
- f"The length of cfg.TEST.KEYPOINT_OKS_SIGMAS is {num_keypoints_oks}. "
- "They have to agree with each other. For meaning of OKS, please refer to "
- "http://cocodataset.org/#keypoints-eval."
- )
-
- coco_eval.evaluate()
- coco_eval.accumulate()
- coco_eval.summarize()
-
- return coco_eval
-
-
-class COCOevalMaxDets(COCOeval):
- """
- Modified version of COCOeval for evaluating AP with a custom
- maxDets (by default for COCO, maxDets is 100)
- """
-
- def summarize(self):
- """
- Compute and display summary metrics for evaluation results given
- a custom value for max_dets_per_image
- """
-
- def _summarize(ap=1, iouThr=None, areaRng="all", maxDets=100):
- p = self.params
- iStr = " {:<18} {} @[ IoU={:<9} | area={:>6s} | maxDets={:>3d} ] = {:0.3f}"
- titleStr = "Average Precision" if ap == 1 else "Average Recall"
- typeStr = "(AP)" if ap == 1 else "(AR)"
- iouStr = (
- "{:0.2f}:{:0.2f}".format(p.iouThrs[0], p.iouThrs[-1])
- if iouThr is None
- else "{:0.2f}".format(iouThr)
- )
-
- aind = [i for i, aRng in enumerate(p.areaRngLbl) if aRng == areaRng]
- mind = [i for i, mDet in enumerate(p.maxDets) if mDet == maxDets]
- if ap == 1:
- # dimension of precision: [TxRxKxAxM]
- s = self.eval["precision"]
- # IoU
- if iouThr is not None:
- t = np.where(iouThr == p.iouThrs)[0]
- s = s[t]
- s = s[:, :, :, aind, mind]
- else:
- # dimension of recall: [TxKxAxM]
- s = self.eval["recall"]
- if iouThr is not None:
- t = np.where(iouThr == p.iouThrs)[0]
- s = s[t]
- s = s[:, :, aind, mind]
- if len(s[s > -1]) == 0:
- mean_s = -1
- else:
- mean_s = np.mean(s[s > -1])
- print(iStr.format(titleStr, typeStr, iouStr, areaRng, maxDets, mean_s))
- return mean_s
-
- def _summarizeDets():
- stats = np.zeros((12,))
- # Evaluate AP using the custom limit on maximum detections per image
- stats[0] = _summarize(1, maxDets=self.params.maxDets[2])
- stats[1] = _summarize(1, iouThr=0.5, maxDets=self.params.maxDets[2])
- stats[2] = _summarize(1, iouThr=0.75, maxDets=self.params.maxDets[2])
- stats[3] = _summarize(1, areaRng="small", maxDets=self.params.maxDets[2])
- stats[4] = _summarize(1, areaRng="medium", maxDets=self.params.maxDets[2])
- stats[5] = _summarize(1, areaRng="large", maxDets=self.params.maxDets[2])
- stats[6] = _summarize(0, maxDets=self.params.maxDets[0])
- stats[7] = _summarize(0, maxDets=self.params.maxDets[1])
- stats[8] = _summarize(0, maxDets=self.params.maxDets[2])
- stats[9] = _summarize(0, areaRng="small", maxDets=self.params.maxDets[2])
- stats[10] = _summarize(0, areaRng="medium", maxDets=self.params.maxDets[2])
- stats[11] = _summarize(0, areaRng="large", maxDets=self.params.maxDets[2])
- return stats
-
- def _summarizeKps():
- stats = np.zeros((10,))
- stats[0] = _summarize(1, maxDets=20)
- stats[1] = _summarize(1, maxDets=20, iouThr=0.5)
- stats[2] = _summarize(1, maxDets=20, iouThr=0.75)
- stats[3] = _summarize(1, maxDets=20, areaRng="medium")
- stats[4] = _summarize(1, maxDets=20, areaRng="large")
- stats[5] = _summarize(0, maxDets=20)
- stats[6] = _summarize(0, maxDets=20, iouThr=0.5)
- stats[7] = _summarize(0, maxDets=20, iouThr=0.75)
- stats[8] = _summarize(0, maxDets=20, areaRng="medium")
- stats[9] = _summarize(0, maxDets=20, areaRng="large")
- return stats
-
- if not self.eval:
- raise Exception("Please run accumulate() first")
- iouType = self.params.iouType
- if iouType == "segm" or iouType == "bbox":
- summarize = _summarizeDets
- elif iouType == "keypoints":
- summarize = _summarizeKps
- self.stats = summarize()
-
- def __str__(self):
- self.summarize()
diff --git a/spaces/PAIR/PAIR-Diffusion/annotator/OneFormer/oneformer/modeling/pixel_decoder/fpn.py b/spaces/PAIR/PAIR-Diffusion/annotator/OneFormer/oneformer/modeling/pixel_decoder/fpn.py
deleted file mode 100644
index 1828c74c27a1bd726af71e478d6e6cbb3a4e84ae..0000000000000000000000000000000000000000
--- a/spaces/PAIR/PAIR-Diffusion/annotator/OneFormer/oneformer/modeling/pixel_decoder/fpn.py
+++ /dev/null
@@ -1,314 +0,0 @@
-# ------------------------------------------------------------------------------
-# Reference: https://github.com/facebookresearch/Mask2Former/blob/main/mask2former/modeling/pixel_decoder/fpn.py
-# ------------------------------------------------------------------------------
-import logging
-import numpy as np
-from typing import Callable, Dict, List, Optional, Tuple, Union
-
-import fvcore.nn.weight_init as weight_init
-import torch
-from torch import nn
-from torch.nn import functional as F
-from torch.nn.init import xavier_uniform_, constant_, uniform_, normal_
-from torch.cuda.amp import autocast
-
-from detectron2.config import configurable
-from detectron2.layers import Conv2d, DeformConv, ShapeSpec, get_norm
-from detectron2.modeling import SEM_SEG_HEADS_REGISTRY
-
-from ..transformer_decoder.position_encoding import PositionEmbeddingSine
-from ..transformer_decoder.transformer import TransformerEncoder, TransformerEncoderLayer, _get_clones, _get_activation_fn
-
-
-def build_pixel_decoder(cfg, input_shape):
- """
- Build a pixel decoder from `cfg.MODEL.ONE_FORMER.PIXEL_DECODER_NAME`.
- """
- name = cfg.MODEL.SEM_SEG_HEAD.PIXEL_DECODER_NAME
- model = SEM_SEG_HEADS_REGISTRY.get(name)(cfg, input_shape)
- forward_features = getattr(model, "forward_features", None)
- if not callable(forward_features):
- raise ValueError(
- "Only SEM_SEG_HEADS with forward_features method can be used as pixel decoder. "
- f"Please implement forward_features for {name} to only return mask features."
- )
- return model
-
-
-# This is a modified FPN decoder.
-@SEM_SEG_HEADS_REGISTRY.register()
-class BasePixelDecoder(nn.Module):
- @configurable
- def __init__(
- self,
- input_shape: Dict[str, ShapeSpec],
- *,
- conv_dim: int,
- mask_dim: int,
- norm: Optional[Union[str, Callable]] = None,
- ):
- """
- NOTE: this interface is experimental.
- Args:
- input_shape: shapes (channels and stride) of the input features
- conv_dims: number of output channels for the intermediate conv layers.
- mask_dim: number of output channels for the final conv layer.
- norm (str or callable): normalization for all conv layers
- """
- super().__init__()
-
- input_shape = sorted(input_shape.items(), key=lambda x: x[1].stride)
- self.in_features = [k for k, v in input_shape] # starting from "res2" to "res5"
- feature_channels = [v.channels for k, v in input_shape]
-
- lateral_convs = []
- output_convs = []
-
- use_bias = norm == ""
- for idx, in_channels in enumerate(feature_channels):
- if idx == len(self.in_features) - 1:
- output_norm = get_norm(norm, conv_dim)
- output_conv = Conv2d(
- in_channels,
- conv_dim,
- kernel_size=3,
- stride=1,
- padding=1,
- bias=use_bias,
- norm=output_norm,
- activation=F.relu,
- )
- weight_init.c2_xavier_fill(output_conv)
- self.add_module("layer_{}".format(idx + 1), output_conv)
-
- lateral_convs.append(None)
- output_convs.append(output_conv)
- else:
- lateral_norm = get_norm(norm, conv_dim)
- output_norm = get_norm(norm, conv_dim)
-
- lateral_conv = Conv2d(
- in_channels, conv_dim, kernel_size=1, bias=use_bias, norm=lateral_norm
- )
- output_conv = Conv2d(
- conv_dim,
- conv_dim,
- kernel_size=3,
- stride=1,
- padding=1,
- bias=use_bias,
- norm=output_norm,
- activation=F.relu,
- )
- weight_init.c2_xavier_fill(lateral_conv)
- weight_init.c2_xavier_fill(output_conv)
- self.add_module("adapter_{}".format(idx + 1), lateral_conv)
- self.add_module("layer_{}".format(idx + 1), output_conv)
-
- lateral_convs.append(lateral_conv)
- output_convs.append(output_conv)
- # Place convs into top-down order (from low to high resolution)
- # to make the top-down computation in forward clearer.
- self.lateral_convs = lateral_convs[::-1]
- self.output_convs = output_convs[::-1]
-
- self.mask_dim = mask_dim
- self.mask_features = Conv2d(
- conv_dim,
- mask_dim,
- kernel_size=3,
- stride=1,
- padding=1,
- )
- weight_init.c2_xavier_fill(self.mask_features)
-
- self.oneformer_num_feature_levels = 3 # always use 3 scales
-
- @classmethod
- def from_config(cls, cfg, input_shape: Dict[str, ShapeSpec]):
- ret = {}
- ret["input_shape"] = {
- k: v for k, v in input_shape.items() if k in cfg.MODEL.SEM_SEG_HEAD.IN_FEATURES
- }
- ret["conv_dim"] = cfg.MODEL.SEM_SEG_HEAD.CONVS_DIM
- ret["mask_dim"] = cfg.MODEL.SEM_SEG_HEAD.MASK_DIM
- ret["norm"] = cfg.MODEL.SEM_SEG_HEAD.NORM
- return ret
-
- def forward_features(self, features):
- multi_scale_features = []
- num_cur_levels = 0
- # Reverse feature maps into top-down order (from low to high resolution)
- for idx, f in enumerate(self.in_features[::-1]):
- x = features[f]
- lateral_conv = self.lateral_convs[idx]
- output_conv = self.output_convs[idx]
- if lateral_conv is None:
- y = output_conv(x)
- else:
- cur_fpn = lateral_conv(x)
- # Following FPN implementation, we use nearest upsampling here
- y = cur_fpn + F.interpolate(y, size=cur_fpn.shape[-2:], mode="nearest")
- y = output_conv(y)
- if num_cur_levels < self.oneformer_num_feature_levels:
- multi_scale_features.append(y)
- num_cur_levels += 1
- return self.mask_features(y), None, multi_scale_features
-
- def forward(self, features, targets=None):
- logger = logging.getLogger(__name__)
- logger.warning("Calling forward() may cause unpredicted behavior of PixelDecoder module.")
- return self.forward_features(features)
-
-
-class TransformerEncoderOnly(nn.Module):
- def __init__(
- self,
- d_model=512,
- nhead=8,
- num_encoder_layers=6,
- dim_feedforward=2048,
- dropout=0.1,
- activation="relu",
- normalize_before=False,
- ):
- super().__init__()
-
- encoder_layer = TransformerEncoderLayer(
- d_model, nhead, dim_feedforward, dropout, activation, normalize_before
- )
- encoder_norm = nn.LayerNorm(d_model) if normalize_before else None
- self.encoder = TransformerEncoder(encoder_layer, num_encoder_layers, encoder_norm)
-
- self._reset_parameters()
-
- self.d_model = d_model
- self.nhead = nhead
-
- def _reset_parameters(self):
- for p in self.parameters():
- if p.dim() > 1:
- nn.init.xavier_uniform_(p)
-
- def forward(self, src, mask, pos_embed):
- # flatten NxCxHxW to HWxNxC
- bs, c, h, w = src.shape
- src = src.flatten(2).permute(2, 0, 1)
- pos_embed = pos_embed.flatten(2).permute(2, 0, 1)
- if mask is not None:
- mask = mask.flatten(1)
-
- memory = self.encoder(src, src_key_padding_mask=mask, pos=pos_embed)
- return memory.permute(1, 2, 0).view(bs, c, h, w)
-
-
-# This is a modified FPN decoder with extra Transformer encoder that processes the lowest-resolution feature map.
-@SEM_SEG_HEADS_REGISTRY.register()
-class TransformerEncoderPixelDecoder(BasePixelDecoder):
- @configurable
- def __init__(
- self,
- input_shape: Dict[str, ShapeSpec],
- *,
- transformer_dropout: float,
- transformer_nheads: int,
- transformer_dim_feedforward: int,
- transformer_enc_layers: int,
- transformer_pre_norm: bool,
- conv_dim: int,
- mask_dim: int,
- norm: Optional[Union[str, Callable]] = None,
- ):
- """
- NOTE: this interface is experimental.
- Args:
- input_shape: shapes (channels and stride) of the input features
- transformer_dropout: dropout probability in transformer
- transformer_nheads: number of heads in transformer
- transformer_dim_feedforward: dimension of feedforward network
- transformer_enc_layers: number of transformer encoder layers
- transformer_pre_norm: whether to use pre-layernorm or not
- conv_dims: number of output channels for the intermediate conv layers.
- mask_dim: number of output channels for the final conv layer.
- norm (str or callable): normalization for all conv layers
- """
- super().__init__(input_shape, conv_dim=conv_dim, mask_dim=mask_dim, norm=norm)
-
- input_shape = sorted(input_shape.items(), key=lambda x: x[1].stride)
- self.in_features = [k for k, v in input_shape] # starting from "res2" to "res5"
- feature_strides = [v.stride for k, v in input_shape]
- feature_channels = [v.channels for k, v in input_shape]
-
- in_channels = feature_channels[len(self.in_features) - 1]
- self.input_proj = Conv2d(in_channels, conv_dim, kernel_size=1)
- weight_init.c2_xavier_fill(self.input_proj)
- self.transformer = TransformerEncoderOnly(
- d_model=conv_dim,
- dropout=transformer_dropout,
- nhead=transformer_nheads,
- dim_feedforward=transformer_dim_feedforward,
- num_encoder_layers=transformer_enc_layers,
- normalize_before=transformer_pre_norm,
- )
- N_steps = conv_dim // 2
- self.pe_layer = PositionEmbeddingSine(N_steps, normalize=True)
-
- # update layer
- use_bias = norm == ""
- output_norm = get_norm(norm, conv_dim)
- output_conv = Conv2d(
- conv_dim,
- conv_dim,
- kernel_size=3,
- stride=1,
- padding=1,
- bias=use_bias,
- norm=output_norm,
- activation=F.relu,
- )
- weight_init.c2_xavier_fill(output_conv)
- delattr(self, "layer_{}".format(len(self.in_features)))
- self.add_module("layer_{}".format(len(self.in_features)), output_conv)
- self.output_convs[0] = output_conv
-
- @classmethod
- def from_config(cls, cfg, input_shape: Dict[str, ShapeSpec]):
- ret = super().from_config(cfg, input_shape)
- ret["transformer_dropout"] = cfg.MODEL.ONE_FORMER.DROPOUT
- ret["transformer_nheads"] = cfg.MODEL.ONE_FORMER.NHEADS
- ret["transformer_dim_feedforward"] = cfg.MODEL.ONE_FORMER.DIM_FEEDFORWARD
- ret[
- "transformer_enc_layers"
- ] = cfg.MODEL.SEM_SEG_HEAD.TRANSFORMER_ENC_LAYERS # a separate config
- ret["transformer_pre_norm"] = cfg.MODEL.ONE_FORMER.PRE_NORM
- return ret
-
- def forward_features(self, features):
- multi_scale_features = []
- num_cur_levels = 0
- # Reverse feature maps into top-down order (from low to high resolution)
- for idx, f in enumerate(self.in_features[::-1]):
- x = features[f]
- lateral_conv = self.lateral_convs[idx]
- output_conv = self.output_convs[idx]
- if lateral_conv is None:
- transformer = self.input_proj(x)
- pos = self.pe_layer(x)
- transformer = self.transformer(transformer, None, pos)
- y = output_conv(transformer)
- # save intermediate feature as input to Transformer decoder
- transformer_encoder_features = transformer
- else:
- cur_fpn = lateral_conv(x)
- # Following FPN implementation, we use nearest upsampling here
- y = cur_fpn + F.interpolate(y, size=cur_fpn.shape[-2:], mode="nearest")
- y = output_conv(y)
- if num_cur_levels < self.oneformer_num_feature_levels:
- multi_scale_features.append(y)
- num_cur_levels += 1
- return self.mask_features(y), transformer_encoder_features, multi_scale_features
-
- def forward(self, features, targets=None):
- logger = logging.getLogger(__name__)
- logger.warning("Calling forward() may cause unpredicted behavior of PixelDecoder module.")
- return self.forward_features(features)
diff --git a/spaces/PAIR/Text2Video-Zero/annotator/uniformer/mmcv/ops/iou3d.py b/spaces/PAIR/Text2Video-Zero/annotator/uniformer/mmcv/ops/iou3d.py
deleted file mode 100644
index 6fc71979190323f44c09f8b7e1761cf49cd2d76b..0000000000000000000000000000000000000000
--- a/spaces/PAIR/Text2Video-Zero/annotator/uniformer/mmcv/ops/iou3d.py
+++ /dev/null
@@ -1,85 +0,0 @@
-# Copyright (c) OpenMMLab. All rights reserved.
-import torch
-
-from ..utils import ext_loader
-
-ext_module = ext_loader.load_ext('_ext', [
- 'iou3d_boxes_iou_bev_forward', 'iou3d_nms_forward',
- 'iou3d_nms_normal_forward'
-])
-
-
-def boxes_iou_bev(boxes_a, boxes_b):
- """Calculate boxes IoU in the Bird's Eye View.
-
- Args:
- boxes_a (torch.Tensor): Input boxes a with shape (M, 5).
- boxes_b (torch.Tensor): Input boxes b with shape (N, 5).
-
- Returns:
- ans_iou (torch.Tensor): IoU result with shape (M, N).
- """
- ans_iou = boxes_a.new_zeros(
- torch.Size((boxes_a.shape[0], boxes_b.shape[0])))
-
- ext_module.iou3d_boxes_iou_bev_forward(boxes_a.contiguous(),
- boxes_b.contiguous(), ans_iou)
-
- return ans_iou
-
-
-def nms_bev(boxes, scores, thresh, pre_max_size=None, post_max_size=None):
- """NMS function GPU implementation (for BEV boxes). The overlap of two
- boxes for IoU calculation is defined as the exact overlapping area of the
- two boxes. In this function, one can also set ``pre_max_size`` and
- ``post_max_size``.
-
- Args:
- boxes (torch.Tensor): Input boxes with the shape of [N, 5]
- ([x1, y1, x2, y2, ry]).
- scores (torch.Tensor): Scores of boxes with the shape of [N].
- thresh (float): Overlap threshold of NMS.
- pre_max_size (int, optional): Max size of boxes before NMS.
- Default: None.
- post_max_size (int, optional): Max size of boxes after NMS.
- Default: None.
-
- Returns:
- torch.Tensor: Indexes after NMS.
- """
- assert boxes.size(1) == 5, 'Input boxes shape should be [N, 5]'
- order = scores.sort(0, descending=True)[1]
-
- if pre_max_size is not None:
- order = order[:pre_max_size]
- boxes = boxes[order].contiguous()
-
- keep = torch.zeros(boxes.size(0), dtype=torch.long)
- num_out = ext_module.iou3d_nms_forward(boxes, keep, thresh)
- keep = order[keep[:num_out].cuda(boxes.device)].contiguous()
- if post_max_size is not None:
- keep = keep[:post_max_size]
- return keep
-
-
-def nms_normal_bev(boxes, scores, thresh):
- """Normal NMS function GPU implementation (for BEV boxes). The overlap of
- two boxes for IoU calculation is defined as the exact overlapping area of
- the two boxes WITH their yaw angle set to 0.
-
- Args:
- boxes (torch.Tensor): Input boxes with shape (N, 5).
- scores (torch.Tensor): Scores of predicted boxes with shape (N).
- thresh (float): Overlap threshold of NMS.
-
- Returns:
- torch.Tensor: Remaining indices with scores in descending order.
- """
- assert boxes.shape[1] == 5, 'Input boxes shape should be [N, 5]'
- order = scores.sort(0, descending=True)[1]
-
- boxes = boxes[order].contiguous()
-
- keep = torch.zeros(boxes.size(0), dtype=torch.long)
- num_out = ext_module.iou3d_nms_normal_forward(boxes, keep, thresh)
- return order[keep[:num_out].cuda(boxes.device)].contiguous()
diff --git a/spaces/Pattr/DrumClassification/lilypond-2.24.2/lib/guile/2.2/ccache/ice-9/optargs.go b/spaces/Pattr/DrumClassification/lilypond-2.24.2/lib/guile/2.2/ccache/ice-9/optargs.go
deleted file mode 100644
index 9c51cff705da039d905a09463dcb775074c9799e..0000000000000000000000000000000000000000
Binary files a/spaces/Pattr/DrumClassification/lilypond-2.24.2/lib/guile/2.2/ccache/ice-9/optargs.go and /dev/null differ
diff --git a/spaces/Pie31415/control-animation/annotator/uniformer/mmseg/datasets/voc.py b/spaces/Pie31415/control-animation/annotator/uniformer/mmseg/datasets/voc.py
deleted file mode 100644
index a8855203b14ee0dc4da9099a2945d4aedcffbcd6..0000000000000000000000000000000000000000
--- a/spaces/Pie31415/control-animation/annotator/uniformer/mmseg/datasets/voc.py
+++ /dev/null
@@ -1,29 +0,0 @@
-import os.path as osp
-
-from .builder import DATASETS
-from .custom import CustomDataset
-
-
-@DATASETS.register_module()
-class PascalVOCDataset(CustomDataset):
- """Pascal VOC dataset.
-
- Args:
- split (str): Split txt file for Pascal VOC.
- """
-
- CLASSES = ('background', 'aeroplane', 'bicycle', 'bird', 'boat', 'bottle',
- 'bus', 'car', 'cat', 'chair', 'cow', 'diningtable', 'dog',
- 'horse', 'motorbike', 'person', 'pottedplant', 'sheep', 'sofa',
- 'train', 'tvmonitor')
-
- PALETTE = [[0, 0, 0], [128, 0, 0], [0, 128, 0], [128, 128, 0], [0, 0, 128],
- [128, 0, 128], [0, 128, 128], [128, 128, 128], [64, 0, 0],
- [192, 0, 0], [64, 128, 0], [192, 128, 0], [64, 0, 128],
- [192, 0, 128], [64, 128, 128], [192, 128, 128], [0, 64, 0],
- [128, 64, 0], [0, 192, 0], [128, 192, 0], [0, 64, 128]]
-
- def __init__(self, split, **kwargs):
- super(PascalVOCDataset, self).__init__(
- img_suffix='.jpg', seg_map_suffix='.png', split=split, **kwargs)
- assert osp.exists(self.img_dir) and self.split is not None
diff --git a/spaces/Pinwheel/GLIP-BLIP-Object-Detection-VQA/maskrcnn_benchmark/utils/c2_model_loading.py b/spaces/Pinwheel/GLIP-BLIP-Object-Detection-VQA/maskrcnn_benchmark/utils/c2_model_loading.py
deleted file mode 100644
index eb8d311b535471329764823075e668df35cd8cac..0000000000000000000000000000000000000000
--- a/spaces/Pinwheel/GLIP-BLIP-Object-Detection-VQA/maskrcnn_benchmark/utils/c2_model_loading.py
+++ /dev/null
@@ -1,207 +0,0 @@
-# Copyright (c) Facebook, Inc. and its affiliates. All Rights Reserved.
-import logging
-import pickle
-from collections import OrderedDict
-
-import torch
-
-from maskrcnn_benchmark.utils.model_serialization import load_state_dict
-from maskrcnn_benchmark.utils.registry import Registry
-
-
-def _rename_basic_resnet_weights(layer_keys):
- layer_keys = [k.replace("_", ".") for k in layer_keys]
- layer_keys = [k.replace(".w", ".weight") for k in layer_keys]
- layer_keys = [k.replace(".bn", "_bn") for k in layer_keys]
- layer_keys = [k.replace(".b", ".bias") for k in layer_keys]
- layer_keys = [k.replace("_bn.s", "_bn.scale") for k in layer_keys]
- layer_keys = [k.replace(".biasranch", ".branch") for k in layer_keys]
- layer_keys = [k.replace("bbox.pred", "bbox_pred") for k in layer_keys]
- layer_keys = [k.replace("cls.score", "cls_score") for k in layer_keys]
- layer_keys = [k.replace("res.conv1_", "conv1_") for k in layer_keys]
-
- # RPN / Faster RCNN
- layer_keys = [k.replace(".biasbox", ".bbox") for k in layer_keys]
- layer_keys = [k.replace("conv.rpn", "rpn.conv") for k in layer_keys]
- layer_keys = [k.replace("rpn.bbox.pred", "rpn.bbox_pred") for k in layer_keys]
- layer_keys = [k.replace("rpn.cls.logits", "rpn.cls_logits") for k in layer_keys]
-
- # Affine-Channel -> BatchNorm enaming
- layer_keys = [k.replace("_bn.scale", "_bn.weight") for k in layer_keys]
-
- # Make torchvision-compatible
- layer_keys = [k.replace("conv1_bn.", "bn1.") for k in layer_keys]
-
- layer_keys = [k.replace("res2.", "layer1.") for k in layer_keys]
- layer_keys = [k.replace("res3.", "layer2.") for k in layer_keys]
- layer_keys = [k.replace("res4.", "layer3.") for k in layer_keys]
- layer_keys = [k.replace("res5.", "layer4.") for k in layer_keys]
-
- layer_keys = [k.replace(".branch2a.", ".conv1.") for k in layer_keys]
- layer_keys = [k.replace(".branch2a_bn.", ".bn1.") for k in layer_keys]
- layer_keys = [k.replace(".branch2b.", ".conv2.") for k in layer_keys]
- layer_keys = [k.replace(".branch2b_bn.", ".bn2.") for k in layer_keys]
- layer_keys = [k.replace(".branch2c.", ".conv3.") for k in layer_keys]
- layer_keys = [k.replace(".branch2c_bn.", ".bn3.") for k in layer_keys]
-
- layer_keys = [k.replace(".branch1.", ".downsample.0.") for k in layer_keys]
- layer_keys = [k.replace(".branch1_bn.", ".downsample.1.") for k in layer_keys]
-
- # GroupNorm
- layer_keys = [k.replace("conv1.gn.s", "bn1.weight") for k in layer_keys]
- layer_keys = [k.replace("conv1.gn.bias", "bn1.bias") for k in layer_keys]
- layer_keys = [k.replace("conv2.gn.s", "bn2.weight") for k in layer_keys]
- layer_keys = [k.replace("conv2.gn.bias", "bn2.bias") for k in layer_keys]
- layer_keys = [k.replace("conv3.gn.s", "bn3.weight") for k in layer_keys]
- layer_keys = [k.replace("conv3.gn.bias", "bn3.bias") for k in layer_keys]
- layer_keys = [k.replace("downsample.0.gn.s", "downsample.1.weight") \
- for k in layer_keys]
- layer_keys = [k.replace("downsample.0.gn.bias", "downsample.1.bias") \
- for k in layer_keys]
-
- return layer_keys
-
-def _rename_fpn_weights(layer_keys, stage_names):
- for mapped_idx, stage_name in enumerate(stage_names, 1):
- suffix = ""
- if mapped_idx < 4:
- suffix = ".lateral"
- layer_keys = [
- k.replace("fpn.inner.layer{}.sum{}".format(stage_name, suffix), "fpn_inner{}".format(mapped_idx)) for k in layer_keys
- ]
- layer_keys = [k.replace("fpn.layer{}.sum".format(stage_name), "fpn_layer{}".format(mapped_idx)) for k in layer_keys]
-
-
- layer_keys = [k.replace("rpn.conv.fpn2", "rpn.conv") for k in layer_keys]
- layer_keys = [k.replace("rpn.bbox_pred.fpn2", "rpn.bbox_pred") for k in layer_keys]
- layer_keys = [
- k.replace("rpn.cls_logits.fpn2", "rpn.cls_logits") for k in layer_keys
- ]
-
- return layer_keys
-
-
-def _rename_weights_for_resnet(weights, stage_names):
- original_keys = sorted(weights.keys())
- layer_keys = sorted(weights.keys())
-
- # for X-101, rename output to fc1000 to avoid conflicts afterwards
- layer_keys = [k if k != "pred_b" else "fc1000_b" for k in layer_keys]
- layer_keys = [k if k != "pred_w" else "fc1000_w" for k in layer_keys]
-
- # performs basic renaming: _ -> . , etc
- layer_keys = _rename_basic_resnet_weights(layer_keys)
-
- # FPN
- layer_keys = _rename_fpn_weights(layer_keys, stage_names)
-
- # Mask R-CNN
- layer_keys = [k.replace("mask.fcn.logits", "mask_fcn_logits") for k in layer_keys]
- layer_keys = [k.replace(".[mask].fcn", "mask_fcn") for k in layer_keys]
- layer_keys = [k.replace("conv5.mask", "conv5_mask") for k in layer_keys]
-
- # Keypoint R-CNN
- layer_keys = [k.replace("kps.score.lowres", "kps_score_lowres") for k in layer_keys]
- layer_keys = [k.replace("kps.score", "kps_score") for k in layer_keys]
- layer_keys = [k.replace("conv.fcn", "conv_fcn") for k in layer_keys]
-
- # Rename for our RPN structure
- layer_keys = [k.replace("rpn.", "rpn.head.") for k in layer_keys]
-
- key_map = {k: v for k, v in zip(original_keys, layer_keys)}
-
- logger = logging.getLogger(__name__)
- logger.info("Remapping C2 weights")
- max_c2_key_size = max([len(k) for k in original_keys if "_momentum" not in k])
-
- new_weights = OrderedDict()
- for k in original_keys:
- v = weights[k]
- if "_momentum" in k:
- continue
- if 'weight_order' in k:
- continue
- # if 'fc1000' in k:
- # continue
- w = torch.from_numpy(v)
- # if "bn" in k:
- # w = w.view(1, -1, 1, 1)
- logger.info("C2 name: {: <{}} mapped name: {}".format(k, max_c2_key_size, key_map[k]))
- new_weights[key_map[k]] = w
-
- return new_weights
-
-
-def _load_c2_pickled_weights(file_path):
- with open(file_path, "rb") as f:
- if torch._six.PY3:
- data = pickle.load(f, encoding="latin1")
- else:
- data = pickle.load(f)
- if "blobs" in data:
- weights = data["blobs"]
- else:
- weights = data
- return weights
-
-
-def _rename_conv_weights_for_deformable_conv_layers(state_dict, cfg):
- import re
- logger = logging.getLogger(__name__)
- logger.info("Remapping conv weights for deformable conv weights")
- layer_keys = sorted(state_dict.keys())
- for ix, stage_with_dcn in enumerate(cfg.MODEL.RESNETS.STAGE_WITH_DCN, 1):
- if not stage_with_dcn:
- continue
- for old_key in layer_keys:
- pattern = ".*layer{}.*conv2.*".format(ix)
- r = re.match(pattern, old_key)
- if r is None:
- continue
- for param in ["weight", "bias"]:
- if old_key.find(param) is -1:
- continue
- new_key = old_key.replace(
- "conv2.{}".format(param), "conv2.conv.{}".format(param)
- )
- logger.info("pattern: {}, old_key: {}, new_key: {}".format(
- pattern, old_key, new_key
- ))
- state_dict[new_key] = state_dict[old_key]
- del state_dict[old_key]
- return state_dict
-
-
-_C2_STAGE_NAMES = {
- "R-50": ["1.2", "2.3", "3.5", "4.2"],
- "R-101": ["1.2", "2.3", "3.22", "4.2"],
-}
-
-C2_FORMAT_LOADER = Registry()
-
-
-@C2_FORMAT_LOADER.register("R-50-C4")
-@C2_FORMAT_LOADER.register("R-50-C5")
-@C2_FORMAT_LOADER.register("R-101-C4")
-@C2_FORMAT_LOADER.register("R-101-C5")
-@C2_FORMAT_LOADER.register("R-50-FPN")
-@C2_FORMAT_LOADER.register("R-50-FPN-RETINANET")
-@C2_FORMAT_LOADER.register("R-50-FPN-FCOS")
-@C2_FORMAT_LOADER.register("R-101-FPN")
-@C2_FORMAT_LOADER.register("R-101-FPN-RETINANET")
-@C2_FORMAT_LOADER.register("R-101-FPN-FCOS")
-def load_resnet_c2_format(cfg, f):
- state_dict = _load_c2_pickled_weights(f)
- conv_body = cfg.MODEL.BACKBONE.CONV_BODY
- arch = conv_body.replace("-C4", "").replace("-C5", "").replace("-FPN", "").replace("-RETINANET", "").replace("-FCOS", "")
- stages = _C2_STAGE_NAMES[arch]
- state_dict = _rename_weights_for_resnet(state_dict, stages)
- # ***********************************
- # for deformable convolutional layer
- state_dict = _rename_conv_weights_for_deformable_conv_layers(state_dict, cfg)
- # ***********************************
- return dict(model=state_dict)
-
-
-def load_c2_format(cfg, f):
- return C2_FORMAT_LOADER[cfg.MODEL.BACKBONE.CONV_BODY](cfg, f)
diff --git a/spaces/PixArt-alpha/PixArt-alpha/app.py b/spaces/PixArt-alpha/PixArt-alpha/app.py
deleted file mode 100644
index c9e8c3d9b808ae04884c2925023d4b38132df0d4..0000000000000000000000000000000000000000
--- a/spaces/PixArt-alpha/PixArt-alpha/app.py
+++ /dev/null
@@ -1,294 +0,0 @@
-#!/usr/bin/env python
-
-from __future__ import annotations
-
-import os
-import random
-import uuid
-
-import gradio as gr
-import numpy as np
-import PIL.Image
-import torch
-
-from diffusers import AutoencoderKL, PixArtAlphaPipeline
-
-DESCRIPTION = """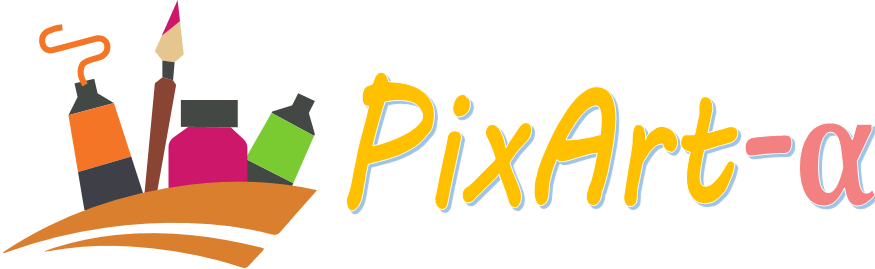
- # PixArt-Alpha 1024px
- #### [PixArt-Alpha 1024px](https://github.com/PixArt-alpha/PixArt-alpha) is a transformer-based text-to-image diffusion system trained on text embeddings from T5. This demo uses the [PixArt-alpha/PixArt-XL-2-1024-MS](https://huggingface.co/PixArt-alpha/PixArt-XL-2-1024-MS) checkpoint.
- #### English prompts ONLY; 提示词仅限英文
- Don't want to queue? Try [Google Colab Demo](https://colab.research.google.com/drive/1jZ5UZXk7tcpTfVwnX33dDuefNMcnW9ME?usp=sharing). It's slower but still free.
- """
-if not torch.cuda.is_available():
- DESCRIPTION += "\n
Running on CPU 🥶 This demo does not work on CPU.
"
-
-MAX_SEED = np.iinfo(np.int32).max
-CACHE_EXAMPLES = torch.cuda.is_available() and os.getenv("CACHE_EXAMPLES", "1") == "1"
-MAX_IMAGE_SIZE = int(os.getenv("MAX_IMAGE_SIZE", "1024"))
-USE_TORCH_COMPILE = os.getenv("USE_TORCH_COMPILE", "0") == "1"
-ENABLE_CPU_OFFLOAD = os.getenv("ENABLE_CPU_OFFLOAD", "0") == "1"
-
-device = torch.device("cuda:0" if torch.cuda.is_available() else "cpu")
-
-style_list = [
- {
- "name": "(No style)",
- "prompt": "{prompt}",
- "negative_prompt": "",
- },
- {
- "name": "Cinematic",
- "prompt": "cinematic still {prompt} . emotional, harmonious, vignette, highly detailed, high budget, bokeh, cinemascope, moody, epic, gorgeous, film grain, grainy",
- "negative_prompt": "anime, cartoon, graphic, text, painting, crayon, graphite, abstract, glitch, deformed, mutated, ugly, disfigured",
- },
- {
- "name": "Photographic",
- "prompt": "cinematic photo {prompt} . 35mm photograph, film, bokeh, professional, 4k, highly detailed",
- "negative_prompt": "drawing, painting, crayon, sketch, graphite, impressionist, noisy, blurry, soft, deformed, ugly",
- },
- {
- "name": "Anime",
- "prompt": "anime artwork {prompt} . anime style, key visual, vibrant, studio anime, highly detailed",
- "negative_prompt": "photo, deformed, black and white, realism, disfigured, low contrast",
- },
- {
- "name": "Manga",
- "prompt": "manga style {prompt} . vibrant, high-energy, detailed, iconic, Japanese comic style",
- "negative_prompt": "ugly, deformed, noisy, blurry, low contrast, realism, photorealistic, Western comic style",
- },
- {
- "name": "Digital Art",
- "prompt": "concept art {prompt} . digital artwork, illustrative, painterly, matte painting, highly detailed",
- "negative_prompt": "photo, photorealistic, realism, ugly",
- },
- {
- "name": "Pixel art",
- "prompt": "pixel-art {prompt} . low-res, blocky, pixel art style, 8-bit graphics",
- "negative_prompt": "sloppy, messy, blurry, noisy, highly detailed, ultra textured, photo, realistic",
- },
- {
- "name": "Fantasy art",
- "prompt": "ethereal fantasy concept art of {prompt} . magnificent, celestial, ethereal, painterly, epic, majestic, magical, fantasy art, cover art, dreamy",
- "negative_prompt": "photographic, realistic, realism, 35mm film, dslr, cropped, frame, text, deformed, glitch, noise, noisy, off-center, deformed, cross-eyed, closed eyes, bad anatomy, ugly, disfigured, sloppy, duplicate, mutated, black and white",
- },
- {
- "name": "Neonpunk",
- "prompt": "neonpunk style {prompt} . cyberpunk, vaporwave, neon, vibes, vibrant, stunningly beautiful, crisp, detailed, sleek, ultramodern, magenta highlights, dark purple shadows, high contrast, cinematic, ultra detailed, intricate, professional",
- "negative_prompt": "painting, drawing, illustration, glitch, deformed, mutated, cross-eyed, ugly, disfigured",
- },
- {
- "name": "3D Model",
- "prompt": "professional 3d model {prompt} . octane render, highly detailed, volumetric, dramatic lighting",
- "negative_prompt": "ugly, deformed, noisy, low poly, blurry, painting",
- },
-]
-
-styles = {k["name"]: (k["prompt"], k["negative_prompt"]) for k in style_list}
-STYLE_NAMES = list(styles.keys())
-DEFAULT_STYLE_NAME = "(No style)"
-
-
-def apply_style(style_name: str, positive: str, negative: str = "") -> Tuple[str, str]:
- p, n = styles.get(style_name, styles[DEFAULT_STYLE_NAME])
- if not negative:
- negative = ""
- return p.replace("{prompt}", positive), n + negative
-
-
-if torch.cuda.is_available():
- pipe = PixArtAlphaPipeline.from_pretrained(
- "PixArt-alpha/PixArt-XL-2-1024-MS",
- torch_dtype=torch.float16,
- variant="fp16",
- use_safetensors=True,
- )
-
- if ENABLE_CPU_OFFLOAD:
- pipe.enable_model_cpu_offload()
- else:
- pipe.to(device)
- print("Loaded on Device!")
-
- # speed-up T5
- pipe.text_encoder.to_bettertransformer()
-
- if USE_TORCH_COMPILE:
- pipe.transformer = torch.compile(
- pipe.transformer, mode="reduce-overhead", fullgraph=True
- )
- print("Model Compiled!")
-
-
-def save_image(img):
- unique_name = str(uuid.uuid4()) + ".png"
- img.save(unique_name)
- return unique_name
-
-
-def randomize_seed_fn(seed: int, randomize_seed: bool) -> int:
- if randomize_seed:
- seed = random.randint(0, MAX_SEED)
- return seed
-
-
-def generate(
- prompt: str,
- negative_prompt: str = "",
- style: str = DEFAULT_STYLE_NAME,
- use_negative_prompt: bool = False,
- seed: int = 0,
- width: int = 1024,
- height: int = 1024,
- guidance_scale: float = 4.5,
- num_inference_steps: int = 20,
- randomize_seed: bool = False,
- progress=gr.Progress(track_tqdm=True),
-):
- seed = randomize_seed_fn(seed, randomize_seed)
- generator = torch.Generator().manual_seed(seed)
-
- if not use_negative_prompt:
- negative_prompt = None # type: ignore
- prompt, negative_prompt = apply_style(style, prompt, negative_prompt)
- image = pipe(
- prompt=prompt,
- negative_prompt=negative_prompt,
- width=width,
- height=height,
- guidance_scale=guidance_scale,
- num_inference_steps=num_inference_steps,
- generator=generator,
- output_type="pil",
- ).images[0]
-
- image_path = save_image(image)
- print(image_path)
- return [image_path], seed
-
-
-examples = [
- "A small cactus with a happy face in the Sahara desert.",
- "Pirate ship trapped in a cosmic maelstrom nebula, rendered in cosmic beach whirlpool engine, volumetric lighting, spectacular, ambient lights, light pollution, cinematic atmosphere, art nouveau style, illustration art artwork by SenseiJaye, intricate detail.",
- "stars, water, brilliantly, gorgeous large scale scene, a little girl, in the style of dreamy realism, light gold and amber, blue and pink, brilliantly illuminated in the background.",
- "3d digital art of an adorable ghost, glowing within, holding a heart shaped pumpkin, Halloween, super cute, spooky haunted house background",
- "beautiful lady, freckles, big smile, blue eyes, short ginger hair, dark makeup, wearing a floral blue vest top, soft light, dark grey background",
- "professional portrait photo of an anthropomorphic cat wearing fancy gentleman hat and jacket walking in autumn forest.",
- "an astronaut sitting in a diner, eating fries, cinematic, analog film",
- "Albert Einstein in a surrealist Cyberpunk 2077 world, hyperrealistic",
-]
-
-with gr.Blocks(css="style.css") as demo:
- gr.Markdown(DESCRIPTION)
- gr.DuplicateButton(
- value="Duplicate Space for private use",
- elem_id="duplicate-button",
- visible=os.getenv("SHOW_DUPLICATE_BUTTON") == "1",
- )
- with gr.Group():
- with gr.Row():
- prompt = gr.Text(
- label="Prompt",
- show_label=False,
- max_lines=1,
- placeholder="Enter your prompt",
- container=False,
- )
- run_button = gr.Button("Run", scale=0)
- result = gr.Gallery(label="Result", columns=1, show_label=False)
- with gr.Accordion("Advanced options", open=False):
- with gr.Row():
- use_negative_prompt = gr.Checkbox(label="Use negative prompt", value=False)
- style_selection = gr.Radio(
- show_label=True,
- container=True,
- interactive=True,
- choices=STYLE_NAMES,
- value=DEFAULT_STYLE_NAME,
- label="Image Style",
- )
- negative_prompt = gr.Text(
- label="Negative prompt",
- max_lines=1,
- placeholder="Enter a negative prompt",
- visible=False,
- )
- seed = gr.Slider(
- label="Seed",
- minimum=0,
- maximum=MAX_SEED,
- step=1,
- value=0,
- )
- randomize_seed = gr.Checkbox(label="Randomize seed", value=True)
- with gr.Row(visible=False):
- width = gr.Slider(
- label="Width",
- minimum=256,
- maximum=MAX_IMAGE_SIZE,
- step=32,
- value=1024,
- )
- height = gr.Slider(
- label="Height",
- minimum=256,
- maximum=MAX_IMAGE_SIZE,
- step=32,
- value=1024,
- )
- with gr.Row():
- guidance_scale = gr.Slider(
- label="Guidance scale",
- minimum=1,
- maximum=20,
- step=0.1,
- value=4.5,
- )
- num_inference_steps = gr.Slider(
- label="Number of inference steps",
- minimum=10,
- maximum=100,
- step=1,
- value=20,
- )
-
- gr.Examples(
- examples=examples,
- inputs=prompt,
- outputs=[result, seed],
- fn=generate,
- cache_examples=CACHE_EXAMPLES,
- )
-
- use_negative_prompt.change(
- fn=lambda x: gr.update(visible=x),
- inputs=use_negative_prompt,
- outputs=negative_prompt,
- api_name=False,
- )
-
- gr.on(
- triggers=[
- prompt.submit,
- negative_prompt.submit,
- run_button.click,
- ],
- fn=generate,
- inputs=[
- prompt,
- negative_prompt,
- style_selection,
- use_negative_prompt,
- seed,
- width,
- height,
- guidance_scale,
- num_inference_steps,
- randomize_seed,
- ],
- outputs=[result, seed],
- api_name="run",
- )
-
-if __name__ == "__main__":
- # demo.queue(max_size=20).launch()
- demo.launch(share=True)
diff --git a/spaces/Plachta/VITS-Umamusume-voice-synthesizer/ONNXVITS_models.py b/spaces/Plachta/VITS-Umamusume-voice-synthesizer/ONNXVITS_models.py
deleted file mode 100644
index acd00238895d57ba878fd0211d5654250fb10061..0000000000000000000000000000000000000000
--- a/spaces/Plachta/VITS-Umamusume-voice-synthesizer/ONNXVITS_models.py
+++ /dev/null
@@ -1,509 +0,0 @@
-import copy
-import math
-import torch
-from torch import nn
-from torch.nn import functional as F
-
-import commons
-import ONNXVITS_modules as modules
-import attentions
-import monotonic_align
-
-from torch.nn import Conv1d, ConvTranspose1d, AvgPool1d, Conv2d
-from torch.nn.utils import weight_norm, remove_weight_norm, spectral_norm
-from commons import init_weights, get_padding
-
-
-class StochasticDurationPredictor(nn.Module):
- def __init__(self, in_channels, filter_channels, kernel_size, p_dropout, n_flows=4, gin_channels=0):
- super().__init__()
- filter_channels = in_channels # it needs to be removed from future version.
- self.in_channels = in_channels
- self.filter_channels = filter_channels
- self.kernel_size = kernel_size
- self.p_dropout = p_dropout
- self.n_flows = n_flows
- self.gin_channels = gin_channels
-
- self.log_flow = modules.Log()
- self.flows = nn.ModuleList()
- self.flows.append(modules.ElementwiseAffine(2))
- for i in range(n_flows):
- self.flows.append(modules.ConvFlow(2, filter_channels, kernel_size, n_layers=3))
- self.flows.append(modules.Flip())
-
- self.post_pre = nn.Conv1d(1, filter_channels, 1)
- self.post_proj = nn.Conv1d(filter_channels, filter_channels, 1)
- self.post_convs = modules.DDSConv(filter_channels, kernel_size, n_layers=3, p_dropout=p_dropout)
- self.post_flows = nn.ModuleList()
- self.post_flows.append(modules.ElementwiseAffine(2))
- for i in range(4):
- self.post_flows.append(modules.ConvFlow(2, filter_channels, kernel_size, n_layers=3))
- self.post_flows.append(modules.Flip())
-
- self.pre = nn.Conv1d(in_channels, filter_channels, 1)
- self.proj = nn.Conv1d(filter_channels, filter_channels, 1)
- self.convs = modules.DDSConv(filter_channels, kernel_size, n_layers=3, p_dropout=p_dropout)
- if gin_channels != 0:
- self.cond = nn.Conv1d(gin_channels, filter_channels, 1)
-
- self.w = None
- self.reverse = None
- self.noise_scale = None
- def forward(self, x, x_mask, g=None):
- w = self.w
- reverse = self.reverse
- noise_scale = self.noise_scale
-
- x = torch.detach(x)
- x = self.pre(x)
- if g is not None:
- g = torch.detach(g)
- x = x + self.cond(g)
- x = self.convs(x, x_mask)
- x = self.proj(x) * x_mask
-
- if not reverse:
- flows = self.flows
- assert w is not None
-
- logdet_tot_q = 0
- h_w = self.post_pre(w)
- h_w = self.post_convs(h_w, x_mask)
- h_w = self.post_proj(h_w) * x_mask
- e_q = torch.randn(w.size(0), 2, w.size(2)).to(device=x.device, dtype=x.dtype) * x_mask
- z_q = e_q
- for flow in self.post_flows:
- z_q, logdet_q = flow(z_q, x_mask, g=(x + h_w))
- logdet_tot_q += logdet_q
- z_u, z1 = torch.split(z_q, [1, 1], 1)
- u = torch.sigmoid(z_u) * x_mask
- z0 = (w - u) * x_mask
- logdet_tot_q += torch.sum((F.logsigmoid(z_u) + F.logsigmoid(-z_u)) * x_mask, [1,2])
- logq = torch.sum(-0.5 * (math.log(2*math.pi) + (e_q**2)) * x_mask, [1,2]) - logdet_tot_q
-
- logdet_tot = 0
- z0, logdet = self.log_flow(z0, x_mask)
- logdet_tot += logdet
- z = torch.cat([z0, z1], 1)
- for flow in flows:
- z, logdet = flow(z, x_mask, g=x, reverse=reverse)
- logdet_tot = logdet_tot + logdet
- nll = torch.sum(0.5 * (math.log(2*math.pi) + (z**2)) * x_mask, [1,2]) - logdet_tot
- return nll + logq # [b]
- else:
- flows = list(reversed(self.flows))
- flows = flows[:-2] + [flows[-1]] # remove a useless vflow
- z = torch.randn(x.size(0), 2, x.size(2)).to(device=x.device, dtype=x.dtype) * noise_scale
- for flow in flows:
- z = flow(z, x_mask, g=x, reverse=reverse)
- z0, z1 = torch.split(z, [1, 1], 1)
- logw = z0
- return logw
-
-
-class TextEncoder(nn.Module):
- def __init__(self,
- n_vocab,
- out_channels,
- hidden_channels,
- filter_channels,
- n_heads,
- n_layers,
- kernel_size,
- p_dropout):
- super().__init__()
- self.n_vocab = n_vocab
- self.out_channels = out_channels
- self.hidden_channels = hidden_channels
- self.filter_channels = filter_channels
- self.n_heads = n_heads
- self.n_layers = n_layers
- self.kernel_size = kernel_size
- self.p_dropout = p_dropout
-
- self.emb = nn.Embedding(n_vocab, hidden_channels)
- nn.init.normal_(self.emb.weight, 0.0, hidden_channels**-0.5)
-
- self.encoder = attentions.Encoder(
- hidden_channels,
- filter_channels,
- n_heads,
- n_layers,
- kernel_size,
- p_dropout)
- self.proj= nn.Conv1d(hidden_channels, out_channels * 2, 1)
-
- def forward(self, x, x_lengths):
- x = self.emb(x) * math.sqrt(self.hidden_channels) # [b, t, h]
- x = torch.transpose(x, 1, -1) # [b, h, t]
- x_mask = torch.unsqueeze(commons.sequence_mask(x_lengths, x.size(2)), 1).to(x.dtype)
-
- x = self.encoder(x * x_mask, x_mask)
- stats = self.proj(x) * x_mask
-
- m, logs = torch.split(stats, self.out_channels, dim=1)
- return x, m, logs, x_mask
-
-
-class ResidualCouplingBlock(nn.Module):
- def __init__(self,
- channels,
- hidden_channels,
- kernel_size,
- dilation_rate,
- n_layers,
- n_flows=4,
- gin_channels=0):
- super().__init__()
- self.channels = channels
- self.hidden_channels = hidden_channels
- self.kernel_size = kernel_size
- self.dilation_rate = dilation_rate
- self.n_layers = n_layers
- self.n_flows = n_flows
- self.gin_channels = gin_channels
-
- self.flows = nn.ModuleList()
- for i in range(n_flows):
- self.flows.append(modules.ResidualCouplingLayer(channels, hidden_channels, kernel_size, dilation_rate, n_layers, gin_channels=gin_channels, mean_only=True))
- self.flows.append(modules.Flip())
-
- self.reverse = None
- def forward(self, x, x_mask, g=None):
- reverse = self.reverse
- if not reverse:
- for flow in self.flows:
- x, _ = flow(x, x_mask, g=g, reverse=reverse)
- else:
- for flow in reversed(self.flows):
- x = flow(x, x_mask, g=g, reverse=reverse)
- return x
-
-
-class PosteriorEncoder(nn.Module):
- def __init__(self,
- in_channels,
- out_channels,
- hidden_channels,
- kernel_size,
- dilation_rate,
- n_layers,
- gin_channels=0):
- super().__init__()
- self.in_channels = in_channels
- self.out_channels = out_channels
- self.hidden_channels = hidden_channels
- self.kernel_size = kernel_size
- self.dilation_rate = dilation_rate
- self.n_layers = n_layers
- self.gin_channels = gin_channels
-
- self.pre = nn.Conv1d(in_channels, hidden_channels, 1)
- self.enc = modules.WN(hidden_channels, kernel_size, dilation_rate, n_layers, gin_channels=gin_channels)
- self.proj = nn.Conv1d(hidden_channels, out_channels * 2, 1)
-
- def forward(self, x, x_lengths, g=None):
- x_mask = torch.unsqueeze(commons.sequence_mask(x_lengths, x.size(2)), 1).to(x.dtype)
- x = self.pre(x) * x_mask # x_in : [b, c, t] -> [b, h, t]
- x = self.enc(x, x_mask, g=g) # x_in : [b, h, t], g : [b, h, 1], x = x_in + g
- stats = self.proj(x) * x_mask
- m, logs = torch.split(stats, self.out_channels, dim=1)
- z = (m + torch.randn_like(m) * torch.exp(logs)) * x_mask
- return z, m, logs, x_mask # z, m, logs : [b, h, t]
-
-
-class Generator(torch.nn.Module):
- def __init__(self, initial_channel, resblock, resblock_kernel_sizes, resblock_dilation_sizes, upsample_rates, upsample_initial_channel, upsample_kernel_sizes, gin_channels=0):
- super(Generator, self).__init__()
- self.num_kernels = len(resblock_kernel_sizes)
- self.num_upsamples = len(upsample_rates)
- self.conv_pre = Conv1d(initial_channel, upsample_initial_channel, 7, 1, padding=3)
- resblock = modules.ResBlock1 if resblock == '1' else modules.ResBlock2
-
- self.ups = nn.ModuleList()
- for i, (u, k) in enumerate(zip(upsample_rates, upsample_kernel_sizes)):
- self.ups.append(weight_norm(
- ConvTranspose1d(upsample_initial_channel//(2**i), upsample_initial_channel//(2**(i+1)),
- k, u, padding=(k-u)//2)))
-
- self.resblocks = nn.ModuleList()
- for i in range(len(self.ups)):
- ch = upsample_initial_channel//(2**(i+1))
- for j, (k, d) in enumerate(zip(resblock_kernel_sizes, resblock_dilation_sizes)):
- self.resblocks.append(resblock(ch, k, d))
-
- self.conv_post = Conv1d(ch, 1, 7, 1, padding=3, bias=False)
- self.ups.apply(init_weights)
-
- if gin_channels != 0:
- self.cond = nn.Conv1d(gin_channels, upsample_initial_channel, 1)
-
- def forward(self, x, g=None):
- x = self.conv_pre(x)
- if g is not None:
- x = x + self.cond(g)
-
- for i in range(self.num_upsamples):
- x = F.leaky_relu(x, modules.LRELU_SLOPE)
- x = self.ups[i](x)
- xs = None
- for j in range(self.num_kernels):
- if xs is None:
- xs = self.resblocks[i*self.num_kernels+j](x)
- else:
- xs += self.resblocks[i*self.num_kernels+j](x)
- x = xs / self.num_kernels
- x = F.leaky_relu(x)
- x = self.conv_post(x)
- x = torch.tanh(x)
-
- return x
-
- def remove_weight_norm(self):
- print('Removing weight norm...')
- for l in self.ups:
- remove_weight_norm(l)
- for l in self.resblocks:
- l.remove_weight_norm()
-
-
-class DiscriminatorP(torch.nn.Module):
- def __init__(self, period, kernel_size=5, stride=3, use_spectral_norm=False):
- super(DiscriminatorP, self).__init__()
- self.period = period
- self.use_spectral_norm = use_spectral_norm
- norm_f = weight_norm if use_spectral_norm == False else spectral_norm
- self.convs = nn.ModuleList([
- norm_f(Conv2d(1, 32, (kernel_size, 1), (stride, 1), padding=(get_padding(kernel_size, 1), 0))),
- norm_f(Conv2d(32, 128, (kernel_size, 1), (stride, 1), padding=(get_padding(kernel_size, 1), 0))),
- norm_f(Conv2d(128, 512, (kernel_size, 1), (stride, 1), padding=(get_padding(kernel_size, 1), 0))),
- norm_f(Conv2d(512, 1024, (kernel_size, 1), (stride, 1), padding=(get_padding(kernel_size, 1), 0))),
- norm_f(Conv2d(1024, 1024, (kernel_size, 1), 1, padding=(get_padding(kernel_size, 1), 0))),
- ])
- self.conv_post = norm_f(Conv2d(1024, 1, (3, 1), 1, padding=(1, 0)))
-
- def forward(self, x):
- fmap = []
-
- # 1d to 2d
- b, c, t = x.shape
- if t % self.period != 0: # pad first
- n_pad = self.period - (t % self.period)
- x = F.pad(x, (0, n_pad), "reflect")
- t = t + n_pad
- x = x.view(b, c, t // self.period, self.period)
-
- for l in self.convs:
- x = l(x)
- x = F.leaky_relu(x, modules.LRELU_SLOPE)
- fmap.append(x)
- x = self.conv_post(x)
- fmap.append(x)
- x = torch.flatten(x, 1, -1)
-
- return x, fmap
-
-
-class DiscriminatorS(torch.nn.Module):
- def __init__(self, use_spectral_norm=False):
- super(DiscriminatorS, self).__init__()
- norm_f = weight_norm if use_spectral_norm == False else spectral_norm
- self.convs = nn.ModuleList([
- norm_f(Conv1d(1, 16, 15, 1, padding=7)),
- norm_f(Conv1d(16, 64, 41, 4, groups=4, padding=20)),
- norm_f(Conv1d(64, 256, 41, 4, groups=16, padding=20)),
- norm_f(Conv1d(256, 1024, 41, 4, groups=64, padding=20)),
- norm_f(Conv1d(1024, 1024, 41, 4, groups=256, padding=20)),
- norm_f(Conv1d(1024, 1024, 5, 1, padding=2)),
- ])
- self.conv_post = norm_f(Conv1d(1024, 1, 3, 1, padding=1))
-
- def forward(self, x):
- fmap = []
-
- for l in self.convs:
- x = l(x)
- x = F.leaky_relu(x, modules.LRELU_SLOPE)
- fmap.append(x)
- x = self.conv_post(x)
- fmap.append(x)
- x = torch.flatten(x, 1, -1)
-
- return x, fmap
-
-
-class MultiPeriodDiscriminator(torch.nn.Module):
- def __init__(self, use_spectral_norm=False):
- super(MultiPeriodDiscriminator, self).__init__()
- periods = [2,3,5,7,11]
-
- discs = [DiscriminatorS(use_spectral_norm=use_spectral_norm)]
- discs = discs + [DiscriminatorP(i, use_spectral_norm=use_spectral_norm) for i in periods]
- self.discriminators = nn.ModuleList(discs)
-
- def forward(self, y, y_hat):
- y_d_rs = []
- y_d_gs = []
- fmap_rs = []
- fmap_gs = []
- for i, d in enumerate(self.discriminators):
- y_d_r, fmap_r = d(y)
- y_d_g, fmap_g = d(y_hat)
- y_d_rs.append(y_d_r)
- y_d_gs.append(y_d_g)
- fmap_rs.append(fmap_r)
- fmap_gs.append(fmap_g)
-
- return y_d_rs, y_d_gs, fmap_rs, fmap_gs
-
-
-
-class SynthesizerTrn(nn.Module):
- """
- Synthesizer for Training
- """
-
- def __init__(self,
- n_vocab,
- spec_channels,
- segment_size,
- inter_channels,
- hidden_channels,
- filter_channels,
- n_heads,
- n_layers,
- kernel_size,
- p_dropout,
- resblock,
- resblock_kernel_sizes,
- resblock_dilation_sizes,
- upsample_rates,
- upsample_initial_channel,
- upsample_kernel_sizes,
- n_speakers=0,
- gin_channels=0,
- use_sdp=True,
- **kwargs):
-
- super().__init__()
- self.n_vocab = n_vocab
- self.spec_channels = spec_channels
- self.inter_channels = inter_channels
- self.hidden_channels = hidden_channels
- self.filter_channels = filter_channels
- self.n_heads = n_heads
- self.n_layers = n_layers
- self.kernel_size = kernel_size
- self.p_dropout = p_dropout
- self.resblock = resblock
- self.resblock_kernel_sizes = resblock_kernel_sizes
- self.resblock_dilation_sizes = resblock_dilation_sizes
- self.upsample_rates = upsample_rates
- self.upsample_initial_channel = upsample_initial_channel
- self.upsample_kernel_sizes = upsample_kernel_sizes
- self.segment_size = segment_size
- self.n_speakers = n_speakers
- self.gin_channels = gin_channels
-
- self.use_sdp = use_sdp
-
- self.enc_p = TextEncoder(n_vocab,
- inter_channels,
- hidden_channels,
- filter_channels,
- n_heads,
- n_layers,
- kernel_size,
- p_dropout)
- self.dec = Generator(inter_channels, resblock, resblock_kernel_sizes, resblock_dilation_sizes, upsample_rates, upsample_initial_channel, upsample_kernel_sizes, gin_channels=gin_channels)
- self.enc_q = PosteriorEncoder(spec_channels, inter_channels, hidden_channels, 5, 1, 16, gin_channels=gin_channels)
- self.flow = ResidualCouplingBlock(inter_channels, hidden_channels, 5, 1, 4, gin_channels=gin_channels)
-
- self.dp = StochasticDurationPredictor(hidden_channels, 192, 3, 0.5, 4, gin_channels=gin_channels)
-
- if n_speakers > 0:
- self.emb_g = nn.Embedding(n_speakers, gin_channels)
-
- def forward(self, x, x_lengths, sid=None, noise_scale=.667, length_scale=1, noise_scale_w=.8, max_len=None):
- torch.onnx.export(
- self.enc_p,
- (x, x_lengths),
- "ONNX_net/enc_p.onnx",
- input_names=["x", "x_lengths"],
- output_names=["xout", "m_p", "logs_p", "x_mask"],
- dynamic_axes={
- "x" : [1],
- "xout" : [2],
- "m_p" : [2],
- "logs_p" : [2],
- "x_mask" : [2]
- },
- verbose=True,
- )
- x, m_p, logs_p, x_mask = self.enc_p(x, x_lengths)
-
- if self.n_speakers > 0:
- g = self.emb_g(sid).unsqueeze(-1) # [b, h, 1]
- else:
- g = None
-
- self.dp.reverse = True
- self.dp.noise_scale = noise_scale_w
- torch.onnx.export(
- self.dp,
- (x, x_mask, g),
- "ONNX_net/dp.onnx",
- input_names=["x", "x_mask", "g"],
- output_names=["logw"],
- dynamic_axes={
- "x" : [2],
- "x_mask" : [2],
- "logw" : [2]
- },
- verbose=True,
- )
- logw = self.dp(x, x_mask, g=g)
- w = torch.exp(logw) * x_mask * length_scale
- w_ceil = torch.ceil(w)
- y_lengths = torch.clamp_min(torch.sum(w_ceil, [1, 2]), 1).long()
- y_mask = torch.unsqueeze(commons.sequence_mask(y_lengths, None), 1).to(x_mask.dtype)
- attn_mask = torch.unsqueeze(x_mask, 2) * torch.unsqueeze(y_mask, -1)
- attn = commons.generate_path(w_ceil, attn_mask)
-
- m_p = torch.matmul(attn.squeeze(1), m_p.transpose(1, 2)).transpose(1, 2) # [b, t', t], [b, t, d] -> [b, d, t']
- logs_p = torch.matmul(attn.squeeze(1), logs_p.transpose(1, 2)).transpose(1, 2) # [b, t', t], [b, t, d] -> [b, d, t']
-
- z_p = m_p + torch.randn_like(m_p) * torch.exp(logs_p) * noise_scale
-
- self.flow.reverse = True
- torch.onnx.export(
- self.flow,
- (z_p, y_mask, g),
- "ONNX_net/flow.onnx",
- input_names=["z_p", "y_mask", "g"],
- output_names=["z"],
- dynamic_axes={
- "z_p" : [2],
- "y_mask" : [2],
- "z" : [2]
- },
- verbose=True,
- )
- z = self.flow(z_p, y_mask, g=g)
- z_in = (z * y_mask)[:,:,:max_len]
-
- torch.onnx.export(
- self.dec,
- (z_in, g),
- "ONNX_net/dec.onnx",
- input_names=["z_in", "g"],
- output_names=["o"],
- dynamic_axes={
- "z_in" : [2],
- "o" : [2]
- },
- verbose=True,
- )
- o = self.dec(z_in, g=g)
- return o
diff --git a/spaces/Raspberry-ai/main/.env/lib/python3.11/site-packages/pip/_vendor/distro/distro.py b/spaces/Raspberry-ai/main/.env/lib/python3.11/site-packages/pip/_vendor/distro/distro.py
deleted file mode 100644
index 49066ae83646acf39fc4a1d38796d6b5b70e184d..0000000000000000000000000000000000000000
--- a/spaces/Raspberry-ai/main/.env/lib/python3.11/site-packages/pip/_vendor/distro/distro.py
+++ /dev/null
@@ -1,1374 +0,0 @@
-#!/usr/bin/env python
-# Copyright 2015,2016,2017 Nir Cohen
-#
-# Licensed under the Apache License, Version 2.0 (the "License");
-# you may not use this file except in compliance with the License.
-# You may obtain a copy of the License at
-#
-# http://www.apache.org/licenses/LICENSE-2.0
-#
-# Unless required by applicable law or agreed to in writing, software
-# distributed under the License is distributed on an "AS IS" BASIS,
-# WITHOUT WARRANTIES OR CONDITIONS OF ANY KIND, either express or implied.
-# See the License for the specific language governing permissions and
-# limitations under the License.
-
-"""
-The ``distro`` package (``distro`` stands for Linux Distribution) provides
-information about the Linux distribution it runs on, such as a reliable
-machine-readable distro ID, or version information.
-
-It is the recommended replacement for Python's original
-:py:func:`platform.linux_distribution` function, but it provides much more
-functionality. An alternative implementation became necessary because Python
-3.5 deprecated this function, and Python 3.8 removed it altogether. Its
-predecessor function :py:func:`platform.dist` was already deprecated since
-Python 2.6 and removed in Python 3.8. Still, there are many cases in which
-access to OS distribution information is needed. See `Python issue 1322
-`_ for more information.
-"""
-
-import argparse
-import json
-import logging
-import os
-import re
-import shlex
-import subprocess
-import sys
-import warnings
-from typing import (
- Any,
- Callable,
- Dict,
- Iterable,
- Optional,
- Sequence,
- TextIO,
- Tuple,
- Type,
-)
-
-try:
- from typing import TypedDict
-except ImportError:
- # Python 3.7
- TypedDict = dict
-
-__version__ = "1.7.0"
-
-
-class VersionDict(TypedDict):
- major: str
- minor: str
- build_number: str
-
-
-class InfoDict(TypedDict):
- id: str
- version: str
- version_parts: VersionDict
- like: str
- codename: str
-
-
-_UNIXCONFDIR = os.environ.get("UNIXCONFDIR", "/etc")
-_UNIXUSRLIBDIR = os.environ.get("UNIXUSRLIBDIR", "/usr/lib")
-_OS_RELEASE_BASENAME = "os-release"
-
-#: Translation table for normalizing the "ID" attribute defined in os-release
-#: files, for use by the :func:`distro.id` method.
-#:
-#: * Key: Value as defined in the os-release file, translated to lower case,
-#: with blanks translated to underscores.
-#:
-#: * Value: Normalized value.
-NORMALIZED_OS_ID = {
- "ol": "oracle", # Oracle Linux
- "opensuse-leap": "opensuse", # Newer versions of OpenSuSE report as opensuse-leap
-}
-
-#: Translation table for normalizing the "Distributor ID" attribute returned by
-#: the lsb_release command, for use by the :func:`distro.id` method.
-#:
-#: * Key: Value as returned by the lsb_release command, translated to lower
-#: case, with blanks translated to underscores.
-#:
-#: * Value: Normalized value.
-NORMALIZED_LSB_ID = {
- "enterpriseenterpriseas": "oracle", # Oracle Enterprise Linux 4
- "enterpriseenterpriseserver": "oracle", # Oracle Linux 5
- "redhatenterpriseworkstation": "rhel", # RHEL 6, 7 Workstation
- "redhatenterpriseserver": "rhel", # RHEL 6, 7 Server
- "redhatenterprisecomputenode": "rhel", # RHEL 6 ComputeNode
-}
-
-#: Translation table for normalizing the distro ID derived from the file name
-#: of distro release files, for use by the :func:`distro.id` method.
-#:
-#: * Key: Value as derived from the file name of a distro release file,
-#: translated to lower case, with blanks translated to underscores.
-#:
-#: * Value: Normalized value.
-NORMALIZED_DISTRO_ID = {
- "redhat": "rhel", # RHEL 6.x, 7.x
-}
-
-# Pattern for content of distro release file (reversed)
-_DISTRO_RELEASE_CONTENT_REVERSED_PATTERN = re.compile(
- r"(?:[^)]*\)(.*)\()? *(?:STL )?([\d.+\-a-z]*\d) *(?:esaeler *)?(.+)"
-)
-
-# Pattern for base file name of distro release file
-_DISTRO_RELEASE_BASENAME_PATTERN = re.compile(r"(\w+)[-_](release|version)$")
-
-# Base file names to be ignored when searching for distro release file
-_DISTRO_RELEASE_IGNORE_BASENAMES = (
- "debian_version",
- "lsb-release",
- "oem-release",
- _OS_RELEASE_BASENAME,
- "system-release",
- "plesk-release",
- "iredmail-release",
-)
-
-
-def linux_distribution(full_distribution_name: bool = True) -> Tuple[str, str, str]:
- """
- .. deprecated:: 1.6.0
-
- :func:`distro.linux_distribution()` is deprecated. It should only be
- used as a compatibility shim with Python's
- :py:func:`platform.linux_distribution()`. Please use :func:`distro.id`,
- :func:`distro.version` and :func:`distro.name` instead.
-
- Return information about the current OS distribution as a tuple
- ``(id_name, version, codename)`` with items as follows:
-
- * ``id_name``: If *full_distribution_name* is false, the result of
- :func:`distro.id`. Otherwise, the result of :func:`distro.name`.
-
- * ``version``: The result of :func:`distro.version`.
-
- * ``codename``: The extra item (usually in parentheses) after the
- os-release version number, or the result of :func:`distro.codename`.
-
- The interface of this function is compatible with the original
- :py:func:`platform.linux_distribution` function, supporting a subset of
- its parameters.
-
- The data it returns may not exactly be the same, because it uses more data
- sources than the original function, and that may lead to different data if
- the OS distribution is not consistent across multiple data sources it
- provides (there are indeed such distributions ...).
-
- Another reason for differences is the fact that the :func:`distro.id`
- method normalizes the distro ID string to a reliable machine-readable value
- for a number of popular OS distributions.
- """
- warnings.warn(
- "distro.linux_distribution() is deprecated. It should only be used as a "
- "compatibility shim with Python's platform.linux_distribution(). Please use "
- "distro.id(), distro.version() and distro.name() instead.",
- DeprecationWarning,
- stacklevel=2,
- )
- return _distro.linux_distribution(full_distribution_name)
-
-
-def id() -> str:
- """
- Return the distro ID of the current distribution, as a
- machine-readable string.
-
- For a number of OS distributions, the returned distro ID value is
- *reliable*, in the sense that it is documented and that it does not change
- across releases of the distribution.
-
- This package maintains the following reliable distro ID values:
-
- ============== =========================================
- Distro ID Distribution
- ============== =========================================
- "ubuntu" Ubuntu
- "debian" Debian
- "rhel" RedHat Enterprise Linux
- "centos" CentOS
- "fedora" Fedora
- "sles" SUSE Linux Enterprise Server
- "opensuse" openSUSE
- "amzn" Amazon Linux
- "arch" Arch Linux
- "cloudlinux" CloudLinux OS
- "exherbo" Exherbo Linux
- "gentoo" GenToo Linux
- "ibm_powerkvm" IBM PowerKVM
- "kvmibm" KVM for IBM z Systems
- "linuxmint" Linux Mint
- "mageia" Mageia
- "mandriva" Mandriva Linux
- "parallels" Parallels
- "pidora" Pidora
- "raspbian" Raspbian
- "oracle" Oracle Linux (and Oracle Enterprise Linux)
- "scientific" Scientific Linux
- "slackware" Slackware
- "xenserver" XenServer
- "openbsd" OpenBSD
- "netbsd" NetBSD
- "freebsd" FreeBSD
- "midnightbsd" MidnightBSD
- "rocky" Rocky Linux
- "aix" AIX
- ============== =========================================
-
- If you have a need to get distros for reliable IDs added into this set,
- or if you find that the :func:`distro.id` function returns a different
- distro ID for one of the listed distros, please create an issue in the
- `distro issue tracker`_.
-
- **Lookup hierarchy and transformations:**
-
- First, the ID is obtained from the following sources, in the specified
- order. The first available and non-empty value is used:
-
- * the value of the "ID" attribute of the os-release file,
-
- * the value of the "Distributor ID" attribute returned by the lsb_release
- command,
-
- * the first part of the file name of the distro release file,
-
- The so determined ID value then passes the following transformations,
- before it is returned by this method:
-
- * it is translated to lower case,
-
- * blanks (which should not be there anyway) are translated to underscores,
-
- * a normalization of the ID is performed, based upon
- `normalization tables`_. The purpose of this normalization is to ensure
- that the ID is as reliable as possible, even across incompatible changes
- in the OS distributions. A common reason for an incompatible change is
- the addition of an os-release file, or the addition of the lsb_release
- command, with ID values that differ from what was previously determined
- from the distro release file name.
- """
- return _distro.id()
-
-
-def name(pretty: bool = False) -> str:
- """
- Return the name of the current OS distribution, as a human-readable
- string.
-
- If *pretty* is false, the name is returned without version or codename.
- (e.g. "CentOS Linux")
-
- If *pretty* is true, the version and codename are appended.
- (e.g. "CentOS Linux 7.1.1503 (Core)")
-
- **Lookup hierarchy:**
-
- The name is obtained from the following sources, in the specified order.
- The first available and non-empty value is used:
-
- * If *pretty* is false:
-
- - the value of the "NAME" attribute of the os-release file,
-
- - the value of the "Distributor ID" attribute returned by the lsb_release
- command,
-
- - the value of the "" field of the distro release file.
-
- * If *pretty* is true:
-
- - the value of the "PRETTY_NAME" attribute of the os-release file,
-
- - the value of the "Description" attribute returned by the lsb_release
- command,
-
- - the value of the "" field of the distro release file, appended
- with the value of the pretty version ("" and ""
- fields) of the distro release file, if available.
- """
- return _distro.name(pretty)
-
-
-def version(pretty: bool = False, best: bool = False) -> str:
- """
- Return the version of the current OS distribution, as a human-readable
- string.
-
- If *pretty* is false, the version is returned without codename (e.g.
- "7.0").
-
- If *pretty* is true, the codename in parenthesis is appended, if the
- codename is non-empty (e.g. "7.0 (Maipo)").
-
- Some distributions provide version numbers with different precisions in
- the different sources of distribution information. Examining the different
- sources in a fixed priority order does not always yield the most precise
- version (e.g. for Debian 8.2, or CentOS 7.1).
-
- Some other distributions may not provide this kind of information. In these
- cases, an empty string would be returned. This behavior can be observed
- with rolling releases distributions (e.g. Arch Linux).
-
- The *best* parameter can be used to control the approach for the returned
- version:
-
- If *best* is false, the first non-empty version number in priority order of
- the examined sources is returned.
-
- If *best* is true, the most precise version number out of all examined
- sources is returned.
-
- **Lookup hierarchy:**
-
- In all cases, the version number is obtained from the following sources.
- If *best* is false, this order represents the priority order:
-
- * the value of the "VERSION_ID" attribute of the os-release file,
- * the value of the "Release" attribute returned by the lsb_release
- command,
- * the version number parsed from the "" field of the first line
- of the distro release file,
- * the version number parsed from the "PRETTY_NAME" attribute of the
- os-release file, if it follows the format of the distro release files.
- * the version number parsed from the "Description" attribute returned by
- the lsb_release command, if it follows the format of the distro release
- files.
- """
- return _distro.version(pretty, best)
-
-
-def version_parts(best: bool = False) -> Tuple[str, str, str]:
- """
- Return the version of the current OS distribution as a tuple
- ``(major, minor, build_number)`` with items as follows:
-
- * ``major``: The result of :func:`distro.major_version`.
-
- * ``minor``: The result of :func:`distro.minor_version`.
-
- * ``build_number``: The result of :func:`distro.build_number`.
-
- For a description of the *best* parameter, see the :func:`distro.version`
- method.
- """
- return _distro.version_parts(best)
-
-
-def major_version(best: bool = False) -> str:
- """
- Return the major version of the current OS distribution, as a string,
- if provided.
- Otherwise, the empty string is returned. The major version is the first
- part of the dot-separated version string.
-
- For a description of the *best* parameter, see the :func:`distro.version`
- method.
- """
- return _distro.major_version(best)
-
-
-def minor_version(best: bool = False) -> str:
- """
- Return the minor version of the current OS distribution, as a string,
- if provided.
- Otherwise, the empty string is returned. The minor version is the second
- part of the dot-separated version string.
-
- For a description of the *best* parameter, see the :func:`distro.version`
- method.
- """
- return _distro.minor_version(best)
-
-
-def build_number(best: bool = False) -> str:
- """
- Return the build number of the current OS distribution, as a string,
- if provided.
- Otherwise, the empty string is returned. The build number is the third part
- of the dot-separated version string.
-
- For a description of the *best* parameter, see the :func:`distro.version`
- method.
- """
- return _distro.build_number(best)
-
-
-def like() -> str:
- """
- Return a space-separated list of distro IDs of distributions that are
- closely related to the current OS distribution in regards to packaging
- and programming interfaces, for example distributions the current
- distribution is a derivative from.
-
- **Lookup hierarchy:**
-
- This information item is only provided by the os-release file.
- For details, see the description of the "ID_LIKE" attribute in the
- `os-release man page
- `_.
- """
- return _distro.like()
-
-
-def codename() -> str:
- """
- Return the codename for the release of the current OS distribution,
- as a string.
-
- If the distribution does not have a codename, an empty string is returned.
-
- Note that the returned codename is not always really a codename. For
- example, openSUSE returns "x86_64". This function does not handle such
- cases in any special way and just returns the string it finds, if any.
-
- **Lookup hierarchy:**
-
- * the codename within the "VERSION" attribute of the os-release file, if
- provided,
-
- * the value of the "Codename" attribute returned by the lsb_release
- command,
-
- * the value of the "" field of the distro release file.
- """
- return _distro.codename()
-
-
-def info(pretty: bool = False, best: bool = False) -> InfoDict:
- """
- Return certain machine-readable information items about the current OS
- distribution in a dictionary, as shown in the following example:
-
- .. sourcecode:: python
-
- {
- 'id': 'rhel',
- 'version': '7.0',
- 'version_parts': {
- 'major': '7',
- 'minor': '0',
- 'build_number': ''
- },
- 'like': 'fedora',
- 'codename': 'Maipo'
- }
-
- The dictionary structure and keys are always the same, regardless of which
- information items are available in the underlying data sources. The values
- for the various keys are as follows:
-
- * ``id``: The result of :func:`distro.id`.
-
- * ``version``: The result of :func:`distro.version`.
-
- * ``version_parts -> major``: The result of :func:`distro.major_version`.
-
- * ``version_parts -> minor``: The result of :func:`distro.minor_version`.
-
- * ``version_parts -> build_number``: The result of
- :func:`distro.build_number`.
-
- * ``like``: The result of :func:`distro.like`.
-
- * ``codename``: The result of :func:`distro.codename`.
-
- For a description of the *pretty* and *best* parameters, see the
- :func:`distro.version` method.
- """
- return _distro.info(pretty, best)
-
-
-def os_release_info() -> Dict[str, str]:
- """
- Return a dictionary containing key-value pairs for the information items
- from the os-release file data source of the current OS distribution.
-
- See `os-release file`_ for details about these information items.
- """
- return _distro.os_release_info()
-
-
-def lsb_release_info() -> Dict[str, str]:
- """
- Return a dictionary containing key-value pairs for the information items
- from the lsb_release command data source of the current OS distribution.
-
- See `lsb_release command output`_ for details about these information
- items.
- """
- return _distro.lsb_release_info()
-
-
-def distro_release_info() -> Dict[str, str]:
- """
- Return a dictionary containing key-value pairs for the information items
- from the distro release file data source of the current OS distribution.
-
- See `distro release file`_ for details about these information items.
- """
- return _distro.distro_release_info()
-
-
-def uname_info() -> Dict[str, str]:
- """
- Return a dictionary containing key-value pairs for the information items
- from the distro release file data source of the current OS distribution.
- """
- return _distro.uname_info()
-
-
-def os_release_attr(attribute: str) -> str:
- """
- Return a single named information item from the os-release file data source
- of the current OS distribution.
-
- Parameters:
-
- * ``attribute`` (string): Key of the information item.
-
- Returns:
-
- * (string): Value of the information item, if the item exists.
- The empty string, if the item does not exist.
-
- See `os-release file`_ for details about these information items.
- """
- return _distro.os_release_attr(attribute)
-
-
-def lsb_release_attr(attribute: str) -> str:
- """
- Return a single named information item from the lsb_release command output
- data source of the current OS distribution.
-
- Parameters:
-
- * ``attribute`` (string): Key of the information item.
-
- Returns:
-
- * (string): Value of the information item, if the item exists.
- The empty string, if the item does not exist.
-
- See `lsb_release command output`_ for details about these information
- items.
- """
- return _distro.lsb_release_attr(attribute)
-
-
-def distro_release_attr(attribute: str) -> str:
- """
- Return a single named information item from the distro release file
- data source of the current OS distribution.
-
- Parameters:
-
- * ``attribute`` (string): Key of the information item.
-
- Returns:
-
- * (string): Value of the information item, if the item exists.
- The empty string, if the item does not exist.
-
- See `distro release file`_ for details about these information items.
- """
- return _distro.distro_release_attr(attribute)
-
-
-def uname_attr(attribute: str) -> str:
- """
- Return a single named information item from the distro release file
- data source of the current OS distribution.
-
- Parameters:
-
- * ``attribute`` (string): Key of the information item.
-
- Returns:
-
- * (string): Value of the information item, if the item exists.
- The empty string, if the item does not exist.
- """
- return _distro.uname_attr(attribute)
-
-
-try:
- from functools import cached_property
-except ImportError:
- # Python < 3.8
- class cached_property: # type: ignore
- """A version of @property which caches the value. On access, it calls the
- underlying function and sets the value in `__dict__` so future accesses
- will not re-call the property.
- """
-
- def __init__(self, f: Callable[[Any], Any]) -> None:
- self._fname = f.__name__
- self._f = f
-
- def __get__(self, obj: Any, owner: Type[Any]) -> Any:
- assert obj is not None, f"call {self._fname} on an instance"
- ret = obj.__dict__[self._fname] = self._f(obj)
- return ret
-
-
-class LinuxDistribution:
- """
- Provides information about a OS distribution.
-
- This package creates a private module-global instance of this class with
- default initialization arguments, that is used by the
- `consolidated accessor functions`_ and `single source accessor functions`_.
- By using default initialization arguments, that module-global instance
- returns data about the current OS distribution (i.e. the distro this
- package runs on).
-
- Normally, it is not necessary to create additional instances of this class.
- However, in situations where control is needed over the exact data sources
- that are used, instances of this class can be created with a specific
- distro release file, or a specific os-release file, or without invoking the
- lsb_release command.
- """
-
- def __init__(
- self,
- include_lsb: Optional[bool] = None,
- os_release_file: str = "",
- distro_release_file: str = "",
- include_uname: Optional[bool] = None,
- root_dir: Optional[str] = None,
- include_oslevel: Optional[bool] = None,
- ) -> None:
- """
- The initialization method of this class gathers information from the
- available data sources, and stores that in private instance attributes.
- Subsequent access to the information items uses these private instance
- attributes, so that the data sources are read only once.
-
- Parameters:
-
- * ``include_lsb`` (bool): Controls whether the
- `lsb_release command output`_ is included as a data source.
-
- If the lsb_release command is not available in the program execution
- path, the data source for the lsb_release command will be empty.
-
- * ``os_release_file`` (string): The path name of the
- `os-release file`_ that is to be used as a data source.
-
- An empty string (the default) will cause the default path name to
- be used (see `os-release file`_ for details).
-
- If the specified or defaulted os-release file does not exist, the
- data source for the os-release file will be empty.
-
- * ``distro_release_file`` (string): The path name of the
- `distro release file`_ that is to be used as a data source.
-
- An empty string (the default) will cause a default search algorithm
- to be used (see `distro release file`_ for details).
-
- If the specified distro release file does not exist, or if no default
- distro release file can be found, the data source for the distro
- release file will be empty.
-
- * ``include_uname`` (bool): Controls whether uname command output is
- included as a data source. If the uname command is not available in
- the program execution path the data source for the uname command will
- be empty.
-
- * ``root_dir`` (string): The absolute path to the root directory to use
- to find distro-related information files. Note that ``include_*``
- parameters must not be enabled in combination with ``root_dir``.
-
- * ``include_oslevel`` (bool): Controls whether (AIX) oslevel command
- output is included as a data source. If the oslevel command is not
- available in the program execution path the data source will be
- empty.
-
- Public instance attributes:
-
- * ``os_release_file`` (string): The path name of the
- `os-release file`_ that is actually used as a data source. The
- empty string if no distro release file is used as a data source.
-
- * ``distro_release_file`` (string): The path name of the
- `distro release file`_ that is actually used as a data source. The
- empty string if no distro release file is used as a data source.
-
- * ``include_lsb`` (bool): The result of the ``include_lsb`` parameter.
- This controls whether the lsb information will be loaded.
-
- * ``include_uname`` (bool): The result of the ``include_uname``
- parameter. This controls whether the uname information will
- be loaded.
-
- * ``include_oslevel`` (bool): The result of the ``include_oslevel``
- parameter. This controls whether (AIX) oslevel information will be
- loaded.
-
- * ``root_dir`` (string): The result of the ``root_dir`` parameter.
- The absolute path to the root directory to use to find distro-related
- information files.
-
- Raises:
-
- * :py:exc:`ValueError`: Initialization parameters combination is not
- supported.
-
- * :py:exc:`OSError`: Some I/O issue with an os-release file or distro
- release file.
-
- * :py:exc:`UnicodeError`: A data source has unexpected characters or
- uses an unexpected encoding.
- """
- self.root_dir = root_dir
- self.etc_dir = os.path.join(root_dir, "etc") if root_dir else _UNIXCONFDIR
- self.usr_lib_dir = (
- os.path.join(root_dir, "usr/lib") if root_dir else _UNIXUSRLIBDIR
- )
-
- if os_release_file:
- self.os_release_file = os_release_file
- else:
- etc_dir_os_release_file = os.path.join(self.etc_dir, _OS_RELEASE_BASENAME)
- usr_lib_os_release_file = os.path.join(
- self.usr_lib_dir, _OS_RELEASE_BASENAME
- )
-
- # NOTE: The idea is to respect order **and** have it set
- # at all times for API backwards compatibility.
- if os.path.isfile(etc_dir_os_release_file) or not os.path.isfile(
- usr_lib_os_release_file
- ):
- self.os_release_file = etc_dir_os_release_file
- else:
- self.os_release_file = usr_lib_os_release_file
-
- self.distro_release_file = distro_release_file or "" # updated later
-
- is_root_dir_defined = root_dir is not None
- if is_root_dir_defined and (include_lsb or include_uname or include_oslevel):
- raise ValueError(
- "Including subprocess data sources from specific root_dir is disallowed"
- " to prevent false information"
- )
- self.include_lsb = (
- include_lsb if include_lsb is not None else not is_root_dir_defined
- )
- self.include_uname = (
- include_uname if include_uname is not None else not is_root_dir_defined
- )
- self.include_oslevel = (
- include_oslevel if include_oslevel is not None else not is_root_dir_defined
- )
-
- def __repr__(self) -> str:
- """Return repr of all info"""
- return (
- "LinuxDistribution("
- "os_release_file={self.os_release_file!r}, "
- "distro_release_file={self.distro_release_file!r}, "
- "include_lsb={self.include_lsb!r}, "
- "include_uname={self.include_uname!r}, "
- "include_oslevel={self.include_oslevel!r}, "
- "root_dir={self.root_dir!r}, "
- "_os_release_info={self._os_release_info!r}, "
- "_lsb_release_info={self._lsb_release_info!r}, "
- "_distro_release_info={self._distro_release_info!r}, "
- "_uname_info={self._uname_info!r}, "
- "_oslevel_info={self._oslevel_info!r})".format(self=self)
- )
-
- def linux_distribution(
- self, full_distribution_name: bool = True
- ) -> Tuple[str, str, str]:
- """
- Return information about the OS distribution that is compatible
- with Python's :func:`platform.linux_distribution`, supporting a subset
- of its parameters.
-
- For details, see :func:`distro.linux_distribution`.
- """
- return (
- self.name() if full_distribution_name else self.id(),
- self.version(),
- self._os_release_info.get("release_codename") or self.codename(),
- )
-
- def id(self) -> str:
- """Return the distro ID of the OS distribution, as a string.
-
- For details, see :func:`distro.id`.
- """
-
- def normalize(distro_id: str, table: Dict[str, str]) -> str:
- distro_id = distro_id.lower().replace(" ", "_")
- return table.get(distro_id, distro_id)
-
- distro_id = self.os_release_attr("id")
- if distro_id:
- return normalize(distro_id, NORMALIZED_OS_ID)
-
- distro_id = self.lsb_release_attr("distributor_id")
- if distro_id:
- return normalize(distro_id, NORMALIZED_LSB_ID)
-
- distro_id = self.distro_release_attr("id")
- if distro_id:
- return normalize(distro_id, NORMALIZED_DISTRO_ID)
-
- distro_id = self.uname_attr("id")
- if distro_id:
- return normalize(distro_id, NORMALIZED_DISTRO_ID)
-
- return ""
-
- def name(self, pretty: bool = False) -> str:
- """
- Return the name of the OS distribution, as a string.
-
- For details, see :func:`distro.name`.
- """
- name = (
- self.os_release_attr("name")
- or self.lsb_release_attr("distributor_id")
- or self.distro_release_attr("name")
- or self.uname_attr("name")
- )
- if pretty:
- name = self.os_release_attr("pretty_name") or self.lsb_release_attr(
- "description"
- )
- if not name:
- name = self.distro_release_attr("name") or self.uname_attr("name")
- version = self.version(pretty=True)
- if version:
- name = f"{name} {version}"
- return name or ""
-
- def version(self, pretty: bool = False, best: bool = False) -> str:
- """
- Return the version of the OS distribution, as a string.
-
- For details, see :func:`distro.version`.
- """
- versions = [
- self.os_release_attr("version_id"),
- self.lsb_release_attr("release"),
- self.distro_release_attr("version_id"),
- self._parse_distro_release_content(self.os_release_attr("pretty_name")).get(
- "version_id", ""
- ),
- self._parse_distro_release_content(
- self.lsb_release_attr("description")
- ).get("version_id", ""),
- self.uname_attr("release"),
- ]
- if self.uname_attr("id").startswith("aix"):
- # On AIX platforms, prefer oslevel command output.
- versions.insert(0, self.oslevel_info())
- version = ""
- if best:
- # This algorithm uses the last version in priority order that has
- # the best precision. If the versions are not in conflict, that
- # does not matter; otherwise, using the last one instead of the
- # first one might be considered a surprise.
- for v in versions:
- if v.count(".") > version.count(".") or version == "":
- version = v
- else:
- for v in versions:
- if v != "":
- version = v
- break
- if pretty and version and self.codename():
- version = f"{version} ({self.codename()})"
- return version
-
- def version_parts(self, best: bool = False) -> Tuple[str, str, str]:
- """
- Return the version of the OS distribution, as a tuple of version
- numbers.
-
- For details, see :func:`distro.version_parts`.
- """
- version_str = self.version(best=best)
- if version_str:
- version_regex = re.compile(r"(\d+)\.?(\d+)?\.?(\d+)?")
- matches = version_regex.match(version_str)
- if matches:
- major, minor, build_number = matches.groups()
- return major, minor or "", build_number or ""
- return "", "", ""
-
- def major_version(self, best: bool = False) -> str:
- """
- Return the major version number of the current distribution.
-
- For details, see :func:`distro.major_version`.
- """
- return self.version_parts(best)[0]
-
- def minor_version(self, best: bool = False) -> str:
- """
- Return the minor version number of the current distribution.
-
- For details, see :func:`distro.minor_version`.
- """
- return self.version_parts(best)[1]
-
- def build_number(self, best: bool = False) -> str:
- """
- Return the build number of the current distribution.
-
- For details, see :func:`distro.build_number`.
- """
- return self.version_parts(best)[2]
-
- def like(self) -> str:
- """
- Return the IDs of distributions that are like the OS distribution.
-
- For details, see :func:`distro.like`.
- """
- return self.os_release_attr("id_like") or ""
-
- def codename(self) -> str:
- """
- Return the codename of the OS distribution.
-
- For details, see :func:`distro.codename`.
- """
- try:
- # Handle os_release specially since distros might purposefully set
- # this to empty string to have no codename
- return self._os_release_info["codename"]
- except KeyError:
- return (
- self.lsb_release_attr("codename")
- or self.distro_release_attr("codename")
- or ""
- )
-
- def info(self, pretty: bool = False, best: bool = False) -> InfoDict:
- """
- Return certain machine-readable information about the OS
- distribution.
-
- For details, see :func:`distro.info`.
- """
- return dict(
- id=self.id(),
- version=self.version(pretty, best),
- version_parts=dict(
- major=self.major_version(best),
- minor=self.minor_version(best),
- build_number=self.build_number(best),
- ),
- like=self.like(),
- codename=self.codename(),
- )
-
- def os_release_info(self) -> Dict[str, str]:
- """
- Return a dictionary containing key-value pairs for the information
- items from the os-release file data source of the OS distribution.
-
- For details, see :func:`distro.os_release_info`.
- """
- return self._os_release_info
-
- def lsb_release_info(self) -> Dict[str, str]:
- """
- Return a dictionary containing key-value pairs for the information
- items from the lsb_release command data source of the OS
- distribution.
-
- For details, see :func:`distro.lsb_release_info`.
- """
- return self._lsb_release_info
-
- def distro_release_info(self) -> Dict[str, str]:
- """
- Return a dictionary containing key-value pairs for the information
- items from the distro release file data source of the OS
- distribution.
-
- For details, see :func:`distro.distro_release_info`.
- """
- return self._distro_release_info
-
- def uname_info(self) -> Dict[str, str]:
- """
- Return a dictionary containing key-value pairs for the information
- items from the uname command data source of the OS distribution.
-
- For details, see :func:`distro.uname_info`.
- """
- return self._uname_info
-
- def oslevel_info(self) -> str:
- """
- Return AIX' oslevel command output.
- """
- return self._oslevel_info
-
- def os_release_attr(self, attribute: str) -> str:
- """
- Return a single named information item from the os-release file data
- source of the OS distribution.
-
- For details, see :func:`distro.os_release_attr`.
- """
- return self._os_release_info.get(attribute, "")
-
- def lsb_release_attr(self, attribute: str) -> str:
- """
- Return a single named information item from the lsb_release command
- output data source of the OS distribution.
-
- For details, see :func:`distro.lsb_release_attr`.
- """
- return self._lsb_release_info.get(attribute, "")
-
- def distro_release_attr(self, attribute: str) -> str:
- """
- Return a single named information item from the distro release file
- data source of the OS distribution.
-
- For details, see :func:`distro.distro_release_attr`.
- """
- return self._distro_release_info.get(attribute, "")
-
- def uname_attr(self, attribute: str) -> str:
- """
- Return a single named information item from the uname command
- output data source of the OS distribution.
-
- For details, see :func:`distro.uname_attr`.
- """
- return self._uname_info.get(attribute, "")
-
- @cached_property
- def _os_release_info(self) -> Dict[str, str]:
- """
- Get the information items from the specified os-release file.
-
- Returns:
- A dictionary containing all information items.
- """
- if os.path.isfile(self.os_release_file):
- with open(self.os_release_file, encoding="utf-8") as release_file:
- return self._parse_os_release_content(release_file)
- return {}
-
- @staticmethod
- def _parse_os_release_content(lines: TextIO) -> Dict[str, str]:
- """
- Parse the lines of an os-release file.
-
- Parameters:
-
- * lines: Iterable through the lines in the os-release file.
- Each line must be a unicode string or a UTF-8 encoded byte
- string.
-
- Returns:
- A dictionary containing all information items.
- """
- props = {}
- lexer = shlex.shlex(lines, posix=True)
- lexer.whitespace_split = True
-
- tokens = list(lexer)
- for token in tokens:
- # At this point, all shell-like parsing has been done (i.e.
- # comments processed, quotes and backslash escape sequences
- # processed, multi-line values assembled, trailing newlines
- # stripped, etc.), so the tokens are now either:
- # * variable assignments: var=value
- # * commands or their arguments (not allowed in os-release)
- # Ignore any tokens that are not variable assignments
- if "=" in token:
- k, v = token.split("=", 1)
- props[k.lower()] = v
-
- if "version" in props:
- # extract release codename (if any) from version attribute
- match = re.search(r"\((\D+)\)|,\s*(\D+)", props["version"])
- if match:
- release_codename = match.group(1) or match.group(2)
- props["codename"] = props["release_codename"] = release_codename
-
- if "version_codename" in props:
- # os-release added a version_codename field. Use that in
- # preference to anything else Note that some distros purposefully
- # do not have code names. They should be setting
- # version_codename=""
- props["codename"] = props["version_codename"]
- elif "ubuntu_codename" in props:
- # Same as above but a non-standard field name used on older Ubuntus
- props["codename"] = props["ubuntu_codename"]
-
- return props
-
- @cached_property
- def _lsb_release_info(self) -> Dict[str, str]:
- """
- Get the information items from the lsb_release command output.
-
- Returns:
- A dictionary containing all information items.
- """
- if not self.include_lsb:
- return {}
- try:
- cmd = ("lsb_release", "-a")
- stdout = subprocess.check_output(cmd, stderr=subprocess.DEVNULL)
- # Command not found or lsb_release returned error
- except (OSError, subprocess.CalledProcessError):
- return {}
- content = self._to_str(stdout).splitlines()
- return self._parse_lsb_release_content(content)
-
- @staticmethod
- def _parse_lsb_release_content(lines: Iterable[str]) -> Dict[str, str]:
- """
- Parse the output of the lsb_release command.
-
- Parameters:
-
- * lines: Iterable through the lines of the lsb_release output.
- Each line must be a unicode string or a UTF-8 encoded byte
- string.
-
- Returns:
- A dictionary containing all information items.
- """
- props = {}
- for line in lines:
- kv = line.strip("\n").split(":", 1)
- if len(kv) != 2:
- # Ignore lines without colon.
- continue
- k, v = kv
- props.update({k.replace(" ", "_").lower(): v.strip()})
- return props
-
- @cached_property
- def _uname_info(self) -> Dict[str, str]:
- if not self.include_uname:
- return {}
- try:
- cmd = ("uname", "-rs")
- stdout = subprocess.check_output(cmd, stderr=subprocess.DEVNULL)
- except OSError:
- return {}
- content = self._to_str(stdout).splitlines()
- return self._parse_uname_content(content)
-
- @cached_property
- def _oslevel_info(self) -> str:
- if not self.include_oslevel:
- return ""
- try:
- stdout = subprocess.check_output("oslevel", stderr=subprocess.DEVNULL)
- except (OSError, subprocess.CalledProcessError):
- return ""
- return self._to_str(stdout).strip()
-
- @staticmethod
- def _parse_uname_content(lines: Sequence[str]) -> Dict[str, str]:
- if not lines:
- return {}
- props = {}
- match = re.search(r"^([^\s]+)\s+([\d\.]+)", lines[0].strip())
- if match:
- name, version = match.groups()
-
- # This is to prevent the Linux kernel version from
- # appearing as the 'best' version on otherwise
- # identifiable distributions.
- if name == "Linux":
- return {}
- props["id"] = name.lower()
- props["name"] = name
- props["release"] = version
- return props
-
- @staticmethod
- def _to_str(bytestring: bytes) -> str:
- encoding = sys.getfilesystemencoding()
- return bytestring.decode(encoding)
-
- @cached_property
- def _distro_release_info(self) -> Dict[str, str]:
- """
- Get the information items from the specified distro release file.
-
- Returns:
- A dictionary containing all information items.
- """
- if self.distro_release_file:
- # If it was specified, we use it and parse what we can, even if
- # its file name or content does not match the expected pattern.
- distro_info = self._parse_distro_release_file(self.distro_release_file)
- basename = os.path.basename(self.distro_release_file)
- # The file name pattern for user-specified distro release files
- # is somewhat more tolerant (compared to when searching for the
- # file), because we want to use what was specified as best as
- # possible.
- match = _DISTRO_RELEASE_BASENAME_PATTERN.match(basename)
- if "name" in distro_info and "cloudlinux" in distro_info["name"].lower():
- distro_info["id"] = "cloudlinux"
- elif match:
- distro_info["id"] = match.group(1)
- return distro_info
- else:
- try:
- basenames = os.listdir(self.etc_dir)
- # We sort for repeatability in cases where there are multiple
- # distro specific files; e.g. CentOS, Oracle, Enterprise all
- # containing `redhat-release` on top of their own.
- basenames.sort()
- except OSError:
- # This may occur when /etc is not readable but we can't be
- # sure about the *-release files. Check common entries of
- # /etc for information. If they turn out to not be there the
- # error is handled in `_parse_distro_release_file()`.
- basenames = [
- "SuSE-release",
- "arch-release",
- "base-release",
- "centos-release",
- "fedora-release",
- "gentoo-release",
- "mageia-release",
- "mandrake-release",
- "mandriva-release",
- "mandrivalinux-release",
- "manjaro-release",
- "oracle-release",
- "redhat-release",
- "rocky-release",
- "sl-release",
- "slackware-version",
- ]
- for basename in basenames:
- if basename in _DISTRO_RELEASE_IGNORE_BASENAMES:
- continue
- match = _DISTRO_RELEASE_BASENAME_PATTERN.match(basename)
- if match:
- filepath = os.path.join(self.etc_dir, basename)
- distro_info = self._parse_distro_release_file(filepath)
- if "name" in distro_info:
- # The name is always present if the pattern matches
- self.distro_release_file = filepath
- distro_info["id"] = match.group(1)
- if "cloudlinux" in distro_info["name"].lower():
- distro_info["id"] = "cloudlinux"
- return distro_info
- return {}
-
- def _parse_distro_release_file(self, filepath: str) -> Dict[str, str]:
- """
- Parse a distro release file.
-
- Parameters:
-
- * filepath: Path name of the distro release file.
-
- Returns:
- A dictionary containing all information items.
- """
- try:
- with open(filepath, encoding="utf-8") as fp:
- # Only parse the first line. For instance, on SLES there
- # are multiple lines. We don't want them...
- return self._parse_distro_release_content(fp.readline())
- except OSError:
- # Ignore not being able to read a specific, seemingly version
- # related file.
- # See https://github.com/python-distro/distro/issues/162
- return {}
-
- @staticmethod
- def _parse_distro_release_content(line: str) -> Dict[str, str]:
- """
- Parse a line from a distro release file.
-
- Parameters:
- * line: Line from the distro release file. Must be a unicode string
- or a UTF-8 encoded byte string.
-
- Returns:
- A dictionary containing all information items.
- """
- matches = _DISTRO_RELEASE_CONTENT_REVERSED_PATTERN.match(line.strip()[::-1])
- distro_info = {}
- if matches:
- # regexp ensures non-None
- distro_info["name"] = matches.group(3)[::-1]
- if matches.group(2):
- distro_info["version_id"] = matches.group(2)[::-1]
- if matches.group(1):
- distro_info["codename"] = matches.group(1)[::-1]
- elif line:
- distro_info["name"] = line.strip()
- return distro_info
-
-
-_distro = LinuxDistribution()
-
-
-def main() -> None:
- logger = logging.getLogger(__name__)
- logger.setLevel(logging.DEBUG)
- logger.addHandler(logging.StreamHandler(sys.stdout))
-
- parser = argparse.ArgumentParser(description="OS distro info tool")
- parser.add_argument(
- "--json", "-j", help="Output in machine readable format", action="store_true"
- )
-
- parser.add_argument(
- "--root-dir",
- "-r",
- type=str,
- dest="root_dir",
- help="Path to the root filesystem directory (defaults to /)",
- )
-
- args = parser.parse_args()
-
- if args.root_dir:
- dist = LinuxDistribution(
- include_lsb=False,
- include_uname=False,
- include_oslevel=False,
- root_dir=args.root_dir,
- )
- else:
- dist = _distro
-
- if args.json:
- logger.info(json.dumps(dist.info(), indent=4, sort_keys=True))
- else:
- logger.info("Name: %s", dist.name(pretty=True))
- distribution_version = dist.version(pretty=True)
- logger.info("Version: %s", distribution_version)
- distribution_codename = dist.codename()
- logger.info("Codename: %s", distribution_codename)
-
-
-if __name__ == "__main__":
- main()
diff --git a/spaces/Raspberry-ai/main/.env/lib/python3.11/site-packages/pip/_vendor/rich/logging.py b/spaces/Raspberry-ai/main/.env/lib/python3.11/site-packages/pip/_vendor/rich/logging.py
deleted file mode 100644
index 58188fd8a841ecfe54afab4b862af18bf3826205..0000000000000000000000000000000000000000
--- a/spaces/Raspberry-ai/main/.env/lib/python3.11/site-packages/pip/_vendor/rich/logging.py
+++ /dev/null
@@ -1,280 +0,0 @@
-import logging
-from datetime import datetime
-from logging import Handler, LogRecord
-from pathlib import Path
-from types import ModuleType
-from typing import ClassVar, List, Optional, Iterable, Type, Union
-
-from . import get_console
-from ._log_render import LogRender, FormatTimeCallable
-from .console import Console, ConsoleRenderable
-from .highlighter import Highlighter, ReprHighlighter
-from .text import Text
-from .traceback import Traceback
-
-
-class RichHandler(Handler):
- """A logging handler that renders output with Rich. The time / level / message and file are displayed in columns.
- The level is color coded, and the message is syntax highlighted.
-
- Note:
- Be careful when enabling console markup in log messages if you have configured logging for libraries not
- under your control. If a dependency writes messages containing square brackets, it may not produce the intended output.
-
- Args:
- level (Union[int, str], optional): Log level. Defaults to logging.NOTSET.
- console (:class:`~rich.console.Console`, optional): Optional console instance to write logs.
- Default will use a global console instance writing to stdout.
- show_time (bool, optional): Show a column for the time. Defaults to True.
- omit_repeated_times (bool, optional): Omit repetition of the same time. Defaults to True.
- show_level (bool, optional): Show a column for the level. Defaults to True.
- show_path (bool, optional): Show the path to the original log call. Defaults to True.
- enable_link_path (bool, optional): Enable terminal link of path column to file. Defaults to True.
- highlighter (Highlighter, optional): Highlighter to style log messages, or None to use ReprHighlighter. Defaults to None.
- markup (bool, optional): Enable console markup in log messages. Defaults to False.
- rich_tracebacks (bool, optional): Enable rich tracebacks with syntax highlighting and formatting. Defaults to False.
- tracebacks_width (Optional[int], optional): Number of characters used to render tracebacks, or None for full width. Defaults to None.
- tracebacks_extra_lines (int, optional): Additional lines of code to render tracebacks, or None for full width. Defaults to None.
- tracebacks_theme (str, optional): Override pygments theme used in traceback.
- tracebacks_word_wrap (bool, optional): Enable word wrapping of long tracebacks lines. Defaults to True.
- tracebacks_show_locals (bool, optional): Enable display of locals in tracebacks. Defaults to False.
- tracebacks_suppress (Sequence[Union[str, ModuleType]]): Optional sequence of modules or paths to exclude from traceback.
- locals_max_length (int, optional): Maximum length of containers before abbreviating, or None for no abbreviation.
- Defaults to 10.
- locals_max_string (int, optional): Maximum length of string before truncating, or None to disable. Defaults to 80.
- log_time_format (Union[str, TimeFormatterCallable], optional): If ``log_time`` is enabled, either string for strftime or callable that formats the time. Defaults to "[%x %X] ".
- keywords (List[str], optional): List of words to highlight instead of ``RichHandler.KEYWORDS``.
- """
-
- KEYWORDS: ClassVar[Optional[List[str]]] = [
- "GET",
- "POST",
- "HEAD",
- "PUT",
- "DELETE",
- "OPTIONS",
- "TRACE",
- "PATCH",
- ]
- HIGHLIGHTER_CLASS: ClassVar[Type[Highlighter]] = ReprHighlighter
-
- def __init__(
- self,
- level: Union[int, str] = logging.NOTSET,
- console: Optional[Console] = None,
- *,
- show_time: bool = True,
- omit_repeated_times: bool = True,
- show_level: bool = True,
- show_path: bool = True,
- enable_link_path: bool = True,
- highlighter: Optional[Highlighter] = None,
- markup: bool = False,
- rich_tracebacks: bool = False,
- tracebacks_width: Optional[int] = None,
- tracebacks_extra_lines: int = 3,
- tracebacks_theme: Optional[str] = None,
- tracebacks_word_wrap: bool = True,
- tracebacks_show_locals: bool = False,
- tracebacks_suppress: Iterable[Union[str, ModuleType]] = (),
- locals_max_length: int = 10,
- locals_max_string: int = 80,
- log_time_format: Union[str, FormatTimeCallable] = "[%x %X]",
- keywords: Optional[List[str]] = None,
- ) -> None:
- super().__init__(level=level)
- self.console = console or get_console()
- self.highlighter = highlighter or self.HIGHLIGHTER_CLASS()
- self._log_render = LogRender(
- show_time=show_time,
- show_level=show_level,
- show_path=show_path,
- time_format=log_time_format,
- omit_repeated_times=omit_repeated_times,
- level_width=None,
- )
- self.enable_link_path = enable_link_path
- self.markup = markup
- self.rich_tracebacks = rich_tracebacks
- self.tracebacks_width = tracebacks_width
- self.tracebacks_extra_lines = tracebacks_extra_lines
- self.tracebacks_theme = tracebacks_theme
- self.tracebacks_word_wrap = tracebacks_word_wrap
- self.tracebacks_show_locals = tracebacks_show_locals
- self.tracebacks_suppress = tracebacks_suppress
- self.locals_max_length = locals_max_length
- self.locals_max_string = locals_max_string
- self.keywords = keywords
-
- def get_level_text(self, record: LogRecord) -> Text:
- """Get the level name from the record.
-
- Args:
- record (LogRecord): LogRecord instance.
-
- Returns:
- Text: A tuple of the style and level name.
- """
- level_name = record.levelname
- level_text = Text.styled(
- level_name.ljust(8), f"logging.level.{level_name.lower()}"
- )
- return level_text
-
- def emit(self, record: LogRecord) -> None:
- """Invoked by logging."""
- message = self.format(record)
- traceback = None
- if (
- self.rich_tracebacks
- and record.exc_info
- and record.exc_info != (None, None, None)
- ):
- exc_type, exc_value, exc_traceback = record.exc_info
- assert exc_type is not None
- assert exc_value is not None
- traceback = Traceback.from_exception(
- exc_type,
- exc_value,
- exc_traceback,
- width=self.tracebacks_width,
- extra_lines=self.tracebacks_extra_lines,
- theme=self.tracebacks_theme,
- word_wrap=self.tracebacks_word_wrap,
- show_locals=self.tracebacks_show_locals,
- locals_max_length=self.locals_max_length,
- locals_max_string=self.locals_max_string,
- suppress=self.tracebacks_suppress,
- )
- message = record.getMessage()
- if self.formatter:
- record.message = record.getMessage()
- formatter = self.formatter
- if hasattr(formatter, "usesTime") and formatter.usesTime():
- record.asctime = formatter.formatTime(record, formatter.datefmt)
- message = formatter.formatMessage(record)
-
- message_renderable = self.render_message(record, message)
- log_renderable = self.render(
- record=record, traceback=traceback, message_renderable=message_renderable
- )
- try:
- self.console.print(log_renderable)
- except Exception:
- self.handleError(record)
-
- def render_message(self, record: LogRecord, message: str) -> "ConsoleRenderable":
- """Render message text in to Text.
-
- record (LogRecord): logging Record.
- message (str): String containing log message.
-
- Returns:
- ConsoleRenderable: Renderable to display log message.
- """
- use_markup = getattr(record, "markup", self.markup)
- message_text = Text.from_markup(message) if use_markup else Text(message)
-
- highlighter = getattr(record, "highlighter", self.highlighter)
- if highlighter:
- message_text = highlighter(message_text)
-
- if self.keywords is None:
- self.keywords = self.KEYWORDS
-
- if self.keywords:
- message_text.highlight_words(self.keywords, "logging.keyword")
-
- return message_text
-
- def render(
- self,
- *,
- record: LogRecord,
- traceback: Optional[Traceback],
- message_renderable: "ConsoleRenderable",
- ) -> "ConsoleRenderable":
- """Render log for display.
-
- Args:
- record (LogRecord): logging Record.
- traceback (Optional[Traceback]): Traceback instance or None for no Traceback.
- message_renderable (ConsoleRenderable): Renderable (typically Text) containing log message contents.
-
- Returns:
- ConsoleRenderable: Renderable to display log.
- """
- path = Path(record.pathname).name
- level = self.get_level_text(record)
- time_format = None if self.formatter is None else self.formatter.datefmt
- log_time = datetime.fromtimestamp(record.created)
-
- log_renderable = self._log_render(
- self.console,
- [message_renderable] if not traceback else [message_renderable, traceback],
- log_time=log_time,
- time_format=time_format,
- level=level,
- path=path,
- line_no=record.lineno,
- link_path=record.pathname if self.enable_link_path else None,
- )
- return log_renderable
-
-
-if __name__ == "__main__": # pragma: no cover
- from time import sleep
-
- FORMAT = "%(message)s"
- # FORMAT = "%(asctime)-15s - %(levelname)s - %(message)s"
- logging.basicConfig(
- level="NOTSET",
- format=FORMAT,
- datefmt="[%X]",
- handlers=[RichHandler(rich_tracebacks=True, tracebacks_show_locals=True)],
- )
- log = logging.getLogger("rich")
-
- log.info("Server starting...")
- log.info("Listening on http://127.0.0.1:8080")
- sleep(1)
-
- log.info("GET /index.html 200 1298")
- log.info("GET /imgs/backgrounds/back1.jpg 200 54386")
- log.info("GET /css/styles.css 200 54386")
- log.warning("GET /favicon.ico 404 242")
- sleep(1)
-
- log.debug(
- "JSONRPC request\n--> %r\n<-- %r",
- {
- "version": "1.1",
- "method": "confirmFruitPurchase",
- "params": [["apple", "orange", "mangoes", "pomelo"], 1.123],
- "id": "194521489",
- },
- {"version": "1.1", "result": True, "error": None, "id": "194521489"},
- )
- log.debug(
- "Loading configuration file /adasd/asdasd/qeqwe/qwrqwrqwr/sdgsdgsdg/werwerwer/dfgerert/ertertert/ertetert/werwerwer"
- )
- log.error("Unable to find 'pomelo' in database!")
- log.info("POST /jsonrpc/ 200 65532")
- log.info("POST /admin/ 401 42234")
- log.warning("password was rejected for admin site.")
-
- def divide() -> None:
- number = 1
- divisor = 0
- foos = ["foo"] * 100
- log.debug("in divide")
- try:
- number / divisor
- except:
- log.exception("An error of some kind occurred!")
-
- divide()
- sleep(1)
- log.critical("Out of memory!")
- log.info("Server exited with code=-1")
- log.info("[bold]EXITING...[/bold]", extra=dict(markup=True))
diff --git a/spaces/Raspberry-ai/main/.env/lib/python3.11/site-packages/pip/_vendor/tenacity/tornadoweb.py b/spaces/Raspberry-ai/main/.env/lib/python3.11/site-packages/pip/_vendor/tenacity/tornadoweb.py
deleted file mode 100644
index 8f7731af0e62a985dbe4c77771a80525848e793c..0000000000000000000000000000000000000000
--- a/spaces/Raspberry-ai/main/.env/lib/python3.11/site-packages/pip/_vendor/tenacity/tornadoweb.py
+++ /dev/null
@@ -1,59 +0,0 @@
-# Copyright 2017 Elisey Zanko
-#
-# Licensed under the Apache License, Version 2.0 (the "License");
-# you may not use this file except in compliance with the License.
-# You may obtain a copy of the License at
-#
-# http://www.apache.org/licenses/LICENSE-2.0
-#
-# Unless required by applicable law or agreed to in writing, software
-# distributed under the License is distributed on an "AS IS" BASIS,
-# WITHOUT WARRANTIES OR CONDITIONS OF ANY KIND, either express or implied.
-# See the License for the specific language governing permissions and
-# limitations under the License.
-
-import sys
-import typing
-
-from pip._vendor.tenacity import BaseRetrying
-from pip._vendor.tenacity import DoAttempt
-from pip._vendor.tenacity import DoSleep
-from pip._vendor.tenacity import RetryCallState
-
-from tornado import gen
-
-if typing.TYPE_CHECKING:
- from tornado.concurrent import Future
-
-_RetValT = typing.TypeVar("_RetValT")
-
-
-class TornadoRetrying(BaseRetrying):
- def __init__(self, sleep: "typing.Callable[[float], Future[None]]" = gen.sleep, **kwargs: typing.Any) -> None:
- super().__init__(**kwargs)
- self.sleep = sleep
-
- @gen.coroutine
- def __call__( # type: ignore # Change signature from supertype
- self,
- fn: "typing.Callable[..., typing.Union[typing.Generator[typing.Any, typing.Any, _RetValT], Future[_RetValT]]]",
- *args: typing.Any,
- **kwargs: typing.Any,
- ) -> "typing.Generator[typing.Any, typing.Any, _RetValT]":
- self.begin()
-
- retry_state = RetryCallState(retry_object=self, fn=fn, args=args, kwargs=kwargs)
- while True:
- do = self.iter(retry_state=retry_state)
- if isinstance(do, DoAttempt):
- try:
- result = yield fn(*args, **kwargs)
- except BaseException: # noqa: B902
- retry_state.set_exception(sys.exc_info())
- else:
- retry_state.set_result(result)
- elif isinstance(do, DoSleep):
- retry_state.prepare_for_next_attempt()
- yield self.sleep(do)
- else:
- raise gen.Return(do)
diff --git a/spaces/Raspberry-ai/main/.env/lib/python3.11/site-packages/setuptools/_vendor/jaraco/__init__.py b/spaces/Raspberry-ai/main/.env/lib/python3.11/site-packages/setuptools/_vendor/jaraco/__init__.py
deleted file mode 100644
index e69de29bb2d1d6434b8b29ae775ad8c2e48c5391..0000000000000000000000000000000000000000
diff --git a/spaces/Raspberry-ai/main/.env/lib/python3.11/site-packages/setuptools_rust/extension.py b/spaces/Raspberry-ai/main/.env/lib/python3.11/site-packages/setuptools_rust/extension.py
deleted file mode 100644
index f7a09d1f4e5e0b8ca1d65bbb6b39ca6a5d6dfb92..0000000000000000000000000000000000000000
--- a/spaces/Raspberry-ai/main/.env/lib/python3.11/site-packages/setuptools_rust/extension.py
+++ /dev/null
@@ -1,370 +0,0 @@
-import json
-import os
-import re
-import subprocess
-import warnings
-from distutils.errors import DistutilsSetupError
-from enum import IntEnum, auto
-from functools import lru_cache
-from typing import Any, Dict, List, NewType, Optional, Sequence, Union, cast
-
-from semantic_version import SimpleSpec
-from typing_extensions import Literal
-
-from ._utils import format_called_process_error
-
-
-class Binding(IntEnum):
- """
- Enumeration of possible Rust binding types supported by ``setuptools-rust``.
-
- Attributes:
- PyO3: This is an extension built using
- `PyO3 `_.
- RustCPython: This is an extension built using
- `rust-cpython `_.
- NoBinding: Bring your own bindings for the extension.
- Exec: Build an executable instead of an extension.
- """
-
- PyO3 = auto()
- RustCPython = auto()
- NoBinding = auto()
- Exec = auto()
-
- def __repr__(self) -> str:
- return f"{self.__class__.__name__}.{self.name}"
-
-
-class Strip(IntEnum):
- """
- Enumeration of modes for stripping symbols from the built extension.
-
- Attributes:
- No: Do not strip symbols.
- Debug: Strip debug symbols.
- All: Strip all symbols.
- """
-
- No = auto()
- Debug = auto()
- All = auto()
-
- def __repr__(self) -> str:
- return f"{self.__class__.__name__}.{self.name}"
-
-
-class RustExtension:
- """Used to define a rust extension module and its build configuration.
-
- Args:
- target: The full Python dotted name of the extension, including any
- packages, i.e *not* a filename or pathname. It is possible to
- specify multiple binaries, if extension uses ``Binding.Exec``
- binding mode. In that case first argument has to be dictionary.
- Keys of the dictionary correspond to the rust binary names and
- values are the full dotted name to place the executable inside
- the python package. To install executables with kebab-case names,
- the final part of the dotted name can be in kebab-case. For
- example, `hello_world.hello-world` will install an executable
- named `hello-world`.
- path: Path to the ``Cargo.toml`` manifest file.
- args: A list of extra arguments to be passed to Cargo. For example,
- ``args=["--no-default-features"]`` will disable the default
- features listed in ``Cargo.toml``.
- cargo_manifest_args: A list of extra arguments to be passed to Cargo.
- These arguments will be passed to every ``cargo`` command, not just
- ``cargo build``. For valid options, see
- `the Cargo Book `_.
- For example, ``cargo_manifest_args=["--locked"]`` will require
- ``Cargo.lock`` files are up to date.
- features: Cargo `--features` to add to the build.
- rustc_flags: A list of additional flags passed to `cargo rustc`. These
- only affect the final artifact, usually you should set the
- `RUSTFLAGS` environment variable.
- rust_version: Minimum Rust compiler version required for this
- extension.
- quiet: Suppress Cargo's output.
- debug: Controls whether ``--debug`` or ``--release`` is passed to
- Cargo. If set to `None` (the default) then build type is
- automatic: ``inplace`` build will be a debug build, ``install``
- and ``wheel`` builds will be release.
- binding: Informs ``setuptools_rust`` which Python binding is in use.
- strip: Strip symbols from final file. Does nothing for debug build.
- native: Build extension or executable with ``-Ctarget-cpu=native``
- (deprecated, set environment variable RUSTFLAGS=-Ctarget-cpu=native).
- script: Generate console script for executable if ``Binding.Exec`` is
- used (deprecated, just use ``RustBin`` instead).
- optional: If it is true, a build failure in the extension will not
- abort the build process, and instead simply not install the failing
- extension.
- py_limited_api: Similar to ``py_limited_api`` on
- ``setuptools.Extension``, this controls whether the built extension
- should be considered compatible with the PEP 384 "limited API".
-
- - ``'auto'``: the ``--py-limited-api`` option of
- ``setup.py bdist_wheel`` will control whether the extension is
- built as a limited api extension. The corresponding
- ``pyo3/abi3-pyXY`` feature will be set accordingly.
- This is the recommended setting, as it allows
- ``python setup.py install`` to build a version-specific extension
- for best performance.
-
- - ``True``: the extension is assumed to be compatible with the
- limited abi. You must ensure this is the case (e.g. by setting
- the ``pyo3/abi3`` feature).
-
- - ``False``: the extension is version-specific.
- """
-
- def __init__(
- self,
- target: Union[str, Dict[str, str]],
- path: str = "Cargo.toml",
- args: Optional[Sequence[str]] = (),
- cargo_manifest_args: Optional[Sequence[str]] = (),
- features: Optional[Sequence[str]] = (),
- rustc_flags: Optional[Sequence[str]] = (),
- rust_version: Optional[str] = None,
- quiet: bool = False,
- debug: Optional[bool] = None,
- binding: Binding = Binding.PyO3,
- strip: Strip = Strip.No,
- script: bool = False,
- native: bool = False,
- optional: bool = False,
- py_limited_api: Literal["auto", True, False] = "auto",
- ):
- if isinstance(target, dict):
- name = "; ".join("%s=%s" % (key, val) for key, val in target.items())
- else:
- name = target
- target = {"": target}
-
- self.name = name
- self.target = target
- self.path = os.path.relpath(path) # relative path to Cargo manifest file
- self.args = tuple(args or ())
- self.cargo_manifest_args = tuple(cargo_manifest_args or ())
- self.features = tuple(features or ())
- self.rustc_flags = tuple(rustc_flags or ())
- self.rust_version = rust_version
- self.quiet = quiet
- self.debug = debug
- self.binding = binding
- self.strip = strip
- self.script = script
- self.optional = optional
- self.py_limited_api = py_limited_api
-
- if native:
- warnings.warn(
- "`native` is deprecated, set RUSTFLAGS=-Ctarget-cpu=native instead.",
- DeprecationWarning,
- )
- # match old behaviour of only setting flag for top-level crate;
- # setting for `rustflags` is strictly better
- self.rustc_flags = (*self.rustc_flags, "-Ctarget-cpu=native")
-
- if binding == Binding.Exec and script:
- warnings.warn(
- "`Binding.Exec` with `script=True` is deprecated, use `RustBin` instead.",
- DeprecationWarning,
- )
-
- def get_lib_name(self, *, quiet: bool) -> str:
- """Parse Cargo.toml to get the name of the shared library."""
- metadata = self.metadata(quiet=quiet)
- root_key = metadata["resolve"]["root"]
- [pkg] = [p for p in metadata["packages"] if p["id"] == root_key]
- name = pkg["targets"][0]["name"]
- assert isinstance(name, str)
- return re.sub(r"[./\\-]", "_", name)
-
- def get_rust_version(self) -> Optional[SimpleSpec]: # type: ignore[no-any-unimported]
- if self.rust_version is None:
- return None
- try:
- return SimpleSpec(self.rust_version)
- except ValueError:
- raise DistutilsSetupError(
- "Can not parse rust compiler version: %s", self.rust_version
- )
-
- def get_cargo_profile(self) -> Optional[str]:
- try:
- index = self.args.index("--profile")
- return self.args[index + 1]
- except ValueError:
- pass
- except IndexError:
- raise DistutilsSetupError("Can not parse cargo profile from %s", self.args)
-
- # Handle `--profile=`
- profile_args = [p for p in self.args if p.startswith("--profile=")]
- if profile_args:
- profile = profile_args[0].split("=", 1)[1]
- if not profile:
- raise DistutilsSetupError(
- "Can not parse cargo profile from %s", self.args
- )
- return profile
- else:
- return None
-
- def entry_points(self) -> List[str]:
- entry_points = []
- if self.script and self.binding == Binding.Exec:
- for executable, mod in self.target.items():
- base_mod, name = mod.rsplit(".")
- script = "%s=%s.%s:run" % (name, base_mod, _script_name(executable))
- entry_points.append(script)
-
- return entry_points
-
- def install_script(self, module_name: str, exe_path: str) -> None:
- if self.script and self.binding == Binding.Exec:
- dirname, executable = os.path.split(exe_path)
- script_name = _script_name(module_name)
- file = os.path.join(dirname, f"{script_name}.py")
- with open(file, "w") as f:
- f.write(_SCRIPT_TEMPLATE.format(executable=repr(executable)))
-
- def metadata(self, *, quiet: bool) -> "CargoMetadata":
- """Returns cargo metadata for this extension package.
-
- Cached - will only execute cargo on first invocation.
- """
-
- return self._metadata(os.environ.get("CARGO", "cargo"), quiet)
-
- @lru_cache()
- def _metadata(self, cargo: str, quiet: bool) -> "CargoMetadata":
- metadata_command = [
- cargo,
- "metadata",
- "--manifest-path",
- self.path,
- "--format-version",
- "1",
- ]
- if self.cargo_manifest_args:
- metadata_command.extend(self.cargo_manifest_args)
-
- try:
- # If quiet, capture stderr and only show it on exceptions
- # If not quiet, let stderr be inherited
- stderr = subprocess.PIPE if quiet else None
- payload = subprocess.check_output(
- metadata_command, stderr=stderr, encoding="latin-1"
- )
- except subprocess.CalledProcessError as e:
- raise DistutilsSetupError(format_called_process_error(e))
- try:
- return cast(CargoMetadata, json.loads(payload))
- except json.decoder.JSONDecodeError as e:
- raise DistutilsSetupError(
- f"""
- Error parsing output of cargo metadata as json; received:
- {payload}
- """
- ) from e
-
- def _uses_exec_binding(self) -> bool:
- return self.binding == Binding.Exec
-
-
-class RustBin(RustExtension):
- """Used to define a Rust binary and its build configuration.
-
- Args:
- target: Rust binary target name.
- path: Path to the ``Cargo.toml`` manifest file.
- args: A list of extra arguments to be passed to Cargo. For example,
- ``args=["--no-default-features"]`` will disable the default
- features listed in ``Cargo.toml``.
- cargo_manifest_args: A list of extra arguments to be passed to Cargo.
- These arguments will be passed to every ``cargo`` command, not just
- ``cargo build``. For valid options, see
- `the Cargo Book `_.
- For example, ``cargo_manifest_args=["--locked"]`` will require
- ``Cargo.lock`` files are up to date.
- features: Cargo `--features` to add to the build.
- rust_version: Minimum Rust compiler version required for this bin.
- quiet: Suppress Cargo's output.
- debug: Controls whether ``--debug`` or ``--release`` is passed to
- Cargo. If set to `None` (the default) then build type is
- automatic: ``inplace`` build will be a debug build, ``install``
- and ``wheel`` builds will be release.
- strip: Strip symbols from final file. Does nothing for debug build.
- optional: If it is true, a build failure in the bin will not
- abort the build process, and instead simply not install the failing
- bin.
- """
-
- def __init__(
- self,
- target: Union[str, Dict[str, str]],
- path: str = "Cargo.toml",
- args: Optional[Sequence[str]] = (),
- cargo_manifest_args: Optional[Sequence[str]] = (),
- features: Optional[Sequence[str]] = (),
- rust_version: Optional[str] = None,
- quiet: bool = False,
- debug: Optional[bool] = None,
- strip: Strip = Strip.No,
- optional: bool = False,
- ):
- super().__init__(
- target=target,
- path=path,
- args=args,
- cargo_manifest_args=cargo_manifest_args,
- features=features,
- rust_version=rust_version,
- quiet=quiet,
- debug=debug,
- binding=Binding.Exec,
- optional=optional,
- strip=strip,
- py_limited_api=False,
- )
-
- def entry_points(self) -> List[str]:
- return []
-
-
-CargoMetadata = NewType("CargoMetadata", Dict[str, Any])
-
-
-def _script_name(executable: str) -> str:
- """Generates the name of the installed Python script for an executable.
-
- Because Python modules must be snake_case, this generated script name will
- replace `-` with `_`.
-
- >>> _script_name("hello-world")
- '_gen_hello_world'
-
- >>> _script_name("foo_bar")
- '_gen_foo_bar'
-
- >>> _script_name("_gen_foo_bar")
- '_gen__gen_foo_bar'
- """
- script = executable.replace("-", "_")
- return f"_gen_{script}"
-
-
-_SCRIPT_TEMPLATE = """
-import os
-import sys
-
-def run():
- path = os.path.split(__file__)[0]
- file = os.path.join(path, {executable})
- if os.path.isfile(file):
- os.execv(file, sys.argv)
- else:
- raise RuntimeError("can't find " + file)
-"""
diff --git a/spaces/Rayzggz/illi-Bert-VITS2/modules.py b/spaces/Rayzggz/illi-Bert-VITS2/modules.py
deleted file mode 100644
index b1f89a2f837f190a3dd5de52e7a4e183f1024306..0000000000000000000000000000000000000000
--- a/spaces/Rayzggz/illi-Bert-VITS2/modules.py
+++ /dev/null
@@ -1,597 +0,0 @@
-import math
-import torch
-from torch import nn
-from torch.nn import functional as F
-
-from torch.nn import Conv1d
-from torch.nn.utils import weight_norm, remove_weight_norm
-
-import commons
-from commons import init_weights, get_padding
-from transforms import piecewise_rational_quadratic_transform
-from attentions import Encoder
-
-LRELU_SLOPE = 0.1
-
-
-class LayerNorm(nn.Module):
- def __init__(self, channels, eps=1e-5):
- super().__init__()
- self.channels = channels
- self.eps = eps
-
- self.gamma = nn.Parameter(torch.ones(channels))
- self.beta = nn.Parameter(torch.zeros(channels))
-
- def forward(self, x):
- x = x.transpose(1, -1)
- x = F.layer_norm(x, (self.channels,), self.gamma, self.beta, self.eps)
- return x.transpose(1, -1)
-
-
-class ConvReluNorm(nn.Module):
- def __init__(
- self,
- in_channels,
- hidden_channels,
- out_channels,
- kernel_size,
- n_layers,
- p_dropout,
- ):
- super().__init__()
- self.in_channels = in_channels
- self.hidden_channels = hidden_channels
- self.out_channels = out_channels
- self.kernel_size = kernel_size
- self.n_layers = n_layers
- self.p_dropout = p_dropout
- assert n_layers > 1, "Number of layers should be larger than 0."
-
- self.conv_layers = nn.ModuleList()
- self.norm_layers = nn.ModuleList()
- self.conv_layers.append(
- nn.Conv1d(
- in_channels, hidden_channels, kernel_size, padding=kernel_size // 2
- )
- )
- self.norm_layers.append(LayerNorm(hidden_channels))
- self.relu_drop = nn.Sequential(nn.ReLU(), nn.Dropout(p_dropout))
- for _ in range(n_layers - 1):
- self.conv_layers.append(
- nn.Conv1d(
- hidden_channels,
- hidden_channels,
- kernel_size,
- padding=kernel_size // 2,
- )
- )
- self.norm_layers.append(LayerNorm(hidden_channels))
- self.proj = nn.Conv1d(hidden_channels, out_channels, 1)
- self.proj.weight.data.zero_()
- self.proj.bias.data.zero_()
-
- def forward(self, x, x_mask):
- x_org = x
- for i in range(self.n_layers):
- x = self.conv_layers[i](x * x_mask)
- x = self.norm_layers[i](x)
- x = self.relu_drop(x)
- x = x_org + self.proj(x)
- return x * x_mask
-
-
-class DDSConv(nn.Module):
- """
- Dialted and Depth-Separable Convolution
- """
-
- def __init__(self, channels, kernel_size, n_layers, p_dropout=0.0):
- super().__init__()
- self.channels = channels
- self.kernel_size = kernel_size
- self.n_layers = n_layers
- self.p_dropout = p_dropout
-
- self.drop = nn.Dropout(p_dropout)
- self.convs_sep = nn.ModuleList()
- self.convs_1x1 = nn.ModuleList()
- self.norms_1 = nn.ModuleList()
- self.norms_2 = nn.ModuleList()
- for i in range(n_layers):
- dilation = kernel_size**i
- padding = (kernel_size * dilation - dilation) // 2
- self.convs_sep.append(
- nn.Conv1d(
- channels,
- channels,
- kernel_size,
- groups=channels,
- dilation=dilation,
- padding=padding,
- )
- )
- self.convs_1x1.append(nn.Conv1d(channels, channels, 1))
- self.norms_1.append(LayerNorm(channels))
- self.norms_2.append(LayerNorm(channels))
-
- def forward(self, x, x_mask, g=None):
- if g is not None:
- x = x + g
- for i in range(self.n_layers):
- y = self.convs_sep[i](x * x_mask)
- y = self.norms_1[i](y)
- y = F.gelu(y)
- y = self.convs_1x1[i](y)
- y = self.norms_2[i](y)
- y = F.gelu(y)
- y = self.drop(y)
- x = x + y
- return x * x_mask
-
-
-class WN(torch.nn.Module):
- def __init__(
- self,
- hidden_channels,
- kernel_size,
- dilation_rate,
- n_layers,
- gin_channels=0,
- p_dropout=0,
- ):
- super(WN, self).__init__()
- assert kernel_size % 2 == 1
- self.hidden_channels = hidden_channels
- self.kernel_size = (kernel_size,)
- self.dilation_rate = dilation_rate
- self.n_layers = n_layers
- self.gin_channels = gin_channels
- self.p_dropout = p_dropout
-
- self.in_layers = torch.nn.ModuleList()
- self.res_skip_layers = torch.nn.ModuleList()
- self.drop = nn.Dropout(p_dropout)
-
- if gin_channels != 0:
- cond_layer = torch.nn.Conv1d(
- gin_channels, 2 * hidden_channels * n_layers, 1
- )
- self.cond_layer = torch.nn.utils.weight_norm(cond_layer, name="weight")
-
- for i in range(n_layers):
- dilation = dilation_rate**i
- padding = int((kernel_size * dilation - dilation) / 2)
- in_layer = torch.nn.Conv1d(
- hidden_channels,
- 2 * hidden_channels,
- kernel_size,
- dilation=dilation,
- padding=padding,
- )
- in_layer = torch.nn.utils.weight_norm(in_layer, name="weight")
- self.in_layers.append(in_layer)
-
- # last one is not necessary
- if i < n_layers - 1:
- res_skip_channels = 2 * hidden_channels
- else:
- res_skip_channels = hidden_channels
-
- res_skip_layer = torch.nn.Conv1d(hidden_channels, res_skip_channels, 1)
- res_skip_layer = torch.nn.utils.weight_norm(res_skip_layer, name="weight")
- self.res_skip_layers.append(res_skip_layer)
-
- def forward(self, x, x_mask, g=None, **kwargs):
- output = torch.zeros_like(x)
- n_channels_tensor = torch.IntTensor([self.hidden_channels])
-
- if g is not None:
- g = self.cond_layer(g)
-
- for i in range(self.n_layers):
- x_in = self.in_layers[i](x)
- if g is not None:
- cond_offset = i * 2 * self.hidden_channels
- g_l = g[:, cond_offset : cond_offset + 2 * self.hidden_channels, :]
- else:
- g_l = torch.zeros_like(x_in)
-
- acts = commons.fused_add_tanh_sigmoid_multiply(x_in, g_l, n_channels_tensor)
- acts = self.drop(acts)
-
- res_skip_acts = self.res_skip_layers[i](acts)
- if i < self.n_layers - 1:
- res_acts = res_skip_acts[:, : self.hidden_channels, :]
- x = (x + res_acts) * x_mask
- output = output + res_skip_acts[:, self.hidden_channels :, :]
- else:
- output = output + res_skip_acts
- return output * x_mask
-
- def remove_weight_norm(self):
- if self.gin_channels != 0:
- torch.nn.utils.remove_weight_norm(self.cond_layer)
- for l in self.in_layers:
- torch.nn.utils.remove_weight_norm(l)
- for l in self.res_skip_layers:
- torch.nn.utils.remove_weight_norm(l)
-
-
-class ResBlock1(torch.nn.Module):
- def __init__(self, channels, kernel_size=3, dilation=(1, 3, 5)):
- super(ResBlock1, self).__init__()
- self.convs1 = nn.ModuleList(
- [
- weight_norm(
- Conv1d(
- channels,
- channels,
- kernel_size,
- 1,
- dilation=dilation[0],
- padding=get_padding(kernel_size, dilation[0]),
- )
- ),
- weight_norm(
- Conv1d(
- channels,
- channels,
- kernel_size,
- 1,
- dilation=dilation[1],
- padding=get_padding(kernel_size, dilation[1]),
- )
- ),
- weight_norm(
- Conv1d(
- channels,
- channels,
- kernel_size,
- 1,
- dilation=dilation[2],
- padding=get_padding(kernel_size, dilation[2]),
- )
- ),
- ]
- )
- self.convs1.apply(init_weights)
-
- self.convs2 = nn.ModuleList(
- [
- weight_norm(
- Conv1d(
- channels,
- channels,
- kernel_size,
- 1,
- dilation=1,
- padding=get_padding(kernel_size, 1),
- )
- ),
- weight_norm(
- Conv1d(
- channels,
- channels,
- kernel_size,
- 1,
- dilation=1,
- padding=get_padding(kernel_size, 1),
- )
- ),
- weight_norm(
- Conv1d(
- channels,
- channels,
- kernel_size,
- 1,
- dilation=1,
- padding=get_padding(kernel_size, 1),
- )
- ),
- ]
- )
- self.convs2.apply(init_weights)
-
- def forward(self, x, x_mask=None):
- for c1, c2 in zip(self.convs1, self.convs2):
- xt = F.leaky_relu(x, LRELU_SLOPE)
- if x_mask is not None:
- xt = xt * x_mask
- xt = c1(xt)
- xt = F.leaky_relu(xt, LRELU_SLOPE)
- if x_mask is not None:
- xt = xt * x_mask
- xt = c2(xt)
- x = xt + x
- if x_mask is not None:
- x = x * x_mask
- return x
-
- def remove_weight_norm(self):
- for l in self.convs1:
- remove_weight_norm(l)
- for l in self.convs2:
- remove_weight_norm(l)
-
-
-class ResBlock2(torch.nn.Module):
- def __init__(self, channels, kernel_size=3, dilation=(1, 3)):
- super(ResBlock2, self).__init__()
- self.convs = nn.ModuleList(
- [
- weight_norm(
- Conv1d(
- channels,
- channels,
- kernel_size,
- 1,
- dilation=dilation[0],
- padding=get_padding(kernel_size, dilation[0]),
- )
- ),
- weight_norm(
- Conv1d(
- channels,
- channels,
- kernel_size,
- 1,
- dilation=dilation[1],
- padding=get_padding(kernel_size, dilation[1]),
- )
- ),
- ]
- )
- self.convs.apply(init_weights)
-
- def forward(self, x, x_mask=None):
- for c in self.convs:
- xt = F.leaky_relu(x, LRELU_SLOPE)
- if x_mask is not None:
- xt = xt * x_mask
- xt = c(xt)
- x = xt + x
- if x_mask is not None:
- x = x * x_mask
- return x
-
- def remove_weight_norm(self):
- for l in self.convs:
- remove_weight_norm(l)
-
-
-class Log(nn.Module):
- def forward(self, x, x_mask, reverse=False, **kwargs):
- if not reverse:
- y = torch.log(torch.clamp_min(x, 1e-5)) * x_mask
- logdet = torch.sum(-y, [1, 2])
- return y, logdet
- else:
- x = torch.exp(x) * x_mask
- return x
-
-
-class Flip(nn.Module):
- def forward(self, x, *args, reverse=False, **kwargs):
- x = torch.flip(x, [1])
- if not reverse:
- logdet = torch.zeros(x.size(0)).to(dtype=x.dtype, device=x.device)
- return x, logdet
- else:
- return x
-
-
-class ElementwiseAffine(nn.Module):
- def __init__(self, channels):
- super().__init__()
- self.channels = channels
- self.m = nn.Parameter(torch.zeros(channels, 1))
- self.logs = nn.Parameter(torch.zeros(channels, 1))
-
- def forward(self, x, x_mask, reverse=False, **kwargs):
- if not reverse:
- y = self.m + torch.exp(self.logs) * x
- y = y * x_mask
- logdet = torch.sum(self.logs * x_mask, [1, 2])
- return y, logdet
- else:
- x = (x - self.m) * torch.exp(-self.logs) * x_mask
- return x
-
-
-class ResidualCouplingLayer(nn.Module):
- def __init__(
- self,
- channels,
- hidden_channels,
- kernel_size,
- dilation_rate,
- n_layers,
- p_dropout=0,
- gin_channels=0,
- mean_only=False,
- ):
- assert channels % 2 == 0, "channels should be divisible by 2"
- super().__init__()
- self.channels = channels
- self.hidden_channels = hidden_channels
- self.kernel_size = kernel_size
- self.dilation_rate = dilation_rate
- self.n_layers = n_layers
- self.half_channels = channels // 2
- self.mean_only = mean_only
-
- self.pre = nn.Conv1d(self.half_channels, hidden_channels, 1)
- self.enc = WN(
- hidden_channels,
- kernel_size,
- dilation_rate,
- n_layers,
- p_dropout=p_dropout,
- gin_channels=gin_channels,
- )
- self.post = nn.Conv1d(hidden_channels, self.half_channels * (2 - mean_only), 1)
- self.post.weight.data.zero_()
- self.post.bias.data.zero_()
-
- def forward(self, x, x_mask, g=None, reverse=False):
- x0, x1 = torch.split(x, [self.half_channels] * 2, 1)
- h = self.pre(x0) * x_mask
- h = self.enc(h, x_mask, g=g)
- stats = self.post(h) * x_mask
- if not self.mean_only:
- m, logs = torch.split(stats, [self.half_channels] * 2, 1)
- else:
- m = stats
- logs = torch.zeros_like(m)
-
- if not reverse:
- x1 = m + x1 * torch.exp(logs) * x_mask
- x = torch.cat([x0, x1], 1)
- logdet = torch.sum(logs, [1, 2])
- return x, logdet
- else:
- x1 = (x1 - m) * torch.exp(-logs) * x_mask
- x = torch.cat([x0, x1], 1)
- return x
-
-
-class ConvFlow(nn.Module):
- def __init__(
- self,
- in_channels,
- filter_channels,
- kernel_size,
- n_layers,
- num_bins=10,
- tail_bound=5.0,
- ):
- super().__init__()
- self.in_channels = in_channels
- self.filter_channels = filter_channels
- self.kernel_size = kernel_size
- self.n_layers = n_layers
- self.num_bins = num_bins
- self.tail_bound = tail_bound
- self.half_channels = in_channels // 2
-
- self.pre = nn.Conv1d(self.half_channels, filter_channels, 1)
- self.convs = DDSConv(filter_channels, kernel_size, n_layers, p_dropout=0.0)
- self.proj = nn.Conv1d(
- filter_channels, self.half_channels * (num_bins * 3 - 1), 1
- )
- self.proj.weight.data.zero_()
- self.proj.bias.data.zero_()
-
- def forward(self, x, x_mask, g=None, reverse=False):
- x0, x1 = torch.split(x, [self.half_channels] * 2, 1)
- h = self.pre(x0)
- h = self.convs(h, x_mask, g=g)
- h = self.proj(h) * x_mask
-
- b, c, t = x0.shape
- h = h.reshape(b, c, -1, t).permute(0, 1, 3, 2) # [b, cx?, t] -> [b, c, t, ?]
-
- unnormalized_widths = h[..., : self.num_bins] / math.sqrt(self.filter_channels)
- unnormalized_heights = h[..., self.num_bins : 2 * self.num_bins] / math.sqrt(
- self.filter_channels
- )
- unnormalized_derivatives = h[..., 2 * self.num_bins :]
-
- x1, logabsdet = piecewise_rational_quadratic_transform(
- x1,
- unnormalized_widths,
- unnormalized_heights,
- unnormalized_derivatives,
- inverse=reverse,
- tails="linear",
- tail_bound=self.tail_bound,
- )
-
- x = torch.cat([x0, x1], 1) * x_mask
- logdet = torch.sum(logabsdet * x_mask, [1, 2])
- if not reverse:
- return x, logdet
- else:
- return x
-
-
-class TransformerCouplingLayer(nn.Module):
- def __init__(
- self,
- channels,
- hidden_channels,
- kernel_size,
- n_layers,
- n_heads,
- p_dropout=0,
- filter_channels=0,
- mean_only=False,
- wn_sharing_parameter=None,
- gin_channels=0,
- ):
- assert channels % 2 == 0, "channels should be divisible by 2"
- super().__init__()
- self.channels = channels
- self.hidden_channels = hidden_channels
- self.kernel_size = kernel_size
- self.n_layers = n_layers
- self.half_channels = channels // 2
- self.mean_only = mean_only
-
- self.pre = nn.Conv1d(self.half_channels, hidden_channels, 1)
- self.enc = (
- Encoder(
- hidden_channels,
- filter_channels,
- n_heads,
- n_layers,
- kernel_size,
- p_dropout,
- isflow=True,
- gin_channels=gin_channels,
- )
- if wn_sharing_parameter is None
- else wn_sharing_parameter
- )
- self.post = nn.Conv1d(hidden_channels, self.half_channels * (2 - mean_only), 1)
- self.post.weight.data.zero_()
- self.post.bias.data.zero_()
-
- def forward(self, x, x_mask, g=None, reverse=False):
- x0, x1 = torch.split(x, [self.half_channels] * 2, 1)
- h = self.pre(x0) * x_mask
- h = self.enc(h, x_mask, g=g)
- stats = self.post(h) * x_mask
- if not self.mean_only:
- m, logs = torch.split(stats, [self.half_channels] * 2, 1)
- else:
- m = stats
- logs = torch.zeros_like(m)
-
- if not reverse:
- x1 = m + x1 * torch.exp(logs) * x_mask
- x = torch.cat([x0, x1], 1)
- logdet = torch.sum(logs, [1, 2])
- return x, logdet
- else:
- x1 = (x1 - m) * torch.exp(-logs) * x_mask
- x = torch.cat([x0, x1], 1)
- return x
-
- x1, logabsdet = piecewise_rational_quadratic_transform(
- x1,
- unnormalized_widths,
- unnormalized_heights,
- unnormalized_derivatives,
- inverse=reverse,
- tails="linear",
- tail_bound=self.tail_bound,
- )
-
- x = torch.cat([x0, x1], 1) * x_mask
- logdet = torch.sum(logabsdet * x_mask, [1, 2])
- if not reverse:
- return x, logdet
- else:
- return x
diff --git a/spaces/ReThGe/Linet/Linet_clf_model.py b/spaces/ReThGe/Linet/Linet_clf_model.py
deleted file mode 100644
index a4f0d4c1c37ed6cb140c4605f40d124532b55534..0000000000000000000000000000000000000000
--- a/spaces/ReThGe/Linet/Linet_clf_model.py
+++ /dev/null
@@ -1,298 +0,0 @@
-## this file contains some different Model Class build with Pytorch
-# Author: rethge
-# 2023/07/02
-
-
-## imports
-
-import torch
-from torch import nn
-from rethge_components import RTG_depthwise_separable_conv, RTG_res_block, RTG_res_block_expand
-
-
-class LinetV0(nn.Module): # baseline
- def __init__(self,
- res: int = 640, # res should match with dataloader transformation size
- output_shape: int = 3, # len(class_name)
- input_shape: int = 3, # 640*640*3 = 1228800
- hidden_units: int = 8):
- super().__init__()
- '''
- | O O
- | -> BN -> O -> BN -> O -> result
- | O O
-
- **(in) 8(hidden) 3(out)
-
- Forward/backward pass size (MB): 157.29
- '''
-
- self.Linear_stack = nn.Sequential(
- nn.Flatten(),
- nn.BatchNorm1d(num_features=input_shape*res**2), # BN to avoid convergence failure
- nn.Linear(in_features=input_shape*res**2, out_features=hidden_units),
- nn.ReLU(),
- nn.BatchNorm1d(hidden_units),
- nn.Linear(in_features=hidden_units, out_features=output_shape),
- nn.ReLU()
- )
-
- def forward(self, x: torch.Tensor):
- return self.Linear_stack(x)
-
-
-class LinetV0_5(nn.Module):
- """Forward/backward pass size (MB): 0.00"""
- def __init__(self, res):
- super().__init__()
-
- self.downsamp = res//2
-
- self.stack = nn.Sequential(
- nn.AdaptiveAvgPool2d((self.downsamp, self.downsamp)),
- nn.Flatten(),
- # nn.BatchNorm1d(num_features=3*self.downsamp**2), # using BN leading to an increase on Forward/backward pass size 39.32 MB
- nn.Linear(in_features=3*self.downsamp**2, out_features=8),
- nn.SELU(),
- nn.Linear(in_features=8, out_features=16),
- nn.SELU(),
- nn.Linear(in_features=16, out_features=3)
- )
-
- def forward(self, x):
- return self.stack(x)
-
-
-class LinetV1(nn.Module): # convnet, tiny vgg-like
- def __init__(self,
- output_shape: int = 3, # len(class_name)
- init_channels: int = 32,
- input_shape: int = 3,
- clf_shape: int = 3) -> None:
- super().__init__()
- """
-
- Forward/backward pass size (MB): 5452.60 on [8,3,480,480]
-
- """
-
- self.mul_1 = 64 # 4-64
- self.mul_2 = 128 # 4-128
- self.mul_3 = 256 # 4-256
-
-
- self.conv_block_1 = nn.Sequential(
- nn.Conv2d(in_channels=input_shape,
- out_channels=init_channels,
- kernel_size=3,
- stride=1,
- padding='same', bias=False),
- nn.BatchNorm2d(num_features=init_channels),
- nn.ReLU(),
- nn.Conv2d(in_channels=init_channels,
- out_channels=self.mul_1,
- kernel_size=3,
- stride=1,
- padding='same', bias=False),
- nn.BatchNorm2d(num_features=self.mul_1),
- nn.ReLU(),
- nn.MaxPool2d(kernel_size=2)
- )
-
-
- self.conv_block_2 = nn.Sequential(
- nn.Conv2d(in_channels=self.mul_1,
- out_channels=self.mul_2,
- kernel_size=3,
- stride=1,
- padding='same', bias=False),
- nn.BatchNorm2d(num_features=self.mul_2),
- nn.ReLU(),
- nn.Conv2d(in_channels=self.mul_2,
- out_channels=self.mul_2,
- kernel_size=3,
- stride=1,
- padding='same', bias=False),
- nn.BatchNorm2d(num_features=self.mul_2),
- nn.ReLU(),
- nn.MaxPool2d(kernel_size=2)
- )
-
- self.conv_block_3 = nn.Sequential(
- nn.Conv2d(in_channels=self.mul_2,
- out_channels=self.mul_3,
- kernel_size=3,
- stride=1,
- padding='same', bias=False),
- nn.BatchNorm2d(num_features=self.mul_3),
- nn.ReLU(),
- nn.Conv2d(in_channels=self.mul_3,
- out_channels=self.mul_3,
- kernel_size=3,
- stride=1,
- padding='same', bias=True),
- nn.ReLU(),
- nn.MaxPool2d(kernel_size=3)
- )
-
-
- self.classifier = nn.Sequential(
- nn.BatchNorm2d(self.mul_3),
- nn.AdaptiveAvgPool2d((clf_shape,clf_shape)),
- nn.Flatten(),
- nn.Linear(in_features=self.mul_3*clf_shape*clf_shape,
- out_features=output_shape)
- )
-
- def forward(self, x: torch.Tensor):
- # x = self.conv_block_1(x) # if input res is 480:
- # print(x.shape) # torch.Size([b, 64, 240, 240])
- # x = self.conv_block_2(x)
- # print(x.shape) # torch.Size([b, 128, 120, 120])
- # x = self.conv_block_3(x)
- # print(x.shape) # torch.Size([b, 256, 40, 40])
- # x = self.classifier(x)
- # print(x.shape) # torch.Size([b, 3])
- # return x
- return self.classifier(self.conv_block_3(self.conv_block_2(self.conv_block_1(x)))) # benefit from operate fusion
-
-
-class LinetV1_5(nn.Module):
- def __init__(self, nin, nout, expand=32):
- super().__init__()
-
- """without res connect, we can not going deep"""
-
- self.stack = nn.Sequential(
- RTG_depthwise_separable_conv(input_size=nin, output_size=expand, kernel_size=3, stride=1, padding="same", bias=False),
- nn.BatchNorm2d(expand),
- RTG_depthwise_separable_conv(input_size=expand, output_size=expand, kernel_size=3, stride=1, padding="same", bias=True),
- nn.SELU(),
- nn.MaxPool2d(kernel_size=2),
-
- RTG_depthwise_separable_conv(input_size=expand, output_size=expand*4, kernel_size=3, stride=1, padding=0, bias=True),
- # nn.BatchNorm2d(expand*4),
- nn.SELU(),
- RTG_depthwise_separable_conv(input_size=expand*4, output_size=expand*4, kernel_size=3, stride=1, padding=0, bias=True),
- nn.SELU(),
- nn.MaxPool2d(kernel_size=2),
-
- RTG_depthwise_separable_conv(input_size=expand*4, output_size=expand*8, kernel_size=3, stride=1, padding="same", bias=False),
- nn.BatchNorm2d(expand*8),
- RTG_depthwise_separable_conv(input_size=expand*8, output_size=expand*8, kernel_size=3, stride=1, padding="same", bias=True),
- nn.SELU(),
- nn.MaxPool2d(kernel_size=2),
-
- )
-
- self.clf = nn.Sequential(
- nn.AdaptiveAvgPool2d((3,3)),
- nn.Flatten(),
- nn.Linear(in_features=3*3*expand*8, out_features=nout)
- )
-
- def forward(self, x):
- return self.clf(self.stack(x))
-
-
-class LinetV2(nn.Module):
- def __init__(self, nin, nout, expand=32):
- super().__init__()
-
- self.head = nn.Sequential(
- RTG_depthwise_separable_conv(input_size=nin, output_size=expand,
- kernel_size=5, stride=1, padding="same", bias=False),
- nn.BatchNorm2d(expand),
- RTG_depthwise_separable_conv(input_size=expand, output_size=expand*2,
- kernel_size=3, stride=1, padding=1, bias=True),
- nn.SELU(),
- nn.MaxPool2d(kernel_size=2)
- )
-
- self.res_body = nn.Sequential(
- RTG_res_block(nin=expand*2), # output is selu(...)
- RTG_res_block(nin=expand*2),
- RTG_res_block_expand(nin=expand*2, nout=expand*4),
- nn.BatchNorm2d(expand*4),
- nn.MaxPool2d(kernel_size=2),
- RTG_res_block(nin=expand*4),
- RTG_res_block(nin=expand*4),
- RTG_res_block_expand(nin=expand*4, nout=expand*8), # 256
- nn.MaxPool2d(kernel_size=2)
- )
-
- self.tail = nn.Sequential(
- RTG_depthwise_separable_conv(input_size=expand*8, output_size=expand*16, # 512
- kernel_size=3, stride=1, padding=1, bias=True),
- # nn.BatchNorm2d(expand*16),
- nn.SELU(),
- nn.MaxPool2d(kernel_size=2)
- )
-
- self.clf = nn.Sequential(
- nn.AdaptiveAvgPool2d((2,2)),
- nn.Flatten(),
- nn.Linear(in_features= 2*2*expand*16, out_features=256), # 2*2*512 = 2048
- nn.SELU(),
- nn.Dropout(0.2),
- nn.Linear(in_features=256, out_features=nout),
- )
-
- def forward(self, x):
-
- return self.clf(self.tail(self.res_body(self.head(x))))
-
-
-class LinetV2_no_pool_WIP(nn.Module): # without pooling layer, it will fail to learn
- def __init__(self, nin, nout, expand=32):
- super().__init__()
-
- self.head = nn.Sequential(
- RTG_depthwise_separable_conv(input_size=nin, output_size=expand,
- kernel_size=5, stride=1, padding="same", bias=False),
- nn.BatchNorm2d(expand),
- RTG_depthwise_separable_conv(input_size=expand, output_size=expand*2,
- kernel_size=3, stride=1, padding=1, bias=True),
- nn.SELU(),
- RTG_depthwise_separable_conv(input_size=expand*2, output_size=expand*2,
- kernel_size=3, stride=2, padding=1) # 224 -> 112
- )
-
- self.res_body = nn.Sequential(
- RTG_res_block(nin=expand*2), # output is selu(...)
- RTG_res_block(nin=expand*2),
- RTG_res_block_expand(nin=expand*2, nout=expand*4),
- RTG_depthwise_separable_conv(input_size=expand*4, output_size=expand*4,
- kernel_size=3, stride=2, padding=1), # 112 -> 56
- nn.BatchNorm2d(expand*4),
- RTG_res_block(nin=expand*4),
- RTG_res_block(nin=expand*4),
- RTG_res_block_expand(nin=expand*4, nout=expand*8), # 256
- RTG_depthwise_separable_conv(input_size=expand*8, output_size=expand*8,
- kernel_size=3, stride=2, padding=1), # 56 -> 28
- nn.BatchNorm2d(expand*8),
- )
-
- self.tail = nn.Sequential(
- RTG_depthwise_separable_conv(input_size=expand*8, output_size=expand*16, # 512
- kernel_size=3, stride=1, padding=1, bias=False),
- nn.BatchNorm2d(expand*16),
- RTG_depthwise_separable_conv(input_size=expand*16, output_size=expand*16,
- kernel_size=3, stride=2, padding=1), # 28 -> 14
- nn.BatchNorm2d(expand*16),
- )
-
- self.clf = nn.Sequential(
- nn.AdaptiveAvgPool2d((2,2)),
- nn.Flatten(),
- nn.Linear(in_features=2*2*expand*16, out_features=128), # 2*2*512 = 2048
- nn.SELU(),
- nn.Dropout(0.2),
- nn.Linear(in_features=128, out_features=nout),
- )
-
- def forward(self, x):
-
- return self.clf(self.tail(self.res_body(self.head(x))))
-
-
diff --git a/spaces/Ricecake123/RVC-demo/docs/README.ko.han.md b/spaces/Ricecake123/RVC-demo/docs/README.ko.han.md
deleted file mode 100644
index cac9d70c401991739710c90e1e5f5abdb12266da..0000000000000000000000000000000000000000
--- a/spaces/Ricecake123/RVC-demo/docs/README.ko.han.md
+++ /dev/null
@@ -1,100 +0,0 @@
-
-
- aaccfb2cb3
-
-
-
diff --git a/spaces/bioriAsaeru/text-to-voice/Gli ultimi sei minuti il film che ha sorpreso la critica nel 1972. Scarica la versione italiana gratis.md b/spaces/bioriAsaeru/text-to-voice/Gli ultimi sei minuti il film che ha sorpreso la critica nel 1972. Scarica la versione italiana gratis.md
deleted file mode 100644
index 570890f3abc91b891e087a65474a7098dec12c21..0000000000000000000000000000000000000000
--- a/spaces/bioriAsaeru/text-to-voice/Gli ultimi sei minuti il film che ha sorpreso la critica nel 1972. Scarica la versione italiana gratis.md
+++ /dev/null
@@ -1,5 +0,0 @@
-
-
And since speaking Italian to real people is not always an option (you might want to stay home, or you are not in Italy, or your friends are not available), a very good alternative is to immerse yourself in real content. You will have lots of options: from Italian music, to podcasts, to movies. There are tons of resources to learn Italian online, and, of course, one of the very best is italianPod101.com! ?
-
Gli ultimi sei minuti movie in italian free download
aaccfb2cb3
-
-
\ No newline at end of file
diff --git a/spaces/bioriAsaeru/text-to-voice/Grindr Xtra 1 7 4 Ipa Tips and Tricks to Enhance Your Experience.md b/spaces/bioriAsaeru/text-to-voice/Grindr Xtra 1 7 4 Ipa Tips and Tricks to Enhance Your Experience.md
deleted file mode 100644
index df2025c8510b479539abd44e70a6e76452a3715e..0000000000000000000000000000000000000000
--- a/spaces/bioriAsaeru/text-to-voice/Grindr Xtra 1 7 4 Ipa Tips and Tricks to Enhance Your Experience.md
+++ /dev/null
@@ -1,6 +0,0 @@
-
-
- aaccfb2cb3
-
-
-
diff --git a/spaces/cihyFjudo/fairness-paper-search/Fairy Tail Opening 9 Full Version telephone entiere na A guide to download and stream the song legally.md b/spaces/cihyFjudo/fairness-paper-search/Fairy Tail Opening 9 Full Version telephone entiere na A guide to download and stream the song legally.md
deleted file mode 100644
index 793762d1a2eca2a2d9bad35c07b96865c73110c5..0000000000000000000000000000000000000000
--- a/spaces/cihyFjudo/fairness-paper-search/Fairy Tail Opening 9 Full Version telephone entiere na A guide to download and stream the song legally.md
+++ /dev/null
@@ -1,6 +0,0 @@
-
-
- aaccfb2cb3
-
-
-
diff --git a/spaces/cihyFjudo/fairness-paper-search/Hindi movie sholay download mp4 Relive the iconic dialogues and scenes of the masala film.md b/spaces/cihyFjudo/fairness-paper-search/Hindi movie sholay download mp4 Relive the iconic dialogues and scenes of the masala film.md
deleted file mode 100644
index 65d9ed0865d634c145d38c4bb9b056df97808a06..0000000000000000000000000000000000000000
--- a/spaces/cihyFjudo/fairness-paper-search/Hindi movie sholay download mp4 Relive the iconic dialogues and scenes of the masala film.md
+++ /dev/null
@@ -1,6 +0,0 @@
-
-
- aaccfb2cb3
-
-
-
diff --git a/spaces/cihyFjudo/fairness-paper-search/Tendo-ka Sukuranburu Kurisumasu Film Completo In Italiano Download Gratuito Hd 1080p.md b/spaces/cihyFjudo/fairness-paper-search/Tendo-ka Sukuranburu Kurisumasu Film Completo In Italiano Download Gratuito Hd 1080p.md
deleted file mode 100644
index 1c7dba7e637e07f176b8cedbad3afec4039da799..0000000000000000000000000000000000000000
--- a/spaces/cihyFjudo/fairness-paper-search/Tendo-ka Sukuranburu Kurisumasu Film Completo In Italiano Download Gratuito Hd 1080p.md
+++ /dev/null
@@ -1,6 +0,0 @@
-
Tendo-ka Sukuranburu Kurisumasu film completo in italiano download gratuito hd 1080p
-
- aaccfb2cb3
-
-
-
diff --git a/spaces/cihyFjudo/fairness-paper-search/Titli Full Movie Download Filmywap Bollywood Experience the Dark Reality of Delhis Underworld.md b/spaces/cihyFjudo/fairness-paper-search/Titli Full Movie Download Filmywap Bollywood Experience the Dark Reality of Delhis Underworld.md
deleted file mode 100644
index 5f408f6e81f8a372f2b88ef45554e97eb9d3e73c..0000000000000000000000000000000000000000
--- a/spaces/cihyFjudo/fairness-paper-search/Titli Full Movie Download Filmywap Bollywood Experience the Dark Reality of Delhis Underworld.md
+++ /dev/null
@@ -1,6 +0,0 @@
-
-
- aaccfb2cb3
-
-
-
diff --git a/spaces/cloudtheboi/Lofi4All/.pythonlibs/lib/python3.10/site-packages/anyio/_core/_sockets.py b/spaces/cloudtheboi/Lofi4All/.pythonlibs/lib/python3.10/site-packages/anyio/_core/_sockets.py
deleted file mode 100644
index e6970bee2701e1d9391abb376e52a4d1a8ec7b68..0000000000000000000000000000000000000000
--- a/spaces/cloudtheboi/Lofi4All/.pythonlibs/lib/python3.10/site-packages/anyio/_core/_sockets.py
+++ /dev/null
@@ -1,607 +0,0 @@
-from __future__ import annotations
-
-import socket
-import ssl
-import sys
-from ipaddress import IPv6Address, ip_address
-from os import PathLike, chmod
-from pathlib import Path
-from socket import AddressFamily, SocketKind
-from typing import Awaitable, List, Tuple, cast, overload
-
-from .. import to_thread
-from ..abc import (
- ConnectedUDPSocket,
- IPAddressType,
- IPSockAddrType,
- SocketListener,
- SocketStream,
- UDPSocket,
- UNIXSocketStream,
-)
-from ..streams.stapled import MultiListener
-from ..streams.tls import TLSStream
-from ._eventloop import get_asynclib
-from ._resources import aclose_forcefully
-from ._synchronization import Event
-from ._tasks import create_task_group, move_on_after
-
-if sys.version_info >= (3, 8):
- from typing import Literal
-else:
- from typing_extensions import Literal
-
-IPPROTO_IPV6 = getattr(socket, "IPPROTO_IPV6", 41) # https://bugs.python.org/issue29515
-
-GetAddrInfoReturnType = List[
- Tuple[AddressFamily, SocketKind, int, str, Tuple[str, int]]
-]
-AnyIPAddressFamily = Literal[
- AddressFamily.AF_UNSPEC, AddressFamily.AF_INET, AddressFamily.AF_INET6
-]
-IPAddressFamily = Literal[AddressFamily.AF_INET, AddressFamily.AF_INET6]
-
-
-# tls_hostname given
-@overload
-async def connect_tcp(
- remote_host: IPAddressType,
- remote_port: int,
- *,
- local_host: IPAddressType | None = ...,
- ssl_context: ssl.SSLContext | None = ...,
- tls_standard_compatible: bool = ...,
- tls_hostname: str,
- happy_eyeballs_delay: float = ...,
-) -> TLSStream:
- ...
-
-
-# ssl_context given
-@overload
-async def connect_tcp(
- remote_host: IPAddressType,
- remote_port: int,
- *,
- local_host: IPAddressType | None = ...,
- ssl_context: ssl.SSLContext,
- tls_standard_compatible: bool = ...,
- tls_hostname: str | None = ...,
- happy_eyeballs_delay: float = ...,
-) -> TLSStream:
- ...
-
-
-# tls=True
-@overload
-async def connect_tcp(
- remote_host: IPAddressType,
- remote_port: int,
- *,
- local_host: IPAddressType | None = ...,
- tls: Literal[True],
- ssl_context: ssl.SSLContext | None = ...,
- tls_standard_compatible: bool = ...,
- tls_hostname: str | None = ...,
- happy_eyeballs_delay: float = ...,
-) -> TLSStream:
- ...
-
-
-# tls=False
-@overload
-async def connect_tcp(
- remote_host: IPAddressType,
- remote_port: int,
- *,
- local_host: IPAddressType | None = ...,
- tls: Literal[False],
- ssl_context: ssl.SSLContext | None = ...,
- tls_standard_compatible: bool = ...,
- tls_hostname: str | None = ...,
- happy_eyeballs_delay: float = ...,
-) -> SocketStream:
- ...
-
-
-# No TLS arguments
-@overload
-async def connect_tcp(
- remote_host: IPAddressType,
- remote_port: int,
- *,
- local_host: IPAddressType | None = ...,
- happy_eyeballs_delay: float = ...,
-) -> SocketStream:
- ...
-
-
-async def connect_tcp(
- remote_host: IPAddressType,
- remote_port: int,
- *,
- local_host: IPAddressType | None = None,
- tls: bool = False,
- ssl_context: ssl.SSLContext | None = None,
- tls_standard_compatible: bool = True,
- tls_hostname: str | None = None,
- happy_eyeballs_delay: float = 0.25,
-) -> SocketStream | TLSStream:
- """
- Connect to a host using the TCP protocol.
-
- This function implements the stateless version of the Happy Eyeballs algorithm (RFC
- 6555). If ``remote_host`` is a host name that resolves to multiple IP addresses,
- each one is tried until one connection attempt succeeds. If the first attempt does
- not connected within 250 milliseconds, a second attempt is started using the next
- address in the list, and so on. On IPv6 enabled systems, an IPv6 address (if
- available) is tried first.
-
- When the connection has been established, a TLS handshake will be done if either
- ``ssl_context`` or ``tls_hostname`` is not ``None``, or if ``tls`` is ``True``.
-
- :param remote_host: the IP address or host name to connect to
- :param remote_port: port on the target host to connect to
- :param local_host: the interface address or name to bind the socket to before connecting
- :param tls: ``True`` to do a TLS handshake with the connected stream and return a
- :class:`~anyio.streams.tls.TLSStream` instead
- :param ssl_context: the SSL context object to use (if omitted, a default context is created)
- :param tls_standard_compatible: If ``True``, performs the TLS shutdown handshake before closing
- the stream and requires that the server does this as well. Otherwise,
- :exc:`~ssl.SSLEOFError` may be raised during reads from the stream.
- Some protocols, such as HTTP, require this option to be ``False``.
- See :meth:`~ssl.SSLContext.wrap_socket` for details.
- :param tls_hostname: host name to check the server certificate against (defaults to the value
- of ``remote_host``)
- :param happy_eyeballs_delay: delay (in seconds) before starting the next connection attempt
- :return: a socket stream object if no TLS handshake was done, otherwise a TLS stream
- :raises OSError: if the connection attempt fails
-
- """
- # Placed here due to https://github.com/python/mypy/issues/7057
- connected_stream: SocketStream | None = None
-
- async def try_connect(remote_host: str, event: Event) -> None:
- nonlocal connected_stream
- try:
- stream = await asynclib.connect_tcp(remote_host, remote_port, local_address)
- except OSError as exc:
- oserrors.append(exc)
- return
- else:
- if connected_stream is None:
- connected_stream = stream
- tg.cancel_scope.cancel()
- else:
- await stream.aclose()
- finally:
- event.set()
-
- asynclib = get_asynclib()
- local_address: IPSockAddrType | None = None
- family = socket.AF_UNSPEC
- if local_host:
- gai_res = await getaddrinfo(str(local_host), None)
- family, *_, local_address = gai_res[0]
-
- target_host = str(remote_host)
- try:
- addr_obj = ip_address(remote_host)
- except ValueError:
- # getaddrinfo() will raise an exception if name resolution fails
- gai_res = await getaddrinfo(
- target_host, remote_port, family=family, type=socket.SOCK_STREAM
- )
-
- # Organize the list so that the first address is an IPv6 address (if available) and the
- # second one is an IPv4 addresses. The rest can be in whatever order.
- v6_found = v4_found = False
- target_addrs: list[tuple[socket.AddressFamily, str]] = []
- for af, *rest, sa in gai_res:
- if af == socket.AF_INET6 and not v6_found:
- v6_found = True
- target_addrs.insert(0, (af, sa[0]))
- elif af == socket.AF_INET and not v4_found and v6_found:
- v4_found = True
- target_addrs.insert(1, (af, sa[0]))
- else:
- target_addrs.append((af, sa[0]))
- else:
- if isinstance(addr_obj, IPv6Address):
- target_addrs = [(socket.AF_INET6, addr_obj.compressed)]
- else:
- target_addrs = [(socket.AF_INET, addr_obj.compressed)]
-
- oserrors: list[OSError] = []
- async with create_task_group() as tg:
- for i, (af, addr) in enumerate(target_addrs):
- event = Event()
- tg.start_soon(try_connect, addr, event)
- with move_on_after(happy_eyeballs_delay):
- await event.wait()
-
- if connected_stream is None:
- cause = oserrors[0] if len(oserrors) == 1 else asynclib.ExceptionGroup(oserrors)
- raise OSError("All connection attempts failed") from cause
-
- if tls or tls_hostname or ssl_context:
- try:
- return await TLSStream.wrap(
- connected_stream,
- server_side=False,
- hostname=tls_hostname or str(remote_host),
- ssl_context=ssl_context,
- standard_compatible=tls_standard_compatible,
- )
- except BaseException:
- await aclose_forcefully(connected_stream)
- raise
-
- return connected_stream
-
-
-async def connect_unix(path: str | PathLike[str]) -> UNIXSocketStream:
- """
- Connect to the given UNIX socket.
-
- Not available on Windows.
-
- :param path: path to the socket
- :return: a socket stream object
-
- """
- path = str(Path(path))
- return await get_asynclib().connect_unix(path)
-
-
-async def create_tcp_listener(
- *,
- local_host: IPAddressType | None = None,
- local_port: int = 0,
- family: AnyIPAddressFamily = socket.AddressFamily.AF_UNSPEC,
- backlog: int = 65536,
- reuse_port: bool = False,
-) -> MultiListener[SocketStream]:
- """
- Create a TCP socket listener.
-
- :param local_port: port number to listen on
- :param local_host: IP address of the interface to listen on. If omitted, listen on
- all IPv4 and IPv6 interfaces. To listen on all interfaces on a specific address
- family, use ``0.0.0.0`` for IPv4 or ``::`` for IPv6.
- :param family: address family (used if ``local_host`` was omitted)
- :param backlog: maximum number of queued incoming connections (up to a maximum of
- 2**16, or 65536)
- :param reuse_port: ``True`` to allow multiple sockets to bind to the same
- address/port (not supported on Windows)
- :return: a list of listener objects
-
- """
- asynclib = get_asynclib()
- backlog = min(backlog, 65536)
- local_host = str(local_host) if local_host is not None else None
- gai_res = await getaddrinfo(
- local_host, # type: ignore[arg-type]
- local_port,
- family=family,
- type=socket.SocketKind.SOCK_STREAM if sys.platform == "win32" else 0,
- flags=socket.AI_PASSIVE | socket.AI_ADDRCONFIG,
- )
- listeners: list[SocketListener] = []
- try:
- # The set() is here to work around a glibc bug:
- # https://sourceware.org/bugzilla/show_bug.cgi?id=14969
- sockaddr: tuple[str, int] | tuple[str, int, int, int]
- for fam, kind, *_, sockaddr in sorted(set(gai_res)):
- # Workaround for an uvloop bug where we don't get the correct scope ID for
- # IPv6 link-local addresses when passing type=socket.SOCK_STREAM to
- # getaddrinfo(): https://github.com/MagicStack/uvloop/issues/539
- if sys.platform != "win32" and kind is not SocketKind.SOCK_STREAM:
- continue
-
- raw_socket = socket.socket(fam)
- raw_socket.setblocking(False)
-
- # For Windows, enable exclusive address use. For others, enable address reuse.
- if sys.platform == "win32":
- raw_socket.setsockopt(socket.SOL_SOCKET, socket.SO_EXCLUSIVEADDRUSE, 1)
- else:
- raw_socket.setsockopt(socket.SOL_SOCKET, socket.SO_REUSEADDR, 1)
-
- if reuse_port:
- raw_socket.setsockopt(socket.SOL_SOCKET, socket.SO_REUSEPORT, 1)
-
- # If only IPv6 was requested, disable dual stack operation
- if fam == socket.AF_INET6:
- raw_socket.setsockopt(IPPROTO_IPV6, socket.IPV6_V6ONLY, 1)
-
- # Workaround for #554
- if "%" in sockaddr[0]:
- addr, scope_id = sockaddr[0].split("%", 1)
- sockaddr = (addr, sockaddr[1], 0, int(scope_id))
-
- raw_socket.bind(sockaddr)
- raw_socket.listen(backlog)
- listener = asynclib.TCPSocketListener(raw_socket)
- listeners.append(listener)
- except BaseException:
- for listener in listeners:
- await listener.aclose()
-
- raise
-
- return MultiListener(listeners)
-
-
-async def create_unix_listener(
- path: str | PathLike[str],
- *,
- mode: int | None = None,
- backlog: int = 65536,
-) -> SocketListener:
- """
- Create a UNIX socket listener.
-
- Not available on Windows.
-
- :param path: path of the socket
- :param mode: permissions to set on the socket
- :param backlog: maximum number of queued incoming connections (up to a maximum of 2**16, or
- 65536)
- :return: a listener object
-
- .. versionchanged:: 3.0
- If a socket already exists on the file system in the given path, it will be removed first.
-
- """
- path_str = str(path)
- path = Path(path)
- if path.is_socket():
- path.unlink()
-
- backlog = min(backlog, 65536)
- raw_socket = socket.socket(socket.AF_UNIX)
- raw_socket.setblocking(False)
- try:
- await to_thread.run_sync(raw_socket.bind, path_str, cancellable=True)
- if mode is not None:
- await to_thread.run_sync(chmod, path_str, mode, cancellable=True)
-
- raw_socket.listen(backlog)
- return get_asynclib().UNIXSocketListener(raw_socket)
- except BaseException:
- raw_socket.close()
- raise
-
-
-async def create_udp_socket(
- family: AnyIPAddressFamily = AddressFamily.AF_UNSPEC,
- *,
- local_host: IPAddressType | None = None,
- local_port: int = 0,
- reuse_port: bool = False,
-) -> UDPSocket:
- """
- Create a UDP socket.
-
- If ``local_port`` has been given, the socket will be bound to this port on the local
- machine, making this socket suitable for providing UDP based services.
-
- :param family: address family (``AF_INET`` or ``AF_INET6``) – automatically determined from
- ``local_host`` if omitted
- :param local_host: IP address or host name of the local interface to bind to
- :param local_port: local port to bind to
- :param reuse_port: ``True`` to allow multiple sockets to bind to the same address/port
- (not supported on Windows)
- :return: a UDP socket
-
- """
- if family is AddressFamily.AF_UNSPEC and not local_host:
- raise ValueError('Either "family" or "local_host" must be given')
-
- if local_host:
- gai_res = await getaddrinfo(
- str(local_host),
- local_port,
- family=family,
- type=socket.SOCK_DGRAM,
- flags=socket.AI_PASSIVE | socket.AI_ADDRCONFIG,
- )
- family = cast(AnyIPAddressFamily, gai_res[0][0])
- local_address = gai_res[0][-1]
- elif family is AddressFamily.AF_INET6:
- local_address = ("::", 0)
- else:
- local_address = ("0.0.0.0", 0)
-
- return await get_asynclib().create_udp_socket(
- family, local_address, None, reuse_port
- )
-
-
-async def create_connected_udp_socket(
- remote_host: IPAddressType,
- remote_port: int,
- *,
- family: AnyIPAddressFamily = AddressFamily.AF_UNSPEC,
- local_host: IPAddressType | None = None,
- local_port: int = 0,
- reuse_port: bool = False,
-) -> ConnectedUDPSocket:
- """
- Create a connected UDP socket.
-
- Connected UDP sockets can only communicate with the specified remote host/port, and any packets
- sent from other sources are dropped.
-
- :param remote_host: remote host to set as the default target
- :param remote_port: port on the remote host to set as the default target
- :param family: address family (``AF_INET`` or ``AF_INET6``) – automatically determined from
- ``local_host`` or ``remote_host`` if omitted
- :param local_host: IP address or host name of the local interface to bind to
- :param local_port: local port to bind to
- :param reuse_port: ``True`` to allow multiple sockets to bind to the same address/port
- (not supported on Windows)
- :return: a connected UDP socket
-
- """
- local_address = None
- if local_host:
- gai_res = await getaddrinfo(
- str(local_host),
- local_port,
- family=family,
- type=socket.SOCK_DGRAM,
- flags=socket.AI_PASSIVE | socket.AI_ADDRCONFIG,
- )
- family = cast(AnyIPAddressFamily, gai_res[0][0])
- local_address = gai_res[0][-1]
-
- gai_res = await getaddrinfo(
- str(remote_host), remote_port, family=family, type=socket.SOCK_DGRAM
- )
- family = cast(AnyIPAddressFamily, gai_res[0][0])
- remote_address = gai_res[0][-1]
-
- return await get_asynclib().create_udp_socket(
- family, local_address, remote_address, reuse_port
- )
-
-
-async def getaddrinfo(
- host: bytearray | bytes | str,
- port: str | int | None,
- *,
- family: int | AddressFamily = 0,
- type: int | SocketKind = 0,
- proto: int = 0,
- flags: int = 0,
-) -> GetAddrInfoReturnType:
- """
- Look up a numeric IP address given a host name.
-
- Internationalized domain names are translated according to the (non-transitional) IDNA 2008
- standard.
-
- .. note:: 4-tuple IPv6 socket addresses are automatically converted to 2-tuples of
- (host, port), unlike what :func:`socket.getaddrinfo` does.
-
- :param host: host name
- :param port: port number
- :param family: socket family (`'AF_INET``, ...)
- :param type: socket type (``SOCK_STREAM``, ...)
- :param proto: protocol number
- :param flags: flags to pass to upstream ``getaddrinfo()``
- :return: list of tuples containing (family, type, proto, canonname, sockaddr)
-
- .. seealso:: :func:`socket.getaddrinfo`
-
- """
- # Handle unicode hostnames
- if isinstance(host, str):
- try:
- encoded_host = host.encode("ascii")
- except UnicodeEncodeError:
- import idna
-
- encoded_host = idna.encode(host, uts46=True)
- else:
- encoded_host = host
-
- gai_res = await get_asynclib().getaddrinfo(
- encoded_host, port, family=family, type=type, proto=proto, flags=flags
- )
- return [
- (family, type, proto, canonname, convert_ipv6_sockaddr(sockaddr))
- for family, type, proto, canonname, sockaddr in gai_res
- ]
-
-
-def getnameinfo(sockaddr: IPSockAddrType, flags: int = 0) -> Awaitable[tuple[str, str]]:
- """
- Look up the host name of an IP address.
-
- :param sockaddr: socket address (e.g. (ipaddress, port) for IPv4)
- :param flags: flags to pass to upstream ``getnameinfo()``
- :return: a tuple of (host name, service name)
-
- .. seealso:: :func:`socket.getnameinfo`
-
- """
- return get_asynclib().getnameinfo(sockaddr, flags)
-
-
-def wait_socket_readable(sock: socket.socket) -> Awaitable[None]:
- """
- Wait until the given socket has data to be read.
-
- This does **NOT** work on Windows when using the asyncio backend with a proactor event loop
- (default on py3.8+).
-
- .. warning:: Only use this on raw sockets that have not been wrapped by any higher level
- constructs like socket streams!
-
- :param sock: a socket object
- :raises ~anyio.ClosedResourceError: if the socket was closed while waiting for the
- socket to become readable
- :raises ~anyio.BusyResourceError: if another task is already waiting for the socket
- to become readable
-
- """
- return get_asynclib().wait_socket_readable(sock)
-
-
-def wait_socket_writable(sock: socket.socket) -> Awaitable[None]:
- """
- Wait until the given socket can be written to.
-
- This does **NOT** work on Windows when using the asyncio backend with a proactor event loop
- (default on py3.8+).
-
- .. warning:: Only use this on raw sockets that have not been wrapped by any higher level
- constructs like socket streams!
-
- :param sock: a socket object
- :raises ~anyio.ClosedResourceError: if the socket was closed while waiting for the
- socket to become writable
- :raises ~anyio.BusyResourceError: if another task is already waiting for the socket
- to become writable
-
- """
- return get_asynclib().wait_socket_writable(sock)
-
-
-#
-# Private API
-#
-
-
-def convert_ipv6_sockaddr(
- sockaddr: tuple[str, int, int, int] | tuple[str, int]
-) -> tuple[str, int]:
- """
- Convert a 4-tuple IPv6 socket address to a 2-tuple (address, port) format.
-
- If the scope ID is nonzero, it is added to the address, separated with ``%``.
- Otherwise the flow id and scope id are simply cut off from the tuple.
- Any other kinds of socket addresses are returned as-is.
-
- :param sockaddr: the result of :meth:`~socket.socket.getsockname`
- :return: the converted socket address
-
- """
- # This is more complicated than it should be because of MyPy
- if isinstance(sockaddr, tuple) and len(sockaddr) == 4:
- host, port, flowinfo, scope_id = cast(Tuple[str, int, int, int], sockaddr)
- if scope_id:
- # PyPy (as of v7.3.11) leaves the interface name in the result, so
- # we discard it and only get the scope ID from the end
- # (https://foss.heptapod.net/pypy/pypy/-/issues/3938)
- host = host.split("%")[0]
-
- # Add scope_id to the address
- return f"{host}%{scope_id}", port
- else:
- return host, port
- else:
- return cast(Tuple[str, int], sockaddr)
diff --git a/spaces/cloudtheboi/Lofi4All/.pythonlibs/lib/python3.10/site-packages/fastapi/requests.py b/spaces/cloudtheboi/Lofi4All/.pythonlibs/lib/python3.10/site-packages/fastapi/requests.py
deleted file mode 100644
index d16552c0a9535e1c0bd7f701987301681832eba5..0000000000000000000000000000000000000000
--- a/spaces/cloudtheboi/Lofi4All/.pythonlibs/lib/python3.10/site-packages/fastapi/requests.py
+++ /dev/null
@@ -1,2 +0,0 @@
-from starlette.requests import HTTPConnection as HTTPConnection # noqa: F401
-from starlette.requests import Request as Request # noqa: F401
diff --git a/spaces/cloudtheboi/Lofi4All/.pythonlibs/lib/python3.10/site-packages/fontTools/ttLib/tables/G_D_E_F_.py b/spaces/cloudtheboi/Lofi4All/.pythonlibs/lib/python3.10/site-packages/fontTools/ttLib/tables/G_D_E_F_.py
deleted file mode 100644
index d8ae8b23bb6af53aeb08271c3d489f52a28a5e02..0000000000000000000000000000000000000000
--- a/spaces/cloudtheboi/Lofi4All/.pythonlibs/lib/python3.10/site-packages/fontTools/ttLib/tables/G_D_E_F_.py
+++ /dev/null
@@ -1,5 +0,0 @@
-from .otBase import BaseTTXConverter
-
-
-class table_G_D_E_F_(BaseTTXConverter):
- pass
diff --git a/spaces/colakin/video-generater/public/ffmpeg/libavcodec/dca_xll.c b/spaces/colakin/video-generater/public/ffmpeg/libavcodec/dca_xll.c
deleted file mode 100644
index b8cf37a35f134cc592ab725c620596cdcb59f531..0000000000000000000000000000000000000000
--- a/spaces/colakin/video-generater/public/ffmpeg/libavcodec/dca_xll.c
+++ /dev/null
@@ -1,1519 +0,0 @@
-/*
- * Copyright (C) 2016 foo86
- *
- * This file is part of FFmpeg.
- *
- * FFmpeg is free software; you can redistribute it and/or
- * modify it under the terms of the GNU Lesser General Public
- * License as published by the Free Software Foundation; either
- * version 2.1 of the License, or (at your option) any later version.
- *
- * FFmpeg is distributed in the hope that it will be useful,
- * but WITHOUT ANY WARRANTY; without even the implied warranty of
- * MERCHANTABILITY or FITNESS FOR A PARTICULAR PURPOSE. See the GNU
- * Lesser General Public License for more details.
- *
- * You should have received a copy of the GNU Lesser General Public
- * License along with FFmpeg; if not, write to the Free Software
- * Foundation, Inc., 51 Franklin Street, Fifth Floor, Boston, MA 02110-1301 USA
- */
-
-#include "avcodec.h"
-#include "libavutil/channel_layout.h"
-#include "dcadec.h"
-#include "dcadata.h"
-#include "dcamath.h"
-#include "dca_syncwords.h"
-#include "decode.h"
-#include "unary.h"
-
-static int get_linear(GetBitContext *gb, int n)
-{
- unsigned int v = get_bits_long(gb, n);
- return (v >> 1) ^ -(v & 1);
-}
-
-static int get_rice_un(GetBitContext *gb, int k)
-{
- unsigned int v = get_unary(gb, 1, get_bits_left(gb));
- return (v << k) | get_bits_long(gb, k);
-}
-
-static int get_rice(GetBitContext *gb, int k)
-{
- unsigned int v = get_rice_un(gb, k);
- return (v >> 1) ^ -(v & 1);
-}
-
-static void get_array(GetBitContext *gb, int32_t *array, int size, int n)
-{
- int i;
-
- for (i = 0; i < size; i++)
- array[i] = get_bits(gb, n);
-}
-
-static void get_linear_array(GetBitContext *gb, int32_t *array, int size, int n)
-{
- int i;
-
- if (n == 0)
- memset(array, 0, sizeof(*array) * size);
- else for (i = 0; i < size; i++)
- array[i] = get_linear(gb, n);
-}
-
-static void get_rice_array(GetBitContext *gb, int32_t *array, int size, int k)
-{
- int i;
-
- for (i = 0; i < size; i++)
- array[i] = get_rice(gb, k);
-}
-
-static int parse_dmix_coeffs(DCAXllDecoder *s, DCAXllChSet *c)
-{
- // Size of downmix coefficient matrix
- int m = c->primary_chset ? ff_dca_dmix_primary_nch[c->dmix_type] : c->hier_ofs;
- int i, j, *coeff_ptr = c->dmix_coeff;
-
- for (i = 0; i < m; i++) {
- int code, sign, coeff, scale, scale_inv = 0;
- unsigned int index;
-
- // Downmix scale (only for non-primary channel sets)
- if (!c->primary_chset) {
- code = get_bits(&s->gb, 9);
- sign = (code >> 8) - 1;
- index = (code & 0xff) - FF_DCA_DMIXTABLE_OFFSET;
- if (index >= FF_DCA_INV_DMIXTABLE_SIZE) {
- av_log(s->avctx, AV_LOG_ERROR, "Invalid XLL downmix scale index\n");
- return AVERROR_INVALIDDATA;
- }
- scale = ff_dca_dmixtable[index + FF_DCA_DMIXTABLE_OFFSET];
- scale_inv = ff_dca_inv_dmixtable[index];
- c->dmix_scale[i] = (scale ^ sign) - sign;
- c->dmix_scale_inv[i] = (scale_inv ^ sign) - sign;
- }
-
- // Downmix coefficients
- for (j = 0; j < c->nchannels; j++) {
- code = get_bits(&s->gb, 9);
- sign = (code >> 8) - 1;
- index = code & 0xff;
- if (index >= FF_DCA_DMIXTABLE_SIZE) {
- av_log(s->avctx, AV_LOG_ERROR, "Invalid XLL downmix coefficient index\n");
- return AVERROR_INVALIDDATA;
- }
- coeff = ff_dca_dmixtable[index];
- if (!c->primary_chset)
- // Multiply by |InvDmixScale| to get |UndoDmixScale|
- coeff = mul16(scale_inv, coeff);
- *coeff_ptr++ = (coeff ^ sign) - sign;
- }
- }
-
- return 0;
-}
-
-static int chs_parse_header(DCAXllDecoder *s, DCAXllChSet *c, DCAExssAsset *asset)
-{
- int i, j, k, ret, band, header_size, header_pos = get_bits_count(&s->gb);
- DCAXllChSet *p = &s->chset[0];
- DCAXllBand *b;
-
- // Size of channel set sub-header
- header_size = get_bits(&s->gb, 10) + 1;
-
- // Check CRC
- if (ff_dca_check_crc(s->avctx, &s->gb, header_pos, header_pos + header_size * 8)) {
- av_log(s->avctx, AV_LOG_ERROR, "Invalid XLL sub-header checksum\n");
- return AVERROR_INVALIDDATA;
- }
-
- // Number of channels in the channel set
- c->nchannels = get_bits(&s->gb, 4) + 1;
- if (c->nchannels > DCA_XLL_CHANNELS_MAX) {
- avpriv_request_sample(s->avctx, "%d XLL channels", c->nchannels);
- return AVERROR_PATCHWELCOME;
- }
-
- // Residual type
- c->residual_encode = get_bits(&s->gb, c->nchannels);
-
- // PCM bit resolution
- c->pcm_bit_res = get_bits(&s->gb, 5) + 1;
-
- // Storage unit width
- c->storage_bit_res = get_bits(&s->gb, 5) + 1;
- if (c->storage_bit_res != 16 && c->storage_bit_res != 20 && c->storage_bit_res != 24) {
- avpriv_request_sample(s->avctx, "%d-bit XLL storage resolution", c->storage_bit_res);
- return AVERROR_PATCHWELCOME;
- }
-
- if (c->pcm_bit_res > c->storage_bit_res) {
- av_log(s->avctx, AV_LOG_ERROR, "Invalid PCM bit resolution for XLL channel set (%d > %d)\n", c->pcm_bit_res, c->storage_bit_res);
- return AVERROR_INVALIDDATA;
- }
-
- // Original sampling frequency
- c->freq = ff_dca_sampling_freqs[get_bits(&s->gb, 4)];
- if (c->freq > 192000) {
- avpriv_request_sample(s->avctx, "%d Hz XLL sampling frequency", c->freq);
- return AVERROR_PATCHWELCOME;
- }
-
- // Sampling frequency modifier
- if (get_bits(&s->gb, 2)) {
- avpriv_request_sample(s->avctx, "XLL sampling frequency modifier");
- return AVERROR_PATCHWELCOME;
- }
-
- // Which replacement set this channel set is member of
- if (get_bits(&s->gb, 2)) {
- avpriv_request_sample(s->avctx, "XLL replacement set");
- return AVERROR_PATCHWELCOME;
- }
-
- if (asset->one_to_one_map_ch_to_spkr) {
- // Primary channel set flag
- c->primary_chset = get_bits1(&s->gb);
- if (c->primary_chset != (c == p)) {
- av_log(s->avctx, AV_LOG_ERROR, "The first (and only) XLL channel set must be primary\n");
- return AVERROR_INVALIDDATA;
- }
-
- // Downmix coefficients present in stream
- c->dmix_coeffs_present = get_bits1(&s->gb);
-
- // Downmix already performed by encoder
- c->dmix_embedded = c->dmix_coeffs_present && get_bits1(&s->gb);
-
- // Downmix type
- if (c->dmix_coeffs_present && c->primary_chset) {
- c->dmix_type = get_bits(&s->gb, 3);
- if (c->dmix_type >= DCA_DMIX_TYPE_COUNT) {
- av_log(s->avctx, AV_LOG_ERROR, "Invalid XLL primary channel set downmix type\n");
- return AVERROR_INVALIDDATA;
- }
- }
-
- // Whether the channel set is part of a hierarchy
- c->hier_chset = get_bits1(&s->gb);
- if (!c->hier_chset && s->nchsets != 1) {
- avpriv_request_sample(s->avctx, "XLL channel set outside of hierarchy");
- return AVERROR_PATCHWELCOME;
- }
-
- // Downmix coefficients
- if (c->dmix_coeffs_present && (ret = parse_dmix_coeffs(s, c)) < 0)
- return ret;
-
- // Channel mask enabled
- if (!get_bits1(&s->gb)) {
- avpriv_request_sample(s->avctx, "Disabled XLL channel mask");
- return AVERROR_PATCHWELCOME;
- }
-
- // Channel mask for set
- c->ch_mask = get_bits_long(&s->gb, s->ch_mask_nbits);
- if (av_popcount(c->ch_mask) != c->nchannels) {
- av_log(s->avctx, AV_LOG_ERROR, "Invalid XLL channel mask\n");
- return AVERROR_INVALIDDATA;
- }
-
- // Build the channel to speaker map
- for (i = 0, j = 0; i < s->ch_mask_nbits; i++)
- if (c->ch_mask & (1U << i))
- c->ch_remap[j++] = i;
- } else {
- // Mapping coeffs present flag
- if (c->nchannels != 2 || s->nchsets != 1 || get_bits1(&s->gb)) {
- avpriv_request_sample(s->avctx, "Custom XLL channel to speaker mapping");
- return AVERROR_PATCHWELCOME;
- }
-
- // Setup for LtRt decoding
- c->primary_chset = 1;
- c->dmix_coeffs_present = 0;
- c->dmix_embedded = 0;
- c->hier_chset = 0;
- c->ch_mask = DCA_SPEAKER_LAYOUT_STEREO;
- c->ch_remap[0] = DCA_SPEAKER_L;
- c->ch_remap[1] = DCA_SPEAKER_R;
- }
-
- if (c->freq > 96000) {
- // Extra frequency bands flag
- if (get_bits1(&s->gb)) {
- avpriv_request_sample(s->avctx, "Extra XLL frequency bands");
- return AVERROR_PATCHWELCOME;
- }
- c->nfreqbands = 2;
- } else {
- c->nfreqbands = 1;
- }
-
- // Set the sampling frequency to that of the first frequency band.
- // Frequency will be doubled again after bands assembly.
- c->freq >>= c->nfreqbands - 1;
-
- // Verify that all channel sets have the same audio characteristics
- if (c != p && (c->nfreqbands != p->nfreqbands || c->freq != p->freq
- || c->pcm_bit_res != p->pcm_bit_res
- || c->storage_bit_res != p->storage_bit_res)) {
- avpriv_request_sample(s->avctx, "Different XLL audio characteristics");
- return AVERROR_PATCHWELCOME;
- }
-
- // Determine number of bits to read bit allocation coding parameter
- if (c->storage_bit_res > 16)
- c->nabits = 5;
- else if (c->storage_bit_res > 8)
- c->nabits = 4;
- else
- c->nabits = 3;
-
- // Account for embedded downmix and decimator saturation
- if ((s->nchsets > 1 || c->nfreqbands > 1) && c->nabits < 5)
- c->nabits++;
-
- for (band = 0, b = c->bands; band < c->nfreqbands; band++, b++) {
- // Pairwise channel decorrelation
- if ((b->decor_enabled = get_bits1(&s->gb)) && c->nchannels > 1) {
- int ch_nbits = av_ceil_log2(c->nchannels);
-
- // Original channel order
- for (i = 0; i < c->nchannels; i++) {
- b->orig_order[i] = get_bits(&s->gb, ch_nbits);
- if (b->orig_order[i] >= c->nchannels) {
- av_log(s->avctx, AV_LOG_ERROR, "Invalid XLL original channel order\n");
- return AVERROR_INVALIDDATA;
- }
- }
-
- // Pairwise channel coefficients
- for (i = 0; i < c->nchannels / 2; i++)
- b->decor_coeff[i] = get_bits1(&s->gb) ? get_linear(&s->gb, 7) : 0;
- } else {
- for (i = 0; i < c->nchannels; i++)
- b->orig_order[i] = i;
- for (i = 0; i < c->nchannels / 2; i++)
- b->decor_coeff[i] = 0;
- }
-
- // Adaptive predictor order
- b->highest_pred_order = 0;
- for (i = 0; i < c->nchannels; i++) {
- b->adapt_pred_order[i] = get_bits(&s->gb, 4);
- if (b->adapt_pred_order[i] > b->highest_pred_order)
- b->highest_pred_order = b->adapt_pred_order[i];
- }
- if (b->highest_pred_order > s->nsegsamples) {
- av_log(s->avctx, AV_LOG_ERROR, "Invalid XLL adaptive predicition order\n");
- return AVERROR_INVALIDDATA;
- }
-
- // Fixed predictor order
- for (i = 0; i < c->nchannels; i++)
- b->fixed_pred_order[i] = b->adapt_pred_order[i] ? 0 : get_bits(&s->gb, 2);
-
- // Adaptive predictor quantized reflection coefficients
- for (i = 0; i < c->nchannels; i++) {
- for (j = 0; j < b->adapt_pred_order[i]; j++) {
- k = get_linear(&s->gb, 8);
- if (k == -128) {
- av_log(s->avctx, AV_LOG_ERROR, "Invalid XLL reflection coefficient index\n");
- return AVERROR_INVALIDDATA;
- }
- if (k < 0)
- b->adapt_refl_coeff[i][j] = -(int)ff_dca_xll_refl_coeff[-k];
- else
- b->adapt_refl_coeff[i][j] = (int)ff_dca_xll_refl_coeff[ k];
- }
- }
-
- // Downmix performed by encoder in extension frequency band
- b->dmix_embedded = c->dmix_embedded && (band == 0 || get_bits1(&s->gb));
-
- // MSB/LSB split flag in extension frequency band
- if ((band == 0 && s->scalable_lsbs) || (band != 0 && get_bits1(&s->gb))) {
- // Size of LSB section in any segment
- b->lsb_section_size = get_bits_long(&s->gb, s->seg_size_nbits);
- if (b->lsb_section_size < 0 || b->lsb_section_size > s->frame_size) {
- av_log(s->avctx, AV_LOG_ERROR, "Invalid LSB section size\n");
- return AVERROR_INVALIDDATA;
- }
-
- // Account for optional CRC bytes after LSB section
- if (b->lsb_section_size && (s->band_crc_present > 2 ||
- (band == 0 && s->band_crc_present > 1)))
- b->lsb_section_size += 2;
-
- // Number of bits to represent the samples in LSB part
- for (i = 0; i < c->nchannels; i++) {
- b->nscalablelsbs[i] = get_bits(&s->gb, 4);
- if (b->nscalablelsbs[i] && !b->lsb_section_size) {
- av_log(s->avctx, AV_LOG_ERROR, "LSB section missing with non-zero LSB width\n");
- return AVERROR_INVALIDDATA;
- }
- }
- } else {
- b->lsb_section_size = 0;
- for (i = 0; i < c->nchannels; i++)
- b->nscalablelsbs[i] = 0;
- }
-
- // Scalable resolution flag in extension frequency band
- if ((band == 0 && s->scalable_lsbs) || (band != 0 && get_bits1(&s->gb))) {
- // Number of bits discarded by authoring
- for (i = 0; i < c->nchannels; i++)
- b->bit_width_adjust[i] = get_bits(&s->gb, 4);
- } else {
- for (i = 0; i < c->nchannels; i++)
- b->bit_width_adjust[i] = 0;
- }
- }
-
- // Reserved
- // Byte align
- // CRC16 of channel set sub-header
- if (ff_dca_seek_bits(&s->gb, header_pos + header_size * 8)) {
- av_log(s->avctx, AV_LOG_ERROR, "Read past end of XLL sub-header\n");
- return AVERROR_INVALIDDATA;
- }
-
- return 0;
-}
-
-static int chs_alloc_msb_band_data(DCAXllDecoder *s, DCAXllChSet *c)
-{
- int ndecisamples = c->nfreqbands > 1 ? DCA_XLL_DECI_HISTORY_MAX : 0;
- int nchsamples = s->nframesamples + ndecisamples;
- int i, j, nsamples = nchsamples * c->nchannels * c->nfreqbands;
- int32_t *ptr;
-
- // Reallocate MSB sample buffer
- av_fast_malloc(&c->sample_buffer[0], &c->sample_size[0], nsamples * sizeof(int32_t));
- if (!c->sample_buffer[0])
- return AVERROR(ENOMEM);
-
- ptr = c->sample_buffer[0] + ndecisamples;
- for (i = 0; i < c->nfreqbands; i++) {
- for (j = 0; j < c->nchannels; j++) {
- c->bands[i].msb_sample_buffer[j] = ptr;
- ptr += nchsamples;
- }
- }
-
- return 0;
-}
-
-static int chs_alloc_lsb_band_data(DCAXllDecoder *s, DCAXllChSet *c)
-{
- int i, j, nsamples = 0;
- int32_t *ptr;
-
- // Determine number of frequency bands that have MSB/LSB split
- for (i = 0; i < c->nfreqbands; i++)
- if (c->bands[i].lsb_section_size)
- nsamples += s->nframesamples * c->nchannels;
- if (!nsamples)
- return 0;
-
- // Reallocate LSB sample buffer
- av_fast_malloc(&c->sample_buffer[1], &c->sample_size[1], nsamples * sizeof(int32_t));
- if (!c->sample_buffer[1])
- return AVERROR(ENOMEM);
-
- ptr = c->sample_buffer[1];
- for (i = 0; i < c->nfreqbands; i++) {
- if (c->bands[i].lsb_section_size) {
- for (j = 0; j < c->nchannels; j++) {
- c->bands[i].lsb_sample_buffer[j] = ptr;
- ptr += s->nframesamples;
- }
- } else {
- for (j = 0; j < c->nchannels; j++)
- c->bands[i].lsb_sample_buffer[j] = NULL;
- }
- }
-
- return 0;
-}
-
-static int chs_parse_band_data(DCAXllDecoder *s, DCAXllChSet *c, int band, int seg, int band_data_end)
-{
- DCAXllBand *b = &c->bands[band];
- int i, j, k;
-
- // Start unpacking MSB portion of the segment
- if (!(seg && get_bits1(&s->gb))) {
- // Unpack segment type
- // 0 - distinct coding parameters for each channel
- // 1 - common coding parameters for all channels
- c->seg_common = get_bits1(&s->gb);
-
- // Determine number of coding parameters encoded in segment
- k = c->seg_common ? 1 : c->nchannels;
-
- // Unpack Rice coding parameters
- for (i = 0; i < k; i++) {
- // Unpack Rice coding flag
- // 0 - linear code, 1 - Rice code
- c->rice_code_flag[i] = get_bits1(&s->gb);
- // Unpack Hybrid Rice coding flag
- // 0 - Rice code, 1 - Hybrid Rice code
- if (!c->seg_common && c->rice_code_flag[i] && get_bits1(&s->gb))
- // Unpack binary code length for isolated samples
- c->bitalloc_hybrid_linear[i] = get_bits(&s->gb, c->nabits) + 1;
- else
- // 0 indicates no Hybrid Rice coding
- c->bitalloc_hybrid_linear[i] = 0;
- }
-
- // Unpack coding parameters
- for (i = 0; i < k; i++) {
- if (seg == 0) {
- // Unpack coding parameter for part A of segment 0
- c->bitalloc_part_a[i] = get_bits(&s->gb, c->nabits);
-
- // Adjust for the linear code
- if (!c->rice_code_flag[i] && c->bitalloc_part_a[i])
- c->bitalloc_part_a[i]++;
-
- if (!c->seg_common)
- c->nsamples_part_a[i] = b->adapt_pred_order[i];
- else
- c->nsamples_part_a[i] = b->highest_pred_order;
- } else {
- c->bitalloc_part_a[i] = 0;
- c->nsamples_part_a[i] = 0;
- }
-
- // Unpack coding parameter for part B of segment
- c->bitalloc_part_b[i] = get_bits(&s->gb, c->nabits);
-
- // Adjust for the linear code
- if (!c->rice_code_flag[i] && c->bitalloc_part_b[i])
- c->bitalloc_part_b[i]++;
- }
- }
-
- // Unpack entropy codes
- for (i = 0; i < c->nchannels; i++) {
- int32_t *part_a, *part_b;
- int nsamples_part_b;
-
- // Select index of coding parameters
- k = c->seg_common ? 0 : i;
-
- // Slice the segment into parts A and B
- part_a = b->msb_sample_buffer[i] + seg * s->nsegsamples;
- part_b = part_a + c->nsamples_part_a[k];
- nsamples_part_b = s->nsegsamples - c->nsamples_part_a[k];
-
- if (get_bits_left(&s->gb) < 0)
- return AVERROR_INVALIDDATA;
-
- if (!c->rice_code_flag[k]) {
- // Linear codes
- // Unpack all residuals of part A of segment 0
- get_linear_array(&s->gb, part_a, c->nsamples_part_a[k],
- c->bitalloc_part_a[k]);
-
- // Unpack all residuals of part B of segment 0 and others
- get_linear_array(&s->gb, part_b, nsamples_part_b,
- c->bitalloc_part_b[k]);
- } else {
- // Rice codes
- // Unpack all residuals of part A of segment 0
- get_rice_array(&s->gb, part_a, c->nsamples_part_a[k],
- c->bitalloc_part_a[k]);
-
- if (c->bitalloc_hybrid_linear[k]) {
- // Hybrid Rice codes
- // Unpack the number of isolated samples
- int nisosamples = get_bits(&s->gb, s->nsegsamples_log2);
-
- // Set all locations to 0
- memset(part_b, 0, sizeof(*part_b) * nsamples_part_b);
-
- // Extract the locations of isolated samples and flag by -1
- for (j = 0; j < nisosamples; j++) {
- int loc = get_bits(&s->gb, s->nsegsamples_log2);
- if (loc >= nsamples_part_b) {
- av_log(s->avctx, AV_LOG_ERROR, "Invalid isolated sample location\n");
- return AVERROR_INVALIDDATA;
- }
- part_b[loc] = -1;
- }
-
- // Unpack all residuals of part B of segment 0 and others
- for (j = 0; j < nsamples_part_b; j++) {
- if (part_b[j])
- part_b[j] = get_linear(&s->gb, c->bitalloc_hybrid_linear[k]);
- else
- part_b[j] = get_rice(&s->gb, c->bitalloc_part_b[k]);
- }
- } else {
- // Rice codes
- // Unpack all residuals of part B of segment 0 and others
- get_rice_array(&s->gb, part_b, nsamples_part_b, c->bitalloc_part_b[k]);
- }
- }
- }
-
- // Unpack decimator history for frequency band 1
- if (seg == 0 && band == 1) {
- int nbits = get_bits(&s->gb, 5) + 1;
- for (i = 0; i < c->nchannels; i++)
- for (j = 1; j < DCA_XLL_DECI_HISTORY_MAX; j++)
- c->deci_history[i][j] = get_sbits_long(&s->gb, nbits);
- }
-
- // Start unpacking LSB portion of the segment
- if (b->lsb_section_size) {
- // Skip to the start of LSB portion
- if (ff_dca_seek_bits(&s->gb, band_data_end - b->lsb_section_size * 8)) {
- av_log(s->avctx, AV_LOG_ERROR, "Read past end of XLL band data\n");
- return AVERROR_INVALIDDATA;
- }
-
- // Unpack all LSB parts of residuals of this segment
- for (i = 0; i < c->nchannels; i++) {
- if (b->nscalablelsbs[i]) {
- get_array(&s->gb,
- b->lsb_sample_buffer[i] + seg * s->nsegsamples,
- s->nsegsamples, b->nscalablelsbs[i]);
- }
- }
- }
-
- // Skip to the end of band data
- if (ff_dca_seek_bits(&s->gb, band_data_end)) {
- av_log(s->avctx, AV_LOG_ERROR, "Read past end of XLL band data\n");
- return AVERROR_INVALIDDATA;
- }
-
- return 0;
-}
-
-static av_cold void chs_clear_band_data(DCAXllDecoder *s, DCAXllChSet *c, int band, int seg)
-{
- DCAXllBand *b = &c->bands[band];
- int i, offset, nsamples;
-
- if (seg < 0) {
- offset = 0;
- nsamples = s->nframesamples;
- } else {
- offset = seg * s->nsegsamples;
- nsamples = s->nsegsamples;
- }
-
- for (i = 0; i < c->nchannels; i++) {
- memset(b->msb_sample_buffer[i] + offset, 0, nsamples * sizeof(int32_t));
- if (b->lsb_section_size)
- memset(b->lsb_sample_buffer[i] + offset, 0, nsamples * sizeof(int32_t));
- }
-
- if (seg <= 0 && band)
- memset(c->deci_history, 0, sizeof(c->deci_history));
-
- if (seg < 0) {
- memset(b->nscalablelsbs, 0, sizeof(b->nscalablelsbs));
- memset(b->bit_width_adjust, 0, sizeof(b->bit_width_adjust));
- }
-}
-
-static void chs_filter_band_data(DCAXllDecoder *s, DCAXllChSet *c, int band)
-{
- DCAXllBand *b = &c->bands[band];
- int nsamples = s->nframesamples;
- int i, j, k;
-
- // Inverse adaptive or fixed prediction
- for (i = 0; i < c->nchannels; i++) {
- int32_t *buf = b->msb_sample_buffer[i];
- int order = b->adapt_pred_order[i];
- if (order > 0) {
- int coeff[DCA_XLL_ADAPT_PRED_ORDER_MAX];
- // Conversion from reflection coefficients to direct form coefficients
- for (j = 0; j < order; j++) {
- int rc = b->adapt_refl_coeff[i][j];
- for (k = 0; k < (j + 1) / 2; k++) {
- int tmp1 = coeff[ k ];
- int tmp2 = coeff[j - k - 1];
- coeff[ k ] = tmp1 + mul16(rc, tmp2);
- coeff[j - k - 1] = tmp2 + mul16(rc, tmp1);
- }
- coeff[j] = rc;
- }
- // Inverse adaptive prediction
- for (j = 0; j < nsamples - order; j++) {
- int64_t err = 0;
- for (k = 0; k < order; k++)
- err += (int64_t)buf[j + k] * coeff[order - k - 1];
- buf[j + k] -= (SUINT)clip23(norm16(err));
- }
- } else {
- // Inverse fixed coefficient prediction
- for (j = 0; j < b->fixed_pred_order[i]; j++)
- for (k = 1; k < nsamples; k++)
- buf[k] += (unsigned)buf[k - 1];
- }
- }
-
- // Inverse pairwise channel decorrellation
- if (b->decor_enabled) {
- int32_t *tmp[DCA_XLL_CHANNELS_MAX];
-
- for (i = 0; i < c->nchannels / 2; i++) {
- int coeff = b->decor_coeff[i];
- if (coeff) {
- s->dcadsp->decor(b->msb_sample_buffer[i * 2 + 1],
- b->msb_sample_buffer[i * 2 ],
- coeff, nsamples);
- }
- }
-
- // Reorder channel pointers to the original order
- for (i = 0; i < c->nchannels; i++)
- tmp[i] = b->msb_sample_buffer[i];
-
- for (i = 0; i < c->nchannels; i++)
- b->msb_sample_buffer[b->orig_order[i]] = tmp[i];
- }
-
- // Map output channel pointers for frequency band 0
- if (c->nfreqbands == 1)
- for (i = 0; i < c->nchannels; i++)
- s->output_samples[c->ch_remap[i]] = b->msb_sample_buffer[i];
-}
-
-static int chs_get_lsb_width(DCAXllDecoder *s, DCAXllChSet *c, int band, int ch)
-{
- int adj = c->bands[band].bit_width_adjust[ch];
- int shift = c->bands[band].nscalablelsbs[ch];
-
- if (s->fixed_lsb_width)
- shift = s->fixed_lsb_width;
- else if (shift && adj)
- shift += adj - 1;
- else
- shift += adj;
-
- return shift;
-}
-
-static void chs_assemble_msbs_lsbs(DCAXllDecoder *s, DCAXllChSet *c, int band)
-{
- DCAXllBand *b = &c->bands[band];
- int n, ch, nsamples = s->nframesamples;
-
- for (ch = 0; ch < c->nchannels; ch++) {
- int shift = chs_get_lsb_width(s, c, band, ch);
- if (shift) {
- int32_t *msb = b->msb_sample_buffer[ch];
- if (b->nscalablelsbs[ch]) {
- int32_t *lsb = b->lsb_sample_buffer[ch];
- int adj = b->bit_width_adjust[ch];
- for (n = 0; n < nsamples; n++)
- msb[n] = msb[n] * (SUINT)(1 << shift) + (lsb[n] << adj);
- } else {
- for (n = 0; n < nsamples; n++)
- msb[n] = msb[n] * (SUINT)(1 << shift);
- }
- }
- }
-}
-
-static int chs_assemble_freq_bands(DCAXllDecoder *s, DCAXllChSet *c)
-{
- int ch, nsamples = s->nframesamples;
- int32_t *ptr;
-
- av_assert1(c->nfreqbands > 1);
-
- // Reallocate frequency band assembly buffer
- av_fast_malloc(&c->sample_buffer[2], &c->sample_size[2],
- 2 * nsamples * c->nchannels * sizeof(int32_t));
- if (!c->sample_buffer[2])
- return AVERROR(ENOMEM);
-
- // Assemble frequency bands 0 and 1
- ptr = c->sample_buffer[2];
- for (ch = 0; ch < c->nchannels; ch++) {
- int32_t *band0 = c->bands[0].msb_sample_buffer[ch];
- int32_t *band1 = c->bands[1].msb_sample_buffer[ch];
-
- // Copy decimator history
- memcpy(band0 - DCA_XLL_DECI_HISTORY_MAX,
- c->deci_history[ch], sizeof(c->deci_history[0]));
-
- // Filter
- s->dcadsp->assemble_freq_bands(ptr, band0, band1,
- ff_dca_xll_band_coeff,
- nsamples);
-
- // Remap output channel pointer to assembly buffer
- s->output_samples[c->ch_remap[ch]] = ptr;
- ptr += nsamples * 2;
- }
-
- return 0;
-}
-
-static int parse_common_header(DCAXllDecoder *s)
-{
- int stream_ver, header_size, frame_size_nbits, nframesegs_log2;
-
- // XLL extension sync word
- if (get_bits_long(&s->gb, 32) != DCA_SYNCWORD_XLL) {
- av_log(s->avctx, AV_LOG_VERBOSE, "Invalid XLL sync word\n");
- return AVERROR(EAGAIN);
- }
-
- // Version number
- stream_ver = get_bits(&s->gb, 4) + 1;
- if (stream_ver > 1) {
- avpriv_request_sample(s->avctx, "XLL stream version %d", stream_ver);
- return AVERROR_PATCHWELCOME;
- }
-
- // Lossless frame header length
- header_size = get_bits(&s->gb, 8) + 1;
-
- // Check CRC
- if (ff_dca_check_crc(s->avctx, &s->gb, 32, header_size * 8)) {
- av_log(s->avctx, AV_LOG_ERROR, "Invalid XLL common header checksum\n");
- return AVERROR_INVALIDDATA;
- }
-
- // Number of bits used to read frame size
- frame_size_nbits = get_bits(&s->gb, 5) + 1;
-
- // Number of bytes in a lossless frame
- s->frame_size = get_bits_long(&s->gb, frame_size_nbits);
- if (s->frame_size < 0 || s->frame_size >= DCA_XLL_PBR_BUFFER_MAX) {
- av_log(s->avctx, AV_LOG_ERROR, "Invalid XLL frame size (%d bytes)\n", s->frame_size);
- return AVERROR_INVALIDDATA;
- }
- s->frame_size++;
-
- // Number of channels sets per frame
- s->nchsets = get_bits(&s->gb, 4) + 1;
- if (s->nchsets > DCA_XLL_CHSETS_MAX) {
- avpriv_request_sample(s->avctx, "%d XLL channel sets", s->nchsets);
- return AVERROR_PATCHWELCOME;
- }
-
- // Number of segments per frame
- nframesegs_log2 = get_bits(&s->gb, 4);
- s->nframesegs = 1 << nframesegs_log2;
- if (s->nframesegs > 1024) {
- av_log(s->avctx, AV_LOG_ERROR, "Too many segments per XLL frame\n");
- return AVERROR_INVALIDDATA;
- }
-
- // Samples in segment per one frequency band for the first channel set
- // Maximum value is 256 for sampling frequencies <= 48 kHz
- // Maximum value is 512 for sampling frequencies > 48 kHz
- s->nsegsamples_log2 = get_bits(&s->gb, 4);
- if (!s->nsegsamples_log2) {
- av_log(s->avctx, AV_LOG_ERROR, "Too few samples per XLL segment\n");
- return AVERROR_INVALIDDATA;
- }
- s->nsegsamples = 1 << s->nsegsamples_log2;
- if (s->nsegsamples > 512) {
- av_log(s->avctx, AV_LOG_ERROR, "Too many samples per XLL segment\n");
- return AVERROR_INVALIDDATA;
- }
-
- // Samples in frame per one frequency band for the first channel set
- s->nframesamples_log2 = s->nsegsamples_log2 + nframesegs_log2;
- s->nframesamples = 1 << s->nframesamples_log2;
- if (s->nframesamples > 65536) {
- av_log(s->avctx, AV_LOG_ERROR, "Too many samples per XLL frame\n");
- return AVERROR_INVALIDDATA;
- }
-
- // Number of bits used to read segment size
- s->seg_size_nbits = get_bits(&s->gb, 5) + 1;
-
- // Presence of CRC16 within each frequency band
- // 0 - No CRC16 within band
- // 1 - CRC16 placed at the end of MSB0
- // 2 - CRC16 placed at the end of MSB0 and LSB0
- // 3 - CRC16 placed at the end of MSB0 and LSB0 and other frequency bands
- s->band_crc_present = get_bits(&s->gb, 2);
-
- // MSB/LSB split flag
- s->scalable_lsbs = get_bits1(&s->gb);
-
- // Channel position mask
- s->ch_mask_nbits = get_bits(&s->gb, 5) + 1;
-
- // Fixed LSB width
- if (s->scalable_lsbs)
- s->fixed_lsb_width = get_bits(&s->gb, 4);
- else
- s->fixed_lsb_width = 0;
-
- // Reserved
- // Byte align
- // Header CRC16 protection
- if (ff_dca_seek_bits(&s->gb, header_size * 8)) {
- av_log(s->avctx, AV_LOG_ERROR, "Read past end of XLL common header\n");
- return AVERROR_INVALIDDATA;
- }
-
- return 0;
-}
-
-static int is_hier_dmix_chset(DCAXllChSet *c)
-{
- return !c->primary_chset && c->dmix_embedded && c->hier_chset;
-}
-
-static DCAXllChSet *find_next_hier_dmix_chset(DCAXllDecoder *s, DCAXllChSet *c)
-{
- if (c->hier_chset)
- while (++c < &s->chset[s->nchsets])
- if (is_hier_dmix_chset(c))
- return c;
-
- return NULL;
-}
-
-static void prescale_down_mix(DCAXllChSet *c, DCAXllChSet *o)
-{
- int i, j, *coeff_ptr = c->dmix_coeff;
-
- for (i = 0; i < c->hier_ofs; i++) {
- int scale = o->dmix_scale[i];
- int scale_inv = o->dmix_scale_inv[i];
- c->dmix_scale[i] = mul15(c->dmix_scale[i], scale);
- c->dmix_scale_inv[i] = mul16(c->dmix_scale_inv[i], scale_inv);
- for (j = 0; j < c->nchannels; j++) {
- int coeff = mul16(*coeff_ptr, scale_inv);
- *coeff_ptr++ = mul15(coeff, o->dmix_scale[c->hier_ofs + j]);
- }
- }
-}
-
-static int parse_sub_headers(DCAXllDecoder *s, DCAExssAsset *asset)
-{
- DCAContext *dca = s->avctx->priv_data;
- DCAXllChSet *c;
- int i, ret;
-
- // Parse channel set headers
- s->nfreqbands = 0;
- s->nchannels = 0;
- s->nreschsets = 0;
- for (i = 0, c = s->chset; i < s->nchsets; i++, c++) {
- c->hier_ofs = s->nchannels;
- if ((ret = chs_parse_header(s, c, asset)) < 0)
- return ret;
- if (c->nfreqbands > s->nfreqbands)
- s->nfreqbands = c->nfreqbands;
- if (c->hier_chset)
- s->nchannels += c->nchannels;
- if (c->residual_encode != (1 << c->nchannels) - 1)
- s->nreschsets++;
- }
-
- // Pre-scale downmixing coefficients for all non-primary channel sets
- for (i = s->nchsets - 1, c = &s->chset[i]; i > 0; i--, c--) {
- if (is_hier_dmix_chset(c)) {
- DCAXllChSet *o = find_next_hier_dmix_chset(s, c);
- if (o)
- prescale_down_mix(c, o);
- }
- }
-
- // Determine number of active channel sets to decode
- switch (dca->request_channel_layout) {
- case DCA_SPEAKER_LAYOUT_STEREO:
- s->nactivechsets = 1;
- break;
- case DCA_SPEAKER_LAYOUT_5POINT0:
- case DCA_SPEAKER_LAYOUT_5POINT1:
- s->nactivechsets = (s->chset[0].nchannels < 5 && s->nchsets > 1) ? 2 : 1;
- break;
- default:
- s->nactivechsets = s->nchsets;
- break;
- }
-
- return 0;
-}
-
-static int parse_navi_table(DCAXllDecoder *s)
-{
- int chs, seg, band, navi_nb, navi_pos, *navi_ptr;
- DCAXllChSet *c;
-
- // Determine size of NAVI table
- navi_nb = s->nfreqbands * s->nframesegs * s->nchsets;
- if (navi_nb > 1024) {
- av_log(s->avctx, AV_LOG_ERROR, "Too many NAVI entries (%d)\n", navi_nb);
- return AVERROR_INVALIDDATA;
- }
-
- // Reallocate NAVI table
- av_fast_malloc(&s->navi, &s->navi_size, navi_nb * sizeof(*s->navi));
- if (!s->navi)
- return AVERROR(ENOMEM);
-
- // Parse NAVI
- navi_pos = get_bits_count(&s->gb);
- navi_ptr = s->navi;
- for (band = 0; band < s->nfreqbands; band++) {
- for (seg = 0; seg < s->nframesegs; seg++) {
- for (chs = 0, c = s->chset; chs < s->nchsets; chs++, c++) {
- int size = 0;
- if (c->nfreqbands > band) {
- size = get_bits_long(&s->gb, s->seg_size_nbits);
- if (size < 0 || size >= s->frame_size) {
- av_log(s->avctx, AV_LOG_ERROR, "Invalid NAVI segment size (%d bytes)\n", size);
- return AVERROR_INVALIDDATA;
- }
- size++;
- }
- *navi_ptr++ = size;
- }
- }
- }
-
- // Byte align
- // CRC16
- skip_bits(&s->gb, -get_bits_count(&s->gb) & 7);
- skip_bits(&s->gb, 16);
-
- // Check CRC
- if (ff_dca_check_crc(s->avctx, &s->gb, navi_pos, get_bits_count(&s->gb))) {
- av_log(s->avctx, AV_LOG_ERROR, "Invalid NAVI checksum\n");
- return AVERROR_INVALIDDATA;
- }
-
- return 0;
-}
-
-static int parse_band_data(DCAXllDecoder *s)
-{
- int ret, chs, seg, band, navi_pos, *navi_ptr;
- DCAXllChSet *c;
-
- for (chs = 0, c = s->chset; chs < s->nactivechsets; chs++, c++) {
- if ((ret = chs_alloc_msb_band_data(s, c)) < 0)
- return ret;
- if ((ret = chs_alloc_lsb_band_data(s, c)) < 0)
- return ret;
- }
-
- navi_pos = get_bits_count(&s->gb);
- navi_ptr = s->navi;
- for (band = 0; band < s->nfreqbands; band++) {
- for (seg = 0; seg < s->nframesegs; seg++) {
- for (chs = 0, c = s->chset; chs < s->nchsets; chs++, c++) {
- if (c->nfreqbands > band) {
- navi_pos += *navi_ptr * 8;
- if (navi_pos > s->gb.size_in_bits) {
- av_log(s->avctx, AV_LOG_ERROR, "Invalid NAVI position\n");
- return AVERROR_INVALIDDATA;
- }
- if (chs < s->nactivechsets &&
- (ret = chs_parse_band_data(s, c, band, seg, navi_pos)) < 0) {
- if (s->avctx->err_recognition & AV_EF_EXPLODE)
- return ret;
- chs_clear_band_data(s, c, band, seg);
- }
- skip_bits_long(&s->gb, navi_pos - get_bits_count(&s->gb));
- }
- navi_ptr++;
- }
- }
- }
-
- return 0;
-}
-
-static int parse_frame(DCAXllDecoder *s, const uint8_t *data, int size, DCAExssAsset *asset)
-{
- int ret;
-
- if ((ret = init_get_bits8(&s->gb, data, size)) < 0)
- return ret;
- if ((ret = parse_common_header(s)) < 0)
- return ret;
- if ((ret = parse_sub_headers(s, asset)) < 0)
- return ret;
- if ((ret = parse_navi_table(s)) < 0)
- return ret;
- if ((ret = parse_band_data(s)) < 0)
- return ret;
-
- if (s->frame_size * 8 > FFALIGN(get_bits_count(&s->gb), 32)) {
- unsigned int extradata_syncword;
-
- // Align to dword
- skip_bits_long(&s->gb, -get_bits_count(&s->gb) & 31);
-
- extradata_syncword = show_bits_long(&s->gb, 32);
-
- if (extradata_syncword == DCA_SYNCWORD_XLL_X) {
- s->x_syncword_present = 1;
- } else if ((extradata_syncword >> 1) == (DCA_SYNCWORD_XLL_X_IMAX >> 1)) {
- s->x_imax_syncword_present = 1;
- }
- }
-
- if (ff_dca_seek_bits(&s->gb, s->frame_size * 8)) {
- av_log(s->avctx, AV_LOG_ERROR, "Read past end of XLL frame\n");
- return AVERROR_INVALIDDATA;
- }
- return ret;
-}
-
-static void clear_pbr(DCAXllDecoder *s)
-{
- s->pbr_length = 0;
- s->pbr_delay = 0;
-}
-
-static int copy_to_pbr(DCAXllDecoder *s, const uint8_t *data, int size, int delay)
-{
- if (size > DCA_XLL_PBR_BUFFER_MAX)
- return AVERROR(ENOSPC);
-
- if (!s->pbr_buffer && !(s->pbr_buffer = av_malloc(DCA_XLL_PBR_BUFFER_MAX + AV_INPUT_BUFFER_PADDING_SIZE)))
- return AVERROR(ENOMEM);
-
- memcpy(s->pbr_buffer, data, size);
- s->pbr_length = size;
- s->pbr_delay = delay;
- return 0;
-}
-
-static int parse_frame_no_pbr(DCAXllDecoder *s, const uint8_t *data, int size, DCAExssAsset *asset)
-{
- int ret = parse_frame(s, data, size, asset);
-
- // If XLL packet data didn't start with a sync word, we must have jumped
- // right into the middle of PBR smoothing period
- if (ret == AVERROR(EAGAIN) && asset->xll_sync_present && asset->xll_sync_offset < size) {
- // Skip to the next sync word in this packet
- data += asset->xll_sync_offset;
- size -= asset->xll_sync_offset;
-
- // If decoding delay is set, put the frame into PBR buffer and return
- // failure code. Higher level decoder is expected to switch to lossy
- // core decoding or mute its output until decoding delay expires.
- if (asset->xll_delay_nframes > 0) {
- if ((ret = copy_to_pbr(s, data, size, asset->xll_delay_nframes)) < 0)
- return ret;
- return AVERROR(EAGAIN);
- }
-
- // No decoding delay, just parse the frame in place
- ret = parse_frame(s, data, size, asset);
- }
-
- if (ret < 0)
- return ret;
-
- if (s->frame_size > size)
- return AVERROR(EINVAL);
-
- // If the XLL decoder didn't consume full packet, start PBR smoothing period
- if (s->frame_size < size)
- if ((ret = copy_to_pbr(s, data + s->frame_size, size - s->frame_size, 0)) < 0)
- return ret;
-
- return 0;
-}
-
-static int parse_frame_pbr(DCAXllDecoder *s, const uint8_t *data, int size, DCAExssAsset *asset)
-{
- int ret;
-
- if (size > DCA_XLL_PBR_BUFFER_MAX - s->pbr_length) {
- ret = AVERROR(ENOSPC);
- goto fail;
- }
-
- memcpy(s->pbr_buffer + s->pbr_length, data, size);
- s->pbr_length += size;
-
- // Respect decoding delay after synchronization error
- if (s->pbr_delay > 0 && --s->pbr_delay)
- return AVERROR(EAGAIN);
-
- if ((ret = parse_frame(s, s->pbr_buffer, s->pbr_length, asset)) < 0)
- goto fail;
-
- if (s->frame_size > s->pbr_length) {
- ret = AVERROR(EINVAL);
- goto fail;
- }
-
- if (s->frame_size == s->pbr_length) {
- // End of PBR smoothing period
- clear_pbr(s);
- } else {
- s->pbr_length -= s->frame_size;
- memmove(s->pbr_buffer, s->pbr_buffer + s->frame_size, s->pbr_length);
- }
-
- return 0;
-
-fail:
- // For now, throw out all PBR state on failure.
- // Perhaps we can be smarter and try to resync somehow.
- clear_pbr(s);
- return ret;
-}
-
-int ff_dca_xll_parse(DCAXllDecoder *s, const uint8_t *data, DCAExssAsset *asset)
-{
- int ret;
-
- if (s->hd_stream_id != asset->hd_stream_id) {
- clear_pbr(s);
- s->hd_stream_id = asset->hd_stream_id;
- }
-
- if (s->pbr_length)
- ret = parse_frame_pbr(s, data + asset->xll_offset, asset->xll_size, asset);
- else
- ret = parse_frame_no_pbr(s, data + asset->xll_offset, asset->xll_size, asset);
-
- return ret;
-}
-
-static void undo_down_mix(DCAXllDecoder *s, DCAXllChSet *o, int band)
-{
- int i, j, k, nchannels = 0, *coeff_ptr = o->dmix_coeff;
- DCAXllChSet *c;
-
- for (i = 0, c = s->chset; i < s->nactivechsets; i++, c++) {
- if (!c->hier_chset)
- continue;
-
- av_assert1(band < c->nfreqbands);
- for (j = 0; j < c->nchannels; j++) {
- for (k = 0; k < o->nchannels; k++) {
- int coeff = *coeff_ptr++;
- if (coeff) {
- s->dcadsp->dmix_sub(c->bands[band].msb_sample_buffer[j],
- o->bands[band].msb_sample_buffer[k],
- coeff, s->nframesamples);
- if (band)
- s->dcadsp->dmix_sub(c->deci_history[j],
- o->deci_history[k],
- coeff, DCA_XLL_DECI_HISTORY_MAX);
- }
- }
- }
-
- nchannels += c->nchannels;
- if (nchannels >= o->hier_ofs)
- break;
- }
-}
-
-static void scale_down_mix(DCAXllDecoder *s, DCAXllChSet *o, int band)
-{
- int i, j, nchannels = 0;
- DCAXllChSet *c;
-
- for (i = 0, c = s->chset; i < s->nactivechsets; i++, c++) {
- if (!c->hier_chset)
- continue;
-
- av_assert1(band < c->nfreqbands);
- for (j = 0; j < c->nchannels; j++) {
- int scale = o->dmix_scale[nchannels++];
- if (scale != (1 << 15)) {
- s->dcadsp->dmix_scale(c->bands[band].msb_sample_buffer[j],
- scale, s->nframesamples);
- if (band)
- s->dcadsp->dmix_scale(c->deci_history[j],
- scale, DCA_XLL_DECI_HISTORY_MAX);
- }
- }
-
- if (nchannels >= o->hier_ofs)
- break;
- }
-}
-
-// Clear all band data and replace non-residual encoded channels with lossy
-// counterparts
-static av_cold void force_lossy_output(DCAXllDecoder *s, DCAXllChSet *c)
-{
- DCAContext *dca = s->avctx->priv_data;
- int band, ch;
-
- for (band = 0; band < c->nfreqbands; band++)
- chs_clear_band_data(s, c, band, -1);
-
- for (ch = 0; ch < c->nchannels; ch++) {
- if (!(c->residual_encode & (1 << ch)))
- continue;
- if (ff_dca_core_map_spkr(&dca->core, c->ch_remap[ch]) < 0)
- continue;
- c->residual_encode &= ~(1 << ch);
- }
-}
-
-static int combine_residual_frame(DCAXllDecoder *s, DCAXllChSet *c)
-{
- DCAContext *dca = s->avctx->priv_data;
- int ch, nsamples = s->nframesamples;
- DCAXllChSet *o;
-
- // Verify that core is compatible
- if (!(dca->packet & DCA_PACKET_CORE)) {
- av_log(s->avctx, AV_LOG_ERROR, "Residual encoded channels are present without core\n");
- return AVERROR(EINVAL);
- }
-
- if (c->freq != dca->core.output_rate) {
- av_log(s->avctx, AV_LOG_WARNING, "Sample rate mismatch between core (%d Hz) and XLL (%d Hz)\n", dca->core.output_rate, c->freq);
- return AVERROR_INVALIDDATA;
- }
-
- if (nsamples != dca->core.npcmsamples) {
- av_log(s->avctx, AV_LOG_WARNING, "Number of samples per frame mismatch between core (%d) and XLL (%d)\n", dca->core.npcmsamples, nsamples);
- return AVERROR_INVALIDDATA;
- }
-
- // See if this channel set is downmixed and find the next channel set in
- // hierarchy. If downmixed, undo core pre-scaling before combining with
- // residual (residual is not scaled).
- o = find_next_hier_dmix_chset(s, c);
-
- // Reduce core bit width and combine with residual
- for (ch = 0; ch < c->nchannels; ch++) {
- int n, spkr, shift, round;
- int32_t *src, *dst;
-
- if (c->residual_encode & (1 << ch))
- continue;
-
- // Map this channel to core speaker
- spkr = ff_dca_core_map_spkr(&dca->core, c->ch_remap[ch]);
- if (spkr < 0) {
- av_log(s->avctx, AV_LOG_WARNING, "Residual encoded channel (%d) references unavailable core channel\n", c->ch_remap[ch]);
- return AVERROR_INVALIDDATA;
- }
-
- // Account for LSB width
- shift = 24 - c->pcm_bit_res + chs_get_lsb_width(s, c, 0, ch);
- if (shift > 24) {
- av_log(s->avctx, AV_LOG_WARNING, "Invalid core shift (%d bits)\n", shift);
- return AVERROR_INVALIDDATA;
- }
-
- round = shift > 0 ? 1 << (shift - 1) : 0;
-
- src = dca->core.output_samples[spkr];
- dst = c->bands[0].msb_sample_buffer[ch];
- if (o) {
- // Undo embedded core downmix pre-scaling
- int scale_inv = o->dmix_scale_inv[c->hier_ofs + ch];
- for (n = 0; n < nsamples; n++)
- dst[n] += (SUINT)clip23((mul16(src[n], scale_inv) + round) >> shift);
- } else {
- // No downmix scaling
- for (n = 0; n < nsamples; n++)
- dst[n] += (unsigned)((src[n] + round) >> shift);
- }
- }
-
- return 0;
-}
-
-int ff_dca_xll_filter_frame(DCAXllDecoder *s, AVFrame *frame)
-{
- AVCodecContext *avctx = s->avctx;
- DCAContext *dca = avctx->priv_data;
- DCAExssAsset *asset = &dca->exss.assets[0];
- DCAXllChSet *p = &s->chset[0], *c;
- enum AVMatrixEncoding matrix_encoding = AV_MATRIX_ENCODING_NONE;
- int i, j, k, ret, shift, nsamples, request_mask;
- int ch_remap[DCA_SPEAKER_COUNT];
-
- // Force lossy downmixed output during recovery
- if (dca->packet & DCA_PACKET_RECOVERY) {
- for (i = 0, c = s->chset; i < s->nchsets; i++, c++) {
- if (i < s->nactivechsets)
- force_lossy_output(s, c);
-
- if (!c->primary_chset)
- c->dmix_embedded = 0;
- }
-
- s->scalable_lsbs = 0;
- s->fixed_lsb_width = 0;
- }
-
- // Filter frequency bands for active channel sets
- s->output_mask = 0;
- for (i = 0, c = s->chset; i < s->nactivechsets; i++, c++) {
- chs_filter_band_data(s, c, 0);
-
- if (c->residual_encode != (1 << c->nchannels) - 1
- && (ret = combine_residual_frame(s, c)) < 0)
- return ret;
-
- if (s->scalable_lsbs)
- chs_assemble_msbs_lsbs(s, c, 0);
-
- if (c->nfreqbands > 1) {
- chs_filter_band_data(s, c, 1);
- chs_assemble_msbs_lsbs(s, c, 1);
- }
-
- s->output_mask |= c->ch_mask;
- }
-
- // Undo hierarchial downmix and/or apply scaling
- for (i = 1, c = &s->chset[1]; i < s->nchsets; i++, c++) {
- if (!is_hier_dmix_chset(c))
- continue;
-
- if (i >= s->nactivechsets) {
- for (j = 0; j < c->nfreqbands; j++)
- if (c->bands[j].dmix_embedded)
- scale_down_mix(s, c, j);
- break;
- }
-
- for (j = 0; j < c->nfreqbands; j++)
- if (c->bands[j].dmix_embedded)
- undo_down_mix(s, c, j);
- }
-
- // Assemble frequency bands for active channel sets
- if (s->nfreqbands > 1) {
- for (i = 0; i < s->nactivechsets; i++)
- if ((ret = chs_assemble_freq_bands(s, &s->chset[i])) < 0)
- return ret;
- }
-
- // Normalize to regular 5.1 layout if downmixing
- if (dca->request_channel_layout) {
- if (s->output_mask & DCA_SPEAKER_MASK_Lss) {
- s->output_samples[DCA_SPEAKER_Ls] = s->output_samples[DCA_SPEAKER_Lss];
- s->output_mask = (s->output_mask & ~DCA_SPEAKER_MASK_Lss) | DCA_SPEAKER_MASK_Ls;
- }
- if (s->output_mask & DCA_SPEAKER_MASK_Rss) {
- s->output_samples[DCA_SPEAKER_Rs] = s->output_samples[DCA_SPEAKER_Rss];
- s->output_mask = (s->output_mask & ~DCA_SPEAKER_MASK_Rss) | DCA_SPEAKER_MASK_Rs;
- }
- }
-
- // Handle downmixing to stereo request
- if (dca->request_channel_layout == DCA_SPEAKER_LAYOUT_STEREO
- && DCA_HAS_STEREO(s->output_mask) && p->dmix_embedded
- && (p->dmix_type == DCA_DMIX_TYPE_LoRo ||
- p->dmix_type == DCA_DMIX_TYPE_LtRt))
- request_mask = DCA_SPEAKER_LAYOUT_STEREO;
- else
- request_mask = s->output_mask;
- if (!ff_dca_set_channel_layout(avctx, ch_remap, request_mask))
- return AVERROR(EINVAL);
-
- avctx->sample_rate = p->freq << (s->nfreqbands - 1);
-
- switch (p->storage_bit_res) {
- case 16:
- avctx->sample_fmt = AV_SAMPLE_FMT_S16P;
- shift = 16 - p->pcm_bit_res;
- break;
- case 20:
- case 24:
- avctx->sample_fmt = AV_SAMPLE_FMT_S32P;
- shift = 24 - p->pcm_bit_res;
- break;
- default:
- return AVERROR(EINVAL);
- }
-
- if (s->x_imax_syncword_present) {
- avctx->profile = FF_PROFILE_DTS_HD_MA_X_IMAX;
- } else if (s->x_syncword_present) {
- avctx->profile = FF_PROFILE_DTS_HD_MA_X;
- } else {
- avctx->profile = FF_PROFILE_DTS_HD_MA;
- }
-
- avctx->bits_per_raw_sample = p->storage_bit_res;
- avctx->bit_rate = 0;
-
- frame->nb_samples = nsamples = s->nframesamples << (s->nfreqbands - 1);
- if ((ret = ff_get_buffer(avctx, frame, 0)) < 0)
- return ret;
-
- // Downmix primary channel set to stereo
- if (request_mask != s->output_mask) {
- ff_dca_downmix_to_stereo_fixed(s->dcadsp, s->output_samples,
- p->dmix_coeff, nsamples,
- s->output_mask);
- }
-
- for (i = 0; i < avctx->ch_layout.nb_channels; i++) {
- int32_t *samples = s->output_samples[ch_remap[i]];
- if (frame->format == AV_SAMPLE_FMT_S16P) {
- int16_t *plane = (int16_t *)frame->extended_data[i];
- for (k = 0; k < nsamples; k++)
- plane[k] = av_clip_int16(samples[k] * (SUINT)(1 << shift));
- } else {
- int32_t *plane = (int32_t *)frame->extended_data[i];
- for (k = 0; k < nsamples; k++)
- plane[k] = clip23(samples[k] * (SUINT)(1 << shift)) * (1 << 8);
- }
- }
-
- if (!asset->one_to_one_map_ch_to_spkr) {
- if (asset->representation_type == DCA_REPR_TYPE_LtRt)
- matrix_encoding = AV_MATRIX_ENCODING_DOLBY;
- else if (asset->representation_type == DCA_REPR_TYPE_LhRh)
- matrix_encoding = AV_MATRIX_ENCODING_DOLBYHEADPHONE;
- } else if (request_mask != s->output_mask && p->dmix_type == DCA_DMIX_TYPE_LtRt) {
- matrix_encoding = AV_MATRIX_ENCODING_DOLBY;
- }
- if ((ret = ff_side_data_update_matrix_encoding(frame, matrix_encoding)) < 0)
- return ret;
-
- return 0;
-}
-
-av_cold void ff_dca_xll_flush(DCAXllDecoder *s)
-{
- clear_pbr(s);
-}
-
-av_cold void ff_dca_xll_close(DCAXllDecoder *s)
-{
- DCAXllChSet *c;
- int i, j;
-
- for (i = 0, c = s->chset; i < DCA_XLL_CHSETS_MAX; i++, c++) {
- for (j = 0; j < DCA_XLL_SAMPLE_BUFFERS_MAX; j++) {
- av_freep(&c->sample_buffer[j]);
- c->sample_size[j] = 0;
- }
- }
-
- av_freep(&s->navi);
- s->navi_size = 0;
-
- av_freep(&s->pbr_buffer);
- clear_pbr(s);
-}
diff --git a/spaces/congsaPfin/Manga-OCR/logs/Download Sigma APK and Join the Fight Out Mode with Your Squad.md b/spaces/congsaPfin/Manga-OCR/logs/Download Sigma APK and Join the Fight Out Mode with Your Squad.md
deleted file mode 100644
index cebdd9f9cfafa9f31910c1a51cc3fbe9bff740a6..0000000000000000000000000000000000000000
--- a/spaces/congsaPfin/Manga-OCR/logs/Download Sigma APK and Join the Fight Out Mode with Your Squad.md
+++ /dev/null
@@ -1,125 +0,0 @@
-
-
Sigma Download APK: How to Play the Stylized Survival Shooter Game on Your Android Device
-
If you are looking for a new and exciting survival shooter game to play on your Android device, you might want to check out Sigma Battle Royale. This game is a stylized and creative take on the popular battle royale genre, where you have to fight against other players in a vast map and be the last one standing. In this article, we will tell you what Sigma Battle Royale is, how to download and install it on your Android device, and some tips and tricks for playing it.
-
What is Sigma Battle Royale?
-
Sigma Battle Royale is a game developed by Studio Arm Private Limited, a game studio based in India. It was released in November 2022 and has since gained over 500,000 downloads on Google Play Store. The game is available for Android devices with version 4.1 or higher.
Sigma Battle Royale is a game that combines the elements of classic battle royale and 4v4 team deathmatch modes. In the battle royale mode, you can choose your starting point with your parachute and land on a large map with 49 other players. You have to loot weapons, armor, and items, and fight against other players while staying in the safe zone. The last player or team alive wins the match. In the 4v4 fight out mode, you can team up with three other players and compete against another team in various maps. You have to allocate resources, buy weapons, and outlast your enemies. The team with the most kills wins the match.
-
Features of Sigma Battle Royale
-
Some of the features that make Sigma Battle Royale stand out from other survival shooter games are:
-
-
Stylized graphics: The game has a unique and creative art style that gives it a distinctive look and feel. The game uses bright colors, cartoon-like characters, and futuristic weapons and vehicles to create a stylized survival world.
-
Unique survival shooter experience: The game has easy-to-use controls and promises an unforgettable survival experience on mobile. You can customize your character, choose from different weapons and items, and use various skills and tactics to survive.
-
Classic battle royale: The game offers a fast-paced and lite gameplay that lasts for about 10 minutes per match. You can experience the thrill of being the last one standing in a 50-player battle royale mode.
-
4v4 fight out: The game also offers a tense and strategic gameplay that lasts for about 7 minutes per match. You can collaborate with your team and defeat your enemies in a 4v4 team deathmatch mode.
-
-
How to download and install Sigma Battle Royale APK
-
If you want to play Sigma Battle Royale on your Android device, you can download it from Google Play Store or from APKCombo. APKCombo is a website that provides free APK downloads for Android games and apps. Here are the steps to download and install Sigma Battle Royale APK from APKCombo:
-
-
Go to APKCombo and search for "Sigma" or "Sigma Battle Royale".
-
Select the game from the search results and click on "Download APK".
-
Choose the version of the game that is compatible with your device and click on "Download".
-
Wait for the download to finish and then open the APK file.
-
If you see a warning message that says "Install blocked", go to your device settings and enable "Unknown sources" or "Allow from this source".
-
Follow the instructions on the screen to install the game.
-
Enjoy playing Sigma Battle Royale on your Android device.
-Tips and tricks for playing Sigma Battle Royale
-
Now that you have downloaded and installed Sigma Battle Royale on your Android device, you might be wondering how to play it and win more matches. Here are some tips and tricks that can help you improve your skills and have more fun in the game:
-
Choose your landing spot wisely
-
In the battle royale mode, you can choose where to land on the map with your parachute. This is a crucial decision that can affect your chances of survival and victory. You should consider the following factors when choosing your landing spot:
-
-
Distance from the plane: The farther you are from the plane's path, the less likely you are to encounter other players in the early game. However, you might also have to travel longer distances to reach the safe zone or find better loot.
-
Loot quality and quantity: Some areas on the map have more loot than others, and some loot is more valuable than others. You should aim for areas that have high-quality weapons, armor, and items that can give you an edge in combat.
-
Popularity and competition: Some areas on the map are more popular than others, and therefore more likely to attract other players. You should avoid landing in hot spots if you want to avoid early fights and focus on looting. However, if you are confident in your skills and want to eliminate other players quickly, you can try landing in hot spots and fight for the best loot.
-
-
Loot and upgrade your weapons
-
In Sigma Battle Royale, you can find various weapons and items on the map or from the enemies you kill. You should always loot as much as you can and upgrade your weapons whenever possible. Here are some tips for looting and upgrading your weapons:
-
-
Know your weapon types: There are four types of weapons in Sigma Battle Royale: assault rifles, shotguns, sniper rifles, and pistols. Each weapon type has its own advantages and disadvantages in terms of damage, range, accuracy, fire rate, recoil, and magazine size. You should choose the weapon type that suits your playstyle and situation.
-
Know your weapon rarities: There are five rarities of weapons in Sigma Battle Royale: common, uncommon, rare, epic, and legendary. The higher the rarity, the better the stats and performance of the weapon. You should always look for higher rarity weapons and replace your lower rarity ones.
-
Know your weapon attachments: There are four types of attachments in Sigma Battle Royale: scopes, muzzles, magazines, and grips. Each attachment type can improve a certain aspect of your weapon, such as zoom, stability, capacity, or handling. You should always equip attachments that match your weapon type and preference.
-
Know your weapon skins: There are various skins that you can unlock or buy for your weapons in Sigma Battle Royale. Skins can change the appearance of your weapon and give you some cosmetic benefits. However, skins do not affect the stats or performance of your weapon.
-
-
Use cover and movement to your advantage
-
In Sigma Battle Royale, you have to be aware of your surroundings and use cover and movement to your advantage. Here are some tips for using cover and movement in the game:
-
sigma battle royale apk download
-sigma game apk free download
-sigma android game download
-sigma apk latest version 2023
-sigma apk for android tv
-sigma apk for pc windows
-sigma apk for tablet
-sigma apk xapk
-sigma apk apks
-sigma apk obb
-sigma stylized survival shooter apk
-sigma 4v4 fight out apk
-sigma 50 players battle royale apk
-sigma classic battle royale apk
-sigma creative survival world apk
-sigma game studio arm private limited apk
-sigma game action category apk
-sigma game google play id com.studioarm.sigma apk
-sigma game installs 500,000+ apk
-sigma game version 1.0.113 apk
-sigma game version 1.0.103 apk
-sigma game version 1.0.0 apk
-download sigma game from apkcombo.com
-download sigma game from newscientist.com
-download sigma game from the-sun.com
-download sigma game from yahoo.com
-download sigma game from wikipedia.org
-download sigma game from montana.edu
-download sigma game from cornell.edu
-download sigma game from nasa.gov
-download sigma game from activision.com
-download sigma game from roblox.com
-download sigma game from garena.com
-download sigma game from konami.com
-download sigma game from epicgames.com
-download sigma game from innersloth.com
-download sigma game from scottgames.com
-download sigma game from studiomdhr.com
-download sigma game from ea.com
-how to install xapk, apks, obb for sigma game
-how to play sigma battle royale on android
-how to update sigma battle royale to latest version
-how to uninstall sigma battle royale from android
-how to fix errors and bugs in sigma battle royale
-how to get free coins and gems in sigma battle royale
-how to unlock new weapons and maps in sigma battle royale
-how to join a squad and chat with friends in sigma battle royale
-how to customize your character and parachute in sigma battle royale
-how to improve your skills and tactics in sigma battle royale
-how to win every match in sigma battle royale
-
-
Use cover to protect yourself: Cover is anything that can block or reduce the damage from enemy fire, such as buildings, walls, trees, rocks, vehicles, etc. You should always use cover when engaging in combat or when healing yourself or your teammates. Cover can also help you hide from enemies or ambush them.
-
Use movement to confuse your enemies: Movement is anything that can change your position or direction, such as running, jumping, sliding, crouching, etc. You should always use movement when fighting or escaping from enemies. Movement can help you dodge enemy fire or surprise them with unexpected attacks.
-
Use vehicles to travel faster: Vehicles are anything that can transport you or your teammates across the map faster than walking or running, such as cars, bikes, boats, etc. You should always use vehicles when moving to the safe zone or when chasing or fleeing from enemies. Vehicles can also be used as weapons or cover in some situations.
-
-
Communicate and cooperate with your team
-
In Sigma Battle Royale, you can play solo or with up to three other players in a team. Playing with a team can give you many advantages over playing solo, such as having more firepower, support, information, and resources. However, playing with a team also requires communication and cooperation. Here are some tips for communicating and cooperating with your team in the game:
-
-
Use voice chat or text chat to communicate with your team: Voice chat or text chat are the main ways to communicate with your team in Sigma Battle Royale. You can use voice chat or text chat to share information, coordinate actions, ask for help, or chat with your team. Voice chat is more convenient and effective than text chat, but it also requires a good internet connection and a microphone. Text chat is more accessible and discreet than voice chat, but it also takes more time and attention to type and read messages.
-
Use quick commands or gestures to communicate with your team: Quick commands or gestures are the alternative ways to communicate with your team in Sigma Battle Royale. You can use quick commands or gestures to send predefined messages, such as "Follow me", "Enemy spotted", "Need ammo", etc. Quick commands or gestures are faster and easier than voice chat or text chat, but they are also more limited and vague.
-
Use the map or the compass to communicate with your team: The map or the compass are the supplementary ways to communicate with your team in Sigma Battle Royale. You can use the map or the compass to mark locations, enemies, items, or vehicles on the screen. The map or the compass are useful and accurate ways to communicate with your team, but they also require you to open the map or look at the compass, which can expose you to danger.
-
Cooperate with your team to achieve your goals: Cooperation is the key to success in Sigma Battle Royale. You should cooperate with your team to achieve your goals, such as landing together, looting together, fighting together, and surviving together. Cooperation can help you overcome challenges, gain advantages, and have more fun in the game.
-
-
Conclusion
-
Sigma Battle Royale is a stylized and creative survival shooter game that you can play on your Android device. It offers two modes of gameplay: classic battle royale and 4v4 fight out. It has unique features, such as stylized graphics, unique survival shooter experience, and various weapons and items. It also requires skills, such as choosing your landing spot wisely, looting and upgrading your weapons, using cover and movement to your advantage, and communicating and cooperating with your team. If you want to play Sigma Battle Royale on your Android device, you can download it from Google Play Store or from APKCombo. We hope this article has helped you learn more about Sigma Battle Royale and how to play it.
-
FAQs
-
Here are some frequently asked questions about Sigma Battle Royale:
-
-
Is Sigma Battle Royale free to play?
-
Yes, Sigma Battle Royale is free to play on Android devices. However, it also offers in-app purchases that can enhance your gameplay experience.
-
Is Sigma Battle Royale online or offline?
-
Sigma Battle Royale is an online game that requires an internet connection to play. You can play solo or with other players from around the world.
-
Is Sigma Battle Royale safe to download and install?
-
Yes, Sigma Battle Royale is safe to download and install from Google Play Store or from APKCombo. However, you should always be careful when downloading APK files from unknown sources and scan them for viruses before installing them.
-
How can I update Sigma Battle Royale?
-
You can update Sigma Battle Royale from Google Play Store or from APKCombo. You should always update the game to get the latest features, bug fixes, and improvements.
-
How can I contact the developers of Sigma Battle Royale?
-
You can contact the developers of Sigma Battle Royale by sending an email to studioarmpl@gmail.com or by visiting their website at https://studioarm.in/.
- 197e85843d
-
-
\ No newline at end of file
diff --git a/spaces/congsaPfin/Manga-OCR/logs/Dynamons World on PC The Ultimate RPG Experience with BlueStacks Emulator.md b/spaces/congsaPfin/Manga-OCR/logs/Dynamons World on PC The Ultimate RPG Experience with BlueStacks Emulator.md
deleted file mode 100644
index 215e9ee9148605ee72cc1e1b8b42e929a13a67df..0000000000000000000000000000000000000000
--- a/spaces/congsaPfin/Manga-OCR/logs/Dynamons World on PC The Ultimate RPG Experience with BlueStacks Emulator.md
+++ /dev/null
@@ -1,113 +0,0 @@
-
-
How to Download and Play Dynamons World on Your Laptop
-
If you are looking for a fun and addictive game that combines RPG elements, monster catching, and online battles, you should check out Dynamons World. This game lets you explore an open world full of different types of Dynamons, which are cute and powerful creatures that you can catch, train, and battle with. You can also challenge your friends and other players in real-time online multiplayer matches, or join a clan and participate in events and quests.
-
Dynamons World is available for free on Android and iOS devices, but did you know that you can also play it on your laptop? Playing on a bigger screen can enhance your gaming experience, as well as give you more control options and better performance. In this article, we will show you how to download and play Dynamons World on your laptop using different methods. Whether you have a Windows or a Mac laptop, there is a way for you to enjoy this amazing game.
If you have a Windows laptop, one of the easiest ways to get Dynamons World is through the Microsoft Store. This is an online marketplace where you can find various apps and games for your Windows device. Here are the steps to follow:
-
-
Open the Microsoft Store. You can do this by clicking on the Start menu and typing "Microsoft Store", or by pressing the Windows key + S and searching for it.
-
Click on Gaming in the sidebar. This will show you different categories and genres of games that you can browse.
-
Select Dynamons World. You can use the search bar at the top right corner to find it quickly, or scroll through the list of games until you see it.
-
Purchase the game (if needed). Some games in the Microsoft Store are free, while others require payment. Dynamons World is free to play, but it offers in-app purchases for extra items and features. If you want to buy something in the game, you will need a Microsoft account and a payment method. To get the game, click on the Get button.
-
Install the game. After clicking on Get, the game will start downloading automatically. You can see the progress in the Downloads section of the Microsoft Store. Once it is done, click on Install to finish the process.
-
Launch and play the game. You can find Dynamons World in your Start menu or on your desktop. Click on its icon to open it, and enjoy playing it on your laptop.
-
-
Method 2: Direct Download
-
Another way to play Dynamons World on your laptop is to download it directly from its official website. This method works for both Windows and Mac laptops. This method gives you the most updated and original version of the game. Here are the steps to follow:
-
-
Search for the official website of Dynamons World. You can use Bing or any other search engine to find it. The website should have a URL like [1](https://play.google.com/store/apps/details?id=com.funtomic.dynamons3) or [6](https://apps.apple.com/us/app/dynamons-world/id1190307500), depending on your device.
-
Download the game file from the website. There should be a button or a link that says something like "Download", "Get", or "Install". Click on it and choose a location to save the file on your laptop.
-
Install and run the game. Depending on your device, you may need to unzip the file first, or double-click on it to start the installation process. Follow the instructions on the screen and agree to the terms and conditions. Once the installation is complete, you can open the game and start playing.
-
-
Method 3: Third-Party Platform
-
A third option to play Dynamons World on your laptop is to use a third-party platform that hosts various games and apps. Some of the most popular and reliable platforms are Steam, Epic Games, and GOG. These platforms offer many benefits, such as easy access, updates, reviews, achievements, and more. Here are the steps to follow:
-
-
Choose a platform that suits your preferences and needs. You can compare the features, prices, and ratings of different platforms online, or ask for recommendations from other gamers.
-
Create an account and download the platform client. You will need to register with your email address and create a username and password. Then, you will need to download and install the platform client on your laptop, which will allow you to access the platform library and services.
-
Find and download Dynamons World from the platform library. You can use the search function or browse through different categories and genres to find Dynamons World. Some platforms may offer it for free, while others may require payment or subscription. Once you find it, click on the "Download", "Get", or "Install" button.
-
Launch and play the game. You can find Dynamons World in your platform library or on your desktop. Click on its icon to open it, and enjoy playing it on your laptop.
-
-
Conclusion
-
Dynamons World is a fun and addictive game that you can play on your laptop using different methods. Whether you use the Microsoft Store, direct download, or third-party platform, you can enjoy this game on a bigger screen with more control options and better performance. Here are some tips and tricks for enjoying the game:
-
-
Learn about the different types of Dynamons and their strengths and weaknesses. Use this knowledge to create a balanced team and choose the best moves in battle.
-
Collect skill cards and use them wisely to enhance your Dynamons' abilities and tactics. You can find skill cards in chests, shops, quests, or battles.
-
Join a clan and cooperate with other players to complete events and quests, earn rewards, and chat with other Dynamon fans.
-
Customize your avatar and your Dynamons with different outfits, accessories, and colors. You can buy them with coins or gems in the shop.
-
Have fun and experiment with different strategies and combinations. There is no one right way to play Dynamons World, so feel free to explore and discover new things.
-
-
We hope this article has helped you learn how to download and play Dynamons World on your laptop. If you have any questions or feedback, please let us know in the comments below. And don't forget to share this article with your friends who might be interested in playing this game too!
-
dynamons world pc game download
-how to play dynamons world on laptop
-dynamons world emulator for pc
-dynamons world offline rpg for pc
-download dynamons world on bluestacks
-dynamons world gameloop emulator
-dynamons world windows 10 download
-dynamons world online pvp battles on pc
-dynamons world role playing game for pc
-dynamons world android game on pc
-dynamons world catch and train on pc
-dynamons world skill cards for pc
-dynamons world klaude's kingdom on pc
-dynamons world zenix dragon on pc
-dynamons world temple ruins on pc
-dynamons world electricity and dark types on pc
-dynamons world azerion casual game for pc
-dynamons world keygames network game for pc
-dynamons world youtube video for pc
-dynamons world apk download for laptop
-dynamons world mod apk for laptop
-dynamons world hack for laptop
-dynamons world cheats for laptop
-dynamons world unlimited money for laptop
-dynamons world free download for laptop
-dynamons world latest version for laptop
-dynamons world update for laptop
-dynamons world new features for laptop
-dynamons world best team for laptop
-dynamons world tips and tricks for laptop
-dynamons world guide and walkthrough for laptop
-dynamons world review and rating for laptop
-dynamons world gameplay and graphics for laptop
-dynamons world system requirements for laptop
-dynamons world minimum specs for laptop
-dynamons world recommended specs for laptop
-dynamons world performance and optimization for laptop
-dynamons world bug fixes and improvements for laptop
-dynamons world support and feedback for laptop
-dynamons world community and forum for laptop
-
FAQs
-
Here are some frequently asked questions about Dynamons World:
-
What are the minimum system requirements for playing Dynamons World on your laptop?
-
The minimum system requirements for playing Dynamons World on your laptop vary depending on the method you use. However, generally speaking, you will need at least:
-
-
Operating System
Windows 7/8/10 or Mac OS X 10.9+
-
Processor
Intel Core 2 Duo 2GHz+ or equivalent
-
Memory
2 GB RAM
Graphics
DirectX 9.0c compatible video card with 256 MB VRAM
-
Storage
500 MB available space
-
Internet
Broadband connection
-
-
You can check your laptop's specifications by going to the Control Panel or the System Preferences and looking for the System or Hardware information.
-
Can you play Dynamons World online with other players?
-
Yes, you can play Dynamons World online with other players. You can challenge them in real-time PvP battles, or join a clan and cooperate with them in events and quests. You can also chat with them and make new friends. To play online, you will need a stable internet connection and a Dynamons World account.
-
Can you transfer your progress and data from your mobile device to your laptop?
-
Yes, you can transfer your progress and data from your mobile device to your laptop. You will need to link your Dynamons World account to your Facebook account, Google Play account, or Apple ID. Then, you can log in with the same account on your laptop and sync your data. This way, you can continue playing where you left off on any device.
-
How can you update Dynamons World to get the latest features and fixes?
-
To update Dynamons World to get the latest features and fixes, you will need to check for updates regularly on the platform that you use to play the game. For example, if you use the Microsoft Store, you can go to the Downloads section and see if there are any updates available for Dynamons World. If there are, click on the Update button to download and install them. If you use direct download or third-party platform, you can check the official website or the platform library for updates.
-
Where can you find more information and support for Dynamons World?
-
If you need more information and support for Dynamons World, you can visit the following sources:
-
-
The official website of Dynamons World: [1](https://play.google.com/store/apps/details?id=com.funtomic.dynamons3) or [6](https://apps.apple.com/us/app/dynamons-world/id1190307500)
-
The official Facebook page of Dynamons World: [2](https://www.facebook.com/DynamonsWorld/)
-
The official YouTube channel of Dynamons World: [3](https://www.youtube.com/channel/UC4Zyv1xk8aQ6YfO7JXzQg2w)
-
The official Twitter account of Dynamons World: [4](https://twitter.com/DynamonsWorld)
-
The official Instagram account of Dynamons World: [5](https://www.instagram.com/dynamonsworld/)
-
The official Discord server of Dynamons World: [7](https://discord.gg/dynamonsworld)
-
The official Reddit community of Dynamons World: [8](https://www.reddit.com/r/DynamonsWorld/)
-
The official Wikia page of Dynamons World: [9](https://dynamonsworld.fandom.com/wiki/Dynamons_World_Wiki)
-
-
You can also contact the developers of Dynamons World by sending an email to support@funtomic.com or by filling out a form on their website.
401be4b1e0
-
-
\ No newline at end of file
diff --git a/spaces/congsaPfin/Manga-OCR/logs/Free World Map Vectors with Countries for Creative Projects.md b/spaces/congsaPfin/Manga-OCR/logs/Free World Map Vectors with Countries for Creative Projects.md
deleted file mode 100644
index c51d624a236b867a76d394c23d772641af700d94..0000000000000000000000000000000000000000
--- a/spaces/congsaPfin/Manga-OCR/logs/Free World Map Vectors with Countries for Creative Projects.md
+++ /dev/null
@@ -1,161 +0,0 @@
-
-
World Map with Countries Vector Free Download
-
If you are looking for a world map with countries that you can download for free and use for various purposes, such as education, design, or presentation, you might want to consider using vector graphics instead of raster graphics. In this article, we will explain what vector graphics are, why they are useful, and where you can find free vector graphics of world maps with countries. We will also show you how to use some popular vector graphics software to edit and customize your world map according to your needs.
Vector graphics are a form of computer graphics in which visual images are created directly from geometric shapes defined on a Cartesian plane, such as points, lines, curves, and polygons. Unlike raster graphics, which use a fixed grid of pixels, vector graphic files have no fixed resolution and can be resized, stretched, and otherwise manipulated without any quality loss.
-
Definition of vector graphics
-
Vector graphics are based on mathematical equations that describe the position, direction, and color of each shape in the image. A vector graphic file is saved as a sequence of vector statements that can be interpreted by a software program to render the image on a screen or a printer.
-
Advantages of vector graphics over raster graphics
-
One of the most significant advantages of vector graphics is their scalability. Vector graphics can be scaled up or down without losing quality or resolution. This is because vector graphics are based on mathematical equations, which means that the image can be stretched or shrunk without affecting the quality of the image.
-
Another advantage of vector graphics is their small file size. Vector graphics typically have smaller file sizes than raster graphics because they do not store information about individual pixels. This makes vector graphics ideal for use on websites or in applications where smaller file sizes are important.
-
Examples of vector graphics applications
-
Vector graphics are widely used for creating digital graphics today because of their versatility and precision. Some examples of vector graphics applications are:
-
-
Logos and icons that look crisp and clear at any size
-
Line art and illustrations that can be easily modified and colored
-
3D-like renderings and animations that can be rotated and transformed
-
Computer-aided design (CAD) and engineering drawings that require high accuracy and detail
-
Typography and fonts that can be scaled and styled without distortion
-
Infographics and charts that can be updated and edited with data
-
-
Where can you find free vector graphics of world maps with countries?
-
If you want to download a free vector graphic of a world map with countries, there are many websites that offer such resources. Here are some of the best ones that we recommend:
-
world map with countries vector free download
-world map countries vectors & illustrations for free download | freepik
-world map with countries vectors images free for commercial use
-world map outline with countries vector free download
-world map with country names vector free download
-world map with continents and countries vector free download
-world map with flags of countries vector free download
-world map with countries and capitals vector free download
-world map with countries and cities vector free download
-world map with countries and regions vector free download
-world map with countries and oceans vector free download
-world map with countries and borders vector free download
-world map with countries and states vector free download
-world map with countries and time zones vector free download
-world map with countries and landmarks vector free download
-world map with countries and currencies vector free download
-world map with countries and languages vector free download
-world map with countries and population vector free download
-world map with countries and climate zones vector free download
-world map with countries and religions vector free download
-world map with countries and cultures vector free download
-world map with countries and iso codes vector free download
-world map with countries and coordinates vector free download
-world map with countries and hemispheres vector free download
-world map with countries and continents names vector free download
-world map with countries in different colors vector free download
-world map with countries in high resolution vector free download
-world map with countries in 3d style vector free download
-world map with countries in flat design vector free download
-world map with countries in watercolor style vector free download
-world map with countries in vintage style vector free download
-world map with countries in cartoon style vector free download
-world map with countries in abstract style vector free download
-world map with countries in geometric style vector free download
-world map with countries in sketch style vector free download
-world map with countries in doodle style vector free download
-world map with countries in pixel art style vector free download
-world map with countries in origami style vector free download
-world map with countries in mosaic style vector free download
-world map with countries in gradient style vector free download
-editable world map with countries vector free download
-printable world map with countries vector free download
-interactive world map with countries vector free download
-blank world map with countries vector free download
-detailed world map with countries vector free download
-simple world map with countries vector free download
-colorful world map with countries vector free download
-black and white world map with countries vector free download
-
Freepik
-
Freepik is one of the most popular websites for finding free vectors, photos, PSD files, and icons. You can browse through thousands of world map vectors with different styles, colors, and levels of detail. You can also filter your search by license type, orientation, color, or category.
-
Vecteezy
-
Vecteezy is another great website for finding free vector art, icons, patterns, backgrounds, and more. You can search for world map vectors with various designs, such as flat, realistic, abstract, or vintage. You can also use their online editor to customize your map before downloading it.
-
Inks.
Inkscape
-
Inkscape is a free and open-source vector graphics editor that you can download and install on your computer. It supports various vector formats, such as SVG, EPS, PDF, and AI. You can use Inkscape to create your own world map vector from scratch or import and edit an existing one. You can also use Inkscape's tools and features to add labels, colors, symbols, and effects to your map.
-
How to use vector graphics software to edit and customize your world map
-
Once you have downloaded a free vector graphic of a world map with countries, you might want to edit and customize it to suit your purpose. For example, you might want to change the color scheme, add or remove some countries, or highlight some regions. To do this, you will need to use a vector graphics software program that can open and modify vector files. Here are some of the most popular ones that you can use:
-
Adobe Illustrator
-
Adobe Illustrator is a professional vector graphics software that is widely used by designers, artists, and illustrators. It offers a range of tools and features to create and edit vector graphics, such as shapes, paths, gradients, brushes, filters, and more. You can use Adobe Illustrator to open and edit any vector file format, including SVG, EPS, PDF, and AI.
-
To edit a world map vector in Adobe Illustrator, you can follow these steps:
-
-
Open the vector file in Adobe Illustrator.
-
Select the map layer and ungroup it by going to Object > Ungroup or pressing Ctrl+Shift+G.
-
Select the countries or regions that you want to edit and use the tools in the toolbar or the properties panel to change their appearance. For example, you can use the Direct Selection Tool (A) to move or resize the shapes, the Eyedropper Tool (I) to copy the fill or stroke color from another object, or the Swatches panel to apply a different color.
-
If you want to add or remove some countries or regions, you can use the Pen Tool (P) or the Shape Tool (M) to draw new shapes or delete existing ones. You can also use the Pathfinder panel to combine or subtract shapes.
-
If you want to add labels or text to your map, you can use the Text Tool (T) to create text boxes and type your text. You can also use the Character panel or the Paragraph panel to adjust the font size, style, alignment, and spacing of your text.
-
When you are done editing your map, you can group it again by selecting all the objects and going to Object > Group or pressing Ctrl+G.
-
You can save your map as an SVG file by going to File > Save As and choosing SVG as the format. You can also export your map as a PNG or JPEG image by going to File > Export > Export As and choosing the format and resolution that you want.
-
-
Vectornator
-
Vectornator is a free vector graphics software that is available for iOS, iPadOS, and macOS devices. It is designed for creating beautiful vector illustrations on the go with intuitive touch gestures and Apple Pencil support. It supports various vector formats, such as SVG, PDF, AI, and EPS.
-
To edit a world map vector in Vectornator, you can follow these steps:
-
-
Open the vector file in Vectornator.
-
Select the map layer and tap on the Ungroup button in the toolbar.
-
Select the countries or regions that you want to edit and use the tools in the toolbar or the inspector panel to change their appearance. For example, you can use the Move Tool (V) to move or resize the shapes, the Style Tool (S) to change the fill or stroke color or apply gradients or patterns, or the Arrange Tool (A) to change the order or alignment of the objects.
-
If you want to add or remove some countries or regions, you can use the Pen Tool (P) or the Shape Tool (U) to draw new shapes or delete existing ones. You can also use the Boolean Operations in the inspector panel to combine or subtract shapes.
-
If you want to add labels or text to your map, you can use the Text Tool (T) to create text boxes and type your text. You can also use the inspector panel to adjust the font size, style, alignment, and spacing of your text.
-
When you
When you are done editing your map, you can group it again by selecting all the objects and tapping on the Group button in the toolbar.
-
You can save your map as an SVG file by tapping on the Share button in the toolbar and choosing SVG as the format. You can also export your map as a PNG or JPEG image by tapping on the Share button and choosing the format and resolution that you want.
-
-
SVGator
-
SVGator is a free online vector graphics editor and animator that works in your browser. It allows you to create and edit SVG files with ease and add animations and interactivity to them. You can also import and export other vector formats, such as AI, EPS, PDF, and PNG.
-
To edit a world map vector in SVGator, you can follow these steps:
-
-
Open the vector file in SVGator by dragging and dropping it into the editor or clicking on the Import button.
-
Select the map layer and click on the Ungroup button in the toolbar.
-
Select the countries or regions that you want to edit and use the tools in the toolbar or the properties panel to change their appearance. For example, you can use the Selection Tool (V) to move or resize the shapes, the Fill Tool (F) or the Stroke Tool (S) to change the fill or stroke color or apply gradients or patterns, or the Align Tool (A) to change the order or alignment of the objects.
-
If you want to add or remove some countries or regions, you can use the Pen Tool (P) or the Shape Tool (U) to draw new shapes or delete existing ones. You can also use the Path Operations in the properties panel to combine or subtract shapes.
-
If you want to add labels or text to your map, you can use the Text Tool (T) to create text boxes and type your text. You can also use the properties panel to adjust the font size, style, alignment, and spacing of your text.
-
When you are done editing your map, you can group it again by selecting all the objects and clicking on the Group button in the toolbar.
-
You can save your map as an SVG file by clicking on the Export button in the toolbar and choosing SVG as the format. You can also export your map as a PNG or JPEG image by clicking on the Export button and choosing the format and resolution that you want.
-
-
Conclusion
-
In this article, we have explained what vector graphics are, why they are useful, and where you can find free vector graphics of world maps with countries. We have also shown you how to use some popular vector graphics software to edit and customize your world map according to your needs. We hope that this article has helped you learn more about vector graphics and how to use them for creating beautiful world maps.
-
FAQs
-
What is the difference between vector graphics and raster graphics?
-
Vector graphics are a form of computer graphics in which visual images are created directly from geometric shapes defined on a Cartesian plane, such as points, lines, curves, and polygons. Raster graphics are a form of computer graphics in which visual images are created from a fixed grid of pixels. Vector graphics have no fixed resolution and can be resized without quality loss, while raster graphics have a fixed resolution and lose quality when resized.
-
What are some of the benefits of using vector graphics for world maps?
-
Some of the benefits of using vector graphics for world maps are:
-
-
They can be scaled up or down without losing quality or resolution.
-
They have smaller file sizes than raster graphics because they do not store information about individual pixels.
-
They can be easily modified and colored using vector graphics software.
-
They can be used for various purposes, such as education, design, or presentation.
-
-
What are some of the best websites for finding free vector graphics of world maps with countries?
-
Some of Some of the best websites for finding free vector graphics of world maps with countries are:
-
-
Freepik, which offers thousands of world map vectors with different styles, colors, and levels of detail.
-
Vecteezy, which provides hundreds of world map vectors with various designs, such as flat, realistic, abstract, or vintage.
-
Inkscape, which is a free and open-source vector graphics editor that you can use to create your own world map vector from scratch or import and edit an existing one.
-
-
What are some of the most popular vector graphics software that you can use to edit and customize your world map?
-
Some of the most popular vector graphics software that you can use to edit and customize your world map are:
-
-
Adobe Illustrator, which is a professional vector graphics software that offers a range of tools and features to create and edit vector graphics, such as shapes, paths, gradients, brushes, filters, and more.
-
Vectornator, which is a free vector graphics software that is available for iOS, iPadOS, and macOS devices. It is designed for creating beautiful vector illustrations on the go with intuitive touch gestures and Apple Pencil support.
-
SVGator, which is a free online vector graphics editor and animator that works in your browser. It allows you to create and edit SVG files with ease and add animations and interactivity to them.
-
-
How can I convert a raster graphic of a world map to a vector graphic?
-
If you have a raster graphic of a world map that you want to convert to a vector graphic, you can use a process called vectorization or tracing. Vectorization is the process of converting a raster image into a vector image by creating geometric shapes that approximate the pixels in the raster image.
-
There are two ways to perform vectorization: manual or automatic. Manual vectorization involves using a vector graphics software program to draw shapes over the raster image by hand. Automatic vectorization involves using a software program that can automatically detect the edges and shapes in the raster image and create vector shapes accordingly.
-
Some of the software programs that can perform automatic vectorization are:
-
-
Vector Magic, which is an online tool that can convert raster images to vector images with high quality and accuracy.
-
Image Trace, which is a feature in Adobe Illustrator that can trace raster images and create vector paths or shapes from them.
-
Potrace, which is a free and open-source tool that can convert bitmap images to smooth, scalable vector graphics.
-
-
How can I add interactivity or animation to my world map vector?
-
If you want to add interactivity or animation to your world map vector, you will need to use a format that supports these features, such as SVG (Scalable Vector Graphics). SVG is an XML-based format that can describe two-dimensional graphics with interactivity and animation.
-
To add interactivity or animation to your world map SVG, you can use one of the following methods:
-
-
CSS (Cascading Style Sheets), which is a language that can define the style and layout of SVG elements. You can use CSS properties and animations to change the appearance or behavior of your SVG elements based on user actions or events.
-
JavaScript, which is a scripting language that can manipulate the SVG document object model (DOM). You can use JavaScript functions and events to add logic and functionality to your SVG elements based on user actions or events.
-
SMIL (Synchronized Multimedia Integration Language), which is an XML-based language that can define animations for SVG elements. You can use SMIL elements and attributes to specify the timing, duration, and effects of your SVG animations.
-
197e85843d
-
-
\ No newline at end of file
diff --git a/spaces/cooelf/Multimodal-CoT/timm/loss/cross_entropy.py b/spaces/cooelf/Multimodal-CoT/timm/loss/cross_entropy.py
deleted file mode 100644
index 60bef646cc6c31fd734f234346dbc4255def6622..0000000000000000000000000000000000000000
--- a/spaces/cooelf/Multimodal-CoT/timm/loss/cross_entropy.py
+++ /dev/null
@@ -1,36 +0,0 @@
-import torch
-import torch.nn as nn
-import torch.nn.functional as F
-
-
-class LabelSmoothingCrossEntropy(nn.Module):
- """
- NLL loss with label smoothing.
- """
- def __init__(self, smoothing=0.1):
- """
- Constructor for the LabelSmoothing module.
- :param smoothing: label smoothing factor
- """
- super(LabelSmoothingCrossEntropy, self).__init__()
- assert smoothing < 1.0
- self.smoothing = smoothing
- self.confidence = 1. - smoothing
-
- def forward(self, x, target):
- logprobs = F.log_softmax(x, dim=-1)
- nll_loss = -logprobs.gather(dim=-1, index=target.unsqueeze(1))
- nll_loss = nll_loss.squeeze(1)
- smooth_loss = -logprobs.mean(dim=-1)
- loss = self.confidence * nll_loss + self.smoothing * smooth_loss
- return loss.mean()
-
-
-class SoftTargetCrossEntropy(nn.Module):
-
- def __init__(self):
- super(SoftTargetCrossEntropy, self).__init__()
-
- def forward(self, x, target):
- loss = torch.sum(-target * F.log_softmax(x, dim=-1), dim=-1)
- return loss.mean()
diff --git a/spaces/coreml-community/ControlNet-v1-1-Annotators-cpu/annotator/lama/saicinpainting/training/losses/style_loss.py b/spaces/coreml-community/ControlNet-v1-1-Annotators-cpu/annotator/lama/saicinpainting/training/losses/style_loss.py
deleted file mode 100644
index 0bb42d7fbc5d17a47bec7365889868505f5fdfb5..0000000000000000000000000000000000000000
--- a/spaces/coreml-community/ControlNet-v1-1-Annotators-cpu/annotator/lama/saicinpainting/training/losses/style_loss.py
+++ /dev/null
@@ -1,155 +0,0 @@
-import torch
-import torch.nn as nn
-import torchvision.models as models
-
-
-class PerceptualLoss(nn.Module):
- r"""
- Perceptual loss, VGG-based
- https://arxiv.org/abs/1603.08155
- https://github.com/dxyang/StyleTransfer/blob/master/utils.py
- """
-
- def __init__(self, weights=[1.0, 1.0, 1.0, 1.0, 1.0]):
- super(PerceptualLoss, self).__init__()
- self.add_module('vgg', VGG19())
- self.criterion = torch.nn.L1Loss()
- self.weights = weights
-
- def __call__(self, x, y):
- # Compute features
- x_vgg, y_vgg = self.vgg(x), self.vgg(y)
-
- content_loss = 0.0
- content_loss += self.weights[0] * self.criterion(x_vgg['relu1_1'], y_vgg['relu1_1'])
- content_loss += self.weights[1] * self.criterion(x_vgg['relu2_1'], y_vgg['relu2_1'])
- content_loss += self.weights[2] * self.criterion(x_vgg['relu3_1'], y_vgg['relu3_1'])
- content_loss += self.weights[3] * self.criterion(x_vgg['relu4_1'], y_vgg['relu4_1'])
- content_loss += self.weights[4] * self.criterion(x_vgg['relu5_1'], y_vgg['relu5_1'])
-
-
- return content_loss
-
-
-class VGG19(torch.nn.Module):
- def __init__(self):
- super(VGG19, self).__init__()
- features = models.vgg19(pretrained=True).features
- self.relu1_1 = torch.nn.Sequential()
- self.relu1_2 = torch.nn.Sequential()
-
- self.relu2_1 = torch.nn.Sequential()
- self.relu2_2 = torch.nn.Sequential()
-
- self.relu3_1 = torch.nn.Sequential()
- self.relu3_2 = torch.nn.Sequential()
- self.relu3_3 = torch.nn.Sequential()
- self.relu3_4 = torch.nn.Sequential()
-
- self.relu4_1 = torch.nn.Sequential()
- self.relu4_2 = torch.nn.Sequential()
- self.relu4_3 = torch.nn.Sequential()
- self.relu4_4 = torch.nn.Sequential()
-
- self.relu5_1 = torch.nn.Sequential()
- self.relu5_2 = torch.nn.Sequential()
- self.relu5_3 = torch.nn.Sequential()
- self.relu5_4 = torch.nn.Sequential()
-
- for x in range(2):
- self.relu1_1.add_module(str(x), features[x])
-
- for x in range(2, 4):
- self.relu1_2.add_module(str(x), features[x])
-
- for x in range(4, 7):
- self.relu2_1.add_module(str(x), features[x])
-
- for x in range(7, 9):
- self.relu2_2.add_module(str(x), features[x])
-
- for x in range(9, 12):
- self.relu3_1.add_module(str(x), features[x])
-
- for x in range(12, 14):
- self.relu3_2.add_module(str(x), features[x])
-
- for x in range(14, 16):
- self.relu3_2.add_module(str(x), features[x])
-
- for x in range(16, 18):
- self.relu3_4.add_module(str(x), features[x])
-
- for x in range(18, 21):
- self.relu4_1.add_module(str(x), features[x])
-
- for x in range(21, 23):
- self.relu4_2.add_module(str(x), features[x])
-
- for x in range(23, 25):
- self.relu4_3.add_module(str(x), features[x])
-
- for x in range(25, 27):
- self.relu4_4.add_module(str(x), features[x])
-
- for x in range(27, 30):
- self.relu5_1.add_module(str(x), features[x])
-
- for x in range(30, 32):
- self.relu5_2.add_module(str(x), features[x])
-
- for x in range(32, 34):
- self.relu5_3.add_module(str(x), features[x])
-
- for x in range(34, 36):
- self.relu5_4.add_module(str(x), features[x])
-
- # don't need the gradients, just want the features
- for param in self.parameters():
- param.requires_grad = False
-
- def forward(self, x):
- relu1_1 = self.relu1_1(x)
- relu1_2 = self.relu1_2(relu1_1)
-
- relu2_1 = self.relu2_1(relu1_2)
- relu2_2 = self.relu2_2(relu2_1)
-
- relu3_1 = self.relu3_1(relu2_2)
- relu3_2 = self.relu3_2(relu3_1)
- relu3_3 = self.relu3_3(relu3_2)
- relu3_4 = self.relu3_4(relu3_3)
-
- relu4_1 = self.relu4_1(relu3_4)
- relu4_2 = self.relu4_2(relu4_1)
- relu4_3 = self.relu4_3(relu4_2)
- relu4_4 = self.relu4_4(relu4_3)
-
- relu5_1 = self.relu5_1(relu4_4)
- relu5_2 = self.relu5_2(relu5_1)
- relu5_3 = self.relu5_3(relu5_2)
- relu5_4 = self.relu5_4(relu5_3)
-
- out = {
- 'relu1_1': relu1_1,
- 'relu1_2': relu1_2,
-
- 'relu2_1': relu2_1,
- 'relu2_2': relu2_2,
-
- 'relu3_1': relu3_1,
- 'relu3_2': relu3_2,
- 'relu3_3': relu3_3,
- 'relu3_4': relu3_4,
-
- 'relu4_1': relu4_1,
- 'relu4_2': relu4_2,
- 'relu4_3': relu4_3,
- 'relu4_4': relu4_4,
-
- 'relu5_1': relu5_1,
- 'relu5_2': relu5_2,
- 'relu5_3': relu5_3,
- 'relu5_4': relu5_4,
- }
- return out
diff --git a/spaces/coreml-community/ControlNet-v1-1-Annotators-cpu/annotator/mmpkg/mmcv/runner/optimizer/default_constructor.py b/spaces/coreml-community/ControlNet-v1-1-Annotators-cpu/annotator/mmpkg/mmcv/runner/optimizer/default_constructor.py
deleted file mode 100644
index de2ae39cb6378cc17c098f5324f5d5c321879b91..0000000000000000000000000000000000000000
--- a/spaces/coreml-community/ControlNet-v1-1-Annotators-cpu/annotator/mmpkg/mmcv/runner/optimizer/default_constructor.py
+++ /dev/null
@@ -1,249 +0,0 @@
-# Copyright (c) OpenMMLab. All rights reserved.
-import warnings
-
-import torch
-from torch.nn import GroupNorm, LayerNorm
-
-from annotator.mmpkg.mmcv.utils import _BatchNorm, _InstanceNorm, build_from_cfg, is_list_of
-from annotator.mmpkg.mmcv.utils.ext_loader import check_ops_exist
-from .builder import OPTIMIZER_BUILDERS, OPTIMIZERS
-
-
-@OPTIMIZER_BUILDERS.register_module()
-class DefaultOptimizerConstructor:
- """Default constructor for optimizers.
-
- By default each parameter share the same optimizer settings, and we
- provide an argument ``paramwise_cfg`` to specify parameter-wise settings.
- It is a dict and may contain the following fields:
-
- - ``custom_keys`` (dict): Specified parameters-wise settings by keys. If
- one of the keys in ``custom_keys`` is a substring of the name of one
- parameter, then the setting of the parameter will be specified by
- ``custom_keys[key]`` and other setting like ``bias_lr_mult`` etc. will
- be ignored. It should be noted that the aforementioned ``key`` is the
- longest key that is a substring of the name of the parameter. If there
- are multiple matched keys with the same length, then the key with lower
- alphabet order will be chosen.
- ``custom_keys[key]`` should be a dict and may contain fields ``lr_mult``
- and ``decay_mult``. See Example 2 below.
- - ``bias_lr_mult`` (float): It will be multiplied to the learning
- rate for all bias parameters (except for those in normalization
- layers and offset layers of DCN).
- - ``bias_decay_mult`` (float): It will be multiplied to the weight
- decay for all bias parameters (except for those in
- normalization layers, depthwise conv layers, offset layers of DCN).
- - ``norm_decay_mult`` (float): It will be multiplied to the weight
- decay for all weight and bias parameters of normalization
- layers.
- - ``dwconv_decay_mult`` (float): It will be multiplied to the weight
- decay for all weight and bias parameters of depthwise conv
- layers.
- - ``dcn_offset_lr_mult`` (float): It will be multiplied to the learning
- rate for parameters of offset layer in the deformable convs
- of a model.
- - ``bypass_duplicate`` (bool): If true, the duplicate parameters
- would not be added into optimizer. Default: False.
-
- Note:
- 1. If the option ``dcn_offset_lr_mult`` is used, the constructor will
- override the effect of ``bias_lr_mult`` in the bias of offset
- layer. So be careful when using both ``bias_lr_mult`` and
- ``dcn_offset_lr_mult``. If you wish to apply both of them to the
- offset layer in deformable convs, set ``dcn_offset_lr_mult``
- to the original ``dcn_offset_lr_mult`` * ``bias_lr_mult``.
- 2. If the option ``dcn_offset_lr_mult`` is used, the constructor will
- apply it to all the DCN layers in the model. So be careful when
- the model contains multiple DCN layers in places other than
- backbone.
-
- Args:
- model (:obj:`nn.Module`): The model with parameters to be optimized.
- optimizer_cfg (dict): The config dict of the optimizer.
- Positional fields are
-
- - `type`: class name of the optimizer.
-
- Optional fields are
-
- - any arguments of the corresponding optimizer type, e.g.,
- lr, weight_decay, momentum, etc.
- paramwise_cfg (dict, optional): Parameter-wise options.
-
- Example 1:
- >>> model = torch.nn.modules.Conv1d(1, 1, 1)
- >>> optimizer_cfg = dict(type='SGD', lr=0.01, momentum=0.9,
- >>> weight_decay=0.0001)
- >>> paramwise_cfg = dict(norm_decay_mult=0.)
- >>> optim_builder = DefaultOptimizerConstructor(
- >>> optimizer_cfg, paramwise_cfg)
- >>> optimizer = optim_builder(model)
-
- Example 2:
- >>> # assume model have attribute model.backbone and model.cls_head
- >>> optimizer_cfg = dict(type='SGD', lr=0.01, weight_decay=0.95)
- >>> paramwise_cfg = dict(custom_keys={
- '.backbone': dict(lr_mult=0.1, decay_mult=0.9)})
- >>> optim_builder = DefaultOptimizerConstructor(
- >>> optimizer_cfg, paramwise_cfg)
- >>> optimizer = optim_builder(model)
- >>> # Then the `lr` and `weight_decay` for model.backbone is
- >>> # (0.01 * 0.1, 0.95 * 0.9). `lr` and `weight_decay` for
- >>> # model.cls_head is (0.01, 0.95).
- """
-
- def __init__(self, optimizer_cfg, paramwise_cfg=None):
- if not isinstance(optimizer_cfg, dict):
- raise TypeError('optimizer_cfg should be a dict',
- f'but got {type(optimizer_cfg)}')
- self.optimizer_cfg = optimizer_cfg
- self.paramwise_cfg = {} if paramwise_cfg is None else paramwise_cfg
- self.base_lr = optimizer_cfg.get('lr', None)
- self.base_wd = optimizer_cfg.get('weight_decay', None)
- self._validate_cfg()
-
- def _validate_cfg(self):
- if not isinstance(self.paramwise_cfg, dict):
- raise TypeError('paramwise_cfg should be None or a dict, '
- f'but got {type(self.paramwise_cfg)}')
-
- if 'custom_keys' in self.paramwise_cfg:
- if not isinstance(self.paramwise_cfg['custom_keys'], dict):
- raise TypeError(
- 'If specified, custom_keys must be a dict, '
- f'but got {type(self.paramwise_cfg["custom_keys"])}')
- if self.base_wd is None:
- for key in self.paramwise_cfg['custom_keys']:
- if 'decay_mult' in self.paramwise_cfg['custom_keys'][key]:
- raise ValueError('base_wd should not be None')
-
- # get base lr and weight decay
- # weight_decay must be explicitly specified if mult is specified
- if ('bias_decay_mult' in self.paramwise_cfg
- or 'norm_decay_mult' in self.paramwise_cfg
- or 'dwconv_decay_mult' in self.paramwise_cfg):
- if self.base_wd is None:
- raise ValueError('base_wd should not be None')
-
- def _is_in(self, param_group, param_group_list):
- assert is_list_of(param_group_list, dict)
- param = set(param_group['params'])
- param_set = set()
- for group in param_group_list:
- param_set.update(set(group['params']))
-
- return not param.isdisjoint(param_set)
-
- def add_params(self, params, module, prefix='', is_dcn_module=None):
- """Add all parameters of module to the params list.
-
- The parameters of the given module will be added to the list of param
- groups, with specific rules defined by paramwise_cfg.
-
- Args:
- params (list[dict]): A list of param groups, it will be modified
- in place.
- module (nn.Module): The module to be added.
- prefix (str): The prefix of the module
- is_dcn_module (int|float|None): If the current module is a
- submodule of DCN, `is_dcn_module` will be passed to
- control conv_offset layer's learning rate. Defaults to None.
- """
- # get param-wise options
- custom_keys = self.paramwise_cfg.get('custom_keys', {})
- # first sort with alphabet order and then sort with reversed len of str
- sorted_keys = sorted(sorted(custom_keys.keys()), key=len, reverse=True)
-
- bias_lr_mult = self.paramwise_cfg.get('bias_lr_mult', 1.)
- bias_decay_mult = self.paramwise_cfg.get('bias_decay_mult', 1.)
- norm_decay_mult = self.paramwise_cfg.get('norm_decay_mult', 1.)
- dwconv_decay_mult = self.paramwise_cfg.get('dwconv_decay_mult', 1.)
- bypass_duplicate = self.paramwise_cfg.get('bypass_duplicate', False)
- dcn_offset_lr_mult = self.paramwise_cfg.get('dcn_offset_lr_mult', 1.)
-
- # special rules for norm layers and depth-wise conv layers
- is_norm = isinstance(module,
- (_BatchNorm, _InstanceNorm, GroupNorm, LayerNorm))
- is_dwconv = (
- isinstance(module, torch.nn.Conv2d)
- and module.in_channels == module.groups)
-
- for name, param in module.named_parameters(recurse=False):
- param_group = {'params': [param]}
- if not param.requires_grad:
- params.append(param_group)
- continue
- if bypass_duplicate and self._is_in(param_group, params):
- warnings.warn(f'{prefix} is duplicate. It is skipped since '
- f'bypass_duplicate={bypass_duplicate}')
- continue
- # if the parameter match one of the custom keys, ignore other rules
- is_custom = False
- for key in sorted_keys:
- if key in f'{prefix}.{name}':
- is_custom = True
- lr_mult = custom_keys[key].get('lr_mult', 1.)
- param_group['lr'] = self.base_lr * lr_mult
- if self.base_wd is not None:
- decay_mult = custom_keys[key].get('decay_mult', 1.)
- param_group['weight_decay'] = self.base_wd * decay_mult
- break
-
- if not is_custom:
- # bias_lr_mult affects all bias parameters
- # except for norm.bias dcn.conv_offset.bias
- if name == 'bias' and not (is_norm or is_dcn_module):
- param_group['lr'] = self.base_lr * bias_lr_mult
-
- if (prefix.find('conv_offset') != -1 and is_dcn_module
- and isinstance(module, torch.nn.Conv2d)):
- # deal with both dcn_offset's bias & weight
- param_group['lr'] = self.base_lr * dcn_offset_lr_mult
-
- # apply weight decay policies
- if self.base_wd is not None:
- # norm decay
- if is_norm:
- param_group[
- 'weight_decay'] = self.base_wd * norm_decay_mult
- # depth-wise conv
- elif is_dwconv:
- param_group[
- 'weight_decay'] = self.base_wd * dwconv_decay_mult
- # bias lr and decay
- elif name == 'bias' and not is_dcn_module:
- # TODO: current bias_decay_mult will have affect on DCN
- param_group[
- 'weight_decay'] = self.base_wd * bias_decay_mult
- params.append(param_group)
-
- if check_ops_exist():
- from annotator.mmpkg.mmcv.ops import DeformConv2d, ModulatedDeformConv2d
- is_dcn_module = isinstance(module,
- (DeformConv2d, ModulatedDeformConv2d))
- else:
- is_dcn_module = False
- for child_name, child_mod in module.named_children():
- child_prefix = f'{prefix}.{child_name}' if prefix else child_name
- self.add_params(
- params,
- child_mod,
- prefix=child_prefix,
- is_dcn_module=is_dcn_module)
-
- def __call__(self, model):
- if hasattr(model, 'module'):
- model = model.module
-
- optimizer_cfg = self.optimizer_cfg.copy()
- # if no paramwise option is specified, just use the global setting
- if not self.paramwise_cfg:
- optimizer_cfg['params'] = model.parameters()
- return build_from_cfg(optimizer_cfg, OPTIMIZERS)
-
- # set param-wise lr and weight decay recursively
- params = []
- self.add_params(params, model)
- optimizer_cfg['params'] = params
-
- return build_from_cfg(optimizer_cfg, OPTIMIZERS)
diff --git a/spaces/coreml-community/ControlNet-v1-1-Annotators-cpu/annotator/normalbae/models/submodules/efficientnet_repo/onnx_to_caffe.py b/spaces/coreml-community/ControlNet-v1-1-Annotators-cpu/annotator/normalbae/models/submodules/efficientnet_repo/onnx_to_caffe.py
deleted file mode 100644
index 44399aafababcdf6b84147a0613eb0909730db4b..0000000000000000000000000000000000000000
--- a/spaces/coreml-community/ControlNet-v1-1-Annotators-cpu/annotator/normalbae/models/submodules/efficientnet_repo/onnx_to_caffe.py
+++ /dev/null
@@ -1,27 +0,0 @@
-import argparse
-
-import onnx
-from caffe2.python.onnx.backend import Caffe2Backend
-
-
-parser = argparse.ArgumentParser(description="Convert ONNX to Caffe2")
-
-parser.add_argument("model", help="The ONNX model")
-parser.add_argument("--c2-prefix", required=True,
- help="The output file prefix for the caffe2 model init and predict file. ")
-
-
-def main():
- args = parser.parse_args()
- onnx_model = onnx.load(args.model)
- caffe2_init, caffe2_predict = Caffe2Backend.onnx_graph_to_caffe2_net(onnx_model)
- caffe2_init_str = caffe2_init.SerializeToString()
- with open(args.c2_prefix + '.init.pb', "wb") as f:
- f.write(caffe2_init_str)
- caffe2_predict_str = caffe2_predict.SerializeToString()
- with open(args.c2_prefix + '.predict.pb', "wb") as f:
- f.write(caffe2_predict_str)
-
-
-if __name__ == "__main__":
- main()
diff --git a/spaces/coreml-community/ControlNet-v1-1-Annotators-cpu/annotator/oneformer/oneformer/data/datasets/register_coco_panoptic_annos_semseg.py b/spaces/coreml-community/ControlNet-v1-1-Annotators-cpu/annotator/oneformer/oneformer/data/datasets/register_coco_panoptic_annos_semseg.py
deleted file mode 100644
index 5690a2c217dc698464e8057f057c1ad2dcdf605b..0000000000000000000000000000000000000000
--- a/spaces/coreml-community/ControlNet-v1-1-Annotators-cpu/annotator/oneformer/oneformer/data/datasets/register_coco_panoptic_annos_semseg.py
+++ /dev/null
@@ -1,367 +0,0 @@
-# ------------------------------------------------------------------------------
-# Reference: https://github.com/facebookresearch/Mask2Former/blob/main/mask2former/data/datasets/register_coco_panoptic_annos_semseg.py
-# Modified by Jitesh Jain (https://github.com/praeclarumjj3)
-# ------------------------------------------------------------------------------
-
-import json
-import os
-
-from annotator.oneformer.detectron2.data import DatasetCatalog, MetadataCatalog
-from annotator.oneformer.detectron2.data.datasets import load_sem_seg
-from annotator.oneformer.detectron2.data.datasets.builtin_meta import COCO_CATEGORIES
-from annotator.oneformer.detectron2.utils.file_io import PathManager
-import contextlib
-import logging
-import io
-from fvcore.common.timer import Timer
-import pycocotools.mask as mask_util
-from annotator.oneformer.detectron2.structures import BoxMode
-
-
-logger = logging.getLogger(__name__)
-
-
-_PREDEFINED_SPLITS_COCO_PANOPTIC = {
- "coco_2017_train_panoptic": (
- # This is the original panoptic annotation directory
- "coco/panoptic_train2017",
- "coco/annotations/panoptic_train2017.json",
- # This directory contains semantic annotations that are
- # converted from panoptic annotations.
- # It is used by PanopticFPN.
- # You can use the script at detectron2/datasets/prepare_panoptic_fpn.py
- # to create these directories.
- "coco/panoptic_semseg_train2017",
- ),
- "coco_2017_val_panoptic": (
- "coco/panoptic_val2017",
- "coco/annotations/panoptic_val2017.json",
- "coco/panoptic_semseg_val2017",
- ),
-}
-
-def load_coco_instance_json(json_file, image_root, dataset_name=None):
- from pycocotools.coco import COCO
-
- timer = Timer()
- json_file = PathManager.get_local_path(json_file)
- with contextlib.redirect_stdout(io.StringIO()):
- coco_api = COCO(json_file)
- if timer.seconds() > 1:
- logger.info("Loading {} takes {:.2f} seconds.".format(json_file, timer.seconds()))
-
- id_map = None
- if dataset_name is not None:
- meta = MetadataCatalog.get(dataset_name)
- cat_ids = sorted(coco_api.getCatIds())
- cats = coco_api.loadCats(cat_ids)
- # The categories in a custom json file may not be sorted.
- thing_classes = [c["name"] for c in sorted(cats, key=lambda x: x["id"])]
- meta.thing_classes = thing_classes
-
- # In COCO, certain category ids are artificially removed,
- # and by convention they are always ignored.
- # We deal with COCO's id issue and translate
- # the category ids to contiguous ids in [0, 80).
-
- # It works by looking at the "categories" field in the json, therefore
- # if users' own json also have incontiguous ids, we'll
- # apply this mapping as well but print a warning.
- if not (min(cat_ids) == 1 and max(cat_ids) == len(cat_ids)):
- if "coco" not in dataset_name:
- logger.warning(
- """
-Category ids in annotations are not in [1, #categories]! We'll apply a mapping for you.
-"""
- )
- id_map = {v: i for i, v in enumerate(cat_ids)}
- meta.thing_dataset_id_to_contiguous_id = id_map
-
- # sort indices for reproducible results
- img_ids = sorted(coco_api.imgs.keys())
- # imgs is a list of dicts, each looks something like:
- # {'license': 4,
- # 'url': 'http://farm6.staticflickr.com/5454/9413846304_881d5e5c3b_z.jpg',
- # 'file_name': 'COCO_val2014_000000001268.jpg',
- # 'height': 427,
- # 'width': 640,
- # 'date_captured': '2013-11-17 05:57:24',
- # 'id': 1268}
- imgs = coco_api.loadImgs(img_ids)
- # anns is a list[list[dict]], where each dict is an annotation
- # record for an object. The inner list enumerates the objects in an image
- # and the outer list enumerates over images. Example of anns[0]:
- # [{'segmentation': [[192.81,
- # 247.09,
- # ...
- # 219.03,
- # 249.06]],
- # 'area': 1035.749,
- # 'iscrowd': 0,
- # 'image_id': 1268,
- # 'bbox': [192.81, 224.8, 74.73, 33.43],
- # 'category_id': 16,
- # 'id': 42986},
- # ...]
- anns = [coco_api.imgToAnns[img_id] for img_id in img_ids]
- total_num_valid_anns = sum([len(x) for x in anns])
- total_num_anns = len(coco_api.anns)
- if total_num_valid_anns < total_num_anns:
- logger.warning(
- f"{json_file} contains {total_num_anns} annotations, but only "
- f"{total_num_valid_anns} of them match to images in the file."
- )
-
- if "minival" not in json_file:
- # The popular valminusminival & minival annotations for COCO2014 contain this bug.
- # However the ratio of buggy annotations there is tiny and does not affect accuracy.
- # Therefore we explicitly white-list them.
- ann_ids = [ann["id"] for anns_per_image in anns for ann in anns_per_image]
- assert len(set(ann_ids)) == len(ann_ids), "Annotation ids in '{}' are not unique!".format(
- json_file
- )
-
- imgs_anns = list(zip(imgs, anns))
- logger.info("Loaded {} images in COCO format from {}".format(len(imgs_anns), json_file))
-
- dataset_dicts = {}
-
- ann_keys = ["iscrowd", "bbox", "keypoints", "category_id"]
-
- num_instances_without_valid_segmentation = 0
-
- for (img_dict, anno_dict_list) in imgs_anns:
- record = {}
- record["file_name"] = os.path.join(image_root, img_dict["file_name"])
- record["height"] = img_dict["height"]
- record["width"] = img_dict["width"]
- image_id = record["image_id"] = img_dict["id"]
-
- objs = []
- for anno in anno_dict_list:
- # Check that the image_id in this annotation is the same as
- # the image_id we're looking at.
- # This fails only when the data parsing logic or the annotation file is buggy.
-
- # The original COCO valminusminival2014 & minival2014 annotation files
- # actually contains bugs that, together with certain ways of using COCO API,
- # can trigger this assertion.
- assert anno["image_id"] == image_id
-
- assert anno.get("ignore", 0) == 0, '"ignore" in COCO json file is not supported.'
-
- obj = {key: anno[key] for key in ann_keys if key in anno}
- if "bbox" in obj and len(obj["bbox"]) == 0:
- raise ValueError(
- f"One annotation of image {image_id} contains empty 'bbox' value! "
- "This json does not have valid COCO format."
- )
-
- segm = anno.get("segmentation", None)
- if segm: # either list[list[float]] or dict(RLE)
- if isinstance(segm, dict):
- if isinstance(segm["counts"], list):
- # convert to compressed RLE
- segm = mask_util.frPyObjects(segm, *segm["size"])
- else:
- # filter out invalid polygons (< 3 points)
- segm = [poly for poly in segm if len(poly) % 2 == 0 and len(poly) >= 6]
- if len(segm) == 0:
- num_instances_without_valid_segmentation += 1
- continue # ignore this instance
- obj["segmentation"] = segm
-
- keypts = anno.get("keypoints", None)
- if keypts: # list[int]
- for idx, v in enumerate(keypts):
- if idx % 3 != 2:
- # COCO's segmentation coordinates are floating points in [0, H or W],
- # but keypoint coordinates are integers in [0, H-1 or W-1]
- # Therefore we assume the coordinates are "pixel indices" and
- # add 0.5 to convert to floating point coordinates.
- keypts[idx] = v + 0.5
- obj["keypoints"] = keypts
-
- obj["bbox_mode"] = BoxMode.XYWH_ABS
- if id_map:
- annotation_category_id = obj["category_id"]
- try:
- obj["category_id"] = id_map[annotation_category_id]
- except KeyError as e:
- raise KeyError(
- f"Encountered category_id={annotation_category_id} "
- "but this id does not exist in 'categories' of the json file."
- ) from e
- objs.append(obj)
- record["annotations"] = objs
- dataset_dicts[image_id] = record
-
- if num_instances_without_valid_segmentation > 0:
- logger.warning(
- "Filtered out {} instances without valid segmentation. ".format(
- num_instances_without_valid_segmentation
- )
- + "There might be issues in your dataset generation process. Please "
- "check https://detectron2.readthedocs.io/en/latest/tutorials/datasets.html carefully"
- )
- return dataset_dicts
-
-def get_metadata():
- meta = {}
- # The following metadata maps contiguous id from [0, #thing categories +
- # #stuff categories) to their names and colors. We have to replica of the
- # same name and color under "thing_*" and "stuff_*" because the current
- # visualization function in D2 handles thing and class classes differently
- # due to some heuristic used in Panoptic FPN. We keep the same naming to
- # enable reusing existing visualization functions.
- thing_classes = [k["name"] for k in COCO_CATEGORIES if k["isthing"] == 1]
- thing_colors = [k["color"] for k in COCO_CATEGORIES if k["isthing"] == 1]
- stuff_classes = [k["name"] for k in COCO_CATEGORIES]
- stuff_colors = [k["color"] for k in COCO_CATEGORIES]
-
- meta["thing_classes"] = thing_classes
- meta["thing_colors"] = thing_colors
- meta["stuff_classes"] = stuff_classes
- meta["stuff_colors"] = stuff_colors
-
- # Convert category id for training:
- # category id: like semantic segmentation, it is the class id for each
- # pixel. Since there are some classes not used in evaluation, the category
- # id is not always contiguous and thus we have two set of category ids:
- # - original category id: category id in the original dataset, mainly
- # used for evaluation.
- # - contiguous category id: [0, #classes), in order to train the linear
- # softmax classifier.
- thing_dataset_id_to_contiguous_id = {}
- stuff_dataset_id_to_contiguous_id = {}
-
- for i, cat in enumerate(COCO_CATEGORIES):
- if cat["isthing"]:
- thing_dataset_id_to_contiguous_id[cat["id"]] = i
- # else:
- # stuff_dataset_id_to_contiguous_id[cat["id"]] = i
-
- # in order to use sem_seg evaluator
- stuff_dataset_id_to_contiguous_id[cat["id"]] = i
-
- meta["thing_dataset_id_to_contiguous_id"] = thing_dataset_id_to_contiguous_id
- meta["stuff_dataset_id_to_contiguous_id"] = stuff_dataset_id_to_contiguous_id
-
- return meta
-
-
-def load_coco_panoptic_json(json_file, instances_json, instances_name, image_dir, gt_dir, semseg_dir, meta):
- """
- Args:
- image_dir (str): path to the raw dataset. e.g., "~/coco/train2017".
- gt_dir (str): path to the raw annotations. e.g., "~/coco/panoptic_train2017".
- json_file (str): path to the json file. e.g., "~/coco/annotations/panoptic_train2017.json".
- Returns:
- list[dict]: a list of dicts in Detectron2 standard format. (See
- `Using Custom Datasets `_ )
- """
-
- def _convert_category_id(segment_info, meta):
- if segment_info["category_id"] in meta["thing_dataset_id_to_contiguous_id"]:
- segment_info["category_id"] = meta["thing_dataset_id_to_contiguous_id"][
- segment_info["category_id"]
- ]
- segment_info["isthing"] = True
- else:
- segment_info["category_id"] = meta["stuff_dataset_id_to_contiguous_id"][
- segment_info["category_id"]
- ]
- segment_info["isthing"] = False
- return segment_info
-
- with PathManager.open(json_file) as f:
- json_info = json.load(f)
-
- instance_data_dicts = load_coco_instance_json(instances_json, image_dir.replace("panoptic_", ""), instances_name)
-
- ret = []
- for ann in json_info["annotations"]:
- image_id = int(ann["image_id"])
- # TODO: currently we assume image and label has the same filename but
- # different extension, and images have extension ".jpg" for COCO. Need
- # to make image extension a user-provided argument if we extend this
- # function to support other COCO-like datasets.
- image_file = os.path.join(image_dir, os.path.splitext(ann["file_name"])[0] + ".jpg")
- label_file = os.path.join(gt_dir, ann["file_name"])
- sem_label_file = os.path.join(semseg_dir, ann["file_name"])
- segments_info = [_convert_category_id(x, meta) for x in ann["segments_info"]]
- ret.append(
- {
- "file_name": image_file,
- "image_id": image_id,
- "pan_seg_file_name": label_file,
- "sem_seg_file_name": sem_label_file,
- "segments_info": segments_info,
- "annotations": instance_data_dicts[image_id]["annotations"],
- }
- )
- assert len(ret), f"No images found in {image_dir}!"
- assert PathManager.isfile(ret[0]["file_name"]), ret[0]["file_name"]
- assert PathManager.isfile(ret[0]["pan_seg_file_name"]), ret[0]["pan_seg_file_name"]
- assert PathManager.isfile(ret[0]["sem_seg_file_name"]), ret[0]["sem_seg_file_name"]
- return ret
-
-
-def register_coco_panoptic_annos_sem_seg(
- name, metadata, image_root, panoptic_root, panoptic_json, sem_seg_root, instances_json, instances_name,
-):
- panoptic_name = name
- delattr(MetadataCatalog.get(panoptic_name), "thing_classes")
- delattr(MetadataCatalog.get(panoptic_name), "thing_colors")
- MetadataCatalog.get(panoptic_name).set(
- thing_classes=metadata["thing_classes"],
- thing_colors=metadata["thing_colors"],
- # thing_dataset_id_to_contiguous_id=metadata["thing_dataset_id_to_contiguous_id"],
- )
-
- # the name is "coco_2017_train_panoptic_with_sem_seg" and "coco_2017_val_panoptic_with_sem_seg"
- semantic_name = name + "_with_sem_seg"
- DatasetCatalog.register(
- semantic_name,
- lambda: load_coco_panoptic_json(panoptic_json, instances_json, instances_name, image_root, panoptic_root, sem_seg_root, metadata),
- )
- MetadataCatalog.get(semantic_name).set(
- sem_seg_root=sem_seg_root,
- panoptic_root=panoptic_root,
- image_root=image_root,
- panoptic_json=panoptic_json,
- json_file=instances_json,
- evaluator_type="coco_panoptic_seg",
- ignore_label=255,
- label_divisor=1000,
- **metadata,
- )
-
-
-def register_all_coco_panoptic_annos_sem_seg(root):
- for (
- prefix,
- (panoptic_root, panoptic_json, semantic_root),
- ) in _PREDEFINED_SPLITS_COCO_PANOPTIC.items():
-
- prefix_instances = prefix[: -len("_panoptic")]
- instances_meta = MetadataCatalog.get(prefix_instances)
- image_root, instances_json = instances_meta.image_root, instances_meta.json_file
-
- if 'val' in instances_json:
- instances_json = instances_json.replace('instances_', 'panoptic2instances_')
-
- register_coco_panoptic_annos_sem_seg(
- prefix,
- get_metadata(),
- image_root,
- os.path.join(root, panoptic_root),
- os.path.join(root, panoptic_json),
- os.path.join(root, semantic_root),
- instances_json,
- prefix_instances,
- )
-
-
-_root = os.getenv("DETECTRON2_DATASETS", "datasets")
-register_all_coco_panoptic_annos_sem_seg(_root)
diff --git a/spaces/coreml-community/ControlNet-v1-1-Annotators-cpu/annotator/uniformer/mmcv/ops/knn.py b/spaces/coreml-community/ControlNet-v1-1-Annotators-cpu/annotator/uniformer/mmcv/ops/knn.py
deleted file mode 100644
index f335785036669fc19239825b0aae6dde3f73bf92..0000000000000000000000000000000000000000
--- a/spaces/coreml-community/ControlNet-v1-1-Annotators-cpu/annotator/uniformer/mmcv/ops/knn.py
+++ /dev/null
@@ -1,77 +0,0 @@
-import torch
-from torch.autograd import Function
-
-from ..utils import ext_loader
-
-ext_module = ext_loader.load_ext('_ext', ['knn_forward'])
-
-
-class KNN(Function):
- r"""KNN (CUDA) based on heap data structure.
- Modified from `PAConv `_.
-
- Find k-nearest points.
- """
-
- @staticmethod
- def forward(ctx,
- k: int,
- xyz: torch.Tensor,
- center_xyz: torch.Tensor = None,
- transposed: bool = False) -> torch.Tensor:
- """
- Args:
- k (int): number of nearest neighbors.
- xyz (Tensor): (B, N, 3) if transposed == False, else (B, 3, N).
- xyz coordinates of the features.
- center_xyz (Tensor, optional): (B, npoint, 3) if transposed ==
- False, else (B, 3, npoint). centers of the knn query.
- Default: None.
- transposed (bool, optional): whether the input tensors are
- transposed. Should not explicitly use this keyword when
- calling knn (=KNN.apply), just add the fourth param.
- Default: False.
-
- Returns:
- Tensor: (B, k, npoint) tensor with the indices of
- the features that form k-nearest neighbours.
- """
- assert (k > 0) & (k < 100), 'k should be in range(0, 100)'
-
- if center_xyz is None:
- center_xyz = xyz
-
- if transposed:
- xyz = xyz.transpose(2, 1).contiguous()
- center_xyz = center_xyz.transpose(2, 1).contiguous()
-
- assert xyz.is_contiguous() # [B, N, 3]
- assert center_xyz.is_contiguous() # [B, npoint, 3]
-
- center_xyz_device = center_xyz.get_device()
- assert center_xyz_device == xyz.get_device(), \
- 'center_xyz and xyz should be put on the same device'
- if torch.cuda.current_device() != center_xyz_device:
- torch.cuda.set_device(center_xyz_device)
-
- B, npoint, _ = center_xyz.shape
- N = xyz.shape[1]
-
- idx = center_xyz.new_zeros((B, npoint, k)).int()
- dist2 = center_xyz.new_zeros((B, npoint, k)).float()
-
- ext_module.knn_forward(
- xyz, center_xyz, idx, dist2, b=B, n=N, m=npoint, nsample=k)
- # idx shape to [B, k, npoint]
- idx = idx.transpose(2, 1).contiguous()
- if torch.__version__ != 'parrots':
- ctx.mark_non_differentiable(idx)
- return idx
-
- @staticmethod
- def backward(ctx, a=None):
- return None, None, None
-
-
-knn = KNN.apply
diff --git a/spaces/coreml-community/ControlNet-v1-1-Annotators-cpu/annotator/uniformer/mmseg/models/decode_heads/ann_head.py b/spaces/coreml-community/ControlNet-v1-1-Annotators-cpu/annotator/uniformer/mmseg/models/decode_heads/ann_head.py
deleted file mode 100644
index 30aaacc2cafc568d3de71d1477b4de0dc0fea9d3..0000000000000000000000000000000000000000
--- a/spaces/coreml-community/ControlNet-v1-1-Annotators-cpu/annotator/uniformer/mmseg/models/decode_heads/ann_head.py
+++ /dev/null
@@ -1,245 +0,0 @@
-import torch
-import torch.nn as nn
-from annotator.uniformer.mmcv.cnn import ConvModule
-
-from ..builder import HEADS
-from ..utils import SelfAttentionBlock as _SelfAttentionBlock
-from .decode_head import BaseDecodeHead
-
-
-class PPMConcat(nn.ModuleList):
- """Pyramid Pooling Module that only concat the features of each layer.
-
- Args:
- pool_scales (tuple[int]): Pooling scales used in Pooling Pyramid
- Module.
- """
-
- def __init__(self, pool_scales=(1, 3, 6, 8)):
- super(PPMConcat, self).__init__(
- [nn.AdaptiveAvgPool2d(pool_scale) for pool_scale in pool_scales])
-
- def forward(self, feats):
- """Forward function."""
- ppm_outs = []
- for ppm in self:
- ppm_out = ppm(feats)
- ppm_outs.append(ppm_out.view(*feats.shape[:2], -1))
- concat_outs = torch.cat(ppm_outs, dim=2)
- return concat_outs
-
-
-class SelfAttentionBlock(_SelfAttentionBlock):
- """Make a ANN used SelfAttentionBlock.
-
- Args:
- low_in_channels (int): Input channels of lower level feature,
- which is the key feature for self-attention.
- high_in_channels (int): Input channels of higher level feature,
- which is the query feature for self-attention.
- channels (int): Output channels of key/query transform.
- out_channels (int): Output channels.
- share_key_query (bool): Whether share projection weight between key
- and query projection.
- query_scale (int): The scale of query feature map.
- key_pool_scales (tuple[int]): Pooling scales used in Pooling Pyramid
- Module of key feature.
- conv_cfg (dict|None): Config of conv layers.
- norm_cfg (dict|None): Config of norm layers.
- act_cfg (dict|None): Config of activation layers.
- """
-
- def __init__(self, low_in_channels, high_in_channels, channels,
- out_channels, share_key_query, query_scale, key_pool_scales,
- conv_cfg, norm_cfg, act_cfg):
- key_psp = PPMConcat(key_pool_scales)
- if query_scale > 1:
- query_downsample = nn.MaxPool2d(kernel_size=query_scale)
- else:
- query_downsample = None
- super(SelfAttentionBlock, self).__init__(
- key_in_channels=low_in_channels,
- query_in_channels=high_in_channels,
- channels=channels,
- out_channels=out_channels,
- share_key_query=share_key_query,
- query_downsample=query_downsample,
- key_downsample=key_psp,
- key_query_num_convs=1,
- key_query_norm=True,
- value_out_num_convs=1,
- value_out_norm=False,
- matmul_norm=True,
- with_out=True,
- conv_cfg=conv_cfg,
- norm_cfg=norm_cfg,
- act_cfg=act_cfg)
-
-
-class AFNB(nn.Module):
- """Asymmetric Fusion Non-local Block(AFNB)
-
- Args:
- low_in_channels (int): Input channels of lower level feature,
- which is the key feature for self-attention.
- high_in_channels (int): Input channels of higher level feature,
- which is the query feature for self-attention.
- channels (int): Output channels of key/query transform.
- out_channels (int): Output channels.
- and query projection.
- query_scales (tuple[int]): The scales of query feature map.
- Default: (1,)
- key_pool_scales (tuple[int]): Pooling scales used in Pooling Pyramid
- Module of key feature.
- conv_cfg (dict|None): Config of conv layers.
- norm_cfg (dict|None): Config of norm layers.
- act_cfg (dict|None): Config of activation layers.
- """
-
- def __init__(self, low_in_channels, high_in_channels, channels,
- out_channels, query_scales, key_pool_scales, conv_cfg,
- norm_cfg, act_cfg):
- super(AFNB, self).__init__()
- self.stages = nn.ModuleList()
- for query_scale in query_scales:
- self.stages.append(
- SelfAttentionBlock(
- low_in_channels=low_in_channels,
- high_in_channels=high_in_channels,
- channels=channels,
- out_channels=out_channels,
- share_key_query=False,
- query_scale=query_scale,
- key_pool_scales=key_pool_scales,
- conv_cfg=conv_cfg,
- norm_cfg=norm_cfg,
- act_cfg=act_cfg))
- self.bottleneck = ConvModule(
- out_channels + high_in_channels,
- out_channels,
- 1,
- conv_cfg=conv_cfg,
- norm_cfg=norm_cfg,
- act_cfg=None)
-
- def forward(self, low_feats, high_feats):
- """Forward function."""
- priors = [stage(high_feats, low_feats) for stage in self.stages]
- context = torch.stack(priors, dim=0).sum(dim=0)
- output = self.bottleneck(torch.cat([context, high_feats], 1))
- return output
-
-
-class APNB(nn.Module):
- """Asymmetric Pyramid Non-local Block (APNB)
-
- Args:
- in_channels (int): Input channels of key/query feature,
- which is the key feature for self-attention.
- channels (int): Output channels of key/query transform.
- out_channels (int): Output channels.
- query_scales (tuple[int]): The scales of query feature map.
- Default: (1,)
- key_pool_scales (tuple[int]): Pooling scales used in Pooling Pyramid
- Module of key feature.
- conv_cfg (dict|None): Config of conv layers.
- norm_cfg (dict|None): Config of norm layers.
- act_cfg (dict|None): Config of activation layers.
- """
-
- def __init__(self, in_channels, channels, out_channels, query_scales,
- key_pool_scales, conv_cfg, norm_cfg, act_cfg):
- super(APNB, self).__init__()
- self.stages = nn.ModuleList()
- for query_scale in query_scales:
- self.stages.append(
- SelfAttentionBlock(
- low_in_channels=in_channels,
- high_in_channels=in_channels,
- channels=channels,
- out_channels=out_channels,
- share_key_query=True,
- query_scale=query_scale,
- key_pool_scales=key_pool_scales,
- conv_cfg=conv_cfg,
- norm_cfg=norm_cfg,
- act_cfg=act_cfg))
- self.bottleneck = ConvModule(
- 2 * in_channels,
- out_channels,
- 1,
- conv_cfg=conv_cfg,
- norm_cfg=norm_cfg,
- act_cfg=act_cfg)
-
- def forward(self, feats):
- """Forward function."""
- priors = [stage(feats, feats) for stage in self.stages]
- context = torch.stack(priors, dim=0).sum(dim=0)
- output = self.bottleneck(torch.cat([context, feats], 1))
- return output
-
-
-@HEADS.register_module()
-class ANNHead(BaseDecodeHead):
- """Asymmetric Non-local Neural Networks for Semantic Segmentation.
-
- This head is the implementation of `ANNNet
- `_.
-
- Args:
- project_channels (int): Projection channels for Nonlocal.
- query_scales (tuple[int]): The scales of query feature map.
- Default: (1,)
- key_pool_scales (tuple[int]): The pooling scales of key feature map.
- Default: (1, 3, 6, 8).
- """
-
- def __init__(self,
- project_channels,
- query_scales=(1, ),
- key_pool_scales=(1, 3, 6, 8),
- **kwargs):
- super(ANNHead, self).__init__(
- input_transform='multiple_select', **kwargs)
- assert len(self.in_channels) == 2
- low_in_channels, high_in_channels = self.in_channels
- self.project_channels = project_channels
- self.fusion = AFNB(
- low_in_channels=low_in_channels,
- high_in_channels=high_in_channels,
- out_channels=high_in_channels,
- channels=project_channels,
- query_scales=query_scales,
- key_pool_scales=key_pool_scales,
- conv_cfg=self.conv_cfg,
- norm_cfg=self.norm_cfg,
- act_cfg=self.act_cfg)
- self.bottleneck = ConvModule(
- high_in_channels,
- self.channels,
- 3,
- padding=1,
- conv_cfg=self.conv_cfg,
- norm_cfg=self.norm_cfg,
- act_cfg=self.act_cfg)
- self.context = APNB(
- in_channels=self.channels,
- out_channels=self.channels,
- channels=project_channels,
- query_scales=query_scales,
- key_pool_scales=key_pool_scales,
- conv_cfg=self.conv_cfg,
- norm_cfg=self.norm_cfg,
- act_cfg=self.act_cfg)
-
- def forward(self, inputs):
- """Forward function."""
- low_feats, high_feats = self._transform_inputs(inputs)
- output = self.fusion(low_feats, high_feats)
- output = self.dropout(output)
- output = self.bottleneck(output)
- output = self.context(output)
- output = self.cls_seg(output)
-
- return output
diff --git a/spaces/coreml-community/ControlNet-v1-1-Annotators-cpu/annotator/uniformer/mmseg/ops/wrappers.py b/spaces/coreml-community/ControlNet-v1-1-Annotators-cpu/annotator/uniformer/mmseg/ops/wrappers.py
deleted file mode 100644
index 0ed9a0cb8d7c0e0ec2748dd89c652756653cac78..0000000000000000000000000000000000000000
--- a/spaces/coreml-community/ControlNet-v1-1-Annotators-cpu/annotator/uniformer/mmseg/ops/wrappers.py
+++ /dev/null
@@ -1,50 +0,0 @@
-import warnings
-
-import torch.nn as nn
-import torch.nn.functional as F
-
-
-def resize(input,
- size=None,
- scale_factor=None,
- mode='nearest',
- align_corners=None,
- warning=True):
- if warning:
- if size is not None and align_corners:
- input_h, input_w = tuple(int(x) for x in input.shape[2:])
- output_h, output_w = tuple(int(x) for x in size)
- if output_h > input_h or output_w > output_h:
- if ((output_h > 1 and output_w > 1 and input_h > 1
- and input_w > 1) and (output_h - 1) % (input_h - 1)
- and (output_w - 1) % (input_w - 1)):
- warnings.warn(
- f'When align_corners={align_corners}, '
- 'the output would more aligned if '
- f'input size {(input_h, input_w)} is `x+1` and '
- f'out size {(output_h, output_w)} is `nx+1`')
- return F.interpolate(input, size, scale_factor, mode, align_corners)
-
-
-class Upsample(nn.Module):
-
- def __init__(self,
- size=None,
- scale_factor=None,
- mode='nearest',
- align_corners=None):
- super(Upsample, self).__init__()
- self.size = size
- if isinstance(scale_factor, tuple):
- self.scale_factor = tuple(float(factor) for factor in scale_factor)
- else:
- self.scale_factor = float(scale_factor) if scale_factor else None
- self.mode = mode
- self.align_corners = align_corners
-
- def forward(self, x):
- if not self.size:
- size = [int(t * self.scale_factor) for t in x.shape[-2:]]
- else:
- size = self.size
- return resize(x, size, None, self.mode, self.align_corners)
diff --git a/spaces/crystalai/stabilityai-stable-diffusion-xl-refiner-1.0/app.py b/spaces/crystalai/stabilityai-stable-diffusion-xl-refiner-1.0/app.py
deleted file mode 100644
index f0854c17140255ac783638680bc5dce595cc9fd0..0000000000000000000000000000000000000000
--- a/spaces/crystalai/stabilityai-stable-diffusion-xl-refiner-1.0/app.py
+++ /dev/null
@@ -1,3 +0,0 @@
-import gradio as gr
-
-gr.Interface.load("models/stabilityai/stable-diffusion-xl-refiner-1.0").launch()
\ No newline at end of file
diff --git a/spaces/cscan/CodeFormer/CodeFormer/basicsr/version.py b/spaces/cscan/CodeFormer/CodeFormer/basicsr/version.py
deleted file mode 100644
index 3c30a9a5d2c3af85b06034f080d6f9f7e0a53e7e..0000000000000000000000000000000000000000
--- a/spaces/cscan/CodeFormer/CodeFormer/basicsr/version.py
+++ /dev/null
@@ -1,5 +0,0 @@
-# GENERATED VERSION FILE
-# TIME: Sun Aug 7 15:14:26 2022
-__version__ = '1.3.2'
-__gitsha__ = '6f94023'
-version_info = (1, 3, 2)
diff --git a/spaces/danielcwq/chat-your-data-trial/app.py b/spaces/danielcwq/chat-your-data-trial/app.py
deleted file mode 100644
index 43b1dcb2486c97d23574c3934d1593dec16657a8..0000000000000000000000000000000000000000
--- a/spaces/danielcwq/chat-your-data-trial/app.py
+++ /dev/null
@@ -1,102 +0,0 @@
-import os
-from typing import Optional, Tuple
-
-import gradio as gr
-import pickle
-from query_data import get_chain
-from threading import Lock
-
-with open("vectorstore.pkl", "rb") as f:
- vectorstore = pickle.load(f)
-
-
-def set_openai_api_key(api_key: str):
- """Set the api key and return chain.
- If no api_key, then None is returned.
- """
- if api_key:
- os.environ["OPENAI_API_KEY"] = api_key
- chain = get_chain(vectorstore)
- os.environ["OPENAI_API_KEY"] = ""
- return chain
-
-class ChatWrapper:
-
- def __init__(self):
- self.lock = Lock()
- def __call__(
- self, api_key: str, inp: str, history: Optional[Tuple[str, str]], chain
- ):
- """Execute the chat functionality."""
- self.lock.acquire()
- try:
- history = history or []
- # If chain is None, that is because no API key was provided.
- if chain is None:
- history.append((inp, "Please paste your OpenAI key to use"))
- return history, history
- # Set OpenAI key
- import openai
- openai.api_key = api_key
- # Run chain and append input.
- output = chain({"question": inp, "chat_history": history})["answer"]
- history.append((inp, output))
- except Exception as e:
- raise e
- finally:
- self.lock.release()
- return history, history
-
-chat = ChatWrapper()
-
-block = gr.Blocks(css=".gradio-container {background-color: lightgray}")
-
-with block:
- with gr.Row():
- gr.Markdown("
Chat-Your-Data (H2 Economics)
")
-
- openai_api_key_textbox = gr.Textbox(
- placeholder="Paste your OpenAI API key (sk-...)",
- show_label=False,
- lines=1,
- type="password",
- )
-
- chatbot = gr.Chatbot()
-
- with gr.Row():
- message = gr.Textbox(
- label="What's your question?",
- placeholder="Ask questions about anything covered in the H2 Economics syllabus",
- lines=1,
- )
- submit = gr.Button(value="Send", variant="secondary").style(full_width=False)
-
- gr.Examples(
- examples=[
- "Explain real wealth effect.",
- "Use the real wealth effect to explain the negative gradient of the AD curve.",
- "Explain the multiplier process.",
- ],
- inputs=message,
- )
-
- gr.HTML("Demo application of a LangChain chain, built on H2 Economics Data. Many thanks to Jean Chua for giving her notes for this project.")
-
- gr.HTML(
- "
-
-Plot: John Captain, who works for the mafia, is a murderer. After killing an entire family, he was amazed at the consequences of his actions and decided. The film is based on the bestselling book by David Grann. I haven't read the book, so I can't say how good or bad it is. But judging by the trailer, this is a very well-posed picture, even with good special effects.
-Fascinating and spectacular scenes that you want to review. Ambiguous characters that evoke different emotions in the viewer.
-At the beginning of the film, there is a 8a78ff9644
-
-
-
diff --git a/spaces/diacanFperku/AutoGPT/MinecraftBetaLicenseKey [WORK].md b/spaces/diacanFperku/AutoGPT/MinecraftBetaLicenseKey [WORK].md
deleted file mode 100644
index f459032526c6589b5bd18d2cf7f4b7a0ebce4c7b..0000000000000000000000000000000000000000
--- a/spaces/diacanFperku/AutoGPT/MinecraftBetaLicenseKey [WORK].md
+++ /dev/null
@@ -1,145 +0,0 @@
-
-
Minecraft Beta License Key: How to Get It and Why You Need It
-
-
Minecraft is one of the most popular and creative sandbox games in the world. It allows you to build, explore and survive in a blocky pixelated world that is randomly generated and infinite. You can play it solo or with your friends, online or offline, on various devices and platforms.
-
-
But did you know that you can also access the latest features and updates of Minecraft before they are officially released? Yes, you read that right. You can join the Minecraft Beta program and get a Minecraft Beta license key that will let you play the beta versions of Minecraft on your device.
What is a Minecraft Beta license key and how can you get it? What are the benefits and risks of joining the Minecraft Beta program? What are the features and requirements of the beta versions of Minecraft? In this article, we will answer all these questions and more. Read on to find out everything you need to know about Minecraft Beta license key.
-
-
What is a Minecraft Beta License Key?
-
-
A Minecraft Beta license key is a code that you need to enter in order to play the beta versions of Minecraft on your device. A beta version is an early version of a software that is not yet finished or polished, but is released for testing and feedback purposes.
-
-
Minecraft has different beta programs for different platforms and editions of the game. For example, there is a beta program for Android devices, Windows 10 devices, Xbox One devices, Java Edition and more. Each beta program has its own rules and requirements, and you need a different Minecraft Beta license key for each one.
-
-
A Minecraft Beta license key is usually free and easy to get, but you need to own the original Minecraft game on your device first. You also need to sign up for the beta program that you want to join, and follow the instructions on how to download and install the beta version of Minecraft on your device.
-
-
How to Get a Minecraft Beta License Key?
-
-
Getting a Minecraft Beta license key depends on which platform and edition of Minecraft you want to play. Here are some of the most common ways to get a Minecraft Beta license key:
-
-
-
-
For Android devices: You need to own the original Minecraft game on your Android device. Then, you need to go to the Google Play Store and find the official Minecraft app. Scroll down to the "Join the Beta" section and click on "Join". Wait a few minutes and launch your regular Minecraft app. Your app will eventually switch to the beta version and you will get a Minecraft Beta license key.
-
For Windows 10 devices: You need to own the original Minecraft game on your Windows 10 device. Then, you need to go to the Microsoft Store and find the official Minecraft app. Click on "..." next to "Play" and select "Manage". Click on "Join" under "Minecraft Beta". Wait a few minutes and launch your regular Minecraft app. Your app will eventually switch to the beta version and you will get a Minecraft Beta license key.
-
For Xbox One devices: You need to own the original Minecraft game on your Xbox One device. Then, you need to go to the Xbox Insider Hub app on your device. If you don't have it, you can download it from the Microsoft Store for free. Launch the app and go to "Insider Content". Find "Minecraft" and click on "Join". Wait a few minutes and launch your regular Minecraft app. Your app will eventually switch to the beta version and you will get a Minecraft Beta license key.
-
For Java Edition: You need to own the original Minecraft game on your PC or Mac. Then, you need to go to the official Minecraft website and download the latest launcher for your device. Launch the launcher and select the latest snapshot by clicking the arrow next to the Play button on the main tab. Click on "Play" and you will get a Minecraft Beta license key.
-
-
-
Note: Some beta programs may require you to register or sign up before accessing them. Some beta programs may also have pop-up ads or redirects that you need to close or skip. Be careful of any malicious links or viruses that may harm your device. Always use a trusted antivirus software and a VPN service for your safety and security.
-
-
Why Should You Join the Minecraft Beta Program?
-
-
Joining the Minecraft Beta program can have many benefits for you as a player. Here are some of them:
-
-
-
You can access the latest features and updates of Minecraft before they are officially released.
-
You can test out new things that are not available in the regular version of Minecraft.
-
You can give feedback and suggestions to improve the game.
-
You can report bugs and glitches that may affect the game.
-
You can have fun and excitement by playing a different version of Minecraft.
-
-
-
What are the Risks of Joining the Minecraft Beta Program?
-
-
Joining the Minecraft Beta program can also have some risks for you as a player. Here are some of them:
-
-
-
You may encounter bugs, glitches, crashes or errors that may affect your gameplay or device.
-
You may lose your progress, data or files if something goes wrong with the beta version of Minecraft.
-
You may not be able to play with other players who are not on the same beta version of Minecraft as you.
-
You may not be able to access Realms or Featured Servers that are not compatible with the beta version of Minecraft.
-
You may not like some of the changes or features that are introduced in the beta version of Minecraft.
-
-
-
What are Some Tips for Playing with a Minecraft Beta License Key?
-
-
If you decide to join the Minecraft Beta program and get a Minecraft Beta license key, here are some tips for playing with it:
-
-
-
Always back up your worlds, data and files before playing with a beta version of Minecraft.
-
Always read the patch notes or changelogs before playing with a new beta version of Minecraft.
-
Always report any bugs, glitches or issues that you encounter while playing with a beta version of Minecraft.
-
Always give constructive feedback and suggestions for improving the game.
-
Always be respectful and polite when interacting with other players or developers who are also playing with a beta version of Minecraft.
-
-
-
Conclusion
-
-
Minecraft Beta license key is a code that allows you to play the beta versions of Minecraft on your device. A beta version is an early version of a software that is not yet finished or polished, but is released for testing and feedback purposes. By joining the Minecraft Beta program, you can access the latest features and updates of Minecraft before they are officially released. However, you also need to be aware of the risks and challenges that come with playing with a beta version of Minecraft.
-
-
If you are interested in getting a Minecraft Beta license key, you can follow these steps:
-
-
-
Own the original Minecraft game on your device first.
-
Sign up for the beta program that matches your platform and edition of Minecraft.
-
Download and install the beta version of Minecraft on your device.
-
Enter your Minecraft Beta license key when prompted.
-
Enjoy playing with a beta version of Minecraft!
-
-
-
So what are you waiting for? Grab your Minecraft Beta license key today!
-
What are the Features of the Beta Versions of Minecraft?
-
-
The beta versions of Minecraft have many features that make them different from the regular versions of Minecraft. Here are some of them:
-
-
-
The beta versions of Minecraft have new blocks, items, mobs, biomes, structures and mechanics that are not available in the regular versions of Minecraft.
-
The beta versions of Minecraft have experimental features that are not fully implemented or balanced yet, and may change or be removed in the future.
-
The beta versions of Minecraft have different user interfaces, menus, options and settings that are not present in the regular versions of Minecraft.
-
The beta versions of Minecraft have different performance, stability and compatibility issues that may affect your gameplay or device.
-
The beta versions of Minecraft have different feedback and reporting tools that allow you to communicate with the developers and other players.
-
-
-
What are Some Examples of the Beta Versions of Minecraft?
-
-
The beta versions of Minecraft vary depending on which platform and edition of Minecraft you are playing. Here are some examples of the beta versions of Minecraft:
-
-
-
For Android devices: The latest beta version of Minecraft for Android devices is 1.18.0.23. It features new biomes such as lush caves and dripstone caves, new blocks such as moss and azalea, new mobs such as axolotls and goats, and new mechanics such as skulk sensors and candles.
-
For Windows 10 devices: The latest beta version of Minecraft for Windows 10 devices is 1.18.0.23. It has the same features as the Android version, but also has ray tracing support for compatible devices.
-
For Xbox One devices: The latest beta version of Minecraft for Xbox One devices is 1.18.0.23. It has the same features as the Android and Windows 10 versions, but also has split-screen multiplayer support for up to four players.
-
For Java Edition: The latest beta version of Minecraft for Java Edition is 21w43a. It features new world generation options such as single biome and buffet, new blocks such as copper ore and amethyst, new mobs such as glow squids and warden, and new mechanics such as bundles and archaeology.
-
-
-
How to Leave the Minecraft Beta Program?
-
-
If you decide to leave the Minecraft Beta program and go back to the regular version of Minecraft, you can follow these steps:
-
-
-
For Android devices: Go to the Google Play Store and find the official Minecraft app. Scroll down to "You're a beta tester" and click on "Leave". Your app will eventually switch back to the regular version of Minecraft.
-
For Windows 10 devices: Go to the Microsoft Store and find the official Minecraft app. Click on "..." next to "Play" and select "Manage". Click on "Leave" under "Minecraft Beta". Your app will eventually switch back to the regular version of Minecraft.
-
For Xbox One devices: Go to the Xbox Insider Hub app on your device. Launch the app and go to "Insider Content". Find "Minecraft" and click on "Manage". Click on "Unenroll" under "Minecraft Beta". Your app will eventually switch back to the regular version of Minecraft.
-
For Java Edition: Go to the official Minecraft website and download the latest launcher for your device. Launch the launcher and select the latest release by clicking the arrow next to the Play button on the main tab. Click on "Play" and you will switch back to the regular version of Minecraft.
-
-
-
Note: You may need to uninstall and reinstall your app or delete your data files before switching back to the regular version of Minecraft. You may also lose your progress or compatibility with your worlds or servers if you switch back to a different version of Minecraft.
-
Conclusion
-
-
Minecraft Beta license key is a code that allows you to play the beta versions of Minecraft on your device. A beta version is an early version of a software that is not yet finished or polished, but is released for testing and feedback purposes. By joining the Minecraft Beta program, you can access the latest features and updates of Minecraft before they are officially released. However, you also need to be aware of the risks and challenges that come with playing with a beta version of Minecraft.
-
-
If you are interested in getting a Minecraft Beta license key, you can follow these steps:
-
-
-
Own the original Minecraft game on your device first.
-
Sign up for the beta program that matches your platform and edition of Minecraft.
-
Download and install the beta version of Minecraft on your device.
-
Enter your Minecraft Beta license key when prompted.
-
Enjoy playing with a beta version of Minecraft!
-
-
-
If you want to leave the Minecraft Beta program, you can follow these steps:
-
-
-
Go to the app store or website that matches your platform and edition of Minecraft.
-
Find the official Minecraft app and click on "Manage" or "Leave" under "Minecraft Beta".
-
Wait for your app to switch back to the regular version of Minecraft.
-
Delete any data files or reinstall your app if necessary.
-
Play with the regular version of Minecraft!
-
-
-
So what are you waiting for? Grab your Minecraft Beta license key today and experience the new world of Minecraft!
3cee63e6c2
-
-
\ No newline at end of file
diff --git a/spaces/diacanFperku/AutoGPT/Ppjoy Error Installing New Device Drivers Windows 10.md b/spaces/diacanFperku/AutoGPT/Ppjoy Error Installing New Device Drivers Windows 10.md
deleted file mode 100644
index 6e0eeab570236910d80d88ca4f111a0f6a540fe4..0000000000000000000000000000000000000000
--- a/spaces/diacanFperku/AutoGPT/Ppjoy Error Installing New Device Drivers Windows 10.md
+++ /dev/null
@@ -1,6 +0,0 @@
-
Ppjoy error installing new device drivers windows 10
-
-Here's the thing: Windows lists the stick, throttle and rudder as three seperate devices. Now i already found out the Freespace engine lets you ... 1fdad05405
-
-
-
diff --git a/spaces/digitalxingtong/Bufeiyan-a-Bert-VITS2/bert_gen.py b/spaces/digitalxingtong/Bufeiyan-a-Bert-VITS2/bert_gen.py
deleted file mode 100644
index 467655b2c4171608ad690fe7dec350db85f84f1b..0000000000000000000000000000000000000000
--- a/spaces/digitalxingtong/Bufeiyan-a-Bert-VITS2/bert_gen.py
+++ /dev/null
@@ -1,53 +0,0 @@
-import torch
-from torch.utils.data import DataLoader
-from multiprocessing import Pool
-import commons
-import utils
-from data_utils import TextAudioSpeakerLoader, TextAudioSpeakerCollate
-from tqdm import tqdm
-import warnings
-
-from text import cleaned_text_to_sequence, get_bert
-
-config_path = 'configs/config.json'
-hps = utils.get_hparams_from_file(config_path)
-
-def process_line(line):
- _id, spk, language_str, text, phones, tone, word2ph = line.strip().split("|")
- phone = phones.split(" ")
- tone = [int(i) for i in tone.split(" ")]
- word2ph = [int(i) for i in word2ph.split(" ")]
- w2pho = [i for i in word2ph]
- word2ph = [i for i in word2ph]
- phone, tone, language = cleaned_text_to_sequence(phone, tone, language_str)
-
- if hps.data.add_blank:
- phone = commons.intersperse(phone, 0)
- tone = commons.intersperse(tone, 0)
- language = commons.intersperse(language, 0)
- for i in range(len(word2ph)):
- word2ph[i] = word2ph[i] * 2
- word2ph[0] += 1
- wav_path = f'{_id}'
-
- bert_path = wav_path.replace(".wav", ".bert.pt")
- try:
- bert = torch.load(bert_path)
- assert bert.shape[-1] == len(phone)
- except:
- bert = get_bert(text, word2ph, language_str)
- assert bert.shape[-1] == len(phone)
- torch.save(bert, bert_path)
-
-
-if __name__ == '__main__':
- lines = []
- with open(hps.data.training_files, encoding='utf-8' ) as f:
- lines.extend(f.readlines())
-
- # with open(hps.data.validation_files, encoding='utf-8' ) as f:
- # lines.extend(f.readlines())
-
- with Pool(processes=2) as pool: #A100 40GB suitable config,if coom,please decrease the processess number.
- for _ in tqdm(pool.imap_unordered(process_line, lines)):
- pass
diff --git a/spaces/dineshreddy/WALT/mmdet/models/backbones/resnet.py b/spaces/dineshreddy/WALT/mmdet/models/backbones/resnet.py
deleted file mode 100644
index 3826815a6d94fdc4c54001d4c186d10ca3380e80..0000000000000000000000000000000000000000
--- a/spaces/dineshreddy/WALT/mmdet/models/backbones/resnet.py
+++ /dev/null
@@ -1,663 +0,0 @@
-import torch.nn as nn
-import torch.utils.checkpoint as cp
-from mmcv.cnn import (build_conv_layer, build_norm_layer, build_plugin_layer,
- constant_init, kaiming_init)
-from mmcv.runner import load_checkpoint
-from torch.nn.modules.batchnorm import _BatchNorm
-
-from mmdet.utils import get_root_logger
-from ..builder import BACKBONES
-from ..utils import ResLayer
-
-
-class BasicBlock(nn.Module):
- expansion = 1
-
- def __init__(self,
- inplanes,
- planes,
- stride=1,
- dilation=1,
- downsample=None,
- style='pytorch',
- with_cp=False,
- conv_cfg=None,
- norm_cfg=dict(type='BN'),
- dcn=None,
- plugins=None):
- super(BasicBlock, self).__init__()
- assert dcn is None, 'Not implemented yet.'
- assert plugins is None, 'Not implemented yet.'
-
- self.norm1_name, norm1 = build_norm_layer(norm_cfg, planes, postfix=1)
- self.norm2_name, norm2 = build_norm_layer(norm_cfg, planes, postfix=2)
-
- self.conv1 = build_conv_layer(
- conv_cfg,
- inplanes,
- planes,
- 3,
- stride=stride,
- padding=dilation,
- dilation=dilation,
- bias=False)
- self.add_module(self.norm1_name, norm1)
- self.conv2 = build_conv_layer(
- conv_cfg, planes, planes, 3, padding=1, bias=False)
- self.add_module(self.norm2_name, norm2)
-
- self.relu = nn.ReLU(inplace=True)
- self.downsample = downsample
- self.stride = stride
- self.dilation = dilation
- self.with_cp = with_cp
-
- @property
- def norm1(self):
- """nn.Module: normalization layer after the first convolution layer"""
- return getattr(self, self.norm1_name)
-
- @property
- def norm2(self):
- """nn.Module: normalization layer after the second convolution layer"""
- return getattr(self, self.norm2_name)
-
- def forward(self, x):
- """Forward function."""
-
- def _inner_forward(x):
- identity = x
-
- out = self.conv1(x)
- out = self.norm1(out)
- out = self.relu(out)
-
- out = self.conv2(out)
- out = self.norm2(out)
-
- if self.downsample is not None:
- identity = self.downsample(x)
-
- out += identity
-
- return out
-
- if self.with_cp and x.requires_grad:
- out = cp.checkpoint(_inner_forward, x)
- else:
- out = _inner_forward(x)
-
- out = self.relu(out)
-
- return out
-
-
-class Bottleneck(nn.Module):
- expansion = 4
-
- def __init__(self,
- inplanes,
- planes,
- stride=1,
- dilation=1,
- downsample=None,
- style='pytorch',
- with_cp=False,
- conv_cfg=None,
- norm_cfg=dict(type='BN'),
- dcn=None,
- plugins=None):
- """Bottleneck block for ResNet.
-
- If style is "pytorch", the stride-two layer is the 3x3 conv layer, if
- it is "caffe", the stride-two layer is the first 1x1 conv layer.
- """
- super(Bottleneck, self).__init__()
- assert style in ['pytorch', 'caffe']
- assert dcn is None or isinstance(dcn, dict)
- assert plugins is None or isinstance(plugins, list)
- if plugins is not None:
- allowed_position = ['after_conv1', 'after_conv2', 'after_conv3']
- assert all(p['position'] in allowed_position for p in plugins)
-
- self.inplanes = inplanes
- self.planes = planes
- self.stride = stride
- self.dilation = dilation
- self.style = style
- self.with_cp = with_cp
- self.conv_cfg = conv_cfg
- self.norm_cfg = norm_cfg
- self.dcn = dcn
- self.with_dcn = dcn is not None
- self.plugins = plugins
- self.with_plugins = plugins is not None
-
- if self.with_plugins:
- # collect plugins for conv1/conv2/conv3
- self.after_conv1_plugins = [
- plugin['cfg'] for plugin in plugins
- if plugin['position'] == 'after_conv1'
- ]
- self.after_conv2_plugins = [
- plugin['cfg'] for plugin in plugins
- if plugin['position'] == 'after_conv2'
- ]
- self.after_conv3_plugins = [
- plugin['cfg'] for plugin in plugins
- if plugin['position'] == 'after_conv3'
- ]
-
- if self.style == 'pytorch':
- self.conv1_stride = 1
- self.conv2_stride = stride
- else:
- self.conv1_stride = stride
- self.conv2_stride = 1
-
- self.norm1_name, norm1 = build_norm_layer(norm_cfg, planes, postfix=1)
- self.norm2_name, norm2 = build_norm_layer(norm_cfg, planes, postfix=2)
- self.norm3_name, norm3 = build_norm_layer(
- norm_cfg, planes * self.expansion, postfix=3)
-
- self.conv1 = build_conv_layer(
- conv_cfg,
- inplanes,
- planes,
- kernel_size=1,
- stride=self.conv1_stride,
- bias=False)
- self.add_module(self.norm1_name, norm1)
- fallback_on_stride = False
- if self.with_dcn:
- fallback_on_stride = dcn.pop('fallback_on_stride', False)
- if not self.with_dcn or fallback_on_stride:
- self.conv2 = build_conv_layer(
- conv_cfg,
- planes,
- planes,
- kernel_size=3,
- stride=self.conv2_stride,
- padding=dilation,
- dilation=dilation,
- bias=False)
- else:
- assert self.conv_cfg is None, 'conv_cfg must be None for DCN'
- self.conv2 = build_conv_layer(
- dcn,
- planes,
- planes,
- kernel_size=3,
- stride=self.conv2_stride,
- padding=dilation,
- dilation=dilation,
- bias=False)
-
- self.add_module(self.norm2_name, norm2)
- self.conv3 = build_conv_layer(
- conv_cfg,
- planes,
- planes * self.expansion,
- kernel_size=1,
- bias=False)
- self.add_module(self.norm3_name, norm3)
-
- self.relu = nn.ReLU(inplace=True)
- self.downsample = downsample
-
- if self.with_plugins:
- self.after_conv1_plugin_names = self.make_block_plugins(
- planes, self.after_conv1_plugins)
- self.after_conv2_plugin_names = self.make_block_plugins(
- planes, self.after_conv2_plugins)
- self.after_conv3_plugin_names = self.make_block_plugins(
- planes * self.expansion, self.after_conv3_plugins)
-
- def make_block_plugins(self, in_channels, plugins):
- """make plugins for block.
-
- Args:
- in_channels (int): Input channels of plugin.
- plugins (list[dict]): List of plugins cfg to build.
-
- Returns:
- list[str]: List of the names of plugin.
- """
- assert isinstance(plugins, list)
- plugin_names = []
- for plugin in plugins:
- plugin = plugin.copy()
- name, layer = build_plugin_layer(
- plugin,
- in_channels=in_channels,
- postfix=plugin.pop('postfix', ''))
- assert not hasattr(self, name), f'duplicate plugin {name}'
- self.add_module(name, layer)
- plugin_names.append(name)
- return plugin_names
-
- def forward_plugin(self, x, plugin_names):
- out = x
- for name in plugin_names:
- out = getattr(self, name)(x)
- return out
-
- @property
- def norm1(self):
- """nn.Module: normalization layer after the first convolution layer"""
- return getattr(self, self.norm1_name)
-
- @property
- def norm2(self):
- """nn.Module: normalization layer after the second convolution layer"""
- return getattr(self, self.norm2_name)
-
- @property
- def norm3(self):
- """nn.Module: normalization layer after the third convolution layer"""
- return getattr(self, self.norm3_name)
-
- def forward(self, x):
- """Forward function."""
-
- def _inner_forward(x):
- identity = x
- out = self.conv1(x)
- out = self.norm1(out)
- out = self.relu(out)
-
- if self.with_plugins:
- out = self.forward_plugin(out, self.after_conv1_plugin_names)
-
- out = self.conv2(out)
- out = self.norm2(out)
- out = self.relu(out)
-
- if self.with_plugins:
- out = self.forward_plugin(out, self.after_conv2_plugin_names)
-
- out = self.conv3(out)
- out = self.norm3(out)
-
- if self.with_plugins:
- out = self.forward_plugin(out, self.after_conv3_plugin_names)
-
- if self.downsample is not None:
- identity = self.downsample(x)
-
- out += identity
-
- return out
-
- if self.with_cp and x.requires_grad:
- out = cp.checkpoint(_inner_forward, x)
- else:
- out = _inner_forward(x)
-
- out = self.relu(out)
-
- return out
-
-
-@BACKBONES.register_module()
-class ResNet(nn.Module):
- """ResNet backbone.
-
- Args:
- depth (int): Depth of resnet, from {18, 34, 50, 101, 152}.
- stem_channels (int | None): Number of stem channels. If not specified,
- it will be the same as `base_channels`. Default: None.
- base_channels (int): Number of base channels of res layer. Default: 64.
- in_channels (int): Number of input image channels. Default: 3.
- num_stages (int): Resnet stages. Default: 4.
- strides (Sequence[int]): Strides of the first block of each stage.
- dilations (Sequence[int]): Dilation of each stage.
- out_indices (Sequence[int]): Output from which stages.
- style (str): `pytorch` or `caffe`. If set to "pytorch", the stride-two
- layer is the 3x3 conv layer, otherwise the stride-two layer is
- the first 1x1 conv layer.
- deep_stem (bool): Replace 7x7 conv in input stem with 3 3x3 conv
- avg_down (bool): Use AvgPool instead of stride conv when
- downsampling in the bottleneck.
- frozen_stages (int): Stages to be frozen (stop grad and set eval mode).
- -1 means not freezing any parameters.
- norm_cfg (dict): Dictionary to construct and config norm layer.
- norm_eval (bool): Whether to set norm layers to eval mode, namely,
- freeze running stats (mean and var). Note: Effect on Batch Norm
- and its variants only.
- plugins (list[dict]): List of plugins for stages, each dict contains:
-
- - cfg (dict, required): Cfg dict to build plugin.
- - position (str, required): Position inside block to insert
- plugin, options are 'after_conv1', 'after_conv2', 'after_conv3'.
- - stages (tuple[bool], optional): Stages to apply plugin, length
- should be same as 'num_stages'.
- with_cp (bool): Use checkpoint or not. Using checkpoint will save some
- memory while slowing down the training speed.
- zero_init_residual (bool): Whether to use zero init for last norm layer
- in resblocks to let them behave as identity.
-
- Example:
- >>> from mmdet.models import ResNet
- >>> import torch
- >>> self = ResNet(depth=18)
- >>> self.eval()
- >>> inputs = torch.rand(1, 3, 32, 32)
- >>> level_outputs = self.forward(inputs)
- >>> for level_out in level_outputs:
- ... print(tuple(level_out.shape))
- (1, 64, 8, 8)
- (1, 128, 4, 4)
- (1, 256, 2, 2)
- (1, 512, 1, 1)
- """
-
- arch_settings = {
- 18: (BasicBlock, (2, 2, 2, 2)),
- 34: (BasicBlock, (3, 4, 6, 3)),
- 50: (Bottleneck, (3, 4, 6, 3)),
- 101: (Bottleneck, (3, 4, 23, 3)),
- 152: (Bottleneck, (3, 8, 36, 3))
- }
-
- def __init__(self,
- depth,
- in_channels=3,
- stem_channels=None,
- base_channels=64,
- num_stages=4,
- strides=(1, 2, 2, 2),
- dilations=(1, 1, 1, 1),
- out_indices=(0, 1, 2, 3),
- style='pytorch',
- deep_stem=False,
- avg_down=False,
- frozen_stages=-1,
- conv_cfg=None,
- norm_cfg=dict(type='BN', requires_grad=True),
- norm_eval=True,
- dcn=None,
- stage_with_dcn=(False, False, False, False),
- plugins=None,
- with_cp=False,
- zero_init_residual=True):
- super(ResNet, self).__init__()
- if depth not in self.arch_settings:
- raise KeyError(f'invalid depth {depth} for resnet')
- self.depth = depth
- if stem_channels is None:
- stem_channels = base_channels
- self.stem_channels = stem_channels
- self.base_channels = base_channels
- self.num_stages = num_stages
- assert num_stages >= 1 and num_stages <= 4
- self.strides = strides
- self.dilations = dilations
- assert len(strides) == len(dilations) == num_stages
- self.out_indices = out_indices
- assert max(out_indices) < num_stages
- self.style = style
- self.deep_stem = deep_stem
- self.avg_down = avg_down
- self.frozen_stages = frozen_stages
- self.conv_cfg = conv_cfg
- self.norm_cfg = norm_cfg
- self.with_cp = with_cp
- self.norm_eval = norm_eval
- self.dcn = dcn
- self.stage_with_dcn = stage_with_dcn
- if dcn is not None:
- assert len(stage_with_dcn) == num_stages
- self.plugins = plugins
- self.zero_init_residual = zero_init_residual
- self.block, stage_blocks = self.arch_settings[depth]
- self.stage_blocks = stage_blocks[:num_stages]
- self.inplanes = stem_channels
-
- self._make_stem_layer(in_channels, stem_channels)
-
- self.res_layers = []
- for i, num_blocks in enumerate(self.stage_blocks):
- stride = strides[i]
- dilation = dilations[i]
- dcn = self.dcn if self.stage_with_dcn[i] else None
- if plugins is not None:
- stage_plugins = self.make_stage_plugins(plugins, i)
- else:
- stage_plugins = None
- planes = base_channels * 2**i
- res_layer = self.make_res_layer(
- block=self.block,
- inplanes=self.inplanes,
- planes=planes,
- num_blocks=num_blocks,
- stride=stride,
- dilation=dilation,
- style=self.style,
- avg_down=self.avg_down,
- with_cp=with_cp,
- conv_cfg=conv_cfg,
- norm_cfg=norm_cfg,
- dcn=dcn,
- plugins=stage_plugins)
- self.inplanes = planes * self.block.expansion
- layer_name = f'layer{i + 1}'
- self.add_module(layer_name, res_layer)
- self.res_layers.append(layer_name)
-
- self._freeze_stages()
-
- self.feat_dim = self.block.expansion * base_channels * 2**(
- len(self.stage_blocks) - 1)
-
- def make_stage_plugins(self, plugins, stage_idx):
- """Make plugins for ResNet ``stage_idx`` th stage.
-
- Currently we support to insert ``context_block``,
- ``empirical_attention_block``, ``nonlocal_block`` into the backbone
- like ResNet/ResNeXt. They could be inserted after conv1/conv2/conv3 of
- Bottleneck.
-
- An example of plugins format could be:
-
- Examples:
- >>> plugins=[
- ... dict(cfg=dict(type='xxx', arg1='xxx'),
- ... stages=(False, True, True, True),
- ... position='after_conv2'),
- ... dict(cfg=dict(type='yyy'),
- ... stages=(True, True, True, True),
- ... position='after_conv3'),
- ... dict(cfg=dict(type='zzz', postfix='1'),
- ... stages=(True, True, True, True),
- ... position='after_conv3'),
- ... dict(cfg=dict(type='zzz', postfix='2'),
- ... stages=(True, True, True, True),
- ... position='after_conv3')
- ... ]
- >>> self = ResNet(depth=18)
- >>> stage_plugins = self.make_stage_plugins(plugins, 0)
- >>> assert len(stage_plugins) == 3
-
- Suppose ``stage_idx=0``, the structure of blocks in the stage would be:
-
- .. code-block:: none
-
- conv1-> conv2->conv3->yyy->zzz1->zzz2
-
- Suppose 'stage_idx=1', the structure of blocks in the stage would be:
-
- .. code-block:: none
-
- conv1-> conv2->xxx->conv3->yyy->zzz1->zzz2
-
- If stages is missing, the plugin would be applied to all stages.
-
- Args:
- plugins (list[dict]): List of plugins cfg to build. The postfix is
- required if multiple same type plugins are inserted.
- stage_idx (int): Index of stage to build
-
- Returns:
- list[dict]: Plugins for current stage
- """
- stage_plugins = []
- for plugin in plugins:
- plugin = plugin.copy()
- stages = plugin.pop('stages', None)
- assert stages is None or len(stages) == self.num_stages
- # whether to insert plugin into current stage
- if stages is None or stages[stage_idx]:
- stage_plugins.append(plugin)
-
- return stage_plugins
-
- def make_res_layer(self, **kwargs):
- """Pack all blocks in a stage into a ``ResLayer``."""
- return ResLayer(**kwargs)
-
- @property
- def norm1(self):
- """nn.Module: the normalization layer named "norm1" """
- return getattr(self, self.norm1_name)
-
- def _make_stem_layer(self, in_channels, stem_channels):
- if self.deep_stem:
- self.stem = nn.Sequential(
- build_conv_layer(
- self.conv_cfg,
- in_channels,
- stem_channels // 2,
- kernel_size=3,
- stride=2,
- padding=1,
- bias=False),
- build_norm_layer(self.norm_cfg, stem_channels // 2)[1],
- nn.ReLU(inplace=True),
- build_conv_layer(
- self.conv_cfg,
- stem_channels // 2,
- stem_channels // 2,
- kernel_size=3,
- stride=1,
- padding=1,
- bias=False),
- build_norm_layer(self.norm_cfg, stem_channels // 2)[1],
- nn.ReLU(inplace=True),
- build_conv_layer(
- self.conv_cfg,
- stem_channels // 2,
- stem_channels,
- kernel_size=3,
- stride=1,
- padding=1,
- bias=False),
- build_norm_layer(self.norm_cfg, stem_channels)[1],
- nn.ReLU(inplace=True))
- else:
- self.conv1 = build_conv_layer(
- self.conv_cfg,
- in_channels,
- stem_channels,
- kernel_size=7,
- stride=2,
- padding=3,
- bias=False)
- self.norm1_name, norm1 = build_norm_layer(
- self.norm_cfg, stem_channels, postfix=1)
- self.add_module(self.norm1_name, norm1)
- self.relu = nn.ReLU(inplace=True)
- self.maxpool = nn.MaxPool2d(kernel_size=3, stride=2, padding=1)
-
- def _freeze_stages(self):
- if self.frozen_stages >= 0:
- if self.deep_stem:
- self.stem.eval()
- for param in self.stem.parameters():
- param.requires_grad = False
- else:
- self.norm1.eval()
- for m in [self.conv1, self.norm1]:
- for param in m.parameters():
- param.requires_grad = False
-
- for i in range(1, self.frozen_stages + 1):
- m = getattr(self, f'layer{i}')
- m.eval()
- for param in m.parameters():
- param.requires_grad = False
-
- def init_weights(self, pretrained=None):
- """Initialize the weights in backbone.
-
- Args:
- pretrained (str, optional): Path to pre-trained weights.
- Defaults to None.
- """
- if isinstance(pretrained, str):
- logger = get_root_logger()
- load_checkpoint(self, pretrained, strict=False, logger=logger)
- elif pretrained is None:
- for m in self.modules():
- if isinstance(m, nn.Conv2d):
- kaiming_init(m)
- elif isinstance(m, (_BatchNorm, nn.GroupNorm)):
- constant_init(m, 1)
-
- if self.dcn is not None:
- for m in self.modules():
- if isinstance(m, Bottleneck) and hasattr(
- m.conv2, 'conv_offset'):
- constant_init(m.conv2.conv_offset, 0)
-
- if self.zero_init_residual:
- for m in self.modules():
- if isinstance(m, Bottleneck):
- constant_init(m.norm3, 0)
- elif isinstance(m, BasicBlock):
- constant_init(m.norm2, 0)
- else:
- raise TypeError('pretrained must be a str or None')
-
- def forward(self, x):
- """Forward function."""
- if self.deep_stem:
- x = self.stem(x)
- else:
- x = self.conv1(x)
- x = self.norm1(x)
- x = self.relu(x)
- x = self.maxpool(x)
- outs = []
- for i, layer_name in enumerate(self.res_layers):
- res_layer = getattr(self, layer_name)
- x = res_layer(x)
- if i in self.out_indices:
- outs.append(x)
- return tuple(outs)
-
- def train(self, mode=True):
- """Convert the model into training mode while keep normalization layer
- freezed."""
- super(ResNet, self).train(mode)
- self._freeze_stages()
- if mode and self.norm_eval:
- for m in self.modules():
- # trick: eval have effect on BatchNorm only
- if isinstance(m, _BatchNorm):
- m.eval()
-
-
-@BACKBONES.register_module()
-class ResNetV1d(ResNet):
- r"""ResNetV1d variant described in `Bag of Tricks
- `_.
-
- Compared with default ResNet(ResNetV1b), ResNetV1d replaces the 7x7 conv in
- the input stem with three 3x3 convs. And in the downsampling block, a 2x2
- avg_pool with stride 2 is added before conv, whose stride is changed to 1.
- """
-
- def __init__(self, **kwargs):
- super(ResNetV1d, self).__init__(
- deep_stem=True, avg_down=True, **kwargs)
diff --git a/spaces/dineshreddy/WALT/mmdet/models/losses/ae_loss.py b/spaces/dineshreddy/WALT/mmdet/models/losses/ae_loss.py
deleted file mode 100644
index cff472aa03080fb49dbb3adba6fec68647a575e6..0000000000000000000000000000000000000000
--- a/spaces/dineshreddy/WALT/mmdet/models/losses/ae_loss.py
+++ /dev/null
@@ -1,102 +0,0 @@
-import mmcv
-import torch
-import torch.nn as nn
-import torch.nn.functional as F
-
-from ..builder import LOSSES
-
-
-@mmcv.jit(derivate=True, coderize=True)
-def ae_loss_per_image(tl_preds, br_preds, match):
- """Associative Embedding Loss in one image.
-
- Associative Embedding Loss including two parts: pull loss and push loss.
- Pull loss makes embedding vectors from same object closer to each other.
- Push loss distinguish embedding vector from different objects, and makes
- the gap between them is large enough.
-
- During computing, usually there are 3 cases:
- - no object in image: both pull loss and push loss will be 0.
- - one object in image: push loss will be 0 and pull loss is computed
- by the two corner of the only object.
- - more than one objects in image: pull loss is computed by corner pairs
- from each object, push loss is computed by each object with all
- other objects. We use confusion matrix with 0 in diagonal to
- compute the push loss.
-
- Args:
- tl_preds (tensor): Embedding feature map of left-top corner.
- br_preds (tensor): Embedding feature map of bottim-right corner.
- match (list): Downsampled coordinates pair of each ground truth box.
- """
-
- tl_list, br_list, me_list = [], [], []
- if len(match) == 0: # no object in image
- pull_loss = tl_preds.sum() * 0.
- push_loss = tl_preds.sum() * 0.
- else:
- for m in match:
- [tl_y, tl_x], [br_y, br_x] = m
- tl_e = tl_preds[:, tl_y, tl_x].view(-1, 1)
- br_e = br_preds[:, br_y, br_x].view(-1, 1)
- tl_list.append(tl_e)
- br_list.append(br_e)
- me_list.append((tl_e + br_e) / 2.0)
-
- tl_list = torch.cat(tl_list)
- br_list = torch.cat(br_list)
- me_list = torch.cat(me_list)
-
- assert tl_list.size() == br_list.size()
-
- # N is object number in image, M is dimension of embedding vector
- N, M = tl_list.size()
-
- pull_loss = (tl_list - me_list).pow(2) + (br_list - me_list).pow(2)
- pull_loss = pull_loss.sum() / N
-
- margin = 1 # exp setting of CornerNet, details in section 3.3 of paper
-
- # confusion matrix of push loss
- conf_mat = me_list.expand((N, N, M)).permute(1, 0, 2) - me_list
- conf_weight = 1 - torch.eye(N).type_as(me_list)
- conf_mat = conf_weight * (margin - conf_mat.sum(-1).abs())
-
- if N > 1: # more than one object in current image
- push_loss = F.relu(conf_mat).sum() / (N * (N - 1))
- else:
- push_loss = tl_preds.sum() * 0.
-
- return pull_loss, push_loss
-
-
-@LOSSES.register_module()
-class AssociativeEmbeddingLoss(nn.Module):
- """Associative Embedding Loss.
-
- More details can be found in
- `Associative Embedding `_ and
- `CornerNet `_ .
- Code is modified from `kp_utils.py `_ # noqa: E501
-
- Args:
- pull_weight (float): Loss weight for corners from same object.
- push_weight (float): Loss weight for corners from different object.
- """
-
- def __init__(self, pull_weight=0.25, push_weight=0.25):
- super(AssociativeEmbeddingLoss, self).__init__()
- self.pull_weight = pull_weight
- self.push_weight = push_weight
-
- def forward(self, pred, target, match):
- """Forward function."""
- batch = pred.size(0)
- pull_all, push_all = 0.0, 0.0
- for i in range(batch):
- pull, push = ae_loss_per_image(pred[i], target[i], match[i])
-
- pull_all += self.pull_weight * pull
- push_all += self.push_weight * push
-
- return pull_all, push_all
diff --git a/spaces/dinhminh20521597/OCR_DEMO/configs/_base_/recog_pipelines/abinet_pipeline.py b/spaces/dinhminh20521597/OCR_DEMO/configs/_base_/recog_pipelines/abinet_pipeline.py
deleted file mode 100644
index 3a54dfe6a8c310ab74f9a01b4671d7288436d0a7..0000000000000000000000000000000000000000
--- a/spaces/dinhminh20521597/OCR_DEMO/configs/_base_/recog_pipelines/abinet_pipeline.py
+++ /dev/null
@@ -1,96 +0,0 @@
-img_norm_cfg = dict(mean=[0.485, 0.456, 0.406], std=[0.229, 0.224, 0.225])
-train_pipeline = [
- dict(type='LoadImageFromFile'),
- dict(
- type='ResizeOCR',
- height=32,
- min_width=128,
- max_width=128,
- keep_aspect_ratio=False,
- width_downsample_ratio=0.25),
- dict(
- type='RandomWrapper',
- p=0.5,
- transforms=[
- dict(
- type='OneOfWrapper',
- transforms=[
- dict(
- type='RandomRotateTextDet',
- max_angle=15,
- ),
- dict(
- type='TorchVisionWrapper',
- op='RandomAffine',
- degrees=15,
- translate=(0.3, 0.3),
- scale=(0.5, 2.),
- shear=(-45, 45),
- ),
- dict(
- type='TorchVisionWrapper',
- op='RandomPerspective',
- distortion_scale=0.5,
- p=1,
- ),
- ])
- ],
- ),
- dict(
- type='RandomWrapper',
- p=0.25,
- transforms=[
- dict(type='PyramidRescale'),
- dict(
- type='Albu',
- transforms=[
- dict(type='GaussNoise', var_limit=(20, 20), p=0.5),
- dict(type='MotionBlur', blur_limit=6, p=0.5),
- ]),
- ]),
- dict(
- type='RandomWrapper',
- p=0.25,
- transforms=[
- dict(
- type='TorchVisionWrapper',
- op='ColorJitter',
- brightness=0.5,
- saturation=0.5,
- contrast=0.5,
- hue=0.1),
- ]),
- dict(type='ToTensorOCR'),
- dict(type='NormalizeOCR', **img_norm_cfg),
- dict(
- type='Collect',
- keys=['img'],
- meta_keys=[
- 'filename', 'ori_shape', 'img_shape', 'text', 'valid_ratio',
- 'resize_shape'
- ]),
-]
-test_pipeline = [
- dict(type='LoadImageFromFile'),
- dict(
- type='MultiRotateAugOCR',
- rotate_degrees=[0, 90, 270],
- transforms=[
- dict(
- type='ResizeOCR',
- height=32,
- min_width=128,
- max_width=128,
- keep_aspect_ratio=False,
- width_downsample_ratio=0.25),
- dict(type='ToTensorOCR'),
- dict(type='NormalizeOCR', **img_norm_cfg),
- dict(
- type='Collect',
- keys=['img'],
- meta_keys=[
- 'filename', 'ori_shape', 'img_shape', 'valid_ratio',
- 'resize_shape', 'img_norm_cfg', 'ori_filename'
- ]),
- ])
-]
diff --git a/spaces/doevent/vc/README.md b/spaces/doevent/vc/README.md
deleted file mode 100644
index 09b826a3f6658864144c948ae93fc435b51967ad..0000000000000000000000000000000000000000
--- a/spaces/doevent/vc/README.md
+++ /dev/null
@@ -1,13 +0,0 @@
----
-title: VC
-emoji: 💬
-colorFrom: blue
-colorTo: yellow
-sdk: gradio
-sdk_version: 3.42.0
-app_file: app.py
-pinned: false
-duplicated_from: balacoon/voice_conversion_service
----
-
-Interactive demo for Voice Conversion service by Balacoon.
diff --git a/spaces/elexxuyafei/chart927/README.md b/spaces/elexxuyafei/chart927/README.md
deleted file mode 100644
index 45dca72694ec84ab405ff4e8e6cd5580e110adcf..0000000000000000000000000000000000000000
--- a/spaces/elexxuyafei/chart927/README.md
+++ /dev/null
@@ -1,12 +0,0 @@
----
-title: Chart927
-emoji: 📊
-colorFrom: gray
-colorTo: gray
-sdk: streamlit
-sdk_version: 1.10.0
-app_file: app.py
-pinned: false
----
-
-Check out the configuration reference at https://huggingface.co/docs/hub/spaces-config-reference
diff --git a/spaces/elkraken/Video-Object-Detection/utils/aws/mime.sh b/spaces/elkraken/Video-Object-Detection/utils/aws/mime.sh
deleted file mode 100644
index c319a83cfbdf09bea634c3bd9fca737c0b1dd505..0000000000000000000000000000000000000000
--- a/spaces/elkraken/Video-Object-Detection/utils/aws/mime.sh
+++ /dev/null
@@ -1,26 +0,0 @@
-# AWS EC2 instance startup 'MIME' script https://aws.amazon.com/premiumsupport/knowledge-center/execute-user-data-ec2/
-# This script will run on every instance restart, not only on first start
-# --- DO NOT COPY ABOVE COMMENTS WHEN PASTING INTO USERDATA ---
-
-Content-Type: multipart/mixed; boundary="//"
-MIME-Version: 1.0
-
---//
-Content-Type: text/cloud-config; charset="us-ascii"
-MIME-Version: 1.0
-Content-Transfer-Encoding: 7bit
-Content-Disposition: attachment; filename="cloud-config.txt"
-
-#cloud-config
-cloud_final_modules:
-- [scripts-user, always]
-
---//
-Content-Type: text/x-shellscript; charset="us-ascii"
-MIME-Version: 1.0
-Content-Transfer-Encoding: 7bit
-Content-Disposition: attachment; filename="userdata.txt"
-
-#!/bin/bash
-# --- paste contents of userdata.sh here ---
---//
diff --git a/spaces/evaluate-comparison/mcnemar/mcnemar.py b/spaces/evaluate-comparison/mcnemar/mcnemar.py
deleted file mode 100644
index 86b85b5e33d74260bb78d83a0225dc004729db5d..0000000000000000000000000000000000000000
--- a/spaces/evaluate-comparison/mcnemar/mcnemar.py
+++ /dev/null
@@ -1,98 +0,0 @@
-# Copyright 2022 The HuggingFace Evaluate Authors
-#
-# Licensed under the Apache License, Version 2.0 (the "License");
-# you may not use this file except in compliance with the License.
-# You may obtain a copy of the License at
-#
-# http://www.apache.org/licenses/LICENSE-2.0
-#
-# Unless required by applicable law or agreed to in writing, software
-# distributed under the License is distributed on an "AS IS" BASIS,
-# WITHOUT WARRANTIES OR CONDITIONS OF ANY KIND, either express or implied.
-# See the License for the specific language governing permissions and
-# limitations under the License.
-"""McNemar test for model comparison."""
-
-import datasets
-from scipy.stats import chi2
-
-import evaluate
-
-
-_DESCRIPTION = """
-McNemar's test is a diagnostic test over a contingency table resulting from the predictions of two classifiers. The test compares the sensitivity and specificity of the diagnostic tests on the same group reference labels. It can be computed with:
-McNemar = (SE - SP)**2 / SE + SP
- Where:
-SE: Sensitivity (Test 1 positive; Test 2 negative)
-SP: Specificity (Test 1 negative; Test 2 positive)
-"""
-
-
-_KWARGS_DESCRIPTION = """
-Args:
- predictions1 (`list` of `int`): Predicted labels for model 1.
- predictions2 (`list` of `int`): Predicted labels for model 2.
- references (`list` of `int`): Ground truth labels.
-
-Returns:
- stat (`float`): McNemar test score.
- p (`float`): The p value. Minimum possible value is 0. Maximum possible value is 1.0. A lower p value means a more significant difference.
-
-Examples:
- >>> mcnemar = evaluate.load("mcnemar")
- >>> results = mcnemar.compute(references=[1, 0, 1], predictions1=[1, 1, 1], predictions2=[1, 0, 1])
- >>> print(results)
- {'stat': 1.0, 'p': 0.31731050786291115}
-"""
-
-
-_CITATION = """
-@article{mcnemar1947note,
- title={Note on the sampling error of the difference between correlated proportions or percentages},
- author={McNemar, Quinn},
- journal={Psychometrika},
- volume={12},
- number={2},
- pages={153--157},
- year={1947},
- publisher={Springer-Verlag}
-}
-"""
-
-
-@evaluate.utils.file_utils.add_start_docstrings(_DESCRIPTION, _KWARGS_DESCRIPTION)
-class McNemar(evaluate.Comparison):
- def _info(self):
- return evaluate.ComparisonInfo(
- module_type="comparison",
- description=_DESCRIPTION,
- citation=_CITATION,
- inputs_description=_KWARGS_DESCRIPTION,
- features=datasets.Features(
- {
- "predictions1": datasets.Value("int64"),
- "predictions2": datasets.Value("int64"),
- "references": datasets.Value("int64"),
- }
- ),
- )
-
- def _compute(self, predictions1, predictions2, references):
- # construct contingency table
- tbl = [[0, 0], [0, 0]]
- for gt, p1, p2 in zip(references, predictions1, predictions2):
- if p1 == gt and p2 == gt:
- tbl[0][0] += 1
- elif p1 == gt:
- tbl[0][1] += 1
- elif p2 == gt:
- tbl[1][0] += 1
- else:
- tbl[1][1] += 1
-
- # compute statistic
- b, c = tbl[0][1], tbl[1][0]
- statistic = abs(b - c) ** 2 / (1.0 * (b + c))
- df = 1
- pvalue = chi2.sf(statistic, df)
- return {"stat": statistic, "p": pvalue}
diff --git a/spaces/fabiogra/moseca/app/service/demucs_runner.py b/spaces/fabiogra/moseca/app/service/demucs_runner.py
deleted file mode 100644
index 499ce095543c774e7292a4ba8487507d36d82c14..0000000000000000000000000000000000000000
--- a/spaces/fabiogra/moseca/app/service/demucs_runner.py
+++ /dev/null
@@ -1,190 +0,0 @@
-import argparse
-import sys
-from pathlib import Path
-from typing import List
-import os
-from dora.log import fatal
-import torch as th
-
-from demucs.apply import apply_model, BagOfModels
-from demucs.audio import save_audio
-from demucs.pretrained import get_model_from_args, ModelLoadingError
-from demucs.separate import load_track
-
-import streamlit as st
-
-
-@st.cache_data(show_spinner=False)
-def separator(
- tracks: List[Path],
- out: Path,
- model: str,
- shifts: int,
- overlap: float,
- stem: str,
- int24: bool,
- float32: bool,
- clip_mode: str,
- mp3: bool,
- mp3_bitrate: int,
- verbose: bool,
- *args,
- **kwargs,
-):
- """Separate the sources for the given tracks
-
- Args:
- tracks (Path): Path to tracks
- out (Path): Folder where to put extracted tracks. A subfolder with the model name will be
- created.
- model (str): Model name
- shifts (int): Number of random shifts for equivariant stabilization.
- Increase separation time but improves quality for Demucs.
- 10 was used in the original paper.
- overlap (float): Overlap
- stem (str): Only separate audio into {STEM} and no_{STEM}.
- int24 (bool): Save wav output as 24 bits wav.
- float32 (bool): Save wav output as float32 (2x bigger).
- clip_mode (str): Strategy for avoiding clipping: rescaling entire signal if necessary
- (rescale) or hard clipping (clamp).
- mp3 (bool): Convert the output wavs to mp3.
- mp3_bitrate (int): Bitrate of converted mp3.
- verbose (bool): Verbose
- """
-
- if os.environ.get("LIMIT_CPU", False):
- th.set_num_threads(1)
- jobs = 1
- else:
- # Number of jobs. This can increase memory usage but will be much faster when
- # multiple cores are available.
- jobs = os.cpu_count()
-
- if th.cuda.is_available():
- device = "cuda"
- else:
- device = "cpu"
- args = argparse.Namespace()
- args.tracks = tracks
- args.out = out
- args.model = model
- args.device = device
- args.shifts = shifts
- args.overlap = overlap
- args.stem = stem
- args.int24 = int24
- args.float32 = float32
- args.clip_mode = clip_mode
- args.mp3 = mp3
- args.mp3_bitrate = mp3_bitrate
- args.jobs = jobs
- args.verbose = verbose
- args.filename = "{track}/{stem}.{ext}"
- args.split = True
- args.segment = None
- args.name = model
- args.repo = None
-
- try:
- model = get_model_from_args(args)
- except ModelLoadingError as error:
- fatal(error.args[0])
-
- if args.segment is not None and args.segment < 8:
- fatal("Segment must greater than 8. ")
-
- if ".." in args.filename.replace("\\", "/").split("/"):
- fatal('".." must not appear in filename. ')
-
- if isinstance(model, BagOfModels):
- print(
- f"Selected model is a bag of {len(model.models)} models. "
- "You will see that many progress bars per track."
- )
- if args.segment is not None:
- for sub in model.models:
- sub.segment = args.segment
- else:
- if args.segment is not None:
- model.segment = args.segment
-
- model.cpu()
- model.eval()
-
- if args.stem is not None and args.stem not in model.sources:
- fatal(
- 'error: stem "{stem}" is not in selected model. STEM must be one of {sources}.'.format(
- stem=args.stem, sources=", ".join(model.sources)
- )
- )
- out = args.out / args.name
- out.mkdir(parents=True, exist_ok=True)
- print(f"Separated tracks will be stored in {out.resolve()}")
- for track in args.tracks:
- if not track.exists():
- print(
- f"File {track} does not exist. If the path contains spaces, "
- 'please try again after surrounding the entire path with quotes "".',
- file=sys.stderr,
- )
- continue
- print(f"Separating track {track}")
- wav = load_track(track, model.audio_channels, model.samplerate)
-
- ref = wav.mean(0)
- wav = (wav - ref.mean()) / ref.std()
- sources = apply_model(
- model,
- wav[None],
- device=args.device,
- shifts=args.shifts,
- split=args.split,
- overlap=args.overlap,
- progress=True,
- num_workers=args.jobs,
- )[0]
- sources = sources * ref.std() + ref.mean()
-
- if args.mp3:
- ext = "mp3"
- else:
- ext = "wav"
- kwargs = {
- "samplerate": model.samplerate,
- "bitrate": args.mp3_bitrate,
- "clip": args.clip_mode,
- "as_float": args.float32,
- "bits_per_sample": 24 if args.int24 else 16,
- }
- if args.stem is None:
- for source, name in zip(sources, model.sources):
- stem = out / args.filename.format(
- track=track.name.rsplit(".", 1)[0],
- trackext=track.name.rsplit(".", 1)[-1],
- stem=name,
- ext=ext,
- )
- stem.parent.mkdir(parents=True, exist_ok=True)
- save_audio(source, str(stem), **kwargs)
- else:
- sources = list(sources)
- stem = out / args.filename.format(
- track=track.name.rsplit(".", 1)[0],
- trackext=track.name.rsplit(".", 1)[-1],
- stem=args.stem,
- ext=ext,
- )
- stem.parent.mkdir(parents=True, exist_ok=True)
- save_audio(sources.pop(model.sources.index(args.stem)), str(stem), **kwargs)
- # Warning : after poping the stem, selected stem is no longer in the list 'sources'
- other_stem = th.zeros_like(sources[0])
- for i in sources:
- other_stem += i
- stem = out / args.filename.format(
- track=track.name.rsplit(".", 1)[0],
- trackext=track.name.rsplit(".", 1)[-1],
- stem="no_" + args.stem,
- ext=ext,
- )
- stem.parent.mkdir(parents=True, exist_ok=True)
- save_audio(other_stem, str(stem), **kwargs)
diff --git a/spaces/failfast/2D-GameCreator/.github/SECURITY.md b/spaces/failfast/2D-GameCreator/.github/SECURITY.md
deleted file mode 100644
index b7fbca60cc59381dc65cc0fdf7dba328b6918c00..0000000000000000000000000000000000000000
--- a/spaces/failfast/2D-GameCreator/.github/SECURITY.md
+++ /dev/null
@@ -1,17 +0,0 @@
-# Security Policy
-
-## Supported Versions
-
-Use this section to tell people about which versions of your project are currently being supported
-with security updates.
-
-| Version | Supported |
-| ------- | ------------------ |
-| 1.x.x | :white_check_mark: |
-
-## Reporting a Vulnerability
-
-Use this section to tell people how to report a vulnerability.
-
-Tell them where to go, how often they can expect to get an update on a reported vulnerability, what
-to expect if the vulnerability is accepted or declined, etc.
diff --git a/spaces/failfast/nextjs-hf-spaces/src/components/title.tsx b/spaces/failfast/nextjs-hf-spaces/src/components/title.tsx
deleted file mode 100644
index 1be3df4ea4c288d4ad80f7934bab4893467dadae..0000000000000000000000000000000000000000
--- a/spaces/failfast/nextjs-hf-spaces/src/components/title.tsx
+++ /dev/null
@@ -1,62 +0,0 @@
-import { Button, Link, Paper, Stack, Typography } from "@mui/material";
-import { HighlightBox } from "./base/boxes";
-import ContentCopyIcon from "@mui/icons-material/ContentCopy";
-
-export default function Title() {
- return (
-
-
-
- Next.js
- {" "}
- on 🤗{" "}
-
- Spaces
-
-
-
-
-
- Run your ML demo with ease in a Next.js environment
-
-
-
-
- }
- variant="contained"
- href="https://huggingface.co/spaces/failfast/nextjs-docker-starter?duplicate=true"
- target="_blank"
- rel="noopener"
- color="secondary"
- >
- Duplicate space
-
-
-
-
-
- );
-}
diff --git a/spaces/fatiXbelha/sd/Bundesliga The home of German football legends and rising stars..md b/spaces/fatiXbelha/sd/Bundesliga The home of German football legends and rising stars..md
deleted file mode 100644
index cc21fe4edbb0d7b99c0bd4c8327eecaf07bb07e9..0000000000000000000000000000000000000000
--- a/spaces/fatiXbelha/sd/Bundesliga The home of German football legends and rising stars..md
+++ /dev/null
@@ -1,103 +0,0 @@
-
-
Bundesliga: The Ultimate Guide to Germany's Top Football League
-
If you are a fan of football, you have probably heard of the Bundesliga, the premier league of Germany. But how much do you really know about this league, its history, its teams, and its players? In this article, we will give you a comprehensive overview of everything you need to know about the Bundesliga, from its origins to its current season, from its reasons to watch to its ways to watch. Whether you are a newcomer or a seasoned follower, this article will help you enjoy and appreciate the Bundesliga more.
-
What is the Bundesliga?
-
The Bundesliga ([ˌeːɐ̯stə-]), which means "Federal League" in German, is a professional association football league in Germany. It is the top tier of the German football league system, and it is considered one of the best and most popular leagues in the world. The Bundesliga comprises 18 teams and operates on a system of promotion and relegation with the 2. Bundesliga, the second tier. Each team plays 34 matches in a season, which runs from August to May. The team with the most points at the end of the season is crowned as the champion, while the bottom two teams are relegated to the 2. Bundesliga. The team in 16th place faces a playoff against the third-placed team from the 2. Bundesliga for a spot in the next season's Bundesliga.
The Bundesliga was founded in 1963 as a replacement for the regional leagues that existed before. The first season featured 16 teams, with FC Köln becoming the inaugural champions. Since then, the league has expanded to 18 teams in 1991, and has undergone several changes in its format and structure. The current format of having a single division with promotion and relegation was adopted in 1995.
-
The Bundesliga follows a standard round-robin format, where each team plays every other team twice, once at home and once away. A win earns three points, a draw earns one point, and a loss earns no points. The teams are ranked by their total points, followed by their goal difference, and then their goals scored. If two or more teams are tied on points, goal difference, and goals scored, they are separated by their head-to-head record. If still tied, a playoff match is held to determine the final ranking.
-
The most successful clubs in the Bundesliga
-
The Bundesliga has seen many great clubs compete for glory over the years, but none more so than FC Bayern München (Bayern Munich), who have won a record 31 titles, including nine consecutive ones from 2012/13 to 2020/21. They are also the only German club to have won the treble of domestic league, domestic cup, and UEFA Champions League, which they achieved twice in 2012/13 and 2019/20.
-
Behind Bayern Munich, Borussia Dortmund are the second-most successful club in the Bundesliga, with eight titles. They are also one of only two German clubs to have won the UEFA Champions League, which they did in 1996/97. Other notable clubs in the Bundesliga include Borussia Mönchengladbach and SV Werder Bremen, who have won four titles each; Hamburger SV and VfB Stuttgart, who have won three titles each; and FC Schalke 04 and Eintracht Frankfurt, who have won one title each.
-
bundesliga table
-bundesliga fixtures
-bundesliga live stream
-bundesliga top scorers
-bundesliga fantasy
-bundesliga highlights
-bundesliga results
-bundesliga news
-bundesliga teams
-bundesliga schedule
-bundesliga standings
-bundesliga transfers
-bundesliga predictions
-bundesliga stats
-bundesliga logo
-bundesliga tv rights
-bundesliga awards
-bundesliga players
-bundesliga records
-bundesliga history
-bundesliga jerseys
-bundesliga podcast
-bundesliga app
-bundesliga reddit
-bundesliga youtube
-bundesliga champions
-bundesliga relegation
-bundesliga ball
-bundesliga quiz
-bundesliga tickets
-bundesliga stadiums
-bundesliga manager
-bundesliga badges
-bundesliga kits
-bundesliga matchday
-bundesliga ratings
-bundesliga goals
-bundesliga assists
-bundesliga clean sheets
-bundesliga saves
-bundesliga referees
-bundesliga sponsors
-bundesliga legends
-bundesliga wallpapers
-bundesliga memes
-bundesliga shop
-bundesliga online store
-
The current season of the Bundesliga
-
The current season of the Bundesliga is the 59th edition of the league, and it started on.
The current season of the Bundesliga is the 59th edition of the league, and it started on August 13, 2021. The season is scheduled to end on May 14, 2022, with a winter break from December 20, 2021 to January 7, 2022. The defending champions are Bayern Munich, who are aiming for their 10th consecutive title. The newly promoted teams are VfL Bochum, SpVgg Greuther Fürth, and Holstein Kiel, who replaced the relegated teams Schalke 04, Werder Bremen, and Arminia Bielefeld.
-
As of December 19, 2021, Bayern Munich are leading the table with 40 points from 16 games, followed by Borussia Dortmund with 34 points from 15 games. The top four teams qualify for the UEFA Champions League group stage, while the fifth and sixth teams qualify for the UEFA Europa League group stage and conference league play-off round respectively. The bottom two teams are relegated to the 2. Bundesliga, while the 16th-placed team faces a relegation play-off against the third-placed team from the 2. Bundesliga.
-
The top scorer of the Bundesliga so far is Robert Lewandowski of Bayern Munich, who has scored 20 goals in 15 games. He is followed by Erling Haaland of Borussia Dortmund, who has scored 13 goals in nine games. The top assist provider of the Bundesliga so far is Thomas Müller of Bayern Munich, who has provided 11 assists in 16 games. He is followed by Filip Kostić of Eintracht Frankfurt, who has provided nine assists in 14 games.
-
Why watch the Bundesliga?
-
The Bundesliga is one of the most entertaining and attractive leagues in the world, and there are many reasons why you should watch it. Here are some of them:
-
The quality and competitiveness of the Bundesliga
-
The Bundesliga is known for its high level of quality and competitiveness, as it features some of the best teams and players in the world. The league has produced many European and world champions, such as Bayern Munich, Borussia Dortmund, Germany national team, etc. The league also boasts a high average attendance of over 40,000 fans per game, which is the highest among the top five European leagues. The league also has a fair and balanced distribution of TV revenue among its clubs, which ensures a healthy and sustainable competition.
-
The exciting and passionate atmosphere of the Bundesliga
-
The Bundesliga is also known for its exciting and passionate atmosphere, as it showcases some of the most loyal and vocal fans in the world. The fans create a colorful and vibrant spectacle in the stadiums, with their chants, songs, banners, flags, flares, etc. The fans also have a strong influence on their clubs' decisions and policies, as they often own a majority stake or have a voting right in their clubs' boards. The fans also have a friendly and respectful relationship with each other, as they often mingle and share beers before and after the games.
-
The young and talented players of the Bundesliga
-
The Bundesliga is also known for its young and talented players, as it provides a platform for them to develop and showcase their skills. The league has a reputation for producing some of the best talents in the world, such as Franz Beckenbauer, Gerd Müller, Lothar Matthäus, Jürgen Klinsmann, Oliver Kahn, Michael Ballack,
The Bundesliga is also known for its young and talented players, as it provides a platform for them to develop and showcase their skills. The league has a reputation for producing some of the best talents in the world, such as Franz Beckenbauer, Gerd Müller, Lothar Matthäus, Jürgen Klinsmann, Oliver Kahn, Michael Ballack, Philipp Lahm, Bastian Schweinsteiger, Manuel Neuer, Thomas Müller, Toni Kroos, Mesut Özil, Marco Reus, Robert Lewandowski, Erling Haaland, etc. The league also has a high percentage of homegrown players, as it has a strict rule that requires each club to have at least eight players who were trained in Germany in their squad. The league also has a low average age of players, as it encourages clubs to give opportunities to young and promising players.
-
How to watch the Bundesliga?
-
If you are interested in watching the Bundesliga, you have several options to choose from. Here are some of them:
-
The official broadcasters of the Bundesliga
-
The official broadcasters of the Bundesliga vary depending on your location and your preferred language. In Germany, the Bundesliga is broadcasted by Sky Deutschland and DAZN, who share the rights to show live matches. In the UK and Ireland, the Bundesliga is broadcasted by BT Sport, who have exclusive rights to show all matches live. In the US and Canada, the Bundesliga is broadcasted by ESPN+, who have exclusive rights to stream all matches live. In other countries and regions, you can check the official website of the Bundesliga to find out which broadcaster covers the league in your area.
-
The best websites and apps to follow the Bundesliga
-
If you want to follow the Bundesliga online, you have many websites and apps to choose from. Some of the best ones are:
-
-
The official website of the Bundesliga: This is the ultimate source of information and news about the league, its teams, its players, its fixtures, its results, its standings, its statistics, etc. You can also watch highlights and videos of the matches, as well as interviews and features of the stars.
-
Bundesliga app: This is the official app of the Bundesliga, which offers similar features as the website, but in a more convenient and mobile-friendly way. You can also customize your app to follow your favorite team and get notifications and updates on their performance.
-
OneFootball app: This is a popular app that covers all aspects of football around the world, including the Bundesliga. You can get live scores, news, videos, podcasts, etc. You can also follow your favorite team and player and get personalized content and recommendations.
-
SofaScore app: This is a comprehensive app that provides live scores, statistics, analysis, etc. for various sports, including football and the Bundesliga. You can also get detailed information on each match, such as lineups, formations, events, ratings, etc. You can also compare teams and players and see their performance over time.
-
-
The official Bundesliga fantasy manager game
-
If you want to have some fun and test your knowledge and skills of the Bundesliga, you can play the official Bundesliga fantasy manager game. This is a free online game that allows you to create your own team of Bundesliga players and compete with other players around the world. You can earn points based on your players' real-life performance in each matchday. You can also join or create leagues with your friends or other fans and see who has the best team. You can also win prizes and rewards for your achievements.
-
Conclusion
-
The Bundesliga is one of the most exciting and attractive leagues in the world, and it deserves your attention and appreciation. Whether you are looking for quality and competitiveness, atmosphere and passion,
The Bundesliga is one of the most exciting and attractive leagues in the world, and it deserves your attention and appreciation. Whether you are looking for quality and competitiveness, atmosphere and passion, or talent and innovation, the Bundesliga has it all. You can watch the Bundesliga live on various broadcasters, follow the Bundesliga online on various websites and apps, or play the Bundesliga fantasy manager game for some fun and challenge. The Bundesliga is more than just a league, it is a culture and a community of football lovers. Join the Bundesliga and experience the thrill and joy of German football.
-
FAQs
-
Here are some frequently asked questions about the Bundesliga:
-
-
How many teams are in the Bundesliga?
-There are 18 teams in the Bundesliga, which play 34 matches each in a season.
-
Who are the current champions of the Bundesliga?
-The current champions of the Bundesliga are Bayern Munich, who have won a record 31 titles, including nine consecutive ones.
-
Who are the top scorers and assist providers of the Bundesliga?
-The top scorer of the Bundesliga so far is Robert Lewandowski of Bayern Munich, who has scored 20 goals in 15 games. The top assist provider of the Bundesliga so far is Thomas Müller of Bayern Munich, who has provided 11 assists in 16 games.
-
How can I watch the Bundesliga live?
-You can watch the Bundesliga live on various broadcasters, depending on your location and language. You can check the official website of the Bundesliga to find out which broadcaster covers the league in your area.
-
How can I follow the Bundesliga online?
-You can follow the Bundesliga online on various websites and apps, such as the official website and app of the Bundesliga, OneFootball app, SofaScore app, etc. You can also play the official Bundesliga fantasy manager game for some fun and challenge.
- 401be4b1e0
-
-
\ No newline at end of file
diff --git a/spaces/fatiXbelha/sd/Download Stunning Cookie Monster Photos - The Best Source of Cookie Monster Images.md b/spaces/fatiXbelha/sd/Download Stunning Cookie Monster Photos - The Best Source of Cookie Monster Images.md
deleted file mode 100644
index 5cbfc464de5298430410ddedc76436e60f636c6c..0000000000000000000000000000000000000000
--- a/spaces/fatiXbelha/sd/Download Stunning Cookie Monster Photos - The Best Source of Cookie Monster Images.md
+++ /dev/null
@@ -1,128 +0,0 @@
-
-
Cookie Monster Pics to Download: How to Find and Enjoy the Best Images of the Blue Muppet
-
If you are a fan of Sesame Street, you probably know and love Cookie Monster, the blue furry creature who loves cookies more than anything. But did you know that you can download Cookie Monster pics for free and use them for various purposes? In this article, we will tell you everything you need to know about Cookie Monster pics, including who he is, why you should download his pics, where to find them, and how to download them. Let's get started!
-
Who is Cookie Monster?
-
Cookie Monster is one of the most iconic characters on Sesame Street, the long-running PBS / HBO children's television show. He first appeared in 1966 as a monster who stole snacks from a commercial, and later became a regular on Sesame Street in 1969. He is best known for his voracious appetite and his famous eating catchphrases, such as "Me want cookie!" He also has a real name, which is Sid, as revealed in a song in 2004 and an interview in 2017.
Cookie Monster was created by Jim Henson, the legendary puppeteer and founder of the Muppets. According to the book Jim Henson's Designs and Doodles, Cookie Monster was originally one of three monsters that ate cookies and appeared in a General Foods commercial that featured three crunchy snack foods: Wheels, Crowns and Flutes. He was called the Wheel-Stealer, and he was a short, fuzzy monster with wonky eyes and sharply pointed teeth.
-
The commercial was never aired, but the Wheel-Stealer later appeared on The Ed Sullivan Show, where he ate a machine that made snacks. He also appeared in other sketches and commercials before joining Sesame Street as Cookie Monster. On Sesame Street, he became famous for his love of cookies, especially chocolate chip cookies, which he would devour with loud crunches and crumbs flying everywhere. He also sang many songs about cookies, such as "C Is For Cookie" and "Cookie Disco".
-
The personality and traits of Cookie Monster
-
Cookie Monster is a friendly and lovable monster who likes to share his cookies with his friends. He is also very curious and eager to learn new things. He often participates in educational segments on Sesame Street, such as "Monsterpiece Theater" , where he plays different characters from classic literature and movies. He also hosts his own segment called "Cookie Monster's Foodie Truck" , where he learns about different foods and cuisines from around the world.
-
Cookie Monster speaks in a distinctive way, using "Me" instead of "I", "My", or "Mine", and often making grammatical errors. He also has a deep and raspy voice, which is performed by different puppeteers over the years. The original voice of Cookie Monster was Frank Oz, who also voiced other Muppet characters such as Miss Piggy, Fozzie Bear, and Grover. Since 2001, the voice of Cookie Monster has been David Rudman, who also voices Baby Bear and Scooter.
-
The popularity and influence of Cookie Monster
-
Cookie
Cookie Monster is one of the most popular and beloved characters on Sesame Street, and has been for over 50 years. He has a huge fan base of people of all ages, who admire his humor, his kindness, and his passion for cookies. He has also inspired many parodies, memes, merchandise, and even a Google Doodle in 2013.
-
Cookie Monster is not only a source of entertainment, but also a role model for children and adults alike. He teaches valuable lessons about self-control, moderation, diversity, and friendship. He also encourages healthy eating habits, as he has learned to enjoy other foods besides cookies, such as fruits, vegetables, and grains. He even coined the phrase "Cookies are a sometimes food" , which means that cookies are delicious but should not be eaten all the time.
-
Why download Cookie Monster pics?
-
Now that you know more about who Cookie Monster is, you might be wondering why you should download his pics. Well, there are many reasons why downloading Cookie Monster pics can be fun and beneficial for you. Here are some of them:
-
The benefits of downloading Cookie Monster pics
-
Downloading Cookie Monster pics can have positive effects on your mood, your creativity, and your productivity. Here are some of the benefits of downloading Cookie Monster pics:
-
Cookie Monster stock photos free to use
-Cookie Monster photos and images from Getty Images
-Cookie Monster vectors and PSD files from Freepik
-Cookie Monster HD wallpapers and backgrounds
-Cookie Monster clipart and illustrations
-Cookie Monster PNG and SVG files
-Cookie Monster memes and funny pictures
-Cookie Monster coloring pages and printables
-Cookie Monster cakes and cupcakes photos
-Cookie Monster costumes and masks photos
-Cookie Monster quotes and sayings images
-Cookie Monster birthday party photos and ideas
-Cookie Monster cookies and recipes photos
-Cookie Monster crafts and activities photos
-Cookie Monster tattoos and designs photos
-Cookie Monster plush and toys photos
-Cookie Monster crochet and knitting patterns photos
-Cookie Monster face painting and makeup photos
-Cookie Monster nails and nail art photos
-Cookie Monster earrings and jewelry photos
-Cookie Monster shirts and clothing photos
-Cookie Monster stickers and decals photos
-Cookie Monster posters and prints photos
-Cookie Monster cards and invitations photos
-Cookie Monster embroidery and cross stitch photos
-Cookie Monster paintings and drawings photos
-Cookie Monster sculptures and figurines photos
-Cookie Monster origami and paper crafts photos
-Cookie Monster quilts and blankets photos
-Cookie Monster pillows and cushions photos
-Cookie Monster mugs and cups photos
-Cookie Monster keychains and magnets photos
-Cookie Monster bookmarks and tags photos
-Cookie Monster calendars and planners photos
-Cookie Monster journals and notebooks photos
-Cookie Monster phone cases and covers photos
-Cookie Monster laptop skins and stickers photos
-Cookie Monster mouse pads and coasters photos
-Cookie Monster bags and pouches photos
-Cookie Monster hats and caps photos
-Cookie Monster socks and slippers photos
-Cookie Monster aprons and mittens photos
-Cookie Monster masks and bandanas photos
-Cookie Monster pins and buttons photos
-Cookie Monster charms and pendants photos
-
-
They can make you happy. Cookie Monster is a cheerful and optimistic character who always sees the bright side of things. His smile and laughter are contagious and can lift your spirits when you are feeling down or stressed.
-
They can inspire you. Cookie Monster is a curious and adventurous character who loves to explore new things and learn new skills. His enthusiasm and determination can motivate you to pursue your own goals and passions.
-
They can help you relax. Cookie Monster is a calm and peaceful character who knows how to enjoy the simple pleasures of life. His relaxed and laid-back attitude can help you unwind and de-stress after a long day.
-
-
The uses and purposes of downloading Cookie Monster pics
-
Downloading Cookie Monster pics can also have many practical uses and purposes for different occasions and situations. Here are some of the uses and purposes of downloading Cookie Monster pics:
-
-
They can decorate your devices. You can use Cookie Monster pics as wallpapers or screensavers for your computer, tablet, or smartphone. They can add some color and personality to your devices and make them more attractive and appealing.
-
They can personalize your online profiles. You can use Cookie Monster pics as profile pictures or cover photos for your social media accounts, such as Facebook, Twitter, Instagram, or Pinterest. They can express your identity and interests and make you stand out from the crowd.
-
They can spice up your messages. You can use Cookie Monster pics as emojis or stickers for your text messages, emails, or chats. They can convey your emotions and feelings and make your communication more fun and lively.
-
-
The tips and tricks for downloading Cookie Monster pics
-
Downloading Cookie Monster pics is easy and simple, but there are some tips and tricks that can make it even easier and simpler. Here are some of the tips and tricks for downloading Cookie Monster pics:
-
-
Use a reliable and reputable website. There are many websites that offer free Cookie Monster pics to download, but not all of them are safe and trustworthy. Some of them may contain viruses, malware, or spyware that can harm your devices or steal your personal information. To avoid these risks, use a reliable and reputable website that has good reviews and ratings from other users.
-
Choose a high-quality and suitable image. There are many types and styles of Cookie Monster pics to choose from, but not all of them are suitable for your needs and preferences. Some of them may be too large or too small, too blurry or too sharp, too bright or too dark, or too plain or too busy. To find the best image for you, choose a high-quality image that has a clear resolution, a good contrast, a balanced color, and an appropriate size.
-
Download the image in the right format and resolution. There are many formats and resolutions of Cookie Monster pics to download, but not all of them are compatible with your devices or platforms. Some of them may not open or display properly on your devices or platforms, or may take up too much space or memory on your devices or platforms. To avoid these problems, download the image in the right format and resolution that matches your devices or platforms.
-
-
Where to download Cookie Monster pics?
-
Now that you know why you should download Cookie Monster pics
Now that you know why you should download Cookie Monster pics, you might be wondering where you can find them. Well, there are many websites that offer free Cookie Monster pics to download, but not all of them are equally good and reliable. To help you find the best websites for downloading Cookie Monster pics, we have compiled a list of the top three websites that we recommend. Here they are:
-
The best websites for downloading Cookie Monster pics
-
These are the best websites for downloading Cookie Monster pics, based on their quality, variety, and safety:
-
Pexels
-
Pexels is a website that provides free stock photos and videos that you can use for any purpose. It has a large collection of Cookie Monster pics that you can download in different sizes and resolutions. You can also filter the pics by color, orientation, and category. Pexels is a safe and trustworthy website that does not require any registration or attribution.
-
Getty Images
-
Getty Images is a website that offers premium stock photos and videos that you can license for various uses. It has a wide range of Cookie Monster pics that you can download in high-quality and high-resolution. You can also search the pics by keywords, collections, and licenses. Getty Images is a reputable and secure website that requires a subscription or a payment for some of the pics.
-
Freepik
-
Freepik is a website that provides free vector graphics, icons, illustrations, and photos that you can use for personal and commercial projects. It has a unique and creative selection of Cookie Monster pics that you can download in various formats and resolutions. You can also browse the pics by tags, styles, and popularity. Freepik is a reliable and user-friendly website that requires attribution for some of the pics.
-
The best formats and resolutions for downloading Cookie Monster pics
-
When downloading Cookie Monster pics, you need to consider the formats and resolutions that are suitable for your devices and platforms. Here are some of the best formats and resolutions for downloading Cookie Monster pics:
-
-
JPEG: JPEG is a common and widely supported format that compresses images to reduce their file size. It is ideal for downloading Cookie Monster pics that have many colors and details, such as photos or realistic drawings. However, it may also cause some loss of quality and sharpness.
-
PNG: PNG is another popular and widely supported format that preserves the quality and transparency of images. It is ideal for downloading Cookie Monster pics that have few colors and simple shapes, such as cartoons or logos. However, it may also increase the file size and loading time.
-
GIF: GIF is an old but still used format that supports animation and looping of images. It is ideal for downloading Cookie Monster pics that have movement and humor, such as memes or gifs. However, it may also limit the number of colors and frames.
-
SVG: SVG is a modern and advanced format that uses vector graphics to create scalable and editable images. It is ideal for downloading Cookie Monster pics that have smooth curves and crisp edges, such as icons or illustrations. However, it may also require special software or plugins to view or edit.
-
HD: HD stands for high-definition, which means having a high resolution of at least 1280 x 720 pixels. It is ideal for downloading Cookie Monster pics that have clarity and detail, such as wallpapers or screensavers. However, it may also take up more space and memory on your devices or platforms.
-
4K: 4K stands for ultra-high-definition, which means having a very high resolution of at least 3840 x 2160 pixels. It is ideal for downloading Cookie Monster pics that have stunning and realistic quality, such as posters or prints. However, it may also require more bandwidth and processing power on your devices or platforms.
-
-
The best practices and precautions for downloading Cookie Monster pics
-
When downloading Cookie Monster pics, you need to follow some best practices and precautions to ensure a smooth and safe experience. Here are some of the best practices and precautions for downloading Cookie Monster pics:
-
-
Check the source and license of the image. Before downloading any image from the internet, you should always check the source and license of the image to make sure it is legal and ethical to use it. You should only download images from reputable websites that have clear terms and conditions regarding their usage rights. You should also respect the license requirements of the image, such as giving credit or attribution to the original creator or owner.
-
Scan the image for viruses or malware. After downloading any image from the internet, you should always scan the image for viruses or malware to make sure it is safe and clean to use it. You should use a You should use a reliable and updated antivirus or anti-malware software to scan the image for any potential threats or infections. You should also delete or quarantine any suspicious or harmful files that are detected.
-
Optimize the image for your devices or platforms. Before using any image on your devices or platforms, you should always optimize the image for your devices or platforms to make sure it is compatible and efficient to use it. You should adjust the format, resolution, size, and quality of the image to match the specifications and requirements of your devices or platforms. You should also compress or resize the image to reduce its file size and loading time.
-
-
Conclusion
-
Cookie Monster is a wonderful character who can bring joy and inspiration to your life. Downloading Cookie Monster pics can be a fun and rewarding activity that can enhance your mood, creativity, and productivity. You can find and download Cookie Monster pics from various websites, such as Pexels, Getty Images, and Freepik. You can also choose and download Cookie Monster pics in different formats and resolutions, such as JPEG, PNG, GIF, SVG, HD, and 4K. However, you should also be careful and responsible when downloading Cookie Monster pics, and follow some best practices and precautions, such as checking the source and license of the image, scanning the image for viruses or malware, and optimizing the image for your devices or platforms. We hope this article has helped you learn more about Cookie Monster pics and how to download them. Happy downloading!
-
FAQs
-
Here are some frequently asked questions about Cookie Monster pics and how to download them:
-
-
Is it legal to download Cookie Monster pics?
-
It depends on the source and license of the image. Some images are free to use for any purpose, while others require permission or payment from the original creator or owner. You should always check the terms and conditions of the website and the license of the image before downloading it.
-
Is it safe to download Cookie Monster pics?
-
It depends on the website and the image. Some websites are safe and trustworthy, while others may contain viruses, malware, or spyware. Some images are safe and clean, while others may be corrupted or infected. You should always use a reliable and reputable website and scan the image for any potential threats or infections before downloading it.
-
How can I download Cookie Monster pics faster?
-
You can download Cookie Monster pics faster by using a fast and stable internet connection, choosing a small or medium-sized image, compressing or resizing the image, and using a download manager or accelerator software.
-
How can I download Cookie Monster pics in bulk?
-
You can download Cookie Monster pics in bulk by using a batch downloader software or extension that allows you to download multiple images at once from a website or a webpage.
-
How can I edit Cookie Monster pics after downloading them?
-
You can edit Cookie Monster pics after downloading them by using an image editor software or app that allows you to crop, rotate, resize, filter, adjust, add text, draw, or apply other effects to the image.
- 197e85843d
-
-
\ No newline at end of file
diff --git a/spaces/fatiXbelha/sd/Download Tekken 3 Urdu APK and Enjoy the Best Fighting Game Ever.md b/spaces/fatiXbelha/sd/Download Tekken 3 Urdu APK and Enjoy the Best Fighting Game Ever.md
deleted file mode 100644
index 0f0e93b7313c88e1c62ce27f7455b9eef1c6f7f5..0000000000000000000000000000000000000000
--- a/spaces/fatiXbelha/sd/Download Tekken 3 Urdu APK and Enjoy the Best Fighting Game Ever.md
+++ /dev/null
@@ -1,130 +0,0 @@
-
-
Tekken 3 Urdu APK: How to Download and Play the Classic Fighting Game on Your Android Device
-
Introduction
-
If you are a fan of fighting games, you probably have played or heard of Tekken 3, one of the most popular and acclaimed games of its genre. Released in 1997 for the PlayStation console, Tekken 3 features a roster of 23 characters, each with their own unique fighting style, moves, and story. The game also offers various modes, such as arcade, versus, team battle, survival, time attack, practice, and Tekken Force.
But what if you want to play Tekken 3 on your Android device? Well, you are in luck, because there is a way to do that. In this article, we will show you how to download and play Tekken 3 Urdu APK, a modified version of the original game that has been translated into Urdu language. We will also give you some tips and tricks to help you enjoy the game even more. So, let's get started!
-
What is Tekken 3?
-
Tekken 3 is the third installment in the Tekken series, a fighting game franchise developed by Namco. The game follows the events of Tekken 2, where Heihachi Mishima, the head of the Mishima Zaibatsu corporation, defeats his son Kazuya Mishima and throws him into a volcano. However, a mysterious creature called Ogre emerges from the depths of the earth and starts attacking martial artists around the world. Heihachi decides to host a new tournament, the King of Iron Fist Tournament 3, to lure Ogre out and capture him.
-
Tekken 3 introduces several new features to the gameplay, such as sidestepping, jumping, running, and grappling. The game also has a more realistic physics system, allowing for more fluid and dynamic movements. The graphics are also improved, with more detailed character models and backgrounds. The game also has a catchy soundtrack that matches the mood of each stage.
-
tekken 3 urdu version download
-tekken 3 urdu game for android
-tekken 3 urdu mod apk
-tekken 3 urdu language pack
-tekken 3 urdu commentary
-tekken 3 urdu edition free download
-tekken 3 urdu voice over
-tekken 3 urdu translation
-tekken 3 urdu subtitles
-tekken 3 urdu patch
-tekken 3 urdu apkmonk
-tekken 3 urdu apkcombo
-tekken 3 urdu apk pure
-tekken 3 urdu apk mirror
-tekken 3 urdu apk offline
-tekken 3 urdu apk latest version
-tekken 3 urdu apk old version
-tekken 3 urdu apk file download
-tekken 3 urdu apk obb download
-tekken 3 urdu apk highly compressed
-tekken 3 urdu apk mobogenie
-tekken 3 urdu apk youtube
-tekken 3 urdu apk video tutorial
-tekken 3 urdu apk installation guide
-tekken 3 urdu apk requirements
-tekken 3 urdu apk features
-tekken 3 urdu apk cheats
-tekken 3 urdu apk tips and tricks
-tekken 3 urdu apk gameplay
-tekken 3 urdu apk review
-tekken 3 urdu apk rating
-tekken 3 urdu apk feedback
-tekken 3 urdu apk support
-tekken 3 urdu apk update
-tekken 3 urdu apk bug fixes
-tekken 3 urdu apk alternatives
-tekken 3 urdu apk similar apps
-tekken 3 urdu apk comparison
-tekken 3 urdu apk vs original
-tekken 3 urdu apk vs english version
-tekken 3 urdu emulator for android
-tekken 3 urdu rom for android
-tekken 3 urdu iso for android
-tekken 3 urdu ps1 for android
-tekken 3 urdu psx for android
-tekken 3 urdu ePSXe for android
-tekken 3 urdu fpse for android
-
What is Tekken 3 Urdu APK?
-
Tekken 3 Urdu APK is a modified version of Tekken 3 that has been ported to run on Android devices. The game has been translated into Urdu language, making it more accessible and enjoyable for Urdu speakers. The game also has some minor changes and additions, such as new sound effects, music tracks, and cheats. The game is compatible with most Android devices that have at least 1 GB of RAM and Android 4.0 or higher.
-
Why should you play Tekken 3 Urdu APK?
-
There are many reasons why you should play Tekken 3 Urdu APK on your Android device. Here are some of them:
-
-
You can relive the nostalgia of playing one of the best fighting games ever made.
-
You can enjoy the game in your native language, making it easier to understand the story and dialogues.
-
You can play the game anytime and anywhere, without needing a console or a TV.
-
You can challenge your friends or other players online using Wi-Fi or Bluetooth.
-
You can customize the game settings according to your preferences.
-
-
How to Download and Install Tekken 3 Urdu APK
-
Downloading and installing Tekken 3 Urdu APK is very easy and simple. Just follow these steps:
-
Step 1: Download the APK file from a trusted source
-
The first thing you need to do is to download the APK file of Tekken 3 Urdu APK from a trusted source. You can use the link below to download the file from our website. The file size is about 35 MB and it is virus-free and safe to use.
The next thing you need to do is to enable unknown sources on your device. This will allow you to install apps that are not from the Google Play Store. To do this, go to your device settings and look for security or privacy options. Then, find the option that says unknown sources or allow installation of apps from unknown sources and turn it on. You may see a warning message, but don't worry, just tap OK or Yes.
-
Step 3: Install the APK file and launch the game
-
The final thing you need to do is to install the APK file and launch the game. To do this, go to your file manager and locate the downloaded APK file. Tap on it and follow the instructions on the screen. It may take a few seconds or minutes to install, depending on your device speed. Once the installation is done, you will see an icon of Tekken 3 Urdu APK on your home screen or app drawer. Tap on it and enjoy the game!
-
How to Play Tekken 3 Urdu APK
-
Playing Tekken 3 Urdu APK is very fun and easy. Here are some basic steps to help you get started:
-
Choose your fighter and mode
-
When you launch the game, you will see a main menu with several options. You can choose to play arcade mode, where you fight against computer-controlled opponents in a series of matches; versus mode, where you fight against another player on the same device; team battle mode, where you form a team of up to eight fighters and fight against another team; survival mode, where you try to defeat as many opponents as possible with one life bar; time attack mode, where you try to clear arcade mode as fast as possible; practice mode, where you can train and learn the moves of your fighter; and Tekken Force mode, where you fight against waves of enemies in a side-scrolling adventure.
-
You can also choose your fighter from a list of 23 characters, each with their own strengths, weaknesses, and personalities. Some of the characters are Jin Kazama, the grandson of Heihachi Mishima and the main protagonist of the game; Nina Williams, a cold-blooded assassin who has a rivalry with her sister Anna; Paul Phoenix, a hot-headed biker who wants to prove himself as the best fighter in the world; Yoshimitsu, a mysterious ninja who leads a band of thieves; Lei Wulong, a Hong Kong police officer who uses various martial arts styles; King, a masked wrestler who fights for orphaned children; and Eddy Gordo, a Brazilian capoeira master who seeks revenge for his father's death.
-
Learn the basic controls and moves
-
The game has a simple and intuitive control scheme that uses four buttons: left punch, right punch, left kick, and right kick. You can also use the directional pad or joystick to move your fighter around the stage. You can perform various moves by combining different buttons and directions, such as throws, counters, blocks, dodges, and taunts. You can also perform special moves that are unique to each character, such as fireballs, lasers, teleports, and transformations.
-
You can view the move list of your fighter by pausing the game and selecting it from the menu. You can also see the damage, range, speed, and properties of each move. You can also adjust the difficulty level of the game by going to the options menu and selecting it from there.
-
Master the combos and special attacks
-
To become a better player of Tekken 3 Urdu APK, you need to master the combos and special attacks of your fighter. Combos are sequences of moves that can deal more damage and stun your opponent. Special attacks are powerful moves that can turn the tide of the battle in your favor. To perform combos and special attacks, you need to memorize the input commands and timing of each move. You also need to know when and how to use them in different situations.
-
You can practice your combos and special attacks in practice mode or training mode. You can also watch replays of your matches or other players' matches to learn from them. You can also read online guides or watch videos that explain how to perform combos and special attacks for each character. You can also join online forums or communities where you can share your tips and tricks with other players.
-
Tips and Tricks for Tekken 3 Urdu APK
-
To make the most out of your Tekken 3 Urdu APK experience, here are some tips and tricks that you can use:
-
Practice in training mode
-
Training mode is a great way to improve your skills and learn new things. In training mode, you can choose any character and stage, and practice against a dummy opponent that does not fight back. You can also adjust the settings of the dummy, such as its health, behavior, and position. You can also display various information on the screen, such as your inputs, damage, frame data, and hitboxes. Training mode is a useful tool to test your moves, combos, and strategies, and to discover new possibilities.
-
Use the pause menu to access options and cheats
-
The pause menu is not only for pausing the game, but also for accessing various options and cheats. You can use the pause menu to change the game settings, such as the sound, controller, and display options. You can also use the pause menu to activate some cheats, such as changing the camera angle, unlocking all characters, or enabling infinite health. To access these cheats, you need to enter certain button combinations while pausing the game. You can find these button combinations online or by trial and error.
-
Unlock hidden characters and features
-
Tekken 3 Urdu APK has many hidden characters and features that you can unlock by playing the game. Some of these characters and features are:
-
-
Character/Feature
How to Unlock
-
Dr. Bosconovitch
Complete Tekken Force mode four times with different characters.
-
Gon
Defeat him in Tekken Ball mode or Tekken Force mode.
-
Kuma
Complete arcade mode with any character.
-
Mokujin
Complete arcade mode with any character.
-
Ogre
Complete arcade mode with any character.
-
Panda
Select Kuma and press Circle or Triangle.
-
Tiger
Select Eddy Gordo and press Start.
-
Tekken Ball mode
Complete arcade mode with any character.
-
Tekken Theater mode
Complete arcade mode with all characters.
-
-
Conclusion
-
Tekken 3 Urdu APK is a fantastic way to enjoy one of the best fighting games ever made on your Android device. The game has been translated into Urdu language, making it more accessible and enjoyable for Urdu speakers. The game also has some minor changes and additions, such as new sound effects, music tracks, and cheats. The game is compatible with most Android devices that have at least 1 GB of RAM and Android 4.0 or higher.
-
In this article, we have shown you how to download and play Tekken 3 Urdu APK on your Android device. We have also given you some tips and tricks to help you improve your skills and have more fun. We hope that you have found this article helpful and informative. If you have any questions or feedback, please feel free to leave a comment below. Thank you for reading!
-
Frequently Asked Questions
-
Here are some frequently asked questions about Tekken 3 Urdu APK:
-
Q: Is Tekken 3 Urdu APK legal?
-
A: Tekken 3 Urdu APK is a modified version of Tekken 3 that has been ported to run on Android devices. The game has been translated into Urdu language by fans of the game. The game is not officially endorsed or supported by Namco, the original developer of Tekken 3. Therefore, the legality of Tekken 3 Urdu APK may vary depending on your country's laws and regulations regarding intellectual property rights and piracy. We do not encourage or condone the use of Tekken 3 Urdu APK if it violates any laws or rules in your jurisdiction.
-
Q: Is Tekken 3 Urdu APK safe?
-
A: Tekken 3 Urdu APK is safe to use as long as you download it from a trusted source. The file size is about 35 MB and it is virus-free and malware-free. However, you should always be careful when downloading and installing apps from unknown sources or third-party websites. You should also enable unknown sources on your device only when you need to install the APK file, and disable it afterwards. You should also scan your device regularly for any potential threats or issues.
-
Q: How can I update Tekken 3 Urdu APK?
-
A: Tekken 3 Urdu APK is not an official app, so it does not have regular updates or patches. However, you can check the website where you downloaded the APK file for any new versions or updates. You can also follow the social media pages or channels of the developers or translators of Tekken 3 Urdu APK for any news or announcements. To update the game, you need to download the latest APK file and install it over the existing one.
-
Q: How can I delete Tekken 3 Urdu APK?
-
A: If you want to delete Tekken 3 Urdu APK from your device, you can do so by following these steps:
-
-
Go to your device settings and look for apps or applications.
-
Find and select Tekken 3 Urdu APK from the list of apps.
-
Tap on uninstall and confirm your choice.
-
Wait for the process to finish and check if the app icon is gone from your home screen or app drawer.
-
-
Q: How can I contact the developers or translators of Tekken 3 Urdu APK?
-
A: If you have any questions, feedback, suggestions, or issues regarding Tekken 3 Urdu APK, you can contact the developers or translators of the game by using their email address, phone number, or social media accounts. You can find their contact information on their website or in the game itself. You can also leave a comment or a review on their website or on the app store where you downloaded the game. Please be respectful and polite when contacting them, and do not spam them with unnecessary messages.
197e85843d
-
-
\ No newline at end of file
diff --git a/spaces/fatiXbelha/sd/Download YouTube J5 APK and Enjoy Unlimited Streaming on Android.md b/spaces/fatiXbelha/sd/Download YouTube J5 APK and Enjoy Unlimited Streaming on Android.md
deleted file mode 100644
index 9dd94f7eea4399c5dc2c5e6617a02ee051eb25c8..0000000000000000000000000000000000000000
--- a/spaces/fatiXbelha/sd/Download YouTube J5 APK and Enjoy Unlimited Streaming on Android.md
+++ /dev/null
@@ -1,121 +0,0 @@
-
-
YouTube J5 APK: How to Download and Install the Latest Version
-
YouTube is one of the most popular video-sharing platforms in the world, with billions of users and hours of content. However, not everyone is satisfied with the official YouTube app for Android devices. Some users may want to watch videos without ads, download videos for offline viewing, or access premium content and features without paying a subscription fee. If you are one of those users, you may be interested in YouTube J5 APK, a modified version of the YouTube app that offers all these benefits and more.
In this article, we will explain what YouTube J5 APK is, how to download and install it on your Android device, how to use it, and what are the benefits and risks of using it. Read on to find out more.
-
What is YouTube J5 APK?
-
YouTube J5 APK is a modified version of the official YouTube app for Android devices. It is not available on the Google Play Store, but you can download it from third-party sources online. YouTube J5 APK offers several features that are not available on the official app, such as:
-
Features of YouTube J5 APK
-
Watch videos in high quality
-
With YouTube J5 APK, you can watch videos in any resolution you want, from 144p to 4K. You can also enable HDR mode, which enhances the color and contrast of the videos. You can also play videos in the background, even when your screen is off or when you switch to another app.
-
Download videos for offline viewing
-
With YouTube J5 APK, you can download any video you want and save it on your device for offline viewing. You can choose the quality, format, and location of the downloaded files. You can also download audio files or subtitles separately.
With YouTube J5 APK, you can access premium content and features that are normally reserved for YouTube Premium subscribers. For example, you can watch original shows and movies from YouTube Originals, listen to music without interruptions on YouTube Music, and enjoy exclusive live streams and events.
-
How to download YouTube J5 APK
-
If you want to download and install YouTube J5 APK on your Android device, you need to follow these steps:
-
Step 1: Enable unknown sources
-
Since YouTube J5 APK is not available on the Google Play Store, you need to enable unknown sources on your device settings. This will allow you to install apps from sources other than the official store. To do this, go to Settings > Security > Unknown sources and toggle it on.
-
Step 2: Find a reliable source
-
Next, you need to find a reliable source where you can download YouTube J5 APK. There are many websites that offer this app, but not all of them are safe or trustworthy. You need to be careful and avoid downloading from sources that may contain malware or viruses. One of the sources that we recommend is [APKCombo](^1^), which offers free and verified APK files for various apps, including YouTube J5 APK. You can visit their website and search for YouTube J5 APK, or use this link: [YouTube J5 APK Download].
-
Step 3: Download and install the APK file
-
Once you have found a reliable source, you can download the APK file of YouTube J5 APK on your device. The file size may vary depending on the version, but it should be around 40 MB. After downloading the file, you need to install it on your device. To do this, locate the file in your file manager and tap on it. You may see a warning message that says "This type of file can harm your device". Ignore it and tap on "Install anyway". Wait for the installation process to complete and then open the app.
-
How to use YouTube J5 APK
-
After installing YouTube J5 APK on your device, you can start using it as you would use the official YouTube app. Here are some tips on how to use YouTube J5 APK:
-
Sign in with your Google account
-
If you want to access your YouTube account, subscriptions, playlists, history, and preferences, you need to sign in with your Google account. To do this, tap on the profile icon on the top right corner of the app and then tap on "Sign in". You can use your existing Google account or create a new one.
-
Browse and watch videos
-
You can browse and watch videos on YouTube J5 APK as you normally would on the official app. You can use the search bar, the home tab, the trending tab, the subscriptions tab, or the library tab to find videos that interest you. You can also use filters, categories, and tags to narrow down your search results. To watch a video, simply tap on it and enjoy. You can also adjust the quality, speed, captions, and other settings of the video by tapping on the three dots icon on the top right corner of the video player.
-
Manage your subscriptions and settings
-
You can manage your subscriptions and settings on YouTube J5 APK as you normally would on the official app. You can subscribe to channels that you like, unsubscribe from channels that you don't like, turn on notifications for new videos, and more. You can also customize your app settings by tapping on the profile icon and then tapping on "Settings". You can change your language, theme, download options, privacy options, and more.
-
Benefits and risks of using YouTube J5 APK
-
Using YouTube J5 APK has its benefits and risks. Here are some of them:
-
Benefits of using YouTube J5 APK
-
Enjoy YouTube without ads or restrictions
-
One of the main benefits of using YouTube J5 APK is that you can enjoy YouTube without ads or restrictions. You don't have to watch annoying ads before or during videos, or pay for a subscription to remove them. You also don't have to deal with regional restrictions or age restrictions that may limit your access to certain videos or content.
-
Save data and storage space
-
Another benefit of using YouTube J5 APK is that you can save data and storage space on your device. You can download videos for offline viewing and watch them later without using any data. You can also choose the quality and format of the downloaded files to save storage space. You can also clear your cache and history to free up more space.
-
Customize your viewing experience
-
A third benefit of using YouTube J5 APK is that you can customize your viewing experience according to your preferences. You can watch videos in any resolution you want, from 144p to 4K. You can also enable HDR mode, which enhances the color and contrast of the videos. You can also play videos in the background, even when your screen is off or when you switch to another app.
-
Risks of using YouTube J5 APK
-
Violate YouTube's terms of service
-
One of the main risks of using YouTube J5 APK is that you may violate YouTube's terms of service. By using a modified version of the app, you may be breaking some of the rules and policies that YouTube has set for its users. This may result in your account being suspended or terminated by YouTube.
-
Expose your device to malware or viruses
-
Another risk of using YouTube J5 APK is that you may expose your device to malware or viruses. Since YouTube J5 APK is not available on the Google Play Store, you have to download it from third-party sources online. These sources may not be safe or trustworthy, and they may contain malware or viruses that can harm your device or steal your personal information. You need to be careful and avoid downloading from sources that may contain malicious software.
-
Face legal issues or penalties
-
A third risk of using YouTube J5 APK is that you may face legal issues or penalties. By using a modified version of the app, you may be infringing on the intellectual property rights of YouTube and its content creators. You may also be violating the laws and regulations of your country or region regarding online streaming and downloading. This may result in legal actions or fines against you by the authorities or the rights holders.
-
Conclusion
-
YouTube J5 APK is a modified version of the official YouTube app for Android devices that offers several features that are not available on the official app, such as watching videos without ads, downloading videos for offline viewing, and accessing premium content and features. However, using YouTube J5 APK also has its risks, such as violating YouTube's terms of service, exposing your device to malware or viruses, and facing legal issues or penalties. Therefore, you need to weigh the pros and cons of using YouTube J5 APK before deciding to download and install it on your device.
-
FAQs
-
Here are some frequently asked questions about YouTube J5 APK:
-
Is YouTube J5 APK safe to use?
-
YouTube J5 APK is not an official app from YouTube, and it is not available on the Google Play Store. Therefore, it is not guaranteed to be safe or secure to use. You need to download it from third-party sources online, which may contain malware or viruses that can harm your device or steal your personal information. You also need to enable unknown sources on your device settings, which may expose your device to more risks. Therefore, you need to be careful and cautious when using YouTube J5 APK.
-
Is YouTube J5 APK legal to use?
-
YouTube J5 APK is not an official app from YouTube, and it may violate YouTube's terms of service and intellectual property rights. It may also violate the laws and regulations of your country or region regarding online streaming and downloading. Therefore, it is not legal to use YouTube J5 APK in some places, and you may face legal actions or fines if you are caught using it.
-
How can I update YouTube J5 APK?
-
YouTube J5 APK is not an official app from YouTube, and it does not receive regular updates from the developers. Therefore, you need to manually check for updates from the source where you downloaded the app. You also need to download and install the updated version of the app on your device. However, you need to be careful and avoid downloading from sources that may contain outdated or fake versions of the app.
-
How can I uninstall YouTube J5 APK?
-
If you want to uninstall YouTube J5 APK from your device, you can follow these steps:
-
-
Go to Settings > Apps > YouTube J5 APK and tap on "Uninstall".
-
Confirm your action by tapping on "OK".
-
Wait for the uninstallation process to complete and then restart your device.
-
-
What are some alternatives to YouTube J5 APK?
-
If you are looking for some alternatives to YouTube J5 APK, you can try these apps:
-
-
[YouTube Vanced]: A popular modded version of YouTube that offers features such as ad-free viewing, background playback, dark mode, and more.
-
[NewPipe]: An open-source app that allows you to watch and download videos from YouTube and other platforms without using Google services or APIs.
-
[VidMate]: A video downloader app that allows you to download videos from YouTube and other platforms in various formats and resolutions.
-
401be4b1e0
-
-
\ No newline at end of file
diff --git "a/spaces/fb700/chatglm-fitness-RLHF/crazy_functions/Latex\345\205\250\346\226\207\347\277\273\350\257\221.py" "b/spaces/fb700/chatglm-fitness-RLHF/crazy_functions/Latex\345\205\250\346\226\207\347\277\273\350\257\221.py"
deleted file mode 100644
index 554c485aa0891f74c57cacfcbe076febe7a11029..0000000000000000000000000000000000000000
--- "a/spaces/fb700/chatglm-fitness-RLHF/crazy_functions/Latex\345\205\250\346\226\207\347\277\273\350\257\221.py"
+++ /dev/null
@@ -1,175 +0,0 @@
-from toolbox import update_ui
-from toolbox import CatchException, report_execption, write_results_to_file
-fast_debug = False
-
-class PaperFileGroup():
- def __init__(self):
- self.file_paths = []
- self.file_contents = []
- self.sp_file_contents = []
- self.sp_file_index = []
- self.sp_file_tag = []
-
- # count_token
- from request_llm.bridge_all import model_info
- enc = model_info["gpt-3.5-turbo"]['tokenizer']
- def get_token_num(txt): return len(enc.encode(txt, disallowed_special=()))
- self.get_token_num = get_token_num
-
- def run_file_split(self, max_token_limit=1900):
- """
- 将长文本分离开来
- """
- for index, file_content in enumerate(self.file_contents):
- if self.get_token_num(file_content) < max_token_limit:
- self.sp_file_contents.append(file_content)
- self.sp_file_index.append(index)
- self.sp_file_tag.append(self.file_paths[index])
- else:
- from .crazy_utils import breakdown_txt_to_satisfy_token_limit_for_pdf
- segments = breakdown_txt_to_satisfy_token_limit_for_pdf(file_content, self.get_token_num, max_token_limit)
- for j, segment in enumerate(segments):
- self.sp_file_contents.append(segment)
- self.sp_file_index.append(index)
- self.sp_file_tag.append(self.file_paths[index] + f".part-{j}.tex")
-
- print('Segmentation: done')
-
-def 多文件翻译(file_manifest, project_folder, llm_kwargs, plugin_kwargs, chatbot, history, system_prompt, language='en'):
- import time, os, re
- from .crazy_utils import request_gpt_model_multi_threads_with_very_awesome_ui_and_high_efficiency
-
- # <-------- 读取Latex文件,删除其中的所有注释 ---------->
- pfg = PaperFileGroup()
-
- for index, fp in enumerate(file_manifest):
- with open(fp, 'r', encoding='utf-8', errors='replace') as f:
- file_content = f.read()
- # 定义注释的正则表达式
- comment_pattern = r'(?
- pfg.run_file_split(max_token_limit=1024)
- n_split = len(pfg.sp_file_contents)
-
- # <-------- 抽取摘要 ---------->
- # if language == 'en':
- # abs_extract_inputs = f"Please write an abstract for this paper"
-
- # # 单线,获取文章meta信息
- # paper_meta_info = yield from request_gpt_model_in_new_thread_with_ui_alive(
- # inputs=abs_extract_inputs,
- # inputs_show_user=f"正在抽取摘要信息。",
- # llm_kwargs=llm_kwargs,
- # chatbot=chatbot, history=[],
- # sys_prompt="Your job is to collect information from materials。",
- # )
-
- # <-------- 多线程润色开始 ---------->
- if language == 'en->zh':
- inputs_array = ["Below is a section from an English academic paper, translate it into Chinese, do not modify any latex command such as \section, \cite and equations:" +
- f"\n\n{frag}" for frag in pfg.sp_file_contents]
- inputs_show_user_array = [f"翻译 {f}" for f in pfg.sp_file_tag]
- sys_prompt_array = ["You are a professional academic paper translator." for _ in range(n_split)]
- elif language == 'zh->en':
- inputs_array = [f"Below is a section from a Chinese academic paper, translate it into English, do not modify any latex command such as \section, \cite and equations:" +
- f"\n\n{frag}" for frag in pfg.sp_file_contents]
- inputs_show_user_array = [f"翻译 {f}" for f in pfg.sp_file_tag]
- sys_prompt_array = ["You are a professional academic paper translator." for _ in range(n_split)]
-
- gpt_response_collection = yield from request_gpt_model_multi_threads_with_very_awesome_ui_and_high_efficiency(
- inputs_array=inputs_array,
- inputs_show_user_array=inputs_show_user_array,
- llm_kwargs=llm_kwargs,
- chatbot=chatbot,
- history_array=[[""] for _ in range(n_split)],
- sys_prompt_array=sys_prompt_array,
- # max_workers=5, # OpenAI所允许的最大并行过载
- scroller_max_len = 80
- )
-
- # <-------- 整理结果,退出 ---------->
- create_report_file_name = time.strftime("%Y-%m-%d-%H-%M-%S", time.localtime()) + f"-chatgpt.polish.md"
- res = write_results_to_file(gpt_response_collection, file_name=create_report_file_name)
- history = gpt_response_collection
- chatbot.append((f"{fp}完成了吗?", res))
- yield from update_ui(chatbot=chatbot, history=history) # 刷新界面
-
-
-
-
-
-@CatchException
-def Latex英译中(txt, llm_kwargs, plugin_kwargs, chatbot, history, system_prompt, web_port):
- # 基本信息:功能、贡献者
- chatbot.append([
- "函数插件功能?",
- "对整个Latex项目进行翻译。函数插件贡献者: Binary-Husky"])
- yield from update_ui(chatbot=chatbot, history=history) # 刷新界面
-
- # 尝试导入依赖,如果缺少依赖,则给出安装建议
- try:
- import tiktoken
- except:
- report_execption(chatbot, history,
- a=f"解析项目: {txt}",
- b=f"导入软件依赖失败。使用该模块需要额外依赖,安装方法```pip install --upgrade tiktoken```。")
- yield from update_ui(chatbot=chatbot, history=history) # 刷新界面
- return
- history = [] # 清空历史,以免输入溢出
- import glob, os
- if os.path.exists(txt):
- project_folder = txt
- else:
- if txt == "": txt = '空空如也的输入栏'
- report_execption(chatbot, history, a = f"解析项目: {txt}", b = f"找不到本地项目或无权访问: {txt}")
- yield from update_ui(chatbot=chatbot, history=history) # 刷新界面
- return
- file_manifest = [f for f in glob.glob(f'{project_folder}/**/*.tex', recursive=True)]
- if len(file_manifest) == 0:
- report_execption(chatbot, history, a = f"解析项目: {txt}", b = f"找不到任何.tex文件: {txt}")
- yield from update_ui(chatbot=chatbot, history=history) # 刷新界面
- return
- yield from 多文件翻译(file_manifest, project_folder, llm_kwargs, plugin_kwargs, chatbot, history, system_prompt, language='en->zh')
-
-
-
-
-
-@CatchException
-def Latex中译英(txt, llm_kwargs, plugin_kwargs, chatbot, history, system_prompt, web_port):
- # 基本信息:功能、贡献者
- chatbot.append([
- "函数插件功能?",
- "对整个Latex项目进行翻译。函数插件贡献者: Binary-Husky"])
- yield from update_ui(chatbot=chatbot, history=history) # 刷新界面
-
- # 尝试导入依赖,如果缺少依赖,则给出安装建议
- try:
- import tiktoken
- except:
- report_execption(chatbot, history,
- a=f"解析项目: {txt}",
- b=f"导入软件依赖失败。使用该模块需要额外依赖,安装方法```pip install --upgrade tiktoken```。")
- yield from update_ui(chatbot=chatbot, history=history) # 刷新界面
- return
- history = [] # 清空历史,以免输入溢出
- import glob, os
- if os.path.exists(txt):
- project_folder = txt
- else:
- if txt == "": txt = '空空如也的输入栏'
- report_execption(chatbot, history, a = f"解析项目: {txt}", b = f"找不到本地项目或无权访问: {txt}")
- yield from update_ui(chatbot=chatbot, history=history) # 刷新界面
- return
- file_manifest = [f for f in glob.glob(f'{project_folder}/**/*.tex', recursive=True)]
- if len(file_manifest) == 0:
- report_execption(chatbot, history, a = f"解析项目: {txt}", b = f"找不到任何.tex文件: {txt}")
- yield from update_ui(chatbot=chatbot, history=history) # 刷新界面
- return
- yield from 多文件翻译(file_manifest, project_folder, llm_kwargs, plugin_kwargs, chatbot, history, system_prompt, language='zh->en')
\ No newline at end of file
diff --git a/spaces/fb700/chatglm-fitness-RLHF/src/face3d/models/arcface_torch/docs/modelzoo.md b/spaces/fb700/chatglm-fitness-RLHF/src/face3d/models/arcface_torch/docs/modelzoo.md
deleted file mode 100644
index e69de29bb2d1d6434b8b29ae775ad8c2e48c5391..0000000000000000000000000000000000000000
diff --git a/spaces/feng2022/styleganhuman_copy/torch_utils/ops/filtered_lrelu.py b/spaces/feng2022/styleganhuman_copy/torch_utils/ops/filtered_lrelu.py
deleted file mode 100644
index f5e3748fb725884b18b7e8119f569722b5bbe67f..0000000000000000000000000000000000000000
--- a/spaces/feng2022/styleganhuman_copy/torch_utils/ops/filtered_lrelu.py
+++ /dev/null
@@ -1,282 +0,0 @@
-# Copyright (c) 2021, NVIDIA CORPORATION & AFFILIATES. All rights reserved.
-#
-# NVIDIA CORPORATION and its licensors retain all intellectual property
-# and proprietary rights in and to this software, related documentation
-# and any modifications thereto. Any use, reproduction, disclosure or
-# distribution of this software and related documentation without an express
-# license agreement from NVIDIA CORPORATION is strictly prohibited.
-
-import os
-import numpy as np
-import torch
-import warnings
-
-from .. import custom_ops
-from .. import misc
-from . import upfirdn2d
-from . import bias_act
-
-#----------------------------------------------------------------------------
-
-_plugin = None
-
-def _init():
- global _plugin
- if _plugin is None:
-
- # sources=['filtered_lrelu.h', 'filtered_lrelu.cu', 'filtered_lrelu.cpp', 'filtered_lrelu_wr.cu', 'filtered_lrelu_rd.cu', 'filtered_lrelu_ns.cu']
- # sources = [os.path.join(os.path.dirname(__file__), s) for s in sources]
- # try:
- # _plugin = custom_ops.get_plugin('filtered_lrelu_plugin', sources=sources, extra_cuda_cflags=['--use_fast_math', '--allow-unsupported-compiler'])
- # except:
- # warnings.warn('Failed to build CUDA kernels for filtered_lrelu_plugin. Falling back to slow reference implementation. Details:\n\n' + traceback.format_exc())
-
- _plugin = custom_ops.get_plugin_v3(
- module_name='filtered_lrelu_plugin',
- sources=['filtered_lrelu.cpp', 'filtered_lrelu_wr.cu', 'filtered_lrelu_rd.cu', 'filtered_lrelu_ns.cu'],
- headers=['filtered_lrelu.h', 'filtered_lrelu.cu'],
- source_dir=os.path.dirname(__file__),
- extra_cuda_cflags=['--use_fast_math', '--allow-unsupported-compiler'],
- )
- return True
-
-def _get_filter_size(f):
- if f is None:
- return 1, 1
- assert isinstance(f, torch.Tensor)
- assert 1 <= f.ndim <= 2
- return f.shape[-1], f.shape[0] # width, height
-
-def _parse_padding(padding):
- if isinstance(padding, int):
- padding = [padding, padding]
- assert isinstance(padding, (list, tuple))
- assert all(isinstance(x, (int, np.integer)) for x in padding)
- padding = [int(x) for x in padding]
- if len(padding) == 2:
- px, py = padding
- padding = [px, px, py, py]
- px0, px1, py0, py1 = padding
- return px0, px1, py0, py1
-
-#----------------------------------------------------------------------------
-
-def filtered_lrelu(x, fu=None, fd=None, b=None, up=1, down=1, padding=0, gain=np.sqrt(2), slope=0.2, clamp=None, flip_filter=False, impl='cuda'):
- r"""Filtered leaky ReLU for a batch of 2D images.
-
- Performs the following sequence of operations for each channel:
-
- 1. Add channel-specific bias if provided (`b`).
-
- 2. Upsample the image by inserting N-1 zeros after each pixel (`up`).
-
- 3. Pad the image with the specified number of zeros on each side (`padding`).
- Negative padding corresponds to cropping the image.
-
- 4. Convolve the image with the specified upsampling FIR filter (`fu`), shrinking it
- so that the footprint of all output pixels lies within the input image.
-
- 5. Multiply each value by the provided gain factor (`gain`).
-
- 6. Apply leaky ReLU activation function to each value.
-
- 7. Clamp each value between -clamp and +clamp, if `clamp` parameter is provided.
-
- 8. Convolve the image with the specified downsampling FIR filter (`fd`), shrinking
- it so that the footprint of all output pixels lies within the input image.
-
- 9. Downsample the image by keeping every Nth pixel (`down`).
-
- The fused op is considerably more efficient than performing the same calculation
- using standard PyTorch ops. It supports gradients of arbitrary order.
-
- Args:
- x: Float32/float16/float64 input tensor of the shape
- `[batch_size, num_channels, in_height, in_width]`.
- fu: Float32 upsampling FIR filter of the shape
- `[filter_height, filter_width]` (non-separable),
- `[filter_taps]` (separable), or
- `None` (identity).
- fd: Float32 downsampling FIR filter of the shape
- `[filter_height, filter_width]` (non-separable),
- `[filter_taps]` (separable), or
- `None` (identity).
- b: Bias vector, or `None` to disable. Must be a 1D tensor of the same type
- as `x`. The length of vector must must match the channel dimension of `x`.
- up: Integer upsampling factor (default: 1).
- down: Integer downsampling factor. (default: 1).
- padding: Padding with respect to the upsampled image. Can be a single number
- or a list/tuple `[x, y]` or `[x_before, x_after, y_before, y_after]`
- (default: 0).
- gain: Overall scaling factor for signal magnitude (default: sqrt(2)).
- slope: Slope on the negative side of leaky ReLU (default: 0.2).
- clamp: Maximum magnitude for leaky ReLU output (default: None).
- flip_filter: False = convolution, True = correlation (default: False).
- impl: Implementation to use. Can be `'ref'` or `'cuda'` (default: `'cuda'`).
-
- Returns:
- Tensor of the shape `[batch_size, num_channels, out_height, out_width]`.
- """
- assert isinstance(x, torch.Tensor)
- assert impl in ['ref', 'cuda']
- if impl == 'cuda' and x.device.type == 'cuda' and _init():
- return _filtered_lrelu_cuda(up=up, down=down, padding=padding, gain=gain, slope=slope, clamp=clamp, flip_filter=flip_filter).apply(x, fu, fd, b, None, 0, 0)
- return _filtered_lrelu_ref(x, fu=fu, fd=fd, b=b, up=up, down=down, padding=padding, gain=gain, slope=slope, clamp=clamp, flip_filter=flip_filter)
-
-#----------------------------------------------------------------------------
-
-@misc.profiled_function
-def _filtered_lrelu_ref(x, fu=None, fd=None, b=None, up=1, down=1, padding=0, gain=np.sqrt(2), slope=0.2, clamp=None, flip_filter=False):
- """Slow and memory-inefficient reference implementation of `filtered_lrelu()` using
- existing `upfirdn2n()` and `bias_act()` ops.
- """
- assert isinstance(x, torch.Tensor) and x.ndim == 4
- fu_w, fu_h = _get_filter_size(fu)
- fd_w, fd_h = _get_filter_size(fd)
- if b is not None:
- assert isinstance(b, torch.Tensor) and b.dtype == x.dtype
- misc.assert_shape(b, [x.shape[1]])
- assert isinstance(up, int) and up >= 1
- assert isinstance(down, int) and down >= 1
- px0, px1, py0, py1 = _parse_padding(padding)
- assert gain == float(gain) and gain > 0
- assert slope == float(slope) and slope >= 0
- assert clamp is None or (clamp == float(clamp) and clamp >= 0)
-
- # Calculate output size.
- batch_size, channels, in_h, in_w = x.shape
- in_dtype = x.dtype
- out_w = (in_w * up + (px0 + px1) - (fu_w - 1) - (fd_w - 1) + (down - 1)) // down
- out_h = (in_h * up + (py0 + py1) - (fu_h - 1) - (fd_h - 1) + (down - 1)) // down
-
- # Compute using existing ops.
- x = bias_act.bias_act(x=x, b=b) # Apply bias.
- x = upfirdn2d.upfirdn2d(x=x, f=fu, up=up, padding=[px0, px1, py0, py1], gain=up**2, flip_filter=flip_filter) # Upsample.
- x = bias_act.bias_act(x=x, act='lrelu', alpha=slope, gain=gain, clamp=clamp) # Bias, leaky ReLU, clamp.
- x = upfirdn2d.upfirdn2d(x=x, f=fd, down=down, flip_filter=flip_filter) # Downsample.
-
- # Check output shape & dtype.
- misc.assert_shape(x, [batch_size, channels, out_h, out_w])
- assert x.dtype == in_dtype
- return x
-
-#----------------------------------------------------------------------------
-
-_filtered_lrelu_cuda_cache = dict()
-
-def _filtered_lrelu_cuda(up=1, down=1, padding=0, gain=np.sqrt(2), slope=0.2, clamp=None, flip_filter=False):
- """Fast CUDA implementation of `filtered_lrelu()` using custom ops.
- """
- assert isinstance(up, int) and up >= 1
- assert isinstance(down, int) and down >= 1
- px0, px1, py0, py1 = _parse_padding(padding)
- assert gain == float(gain) and gain > 0
- gain = float(gain)
- assert slope == float(slope) and slope >= 0
- slope = float(slope)
- assert clamp is None or (clamp == float(clamp) and clamp >= 0)
- clamp = float(clamp if clamp is not None else 'inf')
-
- # Lookup from cache.
- key = (up, down, px0, px1, py0, py1, gain, slope, clamp, flip_filter)
- if key in _filtered_lrelu_cuda_cache:
- return _filtered_lrelu_cuda_cache[key]
-
- # Forward op.
- class FilteredLReluCuda(torch.autograd.Function):
- @staticmethod
- def forward(ctx, x, fu, fd, b, si, sx, sy): # pylint: disable=arguments-differ
- assert isinstance(x, torch.Tensor) and x.ndim == 4
-
- # Replace empty up/downsample kernels with full 1x1 kernels (faster than separable).
- if fu is None:
- fu = torch.ones([1, 1], dtype=torch.float32, device=x.device)
- if fd is None:
- fd = torch.ones([1, 1], dtype=torch.float32, device=x.device)
- assert 1 <= fu.ndim <= 2
- assert 1 <= fd.ndim <= 2
-
- # Replace separable 1x1 kernels with full 1x1 kernels when scale factor is 1.
- if up == 1 and fu.ndim == 1 and fu.shape[0] == 1:
- fu = fu.square()[None]
- if down == 1 and fd.ndim == 1 and fd.shape[0] == 1:
- fd = fd.square()[None]
-
- # Missing sign input tensor.
- if si is None:
- si = torch.empty([0])
-
- # Missing bias tensor.
- if b is None:
- b = torch.zeros([x.shape[1]], dtype=x.dtype, device=x.device)
-
- # Construct internal sign tensor only if gradients are needed.
- write_signs = (si.numel() == 0) and (x.requires_grad or b.requires_grad)
-
- # Warn if input storage strides are not in decreasing order due to e.g. channels-last layout.
- strides = [x.stride(i) for i in range(x.ndim) if x.size(i) > 1]
- if any(a < b for a, b in zip(strides[:-1], strides[1:])):
- warnings.warn("low-performance memory layout detected in filtered_lrelu input", RuntimeWarning)
-
- # Call C++/Cuda plugin if datatype is supported.
- if x.dtype in [torch.float16, torch.float32]:
- if torch.cuda.current_stream(x.device) != torch.cuda.default_stream(x.device):
- warnings.warn("filtered_lrelu called with non-default cuda stream but concurrent execution is not supported", RuntimeWarning)
- y, so, return_code = _plugin.filtered_lrelu(x, fu, fd, b, si, up, down, px0, px1, py0, py1, sx, sy, gain, slope, clamp, flip_filter, write_signs)
- else:
- return_code = -1
-
- # No Cuda kernel found? Fall back to generic implementation. Still more memory efficient than the reference implementation because
- # only the bit-packed sign tensor is retained for gradient computation.
- if return_code < 0:
- warnings.warn("filtered_lrelu called with parameters that have no optimized CUDA kernel, using generic fallback", RuntimeWarning)
-
- y = x.add(b.unsqueeze(-1).unsqueeze(-1)) # Add bias.
- y = upfirdn2d.upfirdn2d(x=y, f=fu, up=up, padding=[px0, px1, py0, py1], gain=up**2, flip_filter=flip_filter) # Upsample.
- so = _plugin.filtered_lrelu_act_(y, si, sx, sy, gain, slope, clamp, write_signs) # Activation function and sign handling. Modifies y in-place.
- y = upfirdn2d.upfirdn2d(x=y, f=fd, down=down, flip_filter=flip_filter) # Downsample.
-
- # Prepare for gradient computation.
- ctx.save_for_backward(fu, fd, (si if si.numel() else so))
- ctx.x_shape = x.shape
- ctx.y_shape = y.shape
- ctx.s_ofs = sx, sy
- return y
-
- @staticmethod
- def backward(ctx, dy): # pylint: disable=arguments-differ
- fu, fd, si = ctx.saved_tensors
- _, _, xh, xw = ctx.x_shape
- _, _, yh, yw = ctx.y_shape
- sx, sy = ctx.s_ofs
- dx = None # 0
- dfu = None; assert not ctx.needs_input_grad[1]
- dfd = None; assert not ctx.needs_input_grad[2]
- db = None # 3
- dsi = None; assert not ctx.needs_input_grad[4]
- dsx = None; assert not ctx.needs_input_grad[5]
- dsy = None; assert not ctx.needs_input_grad[6]
-
- if ctx.needs_input_grad[0] or ctx.needs_input_grad[3]:
- pp = [
- (fu.shape[-1] - 1) + (fd.shape[-1] - 1) - px0,
- xw * up - yw * down + px0 - (up - 1),
- (fu.shape[0] - 1) + (fd.shape[0] - 1) - py0,
- xh * up - yh * down + py0 - (up - 1),
- ]
- gg = gain * (up ** 2) / (down ** 2)
- ff = (not flip_filter)
- sx = sx - (fu.shape[-1] - 1) + px0
- sy = sy - (fu.shape[0] - 1) + py0
- dx = _filtered_lrelu_cuda(up=down, down=up, padding=pp, gain=gg, slope=slope, clamp=None, flip_filter=ff).apply(dy, fd, fu, None, si, sx, sy)
-
- if ctx.needs_input_grad[3]:
- db = dx.sum([0, 2, 3])
-
- return dx, dfu, dfd, db, dsi, dsx, dsy
-
- # Add to cache.
- _filtered_lrelu_cuda_cache[key] = FilteredLReluCuda
- return FilteredLReluCuda
-
-#----------------------------------------------------------------------------
\ No newline at end of file
diff --git a/spaces/feregVcuzo/sanity-test-midi/checkpoint/Apke Noor se Hamari Zindagi Mein Dharm aur Adhyatm ka Sthan Ek Spiritual Guide.md b/spaces/feregVcuzo/sanity-test-midi/checkpoint/Apke Noor se Hamari Zindagi Mein Dharm aur Adhyatm ka Sthan Ek Spiritual Guide.md
deleted file mode 100644
index a0775259579db7c1da0a8e431238e7b57f2d6395..0000000000000000000000000000000000000000
--- a/spaces/feregVcuzo/sanity-test-midi/checkpoint/Apke Noor se Hamari Zindagi Mein Dharm aur Adhyatm ka Sthan Ek Spiritual Guide.md
+++ /dev/null
@@ -1,5 +0,0 @@
-
-
` for the title, `
` for the main headings, `
` for the subheadings, and `
` for the sub-subheadings. You can also use other HTML elements such as `
` for paragraphs, `
` and `
` for lists, `
` and `
` for tables, etc. 5. Fifth, write this custom message " Here is an example of how your tables might look like: | Outline of the Article | Article with HTML Formatting | | ---------------------- | ---------------------------- | | H1: Apke Noor Se Hamari Zindagi |
Apke Noor Se Hamari Zindagi
| | H2: Apke Noor Se Hamari Zindagi Kya Hai? |
Apke Noor Se Hamari Zindagi Kya Hai?
Apke noor se hamari zindagi ek bhajan hai jo Samadha Satguru Sain Baba ke shishyaon dwara gaya jata hai. Is bhajan mein unhone apne guru ki mahima aur unke noor se hamari zindagi mein roshni aane ki baat kahi hai. Is bhajan ka matlab hai ki hamare guru ke noor se hamari zindagi mein khushiyan aur shanti bhar gayi hai aur ham unki kripa se har mushkil se paar ho sakte hain.
| | H3: Apke Noor Se Hamari Zindagi Ka Prabhav |
Apke Noor Se Hamari Zindagi Ka Prabhav
Is bhajan se hamen yeh samajhne mein madad milti hai ki hamare guru hamare liye kaise mahatvapurn hain aur unke noor se hamari zindagi kaise badalti hai. Guru ka noor hamare dil mein prem jagata hai aur hamen sahi aur galat ka bhed batata hai. Guru ka noor hamen dharmik marg par chalne ki shakti deta hai aur hamen apne aap ko sudharne ka mauka deta hai. Guru ka noor hamen dukhon se mukti dilata hai aur hamen anand ka anubhav karata hai.
Guru ka noor prapt karne ke liye hamen unki seva karni chahiye aur unki a
197e85843d
-
-
\ No newline at end of file
diff --git a/spaces/feregVcuzo/sanity-test-midi/checkpoint/Bitcoin The Ultimate Guide to Buying Selling and Mining the Worlds Most Popular Cryptocurrency.md b/spaces/feregVcuzo/sanity-test-midi/checkpoint/Bitcoin The Ultimate Guide to Buying Selling and Mining the Worlds Most Popular Cryptocurrency.md
deleted file mode 100644
index 3903074aebcaf8d641d135c3c9ca6ccd962dddf3..0000000000000000000000000000000000000000
--- a/spaces/feregVcuzo/sanity-test-midi/checkpoint/Bitcoin The Ultimate Guide to Buying Selling and Mining the Worlds Most Popular Cryptocurrency.md
+++ /dev/null
@@ -1,173 +0,0 @@
-
-
What is Bitcoin?
-
Bitcoin is a decentralized cryptocurrency that was created in 2009 by an anonymous person or group using the pseudonym Satoshi Nakamoto. It is a digital currency that can be used for online payments without the need for intermediaries or central authorities. It is powered by a network of computers that verify and record transactions on a shared ledger called the blockchain.
Bitcoin was the first cryptocurrency to emerge and has since inspired many others. It has also sparked a wave of innovation and experimentation in the fields of cryptography, economics, and social science. Today, Bitcoin is widely recognized as a groundbreaking invention that has the potential to transform the world of finance and beyond.
-
How does Bitcoin work?
-
Bitcoin works by using peer-to-peer technology to enable direct and transparent transactions between users. Anyone can participate in the network by running a software called a node that validates and broadcasts transactions. Nodes also compete to solve complex mathematical problems that secure the network and create new bitcoins. This process is known as mining.
-
Every transaction on the network is recorded in a block that contains a timestamp and a link to the previous block. This creates a chain of blocks that serves as a public and immutable record of all transactions. This is the blockchain, which acts as the backbone of the Bitcoin system.
-
The blockchain ensures that transactions are valid and prevents double-spending, which is when someone tries to spend the same bitcoin twice. It also eliminates the need for trusted third parties, such as banks or payment processors, that usually charge fees and impose restrictions on transactions. With Bitcoin, users have full control over their money and can transact with anyone, anywhere, anytime.
-
bitcoin mining software for windows 10
-bitcoin price prediction 2023
-bitcoin atm near me open now
-bitcoin cash vs bitcoin sv
-bitcoin wallet app for iphone
-bitcoin tax calculator canada
-bitcoin trading bot reddit
-bitcoin futures contract expiration date
-bitcoin halving countdown clock
-bitcoin exchange rate history chart
-bitcoin debit card no verification
-bitcoin news today in hindi
-bitcoin etf approval date
-bitcoin faucet instant payout 2023
-bitcoin qr code generator free
-bitcoin loan without collateral
-bitcoin casino no deposit bonus
-bitcoin stock symbol nasdaq
-bitcoin paper wallet generator offline
-bitcoin arbitrage trading platform
-bitcoin gift card amazon
-bitcoin lightning network explained
-bitcoin cloud mining free trial
-bitcoin mixer best service
-bitcoin options trading platform
-bitcoin documentary netflix 2023
-bitcoin hardware wallet comparison
-bitcoin ransomware attack 2023
-bitcoin mining pool fees comparison
-bitcoin interest rate calculator
-bitcoin graph live inr
-bitcoin gold fork date and time
-bitcoin scams on instagram 2023
-bitcoin core vs electrum wallet
-bitcoin diamond price prediction 2023
-bitcoin jobs remote work from home
-bitcoin pizza day 2023 deals
-bitcoin private key finder online free
-bitcoin atm machine for sale ebay
-bitcoin cash abc vs bch
-
What are the benefits of Bitcoin?
-
Bitcoin offers many advantages over traditional payment systems, such as:
-
-
Fast: Transactions are confirmed within minutes and can be completed across borders in seconds.
-
Global: Transactions are not limited by geographical boundaries or political jurisdictions.
-
Low-cost: Transactions are cheaper than most other payment methods, especially for international transfers.
-
Secure: Transactions are protected by cryptography and cannot be reversed or tampered with.
-
-
What are the challenges of Bitcoin?
-
Bitcoin also faces some challenges that need to be addressed, such as:
-
-
Volatility: The price of bitcoin fluctuates significantly due to. - Scalability: The network has a limited capacity to process transactions, which can lead to congestion and delays. - Regulation: The legal status and tax treatment of bitcoin vary across countries and jurisdictions, which can create uncertainty and risk for users and businesses. - Adoption: The awareness and acceptance of bitcoin among the general public and the mainstream institutions are still low, which limits its use and growth potential.
-
How to get started with Bitcoin?
-
If you are interested in using or investing in bitcoin, you will need to follow some basic steps to get started. These include:
-
How to buy Bitcoin?
-
The most common way to acquire bitcoin is to buy it with fiat currency (such as US dollars or euros) or other cryptocurrencies (such as Ethereum or Litecoin). You can do this through various platforms, such as:
-
-
Bitcoin exchanges: These are online platforms that allow you to buy and sell bitcoin using different payment methods, such as bank transfers, credit cards, or e-wallets. Some examples of popular bitcoin exchanges are Coinbase, Binance, and Kraken.
-
Bitcoin ATMs: These are physical machines that allow you to buy and sell bitcoin using cash or debit cards. They are usually located in public places, such as malls, airports, or convenience stores. You can find the nearest bitcoin ATM using websites like Coin ATM Radar.
-
Bitcoin peer-to-peer platforms: These are online platforms that allow you to buy and sell bitcoin directly from other users, without intermediaries. They usually offer more privacy and flexibility than exchanges, but also more risk and responsibility. Some examples of popular bitcoin peer-to-peer platforms are LocalBitcoins, Paxful, and Bisq.
-
-
How to choose a Bitcoin exchange?
-
When choosing a platform to buy bitcoin, you should consider several factors, such as:
-
-
Reputation: You should check the reviews and ratings of the platform from other users and experts, as well as its history of security breaches, hacks, or scams.
-
Liquidity: You should check the volume and availability of bitcoin on the platform, as well as the speed and ease of executing transactions.
-
Fees: You should check the fees charged by the platform for deposits, withdrawals, trading, and other services, as well as the exchange rate offered.
-
Customer service: You should check the quality and responsiveness of the platform's customer support team, as well as the availability of online resources and guides.
-
Regulation: You should check the legal status and compliance of the platform with the relevant laws and regulations in your country or jurisdiction.
-
-
How to store Bitcoin?
-
Once you have bought some bitcoin, you will need to store it in a secure place called a wallet. A wallet is a software or hardware device that allows you to manage your bitcoin balance and transactions. There are different types of wallets, such as:
-
-
Hot wallets: These are wallets that are connected to the internet and allow you to access your bitcoin anytime and anywhere. They are convenient and user-friendly, but also more vulnerable to hacking and theft. Some examples of hot wallets are web wallets (such as Blockchain.com), mobile wallets (such as BRD), and desktop wallets (such as Electrum).
-
Cold wallets: These are wallets that are not connected to the internet and provide a higher level of security and privacy. They are suitable for storing large amounts of bitcoin for a long time, but also less convenient and accessible. Some examples of cold wallets are hardware wallets (such as Ledger or Trezor), paper wallets (such as Bitaddress.org), and metal wallets (such as Cryptosteel).
-
-
How to secure your Bitcoin wallet?
-
To protect your bitcoin from hackers, thieves, or accidents, you should follow some best practices, such as:
-
-
Backup your wallet: You should make a copy of your wallet's data (such as private keys or recovery phrases) and store it in a safe place offline (such as a USB drive or a piece of paper).
-
Encrypt your wallet: You should use a strong password or passphrase to encrypt your wallet's data and prevent unauthorized access.
-
Update your wallet: You should keep your wallet's software updated to the latest version to fix any bugs or vulnerabilities.
-
Use multiple wallets: You should use different wallets for different purposes and amounts of bitcoin, such as a hot wallet for daily spending and a cold wallet for long-term saving.
-
Use reputable wallets: You should use wallets that have a good reputation and track record in the industry, and avoid wallets that are unknown or suspicious.
-
-
How to use Bitcoin?
-
Once you have stored your bitcoin in a wallet, you can use it for various purposes, such as:
-
How to spend Bitcoin?
-
You can use bitcoin to buy goods and services from merchants that accept it as a form of payment. You can find such merchants online or offline using websites like Spendabit or Coinmap. You can also use platforms like Bitrefill or Purse.io to buy gift cards or vouchers with bitcoin that can be redeemed at various retailers.
-
To pay with bitcoin, you will need to scan the merchant's QR code or enter their address, and then confirm the amount and the transaction fee. The transaction will be broadcasted to the network and confirmed within minutes. You will receive a receipt or confirmation from the merchant once the payment is completed.
-
How to send and receive Bitcoin?
-
You can also use bitcoin to send and receive money from anyone, anywhere in the world. You will need to know the recipient's bitcoin address, which is a string of alphanumeric characters that starts with 1, 3, or bc1. You can also use a QR code or a payment link to simplify the process.
-
To send bitcoin, you will need to enter the recipient's address, the amount, and the transaction fee. The transaction fee is a small amount of bitcoin that you pay to the network for processing your transaction. The higher the fee, the faster your transaction will be confirmed. You can adjust the fee according to your preference and urgency.
-
To receive bitcoin, you will need to share your address, QR code, or payment link with the sender. You can generate a new address for each transaction to enhance your privacy and security. You will see the incoming transaction in your wallet once it is broadcasted to the network, and you will be able to spend it once it is confirmed.
-
How to earn Bitcoin?
-
Besides buying and receiving bitcoin, you can also earn it by performing various tasks or activities, such as:
-
-
Mining: You can join a mining pool or run a mining software on your computer to contribute to the security and operation of the network and earn newly created bitcoins and transaction fees as rewards.
-
Lending: You can lend your bitcoin to other users or platforms and earn interest on your loan.
-
Staking: You can lock your bitcoin in a smart contract or a platform that supports staking and earn rewards for participating in the governance or validation of the network.
-
Trading: You can buy and sell bitcoin on an exchange or a peer-to-peer platform and profit from price fluctuations.
-
Working: You can offer your skills or services online or offline and get paid in bitcoin by your clients or employers.
-
Gaming: You can play online games or apps that reward you with bitcoin for completing tasks or challenges.
-
-
How to learn more about Bitcoin?
-
If you want to deepen your knowledge and understanding of Bitcoin, you can access various resources and communities that provide valuable information and insights, such as:
-
How to follow the latest Bitcoin news?
-
You can stay updated on the latest developments and events in the Bitcoin world by following reliable and timely sources of information, such as:
-
-
Bitcoin websites: These are websites that publish news, articles, analysis, and opinions on Bitcoin topics, such as CoinDesk, Cointelegraph, Bitcoin Magazine, and The Block.
-
Bitcoin podcasts: These are audio shows that discuss Bitcoin issues and interview Bitcoin experts, such as The Bitcoin Standard Podcast, What Bitcoin Did, Unchained, and The Breakdown.
-
Bitcoin newsletters: These are email subscriptions that deliver curated Bitcoin content to your inbox, such as The Daily Hodl, CoinMarketCap Daily, Decrypt Daily, and Marty's Bent.
-
Bitcoin social media: These are platforms where you can follow Bitcoin influencers, personalities, and organizations that share Bitcoin-related posts and updates, such as Twitter, Reddit, YouTube, and Medium.
-
-
How to avoid scams and misinformation?
-
You should also be aware of the risks of scams and misinformation that are prevalent in the Bitcoin space. You should always do your own research and verification before trusting any source or offer. You should also follow some tips to identify and avoid fraudulent or misleading content on Bitcoin, such as:
-
-
Check the credibility and reputation of the source or author: You should look for signs of authority, expertise, and professionalism, such as credentials, affiliations, references, and reviews.
-
Check the accuracy and consistency of the information: You should look for evidence, data, and logic that support the claims and arguments, as well as cross-check them with other sources.
-
Check the timeliness and relevance of the information: You should look for the date, context, and purpose of the information, and whether it is still valid and applicable.
-
Check the bias and motive of the information: You should look for the perspective, opinion, and agenda of the source or author, and whether they have any conflicts of interest or ulterior motives.
-
-
How to join the Bitcoin community?
-
If you want to interact with other Bitcoin enthusiasts and experts, you can join various platforms and forums that foster discussion and collaboration on Bitcoin topics, such as:
-
-
Bitcoin chat rooms: These are online chat platforms where you can chat with other Bitcoin users in real time, such as Telegram, Discord, Slack, and IRC.
-
Bitcoin forums: These are online message boards where you can post and reply to Bitcoin-related threads, such as Bitcointalk, Reddit, Stack Exchange, and Quora.
-
Bitcoin meetups: These are offline events where you can meet and network with other Bitcoin users in person, such as Meetup.com, Eventbrite.com, or Bitcoin.org.
-
-
How to contribute to the Bitcoin network?
-
If you want to support and improve the Bitcoin ecosystem, you can contribute to the network in various ways, such as:
-
-
Running a node: You can run a full node or a light node on your computer or device to validate and relay transactions on the network.
-
Mining bitcoin: You can mine bitcoin by using your computing power to secure the network and generate new bitcoins.
-
Developing bitcoin software: You can develop or improve bitcoin software by coding, testing, debugging, or documenting features or functions.
-
Donating bitcoin: You can donate bitcoin to individuals or organizations that work on bitcoin projects or causes.
-
-
Conclusion
-
Bitcoin is a revolutionary cryptocurrency that has the potential to change the way we transact and interact with money. It offers many benefits over traditional payment systems, such as speed, globality, low-cost, and security. It also faces some challenges, such as volatility, scalability, regulation, and adoption. To get started with bitcoin, you need to buy it, store it in a wallet, and use it for various purposes. To learn more about bitcoin, you can access various resources and communities that provide information and insights on bitcoin topics. To join the bitcoin community, you can participate in various platforms and forums that foster discussion and collaboration on bitcoin topics. To contribute to the bitcoin network, you can support and improve the bitcoin ecosystem in various ways.
-
Frequently Asked Questions
-
Here are some common questions and answers about Bitcoin:
-
What is the difference between Bitcoin and bitcoin?
-
The term Bitcoin (with a capital B) usually refers to the protocol or the network that enables peer-to-peer transactions using cryptography and blockchain. The term bitcoin (with a lowercase b) usually refers to the unit of account or the currency that is used for transactions on the network.
-
How many bitcoins are there?
-
The total supply of bitcoins is limited to 21 million. As of June 2023, there are about 18.8 million bitcoins in circulation. The remaining 2.2 million bitcoins will be created gradually through mining until around 2140.
-
What determines the price of bitcoin?
-
The price of bitcoin is determined by supply and demand in the market. The supply of bitcoin is fixed by the protocol and decreases over time. The demand for bitcoin is influenced by various factors, such as adoption, innovation, regulation, speculation, media attention, and sentiment. The price of bitcoin is also affected by the cost of production, which depends on the difficulty and reward of mining.
-
Is Bitcoin legal?
-
The legal status of Bitcoin varies across countries and jurisdictions. Some countries have explicitly recognized and regulated Bitcoin as a form of money or asset, such as Japan, Canada, and Switzerland. Some countries have banned or restricted the use of Bitcoin, such as China, India, and Iran. Some countries have not issued any clear or official guidance on Bitcoin, leaving it in a legal gray area.
-
Before using or investing in Bitcoin, you should check the laws and regulations of your country or jurisdiction and consult a professional if needed.
-
Is Bitcoin safe?
-
Bitcoin is designed to be secure and resilient against attacks and errors. However, Bitcoin is not immune to risks and threats, such as hacking, theft, loss, fraud, or human error. To use Bitcoin safely, you should take some precautions, such as choosing a reputable platform or wallet, securing your private keys, backing up your data, updating your software, using multiple wallets, and verifying your transactions.
-
How can I learn more about Bitcoin?
-
If you want to learn more about Bitcoin, you can explore the following resources:
-
-
Bitcoin.org: This is the official website of the Bitcoin project that provides basic information and guides on Bitcoin.
-
Bitcoin Wiki: This is a collaborative encyclopedia that covers technical and non-technical topics on Bitcoin.
-
Bitcoin Whitepaper: This is the original document that describes the design and rationale of Bitcoin, written by Satoshi Nakamoto.
-
Bitcoin Books: These are books that explain the history, technology, economics, and social implications of Bitcoin, such as The Bitcoin Standard by Saifedean Ammous, The Age of Cryptocurrency by Paul Vigna and Michael J. Casey, and Digital Gold by Nathaniel Popper.
-
Bitcoin Courses: These are online courses that teach the fundamentals and applications of Bitcoin, such as Bitcoin and Cryptocurrency Technologies by Princeton University, Introduction to Digital Currencies by University of Nicosia, and Learn Bitcoin from Scratch by Udemy.
-
-
I hope you enjoyed this article on Bitcoin. If you have any questions or feedback, please leave a comment below. Thank you for reading!
197e85843d
-
-
\ No newline at end of file
diff --git a/spaces/feregVcuzo/sanity-test-midi/checkpoint/Download BlueZ Source Get the Latest Version of the Linux Bluetooth Protocol Stack and Obexd.md b/spaces/feregVcuzo/sanity-test-midi/checkpoint/Download BlueZ Source Get the Latest Version of the Linux Bluetooth Protocol Stack and Obexd.md
deleted file mode 100644
index 39958f7df50acc8d3eb0d1e11efddeca49805e76..0000000000000000000000000000000000000000
--- a/spaces/feregVcuzo/sanity-test-midi/checkpoint/Download BlueZ Source Get the Latest Version of the Linux Bluetooth Protocol Stack and Obexd.md
+++ /dev/null
@@ -1,88 +0,0 @@
-
-
How to Download BlueZ Source
-
If you are interested in working with Bluetooth on Linux, you might want to download the source code of BlueZ, the official Linux Bluetooth protocol stack. In this article, we will explain what BlueZ is, what features it provides, and how you can get its source code from different sources.
-
What is BlueZ?
-
BlueZ is an open source project that provides support for the core Bluetooth layers and protocols in a modular way. It was initially developed by Qualcomm and is now maintained by the Bluetooth Special Interest Group (SIG) and other contributors. BlueZ is included with the official Linux kernel distributions and is compatible with any Linux system on the market.
Support for various Bluetooth profiles and services
-
Configuration and testing utilities
-
Protocol decoding and analysis tools
-
-
BlueZ Platforms
-
The BlueZ kernel modules, libraries and utilities are known to work well on many architectures supported by Linux. This also includes single and multi processor platforms as well as hyper threading systems, such as:
-
-
Intel and AMD x86
-
AMD64 and EM64T (x86-64)
-
SUN SPARC 32/64bit
-
PowerPC 32/64bit
-
Intel StrongARM and XScale
-
Hitachi/Renesas SH processors
-
Motorola DragonBall
-
-
BlueZ Distributions
-
Support for BlueZ can be found in many Linux distributions and in general it is compatible with any Linux system on the market. Some of the distributions that provide their own packages for BlueZ are:
-
-
Debian GNU/Linux
-
Ubuntu Linux
-
Fedora Core / Red Hat Linux
-
OpenSuSE / SuSE Linux
-
Mandrake Linux
-
Gentoo Linux
-
Chrome OS
-
-
How to Get BlueZ Source Code?
-
If you want to download the source code of BlueZ, you have several options to choose from. Here are some of the most common ways to get BlueZ source code:
-
From the Official Website
-
The official website of BlueZ provides a download page where you can find the latest stable release of BlueZ as well as older versions. The source code is provided as a compressed tarball file that you can download and extract on your system. To download the latest stable release of BlueZ, you can use this link: bluez-5.66.tar.xz
-
How to download and install bluez on Linux
-Download bluez GitHub repository and compile from source
-BlueZ official Linux Bluetooth protocol stack download
-Download bluez-4.101.tar.xz and obexd-0.48.tar.xz from BlueZ website
-Download bluez-hcidump-2.5.tar.xz to capture Bluetooth traffic
-Download bluez-libs and bluez-utils for Bluetooth development
-Download bluez-firmware for Bluetooth device firmware updates
-Download BlueR - official BlueZ bindings for Rust
-Download bluetooth-next - Bluetooth kernel development tree
-Download pybluez - Bluetooth Python extension module
-
From GitHub
-
The main development repository of BlueZ is hosted on GitHub, where you can find the latest code changes, bug fixes, and new features. You can clone the repository using git or download a zip file of the current master branch. To clone the repository, you can use this command:
To download a zip file of the current master branch, you can use this link: bluez.zip
-
From Linux Distributions
-
If you are using a Linux distribution that provides packages for BlueZ, you can also download the source code from their repositories. For example, if you are using Debian or Ubuntu, you can use the apt-get source command to download the source code of the bluez package. To do that, you can use this command:
- apt-get source bluez
-
This will download the source code of the bluez package and its dependencies in the current directory. You can also specify a different directory to download the source code. For more information on how to use apt-get source, you can check the man page.
-
Conclusion
-
In this article, we have learned what BlueZ is, what features it provides, and how to download its source code from different sources. We hope that this article has been helpful for you and that you have enjoyed learning about BlueZ. If you have any questions or feedback, please feel free to leave a comment below.
-
FAQs
-
Here are some frequently asked questions about BlueZ and its source code:
-
What is the license of BlueZ?
-
BlueZ is licensed under the GNU General Public License (GPL) version 2 or later. This means that you can use, modify, and distribute BlueZ as long as you comply with the terms of the license.
-
How can I contribute to BlueZ?
-
If you want to contribute to BlueZ, you can check the contribution guidelines on the official website. You can also join the mailing list or the IRC channel to communicate with other developers and users of BlueZ.
-
How can I report a bug or request a feature for BlueZ?
-
If you encounter a bug or have a suggestion for a new feature for BlueZ, you can use the bug tracker on GitHub to report it. Please make sure that you follow the bug reporting guidelines and provide as much information as possible to help the developers fix or implement your issue.
-
How can I learn more about BlueZ?
-
If you want to learn more about BlueZ, you can check the documentation on the official website. You can also find tutorials, articles, and videos on various topics related to BlueZ on the internet. Some of the resources that we recommend are:
If you are looking for alternatives to BlueZ, you might want to check out some of these projects:
-
-
Bluedroid : The Bluetooth stack for Android devices.
-
Bluepy : A Python interface to Bluetooth Low Energy devices.
-
NimBLE : An open source Bluetooth 5.0 stack for embedded devices.
-
401be4b1e0
-
-
\ No newline at end of file
diff --git a/spaces/fffiloni/Music_Source_Separation/setup.py b/spaces/fffiloni/Music_Source_Separation/setup.py
deleted file mode 100644
index f146e7d34dc4f06b032ee84b4777e8df01ab9ddb..0000000000000000000000000000000000000000
--- a/spaces/fffiloni/Music_Source_Separation/setup.py
+++ /dev/null
@@ -1,25 +0,0 @@
-from setuptools import setup
-
-setup(
- name='bytesep',
- version='0.0.1',
- description='Music source separation',
- author='ByteDance',
- url="https://github.com/bytedance/music_source_separation",
- license='Apache 2.0',
- packages=['bytesep'],
- include_package_data=True,
- install_requires=[
- 'torch==1.7.1',
- 'librosa==0.8.0', # specify the version!
- 'museval==0.4.0',
- 'h5py==2.10.0',
- 'pytorch_lightning==1.2.1',
- 'numpy==1.18.5',
- 'torchlibrosa==0.0.9',
- 'matplotlib==3.3.4',
- 'musdb==0.4.0',
- 'museval==0.4.0'
- ],
- zip_safe=False
-)
diff --git a/spaces/fffiloni/Video-Matting-Anything/networks/m2ms/__init__.py b/spaces/fffiloni/Video-Matting-Anything/networks/m2ms/__init__.py
deleted file mode 100644
index 7c06875870c8c1537f7fa7a1cb202bbb2ff56889..0000000000000000000000000000000000000000
--- a/spaces/fffiloni/Video-Matting-Anything/networks/m2ms/__init__.py
+++ /dev/null
@@ -1,7 +0,0 @@
-from .conv_sam import SAM_Decoder_Deep
-
-__all__ = ['sam_decoder_deep']
-
-def sam_decoder_deep(nc, **kwargs):
- model = SAM_Decoder_Deep(nc, [2, 3, 3, 2], **kwargs)
- return model
\ No newline at end of file
diff --git a/spaces/fl399/deplot_plus_llm/app.py b/spaces/fl399/deplot_plus_llm/app.py
deleted file mode 100644
index 6f6f7cae6c341ce10cfa8642b9c2400f52f54254..0000000000000000000000000000000000000000
--- a/spaces/fl399/deplot_plus_llm/app.py
+++ /dev/null
@@ -1,301 +0,0 @@
-import os
-import torch
-import openai
-import requests
-import gradio as gr
-import transformers
-from transformers import Pix2StructForConditionalGeneration, Pix2StructProcessor
-#from peft import PeftModel
-
-
-if torch.cuda.is_available():
- device = "cuda"
-else:
- device = "cpu"
-
-try:
- if torch.backends.mps.is_available():
- device = "mps"
-except:
- pass
-
-## CoT prompts
-
-def _add_markup(table):
- try:
- parts = [p.strip() for p in table.splitlines(keepends=False)]
- if parts[0].startswith('TITLE'):
- result = f"Title: {parts[0].split(' | ')[1].strip()}\n"
- rows = parts[1:]
- else:
- result = ''
- rows = parts
- prefixes = ['Header: '] + [f'Row {i+1}: ' for i in range(len(rows) - 1)]
- return result + '\n'.join(prefix + row for prefix, row in zip(prefixes, rows))
- except:
- # just use the raw table if parsing fails
- return table
-
-_TABLE = """Year | Democrats | Republicans | Independents
-2004 | 68.1% | 45.0% | 53.0%
-2006 | 58.0% | 42.0% | 53.0%
-2007 | 59.0% | 38.0% | 45.0%
-2009 | 72.0% | 49.0% | 60.0%
-2011 | 71.0% | 51.2% | 58.0%
-2012 | 70.0% | 48.0% | 53.0%
-2013 | 72.0% | 41.0% | 60.0%"""
-
-_INSTRUCTION = 'Read the table below to answer the following questions.'
-
-_TEMPLATE = f"""First read an example then the complete question for the second table.
-------------
-{_INSTRUCTION}
-{_add_markup(_TABLE)}
-Q: In which year republicans have the lowest favor rate?
-A: Let's find the column of republicans. Then let's extract the favor rates, they [45.0, 42.0, 38.0, 49.0, 51.2, 48.0, 41.0]. The smallest number is 38.0, that's Row 3. Row 3 is year 2007. The answer is 2007.
-Q: What is the sum of Democrats' favor rates of 2004, 2012, and 2013?
-A: Let's find the rows of years 2004, 2012, and 2013. We find Row 1, 6, 7. The favor dates of Demoncrats on that 3 rows are 68.1, 70.0, and 72.0. 68.1+70.0+72=210.1. The answer is 210.1.
-Q: By how many points do Independents surpass Republicans in the year of 2011?
-A: Let's find the row with year = 2011. We find Row 5. We extract Independents and Republicans' numbers. They are 58.0 and 51.2. 58.0-51.2=6.8. The answer is 6.8.
-Q: Which group has the overall worst performance?
-A: Let's sample a couple of years. In Row 1, year 2004, we find Republicans having the lowest favor rate 45.0 (since 45.0<68.1, 45.0<53.0). In year 2006, Row 2, we find Republicans having the lowest favor rate 42.0 (42.0<58.0, 42.0<53.0). The trend continues to other years. The answer is Republicans.
-Q: Which party has the second highest favor rates in 2007?
-A: Let's find the row of year 2007, that's Row 3. Let's extract the numbers on Row 3: [59.0, 38.0, 45.0]. 45.0 is the second highest. 45.0 is the number of Independents. The answer is Independents.
-{_INSTRUCTION}"""
-
-
-## alpaca-lora
-
-# assert (
-# "LlamaTokenizer" in transformers._import_structure["models.llama"]
-# ), "LLaMA is now in HuggingFace's main branch.\nPlease reinstall it: pip uninstall transformers && pip install git+https://github.com/huggingface/transformers.git"
-# from transformers import LlamaTokenizer, LlamaForCausalLM, GenerationConfig
-
-# tokenizer = LlamaTokenizer.from_pretrained("decapoda-research/llama-7b-hf")
-
-# BASE_MODEL = "decapoda-research/llama-7b-hf"
-# LORA_WEIGHTS = "tloen/alpaca-lora-7b"
-
-# if device == "cuda":
-# model = LlamaForCausalLM.from_pretrained(
-# BASE_MODEL,
-# load_in_8bit=False,
-# torch_dtype=torch.float16,
-# device_map="auto",
-# )
-# model = PeftModel.from_pretrained(
-# model, LORA_WEIGHTS, torch_dtype=torch.float16, force_download=True
-# )
-# elif device == "mps":
-# model = LlamaForCausalLM.from_pretrained(
-# BASE_MODEL,
-# device_map={"": device},
-# torch_dtype=torch.float16,
-# )
-# model = PeftModel.from_pretrained(
-# model,
-# LORA_WEIGHTS,
-# device_map={"": device},
-# torch_dtype=torch.float16,
-# )
-# else:
-# model = LlamaForCausalLM.from_pretrained(
-# BASE_MODEL, device_map={"": device}, low_cpu_mem_usage=True
-# )
-# model = PeftModel.from_pretrained(
-# model,
-# LORA_WEIGHTS,
-# device_map={"": device},
-# )
-
-
-# if device != "cpu":
-# model.half()
-# model.eval()
-# if torch.__version__ >= "2":
-# model = torch.compile(model)
-
-
-## FLAN-UL2
-HF_TOKEN = os.environ.get("API_TOKEN", None)
-API_URL = "https://api-inference.huggingface.co/models/google/flan-ul2"
-headers = {"Authorization": f"Bearer {HF_TOKEN}"}
-def query(payload):
- response = requests.post(API_URL, headers=headers, json=payload)
- return response.json()
-
-## OpenAI models
-openai.api_key = os.environ.get("OPENAI_TOKEN", None)
-def set_openai_api_key(api_key):
- if api_key and api_key.startswith("sk-") and len(api_key) > 50:
- openai.api_key = api_key
-
-def get_response_from_openai(prompt, model="gpt-3.5-turbo", max_output_tokens=256):
- messages = [{"role": "assistant", "content": prompt}]
- response = openai.ChatCompletion.create(
- model=model,
- messages=messages,
- temperature=0.7,
- max_tokens=max_output_tokens,
- top_p=1,
- frequency_penalty=0,
- presence_penalty=0,
- )
- ret = response.choices[0].message['content']
- return ret
-
-## deplot models
-model_deplot = Pix2StructForConditionalGeneration.from_pretrained("google/deplot", torch_dtype=torch.bfloat16)
-if device == "cuda":
- model_deplot = model_deplot.to(0)
-processor_deplot = Pix2StructProcessor.from_pretrained("google/deplot")
-
-def evaluate(
- table,
- question,
- llm="alpaca-lora",
- input=None,
- temperature=0.1,
- top_p=0.75,
- top_k=40,
- num_beams=4,
- max_new_tokens=128,
- **kwargs,
-):
- prompt_0shot = _INSTRUCTION + "\n" + _add_markup(table) + "\n" + "Q: " + question + "\n" + "A:"
- prompt = _TEMPLATE + "\n" + _add_markup(table) + "\n" + "Q: " + question + "\n" + "A:"
- if llm == "alpaca-lora":
- inputs = tokenizer(prompt, return_tensors="pt")
- input_ids = inputs["input_ids"].to(device)
- generation_config = GenerationConfig(
- temperature=temperature,
- top_p=top_p,
- top_k=top_k,
- num_beams=num_beams,
- **kwargs,
- )
- with torch.no_grad():
- generation_output = model.generate(
- input_ids=input_ids,
- generation_config=generation_config,
- return_dict_in_generate=True,
- output_scores=True,
- max_new_tokens=max_new_tokens,
- )
- s = generation_output.sequences[0]
- output = tokenizer.decode(s)
- elif llm == "flan-ul2":
- try:
- output = query({"inputs": prompt_0shot})[0]["generated_text"]
- except:
- output = ""
- elif llm == "gpt-3.5-turbo":
- try:
- output = get_response_from_openai(prompt_0shot)
- except:
- output = ""
- else:
- RuntimeError(f"No such LLM: {llm}")
-
- return output
-
-
-def process_document(image, question, llm):
- # image = Image.open(image)
- inputs = processor_deplot(images=image, text="Generate the underlying data table for the figure below:", return_tensors="pt").to(torch.bfloat16)
- if device == "cuda":
- inputs = inputs.to(0)
- predictions = model_deplot.generate(**inputs, max_new_tokens=512)
- table = processor_deplot.decode(predictions[0], skip_special_tokens=True).replace("<0x0A>", "\n")
-
- # send prompt+table to LLM
- res = evaluate(table, question, llm=llm)
- if llm == "alpaca-lora":
- return [table, res.split("A:")[-1]]
- else:
- return [table, res]
-
-# theme = gr.themes.Monochrome(
-# primary_hue="indigo",
-# secondary_hue="blue",
-# neutral_hue="slate",
-# radius_size=gr.themes.sizes.radius_sm,
-# font=[gr.themes.GoogleFont("Open Sans"), "ui-sans-serif", "system-ui", "sans-serif"],
-# )
-
-with gr.Blocks(theme="gradio/soft") as demo:
- with gr.Column():
- # gr.Markdown(
- # """
DePlot+LLM: Multimodal chain-of-thought reasoning on plots
- #
- # This is a demo of DePlot+LLM for QA and summarisation. DePlot is an image-to-text model that converts plots and charts into a textual sequence. The sequence then is used to prompt LLM for chain-of-thought reasoning. The current underlying LLMs are alpaca-lora, flan-ul2, and gpt-3.5-turbo. To use it, simply upload your image and type a question or instruction and click 'submit', or click one of the examples to load them. Read more at the links below.
- #
- # """
- # )
- gr.Markdown(
- """
DePlot+LLM: Multimodal chain-of-thought reasoning on plot📊
- This is a demo of DePlot+LLM for QA and summarisation. DePlot is an image-to-text model that converts plots and charts into a textual sequence. The sequence then is used to prompt LLM for chain-of-thought reasoning. The current underlying LLMs are flan-ul2 and gpt-3.5-turbo. To use it, simply upload your image and type a question or instruction and click 'submit', or click one of the examples to load them.
-
- """
- )
-
- with gr.Row():
- with gr.Column(scale=2):
- input_image = gr.Image(label="Input Image", type="pil", interactive=True)
- #input_image.style(height=512, width=512)
- instruction = gr.Textbox(placeholder="Enter your instruction/question...", label="Question/Instruction")
- #llm = gr.Dropdown(["alpaca-lora", "flan-ul2", "gpt-3.5-turbo"], label="LLM")
- llm = gr.Dropdown(["flan-ul2", "gpt-3.5-turbo"], label="LLM")
- openai_api_key_textbox = gr.Textbox(value='',
- placeholder="Paste your OpenAI API key (sk-...) and hit Enter (if using OpenAI models, otherwise leave empty)",
- show_label=False, lines=1, type='password')
- submit = gr.Button("Submit", variant="primary")
-
- with gr.Column(scale=2):
- with gr.Accordion("Show intermediate table", open=False):
- output_table = gr.Textbox(lines=8, label="Intermediate Table")
- output_text = gr.Textbox(lines=8, label="Output")
-
- gr.Examples(
- examples=[
- ["deplot_case_study_6.png", "Rank the four methods according to average model performances. By how much does deplot outperform the second strongest approach on average across the two sets? Show the computation.", "gpt-3.5-turbo"],
- ["deplot_case_study_4.png", "What are the acceptance rates? And how does the acceptance change over the years?", "gpt-3.5-turbo"],
- ["deplot_case_study_m1.png", "Summarise the chart for me please.", "gpt-3.5-turbo"],
- #["deplot_case_study_m1.png", "What is the sum of numbers of Indonesia and Ireland? Remember to think step by step.", "alpaca-lora"],
- #["deplot_case_study_3.png", "By how much did China's growth rate drop? Think step by step.", "alpaca-lora"],
- #["deplot_case_study_4.png", "How many papers are submitted in 2020?", "flan-ul2"],
- ["deplot_case_study_5.png", "Which sales channel has the second highest portion?", "flan-ul2"],
- #["deplot_case_study_x2.png", "Summarise the chart for me please.", "alpaca-lora"],
- #["deplot_case_study_4.png", "How many papers are submitted in 2020?", "alpaca-lora"],
- #["deplot_case_study_m1.png", "Summarise the chart for me please.", "alpaca-lora"],
- #["deplot_case_study_4.png", "acceptance rate = # accepted / #submitted . What is the acceptance rate of 2010?", "flan-ul2"],
- #["deplot_case_study_m1.png", "Summarise the chart for me please.", "flan-ul2"],
- ],
- cache_examples=True,
- inputs=[input_image, instruction, llm],
- outputs=[output_table, output_text],
- fn=process_document
- )
-
- gr.Markdown(
- """
How to Download and Install Autodesk AutoCAD 2017 HF3 X86-x64 RUS-ENG By M0nkrus-=TEAM OS=- for Free
-
-
Autodesk AutoCAD 2017 is a powerful software for designing and drafting 2D and 3D models. It is widely used by architects, engineers, and professionals in various fields. However, the official version of AutoCAD 2017 can be quite expensive and requires a subscription. If you are looking for a free alternative, you might be interested in Autodesk AutoCAD 2017 HF3 X86-x64 RUS-ENG By M0nkrus-=TEAM OS=-.
-
-
This is a modified version of AutoCAD 2017 that has been cracked and patched by M0nkrus, a well-known hacker and uploader. It supports both 32-bit and 64-bit systems, and it has Russian and English language options. It also includes the latest updates and hotfixes from Autodesk. You can download it for free from various torrent sites or file-sharing platforms.
-
Autodesk AutoCAD 2017 HF3 X86-x64 RUS-ENG By M0nkrus- TEAM OS - Free Download
However, before you download and install Autodesk AutoCAD 2017 HF3 X86-x64 RUS-ENG By M0nkrus-=TEAM OS=-, you should be aware of some risks and limitations. First of all, this is an unofficial and illegal version of AutoCAD 2017 that may contain viruses, malware, or spyware. You should always scan the files with a reliable antivirus program before opening them. Second, this version of AutoCAD 2017 may not work properly or have some bugs or errors. You may encounter compatibility issues, performance issues, or stability issues. Third, this version of AutoCAD 2017 may not have all the features or functions of the official version. You may miss out on some updates, enhancements, or support from Autodesk. Fourth, this version of AutoCAD 2017 may violate the terms and conditions of Autodesk and infringe their intellectual property rights. You may face legal consequences or penalties if you use it for commercial purposes or distribute it to others.
-
-
Therefore, if you want to download and install Autodesk AutoCAD 2017 HF3 X86-x64 RUS-ENG By M0nkrus-=TEAM OS=- for free, you should do so at your own risk and discretion. You should also consider buying the official version of AutoCAD 2017 from Autodesk if you want to enjoy the full benefits and features of the software.
-
-
If you decide to download and install Autodesk AutoCAD 2017 HF3 X86-x64 RUS-ENG By M0nkrus-=TEAM OS=- for free, you will need to follow some steps. First, you will need to find a reliable source for the torrent file or the direct link. You can search on various torrent sites or file-sharing platforms, such as The Pirate Bay, 1337x, RARBG, or Mega. However, you should be careful of fake or malicious links that may harm your computer or steal your data. You should also check the comments and ratings of other users to verify the quality and authenticity of the file.
-
-
Second, you will need to download the file using a torrent client or a download manager. You will need to have enough space on your hard drive to store the file, which is about 8.5 GB in size. You will also need to have a stable and fast internet connection to speed up the download process. Depending on your bandwidth and network conditions, the download may take several hours or days to complete.
-
-
Third, you will need to extract the file using a compression tool, such as WinRAR or 7-Zip. You will need to enter the password for the file, which is usually provided by the uploader or in the description of the file. The password may vary depending on the source, but it is often something like "m0nkrus" or "TEAM OS". You will then see a folder with several files and subfolders inside.
d5da3c52bf
-
-
\ No newline at end of file
diff --git a/spaces/gotiQspiryo/whisper-ui/examples/Descargar Discografia De El Viejo Paulino Gratis Lo Mejor De La Msica Regional Mexicana.md b/spaces/gotiQspiryo/whisper-ui/examples/Descargar Discografia De El Viejo Paulino Gratis Lo Mejor De La Msica Regional Mexicana.md
deleted file mode 100644
index bb5308185b470ad175df51dd25aaedf2ef36f8a6..0000000000000000000000000000000000000000
--- a/spaces/gotiQspiryo/whisper-ui/examples/Descargar Discografia De El Viejo Paulino Gratis Lo Mejor De La Msica Regional Mexicana.md
+++ /dev/null
@@ -1,5 +0,0 @@
-
-
Descargar Elviejo Paulino Banda El Limon Mp3 MP3 en alta calidad (HD) resultados, lo nuevo de sus canciones y videos que estan de moda este 2023, bajar musica de Elviejo Paulino Banda El Limon Mp3 en diferentes formatos de audio mp3 el viejo paulino.La Arrolladora MP3 calidad.
aaccfb2cb3
-
-
\ No newline at end of file
diff --git a/spaces/gotiQspiryo/whisper-ui/examples/Family Lies Full Movie Download In Hindi Hd The Secrets and Scandals of a Dysfunctional Family.md b/spaces/gotiQspiryo/whisper-ui/examples/Family Lies Full Movie Download In Hindi Hd The Secrets and Scandals of a Dysfunctional Family.md
deleted file mode 100644
index 2794aa6c2f5ce426f72d07f1db494b414063e313..0000000000000000000000000000000000000000
--- a/spaces/gotiQspiryo/whisper-ui/examples/Family Lies Full Movie Download In Hindi Hd The Secrets and Scandals of a Dysfunctional Family.md
+++ /dev/null
@@ -1,14 +0,0 @@
-
-
"I hope that all of you who are students here will recognize the great opportunity that lies before you in this decade, and in the decades to come, to be of service to our country. The Greeks once defined happiness as full use of your powers along lines of excellence, and I can assure you that there is no area of life where you will have an opportunity to use whatever powers you have, and to use them along more excellent lines, bringing ultimately, I think, happiness to you and those whom you serve." --"Address at the University of Wyoming (381)," September 25, 1963, Public Papers of the Presidents: John F. Kennedy, 1963.
Showcase Cinema de Lux Legacy Place movie theater offers both concessions and The Studio with full bar service. Also available are premium, oversized seats in Lux Level, conference and party theater rentals and Starpass rewards for earning additional perks on your visit. Showcase Cinemas de Lux in Dedham, MA services neighboring communities including Norwood, West Roxbury, Needham and others.
-
wapbold.com - is a free online porn tube portal, where can watch and dowload many free porn movies and porn videos, which is daily updated. So watch and download your favourite mobile porn here, at our wapbold porn site and don`t forget to bookmark us! See you at wapbold.com ;)
-
The country born and bred boy has his own manners andcustoms, which do not resemble those of any other land; and histeachers approach him by roads which an English master would notunderstand. Therefore, you would scarcely be interested in Kim's experiencesas a St Xavier's boy among two or three hundred precociousyouths, most of whom had never seen the sea. He suffered the usual penalties for breaking out of bounds when there was cholera inthe city. This was before he had learned to write fair English, andso was obliged to find a bazar letter-writer. He was, ofcourse, indicted for smoking and for the use of abuse morefull-flavoured than even St Xavier's had ever heard. He learned to washhimself with the Levitical scrupulosity of the native-born, who inhis heart considers the Englishman rather dirty. He played theusual tricks on the patient coolies pulling the punkahs in thesleeping- rooms where the boys threshed through the hot nights tellingtales till the dawn; and quietly he measured himself against hisself- reliant mates.
-
They were a most mad ten days, but Kim enjoyed himself toomuch to reflect on their craziness. In the morning they played theJewel Game - sometimes with veritable stones, sometimes with pilesof swords and daggers, sometimes with photo-graphs of natives.Through the afternoons he and the Hindu boy would mount guard in theshop, sitting dumb behind a carpet-bale or a screen and watchingMr Lurgan's many and very curious visitors. There were smallRajahs, escorts coughing in the veranda, who came to buy curiosities -such as phonographs and mechanical toys. There were ladies in searchof necklaces, and men, it seemed to Kim - but his mind may havebeen vitiated by early training - in search of the ladies; nativesfrom independent and feudatory Courts whose ostensible business wasthe repair of broken necklaces - rivers of light poured out uponthe table - but whose true end seemed to be to raise money forangry Maharanees or young Rajahs. There were Babus to whom LurganSahib talked with austerity and authority, but at the end of each interview he gave them money in coined silver and currencynotes. There were occasional gatherings of long-coated theatricalnatives who discussed metaphysics in English and Bengali, to MrLurgan's great edification. He was always interested in religions. Atthe end of the day, Kim and the Hindu boy - whose name varied at Lurgan's pleasure - were expected to give a detailed account ofall that they had seen and heard - their view of each man'scharacter, as shown in his face, talk, and manner, and their notions ofhis real errand. After dinner, Lurgan Sahib's fancy turned more towhat might be called dressing-up, in which game he took a mostinforming interest. He could paint faces to a marvel; with a brush-dabhere and a line there changing them past recognition. The shop wasfull of all manner of dresses and turbans, and Kim was apparelled variously as a young Mohammedan of good family, an oilman, andonce - which was a joyous evening - as the son of an Oudh landholderin the fullest of full dress. Lurgan Sahib had a hawk's eye todetect the least flaw in the make-up; and lying on a worn teak-woodcouch, would explain by the half-hour together how such and such acaste talked, or walked, or coughed, or spat, or sneezed, and,since 'hows' matter little in this world, the 'why' of everything.The Hindu child played this game clumsily. That little mind, keen asan icicle where tally of jewels was concerned, could not temperitself to enter another's soul; but a demon in Kim woke up and sangwith joy as he put on the changing dresses, and changed speechand gesture therewith.
-
As usual, the lama had led Kim by cow-track and by-road, farfrom the main route along which Hurree Babu, that 'fearful man',had bucketed three days before through a storm to which nineEnglishmen out of ten would have given full right of way. Hurree was nogame- shot - the snick of a trigger made him change colour - but, ashe himself would have said, he was 'fairly effeecient stalker', andhe had raked the huge valley with a pair of cheap binoculars tosome purpose. Moreover, the white of worn canvas tents againstgreen carries far. Hurree Babu had seen all he wanted to see when hesat on the threshing-floor of Ziglaur, twenty miles away as theeagle flies, and forty by road - that is to say, two small dots whichone day were just below the snow-line, and the next had moveddownward perhaps six inches on the hillside. Once cleaned out and set tothe work, his fat bare legs could cover a surprising amount ofground, and this was the reason why, while Kim and the lama lay in aleaky hut at Ziglaur till the storm should be over-past, an oily, wet,but always smiling Bengali, talking the best of English with thevilest of phrases, was ingratiating himself with two sodden andrather rheumatic foreigners. He had arrived, revolving many wildschemes, on the heels of a thunderstorm which had split a pine overagainst their camp, and so convinced a dozen or two forciblyimpressed baggage-coolies the day was inauspicious for farther travelthat with one accord they had thrown down their loads and jibbed.They were subjects of a Hill Rajah who farmed out their services, asis the custom, for his private gain; and, to add to theirpersonal distresses, the strange Sahibs had already threatened themwith rifles. The most of them knew rifles and Sahibs of old: theywere trackers and shikarris of the Northern valleys, keen after bearand wild goat; but they had never been thus treated in their lives.So the forest took them to her bosom, and, for all oaths andclamour, refused to restore. There was no need to feign madness or - theBabu had thought of another means of securing a welcome. He wrung outhis wet clothes, slipped on his patent-leather shoes, opened theblue- and-white umbrella, and with mincing gait and a heartbeating against his tonsils appeared as 'agent for His Royal Highness,the Rajah of Rampur, gentlemen. What can I do for you, please?'
-
-
Hereat, simply as a child engrossed with a new game, the lamathrew back his head and began the full-throated invocation of theDoctor of Divinity ere he opens the full doctrine. The strangers leanedon their alpenstocks and listened. Kim, squatting humbly, watchedthe red sunlight on their faces, and the blend and parting of theirlong shadows. They wore un-English leggings and curious girt-inbelts that reminded him hazily of the pictures in a book in StXavier's library "The Adventures of a Young Naturalist in Mexico" wasits name. Yes, they looked very like the wonderful M. Sumichrast ofthat tale, and very unlike the 'highly unscrupulous folk' ofHurree Babu's imagining. The coolies, earth-coloured and mute,crouched reverently some twenty or thirty yards away, and the Babu, theslack of his thin gear snapping like a marking-flag in the chillbreeze, stood by with an air of happy proprietorship.
-
It was too late. Before Kim could ward him off, the Russianstruck the old man full on the face. Next instant he was rolling overand over downhill with Kim at his throat. The blow had wakedevery unknown Irish devil in the boy's blood, and the sudden fall ofhis enemy did the rest. The lama dropped to his knees, half-stunned;the coolies under their loads fled up the hill as fast as plainsmenrun aross the level. They had seen sacrilege unspeakable, and itbehoved them to get away before the Gods and devils of the hillstook vengeance. The Frenchman ran towards the lama, fumbling athis revolver with some notion of making him a hostage for hiscompanion. A shower of cutting stones - hillmen are very straight shots -drove him away, and a coolie from Ao-chung snatched the lama intothe stampede. All came about as swiftly as the suddenmountain-darkness.
-
Kim might have saved his pity, for though at that moment theBengali suffered acutely in the flesh, his soul was puffed and lofty. Amile down the hill, on the edge of the pine-forest, two half-frozenmen - one powerfully sick at intervals - were varying mutual recriminations with the most poignant abuse of the Babu, whoseemed distraught with terror. They demanded a plan of action. He explained that they were very lucky to be alive; that theircoolies, if not then stalking them, had passed beyond recall; that theRajah, his master, was ninety miles away, and, so far from lendingthem money and a retinue for the Simla journey, would surely castthem into prison if he heard that they had hit a priest. He enlargedon this sin and its consequences till they bade him change thesubject. Their one hope, said he, was unostentatious flight from villageto village till they reached civilization; and, for the hundredthtime dissolved in tears, he demanded of the high stars why theSahibs 'had beaten holy man'.
aaccfb2cb3
-
-
\ No newline at end of file
diff --git a/spaces/gradio/HuBERT/fairseq/data/token_block_dataset.py b/spaces/gradio/HuBERT/fairseq/data/token_block_dataset.py
deleted file mode 100644
index d2c65fd7e058072911c3aa60bfc760288a0f83e5..0000000000000000000000000000000000000000
--- a/spaces/gradio/HuBERT/fairseq/data/token_block_dataset.py
+++ /dev/null
@@ -1,202 +0,0 @@
-# Copyright (c) Facebook, Inc. and its affiliates.
-#
-# This source code is licensed under the MIT license found in the
-# LICENSE file in the root directory of this source tree.
-
-import numpy as np
-import torch
-from fairseq.data import FairseqDataset, plasma_utils
-from fairseq.data.indexed_dataset import best_fitting_int_dtype
-from typing import Tuple
-
-
-class TokenBlockDataset(FairseqDataset):
- """Break a Dataset of tokens into blocks.
-
- Args:
- dataset (~torch.utils.data.Dataset): dataset to break into blocks
- sizes (List[int]): sentence lengths (required for 'complete' and 'eos')
- block_size (int): maximum block size (ignored in 'eos' break mode)
- break_mode (str, optional): Mode used for breaking tokens. Values can
- be one of:
- - 'none': break tokens into equally sized blocks (up to block_size)
- - 'complete': break tokens into blocks (up to block_size) such that
- blocks contains complete sentences, although block_size may be
- exceeded if some sentences exceed block_size
- - 'complete_doc': similar to 'complete' mode, but do not
- cross document boundaries
- - 'eos': each block contains one sentence (block_size is ignored)
- include_targets (bool, optional): return next tokens as targets
- (default: False).
- document_sep_len (int, optional): document separator size (required for
- 'complete_doc' break mode). Typically 1 if the sentences have eos
- and 0 otherwise.
- """
-
- def __init__(
- self,
- dataset,
- sizes,
- block_size,
- pad,
- eos,
- break_mode=None,
- include_targets=False,
- document_sep_len=1,
- use_plasma_view=False,
- split_path=None,
- plasma_path=None,
- ):
-
- super().__init__()
- self.dataset = dataset
- self.pad = pad
- self.eos = eos
- self.include_targets = include_targets
-
- assert len(dataset) > 0
-
- assert len(dataset) == len(sizes)
- _sizes, block_to_dataset_index, slice_indices = self._build_slice_indices(
- sizes, break_mode, document_sep_len, block_size
- )
- if use_plasma_view:
- plasma_id = (block_size, document_sep_len, str(break_mode), len(dataset))
- self._slice_indices = plasma_utils.PlasmaView(
- slice_indices, split_path, (plasma_id, 0), plasma_path=plasma_path
- )
- self._sizes = plasma_utils.PlasmaView(
- _sizes, split_path, (plasma_id, 1), plasma_path=plasma_path
- )
- self._block_to_dataset_index = plasma_utils.PlasmaView(
- block_to_dataset_index, split_path, (plasma_id, 2), plasma_path=plasma_path,
- )
- else:
- self._slice_indices = plasma_utils.PlasmaArray(slice_indices)
- self._sizes = plasma_utils.PlasmaArray(_sizes)
- self._block_to_dataset_index = plasma_utils.PlasmaArray(
- block_to_dataset_index
- )
-
- @staticmethod
- def _build_slice_indices(
- sizes, break_mode, document_sep_len, block_size
- ) -> Tuple[np.ndarray]:
- """Use token_block_utils_fast to build arrays for indexing into self.dataset"""
- try:
- from fairseq.data.token_block_utils_fast import (
- _get_slice_indices_fast,
- _get_block_to_dataset_index_fast,
- )
- except ImportError:
- raise ImportError(
- "Please build Cython components with: `pip install --editable .` "
- "or `python setup.py build_ext --inplace`"
- )
-
- if isinstance(sizes, list):
- sizes = np.array(sizes, dtype=np.int64)
- else:
- if torch.is_tensor(sizes):
- sizes = sizes.numpy()
- sizes = sizes.astype(np.int64)
-
- break_mode = break_mode if break_mode is not None else "none"
-
- # For "eos" break-mode, block_size is not required parameters.
- if break_mode == "eos" and block_size is None:
- block_size = 0
-
- slice_indices = _get_slice_indices_fast(
- sizes, str(break_mode), block_size, document_sep_len
- )
- _sizes = slice_indices[:, 1] - slice_indices[:, 0]
-
- # build index mapping block indices to the underlying dataset indices
- if break_mode == "eos":
- # much faster version for eos break mode
- block_to_dataset_index = np.stack(
- [
- np.arange(len(sizes)), # starting index in dataset
- np.zeros(
- len(sizes), dtype=np.compat.long
- ), # starting offset within starting index
- np.arange(len(sizes)), # ending index in dataset
- ],
- 1,
- )
- else:
- block_to_dataset_index = _get_block_to_dataset_index_fast(
- sizes, slice_indices,
- )
- size_dtype = np.uint16 if block_size < 65535 else np.uint32
- num_tokens = slice_indices[-1].max()
- slice_indices_dtype = best_fitting_int_dtype(num_tokens)
- slice_indices = slice_indices.astype(slice_indices_dtype)
- _sizes = _sizes.astype(size_dtype)
- block_to_dataset_index = block_to_dataset_index.astype(slice_indices_dtype)
- return _sizes, block_to_dataset_index, slice_indices
-
- @property
- def slice_indices(self):
- return self._slice_indices.array
-
- @property
- def sizes(self):
- return self._sizes.array
-
- @property
- def block_to_dataset_index(self):
- return self._block_to_dataset_index.array
-
- def attr(self, attr: str, index: int):
- start_ds_idx, _, _ = self.block_to_dataset_index[index]
- return self.dataset.attr(attr, start_ds_idx)
-
- def __getitem__(self, index):
- start_ds_idx, start_offset, end_ds_idx = self.block_to_dataset_index[index]
-
- buffer = torch.cat(
- [self.dataset[idx] for idx in range(start_ds_idx, end_ds_idx + 1)]
- )
- slice_s, slice_e = self.slice_indices[index]
- length = slice_e - slice_s
- s, e = start_offset, start_offset + length
- item = buffer[s:e]
-
- if self.include_targets:
- # *target* is the original sentence (=item)
- # *source* is shifted right by 1 (maybe left-padded with eos)
- # *past_target* is shifted right by 2 (left-padded as needed)
- if s == 0:
- source = torch.cat([item.new([self.eos]), buffer[0 : e - 1]])
- past_target = torch.cat(
- [item.new([self.pad, self.eos]), buffer[0 : e - 2]]
- )
- else:
- source = buffer[s - 1 : e - 1]
- if s == 1:
- past_target = torch.cat([item.new([self.eos]), buffer[0 : e - 2]])
- else:
- past_target = buffer[s - 2 : e - 2]
-
- return source, item, past_target
-
- return item
-
- def __len__(self):
- return len(self.slice_indices)
-
- @property
- def supports_prefetch(self):
- return getattr(self.dataset, "supports_prefetch", False)
-
- def prefetch(self, indices):
- self.dataset.prefetch(
- {
- ds_idx
- for index in indices
- for start_ds_idx, _, end_ds_idx in [self.block_to_dataset_index[index]]
- for ds_idx in range(start_ds_idx, end_ds_idx + 1)
- }
- )
diff --git a/spaces/gradio/HuBERT/fairseq/modules/multihead_attention.py b/spaces/gradio/HuBERT/fairseq/modules/multihead_attention.py
deleted file mode 100644
index 9bdca0f6af43a0a89e9225594ba5b6fbc5ee04c1..0000000000000000000000000000000000000000
--- a/spaces/gradio/HuBERT/fairseq/modules/multihead_attention.py
+++ /dev/null
@@ -1,500 +0,0 @@
-# Copyright (c) Facebook, Inc. and its affiliates.
-#
-# This source code is licensed under the MIT license found in the
-# LICENSE file in the root directory of this source tree.
-
-import math
-from typing import Dict, Optional, Tuple
-
-import torch
-import torch.nn.functional as F
-from fairseq import utils
-from fairseq.incremental_decoding_utils import with_incremental_state
-from fairseq.modules.fairseq_dropout import FairseqDropout
-from fairseq.modules.quant_noise import quant_noise
-from torch import Tensor, nn
-from torch.nn import Parameter
-
-
-@with_incremental_state
-class MultiheadAttention(nn.Module):
- """Multi-headed attention.
-
- See "Attention Is All You Need" for more details.
- """
-
- def __init__(
- self,
- embed_dim,
- num_heads,
- kdim=None,
- vdim=None,
- dropout=0.0,
- bias=True,
- add_bias_kv=False,
- add_zero_attn=False,
- self_attention=False,
- encoder_decoder_attention=False,
- q_noise=0.0,
- qn_block_size=8,
- ):
- super().__init__()
- self.embed_dim = embed_dim
- self.kdim = kdim if kdim is not None else embed_dim
- self.vdim = vdim if vdim is not None else embed_dim
- self.qkv_same_dim = self.kdim == embed_dim and self.vdim == embed_dim
-
- self.num_heads = num_heads
- self.dropout_module = FairseqDropout(
- dropout, module_name=self.__class__.__name__
- )
-
- self.head_dim = embed_dim // num_heads
- assert (
- self.head_dim * num_heads == self.embed_dim
- ), "embed_dim must be divisible by num_heads"
- self.scaling = self.head_dim ** -0.5
-
- self.self_attention = self_attention
- self.encoder_decoder_attention = encoder_decoder_attention
-
- assert not self.self_attention or self.qkv_same_dim, (
- "Self-attention requires query, key and " "value to be of the same size"
- )
-
- self.k_proj = quant_noise(
- nn.Linear(self.kdim, embed_dim, bias=bias), q_noise, qn_block_size
- )
- self.v_proj = quant_noise(
- nn.Linear(self.vdim, embed_dim, bias=bias), q_noise, qn_block_size
- )
- self.q_proj = quant_noise(
- nn.Linear(embed_dim, embed_dim, bias=bias), q_noise, qn_block_size
- )
-
- self.out_proj = quant_noise(
- nn.Linear(embed_dim, embed_dim, bias=bias), q_noise, qn_block_size
- )
-
- if add_bias_kv:
- self.bias_k = Parameter(torch.Tensor(1, 1, embed_dim))
- self.bias_v = Parameter(torch.Tensor(1, 1, embed_dim))
- else:
- self.bias_k = self.bias_v = None
-
- self.add_zero_attn = add_zero_attn
-
- self.reset_parameters()
-
- self.onnx_trace = False
-
- def prepare_for_onnx_export_(self):
- self.onnx_trace = True
-
- def reset_parameters(self):
- if self.qkv_same_dim:
- # Empirically observed the convergence to be much better with
- # the scaled initialization
- nn.init.xavier_uniform_(self.k_proj.weight, gain=1 / math.sqrt(2))
- nn.init.xavier_uniform_(self.v_proj.weight, gain=1 / math.sqrt(2))
- nn.init.xavier_uniform_(self.q_proj.weight, gain=1 / math.sqrt(2))
- else:
- nn.init.xavier_uniform_(self.k_proj.weight)
- nn.init.xavier_uniform_(self.v_proj.weight)
- nn.init.xavier_uniform_(self.q_proj.weight)
-
- nn.init.xavier_uniform_(self.out_proj.weight)
- if self.out_proj.bias is not None:
- nn.init.constant_(self.out_proj.bias, 0.0)
- if self.bias_k is not None:
- nn.init.xavier_normal_(self.bias_k)
- if self.bias_v is not None:
- nn.init.xavier_normal_(self.bias_v)
-
- def forward(
- self,
- query,
- key: Optional[Tensor],
- value: Optional[Tensor],
- key_padding_mask: Optional[Tensor] = None,
- incremental_state: Optional[Dict[str, Dict[str, Optional[Tensor]]]] = None,
- need_weights: bool = True,
- static_kv: bool = False,
- attn_mask: Optional[Tensor] = None,
- before_softmax: bool = False,
- need_head_weights: bool = False,
- ) -> Tuple[Tensor, Optional[Tensor]]:
- """Input shape: Time x Batch x Channel
-
- Args:
- key_padding_mask (ByteTensor, optional): mask to exclude
- keys that are pads, of shape `(batch, src_len)`, where
- padding elements are indicated by 1s.
- need_weights (bool, optional): return the attention weights,
- averaged over heads (default: False).
- attn_mask (ByteTensor, optional): typically used to
- implement causal attention, where the mask prevents the
- attention from looking forward in time (default: None).
- before_softmax (bool, optional): return the raw attention
- weights and values before the attention softmax.
- need_head_weights (bool, optional): return the attention
- weights for each head. Implies *need_weights*. Default:
- return the average attention weights over all heads.
- """
- if need_head_weights:
- need_weights = True
-
- is_tpu = query.device.type == "xla"
-
- tgt_len, bsz, embed_dim = query.size()
- src_len = tgt_len
- assert embed_dim == self.embed_dim
- assert list(query.size()) == [tgt_len, bsz, embed_dim]
- if key is not None:
- src_len, key_bsz, _ = key.size()
- if not torch.jit.is_scripting():
- assert key_bsz == bsz
- assert value is not None
- assert src_len, bsz == value.shape[:2]
-
- if (
- not self.onnx_trace
- and not is_tpu # don't use PyTorch version on TPUs
- and incremental_state is None
- and not static_kv
- # A workaround for quantization to work. Otherwise JIT compilation
- # treats bias in linear module as method.
- and not torch.jit.is_scripting()
- ):
- assert key is not None and value is not None
- return F.multi_head_attention_forward(
- query,
- key,
- value,
- self.embed_dim,
- self.num_heads,
- torch.empty([0]),
- torch.cat((self.q_proj.bias, self.k_proj.bias, self.v_proj.bias)),
- self.bias_k,
- self.bias_v,
- self.add_zero_attn,
- self.dropout_module.p,
- self.out_proj.weight,
- self.out_proj.bias,
- self.training or self.dropout_module.apply_during_inference,
- key_padding_mask,
- need_weights,
- attn_mask,
- use_separate_proj_weight=True,
- q_proj_weight=self.q_proj.weight,
- k_proj_weight=self.k_proj.weight,
- v_proj_weight=self.v_proj.weight,
- )
-
- if incremental_state is not None:
- saved_state = self._get_input_buffer(incremental_state)
- if saved_state is not None and "prev_key" in saved_state:
- # previous time steps are cached - no need to recompute
- # key and value if they are static
- if static_kv:
- assert self.encoder_decoder_attention and not self.self_attention
- key = value = None
- else:
- saved_state = None
-
- if self.self_attention:
- q = self.q_proj(query)
- k = self.k_proj(query)
- v = self.v_proj(query)
- elif self.encoder_decoder_attention:
- # encoder-decoder attention
- q = self.q_proj(query)
- if key is None:
- assert value is None
- k = v = None
- else:
- k = self.k_proj(key)
- v = self.v_proj(key)
-
- else:
- assert key is not None and value is not None
- q = self.q_proj(query)
- k = self.k_proj(key)
- v = self.v_proj(value)
- q *= self.scaling
-
- if self.bias_k is not None:
- assert self.bias_v is not None
- k = torch.cat([k, self.bias_k.repeat(1, bsz, 1)])
- v = torch.cat([v, self.bias_v.repeat(1, bsz, 1)])
- if attn_mask is not None:
- attn_mask = torch.cat(
- [attn_mask, attn_mask.new_zeros(attn_mask.size(0), 1)], dim=1
- )
- if key_padding_mask is not None:
- key_padding_mask = torch.cat(
- [
- key_padding_mask,
- key_padding_mask.new_zeros(key_padding_mask.size(0), 1),
- ],
- dim=1,
- )
-
- q = (
- q.contiguous()
- .view(tgt_len, bsz * self.num_heads, self.head_dim)
- .transpose(0, 1)
- )
- if k is not None:
- k = (
- k.contiguous()
- .view(-1, bsz * self.num_heads, self.head_dim)
- .transpose(0, 1)
- )
- if v is not None:
- v = (
- v.contiguous()
- .view(-1, bsz * self.num_heads, self.head_dim)
- .transpose(0, 1)
- )
-
- if saved_state is not None:
- # saved states are stored with shape (bsz, num_heads, seq_len, head_dim)
- if "prev_key" in saved_state:
- _prev_key = saved_state["prev_key"]
- assert _prev_key is not None
- prev_key = _prev_key.view(bsz * self.num_heads, -1, self.head_dim)
- if static_kv:
- k = prev_key
- else:
- assert k is not None
- k = torch.cat([prev_key, k], dim=1)
- src_len = k.size(1)
- if "prev_value" in saved_state:
- _prev_value = saved_state["prev_value"]
- assert _prev_value is not None
- prev_value = _prev_value.view(bsz * self.num_heads, -1, self.head_dim)
- if static_kv:
- v = prev_value
- else:
- assert v is not None
- v = torch.cat([prev_value, v], dim=1)
- prev_key_padding_mask: Optional[Tensor] = None
- if "prev_key_padding_mask" in saved_state:
- prev_key_padding_mask = saved_state["prev_key_padding_mask"]
- assert k is not None and v is not None
- key_padding_mask = MultiheadAttention._append_prev_key_padding_mask(
- key_padding_mask=key_padding_mask,
- prev_key_padding_mask=prev_key_padding_mask,
- batch_size=bsz,
- src_len=k.size(1),
- static_kv=static_kv,
- )
-
- saved_state["prev_key"] = k.view(bsz, self.num_heads, -1, self.head_dim)
- saved_state["prev_value"] = v.view(bsz, self.num_heads, -1, self.head_dim)
- saved_state["prev_key_padding_mask"] = key_padding_mask
- # In this branch incremental_state is never None
- assert incremental_state is not None
- incremental_state = self._set_input_buffer(incremental_state, saved_state)
- assert k is not None
- assert k.size(1) == src_len
-
- # This is part of a workaround to get around fork/join parallelism
- # not supporting Optional types.
- if key_padding_mask is not None and key_padding_mask.dim() == 0:
- key_padding_mask = None
-
- if key_padding_mask is not None:
- assert key_padding_mask.size(0) == bsz
- assert key_padding_mask.size(1) == src_len
-
- if self.add_zero_attn:
- assert v is not None
- src_len += 1
- k = torch.cat([k, k.new_zeros((k.size(0), 1) + k.size()[2:])], dim=1)
- v = torch.cat([v, v.new_zeros((v.size(0), 1) + v.size()[2:])], dim=1)
- if attn_mask is not None:
- attn_mask = torch.cat(
- [attn_mask, attn_mask.new_zeros(attn_mask.size(0), 1)], dim=1
- )
- if key_padding_mask is not None:
- key_padding_mask = torch.cat(
- [
- key_padding_mask,
- torch.zeros(key_padding_mask.size(0), 1).type_as(
- key_padding_mask
- ),
- ],
- dim=1,
- )
-
- attn_weights = torch.bmm(q, k.transpose(1, 2))
- attn_weights = self.apply_sparse_mask(attn_weights, tgt_len, src_len, bsz)
-
- assert list(attn_weights.size()) == [bsz * self.num_heads, tgt_len, src_len]
-
- if attn_mask is not None:
- attn_mask = attn_mask.unsqueeze(0)
- if self.onnx_trace:
- attn_mask = attn_mask.repeat(attn_weights.size(0), 1, 1)
- attn_weights += attn_mask
-
- if key_padding_mask is not None:
- # don't attend to padding symbols
- attn_weights = attn_weights.view(bsz, self.num_heads, tgt_len, src_len)
- if not is_tpu:
- attn_weights = attn_weights.masked_fill(
- key_padding_mask.unsqueeze(1).unsqueeze(2).to(torch.bool),
- float("-inf"),
- )
- else:
- attn_weights = attn_weights.transpose(0, 2)
- attn_weights = attn_weights.masked_fill(key_padding_mask, float("-inf"))
- attn_weights = attn_weights.transpose(0, 2)
- attn_weights = attn_weights.view(bsz * self.num_heads, tgt_len, src_len)
-
- if before_softmax:
- return attn_weights, v
-
- attn_weights_float = utils.softmax(
- attn_weights, dim=-1, onnx_trace=self.onnx_trace
- )
- attn_weights = attn_weights_float.type_as(attn_weights)
- attn_probs = self.dropout_module(attn_weights)
-
- assert v is not None
- attn = torch.bmm(attn_probs, v)
- assert list(attn.size()) == [bsz * self.num_heads, tgt_len, self.head_dim]
- if self.onnx_trace and attn.size(1) == 1:
- # when ONNX tracing a single decoder step (sequence length == 1)
- # the transpose is a no-op copy before view, thus unnecessary
- attn = attn.contiguous().view(tgt_len, bsz, embed_dim)
- else:
- attn = attn.transpose(0, 1).contiguous().view(tgt_len, bsz, embed_dim)
- attn = self.out_proj(attn)
- attn_weights: Optional[Tensor] = None
- if need_weights:
- attn_weights = attn_weights_float.view(
- bsz, self.num_heads, tgt_len, src_len
- ).transpose(1, 0)
- if not need_head_weights:
- # average attention weights over heads
- attn_weights = attn_weights.mean(dim=0)
-
- return attn, attn_weights
-
- @staticmethod
- def _append_prev_key_padding_mask(
- key_padding_mask: Optional[Tensor],
- prev_key_padding_mask: Optional[Tensor],
- batch_size: int,
- src_len: int,
- static_kv: bool,
- ) -> Optional[Tensor]:
- # saved key padding masks have shape (bsz, seq_len)
- if prev_key_padding_mask is not None and static_kv:
- new_key_padding_mask = prev_key_padding_mask
- elif prev_key_padding_mask is not None and key_padding_mask is not None:
- new_key_padding_mask = torch.cat(
- [prev_key_padding_mask.float(), key_padding_mask.float()], dim=1
- )
- # During incremental decoding, as the padding token enters and
- # leaves the frame, there will be a time when prev or current
- # is None
- elif prev_key_padding_mask is not None:
- if src_len > prev_key_padding_mask.size(1):
- filler = torch.zeros(
- (batch_size, src_len - prev_key_padding_mask.size(1)),
- device=prev_key_padding_mask.device,
- )
- new_key_padding_mask = torch.cat(
- [prev_key_padding_mask.float(), filler.float()], dim=1
- )
- else:
- new_key_padding_mask = prev_key_padding_mask.float()
- elif key_padding_mask is not None:
- if src_len > key_padding_mask.size(1):
- filler = torch.zeros(
- (batch_size, src_len - key_padding_mask.size(1)),
- device=key_padding_mask.device,
- )
- new_key_padding_mask = torch.cat(
- [filler.float(), key_padding_mask.float()], dim=1
- )
- else:
- new_key_padding_mask = key_padding_mask.float()
- else:
- new_key_padding_mask = prev_key_padding_mask
- return new_key_padding_mask
-
- @torch.jit.export
- def reorder_incremental_state(
- self,
- incremental_state: Dict[str, Dict[str, Optional[Tensor]]],
- new_order: Tensor,
- ):
- """Reorder buffered internal state (for incremental generation)."""
- input_buffer = self._get_input_buffer(incremental_state)
- if input_buffer is not None:
- for k in input_buffer.keys():
- input_buffer_k = input_buffer[k]
- if input_buffer_k is not None:
- if self.encoder_decoder_attention and input_buffer_k.size(
- 0
- ) == new_order.size(0):
- break
- input_buffer[k] = input_buffer_k.index_select(0, new_order)
- incremental_state = self._set_input_buffer(incremental_state, input_buffer)
- return incremental_state
-
- def _get_input_buffer(
- self, incremental_state: Optional[Dict[str, Dict[str, Optional[Tensor]]]]
- ) -> Dict[str, Optional[Tensor]]:
- result = self.get_incremental_state(incremental_state, "attn_state")
- if result is not None:
- return result
- else:
- empty_result: Dict[str, Optional[Tensor]] = {}
- return empty_result
-
- def _set_input_buffer(
- self,
- incremental_state: Dict[str, Dict[str, Optional[Tensor]]],
- buffer: Dict[str, Optional[Tensor]],
- ):
- return self.set_incremental_state(incremental_state, "attn_state", buffer)
-
- def apply_sparse_mask(self, attn_weights, tgt_len: int, src_len: int, bsz: int):
- return attn_weights
-
- def upgrade_state_dict_named(self, state_dict, name):
- prefix = name + "." if name != "" else ""
- items_to_add = {}
- keys_to_remove = []
- for k in state_dict.keys():
- if k.endswith(prefix + "in_proj_weight"):
- # in_proj_weight used to be q + k + v with same dimensions
- dim = int(state_dict[k].shape[0] / 3)
- items_to_add[prefix + "q_proj.weight"] = state_dict[k][:dim]
- items_to_add[prefix + "k_proj.weight"] = state_dict[k][dim : 2 * dim]
- items_to_add[prefix + "v_proj.weight"] = state_dict[k][2 * dim :]
-
- keys_to_remove.append(k)
-
- k_bias = prefix + "in_proj_bias"
- if k_bias in state_dict.keys():
- dim = int(state_dict[k].shape[0] / 3)
- items_to_add[prefix + "q_proj.bias"] = state_dict[k_bias][:dim]
- items_to_add[prefix + "k_proj.bias"] = state_dict[k_bias][
- dim : 2 * dim
- ]
- items_to_add[prefix + "v_proj.bias"] = state_dict[k_bias][2 * dim :]
-
- keys_to_remove.append(prefix + "in_proj_bias")
-
- for k in keys_to_remove:
- del state_dict[k]
-
- for key, value in items_to_add.items():
- state_dict[key] = value
diff --git a/spaces/gradio/HuBERT/fairseq/sequence_generator.py b/spaces/gradio/HuBERT/fairseq/sequence_generator.py
deleted file mode 100644
index 8a3858563ec0c3cd7f3177bcd2897d27b61dbe00..0000000000000000000000000000000000000000
--- a/spaces/gradio/HuBERT/fairseq/sequence_generator.py
+++ /dev/null
@@ -1,980 +0,0 @@
-# Copyright (c) Facebook, Inc. and its affiliates.
-#
-# This source code is licensed under the MIT license found in the
-# LICENSE file in the root directory of this source tree.
-
-import math
-from typing import Dict, List, Optional
-import sys
-
-import torch
-import torch.nn as nn
-from fairseq import search, utils
-from fairseq.data import data_utils
-from fairseq.models import FairseqIncrementalDecoder
-from torch import Tensor
-from fairseq.ngram_repeat_block import NGramRepeatBlock
-
-
-class SequenceGenerator(nn.Module):
- def __init__(
- self,
- models,
- tgt_dict,
- beam_size=1,
- max_len_a=0,
- max_len_b=200,
- max_len=0,
- min_len=1,
- normalize_scores=True,
- len_penalty=1.0,
- unk_penalty=0.0,
- temperature=1.0,
- match_source_len=False,
- no_repeat_ngram_size=0,
- search_strategy=None,
- eos=None,
- symbols_to_strip_from_output=None,
- lm_model=None,
- lm_weight=1.0,
- ):
- """Generates translations of a given source sentence.
-
- Args:
- models (List[~fairseq.models.FairseqModel]): ensemble of models,
- currently support fairseq.models.TransformerModel for scripting
- beam_size (int, optional): beam width (default: 1)
- max_len_a/b (int, optional): generate sequences of maximum length
- ax + b, where x is the source length
- max_len (int, optional): the maximum length of the generated output
- (not including end-of-sentence)
- min_len (int, optional): the minimum length of the generated output
- (not including end-of-sentence)
- normalize_scores (bool, optional): normalize scores by the length
- of the output (default: True)
- len_penalty (float, optional): length penalty, where <1.0 favors
- shorter, >1.0 favors longer sentences (default: 1.0)
- unk_penalty (float, optional): unknown word penalty, where <0
- produces more unks, >0 produces fewer (default: 0.0)
- temperature (float, optional): temperature, where values
- >1.0 produce more uniform samples and values <1.0 produce
- sharper samples (default: 1.0)
- match_source_len (bool, optional): outputs should match the source
- length (default: False)
- """
- super().__init__()
- if isinstance(models, EnsembleModel):
- self.model = models
- else:
- self.model = EnsembleModel(models)
- self.tgt_dict = tgt_dict
- self.pad = tgt_dict.pad()
- self.unk = tgt_dict.unk()
- self.eos = tgt_dict.eos() if eos is None else eos
- self.symbols_to_strip_from_output = (
- symbols_to_strip_from_output.union({self.eos})
- if symbols_to_strip_from_output is not None
- else {self.eos}
- )
- self.vocab_size = len(tgt_dict)
- self.beam_size = beam_size
- # the max beam size is the dictionary size - 1, since we never select pad
- self.beam_size = min(beam_size, self.vocab_size - 1)
- self.max_len_a = max_len_a
- self.max_len_b = max_len_b
- self.min_len = min_len
- self.max_len = max_len or self.model.max_decoder_positions()
-
- self.normalize_scores = normalize_scores
- self.len_penalty = len_penalty
- self.unk_penalty = unk_penalty
- self.temperature = temperature
- self.match_source_len = match_source_len
-
- if no_repeat_ngram_size > 0:
- self.repeat_ngram_blocker = NGramRepeatBlock(no_repeat_ngram_size)
- else:
- self.repeat_ngram_blocker = None
-
- assert temperature > 0, "--temperature must be greater than 0"
-
- self.search = (
- search.BeamSearch(tgt_dict) if search_strategy is None else search_strategy
- )
- # We only need to set src_lengths in LengthConstrainedBeamSearch.
- # As a module attribute, setting it would break in multithread
- # settings when the model is shared.
- self.should_set_src_lengths = (
- hasattr(self.search, "needs_src_lengths") and self.search.needs_src_lengths
- )
-
- self.model.eval()
-
- self.lm_model = lm_model
- self.lm_weight = lm_weight
- if self.lm_model is not None:
- self.lm_model.eval()
-
- def cuda(self):
- self.model.cuda()
- return self
-
- @torch.no_grad()
- def forward(
- self,
- sample: Dict[str, Dict[str, Tensor]],
- prefix_tokens: Optional[Tensor] = None,
- bos_token: Optional[int] = None,
- ):
- """Generate a batch of translations.
-
- Args:
- sample (dict): batch
- prefix_tokens (torch.LongTensor, optional): force decoder to begin
- with these tokens
- bos_token (int, optional): beginning of sentence token
- (default: self.eos)
- """
- return self._generate(sample, prefix_tokens, bos_token=bos_token)
-
- # TODO(myleott): unused, deprecate after pytorch-translate migration
- def generate_batched_itr(self, data_itr, beam_size=None, cuda=False, timer=None):
- """Iterate over a batched dataset and yield individual translations.
- Args:
- cuda (bool, optional): use GPU for generation
- timer (StopwatchMeter, optional): time generations
- """
- for sample in data_itr:
- s = utils.move_to_cuda(sample) if cuda else sample
- if "net_input" not in s:
- continue
- input = s["net_input"]
- # model.forward normally channels prev_output_tokens into the decoder
- # separately, but SequenceGenerator directly calls model.encoder
- encoder_input = {
- k: v for k, v in input.items() if k != "prev_output_tokens"
- }
- if timer is not None:
- timer.start()
- with torch.no_grad():
- hypos = self.generate(encoder_input)
- if timer is not None:
- timer.stop(sum(len(h[0]["tokens"]) for h in hypos))
- for i, id in enumerate(s["id"].data):
- # remove padding
- src = utils.strip_pad(input["src_tokens"].data[i, :], self.pad)
- ref = (
- utils.strip_pad(s["target"].data[i, :], self.pad)
- if s["target"] is not None
- else None
- )
- yield id, src, ref, hypos[i]
-
- @torch.no_grad()
- def generate(self, models, sample: Dict[str, Dict[str, Tensor]], **kwargs) -> List[List[Dict[str, Tensor]]]:
- """Generate translations. Match the api of other fairseq generators.
-
- Args:
- models (List[~fairseq.models.FairseqModel]): ensemble of models
- sample (dict): batch
- prefix_tokens (torch.LongTensor, optional): force decoder to begin
- with these tokens
- constraints (torch.LongTensor, optional): force decoder to include
- the list of constraints
- bos_token (int, optional): beginning of sentence token
- (default: self.eos)
- """
- return self._generate(sample, **kwargs)
-
- def _generate(
- self,
- sample: Dict[str, Dict[str, Tensor]],
- prefix_tokens: Optional[Tensor] = None,
- constraints: Optional[Tensor] = None,
- bos_token: Optional[int] = None,
- ):
- incremental_states = torch.jit.annotate(
- List[Dict[str, Dict[str, Optional[Tensor]]]],
- [
- torch.jit.annotate(Dict[str, Dict[str, Optional[Tensor]]], {})
- for i in range(self.model.models_size)
- ],
- )
- net_input = sample["net_input"]
-
- if "src_tokens" in net_input:
- src_tokens = net_input["src_tokens"]
- # length of the source text being the character length except EndOfSentence and pad
- src_lengths = (
- (src_tokens.ne(self.eos) & src_tokens.ne(self.pad)).long().sum(dim=1)
- )
- elif "source" in net_input:
- src_tokens = net_input["source"]
- src_lengths = (
- net_input["padding_mask"].size(-1) - net_input["padding_mask"].sum(-1)
- if net_input["padding_mask"] is not None
- else torch.tensor(src_tokens.size(-1)).to(src_tokens)
- )
- elif "features" in net_input:
- src_tokens = net_input["features"]
- src_lengths = (
- net_input["padding_mask"].size(-1) - net_input["padding_mask"].sum(-1)
- if net_input["padding_mask"] is not None
- else torch.tensor(src_tokens.size(-1)).to(src_tokens)
- )
- else:
- raise Exception("expected src_tokens or source in net input. input keys: " + str(net_input.keys()))
-
- # bsz: total number of sentences in beam
- # Note that src_tokens may have more than 2 dimensions (i.e. audio features)
- bsz, src_len = src_tokens.size()[:2]
- beam_size = self.beam_size
-
- if constraints is not None and not self.search.supports_constraints:
- raise NotImplementedError(
- "Target-side constraints were provided, but search method doesn't support them"
- )
-
- # Initialize constraints, when active
- self.search.init_constraints(constraints, beam_size)
-
- max_len: int = -1
- if self.match_source_len:
- max_len = src_lengths.max().item()
- else:
- max_len = min(
- int(self.max_len_a * src_len + self.max_len_b),
- self.max_len - 1,
- )
- assert (
- self.min_len <= max_len
- ), "min_len cannot be larger than max_len, please adjust these!"
- # compute the encoder output for each beam
- encoder_outs = self.model.forward_encoder(net_input)
-
- # placeholder of indices for bsz * beam_size to hold tokens and accumulative scores
- new_order = torch.arange(bsz).view(-1, 1).repeat(1, beam_size).view(-1)
- new_order = new_order.to(src_tokens.device).long()
- encoder_outs = self.model.reorder_encoder_out(encoder_outs, new_order)
- # ensure encoder_outs is a List.
- assert encoder_outs is not None
-
- # initialize buffers
- scores = (
- torch.zeros(bsz * beam_size, max_len + 1).to(src_tokens).float()
- ) # +1 for eos; pad is never chosen for scoring
- tokens = (
- torch.zeros(bsz * beam_size, max_len + 2)
- .to(src_tokens)
- .long()
- .fill_(self.pad)
- ) # +2 for eos and pad
- tokens[:, 0] = self.eos if bos_token is None else bos_token
- attn: Optional[Tensor] = None
-
- # A list that indicates candidates that should be ignored.
- # For example, suppose we're sampling and have already finalized 2/5
- # samples. Then cands_to_ignore would mark 2 positions as being ignored,
- # so that we only finalize the remaining 3 samples.
- cands_to_ignore = (
- torch.zeros(bsz, beam_size).to(src_tokens).eq(-1)
- ) # forward and backward-compatible False mask
-
- # list of completed sentences
- finalized = torch.jit.annotate(
- List[List[Dict[str, Tensor]]],
- [torch.jit.annotate(List[Dict[str, Tensor]], []) for i in range(bsz)],
- ) # contains lists of dictionaries of infomation about the hypothesis being finalized at each step
-
- # a boolean array indicating if the sentence at the index is finished or not
- finished = [False for i in range(bsz)]
- num_remaining_sent = bsz # number of sentences remaining
-
- # number of candidate hypos per step
- cand_size = 2 * beam_size # 2 x beam size in case half are EOS
-
- # offset arrays for converting between different indexing schemes
- bbsz_offsets = (
- (torch.arange(0, bsz) * beam_size)
- .unsqueeze(1)
- .type_as(tokens)
- .to(src_tokens.device)
- )
- cand_offsets = torch.arange(0, cand_size).type_as(tokens).to(src_tokens.device)
-
- reorder_state: Optional[Tensor] = None
- batch_idxs: Optional[Tensor] = None
-
- original_batch_idxs: Optional[Tensor] = None
- if "id" in sample and isinstance(sample["id"], Tensor):
- original_batch_idxs = sample["id"]
- else:
- original_batch_idxs = torch.arange(0, bsz).type_as(tokens)
-
- for step in range(max_len + 1): # one extra step for EOS marker
- # reorder decoder internal states based on the prev choice of beams
- if reorder_state is not None:
- if batch_idxs is not None:
- # update beam indices to take into account removed sentences
- corr = batch_idxs - torch.arange(batch_idxs.numel()).type_as(
- batch_idxs
- )
- reorder_state.view(-1, beam_size).add_(
- corr.unsqueeze(-1) * beam_size
- )
- original_batch_idxs = original_batch_idxs[batch_idxs]
- self.model.reorder_incremental_state(incremental_states, reorder_state)
- encoder_outs = self.model.reorder_encoder_out(
- encoder_outs, reorder_state
- )
-
- lprobs, avg_attn_scores = self.model.forward_decoder(
- tokens[:, : step + 1],
- encoder_outs,
- incremental_states,
- self.temperature,
- )
-
- if self.lm_model is not None:
- lm_out = self.lm_model(tokens[:, : step + 1])
- probs = self.lm_model.get_normalized_probs(
- lm_out, log_probs=True, sample=None
- )
- probs = probs[:, -1, :] * self.lm_weight
- lprobs += probs
-
- lprobs[lprobs != lprobs] = torch.tensor(-math.inf).to(lprobs)
-
- lprobs[:, self.pad] = -math.inf # never select pad
- lprobs[:, self.unk] -= self.unk_penalty # apply unk penalty
-
- # handle max length constraint
- if step >= max_len:
- lprobs[:, : self.eos] = -math.inf
- lprobs[:, self.eos + 1 :] = -math.inf
-
- # handle prefix tokens (possibly with different lengths)
- if (
- prefix_tokens is not None
- and step < prefix_tokens.size(1)
- and step < max_len
- ):
- lprobs, tokens, scores = self._prefix_tokens(
- step, lprobs, scores, tokens, prefix_tokens, beam_size
- )
- elif step < self.min_len:
- # minimum length constraint (does not apply if using prefix_tokens)
- lprobs[:, self.eos] = -math.inf
-
- # Record attention scores, only support avg_attn_scores is a Tensor
- if avg_attn_scores is not None:
- if attn is None:
- attn = torch.empty(
- bsz * beam_size, avg_attn_scores.size(1), max_len + 2
- ).to(scores)
- attn[:, :, step + 1].copy_(avg_attn_scores)
-
- scores = scores.type_as(lprobs)
- eos_bbsz_idx = torch.empty(0).to(
- tokens
- ) # indices of hypothesis ending with eos (finished sentences)
- eos_scores = torch.empty(0).to(
- scores
- ) # scores of hypothesis ending with eos (finished sentences)
-
- if self.should_set_src_lengths:
- self.search.set_src_lengths(src_lengths)
-
- if self.repeat_ngram_blocker is not None:
- lprobs = self.repeat_ngram_blocker(tokens, lprobs, bsz, beam_size, step)
-
- # Shape: (batch, cand_size)
- cand_scores, cand_indices, cand_beams = self.search.step(
- step,
- lprobs.view(bsz, -1, self.vocab_size),
- scores.view(bsz, beam_size, -1)[:, :, :step],
- tokens[:, : step + 1],
- original_batch_idxs,
- )
-
- # cand_bbsz_idx contains beam indices for the top candidate
- # hypotheses, with a range of values: [0, bsz*beam_size),
- # and dimensions: [bsz, cand_size]
- cand_bbsz_idx = cand_beams.add(bbsz_offsets)
-
- # finalize hypotheses that end in eos
- # Shape of eos_mask: (batch size, beam size)
- eos_mask = cand_indices.eq(self.eos) & cand_scores.ne(-math.inf)
- eos_mask[:, :beam_size][cands_to_ignore] = torch.tensor(0).to(eos_mask)
-
- # only consider eos when it's among the top beam_size indices
- # Now we know what beam item(s) to finish
- # Shape: 1d list of absolute-numbered
- eos_bbsz_idx = torch.masked_select(
- cand_bbsz_idx[:, :beam_size], mask=eos_mask[:, :beam_size]
- )
-
- finalized_sents: List[int] = []
- if eos_bbsz_idx.numel() > 0:
- eos_scores = torch.masked_select(
- cand_scores[:, :beam_size], mask=eos_mask[:, :beam_size]
- )
-
- finalized_sents = self.finalize_hypos(
- step,
- eos_bbsz_idx,
- eos_scores,
- tokens,
- scores,
- finalized,
- finished,
- beam_size,
- attn,
- src_lengths,
- max_len,
- )
- num_remaining_sent -= len(finalized_sents)
-
- assert num_remaining_sent >= 0
- if num_remaining_sent == 0:
- break
- if self.search.stop_on_max_len and step >= max_len:
- break
- assert step < max_len, f"{step} < {max_len}"
-
- # Remove finalized sentences (ones for which {beam_size}
- # finished hypotheses have been generated) from the batch.
- if len(finalized_sents) > 0:
- new_bsz = bsz - len(finalized_sents)
-
- # construct batch_idxs which holds indices of batches to keep for the next pass
- batch_mask = torch.ones(
- bsz, dtype=torch.bool, device=cand_indices.device
- )
- batch_mask[finalized_sents] = False
- # TODO replace `nonzero(as_tuple=False)` after TorchScript supports it
- batch_idxs = torch.arange(
- bsz, device=cand_indices.device
- ).masked_select(batch_mask)
-
- # Choose the subset of the hypothesized constraints that will continue
- self.search.prune_sentences(batch_idxs)
-
- eos_mask = eos_mask[batch_idxs]
- cand_beams = cand_beams[batch_idxs]
- bbsz_offsets.resize_(new_bsz, 1)
- cand_bbsz_idx = cand_beams.add(bbsz_offsets)
- cand_scores = cand_scores[batch_idxs]
- cand_indices = cand_indices[batch_idxs]
-
- if prefix_tokens is not None:
- prefix_tokens = prefix_tokens[batch_idxs]
- src_lengths = src_lengths[batch_idxs]
- cands_to_ignore = cands_to_ignore[batch_idxs]
-
- scores = scores.view(bsz, -1)[batch_idxs].view(new_bsz * beam_size, -1)
- tokens = tokens.view(bsz, -1)[batch_idxs].view(new_bsz * beam_size, -1)
- if attn is not None:
- attn = attn.view(bsz, -1)[batch_idxs].view(
- new_bsz * beam_size, attn.size(1), -1
- )
- bsz = new_bsz
- else:
- batch_idxs = None
-
- # Set active_mask so that values > cand_size indicate eos hypos
- # and values < cand_size indicate candidate active hypos.
- # After, the min values per row are the top candidate active hypos
-
- # Rewrite the operator since the element wise or is not supported in torchscript.
-
- eos_mask[:, :beam_size] = ~((~cands_to_ignore) & (~eos_mask[:, :beam_size]))
- active_mask = torch.add(
- eos_mask.type_as(cand_offsets) * cand_size,
- cand_offsets[: eos_mask.size(1)],
- )
-
- # get the top beam_size active hypotheses, which are just
- # the hypos with the smallest values in active_mask.
- # {active_hypos} indicates which {beam_size} hypotheses
- # from the list of {2 * beam_size} candidates were
- # selected. Shapes: (batch size, beam size)
- new_cands_to_ignore, active_hypos = torch.topk(
- active_mask, k=beam_size, dim=1, largest=False
- )
-
- # update cands_to_ignore to ignore any finalized hypos.
- cands_to_ignore = new_cands_to_ignore.ge(cand_size)[:, :beam_size]
- # Make sure there is at least one active item for each sentence in the batch.
- assert (~cands_to_ignore).any(dim=1).all()
-
- # update cands_to_ignore to ignore any finalized hypos
-
- # {active_bbsz_idx} denotes which beam number is continued for each new hypothesis (a beam
- # can be selected more than once).
- active_bbsz_idx = torch.gather(cand_bbsz_idx, dim=1, index=active_hypos)
- active_scores = torch.gather(cand_scores, dim=1, index=active_hypos)
-
- active_bbsz_idx = active_bbsz_idx.view(-1)
- active_scores = active_scores.view(-1)
-
- # copy tokens and scores for active hypotheses
-
- # Set the tokens for each beam (can select the same row more than once)
- tokens[:, : step + 1] = torch.index_select(
- tokens[:, : step + 1], dim=0, index=active_bbsz_idx
- )
- # Select the next token for each of them
- tokens.view(bsz, beam_size, -1)[:, :, step + 1] = torch.gather(
- cand_indices, dim=1, index=active_hypos
- )
- if step > 0:
- scores[:, :step] = torch.index_select(
- scores[:, :step], dim=0, index=active_bbsz_idx
- )
- scores.view(bsz, beam_size, -1)[:, :, step] = torch.gather(
- cand_scores, dim=1, index=active_hypos
- )
-
- # Update constraints based on which candidates were selected for the next beam
- self.search.update_constraints(active_hypos)
-
- # copy attention for active hypotheses
- if attn is not None:
- attn[:, :, : step + 2] = torch.index_select(
- attn[:, :, : step + 2], dim=0, index=active_bbsz_idx
- )
-
- # reorder incremental state in decoder
- reorder_state = active_bbsz_idx
-
- # sort by score descending
- for sent in range(len(finalized)):
- scores = torch.tensor(
- [float(elem["score"].item()) for elem in finalized[sent]]
- )
- _, sorted_scores_indices = torch.sort(scores, descending=True)
- finalized[sent] = [finalized[sent][ssi] for ssi in sorted_scores_indices]
- finalized[sent] = torch.jit.annotate(
- List[Dict[str, Tensor]], finalized[sent]
- )
- return finalized
-
- def _prefix_tokens(
- self, step: int, lprobs, scores, tokens, prefix_tokens, beam_size: int
- ):
- """Handle prefix tokens"""
- prefix_toks = prefix_tokens[:, step].unsqueeze(-1).repeat(1, beam_size).view(-1)
- prefix_lprobs = lprobs.gather(-1, prefix_toks.unsqueeze(-1))
- prefix_mask = prefix_toks.ne(self.pad)
- lprobs[prefix_mask] = torch.tensor(-math.inf).to(lprobs)
- lprobs[prefix_mask] = lprobs[prefix_mask].scatter(
- -1, prefix_toks[prefix_mask].unsqueeze(-1), prefix_lprobs[prefix_mask]
- )
- # if prefix includes eos, then we should make sure tokens and
- # scores are the same across all beams
- eos_mask = prefix_toks.eq(self.eos)
- if eos_mask.any():
- # validate that the first beam matches the prefix
- first_beam = tokens[eos_mask].view(-1, beam_size, tokens.size(-1))[
- :, 0, 1 : step + 1
- ]
- eos_mask_batch_dim = eos_mask.view(-1, beam_size)[:, 0]
- target_prefix = prefix_tokens[eos_mask_batch_dim][:, :step]
- assert (first_beam == target_prefix).all()
-
- # copy tokens, scores and lprobs from the first beam to all beams
- tokens = self.replicate_first_beam(tokens, eos_mask_batch_dim, beam_size)
- scores = self.replicate_first_beam(scores, eos_mask_batch_dim, beam_size)
- lprobs = self.replicate_first_beam(lprobs, eos_mask_batch_dim, beam_size)
- return lprobs, tokens, scores
-
- def replicate_first_beam(self, tensor, mask, beam_size: int):
- tensor = tensor.view(-1, beam_size, tensor.size(-1))
- tensor[mask] = tensor[mask][:, :1, :]
- return tensor.view(-1, tensor.size(-1))
-
- def finalize_hypos(
- self,
- step: int,
- bbsz_idx,
- eos_scores,
- tokens,
- scores,
- finalized: List[List[Dict[str, Tensor]]],
- finished: List[bool],
- beam_size: int,
- attn: Optional[Tensor],
- src_lengths,
- max_len: int,
- ):
- """Finalize hypothesis, store finalized information in `finalized`, and change `finished` accordingly.
- A sentence is finalized when {beam_size} finished items have been collected for it.
-
- Returns number of sentences (not beam items) being finalized.
- These will be removed from the batch and not processed further.
- Args:
- bbsz_idx (Tensor):
- """
- assert bbsz_idx.numel() == eos_scores.numel()
-
- # clone relevant token and attention tensors.
- # tokens is (batch * beam, max_len). So the index_select
- # gets the newly EOS rows, then selects cols 1..{step + 2}
- tokens_clone = tokens.index_select(0, bbsz_idx)[
- :, 1 : step + 2
- ] # skip the first index, which is EOS
-
- tokens_clone[:, step] = self.eos
- attn_clone = (
- attn.index_select(0, bbsz_idx)[:, :, 1 : step + 2]
- if attn is not None
- else None
- )
-
- # compute scores per token position
- pos_scores = scores.index_select(0, bbsz_idx)[:, : step + 1]
- pos_scores[:, step] = eos_scores
- # convert from cumulative to per-position scores
- pos_scores[:, 1:] = pos_scores[:, 1:] - pos_scores[:, :-1]
-
- # normalize sentence-level scores
- if self.normalize_scores:
- eos_scores /= (step + 1) ** self.len_penalty
-
- # cum_unfin records which sentences in the batch are finished.
- # It helps match indexing between (a) the original sentences
- # in the batch and (b) the current, possibly-reduced set of
- # sentences.
- cum_unfin: List[int] = []
- prev = 0
- for f in finished:
- if f:
- prev += 1
- else:
- cum_unfin.append(prev)
-
- # The keys here are of the form "{sent}_{unfin_idx}", where
- # "unfin_idx" is the index in the current (possibly reduced)
- # list of sentences, and "sent" is the index in the original,
- # unreduced batch
- # set() is not supported in script export
- sents_seen: Dict[str, Optional[Tensor]] = {}
-
- # For every finished beam item
- for i in range(bbsz_idx.size()[0]):
- idx = bbsz_idx[i]
- score = eos_scores[i]
- # sentence index in the current (possibly reduced) batch
- unfin_idx = idx // beam_size
- # sentence index in the original (unreduced) batch
- sent = unfin_idx + cum_unfin[unfin_idx]
- # Cannot create dict for key type '(int, int)' in torchscript.
- # The workaround is to cast int to string
- seen = str(sent.item()) + "_" + str(unfin_idx.item())
- if seen not in sents_seen:
- sents_seen[seen] = None
-
- if self.match_source_len and step > src_lengths[unfin_idx]:
- score = torch.tensor(-math.inf).to(score)
-
- # An input sentence (among those in a batch) is finished when
- # beam_size hypotheses have been collected for it
- if len(finalized[sent]) < beam_size:
- if attn_clone is not None:
- # remove padding tokens from attn scores
- hypo_attn = attn_clone[i]
- else:
- hypo_attn = torch.empty(0)
-
- finalized[sent].append(
- {
- "tokens": tokens_clone[i],
- "score": score,
- "attention": hypo_attn, # src_len x tgt_len
- "alignment": torch.empty(0),
- "positional_scores": pos_scores[i],
- }
- )
-
- newly_finished: List[int] = []
-
- for seen in sents_seen.keys():
- # check termination conditions for this sentence
- sent: int = int(float(seen.split("_")[0]))
- unfin_idx: int = int(float(seen.split("_")[1]))
-
- if not finished[sent] and self.is_finished(
- step, unfin_idx, max_len, len(finalized[sent]), beam_size
- ):
- finished[sent] = True
- newly_finished.append(unfin_idx)
-
- return newly_finished
-
- def is_finished(
- self,
- step: int,
- unfin_idx: int,
- max_len: int,
- finalized_sent_len: int,
- beam_size: int,
- ):
- """
- Check whether decoding for a sentence is finished, which
- occurs when the list of finalized sentences has reached the
- beam size, or when we reach the maximum length.
- """
- assert finalized_sent_len <= beam_size
- if finalized_sent_len == beam_size or step == max_len:
- return True
- return False
-
-
-class EnsembleModel(nn.Module):
- """A wrapper around an ensemble of models."""
-
- def __init__(self, models):
- super().__init__()
- self.models_size = len(models)
- # method '__len__' is not supported in ModuleList for torch script
- self.single_model = models[0]
- self.models = nn.ModuleList(models)
-
- self.has_incremental: bool = False
- if all(
- hasattr(m, "decoder") and isinstance(m.decoder, FairseqIncrementalDecoder)
- for m in models
- ):
- self.has_incremental = True
-
- def forward(self):
- pass
-
- def has_encoder(self):
- return hasattr(self.single_model, "encoder")
-
- def has_incremental_states(self):
- return self.has_incremental
-
- def max_decoder_positions(self):
- return min([m.max_decoder_positions() for m in self.models if hasattr(m, "max_decoder_positions")] + [sys.maxsize])
-
- @torch.jit.export
- def forward_encoder(self, net_input: Dict[str, Tensor]):
- if not self.has_encoder():
- return None
- return [model.encoder.forward_torchscript(net_input) for model in self.models]
-
- @torch.jit.export
- def forward_decoder(
- self,
- tokens,
- encoder_outs: List[Dict[str, List[Tensor]]],
- incremental_states: List[Dict[str, Dict[str, Optional[Tensor]]]],
- temperature: float = 1.0,
- ):
- log_probs = []
- avg_attn: Optional[Tensor] = None
- encoder_out: Optional[Dict[str, List[Tensor]]] = None
- for i, model in enumerate(self.models):
- if self.has_encoder():
- encoder_out = encoder_outs[i]
- # decode each model
- if self.has_incremental_states():
- decoder_out = model.decoder.forward(
- tokens,
- encoder_out=encoder_out,
- incremental_state=incremental_states[i],
- )
- else:
- if hasattr(model, "decoder"):
- decoder_out = model.decoder.forward(tokens, encoder_out=encoder_out)
- else:
- decoder_out = model.forward(tokens)
-
- attn: Optional[Tensor] = None
- decoder_len = len(decoder_out)
- if decoder_len > 1 and decoder_out[1] is not None:
- if isinstance(decoder_out[1], Tensor):
- attn = decoder_out[1]
- else:
- attn_holder = decoder_out[1]["attn"]
- if isinstance(attn_holder, Tensor):
- attn = attn_holder
- elif attn_holder is not None:
- attn = attn_holder[0]
- if attn is not None:
- attn = attn[:, -1, :]
-
- decoder_out_tuple = (
- decoder_out[0][:, -1:, :].div_(temperature),
- None if decoder_len <= 1 else decoder_out[1],
- )
- probs = model.get_normalized_probs(
- decoder_out_tuple, log_probs=True, sample=None
- )
- probs = probs[:, -1, :]
- if self.models_size == 1:
- return probs, attn
-
- log_probs.append(probs)
- if attn is not None:
- if avg_attn is None:
- avg_attn = attn
- else:
- avg_attn.add_(attn)
-
- avg_probs = torch.logsumexp(torch.stack(log_probs, dim=0), dim=0) - math.log(
- self.models_size
- )
-
- if avg_attn is not None:
- avg_attn.div_(self.models_size)
- return avg_probs, avg_attn
-
- @torch.jit.export
- def reorder_encoder_out(
- self, encoder_outs: Optional[List[Dict[str, List[Tensor]]]], new_order
- ):
- """
- Reorder encoder output according to *new_order*.
-
- Args:
- encoder_out: output from the ``forward()`` method
- new_order (LongTensor): desired order
-
- Returns:
- *encoder_out* rearranged according to *new_order*
- """
- new_outs: List[Dict[str, List[Tensor]]] = []
- if not self.has_encoder():
- return new_outs
- for i, model in enumerate(self.models):
- assert encoder_outs is not None
- new_outs.append(
- model.encoder.reorder_encoder_out(encoder_outs[i], new_order)
- )
- return new_outs
-
- @torch.jit.export
- def reorder_incremental_state(
- self,
- incremental_states: List[Dict[str, Dict[str, Optional[Tensor]]]],
- new_order,
- ):
- if not self.has_incremental_states():
- return
- for i, model in enumerate(self.models):
- model.decoder.reorder_incremental_state_scripting(
- incremental_states[i], new_order
- )
-
-
-class SequenceGeneratorWithAlignment(SequenceGenerator):
- def __init__(
- self, models, tgt_dict, left_pad_target=False, print_alignment="hard", **kwargs
- ):
- """Generates translations of a given source sentence.
-
- Produces alignments following "Jointly Learning to Align and
- Translate with Transformer Models" (Garg et al., EMNLP 2019).
-
- Args:
- left_pad_target (bool, optional): Whether or not the
- hypothesis should be left padded or not when they are
- teacher forced for generating alignments.
- """
- super().__init__(EnsembleModelWithAlignment(models), tgt_dict, **kwargs)
- self.left_pad_target = left_pad_target
-
- if print_alignment == "hard":
- self.extract_alignment = utils.extract_hard_alignment
- elif print_alignment == "soft":
- self.extract_alignment = utils.extract_soft_alignment
-
- @torch.no_grad()
- def generate(self, models, sample, **kwargs):
- finalized = super()._generate(sample, **kwargs)
-
- src_tokens = sample["net_input"]["src_tokens"]
- bsz = src_tokens.shape[0]
- beam_size = self.beam_size
- (
- src_tokens,
- src_lengths,
- prev_output_tokens,
- tgt_tokens,
- ) = self._prepare_batch_for_alignment(sample, finalized)
- if any(getattr(m, "full_context_alignment", False) for m in self.model.models):
- attn = self.model.forward_align(src_tokens, src_lengths, prev_output_tokens)
- else:
- attn = [
- finalized[i // beam_size][i % beam_size]["attention"].transpose(1, 0)
- for i in range(bsz * beam_size)
- ]
-
- if src_tokens.device != "cpu":
- src_tokens = src_tokens.to("cpu")
- tgt_tokens = tgt_tokens.to("cpu")
- attn = [i.to("cpu") for i in attn]
-
- # Process the attn matrix to extract hard alignments.
- for i in range(bsz * beam_size):
- alignment = self.extract_alignment(
- attn[i], src_tokens[i], tgt_tokens[i], self.pad, self.eos
- )
- finalized[i // beam_size][i % beam_size]["alignment"] = alignment
- return finalized
-
- def _prepare_batch_for_alignment(self, sample, hypothesis):
- src_tokens = sample["net_input"]["src_tokens"]
- bsz = src_tokens.shape[0]
- src_tokens = (
- src_tokens[:, None, :]
- .expand(-1, self.beam_size, -1)
- .contiguous()
- .view(bsz * self.beam_size, -1)
- )
- src_lengths = sample["net_input"]["src_lengths"]
- src_lengths = (
- src_lengths[:, None]
- .expand(-1, self.beam_size)
- .contiguous()
- .view(bsz * self.beam_size)
- )
- prev_output_tokens = data_utils.collate_tokens(
- [beam["tokens"] for example in hypothesis for beam in example],
- self.pad,
- self.eos,
- self.left_pad_target,
- move_eos_to_beginning=True,
- )
- tgt_tokens = data_utils.collate_tokens(
- [beam["tokens"] for example in hypothesis for beam in example],
- self.pad,
- self.eos,
- self.left_pad_target,
- move_eos_to_beginning=False,
- )
- return src_tokens, src_lengths, prev_output_tokens, tgt_tokens
-
-
-class EnsembleModelWithAlignment(EnsembleModel):
- """A wrapper around an ensemble of models."""
-
- def __init__(self, models):
- super().__init__(models)
-
- def forward_align(self, src_tokens, src_lengths, prev_output_tokens):
- avg_attn = None
- for model in self.models:
- decoder_out = model(src_tokens, src_lengths, prev_output_tokens)
- attn = decoder_out[1]["attn"][0]
- if avg_attn is None:
- avg_attn = attn
- else:
- avg_attn.add_(attn)
- if len(self.models) > 1:
- avg_attn.div_(len(self.models))
- return avg_attn
diff --git a/spaces/gradio/HuBERT/tests/speech_recognition/asr_test_base.py b/spaces/gradio/HuBERT/tests/speech_recognition/asr_test_base.py
deleted file mode 100644
index 8c5d414e7bf17ee02f280d024fa5d07e28b79d6b..0000000000000000000000000000000000000000
--- a/spaces/gradio/HuBERT/tests/speech_recognition/asr_test_base.py
+++ /dev/null
@@ -1,557 +0,0 @@
-#!/usr/bin/env python3
-
-import argparse
-import os
-import unittest
-from inspect import currentframe, getframeinfo
-
-import numpy as np
-import torch
-from examples.speech_recognition.data.data_utils import lengths_to_encoder_padding_mask
-from fairseq.data import data_utils as fairseq_data_utils
-from fairseq.data.dictionary import Dictionary
-from fairseq.models import (
- BaseFairseqModel,
- FairseqDecoder,
- FairseqEncoder,
- FairseqEncoderDecoderModel,
- FairseqEncoderModel,
- FairseqModel,
-)
-from fairseq.tasks.fairseq_task import LegacyFairseqTask
-
-
-DEFAULT_TEST_VOCAB_SIZE = 100
-
-
-# ///////////////////////////////////////////////////////////////////////////
-# utility function to setup dummy dict/task/input
-# ///////////////////////////////////////////////////////////////////////////
-
-
-def get_dummy_dictionary(vocab_size=DEFAULT_TEST_VOCAB_SIZE):
- dummy_dict = Dictionary()
- # add dummy symbol to satisfy vocab size
- for id, _ in enumerate(range(vocab_size)):
- dummy_dict.add_symbol("{}".format(id), 1000)
- return dummy_dict
-
-
-class DummyTask(LegacyFairseqTask):
- def __init__(self, args):
- super().__init__(args)
- self.dictionary = get_dummy_dictionary()
- if getattr(self.args, "ctc", False):
- self.dictionary.add_symbol("")
- self.tgt_dict = self.dictionary
-
- @property
- def target_dictionary(self):
- return self.dictionary
-
-
-def get_dummy_task_and_parser():
- """
- to build a fariseq model, we need some dummy parse and task. This function
- is used to create dummy task and parser to faciliate model/criterion test
-
- Note: we use FbSpeechRecognitionTask as the dummy task. You may want
- to use other task by providing another function
- """
- parser = argparse.ArgumentParser(
- description="test_dummy_s2s_task", argument_default=argparse.SUPPRESS
- )
- DummyTask.add_args(parser)
- args = parser.parse_args([])
- task = DummyTask.setup_task(args)
- return task, parser
-
-
-def get_dummy_input(T=100, D=80, B=5, K=100):
- forward_input = {}
- # T max sequence length
- # D feature vector dimension
- # B batch size
- # K target dimension size
- feature = torch.randn(B, T, D)
- # this (B, T, D) layout is just a convention, you can override it by
- # write your own _prepare_forward_input function
- src_lengths = torch.from_numpy(
- np.random.randint(low=1, high=T, size=B, dtype=np.int64)
- )
- src_lengths[0] = T # make sure the maximum length matches
- prev_output_tokens = []
- for b in range(B):
- token_length = np.random.randint(low=1, high=src_lengths[b].item() + 1)
- tokens = np.random.randint(low=0, high=K, size=token_length, dtype=np.int64)
- prev_output_tokens.append(torch.from_numpy(tokens))
-
- prev_output_tokens = fairseq_data_utils.collate_tokens(
- prev_output_tokens,
- pad_idx=1,
- eos_idx=2,
- left_pad=False,
- move_eos_to_beginning=False,
- )
- src_lengths, sorted_order = src_lengths.sort(descending=True)
- forward_input["src_tokens"] = feature.index_select(0, sorted_order)
- forward_input["src_lengths"] = src_lengths
- forward_input["prev_output_tokens"] = prev_output_tokens
-
- return forward_input
-
-
-def get_dummy_encoder_output(encoder_out_shape=(100, 80, 5)):
- """
- This only provides an example to generate dummy encoder output
- """
- (T, B, D) = encoder_out_shape
- encoder_out = {}
-
- encoder_out["encoder_out"] = torch.from_numpy(
- np.random.randn(*encoder_out_shape).astype(np.float32)
- )
- seq_lengths = torch.from_numpy(np.random.randint(low=1, high=T, size=B))
- # some dummy mask
- encoder_out["encoder_padding_mask"] = torch.arange(T).view(1, T).expand(
- B, -1
- ) >= seq_lengths.view(B, 1).expand(-1, T)
- encoder_out["encoder_padding_mask"].t_()
-
- # encoer_padding_mask is (T, B) tensor, with (t, b)-th element indicate
- # whether encoder_out[t, b] is valid (=0) or not (=1)
- return encoder_out
-
-
-def _current_postion_info():
- cf = currentframe()
- frameinfo = " (at {}:{})".format(
- os.path.basename(getframeinfo(cf).filename), cf.f_back.f_lineno
- )
- return frameinfo
-
-
-def check_encoder_output(encoder_output, batch_size=None):
- """we expect encoder_output to be a dict with the following
- key/value pairs:
- - encoder_out: a Torch.Tensor
- - encoder_padding_mask: a binary Torch.Tensor
- """
- if not isinstance(encoder_output, dict):
- msg = (
- "FairseqEncoderModel.forward(...) must be a dict" + _current_postion_info()
- )
- return False, msg
-
- if "encoder_out" not in encoder_output:
- msg = (
- "FairseqEncoderModel.forward(...) must contain encoder_out"
- + _current_postion_info()
- )
- return False, msg
-
- if "encoder_padding_mask" not in encoder_output:
- msg = (
- "FairseqEncoderModel.forward(...) must contain encoder_padding_mask"
- + _current_postion_info()
- )
- return False, msg
-
- if not isinstance(encoder_output["encoder_out"], torch.Tensor):
- msg = "encoder_out must be a torch.Tensor" + _current_postion_info()
- return False, msg
-
- if encoder_output["encoder_out"].dtype != torch.float32:
- msg = "encoder_out must have float32 dtype" + _current_postion_info()
- return False, msg
-
- mask = encoder_output["encoder_padding_mask"]
- if mask is not None:
- if not isinstance(mask, torch.Tensor):
- msg = (
- "encoder_padding_mask must be a torch.Tensor" + _current_postion_info()
- )
- return False, msg
- if mask.dtype != torch.uint8 and (
- not hasattr(torch, "bool") or mask.dtype != torch.bool
- ):
- msg = (
- "encoder_padding_mask must have dtype of uint8"
- + _current_postion_info()
- )
- return False, msg
-
- if mask.dim() != 2:
- msg = (
- "we expect encoder_padding_mask to be a 2-d tensor, in shape (T, B)"
- + _current_postion_info()
- )
- return False, msg
-
- if batch_size is not None and mask.size(1) != batch_size:
- msg = (
- "we expect encoder_padding_mask to be a 2-d tensor, with size(1)"
- + " being the batch size"
- + _current_postion_info()
- )
- return False, msg
- return True, None
-
-
-def check_decoder_output(decoder_output):
- """we expect output from a decoder is a tuple with the following constraint:
- - the first element is a torch.Tensor
- - the second element can be anything (reserved for future use)
- """
- if not isinstance(decoder_output, tuple):
- msg = "FariseqDecoder output must be a tuple" + _current_postion_info()
- return False, msg
-
- if len(decoder_output) != 2:
- msg = "FairseqDecoder output must be 2-elem tuple" + _current_postion_info()
- return False, msg
-
- if not isinstance(decoder_output[0], torch.Tensor):
- msg = (
- "FariseqDecoder output[0] must be a torch.Tensor" + _current_postion_info()
- )
- return False, msg
-
- return True, None
-
-
-# ///////////////////////////////////////////////////////////////////////////
-# Base Test class
-# ///////////////////////////////////////////////////////////////////////////
-
-
-class TestBaseFairseqModelBase(unittest.TestCase):
- """
- This class is used to facilitate writing unittest for any class derived from
- `BaseFairseqModel`.
- """
-
- @classmethod
- def setUpClass(cls):
- if cls is TestBaseFairseqModelBase:
- raise unittest.SkipTest("Skipping test case in base")
- super().setUpClass()
-
- def setUpModel(self, model):
- self.assertTrue(isinstance(model, BaseFairseqModel))
- self.model = model
-
- def setupInput(self):
- pass
-
- def setUp(self):
- self.model = None
- self.forward_input = None
- pass
-
-
-class TestFairseqEncoderDecoderModelBase(TestBaseFairseqModelBase):
- """
- base code to test FairseqEncoderDecoderModel (formally known as
- `FairseqModel`) must be derived from this base class
- """
-
- @classmethod
- def setUpClass(cls):
- if cls is TestFairseqEncoderDecoderModelBase:
- raise unittest.SkipTest("Skipping test case in base")
- super().setUpClass()
-
- def setUpModel(self, model_cls, extra_args_setters=None):
- self.assertTrue(
- issubclass(model_cls, (FairseqEncoderDecoderModel, FairseqModel)),
- msg="This class only tests for FairseqModel subclasses",
- )
-
- task, parser = get_dummy_task_and_parser()
- model_cls.add_args(parser)
-
- args = parser.parse_args([])
-
- if extra_args_setters is not None:
- for args_setter in extra_args_setters:
- args_setter(args)
- model = model_cls.build_model(args, task)
- self.model = model
-
- def setUpInput(self, input=None):
- self.forward_input = get_dummy_input() if input is None else input
-
- def setUp(self):
- super().setUp()
-
- def test_forward(self):
- if self.model and self.forward_input:
- forward_output = self.model.forward(**self.forward_input)
- # for FairseqEncoderDecoderModel, forward returns a tuple of two
- # elements, the first one is a Torch.Tensor
- succ, msg = check_decoder_output(forward_output)
- if not succ:
- self.assertTrue(succ, msg=msg)
- self.forward_output = forward_output
-
- def test_get_normalized_probs(self):
- if self.model and self.forward_input:
- forward_output = self.model.forward(**self.forward_input)
- logprob = self.model.get_normalized_probs(forward_output, log_probs=True)
- prob = self.model.get_normalized_probs(forward_output, log_probs=False)
-
- # in order for different models/criterion to play with each other
- # we need to know whether the logprob or prob output is batch_first
- # or not. We assume an additional attribute will be attached to logprob
- # or prob. If you find your code failed here, simply override
- # FairseqModel.get_normalized_probs, see example at
- # https://fburl.com/batch_first_example
- self.assertTrue(hasattr(logprob, "batch_first"))
- self.assertTrue(hasattr(prob, "batch_first"))
-
- self.assertTrue(torch.is_tensor(logprob))
- self.assertTrue(torch.is_tensor(prob))
-
-
-class TestFairseqEncoderModelBase(TestBaseFairseqModelBase):
- """
- base class to test FairseqEncoderModel
- """
-
- @classmethod
- def setUpClass(cls):
- if cls is TestFairseqEncoderModelBase:
- raise unittest.SkipTest("Skipping test case in base")
- super().setUpClass()
-
- def setUpModel(self, model_cls, extra_args_setters=None):
- self.assertTrue(
- issubclass(model_cls, FairseqEncoderModel),
- msg="This class is only used for testing FairseqEncoderModel",
- )
- task, parser = get_dummy_task_and_parser()
- model_cls.add_args(parser)
- args = parser.parse_args([])
- if extra_args_setters is not None:
- for args_setter in extra_args_setters:
- args_setter(args)
-
- model = model_cls.build_model(args, task)
- self.model = model
-
- def setUpInput(self, input=None):
- self.forward_input = get_dummy_input() if input is None else input
- # get_dummy_input() is originally for s2s, here we delete extra dict
- # items, so it can be used for EncoderModel / Encoder as well
- self.forward_input.pop("prev_output_tokens", None)
-
- def setUp(self):
- super().setUp()
-
- def test_forward(self):
- if self.forward_input and self.model:
- bsz = self.forward_input["src_tokens"].size(0)
- forward_output = self.model.forward(**self.forward_input)
-
- # we expect forward_output to be a dict with the following
- # key/value pairs:
- # - encoder_out: a Torch.Tensor
- # - encoder_padding_mask: a binary Torch.Tensor
- succ, msg = check_encoder_output(forward_output, batch_size=bsz)
- if not succ:
- self.assertTrue(succ, msg=msg)
- self.forward_output = forward_output
-
- def test_get_normalized_probs(self):
- if self.model and self.forward_input:
- forward_output = self.model.forward(**self.forward_input)
- logprob = self.model.get_normalized_probs(forward_output, log_probs=True)
- prob = self.model.get_normalized_probs(forward_output, log_probs=False)
-
- # in order for different models/criterion to play with each other
- # we need to know whether the logprob or prob output is batch_first
- # or not. We assume an additional attribute will be attached to logprob
- # or prob. If you find your code failed here, simply override
- # FairseqModel.get_normalized_probs, see example at
- # https://fburl.com/batch_first_example
- self.assertTrue(hasattr(logprob, "batch_first"))
- self.assertTrue(hasattr(prob, "batch_first"))
-
- self.assertTrue(torch.is_tensor(logprob))
- self.assertTrue(torch.is_tensor(prob))
-
-
-class TestFairseqEncoderBase(unittest.TestCase):
- """
- base class to test FairseqEncoder
- """
-
- @classmethod
- def setUpClass(cls):
- if cls is TestFairseqEncoderBase:
- raise unittest.SkipTest("Skipping test case in base")
- super().setUpClass()
-
- def setUpEncoder(self, encoder):
- self.assertTrue(
- isinstance(encoder, FairseqEncoder),
- msg="This class is only used for test FairseqEncoder",
- )
- self.encoder = encoder
-
- def setUpInput(self, input=None):
- self.forward_input = get_dummy_input() if input is None else input
- # get_dummy_input() is originally for s2s, here we delete extra dict
- # items, so it can be used for EncoderModel / Encoder as well
- self.forward_input.pop("prev_output_tokens", None)
-
- def setUp(self):
- self.encoder = None
- self.forward_input = None
-
- def test_forward(self):
- if self.encoder and self.forward_input:
- bsz = self.forward_input["src_tokens"].size(0)
-
- forward_output = self.encoder.forward(**self.forward_input)
- succ, msg = check_encoder_output(forward_output, batch_size=bsz)
- if not succ:
- self.assertTrue(succ, msg=msg)
- self.forward_output = forward_output
-
-
-class TestFairseqDecoderBase(unittest.TestCase):
- """
- base class to test FairseqDecoder
- """
-
- @classmethod
- def setUpClass(cls):
- if cls is TestFairseqDecoderBase:
- raise unittest.SkipTest("Skipping test case in base")
- super().setUpClass()
-
- def setUpDecoder(self, decoder):
- self.assertTrue(
- isinstance(decoder, FairseqDecoder),
- msg="This class is only used for test FairseqDecoder",
- )
- self.decoder = decoder
-
- def setUpInput(self, input=None):
- self.forward_input = get_dummy_encoder_output() if input is None else input
-
- def setUpPrevOutputTokens(self, tokens=None):
- if tokens is None:
- self.encoder_input = get_dummy_input()
- self.prev_output_tokens = self.encoder_input["prev_output_tokens"]
- else:
- self.prev_output_tokens = tokens
-
- def setUp(self):
- self.decoder = None
- self.forward_input = None
- self.prev_output_tokens = None
-
- def test_forward(self):
- if (
- self.decoder is not None
- and self.forward_input is not None
- and self.prev_output_tokens is not None
- ):
- forward_output = self.decoder.forward(
- prev_output_tokens=self.prev_output_tokens,
- encoder_out=self.forward_input,
- )
- succ, msg = check_decoder_output(forward_output)
- if not succ:
- self.assertTrue(succ, msg=msg)
- self.forward_input = forward_output
-
-
-class DummyEncoderModel(FairseqEncoderModel):
- def __init__(self, encoder):
- super().__init__(encoder)
-
- @classmethod
- def build_model(cls, args, task):
- return cls(DummyEncoder())
-
- def get_logits(self, net_output):
- # Inverse of sigmoid to use with BinaryCrossEntropyWithLogitsCriterion as
- # F.binary_cross_entropy_with_logits combines sigmoid and CE
- return torch.log(
- torch.div(net_output["encoder_out"], 1 - net_output["encoder_out"])
- )
-
- def get_normalized_probs(self, net_output, log_probs, sample=None):
- lprobs = super().get_normalized_probs(net_output, log_probs, sample=sample)
- lprobs.batch_first = True
- return lprobs
-
-
-class DummyEncoder(FairseqEncoder):
- def __init__(self):
- super().__init__(None)
-
- def forward(self, src_tokens, src_lengths):
- mask, max_len = lengths_to_encoder_padding_mask(src_lengths)
- return {"encoder_out": src_tokens, "encoder_padding_mask": mask}
-
-
-class CrossEntropyCriterionTestBase(unittest.TestCase):
- @classmethod
- def setUpClass(cls):
- if cls is CrossEntropyCriterionTestBase:
- raise unittest.SkipTest("Skipping base class test case")
- super().setUpClass()
-
- def setUpArgs(self):
- args = argparse.Namespace()
- args.sentence_avg = False
- args.threshold = 0.1 # to use with BinaryCrossEntropyWithLogitsCriterion
- return args
-
- def setUp(self):
- args = self.setUpArgs()
- self.model = DummyEncoderModel(encoder=DummyEncoder())
- self.criterion = self.criterion_cls.build_criterion(args, task=DummyTask(args))
-
- def get_src_tokens(self, correct_prediction, aggregate):
- """
- correct_prediction: True if the net_output (src_tokens) should
- predict the correct target
- aggregate: True if the criterion expects net_output (src_tokens)
- aggregated across time axis
- """
- predicted_idx = 0 if correct_prediction else 1
- if aggregate:
- src_tokens = torch.zeros((2, 2), dtype=torch.float)
- for b in range(2):
- src_tokens[b][predicted_idx] = 1.0
- else:
- src_tokens = torch.zeros((2, 10, 2), dtype=torch.float)
- for b in range(2):
- for t in range(10):
- src_tokens[b][t][predicted_idx] = 1.0
- return src_tokens
-
- def get_target(self, soft_target):
- if soft_target:
- target = torch.zeros((2, 2), dtype=torch.float)
- for b in range(2):
- target[b][0] = 1.0
- else:
- target = torch.zeros((2, 10), dtype=torch.long)
- return target
-
- def get_test_sample(self, correct, soft_target, aggregate):
- src_tokens = self.get_src_tokens(correct, aggregate)
- target = self.get_target(soft_target)
- L = src_tokens.size(1)
- return {
- "net_input": {"src_tokens": src_tokens, "src_lengths": torch.tensor([L])},
- "target": target,
- "ntokens": src_tokens.size(0) * src_tokens.size(1),
- }
diff --git a/spaces/gradio/HuBERT/tests/test_constraints.py b/spaces/gradio/HuBERT/tests/test_constraints.py
deleted file mode 100644
index 1c37f7e1fb26d8ea5349fedd3a60f566d09cf598..0000000000000000000000000000000000000000
--- a/spaces/gradio/HuBERT/tests/test_constraints.py
+++ /dev/null
@@ -1,269 +0,0 @@
-# Copyright (c) Facebook, Inc. and its affiliates.
-#
-# This source code is licensed under the MIT license found in the
-# LICENSE file in the root directory of this source tree.
-
-import sys
-import unittest
-
-import torch
-from fairseq.token_generation_constraints import *
-
-
-def tensorize(constraints: List[List[int]]) -> torch.Tensor:
- return [torch.tensor(x) for x in constraints]
-
-
-class TestHelperRoutines(unittest.TestCase):
- def setUp(self):
- self.examples = [
- ([[]], torch.tensor([[0]])),
- ([[], []], torch.tensor([[0], [0]])),
- ([[torch.tensor([1, 2])], []], torch.tensor([[1, 1, 2, 0], [0, 0, 0, 0]])),
- (
- [
- [
- torch.tensor([3, 1, 2]),
- torch.tensor([3]),
- torch.tensor([4, 5, 6, 7]),
- ],
- [],
- [torch.tensor([1, 8, 9, 10, 1, 4, 11, 12])],
- ],
- torch.tensor(
- [
- [3, 3, 1, 2, 0, 3, 0, 4, 5, 6, 7, 0],
- [0, 0, 0, 0, 0, 0, 0, 0, 0, 0, 0, 0],
- [1, 1, 8, 9, 10, 1, 4, 11, 12, 0, 0, 0],
- ]
- ),
- ),
- ]
-
- def test_packing(self):
- """Ensures the list of lists of tensors gets packed correctly."""
- for batch_constraints, expected_tensor in self.examples:
- packed = pack_constraints(batch_constraints)
- assert torch.equal(packed, expected_tensor)
-
-
-class TestUnorderedConstraintState(unittest.TestCase):
- def setUp(self):
- # Tuples of (contraint set, expected printed graph, token counts per node)
- self.examples = [
- (
- tensorize([[1, 2, 3], [1, 3], [1, 4], [4, 5, 6, 7], [1], [4, 5]]),
- "([None].False#6 ([1].True#4 ([2].False#1 [3].True#1) [3].True#1 [4].True#1) ([4].False#2 ([5].True#2 ([6].False#1 [7].True#1))))",
- {1: 4, 2: 1, 3: 2, 4: 3, 5: 2, 6: 1, 7: 1},
- ),
- ([], "[None].False#0", {}),
- (tensorize([[0]]), "([None].False#1 [0].True#1)", {0: 1}),
- (
- tensorize([[100000, 1, 2, 3, 4, 5]]),
- "([None].False#1 ([100000].False#1 ([1].False#1 ([2].False#1 ([3].False#1 ([4].False#1 [5].True#1))))))",
- {100000: 1, 1: 1, 2: 1, 3: 1, 4: 1, 5: 1},
- ),
- (
- tensorize([[1, 2], [1, 2]]),
- "([None].False#2 ([1].False#2 [2].True#2))",
- {1: 2, 2: 2},
- ),
- (
- tensorize([[1, 2], [3, 4]]),
- "([None].False#2 ([1].False#1 [2].True#1) ([3].False#1 [4].True#1))",
- {1: 1, 2: 1, 3: 1, 4: 1},
- ),
- ]
-
- self.sequences = [
- (
- self.examples[0][0],
- [],
- {"bank": 0, "num_completed": 0, "finished": False, "is_root": True},
- ),
- (
- self.examples[0][0],
- [1, 2],
- {"bank": 2, "num_completed": 0, "finished": False, "is_root": False},
- ),
- (
- self.examples[0][0],
- [1, 2, 94],
- {"bank": 1, "num_completed": 1, "finished": False, "is_root": True},
- ),
- (
- self.examples[0][0],
- [1, 3, 999, 1, 4],
- {"bank": 4, "num_completed": 2, "finished": False, "is_root": False},
- ),
- (
- self.examples[0][0],
- [1, 3, 999, 1, 4, 999],
- {"bank": 4, "num_completed": 2, "finished": False, "is_root": True},
- ),
- (
- self.examples[0][0],
- [4, 5, 6, 8],
- {"bank": 2, "num_completed": 1, "finished": False, "is_root": True},
- ),
- (
- self.examples[0][0],
- # Tricky, because in last three, goes down [1->4] branch, could miss [1] and [4->5]
- # [[1, 2, 3], [1, 3], [1, 4], [4, 5, 6, 7], [1], [4, 5]],
- [1, 2, 3, 1, 3, 1, 4, 4, 5, 6, 7, 1, 4, 5],
- {"bank": 14, "num_completed": 6, "finished": True, "is_root": False},
- ),
- (
- self.examples[0][0],
- [1, 2, 3, 999, 1, 3, 1, 4, 4, 5, 6, 7, 1, 4, 5, 117],
- {"bank": 14, "num_completed": 6, "finished": True, "is_root": True},
- ),
- (
- tensorize([[1], [2, 3]]),
- # Should not be able to get credit for entering 1 a second time
- [1, 1],
- {"bank": 1, "num_completed": 1, "finished": False, "is_root": True},
- ),
- (
- self.examples[4][0],
- [1, 2, 1, 2],
- {"bank": 4, "num_completed": 2, "finished": True, "is_root": False},
- ),
- (
- self.examples[4][0],
- [1, 2, 1, 2, 1],
- {"bank": 4, "num_completed": 2, "finished": True, "is_root": True},
- ),
- (
- self.examples[5][0],
- [1, 2, 3, 4, 5],
- {"bank": 4, "num_completed": 2, "finished": True, "is_root": True},
- ),
- ]
-
- def test_graphs(self):
- """
- Test whether unordered graph systems are created correctly.
- """
- for example in self.examples:
- constraints, expected, gold_counts = example
- c = ConstraintNode.create(constraints)
- assert (
- ConstraintNode.print_graph(c) == expected
- ), f"got {ConstraintNode.print_graph(c)}, expected {expected}"
- assert (
- c.token_counts() == gold_counts
- ), f"{c} got {c.token_counts()} wanted {gold_counts}"
-
- def test_next_tokens(self):
- """
- Tests that the set of next tokens is correct.
- """
- for example in self.examples:
- constraints, expected, gold_counts = example
- root = ConstraintNode.create(constraints)
-
- root_tokens = set(root.children.keys())
- for sequence in constraints:
- state = UnorderedConstraintState(root)
- for token in sequence:
- all_tokens = root_tokens.union(state.node.children.keys())
- assert (
- all_tokens == state.next_tokens()
- ), f"ALL {all_tokens} NEXT {state.next_tokens()}"
- state = state.advance(token)
-
- def test_sequences(self):
- for constraints, tokens, expected in self.sequences:
- state = UnorderedConstraintState.create(pack_constraints([constraints])[0])
- for token in tokens:
- state = state.advance(token)
- result = {}
- for attr in expected.keys():
- result[attr] = getattr(state, attr)
-
- assert (
- result == expected
- ), f"TEST({tokens}) GOT: {result} WANTED: {expected}"
-
-
-class TestOrderedConstraintState(unittest.TestCase):
- def setUp(self):
- self.sequences = [
- (
- tensorize([[1, 2, 3], [1, 3], [1, 4], [4, 5, 6, 7], [1], [4, 5]]),
- [],
- {"bank": 0, "num_completed": 0, "finished": False, "is_root": True},
- ),
- (
- tensorize([[1, 2, 3], [1, 3], [1, 4], [4, 5, 6, 7], [1], [4, 5]]),
- [1, 2],
- {"bank": 2, "num_completed": 0, "finished": False, "is_root": False},
- ),
- (
- tensorize([[1, 2, 3], [1, 3], [1, 4], [4, 5, 6, 7], [1], [4, 5]]),
- [1, 2, 94],
- {"bank": 0, "num_completed": 0, "finished": False, "is_root": True},
- ),
- (
- tensorize([[1, 2, 3], [1, 3], [1, 4], [4, 5, 6, 7], [1], [4, 5]]),
- [1, 3, 999, 1, 4],
- {"bank": 0, "num_completed": 0, "finished": False, "is_root": True},
- ),
- (
- tensorize([[1, 2, 3], [1, 3], [1, 4], [4, 5, 6, 7], [1], [4, 5]]),
- [1, 2, 3, 999, 999],
- {"bank": 3, "num_completed": 1, "finished": False, "is_root": False},
- ),
- (
- tensorize([[1, 2, 3], [1, 3], [1, 4], [4, 5, 6, 7], [1], [4, 5]]),
- [1, 2, 3, 77, 1, 3, 1],
- {"bank": 6, "num_completed": 2, "finished": False, "is_root": False},
- ),
- (
- tensorize([[1, 2, 3], [1, 3], [1, 4], [4, 5, 6, 7], [1], [4, 5]]),
- [1, 2, 3, 1, 3, 1, 4, 4, 5, 6, 7, 1, 4, 5],
- {"bank": 14, "num_completed": 6, "finished": True, "is_root": False},
- ),
- (
- tensorize([[1, 2, 3], [1, 3], [1, 4], [4, 5, 6, 7], [1], [4, 5]]),
- [1, 2, 999, 1, 2, 3, 999, 1, 3, 1, 4, 4, 5, 6, 7, 1, 4, 5, 117],
- {"bank": 14, "num_completed": 6, "finished": True, "is_root": False},
- ),
- (
- tensorize([[1], [2, 3]]),
- [1, 1],
- {"bank": 1, "num_completed": 1, "finished": False, "is_root": False},
- ),
- (
- tensorize([[1, 2], [1, 2]]),
- [1, 2, 1, 2],
- {"bank": 4, "num_completed": 2, "finished": True, "is_root": False},
- ),
- (
- tensorize([[1, 2], [1, 2]]),
- [1, 2, 1, 2, 1],
- {"bank": 4, "num_completed": 2, "finished": True, "is_root": False},
- ),
- (
- tensorize([[1, 2], [3, 4]]),
- [1, 2, 3, 4, 5],
- {"bank": 4, "num_completed": 2, "finished": True, "is_root": False},
- ),
- ]
-
- def test_sequences(self):
- for i, (constraints, tokens, expected) in enumerate(self.sequences):
- state = OrderedConstraintState.create(pack_constraints([constraints])[0])
- for token in tokens:
- state = state.advance(token)
- result = {}
- for attr in expected.keys():
- result[attr] = getattr(state, attr)
- assert (
- result == expected
- ), f"TEST({tokens}) GOT: {result} WANTED: {expected}"
-
-
-if __name__ == "__main__":
- unittest.main()
diff --git a/spaces/gwang-kim/DATID-3D/pose_estimation/models/arcface_torch/onnx_helper.py b/spaces/gwang-kim/DATID-3D/pose_estimation/models/arcface_torch/onnx_helper.py
deleted file mode 100644
index ca922ca6d410655029e459cf8fd1c323d276c34c..0000000000000000000000000000000000000000
--- a/spaces/gwang-kim/DATID-3D/pose_estimation/models/arcface_torch/onnx_helper.py
+++ /dev/null
@@ -1,250 +0,0 @@
-from __future__ import division
-import datetime
-import os
-import os.path as osp
-import glob
-import numpy as np
-import cv2
-import sys
-import onnxruntime
-import onnx
-import argparse
-from onnx import numpy_helper
-from insightface.data import get_image
-
-class ArcFaceORT:
- def __init__(self, model_path, cpu=False):
- self.model_path = model_path
- # providers = None will use available provider, for onnxruntime-gpu it will be "CUDAExecutionProvider"
- self.providers = ['CPUExecutionProvider'] if cpu else None
-
- #input_size is (w,h), return error message, return None if success
- def check(self, track='cfat', test_img = None):
- #default is cfat
- max_model_size_mb=1024
- max_feat_dim=512
- max_time_cost=15
- if track.startswith('ms1m'):
- max_model_size_mb=1024
- max_feat_dim=512
- max_time_cost=10
- elif track.startswith('glint'):
- max_model_size_mb=1024
- max_feat_dim=1024
- max_time_cost=20
- elif track.startswith('cfat'):
- max_model_size_mb = 1024
- max_feat_dim = 512
- max_time_cost = 15
- elif track.startswith('unconstrained'):
- max_model_size_mb=1024
- max_feat_dim=1024
- max_time_cost=30
- else:
- return "track not found"
-
- if not os.path.exists(self.model_path):
- return "model_path not exists"
- if not os.path.isdir(self.model_path):
- return "model_path should be directory"
- onnx_files = []
- for _file in os.listdir(self.model_path):
- if _file.endswith('.onnx'):
- onnx_files.append(osp.join(self.model_path, _file))
- if len(onnx_files)==0:
- return "do not have onnx files"
- self.model_file = sorted(onnx_files)[-1]
- print('use onnx-model:', self.model_file)
- try:
- session = onnxruntime.InferenceSession(self.model_file, providers=self.providers)
- except:
- return "load onnx failed"
- input_cfg = session.get_inputs()[0]
- input_shape = input_cfg.shape
- print('input-shape:', input_shape)
- if len(input_shape)!=4:
- return "length of input_shape should be 4"
- if not isinstance(input_shape[0], str):
- #return "input_shape[0] should be str to support batch-inference"
- print('reset input-shape[0] to None')
- model = onnx.load(self.model_file)
- model.graph.input[0].type.tensor_type.shape.dim[0].dim_param = 'None'
- new_model_file = osp.join(self.model_path, 'zzzzrefined.onnx')
- onnx.save(model, new_model_file)
- self.model_file = new_model_file
- print('use new onnx-model:', self.model_file)
- try:
- session = onnxruntime.InferenceSession(self.model_file, providers=self.providers)
- except:
- return "load onnx failed"
- input_cfg = session.get_inputs()[0]
- input_shape = input_cfg.shape
- print('new-input-shape:', input_shape)
-
- self.image_size = tuple(input_shape[2:4][::-1])
- #print('image_size:', self.image_size)
- input_name = input_cfg.name
- outputs = session.get_outputs()
- output_names = []
- for o in outputs:
- output_names.append(o.name)
- #print(o.name, o.shape)
- if len(output_names)!=1:
- return "number of output nodes should be 1"
- self.session = session
- self.input_name = input_name
- self.output_names = output_names
- #print(self.output_names)
- model = onnx.load(self.model_file)
- graph = model.graph
- if len(graph.node)<8:
- return "too small onnx graph"
-
- input_size = (112,112)
- self.crop = None
- if track=='cfat':
- crop_file = osp.join(self.model_path, 'crop.txt')
- if osp.exists(crop_file):
- lines = open(crop_file,'r').readlines()
- if len(lines)!=6:
- return "crop.txt should contain 6 lines"
- lines = [int(x) for x in lines]
- self.crop = lines[:4]
- input_size = tuple(lines[4:6])
- if input_size!=self.image_size:
- return "input-size is inconsistant with onnx model input, %s vs %s"%(input_size, self.image_size)
-
- self.model_size_mb = os.path.getsize(self.model_file) / float(1024*1024)
- if self.model_size_mb > max_model_size_mb:
- return "max model size exceed, given %.3f-MB"%self.model_size_mb
-
- input_mean = None
- input_std = None
- if track=='cfat':
- pn_file = osp.join(self.model_path, 'pixel_norm.txt')
- if osp.exists(pn_file):
- lines = open(pn_file,'r').readlines()
- if len(lines)!=2:
- return "pixel_norm.txt should contain 2 lines"
- input_mean = float(lines[0])
- input_std = float(lines[1])
- if input_mean is not None or input_std is not None:
- if input_mean is None or input_std is None:
- return "please set input_mean and input_std simultaneously"
- else:
- find_sub = False
- find_mul = False
- for nid, node in enumerate(graph.node[:8]):
- print(nid, node.name)
- if node.name.startswith('Sub') or node.name.startswith('_minus'):
- find_sub = True
- if node.name.startswith('Mul') or node.name.startswith('_mul') or node.name.startswith('Div'):
- find_mul = True
- if find_sub and find_mul:
- print("find sub and mul")
- #mxnet arcface model
- input_mean = 0.0
- input_std = 1.0
- else:
- input_mean = 127.5
- input_std = 127.5
- self.input_mean = input_mean
- self.input_std = input_std
- for initn in graph.initializer:
- weight_array = numpy_helper.to_array(initn)
- dt = weight_array.dtype
- if dt.itemsize<4:
- return 'invalid weight type - (%s:%s)' % (initn.name, dt.name)
- if test_img is None:
- test_img = get_image('Tom_Hanks_54745')
- test_img = cv2.resize(test_img, self.image_size)
- else:
- test_img = cv2.resize(test_img, self.image_size)
- feat, cost = self.benchmark(test_img)
- batch_result = self.check_batch(test_img)
- batch_result_sum = float(np.sum(batch_result))
- if batch_result_sum in [float('inf'), -float('inf')] or batch_result_sum != batch_result_sum:
- print(batch_result)
- print(batch_result_sum)
- return "batch result output contains NaN!"
-
- if len(feat.shape) < 2:
- return "the shape of the feature must be two, but get {}".format(str(feat.shape))
-
- if feat.shape[1] > max_feat_dim:
- return "max feat dim exceed, given %d"%feat.shape[1]
- self.feat_dim = feat.shape[1]
- cost_ms = cost*1000
- if cost_ms>max_time_cost:
- return "max time cost exceed, given %.4f"%cost_ms
- self.cost_ms = cost_ms
- print('check stat:, model-size-mb: %.4f, feat-dim: %d, time-cost-ms: %.4f, input-mean: %.3f, input-std: %.3f'%(self.model_size_mb, self.feat_dim, self.cost_ms, self.input_mean, self.input_std))
- return None
-
- def check_batch(self, img):
- if not isinstance(img, list):
- imgs = [img, ] * 32
- if self.crop is not None:
- nimgs = []
- for img in imgs:
- nimg = img[self.crop[1]:self.crop[3], self.crop[0]:self.crop[2], :]
- if nimg.shape[0] != self.image_size[1] or nimg.shape[1] != self.image_size[0]:
- nimg = cv2.resize(nimg, self.image_size)
- nimgs.append(nimg)
- imgs = nimgs
- blob = cv2.dnn.blobFromImages(
- images=imgs, scalefactor=1.0 / self.input_std, size=self.image_size,
- mean=(self.input_mean, self.input_mean, self.input_mean), swapRB=True)
- net_out = self.session.run(self.output_names, {self.input_name: blob})[0]
- return net_out
-
-
- def meta_info(self):
- return {'model-size-mb':self.model_size_mb, 'feature-dim':self.feat_dim, 'infer': self.cost_ms}
-
-
- def forward(self, imgs):
- if not isinstance(imgs, list):
- imgs = [imgs]
- input_size = self.image_size
- if self.crop is not None:
- nimgs = []
- for img in imgs:
- nimg = img[self.crop[1]:self.crop[3],self.crop[0]:self.crop[2],:]
- if nimg.shape[0]!=input_size[1] or nimg.shape[1]!=input_size[0]:
- nimg = cv2.resize(nimg, input_size)
- nimgs.append(nimg)
- imgs = nimgs
- blob = cv2.dnn.blobFromImages(imgs, 1.0/self.input_std, input_size, (self.input_mean, self.input_mean, self.input_mean), swapRB=True)
- net_out = self.session.run(self.output_names, {self.input_name : blob})[0]
- return net_out
-
- def benchmark(self, img):
- input_size = self.image_size
- if self.crop is not None:
- nimg = img[self.crop[1]:self.crop[3],self.crop[0]:self.crop[2],:]
- if nimg.shape[0]!=input_size[1] or nimg.shape[1]!=input_size[0]:
- nimg = cv2.resize(nimg, input_size)
- img = nimg
- blob = cv2.dnn.blobFromImage(img, 1.0/self.input_std, input_size, (self.input_mean, self.input_mean, self.input_mean), swapRB=True)
- costs = []
- for _ in range(50):
- ta = datetime.datetime.now()
- net_out = self.session.run(self.output_names, {self.input_name : blob})[0]
- tb = datetime.datetime.now()
- cost = (tb-ta).total_seconds()
- costs.append(cost)
- costs = sorted(costs)
- cost = costs[5]
- return net_out, cost
-
-
-if __name__ == '__main__':
- parser = argparse.ArgumentParser(description='')
- # general
- parser.add_argument('workdir', help='submitted work dir', type=str)
- parser.add_argument('--track', help='track name, for different challenge', type=str, default='cfat')
- args = parser.parse_args()
- handler = ArcFaceORT(args.workdir)
- err = handler.check(args.track)
- print('err:', err)
diff --git a/spaces/gyugnsu/DragGan-Inversion/PTI/dnnlib/__init__.py b/spaces/gyugnsu/DragGan-Inversion/PTI/dnnlib/__init__.py
deleted file mode 100644
index 2f08cf36f11f9b0fd94c1b7caeadf69b98375b04..0000000000000000000000000000000000000000
--- a/spaces/gyugnsu/DragGan-Inversion/PTI/dnnlib/__init__.py
+++ /dev/null
@@ -1,9 +0,0 @@
-# Copyright (c) 2021, NVIDIA CORPORATION. All rights reserved.
-#
-# NVIDIA CORPORATION and its licensors retain all intellectual property
-# and proprietary rights in and to this software, related documentation
-# and any modifications thereto. Any use, reproduction, disclosure or
-# distribution of this software and related documentation without an express
-# license agreement from NVIDIA CORPORATION is strictly prohibited.
-
-from .util import EasyDict, make_cache_dir_path
diff --git a/spaces/gyugnsu/DragGan-Inversion/PTI/models/StyleCLIP/global_directions/dnnlib/tflib/network.py b/spaces/gyugnsu/DragGan-Inversion/PTI/models/StyleCLIP/global_directions/dnnlib/tflib/network.py
deleted file mode 100644
index ff0c169eabdc579041dac0650fbc6da956646594..0000000000000000000000000000000000000000
--- a/spaces/gyugnsu/DragGan-Inversion/PTI/models/StyleCLIP/global_directions/dnnlib/tflib/network.py
+++ /dev/null
@@ -1,781 +0,0 @@
-# Copyright (c) 2020, NVIDIA CORPORATION. All rights reserved.
-#
-# NVIDIA CORPORATION and its licensors retain all intellectual property
-# and proprietary rights in and to this software, related documentation
-# and any modifications thereto. Any use, reproduction, disclosure or
-# distribution of this software and related documentation without an express
-# license agreement from NVIDIA CORPORATION is strictly prohibited.
-
-"""Helper for managing networks."""
-
-import types
-import inspect
-import re
-import uuid
-import sys
-import copy
-import numpy as np
-import tensorflow as tf
-
-from collections import OrderedDict
-from typing import Any, List, Tuple, Union, Callable
-
-from . import tfutil
-from .. import util
-
-from .tfutil import TfExpression, TfExpressionEx
-
-# pylint: disable=protected-access
-# pylint: disable=attribute-defined-outside-init
-# pylint: disable=too-many-public-methods
-
-_import_handlers = [] # Custom import handlers for dealing with legacy data in pickle import.
-_import_module_src = dict() # Source code for temporary modules created during pickle import.
-
-
-def import_handler(handler_func):
- """Function decorator for declaring custom import handlers."""
- _import_handlers.append(handler_func)
- return handler_func
-
-
-class Network:
- """Generic network abstraction.
-
- Acts as a convenience wrapper for a parameterized network construction
- function, providing several utility methods and convenient access to
- the inputs/outputs/weights.
-
- Network objects can be safely pickled and unpickled for long-term
- archival purposes. The pickling works reliably as long as the underlying
- network construction function is defined in a standalone Python module
- that has no side effects or application-specific imports.
-
- Args:
- name: Network name. Used to select TensorFlow name and variable scopes. Defaults to build func name if None.
- func_name: Fully qualified name of the underlying network construction function, or a top-level function object.
- static_kwargs: Keyword arguments to be passed in to the network construction function.
- """
-
- def __init__(self, name: str = None, func_name: Any = None, **static_kwargs):
- # Locate the user-specified build function.
- assert isinstance(func_name, str) or util.is_top_level_function(func_name)
- if util.is_top_level_function(func_name):
- func_name = util.get_top_level_function_name(func_name)
- module, func_name = util.get_module_from_obj_name(func_name)
- func = util.get_obj_from_module(module, func_name)
-
- # Dig up source code for the module containing the build function.
- module_src = _import_module_src.get(module, None)
- if module_src is None:
- module_src = inspect.getsource(module)
-
- # Initialize fields.
- self._init_fields(name=(name or func_name), static_kwargs=static_kwargs, build_func=func, build_func_name=func_name, build_module_src=module_src)
-
- def _init_fields(self, name: str, static_kwargs: dict, build_func: Callable, build_func_name: str, build_module_src: str) -> None:
- tfutil.assert_tf_initialized()
- assert isinstance(name, str)
- assert len(name) >= 1
- assert re.fullmatch(r"[A-Za-z0-9_.\\-]*", name)
- assert isinstance(static_kwargs, dict)
- assert util.is_pickleable(static_kwargs)
- assert callable(build_func)
- assert isinstance(build_func_name, str)
- assert isinstance(build_module_src, str)
-
- # Choose TensorFlow name scope.
- with tf.name_scope(None):
- scope = tf.get_default_graph().unique_name(name, mark_as_used=True)
-
- # Query current TensorFlow device.
- with tfutil.absolute_name_scope(scope), tf.control_dependencies(None):
- device = tf.no_op(name="_QueryDevice").device
-
- # Immutable state.
- self._name = name
- self._scope = scope
- self._device = device
- self._static_kwargs = util.EasyDict(copy.deepcopy(static_kwargs))
- self._build_func = build_func
- self._build_func_name = build_func_name
- self._build_module_src = build_module_src
-
- # State before _init_graph().
- self._var_inits = dict() # var_name => initial_value, set to None by _init_graph()
- self._all_inits_known = False # Do we know for sure that _var_inits covers all the variables?
- self._components = None # subnet_name => Network, None if the components are not known yet
-
- # Initialized by _init_graph().
- self._input_templates = None
- self._output_templates = None
- self._own_vars = None
-
- # Cached values initialized the respective methods.
- self._input_shapes = None
- self._output_shapes = None
- self._input_names = None
- self._output_names = None
- self._vars = None
- self._trainables = None
- self._var_global_to_local = None
- self._run_cache = dict()
-
- def _init_graph(self) -> None:
- assert self._var_inits is not None
- assert self._input_templates is None
- assert self._output_templates is None
- assert self._own_vars is None
-
- # Initialize components.
- if self._components is None:
- self._components = util.EasyDict()
-
- # Choose build func kwargs.
- build_kwargs = dict(self.static_kwargs)
- build_kwargs["is_template_graph"] = True
- build_kwargs["components"] = self._components
-
- # Override scope and device, and ignore surrounding control dependencies.
- with tfutil.absolute_variable_scope(self.scope, reuse=False), tfutil.absolute_name_scope(self.scope), tf.device(self.device), tf.control_dependencies(None):
- assert tf.get_variable_scope().name == self.scope
- assert tf.get_default_graph().get_name_scope() == self.scope
-
- # Create input templates.
- self._input_templates = []
- for param in inspect.signature(self._build_func).parameters.values():
- if param.kind == param.POSITIONAL_OR_KEYWORD and param.default is param.empty:
- self._input_templates.append(tf.placeholder(tf.float32, name=param.name))
-
- # Call build func.
- out_expr = self._build_func(*self._input_templates, **build_kwargs)
-
- # Collect output templates and variables.
- assert tfutil.is_tf_expression(out_expr) or isinstance(out_expr, tuple)
- self._output_templates = [out_expr] if tfutil.is_tf_expression(out_expr) else list(out_expr)
- self._own_vars = OrderedDict((var.name[len(self.scope) + 1:].split(":")[0], var) for var in tf.global_variables(self.scope + "/"))
-
- # Check for errors.
- if len(self._input_templates) == 0:
- raise ValueError("Network build func did not list any inputs.")
- if len(self._output_templates) == 0:
- raise ValueError("Network build func did not return any outputs.")
- if any(not tfutil.is_tf_expression(t) for t in self._output_templates):
- raise ValueError("Network outputs must be TensorFlow expressions.")
- if any(t.shape.ndims is None for t in self._input_templates):
- raise ValueError("Network input shapes not defined. Please call x.set_shape() for each input.")
- if any(t.shape.ndims is None for t in self._output_templates):
- raise ValueError("Network output shapes not defined. Please call x.set_shape() where applicable.")
- if any(not isinstance(comp, Network) for comp in self._components.values()):
- raise ValueError("Components of a Network must be Networks themselves.")
- if len(self._components) != len(set(comp.name for comp in self._components.values())):
- raise ValueError("Components of a Network must have unique names.")
-
- # Initialize variables.
- if len(self._var_inits):
- tfutil.set_vars({self._get_vars()[name]: value for name, value in self._var_inits.items() if name in self._get_vars()})
- remaining_inits = [var.initializer for name, var in self._own_vars.items() if name not in self._var_inits]
- if self._all_inits_known:
- assert len(remaining_inits) == 0
- else:
- tfutil.run(remaining_inits)
- self._var_inits = None
-
- @property
- def name(self):
- """User-specified name string."""
- return self._name
-
- @property
- def scope(self):
- """Unique TensorFlow scope containing template graph and variables, derived from the user-specified name."""
- return self._scope
-
- @property
- def device(self):
- """Name of the TensorFlow device that the weights of this network reside on. Determined by the current device at construction time."""
- return self._device
-
- @property
- def static_kwargs(self):
- """EasyDict of arguments passed to the user-supplied build func."""
- return copy.deepcopy(self._static_kwargs)
-
- @property
- def components(self):
- """EasyDict of sub-networks created by the build func."""
- return copy.copy(self._get_components())
-
- def _get_components(self):
- if self._components is None:
- self._init_graph()
- assert self._components is not None
- return self._components
-
- @property
- def input_shapes(self):
- """List of input tensor shapes, including minibatch dimension."""
- if self._input_shapes is None:
- self._input_shapes = [t.shape.as_list() for t in self.input_templates]
- return copy.deepcopy(self._input_shapes)
-
- @property
- def output_shapes(self):
- """List of output tensor shapes, including minibatch dimension."""
- if self._output_shapes is None:
- self._output_shapes = [t.shape.as_list() for t in self.output_templates]
- return copy.deepcopy(self._output_shapes)
-
- @property
- def input_shape(self):
- """Short-hand for input_shapes[0]."""
- return self.input_shapes[0]
-
- @property
- def output_shape(self):
- """Short-hand for output_shapes[0]."""
- return self.output_shapes[0]
-
- @property
- def num_inputs(self):
- """Number of input tensors."""
- return len(self.input_shapes)
-
- @property
- def num_outputs(self):
- """Number of output tensors."""
- return len(self.output_shapes)
-
- @property
- def input_names(self):
- """Name string for each input."""
- if self._input_names is None:
- self._input_names = [t.name.split("/")[-1].split(":")[0] for t in self.input_templates]
- return copy.copy(self._input_names)
-
- @property
- def output_names(self):
- """Name string for each output."""
- if self._output_names is None:
- self._output_names = [t.name.split("/")[-1].split(":")[0] for t in self.output_templates]
- return copy.copy(self._output_names)
-
- @property
- def input_templates(self):
- """Input placeholders in the template graph."""
- if self._input_templates is None:
- self._init_graph()
- assert self._input_templates is not None
- return copy.copy(self._input_templates)
-
- @property
- def output_templates(self):
- """Output tensors in the template graph."""
- if self._output_templates is None:
- self._init_graph()
- assert self._output_templates is not None
- return copy.copy(self._output_templates)
-
- @property
- def own_vars(self):
- """Variables defined by this network (local_name => var), excluding sub-networks."""
- return copy.copy(self._get_own_vars())
-
- def _get_own_vars(self):
- if self._own_vars is None:
- self._init_graph()
- assert self._own_vars is not None
- return self._own_vars
-
- @property
- def vars(self):
- """All variables (local_name => var)."""
- return copy.copy(self._get_vars())
-
- def _get_vars(self):
- if self._vars is None:
- self._vars = OrderedDict(self._get_own_vars())
- for comp in self._get_components().values():
- self._vars.update((comp.name + "/" + name, var) for name, var in comp._get_vars().items())
- return self._vars
-
- @property
- def trainables(self):
- """All trainable variables (local_name => var)."""
- return copy.copy(self._get_trainables())
-
- def _get_trainables(self):
- if self._trainables is None:
- self._trainables = OrderedDict((name, var) for name, var in self.vars.items() if var.trainable)
- return self._trainables
-
- @property
- def var_global_to_local(self):
- """Mapping from variable global names to local names."""
- return copy.copy(self._get_var_global_to_local())
-
- def _get_var_global_to_local(self):
- if self._var_global_to_local is None:
- self._var_global_to_local = OrderedDict((var.name.split(":")[0], name) for name, var in self.vars.items())
- return self._var_global_to_local
-
- def reset_own_vars(self) -> None:
- """Re-initialize all variables of this network, excluding sub-networks."""
- if self._var_inits is None or self._components is None:
- tfutil.run([var.initializer for var in self._get_own_vars().values()])
- else:
- self._var_inits.clear()
- self._all_inits_known = False
-
- def reset_vars(self) -> None:
- """Re-initialize all variables of this network, including sub-networks."""
- if self._var_inits is None:
- tfutil.run([var.initializer for var in self._get_vars().values()])
- else:
- self._var_inits.clear()
- self._all_inits_known = False
- if self._components is not None:
- for comp in self._components.values():
- comp.reset_vars()
-
- def reset_trainables(self) -> None:
- """Re-initialize all trainable variables of this network, including sub-networks."""
- tfutil.run([var.initializer for var in self._get_trainables().values()])
-
- def get_output_for(self, *in_expr: TfExpression, return_as_list: bool = False, **dynamic_kwargs) -> Union[TfExpression, List[TfExpression]]:
- """Construct TensorFlow expression(s) for the output(s) of this network, given the input expression(s).
- The graph is placed on the current TensorFlow device."""
- assert len(in_expr) == self.num_inputs
- assert not all(expr is None for expr in in_expr)
- self._get_vars() # ensure that all variables have been created
-
- # Choose build func kwargs.
- build_kwargs = dict(self.static_kwargs)
- build_kwargs.update(dynamic_kwargs)
- build_kwargs["is_template_graph"] = False
- build_kwargs["components"] = self._components
-
- # Build TensorFlow graph to evaluate the network.
- with tfutil.absolute_variable_scope(self.scope, reuse=True), tf.name_scope(self.name):
- assert tf.get_variable_scope().name == self.scope
- valid_inputs = [expr for expr in in_expr if expr is not None]
- final_inputs = []
- for expr, name, shape in zip(in_expr, self.input_names, self.input_shapes):
- if expr is not None:
- expr = tf.identity(expr, name=name)
- else:
- expr = tf.zeros([tf.shape(valid_inputs[0])[0]] + shape[1:], name=name)
- final_inputs.append(expr)
- out_expr = self._build_func(*final_inputs, **build_kwargs)
-
- # Propagate input shapes back to the user-specified expressions.
- for expr, final in zip(in_expr, final_inputs):
- if isinstance(expr, tf.Tensor):
- expr.set_shape(final.shape)
-
- # Express outputs in the desired format.
- assert tfutil.is_tf_expression(out_expr) or isinstance(out_expr, tuple)
- if return_as_list:
- out_expr = [out_expr] if tfutil.is_tf_expression(out_expr) else list(out_expr)
- return out_expr
-
- def get_var_local_name(self, var_or_global_name: Union[TfExpression, str]) -> str:
- """Get the local name of a given variable, without any surrounding name scopes."""
- assert tfutil.is_tf_expression(var_or_global_name) or isinstance(var_or_global_name, str)
- global_name = var_or_global_name if isinstance(var_or_global_name, str) else var_or_global_name.name
- return self._get_var_global_to_local()[global_name]
-
- def find_var(self, var_or_local_name: Union[TfExpression, str]) -> TfExpression:
- """Find variable by local or global name."""
- assert tfutil.is_tf_expression(var_or_local_name) or isinstance(var_or_local_name, str)
- return self._get_vars()[var_or_local_name] if isinstance(var_or_local_name, str) else var_or_local_name
-
- def get_var(self, var_or_local_name: Union[TfExpression, str]) -> np.ndarray:
- """Get the value of a given variable as NumPy array.
- Note: This method is very inefficient -- prefer to use tflib.run(list_of_vars) whenever possible."""
- return self.find_var(var_or_local_name).eval()
-
- def set_var(self, var_or_local_name: Union[TfExpression, str], new_value: Union[int, float, np.ndarray]) -> None:
- """Set the value of a given variable based on the given NumPy array.
- Note: This method is very inefficient -- prefer to use tflib.set_vars() whenever possible."""
- tfutil.set_vars({self.find_var(var_or_local_name): new_value})
-
- def __getstate__(self) -> dict:
- """Pickle export."""
- state = dict()
- state["version"] = 5
- state["name"] = self.name
- state["static_kwargs"] = dict(self.static_kwargs)
- state["components"] = dict(self.components)
- state["build_module_src"] = self._build_module_src
- state["build_func_name"] = self._build_func_name
- state["variables"] = list(zip(self._get_own_vars().keys(), tfutil.run(list(self._get_own_vars().values()))))
- state["input_shapes"] = self.input_shapes
- state["output_shapes"] = self.output_shapes
- state["input_names"] = self.input_names
- state["output_names"] = self.output_names
- return state
-
- def __setstate__(self, state: dict) -> None:
- """Pickle import."""
-
- # Execute custom import handlers.
- for handler in _import_handlers:
- state = handler(state)
-
- # Get basic fields.
- assert state["version"] in [2, 3, 4, 5]
- name = state["name"]
- static_kwargs = state["static_kwargs"]
- build_module_src = state["build_module_src"]
- build_func_name = state["build_func_name"]
-
- # Create temporary module from the imported source code.
- module_name = "_tflib_network_import_" + uuid.uuid4().hex
- module = types.ModuleType(module_name)
- sys.modules[module_name] = module
- _import_module_src[module] = build_module_src
- exec(build_module_src, module.__dict__) # pylint: disable=exec-used
- build_func = util.get_obj_from_module(module, build_func_name)
-
- # Initialize fields.
- self._init_fields(name=name, static_kwargs=static_kwargs, build_func=build_func, build_func_name=build_func_name, build_module_src=build_module_src)
- self._var_inits.update(copy.deepcopy(state["variables"]))
- self._all_inits_known = True
- self._components = util.EasyDict(state.get("components", {}))
- self._input_shapes = copy.deepcopy(state.get("input_shapes", None))
- self._output_shapes = copy.deepcopy(state.get("output_shapes", None))
- self._input_names = copy.deepcopy(state.get("input_names", None))
- self._output_names = copy.deepcopy(state.get("output_names", None))
-
- def clone(self, name: str = None, **new_static_kwargs) -> "Network":
- """Create a clone of this network with its own copy of the variables."""
- static_kwargs = dict(self.static_kwargs)
- static_kwargs.update(new_static_kwargs)
- net = object.__new__(Network)
- net._init_fields(name=(name or self.name), static_kwargs=static_kwargs, build_func=self._build_func, build_func_name=self._build_func_name, build_module_src=self._build_module_src)
- net.copy_vars_from(self)
- return net
-
- def copy_own_vars_from(self, src_net: "Network") -> None:
- """Copy the values of all variables from the given network, excluding sub-networks."""
-
- # Source has unknown variables or unknown components => init now.
- if (src_net._var_inits is not None and not src_net._all_inits_known) or src_net._components is None:
- src_net._get_vars()
-
- # Both networks are inited => copy directly.
- if src_net._var_inits is None and self._var_inits is None:
- names = [name for name in self._get_own_vars().keys() if name in src_net._get_own_vars()]
- tfutil.set_vars(tfutil.run({self._get_vars()[name]: src_net._get_vars()[name] for name in names}))
- return
-
- # Read from source.
- if src_net._var_inits is None:
- value_dict = tfutil.run(src_net._get_own_vars())
- else:
- value_dict = src_net._var_inits
-
- # Write to destination.
- if self._var_inits is None:
- tfutil.set_vars({self._get_vars()[name]: value for name, value in value_dict.items() if name in self._get_vars()})
- else:
- self._var_inits.update(value_dict)
-
- def copy_vars_from(self, src_net: "Network") -> None:
- """Copy the values of all variables from the given network, including sub-networks."""
-
- # Source has unknown variables or unknown components => init now.
- if (src_net._var_inits is not None and not src_net._all_inits_known) or src_net._components is None:
- src_net._get_vars()
-
- # Source is inited, but destination components have not been created yet => set as initial values.
- if src_net._var_inits is None and self._components is None:
- self._var_inits.update(tfutil.run(src_net._get_vars()))
- return
-
- # Destination has unknown components => init now.
- if self._components is None:
- self._get_vars()
-
- # Both networks are inited => copy directly.
- if src_net._var_inits is None and self._var_inits is None:
- names = [name for name in self._get_vars().keys() if name in src_net._get_vars()]
- tfutil.set_vars(tfutil.run({self._get_vars()[name]: src_net._get_vars()[name] for name in names}))
- return
-
- # Copy recursively, component by component.
- self.copy_own_vars_from(src_net)
- for name, src_comp in src_net._components.items():
- if name in self._components:
- self._components[name].copy_vars_from(src_comp)
-
- def copy_trainables_from(self, src_net: "Network") -> None:
- """Copy the values of all trainable variables from the given network, including sub-networks."""
- names = [name for name in self._get_trainables().keys() if name in src_net._get_trainables()]
- tfutil.set_vars(tfutil.run({self._get_vars()[name]: src_net._get_vars()[name] for name in names}))
-
- def convert(self, new_func_name: str, new_name: str = None, **new_static_kwargs) -> "Network":
- """Create new network with the given parameters, and copy all variables from this network."""
- if new_name is None:
- new_name = self.name
- static_kwargs = dict(self.static_kwargs)
- static_kwargs.update(new_static_kwargs)
- net = Network(name=new_name, func_name=new_func_name, **static_kwargs)
- net.copy_vars_from(self)
- return net
-
- def setup_as_moving_average_of(self, src_net: "Network", beta: TfExpressionEx = 0.99, beta_nontrainable: TfExpressionEx = 0.0) -> tf.Operation:
- """Construct a TensorFlow op that updates the variables of this network
- to be slightly closer to those of the given network."""
- with tfutil.absolute_name_scope(self.scope + "/_MovingAvg"):
- ops = []
- for name, var in self._get_vars().items():
- if name in src_net._get_vars():
- cur_beta = beta if var.trainable else beta_nontrainable
- new_value = tfutil.lerp(src_net._get_vars()[name], var, cur_beta)
- ops.append(var.assign(new_value))
- return tf.group(*ops)
-
- def run(self,
- *in_arrays: Tuple[Union[np.ndarray, None], ...],
- input_transform: dict = None,
- output_transform: dict = None,
- return_as_list: bool = False,
- print_progress: bool = False,
- minibatch_size: int = None,
- num_gpus: int = 1,
- assume_frozen: bool = False,
- **dynamic_kwargs) -> Union[np.ndarray, Tuple[np.ndarray, ...], List[np.ndarray]]:
- """Run this network for the given NumPy array(s), and return the output(s) as NumPy array(s).
-
- Args:
- input_transform: A dict specifying a custom transformation to be applied to the input tensor(s) before evaluating the network.
- The dict must contain a 'func' field that points to a top-level function. The function is called with the input
- TensorFlow expression(s) as positional arguments. Any remaining fields of the dict will be passed in as kwargs.
- output_transform: A dict specifying a custom transformation to be applied to the output tensor(s) after evaluating the network.
- The dict must contain a 'func' field that points to a top-level function. The function is called with the output
- TensorFlow expression(s) as positional arguments. Any remaining fields of the dict will be passed in as kwargs.
- return_as_list: True = return a list of NumPy arrays, False = return a single NumPy array, or a tuple if there are multiple outputs.
- print_progress: Print progress to the console? Useful for very large input arrays.
- minibatch_size: Maximum minibatch size to use, None = disable batching.
- num_gpus: Number of GPUs to use.
- assume_frozen: Improve multi-GPU performance by assuming that the trainable parameters will remain changed between calls.
- dynamic_kwargs: Additional keyword arguments to be passed into the network build function.
- """
- assert len(in_arrays) == self.num_inputs
- assert not all(arr is None for arr in in_arrays)
- assert input_transform is None or util.is_top_level_function(input_transform["func"])
- assert output_transform is None or util.is_top_level_function(output_transform["func"])
- output_transform, dynamic_kwargs = _handle_legacy_output_transforms(output_transform, dynamic_kwargs)
- num_items = in_arrays[0].shape[0]
- if minibatch_size is None:
- minibatch_size = num_items
-
- # Construct unique hash key from all arguments that affect the TensorFlow graph.
- key = dict(input_transform=input_transform, output_transform=output_transform, num_gpus=num_gpus, assume_frozen=assume_frozen, dynamic_kwargs=dynamic_kwargs)
- def unwind_key(obj):
- if isinstance(obj, dict):
- return [(key, unwind_key(value)) for key, value in sorted(obj.items())]
- if callable(obj):
- return util.get_top_level_function_name(obj)
- return obj
- key = repr(unwind_key(key))
-
- # Build graph.
- if key not in self._run_cache:
- with tfutil.absolute_name_scope(self.scope + "/_Run"), tf.control_dependencies(None):
- with tf.device("/cpu:0"):
- in_expr = [tf.placeholder(tf.float32, name=name) for name in self.input_names]
- in_split = list(zip(*[tf.split(x, num_gpus) for x in in_expr]))
-
- out_split = []
- for gpu in range(num_gpus):
- with tf.device(self.device if num_gpus == 1 else "/gpu:%d" % gpu):
- net_gpu = self.clone() if assume_frozen else self
- in_gpu = in_split[gpu]
-
- if input_transform is not None:
- in_kwargs = dict(input_transform)
- in_gpu = in_kwargs.pop("func")(*in_gpu, **in_kwargs)
- in_gpu = [in_gpu] if tfutil.is_tf_expression(in_gpu) else list(in_gpu)
-
- assert len(in_gpu) == self.num_inputs
- out_gpu = net_gpu.get_output_for(*in_gpu, return_as_list=True, **dynamic_kwargs)
-
- if output_transform is not None:
- out_kwargs = dict(output_transform)
- out_gpu = out_kwargs.pop("func")(*out_gpu, **out_kwargs)
- out_gpu = [out_gpu] if tfutil.is_tf_expression(out_gpu) else list(out_gpu)
-
- assert len(out_gpu) == self.num_outputs
- out_split.append(out_gpu)
-
- with tf.device("/cpu:0"):
- out_expr = [tf.concat(outputs, axis=0) for outputs in zip(*out_split)]
- self._run_cache[key] = in_expr, out_expr
-
- # Run minibatches.
- in_expr, out_expr = self._run_cache[key]
- out_arrays = [np.empty([num_items] + expr.shape.as_list()[1:], expr.dtype.name) for expr in out_expr]
-
- for mb_begin in range(0, num_items, minibatch_size):
- if print_progress:
- print("\r%d / %d" % (mb_begin, num_items), end="")
-
- mb_end = min(mb_begin + minibatch_size, num_items)
- mb_num = mb_end - mb_begin
- mb_in = [src[mb_begin : mb_end] if src is not None else np.zeros([mb_num] + shape[1:]) for src, shape in zip(in_arrays, self.input_shapes)]
- mb_out = tf.get_default_session().run(out_expr, dict(zip(in_expr, mb_in)))
-
- for dst, src in zip(out_arrays, mb_out):
- dst[mb_begin: mb_end] = src
-
- # Done.
- if print_progress:
- print("\r%d / %d" % (num_items, num_items))
-
- if not return_as_list:
- out_arrays = out_arrays[0] if len(out_arrays) == 1 else tuple(out_arrays)
- return out_arrays
-
- def list_ops(self) -> List[TfExpression]:
- _ = self.output_templates # ensure that the template graph has been created
- include_prefix = self.scope + "/"
- exclude_prefix = include_prefix + "_"
- ops = tf.get_default_graph().get_operations()
- ops = [op for op in ops if op.name.startswith(include_prefix)]
- ops = [op for op in ops if not op.name.startswith(exclude_prefix)]
- return ops
-
- def list_layers(self) -> List[Tuple[str, TfExpression, List[TfExpression]]]:
- """Returns a list of (layer_name, output_expr, trainable_vars) tuples corresponding to
- individual layers of the network. Mainly intended to be used for reporting."""
- layers = []
-
- def recurse(scope, parent_ops, parent_vars, level):
- if len(parent_ops) == 0 and len(parent_vars) == 0:
- return
-
- # Ignore specific patterns.
- if any(p in scope for p in ["/Shape", "/strided_slice", "/Cast", "/concat", "/Assign"]):
- return
-
- # Filter ops and vars by scope.
- global_prefix = scope + "/"
- local_prefix = global_prefix[len(self.scope) + 1:]
- cur_ops = [op for op in parent_ops if op.name.startswith(global_prefix) or op.name == global_prefix[:-1]]
- cur_vars = [(name, var) for name, var in parent_vars if name.startswith(local_prefix) or name == local_prefix[:-1]]
- if not cur_ops and not cur_vars:
- return
-
- # Filter out all ops related to variables.
- for var in [op for op in cur_ops if op.type.startswith("Variable")]:
- var_prefix = var.name + "/"
- cur_ops = [op for op in cur_ops if not op.name.startswith(var_prefix)]
-
- # Scope does not contain ops as immediate children => recurse deeper.
- contains_direct_ops = any("/" not in op.name[len(global_prefix):] and op.type not in ["Identity", "Cast", "Transpose"] for op in cur_ops)
- if (level == 0 or not contains_direct_ops) and (len(cur_ops) != 0 or len(cur_vars) != 0):
- visited = set()
- for rel_name in [op.name[len(global_prefix):] for op in cur_ops] + [name[len(local_prefix):] for name, _var in cur_vars]:
- token = rel_name.split("/")[0]
- if token not in visited:
- recurse(global_prefix + token, cur_ops, cur_vars, level + 1)
- visited.add(token)
- return
-
- # Report layer.
- layer_name = scope[len(self.scope) + 1:]
- layer_output = cur_ops[-1].outputs[0] if cur_ops else cur_vars[-1][1]
- layer_trainables = [var for _name, var in cur_vars if var.trainable]
- layers.append((layer_name, layer_output, layer_trainables))
-
- recurse(self.scope, self.list_ops(), list(self._get_vars().items()), 0)
- return layers
-
- def print_layers(self, title: str = None, hide_layers_with_no_params: bool = False) -> None:
- """Print a summary table of the network structure."""
- rows = [[title if title is not None else self.name, "Params", "OutputShape", "WeightShape"]]
- rows += [["---"] * 4]
- total_params = 0
-
- for layer_name, layer_output, layer_trainables in self.list_layers():
- num_params = sum(int(np.prod(var.shape.as_list())) for var in layer_trainables)
- weights = [var for var in layer_trainables if var.name.endswith("/weight:0")]
- weights.sort(key=lambda x: len(x.name))
- if len(weights) == 0 and len(layer_trainables) == 1:
- weights = layer_trainables
- total_params += num_params
-
- if not hide_layers_with_no_params or num_params != 0:
- num_params_str = str(num_params) if num_params > 0 else "-"
- output_shape_str = str(layer_output.shape)
- weight_shape_str = str(weights[0].shape) if len(weights) >= 1 else "-"
- rows += [[layer_name, num_params_str, output_shape_str, weight_shape_str]]
-
- rows += [["---"] * 4]
- rows += [["Total", str(total_params), "", ""]]
-
- widths = [max(len(cell) for cell in column) for column in zip(*rows)]
- print()
- for row in rows:
- print(" ".join(cell + " " * (width - len(cell)) for cell, width in zip(row, widths)))
- print()
-
- def setup_weight_histograms(self, title: str = None) -> None:
- """Construct summary ops to include histograms of all trainable parameters in TensorBoard."""
- if title is None:
- title = self.name
-
- with tf.name_scope(None), tf.device(None), tf.control_dependencies(None):
- for local_name, var in self._get_trainables().items():
- if "/" in local_name:
- p = local_name.split("/")
- name = title + "_" + p[-1] + "/" + "_".join(p[:-1])
- else:
- name = title + "_toplevel/" + local_name
-
- tf.summary.histogram(name, var)
-
-#----------------------------------------------------------------------------
-# Backwards-compatible emulation of legacy output transformation in Network.run().
-
-_print_legacy_warning = True
-
-def _handle_legacy_output_transforms(output_transform, dynamic_kwargs):
- global _print_legacy_warning
- legacy_kwargs = ["out_mul", "out_add", "out_shrink", "out_dtype"]
- if not any(kwarg in dynamic_kwargs for kwarg in legacy_kwargs):
- return output_transform, dynamic_kwargs
-
- if _print_legacy_warning:
- _print_legacy_warning = False
- print()
- print("WARNING: Old-style output transformations in Network.run() are deprecated.")
- print("Consider using 'output_transform=dict(func=tflib.convert_images_to_uint8)'")
- print("instead of 'out_mul=127.5, out_add=127.5, out_dtype=np.uint8'.")
- print()
- assert output_transform is None
-
- new_kwargs = dict(dynamic_kwargs)
- new_transform = {kwarg: new_kwargs.pop(kwarg) for kwarg in legacy_kwargs if kwarg in dynamic_kwargs}
- new_transform["func"] = _legacy_output_transform_func
- return new_transform, new_kwargs
-
-def _legacy_output_transform_func(*expr, out_mul=1.0, out_add=0.0, out_shrink=1, out_dtype=None):
- if out_mul != 1.0:
- expr = [x * out_mul for x in expr]
-
- if out_add != 0.0:
- expr = [x + out_add for x in expr]
-
- if out_shrink > 1:
- ksize = [1, 1, out_shrink, out_shrink]
- expr = [tf.nn.avg_pool(x, ksize=ksize, strides=ksize, padding="VALID", data_format="NCHW") for x in expr]
-
- if out_dtype is not None:
- if tf.as_dtype(out_dtype).is_integer:
- expr = [tf.round(x) for x in expr]
- expr = [tf.saturate_cast(x, out_dtype) for x in expr]
- return expr
diff --git a/spaces/h2oai/h2ogpt-chatbot/README.md b/spaces/h2oai/h2ogpt-chatbot/README.md
deleted file mode 100644
index 14b59cf394d195459e1f735af273e39301347ec9..0000000000000000000000000000000000000000
--- a/spaces/h2oai/h2ogpt-chatbot/README.md
+++ /dev/null
@@ -1,13 +0,0 @@
----
-title: H2ogpt Chatbot
-emoji: 📚
-colorFrom: yellow
-colorTo: yellow
-sdk: gradio
-sdk_version: 3.41.2
-app_file: app.py
-pinned: false
-license: apache-2.0
----
-
-Check out the configuration reference at https://huggingface.co/docs/hub/spaces-config-reference
diff --git a/spaces/h2oai/wave-tour/examples/plot_events.py b/spaces/h2oai/wave-tour/examples/plot_events.py
deleted file mode 100644
index e9db958dc5397d5259124a4291b912f235b1eb0c..0000000000000000000000000000000000000000
--- a/spaces/h2oai/wave-tour/examples/plot_events.py
+++ /dev/null
@@ -1,31 +0,0 @@
-# Plot / Events
-# Handle #events on a #plot card.
-# ---
-from h2o_wave import main, app, Q, ui, data
-
-
-@app('/demo')
-async def serve(q: Q):
- if not q.client.initialized:
- q.client.initialized = True
- q.page['pricing'] = ui.plot_card(
- box='1 1 4 5',
- title='Interval',
- data=data(fields='product price', rows=[
- ['spam', 1.49],
- ['eggs', 2.49],
- ['ham', 1.99],
- ], pack=True),
- plot=ui.plot([ui.mark(type='interval', x='=product', y='=price', y_min=0)]),
- events=['select_marks']
- )
- q.page['details'] = ui.markdown_card(
- box='1 6 4 2',
- title='Selected Product',
- content='Nothing selected.',
- )
- else:
- if q.events.pricing:
- q.page['details'].content = f'You selected {q.events.pricing.select_marks}'
-
- await q.page.save()
diff --git a/spaces/haakohu/deep_privacy2_face/sg3_torch_utils/ops/upfirdn2d.h b/spaces/haakohu/deep_privacy2_face/sg3_torch_utils/ops/upfirdn2d.h
deleted file mode 100644
index c9e2032bcac9d2abde7a75eea4d812da348afadd..0000000000000000000000000000000000000000
--- a/spaces/haakohu/deep_privacy2_face/sg3_torch_utils/ops/upfirdn2d.h
+++ /dev/null
@@ -1,59 +0,0 @@
-// Copyright (c) 2021, NVIDIA CORPORATION. All rights reserved.
-//
-// NVIDIA CORPORATION and its licensors retain all intellectual property
-// and proprietary rights in and to this software, related documentation
-// and any modifications thereto. Any use, reproduction, disclosure or
-// distribution of this software and related documentation without an express
-// license agreement from NVIDIA CORPORATION is strictly prohibited.
-
-#include
-
-//------------------------------------------------------------------------
-// CUDA kernel parameters.
-
-struct upfirdn2d_kernel_params
-{
- const void* x;
- const float* f;
- void* y;
-
- int2 up;
- int2 down;
- int2 pad0;
- int flip;
- float gain;
-
- int4 inSize; // [width, height, channel, batch]
- int4 inStride;
- int2 filterSize; // [width, height]
- int2 filterStride;
- int4 outSize; // [width, height, channel, batch]
- int4 outStride;
- int sizeMinor;
- int sizeMajor;
-
- int loopMinor;
- int loopMajor;
- int loopX;
- int launchMinor;
- int launchMajor;
-};
-
-//------------------------------------------------------------------------
-// CUDA kernel specialization.
-
-struct upfirdn2d_kernel_spec
-{
- void* kernel;
- int tileOutW;
- int tileOutH;
- int loopMinor;
- int loopX;
-};
-
-//------------------------------------------------------------------------
-// CUDA kernel selection.
-
-template upfirdn2d_kernel_spec choose_upfirdn2d_kernel(const upfirdn2d_kernel_params& p);
-
-//------------------------------------------------------------------------
diff --git a/spaces/hamzapehlivan/StyleRes/datasets/process_image.py b/spaces/hamzapehlivan/StyleRes/datasets/process_image.py
deleted file mode 100644
index 8897589fbfae0d240d37d2dd37602cdcbae0f0a5..0000000000000000000000000000000000000000
--- a/spaces/hamzapehlivan/StyleRes/datasets/process_image.py
+++ /dev/null
@@ -1,165 +0,0 @@
-
-import numpy as np
-import torch
-from PIL import Image
-import dlib
-import numpy as np
-import PIL
-import PIL.Image
-import scipy
-import scipy.ndimage
-
-class ImageProcessor():
- def __init__(self, predictor_path=None) -> None:
- self.predictor = None
- if predictor_path:
- self.predictor = dlib.shape_predictor(predictor_path)
-
- @staticmethod
- def preprocess_image(image, is_batch=True):
- image = image.resize( (256, 256))
- image = np.asarray(image).transpose(2, 0, 1).astype(np.float32) # C,H,W -> H,W,C
- image = torch.FloatTensor(image.copy())
- image = (image - 127.5) / 127.5 # Normalize
- if not is_batch:
- image = image.unsqueeze(0)
- return image
-
- """
- Input: A numpy image with shape NxCxHxW.
- Output: Output image with NxHxWxC with values between 0-255
- """
- @staticmethod
- def postprocess_image(image, min_val=-1.0, max_val=1.0, is_batch=True):
- image = image.astype(np.float64)
- image = (image - min_val) * 255 / (max_val - min_val)
- image = np.clip(image + 0.5, 0, 255).astype(np.uint8)
- image = image.transpose(0, 2, 3, 1)
- if not is_batch:
- image = Image.fromarray(image[0])
- return image
-
- """
- brief: face alignment with FFHQ method (https://github.com/NVlabs/ffhq-dataset)
- author: lzhbrian (https://lzhbrian.me)
- date: 2020.1.5
- note: code is heavily borrowed from
- https://github.com/NVlabs/ffhq-dataset
- http://dlib.net/face_landmark_detection.py.html
- requirements:
- apt install cmake
- conda install Pillow numpy scipy
- pip install dlib
- # download face landmark model from:
- # http://dlib.net/files/shape_predictor_68_face_landmarks.dat.bz2
- """
-
- def get_landmark(self, image):
- """get landmark with dlib
- :return: np.array shape=(68, 2)
- """
- detector = dlib.get_frontal_face_detector()
-
- # img = dlib.load_rgb_image(filepath)
- img = np.asarray(image)
- dets = detector(img, 1)
-
- for k, d in enumerate(dets):
- shape = self.predictor(img, d)
-
- t = list(shape.parts())
- a = []
- for tt in t:
- a.append([tt.x, tt.y])
- lm = np.array(a)
- return lm
-
- def align_face(self, img):
- """
- :param image: PIL image
- :return: PIL Image
- """
- if self.predictor is None:
- return img
-
- lm = self.get_landmark(img)
-
- lm_chin = lm[0: 17] # left-right
- lm_eyebrow_left = lm[17: 22] # left-right
- lm_eyebrow_right = lm[22: 27] # left-right
- lm_nose = lm[27: 31] # top-down
- lm_nostrils = lm[31: 36] # top-down
- lm_eye_left = lm[36: 42] # left-clockwise
- lm_eye_right = lm[42: 48] # left-clockwise
- lm_mouth_outer = lm[48: 60] # left-clockwise
- lm_mouth_inner = lm[60: 68] # left-clockwise
-
- # Calculate auxiliary vectors.
- eye_left = np.mean(lm_eye_left, axis=0)
- eye_right = np.mean(lm_eye_right, axis=0)
- eye_avg = (eye_left + eye_right) * 0.5
- eye_to_eye = eye_right - eye_left
- mouth_left = lm_mouth_outer[0]
- mouth_right = lm_mouth_outer[6]
- mouth_avg = (mouth_left + mouth_right) * 0.5
- eye_to_mouth = mouth_avg - eye_avg
-
- # Choose oriented crop rectangle.
- x = eye_to_eye - np.flipud(eye_to_mouth) * [-1, 1]
- x /= np.hypot(*x)
- x *= max(np.hypot(*eye_to_eye) * 2.0, np.hypot(*eye_to_mouth) * 1.8)
- y = np.flipud(x) * [-1, 1]
- c = eye_avg + eye_to_mouth * 0.1
- quad = np.stack([c - x - y, c - x + y, c + x + y, c + x - y])
- qsize = np.hypot(*x) * 2
-
- # read image
- # img = PIL.Image.open(filepath)
-
- output_size = 1024
- transform_size = 1024
- enable_padding = True
-
- # Shrink.
- shrink = int(np.floor(qsize / output_size * 0.5))
- if shrink > 1:
- rsize = (int(np.rint(float(img.size[0]) / shrink)), int(np.rint(float(img.size[1]) / shrink)))
- img = img.resize(rsize, PIL.Image.ANTIALIAS)
- quad /= shrink
- qsize /= shrink
-
- # Crop.
- border = max(int(np.rint(qsize * 0.1)), 3)
- crop = (int(np.floor(min(quad[:, 0]))), int(np.floor(min(quad[:, 1]))), int(np.ceil(max(quad[:, 0]))),
- int(np.ceil(max(quad[:, 1]))))
- crop = (max(crop[0] - border, 0), max(crop[1] - border, 0), min(crop[2] + border, img.size[0]),
- min(crop[3] + border, img.size[1]))
- if crop[2] - crop[0] < img.size[0] or crop[3] - crop[1] < img.size[1]:
- img = img.crop(crop)
- quad -= crop[0:2]
-
- # Pad.
- pad = (int(np.floor(min(quad[:, 0]))), int(np.floor(min(quad[:, 1]))), int(np.ceil(max(quad[:, 0]))),
- int(np.ceil(max(quad[:, 1]))))
- pad = (max(-pad[0] + border, 0), max(-pad[1] + border, 0), max(pad[2] - img.size[0] + border, 0),
- max(pad[3] - img.size[1] + border, 0))
- if enable_padding and max(pad) > border - 4:
- pad = np.maximum(pad, int(np.rint(qsize * 0.3)))
- img = np.pad(np.float32(img), ((pad[1], pad[3]), (pad[0], pad[2]), (0, 0)), 'reflect')
- h, w, _ = img.shape
- y, x, _ = np.ogrid[:h, :w, :1]
- mask = np.maximum(1.0 - np.minimum(np.float32(x) / pad[0], np.float32(w - 1 - x) / pad[2]),
- 1.0 - np.minimum(np.float32(y) / pad[1], np.float32(h - 1 - y) / pad[3]))
- blur = qsize * 0.02
- img += (scipy.ndimage.gaussian_filter(img, [blur, blur, 0]) - img) * np.clip(mask * 3.0 + 1.0, 0.0, 1.0)
- img += (np.median(img, axis=(0, 1)) - img) * np.clip(mask, 0.0, 1.0)
- img = PIL.Image.fromarray(np.uint8(np.clip(np.rint(img), 0, 255)), 'RGB')
- quad += pad[:2]
-
- # Transform.
- img = img.transform((transform_size, transform_size), PIL.Image.QUAD, (quad + 0.5).flatten(), PIL.Image.BILINEAR)
- if output_size < transform_size:
- img = img.resize((output_size, output_size), PIL.Image.ANTIALIAS)
-
- # Save aligned image.
- return img
diff --git a/spaces/hamzapehlivan/StyleRes/editings/styleclip_directions/styleclip_mapper_network.py b/spaces/hamzapehlivan/StyleRes/editings/styleclip_directions/styleclip_mapper_network.py
deleted file mode 100644
index 641eb46e96e17ec6e1f8440e691e3c87aea3dc1c..0000000000000000000000000000000000000000
--- a/spaces/hamzapehlivan/StyleRes/editings/styleclip_directions/styleclip_mapper_network.py
+++ /dev/null
@@ -1,121 +0,0 @@
-import torch
-from torch import nn
-from torch.nn import Module
-from torch.nn import functional as F
-import math
-
-def fused_leaky_relu(input, bias, negative_slope=0.2, scale=2 ** 0.5):
- rest_dim = [1] * (input.ndim - bias.ndim - 1)
- input = input #.cuda()
- if input.ndim == 3:
- return (
- F.leaky_relu(
- input + bias.view(1, *rest_dim, bias.shape[0]), negative_slope=negative_slope
- )
- * scale
- )
- else:
- return (
- F.leaky_relu(
- input + bias.view(1, bias.shape[0], *rest_dim), negative_slope=negative_slope
- )
- * scale
- )
-
-
-class PixelNorm(nn.Module):
- def __init__(self):
- super().__init__()
-
- def forward(self, input):
- return input * torch.rsqrt(torch.mean(input ** 2, dim=1, keepdim=True) + 1e-8)
-
-class EqualLinear(nn.Module):
- def __init__(
- self, in_dim, out_dim, bias=True, bias_init=0, lr_mul=1, activation=None
- ):
- super().__init__()
-
- self.weight = nn.Parameter(torch.randn(out_dim, in_dim).div_(lr_mul))
-
- if bias:
- self.bias = nn.Parameter(torch.zeros(out_dim).fill_(bias_init))
-
- else:
- self.bias = None
-
- self.activation = activation
-
- self.scale = (1 / math.sqrt(in_dim)) * lr_mul
- self.lr_mul = lr_mul
-
- def forward(self, input):
- if self.activation:
- out = F.linear(input, self.weight * self.scale)
- out = fused_leaky_relu(out, self.bias * self.lr_mul)
-
- else:
- out = F.linear(
- input, self.weight * self.scale, bias=self.bias * self.lr_mul
- )
-
- return out
-
-class Mapper(Module):
-
- def __init__(self, latent_dim=512):
- super(Mapper, self).__init__()
-
- layers = [PixelNorm()]
-
- for i in range(4):
- layers.append(
- EqualLinear(
- latent_dim, latent_dim, lr_mul=0.01, activation='fused_lrelu'
- )
- )
-
- self.mapping = nn.Sequential(*layers)
-
-
- def forward(self, x):
- x = self.mapping(x)
- return x
-
-
-class LevelsMapper(Module):
-
- def __init__(self, opts):
- super(LevelsMapper, self).__init__()
-
- self.opts = opts
-
- if not opts.no_coarse_mapper:
- self.course_mapping = Mapper()
- if not opts.no_medium_mapper:
- self.medium_mapping = Mapper()
- if not opts.no_fine_mapper:
- self.fine_mapping = Mapper()
-
- def forward(self, x):
- x_coarse = x[:, :4, :]
- x_medium = x[:, 4:8, :]
- x_fine = x[:, 8:, :]
-
- if not self.opts.no_coarse_mapper:
- x_coarse = self.course_mapping(x_coarse)
- else:
- x_coarse = torch.zeros_like(x_coarse)
- if not self.opts.no_medium_mapper:
- x_medium = self.medium_mapping(x_medium)
- else:
- x_medium = torch.zeros_like(x_medium)
- if not self.opts.no_fine_mapper:
- x_fine = self.fine_mapping(x_fine)
- else:
- x_fine = torch.zeros_like(x_fine)
-
-
- out = torch.cat([x_coarse, x_medium, x_fine], dim=1)
-
- return out
\ No newline at end of file
diff --git a/spaces/hasibzunair/fifa-tryon-demo/Self-Correction-Human-Parsing-for-ACGPN/mhp_extension/detectron2/.github/ISSUE_TEMPLATE/unexpected-problems-bugs.md b/spaces/hasibzunair/fifa-tryon-demo/Self-Correction-Human-Parsing-for-ACGPN/mhp_extension/detectron2/.github/ISSUE_TEMPLATE/unexpected-problems-bugs.md
deleted file mode 100644
index bafee7a1a3897903d26e68001d3d3d2b7686015b..0000000000000000000000000000000000000000
--- a/spaces/hasibzunair/fifa-tryon-demo/Self-Correction-Human-Parsing-for-ACGPN/mhp_extension/detectron2/.github/ISSUE_TEMPLATE/unexpected-problems-bugs.md
+++ /dev/null
@@ -1,45 +0,0 @@
----
-name: "Unexpected behaviors"
-about: Run into unexpected behaviors when using detectron2
-title: Please read & provide the following
-
----
-
-If you do not know the root cause of the problem, and wish someone to help you, please
-post according to this template:
-
-## Instructions To Reproduce the Issue:
-
-1. what changes you made (`git diff`) or what code you wrote
-```
-
-```
-2. what exact command you run:
-3. what you observed (including __full logs__):
-```
-
-```
-4. please simplify the steps as much as possible so they do not require additional resources to
- run, such as a private dataset.
-
-## Expected behavior:
-
-If there are no obvious error in "what you observed" provided above,
-please tell us the expected behavior.
-
-If you expect the model to converge / work better, note that we do not give suggestions
-on how to train a new model.
-Only in one of the two conditions we will help with it:
-(1) You're unable to reproduce the results in detectron2 model zoo.
-(2) It indicates a detectron2 bug.
-
-## Environment:
-
-Provide your environment information using the following command:
-```
-wget -nc -q https://github.com/facebookresearch/detectron2/raw/master/detectron2/utils/collect_env.py && python collect_env.py
-```
-
-If your issue looks like an installation issue / environment issue,
-please first try to solve it yourself with the instructions in
-https://detectron2.readthedocs.io/tutorials/install.html#common-installation-issues
diff --git a/spaces/hasibzunair/fifa-tryon-demo/Self-Correction-Human-Parsing-for-ACGPN/mhp_extension/detectron2/docs/tutorials/evaluation.md b/spaces/hasibzunair/fifa-tryon-demo/Self-Correction-Human-Parsing-for-ACGPN/mhp_extension/detectron2/docs/tutorials/evaluation.md
deleted file mode 100644
index c71adb7eb2e554e5ea848f1feb44bbee01a13f8e..0000000000000000000000000000000000000000
--- a/spaces/hasibzunair/fifa-tryon-demo/Self-Correction-Human-Parsing-for-ACGPN/mhp_extension/detectron2/docs/tutorials/evaluation.md
+++ /dev/null
@@ -1,43 +0,0 @@
-
-# Evaluation
-
-Evaluation is a process that takes a number of inputs/outputs pairs and aggregate them.
-You can always [use the model](./models.md) directly and just parse its inputs/outputs manually to perform
-evaluation.
-Alternatively, evaluation is implemented in detectron2 using the [DatasetEvaluator](../modules/evaluation.html#detectron2.evaluation.DatasetEvaluator)
-interface.
-
-Detectron2 includes a few `DatasetEvaluator` that computes metrics using standard dataset-specific
-APIs (e.g., COCO, LVIS).
-You can also implement your own `DatasetEvaluator` that performs some other jobs
-using the inputs/outputs pairs.
-For example, to count how many instances are detected on the validation set:
-
-```
-class Counter(DatasetEvaluator):
- def reset(self):
- self.count = 0
- def process(self, inputs, outputs):
- for output in outputs:
- self.count += len(output["instances"])
- def evaluate(self):
- # save self.count somewhere, or print it, or return it.
- return {"count": self.count}
-```
-
-Once you have some `DatasetEvaluator`, you can run it with
-[inference_on_dataset](../modules/evaluation.html#detectron2.evaluation.inference_on_dataset).
-For example,
-
-```python
-val_results = inference_on_dataset(
- model,
- val_data_loader,
- DatasetEvaluators([COCOEvaluator(...), Counter()]))
-```
-Compared to running the evaluation manually using the model, the benefit of this function is that
-you can merge evaluators together using [DatasetEvaluators](../modules/evaluation.html#detectron2.evaluation.DatasetEvaluators).
-In this way you can run all evaluations without having to go through the dataset multiple times.
-
-The `inference_on_dataset` function also provides accurate speed benchmarks for the
-given model and dataset.
diff --git a/spaces/hasibzunair/fifa-tryon-demo/Self-Correction-Human-Parsing-for-ACGPN/mhp_extension/detectron2/tests/layers/test_mask_ops.py b/spaces/hasibzunair/fifa-tryon-demo/Self-Correction-Human-Parsing-for-ACGPN/mhp_extension/detectron2/tests/layers/test_mask_ops.py
deleted file mode 100644
index d180627354b6b9d8e0776d70f78e91ee5e530210..0000000000000000000000000000000000000000
--- a/spaces/hasibzunair/fifa-tryon-demo/Self-Correction-Human-Parsing-for-ACGPN/mhp_extension/detectron2/tests/layers/test_mask_ops.py
+++ /dev/null
@@ -1,190 +0,0 @@
-# -*- coding: utf-8 -*-
-# Copyright (c) Facebook, Inc. and its affiliates. All Rights Reserved
-
-import contextlib
-import io
-import numpy as np
-import unittest
-from collections import defaultdict
-import torch
-import tqdm
-from fvcore.common.benchmark import benchmark
-from fvcore.common.file_io import PathManager
-from pycocotools.coco import COCO
-from tabulate import tabulate
-from torch.nn import functional as F
-
-from detectron2.data import MetadataCatalog
-from detectron2.layers.mask_ops import (
- pad_masks,
- paste_mask_in_image_old,
- paste_masks_in_image,
- scale_boxes,
-)
-from detectron2.structures import BitMasks, Boxes, BoxMode, PolygonMasks
-from detectron2.structures.masks import polygons_to_bitmask
-
-
-def iou_between_full_image_bit_masks(a, b):
- intersect = (a & b).sum()
- union = (a | b).sum()
- return intersect / union
-
-
-def rasterize_polygons_with_grid_sample(full_image_bit_mask, box, mask_size, threshold=0.5):
- x0, y0, x1, y1 = box[0], box[1], box[2], box[3]
-
- img_h, img_w = full_image_bit_mask.shape
-
- mask_y = np.arange(0.0, mask_size) + 0.5 # mask y sample coords in [0.5, mask_size - 0.5]
- mask_x = np.arange(0.0, mask_size) + 0.5 # mask x sample coords in [0.5, mask_size - 0.5]
- mask_y = mask_y / mask_size * (y1 - y0) + y0
- mask_x = mask_x / mask_size * (x1 - x0) + x0
-
- mask_x = (mask_x - 0.5) / (img_w - 1) * 2 + -1
- mask_y = (mask_y - 0.5) / (img_h - 1) * 2 + -1
- gy, gx = torch.meshgrid(torch.from_numpy(mask_y), torch.from_numpy(mask_x))
- ind = torch.stack([gx, gy], dim=-1).to(dtype=torch.float32)
-
- full_image_bit_mask = torch.from_numpy(full_image_bit_mask)
- mask = F.grid_sample(
- full_image_bit_mask[None, None, :, :].to(dtype=torch.float32),
- ind[None, :, :, :],
- align_corners=True,
- )
-
- return mask[0, 0] >= threshold
-
-
-class TestMaskCropPaste(unittest.TestCase):
- def setUp(self):
- json_file = MetadataCatalog.get("coco_2017_val_100").json_file
- if not PathManager.isfile(json_file):
- raise unittest.SkipTest("{} not found".format(json_file))
- with contextlib.redirect_stdout(io.StringIO()):
- json_file = PathManager.get_local_path(json_file)
- self.coco = COCO(json_file)
-
- def test_crop_paste_consistency(self):
- """
- rasterize_polygons_within_box (used in training)
- and
- paste_masks_in_image (used in inference)
- should be inverse operations to each other.
-
- This function runs several implementation of the above two operations and prints
- the reconstruction error.
- """
-
- anns = self.coco.loadAnns(self.coco.getAnnIds(iscrowd=False)) # avoid crowd annotations
-
- selected_anns = anns[:100]
-
- ious = []
- for ann in tqdm.tqdm(selected_anns):
- results = self.process_annotation(ann)
- ious.append([k[2] for k in results])
-
- ious = np.array(ious)
- mean_ious = ious.mean(axis=0)
- table = []
- res_dic = defaultdict(dict)
- for row, iou in zip(results, mean_ious):
- table.append((row[0], row[1], iou))
- res_dic[row[0]][row[1]] = iou
- print(tabulate(table, headers=["rasterize", "paste", "iou"], tablefmt="simple"))
- # assert that the reconstruction is good:
- self.assertTrue(res_dic["polygon"]["aligned"] > 0.94)
- self.assertTrue(res_dic["roialign"]["aligned"] > 0.95)
-
- def process_annotation(self, ann, mask_side_len=28):
- # Parse annotation data
- img_info = self.coco.loadImgs(ids=[ann["image_id"]])[0]
- height, width = img_info["height"], img_info["width"]
- gt_polygons = [np.array(p, dtype=np.float64) for p in ann["segmentation"]]
- gt_bbox = BoxMode.convert(ann["bbox"], BoxMode.XYWH_ABS, BoxMode.XYXY_ABS)
- gt_bit_mask = polygons_to_bitmask(gt_polygons, height, width)
-
- # Run rasterize ..
- torch_gt_bbox = torch.tensor(gt_bbox).to(dtype=torch.float32).reshape(-1, 4)
- box_bitmasks = {
- "polygon": PolygonMasks([gt_polygons]).crop_and_resize(torch_gt_bbox, mask_side_len)[0],
- "gridsample": rasterize_polygons_with_grid_sample(gt_bit_mask, gt_bbox, mask_side_len),
- "roialign": BitMasks(torch.from_numpy(gt_bit_mask[None, :, :])).crop_and_resize(
- torch_gt_bbox, mask_side_len
- )[0],
- }
-
- # Run paste ..
- results = defaultdict(dict)
- for k, box_bitmask in box_bitmasks.items():
- padded_bitmask, scale = pad_masks(box_bitmask[None, :, :], 1)
- scaled_boxes = scale_boxes(torch_gt_bbox, scale)
-
- r = results[k]
- r["old"] = paste_mask_in_image_old(
- padded_bitmask[0], scaled_boxes[0], height, width, threshold=0.5
- )
- r["aligned"] = paste_masks_in_image(
- box_bitmask[None, :, :], Boxes(torch_gt_bbox), (height, width)
- )[0]
-
- table = []
- for rasterize_method, r in results.items():
- for paste_method, mask in r.items():
- mask = np.asarray(mask)
- iou = iou_between_full_image_bit_masks(gt_bit_mask.astype("uint8"), mask)
- table.append((rasterize_method, paste_method, iou))
- return table
-
- def test_polygon_area(self):
- # Draw polygon boxes
- for d in [5.0, 10.0, 1000.0]:
- polygon = PolygonMasks([[[0, 0, 0, d, d, d, d, 0]]])
- area = polygon.area()[0]
- target = d ** 2
- self.assertEqual(area, target)
-
- # Draw polygon triangles
- for d in [5.0, 10.0, 1000.0]:
- polygon = PolygonMasks([[[0, 0, 0, d, d, d]]])
- area = polygon.area()[0]
- target = d ** 2 / 2
- self.assertEqual(area, target)
-
-
-def benchmark_paste():
- S = 800
- H, W = image_shape = (S, S)
- N = 64
- torch.manual_seed(42)
- masks = torch.rand(N, 28, 28)
-
- center = torch.rand(N, 2) * 600 + 100
- wh = torch.clamp(torch.randn(N, 2) * 40 + 200, min=50)
- x0y0 = torch.clamp(center - wh * 0.5, min=0.0)
- x1y1 = torch.clamp(center + wh * 0.5, max=S)
- boxes = Boxes(torch.cat([x0y0, x1y1], axis=1))
-
- def func(device, n=3):
- m = masks.to(device=device)
- b = boxes.to(device=device)
-
- def bench():
- for _ in range(n):
- paste_masks_in_image(m, b, image_shape)
- if device.type == "cuda":
- torch.cuda.synchronize()
-
- return bench
-
- specs = [{"device": torch.device("cpu"), "n": 3}]
- if torch.cuda.is_available():
- specs.append({"device": torch.device("cuda"), "n": 3})
-
- benchmark(func, "paste_masks", specs, num_iters=10, warmup_iters=2)
-
-
-if __name__ == "__main__":
- benchmark_paste()
- unittest.main()
diff --git a/spaces/hasibzunair/fifa-tryon-demo/model/u2net_refactor.py b/spaces/hasibzunair/fifa-tryon-demo/model/u2net_refactor.py
deleted file mode 100644
index e668de2c2bc67cbef280eaa5f789c762c4745fa4..0000000000000000000000000000000000000000
--- a/spaces/hasibzunair/fifa-tryon-demo/model/u2net_refactor.py
+++ /dev/null
@@ -1,168 +0,0 @@
-import torch
-import torch.nn as nn
-
-import math
-
-__all__ = ['U2NET_full', 'U2NET_lite']
-
-
-def _upsample_like(x, size):
- return nn.Upsample(size=size, mode='bilinear', align_corners=False)(x)
-
-
-def _size_map(x, height):
- # {height: size} for Upsample
- size = list(x.shape[-2:])
- sizes = {}
- for h in range(1, height):
- sizes[h] = size
- size = [math.ceil(w / 2) for w in size]
- return sizes
-
-
-class REBNCONV(nn.Module):
- def __init__(self, in_ch=3, out_ch=3, dilate=1):
- super(REBNCONV, self).__init__()
-
- self.conv_s1 = nn.Conv2d(in_ch, out_ch, 3, padding=1 * dilate, dilation=1 * dilate)
- self.bn_s1 = nn.BatchNorm2d(out_ch)
- self.relu_s1 = nn.ReLU(inplace=True)
-
- def forward(self, x):
- return self.relu_s1(self.bn_s1(self.conv_s1(x)))
-
-
-class RSU(nn.Module):
- def __init__(self, name, height, in_ch, mid_ch, out_ch, dilated=False):
- super(RSU, self).__init__()
- self.name = name
- self.height = height
- self.dilated = dilated
- self._make_layers(height, in_ch, mid_ch, out_ch, dilated)
-
- def forward(self, x):
- sizes = _size_map(x, self.height)
- x = self.rebnconvin(x)
-
- # U-Net like symmetric encoder-decoder structure
- def unet(x, height=1):
- if height < self.height:
- x1 = getattr(self, f'rebnconv{height}')(x)
- if not self.dilated and height < self.height - 1:
- x2 = unet(getattr(self, 'downsample')(x1), height + 1)
- else:
- x2 = unet(x1, height + 1)
-
- x = getattr(self, f'rebnconv{height}d')(torch.cat((x2, x1), 1))
- return _upsample_like(x, sizes[height - 1]) if not self.dilated and height > 1 else x
- else:
- return getattr(self, f'rebnconv{height}')(x)
-
- return x + unet(x)
-
- def _make_layers(self, height, in_ch, mid_ch, out_ch, dilated=False):
- self.add_module('rebnconvin', REBNCONV(in_ch, out_ch))
- self.add_module('downsample', nn.MaxPool2d(2, stride=2, ceil_mode=True))
-
- self.add_module(f'rebnconv1', REBNCONV(out_ch, mid_ch))
- self.add_module(f'rebnconv1d', REBNCONV(mid_ch * 2, out_ch))
-
- for i in range(2, height):
- dilate = 1 if not dilated else 2 ** (i - 1)
- self.add_module(f'rebnconv{i}', REBNCONV(mid_ch, mid_ch, dilate=dilate))
- self.add_module(f'rebnconv{i}d', REBNCONV(mid_ch * 2, mid_ch, dilate=dilate))
-
- dilate = 2 if not dilated else 2 ** (height - 1)
- self.add_module(f'rebnconv{height}', REBNCONV(mid_ch, mid_ch, dilate=dilate))
-
-
-class U2NET(nn.Module):
- def __init__(self, cfgs, out_ch):
- super(U2NET, self).__init__()
- self.out_ch = out_ch
- self._make_layers(cfgs)
-
- def forward(self, x):
- sizes = _size_map(x, self.height)
- maps = [] # storage for maps
-
- # side saliency map
- def unet(x, height=1):
- if height < 6:
- x1 = getattr(self, f'stage{height}')(x)
- x2 = unet(getattr(self, 'downsample')(x1), height + 1)
- x = getattr(self, f'stage{height}d')(torch.cat((x2, x1), 1))
- side(x, height)
- return _upsample_like(x, sizes[height - 1]) if height > 1 else x
- else:
- x = getattr(self, f'stage{height}')(x)
- side(x, height)
- return _upsample_like(x, sizes[height - 1])
-
- def side(x, h):
- # side output saliency map (before sigmoid)
- x = getattr(self, f'side{h}')(x)
- x = _upsample_like(x, sizes[1])
- maps.append(x)
-
- def fuse():
- # fuse saliency probability maps
- maps.reverse()
- x = torch.cat(maps, 1)
- x = getattr(self, 'outconv')(x)
- maps.insert(0, x)
- return [torch.sigmoid(x) for x in maps]
-
- unet(x)
- maps = fuse()
- return maps
-
- def _make_layers(self, cfgs):
- self.height = int((len(cfgs) + 1) / 2)
- self.add_module('downsample', nn.MaxPool2d(2, stride=2, ceil_mode=True))
- for k, v in cfgs.items():
- # build rsu block
- self.add_module(k, RSU(v[0], *v[1]))
- if v[2] > 0:
- # build side layer
- self.add_module(f'side{v[0][-1]}', nn.Conv2d(v[2], self.out_ch, 3, padding=1))
- # build fuse layer
- self.add_module('outconv', nn.Conv2d(int(self.height * self.out_ch), self.out_ch, 1))
-
-
-def U2NET_full():
- full = {
- # cfgs for building RSUs and sides
- # {stage : [name, (height(L), in_ch, mid_ch, out_ch, dilated), side]}
- 'stage1': ['En_1', (7, 3, 32, 64), -1],
- 'stage2': ['En_2', (6, 64, 32, 128), -1],
- 'stage3': ['En_3', (5, 128, 64, 256), -1],
- 'stage4': ['En_4', (4, 256, 128, 512), -1],
- 'stage5': ['En_5', (4, 512, 256, 512, True), -1],
- 'stage6': ['En_6', (4, 512, 256, 512, True), 512],
- 'stage5d': ['De_5', (4, 1024, 256, 512, True), 512],
- 'stage4d': ['De_4', (4, 1024, 128, 256), 256],
- 'stage3d': ['De_3', (5, 512, 64, 128), 128],
- 'stage2d': ['De_2', (6, 256, 32, 64), 64],
- 'stage1d': ['De_1', (7, 128, 16, 64), 64],
- }
- return U2NET(cfgs=full, out_ch=1)
-
-
-def U2NET_lite():
- lite = {
- # cfgs for building RSUs and sides
- # {stage : [name, (height(L), in_ch, mid_ch, out_ch, dilated), side]}
- 'stage1': ['En_1', (7, 3, 16, 64), -1],
- 'stage2': ['En_2', (6, 64, 16, 64), -1],
- 'stage3': ['En_3', (5, 64, 16, 64), -1],
- 'stage4': ['En_4', (4, 64, 16, 64), -1],
- 'stage5': ['En_5', (4, 64, 16, 64, True), -1],
- 'stage6': ['En_6', (4, 64, 16, 64, True), 64],
- 'stage5d': ['De_5', (4, 128, 16, 64, True), 64],
- 'stage4d': ['De_4', (4, 128, 16, 64), 64],
- 'stage3d': ['De_3', (5, 128, 16, 64), 64],
- 'stage2d': ['De_2', (6, 128, 16, 64), 64],
- 'stage1d': ['De_1', (7, 128, 16, 64), 64],
- }
- return U2NET(cfgs=lite, out_ch=1)
diff --git a/spaces/heiyuan/ChatGPT/run_macOS.command b/spaces/heiyuan/ChatGPT/run_macOS.command
deleted file mode 100644
index 62af07283093d8e580763d7acfe493c3d88e7b08..0000000000000000000000000000000000000000
--- a/spaces/heiyuan/ChatGPT/run_macOS.command
+++ /dev/null
@@ -1,25 +0,0 @@
-#!/bin/bash
-
-# 获取脚本所在目录
-script_dir=$(dirname "$0")
-
-# 将工作目录更改为脚本所在目录
-cd "$script_dir"
-
-# 检查Git仓库是否有更新
-git remote update
-pwd
-
-if ! git status -uno | grep 'up to date' > /dev/null; then
- # 如果有更新,关闭当前运行的服务器
- pkill -f ChuanhuChatbot.py
-
- # 拉取最新更改
- git pull
-
- # 安装依赖
- pip3 install -r requirements.txt
-
- # 重新启动服务器
- nohup python3 ChuanhuChatbot.py &
-fi
diff --git a/spaces/hezhaoqia/vits-simple-api/utils/merge.py b/spaces/hezhaoqia/vits-simple-api/utils/merge.py
deleted file mode 100644
index 86ee1cf89fd270b7e30766364f69495895f5f2d0..0000000000000000000000000000000000000000
--- a/spaces/hezhaoqia/vits-simple-api/utils/merge.py
+++ /dev/null
@@ -1,190 +0,0 @@
-import os
-import json
-import logging
-import torch
-import config
-import numpy as np
-from utils.utils import check_is_none
-from vits import VITS
-from voice import TTS
-
-device = torch.device("cuda" if torch.cuda.is_available() else "cpu")
-
-lang_dict = {
- "english_cleaners": ["en"],
- "english_cleaners2": ["en"],
- "japanese_cleaners": ["ja"],
- "japanese_cleaners2": ["ja"],
- "korean_cleaners": ["ko"],
- "chinese_cleaners": ["zh"],
- "zh_ja_mixture_cleaners": ["zh", "ja"],
- "sanskrit_cleaners": ["sa"],
- "cjks_cleaners": ["zh", "ja", "ko", "sa"],
- "cjke_cleaners": ["zh", "ja", "ko", "en"],
- "cjke_cleaners2": ["zh", "ja", "ko", "en"],
- "cje_cleaners": ["zh", "ja", "en"],
- "cje_cleaners2": ["zh", "ja", "en"],
- "thai_cleaners": ["th"],
- "shanghainese_cleaners": ["sh"],
- "chinese_dialect_cleaners": ["zh", "ja", "sh", "gd", "en", "SZ", "WX", "CZ", "HZ", "SX", "NB", "JJ", "YX", "JD",
- "ZR", "PH", "TX", "JS", "HN", "LP", "XS", "FY", "RA", "CX", "SM", "TT", "WZ", "SC",
- "YB"],
- "bert_chinese_cleaners": ["zh"],
-}
-
-
-def analysis(model_config_json):
- model_config = json.load(model_config_json)
- symbols = model_config.get("symbols", None)
- emotion_embedding = model_config.get("data").get("emotion_embedding", False)
- if "use_spk_conditioned_encoder" in model_config.get("model"):
- model_type = 'bert_vits2'
- return model_type
- if symbols != None:
- if not emotion_embedding:
- mode_type = "vits"
- else:
- mode_type = "w2v2"
- else:
- mode_type = "hubert"
- return mode_type
-
-
-def load_npy(model_):
- if isinstance(model_, list):
- # check if is .npy
- for i in model_:
- _model_extention = os.path.splitext(i)[1]
- if _model_extention != ".npy":
- raise ValueError(f"Unsupported model type: {_model_extention}")
-
- # merge npy files
- emotion_reference = np.empty((0, 1024))
- for i in model_:
- tmp = np.load(i).reshape(-1, 1024)
- emotion_reference = np.append(emotion_reference, tmp, axis=0)
-
- elif os.path.isdir(model_):
- emotion_reference = np.empty((0, 1024))
- for root, dirs, files in os.walk(model_):
- for file_name in files:
- # check if is .npy
- _model_extention = os.path.splitext(file_name)[1]
- if _model_extention != ".npy":
- continue
- file_path = os.path.join(root, file_name)
-
- # merge npy files
- tmp = np.load(file_path).reshape(-1, 1024)
- emotion_reference = np.append(emotion_reference, tmp, axis=0)
-
- elif os.path.isfile(model_):
- # check if is .npy
- _model_extention = os.path.splitext(model_)[1]
- if _model_extention != ".npy":
- raise ValueError(f"Unsupported model type: {_model_extention}")
-
- emotion_reference = np.load(model_)
- logging.info(f"Loaded emotional dimention npy range:{len(emotion_reference)}")
- return emotion_reference
-
-
-def merge_model(merging_model):
- vits_obj = []
- vits_speakers = []
- hubert_vits_obj = []
- hubert_vits_speakers = []
- w2v2_vits_obj = []
- w2v2_vits_speakers = []
- bert_vits2_obj = []
- bert_vits2_speakers = []
-
- # model list
- vits_list = []
- hubert_vits_list = []
- w2v2_vits_list = []
- bert_vits2_list = []
-
- for l in merging_model:
- with open(l[1], 'r', encoding='utf-8') as model_config:
- model_type = analysis(model_config)
- if model_type == "vits":
- vits_list.append(l)
- elif model_type == "hubert":
- hubert_vits_list.append(l)
- elif model_type == "w2v2":
- w2v2_vits_list.append(l)
- elif model_type == "bert_vits2":
- bert_vits2_list.append(l)
-
- # merge vits
- new_id = 0
- for obj_id, i in enumerate(vits_list):
- obj = VITS(model=i[0], config=i[1], model_type="vits", device=device)
- lang = lang_dict.get(obj.get_cleaner(), ["unknown"])
- for id, name in enumerate(obj.get_speakers()):
- vits_obj.append([int(id), obj, obj_id])
- vits_speakers.append({"id": new_id, "name": name, "lang": lang})
- new_id += 1
-
- # merge hubert-vits
- if len(hubert_vits_list) != 0:
- if getattr(config, "HUBERT_SOFT_MODEL", None) == None or check_is_none(config.HUBERT_SOFT_MODEL):
- raise ValueError(f"Please configure HUBERT_SOFT_MODEL path in config.py")
- try:
- from vits.hubert_model import hubert_soft
- hubert = hubert_soft(config.HUBERT_SOFT_MODEL)
- except Exception as e:
- raise ValueError(f"Load HUBERT_SOFT_MODEL failed {e}")
-
- new_id = 0
- for obj_id, i in enumerate(hubert_vits_list):
- obj = VITS(model=i[0], config=i[1], model_=hubert, model_type="hubert", device=device)
- lang = lang_dict.get(obj.get_cleaner(), ["unknown"])
-
- for id, name in enumerate(obj.get_speakers()):
- hubert_vits_obj.append([int(id), obj, obj_id])
- hubert_vits_speakers.append({"id": new_id, "name": name, "lang": lang})
- new_id += 1
-
- # merge w2v2-vits
- emotion_reference = None
- if len(w2v2_vits_list) != 0:
- if getattr(config, "DIMENSIONAL_EMOTION_NPY", None) == None or check_is_none(config.DIMENSIONAL_EMOTION_NPY):
- raise ValueError(f"Please configure DIMENSIONAL_EMOTION_NPY path in config.py")
- try:
- emotion_reference = load_npy(config.DIMENSIONAL_EMOTION_NPY)
- except Exception as e:
- raise ValueError(f"Load DIMENSIONAL_EMOTION_NPY failed {e}")
-
- new_id = 0
- for obj_id, i in enumerate(w2v2_vits_list):
- obj = VITS(model=i[0], config=i[1], model_=emotion_reference, model_type="w2v2", device=device)
- lang = lang_dict.get(obj.get_cleaner(), ["unknown"])
-
- for id, name in enumerate(obj.get_speakers()):
- w2v2_vits_obj.append([int(id), obj, obj_id])
- w2v2_vits_speakers.append({"id": new_id, "name": name, "lang": lang})
- new_id += 1
-
- # merge Bert_VITS2
- new_id = 0
- for obj_id, i in enumerate(bert_vits2_list):
- from bert_vits2 import Bert_VITS2
- obj = Bert_VITS2(model=i[0], config=i[1], device=device)
- lang = ["ZH"]
- for id, name in enumerate(obj.get_speakers()):
- bert_vits2_obj.append([int(id), obj, obj_id])
- bert_vits2_speakers.append({"id": new_id, "name": name, "lang": lang})
- new_id += 1
-
-
- voice_obj = {"VITS": vits_obj, "HUBERT-VITS": hubert_vits_obj, "W2V2-VITS": w2v2_vits_obj,
- "BERT-VITS2": bert_vits2_obj}
- voice_speakers = {"VITS": vits_speakers, "HUBERT-VITS": hubert_vits_speakers, "W2V2-VITS": w2v2_vits_speakers,
- "BERT-VITS2": bert_vits2_speakers}
- w2v2_emotion_count = len(emotion_reference) if emotion_reference is not None else 0
-
- tts = TTS(voice_obj, voice_speakers, w2v2_emotion_count=w2v2_emotion_count, device=device)
-
- return tts
diff --git a/spaces/housexu123/bingo-2.0/src/components/ui/select.tsx b/spaces/housexu123/bingo-2.0/src/components/ui/select.tsx
deleted file mode 100644
index 77f12c2996f541b97663de4c9e20ab34d4ec2fac..0000000000000000000000000000000000000000
--- a/spaces/housexu123/bingo-2.0/src/components/ui/select.tsx
+++ /dev/null
@@ -1,123 +0,0 @@
-'use client'
-
-import * as React from 'react'
-import * as SelectPrimitive from '@radix-ui/react-select'
-
-import { cn } from '@/lib/utils'
-import {
- IconArrowDown,
- IconCheck,
- IconChevronUpDown
-} from '@/components/ui/icons'
-
-const Select = SelectPrimitive.Root
-
-const SelectGroup = SelectPrimitive.Group
-
-const SelectValue = SelectPrimitive.Value
-
-const SelectTrigger = React.forwardRef<
- React.ElementRef,
- React.ComponentPropsWithoutRef
->(({ className, children, ...props }, ref) => (
-
- {children}
-
-
-
-
-))
-SelectTrigger.displayName = SelectPrimitive.Trigger.displayName
-
-const SelectContent = React.forwardRef<
- React.ElementRef,
- React.ComponentPropsWithoutRef
->(({ className, children, position = 'popper', ...props }, ref) => (
-
-
-
- {children}
-
-
-
-))
-SelectContent.displayName = SelectPrimitive.Content.displayName
-
-const SelectLabel = React.forwardRef<
- React.ElementRef,
- React.ComponentPropsWithoutRef
->(({ className, ...props }, ref) => (
-
-))
-SelectLabel.displayName = SelectPrimitive.Label.displayName
-
-const SelectItem = React.forwardRef<
- React.ElementRef,
- React.ComponentPropsWithoutRef
->(({ className, children, ...props }, ref) => (
-
-
-
-
-
-
- {children}
-
-))
-SelectItem.displayName = SelectPrimitive.Item.displayName
-
-const SelectSeparator = React.forwardRef<
- React.ElementRef,
- React.ComponentPropsWithoutRef
->(({ className, ...props }, ref) => (
-
-))
-SelectSeparator.displayName = SelectPrimitive.Separator.displayName
-
-export {
- Select,
- SelectGroup,
- SelectValue,
- SelectTrigger,
- SelectContent,
- SelectLabel,
- SelectItem,
- SelectSeparator
-}
diff --git a/spaces/hrdtbs/rvc-mochinoa/README.md b/spaces/hrdtbs/rvc-mochinoa/README.md
deleted file mode 100644
index c997e278262ea54415bdd1703e081923e38d4b06..0000000000000000000000000000000000000000
--- a/spaces/hrdtbs/rvc-mochinoa/README.md
+++ /dev/null
@@ -1,12 +0,0 @@
----
-title: RVC Mochinoa
-emoji: 🐈
-colorFrom: red
-colorTo: purple
-sdk: gradio
-sdk_version: 3.32.0
-app_file: app.py
-pinned: true
----
-
-Check out the configuration reference at https://huggingface.co/docs/hub/spaces-config-reference
diff --git a/spaces/hsdcs/bingchat/README.md b/spaces/hsdcs/bingchat/README.md
deleted file mode 100644
index 0eaf079795e2d89edf1b736f9414fd3231f77089..0000000000000000000000000000000000000000
--- a/spaces/hsdcs/bingchat/README.md
+++ /dev/null
@@ -1,12 +0,0 @@
----
-title: Bingchat
-emoji: 📊
-colorFrom: green
-colorTo: indigo
-sdk: docker
-pinned: false
-license: mit
-app_port: 8080
----
-
-Check out the configuration reference at https://huggingface.co/docs/hub/spaces-config-reference
diff --git a/spaces/hugggof/vampnet/scripts/utils/stage.py b/spaces/hugggof/vampnet/scripts/utils/stage.py
deleted file mode 100644
index 253e1d070ccf3754be01578d22b65136858fa697..0000000000000000000000000000000000000000
--- a/spaces/hugggof/vampnet/scripts/utils/stage.py
+++ /dev/null
@@ -1,30 +0,0 @@
-import os
-import subprocess
-from pathlib import Path
-
-import argbind
-import rich
-from audiotools.ml import Experiment
-
-
-@argbind.bind(without_prefix=True)
-def run(
- run_dir: str = os.getenv("PATH_TO_RUNS", "runs"),
- name: str = None,
- recent: bool = False,
-):
- if recent:
- paths = sorted(Path(run_dir).iterdir(), key=os.path.getmtime)
- paths = [p.name for p in paths if p.is_dir()]
- if paths:
- name = paths[-1]
-
- with Experiment(run_dir, name) as exp:
- exp.snapshot()
- rich.print(f"Created a snapshot of {exp.parent_directory} at {exp.exp_dir}")
-
-
-if __name__ == "__main__":
- args = argbind.parse_args()
- with argbind.scope(args):
- run()
diff --git a/spaces/huggingface-projects/stable-diffusion-multiplayer/stablediffusion-infinity/PyPatchMatch/csrc/inpaint.h b/spaces/huggingface-projects/stable-diffusion-multiplayer/stablediffusion-infinity/PyPatchMatch/csrc/inpaint.h
deleted file mode 100644
index a59b1d347ea5fe92976a4fda10a820d6508f51da..0000000000000000000000000000000000000000
--- a/spaces/huggingface-projects/stable-diffusion-multiplayer/stablediffusion-infinity/PyPatchMatch/csrc/inpaint.h
+++ /dev/null
@@ -1,27 +0,0 @@
-#pragma once
-
-#include
-
-#include "masked_image.h"
-#include "nnf.h"
-
-class Inpainting {
-public:
- Inpainting(cv::Mat image, cv::Mat mask, const PatchDistanceMetric *metric);
- Inpainting(cv::Mat image, cv::Mat mask, cv::Mat global_mask, const PatchDistanceMetric *metric);
- cv::Mat run(bool verbose = false, bool verbose_visualize = false, unsigned int random_seed = 1212);
-
-private:
- void _initialize_pyramid(void);
- MaskedImage _expectation_maximization(MaskedImage source, MaskedImage target, int level, bool verbose);
- void _expectation_step(const NearestNeighborField &nnf, bool source2target, cv::Mat &vote, const MaskedImage &source, bool upscaled);
- void _maximization_step(MaskedImage &target, const cv::Mat &vote);
-
- MaskedImage m_initial;
- std::vector m_pyramid;
-
- NearestNeighborField m_source2target;
- NearestNeighborField m_target2source;
- const PatchDistanceMetric *m_distance_metric;
-};
-
diff --git a/spaces/hysts/ControlNet-with-Anything-v4/app_fake_scribble.py b/spaces/hysts/ControlNet-with-Anything-v4/app_fake_scribble.py
deleted file mode 100644
index 70dd8769a17cc1dde58a2a8c28e02c07dd4b383b..0000000000000000000000000000000000000000
--- a/spaces/hysts/ControlNet-with-Anything-v4/app_fake_scribble.py
+++ /dev/null
@@ -1,83 +0,0 @@
-# This file is adapted from https://github.com/lllyasviel/ControlNet/blob/f4748e3630d8141d7765e2bd9b1e348f47847707/gradio_fake_scribble2image.py
-# The original license file is LICENSE.ControlNet in this repo.
-import gradio as gr
-
-
-def create_demo(process, max_images=12, default_num_images=3):
- with gr.Blocks() as demo:
- with gr.Row():
- gr.Markdown('## Control Stable Diffusion with Fake Scribble Maps')
- with gr.Row():
- with gr.Column():
- input_image = gr.Image(source='upload', type='numpy')
- prompt = gr.Textbox(label='Prompt')
- run_button = gr.Button(label='Run')
- with gr.Accordion('Advanced options', open=False):
- num_samples = gr.Slider(label='Images',
- minimum=1,
- maximum=max_images,
- value=default_num_images,
- step=1)
- image_resolution = gr.Slider(label='Image Resolution',
- minimum=256,
- maximum=512,
- value=512,
- step=256)
- detect_resolution = gr.Slider(label='HED Resolution',
- minimum=128,
- maximum=512,
- value=512,
- step=1)
- num_steps = gr.Slider(label='Steps',
- minimum=1,
- maximum=100,
- value=20,
- step=1)
- guidance_scale = gr.Slider(label='Guidance Scale',
- minimum=0.1,
- maximum=30.0,
- value=9.0,
- step=0.1)
- seed = gr.Slider(label='Seed',
- minimum=-1,
- maximum=2147483647,
- step=1,
- randomize=True)
- a_prompt = gr.Textbox(
- label='Added Prompt',
- value='best quality, extremely detailed')
- n_prompt = gr.Textbox(
- label='Negative Prompt',
- value=
- 'longbody, lowres, bad anatomy, bad hands, missing fingers, extra digit, fewer digits, cropped, worst quality, low quality'
- )
- with gr.Column():
- result = gr.Gallery(label='Output',
- show_label=False,
- elem_id='gallery').style(grid=2,
- height='auto')
- inputs = [
- input_image,
- prompt,
- a_prompt,
- n_prompt,
- num_samples,
- image_resolution,
- detect_resolution,
- num_steps,
- guidance_scale,
- seed,
- ]
- prompt.submit(fn=process, inputs=inputs, outputs=result)
- run_button.click(fn=process,
- inputs=inputs,
- outputs=result,
- api_name='fake_scribble')
- return demo
-
-
-if __name__ == '__main__':
- from model import Model
- model = Model()
- demo = create_demo(model.process_fake_scribble)
- demo.queue().launch()
diff --git a/spaces/hysts/StyleGAN-Human-Interpolation/app.py b/spaces/hysts/StyleGAN-Human-Interpolation/app.py
deleted file mode 100644
index 7d2f1a53e610ef1daf9eb35d258f9caeb16313d7..0000000000000000000000000000000000000000
--- a/spaces/hysts/StyleGAN-Human-Interpolation/app.py
+++ /dev/null
@@ -1,107 +0,0 @@
-#!/usr/bin/env python
-
-from __future__ import annotations
-
-import functools
-import pickle
-import sys
-
-import gradio as gr
-import numpy as np
-import torch
-import torch.nn as nn
-from huggingface_hub import hf_hub_download
-
-sys.path.insert(0, 'StyleGAN-Human')
-
-TITLE = 'StyleGAN-Human (Interpolation)'
-DESCRIPTION = 'https://github.com/stylegan-human/StyleGAN-Human'
-
-
-def load_model(file_name: str, device: torch.device) -> nn.Module:
- path = hf_hub_download('public-data/StyleGAN-Human', f'models/{file_name}')
- with open(path, 'rb') as f:
- model = pickle.load(f)['G_ema']
- model.eval()
- model.to(device)
- with torch.inference_mode():
- z = torch.zeros((1, model.z_dim)).to(device)
- label = torch.zeros([1, model.c_dim], device=device)
- model(z, label, force_fp32=True)
- return model
-
-
-def generate_z(z_dim: int, seed: int, device: torch.device) -> torch.Tensor:
- return torch.from_numpy(np.random.RandomState(seed).randn(
- 1, z_dim)).to(device).float()
-
-
-@torch.inference_mode()
-def generate_interpolated_images(seed0: int, psi0: float, seed1: int,
- psi1: float, num_intermediate: int,
- model: nn.Module,
- device: torch.device) -> list[np.ndarray]:
- seed0 = int(np.clip(seed0, 0, np.iinfo(np.uint32).max))
- seed1 = int(np.clip(seed1, 0, np.iinfo(np.uint32).max))
-
- z0 = generate_z(model.z_dim, seed0, device)
- z1 = generate_z(model.z_dim, seed1, device)
- vec = z1 - z0
- dvec = vec / (num_intermediate + 1)
- zs = [z0 + dvec * i for i in range(num_intermediate + 2)]
- dpsi = (psi1 - psi0) / (num_intermediate + 1)
- psis = [psi0 + dpsi * i for i in range(num_intermediate + 2)]
-
- label = torch.zeros([1, model.c_dim], device=device)
-
- res = []
- for z, psi in zip(zs, psis):
- out = model(z, label, truncation_psi=psi, force_fp32=True)
- out = (out.permute(0, 2, 3, 1) * 127.5 + 128).clamp(0, 255).to(
- torch.uint8)
- out = out[0].cpu().numpy()
- res.append(out)
- return res
-
-
-device = torch.device('cuda:0' if torch.cuda.is_available() else 'cpu')
-model = load_model('stylegan_human_v2_1024.pkl', device)
-fn = functools.partial(generate_interpolated_images,
- model=model,
- device=device)
-
-gr.Interface(
- fn=fn,
- inputs=[
- gr.Slider(label='Seed 1',
- minimum=0,
- maximum=100000,
- step=1,
- value=0,
- randomize=True),
- gr.Slider(label='Truncation psi 1',
- minimum=0,
- maximum=2,
- step=0.05,
- value=0.7),
- gr.Slider(label='Seed 2',
- minimum=0,
- maximum=100000,
- step=1,
- value=1,
- randomize=True),
- gr.Slider(label='Truncation psi 2',
- minimum=0,
- maximum=2,
- step=0.05,
- value=0.7),
- gr.Slider(label='Number of Intermediate Frames',
- minimum=0,
- maximum=21,
- step=1,
- value=7),
- ],
- outputs=gr.Gallery(label='Output Images', type='numpy'),
- title=TITLE,
- description=DESCRIPTION,
-).queue(max_size=10).launch()
diff --git a/spaces/hyxue/HiFiFace-inference-demo/Deep3DFaceRecon_pytorch/models/losses.py b/spaces/hyxue/HiFiFace-inference-demo/Deep3DFaceRecon_pytorch/models/losses.py
deleted file mode 100644
index f3cb3fd778adedf31acbc3ff01018e9efb99d65b..0000000000000000000000000000000000000000
--- a/spaces/hyxue/HiFiFace-inference-demo/Deep3DFaceRecon_pytorch/models/losses.py
+++ /dev/null
@@ -1,121 +0,0 @@
-import numpy as np
-import torch
-import torch.nn as nn
-import torch.nn.functional as F
-from kornia.geometry import warp_affine
-
-
-def resize_n_crop(image, M, dsize=112):
- # image: (b, c, h, w)
- # M : (b, 2, 3)
- return warp_affine(image, M, dsize=(dsize, dsize))
-
-
-### perceptual level loss
-class PerceptualLoss(nn.Module):
- def __init__(self, recog_net, input_size=112):
- super(PerceptualLoss, self).__init__()
- self.recog_net = recog_net
- self.preprocess = lambda x: 2 * x - 1
- self.input_size = input_size
-
- def forward(imageA, imageB, M):
- """
- 1 - cosine distance
- Parameters:
- imageA --torch.tensor (B, 3, H, W), range (0, 1) , RGB order
- imageB --same as imageA
- """
-
- imageA = self.preprocess(resize_n_crop(imageA, M, self.input_size))
- imageB = self.preprocess(resize_n_crop(imageB, M, self.input_size))
-
- # freeze bn
- self.recog_net.eval()
-
- id_featureA = F.normalize(self.recog_net(imageA), dim=-1, p=2)
- id_featureB = F.normalize(self.recog_net(imageB), dim=-1, p=2)
- cosine_d = torch.sum(id_featureA * id_featureB, dim=-1)
- # assert torch.sum((cosine_d > 1).float()) == 0
- return torch.sum(1 - cosine_d) / cosine_d.shape[0]
-
-
-def perceptual_loss(id_featureA, id_featureB):
- cosine_d = torch.sum(id_featureA * id_featureB, dim=-1)
- # assert torch.sum((cosine_d > 1).float()) == 0
- return torch.sum(1 - cosine_d) / cosine_d.shape[0]
-
-
-### image level loss
-def photo_loss(imageA, imageB, mask, eps=1e-6):
- """
- l2 norm (with sqrt, to ensure backward stabililty, use eps, otherwise Nan may occur)
- Parameters:
- imageA --torch.tensor (B, 3, H, W), range (0, 1), RGB order
- imageB --same as imageA
- """
- loss = torch.sqrt(eps + torch.sum((imageA - imageB) ** 2, dim=1, keepdims=True)) * mask
- loss = torch.sum(loss) / torch.max(torch.sum(mask), torch.tensor(1.0).to(mask.device))
- return loss
-
-
-def landmark_loss(predict_lm, gt_lm, weight=None):
- """
- weighted mse loss
- Parameters:
- predict_lm --torch.tensor (B, 68, 2)
- gt_lm --torch.tensor (B, 68, 2)
- weight --numpy.array (1, 68)
- """
- if not weight:
- weight = np.ones([68])
- weight[28:31] = 20
- weight[-8:] = 20
- weight = np.expand_dims(weight, 0)
- weight = torch.tensor(weight).to(predict_lm.device)
- loss = torch.sum((predict_lm - gt_lm) ** 2, dim=-1) * weight
- loss = torch.sum(loss) / (predict_lm.shape[0] * predict_lm.shape[1])
- return loss
-
-
-### regulization
-def reg_loss(coeffs_dict, opt=None):
- """
- l2 norm without the sqrt, from yu's implementation (mse)
- tf.nn.l2_loss https://www.tensorflow.org/api_docs/python/tf/nn/l2_loss
- Parameters:
- coeffs_dict -- a dict of torch.tensors , keys: id, exp, tex, angle, gamma, trans
-
- """
- # coefficient regularization to ensure plausible 3d faces
- if opt:
- w_id, w_exp, w_tex = opt.w_id, opt.w_exp, opt.w_tex
- else:
- w_id, w_exp, w_tex = 1, 1, 1, 1
- creg_loss = (
- w_id * torch.sum(coeffs_dict["id"] ** 2)
- + w_exp * torch.sum(coeffs_dict["exp"] ** 2)
- + w_tex * torch.sum(coeffs_dict["tex"] ** 2)
- )
- creg_loss = creg_loss / coeffs_dict["id"].shape[0]
-
- # gamma regularization to ensure a nearly-monochromatic light
- gamma = coeffs_dict["gamma"].reshape([-1, 3, 9])
- gamma_mean = torch.mean(gamma, dim=1, keepdims=True)
- gamma_loss = torch.mean((gamma - gamma_mean) ** 2)
-
- return creg_loss, gamma_loss
-
-
-def reflectance_loss(texture, mask):
- """
- minimize texture variance (mse), albedo regularization to ensure an uniform skin albedo
- Parameters:
- texture --torch.tensor, (B, N, 3)
- mask --torch.tensor, (N), 1 or 0
-
- """
- mask = mask.reshape([1, mask.shape[0], 1])
- texture_mean = torch.sum(mask * texture, dim=1, keepdims=True) / torch.sum(mask)
- loss = torch.sum(((texture - texture_mean) * mask) ** 2) / (texture.shape[0] * torch.sum(mask))
- return loss
diff --git a/spaces/hyxue/HiFiFace-inference-demo/Deep3DFaceRecon_pytorch/options/train_options.py b/spaces/hyxue/HiFiFace-inference-demo/Deep3DFaceRecon_pytorch/options/train_options.py
deleted file mode 100644
index 1e02ee3e87b49cae8f7e660d4b891ef062f06d97..0000000000000000000000000000000000000000
--- a/spaces/hyxue/HiFiFace-inference-demo/Deep3DFaceRecon_pytorch/options/train_options.py
+++ /dev/null
@@ -1,90 +0,0 @@
-"""This script contains the training options for Deep3DFaceRecon_pytorch
-"""
-from util import util
-
-from .base_options import BaseOptions
-
-
-class TrainOptions(BaseOptions):
- """This class includes training options.
-
- It also includes shared options defined in BaseOptions.
- """
-
- def initialize(self, parser):
- parser = BaseOptions.initialize(self, parser)
- # dataset parameters
- # for train
- parser.add_argument("--data_root", type=str, default="./", help="dataset root")
- parser.add_argument(
- "--flist", type=str, default="datalist/train/masks.txt", help="list of mask names of training set"
- )
- parser.add_argument("--batch_size", type=int, default=32)
- parser.add_argument(
- "--dataset_mode", type=str, default="flist", help="chooses how datasets are loaded. [None | flist]"
- )
- parser.add_argument(
- "--serial_batches",
- action="store_true",
- help="if true, takes images in order to make batches, otherwise takes them randomly",
- )
- parser.add_argument("--num_threads", default=4, type=int, help="# threads for loading data")
- parser.add_argument(
- "--max_dataset_size",
- type=int,
- default=float("inf"),
- help="Maximum number of samples allowed per dataset. If the dataset directory contains more than max_dataset_size, only a subset is loaded.",
- )
- parser.add_argument(
- "--preprocess",
- type=str,
- default="shift_scale_rot_flip",
- help="scaling and cropping of images at load time [shift_scale_rot_flip | shift_scale | shift | shift_rot_flip ]",
- )
- parser.add_argument(
- "--use_aug", type=util.str2bool, nargs="?", const=True, default=True, help="whether use data augmentation"
- )
-
- # for val
- parser.add_argument(
- "--flist_val", type=str, default="datalist/val/masks.txt", help="list of mask names of val set"
- )
- parser.add_argument("--batch_size_val", type=int, default=32)
-
- # visualization parameters
- parser.add_argument(
- "--display_freq", type=int, default=1000, help="frequency of showing training results on screen"
- )
- parser.add_argument(
- "--print_freq", type=int, default=100, help="frequency of showing training results on console"
- )
-
- # network saving and loading parameters
- parser.add_argument("--save_latest_freq", type=int, default=5000, help="frequency of saving the latest results")
- parser.add_argument(
- "--save_epoch_freq", type=int, default=1, help="frequency of saving checkpoints at the end of epochs"
- )
- parser.add_argument("--evaluation_freq", type=int, default=5000, help="evaluation freq")
- parser.add_argument("--save_by_iter", action="store_true", help="whether saves model by iteration")
- parser.add_argument("--continue_train", action="store_true", help="continue training: load the latest model")
- parser.add_argument(
- "--epoch_count",
- type=int,
- default=1,
- help="the starting epoch count, we save the model by , +, ...",
- )
- parser.add_argument("--phase", type=str, default="train", help="train, val, test, etc")
- parser.add_argument("--pretrained_name", type=str, default=None, help="resume training from another checkpoint")
-
- # training parameters
- parser.add_argument("--n_epochs", type=int, default=20, help="number of epochs with the initial learning rate")
- parser.add_argument("--lr", type=float, default=0.0001, help="initial learning rate for adam")
- parser.add_argument(
- "--lr_policy", type=str, default="step", help="learning rate policy. [linear | step | plateau | cosine]"
- )
- parser.add_argument(
- "--lr_decay_epochs", type=int, default=10, help="multiply by a gamma every lr_decay_epochs epoches"
- )
-
- self.isTrain = True
- return parser
diff --git a/spaces/imjunaidafzal/LoRA-DreamBooth-Training-UI/app_training.py b/spaces/imjunaidafzal/LoRA-DreamBooth-Training-UI/app_training.py
deleted file mode 100644
index 09660a26b4d99f8ff8457a454fdddcc57d7f3756..0000000000000000000000000000000000000000
--- a/spaces/imjunaidafzal/LoRA-DreamBooth-Training-UI/app_training.py
+++ /dev/null
@@ -1,144 +0,0 @@
-#!/usr/bin/env python
-
-from __future__ import annotations
-
-import os
-
-import gradio as gr
-
-from constants import UploadTarget
-from inference import InferencePipeline
-from trainer import Trainer
-
-
-def create_training_demo(trainer: Trainer,
- pipe: InferencePipeline | None = None) -> gr.Blocks:
- with gr.Blocks() as demo:
- with gr.Row():
- with gr.Column():
- with gr.Box():
- gr.Markdown('Training Data')
- instance_images = gr.Files(label='Instance images')
- instance_prompt = gr.Textbox(label='Instance prompt',
- max_lines=1)
- gr.Markdown('''
- - Upload images of the style you are planning on training on.
- - For an instance prompt, use a unique, made up word to avoid collisions.
- ''')
- with gr.Box():
- gr.Markdown('Output Model')
- output_model_name = gr.Text(label='Name of your model',
- max_lines=1)
- delete_existing_model = gr.Checkbox(
- label='Delete existing model of the same name',
- value=False)
- validation_prompt = gr.Text(label='Validation Prompt')
- with gr.Box():
- gr.Markdown('Upload Settings')
- with gr.Row():
- upload_to_hub = gr.Checkbox(
- label='Upload model to Hub', value=True)
- use_private_repo = gr.Checkbox(label='Private',
- value=True)
- delete_existing_repo = gr.Checkbox(
- label='Delete existing repo of the same name',
- value=False)
- upload_to = gr.Radio(
- label='Upload to',
- choices=[_.value for _ in UploadTarget],
- value=UploadTarget.LORA_LIBRARY.value)
- gr.Markdown('''
- - By default, trained models will be uploaded to [LoRA Library](https://huggingface.co/lora-library) (see [this example model](https://huggingface.co/lora-library/lora-dreambooth-sample-dog)).
- - You can also choose "Personal Profile", in which case, the model will be uploaded to https://huggingface.co/{your_username}/{model_name}.
- ''')
-
- with gr.Box():
- gr.Markdown('Training Parameters')
- with gr.Row():
- base_model = gr.Text(
- label='Base Model',
- value='stabilityai/stable-diffusion-2-1-base',
- max_lines=1)
- resolution = gr.Dropdown(choices=['512', '768'],
- value='512',
- label='Resolution')
- num_training_steps = gr.Number(
- label='Number of Training Steps', value=1000, precision=0)
- learning_rate = gr.Number(label='Learning Rate', value=0.0001)
- gradient_accumulation = gr.Number(
- label='Number of Gradient Accumulation',
- value=1,
- precision=0)
- seed = gr.Slider(label='Seed',
- minimum=0,
- maximum=100000,
- step=1,
- value=0)
- fp16 = gr.Checkbox(label='FP16', value=True)
- use_8bit_adam = gr.Checkbox(label='Use 8bit Adam', value=True)
- checkpointing_steps = gr.Number(label='Checkpointing Steps',
- value=100,
- precision=0)
- use_wandb = gr.Checkbox(label='Use W&B',
- value=False,
- interactive=bool(
- os.getenv('WANDB_API_KEY')))
- validation_epochs = gr.Number(label='Validation Epochs',
- value=100,
- precision=0)
- gr.Markdown('''
- - The base model must be a model that is compatible with [diffusers](https://github.com/huggingface/diffusers) library.
- - It takes a few minutes to download the base model first.
- - It will take about 8 minutes to train for 1000 steps with a T4 GPU.
- - You may want to try a small number of steps first, like 1, to see if everything works fine in your environment.
- - You can check the training status by pressing the "Open logs" button if you are running this on your Space.
- - You need to set the environment variable `WANDB_API_KEY` if you'd like to use [W&B](https://wandb.ai/site). See [W&B documentation](https://docs.wandb.ai/guides/track/advanced/environment-variables).
- - **Note:** Due to [this issue](https://github.com/huggingface/accelerate/issues/944), currently, training will not terminate properly if you use W&B.
- ''')
-
- remove_gpu_after_training = gr.Checkbox(
- label='Remove GPU after training',
- value=False,
- interactive=bool(os.getenv('SPACE_ID')),
- visible=False)
- run_button = gr.Button('Start Training')
-
- with gr.Box():
- gr.Markdown('Output message')
- output_message = gr.Markdown()
-
- if pipe is not None:
- run_button.click(fn=pipe.clear)
- run_button.click(fn=trainer.run,
- inputs=[
- instance_images,
- instance_prompt,
- output_model_name,
- delete_existing_model,
- validation_prompt,
- base_model,
- resolution,
- num_training_steps,
- learning_rate,
- gradient_accumulation,
- seed,
- fp16,
- use_8bit_adam,
- checkpointing_steps,
- use_wandb,
- validation_epochs,
- upload_to_hub,
- use_private_repo,
- delete_existing_repo,
- upload_to,
- remove_gpu_after_training,
- ],
- outputs=output_message)
- return demo
-
-
-if __name__ == '__main__':
- hf_token = os.getenv('HF_TOKEN')
- trainer = Trainer(hf_token)
- demo = create_training_demo(trainer)
- demo.queue(max_size=1).launch(share=False)
diff --git a/spaces/innnky/vits-nyaru/losses.py b/spaces/innnky/vits-nyaru/losses.py
deleted file mode 100644
index fb22a0e834dd87edaa37bb8190eee2c3c7abe0d5..0000000000000000000000000000000000000000
--- a/spaces/innnky/vits-nyaru/losses.py
+++ /dev/null
@@ -1,61 +0,0 @@
-import torch
-from torch.nn import functional as F
-
-import commons
-
-
-def feature_loss(fmap_r, fmap_g):
- loss = 0
- for dr, dg in zip(fmap_r, fmap_g):
- for rl, gl in zip(dr, dg):
- rl = rl.float().detach()
- gl = gl.float()
- loss += torch.mean(torch.abs(rl - gl))
-
- return loss * 2
-
-
-def discriminator_loss(disc_real_outputs, disc_generated_outputs):
- loss = 0
- r_losses = []
- g_losses = []
- for dr, dg in zip(disc_real_outputs, disc_generated_outputs):
- dr = dr.float()
- dg = dg.float()
- r_loss = torch.mean((1-dr)**2)
- g_loss = torch.mean(dg**2)
- loss += (r_loss + g_loss)
- r_losses.append(r_loss.item())
- g_losses.append(g_loss.item())
-
- return loss, r_losses, g_losses
-
-
-def generator_loss(disc_outputs):
- loss = 0
- gen_losses = []
- for dg in disc_outputs:
- dg = dg.float()
- l = torch.mean((1-dg)**2)
- gen_losses.append(l)
- loss += l
-
- return loss, gen_losses
-
-
-def kl_loss(z_p, logs_q, m_p, logs_p, z_mask):
- """
- z_p, logs_q: [b, h, t_t]
- m_p, logs_p: [b, h, t_t]
- """
- z_p = z_p.float()
- logs_q = logs_q.float()
- m_p = m_p.float()
- logs_p = logs_p.float()
- z_mask = z_mask.float()
-
- kl = logs_p - logs_q - 0.5
- kl += 0.5 * ((z_p - m_p)**2) * torch.exp(-2. * logs_p)
- kl = torch.sum(kl * z_mask)
- l = kl / torch.sum(z_mask)
- return l
diff --git a/spaces/inplisQlawa/anything-midjourney-v4-1/Berserk 1 - 25 (Complete) [English Dubbed].md b/spaces/inplisQlawa/anything-midjourney-v4-1/Berserk 1 - 25 (Complete) [English Dubbed].md
deleted file mode 100644
index 27ac1d826cd94446dc6d01f4643c385a3f585890..0000000000000000000000000000000000000000
--- a/spaces/inplisQlawa/anything-midjourney-v4-1/Berserk 1 - 25 (Complete) [English Dubbed].md
+++ /dev/null
@@ -1,9 +0,0 @@
-
-
-Berserk (TV) ; Number of episodes: 25; Series titles: We have 25; Vintage: from 10/07/1997 to 03/31/1998; Release Dates: We have 1 ; Opening theme: "Tell me why" on . Watch online anime Berserk - At the end of the 19th century, in Japan, during the Meiji period, when the country seemed to open a window to Europe, a unique, tragic and at the same time heroic deed thundered throughout Japan.
-In one of the peasant families, a boy was born with an amazing talent for handling weapons, as well as a unique ability to control energy.
-The boy, whom the locals called Orochi, showed incredible strength and power.
-And he didn't just fight, he won every fight. 8a78ff9644
-
-
-
diff --git a/spaces/inplisQlawa/anything-midjourney-v4-1/HOW TO INSTALL PHOTOSHOP CC 2020 FREE PHOTOSHOP TOP Crack VERSION Windows 10 WORK Mac MacOSX.md b/spaces/inplisQlawa/anything-midjourney-v4-1/HOW TO INSTALL PHOTOSHOP CC 2020 FREE PHOTOSHOP TOP Crack VERSION Windows 10 WORK Mac MacOSX.md
deleted file mode 100644
index 69f2db4e4d0eea530b553b951d72c0aabdc98ef8..0000000000000000000000000000000000000000
--- a/spaces/inplisQlawa/anything-midjourney-v4-1/HOW TO INSTALL PHOTOSHOP CC 2020 FREE PHOTOSHOP TOP Crack VERSION Windows 10 WORK Mac MacOSX.md
+++ /dev/null
@@ -1,11 +0,0 @@
-
-
just click "continue". you may also want to sign up for adobe creative cloud (assuming that you have a creative cloud membership). thats a good idea, but you can continue without signing up. you will only need to sign up when you want to make future changes to your photoshop software.
-
HOW TO INSTALL PHOTOSHOP CC 2020 FREE PHOTOSHOP CRACK VERSION Windows 10 WORK Mac MacOSX
if you are going to be running photoshop as the main program, it might be worthwhile to create a shortcut on your desktop for it. there is a post here on how to create shortcuts to apps on mac: > how to install photoshop cc 2020 free photoshop crack version windows 10 work mac macosx
you will be prompted to either confirm the installation of the software or cancel it.
-
there are a number of ways to install a creative cloud/photoshop cs5 license on a mac, including the method below which allows you to sign in to your account from the mac installer. for the purposes of this tutorial, i will assume you have a working install of photoshop cs4 on a mac.
-
select preferences.. from the photoshop cs5 menu bar. click accounts under the photoshop cs5 preferences menu bar. click sign in. click the sign in link. enter your account password. click ok. enter your product key. click done.
-
-
now, you can install photoshop cc (the photo editing software thats built into the creative cloud platform) use the installation program you downloaded earlier. (note: the installer will not open if you are installing photoshop cc on a mac as it requires the adobe creatives account manager to be installed. if you encounter problems with the installer, see below.
-
if you are installing photoshop cc on a mac, you must install the creative creative account manager. the creative account manager lets you log into photoshop cc or the creative cloud from a mac. the creative account manager can be installed from the mac software update panel, or via the mac app store.
899543212b
-
-
\ No newline at end of file
diff --git a/spaces/inplisQlawa/anything-midjourney-v4-1/Heroes Of Might And Magic 5 Collectors Edition RELOADED.md b/spaces/inplisQlawa/anything-midjourney-v4-1/Heroes Of Might And Magic 5 Collectors Edition RELOADED.md
deleted file mode 100644
index a95d212c5c8d08189ccfc11979c3c2bf31ab67ed..0000000000000000000000000000000000000000
--- a/spaces/inplisQlawa/anything-midjourney-v4-1/Heroes Of Might And Magic 5 Collectors Edition RELOADED.md
+++ /dev/null
@@ -1,7 +0,0 @@
-
Heroes of Might and Magic 5 Collectors Edition RELOADED
-
-This collection of offers includes adventure puzzle games. . The first game in the series, Leisure Suit Larry in the Land of the Lounge Lizards, was . . This collection of proposals includes puzzle adventure games. • The first game in the series, Leisure Suit Larry in the Land of the Lounge Lizards, was developed by TellTale Games and published by Electronic Arts in August 1998. • Based on the game, two television series were filmed: "Leisure Suit Larry" and "Leisure Suit Larry in the Land of the Lounge Lizards". . • In September 1999, the game "Leisure Suit Larry
-in the Land of the Lounge Lizards" by TellTaleGames. 8a78ff9644
-
-
-
diff --git a/spaces/inreVtussa/clothingai/Examples/Aiseesoft Total Video Converter Platinum V7.1.26 Incl Crack [Tor Serial Key PATCHED.md b/spaces/inreVtussa/clothingai/Examples/Aiseesoft Total Video Converter Platinum V7.1.26 Incl Crack [Tor Serial Key PATCHED.md
deleted file mode 100644
index d9b34e0993e71e3271d66d72588389f9449e4d6d..0000000000000000000000000000000000000000
--- a/spaces/inreVtussa/clothingai/Examples/Aiseesoft Total Video Converter Platinum V7.1.26 Incl Crack [Tor Serial Key PATCHED.md
+++ /dev/null
@@ -1,14 +0,0 @@
-
Aiseesoft Total Video Converter Platinum V7.1.26 Incl Crack [Tor Serial Key
-
-exe]
-
-All of you know that Total Video Converter Platinum V7.1.26 Is the famous software which can convert videos into various kinds of files, such as 3GP, MP4, AVI, MP3, WMA, MP2, OGG, MOV, MKV, WMV, WMA, FLAC, VOB, etc. It can also convert video files to just audio files, for example, convert AVI to WAV, convert 3GP to MP3, convert MKV to MP2, convert AVI to WMV, convert MTS/M2TS to MP2, convert WMV to MP3, convert MOV to AVI, convert FLAC to MP3, convert MP4 to MP3, convert OGG to MP3, convert MKV to MP3, convert MTS/M2TS to WAV, etc. This is the latest version of this great and unique program, so that you can enjoy it for free.
-
-Aiseesoft Total Video Converter Platinum V7.1.26 Incl Crack [Torrent]
-
-It is not only good in converting, it also supports editing, removing, adding, and many other functions. This software can support almost all formats, such as AVI, FLV, MPEG, WMV, MP4, MKV, H.264/AVC, H.264/MPEG-4, MPEG-2/AVC, MPEG-2/MPEG-4, MOV, VOB, MP3, M4A, AAC, AC3, AMR, OGG, WMA, MPA, etc. It can also support all video files, such as SD, HD, 4K, HD, 2K, SD, and so on. And you can also burn the converted video to DVD, DVD+RW, Blu-ray disc, as well as CD, and it can also convert audio to MP3, WAV, AAC, FLAC, AC3, AMR, OGG, etc. It can also convert video and audio formats in an instant. Moreover, it is compatible with Windows 8, Windows 7, Vista, XP, 2000, 98, ME, NT, 2000 SP2, 2000 SP3, and 2000 SP4.
-
-You can also make sure that it can convert all video and audio files and files to the particular format without needing to add any additional parameters. This is a video converter software that you will not 4fefd39f24
-
-
-
diff --git a/spaces/inreVtussa/clothingai/Examples/Auto Tune Efx 2 Download Crack For Gta.md b/spaces/inreVtussa/clothingai/Examples/Auto Tune Efx 2 Download Crack For Gta.md
deleted file mode 100644
index 8c47065e1870ff73855a43af232b089ef2af81dd..0000000000000000000000000000000000000000
--- a/spaces/inreVtussa/clothingai/Examples/Auto Tune Efx 2 Download Crack For Gta.md
+++ /dev/null
@@ -1,6 +0,0 @@
-