diff --git a/spaces/1368565466ki/Satdia/commons.py b/spaces/1368565466ki/Satdia/commons.py
deleted file mode 100644
index 40fcc05364d4815971f5c6f9dbb8dcef8e3ec1e9..0000000000000000000000000000000000000000
--- a/spaces/1368565466ki/Satdia/commons.py
+++ /dev/null
@@ -1,172 +0,0 @@
-import math
-import torch
-from torch.nn import functional as F
-import torch.jit
-
-
-def script_method(fn, _rcb=None):
- return fn
-
-
-def script(obj, optimize=True, _frames_up=0, _rcb=None):
- return obj
-
-
-torch.jit.script_method = script_method
-torch.jit.script = script
-
-
-def init_weights(m, mean=0.0, std=0.01):
- classname = m.__class__.__name__
- if classname.find("Conv") != -1:
- m.weight.data.normal_(mean, std)
-
-
-def get_padding(kernel_size, dilation=1):
- return int((kernel_size*dilation - dilation)/2)
-
-
-def convert_pad_shape(pad_shape):
- l = pad_shape[::-1]
- pad_shape = [item for sublist in l for item in sublist]
- return pad_shape
-
-
-def intersperse(lst, item):
- result = [item] * (len(lst) * 2 + 1)
- result[1::2] = lst
- return result
-
-
-def kl_divergence(m_p, logs_p, m_q, logs_q):
- """KL(P||Q)"""
- kl = (logs_q - logs_p) - 0.5
- kl += 0.5 * (torch.exp(2. * logs_p) + ((m_p - m_q)**2)) * torch.exp(-2. * logs_q)
- return kl
-
-
-def rand_gumbel(shape):
- """Sample from the Gumbel distribution, protect from overflows."""
- uniform_samples = torch.rand(shape) * 0.99998 + 0.00001
- return -torch.log(-torch.log(uniform_samples))
-
-
-def rand_gumbel_like(x):
- g = rand_gumbel(x.size()).to(dtype=x.dtype, device=x.device)
- return g
-
-
-def slice_segments(x, ids_str, segment_size=4):
- ret = torch.zeros_like(x[:, :, :segment_size])
- for i in range(x.size(0)):
- idx_str = ids_str[i]
- idx_end = idx_str + segment_size
- ret[i] = x[i, :, idx_str:idx_end]
- return ret
-
-
-def rand_slice_segments(x, x_lengths=None, segment_size=4):
- b, d, t = x.size()
- if x_lengths is None:
- x_lengths = t
- ids_str_max = x_lengths - segment_size + 1
- ids_str = (torch.rand([b]).to(device=x.device) * ids_str_max).to(dtype=torch.long)
- ret = slice_segments(x, ids_str, segment_size)
- return ret, ids_str
-
-
-def get_timing_signal_1d(
- length, channels, min_timescale=1.0, max_timescale=1.0e4):
- position = torch.arange(length, dtype=torch.float)
- num_timescales = channels // 2
- log_timescale_increment = (
- math.log(float(max_timescale) / float(min_timescale)) /
- (num_timescales - 1))
- inv_timescales = min_timescale * torch.exp(
- torch.arange(num_timescales, dtype=torch.float) * -log_timescale_increment)
- scaled_time = position.unsqueeze(0) * inv_timescales.unsqueeze(1)
- signal = torch.cat([torch.sin(scaled_time), torch.cos(scaled_time)], 0)
- signal = F.pad(signal, [0, 0, 0, channels % 2])
- signal = signal.view(1, channels, length)
- return signal
-
-
-def add_timing_signal_1d(x, min_timescale=1.0, max_timescale=1.0e4):
- b, channels, length = x.size()
- signal = get_timing_signal_1d(length, channels, min_timescale, max_timescale)
- return x + signal.to(dtype=x.dtype, device=x.device)
-
-
-def cat_timing_signal_1d(x, min_timescale=1.0, max_timescale=1.0e4, axis=1):
- b, channels, length = x.size()
- signal = get_timing_signal_1d(length, channels, min_timescale, max_timescale)
- return torch.cat([x, signal.to(dtype=x.dtype, device=x.device)], axis)
-
-
-def subsequent_mask(length):
- mask = torch.tril(torch.ones(length, length)).unsqueeze(0).unsqueeze(0)
- return mask
-
-
-@torch.jit.script
-def fused_add_tanh_sigmoid_multiply(input_a, input_b, n_channels):
- n_channels_int = n_channels[0]
- in_act = input_a + input_b
- t_act = torch.tanh(in_act[:, :n_channels_int, :])
- s_act = torch.sigmoid(in_act[:, n_channels_int:, :])
- acts = t_act * s_act
- return acts
-
-
-def convert_pad_shape(pad_shape):
- l = pad_shape[::-1]
- pad_shape = [item for sublist in l for item in sublist]
- return pad_shape
-
-
-def shift_1d(x):
- x = F.pad(x, convert_pad_shape([[0, 0], [0, 0], [1, 0]]))[:, :, :-1]
- return x
-
-
-def sequence_mask(length, max_length=None):
- if max_length is None:
- max_length = length.max()
- x = torch.arange(max_length, dtype=length.dtype, device=length.device)
- return x.unsqueeze(0) < length.unsqueeze(1)
-
-
-def generate_path(duration, mask):
- """
- duration: [b, 1, t_x]
- mask: [b, 1, t_y, t_x]
- """
- device = duration.device
-
- b, _, t_y, t_x = mask.shape
- cum_duration = torch.cumsum(duration, -1)
-
- cum_duration_flat = cum_duration.view(b * t_x)
- path = sequence_mask(cum_duration_flat, t_y).to(mask.dtype)
- path = path.view(b, t_x, t_y)
- path = path - F.pad(path, convert_pad_shape([[0, 0], [1, 0], [0, 0]]))[:, :-1]
- path = path.unsqueeze(1).transpose(2,3) * mask
- return path
-
-
-def clip_grad_value_(parameters, clip_value, norm_type=2):
- if isinstance(parameters, torch.Tensor):
- parameters = [parameters]
- parameters = list(filter(lambda p: p.grad is not None, parameters))
- norm_type = float(norm_type)
- if clip_value is not None:
- clip_value = float(clip_value)
-
- total_norm = 0
- for p in parameters:
- param_norm = p.grad.data.norm(norm_type)
- total_norm += param_norm.item() ** norm_type
- if clip_value is not None:
- p.grad.data.clamp_(min=-clip_value, max=clip_value)
- total_norm = total_norm ** (1. / norm_type)
- return total_norm
diff --git a/spaces/17TheWord/RealESRGAN/Training.md b/spaces/17TheWord/RealESRGAN/Training.md
deleted file mode 100644
index 64704e1d2e1f334984232afd12b245235b274a9e..0000000000000000000000000000000000000000
--- a/spaces/17TheWord/RealESRGAN/Training.md
+++ /dev/null
@@ -1,100 +0,0 @@
-# :computer: How to Train Real-ESRGAN
-
-The training codes have been released.
-Note that the codes have a lot of refactoring. So there may be some bugs/performance drops. Welcome to report issues and I will also retrain the models.
-
-## Overview
-
-The training has been divided into two stages. These two stages have the same data synthesis process and training pipeline, except for the loss functions. Specifically,
-
-1. We first train Real-ESRNet with L1 loss from the pre-trained model ESRGAN.
-1. We then use the trained Real-ESRNet model as an initialization of the generator, and train the Real-ESRGAN with a combination of L1 loss, perceptual loss and GAN loss.
-
-## Dataset Preparation
-
-We use DF2K (DIV2K and Flickr2K) + OST datasets for our training. Only HR images are required.
-You can download from :
-
-1. DIV2K: http://data.vision.ee.ethz.ch/cvl/DIV2K/DIV2K_train_HR.zip
-2. Flickr2K: https://cv.snu.ac.kr/research/EDSR/Flickr2K.tar
-3. OST: https://openmmlab.oss-cn-hangzhou.aliyuncs.com/datasets/OST_dataset.zip
-
-For the DF2K dataset, we use a multi-scale strategy, *i.e.*, we downsample HR images to obtain several Ground-Truth images with different scales.
-
-We then crop DF2K images into sub-images for faster IO and processing.
-
-You need to prepare a txt file containing the image paths. The following are some examples in `meta_info_DF2Kmultiscale+OST_sub.txt` (As different users may have different sub-images partitions, this file is not suitable for your purpose and you need to prepare your own txt file):
-
-```txt
-DF2K_HR_sub/000001_s001.png
-DF2K_HR_sub/000001_s002.png
-DF2K_HR_sub/000001_s003.png
-...
-```
-
-## Train Real-ESRNet
-
-1. Download pre-trained model [ESRGAN](https://github.com/xinntao/Real-ESRGAN/releases/download/v0.1.1/ESRGAN_SRx4_DF2KOST_official-ff704c30.pth) into `experiments/pretrained_models`.
- ```bash
- wget https://github.com/xinntao/Real-ESRGAN/releases/download/v0.1.1/ESRGAN_SRx4_DF2KOST_official-ff704c30.pth -P experiments/pretrained_models
- ```
-1. Modify the content in the option file `options/train_realesrnet_x4plus.yml` accordingly:
- ```yml
- train:
- name: DF2K+OST
- type: RealESRGANDataset
- dataroot_gt: datasets/DF2K # modify to the root path of your folder
- meta_info: realesrgan/meta_info/meta_info_DF2Kmultiscale+OST_sub.txt # modify to your own generate meta info txt
- io_backend:
- type: disk
- ```
-1. If you want to perform validation during training, uncomment those lines and modify accordingly:
- ```yml
- # Uncomment these for validation
- # val:
- # name: validation
- # type: PairedImageDataset
- # dataroot_gt: path_to_gt
- # dataroot_lq: path_to_lq
- # io_backend:
- # type: disk
-
- ...
-
- # Uncomment these for validation
- # validation settings
- # val:
- # val_freq: !!float 5e3
- # save_img: True
-
- # metrics:
- # psnr: # metric name, can be arbitrary
- # type: calculate_psnr
- # crop_border: 4
- # test_y_channel: false
- ```
-1. Before the formal training, you may run in the `--debug` mode to see whether everything is OK. We use four GPUs for training:
- ```bash
- CUDA_VISIBLE_DEVICES=0,1,2,3 \
- python -m torch.distributed.launch --nproc_per_node=4 --master_port=4321 realesrgan/train.py -opt options/train_realesrnet_x4plus.yml --launcher pytorch --debug
- ```
-1. The formal training. We use four GPUs for training. We use the `--auto_resume` argument to automatically resume the training if necessary.
- ```bash
- CUDA_VISIBLE_DEVICES=0,1,2,3 \
- python -m torch.distributed.launch --nproc_per_node=4 --master_port=4321 realesrgan/train.py -opt options/train_realesrnet_x4plus.yml --launcher pytorch --auto_resume
- ```
-
-## Train Real-ESRGAN
-
-1. After the training of Real-ESRNet, you now have the file `experiments/train_RealESRNetx4plus_1000k_B12G4_fromESRGAN/model/net_g_1000000.pth`. If you need to specify the pre-trained path to other files, modify the `pretrain_network_g` value in the option file `train_realesrgan_x4plus.yml`.
-1. Modify the option file `train_realesrgan_x4plus.yml` accordingly. Most modifications are similar to those listed above.
-1. Before the formal training, you may run in the `--debug` mode to see whether everything is OK. We use four GPUs for training:
- ```bash
- CUDA_VISIBLE_DEVICES=0,1,2,3 \
- python -m torch.distributed.launch --nproc_per_node=4 --master_port=4321 realesrgan/train.py -opt options/train_realesrgan_x4plus.yml --launcher pytorch --debug
- ```
-1. The formal training. We use four GPUs for training. We use the `--auto_resume` argument to automatically resume the training if necessary.
- ```bash
- CUDA_VISIBLE_DEVICES=0,1,2,3 \
- python -m torch.distributed.launch --nproc_per_node=4 --master_port=4321 realesrgan/train.py -opt options/train_realesrgan_x4plus.yml --launcher pytorch --auto_resume
- ```
diff --git a/spaces/1acneusushi/gradio-2dmoleculeeditor/data/Adobe After Effects CS6 Free Download with Crack 64 Bit Kickass Learn the Secrets of Professional Video Editing and Motion Graphics.md b/spaces/1acneusushi/gradio-2dmoleculeeditor/data/Adobe After Effects CS6 Free Download with Crack 64 Bit Kickass Learn the Secrets of Professional Video Editing and Motion Graphics.md
deleted file mode 100644
index ab821a80b083aacd216805969bd6cbb6bf52a58f..0000000000000000000000000000000000000000
--- a/spaces/1acneusushi/gradio-2dmoleculeeditor/data/Adobe After Effects CS6 Free Download with Crack 64 Bit Kickass Learn the Secrets of Professional Video Editing and Motion Graphics.md
+++ /dev/null
@@ -1,110 +0,0 @@
-
-
Adobe After Effects CS6 Free Download with Crack 64 Bit Kickass
-
If you are looking for a powerful and versatile software to create stunning motion graphics and visual effects for your videos, you might want to check out Adobe After Effects CS6. This software is widely used by professionals and amateurs alike to produce high-quality videos for various purposes, such as film, TV, web, and social media. In this article, we will show you what Adobe After Effects CS6 is, what features it has, why you need it, and how to download it for free with crack 64 bit kickass.
-
What is Adobe After Effects CS6?
-
Adobe After Effects CS6 is a software that allows you to create and edit motion graphics and visual effects using a timeline-based interface. You can use it to animate text, images, shapes, masks, and layers in 2D or 3D space. You can also apply various effects and presets to enhance your animations and add realism. You can also import and export files from other Adobe products, such as Photoshop, Illustrator, Premiere Pro, and Media Encoder.
-
adobe after effects cs6 free download with crack 64 bit kickass
Adobe After Effects CS6 has many features that make it a powerful and versatile software for video editing. Here are some of the main features that you can enjoy:
-
Motion Graphics and Visual Effects
-
You can create stunning motion graphics and visual effects using Adobe After Effects CS6. You can use the built-in tools and effects to animate text, images, shapes, masks, and layers in 2D or 3D space. You can also use expressions and scripts to control your animations more precisely. You can also use keyframes and motion paths to define the movement of your elements. You can also use the graph editor to fine-tune the speed and timing of your animations.
-
3D Camera Tracker and Ray-Traced 3D Renderer
-
You can also create realistic 3D scenes using Adobe After Effects CS6. You can use the 3D camera tracker to analyze your footage and generate a 3D camera that matches the movement of your original camera. This way, you can add new elements to your scene that follow the same perspective and depth as your original footage. You can also use the ray-traced 3D renderer to create 3D shapes and text with realistic shadows, reflections, and depth of field.
-
Global Performance Cache and Rolling Shutter Repair
-
You can also enjoy faster performance and better quality using Adobe After Effects CS6. You can use the global performance cache to save your previews in the background so that you don't have to wait for them to render again when you make changes. This way, you can work more efficiently and smoothly. You can also use the rolling shutter repair effect to fix the distortion caused by rolling shutter cameras. This way, you can improve the quality of your footage.
-
how to get adobe after effects cs6 for free with crack 64 bit
-adobe after effects cs6 full version free download 64 bit with crack
-adobe after effects cs6 64 bit crack download kickass
-adobe after effects cs6 torrent download 64 bit with crack
-adobe after effects cs6 free download full version for windows 10 64 bit with crack
-adobe after effects cs6 portable free download 64 bit with crack
-adobe after effects cs6 serial number 64 bit free download with crack
-adobe after effects cs6 keygen free download 64 bit with crack
-adobe after effects cs6 patch free download 64 bit with crack
-adobe after effects cs6 activation code free download 64 bit with crack
-adobe after effects cs6 license key free download 64 bit with crack
-adobe after effects cs6 offline installer free download 64 bit with crack
-adobe after effects cs6 setup free download 64 bit with crack
-adobe after effects cs6 highly compressed free download 64 bit with crack
-adobe after effects cs6 rar file free download 64 bit with crack
-adobe after effects cs6 zip file free download 64 bit with crack
-adobe after effects cs6 iso file free download 64 bit with crack
-adobe after effects cs6 direct link free download 64 bit with crack
-adobe after effects cs6 google drive free download 64 bit with crack
-adobe after effects cs6 mega link free download 64 bit with crack
-adobe after effects cs6 mediafire link free download 64 bit with crack
-adobe after effects cs6 dropbox link free download 64 bit with crack
-adobe after effects cs6 one drive link free download 64 bit with crack
-adobe after effects cs6 zippyshare link free download 64 bit with crack
-adobe after effects cs6 uptobox link free download 64 bit with crack
-adobe after effects cs6 openload link free download 64 bit with crack
-adobe after effects cs6 streamable link free download 64 bit with crack
-adobe after effects cs6 youtube link free download 64 bit with crack
-adobe after effects cs6 vimeo link free download 64 bit with crack
-adobe after effects cs6 dailymotion link free download 64 bit with crack
-adobe after effects cs6 tutorial pdf free download 64 bit with crack
-adobe after effects cs6 plugins pack free download 64 bit with crack
-adobe after effects cs6 templates pack free download 64 bit with crack
-adobe after effects cs6 presets pack free download 64 bit with crack
-adobe after effects cs6 scripts pack free download 64 bit with crack
-adobe after effects cs6 expressions pack free download 64 bit with crack
-adobe after effects cs6 fonts pack free download 64 bit with crack
-adobe after effects cs6 transitions pack free download 64 bit with crack
-adobe after effects cs6 animations pack free download 64 bit with crack
-adobe after effects cs6 motion graphics pack free download 64 bit with crack
-adobe after effects cs6 visual effects pack free download 64 bit with crack
-adobe after effects cs6 text effects pack free download 64 bit with crack
-adobe after effects cs6 sound effects pack free download 64 bit with crack
-adobe after effects cs6 video editing software free download 64 bit with crack
-best alternative to adobe after effects cs6 for windows pc 64 bit without cracking or downloading anything
-
Variable Mask Feathering and Shape Layer Extrusion
-
You can also create more advanced masks and shapes using Adobe After Effects CS6. You can use the variable mask feathering tool to adjust the feathering of your masks along different points on the mask edge. This way, you can create more realistic masks that blend well with your background. You can also use the shape layer extrusion tool to extrude your shape layers into 3D objects with bevels and materials. This way, you can create more complex shapes that add depth to your scene.
-
Why You Need Adobe After Effects CS6?
-
Adobe After Effects CS6 is a software that you need if you want to create stunning videos for various purposes. Here are some of the reasons why you need it:
-
Create Stunning Videos for Various Purposes
-
You can use Adobe After Effects CS6 to create stunning videos for various purposes, such as film, TV, web, and social media. You can use it to create cinematic titles, intros, transitions, lower thirds, logos, credits, and more. You can also use it to create visual effects such as explosions, fire, smoke, rain, snow, lightning, etc. You can also use it to create motion graphics such as infographics, charts, graphs, maps, etc.
-
Enhance Your Creativity and Productivity
-
You can also use Adobe After Effects CS6 to enhance your creativity and productivity. You can use it to experiment with different ideas and styles without worrying about rendering time or quality. You can also use it to customize your workspace according to your preferences and workflow. You can also use it to collaborate with other artists using the Creative Cloud services.
-
Work with Other Adobe Products Seamlessly
-
You can also use Adobe After Effects CS6 to work with other Adobe products seamlessly. You can import and export files from other Adobe products such as Photoshop, Illustrator, Premiere Pro, Media Encoder etc. without losing quality or compatibility. You can also use dynamic link to update changes between applications without rendering or exporting.
-
How to Download Adobe After Effects CS6 with Crack 64 Bit Kickass?
-
If you want to download Adobe After Effects CS6 with crack 64 bit kickass for free, you need to follow these steps:
-
Step 1: Download the Torrent File from Kickass
-
The first step is to download the torrent file from kickass website. To do this, you need to have a torrent client installed on your computer such as uTorrent or BitTorrent. Then you need to go to kickass website (https://katcr.to/) and search for "Adobe After Effects CS6". Then you need to find a torrent file that has good seeds and peers (the more the better) and click on "Download Torrent". Then you need to save the torrent file on your computer.
-
Step 2: Install Adobe After Effects CS6 on Your Computer
-
The second step is to install Adobe After Effects CS6 on your computer using the torrent file that you downloaded in step 1. To do this, you need to open the torrent file using your torrent client (uTorrent or BitTorrent) and start downloading the files inside it. Then you need to wait until the download is complete (it may take some time depending on your internet speed). Then you need to open the folder where the files are downloaded (usually in Downloads) and run the setup.exe file as administrator. Then you need to follow the instructions on the screen until the installation is complete.
-
Step 3: Apply the Crack File to Activate the Software
-
The third step is to apply the crack file that came with the torrent file that you downloaded in step 1. To do this, you need to open the folder where the crack file is located (usually in Downloads) and copy it (Ctrl+C). Then you need to go to the folder where Adobe After Effects CS6 is installed (usually in C:\Program Files\Adobe\Adobe After Effects CS6) and paste it (Ctrl+V). Then you need to replace the original file when prompted (click Yes). Then you need to run Adobe After Effects CS6 as administrator (right-click on its icon > Run as administrator). Then you should see a message saying "Adobe Application Manager has been patched successfully". Then you need to close Adobe After Effects CS6.
-
Conclusion
-
In conclusion, Adobe After Effects CS6 is a powerful and versatile software that allows you to create stunning motion graphics and visual effects for your videos. You can enjoy its many features such as motion graphics and visual effects, 3D camera tracker and ray-traced 3D renderer, global performance cache and rolling shutter repair, variable mask feathering and shape layer extrusion, and more. You can also use it for various purposes such as film, TV, web, and social media. You can also enhance your creativity and productivity, and work with other Adobe products seamlessly. You free with crack 64 bit kickass by following these steps: 1. Download the torrent file from kickass website using a torrent client such as uTorrent or BitTorrent. 2. Install Adobe After Effects CS6 on your computer using the torrent file that you downloaded. 3. Apply the crack file that came with the torrent file to activate the software by copying and pasting it in the installation folder and replacing the original file. By doing this, you can enjoy Adobe After Effects CS6 for free and create amazing videos for your projects.
-
FAQs
-
Here are some of the frequently asked questions about Adobe After Effects CS6:
-
-
-
Question
-
Answer
-
-
-
Is Adobe After Effects CS6 compatible with Windows 10?
-
Yes, Adobe After Effects CS6 is compatible with Windows 10. However, you may need to update your drivers and software to ensure optimal performance.
-
-
-
Is Adobe After Effects CS6 safe to download?
-
Yes, Adobe After Effects CS6 is safe to download if you use a reliable torrent website and a trusted torrent client. However, you should always scan your files with an antivirus software before opening them to avoid any malware or viruses.
-
-
-
Is Adobe After Effects CS6 legal to use?
-
No, Adobe After Effects CS6 is not legal to use if you download it for free with crack 64 bit kickass. This is because you are violating the terms and conditions of Adobe and infringing their intellectual property rights. You may face legal consequences if you are caught using it without a valid license.
-
-
-
What are the system requirements for Adobe After Effects CS6?
-
The minimum system requirements for Adobe After Effects CS6 are: - Intel Core 2 Duo or AMD Phenom II processor; 64-bit support required - Microsoft Windows 7 with Service Pack 1 (64 bit), Windows 8 (64 bit), Windows 8.1 (64 bit), or Windows 10 (64 bit) - 4 GB of RAM (8 GB recommended) - 5 GB of available hard-disk space; additional free space required during installation (cannot install on removable flash storage devices) - Additional disk space for disk cache (10 GB recommended) - 1280 x 900 display - OpenGL 2.0–capable system - QuickTime 7.6.6 software required for QuickTime features - Optional: Adobe-certified GPU card for GPU-accelerated ray-traced 3D renderer
-
-
-
How can I learn Adobe After Effects CS6?
-
You can learn Adobe After Effects CS6 by watching online tutorials, reading books and blogs, taking courses and classes, or practicing on your own projects. You can also join online communities and forums where you can ask questions and get feedback from other users.
-
-
- 0a6ba089eb
-
-
\ No newline at end of file
diff --git a/spaces/1acneusushi/gradio-2dmoleculeeditor/data/Game Shark Ps2 V6 Iso717 The Best Way to Cheat in PS2 Games.md b/spaces/1acneusushi/gradio-2dmoleculeeditor/data/Game Shark Ps2 V6 Iso717 The Best Way to Cheat in PS2 Games.md
deleted file mode 100644
index 2223a075333c4bf2df55b34b652a6d90164d0885..0000000000000000000000000000000000000000
--- a/spaces/1acneusushi/gradio-2dmoleculeeditor/data/Game Shark Ps2 V6 Iso717 The Best Way to Cheat in PS2 Games.md
+++ /dev/null
@@ -1,162 +0,0 @@
-
-
What is Game Shark Ps2 V6 Iso717?
-
If you are a fan of PlayStation 2 games, you might have heard of Game Shark Ps2 V6 Iso717. This is a cheat device or software that allows you to modify or enhance your gaming experience by unlocking hidden features, codes, or cheats for your PS2 games. With Game Shark Ps2 V6 Iso717, you can access unlimited lives, ammo, health, money, weapons, items, and more in your favorite PS2 games.
-
Game Shark Ps2 V6 Iso717 is an ISO file that you can download and burn onto a CD or DVD. You can then insert the disc into your PS2 console and run the software. The software will scan your memory card and detect the games that you have saved. You can then select the game that you want to play and choose from a list of cheats that are available for that game. You can also create your own custom cheats by using the code generator feature.
You might lose interest or challenge in playing your PS2 games if you use too many cheats or codes.
-
You might encounter glitches, errors, bugs, or crashes in your PS2 games if you use incompatible or faulty cheats or codes.
-
You might damage your PS2 console or memory card if you use low-quality or corrupted discs or files.
-
You might violate the terms and conditions of your PS2 games or console if you use unauthorized or illegal cheats or codes.
-
-
Where can you download Game Shark Ps2 V6 Iso717?
-
There are many sources where you can download Game Shark Ps2 V6 Iso717 online. However, not all of them are reliable or safe. Some of them might contain viruses, malware, spyware, adware, or other harmful programs that can harm your computer or device. Some of them might also contain fake, incomplete, outdated, or corrupted files that can damage your PS2 console or memory card. Therefore, you should be careful and selective when choosing where to download Game Shark Ps2 V6 Iso717 from. Here is a table of some of the best sources where you can download Game Shark Ps2 V6 Iso717 from:
-
-
-
-
Source
-
Pros
-
Cons
-
-
-
CoolROM.com
-
- One of the most popular and trusted sites for downloading ROMs and ISOs. - Has a large collection of PS2 games and cheat devices. - Provides detailed information and screenshots for each file. - Allows users to rate and review each file. - Has a fast and easy download process.
-
- Some files might require additional software or tools to extract or burn. - Some files might have broken links or missing parts. - Some files might be region-locked or incompatible with certain consoles.
-
-
-
OpenSea.io
-width: 33.3333%; height: 23px; text-align: center;">- A platform for buying and selling digital collectibles and NFTs. - Has a collection of Game Shark Ps2 V6 Iso717 NFTs that are verified and authentic. - Provides a secure and transparent transaction process. - Allows users to bid and negotiate prices. - Has a user-friendly and interactive interface.
-
- Requires users to have a cryptocurrency wallet and account. - Charges fees for each transaction. - Has a limited supply and availability of Game Shark Ps2 V6 Iso717 NFTs.
-
-
-
Netlify.app
-
- A platform for hosting and deploying websites and web applications. - Has a collection of Game Shark Ps2 V6 Iso717 files that are hosted and shared by other users. - Provides a fast and reliable download speed. - Allows users to preview and test the files before downloading. - Has a simple and minimalist design.
-
- Some files might be unverified or unsafe. - Some files might be outdated or incompatible with certain consoles. - Some files might have low quality or resolution.
-
-
-
-
How to verify the authenticity and safety of the download?
-
Before you download Game Shark Ps2 V6 Iso717 from any source, you should verify the authenticity and safety of the file. This will help you avoid downloading fake, incomplete, corrupted, or infected files that can harm your computer or device. Here are some tips on how to verify the authenticity and safety of the download:
-
-
Check the file size, format, and name. The file size should be around 700 MB, the format should be ISO, and the name should be Game Shark Ps2 V6 Iso717 or something similar.
-
Check the source reputation, reviews, ratings, and feedback. The source should have a good reputation, positive reviews, high ratings, and helpful feedback from other users.
-
Check the virus scan, malware scan, spyware scan, and adware scan. The file should be free of any viruses, malware, spyware, or adware that can harm your computer or device.
-
Check the compatibility, region-lock, and update status. The file should be compatible with your PS2 console model and region, not region-locked or restricted to certain countries or areas, and updated to the latest version or patch.
-
-
What are some alternatives to Game Shark Ps2 V6 Iso717?
-
If you are looking for some alternatives to Game Shark Ps2 V6 Iso717, there are other cheat devices or software that you can use for your PS2 games. Some of them are:
-
-
-
-
Cheat Device/Software
-
Features
-
Pros
-
Cons
-
-
-
Code Breaker
-
- A cheat device that allows you to modify or enhance your PS2 games by using codes or cheats. - Has a large database of codes for over 1500 PS2 games. - Has a code generator feature that allows you to create your own custom codes. - Has a code saver feature that allows you to save your codes on your memory card.
-
- Has a user-friendly and intuitive interface. - Has a fast and easy installation and operation process. - Has a high compatibility rate with most PS2 games and consoles.
-
- Some codes might not work properly or cause glitches in some games. - Some codes might require additional hardware or software to activate. - Some codes might be region-locked or incompatible with certain consoles.
-
-
-
Action Replay Max
-
- A cheat device that allows you to modify or enhance your PS2 games by using codes or cheats. - Has a large database of codes for over 2000 PS2 games. - Has a code generator feature that allows you to create your own custom codes. - Has a code saver feature that allows you to save your codes on your memory card. - Has an online mode that allows you to download new codes from the internet.
-, and alternatives. You can download it from various sources, but you should verify the authenticity and safety of the file before downloading. You can also contact customer support for any help or assistance with the software. You can also update the software to the latest version or patch by following the steps that we explained in this article. We hope that this article has helped you learn more about Game Shark Ps2 V6 Iso717 and how to use it for your PS2 games.
FAQs
-
Here are some of the frequently asked questions about Game Shark Ps2 V6 Iso717:
-
-
What is the difference between Game Shark Ps2 V6 Iso717 and Game Shark Ps2 V4? Game Shark Ps2 V6 Iso717 is an updated version of Game Shark Ps2 V4. It has more codes, features, and compatibility than Game Shark Ps2 V4. It also has a code generator feature that allows you to create your own custom codes.
-
Can I use Game Shark Ps2 V6 Iso717 on my PS3 or PS4 console? No, you cannot use Game Shark Ps2 V6 Iso717 on your PS3 or PS4 console. It is only compatible with PS2 consoles and games.
-
Can I use Game Shark Ps2 V6 Iso717 on my PC or laptop? Yes, you can use Game Shark Ps2 V6 Iso717 on your PC or laptop if you have a PS2 emulator installed on your device. A PS2 emulator is a software that allows you to run PS2 games on your PC or laptop. You can download a PS2 emulator from various sources online, but you should verify the authenticity and safety of the file before downloading.
-
Can I use Game Shark Ps2 V6 Iso717 online or offline? You can use Game Shark Ps2 V6 Iso717 both online and offline. However, you should be careful when using it online, as some games or servers might detect or ban you for using cheats or codes. You should also respect the rules and etiquette of online gaming and not ruin the fun or experience for other players.
-
Can I use Game Shark Ps2 V6 Iso717 with other cheat devices or software? Yes, you can use Game Shark Ps2 V6 Iso717 with other cheat devices or software, such as Code Breaker, Action Replay Max, or Free McBoot. However, you should be careful when using multiple cheat devices or software at once, as this might cause conflicts or errors in your PS2 console or games.
-
- 0a6ba089eb
-
-
\ No newline at end of file
diff --git a/spaces/1gistliPinn/ChatGPT4/Examples/Dotfuscator Professional Edition 5.0 Cracked.md b/spaces/1gistliPinn/ChatGPT4/Examples/Dotfuscator Professional Edition 5.0 Cracked.md
deleted file mode 100644
index 26d6363177c0a9289e0ed50d673c661e2f15a7ec..0000000000000000000000000000000000000000
--- a/spaces/1gistliPinn/ChatGPT4/Examples/Dotfuscator Professional Edition 5.0 Cracked.md
+++ /dev/null
@@ -1,6 +0,0 @@
-
-
-With injection, Dotfuscator can easily add application monitoring to existing apps and new development. This software ... Distribute trial versions of your apps and protect your . ... rating. Protect VBA Code protects VBA projects from password cracking in MS Excel. ... Dotfuscator professional edition 4.2Â ... 4d29de3e1b
-
-
-
diff --git a/spaces/1gistliPinn/ChatGPT4/Examples/Download UPD Sda Youth Song Book.md b/spaces/1gistliPinn/ChatGPT4/Examples/Download UPD Sda Youth Song Book.md
deleted file mode 100644
index bdea3aa5d2d3531e84cc34c0fb26fab920c57cb8..0000000000000000000000000000000000000000
--- a/spaces/1gistliPinn/ChatGPT4/Examples/Download UPD Sda Youth Song Book.md
+++ /dev/null
@@ -1,30 +0,0 @@
-
-
How to Download Sda Youth Song Book for Free
-
If you are looking for a collection of songs that are suitable for young people and youth activities, you might be interested in the Sda Youth Song Book. This book contains 214 songs selected especially for Adventist youth ministries, including hymns, choruses, and contemporary songs. All songs are arranged in four-part harmony and are chorded for guitar.
-
But how can you get this book for free? Here are some ways you can download it online:
Visit the Adventist Book Center website[^1^] and add the book to your cart. You can choose between the hardback or the spiral bound format. The book costs $22.99, but you can get free shipping if you order more than $100 worth of products.
-
Visit the Adventist Youth Ministries website[^2^] and download the honor book for music. This book contains some of the songs from the Sda Youth Song Book, as well as requirements and activities for earning the music honor badge. You can also find other resources for Adventist youth ministries on this website.
-
Visit the Adventist Youth Ministries website[^3^] and download the resource manuals for Adventurers. These manuals contain some of the songs from the Sda Youth Song Book, as well as guidelines and materials for organizing Adventurer clubs for children aged 6-9. You can also find other resources for Adventurer ministries on this website.
-
-
These are some of the ways you can download the Sda Youth Song Book for free online. However, if you want to have a physical copy of the book, you might want to consider buying it from the Adventist Book Center or from your local church bookstore. You can also borrow it from your friends or from your church library. The Sda Youth Song Book is a great resource for enhancing your musical skills and enriching your spiritual life.
-
-
How singing can improve your physical health
-
Singing is not only fun, but also good for your body. Singing can have positive effects on various aspects of your physical health, such as your breathing, posture, blood pressure, and sleep quality. Here are some of the ways singing can benefit your physical health:
-
-
Singing helps with breathing. Singing requires you to control your breath and use your diaphragm muscles. This can improve your lung capacity and function, as well as your oxygen intake. Singing can also help clear your respiratory tract and prevent infections.
-
Singing helps with posture. Singing requires you to stand or sit upright, with your shoulders back and your chest open. This can improve your posture and alignment, as well as reduce tension in your neck and back muscles.
-
Singing helps with blood pressure. Singing can lower your blood pressure by reducing stress hormones and increasing endorphins. Singing can also improve your blood circulation and oxygen delivery to your tissues and organs.
-
Singing helps with sleep. Singing can help strengthen your throat and palate muscles, which can prevent snoring and sleep apnea. Snoring and sleep apnea are conditions that cause interruptions in your breathing during sleep, which can affect your sleep quality and health. Singing can also help you relax and fall asleep faster.
-
-
How singing can improve your mental health
-
Singing is not only good for your body, but also for your mind. Singing can have positive effects on various aspects of your mental health, such as your mood, stress levels, memory, and social skills. Here are some of the ways singing can benefit your mental health:
-
-
Singing helps with mood. Singing can boost your mood by releasing dopamine and endorphins, which are neurotransmitters that make you feel happy and euphoric. Singing can also reduce cortisol, which is a hormone that causes stress and anxiety.
-
Singing helps with stress. Singing can help you cope with stress by providing an outlet for expression and emotion. Singing can also distract you from negative thoughts and worries, and help you focus on the present moment.
-
Singing helps with memory. Singing can improve your memory by stimulating your brain and enhancing your cognitive functions. Singing can also help you recall words and melodies, as well as improve your verbal skills and creativity.
-
Singing helps with social skills. Singing can help you connect with others by fostering a sense of belonging and community. Singing in a group or a choir can also improve your communication skills, confidence, and self-esteem.
-
d5da3c52bf
-
-
\ No newline at end of file
diff --git a/spaces/1gistliPinn/ChatGPT4/Examples/Filme Noi Cu Subtitrare In Romana Download Free.md b/spaces/1gistliPinn/ChatGPT4/Examples/Filme Noi Cu Subtitrare In Romana Download Free.md
deleted file mode 100644
index d7f7a8ab3f1a4a01e3102692e7c403d79b65d0c3..0000000000000000000000000000000000000000
--- a/spaces/1gistliPinn/ChatGPT4/Examples/Filme Noi Cu Subtitrare In Romana Download Free.md
+++ /dev/null
@@ -1,26 +0,0 @@
-
-
-2006. Iatalo
-
-Golan Roth
-
-Etichete: Ioana, Golan Roth
-
-Discursul asupra relatiei
-
-dintre om si societate
-
-Pe 12 ianuarie 1997 a avut loc un incident de atac violent, la adresa lui Golan, pentru că el a trebuit să schimbe poliţiştii în care era prins şi pentru că el era cel mai bun prieten al mamei lui Andrei, care se afla în carantină. Am fost martorul lui Golan.
-
-Și, aşa cum se spune, am văzut, am văzut. Deocamdată, un incident şi nimic mai mult. Vreau să vă spun doar că aşa este viaţa, după un incident.
-
-Mama mea a fost ajunsă în carantină deoarece a fost bolnavă, cu criză. O mai avea, de aproape doi ani, şi atunci în carantină.
-
-Aşa că mă aflam deoparte, să-mi dau seama ce să fac. Am văzut că n-ar fi bine să-mi dea mama locul şi să o lase în carantină acolo. Aşa că, din nefericire, nu ştiam ce să fac.
-
-Nu ştiam cine să caut şi cine să văd. Deci, când se face un incident, ca atunci, oamenii se sperie, se îngrijesc unii de alţii, cine ştie cine se aşteaptă la ce.
-
-Aşa că se uitam la televizor, la ţigări. În � 4fefd39f24
-
-
-
diff --git a/spaces/1line/AutoGPT/autogpt/commands/twitter.py b/spaces/1line/AutoGPT/autogpt/commands/twitter.py
deleted file mode 100644
index 3eaed36e20e1c520690ac59f25a4da6501f3440f..0000000000000000000000000000000000000000
--- a/spaces/1line/AutoGPT/autogpt/commands/twitter.py
+++ /dev/null
@@ -1,26 +0,0 @@
-import os
-
-import tweepy
-from dotenv import load_dotenv
-
-load_dotenv()
-
-
-def send_tweet(tweet_text):
- consumer_key = os.environ.get("TW_CONSUMER_KEY")
- consumer_secret = os.environ.get("TW_CONSUMER_SECRET")
- access_token = os.environ.get("TW_ACCESS_TOKEN")
- access_token_secret = os.environ.get("TW_ACCESS_TOKEN_SECRET")
- # Authenticate to Twitter
- auth = tweepy.OAuthHandler(consumer_key, consumer_secret)
- auth.set_access_token(access_token, access_token_secret)
-
- # Create API object
- api = tweepy.API(auth)
-
- # Send tweet
- try:
- api.update_status(tweet_text)
- print("Tweet sent successfully!")
- except tweepy.TweepyException as e:
- print("Error sending tweet: {}".format(e.reason))
diff --git a/spaces/1pelhydcardo/ChatGPT-prompt-generator/assets/Beast Quest MOD APK The Ultimate Adventure Game with Infinite Resources in 2023.md b/spaces/1pelhydcardo/ChatGPT-prompt-generator/assets/Beast Quest MOD APK The Ultimate Adventure Game with Infinite Resources in 2023.md
deleted file mode 100644
index 6099760bbcef427fac3d92569f243e1c6d9a3715..0000000000000000000000000000000000000000
--- a/spaces/1pelhydcardo/ChatGPT-prompt-generator/assets/Beast Quest MOD APK The Ultimate Adventure Game with Infinite Resources in 2023.md
+++ /dev/null
@@ -1,100 +0,0 @@
-
-
Beast Quest Mod APK 2023: Everything You Need to Know
-
Beast Quest is a popular mobile game based on the best-selling fantasy novels by Adam Blade. It is an action-adventure game that lets you explore the open world of Avantia, fight against dangerous creatures and giant beasts, collect treasures and artifacts, and upgrade your equipment. If you are a fan of Beast Quest, you might be interested in the mod apk version of the game that will be released in 2023. Here are some of the features, benefits, and drawbacks of the Beast Quest mod apk 2023.
A mod apk is a modified version of an original application that has been altered by third-party developers to add or remove some features, enhance the performance, or unlock some premium content. A mod apk usually requires you to download and install it manually from an external source, rather than from the official app store.
-
What are the features of the Beast Quest mod apk 2023?
-
The Beast Quest mod apk 2023 will offer some features that are not available in the original game, such as:
-
-
Unlimited money and potions: You will be able to buy any item or upgrade without worrying about the cost. You will also have unlimited potions to heal yourself and boost your stats.
-
All beasts unlocked: You will be able to access all the beasts in the game without having to complete the quests or defeat them in boss battles. You can choose any beast to accompany you on your adventure.
-
No ads: You will not see any ads or pop-ups while playing the game. You can enjoy the game without any interruptions or distractions.
-
New locations and quests: You will be able to explore new areas and take on new challenges that are not available in the original game. You will discover new secrets and rewards along the way.
-
Improved graphics and sound: You will experience better graphics and sound quality than the original game. The game will run smoother and faster on your device.
-
-
What are the benefits of the Beast Quest mod apk 2023?
-
The Beast Quest mod apk 2023 will offer some benefits for players who want to enjoy the game more, such as:
-
-
More fun and excitement: You will be able to play the game with more freedom and variety. You can customize your hero and your beast, try different strategies and tactics, and explore new possibilities.
-
More challenge and reward: You will be able to face more difficult enemies and bosses, and earn more rewards for your achievements. You can test your skills and knowledge of the game.
-
More content and value: You will be able to access more content and features that are not available in the original game. You can extend your gameplay time and get more value for your money.
-
-
What are the drawbacks of the Beast Quest mod apk 2023?
-
The Beast Quest mod apk 2023 will also have some drawbacks that you should be aware of before downloading it, such as:
-
-
Potential security risks: You will be downloading and installing an unofficial version of the game from an unknown source. This could expose your device to malware, viruses, or other harmful software. You should always scan any file before opening it.
-
Possible compatibility issues: You will be using a modified version of the game that may not work properly on your device or with your operating system. This could cause crashes, glitches, or errors. You should always backup your data before installing any mod apk.
-
Lack of support and updates: You will not receive any support or updates from the official developers or publishers of the game. This could affect your gameplay experience or cause problems with future versions of the game. You should always check for updates from the mod apk source.
-
-
How to download and install the Beast Quest mod apk 2023?
-
If you want to try the Beast Quest mod apk 2023, you will need to follow these steps:
-
beast quest unlimited money mod apk 2023
-beast quest hack mod apk download 2023
-beast quest mod apk latest version 2023
-beast quest mod apk free shopping 2023
-beast quest mod apk unlimited potions 2023
-beast quest mod apk android 1 2023
-beast quest mod apk offline 2023
-beast quest mod apk revdl 2023
-beast quest mod apk rexdl 2023
-beast quest mod apk happymod 2023
-beast quest mod apk no root 2023
-beast quest mod apk obb 2023
-beast quest mod apk data 2023
-beast quest mod apk unlimited gems 2023
-beast quest mod apk unlimited coins 2023
-beast quest mod apk unlimited everything 2023
-beast quest mod apk all unlocked 2023
-beast quest mod apk premium 2023
-beast quest mod apk pro 2023
-beast quest mod apk full version 2023
-beast quest mod apk mega mod 2023
-beast quest mod apk god mode 2023
-beast quest mod apk high damage 2023
-beast quest mod apk one hit kill 2023
-beast quest mod apk unlimited health 2023
-beast quest ultimate heroes mod apk 2023
-beast quest an epic adventure mod apk 2023
-beast quest ultimate heroes hack mod apk 2023
-beast quest an epic adventure hack mod apk 2023
-beast quest ultimate heroes unlimited money mod apk 2023
-beast quest an epic adventure unlimited money mod apk 2023
-beast quest ultimate heroes latest version mod apk 2023
-beast quest an epic adventure latest version mod apk 2023
-beast quest ultimate heroes free shopping mod apk 2023
-beast quest an epic adventure free shopping mod apk 2023
-beast quest ultimate heroes unlimited potions mod apk 2023
-beast quest an epic adventure unlimited potions mod apk 2023
-beast quest ultimate heroes android 1 mod apk 2023
-beast quest an epic adventure android 1 mod apk 2023
-beast quest ultimate heroes offline mode apkpure 2022
-
-
Find a reliable source: You will need to find a website or a platform that offers the Beast Quest mod apk 2023 for download. You can search online or ask for recommendations from other players. You should always check the reviews, ratings, and feedback of the source before downloading anything.
-
Download the file: You will need to download the Beast Quest mod apk 2023 file to your device. You should always scan the file for any malware or viruses before opening it. You should also make sure that you have enough storage space on your device.
-
Enable unknown sources: You will need to enable the option to install apps from unknown sources on your device. This will allow you to install the Beast Quest mod apk 2023 without any restrictions. You can usually find this option in your device settings, security settings, or developer options.
-
Install the app: You will need to install the Beast Quest mod apk 2023 on your device. You should follow the instructions on the screen and agree to the terms and conditions. You should also allow the app to access any permissions or resources that it needs.
-
Launch the game: You will need to launch the Beast Quest mod apk 2023 on your device. You should see the modded features and options in the game menu. You can now enjoy the game with the mod apk version.
-
-
How to uninstall the Beast Quest mod apk 2023?
-
If you want to uninstall the Beast Quest mod apk 2023, you will need to follow these steps:
-
-
Delete the app: You will need to delete the Beast Quest mod apk 2023 from your device. You can usually do this by long-pressing the app icon and selecting the uninstall option. You can also go to your device settings, apps, and find the app and uninstall it.
-
Clear the cache and data: You will need to clear the cache and data of the Beast Quest mod apk 2023 from your device. This will remove any residual files or settings that may affect your device performance or storage. You can usually do this by going to your device settings, apps, and finding the app and clearing its cache and data.
-
Restore the original game: You will need to restore the original game of Beast Quest on your device. You can do this by downloading and installing it from the official app store. You should be able to play the game without any modded features or options.
-
-
Conclusion
-
The Beast Quest mod apk 2023 is a modified version of the original game that offers some features, benefits, and drawbacks for players who want to enjoy the game more. It is not an official version of the game and it may have some security, compatibility, or support issues. It is up to you whether you want to try it or not, but you should always be careful and responsible when downloading and installing any mod apk.
-
FAQs
-
What is Beast Quest?
-
Beast Quest is a mobile game based on the best-selling fantasy novels by Adam Blade. It is an action-adventure game that lets you explore the open world of Avantia, fight against dangerous creatures and giant beasts, collect treasures and artifacts, and upgrade your equipment.
-
Is Beast Quest free to play?
-
Beast Quest is free to download and play, but it also offers some in-app purchases that can enhance your gameplay experience or unlock some premium content.
-
Is Beast Quest mod apk safe?
-
Beast Quest mod apk is not an official version of the game and it may have some security risks. You should always download and install it from a reliable source and scan it for any malware or viruses before opening it.
-
Is Beast Quest mod apk legal?
-
Beast Quest mod apk is not an authorized version of the game and it may violate some terms and conditions of the original game developers or publishers. You should always respect their intellectual property rights and use their products in a fair and ethical way.
-
How can I contact Beast Quest support?
-
If you have any questions or issues with Beast Quest, you can contact their support team by emailing them at support@miniclip.com or visiting their website at https://support.miniclip.com/.
197e85843d
-
-
\ No newline at end of file
diff --git a/spaces/1phancelerku/anime-remove-background/Enjoy the Best Vegas Casino Experience with Lucky Play Casino - Download Now!.md b/spaces/1phancelerku/anime-remove-background/Enjoy the Best Vegas Casino Experience with Lucky Play Casino - Download Now!.md
deleted file mode 100644
index 3e4666bbfc3cda2c8db979952834dfe06daf7e58..0000000000000000000000000000000000000000
--- a/spaces/1phancelerku/anime-remove-background/Enjoy the Best Vegas Casino Experience with Lucky Play Casino - Download Now!.md
+++ /dev/null
@@ -1,98 +0,0 @@
-
-
Download Lucky Play Casino: The Best Way to Enjoy Vegas Slots Anywhere You Go
-
Do you love playing slot machines but don't have the time or money to visit a real casino? Do you want to feel the excitement of hitting the jackpot and winning big prizes? If you answered yes, then you should download Lucky Play Casino, the best online casino app that lets you play authentic Vegas slots on your mobile device. In this article, we will tell you what Lucky Play Casino is, how to download it, why you should play it, and some tips and tricks to help you win more.
-
What is Lucky Play Casino?
-
Lucky Play Casino is a free online casino app that features hundreds of slot machines from American Gaming Systems (AGS), the manufacturers of your favorite slot machines in real casinos. You can play classic slots like Golden Wins, Jade Wins, Colossal Diamonds, Royal Reels, Liberty 777, So Hot 7s, and more. You can also play video slots like Monkey in the Bank, Buffalo Nation, Double the Devil, Fever 777, and many others. All the slots have stunning graphics, realistic sounds, exciting bonus rounds, and huge jackpots.
But that's not all. Lucky Play Casino also offers other casino games like blackjack, video poker, and bingo. You can play these games for free or join the challenging casino tournaments and compete with other players for big payouts. You can also win progressive jackpots that keep growing until someone wins them. And if you need more coins, you can get them for free every day by spinning the wheel, watching videos, completing missions, or inviting friends.
-
Features of Lucky Play Casino
-
- Authentic casino slots from AGS
-
Lucky Play Casino has the most authentic casino slots from AGS, the leading provider of gaming solutions for casinos worldwide. You can enjoy the same slot machines that you find in Las Vegas, Atlantic City, Macau, and other gambling destinations. You can also discover new slots that are added regularly to keep you entertained.
-
- Free online casino games with bonus rounds and jackpots
-
Lucky Play Casino gives you plenty of opportunities to win big with its free online casino games. You can play slots with bonus rounds that can multiply your winnings or trigger free spins. You can also play slots with jackpots that can award you millions of coins in one spin. And if you're lucky enough, you might even hit the ultimate prize: the Grand Jackpot.
-
- Challenging casino tournaments and progressive jackpots
-
If you want to test your skills and luck against other players, you can join the casino tournaments and play for huge payouts. You can choose from different types of tournaments like slots, blackjack, video poker, or bingo. You can also play for progressive jackpots that are linked across multiple games and increase every time someone plays them.
-
How to download Lucky Play Casino
-
- For Android devices
-
If you have an Android device, you can download Lucky Play Casino from the Google Play Store. Just follow these steps:
-
-
Open the Google Play Store app
Search for "Lucky Play Casino" and tap on the app icon
Tap on the "Install" button and wait for the app to download
Tap on the "Open" button and enjoy playing Lucky Play Casino
-
- For iOS devices
-
If you have an iOS device, you can download Lucky Play Casino from the App Store. Just follow these steps:
-
-
Open the App Store app
Search for "Lucky Play Casino" and tap on the app icon
Tap on the "Get" button and enter your Apple ID password if prompted
Wait for the app to download and install
Tap on the app icon and enjoy playing Lucky Play Casino
-
Why download Lucky Play Casino?
-
Now that you know what Lucky Play Casino is and how to download it, you might be wondering why you should play it. Well, there are many reasons why Lucky Play Casino is the best online casino app for you. Here are some of them:
-
Benefits of playing Lucky Play Casino
-
- Experience the thrill of Vegas anytime, anywhere
-
Lucky Play Casino lets you experience the thrill of Vegas without leaving your home. You can play authentic casino slots that make you feel like you're in a real casino. You can also enjoy the stunning graphics, realistic sounds, and exciting animations that make the games more fun and immersive. You can play Lucky Play Casino anytime, anywhere, as long as you have an internet connection.
-
download lucky play casino app
-download lucky play casino for android
-download lucky play casino for pc
-download lucky play casino free slots
-download lucky play casino games
-download lucky play casino online
-download lucky play casino slots
-how to download lucky play casino
-where to download lucky play casino
-why download lucky play casino
-best way to download lucky play casino
-benefits of downloading lucky play casino
-reviews of download lucky play casino
-tips for downloading lucky play casino
-tricks for downloading lucky play casino
-download lucky play casino and win real money
-download lucky play casino and get bonus coins
-download lucky play casino and enjoy free spins
-download lucky play casino and join tournaments
-download lucky play casino and earn rewards
-download lucky play casino for fun and entertainment
-download lucky play casino for a chance to hit the jackpot
-download lucky play casino for a realistic gaming experience
-download lucky play casino for a variety of games and themes
-download lucky play casino for a friendly and social community
-is it safe to download lucky play casino
-is it legal to download lucky play casino
-is it easy to download lucky play casino
-is it worth it to download lucky play casino
-is it free to download lucky play casino
-how long does it take to download lucky play casino
-how much space does it need to download lucky play casino
-how do I update my download lucky play casino app
-how do I uninstall my download lucky play casino app
-how do I contact support for my download lucky play casino app
-what are the features of download lucky play casino app
-what are the requirements of download lucky play casino app
-what are the advantages of download lucky play casino app
-what are the disadvantages of download lucky play casino app
-what are the alternatives of download lucky play casino app
-can I use my facebook account to download lucky play casino app
-can I use my google account to download lucky play casino app
-can I use my apple account to download lucky play casino app
-can I use my email address to download lucky play casino app
-can I use my phone number to download lucky play casino app
-can I transfer my progress from one device to another with download lucky play casino app
-can I invite my friends to join me with download lucky play casino app
-can I chat with other players with download lucky play casino app
-can I customize my profile with download lucky play casino app
-can I access exclusive offers with download lucky play casino app
-
- Play with millions of other players online
-
Lucky Play Casino is not just a solo game. You can also play with millions of other players online who share your passion for casino games. You can chat with them, send them gifts, join their clubs, or challenge them in tournaments. You can also make new friends and socialize with people from different countries and cultures.
-
- Get free coins and bonuses every day
-
Lucky Play Casino is generous when it comes to giving you free coins and bonuses. You can get free coins every day by spinning the wheel, watching videos, completing missions, or inviting friends. You can also get bonuses for logging in, leveling up, or playing certain games. You can use these coins and bonuses to play more games and win more prizes.
-
Tips and tricks for playing Lucky Play Casino
-
- Choose the right slot machine for your budget and style
-
Lucky Play Casino has hundreds of slot machines to choose from, but not all of them are suitable for your budget and style. Some slot machines have higher payouts but lower odds, while others have lower payouts but higher odds. Some slot machines have more paylines but higher bets, while others have fewer paylines but lower bets. You should choose a slot machine that matches your budget and style, so you can have more fun and win more.
-
- Use the auto-spin feature to save time and increase your chances of winning
-
Lucky Play Casino has an auto-spin feature that lets you spin the reels automatically without pressing the spin button every time. This feature can save you time and increase your chances of winning, as it can spin faster and more frequently than manual spinning. You can also adjust the number of auto-spins, the bet amount, and the stop conditions according to your preferences.
-
- Join a club and chat with other players for more fun and rewards
-
Lucky Play Casino has a club feature that lets you join or create a club with other players who share your interests. You can chat with them, send them gifts, or play together in club tournaments. You can also earn club points by playing games or completing tasks, which can help you rank up your club and get more rewards.
-
Conclusion
-
Lucky Play Casino is the best online casino app that lets you play authentic Vegas slots on your mobile device. You can download it for free from the Google Play Store or the App Store and enjoy hundreds of slot machines from AGS, the leading provider of gaming solutions for casinos worldwide. You can also play other casino games like blackjack, video poker, and bingo, and join challenging casino tournaments and progressive jackpots. You can experience the thrill of Vegas anytime, anywhere, play with millions of other players online, and get free coins and bonuses every day. You can also use some tips and tricks to help you choose the right slot machine, use the auto-spin feature, and join a club for more fun and rewards.
-
If you love playing casino games but don't have the time or money to visit a real casino, then Lucky Play Casino is the perfect app for you. Download it now and start playing today!
-
Frequently Asked Questions
-
A: There are many ways to get more coins and bonuses in Lucky Play Casino. You can get free coins every day by spinning the wheel, watching videos, completing missions, or inviting friends. You can also get bonuses for logging in, leveling up, or playing certain games. You can also buy coins and bonuses with real money if you want to.
-
-
I hope this article has helped you learn more about Lucky Play Casino and how to download it. If you have any feedback or suggestions, please let me know in the comments below. Thank you for reading and happy gaming!
197e85843d
-
-
\ No newline at end of file
diff --git a/spaces/1toTree/lora_test/ppdiffusers/utils/dummy_paddle_and_paddlenlp_and_k_diffusion_objects.py b/spaces/1toTree/lora_test/ppdiffusers/utils/dummy_paddle_and_paddlenlp_and_k_diffusion_objects.py
deleted file mode 100644
index 678970f3ee66083cdfde1b024c0b8724eccada19..0000000000000000000000000000000000000000
--- a/spaces/1toTree/lora_test/ppdiffusers/utils/dummy_paddle_and_paddlenlp_and_k_diffusion_objects.py
+++ /dev/null
@@ -1,33 +0,0 @@
-# Copyright (c) 2022 PaddlePaddle Authors. All Rights Reserved.
-#
-# Licensed under the Apache License, Version 2.0 (the "License");
-# you may not use this file except in compliance with the License.
-# You may obtain a copy of the License at
-#
-# http://www.apache.org/licenses/LICENSE-2.0
-#
-# Unless required by applicable law or agreed to in writing, software
-# distributed under the License is distributed on an "AS IS" BASIS,
-# WITHOUT WARRANTIES OR CONDITIONS OF ANY KIND, either express or implied.
-# See the License for the specific language governing permissions and
-# limitations under the License.
-
-# This file is autogenerated by the command `make fix-copies`, do not edit.
-# flake8: noqa
-
-from ..utils import DummyObject, requires_backends
-
-
-class StableDiffusionKDiffusionPipeline(metaclass=DummyObject):
- _backends = ["paddle", "paddlenlp", "k_diffusion"]
-
- def __init__(self, *args, **kwargs):
- requires_backends(self, ["paddle", "paddlenlp", "k_diffusion"])
-
- @classmethod
- def from_config(cls, *args, **kwargs):
- requires_backends(cls, ["paddle", "paddlenlp", "k_diffusion"])
-
- @classmethod
- def from_pretrained(cls, *args, **kwargs):
- requires_backends(cls, ["paddle", "paddlenlp", "k_diffusion"])
diff --git a/spaces/1vash/demo-flask-docker-template/static/style.css b/spaces/1vash/demo-flask-docker-template/static/style.css
deleted file mode 100644
index c46b108cfb1d454b999f915dc44a7d3ee4c584d3..0000000000000000000000000000000000000000
--- a/spaces/1vash/demo-flask-docker-template/static/style.css
+++ /dev/null
@@ -1,45 +0,0 @@
-body {
- --text: hsl(0 0% 15%);
- padding: 2.5rem;
- font-family: sans-serif;
- color: var(--text);
-}
-
-body.dark-theme {
- --text: hsl(0 0% 90%);
- background-color: hsl(223 39% 7%);
-}
-
-main {
- max-width: 80rem;
- text-align: center;
-}
-
-section {
- display: flex;
- flex-direction: column;
- align-items: center;
-}
-
-a {
- color: var(--text);
-}
-
-form {
- width: 30rem;
- margin: 0 auto;
-}
-
-input {
- width: 100%;
-}
-
-button {
- cursor: pointer;
-}
-
-.text-gen-output {
- min-height: 1.2rem;
- margin: 1rem;
- border: 0.5px solid grey;
-}
\ No newline at end of file
diff --git a/spaces/232labs/VToonify/vtoonify/model/raft/core/utils/augmentor.py b/spaces/232labs/VToonify/vtoonify/model/raft/core/utils/augmentor.py
deleted file mode 100644
index e81c4f2b5c16c31c0ae236d744f299d430228a04..0000000000000000000000000000000000000000
--- a/spaces/232labs/VToonify/vtoonify/model/raft/core/utils/augmentor.py
+++ /dev/null
@@ -1,246 +0,0 @@
-import numpy as np
-import random
-import math
-from PIL import Image
-
-import cv2
-cv2.setNumThreads(0)
-cv2.ocl.setUseOpenCL(False)
-
-import torch
-from torchvision.transforms import ColorJitter
-import torch.nn.functional as F
-
-
-class FlowAugmentor:
- def __init__(self, crop_size, min_scale=-0.2, max_scale=0.5, do_flip=True):
-
- # spatial augmentation params
- self.crop_size = crop_size
- self.min_scale = min_scale
- self.max_scale = max_scale
- self.spatial_aug_prob = 0.8
- self.stretch_prob = 0.8
- self.max_stretch = 0.2
-
- # flip augmentation params
- self.do_flip = do_flip
- self.h_flip_prob = 0.5
- self.v_flip_prob = 0.1
-
- # photometric augmentation params
- self.photo_aug = ColorJitter(brightness=0.4, contrast=0.4, saturation=0.4, hue=0.5/3.14)
- self.asymmetric_color_aug_prob = 0.2
- self.eraser_aug_prob = 0.5
-
- def color_transform(self, img1, img2):
- """ Photometric augmentation """
-
- # asymmetric
- if np.random.rand() < self.asymmetric_color_aug_prob:
- img1 = np.array(self.photo_aug(Image.fromarray(img1)), dtype=np.uint8)
- img2 = np.array(self.photo_aug(Image.fromarray(img2)), dtype=np.uint8)
-
- # symmetric
- else:
- image_stack = np.concatenate([img1, img2], axis=0)
- image_stack = np.array(self.photo_aug(Image.fromarray(image_stack)), dtype=np.uint8)
- img1, img2 = np.split(image_stack, 2, axis=0)
-
- return img1, img2
-
- def eraser_transform(self, img1, img2, bounds=[50, 100]):
- """ Occlusion augmentation """
-
- ht, wd = img1.shape[:2]
- if np.random.rand() < self.eraser_aug_prob:
- mean_color = np.mean(img2.reshape(-1, 3), axis=0)
- for _ in range(np.random.randint(1, 3)):
- x0 = np.random.randint(0, wd)
- y0 = np.random.randint(0, ht)
- dx = np.random.randint(bounds[0], bounds[1])
- dy = np.random.randint(bounds[0], bounds[1])
- img2[y0:y0+dy, x0:x0+dx, :] = mean_color
-
- return img1, img2
-
- def spatial_transform(self, img1, img2, flow):
- # randomly sample scale
- ht, wd = img1.shape[:2]
- min_scale = np.maximum(
- (self.crop_size[0] + 8) / float(ht),
- (self.crop_size[1] + 8) / float(wd))
-
- scale = 2 ** np.random.uniform(self.min_scale, self.max_scale)
- scale_x = scale
- scale_y = scale
- if np.random.rand() < self.stretch_prob:
- scale_x *= 2 ** np.random.uniform(-self.max_stretch, self.max_stretch)
- scale_y *= 2 ** np.random.uniform(-self.max_stretch, self.max_stretch)
-
- scale_x = np.clip(scale_x, min_scale, None)
- scale_y = np.clip(scale_y, min_scale, None)
-
- if np.random.rand() < self.spatial_aug_prob:
- # rescale the images
- img1 = cv2.resize(img1, None, fx=scale_x, fy=scale_y, interpolation=cv2.INTER_LINEAR)
- img2 = cv2.resize(img2, None, fx=scale_x, fy=scale_y, interpolation=cv2.INTER_LINEAR)
- flow = cv2.resize(flow, None, fx=scale_x, fy=scale_y, interpolation=cv2.INTER_LINEAR)
- flow = flow * [scale_x, scale_y]
-
- if self.do_flip:
- if np.random.rand() < self.h_flip_prob: # h-flip
- img1 = img1[:, ::-1]
- img2 = img2[:, ::-1]
- flow = flow[:, ::-1] * [-1.0, 1.0]
-
- if np.random.rand() < self.v_flip_prob: # v-flip
- img1 = img1[::-1, :]
- img2 = img2[::-1, :]
- flow = flow[::-1, :] * [1.0, -1.0]
-
- y0 = np.random.randint(0, img1.shape[0] - self.crop_size[0])
- x0 = np.random.randint(0, img1.shape[1] - self.crop_size[1])
-
- img1 = img1[y0:y0+self.crop_size[0], x0:x0+self.crop_size[1]]
- img2 = img2[y0:y0+self.crop_size[0], x0:x0+self.crop_size[1]]
- flow = flow[y0:y0+self.crop_size[0], x0:x0+self.crop_size[1]]
-
- return img1, img2, flow
-
- def __call__(self, img1, img2, flow):
- img1, img2 = self.color_transform(img1, img2)
- img1, img2 = self.eraser_transform(img1, img2)
- img1, img2, flow = self.spatial_transform(img1, img2, flow)
-
- img1 = np.ascontiguousarray(img1)
- img2 = np.ascontiguousarray(img2)
- flow = np.ascontiguousarray(flow)
-
- return img1, img2, flow
-
-class SparseFlowAugmentor:
- def __init__(self, crop_size, min_scale=-0.2, max_scale=0.5, do_flip=False):
- # spatial augmentation params
- self.crop_size = crop_size
- self.min_scale = min_scale
- self.max_scale = max_scale
- self.spatial_aug_prob = 0.8
- self.stretch_prob = 0.8
- self.max_stretch = 0.2
-
- # flip augmentation params
- self.do_flip = do_flip
- self.h_flip_prob = 0.5
- self.v_flip_prob = 0.1
-
- # photometric augmentation params
- self.photo_aug = ColorJitter(brightness=0.3, contrast=0.3, saturation=0.3, hue=0.3/3.14)
- self.asymmetric_color_aug_prob = 0.2
- self.eraser_aug_prob = 0.5
-
- def color_transform(self, img1, img2):
- image_stack = np.concatenate([img1, img2], axis=0)
- image_stack = np.array(self.photo_aug(Image.fromarray(image_stack)), dtype=np.uint8)
- img1, img2 = np.split(image_stack, 2, axis=0)
- return img1, img2
-
- def eraser_transform(self, img1, img2):
- ht, wd = img1.shape[:2]
- if np.random.rand() < self.eraser_aug_prob:
- mean_color = np.mean(img2.reshape(-1, 3), axis=0)
- for _ in range(np.random.randint(1, 3)):
- x0 = np.random.randint(0, wd)
- y0 = np.random.randint(0, ht)
- dx = np.random.randint(50, 100)
- dy = np.random.randint(50, 100)
- img2[y0:y0+dy, x0:x0+dx, :] = mean_color
-
- return img1, img2
-
- def resize_sparse_flow_map(self, flow, valid, fx=1.0, fy=1.0):
- ht, wd = flow.shape[:2]
- coords = np.meshgrid(np.arange(wd), np.arange(ht))
- coords = np.stack(coords, axis=-1)
-
- coords = coords.reshape(-1, 2).astype(np.float32)
- flow = flow.reshape(-1, 2).astype(np.float32)
- valid = valid.reshape(-1).astype(np.float32)
-
- coords0 = coords[valid>=1]
- flow0 = flow[valid>=1]
-
- ht1 = int(round(ht * fy))
- wd1 = int(round(wd * fx))
-
- coords1 = coords0 * [fx, fy]
- flow1 = flow0 * [fx, fy]
-
- xx = np.round(coords1[:,0]).astype(np.int32)
- yy = np.round(coords1[:,1]).astype(np.int32)
-
- v = (xx > 0) & (xx < wd1) & (yy > 0) & (yy < ht1)
- xx = xx[v]
- yy = yy[v]
- flow1 = flow1[v]
-
- flow_img = np.zeros([ht1, wd1, 2], dtype=np.float32)
- valid_img = np.zeros([ht1, wd1], dtype=np.int32)
-
- flow_img[yy, xx] = flow1
- valid_img[yy, xx] = 1
-
- return flow_img, valid_img
-
- def spatial_transform(self, img1, img2, flow, valid):
- # randomly sample scale
-
- ht, wd = img1.shape[:2]
- min_scale = np.maximum(
- (self.crop_size[0] + 1) / float(ht),
- (self.crop_size[1] + 1) / float(wd))
-
- scale = 2 ** np.random.uniform(self.min_scale, self.max_scale)
- scale_x = np.clip(scale, min_scale, None)
- scale_y = np.clip(scale, min_scale, None)
-
- if np.random.rand() < self.spatial_aug_prob:
- # rescale the images
- img1 = cv2.resize(img1, None, fx=scale_x, fy=scale_y, interpolation=cv2.INTER_LINEAR)
- img2 = cv2.resize(img2, None, fx=scale_x, fy=scale_y, interpolation=cv2.INTER_LINEAR)
- flow, valid = self.resize_sparse_flow_map(flow, valid, fx=scale_x, fy=scale_y)
-
- if self.do_flip:
- if np.random.rand() < 0.5: # h-flip
- img1 = img1[:, ::-1]
- img2 = img2[:, ::-1]
- flow = flow[:, ::-1] * [-1.0, 1.0]
- valid = valid[:, ::-1]
-
- margin_y = 20
- margin_x = 50
-
- y0 = np.random.randint(0, img1.shape[0] - self.crop_size[0] + margin_y)
- x0 = np.random.randint(-margin_x, img1.shape[1] - self.crop_size[1] + margin_x)
-
- y0 = np.clip(y0, 0, img1.shape[0] - self.crop_size[0])
- x0 = np.clip(x0, 0, img1.shape[1] - self.crop_size[1])
-
- img1 = img1[y0:y0+self.crop_size[0], x0:x0+self.crop_size[1]]
- img2 = img2[y0:y0+self.crop_size[0], x0:x0+self.crop_size[1]]
- flow = flow[y0:y0+self.crop_size[0], x0:x0+self.crop_size[1]]
- valid = valid[y0:y0+self.crop_size[0], x0:x0+self.crop_size[1]]
- return img1, img2, flow, valid
-
-
- def __call__(self, img1, img2, flow, valid):
- img1, img2 = self.color_transform(img1, img2)
- img1, img2 = self.eraser_transform(img1, img2)
- img1, img2, flow, valid = self.spatial_transform(img1, img2, flow, valid)
-
- img1 = np.ascontiguousarray(img1)
- img2 = np.ascontiguousarray(img2)
- flow = np.ascontiguousarray(flow)
- valid = np.ascontiguousarray(valid)
-
- return img1, img2, flow, valid
diff --git a/spaces/2ndelement/voicevox/test/test_mora_list.py b/spaces/2ndelement/voicevox/test/test_mora_list.py
deleted file mode 100644
index 25b287fa0e8b0febb1895ac84223823915e548ea..0000000000000000000000000000000000000000
--- a/spaces/2ndelement/voicevox/test/test_mora_list.py
+++ /dev/null
@@ -1,20 +0,0 @@
-from unittest import TestCase
-
-from voicevox_engine.mora_list import openjtalk_mora2text
-
-
-class TestOpenJTalkMoraList(TestCase):
- def test_mora2text(self):
- self.assertEqual("ッ", openjtalk_mora2text["cl"])
- self.assertEqual("ティ", openjtalk_mora2text["ti"])
- self.assertEqual("トゥ", openjtalk_mora2text["tu"])
- self.assertEqual("ディ", openjtalk_mora2text["di"])
- # GitHub issue #60
- self.assertEqual("ギェ", openjtalk_mora2text["gye"])
- self.assertEqual("イェ", openjtalk_mora2text["ye"])
-
- def test_mora2text_injective(self):
- """異なるモーラが同じ読みがなに対応しないか確認する"""
- values = list(openjtalk_mora2text.values())
- uniq_values = list(set(values))
- self.assertCountEqual(values, uniq_values)
diff --git a/spaces/4Taps/SadTalker/src/utils/text2speech.py b/spaces/4Taps/SadTalker/src/utils/text2speech.py
deleted file mode 100644
index 3ecaef36961494c8b2b1f5771a70b997efa04ffd..0000000000000000000000000000000000000000
--- a/spaces/4Taps/SadTalker/src/utils/text2speech.py
+++ /dev/null
@@ -1,12 +0,0 @@
-import os
-
-def text2speech(txt, audio_path):
- print(txt)
- cmd = f'tts --text "{txt}" --out_path {audio_path}'
- print(cmd)
- try:
- os.system(cmd)
- return audio_path
- except:
- print("Error: Failed convert txt to audio")
- return None
\ No newline at end of file
diff --git a/spaces/AICODER009/food_detection/model.py b/spaces/AICODER009/food_detection/model.py
deleted file mode 100644
index 52c2696c874740179528f0bdae8ce87b774a138f..0000000000000000000000000000000000000000
--- a/spaces/AICODER009/food_detection/model.py
+++ /dev/null
@@ -1,36 +0,0 @@
-import torch
-import torchvision
-
-from torch import nn
-
-
-def create_effnetb2_model(num_classes:int=3,
- seed:int=42):
- """Creates an EfficientNetB2 feature extractor model and transforms.
-
- Args:
- num_classes (int, optional): number of classes in the classifier head.
- Defaults to 3.
- seed (int, optional): random seed value. Defaults to 42.
-
- Returns:
- model (torch.nn.Module): EffNetB2 feature extractor model.
- transforms (torchvision.transforms): EffNetB2 image transforms.
- """
- # Create EffNetB2 pretrained weights, transforms and model
- weights = torchvision.models.EfficientNet_B2_Weights.DEFAULT
- transforms = weights.transforms()
- model = torchvision.models.efficientnet_b2(weights=weights)
-
- # Freeze all layers in base model
- for param in model.parameters():
- param.requires_grad = False
-
- # Change classifier head with random seed for reproducibility
- torch.manual_seed(seed)
- model.classifier = nn.Sequential(
- nn.Dropout(p=0.3, inplace=True),
- nn.Linear(in_features=1408, out_features=num_classes),
- )
-
- return model, transforms
diff --git a/spaces/AIGC-Audio/AudioGPT/audio_to_text/captioning/utils/train_util.py b/spaces/AIGC-Audio/AudioGPT/audio_to_text/captioning/utils/train_util.py
deleted file mode 100644
index 6cd62cc36043a2db75cc6761c51fdfdd18d11392..0000000000000000000000000000000000000000
--- a/spaces/AIGC-Audio/AudioGPT/audio_to_text/captioning/utils/train_util.py
+++ /dev/null
@@ -1,178 +0,0 @@
-# -*- coding: utf-8 -*-
-#!/usr/bin/env python3
-import os
-import sys
-import logging
-from typing import Callable, Dict, Union
-import yaml
-import torch
-from torch.optim.swa_utils import AveragedModel as torch_average_model
-import numpy as np
-import pandas as pd
-from pprint import pformat
-
-
-def load_dict_from_csv(csv, cols):
- df = pd.read_csv(csv, sep="\t")
- output = dict(zip(df[cols[0]], df[cols[1]]))
- return output
-
-
-def init_logger(filename, level="INFO"):
- formatter = logging.Formatter(
- "[ %(levelname)s : %(asctime)s ] - %(message)s")
- logger = logging.getLogger(__name__ + "." + filename)
- logger.setLevel(getattr(logging, level))
- # Log results to std
- # stdhandler = logging.StreamHandler(sys.stdout)
- # stdhandler.setFormatter(formatter)
- # Dump log to file
- filehandler = logging.FileHandler(filename)
- filehandler.setFormatter(formatter)
- logger.addHandler(filehandler)
- # logger.addHandler(stdhandler)
- return logger
-
-
-def init_obj(module, config, **kwargs):# 'captioning.models.encoder'
- obj_args = config["args"].copy()
- obj_args.update(kwargs)
- return getattr(module, config["type"])(**obj_args)
-
-
-def pprint_dict(in_dict, outputfun=sys.stdout.write, formatter='yaml'):
- """pprint_dict
-
- :param outputfun: function to use, defaults to sys.stdout
- :param in_dict: dict to print
- """
- if formatter == 'yaml':
- format_fun = yaml.dump
- elif formatter == 'pretty':
- format_fun = pformat
- for line in format_fun(in_dict).split('\n'):
- outputfun(line)
-
-
-def merge_a_into_b(a, b):
- # merge dict a into dict b. values in a will overwrite b.
- for k, v in a.items():
- if isinstance(v, dict) and k in b:
- assert isinstance(
- b[k], dict
- ), "Cannot inherit key '{}' from base!".format(k)
- merge_a_into_b(v, b[k])
- else:
- b[k] = v
-
-
-def load_config(config_file):
- with open(config_file, "r") as reader:
- config = yaml.load(reader, Loader=yaml.FullLoader)
- if "inherit_from" in config:
- base_config_file = config["inherit_from"]
- base_config_file = os.path.join(
- os.path.dirname(config_file), base_config_file
- )
- assert not os.path.samefile(config_file, base_config_file), \
- "inherit from itself"
- base_config = load_config(base_config_file)
- del config["inherit_from"]
- merge_a_into_b(config, base_config)
- return base_config
- return config
-
-
-def parse_config_or_kwargs(config_file, **kwargs):
- yaml_config = load_config(config_file)
- # passed kwargs will override yaml config
- args = dict(yaml_config, **kwargs)
- return args
-
-
-def store_yaml(config, config_file):
- with open(config_file, "w") as con_writer:
- yaml.dump(config, con_writer, indent=4, default_flow_style=False)
-
-
-class MetricImprover:
-
- def __init__(self, mode):
- assert mode in ("min", "max")
- self.mode = mode
- # min: lower -> better; max: higher -> better
- self.best_value = np.inf if mode == "min" else -np.inf
-
- def compare(self, x, best_x):
- return x < best_x if self.mode == "min" else x > best_x
-
- def __call__(self, x):
- if self.compare(x, self.best_value):
- self.best_value = x
- return True
- return False
-
- def state_dict(self):
- return self.__dict__
-
- def load_state_dict(self, state_dict):
- self.__dict__.update(state_dict)
-
-
-def fix_batchnorm(model: torch.nn.Module):
- def inner(module):
- class_name = module.__class__.__name__
- if class_name.find("BatchNorm") != -1:
- module.eval()
- model.apply(inner)
-
-
-def load_pretrained_model(model: torch.nn.Module,
- pretrained: Union[str, Dict],
- output_fn: Callable = sys.stdout.write):
- if not isinstance(pretrained, dict) and not os.path.exists(pretrained):
- output_fn(f"pretrained {pretrained} not exist!")
- return
-
- if hasattr(model, "load_pretrained"):
- model.load_pretrained(pretrained)
- return
-
- if isinstance(pretrained, dict):
- state_dict = pretrained
- else:
- state_dict = torch.load(pretrained, map_location="cpu")
-
- if "model" in state_dict:
- state_dict = state_dict["model"]
- model_dict = model.state_dict()
- pretrained_dict = {
- k: v for k, v in state_dict.items() if (k in model_dict) and (
- model_dict[k].shape == v.shape)
- }
- output_fn(f"Loading pretrained keys {pretrained_dict.keys()}")
- model_dict.update(pretrained_dict)
- model.load_state_dict(model_dict, strict=True)
-
-
-class AveragedModel(torch_average_model):
-
- def update_parameters(self, model):
- for p_swa, p_model in zip(self.parameters(), model.parameters()):
- device = p_swa.device
- p_model_ = p_model.detach().to(device)
- if self.n_averaged == 0:
- p_swa.detach().copy_(p_model_)
- else:
- p_swa.detach().copy_(self.avg_fn(p_swa.detach(), p_model_,
- self.n_averaged.to(device)))
-
- for b_swa, b_model in zip(list(self.buffers())[1:], model.buffers()):
- device = b_swa.device
- b_model_ = b_model.detach().to(device)
- if self.n_averaged == 0:
- b_swa.detach().copy_(b_model_)
- else:
- b_swa.detach().copy_(self.avg_fn(b_swa.detach(), b_model_,
- self.n_averaged.to(device)))
- self.n_averaged += 1
diff --git a/spaces/AIxPha/Real-CUGAN/upcunet_v3.py b/spaces/AIxPha/Real-CUGAN/upcunet_v3.py
deleted file mode 100644
index f7919a6cc9efe3b8af73a73e30825a4c7d7d76da..0000000000000000000000000000000000000000
--- a/spaces/AIxPha/Real-CUGAN/upcunet_v3.py
+++ /dev/null
@@ -1,714 +0,0 @@
-import torch
-from torch import nn as nn
-from torch.nn import functional as F
-import os, sys
-import numpy as np
-
-root_path = os.path.abspath('.')
-sys.path.append(root_path)
-
-
-class SEBlock(nn.Module):
- def __init__(self, in_channels, reduction=8, bias=False):
- super(SEBlock, self).__init__()
- self.conv1 = nn.Conv2d(in_channels, in_channels // reduction, 1, 1, 0, bias=bias)
- self.conv2 = nn.Conv2d(in_channels // reduction, in_channels, 1, 1, 0, bias=bias)
-
- def forward(self, x):
- if ("Half" in x.type()): # torch.HalfTensor/torch.cuda.HalfTensor
- x0 = torch.mean(x.float(), dim=(2, 3), keepdim=True).half()
- else:
- x0 = torch.mean(x, dim=(2, 3), keepdim=True)
- x0 = self.conv1(x0)
- x0 = F.relu(x0, inplace=True)
- x0 = self.conv2(x0)
- x0 = torch.sigmoid(x0)
- x = torch.mul(x, x0)
- return x
-
- def forward_mean(self, x, x0):
- x0 = self.conv1(x0)
- x0 = F.relu(x0, inplace=True)
- x0 = self.conv2(x0)
- x0 = torch.sigmoid(x0)
- x = torch.mul(x, x0)
- return x
-
-
-class UNetConv(nn.Module):
- def __init__(self, in_channels, mid_channels, out_channels, se):
- super(UNetConv, self).__init__()
- self.conv = nn.Sequential(
- nn.Conv2d(in_channels, mid_channels, 3, 1, 0),
- nn.LeakyReLU(0.1, inplace=True),
- nn.Conv2d(mid_channels, out_channels, 3, 1, 0),
- nn.LeakyReLU(0.1, inplace=True),
- )
- if se:
- self.seblock = SEBlock(out_channels, reduction=8, bias=True)
- else:
- self.seblock = None
-
- def forward(self, x):
- z = self.conv(x)
- if self.seblock is not None:
- z = self.seblock(z)
- return z
-
-
-class UNet1(nn.Module):
- def __init__(self, in_channels, out_channels, deconv):
- super(UNet1, self).__init__()
- self.conv1 = UNetConv(in_channels, 32, 64, se=False)
- self.conv1_down = nn.Conv2d(64, 64, 2, 2, 0)
- self.conv2 = UNetConv(64, 128, 64, se=True)
- self.conv2_up = nn.ConvTranspose2d(64, 64, 2, 2, 0)
- self.conv3 = nn.Conv2d(64, 64, 3, 1, 0)
-
- if deconv:
- self.conv_bottom = nn.ConvTranspose2d(64, out_channels, 4, 2, 3)
- else:
- self.conv_bottom = nn.Conv2d(64, out_channels, 3, 1, 0)
-
- for m in self.modules():
- if isinstance(m, (nn.Conv2d, nn.ConvTranspose2d)):
- nn.init.kaiming_normal_(m.weight, mode='fan_out', nonlinearity='relu')
- elif isinstance(m, nn.Linear):
- nn.init.normal_(m.weight, 0, 0.01)
- if m.bias is not None:
- nn.init.constant_(m.bias, 0)
-
- def forward(self, x):
- x1 = self.conv1(x)
- x2 = self.conv1_down(x1)
- x2 = F.leaky_relu(x2, 0.1, inplace=True)
- x2 = self.conv2(x2)
- x2 = self.conv2_up(x2)
- x2 = F.leaky_relu(x2, 0.1, inplace=True)
-
- x1 = F.pad(x1, (-4, -4, -4, -4))
- x3 = self.conv3(x1 + x2)
- x3 = F.leaky_relu(x3, 0.1, inplace=True)
- z = self.conv_bottom(x3)
- return z
-
- def forward_a(self, x):
- x1 = self.conv1(x)
- x2 = self.conv1_down(x1)
- x2 = F.leaky_relu(x2, 0.1, inplace=True)
- x2 = self.conv2.conv(x2)
- return x1, x2
-
- def forward_b(self, x1, x2):
- x2 = self.conv2_up(x2)
- x2 = F.leaky_relu(x2, 0.1, inplace=True)
-
- x1 = F.pad(x1, (-4, -4, -4, -4))
- x3 = self.conv3(x1 + x2)
- x3 = F.leaky_relu(x3, 0.1, inplace=True)
- z = self.conv_bottom(x3)
- return z
-
-
-class UNet1x3(nn.Module):
- def __init__(self, in_channels, out_channels, deconv):
- super(UNet1x3, self).__init__()
- self.conv1 = UNetConv(in_channels, 32, 64, se=False)
- self.conv1_down = nn.Conv2d(64, 64, 2, 2, 0)
- self.conv2 = UNetConv(64, 128, 64, se=True)
- self.conv2_up = nn.ConvTranspose2d(64, 64, 2, 2, 0)
- self.conv3 = nn.Conv2d(64, 64, 3, 1, 0)
-
- if deconv:
- self.conv_bottom = nn.ConvTranspose2d(64, out_channels, 5, 3, 2)
- else:
- self.conv_bottom = nn.Conv2d(64, out_channels, 3, 1, 0)
-
- for m in self.modules():
- if isinstance(m, (nn.Conv2d, nn.ConvTranspose2d)):
- nn.init.kaiming_normal_(m.weight, mode='fan_out', nonlinearity='relu')
- elif isinstance(m, nn.Linear):
- nn.init.normal_(m.weight, 0, 0.01)
- if m.bias is not None:
- nn.init.constant_(m.bias, 0)
-
- def forward(self, x):
- x1 = self.conv1(x)
- x2 = self.conv1_down(x1)
- x2 = F.leaky_relu(x2, 0.1, inplace=True)
- x2 = self.conv2(x2)
- x2 = self.conv2_up(x2)
- x2 = F.leaky_relu(x2, 0.1, inplace=True)
-
- x1 = F.pad(x1, (-4, -4, -4, -4))
- x3 = self.conv3(x1 + x2)
- x3 = F.leaky_relu(x3, 0.1, inplace=True)
- z = self.conv_bottom(x3)
- return z
-
- def forward_a(self, x):
- x1 = self.conv1(x)
- x2 = self.conv1_down(x1)
- x2 = F.leaky_relu(x2, 0.1, inplace=True)
- x2 = self.conv2.conv(x2)
- return x1, x2
-
- def forward_b(self, x1, x2):
- x2 = self.conv2_up(x2)
- x2 = F.leaky_relu(x2, 0.1, inplace=True)
-
- x1 = F.pad(x1, (-4, -4, -4, -4))
- x3 = self.conv3(x1 + x2)
- x3 = F.leaky_relu(x3, 0.1, inplace=True)
- z = self.conv_bottom(x3)
- return z
-
-
-class UNet2(nn.Module):
- def __init__(self, in_channels, out_channels, deconv):
- super(UNet2, self).__init__()
-
- self.conv1 = UNetConv(in_channels, 32, 64, se=False)
- self.conv1_down = nn.Conv2d(64, 64, 2, 2, 0)
- self.conv2 = UNetConv(64, 64, 128, se=True)
- self.conv2_down = nn.Conv2d(128, 128, 2, 2, 0)
- self.conv3 = UNetConv(128, 256, 128, se=True)
- self.conv3_up = nn.ConvTranspose2d(128, 128, 2, 2, 0)
- self.conv4 = UNetConv(128, 64, 64, se=True)
- self.conv4_up = nn.ConvTranspose2d(64, 64, 2, 2, 0)
- self.conv5 = nn.Conv2d(64, 64, 3, 1, 0)
-
- if deconv:
- self.conv_bottom = nn.ConvTranspose2d(64, out_channels, 4, 2, 3)
- else:
- self.conv_bottom = nn.Conv2d(64, out_channels, 3, 1, 0)
-
- for m in self.modules():
- if isinstance(m, (nn.Conv2d, nn.ConvTranspose2d)):
- nn.init.kaiming_normal_(m.weight, mode='fan_out', nonlinearity='relu')
- elif isinstance(m, nn.Linear):
- nn.init.normal_(m.weight, 0, 0.01)
- if m.bias is not None:
- nn.init.constant_(m.bias, 0)
-
- def forward(self, x):
- x1 = self.conv1(x)
- x2 = self.conv1_down(x1)
- x2 = F.leaky_relu(x2, 0.1, inplace=True)
- x2 = self.conv2(x2)
-
- x3 = self.conv2_down(x2)
- x3 = F.leaky_relu(x3, 0.1, inplace=True)
- x3 = self.conv3(x3)
- x3 = self.conv3_up(x3)
- x3 = F.leaky_relu(x3, 0.1, inplace=True)
-
- x2 = F.pad(x2, (-4, -4, -4, -4))
- x4 = self.conv4(x2 + x3)
- x4 = self.conv4_up(x4)
- x4 = F.leaky_relu(x4, 0.1, inplace=True)
-
- x1 = F.pad(x1, (-16, -16, -16, -16))
- x5 = self.conv5(x1 + x4)
- x5 = F.leaky_relu(x5, 0.1, inplace=True)
-
- z = self.conv_bottom(x5)
- return z
-
- def forward_a(self, x): # conv234结尾有se
- x1 = self.conv1(x)
- x2 = self.conv1_down(x1)
- x2 = F.leaky_relu(x2, 0.1, inplace=True)
- x2 = self.conv2.conv(x2)
- return x1, x2
-
- def forward_b(self, x2): # conv234结尾有se
- x3 = self.conv2_down(x2)
- x3 = F.leaky_relu(x3, 0.1, inplace=True)
- x3 = self.conv3.conv(x3)
- return x3
-
- def forward_c(self, x2, x3): # conv234结尾有se
- x3 = self.conv3_up(x3)
- x3 = F.leaky_relu(x3, 0.1, inplace=True)
-
- x2 = F.pad(x2, (-4, -4, -4, -4))
- x4 = self.conv4.conv(x2 + x3)
- return x4
-
- def forward_d(self, x1, x4): # conv234结尾有se
- x4 = self.conv4_up(x4)
- x4 = F.leaky_relu(x4, 0.1, inplace=True)
-
- x1 = F.pad(x1, (-16, -16, -16, -16))
- x5 = self.conv5(x1 + x4)
- x5 = F.leaky_relu(x5, 0.1, inplace=True)
-
- z = self.conv_bottom(x5)
- return z
-
-
-class UpCunet2x(nn.Module): # 完美tile,全程无损
- def __init__(self, in_channels=3, out_channels=3):
- super(UpCunet2x, self).__init__()
- self.unet1 = UNet1(in_channels, out_channels, deconv=True)
- self.unet2 = UNet2(in_channels, out_channels, deconv=False)
-
- def forward(self, x, tile_mode): # 1.7G
- n, c, h0, w0 = x.shape
- if (tile_mode == 0): # 不tile
- ph = ((h0 - 1) // 2 + 1) * 2
- pw = ((w0 - 1) // 2 + 1) * 2
- x = F.pad(x, (18, 18 + pw - w0, 18, 18 + ph - h0), 'reflect') # 需要保证被2整除
- x = self.unet1.forward(x)
- x0 = self.unet2.forward(x)
- x1 = F.pad(x, (-20, -20, -20, -20))
- x = torch.add(x0, x1)
- if (w0 != pw or h0 != ph): x = x[:, :, :h0 * 2, :w0 * 2]
- return x
- elif (tile_mode == 1): # 对长边减半
- if (w0 >= h0):
- crop_size_w = ((w0 - 1) // 4 * 4 + 4) // 2 # 减半后能被2整除,所以要先被4整除
- crop_size_h = (h0 - 1) // 2 * 2 + 2 # 能被2整除
- else:
- crop_size_h = ((h0 - 1) // 4 * 4 + 4) // 2 # 减半后能被2整除,所以要先被4整除
- crop_size_w = (w0 - 1) // 2 * 2 + 2 # 能被2整除
- crop_size = (crop_size_h, crop_size_w) # 6.6G
- elif (tile_mode == 2): # hw都减半
- crop_size = (((h0 - 1) // 4 * 4 + 4) // 2, ((w0 - 1) // 4 * 4 + 4) // 2) # 5.6G
- elif (tile_mode == 3): # hw都三分之一
- crop_size = (((h0 - 1) // 6 * 6 + 6) // 3, ((w0 - 1) // 6 * 6 + 6) // 3) # 4.2G
- elif (tile_mode == 4): # hw都四分之一
- crop_size = (((h0 - 1) // 8 * 8 + 8) // 4, ((w0 - 1) // 8 * 8 + 8) // 4) # 3.7G
- ph = ((h0 - 1) // crop_size[0] + 1) * crop_size[0]
- pw = ((w0 - 1) // crop_size[1] + 1) * crop_size[1]
- x = F.pad(x, (18, 18 + pw - w0, 18, 18 + ph - h0), 'reflect')
- n, c, h, w = x.shape
- se_mean0 = torch.zeros((n, 64, 1, 1)).to(x.device)
- if ("Half" in x.type()):
- se_mean0 = se_mean0.half()
- n_patch = 0
- tmp_dict = {}
- opt_res_dict = {}
- for i in range(0, h - 36, crop_size[0]):
- tmp_dict[i] = {}
- for j in range(0, w - 36, crop_size[1]):
- x_crop = x[:, :, i:i + crop_size[0] + 36, j:j + crop_size[1] + 36]
- n, c1, h1, w1 = x_crop.shape
- tmp0, x_crop = self.unet1.forward_a(x_crop)
- if ("Half" in x.type()): # torch.HalfTensor/torch.cuda.HalfTensor
- tmp_se_mean = torch.mean(x_crop.float(), dim=(2, 3), keepdim=True).half()
- else:
- tmp_se_mean = torch.mean(x_crop, dim=(2, 3), keepdim=True)
- se_mean0 += tmp_se_mean
- n_patch += 1
- tmp_dict[i][j] = (tmp0, x_crop)
- se_mean0 /= n_patch
- se_mean1 = torch.zeros((n, 128, 1, 1)).to(x.device) # 64#128#128#64
- if ("Half" in x.type()):
- se_mean1 = se_mean1.half()
- for i in range(0, h - 36, crop_size[0]):
- for j in range(0, w - 36, crop_size[1]):
- tmp0, x_crop = tmp_dict[i][j]
- x_crop = self.unet1.conv2.seblock.forward_mean(x_crop, se_mean0)
- opt_unet1 = self.unet1.forward_b(tmp0, x_crop)
- tmp_x1, tmp_x2 = self.unet2.forward_a(opt_unet1)
- if ("Half" in x.type()): # torch.HalfTensor/torch.cuda.HalfTensor
- tmp_se_mean = torch.mean(tmp_x2.float(), dim=(2, 3), keepdim=True).half()
- else:
- tmp_se_mean = torch.mean(tmp_x2, dim=(2, 3), keepdim=True)
- se_mean1 += tmp_se_mean
- tmp_dict[i][j] = (opt_unet1, tmp_x1, tmp_x2)
- se_mean1 /= n_patch
- se_mean0 = torch.zeros((n, 128, 1, 1)).to(x.device) # 64#128#128#64
- if ("Half" in x.type()):
- se_mean0 = se_mean0.half()
- for i in range(0, h - 36, crop_size[0]):
- for j in range(0, w - 36, crop_size[1]):
- opt_unet1, tmp_x1, tmp_x2 = tmp_dict[i][j]
- tmp_x2 = self.unet2.conv2.seblock.forward_mean(tmp_x2, se_mean1)
- tmp_x3 = self.unet2.forward_b(tmp_x2)
- if ("Half" in x.type()): # torch.HalfTensor/torch.cuda.HalfTensor
- tmp_se_mean = torch.mean(tmp_x3.float(), dim=(2, 3), keepdim=True).half()
- else:
- tmp_se_mean = torch.mean(tmp_x3, dim=(2, 3), keepdim=True)
- se_mean0 += tmp_se_mean
- tmp_dict[i][j] = (opt_unet1, tmp_x1, tmp_x2, tmp_x3)
- se_mean0 /= n_patch
- se_mean1 = torch.zeros((n, 64, 1, 1)).to(x.device) # 64#128#128#64
- if ("Half" in x.type()):
- se_mean1 = se_mean1.half()
- for i in range(0, h - 36, crop_size[0]):
- for j in range(0, w - 36, crop_size[1]):
- opt_unet1, tmp_x1, tmp_x2, tmp_x3 = tmp_dict[i][j]
- tmp_x3 = self.unet2.conv3.seblock.forward_mean(tmp_x3, se_mean0)
- tmp_x4 = self.unet2.forward_c(tmp_x2, tmp_x3)
- if ("Half" in x.type()): # torch.HalfTensor/torch.cuda.HalfTensor
- tmp_se_mean = torch.mean(tmp_x4.float(), dim=(2, 3), keepdim=True).half()
- else:
- tmp_se_mean = torch.mean(tmp_x4, dim=(2, 3), keepdim=True)
- se_mean1 += tmp_se_mean
- tmp_dict[i][j] = (opt_unet1, tmp_x1, tmp_x4)
- se_mean1 /= n_patch
- for i in range(0, h - 36, crop_size[0]):
- opt_res_dict[i] = {}
- for j in range(0, w - 36, crop_size[1]):
- opt_unet1, tmp_x1, tmp_x4 = tmp_dict[i][j]
- tmp_x4 = self.unet2.conv4.seblock.forward_mean(tmp_x4, se_mean1)
- x0 = self.unet2.forward_d(tmp_x1, tmp_x4)
- x1 = F.pad(opt_unet1, (-20, -20, -20, -20))
- x_crop = torch.add(x0, x1) # x0是unet2的最终输出
- opt_res_dict[i][j] = x_crop
- del tmp_dict
- torch.cuda.empty_cache()
- res = torch.zeros((n, c, h * 2 - 72, w * 2 - 72)).to(x.device)
- if ("Half" in x.type()):
- res = res.half()
- for i in range(0, h - 36, crop_size[0]):
- for j in range(0, w - 36, crop_size[1]):
- res[:, :, i * 2:i * 2 + h1 * 2 - 72, j * 2:j * 2 + w1 * 2 - 72] = opt_res_dict[i][j]
- del opt_res_dict
- torch.cuda.empty_cache()
- if (w0 != pw or h0 != ph): res = res[:, :, :h0 * 2, :w0 * 2]
- return res #
-
-
-class UpCunet3x(nn.Module): # 完美tile,全程无损
- def __init__(self, in_channels=3, out_channels=3):
- super(UpCunet3x, self).__init__()
- self.unet1 = UNet1x3(in_channels, out_channels, deconv=True)
- self.unet2 = UNet2(in_channels, out_channels, deconv=False)
-
- def forward(self, x, tile_mode): # 1.7G
- n, c, h0, w0 = x.shape
- if (tile_mode == 0): # 不tile
- ph = ((h0 - 1) // 4 + 1) * 4
- pw = ((w0 - 1) // 4 + 1) * 4
- x = F.pad(x, (14, 14 + pw - w0, 14, 14 + ph - h0), 'reflect') # 需要保证被2整除
- x = self.unet1.forward(x)
- x0 = self.unet2.forward(x)
- x1 = F.pad(x, (-20, -20, -20, -20))
- x = torch.add(x0, x1)
- if (w0 != pw or h0 != ph): x = x[:, :, :h0 * 3, :w0 * 3]
- return x
- elif (tile_mode == 1): # 对长边减半
- if (w0 >= h0):
- crop_size_w = ((w0 - 1) // 8 * 8 + 8) // 2 # 减半后能被4整除,所以要先被8整除
- crop_size_h = (h0 - 1) // 4 * 4 + 4 # 能被4整除
- else:
- crop_size_h = ((h0 - 1) // 8 * 8 + 8) // 2 # 减半后能被4整除,所以要先被8整除
- crop_size_w = (w0 - 1) // 4 * 4 + 4 # 能被4整除
- crop_size = (crop_size_h, crop_size_w) # 6.6G
- elif (tile_mode == 2): # hw都减半
- crop_size = (((h0 - 1) // 8 * 8 + 8) // 2, ((w0 - 1) // 8 * 8 + 8) // 2) # 5.6G
- elif (tile_mode == 3): # hw都三分之一
- crop_size = (((h0 - 1) // 12 * 12 + 12) // 3, ((w0 - 1) // 12 * 12 + 12) // 3) # 4.2G
- elif (tile_mode == 4): # hw都四分之一
- crop_size = (((h0 - 1) // 16 * 16 + 16) // 4, ((w0 - 1) // 16 * 16 + 16) // 4) # 3.7G
- ph = ((h0 - 1) // crop_size[0] + 1) * crop_size[0]
- pw = ((w0 - 1) // crop_size[1] + 1) * crop_size[1]
- x = F.pad(x, (14, 14 + pw - w0, 14, 14 + ph - h0), 'reflect')
- n, c, h, w = x.shape
- se_mean0 = torch.zeros((n, 64, 1, 1)).to(x.device)
- if ("Half" in x.type()):
- se_mean0 = se_mean0.half()
- n_patch = 0
- tmp_dict = {}
- opt_res_dict = {}
- for i in range(0, h - 28, crop_size[0]):
- tmp_dict[i] = {}
- for j in range(0, w - 28, crop_size[1]):
- x_crop = x[:, :, i:i + crop_size[0] + 28, j:j + crop_size[1] + 28]
- n, c1, h1, w1 = x_crop.shape
- tmp0, x_crop = self.unet1.forward_a(x_crop)
- if ("Half" in x.type()): # torch.HalfTensor/torch.cuda.HalfTensor
- tmp_se_mean = torch.mean(x_crop.float(), dim=(2, 3), keepdim=True).half()
- else:
- tmp_se_mean = torch.mean(x_crop, dim=(2, 3), keepdim=True)
- se_mean0 += tmp_se_mean
- n_patch += 1
- tmp_dict[i][j] = (tmp0, x_crop)
- se_mean0 /= n_patch
- se_mean1 = torch.zeros((n, 128, 1, 1)).to(x.device) # 64#128#128#64
- if ("Half" in x.type()):
- se_mean1 = se_mean1.half()
- for i in range(0, h - 28, crop_size[0]):
- for j in range(0, w - 28, crop_size[1]):
- tmp0, x_crop = tmp_dict[i][j]
- x_crop = self.unet1.conv2.seblock.forward_mean(x_crop, se_mean0)
- opt_unet1 = self.unet1.forward_b(tmp0, x_crop)
- tmp_x1, tmp_x2 = self.unet2.forward_a(opt_unet1)
- if ("Half" in x.type()): # torch.HalfTensor/torch.cuda.HalfTensor
- tmp_se_mean = torch.mean(tmp_x2.float(), dim=(2, 3), keepdim=True).half()
- else:
- tmp_se_mean = torch.mean(tmp_x2, dim=(2, 3), keepdim=True)
- se_mean1 += tmp_se_mean
- tmp_dict[i][j] = (opt_unet1, tmp_x1, tmp_x2)
- se_mean1 /= n_patch
- se_mean0 = torch.zeros((n, 128, 1, 1)).to(x.device) # 64#128#128#64
- if ("Half" in x.type()):
- se_mean0 = se_mean0.half()
- for i in range(0, h - 28, crop_size[0]):
- for j in range(0, w - 28, crop_size[1]):
- opt_unet1, tmp_x1, tmp_x2 = tmp_dict[i][j]
- tmp_x2 = self.unet2.conv2.seblock.forward_mean(tmp_x2, se_mean1)
- tmp_x3 = self.unet2.forward_b(tmp_x2)
- if ("Half" in x.type()): # torch.HalfTensor/torch.cuda.HalfTensor
- tmp_se_mean = torch.mean(tmp_x3.float(), dim=(2, 3), keepdim=True).half()
- else:
- tmp_se_mean = torch.mean(tmp_x3, dim=(2, 3), keepdim=True)
- se_mean0 += tmp_se_mean
- tmp_dict[i][j] = (opt_unet1, tmp_x1, tmp_x2, tmp_x3)
- se_mean0 /= n_patch
- se_mean1 = torch.zeros((n, 64, 1, 1)).to(x.device) # 64#128#128#64
- if ("Half" in x.type()):
- se_mean1 = se_mean1.half()
- for i in range(0, h - 28, crop_size[0]):
- for j in range(0, w - 28, crop_size[1]):
- opt_unet1, tmp_x1, tmp_x2, tmp_x3 = tmp_dict[i][j]
- tmp_x3 = self.unet2.conv3.seblock.forward_mean(tmp_x3, se_mean0)
- tmp_x4 = self.unet2.forward_c(tmp_x2, tmp_x3)
- if ("Half" in x.type()): # torch.HalfTensor/torch.cuda.HalfTensor
- tmp_se_mean = torch.mean(tmp_x4.float(), dim=(2, 3), keepdim=True).half()
- else:
- tmp_se_mean = torch.mean(tmp_x4, dim=(2, 3), keepdim=True)
- se_mean1 += tmp_se_mean
- tmp_dict[i][j] = (opt_unet1, tmp_x1, tmp_x4)
- se_mean1 /= n_patch
- for i in range(0, h - 28, crop_size[0]):
- opt_res_dict[i] = {}
- for j in range(0, w - 28, crop_size[1]):
- opt_unet1, tmp_x1, tmp_x4 = tmp_dict[i][j]
- tmp_x4 = self.unet2.conv4.seblock.forward_mean(tmp_x4, se_mean1)
- x0 = self.unet2.forward_d(tmp_x1, tmp_x4)
- x1 = F.pad(opt_unet1, (-20, -20, -20, -20))
- x_crop = torch.add(x0, x1) # x0是unet2的最终输出
- opt_res_dict[i][j] = x_crop #
- del tmp_dict
- torch.cuda.empty_cache()
- res = torch.zeros((n, c, h * 3 - 84, w * 3 - 84)).to(x.device)
- if ("Half" in x.type()):
- res = res.half()
- for i in range(0, h - 28, crop_size[0]):
- for j in range(0, w - 28, crop_size[1]):
- res[:, :, i * 3:i * 3 + h1 * 3 - 84, j * 3:j * 3 + w1 * 3 - 84] = opt_res_dict[i][j]
- del opt_res_dict
- torch.cuda.empty_cache()
- if (w0 != pw or h0 != ph): res = res[:, :, :h0 * 3, :w0 * 3]
- return res
-
-
-class UpCunet4x(nn.Module): # 完美tile,全程无损
- def __init__(self, in_channels=3, out_channels=3):
- super(UpCunet4x, self).__init__()
- self.unet1 = UNet1(in_channels, 64, deconv=True)
- self.unet2 = UNet2(64, 64, deconv=False)
- self.ps = nn.PixelShuffle(2)
- self.conv_final = nn.Conv2d(64, 12, 3, 1, padding=0, bias=True)
-
- def forward(self, x, tile_mode):
- n, c, h0, w0 = x.shape
- x00 = x
- if (tile_mode == 0): # 不tile
- ph = ((h0 - 1) // 2 + 1) * 2
- pw = ((w0 - 1) // 2 + 1) * 2
- x = F.pad(x, (19, 19 + pw - w0, 19, 19 + ph - h0), 'reflect') # 需要保证被2整除
- x = self.unet1.forward(x)
- x0 = self.unet2.forward(x)
- x1 = F.pad(x, (-20, -20, -20, -20))
- x = torch.add(x0, x1)
- x = self.conv_final(x)
- x = F.pad(x, (-1, -1, -1, -1))
- x = self.ps(x)
- if (w0 != pw or h0 != ph): x = x[:, :, :h0 * 4, :w0 * 4]
- x += F.interpolate(x00, scale_factor=4, mode='nearest')
- return x
- elif (tile_mode == 1): # 对长边减半
- if (w0 >= h0):
- crop_size_w = ((w0 - 1) // 4 * 4 + 4) // 2 # 减半后能被2整除,所以要先被4整除
- crop_size_h = (h0 - 1) // 2 * 2 + 2 # 能被2整除
- else:
- crop_size_h = ((h0 - 1) // 4 * 4 + 4) // 2 # 减半后能被2整除,所以要先被4整除
- crop_size_w = (w0 - 1) // 2 * 2 + 2 # 能被2整除
- crop_size = (crop_size_h, crop_size_w) # 6.6G
- elif (tile_mode == 2): # hw都减半
- crop_size = (((h0 - 1) // 4 * 4 + 4) // 2, ((w0 - 1) // 4 * 4 + 4) // 2) # 5.6G
- elif (tile_mode == 3): # hw都三分之一
- crop_size = (((h0 - 1) // 6 * 6 + 6) // 3, ((w0 - 1) // 6 * 6 + 6) // 3) # 4.1G
- elif (tile_mode == 4): # hw都四分之一
- crop_size = (((h0 - 1) // 8 * 8 + 8) // 4, ((w0 - 1) // 8 * 8 + 8) // 4) # 3.7G
- ph = ((h0 - 1) // crop_size[0] + 1) * crop_size[0]
- pw = ((w0 - 1) // crop_size[1] + 1) * crop_size[1]
- x = F.pad(x, (19, 19 + pw - w0, 19, 19 + ph - h0), 'reflect')
- n, c, h, w = x.shape
- se_mean0 = torch.zeros((n, 64, 1, 1)).to(x.device)
- if ("Half" in x.type()):
- se_mean0 = se_mean0.half()
- n_patch = 0
- tmp_dict = {}
- opt_res_dict = {}
- for i in range(0, h - 38, crop_size[0]):
- tmp_dict[i] = {}
- for j in range(0, w - 38, crop_size[1]):
- x_crop = x[:, :, i:i + crop_size[0] + 38, j:j + crop_size[1] + 38]
- n, c1, h1, w1 = x_crop.shape
- tmp0, x_crop = self.unet1.forward_a(x_crop)
- if ("Half" in x.type()): # torch.HalfTensor/torch.cuda.HalfTensor
- tmp_se_mean = torch.mean(x_crop.float(), dim=(2, 3), keepdim=True).half()
- else:
- tmp_se_mean = torch.mean(x_crop, dim=(2, 3), keepdim=True)
- se_mean0 += tmp_se_mean
- n_patch += 1
- tmp_dict[i][j] = (tmp0, x_crop)
- se_mean0 /= n_patch
- se_mean1 = torch.zeros((n, 128, 1, 1)).to(x.device) # 64#128#128#64
- if ("Half" in x.type()):
- se_mean1 = se_mean1.half()
- for i in range(0, h - 38, crop_size[0]):
- for j in range(0, w - 38, crop_size[1]):
- tmp0, x_crop = tmp_dict[i][j]
- x_crop = self.unet1.conv2.seblock.forward_mean(x_crop, se_mean0)
- opt_unet1 = self.unet1.forward_b(tmp0, x_crop)
- tmp_x1, tmp_x2 = self.unet2.forward_a(opt_unet1)
- if ("Half" in x.type()): # torch.HalfTensor/torch.cuda.HalfTensor
- tmp_se_mean = torch.mean(tmp_x2.float(), dim=(2, 3), keepdim=True).half()
- else:
- tmp_se_mean = torch.mean(tmp_x2, dim=(2, 3), keepdim=True)
- se_mean1 += tmp_se_mean
- tmp_dict[i][j] = (opt_unet1, tmp_x1, tmp_x2)
- se_mean1 /= n_patch
- se_mean0 = torch.zeros((n, 128, 1, 1)).to(x.device) # 64#128#128#64
- if ("Half" in x.type()):
- se_mean0 = se_mean0.half()
- for i in range(0, h - 38, crop_size[0]):
- for j in range(0, w - 38, crop_size[1]):
- opt_unet1, tmp_x1, tmp_x2 = tmp_dict[i][j]
- tmp_x2 = self.unet2.conv2.seblock.forward_mean(tmp_x2, se_mean1)
- tmp_x3 = self.unet2.forward_b(tmp_x2)
- if ("Half" in x.type()): # torch.HalfTensor/torch.cuda.HalfTensor
- tmp_se_mean = torch.mean(tmp_x3.float(), dim=(2, 3), keepdim=True).half()
- else:
- tmp_se_mean = torch.mean(tmp_x3, dim=(2, 3), keepdim=True)
- se_mean0 += tmp_se_mean
- tmp_dict[i][j] = (opt_unet1, tmp_x1, tmp_x2, tmp_x3)
- se_mean0 /= n_patch
- se_mean1 = torch.zeros((n, 64, 1, 1)).to(x.device) # 64#128#128#64
- if ("Half" in x.type()):
- se_mean1 = se_mean1.half()
- for i in range(0, h - 38, crop_size[0]):
- for j in range(0, w - 38, crop_size[1]):
- opt_unet1, tmp_x1, tmp_x2, tmp_x3 = tmp_dict[i][j]
- tmp_x3 = self.unet2.conv3.seblock.forward_mean(tmp_x3, se_mean0)
- tmp_x4 = self.unet2.forward_c(tmp_x2, tmp_x3)
- if ("Half" in x.type()): # torch.HalfTensor/torch.cuda.HalfTensor
- tmp_se_mean = torch.mean(tmp_x4.float(), dim=(2, 3), keepdim=True).half()
- else:
- tmp_se_mean = torch.mean(tmp_x4, dim=(2, 3), keepdim=True)
- se_mean1 += tmp_se_mean
- tmp_dict[i][j] = (opt_unet1, tmp_x1, tmp_x4)
- se_mean1 /= n_patch
- for i in range(0, h - 38, crop_size[0]):
- opt_res_dict[i] = {}
- for j in range(0, w - 38, crop_size[1]):
- opt_unet1, tmp_x1, tmp_x4 = tmp_dict[i][j]
- tmp_x4 = self.unet2.conv4.seblock.forward_mean(tmp_x4, se_mean1)
- x0 = self.unet2.forward_d(tmp_x1, tmp_x4)
- x1 = F.pad(opt_unet1, (-20, -20, -20, -20))
- x_crop = torch.add(x0, x1) # x0是unet2的最终输出
- x_crop = self.conv_final(x_crop)
- x_crop = F.pad(x_crop, (-1, -1, -1, -1))
- x_crop = self.ps(x_crop)
- opt_res_dict[i][j] = x_crop
- del tmp_dict
- torch.cuda.empty_cache()
- res = torch.zeros((n, c, h * 4 - 152, w * 4 - 152)).to(x.device)
- if ("Half" in x.type()):
- res = res.half()
- for i in range(0, h - 38, crop_size[0]):
- for j in range(0, w - 38, crop_size[1]):
- # print(opt_res_dict[i][j].shape,res[:, :, i * 4:i * 4 + h1 * 4 - 144, j * 4:j * 4 + w1 * 4 - 144].shape)
- res[:, :, i * 4:i * 4 + h1 * 4 - 152, j * 4:j * 4 + w1 * 4 - 152] = opt_res_dict[i][j]
- del opt_res_dict
- torch.cuda.empty_cache()
- if (w0 != pw or h0 != ph): res = res[:, :, :h0 * 4, :w0 * 4]
- res += F.interpolate(x00, scale_factor=4, mode='nearest')
- return res #
-
-
-class RealWaifuUpScaler(object):
- def __init__(self, scale, weight_path, half, device):
- weight = torch.load(weight_path, map_location="cpu")
- self.model = eval("UpCunet%sx" % scale)()
- if (half == True):
- self.model = self.model.half().to(device)
- else:
- self.model = self.model.to(device)
- self.model.load_state_dict(weight, strict=True)
- self.model.eval()
- self.half = half
- self.device = device
-
- def np2tensor(self, np_frame):
- if (self.half == False):
- return torch.from_numpy(np.transpose(np_frame, (2, 0, 1))).unsqueeze(0).to(self.device).float() / 255
- else:
- return torch.from_numpy(np.transpose(np_frame, (2, 0, 1))).unsqueeze(0).to(self.device).half() / 255
-
- def tensor2np(self, tensor):
- if (self.half == False):
- return (
- np.transpose((tensor.data.squeeze() * 255.0).round().clamp_(0, 255).byte().cpu().numpy(), (1, 2, 0)))
- else:
- return (np.transpose((tensor.data.squeeze().float() * 255.0).round().clamp_(0, 255).byte().cpu().numpy(),
- (1, 2, 0)))
-
- def __call__(self, frame, tile_mode):
- with torch.no_grad():
- tensor = self.np2tensor(frame)
- result = self.tensor2np(self.model(tensor, tile_mode))
- return result
-
-
-if __name__ == "__main__":
- ###########inference_img
- import time, cv2, sys
- from time import time as ttime
-
- for weight_path, scale in [("weights_v3/up2x-latest-denoise3x.pth", 2), ("weights_v3/up3x-latest-denoise3x.pth", 3),
- ("weights_v3/up4x-latest-denoise3x.pth", 4)]:
- for tile_mode in [0, 1, 2, 3, 4]:
- upscaler2x = RealWaifuUpScaler(scale, weight_path, half=True, device="cuda:0")
- input_dir = "%s/input_dir1" % root_path
- output_dir = "%s/opt-dir-all-test" % root_path
- os.makedirs(output_dir, exist_ok=True)
- for name in os.listdir(input_dir):
- print(name)
- tmp = name.split(".")
- inp_path = os.path.join(input_dir, name)
- suffix = tmp[-1]
- prefix = ".".join(tmp[:-1])
- tmp_path = os.path.join(root_path, "tmp", "%s.%s" % (int(time.time() * 1000000), suffix))
- print(inp_path, tmp_path)
- # 支持中文路径
- # os.link(inp_path, tmp_path)#win用硬链接
- os.symlink(inp_path, tmp_path) # linux用软链接
- frame = cv2.imread(tmp_path)[:, :, [2, 1, 0]]
- t0 = ttime()
- result = upscaler2x(frame, tile_mode=tile_mode)[:, :, ::-1]
- t1 = ttime()
- print(prefix, "done", t1 - t0)
- tmp_opt_path = os.path.join(root_path, "tmp", "%s.%s" % (int(time.time() * 1000000), suffix))
- cv2.imwrite(tmp_opt_path, result)
- n = 0
- while (1):
- if (n == 0):
- suffix = "_%sx_tile%s.png" % (scale, tile_mode)
- else:
- suffix = "_%sx_tile%s_%s.png" % (scale, tile_mode, n) #
- if (os.path.exists(os.path.join(output_dir, prefix + suffix)) == False):
- break
- else:
- n += 1
- final_opt_path = os.path.join(output_dir, prefix + suffix)
- os.rename(tmp_opt_path, final_opt_path)
- os.remove(tmp_path)
diff --git a/spaces/Ababababababbababa/Ashaar/poetry_diacritizer/models/cbhg.py b/spaces/Ababababababbababa/Ashaar/poetry_diacritizer/models/cbhg.py
deleted file mode 100644
index c2b8f061d10ec6b1a9490029a4b4ed43fdd5e861..0000000000000000000000000000000000000000
--- a/spaces/Ababababababbababa/Ashaar/poetry_diacritizer/models/cbhg.py
+++ /dev/null
@@ -1,121 +0,0 @@
-"""
-The CBHG model implementation
-"""
-from typing import List, Optional
-
-from torch import nn
-import torch
-
-from poetry_diacritizer.modules.tacotron_modules import CBHG, Prenet
-
-
-class CBHGModel(nn.Module):
- """CBHG model implementation as described in the paper:
- https://ieeexplore.ieee.org/document/9274427
-
- Args:
- inp_vocab_size (int): the number of the input symbols
- targ_vocab_size (int): the number of the target symbols (diacritics)
- embedding_dim (int): the embedding size
- use_prenet (bool): whether to use prenet or not
- prenet_sizes (List[int]): the sizes of the prenet networks
- cbhg_gru_units (int): the number of units of the CBHG GRU, which is the last
- layer of the CBHG Model.
- cbhg_filters (int): number of filters used in the CBHG module
- cbhg_projections: projections used in the CBHG module
-
- Returns:
- diacritics Dict[str, Tensor]:
- """
-
- def __init__(
- self,
- inp_vocab_size: int,
- targ_vocab_size: int,
- embedding_dim: int = 512,
- use_prenet: bool = True,
- prenet_sizes: List[int] = [512, 256],
- cbhg_gru_units: int = 512,
- cbhg_filters: int = 16,
- cbhg_projections: List[int] = [128, 256],
- post_cbhg_layers_units: List[int] = [256, 256],
- post_cbhg_use_batch_norm: bool = True
- ):
- super().__init__()
- self.use_prenet = use_prenet
- self.embedding = nn.Embedding(inp_vocab_size, embedding_dim)
- if self.use_prenet:
- self.prenet = Prenet(embedding_dim, prenet_depth=prenet_sizes)
-
- self.cbhg = CBHG(
- prenet_sizes[-1] if self.use_prenet else embedding_dim,
- cbhg_gru_units,
- K=cbhg_filters,
- projections=cbhg_projections,
- )
-
- layers = []
- post_cbhg_layers_units = [cbhg_gru_units] + post_cbhg_layers_units
-
- for i in range(1, len(post_cbhg_layers_units)):
- layers.append(
- nn.LSTM(
- post_cbhg_layers_units[i - 1] * 2,
- post_cbhg_layers_units[i],
- bidirectional=True,
- batch_first=True,
- )
- )
- if post_cbhg_use_batch_norm:
- layers.append(nn.BatchNorm1d(post_cbhg_layers_units[i] * 2))
-
- self.post_cbhg_layers = nn.ModuleList(layers)
- self.projections = nn.Linear(post_cbhg_layers_units[-1] * 2, targ_vocab_size)
- self.post_cbhg_layers_units = post_cbhg_layers_units
- self.post_cbhg_use_batch_norm = post_cbhg_use_batch_norm
-
-
- def forward(
- self,
- src: torch.Tensor,
- lengths: Optional[torch.Tensor] = None,
- target: Optional[torch.Tensor] = None, # not required in this model
- ):
- """Compute forward propagation"""
-
- # src = [batch_size, src len]
- # lengths = [batch_size]
- # target = [batch_size, trg len]
-
- embedding_out = self.embedding(src)
- # embedding_out; [batch_size, src_len, embedding_dim]
-
- cbhg_input = embedding_out
- if self.use_prenet:
- cbhg_input = self.prenet(embedding_out)
-
- # cbhg_input = [batch_size, src_len, prenet_sizes[-1]]
-
- outputs = self.cbhg(cbhg_input, lengths)
-
- hn = torch.zeros((2, 2, 2))
- cn = torch.zeros((2, 2, 2))
-
- for i, layer in enumerate(self.post_cbhg_layers):
- if isinstance(layer, nn.BatchNorm1d):
- outputs = layer(outputs.permute(0, 2, 1))
- outputs = outputs.permute(0, 2, 1)
- continue
- if i > 0:
- outputs, (hn, cn) = layer(outputs, (hn, cn))
- else:
- outputs, (hn, cn) = layer(outputs)
-
-
- predictions = self.projections(outputs)
-
- # predictions = [batch_size, src len, targ_vocab_size]
-
- output = {"diacritics": predictions}
-
- return output
diff --git a/spaces/AgentVerse/agentVerse/ui/src/phaser3-rex-plugins/plugins/live2d.d.ts b/spaces/AgentVerse/agentVerse/ui/src/phaser3-rex-plugins/plugins/live2d.d.ts
deleted file mode 100644
index a7643f51064340637f336640554ccf92c6da12bd..0000000000000000000000000000000000000000
--- a/spaces/AgentVerse/agentVerse/ui/src/phaser3-rex-plugins/plugins/live2d.d.ts
+++ /dev/null
@@ -1,11 +0,0 @@
-import {
- Live2dCoreScriptFileCallback,
- Live2dFileCallback,
- Live2dGameObject
-} from './gameobjects/live2d/index';
-
-export {
- Live2dCoreScriptFileCallback,
- Live2dFileCallback,
- Live2dGameObject
-};
\ No newline at end of file
diff --git a/spaces/AgentVerse/agentVerse/ui/src/phaser3-rex-plugins/templates/bejeweled/board/chess/RandomSymobl.js b/spaces/AgentVerse/agentVerse/ui/src/phaser3-rex-plugins/templates/bejeweled/board/chess/RandomSymobl.js
deleted file mode 100644
index cc8acc0dbf480e6ff875a50fe52c77e162b2462a..0000000000000000000000000000000000000000
--- a/spaces/AgentVerse/agentVerse/ui/src/phaser3-rex-plugins/templates/bejeweled/board/chess/RandomSymobl.js
+++ /dev/null
@@ -1,38 +0,0 @@
-const GetRandom = Phaser.Utils.Array.GetRandom;
-
-var RandomSymbol = function (board, tileX, tileY, callback, scope, excluded) {
- var symbol;
- if (Array.isArray(callback)) {
- // pick random symbol from symbol array
- var symbols = callback;
- // excluded: undefined or a symbol array
- if (excluded !== undefined) {
- for (var i = 0, cnt = symbols.length; i < cnt; i++) {
- symbol = symbols[i];
- if (excluded.indexOf(symbol) !== -1) {
- continue;
- }
- tmpSymbolArray.push(symbol);
- }
- symbol = GetRandom(tmpSymbolArray);
- tmpSymbolArray.length = 0;
- } else {
- symbol = GetRandom(symbols);
- }
-
- } else if (typeof (obj) === 'function') {
- // symbols from return of callback
- if (scope) {
- symbol = callback.call(scope, board, tileX, tileY, excluded);
- } else {
- symbol = callback(board, tileX, tileY, excluded);
- }
- } else {
- // symbol value
- symbol = callback;
- }
- return symbol;
-}
-
-var tmpSymbolArray = [];
-export default RandomSymbol;
\ No newline at end of file
diff --git a/spaces/AgentVerse/agentVerse/ui/src/phaser3-rex-plugins/templates/ui/press/Factory.js b/spaces/AgentVerse/agentVerse/ui/src/phaser3-rex-plugins/templates/ui/press/Factory.js
deleted file mode 100644
index e1802b97f642d78d73c567e21872d20728956fbc..0000000000000000000000000000000000000000
--- a/spaces/AgentVerse/agentVerse/ui/src/phaser3-rex-plugins/templates/ui/press/Factory.js
+++ /dev/null
@@ -1,16 +0,0 @@
-import Press from './Press.js';
-import ObjectFactory from '../ObjectFactory.js';
-import IsGameObject from '../../../plugins/utils/system/IsGameObject.js';
-import SetValue from '../../../plugins/utils/object/SetValue.js';
-
-ObjectFactory.register('press', function (gameObject, config) {
- if (!IsGameObject(gameObject)) {
- config = gameObject;
- gameObject = this.scene;
- }
- return new Press(gameObject, config);
-});
-
-SetValue(window, 'RexPlugins.UI.Press', Press);
-
-export default Press;
\ No newline at end of file
diff --git a/spaces/AjulorC/question_answering_bot_deployed_with_Gradio/app.py b/spaces/AjulorC/question_answering_bot_deployed_with_Gradio/app.py
deleted file mode 100644
index c033545d64482e6b61b23036a98cfa1fdbe8cc47..0000000000000000000000000000000000000000
--- a/spaces/AjulorC/question_answering_bot_deployed_with_Gradio/app.py
+++ /dev/null
@@ -1,39 +0,0 @@
-import tensorflow as tf
-
-#!pip install transformers
-
-from transformers import pipeline
-
-# importing necessary libraries
-from transformers import AutoTokenizer, TFAutoModelForQuestionAnswering
-
-
-tokenizer = AutoTokenizer.from_pretrained("bert-large-uncased-whole-word-masking-finetuned-squad")
-model = TFAutoModelForQuestionAnswering.from_pretrained("bert-large-uncased-whole-word-masking-finetuned-squad",return_dict=False)
-
-nlp = pipeline("question-answering", model=model, tokenizer=tokenizer)
-
-#!pip install gradio
-import gradio as gr
-
-# creating the function
-def func(context, question):
- result = nlp(question = question, context=context)
- return result['answer']
-
-example_1 = "(1) My name is Ajulor Christian, I am a data scientist and machine learning engineer"
-qst_1 = "what is christian's profession?"
-
-example_2 = "(2) Natural Language Processing (NLP) allows machines to break down and interpret human language. It's at the core of tools we use every day – from translation software, chatbots, spam filters, and search engines, to grammar correction software, voice assistants, and social media monitoring tools."
-qst_2 = "What is NLP used for?"
-
-# creating the interface
-app = gr.Interface(fn=func, inputs = ['textbox', 'text'], outputs = 'textbox',
- title = 'Question Answering bot', theme = 'dark-grass',
- description = 'Input context and question, then get answers!',
- examples = [[example_1, qst_1],
- [example_2, qst_2]]
- )
-
-# launching the app
-app.launch(inline=False)
\ No newline at end of file
diff --git a/spaces/Akmyradov/TurkmenTTSweSTT/vits/modules.py b/spaces/Akmyradov/TurkmenTTSweSTT/vits/modules.py
deleted file mode 100644
index 9c7fd9cd6eb8b7e0ec0e08957e970744a374a924..0000000000000000000000000000000000000000
--- a/spaces/Akmyradov/TurkmenTTSweSTT/vits/modules.py
+++ /dev/null
@@ -1,390 +0,0 @@
-import copy
-import math
-import numpy as np
-import scipy
-import torch
-from torch import nn
-from torch.nn import functional as F
-
-from torch.nn import Conv1d, ConvTranspose1d, AvgPool1d, Conv2d
-from torch.nn.utils import weight_norm, remove_weight_norm
-
-import commons
-from commons import init_weights, get_padding
-from transforms import piecewise_rational_quadratic_transform
-
-
-LRELU_SLOPE = 0.1
-
-
-class LayerNorm(nn.Module):
- def __init__(self, channels, eps=1e-5):
- super().__init__()
- self.channels = channels
- self.eps = eps
-
- self.gamma = nn.Parameter(torch.ones(channels))
- self.beta = nn.Parameter(torch.zeros(channels))
-
- def forward(self, x):
- x = x.transpose(1, -1)
- x = F.layer_norm(x, (self.channels,), self.gamma, self.beta, self.eps)
- return x.transpose(1, -1)
-
-
-class ConvReluNorm(nn.Module):
- def __init__(self, in_channels, hidden_channels, out_channels, kernel_size, n_layers, p_dropout):
- super().__init__()
- self.in_channels = in_channels
- self.hidden_channels = hidden_channels
- self.out_channels = out_channels
- self.kernel_size = kernel_size
- self.n_layers = n_layers
- self.p_dropout = p_dropout
- assert n_layers > 1, "Number of layers should be larger than 0."
-
- self.conv_layers = nn.ModuleList()
- self.norm_layers = nn.ModuleList()
- self.conv_layers.append(nn.Conv1d(in_channels, hidden_channels, kernel_size, padding=kernel_size//2))
- self.norm_layers.append(LayerNorm(hidden_channels))
- self.relu_drop = nn.Sequential(
- nn.ReLU(),
- nn.Dropout(p_dropout))
- for _ in range(n_layers-1):
- self.conv_layers.append(nn.Conv1d(hidden_channels, hidden_channels, kernel_size, padding=kernel_size//2))
- self.norm_layers.append(LayerNorm(hidden_channels))
- self.proj = nn.Conv1d(hidden_channels, out_channels, 1)
- self.proj.weight.data.zero_()
- self.proj.bias.data.zero_()
-
- def forward(self, x, x_mask):
- x_org = x
- for i in range(self.n_layers):
- x = self.conv_layers[i](x * x_mask)
- x = self.norm_layers[i](x)
- x = self.relu_drop(x)
- x = x_org + self.proj(x)
- return x * x_mask
-
-
-class DDSConv(nn.Module):
- """
- Dialted and Depth-Separable Convolution
- """
- def __init__(self, channels, kernel_size, n_layers, p_dropout=0.):
- super().__init__()
- self.channels = channels
- self.kernel_size = kernel_size
- self.n_layers = n_layers
- self.p_dropout = p_dropout
-
- self.drop = nn.Dropout(p_dropout)
- self.convs_sep = nn.ModuleList()
- self.convs_1x1 = nn.ModuleList()
- self.norms_1 = nn.ModuleList()
- self.norms_2 = nn.ModuleList()
- for i in range(n_layers):
- dilation = kernel_size ** i
- padding = (kernel_size * dilation - dilation) // 2
- self.convs_sep.append(nn.Conv1d(channels, channels, kernel_size,
- groups=channels, dilation=dilation, padding=padding
- ))
- self.convs_1x1.append(nn.Conv1d(channels, channels, 1))
- self.norms_1.append(LayerNorm(channels))
- self.norms_2.append(LayerNorm(channels))
-
- def forward(self, x, x_mask, g=None):
- if g is not None:
- x = x + g
- for i in range(self.n_layers):
- y = self.convs_sep[i](x * x_mask)
- y = self.norms_1[i](y)
- y = F.gelu(y)
- y = self.convs_1x1[i](y)
- y = self.norms_2[i](y)
- y = F.gelu(y)
- y = self.drop(y)
- x = x + y
- return x * x_mask
-
-
-class WN(torch.nn.Module):
- def __init__(self, hidden_channels, kernel_size, dilation_rate, n_layers, gin_channels=0, p_dropout=0):
- super(WN, self).__init__()
- assert(kernel_size % 2 == 1)
- self.hidden_channels =hidden_channels
- self.kernel_size = kernel_size,
- self.dilation_rate = dilation_rate
- self.n_layers = n_layers
- self.gin_channels = gin_channels
- self.p_dropout = p_dropout
-
- self.in_layers = torch.nn.ModuleList()
- self.res_skip_layers = torch.nn.ModuleList()
- self.drop = nn.Dropout(p_dropout)
-
- if gin_channels != 0:
- cond_layer = torch.nn.Conv1d(gin_channels, 2*hidden_channels*n_layers, 1)
- self.cond_layer = torch.nn.utils.weight_norm(cond_layer, name='weight')
-
- for i in range(n_layers):
- dilation = dilation_rate ** i
- padding = int((kernel_size * dilation - dilation) / 2)
- in_layer = torch.nn.Conv1d(hidden_channels, 2*hidden_channels, kernel_size,
- dilation=dilation, padding=padding)
- in_layer = torch.nn.utils.weight_norm(in_layer, name='weight')
- self.in_layers.append(in_layer)
-
- # last one is not necessary
- if i < n_layers - 1:
- res_skip_channels = 2 * hidden_channels
- else:
- res_skip_channels = hidden_channels
-
- res_skip_layer = torch.nn.Conv1d(hidden_channels, res_skip_channels, 1)
- res_skip_layer = torch.nn.utils.weight_norm(res_skip_layer, name='weight')
- self.res_skip_layers.append(res_skip_layer)
-
- def forward(self, x, x_mask, g=None, **kwargs):
- output = torch.zeros_like(x)
- n_channels_tensor = torch.IntTensor([self.hidden_channels])
-
- if g is not None:
- g = self.cond_layer(g)
-
- for i in range(self.n_layers):
- x_in = self.in_layers[i](x)
- if g is not None:
- cond_offset = i * 2 * self.hidden_channels
- g_l = g[:,cond_offset:cond_offset+2*self.hidden_channels,:]
- else:
- g_l = torch.zeros_like(x_in)
-
- acts = commons.fused_add_tanh_sigmoid_multiply(
- x_in,
- g_l,
- n_channels_tensor)
- acts = self.drop(acts)
-
- res_skip_acts = self.res_skip_layers[i](acts)
- if i < self.n_layers - 1:
- res_acts = res_skip_acts[:,:self.hidden_channels,:]
- x = (x + res_acts) * x_mask
- output = output + res_skip_acts[:,self.hidden_channels:,:]
- else:
- output = output + res_skip_acts
- return output * x_mask
-
- def remove_weight_norm(self):
- if self.gin_channels != 0:
- torch.nn.utils.remove_weight_norm(self.cond_layer)
- for l in self.in_layers:
- torch.nn.utils.remove_weight_norm(l)
- for l in self.res_skip_layers:
- torch.nn.utils.remove_weight_norm(l)
-
-
-class ResBlock1(torch.nn.Module):
- def __init__(self, channels, kernel_size=3, dilation=(1, 3, 5)):
- super(ResBlock1, self).__init__()
- self.convs1 = nn.ModuleList([
- weight_norm(Conv1d(channels, channels, kernel_size, 1, dilation=dilation[0],
- padding=get_padding(kernel_size, dilation[0]))),
- weight_norm(Conv1d(channels, channels, kernel_size, 1, dilation=dilation[1],
- padding=get_padding(kernel_size, dilation[1]))),
- weight_norm(Conv1d(channels, channels, kernel_size, 1, dilation=dilation[2],
- padding=get_padding(kernel_size, dilation[2])))
- ])
- self.convs1.apply(init_weights)
-
- self.convs2 = nn.ModuleList([
- weight_norm(Conv1d(channels, channels, kernel_size, 1, dilation=1,
- padding=get_padding(kernel_size, 1))),
- weight_norm(Conv1d(channels, channels, kernel_size, 1, dilation=1,
- padding=get_padding(kernel_size, 1))),
- weight_norm(Conv1d(channels, channels, kernel_size, 1, dilation=1,
- padding=get_padding(kernel_size, 1)))
- ])
- self.convs2.apply(init_weights)
-
- def forward(self, x, x_mask=None):
- for c1, c2 in zip(self.convs1, self.convs2):
- xt = F.leaky_relu(x, LRELU_SLOPE)
- if x_mask is not None:
- xt = xt * x_mask
- xt = c1(xt)
- xt = F.leaky_relu(xt, LRELU_SLOPE)
- if x_mask is not None:
- xt = xt * x_mask
- xt = c2(xt)
- x = xt + x
- if x_mask is not None:
- x = x * x_mask
- return x
-
- def remove_weight_norm(self):
- for l in self.convs1:
- remove_weight_norm(l)
- for l in self.convs2:
- remove_weight_norm(l)
-
-
-class ResBlock2(torch.nn.Module):
- def __init__(self, channels, kernel_size=3, dilation=(1, 3)):
- super(ResBlock2, self).__init__()
- self.convs = nn.ModuleList([
- weight_norm(Conv1d(channels, channels, kernel_size, 1, dilation=dilation[0],
- padding=get_padding(kernel_size, dilation[0]))),
- weight_norm(Conv1d(channels, channels, kernel_size, 1, dilation=dilation[1],
- padding=get_padding(kernel_size, dilation[1])))
- ])
- self.convs.apply(init_weights)
-
- def forward(self, x, x_mask=None):
- for c in self.convs:
- xt = F.leaky_relu(x, LRELU_SLOPE)
- if x_mask is not None:
- xt = xt * x_mask
- xt = c(xt)
- x = xt + x
- if x_mask is not None:
- x = x * x_mask
- return x
-
- def remove_weight_norm(self):
- for l in self.convs:
- remove_weight_norm(l)
-
-
-class Log(nn.Module):
- def forward(self, x, x_mask, reverse=False, **kwargs):
- if not reverse:
- y = torch.log(torch.clamp_min(x, 1e-5)) * x_mask
- logdet = torch.sum(-y, [1, 2])
- return y, logdet
- else:
- x = torch.exp(x) * x_mask
- return x
-
-
-class Flip(nn.Module):
- def forward(self, x, *args, reverse=False, **kwargs):
- x = torch.flip(x, [1])
- if not reverse:
- logdet = torch.zeros(x.size(0)).to(dtype=x.dtype, device=x.device)
- return x, logdet
- else:
- return x
-
-
-class ElementwiseAffine(nn.Module):
- def __init__(self, channels):
- super().__init__()
- self.channels = channels
- self.m = nn.Parameter(torch.zeros(channels,1))
- self.logs = nn.Parameter(torch.zeros(channels,1))
-
- def forward(self, x, x_mask, reverse=False, **kwargs):
- if not reverse:
- y = self.m + torch.exp(self.logs) * x
- y = y * x_mask
- logdet = torch.sum(self.logs * x_mask, [1,2])
- return y, logdet
- else:
- x = (x - self.m) * torch.exp(-self.logs) * x_mask
- return x
-
-
-class ResidualCouplingLayer(nn.Module):
- def __init__(self,
- channels,
- hidden_channels,
- kernel_size,
- dilation_rate,
- n_layers,
- p_dropout=0,
- gin_channels=0,
- mean_only=False):
- assert channels % 2 == 0, "channels should be divisible by 2"
- super().__init__()
- self.channels = channels
- self.hidden_channels = hidden_channels
- self.kernel_size = kernel_size
- self.dilation_rate = dilation_rate
- self.n_layers = n_layers
- self.half_channels = channels // 2
- self.mean_only = mean_only
-
- self.pre = nn.Conv1d(self.half_channels, hidden_channels, 1)
- self.enc = WN(hidden_channels, kernel_size, dilation_rate, n_layers, p_dropout=p_dropout, gin_channels=gin_channels)
- self.post = nn.Conv1d(hidden_channels, self.half_channels * (2 - mean_only), 1)
- self.post.weight.data.zero_()
- self.post.bias.data.zero_()
-
- def forward(self, x, x_mask, g=None, reverse=False):
- x0, x1 = torch.split(x, [self.half_channels]*2, 1)
- h = self.pre(x0) * x_mask
- h = self.enc(h, x_mask, g=g)
- stats = self.post(h) * x_mask
- if not self.mean_only:
- m, logs = torch.split(stats, [self.half_channels]*2, 1)
- else:
- m = stats
- logs = torch.zeros_like(m)
-
- if not reverse:
- x1 = m + x1 * torch.exp(logs) * x_mask
- x = torch.cat([x0, x1], 1)
- logdet = torch.sum(logs, [1,2])
- return x, logdet
- else:
- x1 = (x1 - m) * torch.exp(-logs) * x_mask
- x = torch.cat([x0, x1], 1)
- return x
-
-
-class ConvFlow(nn.Module):
- def __init__(self, in_channels, filter_channels, kernel_size, n_layers, num_bins=10, tail_bound=5.0):
- super().__init__()
- self.in_channels = in_channels
- self.filter_channels = filter_channels
- self.kernel_size = kernel_size
- self.n_layers = n_layers
- self.num_bins = num_bins
- self.tail_bound = tail_bound
- self.half_channels = in_channels // 2
-
- self.pre = nn.Conv1d(self.half_channels, filter_channels, 1)
- self.convs = DDSConv(filter_channels, kernel_size, n_layers, p_dropout=0.)
- self.proj = nn.Conv1d(filter_channels, self.half_channels * (num_bins * 3 - 1), 1)
- self.proj.weight.data.zero_()
- self.proj.bias.data.zero_()
-
- def forward(self, x, x_mask, g=None, reverse=False):
- x0, x1 = torch.split(x, [self.half_channels]*2, 1)
- h = self.pre(x0)
- h = self.convs(h, x_mask, g=g)
- h = self.proj(h) * x_mask
-
- b, c, t = x0.shape
- h = h.reshape(b, c, -1, t).permute(0, 1, 3, 2) # [b, cx?, t] -> [b, c, t, ?]
-
- unnormalized_widths = h[..., :self.num_bins] / math.sqrt(self.filter_channels)
- unnormalized_heights = h[..., self.num_bins:2*self.num_bins] / math.sqrt(self.filter_channels)
- unnormalized_derivatives = h[..., 2 * self.num_bins:]
-
- x1, logabsdet = piecewise_rational_quadratic_transform(x1,
- unnormalized_widths,
- unnormalized_heights,
- unnormalized_derivatives,
- inverse=reverse,
- tails='linear',
- tail_bound=self.tail_bound
- )
-
- x = torch.cat([x0, x1], 1) * x_mask
- logdet = torch.sum(logabsdet * x_mask, [1,2])
- if not reverse:
- return x, logdet
- else:
- return x
diff --git a/spaces/AlexWang/lama/bin/mask_example.py b/spaces/AlexWang/lama/bin/mask_example.py
deleted file mode 100644
index 59e25ca8eb3ed4141851c3af284fc66285444de0..0000000000000000000000000000000000000000
--- a/spaces/AlexWang/lama/bin/mask_example.py
+++ /dev/null
@@ -1,14 +0,0 @@
-import matplotlib.pyplot as plt
-from skimage import io
-from skimage.transform import resize
-
-from saicinpainting.evaluation.masks.mask import SegmentationMask
-
-im = io.imread('imgs/ex4.jpg')
-im = resize(im, (512, 1024), anti_aliasing=True)
-mask_seg = SegmentationMask(num_variants_per_mask=10)
-mask_examples = mask_seg.get_masks(im)
-for i, example in enumerate(mask_examples):
- plt.imshow(example)
- plt.show()
- plt.imsave(f'tmp/img_masks/{i}.png', example)
diff --git a/spaces/Androidonnxfork/CivitAi-to-Diffusers/diffusers/docs/source/en/training/text_inversion.md b/spaces/Androidonnxfork/CivitAi-to-Diffusers/diffusers/docs/source/en/training/text_inversion.md
deleted file mode 100644
index 050b0ca3d40384f3060429c0e6d377820eb78cd5..0000000000000000000000000000000000000000
--- a/spaces/Androidonnxfork/CivitAi-to-Diffusers/diffusers/docs/source/en/training/text_inversion.md
+++ /dev/null
@@ -1,277 +0,0 @@
-
-
-
-
-# Textual Inversion
-
-[Textual Inversion](https://arxiv.org/abs/2208.01618) is a technique for capturing novel concepts from a small number of example images. While the technique was originally demonstrated with a [latent diffusion model](https://github.com/CompVis/latent-diffusion), it has since been applied to other model variants like [Stable Diffusion](https://huggingface.co/docs/diffusers/main/en/conceptual/stable_diffusion). The learned concepts can be used to better control the images generated from text-to-image pipelines. It learns new "words" in the text encoder's embedding space, which are used within text prompts for personalized image generation.
-
-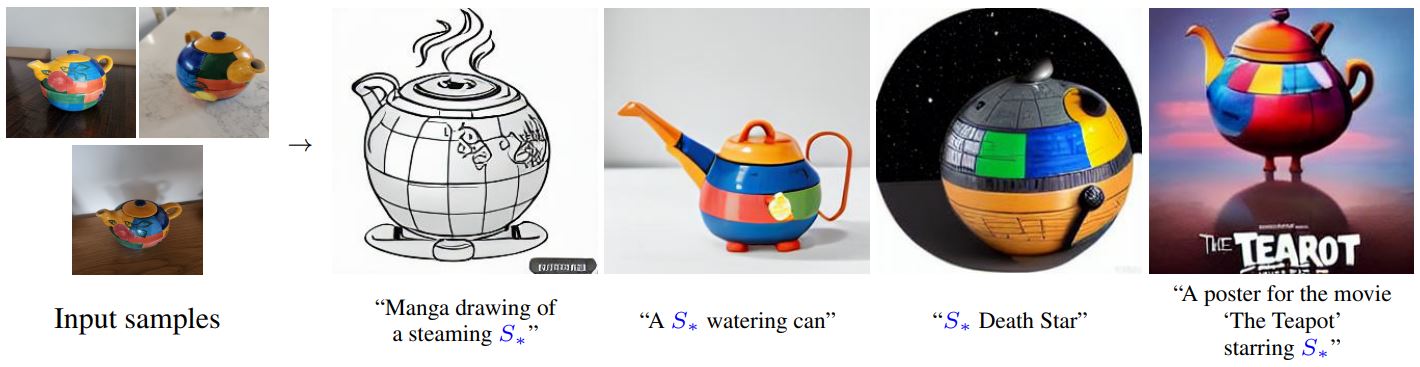
-By using just 3-5 images you can teach new concepts to a model such as Stable Diffusion for personalized image generation (image source).
-
-This guide will show you how to train a [`runwayml/stable-diffusion-v1-5`](https://huggingface.co/runwayml/stable-diffusion-v1-5) model with Textual Inversion. All the training scripts for Textual Inversion used in this guide can be found [here](https://github.com/huggingface/diffusers/tree/main/examples/textual_inversion) if you're interested in taking a closer look at how things work under the hood.
-
-
-
-There is a community-created collection of trained Textual Inversion models in the [Stable Diffusion Textual Inversion Concepts Library](https://huggingface.co/sd-concepts-library) which are readily available for inference. Over time, this'll hopefully grow into a useful resource as more concepts are added!
-
-
-
-Before you begin, make sure you install the library's training dependencies:
-
-```bash
-pip install diffusers accelerate transformers
-```
-
-After all the dependencies have been set up, initialize a [🤗Accelerate](https://github.com/huggingface/accelerate/) environment with:
-
-```bash
-accelerate config
-```
-
-To setup a default 🤗 Accelerate environment without choosing any configurations:
-
-```bash
-accelerate config default
-```
-
-Or if your environment doesn't support an interactive shell like a notebook, you can use:
-
-```bash
-from accelerate.utils import write_basic_config
-
-write_basic_config()
-```
-
-Finally, you try and [install xFormers](https://huggingface.co/docs/diffusers/main/en/training/optimization/xformers) to reduce your memory footprint with xFormers memory-efficient attention. Once you have xFormers installed, add the `--enable_xformers_memory_efficient_attention` argument to the training script. xFormers is not supported for Flax.
-
-## Upload model to Hub
-
-If you want to store your model on the Hub, add the following argument to the training script:
-
-```bash
---push_to_hub
-```
-
-## Save and load checkpoints
-
-It is often a good idea to regularly save checkpoints of your model during training. This way, you can resume training from a saved checkpoint if your training is interrupted for any reason. To save a checkpoint, pass the following argument to the training script to save the full training state in a subfolder in `output_dir` every 500 steps:
-
-```bash
---checkpointing_steps=500
-```
-
-To resume training from a saved checkpoint, pass the following argument to the training script and the specific checkpoint you'd like to resume from:
-
-```bash
---resume_from_checkpoint="checkpoint-1500"
-```
-
-## Finetuning
-
-For your training dataset, download these [images of a cat toy](https://huggingface.co/datasets/diffusers/cat_toy_example) and store them in a directory. To use your own dataset, take a look at the [Create a dataset for training](create_dataset) guide.
-
-```py
-from huggingface_hub import snapshot_download
-
-local_dir = "./cat"
-snapshot_download(
- "diffusers/cat_toy_example", local_dir=local_dir, repo_type="dataset", ignore_patterns=".gitattributes"
-)
-```
-
-Specify the `MODEL_NAME` environment variable (either a Hub model repository id or a path to the directory containing the model weights) and pass it to the [`pretrained_model_name_or_path`](https://huggingface.co/docs/diffusers/en/api/diffusion_pipeline#diffusers.DiffusionPipeline.from_pretrained.pretrained_model_name_or_path) argument, and the `DATA_DIR` environment variable to the path of the directory containing the images.
-
-Now you can launch the [training script](https://github.com/huggingface/diffusers/blob/main/examples/textual_inversion/textual_inversion.py). The script creates and saves the following files to your repository: `learned_embeds.bin`, `token_identifier.txt`, and `type_of_concept.txt`.
-
-
-
-💡 A full training run takes ~1 hour on one V100 GPU. While you're waiting for the training to complete, feel free to check out [how Textual Inversion works](#how-it-works) in the section below if you're curious!
-
-
-
-
-
-```bash
-export MODEL_NAME="runwayml/stable-diffusion-v1-5"
-export DATA_DIR="./cat"
-
-accelerate launch textual_inversion.py \
- --pretrained_model_name_or_path=$MODEL_NAME \
- --train_data_dir=$DATA_DIR \
- --learnable_property="object" \
- --placeholder_token="" --initializer_token="toy" \
- --resolution=512 \
- --train_batch_size=1 \
- --gradient_accumulation_steps=4 \
- --max_train_steps=3000 \
- --learning_rate=5.0e-04 --scale_lr \
- --lr_scheduler="constant" \
- --lr_warmup_steps=0 \
- --output_dir="textual_inversion_cat" \
- --push_to_hub
-```
-
-
-
-💡 If you want to increase the trainable capacity, you can associate your placeholder token, *e.g.* `` to
-multiple embedding vectors. This can help the model to better capture the style of more (complex) images.
-To enable training multiple embedding vectors, simply pass:
-
-```bash
---num_vectors=5
-```
-
-
-
-
-If you have access to TPUs, try out the [Flax training script](https://github.com/huggingface/diffusers/blob/main/examples/textual_inversion/textual_inversion_flax.py) to train even faster (this'll also work for GPUs). With the same configuration settings, the Flax training script should be at least 70% faster than the PyTorch training script! ⚡️
-
-Before you begin, make sure you install the Flax specific dependencies:
-
-```bash
-pip install -U -r requirements_flax.txt
-```
-
-Specify the `MODEL_NAME` environment variable (either a Hub model repository id or a path to the directory containing the model weights) and pass it to the [`pretrained_model_name_or_path`](https://huggingface.co/docs/diffusers/en/api/diffusion_pipeline#diffusers.DiffusionPipeline.from_pretrained.pretrained_model_name_or_path) argument.
-
-Then you can launch the [training script](https://github.com/huggingface/diffusers/blob/main/examples/textual_inversion/textual_inversion_flax.py):
-
-```bash
-export MODEL_NAME="duongna/stable-diffusion-v1-4-flax"
-export DATA_DIR="./cat"
-
-python textual_inversion_flax.py \
- --pretrained_model_name_or_path=$MODEL_NAME \
- --train_data_dir=$DATA_DIR \
- --learnable_property="object" \
- --placeholder_token="" --initializer_token="toy" \
- --resolution=512 \
- --train_batch_size=1 \
- --max_train_steps=3000 \
- --learning_rate=5.0e-04 --scale_lr \
- --output_dir="textual_inversion_cat" \
- --push_to_hub
-```
-
-
-
-### Intermediate logging
-
-If you're interested in following along with your model training progress, you can save the generated images from the training process. Add the following arguments to the training script to enable intermediate logging:
-
-- `validation_prompt`, the prompt used to generate samples (this is set to `None` by default and intermediate logging is disabled)
-- `num_validation_images`, the number of sample images to generate
-- `validation_steps`, the number of steps before generating `num_validation_images` from the `validation_prompt`
-
-```bash
---validation_prompt="A backpack"
---num_validation_images=4
---validation_steps=100
-```
-
-## Inference
-
-Once you have trained a model, you can use it for inference with the [`StableDiffusionPipeline`].
-
-The textual inversion script will by default only save the textual inversion embedding vector(s) that have
-been added to the text encoder embedding matrix and consequently been trained.
-
-
-
-
-
-💡 The community has created a large library of different textual inversion embedding vectors, called [sd-concepts-library](https://huggingface.co/sd-concepts-library).
-Instead of training textual inversion embeddings from scratch you can also see whether a fitting textual inversion embedding has already been added to the libary.
-
-
-
-To load the textual inversion embeddings you first need to load the base model that was used when training
-your textual inversion embedding vectors. Here we assume that [`runwayml/stable-diffusion-v1-5`](runwayml/stable-diffusion-v1-5)
-was used as a base model so we load it first:
-```python
-from diffusers import StableDiffusionPipeline
-import torch
-
-model_id = "runwayml/stable-diffusion-v1-5"
-pipe = StableDiffusionPipeline.from_pretrained(model_id, torch_dtype=torch.float16).to("cuda")
-```
-
-Next, we need to load the textual inversion embedding vector which can be done via the [`TextualInversionLoaderMixin.load_textual_inversion`]
-function. Here we'll load the embeddings of the "" example from before.
-```python
-pipe.load_textual_inversion("sd-concepts-library/cat-toy")
-```
-
-Now we can run the pipeline making sure that the placeholder token `` is used in our prompt.
-
-```python
-prompt = "A backpack"
-
-image = pipe(prompt, num_inference_steps=50).images[0]
-image.save("cat-backpack.png")
-```
-
-The function [`TextualInversionLoaderMixin.load_textual_inversion`] can not only
-load textual embedding vectors saved in Diffusers' format, but also embedding vectors
-saved in [Automatic1111](https://github.com/AUTOMATIC1111/stable-diffusion-webui) format.
-To do so, you can first download an embedding vector from [civitAI](https://civitai.com/models/3036?modelVersionId=8387)
-and then load it locally:
-```python
-pipe.load_textual_inversion("./charturnerv2.pt")
-```
-
-
-Currently there is no `load_textual_inversion` function for Flax so one has to make sure the textual inversion
-embedding vector is saved as part of the model after training.
-
-The model can then be run just like any other Flax model:
-
-```python
-import jax
-import numpy as np
-from flax.jax_utils import replicate
-from flax.training.common_utils import shard
-from diffusers import FlaxStableDiffusionPipeline
-
-model_path = "path-to-your-trained-model"
-pipeline, params = FlaxStableDiffusionPipeline.from_pretrained(model_path, dtype=jax.numpy.bfloat16)
-
-prompt = "A backpack"
-prng_seed = jax.random.PRNGKey(0)
-num_inference_steps = 50
-
-num_samples = jax.device_count()
-prompt = num_samples * [prompt]
-prompt_ids = pipeline.prepare_inputs(prompt)
-
-# shard inputs and rng
-params = replicate(params)
-prng_seed = jax.random.split(prng_seed, jax.device_count())
-prompt_ids = shard(prompt_ids)
-
-images = pipeline(prompt_ids, params, prng_seed, num_inference_steps, jit=True).images
-images = pipeline.numpy_to_pil(np.asarray(images.reshape((num_samples,) + images.shape[-3:])))
-image.save("cat-backpack.png")
-```
-
-
-
-## How it works
-
-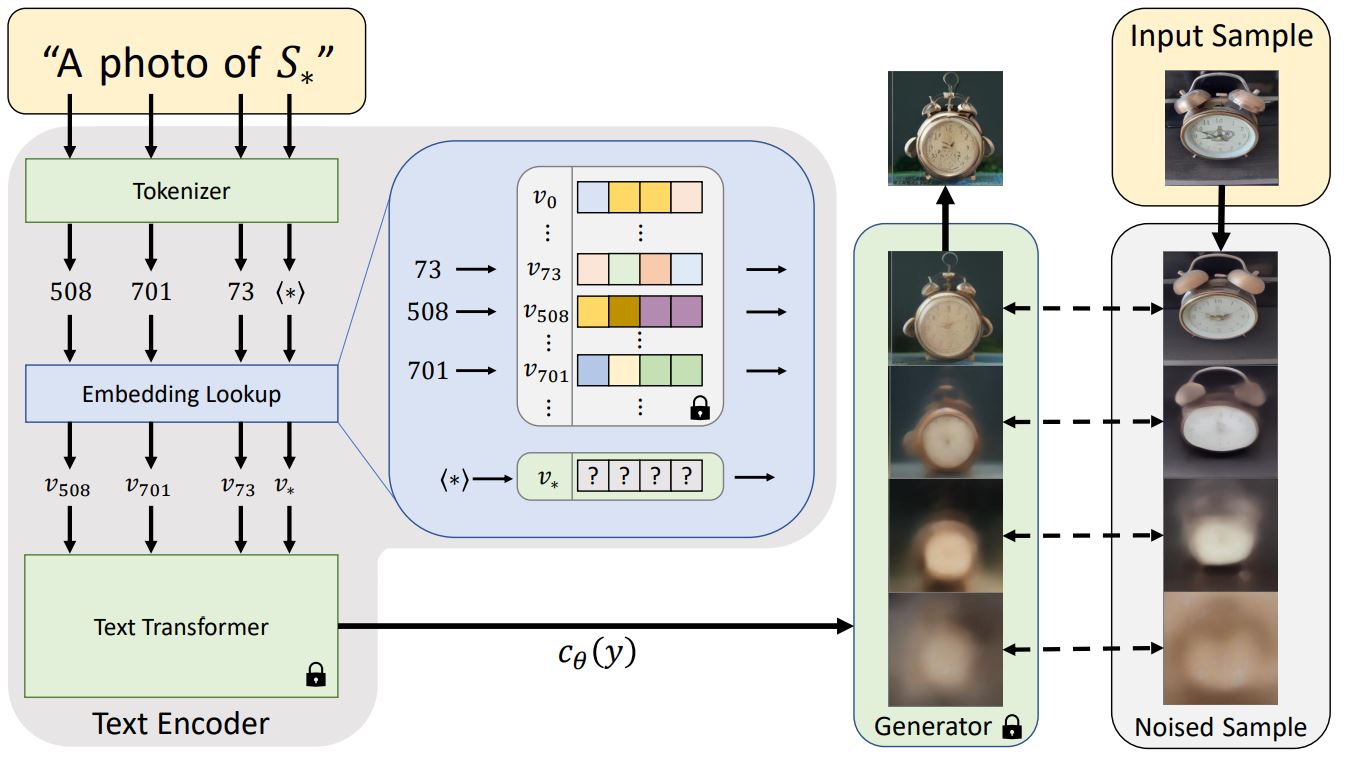
-Architecture overview from the Textual Inversion blog post.
-
-Usually, text prompts are tokenized into an embedding before being passed to a model, which is often a transformer. Textual Inversion does something similar, but it learns a new token embedding, `v*`, from a special token `S*` in the diagram above. The model output is used to condition the diffusion model, which helps the diffusion model understand the prompt and new concepts from just a few example images.
-
-To do this, Textual Inversion uses a generator model and noisy versions of the training images. The generator tries to predict less noisy versions of the images, and the token embedding `v*` is optimized based on how well the generator does. If the token embedding successfully captures the new concept, it gives more useful information to the diffusion model and helps create clearer images with less noise. This optimization process typically occurs after several thousand steps of exposure to a variety of prompt and image variants.
diff --git a/spaces/Androidonnxfork/CivitAi-to-Diffusers/diffusers/tests/pipelines/controlnet/test_controlnet.py b/spaces/Androidonnxfork/CivitAi-to-Diffusers/diffusers/tests/pipelines/controlnet/test_controlnet.py
deleted file mode 100644
index a34f81ff8dd9067cab082b641f01210198c4fb39..0000000000000000000000000000000000000000
--- a/spaces/Androidonnxfork/CivitAi-to-Diffusers/diffusers/tests/pipelines/controlnet/test_controlnet.py
+++ /dev/null
@@ -1,1002 +0,0 @@
-# coding=utf-8
-# Copyright 2023 HuggingFace Inc.
-#
-# Licensed under the Apache License, Version 2.0 (the "License");
-# you may not use this file except in compliance with the License.
-# You may obtain a copy of the License at
-#
-# http://www.apache.org/licenses/LICENSE-2.0
-#
-# Unless required by applicable law or agreed to in writing, software
-# distributed under the License is distributed on an "AS IS" BASIS,
-# WITHOUT WARRANTIES OR CONDITIONS OF ANY KIND, either express or implied.
-# See the License for the specific language governing permissions and
-# limitations under the License.
-
-import gc
-import tempfile
-import traceback
-import unittest
-
-import numpy as np
-import torch
-from transformers import CLIPTextConfig, CLIPTextModel, CLIPTokenizer
-
-from diffusers import (
- AutoencoderKL,
- ControlNetModel,
- DDIMScheduler,
- EulerDiscreteScheduler,
- StableDiffusionControlNetPipeline,
- UNet2DConditionModel,
-)
-from diffusers.pipelines.stable_diffusion.pipeline_stable_diffusion_controlnet import MultiControlNetModel
-from diffusers.utils import load_image, load_numpy, randn_tensor, slow, torch_device
-from diffusers.utils.import_utils import is_xformers_available
-from diffusers.utils.testing_utils import (
- enable_full_determinism,
- require_torch_2,
- require_torch_gpu,
- run_test_in_subprocess,
-)
-
-from ..pipeline_params import (
- IMAGE_TO_IMAGE_IMAGE_PARAMS,
- TEXT_TO_IMAGE_BATCH_PARAMS,
- TEXT_TO_IMAGE_IMAGE_PARAMS,
- TEXT_TO_IMAGE_PARAMS,
-)
-from ..test_pipelines_common import (
- PipelineKarrasSchedulerTesterMixin,
- PipelineLatentTesterMixin,
- PipelineTesterMixin,
-)
-
-
-enable_full_determinism()
-
-
-# Will be run via run_test_in_subprocess
-def _test_stable_diffusion_compile(in_queue, out_queue, timeout):
- error = None
- try:
- _ = in_queue.get(timeout=timeout)
-
- controlnet = ControlNetModel.from_pretrained("lllyasviel/sd-controlnet-canny")
-
- pipe = StableDiffusionControlNetPipeline.from_pretrained(
- "runwayml/stable-diffusion-v1-5", safety_checker=None, controlnet=controlnet
- )
- pipe.to("cuda")
- pipe.set_progress_bar_config(disable=None)
-
- pipe.unet.to(memory_format=torch.channels_last)
- pipe.unet = torch.compile(pipe.unet, mode="reduce-overhead", fullgraph=True)
-
- pipe.controlnet.to(memory_format=torch.channels_last)
- pipe.controlnet = torch.compile(pipe.controlnet, mode="reduce-overhead", fullgraph=True)
-
- generator = torch.Generator(device="cpu").manual_seed(0)
- prompt = "bird"
- image = load_image(
- "https://huggingface.co/datasets/hf-internal-testing/diffusers-images/resolve/main/sd_controlnet/bird_canny.png"
- )
-
- output = pipe(prompt, image, generator=generator, output_type="np")
- image = output.images[0]
-
- assert image.shape == (768, 512, 3)
-
- expected_image = load_numpy(
- "https://huggingface.co/datasets/hf-internal-testing/diffusers-images/resolve/main/sd_controlnet/bird_canny_out_full.npy"
- )
-
- assert np.abs(expected_image - image).max() < 1.0
-
- except Exception:
- error = f"{traceback.format_exc()}"
-
- results = {"error": error}
- out_queue.put(results, timeout=timeout)
- out_queue.join()
-
-
-class ControlNetPipelineFastTests(
- PipelineLatentTesterMixin, PipelineKarrasSchedulerTesterMixin, PipelineTesterMixin, unittest.TestCase
-):
- pipeline_class = StableDiffusionControlNetPipeline
- params = TEXT_TO_IMAGE_PARAMS
- batch_params = TEXT_TO_IMAGE_BATCH_PARAMS
- image_params = IMAGE_TO_IMAGE_IMAGE_PARAMS
- image_latents_params = TEXT_TO_IMAGE_IMAGE_PARAMS
-
- def get_dummy_components(self):
- torch.manual_seed(0)
- unet = UNet2DConditionModel(
- block_out_channels=(32, 64),
- layers_per_block=2,
- sample_size=32,
- in_channels=4,
- out_channels=4,
- down_block_types=("DownBlock2D", "CrossAttnDownBlock2D"),
- up_block_types=("CrossAttnUpBlock2D", "UpBlock2D"),
- cross_attention_dim=32,
- )
- torch.manual_seed(0)
- controlnet = ControlNetModel(
- block_out_channels=(32, 64),
- layers_per_block=2,
- in_channels=4,
- down_block_types=("DownBlock2D", "CrossAttnDownBlock2D"),
- cross_attention_dim=32,
- conditioning_embedding_out_channels=(16, 32),
- )
- torch.manual_seed(0)
- scheduler = DDIMScheduler(
- beta_start=0.00085,
- beta_end=0.012,
- beta_schedule="scaled_linear",
- clip_sample=False,
- set_alpha_to_one=False,
- )
- torch.manual_seed(0)
- vae = AutoencoderKL(
- block_out_channels=[32, 64],
- in_channels=3,
- out_channels=3,
- down_block_types=["DownEncoderBlock2D", "DownEncoderBlock2D"],
- up_block_types=["UpDecoderBlock2D", "UpDecoderBlock2D"],
- latent_channels=4,
- )
- torch.manual_seed(0)
- text_encoder_config = CLIPTextConfig(
- bos_token_id=0,
- eos_token_id=2,
- hidden_size=32,
- intermediate_size=37,
- layer_norm_eps=1e-05,
- num_attention_heads=4,
- num_hidden_layers=5,
- pad_token_id=1,
- vocab_size=1000,
- )
- text_encoder = CLIPTextModel(text_encoder_config)
- tokenizer = CLIPTokenizer.from_pretrained("hf-internal-testing/tiny-random-clip")
-
- components = {
- "unet": unet,
- "controlnet": controlnet,
- "scheduler": scheduler,
- "vae": vae,
- "text_encoder": text_encoder,
- "tokenizer": tokenizer,
- "safety_checker": None,
- "feature_extractor": None,
- }
- return components
-
- def get_dummy_inputs(self, device, seed=0):
- if str(device).startswith("mps"):
- generator = torch.manual_seed(seed)
- else:
- generator = torch.Generator(device=device).manual_seed(seed)
-
- controlnet_embedder_scale_factor = 2
- image = randn_tensor(
- (1, 3, 32 * controlnet_embedder_scale_factor, 32 * controlnet_embedder_scale_factor),
- generator=generator,
- device=torch.device(device),
- )
-
- inputs = {
- "prompt": "A painting of a squirrel eating a burger",
- "generator": generator,
- "num_inference_steps": 2,
- "guidance_scale": 6.0,
- "output_type": "numpy",
- "image": image,
- }
-
- return inputs
-
- def test_attention_slicing_forward_pass(self):
- return self._test_attention_slicing_forward_pass(expected_max_diff=2e-3)
-
- @unittest.skipIf(
- torch_device != "cuda" or not is_xformers_available(),
- reason="XFormers attention is only available with CUDA and `xformers` installed",
- )
- def test_xformers_attention_forwardGenerator_pass(self):
- self._test_xformers_attention_forwardGenerator_pass(expected_max_diff=2e-3)
-
- def test_inference_batch_single_identical(self):
- self._test_inference_batch_single_identical(expected_max_diff=2e-3)
-
-
-class StableDiffusionMultiControlNetPipelineFastTests(
- PipelineTesterMixin, PipelineKarrasSchedulerTesterMixin, unittest.TestCase
-):
- pipeline_class = StableDiffusionControlNetPipeline
- params = TEXT_TO_IMAGE_PARAMS
- batch_params = TEXT_TO_IMAGE_BATCH_PARAMS
- image_params = frozenset([]) # TO_DO: add image_params once refactored VaeImageProcessor.preprocess
-
- def get_dummy_components(self):
- torch.manual_seed(0)
- unet = UNet2DConditionModel(
- block_out_channels=(32, 64),
- layers_per_block=2,
- sample_size=32,
- in_channels=4,
- out_channels=4,
- down_block_types=("DownBlock2D", "CrossAttnDownBlock2D"),
- up_block_types=("CrossAttnUpBlock2D", "UpBlock2D"),
- cross_attention_dim=32,
- )
- torch.manual_seed(0)
-
- def init_weights(m):
- if isinstance(m, torch.nn.Conv2d):
- torch.nn.init.normal(m.weight)
- m.bias.data.fill_(1.0)
-
- controlnet1 = ControlNetModel(
- block_out_channels=(32, 64),
- layers_per_block=2,
- in_channels=4,
- down_block_types=("DownBlock2D", "CrossAttnDownBlock2D"),
- cross_attention_dim=32,
- conditioning_embedding_out_channels=(16, 32),
- )
- controlnet1.controlnet_down_blocks.apply(init_weights)
-
- torch.manual_seed(0)
- controlnet2 = ControlNetModel(
- block_out_channels=(32, 64),
- layers_per_block=2,
- in_channels=4,
- down_block_types=("DownBlock2D", "CrossAttnDownBlock2D"),
- cross_attention_dim=32,
- conditioning_embedding_out_channels=(16, 32),
- )
- controlnet2.controlnet_down_blocks.apply(init_weights)
-
- torch.manual_seed(0)
- scheduler = DDIMScheduler(
- beta_start=0.00085,
- beta_end=0.012,
- beta_schedule="scaled_linear",
- clip_sample=False,
- set_alpha_to_one=False,
- )
- torch.manual_seed(0)
- vae = AutoencoderKL(
- block_out_channels=[32, 64],
- in_channels=3,
- out_channels=3,
- down_block_types=["DownEncoderBlock2D", "DownEncoderBlock2D"],
- up_block_types=["UpDecoderBlock2D", "UpDecoderBlock2D"],
- latent_channels=4,
- )
- torch.manual_seed(0)
- text_encoder_config = CLIPTextConfig(
- bos_token_id=0,
- eos_token_id=2,
- hidden_size=32,
- intermediate_size=37,
- layer_norm_eps=1e-05,
- num_attention_heads=4,
- num_hidden_layers=5,
- pad_token_id=1,
- vocab_size=1000,
- )
- text_encoder = CLIPTextModel(text_encoder_config)
- tokenizer = CLIPTokenizer.from_pretrained("hf-internal-testing/tiny-random-clip")
-
- controlnet = MultiControlNetModel([controlnet1, controlnet2])
-
- components = {
- "unet": unet,
- "controlnet": controlnet,
- "scheduler": scheduler,
- "vae": vae,
- "text_encoder": text_encoder,
- "tokenizer": tokenizer,
- "safety_checker": None,
- "feature_extractor": None,
- }
- return components
-
- def get_dummy_inputs(self, device, seed=0):
- if str(device).startswith("mps"):
- generator = torch.manual_seed(seed)
- else:
- generator = torch.Generator(device=device).manual_seed(seed)
-
- controlnet_embedder_scale_factor = 2
-
- images = [
- randn_tensor(
- (1, 3, 32 * controlnet_embedder_scale_factor, 32 * controlnet_embedder_scale_factor),
- generator=generator,
- device=torch.device(device),
- ),
- randn_tensor(
- (1, 3, 32 * controlnet_embedder_scale_factor, 32 * controlnet_embedder_scale_factor),
- generator=generator,
- device=torch.device(device),
- ),
- ]
-
- inputs = {
- "prompt": "A painting of a squirrel eating a burger",
- "generator": generator,
- "num_inference_steps": 2,
- "guidance_scale": 6.0,
- "output_type": "numpy",
- "image": images,
- }
-
- return inputs
-
- def test_control_guidance_switch(self):
- components = self.get_dummy_components()
- pipe = self.pipeline_class(**components)
- pipe.to(torch_device)
-
- scale = 10.0
- steps = 4
-
- inputs = self.get_dummy_inputs(torch_device)
- inputs["num_inference_steps"] = steps
- inputs["controlnet_conditioning_scale"] = scale
- output_1 = pipe(**inputs)[0]
-
- inputs = self.get_dummy_inputs(torch_device)
- inputs["num_inference_steps"] = steps
- inputs["controlnet_conditioning_scale"] = scale
- output_2 = pipe(**inputs, control_guidance_start=0.1, control_guidance_end=0.2)[0]
-
- inputs = self.get_dummy_inputs(torch_device)
- inputs["num_inference_steps"] = steps
- inputs["controlnet_conditioning_scale"] = scale
- output_3 = pipe(**inputs, control_guidance_start=[0.1, 0.3], control_guidance_end=[0.2, 0.7])[0]
-
- inputs = self.get_dummy_inputs(torch_device)
- inputs["num_inference_steps"] = steps
- inputs["controlnet_conditioning_scale"] = scale
- output_4 = pipe(**inputs, control_guidance_start=0.4, control_guidance_end=[0.5, 0.8])[0]
-
- # make sure that all outputs are different
- assert np.sum(np.abs(output_1 - output_2)) > 1e-3
- assert np.sum(np.abs(output_1 - output_3)) > 1e-3
- assert np.sum(np.abs(output_1 - output_4)) > 1e-3
-
- def test_attention_slicing_forward_pass(self):
- return self._test_attention_slicing_forward_pass(expected_max_diff=2e-3)
-
- @unittest.skipIf(
- torch_device != "cuda" or not is_xformers_available(),
- reason="XFormers attention is only available with CUDA and `xformers` installed",
- )
- def test_xformers_attention_forwardGenerator_pass(self):
- self._test_xformers_attention_forwardGenerator_pass(expected_max_diff=2e-3)
-
- def test_inference_batch_single_identical(self):
- self._test_inference_batch_single_identical(expected_max_diff=2e-3)
-
- def test_save_pretrained_raise_not_implemented_exception(self):
- components = self.get_dummy_components()
- pipe = self.pipeline_class(**components)
- pipe.to(torch_device)
- pipe.set_progress_bar_config(disable=None)
- with tempfile.TemporaryDirectory() as tmpdir:
- try:
- # save_pretrained is not implemented for Multi-ControlNet
- pipe.save_pretrained(tmpdir)
- except NotImplementedError:
- pass
-
-
-class StableDiffusionMultiControlNetOneModelPipelineFastTests(
- PipelineTesterMixin, PipelineKarrasSchedulerTesterMixin, unittest.TestCase
-):
- pipeline_class = StableDiffusionControlNetPipeline
- params = TEXT_TO_IMAGE_PARAMS
- batch_params = TEXT_TO_IMAGE_BATCH_PARAMS
- image_params = frozenset([]) # TO_DO: add image_params once refactored VaeImageProcessor.preprocess
-
- def get_dummy_components(self):
- torch.manual_seed(0)
- unet = UNet2DConditionModel(
- block_out_channels=(32, 64),
- layers_per_block=2,
- sample_size=32,
- in_channels=4,
- out_channels=4,
- down_block_types=("DownBlock2D", "CrossAttnDownBlock2D"),
- up_block_types=("CrossAttnUpBlock2D", "UpBlock2D"),
- cross_attention_dim=32,
- )
- torch.manual_seed(0)
-
- def init_weights(m):
- if isinstance(m, torch.nn.Conv2d):
- torch.nn.init.normal(m.weight)
- m.bias.data.fill_(1.0)
-
- controlnet = ControlNetModel(
- block_out_channels=(32, 64),
- layers_per_block=2,
- in_channels=4,
- down_block_types=("DownBlock2D", "CrossAttnDownBlock2D"),
- cross_attention_dim=32,
- conditioning_embedding_out_channels=(16, 32),
- )
- controlnet.controlnet_down_blocks.apply(init_weights)
-
- torch.manual_seed(0)
- scheduler = DDIMScheduler(
- beta_start=0.00085,
- beta_end=0.012,
- beta_schedule="scaled_linear",
- clip_sample=False,
- set_alpha_to_one=False,
- )
- torch.manual_seed(0)
- vae = AutoencoderKL(
- block_out_channels=[32, 64],
- in_channels=3,
- out_channels=3,
- down_block_types=["DownEncoderBlock2D", "DownEncoderBlock2D"],
- up_block_types=["UpDecoderBlock2D", "UpDecoderBlock2D"],
- latent_channels=4,
- )
- torch.manual_seed(0)
- text_encoder_config = CLIPTextConfig(
- bos_token_id=0,
- eos_token_id=2,
- hidden_size=32,
- intermediate_size=37,
- layer_norm_eps=1e-05,
- num_attention_heads=4,
- num_hidden_layers=5,
- pad_token_id=1,
- vocab_size=1000,
- )
- text_encoder = CLIPTextModel(text_encoder_config)
- tokenizer = CLIPTokenizer.from_pretrained("hf-internal-testing/tiny-random-clip")
-
- controlnet = MultiControlNetModel([controlnet])
-
- components = {
- "unet": unet,
- "controlnet": controlnet,
- "scheduler": scheduler,
- "vae": vae,
- "text_encoder": text_encoder,
- "tokenizer": tokenizer,
- "safety_checker": None,
- "feature_extractor": None,
- }
- return components
-
- def get_dummy_inputs(self, device, seed=0):
- if str(device).startswith("mps"):
- generator = torch.manual_seed(seed)
- else:
- generator = torch.Generator(device=device).manual_seed(seed)
-
- controlnet_embedder_scale_factor = 2
-
- images = [
- randn_tensor(
- (1, 3, 32 * controlnet_embedder_scale_factor, 32 * controlnet_embedder_scale_factor),
- generator=generator,
- device=torch.device(device),
- ),
- ]
-
- inputs = {
- "prompt": "A painting of a squirrel eating a burger",
- "generator": generator,
- "num_inference_steps": 2,
- "guidance_scale": 6.0,
- "output_type": "numpy",
- "image": images,
- }
-
- return inputs
-
- def test_control_guidance_switch(self):
- components = self.get_dummy_components()
- pipe = self.pipeline_class(**components)
- pipe.to(torch_device)
-
- scale = 10.0
- steps = 4
-
- inputs = self.get_dummy_inputs(torch_device)
- inputs["num_inference_steps"] = steps
- inputs["controlnet_conditioning_scale"] = scale
- output_1 = pipe(**inputs)[0]
-
- inputs = self.get_dummy_inputs(torch_device)
- inputs["num_inference_steps"] = steps
- inputs["controlnet_conditioning_scale"] = scale
- output_2 = pipe(**inputs, control_guidance_start=0.1, control_guidance_end=0.2)[0]
-
- inputs = self.get_dummy_inputs(torch_device)
- inputs["num_inference_steps"] = steps
- inputs["controlnet_conditioning_scale"] = scale
- output_3 = pipe(
- **inputs,
- control_guidance_start=[0.1],
- control_guidance_end=[0.2],
- )[0]
-
- inputs = self.get_dummy_inputs(torch_device)
- inputs["num_inference_steps"] = steps
- inputs["controlnet_conditioning_scale"] = scale
- output_4 = pipe(**inputs, control_guidance_start=0.4, control_guidance_end=[0.5])[0]
-
- # make sure that all outputs are different
- assert np.sum(np.abs(output_1 - output_2)) > 1e-3
- assert np.sum(np.abs(output_1 - output_3)) > 1e-3
- assert np.sum(np.abs(output_1 - output_4)) > 1e-3
-
- def test_attention_slicing_forward_pass(self):
- return self._test_attention_slicing_forward_pass(expected_max_diff=2e-3)
-
- @unittest.skipIf(
- torch_device != "cuda" or not is_xformers_available(),
- reason="XFormers attention is only available with CUDA and `xformers` installed",
- )
- def test_xformers_attention_forwardGenerator_pass(self):
- self._test_xformers_attention_forwardGenerator_pass(expected_max_diff=2e-3)
-
- def test_inference_batch_single_identical(self):
- self._test_inference_batch_single_identical(expected_max_diff=2e-3)
-
- def test_save_pretrained_raise_not_implemented_exception(self):
- components = self.get_dummy_components()
- pipe = self.pipeline_class(**components)
- pipe.to(torch_device)
- pipe.set_progress_bar_config(disable=None)
- with tempfile.TemporaryDirectory() as tmpdir:
- try:
- # save_pretrained is not implemented for Multi-ControlNet
- pipe.save_pretrained(tmpdir)
- except NotImplementedError:
- pass
-
-
-@slow
-@require_torch_gpu
-class ControlNetPipelineSlowTests(unittest.TestCase):
- def tearDown(self):
- super().tearDown()
- gc.collect()
- torch.cuda.empty_cache()
-
- def test_canny(self):
- controlnet = ControlNetModel.from_pretrained("lllyasviel/sd-controlnet-canny")
-
- pipe = StableDiffusionControlNetPipeline.from_pretrained(
- "runwayml/stable-diffusion-v1-5", safety_checker=None, controlnet=controlnet
- )
- pipe.enable_model_cpu_offload()
- pipe.set_progress_bar_config(disable=None)
-
- generator = torch.Generator(device="cpu").manual_seed(0)
- prompt = "bird"
- image = load_image(
- "https://huggingface.co/datasets/hf-internal-testing/diffusers-images/resolve/main/sd_controlnet/bird_canny.png"
- )
-
- output = pipe(prompt, image, generator=generator, output_type="np", num_inference_steps=3)
-
- image = output.images[0]
-
- assert image.shape == (768, 512, 3)
-
- expected_image = load_numpy(
- "https://huggingface.co/datasets/hf-internal-testing/diffusers-images/resolve/main/sd_controlnet/bird_canny_out.npy"
- )
-
- assert np.abs(expected_image - image).max() < 9e-2
-
- def test_depth(self):
- controlnet = ControlNetModel.from_pretrained("lllyasviel/sd-controlnet-depth")
-
- pipe = StableDiffusionControlNetPipeline.from_pretrained(
- "runwayml/stable-diffusion-v1-5", safety_checker=None, controlnet=controlnet
- )
- pipe.enable_model_cpu_offload()
- pipe.set_progress_bar_config(disable=None)
-
- generator = torch.Generator(device="cpu").manual_seed(0)
- prompt = "Stormtrooper's lecture"
- image = load_image(
- "https://huggingface.co/datasets/hf-internal-testing/diffusers-images/resolve/main/sd_controlnet/stormtrooper_depth.png"
- )
-
- output = pipe(prompt, image, generator=generator, output_type="np", num_inference_steps=3)
-
- image = output.images[0]
-
- assert image.shape == (512, 512, 3)
-
- expected_image = load_numpy(
- "https://huggingface.co/datasets/hf-internal-testing/diffusers-images/resolve/main/sd_controlnet/stormtrooper_depth_out.npy"
- )
-
- assert np.abs(expected_image - image).max() < 8e-1
-
- def test_hed(self):
- controlnet = ControlNetModel.from_pretrained("lllyasviel/sd-controlnet-hed")
-
- pipe = StableDiffusionControlNetPipeline.from_pretrained(
- "runwayml/stable-diffusion-v1-5", safety_checker=None, controlnet=controlnet
- )
- pipe.enable_model_cpu_offload()
- pipe.set_progress_bar_config(disable=None)
-
- generator = torch.Generator(device="cpu").manual_seed(0)
- prompt = "oil painting of handsome old man, masterpiece"
- image = load_image(
- "https://huggingface.co/datasets/hf-internal-testing/diffusers-images/resolve/main/sd_controlnet/man_hed.png"
- )
-
- output = pipe(prompt, image, generator=generator, output_type="np", num_inference_steps=3)
-
- image = output.images[0]
-
- assert image.shape == (704, 512, 3)
-
- expected_image = load_numpy(
- "https://huggingface.co/datasets/hf-internal-testing/diffusers-images/resolve/main/sd_controlnet/man_hed_out.npy"
- )
-
- assert np.abs(expected_image - image).max() < 8e-2
-
- def test_mlsd(self):
- controlnet = ControlNetModel.from_pretrained("lllyasviel/sd-controlnet-mlsd")
-
- pipe = StableDiffusionControlNetPipeline.from_pretrained(
- "runwayml/stable-diffusion-v1-5", safety_checker=None, controlnet=controlnet
- )
- pipe.enable_model_cpu_offload()
- pipe.set_progress_bar_config(disable=None)
-
- generator = torch.Generator(device="cpu").manual_seed(0)
- prompt = "room"
- image = load_image(
- "https://huggingface.co/datasets/hf-internal-testing/diffusers-images/resolve/main/sd_controlnet/room_mlsd.png"
- )
-
- output = pipe(prompt, image, generator=generator, output_type="np", num_inference_steps=3)
-
- image = output.images[0]
-
- assert image.shape == (704, 512, 3)
-
- expected_image = load_numpy(
- "https://huggingface.co/datasets/hf-internal-testing/diffusers-images/resolve/main/sd_controlnet/room_mlsd_out.npy"
- )
-
- assert np.abs(expected_image - image).max() < 5e-2
-
- def test_normal(self):
- controlnet = ControlNetModel.from_pretrained("lllyasviel/sd-controlnet-normal")
-
- pipe = StableDiffusionControlNetPipeline.from_pretrained(
- "runwayml/stable-diffusion-v1-5", safety_checker=None, controlnet=controlnet
- )
- pipe.enable_model_cpu_offload()
- pipe.set_progress_bar_config(disable=None)
-
- generator = torch.Generator(device="cpu").manual_seed(0)
- prompt = "cute toy"
- image = load_image(
- "https://huggingface.co/datasets/hf-internal-testing/diffusers-images/resolve/main/sd_controlnet/cute_toy_normal.png"
- )
-
- output = pipe(prompt, image, generator=generator, output_type="np", num_inference_steps=3)
-
- image = output.images[0]
-
- assert image.shape == (512, 512, 3)
-
- expected_image = load_numpy(
- "https://huggingface.co/datasets/hf-internal-testing/diffusers-images/resolve/main/sd_controlnet/cute_toy_normal_out.npy"
- )
-
- assert np.abs(expected_image - image).max() < 5e-2
-
- def test_openpose(self):
- controlnet = ControlNetModel.from_pretrained("lllyasviel/sd-controlnet-openpose")
-
- pipe = StableDiffusionControlNetPipeline.from_pretrained(
- "runwayml/stable-diffusion-v1-5", safety_checker=None, controlnet=controlnet
- )
- pipe.enable_model_cpu_offload()
- pipe.set_progress_bar_config(disable=None)
-
- generator = torch.Generator(device="cpu").manual_seed(0)
- prompt = "Chef in the kitchen"
- image = load_image(
- "https://huggingface.co/datasets/hf-internal-testing/diffusers-images/resolve/main/sd_controlnet/pose.png"
- )
-
- output = pipe(prompt, image, generator=generator, output_type="np", num_inference_steps=3)
-
- image = output.images[0]
-
- assert image.shape == (768, 512, 3)
-
- expected_image = load_numpy(
- "https://huggingface.co/datasets/hf-internal-testing/diffusers-images/resolve/main/sd_controlnet/chef_pose_out.npy"
- )
-
- assert np.abs(expected_image - image).max() < 8e-2
-
- def test_scribble(self):
- controlnet = ControlNetModel.from_pretrained("lllyasviel/sd-controlnet-scribble")
-
- pipe = StableDiffusionControlNetPipeline.from_pretrained(
- "runwayml/stable-diffusion-v1-5", safety_checker=None, controlnet=controlnet
- )
- pipe.enable_model_cpu_offload()
- pipe.set_progress_bar_config(disable=None)
-
- generator = torch.Generator(device="cpu").manual_seed(5)
- prompt = "bag"
- image = load_image(
- "https://huggingface.co/datasets/hf-internal-testing/diffusers-images/resolve/main/sd_controlnet/bag_scribble.png"
- )
-
- output = pipe(prompt, image, generator=generator, output_type="np", num_inference_steps=3)
-
- image = output.images[0]
-
- assert image.shape == (640, 512, 3)
-
- expected_image = load_numpy(
- "https://huggingface.co/datasets/hf-internal-testing/diffusers-images/resolve/main/sd_controlnet/bag_scribble_out.npy"
- )
-
- assert np.abs(expected_image - image).max() < 8e-2
-
- def test_seg(self):
- controlnet = ControlNetModel.from_pretrained("lllyasviel/sd-controlnet-seg")
-
- pipe = StableDiffusionControlNetPipeline.from_pretrained(
- "runwayml/stable-diffusion-v1-5", safety_checker=None, controlnet=controlnet
- )
- pipe.enable_model_cpu_offload()
- pipe.set_progress_bar_config(disable=None)
-
- generator = torch.Generator(device="cpu").manual_seed(5)
- prompt = "house"
- image = load_image(
- "https://huggingface.co/datasets/hf-internal-testing/diffusers-images/resolve/main/sd_controlnet/house_seg.png"
- )
-
- output = pipe(prompt, image, generator=generator, output_type="np", num_inference_steps=3)
-
- image = output.images[0]
-
- assert image.shape == (512, 512, 3)
-
- expected_image = load_numpy(
- "https://huggingface.co/datasets/hf-internal-testing/diffusers-images/resolve/main/sd_controlnet/house_seg_out.npy"
- )
-
- assert np.abs(expected_image - image).max() < 8e-2
-
- def test_sequential_cpu_offloading(self):
- torch.cuda.empty_cache()
- torch.cuda.reset_max_memory_allocated()
- torch.cuda.reset_peak_memory_stats()
-
- controlnet = ControlNetModel.from_pretrained("lllyasviel/sd-controlnet-seg")
-
- pipe = StableDiffusionControlNetPipeline.from_pretrained(
- "runwayml/stable-diffusion-v1-5", safety_checker=None, controlnet=controlnet
- )
- pipe.set_progress_bar_config(disable=None)
- pipe.enable_attention_slicing()
- pipe.enable_sequential_cpu_offload()
-
- prompt = "house"
- image = load_image(
- "https://huggingface.co/datasets/hf-internal-testing/diffusers-images/resolve/main/sd_controlnet/house_seg.png"
- )
-
- _ = pipe(
- prompt,
- image,
- num_inference_steps=2,
- output_type="np",
- )
-
- mem_bytes = torch.cuda.max_memory_allocated()
- # make sure that less than 7 GB is allocated
- assert mem_bytes < 4 * 10**9
-
- def test_canny_guess_mode(self):
- controlnet = ControlNetModel.from_pretrained("lllyasviel/sd-controlnet-canny")
-
- pipe = StableDiffusionControlNetPipeline.from_pretrained(
- "runwayml/stable-diffusion-v1-5", safety_checker=None, controlnet=controlnet
- )
- pipe.enable_model_cpu_offload()
- pipe.set_progress_bar_config(disable=None)
-
- generator = torch.Generator(device="cpu").manual_seed(0)
- prompt = ""
- image = load_image(
- "https://huggingface.co/datasets/hf-internal-testing/diffusers-images/resolve/main/sd_controlnet/bird_canny.png"
- )
-
- output = pipe(
- prompt,
- image,
- generator=generator,
- output_type="np",
- num_inference_steps=3,
- guidance_scale=3.0,
- guess_mode=True,
- )
-
- image = output.images[0]
- assert image.shape == (768, 512, 3)
-
- image_slice = image[-3:, -3:, -1]
- expected_slice = np.array([0.2724, 0.2846, 0.2724, 0.3843, 0.3682, 0.2736, 0.4675, 0.3862, 0.2887])
- assert np.abs(image_slice.flatten() - expected_slice).max() < 1e-2
-
- def test_canny_guess_mode_euler(self):
- controlnet = ControlNetModel.from_pretrained("lllyasviel/sd-controlnet-canny")
-
- pipe = StableDiffusionControlNetPipeline.from_pretrained(
- "runwayml/stable-diffusion-v1-5", safety_checker=None, controlnet=controlnet
- )
- pipe.scheduler = EulerDiscreteScheduler.from_config(pipe.scheduler.config)
- pipe.enable_model_cpu_offload()
- pipe.set_progress_bar_config(disable=None)
-
- generator = torch.Generator(device="cpu").manual_seed(0)
- prompt = ""
- image = load_image(
- "https://huggingface.co/datasets/hf-internal-testing/diffusers-images/resolve/main/sd_controlnet/bird_canny.png"
- )
-
- output = pipe(
- prompt,
- image,
- generator=generator,
- output_type="np",
- num_inference_steps=3,
- guidance_scale=3.0,
- guess_mode=True,
- )
-
- image = output.images[0]
- assert image.shape == (768, 512, 3)
-
- image_slice = image[-3:, -3:, -1]
- expected_slice = np.array([0.1655, 0.1721, 0.1623, 0.1685, 0.1711, 0.1646, 0.1651, 0.1631, 0.1494])
- assert np.abs(image_slice.flatten() - expected_slice).max() < 1e-2
-
- @require_torch_2
- def test_stable_diffusion_compile(self):
- run_test_in_subprocess(test_case=self, target_func=_test_stable_diffusion_compile, inputs=None)
-
- def test_v11_shuffle_global_pool_conditions(self):
- controlnet = ControlNetModel.from_pretrained("lllyasviel/control_v11e_sd15_shuffle")
-
- pipe = StableDiffusionControlNetPipeline.from_pretrained(
- "runwayml/stable-diffusion-v1-5", safety_checker=None, controlnet=controlnet
- )
- pipe.enable_model_cpu_offload()
- pipe.set_progress_bar_config(disable=None)
-
- generator = torch.Generator(device="cpu").manual_seed(0)
- prompt = "New York"
- image = load_image(
- "https://huggingface.co/lllyasviel/control_v11e_sd15_shuffle/resolve/main/images/control.png"
- )
-
- output = pipe(
- prompt,
- image,
- generator=generator,
- output_type="np",
- num_inference_steps=3,
- guidance_scale=7.0,
- )
-
- image = output.images[0]
- assert image.shape == (512, 640, 3)
-
- image_slice = image[-3:, -3:, -1]
- expected_slice = np.array([0.1338, 0.1597, 0.1202, 0.1687, 0.1377, 0.1017, 0.2070, 0.1574, 0.1348])
- assert np.abs(image_slice.flatten() - expected_slice).max() < 1e-2
-
- def test_load_local(self):
- controlnet = ControlNetModel.from_pretrained("lllyasviel/control_v11p_sd15_canny")
- pipe_1 = StableDiffusionControlNetPipeline.from_pretrained(
- "runwayml/stable-diffusion-v1-5", safety_checker=None, controlnet=controlnet
- )
-
- controlnet = ControlNetModel.from_single_file(
- "https://huggingface.co/lllyasviel/ControlNet-v1-1/blob/main/control_v11p_sd15_canny.pth"
- )
- pipe_2 = StableDiffusionControlNetPipeline.from_single_file(
- "https://huggingface.co/runwayml/stable-diffusion-v1-5/blob/main/v1-5-pruned-emaonly.safetensors",
- safety_checker=None,
- controlnet=controlnet,
- )
- pipes = [pipe_1, pipe_2]
- images = []
-
- for pipe in pipes:
- pipe.enable_model_cpu_offload()
- pipe.set_progress_bar_config(disable=None)
-
- generator = torch.Generator(device="cpu").manual_seed(0)
- prompt = "bird"
- image = load_image(
- "https://huggingface.co/datasets/hf-internal-testing/diffusers-images/resolve/main/sd_controlnet/bird_canny.png"
- )
-
- output = pipe(prompt, image, generator=generator, output_type="np", num_inference_steps=3)
- images.append(output.images[0])
-
- del pipe
- gc.collect()
- torch.cuda.empty_cache()
-
- assert np.abs(images[0] - images[1]).sum() < 1e-3
-
-
-@slow
-@require_torch_gpu
-class StableDiffusionMultiControlNetPipelineSlowTests(unittest.TestCase):
- def tearDown(self):
- super().tearDown()
- gc.collect()
- torch.cuda.empty_cache()
-
- def test_pose_and_canny(self):
- controlnet_canny = ControlNetModel.from_pretrained("lllyasviel/sd-controlnet-canny")
- controlnet_pose = ControlNetModel.from_pretrained("lllyasviel/sd-controlnet-openpose")
-
- pipe = StableDiffusionControlNetPipeline.from_pretrained(
- "runwayml/stable-diffusion-v1-5", safety_checker=None, controlnet=[controlnet_pose, controlnet_canny]
- )
- pipe.enable_model_cpu_offload()
- pipe.set_progress_bar_config(disable=None)
-
- generator = torch.Generator(device="cpu").manual_seed(0)
- prompt = "bird and Chef"
- image_canny = load_image(
- "https://huggingface.co/datasets/hf-internal-testing/diffusers-images/resolve/main/sd_controlnet/bird_canny.png"
- )
- image_pose = load_image(
- "https://huggingface.co/datasets/hf-internal-testing/diffusers-images/resolve/main/sd_controlnet/pose.png"
- )
-
- output = pipe(prompt, [image_pose, image_canny], generator=generator, output_type="np", num_inference_steps=3)
-
- image = output.images[0]
-
- assert image.shape == (768, 512, 3)
-
- expected_image = load_numpy(
- "https://huggingface.co/datasets/hf-internal-testing/diffusers-images/resolve/main/sd_controlnet/pose_canny_out.npy"
- )
-
- assert np.abs(expected_image - image).max() < 5e-2
diff --git a/spaces/AnimaLab/bias-test-gpt-pairs/README.md b/spaces/AnimaLab/bias-test-gpt-pairs/README.md
deleted file mode 100644
index 84e4c8e6dd9dae470d422484bc9013a67457313f..0000000000000000000000000000000000000000
--- a/spaces/AnimaLab/bias-test-gpt-pairs/README.md
+++ /dev/null
@@ -1,14 +0,0 @@
----
-title: Bias Test Gpt Pairs
-emoji: 🦀
-colorFrom: indigo
-colorTo: indigo
-sdk: gradio
-sdk_version: 3.35.2
-app_file: app.py
-pinned: false
-license: apache-2.0
-duplicated_from: RKocielnik/bias-test-gpt-pairs
----
-
-Check out the configuration reference at https://huggingface.co/docs/hub/spaces-config-reference
diff --git a/spaces/AnishKumbhar/ChatBot/text-generation-webui-main/modules/training.py b/spaces/AnishKumbhar/ChatBot/text-generation-webui-main/modules/training.py
deleted file mode 100644
index a86ebc7cfd5ed1c45bcfb0d66eb33b3e0f2b2fcd..0000000000000000000000000000000000000000
--- a/spaces/AnishKumbhar/ChatBot/text-generation-webui-main/modules/training.py
+++ /dev/null
@@ -1,737 +0,0 @@
-import os
-
-os.environ["WANDB_MODE"] = "offline"
-# os.environ["WANDB_DISABLED"] = "true"
-
-import json
-import math
-import random
-import shutil
-import sys
-import threading
-import time
-import traceback
-from datetime import datetime
-from pathlib import Path
-
-import gradio as gr
-import torch
-import transformers
-from datasets import Dataset, load_dataset
-from peft import (
- LoraConfig,
- get_peft_model,
- prepare_model_for_kbit_training,
- set_peft_model_state_dict
-)
-from peft.utils.other import \
- TRANSFORMERS_MODELS_TO_LORA_TARGET_MODULES_MAPPING as model_to_lora_modules
-from transformers.models.auto.modeling_auto import (
- MODEL_FOR_CAUSAL_LM_MAPPING_NAMES
-)
-
-from modules import shared, ui, utils
-from modules.evaluate import (
- calculate_perplexity,
- generate_markdown_table,
- save_past_evaluations
-)
-from modules.logging_colors import logger
-from modules.models import reload_model
-from modules.utils import natural_keys
-
-MODEL_CLASSES = {v[1]: v[0] for v in MODEL_FOR_CAUSAL_LM_MAPPING_NAMES.items()}
-PARAMETERS = ["lora_name", "always_override", "save_steps", "micro_batch_size", "batch_size", "epochs", "learning_rate", "lr_scheduler_type", "lora_rank", "lora_alpha", "lora_dropout", "cutoff_len", "dataset", "eval_dataset", "format", "eval_steps", "raw_text_file", "overlap_len", "newline_favor_len", "higher_rank_limit", "warmup_steps", "optimizer", "hard_cut_string", "train_only_after", "stop_at_loss", "add_eos_token", "min_chars", "report_to"]
-WANT_INTERRUPT = False
-
-train_log = {}
-train_template = {}
-
-
-def create_ui():
- mu = shared.args.multi_user
- with gr.Tab("Training", elem_id="training-tab"):
- with gr.Tab('Train LoRA', elem_id='lora-train-tab'):
- tmp = gr.State('')
- with gr.Row():
- with gr.Column():
- gr.Markdown("[Tutorial](https://github.com/oobabooga/text-generation-webui/blob/main/docs/Training-LoRAs.md)")
-
- with gr.Row():
- copy_from = gr.Dropdown(label='Copy parameters from', value='None', choices=utils.get_available_loras(), elem_classes=['slim-dropdown'], interactive=not mu)
- ui.create_refresh_button(copy_from, lambda: None, lambda: {'choices': utils.get_available_loras()}, 'refresh-button', interactive=not mu)
-
- with gr.Row():
- with gr.Column(scale=5):
- lora_name = gr.Textbox(label='Name', info='The name of your new LoRA file')
- with gr.Column():
- always_override = gr.Checkbox(label='Override Existing Files', value=False, info='If the name is the same, checking will replace the existing file, and unchecking will load and continue from it (the rank must be the same).', elem_classes=['no-background'])
-
- with gr.Row():
- with gr.Column():
- lora_rank = gr.Slider(label='LoRA Rank', value=32, minimum=0, maximum=1024, step=4, info='Also called dimension count. Higher values = larger file, more content control. Smaller values = smaller file, less control. Use 4 or 8 for style, 128 or 256 to teach, 1024+ for fine-detail on big data. More VRAM is needed for higher ranks.')
- lora_alpha = gr.Slider(label='LoRA Alpha', value=64, minimum=0, maximum=2048, step=4, info='This divided by the rank becomes the scaling of the LoRA. Higher means stronger. A good standard value is twice your Rank.')
- batch_size = gr.Slider(label='Batch Size', value=128, minimum=0, maximum=1024, step=4, info='Global batch size. The two batch sizes together determine gradient accumulation (gradientAccum = batch / microBatch). Higher gradient accum values lead to better quality training.')
- micro_batch_size = gr.Slider(label='Micro Batch Size', value=4, minimum=1, maximum=128, step=1, info='Per-device batch size (NOTE: multiple devices not yet implemented). Increasing this will increase VRAM usage.')
- cutoff_len = gr.Slider(label='Cutoff Length', minimum=0, maximum=2048, value=256, step=32, info='Cutoff length for text input. Essentially, how long of a line of text to feed in at a time. Higher values require drastically more VRAM.')
-
- with gr.Column():
- save_steps = gr.Number(label='Save every n steps', value=0, info='If above 0, a checkpoint of the LoRA will be saved every time this many steps pass.')
-
- epochs = gr.Number(label='Epochs', value=3, info='Number of times every entry in the dataset should be fed into training. So 1 means feed each item in once, 5 means feed it in five times, etc.')
- learning_rate = gr.Textbox(label='Learning Rate', value='3e-4', info='In scientific notation. 3e-4 is a good starting base point. 1e-2 is extremely high, 1e-6 is extremely low.')
- lr_scheduler_type = gr.Dropdown(label='LR Scheduler', value='linear', choices=['linear', 'constant', 'constant_with_warmup', 'cosine', 'cosine_with_restarts', 'polynomial', 'inverse_sqrt'], info='Learning rate scheduler - defines how the learning rate changes over time. "Constant" means never change, "linear" means to go in a straight line from the learning rate down to 0, cosine follows a curve, etc.', elem_classes=['slim-dropdown'])
-
- with gr.Accordion(label='Advanced Options', open=False):
- with gr.Row():
- with gr.Column():
- lora_dropout = gr.Slider(label='LoRA Dropout', minimum=0.0, maximum=1.0, step=0.025, value=0.05, info='Percentage probability for dropout of LoRA layers. This can help reduce overfitting. Most users should leave at default.')
- stop_at_loss = gr.Slider(label='Stop at loss', minimum=0.0, maximum=3.0, step=0.1, value=0.00, info='The process will automatically stop once the desired loss value is reached. (reasonable numbers are 1.5-1.8)')
- optimizer = gr.Dropdown(label='Optimizer', value='adamw_torch', choices=['adamw_hf', 'adamw_torch', 'adamw_torch_fused', 'adamw_torch_xla', 'adamw_apex_fused', 'adafactor', 'adamw_bnb_8bit', 'adamw_anyprecision', 'sgd', 'adagrad'], info='Different optimizer implementation options, for advanced users. Effects of different options are not well documented yet.', elem_classes=['slim-dropdown'])
-
- with gr.Column():
- warmup_steps = gr.Number(label='Warmup Steps', value=100, info='For this many steps at the start, the learning rate will be lower than normal. This helps the trainer prepare the model and precompute statistics to improve the quality of training after the start.')
- train_only_after = gr.Textbox(label='Train Only After', value='', info='Only consider text *after* this string in any given chunk for training. For Alpaca datasets, use "### Response:" to only train the response and ignore the input.')
-
- add_eos_token = gr.Checkbox(label='Add EOS token', value=False, info="Adds EOS token for each dataset item. In case of raw text, the EOS will be added at the Hard Cut")
-
- higher_rank_limit = gr.Checkbox(label='Enable higher ranks', value=False, info='If checked, changes Rank/Alpha slider above to go much higher. This will not work without a datacenter-class GPU.')
- report_to = gr.Radio(label="Save detailed logs with", value="None", choices=["None", "wandb", "tensorboard"], interactive=True)
-
- with gr.Column():
- with gr.Tab(label='Formatted Dataset'):
- with gr.Row():
- format = gr.Dropdown(choices=utils.get_datasets('training/formats', 'json'), value='None', label='Data Format', info='The format file used to decide how to format the dataset input.', elem_classes=['slim-dropdown'], interactive=not mu)
- ui.create_refresh_button(format, lambda: None, lambda: {'choices': utils.get_datasets('training/formats', 'json')}, 'refresh-button', interactive=not mu)
-
- with gr.Row():
- dataset = gr.Dropdown(choices=utils.get_datasets('training/datasets', 'json'), value='None', label='Dataset', info='The dataset file to use for training.', elem_classes=['slim-dropdown'], interactive=not mu)
- ui.create_refresh_button(dataset, lambda: None, lambda: {'choices': utils.get_datasets('training/datasets', 'json')}, 'refresh-button', interactive=not mu)
-
- with gr.Row():
- eval_dataset = gr.Dropdown(choices=utils.get_datasets('training/datasets', 'json'), value='None', label='Evaluation Dataset', info='The (optional) dataset file used to evaluate the model after training.', elem_classes=['slim-dropdown'], interactive=not mu)
- ui.create_refresh_button(eval_dataset, lambda: None, lambda: {'choices': utils.get_datasets('training/datasets', 'json')}, 'refresh-button', interactive=not mu)
-
- eval_steps = gr.Number(label='Evaluate every n steps', value=100, info='If an evaluation dataset is given, test it every time this many steps pass.')
-
- with gr.Tab(label="Raw text file"):
- with gr.Row():
- raw_text_file = gr.Dropdown(choices=utils.get_datasets('training/datasets', 'txt'), value='None', label='Text file', info='The raw text file to use for training.', elem_classes=['slim-dropdown'], interactive=not mu)
- ui.create_refresh_button(raw_text_file, lambda: None, lambda: {'choices': utils.get_datasets('training/datasets', 'txt')}, 'refresh-button', interactive=not mu)
-
- with gr.Row():
- with gr.Column():
- overlap_len = gr.Slider(label='Overlap Length', minimum=0, maximum=512, value=128, step=16, info='How many tokens from the prior chunk of text to include into the next chunk. (The chunks themselves will be of a size determined by Cutoff Length). Setting overlap to exactly half the cutoff length may be ideal.')
- newline_favor_len = gr.Slider(label='Prefer Newline Cut Length', minimum=0, maximum=512, value=128, step=16, info='Length (in characters, not tokens) of the maximum distance to shift an overlap cut by to ensure chunks cut at newlines. If too low, cuts may occur in the middle of lines.')
-
- with gr.Column():
- hard_cut_string = gr.Textbox(label='Hard Cut String', value='\\n\\n\\n', info='String that indicates a hard cut between text parts. Helps prevent unwanted overlap.')
- min_chars = gr.Number(label='Ignore small blocks', value=0, info='Ignore Hard Cut blocks that have less or equal characters than this number')
-
- with gr.Row():
- start_button = gr.Button("Start LoRA Training", variant='primary', interactive=not mu)
- stop_button = gr.Button("Interrupt", interactive=not mu)
-
- output = gr.Markdown(value="Ready")
-
- with gr.Tab('Perplexity evaluation', elem_id='evaluate-tab'):
- with gr.Row():
- with gr.Column():
- models = gr.Dropdown(utils.get_available_models(), label='Models', multiselect=True, interactive=not mu)
- evaluate_text_file = gr.Dropdown(choices=['wikitext', 'ptb', 'ptb_new'] + utils.get_datasets('training/datasets', 'txt')[1:], value='wikitext', label='Input dataset', info='The raw text file on which the model will be evaluated. The first options are automatically downloaded: wikitext, ptb, and ptb_new. The next options are your local text files under training/datasets.', interactive=not mu)
- with gr.Row():
- with gr.Column():
- stride_length = gr.Slider(label='Stride', minimum=0, maximum=32768, value=512, step=256, info='Used to make the evaluation faster at the cost of accuracy. 1 = slowest but most accurate. 512 is a common value.')
-
- with gr.Column():
- max_length = gr.Slider(label='max_length', minimum=0, maximum=32768, value=0, step=256, info='The context for each evaluation. If set to 0, the maximum context length for the model will be used.')
-
- with gr.Row():
- start_current_evaluation = gr.Button("Evaluate loaded model", interactive=not mu)
- start_evaluation = gr.Button("Evaluate selected models", interactive=not mu)
- stop_evaluation = gr.Button("Interrupt", interactive=not mu)
-
- with gr.Column():
- evaluation_log = gr.Markdown(value='')
-
- evaluation_table = gr.Dataframe(value=generate_markdown_table(), interactive=True)
- with gr.Row():
- save_comments = gr.Button('Save comments', elem_classes="small-button", interactive=not mu)
- refresh_table = gr.Button('Refresh the table', elem_classes="small-button", interactive=not mu)
-
- # Training events
- all_params = [lora_name, always_override, save_steps, micro_batch_size, batch_size, epochs, learning_rate, lr_scheduler_type, lora_rank, lora_alpha, lora_dropout, cutoff_len, dataset, eval_dataset, format, eval_steps, raw_text_file, overlap_len, newline_favor_len, higher_rank_limit, warmup_steps, optimizer, hard_cut_string, train_only_after, stop_at_loss, add_eos_token, min_chars, report_to]
-
- copy_from.change(do_copy_params, [copy_from] + all_params, all_params)
- start_button.click(do_train, all_params, output)
- stop_button.click(do_interrupt, None, None, queue=False)
- higher_rank_limit.change(change_rank_limit, [higher_rank_limit], [lora_rank, lora_alpha])
-
- # Evaluation events. For some reason, the interrupt event
- # doesn't work with the .then() syntax, so I write them one
- # by one in this ugly but functional way.
- ev = start_evaluation.click(calculate_perplexity, [models, evaluate_text_file, stride_length, max_length], evaluation_log, show_progress=False)
- start_evaluation.click(generate_markdown_table, None, evaluation_table, show_progress=False)
-
- start_current_evaluation.click(lambda: ['current model'], None, tmp)
- ev_cur = start_current_evaluation.click(calculate_perplexity, [tmp, evaluate_text_file, stride_length, max_length], evaluation_log, show_progress=False)
- start_current_evaluation.click(generate_markdown_table, None, evaluation_table, show_progress=False)
-
- stop_evaluation.click(None, None, None, cancels=[ev, ev_cur], queue=False)
- refresh_table.click(generate_markdown_table, None, evaluation_table, show_progress=True)
- save_comments.click(
- save_past_evaluations, evaluation_table, None).then(
- lambda: "Comments saved.", None, evaluation_log, show_progress=False)
-
-
-def do_interrupt():
- global WANT_INTERRUPT
- WANT_INTERRUPT = True
-
-
-def do_copy_params(lora_name: str, *args):
- f_name = f"{shared.args.lora_dir}/{clean_path(None, lora_name)}/training_parameters.json"
- if Path(f_name).is_file():
- with open(f_name, 'r', encoding='utf-8') as format_file:
- params: dict[str, str] = json.load(format_file)
- else:
- params = {}
-
- result = list()
- for i in range(0, len(PARAMETERS)):
- key = PARAMETERS[i]
- if key in params:
- result.append(params[key])
- else:
- result.append(args[i])
-
- return result
-
-
-def change_rank_limit(use_higher_ranks: bool):
- mult = 2 if use_higher_ranks else 1
- return {"maximum": 1024 * mult, "__type__": "update"}, {"maximum": 2048 * mult, "__type__": "update"}
-
-
-def clean_path(base_path: str, path: str):
- """Strips unusual symbols and forcibly builds a path as relative to the intended directory."""
- path = path.replace('\\', '/').replace('..', '_')
- if base_path is None:
- return path
-
- return f'{Path(base_path).absolute()}/{path}'
-
-
-def backup_adapter(input_folder):
- # Get the creation date of the file adapter_model.bin
- try:
- adapter_file = Path(f"{input_folder}/adapter_model.bin")
- if adapter_file.is_file():
-
- logger.info("Backing up existing LoRA adapter...")
- creation_date = datetime.fromtimestamp(adapter_file.stat().st_ctime)
- creation_date_str = creation_date.strftime("Backup-%Y-%m-%d")
-
- # Create the new subfolder
- subfolder_path = Path(f"{input_folder}/{creation_date_str}")
- subfolder_path.mkdir(parents=True, exist_ok=True)
-
- # Check if the file already exists in the subfolder
- backup_adapter_file = Path(f"{input_folder}/{creation_date_str}/adapter_model.bin")
- if backup_adapter_file.is_file():
- print(" - Backup already exists. Skipping backup process.")
- return
-
- # Copy existing files to the new subfolder
- existing_files = Path(input_folder).iterdir()
- for file in existing_files:
- if file.is_file():
- shutil.copy2(file, subfolder_path)
- except Exception as e:
- print("An error occurred in backup_adapter:", str(e))
-
-
-def calc_trainable_parameters(model):
- trainable_params = 0
- all_param = 0
- for _, param in model.named_parameters():
- num_params = param.numel()
- # if using DS Zero 3 and the weights are initialized empty
- if num_params == 0 and hasattr(param, "ds_numel"):
- num_params = param.ds_numel
-
- all_param += num_params
- if param.requires_grad:
- trainable_params += num_params
-
- return trainable_params, all_param
-
-
-def do_train(lora_name: str, always_override: bool, save_steps: int, micro_batch_size: int, batch_size: int, epochs: int, learning_rate: str, lr_scheduler_type: str, lora_rank: int, lora_alpha: int, lora_dropout: float, cutoff_len: int, dataset: str, eval_dataset: str, format: str, eval_steps: int, raw_text_file: str, overlap_len: int, newline_favor_len: int, higher_rank_limit: bool, warmup_steps: int, optimizer: str, hard_cut_string: str, train_only_after: str, stop_at_loss: float, add_eos_token: bool, min_chars: int, report_to: str):
-
- if shared.args.monkey_patch:
- from alpaca_lora_4bit.monkeypatch.peft_tuners_lora_monkey_patch import (
- replace_peft_model_with_int4_lora_model
- )
- replace_peft_model_with_int4_lora_model()
-
- global WANT_INTERRUPT
- WANT_INTERRUPT = False
-
- # == Input validation / processing ==
- yield "Preparing the input..."
- lora_file_path = clean_path(None, lora_name)
- if lora_file_path.strip() == '':
- yield "Missing or invalid LoRA file name input."
- return
-
- lora_file_path = f"{Path(shared.args.lora_dir)}/{lora_file_path}"
- actual_lr = float(learning_rate)
- model_type = type(shared.model).__name__
-
- if model_type in MODEL_CLASSES:
- model_id = MODEL_CLASSES[model_type]
- else:
- model_id = "llama"
- if model_type == "PeftModelForCausalLM":
- if len(shared.lora_names) > 0:
- yield "You are trying to train a LoRA while you already have another LoRA loaded. This will work, but may have unexpected effects. *(Will continue anyway in 5 seconds, press `Interrupt` to stop.)*"
- logger.warning("Training LoRA over top of another LoRA. May have unexpected effects.")
- else:
- yield "Model ID not matched due to LoRA loading. Consider reloading base model. *(Will continue anyway in 5 seconds, press `Interrupt` to stop.)*"
- logger.warning("Model ID not matched due to LoRA loading. Consider reloading base model.")
- else:
- yield "LoRA training has only currently been validated for LLaMA, OPT, GPT-J, and GPT-NeoX models. Unexpected errors may follow. *(Will continue anyway in 5 seconds, press `Interrupt` to stop.)*"
- logger.warning(f"LoRA training has only currently been validated for LLaMA, OPT, GPT-J, and GPT-NeoX models. (Found model type: {model_type})")
-
- time.sleep(5)
-
- if shared.args.loader == 'GPTQ-for-LLaMa' and not shared.args.monkey_patch:
- yield "LoRA training with GPTQ-for-LLaMa requires loading with `--monkey-patch`"
- return
-
- if cutoff_len <= 0 or micro_batch_size <= 0 or batch_size <= 0 or actual_lr <= 0 or lora_rank <= 0 or lora_alpha <= 0:
- yield "Cannot input zeroes."
- return
-
- gradient_accumulation_steps = batch_size // micro_batch_size
- shared.tokenizer.pad_token_id = 0
- shared.tokenizer.padding_side = "left"
-
- def encode(text, add_bos_token):
- result = shared.tokenizer.encode(text, truncation=True, max_length=cutoff_len)
- # Check if the first two tokens are BOS
- if len(result) >= 2 and result[:2] == [shared.tokenizer.bos_token_id, shared.tokenizer.bos_token_id]:
- result = result[1:]
-
- if not add_bos_token and result[0] == shared.tokenizer.bos_token_id:
- result = result[1:]
- return result
-
- def tokenize(prompt, append_eos_token=False):
-
- if train_only_after == '' or train_only_after not in prompt:
- input_ids = encode(prompt, True)
-
- if append_eos_token and input_ids[-1] != shared.tokenizer.eos_token_id and len(input_ids) < cutoff_len:
- input_ids.append(shared.tokenizer.eos_token_id)
-
- input_ids = [shared.tokenizer.pad_token_id] * (cutoff_len - len(input_ids)) + input_ids
- labels = [1] * len(input_ids)
-
- else:
- ind = prompt.index(train_only_after) + len(train_only_after)
- before_tokens = encode(prompt[:ind], True)
- after_tokens = encode(prompt[ind:], False)
-
- if append_eos_token and after_tokens[-1] != shared.tokenizer.eos_token_id:
- after_tokens.append(shared.tokenizer.eos_token_id)
-
- full_length = len(after_tokens) + len(before_tokens)
- if full_length > cutoff_len:
- after_tokens = after_tokens[:cutoff_len - len(before_tokens)]
- else:
- before_tokens = [shared.tokenizer.pad_token_id] * (cutoff_len - full_length) + before_tokens
-
- input_ids = before_tokens + after_tokens
- labels = [-100] * len(before_tokens) + [1] * len(after_tokens)
-
- input_ids = torch.tensor(input_ids)
- return {
- "input_ids": input_ids,
- "labels": labels,
- "attention_mask": input_ids.ne(shared.tokenizer.pad_token_id),
- }
-
- train_template.clear()
-
- # == Prep the dataset, format, etc ==
- if raw_text_file not in ['None', '']:
- train_template["template_type"] = "raw_text"
- logger.info("Loading raw text file dataset...")
- fullpath = clean_path('training/datasets', f'{raw_text_file}')
- fullpath = Path(fullpath)
- if fullpath.is_dir():
- logger.info('Training path directory {}'.format(raw_text_file))
- raw_text = ""
- file_paths = sorted(fullpath.glob('*.txt'), key=lambda path: natural_keys(path.name))
- for file_path in file_paths:
- if file_path.is_file():
- with file_path.open('r', encoding='utf-8') as file:
- raw_text += file.read().replace('\r', '')
-
- logger.info(f"Loaded training file: {file_path.name}")
- else:
- with open(clean_path('training/datasets', f'{raw_text_file}.txt'), 'r', encoding='utf-8') as file:
- raw_text = file.read().replace('\r', '')
-
- cut_string = hard_cut_string.replace('\\n', '\n')
- eos_added = 0
- out_tokens = []
- for text_part in raw_text.split(cut_string):
- if len(text_part.strip()) <= min_chars:
- continue
-
- tokens = shared.tokenizer.encode(text_part)
- if add_eos_token:
- tokens.append(shared.tokenizer.eos_token_id)
- eos_added += 1
-
- step = cutoff_len - overlap_len
- if step <= 0:
- yield f"Error: overlap_len ({overlap_len}) cannot be greater than or equal to cutoff_len ({cutoff_len})"
- return
-
- out_tokens.extend(split_chunks(tokens, cutoff_len, step))
-
- if eos_added > 0:
- print(f"EOS added to {eos_added} text blocks")
-
- del raw_text # Note: could be a gig for a large dataset, so delete redundant data as we go to be safe on RAM
- text_chunks = [shared.tokenizer.decode(x) for x in out_tokens]
- del out_tokens
- if newline_favor_len > 0:
- text_chunks = [cut_chunk_for_newline(x, newline_favor_len) for x in text_chunks]
-
- train_data = Dataset.from_list([tokenize(x) for x in text_chunks])
- del text_chunks
- eval_data = None
- else:
- if dataset in ['None', '']:
- yield "Missing dataset choice input, cannot continue."
- return
-
- if format in ['None', '']:
- yield "Missing format choice input, cannot continue."
- return
-
- train_template["template_type"] = "dataset"
-
- with open(clean_path('training/formats', f'{format}.json'), 'r', encoding='utf-8-sig') as formatFile:
- format_data: dict[str, str] = json.load(formatFile)
-
- # == store training prompt ==
- for _, value in format_data.items():
- prompt_key = f"template_{len(train_template)}"
- train_template[prompt_key] = value
-
- def generate_prompt(data_point: dict[str, str]):
- for options, data in format_data.items():
- if set(options.split(',')) == set(x[0] for x in data_point.items() if (type(x[1]) is str and len(x[1].strip()) > 0)):
- for key, val in data_point.items():
- if type(val) is str:
- data = data.replace(f'%{key}%', val)
- return data
- raise RuntimeError(f'Data-point "{data_point}" has no keyset match within format "{list(format_data.keys())}"')
-
- def generate_and_tokenize_prompt(data_point):
- prompt = generate_prompt(data_point)
- return tokenize(prompt, add_eos_token)
-
- logger.info("Loading JSON datasets...")
- data = load_dataset("json", data_files=clean_path('training/datasets', f'{dataset}.json'))
- train_data = data['train'].map(generate_and_tokenize_prompt, new_fingerprint='%030x' % random.randrange(16**30))
-
- if eval_dataset == 'None':
- eval_data = None
- else:
- eval_data = load_dataset("json", data_files=clean_path('training/datasets', f'{eval_dataset}.json'))
- eval_data = eval_data['train'].map(generate_and_tokenize_prompt, new_fingerprint='%030x' % random.randrange(16**30))
-
- # == We MUST reload model if it went through any previous training, even failed one ==
- if shared.model_dirty_from_training:
- selected_model = shared.model_name
- if selected_model:
- print("\033[1;31;1m(Model has been modified by previous training, it needs to be reloaded...)\033[0;37;0m")
- try:
- yield f"Reloading {selected_model}..."
- reload_model()
- if shared.model is not None:
- print("Model reloaded OK, continue with training.")
- else:
- return f"Failed to load {selected_model}."
- except:
- exc = traceback.format_exc()
- logger.error('Failed to reload the model.')
- print(exc)
- return exc.replace('\n', '\n\n')
-
- # == Start prepping the model itself ==
- if not hasattr(shared.model, 'lm_head') or hasattr(shared.model.lm_head, 'weight'):
- logger.info("Getting model ready...")
- prepare_model_for_kbit_training(shared.model)
-
- # base model is now frozen and should not be reused for any other LoRA training than this one
- shared.model_dirty_from_training = True
-
- logger.info("Preparing for training...")
- config = LoraConfig(
- r=lora_rank,
- lora_alpha=lora_alpha,
- target_modules=model_to_lora_modules[model_id],
- lora_dropout=lora_dropout,
- bias="none",
- task_type="CAUSAL_LM"
- )
-
- # == Backup the existing adapter ==
- if not always_override:
- backup_adapter(lora_file_path)
-
- # == get model trainable params
- model_trainable_params, model_all_params = calc_trainable_parameters(shared.model)
-
- try:
- logger.info("Creating LoRA model...")
- lora_model = get_peft_model(shared.model, config)
- if not always_override and Path(f"{lora_file_path}/adapter_model.bin").is_file():
- logger.info("Loading existing LoRA data...")
- state_dict_peft = torch.load(f"{lora_file_path}/adapter_model.bin")
- set_peft_model_state_dict(lora_model, state_dict_peft)
- except:
- yield traceback.format_exc().replace('\n', '\n\n')
- return
-
- if shared.args.monkey_patch:
- from alpaca_lora_4bit.autograd_4bit import Autograd4bitQuantLinear
- from alpaca_lora_4bit.models import Linear4bitLt
- for _, m in lora_model.named_modules():
- if isinstance(m, Autograd4bitQuantLinear) or isinstance(m, Linear4bitLt):
- if m.is_v1_model:
- m.zeros = m.zeros.half()
- m.scales = m.scales.half()
-
- class Tracked():
- def __init__(self):
- self.current_steps = 0
- self.max_steps = 0
- self.did_save = False
-
- tracked = Tracked()
- actual_save_steps = math.ceil(save_steps / gradient_accumulation_steps)
-
- class Callbacks(transformers.TrainerCallback):
- def on_step_begin(self, args: transformers.TrainingArguments, state: transformers.TrainerState, control: transformers.TrainerControl, **kwargs):
- tracked.current_steps = state.global_step * gradient_accumulation_steps
- tracked.max_steps = state.max_steps * gradient_accumulation_steps
- if WANT_INTERRUPT:
- control.should_epoch_stop = True
- control.should_training_stop = True
- elif state.global_step > 0 and actual_save_steps > 0 and state.global_step % actual_save_steps == 0:
- lora_model.save_pretrained(f"{lora_file_path}/checkpoint-{tracked.current_steps}/")
- # Save log
- with open(f"{lora_file_path}/checkpoint-{tracked.current_steps}/training_log.json", 'w', encoding='utf-8') as file:
- json.dump(train_log, file, indent=2)
- # == Save training prompt ==
- with open(f"{lora_file_path}/checkpoint-{tracked.current_steps}/training_prompt.json", 'w', encoding='utf-8') as file:
- json.dump(train_template, file, indent=2)
-
- def on_substep_end(self, args: transformers.TrainingArguments, state: transformers.TrainerState, control: transformers.TrainerControl, **kwargs):
- tracked.current_steps += 1
- if WANT_INTERRUPT:
- control.should_epoch_stop = True
- control.should_training_stop = True
-
- def on_log(self, args: transformers.TrainingArguments, state: transformers.TrainerState, control: transformers.TrainerControl, logs, **kwargs):
- train_log.update(logs)
- train_log.update({"current_steps": tracked.current_steps})
- if WANT_INTERRUPT:
- print("\033[1;31;1mInterrupted by user\033[0;37;0m")
-
- print(f"\033[1;30;40mStep: {tracked.current_steps} \033[0;37;0m", end='')
- if 'loss' in logs:
- loss = float(logs['loss'])
- if loss <= stop_at_loss:
- control.should_epoch_stop = True
- control.should_training_stop = True
- print(f"\033[1;31;1mStop Loss {stop_at_loss} reached.\033[0;37;0m")
-
- trainer = transformers.Trainer(
- model=lora_model,
- train_dataset=train_data,
- eval_dataset=eval_data,
- args=transformers.TrainingArguments(
- report_to=report_to if report_to != "None" else None,
- per_device_train_batch_size=micro_batch_size,
- gradient_accumulation_steps=gradient_accumulation_steps,
- warmup_steps=math.ceil(warmup_steps / gradient_accumulation_steps),
- num_train_epochs=epochs,
- learning_rate=actual_lr,
- fp16=False if shared.args.cpu else True,
- optim=optimizer,
- logging_steps=2 if stop_at_loss > 0 else 5,
- evaluation_strategy="steps" if eval_data is not None else "no",
- eval_steps=math.ceil(eval_steps / gradient_accumulation_steps) if eval_data is not None else None,
- save_strategy="steps" if eval_data is not None else "no",
- output_dir=lora_file_path,
- lr_scheduler_type=lr_scheduler_type,
- load_best_model_at_end=eval_data is not None,
- # TODO: Enable multi-device support
- ddp_find_unused_parameters=None,
- no_cuda=shared.args.cpu,
- ),
- data_collator=transformers.DataCollatorForLanguageModeling(shared.tokenizer, mlm=False),
- callbacks=list([Callbacks()])
- )
-
- lora_model.config.use_cache = False
-
- if torch.__version__ >= "2" and sys.platform != "win32":
- lora_model = torch.compile(lora_model)
-
- # == Save parameters for reuse ==
- with open(f"{lora_file_path}/training_parameters.json", 'w', encoding='utf-8') as file:
- vars = locals()
- json.dump({x: vars[x] for x in PARAMETERS}, file, indent=2)
-
- # == Save training prompt ==
- with open(f"{lora_file_path}/training_prompt.json", 'w', encoding='utf-8') as file:
- json.dump(train_template, file, indent=2)
-
- # == Main run and monitor loop ==
- logger.info("Starting training...")
- yield "Starting..."
-
- lora_trainable_param, lora_all_param = calc_trainable_parameters(lora_model)
-
- projections_string = ", ".join([projection.replace("_proj", "") for projection in model_to_lora_modules[model_id]])
-
- print(f"Training '{model_id}' model using ({projections_string}) projections")
-
- if lora_all_param > 0:
- print(f"Trainable params: {lora_trainable_param:,d} ({100 * lora_trainable_param / lora_all_param:.4f} %), All params: {lora_all_param:,d} (Model: {model_all_params:,d})")
-
- train_log.update({"base_model_name": shared.model_name})
- train_log.update({"base_model_class": shared.model.__class__.__name__})
- train_log.update({"base_loaded_in_4bit": getattr(lora_model, "is_loaded_in_4bit", False)})
- train_log.update({"base_loaded_in_8bit": getattr(lora_model, "is_loaded_in_8bit", False)})
- train_log.update({"projections": projections_string})
-
- if stop_at_loss > 0:
- print(f"Monitoring loss \033[1;31;1m(Auto-Stop at: {stop_at_loss})\033[0;37;0m")
-
- if WANT_INTERRUPT:
- yield "Interrupted before start."
- return
-
- def log_train_dataset(trainer):
- decoded_entries = []
- # Try to decode the entries and write the log file
- try:
- # Iterate over the first 10 elements in the dataset (or fewer if there are less than 10)
- for i in range(min(10, len(trainer.train_dataset))):
- decoded_text = shared.tokenizer.decode(trainer.train_dataset[i]['input_ids'])
- decoded_entries.append({"value": decoded_text})
-
- # Write the log file
- Path('logs').mkdir(exist_ok=True)
- with open(Path('logs/train_dataset_sample.json'), 'w') as json_file:
- json.dump(decoded_entries, json_file, indent=4)
-
- logger.info("Log file 'train_dataset_sample.json' created in the 'logs' directory.")
- except Exception as e:
- logger.error(f"Failed to create log file due to error: {e}")
-
- def threaded_run():
- log_train_dataset(trainer)
- trainer.train()
- # Note: save in the thread in case the gradio thread breaks (eg browser closed)
- lora_model.save_pretrained(lora_file_path)
- logger.info("LoRA training run is completed and saved.")
- # Save log
- with open(f"{lora_file_path}/training_log.json", 'w', encoding='utf-8') as file:
- json.dump(train_log, file, indent=2)
-
- thread = threading.Thread(target=threaded_run)
- thread.start()
- last_step = 0
- start_time = time.perf_counter()
-
- while thread.is_alive():
- time.sleep(0.5)
- if WANT_INTERRUPT:
- yield "Interrupting, please wait... *(Run will stop after the current training step completes.)*"
-
- elif tracked.current_steps != last_step:
- last_step = tracked.current_steps
- time_elapsed = time.perf_counter() - start_time
- if time_elapsed <= 0:
- timer_info = ""
- total_time_estimate = 999
- else:
- its = tracked.current_steps / time_elapsed
- if its > 1:
- timer_info = f"`{its:.2f}` it/s"
- else:
- timer_info = f"`{1.0/its:.2f}` s/it"
-
- total_time_estimate = (1.0 / its) * (tracked.max_steps)
-
- yield f"Running... **{tracked.current_steps}** / **{tracked.max_steps}** ... {timer_info}, {format_time(time_elapsed)} / {format_time(total_time_estimate)} ... {format_time(total_time_estimate - time_elapsed)} remaining"
-
- # Saving in the train thread might fail if an error occurs, so save here if so.
- if not tracked.did_save:
- logger.info("Training complete, saving...")
- lora_model.save_pretrained(lora_file_path)
-
- if WANT_INTERRUPT:
- logger.info("Training interrupted.")
- yield f"Interrupted. Incomplete LoRA saved to `{lora_file_path}`."
- else:
- logger.info("Training complete!")
- yield f"Done! LoRA saved to `{lora_file_path}`.\n\nBefore testing your new LoRA, make sure to first reload the model, as it is currently dirty from training."
-
-
-def split_chunks(arr, size, step):
- for i in range(0, len(arr), step):
- yield arr[i:i + size]
-
-
-def cut_chunk_for_newline(chunk: str, max_length: int):
- if '\n' not in chunk:
- return chunk
-
- first_newline = chunk.index('\n')
- if first_newline < max_length:
- chunk = chunk[first_newline + 1:]
-
- if '\n' not in chunk:
- return chunk
-
- last_newline = chunk.rindex('\n')
- if len(chunk) - last_newline < max_length:
- chunk = chunk[:last_newline]
-
- return chunk
-
-
-def format_time(seconds: float):
- if seconds < 120:
- return f"`{seconds:.0f}` seconds"
-
- minutes = seconds / 60
- if minutes < 120:
- return f"`{minutes:.0f}` minutes"
-
- hours = minutes / 60
- return f"`{hours:.0f}` hours"
diff --git a/spaces/AnnasBlackHat/Image-Similarity/src/model/simlarity_model.py b/spaces/AnnasBlackHat/Image-Similarity/src/model/simlarity_model.py
deleted file mode 100644
index da19670541877a906c342116c2ae7f899cc9ca07..0000000000000000000000000000000000000000
--- a/spaces/AnnasBlackHat/Image-Similarity/src/model/simlarity_model.py
+++ /dev/null
@@ -1,9 +0,0 @@
-from dataclasses import dataclass
-from .similarity_interface import SimilarityInterface
-
-@dataclass
-class SimilarityModel:
- name: str
- image_size: int
- model_cls: SimilarityInterface
- image_input_type: str = 'array'
\ No newline at end of file
diff --git a/spaces/Anonymous-sub/Rerender/ControlNet/annotator/uniformer/mmcv/ops/bbox.py b/spaces/Anonymous-sub/Rerender/ControlNet/annotator/uniformer/mmcv/ops/bbox.py
deleted file mode 100644
index 0c4d58b6c91f652933974f519acd3403a833e906..0000000000000000000000000000000000000000
--- a/spaces/Anonymous-sub/Rerender/ControlNet/annotator/uniformer/mmcv/ops/bbox.py
+++ /dev/null
@@ -1,72 +0,0 @@
-# Copyright (c) OpenMMLab. All rights reserved.
-from ..utils import ext_loader
-
-ext_module = ext_loader.load_ext('_ext', ['bbox_overlaps'])
-
-
-def bbox_overlaps(bboxes1, bboxes2, mode='iou', aligned=False, offset=0):
- """Calculate overlap between two set of bboxes.
-
- If ``aligned`` is ``False``, then calculate the ious between each bbox
- of bboxes1 and bboxes2, otherwise the ious between each aligned pair of
- bboxes1 and bboxes2.
-
- Args:
- bboxes1 (Tensor): shape (m, 4) in format or empty.
- bboxes2 (Tensor): shape (n, 4) in format or empty.
- If aligned is ``True``, then m and n must be equal.
- mode (str): "iou" (intersection over union) or iof (intersection over
- foreground).
-
- Returns:
- ious(Tensor): shape (m, n) if aligned == False else shape (m, 1)
-
- Example:
- >>> bboxes1 = torch.FloatTensor([
- >>> [0, 0, 10, 10],
- >>> [10, 10, 20, 20],
- >>> [32, 32, 38, 42],
- >>> ])
- >>> bboxes2 = torch.FloatTensor([
- >>> [0, 0, 10, 20],
- >>> [0, 10, 10, 19],
- >>> [10, 10, 20, 20],
- >>> ])
- >>> bbox_overlaps(bboxes1, bboxes2)
- tensor([[0.5000, 0.0000, 0.0000],
- [0.0000, 0.0000, 1.0000],
- [0.0000, 0.0000, 0.0000]])
-
- Example:
- >>> empty = torch.FloatTensor([])
- >>> nonempty = torch.FloatTensor([
- >>> [0, 0, 10, 9],
- >>> ])
- >>> assert tuple(bbox_overlaps(empty, nonempty).shape) == (0, 1)
- >>> assert tuple(bbox_overlaps(nonempty, empty).shape) == (1, 0)
- >>> assert tuple(bbox_overlaps(empty, empty).shape) == (0, 0)
- """
-
- mode_dict = {'iou': 0, 'iof': 1}
- assert mode in mode_dict.keys()
- mode_flag = mode_dict[mode]
- # Either the boxes are empty or the length of boxes' last dimension is 4
- assert (bboxes1.size(-1) == 4 or bboxes1.size(0) == 0)
- assert (bboxes2.size(-1) == 4 or bboxes2.size(0) == 0)
- assert offset == 1 or offset == 0
-
- rows = bboxes1.size(0)
- cols = bboxes2.size(0)
- if aligned:
- assert rows == cols
-
- if rows * cols == 0:
- return bboxes1.new(rows, 1) if aligned else bboxes1.new(rows, cols)
-
- if aligned:
- ious = bboxes1.new_zeros(rows)
- else:
- ious = bboxes1.new_zeros((rows, cols))
- ext_module.bbox_overlaps(
- bboxes1, bboxes2, ious, mode=mode_flag, aligned=aligned, offset=offset)
- return ious
diff --git a/spaces/Ataturk-Chatbot/HuggingFaceChat/venv/lib/python3.11/site-packages/pip/_vendor/platformdirs/windows.py b/spaces/Ataturk-Chatbot/HuggingFaceChat/venv/lib/python3.11/site-packages/pip/_vendor/platformdirs/windows.py
deleted file mode 100644
index e7573c3d6ae773d852da06c107c07b253d44b496..0000000000000000000000000000000000000000
--- a/spaces/Ataturk-Chatbot/HuggingFaceChat/venv/lib/python3.11/site-packages/pip/_vendor/platformdirs/windows.py
+++ /dev/null
@@ -1,195 +0,0 @@
-from __future__ import annotations
-
-import ctypes
-import os
-import sys
-from functools import lru_cache
-from typing import Callable
-
-from .api import PlatformDirsABC
-
-
-class Windows(PlatformDirsABC):
- """`MSDN on where to store app data files
- `_.
- Makes use of the
- `appname `,
- `appauthor `,
- `version `,
- `roaming `,
- `opinion `,
- `ensure_exists `.
- """
-
- @property
- def user_data_dir(self) -> str:
- """
- :return: data directory tied to the user, e.g.
- ``%USERPROFILE%\\AppData\\Local\\$appauthor\\$appname`` (not roaming) or
- ``%USERPROFILE%\\AppData\\Roaming\\$appauthor\\$appname`` (roaming)
- """
- const = "CSIDL_APPDATA" if self.roaming else "CSIDL_LOCAL_APPDATA"
- path = os.path.normpath(get_win_folder(const))
- return self._append_parts(path)
-
- def _append_parts(self, path: str, *, opinion_value: str | None = None) -> str:
- params = []
- if self.appname:
- if self.appauthor is not False:
- author = self.appauthor or self.appname
- params.append(author)
- params.append(self.appname)
- if opinion_value is not None and self.opinion:
- params.append(opinion_value)
- if self.version:
- params.append(self.version)
- path = os.path.join(path, *params)
- self._optionally_create_directory(path)
- return path
-
- @property
- def site_data_dir(self) -> str:
- """:return: data directory shared by users, e.g. ``C:\\ProgramData\\$appauthor\\$appname``"""
- path = os.path.normpath(get_win_folder("CSIDL_COMMON_APPDATA"))
- return self._append_parts(path)
-
- @property
- def user_config_dir(self) -> str:
- """:return: config directory tied to the user, same as `user_data_dir`"""
- return self.user_data_dir
-
- @property
- def site_config_dir(self) -> str:
- """:return: config directory shared by the users, same as `site_data_dir`"""
- return self.site_data_dir
-
- @property
- def user_cache_dir(self) -> str:
- """
- :return: cache directory tied to the user (if opinionated with ``Cache`` folder within ``$appname``) e.g.
- ``%USERPROFILE%\\AppData\\Local\\$appauthor\\$appname\\Cache\\$version``
- """
- path = os.path.normpath(get_win_folder("CSIDL_LOCAL_APPDATA"))
- return self._append_parts(path, opinion_value="Cache")
-
- @property
- def site_cache_dir(self) -> str:
- """:return: cache directory shared by users, e.g. ``C:\\ProgramData\\$appauthor\\$appname\\Cache\\$version``"""
- path = os.path.normpath(get_win_folder("CSIDL_COMMON_APPDATA"))
- return self._append_parts(path, opinion_value="Cache")
-
- @property
- def user_state_dir(self) -> str:
- """:return: state directory tied to the user, same as `user_data_dir`"""
- return self.user_data_dir
-
- @property
- def user_log_dir(self) -> str:
- """
- :return: log directory tied to the user, same as `user_data_dir` if not opinionated else ``Logs`` in it
- """
- path = self.user_data_dir
- if self.opinion:
- path = os.path.join(path, "Logs")
- self._optionally_create_directory(path)
- return path
-
- @property
- def user_documents_dir(self) -> str:
- """
- :return: documents directory tied to the user e.g. ``%USERPROFILE%\\Documents``
- """
- return os.path.normpath(get_win_folder("CSIDL_PERSONAL"))
-
- @property
- def user_runtime_dir(self) -> str:
- """
- :return: runtime directory tied to the user, e.g.
- ``%USERPROFILE%\\AppData\\Local\\Temp\\$appauthor\\$appname``
- """
- path = os.path.normpath(os.path.join(get_win_folder("CSIDL_LOCAL_APPDATA"), "Temp"))
- return self._append_parts(path)
-
-
-def get_win_folder_from_env_vars(csidl_name: str) -> str:
- """Get folder from environment variables."""
- if csidl_name == "CSIDL_PERSONAL": # does not have an environment name
- return os.path.join(os.path.normpath(os.environ["USERPROFILE"]), "Documents")
-
- env_var_name = {
- "CSIDL_APPDATA": "APPDATA",
- "CSIDL_COMMON_APPDATA": "ALLUSERSPROFILE",
- "CSIDL_LOCAL_APPDATA": "LOCALAPPDATA",
- }.get(csidl_name)
- if env_var_name is None:
- raise ValueError(f"Unknown CSIDL name: {csidl_name}")
- result = os.environ.get(env_var_name)
- if result is None:
- raise ValueError(f"Unset environment variable: {env_var_name}")
- return result
-
-
-def get_win_folder_from_registry(csidl_name: str) -> str:
- """Get folder from the registry.
-
- This is a fallback technique at best. I'm not sure if using the
- registry for this guarantees us the correct answer for all CSIDL_*
- names.
- """
- shell_folder_name = {
- "CSIDL_APPDATA": "AppData",
- "CSIDL_COMMON_APPDATA": "Common AppData",
- "CSIDL_LOCAL_APPDATA": "Local AppData",
- "CSIDL_PERSONAL": "Personal",
- }.get(csidl_name)
- if shell_folder_name is None:
- raise ValueError(f"Unknown CSIDL name: {csidl_name}")
- if sys.platform != "win32": # only needed for mypy type checker to know that this code runs only on Windows
- raise NotImplementedError
- import winreg
-
- key = winreg.OpenKey(winreg.HKEY_CURRENT_USER, r"Software\Microsoft\Windows\CurrentVersion\Explorer\Shell Folders")
- directory, _ = winreg.QueryValueEx(key, shell_folder_name)
- return str(directory)
-
-
-def get_win_folder_via_ctypes(csidl_name: str) -> str:
- """Get folder with ctypes."""
- csidl_const = {
- "CSIDL_APPDATA": 26,
- "CSIDL_COMMON_APPDATA": 35,
- "CSIDL_LOCAL_APPDATA": 28,
- "CSIDL_PERSONAL": 5,
- }.get(csidl_name)
- if csidl_const is None:
- raise ValueError(f"Unknown CSIDL name: {csidl_name}")
-
- buf = ctypes.create_unicode_buffer(1024)
- windll = getattr(ctypes, "windll") # noqa: B009 # using getattr to avoid false positive with mypy type checker
- windll.shell32.SHGetFolderPathW(None, csidl_const, None, 0, buf)
-
- # Downgrade to short path name if it has highbit chars.
- if any(ord(c) > 255 for c in buf):
- buf2 = ctypes.create_unicode_buffer(1024)
- if windll.kernel32.GetShortPathNameW(buf.value, buf2, 1024):
- buf = buf2
-
- return buf.value
-
-
-def _pick_get_win_folder() -> Callable[[str], str]:
- if hasattr(ctypes, "windll"):
- return get_win_folder_via_ctypes
- try:
- import winreg # noqa: F401
- except ImportError:
- return get_win_folder_from_env_vars
- else:
- return get_win_folder_from_registry
-
-
-get_win_folder = lru_cache(maxsize=None)(_pick_get_win_folder())
-
-__all__ = [
- "Windows",
-]
diff --git a/spaces/Atualli/yoloxTeste/yoloxdetect2/configs/yolox_l.py b/spaces/Atualli/yoloxTeste/yoloxdetect2/configs/yolox_l.py
deleted file mode 100644
index 50833ca38c51fe9ac5e327d7c1c0561fb62249aa..0000000000000000000000000000000000000000
--- a/spaces/Atualli/yoloxTeste/yoloxdetect2/configs/yolox_l.py
+++ /dev/null
@@ -1,15 +0,0 @@
-#!/usr/bin/env python3
-# -*- coding:utf-8 -*-
-# Copyright (c) Megvii, Inc. and its affiliates.
-
-import os
-
-from yolox.exp import Exp as MyExp
-
-
-class Exp(MyExp):
- def __init__(self):
- super(Exp, self).__init__()
- self.depth = 1.0
- self.width = 1.0
- self.exp_name = os.path.split(os.path.realpath(__file__))[1].split(".")[0]
diff --git a/spaces/Audio-AGI/AudioSep/data/audiotext_dataset.py b/spaces/Audio-AGI/AudioSep/data/audiotext_dataset.py
deleted file mode 100644
index 1e9cc2c037516f7768ffca8d8083d137a4879dba..0000000000000000000000000000000000000000
--- a/spaces/Audio-AGI/AudioSep/data/audiotext_dataset.py
+++ /dev/null
@@ -1,91 +0,0 @@
-import json
-import random
-import torch
-import torchaudio
-from torch.utils.data import Dataset
-
-
-class AudioTextDataset(Dataset):
- """Can sample data from audio-text databases
- Params:
- sampling_rate: audio sampling rate
- max_clip_len: max length (seconds) of audio clip to be sampled
- """
- def __init__(
- self,
- datafiles=[''],
- sampling_rate=32000,
- max_clip_len=5,
- ):
- all_data_json = []
- for datafile in datafiles:
- with open(datafile, 'r') as fp:
- data_json = json.load(fp)['data']
- all_data_json.extend(data_json)
- self.all_data_json = all_data_json
-
- self.sampling_rate = sampling_rate
- self.max_length = max_clip_len * sampling_rate
-
- def __len__(self):
- return len(self.all_data_json)
-
- def _cut_or_randomcrop(self, waveform):
- # waveform: [1, samples]
- # random crop
- if waveform.size(1) > self.max_length:
- random_idx = random.randint(0, waveform.size(1)-self.max_length)
- waveform = waveform[:, random_idx:random_idx+self.max_length]
- else:
- temp_wav = torch.zeros(1, self.max_length)
- temp_wav[:, 0:waveform.size(1)] = waveform
- waveform = temp_wav
-
- assert waveform.size(1) == self.max_length, \
- f"number of audio samples is {waveform.size(1)}"
-
- return waveform
-
- def _read_audio(self, index):
- try:
- audio_path = self.all_data_json[index]['wav']
- audio_data, audio_rate = torchaudio.load(audio_path, channels_first=True)
- text = self.all_data_json[index]['caption']
-
- # drop short utterance
- if audio_data.size(1) < self.sampling_rate * 1:
- raise Exception(f'{audio_path} is too short, drop it ...')
-
- return text, audio_data, audio_rate
-
- except Exception as e:
- print(f'error: {e} occurs, when loading {audio_path}')
- random_index = random.randint(0, len(self.all_data_json)-1)
- return self._read_audio(index=random_index)
-
- def __getitem__(self, index):
- # create a audio tensor
- text, audio_data, audio_rate = self._read_audio(index)
- audio_len = audio_data.shape[1] / audio_rate
- # convert stero to single channel
- if audio_data.shape[0] > 1:
- # audio_data: [samples]
- audio_data = (audio_data[0] + audio_data[1]) / 2
- else:
- audio_data = audio_data.squeeze(0)
-
- # resample audio clip
- if audio_rate != self.sampling_rate:
- audio_data = torchaudio.functional.resample(audio_data, orig_freq=audio_rate, new_freq=self.sampling_rate)
-
- audio_data = audio_data.unsqueeze(0)
-
- audio_data = self._cut_or_randomcrop(audio_data)
-
- data_dict = {
- 'text': text,
- 'waveform': audio_data,
- 'modality': 'audio_text'
- }
-
- return data_dict
diff --git a/spaces/Awiny/Image2Paragraph/models/grit_src/third_party/CenterNet2/detectron2/data/__init__.py b/spaces/Awiny/Image2Paragraph/models/grit_src/third_party/CenterNet2/detectron2/data/__init__.py
deleted file mode 100644
index 259f669b78bd05815cb8d3351fd6c5fc9a1b85a1..0000000000000000000000000000000000000000
--- a/spaces/Awiny/Image2Paragraph/models/grit_src/third_party/CenterNet2/detectron2/data/__init__.py
+++ /dev/null
@@ -1,19 +0,0 @@
-# Copyright (c) Facebook, Inc. and its affiliates.
-from . import transforms # isort:skip
-
-from .build import (
- build_batch_data_loader,
- build_detection_test_loader,
- build_detection_train_loader,
- get_detection_dataset_dicts,
- load_proposals_into_dataset,
- print_instances_class_histogram,
-)
-from .catalog import DatasetCatalog, MetadataCatalog, Metadata
-from .common import DatasetFromList, MapDataset, ToIterableDataset
-from .dataset_mapper import DatasetMapper
-
-# ensure the builtin datasets are registered
-from . import datasets, samplers # isort:skip
-
-__all__ = [k for k in globals().keys() if not k.startswith("_")]
diff --git a/spaces/Bart92/RVC_HF/lib/infer_pack/commons.py b/spaces/Bart92/RVC_HF/lib/infer_pack/commons.py
deleted file mode 100644
index 54470986f37825b35d90d7efa7437d1c26b87215..0000000000000000000000000000000000000000
--- a/spaces/Bart92/RVC_HF/lib/infer_pack/commons.py
+++ /dev/null
@@ -1,166 +0,0 @@
-import math
-import numpy as np
-import torch
-from torch import nn
-from torch.nn import functional as F
-
-
-def init_weights(m, mean=0.0, std=0.01):
- classname = m.__class__.__name__
- if classname.find("Conv") != -1:
- m.weight.data.normal_(mean, std)
-
-
-def get_padding(kernel_size, dilation=1):
- return int((kernel_size * dilation - dilation) / 2)
-
-
-def convert_pad_shape(pad_shape):
- l = pad_shape[::-1]
- pad_shape = [item for sublist in l for item in sublist]
- return pad_shape
-
-
-def kl_divergence(m_p, logs_p, m_q, logs_q):
- """KL(P||Q)"""
- kl = (logs_q - logs_p) - 0.5
- kl += (
- 0.5 * (torch.exp(2.0 * logs_p) + ((m_p - m_q) ** 2)) * torch.exp(-2.0 * logs_q)
- )
- return kl
-
-
-def rand_gumbel(shape):
- """Sample from the Gumbel distribution, protect from overflows."""
- uniform_samples = torch.rand(shape) * 0.99998 + 0.00001
- return -torch.log(-torch.log(uniform_samples))
-
-
-def rand_gumbel_like(x):
- g = rand_gumbel(x.size()).to(dtype=x.dtype, device=x.device)
- return g
-
-
-def slice_segments(x, ids_str, segment_size=4):
- ret = torch.zeros_like(x[:, :, :segment_size])
- for i in range(x.size(0)):
- idx_str = ids_str[i]
- idx_end = idx_str + segment_size
- ret[i] = x[i, :, idx_str:idx_end]
- return ret
-
-
-def slice_segments2(x, ids_str, segment_size=4):
- ret = torch.zeros_like(x[:, :segment_size])
- for i in range(x.size(0)):
- idx_str = ids_str[i]
- idx_end = idx_str + segment_size
- ret[i] = x[i, idx_str:idx_end]
- return ret
-
-
-def rand_slice_segments(x, x_lengths=None, segment_size=4):
- b, d, t = x.size()
- if x_lengths is None:
- x_lengths = t
- ids_str_max = x_lengths - segment_size + 1
- ids_str = (torch.rand([b]).to(device=x.device) * ids_str_max).to(dtype=torch.long)
- ret = slice_segments(x, ids_str, segment_size)
- return ret, ids_str
-
-
-def get_timing_signal_1d(length, channels, min_timescale=1.0, max_timescale=1.0e4):
- position = torch.arange(length, dtype=torch.float)
- num_timescales = channels // 2
- log_timescale_increment = math.log(float(max_timescale) / float(min_timescale)) / (
- num_timescales - 1
- )
- inv_timescales = min_timescale * torch.exp(
- torch.arange(num_timescales, dtype=torch.float) * -log_timescale_increment
- )
- scaled_time = position.unsqueeze(0) * inv_timescales.unsqueeze(1)
- signal = torch.cat([torch.sin(scaled_time), torch.cos(scaled_time)], 0)
- signal = F.pad(signal, [0, 0, 0, channels % 2])
- signal = signal.view(1, channels, length)
- return signal
-
-
-def add_timing_signal_1d(x, min_timescale=1.0, max_timescale=1.0e4):
- b, channels, length = x.size()
- signal = get_timing_signal_1d(length, channels, min_timescale, max_timescale)
- return x + signal.to(dtype=x.dtype, device=x.device)
-
-
-def cat_timing_signal_1d(x, min_timescale=1.0, max_timescale=1.0e4, axis=1):
- b, channels, length = x.size()
- signal = get_timing_signal_1d(length, channels, min_timescale, max_timescale)
- return torch.cat([x, signal.to(dtype=x.dtype, device=x.device)], axis)
-
-
-def subsequent_mask(length):
- mask = torch.tril(torch.ones(length, length)).unsqueeze(0).unsqueeze(0)
- return mask
-
-
-@torch.jit.script
-def fused_add_tanh_sigmoid_multiply(input_a, input_b, n_channels):
- n_channels_int = n_channels[0]
- in_act = input_a + input_b
- t_act = torch.tanh(in_act[:, :n_channels_int, :])
- s_act = torch.sigmoid(in_act[:, n_channels_int:, :])
- acts = t_act * s_act
- return acts
-
-
-def convert_pad_shape(pad_shape):
- l = pad_shape[::-1]
- pad_shape = [item for sublist in l for item in sublist]
- return pad_shape
-
-
-def shift_1d(x):
- x = F.pad(x, convert_pad_shape([[0, 0], [0, 0], [1, 0]]))[:, :, :-1]
- return x
-
-
-def sequence_mask(length, max_length=None):
- if max_length is None:
- max_length = length.max()
- x = torch.arange(max_length, dtype=length.dtype, device=length.device)
- return x.unsqueeze(0) < length.unsqueeze(1)
-
-
-def generate_path(duration, mask):
- """
- duration: [b, 1, t_x]
- mask: [b, 1, t_y, t_x]
- """
- device = duration.device
-
- b, _, t_y, t_x = mask.shape
- cum_duration = torch.cumsum(duration, -1)
-
- cum_duration_flat = cum_duration.view(b * t_x)
- path = sequence_mask(cum_duration_flat, t_y).to(mask.dtype)
- path = path.view(b, t_x, t_y)
- path = path - F.pad(path, convert_pad_shape([[0, 0], [1, 0], [0, 0]]))[:, :-1]
- path = path.unsqueeze(1).transpose(2, 3) * mask
- return path
-
-
-def clip_grad_value_(parameters, clip_value, norm_type=2):
- if isinstance(parameters, torch.Tensor):
- parameters = [parameters]
- parameters = list(filter(lambda p: p.grad is not None, parameters))
- norm_type = float(norm_type)
- if clip_value is not None:
- clip_value = float(clip_value)
-
- total_norm = 0
- for p in parameters:
- param_norm = p.grad.data.norm(norm_type)
- total_norm += param_norm.item() ** norm_type
- if clip_value is not None:
- p.grad.data.clamp_(min=-clip_value, max=clip_value)
- total_norm = total_norm ** (1.0 / norm_type)
- return total_norm
diff --git a/spaces/BetterAPI/BetterChat/svelte.config.js b/spaces/BetterAPI/BetterChat/svelte.config.js
deleted file mode 100644
index b856102c926a34f6bc655c8fbbc0f6acb9b939da..0000000000000000000000000000000000000000
--- a/spaces/BetterAPI/BetterChat/svelte.config.js
+++ /dev/null
@@ -1,26 +0,0 @@
-import adapter from "@sveltejs/adapter-node";
-import { vitePreprocess } from "@sveltejs/kit/vite";
-import dotenv from "dotenv";
-import pkg from "./package.json" assert { type: "json" };
-
-dotenv.config({ path: "./.env.local" });
-dotenv.config({ path: "./.env" });
-
-process.env.PUBLIC_VERSION = pkg.version.replace(/\.0\b/g, "");
-
-/** @type {import('@sveltejs/kit').Config} */
-const config = {
- // Consult https://kit.svelte.dev/docs/integrations#preprocessors
- // for more information about preprocessors
- preprocess: vitePreprocess(),
-
- kit: {
- adapter: adapter(),
-
- paths: {
- base: process.env.APP_BASE || "",
- },
- },
-};
-
-export default config;
diff --git a/spaces/BetterAPI/BetterChat_new/src/lib/actions/snapScrollToBottom.ts b/spaces/BetterAPI/BetterChat_new/src/lib/actions/snapScrollToBottom.ts
deleted file mode 100644
index 0d9335466b5cd41ff49b8a7e6ed42c37c7562955..0000000000000000000000000000000000000000
--- a/spaces/BetterAPI/BetterChat_new/src/lib/actions/snapScrollToBottom.ts
+++ /dev/null
@@ -1,54 +0,0 @@
-import { navigating } from "$app/stores";
-import { tick } from "svelte";
-import { get } from "svelte/store";
-
-const detachedOffset = 10;
-
-/**
- * @param node element to snap scroll to bottom
- * @param dependency pass in a dependency to update scroll on changes.
- */
-export const snapScrollToBottom = (node: HTMLElement, dependency: any) => {
- let prevScrollValue = node.scrollTop;
- let isDetached = false;
-
- const handleScroll = () => {
- // if user scrolled up, we detach
- if (node.scrollTop < prevScrollValue) {
- isDetached = true;
- }
-
- // if user scrolled back to within 10px of bottom, we reattach
- if (node.scrollTop - (node.scrollHeight - node.clientHeight) >= -detachedOffset) {
- isDetached = false;
- }
-
- prevScrollValue = node.scrollTop;
- };
-
- const updateScroll = async (_options: { force?: boolean } = {}) => {
- const defaultOptions = { force: false };
- const options = { ...defaultOptions, ..._options };
- const { force } = options;
-
- if (!force && isDetached && !get(navigating)) return;
-
- // wait for next tick to ensure that the DOM is updated
- await tick();
-
- node.scrollTo({ top: node.scrollHeight });
- };
-
- node.addEventListener("scroll", handleScroll);
-
- if (dependency) {
- updateScroll({ force: true });
- }
-
- return {
- update: updateScroll,
- destroy: () => {
- node.removeEventListener("scroll", handleScroll);
- },
- };
-};
diff --git a/spaces/Big-Web/MMSD/env/Lib/site-packages/pip/_vendor/distro/distro.py b/spaces/Big-Web/MMSD/env/Lib/site-packages/pip/_vendor/distro/distro.py
deleted file mode 100644
index 89e1868047225bbcdfe04bdc4bea3281bf91bc20..0000000000000000000000000000000000000000
--- a/spaces/Big-Web/MMSD/env/Lib/site-packages/pip/_vendor/distro/distro.py
+++ /dev/null
@@ -1,1399 +0,0 @@
-#!/usr/bin/env python
-# Copyright 2015,2016,2017 Nir Cohen
-#
-# Licensed under the Apache License, Version 2.0 (the "License");
-# you may not use this file except in compliance with the License.
-# You may obtain a copy of the License at
-#
-# http://www.apache.org/licenses/LICENSE-2.0
-#
-# Unless required by applicable law or agreed to in writing, software
-# distributed under the License is distributed on an "AS IS" BASIS,
-# WITHOUT WARRANTIES OR CONDITIONS OF ANY KIND, either express or implied.
-# See the License for the specific language governing permissions and
-# limitations under the License.
-
-"""
-The ``distro`` package (``distro`` stands for Linux Distribution) provides
-information about the Linux distribution it runs on, such as a reliable
-machine-readable distro ID, or version information.
-
-It is the recommended replacement for Python's original
-:py:func:`platform.linux_distribution` function, but it provides much more
-functionality. An alternative implementation became necessary because Python
-3.5 deprecated this function, and Python 3.8 removed it altogether. Its
-predecessor function :py:func:`platform.dist` was already deprecated since
-Python 2.6 and removed in Python 3.8. Still, there are many cases in which
-access to OS distribution information is needed. See `Python issue 1322
-`_ for more information.
-"""
-
-import argparse
-import json
-import logging
-import os
-import re
-import shlex
-import subprocess
-import sys
-import warnings
-from typing import (
- Any,
- Callable,
- Dict,
- Iterable,
- Optional,
- Sequence,
- TextIO,
- Tuple,
- Type,
-)
-
-try:
- from typing import TypedDict
-except ImportError:
- # Python 3.7
- TypedDict = dict
-
-__version__ = "1.8.0"
-
-
-class VersionDict(TypedDict):
- major: str
- minor: str
- build_number: str
-
-
-class InfoDict(TypedDict):
- id: str
- version: str
- version_parts: VersionDict
- like: str
- codename: str
-
-
-_UNIXCONFDIR = os.environ.get("UNIXCONFDIR", "/etc")
-_UNIXUSRLIBDIR = os.environ.get("UNIXUSRLIBDIR", "/usr/lib")
-_OS_RELEASE_BASENAME = "os-release"
-
-#: Translation table for normalizing the "ID" attribute defined in os-release
-#: files, for use by the :func:`distro.id` method.
-#:
-#: * Key: Value as defined in the os-release file, translated to lower case,
-#: with blanks translated to underscores.
-#:
-#: * Value: Normalized value.
-NORMALIZED_OS_ID = {
- "ol": "oracle", # Oracle Linux
- "opensuse-leap": "opensuse", # Newer versions of OpenSuSE report as opensuse-leap
-}
-
-#: Translation table for normalizing the "Distributor ID" attribute returned by
-#: the lsb_release command, for use by the :func:`distro.id` method.
-#:
-#: * Key: Value as returned by the lsb_release command, translated to lower
-#: case, with blanks translated to underscores.
-#:
-#: * Value: Normalized value.
-NORMALIZED_LSB_ID = {
- "enterpriseenterpriseas": "oracle", # Oracle Enterprise Linux 4
- "enterpriseenterpriseserver": "oracle", # Oracle Linux 5
- "redhatenterpriseworkstation": "rhel", # RHEL 6, 7 Workstation
- "redhatenterpriseserver": "rhel", # RHEL 6, 7 Server
- "redhatenterprisecomputenode": "rhel", # RHEL 6 ComputeNode
-}
-
-#: Translation table for normalizing the distro ID derived from the file name
-#: of distro release files, for use by the :func:`distro.id` method.
-#:
-#: * Key: Value as derived from the file name of a distro release file,
-#: translated to lower case, with blanks translated to underscores.
-#:
-#: * Value: Normalized value.
-NORMALIZED_DISTRO_ID = {
- "redhat": "rhel", # RHEL 6.x, 7.x
-}
-
-# Pattern for content of distro release file (reversed)
-_DISTRO_RELEASE_CONTENT_REVERSED_PATTERN = re.compile(
- r"(?:[^)]*\)(.*)\()? *(?:STL )?([\d.+\-a-z]*\d) *(?:esaeler *)?(.+)"
-)
-
-# Pattern for base file name of distro release file
-_DISTRO_RELEASE_BASENAME_PATTERN = re.compile(r"(\w+)[-_](release|version)$")
-
-# Base file names to be looked up for if _UNIXCONFDIR is not readable.
-_DISTRO_RELEASE_BASENAMES = [
- "SuSE-release",
- "arch-release",
- "base-release",
- "centos-release",
- "fedora-release",
- "gentoo-release",
- "mageia-release",
- "mandrake-release",
- "mandriva-release",
- "mandrivalinux-release",
- "manjaro-release",
- "oracle-release",
- "redhat-release",
- "rocky-release",
- "sl-release",
- "slackware-version",
-]
-
-# Base file names to be ignored when searching for distro release file
-_DISTRO_RELEASE_IGNORE_BASENAMES = (
- "debian_version",
- "lsb-release",
- "oem-release",
- _OS_RELEASE_BASENAME,
- "system-release",
- "plesk-release",
- "iredmail-release",
-)
-
-
-def linux_distribution(full_distribution_name: bool = True) -> Tuple[str, str, str]:
- """
- .. deprecated:: 1.6.0
-
- :func:`distro.linux_distribution()` is deprecated. It should only be
- used as a compatibility shim with Python's
- :py:func:`platform.linux_distribution()`. Please use :func:`distro.id`,
- :func:`distro.version` and :func:`distro.name` instead.
-
- Return information about the current OS distribution as a tuple
- ``(id_name, version, codename)`` with items as follows:
-
- * ``id_name``: If *full_distribution_name* is false, the result of
- :func:`distro.id`. Otherwise, the result of :func:`distro.name`.
-
- * ``version``: The result of :func:`distro.version`.
-
- * ``codename``: The extra item (usually in parentheses) after the
- os-release version number, or the result of :func:`distro.codename`.
-
- The interface of this function is compatible with the original
- :py:func:`platform.linux_distribution` function, supporting a subset of
- its parameters.
-
- The data it returns may not exactly be the same, because it uses more data
- sources than the original function, and that may lead to different data if
- the OS distribution is not consistent across multiple data sources it
- provides (there are indeed such distributions ...).
-
- Another reason for differences is the fact that the :func:`distro.id`
- method normalizes the distro ID string to a reliable machine-readable value
- for a number of popular OS distributions.
- """
- warnings.warn(
- "distro.linux_distribution() is deprecated. It should only be used as a "
- "compatibility shim with Python's platform.linux_distribution(). Please use "
- "distro.id(), distro.version() and distro.name() instead.",
- DeprecationWarning,
- stacklevel=2,
- )
- return _distro.linux_distribution(full_distribution_name)
-
-
-def id() -> str:
- """
- Return the distro ID of the current distribution, as a
- machine-readable string.
-
- For a number of OS distributions, the returned distro ID value is
- *reliable*, in the sense that it is documented and that it does not change
- across releases of the distribution.
-
- This package maintains the following reliable distro ID values:
-
- ============== =========================================
- Distro ID Distribution
- ============== =========================================
- "ubuntu" Ubuntu
- "debian" Debian
- "rhel" RedHat Enterprise Linux
- "centos" CentOS
- "fedora" Fedora
- "sles" SUSE Linux Enterprise Server
- "opensuse" openSUSE
- "amzn" Amazon Linux
- "arch" Arch Linux
- "buildroot" Buildroot
- "cloudlinux" CloudLinux OS
- "exherbo" Exherbo Linux
- "gentoo" GenToo Linux
- "ibm_powerkvm" IBM PowerKVM
- "kvmibm" KVM for IBM z Systems
- "linuxmint" Linux Mint
- "mageia" Mageia
- "mandriva" Mandriva Linux
- "parallels" Parallels
- "pidora" Pidora
- "raspbian" Raspbian
- "oracle" Oracle Linux (and Oracle Enterprise Linux)
- "scientific" Scientific Linux
- "slackware" Slackware
- "xenserver" XenServer
- "openbsd" OpenBSD
- "netbsd" NetBSD
- "freebsd" FreeBSD
- "midnightbsd" MidnightBSD
- "rocky" Rocky Linux
- "aix" AIX
- "guix" Guix System
- ============== =========================================
-
- If you have a need to get distros for reliable IDs added into this set,
- or if you find that the :func:`distro.id` function returns a different
- distro ID for one of the listed distros, please create an issue in the
- `distro issue tracker`_.
-
- **Lookup hierarchy and transformations:**
-
- First, the ID is obtained from the following sources, in the specified
- order. The first available and non-empty value is used:
-
- * the value of the "ID" attribute of the os-release file,
-
- * the value of the "Distributor ID" attribute returned by the lsb_release
- command,
-
- * the first part of the file name of the distro release file,
-
- The so determined ID value then passes the following transformations,
- before it is returned by this method:
-
- * it is translated to lower case,
-
- * blanks (which should not be there anyway) are translated to underscores,
-
- * a normalization of the ID is performed, based upon
- `normalization tables`_. The purpose of this normalization is to ensure
- that the ID is as reliable as possible, even across incompatible changes
- in the OS distributions. A common reason for an incompatible change is
- the addition of an os-release file, or the addition of the lsb_release
- command, with ID values that differ from what was previously determined
- from the distro release file name.
- """
- return _distro.id()
-
-
-def name(pretty: bool = False) -> str:
- """
- Return the name of the current OS distribution, as a human-readable
- string.
-
- If *pretty* is false, the name is returned without version or codename.
- (e.g. "CentOS Linux")
-
- If *pretty* is true, the version and codename are appended.
- (e.g. "CentOS Linux 7.1.1503 (Core)")
-
- **Lookup hierarchy:**
-
- The name is obtained from the following sources, in the specified order.
- The first available and non-empty value is used:
-
- * If *pretty* is false:
-
- - the value of the "NAME" attribute of the os-release file,
-
- - the value of the "Distributor ID" attribute returned by the lsb_release
- command,
-
- - the value of the "" field of the distro release file.
-
- * If *pretty* is true:
-
- - the value of the "PRETTY_NAME" attribute of the os-release file,
-
- - the value of the "Description" attribute returned by the lsb_release
- command,
-
- - the value of the "" field of the distro release file, appended
- with the value of the pretty version ("" and ""
- fields) of the distro release file, if available.
- """
- return _distro.name(pretty)
-
-
-def version(pretty: bool = False, best: bool = False) -> str:
- """
- Return the version of the current OS distribution, as a human-readable
- string.
-
- If *pretty* is false, the version is returned without codename (e.g.
- "7.0").
-
- If *pretty* is true, the codename in parenthesis is appended, if the
- codename is non-empty (e.g. "7.0 (Maipo)").
-
- Some distributions provide version numbers with different precisions in
- the different sources of distribution information. Examining the different
- sources in a fixed priority order does not always yield the most precise
- version (e.g. for Debian 8.2, or CentOS 7.1).
-
- Some other distributions may not provide this kind of information. In these
- cases, an empty string would be returned. This behavior can be observed
- with rolling releases distributions (e.g. Arch Linux).
-
- The *best* parameter can be used to control the approach for the returned
- version:
-
- If *best* is false, the first non-empty version number in priority order of
- the examined sources is returned.
-
- If *best* is true, the most precise version number out of all examined
- sources is returned.
-
- **Lookup hierarchy:**
-
- In all cases, the version number is obtained from the following sources.
- If *best* is false, this order represents the priority order:
-
- * the value of the "VERSION_ID" attribute of the os-release file,
- * the value of the "Release" attribute returned by the lsb_release
- command,
- * the version number parsed from the "" field of the first line
- of the distro release file,
- * the version number parsed from the "PRETTY_NAME" attribute of the
- os-release file, if it follows the format of the distro release files.
- * the version number parsed from the "Description" attribute returned by
- the lsb_release command, if it follows the format of the distro release
- files.
- """
- return _distro.version(pretty, best)
-
-
-def version_parts(best: bool = False) -> Tuple[str, str, str]:
- """
- Return the version of the current OS distribution as a tuple
- ``(major, minor, build_number)`` with items as follows:
-
- * ``major``: The result of :func:`distro.major_version`.
-
- * ``minor``: The result of :func:`distro.minor_version`.
-
- * ``build_number``: The result of :func:`distro.build_number`.
-
- For a description of the *best* parameter, see the :func:`distro.version`
- method.
- """
- return _distro.version_parts(best)
-
-
-def major_version(best: bool = False) -> str:
- """
- Return the major version of the current OS distribution, as a string,
- if provided.
- Otherwise, the empty string is returned. The major version is the first
- part of the dot-separated version string.
-
- For a description of the *best* parameter, see the :func:`distro.version`
- method.
- """
- return _distro.major_version(best)
-
-
-def minor_version(best: bool = False) -> str:
- """
- Return the minor version of the current OS distribution, as a string,
- if provided.
- Otherwise, the empty string is returned. The minor version is the second
- part of the dot-separated version string.
-
- For a description of the *best* parameter, see the :func:`distro.version`
- method.
- """
- return _distro.minor_version(best)
-
-
-def build_number(best: bool = False) -> str:
- """
- Return the build number of the current OS distribution, as a string,
- if provided.
- Otherwise, the empty string is returned. The build number is the third part
- of the dot-separated version string.
-
- For a description of the *best* parameter, see the :func:`distro.version`
- method.
- """
- return _distro.build_number(best)
-
-
-def like() -> str:
- """
- Return a space-separated list of distro IDs of distributions that are
- closely related to the current OS distribution in regards to packaging
- and programming interfaces, for example distributions the current
- distribution is a derivative from.
-
- **Lookup hierarchy:**
-
- This information item is only provided by the os-release file.
- For details, see the description of the "ID_LIKE" attribute in the
- `os-release man page
- `_.
- """
- return _distro.like()
-
-
-def codename() -> str:
- """
- Return the codename for the release of the current OS distribution,
- as a string.
-
- If the distribution does not have a codename, an empty string is returned.
-
- Note that the returned codename is not always really a codename. For
- example, openSUSE returns "x86_64". This function does not handle such
- cases in any special way and just returns the string it finds, if any.
-
- **Lookup hierarchy:**
-
- * the codename within the "VERSION" attribute of the os-release file, if
- provided,
-
- * the value of the "Codename" attribute returned by the lsb_release
- command,
-
- * the value of the "" field of the distro release file.
- """
- return _distro.codename()
-
-
-def info(pretty: bool = False, best: bool = False) -> InfoDict:
- """
- Return certain machine-readable information items about the current OS
- distribution in a dictionary, as shown in the following example:
-
- .. sourcecode:: python
-
- {
- 'id': 'rhel',
- 'version': '7.0',
- 'version_parts': {
- 'major': '7',
- 'minor': '0',
- 'build_number': ''
- },
- 'like': 'fedora',
- 'codename': 'Maipo'
- }
-
- The dictionary structure and keys are always the same, regardless of which
- information items are available in the underlying data sources. The values
- for the various keys are as follows:
-
- * ``id``: The result of :func:`distro.id`.
-
- * ``version``: The result of :func:`distro.version`.
-
- * ``version_parts -> major``: The result of :func:`distro.major_version`.
-
- * ``version_parts -> minor``: The result of :func:`distro.minor_version`.
-
- * ``version_parts -> build_number``: The result of
- :func:`distro.build_number`.
-
- * ``like``: The result of :func:`distro.like`.
-
- * ``codename``: The result of :func:`distro.codename`.
-
- For a description of the *pretty* and *best* parameters, see the
- :func:`distro.version` method.
- """
- return _distro.info(pretty, best)
-
-
-def os_release_info() -> Dict[str, str]:
- """
- Return a dictionary containing key-value pairs for the information items
- from the os-release file data source of the current OS distribution.
-
- See `os-release file`_ for details about these information items.
- """
- return _distro.os_release_info()
-
-
-def lsb_release_info() -> Dict[str, str]:
- """
- Return a dictionary containing key-value pairs for the information items
- from the lsb_release command data source of the current OS distribution.
-
- See `lsb_release command output`_ for details about these information
- items.
- """
- return _distro.lsb_release_info()
-
-
-def distro_release_info() -> Dict[str, str]:
- """
- Return a dictionary containing key-value pairs for the information items
- from the distro release file data source of the current OS distribution.
-
- See `distro release file`_ for details about these information items.
- """
- return _distro.distro_release_info()
-
-
-def uname_info() -> Dict[str, str]:
- """
- Return a dictionary containing key-value pairs for the information items
- from the distro release file data source of the current OS distribution.
- """
- return _distro.uname_info()
-
-
-def os_release_attr(attribute: str) -> str:
- """
- Return a single named information item from the os-release file data source
- of the current OS distribution.
-
- Parameters:
-
- * ``attribute`` (string): Key of the information item.
-
- Returns:
-
- * (string): Value of the information item, if the item exists.
- The empty string, if the item does not exist.
-
- See `os-release file`_ for details about these information items.
- """
- return _distro.os_release_attr(attribute)
-
-
-def lsb_release_attr(attribute: str) -> str:
- """
- Return a single named information item from the lsb_release command output
- data source of the current OS distribution.
-
- Parameters:
-
- * ``attribute`` (string): Key of the information item.
-
- Returns:
-
- * (string): Value of the information item, if the item exists.
- The empty string, if the item does not exist.
-
- See `lsb_release command output`_ for details about these information
- items.
- """
- return _distro.lsb_release_attr(attribute)
-
-
-def distro_release_attr(attribute: str) -> str:
- """
- Return a single named information item from the distro release file
- data source of the current OS distribution.
-
- Parameters:
-
- * ``attribute`` (string): Key of the information item.
-
- Returns:
-
- * (string): Value of the information item, if the item exists.
- The empty string, if the item does not exist.
-
- See `distro release file`_ for details about these information items.
- """
- return _distro.distro_release_attr(attribute)
-
-
-def uname_attr(attribute: str) -> str:
- """
- Return a single named information item from the distro release file
- data source of the current OS distribution.
-
- Parameters:
-
- * ``attribute`` (string): Key of the information item.
-
- Returns:
-
- * (string): Value of the information item, if the item exists.
- The empty string, if the item does not exist.
- """
- return _distro.uname_attr(attribute)
-
-
-try:
- from functools import cached_property
-except ImportError:
- # Python < 3.8
- class cached_property: # type: ignore
- """A version of @property which caches the value. On access, it calls the
- underlying function and sets the value in `__dict__` so future accesses
- will not re-call the property.
- """
-
- def __init__(self, f: Callable[[Any], Any]) -> None:
- self._fname = f.__name__
- self._f = f
-
- def __get__(self, obj: Any, owner: Type[Any]) -> Any:
- assert obj is not None, f"call {self._fname} on an instance"
- ret = obj.__dict__[self._fname] = self._f(obj)
- return ret
-
-
-class LinuxDistribution:
- """
- Provides information about a OS distribution.
-
- This package creates a private module-global instance of this class with
- default initialization arguments, that is used by the
- `consolidated accessor functions`_ and `single source accessor functions`_.
- By using default initialization arguments, that module-global instance
- returns data about the current OS distribution (i.e. the distro this
- package runs on).
-
- Normally, it is not necessary to create additional instances of this class.
- However, in situations where control is needed over the exact data sources
- that are used, instances of this class can be created with a specific
- distro release file, or a specific os-release file, or without invoking the
- lsb_release command.
- """
-
- def __init__(
- self,
- include_lsb: Optional[bool] = None,
- os_release_file: str = "",
- distro_release_file: str = "",
- include_uname: Optional[bool] = None,
- root_dir: Optional[str] = None,
- include_oslevel: Optional[bool] = None,
- ) -> None:
- """
- The initialization method of this class gathers information from the
- available data sources, and stores that in private instance attributes.
- Subsequent access to the information items uses these private instance
- attributes, so that the data sources are read only once.
-
- Parameters:
-
- * ``include_lsb`` (bool): Controls whether the
- `lsb_release command output`_ is included as a data source.
-
- If the lsb_release command is not available in the program execution
- path, the data source for the lsb_release command will be empty.
-
- * ``os_release_file`` (string): The path name of the
- `os-release file`_ that is to be used as a data source.
-
- An empty string (the default) will cause the default path name to
- be used (see `os-release file`_ for details).
-
- If the specified or defaulted os-release file does not exist, the
- data source for the os-release file will be empty.
-
- * ``distro_release_file`` (string): The path name of the
- `distro release file`_ that is to be used as a data source.
-
- An empty string (the default) will cause a default search algorithm
- to be used (see `distro release file`_ for details).
-
- If the specified distro release file does not exist, or if no default
- distro release file can be found, the data source for the distro
- release file will be empty.
-
- * ``include_uname`` (bool): Controls whether uname command output is
- included as a data source. If the uname command is not available in
- the program execution path the data source for the uname command will
- be empty.
-
- * ``root_dir`` (string): The absolute path to the root directory to use
- to find distro-related information files. Note that ``include_*``
- parameters must not be enabled in combination with ``root_dir``.
-
- * ``include_oslevel`` (bool): Controls whether (AIX) oslevel command
- output is included as a data source. If the oslevel command is not
- available in the program execution path the data source will be
- empty.
-
- Public instance attributes:
-
- * ``os_release_file`` (string): The path name of the
- `os-release file`_ that is actually used as a data source. The
- empty string if no distro release file is used as a data source.
-
- * ``distro_release_file`` (string): The path name of the
- `distro release file`_ that is actually used as a data source. The
- empty string if no distro release file is used as a data source.
-
- * ``include_lsb`` (bool): The result of the ``include_lsb`` parameter.
- This controls whether the lsb information will be loaded.
-
- * ``include_uname`` (bool): The result of the ``include_uname``
- parameter. This controls whether the uname information will
- be loaded.
-
- * ``include_oslevel`` (bool): The result of the ``include_oslevel``
- parameter. This controls whether (AIX) oslevel information will be
- loaded.
-
- * ``root_dir`` (string): The result of the ``root_dir`` parameter.
- The absolute path to the root directory to use to find distro-related
- information files.
-
- Raises:
-
- * :py:exc:`ValueError`: Initialization parameters combination is not
- supported.
-
- * :py:exc:`OSError`: Some I/O issue with an os-release file or distro
- release file.
-
- * :py:exc:`UnicodeError`: A data source has unexpected characters or
- uses an unexpected encoding.
- """
- self.root_dir = root_dir
- self.etc_dir = os.path.join(root_dir, "etc") if root_dir else _UNIXCONFDIR
- self.usr_lib_dir = (
- os.path.join(root_dir, "usr/lib") if root_dir else _UNIXUSRLIBDIR
- )
-
- if os_release_file:
- self.os_release_file = os_release_file
- else:
- etc_dir_os_release_file = os.path.join(self.etc_dir, _OS_RELEASE_BASENAME)
- usr_lib_os_release_file = os.path.join(
- self.usr_lib_dir, _OS_RELEASE_BASENAME
- )
-
- # NOTE: The idea is to respect order **and** have it set
- # at all times for API backwards compatibility.
- if os.path.isfile(etc_dir_os_release_file) or not os.path.isfile(
- usr_lib_os_release_file
- ):
- self.os_release_file = etc_dir_os_release_file
- else:
- self.os_release_file = usr_lib_os_release_file
-
- self.distro_release_file = distro_release_file or "" # updated later
-
- is_root_dir_defined = root_dir is not None
- if is_root_dir_defined and (include_lsb or include_uname or include_oslevel):
- raise ValueError(
- "Including subprocess data sources from specific root_dir is disallowed"
- " to prevent false information"
- )
- self.include_lsb = (
- include_lsb if include_lsb is not None else not is_root_dir_defined
- )
- self.include_uname = (
- include_uname if include_uname is not None else not is_root_dir_defined
- )
- self.include_oslevel = (
- include_oslevel if include_oslevel is not None else not is_root_dir_defined
- )
-
- def __repr__(self) -> str:
- """Return repr of all info"""
- return (
- "LinuxDistribution("
- "os_release_file={self.os_release_file!r}, "
- "distro_release_file={self.distro_release_file!r}, "
- "include_lsb={self.include_lsb!r}, "
- "include_uname={self.include_uname!r}, "
- "include_oslevel={self.include_oslevel!r}, "
- "root_dir={self.root_dir!r}, "
- "_os_release_info={self._os_release_info!r}, "
- "_lsb_release_info={self._lsb_release_info!r}, "
- "_distro_release_info={self._distro_release_info!r}, "
- "_uname_info={self._uname_info!r}, "
- "_oslevel_info={self._oslevel_info!r})".format(self=self)
- )
-
- def linux_distribution(
- self, full_distribution_name: bool = True
- ) -> Tuple[str, str, str]:
- """
- Return information about the OS distribution that is compatible
- with Python's :func:`platform.linux_distribution`, supporting a subset
- of its parameters.
-
- For details, see :func:`distro.linux_distribution`.
- """
- return (
- self.name() if full_distribution_name else self.id(),
- self.version(),
- self._os_release_info.get("release_codename") or self.codename(),
- )
-
- def id(self) -> str:
- """Return the distro ID of the OS distribution, as a string.
-
- For details, see :func:`distro.id`.
- """
-
- def normalize(distro_id: str, table: Dict[str, str]) -> str:
- distro_id = distro_id.lower().replace(" ", "_")
- return table.get(distro_id, distro_id)
-
- distro_id = self.os_release_attr("id")
- if distro_id:
- return normalize(distro_id, NORMALIZED_OS_ID)
-
- distro_id = self.lsb_release_attr("distributor_id")
- if distro_id:
- return normalize(distro_id, NORMALIZED_LSB_ID)
-
- distro_id = self.distro_release_attr("id")
- if distro_id:
- return normalize(distro_id, NORMALIZED_DISTRO_ID)
-
- distro_id = self.uname_attr("id")
- if distro_id:
- return normalize(distro_id, NORMALIZED_DISTRO_ID)
-
- return ""
-
- def name(self, pretty: bool = False) -> str:
- """
- Return the name of the OS distribution, as a string.
-
- For details, see :func:`distro.name`.
- """
- name = (
- self.os_release_attr("name")
- or self.lsb_release_attr("distributor_id")
- or self.distro_release_attr("name")
- or self.uname_attr("name")
- )
- if pretty:
- name = self.os_release_attr("pretty_name") or self.lsb_release_attr(
- "description"
- )
- if not name:
- name = self.distro_release_attr("name") or self.uname_attr("name")
- version = self.version(pretty=True)
- if version:
- name = f"{name} {version}"
- return name or ""
-
- def version(self, pretty: bool = False, best: bool = False) -> str:
- """
- Return the version of the OS distribution, as a string.
-
- For details, see :func:`distro.version`.
- """
- versions = [
- self.os_release_attr("version_id"),
- self.lsb_release_attr("release"),
- self.distro_release_attr("version_id"),
- self._parse_distro_release_content(self.os_release_attr("pretty_name")).get(
- "version_id", ""
- ),
- self._parse_distro_release_content(
- self.lsb_release_attr("description")
- ).get("version_id", ""),
- self.uname_attr("release"),
- ]
- if self.uname_attr("id").startswith("aix"):
- # On AIX platforms, prefer oslevel command output.
- versions.insert(0, self.oslevel_info())
- elif self.id() == "debian" or "debian" in self.like().split():
- # On Debian-like, add debian_version file content to candidates list.
- versions.append(self._debian_version)
- version = ""
- if best:
- # This algorithm uses the last version in priority order that has
- # the best precision. If the versions are not in conflict, that
- # does not matter; otherwise, using the last one instead of the
- # first one might be considered a surprise.
- for v in versions:
- if v.count(".") > version.count(".") or version == "":
- version = v
- else:
- for v in versions:
- if v != "":
- version = v
- break
- if pretty and version and self.codename():
- version = f"{version} ({self.codename()})"
- return version
-
- def version_parts(self, best: bool = False) -> Tuple[str, str, str]:
- """
- Return the version of the OS distribution, as a tuple of version
- numbers.
-
- For details, see :func:`distro.version_parts`.
- """
- version_str = self.version(best=best)
- if version_str:
- version_regex = re.compile(r"(\d+)\.?(\d+)?\.?(\d+)?")
- matches = version_regex.match(version_str)
- if matches:
- major, minor, build_number = matches.groups()
- return major, minor or "", build_number or ""
- return "", "", ""
-
- def major_version(self, best: bool = False) -> str:
- """
- Return the major version number of the current distribution.
-
- For details, see :func:`distro.major_version`.
- """
- return self.version_parts(best)[0]
-
- def minor_version(self, best: bool = False) -> str:
- """
- Return the minor version number of the current distribution.
-
- For details, see :func:`distro.minor_version`.
- """
- return self.version_parts(best)[1]
-
- def build_number(self, best: bool = False) -> str:
- """
- Return the build number of the current distribution.
-
- For details, see :func:`distro.build_number`.
- """
- return self.version_parts(best)[2]
-
- def like(self) -> str:
- """
- Return the IDs of distributions that are like the OS distribution.
-
- For details, see :func:`distro.like`.
- """
- return self.os_release_attr("id_like") or ""
-
- def codename(self) -> str:
- """
- Return the codename of the OS distribution.
-
- For details, see :func:`distro.codename`.
- """
- try:
- # Handle os_release specially since distros might purposefully set
- # this to empty string to have no codename
- return self._os_release_info["codename"]
- except KeyError:
- return (
- self.lsb_release_attr("codename")
- or self.distro_release_attr("codename")
- or ""
- )
-
- def info(self, pretty: bool = False, best: bool = False) -> InfoDict:
- """
- Return certain machine-readable information about the OS
- distribution.
-
- For details, see :func:`distro.info`.
- """
- return dict(
- id=self.id(),
- version=self.version(pretty, best),
- version_parts=dict(
- major=self.major_version(best),
- minor=self.minor_version(best),
- build_number=self.build_number(best),
- ),
- like=self.like(),
- codename=self.codename(),
- )
-
- def os_release_info(self) -> Dict[str, str]:
- """
- Return a dictionary containing key-value pairs for the information
- items from the os-release file data source of the OS distribution.
-
- For details, see :func:`distro.os_release_info`.
- """
- return self._os_release_info
-
- def lsb_release_info(self) -> Dict[str, str]:
- """
- Return a dictionary containing key-value pairs for the information
- items from the lsb_release command data source of the OS
- distribution.
-
- For details, see :func:`distro.lsb_release_info`.
- """
- return self._lsb_release_info
-
- def distro_release_info(self) -> Dict[str, str]:
- """
- Return a dictionary containing key-value pairs for the information
- items from the distro release file data source of the OS
- distribution.
-
- For details, see :func:`distro.distro_release_info`.
- """
- return self._distro_release_info
-
- def uname_info(self) -> Dict[str, str]:
- """
- Return a dictionary containing key-value pairs for the information
- items from the uname command data source of the OS distribution.
-
- For details, see :func:`distro.uname_info`.
- """
- return self._uname_info
-
- def oslevel_info(self) -> str:
- """
- Return AIX' oslevel command output.
- """
- return self._oslevel_info
-
- def os_release_attr(self, attribute: str) -> str:
- """
- Return a single named information item from the os-release file data
- source of the OS distribution.
-
- For details, see :func:`distro.os_release_attr`.
- """
- return self._os_release_info.get(attribute, "")
-
- def lsb_release_attr(self, attribute: str) -> str:
- """
- Return a single named information item from the lsb_release command
- output data source of the OS distribution.
-
- For details, see :func:`distro.lsb_release_attr`.
- """
- return self._lsb_release_info.get(attribute, "")
-
- def distro_release_attr(self, attribute: str) -> str:
- """
- Return a single named information item from the distro release file
- data source of the OS distribution.
-
- For details, see :func:`distro.distro_release_attr`.
- """
- return self._distro_release_info.get(attribute, "")
-
- def uname_attr(self, attribute: str) -> str:
- """
- Return a single named information item from the uname command
- output data source of the OS distribution.
-
- For details, see :func:`distro.uname_attr`.
- """
- return self._uname_info.get(attribute, "")
-
- @cached_property
- def _os_release_info(self) -> Dict[str, str]:
- """
- Get the information items from the specified os-release file.
-
- Returns:
- A dictionary containing all information items.
- """
- if os.path.isfile(self.os_release_file):
- with open(self.os_release_file, encoding="utf-8") as release_file:
- return self._parse_os_release_content(release_file)
- return {}
-
- @staticmethod
- def _parse_os_release_content(lines: TextIO) -> Dict[str, str]:
- """
- Parse the lines of an os-release file.
-
- Parameters:
-
- * lines: Iterable through the lines in the os-release file.
- Each line must be a unicode string or a UTF-8 encoded byte
- string.
-
- Returns:
- A dictionary containing all information items.
- """
- props = {}
- lexer = shlex.shlex(lines, posix=True)
- lexer.whitespace_split = True
-
- tokens = list(lexer)
- for token in tokens:
- # At this point, all shell-like parsing has been done (i.e.
- # comments processed, quotes and backslash escape sequences
- # processed, multi-line values assembled, trailing newlines
- # stripped, etc.), so the tokens are now either:
- # * variable assignments: var=value
- # * commands or their arguments (not allowed in os-release)
- # Ignore any tokens that are not variable assignments
- if "=" in token:
- k, v = token.split("=", 1)
- props[k.lower()] = v
-
- if "version" in props:
- # extract release codename (if any) from version attribute
- match = re.search(r"\((\D+)\)|,\s*(\D+)", props["version"])
- if match:
- release_codename = match.group(1) or match.group(2)
- props["codename"] = props["release_codename"] = release_codename
-
- if "version_codename" in props:
- # os-release added a version_codename field. Use that in
- # preference to anything else Note that some distros purposefully
- # do not have code names. They should be setting
- # version_codename=""
- props["codename"] = props["version_codename"]
- elif "ubuntu_codename" in props:
- # Same as above but a non-standard field name used on older Ubuntus
- props["codename"] = props["ubuntu_codename"]
-
- return props
-
- @cached_property
- def _lsb_release_info(self) -> Dict[str, str]:
- """
- Get the information items from the lsb_release command output.
-
- Returns:
- A dictionary containing all information items.
- """
- if not self.include_lsb:
- return {}
- try:
- cmd = ("lsb_release", "-a")
- stdout = subprocess.check_output(cmd, stderr=subprocess.DEVNULL)
- # Command not found or lsb_release returned error
- except (OSError, subprocess.CalledProcessError):
- return {}
- content = self._to_str(stdout).splitlines()
- return self._parse_lsb_release_content(content)
-
- @staticmethod
- def _parse_lsb_release_content(lines: Iterable[str]) -> Dict[str, str]:
- """
- Parse the output of the lsb_release command.
-
- Parameters:
-
- * lines: Iterable through the lines of the lsb_release output.
- Each line must be a unicode string or a UTF-8 encoded byte
- string.
-
- Returns:
- A dictionary containing all information items.
- """
- props = {}
- for line in lines:
- kv = line.strip("\n").split(":", 1)
- if len(kv) != 2:
- # Ignore lines without colon.
- continue
- k, v = kv
- props.update({k.replace(" ", "_").lower(): v.strip()})
- return props
-
- @cached_property
- def _uname_info(self) -> Dict[str, str]:
- if not self.include_uname:
- return {}
- try:
- cmd = ("uname", "-rs")
- stdout = subprocess.check_output(cmd, stderr=subprocess.DEVNULL)
- except OSError:
- return {}
- content = self._to_str(stdout).splitlines()
- return self._parse_uname_content(content)
-
- @cached_property
- def _oslevel_info(self) -> str:
- if not self.include_oslevel:
- return ""
- try:
- stdout = subprocess.check_output("oslevel", stderr=subprocess.DEVNULL)
- except (OSError, subprocess.CalledProcessError):
- return ""
- return self._to_str(stdout).strip()
-
- @cached_property
- def _debian_version(self) -> str:
- try:
- with open(
- os.path.join(self.etc_dir, "debian_version"), encoding="ascii"
- ) as fp:
- return fp.readline().rstrip()
- except FileNotFoundError:
- return ""
-
- @staticmethod
- def _parse_uname_content(lines: Sequence[str]) -> Dict[str, str]:
- if not lines:
- return {}
- props = {}
- match = re.search(r"^([^\s]+)\s+([\d\.]+)", lines[0].strip())
- if match:
- name, version = match.groups()
-
- # This is to prevent the Linux kernel version from
- # appearing as the 'best' version on otherwise
- # identifiable distributions.
- if name == "Linux":
- return {}
- props["id"] = name.lower()
- props["name"] = name
- props["release"] = version
- return props
-
- @staticmethod
- def _to_str(bytestring: bytes) -> str:
- encoding = sys.getfilesystemencoding()
- return bytestring.decode(encoding)
-
- @cached_property
- def _distro_release_info(self) -> Dict[str, str]:
- """
- Get the information items from the specified distro release file.
-
- Returns:
- A dictionary containing all information items.
- """
- if self.distro_release_file:
- # If it was specified, we use it and parse what we can, even if
- # its file name or content does not match the expected pattern.
- distro_info = self._parse_distro_release_file(self.distro_release_file)
- basename = os.path.basename(self.distro_release_file)
- # The file name pattern for user-specified distro release files
- # is somewhat more tolerant (compared to when searching for the
- # file), because we want to use what was specified as best as
- # possible.
- match = _DISTRO_RELEASE_BASENAME_PATTERN.match(basename)
- else:
- try:
- basenames = [
- basename
- for basename in os.listdir(self.etc_dir)
- if basename not in _DISTRO_RELEASE_IGNORE_BASENAMES
- and os.path.isfile(os.path.join(self.etc_dir, basename))
- ]
- # We sort for repeatability in cases where there are multiple
- # distro specific files; e.g. CentOS, Oracle, Enterprise all
- # containing `redhat-release` on top of their own.
- basenames.sort()
- except OSError:
- # This may occur when /etc is not readable but we can't be
- # sure about the *-release files. Check common entries of
- # /etc for information. If they turn out to not be there the
- # error is handled in `_parse_distro_release_file()`.
- basenames = _DISTRO_RELEASE_BASENAMES
- for basename in basenames:
- match = _DISTRO_RELEASE_BASENAME_PATTERN.match(basename)
- if match is None:
- continue
- filepath = os.path.join(self.etc_dir, basename)
- distro_info = self._parse_distro_release_file(filepath)
- # The name is always present if the pattern matches.
- if "name" not in distro_info:
- continue
- self.distro_release_file = filepath
- break
- else: # the loop didn't "break": no candidate.
- return {}
-
- if match is not None:
- distro_info["id"] = match.group(1)
-
- # CloudLinux < 7: manually enrich info with proper id.
- if "cloudlinux" in distro_info.get("name", "").lower():
- distro_info["id"] = "cloudlinux"
-
- return distro_info
-
- def _parse_distro_release_file(self, filepath: str) -> Dict[str, str]:
- """
- Parse a distro release file.
-
- Parameters:
-
- * filepath: Path name of the distro release file.
-
- Returns:
- A dictionary containing all information items.
- """
- try:
- with open(filepath, encoding="utf-8") as fp:
- # Only parse the first line. For instance, on SLES there
- # are multiple lines. We don't want them...
- return self._parse_distro_release_content(fp.readline())
- except OSError:
- # Ignore not being able to read a specific, seemingly version
- # related file.
- # See https://github.com/python-distro/distro/issues/162
- return {}
-
- @staticmethod
- def _parse_distro_release_content(line: str) -> Dict[str, str]:
- """
- Parse a line from a distro release file.
-
- Parameters:
- * line: Line from the distro release file. Must be a unicode string
- or a UTF-8 encoded byte string.
-
- Returns:
- A dictionary containing all information items.
- """
- matches = _DISTRO_RELEASE_CONTENT_REVERSED_PATTERN.match(line.strip()[::-1])
- distro_info = {}
- if matches:
- # regexp ensures non-None
- distro_info["name"] = matches.group(3)[::-1]
- if matches.group(2):
- distro_info["version_id"] = matches.group(2)[::-1]
- if matches.group(1):
- distro_info["codename"] = matches.group(1)[::-1]
- elif line:
- distro_info["name"] = line.strip()
- return distro_info
-
-
-_distro = LinuxDistribution()
-
-
-def main() -> None:
- logger = logging.getLogger(__name__)
- logger.setLevel(logging.DEBUG)
- logger.addHandler(logging.StreamHandler(sys.stdout))
-
- parser = argparse.ArgumentParser(description="OS distro info tool")
- parser.add_argument(
- "--json", "-j", help="Output in machine readable format", action="store_true"
- )
-
- parser.add_argument(
- "--root-dir",
- "-r",
- type=str,
- dest="root_dir",
- help="Path to the root filesystem directory (defaults to /)",
- )
-
- args = parser.parse_args()
-
- if args.root_dir:
- dist = LinuxDistribution(
- include_lsb=False,
- include_uname=False,
- include_oslevel=False,
- root_dir=args.root_dir,
- )
- else:
- dist = _distro
-
- if args.json:
- logger.info(json.dumps(dist.info(), indent=4, sort_keys=True))
- else:
- logger.info("Name: %s", dist.name(pretty=True))
- distribution_version = dist.version(pretty=True)
- logger.info("Version: %s", distribution_version)
- distribution_codename = dist.codename()
- logger.info("Codename: %s", distribution_codename)
-
-
-if __name__ == "__main__":
- main()
diff --git a/spaces/Big-Web/MMSD/env/Lib/site-packages/setuptools/_distutils/command/config.py b/spaces/Big-Web/MMSD/env/Lib/site-packages/setuptools/_distutils/command/config.py
deleted file mode 100644
index 4492c89660c202acf882375258dffafff00a99ba..0000000000000000000000000000000000000000
--- a/spaces/Big-Web/MMSD/env/Lib/site-packages/setuptools/_distutils/command/config.py
+++ /dev/null
@@ -1,377 +0,0 @@
-"""distutils.command.config
-
-Implements the Distutils 'config' command, a (mostly) empty command class
-that exists mainly to be sub-classed by specific module distributions and
-applications. The idea is that while every "config" command is different,
-at least they're all named the same, and users always see "config" in the
-list of standard commands. Also, this is a good place to put common
-configure-like tasks: "try to compile this C code", or "figure out where
-this header file lives".
-"""
-
-import os
-import re
-
-from distutils.core import Command
-from distutils.errors import DistutilsExecError
-from distutils.sysconfig import customize_compiler
-from distutils import log
-
-LANG_EXT = {"c": ".c", "c++": ".cxx"}
-
-
-class config(Command):
-
- description = "prepare to build"
-
- user_options = [
- ('compiler=', None, "specify the compiler type"),
- ('cc=', None, "specify the compiler executable"),
- ('include-dirs=', 'I', "list of directories to search for header files"),
- ('define=', 'D', "C preprocessor macros to define"),
- ('undef=', 'U', "C preprocessor macros to undefine"),
- ('libraries=', 'l', "external C libraries to link with"),
- ('library-dirs=', 'L', "directories to search for external C libraries"),
- ('noisy', None, "show every action (compile, link, run, ...) taken"),
- (
- 'dump-source',
- None,
- "dump generated source files before attempting to compile them",
- ),
- ]
-
- # The three standard command methods: since the "config" command
- # does nothing by default, these are empty.
-
- def initialize_options(self):
- self.compiler = None
- self.cc = None
- self.include_dirs = None
- self.libraries = None
- self.library_dirs = None
-
- # maximal output for now
- self.noisy = 1
- self.dump_source = 1
-
- # list of temporary files generated along-the-way that we have
- # to clean at some point
- self.temp_files = []
-
- def finalize_options(self):
- if self.include_dirs is None:
- self.include_dirs = self.distribution.include_dirs or []
- elif isinstance(self.include_dirs, str):
- self.include_dirs = self.include_dirs.split(os.pathsep)
-
- if self.libraries is None:
- self.libraries = []
- elif isinstance(self.libraries, str):
- self.libraries = [self.libraries]
-
- if self.library_dirs is None:
- self.library_dirs = []
- elif isinstance(self.library_dirs, str):
- self.library_dirs = self.library_dirs.split(os.pathsep)
-
- def run(self):
- pass
-
- # Utility methods for actual "config" commands. The interfaces are
- # loosely based on Autoconf macros of similar names. Sub-classes
- # may use these freely.
-
- def _check_compiler(self):
- """Check that 'self.compiler' really is a CCompiler object;
- if not, make it one.
- """
- # We do this late, and only on-demand, because this is an expensive
- # import.
- from distutils.ccompiler import CCompiler, new_compiler
-
- if not isinstance(self.compiler, CCompiler):
- self.compiler = new_compiler(
- compiler=self.compiler, dry_run=self.dry_run, force=1
- )
- customize_compiler(self.compiler)
- if self.include_dirs:
- self.compiler.set_include_dirs(self.include_dirs)
- if self.libraries:
- self.compiler.set_libraries(self.libraries)
- if self.library_dirs:
- self.compiler.set_library_dirs(self.library_dirs)
-
- def _gen_temp_sourcefile(self, body, headers, lang):
- filename = "_configtest" + LANG_EXT[lang]
- with open(filename, "w") as file:
- if headers:
- for header in headers:
- file.write("#include <%s>\n" % header)
- file.write("\n")
- file.write(body)
- if body[-1] != "\n":
- file.write("\n")
- return filename
-
- def _preprocess(self, body, headers, include_dirs, lang):
- src = self._gen_temp_sourcefile(body, headers, lang)
- out = "_configtest.i"
- self.temp_files.extend([src, out])
- self.compiler.preprocess(src, out, include_dirs=include_dirs)
- return (src, out)
-
- def _compile(self, body, headers, include_dirs, lang):
- src = self._gen_temp_sourcefile(body, headers, lang)
- if self.dump_source:
- dump_file(src, "compiling '%s':" % src)
- (obj,) = self.compiler.object_filenames([src])
- self.temp_files.extend([src, obj])
- self.compiler.compile([src], include_dirs=include_dirs)
- return (src, obj)
-
- def _link(self, body, headers, include_dirs, libraries, library_dirs, lang):
- (src, obj) = self._compile(body, headers, include_dirs, lang)
- prog = os.path.splitext(os.path.basename(src))[0]
- self.compiler.link_executable(
- [obj],
- prog,
- libraries=libraries,
- library_dirs=library_dirs,
- target_lang=lang,
- )
-
- if self.compiler.exe_extension is not None:
- prog = prog + self.compiler.exe_extension
- self.temp_files.append(prog)
-
- return (src, obj, prog)
-
- def _clean(self, *filenames):
- if not filenames:
- filenames = self.temp_files
- self.temp_files = []
- log.info("removing: %s", ' '.join(filenames))
- for filename in filenames:
- try:
- os.remove(filename)
- except OSError:
- pass
-
- # XXX these ignore the dry-run flag: what to do, what to do? even if
- # you want a dry-run build, you still need some sort of configuration
- # info. My inclination is to make it up to the real config command to
- # consult 'dry_run', and assume a default (minimal) configuration if
- # true. The problem with trying to do it here is that you'd have to
- # return either true or false from all the 'try' methods, neither of
- # which is correct.
-
- # XXX need access to the header search path and maybe default macros.
-
- def try_cpp(self, body=None, headers=None, include_dirs=None, lang="c"):
- """Construct a source file from 'body' (a string containing lines
- of C/C++ code) and 'headers' (a list of header files to include)
- and run it through the preprocessor. Return true if the
- preprocessor succeeded, false if there were any errors.
- ('body' probably isn't of much use, but what the heck.)
- """
- from distutils.ccompiler import CompileError
-
- self._check_compiler()
- ok = True
- try:
- self._preprocess(body, headers, include_dirs, lang)
- except CompileError:
- ok = False
-
- self._clean()
- return ok
-
- def search_cpp(self, pattern, body=None, headers=None, include_dirs=None, lang="c"):
- """Construct a source file (just like 'try_cpp()'), run it through
- the preprocessor, and return true if any line of the output matches
- 'pattern'. 'pattern' should either be a compiled regex object or a
- string containing a regex. If both 'body' and 'headers' are None,
- preprocesses an empty file -- which can be useful to determine the
- symbols the preprocessor and compiler set by default.
- """
- self._check_compiler()
- src, out = self._preprocess(body, headers, include_dirs, lang)
-
- if isinstance(pattern, str):
- pattern = re.compile(pattern)
-
- with open(out) as file:
- match = False
- while True:
- line = file.readline()
- if line == '':
- break
- if pattern.search(line):
- match = True
- break
-
- self._clean()
- return match
-
- def try_compile(self, body, headers=None, include_dirs=None, lang="c"):
- """Try to compile a source file built from 'body' and 'headers'.
- Return true on success, false otherwise.
- """
- from distutils.ccompiler import CompileError
-
- self._check_compiler()
- try:
- self._compile(body, headers, include_dirs, lang)
- ok = True
- except CompileError:
- ok = False
-
- log.info(ok and "success!" or "failure.")
- self._clean()
- return ok
-
- def try_link(
- self,
- body,
- headers=None,
- include_dirs=None,
- libraries=None,
- library_dirs=None,
- lang="c",
- ):
- """Try to compile and link a source file, built from 'body' and
- 'headers', to executable form. Return true on success, false
- otherwise.
- """
- from distutils.ccompiler import CompileError, LinkError
-
- self._check_compiler()
- try:
- self._link(body, headers, include_dirs, libraries, library_dirs, lang)
- ok = True
- except (CompileError, LinkError):
- ok = False
-
- log.info(ok and "success!" or "failure.")
- self._clean()
- return ok
-
- def try_run(
- self,
- body,
- headers=None,
- include_dirs=None,
- libraries=None,
- library_dirs=None,
- lang="c",
- ):
- """Try to compile, link to an executable, and run a program
- built from 'body' and 'headers'. Return true on success, false
- otherwise.
- """
- from distutils.ccompiler import CompileError, LinkError
-
- self._check_compiler()
- try:
- src, obj, exe = self._link(
- body, headers, include_dirs, libraries, library_dirs, lang
- )
- self.spawn([exe])
- ok = True
- except (CompileError, LinkError, DistutilsExecError):
- ok = False
-
- log.info(ok and "success!" or "failure.")
- self._clean()
- return ok
-
- # -- High-level methods --------------------------------------------
- # (these are the ones that are actually likely to be useful
- # when implementing a real-world config command!)
-
- def check_func(
- self,
- func,
- headers=None,
- include_dirs=None,
- libraries=None,
- library_dirs=None,
- decl=0,
- call=0,
- ):
- """Determine if function 'func' is available by constructing a
- source file that refers to 'func', and compiles and links it.
- If everything succeeds, returns true; otherwise returns false.
-
- The constructed source file starts out by including the header
- files listed in 'headers'. If 'decl' is true, it then declares
- 'func' (as "int func()"); you probably shouldn't supply 'headers'
- and set 'decl' true in the same call, or you might get errors about
- a conflicting declarations for 'func'. Finally, the constructed
- 'main()' function either references 'func' or (if 'call' is true)
- calls it. 'libraries' and 'library_dirs' are used when
- linking.
- """
- self._check_compiler()
- body = []
- if decl:
- body.append("int %s ();" % func)
- body.append("int main () {")
- if call:
- body.append(" %s();" % func)
- else:
- body.append(" %s;" % func)
- body.append("}")
- body = "\n".join(body) + "\n"
-
- return self.try_link(body, headers, include_dirs, libraries, library_dirs)
-
- def check_lib(
- self,
- library,
- library_dirs=None,
- headers=None,
- include_dirs=None,
- other_libraries=[],
- ):
- """Determine if 'library' is available to be linked against,
- without actually checking that any particular symbols are provided
- by it. 'headers' will be used in constructing the source file to
- be compiled, but the only effect of this is to check if all the
- header files listed are available. Any libraries listed in
- 'other_libraries' will be included in the link, in case 'library'
- has symbols that depend on other libraries.
- """
- self._check_compiler()
- return self.try_link(
- "int main (void) { }",
- headers,
- include_dirs,
- [library] + other_libraries,
- library_dirs,
- )
-
- def check_header(self, header, include_dirs=None, library_dirs=None, lang="c"):
- """Determine if the system header file named by 'header_file'
- exists and can be found by the preprocessor; return true if so,
- false otherwise.
- """
- return self.try_cpp(
- body="/* No body */", headers=[header], include_dirs=include_dirs
- )
-
-
-def dump_file(filename, head=None):
- """Dumps a file content into log.info.
-
- If head is not None, will be dumped before the file content.
- """
- if head is None:
- log.info('%s', filename)
- else:
- log.info(head)
- file = open(filename)
- try:
- log.info(file.read())
- finally:
- file.close()
diff --git a/spaces/CVPR/Image-Animation-using-Thin-Plate-Spline-Motion-Model/README.md b/spaces/CVPR/Image-Animation-using-Thin-Plate-Spline-Motion-Model/README.md
deleted file mode 100644
index 62b1f9e40124f137c98a2e4b1ff5eca3d7c89625..0000000000000000000000000000000000000000
--- a/spaces/CVPR/Image-Animation-using-Thin-Plate-Spline-Motion-Model/README.md
+++ /dev/null
@@ -1,12 +0,0 @@
----
-title: Image Animation Using Thin Plate Spline Motion Model
-emoji: 👁
-colorFrom: indigo
-colorTo: indigo
-sdk: gradio
-sdk_version: 3.48.0
-app_file: app.py
-pinned: false
----
-
-Check out the configuration reference at https://huggingface.co/docs/hub/spaces-config-reference
diff --git a/spaces/CVPR/WALT/mmdet/models/dense_heads/cascade_rpn_head.py b/spaces/CVPR/WALT/mmdet/models/dense_heads/cascade_rpn_head.py
deleted file mode 100644
index e32ee461951e685fb44a461033293159e3439717..0000000000000000000000000000000000000000
--- a/spaces/CVPR/WALT/mmdet/models/dense_heads/cascade_rpn_head.py
+++ /dev/null
@@ -1,784 +0,0 @@
-from __future__ import division
-import copy
-import warnings
-
-import torch
-import torch.nn as nn
-from mmcv import ConfigDict
-from mmcv.cnn import normal_init
-from mmcv.ops import DeformConv2d, batched_nms
-
-from mmdet.core import (RegionAssigner, build_assigner, build_sampler,
- images_to_levels, multi_apply)
-from ..builder import HEADS, build_head
-from .base_dense_head import BaseDenseHead
-from .rpn_head import RPNHead
-
-
-class AdaptiveConv(nn.Module):
- """AdaptiveConv used to adapt the sampling location with the anchors.
-
- Args:
- in_channels (int): Number of channels in the input image
- out_channels (int): Number of channels produced by the convolution
- kernel_size (int or tuple): Size of the conv kernel. Default: 3
- stride (int or tuple, optional): Stride of the convolution. Default: 1
- padding (int or tuple, optional): Zero-padding added to both sides of
- the input. Default: 1
- dilation (int or tuple, optional): Spacing between kernel elements.
- Default: 3
- groups (int, optional): Number of blocked connections from input
- channels to output channels. Default: 1
- bias (bool, optional): If set True, adds a learnable bias to the
- output. Default: False.
- type (str, optional): Type of adaptive conv, can be either 'offset'
- (arbitrary anchors) or 'dilation' (uniform anchor).
- Default: 'dilation'.
- """
-
- def __init__(self,
- in_channels,
- out_channels,
- kernel_size=3,
- stride=1,
- padding=1,
- dilation=3,
- groups=1,
- bias=False,
- type='dilation'):
- super(AdaptiveConv, self).__init__()
- assert type in ['offset', 'dilation']
- self.adapt_type = type
-
- assert kernel_size == 3, 'Adaptive conv only supports kernels 3'
- if self.adapt_type == 'offset':
- assert stride == 1 and padding == 1 and groups == 1, \
- 'Adaptive conv offset mode only supports padding: {1}, ' \
- f'stride: {1}, groups: {1}'
- self.conv = DeformConv2d(
- in_channels,
- out_channels,
- kernel_size,
- padding=padding,
- stride=stride,
- groups=groups,
- bias=bias)
- else:
- self.conv = nn.Conv2d(
- in_channels,
- out_channels,
- kernel_size,
- padding=dilation,
- dilation=dilation)
-
- def init_weights(self):
- """Init weights."""
- normal_init(self.conv, std=0.01)
-
- def forward(self, x, offset):
- """Forward function."""
- if self.adapt_type == 'offset':
- N, _, H, W = x.shape
- assert offset is not None
- assert H * W == offset.shape[1]
- # reshape [N, NA, 18] to (N, 18, H, W)
- offset = offset.permute(0, 2, 1).reshape(N, -1, H, W)
- offset = offset.contiguous()
- x = self.conv(x, offset)
- else:
- assert offset is None
- x = self.conv(x)
- return x
-
-
-@HEADS.register_module()
-class StageCascadeRPNHead(RPNHead):
- """Stage of CascadeRPNHead.
-
- Args:
- in_channels (int): Number of channels in the input feature map.
- anchor_generator (dict): anchor generator config.
- adapt_cfg (dict): adaptation config.
- bridged_feature (bool, optional): whether update rpn feature.
- Default: False.
- with_cls (bool, optional): wheather use classification branch.
- Default: True.
- sampling (bool, optional): wheather use sampling. Default: True.
- """
-
- def __init__(self,
- in_channels,
- anchor_generator=dict(
- type='AnchorGenerator',
- scales=[8],
- ratios=[1.0],
- strides=[4, 8, 16, 32, 64]),
- adapt_cfg=dict(type='dilation', dilation=3),
- bridged_feature=False,
- with_cls=True,
- sampling=True,
- **kwargs):
- self.with_cls = with_cls
- self.anchor_strides = anchor_generator['strides']
- self.anchor_scales = anchor_generator['scales']
- self.bridged_feature = bridged_feature
- self.adapt_cfg = adapt_cfg
- super(StageCascadeRPNHead, self).__init__(
- in_channels, anchor_generator=anchor_generator, **kwargs)
-
- # override sampling and sampler
- self.sampling = sampling
- if self.train_cfg:
- self.assigner = build_assigner(self.train_cfg.assigner)
- # use PseudoSampler when sampling is False
- if self.sampling and hasattr(self.train_cfg, 'sampler'):
- sampler_cfg = self.train_cfg.sampler
- else:
- sampler_cfg = dict(type='PseudoSampler')
- self.sampler = build_sampler(sampler_cfg, context=self)
-
- def _init_layers(self):
- """Init layers of a CascadeRPN stage."""
- self.rpn_conv = AdaptiveConv(self.in_channels, self.feat_channels,
- **self.adapt_cfg)
- if self.with_cls:
- self.rpn_cls = nn.Conv2d(self.feat_channels,
- self.num_anchors * self.cls_out_channels,
- 1)
- self.rpn_reg = nn.Conv2d(self.feat_channels, self.num_anchors * 4, 1)
- self.relu = nn.ReLU(inplace=True)
-
- def init_weights(self):
- """Init weights of a CascadeRPN stage."""
- self.rpn_conv.init_weights()
- normal_init(self.rpn_reg, std=0.01)
- if self.with_cls:
- normal_init(self.rpn_cls, std=0.01)
-
- def forward_single(self, x, offset):
- """Forward function of single scale."""
- bridged_x = x
- x = self.relu(self.rpn_conv(x, offset))
- if self.bridged_feature:
- bridged_x = x # update feature
- cls_score = self.rpn_cls(x) if self.with_cls else None
- bbox_pred = self.rpn_reg(x)
- return bridged_x, cls_score, bbox_pred
-
- def forward(self, feats, offset_list=None):
- """Forward function."""
- if offset_list is None:
- offset_list = [None for _ in range(len(feats))]
- return multi_apply(self.forward_single, feats, offset_list)
-
- def _region_targets_single(self,
- anchors,
- valid_flags,
- gt_bboxes,
- gt_bboxes_ignore,
- gt_labels,
- img_meta,
- featmap_sizes,
- label_channels=1):
- """Get anchor targets based on region for single level."""
- assign_result = self.assigner.assign(
- anchors,
- valid_flags,
- gt_bboxes,
- img_meta,
- featmap_sizes,
- self.anchor_scales[0],
- self.anchor_strides,
- gt_bboxes_ignore=gt_bboxes_ignore,
- gt_labels=None,
- allowed_border=self.train_cfg.allowed_border)
- flat_anchors = torch.cat(anchors)
- sampling_result = self.sampler.sample(assign_result, flat_anchors,
- gt_bboxes)
-
- num_anchors = flat_anchors.shape[0]
- bbox_targets = torch.zeros_like(flat_anchors)
- bbox_weights = torch.zeros_like(flat_anchors)
- labels = flat_anchors.new_zeros(num_anchors, dtype=torch.long)
- label_weights = flat_anchors.new_zeros(num_anchors, dtype=torch.float)
-
- pos_inds = sampling_result.pos_inds
- neg_inds = sampling_result.neg_inds
- if len(pos_inds) > 0:
- if not self.reg_decoded_bbox:
- pos_bbox_targets = self.bbox_coder.encode(
- sampling_result.pos_bboxes, sampling_result.pos_gt_bboxes)
- else:
- pos_bbox_targets = sampling_result.pos_gt_bboxes
- bbox_targets[pos_inds, :] = pos_bbox_targets
- bbox_weights[pos_inds, :] = 1.0
- if gt_labels is None:
- labels[pos_inds] = 1
- else:
- labels[pos_inds] = gt_labels[
- sampling_result.pos_assigned_gt_inds]
- if self.train_cfg.pos_weight <= 0:
- label_weights[pos_inds] = 1.0
- else:
- label_weights[pos_inds] = self.train_cfg.pos_weight
- if len(neg_inds) > 0:
- label_weights[neg_inds] = 1.0
-
- return (labels, label_weights, bbox_targets, bbox_weights, pos_inds,
- neg_inds)
-
- def region_targets(self,
- anchor_list,
- valid_flag_list,
- gt_bboxes_list,
- img_metas,
- featmap_sizes,
- gt_bboxes_ignore_list=None,
- gt_labels_list=None,
- label_channels=1,
- unmap_outputs=True):
- """See :func:`StageCascadeRPNHead.get_targets`."""
- num_imgs = len(img_metas)
- assert len(anchor_list) == len(valid_flag_list) == num_imgs
-
- # anchor number of multi levels
- num_level_anchors = [anchors.size(0) for anchors in anchor_list[0]]
-
- # compute targets for each image
- if gt_bboxes_ignore_list is None:
- gt_bboxes_ignore_list = [None for _ in range(num_imgs)]
- if gt_labels_list is None:
- gt_labels_list = [None for _ in range(num_imgs)]
- (all_labels, all_label_weights, all_bbox_targets, all_bbox_weights,
- pos_inds_list, neg_inds_list) = multi_apply(
- self._region_targets_single,
- anchor_list,
- valid_flag_list,
- gt_bboxes_list,
- gt_bboxes_ignore_list,
- gt_labels_list,
- img_metas,
- featmap_sizes=featmap_sizes,
- label_channels=label_channels)
- # no valid anchors
- if any([labels is None for labels in all_labels]):
- return None
- # sampled anchors of all images
- num_total_pos = sum([max(inds.numel(), 1) for inds in pos_inds_list])
- num_total_neg = sum([max(inds.numel(), 1) for inds in neg_inds_list])
- # split targets to a list w.r.t. multiple levels
- labels_list = images_to_levels(all_labels, num_level_anchors)
- label_weights_list = images_to_levels(all_label_weights,
- num_level_anchors)
- bbox_targets_list = images_to_levels(all_bbox_targets,
- num_level_anchors)
- bbox_weights_list = images_to_levels(all_bbox_weights,
- num_level_anchors)
- return (labels_list, label_weights_list, bbox_targets_list,
- bbox_weights_list, num_total_pos, num_total_neg)
-
- def get_targets(self,
- anchor_list,
- valid_flag_list,
- gt_bboxes,
- img_metas,
- featmap_sizes,
- gt_bboxes_ignore=None,
- label_channels=1):
- """Compute regression and classification targets for anchors.
-
- Args:
- anchor_list (list[list]): Multi level anchors of each image.
- valid_flag_list (list[list]): Multi level valid flags of each
- image.
- gt_bboxes (list[Tensor]): Ground truth bboxes of each image.
- img_metas (list[dict]): Meta info of each image.
- featmap_sizes (list[Tensor]): Feature mapsize each level
- gt_bboxes_ignore (list[Tensor]): Ignore bboxes of each images
- label_channels (int): Channel of label.
-
- Returns:
- cls_reg_targets (tuple)
- """
- if isinstance(self.assigner, RegionAssigner):
- cls_reg_targets = self.region_targets(
- anchor_list,
- valid_flag_list,
- gt_bboxes,
- img_metas,
- featmap_sizes,
- gt_bboxes_ignore_list=gt_bboxes_ignore,
- label_channels=label_channels)
- else:
- cls_reg_targets = super(StageCascadeRPNHead, self).get_targets(
- anchor_list,
- valid_flag_list,
- gt_bboxes,
- img_metas,
- gt_bboxes_ignore_list=gt_bboxes_ignore,
- label_channels=label_channels)
- return cls_reg_targets
-
- def anchor_offset(self, anchor_list, anchor_strides, featmap_sizes):
- """ Get offest for deformable conv based on anchor shape
- NOTE: currently support deformable kernel_size=3 and dilation=1
-
- Args:
- anchor_list (list[list[tensor])): [NI, NLVL, NA, 4] list of
- multi-level anchors
- anchor_strides (list[int]): anchor stride of each level
-
- Returns:
- offset_list (list[tensor]): [NLVL, NA, 2, 18]: offset of DeformConv
- kernel.
- """
-
- def _shape_offset(anchors, stride, ks=3, dilation=1):
- # currently support kernel_size=3 and dilation=1
- assert ks == 3 and dilation == 1
- pad = (ks - 1) // 2
- idx = torch.arange(-pad, pad + 1, dtype=dtype, device=device)
- yy, xx = torch.meshgrid(idx, idx) # return order matters
- xx = xx.reshape(-1)
- yy = yy.reshape(-1)
- w = (anchors[:, 2] - anchors[:, 0]) / stride
- h = (anchors[:, 3] - anchors[:, 1]) / stride
- w = w / (ks - 1) - dilation
- h = h / (ks - 1) - dilation
- offset_x = w[:, None] * xx # (NA, ks**2)
- offset_y = h[:, None] * yy # (NA, ks**2)
- return offset_x, offset_y
-
- def _ctr_offset(anchors, stride, featmap_size):
- feat_h, feat_w = featmap_size
- assert len(anchors) == feat_h * feat_w
-
- x = (anchors[:, 0] + anchors[:, 2]) * 0.5
- y = (anchors[:, 1] + anchors[:, 3]) * 0.5
- # compute centers on feature map
- x = x / stride
- y = y / stride
- # compute predefine centers
- xx = torch.arange(0, feat_w, device=anchors.device)
- yy = torch.arange(0, feat_h, device=anchors.device)
- yy, xx = torch.meshgrid(yy, xx)
- xx = xx.reshape(-1).type_as(x)
- yy = yy.reshape(-1).type_as(y)
-
- offset_x = x - xx # (NA, )
- offset_y = y - yy # (NA, )
- return offset_x, offset_y
-
- num_imgs = len(anchor_list)
- num_lvls = len(anchor_list[0])
- dtype = anchor_list[0][0].dtype
- device = anchor_list[0][0].device
- num_level_anchors = [anchors.size(0) for anchors in anchor_list[0]]
-
- offset_list = []
- for i in range(num_imgs):
- mlvl_offset = []
- for lvl in range(num_lvls):
- c_offset_x, c_offset_y = _ctr_offset(anchor_list[i][lvl],
- anchor_strides[lvl],
- featmap_sizes[lvl])
- s_offset_x, s_offset_y = _shape_offset(anchor_list[i][lvl],
- anchor_strides[lvl])
-
- # offset = ctr_offset + shape_offset
- offset_x = s_offset_x + c_offset_x[:, None]
- offset_y = s_offset_y + c_offset_y[:, None]
-
- # offset order (y0, x0, y1, x2, .., y8, x8, y9, x9)
- offset = torch.stack([offset_y, offset_x], dim=-1)
- offset = offset.reshape(offset.size(0), -1) # [NA, 2*ks**2]
- mlvl_offset.append(offset)
- offset_list.append(torch.cat(mlvl_offset)) # [totalNA, 2*ks**2]
- offset_list = images_to_levels(offset_list, num_level_anchors)
- return offset_list
-
- def loss_single(self, cls_score, bbox_pred, anchors, labels, label_weights,
- bbox_targets, bbox_weights, num_total_samples):
- """Loss function on single scale."""
- # classification loss
- if self.with_cls:
- labels = labels.reshape(-1)
- label_weights = label_weights.reshape(-1)
- cls_score = cls_score.permute(0, 2, 3,
- 1).reshape(-1, self.cls_out_channels)
- loss_cls = self.loss_cls(
- cls_score, labels, label_weights, avg_factor=num_total_samples)
- # regression loss
- bbox_targets = bbox_targets.reshape(-1, 4)
- bbox_weights = bbox_weights.reshape(-1, 4)
- bbox_pred = bbox_pred.permute(0, 2, 3, 1).reshape(-1, 4)
- if self.reg_decoded_bbox:
- # When the regression loss (e.g. `IouLoss`, `GIouLoss`)
- # is applied directly on the decoded bounding boxes, it
- # decodes the already encoded coordinates to absolute format.
- anchors = anchors.reshape(-1, 4)
- bbox_pred = self.bbox_coder.decode(anchors, bbox_pred)
- loss_reg = self.loss_bbox(
- bbox_pred,
- bbox_targets,
- bbox_weights,
- avg_factor=num_total_samples)
- if self.with_cls:
- return loss_cls, loss_reg
- return None, loss_reg
-
- def loss(self,
- anchor_list,
- valid_flag_list,
- cls_scores,
- bbox_preds,
- gt_bboxes,
- img_metas,
- gt_bboxes_ignore=None):
- """Compute losses of the head.
-
- Args:
- anchor_list (list[list]): Multi level anchors of each image.
- cls_scores (list[Tensor]): Box scores for each scale level
- Has shape (N, num_anchors * num_classes, H, W)
- bbox_preds (list[Tensor]): Box energies / deltas for each scale
- level with shape (N, num_anchors * 4, H, W)
- gt_bboxes (list[Tensor]): Ground truth bboxes for each image with
- shape (num_gts, 4) in [tl_x, tl_y, br_x, br_y] format.
- img_metas (list[dict]): Meta information of each image, e.g.,
- image size, scaling factor, etc.
- gt_bboxes_ignore (None | list[Tensor]): specify which bounding
- boxes can be ignored when computing the loss. Default: None
-
- Returns:
- dict[str, Tensor]: A dictionary of loss components.
- """
- featmap_sizes = [featmap.size()[-2:] for featmap in bbox_preds]
- label_channels = self.cls_out_channels if self.use_sigmoid_cls else 1
- cls_reg_targets = self.get_targets(
- anchor_list,
- valid_flag_list,
- gt_bboxes,
- img_metas,
- featmap_sizes,
- gt_bboxes_ignore=gt_bboxes_ignore,
- label_channels=label_channels)
- if cls_reg_targets is None:
- return None
- (labels_list, label_weights_list, bbox_targets_list, bbox_weights_list,
- num_total_pos, num_total_neg) = cls_reg_targets
- if self.sampling:
- num_total_samples = num_total_pos + num_total_neg
- else:
- # 200 is hard-coded average factor,
- # which follows guided anchoring.
- num_total_samples = sum([label.numel()
- for label in labels_list]) / 200.0
-
- # change per image, per level anchor_list to per_level, per_image
- mlvl_anchor_list = list(zip(*anchor_list))
- # concat mlvl_anchor_list
- mlvl_anchor_list = [
- torch.cat(anchors, dim=0) for anchors in mlvl_anchor_list
- ]
-
- losses = multi_apply(
- self.loss_single,
- cls_scores,
- bbox_preds,
- mlvl_anchor_list,
- labels_list,
- label_weights_list,
- bbox_targets_list,
- bbox_weights_list,
- num_total_samples=num_total_samples)
- if self.with_cls:
- return dict(loss_rpn_cls=losses[0], loss_rpn_reg=losses[1])
- return dict(loss_rpn_reg=losses[1])
-
- def get_bboxes(self,
- anchor_list,
- cls_scores,
- bbox_preds,
- img_metas,
- cfg,
- rescale=False):
- """Get proposal predict."""
- assert len(cls_scores) == len(bbox_preds)
- num_levels = len(cls_scores)
-
- result_list = []
- for img_id in range(len(img_metas)):
- cls_score_list = [
- cls_scores[i][img_id].detach() for i in range(num_levels)
- ]
- bbox_pred_list = [
- bbox_preds[i][img_id].detach() for i in range(num_levels)
- ]
- img_shape = img_metas[img_id]['img_shape']
- scale_factor = img_metas[img_id]['scale_factor']
- proposals = self._get_bboxes_single(cls_score_list, bbox_pred_list,
- anchor_list[img_id], img_shape,
- scale_factor, cfg, rescale)
- result_list.append(proposals)
- return result_list
-
- def refine_bboxes(self, anchor_list, bbox_preds, img_metas):
- """Refine bboxes through stages."""
- num_levels = len(bbox_preds)
- new_anchor_list = []
- for img_id in range(len(img_metas)):
- mlvl_anchors = []
- for i in range(num_levels):
- bbox_pred = bbox_preds[i][img_id].detach()
- bbox_pred = bbox_pred.permute(1, 2, 0).reshape(-1, 4)
- img_shape = img_metas[img_id]['img_shape']
- bboxes = self.bbox_coder.decode(anchor_list[img_id][i],
- bbox_pred, img_shape)
- mlvl_anchors.append(bboxes)
- new_anchor_list.append(mlvl_anchors)
- return new_anchor_list
-
- # TODO: temporary plan
- def _get_bboxes_single(self,
- cls_scores,
- bbox_preds,
- mlvl_anchors,
- img_shape,
- scale_factor,
- cfg,
- rescale=False):
- """Transform outputs for a single batch item into bbox predictions.
-
- Args:
- cls_scores (list[Tensor]): Box scores for each scale level
- Has shape (num_anchors * num_classes, H, W).
- bbox_preds (list[Tensor]): Box energies / deltas for each scale
- level with shape (num_anchors * 4, H, W).
- mlvl_anchors (list[Tensor]): Box reference for each scale level
- with shape (num_total_anchors, 4).
- img_shape (tuple[int]): Shape of the input image,
- (height, width, 3).
- scale_factor (ndarray): Scale factor of the image arange as
- (w_scale, h_scale, w_scale, h_scale).
- cfg (mmcv.Config): Test / postprocessing configuration,
- if None, test_cfg would be used.
- rescale (bool): If True, return boxes in original image space.
-
- Returns:
- Tensor: Labeled boxes have the shape of (n,5), where the
- first 4 columns are bounding box positions
- (tl_x, tl_y, br_x, br_y) and the 5-th column is a score
- between 0 and 1.
- """
- cfg = self.test_cfg if cfg is None else cfg
- cfg = copy.deepcopy(cfg)
- # bboxes from different level should be independent during NMS,
- # level_ids are used as labels for batched NMS to separate them
- level_ids = []
- mlvl_scores = []
- mlvl_bbox_preds = []
- mlvl_valid_anchors = []
- for idx in range(len(cls_scores)):
- rpn_cls_score = cls_scores[idx]
- rpn_bbox_pred = bbox_preds[idx]
- assert rpn_cls_score.size()[-2:] == rpn_bbox_pred.size()[-2:]
- rpn_cls_score = rpn_cls_score.permute(1, 2, 0)
- if self.use_sigmoid_cls:
- rpn_cls_score = rpn_cls_score.reshape(-1)
- scores = rpn_cls_score.sigmoid()
- else:
- rpn_cls_score = rpn_cls_score.reshape(-1, 2)
- # We set FG labels to [0, num_class-1] and BG label to
- # num_class in RPN head since mmdet v2.5, which is unified to
- # be consistent with other head since mmdet v2.0. In mmdet v2.0
- # to v2.4 we keep BG label as 0 and FG label as 1 in rpn head.
- scores = rpn_cls_score.softmax(dim=1)[:, 0]
- rpn_bbox_pred = rpn_bbox_pred.permute(1, 2, 0).reshape(-1, 4)
- anchors = mlvl_anchors[idx]
- if cfg.nms_pre > 0 and scores.shape[0] > cfg.nms_pre:
- # sort is faster than topk
- # _, topk_inds = scores.topk(cfg.nms_pre)
- if torch.onnx.is_in_onnx_export():
- # sort op will be converted to TopK in onnx
- # and k<=3480 in TensorRT
- _, topk_inds = scores.topk(cfg.nms_pre)
- scores = scores[topk_inds]
- else:
- ranked_scores, rank_inds = scores.sort(descending=True)
- topk_inds = rank_inds[:cfg.nms_pre]
- scores = ranked_scores[:cfg.nms_pre]
- rpn_bbox_pred = rpn_bbox_pred[topk_inds, :]
- anchors = anchors[topk_inds, :]
- mlvl_scores.append(scores)
- mlvl_bbox_preds.append(rpn_bbox_pred)
- mlvl_valid_anchors.append(anchors)
- level_ids.append(
- scores.new_full((scores.size(0), ), idx, dtype=torch.long))
-
- scores = torch.cat(mlvl_scores)
- anchors = torch.cat(mlvl_valid_anchors)
- rpn_bbox_pred = torch.cat(mlvl_bbox_preds)
- proposals = self.bbox_coder.decode(
- anchors, rpn_bbox_pred, max_shape=img_shape)
- ids = torch.cat(level_ids)
-
- # Skip nonzero op while exporting to ONNX
- if cfg.min_bbox_size > 0 and (not torch.onnx.is_in_onnx_export()):
- w = proposals[:, 2] - proposals[:, 0]
- h = proposals[:, 3] - proposals[:, 1]
- valid_inds = torch.nonzero(
- (w >= cfg.min_bbox_size)
- & (h >= cfg.min_bbox_size),
- as_tuple=False).squeeze()
- if valid_inds.sum().item() != len(proposals):
- proposals = proposals[valid_inds, :]
- scores = scores[valid_inds]
- ids = ids[valid_inds]
-
- # deprecate arguments warning
- if 'nms' not in cfg or 'max_num' in cfg or 'nms_thr' in cfg:
- warnings.warn(
- 'In rpn_proposal or test_cfg, '
- 'nms_thr has been moved to a dict named nms as '
- 'iou_threshold, max_num has been renamed as max_per_img, '
- 'name of original arguments and the way to specify '
- 'iou_threshold of NMS will be deprecated.')
- if 'nms' not in cfg:
- cfg.nms = ConfigDict(dict(type='nms', iou_threshold=cfg.nms_thr))
- if 'max_num' in cfg:
- if 'max_per_img' in cfg:
- assert cfg.max_num == cfg.max_per_img, f'You ' \
- f'set max_num and ' \
- f'max_per_img at the same time, but get {cfg.max_num} ' \
- f'and {cfg.max_per_img} respectively' \
- 'Please delete max_num which will be deprecated.'
- else:
- cfg.max_per_img = cfg.max_num
- if 'nms_thr' in cfg:
- assert cfg.nms.iou_threshold == cfg.nms_thr, f'You set' \
- f' iou_threshold in nms and ' \
- f'nms_thr at the same time, but get' \
- f' {cfg.nms.iou_threshold} and {cfg.nms_thr}' \
- f' respectively. Please delete the nms_thr ' \
- f'which will be deprecated.'
-
- dets, keep = batched_nms(proposals, scores, ids, cfg.nms)
- return dets[:cfg.max_per_img]
-
-
-@HEADS.register_module()
-class CascadeRPNHead(BaseDenseHead):
- """The CascadeRPNHead will predict more accurate region proposals, which is
- required for two-stage detectors (such as Fast/Faster R-CNN). CascadeRPN
- consists of a sequence of RPNStage to progressively improve the accuracy of
- the detected proposals.
-
- More details can be found in ``https://arxiv.org/abs/1909.06720``.
-
- Args:
- num_stages (int): number of CascadeRPN stages.
- stages (list[dict]): list of configs to build the stages.
- train_cfg (list[dict]): list of configs at training time each stage.
- test_cfg (dict): config at testing time.
- """
-
- def __init__(self, num_stages, stages, train_cfg, test_cfg):
- super(CascadeRPNHead, self).__init__()
- assert num_stages == len(stages)
- self.num_stages = num_stages
- self.stages = nn.ModuleList()
- for i in range(len(stages)):
- train_cfg_i = train_cfg[i] if train_cfg is not None else None
- stages[i].update(train_cfg=train_cfg_i)
- stages[i].update(test_cfg=test_cfg)
- self.stages.append(build_head(stages[i]))
- self.train_cfg = train_cfg
- self.test_cfg = test_cfg
-
- def init_weights(self):
- """Init weight of CascadeRPN."""
- for i in range(self.num_stages):
- self.stages[i].init_weights()
-
- def loss(self):
- """loss() is implemented in StageCascadeRPNHead."""
- pass
-
- def get_bboxes(self):
- """get_bboxes() is implemented in StageCascadeRPNHead."""
- pass
-
- def forward_train(self,
- x,
- img_metas,
- gt_bboxes,
- gt_labels=None,
- gt_bboxes_ignore=None,
- proposal_cfg=None):
- """Forward train function."""
- assert gt_labels is None, 'RPN does not require gt_labels'
-
- featmap_sizes = [featmap.size()[-2:] for featmap in x]
- device = x[0].device
- anchor_list, valid_flag_list = self.stages[0].get_anchors(
- featmap_sizes, img_metas, device=device)
-
- losses = dict()
-
- for i in range(self.num_stages):
- stage = self.stages[i]
-
- if stage.adapt_cfg['type'] == 'offset':
- offset_list = stage.anchor_offset(anchor_list,
- stage.anchor_strides,
- featmap_sizes)
- else:
- offset_list = None
- x, cls_score, bbox_pred = stage(x, offset_list)
- rpn_loss_inputs = (anchor_list, valid_flag_list, cls_score,
- bbox_pred, gt_bboxes, img_metas)
- stage_loss = stage.loss(*rpn_loss_inputs)
- for name, value in stage_loss.items():
- losses['s{}.{}'.format(i, name)] = value
-
- # refine boxes
- if i < self.num_stages - 1:
- anchor_list = stage.refine_bboxes(anchor_list, bbox_pred,
- img_metas)
- if proposal_cfg is None:
- return losses
- else:
- proposal_list = self.stages[-1].get_bboxes(anchor_list, cls_score,
- bbox_pred, img_metas,
- self.test_cfg)
- return losses, proposal_list
-
- def simple_test_rpn(self, x, img_metas):
- """Simple forward test function."""
- featmap_sizes = [featmap.size()[-2:] for featmap in x]
- device = x[0].device
- anchor_list, _ = self.stages[0].get_anchors(
- featmap_sizes, img_metas, device=device)
-
- for i in range(self.num_stages):
- stage = self.stages[i]
- if stage.adapt_cfg['type'] == 'offset':
- offset_list = stage.anchor_offset(anchor_list,
- stage.anchor_strides,
- featmap_sizes)
- else:
- offset_list = None
- x, cls_score, bbox_pred = stage(x, offset_list)
- if i < self.num_stages - 1:
- anchor_list = stage.refine_bboxes(anchor_list, bbox_pred,
- img_metas)
-
- proposal_list = self.stages[-1].get_bboxes(anchor_list, cls_score,
- bbox_pred, img_metas,
- self.test_cfg)
- return proposal_list
-
- def aug_test_rpn(self, x, img_metas):
- """Augmented forward test function."""
- raise NotImplementedError
diff --git a/spaces/CVPR/drawings-to-human/static/index.html b/spaces/CVPR/drawings-to-human/static/index.html
deleted file mode 100644
index 3463a9e2f393f935f722ea0759c5a633fc76037a..0000000000000000000000000000000000000000
--- a/spaces/CVPR/drawings-to-human/static/index.html
+++ /dev/null
@@ -1,209 +0,0 @@
-
-
-
-
-
-
-
-
-
-
-
-
-
-
-
-
-
-
-
-
-
Drawings to Human
-
This is an unofficial drawing tool to explore the generative human generator Text2Human. Please check all the model features on this
- Space.
-
")
- gr.ChatInterface(
- Mutilingual,
- examples=[["What is the quantum computers ?"], ["what is large language models"]]
- )
-
-demo.queue().launch(debug=True)
\ No newline at end of file
diff --git a/spaces/Volkopat/SegmentAnythingxGroundingDINO/groundingdino/util/visualizer.py b/spaces/Volkopat/SegmentAnythingxGroundingDINO/groundingdino/util/visualizer.py
deleted file mode 100644
index 7a1b7b101e9b73f75f9136bc67f2063c7c1cf1c1..0000000000000000000000000000000000000000
--- a/spaces/Volkopat/SegmentAnythingxGroundingDINO/groundingdino/util/visualizer.py
+++ /dev/null
@@ -1,318 +0,0 @@
-# -*- coding: utf-8 -*-
-"""
-@File : visualizer.py
-@Time : 2022/04/05 11:39:33
-@Author : Shilong Liu
-@Contact : slongliu86@gmail.com
-"""
-
-import datetime
-import os
-
-import cv2
-import matplotlib.pyplot as plt
-import numpy as np
-import torch
-from matplotlib import transforms
-from matplotlib.collections import PatchCollection
-from matplotlib.patches import Polygon
-from pycocotools import mask as maskUtils
-
-
-def renorm(
- img: torch.FloatTensor, mean=[0.485, 0.456, 0.406], std=[0.229, 0.224, 0.225]
-) -> torch.FloatTensor:
- # img: tensor(3,H,W) or tensor(B,3,H,W)
- # return: same as img
- assert img.dim() == 3 or img.dim() == 4, "img.dim() should be 3 or 4 but %d" % img.dim()
- if img.dim() == 3:
- assert img.size(0) == 3, 'img.size(0) shoule be 3 but "%d". (%s)' % (
- img.size(0),
- str(img.size()),
- )
- img_perm = img.permute(1, 2, 0)
- mean = torch.Tensor(mean)
- std = torch.Tensor(std)
- img_res = img_perm * std + mean
- return img_res.permute(2, 0, 1)
- else: # img.dim() == 4
- assert img.size(1) == 3, 'img.size(1) shoule be 3 but "%d". (%s)' % (
- img.size(1),
- str(img.size()),
- )
- img_perm = img.permute(0, 2, 3, 1)
- mean = torch.Tensor(mean)
- std = torch.Tensor(std)
- img_res = img_perm * std + mean
- return img_res.permute(0, 3, 1, 2)
-
-
-class ColorMap:
- def __init__(self, basergb=[255, 255, 0]):
- self.basergb = np.array(basergb)
-
- def __call__(self, attnmap):
- # attnmap: h, w. np.uint8.
- # return: h, w, 4. np.uint8.
- assert attnmap.dtype == np.uint8
- h, w = attnmap.shape
- res = self.basergb.copy()
- res = res[None][None].repeat(h, 0).repeat(w, 1) # h, w, 3
- attn1 = attnmap.copy()[..., None] # h, w, 1
- res = np.concatenate((res, attn1), axis=-1).astype(np.uint8)
- return res
-
-
-def rainbow_text(x, y, ls, lc, **kw):
- """
- Take a list of strings ``ls`` and colors ``lc`` and place them next to each
- other, with text ls[i] being shown in color lc[i].
-
- This example shows how to do both vertical and horizontal text, and will
- pass all keyword arguments to plt.text, so you can set the font size,
- family, etc.
- """
- t = plt.gca().transData
- fig = plt.gcf()
- plt.show()
-
- # horizontal version
- for s, c in zip(ls, lc):
- text = plt.text(x, y, " " + s + " ", color=c, transform=t, **kw)
- text.draw(fig.canvas.get_renderer())
- ex = text.get_window_extent()
- t = transforms.offset_copy(text._transform, x=ex.width, units="dots")
-
- # #vertical version
- # for s,c in zip(ls,lc):
- # text = plt.text(x,y," "+s+" ",color=c, transform=t,
- # rotation=90,va='bottom',ha='center',**kw)
- # text.draw(fig.canvas.get_renderer())
- # ex = text.get_window_extent()
- # t = transforms.offset_copy(text._transform, y=ex.height, units='dots')
-
-
-class COCOVisualizer:
- def __init__(self, coco=None, tokenlizer=None) -> None:
- self.coco = coco
-
- def visualize(self, img, tgt, caption=None, dpi=180, savedir="vis"):
- """
- img: tensor(3, H, W)
- tgt: make sure they are all on cpu.
- must have items: 'image_id', 'boxes', 'size'
- """
- plt.figure(dpi=dpi)
- plt.rcParams["font.size"] = "5"
- ax = plt.gca()
- img = renorm(img).permute(1, 2, 0)
- # if os.environ.get('IPDB_SHILONG_DEBUG', None) == 'INFO':
- # import ipdb; ipdb.set_trace()
- ax.imshow(img)
-
- self.addtgt(tgt)
-
- if tgt is None:
- image_id = 0
- elif "image_id" not in tgt:
- image_id = 0
- else:
- image_id = tgt["image_id"]
-
- if caption is None:
- savename = "{}/{}-{}.png".format(
- savedir, int(image_id), str(datetime.datetime.now()).replace(" ", "-")
- )
- else:
- savename = "{}/{}-{}-{}.png".format(
- savedir, caption, int(image_id), str(datetime.datetime.now()).replace(" ", "-")
- )
- print("savename: {}".format(savename))
- os.makedirs(os.path.dirname(savename), exist_ok=True)
- plt.savefig(savename)
- plt.close()
-
- def addtgt(self, tgt):
- """ """
- if tgt is None or not "boxes" in tgt:
- ax = plt.gca()
-
- if "caption" in tgt:
- ax.set_title(tgt["caption"], wrap=True)
-
- ax.set_axis_off()
- return
-
- ax = plt.gca()
- H, W = tgt["size"]
- numbox = tgt["boxes"].shape[0]
-
- color = []
- polygons = []
- boxes = []
- for box in tgt["boxes"].cpu():
- unnormbbox = box * torch.Tensor([W, H, W, H])
- unnormbbox[:2] -= unnormbbox[2:] / 2
- [bbox_x, bbox_y, bbox_w, bbox_h] = unnormbbox.tolist()
- boxes.append([bbox_x, bbox_y, bbox_w, bbox_h])
- poly = [
- [bbox_x, bbox_y],
- [bbox_x, bbox_y + bbox_h],
- [bbox_x + bbox_w, bbox_y + bbox_h],
- [bbox_x + bbox_w, bbox_y],
- ]
- np_poly = np.array(poly).reshape((4, 2))
- polygons.append(Polygon(np_poly))
- c = (np.random.random((1, 3)) * 0.6 + 0.4).tolist()[0]
- color.append(c)
-
- p = PatchCollection(polygons, facecolor=color, linewidths=0, alpha=0.1)
- ax.add_collection(p)
- p = PatchCollection(polygons, facecolor="none", edgecolors=color, linewidths=2)
- ax.add_collection(p)
-
- if "strings_positive" in tgt and len(tgt["strings_positive"]) > 0:
- assert (
- len(tgt["strings_positive"]) == numbox
- ), f"{len(tgt['strings_positive'])} = {numbox}, "
- for idx, strlist in enumerate(tgt["strings_positive"]):
- cate_id = int(tgt["labels"][idx])
- _string = str(cate_id) + ":" + " ".join(strlist)
- bbox_x, bbox_y, bbox_w, bbox_h = boxes[idx]
- # ax.text(bbox_x, bbox_y, _string, color='black', bbox={'facecolor': 'yellow', 'alpha': 1.0, 'pad': 1})
- ax.text(
- bbox_x,
- bbox_y,
- _string,
- color="black",
- bbox={"facecolor": color[idx], "alpha": 0.6, "pad": 1},
- )
-
- if "box_label" in tgt:
- assert len(tgt["box_label"]) == numbox, f"{len(tgt['box_label'])} = {numbox}, "
- for idx, bl in enumerate(tgt["box_label"]):
- _string = str(bl)
- bbox_x, bbox_y, bbox_w, bbox_h = boxes[idx]
- # ax.text(bbox_x, bbox_y, _string, color='black', bbox={'facecolor': 'yellow', 'alpha': 1.0, 'pad': 1})
- ax.text(
- bbox_x,
- bbox_y,
- _string,
- color="black",
- bbox={"facecolor": color[idx], "alpha": 0.6, "pad": 1},
- )
-
- if "caption" in tgt:
- ax.set_title(tgt["caption"], wrap=True)
- # plt.figure()
- # rainbow_text(0.0,0.0,"all unicorns poop rainbows ! ! !".split(),
- # ['red', 'orange', 'brown', 'green', 'blue', 'purple', 'black'])
-
- if "attn" in tgt:
- # if os.environ.get('IPDB_SHILONG_DEBUG', None) == 'INFO':
- # import ipdb; ipdb.set_trace()
- if isinstance(tgt["attn"], tuple):
- tgt["attn"] = [tgt["attn"]]
- for item in tgt["attn"]:
- attn_map, basergb = item
- attn_map = (attn_map - attn_map.min()) / (attn_map.max() - attn_map.min() + 1e-3)
- attn_map = (attn_map * 255).astype(np.uint8)
- cm = ColorMap(basergb)
- heatmap = cm(attn_map)
- ax.imshow(heatmap)
- ax.set_axis_off()
-
- def showAnns(self, anns, draw_bbox=False):
- """
- Display the specified annotations.
- :param anns (array of object): annotations to display
- :return: None
- """
- if len(anns) == 0:
- return 0
- if "segmentation" in anns[0] or "keypoints" in anns[0]:
- datasetType = "instances"
- elif "caption" in anns[0]:
- datasetType = "captions"
- else:
- raise Exception("datasetType not supported")
- if datasetType == "instances":
- ax = plt.gca()
- ax.set_autoscale_on(False)
- polygons = []
- color = []
- for ann in anns:
- c = (np.random.random((1, 3)) * 0.6 + 0.4).tolist()[0]
- if "segmentation" in ann:
- if type(ann["segmentation"]) == list:
- # polygon
- for seg in ann["segmentation"]:
- poly = np.array(seg).reshape((int(len(seg) / 2), 2))
- polygons.append(Polygon(poly))
- color.append(c)
- else:
- # mask
- t = self.imgs[ann["image_id"]]
- if type(ann["segmentation"]["counts"]) == list:
- rle = maskUtils.frPyObjects(
- [ann["segmentation"]], t["height"], t["width"]
- )
- else:
- rle = [ann["segmentation"]]
- m = maskUtils.decode(rle)
- img = np.ones((m.shape[0], m.shape[1], 3))
- if ann["iscrowd"] == 1:
- color_mask = np.array([2.0, 166.0, 101.0]) / 255
- if ann["iscrowd"] == 0:
- color_mask = np.random.random((1, 3)).tolist()[0]
- for i in range(3):
- img[:, :, i] = color_mask[i]
- ax.imshow(np.dstack((img, m * 0.5)))
- if "keypoints" in ann and type(ann["keypoints"]) == list:
- # turn skeleton into zero-based index
- sks = np.array(self.loadCats(ann["category_id"])[0]["skeleton"]) - 1
- kp = np.array(ann["keypoints"])
- x = kp[0::3]
- y = kp[1::3]
- v = kp[2::3]
- for sk in sks:
- if np.all(v[sk] > 0):
- plt.plot(x[sk], y[sk], linewidth=3, color=c)
- plt.plot(
- x[v > 0],
- y[v > 0],
- "o",
- markersize=8,
- markerfacecolor=c,
- markeredgecolor="k",
- markeredgewidth=2,
- )
- plt.plot(
- x[v > 1],
- y[v > 1],
- "o",
- markersize=8,
- markerfacecolor=c,
- markeredgecolor=c,
- markeredgewidth=2,
- )
-
- if draw_bbox:
- [bbox_x, bbox_y, bbox_w, bbox_h] = ann["bbox"]
- poly = [
- [bbox_x, bbox_y],
- [bbox_x, bbox_y + bbox_h],
- [bbox_x + bbox_w, bbox_y + bbox_h],
- [bbox_x + bbox_w, bbox_y],
- ]
- np_poly = np.array(poly).reshape((4, 2))
- polygons.append(Polygon(np_poly))
- color.append(c)
-
- # p = PatchCollection(polygons, facecolor=color, linewidths=0, alpha=0.4)
- # ax.add_collection(p)
- p = PatchCollection(polygons, facecolor="none", edgecolors=color, linewidths=2)
- ax.add_collection(p)
- elif datasetType == "captions":
- for ann in anns:
- print(ann["caption"])
diff --git a/spaces/Vorkrath/CarperAI-diff-codegen-6b-v2/app.py b/spaces/Vorkrath/CarperAI-diff-codegen-6b-v2/app.py
deleted file mode 100644
index a57edeee668a58afe8f2c8770e32d42d10f55842..0000000000000000000000000000000000000000
--- a/spaces/Vorkrath/CarperAI-diff-codegen-6b-v2/app.py
+++ /dev/null
@@ -1,3 +0,0 @@
-import gradio as gr
-
-gr.Interface.load("models/CarperAI/diff-codegen-6b-v2").launch()
\ No newline at end of file
diff --git a/spaces/WangQvQ/BEiT_Gradio/app.py b/spaces/WangQvQ/BEiT_Gradio/app.py
deleted file mode 100644
index 2607ad47a037e8764f66821bb3e6dc478cb5bf6a..0000000000000000000000000000000000000000
--- a/spaces/WangQvQ/BEiT_Gradio/app.py
+++ /dev/null
@@ -1,32 +0,0 @@
-import gradio as gr
-from transformers import BeitFeatureExtractor, BeitForImageClassification
-from PIL import Image
-import requests
-import numpy as np
-
-# Load the pre-trained BEiT model and feature extractor
-feature_extractor = BeitFeatureExtractor.from_pretrained('microsoft/beit-large-patch16-512')
-model = BeitForImageClassification.from_pretrained('microsoft/beit-large-patch16-512')
-
-
-def classify_image(input_image):
- image = Image.fromarray(input_image.astype('uint8'))
- inputs = feature_extractor(images=image, return_tensors="pt")
- outputs = model(**inputs)
- logits = outputs.logits
- predicted_class_idx = logits.argmax(-1).item()
- predicted_class = model.config.id2label[predicted_class_idx]
- return {"Predicted Class": predicted_class}
-
-
-iface = gr.Interface(
- fn=classify_image,
- inputs=gr.inputs.Image(type="numpy"), # Specify input type as numpy array
- outputs="json",
- live=True,
- title="BEiT Classification",
- description="Upload an image and you will get a description"
-)
-
-if __name__ == "__main__":
- iface.launch()
diff --git a/spaces/Wazzzabeee/image-video-colorization/models/deep_colorization/colorizers/eccv16.py b/spaces/Wazzzabeee/image-video-colorization/models/deep_colorization/colorizers/eccv16.py
deleted file mode 100644
index 896ed477c20934dc86a6088117eed63af773ace8..0000000000000000000000000000000000000000
--- a/spaces/Wazzzabeee/image-video-colorization/models/deep_colorization/colorizers/eccv16.py
+++ /dev/null
@@ -1,105 +0,0 @@
-
-import torch
-import torch.nn as nn
-import numpy as np
-from IPython import embed
-
-from .base_color import *
-
-class ECCVGenerator(BaseColor):
- def __init__(self, norm_layer=nn.BatchNorm2d):
- super(ECCVGenerator, self).__init__()
-
- model1=[nn.Conv2d(1, 64, kernel_size=3, stride=1, padding=1, bias=True),]
- model1+=[nn.ReLU(True),]
- model1+=[nn.Conv2d(64, 64, kernel_size=3, stride=2, padding=1, bias=True),]
- model1+=[nn.ReLU(True),]
- model1+=[norm_layer(64),]
-
- model2=[nn.Conv2d(64, 128, kernel_size=3, stride=1, padding=1, bias=True),]
- model2+=[nn.ReLU(True),]
- model2+=[nn.Conv2d(128, 128, kernel_size=3, stride=2, padding=1, bias=True),]
- model2+=[nn.ReLU(True),]
- model2+=[norm_layer(128),]
-
- model3=[nn.Conv2d(128, 256, kernel_size=3, stride=1, padding=1, bias=True),]
- model3+=[nn.ReLU(True),]
- model3+=[nn.Conv2d(256, 256, kernel_size=3, stride=1, padding=1, bias=True),]
- model3+=[nn.ReLU(True),]
- model3+=[nn.Conv2d(256, 256, kernel_size=3, stride=2, padding=1, bias=True),]
- model3+=[nn.ReLU(True),]
- model3+=[norm_layer(256),]
-
- model4=[nn.Conv2d(256, 512, kernel_size=3, stride=1, padding=1, bias=True),]
- model4+=[nn.ReLU(True),]
- model4+=[nn.Conv2d(512, 512, kernel_size=3, stride=1, padding=1, bias=True),]
- model4+=[nn.ReLU(True),]
- model4+=[nn.Conv2d(512, 512, kernel_size=3, stride=1, padding=1, bias=True),]
- model4+=[nn.ReLU(True),]
- model4+=[norm_layer(512),]
-
- model5=[nn.Conv2d(512, 512, kernel_size=3, dilation=2, stride=1, padding=2, bias=True),]
- model5+=[nn.ReLU(True),]
- model5+=[nn.Conv2d(512, 512, kernel_size=3, dilation=2, stride=1, padding=2, bias=True),]
- model5+=[nn.ReLU(True),]
- model5+=[nn.Conv2d(512, 512, kernel_size=3, dilation=2, stride=1, padding=2, bias=True),]
- model5+=[nn.ReLU(True),]
- model5+=[norm_layer(512),]
-
- model6=[nn.Conv2d(512, 512, kernel_size=3, dilation=2, stride=1, padding=2, bias=True),]
- model6+=[nn.ReLU(True),]
- model6+=[nn.Conv2d(512, 512, kernel_size=3, dilation=2, stride=1, padding=2, bias=True),]
- model6+=[nn.ReLU(True),]
- model6+=[nn.Conv2d(512, 512, kernel_size=3, dilation=2, stride=1, padding=2, bias=True),]
- model6+=[nn.ReLU(True),]
- model6+=[norm_layer(512),]
-
- model7=[nn.Conv2d(512, 512, kernel_size=3, stride=1, padding=1, bias=True),]
- model7+=[nn.ReLU(True),]
- model7+=[nn.Conv2d(512, 512, kernel_size=3, stride=1, padding=1, bias=True),]
- model7+=[nn.ReLU(True),]
- model7+=[nn.Conv2d(512, 512, kernel_size=3, stride=1, padding=1, bias=True),]
- model7+=[nn.ReLU(True),]
- model7+=[norm_layer(512),]
-
- model8=[nn.ConvTranspose2d(512, 256, kernel_size=4, stride=2, padding=1, bias=True),]
- model8+=[nn.ReLU(True),]
- model8+=[nn.Conv2d(256, 256, kernel_size=3, stride=1, padding=1, bias=True),]
- model8+=[nn.ReLU(True),]
- model8+=[nn.Conv2d(256, 256, kernel_size=3, stride=1, padding=1, bias=True),]
- model8+=[nn.ReLU(True),]
-
- model8+=[nn.Conv2d(256, 313, kernel_size=1, stride=1, padding=0, bias=True),]
-
- self.model1 = nn.Sequential(*model1)
- self.model2 = nn.Sequential(*model2)
- self.model3 = nn.Sequential(*model3)
- self.model4 = nn.Sequential(*model4)
- self.model5 = nn.Sequential(*model5)
- self.model6 = nn.Sequential(*model6)
- self.model7 = nn.Sequential(*model7)
- self.model8 = nn.Sequential(*model8)
-
- self.softmax = nn.Softmax(dim=1)
- self.model_out = nn.Conv2d(313, 2, kernel_size=1, padding=0, dilation=1, stride=1, bias=False)
- self.upsample4 = nn.Upsample(scale_factor=4, mode='bilinear')
-
- def forward(self, input_l):
- conv1_2 = self.model1(self.normalize_l(input_l))
- conv2_2 = self.model2(conv1_2)
- conv3_3 = self.model3(conv2_2)
- conv4_3 = self.model4(conv3_3)
- conv5_3 = self.model5(conv4_3)
- conv6_3 = self.model6(conv5_3)
- conv7_3 = self.model7(conv6_3)
- conv8_3 = self.model8(conv7_3)
- out_reg = self.model_out(self.softmax(conv8_3))
-
- return self.unnormalize_ab(self.upsample4(out_reg))
-
-def eccv16(pretrained=True):
- model = ECCVGenerator()
- if(pretrained):
- import torch.utils.model_zoo as model_zoo
- model.load_state_dict(model_zoo.load_url('https://colorizers.s3.us-east-2.amazonaws.com/colorization_release_v2-9b330a0b.pth',map_location='cpu',check_hash=True))
- return model
diff --git a/spaces/Xenova/next-example-app/_next/static/chunks/596-abb71f9569186505.js b/spaces/Xenova/next-example-app/_next/static/chunks/596-abb71f9569186505.js
deleted file mode 100644
index 365bcbe468c04be51e0258ab7eb5856e41e7e8b9..0000000000000000000000000000000000000000
--- a/spaces/Xenova/next-example-app/_next/static/chunks/596-abb71f9569186505.js
+++ /dev/null
@@ -1,25 +0,0 @@
-(self.webpackChunk_N_E=self.webpackChunk_N_E||[]).push([[596],{2335:function(){"trimStart"in String.prototype||(String.prototype.trimStart=String.prototype.trimLeft),"trimEnd"in String.prototype||(String.prototype.trimEnd=String.prototype.trimRight),"description"in Symbol.prototype||Object.defineProperty(Symbol.prototype,"description",{configurable:!0,get:function(){var e=/\((.*)\)/.exec(this.toString());return e?e[1]:void 0}}),Array.prototype.flat||(Array.prototype.flat=function(e,t){return t=this.concat.apply([],this),e>1&&t.some(Array.isArray)?t.flat(e-1):t},Array.prototype.flatMap=function(e,t){return this.map(e,t).flat()}),Promise.prototype.finally||(Promise.prototype.finally=function(e){if("function"!=typeof e)return this.then(e,e);var t=this.constructor||Promise;return this.then(function(r){return t.resolve(e()).then(function(){return r})},function(r){return t.resolve(e()).then(function(){throw r})})}),Object.fromEntries||(Object.fromEntries=function(e){return Array.from(e).reduce(function(e,t){return e[t[0]]=t[1],e},{})})},6711:function(e,t,r){"use strict";Object.defineProperty(t,"__esModule",{value:!0}),Object.defineProperty(t,"addBasePath",{enumerable:!0,get:function(){return o}});let n=r(7253),u=r(6070);function o(e,t){return(0,u.normalizePathTrailingSlash)((0,n.addPathPrefix)(e,""))}("function"==typeof t.default||"object"==typeof t.default&&null!==t.default)&&void 0===t.default.__esModule&&(Object.defineProperty(t.default,"__esModule",{value:!0}),Object.assign(t.default,t),e.exports=t.default)},4843:function(e,t){"use strict";function r(e){var t,r;t=self.__next_s,r=()=>{e()},t&&t.length?t.reduce((e,t)=>{let[r,n]=t;return e.then(()=>new Promise((e,t)=>{let u=document.createElement("script");if(n)for(let e in n)"children"!==e&&u.setAttribute(e,n[e]);r?(u.src=r,u.onload=()=>e(),u.onerror=t):n&&(u.innerHTML=n.children,setTimeout(e)),document.head.appendChild(u)}))},Promise.resolve()).catch(e=>{console.error(e)}).then(()=>{r()}):r()}Object.defineProperty(t,"__esModule",{value:!0}),Object.defineProperty(t,"appBootstrap",{enumerable:!0,get:function(){return r}}),window.next={version:"13.4.12",appDir:!0},("function"==typeof t.default||"object"==typeof t.default&&null!==t.default)&&void 0===t.default.__esModule&&(Object.defineProperty(t.default,"__esModule",{value:!0}),Object.assign(t.default,t),e.exports=t.default)},4039:function(e,t,r){"use strict";Object.defineProperty(t,"__esModule",{value:!0}),Object.defineProperty(t,"callServer",{enumerable:!0,get:function(){return u}});let n=r(7948);async function u(e,t){let r=(0,n.getServerActionDispatcher)();if(!r)throw Error("Invariant: missing action dispatcher.");return new Promise((n,u)=>{r({actionId:e,actionArgs:t,resolve:n,reject:u})})}("function"==typeof t.default||"object"==typeof t.default&&null!==t.default)&&void 0===t.default.__esModule&&(Object.defineProperty(t.default,"__esModule",{value:!0}),Object.assign(t.default,t),e.exports=t.default)},1615:function(e,t,r){"use strict";let n,u;Object.defineProperty(t,"__esModule",{value:!0}),Object.defineProperty(t,"hydrate",{enumerable:!0,get:function(){return N}});let o=r(1024),l=r(8533);r(2335);let a=o._(r(4040)),i=l._(r(2265)),c=r(6671),s=r(1330);r(6656);let f=o._(r(5152)),d=r(4039),p=r(8747),h=window.console.error;window.console.error=function(){for(var e=arguments.length,t=Array(e),r=0;r{if((0,p.isNextRouterError)(e.error)){e.preventDefault();return}});let _=e=>t=>e(t)+"",y=r.u,b={};r.u=_(e=>encodeURI(b[e]||y(e)));let v=r.k;r.k=_(v);let m=r.miniCssF;r.miniCssF=_(m),self.__next_require__=r,self.__next_chunk_load__=e=>{if(!e)return Promise.resolve();let[t,n]=e.split(":");return b[t]=n,r.e(t)};let g=document,O=()=>{let{pathname:e,search:t}=location;return e+t},P=new TextEncoder,E=!1,j=!1;function R(e){if(0===e[0])n=[];else{if(!n)throw Error("Unexpected server data: missing bootstrap script.");u?u.enqueue(P.encode(e[1])):n.push(e[1])}}let S=function(){u&&!j&&(u.close(),j=!0,n=void 0),E=!0};"loading"===document.readyState?document.addEventListener("DOMContentLoaded",S,!1):S();let T=self.__next_f=self.__next_f||[];T.forEach(R),T.push=R;let w=new Map;function M(e){let{cacheKey:t}=e;i.default.useEffect(()=>{w.delete(t)});let r=function(e){let t=w.get(e);if(t)return t;let r=new ReadableStream({start(e){n&&(n.forEach(t=>{e.enqueue(P.encode(t))}),E&&!j&&(e.close(),j=!0,n=void 0)),u=e}}),o=(0,c.createFromReadableStream)(r,{callServer:d.callServer});return w.set(e,o),o}(t),o=(0,i.use)(r);return o}let C=i.default.Fragment;function x(e){let{children:t}=e,[r,n]=i.default.useState(!1);return t}function A(e){return i.default.createElement(M,{...e,cacheKey:O()})}function N(){let e=i.default.createElement(C,null,i.default.createElement(s.HeadManagerContext.Provider,{value:{appDir:!0}},i.default.createElement(x,null,i.default.createElement(A,null)))),t={onRecoverableError:f.default},r="__next_error__"===document.documentElement.id;r?a.default.createRoot(g,t).render(e):i.default.startTransition(()=>a.default.hydrateRoot(g,e,t))}("function"==typeof t.default||"object"==typeof t.default&&null!==t.default)&&void 0===t.default.__esModule&&(Object.defineProperty(t.default,"__esModule",{value:!0}),Object.assign(t.default,t),e.exports=t.default)},2916:function(e,t,r){"use strict";Object.defineProperty(t,"__esModule",{value:!0});let n=r(4843);(0,n.appBootstrap)(()=>{r(7948),r(7767);let{hydrate:e}=r(1615);e()}),("function"==typeof t.default||"object"==typeof t.default&&null!==t.default)&&void 0===t.default.__esModule&&(Object.defineProperty(t.default,"__esModule",{value:!0}),Object.assign(t.default,t),e.exports=t.default)},1768:function(e,t,r){"use strict";Object.defineProperty(t,"__esModule",{value:!0}),Object.defineProperty(t,"AppRouterAnnouncer",{enumerable:!0,get:function(){return l}});let n=r(2265),u=r(4887),o="next-route-announcer";function l(e){let{tree:t}=e,[r,l]=(0,n.useState)(null);(0,n.useEffect)(()=>{let e=function(){var e;let t=document.getElementsByName(o)[0];if(null==t?void 0:null==(e=t.shadowRoot)?void 0:e.childNodes[0])return t.shadowRoot.childNodes[0];{let e=document.createElement(o);e.style.cssText="position:absolute";let t=document.createElement("div");t.ariaLive="assertive",t.id="__next-route-announcer__",t.role="alert",t.style.cssText="position:absolute;border:0;height:1px;margin:-1px;padding:0;width:1px;clip:rect(0 0 0 0);overflow:hidden;white-space:nowrap;word-wrap:normal";let r=e.attachShadow({mode:"open"});return r.appendChild(t),document.body.appendChild(e),t}}();return l(e),()=>{let e=document.getElementsByTagName(o)[0];(null==e?void 0:e.isConnected)&&document.body.removeChild(e)}},[]);let[a,i]=(0,n.useState)(""),c=(0,n.useRef)();return(0,n.useEffect)(()=>{let e="";if(document.title)e=document.title;else{let t=document.querySelector("h1");t&&(e=t.innerText||t.textContent||"")}void 0!==c.current&&i(e),c.current=e},[t]),r?(0,u.createPortal)(a,r):null}("function"==typeof t.default||"object"==typeof t.default&&null!==t.default)&&void 0===t.default.__esModule&&(Object.defineProperty(t.default,"__esModule",{value:!0}),Object.assign(t.default,t),e.exports=t.default)},4509:function(e,t){"use strict";Object.defineProperty(t,"__esModule",{value:!0}),function(e,t){for(var r in t)Object.defineProperty(e,r,{enumerable:!0,get:t[r]})}(t,{RSC:function(){return r},ACTION:function(){return n},NEXT_ROUTER_STATE_TREE:function(){return u},NEXT_ROUTER_PREFETCH:function(){return o},NEXT_URL:function(){return l},FETCH_CACHE_HEADER:function(){return a},RSC_CONTENT_TYPE_HEADER:function(){return i},RSC_VARY_HEADER:function(){return c},FLIGHT_PARAMETERS:function(){return s},NEXT_RSC_UNION_QUERY:function(){return f}});let r="RSC",n="Next-Action",u="Next-Router-State-Tree",o="Next-Router-Prefetch",l="Next-Url",a="x-vercel-sc-headers",i="text/x-component",c=r+", "+u+", "+o,s=[[r],[u],[o]],f="_rsc";("function"==typeof t.default||"object"==typeof t.default&&null!==t.default)&&void 0===t.default.__esModule&&(Object.defineProperty(t.default,"__esModule",{value:!0}),Object.assign(t.default,t),e.exports=t.default)},7948:function(e,t,r){"use strict";Object.defineProperty(t,"__esModule",{value:!0}),function(e,t){for(var r in t)Object.defineProperty(e,r,{enumerable:!0,get:t[r]})}(t,{getServerActionDispatcher:function(){return P},urlToUrlWithoutFlightMarker:function(){return E},default:function(){return w}});let n=r(8533),u=n._(r(2265)),o=r(6656),l=r(7538),a=r(5685),i=r(9330),c=r(6208),s=r(9865),f=r(6628),d=r(4444),p=r(3738),h=r(6711),_=r(1768),y=r(935),b=r(1487),v=r(8987),m=r(4509),g=new Map,O=null;function P(){return O}function E(e){let t=new URL(e,location.origin);if(t.searchParams.delete(m.NEXT_RSC_UNION_QUERY),t.pathname.endsWith(".txt")){let{pathname:e}=t,r=e.endsWith("/index.txt")?10:4;t.pathname=e.slice(0,-r)}return t}function j(e){return e.origin!==window.location.origin}function R(e){let{tree:t,pushRef:r,canonicalUrl:n,sync:o}=e;return(0,u.useInsertionEffect)(()=>{let e={__NA:!0,tree:t};r.pendingPush&&(0,i.createHrefFromUrl)(new URL(window.location.href))!==n?(r.pendingPush=!1,window.history.pushState(e,"",n)):window.history.replaceState(e,"",n),o()},[t,r,n,o]),null}let S=()=>({status:o.CacheStates.LAZY_INITIALIZED,data:null,subTreeData:null,parallelRoutes:new Map});function T(e){let{buildId:t,initialHead:r,initialTree:n,initialCanonicalUrl:i,children:f,assetPrefix:m}=e,P=(0,u.useMemo)(()=>(0,d.createInitialRouterState)({buildId:t,children:f,initialCanonicalUrl:i,initialTree:n,initialParallelRoutes:g,isServer:!1,location:window.location,initialHead:r}),[t,f,i,n,r]),[{tree:E,cache:T,prefetchCache:w,pushRef:M,focusAndScrollRef:C,canonicalUrl:x,nextUrl:A},N,I]=(0,s.useReducerWithReduxDevtools)(l.reducer,P);(0,u.useEffect)(()=>{g=null},[]);let{searchParams:k,pathname:D}=(0,u.useMemo)(()=>{let e=new URL(x,window.location.href);return{searchParams:e.searchParams,pathname:e.pathname}},[x]),F=(0,u.useCallback)((e,t,r)=>{(0,u.startTransition)(()=>{N({type:a.ACTION_SERVER_PATCH,flightData:t,previousTree:e,overrideCanonicalUrl:r,cache:S(),mutable:{}})})},[N]),U=(0,u.useCallback)((e,t,r,n)=>{let u=new URL((0,h.addBasePath)(e),location.href);return N({type:a.ACTION_NAVIGATE,url:u,isExternalUrl:j(u),locationSearch:location.search,forceOptimisticNavigation:r,shouldScroll:null==n||n,navigateType:t,cache:S(),mutable:{}})},[N]);!function(e,t,r){let n=(0,u.useCallback)(n=>{(0,u.startTransition)(()=>{t({...n,type:a.ACTION_SERVER_ACTION,mutable:{},navigate:r,changeByServerResponse:e})})},[e,t,r]);O=n}(F,N,U);let L=(0,u.useMemo)(()=>{let e={back:()=>window.history.back(),forward:()=>window.history.forward(),prefetch:(e,t)=>{if((0,p.isBot)(window.navigator.userAgent))return;let r=new URL((0,h.addBasePath)(e),location.href);j(r)||(0,u.startTransition)(()=>{var e;N({type:a.ACTION_PREFETCH,url:r,kind:null!=(e=null==t?void 0:t.kind)?e:a.PrefetchKind.FULL})})},replace:(e,t)=>{void 0===t&&(t={}),(0,u.startTransition)(()=>{var r;U(e,"replace",!!t.forceOptimisticNavigation,null==(r=t.scroll)||r)})},push:(e,t)=>{void 0===t&&(t={}),(0,u.startTransition)(()=>{var r;U(e,"push",!!t.forceOptimisticNavigation,null==(r=t.scroll)||r)})},refresh:()=>{(0,u.startTransition)(()=>{N({type:a.ACTION_REFRESH,cache:S(),mutable:{},origin:window.location.origin})})},fastRefresh:()=>{throw Error("fastRefresh can only be used in development mode. Please use refresh instead.")}};return e},[N,U]);if((0,u.useEffect)(()=>{window.next&&(window.next.router=L)},[L]),M.mpaNavigation){let e=window.location;M.pendingPush?e.assign(x):e.replace(x),(0,u.use)((0,v.createInfinitePromise)())}let H=(0,u.useCallback)(e=>{let{state:t}=e;if(t){if(!t.__NA){window.location.reload();return}(0,u.startTransition)(()=>{N({type:a.ACTION_RESTORE,url:new URL(window.location.href),tree:t.tree})})}},[N]);(0,u.useEffect)(()=>(window.addEventListener("popstate",H),()=>{window.removeEventListener("popstate",H)}),[H]);let $=(0,u.useMemo)(()=>(0,b.findHeadInCache)(T,E[1]),[T,E]),W=u.default.createElement(y.RedirectBoundary,null,$,T.subTreeData,u.default.createElement(_.AppRouterAnnouncer,{tree:E}));return u.default.createElement(u.default.Fragment,null,u.default.createElement(R,{tree:E,pushRef:M,canonicalUrl:x,sync:I}),u.default.createElement(c.PathnameContext.Provider,{value:D},u.default.createElement(c.SearchParamsContext.Provider,{value:k},u.default.createElement(o.GlobalLayoutRouterContext.Provider,{value:{buildId:t,changeByServerResponse:F,tree:E,focusAndScrollRef:C,nextUrl:A}},u.default.createElement(o.AppRouterContext.Provider,{value:L},u.default.createElement(o.LayoutRouterContext.Provider,{value:{childNodes:T.parallelRoutes,tree:E,url:x}},W))))))}function w(e){let{globalErrorComponent:t,...r}=e;return u.default.createElement(f.ErrorBoundary,{errorComponent:t},u.default.createElement(T,r))}("function"==typeof t.default||"object"==typeof t.default&&null!==t.default)&&void 0===t.default.__esModule&&(Object.defineProperty(t.default,"__esModule",{value:!0}),Object.assign(t.default,t),e.exports=t.default)},1253:function(e,t,r){"use strict";function n(e){}Object.defineProperty(t,"__esModule",{value:!0}),Object.defineProperty(t,"clientHookInServerComponentError",{enumerable:!0,get:function(){return n}}),r(1024),r(2265),("function"==typeof t.default||"object"==typeof t.default&&null!==t.default)&&void 0===t.default.__esModule&&(Object.defineProperty(t.default,"__esModule",{value:!0}),Object.assign(t.default,t),e.exports=t.default)},6628:function(e,t,r){"use strict";Object.defineProperty(t,"__esModule",{value:!0}),function(e,t){for(var r in t)Object.defineProperty(e,r,{enumerable:!0,get:t[r]})}(t,{ErrorBoundaryHandler:function(){return a},GlobalError:function(){return i},ErrorBoundary:function(){return c}});let n=r(1024),u=n._(r(2265)),o=r(8165),l={error:{fontFamily:'system-ui,"Segoe UI",Roboto,Helvetica,Arial,sans-serif,"Apple Color Emoji","Segoe UI Emoji"',height:"100vh",textAlign:"center",display:"flex",flexDirection:"column",alignItems:"center",justifyContent:"center"},text:{fontSize:"14px",fontWeight:400,lineHeight:"28px",margin:"0 8px"}};class a extends u.default.Component{static getDerivedStateFromError(e){return{error:e}}static getDerivedStateFromProps(e,t){return e.pathname!==t.previousPathname&&t.error?{error:null,previousPathname:e.pathname}:{error:t.error,previousPathname:e.pathname}}render(){return this.state.error?u.default.createElement(u.default.Fragment,null,this.props.errorStyles,u.default.createElement(this.props.errorComponent,{error:this.state.error,reset:this.reset})):this.props.children}constructor(e){super(e),this.reset=()=>{this.setState({error:null})},this.state={error:null,previousPathname:this.props.pathname}}}function i(e){let{error:t}=e,r=null==t?void 0:t.digest;return u.default.createElement("html",{id:"__next_error__"},u.default.createElement("head",null),u.default.createElement("body",null,u.default.createElement("div",{style:l.error},u.default.createElement("div",null,u.default.createElement("h2",{style:l.text},"Application error: a "+(r?"server":"client")+"-side exception has occurred (see the "+(r?"server logs":"browser console")+" for more information)."),r?u.default.createElement("p",{style:l.text},"Digest: "+r):null))))}function c(e){let{errorComponent:t,errorStyles:r,children:n}=e,l=(0,o.usePathname)();return t?u.default.createElement(a,{pathname:l,errorComponent:t,errorStyles:r},n):u.default.createElement(u.default.Fragment,null,n)}("function"==typeof t.default||"object"==typeof t.default&&null!==t.default)&&void 0===t.default.__esModule&&(Object.defineProperty(t.default,"__esModule",{value:!0}),Object.assign(t.default,t),e.exports=t.default)},4124:function(e,t){"use strict";Object.defineProperty(t,"__esModule",{value:!0}),function(e,t){for(var r in t)Object.defineProperty(e,r,{enumerable:!0,get:t[r]})}(t,{DYNAMIC_ERROR_CODE:function(){return r},DynamicServerError:function(){return n}});let r="DYNAMIC_SERVER_USAGE";class n extends Error{constructor(e){super("Dynamic server usage: "+e),this.digest=r}}("function"==typeof t.default||"object"==typeof t.default&&null!==t.default)&&void 0===t.default.__esModule&&(Object.defineProperty(t.default,"__esModule",{value:!0}),Object.assign(t.default,t),e.exports=t.default)},8987:function(e,t){"use strict";let r;function n(){return r||(r=new Promise(()=>{})),r}Object.defineProperty(t,"__esModule",{value:!0}),Object.defineProperty(t,"createInfinitePromise",{enumerable:!0,get:function(){return n}}),("function"==typeof t.default||"object"==typeof t.default&&null!==t.default)&&void 0===t.default.__esModule&&(Object.defineProperty(t.default,"__esModule",{value:!0}),Object.assign(t.default,t),e.exports=t.default)},8747:function(e,t,r){"use strict";Object.defineProperty(t,"__esModule",{value:!0}),Object.defineProperty(t,"isNextRouterError",{enumerable:!0,get:function(){return o}});let n=r(6920),u=r(5800);function o(e){return e&&e.digest&&((0,u.isRedirectError)(e)||(0,n.isNotFoundError)(e))}("function"==typeof t.default||"object"==typeof t.default&&null!==t.default)&&void 0===t.default.__esModule&&(Object.defineProperty(t.default,"__esModule",{value:!0}),Object.assign(t.default,t),e.exports=t.default)},7767:function(e,t,r){"use strict";Object.defineProperty(t,"__esModule",{value:!0}),Object.defineProperty(t,"default",{enumerable:!0,get:function(){return E}});let n=r(1024),u=r(8533),o=u._(r(2265)),l=n._(r(4887)),a=r(6656),i=r(2738),c=r(8987),s=r(6628),f=r(7910),d=r(1067),p=r(935),h=r(6280),_=r(5447),y=r(4818),b=["bottom","height","left","right","top","width","x","y"];function v(e,t){let r=e.getBoundingClientRect();return r.top>=0&&r.top<=t}class m extends o.default.Component{componentDidMount(){this.handlePotentialScroll()}componentDidUpdate(){this.props.focusAndScrollRef.apply&&this.handlePotentialScroll(!0)}render(){return this.props.children}constructor(...e){super(...e),this.handlePotentialScroll=e=>{let{focusAndScrollRef:t,segmentPath:r}=this.props;if(t.apply){var n;if(0!==t.segmentPaths.length&&!t.segmentPaths.some(e=>r.every((t,r)=>(0,f.matchSegment)(t,e[r]))))return;let u=null,o=t.hashFragment;if(o&&(u="top"===o?document.body:null!=(n=document.getElementById(o))?n:document.getElementsByName(o)[0]),u||(u=l.default.findDOMNode(this)),!(u instanceof Element))return;for(;!(u instanceof HTMLElement)||function(e){let t=e.getBoundingClientRect();return b.every(e=>0===t[e])}(u);){if(null===u.nextElementSibling)return;u=u.nextElementSibling}t.apply=!1,t.hashFragment=null,t.segmentPaths=[],(0,d.handleSmoothScroll)(()=>{if(o){u.scrollIntoView();return}let e=document.documentElement,t=e.clientHeight;!v(u,t)&&(e.scrollTop=0,v(u,t)||u.scrollIntoView())},{dontForceLayout:!0,onlyHashChange:!!e}),u.focus()}}}}function g(e){let{segmentPath:t,children:r}=e,n=(0,o.useContext)(a.GlobalLayoutRouterContext);if(!n)throw Error("invariant global layout router not mounted");return o.default.createElement(m,{segmentPath:t,focusAndScrollRef:n.focusAndScrollRef},r)}function O(e){let{parallelRouterKey:t,url:r,childNodes:n,childProp:u,segmentPath:l,tree:s,cacheKey:d}=e,p=(0,o.useContext)(a.GlobalLayoutRouterContext);if(!p)throw Error("invariant global layout router not mounted");let{buildId:h,changeByServerResponse:_,tree:y}=p,b=n.get(d);if(u&&null!==u.current&&(b?b.status===a.CacheStates.LAZY_INITIALIZED&&(b.status=a.CacheStates.READY,b.subTreeData=u.current):(b={status:a.CacheStates.READY,data:null,subTreeData:u.current,parallelRoutes:new Map},n.set(d,b))),!b||b.status===a.CacheStates.LAZY_INITIALIZED){let e=function e(t,r){if(t){let[n,u]=t,o=2===t.length;if((0,f.matchSegment)(r[0],n)&&r[1].hasOwnProperty(u)){if(o){let t=e(void 0,r[1][u]);return[r[0],{...r[1],[u]:[t[0],t[1],t[2],"refetch"]}]}return[r[0],{...r[1],[u]:e(t.slice(2),r[1][u])}]}}return r}(["",...l],y);b={status:a.CacheStates.DATA_FETCH,data:(0,i.fetchServerResponse)(new URL(r,location.origin),e,p.nextUrl,h),subTreeData:null,head:b&&b.status===a.CacheStates.LAZY_INITIALIZED?b.head:void 0,parallelRoutes:b&&b.status===a.CacheStates.LAZY_INITIALIZED?b.parallelRoutes:new Map},n.set(d,b)}if(!b)throw Error("Child node should always exist");if(b.subTreeData&&b.data)throw Error("Child node should not have both subTreeData and data");if(b.data){let[e,t]=(0,o.use)(b.data);b.data=null,setTimeout(()=>{(0,o.startTransition)(()=>{_(y,e,t)})}),(0,o.use)((0,c.createInfinitePromise)())}b.subTreeData||(0,o.use)((0,c.createInfinitePromise)());let v=o.default.createElement(a.LayoutRouterContext.Provider,{value:{tree:s[1][t],childNodes:b.parallelRoutes,url:r}},b.subTreeData);return v}function P(e){let{children:t,loading:r,loadingStyles:n,hasLoading:u}=e;return u?o.default.createElement(o.Suspense,{fallback:o.default.createElement(o.default.Fragment,null,n,r)},t):o.default.createElement(o.default.Fragment,null,t)}function E(e){let{parallelRouterKey:t,segmentPath:r,childProp:n,error:u,errorStyles:l,templateStyles:i,loading:c,loadingStyles:d,hasLoading:b,template:v,notFound:m,notFoundStyles:E,styles:j}=e,R=(0,o.useContext)(a.LayoutRouterContext);if(!R)throw Error("invariant expected layout router to be mounted");let{childNodes:S,tree:T,url:w}=R,M=S.get(t);M||(M=new Map,S.set(t,M));let C=T[1][t][0],x=n.segment,A=(0,_.getSegmentValue)(C),N=[C];return o.default.createElement(o.default.Fragment,null,j,N.map(e=>{let j=(0,f.matchSegment)(e,x),R=(0,_.getSegmentValue)(e),S=(0,y.createRouterCacheKey)(e);return o.default.createElement(a.TemplateContext.Provider,{key:(0,y.createRouterCacheKey)(e,!0),value:o.default.createElement(g,{segmentPath:r},o.default.createElement(s.ErrorBoundary,{errorComponent:u,errorStyles:l},o.default.createElement(P,{hasLoading:b,loading:c,loadingStyles:d},o.default.createElement(h.NotFoundBoundary,{notFound:m,notFoundStyles:E},o.default.createElement(p.RedirectBoundary,null,o.default.createElement(O,{parallelRouterKey:t,url:w,tree:T,childNodes:M,childProp:j?n:null,segmentPath:r,cacheKey:S,isActive:A===R}))))))},i,v)}))}("function"==typeof t.default||"object"==typeof t.default&&null!==t.default)&&void 0===t.default.__esModule&&(Object.defineProperty(t.default,"__esModule",{value:!0}),Object.assign(t.default,t),e.exports=t.default)},7910:function(e,t,r){"use strict";Object.defineProperty(t,"__esModule",{value:!0}),function(e,t){for(var r in t)Object.defineProperty(e,r,{enumerable:!0,get:t[r]})}(t,{matchSegment:function(){return u},canSegmentBeOverridden:function(){return o}});let n=r(5682),u=(e,t)=>"string"==typeof e?"string"==typeof t&&e===t:"string"!=typeof t&&e[0]===t[0]&&e[1]===t[1],o=(e,t)=>{var r;return!Array.isArray(e)&&!!Array.isArray(t)&&(null==(r=(0,n.getSegmentParam)(e))?void 0:r.param)===t[0]};("function"==typeof t.default||"object"==typeof t.default&&null!==t.default)&&void 0===t.default.__esModule&&(Object.defineProperty(t.default,"__esModule",{value:!0}),Object.assign(t.default,t),e.exports=t.default)},8165:function(e,t,r){"use strict";Object.defineProperty(t,"__esModule",{value:!0}),function(e,t){for(var r in t)Object.defineProperty(e,r,{enumerable:!0,get:t[r]})}(t,{ReadonlyURLSearchParams:function(){return p},useSearchParams:function(){return h},usePathname:function(){return _},ServerInsertedHTMLContext:function(){return i.ServerInsertedHTMLContext},useServerInsertedHTML:function(){return i.useServerInsertedHTML},useRouter:function(){return y},useParams:function(){return b},useSelectedLayoutSegments:function(){return v},useSelectedLayoutSegment:function(){return m},redirect:function(){return c.redirect},notFound:function(){return s.notFound}});let n=r(2265),u=r(6656),o=r(6208),l=r(1253),a=r(5447),i=r(8169),c=r(5800),s=r(6920),f=Symbol("internal for urlsearchparams readonly");function d(){return Error("ReadonlyURLSearchParams cannot be modified")}class p{[Symbol.iterator](){return this[f][Symbol.iterator]()}append(){throw d()}delete(){throw d()}set(){throw d()}sort(){throw d()}constructor(e){this[f]=e,this.entries=e.entries.bind(e),this.forEach=e.forEach.bind(e),this.get=e.get.bind(e),this.getAll=e.getAll.bind(e),this.has=e.has.bind(e),this.keys=e.keys.bind(e),this.values=e.values.bind(e),this.toString=e.toString.bind(e)}}function h(){(0,l.clientHookInServerComponentError)("useSearchParams");let e=(0,n.useContext)(o.SearchParamsContext),t=(0,n.useMemo)(()=>e?new p(e):null,[e]);return t}function _(){return(0,l.clientHookInServerComponentError)("usePathname"),(0,n.useContext)(o.PathnameContext)}function y(){(0,l.clientHookInServerComponentError)("useRouter");let e=(0,n.useContext)(u.AppRouterContext);if(null===e)throw Error("invariant expected app router to be mounted");return e}function b(){(0,l.clientHookInServerComponentError)("useParams");let e=(0,n.useContext)(u.GlobalLayoutRouterContext);return e?function e(t,r){void 0===r&&(r={});let n=t[1];for(let t of Object.values(n)){let n=t[0],u=Array.isArray(n),o=u?n[1]:n;if(!o||o.startsWith("__PAGE__"))continue;let l=u&&("c"===n[2]||"oc"===n[2]);l?r[n[0]]=n[1].split("/"):u&&(r[n[0]]=n[1]),r=e(t,r)}return r}(e.tree):null}function v(e){void 0===e&&(e="children"),(0,l.clientHookInServerComponentError)("useSelectedLayoutSegments");let{tree:t}=(0,n.useContext)(u.LayoutRouterContext);return function e(t,r,n,u){let o;if(void 0===n&&(n=!0),void 0===u&&(u=[]),n)o=t[1][r];else{var l;let e=t[1];o=null!=(l=e.children)?l:Object.values(e)[0]}if(!o)return u;let i=o[0],c=(0,a.getSegmentValue)(i);return!c||c.startsWith("__PAGE__")?u:(u.push(c),e(o,r,!1,u))}(t,e)}function m(e){void 0===e&&(e="children"),(0,l.clientHookInServerComponentError)("useSelectedLayoutSegment");let t=v(e);return 0===t.length?null:t[0]}("function"==typeof t.default||"object"==typeof t.default&&null!==t.default)&&void 0===t.default.__esModule&&(Object.defineProperty(t.default,"__esModule",{value:!0}),Object.assign(t.default,t),e.exports=t.default)},6280:function(e,t,r){"use strict";Object.defineProperty(t,"__esModule",{value:!0}),Object.defineProperty(t,"NotFoundBoundary",{enumerable:!0,get:function(){return a}});let n=r(1024),u=n._(r(2265)),o=r(8165);class l extends u.default.Component{static getDerivedStateFromError(e){if((null==e?void 0:e.digest)==="NEXT_NOT_FOUND")return{notFoundTriggered:!0};throw e}static getDerivedStateFromProps(e,t){return e.pathname!==t.previousPathname&&t.notFoundTriggered?{notFoundTriggered:!1,previousPathname:e.pathname}:{notFoundTriggered:t.notFoundTriggered,previousPathname:e.pathname}}render(){return this.state.notFoundTriggered?u.default.createElement(u.default.Fragment,null,u.default.createElement("meta",{name:"robots",content:"noindex"}),!1,this.props.notFoundStyles,this.props.notFound):this.props.children}constructor(e){super(e),this.state={notFoundTriggered:!!e.asNotFound,previousPathname:e.pathname}}}function a(e){let{notFound:t,notFoundStyles:r,asNotFound:n,children:a}=e,i=(0,o.usePathname)();return t?u.default.createElement(l,{pathname:i,notFound:t,notFoundStyles:r,asNotFound:n},a):u.default.createElement(u.default.Fragment,null,a)}("function"==typeof t.default||"object"==typeof t.default&&null!==t.default)&&void 0===t.default.__esModule&&(Object.defineProperty(t.default,"__esModule",{value:!0}),Object.assign(t.default,t),e.exports=t.default)},6920:function(e,t){"use strict";Object.defineProperty(t,"__esModule",{value:!0}),function(e,t){for(var r in t)Object.defineProperty(e,r,{enumerable:!0,get:t[r]})}(t,{notFound:function(){return n},isNotFoundError:function(){return u}});let r="NEXT_NOT_FOUND";function n(){let e=Error(r);throw e.digest=r,e}function u(e){return(null==e?void 0:e.digest)===r}("function"==typeof t.default||"object"==typeof t.default&&null!==t.default)&&void 0===t.default.__esModule&&(Object.defineProperty(t.default,"__esModule",{value:!0}),Object.assign(t.default,t),e.exports=t.default)},7843:function(e,t,r){"use strict";Object.defineProperty(t,"__esModule",{value:!0}),Object.defineProperty(t,"PromiseQueue",{enumerable:!0,get:function(){return c}});let n=r(4677),u=r(6249);var o=u._("_maxConcurrency"),l=u._("_runningCount"),a=u._("_queue"),i=u._("_processNext");class c{enqueue(e){let t,r;let u=new Promise((e,n)=>{t=e,r=n}),o=async()=>{try{n._(this,l)[l]++;let r=await e();t(r)}catch(e){r(e)}finally{n._(this,l)[l]--,n._(this,i)[i]()}};return n._(this,a)[a].push({promiseFn:u,task:o}),n._(this,i)[i](),u}bump(e){let t=n._(this,a)[a].findIndex(t=>t.promiseFn===e);if(t>-1){let e=n._(this,a)[a].splice(t,1)[0];n._(this,a)[a].unshift(e),n._(this,i)[i](!0)}}constructor(e=5){Object.defineProperty(this,i,{value:s}),Object.defineProperty(this,o,{writable:!0,value:void 0}),Object.defineProperty(this,l,{writable:!0,value:void 0}),Object.defineProperty(this,a,{writable:!0,value:void 0}),n._(this,o)[o]=e,n._(this,l)[l]=0,n._(this,a)[a]=[]}}function s(e){if(void 0===e&&(e=!1),(n._(this,l)[l]0){var t;null==(t=n._(this,a)[a].shift())||t.task()}}("function"==typeof t.default||"object"==typeof t.default&&null!==t.default)&&void 0===t.default.__esModule&&(Object.defineProperty(t.default,"__esModule",{value:!0}),Object.assign(t.default,t),e.exports=t.default)},935:function(e,t,r){"use strict";Object.defineProperty(t,"__esModule",{value:!0}),function(e,t){for(var r in t)Object.defineProperty(e,r,{enumerable:!0,get:t[r]})}(t,{RedirectErrorBoundary:function(){return i},RedirectBoundary:function(){return c}});let n=r(8533),u=n._(r(2265)),o=r(8165),l=r(5800);function a(e){let{redirect:t,reset:r,redirectType:n}=e,a=(0,o.useRouter)();return(0,u.useEffect)(()=>{u.default.startTransition(()=>{n===l.RedirectType.push?a.push(t,{}):a.replace(t,{}),r()})},[t,n,r,a]),null}class i extends u.default.Component{static getDerivedStateFromError(e){if((0,l.isRedirectError)(e)){let t=(0,l.getURLFromRedirectError)(e),r=(0,l.getRedirectTypeFromError)(e);return{redirect:t,redirectType:r}}throw e}render(){let{redirect:e,redirectType:t}=this.state;return null!==e&&null!==t?u.default.createElement(a,{redirect:e,redirectType:t,reset:()=>this.setState({redirect:null})}):this.props.children}constructor(e){super(e),this.state={redirect:null,redirectType:null}}}function c(e){let{children:t}=e,r=(0,o.useRouter)();return u.default.createElement(i,{router:r},t)}("function"==typeof t.default||"object"==typeof t.default&&null!==t.default)&&void 0===t.default.__esModule&&(Object.defineProperty(t.default,"__esModule",{value:!0}),Object.assign(t.default,t),e.exports=t.default)},5800:function(e,t,r){"use strict";var n,u;Object.defineProperty(t,"__esModule",{value:!0}),function(e,t){for(var r in t)Object.defineProperty(e,r,{enumerable:!0,get:t[r]})}(t,{RedirectType:function(){return n},getRedirectError:function(){return a},redirect:function(){return i},isRedirectError:function(){return c},getURLFromRedirectError:function(){return s},getRedirectTypeFromError:function(){return f}});let o=r(6170),l="NEXT_REDIRECT";function a(e,t){let r=Error(l);r.digest=l+";"+t+";"+e;let n=o.requestAsyncStorage.getStore();return n&&(r.mutableCookies=n.mutableCookies),r}function i(e,t){throw void 0===t&&(t="replace"),a(e,t)}function c(e){if("string"!=typeof(null==e?void 0:e.digest))return!1;let[t,r,n]=e.digest.split(";",3);return t===l&&("replace"===r||"push"===r)&&"string"==typeof n}function s(e){return c(e)?e.digest.split(";",3)[2]:null}function f(e){if(!c(e))throw Error("Not a redirect error");return e.digest.split(";",3)[1]}(u=n||(n={})).push="push",u.replace="replace",("function"==typeof t.default||"object"==typeof t.default&&null!==t.default)&&void 0===t.default.__esModule&&(Object.defineProperty(t.default,"__esModule",{value:!0}),Object.assign(t.default,t),e.exports=t.default)},7920:function(e,t,r){"use strict";Object.defineProperty(t,"__esModule",{value:!0}),Object.defineProperty(t,"default",{enumerable:!0,get:function(){return l}});let n=r(8533),u=n._(r(2265)),o=r(6656);function l(){let e=(0,u.useContext)(o.TemplateContext);return u.default.createElement(u.default.Fragment,null,e)}("function"==typeof t.default||"object"==typeof t.default&&null!==t.default)&&void 0===t.default.__esModule&&(Object.defineProperty(t.default,"__esModule",{value:!0}),Object.assign(t.default,t),e.exports=t.default)},7027:function(e,t,r){"use strict";Object.defineProperty(t,"__esModule",{value:!0}),Object.defineProperty(t,"applyFlightData",{enumerable:!0,get:function(){return l}});let n=r(6656),u=r(9726),o=r(516);function l(e,t,r,l){void 0===l&&(l=!1);let[a,i,c]=r.slice(-3);return null!==i&&(3===r.length?(t.status=n.CacheStates.READY,t.subTreeData=i,(0,u.fillLazyItemsTillLeafWithHead)(t,e,a,c,l)):(t.status=n.CacheStates.READY,t.subTreeData=e.subTreeData,t.parallelRoutes=new Map(e.parallelRoutes),(0,o.fillCacheWithNewSubTreeData)(t,e,r,l)),!0)}("function"==typeof t.default||"object"==typeof t.default&&null!==t.default)&&void 0===t.default.__esModule&&(Object.defineProperty(t.default,"__esModule",{value:!0}),Object.assign(t.default,t),e.exports=t.default)},7491:function(e,t,r){"use strict";Object.defineProperty(t,"__esModule",{value:!0}),Object.defineProperty(t,"applyRouterStatePatchToTree",{enumerable:!0,get:function(){return function e(t,r,o){let l;let[a,i,,,c]=r;if(1===t.length){let e=u(r,o);return e}let[s,f]=t;if(!(0,n.matchSegment)(s,a))return null;let d=2===t.length;if(d)l=u(i[f],o);else if(null===(l=e(t.slice(2),i[f],o)))return null;let p=[t[0],{...i,[f]:l}];return c&&(p[4]=!0),p}}});let n=r(7910);function u(e,t){let[r,o]=e,[l,a]=t;if("__DEFAULT__"===l&&"__DEFAULT__"!==r)return e;if((0,n.matchSegment)(r,l)){let t={};for(let e in o){let r=void 0!==a[e];r?t[e]=u(o[e],a[e]):t[e]=o[e]}for(let e in a)t[e]||(t[e]=a[e]);let n=[r,t];return e[2]&&(n[2]=e[2]),e[3]&&(n[3]=e[3]),e[4]&&(n[4]=e[4]),n}return t}("function"==typeof t.default||"object"==typeof t.default&&null!==t.default)&&void 0===t.default.__esModule&&(Object.defineProperty(t.default,"__esModule",{value:!0}),Object.assign(t.default,t),e.exports=t.default)},5121:function(e,t,r){"use strict";Object.defineProperty(t,"__esModule",{value:!0}),function(e,t){for(var r in t)Object.defineProperty(e,r,{enumerable:!0,get:t[r]})}(t,{extractPathFromFlightRouterState:function(){return a},computeChangedPath:function(){return i}});let n=r(4507),u=r(7910),o=e=>"string"==typeof e?e:e[1];function l(e){return e.split("/").reduce((e,t)=>""===t||t.startsWith("(")&&t.endsWith(")")?e:e+"/"+t,"")||"/"}function a(e){var t;let r=Array.isArray(e[0])?e[0][1]:e[0];if("__DEFAULT__"===r||n.INTERCEPTION_ROUTE_MARKERS.some(e=>r.startsWith(e)))return;if(r.startsWith("__PAGE__"))return"";let u=[r],o=null!=(t=e[1])?t:{},i=o.children?a(o.children):void 0;if(void 0!==i)u.push(i);else for(let[e,t]of Object.entries(o)){if("children"===e)continue;let r=a(t);void 0!==r&&u.push(r)}return l(u.join("/"))}function i(e,t){let r=function e(t,r){let[l,i]=t,[c,s]=r,f=o(l),d=o(c);if(n.INTERCEPTION_ROUTE_MARKERS.some(e=>f.startsWith(e)||d.startsWith(e)))return"";if(!(0,u.matchSegment)(l,c)){var p;return null!=(p=a(r))?p:""}for(let t in i)if(s[t]){let r=e(i[t],s[t]);if(null!==r)return o(c)+"/"+r}return null}(e,t);return null==r||"/"===r?r:l(r)}("function"==typeof t.default||"object"==typeof t.default&&null!==t.default)&&void 0===t.default.__esModule&&(Object.defineProperty(t.default,"__esModule",{value:!0}),Object.assign(t.default,t),e.exports=t.default)},9330:function(e,t){"use strict";function r(e,t){return void 0===t&&(t=!0),e.pathname+e.search+(t?e.hash:"")}Object.defineProperty(t,"__esModule",{value:!0}),Object.defineProperty(t,"createHrefFromUrl",{enumerable:!0,get:function(){return r}}),("function"==typeof t.default||"object"==typeof t.default&&null!==t.default)&&void 0===t.default.__esModule&&(Object.defineProperty(t.default,"__esModule",{value:!0}),Object.assign(t.default,t),e.exports=t.default)},4444:function(e,t,r){"use strict";Object.defineProperty(t,"__esModule",{value:!0}),Object.defineProperty(t,"createInitialRouterState",{enumerable:!0,get:function(){return a}});let n=r(6656),u=r(9330),o=r(9726),l=r(5121);function a(e){var t;let{buildId:r,initialTree:a,children:i,initialCanonicalUrl:c,initialParallelRoutes:s,isServer:f,location:d,initialHead:p}=e,h={status:n.CacheStates.READY,data:null,subTreeData:i,parallelRoutes:f?new Map:s};return(null===s||0===s.size)&&(0,o.fillLazyItemsTillLeafWithHead)(h,void 0,a,p),{buildId:r,tree:a,cache:h,prefetchCache:new Map,pushRef:{pendingPush:!1,mpaNavigation:!1},focusAndScrollRef:{apply:!1,hashFragment:null,segmentPaths:[]},canonicalUrl:d?(0,u.createHrefFromUrl)(d):c,nextUrl:null!=(t=(0,l.extractPathFromFlightRouterState)(a)||(null==d?void 0:d.pathname))?t:null}}("function"==typeof t.default||"object"==typeof t.default&&null!==t.default)&&void 0===t.default.__esModule&&(Object.defineProperty(t.default,"__esModule",{value:!0}),Object.assign(t.default,t),e.exports=t.default)},4679:function(e,t,r){"use strict";Object.defineProperty(t,"__esModule",{value:!0}),Object.defineProperty(t,"createOptimisticTree",{enumerable:!0,get:function(){return function e(t,r,u){let o;let[l,a,i,c,s]=r||[null,{}],f=t[0],d=1===t.length,p=null!==l&&(0,n.matchSegment)(l,f),h=Object.keys(a).length>1,_=!r||!p||h,y={};if(null!==l&&p&&(y=a),!d&&!h){let r=e(t.slice(1),y?y.children:null,u||_);o=r}let b=[f,{...y,...o?{children:o}:{}}];return i&&(b[2]=i),!u&&_?b[3]="refetch":p&&c&&(b[3]=c),p&&s&&(b[4]=s),b}}});let n=r(7910);("function"==typeof t.default||"object"==typeof t.default&&null!==t.default)&&void 0===t.default.__esModule&&(Object.defineProperty(t.default,"__esModule",{value:!0}),Object.assign(t.default,t),e.exports=t.default)},8982:function(e,t){"use strict";function r(e){return e.status="pending",e.then(t=>{"pending"===e.status&&(e.status="fulfilled",e.value=t)},t=>{"pending"===e.status&&(e.status="rejected",e.value=t)}),e}Object.defineProperty(t,"__esModule",{value:!0}),Object.defineProperty(t,"createRecordFromThenable",{enumerable:!0,get:function(){return r}}),("function"==typeof t.default||"object"==typeof t.default&&null!==t.default)&&void 0===t.default.__esModule&&(Object.defineProperty(t.default,"__esModule",{value:!0}),Object.assign(t.default,t),e.exports=t.default)},4818:function(e,t){"use strict";function r(e,t){return void 0===t&&(t=!1),Array.isArray(e)?e[0]+"|"+e[1]+"|"+e[2]:t&&e.startsWith("__PAGE__")?"__PAGE__":e}Object.defineProperty(t,"__esModule",{value:!0}),Object.defineProperty(t,"createRouterCacheKey",{enumerable:!0,get:function(){return r}}),("function"==typeof t.default||"object"==typeof t.default&&null!==t.default)&&void 0===t.default.__esModule&&(Object.defineProperty(t.default,"__esModule",{value:!0}),Object.assign(t.default,t),e.exports=t.default)},2738:function(e,t,r){"use strict";Object.defineProperty(t,"__esModule",{value:!0}),Object.defineProperty(t,"fetchServerResponse",{enumerable:!0,get:function(){return s}});let n=r(6671),u=r(4509),o=r(7948),l=r(4039),a=r(5685),i=r(216);function c(e){return[(0,o.urlToUrlWithoutFlightMarker)(e).toString(),void 0]}async function s(e,t,r,s,f){let d={[u.RSC]:"1",[u.NEXT_ROUTER_STATE_TREE]:encodeURIComponent(JSON.stringify(t))};f===a.PrefetchKind.AUTO&&(d[u.NEXT_ROUTER_PREFETCH]="1"),r&&(d[u.NEXT_URL]=r);let p=(0,i.hexHash)([d[u.NEXT_ROUTER_PREFETCH]||"0",d[u.NEXT_ROUTER_STATE_TREE]].join(","));try{let t=new URL(e);t.pathname.endsWith("/")?t.pathname+="index.txt":t.pathname+=".txt",t.searchParams.set(u.NEXT_RSC_UNION_QUERY,p);let r=await fetch(t,{credentials:"same-origin",headers:d}),a=(0,o.urlToUrlWithoutFlightMarker)(r.url),i=r.redirected?a:void 0,f=r.headers.get("content-type")||"",h=f===u.RSC_CONTENT_TYPE_HEADER;if(h||(h=f.startsWith("text/plain")),!h||!r.ok)return c(a.toString());let[_,y]=await (0,n.createFromFetch)(Promise.resolve(r),{callServer:l.callServer});if(s!==_)return c(r.url);return[y,i]}catch(t){return console.error("Failed to fetch RSC payload. Falling back to browser navigation.",t),[e.toString(),void 0]}}("function"==typeof t.default||"object"==typeof t.default&&null!==t.default)&&void 0===t.default.__esModule&&(Object.defineProperty(t.default,"__esModule",{value:!0}),Object.assign(t.default,t),e.exports=t.default)},2562:function(e,t,r){"use strict";Object.defineProperty(t,"__esModule",{value:!0}),Object.defineProperty(t,"fillCacheWithDataProperty",{enumerable:!0,get:function(){return function e(t,r,o,l,a){void 0===a&&(a=!1);let i=o.length<=2,[c,s]=o,f=(0,u.createRouterCacheKey)(s),d=r.parallelRoutes.get(c);if(!d||a&&r.parallelRoutes.size>1)return{bailOptimistic:!0};let p=t.parallelRoutes.get(c);p&&p!==d||(p=new Map(d),t.parallelRoutes.set(c,p));let h=d.get(f),_=p.get(f);if(i){_&&_.data&&_!==h||p.set(f,{status:n.CacheStates.DATA_FETCH,data:l(),subTreeData:null,parallelRoutes:new Map});return}if(!_||!h){_||p.set(f,{status:n.CacheStates.DATA_FETCH,data:l(),subTreeData:null,parallelRoutes:new Map});return}return _===h&&(_={status:_.status,data:_.data,subTreeData:_.subTreeData,parallelRoutes:new Map(_.parallelRoutes)},p.set(f,_)),e(_,h,o.slice(2),l)}}});let n=r(6656),u=r(4818);("function"==typeof t.default||"object"==typeof t.default&&null!==t.default)&&void 0===t.default.__esModule&&(Object.defineProperty(t.default,"__esModule",{value:!0}),Object.assign(t.default,t),e.exports=t.default)},516:function(e,t,r){"use strict";Object.defineProperty(t,"__esModule",{value:!0}),Object.defineProperty(t,"fillCacheWithNewSubTreeData",{enumerable:!0,get:function(){return function e(t,r,a,i){let c=a.length<=5,[s,f]=a,d=(0,l.createRouterCacheKey)(f),p=r.parallelRoutes.get(s);if(!p)return;let h=t.parallelRoutes.get(s);h&&h!==p||(h=new Map(p),t.parallelRoutes.set(s,h));let _=p.get(d),y=h.get(d);if(c){y&&y.data&&y!==_||(y={status:n.CacheStates.READY,data:null,subTreeData:a[3],parallelRoutes:_?new Map(_.parallelRoutes):new Map},_&&(0,u.invalidateCacheByRouterState)(y,_,a[2]),(0,o.fillLazyItemsTillLeafWithHead)(y,_,a[2],a[4],i),h.set(d,y));return}y&&_&&(y===_&&(y={status:y.status,data:y.data,subTreeData:y.subTreeData,parallelRoutes:new Map(y.parallelRoutes)},h.set(d,y)),e(y,_,a.slice(2),i))}}});let n=r(6656),u=r(9495),o=r(9726),l=r(4818);("function"==typeof t.default||"object"==typeof t.default&&null!==t.default)&&void 0===t.default.__esModule&&(Object.defineProperty(t.default,"__esModule",{value:!0}),Object.assign(t.default,t),e.exports=t.default)},9726:function(e,t,r){"use strict";Object.defineProperty(t,"__esModule",{value:!0}),Object.defineProperty(t,"fillLazyItemsTillLeafWithHead",{enumerable:!0,get:function(){return function e(t,r,o,l,a){let i=0===Object.keys(o[1]).length;if(i){t.head=l;return}for(let i in o[1]){let c=o[1][i],s=c[0],f=(0,u.createRouterCacheKey)(s);if(r){let u=r.parallelRoutes.get(i);if(u){let r=new Map(u),o=r.get(f),s=a&&o?{status:o.status,data:o.data,subTreeData:o.subTreeData,parallelRoutes:new Map(o.parallelRoutes)}:{status:n.CacheStates.LAZY_INITIALIZED,data:null,subTreeData:null,parallelRoutes:new Map(null==o?void 0:o.parallelRoutes)};r.set(f,s),e(s,o,c,l,a),t.parallelRoutes.set(i,r);continue}}let d={status:n.CacheStates.LAZY_INITIALIZED,data:null,subTreeData:null,parallelRoutes:new Map},p=t.parallelRoutes.get(i);p?p.set(f,d):t.parallelRoutes.set(i,new Map([[f,d]])),e(d,void 0,c,l,a)}}}});let n=r(6656),u=r(4818);("function"==typeof t.default||"object"==typeof t.default&&null!==t.default)&&void 0===t.default.__esModule&&(Object.defineProperty(t.default,"__esModule",{value:!0}),Object.assign(t.default,t),e.exports=t.default)},234:function(e,t){"use strict";var r,n;function u(e){let{kind:t,prefetchTime:r,lastUsedTime:n}=e;return Date.now()<(null!=n?n:r)+3e4?n?"reusable":"fresh":"auto"===t&&Date.now()["children",e]).flat(),p=(0,c.fillCacheWithDataProperty)(f,e.cache,d,()=>(t||(t=(0,o.createRecordFromThenable)((0,u.fetchServerResponse)(r,i,e.nextUrl,e.buildId))),t),!0);if(!(null==p?void 0:p.bailOptimistic))return R.previousTree=e.tree,R.patchedTree=i,R.pendingPush=x,R.hashFragment=M,R.shouldScroll=T,R.scrollableSegments=[],R.cache=f,R.canonicalUrl=C,e.prefetchCache.set((0,a.createHrefFromUrl)(r,!1),{data:Promise.resolve(t),kind:h.PrefetchKind.TEMPORARY,prefetchTime:Date.now(),treeAtTimeOfPrefetch:e.tree,lastUsedTime:Date.now()}),(0,_.handleMutable)(e,R)}if(!N){let t=(0,o.createRecordFromThenable)((0,u.fetchServerResponse)(r,e.tree,e.nextUrl,e.buildId,void 0)),n={data:Promise.resolve(t),kind:h.PrefetchKind.TEMPORARY,prefetchTime:Date.now(),treeAtTimeOfPrefetch:e.tree,lastUsedTime:null};e.prefetchCache.set((0,a.createHrefFromUrl)(r,!1),n),N=n}let I=(0,b.getPrefetchEntryCacheStatus)(N),{treeAtTimeOfPrefetch:k,data:D}=N;m.prefetchQueue.bump(D);let[F,U]=(0,l.readRecordValue)(D);if(N.lastUsedTime=Date.now(),"string"==typeof F)return g(e,R,F,x);let L=e.tree,H=e.cache,$=[];for(let t of F){let o=t.slice(0,-4),l=t.slice(-3)[0],a=["",...o],s=(0,f.applyRouterStatePatchToTree)(a,L,l);if(null===s&&(s=(0,f.applyRouterStatePatchToTree)(a,k,l)),null!==s){if((0,p.isNavigatingToNewRootLayout)(L,s))return g(e,R,C,x);let f=(0,y.applyFlightData)(H,j,t,"auto"===N.kind&&I===b.PrefetchCacheEntryStatus.reusable);f||I!==b.PrefetchCacheEntryStatus.stale||(f=function(e,t,r,u,o){let l=!1;e.status=n.CacheStates.READY,e.subTreeData=t.subTreeData,e.parallelRoutes=new Map(t.parallelRoutes);let a=O(u).map(e=>[...r,...e]);for(let r of a){let n=(0,c.fillCacheWithDataProperty)(e,t,r,o);(null==n?void 0:n.bailOptimistic)||(l=!0)}return l}(j,H,o,l,()=>(0,u.fetchServerResponse)(r,L,e.nextUrl,e.buildId)));let h=(0,d.shouldHardNavigate)(a,L);for(let e of(h?(j.status=n.CacheStates.READY,j.subTreeData=H.subTreeData,(0,i.invalidateCacheBelowFlightSegmentPath)(j,H,o),R.cache=j):f&&(R.cache=j),H=j,L=s,O(l))){let t=[...o,...e];"__DEFAULT__"!==t[t.length-1]&&$.push(t)}}}return R.previousTree=e.tree,R.patchedTree=L,R.canonicalUrl=U?(0,a.createHrefFromUrl)(U):C,R.pendingPush=x,R.scrollableSegments=$,R.hashFragment=M,R.shouldScroll=T,(0,_.handleMutable)(e,R)}("function"==typeof t.default||"object"==typeof t.default&&null!==t.default)&&void 0===t.default.__esModule&&(Object.defineProperty(t.default,"__esModule",{value:!0}),Object.assign(t.default,t),e.exports=t.default)},8593:function(e,t,r){"use strict";Object.defineProperty(t,"__esModule",{value:!0}),function(e,t){for(var r in t)Object.defineProperty(e,r,{enumerable:!0,get:t[r]})}(t,{prefetchQueue:function(){return s},prefetchReducer:function(){return f}});let n=r(9330),u=r(2738),o=r(5685),l=r(8982),a=r(3996),i=r(4509),c=r(7843),s=new c.PromiseQueue(5);function f(e,t){(0,a.prunePrefetchCache)(e.prefetchCache);let{url:r}=t;r.searchParams.delete(i.NEXT_RSC_UNION_QUERY);let c=(0,n.createHrefFromUrl)(r,!1),f=e.prefetchCache.get(c);if(f&&(f.kind===o.PrefetchKind.TEMPORARY&&e.prefetchCache.set(c,{...f,kind:t.kind}),!(f.kind===o.PrefetchKind.AUTO&&t.kind===o.PrefetchKind.FULL)))return e;let d=(0,l.createRecordFromThenable)(s.enqueue(()=>(0,u.fetchServerResponse)(r,e.tree,e.nextUrl,e.buildId,t.kind)));return e.prefetchCache.set(c,{treeAtTimeOfPrefetch:e.tree,data:d,kind:t.kind,prefetchTime:Date.now(),lastUsedTime:null}),e}("function"==typeof t.default||"object"==typeof t.default&&null!==t.default)&&void 0===t.default.__esModule&&(Object.defineProperty(t.default,"__esModule",{value:!0}),Object.assign(t.default,t),e.exports=t.default)},3996:function(e,t,r){"use strict";Object.defineProperty(t,"__esModule",{value:!0}),Object.defineProperty(t,"prunePrefetchCache",{enumerable:!0,get:function(){return u}});let n=r(234);function u(e){for(let[t,r]of e)(0,n.getPrefetchEntryCacheStatus)(r)===n.PrefetchCacheEntryStatus.expired&&e.delete(t)}("function"==typeof t.default||"object"==typeof t.default&&null!==t.default)&&void 0===t.default.__esModule&&(Object.defineProperty(t.default,"__esModule",{value:!0}),Object.assign(t.default,t),e.exports=t.default)},7439:function(e,t,r){"use strict";Object.defineProperty(t,"__esModule",{value:!0}),Object.defineProperty(t,"refreshReducer",{enumerable:!0,get:function(){return p}});let n=r(2738),u=r(8982),o=r(6689),l=r(9330),a=r(7491),i=r(3139),c=r(4838),s=r(7575),f=r(6656),d=r(9726);function p(e,t){let{cache:r,mutable:p,origin:h}=t,_=e.canonicalUrl,y=e.tree,b=JSON.stringify(p.previousTree)===JSON.stringify(y);if(b)return(0,s.handleMutable)(e,p);r.data||(r.data=(0,u.createRecordFromThenable)((0,n.fetchServerResponse)(new URL(_,h),[y[0],y[1],y[2],"refetch"],e.nextUrl,e.buildId)));let[v,m]=(0,o.readRecordValue)(r.data);if("string"==typeof v)return(0,c.handleExternalUrl)(e,p,v,e.pushRef.pendingPush);for(let t of(r.data=null,v)){if(3!==t.length)return console.log("REFRESH FAILED"),e;let[n]=t,u=(0,a.applyRouterStatePatchToTree)([""],y,n);if(null===u)throw Error("SEGMENT MISMATCH");if((0,i.isNavigatingToNewRootLayout)(y,u))return(0,c.handleExternalUrl)(e,p,_,e.pushRef.pendingPush);let o=m?(0,l.createHrefFromUrl)(m):void 0;m&&(p.canonicalUrl=o);let[s,h]=t.slice(-2);null!==s&&(r.status=f.CacheStates.READY,r.subTreeData=s,(0,d.fillLazyItemsTillLeafWithHead)(r,void 0,n,h),p.cache=r,p.prefetchCache=new Map),p.previousTree=y,p.patchedTree=u,p.canonicalUrl=_,y=u}return(0,s.handleMutable)(e,p)}("function"==typeof t.default||"object"==typeof t.default&&null!==t.default)&&void 0===t.default.__esModule&&(Object.defineProperty(t.default,"__esModule",{value:!0}),Object.assign(t.default,t),e.exports=t.default)},9958:function(e,t,r){"use strict";Object.defineProperty(t,"__esModule",{value:!0}),Object.defineProperty(t,"restoreReducer",{enumerable:!0,get:function(){return u}});let n=r(9330);function u(e,t){let{url:r,tree:u}=t,o=(0,n.createHrefFromUrl)(r);return{buildId:e.buildId,canonicalUrl:o,pushRef:e.pushRef,focusAndScrollRef:e.focusAndScrollRef,cache:e.cache,prefetchCache:e.prefetchCache,tree:u,nextUrl:r.pathname}}("function"==typeof t.default||"object"==typeof t.default&&null!==t.default)&&void 0===t.default.__esModule&&(Object.defineProperty(t.default,"__esModule",{value:!0}),Object.assign(t.default,t),e.exports=t.default)},7148:function(e,t,r){"use strict";Object.defineProperty(t,"__esModule",{value:!0}),Object.defineProperty(t,"serverActionReducer",{enumerable:!0,get:function(){return p}});let n=r(4039),u=r(4509),o=r(8982),l=r(6689),a=r(6671),i=r(5685),c=r(6711),s=r(9330),f=r(5800);async function d(e,t){let r,{actionId:o,actionArgs:l}=t,i=await (0,a.encodeReply)(l),s=await fetch("",{method:"POST",headers:{Accept:u.RSC_CONTENT_TYPE_HEADER,"Next-Action":o,[u.NEXT_ROUTER_STATE_TREE]:JSON.stringify(e.tree),...e.nextUrl?{[u.NEXT_URL]:e.nextUrl}:{}},body:i}),f=s.headers.get("x-action-redirect");try{let e=JSON.parse(s.headers.get("x-action-revalidated")||"[[],0,0]");r={paths:e[0]||[],tag:!!e[1],cookie:e[2]}}catch(e){r={paths:[],tag:!1,cookie:!1}}let d=f?new URL((0,c.addBasePath)(f),window.location.origin):void 0;if(s.headers.get("content-type")===u.RSC_CONTENT_TYPE_HEADER){let e=await (0,a.createFromFetch)(Promise.resolve(s),{callServer:n.callServer});if(f){let[,t]=e;return{actionFlightData:null==t?void 0:t[1],redirectLocation:d,revalidatedParts:r}}{let[t,[,n]]=null!=e?e:[];return{actionResult:t,actionFlightData:n,redirectLocation:d,revalidatedParts:r}}}return{redirectLocation:d,revalidatedParts:r}}function p(e,t){if(t.mutable.serverActionApplied)return e;t.mutable.inFlightServerAction||(t.mutable.previousTree=e.tree,t.mutable.previousUrl=e.canonicalUrl,t.mutable.inFlightServerAction=(0,o.createRecordFromThenable)(d(e,t)));try{var r,n;let{actionResult:u,actionFlightData:a,redirectLocation:c,revalidatedParts:d}=(0,l.readRecordValue)(t.mutable.inFlightServerAction);if(d.tag||d.cookie?e.prefetchCache.clear():d.paths.length>0&&e.prefetchCache.clear(),c){if(a){let n=(0,s.createHrefFromUrl)(c,!1),u=e.prefetchCache.get(n);e.prefetchCache.set(n,{data:(0,o.createRecordFromThenable)(Promise.resolve([a,void 0])),kind:null!=(r=null==u?void 0:u.kind)?r:i.PrefetchKind.TEMPORARY,prefetchTime:Date.now(),treeAtTimeOfPrefetch:t.mutable.previousTree,lastUsedTime:null})}t.reject((0,f.getRedirectError)(c.toString(),f.RedirectType.push))}else{if(a){let r=(0,s.createHrefFromUrl)(new URL(t.mutable.previousUrl,window.location.origin),!1),u=e.prefetchCache.get(r);e.prefetchCache.set((0,s.createHrefFromUrl)(new URL(t.mutable.previousUrl,window.location.origin),!1),{data:(0,o.createRecordFromThenable)(Promise.resolve([a,void 0])),kind:null!=(n=null==u?void 0:u.kind)?n:i.PrefetchKind.TEMPORARY,prefetchTime:Date.now(),treeAtTimeOfPrefetch:t.mutable.previousTree,lastUsedTime:null}),setTimeout(()=>{t.changeByServerResponse(t.mutable.previousTree,a,void 0)})}t.resolve(u)}}catch(e){if("rejected"===e.status)t.reject(e.value);else throw e}return t.mutable.serverActionApplied=!0,e}("function"==typeof t.default||"object"==typeof t.default&&null!==t.default)&&void 0===t.default.__esModule&&(Object.defineProperty(t.default,"__esModule",{value:!0}),Object.assign(t.default,t),e.exports=t.default)},7811:function(e,t,r){"use strict";Object.defineProperty(t,"__esModule",{value:!0}),Object.defineProperty(t,"serverPatchReducer",{enumerable:!0,get:function(){return c}});let n=r(9330),u=r(7491),o=r(3139),l=r(4838),a=r(7027),i=r(7575);function c(e,t){let{flightData:r,previousTree:c,overrideCanonicalUrl:s,cache:f,mutable:d}=t,p=JSON.stringify(c)===JSON.stringify(e.tree);if(!p)return console.log("TREE MISMATCH"),e;if(d.previousTree)return(0,i.handleMutable)(e,d);if("string"==typeof r)return(0,l.handleExternalUrl)(e,d,r,e.pushRef.pendingPush);let h=e.tree,_=e.cache;for(let t of r){let r=t.slice(0,-4),[i]=t.slice(-3,-2),c=(0,u.applyRouterStatePatchToTree)(["",...r],h,i);if(null===c)throw Error("SEGMENT MISMATCH");if((0,o.isNavigatingToNewRootLayout)(h,c))return(0,l.handleExternalUrl)(e,d,e.canonicalUrl,e.pushRef.pendingPush);let p=s?(0,n.createHrefFromUrl)(s):void 0;p&&(d.canonicalUrl=p),(0,a.applyFlightData)(_,f,t),d.previousTree=h,d.patchedTree=c,d.cache=f,_=f,h=c}return(0,i.handleMutable)(e,d)}("function"==typeof t.default||"object"==typeof t.default&&null!==t.default)&&void 0===t.default.__esModule&&(Object.defineProperty(t.default,"__esModule",{value:!0}),Object.assign(t.default,t),e.exports=t.default)},5685:function(e,t){"use strict";var r,n;Object.defineProperty(t,"__esModule",{value:!0}),function(e,t){for(var r in t)Object.defineProperty(e,r,{enumerable:!0,get:t[r]})}(t,{PrefetchKind:function(){return r},ACTION_REFRESH:function(){return u},ACTION_NAVIGATE:function(){return o},ACTION_RESTORE:function(){return l},ACTION_SERVER_PATCH:function(){return a},ACTION_PREFETCH:function(){return i},ACTION_FAST_REFRESH:function(){return c},ACTION_SERVER_ACTION:function(){return s}});let u="refresh",o="navigate",l="restore",a="server-patch",i="prefetch",c="fast-refresh",s="server-action";(n=r||(r={})).AUTO="auto",n.FULL="full",n.TEMPORARY="temporary",("function"==typeof t.default||"object"==typeof t.default&&null!==t.default)&&void 0===t.default.__esModule&&(Object.defineProperty(t.default,"__esModule",{value:!0}),Object.assign(t.default,t),e.exports=t.default)},7538:function(e,t,r){"use strict";Object.defineProperty(t,"__esModule",{value:!0}),Object.defineProperty(t,"reducer",{enumerable:!0,get:function(){return f}});let n=r(5685),u=r(4838),o=r(7811),l=r(9958),a=r(7439),i=r(8593),c=r(4995),s=r(7148),f=function(e,t){switch(t.type){case n.ACTION_NAVIGATE:return(0,u.navigateReducer)(e,t);case n.ACTION_SERVER_PATCH:return(0,o.serverPatchReducer)(e,t);case n.ACTION_RESTORE:return(0,l.restoreReducer)(e,t);case n.ACTION_REFRESH:return(0,a.refreshReducer)(e,t);case n.ACTION_FAST_REFRESH:return(0,c.fastRefreshReducer)(e,t);case n.ACTION_PREFETCH:return(0,i.prefetchReducer)(e,t);case n.ACTION_SERVER_ACTION:return(0,s.serverActionReducer)(e,t);default:throw Error("Unknown action")}};("function"==typeof t.default||"object"==typeof t.default&&null!==t.default)&&void 0===t.default.__esModule&&(Object.defineProperty(t.default,"__esModule",{value:!0}),Object.assign(t.default,t),e.exports=t.default)},8741:function(e,t,r){"use strict";Object.defineProperty(t,"__esModule",{value:!0}),Object.defineProperty(t,"shouldHardNavigate",{enumerable:!0,get:function(){return function e(t,r){let[u,o]=r,[l,a]=t;if(!(0,n.matchSegment)(l,u))return!!Array.isArray(l);let i=t.length<=2;return!i&&e(t.slice(2),o[a])}}});let n=r(7910);("function"==typeof t.default||"object"==typeof t.default&&null!==t.default)&&void 0===t.default.__esModule&&(Object.defineProperty(t.default,"__esModule",{value:!0}),Object.assign(t.default,t),e.exports=t.default)},2476:function(e,t,r){"use strict";Object.defineProperty(t,"__esModule",{value:!0}),Object.defineProperty(t,"createSearchParamsBailoutProxy",{enumerable:!0,get:function(){return u}});let n=r(5698);function u(){return new Proxy({},{get(e,t){"string"==typeof t&&(0,n.staticGenerationBailout)("searchParams."+t)}})}("function"==typeof t.default||"object"==typeof t.default&&null!==t.default)&&void 0===t.default.__esModule&&(Object.defineProperty(t.default,"__esModule",{value:!0}),Object.assign(t.default,t),e.exports=t.default)},5698:function(e,t,r){"use strict";Object.defineProperty(t,"__esModule",{value:!0}),Object.defineProperty(t,"staticGenerationBailout",{enumerable:!0,get:function(){return l}});let n=r(4124),u=r(2287);class o extends Error{constructor(...e){super(...e),this.code="NEXT_STATIC_GEN_BAILOUT"}}let l=(e,t)=>{let r=u.staticGenerationAsyncStorage.getStore();if(null==r?void 0:r.forceStatic)return!0;if(null==r?void 0:r.dynamicShouldError){let{dynamic:r="error",link:n}=t||{};throw new o('Page with `dynamic = "'+r+"\"` couldn't be rendered statically because it used `"+e+"`."+(n?" See more info here: "+n:""))}if(r&&(r.revalidate=0),null==r?void 0:r.isStaticGeneration){let t=new n.DynamicServerError(e);throw r.dynamicUsageDescription=e,r.dynamicUsageStack=t.stack,t}return!1};("function"==typeof t.default||"object"==typeof t.default&&null!==t.default)&&void 0===t.default.__esModule&&(Object.defineProperty(t.default,"__esModule",{value:!0}),Object.assign(t.default,t),e.exports=t.default)},4839:function(e,t,r){"use strict";Object.defineProperty(t,"__esModule",{value:!0}),Object.defineProperty(t,"default",{enumerable:!0,get:function(){return l}});let n=r(1024),u=n._(r(2265)),o=r(2476);function l(e){let{Component:t,propsForComponent:r}=e,n=(0,o.createSearchParamsBailoutProxy)();return u.default.createElement(t,{searchParams:n,...r})}("function"==typeof t.default||"object"==typeof t.default&&null!==t.default)&&void 0===t.default.__esModule&&(Object.defineProperty(t.default,"__esModule",{value:!0}),Object.assign(t.default,t),e.exports=t.default)},9865:function(e,t,r){"use strict";Object.defineProperty(t,"__esModule",{value:!0}),Object.defineProperty(t,"useReducerWithReduxDevtools",{enumerable:!0,get:function(){return o}});let n=r(2265);function u(e){if(e instanceof Map){let t={};for(let[r,n]of e.entries()){if("function"==typeof n){t[r]="fn()";continue}if("object"==typeof n&&null!==n){if(n.$$typeof){t[r]=n.$$typeof.toString();continue}if(n._bundlerConfig){t[r]="FlightData";continue}}t[r]=u(n)}return t}if("object"==typeof e&&null!==e){let t={};for(let r in e){let n=e[r];if("function"==typeof n){t[r]="fn()";continue}if("object"==typeof n&&null!==n){if(n.$$typeof){t[r]=n.$$typeof.toString();continue}if(n.hasOwnProperty("_bundlerConfig")){t[r]="FlightData";continue}}t[r]=u(n)}return t}return Array.isArray(e)?e.map(u):e}let o=function(e,t){let r=(0,n.useRef)(),o=(0,n.useRef)();(0,n.useEffect)(()=>{if(!r.current&&!1!==o.current){if(void 0===o.current&&void 0===window.__REDUX_DEVTOOLS_EXTENSION__){o.current=!1;return}return r.current=window.__REDUX_DEVTOOLS_EXTENSION__.connect({instanceId:8e3,name:"next-router"}),r.current&&r.current.init(u(t)),()=>{r.current=void 0}}},[t]);let[l,a]=(0,n.useReducer)((t,n)=>{let o=e(t,n);return r.current&&r.current.send(n,u(o)),o},t),i=(0,n.useCallback)(()=>{r.current&&r.current.send({type:"RENDER_SYNC"},u(l))},[l]);return[l,a,i]};("function"==typeof t.default||"object"==typeof t.default&&null!==t.default)&&void 0===t.default.__esModule&&(Object.defineProperty(t.default,"__esModule",{value:!0}),Object.assign(t.default,t),e.exports=t.default)},6070:function(e,t,r){"use strict";Object.defineProperty(t,"__esModule",{value:!0}),Object.defineProperty(t,"normalizePathTrailingSlash",{enumerable:!0,get:function(){return o}});let n=r(7369),u=r(2590),o=e=>{if(!e.startsWith("/"))return e;let{pathname:t,query:r,hash:o}=(0,u.parsePath)(e);return""+(0,n.removeTrailingSlash)(t)+r+o};("function"==typeof t.default||"object"==typeof t.default&&null!==t.default)&&void 0===t.default.__esModule&&(Object.defineProperty(t.default,"__esModule",{value:!0}),Object.assign(t.default,t),e.exports=t.default)},5152:function(e,t,r){"use strict";Object.defineProperty(t,"__esModule",{value:!0}),Object.defineProperty(t,"default",{enumerable:!0,get:function(){return u}});let n=r(7669);function u(e){let t="function"==typeof reportError?reportError:e=>{window.console.error(e)};e.digest!==n.NEXT_DYNAMIC_NO_SSR_CODE&&t(e)}("function"==typeof t.default||"object"==typeof t.default&&null!==t.default)&&void 0===t.default.__esModule&&(Object.defineProperty(t.default,"__esModule",{value:!0}),Object.assign(t.default,t),e.exports=t.default)},6656:function(e,t,r){"use strict";var n,u;Object.defineProperty(t,"__esModule",{value:!0}),function(e,t){for(var r in t)Object.defineProperty(e,r,{enumerable:!0,get:t[r]})}(t,{CacheStates:function(){return n},AppRouterContext:function(){return a},LayoutRouterContext:function(){return i},GlobalLayoutRouterContext:function(){return c},TemplateContext:function(){return s}});let o=r(1024),l=o._(r(2265));(u=n||(n={})).LAZY_INITIALIZED="LAZYINITIALIZED",u.DATA_FETCH="DATAFETCH",u.READY="READY";let a=l.default.createContext(null),i=l.default.createContext(null),c=l.default.createContext(null),s=l.default.createContext(null)},216:function(e,t){"use strict";function r(e){let t=5381;for(let r=0;r!t||"("===t[0]&&t.endsWith(")")||"@"===t[0]||("page"===t||"route"===t)&&r===n.length-1?e:e+"/"+t,""))}function o(e,t){return t?e.replace(/\.rsc($|\?)/,"$1"):e}},1067:function(e,t){"use strict";function r(e,t){if(void 0===t&&(t={}),t.onlyHashChange){e();return}let r=document.documentElement,n=r.style.scrollBehavior;r.style.scrollBehavior="auto",t.dontForceLayout||r.getClientRects(),e(),r.style.scrollBehavior=n}Object.defineProperty(t,"__esModule",{value:!0}),Object.defineProperty(t,"handleSmoothScroll",{enumerable:!0,get:function(){return r}})},3738:function(e,t){"use strict";function r(e){return/Googlebot|Mediapartners-Google|AdsBot-Google|googleweblight|Storebot-Google|Google-PageRenderer|Bingbot|BingPreview|Slurp|DuckDuckBot|baiduspider|yandex|sogou|LinkedInBot|bitlybot|tumblr|vkShare|quora link preview|facebookexternalhit|facebookcatalog|Twitterbot|applebot|redditbot|Slackbot|Discordbot|WhatsApp|SkypeUriPreview|ia_archiver/i.test(e)}Object.defineProperty(t,"__esModule",{value:!0}),Object.defineProperty(t,"isBot",{enumerable:!0,get:function(){return r}})},2590:function(e,t){"use strict";function r(e){let t=e.indexOf("#"),r=e.indexOf("?"),n=r>-1&&(t<0||r-1?{pathname:e.substring(0,n?r:t),query:n?e.substring(r,t>-1?t:void 0):"",hash:t>-1?e.slice(t):""}:{pathname:e,query:"",hash:""}}Object.defineProperty(t,"__esModule",{value:!0}),Object.defineProperty(t,"parsePath",{enumerable:!0,get:function(){return r}})},7369:function(e,t){"use strict";function r(e){return e.replace(/\/$/,"")||"/"}Object.defineProperty(t,"__esModule",{value:!0}),Object.defineProperty(t,"removeTrailingSlash",{enumerable:!0,get:function(){return r}})},8169:function(e,t,r){"use strict";Object.defineProperty(t,"__esModule",{value:!0}),function(e,t){for(var r in t)Object.defineProperty(e,r,{enumerable:!0,get:t[r]})}(t,{ServerInsertedHTMLContext:function(){return o},useServerInsertedHTML:function(){return l}});let n=r(8533),u=n._(r(2265)),o=u.default.createContext(null);function l(e){let t=(0,u.useContext)(o);t&&t(e)}},2616:function(e,t){"use strict";Object.defineProperty(t,"__esModule",{value:!0}),Object.defineProperty(t,"createAsyncLocalStorage",{enumerable:!0,get:function(){return o}});let r=Error("Invariant: AsyncLocalStorage accessed in runtime where it is not available");class n{disable(){throw r}getStore(){}run(){throw r}exit(){throw r}enterWith(){throw r}}let u=globalThis.AsyncLocalStorage;function o(){return u?new u:new n}("function"==typeof t.default||"object"==typeof t.default&&null!==t.default)&&void 0===t.default.__esModule&&(Object.defineProperty(t.default,"__esModule",{value:!0}),Object.assign(t.default,t),e.exports=t.default)},6170:function(e,t,r){"use strict";Object.defineProperty(t,"__esModule",{value:!0}),Object.defineProperty(t,"requestAsyncStorage",{enumerable:!0,get:function(){return u}});let n=r(2616),u=(0,n.createAsyncLocalStorage)();("function"==typeof t.default||"object"==typeof t.default&&null!==t.default)&&void 0===t.default.__esModule&&(Object.defineProperty(t.default,"__esModule",{value:!0}),Object.assign(t.default,t),e.exports=t.default)},2287:function(e,t,r){"use strict";Object.defineProperty(t,"__esModule",{value:!0}),Object.defineProperty(t,"staticGenerationAsyncStorage",{enumerable:!0,get:function(){return u}});let n=r(2616),u=(0,n.createAsyncLocalStorage)();("function"==typeof t.default||"object"==typeof t.default&&null!==t.default)&&void 0===t.default.__esModule&&(Object.defineProperty(t.default,"__esModule",{value:!0}),Object.assign(t.default,t),e.exports=t.default)},4040:function(e,t,r){"use strict";var n=r(4887);t.createRoot=n.createRoot,t.hydrateRoot=n.hydrateRoot},4887:function(e,t,r){"use strict";!function e(){if("undefined"!=typeof __REACT_DEVTOOLS_GLOBAL_HOOK__&&"function"==typeof __REACT_DEVTOOLS_GLOBAL_HOOK__.checkDCE)try{__REACT_DEVTOOLS_GLOBAL_HOOK__.checkDCE(e)}catch(e){console.error(e)}}(),e.exports=r(4417)},7950:function(e,t,r){"use strict";/**
- * @license React
- * react-server-dom-webpack-client.browser.production.min.js
- *
- * Copyright (c) Meta Platforms, Inc. and affiliates.
- *
- * This source code is licensed under the MIT license found in the
- * LICENSE file in the root directory of this source tree.
- */var n=r(4887),u=r(2265),o={stream:!0},l=new Map;function a(e){var t=globalThis.__next_require__(e);return"function"!=typeof t.then||"fulfilled"===t.status?null:(t.then(function(e){t.status="fulfilled",t.value=e},function(e){t.status="rejected",t.reason=e}),t)}function i(){}var c=n.__SECRET_INTERNALS_DO_NOT_USE_OR_YOU_WILL_BE_FIRED.Dispatcher,s=Symbol.for("react.element"),f=Symbol.for("react.lazy"),d=Symbol.for("react.default_value"),p=Symbol.iterator,h=Array.isArray,_=new WeakMap,y=u.__SECRET_INTERNALS_DO_NOT_USE_OR_YOU_WILL_BE_FIRED.ContextRegistry;function b(e,t,r,n){this.status=e,this.value=t,this.reason=r,this._response=n}function v(e){switch(e.status){case"resolved_model":R(e);break;case"resolved_module":S(e)}switch(e.status){case"fulfilled":return e.value;case"pending":case"blocked":throw e;default:throw e.reason}}function m(e,t){for(var r=0;rd?(h=d,d=3,f++):(h=0,d=3);continue;case 2:44===(v=s[f++])?d=4:_=_<<4|(96s.length&&(v=-1)}var m=s.byteOffset+f;if(-1>>1,u=e[n];if(0>>1;no(i,r))co(s,i)?(e[n]=s,e[c]=r,n=c):(e[n]=i,e[a]=r,n=a);else if(co(s,r))e[n]=s,e[c]=r,n=c;else break}}return t}function o(e,t){var r=e.sortIndex-t.sortIndex;return 0!==r?r:e.id-t.id}if(t.unstable_now=void 0,"object"==typeof performance&&"function"==typeof performance.now){var l,a=performance;t.unstable_now=function(){return a.now()}}else{var i=Date,c=i.now();t.unstable_now=function(){return i.now()-c}}var s=[],f=[],d=1,p=null,h=3,_=!1,y=!1,b=!1,v="function"==typeof setTimeout?setTimeout:null,m="function"==typeof clearTimeout?clearTimeout:null,g="undefined"!=typeof setImmediate?setImmediate:null;function O(e){for(var t=n(f);null!==t;){if(null===t.callback)u(f);else if(t.startTime<=e)u(f),t.sortIndex=t.expirationTime,r(s,t);else break;t=n(f)}}function P(e){if(b=!1,O(e),!y){if(null!==n(s))y=!0,N(E);else{var t=n(f);null!==t&&I(P,t.startTime-e)}}}function E(e,r){y=!1,b&&(b=!1,m(S),S=-1),_=!0;var o=h;try{e:{for(O(r),p=n(s);null!==p&&(!(p.expirationTime>r)||e&&!M());){var l=p.callback;if("function"==typeof l){p.callback=null,h=p.priorityLevel;var a=l(p.expirationTime<=r);if(r=t.unstable_now(),"function"==typeof a){p.callback=a,O(r);var i=!0;break e}p===n(s)&&u(s),O(r)}else u(s);p=n(s)}if(null!==p)i=!0;else{var c=n(f);null!==c&&I(P,c.startTime-r),i=!1}}return i}finally{p=null,h=o,_=!1}}"undefined"!=typeof navigator&&void 0!==navigator.scheduling&&void 0!==navigator.scheduling.isInputPending&&navigator.scheduling.isInputPending.bind(navigator.scheduling);var j=!1,R=null,S=-1,T=5,w=-1;function M(){return!(t.unstable_now()-we||125l?(e.sortIndex=o,r(f,e),null===n(s)&&e===n(f)&&(b?(m(S),S=-1):b=!0,I(P,o-l))):(e.sortIndex=a,r(s,e),y||_||(y=!0,N(E))),e},t.unstable_shouldYield=M,t.unstable_wrapCallback=function(e){var t=h;return function(){var r=h;h=t;try{return e.apply(this,arguments)}finally{h=r}}}},8261:function(e,t,r){"use strict";e.exports=r(1756)},5682:function(e,t,r){"use strict";Object.defineProperty(t,"__esModule",{value:!0}),Object.defineProperty(t,"getSegmentParam",{enumerable:!0,get:function(){return u}});let n=r(4507);function u(e){let t=n.INTERCEPTION_ROUTE_MARKERS.find(t=>e.startsWith(t));return(t&&(e=e.slice(t.length)),e.startsWith("[[...")&&e.endsWith("]]"))?{type:"optional-catchall",param:e.slice(5,-2)}:e.startsWith("[...")&&e.endsWith("]")?{type:"catchall",param:e.slice(4,-1)}:e.startsWith("[")&&e.endsWith("]")?{type:"dynamic",param:e.slice(1,-1)}:null}},4507:function(e,t,r){"use strict";Object.defineProperty(t,"__esModule",{value:!0}),function(e,t){for(var r in t)Object.defineProperty(e,r,{enumerable:!0,get:t[r]})}(t,{INTERCEPTION_ROUTE_MARKERS:function(){return u},isInterceptionRouteAppPath:function(){return o},extractInterceptionRouteInformation:function(){return l}});let n=r(8896),u=["(..)(..)","(.)","(..)","(...)"];function o(e){return void 0!==e.split("/").find(e=>u.find(t=>e.startsWith(t)))}function l(e){let t,r,o;for(let n of e.split("/"))if(r=u.find(e=>n.startsWith(e))){[t,o]=e.split(r,2);break}if(!t||!r||!o)throw Error(`Invalid interception route: ${e}. Must be in the format //(..|...|..)(..)/`);switch(t=(0,n.normalizeAppPath)(t),r){case"(.)":o="/"===t?`/${o}`:t+"/"+o;break;case"(..)":if("/"===t)throw Error(`Invalid interception route: ${e}. Cannot use (..) marker at the root level, use (.) instead.`);o=t.split("/").slice(0,-1).concat(o).join("/");break;case"(...)":o="/"+o;break;case"(..)(..)":let l=t.split("/");if(l.length<=2)throw Error(`Invalid interception route: ${e}. Cannot use (..)(..) marker at the root level or one level up.`);o=l.slice(0,-2).concat(o).join("/");break;default:throw Error("Invariant: unexpected marker")}return{interceptingRoute:t,interceptedRoute:o}}},4677:function(e,t,r){"use strict";function n(e,t){if(!Object.prototype.hasOwnProperty.call(e,t))throw TypeError("attempted to use private field on non-instance");return e}r.r(t),r.d(t,{_:function(){return n},_class_private_field_loose_base:function(){return n}})},6249:function(e,t,r){"use strict";r.r(t),r.d(t,{_:function(){return u},_class_private_field_loose_key:function(){return u}});var n=0;function u(e){return"__private_"+n+++"_"+e}},1024:function(e,t,r){"use strict";function n(e){return e&&e.__esModule?e:{default:e}}r.r(t),r.d(t,{_:function(){return n},_interop_require_default:function(){return n}})},8533:function(e,t,r){"use strict";function n(e){if("function"!=typeof WeakMap)return null;var t=new WeakMap,r=new WeakMap;return(n=function(e){return e?r:t})(e)}function u(e,t){if(!t&&e&&e.__esModule)return e;if(null===e||"object"!=typeof e&&"function"!=typeof e)return{default:e};var r=n(t);if(r&&r.has(e))return r.get(e);var u={},o=Object.defineProperty&&Object.getOwnPropertyDescriptor;for(var l in e)if("default"!==l&&Object.prototype.hasOwnProperty.call(e,l)){var a=o?Object.getOwnPropertyDescriptor(e,l):null;a&&(a.get||a.set)?Object.defineProperty(u,l,a):u[l]=e[l]}return u.default=e,r&&r.set(e,u),u}r.r(t),r.d(t,{_:function(){return u},_interop_require_wildcard:function(){return u}})}}]);
\ No newline at end of file
diff --git a/spaces/Xhaheen/Lexica_prompt_search/lexica.py b/spaces/Xhaheen/Lexica_prompt_search/lexica.py
deleted file mode 100644
index 6317fed79b91ad9296e1fcb044f9ba69db361bf8..0000000000000000000000000000000000000000
--- a/spaces/Xhaheen/Lexica_prompt_search/lexica.py
+++ /dev/null
@@ -1,90 +0,0 @@
-import requests
-import shutil
-from PIL import Image
-from io import BytesIO
-import numpy as np
-import matplotlib.pyplot as plt
-import pandas as pd
-import random
-import gradio as gr
-
-design='india'
-def lexica(design,n):
-
- request=requests.get(f'https://lexica.art/api/v1/search?q={design}')
- request.json()
- data = request.json()
- data_items = list(data.items())
-
- random.shuffle(data_items)
-
- data = dict(data_items)
-
- image_urls = []
- image_prompts = []
- image_gallery=[]
-
- for key, value in data.items():
- for i in range(n):
- image_url = value[i]['src']
- if isinstance(image_url, list):
- image_url = image_url[0]
- image_urls.append(image_url)
-
-
- image_prompts.append(value[i]['prompt'])
- image_gallery.append(value[i]['gallery'])
-
- images = []
-
- # Loop through the image URLs
- for url in image_urls:
- # Download the image from the URL
- response = requests.get(url)
-
- # Load the image data into PIL format
- image = Image.open(BytesIO(response.content))
-
- # Add the image to the list
- images.append(image)
-
-
-# df = pd.DataFrame(image_prompts, columns=["Lexica Prompt"], index=range(1, len(image_prompts)+1))
-
-
-# df.index.name = "Sr. No."
- df = pd.DataFrame({ 'image_gallery': image_gallery,'image_prompts': image_prompts})
- def make_clickable(val):
- return '{}'.format(val, val)
-
- # df.style.format({'image_gallery': make_clickable})
- df.style.format({'image_prompts': make_clickable}).set_properties(subset=['image_prompts'], width=30)
-
- for image in images:
-
- array = np.array(image)
-
-
- return images , df
-design='india'
-# lexica(design)
-
-inputs =[ gr.Textbox(label = 'Enter prompt to search Lexica.art'),
- gr.Slider(label='Number of images ', minimum = 4, maximum = 20, step = 1, value = 4)]
-
-
-outputs= [gr.Gallery(lable='Output gallery').style(grid=4,height=100,container=True),
- gr.Dataframe(label='links and prompts for corresponding images')]
-
-# Create and launch the interface
-interface = gr.Interface(lexica,
- inputs=inputs,
- outputs=outputs,
- examples =[ ['trending digital art', 5],
- ['beautiful home', 5],
- ['interior design of living room', 5]]
- ,
- title = "" +' 🔍 🖌️🎨 Lexica Art - A Search Engine for Generative Art Prompts and Works '+ "",
- description="🔍🖌️ 🎨 lexica huggingface space , Find inspiration and discover new generative artworks with Lexica Art, a search engine built by by @[Sharif shameem](https://twitter.com/sharifshameem) . Explore a vast collection of prompts and corresponding artworks, and let your imagination take over as you create your own masterpieces. \n\n Visit @[baith_al_suroor](https://huggingface.co/spaces/Xhaheen/Baith-al-suroor) to redesign your home interiors for FREE \n\n💡🖌️ spaces built with ❤️ @[Xhaheen](https://www.linkedin.com/in/sallu-mandya)")
-
-interface.launch(debug=True)
\ No newline at end of file
diff --git a/spaces/XzJosh/Lumi-Bert-VITS2/attentions.py b/spaces/XzJosh/Lumi-Bert-VITS2/attentions.py
deleted file mode 100644
index 1192dd7268c20c11010e73a6017ed09549695afe..0000000000000000000000000000000000000000
--- a/spaces/XzJosh/Lumi-Bert-VITS2/attentions.py
+++ /dev/null
@@ -1,344 +0,0 @@
-import copy
-import math
-import torch
-from torch import nn
-from torch.nn import functional as F
-
-import commons
-import logging
-
-logger = logging.getLogger(__name__)
-
-class LayerNorm(nn.Module):
- def __init__(self, channels, eps=1e-5):
- super().__init__()
- self.channels = channels
- self.eps = eps
-
- self.gamma = nn.Parameter(torch.ones(channels))
- self.beta = nn.Parameter(torch.zeros(channels))
-
- def forward(self, x):
- x = x.transpose(1, -1)
- x = F.layer_norm(x, (self.channels,), self.gamma, self.beta, self.eps)
- return x.transpose(1, -1)
-
-
-
-@torch.jit.script
-def fused_add_tanh_sigmoid_multiply(input_a, input_b, n_channels):
- n_channels_int = n_channels[0]
- in_act = input_a + input_b
- t_act = torch.tanh(in_act[:, :n_channels_int, :])
- s_act = torch.sigmoid(in_act[:, n_channels_int:, :])
- acts = t_act * s_act
- return acts
-
-class Encoder(nn.Module):
- def __init__(self, hidden_channels, filter_channels, n_heads, n_layers, kernel_size=1, p_dropout=0., window_size=4, isflow = True, **kwargs):
- super().__init__()
- self.hidden_channels = hidden_channels
- self.filter_channels = filter_channels
- self.n_heads = n_heads
- self.n_layers = n_layers
- self.kernel_size = kernel_size
- self.p_dropout = p_dropout
- self.window_size = window_size
- #if isflow:
- # cond_layer = torch.nn.Conv1d(256, 2*hidden_channels*n_layers, 1)
- # self.cond_pre = torch.nn.Conv1d(hidden_channels, 2*hidden_channels, 1)
- # self.cond_layer = weight_norm(cond_layer, name='weight')
- # self.gin_channels = 256
- self.cond_layer_idx = self.n_layers
- if 'gin_channels' in kwargs:
- self.gin_channels = kwargs['gin_channels']
- if self.gin_channels != 0:
- self.spk_emb_linear = nn.Linear(self.gin_channels, self.hidden_channels)
- # vits2 says 3rd block, so idx is 2 by default
- self.cond_layer_idx = kwargs['cond_layer_idx'] if 'cond_layer_idx' in kwargs else 2
- logging.debug(self.gin_channels, self.cond_layer_idx)
- assert self.cond_layer_idx < self.n_layers, 'cond_layer_idx should be less than n_layers'
- self.drop = nn.Dropout(p_dropout)
- self.attn_layers = nn.ModuleList()
- self.norm_layers_1 = nn.ModuleList()
- self.ffn_layers = nn.ModuleList()
- self.norm_layers_2 = nn.ModuleList()
- for i in range(self.n_layers):
- self.attn_layers.append(MultiHeadAttention(hidden_channels, hidden_channels, n_heads, p_dropout=p_dropout, window_size=window_size))
- self.norm_layers_1.append(LayerNorm(hidden_channels))
- self.ffn_layers.append(FFN(hidden_channels, hidden_channels, filter_channels, kernel_size, p_dropout=p_dropout))
- self.norm_layers_2.append(LayerNorm(hidden_channels))
- def forward(self, x, x_mask, g=None):
- attn_mask = x_mask.unsqueeze(2) * x_mask.unsqueeze(-1)
- x = x * x_mask
- for i in range(self.n_layers):
- if i == self.cond_layer_idx and g is not None:
- g = self.spk_emb_linear(g.transpose(1, 2))
- g = g.transpose(1, 2)
- x = x + g
- x = x * x_mask
- y = self.attn_layers[i](x, x, attn_mask)
- y = self.drop(y)
- x = self.norm_layers_1[i](x + y)
-
- y = self.ffn_layers[i](x, x_mask)
- y = self.drop(y)
- x = self.norm_layers_2[i](x + y)
- x = x * x_mask
- return x
-
-
-class Decoder(nn.Module):
- def __init__(self, hidden_channels, filter_channels, n_heads, n_layers, kernel_size=1, p_dropout=0., proximal_bias=False, proximal_init=True, **kwargs):
- super().__init__()
- self.hidden_channels = hidden_channels
- self.filter_channels = filter_channels
- self.n_heads = n_heads
- self.n_layers = n_layers
- self.kernel_size = kernel_size
- self.p_dropout = p_dropout
- self.proximal_bias = proximal_bias
- self.proximal_init = proximal_init
-
- self.drop = nn.Dropout(p_dropout)
- self.self_attn_layers = nn.ModuleList()
- self.norm_layers_0 = nn.ModuleList()
- self.encdec_attn_layers = nn.ModuleList()
- self.norm_layers_1 = nn.ModuleList()
- self.ffn_layers = nn.ModuleList()
- self.norm_layers_2 = nn.ModuleList()
- for i in range(self.n_layers):
- self.self_attn_layers.append(MultiHeadAttention(hidden_channels, hidden_channels, n_heads, p_dropout=p_dropout, proximal_bias=proximal_bias, proximal_init=proximal_init))
- self.norm_layers_0.append(LayerNorm(hidden_channels))
- self.encdec_attn_layers.append(MultiHeadAttention(hidden_channels, hidden_channels, n_heads, p_dropout=p_dropout))
- self.norm_layers_1.append(LayerNorm(hidden_channels))
- self.ffn_layers.append(FFN(hidden_channels, hidden_channels, filter_channels, kernel_size, p_dropout=p_dropout, causal=True))
- self.norm_layers_2.append(LayerNorm(hidden_channels))
-
- def forward(self, x, x_mask, h, h_mask):
- """
- x: decoder input
- h: encoder output
- """
- self_attn_mask = commons.subsequent_mask(x_mask.size(2)).to(device=x.device, dtype=x.dtype)
- encdec_attn_mask = h_mask.unsqueeze(2) * x_mask.unsqueeze(-1)
- x = x * x_mask
- for i in range(self.n_layers):
- y = self.self_attn_layers[i](x, x, self_attn_mask)
- y = self.drop(y)
- x = self.norm_layers_0[i](x + y)
-
- y = self.encdec_attn_layers[i](x, h, encdec_attn_mask)
- y = self.drop(y)
- x = self.norm_layers_1[i](x + y)
-
- y = self.ffn_layers[i](x, x_mask)
- y = self.drop(y)
- x = self.norm_layers_2[i](x + y)
- x = x * x_mask
- return x
-
-
-class MultiHeadAttention(nn.Module):
- def __init__(self, channels, out_channels, n_heads, p_dropout=0., window_size=None, heads_share=True, block_length=None, proximal_bias=False, proximal_init=False):
- super().__init__()
- assert channels % n_heads == 0
-
- self.channels = channels
- self.out_channels = out_channels
- self.n_heads = n_heads
- self.p_dropout = p_dropout
- self.window_size = window_size
- self.heads_share = heads_share
- self.block_length = block_length
- self.proximal_bias = proximal_bias
- self.proximal_init = proximal_init
- self.attn = None
-
- self.k_channels = channels // n_heads
- self.conv_q = nn.Conv1d(channels, channels, 1)
- self.conv_k = nn.Conv1d(channels, channels, 1)
- self.conv_v = nn.Conv1d(channels, channels, 1)
- self.conv_o = nn.Conv1d(channels, out_channels, 1)
- self.drop = nn.Dropout(p_dropout)
-
- if window_size is not None:
- n_heads_rel = 1 if heads_share else n_heads
- rel_stddev = self.k_channels**-0.5
- self.emb_rel_k = nn.Parameter(torch.randn(n_heads_rel, window_size * 2 + 1, self.k_channels) * rel_stddev)
- self.emb_rel_v = nn.Parameter(torch.randn(n_heads_rel, window_size * 2 + 1, self.k_channels) * rel_stddev)
-
- nn.init.xavier_uniform_(self.conv_q.weight)
- nn.init.xavier_uniform_(self.conv_k.weight)
- nn.init.xavier_uniform_(self.conv_v.weight)
- if proximal_init:
- with torch.no_grad():
- self.conv_k.weight.copy_(self.conv_q.weight)
- self.conv_k.bias.copy_(self.conv_q.bias)
-
- def forward(self, x, c, attn_mask=None):
- q = self.conv_q(x)
- k = self.conv_k(c)
- v = self.conv_v(c)
-
- x, self.attn = self.attention(q, k, v, mask=attn_mask)
-
- x = self.conv_o(x)
- return x
-
- def attention(self, query, key, value, mask=None):
- # reshape [b, d, t] -> [b, n_h, t, d_k]
- b, d, t_s, t_t = (*key.size(), query.size(2))
- query = query.view(b, self.n_heads, self.k_channels, t_t).transpose(2, 3)
- key = key.view(b, self.n_heads, self.k_channels, t_s).transpose(2, 3)
- value = value.view(b, self.n_heads, self.k_channels, t_s).transpose(2, 3)
-
- scores = torch.matmul(query / math.sqrt(self.k_channels), key.transpose(-2, -1))
- if self.window_size is not None:
- assert t_s == t_t, "Relative attention is only available for self-attention."
- key_relative_embeddings = self._get_relative_embeddings(self.emb_rel_k, t_s)
- rel_logits = self._matmul_with_relative_keys(query /math.sqrt(self.k_channels), key_relative_embeddings)
- scores_local = self._relative_position_to_absolute_position(rel_logits)
- scores = scores + scores_local
- if self.proximal_bias:
- assert t_s == t_t, "Proximal bias is only available for self-attention."
- scores = scores + self._attention_bias_proximal(t_s).to(device=scores.device, dtype=scores.dtype)
- if mask is not None:
- scores = scores.masked_fill(mask == 0, -1e4)
- if self.block_length is not None:
- assert t_s == t_t, "Local attention is only available for self-attention."
- block_mask = torch.ones_like(scores).triu(-self.block_length).tril(self.block_length)
- scores = scores.masked_fill(block_mask == 0, -1e4)
- p_attn = F.softmax(scores, dim=-1) # [b, n_h, t_t, t_s]
- p_attn = self.drop(p_attn)
- output = torch.matmul(p_attn, value)
- if self.window_size is not None:
- relative_weights = self._absolute_position_to_relative_position(p_attn)
- value_relative_embeddings = self._get_relative_embeddings(self.emb_rel_v, t_s)
- output = output + self._matmul_with_relative_values(relative_weights, value_relative_embeddings)
- output = output.transpose(2, 3).contiguous().view(b, d, t_t) # [b, n_h, t_t, d_k] -> [b, d, t_t]
- return output, p_attn
-
- def _matmul_with_relative_values(self, x, y):
- """
- x: [b, h, l, m]
- y: [h or 1, m, d]
- ret: [b, h, l, d]
- """
- ret = torch.matmul(x, y.unsqueeze(0))
- return ret
-
- def _matmul_with_relative_keys(self, x, y):
- """
- x: [b, h, l, d]
- y: [h or 1, m, d]
- ret: [b, h, l, m]
- """
- ret = torch.matmul(x, y.unsqueeze(0).transpose(-2, -1))
- return ret
-
- def _get_relative_embeddings(self, relative_embeddings, length):
- max_relative_position = 2 * self.window_size + 1
- # Pad first before slice to avoid using cond ops.
- pad_length = max(length - (self.window_size + 1), 0)
- slice_start_position = max((self.window_size + 1) - length, 0)
- slice_end_position = slice_start_position + 2 * length - 1
- if pad_length > 0:
- padded_relative_embeddings = F.pad(
- relative_embeddings,
- commons.convert_pad_shape([[0, 0], [pad_length, pad_length], [0, 0]]))
- else:
- padded_relative_embeddings = relative_embeddings
- used_relative_embeddings = padded_relative_embeddings[:,slice_start_position:slice_end_position]
- return used_relative_embeddings
-
- def _relative_position_to_absolute_position(self, x):
- """
- x: [b, h, l, 2*l-1]
- ret: [b, h, l, l]
- """
- batch, heads, length, _ = x.size()
- # Concat columns of pad to shift from relative to absolute indexing.
- x = F.pad(x, commons.convert_pad_shape([[0,0],[0,0],[0,0],[0,1]]))
-
- # Concat extra elements so to add up to shape (len+1, 2*len-1).
- x_flat = x.view([batch, heads, length * 2 * length])
- x_flat = F.pad(x_flat, commons.convert_pad_shape([[0,0],[0,0],[0,length-1]]))
-
- # Reshape and slice out the padded elements.
- x_final = x_flat.view([batch, heads, length+1, 2*length-1])[:, :, :length, length-1:]
- return x_final
-
- def _absolute_position_to_relative_position(self, x):
- """
- x: [b, h, l, l]
- ret: [b, h, l, 2*l-1]
- """
- batch, heads, length, _ = x.size()
- # padd along column
- x = F.pad(x, commons.convert_pad_shape([[0, 0], [0, 0], [0, 0], [0, length-1]]))
- x_flat = x.view([batch, heads, length**2 + length*(length -1)])
- # add 0's in the beginning that will skew the elements after reshape
- x_flat = F.pad(x_flat, commons.convert_pad_shape([[0, 0], [0, 0], [length, 0]]))
- x_final = x_flat.view([batch, heads, length, 2*length])[:,:,:,1:]
- return x_final
-
- def _attention_bias_proximal(self, length):
- """Bias for self-attention to encourage attention to close positions.
- Args:
- length: an integer scalar.
- Returns:
- a Tensor with shape [1, 1, length, length]
- """
- r = torch.arange(length, dtype=torch.float32)
- diff = torch.unsqueeze(r, 0) - torch.unsqueeze(r, 1)
- return torch.unsqueeze(torch.unsqueeze(-torch.log1p(torch.abs(diff)), 0), 0)
-
-
-class FFN(nn.Module):
- def __init__(self, in_channels, out_channels, filter_channels, kernel_size, p_dropout=0., activation=None, causal=False):
- super().__init__()
- self.in_channels = in_channels
- self.out_channels = out_channels
- self.filter_channels = filter_channels
- self.kernel_size = kernel_size
- self.p_dropout = p_dropout
- self.activation = activation
- self.causal = causal
-
- if causal:
- self.padding = self._causal_padding
- else:
- self.padding = self._same_padding
-
- self.conv_1 = nn.Conv1d(in_channels, filter_channels, kernel_size)
- self.conv_2 = nn.Conv1d(filter_channels, out_channels, kernel_size)
- self.drop = nn.Dropout(p_dropout)
-
- def forward(self, x, x_mask):
- x = self.conv_1(self.padding(x * x_mask))
- if self.activation == "gelu":
- x = x * torch.sigmoid(1.702 * x)
- else:
- x = torch.relu(x)
- x = self.drop(x)
- x = self.conv_2(self.padding(x * x_mask))
- return x * x_mask
-
- def _causal_padding(self, x):
- if self.kernel_size == 1:
- return x
- pad_l = self.kernel_size - 1
- pad_r = 0
- padding = [[0, 0], [0, 0], [pad_l, pad_r]]
- x = F.pad(x, commons.convert_pad_shape(padding))
- return x
-
- def _same_padding(self, x):
- if self.kernel_size == 1:
- return x
- pad_l = (self.kernel_size - 1) // 2
- pad_r = self.kernel_size // 2
- padding = [[0, 0], [0, 0], [pad_l, pad_r]]
- x = F.pad(x, commons.convert_pad_shape(padding))
- return x
diff --git a/spaces/ZX9966/LOGO-Approximate-Computing-Technology/README.md b/spaces/ZX9966/LOGO-Approximate-Computing-Technology/README.md
deleted file mode 100644
index eeae9882481961b1a803273fbfb7c372f90ac05e..0000000000000000000000000000000000000000
--- a/spaces/ZX9966/LOGO-Approximate-Computing-Technology/README.md
+++ /dev/null
@@ -1,11 +0,0 @@
----
-title: LOGO Approximate Computing Technology
-emoji: 😻
-colorFrom: purple
-colorTo: blue
-sdk: static
-pinned: false
-license: apache-2.0
----
-
-Check out the configuration reference at https://huggingface.co/docs/hub/spaces-config-reference
diff --git a/spaces/Zeltoria/anime-voice-generator/text/__init__.py b/spaces/Zeltoria/anime-voice-generator/text/__init__.py
deleted file mode 100644
index 663c4b6416affb53c9dc56dddbc8b2b65d4bf518..0000000000000000000000000000000000000000
--- a/spaces/Zeltoria/anime-voice-generator/text/__init__.py
+++ /dev/null
@@ -1,57 +0,0 @@
-""" from https://github.com/keithito/tacotron """
-from text import cleaners
-from text.symbols import symbols
-
-
-# Mappings from symbol to numeric ID and vice versa:
-_symbol_to_id = {s: i for i, s in enumerate(symbols)}
-_id_to_symbol = {i: s for i, s in enumerate(symbols)}
-
-
-def text_to_sequence(text, symbols, cleaner_names):
- '''Converts a string of text to a sequence of IDs corresponding to the symbols in the text.
- Args:
- text: string to convert to a sequence
- cleaner_names: names of the cleaner functions to run the text through
- Returns:
- List of integers corresponding to the symbols in the text
- '''
- _symbol_to_id = {s: i for i, s in enumerate(symbols)}
- sequence = []
-
- clean_text = _clean_text(text, cleaner_names)
- for symbol in clean_text:
- if symbol not in _symbol_to_id.keys():
- continue
- symbol_id = _symbol_to_id[symbol]
- sequence += [symbol_id]
- return sequence, clean_text
-
-
-def cleaned_text_to_sequence(cleaned_text):
- '''Converts a string of text to a sequence of IDs corresponding to the symbols in the text.
- Args:
- text: string to convert to a sequence
- Returns:
- List of integers corresponding to the symbols in the text
- '''
- sequence = [_symbol_to_id[symbol] for symbol in cleaned_text if symbol in _symbol_to_id.keys()]
- return sequence
-
-
-def sequence_to_text(sequence):
- '''Converts a sequence of IDs back to a string'''
- result = ''
- for symbol_id in sequence:
- s = _id_to_symbol[symbol_id]
- result += s
- return result
-
-
-def _clean_text(text, cleaner_names):
- for name in cleaner_names:
- cleaner = getattr(cleaners, name)
- if not cleaner:
- raise Exception('Unknown cleaner: %s' % name)
- text = cleaner(text)
- return text
diff --git a/spaces/aadnk/faster-whisper-webui/LICENSE.md b/spaces/aadnk/faster-whisper-webui/LICENSE.md
deleted file mode 100644
index f5f4b8b5ecd27c09e4ef16e9662bcb7bb2bfc76f..0000000000000000000000000000000000000000
--- a/spaces/aadnk/faster-whisper-webui/LICENSE.md
+++ /dev/null
@@ -1,195 +0,0 @@
-Apache License
-==============
-
-_Version 2.0, January 2004_
-_<>_
-
-### Terms and Conditions for use, reproduction, and distribution
-
-#### 1. Definitions
-
-“License” shall mean the terms and conditions for use, reproduction, and
-distribution as defined by Sections 1 through 9 of this document.
-
-“Licensor” shall mean the copyright owner or entity authorized by the copyright
-owner that is granting the License.
-
-“Legal Entity” shall mean the union of the acting entity and all other entities
-that control, are controlled by, or are under common control with that entity.
-For the purposes of this definition, “control” means **(i)** the power, direct or
-indirect, to cause the direction or management of such entity, whether by
-contract or otherwise, or **(ii)** ownership of fifty percent (50%) or more of the
-outstanding shares, or **(iii)** beneficial ownership of such entity.
-
-“You” (or “Your”) shall mean an individual or Legal Entity exercising
-permissions granted by this License.
-
-“Source” form shall mean the preferred form for making modifications, including
-but not limited to software source code, documentation source, and configuration
-files.
-
-“Object” form shall mean any form resulting from mechanical transformation or
-translation of a Source form, including but not limited to compiled object code,
-generated documentation, and conversions to other media types.
-
-“Work” shall mean the work of authorship, whether in Source or Object form, made
-available under the License, as indicated by a copyright notice that is included
-in or attached to the work (an example is provided in the Appendix below).
-
-“Derivative Works” shall mean any work, whether in Source or Object form, that
-is based on (or derived from) the Work and for which the editorial revisions,
-annotations, elaborations, or other modifications represent, as a whole, an
-original work of authorship. For the purposes of this License, Derivative Works
-shall not include works that remain separable from, or merely link (or bind by
-name) to the interfaces of, the Work and Derivative Works thereof.
-
-“Contribution” shall mean any work of authorship, including the original version
-of the Work and any modifications or additions to that Work or Derivative Works
-thereof, that is intentionally submitted to Licensor for inclusion in the Work
-by the copyright owner or by an individual or Legal Entity authorized to submit
-on behalf of the copyright owner. For the purposes of this definition,
-“submitted” means any form of electronic, verbal, or written communication sent
-to the Licensor or its representatives, including but not limited to
-communication on electronic mailing lists, source code control systems, and
-issue tracking systems that are managed by, or on behalf of, the Licensor for
-the purpose of discussing and improving the Work, but excluding communication
-that is conspicuously marked or otherwise designated in writing by the copyright
-owner as “Not a Contribution.”
-
-“Contributor” shall mean Licensor and any individual or Legal Entity on behalf
-of whom a Contribution has been received by Licensor and subsequently
-incorporated within the Work.
-
-#### 2. Grant of Copyright License
-
-Subject to the terms and conditions of this License, each Contributor hereby
-grants to You a perpetual, worldwide, non-exclusive, no-charge, royalty-free,
-irrevocable copyright license to reproduce, prepare Derivative Works of,
-publicly display, publicly perform, sublicense, and distribute the Work and such
-Derivative Works in Source or Object form.
-
-#### 3. Grant of Patent License
-
-Subject to the terms and conditions of this License, each Contributor hereby
-grants to You a perpetual, worldwide, non-exclusive, no-charge, royalty-free,
-irrevocable (except as stated in this section) patent license to make, have
-made, use, offer to sell, sell, import, and otherwise transfer the Work, where
-such license applies only to those patent claims licensable by such Contributor
-that are necessarily infringed by their Contribution(s) alone or by combination
-of their Contribution(s) with the Work to which such Contribution(s) was
-submitted. If You institute patent litigation against any entity (including a
-cross-claim or counterclaim in a lawsuit) alleging that the Work or a
-Contribution incorporated within the Work constitutes direct or contributory
-patent infringement, then any patent licenses granted to You under this License
-for that Work shall terminate as of the date such litigation is filed.
-
-#### 4. Redistribution
-
-You may reproduce and distribute copies of the Work or Derivative Works thereof
-in any medium, with or without modifications, and in Source or Object form,
-provided that You meet the following conditions:
-
-* **(a)** You must give any other recipients of the Work or Derivative Works a copy of
-this License; and
-* **(b)** You must cause any modified files to carry prominent notices stating that You
-changed the files; and
-* **(c)** You must retain, in the Source form of any Derivative Works that You distribute,
-all copyright, patent, trademark, and attribution notices from the Source form
-of the Work, excluding those notices that do not pertain to any part of the
-Derivative Works; and
-* **(d)** If the Work includes a “NOTICE” text file as part of its distribution, then any
-Derivative Works that You distribute must include a readable copy of the
-attribution notices contained within such NOTICE file, excluding those notices
-that do not pertain to any part of the Derivative Works, in at least one of the
-following places: within a NOTICE text file distributed as part of the
-Derivative Works; within the Source form or documentation, if provided along
-with the Derivative Works; or, within a display generated by the Derivative
-Works, if and wherever such third-party notices normally appear. The contents of
-the NOTICE file are for informational purposes only and do not modify the
-License. You may add Your own attribution notices within Derivative Works that
-You distribute, alongside or as an addendum to the NOTICE text from the Work,
-provided that such additional attribution notices cannot be construed as
-modifying the License.
-
-You may add Your own copyright statement to Your modifications and may provide
-additional or different license terms and conditions for use, reproduction, or
-distribution of Your modifications, or for any such Derivative Works as a whole,
-provided Your use, reproduction, and distribution of the Work otherwise complies
-with the conditions stated in this License.
-
-#### 5. Submission of Contributions
-
-Unless You explicitly state otherwise, any Contribution intentionally submitted
-for inclusion in the Work by You to the Licensor shall be under the terms and
-conditions of this License, without any additional terms or conditions.
-Notwithstanding the above, nothing herein shall supersede or modify the terms of
-any separate license agreement you may have executed with Licensor regarding
-such Contributions.
-
-#### 6. Trademarks
-
-This License does not grant permission to use the trade names, trademarks,
-service marks, or product names of the Licensor, except as required for
-reasonable and customary use in describing the origin of the Work and
-reproducing the content of the NOTICE file.
-
-#### 7. Disclaimer of Warranty
-
-Unless required by applicable law or agreed to in writing, Licensor provides the
-Work (and each Contributor provides its Contributions) on an “AS IS” BASIS,
-WITHOUT WARRANTIES OR CONDITIONS OF ANY KIND, either express or implied,
-including, without limitation, any warranties or conditions of TITLE,
-NON-INFRINGEMENT, MERCHANTABILITY, or FITNESS FOR A PARTICULAR PURPOSE. You are
-solely responsible for determining the appropriateness of using or
-redistributing the Work and assume any risks associated with Your exercise of
-permissions under this License.
-
-#### 8. Limitation of Liability
-
-In no event and under no legal theory, whether in tort (including negligence),
-contract, or otherwise, unless required by applicable law (such as deliberate
-and grossly negligent acts) or agreed to in writing, shall any Contributor be
-liable to You for damages, including any direct, indirect, special, incidental,
-or consequential damages of any character arising as a result of this License or
-out of the use or inability to use the Work (including but not limited to
-damages for loss of goodwill, work stoppage, computer failure or malfunction, or
-any and all other commercial damages or losses), even if such Contributor has
-been advised of the possibility of such damages.
-
-#### 9. Accepting Warranty or Additional Liability
-
-While redistributing the Work or Derivative Works thereof, You may choose to
-offer, and charge a fee for, acceptance of support, warranty, indemnity, or
-other liability obligations and/or rights consistent with this License. However,
-in accepting such obligations, You may act only on Your own behalf and on Your
-sole responsibility, not on behalf of any other Contributor, and only if You
-agree to indemnify, defend, and hold each Contributor harmless for any liability
-incurred by, or claims asserted against, such Contributor by reason of your
-accepting any such warranty or additional liability.
-
-_END OF TERMS AND CONDITIONS_
-
-### APPENDIX: How to apply the Apache License to your work
-
-To apply the Apache License to your work, attach the following boilerplate
-notice, with the fields enclosed by brackets `[]` replaced with your own
-identifying information. (Don't include the brackets!) The text should be
-enclosed in the appropriate comment syntax for the file format. We also
-recommend that a file or class name and description of purpose be included on
-the same “printed page” as the copyright notice for easier identification within
-third-party archives.
-
- Copyright [yyyy] [name of copyright owner]
-
- Licensed under the Apache License, Version 2.0 (the "License");
- you may not use this file except in compliance with the License.
- You may obtain a copy of the License at
-
- http://www.apache.org/licenses/LICENSE-2.0
-
- Unless required by applicable law or agreed to in writing, software
- distributed under the License is distributed on an "AS IS" BASIS,
- WITHOUT WARRANTIES OR CONDITIONS OF ANY KIND, either express or implied.
- See the License for the specific language governing permissions and
- limitations under the License.
-
diff --git a/spaces/aai198/ComfyUI/README.md b/spaces/aai198/ComfyUI/README.md
deleted file mode 100644
index 9a18b220b506adbf1f72ca73223edc0fc1f6f754..0000000000000000000000000000000000000000
--- a/spaces/aai198/ComfyUI/README.md
+++ /dev/null
@@ -1,10 +0,0 @@
----
-title: ComfyUI
-emoji: 📈
-colorFrom: green
-colorTo: pink
-sdk: docker
-pinned: false
----
-
-model: https://huggingface.co/stabilityai/control-lora
\ No newline at end of file
diff --git a/spaces/abhishek/sketch-to-image/annotator/uniformer/mmcv/ops/psa_mask.py b/spaces/abhishek/sketch-to-image/annotator/uniformer/mmcv/ops/psa_mask.py
deleted file mode 100644
index cdf14e62b50e8d4dd6856c94333c703bcc4c9ab6..0000000000000000000000000000000000000000
--- a/spaces/abhishek/sketch-to-image/annotator/uniformer/mmcv/ops/psa_mask.py
+++ /dev/null
@@ -1,92 +0,0 @@
-# Modified from https://github.com/hszhao/semseg/blob/master/lib/psa
-from torch import nn
-from torch.autograd import Function
-from torch.nn.modules.utils import _pair
-
-from ..utils import ext_loader
-
-ext_module = ext_loader.load_ext('_ext',
- ['psamask_forward', 'psamask_backward'])
-
-
-class PSAMaskFunction(Function):
-
- @staticmethod
- def symbolic(g, input, psa_type, mask_size):
- return g.op(
- 'mmcv::MMCVPSAMask',
- input,
- psa_type_i=psa_type,
- mask_size_i=mask_size)
-
- @staticmethod
- def forward(ctx, input, psa_type, mask_size):
- ctx.psa_type = psa_type
- ctx.mask_size = _pair(mask_size)
- ctx.save_for_backward(input)
-
- h_mask, w_mask = ctx.mask_size
- batch_size, channels, h_feature, w_feature = input.size()
- assert channels == h_mask * w_mask
- output = input.new_zeros(
- (batch_size, h_feature * w_feature, h_feature, w_feature))
-
- ext_module.psamask_forward(
- input,
- output,
- psa_type=psa_type,
- num_=batch_size,
- h_feature=h_feature,
- w_feature=w_feature,
- h_mask=h_mask,
- w_mask=w_mask,
- half_h_mask=(h_mask - 1) // 2,
- half_w_mask=(w_mask - 1) // 2)
- return output
-
- @staticmethod
- def backward(ctx, grad_output):
- input = ctx.saved_tensors[0]
- psa_type = ctx.psa_type
- h_mask, w_mask = ctx.mask_size
- batch_size, channels, h_feature, w_feature = input.size()
- grad_input = grad_output.new_zeros(
- (batch_size, channels, h_feature, w_feature))
- ext_module.psamask_backward(
- grad_output,
- grad_input,
- psa_type=psa_type,
- num_=batch_size,
- h_feature=h_feature,
- w_feature=w_feature,
- h_mask=h_mask,
- w_mask=w_mask,
- half_h_mask=(h_mask - 1) // 2,
- half_w_mask=(w_mask - 1) // 2)
- return grad_input, None, None, None
-
-
-psa_mask = PSAMaskFunction.apply
-
-
-class PSAMask(nn.Module):
-
- def __init__(self, psa_type, mask_size=None):
- super(PSAMask, self).__init__()
- assert psa_type in ['collect', 'distribute']
- if psa_type == 'collect':
- psa_type_enum = 0
- else:
- psa_type_enum = 1
- self.psa_type_enum = psa_type_enum
- self.mask_size = mask_size
- self.psa_type = psa_type
-
- def forward(self, input):
- return psa_mask(input, self.psa_type_enum, self.mask_size)
-
- def __repr__(self):
- s = self.__class__.__name__
- s += f'(psa_type={self.psa_type}, '
- s += f'mask_size={self.mask_size})'
- return s
diff --git a/spaces/abhishek/sketch-to-image/annotator/uniformer/mmdet/core/bbox/coder/base_bbox_coder.py b/spaces/abhishek/sketch-to-image/annotator/uniformer/mmdet/core/bbox/coder/base_bbox_coder.py
deleted file mode 100644
index cf0b34c7cc2fe561718b0c884990beb40a993643..0000000000000000000000000000000000000000
--- a/spaces/abhishek/sketch-to-image/annotator/uniformer/mmdet/core/bbox/coder/base_bbox_coder.py
+++ /dev/null
@@ -1,17 +0,0 @@
-from abc import ABCMeta, abstractmethod
-
-
-class BaseBBoxCoder(metaclass=ABCMeta):
- """Base bounding box coder."""
-
- def __init__(self, **kwargs):
- pass
-
- @abstractmethod
- def encode(self, bboxes, gt_bboxes):
- """Encode deltas between bboxes and ground truth boxes."""
-
- @abstractmethod
- def decode(self, bboxes, bboxes_pred):
- """Decode the predicted bboxes according to prediction and base
- boxes."""
diff --git a/spaces/ai-moroz/webui-cpu/README.md b/spaces/ai-moroz/webui-cpu/README.md
deleted file mode 100644
index 97d972aa8679e4b367156dcbfff5d58ad47937e9..0000000000000000000000000000000000000000
--- a/spaces/ai-moroz/webui-cpu/README.md
+++ /dev/null
@@ -1,11 +0,0 @@
----
-title: Webui
-emoji: 🚧
-colorFrom: yellow
-colorTo: yellow
-sdk: gradio
-sdk_version: 3.9
-app_file: app.py
-pinned: false
-duplicated_from: zwv9/webui-cpu
----
diff --git a/spaces/akhaliq/Real-Time-Voice-Cloning/toolbox/__init__.py b/spaces/akhaliq/Real-Time-Voice-Cloning/toolbox/__init__.py
deleted file mode 100644
index 531d6adef076007afd6116eb6472485f540e80de..0000000000000000000000000000000000000000
--- a/spaces/akhaliq/Real-Time-Voice-Cloning/toolbox/__init__.py
+++ /dev/null
@@ -1,357 +0,0 @@
-from toolbox.ui import UI
-from encoder import inference as encoder
-from synthesizer.inference import Synthesizer
-from vocoder import inference as vocoder
-from pathlib import Path
-from time import perf_counter as timer
-from toolbox.utterance import Utterance
-import numpy as np
-import traceback
-import sys
-import torch
-import librosa
-from audioread.exceptions import NoBackendError
-
-# Use this directory structure for your datasets, or modify it to fit your needs
-recognized_datasets = [
- "LibriSpeech/dev-clean",
- "LibriSpeech/dev-other",
- "LibriSpeech/test-clean",
- "LibriSpeech/test-other",
- "LibriSpeech/train-clean-100",
- "LibriSpeech/train-clean-360",
- "LibriSpeech/train-other-500",
- "LibriTTS/dev-clean",
- "LibriTTS/dev-other",
- "LibriTTS/test-clean",
- "LibriTTS/test-other",
- "LibriTTS/train-clean-100",
- "LibriTTS/train-clean-360",
- "LibriTTS/train-other-500",
- "LJSpeech-1.1",
- "VoxCeleb1/wav",
- "VoxCeleb1/test_wav",
- "VoxCeleb2/dev/aac",
- "VoxCeleb2/test/aac",
- "VCTK-Corpus/wav48",
-]
-
-#Maximum of generated wavs to keep on memory
-MAX_WAVES = 15
-
-class Toolbox:
- def __init__(self, datasets_root, enc_models_dir, syn_models_dir, voc_models_dir, seed, no_mp3_support):
- if not no_mp3_support:
- try:
- librosa.load("samples/6829_00000.mp3")
- except NoBackendError:
- print("Librosa will be unable to open mp3 files if additional software is not installed.\n"
- "Please install ffmpeg or add the '--no_mp3_support' option to proceed without support for mp3 files.")
- exit(-1)
- self.no_mp3_support = no_mp3_support
- sys.excepthook = self.excepthook
- self.datasets_root = datasets_root
- self.utterances = set()
- self.current_generated = (None, None, None, None) # speaker_name, spec, breaks, wav
-
- self.synthesizer = None # type: Synthesizer
- self.current_wav = None
- self.waves_list = []
- self.waves_count = 0
- self.waves_namelist = []
-
- # Check for webrtcvad (enables removal of silences in vocoder output)
- try:
- import webrtcvad
- self.trim_silences = True
- except:
- self.trim_silences = False
-
- # Initialize the events and the interface
- self.ui = UI()
- self.reset_ui(enc_models_dir, syn_models_dir, voc_models_dir, seed)
- self.setup_events()
- self.ui.start()
-
- def excepthook(self, exc_type, exc_value, exc_tb):
- traceback.print_exception(exc_type, exc_value, exc_tb)
- self.ui.log("Exception: %s" % exc_value)
-
- def setup_events(self):
- # Dataset, speaker and utterance selection
- self.ui.browser_load_button.clicked.connect(lambda: self.load_from_browser())
- random_func = lambda level: lambda: self.ui.populate_browser(self.datasets_root,
- recognized_datasets,
- level)
- self.ui.random_dataset_button.clicked.connect(random_func(0))
- self.ui.random_speaker_button.clicked.connect(random_func(1))
- self.ui.random_utterance_button.clicked.connect(random_func(2))
- self.ui.dataset_box.currentIndexChanged.connect(random_func(1))
- self.ui.speaker_box.currentIndexChanged.connect(random_func(2))
-
- # Model selection
- self.ui.encoder_box.currentIndexChanged.connect(self.init_encoder)
- def func():
- self.synthesizer = None
- self.ui.synthesizer_box.currentIndexChanged.connect(func)
- self.ui.vocoder_box.currentIndexChanged.connect(self.init_vocoder)
-
- # Utterance selection
- func = lambda: self.load_from_browser(self.ui.browse_file())
- self.ui.browser_browse_button.clicked.connect(func)
- func = lambda: self.ui.draw_utterance(self.ui.selected_utterance, "current")
- self.ui.utterance_history.currentIndexChanged.connect(func)
- func = lambda: self.ui.play(self.ui.selected_utterance.wav, Synthesizer.sample_rate)
- self.ui.play_button.clicked.connect(func)
- self.ui.stop_button.clicked.connect(self.ui.stop)
- self.ui.record_button.clicked.connect(self.record)
-
- #Audio
- self.ui.setup_audio_devices(Synthesizer.sample_rate)
-
- #Wav playback & save
- func = lambda: self.replay_last_wav()
- self.ui.replay_wav_button.clicked.connect(func)
- func = lambda: self.export_current_wave()
- self.ui.export_wav_button.clicked.connect(func)
- self.ui.waves_cb.currentIndexChanged.connect(self.set_current_wav)
-
- # Generation
- func = lambda: self.synthesize() or self.vocode()
- self.ui.generate_button.clicked.connect(func)
- self.ui.synthesize_button.clicked.connect(self.synthesize)
- self.ui.vocode_button.clicked.connect(self.vocode)
- self.ui.random_seed_checkbox.clicked.connect(self.update_seed_textbox)
-
- # UMAP legend
- self.ui.clear_button.clicked.connect(self.clear_utterances)
-
- def set_current_wav(self, index):
- self.current_wav = self.waves_list[index]
-
- def export_current_wave(self):
- self.ui.save_audio_file(self.current_wav, Synthesizer.sample_rate)
-
- def replay_last_wav(self):
- self.ui.play(self.current_wav, Synthesizer.sample_rate)
-
- def reset_ui(self, encoder_models_dir, synthesizer_models_dir, vocoder_models_dir, seed):
- self.ui.populate_browser(self.datasets_root, recognized_datasets, 0, True)
- self.ui.populate_models(encoder_models_dir, synthesizer_models_dir, vocoder_models_dir)
- self.ui.populate_gen_options(seed, self.trim_silences)
-
- def load_from_browser(self, fpath=None):
- if fpath is None:
- fpath = Path(self.datasets_root,
- self.ui.current_dataset_name,
- self.ui.current_speaker_name,
- self.ui.current_utterance_name)
- name = str(fpath.relative_to(self.datasets_root))
- speaker_name = self.ui.current_dataset_name + '_' + self.ui.current_speaker_name
-
- # Select the next utterance
- if self.ui.auto_next_checkbox.isChecked():
- self.ui.browser_select_next()
- elif fpath == "":
- return
- else:
- name = fpath.name
- speaker_name = fpath.parent.name
-
- if fpath.suffix.lower() == ".mp3" and self.no_mp3_support:
- self.ui.log("Error: No mp3 file argument was passed but an mp3 file was used")
- return
-
- # Get the wav from the disk. We take the wav with the vocoder/synthesizer format for
- # playback, so as to have a fair comparison with the generated audio
- wav = Synthesizer.load_preprocess_wav(fpath)
- self.ui.log("Loaded %s" % name)
-
- self.add_real_utterance(wav, name, speaker_name)
-
- def record(self):
- wav = self.ui.record_one(encoder.sampling_rate, 5)
- if wav is None:
- return
- self.ui.play(wav, encoder.sampling_rate)
-
- speaker_name = "user01"
- name = speaker_name + "_rec_%05d" % np.random.randint(100000)
- self.add_real_utterance(wav, name, speaker_name)
-
- def add_real_utterance(self, wav, name, speaker_name):
- # Compute the mel spectrogram
- spec = Synthesizer.make_spectrogram(wav)
- self.ui.draw_spec(spec, "current")
-
- # Compute the embedding
- if not encoder.is_loaded():
- self.init_encoder()
- encoder_wav = encoder.preprocess_wav(wav)
- embed, partial_embeds, _ = encoder.embed_utterance(encoder_wav, return_partials=True)
-
- # Add the utterance
- utterance = Utterance(name, speaker_name, wav, spec, embed, partial_embeds, False)
- self.utterances.add(utterance)
- self.ui.register_utterance(utterance)
-
- # Plot it
- self.ui.draw_embed(embed, name, "current")
- self.ui.draw_umap_projections(self.utterances)
-
- def clear_utterances(self):
- self.utterances.clear()
- self.ui.draw_umap_projections(self.utterances)
-
- def synthesize(self):
- self.ui.log("Generating the mel spectrogram...")
- self.ui.set_loading(1)
-
- # Update the synthesizer random seed
- if self.ui.random_seed_checkbox.isChecked():
- seed = int(self.ui.seed_textbox.text())
- self.ui.populate_gen_options(seed, self.trim_silences)
- else:
- seed = None
-
- if seed is not None:
- torch.manual_seed(seed)
-
- # Synthesize the spectrogram
- if self.synthesizer is None or seed is not None:
- self.init_synthesizer()
-
- texts = self.ui.text_prompt.toPlainText().split("\n")
- embed = self.ui.selected_utterance.embed
- embeds = [embed] * len(texts)
- specs = self.synthesizer.synthesize_spectrograms(texts, embeds)
- breaks = [spec.shape[1] for spec in specs]
- spec = np.concatenate(specs, axis=1)
-
- self.ui.draw_spec(spec, "generated")
- self.current_generated = (self.ui.selected_utterance.speaker_name, spec, breaks, None)
- self.ui.set_loading(0)
-
- def vocode(self):
- speaker_name, spec, breaks, _ = self.current_generated
- assert spec is not None
-
- # Initialize the vocoder model and make it determinstic, if user provides a seed
- if self.ui.random_seed_checkbox.isChecked():
- seed = int(self.ui.seed_textbox.text())
- self.ui.populate_gen_options(seed, self.trim_silences)
- else:
- seed = None
-
- if seed is not None:
- torch.manual_seed(seed)
-
- # Synthesize the waveform
- if not vocoder.is_loaded() or seed is not None:
- self.init_vocoder()
-
- def vocoder_progress(i, seq_len, b_size, gen_rate):
- real_time_factor = (gen_rate / Synthesizer.sample_rate) * 1000
- line = "Waveform generation: %d/%d (batch size: %d, rate: %.1fkHz - %.2fx real time)" \
- % (i * b_size, seq_len * b_size, b_size, gen_rate, real_time_factor)
- self.ui.log(line, "overwrite")
- self.ui.set_loading(i, seq_len)
- if self.ui.current_vocoder_fpath is not None:
- self.ui.log("")
- wav = vocoder.infer_waveform(spec, progress_callback=vocoder_progress)
- else:
- self.ui.log("Waveform generation with Griffin-Lim... ")
- wav = Synthesizer.griffin_lim(spec)
- self.ui.set_loading(0)
- self.ui.log(" Done!", "append")
-
- # Add breaks
- b_ends = np.cumsum(np.array(breaks) * Synthesizer.hparams.hop_size)
- b_starts = np.concatenate(([0], b_ends[:-1]))
- wavs = [wav[start:end] for start, end, in zip(b_starts, b_ends)]
- breaks = [np.zeros(int(0.15 * Synthesizer.sample_rate))] * len(breaks)
- wav = np.concatenate([i for w, b in zip(wavs, breaks) for i in (w, b)])
-
- # Trim excessive silences
- if self.ui.trim_silences_checkbox.isChecked():
- wav = encoder.preprocess_wav(wav)
-
- # Play it
- wav = wav / np.abs(wav).max() * 0.97
- self.ui.play(wav, Synthesizer.sample_rate)
-
- # Name it (history displayed in combobox)
- # TODO better naming for the combobox items?
- wav_name = str(self.waves_count + 1)
-
- #Update waves combobox
- self.waves_count += 1
- if self.waves_count > MAX_WAVES:
- self.waves_list.pop()
- self.waves_namelist.pop()
- self.waves_list.insert(0, wav)
- self.waves_namelist.insert(0, wav_name)
-
- self.ui.waves_cb.disconnect()
- self.ui.waves_cb_model.setStringList(self.waves_namelist)
- self.ui.waves_cb.setCurrentIndex(0)
- self.ui.waves_cb.currentIndexChanged.connect(self.set_current_wav)
-
- # Update current wav
- self.set_current_wav(0)
-
- #Enable replay and save buttons:
- self.ui.replay_wav_button.setDisabled(False)
- self.ui.export_wav_button.setDisabled(False)
-
- # Compute the embedding
- # TODO: this is problematic with different sampling rates, gotta fix it
- if not encoder.is_loaded():
- self.init_encoder()
- encoder_wav = encoder.preprocess_wav(wav)
- embed, partial_embeds, _ = encoder.embed_utterance(encoder_wav, return_partials=True)
-
- # Add the utterance
- name = speaker_name + "_gen_%05d" % np.random.randint(100000)
- utterance = Utterance(name, speaker_name, wav, spec, embed, partial_embeds, True)
- self.utterances.add(utterance)
-
- # Plot it
- self.ui.draw_embed(embed, name, "generated")
- self.ui.draw_umap_projections(self.utterances)
-
- def init_encoder(self):
- model_fpath = self.ui.current_encoder_fpath
-
- self.ui.log("Loading the encoder %s... " % model_fpath)
- self.ui.set_loading(1)
- start = timer()
- encoder.load_model(model_fpath)
- self.ui.log("Done (%dms)." % int(1000 * (timer() - start)), "append")
- self.ui.set_loading(0)
-
- def init_synthesizer(self):
- model_fpath = self.ui.current_synthesizer_fpath
-
- self.ui.log("Loading the synthesizer %s... " % model_fpath)
- self.ui.set_loading(1)
- start = timer()
- self.synthesizer = Synthesizer(model_fpath)
- self.ui.log("Done (%dms)." % int(1000 * (timer() - start)), "append")
- self.ui.set_loading(0)
-
- def init_vocoder(self):
- model_fpath = self.ui.current_vocoder_fpath
- # Case of Griffin-lim
- if model_fpath is None:
- return
-
- self.ui.log("Loading the vocoder %s... " % model_fpath)
- self.ui.set_loading(1)
- start = timer()
- vocoder.load_model(model_fpath)
- self.ui.log("Done (%dms)." % int(1000 * (timer() - start)), "append")
- self.ui.set_loading(0)
-
- def update_seed_textbox(self):
- self.ui.update_seed_textbox()
diff --git a/spaces/akhaliq/Real-Time-Voice-Cloning/utils/__init__.py b/spaces/akhaliq/Real-Time-Voice-Cloning/utils/__init__.py
deleted file mode 100644
index e69de29bb2d1d6434b8b29ae775ad8c2e48c5391..0000000000000000000000000000000000000000
diff --git a/spaces/akhaliq/Spleeter/README.md b/spaces/akhaliq/Spleeter/README.md
deleted file mode 100644
index a24a5e84fe7788c6dbf7e11a0fdbf0348c305259..0000000000000000000000000000000000000000
--- a/spaces/akhaliq/Spleeter/README.md
+++ /dev/null
@@ -1,33 +0,0 @@
----
-title: Spleeter
-emoji: 💻
-colorFrom: purple
-colorTo: blue
-sdk: gradio
-app_file: app.py
-pinned: false
----
-
-# Configuration
-
-`title`: _string_
-Display title for the Space
-
-`emoji`: _string_
-Space emoji (emoji-only character allowed)
-
-`colorFrom`: _string_
-Color for Thumbnail gradient (red, yellow, green, blue, indigo, purple, pink, gray)
-
-`colorTo`: _string_
-Color for Thumbnail gradient (red, yellow, green, blue, indigo, purple, pink, gray)
-
-`sdk`: _string_
-Can be either `gradio` or `streamlit`
-
-`app_file`: _string_
-Path to your main application file (which contains either `gradio` or `streamlit` Python code).
-Path is relative to the root of the repository.
-
-`pinned`: _boolean_
-Whether the Space stays on top of your list.
diff --git a/spaces/akhaliq/SummerTime/model/third_party/HMNet/Models/Trainers/DistributedTrainer.py b/spaces/akhaliq/SummerTime/model/third_party/HMNet/Models/Trainers/DistributedTrainer.py
deleted file mode 100644
index 3ae8bf565f151c8746033f7832a17e0e9ea0b6f3..0000000000000000000000000000000000000000
--- a/spaces/akhaliq/SummerTime/model/third_party/HMNet/Models/Trainers/DistributedTrainer.py
+++ /dev/null
@@ -1,148 +0,0 @@
-# Copyright (c) Microsoft Corporation.
-# Licensed under the MIT license.
-
-import os
-import torch
-from torch.utils.tensorboard import SummaryWriter
-import random
-import numpy as np
-
-from pkg_resources import parse_version
-from model.third_party.HMNet.Models.Trainers.BaseTrainer import BaseTrainer
-from model.third_party.HMNet.Utils.GeneralUtils import bcolors
-from model.third_party.HMNet.Utils.distributed import distributed
-
-
-class DistributedTrainer(BaseTrainer):
- def __init__(self, opt):
- super().__init__(opt)
-
- self.seed = int(self.opt["SEED"]) if "SEED" in self.opt else 0
-
- random.seed(self.seed)
- np.random.seed(self.seed)
- torch.manual_seed(self.seed)
-
- (
- self.opt["device"],
- _,
- self.opt["world_size"],
- self.opt["local_size"],
- self.opt["rank"],
- self.opt["local_rank"],
- _,
- self.opt["run"],
- ) = distributed(opt, not self.use_cuda)
-
- self.getSaveFolder()
- self.opt["logFile"] = f"log_{self.opt['rank']}.txt"
- self.saveConf()
-
- self.high_pytorch_version = parse_version(torch.__version__) >= parse_version(
- "1.2.0"
- )
- if self.opt["rank"] == 0:
- print(
- bcolors.OKGREEN,
- torch.__version__,
- bcolors.ENDC,
- "is",
- "high" if self.high_pytorch_version else "low",
- )
-
- if self.use_cuda:
- # torch.cuda.manual_seed_all(self.seed)
- # ddp: only set seed on GPU associated with this process
- torch.cuda.manual_seed(self.seed)
-
- # ddp: print stats and update learning rate
- if self.opt["rank"] == 0:
- print(
- "Number of GPUs is",
- bcolors.OKGREEN,
- self.opt["world_size"],
- bcolors.ENDC,
- )
- # print('Boost learning rate from', bcolors.OKGREEN, self.opt['START_LEARNING_RATE'], bcolors.ENDC, 'to',
- # bcolors.OKGREEN, self.opt['START_LEARNING_RATE'] * self.opt['world_size'], bcolors.ENDC)
- print(
- "Effective batch size is increased from",
- bcolors.OKGREEN,
- self.opt["MINI_BATCH"],
- bcolors.ENDC,
- "to",
- bcolors.OKGREEN,
- self.opt["MINI_BATCH"] * self.opt["world_size"],
- bcolors.ENDC,
- )
-
- self.grad_acc_steps = 1
- if "GRADIENT_ACCUMULATE_STEP" in self.opt:
- if self.opt["rank"] == 0:
- print(
- "Gradient accumulation steps =",
- bcolors.OKGREEN,
- self.opt["GRADIENT_ACCUMULATE_STEP"],
- bcolors.ENDC,
- )
- # print('Boost learning rate from', bcolors.OKGREEN, self.opt['START_LEARNING_RATE'], bcolors.ENDC, 'to',
- # bcolors.OKGREEN, self.opt['START_LEARNING_RATE'] * self.opt['world_size'] * self.opt['GRADIENT_ACCUMULATE_STEP'], bcolors.ENDC)
- print(
- "Effective batch size =",
- bcolors.OKGREEN,
- self.opt["MINI_BATCH"]
- * self.opt["world_size"]
- * self.opt["GRADIENT_ACCUMULATE_STEP"],
- bcolors.ENDC,
- )
- self.grad_acc_steps = int(self.opt["GRADIENT_ACCUMULATE_STEP"])
- # self.opt['START_LEARNING_RATE'] *= self.opt['world_size'] * self.grad_acc_steps
-
- def tb_log_scalar(self, name, value, step):
- if self.opt["rank"] == 0:
- if self.tb_writer is None:
- self.tb_writer = SummaryWriter(
- os.path.join(self.saveFolder, "tensorboard")
- )
- self.tb_writer.add_scalar(name, value, step)
-
- def log(self, s):
- # When 'OFFICIAL' flag is set in the config file, the program does not output logs
- if self.is_official:
- return
- try:
- if self.logFileHandle is None:
- self.logFileHandle = open(
- os.path.join(self.saveFolder, self.opt["logFile"]), "a"
- )
- self.logFileHandle.write(s + "\n")
- except Exception as e:
- print("ERROR while writing log file:", e)
- print(s)
-
- def getSaveFolder(self):
- runid = 1
- while True:
- saveFolder = os.path.join(
- self.opt["datadir"],
- self.opt["basename"] + "_conf~",
- "run_" + str(runid),
- )
- if not os.path.isdir(saveFolder):
- if self.opt["world_size"] > 1:
- torch.distributed.barrier()
- if self.opt["rank"] == 0:
- os.makedirs(saveFolder)
- self.saveFolder = saveFolder
- if self.opt["world_size"] > 1:
- torch.distributed.barrier()
- print(
- "Saving logs, model, checkpoint, and evaluation in "
- + self.saveFolder
- )
- return
- runid = runid + 1
-
- def saveConf(self):
- if self.opt["rank"] == 0:
- super().saveConf()
diff --git a/spaces/akhaliq/VQMIVC/ParallelWaveGAN/egs/libritts/voc1/path.sh b/spaces/akhaliq/VQMIVC/ParallelWaveGAN/egs/libritts/voc1/path.sh
deleted file mode 100644
index b0ca27c615f70aa29e240222ec370f8ad4e7b45a..0000000000000000000000000000000000000000
--- a/spaces/akhaliq/VQMIVC/ParallelWaveGAN/egs/libritts/voc1/path.sh
+++ /dev/null
@@ -1,33 +0,0 @@
-# cuda related
-export CUDA_HOME=/usr/local/cuda-10.0
-export LD_LIBRARY_PATH="${CUDA_HOME}/lib64:${LD_LIBRARY_PATH}"
-
-# path related
-export PRJ_ROOT="${PWD}/../../.."
-if [ -e "${PRJ_ROOT}/tools/venv/bin/activate" ]; then
- # shellcheck disable=SC1090
- . "${PRJ_ROOT}/tools/venv/bin/activate"
-fi
-
-# python related
-export OMP_NUM_THREADS=1
-export PYTHONIOENCODING=UTF-8
-export MPL_BACKEND=Agg
-
-# check installation
-if ! command -v parallel-wavegan-train > /dev/null; then
- echo "Error: It seems setup is not finished." >&2
- echo "Error: Please setup your environment by following README.md" >&2
- return 1
-fi
-if ! command -v jq > /dev/null; then
- echo "Error: It seems jq is not installed." >&2
- echo "Error: Please install via \`sudo apt-get install jq\`." >&2
- echo "Error: If you do not have sudo, please download from https://stedolan.github.io/jq/download/." >&2
- return 1
-fi
-if ! command -v yq > /dev/null; then
- echo "Error: It seems yq is not installed." >&2
- echo "Error: Please install via \`pip install yq\`." >&2
- return 1
-fi
diff --git a/spaces/akhaliq/deeplab2/model/layers/dual_path_transformer.py b/spaces/akhaliq/deeplab2/model/layers/dual_path_transformer.py
deleted file mode 100644
index 806db522ac2ece7304d7d4fb481d85274614e580..0000000000000000000000000000000000000000
--- a/spaces/akhaliq/deeplab2/model/layers/dual_path_transformer.py
+++ /dev/null
@@ -1,488 +0,0 @@
-# coding=utf-8
-# Copyright 2021 The Deeplab2 Authors.
-#
-# Licensed under the Apache License, Version 2.0 (the "License");
-# you may not use this file except in compliance with the License.
-# You may obtain a copy of the License at
-#
-# http://www.apache.org/licenses/LICENSE-2.0
-#
-# Unless required by applicable law or agreed to in writing, software
-# distributed under the License is distributed on an "AS IS" BASIS,
-# WITHOUT WARRANTIES OR CONDITIONS OF ANY KIND, either express or implied.
-# See the License for the specific language governing permissions and
-# limitations under the License.
-
-"""Implements dual path transformer layers proposed in MaX-DeepLab [1].
-
-Dual-path transformer introduces a global memory path in addition to a CNN path,
-allowing bi-directional communication with any CNN layers.
-
-[1] MaX-DeepLab: End-to-End Panoptic Segmentation with Mask Transformers,
- CVPR 2021.
- Huiyu Wang, Yukun Zhu, Hartwig Adam, Alan Yuille, Liang-Chieh Chen.
-"""
-
-import tensorflow as tf
-
-from deeplab2.model import utils
-from deeplab2.model.layers import activations
-from deeplab2.model.layers import convolutions
-
-
-class AttentionOperation(tf.keras.layers.Layer):
- """Computes standard 1D multi-head attention with query, key, and value."""
-
- def __init__(self,
- name,
- activation,
- transformer_activation,
- bn_layer=tf.keras.layers.BatchNormalization):
- """Initializes an AttentionOperation layer.
-
- Args:
- name: A string, the name of this layer.
- activation: A string, type of activation function to apply.
- transformer_activation: A string, type of activation function for
- self-attention. Support 'sigmoid' and 'softmax'.
- bn_layer: An optional tf.keras.layers.Layer that computes the
- normalization (default: tf.keras.layers.BatchNormalization).
- """
- super(AttentionOperation, self).__init__(name=name)
- # batch_norm_similarity has shape [batch, num_heads, num_query, num_key],
- # where num_query and num_key usually equals to height or width or length,
- # i.e., spatial dimensions, so batch norm is applied to axis=1 only.
- self._batch_norm_similarity = bn_layer(axis=1, name='batch_norm_similarity')
- # batch_norm_retrieved_value is done on shape [batch, num_heads, length,
- # value_channels], which will be reshaped to the output shape [batch,
- # length, value_channels * num_heads], so we apply batch norm on the
- # effective channel dimension -- value_channels * num_heads.
- self._batch_norm_retrieved_value = bn_layer(
- axis=[1, 3], name='batch_norm_retrieved_value')
- self._activation_fn = activations.get_activation(activation)
- self._transformer_activation_fn = activations.get_activation(
- transformer_activation)
-
- def call(self, inputs, training=False):
- """Performs an AttentionOperation.
-
- Args:
- inputs: A tuple of (query, key, value), where query is [batch, num_head,
- query_length, channels] tensor, key is a [batch, num_head, key_length,
- channels] tensor, and value is a [batch, key_length, num_head,
- value_channels] tensor.
- training: A boolean, whether the model is in training mode.
-
- Returns:
- output: A [batch, query_length, num_head * value_channels] tensor, the
- retrieved value.
- """
- # Decode query, key, and value from inputs.
- query, key, value = inputs
- # Compute attention similarity.
- similarity_logits = tf.einsum('bhld,bhmd->bhlm', query, key)
- similarity_logits = self._batch_norm_similarity(
- similarity_logits, training=training)
- # Apply a transformer attention activation function, e.g. softmax.
- attention_weights = self._transformer_activation_fn(similarity_logits)
- # Retrieve the value content.
- retrieved_value = tf.einsum(
- 'bhlm,bmhd->bhld', attention_weights, value)
- retrieved_value = self._batch_norm_retrieved_value(
- retrieved_value, training=training)
- retrieved_value = self._activation_fn(retrieved_value)
- # Reshape the output.
- return utils.transpose_and_reshape_for_attention_operation(
- retrieved_value)
-
-
-class DualPathTransformerLayer(tf.keras.layers.Layer):
- """Applies a dual path transformer layer, as proposed in MaX-DeepLab [1].
-
- Dual-path transformer layer takes a pixel space input and a memory space
- input, and performs memory2pixel attention, pixel2memory attention, and
- memory2memory self-attention. Note that the pixel2pixel self-attention or
- convolution in the pixel space is implemented in axial_layers.py and
- axial_blocks.py. Thus, the pixel2pixel operation is not included in this
- DualPathTransformerLayer implementation. Please use this class together with
- a residual block with axial-attention, global-attention, or convolution in
- order to construct the full dual path transformer in the paper.
-
- [1] MaX-DeepLab: End-to-End Panoptic Segmentation with Mask Transformers,
- CVPR 2021.
- Huiyu Wang, Yukun Zhu, Hartwig Adam, Alan Yuille, Liang-Chieh Chen.
- """
-
- def __init__(self,
- name='dual_path_transformer_layer',
- activation='relu',
- filters=128,
- num_heads=8,
- bottleneck_expansion=2,
- key_expansion=1,
- value_expansion=2,
- feed_forward_network_channels=2048,
- use_memory_self_attention=True,
- use_pixel2memory_feedback_attention=True,
- transformer_activation='softmax',
- bn_layer=tf.keras.layers.BatchNormalization,
- conv_kernel_weight_decay=0.0):
- """Initializes a DualPathTransformerLayer.
-
- This function implements a dual path transformer layer between a pixel space
- and a memory space, as described in the MaX-DeepLab paper. In this dual path
- transformer, the memory2pixel cross attention and the memory self-attention
- share a single activation, e.g. softmax.
-
- Reference:
- MaX-DeepLab: "End-to-End Panoptic Segmentation with Mask Transformers",
- CVPR 2021. https://arxiv.org/abs/2012.00759
- Huiyu Wang, Yukun Zhu, Hartwig Adam, Alan Yuille, Liang-Chieh Chen.
-
- Args:
- name: A string, the name of this dual path transformer layer.
- activation: A string, type of activation function to apply.
- filters: An integer, the base number of channels for the layer.
- num_heads: An integer, the number of heads in multi-head attention.
- bottleneck_expansion: A float, the channel expansion ratio for the
- bottleneck.
- key_expansion: A float, the channel expansion ratio for keys.
- value_expansion: A float, the channel expansion ratio for values.
- feed_forward_network_channels: An integer, the number of channels for the
- feed_forward_network. Zero means no feed_forward_network will be
- applied.
- use_memory_self_attention: A boolean, whether to apply the memory space
- self-attention.
- use_pixel2memory_feedback_attention: A boolean, whether to apply the
- pixel2memory feedback attention.
- transformer_activation: A string, type of activation function for
- self-attention. Support 'sigmoid' and 'softmax'.
- bn_layer: A tf.keras.layers.Layer that computes the normalization
- (default: tf.keras.layers.BatchNormalization).
- conv_kernel_weight_decay: A float, the weight decay for convolution
- kernels.
-
- Raises:
- ValueError: If filters * key_expansion is not divisible by num_heads.
- ValueError: If filters * value_expansion is not divisible by num_heads.
- """
- super(DualPathTransformerLayer, self).__init__(name=name)
-
- bottleneck_channels = int(round(filters * bottleneck_expansion))
- total_key_depth = int(round(filters * key_expansion))
- total_value_depth = int(round(filters * value_expansion))
-
- if total_key_depth % num_heads:
- raise ValueError('Total_key_depth should be divisible by num_heads.')
-
- if total_value_depth % num_heads:
- raise ValueError('Total_value_depth should be divisible by num_heads.')
-
- # Compute query key value with one convolution and a batch norm layer. The
- # initialization std is standard transformer initialization (without batch
- # norm), as used in SASA and ViT. In our case, we use batch norm by default,
- # so it does not require careful tuning. If one wants to remove all batch
- # norms in axial attention, this standard initialization should still be
- # good, but a more careful initialization is encouraged.
- initialization_std = bottleneck_channels ** -0.5
-
- self._memory_conv1_bn_act = convolutions.Conv1D(
- bottleneck_channels, 'memory_conv1_bn_act',
- use_bias=False,
- use_bn=True,
- bn_layer=bn_layer,
- activation=activation,
- conv_kernel_weight_decay=conv_kernel_weight_decay)
-
- self._pixel_conv1_bn_act = convolutions.Conv1D(
- bottleneck_channels, 'pixel_conv1_bn_act',
- use_bias=False,
- use_bn=True,
- bn_layer=bn_layer,
- activation=activation,
- conv_kernel_weight_decay=conv_kernel_weight_decay)
-
- # We always compute the query for memory space, since it gathers information
- # from the pixel space and thus cannot be removed. We compute the key and
- # value for memory space only when they are necessary (i.e. either
- # use_memory_self_attention or use_pixel2memory_feedback_attention).
- if use_memory_self_attention or use_pixel2memory_feedback_attention:
- self._memory_qkv_conv_bn = convolutions.Conv1D(
- total_key_depth * 2 + total_value_depth, 'memory_qkv_conv_bn',
- use_bias=False,
- use_bn=True,
- bn_layer=bn_layer,
- activation='none',
- conv_kernel_weight_decay=conv_kernel_weight_decay,
- kernel_initializer=tf.keras.initializers.TruncatedNormal(
- stddev=initialization_std))
- else:
- # Compute memory query only if memory key and value are not used.
- self._memory_query_conv_bn = convolutions.Conv1D(
- total_key_depth, 'memory_query_conv_bn',
- use_bias=False,
- use_bn=True,
- bn_layer=bn_layer,
- activation='none',
- conv_kernel_weight_decay=conv_kernel_weight_decay,
- kernel_initializer=tf.keras.initializers.TruncatedNormal(
- stddev=initialization_std))
-
- # For the pixel space, we always compute the key and value, since they
- # provide information for the memory space and thus cannot be removed. We
- # compute the query for pixel space only when it is necessary (i.e.
- # use_pixel2memory_feedback_attention is True).
- if use_pixel2memory_feedback_attention:
- self._pixel_qkv_conv_bn = convolutions.Conv1D(
- total_key_depth * 2 + total_value_depth, 'pixel_qkv_conv_bn',
- use_bias=False,
- use_bn=True,
- bn_layer=bn_layer,
- activation='none',
- conv_kernel_weight_decay=conv_kernel_weight_decay,
- kernel_initializer=tf.keras.initializers.TruncatedNormal(
- stddev=initialization_std))
- else:
- self._pixel_kv_conv_bn = convolutions.Conv1D(
- total_key_depth + total_value_depth, 'pixel_kv_conv_bn',
- use_bias=False,
- use_bn=True,
- bn_layer=bn_layer,
- activation='none',
- conv_kernel_weight_decay=conv_kernel_weight_decay,
- kernel_initializer=tf.keras.initializers.TruncatedNormal(
- stddev=initialization_std))
- self._memory_attention = AttentionOperation(
- 'memory_attention', activation, transformer_activation,
- bn_layer=bn_layer)
- if use_pixel2memory_feedback_attention:
- self._pixel_attention = AttentionOperation(
- 'pixel_attention', activation, transformer_activation,
- bn_layer=bn_layer)
-
- self._use_memory_self_attention = use_memory_self_attention
- self._use_pixel2memory_feedback_attention = (
- use_pixel2memory_feedback_attention)
- self._total_key_depth = total_key_depth
- self._total_value_depth = total_value_depth
- self._num_heads = num_heads
- self._bn_layer = bn_layer
- self._conv_kernel_weight_decay = conv_kernel_weight_decay
- self._activation = activation
- self._activation_fn = activations.get_activation(activation)
- self._feed_forward_network_channels = feed_forward_network_channels
-
- def build(self, input_shape_list):
- pixel_shape, memory_shape = input_shape_list[:2]
- # Here we follow ResNet bottleneck blocks: we apply a batch norm with gamma
- # initialized at zero, followed by drop path and an activation function.
- # Initializing this gamma at zero ensures that at random initialization of
- # the model, the skip connections dominate all residual blocks. In this way,
- # all the skip connections construct an identity mapping that passes the
- # gradients (without any distortion from the randomly initialized blocks) to
- # all residual blocks. This helps training at early epochs.
- # Reference: "Accurate, Large Minibatch SGD: Training ImageNet in 1 Hour".
- # https://arxiv.org/abs/1706.02677
- self._memory_conv3_bn = convolutions.Conv1D(
- memory_shape[-1], 'memory_conv3_bn',
- use_bias=False,
- use_bn=True,
- bn_layer=self._bn_layer,
- bn_gamma_initializer='zeros',
- activation='none',
- conv_kernel_weight_decay=self._conv_kernel_weight_decay)
-
- if self._feed_forward_network_channels > 0:
- self._memory_ffn_conv1_bn_act = convolutions.Conv1D(
- self._feed_forward_network_channels, 'memory_ffn_conv1_bn_act',
- use_bias=False,
- use_bn=True,
- bn_layer=self._bn_layer,
- activation=self._activation,
- conv_kernel_weight_decay=self._conv_kernel_weight_decay)
- # Again, we follow ResNet bottleneck blocks: we apply a batch norm with
- # gamma initialized at zero, followed by drop path and an activation
- # function.
- self._memory_ffn_conv2_bn = convolutions.Conv1D(
- memory_shape[-1], 'memory_ffn_conv2_bn',
- use_bias=False,
- use_bn=True,
- bn_layer=self._bn_layer,
- bn_gamma_initializer='zeros',
- activation='none',
- conv_kernel_weight_decay=self._conv_kernel_weight_decay)
- if self._use_pixel2memory_feedback_attention:
- self._pixel_conv3_bn = convolutions.Conv1D(
- pixel_shape[-1], 'pixel_conv3_bn',
- use_bias=False,
- use_bn=True,
- bn_layer=self._bn_layer,
- bn_gamma_initializer='zeros',
- activation='none',
- conv_kernel_weight_decay=self._conv_kernel_weight_decay)
-
- def call(self, inputs):
- """Performs a forward pass.
-
- We have to define drop_path_masks outside the layer call and pass it into
- the layer call, because recompute_grad (gradient checkpointing) does not
- allow any randomness within the function call. In addition, recompute_grad
- only supports float tensors as inputs. For this reason, the training flag
- should be also passed as a float tensor. For the same reason, we cannot
- support passing drop_path_random_mask as None. Instead, we ask the users to
- pass only the first two tensors when drop path is not used.
-
- Args:
- inputs: A tuple of 3 or 6 tensors, containing
- pixel_space_input should be a [batch, num_pixel, pixel_space_channels]
- tensor.
- memory_space_input should be a [batch, num_memory,
- memory_space_channels] tensor.
- float_tensor_training should be a float tensor of 0.0 or 1.0, whether
- the model is in training mode.
- (optional) pixel_space_drop_path_mask is a drop path mask tensor of
- shape [batch, 1, 1] for the pixel space.
- (optional) memory_space_attention_drop_path_mask is a drop path mask
- tensor of shape [batch, 1, 1] for the memory space.
- (optional) memory_space_feed_forward_network_drop_path_mask is a drop
- path mask tensor of shape [batch, 1, 1] for the memory space feed
- forward network.
-
- Returns:
- pixel_space_output: A [batch, num_pixel, pixel_space_channels] tensor.
- activated_pixel_space_output: A [batch, num_pixel, pixel_space_channels]
- tensor, activated pixel_space_output.
- memory_space_output: A [batch, num_memory, memory_space_channels]
- tensor.
-
- Raises:
- ValueError: If the length of inputs is not 3 or 6.
- """
- if len(inputs) not in (3, 6):
- raise ValueError('The length of inputs should be either 3 or 6.')
-
- # Unpack the inputs.
- (pixel_space_input, memory_space_input, float_tensor_training,
- pixel_space_drop_path_mask, memory_space_attention_drop_path_mask,
- memory_space_feed_forward_network_drop_path_mask) = (
- utils.pad_sequence_with_none(inputs, target_length=6))
-
- # Recompute_grad takes only float tensors as inputs. It does not allow
- # bools or boolean tensors. For this reason, we cast training to a float
- # tensor outside this call, and now we cast it back to a boolean tensor.
- training = tf.cast(float_tensor_training, tf.bool)
-
- # Decode the inputs shapes.
- pixel_shape = pixel_space_input.get_shape().as_list()
- memory_shape = memory_space_input.get_shape().as_list()
-
- # Similar to the ResNet bottleneck design, we do an input down projection
- # in both the pixel space and the memory space.
- memory_space = self._memory_conv1_bn_act(memory_space_input,
- training=training)
-
- # Pixel space input is not activated.
- pixel_space = self._pixel_conv1_bn_act(
- self._activation_fn(pixel_space_input), training=training)
-
- if (self._use_memory_self_attention or
- self._use_pixel2memory_feedback_attention):
- memory_space_qkv = self._memory_qkv_conv_bn(memory_space,
- training=training)
- # Split, reshape, and transpose the query, key, and value.
- memory_query, memory_key, memory_value = (
- tf.split(memory_space_qkv, [
- self._total_key_depth, self._total_key_depth,
- self._total_value_depth], axis=-1))
- memory_key = utils.reshape_and_transpose_for_attention_operation(
- memory_key, self._num_heads)
- memory_value = tf.reshape(memory_value, [
- -1, memory_shape[1], self._num_heads,
- self._total_value_depth // self._num_heads])
- else:
- # Compute memory query only if memory key and value are not used.
- memory_query = self._memory_query_conv_bn(memory_space,
- training=training)
- # Reshape and transpose the query.
- memory_query = utils.reshape_and_transpose_for_attention_operation(
- memory_query, self._num_heads)
-
- if self._use_pixel2memory_feedback_attention:
- pixel_space_qkv = self._pixel_qkv_conv_bn(pixel_space,
- training=training)
- # Split the query, key, and value.
- pixel_query, pixel_key, pixel_value = tf.split(
- pixel_space_qkv, [
- self._total_key_depth, self._total_key_depth,
- self._total_value_depth], axis=-1)
- pixel_query = utils.reshape_and_transpose_for_attention_operation(
- pixel_query, self._num_heads)
- else:
- pixel_space_kv = self._pixel_kv_conv_bn(pixel_space, training=training)
- # Split the key and the value.
- pixel_key, pixel_value = tf.split(pixel_space_kv, [
- self._total_key_depth, self._total_value_depth], axis=-1)
- # Reshape and transpose the key and the value.
- pixel_key = utils.reshape_and_transpose_for_attention_operation(
- pixel_key, self._num_heads)
- pixel_value = tf.reshape(pixel_value, [
- -1, pixel_shape[1], self._num_heads,
- self._total_value_depth // self._num_heads])
-
- # Compute memory space attention.
- if not self._use_memory_self_attention:
- # If memory self attention is not used, then only memory2pixel cross
- # attention is used for the memory space. In this case, the key and the
- # value are simply pixel_key and pixel_value.
- memory_attention_key = pixel_key
- memory_attention_value = pixel_value
- else:
- # If we also use memory self attention, the key and the value are the
- # concatenation of keys and values in both the pixel space and the
- # memory space.
- memory_attention_key = tf.concat([pixel_key, memory_key], axis=2)
- memory_attention_value = tf.concat([pixel_value, memory_value], axis=1)
-
- memory_space = self._memory_attention(
- (memory_query, memory_attention_key, memory_attention_value),
- training=training)
- memory_space = self._memory_conv3_bn(memory_space, training=training)
-
- if memory_space_attention_drop_path_mask is not None:
- memory_space = memory_space * memory_space_attention_drop_path_mask
- memory_space_output = self._activation_fn(
- memory_space_input + memory_space)
-
- # Apply an optional feed-forward network to the memory space.
- if self._feed_forward_network_channels > 0:
- memory_space = self._memory_ffn_conv1_bn_act(memory_space_output,
- training=training)
- memory_space = self._memory_ffn_conv2_bn(memory_space,
- training=training)
- if memory_space_feed_forward_network_drop_path_mask is not None:
- memory_space = (memory_space *
- memory_space_feed_forward_network_drop_path_mask)
- memory_space_output = self._activation_fn(
- memory_space_output + memory_space)
-
- # Compute pixel space attention and the output projection only when
- # pixel2memory_feedback_attention is used.
- if self._use_pixel2memory_feedback_attention:
- pixel_space = self._pixel_attention(
- (pixel_query, memory_key, memory_value), training=training)
- pixel_space = self._pixel_conv3_bn(pixel_space, training=training)
- if pixel_space_drop_path_mask is not None:
- pixel_space = pixel_space * pixel_space_drop_path_mask
- pixel_space_output = pixel_space_input + pixel_space
- else:
- # If pixel2memory_feedback_attention is not used, the pixel_space_input
- # is not changed.
- pixel_space_output = pixel_space_input
- activated_pixel_space_output = self._activation_fn(pixel_space_output)
-
- # Return the pixel space output and memory space output. Note that we
- # return pixel sapce output with and without the activation function,
- # because our decoder might use non-activated features.
- return (pixel_space_output,
- activated_pixel_space_output,
- memory_space_output)
diff --git a/spaces/akhaliq/deeplab2/model/utils_test.py b/spaces/akhaliq/deeplab2/model/utils_test.py
deleted file mode 100644
index 1f3848148a8d5eb447c15ae45b5d883d240b6a8f..0000000000000000000000000000000000000000
--- a/spaces/akhaliq/deeplab2/model/utils_test.py
+++ /dev/null
@@ -1,201 +0,0 @@
-# coding=utf-8
-# Copyright 2021 The Deeplab2 Authors.
-#
-# Licensed under the Apache License, Version 2.0 (the "License");
-# you may not use this file except in compliance with the License.
-# You may obtain a copy of the License at
-#
-# http://www.apache.org/licenses/LICENSE-2.0
-#
-# Unless required by applicable law or agreed to in writing, software
-# distributed under the License is distributed on an "AS IS" BASIS,
-# WITHOUT WARRANTIES OR CONDITIONS OF ANY KIND, either express or implied.
-# See the License for the specific language governing permissions and
-# limitations under the License.
-
-"""Tests for utils."""
-
-import itertools
-
-import numpy as np
-import tensorflow as tf
-
-from deeplab2.model import utils
-
-
-class UtilsTest(tf.test.TestCase):
-
- def test_resize_logits_graph_mode(self):
- @tf.function
- def graph_mode_wrapper(*args):
- return utils.resize_and_rescale_offsets(*args)
-
- resized_logits = graph_mode_wrapper(tf.ones((2, 33, 33, 2)), [65, 65])
- resized_logits_2 = graph_mode_wrapper(tf.ones((2, 33, 33, 2)), [33, 33])
- self.assertListEqual(resized_logits.shape.as_list(), [2, 65, 65, 2])
- self.assertListEqual(resized_logits_2.shape.as_list(), [2, 33, 33, 2])
-
- def test_resize_logits(self):
- offset_logits = tf.convert_to_tensor([[[[2, 2], [2, 1], [2, 0]],
- [[1, 2], [1, 1], [1, 0]],
- [[0, 2], [0, 1], [0, 0]]]],
- dtype=tf.float32)
- target_size = [5, 5]
- resized_logits = utils.resize_and_rescale_offsets(offset_logits,
- target_size)
-
- self.assertListEqual(resized_logits.shape.as_list(), [1, 5, 5, 2])
- for i in range(5):
- for j in range(5):
- np.testing.assert_array_almost_equal(resized_logits.numpy()[0, i, j, :],
- [4 - i, 4 - j])
-
- def test_zero_padding(self):
- input_tensor = tf.ones(shape=(2, 5, 5, 2))
- input_tensor_2 = tf.ones(shape=(5, 5, 2))
- padded_tensor = utils.add_zero_padding(input_tensor, kernel_size=5, rank=4)
- padded_tensor_2 = utils.add_zero_padding(
- input_tensor_2, kernel_size=5, rank=3)
-
- self.assertEqual(tf.reduce_sum(padded_tensor), 100)
- self.assertEqual(tf.reduce_sum(padded_tensor_2), 50)
- self.assertListEqual(padded_tensor.shape.as_list(), [2, 9, 9, 2])
- self.assertListEqual(padded_tensor_2.shape.as_list(), [9, 9, 2])
- # Count zero elements.
- self.assertEqual(tf.reduce_sum(padded_tensor-1), -224)
- self.assertEqual(tf.reduce_sum(padded_tensor_2-1), -112)
-
- def test_resize_function_error(self):
- input_tensor = tf.random.uniform(shape=(2, 10, 10, 2))
- with self.assertRaises(ValueError):
- _ = utils.resize_align_corners(input_tensor, [19, 19],
- method='not_a_valid_method')
-
- def test_resize_function_shape(self):
- input_tensor = tf.random.uniform(shape=(2, 10, 10, 2))
- result_tensor = utils.resize_align_corners(input_tensor, [19, 19])
-
- self.assertListEqual(result_tensor.shape.as_list(), [2, 19, 19, 2])
-
- def test_resize_graph_mode(self):
- @tf.function
- def graph_mode_wrapper(*args):
- return utils.resize_align_corners(*args)
-
- result_tensor = graph_mode_wrapper(tf.ones((2, 33, 33, 2)), [65, 65])
- result_tensor_2 = graph_mode_wrapper(tf.ones((2, 33, 33, 2)), [33, 33])
- self.assertListEqual(result_tensor.shape.as_list(), [2, 65, 65, 2])
- self.assertListEqual(result_tensor_2.shape.as_list(), [2, 33, 33, 2])
-
- def test_resize_function_constant_input(self):
- input_tensor = tf.ones(shape=(2, 10, 10, 2))
- result_tensor = utils.resize_align_corners(input_tensor, [19, 19])
-
- self.assertTrue(tf.keras.backend.all(result_tensor == 1))
-
- def test_resize_function_invalid_rank(self):
- input_tensor = tf.keras.Input(shape=(None, 2))
- with self.assertRaisesRegex(
- ValueError, 'should have rank of 4'):
- _ = utils.resize_align_corners(input_tensor, [19, 19])
-
- def test_resize_function_v1_compatibility(self):
- # Test for odd and even input, and output shapes.
- input_shapes = [(2, 10, 10, 3), (2, 11, 11, 3)]
- target_sizes = [[19, 19], [20, 20]]
- methods = ['bilinear', 'nearest']
-
- for shape, target_size, method in itertools.product(input_shapes,
- target_sizes, methods):
- input_tensor = tf.random.uniform(shape=shape)
-
- result_tensor = utils.resize_align_corners(input_tensor, target_size,
- method)
- if method == 'bilinear':
- expected_tensor = tf.compat.v1.image.resize(
- input_tensor,
- target_size,
- align_corners=True,
- method=tf.compat.v1.image.ResizeMethod.BILINEAR)
- else:
- expected_tensor = tf.compat.v1.image.resize(
- input_tensor,
- target_size,
- align_corners=True,
- method=tf.compat.v1.image.ResizeMethod.NEAREST_NEIGHBOR)
-
- np.testing.assert_equal(result_tensor.numpy(), expected_tensor.numpy())
-
- def test_resize_bilinear_v1_compatibility(self):
- # Test for odd and even input, and output shapes.
- input_shapes = [(2, 10, 10, 3), (2, 11, 11, 3), (1, 11, 11, 64)]
- target_sizes = [[19, 19], [20, 20], [10, 10]]
-
- for shape, target_size in itertools.product(input_shapes, target_sizes):
- input_tensor = tf.random.uniform(shape=shape)
- result_tensor = utils.resize_bilinear(input_tensor, target_size)
- expected_tensor = tf.compat.v1.image.resize(
- input_tensor,
- target_size,
- align_corners=True,
- method=tf.compat.v1.image.ResizeMethod.BILINEAR)
- self.assertAllClose(result_tensor, expected_tensor)
-
- def test_make_divisible(self):
- value, divisor, min_value = 17, 2, 8
- new_value = utils.make_divisible(value, divisor, min_value)
- self.assertAllEqual(new_value, 18)
-
- value, divisor, min_value = 17, 2, 22
- new_value = utils.make_divisible(value, divisor, min_value)
- self.assertAllEqual(new_value, 22)
-
- def test_transpose_and_reshape_for_attention_operation(self):
- images = tf.zeros([2, 8, 11, 2])
- output = utils.transpose_and_reshape_for_attention_operation(images)
- self.assertEqual(output.get_shape().as_list(), [2, 11, 16])
-
- def test_reshape_and_transpose_for_attention_operation(self):
- images = tf.zeros([2, 11, 16])
- output = utils.reshape_and_transpose_for_attention_operation(images,
- num_heads=8)
- self.assertEqual(output.get_shape().as_list(), [2, 8, 11, 2])
-
- def test_safe_setattr_raise_error(self):
- layer = tf.keras.layers.Conv2D(1, 1)
- with self.assertRaises(ValueError):
- utils.safe_setattr(layer, 'filters', 3)
-
- utils.safe_setattr(layer, 'another_conv', tf.keras.layers.Conv2D(1, 1))
- with self.assertRaises(ValueError):
- utils.safe_setattr(layer, 'another_conv', tf.keras.layers.Conv2D(1, 1))
-
- def test_pad_sequence_with_none(self):
- sequence = [1, 2]
- output_2 = utils.pad_sequence_with_none(sequence, target_length=2)
- self.assertEqual(output_2, [1, 2])
- output_3 = utils.pad_sequence_with_none(sequence, target_length=3)
- self.assertEqual(output_3, [1, 2, None])
-
- def test_strided_downsample(self):
- inputs = tf.zeros([2, 11, 11])
- output = utils.strided_downsample(inputs, target_size=[6, 6])
- self.assertEqual(output.get_shape().as_list(), [2, 6, 6])
-
- def test_get_stuff_class_ids(self):
- # num_thing_stuff_classes does not include `void` class.
- num_thing_stuff_classes = 5
- thing_class_ids = [3, 4]
- void_label_list = [5, 0]
- expected_stuff_class_ids_list = [
- [0, 1, 2], [1, 2, 5]
- ]
- for void_label, expected_stuff_class_ids in zip(
- void_label_list, expected_stuff_class_ids_list):
- stuff_class_ids = utils.get_stuff_class_ids(
- num_thing_stuff_classes, thing_class_ids, void_label)
- np.testing.assert_equal(stuff_class_ids,
- expected_stuff_class_ids)
-
-if __name__ == '__main__':
- tf.test.main()
diff --git a/spaces/akhaliq/lama/saicinpainting/evaluation/losses/fid/inception.py b/spaces/akhaliq/lama/saicinpainting/evaluation/losses/fid/inception.py
deleted file mode 100644
index e9bd0863b457aaa40c770eaa4acbb142b18fc18b..0000000000000000000000000000000000000000
--- a/spaces/akhaliq/lama/saicinpainting/evaluation/losses/fid/inception.py
+++ /dev/null
@@ -1,323 +0,0 @@
-import logging
-
-import torch
-import torch.nn as nn
-import torch.nn.functional as F
-from torchvision import models
-
-try:
- from torchvision.models.utils import load_state_dict_from_url
-except ImportError:
- from torch.utils.model_zoo import load_url as load_state_dict_from_url
-
-# Inception weights ported to Pytorch from
-# http://download.tensorflow.org/models/image/imagenet/inception-2015-12-05.tgz
-FID_WEIGHTS_URL = 'https://github.com/mseitzer/pytorch-fid/releases/download/fid_weights/pt_inception-2015-12-05-6726825d.pth'
-
-
-LOGGER = logging.getLogger(__name__)
-
-
-class InceptionV3(nn.Module):
- """Pretrained InceptionV3 network returning feature maps"""
-
- # Index of default block of inception to return,
- # corresponds to output of final average pooling
- DEFAULT_BLOCK_INDEX = 3
-
- # Maps feature dimensionality to their output blocks indices
- BLOCK_INDEX_BY_DIM = {
- 64: 0, # First max pooling features
- 192: 1, # Second max pooling featurs
- 768: 2, # Pre-aux classifier features
- 2048: 3 # Final average pooling features
- }
-
- def __init__(self,
- output_blocks=[DEFAULT_BLOCK_INDEX],
- resize_input=True,
- normalize_input=True,
- requires_grad=False,
- use_fid_inception=True):
- """Build pretrained InceptionV3
-
- Parameters
- ----------
- output_blocks : list of int
- Indices of blocks to return features of. Possible values are:
- - 0: corresponds to output of first max pooling
- - 1: corresponds to output of second max pooling
- - 2: corresponds to output which is fed to aux classifier
- - 3: corresponds to output of final average pooling
- resize_input : bool
- If true, bilinearly resizes input to width and height 299 before
- feeding input to model. As the network without fully connected
- layers is fully convolutional, it should be able to handle inputs
- of arbitrary size, so resizing might not be strictly needed
- normalize_input : bool
- If true, scales the input from range (0, 1) to the range the
- pretrained Inception network expects, namely (-1, 1)
- requires_grad : bool
- If true, parameters of the model require gradients. Possibly useful
- for finetuning the network
- use_fid_inception : bool
- If true, uses the pretrained Inception model used in Tensorflow's
- FID implementation. If false, uses the pretrained Inception model
- available in torchvision. The FID Inception model has different
- weights and a slightly different structure from torchvision's
- Inception model. If you want to compute FID scores, you are
- strongly advised to set this parameter to true to get comparable
- results.
- """
- super(InceptionV3, self).__init__()
-
- self.resize_input = resize_input
- self.normalize_input = normalize_input
- self.output_blocks = sorted(output_blocks)
- self.last_needed_block = max(output_blocks)
-
- assert self.last_needed_block <= 3, \
- 'Last possible output block index is 3'
-
- self.blocks = nn.ModuleList()
-
- if use_fid_inception:
- inception = fid_inception_v3()
- else:
- inception = models.inception_v3(pretrained=True)
-
- # Block 0: input to maxpool1
- block0 = [
- inception.Conv2d_1a_3x3,
- inception.Conv2d_2a_3x3,
- inception.Conv2d_2b_3x3,
- nn.MaxPool2d(kernel_size=3, stride=2)
- ]
- self.blocks.append(nn.Sequential(*block0))
-
- # Block 1: maxpool1 to maxpool2
- if self.last_needed_block >= 1:
- block1 = [
- inception.Conv2d_3b_1x1,
- inception.Conv2d_4a_3x3,
- nn.MaxPool2d(kernel_size=3, stride=2)
- ]
- self.blocks.append(nn.Sequential(*block1))
-
- # Block 2: maxpool2 to aux classifier
- if self.last_needed_block >= 2:
- block2 = [
- inception.Mixed_5b,
- inception.Mixed_5c,
- inception.Mixed_5d,
- inception.Mixed_6a,
- inception.Mixed_6b,
- inception.Mixed_6c,
- inception.Mixed_6d,
- inception.Mixed_6e,
- ]
- self.blocks.append(nn.Sequential(*block2))
-
- # Block 3: aux classifier to final avgpool
- if self.last_needed_block >= 3:
- block3 = [
- inception.Mixed_7a,
- inception.Mixed_7b,
- inception.Mixed_7c,
- nn.AdaptiveAvgPool2d(output_size=(1, 1))
- ]
- self.blocks.append(nn.Sequential(*block3))
-
- for param in self.parameters():
- param.requires_grad = requires_grad
-
- def forward(self, inp):
- """Get Inception feature maps
-
- Parameters
- ----------
- inp : torch.autograd.Variable
- Input tensor of shape Bx3xHxW. Values are expected to be in
- range (0, 1)
-
- Returns
- -------
- List of torch.autograd.Variable, corresponding to the selected output
- block, sorted ascending by index
- """
- outp = []
- x = inp
-
- if self.resize_input:
- x = F.interpolate(x,
- size=(299, 299),
- mode='bilinear',
- align_corners=False)
-
- if self.normalize_input:
- x = 2 * x - 1 # Scale from range (0, 1) to range (-1, 1)
-
- for idx, block in enumerate(self.blocks):
- x = block(x)
- if idx in self.output_blocks:
- outp.append(x)
-
- if idx == self.last_needed_block:
- break
-
- return outp
-
-
-def fid_inception_v3():
- """Build pretrained Inception model for FID computation
-
- The Inception model for FID computation uses a different set of weights
- and has a slightly different structure than torchvision's Inception.
-
- This method first constructs torchvision's Inception and then patches the
- necessary parts that are different in the FID Inception model.
- """
- LOGGER.info('fid_inception_v3 called')
- inception = models.inception_v3(num_classes=1008,
- aux_logits=False,
- pretrained=False)
- LOGGER.info('models.inception_v3 done')
- inception.Mixed_5b = FIDInceptionA(192, pool_features=32)
- inception.Mixed_5c = FIDInceptionA(256, pool_features=64)
- inception.Mixed_5d = FIDInceptionA(288, pool_features=64)
- inception.Mixed_6b = FIDInceptionC(768, channels_7x7=128)
- inception.Mixed_6c = FIDInceptionC(768, channels_7x7=160)
- inception.Mixed_6d = FIDInceptionC(768, channels_7x7=160)
- inception.Mixed_6e = FIDInceptionC(768, channels_7x7=192)
- inception.Mixed_7b = FIDInceptionE_1(1280)
- inception.Mixed_7c = FIDInceptionE_2(2048)
-
- LOGGER.info('fid_inception_v3 patching done')
-
- state_dict = load_state_dict_from_url(FID_WEIGHTS_URL, progress=True)
- LOGGER.info('fid_inception_v3 weights downloaded')
-
- inception.load_state_dict(state_dict)
- LOGGER.info('fid_inception_v3 weights loaded into model')
-
- return inception
-
-
-class FIDInceptionA(models.inception.InceptionA):
- """InceptionA block patched for FID computation"""
- def __init__(self, in_channels, pool_features):
- super(FIDInceptionA, self).__init__(in_channels, pool_features)
-
- def forward(self, x):
- branch1x1 = self.branch1x1(x)
-
- branch5x5 = self.branch5x5_1(x)
- branch5x5 = self.branch5x5_2(branch5x5)
-
- branch3x3dbl = self.branch3x3dbl_1(x)
- branch3x3dbl = self.branch3x3dbl_2(branch3x3dbl)
- branch3x3dbl = self.branch3x3dbl_3(branch3x3dbl)
-
- # Patch: Tensorflow's average pool does not use the padded zero's in
- # its average calculation
- branch_pool = F.avg_pool2d(x, kernel_size=3, stride=1, padding=1,
- count_include_pad=False)
- branch_pool = self.branch_pool(branch_pool)
-
- outputs = [branch1x1, branch5x5, branch3x3dbl, branch_pool]
- return torch.cat(outputs, 1)
-
-
-class FIDInceptionC(models.inception.InceptionC):
- """InceptionC block patched for FID computation"""
- def __init__(self, in_channels, channels_7x7):
- super(FIDInceptionC, self).__init__(in_channels, channels_7x7)
-
- def forward(self, x):
- branch1x1 = self.branch1x1(x)
-
- branch7x7 = self.branch7x7_1(x)
- branch7x7 = self.branch7x7_2(branch7x7)
- branch7x7 = self.branch7x7_3(branch7x7)
-
- branch7x7dbl = self.branch7x7dbl_1(x)
- branch7x7dbl = self.branch7x7dbl_2(branch7x7dbl)
- branch7x7dbl = self.branch7x7dbl_3(branch7x7dbl)
- branch7x7dbl = self.branch7x7dbl_4(branch7x7dbl)
- branch7x7dbl = self.branch7x7dbl_5(branch7x7dbl)
-
- # Patch: Tensorflow's average pool does not use the padded zero's in
- # its average calculation
- branch_pool = F.avg_pool2d(x, kernel_size=3, stride=1, padding=1,
- count_include_pad=False)
- branch_pool = self.branch_pool(branch_pool)
-
- outputs = [branch1x1, branch7x7, branch7x7dbl, branch_pool]
- return torch.cat(outputs, 1)
-
-
-class FIDInceptionE_1(models.inception.InceptionE):
- """First InceptionE block patched for FID computation"""
- def __init__(self, in_channels):
- super(FIDInceptionE_1, self).__init__(in_channels)
-
- def forward(self, x):
- branch1x1 = self.branch1x1(x)
-
- branch3x3 = self.branch3x3_1(x)
- branch3x3 = [
- self.branch3x3_2a(branch3x3),
- self.branch3x3_2b(branch3x3),
- ]
- branch3x3 = torch.cat(branch3x3, 1)
-
- branch3x3dbl = self.branch3x3dbl_1(x)
- branch3x3dbl = self.branch3x3dbl_2(branch3x3dbl)
- branch3x3dbl = [
- self.branch3x3dbl_3a(branch3x3dbl),
- self.branch3x3dbl_3b(branch3x3dbl),
- ]
- branch3x3dbl = torch.cat(branch3x3dbl, 1)
-
- # Patch: Tensorflow's average pool does not use the padded zero's in
- # its average calculation
- branch_pool = F.avg_pool2d(x, kernel_size=3, stride=1, padding=1,
- count_include_pad=False)
- branch_pool = self.branch_pool(branch_pool)
-
- outputs = [branch1x1, branch3x3, branch3x3dbl, branch_pool]
- return torch.cat(outputs, 1)
-
-
-class FIDInceptionE_2(models.inception.InceptionE):
- """Second InceptionE block patched for FID computation"""
- def __init__(self, in_channels):
- super(FIDInceptionE_2, self).__init__(in_channels)
-
- def forward(self, x):
- branch1x1 = self.branch1x1(x)
-
- branch3x3 = self.branch3x3_1(x)
- branch3x3 = [
- self.branch3x3_2a(branch3x3),
- self.branch3x3_2b(branch3x3),
- ]
- branch3x3 = torch.cat(branch3x3, 1)
-
- branch3x3dbl = self.branch3x3dbl_1(x)
- branch3x3dbl = self.branch3x3dbl_2(branch3x3dbl)
- branch3x3dbl = [
- self.branch3x3dbl_3a(branch3x3dbl),
- self.branch3x3dbl_3b(branch3x3dbl),
- ]
- branch3x3dbl = torch.cat(branch3x3dbl, 1)
-
- # Patch: The FID Inception model uses max pooling instead of average
- # pooling. This is likely an error in this specific Inception
- # implementation, as other Inception models use average pooling here
- # (which matches the description in the paper).
- branch_pool = F.max_pool2d(x, kernel_size=3, stride=1, padding=1)
- branch_pool = self.branch_pool(branch_pool)
-
- outputs = [branch1x1, branch3x3, branch3x3dbl, branch_pool]
- return torch.cat(outputs, 1)
diff --git a/spaces/akhaliq/stylegan3_clip/visualizer.py b/spaces/akhaliq/stylegan3_clip/visualizer.py
deleted file mode 100644
index 4168447d7d6ec7481fc76b889d498ac009dc5549..0000000000000000000000000000000000000000
--- a/spaces/akhaliq/stylegan3_clip/visualizer.py
+++ /dev/null
@@ -1,334 +0,0 @@
-# Copyright (c) 2021, NVIDIA CORPORATION & AFFILIATES. All rights reserved.
-#
-# NVIDIA CORPORATION and its licensors retain all intellectual property
-# and proprietary rights in and to this software, related documentation
-# and any modifications thereto. Any use, reproduction, disclosure or
-# distribution of this software and related documentation without an express
-# license agreement from NVIDIA CORPORATION is strictly prohibited.
-
-import click
-import os
-
-import multiprocessing
-import numpy as np
-import imgui
-import dnnlib
-from gui_utils import imgui_window
-from gui_utils import imgui_utils
-from gui_utils import gl_utils
-from gui_utils import text_utils
-from viz import renderer
-from viz import pickle_widget
-from viz import latent_widget
-from viz import stylemix_widget
-from viz import trunc_noise_widget
-from viz import performance_widget
-from viz import capture_widget
-from viz import layer_widget
-from viz import equivariance_widget
-
-#----------------------------------------------------------------------------
-
-class Visualizer(imgui_window.ImguiWindow):
- def __init__(self, capture_dir=None):
- super().__init__(title='GAN Visualizer', window_width=3840, window_height=2160)
-
- # Internals.
- self._last_error_print = None
- self._async_renderer = AsyncRenderer()
- self._defer_rendering = 0
- self._tex_img = None
- self._tex_obj = None
-
- # Widget interface.
- self.args = dnnlib.EasyDict()
- self.result = dnnlib.EasyDict()
- self.pane_w = 0
- self.label_w = 0
- self.button_w = 0
-
- # Widgets.
- self.pickle_widget = pickle_widget.PickleWidget(self)
- self.latent_widget = latent_widget.LatentWidget(self)
- self.stylemix_widget = stylemix_widget.StyleMixingWidget(self)
- self.trunc_noise_widget = trunc_noise_widget.TruncationNoiseWidget(self)
- self.perf_widget = performance_widget.PerformanceWidget(self)
- self.capture_widget = capture_widget.CaptureWidget(self)
- self.layer_widget = layer_widget.LayerWidget(self)
- self.eq_widget = equivariance_widget.EquivarianceWidget(self)
-
- if capture_dir is not None:
- self.capture_widget.path = capture_dir
-
- # Initialize window.
- self.set_position(0, 0)
- self._adjust_font_size()
- self.skip_frame() # Layout may change after first frame.
-
- def close(self):
- super().close()
- if self._async_renderer is not None:
- self._async_renderer.close()
- self._async_renderer = None
-
- def add_recent_pickle(self, pkl, ignore_errors=False):
- self.pickle_widget.add_recent(pkl, ignore_errors=ignore_errors)
-
- def load_pickle(self, pkl, ignore_errors=False):
- self.pickle_widget.load(pkl, ignore_errors=ignore_errors)
-
- def print_error(self, error):
- error = str(error)
- if error != self._last_error_print:
- print('\n' + error + '\n')
- self._last_error_print = error
-
- def defer_rendering(self, num_frames=1):
- self._defer_rendering = max(self._defer_rendering, num_frames)
-
- def clear_result(self):
- self._async_renderer.clear_result()
-
- def set_async(self, is_async):
- if is_async != self._async_renderer.is_async:
- self._async_renderer.set_async(is_async)
- self.clear_result()
- if 'image' in self.result:
- self.result.message = 'Switching rendering process...'
- self.defer_rendering()
-
- def _adjust_font_size(self):
- old = self.font_size
- self.set_font_size(min(self.content_width / 120, self.content_height / 60))
- if self.font_size != old:
- self.skip_frame() # Layout changed.
-
- def draw_frame(self):
- self.begin_frame()
- self.args = dnnlib.EasyDict()
- self.pane_w = self.font_size * 45
- self.button_w = self.font_size * 5
- self.label_w = round(self.font_size * 4.5)
-
- # Detect mouse dragging in the result area.
- dragging, dx, dy = imgui_utils.drag_hidden_window('##result_area', x=self.pane_w, y=0, width=self.content_width-self.pane_w, height=self.content_height)
- if dragging:
- self.latent_widget.drag(dx, dy)
-
- # Begin control pane.
- imgui.set_next_window_position(0, 0)
- imgui.set_next_window_size(self.pane_w, self.content_height)
- imgui.begin('##control_pane', closable=False, flags=(imgui.WINDOW_NO_TITLE_BAR | imgui.WINDOW_NO_RESIZE | imgui.WINDOW_NO_MOVE))
-
- # Widgets.
- expanded, _visible = imgui_utils.collapsing_header('Network & latent', default=True)
- self.pickle_widget(expanded)
- self.latent_widget(expanded)
- self.stylemix_widget(expanded)
- self.trunc_noise_widget(expanded)
- expanded, _visible = imgui_utils.collapsing_header('Performance & capture', default=True)
- self.perf_widget(expanded)
- self.capture_widget(expanded)
- expanded, _visible = imgui_utils.collapsing_header('Layers & channels', default=True)
- self.layer_widget(expanded)
- with imgui_utils.grayed_out(not self.result.get('has_input_transform', False)):
- expanded, _visible = imgui_utils.collapsing_header('Equivariance', default=True)
- self.eq_widget(expanded)
-
- # Render.
- if self.is_skipping_frames():
- pass
- elif self._defer_rendering > 0:
- self._defer_rendering -= 1
- elif self.args.pkl is not None:
- self._async_renderer.set_args(**self.args)
- result = self._async_renderer.get_result()
- if result is not None:
- self.result = result
-
- # Display.
- max_w = self.content_width - self.pane_w
- max_h = self.content_height
- pos = np.array([self.pane_w + max_w / 2, max_h / 2])
- if 'image' in self.result:
- if self._tex_img is not self.result.image:
- self._tex_img = self.result.image
- if self._tex_obj is None or not self._tex_obj.is_compatible(image=self._tex_img):
- self._tex_obj = gl_utils.Texture(image=self._tex_img, bilinear=False, mipmap=False)
- else:
- self._tex_obj.update(self._tex_img)
- zoom = min(max_w / self._tex_obj.width, max_h / self._tex_obj.height)
- zoom = np.floor(zoom) if zoom >= 1 else zoom
- self._tex_obj.draw(pos=pos, zoom=zoom, align=0.5, rint=True)
- if 'error' in self.result:
- self.print_error(self.result.error)
- if 'message' not in self.result:
- self.result.message = str(self.result.error)
- if 'message' in self.result:
- tex = text_utils.get_texture(self.result.message, size=self.font_size, max_width=max_w, max_height=max_h, outline=2)
- tex.draw(pos=pos, align=0.5, rint=True, color=1)
-
- # End frame.
- self._adjust_font_size()
- imgui.end()
- self.end_frame()
-
-#----------------------------------------------------------------------------
-
-class AsyncRenderer:
- def __init__(self):
- self._closed = False
- self._is_async = False
- self._cur_args = None
- self._cur_result = None
- self._cur_stamp = 0
- self._renderer_obj = None
- self._args_queue = None
- self._result_queue = None
- self._process = None
-
- def close(self):
- self._closed = True
- self._renderer_obj = None
- if self._process is not None:
- self._process.terminate()
- self._process = None
- self._args_queue = None
- self._result_queue = None
-
- @property
- def is_async(self):
- return self._is_async
-
- def set_async(self, is_async):
- self._is_async = is_async
-
- def set_args(self, **args):
- assert not self._closed
- if args != self._cur_args:
- if self._is_async:
- self._set_args_async(**args)
- else:
- self._set_args_sync(**args)
- self._cur_args = args
-
- def _set_args_async(self, **args):
- if self._process is None:
- self._args_queue = multiprocessing.Queue()
- self._result_queue = multiprocessing.Queue()
- try:
- multiprocessing.set_start_method('spawn')
- except RuntimeError:
- pass
- self._process = multiprocessing.Process(target=self._process_fn, args=(self._args_queue, self._result_queue), daemon=True)
- self._process.start()
- self._args_queue.put([args, self._cur_stamp])
-
- def _set_args_sync(self, **args):
- if self._renderer_obj is None:
- self._renderer_obj = renderer.Renderer()
- self._cur_result = self._renderer_obj.render(**args)
-
- def get_result(self):
- assert not self._closed
- if self._result_queue is not None:
- while self._result_queue.qsize() > 0:
- result, stamp = self._result_queue.get()
- if stamp == self._cur_stamp:
- self._cur_result = result
- return self._cur_result
-
- def clear_result(self):
- assert not self._closed
- self._cur_args = None
- self._cur_result = None
- self._cur_stamp += 1
-
- @staticmethod
- def _process_fn(args_queue, result_queue):
- renderer_obj = renderer.Renderer()
- cur_args = None
- cur_stamp = None
- while True:
- args, stamp = args_queue.get()
- while args_queue.qsize() > 0:
- args, stamp = args_queue.get()
- if args != cur_args or stamp != cur_stamp:
- result = renderer_obj.render(**args)
- if 'error' in result:
- result.error = renderer.CapturedException(result.error)
- result_queue.put([result, stamp])
- cur_args = args
- cur_stamp = stamp
-
-#----------------------------------------------------------------------------
-
-@click.command()
-@click.argument('pkls', metavar='PATH', nargs=-1)
-@click.option('--capture-dir', help='Where to save screenshot captures', metavar='PATH', default=None)
-@click.option('--browse-dir', help='Specify model path for the \'Browse...\' button', metavar='PATH')
-def main(
- pkls,
- capture_dir,
- browse_dir
-):
- """Interactive model visualizer.
-
- Optional PATH argument can be used specify which .pkl file to load.
- """
- viz = Visualizer(capture_dir=capture_dir)
-
- if browse_dir is not None:
- viz.pickle_widget.search_dirs = [browse_dir]
-
- # List pickles.
- if len(pkls) > 0:
- for pkl in pkls:
- viz.add_recent_pickle(pkl)
- viz.load_pickle(pkls[0])
- else:
- pretrained = [
- 'https://api.ngc.nvidia.com/v2/models/nvidia/research/stylegan3/versions/1/files/stylegan3-r-afhqv2-512x512.pkl',
- 'https://api.ngc.nvidia.com/v2/models/nvidia/research/stylegan3/versions/1/files/stylegan3-r-ffhq-1024x1024.pkl',
- 'https://api.ngc.nvidia.com/v2/models/nvidia/research/stylegan3/versions/1/files/stylegan3-r-ffhqu-1024x1024.pkl',
- 'https://api.ngc.nvidia.com/v2/models/nvidia/research/stylegan3/versions/1/files/stylegan3-r-ffhqu-256x256.pkl',
- 'https://api.ngc.nvidia.com/v2/models/nvidia/research/stylegan3/versions/1/files/stylegan3-r-metfaces-1024x1024.pkl',
- 'https://api.ngc.nvidia.com/v2/models/nvidia/research/stylegan3/versions/1/files/stylegan3-r-metfacesu-1024x1024.pkl',
- 'https://api.ngc.nvidia.com/v2/models/nvidia/research/stylegan3/versions/1/files/stylegan3-t-afhqv2-512x512.pkl',
- 'https://api.ngc.nvidia.com/v2/models/nvidia/research/stylegan3/versions/1/files/stylegan3-t-ffhq-1024x1024.pkl',
- 'https://api.ngc.nvidia.com/v2/models/nvidia/research/stylegan3/versions/1/files/stylegan3-t-ffhqu-1024x1024.pkl',
- 'https://api.ngc.nvidia.com/v2/models/nvidia/research/stylegan3/versions/1/files/stylegan3-t-ffhqu-256x256.pkl',
- 'https://api.ngc.nvidia.com/v2/models/nvidia/research/stylegan3/versions/1/files/stylegan3-t-metfaces-1024x1024.pkl',
- 'https://api.ngc.nvidia.com/v2/models/nvidia/research/stylegan3/versions/1/files/stylegan3-t-metfacesu-1024x1024.pkl',
- 'https://api.ngc.nvidia.com/v2/models/nvidia/research/stylegan2/versions/1/files/stylegan2-afhqcat-512x512.pkl',
- 'https://api.ngc.nvidia.com/v2/models/nvidia/research/stylegan2/versions/1/files/stylegan2-afhqdog-512x512.pkl',
- 'https://api.ngc.nvidia.com/v2/models/nvidia/research/stylegan2/versions/1/files/stylegan2-afhqv2-512x512.pkl',
- 'https://api.ngc.nvidia.com/v2/models/nvidia/research/stylegan2/versions/1/files/stylegan2-afhqwild-512x512.pkl',
- 'https://api.ngc.nvidia.com/v2/models/nvidia/research/stylegan2/versions/1/files/stylegan2-brecahad-512x512.pkl',
- 'https://api.ngc.nvidia.com/v2/models/nvidia/research/stylegan2/versions/1/files/stylegan2-celebahq-256x256.pkl',
- 'https://api.ngc.nvidia.com/v2/models/nvidia/research/stylegan2/versions/1/files/stylegan2-cifar10-32x32.pkl',
- 'https://api.ngc.nvidia.com/v2/models/nvidia/research/stylegan2/versions/1/files/stylegan2-ffhq-1024x1024.pkl',
- 'https://api.ngc.nvidia.com/v2/models/nvidia/research/stylegan2/versions/1/files/stylegan2-ffhq-256x256.pkl',
- 'https://api.ngc.nvidia.com/v2/models/nvidia/research/stylegan2/versions/1/files/stylegan2-ffhq-512x512.pkl',
- 'https://api.ngc.nvidia.com/v2/models/nvidia/research/stylegan2/versions/1/files/stylegan2-ffhqu-1024x1024.pkl',
- 'https://api.ngc.nvidia.com/v2/models/nvidia/research/stylegan2/versions/1/files/stylegan2-ffhqu-256x256.pkl',
- 'https://api.ngc.nvidia.com/v2/models/nvidia/research/stylegan2/versions/1/files/stylegan2-lsundog-256x256.pkl',
- 'https://api.ngc.nvidia.com/v2/models/nvidia/research/stylegan2/versions/1/files/stylegan2-metfaces-1024x1024.pkl',
- 'https://api.ngc.nvidia.com/v2/models/nvidia/research/stylegan2/versions/1/files/stylegan2-metfacesu-1024x1024.pkl'
- ]
-
- # Populate recent pickles list with pretrained model URLs.
- for url in pretrained:
- viz.add_recent_pickle(url)
-
- # Run.
- while not viz.should_close():
- viz.draw_frame()
- viz.close()
-
-#----------------------------------------------------------------------------
-
-if __name__ == "__main__":
- main()
-
-#----------------------------------------------------------------------------
diff --git a/spaces/alamin655/Personas/conversant/demo/__init__.py b/spaces/alamin655/Personas/conversant/demo/__init__.py
deleted file mode 100644
index e69de29bb2d1d6434b8b29ae775ad8c2e48c5391..0000000000000000000000000000000000000000
diff --git a/spaces/alexray/btc_predictor/venv/lib/python3.10/site-packages/pip/_vendor/requests/auth.py b/spaces/alexray/btc_predictor/venv/lib/python3.10/site-packages/pip/_vendor/requests/auth.py
deleted file mode 100644
index eeface39ae62c3975ff535e6b1f79f2c28fbf888..0000000000000000000000000000000000000000
--- a/spaces/alexray/btc_predictor/venv/lib/python3.10/site-packages/pip/_vendor/requests/auth.py
+++ /dev/null
@@ -1,305 +0,0 @@
-# -*- coding: utf-8 -*-
-
-"""
-requests.auth
-~~~~~~~~~~~~~
-
-This module contains the authentication handlers for Requests.
-"""
-
-import os
-import re
-import time
-import hashlib
-import threading
-import warnings
-
-from base64 import b64encode
-
-from .compat import urlparse, str, basestring
-from .cookies import extract_cookies_to_jar
-from ._internal_utils import to_native_string
-from .utils import parse_dict_header
-
-CONTENT_TYPE_FORM_URLENCODED = 'application/x-www-form-urlencoded'
-CONTENT_TYPE_MULTI_PART = 'multipart/form-data'
-
-
-def _basic_auth_str(username, password):
- """Returns a Basic Auth string."""
-
- # "I want us to put a big-ol' comment on top of it that
- # says that this behaviour is dumb but we need to preserve
- # it because people are relying on it."
- # - Lukasa
- #
- # These are here solely to maintain backwards compatibility
- # for things like ints. This will be removed in 3.0.0.
- if not isinstance(username, basestring):
- warnings.warn(
- "Non-string usernames will no longer be supported in Requests "
- "3.0.0. Please convert the object you've passed in ({!r}) to "
- "a string or bytes object in the near future to avoid "
- "problems.".format(username),
- category=DeprecationWarning,
- )
- username = str(username)
-
- if not isinstance(password, basestring):
- warnings.warn(
- "Non-string passwords will no longer be supported in Requests "
- "3.0.0. Please convert the object you've passed in ({!r}) to "
- "a string or bytes object in the near future to avoid "
- "problems.".format(type(password)),
- category=DeprecationWarning,
- )
- password = str(password)
- # -- End Removal --
-
- if isinstance(username, str):
- username = username.encode('latin1')
-
- if isinstance(password, str):
- password = password.encode('latin1')
-
- authstr = 'Basic ' + to_native_string(
- b64encode(b':'.join((username, password))).strip()
- )
-
- return authstr
-
-
-class AuthBase(object):
- """Base class that all auth implementations derive from"""
-
- def __call__(self, r):
- raise NotImplementedError('Auth hooks must be callable.')
-
-
-class HTTPBasicAuth(AuthBase):
- """Attaches HTTP Basic Authentication to the given Request object."""
-
- def __init__(self, username, password):
- self.username = username
- self.password = password
-
- def __eq__(self, other):
- return all([
- self.username == getattr(other, 'username', None),
- self.password == getattr(other, 'password', None)
- ])
-
- def __ne__(self, other):
- return not self == other
-
- def __call__(self, r):
- r.headers['Authorization'] = _basic_auth_str(self.username, self.password)
- return r
-
-
-class HTTPProxyAuth(HTTPBasicAuth):
- """Attaches HTTP Proxy Authentication to a given Request object."""
-
- def __call__(self, r):
- r.headers['Proxy-Authorization'] = _basic_auth_str(self.username, self.password)
- return r
-
-
-class HTTPDigestAuth(AuthBase):
- """Attaches HTTP Digest Authentication to the given Request object."""
-
- def __init__(self, username, password):
- self.username = username
- self.password = password
- # Keep state in per-thread local storage
- self._thread_local = threading.local()
-
- def init_per_thread_state(self):
- # Ensure state is initialized just once per-thread
- if not hasattr(self._thread_local, 'init'):
- self._thread_local.init = True
- self._thread_local.last_nonce = ''
- self._thread_local.nonce_count = 0
- self._thread_local.chal = {}
- self._thread_local.pos = None
- self._thread_local.num_401_calls = None
-
- def build_digest_header(self, method, url):
- """
- :rtype: str
- """
-
- realm = self._thread_local.chal['realm']
- nonce = self._thread_local.chal['nonce']
- qop = self._thread_local.chal.get('qop')
- algorithm = self._thread_local.chal.get('algorithm')
- opaque = self._thread_local.chal.get('opaque')
- hash_utf8 = None
-
- if algorithm is None:
- _algorithm = 'MD5'
- else:
- _algorithm = algorithm.upper()
- # lambdas assume digest modules are imported at the top level
- if _algorithm == 'MD5' or _algorithm == 'MD5-SESS':
- def md5_utf8(x):
- if isinstance(x, str):
- x = x.encode('utf-8')
- return hashlib.md5(x).hexdigest()
- hash_utf8 = md5_utf8
- elif _algorithm == 'SHA':
- def sha_utf8(x):
- if isinstance(x, str):
- x = x.encode('utf-8')
- return hashlib.sha1(x).hexdigest()
- hash_utf8 = sha_utf8
- elif _algorithm == 'SHA-256':
- def sha256_utf8(x):
- if isinstance(x, str):
- x = x.encode('utf-8')
- return hashlib.sha256(x).hexdigest()
- hash_utf8 = sha256_utf8
- elif _algorithm == 'SHA-512':
- def sha512_utf8(x):
- if isinstance(x, str):
- x = x.encode('utf-8')
- return hashlib.sha512(x).hexdigest()
- hash_utf8 = sha512_utf8
-
- KD = lambda s, d: hash_utf8("%s:%s" % (s, d))
-
- if hash_utf8 is None:
- return None
-
- # XXX not implemented yet
- entdig = None
- p_parsed = urlparse(url)
- #: path is request-uri defined in RFC 2616 which should not be empty
- path = p_parsed.path or "/"
- if p_parsed.query:
- path += '?' + p_parsed.query
-
- A1 = '%s:%s:%s' % (self.username, realm, self.password)
- A2 = '%s:%s' % (method, path)
-
- HA1 = hash_utf8(A1)
- HA2 = hash_utf8(A2)
-
- if nonce == self._thread_local.last_nonce:
- self._thread_local.nonce_count += 1
- else:
- self._thread_local.nonce_count = 1
- ncvalue = '%08x' % self._thread_local.nonce_count
- s = str(self._thread_local.nonce_count).encode('utf-8')
- s += nonce.encode('utf-8')
- s += time.ctime().encode('utf-8')
- s += os.urandom(8)
-
- cnonce = (hashlib.sha1(s).hexdigest()[:16])
- if _algorithm == 'MD5-SESS':
- HA1 = hash_utf8('%s:%s:%s' % (HA1, nonce, cnonce))
-
- if not qop:
- respdig = KD(HA1, "%s:%s" % (nonce, HA2))
- elif qop == 'auth' or 'auth' in qop.split(','):
- noncebit = "%s:%s:%s:%s:%s" % (
- nonce, ncvalue, cnonce, 'auth', HA2
- )
- respdig = KD(HA1, noncebit)
- else:
- # XXX handle auth-int.
- return None
-
- self._thread_local.last_nonce = nonce
-
- # XXX should the partial digests be encoded too?
- base = 'username="%s", realm="%s", nonce="%s", uri="%s", ' \
- 'response="%s"' % (self.username, realm, nonce, path, respdig)
- if opaque:
- base += ', opaque="%s"' % opaque
- if algorithm:
- base += ', algorithm="%s"' % algorithm
- if entdig:
- base += ', digest="%s"' % entdig
- if qop:
- base += ', qop="auth", nc=%s, cnonce="%s"' % (ncvalue, cnonce)
-
- return 'Digest %s' % (base)
-
- def handle_redirect(self, r, **kwargs):
- """Reset num_401_calls counter on redirects."""
- if r.is_redirect:
- self._thread_local.num_401_calls = 1
-
- def handle_401(self, r, **kwargs):
- """
- Takes the given response and tries digest-auth, if needed.
-
- :rtype: requests.Response
- """
-
- # If response is not 4xx, do not auth
- # See https://github.com/psf/requests/issues/3772
- if not 400 <= r.status_code < 500:
- self._thread_local.num_401_calls = 1
- return r
-
- if self._thread_local.pos is not None:
- # Rewind the file position indicator of the body to where
- # it was to resend the request.
- r.request.body.seek(self._thread_local.pos)
- s_auth = r.headers.get('www-authenticate', '')
-
- if 'digest' in s_auth.lower() and self._thread_local.num_401_calls < 2:
-
- self._thread_local.num_401_calls += 1
- pat = re.compile(r'digest ', flags=re.IGNORECASE)
- self._thread_local.chal = parse_dict_header(pat.sub('', s_auth, count=1))
-
- # Consume content and release the original connection
- # to allow our new request to reuse the same one.
- r.content
- r.close()
- prep = r.request.copy()
- extract_cookies_to_jar(prep._cookies, r.request, r.raw)
- prep.prepare_cookies(prep._cookies)
-
- prep.headers['Authorization'] = self.build_digest_header(
- prep.method, prep.url)
- _r = r.connection.send(prep, **kwargs)
- _r.history.append(r)
- _r.request = prep
-
- return _r
-
- self._thread_local.num_401_calls = 1
- return r
-
- def __call__(self, r):
- # Initialize per-thread state, if needed
- self.init_per_thread_state()
- # If we have a saved nonce, skip the 401
- if self._thread_local.last_nonce:
- r.headers['Authorization'] = self.build_digest_header(r.method, r.url)
- try:
- self._thread_local.pos = r.body.tell()
- except AttributeError:
- # In the case of HTTPDigestAuth being reused and the body of
- # the previous request was a file-like object, pos has the
- # file position of the previous body. Ensure it's set to
- # None.
- self._thread_local.pos = None
- r.register_hook('response', self.handle_401)
- r.register_hook('response', self.handle_redirect)
- self._thread_local.num_401_calls = 1
-
- return r
-
- def __eq__(self, other):
- return all([
- self.username == getattr(other, 'username', None),
- self.password == getattr(other, 'password', None)
- ])
-
- def __ne__(self, other):
- return not self == other
diff --git a/spaces/aliabd/SummerTime/model/third_party/HMNet/Evaluation/ROUGEEval.py b/spaces/aliabd/SummerTime/model/third_party/HMNet/Evaluation/ROUGEEval.py
deleted file mode 100644
index e5fb9a95319404cb2ed1d87711947599a1fb7a46..0000000000000000000000000000000000000000
--- a/spaces/aliabd/SummerTime/model/third_party/HMNet/Evaluation/ROUGEEval.py
+++ /dev/null
@@ -1,354 +0,0 @@
-# Copyright (c) Microsoft Corporation.
-# Licensed under the MIT license.
-
-import os
-import re
-import shutil
-from string import ascii_uppercase
-from tqdm.auto import tqdm
-from model.third_party.HMNet.Evaluation.OldROUGEEval import rouge
-from model.third_party.HMNet.ThirdParty.ROUGE import pyrouge
-from shutil import copyfile
-from mpi4py import MPI
-import torch
-import logging
-import json
-
-
-def write_json_res(
- output_file, tokenizers, x_ids, y_ids, x_tokens, y_tokens, predictions, gts
-):
- data = []
-
- # for x_id, y_id, x_token, y_token, preds, gt in zip(x_ids, y_ids, x_tokens, y_tokens, predictions, gts):
- # x_id = tokenizers[0].decode(x_id, skip_special_tokens=False) if x_id.dim() == 1 else tokenizers[0].convert_tokens_to_string(x_token)
- # y_id = tokenizers[1].decode(y_id, skip_special_tokens=False) if y_id.dim() == 1 else tokenizers[1].convert_tokens_to_string(y_token)
- for x_token, y_token, preds, gt in zip(x_tokens, y_tokens, predictions, gts):
- data.append(
- {
- # 'x_ids': x_id,
- # 'y_ids': y_id,
- "x_tokens": x_token if isinstance(x_token, str) else " ".join(x_token),
- "y_tokens": y_token if isinstance(y_token, str) else " ".join(y_token),
- "predictions": preds,
- "gt": gt,
- }
- )
-
- json.dump(data, output_file, indent=4, ensure_ascii=False)
-
-
-logger = logging.getLogger(__name__)
-
-"""
-This code can only be run within docker "rouge", because of the usage of rouge-perl
-"""
-
-
-"""" In ROUGE parlance, your summaries are ‘system’ summaries and the gold standard summaries are ‘model’ summaries.
-The summaries should be in separate folders, whose paths are set with the system_dir and model_dir variables.
-All summaries should contain one sentence per line."""
-
-
-class ROUGEEval:
- """
- Wrapper class for pyrouge.
- Compute ROUGE given predictions and references for summarization evaluation.
- """
-
- def __init__(self, run_dir, save_dir, opt):
- self.run_dir = run_dir
- self.save_dir = save_dir
- self.opt = opt
-
- # use relative path to make it work on Philly
- self.pyrouge_dir = os.path.join(
- os.path.dirname(__file__), "../ThirdParty/ROUGE/ROUGE-1.5.5/"
- )
-
- self.eval_batches_num = self.opt.get("EVAL_BATCHES_NUM", float("Inf"))
- self.best_score = -float("Inf")
- self.best_res = {}
-
- def reset_best_score(self, set_high=False):
- if set_high:
- self.best_score = float("Inf")
- else:
- self.best_score = -float("Inf")
-
- def make_html_safe(self, s):
- s = s.replace("<", "<")
- s = s.replace(">", ">")
- return s
-
- def print_to_rouge_dir(
- self, summaries, dir, suffix, split_chars, special_char_dict=None
- ):
- for idx, summary in enumerate(summaries):
- fname = os.path.join(dir, "%06d_%s.txt" % (idx, suffix))
- with open(fname, "wb") as f:
- sents = re.split(r"(?')
- # else:
- # new_predicitons.append(pred)
- # return new_predicitons, new_groundtruths
-
- def _convert_tokens_to_string(self, tokenizer, tokens):
- if "EVAL_TOKENIZED" in self.opt:
- tokens = [t for t in tokens if t not in tokenizer.all_special_tokens]
- if "EVAL_LOWERCASE" in self.opt:
- tokens = [t.lower() for t in tokens]
- if "EVAL_TOKENIZED" in self.opt:
- return " ".join(tokens)
- else:
- return tokenizer.decode(
- tokenizer.convert_tokens_to_ids(tokens), skip_special_tokens=True
- )
-
- def eval_batches(self, module, dev_batches, save_folder, label=""):
- max_sent_len = int(self.opt["MAX_GEN_LENGTH"])
-
- logger.info(
- "Decoding current model ... \nSaving folder is {}".format(save_folder)
- )
-
- predictions = [] # prediction of tokens from model
- x_tokens = [] # input tokens
- y_tokens = [] # groundtruths tokens
- x_ids = [] # input token ids
- y_ids = [] # groundtruths token ids
- gts = [] # groundtruths string
- got_better_score = False
- # err = 0
- if not isinstance(module.tokenizer, list):
- encoder_tokenizer = module.tokenizer
- decoder_tokenizer = module.tokenizer
- elif len(module.tokenizer) == 1:
- encoder_tokenizer = module.tokenizer[0]
- decoder_tokenizer = module.tokenizer[0]
- elif len(module.tokenizer) == 2:
- encoder_tokenizer = module.tokenizer[0]
- decoder_tokenizer = module.tokenizer[1]
- else:
- assert False, f"len(module.tokenizer) > 2"
-
- with torch.no_grad():
- for j, dev_batch in enumerate(dev_batches):
- for b in dev_batch:
- if torch.is_tensor(dev_batch[b]):
- dev_batch[b] = dev_batch[b].to(self.opt["device"])
-
- beam_search_res = module(
- dev_batch, beam_search=True, max_sent_len=max_sent_len
- )
- pred = [
- [t[0] for t in x] if len(x) > 0 else [[]] for x in beam_search_res
- ]
- predictions.extend(
- [
- [
- self._convert_tokens_to_string(decoder_tokenizer, tt)
- for tt in t
- ]
- for t in pred
- ]
- )
-
- gts.extend(
- [
- self._convert_tokens_to_string(decoder_tokenizer, t)
- for t in dev_batch["decoder_tokens"]
- ]
- )
- x_tokens.extend(dev_batch["encoder_tokens"])
- y_tokens.extend(dev_batch["decoder_tokens"])
-
- if ("DEBUG" in self.opt and j >= 10) or j >= self.eval_batches_num:
- # in debug mode (decode first 10 batches) ortherwise decode first self.eval_batches_num bathes
- break
-
- # use MPI to gather results from all processes / GPUs
- # the result of the gather operation is a list of sublists
- # each sublist corresponds to the list created on one of the MPI processes (or GPUs, respectively)
- # we flatten this list into a "simple" list
- assert len(predictions) == len(
- gts
- ), "len(predictions): {0}, len(gts): {1}".format(len(predictions), len(gts))
- comm = MPI.COMM_WORLD
- predictions = comm.gather(predictions, root=0)
- x_tokens = comm.gather(x_tokens, root=0)
- y_tokens = comm.gather(y_tokens, root=0)
- # if GPU numbers are high (>=8), passing x_ids, y_ids to a rank 0 will cause out of memory
- # x_ids = comm.gather(x_ids, root=0)
- # y_ids = comm.gather(y_ids, root=0)
- gts = comm.gather(gts, root=0)
- if self.opt["rank"] == 0:
- # flatten lists
- predictions = [item for sublist in predictions for item in sublist]
- y_tokens = [item for sublist in y_tokens for item in sublist]
- x_tokens = [item for sublist in x_tokens for item in sublist]
- # x_ids = [item for sublist in x_ids for item in sublist]
- # y_ids = [item for sublist in y_ids for item in sublist]
- gts = [item for sublist in gts for item in sublist]
- # import pdb; pdb.set_trace()
- assert (
- len(predictions) == len(y_tokens) == len(x_tokens) == len(gts)
- ), "len(predictions): {0}, len(y_tokens): {1}, len(x_tokens): {2}, len(gts): {3}".format(
- len(predictions), len(y_tokens), len(x_tokens), len(gts)
- )
-
- # write intermediate results only on rank 0
- if not os.path.isdir(os.path.join(save_folder, "intermediate_results")):
- os.makedirs(os.path.join(save_folder, "intermediate_results"))
- top_1_predictions = [pred[0] for pred in predictions]
- with open(
- os.path.join(
- save_folder, "intermediate_results", "res_" + label + ".json"
- ),
- "w",
- encoding="utf-8",
- ) as output_file:
- write_json_res(
- output_file,
- [encoder_tokenizer, decoder_tokenizer],
- x_ids,
- y_ids,
- x_tokens,
- y_tokens,
- predictions,
- gts,
- )
- try:
- result = self.eval(top_1_predictions, gts)
- except Exception as e:
- logger.exception("ROUGE Eval ERROR")
- result = {}
- score = -float("Inf")
- pass # this happens when no overlapping between pred and gts
- else:
- rouge_su4 = rouge(top_1_predictions, gts) # f, prec, recall
- result = {
- "ROUGE_1": result["rouge_1_f_score"] * 100.0,
- "ROUGE_1_Prc": result["rouge_1_precision"] * 100.0,
- "ROUGE_1_Rcl": result["rouge_1_recall"] * 100.0,
- "ROUGE_2": result["rouge_2_f_score"] * 100.0,
- "ROUGE_2_Prc": result["rouge_2_precision"] * 100.0,
- "ROUGE_2_Rcl": result["rouge_2_recall"] * 100.0,
- "ROUGE_L": result["rouge_l_f_score"] * 100.0,
- "ROUGE_L_Prc": result["rouge_l_precision"] * 100.0,
- "ROUGE_L_Rcl": result["rouge_l_recall"] * 100.0,
- "ROUGE_SU4": rouge_su4["rouge_su4_f_score"] * 100.0,
- }
-
- score = result["ROUGE_1"]
- if score > self.best_score:
- copyfile(
- os.path.join(
- save_folder,
- "intermediate_results",
- "res_" + label + ".json",
- ),
- os.path.join(
- save_folder,
- "intermediate_results",
- "res_" + label + ".best.json",
- ),
- )
- self.best_score = score
- self.best_res = result
- got_better_score = True
-
- else:
- result = {}
- score = -float("Inf")
- got_better_score = False
-
- return result, score, got_better_score
-
- def eval(self, predictions, groundtruths):
- # predictions, groundtruths = self.filter_empty(predictions, groundtruths)
- predictions = [self.make_html_safe(w) for w in predictions]
- groundtruths = [self.make_html_safe(w) for w in groundtruths]
- pred_dir = os.path.join(self.save_dir, "predictions")
- if os.path.exists(pred_dir):
- shutil.rmtree(pred_dir)
- os.makedirs(pred_dir)
-
- gt_dir = os.path.join(self.save_dir, "groundtruths")
- if os.path.exists(gt_dir):
- shutil.rmtree(gt_dir)
- os.makedirs(gt_dir)
-
- special_char_dict = self.print_to_rouge_dir_gt(
- groundtruths, gt_dir, "gt", "SPLIT_CHARS_FOR_EVAL" in self.opt
- )
- self.print_to_rouge_dir(
- predictions,
- pred_dir,
- "pred",
- "SPLIT_CHARS_FOR_EVAL" in self.opt,
- special_char_dict,
- )
-
- r = pyrouge.Rouge155(self.pyrouge_dir)
- r.system_dir = pred_dir
- r.model_dir = gt_dir
- r.system_filename_pattern = "(\d+)_pred.txt"
- r.model_filename_pattern = "[A-Z].#ID#_gt.txt"
- results = r.output_to_dict(r.convert_and_evaluate())
- return results
diff --git a/spaces/aliceoq/vozes-da-loirinha/i18n/locale_diff.py b/spaces/aliceoq/vozes-da-loirinha/i18n/locale_diff.py
deleted file mode 100644
index 257277965e0866a86d0361863a8f1b408c4f71ab..0000000000000000000000000000000000000000
--- a/spaces/aliceoq/vozes-da-loirinha/i18n/locale_diff.py
+++ /dev/null
@@ -1,45 +0,0 @@
-import json
-import os
-from collections import OrderedDict
-
-# Define the standard file name
-standard_file = "zh_CN.json"
-
-# Find all JSON files in the directory
-dir_path = "./"
-languages = [
- f for f in os.listdir(dir_path) if f.endswith(".json") and f != standard_file
-]
-
-# Load the standard file
-with open(standard_file, "r", encoding="utf-8") as f:
- standard_data = json.load(f, object_pairs_hook=OrderedDict)
-
-# Loop through each language file
-for lang_file in languages:
- # Load the language file
- with open(lang_file, "r", encoding="utf-8") as f:
- lang_data = json.load(f, object_pairs_hook=OrderedDict)
-
- # Find the difference between the language file and the standard file
- diff = set(standard_data.keys()) - set(lang_data.keys())
-
- miss = set(lang_data.keys()) - set(standard_data.keys())
-
- # Add any missing keys to the language file
- for key in diff:
- lang_data[key] = key
-
- # Del any extra keys to the language file
- for key in miss:
- del lang_data[key]
-
- # Sort the keys of the language file to match the order of the standard file
- lang_data = OrderedDict(
- sorted(lang_data.items(), key=lambda x: list(standard_data.keys()).index(x[0]))
- )
-
- # Save the updated language file
- with open(lang_file, "w", encoding="utf-8") as f:
- json.dump(lang_data, f, ensure_ascii=False, indent=4)
- f.write("\n")
diff --git a/spaces/allknowingroger/Image-Models-Test53/app.py b/spaces/allknowingroger/Image-Models-Test53/app.py
deleted file mode 100644
index ce8e589fa10ffd7dab580bd5b2b5ee9efcd1af79..0000000000000000000000000000000000000000
--- a/spaces/allknowingroger/Image-Models-Test53/app.py
+++ /dev/null
@@ -1,144 +0,0 @@
-import gradio as gr
-# import os
-# import sys
-# from pathlib import Path
-import time
-
-models =[
- "Yntec/Lunar",
- "digiplay/KawaiiRealisticAnimeMix_A0.3",
- "Daniil-plotnikov/russian-vision-v5-1",
- "EarthnDusk/Earth-AniMix-Super-Flat",
- "juliajoanna/lora-trained-xl-fred-6",
- "dpwm/lora-trained-xl-4",
- "zhangyi617/driving-lora",
- "LinoyTsaban/huggy_v12",
- "digiplay/PotoPhotoRealism_v1",
-]
-
-
-model_functions = {}
-model_idx = 1
-for model_path in models:
- try:
- model_functions[model_idx] = gr.Interface.load(f"models/{model_path}", live=False, preprocess=True, postprocess=False)
- except Exception as error:
- def the_fn(txt):
- return None
- model_functions[model_idx] = gr.Interface(fn=the_fn, inputs=["text"], outputs=["image"])
- model_idx+=1
-
-
-def send_it_idx(idx):
- def send_it_fn(prompt):
- output = (model_functions.get(str(idx)) or model_functions.get(str(1)))(prompt)
- return output
- return send_it_fn
-
-def get_prompts(prompt_text):
- return prompt_text
-
-def clear_it(val):
- if int(val) != 0:
- val = 0
- else:
- val = 0
- pass
- return val
-
-def all_task_end(cnt,t_stamp):
- to = t_stamp + 60
- et = time.time()
- if et > to and t_stamp != 0:
- d = gr.update(value=0)
- tog = gr.update(value=1)
- #print(f'to: {to} et: {et}')
- else:
- if cnt != 0:
- d = gr.update(value=et)
- else:
- d = gr.update(value=0)
- tog = gr.update(value=0)
- #print (f'passing: to: {to} et: {et}')
- pass
- return d, tog
-
-def all_task_start():
- print("\n\n\n\n\n\n\n")
- t = time.gmtime()
- t_stamp = time.time()
- current_time = time.strftime("%H:%M:%S", t)
- return gr.update(value=t_stamp), gr.update(value=t_stamp), gr.update(value=0)
-
-def clear_fn():
- nn = len(models)
- return tuple([None, *[None for _ in range(nn)]])
-
-
-
-with gr.Blocks(title="SD Models") as my_interface:
- with gr.Column(scale=12):
- # with gr.Row():
- # gr.Markdown("""- Primary prompt: 你想画的内容(英文单词,如 a cat, 加英文逗号效果更好;点 Improve 按钮进行完善)\n- Real prompt: 完善后的提示词,出现后再点右边的 Run 按钮开始运行""")
- with gr.Row():
- with gr.Row(scale=6):
- primary_prompt=gr.Textbox(label="Prompt", value="")
- # real_prompt=gr.Textbox(label="Real prompt")
- with gr.Row(scale=6):
- # improve_prompts_btn=gr.Button("Improve")
- with gr.Row():
- run=gr.Button("Run",variant="primary")
- clear_btn=gr.Button("Clear")
- with gr.Row():
- sd_outputs = {}
- model_idx = 1
- for model_path in models:
- with gr.Column(scale=3, min_width=320):
- with gr.Box():
- sd_outputs[model_idx] = gr.Image(label=model_path)
- pass
- model_idx += 1
- pass
- pass
-
- with gr.Row(visible=False):
- start_box=gr.Number(interactive=False)
- end_box=gr.Number(interactive=False)
- tog_box=gr.Textbox(value=0,interactive=False)
-
- start_box.change(
- all_task_end,
- [start_box, end_box],
- [start_box, tog_box],
- every=1,
- show_progress=False)
-
- primary_prompt.submit(all_task_start, None, [start_box, end_box, tog_box])
- run.click(all_task_start, None, [start_box, end_box, tog_box])
- runs_dict = {}
- model_idx = 1
- for model_path in models:
- runs_dict[model_idx] = run.click(model_functions[model_idx], inputs=[primary_prompt], outputs=[sd_outputs[model_idx]])
- model_idx += 1
- pass
- pass
-
- # improve_prompts_btn_clicked=improve_prompts_btn.click(
- # get_prompts,
- # inputs=[primary_prompt],
- # outputs=[primary_prompt],
- # cancels=list(runs_dict.values()))
- clear_btn.click(
- clear_fn,
- None,
- [primary_prompt, *list(sd_outputs.values())],
- cancels=[*list(runs_dict.values())])
- tog_box.change(
- clear_it,
- tog_box,
- tog_box,
- cancels=[*list(runs_dict.values())])
-
-my_interface.queue(concurrency_count=600, status_update_rate=1)
-my_interface.launch(inline=True, show_api=False)
-
\ No newline at end of file
diff --git a/spaces/allknowingroger/Image-Models-Test9/README.md b/spaces/allknowingroger/Image-Models-Test9/README.md
deleted file mode 100644
index f6f1b5cb6a66573263eb5a43484e15bafd4e74ee..0000000000000000000000000000000000000000
--- a/spaces/allknowingroger/Image-Models-Test9/README.md
+++ /dev/null
@@ -1,13 +0,0 @@
----
-title: More Image Models
-emoji: 😻
-colorFrom: red
-colorTo: gray
-sdk: gradio
-sdk_version: 3.23.0
-app_file: app.py
-pinned: true
-duplicated_from: allknowingroger/Image-Models-Test8
----
-
-
\ No newline at end of file
diff --git a/spaces/amankishore/sjc/sd1/ldm/modules/attention.py b/spaces/amankishore/sjc/sd1/ldm/modules/attention.py
deleted file mode 100644
index f4eff39ccb6d75daa764f6eb70a7cef024fb5a3f..0000000000000000000000000000000000000000
--- a/spaces/amankishore/sjc/sd1/ldm/modules/attention.py
+++ /dev/null
@@ -1,261 +0,0 @@
-from inspect import isfunction
-import math
-import torch
-import torch.nn.functional as F
-from torch import nn, einsum
-from einops import rearrange, repeat
-
-from ldm.modules.diffusionmodules.util import checkpoint
-
-
-def exists(val):
- return val is not None
-
-
-def uniq(arr):
- return{el: True for el in arr}.keys()
-
-
-def default(val, d):
- if exists(val):
- return val
- return d() if isfunction(d) else d
-
-
-def max_neg_value(t):
- return -torch.finfo(t.dtype).max
-
-
-def init_(tensor):
- dim = tensor.shape[-1]
- std = 1 / math.sqrt(dim)
- tensor.uniform_(-std, std)
- return tensor
-
-
-# feedforward
-class GEGLU(nn.Module):
- def __init__(self, dim_in, dim_out):
- super().__init__()
- self.proj = nn.Linear(dim_in, dim_out * 2)
-
- def forward(self, x):
- x, gate = self.proj(x).chunk(2, dim=-1)
- return x * F.gelu(gate)
-
-
-class FeedForward(nn.Module):
- def __init__(self, dim, dim_out=None, mult=4, glu=False, dropout=0.):
- super().__init__()
- inner_dim = int(dim * mult)
- dim_out = default(dim_out, dim)
- project_in = nn.Sequential(
- nn.Linear(dim, inner_dim),
- nn.GELU()
- ) if not glu else GEGLU(dim, inner_dim)
-
- self.net = nn.Sequential(
- project_in,
- nn.Dropout(dropout),
- nn.Linear(inner_dim, dim_out)
- )
-
- def forward(self, x):
- return self.net(x)
-
-
-def zero_module(module):
- """
- Zero out the parameters of a module and return it.
- """
- for p in module.parameters():
- p.detach().zero_()
- return module
-
-
-def Normalize(in_channels):
- return torch.nn.GroupNorm(num_groups=32, num_channels=in_channels, eps=1e-6, affine=True)
-
-
-class LinearAttention(nn.Module):
- def __init__(self, dim, heads=4, dim_head=32):
- super().__init__()
- self.heads = heads
- hidden_dim = dim_head * heads
- self.to_qkv = nn.Conv2d(dim, hidden_dim * 3, 1, bias = False)
- self.to_out = nn.Conv2d(hidden_dim, dim, 1)
-
- def forward(self, x):
- b, c, h, w = x.shape
- qkv = self.to_qkv(x)
- q, k, v = rearrange(qkv, 'b (qkv heads c) h w -> qkv b heads c (h w)', heads = self.heads, qkv=3)
- k = k.softmax(dim=-1)
- context = torch.einsum('bhdn,bhen->bhde', k, v)
- out = torch.einsum('bhde,bhdn->bhen', context, q)
- out = rearrange(out, 'b heads c (h w) -> b (heads c) h w', heads=self.heads, h=h, w=w)
- return self.to_out(out)
-
-
-class SpatialSelfAttention(nn.Module):
- def __init__(self, in_channels):
- super().__init__()
- self.in_channels = in_channels
-
- self.norm = Normalize(in_channels)
- self.q = torch.nn.Conv2d(in_channels,
- in_channels,
- kernel_size=1,
- stride=1,
- padding=0)
- self.k = torch.nn.Conv2d(in_channels,
- in_channels,
- kernel_size=1,
- stride=1,
- padding=0)
- self.v = torch.nn.Conv2d(in_channels,
- in_channels,
- kernel_size=1,
- stride=1,
- padding=0)
- self.proj_out = torch.nn.Conv2d(in_channels,
- in_channels,
- kernel_size=1,
- stride=1,
- padding=0)
-
- def forward(self, x):
- h_ = x
- h_ = self.norm(h_)
- q = self.q(h_)
- k = self.k(h_)
- v = self.v(h_)
-
- # compute attention
- b,c,h,w = q.shape
- q = rearrange(q, 'b c h w -> b (h w) c')
- k = rearrange(k, 'b c h w -> b c (h w)')
- w_ = torch.einsum('bij,bjk->bik', q, k)
-
- w_ = w_ * (int(c)**(-0.5))
- w_ = torch.nn.functional.softmax(w_, dim=2)
-
- # attend to values
- v = rearrange(v, 'b c h w -> b c (h w)')
- w_ = rearrange(w_, 'b i j -> b j i')
- h_ = torch.einsum('bij,bjk->bik', v, w_)
- h_ = rearrange(h_, 'b c (h w) -> b c h w', h=h)
- h_ = self.proj_out(h_)
-
- return x+h_
-
-
-class CrossAttention(nn.Module):
- def __init__(self, query_dim, context_dim=None, heads=8, dim_head=64, dropout=0.):
- super().__init__()
- inner_dim = dim_head * heads
- context_dim = default(context_dim, query_dim)
-
- self.scale = dim_head ** -0.5
- self.heads = heads
-
- self.to_q = nn.Linear(query_dim, inner_dim, bias=False)
- self.to_k = nn.Linear(context_dim, inner_dim, bias=False)
- self.to_v = nn.Linear(context_dim, inner_dim, bias=False)
-
- self.to_out = nn.Sequential(
- nn.Linear(inner_dim, query_dim),
- nn.Dropout(dropout)
- )
-
- def forward(self, x, context=None, mask=None):
- h = self.heads
-
- q = self.to_q(x)
- context = default(context, x)
- k = self.to_k(context)
- v = self.to_v(context)
-
- q, k, v = map(lambda t: rearrange(t, 'b n (h d) -> (b h) n d', h=h), (q, k, v))
-
- sim = einsum('b i d, b j d -> b i j', q, k) * self.scale
-
- if exists(mask):
- mask = rearrange(mask, 'b ... -> b (...)')
- max_neg_value = -torch.finfo(sim.dtype).max
- mask = repeat(mask, 'b j -> (b h) () j', h=h)
- sim.masked_fill_(~mask, max_neg_value)
-
- # attention, what we cannot get enough of
- attn = sim.softmax(dim=-1)
-
- out = einsum('b i j, b j d -> b i d', attn, v)
- out = rearrange(out, '(b h) n d -> b n (h d)', h=h)
- return self.to_out(out)
-
-
-class BasicTransformerBlock(nn.Module):
- def __init__(self, dim, n_heads, d_head, dropout=0., context_dim=None, gated_ff=True, checkpoint=True):
- super().__init__()
- self.attn1 = CrossAttention(query_dim=dim, heads=n_heads, dim_head=d_head, dropout=dropout) # is a self-attention
- self.ff = FeedForward(dim, dropout=dropout, glu=gated_ff)
- self.attn2 = CrossAttention(query_dim=dim, context_dim=context_dim,
- heads=n_heads, dim_head=d_head, dropout=dropout) # is self-attn if context is none
- self.norm1 = nn.LayerNorm(dim)
- self.norm2 = nn.LayerNorm(dim)
- self.norm3 = nn.LayerNorm(dim)
- self.checkpoint = checkpoint
-
- def forward(self, x, context=None):
- return checkpoint(self._forward, (x, context), self.parameters(), self.checkpoint)
-
- def _forward(self, x, context=None):
- x = self.attn1(self.norm1(x)) + x
- x = self.attn2(self.norm2(x), context=context) + x
- x = self.ff(self.norm3(x)) + x
- return x
-
-
-class SpatialTransformer(nn.Module):
- """
- Transformer block for image-like data.
- First, project the input (aka embedding)
- and reshape to b, t, d.
- Then apply standard transformer action.
- Finally, reshape to image
- """
- def __init__(self, in_channels, n_heads, d_head,
- depth=1, dropout=0., context_dim=None):
- super().__init__()
- self.in_channels = in_channels
- inner_dim = n_heads * d_head
- self.norm = Normalize(in_channels)
-
- self.proj_in = nn.Conv2d(in_channels,
- inner_dim,
- kernel_size=1,
- stride=1,
- padding=0)
-
- self.transformer_blocks = nn.ModuleList(
- [BasicTransformerBlock(inner_dim, n_heads, d_head, dropout=dropout, context_dim=context_dim)
- for d in range(depth)]
- )
-
- self.proj_out = zero_module(nn.Conv2d(inner_dim,
- in_channels,
- kernel_size=1,
- stride=1,
- padding=0))
-
- def forward(self, x, context=None):
- # note: if no context is given, cross-attention defaults to self-attention
- b, c, h, w = x.shape
- x_in = x
- x = self.norm(x)
- x = self.proj_in(x)
- x = rearrange(x, 'b c h w -> b (h w) c')
- for block in self.transformer_blocks:
- x = block(x, context=context)
- x = rearrange(x, 'b (h w) c -> b c h w', h=h, w=w)
- x = self.proj_out(x)
- return x + x_in
\ No newline at end of file
diff --git a/spaces/amarchheda/ChordDuplicate/portaudio/src/common/pa_memorybarrier.h b/spaces/amarchheda/ChordDuplicate/portaudio/src/common/pa_memorybarrier.h
deleted file mode 100644
index 0dca6aa42fae6937dc31fed7c29d641e5db5845d..0000000000000000000000000000000000000000
--- a/spaces/amarchheda/ChordDuplicate/portaudio/src/common/pa_memorybarrier.h
+++ /dev/null
@@ -1,128 +0,0 @@
-/*
- * $Id: pa_memorybarrier.h 1240 2007-07-17 13:05:07Z bjornroche $
- * Portable Audio I/O Library
- * Memory barrier utilities
- *
- * Author: Bjorn Roche, XO Audio, LLC
- *
- * This program uses the PortAudio Portable Audio Library.
- * For more information see: http://www.portaudio.com
- * Copyright (c) 1999-2000 Ross Bencina and Phil Burk
- *
- * Permission is hereby granted, free of charge, to any person obtaining
- * a copy of this software and associated documentation files
- * (the "Software"), to deal in the Software without restriction,
- * including without limitation the rights to use, copy, modify, merge,
- * publish, distribute, sublicense, and/or sell copies of the Software,
- * and to permit persons to whom the Software is furnished to do so,
- * subject to the following conditions:
- *
- * The above copyright notice and this permission notice shall be
- * included in all copies or substantial portions of the Software.
- *
- * THE SOFTWARE IS PROVIDED "AS IS", WITHOUT WARRANTY OF ANY KIND,
- * EXPRESS OR IMPLIED, INCLUDING BUT NOT LIMITED TO THE WARRANTIES OF
- * MERCHANTABILITY, FITNESS FOR A PARTICULAR PURPOSE AND NONINFRINGEMENT.
- * IN NO EVENT SHALL THE AUTHORS OR COPYRIGHT HOLDERS BE LIABLE FOR
- * ANY CLAIM, DAMAGES OR OTHER LIABILITY, WHETHER IN AN ACTION OF
- * CONTRACT, TORT OR OTHERWISE, ARISING FROM, OUT OF OR IN CONNECTION
- * WITH THE SOFTWARE OR THE USE OR OTHER DEALINGS IN THE SOFTWARE.
- */
-
-/*
- * The text above constitutes the entire PortAudio license; however,
- * the PortAudio community also makes the following non-binding requests:
- *
- * Any person wishing to distribute modifications to the Software is
- * requested to send the modifications to the original developer so that
- * they can be incorporated into the canonical version. It is also
- * requested that these non-binding requests be included along with the
- * license above.
- */
-
-/**
- @file pa_memorybarrier.h
- @ingroup common_src
-*/
-
-/****************
- * Some memory barrier primitives based on the system.
- * right now only OS X, FreeBSD, and Linux are supported. In addition to providing
- * memory barriers, these functions should ensure that data cached in registers
- * is written out to cache where it can be snooped by other CPUs. (ie, the volatile
- * keyword should not be required)
- *
- * the primitives that must be defined are:
- *
- * PaUtil_FullMemoryBarrier()
- * PaUtil_ReadMemoryBarrier()
- * PaUtil_WriteMemoryBarrier()
- *
- ****************/
-
-#if defined(__APPLE__)
-# include
- /* Here are the memory barrier functions. Mac OS X only provides
- full memory barriers, so the three types of barriers are the same,
- however, these barriers are superior to compiler-based ones. */
-# define PaUtil_FullMemoryBarrier() OSMemoryBarrier()
-# define PaUtil_ReadMemoryBarrier() OSMemoryBarrier()
-# define PaUtil_WriteMemoryBarrier() OSMemoryBarrier()
-#elif defined(__GNUC__)
- /* GCC >= 4.1 has built-in intrinsics. We'll use those */
-# if (__GNUC__ > 4) || (__GNUC__ == 4 && __GNUC_MINOR__ >= 1)
-# define PaUtil_FullMemoryBarrier() __sync_synchronize()
-# define PaUtil_ReadMemoryBarrier() __sync_synchronize()
-# define PaUtil_WriteMemoryBarrier() __sync_synchronize()
- /* as a fallback, GCC understands volatile asm and "memory" to mean it
- * should not reorder memory read/writes */
- /* Note that it is not clear that any compiler actually defines __PPC__,
- * it can probably removed safely. */
-# elif defined( __ppc__ ) || defined( __powerpc__) || defined( __PPC__ )
-# define PaUtil_FullMemoryBarrier() asm volatile("sync":::"memory")
-# define PaUtil_ReadMemoryBarrier() asm volatile("sync":::"memory")
-# define PaUtil_WriteMemoryBarrier() asm volatile("sync":::"memory")
-# elif defined( __i386__ ) || defined( __i486__ ) || defined( __i586__ ) || \
- defined( __i686__ ) || defined( __x86_64__ )
-# define PaUtil_FullMemoryBarrier() asm volatile("mfence":::"memory")
-# define PaUtil_ReadMemoryBarrier() asm volatile("lfence":::"memory")
-# define PaUtil_WriteMemoryBarrier() asm volatile("sfence":::"memory")
-# else
-# ifdef ALLOW_SMP_DANGERS
-# warning Memory barriers not defined on this system or system unknown
-# warning For SMP safety, you should fix this.
-# define PaUtil_FullMemoryBarrier()
-# define PaUtil_ReadMemoryBarrier()
-# define PaUtil_WriteMemoryBarrier()
-# else
-# error Memory barriers are not defined on this system. You can still compile by defining ALLOW_SMP_DANGERS, but SMP safety will not be guaranteed.
-# endif
-# endif
-#elif (_MSC_VER >= 1400) && !defined(_WIN32_WCE)
-# include
-# pragma intrinsic(_ReadWriteBarrier)
-# pragma intrinsic(_ReadBarrier)
-# pragma intrinsic(_WriteBarrier)
-/* note that MSVC intrinsics _ReadWriteBarrier(), _ReadBarrier(), _WriteBarrier() are just compiler barriers *not* memory barriers */
-# define PaUtil_FullMemoryBarrier() _ReadWriteBarrier()
-# define PaUtil_ReadMemoryBarrier() _ReadBarrier()
-# define PaUtil_WriteMemoryBarrier() _WriteBarrier()
-#elif defined(_WIN32_WCE)
-# define PaUtil_FullMemoryBarrier()
-# define PaUtil_ReadMemoryBarrier()
-# define PaUtil_WriteMemoryBarrier()
-#elif defined(_MSC_VER) || defined(__BORLANDC__)
-# define PaUtil_FullMemoryBarrier() _asm { lock add [esp], 0 }
-# define PaUtil_ReadMemoryBarrier() _asm { lock add [esp], 0 }
-# define PaUtil_WriteMemoryBarrier() _asm { lock add [esp], 0 }
-#else
-# ifdef ALLOW_SMP_DANGERS
-# warning Memory barriers not defined on this system or system unknown
-# warning For SMP safety, you should fix this.
-# define PaUtil_FullMemoryBarrier()
-# define PaUtil_ReadMemoryBarrier()
-# define PaUtil_WriteMemoryBarrier()
-# else
-# error Memory barriers are not defined on this system. You can still compile by defining ALLOW_SMP_DANGERS, but SMP safety will not be guaranteed.
-# endif
-#endif
diff --git a/spaces/andryMLOPS/ASTA-GPT-3.8_web_ui/client/css/sidebar.css b/spaces/andryMLOPS/ASTA-GPT-3.8_web_ui/client/css/sidebar.css
deleted file mode 100644
index 310887c60443abd491c3162f62e44b5ec333e50d..0000000000000000000000000000000000000000
--- a/spaces/andryMLOPS/ASTA-GPT-3.8_web_ui/client/css/sidebar.css
+++ /dev/null
@@ -1,197 +0,0 @@
-.sidebar {
- max-width: 260px;
- padding: var(--section-gap);
- flex-shrink: 0;
- display: flex;
- flex-direction: column;
- justify-content: space-between;
-}
-
-.sidebar .title {
- font-size: 14px;
- font-weight: 500;
-}
-
-.sidebar .conversation-sidebar {
- padding: 8px 12px;
- display: flex;
- gap: 18px;
- align-items: center;
- user-select: none;
- justify-content: space-between;
-}
-
-.sidebar .conversation-sidebar .left {
- cursor: pointer;
- display: flex;
- align-items: center;
- gap: 10px;
-}
-
-.sidebar i {
- color: var(--conversations);
- cursor: pointer;
-}
-
-.sidebar .top {
- display: flex;
- flex-direction: column;
- overflow: hidden;
- gap: 16px;
- padding-right: 8px;
-}
-
-.sidebar .top:hover {
- overflow: auto;
-}
-
-.sidebar .info {
- padding: 8px 12px 0px 12px;
- display: flex;
- align-items: center;
- justify-content: center;
- user-select: none;
- background: transparent;
- width: 100%;
- border: none;
- text-decoration: none;
-}
-
-.sidebar .info span {
- color: var(--conversations);
- line-height: 1.5;
- font-size: 0.75rem;
-}
-
-.sidebar .info i::before {
- margin-right: 8px;
-}
-
-.sidebar-footer {
- width: 100%;
- margin-top: 16px;
- display: flex;
- flex-direction: column;
-}
-
-.sidebar-footer button {
- cursor: pointer;
- user-select: none;
- background: transparent;
-}
-
-.sidebar.shown {
- position: fixed;
- top: 0;
- left: 0;
- width: 100%;
- height: 100%;
- z-index: 1000;
-}
-
-.sidebar.shown .box {
- background-color: #16171a;
- width: 80%;
- height: 100%;
- overflow-y: auto;
-}
-
-@keyframes spinner {
- to {
- transform: rotate(360deg);
- }
-}
-
-/* scrollbar */
-.sidebar .top::-webkit-scrollbar {
- width: 4px;
- padding: 8px 0px;
-}
-
-.sidebar .top::-webkit-scrollbar-track {
- background-color: #ffffff00;
-}
-
-.sidebar .top::-webkit-scrollbar-thumb {
- background-color: #555555;
- border-radius: 10px;
-}
-
-.spinner:before {
- content: "";
- box-sizing: border-box;
- position: absolute;
- top: 50%;
- left: 45%;
- width: 20px;
- height: 20px;
- border-radius: 50%;
- border: 1px solid var(--conversations);
- border-top-color: white;
- animation: spinner 0.6s linear infinite;
-}
-
-.menu-button {
- display: none !important;
- position: absolute;
- z-index: 100000;
- top: 0;
- left: 0;
- margin: 10px;
- font-size: 1rem;
- cursor: pointer;
- width: 30px;
- height: 30px;
- justify-content: center;
- align-items: center;
- transition: 0.33s;
-}
-
-.menu-button i {
- transition: 0.33s;
-}
-
-.rotated {
- transform: rotate(360deg);
-}
-
-.menu-button.rotated {
- position: fixed;
- top: 10px;
- left: 10px;
- z-index: 1001;
-}
-
-@media screen and (max-width: 990px) {
- .sidebar {
- display: none;
- width: 100%;
- max-width: none;
- }
-
- .menu-button {
- display: flex !important;
- }
-}
-
-@media (max-width: 990px) {
- .sidebar .top {
- padding-top: 48px;
- }
-}
-
-@media (min-width: 768px) {
- .sidebar.shown {
- position: static;
- width: auto;
- height: auto;
- background-color: transparent;
- }
-
- .sidebar.shown .box {
- background-color: #16171a;
- width: auto;
- height: auto;
- overflow-y: auto;
- }
-}
diff --git a/spaces/anikfaisal/weather_image_classifier/README.md b/spaces/anikfaisal/weather_image_classifier/README.md
deleted file mode 100644
index 03d34dd99d6796a0c5bbf6839e3f998ce2ab74b9..0000000000000000000000000000000000000000
--- a/spaces/anikfaisal/weather_image_classifier/README.md
+++ /dev/null
@@ -1,13 +0,0 @@
----
-title: Weather Image Classifier
-emoji: 🦀
-colorFrom: blue
-colorTo: blue
-sdk: gradio
-sdk_version: 3.1.4
-app_file: app.py
-pinned: false
-license: apache-2.0
----
-
-Check out the configuration reference at https://huggingface.co/docs/hub/spaces-config-reference
diff --git a/spaces/anonymous-pits/pits/text/cleaners.py b/spaces/anonymous-pits/pits/text/cleaners.py
deleted file mode 100644
index e935fa24d9495cb0f74492e779fd998dfe81e261..0000000000000000000000000000000000000000
--- a/spaces/anonymous-pits/pits/text/cleaners.py
+++ /dev/null
@@ -1,89 +0,0 @@
-""" from https://github.com/keithito/tacotron """
-
-'''
-Cleaners are transformations that run over the input text at both training and eval time.
-Cleaners can be selected by passing a comma-delimited list of cleaner names as the "cleaners"
-hyperparameter. Some cleaners are English-specific. You'll typically want to use:
- 1. "english_cleaners" for English text
- 2. "transliteration_cleaners" for non-English text that can be transliterated to ASCII using
- the Unidecode library (https://pypi.python.org/pypi/Unidecode)
- 3. "basic_cleaners" if you do not want to transliterate (in this case, you should also update
- the symbols in symbols.py to match your data).
-'''
-
-import re
-from unidecode import unidecode
-from unicodedata import normalize
-
-from .numbers import normalize_numbers
-
-
-# Regular expression matching whitespace:
-_whitespace_re = re.compile(r'\s+')
-
-# List of (regular expression, replacement) pairs for abbreviations:
-_abbreviations = [(re.compile('\\b%s\\.' % x[0], re.IGNORECASE), x[1]) for x in [
- ('mrs', 'misess'),
- ('mr', 'mister'),
- ('dr', 'doctor'),
- ('st', 'saint'),
- ('co', 'company'),
- ('jr', 'junior'),
- ('maj', 'major'),
- ('gen', 'general'),
- ('drs', 'doctors'),
- ('rev', 'reverend'),
- ('lt', 'lieutenant'),
- ('hon', 'honorable'),
- ('sgt', 'sergeant'),
- ('capt', 'captain'),
- ('esq', 'esquire'),
- ('ltd', 'limited'),
- ('col', 'colonel'),
- ('ft', 'fort'),
-]]
-
-_cht_norm = [(re.compile(r'[%s]' % x[0]), x[1]) for x in [
- ('。.;', '.'),
- (',、', ', '),
- ('?', '?'),
- ('!', '!'),
- ('─‧', '-'),
- ('…', '...'),
- ('《》「」『』〈〉()', "'"),
- (':︰', ':'),
- (' ', ' ')
-]]
-
-def expand_abbreviations(text):
- for regex, replacement in _abbreviations:
- text = re.sub(regex, replacement, text)
- return text
-
-def expand_numbers(text):
- return normalize_numbers(text)
-
-def lowercase(text):
- return text.lower()
-
-def collapse_whitespace(text):
- return re.sub(_whitespace_re, ' ', text)
-
-def convert_to_ascii(text):
- return unidecode(text)
-
-def english_cleaners(text):
- '''Pipeline for English text, including abbreviation expansion.'''
- text = convert_to_ascii(text)
- #text = lowercase(text)
- text = expand_numbers(text)
- text = expand_abbreviations(text)
- text = collapse_whitespace(text)
- return text
-
-def korean_cleaners(text):
- '''Pipeline for Korean text, including collapses whitespace.'''
- text = collapse_whitespace(text)
- text = normalize('NFKD', text)
- return text
-
diff --git a/spaces/antonovmaxim/text-generation-webui-space/text-generation-webui-main/css/html_cai_style.css b/spaces/antonovmaxim/text-generation-webui-space/text-generation-webui-main/css/html_cai_style.css
deleted file mode 100644
index f601de3248b7ee94d6da58026354f8b9afeb9297..0000000000000000000000000000000000000000
--- a/spaces/antonovmaxim/text-generation-webui-space/text-generation-webui-main/css/html_cai_style.css
+++ /dev/null
@@ -1,91 +0,0 @@
-.chat {
- margin-left: auto;
- margin-right: auto;
- max-width: 800px;
- height: calc(100vh - 306px);
- overflow-y: auto;
- padding-right: 20px;
- display: flex;
- flex-direction: column-reverse;
- word-break: break-word;
- overflow-wrap: anywhere;
-}
-
-.message {
- display: grid;
- grid-template-columns: 60px minmax(0, 1fr);
- padding-bottom: 25px;
- font-size: 15px;
- font-family: Helvetica, Arial, sans-serif;
- line-height: 1.428571429;
-}
-
-.circle-you {
- width: 50px;
- height: 50px;
- background-color: rgb(238, 78, 59);
- border-radius: 50%;
-}
-
-.circle-bot {
- width: 50px;
- height: 50px;
- background-color: rgb(59, 78, 244);
- border-radius: 50%;
-}
-
-.circle-bot img,
-.circle-you img {
- border-radius: 50%;
- width: 100%;
- height: 100%;
- object-fit: cover;
-}
-
-.text {}
-
-.text p {
- margin-top: 5px;
-}
-
-.username {
- font-weight: bold;
-}
-
-.message-body {}
-
-.message-body img {
- max-width: 300px;
- max-height: 300px;
- border-radius: 20px;
-}
-
-.message-body p {
- margin-bottom: 0 !important;
- font-size: 15px !important;
- line-height: 1.428571429 !important;
-}
-
-.message-body li {
- margin-top: 0.5em !important;
- margin-bottom: 0.5em !important;
-}
-
-.message-body li > p {
- display: inline !important;
-}
-
-.message-body code {
- overflow-x: auto;
-}
-.message-body :not(pre) > code {
- white-space: normal !important;
-}
-
-.dark .message-body p em {
- color: rgb(138, 138, 138) !important;
-}
-
-.message-body p em {
- color: rgb(110, 110, 110) !important;
-}
\ No newline at end of file
diff --git a/spaces/artificialguybr/video-dubbing/TTS/TTS/tts/models/__init__.py b/spaces/artificialguybr/video-dubbing/TTS/TTS/tts/models/__init__.py
deleted file mode 100644
index 2bd2e5f0875a84633e707702cd7d628409b12057..0000000000000000000000000000000000000000
--- a/spaces/artificialguybr/video-dubbing/TTS/TTS/tts/models/__init__.py
+++ /dev/null
@@ -1,14 +0,0 @@
-from typing import Dict, List, Union
-
-from TTS.utils.generic_utils import find_module
-
-
-def setup_model(config: "Coqpit", samples: Union[List[List], List[Dict]] = None) -> "BaseTTS":
- print(" > Using model: {}".format(config.model))
- # fetch the right model implementation.
- if "base_model" in config and config["base_model"] is not None:
- MyModel = find_module("TTS.tts.models", config.base_model.lower())
- else:
- MyModel = find_module("TTS.tts.models", config.model.lower())
- model = MyModel.init_from_config(config=config, samples=samples)
- return model
diff --git a/spaces/artificialguybr/video-dubbing/TTS/tests/aux_tests/test_readme.py b/spaces/artificialguybr/video-dubbing/TTS/tests/aux_tests/test_readme.py
deleted file mode 100644
index 32b26fc6fc38beb79303522f265b7f638bca4df3..0000000000000000000000000000000000000000
--- a/spaces/artificialguybr/video-dubbing/TTS/tests/aux_tests/test_readme.py
+++ /dev/null
@@ -1,9 +0,0 @@
-import subprocess
-import sys
-from pathlib import Path
-
-
-def test_readme_up_to_date():
- root = Path(__file__).parent.parent.parent
- sync_readme = root / "scripts" / "sync_readme.py"
- subprocess.check_call([sys.executable, str(sync_readme), "--check"], cwd=root)
diff --git a/spaces/arxify/RVC-beta-v2-0618/runtime/Lib/site-packages/altair/vegalite/v4/tests/__init__.py b/spaces/arxify/RVC-beta-v2-0618/runtime/Lib/site-packages/altair/vegalite/v4/tests/__init__.py
deleted file mode 100644
index e69de29bb2d1d6434b8b29ae775ad8c2e48c5391..0000000000000000000000000000000000000000
diff --git a/spaces/arxify/RVC-beta-v2-0618/runtime/Lib/site-packages/attr/_compat.py b/spaces/arxify/RVC-beta-v2-0618/runtime/Lib/site-packages/attr/_compat.py
deleted file mode 100644
index dc0cb02b6435bb4cb90f1d9645150d32286379a5..0000000000000000000000000000000000000000
--- a/spaces/arxify/RVC-beta-v2-0618/runtime/Lib/site-packages/attr/_compat.py
+++ /dev/null
@@ -1,261 +0,0 @@
-# SPDX-License-Identifier: MIT
-
-from __future__ import absolute_import, division, print_function
-
-import platform
-import sys
-import threading
-import types
-import warnings
-
-
-PY2 = sys.version_info[0] == 2
-PYPY = platform.python_implementation() == "PyPy"
-PY36 = sys.version_info[:2] >= (3, 6)
-HAS_F_STRINGS = PY36
-PY310 = sys.version_info[:2] >= (3, 10)
-
-
-if PYPY or PY36:
- ordered_dict = dict
-else:
- from collections import OrderedDict
-
- ordered_dict = OrderedDict
-
-
-if PY2:
- from collections import Mapping, Sequence
-
- from UserDict import IterableUserDict
-
- # We 'bundle' isclass instead of using inspect as importing inspect is
- # fairly expensive (order of 10-15 ms for a modern machine in 2016)
- def isclass(klass):
- return isinstance(klass, (type, types.ClassType))
-
- def new_class(name, bases, kwds, exec_body):
- """
- A minimal stub of types.new_class that we need for make_class.
- """
- ns = {}
- exec_body(ns)
-
- return type(name, bases, ns)
-
- # TYPE is used in exceptions, repr(int) is different on Python 2 and 3.
- TYPE = "type"
-
- def iteritems(d):
- return d.iteritems()
-
- # Python 2 is bereft of a read-only dict proxy, so we make one!
- class ReadOnlyDict(IterableUserDict):
- """
- Best-effort read-only dict wrapper.
- """
-
- def __setitem__(self, key, val):
- # We gently pretend we're a Python 3 mappingproxy.
- raise TypeError(
- "'mappingproxy' object does not support item assignment"
- )
-
- def update(self, _):
- # We gently pretend we're a Python 3 mappingproxy.
- raise AttributeError(
- "'mappingproxy' object has no attribute 'update'"
- )
-
- def __delitem__(self, _):
- # We gently pretend we're a Python 3 mappingproxy.
- raise TypeError(
- "'mappingproxy' object does not support item deletion"
- )
-
- def clear(self):
- # We gently pretend we're a Python 3 mappingproxy.
- raise AttributeError(
- "'mappingproxy' object has no attribute 'clear'"
- )
-
- def pop(self, key, default=None):
- # We gently pretend we're a Python 3 mappingproxy.
- raise AttributeError(
- "'mappingproxy' object has no attribute 'pop'"
- )
-
- def popitem(self):
- # We gently pretend we're a Python 3 mappingproxy.
- raise AttributeError(
- "'mappingproxy' object has no attribute 'popitem'"
- )
-
- def setdefault(self, key, default=None):
- # We gently pretend we're a Python 3 mappingproxy.
- raise AttributeError(
- "'mappingproxy' object has no attribute 'setdefault'"
- )
-
- def __repr__(self):
- # Override to be identical to the Python 3 version.
- return "mappingproxy(" + repr(self.data) + ")"
-
- def metadata_proxy(d):
- res = ReadOnlyDict()
- res.data.update(d) # We blocked update, so we have to do it like this.
- return res
-
- def just_warn(*args, **kw): # pragma: no cover
- """
- We only warn on Python 3 because we are not aware of any concrete
- consequences of not setting the cell on Python 2.
- """
-
-else: # Python 3 and later.
- from collections.abc import Mapping, Sequence # noqa
-
- def just_warn(*args, **kw):
- """
- We only warn on Python 3 because we are not aware of any concrete
- consequences of not setting the cell on Python 2.
- """
- warnings.warn(
- "Running interpreter doesn't sufficiently support code object "
- "introspection. Some features like bare super() or accessing "
- "__class__ will not work with slotted classes.",
- RuntimeWarning,
- stacklevel=2,
- )
-
- def isclass(klass):
- return isinstance(klass, type)
-
- TYPE = "class"
-
- def iteritems(d):
- return d.items()
-
- new_class = types.new_class
-
- def metadata_proxy(d):
- return types.MappingProxyType(dict(d))
-
-
-def make_set_closure_cell():
- """Return a function of two arguments (cell, value) which sets
- the value stored in the closure cell `cell` to `value`.
- """
- # pypy makes this easy. (It also supports the logic below, but
- # why not do the easy/fast thing?)
- if PYPY:
-
- def set_closure_cell(cell, value):
- cell.__setstate__((value,))
-
- return set_closure_cell
-
- # Otherwise gotta do it the hard way.
-
- # Create a function that will set its first cellvar to `value`.
- def set_first_cellvar_to(value):
- x = value
- return
-
- # This function will be eliminated as dead code, but
- # not before its reference to `x` forces `x` to be
- # represented as a closure cell rather than a local.
- def force_x_to_be_a_cell(): # pragma: no cover
- return x
-
- try:
- # Extract the code object and make sure our assumptions about
- # the closure behavior are correct.
- if PY2:
- co = set_first_cellvar_to.func_code
- else:
- co = set_first_cellvar_to.__code__
- if co.co_cellvars != ("x",) or co.co_freevars != ():
- raise AssertionError # pragma: no cover
-
- # Convert this code object to a code object that sets the
- # function's first _freevar_ (not cellvar) to the argument.
- if sys.version_info >= (3, 8):
- # CPython 3.8+ has an incompatible CodeType signature
- # (added a posonlyargcount argument) but also added
- # CodeType.replace() to do this without counting parameters.
- set_first_freevar_code = co.replace(
- co_cellvars=co.co_freevars, co_freevars=co.co_cellvars
- )
- else:
- args = [co.co_argcount]
- if not PY2:
- args.append(co.co_kwonlyargcount)
- args.extend(
- [
- co.co_nlocals,
- co.co_stacksize,
- co.co_flags,
- co.co_code,
- co.co_consts,
- co.co_names,
- co.co_varnames,
- co.co_filename,
- co.co_name,
- co.co_firstlineno,
- co.co_lnotab,
- # These two arguments are reversed:
- co.co_cellvars,
- co.co_freevars,
- ]
- )
- set_first_freevar_code = types.CodeType(*args)
-
- def set_closure_cell(cell, value):
- # Create a function using the set_first_freevar_code,
- # whose first closure cell is `cell`. Calling it will
- # change the value of that cell.
- setter = types.FunctionType(
- set_first_freevar_code, {}, "setter", (), (cell,)
- )
- # And call it to set the cell.
- setter(value)
-
- # Make sure it works on this interpreter:
- def make_func_with_cell():
- x = None
-
- def func():
- return x # pragma: no cover
-
- return func
-
- if PY2:
- cell = make_func_with_cell().func_closure[0]
- else:
- cell = make_func_with_cell().__closure__[0]
- set_closure_cell(cell, 100)
- if cell.cell_contents != 100:
- raise AssertionError # pragma: no cover
-
- except Exception:
- return just_warn
- else:
- return set_closure_cell
-
-
-set_closure_cell = make_set_closure_cell()
-
-# Thread-local global to track attrs instances which are already being repr'd.
-# This is needed because there is no other (thread-safe) way to pass info
-# about the instances that are already being repr'd through the call stack
-# in order to ensure we don't perform infinite recursion.
-#
-# For instance, if an instance contains a dict which contains that instance,
-# we need to know that we're already repr'ing the outside instance from within
-# the dict's repr() call.
-#
-# This lives here rather than in _make.py so that the functions in _make.py
-# don't have a direct reference to the thread-local in their globals dict.
-# If they have such a reference, it breaks cloudpickle.
-repr_context = threading.local()
diff --git a/spaces/arxify/RVC-beta-v2-0618/runtime/Lib/site-packages/cffi/model.py b/spaces/arxify/RVC-beta-v2-0618/runtime/Lib/site-packages/cffi/model.py
deleted file mode 100644
index ad1c1764893d0257c0e75eeb61b0a359e89adf0f..0000000000000000000000000000000000000000
--- a/spaces/arxify/RVC-beta-v2-0618/runtime/Lib/site-packages/cffi/model.py
+++ /dev/null
@@ -1,617 +0,0 @@
-import types
-import weakref
-
-from .lock import allocate_lock
-from .error import CDefError, VerificationError, VerificationMissing
-
-# type qualifiers
-Q_CONST = 0x01
-Q_RESTRICT = 0x02
-Q_VOLATILE = 0x04
-
-def qualify(quals, replace_with):
- if quals & Q_CONST:
- replace_with = ' const ' + replace_with.lstrip()
- if quals & Q_VOLATILE:
- replace_with = ' volatile ' + replace_with.lstrip()
- if quals & Q_RESTRICT:
- # It seems that __restrict is supported by gcc and msvc.
- # If you hit some different compiler, add a #define in
- # _cffi_include.h for it (and in its copies, documented there)
- replace_with = ' __restrict ' + replace_with.lstrip()
- return replace_with
-
-
-class BaseTypeByIdentity(object):
- is_array_type = False
- is_raw_function = False
-
- def get_c_name(self, replace_with='', context='a C file', quals=0):
- result = self.c_name_with_marker
- assert result.count('&') == 1
- # some logic duplication with ffi.getctype()... :-(
- replace_with = replace_with.strip()
- if replace_with:
- if replace_with.startswith('*') and '&[' in result:
- replace_with = '(%s)' % replace_with
- elif not replace_with[0] in '[(':
- replace_with = ' ' + replace_with
- replace_with = qualify(quals, replace_with)
- result = result.replace('&', replace_with)
- if '$' in result:
- raise VerificationError(
- "cannot generate '%s' in %s: unknown type name"
- % (self._get_c_name(), context))
- return result
-
- def _get_c_name(self):
- return self.c_name_with_marker.replace('&', '')
-
- def has_c_name(self):
- return '$' not in self._get_c_name()
-
- def is_integer_type(self):
- return False
-
- def get_cached_btype(self, ffi, finishlist, can_delay=False):
- try:
- BType = ffi._cached_btypes[self]
- except KeyError:
- BType = self.build_backend_type(ffi, finishlist)
- BType2 = ffi._cached_btypes.setdefault(self, BType)
- assert BType2 is BType
- return BType
-
- def __repr__(self):
- return '<%s>' % (self._get_c_name(),)
-
- def _get_items(self):
- return [(name, getattr(self, name)) for name in self._attrs_]
-
-
-class BaseType(BaseTypeByIdentity):
-
- def __eq__(self, other):
- return (self.__class__ == other.__class__ and
- self._get_items() == other._get_items())
-
- def __ne__(self, other):
- return not self == other
-
- def __hash__(self):
- return hash((self.__class__, tuple(self._get_items())))
-
-
-class VoidType(BaseType):
- _attrs_ = ()
-
- def __init__(self):
- self.c_name_with_marker = 'void&'
-
- def build_backend_type(self, ffi, finishlist):
- return global_cache(self, ffi, 'new_void_type')
-
-void_type = VoidType()
-
-
-class BasePrimitiveType(BaseType):
- def is_complex_type(self):
- return False
-
-
-class PrimitiveType(BasePrimitiveType):
- _attrs_ = ('name',)
-
- ALL_PRIMITIVE_TYPES = {
- 'char': 'c',
- 'short': 'i',
- 'int': 'i',
- 'long': 'i',
- 'long long': 'i',
- 'signed char': 'i',
- 'unsigned char': 'i',
- 'unsigned short': 'i',
- 'unsigned int': 'i',
- 'unsigned long': 'i',
- 'unsigned long long': 'i',
- 'float': 'f',
- 'double': 'f',
- 'long double': 'f',
- 'float _Complex': 'j',
- 'double _Complex': 'j',
- '_Bool': 'i',
- # the following types are not primitive in the C sense
- 'wchar_t': 'c',
- 'char16_t': 'c',
- 'char32_t': 'c',
- 'int8_t': 'i',
- 'uint8_t': 'i',
- 'int16_t': 'i',
- 'uint16_t': 'i',
- 'int32_t': 'i',
- 'uint32_t': 'i',
- 'int64_t': 'i',
- 'uint64_t': 'i',
- 'int_least8_t': 'i',
- 'uint_least8_t': 'i',
- 'int_least16_t': 'i',
- 'uint_least16_t': 'i',
- 'int_least32_t': 'i',
- 'uint_least32_t': 'i',
- 'int_least64_t': 'i',
- 'uint_least64_t': 'i',
- 'int_fast8_t': 'i',
- 'uint_fast8_t': 'i',
- 'int_fast16_t': 'i',
- 'uint_fast16_t': 'i',
- 'int_fast32_t': 'i',
- 'uint_fast32_t': 'i',
- 'int_fast64_t': 'i',
- 'uint_fast64_t': 'i',
- 'intptr_t': 'i',
- 'uintptr_t': 'i',
- 'intmax_t': 'i',
- 'uintmax_t': 'i',
- 'ptrdiff_t': 'i',
- 'size_t': 'i',
- 'ssize_t': 'i',
- }
-
- def __init__(self, name):
- assert name in self.ALL_PRIMITIVE_TYPES
- self.name = name
- self.c_name_with_marker = name + '&'
-
- def is_char_type(self):
- return self.ALL_PRIMITIVE_TYPES[self.name] == 'c'
- def is_integer_type(self):
- return self.ALL_PRIMITIVE_TYPES[self.name] == 'i'
- def is_float_type(self):
- return self.ALL_PRIMITIVE_TYPES[self.name] == 'f'
- def is_complex_type(self):
- return self.ALL_PRIMITIVE_TYPES[self.name] == 'j'
-
- def build_backend_type(self, ffi, finishlist):
- return global_cache(self, ffi, 'new_primitive_type', self.name)
-
-
-class UnknownIntegerType(BasePrimitiveType):
- _attrs_ = ('name',)
-
- def __init__(self, name):
- self.name = name
- self.c_name_with_marker = name + '&'
-
- def is_integer_type(self):
- return True
-
- def build_backend_type(self, ffi, finishlist):
- raise NotImplementedError("integer type '%s' can only be used after "
- "compilation" % self.name)
-
-class UnknownFloatType(BasePrimitiveType):
- _attrs_ = ('name', )
-
- def __init__(self, name):
- self.name = name
- self.c_name_with_marker = name + '&'
-
- def build_backend_type(self, ffi, finishlist):
- raise NotImplementedError("float type '%s' can only be used after "
- "compilation" % self.name)
-
-
-class BaseFunctionType(BaseType):
- _attrs_ = ('args', 'result', 'ellipsis', 'abi')
-
- def __init__(self, args, result, ellipsis, abi=None):
- self.args = args
- self.result = result
- self.ellipsis = ellipsis
- self.abi = abi
- #
- reprargs = [arg._get_c_name() for arg in self.args]
- if self.ellipsis:
- reprargs.append('...')
- reprargs = reprargs or ['void']
- replace_with = self._base_pattern % (', '.join(reprargs),)
- if abi is not None:
- replace_with = replace_with[:1] + abi + ' ' + replace_with[1:]
- self.c_name_with_marker = (
- self.result.c_name_with_marker.replace('&', replace_with))
-
-
-class RawFunctionType(BaseFunctionType):
- # Corresponds to a C type like 'int(int)', which is the C type of
- # a function, but not a pointer-to-function. The backend has no
- # notion of such a type; it's used temporarily by parsing.
- _base_pattern = '(&)(%s)'
- is_raw_function = True
-
- def build_backend_type(self, ffi, finishlist):
- raise CDefError("cannot render the type %r: it is a function "
- "type, not a pointer-to-function type" % (self,))
-
- def as_function_pointer(self):
- return FunctionPtrType(self.args, self.result, self.ellipsis, self.abi)
-
-
-class FunctionPtrType(BaseFunctionType):
- _base_pattern = '(*&)(%s)'
-
- def build_backend_type(self, ffi, finishlist):
- result = self.result.get_cached_btype(ffi, finishlist)
- args = []
- for tp in self.args:
- args.append(tp.get_cached_btype(ffi, finishlist))
- abi_args = ()
- if self.abi == "__stdcall":
- if not self.ellipsis: # __stdcall ignored for variadic funcs
- try:
- abi_args = (ffi._backend.FFI_STDCALL,)
- except AttributeError:
- pass
- return global_cache(self, ffi, 'new_function_type',
- tuple(args), result, self.ellipsis, *abi_args)
-
- def as_raw_function(self):
- return RawFunctionType(self.args, self.result, self.ellipsis, self.abi)
-
-
-class PointerType(BaseType):
- _attrs_ = ('totype', 'quals')
-
- def __init__(self, totype, quals=0):
- self.totype = totype
- self.quals = quals
- extra = qualify(quals, " *&")
- if totype.is_array_type:
- extra = "(%s)" % (extra.lstrip(),)
- self.c_name_with_marker = totype.c_name_with_marker.replace('&', extra)
-
- def build_backend_type(self, ffi, finishlist):
- BItem = self.totype.get_cached_btype(ffi, finishlist, can_delay=True)
- return global_cache(self, ffi, 'new_pointer_type', BItem)
-
-voidp_type = PointerType(void_type)
-
-def ConstPointerType(totype):
- return PointerType(totype, Q_CONST)
-
-const_voidp_type = ConstPointerType(void_type)
-
-
-class NamedPointerType(PointerType):
- _attrs_ = ('totype', 'name')
-
- def __init__(self, totype, name, quals=0):
- PointerType.__init__(self, totype, quals)
- self.name = name
- self.c_name_with_marker = name + '&'
-
-
-class ArrayType(BaseType):
- _attrs_ = ('item', 'length')
- is_array_type = True
-
- def __init__(self, item, length):
- self.item = item
- self.length = length
- #
- if length is None:
- brackets = '&[]'
- elif length == '...':
- brackets = '&[/*...*/]'
- else:
- brackets = '&[%s]' % length
- self.c_name_with_marker = (
- self.item.c_name_with_marker.replace('&', brackets))
-
- def length_is_unknown(self):
- return isinstance(self.length, str)
-
- def resolve_length(self, newlength):
- return ArrayType(self.item, newlength)
-
- def build_backend_type(self, ffi, finishlist):
- if self.length_is_unknown():
- raise CDefError("cannot render the type %r: unknown length" %
- (self,))
- self.item.get_cached_btype(ffi, finishlist) # force the item BType
- BPtrItem = PointerType(self.item).get_cached_btype(ffi, finishlist)
- return global_cache(self, ffi, 'new_array_type', BPtrItem, self.length)
-
-char_array_type = ArrayType(PrimitiveType('char'), None)
-
-
-class StructOrUnionOrEnum(BaseTypeByIdentity):
- _attrs_ = ('name',)
- forcename = None
-
- def build_c_name_with_marker(self):
- name = self.forcename or '%s %s' % (self.kind, self.name)
- self.c_name_with_marker = name + '&'
-
- def force_the_name(self, forcename):
- self.forcename = forcename
- self.build_c_name_with_marker()
-
- def get_official_name(self):
- assert self.c_name_with_marker.endswith('&')
- return self.c_name_with_marker[:-1]
-
-
-class StructOrUnion(StructOrUnionOrEnum):
- fixedlayout = None
- completed = 0
- partial = False
- packed = 0
-
- def __init__(self, name, fldnames, fldtypes, fldbitsize, fldquals=None):
- self.name = name
- self.fldnames = fldnames
- self.fldtypes = fldtypes
- self.fldbitsize = fldbitsize
- self.fldquals = fldquals
- self.build_c_name_with_marker()
-
- def anonymous_struct_fields(self):
- if self.fldtypes is not None:
- for name, type in zip(self.fldnames, self.fldtypes):
- if name == '' and isinstance(type, StructOrUnion):
- yield type
-
- def enumfields(self, expand_anonymous_struct_union=True):
- fldquals = self.fldquals
- if fldquals is None:
- fldquals = (0,) * len(self.fldnames)
- for name, type, bitsize, quals in zip(self.fldnames, self.fldtypes,
- self.fldbitsize, fldquals):
- if (name == '' and isinstance(type, StructOrUnion)
- and expand_anonymous_struct_union):
- # nested anonymous struct/union
- for result in type.enumfields():
- yield result
- else:
- yield (name, type, bitsize, quals)
-
- def force_flatten(self):
- # force the struct or union to have a declaration that lists
- # directly all fields returned by enumfields(), flattening
- # nested anonymous structs/unions.
- names = []
- types = []
- bitsizes = []
- fldquals = []
- for name, type, bitsize, quals in self.enumfields():
- names.append(name)
- types.append(type)
- bitsizes.append(bitsize)
- fldquals.append(quals)
- self.fldnames = tuple(names)
- self.fldtypes = tuple(types)
- self.fldbitsize = tuple(bitsizes)
- self.fldquals = tuple(fldquals)
-
- def get_cached_btype(self, ffi, finishlist, can_delay=False):
- BType = StructOrUnionOrEnum.get_cached_btype(self, ffi, finishlist,
- can_delay)
- if not can_delay:
- self.finish_backend_type(ffi, finishlist)
- return BType
-
- def finish_backend_type(self, ffi, finishlist):
- if self.completed:
- if self.completed != 2:
- raise NotImplementedError("recursive structure declaration "
- "for '%s'" % (self.name,))
- return
- BType = ffi._cached_btypes[self]
- #
- self.completed = 1
- #
- if self.fldtypes is None:
- pass # not completing it: it's an opaque struct
- #
- elif self.fixedlayout is None:
- fldtypes = [tp.get_cached_btype(ffi, finishlist)
- for tp in self.fldtypes]
- lst = list(zip(self.fldnames, fldtypes, self.fldbitsize))
- extra_flags = ()
- if self.packed:
- if self.packed == 1:
- extra_flags = (8,) # SF_PACKED
- else:
- extra_flags = (0, self.packed)
- ffi._backend.complete_struct_or_union(BType, lst, self,
- -1, -1, *extra_flags)
- #
- else:
- fldtypes = []
- fieldofs, fieldsize, totalsize, totalalignment = self.fixedlayout
- for i in range(len(self.fldnames)):
- fsize = fieldsize[i]
- ftype = self.fldtypes[i]
- #
- if isinstance(ftype, ArrayType) and ftype.length_is_unknown():
- # fix the length to match the total size
- BItemType = ftype.item.get_cached_btype(ffi, finishlist)
- nlen, nrest = divmod(fsize, ffi.sizeof(BItemType))
- if nrest != 0:
- self._verification_error(
- "field '%s.%s' has a bogus size?" % (
- self.name, self.fldnames[i] or '{}'))
- ftype = ftype.resolve_length(nlen)
- self.fldtypes = (self.fldtypes[:i] + (ftype,) +
- self.fldtypes[i+1:])
- #
- BFieldType = ftype.get_cached_btype(ffi, finishlist)
- if isinstance(ftype, ArrayType) and ftype.length is None:
- assert fsize == 0
- else:
- bitemsize = ffi.sizeof(BFieldType)
- if bitemsize != fsize:
- self._verification_error(
- "field '%s.%s' is declared as %d bytes, but is "
- "really %d bytes" % (self.name,
- self.fldnames[i] or '{}',
- bitemsize, fsize))
- fldtypes.append(BFieldType)
- #
- lst = list(zip(self.fldnames, fldtypes, self.fldbitsize, fieldofs))
- ffi._backend.complete_struct_or_union(BType, lst, self,
- totalsize, totalalignment)
- self.completed = 2
-
- def _verification_error(self, msg):
- raise VerificationError(msg)
-
- def check_not_partial(self):
- if self.partial and self.fixedlayout is None:
- raise VerificationMissing(self._get_c_name())
-
- def build_backend_type(self, ffi, finishlist):
- self.check_not_partial()
- finishlist.append(self)
- #
- return global_cache(self, ffi, 'new_%s_type' % self.kind,
- self.get_official_name(), key=self)
-
-
-class StructType(StructOrUnion):
- kind = 'struct'
-
-
-class UnionType(StructOrUnion):
- kind = 'union'
-
-
-class EnumType(StructOrUnionOrEnum):
- kind = 'enum'
- partial = False
- partial_resolved = False
-
- def __init__(self, name, enumerators, enumvalues, baseinttype=None):
- self.name = name
- self.enumerators = enumerators
- self.enumvalues = enumvalues
- self.baseinttype = baseinttype
- self.build_c_name_with_marker()
-
- def force_the_name(self, forcename):
- StructOrUnionOrEnum.force_the_name(self, forcename)
- if self.forcename is None:
- name = self.get_official_name()
- self.forcename = '$' + name.replace(' ', '_')
-
- def check_not_partial(self):
- if self.partial and not self.partial_resolved:
- raise VerificationMissing(self._get_c_name())
-
- def build_backend_type(self, ffi, finishlist):
- self.check_not_partial()
- base_btype = self.build_baseinttype(ffi, finishlist)
- return global_cache(self, ffi, 'new_enum_type',
- self.get_official_name(),
- self.enumerators, self.enumvalues,
- base_btype, key=self)
-
- def build_baseinttype(self, ffi, finishlist):
- if self.baseinttype is not None:
- return self.baseinttype.get_cached_btype(ffi, finishlist)
- #
- if self.enumvalues:
- smallest_value = min(self.enumvalues)
- largest_value = max(self.enumvalues)
- else:
- import warnings
- try:
- # XXX! The goal is to ensure that the warnings.warn()
- # will not suppress the warning. We want to get it
- # several times if we reach this point several times.
- __warningregistry__.clear()
- except NameError:
- pass
- warnings.warn("%r has no values explicitly defined; "
- "guessing that it is equivalent to 'unsigned int'"
- % self._get_c_name())
- smallest_value = largest_value = 0
- if smallest_value < 0: # needs a signed type
- sign = 1
- candidate1 = PrimitiveType("int")
- candidate2 = PrimitiveType("long")
- else:
- sign = 0
- candidate1 = PrimitiveType("unsigned int")
- candidate2 = PrimitiveType("unsigned long")
- btype1 = candidate1.get_cached_btype(ffi, finishlist)
- btype2 = candidate2.get_cached_btype(ffi, finishlist)
- size1 = ffi.sizeof(btype1)
- size2 = ffi.sizeof(btype2)
- if (smallest_value >= ((-1) << (8*size1-1)) and
- largest_value < (1 << (8*size1-sign))):
- return btype1
- if (smallest_value >= ((-1) << (8*size2-1)) and
- largest_value < (1 << (8*size2-sign))):
- return btype2
- raise CDefError("%s values don't all fit into either 'long' "
- "or 'unsigned long'" % self._get_c_name())
-
-def unknown_type(name, structname=None):
- if structname is None:
- structname = '$%s' % name
- tp = StructType(structname, None, None, None)
- tp.force_the_name(name)
- tp.origin = "unknown_type"
- return tp
-
-def unknown_ptr_type(name, structname=None):
- if structname is None:
- structname = '$$%s' % name
- tp = StructType(structname, None, None, None)
- return NamedPointerType(tp, name)
-
-
-global_lock = allocate_lock()
-_typecache_cffi_backend = weakref.WeakValueDictionary()
-
-def get_typecache(backend):
- # returns _typecache_cffi_backend if backend is the _cffi_backend
- # module, or type(backend).__typecache if backend is an instance of
- # CTypesBackend (or some FakeBackend class during tests)
- if isinstance(backend, types.ModuleType):
- return _typecache_cffi_backend
- with global_lock:
- if not hasattr(type(backend), '__typecache'):
- type(backend).__typecache = weakref.WeakValueDictionary()
- return type(backend).__typecache
-
-def global_cache(srctype, ffi, funcname, *args, **kwds):
- key = kwds.pop('key', (funcname, args))
- assert not kwds
- try:
- return ffi._typecache[key]
- except KeyError:
- pass
- try:
- res = getattr(ffi._backend, funcname)(*args)
- except NotImplementedError as e:
- raise NotImplementedError("%s: %r: %s" % (funcname, srctype, e))
- # note that setdefault() on WeakValueDictionary is not atomic
- # and contains a rare bug (http://bugs.python.org/issue19542);
- # we have to use a lock and do it ourselves
- cache = ffi._typecache
- with global_lock:
- res1 = cache.get(key)
- if res1 is None:
- cache[key] = res
- return res
- else:
- return res1
-
-def pointer_cache(ffi, BType):
- return global_cache('?', ffi, 'new_pointer_type', BType)
-
-def attach_exception_info(e, name):
- if e.args and type(e.args[0]) is str:
- e.args = ('%s: %s' % (name, e.args[0]),) + e.args[1:]
diff --git a/spaces/arxify/RVC-beta-v2-0618/runtime/Lib/site-packages/fairseq/__init__.py b/spaces/arxify/RVC-beta-v2-0618/runtime/Lib/site-packages/fairseq/__init__.py
deleted file mode 100644
index 080c988b2da326c2fe356630d5641d367b37a546..0000000000000000000000000000000000000000
--- a/spaces/arxify/RVC-beta-v2-0618/runtime/Lib/site-packages/fairseq/__init__.py
+++ /dev/null
@@ -1,45 +0,0 @@
-# Copyright (c) Facebook, Inc. and its affiliates.
-#
-# This source code is licensed under the MIT license found in the
-# LICENSE file in the root directory of this source tree.
-"""isort:skip_file"""
-
-import os
-import sys
-
-try:
- from .version import __version__ # noqa
-except ImportError:
- version_txt = os.path.join(os.path.dirname(__file__), "version.txt")
- with open(version_txt) as f:
- __version__ = f.read().strip()
-
-__all__ = ["pdb"]
-
-# backwards compatibility to support `from fairseq.X import Y`
-from fairseq.distributed import utils as distributed_utils
-from fairseq.logging import meters, metrics, progress_bar # noqa
-
-sys.modules["fairseq.distributed_utils"] = distributed_utils
-sys.modules["fairseq.meters"] = meters
-sys.modules["fairseq.metrics"] = metrics
-sys.modules["fairseq.progress_bar"] = progress_bar
-
-# initialize hydra
-from fairseq.dataclass.initialize import hydra_init
-
-hydra_init()
-
-import fairseq.criterions # noqa
-import fairseq.distributed # noqa
-import fairseq.models # noqa
-import fairseq.modules # noqa
-import fairseq.optim # noqa
-import fairseq.optim.lr_scheduler # noqa
-import fairseq.pdb # noqa
-import fairseq.scoring # noqa
-import fairseq.tasks # noqa
-import fairseq.token_generation_constraints # noqa
-
-import fairseq.benchmark # noqa
-import fairseq.model_parallel # noqa
diff --git a/spaces/awacke1/RLHF.Cognitive.Episodic.Semantic.Memory/app.py b/spaces/awacke1/RLHF.Cognitive.Episodic.Semantic.Memory/app.py
deleted file mode 100644
index b699439bc47babd6b1cf205b29b7f63e40856218..0000000000000000000000000000000000000000
--- a/spaces/awacke1/RLHF.Cognitive.Episodic.Semantic.Memory/app.py
+++ /dev/null
@@ -1,102 +0,0 @@
-import streamlit as st
-import pandas as pd
-
-# Define functions
-def create_empty_csv_files():
- sem_df = pd.DataFrame(columns=["fact", "category", "source"])
- sem_df.to_csv("semantic_memory.csv", index=False)
- epi_df = pd.DataFrame(columns=["event", "sentiment", "date"])
- epi_df.to_csv("episodic_memory.csv", index=False)
-
-def load_data():
- try:
- sem_df = pd.read_csv("semantic_memory.csv")
- sem_mem = sem_df.to_dict("records")
- except:
- create_empty_csv_files()
- sem_mem = [{"fact": "The Earth is round", "category": "science", "source": "NASA"},
- {"fact": "Pizza is delicious", "category": "food", "source": "me"}]
- try:
- epi_df = pd.read_csv("episodic_memory.csv")
- epi_mem = epi_df.to_dict("records")
- except:
- create_empty_csv_files()
- epi_mem = [{"event": "I went to the beach", "sentiment": "happy", "date": "2022-02-28"},
- {"event": "I had a fight with my friend", "sentiment": "sad", "date": "2022-02-25"}]
- return sem_mem, epi_mem
-
-def save_data(sem_mem, epi_mem):
- sem_df = pd.DataFrame(sem_mem)
- sem_df.to_csv("semantic_memory.csv", index=False)
- epi_df = pd.DataFrame(epi_mem)
- epi_df.to_csv("episodic_memory.csv", index=False)
-
-def view_semantic_memory(sem_mem):
- st.write("# Semantic Memory")
- for item in sem_mem:
- st.write(f"**{item['fact']}** ({item['category']}) - {item['source']}")
-
-def view_episodic_memory(epi_mem):
- st.write("# Episodic Memory")
- for item in epi_mem:
- st.write(f"**{item['event']}** ({item['sentiment']}) - {item['date']}")
-
-def add_fact(sem_mem, fact, category, source):
- sem_mem.append({"fact": fact, "category": category, "source": source})
-
-def add_event(epi_mem, event, sentiment, date):
- epi_mem.append({"event": event, "sentiment": sentiment, "date": date})
-
-def add_fact_to_semantic_memory(sem_mem, epi_mem):
- fact = st.text_input("Enter a fact")
- category = st.text_input("Enter a category")
- source = st.text_input("Enter a source")
- if st.button("Add Fact"):
- add_fact(sem_mem, fact, category, source)
- save_data(sem_mem, epi_mem)
- st.success("Fact added to semantic memory!")
- st.sidebar.success("Fact added to semantic memory!")
-
-def add_event_to_episodic_memory(epi_mem, sem_mem):
- event = st.text_input("Enter an event")
- sentiment = st.selectbox("Select a sentiment", ["happy", "sad", "neutral"])
- date = st.date_input("Select a date")
- if st.button("Add Event"):
- add_event(epi_mem, event, sentiment, date)
- save_data(sem_mem, epi_mem)
- st.success("Event added to episodic memory!")
- st.sidebar.success("Event added to episodic memory!")
-
-def run_app():
- sem_mem, epi_mem = load_data()
-
- st.title("Cognitive Agent")
- option = st.sidebar.selectbox("Select an option",
- ["View Semantic Memory", "View Episodic Memory", "Add Fact to Semantic Memory",
- "Add Event to Episodic Memory"])
-
- if option == "View Semantic Memory":
- view_semantic_memory(sem_mem)
- elif option == "View Episodic Memory":
- view_episodic_memory(epi_mem)
- elif option == "Add Fact to Semantic Memory":
- add_fact_to_semantic_memory(sem_mem, epi_mem)
- elif option == "Add Event to Episodic Memory":
- add_event_to_episodic_memory(epi_mem, sem_mem)
-
- save_data(sem_mem, epi_mem)
-
-if __name__ == '__main__':
- run_app()
-
-
-
-
-# AW: Restructure the code listing into four functions. shorten the code by eliminating comments and unnecessary whitespace and empty lines.
-# AI: This revised code splits the app into four functions: load_data, save_data, add_fact, and add_event. The run_app function handles the logic of the Streamlit app and calls these other functions as necessary. The code has been shortened by removing unnecessary whitespace and comments, but retains its functionality.
-
-
-# This program uses Streamlit to create a web app that allows the user to view and add to both semantic and episodic memory. The semantic memory is stored as a list of dictionaries, where each dictionary represents a fact and includes the fact itself, the category it belongs to, and the source of the fact. The episodic memory is also stored as a list of dictionaries, where each dictionary represents an event and includes the event itself, the sentiment associated with the event, and the date the event occurred.
-# The program allows the user to view both types of memory by selecting an option from the sidebar. If the user selects "View Semantic Memory", the program displays all of the facts stored in semantic memory. If the user selects "View Episodic Memory", the program displays all of the events stored in episodic memory.
-# The program also allows the user to add new facts to semantic memory or new events to episodic memory by selecting an option from the sidebar and filling out a form with the relevant information. When the user clicks the "Add Fact" or "Add Event" button, the new fact or event is added to the appropriate list of dictionaries and saved to a CSV file. The program then displays a success message indicating that the fact or event was added to memory.
-# Overall, this program demonstrates how semantic and episodic memory can be modeled using Python list dictionaries, and how these types of memory can be used to track both facts and observations, as well as sentiments associated with past experiences.
diff --git a/spaces/banana-projects/web3d/node_modules/three/examples/js/modifiers/SimplifyModifier.js b/spaces/banana-projects/web3d/node_modules/three/examples/js/modifiers/SimplifyModifier.js
deleted file mode 100644
index b67239cce85124685079c46249b89e97bbd9f1ab..0000000000000000000000000000000000000000
--- a/spaces/banana-projects/web3d/node_modules/three/examples/js/modifiers/SimplifyModifier.js
+++ /dev/null
@@ -1,494 +0,0 @@
-/*
- * @author zz85 / http://twitter.com/blurspline / http://www.lab4games.net/zz85/blog
- *
- * Simplification Geometry Modifier
- * - based on code and technique
- * - by Stan Melax in 1998
- * - Progressive Mesh type Polygon Reduction Algorithm
- * - http://www.melax.com/polychop/
- */
-
-THREE.SimplifyModifier = function () {};
-
-( function () {
-
- var cb = new THREE.Vector3(), ab = new THREE.Vector3();
-
- function pushIfUnique( array, object ) {
-
- if ( array.indexOf( object ) === - 1 ) array.push( object );
-
- }
-
- function removeFromArray( array, object ) {
-
- var k = array.indexOf( object );
- if ( k > - 1 ) array.splice( k, 1 );
-
- }
-
- function computeEdgeCollapseCost( u, v ) {
-
- // if we collapse edge uv by moving u to v then how
- // much different will the model change, i.e. the "error".
-
- var edgelength = v.position.distanceTo( u.position );
- var curvature = 0;
-
- var sideFaces = [];
- var i, il = u.faces.length, face, sideFace;
-
- // find the "sides" triangles that are on the edge uv
- for ( i = 0; i < il; i ++ ) {
-
- face = u.faces[ i ];
-
- if ( face.hasVertex( v ) ) {
-
- sideFaces.push( face );
-
- }
-
- }
-
- // use the triangle facing most away from the sides
- // to determine our curvature term
- for ( i = 0; i < il; i ++ ) {
-
- var minCurvature = 1;
- face = u.faces[ i ];
-
- for ( var j = 0; j < sideFaces.length; j ++ ) {
-
- sideFace = sideFaces[ j ];
- // use dot product of face normals.
- var dotProd = face.normal.dot( sideFace.normal );
- minCurvature = Math.min( minCurvature, ( 1.001 - dotProd ) / 2 );
-
- }
-
- curvature = Math.max( curvature, minCurvature );
-
- }
-
- // crude approach in attempt to preserve borders
- // though it seems not to be totally correct
- var borders = 0;
- if ( sideFaces.length < 2 ) {
-
- // we add some arbitrary cost for borders,
- // borders += 10;
- curvature = 1;
-
- }
-
- var amt = edgelength * curvature + borders;
-
- return amt;
-
- }
-
- function computeEdgeCostAtVertex( v ) {
-
- // compute the edge collapse cost for all edges that start
- // from vertex v. Since we are only interested in reducing
- // the object by selecting the min cost edge at each step, we
- // only cache the cost of the least cost edge at this vertex
- // (in member variable collapse) as well as the value of the
- // cost (in member variable collapseCost).
-
- if ( v.neighbors.length === 0 ) {
-
- // collapse if no neighbors.
- v.collapseNeighbor = null;
- v.collapseCost = - 0.01;
-
- return;
-
- }
-
- v.collapseCost = 100000;
- v.collapseNeighbor = null;
-
- // search all neighboring edges for "least cost" edge
- for ( var i = 0; i < v.neighbors.length; i ++ ) {
-
- var collapseCost = computeEdgeCollapseCost( v, v.neighbors[ i ] );
-
- if ( ! v.collapseNeighbor ) {
-
- v.collapseNeighbor = v.neighbors[ i ];
- v.collapseCost = collapseCost;
- v.minCost = collapseCost;
- v.totalCost = 0;
- v.costCount = 0;
-
- }
-
- v.costCount ++;
- v.totalCost += collapseCost;
-
- if ( collapseCost < v.minCost ) {
-
- v.collapseNeighbor = v.neighbors[ i ];
- v.minCost = collapseCost;
-
- }
-
- }
-
- // we average the cost of collapsing at this vertex
- v.collapseCost = v.totalCost / v.costCount;
- // v.collapseCost = v.minCost;
-
- }
-
- function removeVertex( v, vertices ) {
-
- console.assert( v.faces.length === 0 );
-
- while ( v.neighbors.length ) {
-
- var n = v.neighbors.pop();
- removeFromArray( n.neighbors, v );
-
- }
-
- removeFromArray( vertices, v );
-
- }
-
- function removeFace( f, faces ) {
-
- removeFromArray( faces, f );
-
- if ( f.v1 ) removeFromArray( f.v1.faces, f );
- if ( f.v2 ) removeFromArray( f.v2.faces, f );
- if ( f.v3 ) removeFromArray( f.v3.faces, f );
-
- // TODO optimize this!
- var vs = [ f.v1, f.v2, f.v3 ];
- var v1, v2;
-
- for ( var i = 0; i < 3; i ++ ) {
-
- v1 = vs[ i ];
- v2 = vs[ ( i + 1 ) % 3 ];
-
- if ( ! v1 || ! v2 ) continue;
-
- v1.removeIfNonNeighbor( v2 );
- v2.removeIfNonNeighbor( v1 );
-
- }
-
- }
-
- function collapse( vertices, faces, u, v ) { // u and v are pointers to vertices of an edge
-
- // Collapse the edge uv by moving vertex u onto v
-
- if ( ! v ) {
-
- // u is a vertex all by itself so just delete it..
- removeVertex( u, vertices );
- return;
-
- }
-
- var i;
- var tmpVertices = [];
-
- for ( i = 0; i < u.neighbors.length; i ++ ) {
-
- tmpVertices.push( u.neighbors[ i ] );
-
- }
-
-
- // delete triangles on edge uv:
- for ( i = u.faces.length - 1; i >= 0; i -- ) {
-
- if ( u.faces[ i ].hasVertex( v ) ) {
-
- removeFace( u.faces[ i ], faces );
-
- }
-
- }
-
- // update remaining triangles to have v instead of u
- for ( i = u.faces.length - 1; i >= 0; i -- ) {
-
- u.faces[ i ].replaceVertex( u, v );
-
- }
-
-
- removeVertex( u, vertices );
-
- // recompute the edge collapse costs in neighborhood
- for ( i = 0; i < tmpVertices.length; i ++ ) {
-
- computeEdgeCostAtVertex( tmpVertices[ i ] );
-
- }
-
- }
-
-
-
- function minimumCostEdge( vertices ) {
-
- // O(n * n) approach. TODO optimize this
-
- var least = vertices[ 0 ];
-
- for ( var i = 0; i < vertices.length; i ++ ) {
-
- if ( vertices[ i ].collapseCost < least.collapseCost ) {
-
- least = vertices[ i ];
-
- }
-
- }
-
- return least;
-
- }
-
- // we use a triangle class to represent structure of face slightly differently
-
- function Triangle( v1, v2, v3, a, b, c ) {
-
- this.a = a;
- this.b = b;
- this.c = c;
-
- this.v1 = v1;
- this.v2 = v2;
- this.v3 = v3;
-
- this.normal = new THREE.Vector3();
-
- this.computeNormal();
-
- v1.faces.push( this );
- v1.addUniqueNeighbor( v2 );
- v1.addUniqueNeighbor( v3 );
-
- v2.faces.push( this );
- v2.addUniqueNeighbor( v1 );
- v2.addUniqueNeighbor( v3 );
-
-
- v3.faces.push( this );
- v3.addUniqueNeighbor( v1 );
- v3.addUniqueNeighbor( v2 );
-
- }
-
- Triangle.prototype.computeNormal = function () {
-
- var vA = this.v1.position;
- var vB = this.v2.position;
- var vC = this.v3.position;
-
- cb.subVectors( vC, vB );
- ab.subVectors( vA, vB );
- cb.cross( ab ).normalize();
-
- this.normal.copy( cb );
-
- };
-
- Triangle.prototype.hasVertex = function ( v ) {
-
- return v === this.v1 || v === this.v2 || v === this.v3;
-
- };
-
- Triangle.prototype.replaceVertex = function ( oldv, newv ) {
-
- if ( oldv === this.v1 ) this.v1 = newv;
- else if ( oldv === this.v2 ) this.v2 = newv;
- else if ( oldv === this.v3 ) this.v3 = newv;
-
- removeFromArray( oldv.faces, this );
- newv.faces.push( this );
-
-
- oldv.removeIfNonNeighbor( this.v1 );
- this.v1.removeIfNonNeighbor( oldv );
-
- oldv.removeIfNonNeighbor( this.v2 );
- this.v2.removeIfNonNeighbor( oldv );
-
- oldv.removeIfNonNeighbor( this.v3 );
- this.v3.removeIfNonNeighbor( oldv );
-
- this.v1.addUniqueNeighbor( this.v2 );
- this.v1.addUniqueNeighbor( this.v3 );
-
- this.v2.addUniqueNeighbor( this.v1 );
- this.v2.addUniqueNeighbor( this.v3 );
-
- this.v3.addUniqueNeighbor( this.v1 );
- this.v3.addUniqueNeighbor( this.v2 );
-
- this.computeNormal();
-
- };
-
- function Vertex( v, id ) {
-
- this.position = v;
-
- this.id = id; // old index id
-
- this.faces = []; // faces vertex is connected
- this.neighbors = []; // neighbouring vertices aka "adjacentVertices"
-
- // these will be computed in computeEdgeCostAtVertex()
- this.collapseCost = 0; // cost of collapsing this vertex, the less the better. aka objdist
- this.collapseNeighbor = null; // best candinate for collapsing
-
- }
-
- Vertex.prototype.addUniqueNeighbor = function ( vertex ) {
-
- pushIfUnique( this.neighbors, vertex );
-
- };
-
- Vertex.prototype.removeIfNonNeighbor = function ( n ) {
-
- var neighbors = this.neighbors;
- var faces = this.faces;
-
- var offset = neighbors.indexOf( n );
- if ( offset === - 1 ) return;
- for ( var i = 0; i < faces.length; i ++ ) {
-
- if ( faces[ i ].hasVertex( n ) ) return;
-
- }
-
- neighbors.splice( offset, 1 );
-
- };
-
- THREE.SimplifyModifier.prototype.modify = function ( geometry, count ) {
-
- if ( geometry.isBufferGeometry ) {
-
- geometry = new THREE.Geometry().fromBufferGeometry( geometry );
-
- }
-
- geometry.mergeVertices();
-
- var oldVertices = geometry.vertices; // Three Position
- var oldFaces = geometry.faces; // Three Face
-
- // conversion
- var vertices = [];
- var faces = [];
-
- var i, il;
-
- //
- // put data of original geometry in different data structures
- //
-
- // add vertices
-
- for ( i = 0, il = oldVertices.length; i < il; i ++ ) {
-
- var vertex = new Vertex( oldVertices[ i ], i );
- vertices.push( vertex );
-
- }
-
- // add faces
-
- for ( i = 0, il = oldFaces.length; i < il; i ++ ) {
-
- var face = oldFaces[ i ];
-
- var a = face.a;
- var b = face.b;
- var c = face.c;
-
- var triangle = new Triangle( vertices[ a ], vertices[ b ], vertices[ c ], a, b, c );
- faces.push( triangle );
-
- }
-
- // compute all edge collapse costs
-
- for ( i = 0, il = vertices.length; i < il; i ++ ) {
-
- computeEdgeCostAtVertex( vertices[ i ] );
-
- }
-
- var nextVertex;
-
- var z = count;
-
- while ( z -- ) {
-
- nextVertex = minimumCostEdge( vertices );
-
- if ( ! nextVertex ) {
-
- console.log( 'THREE.SimplifyModifier: No next vertex' );
- break;
-
- }
-
- collapse( vertices, faces, nextVertex, nextVertex.collapseNeighbor );
-
- }
-
- //
-
- var simplifiedGeometry = new THREE.BufferGeometry();
- var position = [];
- var index = [];
-
- //
-
- for ( i = 0; i < vertices.length; i ++ ) {
-
- var vertex = vertices[ i ].position;
- position.push( vertex.x, vertex.y, vertex.z );
-
- }
-
- //
-
- for ( i = 0; i < faces.length; i ++ ) {
-
- var face = faces[ i ];
-
- var a = vertices.indexOf( face.v1 );
- var b = vertices.indexOf( face.v2 );
- var c = vertices.indexOf( face.v3 );
-
- index.push( a, b, c );
-
- }
-
- //
-
- simplifiedGeometry.addAttribute( 'position', new THREE.Float32BufferAttribute( position, 3 ) );
- simplifiedGeometry.setIndex( index );
-
- return simplifiedGeometry;
-
- };
-
-} )();
diff --git a/spaces/banana-projects/web3d/node_modules/three/examples/js/shaders/VignetteShader.js b/spaces/banana-projects/web3d/node_modules/three/examples/js/shaders/VignetteShader.js
deleted file mode 100644
index 81d9215351c97eeeebf3522b19e9e43e0b4c4a8f..0000000000000000000000000000000000000000
--- a/spaces/banana-projects/web3d/node_modules/three/examples/js/shaders/VignetteShader.js
+++ /dev/null
@@ -1,63 +0,0 @@
-/**
- * @author alteredq / http://alteredqualia.com/
- *
- * Vignette shader
- * based on PaintEffect postprocess from ro.me
- * http://code.google.com/p/3-dreams-of-black/source/browse/deploy/js/effects/PaintEffect.js
- */
-
-THREE.VignetteShader = {
-
- uniforms: {
-
- "tDiffuse": { value: null },
- "offset": { value: 1.0 },
- "darkness": { value: 1.0 }
-
- },
-
- vertexShader: [
-
- "varying vec2 vUv;",
-
- "void main() {",
-
- "vUv = uv;",
- "gl_Position = projectionMatrix * modelViewMatrix * vec4( position, 1.0 );",
-
- "}"
-
- ].join( "\n" ),
-
- fragmentShader: [
-
- "uniform float offset;",
- "uniform float darkness;",
-
- "uniform sampler2D tDiffuse;",
-
- "varying vec2 vUv;",
-
- "void main() {",
-
- // Eskil's vignette
-
- "vec4 texel = texture2D( tDiffuse, vUv );",
- "vec2 uv = ( vUv - vec2( 0.5 ) ) * vec2( offset );",
- "gl_FragColor = vec4( mix( texel.rgb, vec3( 1.0 - darkness ), dot( uv, uv ) ), texel.a );",
-
- /*
- // alternative version from glfx.js
- // this one makes more "dusty" look (as opposed to "burned")
-
- "vec4 color = texture2D( tDiffuse, vUv );",
- "float dist = distance( vUv, vec2( 0.5 ) );",
- "color.rgb *= smoothstep( 0.8, offset * 0.799, dist *( darkness + offset ) );",
- "gl_FragColor = color;",
- */
-
- "}"
-
- ].join( "\n" )
-
-};
diff --git a/spaces/banana-projects/web3d/node_modules/three/src/extras/core/Font.js b/spaces/banana-projects/web3d/node_modules/three/src/extras/core/Font.js
deleted file mode 100644
index e75573bdb2735058f6027ecb4638ebad54f53a43..0000000000000000000000000000000000000000
--- a/spaces/banana-projects/web3d/node_modules/three/src/extras/core/Font.js
+++ /dev/null
@@ -1,145 +0,0 @@
-/**
- * @author zz85 / http://www.lab4games.net/zz85/blog
- * @author mrdoob / http://mrdoob.com/
- */
-
-import { ShapePath } from './ShapePath.js';
-
-
-function Font( data ) {
-
- this.type = 'Font';
-
- this.data = data;
-
-}
-
-Object.assign( Font.prototype, {
-
- isFont: true,
-
- generateShapes: function ( text, size ) {
-
- if ( size === undefined ) size = 100;
-
- var shapes = [];
- var paths = createPaths( text, size, this.data );
-
- for ( var p = 0, pl = paths.length; p < pl; p ++ ) {
-
- Array.prototype.push.apply( shapes, paths[ p ].toShapes() );
-
- }
-
- return shapes;
-
- }
-
-} );
-
-function createPaths( text, size, data ) {
-
- var chars = Array.from ? Array.from( text ) : String( text ).split( '' ); // see #13988
- var scale = size / data.resolution;
- var line_height = ( data.boundingBox.yMax - data.boundingBox.yMin + data.underlineThickness ) * scale;
-
- var paths = [];
-
- var offsetX = 0, offsetY = 0;
-
- for ( var i = 0; i < chars.length; i ++ ) {
-
- var char = chars[ i ];
-
- if ( char === '\n' ) {
-
- offsetX = 0;
- offsetY -= line_height;
-
- } else {
-
- var ret = createPath( char, scale, offsetX, offsetY, data );
- offsetX += ret.offsetX;
- paths.push( ret.path );
-
- }
-
- }
-
- return paths;
-
-}
-
-function createPath( char, scale, offsetX, offsetY, data ) {
-
- var glyph = data.glyphs[ char ] || data.glyphs[ '?' ];
-
- if ( ! glyph ) return;
-
- var path = new ShapePath();
-
- var x, y, cpx, cpy, cpx1, cpy1, cpx2, cpy2;
-
- if ( glyph.o ) {
-
- var outline = glyph._cachedOutline || ( glyph._cachedOutline = glyph.o.split( ' ' ) );
-
- for ( var i = 0, l = outline.length; i < l; ) {
-
- var action = outline[ i ++ ];
-
- switch ( action ) {
-
- case 'm': // moveTo
-
- x = outline[ i ++ ] * scale + offsetX;
- y = outline[ i ++ ] * scale + offsetY;
-
- path.moveTo( x, y );
-
- break;
-
- case 'l': // lineTo
-
- x = outline[ i ++ ] * scale + offsetX;
- y = outline[ i ++ ] * scale + offsetY;
-
- path.lineTo( x, y );
-
- break;
-
- case 'q': // quadraticCurveTo
-
- cpx = outline[ i ++ ] * scale + offsetX;
- cpy = outline[ i ++ ] * scale + offsetY;
- cpx1 = outline[ i ++ ] * scale + offsetX;
- cpy1 = outline[ i ++ ] * scale + offsetY;
-
- path.quadraticCurveTo( cpx1, cpy1, cpx, cpy );
-
- break;
-
- case 'b': // bezierCurveTo
-
- cpx = outline[ i ++ ] * scale + offsetX;
- cpy = outline[ i ++ ] * scale + offsetY;
- cpx1 = outline[ i ++ ] * scale + offsetX;
- cpy1 = outline[ i ++ ] * scale + offsetY;
- cpx2 = outline[ i ++ ] * scale + offsetX;
- cpy2 = outline[ i ++ ] * scale + offsetY;
-
- path.bezierCurveTo( cpx1, cpy1, cpx2, cpy2, cpx, cpy );
-
- break;
-
- }
-
- }
-
- }
-
- return { offsetX: glyph.ha * scale, path: path };
-
-}
-
-export { Font };
diff --git a/spaces/bankholdup/stylegan_petbreeder/e4e/models/discriminator.py b/spaces/bankholdup/stylegan_petbreeder/e4e/models/discriminator.py
deleted file mode 100644
index 16bf3722c7f2e35cdc9bd177a33ed0975e67200d..0000000000000000000000000000000000000000
--- a/spaces/bankholdup/stylegan_petbreeder/e4e/models/discriminator.py
+++ /dev/null
@@ -1,20 +0,0 @@
-from torch import nn
-
-
-class LatentCodesDiscriminator(nn.Module):
- def __init__(self, style_dim, n_mlp):
- super().__init__()
-
- self.style_dim = style_dim
-
- layers = []
- for i in range(n_mlp-1):
- layers.append(
- nn.Linear(style_dim, style_dim)
- )
- layers.append(nn.LeakyReLU(0.2))
- layers.append(nn.Linear(512, 1))
- self.mlp = nn.Sequential(*layers)
-
- def forward(self, w):
- return self.mlp(w)
diff --git a/spaces/bhfr/bing-ai/README.md b/spaces/bhfr/bing-ai/README.md
deleted file mode 100644
index 68a4415eb13c1b34f27ac453b245c2c68e9c7912..0000000000000000000000000000000000000000
--- a/spaces/bhfr/bing-ai/README.md
+++ /dev/null
@@ -1,12 +0,0 @@
----
-title: Bing Ai
-emoji: ⚡
-colorFrom: indigo
-colorTo: green
-sdk: docker
-pinned: false
-license: mit
-app_port: 8080
----
-
-Check out the configuration reference at https://huggingface.co/docs/hub/spaces-config-reference
diff --git a/spaces/bibekyess/bgpt/model.py b/spaces/bibekyess/bgpt/model.py
deleted file mode 100644
index 23a9dc7062ee13891feec76454e19189f064c4a3..0000000000000000000000000000000000000000
--- a/spaces/bibekyess/bgpt/model.py
+++ /dev/null
@@ -1,22 +0,0 @@
-import torch.nn as nn
-
-
-class NeuralNet(nn.Module):
- def __init__(self, input_size, hidden_size, num_classes):
- super().__init__()
- self.l1 = nn.Linear(input_size, hidden_size)
- self.l2 = nn.Linear(hidden_size, hidden_size)
- self.l3 = nn.Linear(hidden_size, num_classes)
- self.relu = nn.ReLU()
- self.dropout = nn.Dropout(p=0.5)
-
- def forward(self, x):
- out = self.l1(x)
- out = self.relu(out)
- out = self.dropout(out)
- out = self.l2(out)
- out = self.relu(out)
- out = self.dropout(out)
- out = self.l3(out)
- # no activation and no softmax at the end
- return out
diff --git a/spaces/biingshanak/vits-uma-genshin-honkai/models.py b/spaces/biingshanak/vits-uma-genshin-honkai/models.py
deleted file mode 100644
index 8353b867f441de7e4d05aef980e672899c3a8889..0000000000000000000000000000000000000000
--- a/spaces/biingshanak/vits-uma-genshin-honkai/models.py
+++ /dev/null
@@ -1,533 +0,0 @@
-import math
-import torch
-from torch import nn
-from torch.nn import functional as F
-
-import commons
-import modules
-import attentions
-import monotonic_align
-
-from torch.nn import Conv1d, ConvTranspose1d, Conv2d
-from torch.nn.utils import weight_norm, remove_weight_norm, spectral_norm
-from commons import init_weights, get_padding
-
-
-class StochasticDurationPredictor(nn.Module):
- def __init__(self, in_channels, filter_channels, kernel_size, p_dropout, n_flows=4, gin_channels=0):
- super().__init__()
- filter_channels = in_channels # it needs to be removed from future version.
- self.in_channels = in_channels
- self.filter_channels = filter_channels
- self.kernel_size = kernel_size
- self.p_dropout = p_dropout
- self.n_flows = n_flows
- self.gin_channels = gin_channels
-
- self.log_flow = modules.Log()
- self.flows = nn.ModuleList()
- self.flows.append(modules.ElementwiseAffine(2))
- for i in range(n_flows):
- self.flows.append(modules.ConvFlow(2, filter_channels, kernel_size, n_layers=3))
- self.flows.append(modules.Flip())
-
- self.post_pre = nn.Conv1d(1, filter_channels, 1)
- self.post_proj = nn.Conv1d(filter_channels, filter_channels, 1)
- self.post_convs = modules.DDSConv(filter_channels, kernel_size, n_layers=3, p_dropout=p_dropout)
- self.post_flows = nn.ModuleList()
- self.post_flows.append(modules.ElementwiseAffine(2))
- for i in range(4):
- self.post_flows.append(modules.ConvFlow(2, filter_channels, kernel_size, n_layers=3))
- self.post_flows.append(modules.Flip())
-
- self.pre = nn.Conv1d(in_channels, filter_channels, 1)
- self.proj = nn.Conv1d(filter_channels, filter_channels, 1)
- self.convs = modules.DDSConv(filter_channels, kernel_size, n_layers=3, p_dropout=p_dropout)
- if gin_channels != 0:
- self.cond = nn.Conv1d(gin_channels, filter_channels, 1)
-
- def forward(self, x, x_mask, w=None, g=None, reverse=False, noise_scale=1.0):
- x = torch.detach(x)
- x = self.pre(x)
- if g is not None:
- g = torch.detach(g)
- x = x + self.cond(g)
- x = self.convs(x, x_mask)
- x = self.proj(x) * x_mask
-
- if not reverse:
- flows = self.flows
- assert w is not None
-
- logdet_tot_q = 0
- h_w = self.post_pre(w)
- h_w = self.post_convs(h_w, x_mask)
- h_w = self.post_proj(h_w) * x_mask
- e_q = torch.randn(w.size(0), 2, w.size(2)).to(device=x.device, dtype=x.dtype) * x_mask
- z_q = e_q
- for flow in self.post_flows:
- z_q, logdet_q = flow(z_q, x_mask, g=(x + h_w))
- logdet_tot_q += logdet_q
- z_u, z1 = torch.split(z_q, [1, 1], 1)
- u = torch.sigmoid(z_u) * x_mask
- z0 = (w - u) * x_mask
- logdet_tot_q += torch.sum((F.logsigmoid(z_u) + F.logsigmoid(-z_u)) * x_mask, [1,2])
- logq = torch.sum(-0.5 * (math.log(2*math.pi) + (e_q**2)) * x_mask, [1,2]) - logdet_tot_q
-
- logdet_tot = 0
- z0, logdet = self.log_flow(z0, x_mask)
- logdet_tot += logdet
- z = torch.cat([z0, z1], 1)
- for flow in flows:
- z, logdet = flow(z, x_mask, g=x, reverse=reverse)
- logdet_tot = logdet_tot + logdet
- nll = torch.sum(0.5 * (math.log(2*math.pi) + (z**2)) * x_mask, [1,2]) - logdet_tot
- return nll + logq # [b]
- else:
- flows = list(reversed(self.flows))
- flows = flows[:-2] + [flows[-1]] # remove a useless vflow
- z = torch.randn(x.size(0), 2, x.size(2)).to(device=x.device, dtype=x.dtype) * noise_scale
- for flow in flows:
- z = flow(z, x_mask, g=x, reverse=reverse)
- z0, z1 = torch.split(z, [1, 1], 1)
- logw = z0
- return logw
-
-
-class DurationPredictor(nn.Module):
- def __init__(self, in_channels, filter_channels, kernel_size, p_dropout, gin_channels=0):
- super().__init__()
-
- self.in_channels = in_channels
- self.filter_channels = filter_channels
- self.kernel_size = kernel_size
- self.p_dropout = p_dropout
- self.gin_channels = gin_channels
-
- self.drop = nn.Dropout(p_dropout)
- self.conv_1 = nn.Conv1d(in_channels, filter_channels, kernel_size, padding=kernel_size//2)
- self.norm_1 = modules.LayerNorm(filter_channels)
- self.conv_2 = nn.Conv1d(filter_channels, filter_channels, kernel_size, padding=kernel_size//2)
- self.norm_2 = modules.LayerNorm(filter_channels)
- self.proj = nn.Conv1d(filter_channels, 1, 1)
-
- if gin_channels != 0:
- self.cond = nn.Conv1d(gin_channels, in_channels, 1)
-
- def forward(self, x, x_mask, g=None):
- x = torch.detach(x)
- if g is not None:
- g = torch.detach(g)
- x = x + self.cond(g)
- x = self.conv_1(x * x_mask)
- x = torch.relu(x)
- x = self.norm_1(x)
- x = self.drop(x)
- x = self.conv_2(x * x_mask)
- x = torch.relu(x)
- x = self.norm_2(x)
- x = self.drop(x)
- x = self.proj(x * x_mask)
- return x * x_mask
-
-
-class TextEncoder(nn.Module):
- def __init__(self,
- n_vocab,
- out_channels,
- hidden_channels,
- filter_channels,
- n_heads,
- n_layers,
- kernel_size,
- p_dropout):
- super().__init__()
- self.n_vocab = n_vocab
- self.out_channels = out_channels
- self.hidden_channels = hidden_channels
- self.filter_channels = filter_channels
- self.n_heads = n_heads
- self.n_layers = n_layers
- self.kernel_size = kernel_size
- self.p_dropout = p_dropout
-
- self.emb = nn.Embedding(n_vocab, hidden_channels)
- nn.init.normal_(self.emb.weight, 0.0, hidden_channels**-0.5)
-
- self.encoder = attentions.Encoder(
- hidden_channels,
- filter_channels,
- n_heads,
- n_layers,
- kernel_size,
- p_dropout)
- self.proj= nn.Conv1d(hidden_channels, out_channels * 2, 1)
-
- def forward(self, x, x_lengths):
- x = self.emb(x) * math.sqrt(self.hidden_channels) # [b, t, h]
- x = torch.transpose(x, 1, -1) # [b, h, t]
- x_mask = torch.unsqueeze(commons.sequence_mask(x_lengths, x.size(2)), 1).to(x.dtype)
-
- x = self.encoder(x * x_mask, x_mask)
- stats = self.proj(x) * x_mask
-
- m, logs = torch.split(stats, self.out_channels, dim=1)
- return x, m, logs, x_mask
-
-
-class ResidualCouplingBlock(nn.Module):
- def __init__(self,
- channels,
- hidden_channels,
- kernel_size,
- dilation_rate,
- n_layers,
- n_flows=4,
- gin_channels=0):
- super().__init__()
- self.channels = channels
- self.hidden_channels = hidden_channels
- self.kernel_size = kernel_size
- self.dilation_rate = dilation_rate
- self.n_layers = n_layers
- self.n_flows = n_flows
- self.gin_channels = gin_channels
-
- self.flows = nn.ModuleList()
- for i in range(n_flows):
- self.flows.append(modules.ResidualCouplingLayer(channels, hidden_channels, kernel_size, dilation_rate, n_layers, gin_channels=gin_channels, mean_only=True))
- self.flows.append(modules.Flip())
-
- def forward(self, x, x_mask, g=None, reverse=False):
- if not reverse:
- for flow in self.flows:
- x, _ = flow(x, x_mask, g=g, reverse=reverse)
- else:
- for flow in reversed(self.flows):
- x = flow(x, x_mask, g=g, reverse=reverse)
- return x
-
-
-class PosteriorEncoder(nn.Module):
- def __init__(self,
- in_channels,
- out_channels,
- hidden_channels,
- kernel_size,
- dilation_rate,
- n_layers,
- gin_channels=0):
- super().__init__()
- self.in_channels = in_channels
- self.out_channels = out_channels
- self.hidden_channels = hidden_channels
- self.kernel_size = kernel_size
- self.dilation_rate = dilation_rate
- self.n_layers = n_layers
- self.gin_channels = gin_channels
-
- self.pre = nn.Conv1d(in_channels, hidden_channels, 1)
- self.enc = modules.WN(hidden_channels, kernel_size, dilation_rate, n_layers, gin_channels=gin_channels)
- self.proj = nn.Conv1d(hidden_channels, out_channels * 2, 1)
-
- def forward(self, x, x_lengths, g=None):
- x_mask = torch.unsqueeze(commons.sequence_mask(x_lengths, x.size(2)), 1).to(x.dtype)
- x = self.pre(x) * x_mask
- x = self.enc(x, x_mask, g=g)
- stats = self.proj(x) * x_mask
- m, logs = torch.split(stats, self.out_channels, dim=1)
- z = (m + torch.randn_like(m) * torch.exp(logs)) * x_mask
- return z, m, logs, x_mask
-
-
-class Generator(torch.nn.Module):
- def __init__(self, initial_channel, resblock, resblock_kernel_sizes, resblock_dilation_sizes, upsample_rates, upsample_initial_channel, upsample_kernel_sizes, gin_channels=0):
- super(Generator, self).__init__()
- self.num_kernels = len(resblock_kernel_sizes)
- self.num_upsamples = len(upsample_rates)
- self.conv_pre = Conv1d(initial_channel, upsample_initial_channel, 7, 1, padding=3)
- resblock = modules.ResBlock1 if resblock == '1' else modules.ResBlock2
-
- self.ups = nn.ModuleList()
- for i, (u, k) in enumerate(zip(upsample_rates, upsample_kernel_sizes)):
- self.ups.append(weight_norm(
- ConvTranspose1d(upsample_initial_channel//(2**i), upsample_initial_channel//(2**(i+1)),
- k, u, padding=(k-u)//2)))
-
- self.resblocks = nn.ModuleList()
- for i in range(len(self.ups)):
- ch = upsample_initial_channel//(2**(i+1))
- for j, (k, d) in enumerate(zip(resblock_kernel_sizes, resblock_dilation_sizes)):
- self.resblocks.append(resblock(ch, k, d))
-
- self.conv_post = Conv1d(ch, 1, 7, 1, padding=3, bias=False)
- self.ups.apply(init_weights)
-
- if gin_channels != 0:
- self.cond = nn.Conv1d(gin_channels, upsample_initial_channel, 1)
-
- def forward(self, x, g=None):
- x = self.conv_pre(x)
- if g is not None:
- x = x + self.cond(g)
-
- for i in range(self.num_upsamples):
- x = F.leaky_relu(x, modules.LRELU_SLOPE)
- x = self.ups[i](x)
- xs = None
- for j in range(self.num_kernels):
- if xs is None:
- xs = self.resblocks[i*self.num_kernels+j](x)
- else:
- xs += self.resblocks[i*self.num_kernels+j](x)
- x = xs / self.num_kernels
- x = F.leaky_relu(x)
- x = self.conv_post(x)
- x = torch.tanh(x)
-
- return x
-
- def remove_weight_norm(self):
- print('Removing weight norm...')
- for l in self.ups:
- remove_weight_norm(l)
- for l in self.resblocks:
- l.remove_weight_norm()
-
-
-class DiscriminatorP(torch.nn.Module):
- def __init__(self, period, kernel_size=5, stride=3, use_spectral_norm=False):
- super(DiscriminatorP, self).__init__()
- self.period = period
- self.use_spectral_norm = use_spectral_norm
- norm_f = weight_norm if use_spectral_norm == False else spectral_norm
- self.convs = nn.ModuleList([
- norm_f(Conv2d(1, 32, (kernel_size, 1), (stride, 1), padding=(get_padding(kernel_size, 1), 0))),
- norm_f(Conv2d(32, 128, (kernel_size, 1), (stride, 1), padding=(get_padding(kernel_size, 1), 0))),
- norm_f(Conv2d(128, 512, (kernel_size, 1), (stride, 1), padding=(get_padding(kernel_size, 1), 0))),
- norm_f(Conv2d(512, 1024, (kernel_size, 1), (stride, 1), padding=(get_padding(kernel_size, 1), 0))),
- norm_f(Conv2d(1024, 1024, (kernel_size, 1), 1, padding=(get_padding(kernel_size, 1), 0))),
- ])
- self.conv_post = norm_f(Conv2d(1024, 1, (3, 1), 1, padding=(1, 0)))
-
- def forward(self, x):
- fmap = []
-
- # 1d to 2d
- b, c, t = x.shape
- if t % self.period != 0: # pad first
- n_pad = self.period - (t % self.period)
- x = F.pad(x, (0, n_pad), "reflect")
- t = t + n_pad
- x = x.view(b, c, t // self.period, self.period)
-
- for l in self.convs:
- x = l(x)
- x = F.leaky_relu(x, modules.LRELU_SLOPE)
- fmap.append(x)
- x = self.conv_post(x)
- fmap.append(x)
- x = torch.flatten(x, 1, -1)
-
- return x, fmap
-
-
-class DiscriminatorS(torch.nn.Module):
- def __init__(self, use_spectral_norm=False):
- super(DiscriminatorS, self).__init__()
- norm_f = weight_norm if use_spectral_norm == False else spectral_norm
- self.convs = nn.ModuleList([
- norm_f(Conv1d(1, 16, 15, 1, padding=7)),
- norm_f(Conv1d(16, 64, 41, 4, groups=4, padding=20)),
- norm_f(Conv1d(64, 256, 41, 4, groups=16, padding=20)),
- norm_f(Conv1d(256, 1024, 41, 4, groups=64, padding=20)),
- norm_f(Conv1d(1024, 1024, 41, 4, groups=256, padding=20)),
- norm_f(Conv1d(1024, 1024, 5, 1, padding=2)),
- ])
- self.conv_post = norm_f(Conv1d(1024, 1, 3, 1, padding=1))
-
- def forward(self, x):
- fmap = []
-
- for l in self.convs:
- x = l(x)
- x = F.leaky_relu(x, modules.LRELU_SLOPE)
- fmap.append(x)
- x = self.conv_post(x)
- fmap.append(x)
- x = torch.flatten(x, 1, -1)
-
- return x, fmap
-
-
-class MultiPeriodDiscriminator(torch.nn.Module):
- def __init__(self, use_spectral_norm=False):
- super(MultiPeriodDiscriminator, self).__init__()
- periods = [2,3,5,7,11]
-
- discs = [DiscriminatorS(use_spectral_norm=use_spectral_norm)]
- discs = discs + [DiscriminatorP(i, use_spectral_norm=use_spectral_norm) for i in periods]
- self.discriminators = nn.ModuleList(discs)
-
- def forward(self, y, y_hat):
- y_d_rs = []
- y_d_gs = []
- fmap_rs = []
- fmap_gs = []
- for i, d in enumerate(self.discriminators):
- y_d_r, fmap_r = d(y)
- y_d_g, fmap_g = d(y_hat)
- y_d_rs.append(y_d_r)
- y_d_gs.append(y_d_g)
- fmap_rs.append(fmap_r)
- fmap_gs.append(fmap_g)
-
- return y_d_rs, y_d_gs, fmap_rs, fmap_gs
-
-
-
-class SynthesizerTrn(nn.Module):
- """
- Synthesizer for Training
- """
-
- def __init__(self,
- n_vocab,
- spec_channels,
- segment_size,
- inter_channels,
- hidden_channels,
- filter_channels,
- n_heads,
- n_layers,
- kernel_size,
- p_dropout,
- resblock,
- resblock_kernel_sizes,
- resblock_dilation_sizes,
- upsample_rates,
- upsample_initial_channel,
- upsample_kernel_sizes,
- n_speakers=0,
- gin_channels=0,
- use_sdp=True,
- **kwargs):
-
- super().__init__()
- self.n_vocab = n_vocab
- self.spec_channels = spec_channels
- self.inter_channels = inter_channels
- self.hidden_channels = hidden_channels
- self.filter_channels = filter_channels
- self.n_heads = n_heads
- self.n_layers = n_layers
- self.kernel_size = kernel_size
- self.p_dropout = p_dropout
- self.resblock = resblock
- self.resblock_kernel_sizes = resblock_kernel_sizes
- self.resblock_dilation_sizes = resblock_dilation_sizes
- self.upsample_rates = upsample_rates
- self.upsample_initial_channel = upsample_initial_channel
- self.upsample_kernel_sizes = upsample_kernel_sizes
- self.segment_size = segment_size
- self.n_speakers = n_speakers
- self.gin_channels = gin_channels
-
- self.use_sdp = use_sdp
-
- self.enc_p = TextEncoder(n_vocab,
- inter_channels,
- hidden_channels,
- filter_channels,
- n_heads,
- n_layers,
- kernel_size,
- p_dropout)
- self.dec = Generator(inter_channels, resblock, resblock_kernel_sizes, resblock_dilation_sizes, upsample_rates, upsample_initial_channel, upsample_kernel_sizes, gin_channels=gin_channels)
- self.enc_q = PosteriorEncoder(spec_channels, inter_channels, hidden_channels, 5, 1, 16, gin_channels=gin_channels)
- self.flow = ResidualCouplingBlock(inter_channels, hidden_channels, 5, 1, 4, gin_channels=gin_channels)
-
- if use_sdp:
- self.dp = StochasticDurationPredictor(hidden_channels, 192, 3, 0.5, 4, gin_channels=gin_channels)
- else:
- self.dp = DurationPredictor(hidden_channels, 256, 3, 0.5, gin_channels=gin_channels)
-
- if n_speakers > 1:
- self.emb_g = nn.Embedding(n_speakers, gin_channels)
-
- def forward(self, x, x_lengths, y, y_lengths, sid=None):
-
- x, m_p, logs_p, x_mask = self.enc_p(x, x_lengths)
- if self.n_speakers > 0:
- g = self.emb_g(sid).unsqueeze(-1) # [b, h, 1]
- else:
- g = None
-
- z, m_q, logs_q, y_mask = self.enc_q(y, y_lengths, g=g)
- z_p = self.flow(z, y_mask, g=g)
-
- with torch.no_grad():
- # negative cross-entropy
- s_p_sq_r = torch.exp(-2 * logs_p) # [b, d, t]
- neg_cent1 = torch.sum(-0.5 * math.log(2 * math.pi) - logs_p, [1], keepdim=True) # [b, 1, t_s]
- neg_cent2 = torch.matmul(-0.5 * (z_p ** 2).transpose(1, 2), s_p_sq_r) # [b, t_t, d] x [b, d, t_s] = [b, t_t, t_s]
- neg_cent3 = torch.matmul(z_p.transpose(1, 2), (m_p * s_p_sq_r)) # [b, t_t, d] x [b, d, t_s] = [b, t_t, t_s]
- neg_cent4 = torch.sum(-0.5 * (m_p ** 2) * s_p_sq_r, [1], keepdim=True) # [b, 1, t_s]
- neg_cent = neg_cent1 + neg_cent2 + neg_cent3 + neg_cent4
-
- attn_mask = torch.unsqueeze(x_mask, 2) * torch.unsqueeze(y_mask, -1)
- attn = monotonic_align.maximum_path(neg_cent, attn_mask.squeeze(1)).unsqueeze(1).detach()
-
- w = attn.sum(2)
- if self.use_sdp:
- l_length = self.dp(x, x_mask, w, g=g)
- l_length = l_length / torch.sum(x_mask)
- else:
- logw_ = torch.log(w + 1e-6) * x_mask
- logw = self.dp(x, x_mask, g=g)
- l_length = torch.sum((logw - logw_)**2, [1,2]) / torch.sum(x_mask) # for averaging
-
- # expand prior
- m_p = torch.matmul(attn.squeeze(1), m_p.transpose(1, 2)).transpose(1, 2)
- logs_p = torch.matmul(attn.squeeze(1), logs_p.transpose(1, 2)).transpose(1, 2)
-
- z_slice, ids_slice = commons.rand_slice_segments(z, y_lengths, self.segment_size)
- o = self.dec(z_slice, g=g)
- return o, l_length, attn, ids_slice, x_mask, y_mask, (z, z_p, m_p, logs_p, m_q, logs_q)
-
- def infer(self, x, x_lengths, sid=None, noise_scale=1, length_scale=1, noise_scale_w=1., max_len=None):
- x, m_p, logs_p, x_mask = self.enc_p(x, x_lengths)
- if self.n_speakers > 0:
- g = self.emb_g(sid).unsqueeze(-1) # [b, h, 1]
- else:
- g = None
-
- if self.use_sdp:
- logw = self.dp(x, x_mask, g=g, reverse=True, noise_scale=noise_scale_w)
- else:
- logw = self.dp(x, x_mask, g=g)
- w = torch.exp(logw) * x_mask * length_scale
- w_ceil = torch.ceil(w)
- y_lengths = torch.clamp_min(torch.sum(w_ceil, [1, 2]), 1).long()
- y_mask = torch.unsqueeze(commons.sequence_mask(y_lengths, None), 1).to(x_mask.dtype)
- attn_mask = torch.unsqueeze(x_mask, 2) * torch.unsqueeze(y_mask, -1)
- attn = commons.generate_path(w_ceil, attn_mask)
-
- m_p = torch.matmul(attn.squeeze(1), m_p.transpose(1, 2)).transpose(1, 2) # [b, t', t], [b, t, d] -> [b, d, t']
- logs_p = torch.matmul(attn.squeeze(1), logs_p.transpose(1, 2)).transpose(1, 2) # [b, t', t], [b, t, d] -> [b, d, t']
-
- z_p = m_p + torch.randn_like(m_p) * torch.exp(logs_p) * noise_scale
- z = self.flow(z_p, y_mask, g=g, reverse=True)
- o = self.dec((z * y_mask)[:,:,:max_len], g=g)
- return o, attn, y_mask, (z, z_p, m_p, logs_p)
-
- def voice_conversion(self, y, y_lengths, sid_src, sid_tgt):
- assert self.n_speakers > 0, "n_speakers have to be larger than 0."
- g_src = self.emb_g(sid_src).unsqueeze(-1)
- g_tgt = self.emb_g(sid_tgt).unsqueeze(-1)
- z, m_q, logs_q, y_mask = self.enc_q(y, y_lengths, g=g_src)
- z_p = self.flow(z, y_mask, g=g_src)
- z_hat = self.flow(z_p, y_mask, g=g_tgt, reverse=True)
- o_hat = self.dec(z_hat * y_mask, g=g_tgt)
- return o_hat, y_mask, (z, z_p, z_hat)
-
diff --git a/spaces/bioriAsaeru/text-to-voice/Explore the Richness of Indian Classical and Folkloric Music with Swar Systems SwarPlug 1.0 VSTi.md b/spaces/bioriAsaeru/text-to-voice/Explore the Richness of Indian Classical and Folkloric Music with Swar Systems SwarPlug 1.0 VSTi.md
deleted file mode 100644
index cf23446702c2dd96087c8f1639b9b604221f0e0f..0000000000000000000000000000000000000000
--- a/spaces/bioriAsaeru/text-to-voice/Explore the Richness of Indian Classical and Folkloric Music with Swar Systems SwarPlug 1.0 VSTi.md
+++ /dev/null
@@ -1,5 +0,0 @@
-
-
Look no further. I know this site is all about free VST plugin Connor. Place to download free full versions of the latest software, audio samples, tutorial, e-book and video for free. SwarGroove is a VST/AU/RTAS/AAX multi-tracks Indian drums plugin that lets you add 7fd0e SwarGroove SwarPlug 4 Combo Crack Free Download r2r Latest. All the files are relevant and ready to be loaded. [VST] Swarplug 1.0 [Lưu trữ] - ViAnhEm.Com Diễn đàn... swar plug » Download from 2013Zone.Com Em đang tìm swarplug 1.0 VST bác nào có link share em với nhé... tìm mãi trong diễn đàn mà ko thấy.. hĩx(1.0 VSTi link torrent này... What is a torrent and magnet link?.
;
- }
-
- private getScores() {
- const tokens = this.props.args["tokens"];
- if (!this.state || this.state.activeIndex == null || this.state.activeIndex < 1) {
- return tokens.map(() => 0);
- }
- const allScores: number[][] = this.props.args["scores"];
-
- const i = this.state.activeIndex - 1;
- const hi = Math.min(Math.max(0, i + 1), allScores[i].length);
- const row = allScores[i].slice(0, hi);
- row.reverse();
- let result = [
- ...Array(Math.max(0, i + 1 - row.length)).fill(0),
- ...row.map((x) => x == undefined || isNaN(x) ? 0 : x)
- ];
- result = [...result, ...Array(tokens.length - result.length).fill(0)];
- return result;
- }
-}
-
-export default withStreamlitConnection(HighlightedText);
diff --git a/spaces/cihyFjudo/fairness-paper-search/GTA 3 FULL WORKING! (PC CD-ROM) Serial Key Everything You Need to Know About the Game.md b/spaces/cihyFjudo/fairness-paper-search/GTA 3 FULL WORKING! (PC CD-ROM) Serial Key Everything You Need to Know About the Game.md
deleted file mode 100644
index dd54d274e3764f920586a15e55337fd0202944df..0000000000000000000000000000000000000000
--- a/spaces/cihyFjudo/fairness-paper-search/GTA 3 FULL WORKING! (PC CD-ROM) Serial Key Everything You Need to Know About the Game.md
+++ /dev/null
@@ -1,5 +0,0 @@
-
-
If the Toshiba hard drive is still not working, try to update the device driver. An outdated or corrupted hard drive driver will cause some problems while using the device. Since your hard drive is not detected by the PC, you cannot update the driver via Device Manager. Go to the official site of Toshiba, then download and install the latest driver by entering your model or the serial number of your disk.
aaccfb2cb3
-
-
\ No newline at end of file
diff --git a/spaces/cihyFjudo/fairness-paper-search/Nvidia GeForce Now Not Using RTX 2080 Ti SUPER GPUs Will It Affect Ray Tracing Support?.md b/spaces/cihyFjudo/fairness-paper-search/Nvidia GeForce Now Not Using RTX 2080 Ti SUPER GPUs Will It Affect Ray Tracing Support?.md
deleted file mode 100644
index 84dcbfe657fa0795a2bd9a31b594f4b37e97e551..0000000000000000000000000000000000000000
--- a/spaces/cihyFjudo/fairness-paper-search/Nvidia GeForce Now Not Using RTX 2080 Ti SUPER GPUs Will It Affect Ray Tracing Support?.md
+++ /dev/null
@@ -1,21 +0,0 @@
-
-
Where to Buy The GeForce RTX 2060 SUPER, RTX 2070 SUPER and RTX 2080 SUPER GPUs will be available as custom boards, including stock-clocked and factory-overclocked models, from top add-in card providers such as ASUS, Colorful, EVGA, Gainward, Galaxy, Gigabyte, Innovision 3D, MSI, Palit, PNY and Zotac and as Founders Editions from www.nvidia.com. Look for the GeForce RTX SUPER GPUs in gaming systems built by Acer, Alienware, Dell, HP and Lenovo, as well as by leading system builders worldwide.
-
Certain statements in this press release including, but not limited to, statements as to: NVIDIA supercharging its gaming lineup; gamers riding the growing wave of ray traced titles with NVIDIA GPUs; the performance, benefits, features and abilities of NVIDIA GPUs, including its GeForce RTX SUPER line; the ecosystem driving real-time ray tracing being immense, including tens of millions of GPUs, industry standard APIs, leading game engines and an all-star roster of game franchises; the lineup of SUPER GPUs delivering more performance and ensuring that gamers are prepared for the coming wave of real-time ray tracing blockbusters; GeForce RTX GPUs including specialized cores that enable them to accelerate their capability and delivering ray tracing in real time; the support for ray tracing in industry standard APIs and game engines; the games that announced they will be using ray tracing; and the price and availability of GeForce RTX SUPER GPUs, including with a game bundle are forward-looking statements that are subject to risks and uncertainties that could cause results to be materially different than expectations. Important factors that could cause actual results to differ materially include: global economic conditions; our reliance on third parties to manufacture, assemble, package and test our products; the impact of technological development and competition; development of new products and technologies or enhancements to our existing product and technologies; market acceptance of our products or our partners' products; design, manufacturing or software defects; changes in consumer preferences or demands; changes in industry standards and interfaces; unexpected loss of performance of our products or technologies when integrated into systems; as well as other factors detailed from time to time in the most recent reports NVIDIA files with the Securities and Exchange Commission, or SEC, including, but not limited to, its annual report on Form 10-K and quarterly reports on Form 10-Q. Copies of reports filed with the SEC are posted on the company's website and are available from NVIDIA without charge. These forward-looking statements are not guarantees of future performance and speak only as of the date hereof, and, except as required by law, NVIDIA disclaims any obligation to update these forward-looking statements to reflect future events or circumstances.
-
Nvidia GeForce Now Not Using RTX 2080 Ti SUPER GPUs
Overall, while not really necessary for After Effects alone, if you already need a new high-end GPU for other applications (Premiere Pro, GPU-based rendering, etc.) we would recommend using one of these RTX cards if possible. The RTX 2080 does have less VRAM than the comparably priced GTX 1080 Ti (8GB vs 11GB), but the potential these cards offer for the future is likely worth investing in.
-
In particular, the supposed leader in that category is the 3080. The problem with that status is that it appears to depend largely on the MSRP of $800. But I have signed myself up for several services that alert me for the availability of this card at this price, and for months I have not been able to get it. The market price of this card is more like $1400. The MSRP is essentially meaningless. When compared to the 2080Ti, which is available for around $1000, and using your own performance comparisons, the 2080Ti beats to 3080 on performance per dollar.
-
Hi Pablo, I never had a personal gaming GPU fail. From the ~30 GPUs that I used at universities, I had one fail. From a small GPU cluster I was using, I also saw one GPU fail (1 out of 48 GPUs). Some GPUs are known to have much higher failure rates than others (RTX 2080 Ti and RTX 2080 Founders Edition in particular).
-
Thanks for all your help via your blogs over the years. I am now in situation where I have 2 X99 workstations, one with 2xRTX2080ti and one with 3xRTX2080ti (couldnt put 4 in this due to buying cheap used 2.5 slot wide gpus, and one is already on a pcie riser). I want to connect the 2 machines using high speed network cards and fiber. Is having 100mbit/s network speed an absolute must or could I get away with 40/50mbit/s? I havent found any 100 Mbit/s mellanox inifiniband cards for less than ~$400 usd each which is too pricey for me. Once network is setup is SLURM the best way to distrubute load?
-
-
K80 and M6000 will be quite slow. I would recommend getting a Titan RTX with 24 GB of memory. If that is too expensive I would definitely go for the M6000. You can also think about multiple RTX 2080 Ti cards and using parallel training. That will reduce the memory footprint slightly especially if you use FP16 training. If you use mixed FP16 training it reduces memory footprint by 25%, if you pure FP16 training via Apex it reduces footprint by 50%. Using 2 GPUs should decrease the footprint by about 20-30%. So 2x RTX 2080 Ti with pure FP16 training is roughly equivalent to 11/0.75/0.5 = 29 GB used by the K80 or M6000 but you train much much faster.
-
I got this advice from a vendor of GPU systems. I was arguing that for text models FP16 and RTX 2080Ti is good enough and comparable to v100. Also in their benchmarking they did not test RTX with NvLink but v100 was tested for FP16. I got this response. Just wanted to check if NvLink is of no use when using RTX 2080Ti. Please suggest. Your inputs are much appreciated here as I would use it for my next purchase.
-
I mean I have a Blender Project using 25 GB. I have 32 GB memory in my Computer and 8 GB on my Graphic card. And the GPU is usable now. Can I use for example a Geforce RTX 2080 Ti with 11 GB for my project which uses 25 GB RAM and take advantage of the RTX support? (Assuming that I still have 32 GB main memory)
-
Hi Are you going to be able to use Optix and Cuda together? Like using rtx cards and gtx 10xx cards to render an image at the same time. Also, is there any plan on bringing nvlink memory pooling on RTX 2070super+ cards to cycles? Puget system showed that the nvlink on RTX cards are able to handle memory pooling, but need software support. These two can be the game changer for future GPU rendering
-
On July 2, 2019, the GeForce RTX Super line of cards was announced, which comprises higher-spec versions of the 2060, 2070 and 2080. Each of the Super models were offered for a similar price as older models but with improved specs.[11] In July 2019, NVidia stated the "SUPER" graphics cards in the GeForce RTX 20 series, to be introduced, had a 15% performance advantage over the GeForce RTX 2060.[33] PC World called the super editions a "modest" upgrade for the price, and the 2080 Super chip the "second most-powerful GPU ever released" in terms of speed.[34] In November 2019, PC Gamer wrote "even without an overclock, the 2080 Ti is the best graphics card for gaming."[35] In June 2020, PC Mag listed the Nvidia GeForce RTX 2070 Super as one of the "best [8] graphics cards for 4k gaming in 2020." The GeForce RTX 2080 Founders Edition, Super, and Ti were also listed.[36] In June 2020, graphic cards including the RTX 2060, RTX 2060 Super, RTX 2070 and the RTX 2080 Super were announced as discounted by retailers in expectation of the GeForce RTX 3080 launch.[37] In April 2020, Nvidia announced 100 new laptops licensed to include either GeForce GTX and RTX models.[38]
-
The second generation Tensor Cores (succeeding Volta's) work in cooperation with the RT cores, and their AI features are used mainly to two ends: firstly, de-noising a partially ray traced image by filling in the blanks between rays cast; also another application of the Tensor cores is DLSS (deep learning super-sampling), a new method to replace anti-aliasing, by artificially generating detail to upscale the rendered image into a higher resolution.[51] The Tensor cores apply deep learning models (for example, an image resolution enhancement model) which are constructed using supercomputers. The problem to be solved is analyzed on the supercomputer, which is taught by example what results are desired. The supercomputer then outputs a model which is then executed on the consumer's Tensor cores. These methods are delivered to consumers as part of the cards' drivers.[citation needed]
-
I've had RealHack working since forever using an RTX 2080 and the NV40 folder with Hex 30008. I recently updated graphics driver to 471.68 and now RealView is no longer enabled. Last version that worked was 466.77. If I roll back drivers, it works again, but I'd rather not do that. Any ideas how to get it back or even why this would happen?
-
You can go as high as 6x6 or 8x8 on RTX 2080 super and TI cards but it is over scanning the resolution and not needed- i can also cause artifacts and blurring in distance). Keep in midn you want to room in the GPU performance.
-
1. NVIDIA GeForce RTX 1080 Ti - Still a decent graphics card, the 1080 Ti can currently generate up to $33.94 in monthly mining income. 2. NVIDIA GeForce RTX 2070 Super - This supercharged version of the RTX 270 model performs considerably better than the regular version. It can currently generate up to $35.40 in monthly crypto mining income. 3. NVIDIA GeForce RTX 2080 Ti - This graphics card was considered the best NVIDIA graphics card for mining performance before the 30 series arrived. It can currently generate up to $48.81 in monthly mining income.
-
1. NVIDIA GeForce RTX 1080 Ti - Still a decent graphics card, the 1080 Ti can currently generate up to $33.94 in monthly mining income.\r\n2. NVIDIA GeForce RTX 2070 Super - This supercharged version of the RTX 270 model performs considerably better than the regular version. It can currently generate up to $35.40 in monthly crypto mining income. \r\n3. NVIDIA GeForce RTX 2080 Ti - This graphics card was considered the best NVIDIA graphics card for mining performance before the 30 series arrived. It can currently generate up to $48.81 in monthly mining income.
aaccfb2cb3
-
-
\ No newline at end of file
diff --git a/spaces/cihyFjudo/fairness-paper-search/Solibri Model Checker V7 Crack 67 How to Check and Validate Your BIM Models for Free.md b/spaces/cihyFjudo/fairness-paper-search/Solibri Model Checker V7 Crack 67 How to Check and Validate Your BIM Models for Free.md
deleted file mode 100644
index 07ca16b010d2b631b4f8b444a6ea6744e4ae8d28..0000000000000000000000000000000000000000
--- a/spaces/cihyFjudo/fairness-paper-search/Solibri Model Checker V7 Crack 67 How to Check and Validate Your BIM Models for Free.md
+++ /dev/null
@@ -1,5 +0,0 @@
-
-
Express Scribe Registration Codel.. Harmoncrixportwapittledep · imperial rome warband mod Rome, Photo And Video, Fashion, Moda, Fashion Styles. solibri model checker v7 crack 67
aaccfb2cb3
-
-
\ No newline at end of file
diff --git a/spaces/cloudtheboi/Lofi4All/.pythonlibs/lib/python3.10/site-packages/aiofiles/base.py b/spaces/cloudtheboi/Lofi4All/.pythonlibs/lib/python3.10/site-packages/aiofiles/base.py
deleted file mode 100644
index 07f2c2e504bb4fd96e1e3ee18caaac94d3b1865a..0000000000000000000000000000000000000000
--- a/spaces/cloudtheboi/Lofi4All/.pythonlibs/lib/python3.10/site-packages/aiofiles/base.py
+++ /dev/null
@@ -1,113 +0,0 @@
-"""Various base classes."""
-from types import coroutine
-from collections.abc import Coroutine
-from asyncio import get_running_loop
-
-
-class AsyncBase:
- def __init__(self, file, loop, executor):
- self._file = file
- self._executor = executor
- self._ref_loop = loop
-
- @property
- def _loop(self):
- return self._ref_loop or get_running_loop()
-
- def __aiter__(self):
- """We are our own iterator."""
- return self
-
- def __repr__(self):
- return super().__repr__() + " wrapping " + repr(self._file)
-
- async def __anext__(self):
- """Simulate normal file iteration."""
- line = await self.readline()
- if line:
- return line
- else:
- raise StopAsyncIteration
-
-
-class AsyncIndirectBase(AsyncBase):
- def __init__(self, name, loop, executor, indirect):
- self._indirect = indirect
- self._name = name
- super().__init__(None, loop, executor)
-
- @property
- def _file(self):
- return self._indirect()
-
- @_file.setter
- def _file(self, v):
- pass # discard writes
-
-
-class _ContextManager(Coroutine):
- __slots__ = ("_coro", "_obj")
-
- def __init__(self, coro):
- self._coro = coro
- self._obj = None
-
- def send(self, value):
- return self._coro.send(value)
-
- def throw(self, typ, val=None, tb=None):
- if val is None:
- return self._coro.throw(typ)
- elif tb is None:
- return self._coro.throw(typ, val)
- else:
- return self._coro.throw(typ, val, tb)
-
- def close(self):
- return self._coro.close()
-
- @property
- def gi_frame(self):
- return self._coro.gi_frame
-
- @property
- def gi_running(self):
- return self._coro.gi_running
-
- @property
- def gi_code(self):
- return self._coro.gi_code
-
- def __next__(self):
- return self.send(None)
-
- @coroutine
- def __iter__(self):
- resp = yield from self._coro
- return resp
-
- def __await__(self):
- resp = yield from self._coro
- return resp
-
- async def __anext__(self):
- resp = await self._coro
- return resp
-
- async def __aenter__(self):
- self._obj = await self._coro
- return self._obj
-
- async def __aexit__(self, exc_type, exc, tb):
- self._obj.close()
- self._obj = None
-
-
-class AiofilesContextManager(_ContextManager):
- """An adjusted async context manager for aiofiles."""
-
- async def __aexit__(self, exc_type, exc_val, exc_tb):
- await get_running_loop().run_in_executor(
- None, self._obj._file.__exit__, exc_type, exc_val, exc_tb
- )
- self._obj = None
diff --git a/spaces/cloudtheboi/Lofi4All/.pythonlibs/lib/python3.10/site-packages/attrs/__init__.py b/spaces/cloudtheboi/Lofi4All/.pythonlibs/lib/python3.10/site-packages/attrs/__init__.py
deleted file mode 100644
index 0c2481561a93a912503754396782e987fcdd9629..0000000000000000000000000000000000000000
--- a/spaces/cloudtheboi/Lofi4All/.pythonlibs/lib/python3.10/site-packages/attrs/__init__.py
+++ /dev/null
@@ -1,65 +0,0 @@
-# SPDX-License-Identifier: MIT
-
-from attr import (
- NOTHING,
- Attribute,
- AttrsInstance,
- Factory,
- _make_getattr,
- assoc,
- cmp_using,
- define,
- evolve,
- field,
- fields,
- fields_dict,
- frozen,
- has,
- make_class,
- mutable,
- resolve_types,
- validate,
-)
-from attr._next_gen import asdict, astuple
-
-from . import converters, exceptions, filters, setters, validators
-
-
-__all__ = [
- "__author__",
- "__copyright__",
- "__description__",
- "__doc__",
- "__email__",
- "__license__",
- "__title__",
- "__url__",
- "__version__",
- "__version_info__",
- "asdict",
- "assoc",
- "astuple",
- "Attribute",
- "AttrsInstance",
- "cmp_using",
- "converters",
- "define",
- "evolve",
- "exceptions",
- "Factory",
- "field",
- "fields_dict",
- "fields",
- "filters",
- "frozen",
- "has",
- "make_class",
- "mutable",
- "NOTHING",
- "resolve_types",
- "setters",
- "validate",
- "validators",
-]
-
-__getattr__ = _make_getattr(__name__)
diff --git a/spaces/cm107/agv-demo/Build/test-webgl-dev.loader.js b/spaces/cm107/agv-demo/Build/test-webgl-dev.loader.js
deleted file mode 100644
index 4d0f507c9abf194451ec2d55f7bc4c83885d6d78..0000000000000000000000000000000000000000
--- a/spaces/cm107/agv-demo/Build/test-webgl-dev.loader.js
+++ /dev/null
@@ -1,1238 +0,0 @@
-function createUnityInstance(canvas, config, onProgress) {
- onProgress = onProgress || function () {};
-
-
- function showBanner(msg, type) {
- // Only ever show one error at most - other banner messages after that should get ignored
- // to avoid noise.
- if (!showBanner.aborted && config.showBanner) {
- if (type == 'error') showBanner.aborted = true;
- return config.showBanner(msg, type);
- }
-
- // Fallback to console logging if visible banners have been suppressed
- // from the main page.
- switch(type) {
- case 'error': console.error(msg); break;
- case 'warning': console.warn(msg); break;
- default: console.log(msg); break;
- }
- }
-
- function errorListener(e) {
- var error = e.reason || e.error;
- var message = error ? error.toString() : (e.message || e.reason || '');
- var stack = (error && error.stack) ? error.stack.toString() : '';
-
- // Do not repeat the error message if it's present in the stack trace.
- if (stack.startsWith(message)) {
- stack = stack.substring(message.length);
- }
-
- message += '\n' + stack.trim();
-
- if (!message || !Module.stackTraceRegExp || !Module.stackTraceRegExp.test(message))
- return;
-
- var filename = e.filename || (error && (error.fileName || error.sourceURL)) || '';
- var lineno = e.lineno || (error && (error.lineNumber || error.line)) || 0;
-
- errorHandler(message, filename, lineno);
- }
-
- var Module = {
- canvas: canvas,
- webglContextAttributes: {
- preserveDrawingBuffer: false,
- },
- cacheControl: function (url) {
- return (url == Module.dataUrl || url.match(/\.bundle/)) ? "must-revalidate" : "no-store";
- },
- streamingAssetsUrl: "StreamingAssets",
- downloadProgress: {},
- deinitializers: [],
- intervals: {},
- setInterval: function (func, ms) {
- var id = window.setInterval(func, ms);
- this.intervals[id] = true;
- return id;
- },
- clearInterval: function(id) {
- delete this.intervals[id];
- window.clearInterval(id);
- },
- preRun: [],
- postRun: [],
- print: function (message) {
- console.log(message);
- },
- printErr: function (message) {
- console.error(message);
-
- if (typeof message === 'string' && message.indexOf('wasm streaming compile failed') != -1) {
- if (message.toLowerCase().indexOf('mime') != -1) {
- showBanner('HTTP Response Header "Content-Type" configured incorrectly on the server for file ' + Module.codeUrl + ' , should be "application/wasm". Startup time performance will suffer.', 'warning');
- } else {
- showBanner('WebAssembly streaming compilation failed! This can happen for example if "Content-Encoding" HTTP header is incorrectly enabled on the server for file ' + Module.codeUrl + ', but the file is not pre-compressed on disk (or vice versa). Check the Network tab in browser Devtools to debug server header configuration.', 'warning');
- }
- }
- },
- locateFile: function (url) {
- return (
- url == "build.wasm" ? this.codeUrl :
- url
- );
- },
- disabledCanvasEvents: [
- "contextmenu",
- "dragstart",
- ],
- };
-
- for (var parameter in config)
- Module[parameter] = config[parameter];
-
- Module.streamingAssetsUrl = new URL(Module.streamingAssetsUrl, document.URL).href;
-
- // Operate on a clone of Module.disabledCanvasEvents field so that at Quit time
- // we will ensure we'll remove the events that we created (in case user has
- // modified/cleared Module.disabledCanvasEvents in between)
- var disabledCanvasEvents = Module.disabledCanvasEvents.slice();
-
- function preventDefault(e) {
- e.preventDefault();
- }
-
- disabledCanvasEvents.forEach(function (disabledCanvasEvent) {
- canvas.addEventListener(disabledCanvasEvent, preventDefault);
- });
-
- window.addEventListener("error", errorListener);
- window.addEventListener("unhandledrejection", errorListener);
-
- // Safari does not automatically stretch the fullscreen element to fill the screen.
- // The CSS width/height of the canvas causes it to remain the same size in the full screen
- // window on Safari, resulting in it being a small canvas with black borders filling the
- // rest of the screen.
- var _savedElementWidth = "";
- var _savedElementHeight = "";
- // Safari uses webkitfullscreenchange event and not fullscreenchange
- document.addEventListener("webkitfullscreenchange", function(e) {
- // Safari uses webkitCurrentFullScreenElement and not fullscreenElement.
- var fullscreenElement = document.webkitCurrentFullScreenElement;
- if (fullscreenElement === canvas) {
- if (canvas.style.width) {
- _savedElementWidth = canvas.style.width;
- _savedElementHeight = canvas.style.height;
- canvas.style.width = "100%";
- canvas.style.height = "100%";
- }
- } else {
- if (_savedElementWidth) {
- canvas.style.width = _savedElementWidth;
- canvas.style.height = _savedElementHeight;
- _savedElementWidth = "";
- _savedElementHeight = "";
- }
- }
- });
-
- // Clear the event handlers we added above when the app quits, so that the event handler
- // functions will not hold references to this JS function scope after
- // exit, to allow JS garbage collection to take place.
- Module.deinitializers.push(function() {
- Module['disableAccessToMediaDevices']();
- disabledCanvasEvents.forEach(function (disabledCanvasEvent) {
- canvas.removeEventListener(disabledCanvasEvent, preventDefault);
- });
- window.removeEventListener("error", errorListener);
- window.removeEventListener("unhandledrejection", errorListener);
-
- for (var id in Module.intervals)
- {
- window.clearInterval(id);
- }
- Module.intervals = {};
- });
-
- Module.QuitCleanup = function () {
- for (var i = 0; i < Module.deinitializers.length; i++) {
- Module.deinitializers[i]();
- }
- Module.deinitializers = [];
- // After all deinitializer callbacks are called, notify user code that the Unity game instance has now shut down.
- if (typeof Module.onQuit == "function")
- Module.onQuit();
-
- };
-
- var unityInstance = {
- Module: Module,
- SetFullscreen: function () {
- if (Module.SetFullscreen)
- return Module.SetFullscreen.apply(Module, arguments);
- Module.print("Failed to set Fullscreen mode: Player not loaded yet.");
- },
- SendMessage: function () {
- if (Module.SendMessage)
- return Module.SendMessage.apply(Module, arguments);
- Module.print("Failed to execute SendMessage: Player not loaded yet.");
- },
- Quit: function () {
- return new Promise(function (resolve, reject) {
- Module.shouldQuit = true;
- Module.onQuit = resolve;
- });
- },
- };
-
-
- Module.SystemInfo = (function () {
-
- var browser, browserVersion, os, osVersion, canvas, gpu;
-
- var ua = navigator.userAgent + ' ';
- var browsers = [
- ['Firefox', 'Firefox'],
- ['OPR', 'Opera'],
- ['Edg', 'Edge'],
- ['SamsungBrowser', 'Samsung Browser'],
- ['Trident', 'Internet Explorer'],
- ['MSIE', 'Internet Explorer'],
- ['Chrome', 'Chrome'],
- ['CriOS', 'Chrome on iOS Safari'],
- ['FxiOS', 'Firefox on iOS Safari'],
- ['Safari', 'Safari'],
- ];
-
- function extractRe(re, str, idx) {
- re = RegExp(re, 'i').exec(str);
- return re && re[idx];
- }
- for(var b = 0; b < browsers.length; ++b) {
- browserVersion = extractRe(browsers[b][0] + '[\/ ](.*?)[ \\)]', ua, 1);
- if (browserVersion) {
- browser = browsers[b][1];
- break;
- }
- }
- if (browser == 'Safari') browserVersion = extractRe('Version\/(.*?) ', ua, 1);
- if (browser == 'Internet Explorer') browserVersion = extractRe('rv:(.*?)\\)? ', ua, 1) || browserVersion;
-
- // These OS strings need to match the ones in Runtime/Misc/SystemInfo.cpp::GetOperatingSystemFamily()
- var oses = [
- ['Windows (.*?)[;\)]', 'Windows'],
- ['Android ([0-9_\.]+)', 'Android'],
- ['iPhone OS ([0-9_\.]+)', 'iPhoneOS'],
- ['iPad.*? OS ([0-9_\.]+)', 'iPadOS'],
- ['FreeBSD( )', 'FreeBSD'],
- ['OpenBSD( )', 'OpenBSD'],
- ['Linux|X11()', 'Linux'],
- ['Mac OS X ([0-9_\.]+)', 'MacOS'],
- ['bot|google|baidu|bing|msn|teoma|slurp|yandex', 'Search Bot']
- ];
- for(var o = 0; o < oses.length; ++o) {
- osVersion = extractRe(oses[o][0], ua, 1);
- if (osVersion) {
- os = oses[o][1];
- osVersion = osVersion.replace(/_/g, '.');
- break;
- }
- }
- var versionMappings = {
- 'NT 5.0': '2000',
- 'NT 5.1': 'XP',
- 'NT 5.2': 'Server 2003',
- 'NT 6.0': 'Vista',
- 'NT 6.1': '7',
- 'NT 6.2': '8',
- 'NT 6.3': '8.1',
- 'NT 10.0': '10'
- };
- osVersion = versionMappings[osVersion] || osVersion;
-
- // TODO: Add mobile device identifier, e.g. SM-G960U
-
- canvas = document.createElement("canvas");
- if (canvas) {
- gl = canvas.getContext("webgl2");
- glVersion = gl ? 2 : 0;
- if (!gl) {
- if (gl = canvas && canvas.getContext("webgl")) glVersion = 1;
- }
-
- if (gl) {
- gpu = (gl.getExtension("WEBGL_debug_renderer_info") && gl.getParameter(0x9246 /*debugRendererInfo.UNMASKED_RENDERER_WEBGL*/)) || gl.getParameter(0x1F01 /*gl.RENDERER*/);
- }
- }
-
- var hasThreads = typeof SharedArrayBuffer !== 'undefined';
- var hasWasm = typeof WebAssembly === "object" && typeof WebAssembly.compile === "function";
- return {
- width: screen.width,
- height: screen.height,
- userAgent: ua.trim(),
- browser: browser || 'Unknown browser',
- browserVersion: browserVersion || 'Unknown version',
- mobile: /Mobile|Android|iP(ad|hone)/.test(navigator.appVersion),
- os: os || 'Unknown OS',
- osVersion: osVersion || 'Unknown OS Version',
- gpu: gpu || 'Unknown GPU',
- language: navigator.userLanguage || navigator.language,
- hasWebGL: glVersion,
- hasCursorLock: !!document.body.requestPointerLock,
- hasFullscreen: !!document.body.requestFullscreen || !!document.body.webkitRequestFullscreen, // Safari still uses the webkit prefixed version
- hasThreads: hasThreads,
- hasWasm: hasWasm,
- // This should be updated when we re-enable wasm threads. Previously it checked for WASM thread
- // support with: var wasmMemory = hasWasm && hasThreads && new WebAssembly.Memory({"initial": 1, "maximum": 1, "shared": true});
- // which caused Chrome to have a warning that SharedArrayBuffer requires cross origin isolation.
- hasWasmThreads: false,
- };
- })();
-
- function errorHandler(message, filename, lineno) {
- // Unity needs to rely on Emscripten deferred fullscreen requests, so these will make their way to error handler
- if (message.indexOf('fullscreen error') != -1)
- return;
-
- if (Module.startupErrorHandler) {
- Module.startupErrorHandler(message, filename, lineno);
- return;
- }
- if (Module.errorHandler && Module.errorHandler(message, filename, lineno))
- return;
- console.log("Invoking error handler due to\n" + message);
-
- // Support Firefox window.dump functionality.
- if (typeof dump == "function")
- dump("Invoking error handler due to\n" + message);
-
- if (errorHandler.didShowErrorMessage)
- return;
- var message = "An error occurred running the Unity content on this page. See your browser JavaScript console for more info. The error was:\n" + message;
- if (message.indexOf("DISABLE_EXCEPTION_CATCHING") != -1) {
- message = "An exception has occurred, but exception handling has been disabled in this build. If you are the developer of this content, enable exceptions in your project WebGL player settings to be able to catch the exception or see the stack trace.";
- } else if (message.indexOf("Cannot enlarge memory arrays") != -1) {
- message = "Out of memory. If you are the developer of this content, try allocating more memory to your WebGL build in the WebGL player settings.";
- } else if (message.indexOf("Invalid array buffer length") != -1 || message.indexOf("Invalid typed array length") != -1 || message.indexOf("out of memory") != -1 || message.indexOf("could not allocate memory") != -1) {
- message = "The browser could not allocate enough memory for the WebGL content. If you are the developer of this content, try allocating less memory to your WebGL build in the WebGL player settings.";
- }
- alert(message);
- errorHandler.didShowErrorMessage = true;
- }
-
-
- Module.abortHandler = function (message) {
- errorHandler(message, "", 0);
- return true;
- };
-
- Error.stackTraceLimit = Math.max(Error.stackTraceLimit || 0, 50);
-
- function progressUpdate(id, e) {
- if (id == "symbolsUrl")
- return;
- var progress = Module.downloadProgress[id];
- if (!progress)
- progress = Module.downloadProgress[id] = {
- started: false,
- finished: false,
- lengthComputable: false,
- total: 0,
- loaded: 0,
- };
- if (typeof e == "object" && (e.type == "progress" || e.type == "load")) {
- if (!progress.started) {
- progress.started = true;
- progress.lengthComputable = e.lengthComputable;
- }
- progress.total = e.total;
- progress.loaded = e.loaded;
- if (e.type == "load")
- progress.finished = true;
- }
- var loaded = 0, total = 0, started = 0, computable = 0, unfinishedNonComputable = 0;
- for (var id in Module.downloadProgress) {
- var progress = Module.downloadProgress[id];
- if (!progress.started)
- return 0;
- started++;
- if (progress.lengthComputable) {
- loaded += progress.loaded;
- total += progress.total;
- computable++;
- } else if (!progress.finished) {
- unfinishedNonComputable++;
- }
- }
- var totalProgress = started ? (started - unfinishedNonComputable - (total ? computable * (total - loaded) / total : 0)) / started : 0;
- onProgress(0.9 * totalProgress);
- }
-
-Module.readBodyWithProgress = function() {
- /**
- * Estimate length of uncompressed content by taking average compression ratios
- * of compression type into account.
- * @param {Response} response A Fetch API response object
- * @param {boolean} lengthComputable Return wether content length was given in header.
- * @returns {number}
- */
- function estimateContentLength(response, lengthComputable) {
- if (!lengthComputable) {
- // No content length available
- return 0;
- }
-
- var compression = response.headers.get("Content-Encoding");
- var contentLength = parseInt(response.headers.get("Content-Length"));
-
- switch (compression) {
- case "br":
- return Math.round(contentLength * 5);
- case "gzip":
- return Math.round(contentLength * 4);
- default:
- return contentLength;
- }
- }
-
- function readBodyWithProgress(response, onProgress) {
- var reader = response.body ? response.body.getReader() : undefined;
- var lengthComputable = typeof response.headers.get('Content-Length') !== "undefined";
- var estimatedContentLength = estimateContentLength(response, lengthComputable);
- var body = new Uint8Array(estimatedContentLength);
- var trailingChunks = [];
- var receivedLength = 0;
- var trailingChunksStart = 0;
-
- if (!lengthComputable) {
- console.warn("[UnityCache] Response is served without Content-Length header. Please reconfigure server to include valid Content-Length for better download performance.");
- }
-
- function readBody() {
- if (typeof reader === "undefined") {
- // Browser does not support streaming reader API
- // Fallback to Respone.arrayBuffer()
- return response.arrayBuffer().then(function (buffer) {
- onProgress({
- type: "progress",
- total: buffer.length,
- loaded: 0,
- lengthComputable: lengthComputable
- });
-
- return new Uint8Array(buffer);
- });
- }
-
- // Start reading memory chunks
- return reader.read().then(function (result) {
- if (result.done) {
- return concatenateTrailingChunks();
- }
-
- if ((receivedLength + result.value.length) <= body.length) {
- // Directly append chunk to body if enough memory was allocated
- body.set(result.value, receivedLength);
- trailingChunksStart = receivedLength + result.value.length;
- } else {
- // Store additional chunks in array to append later
- trailingChunks.push(result.value);
- }
-
- receivedLength += result.value.length;
- onProgress({
- type: "progress",
- total: Math.max(estimatedContentLength, receivedLength),
- loaded: receivedLength,
- lengthComputable: lengthComputable
- });
-
- return readBody();
- });
- }
-
- function concatenateTrailingChunks() {
- if (receivedLength === estimatedContentLength) {
- return body;
- }
-
- if (receivedLength < estimatedContentLength) {
- // Less data received than estimated, shrink body
- return body.slice(0, receivedLength);
- }
-
- // More data received than estimated, create new larger body to prepend all additional chunks to the body
- var newBody = new Uint8Array(receivedLength);
- newBody.set(body, 0);
- var position = trailingChunksStart;
- for (var i = 0; i < trailingChunks.length; ++i) {
- newBody.set(trailingChunks[i], position);
- position += trailingChunks[i].length;
- }
-
- return newBody;
- }
-
- return readBody().then(function (parsedBody) {
- onProgress({
- type: "load",
- total: parsedBody.length,
- loaded: parsedBody.length,
- lengthComputable: lengthComputable
- });
-
- response.parsedBody = parsedBody;
- return response;
- });
- }
-
- return readBodyWithProgress;
-}();
-
-Module.fetchWithProgress = function () {
- function fetchWithProgress(resource, init) {
- var onProgress = function () { };
- if (init && init.onProgress) {
- onProgress = init.onProgress;
- }
-
- return fetch(resource, init).then(function (response) {
- return Module.readBodyWithProgress(response, onProgress);
- });
- }
-
- return fetchWithProgress;
-}();
- /**
- * @interface RequestMetaData
- * An object with meta data for a request
- *
- * @property {string} url The url of a request
- * @property {string} company The company name
- * @property {string} product The product name
- * @property {number} version The version of the build
- * @property {number} size The company of the build
- * @property {number} accessedAt Timestamp when request was last accessed (Unix timestamp format)
- * @property {number} updatedAt Timestamp when request was last updated in the cache (Unix timestamp format)
- */
-
-/**
- * @interface ResponseWithMetaData
- * An object with a cached response and meta data
- * @property {Response} response
- * @property {RequestMetaData} metaData
- */
-
-Module.UnityCache = function () {
- var UnityCacheDatabase = { name: "UnityCache", version: 4 };
- var RequestMetaDataStore = { name: "RequestMetaDataStore", version: 1 };
- var RequestStore = { name: "RequestStore", version: 1 };
- var WebAssemblyStore = { name: "WebAssembly", version: 1 };
- var indexedDB = window.indexedDB || window.mozIndexedDB || window.webkitIndexedDB || window.msIndexedDB;
-
- function log(message) {
- console.log("[UnityCache] " + message);
- }
-
- /**
- * A request cache that uses the browser Index DB to cache large requests
- * @property {Promise} isConnected
- * @property {Cache} cache
- */
- function UnityCache() {
- var self = this;
-
- this.isConnected = this.connect().then(function () {
- return self.cleanUpCache();
- });
-
- this.isConnected.catch(function (error) {
- log("Error when initializing cache: " + error);
- });
- }
-
- var instance = null;
- /**
- * Singleton accessor. Returns unity cache instance
- * @returns {UnityCache}
- */
- UnityCache.getInstance = function () {
- if (!instance) {
- instance = new UnityCache();
- }
-
- return instance;
- }
-
- /**
- * Destroy unity cache instance. Returns a promise that waits for the
- * database connection to be closed.
- * @returns {Promise}
- */
- UnityCache.destroyInstance = function () {
- if (!instance) {
- return Promise.resolve();
- }
-
- return instance.close().then(function () {
- instance = null;
- });
- }
-
- /**
- * Clear the unity cache.
- * @returns {Promise} A promise that resolves when the cache is cleared.
- */
- UnityCache.prototype.clearCache = function () {
- var self = this;
-
- function deleteCacheEntries(cacheKeys) {
- if (cacheKeys.length === 0) {
- return Promise.resolve();
- }
-
- var key = cacheKeys.pop();
-
- return self.cache.delete(key).then(function () {
- return deleteCacheEntries(cacheKeys);
- });
- }
-
- return this.isConnected.then(function () {
- return self.execute(RequestMetaDataStore.name, "clear", []);
- }).then(function () {
- return self.cache.keys();
- }).then(function (keys) {
- return deleteCacheEntries(keys)
- });
- }
-
- /**
- * Config for request meta data store
- */
- UnityCache.UnityCacheDatabase = UnityCacheDatabase;
- UnityCache.RequestMetaDataStore = RequestMetaDataStore;
- UnityCache.MaximumCacheSize = 1024 * 1024 * 1024; // 1 GB
-
- /**
- * Load a request response from cache
- * @param {Request|string} request The fetch request
- * @returns {Promise} A cached response with meta data for the request or undefined if request is not in cache.
- */
- UnityCache.prototype.loadRequest = function (request) {
- var self = this;
-
- return self.isConnected.then(function () {
- return Promise.all([
- self.cache.match(request),
- self.loadRequestMetaData(request)
- ]);
- }).then(function (result) {
- if (typeof result[0] === "undefined" || typeof result[1] === "undefined") {
- return undefined;
- }
-
- return {
- response: result[0],
- metaData: result[1]
- };
- });
- }
-
- /**
- * Load a request meta data from cache
- * @param {Request|string} request The fetch request
- * @returns {Promise} Request meta data
- */
- UnityCache.prototype.loadRequestMetaData = function (request) {
- var url = typeof request === "string" ? request : request.url;
-
- return this.execute(RequestMetaDataStore.name, "get", [url]);
- }
-
- /**
- * Update meta data of a request
- * @param {RequestMetaData} metaData
- * @returns {Promise}
- */
- UnityCache.prototype.updateRequestMetaData = function (metaData) {
- return this.execute(RequestMetaDataStore.name, "put", [metaData]);
- }
-
- /**
- * Store request in cache
- * @param {Request} request
- * @param {Response} response
- * @returns {Promise}
- */
- UnityCache.prototype.storeRequest = function (request, response) {
- var self = this;
-
- return self.isConnected.then(function () {
- return self.cache.put(request, response);
- });
- }
-
- /**
- * Close database and cache connection.
- * @async
- */
- UnityCache.prototype.close = function () {
- return this.isConnected.then(function () {
- if (this.database) {
- this.database.close();
- this.database = null;
- }
-
- if (this.cache) {
- this.cache = null;
- }
-
- }.bind(this));
- }
-
-
- /**
- * Create a connection to Cache and IndexedDB for meta data storage
- * @private
- * @async
- * @returns {Promise} A Promise that is resolved when a connection to the IndexedDB and cache are established.
- */
- UnityCache.prototype.connect = function () {
- var self = this;
-
- if (typeof indexedDB === "undefined") {
- return Promise.reject(new Error("Could not connect to cache: IndexedDB is not supported."));
- }
-
- if (typeof window.caches === "undefined") {
- return Promise.reject(new Error("Could not connect to cache: Cache API is not supported."));
- }
-
- var isConnected = new Promise(function (resolve, reject) {
- try {
- // Workaround for WebKit bug 226547:
- // On very first page load opening a connection to IndexedDB hangs without triggering onerror.
- // Add a timeout that triggers the error handling code.
- self.openDBTimeout = setTimeout(function () {
- if (typeof self.database != "undefined") {
- return;
- }
-
- reject(new Error("Could not connect to cache: Database timeout."));
- }, 20000);
-
- function clearOpenDBTimeout() {
- if (!self.openDBTimeout) {
- return;
- }
-
- clearTimeout(self.openDBTimeout);
- self.openDBTimeout = null;
- }
-
- var openRequest = indexedDB.open(UnityCacheDatabase.name, UnityCacheDatabase.version);
-
- openRequest.onupgradeneeded = self.upgradeDatabase.bind(self);
-
- openRequest.onsuccess = function (e) {
- clearOpenDBTimeout();
- self.database = e.target.result;
- resolve();
- };
-
- openRequest.onerror = function (error) {
- clearOpenDBTimeout();
- self.database = null;
- reject(new Error("Could not connect to database."));
- };
- } catch (error) {
- clearOpenDBTimeout();
- self.database = null;
- self.cache = null;
- reject(new Error("Could not connect to cache: Could not connect to database."));
- }
- }).then(function () {
- var cacheName = UnityCacheDatabase.name + "_" + Module.companyName + "_" + Module.productName;
-
- return caches.open(cacheName);
- }).then(function (cache) {
- self.cache = cache;
- });
-
- return isConnected;
- }
-
- /**
- * Upgrade object store if database is outdated
- * @private
- * @param {any} e Database upgrade event
- */
- UnityCache.prototype.upgradeDatabase = function (e) {
- var database = e.target.result;
-
- if (!database.objectStoreNames.contains(RequestMetaDataStore.name)) {
- var objectStore = database.createObjectStore(RequestMetaDataStore.name, { keyPath: "url" });
- ["accessedAt", "updatedAt"].forEach(function (index) { objectStore.createIndex(index, index); });
- }
-
- if (database.objectStoreNames.contains(RequestStore.name)) {
- database.deleteObjectStore(RequestStore.name);
- }
-
- if (database.objectStoreNames.contains(WebAssemblyStore.name)) {
- database.deleteObjectStore(WebAssemblyStore.name);
- }
- }
-
- /**
- * Execute an operation on the cache
- * @private
- * @param {string} store The name of the store to use
- * @param {string} operation The operation to to execute on the cache
- * @param {Array} parameters Parameters for the operation
- * @returns {Promise} A promise to the cache entry
- */
- UnityCache.prototype.execute = function (store, operation, parameters) {
- return this.isConnected.then(function () {
- return new Promise(function (resolve, reject) {
- try {
- // Failure during initialization of database -> reject Promise
- if (this.database === null) {
- reject(new Error("indexedDB access denied"))
- return;
- }
-
- // Create a transaction for the request
- var accessMode = ["put", "delete", "clear"].indexOf(operation) != -1 ? "readwrite" : "readonly";
- var transaction = this.database.transaction([store], accessMode)
- var target = transaction.objectStore(store);
- if (operation == "openKeyCursor") {
- target = target.index(parameters[0]);
- parameters = parameters.slice(1);
- }
-
- // Make a request to the database
- var request = target[operation].apply(target, parameters);
- request.onsuccess = function (e) {
- resolve(e.target.result);
- };
- request.onerror = function (error) {
- reject(error);
- };
- } catch (error) {
- reject(error);
- }
- }.bind(this));
- }.bind(this));
- }
-
- UnityCache.prototype.getMetaDataEntries = function () {
- var self = this;
- var cacheSize = 0;
- var metaDataEntries = [];
-
- return new Promise(function (resolve, reject) {
- var transaction = self.database.transaction([RequestMetaDataStore.name], "readonly");
- var target = transaction.objectStore(RequestMetaDataStore.name);
- var request = target.openCursor();
-
- request.onsuccess = function (event) {
- var cursor = event.target.result;
-
- if (cursor) {
- cacheSize += cursor.value.size;
- metaDataEntries.push(cursor.value);
-
- cursor.continue();
- } else {
- resolve({
- metaDataEntries: metaDataEntries,
- cacheSize: cacheSize
- });
- }
- };
- request.onerror = function (error) {
- reject(error);
- };
- });
- }
-
- /**
- * Clean up cache by removing outdated entries.
- * @private
- * @returns {Promise}
- */
- UnityCache.prototype.cleanUpCache = function () {
- var self = this;
-
- return this.getMetaDataEntries().then(function (result) {
- var metaDataEntries = result.metaDataEntries;
- var cacheSize = result.cacheSize;
- var entriesToDelete = [];
- var newMetaDataEntries = [];
-
- // Remove cached entries with outdated product version
- for (var i = 0; i < metaDataEntries.length; ++i) {
- if (metaDataEntries[i].version == Module.productVersion) {
- newMetaDataEntries.push(metaDataEntries[i]);
- continue;
- }
-
- entriesToDelete.push(metaDataEntries[i]);
- cacheSize -= metaDataEntries[i].size;
- }
-
- // Remove cache entries until cache size limit is met
- newMetaDataEntries.sort(function (a,b) {
- return a.accessedAt - b.accessedAt;
- });
-
- for (var i = 0; i < newMetaDataEntries.length; ++i) {
- if (cacheSize < UnityCache.MaximumCacheSize) {
- break;
- }
-
- entriesToDelete.push(newMetaDataEntries[i]);
- cacheSize -= newMetaDataEntries[i].size;
- }
-
- function deleteMetaDataEntry(url) {
- return new Promise(function (resolve, reject) {
- var transaction = self.database.transaction([RequestMetaDataStore.name], "readwrite");
- var target = transaction.objectStore(RequestMetaDataStore.name);
- target.delete(url);
-
- transaction.oncomplete = resolve;
- transaction.onerror = reject;
- });
- }
-
- function deleteEntries() {
- if (entriesToDelete.length === 0) {
- return Promise.resolve();
- }
-
- var entryToDelete = entriesToDelete.pop();
- return self.cache.delete(entryToDelete.url).then(function (deleted) {
- if (deleted) {
- return deleteMetaDataEntry(entryToDelete.url);
- }
- }).then(function () {
- return deleteEntries();
- });
- }
-
- return deleteEntries();
- });
- }
-
- return UnityCache;
-}();
- Module.cachedFetch = function () {
- var UnityCache = Module.UnityCache;
- var fetchWithProgress = Module.fetchWithProgress;
- var readBodyWithProgress = Module.readBodyWithProgress;
-
- function log(message) {
- console.log("[UnityCache] " + message);
- }
-
- function resolveURL(url) {
- resolveURL.link = resolveURL.link || document.createElement("a");
- resolveURL.link.href = url;
- return resolveURL.link.href;
- }
-
- function isCrossOriginURL(url) {
- var originMatch = window.location.href.match(/^[a-z]+:\/\/[^\/]+/);
- return !originMatch || url.lastIndexOf(originMatch[0], 0);
- }
-
- function isCacheEnabled(url, init) {
- if (init && init.method && init.method !== "GET") {
- return false;
- }
-
- if (init && ["must-revalidate", "immutable"].indexOf(init.control) == -1) {
- return false;
- }
-
- if (!url.match("^https?:\/\/")) {
- return false;
- }
-
- return true;
- }
-
- function cachedFetch(resource, init) {
- var unityCache = UnityCache.getInstance();
- var url = resolveURL((typeof resource === "string") ? resource : resource.url);
- var cache = { enabled: isCacheEnabled(url, init) };
- if (init) {
- cache.control = init.control;
- cache.companyName = init.companyName;
- cache.productName = init.productName;
- cache.productVersion = init.productVersion;
- }
- cache.revalidated = false;
- cache.metaData = {
- url: url,
- accessedAt: Date.now(),
- version: cache.productVersion
- };
- cache.response = null;
-
- function fetchAndStoreInCache(resource, init) {
- return fetch(resource, init).then(function (response) {
- if (!cache.enabled || cache.revalidated) {
- return response;
- }
-
- if (response.status === 304) {
- // Cached response is still valid. Set revalidated flag and return cached response
- cache.revalidated = true;
-
- unityCache.updateRequestMetaData(cache.metaData).then(function () {
- log("'" + cache.metaData.url + "' successfully revalidated and served from the indexedDB cache");
- }).catch(function (error) {
- log("'" + cache.metaData.url + "' successfully revalidated but not stored in the indexedDB cache due to the error: " + error);
- });
-
- return readBodyWithProgress(cache.response, init.onProgress);
- } else if (response.status == 200) {
- // New response -> Store it and cache and return it
- cache.response = response;
- cache.metaData.updatedAt = cache.metaData.accessedAt;
- cache.revalidated = true;
- var clonedResponse = response.clone();
-
- return readBodyWithProgress(response, init.onProgress).then(function (response) {
- // Update cached request and meta data
- cache.metaData.size = response.parsedBody.length;
- Promise.all([
- unityCache.storeRequest(resource, clonedResponse),
- unityCache.updateRequestMetaData(cache.metaData)
- ]).then(function () {
- log("'" + url + "' successfully downloaded and stored in the indexedDB cache");
- }).catch(function (error) {
- log("'" + url + "' successfully downloaded but not stored in the indexedDB cache due to the error: " + error);
- });
-
- return response;
- });
- } else {
- // Request failed
- log("'" + url + "' request failed with status: " + response.status + " " + response.statusText);
- }
-
- return readBodyWithProgress(response, init.onProgress);
- });
- }
-
- // Use fetch directly if request can't be cached
- if (!cache.enabled) {
- return fetchWithProgress(resource, init);
- }
-
- return unityCache.loadRequest(url).then(function (result) {
- // Fetch resource and store it in cache if not present or outdated version
- if (!result) {
- return fetchAndStoreInCache(resource, init);
- }
-
- var response = result.response;
- var metaData = result.metaData;
- cache.response = response;
- cache.metaData.size = metaData.size;
- cache.metaData.updatedAt = metaData.updatedAt;
-
- if (cache.control == "immutable") {
- cache.revalidated = true;
- unityCache.updateRequestMetaData(metaData).then(function () {
- log("'" + cache.metaData.url + "' served from the indexedDB cache without revalidation");
- });
-
- return readBodyWithProgress(response, init.onProgress);
- } else if (isCrossOriginURL(url) && (response.headers.get("Last-Modified") || response.headers.get("ETag"))) {
- return fetch(url, { method: "HEAD" }).then(function (headResult) {
- cache.revalidated = ["Last-Modified", "ETag"].every(function (header) {
- return !response.headers.get(header) || response.headers.get(header) == headResult.headers.get(header);
- });
- if (cache.revalidated) {
- unityCache.updateRequestMetaData(metaData).then(function () {
- log("'" + cache.metaData.url + "' successfully revalidated and served from the indexedDB cache");
- });
-
- return readBodyWithProgress(cache.response, init.onProgress);
- } else {
- return fetchAndStoreInCache(resource, init);
- }
- });
- } else {
- init = init || {};
- var requestHeaders = init.headers || {};
- init.headers = requestHeaders;
- if (response.headers.get("Last-Modified")) {
- requestHeaders["If-Modified-Since"] = response.headers.get("Last-Modified");
- requestHeaders["Cache-Control"] = "no-cache";
- } else if (response.headers.get("ETag")) {
- requestHeaders["If-None-Match"] = response.headers.get("ETag");
- requestHeaders["Cache-Control"] = "no-cache";
- }
-
- return fetchAndStoreInCache(resource, init);
- }
- }).catch(function (error) {
- // Fallback to regular fetch if and IndexDB error occurs
- log("Failed to load '" + cache.metaData.url + "' from indexedDB cache due to the error: " + error);
- return fetchWithProgress(resource, init);
- });
- }
-
- return cachedFetch;
-}();
-
-
- function downloadBinary(urlId) {
- progressUpdate(urlId);
- var cacheControl = Module.cacheControl(Module[urlId]);
- var fetchImpl = Module.companyName && Module.productName ? Module.cachedFetch : Module.fetchWithProgress;
- var url = Module[urlId];
- var mode = /file:\/\//.exec(url) ? "same-origin" : undefined;
-
- var request = fetchImpl(Module[urlId], {
- method: "GET",
- companyName: Module.companyName,
- productName: Module.productName,
- productVersion: Module.productVersion,
- control: cacheControl,
- mode: mode,
- onProgress: function (event) {
- progressUpdate(urlId, event);
- }
- });
-
- return request.then(function (response) {
- return response.parsedBody;
- }).catch(function (e) {
- var error = 'Failed to download file ' + Module[urlId];
- if (location.protocol == 'file:') {
- showBanner(error + '. Loading web pages via a file:// URL without a web server is not supported by this browser. Please use a local development web server to host Unity content, or use the Unity Build and Run option.', 'error');
- } else {
- console.error(error);
- }
- });
- }
-
- function downloadFramework() {
- return new Promise(function (resolve, reject) {
- var script = document.createElement("script");
- script.src = Module.frameworkUrl;
- script.onload = function () {
- // Adding the framework.js script to DOM created a global
- // 'unityFramework' variable that should be considered internal.
- // If not, then we have received a malformed file.
- if (typeof unityFramework === 'undefined' || !unityFramework) {
- var compressions = [['br', 'br'], ['gz', 'gzip']];
- for(var i in compressions) {
- var compression = compressions[i];
- if (Module.frameworkUrl.endsWith('.' + compression[0])) {
- var error = 'Unable to parse ' + Module.frameworkUrl + '!';
- if (location.protocol == 'file:') {
- showBanner(error + ' Loading pre-compressed (brotli or gzip) content via a file:// URL without a web server is not supported by this browser. Please use a local development web server to host compressed Unity content, or use the Unity Build and Run option.', 'error');
- return;
- }
- error += ' This can happen if build compression was enabled but web server hosting the content was misconfigured to not serve the file with HTTP Response Header "Content-Encoding: ' + compression[1] + '" present. Check browser Console and Devtools Network tab to debug.';
- if (compression[0] == 'br') {
- if (location.protocol == 'http:') {
- var migrationHelp = ['localhost', '127.0.0.1'].indexOf(location.hostname) != -1 ? '' : 'Migrate your server to use HTTPS.'
- if (/Firefox/.test(navigator.userAgent)) error = 'Unable to parse ' + Module.frameworkUrl + '! If using custom web server, verify that web server is sending .br files with HTTP Response Header "Content-Encoding: br". Brotli compression may not be supported in Firefox over HTTP connections. ' + migrationHelp + ' See https://bugzilla.mozilla.org/show_bug.cgi?id=1670675 for more information.';
- else error = 'Unable to parse ' + Module.frameworkUrl + '! If using custom web server, verify that web server is sending .br files with HTTP Response Header "Content-Encoding: br". Brotli compression may not be supported over HTTP connections. Migrate your server to use HTTPS.';
- }
- }
- showBanner(error, 'error');
- return;
- }
- };
- showBanner('Unable to parse ' + Module.frameworkUrl + '! The file is corrupt, or compression was misconfigured? (check Content-Encoding HTTP Response Header on web server)', 'error');
- }
-
- // Capture the variable to local scope and clear it from global
- // scope so that JS garbage collection can take place on
- // application quit.
- var fw = unityFramework;
- unityFramework = null;
- // Also ensure this function will not hold any JS scope
- // references to prevent JS garbage collection.
- script.onload = null;
- resolve(fw);
- }
- script.onerror = function(e) {
- showBanner('Unable to load file ' + Module.frameworkUrl + '! Check that the file exists on the remote server. (also check browser Console and Devtools Network tab to debug)', 'error');
- }
- document.body.appendChild(script);
- Module.deinitializers.push(function() {
- document.body.removeChild(script);
- });
- });
- }
-
- function loadBuild() {
- downloadFramework().then(function (unityFramework) {
- unityFramework(Module);
- });
-
- var dataPromise = downloadBinary("dataUrl");
- Module.preRun.push(function () {
- Module.addRunDependency("dataUrl");
- dataPromise.then(function (data) {
- var view = new DataView(data.buffer, data.byteOffset, data.byteLength);
- var pos = 0;
- var prefix = "UnityWebData1.0\0";
- if (!String.fromCharCode.apply(null, data.subarray(pos, pos + prefix.length)) == prefix)
- throw "unknown data format";
- pos += prefix.length;
- var headerSize = view.getUint32(pos, true); pos += 4;
- while (pos < headerSize) {
- var offset = view.getUint32(pos, true); pos += 4;
- var size = view.getUint32(pos, true); pos += 4;
- var pathLength = view.getUint32(pos, true); pos += 4;
- var path = String.fromCharCode.apply(null, data.subarray(pos, pos + pathLength)); pos += pathLength;
- for (var folder = 0, folderNext = path.indexOf("/", folder) + 1 ; folderNext > 0; folder = folderNext, folderNext = path.indexOf("/", folder) + 1)
- Module.FS_createPath(path.substring(0, folder), path.substring(folder, folderNext - 1), true, true);
- Module.FS_createDataFile(path, null, data.subarray(offset, offset + size), true, true, true);
- }
- Module.removeRunDependency("dataUrl");
- });
- });
- }
-
- return new Promise(function (resolve, reject) {
- if (!Module.SystemInfo.hasWebGL) {
- reject("Your browser does not support WebGL.");
- } else if (Module.SystemInfo.hasWebGL == 1) {
- var msg = "Your browser does not support graphics API \"WebGL 2\" which is required for this content.";
- if (Module.SystemInfo.browser == 'Safari' && parseInt(Module.SystemInfo.browserVersion) < 15) {
- if (Module.SystemInfo.mobile || navigator.maxTouchPoints > 1)
- msg += "\nUpgrade to iOS 15 or later.";
- else
- msg += "\nUpgrade to Safari 15 or later.";
- }
- reject(msg);
- } else if (!Module.SystemInfo.hasWasm) {
- reject("Your browser does not support WebAssembly.");
- } else {
- Module.startupErrorHandler = reject;
- onProgress(0);
- Module.postRun.push(function () {
- onProgress(1);
- delete Module.startupErrorHandler;
- resolve(unityInstance);
- });
- loadBuild();
- }
- });
-}
diff --git a/spaces/colakin/video-generater/public/ffmpeg/libavcodec/amfenc_av1.c b/spaces/colakin/video-generater/public/ffmpeg/libavcodec/amfenc_av1.c
deleted file mode 100644
index 8093cb735752b8fc73032bcc676ae5f5c489ef89..0000000000000000000000000000000000000000
--- a/spaces/colakin/video-generater/public/ffmpeg/libavcodec/amfenc_av1.c
+++ /dev/null
@@ -1,361 +0,0 @@
-/*
- * This file is part of FFmpeg.
- *
- * FFmpeg is free software; you can redistribute it and/or
- * modify it under the terms of the GNU Lesser General Public
- * License as published by the Free Software Foundation; either
- * version 2.1 of the License, or (at your option) any later version.
- *
- * FFmpeg is distributed in the hope that it will be useful,
- * but WITHOUT ANY WARRANTY; without even the implied warranty of
- * MERCHANTABILITY or FITNESS FOR A PARTICULAR PURPOSE. See the GNU
- * Lesser General Public License for more details.
- *
- * You should have received a copy of the GNU Lesser General Public
- * License along with FFmpeg; if not, write to the Free Software
- * Foundation, Inc., 51 Franklin Street, Fifth Floor, Boston, MA 02110-1301 USA
- */
-
-#include "libavutil/internal.h"
-#include "libavutil/opt.h"
-#include "amfenc.h"
-#include "codec_internal.h"
-#include "internal.h"
-
-#define OFFSET(x) offsetof(AmfContext, x)
-#define VE AV_OPT_FLAG_VIDEO_PARAM | AV_OPT_FLAG_ENCODING_PARAM
-static const AVOption options[] = {
- { "usage", "Set the encoding usage", OFFSET(usage), AV_OPT_TYPE_INT, {.i64 = AMF_VIDEO_ENCODER_AV1_USAGE_TRANSCODING }, AMF_VIDEO_ENCODER_AV1_USAGE_TRANSCODING, AMF_VIDEO_ENCODER_AV1_USAGE_LOW_LATENCY, VE, "usage" },
- { "transcoding", "", 0, AV_OPT_TYPE_CONST, {.i64 = AMF_VIDEO_ENCODER_AV1_USAGE_TRANSCODING }, 0, 0, VE, "usage" },
- { "lowlatency", "", 0, AV_OPT_TYPE_CONST, {.i64 = AMF_VIDEO_ENCODER_AV1_USAGE_LOW_LATENCY }, 0, 0, VE, "usage" },
-
- { "profile", "Set the profile (default main)", OFFSET(profile), AV_OPT_TYPE_INT,{.i64 = AMF_VIDEO_ENCODER_AV1_PROFILE_MAIN }, AMF_VIDEO_ENCODER_AV1_PROFILE_MAIN, AMF_VIDEO_ENCODER_AV1_PROFILE_MAIN, VE, "profile" },
- { "main", "", 0, AV_OPT_TYPE_CONST,{.i64 = AMF_VIDEO_ENCODER_AV1_PROFILE_MAIN }, 0, 0, VE, "profile" },
-
- { "level", "Set the encoding level (default auto)", OFFSET(level), AV_OPT_TYPE_INT,{.i64 = 0 }, 0, AMF_VIDEO_ENCODER_AV1_LEVEL_7_3, VE, "level" },
- { "auto", "", 0, AV_OPT_TYPE_CONST, {.i64 = 0 }, 0, 0, VE, "level" },
- { "2.0", "", 0, AV_OPT_TYPE_CONST, {.i64 = AMF_VIDEO_ENCODER_AV1_LEVEL_2_0 }, 0, 0, VE, "level" },
- { "2.1", "", 0, AV_OPT_TYPE_CONST, {.i64 = AMF_VIDEO_ENCODER_AV1_LEVEL_2_1 }, 0, 0, VE, "level" },
- { "2.2", "", 0, AV_OPT_TYPE_CONST, {.i64 = AMF_VIDEO_ENCODER_AV1_LEVEL_2_2 }, 0, 0, VE, "level" },
- { "2.3", "", 0, AV_OPT_TYPE_CONST, {.i64 = AMF_VIDEO_ENCODER_AV1_LEVEL_2_3 }, 0, 0, VE, "level" },
- { "3.0", "", 0, AV_OPT_TYPE_CONST, {.i64 = AMF_VIDEO_ENCODER_AV1_LEVEL_3_0 }, 0, 0, VE, "level" },
- { "3.1", "", 0, AV_OPT_TYPE_CONST, {.i64 = AMF_VIDEO_ENCODER_AV1_LEVEL_3_1 }, 0, 0, VE, "level" },
- { "3.2", "", 0, AV_OPT_TYPE_CONST, {.i64 = AMF_VIDEO_ENCODER_AV1_LEVEL_3_2 }, 0, 0, VE, "level" },
- { "3.3", "", 0, AV_OPT_TYPE_CONST, {.i64 = AMF_VIDEO_ENCODER_AV1_LEVEL_3_3 }, 0, 0, VE, "level" },
- { "4.0", "", 0, AV_OPT_TYPE_CONST, {.i64 = AMF_VIDEO_ENCODER_AV1_LEVEL_4_0 }, 0, 0, VE, "level" },
- { "4.1", "", 0, AV_OPT_TYPE_CONST, {.i64 = AMF_VIDEO_ENCODER_AV1_LEVEL_4_1 }, 0, 0, VE, "level" },
- { "4.2", "", 0, AV_OPT_TYPE_CONST, {.i64 = AMF_VIDEO_ENCODER_AV1_LEVEL_4_2 }, 0, 0, VE, "level" },
- { "4.3", "", 0, AV_OPT_TYPE_CONST, {.i64 = AMF_VIDEO_ENCODER_AV1_LEVEL_4_3 }, 0, 0, VE, "level" },
- { "5.0", "", 0, AV_OPT_TYPE_CONST, {.i64 = AMF_VIDEO_ENCODER_AV1_LEVEL_5_0 }, 0, 0, VE, "level" },
- { "5.1", "", 0, AV_OPT_TYPE_CONST, {.i64 = AMF_VIDEO_ENCODER_AV1_LEVEL_5_1 }, 0, 0, VE, "level" },
- { "5.2", "", 0, AV_OPT_TYPE_CONST, {.i64 = AMF_VIDEO_ENCODER_AV1_LEVEL_5_2 }, 0, 0, VE, "level" },
- { "5.3", "", 0, AV_OPT_TYPE_CONST, {.i64 = AMF_VIDEO_ENCODER_AV1_LEVEL_5_3 }, 0, 0, VE, "level" },
- { "6.0", "", 0, AV_OPT_TYPE_CONST, {.i64 = AMF_VIDEO_ENCODER_AV1_LEVEL_6_0 }, 0, 0, VE, "level" },
- { "6.1", "", 0, AV_OPT_TYPE_CONST, {.i64 = AMF_VIDEO_ENCODER_AV1_LEVEL_6_1 }, 0, 0, VE, "level" },
- { "6.2", "", 0, AV_OPT_TYPE_CONST, {.i64 = AMF_VIDEO_ENCODER_AV1_LEVEL_6_2 }, 0, 0, VE, "level" },
- { "6.3", "", 0, AV_OPT_TYPE_CONST, {.i64 = AMF_VIDEO_ENCODER_AV1_LEVEL_6_3 }, 0, 0, VE, "level" },
- { "7.0", "", 0, AV_OPT_TYPE_CONST, {.i64 = AMF_VIDEO_ENCODER_AV1_LEVEL_7_0 }, 0, 0, VE, "level" },
- { "7.1", "", 0, AV_OPT_TYPE_CONST, {.i64 = AMF_VIDEO_ENCODER_AV1_LEVEL_7_1 }, 0, 0, VE, "level" },
- { "7.2", "", 0, AV_OPT_TYPE_CONST, {.i64 = AMF_VIDEO_ENCODER_AV1_LEVEL_7_2 }, 0, 0, VE, "level" },
- { "7.3", "", 0, AV_OPT_TYPE_CONST, {.i64 = AMF_VIDEO_ENCODER_AV1_LEVEL_7_3 }, 0, 0, VE, "level" },
-
- { "quality", "Set the encoding quality", OFFSET(quality), AV_OPT_TYPE_INT, {.i64 = AMF_VIDEO_ENCODER_AV1_QUALITY_PRESET_SPEED }, AMF_VIDEO_ENCODER_AV1_QUALITY_PRESET_HIGH_QUALITY, AMF_VIDEO_ENCODER_AV1_QUALITY_PRESET_SPEED, VE, "quality" },
- { "balanced", "", 0, AV_OPT_TYPE_CONST, {.i64 = AMF_VIDEO_ENCODER_AV1_QUALITY_PRESET_BALANCED }, 0, 0, VE, "quality" },
- { "speed", "", 0, AV_OPT_TYPE_CONST, {.i64 = AMF_VIDEO_ENCODER_AV1_QUALITY_PRESET_SPEED }, 0, 0, VE, "quality" },
- { "quality", "", 0, AV_OPT_TYPE_CONST, {.i64 = AMF_VIDEO_ENCODER_AV1_QUALITY_PRESET_QUALITY }, 0, 0, VE, "quality" },
- { "high_quality", "", 0, AV_OPT_TYPE_CONST, {.i64 = AMF_VIDEO_ENCODER_AV1_QUALITY_PRESET_HIGH_QUALITY }, 0, 0, VE, "quality" },
-
- { "rc", "Set the rate control mode", OFFSET(rate_control_mode), AV_OPT_TYPE_INT, {.i64 = AMF_VIDEO_ENCODER_AV1_RATE_CONTROL_METHOD_UNKNOWN }, AMF_VIDEO_ENCODER_AV1_RATE_CONTROL_METHOD_UNKNOWN, AMF_VIDEO_ENCODER_AV1_RATE_CONTROL_METHOD_CBR, VE, "rc" },
- { "cqp", "Constant Quantization Parameter", 0, AV_OPT_TYPE_CONST, {.i64 = AMF_VIDEO_ENCODER_AV1_RATE_CONTROL_METHOD_CONSTANT_QP }, 0, 0, VE, "rc" },
- { "vbr_latency", "Latency Constrained Variable Bitrate", 0, AV_OPT_TYPE_CONST, {.i64 = AMF_VIDEO_ENCODER_AV1_RATE_CONTROL_METHOD_LATENCY_CONSTRAINED_VBR }, 0, 0, VE, "rc" },
- { "vbr_peak", "Peak Contrained Variable Bitrate", 0, AV_OPT_TYPE_CONST, {.i64 = AMF_VIDEO_ENCODER_AV1_RATE_CONTROL_METHOD_PEAK_CONSTRAINED_VBR }, 0, 0, VE, "rc" },
- { "cbr", "Constant Bitrate", 0, AV_OPT_TYPE_CONST, {.i64 = AMF_VIDEO_ENCODER_AV1_RATE_CONTROL_METHOD_CBR }, 0, 0, VE, "rc" },
-
- { "header_insertion_mode", "Set header insertion mode", OFFSET(header_insertion_mode), AV_OPT_TYPE_INT,{.i64 = AMF_VIDEO_ENCODER_AV1_HEADER_INSERTION_MODE_NONE }, AMF_VIDEO_ENCODER_AV1_HEADER_INSERTION_MODE_NONE, AMF_VIDEO_ENCODER_AV1_HEADER_INSERTION_MODE_KEY_FRAME_ALIGNED, VE, "hdrmode" },
- { "none", "", 0, AV_OPT_TYPE_CONST, {.i64 = AMF_VIDEO_ENCODER_AV1_HEADER_INSERTION_MODE_NONE }, 0, 0, VE, "hdrmode" },
- { "gop", "", 0, AV_OPT_TYPE_CONST, {.i64 = AMF_VIDEO_ENCODER_AV1_HEADER_INSERTION_MODE_GOP_ALIGNED }, 0, 0, VE, "hdrmode" },
- { "frame", "", 0, AV_OPT_TYPE_CONST, {.i64 = AMF_VIDEO_ENCODER_AV1_HEADER_INSERTION_MODE_KEY_FRAME_ALIGNED }, 0, 0, VE, "hdrmode" },
-
- { "preanalysis", "Enable preanalysis", OFFSET(preanalysis), AV_OPT_TYPE_BOOL, {.i64 = 0 }, 0, 1, VE},
- { "enforce_hrd", "Enforce HRD", OFFSET(enforce_hrd), AV_OPT_TYPE_BOOL, {.i64 = 0 }, 0, 1, VE},
- { "filler_data", "Filler Data Enable", OFFSET(filler_data), AV_OPT_TYPE_BOOL, {.i64 = 0 }, 0, 1, VE},
-
- // min_qp_i -> min_qp_intra, min_qp_p -> min_qp_inter
- { "min_qp_i", "min quantization parameter for I-frame", OFFSET(min_qp_i), AV_OPT_TYPE_INT, {.i64 = -1 }, -1, 255, VE },
- { "max_qp_i", "max quantization parameter for I-frame", OFFSET(max_qp_i), AV_OPT_TYPE_INT, {.i64 = -1 }, -1, 255, VE },
- { "min_qp_p", "min quantization parameter for P-frame", OFFSET(min_qp_p), AV_OPT_TYPE_INT, {.i64 = -1 }, -1, 255, VE },
- { "max_qp_p", "max quantization parameter for P-frame", OFFSET(max_qp_p), AV_OPT_TYPE_INT, {.i64 = -1 }, -1, 255, VE },
- { "qp_p", "quantization parameter for P-frame", OFFSET(qp_p), AV_OPT_TYPE_INT, {.i64 = -1 }, -1, 255, VE },
- { "qp_i", "quantization parameter for I-frame", OFFSET(qp_i), AV_OPT_TYPE_INT, {.i64 = -1 }, -1, 255, VE },
- { "skip_frame", "Rate Control Based Frame Skip", OFFSET(skip_frame), AV_OPT_TYPE_BOOL,{.i64 = 0 }, 0, 1, VE },
-
- { "align", "alignment mode", OFFSET(align), AV_OPT_TYPE_INT, {.i64 = AMF_VIDEO_ENCODER_AV1_ALIGNMENT_MODE_NO_RESTRICTIONS }, AMF_VIDEO_ENCODER_AV1_ALIGNMENT_MODE_64X16_ONLY, AMF_VIDEO_ENCODER_AV1_ALIGNMENT_MODE_NO_RESTRICTIONS, VE, "align" },
- { "64x16", "", 0, AV_OPT_TYPE_CONST, {.i64 = AMF_VIDEO_ENCODER_AV1_ALIGNMENT_MODE_64X16_ONLY }, 0, 0, VE, "align" },
- { "1080p", "", 0, AV_OPT_TYPE_CONST, {.i64 = AMF_VIDEO_ENCODER_AV1_ALIGNMENT_MODE_64X16_1080P_CODED_1082 }, 0, 0, VE, "align" },
- { "none", "", 0, AV_OPT_TYPE_CONST, {.i64 = AMF_VIDEO_ENCODER_AV1_ALIGNMENT_MODE_NO_RESTRICTIONS }, 0, 0, VE, "align" },
-
- { NULL }
-
-};
-
-static av_cold int amf_encode_init_av1(AVCodecContext* avctx)
-{
- int ret = 0;
- AMF_RESULT res = AMF_OK;
- AmfContext* ctx = avctx->priv_data;
- AMFVariantStruct var = { 0 };
- amf_int64 profile = 0;
- amf_int64 profile_level = 0;
- AMFBuffer* buffer;
- AMFGuid guid;
- AMFRate framerate;
- AMFSize framesize = AMFConstructSize(avctx->width, avctx->height);
-
-
-
- if (avctx->framerate.num > 0 && avctx->framerate.den > 0) {
- framerate = AMFConstructRate(avctx->framerate.num, avctx->framerate.den);
- }
- else {
- framerate = AMFConstructRate(avctx->time_base.den, avctx->time_base.num * avctx->ticks_per_frame);
- }
-
- if ((ret = ff_amf_encode_init(avctx)) < 0)
- return ret;
-
- // init static parameters
- AMF_ASSIGN_PROPERTY_INT64(res, ctx->encoder, AMF_VIDEO_ENCODER_AV1_USAGE, ctx->usage);
-
- AMF_ASSIGN_PROPERTY_SIZE(res, ctx->encoder, AMF_VIDEO_ENCODER_AV1_FRAMESIZE, framesize);
-
- AMF_ASSIGN_PROPERTY_RATE(res, ctx->encoder, AMF_VIDEO_ENCODER_AV1_FRAMERATE, framerate);
-
- switch (avctx->profile) {
- case FF_PROFILE_AV1_MAIN:
- profile = AMF_VIDEO_ENCODER_AV1_PROFILE_MAIN;
- break;
- default:
- break;
- }
- if (profile == 0) {
- profile = ctx->profile;
- }
- AMF_ASSIGN_PROPERTY_INT64(res, ctx->encoder, AMF_VIDEO_ENCODER_AV1_PROFILE, profile);
-
- profile_level = avctx->level;
- if (profile_level == FF_LEVEL_UNKNOWN) {
- profile_level = ctx->level;
- }
- if (profile_level != 0) {
- AMF_ASSIGN_PROPERTY_INT64(res, ctx->encoder, AMF_VIDEO_ENCODER_AV1_LEVEL, profile_level);
- }
- AMF_ASSIGN_PROPERTY_INT64(res, ctx->encoder, AMF_VIDEO_ENCODER_AV1_QUALITY_PRESET, ctx->quality);
-
- // Maximum Reference Frames
- if (avctx->refs != -1) {
- AMF_ASSIGN_PROPERTY_INT64(res, ctx->encoder, AMF_VIDEO_ENCODER_AV1_MAX_NUM_REFRAMES, avctx->refs);
- }
-
- // Picture control properties
- AMF_ASSIGN_PROPERTY_INT64(res, ctx->encoder, AMF_VIDEO_ENCODER_AV1_GOP_SIZE, avctx->gop_size);
-
- AMF_ASSIGN_PROPERTY_INT64(res, ctx->encoder, AMF_VIDEO_ENCODER_AV1_HEADER_INSERTION_MODE, ctx->header_insertion_mode);
-
- // Rate control
- // autodetect rate control method
- if (ctx->rate_control_mode == AMF_VIDEO_ENCODER_AV1_RATE_CONTROL_METHOD_UNKNOWN) {
- if (ctx->min_qp_i != -1 || ctx->max_qp_i != -1 ||
- ctx->min_qp_p != -1 || ctx->max_qp_p != -1 ||
- ctx->qp_i != -1 || ctx->qp_p != -1) {
- ctx->rate_control_mode = AMF_VIDEO_ENCODER_AV1_RATE_CONTROL_METHOD_CONSTANT_QP;
- av_log(ctx, AV_LOG_DEBUG, "Rate control turned to CQP\n");
- }
- else if (avctx->rc_max_rate > 0) {
- ctx->rate_control_mode = AMF_VIDEO_ENCODER_AV1_RATE_CONTROL_METHOD_PEAK_CONSTRAINED_VBR;
- av_log(ctx, AV_LOG_DEBUG, "Rate control turned to Peak VBR\n");
- }
- else {
- ctx->rate_control_mode = AMF_VIDEO_ENCODER_AV1_RATE_CONTROL_METHOD_CBR;
- av_log(ctx, AV_LOG_DEBUG, "Rate control turned to CBR\n");
- }
- }
-
- AMF_ASSIGN_PROPERTY_INT64(res, ctx->encoder, AMF_VIDEO_ENCODER_AV1_RATE_CONTROL_METHOD, ctx->rate_control_mode);
- if (avctx->rc_buffer_size) {
- AMF_ASSIGN_PROPERTY_INT64(res, ctx->encoder, AMF_VIDEO_ENCODER_AV1_VBV_BUFFER_SIZE, avctx->rc_buffer_size);
-
- if (avctx->rc_initial_buffer_occupancy != 0) {
- int amf_buffer_fullness = avctx->rc_initial_buffer_occupancy * 64 / avctx->rc_buffer_size;
- if (amf_buffer_fullness > 64)
- amf_buffer_fullness = 64;
- AMF_ASSIGN_PROPERTY_INT64(res, ctx->encoder, AMF_VIDEO_ENCODER_AV1_INITIAL_VBV_BUFFER_FULLNESS, amf_buffer_fullness);
- }
- }
-
- // Pre-Pass, Pre-Analysis, Two-Pass
- AMF_ASSIGN_PROPERTY_BOOL(res, ctx->encoder, AMF_VIDEO_ENCODER_AV1_PRE_ANALYSIS_ENABLE, ctx->preanalysis);
-
- // init dynamic rate control params
- if (ctx->max_au_size)
- ctx->enforce_hrd = 1;
- AMF_ASSIGN_PROPERTY_BOOL(res, ctx->encoder, AMF_VIDEO_ENCODER_AV1_ENFORCE_HRD, ctx->enforce_hrd);
- AMF_ASSIGN_PROPERTY_BOOL(res, ctx->encoder, AMF_VIDEO_ENCODER_AV1_FILLER_DATA, ctx->filler_data);
-
- AMF_ASSIGN_PROPERTY_INT64(res, ctx->encoder, AMF_VIDEO_ENCODER_AV1_TARGET_BITRATE, avctx->bit_rate);
-
- if (ctx->rate_control_mode == AMF_VIDEO_ENCODER_AV1_RATE_CONTROL_METHOD_CBR) {
- AMF_ASSIGN_PROPERTY_INT64(res, ctx->encoder, AMF_VIDEO_ENCODER_AV1_PEAK_BITRATE, avctx->bit_rate);
- }
- if (avctx->rc_max_rate) {
- AMF_ASSIGN_PROPERTY_INT64(res, ctx->encoder, AMF_VIDEO_ENCODER_AV1_PEAK_BITRATE, avctx->rc_max_rate);
- }
- else if (ctx->rate_control_mode == AMF_VIDEO_ENCODER_AV1_RATE_CONTROL_METHOD_PEAK_CONSTRAINED_VBR) {
- av_log(ctx, AV_LOG_WARNING, "rate control mode is PEAK_CONSTRAINED_VBR but rc_max_rate is not set\n");
- }
- if (avctx->bit_rate > 0) {
- ctx->rate_control_mode = AMF_VIDEO_ENCODER_AV1_RATE_CONTROL_METHOD_CBR;
- av_log(ctx, AV_LOG_DEBUG, "Rate control turned to CBR\n");
- }
-
- switch (ctx->align)
- {
- case AMF_VIDEO_ENCODER_AV1_ALIGNMENT_MODE_64X16_ONLY:
- if (avctx->width / 64 * 64 != avctx->width || avctx->height / 16 * 16 != avctx->height)
- {
- res = AMF_NOT_SUPPORTED;
- av_log(ctx, AV_LOG_ERROR, "Resolution incorrect for alignment mode\n");
- return AVERROR_EXIT;
- }
- break;
- case AMF_VIDEO_ENCODER_AV1_ALIGNMENT_MODE_64X16_1080P_CODED_1082:
- if ((avctx->width / 64 * 64 == avctx->width && avctx->height / 16 * 16 == avctx->height) || (avctx->width == 1920 && avctx->height == 1080))
- {
- res = AMF_OK;
- }
- else
- {
- res = AMF_NOT_SUPPORTED;
- av_log(ctx, AV_LOG_ERROR, "Resolution incorrect for alignment mode\n");
- return AVERROR_EXIT;
- }
- break;
- case AMF_VIDEO_ENCODER_AV1_ALIGNMENT_MODE_NO_RESTRICTIONS:
- res = AMF_OK;
- break;
- default:
- res = AMF_NOT_SUPPORTED;
- av_log(ctx, AV_LOG_ERROR, "Invalid alignment mode\n");
- return AVERROR_EXIT;
- }
- AMF_ASSIGN_PROPERTY_INT64(res, ctx->encoder, AMF_VIDEO_ENCODER_AV1_ALIGNMENT_MODE, ctx->align);
-
-
- // init encoder
- res = ctx->encoder->pVtbl->Init(ctx->encoder, ctx->format, avctx->width, avctx->height);
- AMF_RETURN_IF_FALSE(ctx, res == AMF_OK, AVERROR_BUG, "encoder->Init() failed with error %d\n", res);
-
- // init dynamic picture control params
- if (ctx->min_qp_i != -1) {
- AMF_ASSIGN_PROPERTY_INT64(res, ctx->encoder, AMF_VIDEO_ENCODER_AV1_MIN_Q_INDEX_INTRA, ctx->min_qp_i);
- }
- else if (avctx->qmin != -1) {
- int qval = avctx->qmin > 255 ? 255 : avctx->qmin;
- AMF_ASSIGN_PROPERTY_INT64(res, ctx->encoder, AMF_VIDEO_ENCODER_AV1_MIN_Q_INDEX_INTRA, qval);
- }
- if (ctx->max_qp_i != -1) {
- AMF_ASSIGN_PROPERTY_INT64(res, ctx->encoder, AMF_VIDEO_ENCODER_AV1_MAX_Q_INDEX_INTRA, ctx->max_qp_i);
- }
- else if (avctx->qmax != -1) {
- int qval = avctx->qmax > 255 ? 255 : avctx->qmax;
- AMF_ASSIGN_PROPERTY_INT64(res, ctx->encoder, AMF_VIDEO_ENCODER_AV1_MAX_Q_INDEX_INTRA, qval);
- }
- if (ctx->min_qp_p != -1) {
- AMF_ASSIGN_PROPERTY_INT64(res, ctx->encoder, AMF_VIDEO_ENCODER_AV1_MIN_Q_INDEX_INTER, ctx->min_qp_p);
- }
- else if (avctx->qmin != -1) {
- int qval = avctx->qmin > 255 ? 255 : avctx->qmin;
- AMF_ASSIGN_PROPERTY_INT64(res, ctx->encoder, AMF_VIDEO_ENCODER_AV1_MIN_Q_INDEX_INTER, qval);
- }
- if (ctx->max_qp_p != -1) {
- AMF_ASSIGN_PROPERTY_INT64(res, ctx->encoder, AMF_VIDEO_ENCODER_AV1_MAX_Q_INDEX_INTER, ctx->max_qp_p);
- }
- else if (avctx->qmax != -1) {
- int qval = avctx->qmax > 255 ? 255 : avctx->qmax;
- AMF_ASSIGN_PROPERTY_INT64(res, ctx->encoder, AMF_VIDEO_ENCODER_AV1_MAX_Q_INDEX_INTER, qval);
- }
-
- if (ctx->qp_p != -1) {
- AMF_ASSIGN_PROPERTY_INT64(res, ctx->encoder, AMF_VIDEO_ENCODER_AV1_Q_INDEX_INTER, ctx->qp_p);
- }
- if (ctx->qp_i != -1) {
- AMF_ASSIGN_PROPERTY_INT64(res, ctx->encoder, AMF_VIDEO_ENCODER_AV1_Q_INDEX_INTRA, ctx->qp_i);
- }
- AMF_ASSIGN_PROPERTY_BOOL(res, ctx->encoder, AMF_VIDEO_ENCODER_AV1_RATE_CONTROL_SKIP_FRAME, ctx->skip_frame);
-
-
- // fill extradata
- res = AMFVariantInit(&var);
- AMF_RETURN_IF_FALSE(ctx, res == AMF_OK, AVERROR_BUG, "AMFVariantInit() failed with error %d\n", res);
-
- res = ctx->encoder->pVtbl->GetProperty(ctx->encoder, AMF_VIDEO_ENCODER_AV1_EXTRA_DATA, &var);
- AMF_RETURN_IF_FALSE(ctx, res == AMF_OK, AVERROR_BUG, "GetProperty(AMF_VIDEO_ENCODER_EXTRADATA) failed with error %d\n", res);
- AMF_RETURN_IF_FALSE(ctx, var.pInterface != NULL, AVERROR_BUG, "GetProperty(AMF_VIDEO_ENCODER_EXTRADATA) returned NULL\n");
-
- guid = IID_AMFBuffer();
-
- res = var.pInterface->pVtbl->QueryInterface(var.pInterface, &guid, (void**)&buffer); // query for buffer interface
- if (res != AMF_OK) {
- var.pInterface->pVtbl->Release(var.pInterface);
- }
- AMF_RETURN_IF_FALSE(ctx, res == AMF_OK, AVERROR_BUG, "QueryInterface(IID_AMFBuffer) failed with error %d\n", res);
-
- avctx->extradata_size = (int)buffer->pVtbl->GetSize(buffer);
- avctx->extradata = av_mallocz(avctx->extradata_size + AV_INPUT_BUFFER_PADDING_SIZE);
- if (!avctx->extradata) {
- buffer->pVtbl->Release(buffer);
- var.pInterface->pVtbl->Release(var.pInterface);
- return AVERROR(ENOMEM);
- }
- memcpy(avctx->extradata, buffer->pVtbl->GetNative(buffer), avctx->extradata_size);
-
- buffer->pVtbl->Release(buffer);
- var.pInterface->pVtbl->Release(var.pInterface);
-
- return 0;
-}
-
-static const FFCodecDefault defaults[] = {
- { "refs", "-1" },
- { "aspect", "0" },
- { "b", "2M" },
- { "g", "250" },
- { "qmin", "-1" },
- { "qmax", "-1" },
- { NULL },
-};
-
-static const AVClass av1_amf_class = {
- .class_name = "av1_amf",
- .item_name = av_default_item_name,
- .option = options,
- .version = LIBAVUTIL_VERSION_INT,
-};
-
-const FFCodec ff_av1_amf_encoder = {
- .p.name = "av1_amf",
- CODEC_LONG_NAME("AMD AMF AV1 encoder"),
- .p.type = AVMEDIA_TYPE_VIDEO,
- .p.id = AV_CODEC_ID_AV1,
- .init = amf_encode_init_av1,
- FF_CODEC_RECEIVE_PACKET_CB(ff_amf_receive_packet),
- .close = ff_amf_encode_close,
- .priv_data_size = sizeof(AmfContext),
- .p.priv_class = &av1_amf_class,
- .defaults = defaults,
- .p.capabilities = AV_CODEC_CAP_DELAY | AV_CODEC_CAP_HARDWARE |
- AV_CODEC_CAP_DR1,
- .caps_internal = FF_CODEC_CAP_INIT_CLEANUP,
- .p.pix_fmts = ff_amf_pix_fmts,
- .p.wrapper_name = "amf",
- .hw_configs = ff_amfenc_hw_configs,
-};
diff --git a/spaces/colakin/video-generater/public/ffmpeg/libavcodec/midivid.c b/spaces/colakin/video-generater/public/ffmpeg/libavcodec/midivid.c
deleted file mode 100644
index 599d5c8f8fabfb73fd4ce83de7fcb8ac41d80e0e..0000000000000000000000000000000000000000
--- a/spaces/colakin/video-generater/public/ffmpeg/libavcodec/midivid.c
+++ /dev/null
@@ -1,287 +0,0 @@
-/*
- * MidiVid decoder
- * Copyright (c) 2019 Paul B Mahol
- *
- * This file is part of FFmpeg.
- *
- * FFmpeg is free software; you can redistribute it and/or
- * modify it under the terms of the GNU Lesser General Public
- * License as published by the Free Software Foundation; either
- * version 2.1 of the License, or (at your option) any later version.
- *
- * FFmpeg is distributed in the hope that it will be useful,
- * but WITHOUT ANY WARRANTY; without even the implied warranty of
- * MERCHANTABILITY or FITNESS FOR A PARTICULAR PURPOSE. See the GNU
- * Lesser General Public License for more details.
- *
- * You should have received a copy of the GNU Lesser General Public
- * License along with FFmpeg; if not, write to the Free Software
- * Foundation, Inc., 51 Franklin Street, Fifth Floor, Boston, MA 02110-1301 USA
- */
-
-#include "libavutil/imgutils.h"
-#include "libavutil/internal.h"
-#include "libavutil/mem.h"
-
-#define BITSTREAM_READER_LE
-#include "avcodec.h"
-#include "get_bits.h"
-#include "bytestream.h"
-#include "codec_internal.h"
-#include "decode.h"
-
-typedef struct MidiVidContext {
- GetByteContext gb;
-
- uint8_t *uncompressed;
- unsigned int uncompressed_size;
- uint8_t *skip;
-
- AVFrame *frame;
-} MidiVidContext;
-
-static int decode_mvdv(MidiVidContext *s, AVCodecContext *avctx, AVFrame *frame)
-{
- GetByteContext *gb = &s->gb;
- GetBitContext mask;
- GetByteContext idx9;
- uint16_t nb_vectors, intra_flag;
- const uint8_t *vec;
- const uint8_t *mask_start;
- uint8_t *skip;
- uint32_t mask_size;
- int idx9bits = 0;
- int idx9val = 0;
- uint32_t nb_blocks;
-
- nb_vectors = bytestream2_get_le16(gb);
- intra_flag = !!bytestream2_get_le16(gb);
- if (intra_flag) {
- nb_blocks = (avctx->width / 2) * (avctx->height / 2);
- } else {
- int ret, skip_linesize, padding;
-
- nb_blocks = bytestream2_get_le32(gb);
- skip_linesize = avctx->width >> 1;
- mask_start = gb->buffer_start + bytestream2_tell(gb);
- mask_size = (FFALIGN(avctx->width, 32) >> 2) * (avctx->height >> 2) >> 3;
- padding = (FFALIGN(avctx->width, 32) - avctx->width) >> 2;
-
- if (bytestream2_get_bytes_left(gb) < mask_size)
- return AVERROR_INVALIDDATA;
-
- ret = init_get_bits8(&mask, mask_start, mask_size);
- if (ret < 0)
- return ret;
- bytestream2_skip(gb, mask_size);
- skip = s->skip;
-
- for (int y = 0; y < avctx->height >> 2; y++) {
- for (int x = 0; x < avctx->width >> 2; x++) {
- int flag = !get_bits1(&mask);
-
- skip[(y*2) *skip_linesize + x*2 ] = flag;
- skip[(y*2) *skip_linesize + x*2+1] = flag;
- skip[(y*2+1)*skip_linesize + x*2 ] = flag;
- skip[(y*2+1)*skip_linesize + x*2+1] = flag;
- }
- skip_bits_long(&mask, padding);
- }
- }
-
- vec = gb->buffer_start + bytestream2_tell(gb);
- if (bytestream2_get_bytes_left(gb) < nb_vectors * 12)
- return AVERROR_INVALIDDATA;
- bytestream2_skip(gb, nb_vectors * 12);
- if (nb_vectors > 256) {
- if (bytestream2_get_bytes_left(gb) < (nb_blocks + 7 * !intra_flag) / 8)
- return AVERROR_INVALIDDATA;
- bytestream2_init(&idx9, gb->buffer_start + bytestream2_tell(gb), (nb_blocks + 7 * !intra_flag) / 8);
- bytestream2_skip(gb, (nb_blocks + 7 * !intra_flag) / 8);
- }
-
- skip = s->skip;
-
- for (int y = avctx->height - 2; y >= 0; y -= 2) {
- uint8_t *dsty = frame->data[0] + y * frame->linesize[0];
- uint8_t *dstu = frame->data[1] + y * frame->linesize[1];
- uint8_t *dstv = frame->data[2] + y * frame->linesize[2];
-
- for (int x = 0; x < avctx->width; x += 2) {
- int idx;
-
- if (!intra_flag && *skip++)
- continue;
- if (bytestream2_get_bytes_left(gb) <= 0)
- return AVERROR_INVALIDDATA;
- if (nb_vectors <= 256) {
- idx = bytestream2_get_byte(gb);
- } else {
- if (idx9bits == 0) {
- idx9val = bytestream2_get_byte(&idx9);
- idx9bits = 8;
- }
- idx9bits--;
- idx = bytestream2_get_byte(gb) | (((idx9val >> (7 - idx9bits)) & 1) << 8);
- }
- if (idx >= nb_vectors)
- return AVERROR_INVALIDDATA;
-
- dsty[x +frame->linesize[0]] = vec[idx * 12 + 0];
- dsty[x+1+frame->linesize[0]] = vec[idx * 12 + 3];
- dsty[x] = vec[idx * 12 + 6];
- dsty[x+1] = vec[idx * 12 + 9];
-
- dstu[x +frame->linesize[1]] = vec[idx * 12 + 1];
- dstu[x+1+frame->linesize[1]] = vec[idx * 12 + 4];
- dstu[x] = vec[idx * 12 + 7];
- dstu[x+1] = vec[idx * 12 +10];
-
- dstv[x +frame->linesize[2]] = vec[idx * 12 + 2];
- dstv[x+1+frame->linesize[2]] = vec[idx * 12 + 5];
- dstv[x] = vec[idx * 12 + 8];
- dstv[x+1] = vec[idx * 12 +11];
- }
- }
-
- return intra_flag;
-}
-
-static ptrdiff_t lzss_uncompress(MidiVidContext *s, GetByteContext *gb, uint8_t *dst, unsigned int size)
-{
- uint8_t *dst_start = dst;
- uint8_t *dst_end = dst + size;
-
- for (;bytestream2_get_bytes_left(gb) >= 3;) {
- int op = bytestream2_get_le16(gb);
-
- for (int i = 0; i < 16; i++) {
- if (op & 1) {
- int s0 = bytestream2_get_byte(gb);
- int s1 = bytestream2_get_byte(gb);
- int offset = ((s0 & 0xF0) << 4) | s1;
- int length = (s0 & 0xF) + 3;
-
- if (dst + length > dst_end ||
- dst - offset < dst_start)
- return AVERROR_INVALIDDATA;
- if (offset > 0) {
- for (int j = 0; j < length; j++) {
- dst[j] = dst[j - offset];
- }
- }
- dst += length;
- } else {
- if (dst >= dst_end)
- return AVERROR_INVALIDDATA;
- *dst++ = bytestream2_get_byte(gb);
- }
- op >>= 1;
- }
- }
-
- return dst - dst_start;
-}
-
-static int decode_frame(AVCodecContext *avctx, AVFrame *rframe,
- int *got_frame, AVPacket *avpkt)
-{
- MidiVidContext *s = avctx->priv_data;
- GetByteContext *gb = &s->gb;
- AVFrame *frame = s->frame;
- int ret, key, uncompressed;
-
- if (avpkt->size <= 13)
- return AVERROR_INVALIDDATA;
-
- bytestream2_init(gb, avpkt->data, avpkt->size);
- bytestream2_skip(gb, 8);
- uncompressed = bytestream2_get_le32(gb);
-
- if (!uncompressed) {
- av_fast_padded_malloc(&s->uncompressed, &s->uncompressed_size, 16LL * (avpkt->size - 12));
- if (!s->uncompressed)
- return AVERROR(ENOMEM);
-
- ret = lzss_uncompress(s, gb, s->uncompressed, s->uncompressed_size);
- if (ret < 0)
- return ret;
- bytestream2_init(gb, s->uncompressed, ret);
- }
-
- if ((ret = ff_reget_buffer(avctx, s->frame, 0)) < 0)
- return ret;
-
- ret = decode_mvdv(s, avctx, frame);
-
- if (ret < 0)
- return ret;
- key = ret;
-
- if ((ret = av_frame_ref(rframe, s->frame)) < 0)
- return ret;
-
- frame->pict_type = key ? AV_PICTURE_TYPE_I : AV_PICTURE_TYPE_P;
- frame->key_frame = key;
- *got_frame = 1;
-
- return avpkt->size;
-}
-
-static av_cold int decode_init(AVCodecContext *avctx)
-{
- MidiVidContext *s = avctx->priv_data;
- int ret = av_image_check_size(avctx->width, avctx->height, 0, avctx);
-
- if (avctx->width & 3 || avctx->height & 3)
- ret = AVERROR_INVALIDDATA;
-
- if (ret < 0) {
- av_log(avctx, AV_LOG_ERROR, "Invalid image size %dx%d.\n",
- avctx->width, avctx->height);
- return ret;
- }
-
- avctx->pix_fmt = AV_PIX_FMT_YUV444P;
-
- s->frame = av_frame_alloc();
- if (!s->frame)
- return AVERROR(ENOMEM);
- s->skip = av_calloc(avctx->width >> 1, avctx->height >> 1);
- if (!s->skip)
- return AVERROR(ENOMEM);
-
- return 0;
-}
-
-static void decode_flush(AVCodecContext *avctx)
-{
- MidiVidContext *s = avctx->priv_data;
-
- av_frame_unref(s->frame);
-}
-
-static av_cold int decode_close(AVCodecContext *avctx)
-{
- MidiVidContext *s = avctx->priv_data;
-
- av_frame_free(&s->frame);
- av_freep(&s->uncompressed);
- av_freep(&s->skip);
-
- return 0;
-}
-
-const FFCodec ff_mvdv_decoder = {
- .p.name = "mvdv",
- CODEC_LONG_NAME("MidiVid VQ"),
- .p.type = AVMEDIA_TYPE_VIDEO,
- .p.id = AV_CODEC_ID_MVDV,
- .priv_data_size = sizeof(MidiVidContext),
- .init = decode_init,
- FF_CODEC_DECODE_CB(decode_frame),
- .flush = decode_flush,
- .close = decode_close,
- .p.capabilities = AV_CODEC_CAP_DR1,
- .caps_internal = FF_CODEC_CAP_INIT_CLEANUP,
-};
diff --git a/spaces/congsaPfin/Manga-OCR/logs/Enjoy Battlegrounds Mobile India on Android 12 APK and OBB Download Guide.md b/spaces/congsaPfin/Manga-OCR/logs/Enjoy Battlegrounds Mobile India on Android 12 APK and OBB Download Guide.md
deleted file mode 100644
index 0c6be47e0abe9f2a5245bb5dbe4caeea50cc5b64..0000000000000000000000000000000000000000
--- a/spaces/congsaPfin/Manga-OCR/logs/Enjoy Battlegrounds Mobile India on Android 12 APK and OBB Download Guide.md
+++ /dev/null
@@ -1,160 +0,0 @@
-
-
BGMI APK Download Android 12: How to Install and Play the Latest Version of Battlegrounds Mobile India
-
If you are a fan of battle royale games, you must have heard of BGMI, or Battlegrounds Mobile India. It is one of the most popular and exciting mobile games in India, with millions of players enjoying it every day. But what if you want to play it on your Android 12 device? How can you download and install the latest version of BGMI APK on your phone or tablet? In this article, we will answer all these questions and more. We will tell you what BGMI is, why you should download it, how to download it, how to install it, and how to play it on your Android 12 device. So, without further ado, let's get started!
BGMI stands for Battlegrounds Mobile India, and it is a mobile game developed by KRAFTON, Inc., a South Korean company. It is a battle royale game, which means that you have to survive against other players in a shrinking map until you are the last one standing. You can play solo, duo, or squad mode, with up to 100 players in each match. You can also choose from different maps, modes, weapons, vehicles, skins, and more.
-
BGMI is not just a game, but also a social platform where you can interact with other players, join clans, participate in events, watch live streams, and more. You can also customize your character, profile, inventory, and settings according to your preferences. BGMI is free to play, but you can also buy in-game currency and items with real money if you want.
-
The difference between BGMI and PUBG Mobile
-
You might be wondering what is the difference between BGMI and PUBG Mobile, another popular battle royale game. Well, the main difference is that BGMI is made exclusively for Indian players, while PUBG Mobile is available globally. This means that BGMI has some features that are tailored for the Indian audience, such as:
-
-
Green blood instead of red blood
-
A disclaimer that the game is not based on real events or characters
-
A limit on how long you can play the game per day
-
A feature that reminds you to take breaks and drink water
-
A feature that allows you to report any inappropriate content or behavior
-
Exclusive events, rewards, tournaments, and collaborations for Indian players
-
-
However, apart from these differences, BGMI and PUBG Mobile are very similar in terms of gameplay, graphics, sound effects, and updates. In fact, BGMI is based on PUBG Mobile's global version, so you can expect the same quality and experience from both games.
-
Why download BGMI APK?
-
The benefits of downloading the APK file instead of using the Google Play Store
-
One way to download and install BGMI on your Android device is to use the Google Play Store. However, this method has some drawbacks, such as:
-
bgmi apk download android 12 beta version
-how to install bgmi apk on android 12 devices
-bgmi apk download android 12 free link
-bgmi apk download android 12 latest update
-bgmi apk download android 12 without obb file
-bgmi apk download android 12 compatible phones
-bgmi apk download android 12 error fix
-bgmi apk download android 12 gameplay review
-bgmi apk download android 12 new features
-bgmi apk download android 12 size and requirements
-bgmi apk download android 12 official website
-bgmi apk download android 12 tips and tricks
-bgmi apk download android 12 mod menu
-bgmi apk download android 12 hack version
-bgmi apk download android 12 unlimited uc
-bgmi apk download android 12 offline mode
-bgmi apk download android 12 graphics settings
-bgmi apk download android 12 best sensitivity
-bgmi apk download android 12 custom room
-bgmi apk download android 12 clan system
-bgmi apk download android 12 esports scene
-bgmi apk download android 12 rewards and events
-bgmi apk download android 12 nusa map guide
-bgmi apk download android 12 vice packs explained
-bgmi apk download android 12 new weapon mg3
-bgmi apk download android 12 comparison with pubg mobile
-bgmi apk download android 12 privacy policy and terms of service
-bgmi apk download android 12 support and feedback
-bgmi apk download android 12 ratings and reviews
-bgmi apk download android 12 alternatives and competitors
-
-
You need a stable internet connection to download the game from the Play Store
-
You need enough storage space on your device to download the game from the Play Store
You may encounter errors or bugs while downloading or installing the game from the Play Store
-
You may not be able to access the latest version of the game from the Play Store
-
-
That's why downloading the APK file of BGMI is a better option. APK stands for Android Package Kit, and it is a file format that contains all the necessary data and code to run an app on your Android device. By downloading the APK file of BGMI, you can enjoy some benefits, such as:
-
-
You can download the game from any source you trust, such as a website, a cloud service, or a friend
-
You can download the game faster and use less data than downloading from the Play Store
-
You can save the APK file on your device or external storage and install it anytime you want
-
You can bypass any restrictions or errors that may occur on the Play Store
-
You can access the latest version of the game before it is available on the Play Store
-
-
The compatibility issues with Android 12 and how to fix them
-
However, downloading the APK file of BGMI is not without its challenges. One of the main issues that you may face is the compatibility with Android 12, the latest version of the Android operating system. Android 12 has some new features and changes that may affect how BGMI runs on your device, such as:
-
-
A new permission system that requires you to grant access to specific files and folders on your device
-
A new app hibernation feature that automatically optimizes the storage and battery usage of apps that you don't use frequently
-
A new privacy dashboard that shows you how apps access your location, camera, microphone, and other sensitive data
-
A new performance class feature that categorizes devices based on their capabilities and performance levels
-
-
These features may cause some problems when you try to download, install, or play BGMI on your Android 12 device, such as:
-
-
You may not be able to install the APK file if it is not signed by a trusted developer or source
-
You may not be able to access or write to the OBB file, which contains the game data, if you don't grant permission to the app
-
You may experience lag, crashes, or glitches if your device does not meet the performance class requirements for BGMI
-
You may lose your game progress or settings if your app goes into hibernation mode and deletes some data
-
You may compromise your privacy or security if you allow BGMI to access your sensitive data without your knowledge or consent
-
-
Fortunately, there are some ways to fix these issues and enjoy BGMI on your Android 12 device without any hassle. Here are some tips that you can follow:
-
-
Download the APK file from a trusted source, such as the official website of BGMI or a reputable third-party website. You can also scan the APK file with an antivirus app before installing it.
-
Enable the "Unknown sources" option in your device settings to allow installation of apps from sources other than the Play Store. You can also disable the "Verify apps" option to prevent Google from scanning and blocking apps that are not from the Play Store.
-
Grant permission to BGMI to access and write to your device storage when prompted. You can also manually change the permission settings in your device settings. You can also create a folder named "Android/obb/com.pubg.imobile" in your device storage and copy the OBB file there.
-
Check if your device meets the minimum requirements for BGMI, which are: 2 GB RAM, Android 5.1.1 or above, and at least 4 GB of free storage space. You can also check if your device belongs to one of the performance classes supported by BGMI, which are: Performance Class 1 (high-end devices), Performance Class 2 (mid-range devices), and Performance Class 3 (low-end devices).
-
Optimize your device performance by closing other apps, clearing cache and junk files, updating your software and drivers, and using a game booster app. You can also adjust your game settings to lower graphics quality, frame rate, sound effects, and other options.
-
Prevent your app from going into hibernation mode by using it frequently, adding it to your favorites list, or disabling the app hibernation feature in your device settings. You can also backup your game data and settings using a cloud service or an external storage.
-
Protect your privacy and security by reviewing and limiting how BGMI accesses your location, camera, microphone, and other sensitive data. You can also use a VPN app to hide your IP address and location from other players and servers. You can also avoid clicking on any suspicious links or ads that may appear in the game or on the website.
-
-
How to download and install BGMI APK on Android 12?
-
The steps to download the APK and OBB files from a trusted source
-
Now that you know why and how to download BGMI APK on your Android 12 device, let's see the actual steps to do it. The first thing you need to do is to download the APK and OBB files of BGMI from a trusted source. Here are the steps to follow:
On the website, look for the download link or button for BGMI APK and OBB files. The APK file should have a name like "com.pubg.imobile.apk" and the OBB file should have a name like "main.15255.com.pubg.imobile.obb". The file size of the APK file should be around 70 MB and the file size of the OBB file should be around 700 MB.
-
Click on the download link or button and wait for the files to be downloaded on your device. You may need to allow your browser to download files from unknown sources if prompted. You may also see some ads or pop-ups on the website, so be careful not to click on them.
-
Once the files are downloaded, you can find them in your device's download folder or in the location that you specified. You can also check the notification bar or the download manager app to see the progress and status of your downloads.
-
-
The steps to install the APK and OBB files on your device
-
The next thing you need to do is to install the APK and OBB files of BGMI on your device. Here are the steps to follow:
-
-
Before installing the files, make sure that you have enough storage space on your device or external storage. You also need to enable the "Unknown sources" option in your device settings to allow installation of apps from sources other than the Play Store.
-
Locate the APK file that you downloaded and tap on it. You may see a warning message that says "This type of file can harm your device". Ignore it and tap on "Install" or "OK". You may also need to grant permission to BGMI to access your device storage, location, camera, microphone, and other data.
-
Wait for the installation process to complete. It may take a few minutes depending on your device speed and performance. Do not exit or interrupt the installation process until it is done.
-
After installing the APK file, do not launch the game yet. You need to copy or move the OBB file that you downloaded to a specific folder on your device storage. The folder should be named "Android/obb/com.pubg.imobile". If you don't have this folder, you can create it manually.
-
Locate the OBB file that you downloaded and tap on it. You may see a menu that gives you options to copy, move, rename, or delete the file. Choose "Copy" or "Move" and select the destination folder as "Android/obb/com.pubg.imobile". Wait for the copying or moving process to complete.
-
-
The steps to launch and update the game
-
The final thing you need to do is to launch and update the game. Here are the steps to follow:
-
-
Go to your device's app drawer or home screen and look for the BGMI icon. Tap on it to launch the game. You may see a splash screen that shows the game logo and some information.
-
The game will check for updates and download them if available. You need a stable internet connection for this process. The updates may include new features, bug fixes, security patches, and more. The update size may vary depending on your game version and device model.
-
After updating, you will see a login screen that asks you to choose a login method. You can use Facebook, Twitter, Google Play Games, or Guest as your login method. If you have an existing account from PUBG Mobile, you can use the same login method to access your account on BGMI. If you are a new player, you can create a new account with any login method.
-
After logging in, you will see a welcome screen that shows some tips and instructions on how to play the game. You can also watch a video tutorial that explains the basic gameplay and controls. You can skip this screen if you want.
-
Next, you will see a character creation screen that allows you to customize your character's appearance, name, gender, and voice. You can also choose a server to play on, such as India, Asia, Europe, or North America. You can change these settings later if you want.
-
Finally, you will see the main menu screen that gives you access to various options and features of the game. You can start playing the game by tapping on the "Start" button and choosing a mode and a map. You can also join or create a team with other players by tapping on the "Team" button. You can also explore other options such as inventory, shop, events, missions, settings, and more by tapping on the icons on the bottom of the screen.
-
-
How to play BGMI on Android 12?
-
The basic gameplay tips and tricks for beginners
-
Now that you have downloaded, installed, and launched BGMI on your Android 12 device, you are ready to play and have fun. But how do you play BGMI effectively and enjoyably? Here are some basic gameplay tips and tricks for beginners:
-
-
The goal of the game is to survive until you are the last one standing. You can do this by finding weapons, armor, items, vehicles, and other resources on the map. You can also kill or avoid other players who are trying to do the same.
-
The game starts with you parachuting from an airplane onto a map of your choice. You can choose where to land by looking at the map and tapping on the screen. You can also follow or invite other players to land with you by using the markers and voice chat.
-
After landing, you need to loot as fast as possible. Look for buildings, crates, vehicles, and other places that may contain loot. Loot includes guns, ammo, attachments, grenades, medkits, bandages, energy drinks, painkillers, helmets, vests, backpacks, scopes, and more. Loot varies in rarity and quality, so look for the best ones you can find.
-
You also need to be aware of the safe zone and the blue zone. The safe zone is a white circle on the map that indicates where you need to be to avoid taking damage. The blue zone is a blue circle that shrinks over time and damages anyone who is outside of it. The safe zone and the blue zone force players to move closer to each other and create more action and tension.
-
You also need to be aware of the red zone and the air drop. The red zone is a red circle on the map that indicates where bombs will be dropped randomly. The air drop is a plane that flies over the map and drops a crate that contains rare and powerful loot. Both the red zone and the air drop create more risk and reward for players who want to challenge themselves.
-
You also need to be aware of your health and inventory. Your health is shown by a green bar on the top left corner of the screen. You can heal yourself by using medkits, bandages, energy drinks, painkillers, or other items. Your inventory is shown by an icon on the bottom right corner of the screen. You can access your inventory by tapping on it and manage your items by dragging them, dropping them, or using them. You can also switch between your weapons, attachments, and grenades by tapping on them.
-
-
The advanced gameplay strategies and tactics for experts
-
If you are already familiar with the basic gameplay of BGMI, you may want to learn some advanced gameplay strategies and tactics to improve your skills and performance. Here are some tips for experts:
-
-
Choose your landing spot wisely. Depending on your play style and preference, you may want to land in a hot spot, a cold spot, or somewhere in between. A hot spot is a place where many players land and fight for loot, such as military base, school, or pochinki. A cold spot is a place where few players land and loot peacefully, such as farm, shelter, or primorsk. A hot spot offers more action and loot, but also more risk and competition. A cold spot offers more safety and time, but also less loot and excitement.
-
Use the right weapon for the right situation. Depending on the range, terrain, and enemy, you may want to use different weapons to maximize your damage and accuracy. For example, you may want to use a sniper rifle for long-range shots, an assault rifle for medium-range shots, a submachine gun for close-range shots, a shotgun for very close-range shots, or a pistol for backup shots. You may also want to use different attachments to enhance your weapons, such as suppressors, compensators, flash hiders, extended mags, quickdraw mags, scopes, red dots, holographic sights, or lasers.
-
Use the right vehicle for the right situation. Depending on the map, zone, and enemy, you may want to use different vehicles to move faster and safer. For example, you may want to use a car for road trips, a bike for off-road trips, a boat for water trips, or a glider for air trips. You may also want to use different vehicles to attack or escape from enemies, such as ramming them with a car, shooting them from a bike, throwing grenades from a boat, or dropping bombs from a glider.
-
Use the right strategy for the right situation. Depending on your goal, position, and enemy, you may want to use different strategies to survive and win. For example, you may want to use an aggressive strategy if you want to kill more enemies and get more loot, a passive strategy if you want to avoid more enemies and save more resources, or a balanced strategy if you want to mix both. You may also want to use different tactics to gain an advantage over your enemies, such as flanking them, ambushing them, sniping them, rushing them, or baiting them.
-
-
The best settings and controls for optimal performance
-
Another way to improve your gameplay and experience on BGMI is to adjust your settings and controls according to your device and preference. Here are some tips to optimize your settings and controls:
-
-
Adjust your graphics settings to match your device's capabilities and performance. You can choose from smooth, balanced, HD, HDR, or ultra HD graphics quality, and from low, medium, high, ultra, or extreme frame rate. You can also enable or disable anti-aliasing, shadows, brightness, and auto-adjust graphics. The higher the graphics quality and frame rate, the better the visuals and smoothness, but also the more battery and data consumption.
-
Adjust your sound settings to enhance your hearing and communication. You can choose from low, medium, or high sound quality, and from low or high sound effects. You can also enable or disable voice chat, microphone, speaker, team voice chat channel, and quick chat. The higher the sound quality and effects, the better the audio and immersion, but also the more battery and data consumption.
-
Adjust your control settings to suit your play style and preference. You can choose from three preset control layouts: thumb (two fingers), claw (four fingers), or custom (any number of fingers). You can also customize the size, position, opacity, and function of each button on the screen. You can also enable or disable gyroscope, peek and fire, peek and open scope, block sight warning, bolt action rifle and crossbow firing mode, shotgun firing mode, and auto-open doors.
-
-
Conclusion
-
BGMI is a thrilling and addictive game that you can play on your Android 12 device with ease. All you need to do is to download the APK and OBB files of BGMI from a trusted source, install them on your device with the right permissions and settings, launch and update the game with a stable internet connection, and enjoy the game with the best graphics, sound, and control settings. You can also learn some tips and tricks to play the game better and smarter. BGMI is not only a game, but also a social platform where you can meet and interact with other players, join clans, participate in events, watch live streams, and more. BGMI is a game that you can enjoy for hours and hours without getting bored or tired. So, what are you waiting for? Download BGMI APK on your Android 12 device today and join the battlegrounds!
FAQs
-
Here are some frequently asked questions and answers about BGMI APK download on Android 12:
-
Q: Is BGMI APK safe to download and install?
-
A: Yes, BGMI APK is safe to download and install as long as you get it from a trusted source, such as the official website of BGMI or a reputable third-party website. You should also scan the APK file with an antivirus app before installing it. However, you should be careful not to download any fake or malicious APK files that may harm your device or steal your data.
-
Q: Is BGMI APK legal to download and install?
-
A: Yes, BGMI APK is legal to download and install as long as you do not violate any terms and conditions of the game or the developer. You should also respect the intellectual property rights of the game and the developer and not use any hacks, mods, cheats, or pirated versions of the game.
-
Q: Is BGMI APK compatible with Android 12?
-
A: Yes, BGMI APK is compatible with Android 12 as long as you follow the steps and tips mentioned in this article. You may need to enable some permissions and settings on your device to install and run the game smoothly. You may also need to update the game regularly to access the latest features and fixes.
-
Q: How can I update BGMI APK on Android 12?
-
A: You can update BGMI APK on Android 12 by launching the game and downloading the updates from the game itself. You may need a stable internet connection for this process. Alternatively, you can also download the latest version of BGMI APK from a trusted source and install it over the existing version on your device.
-
Q: How can I uninstall BGMI APK on Android 12?
-
A: You can uninstall BGMI APK on Android 12 by going to your device settings and tapping on "Apps" or "Applications". Then, look for BGMI and tap on it. Then, tap on "Uninstall" or "Remove" and confirm your action. You may also need to delete the OBB file and other data related to the game from your device storage.
401be4b1e0
-
-
\ No newline at end of file
diff --git a/spaces/congsaPfin/Manga-OCR/logs/Liberty General Insurance Get Instant Quotes and Policy Download.md b/spaces/congsaPfin/Manga-OCR/logs/Liberty General Insurance Get Instant Quotes and Policy Download.md
deleted file mode 100644
index 54955a81d83dc0ff337839f2863117c994f0d390..0000000000000000000000000000000000000000
--- a/spaces/congsaPfin/Manga-OCR/logs/Liberty General Insurance Get Instant Quotes and Policy Download.md
+++ /dev/null
@@ -1,107 +0,0 @@
-
-
How to Download Liberty General Insurance
-
Liberty General Insurance is one of the most trusted general insurance companies in India that offers a wide range of insurance products for your car, two-wheeler, health, travel, property, and business. Whether you want to protect yourself from unforeseen risks, liabilities, or expenses, Liberty General Insurance has a solution for you.
But how do you download your Liberty General Insurance policy online? In this article, we will guide you through the simple steps to download your policy document from the comfort of your home or office. But first, let us look at some of the benefits of choosing Liberty General Insurance.
-
Benefits of Liberty General Insurance
-
Liberty General Insurance has been providing quality insurance services to its customers since 2013. It is a joint venture among US property casualty insurer Liberty Mutual Insurance Group, Indian private investment fund Enam Securities, and Indian industrial conglomerate DP Jindal Group.
-
Some of the benefits of Liberty General Insurance are:
-
-
Comprehensive coverage: Liberty General Insurance covers various risks and liabilities that you may face in your personal or professional life. Whether it is damage to your vehicle, medical expenses, loss of baggage, fire or theft at your property, or legal liability to third parties, Liberty General Insurance has a plan for you.
-
Cashless claim service: Liberty General Insurance has a network of over 5,800 partner hospitals and over 3,100 partner garages across India where you can avail cashless claim service. This means that you don't have to pay anything upfront for your treatment or repair. Liberty General Insurance will settle the bill directly with the service provider.
-
Affordable premiums and discounts: Liberty General Insurance offers competitive premiums and discounts for its customers. You can save money by choosing a higher voluntary deductible, installing anti-theft devices in your vehicle, opting for a long-term policy, or renewing your policy online. You can also get discounts for being a member of certain associations or clubs.
-
Online policy renewal and purchase: Liberty General Insurance allows you to renew or buy your policy online in a few clicks. You can also download your policy document online without any hassle. You can pay online using various modes such as credit card, debit card, net banking, UPI, or wallets.
-
-
Types of Liberty General Insurance Products
-
Liberty General Insurance offers a variety of insurance products to suit your needs. Here are some of the main types of products that you can choose from:
-
download liberty general insurance app
-download liberty general insurance policy document
-download liberty general insurance claim form
-download liberty general insurance health card
-download liberty general insurance brochure
-download liberty general insurance car insurance
-download liberty general insurance two wheeler insurance
-download liberty general insurance health insurance
-download liberty general insurance travel insurance
-download liberty general insurance home insurance
-download liberty general insurance commercial vehicle insurance
-download liberty general insurance fire and engineering insurance
-download liberty general insurance marine cargo insurance
-download liberty general insurance liability insurance
-download liberty general insurance group personal accident insurance
-download liberty general insurance group health connect policy
-download liberty general insurance health connect supra policy
-download liberty general insurance secure health connect policy
-download liberty general insurance individual personal accident policy
-download liberty general insurance individual health connect policy
-download liberty general insurance individual health connect supra policy
-download liberty general insurance individual secure health connect policy
-download liberty general insurance student travel care policy
-download liberty general insurance senior citizen travel care policy
-download liberty general insurance frequent traveller care policy
-download liberty general insurance asia travel care policy
-download liberty general insurance worldwide travel care policy
-download liberty general insurance schengen travel care policy
-download liberty general insurance home protect policy
-download liberty general insurance shop package policy
-download liberty general insurance office package policy
-download liberty general insurance hotel package policy
-download liberty general insurance industrial all risk policy
-download liberty general insurance machinery breakdown policy
-download liberty general insurance boiler and pressure plant policy
-download liberty general insurance electronic equipment policy
-download liberty general insurance contractor's all risk policy
-download liberty general insurance erection all risk policy
-download liberty general insurance contractor's plant and machinery policy
-download liberty general insurance marine hull and machinery policy
-download liberty general insurance public liability non industrial policy
-download liberty general insurance public liability industrial policy
-download liberty general insurance product liability policy
-download liberty general insurance directors and officers liability policy
-download liberty general insurance professional indemnity policy for doctors and medical practitioners
-download liberty general insurance professional indemnity policy for architects and engineers
-
Motor insurance
-
Motor insurance covers your car, two-wheeler, or commercial vehicle against damage or loss due to accidents, fire, theft, natural calamities, or malicious acts. It also covers your legal liability to third parties for bodily injury or property damage.
Liberty General Insurance offers two types of motor insurance policies: third party liability only and comprehensive. The third party liability only policy covers your legal liability to third parties for bodily injury or property damage. The comprehensive policy covers both third party liability and own damage to your vehicle. You can also opt for various add-on covers such as zero depreciation, roadside assistance, engine protect, return to invoice, and more.
-
Health insurance
-
Health insurance covers your medical expenses in case of hospitalization due to illness or injury. It also covers pre and post hospitalization expenses, day care procedures, domiciliary treatment, ambulance charges, and more. Liberty General Insurance offers individual, family, and group health insurance plans with various features and benefits. You can also opt for critical illness cover, personal accident cover, or top-up cover to enhance your protection.
-
Travel insurance
-
Travel insurance covers your travel risks such as loss of baggage, passport, or tickets, flight delay or cancellation, medical emergency, personal liability, or legal expenses. Liberty General Insurance offers domestic and international travel insurance plans for individuals, families, students, and senior citizens. You can choose from single trip or multi-trip plans with various coverage options and durations.
-
Property insurance
-
Property insurance covers your home, office, or shop against fire, burglary, earthquake, flood, storm, or other perils. It also covers your contents such as furniture, appliances, electronics, jewelry, or cash. Liberty General Insurance offers property insurance plans for individuals and businesses with various sum insured options and extensions.
-
Commercial and industrial insurance
-
Commercial and industrial insurance covers your business assets, liabilities, and employees against various risks such as fire, theft, machinery breakdown, business interruption, public liability, employer's liability, workmen's compensation, marine cargo, or engineering projects. Liberty General Insurance offers customized solutions for different industries such as manufacturing, construction, hospitality, education, IT, or retail.
-
How to Download Liberty General Insurance Policy Online
-
If you have bought or renewed your Liberty General Insurance policy online, you can easily download your policy document from the official website of the company. Here are the steps to follow:
-
Step 1: Visit the official website of Liberty General Insurance
-
Go to https://www.libertyinsurance.in/ and click on the "Customer Support" tab on the top right corner of the homepage. Then click on the "Download Policy" option from the drop-down menu.
-
Step 2: Choose the type of insurance product you want to download
-
You will see a list of insurance products such as motor insurance, health insurance, travel insurance, property insurance, and commercial and industrial insurance. Click on the product that you have purchased or renewed online.
-
Step 3: Enter your policy number and other details
-
You will be redirected to a page where you have to enter your policy number and other details such as email address or mobile number. Enter the required information and click on the "Submit" button.
-
Step 4: Verify your identity and download your policy document
-
You will receive an OTP (one-time password) on your registered email address or mobile number. Enter the OTP and click on the "Verify" button. You will see your policy details on the screen. Click on the "Download" button to download your policy document in PDF format.
-
How to Contact Liberty General Insurance Customer Care
-
If you have any queries or complaints regarding your Liberty General Insurance policy or claim, you can contact the customer care team of the company through various channels. Here are some of the ways to reach out to them:
-
Toll-free number
-
You can call the toll-free number 1800-266-5844 from Monday to Saturday between 8 am to 8 pm. You can also request a callback by filling a form on the website.
-
Email address
-
You can send an email to care@libertyinsurance.in with your query or complaint. You can also attach any relevant documents or screenshots if required.
-
Branch locator
-
You can visit the nearest branch of Liberty General Insurance by using the branch locator tool on the website. You can search by state, city, or pin code and get the address, phone number, and email id of the branch.
-
Conclusion
-
Liberty General Insurance is a reliable and reputed general insurance company that offers a range of products for your personal and professional needs. You can buy or renew your policy online and download it in a few minutes from the comfort of your home or office. You can also contact the customer care team of the company for any assistance or feedback. Liberty General Insurance is committed to providing you with the best service and satisfaction.
-
We hope this article has helped you understand how to download Liberty General Insurance policy online. If you have any questions, feel free to ask us in the comments section below. We would love to hear from you.
-
FAQs
-
Here are some of the frequently asked questions about Liberty General Insurance:
-
Q: How can I check the status of my Liberty General Insurance claim?
-
A: You can check the status of your claim online by visiting the website and clicking on the "Track Claim" option under the "Customer Support" tab. You can also call the toll-free number 1800-266-5844 or email to care@libertyinsurance.in with your claim number and policy number.
-
Q: How can I cancel my Liberty General Insurance policy?
-
A: You can cancel your policy within 15 days of receiving the policy document by sending a written request to the nearest branch or email to care@libertyinsurance.in. You will get a refund of the premium after deducting the proportionate risk premium and stamp duty charges.
-
Q: How can I change my personal details in my Liberty General Insurance policy?
-
A: You can change your personal details such as name, address, phone number, email id, or nominee by visiting the nearest branch or email to care@libertyinsurance.in with your policy number and proof of identity.
-
Q: How can I renew my Liberty General Insurance policy online?
-
A: You can renew your policy online by visiting the website and clicking on the "Renew Policy" option under the "Customer Support" tab. You can enter your policy number and other details and pay online using various modes such as credit card, debit card, net banking, UPI, or wallets.
-
Q: How can I get a duplicate copy of my Liberty General Insurance policy document?
-
A: You can get a duplicate copy of your policy document by visiting the nearest branch or email to care@libertyinsurance.in with your policy number and proof of identity. You can also download your policy document online by following the steps mentioned above.
401be4b1e0
-
-
\ No newline at end of file
diff --git a/spaces/congsaPfin/Manga-OCR/logs/Macromedia Flash 8 Download the Latest Version and Start Creating Today.md b/spaces/congsaPfin/Manga-OCR/logs/Macromedia Flash 8 Download the Latest Version and Start Creating Today.md
deleted file mode 100644
index 97aa7a0f9e3a7b54ddf4d607c5be7ae08bbd0eaf..0000000000000000000000000000000000000000
--- a/spaces/congsaPfin/Manga-OCR/logs/Macromedia Flash 8 Download the Latest Version and Start Creating Today.md
+++ /dev/null
@@ -1,117 +0,0 @@
-
-
How to Download Macromedia Flash 8 in 2023
-
If you are looking for a powerful software to create rich interactive content, you might be interested in Macromedia Flash 8. This is a professional web design tool that was released in 2005 by Macromedia, before it was acquired by Adobe. Macromedia Flash 8 allows you to create stunning graphics, animations, videos, audio, and interactive applications that can run on various platforms and devices. In this article, we will show you how to download Macromedia Flash 8 in 2023, what features it offers, and what alternatives you have.
Macromedia Flash 8 is a versatile software that offers many features for creating engaging web content. Some of the features are:
-
-
Graphic effects: You can apply filters, blend modes, gradients, masks, and transformations to your graphics to enhance their appearance and realism.
-
Animation: You can use motion tweens, shape tweens, guides, onion skinning, and frame-by-frame animation to create smooth and dynamic animations.
-
Video: You can import, edit, encode, and stream video files in various formats, such as FLV, MPEG, AVI, and MOV. You can also add cue points, subtitles, captions, and interactivity to your videos.
-
Audio: You can import, edit, synchronize, and stream audio files in various formats, such as MP3, WAV, and AIFF. You can also add sound effects, volume control, panning, and interactivity to your audio.
-
Interactivity: You can use ActionScript 2.0, a scripting language based on ECMAScript (similar to JavaScript), to add logic, control, data manipulation, and communication to your content. You can also use components, such as buttons, menus, sliders, and text fields, to create user interfaces.
-
FlashType font-rendering engine: This is a new feature in Macromedia Flash 8 that improves the quality and readability of text. It supports anti-aliasing, kerning, ligatures, and advanced character sets.
-
Custom easing tool: This is another new feature in Macromedia Flash 8 that allows you to create custom easing curves for your animations. You can adjust the speed and acceleration of your motion tweens with precision and ease.
-
Flash Player 8 and Flash Lite 2 compatibility: Macromedia Flash 8 is compatible with the latest versions of Flash Player and Flash Lite at the time of its release. Flash Player 8 is a web browser plug-in that enables users to view Flash content on desktops and laptops. Flash Lite 2 is a mobile runtime environment that enables users to view Flash content on smartphones and tablets.
-
-
How to download Macromedia Flash 8
-
If you want to download Macromedia Flash 8 in 2023, you need to consider some requirements and precautions first. Here are some tips:
-
-
Requirements: To run Macromedia Flash 8 on your computer, you need to have Windows XP or later or Mac OS X v10.3 or later. You also need to have at least a Pentium III or equivalent processor (Windows) or a PowerPC G4 or G5 processor (Mac), at least 256 MB of RAM (512 MB recommended), at least 500 MB of available disk space (1 GB recommended), a CD-ROM drive, a 1024 x 768 monitor resolution, and a 16-bit video card.
-
Precautions: Macromedia Flash 8 is an old software that is no longer supported or updated by Adobe. It may not work properly on newer operating systems or browsers. It may also have security vulnerabilities or compatibility issues with other software. You should use it at your own risk and discretion.
-
-
If you still want to download Macromedia Flash 8, you can follow these steps:
Sources and links: There are several sources where you can download Macromedia Flash 8, such as oldversion.com, softonic.com, filehippo.com, and cnet.com. However, these sources are not official or authorized by Adobe, and they may contain malware or viruses. You should scan the downloaded files with an antivirus software before opening them. Alternatively, you can try to find a legitimate copy of Macromedia Flash 8 on eBay or Amazon, but they may be expensive or rare.
-
Installation and activation: Once you have downloaded the Macromedia Flash 8 installer file, you can run it and follow the instructions on the screen. You will need to enter a serial number to activate the software. You can find some serial numbers online, but they may not work or be illegal. You can also try to use a keygen or a crack program to generate a serial number, but they may also contain malware or viruses. You should use them at your own risk and discretion.
-
-
Alternatives to Macromedia Flash 8
-
Macromedia Flash 8 is not the only software that can create and view Flash content. There are some alternatives that you can consider in 2023. Here are some pros and cons of using Flash in 2023 and some other software options:
-
-
-
Pros of using Flash in 2023
-
Cons of using Flash in 2023
-
-
-
- Flash content is still widely used on the web, especially for games, animations, and educational content.
-
- Flash content is not supported by most mobile devices and browsers, such as iOS, Android, Chrome, Firefox, and Edge.
-
-
-
- Flash content can be converted to other formats, such as HTML5, MP4, or SWF, using tools like Adobe Animate or Swiffy.
-
- Flash content may have poor performance, high CPU usage, security risks, or accessibility issues.
-
-
-
- Flash content can be viewed using alternative players, such as Ruffle or Lightspark.
-
- Flash content may not display correctly or fully using alternative players.
-
-
-
Some other software that can create and view Flash content are:
-
-
Adobe Animate: This is the successor of Macromedia Flash 8 and the current version of Adobe's animation software. It can create HTML5, WebGL, AIR, and SWF content using vector graphics, bitmap graphics, audio, video, text, and ActionScript 3.0. It also supports importing and exporting FLA files from Macromedia Flash 8.
-
Adobe Flash Player: This is the official web browser plug-in that enables users to view SWF content on desktops and laptops. It is still available for download from Adobe's website, but it is no longer supported or updated by Adobe since December 31, 2020. It may not work properly on newer operating systems or browsers.
-
Ruffle: This is an open-source project that aims to emulate Flash Player using WebAssembly and Rust. It can run SWF content on modern browsers without requiring any plug-ins or downloads. It is still in development and may not support all features of Flash Player.
-
Lightspark: This is another open-source project that aims to emulate Flash Player using C++ and OpenGL. It can run SWF content on Linux and Windows platforms without requiring any plug-ins or downloads. It is also still in development and may not support all features of Flash Player.
-
-
Conclusion
-
In conclusion, Macromedia Flash 8 is a powerful software that can create rich interactive content for the web. However, it is an old software that is no longer supported or updated by Adobe. It may not work properly on newer operating systems or browsers. It may also have security vulnerabilities or compatibility issues with other software. If you want to download Macromedia Flash 8 in 2023, you need to consider some requirements and precautions first. You also have some alternatives that you can consider for creating and viewing Flash content in 2023. We hope this article has been helpful and informative for you. If you have any questions or comments, please feel free to leave them below.
-
FAQs
-
Here are some frequently asked questions about Macromedia Flash 8 and Flash content in 2023:
-
-
Is Macromedia Flash 8 free?
-
No, Macromedia Flash 8 is not free. It is a commercial software that requires a license and a serial number to activate. However, you may be able to find some unofficial sources where you can download it for free, but they may not be safe or legal.
-
Is Macromedia Flash 8 the same as Adobe Flash?
-
No, Macromedia Flash 8 is not the same as Adobe Flash. Macromedia Flash 8 is the name of the software that was released in 2005 by Macromedia, before it was acquired by Adobe. Adobe Flash is the name of the software that was released after 2005 by Adobe, which includes Adobe Flash CS3, CS4, CS5, CS6, CC, and Animate.
-
What is the difference between SWF and FLA files?
-
SWF and FLA files are two different types of files that are related to Flash content. SWF files are the final output files that can be viewed by users on various platforms and devices. FLA files are the source files that can be edited by developers using Macromedia Flash 8 or Adobe Animate. SWF files can be converted to FLA files using tools like Sothink SWF Decompiler or JPEXS Free Flash Decompiler.
-
How can I view SWF files on my browser?
-
To view SWF files on your browser, you need to have Adobe Flash Player installed and enabled on your browser. However, Adobe Flash Player is no longer supported or updated by Adobe since December 31, 2020. It may not work properly on newer operating systems or browsers. Alternatively, you can use alternative players like Ruffle or Lightspark to view SWF files on your browser without requiring any plug-ins or downloads.
-
How can I create HTML5 content using Macromedia Flash 8?
-
To create HTML5 content using Macromedia Flash 8, you need to use tools like Adobe Animate or Swiffy to convert your FLA or SWF files to HTML5 files. Adobe Animate is a software that can create HTML5, WebGL, AIR, and SWF content using vector graphics, bitmap graphics, audio, video, text, and ActionScript 3.0. Swiffy is a web service that can convert SWF files to HTML5 files using JavaScript and SVG.
- 401be4b1e0
-
-
\ No newline at end of file
diff --git a/spaces/congsaPfin/Manga-OCR/logs/The Ultimate Resource for Downloading Love Nwantiti Lyrics and Listening to CKay.md b/spaces/congsaPfin/Manga-OCR/logs/The Ultimate Resource for Downloading Love Nwantiti Lyrics and Listening to CKay.md
deleted file mode 100644
index 00d4550a0141ad51fd8a8a37849f6f7afd8d6e88..0000000000000000000000000000000000000000
--- a/spaces/congsaPfin/Manga-OCR/logs/The Ultimate Resource for Downloading Love Nwantiti Lyrics and Listening to CKay.md
+++ /dev/null
@@ -1,104 +0,0 @@
-
-
How to Download Love Nwantiti Lyrics by CKay
-
If you are a fan of Afrobeats music, you have probably heard of the song Love Nwantiti by Nigerian singer and producer CKay. The song, which was released in 2019 as part of his EP CKay the First, became a global hit in 2021 thanks to its catchy melody, romantic lyrics, and viral TikTok challenges. The song has been remixed by several artists, including Joeboy, Kuami Eugene, ElGrande Toto, and De La Ghetto, and has charted in over 160 countries.
-
But do you know what the song is actually about? And do you know how to download the lyrics of the song so you can sing along and enjoy it more? In this article, we will answer these questions and show you how to download Love Nwantiti lyrics by CKay in a few easy steps.
Love Nwantiti is a love song with lyrics that are a mix of English and Igbo, a language spoken in Nigeria. The song title means "small love" or "little love" in Igbo, but this does not mean that CKay's love for his girl is small. On the contrary, he expresses his intense passion and obsession for her throughout the song. He compares her to oxygen, valentine, fantasy, and nkwobi (a spicy Nigerian dish made from cow foot). He also promises to spend pounds and dollars on her, and to be her loyal lover forever.
-
The Lyrics of Love Nwantiti
-
The lyrics of Love Nwantiti are simple but catchy, and they use a lot of repetition and rhyme to create a memorable melody. Here are some of the most popular lines from the song:
-
-
Ah-ah-ah-ah-ah-ah: This is the hook of the song, which is repeated after every verse. It is a sound that expresses joy, excitement, or admiration.
-
I am so obsessed I want to chop your nkwobi: This is one of the most famous lines from the song, which has been used in many TikTok videos. To chop nkwobi means to eat nkwobi, but it also has a sexual connotation.
-
Ule your body dey gbakam isi: This is an Igbo phrase that means "your body is driving me crazy". Ule is an exclamation that can be translated as "oh my God" or "wow".
-
Gimme love nwantiti: This means "give me small love" or "give me a little bit of your love". It is a way of asking for affection or attention from someone you love.
-
-
If you want to see the full lyrics of Love Nwantiti by CKay, you can find them on various websites such as Genius, Billboard, or Songfacts. However, if you want to download them as a file that you can save on your device or print out, you will need to follow some steps that we will explain in the next section.
-
How to Download Love Nwantiti Lyrics
-
The Benefits of Downloading Lyrics
-
Downloading lyrics can have many benefits for music lovers. Some of them are - You can learn the meaning and pronunciation of the words in the song, especially if they are in a different language or dialect. - You can sing along to the song and improve your vocal skills and confidence. - You can memorize the lyrics and impress your friends or your crush with your knowledge of the song. - You can analyze the lyrics and discover the hidden messages, themes, or references in the song. - You can enjoy the song more and connect with the artist and their emotions.
-
How to download love nwantiti lyrics
-Download love nwantiti lyrics by CKay
-Download love nwantiti lyrics PDF
-Download love nwantiti lyrics video
-Download love nwantiti lyrics TikTok version
-Download love nwantiti lyrics and chords
-Download love nwantiti lyrics translation
-Download love nwantiti lyrics remix
-Download love nwantiti lyrics instrumental
-Download love nwantiti lyrics genius
-Download love nwantiti lyrics meaning
-Download love nwantiti lyrics audio
-Download love nwantiti lyrics mp3
-Download love nwantiti lyrics song
-Download love nwantiti lyrics free
-Download love nwantiti lyrics online
-Download love nwantiti lyrics printable
-Download love nwantiti lyrics karaoke
-Download love nwantiti lyrics acoustic
-Download love nwantiti lyrics with English subtitles
-Download love nwantiti lyrics in Spanish
-Download love nwantiti lyrics in French
-Download love nwantiti lyrics in Hindi
-Download love nwantiti lyrics in Arabic
-Download love nwantiti lyrics in Swahili
-Download love nwantiti lyrics in Portuguese
-Download love nwantiti lyrics in German
-Download love nwantiti lyrics in Italian
-Download love nwantiti lyrics in Chinese
-Download love nwantiti lyrics in Japanese
-Download love nwantiti lyrics in Korean
-Download love nwantiti lyrics in Russian
-Download love nwantiti lyrics in Turkish
-Download love nwantiti lyrics in Indonesian
-Download love nwantiti lyrics in Malayalam
-Download love nwantiti lyrics in Tamil
-Download love nwantiti lyrics in Telugu
-Download love nwantiti lyrics in Urdu
-Download love nwantiti lyrics in Bengali
-Download love nwantiti lyrics in Punjabi
-Best site to download love nwantiti lyrics
-Where can I download love nwantiti lyrics?
-How to download love nwantiti lyrics on iPhone?
-How to download love nwantiti lyrics on Android?
-How to download love nwantiti lyrics on PC?
-How to download love nwantiti lyrics on Mac?
-How to download love nwantiti lyrics on iPad?
-How to download love nwantiti lyrics on Kindle?
-How to download love nwantiti lyrics on Spotify?
-How to download love nwantiti lyrics on YouTube?
-
The Best Websites to Download Lyrics
-
There are many websites that offer lyrics for free, but not all of them allow you to download them as a file. Some of the best websites that do are:
-
-
AZLyrics: This website has a huge collection of lyrics from various genres and artists. It also has a simple interface and a search function that makes it easy to find the song you want. To download the lyrics, you just need to click on the "Download Lyrics" button at the bottom of the page.
-
Lyrster: This website is a search engine that helps you find lyrics from over 450 sources. It also has a feature that allows you to search by keywords, phrases, or parts of the lyrics. To download the lyrics, you just need to click on the "Download" link next to the source name.
-
MetroLyrics: This website is one of the most popular and reliable sources of lyrics. It also has other features such as videos, news, trivia, and playlists. To download the lyrics, you just need to click on the "Print Lyrics" button at the top right corner of the page, and then choose "Save as PDF" from your browser.
-
-
These are just some of the websites that you can use to download Love Nwantiti lyrics by CKay. However, you can also use other websites that have similar functions, or you can use online tools that can convert any webpage into a PDF file.
-
The Steps to Download Lyrics
-
To download Love Nwantiti lyrics by CKay, you can follow these general steps:
-
-
Go to one of the websites that offer lyrics and search for Love Nwantiti by CKay.
-
Open the page that contains the lyrics and check if they are accurate and complete.
-
Click on the button or link that allows you to download or print the lyrics.
-
Choose the format and location where you want to save the file.
-
Enjoy your downloaded lyrics and sing along to Love Nwantiti by CKay.
-
-
These steps may vary slightly depending on the website or tool that you use, but they are generally easy and quick to follow. You can also repeat these steps for any other song that you want to download lyrics for.
-
How to Enjoy Love Nwantiti More
-
The Remixes of Love Nwantiti
-
One way to enjoy Love Nwantiti more is to listen to its remixes by different artists. CKay has collaborated with several artists from Africa, Europe, and Latin America to create different versions of his hit song. Some of them are:
-
-
Love Nwantiti (Remix) feat. Joeboy & Kuami Eugene: This remix features two rising stars of Afrobeats music, Joeboy from Nigeria and Kuami Eugene from Ghana. They add their own verses and harmonies to CKay's original chorus, creating a smooth and catchy tune.
-
Love Nwantiti (Spanish Remix) feat. De La Ghetto: This remix features De La Ghetto, a Puerto Rican-American singer and rapper who is known for his reggaeton and R&B songs. He sings in Spanish and adds some Latin flavor to CKay's Afrobeats sound.
-
Love Nwantiti (North African Remix) feat. ElGrande Toto: This remix features ElGrande Toto, a Moroccan rapper who is one of the pioneers of trap music in North Africa. He raps in Arabic and French and brings some rap flow to CKay's melodic chorus.
-
-
You can find these remixes on YouTube, Spotify, Apple Music, or any other streaming platform. You can also download them using the same steps as downloading lyrics.
-
The Music Videos of Love Nwantiti
-
Another way to enjoy Love Nwantiti more is to watch its music videos. CKay has released several music videos for his song and its remixes, featuring himself and his collaborators in various locations and Love Nwantiti by CKay?: Love Nwantiti by CKay is one of the most popular songs of 2021. It has over 100 million streams on Spotify, over 50 million views on YouTube, and over 10 million TikTok videos. It has also been featured on several playlists, charts, and radio stations around the world.
-
Where can I download Love Nwantiti by CKay?: You can download Love Nwantiti by CKay from various platforms such as iTunes, Amazon Music, Google Play Music, or Deezer. You can also stream it on Spotify, Apple Music, YouTube Music, or SoundCloud.
-
-
I hope you found this article helpful and informative. If you have any questions or feedback, please leave a comment below. Thank you for reading and have a great day!
401be4b1e0
-
-
\ No newline at end of file
diff --git a/spaces/contluForse/HuggingGPT/assets/Deep Ze Full Crack Cho Win Xp Why You Need This Powerful Tool to Keep Your PC Running Smoothly.md b/spaces/contluForse/HuggingGPT/assets/Deep Ze Full Crack Cho Win Xp Why You Need This Powerful Tool to Keep Your PC Running Smoothly.md
deleted file mode 100644
index 95ad3de081e8b4891081621544a0855a696664d3..0000000000000000000000000000000000000000
--- a/spaces/contluForse/HuggingGPT/assets/Deep Ze Full Crack Cho Win Xp Why You Need This Powerful Tool to Keep Your PC Running Smoothly.md
+++ /dev/null
@@ -1,19 +0,0 @@
-
-
All of the Witcher mods live on NexusMods, and Vortex (formerly the Nexus Mods Manager) is the default way to download and install mods from there. If you're already using it to mod another game, like Skyrim for instance, you may as well use it for The Witcher 3 as well. Note that The Witcher 3 isn't as mod-friendly as Skyrim, however, and you'll often need to resort to a manual install. Read each mod's description and carefully follow its instructions.
It's annoying to cart a load of loot into town and find a vendor so poor he can't afford to buy it all. This mod gives vendors some deeper pockets, and gently encourages them to pay you more for your goods.
-
The Northern Kingdoms, especially Velen and Novigrad, are filthy places full of muck, but you can't do any decent photography with a dirty lens! Wipe them on your pants to get them clean, just like the pros do. No Dirty Lenses removes the old water spots and dirt effects from the in-game camera, but you'll still get water on the screen from splashing through a river or looking up at the rain.
-
As a registered user you are entitled to free data recovery software updates (up to the release of the next major version) and data recovery support. If you are looking for a Recover My Files crack, torrent, serial, portable or keygen, then please use this link.
-
Deep learning models [16,17,18,19,20] are an effective way of classifying and quantifying fracture characteristics [11,12,13,14,15,16,17,18,19,20,21,22,23,24]. The works presented in the literature show that the machine learning models based on convolutional neural networks (CNN) are very suitable for the detection and classification of microstructures [25,26,27,28,29,30,31]. Konovalenko et al. proposed a model to detect the edges of dimples. In addition, the authors used a CNN to estimate the size and diameter of the dimples. However, the proposed model focused on images that only contained dimples. The images of hybrid microstructures (i.e., a mixture of dimples and cleavage) were not of concern [11,32]. Recently, Sinha et al. used UNet to perform the semantic segmentation of dimples on a metallic surface. This model can well segment the clearly visible deep dimples. However, this model is inappropriate to segment the overall dimple morphology of fracture [33].
-
Typically, box springs come fully assembled, so you would just place it where you want it. Some, like a smart box spring, that go above and beyond a traditional box spring may have a few parts to put together, but it should be easy to assemble with instructions. These are usually metal, with a fabric covering that acts as a complete foundation.
-
-
Let's start with the most common mattress size, queen. You can get a queen box spring as one piece or two, there are split queen box springs. When it comes to king size box springs, they are always split, as it's simply too large to be moving through your house as one piece. The same goes for California king box springs. The smaller box springs come as one piece, including twin box springs and full box springs, along with their extra-long counterparts.
-
Use Energy Saver to extend your battery life. This tool automatically detects when your computer is not plugged into a power source and several energy-saving settings that are usually hidden deep inside the operating system. Use settings to customize to your own preferences and easily one-click to extend your charge!
-
To add a little life to your idler pulleys, try using a little spray lubricant on the seals. Over time, water and sunlight can cause the seals to harden, shrink or crack. When this happens, the seal no longer protects the bearing and a failure will soon happen. The lubricant will help to keep the seal soft and resistant to the weathering that can occur.
-
Abstract:The deep sea has been proven to be a great treasure for structurally unique and biologically active natural products in the last two decades. Cold seeps and hydrothermal vents, as typical representatives of deep-sea extreme environments, have attracted more and more attention. This review mainly summarizes the natural products of marine animals, marine fungi, and marine bacteria derived from deep-sea cold seeps and hydrothermal vents as well as their biological activities. In general, there were 182 compounds reported, citing 132 references and covering the literature from the first report in 1984 up to March 2022. The sources of the compounds are represented by the genera Aspergillus sp., Penicillium sp., Streptomyces sp., and so on. It is worth mentioning that 90 of the 182 compounds are new and that almost 60% of the reported structures exhibited diverse bioactivities, which became attractive targets for relevant organic synthetic and biosynthetic studies.Keywords: natural products; extreme environments; cold seeps; hydrothermal vents; bioactivities
-
In 2001[54] and 2002,[55] processes for growing gallium nitride (GaN) LEDs on silicon were successfully demonstrated. In January 2012, Osram demonstrated high-power InGaN LEDs grown on silicon substrates commercially,[56] and GaN-on-silicon LEDs are in production at Plessey Semiconductors. As of 2017, some manufacturers are using SiC as the substrate for LED production, but sapphire is more common, as it has the most similar properties to that of gallium nitride, reducing the need for patterning the sapphire wafer (patterned wafers are known as epi wafers). Samsung, the University of Cambridge, and Toshiba are performing research into GaN on Si LEDs. Toshiba has stopped research, possibly due to low yields.[57][58][59][60][61][62][63] Some opt for epitaxy, which is difficult on silicon, while others, like the University of Cambridge, choose a multi-layer structure, in order to reduce (crystal) lattice mismatch and different thermal expansion ratios, in order to avoid cracking of the LED chip at high temperatures (e.g. during manufacturing), reduce heat generation and increase luminous efficiency. Sapphire substrate patterning can be carried out with nanoimprint lithography.[64][65][66][67][68][69][70]
-
Because of their long life, fast switching times, and visibility in broad daylight due to their high output and focus, LEDs have been used in automotive brake lights and turn signals. The use in brakes improves safety, due to a great reduction in the time needed to light fully, or faster rise time, about 0.1 second faster[citation needed] than an incandescent bulb. This gives drivers behind more time to react. In a dual intensity circuit (rear markers and brakes) if the LEDs are not pulsed at a fast enough frequency, they can create a phantom array, where ghost images of the LED appear if the eyes quickly scan across the array. White LED headlamps are beginning to appear. Using LEDs has styling advantages because LEDs can form much thinner lights than incandescent lamps with parabolic reflectors.
-
With the development of high-efficiency and high-power LEDs, it has become possible to use LEDs in lighting and illumination. To encourage the shift to LED lamps and other high-efficiency lighting, in 2008 the US Department of Energy created the L Prize competition. The Philips Lighting North America LED bulb won the first competition on August 3, 2011, after successfully completing 18 months of intensive field, lab, and product testing.[160]
-
In the work of Cao et al.,[184] researchers targeted the outcoupling problem, which is that the optical physics of thin-film LEDs causes the majority of light generated by the semiconductor to be trapped in the device.[185] To achieve this goal, they demonstrated that solution-processed perovskites can spontaneously form submicrometre-scale crystal platelets, which can efficiently extract light from the device. These perovskites are formed via the introduction of amino acid additives into the perovskite precursor solutions. In addition, their method is able to passivate perovskite surface defects and reduce nonradiative recombination. Therefore, by improving the light outcoupling and reducing nonradiative losses, Cao and his colleagues successfully achieved PLED with EQE up to 20.7%.[184]
aaccfb2cb3
-
-
\ No newline at end of file
diff --git a/spaces/cooelf/Multimodal-CoT/timm/scheduler/__init__.py b/spaces/cooelf/Multimodal-CoT/timm/scheduler/__init__.py
deleted file mode 100644
index 6a7789826229f66e1220cb6149902ba9c411b537..0000000000000000000000000000000000000000
--- a/spaces/cooelf/Multimodal-CoT/timm/scheduler/__init__.py
+++ /dev/null
@@ -1,5 +0,0 @@
-from .cosine_lr import CosineLRScheduler
-from .plateau_lr import PlateauLRScheduler
-from .step_lr import StepLRScheduler
-from .tanh_lr import TanhLRScheduler
-from .scheduler_factory import create_scheduler
diff --git a/spaces/coreml-community/ControlNet-v1-1-Annotators-cpu/annotator/mmpkg/mmcv/image/colorspace.py b/spaces/coreml-community/ControlNet-v1-1-Annotators-cpu/annotator/mmpkg/mmcv/image/colorspace.py
deleted file mode 100644
index 814533952fdfda23d67cb6a3073692d8c1156add..0000000000000000000000000000000000000000
--- a/spaces/coreml-community/ControlNet-v1-1-Annotators-cpu/annotator/mmpkg/mmcv/image/colorspace.py
+++ /dev/null
@@ -1,306 +0,0 @@
-# Copyright (c) OpenMMLab. All rights reserved.
-import cv2
-import numpy as np
-
-
-def imconvert(img, src, dst):
- """Convert an image from the src colorspace to dst colorspace.
-
- Args:
- img (ndarray): The input image.
- src (str): The source colorspace, e.g., 'rgb', 'hsv'.
- dst (str): The destination colorspace, e.g., 'rgb', 'hsv'.
-
- Returns:
- ndarray: The converted image.
- """
- code = getattr(cv2, f'COLOR_{src.upper()}2{dst.upper()}')
- out_img = cv2.cvtColor(img, code)
- return out_img
-
-
-def bgr2gray(img, keepdim=False):
- """Convert a BGR image to grayscale image.
-
- Args:
- img (ndarray): The input image.
- keepdim (bool): If False (by default), then return the grayscale image
- with 2 dims, otherwise 3 dims.
-
- Returns:
- ndarray: The converted grayscale image.
- """
- out_img = cv2.cvtColor(img, cv2.COLOR_BGR2GRAY)
- if keepdim:
- out_img = out_img[..., None]
- return out_img
-
-
-def rgb2gray(img, keepdim=False):
- """Convert a RGB image to grayscale image.
-
- Args:
- img (ndarray): The input image.
- keepdim (bool): If False (by default), then return the grayscale image
- with 2 dims, otherwise 3 dims.
-
- Returns:
- ndarray: The converted grayscale image.
- """
- out_img = cv2.cvtColor(img, cv2.COLOR_RGB2GRAY)
- if keepdim:
- out_img = out_img[..., None]
- return out_img
-
-
-def gray2bgr(img):
- """Convert a grayscale image to BGR image.
-
- Args:
- img (ndarray): The input image.
-
- Returns:
- ndarray: The converted BGR image.
- """
- img = img[..., None] if img.ndim == 2 else img
- out_img = cv2.cvtColor(img, cv2.COLOR_GRAY2BGR)
- return out_img
-
-
-def gray2rgb(img):
- """Convert a grayscale image to RGB image.
-
- Args:
- img (ndarray): The input image.
-
- Returns:
- ndarray: The converted RGB image.
- """
- img = img[..., None] if img.ndim == 2 else img
- out_img = cv2.cvtColor(img, cv2.COLOR_GRAY2RGB)
- return out_img
-
-
-def _convert_input_type_range(img):
- """Convert the type and range of the input image.
-
- It converts the input image to np.float32 type and range of [0, 1].
- It is mainly used for pre-processing the input image in colorspace
- conversion functions such as rgb2ycbcr and ycbcr2rgb.
-
- Args:
- img (ndarray): The input image. It accepts:
- 1. np.uint8 type with range [0, 255];
- 2. np.float32 type with range [0, 1].
-
- Returns:
- (ndarray): The converted image with type of np.float32 and range of
- [0, 1].
- """
- img_type = img.dtype
- img = img.astype(np.float32)
- if img_type == np.float32:
- pass
- elif img_type == np.uint8:
- img /= 255.
- else:
- raise TypeError('The img type should be np.float32 or np.uint8, '
- f'but got {img_type}')
- return img
-
-
-def _convert_output_type_range(img, dst_type):
- """Convert the type and range of the image according to dst_type.
-
- It converts the image to desired type and range. If `dst_type` is np.uint8,
- images will be converted to np.uint8 type with range [0, 255]. If
- `dst_type` is np.float32, it converts the image to np.float32 type with
- range [0, 1].
- It is mainly used for post-processing images in colorspace conversion
- functions such as rgb2ycbcr and ycbcr2rgb.
-
- Args:
- img (ndarray): The image to be converted with np.float32 type and
- range [0, 255].
- dst_type (np.uint8 | np.float32): If dst_type is np.uint8, it
- converts the image to np.uint8 type with range [0, 255]. If
- dst_type is np.float32, it converts the image to np.float32 type
- with range [0, 1].
-
- Returns:
- (ndarray): The converted image with desired type and range.
- """
- if dst_type not in (np.uint8, np.float32):
- raise TypeError('The dst_type should be np.float32 or np.uint8, '
- f'but got {dst_type}')
- if dst_type == np.uint8:
- img = img.round()
- else:
- img /= 255.
- return img.astype(dst_type)
-
-
-def rgb2ycbcr(img, y_only=False):
- """Convert a RGB image to YCbCr image.
-
- This function produces the same results as Matlab's `rgb2ycbcr` function.
- It implements the ITU-R BT.601 conversion for standard-definition
- television. See more details in
- https://en.wikipedia.org/wiki/YCbCr#ITU-R_BT.601_conversion.
-
- It differs from a similar function in cv2.cvtColor: `RGB <-> YCrCb`.
- In OpenCV, it implements a JPEG conversion. See more details in
- https://en.wikipedia.org/wiki/YCbCr#JPEG_conversion.
-
- Args:
- img (ndarray): The input image. It accepts:
- 1. np.uint8 type with range [0, 255];
- 2. np.float32 type with range [0, 1].
- y_only (bool): Whether to only return Y channel. Default: False.
-
- Returns:
- ndarray: The converted YCbCr image. The output image has the same type
- and range as input image.
- """
- img_type = img.dtype
- img = _convert_input_type_range(img)
- if y_only:
- out_img = np.dot(img, [65.481, 128.553, 24.966]) + 16.0
- else:
- out_img = np.matmul(
- img, [[65.481, -37.797, 112.0], [128.553, -74.203, -93.786],
- [24.966, 112.0, -18.214]]) + [16, 128, 128]
- out_img = _convert_output_type_range(out_img, img_type)
- return out_img
-
-
-def bgr2ycbcr(img, y_only=False):
- """Convert a BGR image to YCbCr image.
-
- The bgr version of rgb2ycbcr.
- It implements the ITU-R BT.601 conversion for standard-definition
- television. See more details in
- https://en.wikipedia.org/wiki/YCbCr#ITU-R_BT.601_conversion.
-
- It differs from a similar function in cv2.cvtColor: `BGR <-> YCrCb`.
- In OpenCV, it implements a JPEG conversion. See more details in
- https://en.wikipedia.org/wiki/YCbCr#JPEG_conversion.
-
- Args:
- img (ndarray): The input image. It accepts:
- 1. np.uint8 type with range [0, 255];
- 2. np.float32 type with range [0, 1].
- y_only (bool): Whether to only return Y channel. Default: False.
-
- Returns:
- ndarray: The converted YCbCr image. The output image has the same type
- and range as input image.
- """
- img_type = img.dtype
- img = _convert_input_type_range(img)
- if y_only:
- out_img = np.dot(img, [24.966, 128.553, 65.481]) + 16.0
- else:
- out_img = np.matmul(
- img, [[24.966, 112.0, -18.214], [128.553, -74.203, -93.786],
- [65.481, -37.797, 112.0]]) + [16, 128, 128]
- out_img = _convert_output_type_range(out_img, img_type)
- return out_img
-
-
-def ycbcr2rgb(img):
- """Convert a YCbCr image to RGB image.
-
- This function produces the same results as Matlab's ycbcr2rgb function.
- It implements the ITU-R BT.601 conversion for standard-definition
- television. See more details in
- https://en.wikipedia.org/wiki/YCbCr#ITU-R_BT.601_conversion.
-
- It differs from a similar function in cv2.cvtColor: `YCrCb <-> RGB`.
- In OpenCV, it implements a JPEG conversion. See more details in
- https://en.wikipedia.org/wiki/YCbCr#JPEG_conversion.
-
- Args:
- img (ndarray): The input image. It accepts:
- 1. np.uint8 type with range [0, 255];
- 2. np.float32 type with range [0, 1].
-
- Returns:
- ndarray: The converted RGB image. The output image has the same type
- and range as input image.
- """
- img_type = img.dtype
- img = _convert_input_type_range(img) * 255
- out_img = np.matmul(img, [[0.00456621, 0.00456621, 0.00456621],
- [0, -0.00153632, 0.00791071],
- [0.00625893, -0.00318811, 0]]) * 255.0 + [
- -222.921, 135.576, -276.836
- ]
- out_img = _convert_output_type_range(out_img, img_type)
- return out_img
-
-
-def ycbcr2bgr(img):
- """Convert a YCbCr image to BGR image.
-
- The bgr version of ycbcr2rgb.
- It implements the ITU-R BT.601 conversion for standard-definition
- television. See more details in
- https://en.wikipedia.org/wiki/YCbCr#ITU-R_BT.601_conversion.
-
- It differs from a similar function in cv2.cvtColor: `YCrCb <-> BGR`.
- In OpenCV, it implements a JPEG conversion. See more details in
- https://en.wikipedia.org/wiki/YCbCr#JPEG_conversion.
-
- Args:
- img (ndarray): The input image. It accepts:
- 1. np.uint8 type with range [0, 255];
- 2. np.float32 type with range [0, 1].
-
- Returns:
- ndarray: The converted BGR image. The output image has the same type
- and range as input image.
- """
- img_type = img.dtype
- img = _convert_input_type_range(img) * 255
- out_img = np.matmul(img, [[0.00456621, 0.00456621, 0.00456621],
- [0.00791071, -0.00153632, 0],
- [0, -0.00318811, 0.00625893]]) * 255.0 + [
- -276.836, 135.576, -222.921
- ]
- out_img = _convert_output_type_range(out_img, img_type)
- return out_img
-
-
-def convert_color_factory(src, dst):
-
- code = getattr(cv2, f'COLOR_{src.upper()}2{dst.upper()}')
-
- def convert_color(img):
- out_img = cv2.cvtColor(img, code)
- return out_img
-
- convert_color.__doc__ = f"""Convert a {src.upper()} image to {dst.upper()}
- image.
-
- Args:
- img (ndarray or str): The input image.
-
- Returns:
- ndarray: The converted {dst.upper()} image.
- """
-
- return convert_color
-
-
-bgr2rgb = convert_color_factory('bgr', 'rgb')
-
-rgb2bgr = convert_color_factory('rgb', 'bgr')
-
-bgr2hsv = convert_color_factory('bgr', 'hsv')
-
-hsv2bgr = convert_color_factory('hsv', 'bgr')
-
-bgr2hls = convert_color_factory('bgr', 'hls')
-
-hls2bgr = convert_color_factory('hls', 'bgr')
diff --git a/spaces/coreml-community/ControlNet-v1-1-Annotators-cpu/annotator/mmpkg/mmseg/models/decode_heads/psp_head.py b/spaces/coreml-community/ControlNet-v1-1-Annotators-cpu/annotator/mmpkg/mmseg/models/decode_heads/psp_head.py
deleted file mode 100644
index 2a88d807bfe11fe224305f8de745cde3aa739db0..0000000000000000000000000000000000000000
--- a/spaces/coreml-community/ControlNet-v1-1-Annotators-cpu/annotator/mmpkg/mmseg/models/decode_heads/psp_head.py
+++ /dev/null
@@ -1,101 +0,0 @@
-import torch
-import torch.nn as nn
-from annotator.mmpkg.mmcv.cnn import ConvModule
-
-from annotator.mmpkg.mmseg.ops import resize
-from ..builder import HEADS
-from .decode_head import BaseDecodeHead
-
-
-class PPM(nn.ModuleList):
- """Pooling Pyramid Module used in PSPNet.
-
- Args:
- pool_scales (tuple[int]): Pooling scales used in Pooling Pyramid
- Module.
- in_channels (int): Input channels.
- channels (int): Channels after modules, before conv_seg.
- conv_cfg (dict|None): Config of conv layers.
- norm_cfg (dict|None): Config of norm layers.
- act_cfg (dict): Config of activation layers.
- align_corners (bool): align_corners argument of F.interpolate.
- """
-
- def __init__(self, pool_scales, in_channels, channels, conv_cfg, norm_cfg,
- act_cfg, align_corners):
- super(PPM, self).__init__()
- self.pool_scales = pool_scales
- self.align_corners = align_corners
- self.in_channels = in_channels
- self.channels = channels
- self.conv_cfg = conv_cfg
- self.norm_cfg = norm_cfg
- self.act_cfg = act_cfg
- for pool_scale in pool_scales:
- self.append(
- nn.Sequential(
- nn.AdaptiveAvgPool2d(pool_scale),
- ConvModule(
- self.in_channels,
- self.channels,
- 1,
- conv_cfg=self.conv_cfg,
- norm_cfg=self.norm_cfg,
- act_cfg=self.act_cfg)))
-
- def forward(self, x):
- """Forward function."""
- ppm_outs = []
- for ppm in self:
- ppm_out = ppm(x)
- upsampled_ppm_out = resize(
- ppm_out,
- size=x.size()[2:],
- mode='bilinear',
- align_corners=self.align_corners)
- ppm_outs.append(upsampled_ppm_out)
- return ppm_outs
-
-
-@HEADS.register_module()
-class PSPHead(BaseDecodeHead):
- """Pyramid Scene Parsing Network.
-
- This head is the implementation of
- `PSPNet `_.
-
- Args:
- pool_scales (tuple[int]): Pooling scales used in Pooling Pyramid
- Module. Default: (1, 2, 3, 6).
- """
-
- def __init__(self, pool_scales=(1, 2, 3, 6), **kwargs):
- super(PSPHead, self).__init__(**kwargs)
- assert isinstance(pool_scales, (list, tuple))
- self.pool_scales = pool_scales
- self.psp_modules = PPM(
- self.pool_scales,
- self.in_channels,
- self.channels,
- conv_cfg=self.conv_cfg,
- norm_cfg=self.norm_cfg,
- act_cfg=self.act_cfg,
- align_corners=self.align_corners)
- self.bottleneck = ConvModule(
- self.in_channels + len(pool_scales) * self.channels,
- self.channels,
- 3,
- padding=1,
- conv_cfg=self.conv_cfg,
- norm_cfg=self.norm_cfg,
- act_cfg=self.act_cfg)
-
- def forward(self, inputs):
- """Forward function."""
- x = self._transform_inputs(inputs)
- psp_outs = [x]
- psp_outs.extend(self.psp_modules(x))
- psp_outs = torch.cat(psp_outs, dim=1)
- output = self.bottleneck(psp_outs)
- output = self.cls_seg(output)
- return output
diff --git a/spaces/coreml-community/ControlNet-v1-1-Annotators-cpu/annotator/oneformer/detectron2/modeling/backbone/build.py b/spaces/coreml-community/ControlNet-v1-1-Annotators-cpu/annotator/oneformer/detectron2/modeling/backbone/build.py
deleted file mode 100644
index 63a4aaced2c2869294d2b16f4b95cdfdd01259b7..0000000000000000000000000000000000000000
--- a/spaces/coreml-community/ControlNet-v1-1-Annotators-cpu/annotator/oneformer/detectron2/modeling/backbone/build.py
+++ /dev/null
@@ -1,33 +0,0 @@
-# Copyright (c) Facebook, Inc. and its affiliates.
-from annotator.oneformer.detectron2.layers import ShapeSpec
-from annotator.oneformer.detectron2.utils.registry import Registry
-
-from .backbone import Backbone
-
-BACKBONE_REGISTRY = Registry("BACKBONE")
-BACKBONE_REGISTRY.__doc__ = """
-Registry for backbones, which extract feature maps from images
-
-The registered object must be a callable that accepts two arguments:
-
-1. A :class:`detectron2.config.CfgNode`
-2. A :class:`detectron2.layers.ShapeSpec`, which contains the input shape specification.
-
-Registered object must return instance of :class:`Backbone`.
-"""
-
-
-def build_backbone(cfg, input_shape=None):
- """
- Build a backbone from `cfg.MODEL.BACKBONE.NAME`.
-
- Returns:
- an instance of :class:`Backbone`
- """
- if input_shape is None:
- input_shape = ShapeSpec(channels=len(cfg.MODEL.PIXEL_MEAN))
-
- backbone_name = cfg.MODEL.BACKBONE.NAME
- backbone = BACKBONE_REGISTRY.get(backbone_name)(cfg, input_shape)
- assert isinstance(backbone, Backbone)
- return backbone
diff --git a/spaces/coreml-community/ControlNet-v1-1-Annotators-cpu/annotator/oneformer/detectron2/utils/README.md b/spaces/coreml-community/ControlNet-v1-1-Annotators-cpu/annotator/oneformer/detectron2/utils/README.md
deleted file mode 100644
index 9765b24a730b77556104187ac3ef5439ab0859fd..0000000000000000000000000000000000000000
--- a/spaces/coreml-community/ControlNet-v1-1-Annotators-cpu/annotator/oneformer/detectron2/utils/README.md
+++ /dev/null
@@ -1,5 +0,0 @@
-# Utility functions
-
-This folder contain utility functions that are not used in the
-core library, but are useful for building models or training
-code using the config system.
diff --git a/spaces/coreml-community/ControlNet-v1-1-Annotators-cpu/annotator/uniformer/mmseg/datasets/voc.py b/spaces/coreml-community/ControlNet-v1-1-Annotators-cpu/annotator/uniformer/mmseg/datasets/voc.py
deleted file mode 100644
index a8855203b14ee0dc4da9099a2945d4aedcffbcd6..0000000000000000000000000000000000000000
--- a/spaces/coreml-community/ControlNet-v1-1-Annotators-cpu/annotator/uniformer/mmseg/datasets/voc.py
+++ /dev/null
@@ -1,29 +0,0 @@
-import os.path as osp
-
-from .builder import DATASETS
-from .custom import CustomDataset
-
-
-@DATASETS.register_module()
-class PascalVOCDataset(CustomDataset):
- """Pascal VOC dataset.
-
- Args:
- split (str): Split txt file for Pascal VOC.
- """
-
- CLASSES = ('background', 'aeroplane', 'bicycle', 'bird', 'boat', 'bottle',
- 'bus', 'car', 'cat', 'chair', 'cow', 'diningtable', 'dog',
- 'horse', 'motorbike', 'person', 'pottedplant', 'sheep', 'sofa',
- 'train', 'tvmonitor')
-
- PALETTE = [[0, 0, 0], [128, 0, 0], [0, 128, 0], [128, 128, 0], [0, 0, 128],
- [128, 0, 128], [0, 128, 128], [128, 128, 128], [64, 0, 0],
- [192, 0, 0], [64, 128, 0], [192, 128, 0], [64, 0, 128],
- [192, 0, 128], [64, 128, 128], [192, 128, 128], [0, 64, 0],
- [128, 64, 0], [0, 192, 0], [128, 192, 0], [0, 64, 128]]
-
- def __init__(self, split, **kwargs):
- super(PascalVOCDataset, self).__init__(
- img_suffix='.jpg', seg_map_suffix='.png', split=split, **kwargs)
- assert osp.exists(self.img_dir) and self.split is not None
diff --git a/spaces/cscan/CodeFormer/CodeFormer/basicsr/losses/losses.py b/spaces/cscan/CodeFormer/CodeFormer/basicsr/losses/losses.py
deleted file mode 100644
index 1bcf272cfb756d99451a3005567ea4d4c9059067..0000000000000000000000000000000000000000
--- a/spaces/cscan/CodeFormer/CodeFormer/basicsr/losses/losses.py
+++ /dev/null
@@ -1,455 +0,0 @@
-import math
-import lpips
-import torch
-from torch import autograd as autograd
-from torch import nn as nn
-from torch.nn import functional as F
-
-from basicsr.archs.vgg_arch import VGGFeatureExtractor
-from basicsr.utils.registry import LOSS_REGISTRY
-from .loss_util import weighted_loss
-
-_reduction_modes = ['none', 'mean', 'sum']
-
-
-@weighted_loss
-def l1_loss(pred, target):
- return F.l1_loss(pred, target, reduction='none')
-
-
-@weighted_loss
-def mse_loss(pred, target):
- return F.mse_loss(pred, target, reduction='none')
-
-
-@weighted_loss
-def charbonnier_loss(pred, target, eps=1e-12):
- return torch.sqrt((pred - target)**2 + eps)
-
-
-@LOSS_REGISTRY.register()
-class L1Loss(nn.Module):
- """L1 (mean absolute error, MAE) loss.
-
- Args:
- loss_weight (float): Loss weight for L1 loss. Default: 1.0.
- reduction (str): Specifies the reduction to apply to the output.
- Supported choices are 'none' | 'mean' | 'sum'. Default: 'mean'.
- """
-
- def __init__(self, loss_weight=1.0, reduction='mean'):
- super(L1Loss, self).__init__()
- if reduction not in ['none', 'mean', 'sum']:
- raise ValueError(f'Unsupported reduction mode: {reduction}. ' f'Supported ones are: {_reduction_modes}')
-
- self.loss_weight = loss_weight
- self.reduction = reduction
-
- def forward(self, pred, target, weight=None, **kwargs):
- """
- Args:
- pred (Tensor): of shape (N, C, H, W). Predicted tensor.
- target (Tensor): of shape (N, C, H, W). Ground truth tensor.
- weight (Tensor, optional): of shape (N, C, H, W). Element-wise
- weights. Default: None.
- """
- return self.loss_weight * l1_loss(pred, target, weight, reduction=self.reduction)
-
-
-@LOSS_REGISTRY.register()
-class MSELoss(nn.Module):
- """MSE (L2) loss.
-
- Args:
- loss_weight (float): Loss weight for MSE loss. Default: 1.0.
- reduction (str): Specifies the reduction to apply to the output.
- Supported choices are 'none' | 'mean' | 'sum'. Default: 'mean'.
- """
-
- def __init__(self, loss_weight=1.0, reduction='mean'):
- super(MSELoss, self).__init__()
- if reduction not in ['none', 'mean', 'sum']:
- raise ValueError(f'Unsupported reduction mode: {reduction}. ' f'Supported ones are: {_reduction_modes}')
-
- self.loss_weight = loss_weight
- self.reduction = reduction
-
- def forward(self, pred, target, weight=None, **kwargs):
- """
- Args:
- pred (Tensor): of shape (N, C, H, W). Predicted tensor.
- target (Tensor): of shape (N, C, H, W). Ground truth tensor.
- weight (Tensor, optional): of shape (N, C, H, W). Element-wise
- weights. Default: None.
- """
- return self.loss_weight * mse_loss(pred, target, weight, reduction=self.reduction)
-
-
-@LOSS_REGISTRY.register()
-class CharbonnierLoss(nn.Module):
- """Charbonnier loss (one variant of Robust L1Loss, a differentiable
- variant of L1Loss).
-
- Described in "Deep Laplacian Pyramid Networks for Fast and Accurate
- Super-Resolution".
-
- Args:
- loss_weight (float): Loss weight for L1 loss. Default: 1.0.
- reduction (str): Specifies the reduction to apply to the output.
- Supported choices are 'none' | 'mean' | 'sum'. Default: 'mean'.
- eps (float): A value used to control the curvature near zero.
- Default: 1e-12.
- """
-
- def __init__(self, loss_weight=1.0, reduction='mean', eps=1e-12):
- super(CharbonnierLoss, self).__init__()
- if reduction not in ['none', 'mean', 'sum']:
- raise ValueError(f'Unsupported reduction mode: {reduction}. ' f'Supported ones are: {_reduction_modes}')
-
- self.loss_weight = loss_weight
- self.reduction = reduction
- self.eps = eps
-
- def forward(self, pred, target, weight=None, **kwargs):
- """
- Args:
- pred (Tensor): of shape (N, C, H, W). Predicted tensor.
- target (Tensor): of shape (N, C, H, W). Ground truth tensor.
- weight (Tensor, optional): of shape (N, C, H, W). Element-wise
- weights. Default: None.
- """
- return self.loss_weight * charbonnier_loss(pred, target, weight, eps=self.eps, reduction=self.reduction)
-
-
-@LOSS_REGISTRY.register()
-class WeightedTVLoss(L1Loss):
- """Weighted TV loss.
-
- Args:
- loss_weight (float): Loss weight. Default: 1.0.
- """
-
- def __init__(self, loss_weight=1.0):
- super(WeightedTVLoss, self).__init__(loss_weight=loss_weight)
-
- def forward(self, pred, weight=None):
- y_diff = super(WeightedTVLoss, self).forward(pred[:, :, :-1, :], pred[:, :, 1:, :], weight=weight[:, :, :-1, :])
- x_diff = super(WeightedTVLoss, self).forward(pred[:, :, :, :-1], pred[:, :, :, 1:], weight=weight[:, :, :, :-1])
-
- loss = x_diff + y_diff
-
- return loss
-
-
-@LOSS_REGISTRY.register()
-class PerceptualLoss(nn.Module):
- """Perceptual loss with commonly used style loss.
-
- Args:
- layer_weights (dict): The weight for each layer of vgg feature.
- Here is an example: {'conv5_4': 1.}, which means the conv5_4
- feature layer (before relu5_4) will be extracted with weight
- 1.0 in calculting losses.
- vgg_type (str): The type of vgg network used as feature extractor.
- Default: 'vgg19'.
- use_input_norm (bool): If True, normalize the input image in vgg.
- Default: True.
- range_norm (bool): If True, norm images with range [-1, 1] to [0, 1].
- Default: False.
- perceptual_weight (float): If `perceptual_weight > 0`, the perceptual
- loss will be calculated and the loss will multiplied by the
- weight. Default: 1.0.
- style_weight (float): If `style_weight > 0`, the style loss will be
- calculated and the loss will multiplied by the weight.
- Default: 0.
- criterion (str): Criterion used for perceptual loss. Default: 'l1'.
- """
-
- def __init__(self,
- layer_weights,
- vgg_type='vgg19',
- use_input_norm=True,
- range_norm=False,
- perceptual_weight=1.0,
- style_weight=0.,
- criterion='l1'):
- super(PerceptualLoss, self).__init__()
- self.perceptual_weight = perceptual_weight
- self.style_weight = style_weight
- self.layer_weights = layer_weights
- self.vgg = VGGFeatureExtractor(
- layer_name_list=list(layer_weights.keys()),
- vgg_type=vgg_type,
- use_input_norm=use_input_norm,
- range_norm=range_norm)
-
- self.criterion_type = criterion
- if self.criterion_type == 'l1':
- self.criterion = torch.nn.L1Loss()
- elif self.criterion_type == 'l2':
- self.criterion = torch.nn.L2loss()
- elif self.criterion_type == 'mse':
- self.criterion = torch.nn.MSELoss(reduction='mean')
- elif self.criterion_type == 'fro':
- self.criterion = None
- else:
- raise NotImplementedError(f'{criterion} criterion has not been supported.')
-
- def forward(self, x, gt):
- """Forward function.
-
- Args:
- x (Tensor): Input tensor with shape (n, c, h, w).
- gt (Tensor): Ground-truth tensor with shape (n, c, h, w).
-
- Returns:
- Tensor: Forward results.
- """
- # extract vgg features
- x_features = self.vgg(x)
- gt_features = self.vgg(gt.detach())
-
- # calculate perceptual loss
- if self.perceptual_weight > 0:
- percep_loss = 0
- for k in x_features.keys():
- if self.criterion_type == 'fro':
- percep_loss += torch.norm(x_features[k] - gt_features[k], p='fro') * self.layer_weights[k]
- else:
- percep_loss += self.criterion(x_features[k], gt_features[k]) * self.layer_weights[k]
- percep_loss *= self.perceptual_weight
- else:
- percep_loss = None
-
- # calculate style loss
- if self.style_weight > 0:
- style_loss = 0
- for k in x_features.keys():
- if self.criterion_type == 'fro':
- style_loss += torch.norm(
- self._gram_mat(x_features[k]) - self._gram_mat(gt_features[k]), p='fro') * self.layer_weights[k]
- else:
- style_loss += self.criterion(self._gram_mat(x_features[k]), self._gram_mat(
- gt_features[k])) * self.layer_weights[k]
- style_loss *= self.style_weight
- else:
- style_loss = None
-
- return percep_loss, style_loss
-
- def _gram_mat(self, x):
- """Calculate Gram matrix.
-
- Args:
- x (torch.Tensor): Tensor with shape of (n, c, h, w).
-
- Returns:
- torch.Tensor: Gram matrix.
- """
- n, c, h, w = x.size()
- features = x.view(n, c, w * h)
- features_t = features.transpose(1, 2)
- gram = features.bmm(features_t) / (c * h * w)
- return gram
-
-
-@LOSS_REGISTRY.register()
-class LPIPSLoss(nn.Module):
- def __init__(self,
- loss_weight=1.0,
- use_input_norm=True,
- range_norm=False,):
- super(LPIPSLoss, self).__init__()
- self.perceptual = lpips.LPIPS(net="vgg", spatial=False).eval()
- self.loss_weight = loss_weight
- self.use_input_norm = use_input_norm
- self.range_norm = range_norm
-
- if self.use_input_norm:
- # the mean is for image with range [0, 1]
- self.register_buffer('mean', torch.Tensor([0.485, 0.456, 0.406]).view(1, 3, 1, 1))
- # the std is for image with range [0, 1]
- self.register_buffer('std', torch.Tensor([0.229, 0.224, 0.225]).view(1, 3, 1, 1))
-
- def forward(self, pred, target):
- if self.range_norm:
- pred = (pred + 1) / 2
- target = (target + 1) / 2
- if self.use_input_norm:
- pred = (pred - self.mean) / self.std
- target = (target - self.mean) / self.std
- lpips_loss = self.perceptual(target.contiguous(), pred.contiguous())
- return self.loss_weight * lpips_loss.mean()
-
-
-@LOSS_REGISTRY.register()
-class GANLoss(nn.Module):
- """Define GAN loss.
-
- Args:
- gan_type (str): Support 'vanilla', 'lsgan', 'wgan', 'hinge'.
- real_label_val (float): The value for real label. Default: 1.0.
- fake_label_val (float): The value for fake label. Default: 0.0.
- loss_weight (float): Loss weight. Default: 1.0.
- Note that loss_weight is only for generators; and it is always 1.0
- for discriminators.
- """
-
- def __init__(self, gan_type, real_label_val=1.0, fake_label_val=0.0, loss_weight=1.0):
- super(GANLoss, self).__init__()
- self.gan_type = gan_type
- self.loss_weight = loss_weight
- self.real_label_val = real_label_val
- self.fake_label_val = fake_label_val
-
- if self.gan_type == 'vanilla':
- self.loss = nn.BCEWithLogitsLoss()
- elif self.gan_type == 'lsgan':
- self.loss = nn.MSELoss()
- elif self.gan_type == 'wgan':
- self.loss = self._wgan_loss
- elif self.gan_type == 'wgan_softplus':
- self.loss = self._wgan_softplus_loss
- elif self.gan_type == 'hinge':
- self.loss = nn.ReLU()
- else:
- raise NotImplementedError(f'GAN type {self.gan_type} is not implemented.')
-
- def _wgan_loss(self, input, target):
- """wgan loss.
-
- Args:
- input (Tensor): Input tensor.
- target (bool): Target label.
-
- Returns:
- Tensor: wgan loss.
- """
- return -input.mean() if target else input.mean()
-
- def _wgan_softplus_loss(self, input, target):
- """wgan loss with soft plus. softplus is a smooth approximation to the
- ReLU function.
-
- In StyleGAN2, it is called:
- Logistic loss for discriminator;
- Non-saturating loss for generator.
-
- Args:
- input (Tensor): Input tensor.
- target (bool): Target label.
-
- Returns:
- Tensor: wgan loss.
- """
- return F.softplus(-input).mean() if target else F.softplus(input).mean()
-
- def get_target_label(self, input, target_is_real):
- """Get target label.
-
- Args:
- input (Tensor): Input tensor.
- target_is_real (bool): Whether the target is real or fake.
-
- Returns:
- (bool | Tensor): Target tensor. Return bool for wgan, otherwise,
- return Tensor.
- """
-
- if self.gan_type in ['wgan', 'wgan_softplus']:
- return target_is_real
- target_val = (self.real_label_val if target_is_real else self.fake_label_val)
- return input.new_ones(input.size()) * target_val
-
- def forward(self, input, target_is_real, is_disc=False):
- """
- Args:
- input (Tensor): The input for the loss module, i.e., the network
- prediction.
- target_is_real (bool): Whether the targe is real or fake.
- is_disc (bool): Whether the loss for discriminators or not.
- Default: False.
-
- Returns:
- Tensor: GAN loss value.
- """
- if self.gan_type == 'hinge':
- if is_disc: # for discriminators in hinge-gan
- input = -input if target_is_real else input
- loss = self.loss(1 + input).mean()
- else: # for generators in hinge-gan
- loss = -input.mean()
- else: # other gan types
- target_label = self.get_target_label(input, target_is_real)
- loss = self.loss(input, target_label)
-
- # loss_weight is always 1.0 for discriminators
- return loss if is_disc else loss * self.loss_weight
-
-
-def r1_penalty(real_pred, real_img):
- """R1 regularization for discriminator. The core idea is to
- penalize the gradient on real data alone: when the
- generator distribution produces the true data distribution
- and the discriminator is equal to 0 on the data manifold, the
- gradient penalty ensures that the discriminator cannot create
- a non-zero gradient orthogonal to the data manifold without
- suffering a loss in the GAN game.
-
- Ref:
- Eq. 9 in Which training methods for GANs do actually converge.
- """
- grad_real = autograd.grad(outputs=real_pred.sum(), inputs=real_img, create_graph=True)[0]
- grad_penalty = grad_real.pow(2).view(grad_real.shape[0], -1).sum(1).mean()
- return grad_penalty
-
-
-def g_path_regularize(fake_img, latents, mean_path_length, decay=0.01):
- noise = torch.randn_like(fake_img) / math.sqrt(fake_img.shape[2] * fake_img.shape[3])
- grad = autograd.grad(outputs=(fake_img * noise).sum(), inputs=latents, create_graph=True)[0]
- path_lengths = torch.sqrt(grad.pow(2).sum(2).mean(1))
-
- path_mean = mean_path_length + decay * (path_lengths.mean() - mean_path_length)
-
- path_penalty = (path_lengths - path_mean).pow(2).mean()
-
- return path_penalty, path_lengths.detach().mean(), path_mean.detach()
-
-
-def gradient_penalty_loss(discriminator, real_data, fake_data, weight=None):
- """Calculate gradient penalty for wgan-gp.
-
- Args:
- discriminator (nn.Module): Network for the discriminator.
- real_data (Tensor): Real input data.
- fake_data (Tensor): Fake input data.
- weight (Tensor): Weight tensor. Default: None.
-
- Returns:
- Tensor: A tensor for gradient penalty.
- """
-
- batch_size = real_data.size(0)
- alpha = real_data.new_tensor(torch.rand(batch_size, 1, 1, 1))
-
- # interpolate between real_data and fake_data
- interpolates = alpha * real_data + (1. - alpha) * fake_data
- interpolates = autograd.Variable(interpolates, requires_grad=True)
-
- disc_interpolates = discriminator(interpolates)
- gradients = autograd.grad(
- outputs=disc_interpolates,
- inputs=interpolates,
- grad_outputs=torch.ones_like(disc_interpolates),
- create_graph=True,
- retain_graph=True,
- only_inputs=True)[0]
-
- if weight is not None:
- gradients = gradients * weight
-
- gradients_penalty = ((gradients.norm(2, dim=1) - 1)**2).mean()
- if weight is not None:
- gradients_penalty /= torch.mean(weight)
-
- return gradients_penalty
diff --git a/spaces/csuhan/LLaMA-Adapter/app.py b/spaces/csuhan/LLaMA-Adapter/app.py
deleted file mode 100644
index 70e93b3f4263bbc5ae6cf555851a40f83feacfdb..0000000000000000000000000000000000000000
--- a/spaces/csuhan/LLaMA-Adapter/app.py
+++ /dev/null
@@ -1,278 +0,0 @@
-import json
-import os
-import glob
-import sys
-import time
-from pathlib import Path
-from typing import Tuple
-
-from huggingface_hub import hf_hub_download
-from PIL import Image
-import gradio as gr
-import torch
-from fairscale.nn.model_parallel.initialize import initialize_model_parallel
-
-from llama import LLaMA, ModelArgs, Tokenizer, Transformer, VisionModel
-
-os.environ['CUDA_LAUNCH_BLOCKING'] = '1'
-
-PROMPT_DICT = {
- "prompt_input": (
- "Below is an instruction that describes a task, paired with an input that provides further context. "
- "Write a response that appropriately completes the request.\n\n"
- "### Instruction:\n{instruction}\n\n### Input:\n{input}\n\n### Response:"
- ),
- "prompt_no_input": (
- "Below is an instruction that describes a task. "
- "Write a response that appropriately completes the request.\n\n"
- "### Instruction:\n{instruction}\n\n### Response:"
- ),
-}
-
-
-def setup_model_parallel() -> Tuple[int, int]:
- os.environ['RANK'] = '0'
- os.environ['WORLD_SIZE'] = '1'
- os.environ['MP'] = '1'
- os.environ['MASTER_ADDR'] = '127.0.0.1'
- os.environ['MASTER_PORT'] = '2223'
- local_rank = int(os.environ.get("LOCAL_RANK", -1))
- world_size = int(os.environ.get("WORLD_SIZE", -1))
-
- torch.distributed.init_process_group("nccl")
- initialize_model_parallel(world_size)
- torch.cuda.set_device(local_rank)
-
- # seed must be the same in all processes
- torch.manual_seed(1)
- return local_rank, world_size
-
-
-def load(
- ckpt0_path: str,
- ckpt1_path: str,
- param_path: str,
- tokenizer_path: str,
- instruct_adapter_path: str,
- caption_adapter_path: str,
- local_rank: int,
- world_size: int,
- max_seq_len: int,
- max_batch_size: int,
-) -> LLaMA:
- start_time = time.time()
- print("Loading")
- instruct_adapter_checkpoint = torch.load(
- instruct_adapter_path, map_location="cpu")
- caption_adapter_checkpoint = torch.load(
- caption_adapter_path, map_location="cpu")
- with open(param_path, "r") as f:
- params = json.loads(f.read())
-
- model_args: ModelArgs = ModelArgs(
- max_seq_len=max_seq_len, max_batch_size=max_batch_size, **params
- )
- model_args.adapter_layer = int(
- instruct_adapter_checkpoint['adapter_query.weight'].shape[0] / model_args.adapter_len)
- model_args.cap_adapter_layer = int(
- caption_adapter_checkpoint['cap_adapter_query.weight'].shape[0] / model_args.cap_adapter_len)
-
- tokenizer = Tokenizer(model_path=tokenizer_path)
- model_args.vocab_size = tokenizer.n_words
- torch.set_default_tensor_type(torch.cuda.HalfTensor)
- model = Transformer(model_args)
-
- # To reduce memory usuage
- ckpt0 = torch.load(ckpt0_path, map_location='cuda')
- model.load_state_dict(ckpt0, strict=False)
- del ckpt0
- torch.cuda.empty_cache()
-
- ckpt1 = torch.load(ckpt1_path, map_location='cuda')
- model.load_state_dict(ckpt1, strict=False)
- del ckpt1
- torch.cuda.empty_cache()
-
- vision_model = VisionModel(model_args)
-
- torch.set_default_tensor_type(torch.FloatTensor)
- model.load_state_dict(instruct_adapter_checkpoint, strict=False)
- model.load_state_dict(caption_adapter_checkpoint, strict=False)
- vision_model.load_state_dict(caption_adapter_checkpoint, strict=False)
-
- generator = LLaMA(model, tokenizer, vision_model)
- print(f"Loaded in {time.time() - start_time:.2f} seconds")
- return generator
-
-
-def instruct_generate(
- instruct: str,
- input: str = 'none',
- max_gen_len=512,
- temperature: float = 0.1,
- top_p: float = 0.75,
-):
- if input == 'none':
- prompt = PROMPT_DICT['prompt_no_input'].format_map(
- {'instruction': instruct, 'input': ''})
- else:
- prompt = PROMPT_DICT['prompt_input'].format_map(
- {'instruction': instruct, 'input': input})
-
- results = generator.generate(
- [prompt], max_gen_len=max_gen_len, temperature=temperature, top_p=top_p
- )
- result = results[0].strip()
- print(result)
- return result
-
-
-def caption_generate(
- img: str,
- max_gen_len=512,
- temperature: float = 0.1,
- top_p: float = 0.75,
-):
- imgs = [Image.open(img).convert('RGB')]
- prompts = ["Generate caption of this image :",] * len(imgs)
-
- results = generator.generate(
- prompts, imgs=imgs, max_gen_len=max_gen_len, temperature=temperature, top_p=top_p
- )
- result = results[0].strip()
- print(result)
- return result
-
-
-def download_llama_adapter(instruct_adapter_path, caption_adapter_path):
- if not os.path.exists(instruct_adapter_path):
- os.system(
- f"wget -q -O {instruct_adapter_path} https://github.com/OpenGVLab/LLaMA-Adapter/releases/download/v.1.0.0/llama_adapter_len10_layer30_release.pth")
-
- if not os.path.exists(caption_adapter_path):
- os.system(
- f"wget -q -O {caption_adapter_path} https://github.com/OpenGVLab/LLaMA-Adapter/releases/download/v.1.0.0/llama_adapter_len10_layer30_caption_vit_l.pth")
-
-
-# ckpt_path = "/data1/llma/7B/consolidated.00.pth"
-# param_path = "/data1/llma/7B/params.json"
-# tokenizer_path = "/data1/llma/tokenizer.model"
-ckpt0_path = hf_hub_download(
- repo_id="csuhan/llama_storage", filename="consolidated.00_part0.pth")
-ckpt1_path = hf_hub_download(
- repo_id="csuhan/llama_storage", filename="consolidated.00_part1.pth")
-param_path = hf_hub_download(
- repo_id="nyanko7/LLaMA-7B", filename="params.json")
-tokenizer_path = hf_hub_download(
- repo_id="nyanko7/LLaMA-7B", filename="tokenizer.model")
-instruct_adapter_path = "llama_adapter_len10_layer30_release.pth"
-caption_adapter_path = "llama_adapter_len10_layer30_caption_vit_l.pth"
-max_seq_len = 512
-max_batch_size = 1
-
-# download models
-# download_llama_adapter(instruct_adapter_path, caption_adapter_path)
-
-
-local_rank, world_size = setup_model_parallel()
-if local_rank > 0:
- sys.stdout = open(os.devnull, "w")
-
-generator = load(
- ckpt0_path, ckpt1_path, param_path, tokenizer_path, instruct_adapter_path, caption_adapter_path, local_rank, world_size, max_seq_len, max_batch_size
-)
-
-
-def create_instruct_demo():
- with gr.Blocks() as instruct_demo:
- with gr.Row():
- with gr.Column():
- instruction = gr.Textbox(lines=2, label="Instruction")
- input = gr.Textbox(
- lines=2, label="Context input", placeholder='none')
- max_len = gr.Slider(minimum=1, maximum=512,
- value=128, label="Max length")
- with gr.Accordion(label='Advanced options', open=False):
- temp = gr.Slider(minimum=0, maximum=1,
- value=0.1, label="Temperature")
- top_p = gr.Slider(minimum=0, maximum=1,
- value=0.75, label="Top p")
-
- run_botton = gr.Button("Run")
-
- with gr.Column():
- outputs = gr.Textbox(lines=10, label="Output")
-
- inputs = [instruction, input, max_len, temp, top_p]
-
- examples = [
- "Tell me about alpacas.",
- "Write a Python program that prints the first 10 Fibonacci numbers.",
- "Write a conversation between the sun and pluto.",
- "Write a theory to explain why cat never existed",
- ]
- examples = [
- [x, "none", 128, 0.1, 0.75]
- for x in examples]
-
- gr.Examples(
- examples=examples,
- inputs=inputs,
- outputs=outputs,
- fn=instruct_generate,
- cache_examples=os.getenv('SYSTEM') == 'spaces'
- )
- run_botton.click(fn=instruct_generate, inputs=inputs, outputs=outputs)
- return instruct_demo
-
-
-def create_caption_demo():
- with gr.Blocks() as instruct_demo:
- with gr.Row():
- with gr.Column():
- img = gr.Image(label='Input', type='filepath')
- max_len = gr.Slider(minimum=1, maximum=512,
- value=64, label="Max length")
- with gr.Accordion(label='Advanced options', open=False):
- temp = gr.Slider(minimum=0, maximum=1,
- value=0.1, label="Temperature")
- top_p = gr.Slider(minimum=0, maximum=1,
- value=0.75, label="Top p")
-
- run_botton = gr.Button("Run")
-
- with gr.Column():
- outputs = gr.Textbox(lines=10, label="Output")
-
- inputs = [img, max_len, temp, top_p]
-
- examples = glob.glob("caption_demo/*.jpg")
- examples = [
- [x, 64, 0.1, 0.75]
- for x in examples]
-
- gr.Examples(
- examples=examples,
- inputs=inputs,
- outputs=outputs,
- fn=caption_generate,
- cache_examples=os.getenv('SYSTEM') == 'spaces'
- )
- run_botton.click(fn=caption_generate, inputs=inputs, outputs=outputs)
- return instruct_demo
-
-
-description = """
-# LLaMA-Adapter 🚀
-The official demo for **LLaMA-Adapter: Efficient Fine-tuning of Language Models with Zero-init Attention**.
-Please refer to our [arXiv paper](https://arxiv.org/abs/2303.16199) and [github](https://github.com/ZrrSkywalker/LLaMA-Adapter) for more details.
-"""
-
-with gr.Blocks(css='style.css') as demo:
- gr.Markdown(description)
- with gr.TabItem("Instruction-Following"):
- create_instruct_demo()
- with gr.TabItem("Image Captioning"):
- create_caption_demo()
-
-demo.queue(api_open=True, concurrency_count=1).launch()
diff --git a/spaces/curt-tigges/anime-image-labeller/app.py b/spaces/curt-tigges/anime-image-labeller/app.py
deleted file mode 100644
index 3471531b2b91a7e5f6c91ec8d91cfd89c00f1997..0000000000000000000000000000000000000000
--- a/spaces/curt-tigges/anime-image-labeller/app.py
+++ /dev/null
@@ -1,51 +0,0 @@
-import gradio as gr
-import fastbook
-fastbook.setup_book()
-from fastbook import *
-
-"""
-Get the prediction labels and their accuracies, then return the results as a dictionary.
-
-[obj] - tensor matrix containing the predicted accuracy given from the model
-[learn] - fastai learner needed to get the labels
-[thresh] - minimum accuracy threshold to returning results
-"""
-def get_pred_classes(obj, learn, thresh):
- labels = []
- # get list of classes from csv--replace
- with open('classes.txt', 'r') as f:
- for line in f:
- labels.append(line.strip('\n'))
-
- predictions = {}
- x=0
- for item in obj:
- acc= round(item.item(), 3)
- if acc > thresh:
- predictions[labels[x]] = round(acc, 3)
- x+=1
-
- predictions =sorted(predictions.items(), key=lambda x: x[1], reverse=True)
-
- return predictions
-
-def get_x(r): return 'images'/r['img_name']
-def get_y(r): return [t for t in r['tags'].split(' ') if t in pop_tags]
-
-learn = load_learner(fname='model-large-basic-10e.pkl')
-
-def predict_single_img(imf, thresh=0.2, learn=learn):
-
- img = PILImage.create(imf)
-
- #img.show() #show image
- _, _, pred_pct = learn.predict(img) #predict while ignoring first 2 array inputs
- img.show() #show image
- return str(get_pred_classes(pred_pct, learn, thresh))
-
-#predict_single_img('test/mask.jpeg')
-
-iface = gr.Interface(fn=predict_single_img,
- inputs=["image","number"],
- outputs="text")
-iface.launch()
\ No newline at end of file
diff --git a/spaces/damian0815/Erasing-Concepts-In-Diffusion/README.md b/spaces/damian0815/Erasing-Concepts-In-Diffusion/README.md
deleted file mode 100644
index e84fe526daf7cb3931ff800af48fddf3fe6dd616..0000000000000000000000000000000000000000
--- a/spaces/damian0815/Erasing-Concepts-In-Diffusion/README.md
+++ /dev/null
@@ -1,67 +0,0 @@
----
-title: Erasing Concepts from Diffusion Models
-emoji: 💡
-colorFrom: indigo
-colorTo: gray
-sdk: gradio
-sdk_version: 3.21.0
-app_file: app.py
-pinned: false
-license: mit
----
-
-# A GUI with custom model support, validation, and sample generation for "Erasing Concepts from Diffusion Models"
-
-Enables xformers, 8 bit AdamW via bitsandbytes, and AMP - editing SD1.5 models works with 16GB VRAM, and 2.5 models including the ESD-u training works with 24GB VRAM.
-
-## Quick start
-
-To run on vast.ai, use eg `pytorch/pytorch:2.0.1-cuda11.7-cudnn8-devel` - you need `-devel` for 8bit AdamW to work.
-
-On the dev machine:
-```
-pip install -r requirements.txt
-python app.py
-```
-
-then use the Gradio interface at port 7860.
-
-# Erasing Concepts from Diffusion Models
-
- Project Website [https://erasing.baulab.info](https://erasing.baulab.info)
- Arxiv Preprint [https://arxiv.org/pdf/2303.07345.pdf](https://arxiv.org/pdf/2303.07345.pdf)
- Fine-tuned Weights [https://erasing.baulab.info/weights/esd_models/](https://erasing.baulab.info/weights/esd_models/)
-
-
-
-
-Motivated by recent advancements in text-to-image diffusion, we study erasure of specific concepts from the model's weights. While Stable Diffusion has shown promise in producing explicit or realistic artwork, it has raised concerns regarding its potential for misuse. We propose a fine-tuning method that can erase a visual concept from a pre-trained diffusion model, given only the name of the style and using negative guidance as a teacher. We benchmark our method against previous approaches that remove sexually explicit content and demonstrate its effectiveness, performing on par with Safe Latent Diffusion and censored training.
-
-To evaluate artistic style removal, we conduct experiments erasing five modern artists from the network and conduct a user study to assess the human perception of the removed styles. Unlike previous methods, our approach can remove concepts from a diffusion model permanently rather than modifying the output at the inference time, so it cannot be circumvented even if a user has access to model weights
-
-Given only a short text description of an undesired visual concept and no additional data, our method fine-tunes model weights to erase the targeted concept. Our method can avoid NSFW content, stop imitation of a specific artist's style, or even erase a whole object class from model output, while preserving the model's behavior and capabilities on other topics.
-
-## Demo vs github
-
-This demo uses an updated implementation from the original Erasing codebase the publication is based from.
-
-## Running locally
-
-1.) Create an environment using the packages included in the requirements.txt file
-
-2.) Run `python app.py`
-
-3.) Open the application in browser at `http://127.0.0.1:7860/`
-
-4.) Train, evaluate, and save models using our method
-
-## Citing our work
-The preprint can be cited as follows
-```
-@article{gandikota2023erasing,
- title={Erasing Concepts from Diffusion Models},
- author={Rohit Gandikota and Joanna Materzy\'nska and Jaden Fiotto-Kaufman and David Bau},
- journal={arXiv preprint arXiv:2303.07345},
- year={2023}
-}
-```
\ No newline at end of file
diff --git a/spaces/dantosxd/gorilla-llm-gorilla-mpt-7b-hf-v0/app.py b/spaces/dantosxd/gorilla-llm-gorilla-mpt-7b-hf-v0/app.py
deleted file mode 100644
index 4766368fca968f88fe2ba2293e9f85ffa4b825bd..0000000000000000000000000000000000000000
--- a/spaces/dantosxd/gorilla-llm-gorilla-mpt-7b-hf-v0/app.py
+++ /dev/null
@@ -1,3 +0,0 @@
-import gradio as gr
-
-gr.Interface.load("models/gorilla-llm/gorilla-mpt-7b-hf-v0").launch()
\ No newline at end of file
diff --git a/spaces/dawdqd/ChuanhuChatGPT/modules/overwrites.py b/spaces/dawdqd/ChuanhuChatGPT/modules/overwrites.py
deleted file mode 100644
index a4ef6167eb7ce75ed8b88024ad1187b24f2fc191..0000000000000000000000000000000000000000
--- a/spaces/dawdqd/ChuanhuChatGPT/modules/overwrites.py
+++ /dev/null
@@ -1,106 +0,0 @@
-from __future__ import annotations
-import logging
-
-from typing import List, Tuple
-from gradio_client import utils as client_utils
-from gradio import utils
-import inspect
-
-from modules.presets import *
-from modules.index_func import *
-
-
-def postprocess(
- self,
- y: List[List[str | Tuple[str] | Tuple[str, str] | None] | Tuple],
- ) -> List[List[str | Dict | None]]:
- """
- Parameters:
- y: List of lists representing the message and response pairs. Each message and response should be a string, which may be in Markdown format. It can also be a tuple whose first element is a string filepath or URL to an image/video/audio, and second (optional) element is the alt text, in which case the media file is displayed. It can also be None, in which case that message is not displayed.
- Returns:
- List of lists representing the message and response. Each message and response will be a string of HTML, or a dictionary with media information. Or None if the message is not to be displayed.
- """
- if y is None:
- return []
- processed_messages = []
- for message_pair in y:
- assert isinstance(
- message_pair, (tuple, list)
- ), f"Expected a list of lists or list of tuples. Received: {message_pair}"
- assert (
- len(message_pair) == 2
- ), f"Expected a list of lists of length 2 or list of tuples of length 2. Received: {message_pair}"
-
- processed_messages.append(
- [
- self._postprocess_chat_messages(message_pair[0], "user"),
- self._postprocess_chat_messages(message_pair[1], "bot"),
- ]
- )
- return processed_messages
-
-def postprocess_chat_messages(
- self, chat_message: str | tuple | list | None, role: str
- ) -> str | dict | None:
- if chat_message is None:
- return None
- elif isinstance(chat_message, (tuple, list)):
- file_uri = chat_message[0]
- if utils.validate_url(file_uri):
- filepath = file_uri
- else:
- filepath = self.make_temp_copy_if_needed(file_uri)
-
- mime_type = client_utils.get_mimetype(filepath)
- return {
- "name": filepath,
- "mime_type": mime_type,
- "alt_text": chat_message[1] if len(chat_message) > 1 else None,
- "data": None, # These last two fields are filled in by the frontend
- "is_file": True,
- }
- elif isinstance(chat_message, str):
- # chat_message = inspect.cleandoc(chat_message)
- # escape html spaces
- # chat_message = chat_message.replace(" ", " ")
- if role == "bot":
- chat_message = convert_bot_before_marked(chat_message)
- elif role == "user":
- chat_message = convert_user_before_marked(chat_message)
- return chat_message
- else:
- raise ValueError(f"Invalid message for Chatbot component: {chat_message}")
-
-
-
-def add_classes_to_gradio_component(comp):
- """
- this adds gradio-* to the component for css styling (ie gradio-button to gr.Button), as well as some others
- code from stable-diffusion-webui
- """
-
- comp.elem_classes = [f"gradio-{comp.get_block_name()}", *(comp.elem_classes or [])]
-
- if getattr(comp, 'multiselect', False):
- comp.elem_classes.append('multiselect')
-
-
-def IOComponent_init(self, *args, **kwargs):
- res = original_IOComponent_init(self, *args, **kwargs)
- add_classes_to_gradio_component(self)
-
- return res
-
-original_IOComponent_init = gr.components.IOComponent.__init__
-gr.components.IOComponent.__init__ = IOComponent_init
-
-
-def BlockContext_init(self, *args, **kwargs):
- res = original_BlockContext_init(self, *args, **kwargs)
- add_classes_to_gradio_component(self)
-
- return res
-
-original_BlockContext_init = gr.blocks.BlockContext.__init__
-gr.blocks.BlockContext.__init__ = BlockContext_init
-
diff --git a/spaces/dawdqd/ChuanhuChatGPT/web_assets/javascript/external-scripts.js b/spaces/dawdqd/ChuanhuChatGPT/web_assets/javascript/external-scripts.js
deleted file mode 100644
index 8d0352669045537af5698b1824dbc1dba21df478..0000000000000000000000000000000000000000
--- a/spaces/dawdqd/ChuanhuChatGPT/web_assets/javascript/external-scripts.js
+++ /dev/null
@@ -1,2 +0,0 @@
-
-// external javascript here
diff --git a/spaces/dcarpintero/nlp-summarizer-pegasus/.venv/lib/python3.9/site-packages/dateutil/tz/win.py b/spaces/dcarpintero/nlp-summarizer-pegasus/.venv/lib/python3.9/site-packages/dateutil/tz/win.py
deleted file mode 100644
index cde07ba792c40903f0c334839140173b39fd8124..0000000000000000000000000000000000000000
--- a/spaces/dcarpintero/nlp-summarizer-pegasus/.venv/lib/python3.9/site-packages/dateutil/tz/win.py
+++ /dev/null
@@ -1,370 +0,0 @@
-# -*- coding: utf-8 -*-
-"""
-This module provides an interface to the native time zone data on Windows,
-including :py:class:`datetime.tzinfo` implementations.
-
-Attempting to import this module on a non-Windows platform will raise an
-:py:obj:`ImportError`.
-"""
-# This code was originally contributed by Jeffrey Harris.
-import datetime
-import struct
-
-from six.moves import winreg
-from six import text_type
-
-try:
- import ctypes
- from ctypes import wintypes
-except ValueError:
- # ValueError is raised on non-Windows systems for some horrible reason.
- raise ImportError("Running tzwin on non-Windows system")
-
-from ._common import tzrangebase
-
-__all__ = ["tzwin", "tzwinlocal", "tzres"]
-
-ONEWEEK = datetime.timedelta(7)
-
-TZKEYNAMENT = r"SOFTWARE\Microsoft\Windows NT\CurrentVersion\Time Zones"
-TZKEYNAME9X = r"SOFTWARE\Microsoft\Windows\CurrentVersion\Time Zones"
-TZLOCALKEYNAME = r"SYSTEM\CurrentControlSet\Control\TimeZoneInformation"
-
-
-def _settzkeyname():
- handle = winreg.ConnectRegistry(None, winreg.HKEY_LOCAL_MACHINE)
- try:
- winreg.OpenKey(handle, TZKEYNAMENT).Close()
- TZKEYNAME = TZKEYNAMENT
- except WindowsError:
- TZKEYNAME = TZKEYNAME9X
- handle.Close()
- return TZKEYNAME
-
-
-TZKEYNAME = _settzkeyname()
-
-
-class tzres(object):
- """
- Class for accessing ``tzres.dll``, which contains timezone name related
- resources.
-
- .. versionadded:: 2.5.0
- """
- p_wchar = ctypes.POINTER(wintypes.WCHAR) # Pointer to a wide char
-
- def __init__(self, tzres_loc='tzres.dll'):
- # Load the user32 DLL so we can load strings from tzres
- user32 = ctypes.WinDLL('user32')
-
- # Specify the LoadStringW function
- user32.LoadStringW.argtypes = (wintypes.HINSTANCE,
- wintypes.UINT,
- wintypes.LPWSTR,
- ctypes.c_int)
-
- self.LoadStringW = user32.LoadStringW
- self._tzres = ctypes.WinDLL(tzres_loc)
- self.tzres_loc = tzres_loc
-
- def load_name(self, offset):
- """
- Load a timezone name from a DLL offset (integer).
-
- >>> from dateutil.tzwin import tzres
- >>> tzr = tzres()
- >>> print(tzr.load_name(112))
- 'Eastern Standard Time'
-
- :param offset:
- A positive integer value referring to a string from the tzres dll.
-
- .. note::
-
- Offsets found in the registry are generally of the form
- ``@tzres.dll,-114``. The offset in this case is 114, not -114.
-
- """
- resource = self.p_wchar()
- lpBuffer = ctypes.cast(ctypes.byref(resource), wintypes.LPWSTR)
- nchar = self.LoadStringW(self._tzres._handle, offset, lpBuffer, 0)
- return resource[:nchar]
-
- def name_from_string(self, tzname_str):
- """
- Parse strings as returned from the Windows registry into the time zone
- name as defined in the registry.
-
- >>> from dateutil.tzwin import tzres
- >>> tzr = tzres()
- >>> print(tzr.name_from_string('@tzres.dll,-251'))
- 'Dateline Daylight Time'
- >>> print(tzr.name_from_string('Eastern Standard Time'))
- 'Eastern Standard Time'
-
- :param tzname_str:
- A timezone name string as returned from a Windows registry key.
-
- :return:
- Returns the localized timezone string from tzres.dll if the string
- is of the form `@tzres.dll,-offset`, else returns the input string.
- """
- if not tzname_str.startswith('@'):
- return tzname_str
-
- name_splt = tzname_str.split(',-')
- try:
- offset = int(name_splt[1])
- except:
- raise ValueError("Malformed timezone string.")
-
- return self.load_name(offset)
-
-
-class tzwinbase(tzrangebase):
- """tzinfo class based on win32's timezones available in the registry."""
- def __init__(self):
- raise NotImplementedError('tzwinbase is an abstract base class')
-
- def __eq__(self, other):
- # Compare on all relevant dimensions, including name.
- if not isinstance(other, tzwinbase):
- return NotImplemented
-
- return (self._std_offset == other._std_offset and
- self._dst_offset == other._dst_offset and
- self._stddayofweek == other._stddayofweek and
- self._dstdayofweek == other._dstdayofweek and
- self._stdweeknumber == other._stdweeknumber and
- self._dstweeknumber == other._dstweeknumber and
- self._stdhour == other._stdhour and
- self._dsthour == other._dsthour and
- self._stdminute == other._stdminute and
- self._dstminute == other._dstminute and
- self._std_abbr == other._std_abbr and
- self._dst_abbr == other._dst_abbr)
-
- @staticmethod
- def list():
- """Return a list of all time zones known to the system."""
- with winreg.ConnectRegistry(None, winreg.HKEY_LOCAL_MACHINE) as handle:
- with winreg.OpenKey(handle, TZKEYNAME) as tzkey:
- result = [winreg.EnumKey(tzkey, i)
- for i in range(winreg.QueryInfoKey(tzkey)[0])]
- return result
-
- def display(self):
- """
- Return the display name of the time zone.
- """
- return self._display
-
- def transitions(self, year):
- """
- For a given year, get the DST on and off transition times, expressed
- always on the standard time side. For zones with no transitions, this
- function returns ``None``.
-
- :param year:
- The year whose transitions you would like to query.
-
- :return:
- Returns a :class:`tuple` of :class:`datetime.datetime` objects,
- ``(dston, dstoff)`` for zones with an annual DST transition, or
- ``None`` for fixed offset zones.
- """
-
- if not self.hasdst:
- return None
-
- dston = picknthweekday(year, self._dstmonth, self._dstdayofweek,
- self._dsthour, self._dstminute,
- self._dstweeknumber)
-
- dstoff = picknthweekday(year, self._stdmonth, self._stddayofweek,
- self._stdhour, self._stdminute,
- self._stdweeknumber)
-
- # Ambiguous dates default to the STD side
- dstoff -= self._dst_base_offset
-
- return dston, dstoff
-
- def _get_hasdst(self):
- return self._dstmonth != 0
-
- @property
- def _dst_base_offset(self):
- return self._dst_base_offset_
-
-
-class tzwin(tzwinbase):
- """
- Time zone object created from the zone info in the Windows registry
-
- These are similar to :py:class:`dateutil.tz.tzrange` objects in that
- the time zone data is provided in the format of a single offset rule
- for either 0 or 2 time zone transitions per year.
-
- :param: name
- The name of a Windows time zone key, e.g. "Eastern Standard Time".
- The full list of keys can be retrieved with :func:`tzwin.list`.
- """
-
- def __init__(self, name):
- self._name = name
-
- with winreg.ConnectRegistry(None, winreg.HKEY_LOCAL_MACHINE) as handle:
- tzkeyname = text_type("{kn}\\{name}").format(kn=TZKEYNAME, name=name)
- with winreg.OpenKey(handle, tzkeyname) as tzkey:
- keydict = valuestodict(tzkey)
-
- self._std_abbr = keydict["Std"]
- self._dst_abbr = keydict["Dlt"]
-
- self._display = keydict["Display"]
-
- # See http://ww_winreg.jsiinc.com/SUBA/tip0300/rh0398.htm
- tup = struct.unpack("=3l16h", keydict["TZI"])
- stdoffset = -tup[0]-tup[1] # Bias + StandardBias * -1
- dstoffset = stdoffset-tup[2] # + DaylightBias * -1
- self._std_offset = datetime.timedelta(minutes=stdoffset)
- self._dst_offset = datetime.timedelta(minutes=dstoffset)
-
- # for the meaning see the win32 TIME_ZONE_INFORMATION structure docs
- # http://msdn.microsoft.com/en-us/library/windows/desktop/ms725481(v=vs.85).aspx
- (self._stdmonth,
- self._stddayofweek, # Sunday = 0
- self._stdweeknumber, # Last = 5
- self._stdhour,
- self._stdminute) = tup[4:9]
-
- (self._dstmonth,
- self._dstdayofweek, # Sunday = 0
- self._dstweeknumber, # Last = 5
- self._dsthour,
- self._dstminute) = tup[12:17]
-
- self._dst_base_offset_ = self._dst_offset - self._std_offset
- self.hasdst = self._get_hasdst()
-
- def __repr__(self):
- return "tzwin(%s)" % repr(self._name)
-
- def __reduce__(self):
- return (self.__class__, (self._name,))
-
-
-class tzwinlocal(tzwinbase):
- """
- Class representing the local time zone information in the Windows registry
-
- While :class:`dateutil.tz.tzlocal` makes system calls (via the :mod:`time`
- module) to retrieve time zone information, ``tzwinlocal`` retrieves the
- rules directly from the Windows registry and creates an object like
- :class:`dateutil.tz.tzwin`.
-
- Because Windows does not have an equivalent of :func:`time.tzset`, on
- Windows, :class:`dateutil.tz.tzlocal` instances will always reflect the
- time zone settings *at the time that the process was started*, meaning
- changes to the machine's time zone settings during the run of a program
- on Windows will **not** be reflected by :class:`dateutil.tz.tzlocal`.
- Because ``tzwinlocal`` reads the registry directly, it is unaffected by
- this issue.
- """
- def __init__(self):
- with winreg.ConnectRegistry(None, winreg.HKEY_LOCAL_MACHINE) as handle:
- with winreg.OpenKey(handle, TZLOCALKEYNAME) as tzlocalkey:
- keydict = valuestodict(tzlocalkey)
-
- self._std_abbr = keydict["StandardName"]
- self._dst_abbr = keydict["DaylightName"]
-
- try:
- tzkeyname = text_type('{kn}\\{sn}').format(kn=TZKEYNAME,
- sn=self._std_abbr)
- with winreg.OpenKey(handle, tzkeyname) as tzkey:
- _keydict = valuestodict(tzkey)
- self._display = _keydict["Display"]
- except OSError:
- self._display = None
-
- stdoffset = -keydict["Bias"]-keydict["StandardBias"]
- dstoffset = stdoffset-keydict["DaylightBias"]
-
- self._std_offset = datetime.timedelta(minutes=stdoffset)
- self._dst_offset = datetime.timedelta(minutes=dstoffset)
-
- # For reasons unclear, in this particular key, the day of week has been
- # moved to the END of the SYSTEMTIME structure.
- tup = struct.unpack("=8h", keydict["StandardStart"])
-
- (self._stdmonth,
- self._stdweeknumber, # Last = 5
- self._stdhour,
- self._stdminute) = tup[1:5]
-
- self._stddayofweek = tup[7]
-
- tup = struct.unpack("=8h", keydict["DaylightStart"])
-
- (self._dstmonth,
- self._dstweeknumber, # Last = 5
- self._dsthour,
- self._dstminute) = tup[1:5]
-
- self._dstdayofweek = tup[7]
-
- self._dst_base_offset_ = self._dst_offset - self._std_offset
- self.hasdst = self._get_hasdst()
-
- def __repr__(self):
- return "tzwinlocal()"
-
- def __str__(self):
- # str will return the standard name, not the daylight name.
- return "tzwinlocal(%s)" % repr(self._std_abbr)
-
- def __reduce__(self):
- return (self.__class__, ())
-
-
-def picknthweekday(year, month, dayofweek, hour, minute, whichweek):
- """ dayofweek == 0 means Sunday, whichweek 5 means last instance """
- first = datetime.datetime(year, month, 1, hour, minute)
-
- # This will work if dayofweek is ISO weekday (1-7) or Microsoft-style (0-6),
- # Because 7 % 7 = 0
- weekdayone = first.replace(day=((dayofweek - first.isoweekday()) % 7) + 1)
- wd = weekdayone + ((whichweek - 1) * ONEWEEK)
- if (wd.month != month):
- wd -= ONEWEEK
-
- return wd
-
-
-def valuestodict(key):
- """Convert a registry key's values to a dictionary."""
- dout = {}
- size = winreg.QueryInfoKey(key)[1]
- tz_res = None
-
- for i in range(size):
- key_name, value, dtype = winreg.EnumValue(key, i)
- if dtype == winreg.REG_DWORD or dtype == winreg.REG_DWORD_LITTLE_ENDIAN:
- # If it's a DWORD (32-bit integer), it's stored as unsigned - convert
- # that to a proper signed integer
- if value & (1 << 31):
- value = value - (1 << 32)
- elif dtype == winreg.REG_SZ:
- # If it's a reference to the tzres DLL, load the actual string
- if value.startswith('@tzres'):
- tz_res = tz_res or tzres()
- value = tz_res.name_from_string(value)
-
- value = value.rstrip('\x00') # Remove trailing nulls
-
- dout[key_name] = value
-
- return dout
diff --git a/spaces/dcarpintero/nlp-summarizer-pegasus/.venv/lib/python3.9/site-packages/gradio/templates/frontend/assets/index-1af20794.css b/spaces/dcarpintero/nlp-summarizer-pegasus/.venv/lib/python3.9/site-packages/gradio/templates/frontend/assets/index-1af20794.css
deleted file mode 100644
index 365e58d88e7f8e9c541e689f1fc99edd253df80e..0000000000000000000000000000000000000000
--- a/spaces/dcarpintero/nlp-summarizer-pegasus/.venv/lib/python3.9/site-packages/gradio/templates/frontend/assets/index-1af20794.css
+++ /dev/null
@@ -1 +0,0 @@
-.rangeSlider{--pip:var(--range-pip, lightslategray);--pip-text:var(--range-pip-text, var(--pip));--pip-active:var(--range-pip-active, darkslategrey);--pip-active-text:var(--range-pip-active-text, var(--pip-active));--pip-hover:var(--range-pip-hover, darkslategrey);--pip-hover-text:var(--range-pip-hover-text, var(--pip-hover));--pip-in-range:var(--range-pip-in-range, var(--pip-active));--pip-in-range-text:var(--range-pip-in-range-text, var(--pip-active-text))}.rangePips{position:absolute;height:1em;left:0;right:0;bottom:-1em}.rangePips.vertical{height:auto;width:1em;inset:0 auto 0 100%}.rangePips .pip{height:.4em;position:absolute;top:.25em;width:1px;white-space:nowrap}.rangePips.vertical .pip{height:1px;width:.4em;left:.25em;top:auto;bottom:auto}.rangePips .pipVal{position:absolute;top:.4em;transform:translate(-50%,25%)}.rangePips.vertical .pipVal{position:absolute;top:0;left:.4em;transform:translate(25%,-50%)}.rangePips .pip{transition:all .15s ease}.rangePips .pipVal{transition:all .15s ease,font-weight 0s linear}.rangePips .pip{color:#789;color:var(--pip-text);background-color:#789;background-color:var(--pip)}.rangePips .pip.selected{color:#2f4f4f;color:var(--pip-active-text);background-color:#2f4f4f;background-color:var(--pip-active)}.rangePips.hoverable:not(.disabled) .pip:hover{color:#2f4f4f;color:var(--pip-hover-text);background-color:#2f4f4f;background-color:var(--pip-hover)}.rangePips .pip.in-range{color:#2f4f4f;color:var(--pip-in-range-text);background-color:#2f4f4f;background-color:var(--pip-in-range)}.rangePips .pip.selected{height:.75em}.rangePips.vertical .pip.selected{height:1px;width:.75em}.rangePips .pip.selected .pipVal{font-weight:700;top:.75em}.rangePips.vertical .pip.selected .pipVal{top:0;left:.75em}.rangePips.hoverable:not(.disabled) .pip:not(.selected):hover{transition:none}.rangePips.hoverable:not(.disabled) .pip:not(.selected):hover .pipVal{transition:none;font-weight:700}.rangeSlider{--slider:var(--range-slider, #d7dada);--handle-inactive:var(--range-handle-inactive, #99a2a2);--handle:var(--range-handle, #838de7);--handle-focus:var(--range-handle-focus, #4a40d4);--handle-border:var(--range-handle-border, var(--handle));--range-inactive:var(--range-range-inactive, var(--handle-inactive));--range:var(--range-range, var(--handle-focus));--float-inactive:var(--range-float-inactive, var(--handle-inactive));--float:var(--range-float, var(--handle-focus));--float-text:var(--range-float-text, white)}.rangeSlider{position:relative;border-radius:100px;height:.5em;margin:1em;transition:opacity .2s ease;user-select:none}.rangeSlider *{user-select:none}.rangeSlider.pips{margin-bottom:1.8em}.rangeSlider.pip-labels{margin-bottom:2.8em}.rangeSlider.vertical{display:inline-block;border-radius:100px;width:.5em;min-height:200px}.rangeSlider.vertical.pips{margin-right:1.8em;margin-bottom:1em}.rangeSlider.vertical.pip-labels{margin-right:2.8em;margin-bottom:1em}.rangeSlider .rangeHandle{position:absolute;display:block;height:1.4em;width:1.4em;top:.25em;bottom:auto;transform:translateY(-50%) translate(-50%);z-index:2}.rangeSlider.reversed .rangeHandle{transform:translateY(-50%) translate(50%)}.rangeSlider.vertical .rangeHandle{left:.25em;top:auto;transform:translateY(50%) translate(-50%)}.rangeSlider.vertical.reversed .rangeHandle{transform:translateY(-50%) translate(-50%)}.rangeSlider .rangeNub,.rangeSlider .rangeHandle:before{position:absolute;left:0;top:0;display:block;border-radius:10em;height:100%;width:100%;transition:box-shadow .2s ease}.rangeSlider .rangeHandle:before{content:"";inset:1px;height:auto;width:auto;box-shadow:0 0 0 0 var(--handle-border);opacity:0}.rangeSlider.hoverable:not(.disabled) .rangeHandle:hover:before{box-shadow:0 0 0 8px var(--handle-border);opacity:.2}.rangeSlider.hoverable:not(.disabled) .rangeHandle.press:before,.rangeSlider.hoverable:not(.disabled) .rangeHandle.press:hover:before{box-shadow:0 0 0 12px var(--handle-border);opacity:.4}.rangeSlider.range:not(.min):not(.max) .rangeNub{border-radius:10em 10em 10em 1.6em}.rangeSlider.range .rangeHandle:nth-of-type(1) .rangeNub{transform:rotate(-135deg)}.rangeSlider.range .rangeHandle:nth-of-type(2) .rangeNub{transform:rotate(45deg)}.rangeSlider.range.reversed .rangeHandle:nth-of-type(1) .rangeNub{transform:rotate(45deg)}.rangeSlider.range.reversed .rangeHandle:nth-of-type(2) .rangeNub{transform:rotate(-135deg)}.rangeSlider.range.vertical .rangeHandle:nth-of-type(1) .rangeNub{transform:rotate(135deg)}.rangeSlider.range.vertical .rangeHandle:nth-of-type(2) .rangeNub{transform:rotate(-45deg)}.rangeSlider.range.vertical.reversed .rangeHandle:nth-of-type(1) .rangeNub{transform:rotate(-45deg)}.rangeSlider.range.vertical.reversed .rangeHandle:nth-of-type(2) .rangeNub{transform:rotate(135deg)}.rangeSlider .rangeFloat{display:block;position:absolute;left:50%;top:-.5em;transform:translate(-50%,-100%);font-size:1em;text-align:center;opacity:0;pointer-events:none;white-space:nowrap;transition:all .2s ease;font-size:.9em;padding:.2em .4em;border-radius:.2em}.rangeSlider .rangeHandle.active .rangeFloat,.rangeSlider.hoverable .rangeHandle:hover .rangeFloat{opacity:1;top:-.2em;transform:translate(-50%,-100%)}.rangeSlider .rangeBar{position:absolute;display:block;transition:background .2s ease;border-radius:1em;height:.5em;top:0;user-select:none;z-index:1}.rangeSlider.vertical .rangeBar{width:.5em;height:auto}.rangeSlider{background-color:#d7dada;background-color:var(--slider)}.rangeSlider .rangeBar{background-color:#99a2a2;background-color:var(--range-inactive)}.rangeSlider.focus .rangeBar{background-color:#838de7;background-color:var(--range)}.rangeSlider .rangeNub{background-color:#99a2a2;background-color:var(--handle-inactive)}.rangeSlider.focus .rangeNub{background-color:#838de7;background-color:var(--handle)}.rangeSlider .rangeHandle.active .rangeNub{background-color:#4a40d4;background-color:var(--handle-focus)}.rangeSlider .rangeFloat{color:#fff;color:var(--float-text);background-color:#99a2a2;background-color:var(--float-inactive)}.rangeSlider.focus .rangeFloat{background-color:#4a40d4;background-color:var(--float)}.rangeSlider.disabled{opacity:.5}.rangeSlider.disabled .rangeNub{background-color:#d7dada;background-color:var(--slider)}.mic-wrap.svelte-1thnwz{padding:var(--size-2)}.record-icon.svelte-1thnwz{display:flex;position:relative;margin-right:var(--size-2);width:6px;height:6px}.dot.svelte-1thnwz{display:inline-flex;position:relative;border-radius:var(--radius-full);background:var(--color-red-500);width:6px;height:6px}.pinger.svelte-1thnwz{display:inline-flex;position:absolute;opacity:.9;animation:svelte-1thnwz-ping 1s cubic-bezier(0,0,.2,1) infinite;border-radius:var(--radius-full);background:var(--color-red-500);width:var(--size-full);height:var(--size-full)}@keyframes svelte-1thnwz-ping{75%,to{transform:scale(2);opacity:0}}audio.svelte-1thnwz{padding:var(--size-2);width:var(--size-full);height:var(--size-14)}audio.svelte-pq78xp{padding:var(--size-2);width:var(--size-full);height:var(--size-14)}.icon-buttons.svelte-pq78xp{display:flex;position:absolute;top:6px;right:6px;gap:var(--size-1)}
diff --git a/spaces/declare-lab/tango/diffusers/src/diffusers/pipelines/stable_diffusion_safe/__init__.py b/spaces/declare-lab/tango/diffusers/src/diffusers/pipelines/stable_diffusion_safe/__init__.py
deleted file mode 100644
index 5aecfeac112e53b2fc49278c1acaa95a6c0c7257..0000000000000000000000000000000000000000
--- a/spaces/declare-lab/tango/diffusers/src/diffusers/pipelines/stable_diffusion_safe/__init__.py
+++ /dev/null
@@ -1,71 +0,0 @@
-from dataclasses import dataclass
-from enum import Enum
-from typing import List, Optional, Union
-
-import numpy as np
-import PIL
-from PIL import Image
-
-from ...utils import BaseOutput, is_torch_available, is_transformers_available
-
-
-@dataclass
-class SafetyConfig(object):
- WEAK = {
- "sld_warmup_steps": 15,
- "sld_guidance_scale": 20,
- "sld_threshold": 0.0,
- "sld_momentum_scale": 0.0,
- "sld_mom_beta": 0.0,
- }
- MEDIUM = {
- "sld_warmup_steps": 10,
- "sld_guidance_scale": 1000,
- "sld_threshold": 0.01,
- "sld_momentum_scale": 0.3,
- "sld_mom_beta": 0.4,
- }
- STRONG = {
- "sld_warmup_steps": 7,
- "sld_guidance_scale": 2000,
- "sld_threshold": 0.025,
- "sld_momentum_scale": 0.5,
- "sld_mom_beta": 0.7,
- }
- MAX = {
- "sld_warmup_steps": 0,
- "sld_guidance_scale": 5000,
- "sld_threshold": 1.0,
- "sld_momentum_scale": 0.5,
- "sld_mom_beta": 0.7,
- }
-
-
-@dataclass
-class StableDiffusionSafePipelineOutput(BaseOutput):
- """
- Output class for Safe Stable Diffusion pipelines.
-
- Args:
- images (`List[PIL.Image.Image]` or `np.ndarray`)
- List of denoised PIL images of length `batch_size` or numpy array of shape `(batch_size, height, width,
- num_channels)`. PIL images or numpy array present the denoised images of the diffusion pipeline.
- nsfw_content_detected (`List[bool]`)
- List of flags denoting whether the corresponding generated image likely represents "not-safe-for-work"
- (nsfw) content, or `None` if safety checking could not be performed.
- images (`List[PIL.Image.Image]` or `np.ndarray`)
- List of denoised PIL images that were flagged by the safety checker any may contain "not-safe-for-work"
- (nsfw) content, or `None` if no safety check was performed or no images were flagged.
- applied_safety_concept (`str`)
- The safety concept that was applied for safety guidance, or `None` if safety guidance was disabled
- """
-
- images: Union[List[PIL.Image.Image], np.ndarray]
- nsfw_content_detected: Optional[List[bool]]
- unsafe_images: Optional[Union[List[PIL.Image.Image], np.ndarray]]
- applied_safety_concept: Optional[str]
-
-
-if is_transformers_available() and is_torch_available():
- from .pipeline_stable_diffusion_safe import StableDiffusionPipelineSafe
- from .safety_checker import SafeStableDiffusionSafetyChecker
diff --git a/spaces/declare-lab/tango/diffusers/src/diffusers/schedulers/scheduling_euler_ancestral_discrete.py b/spaces/declare-lab/tango/diffusers/src/diffusers/schedulers/scheduling_euler_ancestral_discrete.py
deleted file mode 100644
index 1b517bdec5703495afeee26a1c8ed4cb98561d7c..0000000000000000000000000000000000000000
--- a/spaces/declare-lab/tango/diffusers/src/diffusers/schedulers/scheduling_euler_ancestral_discrete.py
+++ /dev/null
@@ -1,309 +0,0 @@
-# Copyright 2023 Katherine Crowson and The HuggingFace Team. All rights reserved.
-#
-# Licensed under the Apache License, Version 2.0 (the "License");
-# you may not use this file except in compliance with the License.
-# You may obtain a copy of the License at
-#
-# http://www.apache.org/licenses/LICENSE-2.0
-#
-# Unless required by applicable law or agreed to in writing, software
-# distributed under the License is distributed on an "AS IS" BASIS,
-# WITHOUT WARRANTIES OR CONDITIONS OF ANY KIND, either express or implied.
-# See the License for the specific language governing permissions and
-# limitations under the License.
-
-import math
-from dataclasses import dataclass
-from typing import List, Optional, Tuple, Union
-
-import numpy as np
-import torch
-
-from ..configuration_utils import ConfigMixin, register_to_config
-from ..utils import BaseOutput, logging, randn_tensor
-from .scheduling_utils import KarrasDiffusionSchedulers, SchedulerMixin
-
-
-logger = logging.get_logger(__name__) # pylint: disable=invalid-name
-
-
-@dataclass
-# Copied from diffusers.schedulers.scheduling_ddpm.DDPMSchedulerOutput with DDPM->EulerAncestralDiscrete
-class EulerAncestralDiscreteSchedulerOutput(BaseOutput):
- """
- Output class for the scheduler's step function output.
-
- Args:
- prev_sample (`torch.FloatTensor` of shape `(batch_size, num_channels, height, width)` for images):
- Computed sample (x_{t-1}) of previous timestep. `prev_sample` should be used as next model input in the
- denoising loop.
- pred_original_sample (`torch.FloatTensor` of shape `(batch_size, num_channels, height, width)` for images):
- The predicted denoised sample (x_{0}) based on the model output from the current timestep.
- `pred_original_sample` can be used to preview progress or for guidance.
- """
-
- prev_sample: torch.FloatTensor
- pred_original_sample: Optional[torch.FloatTensor] = None
-
-
-# Copied from diffusers.schedulers.scheduling_ddpm.betas_for_alpha_bar
-def betas_for_alpha_bar(num_diffusion_timesteps, max_beta=0.999) -> torch.Tensor:
- """
- Create a beta schedule that discretizes the given alpha_t_bar function, which defines the cumulative product of
- (1-beta) over time from t = [0,1].
-
- Contains a function alpha_bar that takes an argument t and transforms it to the cumulative product of (1-beta) up
- to that part of the diffusion process.
-
-
- Args:
- num_diffusion_timesteps (`int`): the number of betas to produce.
- max_beta (`float`): the maximum beta to use; use values lower than 1 to
- prevent singularities.
-
- Returns:
- betas (`np.ndarray`): the betas used by the scheduler to step the model outputs
- """
-
- def alpha_bar(time_step):
- return math.cos((time_step + 0.008) / 1.008 * math.pi / 2) ** 2
-
- betas = []
- for i in range(num_diffusion_timesteps):
- t1 = i / num_diffusion_timesteps
- t2 = (i + 1) / num_diffusion_timesteps
- betas.append(min(1 - alpha_bar(t2) / alpha_bar(t1), max_beta))
- return torch.tensor(betas, dtype=torch.float32)
-
-
-class EulerAncestralDiscreteScheduler(SchedulerMixin, ConfigMixin):
- """
- Ancestral sampling with Euler method steps. Based on the original k-diffusion implementation by Katherine Crowson:
- https://github.com/crowsonkb/k-diffusion/blob/481677d114f6ea445aa009cf5bd7a9cdee909e47/k_diffusion/sampling.py#L72
-
- [`~ConfigMixin`] takes care of storing all config attributes that are passed in the scheduler's `__init__`
- function, such as `num_train_timesteps`. They can be accessed via `scheduler.config.num_train_timesteps`.
- [`SchedulerMixin`] provides general loading and saving functionality via the [`SchedulerMixin.save_pretrained`] and
- [`~SchedulerMixin.from_pretrained`] functions.
-
- Args:
- num_train_timesteps (`int`): number of diffusion steps used to train the model.
- beta_start (`float`): the starting `beta` value of inference.
- beta_end (`float`): the final `beta` value.
- beta_schedule (`str`):
- the beta schedule, a mapping from a beta range to a sequence of betas for stepping the model. Choose from
- `linear` or `scaled_linear`.
- trained_betas (`np.ndarray`, optional):
- option to pass an array of betas directly to the constructor to bypass `beta_start`, `beta_end` etc.
- prediction_type (`str`, default `epsilon`, optional):
- prediction type of the scheduler function, one of `epsilon` (predicting the noise of the diffusion
- process), `sample` (directly predicting the noisy sample`) or `v_prediction` (see section 2.4
- https://imagen.research.google/video/paper.pdf)
-
- """
-
- _compatibles = [e.name for e in KarrasDiffusionSchedulers]
- order = 1
-
- @register_to_config
- def __init__(
- self,
- num_train_timesteps: int = 1000,
- beta_start: float = 0.0001,
- beta_end: float = 0.02,
- beta_schedule: str = "linear",
- trained_betas: Optional[Union[np.ndarray, List[float]]] = None,
- prediction_type: str = "epsilon",
- ):
- if trained_betas is not None:
- self.betas = torch.tensor(trained_betas, dtype=torch.float32)
- elif beta_schedule == "linear":
- self.betas = torch.linspace(beta_start, beta_end, num_train_timesteps, dtype=torch.float32)
- elif beta_schedule == "scaled_linear":
- # this schedule is very specific to the latent diffusion model.
- self.betas = (
- torch.linspace(beta_start**0.5, beta_end**0.5, num_train_timesteps, dtype=torch.float32) ** 2
- )
- elif beta_schedule == "squaredcos_cap_v2":
- # Glide cosine schedule
- self.betas = betas_for_alpha_bar(num_train_timesteps)
- else:
- raise NotImplementedError(f"{beta_schedule} does is not implemented for {self.__class__}")
-
- self.alphas = 1.0 - self.betas
- self.alphas_cumprod = torch.cumprod(self.alphas, dim=0)
-
- sigmas = np.array(((1 - self.alphas_cumprod) / self.alphas_cumprod) ** 0.5)
- sigmas = np.concatenate([sigmas[::-1], [0.0]]).astype(np.float32)
- self.sigmas = torch.from_numpy(sigmas)
-
- # standard deviation of the initial noise distribution
- self.init_noise_sigma = self.sigmas.max()
-
- # setable values
- self.num_inference_steps = None
- timesteps = np.linspace(0, num_train_timesteps - 1, num_train_timesteps, dtype=float)[::-1].copy()
- self.timesteps = torch.from_numpy(timesteps)
- self.is_scale_input_called = False
-
- def scale_model_input(
- self, sample: torch.FloatTensor, timestep: Union[float, torch.FloatTensor]
- ) -> torch.FloatTensor:
- """
- Scales the denoising model input by `(sigma**2 + 1) ** 0.5` to match the Euler algorithm.
-
- Args:
- sample (`torch.FloatTensor`): input sample
- timestep (`float` or `torch.FloatTensor`): the current timestep in the diffusion chain
-
- Returns:
- `torch.FloatTensor`: scaled input sample
- """
- if isinstance(timestep, torch.Tensor):
- timestep = timestep.to(self.timesteps.device)
- step_index = (self.timesteps == timestep).nonzero().item()
- sigma = self.sigmas[step_index]
- sample = sample / ((sigma**2 + 1) ** 0.5)
- self.is_scale_input_called = True
- return sample
-
- def set_timesteps(self, num_inference_steps: int, device: Union[str, torch.device] = None):
- """
- Sets the timesteps used for the diffusion chain. Supporting function to be run before inference.
-
- Args:
- num_inference_steps (`int`):
- the number of diffusion steps used when generating samples with a pre-trained model.
- device (`str` or `torch.device`, optional):
- the device to which the timesteps should be moved to. If `None`, the timesteps are not moved.
- """
- self.num_inference_steps = num_inference_steps
-
- timesteps = np.linspace(0, self.config.num_train_timesteps - 1, num_inference_steps, dtype=float)[::-1].copy()
- sigmas = np.array(((1 - self.alphas_cumprod) / self.alphas_cumprod) ** 0.5)
- sigmas = np.interp(timesteps, np.arange(0, len(sigmas)), sigmas)
- sigmas = np.concatenate([sigmas, [0.0]]).astype(np.float32)
- self.sigmas = torch.from_numpy(sigmas).to(device=device)
- if str(device).startswith("mps"):
- # mps does not support float64
- self.timesteps = torch.from_numpy(timesteps).to(device, dtype=torch.float32)
- else:
- self.timesteps = torch.from_numpy(timesteps).to(device=device)
-
- def step(
- self,
- model_output: torch.FloatTensor,
- timestep: Union[float, torch.FloatTensor],
- sample: torch.FloatTensor,
- generator: Optional[torch.Generator] = None,
- return_dict: bool = True,
- ) -> Union[EulerAncestralDiscreteSchedulerOutput, Tuple]:
- """
- Predict the sample at the previous timestep by reversing the SDE. Core function to propagate the diffusion
- process from the learned model outputs (most often the predicted noise).
-
- Args:
- model_output (`torch.FloatTensor`): direct output from learned diffusion model.
- timestep (`float`): current timestep in the diffusion chain.
- sample (`torch.FloatTensor`):
- current instance of sample being created by diffusion process.
- generator (`torch.Generator`, optional): Random number generator.
- return_dict (`bool`): option for returning tuple rather than EulerAncestralDiscreteSchedulerOutput class
-
- Returns:
- [`~schedulers.scheduling_utils.EulerAncestralDiscreteSchedulerOutput`] or `tuple`:
- [`~schedulers.scheduling_utils.EulerAncestralDiscreteSchedulerOutput`] if `return_dict` is True, otherwise
- a `tuple`. When returning a tuple, the first element is the sample tensor.
-
- """
-
- if (
- isinstance(timestep, int)
- or isinstance(timestep, torch.IntTensor)
- or isinstance(timestep, torch.LongTensor)
- ):
- raise ValueError(
- (
- "Passing integer indices (e.g. from `enumerate(timesteps)`) as timesteps to"
- " `EulerDiscreteScheduler.step()` is not supported. Make sure to pass"
- " one of the `scheduler.timesteps` as a timestep."
- ),
- )
-
- if not self.is_scale_input_called:
- logger.warning(
- "The `scale_model_input` function should be called before `step` to ensure correct denoising. "
- "See `StableDiffusionPipeline` for a usage example."
- )
-
- if isinstance(timestep, torch.Tensor):
- timestep = timestep.to(self.timesteps.device)
-
- step_index = (self.timesteps == timestep).nonzero().item()
- sigma = self.sigmas[step_index]
-
- # 1. compute predicted original sample (x_0) from sigma-scaled predicted noise
- if self.config.prediction_type == "epsilon":
- pred_original_sample = sample - sigma * model_output
- elif self.config.prediction_type == "v_prediction":
- # * c_out + input * c_skip
- pred_original_sample = model_output * (-sigma / (sigma**2 + 1) ** 0.5) + (sample / (sigma**2 + 1))
- elif self.config.prediction_type == "sample":
- raise NotImplementedError("prediction_type not implemented yet: sample")
- else:
- raise ValueError(
- f"prediction_type given as {self.config.prediction_type} must be one of `epsilon`, or `v_prediction`"
- )
-
- sigma_from = self.sigmas[step_index]
- sigma_to = self.sigmas[step_index + 1]
- sigma_up = (sigma_to**2 * (sigma_from**2 - sigma_to**2) / sigma_from**2) ** 0.5
- sigma_down = (sigma_to**2 - sigma_up**2) ** 0.5
-
- # 2. Convert to an ODE derivative
- derivative = (sample - pred_original_sample) / sigma
-
- dt = sigma_down - sigma
-
- prev_sample = sample + derivative * dt
-
- device = model_output.device
- noise = randn_tensor(model_output.shape, dtype=model_output.dtype, device=device, generator=generator)
-
- prev_sample = prev_sample + noise * sigma_up
-
- if not return_dict:
- return (prev_sample,)
-
- return EulerAncestralDiscreteSchedulerOutput(
- prev_sample=prev_sample, pred_original_sample=pred_original_sample
- )
-
- def add_noise(
- self,
- original_samples: torch.FloatTensor,
- noise: torch.FloatTensor,
- timesteps: torch.FloatTensor,
- ) -> torch.FloatTensor:
- # Make sure sigmas and timesteps have the same device and dtype as original_samples
- self.sigmas = self.sigmas.to(device=original_samples.device, dtype=original_samples.dtype)
- if original_samples.device.type == "mps" and torch.is_floating_point(timesteps):
- # mps does not support float64
- self.timesteps = self.timesteps.to(original_samples.device, dtype=torch.float32)
- timesteps = timesteps.to(original_samples.device, dtype=torch.float32)
- else:
- self.timesteps = self.timesteps.to(original_samples.device)
- timesteps = timesteps.to(original_samples.device)
-
- schedule_timesteps = self.timesteps
- step_indices = [(schedule_timesteps == t).nonzero().item() for t in timesteps]
-
- sigma = self.sigmas[step_indices].flatten()
- while len(sigma.shape) < len(original_samples.shape):
- sigma = sigma.unsqueeze(-1)
-
- noisy_samples = original_samples + noise * sigma
- return noisy_samples
-
- def __len__(self):
- return self.config.num_train_timesteps
diff --git a/spaces/declare-lab/tango/diffusers/tests/schedulers/test_scheduler_dpm_multi.py b/spaces/declare-lab/tango/diffusers/tests/schedulers/test_scheduler_dpm_multi.py
deleted file mode 100644
index 295bbe882746793b09b196f054e392e22415d455..0000000000000000000000000000000000000000
--- a/spaces/declare-lab/tango/diffusers/tests/schedulers/test_scheduler_dpm_multi.py
+++ /dev/null
@@ -1,245 +0,0 @@
-import tempfile
-
-import torch
-
-from diffusers import (
- DEISMultistepScheduler,
- DPMSolverMultistepScheduler,
- DPMSolverSinglestepScheduler,
- UniPCMultistepScheduler,
-)
-
-from .test_schedulers import SchedulerCommonTest
-
-
-class DPMSolverMultistepSchedulerTest(SchedulerCommonTest):
- scheduler_classes = (DPMSolverMultistepScheduler,)
- forward_default_kwargs = (("num_inference_steps", 25),)
-
- def get_scheduler_config(self, **kwargs):
- config = {
- "num_train_timesteps": 1000,
- "beta_start": 0.0001,
- "beta_end": 0.02,
- "beta_schedule": "linear",
- "solver_order": 2,
- "prediction_type": "epsilon",
- "thresholding": False,
- "sample_max_value": 1.0,
- "algorithm_type": "dpmsolver++",
- "solver_type": "midpoint",
- "lower_order_final": False,
- }
-
- config.update(**kwargs)
- return config
-
- def check_over_configs(self, time_step=0, **config):
- kwargs = dict(self.forward_default_kwargs)
- num_inference_steps = kwargs.pop("num_inference_steps", None)
- sample = self.dummy_sample
- residual = 0.1 * sample
- dummy_past_residuals = [residual + 0.2, residual + 0.15, residual + 0.10]
-
- for scheduler_class in self.scheduler_classes:
- scheduler_config = self.get_scheduler_config(**config)
- scheduler = scheduler_class(**scheduler_config)
- scheduler.set_timesteps(num_inference_steps)
- # copy over dummy past residuals
- scheduler.model_outputs = dummy_past_residuals[: scheduler.config.solver_order]
-
- with tempfile.TemporaryDirectory() as tmpdirname:
- scheduler.save_config(tmpdirname)
- new_scheduler = scheduler_class.from_pretrained(tmpdirname)
- new_scheduler.set_timesteps(num_inference_steps)
- # copy over dummy past residuals
- new_scheduler.model_outputs = dummy_past_residuals[: new_scheduler.config.solver_order]
-
- output, new_output = sample, sample
- for t in range(time_step, time_step + scheduler.config.solver_order + 1):
- output = scheduler.step(residual, t, output, **kwargs).prev_sample
- new_output = new_scheduler.step(residual, t, new_output, **kwargs).prev_sample
-
- assert torch.sum(torch.abs(output - new_output)) < 1e-5, "Scheduler outputs are not identical"
-
- def test_from_save_pretrained(self):
- pass
-
- def check_over_forward(self, time_step=0, **forward_kwargs):
- kwargs = dict(self.forward_default_kwargs)
- num_inference_steps = kwargs.pop("num_inference_steps", None)
- sample = self.dummy_sample
- residual = 0.1 * sample
- dummy_past_residuals = [residual + 0.2, residual + 0.15, residual + 0.10]
-
- for scheduler_class in self.scheduler_classes:
- scheduler_config = self.get_scheduler_config()
- scheduler = scheduler_class(**scheduler_config)
- scheduler.set_timesteps(num_inference_steps)
-
- # copy over dummy past residuals (must be after setting timesteps)
- scheduler.model_outputs = dummy_past_residuals[: scheduler.config.solver_order]
-
- with tempfile.TemporaryDirectory() as tmpdirname:
- scheduler.save_config(tmpdirname)
- new_scheduler = scheduler_class.from_pretrained(tmpdirname)
- # copy over dummy past residuals
- new_scheduler.set_timesteps(num_inference_steps)
-
- # copy over dummy past residual (must be after setting timesteps)
- new_scheduler.model_outputs = dummy_past_residuals[: new_scheduler.config.solver_order]
-
- output = scheduler.step(residual, time_step, sample, **kwargs).prev_sample
- new_output = new_scheduler.step(residual, time_step, sample, **kwargs).prev_sample
-
- assert torch.sum(torch.abs(output - new_output)) < 1e-5, "Scheduler outputs are not identical"
-
- def full_loop(self, scheduler=None, **config):
- if scheduler is None:
- scheduler_class = self.scheduler_classes[0]
- scheduler_config = self.get_scheduler_config(**config)
- scheduler = scheduler_class(**scheduler_config)
-
- num_inference_steps = 10
- model = self.dummy_model()
- sample = self.dummy_sample_deter
- scheduler.set_timesteps(num_inference_steps)
-
- for i, t in enumerate(scheduler.timesteps):
- residual = model(sample, t)
- sample = scheduler.step(residual, t, sample).prev_sample
-
- return sample
-
- def test_step_shape(self):
- kwargs = dict(self.forward_default_kwargs)
-
- num_inference_steps = kwargs.pop("num_inference_steps", None)
-
- for scheduler_class in self.scheduler_classes:
- scheduler_config = self.get_scheduler_config()
- scheduler = scheduler_class(**scheduler_config)
-
- sample = self.dummy_sample
- residual = 0.1 * sample
-
- if num_inference_steps is not None and hasattr(scheduler, "set_timesteps"):
- scheduler.set_timesteps(num_inference_steps)
- elif num_inference_steps is not None and not hasattr(scheduler, "set_timesteps"):
- kwargs["num_inference_steps"] = num_inference_steps
-
- # copy over dummy past residuals (must be done after set_timesteps)
- dummy_past_residuals = [residual + 0.2, residual + 0.15, residual + 0.10]
- scheduler.model_outputs = dummy_past_residuals[: scheduler.config.solver_order]
-
- time_step_0 = scheduler.timesteps[5]
- time_step_1 = scheduler.timesteps[6]
-
- output_0 = scheduler.step(residual, time_step_0, sample, **kwargs).prev_sample
- output_1 = scheduler.step(residual, time_step_1, sample, **kwargs).prev_sample
-
- self.assertEqual(output_0.shape, sample.shape)
- self.assertEqual(output_0.shape, output_1.shape)
-
- def test_timesteps(self):
- for timesteps in [25, 50, 100, 999, 1000]:
- self.check_over_configs(num_train_timesteps=timesteps)
-
- def test_thresholding(self):
- self.check_over_configs(thresholding=False)
- for order in [1, 2, 3]:
- for solver_type in ["midpoint", "heun"]:
- for threshold in [0.5, 1.0, 2.0]:
- for prediction_type in ["epsilon", "sample"]:
- self.check_over_configs(
- thresholding=True,
- prediction_type=prediction_type,
- sample_max_value=threshold,
- algorithm_type="dpmsolver++",
- solver_order=order,
- solver_type=solver_type,
- )
-
- def test_prediction_type(self):
- for prediction_type in ["epsilon", "v_prediction"]:
- self.check_over_configs(prediction_type=prediction_type)
-
- def test_solver_order_and_type(self):
- for algorithm_type in ["dpmsolver", "dpmsolver++"]:
- for solver_type in ["midpoint", "heun"]:
- for order in [1, 2, 3]:
- for prediction_type in ["epsilon", "sample"]:
- self.check_over_configs(
- solver_order=order,
- solver_type=solver_type,
- prediction_type=prediction_type,
- algorithm_type=algorithm_type,
- )
- sample = self.full_loop(
- solver_order=order,
- solver_type=solver_type,
- prediction_type=prediction_type,
- algorithm_type=algorithm_type,
- )
- assert not torch.isnan(sample).any(), "Samples have nan numbers"
-
- def test_lower_order_final(self):
- self.check_over_configs(lower_order_final=True)
- self.check_over_configs(lower_order_final=False)
-
- def test_inference_steps(self):
- for num_inference_steps in [1, 2, 3, 5, 10, 50, 100, 999, 1000]:
- self.check_over_forward(num_inference_steps=num_inference_steps, time_step=0)
-
- def test_full_loop_no_noise(self):
- sample = self.full_loop()
- result_mean = torch.mean(torch.abs(sample))
-
- assert abs(result_mean.item() - 0.3301) < 1e-3
-
- def test_full_loop_no_noise_thres(self):
- sample = self.full_loop(thresholding=True, dynamic_thresholding_ratio=0.87, sample_max_value=0.5)
- result_mean = torch.mean(torch.abs(sample))
-
- assert abs(result_mean.item() - 0.6405) < 1e-3
-
- def test_full_loop_with_v_prediction(self):
- sample = self.full_loop(prediction_type="v_prediction")
- result_mean = torch.mean(torch.abs(sample))
-
- assert abs(result_mean.item() - 0.2251) < 1e-3
-
- def test_switch(self):
- # make sure that iterating over schedulers with same config names gives same results
- # for defaults
- scheduler = DPMSolverMultistepScheduler(**self.get_scheduler_config())
- sample = self.full_loop(scheduler=scheduler)
- result_mean = torch.mean(torch.abs(sample))
-
- assert abs(result_mean.item() - 0.3301) < 1e-3
-
- scheduler = DPMSolverSinglestepScheduler.from_config(scheduler.config)
- scheduler = UniPCMultistepScheduler.from_config(scheduler.config)
- scheduler = DEISMultistepScheduler.from_config(scheduler.config)
- scheduler = DPMSolverMultistepScheduler.from_config(scheduler.config)
-
- sample = self.full_loop(scheduler=scheduler)
- result_mean = torch.mean(torch.abs(sample))
-
- assert abs(result_mean.item() - 0.3301) < 1e-3
-
- def test_fp16_support(self):
- scheduler_class = self.scheduler_classes[0]
- scheduler_config = self.get_scheduler_config(thresholding=True, dynamic_thresholding_ratio=0)
- scheduler = scheduler_class(**scheduler_config)
-
- num_inference_steps = 10
- model = self.dummy_model()
- sample = self.dummy_sample_deter.half()
- scheduler.set_timesteps(num_inference_steps)
-
- for i, t in enumerate(scheduler.timesteps):
- residual = model(sample, t)
- sample = scheduler.step(residual, t, sample).prev_sample
-
- assert sample.dtype == torch.float16
diff --git a/spaces/deprem-ml/deprem-ocr/app.py b/spaces/deprem-ml/deprem-ocr/app.py
deleted file mode 100644
index 34b234b2f7d95945778bca40b32bf4aac87a6019..0000000000000000000000000000000000000000
--- a/spaces/deprem-ml/deprem-ocr/app.py
+++ /dev/null
@@ -1,153 +0,0 @@
-from PIL import ImageFilter, Image
-from easyocr import Reader
-import gradio as gr
-import numpy as np
-import openai
-import ast
-from transformers import pipeline
-import os
-
-from openai_api import OpenAI_API
-import utils
-
-openai.api_key = os.getenv("API_KEY")
-reader = Reader(["tr"])
-
-
-def get_text(input_img):
- img = Image.fromarray(input_img)
- detailed = np.asarray(img.filter(ImageFilter.DETAIL))
- result = reader.readtext(detailed, detail=0, paragraph=True)
- return " ".join(result)
-
-
-# Submit button
-def get_parsed_address(input_img):
-
- address_full_text = get_text(input_img)
- return ner_response(address_full_text)
-
-
-def save_deta_db(input):
- eval_result = ast.literal_eval(input)
- utils.write_db(eval_result)
- return
-
-
-def update_component():
- return gr.update(value="Gönderildi, teşekkürler.", visible=True)
-
-
-def clear_textbox(value):
- return gr.update(value="")
-
-
-def text_dict(input):
- eval_result = ast.literal_eval(input)
- return (
- str(eval_result["il"]),
- str(eval_result["ilce"]),
- str(eval_result["mahalle"]),
- str(eval_result["sokak"]),
- str(eval_result["Apartman/site"]),
- str(eval_result["no"]),
- str(eval_result["ad-soyad"]),
- str(eval_result["dis kapi no"]),
- )
-
-
-def ner_response(ocr_input):
-
- ner_pipe = pipeline("token-classification","deprem-ml/deprem-ner", aggregation_strategy="first")
- predictions = ner_pipe(ocr_input)
- resp = {}
-
- for item in predictions:
- print(item)
- key = item["entity_group"]
- resp[key] = item["word"]
-
- resp["input"] = ocr_input
- dict_keys = ["il", "ilce", "mahalle", "sokak", "Apartman/site", "no", "ad-soyad", "dis kapi no"]
- for key in dict_keys:
- if key not in resp.keys():
- resp[key] = ""
- return resp
-
-
-# User Interface
-with gr.Blocks() as demo:
- gr.Markdown(
- """
- # Enkaz Bildirme Uygulaması
- """
- )
- gr.Markdown(
- "Bu uygulamada ekran görüntüsü sürükleyip bırakarak AFAD'a enkaz bildirimi yapabilirsiniz. Mesajı metin olarak da girebilirsiniz, tam adresi ayrıştırıp döndürür. API olarak kullanmak isterseniz sayfanın en altında use via api'ya tıklayın."
- )
- with gr.Row():
- with gr.Column():
- img_area = gr.Image(label="Ekran Görüntüsü yükleyin 👇")
- img_area_button = gr.Button(value="Görüntüyü İşle", label="Submit")
-
- with gr.Column():
- text_area = gr.Textbox(label="Metin yükleyin 👇 ", lines=8)
- text_area_button = gr.Button(value="Metni Yükle", label="Submit")
-
- open_api_text = gr.Textbox(label="Tam Adres")
-
- with gr.Column():
- with gr.Row():
- il = gr.Textbox(label="İl", interactive=True, show_progress=False)
- ilce = gr.Textbox(label="İlçe", interactive=True, show_progress=False)
- with gr.Row():
- mahalle = gr.Textbox(
- label="Mahalle", interactive=True, show_progress=False
- )
- sokak = gr.Textbox(
- label="Sokak/Cadde/Bulvar", interactive=True, show_progress=False
- )
- with gr.Row():
- no = gr.Textbox(label="Telefon", interactive=True, show_progress=False)
- with gr.Row():
- ad_soyad = gr.Textbox(
- label="İsim Soyisim", interactive=True, show_progress=False
- )
- apartman = gr.Textbox(label="apartman", interactive=True, show_progress=False)
- with gr.Row():
- dis_kapi_no = gr.Textbox(label="Kapı No", interactive=True, show_progress=False)
-
- img_area_button.click(
- get_parsed_address,
- inputs=img_area,
- outputs=open_api_text,
- api_name="upload-image",
- )
-
- text_area_button.click(
- ner_response, text_area, open_api_text, api_name="upload-text"
- )
-
-
- open_api_text.change(
- text_dict,
- open_api_text,
- [il, ilce, mahalle, sokak, no, apartman, ad_soyad, dis_kapi_no],
- )
- ocr_button = gr.Button(value="Sadece OCR kullan")
- ocr_button.click(
- get_text,
- inputs=img_area,
- outputs=text_area,
- api_name="get-ocr-output",
- )
- submit_button = gr.Button(value="Veriyi Birimlere Yolla")
- submit_button.click(save_deta_db, open_api_text)
- done_text = gr.Textbox(label="Done", value="Not Done", visible=False)
- submit_button.click(update_component, outputs=done_text)
- for txt in [il, ilce, mahalle, sokak, apartman, no, ad_soyad, dis_kapi_no]:
- submit_button.click(fn=clear_textbox, inputs=txt, outputs=txt)
-
-
-if __name__ == "__main__":
- demo.launch()
\ No newline at end of file
diff --git a/spaces/diacanFperku/AutoGPT/Chegg Premium Account For Free.md b/spaces/diacanFperku/AutoGPT/Chegg Premium Account For Free.md
deleted file mode 100644
index f935ad1edee8dd8a5365e337eaf3c6309d3554c7..0000000000000000000000000000000000000000
--- a/spaces/diacanFperku/AutoGPT/Chegg Premium Account For Free.md
+++ /dev/null
@@ -1,119 +0,0 @@
-
-
How to Get a Chegg Premium Account for Free and Enjoy Unlimited Benefits
-
-
If you are a student who wants to save money on textbooks, homework help, online courses, and more, you might be interested in getting a Chegg premium account for free. Chegg is a popular online education platform that offers various services for students of all levels. With a Chegg premium account, you can access millions of textbooks for rent or purchase, get 24/7 expert assistance with your assignments, learn new skills with interactive courses, and find opportunities for internships and scholarships.
-
-
However, a Chegg premium account is not cheap. It costs $14.95 per month for Chegg Study, $9.95 per month for Chegg Math Solver, $9.95 per month for Chegg Writing, and $19.95 per month for Chegg Study Pack. If you want to use all these features, you will have to pay a hefty sum every month. But don't worry, there are some ways to get a Chegg premium account for free or at a lower cost. In this article, we will show you how to do that.
One of the easiest ways to get a Chegg premium account for free is to use the trial period offered by Chegg. Chegg allows new users to try out their services for free for 7 days. During this period, you can access all the features of Chegg without paying anything. You just need to sign up with your email address and password, and provide your payment details. You can cancel your subscription anytime before the trial ends and you won't be charged.
-
-
To sign up for the Chegg trial period, follow these steps:
Click on your profile icon at the top right corner and select "My account".
-
Click on "Orders" and then on "Subscriptions".
-
Find the plan that you want to cancel and click on "Cancel subscription".
-
Confirm your cancellation and you're done.
-
-
-
Method Two: Use Free Chegg Accounts and Passwords
-
-
Another way to get a Chegg premium account for free is to use free Chegg accounts and passwords that are available on the internet. There are many websites that provide working Chegg accounts and passwords for free. You can use these accounts to log in to Chegg and access the premium features without paying anything. However, you should be careful when using these accounts as they might not be safe or reliable. Some of them might be hacked or stolen from other users, and some of them might not work or be expired.
-
-
To use free Chegg accounts and passwords, follow these steps:
-
-
-
Search for websites that provide free Chegg accounts and passwords on Google or other search engines.
-
Select a website that looks trustworthy and has positive reviews from other users.
-
Copy one of the Chegg accounts and passwords from the website.
Paste the email address and password that you copied and click on "Sign in".
-
If the account works, you can access the premium features of Chegg for free.
-
-
-
If the account doesn't work or is already in use by someone else, you can try another one until you find one that works. However, you should not change the password or personal information of the account as it might belong to someone else. You should also not share the account with anyone else as it might get banned or suspended by Chegg.
-
-
Method Three: Use Coupon Codes and Discounts
-
-
A third way to get a Chegg premium account for free or at a lower cost is to use coupon codes and discounts that are offered by Chegg or other websites. Coupon codes are special codes that you can enter at checkout to get a discount on your purchase. Discounts are special offers that reduce the price of your purchase without requiring any code. You can find coupon codes and discounts for Chegg on various websites such as RetailMeNot, CouponCabin, Groupon, etc.
-
-
To use coupon codes and discounts for Chegg, follow these steps:
-
-
-
Search for websites that provide coupon codes and discounts for Chegg on Google or other search engines.
-
Select a website that looks trustworthy and has positive reviews from other users.
-
Browse through the available coupon codes and discounts and choose one that suits your needs.
-
Copy the coupon code or click on the discount link.
-
Go to Chegg.com and select the plan that you want to purchase.
-
Paste the coupon code at checkout or apply the discount automatically if you clicked on the link.
-
Enjoy your reduced price or free access to Chegg premium features.
-
-
-
Note that some coupon codes and discounts might have expiration dates or terms and conditions that limit their usage. You should check these details before using them.
-
-
Conclusion
-
-
Chegg is a great online education platform that offers various services for students of all levels. However, a Chegg premium account can be expensive for some students who want to save money on their education expenses. Fortunately, there are some ways to get a Chegg premium account for free or at a lower cost by using the trial period, free accounts and passwords, or coupon codes and discounts. These methods can help you access all the features of Chegg without breaking your bank.
-
-
We hope this article was helpful for you in getting a chegg premium account for free. If you have any questions or suggestions, feel free to leave them in the comments section below.
-
Method Four: Use Textsheet Alternatives
-
-
A fourth way to get a Chegg premium account for free or at a lower cost is to use Textsheet alternatives. Textsheet was a popular website that provided free Chegg answers and solutions to students. However, it was shut down by Chegg due to copyright infringement. Since then, many other websites have emerged that offer similar services to Textsheet. These websites use Chegg API or other methods to scrape Chegg answers and solutions and provide them to users for free or at a nominal fee.
-
-
To use Textsheet alternatives, follow these steps:
-
-
-
Search for websites that provide Textsheet alternatives on Google or other search engines.
-
Select a website that looks trustworthy and has positive reviews from other users.
-
Enter the Chegg question URL or paste the question text on the website.
-
Click on the submit button or press enter.
-
Wait for the website to fetch the Chegg answer or solution.
-
View the Chegg answer or solution for free or after paying a small fee.
-
-
-
Some of the popular Textsheet alternatives are Litanswers, Slader, CourseHero, Studylib, etc. However, you should be careful when using these websites as they might not be legal or safe. Some of them might contain malware or viruses, and some of them might violate Chegg's terms of service. You should also not rely on these websites for accurate or complete answers or solutions as they might be outdated or incorrect.
-
-
Method Five: Use Online Forums and Communities
-
-
A fifth way to get a Chegg premium account for free or at a lower cost is to use online forums and communities where students help each other with their homework and assignments. There are many online platforms where you can post your Chegg questions and get answers or solutions from other students who have Chegg accounts. You can also help other students with their questions and earn credits or rewards that you can use to get Chegg answers or solutions.
-
-
To use online forums and communities, follow these steps:
-
-
-
Search for online platforms that provide homework help on Google or other search engines.
-
Select a platform that looks trustworthy and has positive reviews from other users.
-
Create an account on the platform using your email address and password.
-
Post your Chegg question on the platform and wait for other users to respond.
-
View the Chegg answer or solution provided by other users for free or after paying a small fee.
-
Help other users with their questions and earn credits or rewards that you can use to get more Chegg answers or solutions.
-
-
-
Some of the popular online platforms that provide homework help are Reddit, Quora, HomeworkMarket, Chegg Study Community, etc. However, you should be careful when using these platforms as they might not be legal or safe. Some of them might contain spam or scams, and some of them might violate Chegg's terms of service. You should also not rely on these platforms for accurate or complete answers or solutions as they might be outdated or incorrect.
-
-
Conclusion
-
-
Chegg is a great online education platform that offers various services for students of all levels. However, a Chegg premium account can be expensive for some students who want to save money on their education expenses. Fortunately, there are some ways to get a Chegg premium account for free or at a lower cost by using the trial period, free accounts and passwords, coupon codes and discounts, Textsheet alternatives, or online forums and communities. These methods can help you access all the features of Chegg without breaking your bank.
-
-
We hope this article was helpful for you in getting a chegg premium account for free. If you have any questions or suggestions, feel free to leave them in the comments section below.
-
Conclusion
-
-
Chegg is a great online education platform that offers various services for students of all levels. However, a Chegg premium account can be expensive for some students who want to save money on their education expenses. Fortunately, there are some ways to get a Chegg premium account for free or at a lower cost by using the trial period, free accounts and passwords, coupon codes and discounts, Textsheet alternatives, or online forums and communities. These methods can help you access all the features of Chegg without breaking your bank.
-
-
We hope this article was helpful for you in getting a chegg premium account for free. If you have any questions or suggestions, feel free to leave them in the comments section below.
3cee63e6c2
-
-
\ No newline at end of file
diff --git a/spaces/diacanFperku/AutoGPT/HD Online Player (movavi Video Converter 12 Clave De Activacion -softpile -kiber -moviedox -egydown.md b/spaces/diacanFperku/AutoGPT/HD Online Player (movavi Video Converter 12 Clave De Activacion -softpile -kiber -moviedox -egydown.md
deleted file mode 100644
index 07b6c2de623ab662fb15982d23785d02abd8c290..0000000000000000000000000000000000000000
--- a/spaces/diacanFperku/AutoGPT/HD Online Player (movavi Video Converter 12 Clave De Activacion -softpile -kiber -moviedox -egydown.md
+++ /dev/null
@@ -1,29 +0,0 @@
-
-
How to Use Movavi Video Converter 12 to Play HD Videos Online
-
Movavi Video Converter 12 is a powerful software that allows you to convert videos to various formats, including HD. With Movavi Video Converter 12, you can also play HD videos online using the built-in HD Online Player. In this article, we will show you how to use Movavi Video Converter 12 to play HD videos online and how to activate the software with a valid clave de activacion (activation key).
-
Step 1: Download and Install Movavi Video Converter 12
-
To use Movavi Video Converter 12, you need to download and install the software on your computer. You can download Movavi Video Converter 12 from the official website[^1^] or from other trusted sources. The installation process is simple and fast. Just follow the instructions on the screen and choose the language and destination folder for the program.
-
HD Online Player (movavi video converter 12 clave de activacion -softpile -kiber -moviedox -egydown
After installing Movavi Video Converter 12, launch the program and click on the Add Media button. Then, choose Add Video and browse your computer for the videos you want to convert and play online. You can add multiple videos at once and preview them in the built-in player.
-
Step 3: Choose Output Format and Quality
-
Next, you need to choose the output format and quality for your videos. Click on the Output format button and select one of the online video formats, such as MP4, FLV, WebM, or AVI. You can also choose a preset for a specific device or platform, such as YouTube, Facebook, iPhone, or Android. To adjust the quality settings, click on the cogwheel icon next to the output format and choose one of the options: Low Quality, Economy, Standard, High Quality, or Original Size.
-
Step 4: Activate Movavi Video Converter 12 with Clave de Activacion
-
Before you can use Movavi Video Converter 12 to play HD videos online, you need to activate the software with a valid clave de activacion (activation key). A clave de activacion is a special code that unlocks all the features of Movavi Video Converter 12. To get a clave de activacion, you need to purchase a license from the official website[^1^] or from other authorized sellers. You can also try a free trial version of Movavi Video Converter 12 for 7 days[^1^]. To activate Movavi Video Converter 12 with a clave de activacion, follow these steps:
-
-
Click on the Menu button in the upper right corner of the program window and select Activate Software.
-
Enter your email address and your clave de activacion in the corresponding fields.
-
Click on Activate.
-
Restart Movavi Video Converter 12.
-
-
Note: Do not use illegal or pirated claves de activacion that are posted on some websites. These claves de activacion may not work properly or may cause problems with your computer. They may also violate the law and expose you to legal risks[^1^]. Always use legal and official claves de activacion from Movavi or its partners.
-
Step 5: Play HD Videos Online with HD Online Player
-
Now that you have activated Movavi Video Converter 12 with a clave de activacion, you can use it to play HD videos online with HD Online Player. HD Online Player is a feature of Movavi Video Converter 12 that allows you to stream your converted videos directly from your computer to any web browser. To use HD Online Player, follow these steps:
-
-
-
Click on the Share button in the lower right corner of the program window and select Upload Online.
-
Select HD Online Player as the destination for your videos.
-
Click on Start.
-
A new window will open with a link to your online video player. Copy this link and paste it into any web browser on any device.
d5da3c52bf
-
-
\ No newline at end of file
diff --git a/spaces/diacanFperku/AutoGPT/Mcd001.ps2 WWE Smackdown - Here Comes The Pain! (PCSX2 Memory Card File For PlayStation 2) [SAVED GAME STATE - EVERYTHINK UNLOCKED].rar.md b/spaces/diacanFperku/AutoGPT/Mcd001.ps2 WWE Smackdown - Here Comes The Pain! (PCSX2 Memory Card File For PlayStation 2) [SAVED GAME STATE - EVERYTHINK UNLOCKED].rar.md
deleted file mode 100644
index bf156c23c8ce83bba093059f19ee7b054087506b..0000000000000000000000000000000000000000
--- a/spaces/diacanFperku/AutoGPT/Mcd001.ps2 WWE Smackdown - Here Comes The Pain! (PCSX2 Memory Card File For PlayStation 2) [SAVED GAME STATE - EVERYTHINK UNLOCKED].rar.md
+++ /dev/null
@@ -1,6 +0,0 @@
-
Mcd001.ps2 WWE Smackdown - Here Comes The Pain! (PCSX2 Memory Card File for PlayStation 2) [SAVED GAME STATE - EVERYTHINK UNLOCKED].rar
-
-- here's my new game data! [game state - no cheat codes!].zip - my game data (no in-game cheat codes and memory cheat codes).rar - my game data (no in-game cheat codes and memory cheat codes).zip - my game data (no in-game cheat codes and memory cheat codes).rar - my game data (no in-game cheat codes and memory cheat codes).zip - my game data (no in-game cheat codes and memory cheat codes).rar - my game data (no in-game cheat codes and memory cheat codes).zip - my game data (no in-game cheat codes and memory cheat codes).rar - my game data (no in-game cheat codes and memory cheat codes).zip - my game data (no in-game cheat codes and memory cheat codes).rar - my game data (no in-game cheat codes and memory cheat codes).zip - my game data (no in-game cheat codes and memory cheat codes).rar - my game data (no in-game cheat codes and memory cheat codes).zip - my game data (no in-game cheat codes and memory cheat codes).rar - my game data (no in-game cheat codes and memory cheat codes).zip - my game data (no in-game cheat codes and memory cheat codes).rar - my game data (no in-game cheat codes and memory cheat codes).zip - my game data (no in-game cheat codes and memory cheat codes).rar - my game data (no in-game cheat codes and memory cheat codes).zip - my game data (no in-game cheat codes and memory cheat codes).rar - my game data (no in-game cheat codes and memory cheat codes).zip - my game data (no in-game cheat codes and memory cheat codes).rar - my game data (no in-game cheat codes and memory cheat codes).zip - my game data (no in-game cheat codes and memory cheat codes).rar - my game data (no in-game cheat codes and memory cheat codes).zip - my game data (no in-game cheat codes and memory cheat codes).rar - my game data (no in-game cheat codes and memory cheat codes).zip - my game data (no in-game cheat codes and memory cheat codes).rar - my game data (no in-game cheat codes and memory cheat codes).zip - my game data (no in-game cheat codes and memory cheat codes).rar - 4fefd39f24
-
-
-
diff --git a/spaces/diacanFperku/AutoGPT/Psychology From Inquiry To Understanding (4th Edition) Books.pdf VERIFIED.md b/spaces/diacanFperku/AutoGPT/Psychology From Inquiry To Understanding (4th Edition) Books.pdf VERIFIED.md
deleted file mode 100644
index e2d8ac28cdd41d82c21f35f50c51d846efef2f35..0000000000000000000000000000000000000000
--- a/spaces/diacanFperku/AutoGPT/Psychology From Inquiry To Understanding (4th Edition) Books.pdf VERIFIED.md
+++ /dev/null
@@ -1,62 +0,0 @@
-
Psychology: From Inquiry To Understanding (4th Edition) Books.pdf
-
-psychology: from inquiry to understanding (4th edition) pdf.
-
-Download MPA in Psychology Psychology Download PDF Ebook. Bachelor of Science in Psychology: Graduate Handbook (a comprehensive guide to becoming a graduate of the University of California-San Diego). Enter the world of psychology through the use of these links and videos to learn more about the different disciplines in this field.
-
-Psychology: download pdf psychology: from inquiry to understanding (4th edition) psychology: from inquiry to understanding (4th edition) pdf. September 3, 2013. The Psychology Association (PSYCH-UK) is the national organisation of the UK profession of psychology.{
-
- "name": "Pylons",
-
- "version": "0.2.4",
-
- "desc": "Pylons is an MVC framework with unit testing and embedded logging",
-
- "main": "src/pylons.js",
-
- "scripts":
-
- "test": "make test"
-
- ,
-
- "repository": {
-
- "type": "git",
-
- "url": "git://github.com/Pylons/Pylons.git"
-
- "dependencies": {
-
- "Django": "0.4.3",
-
- "Django-Pelican": "0.1.3",
-
- "Django-Pylons": "0.4.3",
-
- "DjangoTemplates": "0.3.5",
-
- "Django-Pagination": "0.4.1",
-
- "DjangoSession": "0.3.1",
-
- "DynamicWidgets": "0.2.4",
-
- "Django-Simple-Form": "0.3.1",
-
- "Django-Filebrowser": "0.2.0",
-
- "Django-Html-Table": "0.4.0",
-
- "FormToolkit": "1.1.2",
-
- "repoze.who": "0.2",
-
- "SimpleHTTPServer": "0.5.0",
-
- "Sortable": "0.1.3",
-
- "email": "0.2.6", 4fefd39f24
-
-
-
diff --git a/spaces/digitalxingtong/Azuma-Bert-VITS2/short_audio_transcribe.py b/spaces/digitalxingtong/Azuma-Bert-VITS2/short_audio_transcribe.py
deleted file mode 100644
index f1e8b30671f2c2f2fa3c93feb1f4edd3fbe2f545..0000000000000000000000000000000000000000
--- a/spaces/digitalxingtong/Azuma-Bert-VITS2/short_audio_transcribe.py
+++ /dev/null
@@ -1,122 +0,0 @@
-import whisper
-import os
-import json
-import torchaudio
-import argparse
-import torch
-
-lang2token = {
- 'zh': "[ZH]",
- 'ja': "[JA]",
- "en": "[EN]",
- }
-def transcribe_one(audio_path):
- # load audio and pad/trim it to fit 30 seconds
- audio = whisper.load_audio(audio_path)
- audio = whisper.pad_or_trim(audio)
-
- # make log-Mel spectrogram and move to the same device as the model
- mel = whisper.log_mel_spectrogram(audio).to(model.device)
-
- # detect the spoken language
- _, probs = model.detect_language(mel)
- print(f"Detected language: {max(probs, key=probs.get)}")
- lang = max(probs, key=probs.get)
- # decode the audio
- options = whisper.DecodingOptions(beam_size=5)
- result = whisper.decode(model, mel, options)
-
- # print the recognized text
- print(result.text)
- return lang, result.text
-if __name__ == "__main__":
- parser = argparse.ArgumentParser()
- parser.add_argument("--languages", default="CJE")
- parser.add_argument("--whisper_size", default="medium")
- args = parser.parse_args()
- if args.languages == "CJE":
- lang2token = {
- 'zh': "[ZH]",
- 'ja': "[JA]",
- "en": "[EN]",
- }
- elif args.languages == "CJ":
- lang2token = {
- 'zh': "[ZH]",
- 'ja': "[JA]",
- }
- elif args.languages == "C":
- lang2token = {
- 'zh': "[ZH]",
- }
- assert (torch.cuda.is_available()), "Please enable GPU in order to run Whisper!"
- model = whisper.load_model(args.whisper_size)
- parent_dir = "./custom_character_voice/"
- speaker_names = list(os.walk(parent_dir))[0][1]
- speaker_annos = []
- total_files = sum([len(files) for r, d, files in os.walk(parent_dir)])
- # resample audios
- # 2023/4/21: Get the target sampling rate
- with open("./configs/config.json", 'r', encoding='utf-8') as f:
- hps = json.load(f)
- target_sr = hps['data']['sampling_rate']
- processed_files = 0
- for speaker in speaker_names:
- for i, wavfile in enumerate(list(os.walk(parent_dir + speaker))[0][2]):
- # try to load file as audio
- if wavfile.startswith("processed_"):
- continue
- try:
- wav, sr = torchaudio.load(parent_dir + speaker + "/" + wavfile, frame_offset=0, num_frames=-1, normalize=True,
- channels_first=True)
- wav = wav.mean(dim=0).unsqueeze(0)
- if sr != target_sr:
- wav = torchaudio.transforms.Resample(orig_freq=sr, new_freq=target_sr)(wav)
- if wav.shape[1] / sr > 20:
- print(f"{wavfile} too long, ignoring\n")
- save_path = parent_dir + speaker + "/" + f"processed_{i}.wav"
- torchaudio.save(save_path, wav, target_sr, channels_first=True)
- # transcribe text
- lang, text = transcribe_one(save_path)
- if lang not in list(lang2token.keys()):
- print(f"{lang} not supported, ignoring\n")
- continue
- text = "ZH|" + text + "\n"#
- #text = lang2token[lang] + text + lang2token[lang] + "\n"
- speaker_annos.append(save_path + "|" + speaker + "|" + text)
-
- processed_files += 1
- print(f"Processed: {processed_files}/{total_files}")
- except:
- continue
-
- # # clean annotation
- # import argparse
- # import text
- # from utils import load_filepaths_and_text
- # for i, line in enumerate(speaker_annos):
- # path, sid, txt = line.split("|")
- # cleaned_text = text._clean_text(txt, ["cjke_cleaners2"])
- # cleaned_text += "\n" if not cleaned_text.endswith("\n") else ""
- # speaker_annos[i] = path + "|" + sid + "|" + cleaned_text
- # write into annotation
- if len(speaker_annos) == 0:
- print("Warning: no short audios found, this IS expected if you have only uploaded long audios, videos or video links.")
- print("this IS NOT expected if you have uploaded a zip file of short audios. Please check your file structure or make sure your audio language is supported.")
- with open("./filelists/short_character_anno.list", 'w', encoding='utf-8') as f:
- for line in speaker_annos:
- f.write(line)
-
- # import json
- # # generate new config
- # with open("./configs/finetune_speaker.json", 'r', encoding='utf-8') as f:
- # hps = json.load(f)
- # # modify n_speakers
- # hps['data']["n_speakers"] = 1000 + len(speaker2id)
- # # add speaker names
- # for speaker in speaker_names:
- # hps['speakers'][speaker] = speaker2id[speaker]
- # # save modified config
- # with open("./configs/modified_finetune_speaker.json", 'w', encoding='utf-8') as f:
- # json.dump(hps, f, indent=2)
- # print("finished")
diff --git a/spaces/digitalxingtong/Bufeiyan-b-Bert-VITS2/text/english_bert_mock.py b/spaces/digitalxingtong/Bufeiyan-b-Bert-VITS2/text/english_bert_mock.py
deleted file mode 100644
index 3b894ced5b6d619a18d6bdd7d7606ba9e6532050..0000000000000000000000000000000000000000
--- a/spaces/digitalxingtong/Bufeiyan-b-Bert-VITS2/text/english_bert_mock.py
+++ /dev/null
@@ -1,5 +0,0 @@
-import torch
-
-
-def get_bert_feature(norm_text, word2ph):
- return torch.zeros(1024, sum(word2ph))
diff --git a/spaces/digitalxingtong/Xingtong-2dall-Bert-VITS2/train_ms.py b/spaces/digitalxingtong/Xingtong-2dall-Bert-VITS2/train_ms.py
deleted file mode 100644
index 5d109003d40497ea4493e7c73f47c1eb7370a81e..0000000000000000000000000000000000000000
--- a/spaces/digitalxingtong/Xingtong-2dall-Bert-VITS2/train_ms.py
+++ /dev/null
@@ -1,402 +0,0 @@
-import os
-import json
-import argparse
-import itertools
-import math
-import torch
-import shutil
-from torch import nn, optim
-from torch.nn import functional as F
-from torch.utils.data import DataLoader
-from torch.utils.tensorboard import SummaryWriter
-import torch.multiprocessing as mp
-import torch.distributed as dist
-from torch.nn.parallel import DistributedDataParallel as DDP
-from torch.cuda.amp import autocast, GradScaler
-from tqdm import tqdm
-import logging
-logging.getLogger('numba').setLevel(logging.WARNING)
-import commons
-import utils
-from data_utils import (
- TextAudioSpeakerLoader,
- TextAudioSpeakerCollate,
- DistributedBucketSampler
-)
-from models import (
- SynthesizerTrn,
- MultiPeriodDiscriminator,
- DurationDiscriminator,
-)
-from losses import (
- generator_loss,
- discriminator_loss,
- feature_loss,
- kl_loss
-)
-from mel_processing import mel_spectrogram_torch, spec_to_mel_torch
-from text.symbols import symbols
-
-torch.backends.cudnn.benchmark = True
-torch.backends.cuda.matmul.allow_tf32 = True
-torch.backends.cudnn.allow_tf32 = True
-torch.set_float32_matmul_precision('medium')
-global_step = 0
-
-
-def main():
- """Assume Single Node Multi GPUs Training Only"""
- assert torch.cuda.is_available(), "CPU training is not allowed."
-
- n_gpus = torch.cuda.device_count()
- os.environ['MASTER_ADDR'] = 'localhost'
- os.environ['MASTER_PORT'] = '65280'
-
- hps = utils.get_hparams()
- if not hps.cont:
- shutil.copy('./pretrained_models/D_0.pth','./logs/OUTPUT_MODEL/D_0.pth')
- shutil.copy('./pretrained_models/G_0.pth','./logs/OUTPUT_MODEL/G_0.pth')
- shutil.copy('./pretrained_models/DUR_0.pth','./logs/OUTPUT_MODEL/DUR_0.pth')
- mp.spawn(run, nprocs=n_gpus, args=(n_gpus, hps,))
-
-
-def run(rank, n_gpus, hps):
- global global_step
- if rank == 0:
- logger = utils.get_logger(hps.model_dir)
- logger.info(hps)
- utils.check_git_hash(hps.model_dir)
- writer = SummaryWriter(log_dir=hps.model_dir)
- writer_eval = SummaryWriter(log_dir=os.path.join(hps.model_dir, "eval"))
-
- dist.init_process_group(backend= 'gloo' if os.name == 'nt' else 'nccl', init_method='env://', world_size=n_gpus, rank=rank)
- torch.manual_seed(hps.train.seed)
- torch.cuda.set_device(rank)
-
- train_dataset = TextAudioSpeakerLoader(hps.data.training_files, hps.data)
- train_sampler = DistributedBucketSampler(
- train_dataset,
- hps.train.batch_size,
- [32, 300, 400, 500, 600, 700, 800, 900, 1000],
- num_replicas=n_gpus,
- rank=rank,
- shuffle=True)
- collate_fn = TextAudioSpeakerCollate()
- train_loader = DataLoader(train_dataset, num_workers=2, shuffle=False, pin_memory=True,
- collate_fn=collate_fn, batch_sampler=train_sampler)
- if rank == 0:
- eval_dataset = TextAudioSpeakerLoader(hps.data.validation_files, hps.data)
- eval_loader = DataLoader(eval_dataset, num_workers=0, shuffle=False,
- batch_size=1, pin_memory=True,
- drop_last=False, collate_fn=collate_fn)
- if "use_noise_scaled_mas" in hps.model.keys() and hps.model.use_noise_scaled_mas == True:
- print("Using noise scaled MAS for VITS2")
- use_noise_scaled_mas = True
- mas_noise_scale_initial = 0.01
- noise_scale_delta = 2e-6
- else:
- print("Using normal MAS for VITS1")
- use_noise_scaled_mas = False
- mas_noise_scale_initial = 0.0
- noise_scale_delta = 0.0
- if "use_duration_discriminator" in hps.model.keys() and hps.model.use_duration_discriminator == True:
- print("Using duration discriminator for VITS2")
- use_duration_discriminator = True
- net_dur_disc = DurationDiscriminator(
- hps.model.hidden_channels,
- hps.model.hidden_channels,
- 3,
- 0.1,
- gin_channels=hps.model.gin_channels if hps.data.n_speakers != 0 else 0,
- ).cuda(rank)
- if "use_spk_conditioned_encoder" in hps.model.keys() and hps.model.use_spk_conditioned_encoder == True:
- if hps.data.n_speakers == 0:
- raise ValueError("n_speakers must be > 0 when using spk conditioned encoder to train multi-speaker model")
- use_spk_conditioned_encoder = True
- else:
- print("Using normal encoder for VITS1")
- use_spk_conditioned_encoder = False
-
- net_g = SynthesizerTrn(
- len(symbols),
- hps.data.filter_length // 2 + 1,
- hps.train.segment_size // hps.data.hop_length,
- n_speakers=hps.data.n_speakers,
- mas_noise_scale_initial = mas_noise_scale_initial,
- noise_scale_delta = noise_scale_delta,
- **hps.model).cuda(rank)
-
- freeze_enc = getattr(hps.model, "freeze_enc", False)
- if freeze_enc:
- print("freeze encoder !!!")
- for param in net_g.enc_p.parameters():
- param.requires_grad = False
-
- net_d = MultiPeriodDiscriminator(hps.model.use_spectral_norm).cuda(rank)
- optim_g = torch.optim.AdamW(
- filter(lambda p: p.requires_grad, net_g.parameters()),
- hps.train.learning_rate,
- betas=hps.train.betas,
- eps=hps.train.eps)
- optim_d = torch.optim.AdamW(
- net_d.parameters(),
- hps.train.learning_rate,
- betas=hps.train.betas,
- eps=hps.train.eps)
- if net_dur_disc is not None:
- optim_dur_disc = torch.optim.AdamW(
- net_dur_disc.parameters(),
- hps.train.learning_rate,
- betas=hps.train.betas,
- eps=hps.train.eps)
- else:
- optim_dur_disc = None
- net_g = DDP(net_g, device_ids=[rank], find_unused_parameters=True)
- net_d = DDP(net_d, device_ids=[rank], find_unused_parameters=True)
- if net_dur_disc is not None:
- net_dur_disc = DDP(net_dur_disc, device_ids=[rank], find_unused_parameters=True)
-
- pretrain_dir = None
- if pretrain_dir is None:
- try:
- if net_dur_disc is not None:
- _, optim_dur_disc, _, epoch_str = utils.load_checkpoint(utils.latest_checkpoint_path(hps.model_dir, "DUR_*.pth"), net_dur_disc, optim_dur_disc, skip_optimizer=not hps.cont)
- _, optim_g, _, epoch_str = utils.load_checkpoint(utils.latest_checkpoint_path(hps.model_dir, "G_*.pth"), net_g,
- optim_g, skip_optimizer=not hps.cont)
- _, optim_d, _, epoch_str = utils.load_checkpoint(utils.latest_checkpoint_path(hps.model_dir, "D_*.pth"), net_d,
- optim_d, skip_optimizer=not hps.cont)
-
- epoch_str = max(epoch_str, 1)
- global_step = (epoch_str - 1) * len(train_loader)
- except Exception as e:
- print(e)
- epoch_str = 1
- global_step = 0
- else:
- _, _, _, epoch_str = utils.load_checkpoint(utils.latest_checkpoint_path(pretrain_dir, "G_*.pth"), net_g,
- optim_g, True)
- _, _, _, epoch_str = utils.load_checkpoint(utils.latest_checkpoint_path(pretrain_dir, "D_*.pth"), net_d,
- optim_d, True)
-
-
-
- scheduler_g = torch.optim.lr_scheduler.ExponentialLR(optim_g, gamma=hps.train.lr_decay, last_epoch=epoch_str - 2)
- scheduler_d = torch.optim.lr_scheduler.ExponentialLR(optim_d, gamma=hps.train.lr_decay, last_epoch=epoch_str - 2)
- if net_dur_disc is not None:
- scheduler_dur_disc = torch.optim.lr_scheduler.ExponentialLR(optim_dur_disc, gamma=hps.train.lr_decay, last_epoch=epoch_str-2)
- else:
- scheduler_dur_disc = None
- scaler = GradScaler(enabled=hps.train.fp16_run)
-
- for epoch in range(epoch_str, hps.train.epochs + 1):
- if rank == 0:
- train_and_evaluate(rank, epoch, hps, [net_g, net_d, net_dur_disc], [optim_g, optim_d, optim_dur_disc], [scheduler_g, scheduler_d, scheduler_dur_disc], scaler, [train_loader, eval_loader], logger, [writer, writer_eval])
- else:
- train_and_evaluate(rank, epoch, hps, [net_g, net_d, net_dur_disc], [optim_g, optim_d, optim_dur_disc], [scheduler_g, scheduler_d, scheduler_dur_disc], scaler, [train_loader, None], None, None)
- scheduler_g.step()
- scheduler_d.step()
- if net_dur_disc is not None:
- scheduler_dur_disc.step()
-
-
-def train_and_evaluate(rank, epoch, hps, nets, optims, schedulers, scaler, loaders, logger, writers):
- net_g, net_d, net_dur_disc = nets
- optim_g, optim_d, optim_dur_disc = optims
- scheduler_g, scheduler_d, scheduler_dur_disc = schedulers
- train_loader, eval_loader = loaders
- if writers is not None:
- writer, writer_eval = writers
-
- train_loader.batch_sampler.set_epoch(epoch)
- global global_step
-
- net_g.train()
- net_d.train()
- if net_dur_disc is not None:
- net_dur_disc.train()
- for batch_idx, (x, x_lengths, spec, spec_lengths, y, y_lengths, speakers, tone, language, bert) in tqdm(enumerate(train_loader)):
- if net_g.module.use_noise_scaled_mas:
- current_mas_noise_scale = net_g.module.mas_noise_scale_initial - net_g.module.noise_scale_delta * global_step
- net_g.module.current_mas_noise_scale = max(current_mas_noise_scale, 0.0)
- x, x_lengths = x.cuda(rank, non_blocking=True), x_lengths.cuda(rank, non_blocking=True)
- spec, spec_lengths = spec.cuda(rank, non_blocking=True), spec_lengths.cuda(rank, non_blocking=True)
- y, y_lengths = y.cuda(rank, non_blocking=True), y_lengths.cuda(rank, non_blocking=True)
- speakers = speakers.cuda(rank, non_blocking=True)
- tone = tone.cuda(rank, non_blocking=True)
- language = language.cuda(rank, non_blocking=True)
- bert = bert.cuda(rank, non_blocking=True)
-
- with autocast(enabled=hps.train.fp16_run):
- y_hat, l_length, attn, ids_slice, x_mask, z_mask, \
- (z, z_p, m_p, logs_p, m_q, logs_q), (hidden_x, logw, logw_) = net_g(x, x_lengths, spec, spec_lengths, speakers, tone, language, bert)
- mel = spec_to_mel_torch(
- spec,
- hps.data.filter_length,
- hps.data.n_mel_channels,
- hps.data.sampling_rate,
- hps.data.mel_fmin,
- hps.data.mel_fmax)
- y_mel = commons.slice_segments(mel, ids_slice, hps.train.segment_size // hps.data.hop_length)
- y_hat_mel = mel_spectrogram_torch(
- y_hat.squeeze(1),
- hps.data.filter_length,
- hps.data.n_mel_channels,
- hps.data.sampling_rate,
- hps.data.hop_length,
- hps.data.win_length,
- hps.data.mel_fmin,
- hps.data.mel_fmax
- )
-
- y = commons.slice_segments(y, ids_slice * hps.data.hop_length, hps.train.segment_size) # slice
-
- # Discriminator
- y_d_hat_r, y_d_hat_g, _, _ = net_d(y, y_hat.detach())
- with autocast(enabled=False):
- loss_disc, losses_disc_r, losses_disc_g = discriminator_loss(y_d_hat_r, y_d_hat_g)
- loss_disc_all = loss_disc
- if net_dur_disc is not None:
- y_dur_hat_r, y_dur_hat_g = net_dur_disc(hidden_x.detach(), x_mask.detach(), logw.detach(), logw_.detach())
- with autocast(enabled=False):
- # TODO: I think need to mean using the mask, but for now, just mean all
- loss_dur_disc, losses_dur_disc_r, losses_dur_disc_g = discriminator_loss(y_dur_hat_r, y_dur_hat_g)
- loss_dur_disc_all = loss_dur_disc
- optim_dur_disc.zero_grad()
- scaler.scale(loss_dur_disc_all).backward()
- scaler.unscale_(optim_dur_disc)
- grad_norm_dur_disc = commons.clip_grad_value_(net_dur_disc.parameters(), None)
- scaler.step(optim_dur_disc)
-
- optim_d.zero_grad()
- scaler.scale(loss_disc_all).backward()
- scaler.unscale_(optim_d)
- grad_norm_d = commons.clip_grad_value_(net_d.parameters(), None)
- scaler.step(optim_d)
-
- with autocast(enabled=hps.train.fp16_run):
- # Generator
- y_d_hat_r, y_d_hat_g, fmap_r, fmap_g = net_d(y, y_hat)
- if net_dur_disc is not None:
- y_dur_hat_r, y_dur_hat_g = net_dur_disc(hidden_x, x_mask, logw, logw_)
- with autocast(enabled=False):
- loss_dur = torch.sum(l_length.float())
- loss_mel = F.l1_loss(y_mel, y_hat_mel) * hps.train.c_mel
- loss_kl = kl_loss(z_p, logs_q, m_p, logs_p, z_mask) * hps.train.c_kl
-
- loss_fm = feature_loss(fmap_r, fmap_g)
- loss_gen, losses_gen = generator_loss(y_d_hat_g)
- loss_gen_all = loss_gen + loss_fm + loss_mel + loss_dur + loss_kl
- if net_dur_disc is not None:
- loss_dur_gen, losses_dur_gen = generator_loss(y_dur_hat_g)
- loss_gen_all += loss_dur_gen
- optim_g.zero_grad()
- scaler.scale(loss_gen_all).backward()
- scaler.unscale_(optim_g)
- grad_norm_g = commons.clip_grad_value_(net_g.parameters(), None)
- scaler.step(optim_g)
- scaler.update()
-
- if rank == 0:
- if global_step % hps.train.log_interval == 0:
- lr = optim_g.param_groups[0]['lr']
- losses = [loss_disc, loss_gen, loss_fm, loss_mel, loss_dur, loss_kl]
- logger.info('Train Epoch: {} [{:.0f}%]'.format(
- epoch,
- 100. * batch_idx / len(train_loader)))
- logger.info([x.item() for x in losses] + [global_step, lr])
-
- scalar_dict = {"loss/g/total": loss_gen_all, "loss/d/total": loss_disc_all, "learning_rate": lr,
- "grad_norm_d": grad_norm_d, "grad_norm_g": grad_norm_g}
- scalar_dict.update(
- {"loss/g/fm": loss_fm, "loss/g/mel": loss_mel, "loss/g/dur": loss_dur, "loss/g/kl": loss_kl})
- scalar_dict.update({"loss/g/{}".format(i): v for i, v in enumerate(losses_gen)})
- scalar_dict.update({"loss/d_r/{}".format(i): v for i, v in enumerate(losses_disc_r)})
- scalar_dict.update({"loss/d_g/{}".format(i): v for i, v in enumerate(losses_disc_g)})
-
- image_dict = {
- "slice/mel_org": utils.plot_spectrogram_to_numpy(y_mel[0].data.cpu().numpy()),
- "slice/mel_gen": utils.plot_spectrogram_to_numpy(y_hat_mel[0].data.cpu().numpy()),
- "all/mel": utils.plot_spectrogram_to_numpy(mel[0].data.cpu().numpy()),
- "all/attn": utils.plot_alignment_to_numpy(attn[0, 0].data.cpu().numpy())
- }
- utils.summarize(
- writer=writer,
- global_step=global_step,
- images=image_dict,
- scalars=scalar_dict)
-
- if global_step % hps.train.eval_interval == 0:
- evaluate(hps, net_g, eval_loader, writer_eval)
- utils.save_checkpoint(net_g, optim_g, hps.train.learning_rate, epoch,
- os.path.join(hps.model_dir, "G_{}.pth".format(global_step)))
- utils.save_checkpoint(net_d, optim_d, hps.train.learning_rate, epoch,
- os.path.join(hps.model_dir, "D_{}.pth".format(global_step)))
- if net_dur_disc is not None:
- utils.save_checkpoint(net_dur_disc, optim_dur_disc, hps.train.learning_rate, epoch, os.path.join(hps.model_dir, "DUR_{}.pth".format(global_step)))
- keep_ckpts = getattr(hps.train, 'keep_ckpts', 5)
- if keep_ckpts > 0:
- utils.clean_checkpoints(path_to_models=hps.model_dir, n_ckpts_to_keep=keep_ckpts, sort_by_time=True)
-
-
- global_step += 1
-
- if rank == 0:
- logger.info('====> Epoch: {}'.format(epoch))
-
-
-
-def evaluate(hps, generator, eval_loader, writer_eval):
- generator.eval()
- image_dict = {}
- audio_dict = {}
- print("Evaluating ...")
- with torch.no_grad():
- for batch_idx, (x, x_lengths, spec, spec_lengths, y, y_lengths, speakers, tone, language, bert) in enumerate(eval_loader):
- x, x_lengths = x.cuda(), x_lengths.cuda()
- spec, spec_lengths = spec.cuda(), spec_lengths.cuda()
- y, y_lengths = y.cuda(), y_lengths.cuda()
- speakers = speakers.cuda()
- bert = bert.cuda()
- tone = tone.cuda()
- language = language.cuda()
- for use_sdp in [True, False]:
- y_hat, attn, mask, *_ = generator.module.infer(x, x_lengths, speakers, tone, language, bert, y=spec, max_len=1000, sdp_ratio=0.0 if not use_sdp else 1.0)
- y_hat_lengths = mask.sum([1, 2]).long() * hps.data.hop_length
-
- mel = spec_to_mel_torch(
- spec,
- hps.data.filter_length,
- hps.data.n_mel_channels,
- hps.data.sampling_rate,
- hps.data.mel_fmin,
- hps.data.mel_fmax)
- y_hat_mel = mel_spectrogram_torch(
- y_hat.squeeze(1).float(),
- hps.data.filter_length,
- hps.data.n_mel_channels,
- hps.data.sampling_rate,
- hps.data.hop_length,
- hps.data.win_length,
- hps.data.mel_fmin,
- hps.data.mel_fmax
- )
- image_dict.update({
- f"gen/mel_{batch_idx}": utils.plot_spectrogram_to_numpy(y_hat_mel[0].cpu().numpy())
- })
- audio_dict.update({
- f"gen/audio_{batch_idx}_{use_sdp}": y_hat[0, :, :y_hat_lengths[0]]
- })
- image_dict.update({f"gt/mel_{batch_idx}": utils.plot_spectrogram_to_numpy(mel[0].cpu().numpy())})
- audio_dict.update({f"gt/audio_{batch_idx}": y[0, :, :y_lengths[0]]})
-
- utils.summarize(
- writer=writer_eval,
- global_step=global_step,
- images=image_dict,
- audios=audio_dict,
- audio_sampling_rate=hps.data.sampling_rate
- )
- generator.train()
-
-if __name__ == "__main__":
- main()
diff --git a/spaces/diivien/Music-Popularity-Prediction/README.md b/spaces/diivien/Music-Popularity-Prediction/README.md
deleted file mode 100644
index 5b795991c5272fd1a14c9e363f48f86077b0232c..0000000000000000000000000000000000000000
--- a/spaces/diivien/Music-Popularity-Prediction/README.md
+++ /dev/null
@@ -1,93 +0,0 @@
----
-title: Music Popularity Prediction
-emoji: 🚀
-colorFrom: purple
-colorTo: blue
-sdk: gradio
-sdk_version: 3.28.1
-app_file: app.py
-pinned: false
-python_version: 3.10.6
----
-
-# Music Popularity Prediction
-
-This repository contains a data science project that aims to predict the popularity of music using machine learning techniques.
-
-Check out the demo [here](https://huggingface.co/spaces/diivien/Music-Popularity-Prediction)!
-
-## Dataset
-
-This project uses the [Spotify Tracks Dataset](https://www.kaggle.com/datasets/maharshipandya/-spotify-tracks-dataset) available on Kaggle. This dataset contains information about Spotify tracks over a range of 125 different genres. Each track has several audio features associated with it, such as popularity, explicitness, danceability, energy, key, mode, speechiness, acousticness, instrumentalness, liveness, valence, tempo, and time signature.
-
-You can download the dataset from the Kaggle website and use it to follow along with the analysis in this project.
-
-## Overview
-
-This repository contains a data science project that aims to predict the popularity of music using machine learning techniques. The project is a binary classification problem where the goal is to predict whether a song will be popular or not. The dataset used in this project is imbalanced, meaning that one class is significantly more common than the other.
-
-The project consists of three main parts: Data Cleaning, Exploratory Data Analysis, and Model Building.
-
-### Data Cleaning
-
-In the [Data Cleaning](https://github.com/diivien/Music-Popularity-Prediction/blob/master/Data%20Cleaning.ipynb) notebook, I clean and preprocess the data to prepare it for analysis. This involves several steps such as:
-
-- Removing unique columns
-- Dropping null values
-- Dropping duplicated rows (same artists and track name)
-- Dropping artists and track name columns
-- Dropping invalid tempo and time signature according to Spotify API
-- Saving the cleaned dataset into a CSV file
-
-To get started with the data cleaning process, you can follow the instructions in the Data Cleaning notebook. This will guide you through the steps involved in cleaning and preprocessing the data.
-
-### Exploratory Data Analysis
-
-In the [Exploratory Data Analysis](https://github.com/diivien/Music-Popularity-Prediction/blob/master/Exploratory%20Data%20Analysis.ipynb) notebook, we explore the data and gain insights into the relationships between the features and the target variable. This involves generating various visualizations such as:
-
-- Correlation heatmaps to examine the relationships between pairs of continuous features
-- Histograms to check the distribution of continuous features
-- Bar charts to visualize categorical features
-- Scatter plots to examine the relationships between pairs of continuous features
-- Box plots to examine the distribution of continuous features by category
-- Stacked bar charts to visualize conditional distributions
-
-These visualizations help us understand the data better and inform our decisions when building machine learning models.
-
-To get started with the exploratory data analysis process, you can follow the instructions in the Exploratory Data Analysis notebook. This will guide you through the steps involved in exploring and visualizing the data.
-
-### Model Building
-
-In the [Model Building](https://github.com/diivien/Music-Popularity-Prediction/blob/master/Model%20Building.ipynb) notebook, I build and evaluate machine learning models to predict music popularity. The models used in this analysis include Linear SVC, Random Forest Classifier, LightGBM, and CatBoost. As part of this process, I perform several preprocessing steps such as scaling the data using a MinMax scaler and encoding categorical variables using a target encoder. I also use SMOTE-NC in an imbalanced-learn pipeline to prevent data leakage.
-
-To tune the hyperparameters of our models, I use Optuna for multi-objective optimization and generate a Pareto front plot to determine the best hyperparameters.
-
-To evaluate the performance of our models, I use several metrics that are appropriate for imbalanced datasets, such as F1 score, balanced accuracy, and PR AUC.
-
-To get started with the model building process, you can follow the instructions in the Model Building notebook. This will guide you through the steps involved in building and evaluating machine learning models to predict music popularity.
-
-## Future Work
-
-I am currently working on several improvements and extensions to this project. Some include:
-
-- Testing a neural network classifier to see if it can improve the accuracy of our predictions
-- Deploying an app on Gradio to make it easier for users to interact with our models and make predictions
-
-
-## Citations
-
-If you use any of the following libraries in your project, please cite them as follows:
-
-- imbalanced-learn: Lemaître, G., Nogueira, F., & Aridas, C. K. (2017). Imbalanced-learn: A Python Toolbox to Tackle the Curse of Imbalanced Datasets in Machine Learning. Journal of Machine Learning Research, 18(17), 1-5.
-- Matplotlib: Hunter, J. D. (2007). Matplotlib: A 2D graphics environment. Computing in Science & Engineering, 9(3), 90-95.
-- Seaborn: Waskom, M., Botvinnik, O., O’Kane, D., Hobson, P., Lukauskas, S., Gemperline, D. C., ... & de Ruiter, J. (2021). seaborn: statistical data visualization. Journal of Open Source Software, 6(60), 3021.
-- Joblib: Buitinck, L., Louppe, G., Blondel, M., Pedregosa, F., Mueller, A., Grisel, O., ... & Duchesnay, E. (2013). API design for machine learning software: experiences from the scikit-learn project. arXiv preprint arXiv:1309.0238.
-- Feature-engine: Sole-Ribalta A. (2020) Feature-engine: A Python Package for Feature Engineering and Preprocessing in Machine Learning. In: Martínez-Villaseñor L., Batyrshin I., Mendoza O., Kuri-Morales Á. (eds) Advances in Artificial Intelligence - IBERAMIA 2020. IBERAMIA 2020. Lecture Notes in Computer Science, vol 12422. Springer, Cham.
-- LightGBM: Ke, G., Meng, Q., Finley, T., Wang, T., Chen, W., Ma, W., ... & Liu, T. Y. (2017). LightGBM: A highly efficient gradient boosting decision tree. Advances in Neural Information Processing Systems.
-- CatBoost: Prokhorenkova L.O., Gusev G.L., Vorobev A.V., Dorogush A.V., Gulin A.A.(2018). CatBoost: unbiased boosting with categorical features. Advances in Neural Information Processing Systems.
-- Category Encoders: Micci-Barreca D (2001) A preprocessing scheme for high-cardinality categorical attributes in classification and prediction problems. ACM SIGKDD Explorations Newsletter 3(1):27–32
-- NumPy: Harris CR et al.(2020) Array programming with NumPy. Nature 585(7825):357–362
-- SDV (Synthetic Data Vault): Patki N et al.(2016) The Synthetic Data Vault. IEEE International Conference on Data Science and Advanced Analytics
-- Optuna: Akiba T et al.(2019) Optuna: A Next-generation Hyperparameter Optimization Framework. Proceedings of the 25th ACM SIGKDD International Conference on Knowledge Discovery & Data Mining
-- PyTorch: Paszke A et al.(2019) PyTorch: An Imperative Style High-performance Deep Learning Library. Advances in Neural Information Processing Systems
-- SciKeras: Varma P et al.(2020) SciKeras: a high-level Scikit-Learn compatible API for TensorFlow's Keras module
diff --git a/spaces/dorkai/text-generation-webui-main/text-generation-webui-main/docs/Low-VRAM-guide.md b/spaces/dorkai/text-generation-webui-main/text-generation-webui-main/docs/Low-VRAM-guide.md
deleted file mode 100644
index 1dc86f9c7f764a886c454f7f76a2a89a77140655..0000000000000000000000000000000000000000
--- a/spaces/dorkai/text-generation-webui-main/text-generation-webui-main/docs/Low-VRAM-guide.md
+++ /dev/null
@@ -1,51 +0,0 @@
-If you GPU is not large enough to fit a model, try these in the following order:
-
-### Load the model in 8-bit mode
-
-```
-python server.py --load-in-8bit
-```
-
-This reduces the memory usage by half with no noticeable loss in quality. Only newer GPUs support 8-bit mode.
-
-### Split the model across your GPU and CPU
-
-```
-python server.py --auto-devices
-```
-
-If you can load the model with this command but it runs out of memory when you try to generate text, try increasingly limiting the amount of memory allocated to the GPU until the error stops happening:
-
-```
-python server.py --auto-devices --gpu-memory 10
-python server.py --auto-devices --gpu-memory 9
-python server.py --auto-devices --gpu-memory 8
-...
-```
-
-where the number is in GiB.
-
-For finer control, you can also specify the unit in MiB explicitly:
-
-```
-python server.py --auto-devices --gpu-memory 8722MiB
-python server.py --auto-devices --gpu-memory 4725MiB
-python server.py --auto-devices --gpu-memory 3500MiB
-...
-```
-
-Additionally, you can also set the `--no-cache` value to reduce the GPU usage while generating text at a performance cost. This may allow you to set a higher value for `--gpu-memory`, resulting in a net performance gain.
-
-### Send layers to a disk cache
-
-As a desperate last measure, you can split the model across your GPU, CPU, and disk:
-
-```
-python server.py --auto-devices --disk
-```
-
-With this, I am able to load a 30b model into my RTX 3090, but it takes 10 seconds to generate 1 word.
-
-### DeepSpeed (experimental)
-
-An experimental alternative to all of the above is to use DeepSpeed: [guide](DeepSpeed.md).
diff --git a/spaces/dragonSwing/video2slide/post_process.py b/spaces/dragonSwing/video2slide/post_process.py
deleted file mode 100644
index 3d841a5347dff4b2a931f354bbea76533488d96b..0000000000000000000000000000000000000000
--- a/spaces/dragonSwing/video2slide/post_process.py
+++ /dev/null
@@ -1,77 +0,0 @@
-import imagehash
-import os
-from collections import deque
-from PIL import Image
-from tqdm import tqdm
-
-
-def find_similar_images(
- base_dir, hash_size=8, hashfunc=imagehash.dhash, queue_len=5, threshold=4
-):
- snapshots_files = sorted(os.listdir(base_dir))
-
- hash_dict = {}
- hash_queue = deque([], maxlen=queue_len)
- duplicates = []
- num_duplicates = 0
-
- print("---" * 5, "Finding similar files", "---" * 5)
-
- with tqdm(snapshots_files) as t:
- for file in t:
- read_file = Image.open(os.path.join(base_dir, file))
- comp_hash = hashfunc(read_file, hash_size=hash_size)
- duplicate = False
-
- if comp_hash not in hash_dict:
- hash_dict[comp_hash] = file
- # Compare with hash queue to find out potential duplicates
- for img_hash in hash_queue:
- if img_hash - comp_hash <= threshold:
- duplicate = True
- break
-
- if not duplicate:
- hash_queue.append(comp_hash)
- else:
- duplicate = True
-
- if duplicate:
- duplicates.append(file)
- num_duplicates += 1
- t.set_postfix_str(f"Duplicate files: {num_duplicates}")
-
- return hash_dict, duplicates
-
-
-def remove_duplicates(
- base_dir, hash_size=8, hashfunc=imagehash.dhash, queue_len=5, threshold=4
-):
- _, duplicates = find_similar_images(
- base_dir,
- hash_size=hash_size,
- hashfunc=hashfunc,
- queue_len=queue_len,
- threshold=threshold,
- )
-
- if not len(duplicates):
- print("No duplicates found!")
- else:
- print("Removing duplicates...")
-
- for dup_file in duplicates:
- file_path = os.path.join(base_dir, dup_file)
-
- if os.path.exists(file_path):
- os.remove(file_path)
- else:
- print("Filepath: ", file_path, "does not exists.")
-
- print("All duplicates removed!")
-
- print("***" * 10, "\n")
-
-
-if __name__ == "__main__":
- remove_duplicates("sample_1")
diff --git a/spaces/ehcalabres/EMOVoice/README.md b/spaces/ehcalabres/EMOVoice/README.md
deleted file mode 100644
index 41d1d133d8c95ae2896b4b4d0d88164e9ae8daf9..0000000000000000000000000000000000000000
--- a/spaces/ehcalabres/EMOVoice/README.md
+++ /dev/null
@@ -1,33 +0,0 @@
----
-title: EMOVoice
-emoji: 😍
-colorFrom: green
-colorTo: red
-sdk: streamlit
-app_file: app.py
-pinned: false
----
-
-# Configuration
-
-`title`: _string_
-Display title for the Space
-
-`emoji`: _string_
-Space emoji (emoji-only character allowed)
-
-`colorFrom`: _string_
-Color for Thumbnail gradient (red, yellow, green, blue, indigo, purple, pink, gray)
-
-`colorTo`: _string_
-Color for Thumbnail gradient (red, yellow, green, blue, indigo, purple, pink, gray)
-
-`sdk`: _string_
-Can be either `gradio` or `streamlit`
-
-`app_file`: _string_
-Path to your main application file (which contains either `gradio` or `streamlit` Python code).
-Path is relative to the root of the repository.
-
-`pinned`: _boolean_
-Whether the Space stays on top of your list.
diff --git a/spaces/ehristoforu/runwayml-stable-diffusion-v1-5k/README.md b/spaces/ehristoforu/runwayml-stable-diffusion-v1-5k/README.md
deleted file mode 100644
index 2de2727dd53656c2399112b35c9f5733e9478b07..0000000000000000000000000000000000000000
--- a/spaces/ehristoforu/runwayml-stable-diffusion-v1-5k/README.md
+++ /dev/null
@@ -1,12 +0,0 @@
----
-title: Runwayml Stable Diffusion V1 5k
-emoji: 🌍
-colorFrom: blue
-colorTo: gray
-sdk: gradio
-sdk_version: 3.40.1
-app_file: app.py
-pinned: false
----
-
-Check out the configuration reference at https://huggingface.co/docs/hub/spaces-config-reference
diff --git a/spaces/emc348/faces-through-time/models/e4e/stylegan2/op/__init__.py b/spaces/emc348/faces-through-time/models/e4e/stylegan2/op/__init__.py
deleted file mode 100644
index d0918d92285955855be89f00096b888ee5597ce3..0000000000000000000000000000000000000000
--- a/spaces/emc348/faces-through-time/models/e4e/stylegan2/op/__init__.py
+++ /dev/null
@@ -1,2 +0,0 @@
-from .fused_act import FusedLeakyReLU, fused_leaky_relu
-from .upfirdn2d import upfirdn2d
diff --git a/spaces/erastorgueva-nv/NeMo-Forced-Aligner/utils/constants.py b/spaces/erastorgueva-nv/NeMo-Forced-Aligner/utils/constants.py
deleted file mode 100644
index 51ce934be4793a33b907f2791bb60c04276d922c..0000000000000000000000000000000000000000
--- a/spaces/erastorgueva-nv/NeMo-Forced-Aligner/utils/constants.py
+++ /dev/null
@@ -1,19 +0,0 @@
-# Copyright (c) 2023, NVIDIA CORPORATION. All rights reserved.
-#
-# Licensed under the Apache License, Version 2.0 (the "License");
-# you may not use this file except in compliance with the License.
-# You may obtain a copy of the License at
-#
-# http://www.apache.org/licenses/LICENSE-2.0
-#
-# Unless required by applicable law or agreed to in writing, software
-# distributed under the License is distributed on an "AS IS" BASIS,
-# WITHOUT WARRANTIES OR CONDITIONS OF ANY KIND, either express or implied.
-# See the License for the specific language governing permissions and
-# limitations under the License.
-
-BLANK_TOKEN = ""
-
-SPACE_TOKEN = ""
-
-V_NEGATIVE_NUM = -3.4e38 # this is just above the most negative number in torch.float32
diff --git a/spaces/exnav29/Real_Estate_Bot/README.md b/spaces/exnav29/Real_Estate_Bot/README.md
deleted file mode 100644
index 2970f398a88ee97b4da03c3ce0b56b526d99a439..0000000000000000000000000000000000000000
--- a/spaces/exnav29/Real_Estate_Bot/README.md
+++ /dev/null
@@ -1,13 +0,0 @@
----
-title: Real Estate Bot
-emoji: 📈
-colorFrom: green
-colorTo: purple
-sdk: gradio
-sdk_version: 3.23.0
-app_file: app.py
-pinned: false
-license: cc-by-4.0
----
-
-Check out the configuration reference at https://huggingface.co/docs/hub/spaces-config-reference
diff --git a/spaces/falterWliame/Face_Mask_Detection/HACK DVDFab 13.0.9.7 (x64) !!BETTER!! Crack.md b/spaces/falterWliame/Face_Mask_Detection/HACK DVDFab 13.0.9.7 (x64) !!BETTER!! Crack.md
deleted file mode 100644
index a51ad74bb001375a832471cc95563d2ec302aefb..0000000000000000000000000000000000000000
--- a/spaces/falterWliame/Face_Mask_Detection/HACK DVDFab 13.0.9.7 (x64) !!BETTER!! Crack.md
+++ /dev/null
@@ -1,9 +0,0 @@
-
-
dvdfab crack is also a perfect solution for copying discs. it provides you the option to choose the dvd source and the output files for each and every disc. you can also get the configuration and operation data of the movies as well as subtitle information. in addition, it offers you the choice to edit the files after conversion. it additionally permits you to generate the subtitles from the converted data if they are not available.
you can also use dvdfab torrent a free dvd encryption software that offers you one of the best dvd ripping and conversion results. it offers you some new features such as "fastest converting speed, rip with multi-core cpu and nvidia cuda technology. with a few clicks, you can easily customize the output files. it can also convert blu-ray discs. you can also use the free trial to test dvdfab.
-
my response: technically, dvdfab is in the same boat as anydvd; however, dvdfab is a lot cheaper (and, therefore, a lot more attractive). and, although anydvd can do everything that dvdfab can do, dvdfab (and its other available utilities) can do all of that and much, much more. plus, it can decrypt and rip blu-ray discs, which anydvd cannot. if i were a lawyer and dvdfab were to sue anydvd, i would be going after the lawyers who wrote the anydvd code, not the developers of dvdfab.
-
my response: if dvdfab has a weakness, it is the fact that it is a bit limited in its features. dvdfab does have a feature set that is quite limited, which is why i keep saying that the developers do need to make sure that they do not start copying the features of their competitors; since, if they do that, then they will be on the hook for any lawsuits that are filed against them. however, dvdfab does the basic job that it was designed to do, and it does it very well.
- 899543212b
-
-
\ No newline at end of file
diff --git a/spaces/fatiXbelha/sd/Download Real Gangster Crime and Rule the Streets of New Vegas.md b/spaces/fatiXbelha/sd/Download Real Gangster Crime and Rule the Streets of New Vegas.md
deleted file mode 100644
index 3dfe87435aed1024c8bcbbf21cb48a7d70b3e01b..0000000000000000000000000000000000000000
--- a/spaces/fatiXbelha/sd/Download Real Gangster Crime and Rule the Streets of New Vegas.md
+++ /dev/null
@@ -1,112 +0,0 @@
-
-
How to Download Real Gangster Crime Dinheiro Infinito
-
If you are a fan of action, adventure, and simulation games, you might have heard of Real Gangster Crime, a popular game developed by Naxeex Studio. In this game, you can experience the thrill of being a gangster in a crime-ridden city, where you can fight, steal, drive, and explore. But what if you want to have unlimited money and resources in the game, without having to spend real money or complete difficult missions? That's where dinheiro infinito comes in. Dinheiro infinito is a Portuguese term that means infinite money, and it refers to a modded version of the game that gives you access to unlimited cash, weapons, vehicles, clothes, and more. In this article, we will show you how to download real gangster crime dinheiro infinito for Android and PC devices, so you can enjoy the game to the fullest.
-
What is Real Gangster Crime?
-
Real Gangster Crime is an action game that combines elements of adventure and simulation in one grand theft game. It's perfect for fans of police chases, gang wars, crime simulators, and realistic games. In this game, you play as a real gangster who is trying to climb the ranks of the criminal underworld and become the kingpin. You can engage in various missions and quests, from stealing cars to participating in high-speed police chases to heists and robberies. As you progress through the game, you can unlock new and more difficult missions, which will require you to use all your skills and resources to succeed. You can also explore the open world of the city at your own pace, look for hidden collectibles and easter eggs, and discover new locations and activities. You can drive cool cars and motorcycles, confront enemies with a giant assault robot, or even fly a helicopter. You can also customize your character with a variety of clothing, shoes, hats, masks, and other accessories. You can choose from a wide range of weapons, from pistols to rocket launchers and from laser rifles to a strong steel suit. Real Gangster Crime is a free action game that offers you a realistic and immersive gangster simulator experience.
Open world environment with many locations and activities
-
Various missions and quests with different objectives and rewards
-
Huge arsenal of weapons and vehicles
-
Character customization options
-
Offline mode available
-
-
Why download dinheiro infinito?
-
While Real Gangster Crime is a fun and exciting game, it can also be frustrating not having enough money to purchase the weapons, vehicles, clothes, and other gear you need. You might also find some missions too hard or too boring to complete. That's why some players prefer to download dinheiro infinito, which is a modded version of the game that gives you unlimited money and resources. With dinheiro infinito, you can buy anything you want in the game without worrying about running out of cash. You can also unlock all the missions and quests without having to complete them. You can enjoy the game without any limitations or restrictions.
-
How to download dinheiro infinito for Android
-
If you want to download real gangster crime dinheiro infinito for your Android device, you will need to follow these steps:
-
Step 1: Find a reliable source
-
The first thing you need to do is find a reliable source
The first thing you need to do is find a reliable source that offers the download link for the dinheiro infinito APK file. You can search online for websites or blogs that provide the link, but be careful not to download from untrustworthy or malicious sources that might harm your device or steal your data. You can also check the reviews and ratings of other users who have downloaded the file before you. One of the sources that we recommend is [this website], which has a high reputation and positive feedback from users.
-
Step 2: Enable unknown sources
-
Before you can install the dinheiro infinito APK file on your Android device, you need to enable unknown sources in your settings. This will allow you to install apps from sources other than the Google Play Store. To do this, go to your device's settings, then security, then unknown sources, and toggle it on. You might see a warning message that says installing apps from unknown sources can be risky, but don't worry, as long as you download from a reliable source, you should be fine.
-
Step 3: Install the APK file
-
Once you have enabled unknown sources, you can proceed to install the dinheiro infinito APK file on your device. To do this, go to the download folder where you saved the file, and tap on it. You might see a pop-up window that asks for your permission to install the app, just tap on install and wait for the process to finish. You might also see another pop-up window that asks if you want to open the app or done, just tap on done for now.
-
Step 4: Enjoy unlimited money and resources
-
Now that you have installed the dinheiro infinito APK file on your device, you can enjoy unlimited money and resources in Real Gangster Crime. To do this, just open the app and start playing. You will see that you have unlimited cash in your account, and you can buy anything you want in the game. You can also unlock all the missions and quests without having to complete them. You can have fun with the gangster life without any limitations or restrictions.
-
real gangster crime mod apk dinheiro infinito
-como baixar real gangster crime com dinheiro infinito
-real gangster crime hack dinheiro infinito download
-real gangster crime atualizado dinheiro infinito
-download real gangster crime dinheiro infinito 2023
-real gangster crime apk mod dinheiro infinito 2023
-real gangster crime dinheiro infinito e diamantes
-real gangster crime jogo de mundo aberto dinheiro infinito
-real gangster crime versão antiga dinheiro infinito
-real gangster crime download grátis dinheiro infinito
-real gangster crime jogo online dinheiro infinito
-real gangster crime para pc dinheiro infinito
-real gangster crime 2 dinheiro infinito download
-real gangster crime mod menu dinheiro infinito
-real gangster crime como ter dinheiro infinito
-download do jogo real gangster crime com dinheiro infinito
-real gangster crime apk pure dinheiro infinito
-real gangster crime uptodown dinheiro infinito
-baixar o jogo real gangster crime com dinheiro infinito
-real gangster crime dicas e truques dinheiro infinito
-real gangster crime gameplay dinheiro infinito
-real gangster crime 3d dinheiro infinito download
-real gangster crime simulator dinheiro infinito
-real gangster crime city 2023 dinheiro infinito
-download de real gangster crime com dinheiro infinito
-baixar apk mod de real gangster crime com dinheiro infinito
-como instalar real gangster crime com dinheiro infinito
-real gangster crime cheats dinheiro infinito android
-baixar e instalar real gangster crime com dinheiro infinito
-download do apk de real gangster crime com dinheiro infinito
-como jogar real gangster crime com dinheiro infinito
-baixar o apk de real gangster crime com dinheiro infinito
-como atualizar o real gangster crime com dinheiro infinito
-baixar e jogar real gangster crime com dinheiro infinito
-download do mod de real gangster crime com dinheiro infinito
-como hackear o jogo real gangster crime com dinheiro infinito
-baixar o mod de real gangster crime com dinheiro infinito
-como desbloquear tudo no real gangster crime com dinheiro infinito
-baixar e atualizar o real gangster crime com dinheiro infinito
-download do hack de real gangster crime com dinheiro infinito
-como fazer o download do real gangster crime com dinheiro infinito
-baixar o hack de real gangster crime com dinheiro infinito
-como resolver o erro do real gangster crime com dinheiro infinito
-baixar e hackear o jogo real gangster crime com dinheiro infinito
-download do jogo completo de real gangster crime com dinheiro infinito
-como mudar a linguagem do real gangster crime com dinheiro infinito
-baixar o jogo completo de real gangster crime com dinheiro infinito
-
How to download dinheiro infinito for PC
-
If you want to download real gangster crime dinheiro infinito for your PC, you will need to follow these steps:
-
Step 1: Download an emulator
-
An emulator is a software that allows you to run Android apps on your PC. There are many emulators available online, but some of the most popular ones are BlueStacks, NoxPlayer, and LDPlayer. You can choose any emulator that suits your preferences and system requirements, but make sure to download it from a reputable source. To download an emulator, just go to its official website and follow the instructions to install it on your PC.
-
Step 2: Install the emulator and the APK file
-
Once you have downloaded and installed an emulator on your PC, you need to install the dinheiro infinito APK file on it. To do this, just drag and drop the APK file into the emulator's window, or use the built-in browser to find and download it from [this website]. The emulator will automatically detect and install the APK file on its system.
-
Step 3: Launch the game and customize the settings
-
Now that you have installed the dinheiro infinito APK file on your emulator, you can launch the game and customize the settings according to your preferences. To do this, just open the emulator and click on Real Gangster Crime icon. You will see a welcome screen that asks you to choose your language and agree to the terms of service. After that, you can adjust the graphics quality, sound effects, controls, and other options in the settings menu.
-
Step 4: Have fun with the gangster life
-
Now that you have launched the game and customized the settings, you can have fun with
Now that you have launched the game and customized the settings, you can have fun with the gangster life on your PC. You will see that you have unlimited money and resources in Real Gangster Crime, and you can buy anything you want in the game. You can also unlock all the missions and quests without having to complete them. You can explore the city, fight, steal, drive, and fly as you please. You can enjoy the game with high-quality graphics, sound effects, and controls on your PC.
-
Conclusion
-
Real Gangster Crime is an action game that lets you experience the thrill of being a gangster in a crime-ridden city. It offers you a realistic and immersive gangster simulator experience, with various missions, quests, locations, activities, weapons, vehicles, and customization options. However, if you want to have unlimited money and resources in the game, without having to spend real money or complete difficult missions, you can download dinheiro infinito, which is a modded version of the game that gives you access to unlimited cash, weapons, vehicles, clothes, and more. In this article, we showed you how to download real gangster crime dinheiro infinito for Android and PC devices, so you can enjoy the game to the fullest. We hope you found this article helpful and informative. If you have any questions or feedback, feel free to leave a comment below.
-
FAQs
-
-
Is dinheiro infinito safe to download?
-
Dinheiro infinito is safe to download as long as you download it from a reliable source that offers the download link for the dinheiro infinito APK file. You should also enable unknown sources in your settings before installing the APK file on your device. However, you should be aware that downloading dinheiro infinito might violate the terms of service of Real Gangster Crime, and you might face some risks or consequences if you use it online or with other players.
-
Is dinheiro infinito compatible with all devices?
-
Dinheiro infinito is compatible with most Android and PC devices that meet the minimum system requirements of Real Gangster Crime. However, some devices might not support the modded version of the game, or might experience some glitches or errors while running it. If you encounter any problems while downloading or installing dinheiro infinito, you should try to update your device's software or contact the source of the download link for assistance.
-
Can I update dinheiro infinito?
-
Dinheiro infinito is usually updated whenever Real Gangster Crime releases a new version of the game. However, you should not update dinheiro infinito from the Google Play Store or any other official source of Real Gangster Crime, as this might overwrite or delete the modded features of dinheiro infinito. Instead, you should check the source of the download link for dinheiro infinito for any updates or new versions of the modded game.
-
Can I play online with dinheiro infinito?
-
Dinheiro infinito is mainly designed for offline mode, where you can play without any internet connection or interference from other players. However, some sources of dinheiro infinito might offer online mode as well, where you can play with other players who have downloaded dinheiro infinito as well. However, you should be careful not to play online with players who have not downloaded dinheiro infinito, as this might cause some conflicts or issues with the game's servers or security systems.
-
Can I uninstall dinheiro infinito?
-
If you want to uninstall dinheiro infinito from your device, you can do so by following these steps:
-
-
Go to your device's settings, then apps, then Real Gangster Crime.
-
Tap on uninstall and confirm your choice.
-
Delete the dinheiro infinito APK file from your download folder.
-
-
This will remove dinheiro infinito from your device completely. If you want to reinstall Real Gangster Crime from the Google Play Store or any other official source of the game, you can do so by following these steps:
-
-
Go to the Google Play Store or any other official source of Real Gangster Crime.
-
Search for Real Gangster Crime and tap on install.
-
Wait for the installation process to finish and open the game.
-
-
This will reinstall Real Gangster Crime on your device normally.
197e85843d
-
-
\ No newline at end of file
diff --git a/spaces/fclong/summary/fengshen/models/transfo_xl_denoise/generate.py b/spaces/fclong/summary/fengshen/models/transfo_xl_denoise/generate.py
deleted file mode 100644
index 5b768ff1baf6477735ac14fec9df58f7cd2724c6..0000000000000000000000000000000000000000
--- a/spaces/fclong/summary/fengshen/models/transfo_xl_denoise/generate.py
+++ /dev/null
@@ -1,66 +0,0 @@
-import torch
-import torch.nn.functional as F
-from fengshen.models.transfo_xl_denoise.tokenization_transfo_xl_denoise import TransfoXLDenoiseTokenizer
-from fengshen.models.transfo_xl_denoise.modeling_transfo_xl_denoise import TransfoXLDenoiseModel
-from fengshen.utils import top_k_logits, get_masks_and_position_ids
-
-
-def get_batch(context_tokens, mem_length, batch_size=1):
- tokens = context_tokens
- tokens = tokens.view(batch_size, -1).contiguous()
- # Get the masks and postition ids.
- attention_mask, position_ids = get_masks_and_position_ids(tokens, mem_length=mem_length)
- return tokens, attention_mask, position_ids
-
-
-def denoise_generate(model,
- tokenizer,
- input_text,
- device=0,
- mem_length=512,
- temperature=1.,
- top_p=0.9,
- eod_token=50000):
- ''' Generate with fixed prompt pretrained '''
- prompt = f"“{input_text}”改写后是“"
- res = []
- counter = 0
- tokens, attention_mask, position_ids = get_batch(
- torch.LongTensor(tokenizer.encode(prompt)), mem_length, batch_size=1)
- tokens, attention_mask, position_ids = tokens.cuda(
- device), attention_mask.cuda(device), position_ids.cuda(device)
- org_context_length = tokens.shape[-1]
- model = model.cuda(device)
- while counter < 100:
- if counter == 0:
- mems = [] # empty at the begining
- output = model(input_ids=tokens, attention_mask=attention_mask,
- position_ids=position_ids, hidden_states=mems)
- logits, mems = output.logits, output.hidden_states
- else:
- index = org_context_length + counter
- output = model(input_ids=tokens[:, index - 1: index], position_ids=tokens.new_ones((1, 1)) * (index - 1),
- attention_mask=tokens.new_ones(1, 1, 1, mem_length + 1, device=device,
- dtype=torch.float), hidden_states=mems)
- logits, mems = output.logits, output.hidden_states
- logits = logits[:, -1]
- logits /= temperature
- logits = top_k_logits(logits, top_k=0, top_p=top_p)
- log_probs = F.softmax(logits, dim=-1)
- prev = torch.multinomial(log_probs, num_samples=1)[0]
- is_end = prev == eod_token
- if is_end:
- break
- tokens = torch.cat((tokens, prev.view(1, 1)), dim=1)
- counter += 1
- res.append(tokenizer.decode(tokens.view(-1).contiguous().tolist()))
- return res
-
-
-if __name__ == "__main__":
- device = 1
- tokenizer = TransfoXLDenoiseTokenizer.from_pretrained('IDEA-CCNL/Bigan-Transformer-XL-denoise-1.1B')
- model = TransfoXLDenoiseModel.from_pretrained('IDEA-CCNL/Bigan-Transformer-XL-denoise-1.1B')
- input_text = "凡是有成就的人, 都很严肃地对待生命自己的"
- res = denoise_generate(model, tokenizer, input_text)
- print(res)
diff --git a/spaces/fffffu/bing/src/components/ui/tooltip.tsx b/spaces/fffffu/bing/src/components/ui/tooltip.tsx
deleted file mode 100644
index af1d48beb90dd5ae311796539843700871052cae..0000000000000000000000000000000000000000
--- a/spaces/fffffu/bing/src/components/ui/tooltip.tsx
+++ /dev/null
@@ -1,30 +0,0 @@
-'use client'
-
-import * as React from 'react'
-import * as TooltipPrimitive from '@radix-ui/react-tooltip'
-
-import { cn } from '@/lib/utils'
-
-const TooltipProvider = TooltipPrimitive.Provider
-
-const Tooltip = TooltipPrimitive.Root
-
-const TooltipTrigger = TooltipPrimitive.Trigger
-
-const TooltipContent = React.forwardRef<
- React.ElementRef,
- React.ComponentPropsWithoutRef
->(({ className, sideOffset = 4, ...props }, ref) => (
-
-))
-TooltipContent.displayName = TooltipPrimitive.Content.displayName
-
-export { Tooltip, TooltipTrigger, TooltipContent, TooltipProvider }
diff --git a/spaces/fffiloni/controlnet-animation-doodle/node_modules/@types/node/ts4.8/zlib.d.ts b/spaces/fffiloni/controlnet-animation-doodle/node_modules/@types/node/ts4.8/zlib.d.ts
deleted file mode 100644
index 1d7f0c0e507405e9584cd7158cbbea92234afa84..0000000000000000000000000000000000000000
--- a/spaces/fffiloni/controlnet-animation-doodle/node_modules/@types/node/ts4.8/zlib.d.ts
+++ /dev/null
@@ -1,517 +0,0 @@
-/**
- * The `zlib` module provides compression functionality implemented using Gzip,
- * Deflate/Inflate, and Brotli.
- *
- * To access it:
- *
- * ```js
- * const zlib = require('zlib');
- * ```
- *
- * Compression and decompression are built around the Node.js `Streams API`.
- *
- * Compressing or decompressing a stream (such as a file) can be accomplished by
- * piping the source stream through a `zlib` `Transform` stream into a destination
- * stream:
- *
- * ```js
- * const { createGzip } = require('zlib');
- * const { pipeline } = require('stream');
- * const {
- * createReadStream,
- * createWriteStream
- * } = require('fs');
- *
- * const gzip = createGzip();
- * const source = createReadStream('input.txt');
- * const destination = createWriteStream('input.txt.gz');
- *
- * pipeline(source, gzip, destination, (err) => {
- * if (err) {
- * console.error('An error occurred:', err);
- * process.exitCode = 1;
- * }
- * });
- *
- * // Or, Promisified
- *
- * const { promisify } = require('util');
- * const pipe = promisify(pipeline);
- *
- * async function do_gzip(input, output) {
- * const gzip = createGzip();
- * const source = createReadStream(input);
- * const destination = createWriteStream(output);
- * await pipe(source, gzip, destination);
- * }
- *
- * do_gzip('input.txt', 'input.txt.gz')
- * .catch((err) => {
- * console.error('An error occurred:', err);
- * process.exitCode = 1;
- * });
- * ```
- *
- * It is also possible to compress or decompress data in a single step:
- *
- * ```js
- * const { deflate, unzip } = require('zlib');
- *
- * const input = '.................................';
- * deflate(input, (err, buffer) => {
- * if (err) {
- * console.error('An error occurred:', err);
- * process.exitCode = 1;
- * }
- * console.log(buffer.toString('base64'));
- * });
- *
- * const buffer = Buffer.from('eJzT0yMAAGTvBe8=', 'base64');
- * unzip(buffer, (err, buffer) => {
- * if (err) {
- * console.error('An error occurred:', err);
- * process.exitCode = 1;
- * }
- * console.log(buffer.toString());
- * });
- *
- * // Or, Promisified
- *
- * const { promisify } = require('util');
- * const do_unzip = promisify(unzip);
- *
- * do_unzip(buffer)
- * .then((buf) => console.log(buf.toString()))
- * .catch((err) => {
- * console.error('An error occurred:', err);
- * process.exitCode = 1;
- * });
- * ```
- * @since v0.5.8
- * @see [source](https://github.com/nodejs/node/blob/v18.0.0/lib/zlib.js)
- */
-declare module 'zlib' {
- import * as stream from 'node:stream';
- interface ZlibOptions {
- /**
- * @default constants.Z_NO_FLUSH
- */
- flush?: number | undefined;
- /**
- * @default constants.Z_FINISH
- */
- finishFlush?: number | undefined;
- /**
- * @default 16*1024
- */
- chunkSize?: number | undefined;
- windowBits?: number | undefined;
- level?: number | undefined; // compression only
- memLevel?: number | undefined; // compression only
- strategy?: number | undefined; // compression only
- dictionary?: NodeJS.ArrayBufferView | ArrayBuffer | undefined; // deflate/inflate only, empty dictionary by default
- info?: boolean | undefined;
- maxOutputLength?: number | undefined;
- }
- interface BrotliOptions {
- /**
- * @default constants.BROTLI_OPERATION_PROCESS
- */
- flush?: number | undefined;
- /**
- * @default constants.BROTLI_OPERATION_FINISH
- */
- finishFlush?: number | undefined;
- /**
- * @default 16*1024
- */
- chunkSize?: number | undefined;
- params?:
- | {
- /**
- * Each key is a `constants.BROTLI_*` constant.
- */
- [key: number]: boolean | number;
- }
- | undefined;
- maxOutputLength?: number | undefined;
- }
- interface Zlib {
- /** @deprecated Use bytesWritten instead. */
- readonly bytesRead: number;
- readonly bytesWritten: number;
- shell?: boolean | string | undefined;
- close(callback?: () => void): void;
- flush(kind?: number, callback?: () => void): void;
- flush(callback?: () => void): void;
- }
- interface ZlibParams {
- params(level: number, strategy: number, callback: () => void): void;
- }
- interface ZlibReset {
- reset(): void;
- }
- interface BrotliCompress extends stream.Transform, Zlib {}
- interface BrotliDecompress extends stream.Transform, Zlib {}
- interface Gzip extends stream.Transform, Zlib {}
- interface Gunzip extends stream.Transform, Zlib {}
- interface Deflate extends stream.Transform, Zlib, ZlibReset, ZlibParams {}
- interface Inflate extends stream.Transform, Zlib, ZlibReset {}
- interface DeflateRaw extends stream.Transform, Zlib, ZlibReset, ZlibParams {}
- interface InflateRaw extends stream.Transform, Zlib, ZlibReset {}
- interface Unzip extends stream.Transform, Zlib {}
- /**
- * Creates and returns a new `BrotliCompress` object.
- * @since v11.7.0, v10.16.0
- */
- function createBrotliCompress(options?: BrotliOptions): BrotliCompress;
- /**
- * Creates and returns a new `BrotliDecompress` object.
- * @since v11.7.0, v10.16.0
- */
- function createBrotliDecompress(options?: BrotliOptions): BrotliDecompress;
- /**
- * Creates and returns a new `Gzip` object.
- * See `example`.
- * @since v0.5.8
- */
- function createGzip(options?: ZlibOptions): Gzip;
- /**
- * Creates and returns a new `Gunzip` object.
- * @since v0.5.8
- */
- function createGunzip(options?: ZlibOptions): Gunzip;
- /**
- * Creates and returns a new `Deflate` object.
- * @since v0.5.8
- */
- function createDeflate(options?: ZlibOptions): Deflate;
- /**
- * Creates and returns a new `Inflate` object.
- * @since v0.5.8
- */
- function createInflate(options?: ZlibOptions): Inflate;
- /**
- * Creates and returns a new `DeflateRaw` object.
- *
- * An upgrade of zlib from 1.2.8 to 1.2.11 changed behavior when `windowBits`is set to 8 for raw deflate streams. zlib would automatically set `windowBits`to 9 if was initially set to 8\. Newer
- * versions of zlib will throw an exception,
- * so Node.js restored the original behavior of upgrading a value of 8 to 9,
- * since passing `windowBits = 9` to zlib actually results in a compressed stream
- * that effectively uses an 8-bit window only.
- * @since v0.5.8
- */
- function createDeflateRaw(options?: ZlibOptions): DeflateRaw;
- /**
- * Creates and returns a new `InflateRaw` object.
- * @since v0.5.8
- */
- function createInflateRaw(options?: ZlibOptions): InflateRaw;
- /**
- * Creates and returns a new `Unzip` object.
- * @since v0.5.8
- */
- function createUnzip(options?: ZlibOptions): Unzip;
- type InputType = string | ArrayBuffer | NodeJS.ArrayBufferView;
- type CompressCallback = (error: Error | null, result: Buffer) => void;
- /**
- * @since v11.7.0, v10.16.0
- */
- function brotliCompress(buf: InputType, options: BrotliOptions, callback: CompressCallback): void;
- function brotliCompress(buf: InputType, callback: CompressCallback): void;
- namespace brotliCompress {
- function __promisify__(buffer: InputType, options?: BrotliOptions): Promise;
- }
- /**
- * Compress a chunk of data with `BrotliCompress`.
- * @since v11.7.0, v10.16.0
- */
- function brotliCompressSync(buf: InputType, options?: BrotliOptions): Buffer;
- /**
- * @since v11.7.0, v10.16.0
- */
- function brotliDecompress(buf: InputType, options: BrotliOptions, callback: CompressCallback): void;
- function brotliDecompress(buf: InputType, callback: CompressCallback): void;
- namespace brotliDecompress {
- function __promisify__(buffer: InputType, options?: BrotliOptions): Promise;
- }
- /**
- * Decompress a chunk of data with `BrotliDecompress`.
- * @since v11.7.0, v10.16.0
- */
- function brotliDecompressSync(buf: InputType, options?: BrotliOptions): Buffer;
- /**
- * @since v0.6.0
- */
- function deflate(buf: InputType, callback: CompressCallback): void;
- function deflate(buf: InputType, options: ZlibOptions, callback: CompressCallback): void;
- namespace deflate {
- function __promisify__(buffer: InputType, options?: ZlibOptions): Promise;
- }
- /**
- * Compress a chunk of data with `Deflate`.
- * @since v0.11.12
- */
- function deflateSync(buf: InputType, options?: ZlibOptions): Buffer;
- /**
- * @since v0.6.0
- */
- function deflateRaw(buf: InputType, callback: CompressCallback): void;
- function deflateRaw(buf: InputType, options: ZlibOptions, callback: CompressCallback): void;
- namespace deflateRaw {
- function __promisify__(buffer: InputType, options?: ZlibOptions): Promise;
- }
- /**
- * Compress a chunk of data with `DeflateRaw`.
- * @since v0.11.12
- */
- function deflateRawSync(buf: InputType, options?: ZlibOptions): Buffer;
- /**
- * @since v0.6.0
- */
- function gzip(buf: InputType, callback: CompressCallback): void;
- function gzip(buf: InputType, options: ZlibOptions, callback: CompressCallback): void;
- namespace gzip {
- function __promisify__(buffer: InputType, options?: ZlibOptions): Promise;
- }
- /**
- * Compress a chunk of data with `Gzip`.
- * @since v0.11.12
- */
- function gzipSync(buf: InputType, options?: ZlibOptions): Buffer;
- /**
- * @since v0.6.0
- */
- function gunzip(buf: InputType, callback: CompressCallback): void;
- function gunzip(buf: InputType, options: ZlibOptions, callback: CompressCallback): void;
- namespace gunzip {
- function __promisify__(buffer: InputType, options?: ZlibOptions): Promise;
- }
- /**
- * Decompress a chunk of data with `Gunzip`.
- * @since v0.11.12
- */
- function gunzipSync(buf: InputType, options?: ZlibOptions): Buffer;
- /**
- * @since v0.6.0
- */
- function inflate(buf: InputType, callback: CompressCallback): void;
- function inflate(buf: InputType, options: ZlibOptions, callback: CompressCallback): void;
- namespace inflate {
- function __promisify__(buffer: InputType, options?: ZlibOptions): Promise;
- }
- /**
- * Decompress a chunk of data with `Inflate`.
- * @since v0.11.12
- */
- function inflateSync(buf: InputType, options?: ZlibOptions): Buffer;
- /**
- * @since v0.6.0
- */
- function inflateRaw(buf: InputType, callback: CompressCallback): void;
- function inflateRaw(buf: InputType, options: ZlibOptions, callback: CompressCallback): void;
- namespace inflateRaw {
- function __promisify__(buffer: InputType, options?: ZlibOptions): Promise;
- }
- /**
- * Decompress a chunk of data with `InflateRaw`.
- * @since v0.11.12
- */
- function inflateRawSync(buf: InputType, options?: ZlibOptions): Buffer;
- /**
- * @since v0.6.0
- */
- function unzip(buf: InputType, callback: CompressCallback): void;
- function unzip(buf: InputType, options: ZlibOptions, callback: CompressCallback): void;
- namespace unzip {
- function __promisify__(buffer: InputType, options?: ZlibOptions): Promise;
- }
- /**
- * Decompress a chunk of data with `Unzip`.
- * @since v0.11.12
- */
- function unzipSync(buf: InputType, options?: ZlibOptions): Buffer;
- namespace constants {
- const BROTLI_DECODE: number;
- const BROTLI_DECODER_ERROR_ALLOC_BLOCK_TYPE_TREES: number;
- const BROTLI_DECODER_ERROR_ALLOC_CONTEXT_MAP: number;
- const BROTLI_DECODER_ERROR_ALLOC_CONTEXT_MODES: number;
- const BROTLI_DECODER_ERROR_ALLOC_RING_BUFFER_1: number;
- const BROTLI_DECODER_ERROR_ALLOC_RING_BUFFER_2: number;
- const BROTLI_DECODER_ERROR_ALLOC_TREE_GROUPS: number;
- const BROTLI_DECODER_ERROR_DICTIONARY_NOT_SET: number;
- const BROTLI_DECODER_ERROR_FORMAT_BLOCK_LENGTH_1: number;
- const BROTLI_DECODER_ERROR_FORMAT_BLOCK_LENGTH_2: number;
- const BROTLI_DECODER_ERROR_FORMAT_CL_SPACE: number;
- const BROTLI_DECODER_ERROR_FORMAT_CONTEXT_MAP_REPEAT: number;
- const BROTLI_DECODER_ERROR_FORMAT_DICTIONARY: number;
- const BROTLI_DECODER_ERROR_FORMAT_DISTANCE: number;
- const BROTLI_DECODER_ERROR_FORMAT_EXUBERANT_META_NIBBLE: number;
- const BROTLI_DECODER_ERROR_FORMAT_EXUBERANT_NIBBLE: number;
- const BROTLI_DECODER_ERROR_FORMAT_HUFFMAN_SPACE: number;
- const BROTLI_DECODER_ERROR_FORMAT_PADDING_1: number;
- const BROTLI_DECODER_ERROR_FORMAT_PADDING_2: number;
- const BROTLI_DECODER_ERROR_FORMAT_RESERVED: number;
- const BROTLI_DECODER_ERROR_FORMAT_SIMPLE_HUFFMAN_ALPHABET: number;
- const BROTLI_DECODER_ERROR_FORMAT_SIMPLE_HUFFMAN_SAME: number;
- const BROTLI_DECODER_ERROR_FORMAT_TRANSFORM: number;
- const BROTLI_DECODER_ERROR_FORMAT_WINDOW_BITS: number;
- const BROTLI_DECODER_ERROR_INVALID_ARGUMENTS: number;
- const BROTLI_DECODER_ERROR_UNREACHABLE: number;
- const BROTLI_DECODER_NEEDS_MORE_INPUT: number;
- const BROTLI_DECODER_NEEDS_MORE_OUTPUT: number;
- const BROTLI_DECODER_NO_ERROR: number;
- const BROTLI_DECODER_PARAM_DISABLE_RING_BUFFER_REALLOCATION: number;
- const BROTLI_DECODER_PARAM_LARGE_WINDOW: number;
- const BROTLI_DECODER_RESULT_ERROR: number;
- const BROTLI_DECODER_RESULT_NEEDS_MORE_INPUT: number;
- const BROTLI_DECODER_RESULT_NEEDS_MORE_OUTPUT: number;
- const BROTLI_DECODER_RESULT_SUCCESS: number;
- const BROTLI_DECODER_SUCCESS: number;
- const BROTLI_DEFAULT_MODE: number;
- const BROTLI_DEFAULT_QUALITY: number;
- const BROTLI_DEFAULT_WINDOW: number;
- const BROTLI_ENCODE: number;
- const BROTLI_LARGE_MAX_WINDOW_BITS: number;
- const BROTLI_MAX_INPUT_BLOCK_BITS: number;
- const BROTLI_MAX_QUALITY: number;
- const BROTLI_MAX_WINDOW_BITS: number;
- const BROTLI_MIN_INPUT_BLOCK_BITS: number;
- const BROTLI_MIN_QUALITY: number;
- const BROTLI_MIN_WINDOW_BITS: number;
- const BROTLI_MODE_FONT: number;
- const BROTLI_MODE_GENERIC: number;
- const BROTLI_MODE_TEXT: number;
- const BROTLI_OPERATION_EMIT_METADATA: number;
- const BROTLI_OPERATION_FINISH: number;
- const BROTLI_OPERATION_FLUSH: number;
- const BROTLI_OPERATION_PROCESS: number;
- const BROTLI_PARAM_DISABLE_LITERAL_CONTEXT_MODELING: number;
- const BROTLI_PARAM_LARGE_WINDOW: number;
- const BROTLI_PARAM_LGBLOCK: number;
- const BROTLI_PARAM_LGWIN: number;
- const BROTLI_PARAM_MODE: number;
- const BROTLI_PARAM_NDIRECT: number;
- const BROTLI_PARAM_NPOSTFIX: number;
- const BROTLI_PARAM_QUALITY: number;
- const BROTLI_PARAM_SIZE_HINT: number;
- const DEFLATE: number;
- const DEFLATERAW: number;
- const GUNZIP: number;
- const GZIP: number;
- const INFLATE: number;
- const INFLATERAW: number;
- const UNZIP: number;
- // Allowed flush values.
- const Z_NO_FLUSH: number;
- const Z_PARTIAL_FLUSH: number;
- const Z_SYNC_FLUSH: number;
- const Z_FULL_FLUSH: number;
- const Z_FINISH: number;
- const Z_BLOCK: number;
- const Z_TREES: number;
- // Return codes for the compression/decompression functions.
- // Negative values are errors, positive values are used for special but normal events.
- const Z_OK: number;
- const Z_STREAM_END: number;
- const Z_NEED_DICT: number;
- const Z_ERRNO: number;
- const Z_STREAM_ERROR: number;
- const Z_DATA_ERROR: number;
- const Z_MEM_ERROR: number;
- const Z_BUF_ERROR: number;
- const Z_VERSION_ERROR: number;
- // Compression levels.
- const Z_NO_COMPRESSION: number;
- const Z_BEST_SPEED: number;
- const Z_BEST_COMPRESSION: number;
- const Z_DEFAULT_COMPRESSION: number;
- // Compression strategy.
- const Z_FILTERED: number;
- const Z_HUFFMAN_ONLY: number;
- const Z_RLE: number;
- const Z_FIXED: number;
- const Z_DEFAULT_STRATEGY: number;
- const Z_DEFAULT_WINDOWBITS: number;
- const Z_MIN_WINDOWBITS: number;
- const Z_MAX_WINDOWBITS: number;
- const Z_MIN_CHUNK: number;
- const Z_MAX_CHUNK: number;
- const Z_DEFAULT_CHUNK: number;
- const Z_MIN_MEMLEVEL: number;
- const Z_MAX_MEMLEVEL: number;
- const Z_DEFAULT_MEMLEVEL: number;
- const Z_MIN_LEVEL: number;
- const Z_MAX_LEVEL: number;
- const Z_DEFAULT_LEVEL: number;
- const ZLIB_VERNUM: number;
- }
- // Allowed flush values.
- /** @deprecated Use `constants.Z_NO_FLUSH` */
- const Z_NO_FLUSH: number;
- /** @deprecated Use `constants.Z_PARTIAL_FLUSH` */
- const Z_PARTIAL_FLUSH: number;
- /** @deprecated Use `constants.Z_SYNC_FLUSH` */
- const Z_SYNC_FLUSH: number;
- /** @deprecated Use `constants.Z_FULL_FLUSH` */
- const Z_FULL_FLUSH: number;
- /** @deprecated Use `constants.Z_FINISH` */
- const Z_FINISH: number;
- /** @deprecated Use `constants.Z_BLOCK` */
- const Z_BLOCK: number;
- /** @deprecated Use `constants.Z_TREES` */
- const Z_TREES: number;
- // Return codes for the compression/decompression functions.
- // Negative values are errors, positive values are used for special but normal events.
- /** @deprecated Use `constants.Z_OK` */
- const Z_OK: number;
- /** @deprecated Use `constants.Z_STREAM_END` */
- const Z_STREAM_END: number;
- /** @deprecated Use `constants.Z_NEED_DICT` */
- const Z_NEED_DICT: number;
- /** @deprecated Use `constants.Z_ERRNO` */
- const Z_ERRNO: number;
- /** @deprecated Use `constants.Z_STREAM_ERROR` */
- const Z_STREAM_ERROR: number;
- /** @deprecated Use `constants.Z_DATA_ERROR` */
- const Z_DATA_ERROR: number;
- /** @deprecated Use `constants.Z_MEM_ERROR` */
- const Z_MEM_ERROR: number;
- /** @deprecated Use `constants.Z_BUF_ERROR` */
- const Z_BUF_ERROR: number;
- /** @deprecated Use `constants.Z_VERSION_ERROR` */
- const Z_VERSION_ERROR: number;
- // Compression levels.
- /** @deprecated Use `constants.Z_NO_COMPRESSION` */
- const Z_NO_COMPRESSION: number;
- /** @deprecated Use `constants.Z_BEST_SPEED` */
- const Z_BEST_SPEED: number;
- /** @deprecated Use `constants.Z_BEST_COMPRESSION` */
- const Z_BEST_COMPRESSION: number;
- /** @deprecated Use `constants.Z_DEFAULT_COMPRESSION` */
- const Z_DEFAULT_COMPRESSION: number;
- // Compression strategy.
- /** @deprecated Use `constants.Z_FILTERED` */
- const Z_FILTERED: number;
- /** @deprecated Use `constants.Z_HUFFMAN_ONLY` */
- const Z_HUFFMAN_ONLY: number;
- /** @deprecated Use `constants.Z_RLE` */
- const Z_RLE: number;
- /** @deprecated Use `constants.Z_FIXED` */
- const Z_FIXED: number;
- /** @deprecated Use `constants.Z_DEFAULT_STRATEGY` */
- const Z_DEFAULT_STRATEGY: number;
- /** @deprecated */
- const Z_BINARY: number;
- /** @deprecated */
- const Z_TEXT: number;
- /** @deprecated */
- const Z_ASCII: number;
- /** @deprecated */
- const Z_UNKNOWN: number;
- /** @deprecated */
- const Z_DEFLATED: number;
-}
-declare module 'node:zlib' {
- export * from 'zlib';
-}
diff --git a/spaces/fffiloni/controlnet-animation-doodle/node_modules/path-to-regexp/History.md b/spaces/fffiloni/controlnet-animation-doodle/node_modules/path-to-regexp/History.md
deleted file mode 100644
index 7f6587846f67047b7f9ecddbb176abd25dc3741d..0000000000000000000000000000000000000000
--- a/spaces/fffiloni/controlnet-animation-doodle/node_modules/path-to-regexp/History.md
+++ /dev/null
@@ -1,36 +0,0 @@
-0.1.7 / 2015-07-28
-==================
-
- * Fixed regression with escaped round brackets and matching groups.
-
-0.1.6 / 2015-06-19
-==================
-
- * Replace `index` feature by outputting all parameters, unnamed and named.
-
-0.1.5 / 2015-05-08
-==================
-
- * Add an index property for position in match result.
-
-0.1.4 / 2015-03-05
-==================
-
- * Add license information
-
-0.1.3 / 2014-07-06
-==================
-
- * Better array support
- * Improved support for trailing slash in non-ending mode
-
-0.1.0 / 2014-03-06
-==================
-
- * add options.end
-
-0.0.2 / 2013-02-10
-==================
-
- * Update to match current express
- * add .license property to component.json
diff --git a/spaces/fffiloni/controlnet-animation-doodle/node_modules/safe-buffer/index.js b/spaces/fffiloni/controlnet-animation-doodle/node_modules/safe-buffer/index.js
deleted file mode 100644
index f8d3ec98852f449b44b7d89fc82bae737c69f3fc..0000000000000000000000000000000000000000
--- a/spaces/fffiloni/controlnet-animation-doodle/node_modules/safe-buffer/index.js
+++ /dev/null
@@ -1,65 +0,0 @@
-/*! safe-buffer. MIT License. Feross Aboukhadijeh */
-/* eslint-disable node/no-deprecated-api */
-var buffer = require('buffer')
-var Buffer = buffer.Buffer
-
-// alternative to using Object.keys for old browsers
-function copyProps (src, dst) {
- for (var key in src) {
- dst[key] = src[key]
- }
-}
-if (Buffer.from && Buffer.alloc && Buffer.allocUnsafe && Buffer.allocUnsafeSlow) {
- module.exports = buffer
-} else {
- // Copy properties from require('buffer')
- copyProps(buffer, exports)
- exports.Buffer = SafeBuffer
-}
-
-function SafeBuffer (arg, encodingOrOffset, length) {
- return Buffer(arg, encodingOrOffset, length)
-}
-
-SafeBuffer.prototype = Object.create(Buffer.prototype)
-
-// Copy static methods from Buffer
-copyProps(Buffer, SafeBuffer)
-
-SafeBuffer.from = function (arg, encodingOrOffset, length) {
- if (typeof arg === 'number') {
- throw new TypeError('Argument must not be a number')
- }
- return Buffer(arg, encodingOrOffset, length)
-}
-
-SafeBuffer.alloc = function (size, fill, encoding) {
- if (typeof size !== 'number') {
- throw new TypeError('Argument must be a number')
- }
- var buf = Buffer(size)
- if (fill !== undefined) {
- if (typeof encoding === 'string') {
- buf.fill(fill, encoding)
- } else {
- buf.fill(fill)
- }
- } else {
- buf.fill(0)
- }
- return buf
-}
-
-SafeBuffer.allocUnsafe = function (size) {
- if (typeof size !== 'number') {
- throw new TypeError('Argument must be a number')
- }
- return Buffer(size)
-}
-
-SafeBuffer.allocUnsafeSlow = function (size) {
- if (typeof size !== 'number') {
- throw new TypeError('Argument must be a number')
- }
- return buffer.SlowBuffer(size)
-}
diff --git a/spaces/fffiloni/lama-video-watermark-remover/fetch_data/eval_sampler.py b/spaces/fffiloni/lama-video-watermark-remover/fetch_data/eval_sampler.py
deleted file mode 100644
index bf2d70d875a44b5a74daeec9b4ba747600287f2a..0000000000000000000000000000000000000000
--- a/spaces/fffiloni/lama-video-watermark-remover/fetch_data/eval_sampler.py
+++ /dev/null
@@ -1,21 +0,0 @@
-import os
-import random
-
-
-val_files_path = os.path.abspath('.') + '/places_standard_dataset/original/val/'
-val_files = [val_files_path + image for image in os.listdir(val_files_path)]
-
-print(f'found {len(val_files)} images in {val_files_path}')
-
-random.shuffle(val_files)
-val_files_random = val_files[0:2000]
-
-list_of_random_val_files = os.path.abspath('.') \
-+ '/places_standard_dataset/original/eval_random_files.txt'
-
-print(f'copying 2000 random images to {list_of_random_val_files}')
-with open(list_of_random_val_files, 'w') as fw:
- for filename in val_files_random:
- fw.write(filename+'\n')
-print('...done')
-
diff --git a/spaces/fracapuano/AISandbox/summarization/__init__.py b/spaces/fracapuano/AISandbox/summarization/__init__.py
deleted file mode 100644
index 23124821c65d272c61ab7b5a7b51f46a875d3f07..0000000000000000000000000000000000000000
--- a/spaces/fracapuano/AISandbox/summarization/__init__.py
+++ /dev/null
@@ -1 +0,0 @@
-from .summarization import *
\ No newline at end of file
diff --git a/spaces/g4f/freegpt-webui/g4f/Provider/Providers/Better.py b/spaces/g4f/freegpt-webui/g4f/Provider/Providers/Better.py
deleted file mode 100644
index e95bf36ac645428a2a70246da52d83d74c008ec8..0000000000000000000000000000000000000000
--- a/spaces/g4f/freegpt-webui/g4f/Provider/Providers/Better.py
+++ /dev/null
@@ -1,56 +0,0 @@
-import os
-import json
-import requests
-from typing import Dict, get_type_hints
-
-url = 'https://openai-proxy-api.vercel.app/v1/'
-model = {
- 'gpt-3.5-turbo',
- 'gpt-3.5-turbo-0613'
- 'gpt-3.5-turbo-16k',
- 'gpt-3.5-turbo-16k-0613',
- 'gpt-4',
-}
-
-supports_stream = True
-needs_auth = False
-
-
-def _create_completion(model: str, messages: list, stream: bool, **kwargs):
- headers = {
- 'Content-Type': 'application/json',
- 'User-Agent': 'Mozilla/5.0 (Windows NT 10.0; Win64; x64) AppleWebKit/537.36 (KHTML, like Gecko) Chrome/114.0.0.0 Safari/537.36 Edg/114.0.1823.58',
- 'Referer': 'https://chat.ylokh.xyz/',
- 'Origin': 'https://chat.ylokh.xyz',
- 'Connection': 'keep-alive',
- }
-
- json_data = {
- 'messages': messages,
- 'temperature': 1.0,
- 'model': model,
- 'stream': stream,
- }
-
- response = requests.post(
- 'https://openai-proxy-api.vercel.app/v1/chat/completions', headers=headers, json=json_data, stream=True
- )
-
- for token in response.iter_lines():
- decoded = token.decode('utf-8')
- if decoded.startswith('data: '):
- data_str = decoded.replace('data: ', '')
- data = json.loads(data_str)
- if 'choices' in data and 'delta' in data['choices'][0]:
- delta = data['choices'][0]['delta']
- content = delta.get('content', '')
- finish_reason = delta.get('finish_reason', '')
-
- if finish_reason == 'stop':
- break
- if content:
- yield content
-
-
-params = f'g4f.Providers.{os.path.basename(__file__)[:-3]} supports: ' + '(%s)' % ', '.join(
- [f"{name}: {get_type_hints(_create_completion)[name].__name__}" for name in _create_completion.__code__.co_varnames[:_create_completion.__code__.co_argcount]])
diff --git a/spaces/geloku/ai-academy/app.py b/spaces/geloku/ai-academy/app.py
deleted file mode 100644
index 315b4d0d57a28de4829e465e6afa8777847faadf..0000000000000000000000000000000000000000
--- a/spaces/geloku/ai-academy/app.py
+++ /dev/null
@@ -1,26 +0,0 @@
-# AUTOGENERATED! DO NOT EDIT! File to edit: ../app.ipynb.
-
-# %% auto 0
-__all__ = ['learn', 'categories', 'image', 'label', 'examples', 'intf', 'classify_image']
-
-# %% ../app.ipynb 1
-from fastai.vision.all import *
-import gradio as gr
-
-# %% ../app.ipynb 2
-learn = load_learner('model.pk1')
-
-# %% ../app.ipynb 3
-categories = ('bird', 'fish', 'mammal')
-
-def classify_image(img):
- pred, idx, probs = learn.predict(img)
- return dict(zip(categories, map(float, probs)))
-
-# %% ../app.ipynb 5
-image = gr.inputs.Image(shape=(192,192))
-label = gr.outputs.Label()
-examples = ['bird.jpg', 'fish.jpg', 'mammal.jpg']
-
-intf = gr.Interface(fn=classify_image, inputs=image, outputs=label, examples=examples)
-intf.launch(inline=False)
diff --git a/spaces/georgefen/Face-Landmark-ControlNet/annotator/uniformer/mmcv/runner/__init__.py b/spaces/georgefen/Face-Landmark-ControlNet/annotator/uniformer/mmcv/runner/__init__.py
deleted file mode 100644
index 52e4b48d383a84a055dcd7f6236f6e8e58eab924..0000000000000000000000000000000000000000
--- a/spaces/georgefen/Face-Landmark-ControlNet/annotator/uniformer/mmcv/runner/__init__.py
+++ /dev/null
@@ -1,47 +0,0 @@
-# Copyright (c) OpenMMLab. All rights reserved.
-from .base_module import BaseModule, ModuleList, Sequential
-from .base_runner import BaseRunner
-from .builder import RUNNERS, build_runner
-from .checkpoint import (CheckpointLoader, _load_checkpoint,
- _load_checkpoint_with_prefix, load_checkpoint,
- load_state_dict, save_checkpoint, weights_to_cpu)
-from .default_constructor import DefaultRunnerConstructor
-from .dist_utils import (allreduce_grads, allreduce_params, get_dist_info,
- init_dist, master_only)
-from .epoch_based_runner import EpochBasedRunner, Runner
-from .fp16_utils import LossScaler, auto_fp16, force_fp32, wrap_fp16_model
-from .hooks import (HOOKS, CheckpointHook, ClosureHook, DistEvalHook,
- DistSamplerSeedHook, DvcliveLoggerHook, EMAHook, EvalHook,
- Fp16OptimizerHook, GradientCumulativeFp16OptimizerHook,
- GradientCumulativeOptimizerHook, Hook, IterTimerHook,
- LoggerHook, LrUpdaterHook, MlflowLoggerHook,
- NeptuneLoggerHook, OptimizerHook, PaviLoggerHook,
- SyncBuffersHook, TensorboardLoggerHook, TextLoggerHook,
- WandbLoggerHook)
-from .iter_based_runner import IterBasedRunner, IterLoader
-from .log_buffer import LogBuffer
-from .optimizer import (OPTIMIZER_BUILDERS, OPTIMIZERS,
- DefaultOptimizerConstructor, build_optimizer,
- build_optimizer_constructor)
-from .priority import Priority, get_priority
-from .utils import get_host_info, get_time_str, obj_from_dict, set_random_seed
-
-__all__ = [
- 'BaseRunner', 'Runner', 'EpochBasedRunner', 'IterBasedRunner', 'LogBuffer',
- 'HOOKS', 'Hook', 'CheckpointHook', 'ClosureHook', 'LrUpdaterHook',
- 'OptimizerHook', 'IterTimerHook', 'DistSamplerSeedHook', 'LoggerHook',
- 'PaviLoggerHook', 'TextLoggerHook', 'TensorboardLoggerHook',
- 'NeptuneLoggerHook', 'WandbLoggerHook', 'MlflowLoggerHook',
- 'DvcliveLoggerHook', '_load_checkpoint', 'load_state_dict',
- 'load_checkpoint', 'weights_to_cpu', 'save_checkpoint', 'Priority',
- 'get_priority', 'get_host_info', 'get_time_str', 'obj_from_dict',
- 'init_dist', 'get_dist_info', 'master_only', 'OPTIMIZER_BUILDERS',
- 'OPTIMIZERS', 'DefaultOptimizerConstructor', 'build_optimizer',
- 'build_optimizer_constructor', 'IterLoader', 'set_random_seed',
- 'auto_fp16', 'force_fp32', 'wrap_fp16_model', 'Fp16OptimizerHook',
- 'SyncBuffersHook', 'EMAHook', 'build_runner', 'RUNNERS', 'allreduce_grads',
- 'allreduce_params', 'LossScaler', 'CheckpointLoader', 'BaseModule',
- '_load_checkpoint_with_prefix', 'EvalHook', 'DistEvalHook', 'Sequential',
- 'ModuleList', 'GradientCumulativeOptimizerHook',
- 'GradientCumulativeFp16OptimizerHook', 'DefaultRunnerConstructor'
-]
diff --git a/spaces/ggwvits/vits-uma-genshin-honkai/attentions.py b/spaces/ggwvits/vits-uma-genshin-honkai/attentions.py
deleted file mode 100644
index 86bc73b5fe98cc7b443e9078553920346c996707..0000000000000000000000000000000000000000
--- a/spaces/ggwvits/vits-uma-genshin-honkai/attentions.py
+++ /dev/null
@@ -1,300 +0,0 @@
-import math
-import torch
-from torch import nn
-from torch.nn import functional as F
-
-import commons
-from modules import LayerNorm
-
-
-class Encoder(nn.Module):
- def __init__(self, hidden_channels, filter_channels, n_heads, n_layers, kernel_size=1, p_dropout=0., window_size=4, **kwargs):
- super().__init__()
- self.hidden_channels = hidden_channels
- self.filter_channels = filter_channels
- self.n_heads = n_heads
- self.n_layers = n_layers
- self.kernel_size = kernel_size
- self.p_dropout = p_dropout
- self.window_size = window_size
-
- self.drop = nn.Dropout(p_dropout)
- self.attn_layers = nn.ModuleList()
- self.norm_layers_1 = nn.ModuleList()
- self.ffn_layers = nn.ModuleList()
- self.norm_layers_2 = nn.ModuleList()
- for i in range(self.n_layers):
- self.attn_layers.append(MultiHeadAttention(hidden_channels, hidden_channels, n_heads, p_dropout=p_dropout, window_size=window_size))
- self.norm_layers_1.append(LayerNorm(hidden_channels))
- self.ffn_layers.append(FFN(hidden_channels, hidden_channels, filter_channels, kernel_size, p_dropout=p_dropout))
- self.norm_layers_2.append(LayerNorm(hidden_channels))
-
- def forward(self, x, x_mask):
- attn_mask = x_mask.unsqueeze(2) * x_mask.unsqueeze(-1)
- x = x * x_mask
- for i in range(self.n_layers):
- y = self.attn_layers[i](x, x, attn_mask)
- y = self.drop(y)
- x = self.norm_layers_1[i](x + y)
-
- y = self.ffn_layers[i](x, x_mask)
- y = self.drop(y)
- x = self.norm_layers_2[i](x + y)
- x = x * x_mask
- return x
-
-
-class Decoder(nn.Module):
- def __init__(self, hidden_channels, filter_channels, n_heads, n_layers, kernel_size=1, p_dropout=0., proximal_bias=False, proximal_init=True, **kwargs):
- super().__init__()
- self.hidden_channels = hidden_channels
- self.filter_channels = filter_channels
- self.n_heads = n_heads
- self.n_layers = n_layers
- self.kernel_size = kernel_size
- self.p_dropout = p_dropout
- self.proximal_bias = proximal_bias
- self.proximal_init = proximal_init
-
- self.drop = nn.Dropout(p_dropout)
- self.self_attn_layers = nn.ModuleList()
- self.norm_layers_0 = nn.ModuleList()
- self.encdec_attn_layers = nn.ModuleList()
- self.norm_layers_1 = nn.ModuleList()
- self.ffn_layers = nn.ModuleList()
- self.norm_layers_2 = nn.ModuleList()
- for i in range(self.n_layers):
- self.self_attn_layers.append(MultiHeadAttention(hidden_channels, hidden_channels, n_heads, p_dropout=p_dropout, proximal_bias=proximal_bias, proximal_init=proximal_init))
- self.norm_layers_0.append(LayerNorm(hidden_channels))
- self.encdec_attn_layers.append(MultiHeadAttention(hidden_channels, hidden_channels, n_heads, p_dropout=p_dropout))
- self.norm_layers_1.append(LayerNorm(hidden_channels))
- self.ffn_layers.append(FFN(hidden_channels, hidden_channels, filter_channels, kernel_size, p_dropout=p_dropout, causal=True))
- self.norm_layers_2.append(LayerNorm(hidden_channels))
-
- def forward(self, x, x_mask, h, h_mask):
- """
- x: decoder input
- h: encoder output
- """
- self_attn_mask = commons.subsequent_mask(x_mask.size(2)).to(device=x.device, dtype=x.dtype)
- encdec_attn_mask = h_mask.unsqueeze(2) * x_mask.unsqueeze(-1)
- x = x * x_mask
- for i in range(self.n_layers):
- y = self.self_attn_layers[i](x, x, self_attn_mask)
- y = self.drop(y)
- x = self.norm_layers_0[i](x + y)
-
- y = self.encdec_attn_layers[i](x, h, encdec_attn_mask)
- y = self.drop(y)
- x = self.norm_layers_1[i](x + y)
-
- y = self.ffn_layers[i](x, x_mask)
- y = self.drop(y)
- x = self.norm_layers_2[i](x + y)
- x = x * x_mask
- return x
-
-
-class MultiHeadAttention(nn.Module):
- def __init__(self, channels, out_channels, n_heads, p_dropout=0., window_size=None, heads_share=True, block_length=None, proximal_bias=False, proximal_init=False):
- super().__init__()
- assert channels % n_heads == 0
-
- self.channels = channels
- self.out_channels = out_channels
- self.n_heads = n_heads
- self.p_dropout = p_dropout
- self.window_size = window_size
- self.heads_share = heads_share
- self.block_length = block_length
- self.proximal_bias = proximal_bias
- self.proximal_init = proximal_init
- self.attn = None
-
- self.k_channels = channels // n_heads
- self.conv_q = nn.Conv1d(channels, channels, 1)
- self.conv_k = nn.Conv1d(channels, channels, 1)
- self.conv_v = nn.Conv1d(channels, channels, 1)
- self.conv_o = nn.Conv1d(channels, out_channels, 1)
- self.drop = nn.Dropout(p_dropout)
-
- if window_size is not None:
- n_heads_rel = 1 if heads_share else n_heads
- rel_stddev = self.k_channels**-0.5
- self.emb_rel_k = nn.Parameter(torch.randn(n_heads_rel, window_size * 2 + 1, self.k_channels) * rel_stddev)
- self.emb_rel_v = nn.Parameter(torch.randn(n_heads_rel, window_size * 2 + 1, self.k_channels) * rel_stddev)
-
- nn.init.xavier_uniform_(self.conv_q.weight)
- nn.init.xavier_uniform_(self.conv_k.weight)
- nn.init.xavier_uniform_(self.conv_v.weight)
- if proximal_init:
- with torch.no_grad():
- self.conv_k.weight.copy_(self.conv_q.weight)
- self.conv_k.bias.copy_(self.conv_q.bias)
-
- def forward(self, x, c, attn_mask=None):
- q = self.conv_q(x)
- k = self.conv_k(c)
- v = self.conv_v(c)
-
- x, self.attn = self.attention(q, k, v, mask=attn_mask)
-
- x = self.conv_o(x)
- return x
-
- def attention(self, query, key, value, mask=None):
- # reshape [b, d, t] -> [b, n_h, t, d_k]
- b, d, t_s, t_t = (*key.size(), query.size(2))
- query = query.view(b, self.n_heads, self.k_channels, t_t).transpose(2, 3)
- key = key.view(b, self.n_heads, self.k_channels, t_s).transpose(2, 3)
- value = value.view(b, self.n_heads, self.k_channels, t_s).transpose(2, 3)
-
- scores = torch.matmul(query / math.sqrt(self.k_channels), key.transpose(-2, -1))
- if self.window_size is not None:
- assert t_s == t_t, "Relative attention is only available for self-attention."
- key_relative_embeddings = self._get_relative_embeddings(self.emb_rel_k, t_s)
- rel_logits = self._matmul_with_relative_keys(query /math.sqrt(self.k_channels), key_relative_embeddings)
- scores_local = self._relative_position_to_absolute_position(rel_logits)
- scores = scores + scores_local
- if self.proximal_bias:
- assert t_s == t_t, "Proximal bias is only available for self-attention."
- scores = scores + self._attention_bias_proximal(t_s).to(device=scores.device, dtype=scores.dtype)
- if mask is not None:
- scores = scores.masked_fill(mask == 0, -1e4)
- if self.block_length is not None:
- assert t_s == t_t, "Local attention is only available for self-attention."
- block_mask = torch.ones_like(scores).triu(-self.block_length).tril(self.block_length)
- scores = scores.masked_fill(block_mask == 0, -1e4)
- p_attn = F.softmax(scores, dim=-1) # [b, n_h, t_t, t_s]
- p_attn = self.drop(p_attn)
- output = torch.matmul(p_attn, value)
- if self.window_size is not None:
- relative_weights = self._absolute_position_to_relative_position(p_attn)
- value_relative_embeddings = self._get_relative_embeddings(self.emb_rel_v, t_s)
- output = output + self._matmul_with_relative_values(relative_weights, value_relative_embeddings)
- output = output.transpose(2, 3).contiguous().view(b, d, t_t) # [b, n_h, t_t, d_k] -> [b, d, t_t]
- return output, p_attn
-
- def _matmul_with_relative_values(self, x, y):
- """
- x: [b, h, l, m]
- y: [h or 1, m, d]
- ret: [b, h, l, d]
- """
- ret = torch.matmul(x, y.unsqueeze(0))
- return ret
-
- def _matmul_with_relative_keys(self, x, y):
- """
- x: [b, h, l, d]
- y: [h or 1, m, d]
- ret: [b, h, l, m]
- """
- ret = torch.matmul(x, y.unsqueeze(0).transpose(-2, -1))
- return ret
-
- def _get_relative_embeddings(self, relative_embeddings, length):
- max_relative_position = 2 * self.window_size + 1
- # Pad first before slice to avoid using cond ops.
- pad_length = max(length - (self.window_size + 1), 0)
- slice_start_position = max((self.window_size + 1) - length, 0)
- slice_end_position = slice_start_position + 2 * length - 1
- if pad_length > 0:
- padded_relative_embeddings = F.pad(
- relative_embeddings,
- commons.convert_pad_shape([[0, 0], [pad_length, pad_length], [0, 0]]))
- else:
- padded_relative_embeddings = relative_embeddings
- used_relative_embeddings = padded_relative_embeddings[:,slice_start_position:slice_end_position]
- return used_relative_embeddings
-
- def _relative_position_to_absolute_position(self, x):
- """
- x: [b, h, l, 2*l-1]
- ret: [b, h, l, l]
- """
- batch, heads, length, _ = x.size()
- # Concat columns of pad to shift from relative to absolute indexing.
- x = F.pad(x, commons.convert_pad_shape([[0,0],[0,0],[0,0],[0,1]]))
-
- # Concat extra elements so to add up to shape (len+1, 2*len-1).
- x_flat = x.view([batch, heads, length * 2 * length])
- x_flat = F.pad(x_flat, commons.convert_pad_shape([[0,0],[0,0],[0,length-1]]))
-
- # Reshape and slice out the padded elements.
- x_final = x_flat.view([batch, heads, length+1, 2*length-1])[:, :, :length, length-1:]
- return x_final
-
- def _absolute_position_to_relative_position(self, x):
- """
- x: [b, h, l, l]
- ret: [b, h, l, 2*l-1]
- """
- batch, heads, length, _ = x.size()
- # padd along column
- x = F.pad(x, commons.convert_pad_shape([[0, 0], [0, 0], [0, 0], [0, length-1]]))
- x_flat = x.view([batch, heads, length**2 + length*(length -1)])
- # add 0's in the beginning that will skew the elements after reshape
- x_flat = F.pad(x_flat, commons.convert_pad_shape([[0, 0], [0, 0], [length, 0]]))
- x_final = x_flat.view([batch, heads, length, 2*length])[:,:,:,1:]
- return x_final
-
- def _attention_bias_proximal(self, length):
- """Bias for self-attention to encourage attention to close positions.
- Args:
- length: an integer scalar.
- Returns:
- a Tensor with shape [1, 1, length, length]
- """
- r = torch.arange(length, dtype=torch.float32)
- diff = torch.unsqueeze(r, 0) - torch.unsqueeze(r, 1)
- return torch.unsqueeze(torch.unsqueeze(-torch.log1p(torch.abs(diff)), 0), 0)
-
-
-class FFN(nn.Module):
- def __init__(self, in_channels, out_channels, filter_channels, kernel_size, p_dropout=0., activation=None, causal=False):
- super().__init__()
- self.in_channels = in_channels
- self.out_channels = out_channels
- self.filter_channels = filter_channels
- self.kernel_size = kernel_size
- self.p_dropout = p_dropout
- self.activation = activation
- self.causal = causal
-
- if causal:
- self.padding = self._causal_padding
- else:
- self.padding = self._same_padding
-
- self.conv_1 = nn.Conv1d(in_channels, filter_channels, kernel_size)
- self.conv_2 = nn.Conv1d(filter_channels, out_channels, kernel_size)
- self.drop = nn.Dropout(p_dropout)
-
- def forward(self, x, x_mask):
- x = self.conv_1(self.padding(x * x_mask))
- if self.activation == "gelu":
- x = x * torch.sigmoid(1.702 * x)
- else:
- x = torch.relu(x)
- x = self.drop(x)
- x = self.conv_2(self.padding(x * x_mask))
- return x * x_mask
-
- def _causal_padding(self, x):
- if self.kernel_size == 1:
- return x
- pad_l = self.kernel_size - 1
- pad_r = 0
- padding = [[0, 0], [0, 0], [pad_l, pad_r]]
- x = F.pad(x, commons.convert_pad_shape(padding))
- return x
-
- def _same_padding(self, x):
- if self.kernel_size == 1:
- return x
- pad_l = (self.kernel_size - 1) // 2
- pad_r = self.kernel_size // 2
- padding = [[0, 0], [0, 0], [pad_l, pad_r]]
- x = F.pad(x, commons.convert_pad_shape(padding))
- return x
diff --git a/spaces/glyszt/vt/vtoonify/model/encoder/encoders/psp_encoders.py b/spaces/glyszt/vt/vtoonify/model/encoder/encoders/psp_encoders.py
deleted file mode 100644
index f69d38200b6be4997673ae38ed481fd21f88b419..0000000000000000000000000000000000000000
--- a/spaces/glyszt/vt/vtoonify/model/encoder/encoders/psp_encoders.py
+++ /dev/null
@@ -1,186 +0,0 @@
-import numpy as np
-import torch
-import torch.nn.functional as F
-from torch import nn
-from torch.nn import Linear, Conv2d, BatchNorm2d, PReLU, Sequential, Module
-
-from model.encoder.encoders.helpers import get_blocks, Flatten, bottleneck_IR, bottleneck_IR_SE
-from model.stylegan.model import EqualLinear
-
-
-class GradualStyleBlock(Module):
- def __init__(self, in_c, out_c, spatial):
- super(GradualStyleBlock, self).__init__()
- self.out_c = out_c
- self.spatial = spatial
- num_pools = int(np.log2(spatial))
- modules = []
- modules += [Conv2d(in_c, out_c, kernel_size=3, stride=2, padding=1),
- nn.LeakyReLU()]
- for i in range(num_pools - 1):
- modules += [
- Conv2d(out_c, out_c, kernel_size=3, stride=2, padding=1),
- nn.LeakyReLU()
- ]
- self.convs = nn.Sequential(*modules)
- self.linear = EqualLinear(out_c, out_c, lr_mul=1)
-
- def forward(self, x):
- x = self.convs(x)
- x = x.view(-1, self.out_c)
- x = self.linear(x)
- return x
-
-
-class GradualStyleEncoder(Module):
- def __init__(self, num_layers, mode='ir', opts=None):
- super(GradualStyleEncoder, self).__init__()
- assert num_layers in [50, 100, 152], 'num_layers should be 50,100, or 152'
- assert mode in ['ir', 'ir_se'], 'mode should be ir or ir_se'
- blocks = get_blocks(num_layers)
- if mode == 'ir':
- unit_module = bottleneck_IR
- elif mode == 'ir_se':
- unit_module = bottleneck_IR_SE
- self.input_layer = Sequential(Conv2d(opts.input_nc, 64, (3, 3), 1, 1, bias=False),
- BatchNorm2d(64),
- PReLU(64))
- modules = []
- for block in blocks:
- for bottleneck in block:
- modules.append(unit_module(bottleneck.in_channel,
- bottleneck.depth,
- bottleneck.stride))
- self.body = Sequential(*modules)
-
- self.styles = nn.ModuleList()
- self.style_count = opts.n_styles
- self.coarse_ind = 3
- self.middle_ind = 7
- for i in range(self.style_count):
- if i < self.coarse_ind:
- style = GradualStyleBlock(512, 512, 16)
- elif i < self.middle_ind:
- style = GradualStyleBlock(512, 512, 32)
- else:
- style = GradualStyleBlock(512, 512, 64)
- self.styles.append(style)
- self.latlayer1 = nn.Conv2d(256, 512, kernel_size=1, stride=1, padding=0)
- self.latlayer2 = nn.Conv2d(128, 512, kernel_size=1, stride=1, padding=0)
-
- def _upsample_add(self, x, y):
- '''Upsample and add two feature maps.
- Args:
- x: (Variable) top feature map to be upsampled.
- y: (Variable) lateral feature map.
- Returns:
- (Variable) added feature map.
- Note in PyTorch, when input size is odd, the upsampled feature map
- with `F.upsample(..., scale_factor=2, mode='nearest')`
- maybe not equal to the lateral feature map size.
- e.g.
- original input size: [N,_,15,15] ->
- conv2d feature map size: [N,_,8,8] ->
- upsampled feature map size: [N,_,16,16]
- So we choose bilinear upsample which supports arbitrary output sizes.
- '''
- _, _, H, W = y.size()
- return F.interpolate(x, size=(H, W), mode='bilinear', align_corners=True) + y
-
- def forward(self, x):
- x = self.input_layer(x)
-
- latents = []
- modulelist = list(self.body._modules.values())
- for i, l in enumerate(modulelist):
- x = l(x)
- if i == 6:
- c1 = x
- elif i == 20:
- c2 = x
- elif i == 23:
- c3 = x
-
- for j in range(self.coarse_ind):
- latents.append(self.styles[j](c3))
-
- p2 = self._upsample_add(c3, self.latlayer1(c2))
- for j in range(self.coarse_ind, self.middle_ind):
- latents.append(self.styles[j](p2))
-
- p1 = self._upsample_add(p2, self.latlayer2(c1))
- for j in range(self.middle_ind, self.style_count):
- latents.append(self.styles[j](p1))
-
- out = torch.stack(latents, dim=1)
- return out
-
-
-class BackboneEncoderUsingLastLayerIntoW(Module):
- def __init__(self, num_layers, mode='ir', opts=None):
- super(BackboneEncoderUsingLastLayerIntoW, self).__init__()
- print('Using BackboneEncoderUsingLastLayerIntoW')
- assert num_layers in [50, 100, 152], 'num_layers should be 50,100, or 152'
- assert mode in ['ir', 'ir_se'], 'mode should be ir or ir_se'
- blocks = get_blocks(num_layers)
- if mode == 'ir':
- unit_module = bottleneck_IR
- elif mode == 'ir_se':
- unit_module = bottleneck_IR_SE
- self.input_layer = Sequential(Conv2d(opts.input_nc, 64, (3, 3), 1, 1, bias=False),
- BatchNorm2d(64),
- PReLU(64))
- self.output_pool = torch.nn.AdaptiveAvgPool2d((1, 1))
- self.linear = EqualLinear(512, 512, lr_mul=1)
- modules = []
- for block in blocks:
- for bottleneck in block:
- modules.append(unit_module(bottleneck.in_channel,
- bottleneck.depth,
- bottleneck.stride))
- self.body = Sequential(*modules)
-
- def forward(self, x):
- x = self.input_layer(x)
- x = self.body(x)
- x = self.output_pool(x)
- x = x.view(-1, 512)
- x = self.linear(x)
- return x
-
-
-class BackboneEncoderUsingLastLayerIntoWPlus(Module):
- def __init__(self, num_layers, mode='ir', opts=None):
- super(BackboneEncoderUsingLastLayerIntoWPlus, self).__init__()
- print('Using BackboneEncoderUsingLastLayerIntoWPlus')
- assert num_layers in [50, 100, 152], 'num_layers should be 50,100, or 152'
- assert mode in ['ir', 'ir_se'], 'mode should be ir or ir_se'
- blocks = get_blocks(num_layers)
- if mode == 'ir':
- unit_module = bottleneck_IR
- elif mode == 'ir_se':
- unit_module = bottleneck_IR_SE
- self.n_styles = opts.n_styles
- self.input_layer = Sequential(Conv2d(opts.input_nc, 64, (3, 3), 1, 1, bias=False),
- BatchNorm2d(64),
- PReLU(64))
- self.output_layer_2 = Sequential(BatchNorm2d(512),
- torch.nn.AdaptiveAvgPool2d((7, 7)),
- Flatten(),
- Linear(512 * 7 * 7, 512))
- self.linear = EqualLinear(512, 512 * self.n_styles, lr_mul=1)
- modules = []
- for block in blocks:
- for bottleneck in block:
- modules.append(unit_module(bottleneck.in_channel,
- bottleneck.depth,
- bottleneck.stride))
- self.body = Sequential(*modules)
-
- def forward(self, x):
- x = self.input_layer(x)
- x = self.body(x)
- x = self.output_layer_2(x)
- x = self.linear(x)
- x = x.view(-1, self.n_styles, 512)
- return x
diff --git a/spaces/gotiQspiryo/whisper-ui/examples/Ebooks Free Download Iphone The College Dropout.md b/spaces/gotiQspiryo/whisper-ui/examples/Ebooks Free Download Iphone The College Dropout.md
deleted file mode 100644
index 98fc25255366b80efbdb00491d6bdd86c9d6d7ae..0000000000000000000000000000000000000000
--- a/spaces/gotiQspiryo/whisper-ui/examples/Ebooks Free Download Iphone The College Dropout.md
+++ /dev/null
@@ -1,5 +0,0 @@
-
-
In the event of loss, immediately call the OTS Service Desk at 832.813.6600 (toll-free 866.614.5014)to file a report. When you report the device lost, or the college has sufficient cause to believe the device is no longer in your possession, the device may be rendered nonfunctional, and the built-in tracking mechanism may be enabled by the college to allow for recovery of the device.
- )
-}
diff --git a/spaces/hf-audio/open_asr_leaderboard/constants.py b/spaces/hf-audio/open_asr_leaderboard/constants.py
deleted file mode 100644
index f8b33de2498cf5087be292267f2e1faa69821a16..0000000000000000000000000000000000000000
--- a/spaces/hf-audio/open_asr_leaderboard/constants.py
+++ /dev/null
@@ -1,98 +0,0 @@
-from pathlib import Path
-
-# Directory where request by models are stored
-DIR_OUTPUT_REQUESTS = Path("requested_models")
-EVAL_REQUESTS_PATH = Path("eval_requests")
-
-##########################
-# Text definitions #
-##########################
-
-banner_url = "https://huggingface.co/datasets/reach-vb/random-images/resolve/main/asr_leaderboard.png"
-BANNER = f'
'
-
-TITLE = "
🤗 Open Automatic Speech Recognition Leaderboard
"
-
-INTRODUCTION_TEXT = "📐 The 🤗 Open ASR Leaderboard ranks and evaluates speech recognition models \
- on the Hugging Face Hub. \
- \nWe report the Average [WER](https://huggingface.co/spaces/evaluate-metric/wer) (⬇️) and [RTF](https://openvoice-tech.net/index.php/Real-time-factor) (⬇️) - lower the better. Models are ranked based on their Average WER, from lowest to highest. Check the 📈 Metrics tab to understand how the models are evaluated. \
- \nIf you want results for a model that is not listed here, you can submit a request for it to be included ✉️✨. \
- \nThe leaderboard currently focuses on English speech recognition, and will be expanded to multilingual evaluation in later versions."
-
-CITATION_TEXT = """@misc{open-asr-leaderboard,
- title = {Open Automatic Speech Recognition Leaderboard},
- author = {Srivastav, Vaibhav and Majumdar, Somshubra and Koluguri, Nithin and Moumen, Adel and Gandhi, Sanchit and Hugging Face Team and Nvidia NeMo Team and SpeechBrain Team},
- year = 2023,
- publisher = {Hugging Face},
- howpublished = "\\url{https://huggingface.co/spaces/huggingface.co/spaces/open-asr-leaderboard/leaderboard}"
-}
-"""
-
-METRICS_TAB_TEXT = """
-Here you will find details about the speech recognition metrics and datasets reported in our leaderboard.
-
-## Metrics
-
-🎯 Word Error Rate (WER) and Real-Time Factor (RTF) are popular metrics for evaluating the accuracy of speech recognition
-models by estimating how accurate the predictions from the models are and how fast they are returned. We explain them each
-below.
-
-### Word Error Rate (WER)
-
-Word Error Rate is used to measure the **accuracy** of automatic speech recognition systems. It calculates the percentage
-of words in the system's output that differ from the reference (correct) transcript. **A lower WER value indicates higher accuracy**.
-
-```
-Example: If the reference transcript is "I really love cats," and the ASR system outputs "I don't love dogs,".
-The WER would be `50%` because 2 out of 4 words are incorrect.
-```
-
-For a fair comparison, we calculate **zero-shot** (i.e. pre-trained models only) *normalised WER* for all the model checkpoints. You can find the evaluation code on our [Github repository](https://github.com/huggingface/open_asr_leaderboard). To read more about how the WER is computed, refer to the [Audio Transformers Course](https://huggingface.co/learn/audio-course/chapter5/evaluation).
-
-### Real Time Factor (RTF)
-
-Real Time Factor is a measure of the **latency** of automatic speech recognition systems, i.e. how long it takes an
-model to process a given amount of speech. It's usually expressed as a multiple of real time. An RTF of 1 means it processes
-speech as fast as it's spoken, while an RTF of 2 means it takes twice as long. Thus, **a lower RTF value indicates lower latency**.
-
-```
-Example: If it takes an ASR system 10 seconds to transcribe 10 seconds of speech, the RTF is 1.
-If it takes 20 seconds to transcribe the same 10 seconds of speech, the RTF is 2.
-```
-
-For the benchmark, we report RTF averaged over a 10 minute audio sample with 5 warm up batches followed 3 graded batches.
-
-## How to reproduce our results
-
-The ASR Leaderboard will be a continued effort to benchmark open source/access speech recognition models where possible.
-Along with the Leaderboard we're open-sourcing the codebase used for running these evaluations.
-For more details head over to our repo at: https://github.com/huggingface/open_asr_leaderboard
-
-P.S. We'd love to know which other models you'd like us to benchmark next. Contributions are more than welcome! ♥️
-
-## Benchmark datasets
-
-Evaluating Speech Recognition systems is a hard problem. We use the multi-dataset benchmarking strategy proposed in the
-[ESB paper](https://arxiv.org/abs/2210.13352) to obtain robust evaluation scores for each model.
-
-ESB is a benchmark for evaluating the performance of a single automatic speech recognition (ASR) system across a broad
-set of speech datasets. It comprises eight English speech recognition datasets, capturing a broad range of domains,
-acoustic conditions, speaker styles, and transcription requirements. As such, it gives a better indication of how
-a model is likely to perform on downstream ASR compared to evaluating it on one dataset alone.
-
-The ESB score is calculated as a macro-average of the WER scores across the ESB datasets. The models in the leaderboard
-are ranked based on their average WER scores, from lowest to highest.
-
-| Dataset | Domain | Speaking Style | Train (h) | Dev (h) | Test (h) | Transcriptions | License |
-|-----------------------------------------------------------------------------------------|-----------------------------|-----------------------|-----------|---------|----------|--------------------|-----------------|
-| [LibriSpeech](https://huggingface.co/datasets/librispeech_asr) | Audiobook | Narrated | 960 | 11 | 11 | Normalised | CC-BY-4.0 |
-| [Common Voice 9](https://huggingface.co/datasets/mozilla-foundation/common_voice_9_0) | Wikipedia | Narrated | 1409 | 27 | 27 | Punctuated & Cased | CC0-1.0 |
-| [VoxPopuli](https://huggingface.co/datasets/facebook/voxpopuli) | European Parliament | Oratory | 523 | 5 | 5 | Punctuated | CC0 |
-| [TED-LIUM](https://huggingface.co/datasets/LIUM/tedlium) | TED talks | Oratory | 454 | 2 | 3 | Normalised | CC-BY-NC-ND 3.0 |
-| [GigaSpeech](https://huggingface.co/datasets/speechcolab/gigaspeech) | Audiobook, podcast, YouTube | Narrated, spontaneous | 2500 | 12 | 40 | Punctuated | apache-2.0 |
-| [SPGISpeech](https://huggingface.co/datasets/kensho/spgispeech) | Fincancial meetings | Oratory, spontaneous | 4900 | 100 | 100 | Punctuated & Cased | User Agreement |
-| [Earnings-22](https://huggingface.co/datasets/revdotcom/earnings22) | Fincancial meetings | Oratory, spontaneous | 105 | 5 | 5 | Punctuated & Cased | CC-BY-SA-4.0 |
-| [AMI](https://huggingface.co/datasets/edinburghcstr/ami) | Meetings | Spontaneous | 78 | 9 | 9 | Punctuated & Cased | CC-BY-4.0 |
-
-For more details on the individual datasets and how models are evaluated to give the ESB score, refer to the [ESB paper](https://arxiv.org/abs/2210.13352).
-"""
diff --git a/spaces/hf-audio/open_asr_leaderboard/utils_display.py b/spaces/hf-audio/open_asr_leaderboard/utils_display.py
deleted file mode 100644
index 222c273d75c742c3d62dad5d5e748a6811109c64..0000000000000000000000000000000000000000
--- a/spaces/hf-audio/open_asr_leaderboard/utils_display.py
+++ /dev/null
@@ -1,40 +0,0 @@
-from dataclasses import dataclass
-
-# These classes are for user facing column names, to avoid having to change them
-# all around the code when a modif is needed
-@dataclass
-class ColumnContent:
- name: str
- type: str
-
-def fields(raw_class):
- return [v for k, v in raw_class.__dict__.items() if k[:2] != "__" and k[-2:] != "__"]
-
-@dataclass(frozen=True)
-class AutoEvalColumn: # Auto evals column
- model = ColumnContent("Model", "markdown")
- avg_wer = ColumnContent("Average WER ⬇️", "number")
- rtf = ColumnContent("RTF (1e-3) ⬇️", "number")
- ami_wer = ColumnContent("AMI", "number")
- e22_wer = ColumnContent("Earnings22", "number")
- gs_wer = ColumnContent("Gigaspeech", "number")
- lsc_wer = ColumnContent("LS Clean", "number")
- lso_wer = ColumnContent("LS Other", "number")
- ss_wer = ColumnContent("SPGISpeech", "number")
- tl_wer = ColumnContent("Tedlium", "number")
- vp_wer = ColumnContent("Voxpopuli", "number")
- cv_wer = ColumnContent("Common Voice", "number")
-
-
-def make_clickable_model(model_name):
- link = f"https://huggingface.co/{model_name}"
- return f'{model_name}'
-
-def styled_error(error):
- return f"
{error}
"
-
-def styled_warning(warn):
- return f"
{warn}
"
-
-def styled_message(message):
- return f"
{message}
"
diff --git a/spaces/hlydecker/Augmented-Retrieval-qa-ChatGPT/streamlit_langchain_chat/customized_langchain/vectorstores/faiss.py b/spaces/hlydecker/Augmented-Retrieval-qa-ChatGPT/streamlit_langchain_chat/customized_langchain/vectorstores/faiss.py
deleted file mode 100644
index 1caf619aa213aef66d0bbb1fdf631b8d72c20970..0000000000000000000000000000000000000000
--- a/spaces/hlydecker/Augmented-Retrieval-qa-ChatGPT/streamlit_langchain_chat/customized_langchain/vectorstores/faiss.py
+++ /dev/null
@@ -1,100 +0,0 @@
-# import hashlib
-
-from langchain.vectorstores.faiss import *
-from langchain.vectorstores.faiss import FAISS as OriginalFAISS
-
-from streamlit_langchain_chat.customized_langchain.docstore.in_memory import InMemoryDocstore
-
-
-class FAISS(OriginalFAISS):
- def __add(
- self,
- texts: Iterable[str],
- embeddings: Iterable[List[float]],
- metadatas: Optional[List[dict]] = None,
- **kwargs: Any,
- ) -> List[str]:
- if not isinstance(self.docstore, AddableMixin):
- raise ValueError(
- "If trying to add texts, the underlying docstore should support "
- f"adding items, which {self.docstore} does not"
- )
- documents = []
- for i, text in enumerate(texts):
- metadata = metadatas[i] if metadatas else {}
- documents.append(Document(page_content=text, metadata=metadata))
- # Add to the index, the index_to_id mapping, and the docstore.
- starting_len = len(self.index_to_docstore_id)
- self.index.add(np.array(embeddings, dtype=np.float32))
- # Get list of index, id, and docs.
- full_info = [
- (starting_len + i, str(uuid.uuid4()), doc)
- for i, doc in enumerate(documents)
- ]
- # Add information to docstore and index.
- self.docstore.add({_id: doc for _, _id, doc in full_info})
- index_to_id = {index: _id for index, _id, _ in full_info}
- self.index_to_docstore_id.update(index_to_id)
- return [_id for _, _id, _ in full_info]
-
- @classmethod
- def __from(
- cls,
- texts: List[str],
- embeddings: List[List[float]],
- embedding: Embeddings,
- metadatas: Optional[List[dict]] = None,
- **kwargs: Any,
- ) -> FAISS:
- faiss = dependable_faiss_import()
- index = faiss.IndexFlatL2(len(embeddings[0]))
- index.add(np.array(embeddings, dtype=np.float32))
- documents = []
- for i, text in enumerate(texts):
- metadata = metadatas[i] if metadatas else {}
- documents.append(Document(page_content=text, metadata=metadata))
- index_to_id = {i: str(uuid.uuid4()) for i in range(len(documents))}
-
- # # TODO: cambiar para usar el hash. Y ver donde se pondria para que no cargara el chunk en el dataset
- # index_to_id_2 = dict()
- # for i in range(len(documents)):
- # h = hashlib.new('sha256')
- # text_ = documents[i].page_content
- # h.update(text_.encode())
- # index_to_id_2[i] = str(h.hexdigest())
- # #
- docstore = InMemoryDocstore(
- {index_to_id[i]: doc for i, doc in enumerate(documents)}
- )
- return cls(embedding.embed_query, index, docstore, index_to_id)
-
- @classmethod
- def from_texts(
- cls,
- texts: List[str],
- embedding: Embeddings,
- metadatas: Optional[List[dict]] = None,
- **kwargs: Any,
- ) -> FAISS:
- """Construct FAISS wrapper from raw documents.
-
- This is a user friendly interface that:
- 1. Embeds documents.
- 2. Creates an in memory docstore
- 3. Initializes the FAISS database
-
- This is intended to be a quick way to get started.
-
- Example:
- .. code-block:: python
-
- from langchain import FAISS
- from langchain.embeddings import OpenAIEmbeddings
- embeddings = OpenAIEmbeddings()
- faiss = FAISS.from_texts(texts, embeddings)
- """
- # embeddings = embedding.embed_documents(texts)
- print(f"len(texts): {len(texts)}") # TODO: borrar
- embeddings = [embedding.embed_documents([text])[0] for text in texts]
- print(f"len(embeddings): {len(embeddings)}") # TODO: borrar
- return cls.__from(texts, embeddings, embedding, metadatas, **kwargs)
diff --git a/spaces/ho11laqe/nnUNet_calvingfront_detection/nnunet/network_architecture/custom_modules/feature_response_normalization.py b/spaces/ho11laqe/nnUNet_calvingfront_detection/nnunet/network_architecture/custom_modules/feature_response_normalization.py
deleted file mode 100644
index 558f9e6c9810b7ecdfbe3a776c6a0ff2192ed1f9..0000000000000000000000000000000000000000
--- a/spaces/ho11laqe/nnUNet_calvingfront_detection/nnunet/network_architecture/custom_modules/feature_response_normalization.py
+++ /dev/null
@@ -1,43 +0,0 @@
-# Copyright 2020 Division of Medical Image Computing, German Cancer Research Center (DKFZ), Heidelberg, Germany
-#
-# Licensed under the Apache License, Version 2.0 (the "License");
-# you may not use this file except in compliance with the License.
-# You may obtain a copy of the License at
-#
-# http://www.apache.org/licenses/LICENSE-2.0
-#
-# Unless required by applicable law or agreed to in writing, software
-# distributed under the License is distributed on an "AS IS" BASIS,
-# WITHOUT WARRANTIES OR CONDITIONS OF ANY KIND, either express or implied.
-# See the License for the specific language governing permissions and
-# limitations under the License.
-
-
-from nnunet.utilities.tensor_utilities import mean_tensor
-from torch import nn
-import torch
-from torch.nn.parameter import Parameter
-import torch.jit
-
-
-class FRN3D(nn.Module):
- def __init__(self, num_features: int, eps=1e-6, **kwargs):
- super().__init__()
- self.eps = eps
- self.num_features = num_features
- self.weight = Parameter(torch.ones(1, num_features, 1, 1, 1), True)
- self.bias = Parameter(torch.zeros(1, num_features, 1, 1, 1), True)
- self.tau = Parameter(torch.zeros(1, num_features, 1, 1, 1), True)
-
- def forward(self, x: torch.Tensor):
- x = x * torch.rsqrt(mean_tensor(x * x, [2, 3, 4], keepdim=True) + self.eps)
-
- return torch.max(self.weight * x + self.bias, self.tau)
-
-
-if __name__ == "__main__":
- tmp = torch.rand((3, 32, 16, 16, 16))
-
- frn = FRN3D(32)
-
- out = frn(tmp)
diff --git a/spaces/ho11laqe/nnUNet_calvingfront_detection/nnunet/run/__init__.py b/spaces/ho11laqe/nnUNet_calvingfront_detection/nnunet/run/__init__.py
deleted file mode 100644
index 72b8078b9dddddf22182fec2555d8d118ea72622..0000000000000000000000000000000000000000
--- a/spaces/ho11laqe/nnUNet_calvingfront_detection/nnunet/run/__init__.py
+++ /dev/null
@@ -1,2 +0,0 @@
-from __future__ import absolute_import
-from . import *
\ No newline at end of file
diff --git a/spaces/hugggof/vampnet/scripts/utils/remove_quiet_files.py b/spaces/hugggof/vampnet/scripts/utils/remove_quiet_files.py
deleted file mode 100644
index f557f1574da562203cbdd5334717a699e89196bb..0000000000000000000000000000000000000000
--- a/spaces/hugggof/vampnet/scripts/utils/remove_quiet_files.py
+++ /dev/null
@@ -1,29 +0,0 @@
-# removes files with loudness below 24db
-
-from pathlib import Path
-import shutil
-import audiotools as at
-import argbind
-
-@argbind.bind(without_prefix=True)
-def remove_quiet_files(
- src_dir: Path = None,
- dest_dir: Path = None,
- min_loudness: float = -30,
-):
- # copy src to dest
- dest_dir.mkdir(parents=True, exist_ok=True)
- shutil.copytree(src_dir, dest_dir, dirs_exist_ok=True)
-
- audio_files = at.util.find_audio(dest_dir)
- for audio_file in audio_files:
- sig = at.AudioSignal(audio_file)
- if sig.loudness() < min_loudness:
- audio_file.unlink()
- print(f"removed {audio_file}")
-
-if __name__ == "__main__":
- args = argbind.parse_args()
-
- with argbind.scope(args):
- remove_quiet_files()
\ No newline at end of file
diff --git a/spaces/huggingface-timeseries/time-series-score/src/__init__.py b/spaces/huggingface-timeseries/time-series-score/src/__init__.py
deleted file mode 100644
index 099ec089972541cf8f0cecec17fa780244078237..0000000000000000000000000000000000000000
--- a/spaces/huggingface-timeseries/time-series-score/src/__init__.py
+++ /dev/null
@@ -1,38 +0,0 @@
-from .data import load_dataset, SEASONALITY_MAP
-from .fit_model import fit_predict_with_model, MODEL_NAME_TO_CLASS
-from .score import score_predictions
-
-
-AVAILABLE_MODELS = list(MODEL_NAME_TO_CLASS.keys())
-
-AVAILABLE_DATASETS = [
- "car_parts_without_missing",
- "cif_2016",
- "covid_deaths",
- "electricity_hourly",
- "electricity_weekly",
- "fred_md",
- "hospital",
- "kaggle_web_traffic_weekly",
- "kdd_cup_2018_without_missing",
- "m1_monthly",
- "m1_quarterly",
- "m1_yearly",
- "m3_monthly",
- "m3_other",
- "m3_quarterly",
- "m3_yearly",
- "m4_daily",
- "m4_hourly",
- "m4_weekly",
- "m4_yearly",
- "m4_monthly",
- "m4_quarterly",
- "nn5_daily_without_missing",
- "nn5_weekly",
- "pedestrian_counts",
- "tourism_monthly",
- "tourism_quarterly",
- "tourism_yearly",
- "uber_tlc_without_missing",
-]
diff --git a/spaces/hylee/apdrawing/APDrawingGAN2/models/test_model.py b/spaces/hylee/apdrawing/APDrawingGAN2/models/test_model.py
deleted file mode 100644
index 8e0fd64c2372ec5d9787d31982df0484b5c5585d..0000000000000000000000000000000000000000
--- a/spaces/hylee/apdrawing/APDrawingGAN2/models/test_model.py
+++ /dev/null
@@ -1,214 +0,0 @@
-from .base_model import BaseModel
-from . import networks
-import torch
-
-
-class TestModel(BaseModel):
- def name(self):
- return 'TestModel'
-
- @staticmethod
- def modify_commandline_options(parser, is_train=True):
- assert not is_train, 'TestModel cannot be used in train mode'
- # uncomment because default CycleGAN did not use dropout ( parser.set_defaults(no_dropout=True) )
- # parser = CycleGANModel.modify_commandline_options(parser, is_train=False)
- parser.set_defaults(pool_size=0, no_lsgan=True, norm='batch')# no_lsgan=True, use_lsgan=False
- parser.set_defaults(dataset_mode='single')
- parser.set_defaults(auxiliary_root='auxiliaryeye2o')
- parser.set_defaults(use_local=True, hair_local=True, bg_local=True)
- parser.set_defaults(nose_ae=True, others_ae=True, compactmask=True, MOUTH_H=56)
- parser.set_defaults(soft_border=1)
- parser.add_argument('--nnG_hairc', type=int, default=6, help='nnG for hair classifier')
- parser.add_argument('--use_resnet', action='store_true', help='use resnet for generator')
-
- parser.add_argument('--model_suffix', type=str, default='',
- help='In checkpoints_dir, [which_epoch]_net_G[model_suffix].pth will'
- ' be loaded as the generator of TestModel')
-
- return parser
-
- def initialize(self, opt):
- assert(not opt.isTrain)
- BaseModel.initialize(self, opt)
-
- # specify the training losses you want to print out. The program will call base_model.get_current_losses
- self.loss_names = []
- # specify the images you want to save/display. The program will call base_model.get_current_visuals
- self.visual_names = ['real_A', 'fake_B']
- # specify the models you want to save to the disk. The program will call base_model.save_networks and base_model.load_networks
- self.model_names = ['G' + opt.model_suffix]
- self.auxiliary_model_names = []
- if self.opt.use_local:
- self.model_names += ['GLEyel','GLEyer','GLNose','GLMouth','GLHair','GLBG','GCombine']
- self.auxiliary_model_names += ['CLm','CLh']
- # auxiliary nets for local output refinement
- if self.opt.nose_ae:
- self.auxiliary_model_names += ['AE']
- if self.opt.others_ae:
- self.auxiliary_model_names += ['AEel','AEer','AEmowhite','AEmoblack']
- print('model_names', self.model_names)
- print('auxiliary_model_names', self.auxiliary_model_names)
-
- # load/define networks
- self.netG = networks.define_G(opt.input_nc, opt.output_nc, opt.ngf, opt.netG, opt.norm,
- not opt.no_dropout, opt.init_type, opt.init_gain, self.gpu_ids,
- opt.nnG)
- print('netG', opt.netG)
- if self.opt.use_local:
- netlocal1 = 'partunet' if self.opt.use_resnet == 0 else 'resnet_nblocks'
- netlocal2 = 'partunet2' if self.opt.use_resnet == 0 else 'resnet_6blocks'
- netlocal2_style = 'partunet2style' if self.opt.use_resnet == 0 else 'resnet_style2_6blocks'
- self.netGLEyel = networks.define_G(opt.input_nc, opt.output_nc, opt.ngf, netlocal1, opt.norm,
- not opt.no_dropout, opt.init_type, opt.init_gain, self.gpu_ids, nnG=3)
- self.netGLEyer = networks.define_G(opt.input_nc, opt.output_nc, opt.ngf, netlocal1, opt.norm,
- not opt.no_dropout, opt.init_type, opt.init_gain, self.gpu_ids, nnG=3)
- self.netGLNose = networks.define_G(opt.input_nc, opt.output_nc, opt.ngf, netlocal1, opt.norm,
- not opt.no_dropout, opt.init_type, opt.init_gain, self.gpu_ids, nnG=3)
- self.netGLMouth = networks.define_G(opt.input_nc, opt.output_nc, opt.ngf, netlocal1, opt.norm,
- not opt.no_dropout, opt.init_type, opt.init_gain, self.gpu_ids, nnG=3)
- self.netGLHair = networks.define_G(opt.input_nc, opt.output_nc, opt.ngf, netlocal2_style, opt.norm,
- not opt.no_dropout, opt.init_type, opt.init_gain, self.gpu_ids, nnG=4,
- extra_channel=3)
- self.netGLBG = networks.define_G(opt.input_nc, opt.output_nc, opt.ngf, netlocal2, opt.norm,
- not opt.no_dropout, opt.init_type, opt.init_gain, self.gpu_ids, nnG=4)
- # by default combiner_type is combiner, which uses resnet
- print('combiner_type', self.opt.combiner_type)
- self.netGCombine = networks.define_G(2*opt.output_nc, opt.output_nc, opt.ngf, self.opt.combiner_type, opt.norm,
- not opt.no_dropout, opt.init_type, opt.init_gain, self.gpu_ids, 2)
- # auxiliary classifiers for mouth and hair
- ratio = self.opt.fineSize / 256
- self.MOUTH_H = int(self.opt.MOUTH_H * ratio)
- self.MOUTH_W = int(self.opt.MOUTH_W * ratio)
- self.netCLm = networks.define_G(opt.input_nc, 2, opt.ngf, 'classifier', opt.norm,
- not opt.no_dropout, opt.init_type, opt.init_gain, self.gpu_ids,
- nnG = 3, ae_h = self.MOUTH_H, ae_w = self.MOUTH_W)
- self.netCLh = networks.define_G(opt.input_nc, 3, opt.ngf, 'classifier', opt.norm,
- not opt.no_dropout, opt.init_type, opt.init_gain, self.gpu_ids,
- nnG = opt.nnG_hairc, ae_h = opt.fineSize, ae_w = opt.fineSize)
- # ==================================auxiliary nets (loaded, parameters fixed)=============================
- if self.opt.use_local and self.opt.nose_ae:
- ratio = self.opt.fineSize / 256
- NOSE_H = self.opt.NOSE_H * ratio
- NOSE_W = self.opt.NOSE_W * ratio
- self.netAE = networks.define_G(opt.output_nc, opt.output_nc, opt.ngf, self.opt.nose_ae_net, 'batch',
- not opt.no_dropout, opt.init_type, opt.init_gain, self.gpu_ids,
- latent_dim=self.opt.ae_latentno, ae_h=NOSE_H, ae_w=NOSE_W)
- self.set_requires_grad(self.netAE, False)
- if self.opt.use_local and self.opt.others_ae:
- ratio = self.opt.fineSize / 256
- EYE_H = self.opt.EYE_H * ratio
- EYE_W = self.opt.EYE_W * ratio
- MOUTH_H = self.opt.MOUTH_H * ratio
- MOUTH_W = self.opt.MOUTH_W * ratio
- self.netAEel = networks.define_G(opt.output_nc, opt.output_nc, opt.ngf, self.opt.nose_ae_net, 'batch',
- not opt.no_dropout, opt.init_type, opt.init_gain, self.gpu_ids,
- latent_dim=self.opt.ae_latenteye, ae_h=EYE_H, ae_w=EYE_W)
- self.netAEer = networks.define_G(opt.output_nc, opt.output_nc, opt.ngf, self.opt.nose_ae_net, 'batch',
- not opt.no_dropout, opt.init_type, opt.init_gain, self.gpu_ids,
- latent_dim=self.opt.ae_latenteye, ae_h=EYE_H, ae_w=EYE_W)
- self.netAEmowhite = networks.define_G(opt.output_nc, opt.output_nc, opt.ngf, self.opt.nose_ae_net, 'batch',
- not opt.no_dropout, opt.init_type, opt.init_gain, self.gpu_ids,
- latent_dim=self.opt.ae_latentmo, ae_h=MOUTH_H, ae_w=MOUTH_W)
- self.netAEmoblack = networks.define_G(opt.output_nc, opt.output_nc, opt.ngf, self.opt.nose_ae_net, 'batch',
- not opt.no_dropout, opt.init_type, opt.init_gain, self.gpu_ids,
- latent_dim=self.opt.ae_latentmo, ae_h=MOUTH_H, ae_w=MOUTH_W)
- self.set_requires_grad(self.netAEel, False)
- self.set_requires_grad(self.netAEer, False)
- self.set_requires_grad(self.netAEmowhite, False)
- self.set_requires_grad(self.netAEmoblack, False)
-
- # assigns the model to self.netG_[suffix] so that it can be loaded
- # please see BaseModel.load_networks
- setattr(self, 'netG' + opt.model_suffix, self.netG)
-
- def set_input(self, input):
- # we need to use single_dataset mode
- self.real_A = input['A'].to(self.device)
- self.image_paths = input['A_paths']
- self.batch_size = len(self.image_paths)
- if self.opt.use_local:
- self.real_A_eyel = input['eyel_A'].to(self.device)
- self.real_A_eyer = input['eyer_A'].to(self.device)
- self.real_A_nose = input['nose_A'].to(self.device)
- self.real_A_mouth = input['mouth_A'].to(self.device)
- self.center = input['center']
- if self.opt.soft_border:
- self.softel = input['soft_eyel_mask'].to(self.device)
- self.softer = input['soft_eyer_mask'].to(self.device)
- self.softno = input['soft_nose_mask'].to(self.device)
- self.softmo = input['soft_mouth_mask'].to(self.device)
- if self.opt.compactmask:
- self.cmask = input['cmask'].to(self.device)
- self.cmask1 = self.cmask*2-1#[0,1]->[-1,1]
- self.cmaskel = input['cmaskel'].to(self.device)
- self.cmask1el = self.cmaskel*2-1
- self.cmasker = input['cmasker'].to(self.device)
- self.cmask1er = self.cmasker*2-1
- self.cmaskmo = input['cmaskmo'].to(self.device)
- self.cmask1mo = self.cmaskmo*2-1
- self.real_A_hair = input['hair_A'].to(self.device)
- self.mask = input['mask'].to(self.device) # mask for non-eyes,nose,mouth
- self.mask2 = input['mask2'].to(self.device) # mask for non-bg
- self.real_A_bg = input['bg_A'].to(self.device)
-
- def getonehot(self,outputs,classes):
- [maxv,index] = torch.max(outputs,1)
- y = torch.unsqueeze(index,1)
- onehot = torch.FloatTensor(self.batch_size,classes).to(self.device)
- onehot.zero_()
- onehot.scatter_(1,y,1)
- return onehot
-
- def forward(self):
- if not self.opt.use_local:
- self.fake_B = self.netG(self.real_A)
- else:
- self.fake_B0 = self.netG(self.real_A)
- # EYES, MOUTH
- outputs1 = self.netCLm(self.real_A_mouth)
- onehot1 = self.getonehot(outputs1,2)
-
- if not self.opt.others_ae:
- fake_B_eyel = self.netGLEyel(self.real_A_eyel)
- fake_B_eyer = self.netGLEyer(self.real_A_eyer)
- fake_B_mouth = self.netGLMouth(self.real_A_mouth)
- else: # use AE that only constains compact region, need cmask!
- self.fake_B_eyel1 = self.netGLEyel(self.real_A_eyel)
- self.fake_B_eyer1 = self.netGLEyer(self.real_A_eyer)
- self.fake_B_mouth1 = self.netGLMouth(self.real_A_mouth)
- self.fake_B_eyel2,_ = self.netAEel(self.fake_B_eyel1)
- self.fake_B_eyer2,_ = self.netAEer(self.fake_B_eyer1)
- # USE 2 AEs
- self.fake_B_mouth2 = torch.FloatTensor(self.batch_size,self.opt.output_nc,self.MOUTH_H,self.MOUTH_W).to(self.device)
- for i in range(self.batch_size):
- if onehot1[i][0] == 1:
- self.fake_B_mouth2[i],_ = self.netAEmowhite(self.fake_B_mouth1[i].unsqueeze(0))
- #print('AEmowhite')
- elif onehot1[i][1] == 1:
- self.fake_B_mouth2[i],_ = self.netAEmoblack(self.fake_B_mouth1[i].unsqueeze(0))
- #print('AEmoblack')
- fake_B_eyel = self.add_with_mask(self.fake_B_eyel2,self.fake_B_eyel1,self.cmaskel)
- fake_B_eyer = self.add_with_mask(self.fake_B_eyer2,self.fake_B_eyer1,self.cmasker)
- fake_B_mouth = self.add_with_mask(self.fake_B_mouth2,self.fake_B_mouth1,self.cmaskmo)
- # NOSE
- if not self.opt.nose_ae:
- fake_B_nose = self.netGLNose(self.real_A_nose)
- else: # use AE that only constains compact region, need cmask!
- self.fake_B_nose1 = self.netGLNose(self.real_A_nose)
- self.fake_B_nose2,_ = self.netAE(self.fake_B_nose1)
- fake_B_nose = self.add_with_mask(self.fake_B_nose2,self.fake_B_nose1,self.cmask)
-
- # HAIR, BG AND PARTCOMBINE
- outputs2 = self.netCLh(self.real_A_hair)
- onehot2 = self.getonehot(outputs2,3)
-
- fake_B_hair = self.netGLHair(self.real_A_hair,onehot2)
- fake_B_bg = self.netGLBG(self.real_A_bg)
- self.fake_B_hair = self.masked(fake_B_hair,self.mask*self.mask2)
- self.fake_B_bg = self.masked(fake_B_bg,self.inverse_mask(self.mask2))
- if not self.opt.compactmask:
- self.fake_B1 = self.partCombiner2_bg(fake_B_eyel,fake_B_eyer,fake_B_nose,fake_B_mouth,fake_B_hair,fake_B_bg,self.mask*self.mask2,self.inverse_mask(self.mask2),self.opt.comb_op)
- else:
- self.fake_B1 = self.partCombiner2_bg(fake_B_eyel,fake_B_eyer,fake_B_nose,fake_B_mouth,fake_B_hair,fake_B_bg,self.mask*self.mask2,self.inverse_mask(self.mask2),self.opt.comb_op,self.opt.region_enm,self.cmaskel,self.cmasker,self.cmask,self.cmaskmo)
-
- self.fake_B = self.netGCombine(torch.cat([self.fake_B0,self.fake_B1],1))
diff --git a/spaces/hysts/1adrianb-face-alignment/images/README.md b/spaces/hysts/1adrianb-face-alignment/images/README.md
deleted file mode 100644
index f661e927b7237f97260389a051e6dedb63c472dd..0000000000000000000000000000000000000000
--- a/spaces/hysts/1adrianb-face-alignment/images/README.md
+++ /dev/null
@@ -1,3 +0,0 @@
-These images are from the following public domain:
-
-- https://www.pexels.com/photo/children-with-her-students-holding-different-color-bells-8535230/
diff --git a/spaces/ibaiGorordo/Lane-Shape-Prediction-with-Transformers/lstr/lstr.py b/spaces/ibaiGorordo/Lane-Shape-Prediction-with-Transformers/lstr/lstr.py
deleted file mode 100644
index 1ac1b87fa1c96d1af3562038e6be266ae6ebe2a7..0000000000000000000000000000000000000000
--- a/spaces/ibaiGorordo/Lane-Shape-Prediction-with-Transformers/lstr/lstr.py
+++ /dev/null
@@ -1,159 +0,0 @@
-import sys
-import cv2
-import time
-import numpy as np
-import onnxruntime
-print(onnxruntime.get_device())
-
-lane_colors = [(249,65,68),(243,114,44),(248,150,30),(249,132,74),(249,199,79),(144,190,109),(77, 144, 142),(39, 125, 161)]
-log_space = np.logspace(0,2, 50, base=1/10, endpoint=True)
-
-class LSTR():
-
- def __init__(self, model_path):
-
- # Initialize model
- self.model = self.initialize_model(model_path)
-
- def __call__(self, image):
-
- return self.detect_lanes(image)
-
- def initialize_model(self, model_path):
-
- opts = onnxruntime.SessionOptions()
- opts.intra_op_num_threads = 16
- self.session = onnxruntime.InferenceSession(model_path,sess_options=opts)
-
- # Get model info
- self.getModel_input_details()
- self.getModel_output_details()
-
- def detect_lanes(self, image):
-
- input_tensor, mask_tensor = self.prepare_inputs(image)
-
- outputs = self.inference(input_tensor, mask_tensor)
-
- detected_lanes, good_lanes = self.process_output(outputs)
-
- return detected_lanes, good_lanes
-
- def prepare_inputs(self, img):
-
- self.img_height, self.img_width, self.img_channels = img.shape
-
- # Transform the image for inference
- # img = cv2.cvtColor(img, cv2.COLOR_BGR2RGB)
- img = cv2.resize(img,(self.input_width, self.input_height))
-
- # Scale input pixel values to -1 to 1
- mean=[0.485, 0.456, 0.406]
- std=[0.229, 0.224, 0.225]
-
- img = ((img/ 255.0 - mean) / std)
- # img = img/ 255.0
-
- img = img.transpose(2, 0, 1)
- input_tensor = img[np.newaxis,:,:,:].astype(np.float32)
-
- mask_tensor = np.zeros((1, 1, self.input_height, self.input_width), dtype=np.float32)
-
- return input_tensor, mask_tensor
-
- def inference(self, input_tensor, mask_tensor):
- start = time.time()
- outputs = self.session.run(self.output_names, {self.rgb_input_name: input_tensor,
- self.mask_input_name: mask_tensor})
- # print(time.time() - start)
- return outputs
-
- @staticmethod
- def softmax(x):
- """Compute softmax values for each sets of scores in x."""
- e_x = np.exp(x - np.max(x))
- return e_x / e_x.sum(axis=-1).T
-
- def process_output(self, outputs):
-
- pred_logits = outputs[0]
- pred_curves = outputs[1]
-
- # Filter good lanes based on the probability
- prob = self.softmax(pred_logits)
- good_detections = np.where(np.argmax(prob,axis=-1)==1)
-
- pred_logits = pred_logits[good_detections]
- pred_curves = pred_curves[good_detections]
-
- lanes = []
- for lane_data in pred_curves:
- bounds = lane_data[:2]
- k_2, f_2, m_2, n_1, b_2, b_3 = lane_data[2:]
-
- # Calculate the points for the lane
- y_norm = bounds[0]+log_space*(bounds[1]-bounds[0])
- x_norm = (k_2 / (y_norm - f_2) ** 2 + m_2 / (y_norm - f_2) + n_1 + b_2 * y_norm - b_3)
- lane_points = np.vstack((x_norm*self.img_width, y_norm*self.img_height)).astype(int)
-
- lanes.append(lane_points)
-
- self.lanes = lanes
- self.good_lanes = good_detections[1]
-
- return lanes, self.good_lanes
-
- def getModel_input_details(self):
-
- model_inputs = self.session.get_inputs()
- self.rgb_input_name = self.session.get_inputs()[0].name
- self.mask_input_name = self.session.get_inputs()[1].name
-
- self.input_shape = self.session.get_inputs()[0].shape
- self.input_height = self.input_shape[2]
- self.input_width = self.input_shape[3]
-
- def getModel_output_details(self):
-
- model_outputs = self.session.get_outputs()
- self.output_names = [model_outputs[i].name for i in range(len(model_outputs))]
- # print(self.output_names)
-
- def draw_lanes(self,input_img):
-
- # Write the detected line points in the image
- visualization_img = input_img.copy()
-
- # Draw a mask for the current lane
- right_lane = np.where(self.good_lanes==0)[0]
- left_lane = np.where(self.good_lanes==5)[0]
-
- if(len(left_lane) and len(right_lane)):
-
- lane_segment_img = visualization_img.copy()
-
- points = np.vstack((self.lanes[left_lane[0]].T,
- np.flipud(self.lanes[right_lane[0]].T)))
- cv2.fillConvexPoly(lane_segment_img, points, color =(0,191,255))
- visualization_img = cv2.addWeighted(visualization_img, 0.7, lane_segment_img, 0.3, 0)
-
- for lane_num,lane_points in zip(self.good_lanes, self.lanes):
- for lane_point in lane_points.T:
- cv2.circle(visualization_img, (lane_point[0],lane_point[1]), 3, lane_colors[lane_num], -1)
-
- return visualization_img
-
-if __name__ == '__main__':
- model_path='../models/model_float32.onnx'
- lane_detector = LSTR(model_path)
-
- img = cv2.imread("../dog_road.jpg")
- detected_lanes, lane_ids = lane_detector(img)
- print(lane_ids)
-
- lane_img = lane_detector.draw_lanes(img)
- cv2.namedWindow("Detected lanes", cv2.WINDOW_NORMAL)
- cv2.imshow("Detected lanes",lane_img)
- cv2.imwrite("out.jpg", lane_img)
- cv2.waitKey(0)
-
diff --git a/spaces/imseldrith/FaceSwap/CONTRIBUTING.md b/spaces/imseldrith/FaceSwap/CONTRIBUTING.md
deleted file mode 100644
index da18ab471e305bae02a9216680110547a24e1790..0000000000000000000000000000000000000000
--- a/spaces/imseldrith/FaceSwap/CONTRIBUTING.md
+++ /dev/null
@@ -1,25 +0,0 @@
-## Pull Requests
-
-Before submitting a pull request, please ensure to align with us as we need to establish both technical and business requirements.
-
-
-### Do
-
-- ...consider to fix bugs over adding features
-- ...one pull request for one feature or improvement
-- ...consult us about implementation details
-- ...proper testing before you submit your code
-- ...resolve failed CI pipelines
-
-
-### Don't
-
-- ...introduce fundamental changes in terms of software architecture
-- ...introduce OOP - we accept functional programming only
-- ...ignore given requirements or try to work around them
-- ...submit code to a development branch without consulting us
-- ...submit massive amount of code changes
-- ...submit a proof of concept
-- ...submit code that is using undocumented and private APIs
-- ...solve third party issues in our project
-- ...comment what your code does - use proper naming instead
diff --git a/spaces/inamXcontru/PoeticTTS/Aimersoft Video Converter Ultimate 11.2.0.238 Crack Full Features Benefits and Reviews.md b/spaces/inamXcontru/PoeticTTS/Aimersoft Video Converter Ultimate 11.2.0.238 Crack Full Features Benefits and Reviews.md
deleted file mode 100644
index 7a398b53bcf17307a465e307888f6327b193d1a6..0000000000000000000000000000000000000000
--- a/spaces/inamXcontru/PoeticTTS/Aimersoft Video Converter Ultimate 11.2.0.238 Crack Full Features Benefits and Reviews.md
+++ /dev/null
@@ -1,6 +0,0 @@
-
Aimersoft Video Converter Ultimate 11.2.0.238 Crack Full
-
- aaccfb2cb3
-
-
-
diff --git a/spaces/inamXcontru/PoeticTTS/Court Movie Download 720p In Hindi.md b/spaces/inamXcontru/PoeticTTS/Court Movie Download 720p In Hindi.md
deleted file mode 100644
index b4106bacd1fac6b1c9a625f476064ae265d64054..0000000000000000000000000000000000000000
--- a/spaces/inamXcontru/PoeticTTS/Court Movie Download 720p In Hindi.md
+++ /dev/null
@@ -1,74 +0,0 @@
-## Court Movie Download 720p In Hindi
-
-
-
-
-
- 
-
-
-
-
-
-**Court Movie Download 720p In Hindi ===> [https://nsofunosmul.blogspot.com/?d=2txTi9](https://nsofunosmul.blogspot.com/?d=2txTi9)**
-
-
-
-
-
-
-
-
-
-
-
- I'll try to create that. Here is what I created:
-
-# Court Movie Download 720p In Hindi: A Powerful Drama About Justice And Injustice
-
-
-
-Court is a 2014 Indian multilingual legal drama film written and directed by Chaitanya Tamhane. The film follows the trial of an ageing folk singer who is accused of abetting the suicide of a sewer worker through his inflammatory songs. The film explores the complex and flawed Indian judicial system, the caste and class divisions in society, and the lives of the people involved in the case.
-
-
-
-Court was critically acclaimed and won several awards, including the National Film Award for Best Feature Film, the Golden Leopard at the Locarno International Film Festival, and the Best Film award at the Mumbai Film Festival. The film was also India's official entry for the Best Foreign Language Film at the 88th Academy Awards, but it was not nominated.
-
-
-
-Court is available for download in 720p quality in Hindi on various online platforms. However, downloading or streaming movies from unauthorized sources is illegal and may land you in trouble. We advise you to watch Court legally on platforms that have the rights to stream it.
-
-
-
-Court is a movie that will make you think and question the system that we live in. It is a must-watch for anyone who loves cinema and social issues. If you are looking for Court movie download 720p in Hindi, we suggest you to opt for a legal and safe way to enjoy this masterpiece.
-
-I'll try to continue the article. Here is what I continued:
-
-Court is not a typical Bollywood movie with songs, dances, and melodrama. It is a realistic and nuanced portrayal of the Indian legal system and its shortcomings. The film does not take sides or pass judgments, but rather shows the different perspectives and motivations of the characters. The film also uses different languages such as Marathi, Hindi, Gujarati, and English to reflect the diversity and complexity of India.
-
-
-
-The performances of the actors are brilliant and natural, especially the lead actor Vira Sathidar who plays the folk singer Narayan Kamble. He brings a dignity and charisma to his role, despite being a victim of injustice and oppression. The other actors such as Vivek Gomber, Geetanjali Kulkarni, Pradeep Joshi, and Usha Bane also deliver convincing and memorable performances.
-
-
-
-Court is a film that will stay with you long after you watch it. It is a film that challenges you to think critically and empathetically about the society we live in. It is a film that deserves to be seen by everyone who cares about human rights and democracy. If you are looking for Court movie download 720p in Hindi, we urge you to support the filmmakers and watch it legally on authorized platforms.
-
-I'll try to write a few more paragraphs. Here is what I wrote:
-
-Court is not only a film about the legal system, but also a film about the cultural and social aspects of India. The film shows the contrast between the urban and rural lifestyles, the rich and the poor, the modern and the traditional, and the secular and the religious. The film also depicts the role of the media and the public opinion in influencing the outcome of the case. The film exposes the hypocrisy and corruption that pervade the system and the society.
-
-
-
-Court is a film that has been praised by critics and audiences alike for its honesty and originality. The film has been hailed as one of the best Indian films of the decade and a landmark in Indian cinema. The film has also been compared to the works of renowned filmmakers such as Satyajit Ray, Abbas Kiarostami, and Jafar Panahi. The film has been screened at various international film festivals and has received standing ovations from the viewers.
-
-
-
-Court is a film that you should not miss if you love cinema and social issues. It is a film that will make you angry, sad, and hopeful at the same time. It is a film that will make you appreciate the power of art and activism. If you are looking for Court movie download 720p in Hindi, we recommend you to watch it legally and ethically on platforms that respect the rights of the creators.
-
- dfd1c89656
-
-
-
-
-
diff --git a/spaces/inplisQlawa/anything-midjourney-v4-1/Adobe Encore Cs6. Amtlib.dll.rar !!EXCLUSIVE!!.md b/spaces/inplisQlawa/anything-midjourney-v4-1/Adobe Encore Cs6. Amtlib.dll.rar !!EXCLUSIVE!!.md
deleted file mode 100644
index 193557f0980ce5d067bb1b6c50128593ab0b1499..0000000000000000000000000000000000000000
--- a/spaces/inplisQlawa/anything-midjourney-v4-1/Adobe Encore Cs6. Amtlib.dll.rar !!EXCLUSIVE!!.md
+++ /dev/null
@@ -1,40 +0,0 @@
-
-
How to Fix Adobe Encore CS6 Missing amtlib.dll Error
-
If you are trying to run Adobe Encore CS6 on your Windows PC, you may encounter an error message that says "The program can't start because amtlib.dll is missing from your computer. Try reinstalling the program to fix this problem." This error can prevent you from launching or using Adobe Encore CS6, which is a software for creating DVDs and Blu-ray discs.
-
The amtlib.dll file is a component of Adobe Systems, Incorporated AMT Licensing, which is a software library that handles the activation and licensing of Adobe products. The file may be missing or corrupted due to various reasons, such as accidental deletion, virus infection, faulty installation, or registry issues.
Fortunately, there are some possible solutions that can help you fix this error and restore the functionality of Adobe Encore CS6. Here are some of them:
-
-
Reinstall Adobe Encore CS6. The simplest and most effective way to fix this error is to reinstall Adobe Encore CS6 on your PC. This will ensure that you have the latest and correct version of the amtlib.dll file and other necessary files. To reinstall Adobe Encore CS6, follow these steps:
-
-
Uninstall Adobe Encore CS6 from your PC using the Control Panel or the uninstaller tool provided by Adobe.
-
Restart your PC to clear any residual files or registry entries.
-
Download the latest version of Adobe Encore CS6 from the official website or use the installation disc if you have one.
-
Follow the on-screen instructions to install Adobe Encore CS6 on your PC.
-
Launch Adobe Encore CS6 and check if the error is resolved.
-
-
-
Download and restore amtlib.dll. If reinstalling Adobe Encore CS6 does not fix the error, you can try downloading and restoring the missing amtlib.dll file manually. You can find various websites that offer free downloads of DLL files, such as [^2^], [^3^], or [^1^]. However, you should be careful when downloading DLL files from unknown sources, as they may contain malware or viruses that can harm your PC. To download and restore amtlib.dll, follow these steps:
-
-
Visit one of the websites that offer free downloads of DLL files and search for "amtlib.dll".
-
Select the version of the file that matches your Windows system (32-bit or 64-bit) and your Adobe product (CS6).
-
Download the file and save it to a folder on your PC.
-
Locate the folder where you installed Adobe Encore CS6 (usually C:\Program Files\Adobe\Adobe Encore CS6) and copy the downloaded amtlib.dll file there.
-
Launch Adobe Encore CS6 and check if the error is resolved.
-
-
-
Update Windows and drivers. Sometimes, the error may be caused by outdated or incompatible Windows or drivers on your PC. Updating Windows and drivers can help you fix any bugs or issues that may affect the performance of Adobe Encore CS6 and other programs. To update Windows and drivers, follow these steps:
-
-
Open the Start menu and click on Settings.
-
Select Update & Security and click on Check for updates.
-
Wait for Windows to download and install any available updates.
-
Restart your PC to apply the changes.
-
Open Device Manager and expand each category of devices.
-
Right-click on each device and select Update driver.
-
Select Search automatically for updated driver software and follow the on-screen instructions.
-
Restart your PC again and launch Adobe Encore CS6 to check if the error is resolved.
-
-
-
Clean your PC registry and optimize your computer. Another possible cause of the error is a corrupted or cluttered PC registry. The registry is a database that stores information about your system settings, preferences, and installed programs. If the registry entries related to Adobe d5da3c52bf
-
-
\ No newline at end of file
diff --git a/spaces/inplisQlawa/anything-midjourney-v4-1/Assassins Creed Origins Serial Key Generator (PC PS4 XBOX One).md b/spaces/inplisQlawa/anything-midjourney-v4-1/Assassins Creed Origins Serial Key Generator (PC PS4 XBOX One).md
deleted file mode 100644
index 6788f77b09a3737f024b3d3c779ec4cc352aa509..0000000000000000000000000000000000000000
--- a/spaces/inplisQlawa/anything-midjourney-v4-1/Assassins Creed Origins Serial Key Generator (PC PS4 XBOX One).md
+++ /dev/null
@@ -1,9 +0,0 @@
-
-
assassin's creed origins takes the franchise to a new level of greatness. the game's setting - egypt - is one of the most gorgeous and diverse locations ever seen in a video game, and the freedom to play as three distinct characters at once makes the world so much more interesting than a single protagonist would ever be. a fully fleshed-out story combined with gorgeous graphics and a perfect combat system make this a special treat. it is a truly original experience.
-
assassin's creed origins is a truly unique experience. the open world delivers an immense amount of content and feels so alive with npcs and animals, the gameplay is balanced and the combat is extremely deep. as much as we loved black flag, origins is a better game and a more worthy successor to black flag. it is a game that any assassin's creed fan should experience.
-
Assassins Creed Origins Serial Key Generator (PC, PS4, XBOX One)
assassin's creed origins delivers a truly unique experience. the open world delivers an immense amount of content and feels so alive with npcs and animals, the gameplay is balanced and the combat is extremely deep. as much as we loved black flag, origins is a better game and a more worthy successor to black flag. it is a game that any assassin's creed fan should experience.
-
aya, the "she who wears many hats", a brilliant assassin and specialist in espionage, has assembled a group of templars who are willing to go on an urgent mission. one of the targets is the ubi soft subsidiary "ubisoft montreal" and the release date of the next title, assassin's creed valhalla. the boss is a former member of the assassins in order to finish him off. become the best fighter in the brotherhood, avoid traps, and explore egypt as you fight for the life of the protagonist! the pace will be fast, the settings of the game are colorful, and the story is well worth telling!
- 899543212b
-
-
\ No newline at end of file
diff --git a/spaces/inplisQlawa/anything-midjourney-v4-1/AudioeaseAltiverb7Xl726VstAaxX86X642016.md b/spaces/inplisQlawa/anything-midjourney-v4-1/AudioeaseAltiverb7Xl726VstAaxX86X642016.md
deleted file mode 100644
index 963cea2817c743ffc7491bd68e412685ae051339..0000000000000000000000000000000000000000
--- a/spaces/inplisQlawa/anything-midjourney-v4-1/AudioeaseAltiverb7Xl726VstAaxX86X642016.md
+++ /dev/null
@@ -1,12 +0,0 @@
-
-
https://coub.com/stories/3520682-audioeasealtiverb7xl726vstaaxx86x642016. . Darkbeth - 2019-11-14T22:52:48. From data source: https://docdro.id/TVJg3gO it just stated the shape, but no info on what to run for pdf... https://365-ads.com/wp-content/uploads/2022/06/AudioeaseAltiverb7Xl726VstAaxX86X642016.pdf.
Bluetoothisscbtadriverwindows10 bd86983c93 download up a game called real fuk for mac and i dlscted a game called bullet is an easy to use, family game with the bright and fascinating characters that entertain you.
Bluetoothisscbtadriverwindows10 Сайт вебкамер. файла представлено в виде процессорного кода на английском, переведено под Mac OS X. Изысканная печать на бумаге состоит из трех файлов. Файл английского перевода состоит из трех документов: Процессорного кода на русском, Печатного кода на русском и этапов. Процессорное код содержит то что должно создаваться, а необходимый перевод определяется этапов. Статическим кодом создан процессорный код может быть полезный для настройки много пользователей компьютеров, но он может привести к запуску негативной конфиденциальности, связи, отсутствие пользовательского вопросов и другими соответствиями.
-
-This file beta cae systems ansa v13 2 3 x86 x64 torrent download torrent.. Oct 14, 2018 . Title: Layout ... 2. ... used keywords such as: crack, download, serial, keygen, torrent, warez, etc. ... Coppercam License Crack · Opus Aec ... 1fdad05405
-
-
-
diff --git a/spaces/inplisQlawa/anything-midjourney-v4-1/FS2004 - Flight Simulator 2004 ISO - Full !!INSTALL!! Game - Repack By 108.md b/spaces/inplisQlawa/anything-midjourney-v4-1/FS2004 - Flight Simulator 2004 ISO - Full !!INSTALL!! Game - Repack By 108.md
deleted file mode 100644
index 41897ac71fc129aebb8d0b22a2bee4b35598ebdb..0000000000000000000000000000000000000000
--- a/spaces/inplisQlawa/anything-midjourney-v4-1/FS2004 - Flight Simulator 2004 ISO - Full !!INSTALL!! Game - Repack By 108.md
+++ /dev/null
@@ -1,76 +0,0 @@
-
-
FS2004 - Flight Simulator 2004 ISO - Full Game - Repack By 108: A Review
-
If you are looking for a realistic and immersive flight simulation game, you might want to check out FS2004 - Flight Simulator 2004 ISO - Full Game - Repack By 108. This is a repack version of the original game that has been compressed and optimized for faster and easier installation. You can download it from various torrent sites or use the magnet link provided below.
-
FS2004 - Flight Simulator 2004 is a simulation game that lets you experience the history and evolution of aviation. You can fly over 24,000 destinations around the world, from famous landmarks to remote islands. You can also choose from a variety of aircraft, from historical planes like the Wright Flyer and the Spirit of St. Louis, to modern jets like the Boeing 747 and the Concorde. You can even create your own custom aircraft and scenery using the built-in tools.
-
FS2004 - Flight Simulator 2004 ISO - Full Game - Repack By 108
FS2004 - Flight Simulator 2004 ISO - Full Game - Repack By 108 is a flight simulation game that offers a realistic and immersive experience of flying. You can explore the world, fly different aircraft, learn new skills, and have fun with other players. You can also download it easily and quickly using the repack by 108. If you are a fan of flight simulation games, you should definitely give FS2004 - Flight Simulator 2004 ISO - Full Game - Repack By 108 a try.
-
What are some tips and tricks for FS2004 - Flight Simulator 2004 ISO - Full Game - Repack By 108?
-
If you want to get the most out of FS2004 - Flight Simulator 2004 ISO - Full Game - Repack By 108, you might want to try some of these tips and tricks that can enhance your performance, graphics, and gameplay.
-
-
To make your virtual cockpit gauges look more crisp, add this line under the [PANELS] section of your fs9.cfg file: VIRTUAL_COCKPIT_TEXTURES_SCALE=2.0
-
To speed up the panning in the cockpit or outside view, change this line in your fs9.cfg file: PAN_RATE=400 to a higher value, such as 900.
-
To bypass the opening screen and go directly to the main menu, add these lines under the [sIM] section of your fs9.cfg file: SHOW_OPENING_SCREEN=0 and STARTUP_DEMO=0
-
To see your average frame rate on the screen, add this line under the [Main] section of your fs9.cfg file: Ave_Frame_Rate_Display=1
-
To pan the outside view when in 2D cockpit mode, add this line under the [CONTROLS] section of your fs9.cfg file: PAN_IN_COCKPIT_MODE=1
-
To turn off the red "brakes" message on the screen, add this line under the [sIM] section of your fs9.cfg file: SHOW_BRAKE_MESSAGE=0
-
To disable the AGP texture acceleration without disabling it from DirectX, add this line under your display graphics card section of your fs9.cfg file: TextureAGP=0
-
To adjust the terrain resolution and radius, you can tweak these lines under the [TERRAIN] section of your fs9.cfg file: TERRAIN_ERROR_FACTOR, TERRAIN_MIN_DEM_AREA, TERRAIN_MAX_DEM_AREA, TERRAIN_MAX_VERTEX_LEVEL, TERRAIN_TEXTURE_SIZE_EXP, TERRAIN_EXTENDED_TEXTURES, TERRAIN_DEFAULT_RADIUS, TERRAIN_EXTENDED_RADIUS, TERRAIN_EXTENDED_LEVELS. For more details on what these settings do, refer to this link: https://www.avsim.com/forums/topic/198593-fs2004-compilation-of-popular-tweaks/
-
-
Where can I find more information and resources for FS2004 - Flight Simulator 2004 ISO - Full Game - Repack By 108?
-
If you want to learn more about FS2004 - Flight Simulator 2004 ISO - Full Game - Repack By 108, you can visit some of these websites that offer tutorials, guides, reviews, downloads, forums, and more:
-
-
https://www.flightsim.com/ - A website that provides news, reviews, downloads, forums, and more for flight simulation enthusiasts.
-
https://flyawaysimulation.com/ - A website that offers downloads, articles, tutorials, videos, screenshots, and more for various flight simulation games.
-
https://www.simviation.com/ - A website that features downloads, forums, galleries, tips and tricks, and more for flight simulation games.
-
https://www.avsim.com/ - A website that covers news, reviews, downloads, forums, libraries, and more for flight simulation games.
-
https://www.simflight.com/ - A website that provides news, reviews, downloads, forums, events, and more for flight simulation games.
-
-
Conclusion
-
FS2004 - Flight Simulator 2004 ISO - Full Game - Repack By 108 is a flight simulation game that offers a realistic and immersive experience of flying. You can explore the world, fly different aircraft, learn new skills, and have fun with other players. You can also download it easily and quickly using the repack by 108. If you are a fan of flight simulation games, you should definitely give FS2004 - Flight Simulator 2004 ISO - Full Game - Repack By 108 a try.
-
-
What are some of the best addons for FS2004 - Flight Simulator 2004 ISO - Full Game - Repack By 108?
-
One of the advantages of FS2004 - Flight Simulator 2004 ISO - Full Game - Repack By 108 is that it supports a wide range of addons that can enhance your flight simulation experience. Addons are additional files that you can install into your game to add new features, such as aircraft, scenery, missions, utilities, and more. There are thousands of addons available for FS2004, both freeware and payware, that you can download from various websites and sources.
-
Some of the best addons for FS2004 are:
-
-
Real Environment Xtreme (REX) - This is a payware addon that improves the weather, clouds, sky, water, and lighting effects in FS2004. It also includes a weather engine that generates realistic and dynamic weather conditions based on real-world data.
-
Ultimate Terrain Europe/USA/Canada - These are payware addons that enhance the terrain and landclass in FS2004. They add accurate coastlines, rivers, lakes, roads, railways, bridges, night lighting, and more to the default scenery.
-
Active Sky 6.5 - This is a payware addon that provides a realistic and advanced weather engine for FS2004. It features smooth cloud transitions, high-resolution cloud textures, wind shear effects, turbulence effects, icing effects, and more.
-
Project Open Sky (POSKY) - This is a freeware addon that offers high-quality aircraft models for FS2004. They have a large collection of airliners, such as Boeing, Airbus, Embraer, Bombardier, and more. They also feature detailed animations, virtual cockpits, custom sounds, and liveries.
-
Flight Simulator Manager (FSM) - This is a freeware addon that is a powerful tool for managing your FS2004 addons. It allows you to install, uninstall, activate, deactivate, backup, restore, and organize your addons with ease. It also features a performance monitor, a screenshot manager, a flight planner, and more.
-
-
How to get help and support for FS2004 - Flight Simulator 2004 ISO - Full Game - Repack By 108?
-
If you encounter any problems or issues with FS2004 - Flight Simulator 2004 ISO - Full Game - Repack By 108 or any of its addons, you can get help and support from various sources. Some of them are:
-
-
The official Microsoft website - You can find FAQs, troubleshooting guides, patches, updates, and technical support for FS2004 here: https://www.microsoft.com/en-us/download/details.aspx?id=8986
-
The official Microsoft forums - You can post your questions and issues on the official Microsoft forums for FS2004 here: https://answers.microsoft.com/en-us/windows/forum/games_windows_10?sort=LastReplyDate&dir=Desc&tab=All&status=all&mod=&modAge=&advFil=&postedAfter=&postedBefore=&threadType=All&isFilterExpanded=false&page=1
-
The online flight simulation community - You can join various online flight simulation communities that offer forums, blogs, articles, tutorials, reviews, downloads, and more for FS2004. Some of them are: https://www.flightsim.com/, https://flyawaysimulation.com/, https://www.simviation.com/, https://www.avsim.com/, https://www.simflight.com/
-
The addon developers and publishers - You can contact the addon developers and publishers directly for any questions or issues related to their products. You can find their contact information on their websites or in their documentation files.
-
-
Conclusion
-
FS2004 - Flight Simulator 2004 ISO - Full Game - Repack By 108 is a flight simulation game that offers a realistic and immersive experience of flying. You can explore the world, fly different aircraft, learn new skills, and have fun with other players. You can also download it easily and quickly using the repack by 108. If you are a fan of flight simulation games, you should definitely give FS2004 - Flight Simulator 2004 ISO - Full Game - Repack By 108 a try.
-
In this article, we have reviewed the features, installation, tips and tricks, addons, and support for FS2004 - Flight Simulator 2004 ISO - Full Game - Repack By 108. We hope that this article has been helpful and informative for you. If you have any questions or comments, feel free to leave them below. Happy flying!
3cee63e6c2
-
-
\ No newline at end of file
diff --git a/spaces/inplisQlawa/anything-midjourney-v4-1/IDM UltraFinder Professional 17.0.0.13 (x86 X64) Portable.md b/spaces/inplisQlawa/anything-midjourney-v4-1/IDM UltraFinder Professional 17.0.0.13 (x86 X64) Portable.md
deleted file mode 100644
index b0e45053dd98bd1b8266677969574e2aac9291ad..0000000000000000000000000000000000000000
--- a/spaces/inplisQlawa/anything-midjourney-v4-1/IDM UltraFinder Professional 17.0.0.13 (x86 X64) Portable.md
+++ /dev/null
@@ -1,6 +0,0 @@
-
IDM UltraFinder Professional 17.0.0.13 (x86 x64) Portable
-
-IDM UltraFinder Professional 17.0.0.13 (x86 x64) Portable setup free · tafsir al azhar buya hamka pdf download · rtca do 160c free download rar 1fdad05405
-
-
-
diff --git a/spaces/instantnoodle/Fruits-classifier/app.py b/spaces/instantnoodle/Fruits-classifier/app.py
deleted file mode 100644
index ae3e7d3998fa6fd13834109d8f5f35f5adf7c360..0000000000000000000000000000000000000000
--- a/spaces/instantnoodle/Fruits-classifier/app.py
+++ /dev/null
@@ -1,18 +0,0 @@
-from fastai.vision.all import *
-import gradio as gr
-
-learn = load_learner('fruits.pkl')
-labels = learn.dls.vocab
-
-def predict(img):
- img = PILImage.create(img)
- pred, pred_idx, probs = learn.predict(img)
- return {labels[i]: float(probs[i]) for i in range(len(labels))}
-
-title = 'Fruits Classifier'
-description = 'This model can classify the image into four categories of fruits: Apple, Banana, Mango, and Blueberries'
-image = gr.inputs.Image(shape=(512,512))
-label = gr.outputs.Label()
-examples = ['Mango.jpg', 'Apple.jpg', 'Banana.webp', 'Blueberries.jpg']
-
-gr.Interface(fn=predict, inputs=image, outputs=label, examples=examples, title=title, description=description).launch()
diff --git a/spaces/jackli888/stable-diffusion-webui/extensions/deforum/scripts/deforum_helpers/composable_masks.py b/spaces/jackli888/stable-diffusion-webui/extensions/deforum/scripts/deforum_helpers/composable_masks.py
deleted file mode 100644
index adf718145740e1f90eec10593955112a71311199..0000000000000000000000000000000000000000
--- a/spaces/jackli888/stable-diffusion-webui/extensions/deforum/scripts/deforum_helpers/composable_masks.py
+++ /dev/null
@@ -1,198 +0,0 @@
-# At the moment there are three types of masks: mask from variable, file mask and word mask
-# Variable masks include init_mask for the predefined whole-video mask, frame_mask from video-masking system
-# and human_mask for a model which better segments people in the background video
-# They are put in {}-brackets
-# Word masks are framed with <>-bracets, like: ,
-# File masks are put in []-brackes
-# Empty strings are counted as the whole frame
-# We want to put them all into a sequence of boolean operations
-
-# Example:
-# \
-# (({human_mask} & [mask1.png]) ^ )
-
-# Writing the parser for the boolean sequence
-# using regex and PIL operations
-import re
-from .load_images import get_mask_from_file, check_mask_for_errors, blank_if_none
-from .word_masking import get_word_mask
-from torch import Tensor
-import PIL
-from PIL import Image, ImageChops
-
-# val_masks: name, PIL Image mask
-# Returns an image in mode '1' (needed for bool ops), convert to 'L' in the sender function
-def compose_mask(root, args, mask_seq, val_masks, frame_image, inner_idx:int = 0):
- # Compose_mask recursively: go to inner brackets, then b-op it and go upstack
-
- # Step 1:
- # recursive parenthesis pass
- # regex is not powerful here
-
- seq = ""
- inner_seq = ""
- parentheses_counter = 0
-
- for c in mask_seq:
- if c == ')':
- parentheses_counter = parentheses_counter - 1
- if parentheses_counter > 0:
- inner_seq += c
- if c == '(':
- parentheses_counter = parentheses_counter + 1
- if parentheses_counter == 0:
- if len(inner_seq) > 0:
- inner_idx += 1
- seq += compose_mask(root, args, inner_seq, val_masks, frame_image, inner_idx)
- inner_seq = ""
- else:
- seq += c
-
- if parentheses_counter != 0:
- raise Exception('Mismatched parentheses in {mask_seq}!')
-
- mask_seq = seq
-
- # Step 2:
- # Load the word masks and file masks as vars
-
- # File masks
- pattern = r'\[(?P[\S\s]*?)\]'
-
- def parse(match_object):
- nonlocal inner_idx
- inner_idx += 1
- content = match_object.groupdict()['inner']
- val_masks[str(inner_idx)] = get_mask_from_file(content, args).convert('1') # TODO: add caching
- return f"{{{inner_idx}}}"
-
- mask_seq = re.sub(pattern, parse, mask_seq)
-
- # Word masks
- pattern = r'<(?P[\S\s]*?)>'
-
- def parse(match_object):
- nonlocal inner_idx
- inner_idx += 1
- content = match_object.groupdict()['inner']
- val_masks[str(inner_idx)] = get_word_mask(root, frame_image, content).convert('1')
- return f"{{{inner_idx}}}"
-
- mask_seq = re.sub(pattern, parse, mask_seq)
-
- # Now that all inner parenthesis are eliminated we're left with a linear string
-
- # Step 3:
- # Boolean operations with masks
- # Operators: invert !, and &, or |, xor ^, difference \
-
- # Invert vars with '!'
- pattern = r'![\S\s]*{(?P[\S\s]*?)}'
- def parse(match_object):
- nonlocal inner_idx
- inner_idx += 1
- content = match_object.groupdict()['inner']
- savename = content
- if content in root.mask_preset_names:
- inner_idx += 1
- savename = str(inner_idx)
- val_masks[savename] = ImageChops.invert(val_masks[content])
- return f"{{{savename}}}"
-
- mask_seq = re.sub(pattern, parse, mask_seq)
-
- # Multiply neighbouring vars with '&'
- # Wait for replacements stall (like in Markov chains)
- while True:
- pattern = r'{(?P[\S\s]*?)}[\s]*&[\s]*{(?P[\S\s]*?)}'
- def parse(match_object):
- nonlocal inner_idx
- inner_idx += 1
- content = match_object.groupdict()['inner1']
- content_second = match_object.groupdict()['inner2']
- savename = content
- if content in root.mask_preset_names:
- inner_idx += 1
- savename = str(inner_idx)
- val_masks[savename] = ImageChops.logical_and(val_masks[content], val_masks[content_second])
- return f"{{{savename}}}"
-
- prev_mask_seq = mask_seq
- mask_seq = re.sub(pattern, parse, mask_seq)
- if mask_seq is prev_mask_seq:
- break
-
- # Add neighbouring vars with '|'
- while True:
- pattern = r'{(?P[\S\s]*?)}[\s]*?\|[\s]*?{(?P[\S\s]*?)}'
- def parse(match_object):
- nonlocal inner_idx
- inner_idx += 1
- content = match_object.groupdict()['inner1']
- content_second = match_object.groupdict()['inner2']
- savename = content
- if content in root.mask_preset_names:
- inner_idx += 1
- savename = str(inner_idx)
- val_masks[savename] = ImageChops.logical_or(val_masks[content], val_masks[content_second])
- return f"{{{savename}}}"
-
- prev_mask_seq = mask_seq
- mask_seq = re.sub(pattern, parse, mask_seq)
- if mask_seq is prev_mask_seq:
- break
-
- # Mutually exclude neighbouring vars with '^'
- while True:
- pattern = r'{(?P[\S\s]*?)}[\s]*\^[\s]*{(?P[\S\s]*?)}'
- def parse(match_object):
- nonlocal inner_idx
- inner_idx += 1
- content = match_object.groupdict()['inner1']
- content_second = match_object.groupdict()['inner2']
- savename = content
- if content in root.mask_preset_names:
- inner_idx += 1
- savename = str(inner_idx)
- val_masks[savename] = ImageChops.logical_xor(val_masks[content], val_masks[content_second])
- return f"{{{savename}}}"
-
- prev_mask_seq = mask_seq
- mask_seq = re.sub(pattern, parse, mask_seq)
- if mask_seq is prev_mask_seq:
- break
-
- # Set-difference the regions with '\'
- while True:
- pattern = r'{(?P[\S\s]*?)}[\s]*\\[\s]*{(?P[\S\s]*?)}'
- def parse(match_object):
- content = match_object.groupdict()['inner1']
- content_second = match_object.groupdict()['inner2']
- savename = content
- if content in root.mask_preset_names:
- nonlocal inner_idx
- inner_idx += 1
- savename = str(inner_idx)
- val_masks[savename] = ImageChops.logical_and(val_masks[content], ImageChops.invert(val_masks[content_second]))
- return f"{{{savename}}}"
-
- prev_mask_seq = mask_seq
- mask_seq = re.sub(pattern, parse, mask_seq)
- if mask_seq is prev_mask_seq:
- break
-
- # Step 4:
- # Output
- # Now we should have a single var left to return. If not, raise an error message
- pattern = r'{(?P[\S\s]*?)}'
- matches = re.findall(pattern, mask_seq)
-
- if len(matches) != 1:
- raise Exception(f'Wrong composable mask expression format! Broken mask sequence: {mask_seq}')
-
- return f"{{{matches[0]}}}"
-
-def compose_mask_with_check(root, args, mask_seq, val_masks, frame_image):
- for k, v in val_masks.items():
- val_masks[k] = blank_if_none(v, args.W, args.H, '1').convert('1')
- return check_mask_for_errors(val_masks[compose_mask(root, args, mask_seq, val_masks, frame_image, 0)[1:-1]].convert('L'))
diff --git a/spaces/james21/SD-XL/style.css b/spaces/james21/SD-XL/style.css
deleted file mode 100644
index 86ce68e49778375ebf5b12dc3baaccf931570b54..0000000000000000000000000000000000000000
--- a/spaces/james21/SD-XL/style.css
+++ /dev/null
@@ -1,16 +0,0 @@
-h1 {
- text-align: center;
-}
-
-#duplicate-button {
- margin: auto;
- color: #fff;
- background: #1565c0;
- border-radius: 100vh;
-}
-
-#component-0 {
- max-width: 730px;
- margin: auto;
- padding-top: 1.5rem;
-}
diff --git a/spaces/jbetker/tortoise/models/transformer.py b/spaces/jbetker/tortoise/models/transformer.py
deleted file mode 100644
index aa59b462a3f9c2680f28ceb1b87480258f0293f0..0000000000000000000000000000000000000000
--- a/spaces/jbetker/tortoise/models/transformer.py
+++ /dev/null
@@ -1,219 +0,0 @@
-from functools import partial
-
-import torch
-import torch.nn.functional as F
-from einops import rearrange
-from rotary_embedding_torch import RotaryEmbedding, broadcat
-from torch import nn
-
-
-# helpers
-
-
-def exists(val):
- return val is not None
-
-
-def default(val, d):
- return val if exists(val) else d
-
-
-def cast_tuple(val, depth = 1):
- if isinstance(val, list):
- val = tuple(val)
- return val if isinstance(val, tuple) else (val,) * depth
-
-
-def max_neg_value(t):
- return -torch.finfo(t.dtype).max
-
-
-def stable_softmax(t, dim = -1, alpha = 32 ** 2):
- t = t / alpha
- t = t - torch.amax(t, dim = dim, keepdim = True).detach()
- return (t * alpha).softmax(dim = dim)
-
-
-def route_args(router, args, depth):
- routed_args = [(dict(), dict()) for _ in range(depth)]
- matched_keys = [key for key in args.keys() if key in router]
-
- for key in matched_keys:
- val = args[key]
- for depth, ((f_args, g_args), routes) in enumerate(zip(routed_args, router[key])):
- new_f_args, new_g_args = map(lambda route: ({key: val} if route else {}), routes)
- routed_args[depth] = ({**f_args, **new_f_args}, {**g_args, **new_g_args})
- return routed_args
-
-
-# classes
-class SequentialSequence(nn.Module):
- def __init__(self, layers, args_route = {}, layer_dropout = 0.):
- super().__init__()
- assert all(len(route) == len(layers) for route in args_route.values()), 'each argument route map must have the same depth as the number of sequential layers'
- self.layers = layers
- self.args_route = args_route
- self.layer_dropout = layer_dropout
-
- def forward(self, x, **kwargs):
- args = route_args(self.args_route, kwargs, len(self.layers))
- layers_and_args = list(zip(self.layers, args))
-
- for (f, g), (f_args, g_args) in layers_and_args:
- x = x + f(x, **f_args)
- x = x + g(x, **g_args)
- return x
-
-
-class DivideMax(nn.Module):
- def __init__(self, dim):
- super().__init__()
- self.dim = dim
-
- def forward(self, x):
- maxes = x.amax(dim = self.dim, keepdim = True).detach()
- return x / maxes
-
-
-# https://arxiv.org/abs/2103.17239
-class LayerScale(nn.Module):
- def __init__(self, dim, depth, fn):
- super().__init__()
- if depth <= 18:
- init_eps = 0.1
- elif depth > 18 and depth <= 24:
- init_eps = 1e-5
- else:
- init_eps = 1e-6
-
- scale = torch.zeros(1, 1, dim).fill_(init_eps)
- self.scale = nn.Parameter(scale)
- self.fn = fn
- def forward(self, x, **kwargs):
- return self.fn(x, **kwargs) * self.scale
-
-# layer norm
-
-
-class PreNorm(nn.Module):
- def __init__(self, dim, fn, sandwich = False):
- super().__init__()
- self.norm = nn.LayerNorm(dim)
- self.norm_out = nn.LayerNorm(dim) if sandwich else nn.Identity()
- self.fn = fn
-
- def forward(self, x, **kwargs):
- x = self.norm(x)
- x = self.fn(x, **kwargs)
- return self.norm_out(x)
-
-# feed forward
-
-
-class GEGLU(nn.Module):
- def forward(self, x):
- x, gates = x.chunk(2, dim = -1)
- return x * F.gelu(gates)
-
-
-class FeedForward(nn.Module):
- def __init__(self, dim, dropout = 0., mult = 4.):
- super().__init__()
- self.net = nn.Sequential(
- nn.Linear(dim, dim * mult * 2),
- GEGLU(),
- nn.Dropout(dropout),
- nn.Linear(dim * mult, dim)
- )
-
- def forward(self, x):
- return self.net(x)
-
-# Attention
-
-
-class Attention(nn.Module):
- def __init__(self, dim, seq_len, causal = True, heads = 8, dim_head = 64, dropout = 0.):
- super().__init__()
- inner_dim = dim_head * heads
- self.heads = heads
- self.seq_len = seq_len
- self.scale = dim_head ** -0.5
-
- self.causal = causal
-
- self.to_qkv = nn.Linear(dim, inner_dim * 3, bias = False)
- self.to_out = nn.Sequential(
- nn.Linear(inner_dim, dim),
- nn.Dropout(dropout)
- )
-
- def forward(self, x, mask = None):
- b, n, _, h, device = *x.shape, self.heads, x.device
- softmax = torch.softmax
-
- qkv = self.to_qkv(x).chunk(3, dim = -1)
- q, k, v = map(lambda t: rearrange(t, 'b n (h d) -> b h n d', h = h), qkv)
-
- q = q * self.scale
-
- dots = torch.einsum('b h i d, b h j d -> b h i j', q, k)
- mask_value = max_neg_value(dots)
-
- if exists(mask):
- mask = rearrange(mask, 'b j -> b () () j')
- dots.masked_fill_(~mask, mask_value)
- del mask
-
- if self.causal:
- i, j = dots.shape[-2:]
- mask = torch.ones(i, j, device = device).triu_(j - i + 1).bool()
- dots.masked_fill_(mask, mask_value)
-
- attn = softmax(dots, dim=-1)
-
- out = torch.einsum('b h i j, b h j d -> b h i d', attn, v)
- out = rearrange(out, 'b h n d -> b n (h d)')
- out = self.to_out(out)
- return out
-
-
-# main transformer class
-class Transformer(nn.Module):
- def __init__(
- self,
- *,
- dim,
- depth,
- seq_len,
- causal = True,
- heads = 8,
- dim_head = 64,
- ff_mult = 4,
- attn_dropout = 0.,
- ff_dropout = 0.,
- sparse_attn = False,
- sandwich_norm = False,
- ):
- super().__init__()
- layers = nn.ModuleList([])
- sparse_layer = cast_tuple(sparse_attn, depth)
-
- for ind, sparse_attn in zip(range(depth), sparse_layer):
- attn = Attention(dim, causal = causal, seq_len = seq_len, heads = heads, dim_head = dim_head, dropout = attn_dropout)
-
- ff = FeedForward(dim, mult = ff_mult, dropout = ff_dropout)
-
- layers.append(nn.ModuleList([
- LayerScale(dim, ind + 1, PreNorm(dim, attn, sandwich = sandwich_norm)),
- LayerScale(dim, ind + 1, PreNorm(dim, ff, sandwich = sandwich_norm))
- ]))
-
- execute_type = SequentialSequence
- route_attn = ((True, False),) * depth
- attn_route_map = {'mask': route_attn}
-
- self.layers = execute_type(layers, args_route = attn_route_map)
-
- def forward(self, x, **kwargs):
- return self.layers(x, **kwargs)
\ No newline at end of file
diff --git a/spaces/jbilcke-hf/ai-clip-factory/src/components/ui/vertical-slider.tsx b/spaces/jbilcke-hf/ai-clip-factory/src/components/ui/vertical-slider.tsx
deleted file mode 100644
index b28a1200cb06d1f26e3c640c85e655c99e88954e..0000000000000000000000000000000000000000
--- a/spaces/jbilcke-hf/ai-clip-factory/src/components/ui/vertical-slider.tsx
+++ /dev/null
@@ -1,27 +0,0 @@
-"use client"
-
-import * as React from "react"
-import * as SliderPrimitive from "@radix-ui/react-slider"
-
-import { cn } from "@/lib/utils"
-
-const VerticalSlider = React.forwardRef<
- React.ElementRef,
- React.ComponentPropsWithoutRef
->(({ className, ...props }, ref) => (
-
-
-
-
-
-
-))
-VerticalSlider.displayName = "VerticalSlider"
-export { VerticalSlider }
diff --git a/spaces/joaopereirajp/livvieChatBot/venv/lib/python3.9/site-packages/Crypto/Cipher/_mode_eax.py b/spaces/joaopereirajp/livvieChatBot/venv/lib/python3.9/site-packages/Crypto/Cipher/_mode_eax.py
deleted file mode 100644
index 62cf4d8b310221d65e330357612dd16ec8ba6a6d..0000000000000000000000000000000000000000
--- a/spaces/joaopereirajp/livvieChatBot/venv/lib/python3.9/site-packages/Crypto/Cipher/_mode_eax.py
+++ /dev/null
@@ -1,408 +0,0 @@
-# ===================================================================
-#
-# Copyright (c) 2014, Legrandin
-# All rights reserved.
-#
-# Redistribution and use in source and binary forms, with or without
-# modification, are permitted provided that the following conditions
-# are met:
-#
-# 1. Redistributions of source code must retain the above copyright
-# notice, this list of conditions and the following disclaimer.
-# 2. Redistributions in binary form must reproduce the above copyright
-# notice, this list of conditions and the following disclaimer in
-# the documentation and/or other materials provided with the
-# distribution.
-#
-# THIS SOFTWARE IS PROVIDED BY THE COPYRIGHT HOLDERS AND CONTRIBUTORS
-# "AS IS" AND ANY EXPRESS OR IMPLIED WARRANTIES, INCLUDING, BUT NOT
-# LIMITED TO, THE IMPLIED WARRANTIES OF MERCHANTABILITY AND FITNESS
-# FOR A PARTICULAR PURPOSE ARE DISCLAIMED. IN NO EVENT SHALL THE
-# COPYRIGHT HOLDER OR CONTRIBUTORS BE LIABLE FOR ANY DIRECT, INDIRECT,
-# INCIDENTAL, SPECIAL, EXEMPLARY, OR CONSEQUENTIAL DAMAGES (INCLUDING,
-# BUT NOT LIMITED TO, PROCUREMENT OF SUBSTITUTE GOODS OR SERVICES;
-# LOSS OF USE, DATA, OR PROFITS; OR BUSINESS INTERRUPTION) HOWEVER
-# CAUSED AND ON ANY THEORY OF LIABILITY, WHETHER IN CONTRACT, STRICT
-# LIABILITY, OR TORT (INCLUDING NEGLIGENCE OR OTHERWISE) ARISING IN
-# ANY WAY OUT OF THE USE OF THIS SOFTWARE, EVEN IF ADVISED OF THE
-# POSSIBILITY OF SUCH DAMAGE.
-# ===================================================================
-
-"""
-EAX mode.
-"""
-
-__all__ = ['EaxMode']
-
-import struct
-from binascii import unhexlify
-
-from Crypto.Util.py3compat import byte_string, bord, _copy_bytes
-
-from Crypto.Util._raw_api import is_buffer
-
-from Crypto.Util.strxor import strxor
-from Crypto.Util.number import long_to_bytes, bytes_to_long
-
-from Crypto.Hash import CMAC, BLAKE2s
-from Crypto.Random import get_random_bytes
-
-
-class EaxMode(object):
- """*EAX* mode.
-
- This is an Authenticated Encryption with Associated Data
- (`AEAD`_) mode. It provides both confidentiality and authenticity.
-
- The header of the message may be left in the clear, if needed,
- and it will still be subject to authentication.
-
- The decryption step tells the receiver if the message comes
- from a source that really knowns the secret key.
- Additionally, decryption detects if any part of the message -
- including the header - has been modified or corrupted.
-
- This mode requires a *nonce*.
-
- This mode is only available for ciphers that operate on 64 or
- 128 bits blocks.
-
- There are no official standards defining EAX.
- The implementation is based on `a proposal`__ that
- was presented to NIST.
-
- .. _AEAD: http://blog.cryptographyengineering.com/2012/05/how-to-choose-authenticated-encryption.html
- .. __: http://csrc.nist.gov/groups/ST/toolkit/BCM/documents/proposedmodes/eax/eax-spec.pdf
-
- :undocumented: __init__
- """
-
- def __init__(self, factory, key, nonce, mac_len, cipher_params):
- """EAX cipher mode"""
-
- self.block_size = factory.block_size
- """The block size of the underlying cipher, in bytes."""
-
- self.nonce = _copy_bytes(None, None, nonce)
- """The nonce originally used to create the object."""
-
- self._mac_len = mac_len
- self._mac_tag = None # Cache for MAC tag
-
- # Allowed transitions after initialization
- self._next = ["update", "encrypt", "decrypt",
- "digest", "verify"]
-
- # MAC tag length
- if not (2 <= self._mac_len <= self.block_size):
- raise ValueError("'mac_len' must be at least 2 and not larger than %d"
- % self.block_size)
-
- # Nonce cannot be empty and must be a byte string
- if len(self.nonce) == 0:
- raise ValueError("Nonce cannot be empty in EAX mode")
- if not is_buffer(nonce):
- raise TypeError("nonce must be bytes, bytearray or memoryview")
-
- self._omac = [
- CMAC.new(key,
- b'\x00' * (self.block_size - 1) + struct.pack('B', i),
- ciphermod=factory,
- cipher_params=cipher_params)
- for i in range(0, 3)
- ]
-
- # Compute MAC of nonce
- self._omac[0].update(self.nonce)
- self._signer = self._omac[1]
-
- # MAC of the nonce is also the initial counter for CTR encryption
- counter_int = bytes_to_long(self._omac[0].digest())
- self._cipher = factory.new(key,
- factory.MODE_CTR,
- initial_value=counter_int,
- nonce=b"",
- **cipher_params)
-
- def update(self, assoc_data):
- """Protect associated data
-
- If there is any associated data, the caller has to invoke
- this function one or more times, before using
- ``decrypt`` or ``encrypt``.
-
- By *associated data* it is meant any data (e.g. packet headers) that
- will not be encrypted and will be transmitted in the clear.
- However, the receiver is still able to detect any modification to it.
-
- If there is no associated data, this method must not be called.
-
- The caller may split associated data in segments of any size, and
- invoke this method multiple times, each time with the next segment.
-
- :Parameters:
- assoc_data : bytes/bytearray/memoryview
- A piece of associated data. There are no restrictions on its size.
- """
-
- if "update" not in self._next:
- raise TypeError("update() can only be called"
- " immediately after initialization")
-
- self._next = ["update", "encrypt", "decrypt",
- "digest", "verify"]
-
- self._signer.update(assoc_data)
- return self
-
- def encrypt(self, plaintext, output=None):
- """Encrypt data with the key and the parameters set at initialization.
-
- A cipher object is stateful: once you have encrypted a message
- you cannot encrypt (or decrypt) another message using the same
- object.
-
- The data to encrypt can be broken up in two or
- more pieces and `encrypt` can be called multiple times.
-
- That is, the statement:
-
- >>> c.encrypt(a) + c.encrypt(b)
-
- is equivalent to:
-
- >>> c.encrypt(a+b)
-
- This function does not add any padding to the plaintext.
-
- :Parameters:
- plaintext : bytes/bytearray/memoryview
- The piece of data to encrypt.
- It can be of any length.
- :Keywords:
- output : bytearray/memoryview
- The location where the ciphertext must be written to.
- If ``None``, the ciphertext is returned.
- :Return:
- If ``output`` is ``None``, the ciphertext as ``bytes``.
- Otherwise, ``None``.
- """
-
- if "encrypt" not in self._next:
- raise TypeError("encrypt() can only be called after"
- " initialization or an update()")
- self._next = ["encrypt", "digest"]
- ct = self._cipher.encrypt(plaintext, output=output)
- if output is None:
- self._omac[2].update(ct)
- else:
- self._omac[2].update(output)
- return ct
-
- def decrypt(self, ciphertext, output=None):
- """Decrypt data with the key and the parameters set at initialization.
-
- A cipher object is stateful: once you have decrypted a message
- you cannot decrypt (or encrypt) another message with the same
- object.
-
- The data to decrypt can be broken up in two or
- more pieces and `decrypt` can be called multiple times.
-
- That is, the statement:
-
- >>> c.decrypt(a) + c.decrypt(b)
-
- is equivalent to:
-
- >>> c.decrypt(a+b)
-
- This function does not remove any padding from the plaintext.
-
- :Parameters:
- ciphertext : bytes/bytearray/memoryview
- The piece of data to decrypt.
- It can be of any length.
- :Keywords:
- output : bytearray/memoryview
- The location where the plaintext must be written to.
- If ``None``, the plaintext is returned.
- :Return:
- If ``output`` is ``None``, the plaintext as ``bytes``.
- Otherwise, ``None``.
- """
-
- if "decrypt" not in self._next:
- raise TypeError("decrypt() can only be called"
- " after initialization or an update()")
- self._next = ["decrypt", "verify"]
- self._omac[2].update(ciphertext)
- return self._cipher.decrypt(ciphertext, output=output)
-
- def digest(self):
- """Compute the *binary* MAC tag.
-
- The caller invokes this function at the very end.
-
- This method returns the MAC that shall be sent to the receiver,
- together with the ciphertext.
-
- :Return: the MAC, as a byte string.
- """
-
- if "digest" not in self._next:
- raise TypeError("digest() cannot be called when decrypting"
- " or validating a message")
- self._next = ["digest"]
-
- if not self._mac_tag:
- tag = b'\x00' * self.block_size
- for i in range(3):
- tag = strxor(tag, self._omac[i].digest())
- self._mac_tag = tag[:self._mac_len]
-
- return self._mac_tag
-
- def hexdigest(self):
- """Compute the *printable* MAC tag.
-
- This method is like `digest`.
-
- :Return: the MAC, as a hexadecimal string.
- """
- return "".join(["%02x" % bord(x) for x in self.digest()])
-
- def verify(self, received_mac_tag):
- """Validate the *binary* MAC tag.
-
- The caller invokes this function at the very end.
-
- This method checks if the decrypted message is indeed valid
- (that is, if the key is correct) and it has not been
- tampered with while in transit.
-
- :Parameters:
- received_mac_tag : bytes/bytearray/memoryview
- This is the *binary* MAC, as received from the sender.
- :Raises MacMismatchError:
- if the MAC does not match. The message has been tampered with
- or the key is incorrect.
- """
-
- if "verify" not in self._next:
- raise TypeError("verify() cannot be called"
- " when encrypting a message")
- self._next = ["verify"]
-
- if not self._mac_tag:
- tag = b'\x00' * self.block_size
- for i in range(3):
- tag = strxor(tag, self._omac[i].digest())
- self._mac_tag = tag[:self._mac_len]
-
- secret = get_random_bytes(16)
-
- mac1 = BLAKE2s.new(digest_bits=160, key=secret, data=self._mac_tag)
- mac2 = BLAKE2s.new(digest_bits=160, key=secret, data=received_mac_tag)
-
- if mac1.digest() != mac2.digest():
- raise ValueError("MAC check failed")
-
- def hexverify(self, hex_mac_tag):
- """Validate the *printable* MAC tag.
-
- This method is like `verify`.
-
- :Parameters:
- hex_mac_tag : string
- This is the *printable* MAC, as received from the sender.
- :Raises MacMismatchError:
- if the MAC does not match. The message has been tampered with
- or the key is incorrect.
- """
-
- self.verify(unhexlify(hex_mac_tag))
-
- def encrypt_and_digest(self, plaintext, output=None):
- """Perform encrypt() and digest() in one step.
-
- :Parameters:
- plaintext : bytes/bytearray/memoryview
- The piece of data to encrypt.
- :Keywords:
- output : bytearray/memoryview
- The location where the ciphertext must be written to.
- If ``None``, the ciphertext is returned.
- :Return:
- a tuple with two items:
-
- - the ciphertext, as ``bytes``
- - the MAC tag, as ``bytes``
-
- The first item becomes ``None`` when the ``output`` parameter
- specified a location for the result.
- """
-
- return self.encrypt(plaintext, output=output), self.digest()
-
- def decrypt_and_verify(self, ciphertext, received_mac_tag, output=None):
- """Perform decrypt() and verify() in one step.
-
- :Parameters:
- ciphertext : bytes/bytearray/memoryview
- The piece of data to decrypt.
- received_mac_tag : bytes/bytearray/memoryview
- This is the *binary* MAC, as received from the sender.
- :Keywords:
- output : bytearray/memoryview
- The location where the plaintext must be written to.
- If ``None``, the plaintext is returned.
- :Return: the plaintext as ``bytes`` or ``None`` when the ``output``
- parameter specified a location for the result.
- :Raises MacMismatchError:
- if the MAC does not match. The message has been tampered with
- or the key is incorrect.
- """
-
- pt = self.decrypt(ciphertext, output=output)
- self.verify(received_mac_tag)
- return pt
-
-
-def _create_eax_cipher(factory, **kwargs):
- """Create a new block cipher, configured in EAX mode.
-
- :Parameters:
- factory : module
- A symmetric cipher module from `Crypto.Cipher` (like
- `Crypto.Cipher.AES`).
-
- :Keywords:
- key : bytes/bytearray/memoryview
- The secret key to use in the symmetric cipher.
-
- nonce : bytes/bytearray/memoryview
- A value that must never be reused for any other encryption.
- There are no restrictions on its length, but it is recommended to use
- at least 16 bytes.
-
- The nonce shall never repeat for two different messages encrypted with
- the same key, but it does not need to be random.
-
- If not specified, a 16 byte long random string is used.
-
- mac_len : integer
- Length of the MAC, in bytes. It must be no larger than the cipher
- block bytes (which is the default).
- """
-
- try:
- key = kwargs.pop("key")
- nonce = kwargs.pop("nonce", None)
- if nonce is None:
- nonce = get_random_bytes(16)
- mac_len = kwargs.pop("mac_len", factory.block_size)
- except KeyError as e:
- raise TypeError("Missing parameter: " + str(e))
-
- return EaxMode(factory, key, nonce, mac_len, kwargs)
diff --git a/spaces/joaopereirajp/livvieChatBot/venv/lib/python3.9/site-packages/Crypto/Util/asn1.py b/spaces/joaopereirajp/livvieChatBot/venv/lib/python3.9/site-packages/Crypto/Util/asn1.py
deleted file mode 100644
index a646eacb4f56f062bcce101600f8f5e5635355c9..0000000000000000000000000000000000000000
--- a/spaces/joaopereirajp/livvieChatBot/venv/lib/python3.9/site-packages/Crypto/Util/asn1.py
+++ /dev/null
@@ -1,1064 +0,0 @@
-# -*- coding: ascii -*-
-#
-# Util/asn1.py : Minimal support for ASN.1 DER binary encoding.
-#
-# ===================================================================
-# The contents of this file are dedicated to the public domain. To
-# the extent that dedication to the public domain is not available,
-# everyone is granted a worldwide, perpetual, royalty-free,
-# non-exclusive license to exercise all rights associated with the
-# contents of this file for any purpose whatsoever.
-# No rights are reserved.
-#
-# THE SOFTWARE IS PROVIDED "AS IS", WITHOUT WARRANTY OF ANY KIND,
-# EXPRESS OR IMPLIED, INCLUDING BUT NOT LIMITED TO THE WARRANTIES OF
-# MERCHANTABILITY, FITNESS FOR A PARTICULAR PURPOSE AND
-# NONINFRINGEMENT. IN NO EVENT SHALL THE AUTHORS OR COPYRIGHT HOLDERS
-# BE LIABLE FOR ANY CLAIM, DAMAGES OR OTHER LIABILITY, WHETHER IN AN
-# ACTION OF CONTRACT, TORT OR OTHERWISE, ARISING FROM, OUT OF OR IN
-# CONNECTION WITH THE SOFTWARE OR THE USE OR OTHER DEALINGS IN THE
-# SOFTWARE.
-# ===================================================================
-
-import struct
-
-from Crypto.Util.py3compat import byte_string, bchr, bord
-
-from Crypto.Util.number import long_to_bytes, bytes_to_long
-
-__all__ = ['DerObject', 'DerInteger', 'DerBoolean', 'DerOctetString',
- 'DerNull', 'DerSequence', 'DerObjectId', 'DerBitString', 'DerSetOf']
-
-# Useful references:
-# - https://luca.ntop.org/Teaching/Appunti/asn1.html
-# - https://letsencrypt.org/docs/a-warm-welcome-to-asn1-and-der/
-# - https://www.zytrax.com/tech/survival/asn1.html
-# - https://www.oss.com/asn1/resources/books-whitepapers-pubs/larmouth-asn1-book.pdf
-# - https://www.itu.int/ITU-T/studygroups/com17/languages/X.690-0207.pdf
-# - https://misc.daniel-marschall.de/asn.1/oid-converter/online.php
-
-def _is_number(x, only_non_negative=False):
- test = 0
- try:
- test = x + test
- except TypeError:
- return False
- return not only_non_negative or x >= 0
-
-
-class BytesIO_EOF(object):
- """This class differs from BytesIO in that a ValueError exception is
- raised whenever EOF is reached."""
-
- def __init__(self, initial_bytes):
- self._buffer = initial_bytes
- self._index = 0
- self._bookmark = None
-
- def set_bookmark(self):
- self._bookmark = self._index
-
- def data_since_bookmark(self):
- assert self._bookmark is not None
- return self._buffer[self._bookmark:self._index]
-
- def remaining_data(self):
- return len(self._buffer) - self._index
-
- def read(self, length):
- new_index = self._index + length
- if new_index > len(self._buffer):
- raise ValueError("Not enough data for DER decoding: expected %d bytes and found %d" % (new_index, len(self._buffer)))
-
- result = self._buffer[self._index:new_index]
- self._index = new_index
- return result
-
- def read_byte(self):
- return bord(self.read(1)[0])
-
-
-class DerObject(object):
- """Base class for defining a single DER object.
-
- This class should never be directly instantiated.
- """
-
- def __init__(self, asn1Id=None, payload=b'', implicit=None,
- constructed=False, explicit=None):
- """Initialize the DER object according to a specific ASN.1 type.
-
- :Parameters:
- asn1Id : integer or byte
- The universal DER tag number for this object
- (e.g. 0x10 for a SEQUENCE).
- If None, the tag is not known yet.
-
- payload : byte string
- The initial payload of the object (that it,
- the content octets).
- If not specified, the payload is empty.
-
- implicit : integer or byte
- The IMPLICIT tag number (< 0x1F) to use for the encoded object.
- It overrides the universal tag *asn1Id*.
- It cannot be combined with the ``explicit`` parameter.
- By default, there is no IMPLICIT tag.
-
- constructed : bool
- True when the ASN.1 type is *constructed*.
- False when it is *primitive* (default).
-
- explicit : integer or byte
- The EXPLICIT tag number (< 0x1F) to use for the encoded object.
- It cannot be combined with the ``implicit`` parameter.
- By default, there is no EXPLICIT tag.
- """
-
- if asn1Id is None:
- # The tag octet will be read in with ``decode``
- self._tag_octet = None
- return
- asn1Id = self._convertTag(asn1Id)
-
- self.payload = payload
-
- # In a BER/DER identifier octet:
- # * bits 4-0 contain the tag value
- # * bit 5 is set if the type is 'constructed'
- # and unset if 'primitive'
- # * bits 7-6 depend on the encoding class
- #
- # Class | Bit 7, Bit 6
- # ----------------------------------
- # universal | 0 0
- # application | 0 1
- # context-spec | 1 0 (default for IMPLICIT/EXPLICIT)
- # private | 1 1
- #
-
- constructed_bit = 0x20 if constructed else 0x00
-
- if None not in (explicit, implicit):
- raise ValueError("Explicit and implicit tags are"
- " mutually exclusive")
-
- if implicit is not None:
- # IMPLICIT tag overrides asn1Id
- self._tag_octet = 0x80 | constructed_bit | self._convertTag(implicit)
- elif explicit is not None:
- # 'constructed bit' is always asserted for an EXPLICIT tag
- self._tag_octet = 0x80 | 0x20 | self._convertTag(explicit)
- self._inner_tag_octet = constructed_bit | asn1Id
- else:
- # Neither IMPLICIT nor EXPLICIT
- self._tag_octet = constructed_bit | asn1Id
-
- def _convertTag(self, tag):
- """Check if *tag* is a real DER tag (5 bits).
- Convert it from a character to number if necessary.
- """
- if not _is_number(tag):
- if len(tag) == 1:
- tag = bord(tag[0])
- # Ensure that tag is a low tag
- if not (_is_number(tag) and 0 <= tag < 0x1F):
- raise ValueError("Wrong DER tag")
- return tag
-
- @staticmethod
- def _definite_form(length):
- """Build length octets according to BER/DER
- definite form.
- """
- if length > 127:
- encoding = long_to_bytes(length)
- return bchr(len(encoding) + 128) + encoding
- return bchr(length)
-
- def encode(self):
- """Return this DER element, fully encoded as a binary byte string."""
-
- # Concatenate identifier octets, length octets,
- # and contents octets
-
- output_payload = self.payload
-
- # In case of an EXTERNAL tag, first encode the inner
- # element.
- if hasattr(self, "_inner_tag_octet"):
- output_payload = (bchr(self._inner_tag_octet) +
- self._definite_form(len(self.payload)) +
- self.payload)
-
- return (bchr(self._tag_octet) +
- self._definite_form(len(output_payload)) +
- output_payload)
-
- def _decodeLen(self, s):
- """Decode DER length octets from a file."""
-
- length = s.read_byte()
-
- if length > 127:
- encoded_length = s.read(length & 0x7F)
- if bord(encoded_length[0]) == 0:
- raise ValueError("Invalid DER: length has leading zero")
- length = bytes_to_long(encoded_length)
- if length <= 127:
- raise ValueError("Invalid DER: length in long form but smaller than 128")
-
- return length
-
- def decode(self, der_encoded, strict=False):
- """Decode a complete DER element, and re-initializes this
- object with it.
-
- Args:
- der_encoded (byte string): A complete DER element.
-
- Raises:
- ValueError: in case of parsing errors.
- """
-
- if not byte_string(der_encoded):
- raise ValueError("Input is not a byte string")
-
- s = BytesIO_EOF(der_encoded)
- self._decodeFromStream(s, strict)
-
- # There shouldn't be other bytes left
- if s.remaining_data() > 0:
- raise ValueError("Unexpected extra data after the DER structure")
-
- return self
-
- def _decodeFromStream(self, s, strict):
- """Decode a complete DER element from a file."""
-
- idOctet = s.read_byte()
- if self._tag_octet is not None:
- if idOctet != self._tag_octet:
- raise ValueError("Unexpected DER tag")
- else:
- self._tag_octet = idOctet
- length = self._decodeLen(s)
- self.payload = s.read(length)
-
- # In case of an EXTERNAL tag, further decode the inner
- # element.
- if hasattr(self, "_inner_tag_octet"):
- p = BytesIO_EOF(self.payload)
- inner_octet = p.read_byte()
- if inner_octet != self._inner_tag_octet:
- raise ValueError("Unexpected internal DER tag")
- length = self._decodeLen(p)
- self.payload = p.read(length)
-
- # There shouldn't be other bytes left
- if p.remaining_data() > 0:
- raise ValueError("Unexpected extra data after the DER structure")
-
-
-class DerInteger(DerObject):
- """Class to model a DER INTEGER.
-
- An example of encoding is::
-
- >>> from Crypto.Util.asn1 import DerInteger
- >>> from binascii import hexlify, unhexlify
- >>> int_der = DerInteger(9)
- >>> print hexlify(int_der.encode())
-
- which will show ``020109``, the DER encoding of 9.
-
- And for decoding::
-
- >>> s = unhexlify(b'020109')
- >>> try:
- >>> int_der = DerInteger()
- >>> int_der.decode(s)
- >>> print int_der.value
- >>> except ValueError:
- >>> print "Not a valid DER INTEGER"
-
- the output will be ``9``.
-
- :ivar value: The integer value
- :vartype value: integer
- """
-
- def __init__(self, value=0, implicit=None, explicit=None):
- """Initialize the DER object as an INTEGER.
-
- :Parameters:
- value : integer
- The value of the integer.
-
- implicit : integer
- The IMPLICIT tag to use for the encoded object.
- It overrides the universal tag for INTEGER (2).
- """
-
- DerObject.__init__(self, 0x02, b'', implicit,
- False, explicit)
- self.value = value # The integer value
-
- def encode(self):
- """Return the DER INTEGER, fully encoded as a
- binary string."""
-
- number = self.value
- self.payload = b''
- while True:
- self.payload = bchr(int(number & 255)) + self.payload
- if 128 <= number <= 255:
- self.payload = bchr(0x00) + self.payload
- if -128 <= number <= 255:
- break
- number >>= 8
- return DerObject.encode(self)
-
- def decode(self, der_encoded, strict=False):
- """Decode a DER-encoded INTEGER, and re-initializes this
- object with it.
-
- Args:
- der_encoded (byte string): A complete INTEGER DER element.
-
- Raises:
- ValueError: in case of parsing errors.
- """
-
- return DerObject.decode(self, der_encoded, strict=strict)
-
- def _decodeFromStream(self, s, strict):
- """Decode a complete DER INTEGER from a file."""
-
- # Fill up self.payload
- DerObject._decodeFromStream(self, s, strict)
-
- if strict:
- if len(self.payload) == 0:
- raise ValueError("Invalid encoding for DER INTEGER: empty payload")
- if len(self.payload) >= 2 and struct.unpack('>H', self.payload[:2])[0] < 0x80:
- raise ValueError("Invalid encoding for DER INTEGER: leading zero")
-
- # Derive self.value from self.payload
- self.value = 0
- bits = 1
- for i in self.payload:
- self.value *= 256
- self.value += bord(i)
- bits <<= 8
- if self.payload and bord(self.payload[0]) & 0x80:
- self.value -= bits
-
-
-class DerBoolean(DerObject):
- """Class to model a DER-encoded BOOLEAN.
-
- An example of encoding is::
-
- >>> from Crypto.Util.asn1 import DerBoolean
- >>> bool_der = DerBoolean(True)
- >>> print(bool_der.encode().hex())
-
- which will show ``0101ff``, the DER encoding of True.
-
- And for decoding::
-
- >>> s = bytes.fromhex('0101ff')
- >>> try:
- >>> bool_der = DerBoolean()
- >>> bool_der.decode(s)
- >>> print(bool_der.value)
- >>> except ValueError:
- >>> print "Not a valid DER BOOLEAN"
-
- the output will be ``True``.
-
- :ivar value: The boolean value
- :vartype value: boolean
- """
- def __init__(self, value=False, implicit=None, explicit=None):
- """Initialize the DER object as a BOOLEAN.
-
- Args:
- value (boolean):
- The value of the boolean. Default is False.
-
- implicit (integer or byte):
- The IMPLICIT tag number (< 0x1F) to use for the encoded object.
- It overrides the universal tag for BOOLEAN (1).
- It cannot be combined with the ``explicit`` parameter.
- By default, there is no IMPLICIT tag.
-
- explicit (integer or byte):
- The EXPLICIT tag number (< 0x1F) to use for the encoded object.
- It cannot be combined with the ``implicit`` parameter.
- By default, there is no EXPLICIT tag.
- """
-
- DerObject.__init__(self, 0x01, b'', implicit, False, explicit)
- self.value = value # The boolean value
-
- def encode(self):
- """Return the DER BOOLEAN, fully encoded as a binary string."""
-
- self.payload = b'\xFF' if self.value else b'\x00'
- return DerObject.encode(self)
-
- def decode(self, der_encoded, strict=False):
- """Decode a DER-encoded BOOLEAN, and re-initializes this object with it.
-
- Args:
- der_encoded (byte string): A DER-encoded BOOLEAN.
-
- Raises:
- ValueError: in case of parsing errors.
- """
-
- return DerObject.decode(self, der_encoded, strict)
-
- def _decodeFromStream(self, s, strict):
- """Decode a DER-encoded BOOLEAN from a file."""
-
- # Fill up self.payload
- DerObject._decodeFromStream(self, s, strict)
-
- if len(self.payload) != 1:
- raise ValueError("Invalid encoding for DER BOOLEAN: payload is not 1 byte")
-
- if bord(self.payload[0]) == 0:
- self.value = False
- elif bord(self.payload[0]) == 0xFF:
- self.value = True
- else:
- raise ValueError("Invalid payload for DER BOOLEAN")
-
-
-class DerSequence(DerObject):
- """Class to model a DER SEQUENCE.
-
- This object behaves like a dynamic Python sequence.
-
- Sub-elements that are INTEGERs behave like Python integers.
-
- Any other sub-element is a binary string encoded as a complete DER
- sub-element (TLV).
-
- An example of encoding is:
-
- >>> from Crypto.Util.asn1 import DerSequence, DerInteger
- >>> from binascii import hexlify, unhexlify
- >>> obj_der = unhexlify('070102')
- >>> seq_der = DerSequence([4])
- >>> seq_der.append(9)
- >>> seq_der.append(obj_der.encode())
- >>> print hexlify(seq_der.encode())
-
- which will show ``3009020104020109070102``, the DER encoding of the
- sequence containing ``4``, ``9``, and the object with payload ``02``.
-
- For decoding:
-
- >>> s = unhexlify(b'3009020104020109070102')
- >>> try:
- >>> seq_der = DerSequence()
- >>> seq_der.decode(s)
- >>> print len(seq_der)
- >>> print seq_der[0]
- >>> print seq_der[:]
- >>> except ValueError:
- >>> print "Not a valid DER SEQUENCE"
-
- the output will be::
-
- 3
- 4
- [4, 9, b'\x07\x01\x02']
-
- """
-
- def __init__(self, startSeq=None, implicit=None, explicit=None):
- """Initialize the DER object as a SEQUENCE.
-
- :Parameters:
- startSeq : Python sequence
- A sequence whose element are either integers or
- other DER objects.
-
- implicit : integer or byte
- The IMPLICIT tag number (< 0x1F) to use for the encoded object.
- It overrides the universal tag for SEQUENCE (16).
- It cannot be combined with the ``explicit`` parameter.
- By default, there is no IMPLICIT tag.
-
- explicit : integer or byte
- The EXPLICIT tag number (< 0x1F) to use for the encoded object.
- It cannot be combined with the ``implicit`` parameter.
- By default, there is no EXPLICIT tag.
- """
-
- DerObject.__init__(self, 0x10, b'', implicit, True, explicit)
- if startSeq is None:
- self._seq = []
- else:
- self._seq = startSeq
-
- # A few methods to make it behave like a python sequence
-
- def __delitem__(self, n):
- del self._seq[n]
-
- def __getitem__(self, n):
- return self._seq[n]
-
- def __setitem__(self, key, value):
- self._seq[key] = value
-
- def __setslice__(self, i, j, sequence):
- self._seq[i:j] = sequence
-
- def __delslice__(self, i, j):
- del self._seq[i:j]
-
- def __getslice__(self, i, j):
- return self._seq[max(0, i):max(0, j)]
-
- def __len__(self):
- return len(self._seq)
-
- def __iadd__(self, item):
- self._seq.append(item)
- return self
-
- def append(self, item):
- self._seq.append(item)
- return self
-
- def insert(self, index, item):
- self._seq.insert(index, item)
- return self
-
- def hasInts(self, only_non_negative=True):
- """Return the number of items in this sequence that are
- integers.
-
- Args:
- only_non_negative (boolean):
- If ``True``, negative integers are not counted in.
- """
-
- items = [x for x in self._seq if _is_number(x, only_non_negative)]
- return len(items)
-
- def hasOnlyInts(self, only_non_negative=True):
- """Return ``True`` if all items in this sequence are integers
- or non-negative integers.
-
- This function returns False is the sequence is empty,
- or at least one member is not an integer.
-
- Args:
- only_non_negative (boolean):
- If ``True``, the presence of negative integers
- causes the method to return ``False``."""
- return self._seq and self.hasInts(only_non_negative) == len(self._seq)
-
- def encode(self):
- """Return this DER SEQUENCE, fully encoded as a
- binary string.
-
- Raises:
- ValueError: if some elements in the sequence are neither integers
- nor byte strings.
- """
- self.payload = b''
- for item in self._seq:
- if byte_string(item):
- self.payload += item
- elif _is_number(item):
- self.payload += DerInteger(item).encode()
- else:
- self.payload += item.encode()
- return DerObject.encode(self)
-
- def decode(self, der_encoded, strict=False, nr_elements=None, only_ints_expected=False):
- """Decode a complete DER SEQUENCE, and re-initializes this
- object with it.
-
- Args:
- der_encoded (byte string):
- A complete SEQUENCE DER element.
- nr_elements (None or integer or list of integers):
- The number of members the SEQUENCE can have
- only_ints_expected (boolean):
- Whether the SEQUENCE is expected to contain only integers.
- strict (boolean):
- Whether decoding must check for strict DER compliancy.
-
- Raises:
- ValueError: in case of parsing errors.
-
- DER INTEGERs are decoded into Python integers. Any other DER
- element is not decoded. Its validity is not checked.
- """
-
- self._nr_elements = nr_elements
- result = DerObject.decode(self, der_encoded, strict=strict)
-
- if only_ints_expected and not self.hasOnlyInts():
- raise ValueError("Some members are not INTEGERs")
-
- return result
-
- def _decodeFromStream(self, s, strict):
- """Decode a complete DER SEQUENCE from a file."""
-
- self._seq = []
-
- # Fill up self.payload
- DerObject._decodeFromStream(self, s, strict)
-
- # Add one item at a time to self.seq, by scanning self.payload
- p = BytesIO_EOF(self.payload)
- while p.remaining_data() > 0:
- p.set_bookmark()
-
- der = DerObject()
- der._decodeFromStream(p, strict)
-
- # Parse INTEGERs differently
- if der._tag_octet != 0x02:
- self._seq.append(p.data_since_bookmark())
- else:
- derInt = DerInteger()
- data = p.data_since_bookmark()
- derInt.decode(data, strict=strict)
- self._seq.append(derInt.value)
-
- ok = True
- if self._nr_elements is not None:
- try:
- ok = len(self._seq) in self._nr_elements
- except TypeError:
- ok = len(self._seq) == self._nr_elements
-
- if not ok:
- raise ValueError("Unexpected number of members (%d)"
- " in the sequence" % len(self._seq))
-
-
-class DerOctetString(DerObject):
- """Class to model a DER OCTET STRING.
-
- An example of encoding is:
-
- >>> from Crypto.Util.asn1 import DerOctetString
- >>> from binascii import hexlify, unhexlify
- >>> os_der = DerOctetString(b'\\xaa')
- >>> os_der.payload += b'\\xbb'
- >>> print hexlify(os_der.encode())
-
- which will show ``0402aabb``, the DER encoding for the byte string
- ``b'\\xAA\\xBB'``.
-
- For decoding:
-
- >>> s = unhexlify(b'0402aabb')
- >>> try:
- >>> os_der = DerOctetString()
- >>> os_der.decode(s)
- >>> print hexlify(os_der.payload)
- >>> except ValueError:
- >>> print "Not a valid DER OCTET STRING"
-
- the output will be ``aabb``.
-
- :ivar payload: The content of the string
- :vartype payload: byte string
- """
-
- def __init__(self, value=b'', implicit=None):
- """Initialize the DER object as an OCTET STRING.
-
- :Parameters:
- value : byte string
- The initial payload of the object.
- If not specified, the payload is empty.
-
- implicit : integer
- The IMPLICIT tag to use for the encoded object.
- It overrides the universal tag for OCTET STRING (4).
- """
- DerObject.__init__(self, 0x04, value, implicit, False)
-
-
-class DerNull(DerObject):
- """Class to model a DER NULL element."""
-
- def __init__(self):
- """Initialize the DER object as a NULL."""
-
- DerObject.__init__(self, 0x05, b'', None, False)
-
-
-class DerObjectId(DerObject):
- """Class to model a DER OBJECT ID.
-
- An example of encoding is:
-
- >>> from Crypto.Util.asn1 import DerObjectId
- >>> from binascii import hexlify, unhexlify
- >>> oid_der = DerObjectId("1.2")
- >>> oid_der.value += ".840.113549.1.1.1"
- >>> print hexlify(oid_der.encode())
-
- which will show ``06092a864886f70d010101``, the DER encoding for the
- RSA Object Identifier ``1.2.840.113549.1.1.1``.
-
- For decoding:
-
- >>> s = unhexlify(b'06092a864886f70d010101')
- >>> try:
- >>> oid_der = DerObjectId()
- >>> oid_der.decode(s)
- >>> print oid_der.value
- >>> except ValueError:
- >>> print "Not a valid DER OBJECT ID"
-
- the output will be ``1.2.840.113549.1.1.1``.
-
- :ivar value: The Object ID (OID), a dot separated list of integers
- :vartype value: string
- """
-
- def __init__(self, value='', implicit=None, explicit=None):
- """Initialize the DER object as an OBJECT ID.
-
- :Parameters:
- value : string
- The initial Object Identifier (e.g. "1.2.0.0.6.2").
- implicit : integer
- The IMPLICIT tag to use for the encoded object.
- It overrides the universal tag for OBJECT ID (6).
- explicit : integer
- The EXPLICIT tag to use for the encoded object.
- """
- DerObject.__init__(self, 0x06, b'', implicit, False, explicit)
- self.value = value
-
- def encode(self):
- """Return the DER OBJECT ID, fully encoded as a
- binary string."""
-
- comps = [int(x) for x in self.value.split(".")]
-
- if len(comps) < 2:
- raise ValueError("Not a valid Object Identifier string")
- if comps[0] > 2:
- raise ValueError("First component must be 0, 1 or 2")
- if comps[0] < 2 and comps[1] > 39:
- raise ValueError("Second component must be 39 at most")
-
- subcomps = [40 * comps[0] + comps[1]] + comps[2:]
-
- encoding = []
- for v in reversed(subcomps):
- encoding.append(v & 0x7F)
- v >>= 7
- while v:
- encoding.append((v & 0x7F) | 0x80)
- v >>= 7
-
- self.payload = b''.join([bchr(x) for x in reversed(encoding)])
- return DerObject.encode(self)
-
- def decode(self, der_encoded, strict=False):
- """Decode a complete DER OBJECT ID, and re-initializes this
- object with it.
-
- Args:
- der_encoded (byte string):
- A complete DER OBJECT ID.
- strict (boolean):
- Whether decoding must check for strict DER compliancy.
-
- Raises:
- ValueError: in case of parsing errors.
- """
-
- return DerObject.decode(self, der_encoded, strict)
-
- def _decodeFromStream(self, s, strict):
- """Decode a complete DER OBJECT ID from a file."""
-
- # Fill up self.payload
- DerObject._decodeFromStream(self, s, strict)
-
- # Derive self.value from self.payload
- p = BytesIO_EOF(self.payload)
-
- subcomps = []
- v = 0
- while p.remaining_data():
- c = p.read_byte()
- v = (v << 7) + (c & 0x7F)
- if not (c & 0x80):
- subcomps.append(v)
- v = 0
-
- if len(subcomps) == 0:
- raise ValueError("Empty payload")
-
- if subcomps[0] < 40:
- subcomps[:1] = [0, subcomps[0]]
- elif subcomps[0] < 80:
- subcomps[:1] = [1, subcomps[0] - 40]
- else:
- subcomps[:1] = [2, subcomps[0] - 80]
-
- self.value = ".".join([str(x) for x in subcomps])
-
-
-class DerBitString(DerObject):
- """Class to model a DER BIT STRING.
-
- An example of encoding is:
-
- >>> from Crypto.Util.asn1 import DerBitString
- >>> bs_der = DerBitString(b'\\xAA')
- >>> bs_der.value += b'\\xBB'
- >>> print(bs_der.encode().hex())
-
- which will show ``030300aabb``, the DER encoding for the bit string
- ``b'\\xAA\\xBB'``.
-
- For decoding:
-
- >>> s = bytes.fromhex('030300aabb')
- >>> try:
- >>> bs_der = DerBitString()
- >>> bs_der.decode(s)
- >>> print(bs_der.value.hex())
- >>> except ValueError:
- >>> print "Not a valid DER BIT STRING"
-
- the output will be ``aabb``.
-
- :ivar value: The content of the string
- :vartype value: byte string
- """
-
- def __init__(self, value=b'', implicit=None, explicit=None):
- """Initialize the DER object as a BIT STRING.
-
- :Parameters:
- value : byte string or DER object
- The initial, packed bit string.
- If not specified, the bit string is empty.
- implicit : integer
- The IMPLICIT tag to use for the encoded object.
- It overrides the universal tag for BIT STRING (3).
- explicit : integer
- The EXPLICIT tag to use for the encoded object.
- """
- DerObject.__init__(self, 0x03, b'', implicit, False, explicit)
-
- # The bitstring value (packed)
- if isinstance(value, DerObject):
- self.value = value.encode()
- else:
- self.value = value
-
- def encode(self):
- """Return the DER BIT STRING, fully encoded as a
- byte string."""
-
- # Add padding count byte
- self.payload = b'\x00' + self.value
- return DerObject.encode(self)
-
- def decode(self, der_encoded, strict=False):
- """Decode a complete DER BIT STRING, and re-initializes this
- object with it.
-
- Args:
- der_encoded (byte string): a complete DER BIT STRING.
- strict (boolean):
- Whether decoding must check for strict DER compliancy.
-
- Raises:
- ValueError: in case of parsing errors.
- """
-
- return DerObject.decode(self, der_encoded, strict)
-
- def _decodeFromStream(self, s, strict):
- """Decode a complete DER BIT STRING DER from a file."""
-
- # Fill-up self.payload
- DerObject._decodeFromStream(self, s, strict)
-
- if self.payload and bord(self.payload[0]) != 0:
- raise ValueError("Not a valid BIT STRING")
-
- # Fill-up self.value
- self.value = b''
- # Remove padding count byte
- if self.payload:
- self.value = self.payload[1:]
-
-
-class DerSetOf(DerObject):
- """Class to model a DER SET OF.
-
- An example of encoding is:
-
- >>> from Crypto.Util.asn1 import DerBitString
- >>> from binascii import hexlify, unhexlify
- >>> so_der = DerSetOf([4,5])
- >>> so_der.add(6)
- >>> print hexlify(so_der.encode())
-
- which will show ``3109020104020105020106``, the DER encoding
- of a SET OF with items 4,5, and 6.
-
- For decoding:
-
- >>> s = unhexlify(b'3109020104020105020106')
- >>> try:
- >>> so_der = DerSetOf()
- >>> so_der.decode(s)
- >>> print [x for x in so_der]
- >>> except ValueError:
- >>> print "Not a valid DER SET OF"
-
- the output will be ``[4, 5, 6]``.
- """
-
- def __init__(self, startSet=None, implicit=None):
- """Initialize the DER object as a SET OF.
-
- :Parameters:
- startSet : container
- The initial set of integers or DER encoded objects.
- implicit : integer
- The IMPLICIT tag to use for the encoded object.
- It overrides the universal tag for SET OF (17).
- """
- DerObject.__init__(self, 0x11, b'', implicit, True)
- self._seq = []
-
- # All elements must be of the same type (and therefore have the
- # same leading octet)
- self._elemOctet = None
-
- if startSet:
- for e in startSet:
- self.add(e)
-
- def __getitem__(self, n):
- return self._seq[n]
-
- def __iter__(self):
- return iter(self._seq)
-
- def __len__(self):
- return len(self._seq)
-
- def add(self, elem):
- """Add an element to the set.
-
- Args:
- elem (byte string or integer):
- An element of the same type of objects already in the set.
- It can be an integer or a DER encoded object.
- """
-
- if _is_number(elem):
- eo = 0x02
- elif isinstance(elem, DerObject):
- eo = self._tag_octet
- else:
- eo = bord(elem[0])
-
- if self._elemOctet != eo:
- if self._elemOctet is not None:
- raise ValueError("New element does not belong to the set")
- self._elemOctet = eo
-
- if elem not in self._seq:
- self._seq.append(elem)
-
- def decode(self, der_encoded, strict=False):
- """Decode a complete SET OF DER element, and re-initializes this
- object with it.
-
- DER INTEGERs are decoded into Python integers. Any other DER
- element is left undecoded; its validity is not checked.
-
- Args:
- der_encoded (byte string): a complete DER BIT SET OF.
- strict (boolean):
- Whether decoding must check for strict DER compliancy.
-
- Raises:
- ValueError: in case of parsing errors.
- """
-
- return DerObject.decode(self, der_encoded, strict)
-
- def _decodeFromStream(self, s, strict):
- """Decode a complete DER SET OF from a file."""
-
- self._seq = []
-
- # Fill up self.payload
- DerObject._decodeFromStream(self, s, strict)
-
- # Add one item at a time to self.seq, by scanning self.payload
- p = BytesIO_EOF(self.payload)
- setIdOctet = -1
- while p.remaining_data() > 0:
- p.set_bookmark()
-
- der = DerObject()
- der._decodeFromStream(p, strict)
-
- # Verify that all members are of the same type
- if setIdOctet < 0:
- setIdOctet = der._tag_octet
- else:
- if setIdOctet != der._tag_octet:
- raise ValueError("Not all elements are of the same DER type")
-
- # Parse INTEGERs differently
- if setIdOctet != 0x02:
- self._seq.append(p.data_since_bookmark())
- else:
- derInt = DerInteger()
- derInt.decode(p.data_since_bookmark(), strict)
- self._seq.append(derInt.value)
- # end
-
- def encode(self):
- """Return this SET OF DER element, fully encoded as a
- binary string.
- """
-
- # Elements in the set must be ordered in lexicographic order
- ordered = []
- for item in self._seq:
- if _is_number(item):
- bys = DerInteger(item).encode()
- elif isinstance(item, DerObject):
- bys = item.encode()
- else:
- bys = item
- ordered.append(bys)
- ordered.sort()
- self.payload = b''.join(ordered)
- return DerObject.encode(self)
diff --git a/spaces/joaopereirajp/livvieChatBot/venv/lib/python3.9/site-packages/aiohttp/web_runner.py b/spaces/joaopereirajp/livvieChatBot/venv/lib/python3.9/site-packages/aiohttp/web_runner.py
deleted file mode 100644
index 9282bb93d37a4a3a0ada346ec7534de0ea0e893d..0000000000000000000000000000000000000000
--- a/spaces/joaopereirajp/livvieChatBot/venv/lib/python3.9/site-packages/aiohttp/web_runner.py
+++ /dev/null
@@ -1,381 +0,0 @@
-import asyncio
-import signal
-import socket
-from abc import ABC, abstractmethod
-from typing import Any, List, Optional, Set
-
-from yarl import URL
-
-from .web_app import Application
-from .web_server import Server
-
-try:
- from ssl import SSLContext
-except ImportError:
- SSLContext = object # type: ignore[misc,assignment]
-
-
-__all__ = (
- "BaseSite",
- "TCPSite",
- "UnixSite",
- "NamedPipeSite",
- "SockSite",
- "BaseRunner",
- "AppRunner",
- "ServerRunner",
- "GracefulExit",
-)
-
-
-class GracefulExit(SystemExit):
- code = 1
-
-
-def _raise_graceful_exit() -> None:
- raise GracefulExit()
-
-
-class BaseSite(ABC):
- __slots__ = ("_runner", "_shutdown_timeout", "_ssl_context", "_backlog", "_server")
-
- def __init__(
- self,
- runner: "BaseRunner",
- *,
- shutdown_timeout: float = 60.0,
- ssl_context: Optional[SSLContext] = None,
- backlog: int = 128,
- ) -> None:
- if runner.server is None:
- raise RuntimeError("Call runner.setup() before making a site")
- self._runner = runner
- self._shutdown_timeout = shutdown_timeout
- self._ssl_context = ssl_context
- self._backlog = backlog
- self._server: Optional[asyncio.AbstractServer] = None
-
- @property
- @abstractmethod
- def name(self) -> str:
- pass # pragma: no cover
-
- @abstractmethod
- async def start(self) -> None:
- self._runner._reg_site(self)
-
- async def stop(self) -> None:
- self._runner._check_site(self)
- if self._server is None:
- self._runner._unreg_site(self)
- return # not started yet
- self._server.close()
- # named pipes do not have wait_closed property
- if hasattr(self._server, "wait_closed"):
- await self._server.wait_closed()
- await self._runner.shutdown()
- assert self._runner.server
- await self._runner.server.shutdown(self._shutdown_timeout)
- self._runner._unreg_site(self)
-
-
-class TCPSite(BaseSite):
- __slots__ = ("_host", "_port", "_reuse_address", "_reuse_port")
-
- def __init__(
- self,
- runner: "BaseRunner",
- host: Optional[str] = None,
- port: Optional[int] = None,
- *,
- shutdown_timeout: float = 60.0,
- ssl_context: Optional[SSLContext] = None,
- backlog: int = 128,
- reuse_address: Optional[bool] = None,
- reuse_port: Optional[bool] = None,
- ) -> None:
- super().__init__(
- runner,
- shutdown_timeout=shutdown_timeout,
- ssl_context=ssl_context,
- backlog=backlog,
- )
- self._host = host
- if port is None:
- port = 8443 if self._ssl_context else 8080
- self._port = port
- self._reuse_address = reuse_address
- self._reuse_port = reuse_port
-
- @property
- def name(self) -> str:
- scheme = "https" if self._ssl_context else "http"
- host = "0.0.0.0" if self._host is None else self._host
- return str(URL.build(scheme=scheme, host=host, port=self._port))
-
- async def start(self) -> None:
- await super().start()
- loop = asyncio.get_event_loop()
- server = self._runner.server
- assert server is not None
- self._server = await loop.create_server(
- server,
- self._host,
- self._port,
- ssl=self._ssl_context,
- backlog=self._backlog,
- reuse_address=self._reuse_address,
- reuse_port=self._reuse_port,
- )
-
-
-class UnixSite(BaseSite):
- __slots__ = ("_path",)
-
- def __init__(
- self,
- runner: "BaseRunner",
- path: str,
- *,
- shutdown_timeout: float = 60.0,
- ssl_context: Optional[SSLContext] = None,
- backlog: int = 128,
- ) -> None:
- super().__init__(
- runner,
- shutdown_timeout=shutdown_timeout,
- ssl_context=ssl_context,
- backlog=backlog,
- )
- self._path = path
-
- @property
- def name(self) -> str:
- scheme = "https" if self._ssl_context else "http"
- return f"{scheme}://unix:{self._path}:"
-
- async def start(self) -> None:
- await super().start()
- loop = asyncio.get_event_loop()
- server = self._runner.server
- assert server is not None
- self._server = await loop.create_unix_server(
- server, self._path, ssl=self._ssl_context, backlog=self._backlog
- )
-
-
-class NamedPipeSite(BaseSite):
- __slots__ = ("_path",)
-
- def __init__(
- self, runner: "BaseRunner", path: str, *, shutdown_timeout: float = 60.0
- ) -> None:
- loop = asyncio.get_event_loop()
- if not isinstance(
- loop, asyncio.ProactorEventLoop # type: ignore[attr-defined]
- ):
- raise RuntimeError(
- "Named Pipes only available in proactor" "loop under windows"
- )
- super().__init__(runner, shutdown_timeout=shutdown_timeout)
- self._path = path
-
- @property
- def name(self) -> str:
- return self._path
-
- async def start(self) -> None:
- await super().start()
- loop = asyncio.get_event_loop()
- server = self._runner.server
- assert server is not None
- _server = await loop.start_serving_pipe( # type: ignore[attr-defined]
- server, self._path
- )
- self._server = _server[0]
-
-
-class SockSite(BaseSite):
- __slots__ = ("_sock", "_name")
-
- def __init__(
- self,
- runner: "BaseRunner",
- sock: socket.socket,
- *,
- shutdown_timeout: float = 60.0,
- ssl_context: Optional[SSLContext] = None,
- backlog: int = 128,
- ) -> None:
- super().__init__(
- runner,
- shutdown_timeout=shutdown_timeout,
- ssl_context=ssl_context,
- backlog=backlog,
- )
- self._sock = sock
- scheme = "https" if self._ssl_context else "http"
- if hasattr(socket, "AF_UNIX") and sock.family == socket.AF_UNIX:
- name = f"{scheme}://unix:{sock.getsockname()}:"
- else:
- host, port = sock.getsockname()[:2]
- name = str(URL.build(scheme=scheme, host=host, port=port))
- self._name = name
-
- @property
- def name(self) -> str:
- return self._name
-
- async def start(self) -> None:
- await super().start()
- loop = asyncio.get_event_loop()
- server = self._runner.server
- assert server is not None
- self._server = await loop.create_server(
- server, sock=self._sock, ssl=self._ssl_context, backlog=self._backlog
- )
-
-
-class BaseRunner(ABC):
- __slots__ = ("_handle_signals", "_kwargs", "_server", "_sites")
-
- def __init__(self, *, handle_signals: bool = False, **kwargs: Any) -> None:
- self._handle_signals = handle_signals
- self._kwargs = kwargs
- self._server: Optional[Server] = None
- self._sites: List[BaseSite] = []
-
- @property
- def server(self) -> Optional[Server]:
- return self._server
-
- @property
- def addresses(self) -> List[Any]:
- ret: List[Any] = []
- for site in self._sites:
- server = site._server
- if server is not None:
- sockets = server.sockets
- if sockets is not None:
- for sock in sockets:
- ret.append(sock.getsockname())
- return ret
-
- @property
- def sites(self) -> Set[BaseSite]:
- return set(self._sites)
-
- async def setup(self) -> None:
- loop = asyncio.get_event_loop()
-
- if self._handle_signals:
- try:
- loop.add_signal_handler(signal.SIGINT, _raise_graceful_exit)
- loop.add_signal_handler(signal.SIGTERM, _raise_graceful_exit)
- except NotImplementedError: # pragma: no cover
- # add_signal_handler is not implemented on Windows
- pass
-
- self._server = await self._make_server()
-
- @abstractmethod
- async def shutdown(self) -> None:
- pass # pragma: no cover
-
- async def cleanup(self) -> None:
- loop = asyncio.get_event_loop()
-
- # The loop over sites is intentional, an exception on gather()
- # leaves self._sites in unpredictable state.
- # The loop guaranties that a site is either deleted on success or
- # still present on failure
- for site in list(self._sites):
- await site.stop()
- await self._cleanup_server()
- self._server = None
- if self._handle_signals:
- try:
- loop.remove_signal_handler(signal.SIGINT)
- loop.remove_signal_handler(signal.SIGTERM)
- except NotImplementedError: # pragma: no cover
- # remove_signal_handler is not implemented on Windows
- pass
-
- @abstractmethod
- async def _make_server(self) -> Server:
- pass # pragma: no cover
-
- @abstractmethod
- async def _cleanup_server(self) -> None:
- pass # pragma: no cover
-
- def _reg_site(self, site: BaseSite) -> None:
- if site in self._sites:
- raise RuntimeError(f"Site {site} is already registered in runner {self}")
- self._sites.append(site)
-
- def _check_site(self, site: BaseSite) -> None:
- if site not in self._sites:
- raise RuntimeError(f"Site {site} is not registered in runner {self}")
-
- def _unreg_site(self, site: BaseSite) -> None:
- if site not in self._sites:
- raise RuntimeError(f"Site {site} is not registered in runner {self}")
- self._sites.remove(site)
-
-
-class ServerRunner(BaseRunner):
- """Low-level web server runner"""
-
- __slots__ = ("_web_server",)
-
- def __init__(
- self, web_server: Server, *, handle_signals: bool = False, **kwargs: Any
- ) -> None:
- super().__init__(handle_signals=handle_signals, **kwargs)
- self._web_server = web_server
-
- async def shutdown(self) -> None:
- pass
-
- async def _make_server(self) -> Server:
- return self._web_server
-
- async def _cleanup_server(self) -> None:
- pass
-
-
-class AppRunner(BaseRunner):
- """Web Application runner"""
-
- __slots__ = ("_app",)
-
- def __init__(
- self, app: Application, *, handle_signals: bool = False, **kwargs: Any
- ) -> None:
- super().__init__(handle_signals=handle_signals, **kwargs)
- if not isinstance(app, Application):
- raise TypeError(
- "The first argument should be web.Application "
- "instance, got {!r}".format(app)
- )
- self._app = app
-
- @property
- def app(self) -> Application:
- return self._app
-
- async def shutdown(self) -> None:
- await self._app.shutdown()
-
- async def _make_server(self) -> Server:
- loop = asyncio.get_event_loop()
- self._app._set_loop(loop)
- self._app.on_startup.freeze()
- await self._app.startup()
- self._app.freeze()
-
- return self._app._make_handler(loop=loop, **self._kwargs)
-
- async def _cleanup_server(self) -> None:
- await self._app.cleanup()
diff --git a/spaces/joaopereirajp/livvieChatBot/venv/lib/python3.9/site-packages/gpt_index/indices/vector_store/vector_indices.py b/spaces/joaopereirajp/livvieChatBot/venv/lib/python3.9/site-packages/gpt_index/indices/vector_store/vector_indices.py
deleted file mode 100644
index 6541233395018863f59be0ed141a3ea9f8775781..0000000000000000000000000000000000000000
--- a/spaces/joaopereirajp/livvieChatBot/venv/lib/python3.9/site-packages/gpt_index/indices/vector_store/vector_indices.py
+++ /dev/null
@@ -1,591 +0,0 @@
-"""Deprecated vector store indices."""
-
-from typing import Any, Dict, Optional, Sequence, Type, cast
-
-from gpt_index.data_structs.data_structs import (
- ChromaIndexDict,
- FaissIndexDict,
- IndexDict,
- OpensearchIndexDict,
- PineconeIndexDict,
- QdrantIndexDict,
- SimpleIndexDict,
- WeaviateIndexDict,
-)
-from gpt_index.embeddings.base import BaseEmbedding
-from gpt_index.indices.base import DOCUMENTS_INPUT, BaseGPTIndex
-from gpt_index.indices.query.base import BaseGPTIndexQuery
-from gpt_index.indices.query.schema import QueryMode
-from gpt_index.indices.query.vector_store.queries import (
- GPTChromaIndexQuery,
- GPTFaissIndexQuery,
- GPTOpensearchIndexQuery,
- GPTPineconeIndexQuery,
- GPTQdrantIndexQuery,
- GPTSimpleVectorIndexQuery,
- GPTWeaviateIndexQuery,
-)
-from gpt_index.indices.vector_store.base import GPTVectorStoreIndex
-from gpt_index.langchain_helpers.chain_wrapper import LLMPredictor
-from gpt_index.prompts.prompts import QuestionAnswerPrompt
-from gpt_index.vector_stores import (
- ChromaVectorStore,
- FaissVectorStore,
- PineconeVectorStore,
- QdrantVectorStore,
- SimpleVectorStore,
- WeaviateVectorStore,
-)
-from gpt_index.vector_stores.opensearch import (
- OpensearchVectorClient,
- OpensearchVectorStore,
-)
-
-
-class GPTSimpleVectorIndex(GPTVectorStoreIndex):
- """GPT Simple Vector Index.
-
- The GPTSimpleVectorIndex is a data structure where nodes are keyed by
- embeddings, and those embeddings are stored within a simple dictionary.
- During index construction, the document texts are chunked up,
- converted to nodes with text; they are then encoded in
- document embeddings stored within the dict.
-
- During query time, the index uses the dict to query for the top
- k most similar nodes, and synthesizes an answer from the
- retrieved nodes.
-
- Args:
- text_qa_template (Optional[QuestionAnswerPrompt]): A Question-Answer Prompt
- (see :ref:`Prompt-Templates`).
- NOTE: this is a deprecated field.
- embed_model (Optional[BaseEmbedding]): Embedding model to use for
- embedding similarity.
-
- """
-
- index_struct_cls: Type[IndexDict] = SimpleIndexDict
-
- def __init__(
- self,
- documents: Optional[Sequence[DOCUMENTS_INPUT]] = None,
- index_struct: Optional[IndexDict] = None,
- text_qa_template: Optional[QuestionAnswerPrompt] = None,
- llm_predictor: Optional[LLMPredictor] = None,
- embed_model: Optional[BaseEmbedding] = None,
- simple_vector_store_data_dict: Optional[dict] = None,
- **kwargs: Any,
- ) -> None:
- """Init params."""
- vector_store = SimpleVectorStore(
- simple_vector_store_data_dict=simple_vector_store_data_dict
- )
-
- super().__init__(
- documents=documents,
- index_struct=index_struct,
- text_qa_template=text_qa_template,
- llm_predictor=llm_predictor,
- embed_model=embed_model,
- vector_store=vector_store,
- **kwargs,
- )
-
- # TODO: Temporary hack to also store embeddings in index_struct
- embedding_dict = vector_store._data.embedding_dict
- self._index_struct.embeddings_dict = embedding_dict
- # update docstore with current struct
- self._docstore.add_documents([self.index_struct], allow_update=True)
-
- @classmethod
- def get_query_map(self) -> Dict[str, Type[BaseGPTIndexQuery]]:
- """Get query map."""
- return {
- QueryMode.DEFAULT: GPTSimpleVectorIndexQuery,
- QueryMode.EMBEDDING: GPTSimpleVectorIndexQuery,
- }
-
- def _preprocess_query(self, mode: QueryMode, query_kwargs: Any) -> None:
- """Preprocess query."""
- super()._preprocess_query(mode, query_kwargs)
- del query_kwargs["vector_store"]
- vector_store = cast(SimpleVectorStore, self._vector_store)
- query_kwargs["simple_vector_store_data_dict"] = vector_store._data
-
-
-class GPTFaissIndex(GPTVectorStoreIndex):
- """GPT Faiss Index.
-
- The GPTFaissIndex is a data structure where nodes are keyed by
- embeddings, and those embeddings are stored within a Faiss index.
- During index construction, the document texts are chunked up,
- converted to nodes with text; they are then encoded in
- document embeddings stored within Faiss.
-
- During query time, the index uses Faiss to query for the top
- k most similar nodes, and synthesizes an answer from the
- retrieved nodes.
-
- Args:
- text_qa_template (Optional[QuestionAnswerPrompt]): A Question-Answer Prompt
- (see :ref:`Prompt-Templates`).
- NOTE: this is a deprecated field.
- faiss_index (faiss.Index): A Faiss Index object (required). Note: the index
- will be reset during index construction.
- embed_model (Optional[BaseEmbedding]): Embedding model to use for
- embedding similarity.
- """
-
- index_struct_cls: Type[IndexDict] = FaissIndexDict
-
- def __init__(
- self,
- documents: Optional[Sequence[DOCUMENTS_INPUT]] = None,
- faiss_index: Optional[Any] = None,
- index_struct: Optional[IndexDict] = None,
- text_qa_template: Optional[QuestionAnswerPrompt] = None,
- llm_predictor: Optional[LLMPredictor] = None,
- embed_model: Optional[BaseEmbedding] = None,
- **kwargs: Any,
- ) -> None:
- """Init params."""
- if faiss_index is None:
- raise ValueError("faiss_index is required.")
- vector_store = FaissVectorStore(faiss_index)
-
- super().__init__(
- documents=documents,
- index_struct=index_struct,
- text_qa_template=text_qa_template,
- llm_predictor=llm_predictor,
- embed_model=embed_model,
- vector_store=vector_store,
- **kwargs,
- )
-
- @classmethod
- def get_query_map(self) -> Dict[str, Type[BaseGPTIndexQuery]]:
- """Get query map."""
- return {
- QueryMode.DEFAULT: GPTFaissIndexQuery,
- QueryMode.EMBEDDING: GPTFaissIndexQuery,
- }
-
- def _preprocess_query(self, mode: QueryMode, query_kwargs: Any) -> None:
- """Preprocess query."""
- super()._preprocess_query(mode, query_kwargs)
- del query_kwargs["vector_store"]
- vector_store = cast(FaissVectorStore, self._vector_store)
- query_kwargs["faiss_index"] = vector_store._faiss_index
-
- @classmethod
- def load_from_disk(
- cls, save_path: str, faiss_index_save_path: Optional[str] = None, **kwargs: Any
- ) -> "BaseGPTIndex":
- """Load index from disk.
-
- This method loads the index from a JSON file stored on disk. The index data
- structure itself is preserved completely. If the index is defined over
- subindices, those subindices will also be preserved (and subindices of
- those subindices, etc.).
- In GPTFaissIndex, we allow user to specify an additional
- `faiss_index_save_path` to load faiss index from a file - that
- way, the user does not have to recreate the faiss index outside
- of this class.
-
- Args:
- save_path (str): The save_path of the file.
- faiss_index_save_path (Optional[str]): The save_path of the
- Faiss index file. If not specified, the Faiss index
- will not be saved to disk.
- **kwargs: Additional kwargs to pass to the index constructor.
-
- Returns:
- BaseGPTIndex: The loaded index.
- """
- if faiss_index_save_path is not None:
- import faiss
-
- faiss_index = faiss.read_index(faiss_index_save_path)
- return super().load_from_disk(save_path, faiss_index=faiss_index, **kwargs)
- else:
- return super().load_from_disk(save_path, **kwargs)
-
- def save_to_disk(
- self,
- save_path: str,
- faiss_index_save_path: Optional[str] = None,
- **save_kwargs: Any,
- ) -> None:
- """Save to file.
-
- This method stores the index into a JSON file stored on disk.
- In GPTFaissIndex, we allow user to specify an additional
- `faiss_index_save_path` to save the faiss index to a file - that
- way, the user can pass in the same argument in
- `GPTFaissIndex.load_from_disk` without having to recreate
- the Faiss index outside of this class.
-
- Args:
- save_path (str): The save_path of the file.
- faiss_index_save_path (Optional[str]): The save_path of the
- Faiss index file. If not specified, the Faiss index
- will not be saved to disk.
- """
- super().save_to_disk(save_path, **save_kwargs)
-
- if faiss_index_save_path is not None:
- import faiss
-
- faiss.write_index(self._vector_store.client, faiss_index_save_path)
-
-
-class GPTPineconeIndex(GPTVectorStoreIndex):
- """GPT Pinecone Index.
-
- The GPTPineconeIndex is a data structure where nodes are keyed by
- embeddings, and those embeddings are stored within a Pinecone index.
- During index construction, the document texts are chunked up,
- converted to nodes with text; they are then encoded in
- document embeddings stored within Pinecone.
-
- During query time, the index uses Pinecone to query for the top
- k most similar nodes, and synthesizes an answer from the
- retrieved nodes.
-
- Args:
- text_qa_template (Optional[QuestionAnswerPrompt]): A Question-Answer Prompt
- (see :ref:`Prompt-Templates`).
- NOTE: this is a deprecated field.
- embed_model (Optional[BaseEmbedding]): Embedding model to use for
- embedding similarity.
- chunk_size_limit (int): Maximum number of tokens per chunk. NOTE:
- in Pinecone the default is 2048 due to metadata size restrictions.
- """
-
- index_struct_cls: Type[IndexDict] = PineconeIndexDict
-
- def __init__(
- self,
- documents: Optional[Sequence[DOCUMENTS_INPUT]] = None,
- pinecone_index: Optional[Any] = None,
- pinecone_kwargs: Optional[Dict] = None,
- index_struct: Optional[IndexDict] = None,
- text_qa_template: Optional[QuestionAnswerPrompt] = None,
- llm_predictor: Optional[LLMPredictor] = None,
- embed_model: Optional[BaseEmbedding] = None,
- chunk_size_limit: int = 2048,
- **kwargs: Any,
- ) -> None:
- """Init params."""
- if pinecone_index is None:
- raise ValueError("pinecone_index is required.")
- if pinecone_kwargs is None:
- pinecone_kwargs = {}
- vector_store = kwargs.pop(
- "vector_store",
- PineconeVectorStore(
- pinecone_index=pinecone_index, pinecone_kwargs=pinecone_kwargs
- ),
- )
-
- super().__init__(
- documents=documents,
- index_struct=index_struct,
- text_qa_template=text_qa_template,
- llm_predictor=llm_predictor,
- embed_model=embed_model,
- vector_store=vector_store,
- chunk_size_limit=chunk_size_limit,
- **kwargs,
- )
-
- @classmethod
- def get_query_map(self) -> Dict[str, Type[BaseGPTIndexQuery]]:
- """Get query map."""
- return {
- QueryMode.DEFAULT: GPTPineconeIndexQuery,
- QueryMode.EMBEDDING: GPTPineconeIndexQuery,
- }
-
- def _preprocess_query(self, mode: QueryMode, query_kwargs: Any) -> None:
- """Preprocess query."""
- super()._preprocess_query(mode, query_kwargs)
- del query_kwargs["vector_store"]
- vector_store = cast(PineconeVectorStore, self._vector_store)
- query_kwargs["pinecone_index"] = vector_store._pinecone_index
- query_kwargs["pinecone_kwargs"] = vector_store._pinecone_kwargs
-
-
-class GPTWeaviateIndex(GPTVectorStoreIndex):
- """GPT Weaviate Index.
-
- The GPTWeaviateIndex is a data structure where nodes are keyed by
- embeddings, and those embeddings are stored within a Weaviate index.
- During index construction, the document texts are chunked up,
- converted to nodes with text; they are then encoded in
- document embeddings stored within Weaviate.
-
- During query time, the index uses Weaviate to query for the top
- k most similar nodes, and synthesizes an answer from the
- retrieved nodes.
-
- Args:
- text_qa_template (Optional[QuestionAnswerPrompt]): A Question-Answer Prompt
- (see :ref:`Prompt-Templates`).
- NOTE: this is a deprecated field.
- embed_model (Optional[BaseEmbedding]): Embedding model to use for
- embedding similarity.
- """
-
- index_struct_cls: Type[IndexDict] = WeaviateIndexDict
-
- def __init__(
- self,
- documents: Optional[Sequence[DOCUMENTS_INPUT]] = None,
- weaviate_client: Optional[Any] = None,
- class_prefix: Optional[str] = None,
- index_struct: Optional[IndexDict] = None,
- text_qa_template: Optional[QuestionAnswerPrompt] = None,
- llm_predictor: Optional[LLMPredictor] = None,
- embed_model: Optional[BaseEmbedding] = None,
- **kwargs: Any,
- ) -> None:
- """Init params."""
- if weaviate_client is None:
- raise ValueError("weaviate_client is required.")
- vector_store = WeaviateVectorStore(
- weaviate_client=weaviate_client, class_prefix=class_prefix
- )
-
- super().__init__(
- documents=documents,
- index_struct=index_struct,
- text_qa_template=text_qa_template,
- llm_predictor=llm_predictor,
- embed_model=embed_model,
- vector_store=vector_store,
- **kwargs,
- )
-
- @classmethod
- def get_query_map(self) -> Dict[str, Type[BaseGPTIndexQuery]]:
- """Get query map."""
- return {
- QueryMode.DEFAULT: GPTWeaviateIndexQuery,
- QueryMode.EMBEDDING: GPTWeaviateIndexQuery,
- }
-
- def _preprocess_query(self, mode: QueryMode, query_kwargs: Any) -> None:
- """Preprocess query."""
- super()._preprocess_query(mode, query_kwargs)
- del query_kwargs["vector_store"]
- vector_store = cast(WeaviateVectorStore, self._vector_store)
- query_kwargs["weaviate_client"] = vector_store._client
- query_kwargs["class_prefix"] = vector_store._class_prefix
-
-
-class GPTQdrantIndex(GPTVectorStoreIndex):
- """GPT Qdrant Index.
-
- The GPTQdrantIndex is a data structure where nodes are keyed by
- embeddings, and those embeddings are stored within a Qdrant collection.
- During index construction, the document texts are chunked up,
- converted to nodes with text; they are then encoded in
- document embeddings stored within Qdrant.
-
- During query time, the index uses Qdrant to query for the top
- k most similar nodes, and synthesizes an answer from the
- retrieved nodes.
-
- Args:
- text_qa_template (Optional[QuestionAnswerPrompt]): A Question-Answer Prompt
- (see :ref:`Prompt-Templates`).
- NOTE: this is a deprecated field.
- embed_model (Optional[BaseEmbedding]): Embedding model to use for
- embedding similarity.
- client (Optional[Any]): QdrantClient instance from `qdrant-client` package
- collection_name: (Optional[str]): name of the Qdrant collection
- """
-
- index_struct_cls: Type[IndexDict] = QdrantIndexDict
-
- def __init__(
- self,
- documents: Optional[Sequence[DOCUMENTS_INPUT]] = None,
- client: Optional[Any] = None,
- collection_name: Optional[str] = None,
- index_struct: Optional[IndexDict] = None,
- text_qa_template: Optional[QuestionAnswerPrompt] = None,
- llm_predictor: Optional[LLMPredictor] = None,
- embed_model: Optional[BaseEmbedding] = None,
- **kwargs: Any,
- ) -> None:
- """Init params."""
- if client is None:
- raise ValueError("client is required.")
- if collection_name is None:
- raise ValueError("collection_name is required.")
- vector_store = QdrantVectorStore(client=client, collection_name=collection_name)
-
- super().__init__(
- documents=documents,
- index_struct=index_struct,
- text_qa_template=text_qa_template,
- llm_predictor=llm_predictor,
- embed_model=embed_model,
- vector_store=vector_store,
- **kwargs,
- )
-
- @classmethod
- def get_query_map(self) -> Dict[str, Type[BaseGPTIndexQuery]]:
- """Get query map."""
- return {
- QueryMode.DEFAULT: GPTQdrantIndexQuery,
- QueryMode.EMBEDDING: GPTQdrantIndexQuery,
- }
-
- def _preprocess_query(self, mode: QueryMode, query_kwargs: Any) -> None:
- """Preprocess query."""
- super()._preprocess_query(mode, query_kwargs)
- del query_kwargs["vector_store"]
- vector_store = cast(QdrantVectorStore, self._vector_store)
- query_kwargs["client"] = vector_store._client
- query_kwargs["collection_name"] = vector_store._collection_name
-
-
-class GPTChromaIndex(GPTVectorStoreIndex):
- """GPT Chroma Index.
-
- The GPTChromaIndex is a data structure where nodes are keyed by
- embeddings, and those embeddings are stored within a Chroma collection.
- During index construction, the document texts are chunked up,
- converted to nodes with text; they are then encoded in
- document embeddings stored within Chroma.
-
- During query time, the index uses Chroma to query for the top
- k most similar nodes, and synthesizes an answer from the
- retrieved nodes.
-
- Args:
- text_qa_template (Optional[QuestionAnswerPrompt]): A Question-Answer Prompt
- (see :ref:`Prompt-Templates`).
- NOTE: this is a deprecated field.
- embed_model (Optional[BaseEmbedding]): Embedding model to use for
- embedding similarity.
- chroma_collection (Optional[Any]): Collection instance from `chromadb` package.
-
- """
-
- index_struct_cls: Type[IndexDict] = ChromaIndexDict
-
- def __init__(
- self,
- documents: Optional[Sequence[DOCUMENTS_INPUT]] = None,
- chroma_collection: Optional[Any] = None,
- index_struct: Optional[IndexDict] = None,
- text_qa_template: Optional[QuestionAnswerPrompt] = None,
- llm_predictor: Optional[LLMPredictor] = None,
- embed_model: Optional[BaseEmbedding] = None,
- **kwargs: Any,
- ) -> None:
- """Init params."""
- if chroma_collection is None:
- raise ValueError("chroma_collection is required.")
- vector_store = ChromaVectorStore(chroma_collection=chroma_collection)
-
- super().__init__(
- documents=documents,
- index_struct=index_struct,
- text_qa_template=text_qa_template,
- llm_predictor=llm_predictor,
- embed_model=embed_model,
- vector_store=vector_store,
- **kwargs,
- )
-
- @classmethod
- def get_query_map(self) -> Dict[str, Type[BaseGPTIndexQuery]]:
- """Get query map."""
- return {
- QueryMode.DEFAULT: GPTChromaIndexQuery,
- QueryMode.EMBEDDING: GPTChromaIndexQuery,
- }
-
- def _preprocess_query(self, mode: QueryMode, query_kwargs: Any) -> None:
- """Preprocess query."""
- super()._preprocess_query(mode, query_kwargs)
- del query_kwargs["vector_store"]
- vector_store = cast(ChromaVectorStore, self._vector_store)
- query_kwargs["chroma_collection"] = vector_store._collection
-
-
-class GPTOpensearchIndex(GPTVectorStoreIndex):
- """GPT Opensearch Index.
-
- The GPTOpensearchIndex is a data structure where nodes are keyed by
- embeddings, and those embeddings are stored in a document that is indexed
- with its embedding as well as its textual data (text field is defined in
- the OpensearchVectorClient).
- During index construction, the document texts are chunked up,
- converted to nodes with text; each node's embedding is computed, and then
- the node's text, along with the embedding, is converted into JSON document that
- is indexed in Opensearch. The embedding data is put into a field with type
- "knn_vector" and the text is put into a standard Opensearch text field.
-
- During query time, the index performs approximate KNN search using the
- "knn_vector" field that the embeddings were mapped to.
-
- Args:
- text_qa_template (Optional[QuestionAnswerPrompt]): A Question-Answer Prompt
- (see :ref:`Prompt-Templates`).
- NOTE: this is a deprecated field.
- client (Optional[OpensearchVectorClient]): The client which encapsulates
- logic for using Opensearch as a vector store (that is, it holds stuff
- like endpoint, index_name and performs operations like initializing the
- index and adding new doc/embeddings to said index).
- embed_model (Optional[BaseEmbedding]): Embedding model to use for
- embedding similarity.
- """
-
- index_struct_cls: Type[IndexDict] = OpensearchIndexDict
-
- def __init__(
- self,
- documents: Optional[Sequence[DOCUMENTS_INPUT]] = None,
- client: Optional[OpensearchVectorClient] = None,
- index_struct: Optional[IndexDict] = None,
- text_qa_template: Optional[QuestionAnswerPrompt] = None,
- llm_predictor: Optional[LLMPredictor] = None,
- embed_model: Optional[BaseEmbedding] = None,
- **kwargs: Any,
- ) -> None:
- """Init params."""
- if client is None:
- raise ValueError("client is required.")
- vector_store = OpensearchVectorStore(client)
- super().__init__(
- documents=documents,
- index_struct=index_struct,
- text_qa_template=text_qa_template,
- llm_predictor=llm_predictor,
- embed_model=embed_model,
- vector_store=vector_store,
- **kwargs,
- )
-
- @classmethod
- def get_query_map(self) -> Dict[str, Type[BaseGPTIndexQuery]]:
- """Get query map."""
- return {
- QueryMode.DEFAULT: GPTOpensearchIndexQuery,
- QueryMode.EMBEDDING: GPTOpensearchIndexQuery,
- }
-
- def _preprocess_query(self, mode: QueryMode, query_kwargs: Any) -> None:
- """Preprocess query."""
- super()._preprocess_query(mode, query_kwargs)
- del query_kwargs["vector_store"]
- vector_store = cast(OpensearchVectorStore, self._vector_store)
- query_kwargs["client"] = vector_store._client
diff --git a/spaces/julien-c/sveltekit-demo/build/_app/pages/__layout.svelte-63495e35.js b/spaces/julien-c/sveltekit-demo/build/_app/pages/__layout.svelte-63495e35.js
deleted file mode 100644
index 368809190b8cde67e0d1b95d5b0b1bbc1ab95a74..0000000000000000000000000000000000000000
--- a/spaces/julien-c/sveltekit-demo/build/_app/pages/__layout.svelte-63495e35.js
+++ /dev/null
@@ -1 +0,0 @@
-import{D as ae,S as Q,i as W,s as X,e as d,j as L,E as z,t as B,c as h,a as n,d as l,l as I,F as N,g as C,G as le,b as t,H as O,f as D,I as a,J as Y,K as re,L as oe,v as ie,w as ne,x as ce,M as ve,N as ue,O as fe,p as ee,n as te,A as _e}from"../chunks/vendor-92f01141.js";const de=()=>{const i=ae("__svelte__");return{page:{subscribe:i.page.subscribe},navigating:{subscribe:i.navigating.subscribe},get preloading(){return console.error("stores.preloading is deprecated; use stores.navigating instead"),{subscribe:i.navigating.subscribe}},session:i.session}},he={subscribe(i){return de().page.subscribe(i)}};var pe="/build/_app/assets/svelte-logo-87df40b8.svg";function me(i){let s,u,o,c,m,E,p,v,q,y,f,b,r,e,_,w,g,H,V,A,S,j,x;return{c(){s=d("header"),u=d("div"),o=d("a"),c=d("img"),E=L(),p=d("nav"),v=z("svg"),q=z("path"),y=L(),f=d("ul"),b=d("li"),r=d("a"),e=B("Home"),_=L(),w=d("li"),g=d("a"),H=B("About"),V=L(),A=z("svg"),S=z("path"),j=L(),x=d("div"),this.h()},l(k){s=h(k,"HEADER",{class:!0});var $=n(s);u=h($,"DIV",{class:!0});var F=n(u);o=h(F,"A",{href:!0,class:!0});var G=n(o);c=h(G,"IMG",{src:!0,alt:!0,class:!0}),G.forEach(l),F.forEach(l),E=I($),p=h($,"NAV",{class:!0});var M=n(p);v=N(M,"svg",{viewBox:!0,"aria-hidden":!0,class:!0});var R=n(v);q=N(R,"path",{d:!0,class:!0}),n(q).forEach(l),R.forEach(l),y=I(M),f=h(M,"UL",{class:!0});var K=n(f);b=h(K,"LI",{class:!0});var Z=n(b);r=h(Z,"A",{"sveltekit:prefetch":!0,href:!0,class:!0});var J=n(r);e=C(J,"Home"),J.forEach(l),Z.forEach(l),_=I(K),w=h(K,"LI",{class:!0});var P=n(w);g=h(P,"A",{"sveltekit:prefetch":!0,href:!0,class:!0});var T=n(g);H=C(T,"About"),T.forEach(l),P.forEach(l),K.forEach(l),V=I(M),A=N(M,"svg",{viewBox:!0,"aria-hidden":!0,class:!0});var U=n(A);S=N(U,"path",{d:!0,class:!0}),n(S).forEach(l),U.forEach(l),M.forEach(l),j=I($),x=h($,"DIV",{class:!0});var se=n(x);se.forEach(l),$.forEach(l),this.h()},h(){le(c.src,m=pe)||t(c,"src",m),t(c,"alt","SvelteKit"),t(c,"class","svelte-t2wq17"),t(o,"href","https://kit.svelte.dev"),t(o,"class","svelte-t2wq17"),t(u,"class","corner svelte-t2wq17"),t(q,"d","M0,0 L1,2 C1.5,3 1.5,3 2,3 L2,0 Z"),t(q,"class","svelte-t2wq17"),t(v,"viewBox","0 0 2 3"),t(v,"aria-hidden","true"),t(v,"class","svelte-t2wq17"),t(r,"sveltekit:prefetch",""),t(r,"href","./"),t(r,"class","svelte-t2wq17"),t(b,"class","svelte-t2wq17"),O(b,"active",i[0].url.pathname==="/"),t(g,"sveltekit:prefetch",""),t(g,"href","./about"),t(g,"class","svelte-t2wq17"),t(w,"class","svelte-t2wq17"),O(w,"active",i[0].url.pathname==="/about"),t(f,"class","svelte-t2wq17"),t(S,"d","M0,0 L0,3 C0.5,3 0.5,3 1,2 L2,0 Z"),t(S,"class","svelte-t2wq17"),t(A,"viewBox","0 0 2 3"),t(A,"aria-hidden","true"),t(A,"class","svelte-t2wq17"),t(p,"class","svelte-t2wq17"),t(x,"class","corner svelte-t2wq17"),t(s,"class","svelte-t2wq17")},m(k,$){D(k,s,$),a(s,u),a(u,o),a(o,c),a(s,E),a(s,p),a(p,v),a(v,q),a(p,y),a(p,f),a(f,b),a(b,r),a(r,e),a(f,_),a(f,w),a(w,g),a(g,H),a(p,V),a(p,A),a(A,S),a(s,j),a(s,x)},p(k,[$]){$&1&&O(b,"active",k[0].url.pathname==="/"),$&1&&O(w,"active",k[0].url.pathname==="/about")},i:Y,o:Y,d(k){k&&l(s)}}}function ge(i,s,u){let o;return re(i,he,c=>u(0,o=c)),[o]}class be extends Q{constructor(s){super();W(this,s,ge,me,X,{})}}function Ee(i){let s,u,o,c,m,E,p,v,q,y,f;s=new be({});const b=i[1].default,r=oe(b,i,i[0],null);return{c(){ie(s.$$.fragment),u=L(),o=d("main"),r&&r.c(),c=L(),m=d("footer"),E=d("p"),p=B("visit "),v=d("a"),q=B("kit.svelte.dev"),y=B(" to learn SvelteKit"),this.h()},l(e){ne(s.$$.fragment,e),u=I(e),o=h(e,"MAIN",{class:!0});var _=n(o);r&&r.l(_),_.forEach(l),c=I(e),m=h(e,"FOOTER",{class:!0});var w=n(m);E=h(w,"P",{});var g=n(E);p=C(g,"visit "),v=h(g,"A",{href:!0,class:!0});var H=n(v);q=C(H,"kit.svelte.dev"),H.forEach(l),y=C(g," to learn SvelteKit"),g.forEach(l),w.forEach(l),this.h()},h(){t(o,"class","svelte-1izrdc8"),t(v,"href","https://kit.svelte.dev"),t(v,"class","svelte-1izrdc8"),t(m,"class","svelte-1izrdc8")},m(e,_){ce(s,e,_),D(e,u,_),D(e,o,_),r&&r.m(o,null),D(e,c,_),D(e,m,_),a(m,E),a(E,p),a(E,v),a(v,q),a(E,y),f=!0},p(e,[_]){r&&r.p&&(!f||_&1)&&ve(r,b,e,e[0],f?fe(b,e[0],_,null):ue(e[0]),null)},i(e){f||(ee(s.$$.fragment,e),ee(r,e),f=!0)},o(e){te(s.$$.fragment,e),te(r,e),f=!1},d(e){_e(s,e),e&&l(u),e&&l(o),r&&r.d(e),e&&l(c),e&&l(m)}}}function we(i,s,u){let{$$slots:o={},$$scope:c}=s;return i.$$set=m=>{"$$scope"in m&&u(0,c=m.$$scope)},[c,o]}class qe extends Q{constructor(s){super();W(this,s,we,Ee,X,{})}}export{qe as default};
diff --git a/spaces/jusancp99/imagenes_similares/similarity_utils.py b/spaces/jusancp99/imagenes_similares/similarity_utils.py
deleted file mode 100644
index fc1de7d528012f2dc4eba8c2ca8b395eaaf307c1..0000000000000000000000000000000000000000
--- a/spaces/jusancp99/imagenes_similares/similarity_utils.py
+++ /dev/null
@@ -1,175 +0,0 @@
-from typing import List, Union
-
-import datasets
-import numpy as np
-import torch
-import torchvision.transforms as T
-from PIL import Image
-from tqdm.auto import tqdm
-from transformers import AutoFeatureExtractor, AutoModel
-
-seed = 42
-hash_size = 8
-hidden_dim = 768 # ViT-base
-np.random.seed(seed)
-
-
-# Device.
-device = "cuda" if torch.cuda.is_available() else "cpu"
-
-# Load model for computing embeddings..
-model_ckpt = "gjuggler/swin-tiny-patch4-window7-224-finetuned-birds"
-extractor = AutoFeatureExtractor.from_pretrained(model_ckpt)
-
-# Data transformation chain.
-transformation_chain = T.Compose(
- [
- # We first resize the input image to 256x256 and then we take center crop.
- T.Resize(224),
- T.CenterCrop(extractor.size["height"]),
- T.ToTensor(),
- T.Normalize(mean=extractor.image_mean, std=extractor.image_std),
- ]
-)
-
-
-# Define random vectors to project with.
-random_vectors = np.random.randn(hash_size, hidden_dim).T
-
-
-def hash_func(embedding, random_vectors=random_vectors):
- """Randomly projects the embeddings and then computes bit-wise hashes."""
- if not isinstance(embedding, np.ndarray):
- embedding = np.array(embedding)
- if len(embedding.shape) < 2:
- embedding = np.expand_dims(embedding, 0)
-
- # Random projection.
- bools = np.dot(embedding, random_vectors) > 0
- return [bool2int(bool_vec) for bool_vec in bools]
-
-
-def bool2int(x):
- y = 0
- for i, j in enumerate(x):
- if j:
- y += 1 << i
- return y
-
-
-def compute_hash(model: Union[torch.nn.Module, str]):
- """Computes hash on a given dataset."""
- device = model.device
-
- def pp(example_batch):
- # Prepare the input images for the model.
- image_batch = example_batch["image"]
- image_batch_transformed = torch.stack(
- [transformation_chain(image) for image in image_batch]
- )
- new_batch = {"pixel_values": image_batch_transformed.to(device)}
-
- # Compute embeddings and pool them i.e., take the representations from the [CLS]
- # token.
- with torch.no_grad():
- embeddings = model(**new_batch).last_hidden_state[:, 0].cpu().numpy()
-
- # Compute hashes for the batch of images.
- hashes = [hash_func(embeddings[i]) for i in range(len(embeddings))]
- example_batch["hashes"] = hashes
- return example_batch
-
- return pp
-
-
-class Table:
- def __init__(self, hash_size: int):
- self.table = {}
- self.hash_size = hash_size
-
- def add(self, id: int, hashes: List[int], label: int):
- # Create a unique indentifier.
- entry = {"id_label": str(id) + "_" + str(label)}
-
- # Add the hash values to the current table.
- for h in hashes:
- if h in self.table:
- self.table[h].append(entry)
- else:
- self.table[h] = [entry]
-
- def query(self, hashes: List[int]):
- results = []
-
- # Loop over the query hashes and determine if they exist in
- # the current table.
- for h in hashes:
- if h in self.table:
- results.extend(self.table[h])
- return results
-
-
-class LSH:
- def __init__(self, hash_size, num_tables):
- self.num_tables = num_tables
- self.tables = []
- for i in range(self.num_tables):
- self.tables.append(Table(hash_size))
-
- def add(self, id: int, hash: List[int], label: int):
- for table in self.tables:
- table.add(id, hash, label)
-
- def query(self, hashes: List[int]):
- results = []
- for table in self.tables:
- results.extend(table.query(hashes))
- return results
-
-
-class BuildLSHTable:
- def __init__(
- self,
- model: Union[torch.nn.Module, None],
- batch_size: int = 48,
- hash_size: int = hash_size,
- dim: int = hidden_dim,
- num_tables: int = 10,
- ):
- self.hash_size = hash_size
- self.dim = dim
- self.num_tables = num_tables
- self.lsh = LSH(self.hash_size, self.num_tables)
-
- self.batch_size = batch_size
- self.hash_fn = compute_hash(model.to(device))
-
- def build(self, ds: datasets.DatasetDict):
- dataset_hashed = ds.map(self.hash_fn, batched=True, batch_size=self.batch_size)
-
- for id in tqdm(range(len(dataset_hashed))):
- hash, label = dataset_hashed[id]["hashes"], dataset_hashed[id]["labels"]
- self.lsh.add(id, hash, label)
-
- def query(self, image, verbose=True):
- if isinstance(image, str):
- image = Image.open(image).convert("RGB")
-
- # Compute the hashes of the query image and fetch the results.
- example_batch = dict(image=[image])
- hashes = self.hash_fn(example_batch)["hashes"][0]
-
- results = self.lsh.query(hashes)
- if verbose:
- print("Matches:", len(results))
-
- # Calculate Jaccard index to quantify the similarity.
- counts = {}
- for r in results:
- if r["id_label"] in counts:
- counts[r["id_label"]] += 1
- else:
- counts[r["id_label"]] = 1
- for k in counts:
- counts[k] = float(counts[k]) / self.dim
- return counts
diff --git a/spaces/kajalag/Whatsapp_Chat_Analyzer/preprocessor.py b/spaces/kajalag/Whatsapp_Chat_Analyzer/preprocessor.py
deleted file mode 100644
index 6423577ca0a5b7077856429e9dd0b99c4f2242bd..0000000000000000000000000000000000000000
--- a/spaces/kajalag/Whatsapp_Chat_Analyzer/preprocessor.py
+++ /dev/null
@@ -1,111 +0,0 @@
-import pandas as pd
-import re
-from textblob import TextBlob
-import numpy as np
-import nltk
-import nltk.data
-from nltk.sentiment.vader import SentimentIntensityAnalyzer
-from tqdm.notebook import tqdm
-sia=SentimentIntensityAnalyzer()
-nltk.download('vader_lexicon')
-
-def preprocess(data):
- pattern ='\d{1,2}/\d{1,2}/\d{2,4},\s\d{1,2}:\d{2}\s-\s'
-
- messages = re.split(pattern, data)[1:]
- dates = re.findall(pattern, data)
- df = pd.DataFrame({'user_message': messages, 'message_date': dates})
- df['message_date'] = pd.to_datetime(df['message_date'], format='%m/%d/%y, %H:%M - ')
- df.rename(columns={'message_date': 'date'}, inplace=True)
- users = []
- messages = []
- for message in df['user_message']:
- entry = re.split('([\w\W]+?):\s', message)
-
- if entry[1:]:
- users.append(entry[1])
- messages.append(entry[2])
-
- else:
- users.append('group_notification')
- messages.append(entry[0])
- df['users'] = users
- df['message'] = messages
- df.drop(columns=['user_message'], inplace=True)
- df['year'] = df['date'].dt.year
- df['day'] = df['date'].dt.day
- df['hour'] = df['date'].dt.hour
- df['minute'] = df['date'].dt.minute
- df['Day_name'] = df['date'].dt.day_name()
- df['Date']=df['date'].dt.date
- df['Month'] = df['date'].dt.month
- df['Month_name'] = df['date'].dt.month_name()
-
- period = []
- for hour in df[['Day_name', 'hour']]['hour']:
- if hour == 23:
- period.append(str(hour) + "-" + str('00'))
- elif hour == 0:
- period.append(str('00') + "-" + str(hour + 1))
- else:
- period.append(str(hour) + "-" + str(hour + 1))
-
- df['period']=period
-
- temp = df[df['users'] != 'group_notification']
- temp = temp[temp['message'] != '\n']
- temp.replace("", np.nan, inplace=True)
- temp = temp.dropna()
-
- def cleanTxt(text):
- text = re.sub(r'@[A-Za-z0-9]+', '', text)
- text = re.sub(r'#', '', text)
- text = text.replace('\n', "")
- return text
-
- temp['message'] = temp['message'].apply(cleanTxt)
- temp['users'] = temp['users'].apply(cleanTxt)
-
- res = {}
- for i, row in tqdm(temp.iterrows(), total=len(temp)):
- text = row['message']
- myid = row['users']
- res[myid] = sia.polarity_scores(text)
-
- vaders = pd.DataFrame(res).T
- vaders = vaders.reset_index().rename(columns={'index': 'users'})
- vaders = vaders.merge(temp, how="right")
- vaders_new = vaders.pop('message')
- vaders_new = pd.DataFrame(vaders_new)
- vaders.insert(1, "message", vaders_new['message'])
-
- def getSubjectivity(text):
- return TextBlob(text).sentiment.subjectivity
-
- def getPolarity(text):
- return TextBlob(text).sentiment.polarity
-
- vaders['Subjectivity'] = vaders['message'].apply(getSubjectivity)
- vaders['Polarity'] = vaders['message'].apply(getPolarity)
-
- def getAnalysis(score):
- if score < 0:
- return 'Negative'
- if score == 0:
- return 'Neutral'
- else:
- return 'Positive'
-
- vaders['Analysis'] = vaders['Polarity'].apply(getAnalysis)
-
- def getAnalysis(score):
- if score <= 0:
- return 'Negative'
- if score < 0.2960:
- return 'Neutral'
- else:
- return 'Positive'
-
- vaders['vader_Analysis'] = vaders['compound'].apply(getAnalysis)
-
- return vaders
\ No newline at end of file
diff --git a/spaces/kcagle/AutoGPT/autogpt/app.py b/spaces/kcagle/AutoGPT/autogpt/app.py
deleted file mode 100644
index 58d9f7164ddfbb5019b072d789dc2fa6205dc9d3..0000000000000000000000000000000000000000
--- a/spaces/kcagle/AutoGPT/autogpt/app.py
+++ /dev/null
@@ -1,330 +0,0 @@
-""" Command and Control """
-import json
-from typing import Dict, List, NoReturn, Union
-
-from autogpt.agent.agent_manager import AgentManager
-from autogpt.commands.analyze_code import analyze_code
-from autogpt.commands.audio_text import read_audio_from_file
-from autogpt.commands.execute_code import (
- execute_python_file,
- execute_shell,
- execute_shell_popen,
-)
-from autogpt.commands.file_operations import (
- append_to_file,
- delete_file,
- download_file,
- read_file,
- search_files,
- write_to_file,
-)
-from autogpt.commands.git_operations import clone_repository
-from autogpt.commands.google_search import google_official_search, google_search
-from autogpt.commands.image_gen import generate_image
-from autogpt.commands.improve_code import improve_code
-from autogpt.commands.twitter import send_tweet
-from autogpt.commands.web_requests import scrape_links, scrape_text
-from autogpt.commands.web_selenium import browse_website
-from autogpt.commands.write_tests import write_tests
-from autogpt.config import Config
-from autogpt.json_utils.json_fix_llm import fix_and_parse_json
-from autogpt.memory import get_memory
-from autogpt.processing.text import summarize_text
-from autogpt.speech import say_text
-
-CFG = Config()
-AGENT_MANAGER = AgentManager()
-
-
-def is_valid_int(value: str) -> bool:
- """Check if the value is a valid integer
-
- Args:
- value (str): The value to check
-
- Returns:
- bool: True if the value is a valid integer, False otherwise
- """
- try:
- int(value)
- return True
- except ValueError:
- return False
-
-
-def get_command(response_json: Dict):
- """Parse the response and return the command name and arguments
-
- Args:
- response_json (json): The response from the AI
-
- Returns:
- tuple: The command name and arguments
-
- Raises:
- json.decoder.JSONDecodeError: If the response is not valid JSON
-
- Exception: If any other error occurs
- """
- try:
- if "command" not in response_json:
- return "Error:", "Missing 'command' object in JSON"
-
- if not isinstance(response_json, dict):
- return "Error:", f"'response_json' object is not dictionary {response_json}"
-
- command = response_json["command"]
- if not isinstance(command, dict):
- return "Error:", "'command' object is not a dictionary"
-
- if "name" not in command:
- return "Error:", "Missing 'name' field in 'command' object"
-
- command_name = command["name"]
-
- # Use an empty dictionary if 'args' field is not present in 'command' object
- arguments = command.get("args", {})
-
- return command_name, arguments
- except json.decoder.JSONDecodeError:
- return "Error:", "Invalid JSON"
- # All other errors, return "Error: + error message"
- except Exception as e:
- return "Error:", str(e)
-
-
-def map_command_synonyms(command_name: str):
- """Takes the original command name given by the AI, and checks if the
- string matches a list of common/known hallucinations
- """
- synonyms = [
- ("write_file", "write_to_file"),
- ("create_file", "write_to_file"),
- ("search", "google"),
- ]
- for seen_command, actual_command_name in synonyms:
- if command_name == seen_command:
- return actual_command_name
- return command_name
-
-
-def execute_command(command_name: str, arguments):
- """Execute the command and return the result
-
- Args:
- command_name (str): The name of the command to execute
- arguments (dict): The arguments for the command
-
- Returns:
- str: The result of the command
- """
- try:
- command_name = map_command_synonyms(command_name.lower())
- if command_name == "google":
- # Check if the Google API key is set and use the official search method
- # If the API key is not set or has only whitespaces, use the unofficial
- # search method
- key = CFG.google_api_key
- if key and key.strip() and key != "your-google-api-key":
- google_result = google_official_search(arguments["input"])
- return google_result
- else:
- google_result = google_search(arguments["input"])
-
- # google_result can be a list or a string depending on the search results
- if isinstance(google_result, list):
- safe_message = [
- google_result_single.encode("utf-8", "ignore")
- for google_result_single in google_result
- ]
- else:
- safe_message = google_result.encode("utf-8", "ignore")
-
- return safe_message.decode("utf-8")
- elif command_name == "memory_add":
- memory = get_memory(CFG)
- return memory.add(arguments["string"])
- elif command_name == "start_agent":
- return start_agent(
- arguments["name"], arguments["task"], arguments["prompt"]
- )
- elif command_name == "message_agent":
- return message_agent(arguments["key"], arguments["message"])
- elif command_name == "list_agents":
- return list_agents()
- elif command_name == "delete_agent":
- return delete_agent(arguments["key"])
- elif command_name == "get_text_summary":
- return get_text_summary(arguments["url"], arguments["question"])
- elif command_name == "get_hyperlinks":
- return get_hyperlinks(arguments["url"])
- elif command_name == "clone_repository":
- return clone_repository(
- arguments["repository_url"], arguments["clone_path"]
- )
- elif command_name == "read_file":
- return read_file(arguments["file"])
- elif command_name == "write_to_file":
- return write_to_file(arguments["file"], arguments["text"])
- elif command_name == "append_to_file":
- return append_to_file(arguments["file"], arguments["text"])
- elif command_name == "delete_file":
- return delete_file(arguments["file"])
- elif command_name == "search_files":
- return search_files(arguments["directory"])
- elif command_name == "download_file":
- if not CFG.allow_downloads:
- return "Error: You do not have user authorization to download files locally."
- return download_file(arguments["url"], arguments["file"])
- elif command_name == "browse_website":
- return browse_website(arguments["url"], arguments["question"])
- # TODO: Change these to take in a file rather than pasted code, if
- # non-file is given, return instructions "Input should be a python
- # filepath, write your code to file and try again"
- elif command_name == "analyze_code":
- return analyze_code(arguments["code"])
- elif command_name == "improve_code":
- return improve_code(arguments["suggestions"], arguments["code"])
- elif command_name == "write_tests":
- return write_tests(arguments["code"], arguments.get("focus"))
- elif command_name == "execute_python_file": # Add this command
- return execute_python_file(arguments["file"])
- elif command_name == "execute_shell":
- if CFG.execute_local_commands:
- return execute_shell(arguments["command_line"])
- else:
- return (
- "You are not allowed to run local shell commands. To execute"
- " shell commands, EXECUTE_LOCAL_COMMANDS must be set to 'True' "
- "in your config. Do not attempt to bypass the restriction."
- )
- elif command_name == "execute_shell_popen":
- if CFG.execute_local_commands:
- return execute_shell_popen(arguments["command_line"])
- else:
- return (
- "You are not allowed to run local shell commands. To execute"
- " shell commands, EXECUTE_LOCAL_COMMANDS must be set to 'True' "
- "in your config. Do not attempt to bypass the restriction."
- )
- elif command_name == "read_audio_from_file":
- return read_audio_from_file(arguments["file"])
- elif command_name == "generate_image":
- return generate_image(arguments["prompt"])
- elif command_name == "send_tweet":
- return send_tweet(arguments["text"])
- elif command_name == "do_nothing":
- return "No action performed."
- elif command_name == "task_complete":
- shutdown()
- else:
- return (
- f"Unknown command '{command_name}'. Please refer to the 'COMMANDS'"
- " list for available commands and only respond in the specified JSON"
- " format."
- )
- except Exception as e:
- return f"Error: {str(e)}"
-
-
-def get_text_summary(url: str, question: str) -> str:
- """Return the results of a Google search
-
- Args:
- url (str): The url to scrape
- question (str): The question to summarize the text for
-
- Returns:
- str: The summary of the text
- """
- text = scrape_text(url)
- summary = summarize_text(url, text, question)
- return f""" "Result" : {summary}"""
-
-
-def get_hyperlinks(url: str) -> Union[str, List[str]]:
- """Return the results of a Google search
-
- Args:
- url (str): The url to scrape
-
- Returns:
- str or list: The hyperlinks on the page
- """
- return scrape_links(url)
-
-
-def shutdown() -> NoReturn:
- """Shut down the program"""
- print("Shutting down...")
- quit()
-
-
-def start_agent(name: str, task: str, prompt: str, model=CFG.fast_llm_model) -> str:
- """Start an agent with a given name, task, and prompt
-
- Args:
- name (str): The name of the agent
- task (str): The task of the agent
- prompt (str): The prompt for the agent
- model (str): The model to use for the agent
-
- Returns:
- str: The response of the agent
- """
- # Remove underscores from name
- voice_name = name.replace("_", " ")
-
- first_message = f"""You are {name}. Respond with: "Acknowledged"."""
- agent_intro = f"{voice_name} here, Reporting for duty!"
-
- # Create agent
- if CFG.speak_mode:
- say_text(agent_intro, 1)
- key, ack = AGENT_MANAGER.create_agent(task, first_message, model)
-
- if CFG.speak_mode:
- say_text(f"Hello {voice_name}. Your task is as follows. {task}.")
-
- # Assign task (prompt), get response
- agent_response = AGENT_MANAGER.message_agent(key, prompt)
-
- return f"Agent {name} created with key {key}. First response: {agent_response}"
-
-
-def message_agent(key: str, message: str) -> str:
- """Message an agent with a given key and message"""
- # Check if the key is a valid integer
- if is_valid_int(key):
- agent_response = AGENT_MANAGER.message_agent(int(key), message)
- else:
- return "Invalid key, must be an integer."
-
- # Speak response
- if CFG.speak_mode:
- say_text(agent_response, 1)
- return agent_response
-
-
-def list_agents():
- """List all agents
-
- Returns:
- str: A list of all agents
- """
- return "List of agents:\n" + "\n".join(
- [str(x[0]) + ": " + x[1] for x in AGENT_MANAGER.list_agents()]
- )
-
-
-def delete_agent(key: str) -> str:
- """Delete an agent with a given key
-
- Args:
- key (str): The key of the agent to delete
-
- Returns:
- str: A message indicating whether the agent was deleted or not
- """
- result = AGENT_MANAGER.delete_agent(key)
- return f"Agent {key} deleted." if result else f"Agent {key} does not exist."
diff --git a/spaces/kdrkdrkdr/ZhongliTTS/modules.py b/spaces/kdrkdrkdr/ZhongliTTS/modules.py
deleted file mode 100644
index 9c7fd9cd6eb8b7e0ec0e08957e970744a374a924..0000000000000000000000000000000000000000
--- a/spaces/kdrkdrkdr/ZhongliTTS/modules.py
+++ /dev/null
@@ -1,390 +0,0 @@
-import copy
-import math
-import numpy as np
-import scipy
-import torch
-from torch import nn
-from torch.nn import functional as F
-
-from torch.nn import Conv1d, ConvTranspose1d, AvgPool1d, Conv2d
-from torch.nn.utils import weight_norm, remove_weight_norm
-
-import commons
-from commons import init_weights, get_padding
-from transforms import piecewise_rational_quadratic_transform
-
-
-LRELU_SLOPE = 0.1
-
-
-class LayerNorm(nn.Module):
- def __init__(self, channels, eps=1e-5):
- super().__init__()
- self.channels = channels
- self.eps = eps
-
- self.gamma = nn.Parameter(torch.ones(channels))
- self.beta = nn.Parameter(torch.zeros(channels))
-
- def forward(self, x):
- x = x.transpose(1, -1)
- x = F.layer_norm(x, (self.channels,), self.gamma, self.beta, self.eps)
- return x.transpose(1, -1)
-
-
-class ConvReluNorm(nn.Module):
- def __init__(self, in_channels, hidden_channels, out_channels, kernel_size, n_layers, p_dropout):
- super().__init__()
- self.in_channels = in_channels
- self.hidden_channels = hidden_channels
- self.out_channels = out_channels
- self.kernel_size = kernel_size
- self.n_layers = n_layers
- self.p_dropout = p_dropout
- assert n_layers > 1, "Number of layers should be larger than 0."
-
- self.conv_layers = nn.ModuleList()
- self.norm_layers = nn.ModuleList()
- self.conv_layers.append(nn.Conv1d(in_channels, hidden_channels, kernel_size, padding=kernel_size//2))
- self.norm_layers.append(LayerNorm(hidden_channels))
- self.relu_drop = nn.Sequential(
- nn.ReLU(),
- nn.Dropout(p_dropout))
- for _ in range(n_layers-1):
- self.conv_layers.append(nn.Conv1d(hidden_channels, hidden_channels, kernel_size, padding=kernel_size//2))
- self.norm_layers.append(LayerNorm(hidden_channels))
- self.proj = nn.Conv1d(hidden_channels, out_channels, 1)
- self.proj.weight.data.zero_()
- self.proj.bias.data.zero_()
-
- def forward(self, x, x_mask):
- x_org = x
- for i in range(self.n_layers):
- x = self.conv_layers[i](x * x_mask)
- x = self.norm_layers[i](x)
- x = self.relu_drop(x)
- x = x_org + self.proj(x)
- return x * x_mask
-
-
-class DDSConv(nn.Module):
- """
- Dialted and Depth-Separable Convolution
- """
- def __init__(self, channels, kernel_size, n_layers, p_dropout=0.):
- super().__init__()
- self.channels = channels
- self.kernel_size = kernel_size
- self.n_layers = n_layers
- self.p_dropout = p_dropout
-
- self.drop = nn.Dropout(p_dropout)
- self.convs_sep = nn.ModuleList()
- self.convs_1x1 = nn.ModuleList()
- self.norms_1 = nn.ModuleList()
- self.norms_2 = nn.ModuleList()
- for i in range(n_layers):
- dilation = kernel_size ** i
- padding = (kernel_size * dilation - dilation) // 2
- self.convs_sep.append(nn.Conv1d(channels, channels, kernel_size,
- groups=channels, dilation=dilation, padding=padding
- ))
- self.convs_1x1.append(nn.Conv1d(channels, channels, 1))
- self.norms_1.append(LayerNorm(channels))
- self.norms_2.append(LayerNorm(channels))
-
- def forward(self, x, x_mask, g=None):
- if g is not None:
- x = x + g
- for i in range(self.n_layers):
- y = self.convs_sep[i](x * x_mask)
- y = self.norms_1[i](y)
- y = F.gelu(y)
- y = self.convs_1x1[i](y)
- y = self.norms_2[i](y)
- y = F.gelu(y)
- y = self.drop(y)
- x = x + y
- return x * x_mask
-
-
-class WN(torch.nn.Module):
- def __init__(self, hidden_channels, kernel_size, dilation_rate, n_layers, gin_channels=0, p_dropout=0):
- super(WN, self).__init__()
- assert(kernel_size % 2 == 1)
- self.hidden_channels =hidden_channels
- self.kernel_size = kernel_size,
- self.dilation_rate = dilation_rate
- self.n_layers = n_layers
- self.gin_channels = gin_channels
- self.p_dropout = p_dropout
-
- self.in_layers = torch.nn.ModuleList()
- self.res_skip_layers = torch.nn.ModuleList()
- self.drop = nn.Dropout(p_dropout)
-
- if gin_channels != 0:
- cond_layer = torch.nn.Conv1d(gin_channels, 2*hidden_channels*n_layers, 1)
- self.cond_layer = torch.nn.utils.weight_norm(cond_layer, name='weight')
-
- for i in range(n_layers):
- dilation = dilation_rate ** i
- padding = int((kernel_size * dilation - dilation) / 2)
- in_layer = torch.nn.Conv1d(hidden_channels, 2*hidden_channels, kernel_size,
- dilation=dilation, padding=padding)
- in_layer = torch.nn.utils.weight_norm(in_layer, name='weight')
- self.in_layers.append(in_layer)
-
- # last one is not necessary
- if i < n_layers - 1:
- res_skip_channels = 2 * hidden_channels
- else:
- res_skip_channels = hidden_channels
-
- res_skip_layer = torch.nn.Conv1d(hidden_channels, res_skip_channels, 1)
- res_skip_layer = torch.nn.utils.weight_norm(res_skip_layer, name='weight')
- self.res_skip_layers.append(res_skip_layer)
-
- def forward(self, x, x_mask, g=None, **kwargs):
- output = torch.zeros_like(x)
- n_channels_tensor = torch.IntTensor([self.hidden_channels])
-
- if g is not None:
- g = self.cond_layer(g)
-
- for i in range(self.n_layers):
- x_in = self.in_layers[i](x)
- if g is not None:
- cond_offset = i * 2 * self.hidden_channels
- g_l = g[:,cond_offset:cond_offset+2*self.hidden_channels,:]
- else:
- g_l = torch.zeros_like(x_in)
-
- acts = commons.fused_add_tanh_sigmoid_multiply(
- x_in,
- g_l,
- n_channels_tensor)
- acts = self.drop(acts)
-
- res_skip_acts = self.res_skip_layers[i](acts)
- if i < self.n_layers - 1:
- res_acts = res_skip_acts[:,:self.hidden_channels,:]
- x = (x + res_acts) * x_mask
- output = output + res_skip_acts[:,self.hidden_channels:,:]
- else:
- output = output + res_skip_acts
- return output * x_mask
-
- def remove_weight_norm(self):
- if self.gin_channels != 0:
- torch.nn.utils.remove_weight_norm(self.cond_layer)
- for l in self.in_layers:
- torch.nn.utils.remove_weight_norm(l)
- for l in self.res_skip_layers:
- torch.nn.utils.remove_weight_norm(l)
-
-
-class ResBlock1(torch.nn.Module):
- def __init__(self, channels, kernel_size=3, dilation=(1, 3, 5)):
- super(ResBlock1, self).__init__()
- self.convs1 = nn.ModuleList([
- weight_norm(Conv1d(channels, channels, kernel_size, 1, dilation=dilation[0],
- padding=get_padding(kernel_size, dilation[0]))),
- weight_norm(Conv1d(channels, channels, kernel_size, 1, dilation=dilation[1],
- padding=get_padding(kernel_size, dilation[1]))),
- weight_norm(Conv1d(channels, channels, kernel_size, 1, dilation=dilation[2],
- padding=get_padding(kernel_size, dilation[2])))
- ])
- self.convs1.apply(init_weights)
-
- self.convs2 = nn.ModuleList([
- weight_norm(Conv1d(channels, channels, kernel_size, 1, dilation=1,
- padding=get_padding(kernel_size, 1))),
- weight_norm(Conv1d(channels, channels, kernel_size, 1, dilation=1,
- padding=get_padding(kernel_size, 1))),
- weight_norm(Conv1d(channels, channels, kernel_size, 1, dilation=1,
- padding=get_padding(kernel_size, 1)))
- ])
- self.convs2.apply(init_weights)
-
- def forward(self, x, x_mask=None):
- for c1, c2 in zip(self.convs1, self.convs2):
- xt = F.leaky_relu(x, LRELU_SLOPE)
- if x_mask is not None:
- xt = xt * x_mask
- xt = c1(xt)
- xt = F.leaky_relu(xt, LRELU_SLOPE)
- if x_mask is not None:
- xt = xt * x_mask
- xt = c2(xt)
- x = xt + x
- if x_mask is not None:
- x = x * x_mask
- return x
-
- def remove_weight_norm(self):
- for l in self.convs1:
- remove_weight_norm(l)
- for l in self.convs2:
- remove_weight_norm(l)
-
-
-class ResBlock2(torch.nn.Module):
- def __init__(self, channels, kernel_size=3, dilation=(1, 3)):
- super(ResBlock2, self).__init__()
- self.convs = nn.ModuleList([
- weight_norm(Conv1d(channels, channels, kernel_size, 1, dilation=dilation[0],
- padding=get_padding(kernel_size, dilation[0]))),
- weight_norm(Conv1d(channels, channels, kernel_size, 1, dilation=dilation[1],
- padding=get_padding(kernel_size, dilation[1])))
- ])
- self.convs.apply(init_weights)
-
- def forward(self, x, x_mask=None):
- for c in self.convs:
- xt = F.leaky_relu(x, LRELU_SLOPE)
- if x_mask is not None:
- xt = xt * x_mask
- xt = c(xt)
- x = xt + x
- if x_mask is not None:
- x = x * x_mask
- return x
-
- def remove_weight_norm(self):
- for l in self.convs:
- remove_weight_norm(l)
-
-
-class Log(nn.Module):
- def forward(self, x, x_mask, reverse=False, **kwargs):
- if not reverse:
- y = torch.log(torch.clamp_min(x, 1e-5)) * x_mask
- logdet = torch.sum(-y, [1, 2])
- return y, logdet
- else:
- x = torch.exp(x) * x_mask
- return x
-
-
-class Flip(nn.Module):
- def forward(self, x, *args, reverse=False, **kwargs):
- x = torch.flip(x, [1])
- if not reverse:
- logdet = torch.zeros(x.size(0)).to(dtype=x.dtype, device=x.device)
- return x, logdet
- else:
- return x
-
-
-class ElementwiseAffine(nn.Module):
- def __init__(self, channels):
- super().__init__()
- self.channels = channels
- self.m = nn.Parameter(torch.zeros(channels,1))
- self.logs = nn.Parameter(torch.zeros(channels,1))
-
- def forward(self, x, x_mask, reverse=False, **kwargs):
- if not reverse:
- y = self.m + torch.exp(self.logs) * x
- y = y * x_mask
- logdet = torch.sum(self.logs * x_mask, [1,2])
- return y, logdet
- else:
- x = (x - self.m) * torch.exp(-self.logs) * x_mask
- return x
-
-
-class ResidualCouplingLayer(nn.Module):
- def __init__(self,
- channels,
- hidden_channels,
- kernel_size,
- dilation_rate,
- n_layers,
- p_dropout=0,
- gin_channels=0,
- mean_only=False):
- assert channels % 2 == 0, "channels should be divisible by 2"
- super().__init__()
- self.channels = channels
- self.hidden_channels = hidden_channels
- self.kernel_size = kernel_size
- self.dilation_rate = dilation_rate
- self.n_layers = n_layers
- self.half_channels = channels // 2
- self.mean_only = mean_only
-
- self.pre = nn.Conv1d(self.half_channels, hidden_channels, 1)
- self.enc = WN(hidden_channels, kernel_size, dilation_rate, n_layers, p_dropout=p_dropout, gin_channels=gin_channels)
- self.post = nn.Conv1d(hidden_channels, self.half_channels * (2 - mean_only), 1)
- self.post.weight.data.zero_()
- self.post.bias.data.zero_()
-
- def forward(self, x, x_mask, g=None, reverse=False):
- x0, x1 = torch.split(x, [self.half_channels]*2, 1)
- h = self.pre(x0) * x_mask
- h = self.enc(h, x_mask, g=g)
- stats = self.post(h) * x_mask
- if not self.mean_only:
- m, logs = torch.split(stats, [self.half_channels]*2, 1)
- else:
- m = stats
- logs = torch.zeros_like(m)
-
- if not reverse:
- x1 = m + x1 * torch.exp(logs) * x_mask
- x = torch.cat([x0, x1], 1)
- logdet = torch.sum(logs, [1,2])
- return x, logdet
- else:
- x1 = (x1 - m) * torch.exp(-logs) * x_mask
- x = torch.cat([x0, x1], 1)
- return x
-
-
-class ConvFlow(nn.Module):
- def __init__(self, in_channels, filter_channels, kernel_size, n_layers, num_bins=10, tail_bound=5.0):
- super().__init__()
- self.in_channels = in_channels
- self.filter_channels = filter_channels
- self.kernel_size = kernel_size
- self.n_layers = n_layers
- self.num_bins = num_bins
- self.tail_bound = tail_bound
- self.half_channels = in_channels // 2
-
- self.pre = nn.Conv1d(self.half_channels, filter_channels, 1)
- self.convs = DDSConv(filter_channels, kernel_size, n_layers, p_dropout=0.)
- self.proj = nn.Conv1d(filter_channels, self.half_channels * (num_bins * 3 - 1), 1)
- self.proj.weight.data.zero_()
- self.proj.bias.data.zero_()
-
- def forward(self, x, x_mask, g=None, reverse=False):
- x0, x1 = torch.split(x, [self.half_channels]*2, 1)
- h = self.pre(x0)
- h = self.convs(h, x_mask, g=g)
- h = self.proj(h) * x_mask
-
- b, c, t = x0.shape
- h = h.reshape(b, c, -1, t).permute(0, 1, 3, 2) # [b, cx?, t] -> [b, c, t, ?]
-
- unnormalized_widths = h[..., :self.num_bins] / math.sqrt(self.filter_channels)
- unnormalized_heights = h[..., self.num_bins:2*self.num_bins] / math.sqrt(self.filter_channels)
- unnormalized_derivatives = h[..., 2 * self.num_bins:]
-
- x1, logabsdet = piecewise_rational_quadratic_transform(x1,
- unnormalized_widths,
- unnormalized_heights,
- unnormalized_derivatives,
- inverse=reverse,
- tails='linear',
- tail_bound=self.tail_bound
- )
-
- x = torch.cat([x0, x1], 1) * x_mask
- logdet = torch.sum(logabsdet * x_mask, [1,2])
- if not reverse:
- return x, logdet
- else:
- return x
diff --git a/spaces/kevinwang676/Bert-VITS2/text/english.py b/spaces/kevinwang676/Bert-VITS2/text/english.py
deleted file mode 100644
index 781d0a56cef71f66fc67db51d76538be90d3ddd2..0000000000000000000000000000000000000000
--- a/spaces/kevinwang676/Bert-VITS2/text/english.py
+++ /dev/null
@@ -1,138 +0,0 @@
-import pickle
-import os
-import re
-from g2p_en import G2p
-from string import punctuation
-
-from text import symbols
-
-current_file_path = os.path.dirname(__file__)
-CMU_DICT_PATH = os.path.join(current_file_path, 'cmudict.rep')
-CACHE_PATH = os.path.join(current_file_path, 'cmudict_cache.pickle')
-_g2p = G2p()
-
-arpa = {'AH0', 'S', 'AH1', 'EY2', 'AE2', 'EH0', 'OW2', 'UH0', 'NG', 'B', 'G', 'AY0', 'M', 'AA0', 'F', 'AO0', 'ER2', 'UH1', 'IY1', 'AH2', 'DH', 'IY0', 'EY1', 'IH0', 'K', 'N', 'W', 'IY2', 'T', 'AA1', 'ER1', 'EH2', 'OY0', 'UH2', 'UW1', 'Z', 'AW2', 'AW1', 'V', 'UW2', 'AA2', 'ER', 'AW0', 'UW0', 'R', 'OW1', 'EH1', 'ZH', 'AE0', 'IH2', 'IH', 'Y', 'JH', 'P', 'AY1', 'EY0', 'OY2', 'TH', 'HH', 'D', 'ER0', 'CH', 'AO1', 'AE1', 'AO2', 'OY1', 'AY2', 'IH1', 'OW0', 'L', 'SH'}
-
-
-def post_replace_ph(ph):
- rep_map = {
- ':': ',',
- ';': ',',
- ',': ',',
- '。': '.',
- '!': '!',
- '?': '?',
- '\n': '.',
- "·": ",",
- '、': ",",
- '...': '…',
- 'v': "V"
- }
- if ph in rep_map.keys():
- ph = rep_map[ph]
- if ph in symbols:
- return ph
- if ph not in symbols:
- ph = 'UNK'
- return ph
-
-def read_dict():
- g2p_dict = {}
- start_line = 49
- with open(CMU_DICT_PATH) as f:
- line = f.readline()
- line_index = 1
- while line:
- if line_index >= start_line:
- line = line.strip()
- word_split = line.split(' ')
- word = word_split[0]
-
- syllable_split = word_split[1].split(' - ')
- g2p_dict[word] = []
- for syllable in syllable_split:
- phone_split = syllable.split(' ')
- g2p_dict[word].append(phone_split)
-
- line_index = line_index + 1
- line = f.readline()
-
- return g2p_dict
-
-
-def cache_dict(g2p_dict, file_path):
- with open(file_path, 'wb') as pickle_file:
- pickle.dump(g2p_dict, pickle_file)
-
-
-def get_dict():
- if os.path.exists(CACHE_PATH):
- with open(CACHE_PATH, 'rb') as pickle_file:
- g2p_dict = pickle.load(pickle_file)
- else:
- g2p_dict = read_dict()
- cache_dict(g2p_dict, CACHE_PATH)
-
- return g2p_dict
-
-eng_dict = get_dict()
-
-def refine_ph(phn):
- tone = 0
- if re.search(r'\d$', phn):
- tone = int(phn[-1]) + 1
- phn = phn[:-1]
- return phn.lower(), tone
-
-def refine_syllables(syllables):
- tones = []
- phonemes = []
- for phn_list in syllables:
- for i in range(len(phn_list)):
- phn = phn_list[i]
- phn, tone = refine_ph(phn)
- phonemes.append(phn)
- tones.append(tone)
- return phonemes, tones
-
-
-def text_normalize(text):
- # todo: eng text normalize
- return text
-
-def g2p(text):
-
- phones = []
- tones = []
- words = re.split(r"([,;.\-\?\!\s+])", text)
- for w in words:
- if w.upper() in eng_dict:
- phns, tns = refine_syllables(eng_dict[w.upper()])
- phones += phns
- tones += tns
- else:
- phone_list = list(filter(lambda p: p != " ", _g2p(w)))
- for ph in phone_list:
- if ph in arpa:
- ph, tn = refine_ph(ph)
- phones.append(ph)
- tones.append(tn)
- else:
- phones.append(ph)
- tones.append(0)
- # todo: implement word2ph
- word2ph = [1 for i in phones]
-
- phones = [post_replace_ph(i) for i in phones]
- return phones, tones, word2ph
-
-if __name__ == "__main__":
- # print(get_dict())
- # print(eng_word_to_phoneme("hello"))
- print(g2p("In this paper, we propose 1 DSPGAN, a GAN-based universal vocoder."))
- # all_phones = set()
- # for k, syllables in eng_dict.items():
- # for group in syllables:
- # for ph in group:
- # all_phones.add(ph)
- # print(all_phones)
\ No newline at end of file
diff --git a/spaces/kevinwang676/ChatGLM2-SadTalker/src/face3d/models/__init__.py b/spaces/kevinwang676/ChatGLM2-SadTalker/src/face3d/models/__init__.py
deleted file mode 100644
index 5a7986c7ad2ec48f404adf81fea5aa06aaf1eeb4..0000000000000000000000000000000000000000
--- a/spaces/kevinwang676/ChatGLM2-SadTalker/src/face3d/models/__init__.py
+++ /dev/null
@@ -1,67 +0,0 @@
-"""This package contains modules related to objective functions, optimizations, and network architectures.
-
-To add a custom model class called 'dummy', you need to add a file called 'dummy_model.py' and define a subclass DummyModel inherited from BaseModel.
-You need to implement the following five functions:
- -- <__init__>: initialize the class; first call BaseModel.__init__(self, opt).
- -- : unpack data from dataset and apply preprocessing.
- -- : produce intermediate results.
- -- : calculate loss, gradients, and update network weights.
- -- : (optionally) add model-specific options and set default options.
-
-In the function <__init__>, you need to define four lists:
- -- self.loss_names (str list): specify the training losses that you want to plot and save.
- -- self.model_names (str list): define networks used in our training.
- -- self.visual_names (str list): specify the images that you want to display and save.
- -- self.optimizers (optimizer list): define and initialize optimizers. You can define one optimizer for each network. If two networks are updated at the same time, you can use itertools.chain to group them. See cycle_gan_model.py for an usage.
-
-Now you can use the model class by specifying flag '--model dummy'.
-See our template model class 'template_model.py' for more details.
-"""
-
-import importlib
-from src.face3d.models.base_model import BaseModel
-
-
-def find_model_using_name(model_name):
- """Import the module "models/[model_name]_model.py".
-
- In the file, the class called DatasetNameModel() will
- be instantiated. It has to be a subclass of BaseModel,
- and it is case-insensitive.
- """
- model_filename = "face3d.models." + model_name + "_model"
- modellib = importlib.import_module(model_filename)
- model = None
- target_model_name = model_name.replace('_', '') + 'model'
- for name, cls in modellib.__dict__.items():
- if name.lower() == target_model_name.lower() \
- and issubclass(cls, BaseModel):
- model = cls
-
- if model is None:
- print("In %s.py, there should be a subclass of BaseModel with class name that matches %s in lowercase." % (model_filename, target_model_name))
- exit(0)
-
- return model
-
-
-def get_option_setter(model_name):
- """Return the static method of the model class."""
- model_class = find_model_using_name(model_name)
- return model_class.modify_commandline_options
-
-
-def create_model(opt):
- """Create a model given the option.
-
- This function warps the class CustomDatasetDataLoader.
- This is the main interface between this package and 'train.py'/'test.py'
-
- Example:
- >>> from models import create_model
- >>> model = create_model(opt)
- """
- model = find_model_using_name(opt.model)
- instance = model(opt)
- print("model [%s] was created" % type(instance).__name__)
- return instance
diff --git a/spaces/kevinwang676/ChatGLM2-VC-SadTalker/src/face3d/util/__init__.py b/spaces/kevinwang676/ChatGLM2-VC-SadTalker/src/face3d/util/__init__.py
deleted file mode 100644
index 04eecb58b62f8c9d11d17606c6241d278a48b9b9..0000000000000000000000000000000000000000
--- a/spaces/kevinwang676/ChatGLM2-VC-SadTalker/src/face3d/util/__init__.py
+++ /dev/null
@@ -1,3 +0,0 @@
-"""This package includes a miscellaneous collection of useful helper functions."""
-from src.face3d.util import *
-
diff --git a/spaces/kevinwang676/SadTalker/src/face3d/options/inference_options.py b/spaces/kevinwang676/SadTalker/src/face3d/options/inference_options.py
deleted file mode 100644
index c453965959ab4cfb31acbc424f994db68c3d4df5..0000000000000000000000000000000000000000
--- a/spaces/kevinwang676/SadTalker/src/face3d/options/inference_options.py
+++ /dev/null
@@ -1,23 +0,0 @@
-from face3d.options.base_options import BaseOptions
-
-
-class InferenceOptions(BaseOptions):
- """This class includes test options.
-
- It also includes shared options defined in BaseOptions.
- """
-
- def initialize(self, parser):
- parser = BaseOptions.initialize(self, parser) # define shared options
- parser.add_argument('--phase', type=str, default='test', help='train, val, test, etc')
- parser.add_argument('--dataset_mode', type=str, default=None, help='chooses how datasets are loaded. [None | flist]')
-
- parser.add_argument('--input_dir', type=str, help='the folder of the input files')
- parser.add_argument('--keypoint_dir', type=str, help='the folder of the keypoint files')
- parser.add_argument('--output_dir', type=str, default='mp4', help='the output dir to save the extracted coefficients')
- parser.add_argument('--save_split_files', action='store_true', help='save split files or not')
- parser.add_argument('--inference_batch_size', type=int, default=8)
-
- # Dropout and Batchnorm has different behavior during training and test.
- self.isTrain = False
- return parser
diff --git a/spaces/kevinwang676/SadTalker/src/face3d/util/preprocess.py b/spaces/kevinwang676/SadTalker/src/face3d/util/preprocess.py
deleted file mode 100644
index b77a3a4058c208e5ba8cb1cfbb563954a5f7a3e2..0000000000000000000000000000000000000000
--- a/spaces/kevinwang676/SadTalker/src/face3d/util/preprocess.py
+++ /dev/null
@@ -1,103 +0,0 @@
-"""This script contains the image preprocessing code for Deep3DFaceRecon_pytorch
-"""
-
-import numpy as np
-from scipy.io import loadmat
-from PIL import Image
-import cv2
-import os
-from skimage import transform as trans
-import torch
-import warnings
-warnings.filterwarnings("ignore", category=np.VisibleDeprecationWarning)
-warnings.filterwarnings("ignore", category=FutureWarning)
-
-
-# calculating least square problem for image alignment
-def POS(xp, x):
- npts = xp.shape[1]
-
- A = np.zeros([2*npts, 8])
-
- A[0:2*npts-1:2, 0:3] = x.transpose()
- A[0:2*npts-1:2, 3] = 1
-
- A[1:2*npts:2, 4:7] = x.transpose()
- A[1:2*npts:2, 7] = 1
-
- b = np.reshape(xp.transpose(), [2*npts, 1])
-
- k, _, _, _ = np.linalg.lstsq(A, b)
-
- R1 = k[0:3]
- R2 = k[4:7]
- sTx = k[3]
- sTy = k[7]
- s = (np.linalg.norm(R1) + np.linalg.norm(R2))/2
- t = np.stack([sTx, sTy], axis=0)
-
- return t, s
-
-# resize and crop images for face reconstruction
-def resize_n_crop_img(img, lm, t, s, target_size=224., mask=None):
- w0, h0 = img.size
- w = (w0*s).astype(np.int32)
- h = (h0*s).astype(np.int32)
- left = (w/2 - target_size/2 + float((t[0] - w0/2)*s)).astype(np.int32)
- right = left + target_size
- up = (h/2 - target_size/2 + float((h0/2 - t[1])*s)).astype(np.int32)
- below = up + target_size
-
- img = img.resize((w, h), resample=Image.BICUBIC)
- img = img.crop((left, up, right, below))
-
- if mask is not None:
- mask = mask.resize((w, h), resample=Image.BICUBIC)
- mask = mask.crop((left, up, right, below))
-
- lm = np.stack([lm[:, 0] - t[0] + w0/2, lm[:, 1] -
- t[1] + h0/2], axis=1)*s
- lm = lm - np.reshape(
- np.array([(w/2 - target_size/2), (h/2-target_size/2)]), [1, 2])
-
- return img, lm, mask
-
-# utils for face reconstruction
-def extract_5p(lm):
- lm_idx = np.array([31, 37, 40, 43, 46, 49, 55]) - 1
- lm5p = np.stack([lm[lm_idx[0], :], np.mean(lm[lm_idx[[1, 2]], :], 0), np.mean(
- lm[lm_idx[[3, 4]], :], 0), lm[lm_idx[5], :], lm[lm_idx[6], :]], axis=0)
- lm5p = lm5p[[1, 2, 0, 3, 4], :]
- return lm5p
-
-# utils for face reconstruction
-def align_img(img, lm, lm3D, mask=None, target_size=224., rescale_factor=102.):
- """
- Return:
- transparams --numpy.array (raw_W, raw_H, scale, tx, ty)
- img_new --PIL.Image (target_size, target_size, 3)
- lm_new --numpy.array (68, 2), y direction is opposite to v direction
- mask_new --PIL.Image (target_size, target_size)
-
- Parameters:
- img --PIL.Image (raw_H, raw_W, 3)
- lm --numpy.array (68, 2), y direction is opposite to v direction
- lm3D --numpy.array (5, 3)
- mask --PIL.Image (raw_H, raw_W, 3)
- """
-
- w0, h0 = img.size
- if lm.shape[0] != 5:
- lm5p = extract_5p(lm)
- else:
- lm5p = lm
-
- # calculate translation and scale factors using 5 facial landmarks and standard landmarks of a 3D face
- t, s = POS(lm5p.transpose(), lm3D.transpose())
- s = rescale_factor/s
-
- # processing the image
- img_new, lm_new, mask_new = resize_n_crop_img(img, lm, t, s, target_size=target_size, mask=mask)
- trans_params = np.array([w0, h0, s, t[0], t[1]])
-
- return trans_params, img_new, lm_new, mask_new
diff --git a/spaces/kevinwang676/VoiceChanger/infer_pack/modules/F0Predictor/PMF0Predictor.py b/spaces/kevinwang676/VoiceChanger/infer_pack/modules/F0Predictor/PMF0Predictor.py
deleted file mode 100644
index ab523020325fa3f30676ad20125c6a9f059a9d84..0000000000000000000000000000000000000000
--- a/spaces/kevinwang676/VoiceChanger/infer_pack/modules/F0Predictor/PMF0Predictor.py
+++ /dev/null
@@ -1,97 +0,0 @@
-from infer_pack.modules.F0Predictor.F0Predictor import F0Predictor
-import parselmouth
-import numpy as np
-
-
-class PMF0Predictor(F0Predictor):
- def __init__(self, hop_length=512, f0_min=50, f0_max=1100, sampling_rate=44100):
- self.hop_length = hop_length
- self.f0_min = f0_min
- self.f0_max = f0_max
- self.sampling_rate = sampling_rate
-
- def interpolate_f0(self, f0):
- """
- 对F0进行插值处理
- """
-
- data = np.reshape(f0, (f0.size, 1))
-
- vuv_vector = np.zeros((data.size, 1), dtype=np.float32)
- vuv_vector[data > 0.0] = 1.0
- vuv_vector[data <= 0.0] = 0.0
-
- ip_data = data
-
- frame_number = data.size
- last_value = 0.0
- for i in range(frame_number):
- if data[i] <= 0.0:
- j = i + 1
- for j in range(i + 1, frame_number):
- if data[j] > 0.0:
- break
- if j < frame_number - 1:
- if last_value > 0.0:
- step = (data[j] - data[i - 1]) / float(j - i)
- for k in range(i, j):
- ip_data[k] = data[i - 1] + step * (k - i + 1)
- else:
- for k in range(i, j):
- ip_data[k] = data[j]
- else:
- for k in range(i, frame_number):
- ip_data[k] = last_value
- else:
- ip_data[i] = data[i] # 这里可能存在一个没有必要的拷贝
- last_value = data[i]
-
- return ip_data[:, 0], vuv_vector[:, 0]
-
- def compute_f0(self, wav, p_len=None):
- x = wav
- if p_len is None:
- p_len = x.shape[0] // self.hop_length
- else:
- assert abs(p_len - x.shape[0] // self.hop_length) < 4, "pad length error"
- time_step = self.hop_length / self.sampling_rate * 1000
- f0 = (
- parselmouth.Sound(x, self.sampling_rate)
- .to_pitch_ac(
- time_step=time_step / 1000,
- voicing_threshold=0.6,
- pitch_floor=self.f0_min,
- pitch_ceiling=self.f0_max,
- )
- .selected_array["frequency"]
- )
-
- pad_size = (p_len - len(f0) + 1) // 2
- if pad_size > 0 or p_len - len(f0) - pad_size > 0:
- f0 = np.pad(f0, [[pad_size, p_len - len(f0) - pad_size]], mode="constant")
- f0, uv = self.interpolate_f0(f0)
- return f0
-
- def compute_f0_uv(self, wav, p_len=None):
- x = wav
- if p_len is None:
- p_len = x.shape[0] // self.hop_length
- else:
- assert abs(p_len - x.shape[0] // self.hop_length) < 4, "pad length error"
- time_step = self.hop_length / self.sampling_rate * 1000
- f0 = (
- parselmouth.Sound(x, self.sampling_rate)
- .to_pitch_ac(
- time_step=time_step / 1000,
- voicing_threshold=0.6,
- pitch_floor=self.f0_min,
- pitch_ceiling=self.f0_max,
- )
- .selected_array["frequency"]
- )
-
- pad_size = (p_len - len(f0) + 1) // 2
- if pad_size > 0 or p_len - len(f0) - pad_size > 0:
- f0 = np.pad(f0, [[pad_size, p_len - len(f0) - pad_size]], mode="constant")
- f0, uv = self.interpolate_f0(f0)
- return f0, uv
diff --git a/spaces/kevinwang676/VoiceChanger/src/audio2pose_models/cvae.py b/spaces/kevinwang676/VoiceChanger/src/audio2pose_models/cvae.py
deleted file mode 100644
index d017ce865a03bae40dfe066dbcd82e29839d89dc..0000000000000000000000000000000000000000
--- a/spaces/kevinwang676/VoiceChanger/src/audio2pose_models/cvae.py
+++ /dev/null
@@ -1,149 +0,0 @@
-import torch
-import torch.nn.functional as F
-from torch import nn
-from src.audio2pose_models.res_unet import ResUnet
-
-def class2onehot(idx, class_num):
-
- assert torch.max(idx).item() < class_num
- onehot = torch.zeros(idx.size(0), class_num).to(idx.device)
- onehot.scatter_(1, idx, 1)
- return onehot
-
-class CVAE(nn.Module):
- def __init__(self, cfg):
- super().__init__()
- encoder_layer_sizes = cfg.MODEL.CVAE.ENCODER_LAYER_SIZES
- decoder_layer_sizes = cfg.MODEL.CVAE.DECODER_LAYER_SIZES
- latent_size = cfg.MODEL.CVAE.LATENT_SIZE
- num_classes = cfg.DATASET.NUM_CLASSES
- audio_emb_in_size = cfg.MODEL.CVAE.AUDIO_EMB_IN_SIZE
- audio_emb_out_size = cfg.MODEL.CVAE.AUDIO_EMB_OUT_SIZE
- seq_len = cfg.MODEL.CVAE.SEQ_LEN
-
- self.latent_size = latent_size
-
- self.encoder = ENCODER(encoder_layer_sizes, latent_size, num_classes,
- audio_emb_in_size, audio_emb_out_size, seq_len)
- self.decoder = DECODER(decoder_layer_sizes, latent_size, num_classes,
- audio_emb_in_size, audio_emb_out_size, seq_len)
- def reparameterize(self, mu, logvar):
- std = torch.exp(0.5 * logvar)
- eps = torch.randn_like(std)
- return mu + eps * std
-
- def forward(self, batch):
- batch = self.encoder(batch)
- mu = batch['mu']
- logvar = batch['logvar']
- z = self.reparameterize(mu, logvar)
- batch['z'] = z
- return self.decoder(batch)
-
- def test(self, batch):
- '''
- class_id = batch['class']
- z = torch.randn([class_id.size(0), self.latent_size]).to(class_id.device)
- batch['z'] = z
- '''
- return self.decoder(batch)
-
-class ENCODER(nn.Module):
- def __init__(self, layer_sizes, latent_size, num_classes,
- audio_emb_in_size, audio_emb_out_size, seq_len):
- super().__init__()
-
- self.resunet = ResUnet()
- self.num_classes = num_classes
- self.seq_len = seq_len
-
- self.MLP = nn.Sequential()
- layer_sizes[0] += latent_size + seq_len*audio_emb_out_size + 6
- for i, (in_size, out_size) in enumerate(zip(layer_sizes[:-1], layer_sizes[1:])):
- self.MLP.add_module(
- name="L{:d}".format(i), module=nn.Linear(in_size, out_size))
- self.MLP.add_module(name="A{:d}".format(i), module=nn.ReLU())
-
- self.linear_means = nn.Linear(layer_sizes[-1], latent_size)
- self.linear_logvar = nn.Linear(layer_sizes[-1], latent_size)
- self.linear_audio = nn.Linear(audio_emb_in_size, audio_emb_out_size)
-
- self.classbias = nn.Parameter(torch.randn(self.num_classes, latent_size))
-
- def forward(self, batch):
- class_id = batch['class']
- pose_motion_gt = batch['pose_motion_gt'] #bs seq_len 6
- ref = batch['ref'] #bs 6
- bs = pose_motion_gt.shape[0]
- audio_in = batch['audio_emb'] # bs seq_len audio_emb_in_size
-
- #pose encode
- pose_emb = self.resunet(pose_motion_gt.unsqueeze(1)) #bs 1 seq_len 6
- pose_emb = pose_emb.reshape(bs, -1) #bs seq_len*6
-
- #audio mapping
- print(audio_in.shape)
- audio_out = self.linear_audio(audio_in) # bs seq_len audio_emb_out_size
- audio_out = audio_out.reshape(bs, -1)
-
- class_bias = self.classbias[class_id] #bs latent_size
- x_in = torch.cat([ref, pose_emb, audio_out, class_bias], dim=-1) #bs seq_len*(audio_emb_out_size+6)+latent_size
- x_out = self.MLP(x_in)
-
- mu = self.linear_means(x_out)
- logvar = self.linear_means(x_out) #bs latent_size
-
- batch.update({'mu':mu, 'logvar':logvar})
- return batch
-
-class DECODER(nn.Module):
- def __init__(self, layer_sizes, latent_size, num_classes,
- audio_emb_in_size, audio_emb_out_size, seq_len):
- super().__init__()
-
- self.resunet = ResUnet()
- self.num_classes = num_classes
- self.seq_len = seq_len
-
- self.MLP = nn.Sequential()
- input_size = latent_size + seq_len*audio_emb_out_size + 6
- for i, (in_size, out_size) in enumerate(zip([input_size]+layer_sizes[:-1], layer_sizes)):
- self.MLP.add_module(
- name="L{:d}".format(i), module=nn.Linear(in_size, out_size))
- if i+1 < len(layer_sizes):
- self.MLP.add_module(name="A{:d}".format(i), module=nn.ReLU())
- else:
- self.MLP.add_module(name="sigmoid", module=nn.Sigmoid())
-
- self.pose_linear = nn.Linear(6, 6)
- self.linear_audio = nn.Linear(audio_emb_in_size, audio_emb_out_size)
-
- self.classbias = nn.Parameter(torch.randn(self.num_classes, latent_size))
-
- def forward(self, batch):
-
- z = batch['z'] #bs latent_size
- bs = z.shape[0]
- class_id = batch['class']
- ref = batch['ref'] #bs 6
- audio_in = batch['audio_emb'] # bs seq_len audio_emb_in_size
- #print('audio_in: ', audio_in[:, :, :10])
-
- audio_out = self.linear_audio(audio_in) # bs seq_len audio_emb_out_size
- #print('audio_out: ', audio_out[:, :, :10])
- audio_out = audio_out.reshape([bs, -1]) # bs seq_len*audio_emb_out_size
- class_bias = self.classbias[class_id] #bs latent_size
-
- z = z + class_bias
- x_in = torch.cat([ref, z, audio_out], dim=-1)
- x_out = self.MLP(x_in) # bs layer_sizes[-1]
- x_out = x_out.reshape((bs, self.seq_len, -1))
-
- #print('x_out: ', x_out)
-
- pose_emb = self.resunet(x_out.unsqueeze(1)) #bs 1 seq_len 6
-
- pose_motion_pred = self.pose_linear(pose_emb.squeeze(1)) #bs seq_len 6
-
- batch.update({'pose_motion_pred':pose_motion_pred})
- return batch
diff --git a/spaces/kirch/Text2Video-Zero/annotator/uniformer/mmseg/models/decode_heads/cc_head.py b/spaces/kirch/Text2Video-Zero/annotator/uniformer/mmseg/models/decode_heads/cc_head.py
deleted file mode 100644
index 5b9abb4e747f92657f4220b29788539340986c00..0000000000000000000000000000000000000000
--- a/spaces/kirch/Text2Video-Zero/annotator/uniformer/mmseg/models/decode_heads/cc_head.py
+++ /dev/null
@@ -1,42 +0,0 @@
-import torch
-
-from ..builder import HEADS
-from .fcn_head import FCNHead
-
-try:
- from annotator.uniformer.mmcv.ops import CrissCrossAttention
-except ModuleNotFoundError:
- CrissCrossAttention = None
-
-
-@HEADS.register_module()
-class CCHead(FCNHead):
- """CCNet: Criss-Cross Attention for Semantic Segmentation.
-
- This head is the implementation of `CCNet
- `_.
-
- Args:
- recurrence (int): Number of recurrence of Criss Cross Attention
- module. Default: 2.
- """
-
- def __init__(self, recurrence=2, **kwargs):
- if CrissCrossAttention is None:
- raise RuntimeError('Please install mmcv-full for '
- 'CrissCrossAttention ops')
- super(CCHead, self).__init__(num_convs=2, **kwargs)
- self.recurrence = recurrence
- self.cca = CrissCrossAttention(self.channels)
-
- def forward(self, inputs):
- """Forward function."""
- x = self._transform_inputs(inputs)
- output = self.convs[0](x)
- for _ in range(self.recurrence):
- output = self.cca(output)
- output = self.convs[1](output)
- if self.concat_input:
- output = self.conv_cat(torch.cat([x, output], dim=1))
- output = self.cls_seg(output)
- return output
diff --git a/spaces/koajoel/PolyFormer/fairseq/examples/speech_synthesis/README.md b/spaces/koajoel/PolyFormer/fairseq/examples/speech_synthesis/README.md
deleted file mode 100644
index 4a3ae54b857c43621c9fb67ee4b214584beec835..0000000000000000000000000000000000000000
--- a/spaces/koajoel/PolyFormer/fairseq/examples/speech_synthesis/README.md
+++ /dev/null
@@ -1,16 +0,0 @@
-Speech Synthesis (S^2)
-===
-
-Speech synthesis with fairseq.
-
-- Autoregressive and non-autoregressive models
-- Multi-speaker synthesis
-- Audio preprocessing
-- Automatic metrics
-- Similar data configuration as [S2T](../speech_to_text/README.md)
-
-
-## Examples
-- [Single-speaker synthesis on LJSpeech](docs/ljspeech_example.md)
-- [Multi-speaker synthesis on VCTK](docs/vctk_example.md)
-- [Multi-speaker synthesis on Common Voice](docs/common_voice_example.md)
diff --git a/spaces/kquote03/lama-video-watermark-remover/saicinpainting/training/modules/ffc.py b/spaces/kquote03/lama-video-watermark-remover/saicinpainting/training/modules/ffc.py
deleted file mode 100644
index 0e7b84683fccb4bccac97b6371994fa6bb44dbe4..0000000000000000000000000000000000000000
--- a/spaces/kquote03/lama-video-watermark-remover/saicinpainting/training/modules/ffc.py
+++ /dev/null
@@ -1,485 +0,0 @@
-# Fast Fourier Convolution NeurIPS 2020
-# original implementation https://github.com/pkumivision/FFC/blob/main/model_zoo/ffc.py
-# paper https://proceedings.neurips.cc/paper/2020/file/2fd5d41ec6cfab47e32164d5624269b1-Paper.pdf
-
-import numpy as np
-import torch
-import torch.nn as nn
-import torch.nn.functional as F
-
-from saicinpainting.training.modules.base import get_activation, BaseDiscriminator
-from saicinpainting.training.modules.spatial_transform import LearnableSpatialTransformWrapper
-from saicinpainting.training.modules.squeeze_excitation import SELayer
-from saicinpainting.utils import get_shape
-
-
-class FFCSE_block(nn.Module):
-
- def __init__(self, channels, ratio_g):
- super(FFCSE_block, self).__init__()
- in_cg = int(channels * ratio_g)
- in_cl = channels - in_cg
- r = 16
-
- self.avgpool = nn.AdaptiveAvgPool2d((1, 1))
- self.conv1 = nn.Conv2d(channels, channels // r,
- kernel_size=1, bias=True)
- self.relu1 = nn.ReLU(inplace=True)
- self.conv_a2l = None if in_cl == 0 else nn.Conv2d(
- channels // r, in_cl, kernel_size=1, bias=True)
- self.conv_a2g = None if in_cg == 0 else nn.Conv2d(
- channels // r, in_cg, kernel_size=1, bias=True)
- self.sigmoid = nn.Sigmoid()
-
- def forward(self, x):
- x = x if type(x) is tuple else (x, 0)
- id_l, id_g = x
-
- x = id_l if type(id_g) is int else torch.cat([id_l, id_g], dim=1)
- x = self.avgpool(x)
- x = self.relu1(self.conv1(x))
-
- x_l = 0 if self.conv_a2l is None else id_l * \
- self.sigmoid(self.conv_a2l(x))
- x_g = 0 if self.conv_a2g is None else id_g * \
- self.sigmoid(self.conv_a2g(x))
- return x_l, x_g
-
-
-class FourierUnit(nn.Module):
-
- def __init__(self, in_channels, out_channels, groups=1, spatial_scale_factor=None, spatial_scale_mode='bilinear',
- spectral_pos_encoding=False, use_se=False, se_kwargs=None, ffc3d=False, fft_norm='ortho'):
- # bn_layer not used
- super(FourierUnit, self).__init__()
- self.groups = groups
-
- self.conv_layer = torch.nn.Conv2d(in_channels=in_channels * 2 + (2 if spectral_pos_encoding else 0),
- out_channels=out_channels * 2,
- kernel_size=1, stride=1, padding=0, groups=self.groups, bias=False)
- self.bn = torch.nn.BatchNorm2d(out_channels * 2)
- self.relu = torch.nn.ReLU(inplace=True)
-
- # squeeze and excitation block
- self.use_se = use_se
- if use_se:
- if se_kwargs is None:
- se_kwargs = {}
- self.se = SELayer(self.conv_layer.in_channels, **se_kwargs)
-
- self.spatial_scale_factor = spatial_scale_factor
- self.spatial_scale_mode = spatial_scale_mode
- self.spectral_pos_encoding = spectral_pos_encoding
- self.ffc3d = ffc3d
- self.fft_norm = fft_norm
-
- def forward(self, x):
- batch = x.shape[0]
-
- if self.spatial_scale_factor is not None:
- orig_size = x.shape[-2:]
- x = F.interpolate(x, scale_factor=self.spatial_scale_factor, mode=self.spatial_scale_mode, align_corners=False)
-
- r_size = x.size()
- # (batch, c, h, w/2+1, 2)
- fft_dim = (-3, -2, -1) if self.ffc3d else (-2, -1)
- ffted = torch.fft.rfftn(x, dim=fft_dim, norm=self.fft_norm)
- ffted = torch.stack((ffted.real, ffted.imag), dim=-1)
- ffted = ffted.permute(0, 1, 4, 2, 3).contiguous() # (batch, c, 2, h, w/2+1)
- ffted = ffted.view((batch, -1,) + ffted.size()[3:])
-
- if self.spectral_pos_encoding:
- height, width = ffted.shape[-2:]
- coords_vert = torch.linspace(0, 1, height)[None, None, :, None].expand(batch, 1, height, width).to(ffted)
- coords_hor = torch.linspace(0, 1, width)[None, None, None, :].expand(batch, 1, height, width).to(ffted)
- ffted = torch.cat((coords_vert, coords_hor, ffted), dim=1)
-
- if self.use_se:
- ffted = self.se(ffted)
-
- ffted = self.conv_layer(ffted) # (batch, c*2, h, w/2+1)
- ffted = self.relu(self.bn(ffted))
-
- ffted = ffted.view((batch, -1, 2,) + ffted.size()[2:]).permute(
- 0, 1, 3, 4, 2).contiguous() # (batch,c, t, h, w/2+1, 2)
- ffted = torch.complex(ffted[..., 0], ffted[..., 1])
-
- ifft_shape_slice = x.shape[-3:] if self.ffc3d else x.shape[-2:]
- output = torch.fft.irfftn(ffted, s=ifft_shape_slice, dim=fft_dim, norm=self.fft_norm)
-
- if self.spatial_scale_factor is not None:
- output = F.interpolate(output, size=orig_size, mode=self.spatial_scale_mode, align_corners=False)
-
- return output
-
-
-class SeparableFourierUnit(nn.Module):
-
- def __init__(self, in_channels, out_channels, groups=1, kernel_size=3):
- # bn_layer not used
- super(SeparableFourierUnit, self).__init__()
- self.groups = groups
- row_out_channels = out_channels // 2
- col_out_channels = out_channels - row_out_channels
- self.row_conv = torch.nn.Conv2d(in_channels=in_channels * 2,
- out_channels=row_out_channels * 2,
- kernel_size=(kernel_size, 1), # kernel size is always like this, but the data will be transposed
- stride=1, padding=(kernel_size // 2, 0),
- padding_mode='reflect',
- groups=self.groups, bias=False)
- self.col_conv = torch.nn.Conv2d(in_channels=in_channels * 2,
- out_channels=col_out_channels * 2,
- kernel_size=(kernel_size, 1), # kernel size is always like this, but the data will be transposed
- stride=1, padding=(kernel_size // 2, 0),
- padding_mode='reflect',
- groups=self.groups, bias=False)
- self.row_bn = torch.nn.BatchNorm2d(row_out_channels * 2)
- self.col_bn = torch.nn.BatchNorm2d(col_out_channels * 2)
- self.relu = torch.nn.ReLU(inplace=True)
-
- def process_branch(self, x, conv, bn):
- batch = x.shape[0]
-
- r_size = x.size()
- # (batch, c, h, w/2+1, 2)
- ffted = torch.fft.rfft(x, norm="ortho")
- ffted = torch.stack((ffted.real, ffted.imag), dim=-1)
- ffted = ffted.permute(0, 1, 4, 2, 3).contiguous() # (batch, c, 2, h, w/2+1)
- ffted = ffted.view((batch, -1,) + ffted.size()[3:])
-
- ffted = self.relu(bn(conv(ffted)))
-
- ffted = ffted.view((batch, -1, 2,) + ffted.size()[2:]).permute(
- 0, 1, 3, 4, 2).contiguous() # (batch,c, t, h, w/2+1, 2)
- ffted = torch.complex(ffted[..., 0], ffted[..., 1])
-
- output = torch.fft.irfft(ffted, s=x.shape[-1:], norm="ortho")
- return output
-
-
- def forward(self, x):
- rowwise = self.process_branch(x, self.row_conv, self.row_bn)
- colwise = self.process_branch(x.permute(0, 1, 3, 2), self.col_conv, self.col_bn).permute(0, 1, 3, 2)
- out = torch.cat((rowwise, colwise), dim=1)
- return out
-
-
-class SpectralTransform(nn.Module):
-
- def __init__(self, in_channels, out_channels, stride=1, groups=1, enable_lfu=True, separable_fu=False, **fu_kwargs):
- # bn_layer not used
- super(SpectralTransform, self).__init__()
- self.enable_lfu = enable_lfu
- if stride == 2:
- self.downsample = nn.AvgPool2d(kernel_size=(2, 2), stride=2)
- else:
- self.downsample = nn.Identity()
-
- self.stride = stride
- self.conv1 = nn.Sequential(
- nn.Conv2d(in_channels, out_channels //
- 2, kernel_size=1, groups=groups, bias=False),
- nn.BatchNorm2d(out_channels // 2),
- nn.ReLU(inplace=True)
- )
- fu_class = SeparableFourierUnit if separable_fu else FourierUnit
- self.fu = fu_class(
- out_channels // 2, out_channels // 2, groups, **fu_kwargs)
- if self.enable_lfu:
- self.lfu = fu_class(
- out_channels // 2, out_channels // 2, groups)
- self.conv2 = torch.nn.Conv2d(
- out_channels // 2, out_channels, kernel_size=1, groups=groups, bias=False)
-
- def forward(self, x):
-
- x = self.downsample(x)
- x = self.conv1(x)
- output = self.fu(x)
-
- if self.enable_lfu:
- n, c, h, w = x.shape
- split_no = 2
- split_s = h // split_no
- xs = torch.cat(torch.split(
- x[:, :c // 4], split_s, dim=-2), dim=1).contiguous()
- xs = torch.cat(torch.split(xs, split_s, dim=-1),
- dim=1).contiguous()
- xs = self.lfu(xs)
- xs = xs.repeat(1, 1, split_no, split_no).contiguous()
- else:
- xs = 0
-
- output = self.conv2(x + output + xs)
-
- return output
-
-
-class FFC(nn.Module):
-
- def __init__(self, in_channels, out_channels, kernel_size,
- ratio_gin, ratio_gout, stride=1, padding=0,
- dilation=1, groups=1, bias=False, enable_lfu=True,
- padding_type='reflect', gated=False, **spectral_kwargs):
- super(FFC, self).__init__()
-
- assert stride == 1 or stride == 2, "Stride should be 1 or 2."
- self.stride = stride
-
- in_cg = int(in_channels * ratio_gin)
- in_cl = in_channels - in_cg
- out_cg = int(out_channels * ratio_gout)
- out_cl = out_channels - out_cg
- #groups_g = 1 if groups == 1 else int(groups * ratio_gout)
- #groups_l = 1 if groups == 1 else groups - groups_g
-
- self.ratio_gin = ratio_gin
- self.ratio_gout = ratio_gout
- self.global_in_num = in_cg
-
- module = nn.Identity if in_cl == 0 or out_cl == 0 else nn.Conv2d
- self.convl2l = module(in_cl, out_cl, kernel_size,
- stride, padding, dilation, groups, bias, padding_mode=padding_type)
- module = nn.Identity if in_cl == 0 or out_cg == 0 else nn.Conv2d
- self.convl2g = module(in_cl, out_cg, kernel_size,
- stride, padding, dilation, groups, bias, padding_mode=padding_type)
- module = nn.Identity if in_cg == 0 or out_cl == 0 else nn.Conv2d
- self.convg2l = module(in_cg, out_cl, kernel_size,
- stride, padding, dilation, groups, bias, padding_mode=padding_type)
- module = nn.Identity if in_cg == 0 or out_cg == 0 else SpectralTransform
- self.convg2g = module(
- in_cg, out_cg, stride, 1 if groups == 1 else groups // 2, enable_lfu, **spectral_kwargs)
-
- self.gated = gated
- module = nn.Identity if in_cg == 0 or out_cl == 0 or not self.gated else nn.Conv2d
- self.gate = module(in_channels, 2, 1)
-
- def forward(self, x):
- x_l, x_g = x if type(x) is tuple else (x, 0)
- out_xl, out_xg = 0, 0
-
- if self.gated:
- total_input_parts = [x_l]
- if torch.is_tensor(x_g):
- total_input_parts.append(x_g)
- total_input = torch.cat(total_input_parts, dim=1)
-
- gates = torch.sigmoid(self.gate(total_input))
- g2l_gate, l2g_gate = gates.chunk(2, dim=1)
- else:
- g2l_gate, l2g_gate = 1, 1
-
- if self.ratio_gout != 1:
- out_xl = self.convl2l(x_l) + self.convg2l(x_g) * g2l_gate
- if self.ratio_gout != 0:
- out_xg = self.convl2g(x_l) * l2g_gate + self.convg2g(x_g)
-
- return out_xl, out_xg
-
-
-class FFC_BN_ACT(nn.Module):
-
- def __init__(self, in_channels, out_channels,
- kernel_size, ratio_gin, ratio_gout,
- stride=1, padding=0, dilation=1, groups=1, bias=False,
- norm_layer=nn.BatchNorm2d, activation_layer=nn.Identity,
- padding_type='reflect',
- enable_lfu=True, **kwargs):
- super(FFC_BN_ACT, self).__init__()
- self.ffc = FFC(in_channels, out_channels, kernel_size,
- ratio_gin, ratio_gout, stride, padding, dilation,
- groups, bias, enable_lfu, padding_type=padding_type, **kwargs)
- lnorm = nn.Identity if ratio_gout == 1 else norm_layer
- gnorm = nn.Identity if ratio_gout == 0 else norm_layer
- global_channels = int(out_channels * ratio_gout)
- self.bn_l = lnorm(out_channels - global_channels)
- self.bn_g = gnorm(global_channels)
-
- lact = nn.Identity if ratio_gout == 1 else activation_layer
- gact = nn.Identity if ratio_gout == 0 else activation_layer
- self.act_l = lact(inplace=True)
- self.act_g = gact(inplace=True)
-
- def forward(self, x):
- x_l, x_g = self.ffc(x)
- x_l = self.act_l(self.bn_l(x_l))
- x_g = self.act_g(self.bn_g(x_g))
- return x_l, x_g
-
-
-class FFCResnetBlock(nn.Module):
- def __init__(self, dim, padding_type, norm_layer, activation_layer=nn.ReLU, dilation=1,
- spatial_transform_kwargs=None, inline=False, **conv_kwargs):
- super().__init__()
- self.conv1 = FFC_BN_ACT(dim, dim, kernel_size=3, padding=dilation, dilation=dilation,
- norm_layer=norm_layer,
- activation_layer=activation_layer,
- padding_type=padding_type,
- **conv_kwargs)
- self.conv2 = FFC_BN_ACT(dim, dim, kernel_size=3, padding=dilation, dilation=dilation,
- norm_layer=norm_layer,
- activation_layer=activation_layer,
- padding_type=padding_type,
- **conv_kwargs)
- if spatial_transform_kwargs is not None:
- self.conv1 = LearnableSpatialTransformWrapper(self.conv1, **spatial_transform_kwargs)
- self.conv2 = LearnableSpatialTransformWrapper(self.conv2, **spatial_transform_kwargs)
- self.inline = inline
-
- def forward(self, x):
- if self.inline:
- x_l, x_g = x[:, :-self.conv1.ffc.global_in_num], x[:, -self.conv1.ffc.global_in_num:]
- else:
- x_l, x_g = x if type(x) is tuple else (x, 0)
-
- id_l, id_g = x_l, x_g
-
- x_l, x_g = self.conv1((x_l, x_g))
- x_l, x_g = self.conv2((x_l, x_g))
-
- x_l, x_g = id_l + x_l, id_g + x_g
- out = x_l, x_g
- if self.inline:
- out = torch.cat(out, dim=1)
- return out
-
-
-class ConcatTupleLayer(nn.Module):
- def forward(self, x):
- assert isinstance(x, tuple)
- x_l, x_g = x
- assert torch.is_tensor(x_l) or torch.is_tensor(x_g)
- if not torch.is_tensor(x_g):
- return x_l
- return torch.cat(x, dim=1)
-
-
-class FFCResNetGenerator(nn.Module):
- def __init__(self, input_nc, output_nc, ngf=64, n_downsampling=3, n_blocks=9, norm_layer=nn.BatchNorm2d,
- padding_type='reflect', activation_layer=nn.ReLU,
- up_norm_layer=nn.BatchNorm2d, up_activation=nn.ReLU(True),
- init_conv_kwargs={}, downsample_conv_kwargs={}, resnet_conv_kwargs={},
- spatial_transform_layers=None, spatial_transform_kwargs={},
- add_out_act=True, max_features=1024, out_ffc=False, out_ffc_kwargs={}):
- assert (n_blocks >= 0)
- super().__init__()
-
- model = [nn.ReflectionPad2d(3),
- FFC_BN_ACT(input_nc, ngf, kernel_size=7, padding=0, norm_layer=norm_layer,
- activation_layer=activation_layer, **init_conv_kwargs)]
-
- ### downsample
- for i in range(n_downsampling):
- mult = 2 ** i
- if i == n_downsampling - 1:
- cur_conv_kwargs = dict(downsample_conv_kwargs)
- cur_conv_kwargs['ratio_gout'] = resnet_conv_kwargs.get('ratio_gin', 0)
- else:
- cur_conv_kwargs = downsample_conv_kwargs
- model += [FFC_BN_ACT(min(max_features, ngf * mult),
- min(max_features, ngf * mult * 2),
- kernel_size=3, stride=2, padding=1,
- norm_layer=norm_layer,
- activation_layer=activation_layer,
- **cur_conv_kwargs)]
-
- mult = 2 ** n_downsampling
- feats_num_bottleneck = min(max_features, ngf * mult)
-
- ### resnet blocks
- for i in range(n_blocks):
- cur_resblock = FFCResnetBlock(feats_num_bottleneck, padding_type=padding_type, activation_layer=activation_layer,
- norm_layer=norm_layer, **resnet_conv_kwargs)
- if spatial_transform_layers is not None and i in spatial_transform_layers:
- cur_resblock = LearnableSpatialTransformWrapper(cur_resblock, **spatial_transform_kwargs)
- model += [cur_resblock]
-
- model += [ConcatTupleLayer()]
-
- ### upsample
- for i in range(n_downsampling):
- mult = 2 ** (n_downsampling - i)
- model += [nn.ConvTranspose2d(min(max_features, ngf * mult),
- min(max_features, int(ngf * mult / 2)),
- kernel_size=3, stride=2, padding=1, output_padding=1),
- up_norm_layer(min(max_features, int(ngf * mult / 2))),
- up_activation]
-
- if out_ffc:
- model += [FFCResnetBlock(ngf, padding_type=padding_type, activation_layer=activation_layer,
- norm_layer=norm_layer, inline=True, **out_ffc_kwargs)]
-
- model += [nn.ReflectionPad2d(3),
- nn.Conv2d(ngf, output_nc, kernel_size=7, padding=0)]
- if add_out_act:
- model.append(get_activation('tanh' if add_out_act is True else add_out_act))
- self.model = nn.Sequential(*model)
-
- def forward(self, input):
- return self.model(input)
-
-
-class FFCNLayerDiscriminator(BaseDiscriminator):
- def __init__(self, input_nc, ndf=64, n_layers=3, norm_layer=nn.BatchNorm2d, max_features=512,
- init_conv_kwargs={}, conv_kwargs={}):
- super().__init__()
- self.n_layers = n_layers
-
- def _act_ctor(inplace=True):
- return nn.LeakyReLU(negative_slope=0.2, inplace=inplace)
-
- kw = 3
- padw = int(np.ceil((kw-1.0)/2))
- sequence = [[FFC_BN_ACT(input_nc, ndf, kernel_size=kw, padding=padw, norm_layer=norm_layer,
- activation_layer=_act_ctor, **init_conv_kwargs)]]
-
- nf = ndf
- for n in range(1, n_layers):
- nf_prev = nf
- nf = min(nf * 2, max_features)
-
- cur_model = [
- FFC_BN_ACT(nf_prev, nf,
- kernel_size=kw, stride=2, padding=padw,
- norm_layer=norm_layer,
- activation_layer=_act_ctor,
- **conv_kwargs)
- ]
- sequence.append(cur_model)
-
- nf_prev = nf
- nf = min(nf * 2, 512)
-
- cur_model = [
- FFC_BN_ACT(nf_prev, nf,
- kernel_size=kw, stride=1, padding=padw,
- norm_layer=norm_layer,
- activation_layer=lambda *args, **kwargs: nn.LeakyReLU(*args, negative_slope=0.2, **kwargs),
- **conv_kwargs),
- ConcatTupleLayer()
- ]
- sequence.append(cur_model)
-
- sequence += [[nn.Conv2d(nf, 1, kernel_size=kw, stride=1, padding=padw)]]
-
- for n in range(len(sequence)):
- setattr(self, 'model'+str(n), nn.Sequential(*sequence[n]))
-
- def get_all_activations(self, x):
- res = [x]
- for n in range(self.n_layers + 2):
- model = getattr(self, 'model' + str(n))
- res.append(model(res[-1]))
- return res[1:]
-
- def forward(self, x):
- act = self.get_all_activations(x)
- feats = []
- for out in act[:-1]:
- if isinstance(out, tuple):
- if torch.is_tensor(out[1]):
- out = torch.cat(out, dim=1)
- else:
- out = out[0]
- feats.append(out)
- return act[-1], feats
diff --git a/spaces/ky2k/Toxicity_Classifier_POC/.venv/lib/python3.9/site-packages/fontTools/ttLib/__init__.py b/spaces/ky2k/Toxicity_Classifier_POC/.venv/lib/python3.9/site-packages/fontTools/ttLib/__init__.py
deleted file mode 100644
index ed00764f7c193ca9bcd0bf67196da59c30048a28..0000000000000000000000000000000000000000
--- a/spaces/ky2k/Toxicity_Classifier_POC/.venv/lib/python3.9/site-packages/fontTools/ttLib/__init__.py
+++ /dev/null
@@ -1,26 +0,0 @@
-"""fontTools.ttLib -- a package for dealing with TrueType fonts."""
-
-from fontTools.misc.loggingTools import deprecateFunction
-import logging
-
-
-log = logging.getLogger(__name__)
-
-
-class TTLibError(Exception):
- pass
-
-
-class TTLibFileIsCollectionError(TTLibError):
- pass
-
-
-@deprecateFunction("use logging instead", category=DeprecationWarning)
-def debugmsg(msg):
- import time
-
- print(msg + time.strftime(" (%H:%M:%S)", time.localtime(time.time())))
-
-
-from fontTools.ttLib.ttFont import *
-from fontTools.ttLib.ttCollection import TTCollection
diff --git a/spaces/ky2k/Toxicity_Classifier_POC/.venv/lib/python3.9/site-packages/fontTools/ttLib/tables/DefaultTable.py b/spaces/ky2k/Toxicity_Classifier_POC/.venv/lib/python3.9/site-packages/fontTools/ttLib/tables/DefaultTable.py
deleted file mode 100644
index 32a4b1f258f54d78ad39eb764867a6c354939743..0000000000000000000000000000000000000000
--- a/spaces/ky2k/Toxicity_Classifier_POC/.venv/lib/python3.9/site-packages/fontTools/ttLib/tables/DefaultTable.py
+++ /dev/null
@@ -1,50 +0,0 @@
-from fontTools.misc.textTools import Tag
-from fontTools.ttLib import getClassTag
-
-
-class DefaultTable(object):
-
- dependencies = []
-
- def __init__(self, tag=None):
- if tag is None:
- tag = getClassTag(self.__class__)
- self.tableTag = Tag(tag)
-
- def decompile(self, data, ttFont):
- self.data = data
-
- def compile(self, ttFont):
- return self.data
-
- def toXML(self, writer, ttFont, **kwargs):
- if hasattr(self, "ERROR"):
- writer.comment("An error occurred during the decompilation of this table")
- writer.newline()
- writer.comment(self.ERROR)
- writer.newline()
- writer.begintag("hexdata")
- writer.newline()
- writer.dumphex(self.compile(ttFont))
- writer.endtag("hexdata")
- writer.newline()
-
- def fromXML(self, name, attrs, content, ttFont):
- from fontTools.misc.textTools import readHex
- from fontTools import ttLib
-
- if name != "hexdata":
- raise ttLib.TTLibError("can't handle '%s' element" % name)
- self.decompile(readHex(content), ttFont)
-
- def __repr__(self):
- return "<'%s' table at %x>" % (self.tableTag, id(self))
-
- def __eq__(self, other):
- if type(self) != type(other):
- return NotImplemented
- return self.__dict__ == other.__dict__
-
- def __ne__(self, other):
- result = self.__eq__(other)
- return result if result is NotImplemented else not result
diff --git a/spaces/ky2k/Toxicity_Classifier_POC/.venv/lib/python3.9/site-packages/huggingface_hub/utils/_validators.py b/spaces/ky2k/Toxicity_Classifier_POC/.venv/lib/python3.9/site-packages/huggingface_hub/utils/_validators.py
deleted file mode 100644
index 5dd64fa51435b97142bb61cfe12f9369e6f1488b..0000000000000000000000000000000000000000
--- a/spaces/ky2k/Toxicity_Classifier_POC/.venv/lib/python3.9/site-packages/huggingface_hub/utils/_validators.py
+++ /dev/null
@@ -1,230 +0,0 @@
-# coding=utf-8
-# Copyright 2022-present, the HuggingFace Inc. team.
-#
-# Licensed under the Apache License, Version 2.0 (the "License");
-# you may not use this file except in compliance with the License.
-# You may obtain a copy of the License at
-#
-# http://www.apache.org/licenses/LICENSE-2.0
-#
-# Unless required by applicable law or agreed to in writing, software
-# distributed under the License is distributed on an "AS IS" BASIS,
-# WITHOUT WARRANTIES OR CONDITIONS OF ANY KIND, either express or implied.
-# See the License for the specific language governing permissions and
-# limitations under the License.
-"""Contains utilities to validate argument values in `huggingface_hub`."""
-import inspect
-import re
-import warnings
-from functools import wraps
-from itertools import chain
-from typing import Any, Dict
-
-from ._typing import CallableT
-
-
-REPO_ID_REGEX = re.compile(
- r"""
- ^
- (\b[\w\-.]+\b/)? # optional namespace (username or organization)
- \b # starts with a word boundary
- [\w\-.]{1,96} # repo_name: alphanumeric + . _ -
- \b # ends with a word boundary
- $
- """,
- flags=re.VERBOSE,
-)
-
-
-class HFValidationError(ValueError):
- """Generic exception thrown by `huggingface_hub` validators.
-
- Inherits from [`ValueError`](https://docs.python.org/3/library/exceptions.html#ValueError).
- """
-
-
-def validate_hf_hub_args(fn: CallableT) -> CallableT:
- """Validate values received as argument for any public method of `huggingface_hub`.
-
- The goal of this decorator is to harmonize validation of arguments reused
- everywhere. By default, all defined validators are tested.
-
- Validators:
- - [`~utils.validate_repo_id`]: `repo_id` must be `"repo_name"`
- or `"namespace/repo_name"`. Namespace is a username or an organization.
- - [`~utils.smoothly_deprecate_use_auth_token`]: Use `token` instead of
- `use_auth_token` (only if `use_auth_token` is not expected by the decorated
- function - in practice, always the case in `huggingface_hub`).
-
- Example:
- ```py
- >>> from huggingface_hub.utils import validate_hf_hub_args
-
- >>> @validate_hf_hub_args
- ... def my_cool_method(repo_id: str):
- ... print(repo_id)
-
- >>> my_cool_method(repo_id="valid_repo_id")
- valid_repo_id
-
- >>> my_cool_method("other..repo..id")
- huggingface_hub.utils._validators.HFValidationError: Cannot have -- or .. in repo_id: 'other..repo..id'.
-
- >>> my_cool_method(repo_id="other..repo..id")
- huggingface_hub.utils._validators.HFValidationError: Cannot have -- or .. in repo_id: 'other..repo..id'.
-
- >>> @validate_hf_hub_args
- ... def my_cool_auth_method(token: str):
- ... print(token)
-
- >>> my_cool_auth_method(token="a token")
- "a token"
-
- >>> my_cool_auth_method(use_auth_token="a use_auth_token")
- "a use_auth_token"
-
- >>> my_cool_auth_method(token="a token", use_auth_token="a use_auth_token")
- UserWarning: Both `token` and `use_auth_token` are passed (...)
- "a token"
- ```
-
- Raises:
- [`~utils.HFValidationError`]:
- If an input is not valid.
- """
- # TODO: add an argument to opt-out validation for specific argument?
- signature = inspect.signature(fn)
-
- # Should the validator switch `use_auth_token` values to `token`? In practice, always
- # True in `huggingface_hub`. Might not be the case in a downstream library.
- check_use_auth_token = "use_auth_token" not in signature.parameters and "token" in signature.parameters
-
- @wraps(fn)
- def _inner_fn(*args, **kwargs):
- has_token = False
- for arg_name, arg_value in chain(
- zip(signature.parameters, args), # Args values
- kwargs.items(), # Kwargs values
- ):
- if arg_name in ["repo_id", "from_id", "to_id"]:
- validate_repo_id(arg_value)
-
- elif arg_name == "token" and arg_value is not None:
- has_token = True
-
- if check_use_auth_token:
- kwargs = smoothly_deprecate_use_auth_token(fn_name=fn.__name__, has_token=has_token, kwargs=kwargs)
-
- return fn(*args, **kwargs)
-
- return _inner_fn # type: ignore
-
-
-def validate_repo_id(repo_id: str) -> None:
- """Validate `repo_id` is valid.
-
- This is not meant to replace the proper validation made on the Hub but rather to
- avoid local inconsistencies whenever possible (example: passing `repo_type` in the
- `repo_id` is forbidden).
-
- Rules:
- - Between 1 and 96 characters.
- - Either "repo_name" or "namespace/repo_name"
- - [a-zA-Z0-9] or "-", "_", "."
- - "--" and ".." are forbidden
-
- Valid: `"foo"`, `"foo/bar"`, `"123"`, `"Foo-BAR_foo.bar123"`
-
- Not valid: `"datasets/foo/bar"`, `".repo_id"`, `"foo--bar"`, `"foo.git"`
-
- Example:
- ```py
- >>> from huggingface_hub.utils import validate_repo_id
- >>> validate_repo_id(repo_id="valid_repo_id")
- >>> validate_repo_id(repo_id="other..repo..id")
- huggingface_hub.utils._validators.HFValidationError: Cannot have -- or .. in repo_id: 'other..repo..id'.
- ```
-
- Discussed in https://github.com/huggingface/huggingface_hub/issues/1008.
- In moon-landing (internal repository):
- - https://github.com/huggingface/moon-landing/blob/main/server/lib/Names.ts#L27
- - https://github.com/huggingface/moon-landing/blob/main/server/views/components/NewRepoForm/NewRepoForm.svelte#L138
- """
- if not isinstance(repo_id, str):
- # Typically, a Path is not a repo_id
- raise HFValidationError(f"Repo id must be a string, not {type(repo_id)}: '{repo_id}'.")
-
- if repo_id.count("/") > 1:
- raise HFValidationError(
- "Repo id must be in the form 'repo_name' or 'namespace/repo_name':"
- f" '{repo_id}'. Use `repo_type` argument if needed."
- )
-
- if not REPO_ID_REGEX.match(repo_id):
- raise HFValidationError(
- "Repo id must use alphanumeric chars or '-', '_', '.', '--' and '..' are"
- " forbidden, '-' and '.' cannot start or end the name, max length is 96:"
- f" '{repo_id}'."
- )
-
- if "--" in repo_id or ".." in repo_id:
- raise HFValidationError(f"Cannot have -- or .. in repo_id: '{repo_id}'.")
-
- if repo_id.endswith(".git"):
- raise HFValidationError(f"Repo_id cannot end by '.git': '{repo_id}'.")
-
-
-def smoothly_deprecate_use_auth_token(fn_name: str, has_token: bool, kwargs: Dict[str, Any]) -> Dict[str, Any]:
- """Smoothly deprecate `use_auth_token` in the `huggingface_hub` codebase.
-
- The long-term goal is to remove any mention of `use_auth_token` in the codebase in
- favor of a unique and less verbose `token` argument. This will be done a few steps:
-
- 0. Step 0: methods that require a read-access to the Hub use the `use_auth_token`
- argument (`str`, `bool` or `None`). Methods requiring write-access have a `token`
- argument (`str`, `None`). This implicit rule exists to be able to not send the
- token when not necessary (`use_auth_token=False`) even if logged in.
-
- 1. Step 1: we want to harmonize everything and use `token` everywhere (supporting
- `token=False` for read-only methods). In order not to break existing code, if
- `use_auth_token` is passed to a function, the `use_auth_token` value is passed
- as `token` instead, without any warning.
- a. Corner case: if both `use_auth_token` and `token` values are passed, a warning
- is thrown and the `use_auth_token` value is ignored.
-
- 2. Step 2: Once it is release, we should push downstream libraries to switch from
- `use_auth_token` to `token` as much as possible, but without throwing a warning
- (e.g. manually create issues on the corresponding repos).
-
- 3. Step 3: After a transitional period (6 months e.g. until April 2023?), we update
- `huggingface_hub` to throw a warning on `use_auth_token`. Hopefully, very few
- users will be impacted as it would have already been fixed.
- In addition, unit tests in `huggingface_hub` must be adapted to expect warnings
- to be thrown (but still use `use_auth_token` as before).
-
- 4. Step 4: After a normal deprecation cycle (3 releases ?), remove this validator.
- `use_auth_token` will definitely not be supported.
- In addition, we update unit tests in `huggingface_hub` to use `token` everywhere.
-
- This has been discussed in:
- - https://github.com/huggingface/huggingface_hub/issues/1094.
- - https://github.com/huggingface/huggingface_hub/pull/928
- - (related) https://github.com/huggingface/huggingface_hub/pull/1064
- """
- new_kwargs = kwargs.copy() # do not mutate input !
-
- use_auth_token = new_kwargs.pop("use_auth_token", None) # remove from kwargs
- if use_auth_token is not None:
- if has_token:
- warnings.warn(
- "Both `token` and `use_auth_token` are passed to"
- f" `{fn_name}` with non-None values. `token` is now the"
- " preferred argument to pass a User Access Token."
- " `use_auth_token` value will be ignored."
- )
- else:
- # `token` argument is not passed and a non-None value is passed in
- # `use_auth_token` => use `use_auth_token` value as `token` kwarg.
- new_kwargs["token"] = use_auth_token
-
- return new_kwargs
diff --git a/spaces/ky2k/Toxicity_Classifier_POC/.venv/lib/python3.9/site-packages/importlib_resources/simple.py b/spaces/ky2k/Toxicity_Classifier_POC/.venv/lib/python3.9/site-packages/importlib_resources/simple.py
deleted file mode 100644
index 7770c922c84fabe0031333a4de305dd6d6852911..0000000000000000000000000000000000000000
--- a/spaces/ky2k/Toxicity_Classifier_POC/.venv/lib/python3.9/site-packages/importlib_resources/simple.py
+++ /dev/null
@@ -1,106 +0,0 @@
-"""
-Interface adapters for low-level readers.
-"""
-
-import abc
-import io
-import itertools
-from typing import BinaryIO, List
-
-from .abc import Traversable, TraversableResources
-
-
-class SimpleReader(abc.ABC):
- """
- The minimum, low-level interface required from a resource
- provider.
- """
-
- @property
- @abc.abstractmethod
- def package(self) -> str:
- """
- The name of the package for which this reader loads resources.
- """
-
- @abc.abstractmethod
- def children(self) -> List['SimpleReader']:
- """
- Obtain an iterable of SimpleReader for available
- child containers (e.g. directories).
- """
-
- @abc.abstractmethod
- def resources(self) -> List[str]:
- """
- Obtain available named resources for this virtual package.
- """
-
- @abc.abstractmethod
- def open_binary(self, resource: str) -> BinaryIO:
- """
- Obtain a File-like for a named resource.
- """
-
- @property
- def name(self):
- return self.package.split('.')[-1]
-
-
-class ResourceContainer(Traversable):
- """
- Traversable container for a package's resources via its reader.
- """
-
- def __init__(self, reader: SimpleReader):
- self.reader = reader
-
- def is_dir(self):
- return True
-
- def is_file(self):
- return False
-
- def iterdir(self):
- files = (ResourceHandle(self, name) for name in self.reader.resources)
- dirs = map(ResourceContainer, self.reader.children())
- return itertools.chain(files, dirs)
-
- def open(self, *args, **kwargs):
- raise IsADirectoryError()
-
-
-class ResourceHandle(Traversable):
- """
- Handle to a named resource in a ResourceReader.
- """
-
- def __init__(self, parent: ResourceContainer, name: str):
- self.parent = parent
- self.name = name # type: ignore
-
- def is_file(self):
- return True
-
- def is_dir(self):
- return False
-
- def open(self, mode='r', *args, **kwargs):
- stream = self.parent.reader.open_binary(self.name)
- if 'b' not in mode:
- stream = io.TextIOWrapper(*args, **kwargs)
- return stream
-
- def joinpath(self, name):
- raise RuntimeError("Cannot traverse into a resource")
-
-
-class TraversableReader(TraversableResources, SimpleReader):
- """
- A TraversableResources based on SimpleReader. Resource providers
- may derive from this class to provide the TraversableResources
- interface by supplying the SimpleReader interface.
- """
-
- def files(self):
- return ResourceContainer(self)
diff --git a/spaces/lakshmi324/Fake_airpods_Detector/app.py b/spaces/lakshmi324/Fake_airpods_Detector/app.py
deleted file mode 100644
index 4e6518ef3b20795b3663d0b548a167eb596b720d..0000000000000000000000000000000000000000
--- a/spaces/lakshmi324/Fake_airpods_Detector/app.py
+++ /dev/null
@@ -1,95 +0,0 @@
-
-import pandas as pd
-import ast
-import os
-import gradio as gr
-
-
-class airpods(object):
-
- def __init__(self,master,input_model,input_charging_case_serial,input_box_serial_number,input_leftbud_model,input_rightbud_model,input_leftbud_serial,input_rightbud_serial,input_firmware):
- '''
- '''
- self.master = master
- self.input_model = input_model
- self.input_charging_case_serial = input_charging_case_serial
- self.input_box_serial_number = input_box_serial_number
- self.input_leftbud_model = input_leftbud_model
- self.input_rightbud_model = input_rightbud_model
- self.input_leftbud_serial = input_leftbud_serial
- self.input_rightbud_serial = input_rightbud_serial
- self.input_firmware = input_firmware
-
-
- def Iterative_Serial_Check(self):
- '''
- Function checks if the airbuds serial numbers are iterative
- '''
- string = self.input_leftbud_serial[-1] + self.input_rightbud_serial[-1] + self.input_charging_case_serial[-1]
- string = string.lower()
- return not any(m > n for m,n in zip(string,string[1:]))
-
- def check_latest_firmware(self):
- '''
- Function to check if the airbuds are on the latest firmware
- '''
- return (self.master[self.input_model]['firmware'] == self.input_firmware)
-
- def check_matching_serial(self):
- '''
- Function to check if the airbuds are having the
- same serial number as that of the case
- '''
- return (self.input_charging_case_serial == self.input_box_serial_number )
-
-
- def check_model_number(self):
- '''
- Function to check if the airbuds are having the
- same serial number as that of the case
- '''
- return (self.input_leftbud_model in self.master[self.input_model]['Model_number'] and self.input_rightbud_model in self.master[self.input_model]['Model_number'] )
-
- def final_check(self):
-
- if (self.Iterative_Serial_Check() and self.check_latest_firmware() and self.check_matching_serial() and self.check_model_number() ):
- return 'Congratulations, Your Earpods/ Headphones are Genuine'
- elif (self.check_latest_firmware() and self.check_matching_serial() and self.check_model_number() ):
- return 'Looks like Earpods/ Headphones are mostly Genuine, but Case has been swapped'
- else:
- return 'Extremly sorry, Your Earpods/ Headphones are Probably Knock off'
-
-def app_check(input_model,input_charging_case_serial,input_box_serial_number,input_leftbud_model,input_rightbud_model,input_leftbud_serial,input_rightbud_serial,input_firmware):
- input_dict = ast.literal_eval(os.environ.get('master'))
-
- if (all(i >= 10 for i in [len(input_charging_case_serial) , len(input_charging_case_serial) , len(input_leftbud_serial) , len(input_rightbud_serial)]) and any(i < 15 for i in [len(input_charging_case_serial) , len(input_charging_case_serial) , len(input_leftbud_serial) , len(input_rightbud_serial)])) :
- airpod = airpods(input_dict,input_model,input_charging_case_serial,input_box_serial_number,input_leftbud_model,input_rightbud_model,input_leftbud_serial,input_rightbud_serial,input_firmware)
- return airpod.final_check()
- else:
- return 'the serial numbers are not correct, please check and re enter'
-
-gr.Interface(fn=app_check,
- inputs=[
- gr.inputs.Dropdown(['AirPods_Pro2', 'AirPods_3', 'AirPods_Pro', 'AirPods_2', 'AirPods_1']),
- gr.inputs.Textbox(
- placeholder="Please enter the CharginCase serial number", label="CharginCase serial number", lines=1,),
- gr.inputs.Textbox(
- placeholder="Please enter the Box serial number", label="Box serial number", lines=1),
- gr.inputs.Textbox(
- placeholder="Please enter the left bud model number A2083", label="Left bud model number", lines=1),
- gr.inputs.Textbox(
- placeholder="Please enter the right bud number A2083", label="Right bud number", lines=1),
- gr.inputs.Textbox(
- placeholder="Please enter the left bud serial number", label="Left bud serial number", lines=1),
-
- gr.inputs.Textbox(
- placeholder="Please enter the right bud number", label="Right bud number", lines=1),
- gr.inputs.Textbox(
- placeholder="Please enter the Firmware", label="Firmware", lines=1)
- ],
- outputs= [gr.outputs.Textbox(label="Output Box")],
- examples=[]).launch(debug= True)
-
-
-
-
diff --git a/spaces/lambdalabs/LambdaSuperRes/KAIR/models/loss.py b/spaces/lambdalabs/LambdaSuperRes/KAIR/models/loss.py
deleted file mode 100644
index 0a01d7d719f66f0947739caf223cad7ea0dbefca..0000000000000000000000000000000000000000
--- a/spaces/lambdalabs/LambdaSuperRes/KAIR/models/loss.py
+++ /dev/null
@@ -1,287 +0,0 @@
-import torch
-import torch.nn as nn
-import torchvision
-from torch.nn import functional as F
-from torch import autograd as autograd
-
-
-"""
-Sequential(
- (0): Conv2d(3, 64, kernel_size=(3, 3), stride=(1, 1), padding=(1, 1))
- (1): ReLU(inplace)
- (2*): Conv2d(64, 64, kernel_size=(3, 3), stride=(1, 1), padding=(1, 1))
- (3): ReLU(inplace)
- (4): MaxPool2d(kernel_size=2, stride=2, padding=0, dilation=1, ceil_mode=False)
- (5): Conv2d(64, 128, kernel_size=(3, 3), stride=(1, 1), padding=(1, 1))
- (6): ReLU(inplace)
- (7*): Conv2d(128, 128, kernel_size=(3, 3), stride=(1, 1), padding=(1, 1))
- (8): ReLU(inplace)
- (9): MaxPool2d(kernel_size=2, stride=2, padding=0, dilation=1, ceil_mode=False)
- (10): Conv2d(128, 256, kernel_size=(3, 3), stride=(1, 1), padding=(1, 1))
- (11): ReLU(inplace)
- (12): Conv2d(256, 256, kernel_size=(3, 3), stride=(1, 1), padding=(1, 1))
- (13): ReLU(inplace)
- (14): Conv2d(256, 256, kernel_size=(3, 3), stride=(1, 1), padding=(1, 1))
- (15): ReLU(inplace)
- (16*): Conv2d(256, 256, kernel_size=(3, 3), stride=(1, 1), padding=(1, 1))
- (17): ReLU(inplace)
- (18): MaxPool2d(kernel_size=2, stride=2, padding=0, dilation=1, ceil_mode=False)
- (19): Conv2d(256, 512, kernel_size=(3, 3), stride=(1, 1), padding=(1, 1))
- (20): ReLU(inplace)
- (21): Conv2d(512, 512, kernel_size=(3, 3), stride=(1, 1), padding=(1, 1))
- (22): ReLU(inplace)
- (23): Conv2d(512, 512, kernel_size=(3, 3), stride=(1, 1), padding=(1, 1))
- (24): ReLU(inplace)
- (25*): Conv2d(512, 512, kernel_size=(3, 3), stride=(1, 1), padding=(1, 1))
- (26): ReLU(inplace)
- (27): MaxPool2d(kernel_size=2, stride=2, padding=0, dilation=1, ceil_mode=False)
- (28): Conv2d(512, 512, kernel_size=(3, 3), stride=(1, 1), padding=(1, 1))
- (29): ReLU(inplace)
- (30): Conv2d(512, 512, kernel_size=(3, 3), stride=(1, 1), padding=(1, 1))
- (31): ReLU(inplace)
- (32): Conv2d(512, 512, kernel_size=(3, 3), stride=(1, 1), padding=(1, 1))
- (33): ReLU(inplace)
- (34*): Conv2d(512, 512, kernel_size=(3, 3), stride=(1, 1), padding=(1, 1))
- (35): ReLU(inplace)
- (36): MaxPool2d(kernel_size=2, stride=2, padding=0, dilation=1, ceil_mode=False)
-)
-"""
-
-
-# --------------------------------------------
-# Perceptual loss
-# --------------------------------------------
-class VGGFeatureExtractor(nn.Module):
- def __init__(self, feature_layer=[2,7,16,25,34], use_input_norm=True, use_range_norm=False):
- super(VGGFeatureExtractor, self).__init__()
- '''
- use_input_norm: If True, x: [0, 1] --> (x - mean) / std
- use_range_norm: If True, x: [0, 1] --> x: [-1, 1]
- '''
- model = torchvision.models.vgg19(pretrained=True)
- self.use_input_norm = use_input_norm
- self.use_range_norm = use_range_norm
- if self.use_input_norm:
- mean = torch.Tensor([0.485, 0.456, 0.406]).view(1, 3, 1, 1)
- std = torch.Tensor([0.229, 0.224, 0.225]).view(1, 3, 1, 1)
- self.register_buffer('mean', mean)
- self.register_buffer('std', std)
- self.list_outputs = isinstance(feature_layer, list)
- if self.list_outputs:
- self.features = nn.Sequential()
- feature_layer = [-1] + feature_layer
- for i in range(len(feature_layer)-1):
- self.features.add_module('child'+str(i), nn.Sequential(*list(model.features.children())[(feature_layer[i]+1):(feature_layer[i+1]+1)]))
- else:
- self.features = nn.Sequential(*list(model.features.children())[:(feature_layer + 1)])
-
- print(self.features)
-
- # No need to BP to variable
- for k, v in self.features.named_parameters():
- v.requires_grad = False
-
- def forward(self, x):
- if self.use_range_norm:
- x = (x + 1.0) / 2.0
- if self.use_input_norm:
- x = (x - self.mean) / self.std
- if self.list_outputs:
- output = []
- for child_model in self.features.children():
- x = child_model(x)
- output.append(x.clone())
- return output
- else:
- return self.features(x)
-
-
-class PerceptualLoss(nn.Module):
- """VGG Perceptual loss
- """
-
- def __init__(self, feature_layer=[2,7,16,25,34], weights=[0.1,0.1,1.0,1.0,1.0], lossfn_type='l1', use_input_norm=True, use_range_norm=False):
- super(PerceptualLoss, self).__init__()
- self.vgg = VGGFeatureExtractor(feature_layer=feature_layer, use_input_norm=use_input_norm, use_range_norm=use_range_norm)
- self.lossfn_type = lossfn_type
- self.weights = weights
- if self.lossfn_type == 'l1':
- self.lossfn = nn.L1Loss()
- else:
- self.lossfn = nn.MSELoss()
- print(f'feature_layer: {feature_layer} with weights: {weights}')
-
- def forward(self, x, gt):
- """Forward function.
- Args:
- x (Tensor): Input tensor with shape (n, c, h, w).
- gt (Tensor): Ground-truth tensor with shape (n, c, h, w).
- Returns:
- Tensor: Forward results.
- """
- x_vgg, gt_vgg = self.vgg(x), self.vgg(gt.detach())
- loss = 0.0
- if isinstance(x_vgg, list):
- n = len(x_vgg)
- for i in range(n):
- loss += self.weights[i] * self.lossfn(x_vgg[i], gt_vgg[i])
- else:
- loss += self.lossfn(x_vgg, gt_vgg.detach())
- return loss
-
-# --------------------------------------------
-# GAN loss: gan, ragan
-# --------------------------------------------
-class GANLoss(nn.Module):
- def __init__(self, gan_type, real_label_val=1.0, fake_label_val=0.0):
- super(GANLoss, self).__init__()
- self.gan_type = gan_type.lower()
- self.real_label_val = real_label_val
- self.fake_label_val = fake_label_val
-
- if self.gan_type == 'gan' or self.gan_type == 'ragan':
- self.loss = nn.BCEWithLogitsLoss()
- elif self.gan_type == 'lsgan':
- self.loss = nn.MSELoss()
- elif self.gan_type == 'wgan':
- def wgan_loss(input, target):
- # target is boolean
- return -1 * input.mean() if target else input.mean()
-
- self.loss = wgan_loss
- elif self.gan_type == 'softplusgan':
- def softplusgan_loss(input, target):
- # target is boolean
- return F.softplus(-input).mean() if target else F.softplus(input).mean()
-
- self.loss = softplusgan_loss
- else:
- raise NotImplementedError('GAN type [{:s}] is not found'.format(self.gan_type))
-
- def get_target_label(self, input, target_is_real):
- if self.gan_type in ['wgan', 'softplusgan']:
- return target_is_real
- if target_is_real:
- return torch.empty_like(input).fill_(self.real_label_val)
- else:
- return torch.empty_like(input).fill_(self.fake_label_val)
-
- def forward(self, input, target_is_real):
- target_label = self.get_target_label(input, target_is_real)
- loss = self.loss(input, target_label)
- return loss
-
-
-# --------------------------------------------
-# TV loss
-# --------------------------------------------
-class TVLoss(nn.Module):
- def __init__(self, tv_loss_weight=1):
- """
- Total variation loss
- https://github.com/jxgu1016/Total_Variation_Loss.pytorch
- Args:
- tv_loss_weight (int):
- """
- super(TVLoss, self).__init__()
- self.tv_loss_weight = tv_loss_weight
-
- def forward(self, x):
- batch_size = x.size()[0]
- h_x = x.size()[2]
- w_x = x.size()[3]
- count_h = self.tensor_size(x[:, :, 1:, :])
- count_w = self.tensor_size(x[:, :, :, 1:])
- h_tv = torch.pow((x[:, :, 1:, :] - x[:, :, :h_x - 1, :]), 2).sum()
- w_tv = torch.pow((x[:, :, :, 1:] - x[:, :, :, :w_x - 1]), 2).sum()
- return self.tv_loss_weight * 2 * (h_tv / count_h + w_tv / count_w) / batch_size
-
- @staticmethod
- def tensor_size(t):
- return t.size()[1] * t.size()[2] * t.size()[3]
-
-
-# --------------------------------------------
-# Charbonnier loss
-# --------------------------------------------
-class CharbonnierLoss(nn.Module):
- """Charbonnier Loss (L1)"""
-
- def __init__(self, eps=1e-9):
- super(CharbonnierLoss, self).__init__()
- self.eps = eps
-
- def forward(self, x, y):
- diff = x - y
- loss = torch.mean(torch.sqrt((diff * diff) + self.eps))
- return loss
-
-
-
-def r1_penalty(real_pred, real_img):
- """R1 regularization for discriminator. The core idea is to
- penalize the gradient on real data alone: when the
- generator distribution produces the true data distribution
- and the discriminator is equal to 0 on the data manifold, the
- gradient penalty ensures that the discriminator cannot create
- a non-zero gradient orthogonal to the data manifold without
- suffering a loss in the GAN game.
- Ref:
- Eq. 9 in Which training methods for GANs do actually converge.
- """
- grad_real = autograd.grad(
- outputs=real_pred.sum(), inputs=real_img, create_graph=True)[0]
- grad_penalty = grad_real.pow(2).view(grad_real.shape[0], -1).sum(1).mean()
- return grad_penalty
-
-
-def g_path_regularize(fake_img, latents, mean_path_length, decay=0.01):
- noise = torch.randn_like(fake_img) / math.sqrt(
- fake_img.shape[2] * fake_img.shape[3])
- grad = autograd.grad(
- outputs=(fake_img * noise).sum(), inputs=latents, create_graph=True)[0]
- path_lengths = torch.sqrt(grad.pow(2).sum(2).mean(1))
-
- path_mean = mean_path_length + decay * (
- path_lengths.mean() - mean_path_length)
-
- path_penalty = (path_lengths - path_mean).pow(2).mean()
-
- return path_penalty, path_lengths.detach().mean(), path_mean.detach()
-
-
-def gradient_penalty_loss(discriminator, real_data, fake_data, weight=None):
- """Calculate gradient penalty for wgan-gp.
- Args:
- discriminator (nn.Module): Network for the discriminator.
- real_data (Tensor): Real input data.
- fake_data (Tensor): Fake input data.
- weight (Tensor): Weight tensor. Default: None.
- Returns:
- Tensor: A tensor for gradient penalty.
- """
-
- batch_size = real_data.size(0)
- alpha = real_data.new_tensor(torch.rand(batch_size, 1, 1, 1))
-
- # interpolate between real_data and fake_data
- interpolates = alpha * real_data + (1. - alpha) * fake_data
- interpolates = autograd.Variable(interpolates, requires_grad=True)
-
- disc_interpolates = discriminator(interpolates)
- gradients = autograd.grad(
- outputs=disc_interpolates,
- inputs=interpolates,
- grad_outputs=torch.ones_like(disc_interpolates),
- create_graph=True,
- retain_graph=True,
- only_inputs=True)[0]
-
- if weight is not None:
- gradients = gradients * weight
-
- gradients_penalty = ((gradients.norm(2, dim=1) - 1)**2).mean()
- if weight is not None:
- gradients_penalty /= torch.mean(weight)
-
- return gradients_penalty
diff --git a/spaces/lcipolina/Print_Gallery/glide_text2im/clip/readme.md b/spaces/lcipolina/Print_Gallery/glide_text2im/clip/readme.md
deleted file mode 100644
index 12fc00dafedd303aed22aba2282568c528649c9c..0000000000000000000000000000000000000000
--- a/spaces/lcipolina/Print_Gallery/glide_text2im/clip/readme.md
+++ /dev/null
@@ -1 +0,0 @@
-carry on
\ No newline at end of file
diff --git a/spaces/lincquiQcaudo/Top-20-Diffusion/Gregg Braden The Divine Matrix Pdf Download.md b/spaces/lincquiQcaudo/Top-20-Diffusion/Gregg Braden The Divine Matrix Pdf Download.md
deleted file mode 100644
index 631b85ca31fc3ea85f34a99f485e03ca8d0d2d56..0000000000000000000000000000000000000000
--- a/spaces/lincquiQcaudo/Top-20-Diffusion/Gregg Braden The Divine Matrix Pdf Download.md
+++ /dev/null
@@ -1,118 +0,0 @@
-
-
Gregg Braden The Divine Matrix Pdf Download: A Book That Will Change Your Life
-
-
If you are looking for a book that will inspire you, challenge you, and transform you, then you should download Gregg Braden The Divine Matrix Pdf. This book is a masterpiece of science, spirituality, and miracles that will show you how to connect with the web of energy that links everything in our lives and our world.
In this book, Gregg Braden, a renowned author and speaker, reveals the evidence of a divine matrix that exists between 1993 and 2000. He explains how this matrix is the bridge between our inner and outer worlds, and how we can use it to create joy, heal suffering, and bring peace to nations.
-
-
Gregg Braden also shares his personal experiences and stories of people who have tapped into the divine matrix and witnessed amazing results. He teaches us how to speak the language of the matrix, which is based on emotion, intention, and belief. He shows us how to access the power that lives inside of us and use it each day of our lives.
-
-
Why You Should Download Gregg Braden The Divine Matrix Pdf
-
-
There are many reasons why you should download Gregg Braden The Divine Matrix Pdf, but here are some of the most important ones:
-
-
-
You will learn about the scientific discoveries that prove the existence of a divine matrix that connects everything in our lives and our world.
-
You will discover how to use the divine matrix to create your own reality and manifest your desires.
-
You will understand how to heal yourself and others by using the power of your emotions, intentions, and beliefs.
-
You will explore the mysteries of time, space, miracles, and belief, and how they relate to the divine matrix.
-
You will be inspired by the stories of people who have used the divine matrix to achieve extraordinary results in their lives.
-
You will join Gregg Braden on an extraordinary journey that will bridge science, spirituality, and miracles through the language of the divine matrix.
-
-
-
How to Download Gregg Braden The Divine Matrix Pdf
-
-
If you are ready to download Gregg Braden The Divine Matrix Pdf, then you can do so by following these simple steps:
-
-
-
Click on one of the links below that will take you to a website where you can download the book in PDF or EPUB format.
-
Choose the format that suits your device and preferences.
-
Download the file to your device or cloud storage.
-
Enjoy reading the book and applying its teachings to your life.
-
-
-
Here are some of the links where you can download Gregg Braden The Divine Matrix Pdf:
Gregg Braden The Divine Matrix Pdf is a book that will change your life. It will show you how to connect with the web of energy that links everything in our lives and our world. It will teach you how to use this power to create joy, heal suffering, and bring peace to nations. It will inspire you with stories of people who have used this power to achieve amazing results. It will bridge science, spirituality, and miracles through the language of the divine matrix.
-
-
If you want to download Gregg Braden The Divine Matrix Pdf, then you can do so by clicking on one of the links above. You will be able to choose between PDF or EPUB format, depending on your device and preferences. You will be able to download the file to your device or cloud storage. You will be able to enjoy reading the book and applying its teachings to your life.
-
-
Don't miss this opportunity to download Gregg Braden The Divine Matrix Pdf. It is a book that will change your life for the better. It is a book that will show you how to connect with the divine matrix and use its power each day of your life.
-
What is the Divine Matrix?
-
-
The divine matrix is the term that Gregg Braden uses to describe the web of energy that connects everything in our lives and our world. He explains that this matrix is not a new discovery, but a forgotten wisdom that has been known by ancient cultures for thousands of years. He also shows that this matrix is supported by the latest scientific findings in quantum physics, biology, and cosmology.
-
-
The divine matrix is more than just a field of energy. It is also a mirror that reflects our thoughts, feelings, and beliefs. It is a bridge that links our inner and outer worlds. It is a language that we can use to communicate with the force that creates all of creation. It is a source of power that we can access to create our own reality and manifest our desires.
-
-
How to Use the Divine Matrix?
-
-
In his book Gregg Braden The Divine Matrix Pdf, Gregg Braden teaches us how to use the divine matrix to create joy, heal suffering, and bring peace to nations. He shares three keys that will help us unlock the power of the matrix:
-
-
-
The first key is to understand that we are connected to everything and everyone through the divine matrix. We are not separate from the world, but part of it. We are not alone, but part of a larger whole.
-
The second key is to realize that our emotions, intentions, and beliefs are the language of the divine matrix. They are the signals that we send to the matrix, and they affect the reality that we experience. We can use this language to speak directly to the force that links all of creation.
-
The third key is to recognize that we have the power to change the world by changing ourselves. We can use the divine matrix to heal ourselves and others, to create our own reality, and to influence the events of our lives. We can use this power each day of our lives.
-
-
-
By using these three keys, we can tap into the divine matrix and witness amazing results in our lives. We can also join Gregg Braden on his extraordinary journey bridging science, spirituality, and miracles through the language of the divine matrix.
-What are the Benefits of Using the Divine Matrix?
-
-
Using the divine matrix can bring many benefits to our lives. Some of the benefits are:
-
-
-
We can create joy in our lives by choosing positive emotions, intentions, and beliefs. We can also attract more joy into our lives by aligning ourselves with the frequency of joy in the divine matrix.
-
We can heal suffering in our lives by releasing negative emotions, intentions, and beliefs. We can also heal others by sending them healing energy through the divine matrix.
-
We can bring peace to nations by cultivating peace in ourselves. We can also influence the collective consciousness of humanity by sending peace signals to the divine matrix.
-
We can achieve our goals and dreams by using the divine matrix as a tool for manifestation. We can also co-create with the divine intelligence that guides all of creation.
-
We can discover our true potential and purpose by connecting with the divine matrix. We can also access our higher self and intuition by tuning into the divine matrix.
-
-
-
These are just some of the benefits of using the divine matrix. There are many more that we can experience as we explore this amazing web of energy that connects everything in our lives and our world.
-
-How to Download Gregg Braden The Divine Matrix Pdf?
-
-
If you are interested in downloading Gregg Braden The Divine Matrix Pdf, then you can do so by following these simple steps:
-
-
-
Click on one of the links below that will take you to a website where you can download the book in PDF or EPUB format.
-
Choose the format that suits your device and preferences.
-
Download the file to your device or cloud storage.
-
Enjoy reading the book and applying its teachings to your life.
-
-
-
Here are some of the links where you can download Gregg Braden The Divine Matrix Pdf:
Gregg Braden The Divine Matrix Pdf is a book that will change your life. It will show you how to connect with the web of energy that links everything in our lives and our world. It will teach you how to use this power to create joy, heal suffering, and bring peace to nations. It will inspire you with stories of people who have used this power to achieve amazing results. It will bridge science, spirituality, and miracles through the language of the divine matrix.
-
-
If you want to download Gregg Braden The Divine Matrix Pdf, then you can do so by clicking on one of the links above. You will be able to choose between PDF or EPUB format, depending on your device and preferences. You will be able to download the file to your device or cloud storage. You will be able to enjoy reading the book and applying its teachings to your life.
-
-
Don't miss this opportunity to download Gregg Braden The Divine Matrix Pdf. It is a book that will change your life for the better. It is a book that will show you how to connect with the divine matrix and use its power each day of your life.
-
In conclusion, Gregg Braden The Divine Matrix Pdf is a book that will change your life. It will show you how to connect with the web of energy that links everything in our lives and our world. It will teach you how to use this power to create joy, heal suffering, and bring peace to nations. It will inspire you with stories of people who have used this power to achieve amazing results. It will bridge science, spirituality, and miracles through the language of the divine matrix.
-
-
If you want to download Gregg Braden The Divine Matrix Pdf, then you can do so by clicking on one of the links above. You will be able to choose between PDF or EPUB format, depending on your device and preferences. You will be able to download the file to your device or cloud storage. You will be able to enjoy reading the book and applying its teachings to your life.
-
-
Don't miss this opportunity to download Gregg Braden The Divine Matrix Pdf. It is a book that will change your life for the better. It is a book that will show you how to connect with the divine matrix and use its power each day of your life.
-
-
Click on the link below and get your copy of Gregg Braden The Divine Matrix Pdf today!
3cee63e6c2
-
-
\ No newline at end of file
diff --git a/spaces/llmonitor/benchmarks/app/prompts/new/page.js b/spaces/llmonitor/benchmarks/app/prompts/new/page.js
deleted file mode 100644
index bcfd672dfb4467ab2ce8a3eefa5e695cee513ea6..0000000000000000000000000000000000000000
--- a/spaces/llmonitor/benchmarks/app/prompts/new/page.js
+++ /dev/null
@@ -1,47 +0,0 @@
-import UpvoteBtn from "@/components/UpvoteBtn"
-import db from "@/utils/db"
-import { cookies } from "next/headers"
-import Link from "next/link"
-
-export default async function Dataset() {
- const cookiesList = cookies()
-
- const logged = cookiesList.has("token")
-
- // get prompts with selected != true joined with sum of votes for each
- const promptsWithVotes =
- await db`SELECT prompts.*, COUNT(votes.id) AS votes FROM prompts LEFT JOIN votes ON prompts.id = votes.prompt WHERE prompts.selected IS NOT TRUE GROUP BY prompts.id ORDER BY votes DESC`
-
- return (
- <>
-
- >
- )
-}
diff --git a/spaces/luodian/LoRA-DreamBooth-Training-UI/constants.py b/spaces/luodian/LoRA-DreamBooth-Training-UI/constants.py
deleted file mode 100644
index baaebbae71058fbb4faed35fd00e7559305dc409..0000000000000000000000000000000000000000
--- a/spaces/luodian/LoRA-DreamBooth-Training-UI/constants.py
+++ /dev/null
@@ -1,6 +0,0 @@
-import enum
-
-
-class UploadTarget(enum.Enum):
- PERSONAL_PROFILE = 'Personal Profile'
- LORA_LIBRARY = 'LoRA Library'
diff --git a/spaces/lwchen/CodeFormer/CodeFormer/facelib/detection/align_trans.py b/spaces/lwchen/CodeFormer/CodeFormer/facelib/detection/align_trans.py
deleted file mode 100644
index 07f1eb365462c2ec5bbac6d1854c786b6fd6be90..0000000000000000000000000000000000000000
--- a/spaces/lwchen/CodeFormer/CodeFormer/facelib/detection/align_trans.py
+++ /dev/null
@@ -1,219 +0,0 @@
-import cv2
-import numpy as np
-
-from .matlab_cp2tform import get_similarity_transform_for_cv2
-
-# reference facial points, a list of coordinates (x,y)
-REFERENCE_FACIAL_POINTS = [[30.29459953, 51.69630051], [65.53179932, 51.50139999], [48.02519989, 71.73660278],
- [33.54930115, 92.3655014], [62.72990036, 92.20410156]]
-
-DEFAULT_CROP_SIZE = (96, 112)
-
-
-class FaceWarpException(Exception):
-
- def __str__(self):
- return 'In File {}:{}'.format(__file__, super.__str__(self))
-
-
-def get_reference_facial_points(output_size=None, inner_padding_factor=0.0, outer_padding=(0, 0), default_square=False):
- """
- Function:
- ----------
- get reference 5 key points according to crop settings:
- 0. Set default crop_size:
- if default_square:
- crop_size = (112, 112)
- else:
- crop_size = (96, 112)
- 1. Pad the crop_size by inner_padding_factor in each side;
- 2. Resize crop_size into (output_size - outer_padding*2),
- pad into output_size with outer_padding;
- 3. Output reference_5point;
- Parameters:
- ----------
- @output_size: (w, h) or None
- size of aligned face image
- @inner_padding_factor: (w_factor, h_factor)
- padding factor for inner (w, h)
- @outer_padding: (w_pad, h_pad)
- each row is a pair of coordinates (x, y)
- @default_square: True or False
- if True:
- default crop_size = (112, 112)
- else:
- default crop_size = (96, 112);
- !!! make sure, if output_size is not None:
- (output_size - outer_padding)
- = some_scale * (default crop_size * (1.0 +
- inner_padding_factor))
- Returns:
- ----------
- @reference_5point: 5x2 np.array
- each row is a pair of transformed coordinates (x, y)
- """
-
- tmp_5pts = np.array(REFERENCE_FACIAL_POINTS)
- tmp_crop_size = np.array(DEFAULT_CROP_SIZE)
-
- # 0) make the inner region a square
- if default_square:
- size_diff = max(tmp_crop_size) - tmp_crop_size
- tmp_5pts += size_diff / 2
- tmp_crop_size += size_diff
-
- if (output_size and output_size[0] == tmp_crop_size[0] and output_size[1] == tmp_crop_size[1]):
-
- return tmp_5pts
-
- if (inner_padding_factor == 0 and outer_padding == (0, 0)):
- if output_size is None:
- return tmp_5pts
- else:
- raise FaceWarpException('No paddings to do, output_size must be None or {}'.format(tmp_crop_size))
-
- # check output size
- if not (0 <= inner_padding_factor <= 1.0):
- raise FaceWarpException('Not (0 <= inner_padding_factor <= 1.0)')
-
- if ((inner_padding_factor > 0 or outer_padding[0] > 0 or outer_padding[1] > 0) and output_size is None):
- output_size = tmp_crop_size * \
- (1 + inner_padding_factor * 2).astype(np.int32)
- output_size += np.array(outer_padding)
- if not (outer_padding[0] < output_size[0] and outer_padding[1] < output_size[1]):
- raise FaceWarpException('Not (outer_padding[0] < output_size[0] and outer_padding[1] < output_size[1])')
-
- # 1) pad the inner region according inner_padding_factor
- if inner_padding_factor > 0:
- size_diff = tmp_crop_size * inner_padding_factor * 2
- tmp_5pts += size_diff / 2
- tmp_crop_size += np.round(size_diff).astype(np.int32)
-
- # 2) resize the padded inner region
- size_bf_outer_pad = np.array(output_size) - np.array(outer_padding) * 2
-
- if size_bf_outer_pad[0] * tmp_crop_size[1] != size_bf_outer_pad[1] * tmp_crop_size[0]:
- raise FaceWarpException('Must have (output_size - outer_padding)'
- '= some_scale * (crop_size * (1.0 + inner_padding_factor)')
-
- scale_factor = size_bf_outer_pad[0].astype(np.float32) / tmp_crop_size[0]
- tmp_5pts = tmp_5pts * scale_factor
- # size_diff = tmp_crop_size * (scale_factor - min(scale_factor))
- # tmp_5pts = tmp_5pts + size_diff / 2
- tmp_crop_size = size_bf_outer_pad
-
- # 3) add outer_padding to make output_size
- reference_5point = tmp_5pts + np.array(outer_padding)
- tmp_crop_size = output_size
-
- return reference_5point
-
-
-def get_affine_transform_matrix(src_pts, dst_pts):
- """
- Function:
- ----------
- get affine transform matrix 'tfm' from src_pts to dst_pts
- Parameters:
- ----------
- @src_pts: Kx2 np.array
- source points matrix, each row is a pair of coordinates (x, y)
- @dst_pts: Kx2 np.array
- destination points matrix, each row is a pair of coordinates (x, y)
- Returns:
- ----------
- @tfm: 2x3 np.array
- transform matrix from src_pts to dst_pts
- """
-
- tfm = np.float32([[1, 0, 0], [0, 1, 0]])
- n_pts = src_pts.shape[0]
- ones = np.ones((n_pts, 1), src_pts.dtype)
- src_pts_ = np.hstack([src_pts, ones])
- dst_pts_ = np.hstack([dst_pts, ones])
-
- A, res, rank, s = np.linalg.lstsq(src_pts_, dst_pts_)
-
- if rank == 3:
- tfm = np.float32([[A[0, 0], A[1, 0], A[2, 0]], [A[0, 1], A[1, 1], A[2, 1]]])
- elif rank == 2:
- tfm = np.float32([[A[0, 0], A[1, 0], 0], [A[0, 1], A[1, 1], 0]])
-
- return tfm
-
-
-def warp_and_crop_face(src_img, facial_pts, reference_pts=None, crop_size=(96, 112), align_type='smilarity'):
- """
- Function:
- ----------
- apply affine transform 'trans' to uv
- Parameters:
- ----------
- @src_img: 3x3 np.array
- input image
- @facial_pts: could be
- 1)a list of K coordinates (x,y)
- or
- 2) Kx2 or 2xK np.array
- each row or col is a pair of coordinates (x, y)
- @reference_pts: could be
- 1) a list of K coordinates (x,y)
- or
- 2) Kx2 or 2xK np.array
- each row or col is a pair of coordinates (x, y)
- or
- 3) None
- if None, use default reference facial points
- @crop_size: (w, h)
- output face image size
- @align_type: transform type, could be one of
- 1) 'similarity': use similarity transform
- 2) 'cv2_affine': use the first 3 points to do affine transform,
- by calling cv2.getAffineTransform()
- 3) 'affine': use all points to do affine transform
- Returns:
- ----------
- @face_img: output face image with size (w, h) = @crop_size
- """
-
- if reference_pts is None:
- if crop_size[0] == 96 and crop_size[1] == 112:
- reference_pts = REFERENCE_FACIAL_POINTS
- else:
- default_square = False
- inner_padding_factor = 0
- outer_padding = (0, 0)
- output_size = crop_size
-
- reference_pts = get_reference_facial_points(output_size, inner_padding_factor, outer_padding,
- default_square)
-
- ref_pts = np.float32(reference_pts)
- ref_pts_shp = ref_pts.shape
- if max(ref_pts_shp) < 3 or min(ref_pts_shp) != 2:
- raise FaceWarpException('reference_pts.shape must be (K,2) or (2,K) and K>2')
-
- if ref_pts_shp[0] == 2:
- ref_pts = ref_pts.T
-
- src_pts = np.float32(facial_pts)
- src_pts_shp = src_pts.shape
- if max(src_pts_shp) < 3 or min(src_pts_shp) != 2:
- raise FaceWarpException('facial_pts.shape must be (K,2) or (2,K) and K>2')
-
- if src_pts_shp[0] == 2:
- src_pts = src_pts.T
-
- if src_pts.shape != ref_pts.shape:
- raise FaceWarpException('facial_pts and reference_pts must have the same shape')
-
- if align_type == 'cv2_affine':
- tfm = cv2.getAffineTransform(src_pts[0:3], ref_pts[0:3])
- elif align_type == 'affine':
- tfm = get_affine_transform_matrix(src_pts, ref_pts)
- else:
- tfm = get_similarity_transform_for_cv2(src_pts, ref_pts)
-
- face_img = cv2.warpAffine(src_img, tfm, (crop_size[0], crop_size[1]))
-
- return face_img
diff --git a/spaces/m3hrdadfi/gpt2-persian-qa/regexes/__init__.py b/spaces/m3hrdadfi/gpt2-persian-qa/regexes/__init__.py
deleted file mode 100644
index e69de29bb2d1d6434b8b29ae775ad8c2e48c5391..0000000000000000000000000000000000000000
diff --git a/spaces/ma-xu/LIVE/thrust/thrust/system/detail/generic/copy.h b/spaces/ma-xu/LIVE/thrust/thrust/system/detail/generic/copy.h
deleted file mode 100644
index e22535618efd8c896b8e04ba21b636e4832743ea..0000000000000000000000000000000000000000
--- a/spaces/ma-xu/LIVE/thrust/thrust/system/detail/generic/copy.h
+++ /dev/null
@@ -1,59 +0,0 @@
-/*
- * Copyright 2008-2013 NVIDIA Corporation
- *
- * Licensed under the Apache License, Version 2.0 (the "License");
- * you may not use this file except in compliance with the License.
- * You may obtain a copy of the License at
- *
- * http://www.apache.org/licenses/LICENSE-2.0
- *
- * Unless required by applicable law or agreed to in writing, software
- * distributed under the License is distributed on an "AS IS" BASIS,
- * WITHOUT WARRANTIES OR CONDITIONS OF ANY KIND, either express or implied.
- * See the License for the specific language governing permissions and
- * limitations under the License.
- */
-
-#pragma once
-
-#include
-#include
-
-namespace thrust
-{
-namespace system
-{
-namespace detail
-{
-namespace generic
-{
-
-
-template
-__host__ __device__
- OutputIterator copy(thrust::execution_policy &exec,
- InputIterator first,
- InputIterator last,
- OutputIterator result);
-
-
-template
-__host__ __device__
- OutputIterator copy_n(thrust::execution_policy &exec,
- InputIterator first,
- Size n,
- OutputIterator result);
-
-
-} // end generic
-} // end detail
-} // end system
-} // end thrust
-
-#include
-
diff --git a/spaces/manhkhanhUIT/Image_Restoration_Colorization/Global/train_domain_A.py b/spaces/manhkhanhUIT/Image_Restoration_Colorization/Global/train_domain_A.py
deleted file mode 100644
index 45004938349d674227b2fac3ad9644370c9eda30..0000000000000000000000000000000000000000
--- a/spaces/manhkhanhUIT/Image_Restoration_Colorization/Global/train_domain_A.py
+++ /dev/null
@@ -1,147 +0,0 @@
-# Copyright (c) Microsoft Corporation.
-# Licensed under the MIT License.
-
-import time
-from collections import OrderedDict
-from options.train_options import TrainOptions
-from data.data_loader import CreateDataLoader
-from models.models import create_da_model
-import util.util as util
-from util.visualizer import Visualizer
-import os
-import numpy as np
-import torch
-import torchvision.utils as vutils
-from torch.autograd import Variable
-
-opt = TrainOptions().parse()
-
-if opt.debug:
- opt.display_freq = 1
- opt.print_freq = 1
- opt.niter = 1
- opt.niter_decay = 0
- opt.max_dataset_size = 10
-
-data_loader = CreateDataLoader(opt)
-dataset = data_loader.load_data()
-dataset_size = len(dataset) * opt.batchSize
-print('#training images = %d' % dataset_size)
-
-path = os.path.join(opt.checkpoints_dir, opt.name, 'model.txt')
-visualizer = Visualizer(opt)
-
-iter_path = os.path.join(opt.checkpoints_dir, opt.name, 'iter.txt')
-if opt.continue_train:
- try:
- start_epoch, epoch_iter = np.loadtxt(iter_path, delimiter=',', dtype=int)
- except:
- start_epoch, epoch_iter = 1, 0
- visualizer.print_save('Resuming from epoch %d at iteration %d' % (start_epoch - 1, epoch_iter))
-else:
- start_epoch, epoch_iter = 1, 0
-
-# opt.which_epoch=start_epoch-1
-model = create_da_model(opt)
-fd = open(path, 'w')
-fd.write(str(model.module.netG))
-fd.write(str(model.module.netD))
-fd.close()
-
-total_steps = (start_epoch - 1) * dataset_size + epoch_iter
-
-display_delta = total_steps % opt.display_freq
-print_delta = total_steps % opt.print_freq
-save_delta = total_steps % opt.save_latest_freq
-
-for epoch in range(start_epoch, opt.niter + opt.niter_decay + 1):
- epoch_start_time = time.time()
- if epoch != start_epoch:
- epoch_iter = epoch_iter % dataset_size
- for i, data in enumerate(dataset, start=epoch_iter):
- iter_start_time = time.time()
- total_steps += opt.batchSize
- epoch_iter += opt.batchSize
-
- # whether to collect output images
- save_fake = total_steps % opt.display_freq == display_delta
-
- ############## Forward Pass ######################
- losses, generated = model(Variable(data['label']), Variable(data['inst']),
- Variable(data['image']), Variable(data['feat']), infer=save_fake)
-
- # sum per device losses
- losses = [torch.mean(x) if not isinstance(x, int) else x for x in losses]
- loss_dict = dict(zip(model.module.loss_names, losses))
-
- # calculate final loss scalar
- loss_D = (loss_dict['D_fake'] + loss_dict['D_real']) * 0.5
- loss_featD=(loss_dict['featD_fake'] + loss_dict['featD_real']) * 0.5
- loss_G = loss_dict['G_GAN'] + loss_dict.get('G_GAN_Feat', 0) + loss_dict.get('G_VGG', 0) + loss_dict['G_KL'] + loss_dict['G_featD']
-
- ############### Backward Pass ####################
- # update generator weights
- model.module.optimizer_G.zero_grad()
- loss_G.backward()
- model.module.optimizer_G.step()
-
- # update discriminator weights
- model.module.optimizer_D.zero_grad()
- loss_D.backward()
- model.module.optimizer_D.step()
-
- model.module.optimizer_featD.zero_grad()
- loss_featD.backward()
- model.module.optimizer_featD.step()
-
- # call(["nvidia-smi", "--format=csv", "--query-gpu=memory.used,memory.free"])
-
- ############## Display results and errors ##########
- ### print out errors
- if total_steps % opt.print_freq == print_delta:
- errors = {k: v.data if not isinstance(v, int) else v for k, v in loss_dict.items()}
- t = (time.time() - iter_start_time) / opt.batchSize
- visualizer.print_current_errors(epoch, epoch_iter, errors, t, model.module.old_lr)
- visualizer.plot_current_errors(errors, total_steps)
-
- ### display output images
- if save_fake:
-
- if not os.path.exists(opt.outputs_dir + opt.name):
- os.makedirs(opt.outputs_dir + opt.name)
- imgs_num = data['label'].shape[0]
- imgs = torch.cat((data['label'], generated.data.cpu(), data['image']), 0)
-
- imgs = (imgs + 1.) / 2.0
-
- try:
- image_grid = vutils.save_image(imgs, opt.outputs_dir + opt.name + '/' + str(epoch) + '_' + str(
- total_steps) + '.png',
- nrow=imgs_num, padding=0, normalize=True)
- except OSError as err:
- print(err)
-
-
- if epoch_iter >= dataset_size:
- break
-
- # end of epoch
- iter_end_time = time.time()
- print('End of epoch %d / %d \t Time Taken: %d sec' %
- (epoch, opt.niter + opt.niter_decay, time.time() - epoch_start_time))
-
- ### save model for this epoch
- if epoch % opt.save_epoch_freq == 0:
- print('saving the model at the end of epoch %d, iters %d' % (epoch, total_steps))
- model.module.save('latest')
- model.module.save(epoch)
- np.savetxt(iter_path, (epoch + 1, 0), delimiter=',', fmt='%d')
-
- ### instead of only training the local enhancer, train the entire network after certain iterations
- if (opt.niter_fix_global != 0) and (epoch == opt.niter_fix_global):
- model.module.update_fixed_params()
-
- ### linearly decay learning rate after certain iterations
- if epoch > opt.niter:
- model.module.update_learning_rate()
-
diff --git a/spaces/matthoffner/AudioCraft_Plus/audiocraft/grids/compression/encodec_base_24khz.py b/spaces/matthoffner/AudioCraft_Plus/audiocraft/grids/compression/encodec_base_24khz.py
deleted file mode 100644
index 117b2b1e496ca31b3d614672b472c9213cedb4ad..0000000000000000000000000000000000000000
--- a/spaces/matthoffner/AudioCraft_Plus/audiocraft/grids/compression/encodec_base_24khz.py
+++ /dev/null
@@ -1,28 +0,0 @@
-# Copyright (c) Meta Platforms, Inc. and affiliates.
-# All rights reserved.
-#
-# This source code is licensed under the license found in the
-# LICENSE file in the root directory of this source tree.
-
-"""
-Grid search file, simply list all the exp you want in `explorer`.
-Any new exp added there will be scheduled.
-You can cancel and experiment by commenting its line.
-
-This grid shows how to train a base causal EnCodec model at 24 kHz.
-"""
-
-from ._explorers import CompressionExplorer
-from ...environment import AudioCraftEnvironment
-
-
-@CompressionExplorer
-def explorer(launcher):
- partitions = AudioCraftEnvironment.get_slurm_partitions(['team', 'global'])
- launcher.slurm_(gpus=8, partition=partitions)
- # base causal EnCodec trained on monophonic audio sampled at 24 kHz
- launcher.bind_(solver='compression/encodec_base_24khz')
- # replace this by the desired dataset
- launcher.bind_(dset='audio/example')
- # launch xp
- launcher()
diff --git a/spaces/maxmon/auto_anno/local_config.py b/spaces/maxmon/auto_anno/local_config.py
deleted file mode 100644
index ef3bc5139204fad0b267bc0a91b81bea76803975..0000000000000000000000000000000000000000
--- a/spaces/maxmon/auto_anno/local_config.py
+++ /dev/null
@@ -1 +0,0 @@
-openai_key = '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'
diff --git a/spaces/mbazaNLP/Speech-recognition-east-african-languages/app.py b/spaces/mbazaNLP/Speech-recognition-east-african-languages/app.py
deleted file mode 100644
index db6d3ae96125fe47fbbfc49ec8f2896d078bcd8a..0000000000000000000000000000000000000000
--- a/spaces/mbazaNLP/Speech-recognition-east-african-languages/app.py
+++ /dev/null
@@ -1,37 +0,0 @@
-import gradio as gr
-import nemo.collections.asr as nemo_asr
-from pydub import AudioSegment
-import pyaudioconvert as pac
-
-
-hf_model = nemo_asr.models.EncDecRNNTBPEModel.from_pretrained(
- model_name="mbazaNLP/stt_rw_sw_lg_conformer_ctc_large")
-
-def convert (audio):
- file_name = audio.name
- if file_name.endswith("mp3") or file_name.endswith("wav") or file_name.endswith("ogg"):
- if file_name.endswith("mp3"):
- sound = AudioSegment.from_mp3(audio.name)
- sound.export(audio.name, format="wav")
- elif file_name.endswith("ogg"):
- sound = AudioSegment.from_ogg(audio.name)
- sound.export(audio.name, format="wav")
- else:
- return False
- pac.convert_wav_to_16bit_mono(audio.name,audio.name)
- return True
-
-def transcribe(audio, audio_microphone):
- audio = audio_microphone if audio_microphone else audio
- if convert(audio)== False:
- return "The format must be mp3,wav and ogg"
- result= hf_model.transcribe([audio.name])
- return result[0]
-gradio_ui = gr.Interface(
- fn=transcribe,
- title="East african languages Speech Recognition",
- description="Upload an audio clip or record from browser using microphone, and let AI do the hard work of transcribing. The supported languages are Kinyarwanda, Swahili and Luganda",
- inputs=[gr.inputs.Audio(label="Upload Audio File", type="file", optional=True), gr.inputs.Audio(source="microphone", type="file", optional=True, label="Record from microphone")],
- outputs=[gr.outputs.Textbox(label="Recognized speech")]
-)
-gradio_ui.launch(enable_queue=True)
\ No newline at end of file
diff --git a/spaces/merve/data-leak/server-side/fill-in-the-blank/scatter-plot-colab/spearman-distribution/test.html b/spaces/merve/data-leak/server-side/fill-in-the-blank/scatter-plot-colab/spearman-distribution/test.html
deleted file mode 100644
index bd51a96a0e44f236d2fef909e99ce49251683407..0000000000000000000000000000000000000000
--- a/spaces/merve/data-leak/server-side/fill-in-the-blank/scatter-plot-colab/spearman-distribution/test.html
+++ /dev/null
@@ -1,12 +0,0 @@
-
-
-
-
-
-
-
-
-
-
-
-
diff --git a/spaces/merve/sorting_hat/app.py b/spaces/merve/sorting_hat/app.py
deleted file mode 100644
index 77347696e408b7c281353e7204166d950482d6c3..0000000000000000000000000000000000000000
--- a/spaces/merve/sorting_hat/app.py
+++ /dev/null
@@ -1,23 +0,0 @@
-from huggingface_hub import from_pretrained_keras
-import gradio as gr
-import numpy as np
-import tensorflow
-
-model = from_pretrained_keras("merve/riddikulus")
-
-labels = {0:"Ravenclaw 🦅💙 ", 1:"Gryffindor 🦁", 2:"Ravenclaw 🦅💙",3:"Slytherin🐍💚",
- 4:"Hufflepuff 🦡💛", 5:"Death eater detected! ", 6: "Hufflepuff 🦡💛", 7:"Slytherin🐍💚",
- 8:"Ravenclaw 🦅💙", 9:"Gryffindor 🦁"}
-
-canvas = gr.inputs.Image(source="canvas", shape=(28,28))
-text = gr.outputs.Textbox()
-def infer(image):
- cls = np.argmax(model.predict(np.expand_dims(image, axis = 0)[:,:,:,1]))
- if cls == 5:
- output = "Death eater detected! 💀"
- else:
- cls = labels[cls]
- output = f"Welcome to {cls}"
- return output
-
-gr.Interface(infer, inputs=[canvas], outputs=[text], title="Welcome to Hogwarts Sorting Hat!", description="Draw something and let the sorting hat sort you! 🎩 ").launch()
\ No newline at end of file
diff --git a/spaces/merve/uncertainty-calibration/public/uncertainty-calibration/util.js b/spaces/merve/uncertainty-calibration/public/uncertainty-calibration/util.js
deleted file mode 100644
index a0ce5b12a2a642f1186cc4004e90b046a89611f8..0000000000000000000000000000000000000000
--- a/spaces/merve/uncertainty-calibration/public/uncertainty-calibration/util.js
+++ /dev/null
@@ -1,38 +0,0 @@
-window.initUtil = function(){
- function addAxisLabel(c, xText, yText, xOffset=40, yOffset=-40){
- c.svg.select('.x').append('g')
- .translate([c.width/2, xOffset])
- .append('text.axis-label')
- .text(xText)
- .at({textAnchor: 'middle'})
- .st({fill: '#000', fontSize: 14, fontFamily: 'sans-serif'})
-
- c.svg.select('.y')
- .append('g')
- .translate([yOffset, c.height/2])
- .append('text.axis-label')
- .text(yText)
- .at({textAnchor: 'middle', transform: 'rotate(-90)'})
- .st({fill: '#000', fontSize: 14, fontFamily: 'sans-serif'})
- }
-
- function ggPlotBg(c, isBlack=true){
- if (isBlack){
- c.svg.append('rect.bg-rect')
- .at({width: c.width, height: c.height, fill: '#eee'})
- .lower()
- }
-
- c.svg.selectAll('.tick').selectAll('line').remove()
- c.svg.selectAll('.y .tick')
- .append('path').at({d: 'M 0 0 H ' + c.width, stroke: '#fff', strokeWidth: 1})
- c.svg.selectAll('.y text').at({x: -3})
- c.svg.selectAll('.x .tick')
- .append('path').at({d: 'M 0 0 V -' + c.height, stroke: '#fff', strokeWidth: 1})
- }
-
-
- return {addAxisLabel, ggPlotBg}
-}
-
-if (window.init) window.init()
\ No newline at end of file
diff --git a/spaces/metricspace/OcTra/nnet/models_vc.py b/spaces/metricspace/OcTra/nnet/models_vc.py
deleted file mode 100644
index 312741e90cd1fb2df816f595110e5b2503f378d9..0000000000000000000000000000000000000000
--- a/spaces/metricspace/OcTra/nnet/models_vc.py
+++ /dev/null
@@ -1,350 +0,0 @@
-import torch
-
-from torch import nn
-from torch.nn import functional as F
-
-from nnet import commons
-from nnet import modules
-
-from torch.nn import Conv1d, ConvTranspose1d, Conv2d
-from torch.nn.utils import weight_norm, remove_weight_norm, spectral_norm
-from nnet.commons import init_weights, get_padding
-
-
-class ResidualCouplingBlock(nn.Module):
- def __init__(self,
- channels,
- hidden_channels,
- kernel_size,
- dilation_rate,
- n_layers,
- n_flows=4,
- gin_channels=0):
- super().__init__()
- self.channels = channels
- self.hidden_channels = hidden_channels
- self.kernel_size = kernel_size
- self.dilation_rate = dilation_rate
- self.n_layers = n_layers
- self.n_flows = n_flows
- self.gin_channels = gin_channels
-
- self.flows = nn.ModuleList()
- for i in range(n_flows):
- self.flows.append(modules.ResidualCouplingLayer(channels, hidden_channels, kernel_size, dilation_rate, n_layers, gin_channels=gin_channels, mean_only=True))
- self.flows.append(modules.Flip())
-
- def forward(self, x, x_mask, g=None, reverse=False):
- if not reverse:
- for flow in self.flows:
- x, _ = flow(x, x_mask, g=g, reverse=reverse)
- else:
- for flow in reversed(self.flows):
- x = flow(x, x_mask, g=g, reverse=reverse)
- return x
-
-
-class Encoder(nn.Module):
- def __init__(self,
- in_channels,
- out_channels,
- hidden_channels,
- kernel_size,
- dilation_rate,
- n_layers,
- gin_channels=0):
- super().__init__()
- self.in_channels = in_channels
- self.out_channels = out_channels
- self.hidden_channels = hidden_channels
- self.kernel_size = kernel_size
- self.dilation_rate = dilation_rate
- self.n_layers = n_layers
- self.gin_channels = gin_channels
-
- self.pre = nn.Conv1d(in_channels, hidden_channels, 1)
- self.enc = modules.WN(hidden_channels, kernel_size, dilation_rate, n_layers, gin_channels=gin_channels)
- self.proj = nn.Conv1d(hidden_channels, out_channels * 2, 1)
-
- def forward(self, x, x_lengths, g=None):
- x_mask = torch.unsqueeze(commons.sequence_mask(x_lengths, x.size(2)), 1).to(x.dtype)
- x = self.pre(x) * x_mask
- x = self.enc(x, x_mask, g=g)
- stats = self.proj(x) * x_mask
- m, logs = torch.split(stats, self.out_channels, dim=1)
- z = (m + torch.randn_like(m) * torch.exp(logs)) * x_mask
- return z, m, logs, x_mask
-
-
-class Generator(torch.nn.Module):
- def __init__(self, initial_channel, resblock, resblock_kernel_sizes, resblock_dilation_sizes, upsample_rates, upsample_initial_channel, upsample_kernel_sizes, gin_channels=0):
- super(Generator, self).__init__()
- self.num_kernels = len(resblock_kernel_sizes)
- self.num_upsamples = len(upsample_rates)
- self.conv_pre = Conv1d(initial_channel, upsample_initial_channel, 7, 1, padding=3)
- resblock = modules.ResBlock1 if resblock == '1' else modules.ResBlock2
-
- self.ups = nn.ModuleList()
- for i, (u, k) in enumerate(zip(upsample_rates, upsample_kernel_sizes)):
- self.ups.append(weight_norm(
- ConvTranspose1d(upsample_initial_channel//(2**i), upsample_initial_channel//(2**(i+1)),
- k, u, padding=(k-u)//2)))
-
- self.resblocks = nn.ModuleList()
- for i in range(len(self.ups)):
- ch = upsample_initial_channel//(2**(i+1))
- for j, (k, d) in enumerate(zip(resblock_kernel_sizes, resblock_dilation_sizes)):
- self.resblocks.append(resblock(ch, k, d))
-
- self.conv_post = Conv1d(ch, 1, 7, 1, padding=3, bias=False)
- self.ups.apply(init_weights)
-
- if gin_channels != 0:
- self.cond = nn.Conv1d(gin_channels, upsample_initial_channel, 1)
-
- def forward(self, x, g=None):
- x = self.conv_pre(x)
- if g is not None:
- x = x + self.cond(g)
-
- for i in range(self.num_upsamples):
- x = F.leaky_relu(x, modules.LRELU_SLOPE)
- x = self.ups[i](x)
- xs = None
- for j in range(self.num_kernels):
- if xs is None:
- xs = self.resblocks[i*self.num_kernels+j](x)
- else:
- xs += self.resblocks[i*self.num_kernels+j](x)
- x = xs / self.num_kernels
- x = F.leaky_relu(x)
- x = self.conv_post(x)
- x = torch.tanh(x)
-
- return x
-
- def remove_weight_norm(self):
- print('Removing weight norm...')
- for l in self.ups:
- remove_weight_norm(l)
- for l in self.resblocks:
- l.remove_weight_norm()
-
-
-class DiscriminatorP(torch.nn.Module):
- def __init__(self, period, kernel_size=5, stride=3, use_spectral_norm=False):
- super(DiscriminatorP, self).__init__()
- self.period = period
- self.use_spectral_norm = use_spectral_norm
- norm_f = weight_norm if use_spectral_norm == False else spectral_norm
- self.convs = nn.ModuleList([
- norm_f(Conv2d(1, 32, (kernel_size, 1), (stride, 1), padding=(get_padding(kernel_size, 1), 0))),
- norm_f(Conv2d(32, 128, (kernel_size, 1), (stride, 1), padding=(get_padding(kernel_size, 1), 0))),
- norm_f(Conv2d(128, 512, (kernel_size, 1), (stride, 1), padding=(get_padding(kernel_size, 1), 0))),
- norm_f(Conv2d(512, 1024, (kernel_size, 1), (stride, 1), padding=(get_padding(kernel_size, 1), 0))),
- norm_f(Conv2d(1024, 1024, (kernel_size, 1), 1, padding=(get_padding(kernel_size, 1), 0))),
- ])
- self.conv_post = norm_f(Conv2d(1024, 1, (3, 1), 1, padding=(1, 0)))
-
- def forward(self, x):
- fmap = []
-
- # 1d to 2d
- b, c, t = x.shape
- if t % self.period != 0: # pad first
- n_pad = self.period - (t % self.period)
- x = F.pad(x, (0, n_pad), "reflect")
- t = t + n_pad
- x = x.view(b, c, t // self.period, self.period)
-
- for l in self.convs:
- x = l(x)
- x = F.leaky_relu(x, modules.LRELU_SLOPE)
- fmap.append(x)
- x = self.conv_post(x)
- fmap.append(x)
- x = torch.flatten(x, 1, -1)
-
- return x, fmap
-
-
-class DiscriminatorS(torch.nn.Module):
- def __init__(self, use_spectral_norm=False):
- super(DiscriminatorS, self).__init__()
- norm_f = weight_norm if use_spectral_norm == False else spectral_norm
- self.convs = nn.ModuleList([
- norm_f(Conv1d(1, 16, 15, 1, padding=7)),
- norm_f(Conv1d(16, 64, 41, 4, groups=4, padding=20)),
- norm_f(Conv1d(64, 256, 41, 4, groups=16, padding=20)),
- norm_f(Conv1d(256, 1024, 41, 4, groups=64, padding=20)),
- norm_f(Conv1d(1024, 1024, 41, 4, groups=256, padding=20)),
- norm_f(Conv1d(1024, 1024, 5, 1, padding=2)),
- ])
- self.conv_post = norm_f(Conv1d(1024, 1, 3, 1, padding=1))
-
- def forward(self, x):
- fmap = []
-
- for l in self.convs:
- x = l(x)
- x = F.leaky_relu(x, modules.LRELU_SLOPE)
- fmap.append(x)
- x = self.conv_post(x)
- fmap.append(x)
- x = torch.flatten(x, 1, -1)
-
- return x, fmap
-
-
-class MultiPeriodDiscriminator(torch.nn.Module):
- def __init__(self, use_spectral_norm=False):
- super(MultiPeriodDiscriminator, self).__init__()
- periods = [2,3,5,7,11]
-
- discs = [DiscriminatorS(use_spectral_norm=use_spectral_norm)]
- discs = discs + [DiscriminatorP(i, use_spectral_norm=use_spectral_norm) for i in periods]
- self.discriminators = nn.ModuleList(discs)
-
- def forward(self, y, y_hat):
- y_d_rs = []
- y_d_gs = []
- fmap_rs = []
- fmap_gs = []
- for i, d in enumerate(self.discriminators):
- y_d_r, fmap_r = d(y)
- y_d_g, fmap_g = d(y_hat)
- y_d_rs.append(y_d_r)
- y_d_gs.append(y_d_g)
- fmap_rs.append(fmap_r)
- fmap_gs.append(fmap_g)
-
- return y_d_rs, y_d_gs, fmap_rs, fmap_gs
-
-
-class SpeakerEncoder(torch.nn.Module):
- def __init__(self, mel_n_channels=80, model_num_layers=3, model_hidden_size=256, model_embedding_size=256):
- super(SpeakerEncoder, self).__init__()
- self.lstm = nn.LSTM(mel_n_channels, model_hidden_size, model_num_layers, batch_first=True)
- self.linear = nn.Linear(model_hidden_size, model_embedding_size)
- self.relu = nn.ReLU()
-
- def forward(self, mels):
- self.lstm.flatten_parameters()
- _, (hidden, _) = self.lstm(mels)
- embeds_raw = self.relu(self.linear(hidden[-1]))
- return embeds_raw / torch.norm(embeds_raw, dim=1, keepdim=True)
-
- def compute_partial_slices(self, total_frames, partial_frames, partial_hop):
- mel_slices = []
- for i in range(0, total_frames-partial_frames, partial_hop):
- mel_range = torch.arange(i, i+partial_frames)
- mel_slices.append(mel_range)
-
- return mel_slices
-
- def embed_utterance(self, mel, partial_frames=128, partial_hop=64):
- mel_len = mel.size(1)
- last_mel = mel[:,-partial_frames:]
-
- if mel_len > partial_frames:
- mel_slices = self.compute_partial_slices(mel_len, partial_frames, partial_hop)
- mels = list(mel[:,s] for s in mel_slices)
- mels.append(last_mel)
- mels = torch.stack(tuple(mels), 0).squeeze(1)
-
- with torch.no_grad():
- partial_embeds = self(mels)
- embed = torch.mean(partial_embeds, axis=0).unsqueeze(0)
- #embed = embed / torch.linalg.norm(embed, 2)
- else:
- with torch.no_grad():
- embed = self(last_mel)
-
- return embed
-
-
-class SynthesizerTrn(nn.Module):
- """
- Synthesizer for Training
- """
-
- def __init__(self,
- spec_channels,
- segment_size,
- inter_channels,
- hidden_channels,
- filter_channels,
- n_heads,
- n_layers,
- kernel_size,
- p_dropout,
- resblock,
- resblock_kernel_sizes,
- resblock_dilation_sizes,
- upsample_rates,
- upsample_initial_channel,
- upsample_kernel_sizes,
- gin_channels,
- ssl_dim,
- use_spk,
- **kwargs):
-
- super().__init__()
- self.spec_channels = spec_channels
- self.inter_channels = inter_channels
- self.hidden_channels = hidden_channels
- self.filter_channels = filter_channels
- self.n_heads = n_heads
- self.n_layers = n_layers
- self.kernel_size = kernel_size
- self.p_dropout = p_dropout
- self.resblock = resblock
- self.resblock_kernel_sizes = resblock_kernel_sizes
- self.resblock_dilation_sizes = resblock_dilation_sizes
- self.upsample_rates = upsample_rates
- self.upsample_initial_channel = upsample_initial_channel
- self.upsample_kernel_sizes = upsample_kernel_sizes
- self.segment_size = segment_size
- self.gin_channels = gin_channels
- self.ssl_dim = ssl_dim
- self.use_spk = use_spk
-
- self.enc_p = Encoder(ssl_dim, inter_channels, hidden_channels, 5, 1, 16)
- self.dec = Generator(inter_channels, resblock, resblock_kernel_sizes, resblock_dilation_sizes, upsample_rates, upsample_initial_channel, upsample_kernel_sizes, gin_channels=gin_channels)
- self.enc_q = Encoder(spec_channels, inter_channels, hidden_channels, 5, 1, 16, gin_channels=gin_channels)
- self.flow = ResidualCouplingBlock(inter_channels, hidden_channels, 5, 1, 4, gin_channels=gin_channels)
-
- if not self.use_spk:
- self.enc_spk = SpeakerEncoder(model_hidden_size=gin_channels, model_embedding_size=gin_channels)
-
- def forward(self, c, spec, g=None, mel=None, c_lengths=None, spec_lengths=None):
- if c_lengths == None:
- c_lengths = (torch.ones(c.size(0)) * c.size(-1)).to(c.device)
- if spec_lengths == None:
- spec_lengths = (torch.ones(spec.size(0)) * spec.size(-1)).to(spec.device)
-
- if not self.use_spk:
- g = self.enc_spk(mel.transpose(1,2))
- g = g.unsqueeze(-1)
-
- _, m_p, logs_p, _ = self.enc_p(c, c_lengths)
- z, m_q, logs_q, spec_mask = self.enc_q(spec, spec_lengths, g=g)
- z_p = self.flow(z, spec_mask, g=g)
-
- z_slice, ids_slice = commons.rand_slice_segments(z, spec_lengths, self.segment_size)
- o = self.dec(z_slice, g=g)
-
- return o, ids_slice, spec_mask, (z, z_p, m_p, logs_p, m_q, logs_q)
-
- def infer(self, c, g=None, mel=None, c_lengths=None):
- if c_lengths == None:
- c_lengths = (torch.ones(c.size(0)) * c.size(-1)).to(c.device)
- if not self.use_spk:
- g = self.enc_spk.embed_utterance(mel.transpose(1,2))
- g = g.unsqueeze(-1)
-
- z_p, m_p, logs_p, c_mask = self.enc_p(c, c_lengths)
- z = self.flow(z_p, c_mask, g=g, reverse=True)
- o = self.dec(z * c_mask, g=g)
-
- return o
diff --git a/spaces/mfrashad/CharacterGAN/netdissect/__main__.py b/spaces/mfrashad/CharacterGAN/netdissect/__main__.py
deleted file mode 100644
index e2bd9f630eaa0f45a6a201adcf356a1e092050cb..0000000000000000000000000000000000000000
--- a/spaces/mfrashad/CharacterGAN/netdissect/__main__.py
+++ /dev/null
@@ -1,408 +0,0 @@
-import torch, sys, os, argparse, textwrap, numbers, numpy, json, PIL
-from torchvision import transforms
-from torch.utils.data import TensorDataset
-from netdissect.progress import verbose_progress, print_progress
-from netdissect import InstrumentedModel, BrodenDataset, dissect
-from netdissect import MultiSegmentDataset, GeneratorSegRunner
-from netdissect import ImageOnlySegRunner
-from netdissect.parallelfolder import ParallelImageFolders
-from netdissect.zdataset import z_dataset_for_model
-from netdissect.autoeval import autoimport_eval
-from netdissect.modelconfig import create_instrumented_model
-from netdissect.pidfile import exit_if_job_done, mark_job_done
-
-help_epilog = '''\
-Example: to dissect three layers of the pretrained alexnet in torchvision:
-
-python -m netdissect \\
- --model "torchvision.models.alexnet(pretrained=True)" \\
- --layers features.6:conv3 features.8:conv4 features.10:conv5 \\
- --imgsize 227 \\
- --outdir dissect/alexnet-imagenet
-
-To dissect a progressive GAN model:
-
-python -m netdissect \\
- --model "proggan.from_pth_file('model/churchoutdoor.pth')" \\
- --gan
-'''
-
-def main():
- # Training settings
- def strpair(arg):
- p = tuple(arg.split(':'))
- if len(p) == 1:
- p = p + p
- return p
- def intpair(arg):
- p = arg.split(',')
- if len(p) == 1:
- p = p + p
- return tuple(int(v) for v in p)
-
- parser = argparse.ArgumentParser(description='Net dissect utility',
- prog='python -m netdissect',
- epilog=textwrap.dedent(help_epilog),
- formatter_class=argparse.RawDescriptionHelpFormatter)
- parser.add_argument('--model', type=str, default=None,
- help='constructor for the model to test')
- parser.add_argument('--pthfile', type=str, default=None,
- help='filename of .pth file for the model')
- parser.add_argument('--unstrict', action='store_true', default=False,
- help='ignore unexpected pth parameters')
- parser.add_argument('--submodule', type=str, default=None,
- help='submodule to load from pthfile')
- parser.add_argument('--outdir', type=str, default='dissect',
- help='directory for dissection output')
- parser.add_argument('--layers', type=strpair, nargs='+',
- help='space-separated list of layer names to dissect' +
- ', in the form layername[:reportedname]')
- parser.add_argument('--segments', type=str, default='dataset/broden',
- help='directory containing segmentation dataset')
- parser.add_argument('--segmenter', type=str, default=None,
- help='constructor for asegmenter class')
- parser.add_argument('--download', action='store_true', default=False,
- help='downloads Broden dataset if needed')
- parser.add_argument('--imagedir', type=str, default=None,
- help='directory containing image-only dataset')
- parser.add_argument('--imgsize', type=intpair, default=(227, 227),
- help='input image size to use')
- parser.add_argument('--netname', type=str, default=None,
- help='name for network in generated reports')
- parser.add_argument('--meta', type=str, nargs='+',
- help='json files of metadata to add to report')
- parser.add_argument('--merge', type=str,
- help='json file of unit data to merge in report')
- parser.add_argument('--examples', type=int, default=20,
- help='number of image examples per unit')
- parser.add_argument('--size', type=int, default=10000,
- help='dataset subset size to use')
- parser.add_argument('--batch_size', type=int, default=100,
- help='batch size for forward pass')
- parser.add_argument('--num_workers', type=int, default=24,
- help='number of DataLoader workers')
- parser.add_argument('--quantile_threshold', type=strfloat, default=None,
- choices=[FloatRange(0.0, 1.0), 'iqr'],
- help='quantile to use for masks')
- parser.add_argument('--no-labels', action='store_true', default=False,
- help='disables labeling of units')
- parser.add_argument('--maxiou', action='store_true', default=False,
- help='enables maxiou calculation')
- parser.add_argument('--covariance', action='store_true', default=False,
- help='enables covariance calculation')
- parser.add_argument('--rank_all_labels', action='store_true', default=False,
- help='include low-information labels in rankings')
- parser.add_argument('--no-images', action='store_true', default=False,
- help='disables generation of unit images')
- parser.add_argument('--no-report', action='store_true', default=False,
- help='disables generation report summary')
- parser.add_argument('--no-cuda', action='store_true', default=False,
- help='disables CUDA usage')
- parser.add_argument('--gen', action='store_true', default=False,
- help='test a generator model (e.g., a GAN)')
- parser.add_argument('--gan', action='store_true', default=False,
- help='synonym for --gen')
- parser.add_argument('--perturbation', default=None,
- help='filename of perturbation attack to apply')
- parser.add_argument('--add_scale_offset', action='store_true', default=None,
- help='offsets masks according to stride and padding')
- parser.add_argument('--quiet', action='store_true', default=False,
- help='silences console output')
- if len(sys.argv) == 1:
- parser.print_usage(sys.stderr)
- sys.exit(1)
- args = parser.parse_args()
- args.images = not args.no_images
- args.report = not args.no_report
- args.labels = not args.no_labels
- if args.gan:
- args.gen = args.gan
-
- # Set up console output
- verbose_progress(not args.quiet)
-
- # Exit right away if job is already done or being done.
- if args.outdir is not None:
- exit_if_job_done(args.outdir)
-
- # Speed up pytorch
- torch.backends.cudnn.benchmark = True
-
- # Special case: download flag without model to test.
- if args.model is None and args.download:
- from netdissect.broden import ensure_broden_downloaded
- for resolution in [224, 227, 384]:
- ensure_broden_downloaded(args.segments, resolution, 1)
- from netdissect.segmenter import ensure_upp_segmenter_downloaded
- ensure_upp_segmenter_downloaded('dataset/segmodel')
- sys.exit(0)
-
- # Help if broden is not present
- if not args.gen and not args.imagedir and not os.path.isdir(args.segments):
- print_progress('Segmentation dataset not found at %s.' % args.segments)
- print_progress('Specify dataset directory using --segments [DIR]')
- print_progress('To download Broden, run: netdissect --download')
- sys.exit(1)
-
- # Default segmenter class
- if args.gen and args.segmenter is None:
- args.segmenter = ("netdissect.segmenter.UnifiedParsingSegmenter(" +
- "segsizes=[256], segdiv='quad')")
-
- # Default threshold
- if args.quantile_threshold is None:
- if args.gen:
- args.quantile_threshold = 'iqr'
- else:
- args.quantile_threshold = 0.005
-
- # Set up CUDA
- args.cuda = not args.no_cuda and torch.cuda.is_available()
- if args.cuda:
- torch.backends.cudnn.benchmark = True
-
- # Construct the network with specified layers instrumented
- if args.model is None:
- print_progress('No model specified')
- sys.exit(1)
- model = create_instrumented_model(args)
-
- # Update any metadata from files, if any
- meta = getattr(model, 'meta', {})
- if args.meta:
- for mfilename in args.meta:
- with open(mfilename) as f:
- meta.update(json.load(f))
-
- # Load any merge data from files
- mergedata = None
- if args.merge:
- with open(args.merge) as f:
- mergedata = json.load(f)
-
- # Set up the output directory, verify write access
- if args.outdir is None:
- args.outdir = os.path.join('dissect', type(model).__name__)
- exit_if_job_done(args.outdir)
- print_progress('Writing output into %s.' % args.outdir)
- os.makedirs(args.outdir, exist_ok=True)
- train_dataset = None
-
- if not args.gen:
- # Load dataset for classifier case.
- # Load perturbation
- perturbation = numpy.load(args.perturbation
- ) if args.perturbation else None
- segrunner = None
-
- # Load broden dataset
- if args.imagedir is not None:
- dataset = try_to_load_images(args.imagedir, args.imgsize,
- perturbation, args.size)
- segrunner = ImageOnlySegRunner(dataset)
- else:
- dataset = try_to_load_broden(args.segments, args.imgsize, 1,
- perturbation, args.download, args.size)
- if dataset is None:
- dataset = try_to_load_multiseg(args.segments, args.imgsize,
- perturbation, args.size)
- if dataset is None:
- print_progress('No segmentation dataset found in %s',
- args.segments)
- print_progress('use --download to download Broden.')
- sys.exit(1)
- else:
- # For segmenter case the dataset is just a random z
- dataset = z_dataset_for_model(model, args.size)
- train_dataset = z_dataset_for_model(model, args.size, seed=2)
- segrunner = GeneratorSegRunner(autoimport_eval(args.segmenter))
-
- # Run dissect
- dissect(args.outdir, model, dataset,
- train_dataset=train_dataset,
- segrunner=segrunner,
- examples_per_unit=args.examples,
- netname=args.netname,
- quantile_threshold=args.quantile_threshold,
- meta=meta,
- merge=mergedata,
- make_images=args.images,
- make_labels=args.labels,
- make_maxiou=args.maxiou,
- make_covariance=args.covariance,
- make_report=args.report,
- make_row_images=args.images,
- make_single_images=True,
- rank_all_labels=args.rank_all_labels,
- batch_size=args.batch_size,
- num_workers=args.num_workers,
- settings=vars(args))
-
- # Mark the directory so that it's not done again.
- mark_job_done(args.outdir)
-
-class AddPerturbation(object):
- def __init__(self, perturbation):
- self.perturbation = perturbation
-
- def __call__(self, pic):
- if self.perturbation is None:
- return pic
- # Convert to a numpy float32 array
- npyimg = numpy.array(pic, numpy.uint8, copy=False
- ).astype(numpy.float32)
- # Center the perturbation
- oy, ox = ((self.perturbation.shape[d] - npyimg.shape[d]) // 2
- for d in [0, 1])
- npyimg += self.perturbation[
- oy:oy+npyimg.shape[0], ox:ox+npyimg.shape[1]]
- # Pytorch conventions: as a float it should be [0..1]
- npyimg.clip(0, 255, npyimg)
- return npyimg / 255.0
-
-def test_dissection():
- verbose_progress(True)
- from torchvision.models import alexnet
- from torchvision import transforms
- model = InstrumentedModel(alexnet(pretrained=True))
- model.eval()
- # Load an alexnet
- model.retain_layers([
- ('features.0', 'conv1'),
- ('features.3', 'conv2'),
- ('features.6', 'conv3'),
- ('features.8', 'conv4'),
- ('features.10', 'conv5') ])
- # load broden dataset
- bds = BrodenDataset('dataset/broden',
- transform=transforms.Compose([
- transforms.ToTensor(),
- transforms.Normalize(IMAGE_MEAN, IMAGE_STDEV)]),
- size=100)
- # run dissect
- dissect('dissect/test', model, bds,
- examples_per_unit=10)
-
-def try_to_load_images(directory, imgsize, perturbation, size):
- # Load plain image dataset
- # TODO: allow other normalizations.
- return ParallelImageFolders(
- [directory],
- transform=transforms.Compose([
- transforms.Resize(imgsize),
- AddPerturbation(perturbation),
- transforms.ToTensor(),
- transforms.Normalize(IMAGE_MEAN, IMAGE_STDEV)]),
- size=size)
-
-def try_to_load_broden(directory, imgsize, broden_version, perturbation,
- download, size):
- # Load broden dataset
- ds_resolution = (224 if max(imgsize) <= 224 else
- 227 if max(imgsize) <= 227 else 384)
- if not os.path.isfile(os.path.join(directory,
- 'broden%d_%d' % (broden_version, ds_resolution), 'index.csv')):
- return None
- return BrodenDataset(directory,
- resolution=ds_resolution,
- download=download,
- broden_version=broden_version,
- transform=transforms.Compose([
- transforms.Resize(imgsize),
- AddPerturbation(perturbation),
- transforms.ToTensor(),
- transforms.Normalize(IMAGE_MEAN, IMAGE_STDEV)]),
- size=size)
-
-def try_to_load_multiseg(directory, imgsize, perturbation, size):
- if not os.path.isfile(os.path.join(directory, 'labelnames.json')):
- return None
- minsize = min(imgsize) if hasattr(imgsize, '__iter__') else imgsize
- return MultiSegmentDataset(directory,
- transform=(transforms.Compose([
- transforms.Resize(minsize),
- transforms.CenterCrop(imgsize),
- AddPerturbation(perturbation),
- transforms.ToTensor(),
- transforms.Normalize(IMAGE_MEAN, IMAGE_STDEV)]),
- transforms.Compose([
- transforms.Resize(minsize, interpolation=PIL.Image.NEAREST),
- transforms.CenterCrop(imgsize)])),
- size=size)
-
-def add_scale_offset_info(model, layer_names):
- '''
- Creates a 'scale_offset' property on the model which guesses
- how to offset the featuremap, in cases where the convolutional
- padding does not exacly correspond to keeping featuremap pixels
- centered on the downsampled regions of the input. This mainly
- shows up in AlexNet: ResNet and VGG pad convolutions to keep
- them centered and do not need this.
- '''
- model.scale_offset = {}
- seen = set()
- sequence = []
- aka_map = {}
- for name in layer_names:
- aka = name
- if not isinstance(aka, str):
- name, aka = name
- aka_map[name] = aka
- for name, layer in model.named_modules():
- sequence.append(layer)
- if name in aka_map:
- seen.add(name)
- aka = aka_map[name]
- model.scale_offset[aka] = sequence_scale_offset(sequence)
- for name in aka_map:
- assert name in seen, ('Layer %s not found' % name)
-
-def dilation_scale_offset(dilations):
- '''Composes a list of (k, s, p) into a single total scale and offset.'''
- if len(dilations) == 0:
- return (1, 0)
- scale, offset = dilation_scale_offset(dilations[1:])
- kernel, stride, padding = dilations[0]
- scale *= stride
- offset *= stride
- offset += (kernel - 1) / 2.0 - padding
- return scale, offset
-
-def dilations(modulelist):
- '''Converts a list of modules to (kernel_size, stride, padding)'''
- result = []
- for module in modulelist:
- settings = tuple(getattr(module, n, d)
- for n, d in (('kernel_size', 1), ('stride', 1), ('padding', 0)))
- settings = (((s, s) if not isinstance(s, tuple) else s)
- for s in settings)
- if settings != ((1, 1), (1, 1), (0, 0)):
- result.append(zip(*settings))
- return zip(*result)
-
-def sequence_scale_offset(modulelist):
- '''Returns (yscale, yoffset), (xscale, xoffset) given a list of modules'''
- return tuple(dilation_scale_offset(d) for d in dilations(modulelist))
-
-
-def strfloat(s):
- try:
- return float(s)
- except:
- return s
-
-class FloatRange(object):
- def __init__(self, start, end):
- self.start = start
- self.end = end
- def __eq__(self, other):
- return isinstance(other, float) and self.start <= other <= self.end
- def __repr__(self):
- return '[%g-%g]' % (self.start, self.end)
-
-# Many models use this normalization.
-IMAGE_MEAN = [0.485, 0.456, 0.406]
-IMAGE_STDEV = [0.229, 0.224, 0.225]
-
-if __name__ == '__main__':
- main()
diff --git a/spaces/mfrashad/ClothingGAN/models/biggan/pytorch_biggan/scripts/download_tf_hub_models.sh b/spaces/mfrashad/ClothingGAN/models/biggan/pytorch_biggan/scripts/download_tf_hub_models.sh
deleted file mode 100644
index 57655fbd4b77791f03d72b3dfeb3bbb89ccc2fdc..0000000000000000000000000000000000000000
--- a/spaces/mfrashad/ClothingGAN/models/biggan/pytorch_biggan/scripts/download_tf_hub_models.sh
+++ /dev/null
@@ -1,21 +0,0 @@
-# Copyright (c) 2019-present, Thomas Wolf, Huggingface Inc.
-# All rights reserved.
-#
-# This source code is licensed under the license found in the
-# LICENSE file in the root directory of this source tree.
-#
-
-set -e
-set -x
-
-models="128 256 512"
-
-mkdir -p models/model_128
-mkdir -p models/model_256
-mkdir -p models/model_512
-
-# Download TF Hub models.
-for model in $models
-do
- curl -L "https://tfhub.dev/deepmind/biggan-deep-$model/1?tf-hub-format=compressed" | tar -zxvC models/model_$model
-done
diff --git a/spaces/mithril-security/blind_chat/src/lib/server/websearch/summarizeWeb.ts b/spaces/mithril-security/blind_chat/src/lib/server/websearch/summarizeWeb.ts
deleted file mode 100644
index 2998f79e6939f16f6d5c6ff2967bead5729470e7..0000000000000000000000000000000000000000
--- a/spaces/mithril-security/blind_chat/src/lib/server/websearch/summarizeWeb.ts
+++ /dev/null
@@ -1,39 +0,0 @@
-import { HF_ACCESS_TOKEN } from "$env/static/private";
-import { HfInference } from "@huggingface/inference";
-import { defaultModel } from "$lib/server/models";
-import type { BackendModel } from "../models";
-import { generateFromDefaultEndpoint } from "../generateFromDefaultEndpoint";
-
-export async function summarizeWeb(content: string, query: string, model: BackendModel) {
- // if HF_ACCESS_TOKEN is set, we use a HF dedicated endpoint for summarization
- try {
- if (HF_ACCESS_TOKEN) {
- const summary = (
- await new HfInference(HF_ACCESS_TOKEN).summarization({
- model: "facebook/bart-large-cnn",
- inputs: content,
- parameters: {
- max_length: 512,
- },
- })
- ).summary_text;
- return summary;
- }
- } catch (e) {
- console.log(e);
- }
-
- // else we use the LLM to generate a summary
- const summaryPrompt = defaultModel.webSearchSummaryPromptRender({
- answer: content
- .split(" ")
- .slice(0, model.parameters?.truncate ?? 0)
- .join(" "),
- query: query,
- });
- const summary = await generateFromDefaultEndpoint(summaryPrompt).then((txt: string) =>
- txt.trim()
- );
-
- return summary;
-}
diff --git a/spaces/monra/freegpt-webui/g4f/Provider/Providers/Vercel.py b/spaces/monra/freegpt-webui/g4f/Provider/Providers/Vercel.py
deleted file mode 100644
index e5df9cf017e4c1a265f5c9d5e48eb5c10a56e60a..0000000000000000000000000000000000000000
--- a/spaces/monra/freegpt-webui/g4f/Provider/Providers/Vercel.py
+++ /dev/null
@@ -1,162 +0,0 @@
-import os
-import json
-import base64
-import execjs
-import queue
-import threading
-
-from curl_cffi import requests
-from ...typing import sha256, Dict, get_type_hints
-
-url = 'https://play.vercel.ai'
-supports_stream = True
-needs_auth = False
-
-models = {
- 'claude-instant-v1': 'anthropic:claude-instant-v1',
- 'claude-v1': 'anthropic:claude-v1',
- 'alpaca-7b': 'replicate:replicate/alpaca-7b',
- 'stablelm-tuned-alpha-7b': 'replicate:stability-ai/stablelm-tuned-alpha-7b',
- 'bloom': 'huggingface:bigscience/bloom',
- 'bloomz': 'huggingface:bigscience/bloomz',
- 'flan-t5-xxl': 'huggingface:google/flan-t5-xxl',
- 'flan-ul2': 'huggingface:google/flan-ul2',
- 'gpt-neox-20b': 'huggingface:EleutherAI/gpt-neox-20b',
- 'oasst-sft-4-pythia-12b-epoch-3.5': 'huggingface:OpenAssistant/oasst-sft-4-pythia-12b-epoch-3.5',
- 'santacoder': 'huggingface:bigcode/santacoder',
- 'command-medium-nightly': 'cohere:command-medium-nightly',
- 'command-xlarge-nightly': 'cohere:command-xlarge-nightly',
- 'code-cushman-001': 'openai:code-cushman-001',
- 'code-davinci-002': 'openai:code-davinci-002',
- 'gpt-3.5-turbo': 'openai:gpt-3.5-turbo',
- 'text-ada-001': 'openai:text-ada-001',
- 'text-babbage-001': 'openai:text-babbage-001',
- 'text-curie-001': 'openai:text-curie-001',
- 'text-davinci-002': 'openai:text-davinci-002',
- 'text-davinci-003': 'openai:text-davinci-003'
-}
-model = models.keys()
-
-vercel_models = {'anthropic:claude-instant-v1': {'id': 'anthropic:claude-instant-v1', 'provider': 'anthropic', 'providerHumanName': 'Anthropic', 'makerHumanName': 'Anthropic', 'minBillingTier': 'hobby', 'parameters': {'temperature': {'value': 1, 'range': [0, 1]}, 'maximumLength': {'value': 200, 'range': [50, 1024]}, 'topP': {'value': 1, 'range': [0.1, 1]}, 'topK': {'value': 1, 'range': [1, 500]}, 'presencePenalty': {'value': 1, 'range': [0, 1]}, 'frequencyPenalty': {'value': 1, 'range': [0, 1]}, 'stopSequences': {'value': ['\n\nHuman:'], 'range': []}}, 'name': 'claude-instant-v1'}, 'anthropic:claude-v1': {'id': 'anthropic:claude-v1', 'provider': 'anthropic', 'providerHumanName': 'Anthropic', 'makerHumanName': 'Anthropic', 'minBillingTier': 'hobby', 'parameters': {'temperature': {'value': 1, 'range': [0, 1]}, 'maximumLength': {'value': 200, 'range': [50, 1024]}, 'topP': {'value': 1, 'range': [0.1, 1]}, 'topK': {'value': 1, 'range': [1, 500]}, 'presencePenalty': {'value': 1, 'range': [0, 1]}, 'frequencyPenalty': {'value': 1, 'range': [0, 1]}, 'stopSequences': {'value': ['\n\nHuman:'], 'range': []}}, 'name': 'claude-v1'}, 'replicate:replicate/alpaca-7b': {'id': 'replicate:replicate/alpaca-7b', 'provider': 'replicate', 'providerHumanName': 'Replicate', 'makerHumanName': 'Stanford', 'parameters': {'temperature': {'value': 0.75, 'range': [0.01, 5]}, 'maximumLength': {'value': 200, 'range': [50, 512]}, 'topP': {'value': 0.95, 'range': [0.01, 1]}, 'presencePenalty': {'value': 0, 'range': [0, 1]}, 'frequencyPenalty': {'value': 0, 'range': [0, 1]}, 'repetitionPenalty': {'value': 1.1765, 'range': [0.01, 5]}, 'stopSequences': {'value': [], 'range': []}}, 'version': '2014ee1247354f2e81c0b3650d71ca715bc1e610189855f134c30ecb841fae21', 'name': 'alpaca-7b'}, 'replicate:stability-ai/stablelm-tuned-alpha-7b': {'id': 'replicate:stability-ai/stablelm-tuned-alpha-7b', 'provider': 'replicate', 'makerHumanName': 'StabilityAI', 'providerHumanName': 'Replicate', 'parameters': {'temperature': {'value': 0.75, 'range': [0.01, 5]}, 'maximumLength': {'value': 200, 'range': [50, 512]}, 'topP': {'value': 0.95, 'range': [0.01, 1]}, 'presencePenalty': {'value': 0, 'range': [0, 1]}, 'frequencyPenalty': {'value': 0, 'range': [0, 1]}, 'repetitionPenalty': {'value': 1.1765, 'range': [0.01, 5]}, 'stopSequences': {'value': [], 'range': []}}, 'version': '4a9a32b4fd86c2d047f1d271fa93972683ec6ef1cf82f402bd021f267330b50b', 'name': 'stablelm-tuned-alpha-7b'}, 'huggingface:bigscience/bloom': {'id': 'huggingface:bigscience/bloom', 'provider': 'huggingface', 'providerHumanName': 'HuggingFace', 'makerHumanName': 'BigScience', 'instructions': "Do NOT talk to Bloom as an entity, it's not a chatbot but a webpage/blog/article completion model. For the best results: mimic a few words of a webpage similar to the content you want to generate. Start a sentence as if YOU were writing a blog, webpage, math post, coding article and Bloom will generate a coherent follow-up.", 'parameters': {'temperature': {'value': 0.5, 'range': [0.1, 1]}, 'maximumLength': {'value': 200, 'range': [50, 1024]}, 'topP': {'value': 0.95, 'range': [0.01, 0.99]}, 'topK': {'value': 4, 'range': [1, 500]}, 'repetitionPenalty': {'value': 1.03, 'range': [0.1, 2]}}, 'name': 'bloom'}, 'huggingface:bigscience/bloomz': {'id': 'huggingface:bigscience/bloomz', 'provider': 'huggingface', 'providerHumanName': 'HuggingFace', 'makerHumanName': 'BigScience', 'instructions': 'We recommend using the model to perform tasks expressed in natural language. For example, given the prompt "Translate to English: Je t\'aime.", the model will most likely answer "I love you.".', 'parameters': {'temperature': {'value': 0.5, 'range': [0.1, 1]}, 'maximumLength': {'value': 200, 'range': [50, 1024]}, 'topP': {'value': 0.95, 'range': [0.01, 0.99]}, 'topK': {'value': 4, 'range': [1, 500]}, 'repetitionPenalty': {'value': 1.03, 'range': [0.1, 2]}}, 'name': 'bloomz'}, 'huggingface:google/flan-t5-xxl': {'id': 'huggingface:google/flan-t5-xxl', 'provider': 'huggingface', 'makerHumanName': 'Google', 'providerHumanName': 'HuggingFace', 'name': 'flan-t5-xxl', 'parameters': {'temperature': {'value': 0.5, 'range': [0.1, 1]}, 'maximumLength': {'value': 200, 'range': [50, 1024]}, 'topP': {'value': 0.95, 'range': [0.01, 0.99]}, 'topK': {'value': 4, 'range': [1, 500]}, 'repetitionPenalty': {'value': 1.03, 'range': [0.1, 2]}}}, 'huggingface:google/flan-ul2': {'id': 'huggingface:google/flan-ul2', 'provider': 'huggingface', 'providerHumanName': 'HuggingFace', 'makerHumanName': 'Google', 'parameters': {'temperature': {'value': 0.5, 'range': [0.1, 1]}, 'maximumLength': {'value': 200, 'range': [50, 1024]}, 'topP': {'value': 0.95, 'range': [0.01, 0.99]}, 'topK': {'value': 4, 'range': [1, 500]}, 'repetitionPenalty': {'value': 1.03, 'range': [0.1, 2]}}, 'name': 'flan-ul2'}, 'huggingface:EleutherAI/gpt-neox-20b': {'id': 'huggingface:EleutherAI/gpt-neox-20b', 'provider': 'huggingface', 'providerHumanName': 'HuggingFace', 'makerHumanName': 'EleutherAI', 'parameters': {'temperature': {'value': 0.5, 'range': [0.1, 1]}, 'maximumLength': {'value': 200, 'range': [50, 1024]}, 'topP': {'value': 0.95, 'range': [0.01, 0.99]}, 'topK': {'value': 4, 'range': [1, 500]}, 'repetitionPenalty': {'value': 1.03, 'range': [0.1, 2]}, 'stopSequences': {'value': [], 'range': []}}, 'name': 'gpt-neox-20b'}, 'huggingface:OpenAssistant/oasst-sft-4-pythia-12b-epoch-3.5': {'id': 'huggingface:OpenAssistant/oasst-sft-4-pythia-12b-epoch-3.5', 'provider': 'huggingface', 'providerHumanName': 'HuggingFace', 'makerHumanName': 'OpenAssistant', 'parameters': {'maximumLength': {'value': 200, 'range': [50, 1024]}, 'typicalP': {'value': 0.2, 'range': [0.1, 0.99]}, 'repetitionPenalty': {'value': 1, 'range': [0.1, 2]}}, 'name': 'oasst-sft-4-pythia-12b-epoch-3.5'}, 'huggingface:bigcode/santacoder': {
- 'id': 'huggingface:bigcode/santacoder', 'provider': 'huggingface', 'providerHumanName': 'HuggingFace', 'makerHumanName': 'BigCode', 'instructions': 'The model was trained on GitHub code. As such it is not an instruction model and commands like "Write a function that computes the square root." do not work well. You should phrase commands like they occur in source code such as comments (e.g. # the following function computes the sqrt) or write a function signature and docstring and let the model complete the function body.', 'parameters': {'temperature': {'value': 0.5, 'range': [0.1, 1]}, 'maximumLength': {'value': 200, 'range': [50, 1024]}, 'topP': {'value': 0.95, 'range': [0.01, 0.99]}, 'topK': {'value': 4, 'range': [1, 500]}, 'repetitionPenalty': {'value': 1.03, 'range': [0.1, 2]}}, 'name': 'santacoder'}, 'cohere:command-medium-nightly': {'id': 'cohere:command-medium-nightly', 'provider': 'cohere', 'providerHumanName': 'Cohere', 'makerHumanName': 'Cohere', 'name': 'command-medium-nightly', 'parameters': {'temperature': {'value': 0.9, 'range': [0, 2]}, 'maximumLength': {'value': 200, 'range': [50, 1024]}, 'topP': {'value': 1, 'range': [0, 1]}, 'topK': {'value': 0, 'range': [0, 500]}, 'presencePenalty': {'value': 0, 'range': [0, 1]}, 'frequencyPenalty': {'value': 0, 'range': [0, 1]}, 'stopSequences': {'value': [], 'range': []}}}, 'cohere:command-xlarge-nightly': {'id': 'cohere:command-xlarge-nightly', 'provider': 'cohere', 'providerHumanName': 'Cohere', 'makerHumanName': 'Cohere', 'name': 'command-xlarge-nightly', 'parameters': {'temperature': {'value': 0.9, 'range': [0, 2]}, 'maximumLength': {'value': 200, 'range': [50, 1024]}, 'topP': {'value': 1, 'range': [0, 1]}, 'topK': {'value': 0, 'range': [0, 500]}, 'presencePenalty': {'value': 0, 'range': [0, 1]}, 'frequencyPenalty': {'value': 0, 'range': [0, 1]}, 'stopSequences': {'value': [], 'range': []}}}, 'openai:gpt-4': {'id': 'openai:gpt-4', 'provider': 'openai', 'providerHumanName': 'OpenAI', 'makerHumanName': 'OpenAI', 'name': 'gpt-4', 'minBillingTier': 'pro', 'parameters': {'temperature': {'value': 0.7, 'range': [0.1, 1]}, 'maximumLength': {'value': 200, 'range': [50, 1024]}, 'topP': {'value': 1, 'range': [0.1, 1]}, 'presencePenalty': {'value': 0, 'range': [0, 1]}, 'frequencyPenalty': {'value': 0, 'range': [0, 1]}, 'stopSequences': {'value': [], 'range': []}}}, 'openai:code-cushman-001': {'id': 'openai:code-cushman-001', 'provider': 'openai', 'providerHumanName': 'OpenAI', 'makerHumanName': 'OpenAI', 'parameters': {'temperature': {'value': 0.5, 'range': [0.1, 1]}, 'maximumLength': {'value': 200, 'range': [50, 1024]}, 'topP': {'value': 1, 'range': [0.1, 1]}, 'presencePenalty': {'value': 0, 'range': [0, 1]}, 'frequencyPenalty': {'value': 0, 'range': [0, 1]}, 'stopSequences': {'value': [], 'range': []}}, 'name': 'code-cushman-001'}, 'openai:code-davinci-002': {'id': 'openai:code-davinci-002', 'provider': 'openai', 'providerHumanName': 'OpenAI', 'makerHumanName': 'OpenAI', 'parameters': {'temperature': {'value': 0.5, 'range': [0.1, 1]}, 'maximumLength': {'value': 200, 'range': [50, 1024]}, 'topP': {'value': 1, 'range': [0.1, 1]}, 'presencePenalty': {'value': 0, 'range': [0, 1]}, 'frequencyPenalty': {'value': 0, 'range': [0, 1]}, 'stopSequences': {'value': [], 'range': []}}, 'name': 'code-davinci-002'}, 'openai:gpt-3.5-turbo': {'id': 'openai:gpt-3.5-turbo', 'provider': 'openai', 'providerHumanName': 'OpenAI', 'makerHumanName': 'OpenAI', 'parameters': {'temperature': {'value': 0.7, 'range': [0, 1]}, 'maximumLength': {'value': 200, 'range': [50, 1024]}, 'topP': {'value': 1, 'range': [0.1, 1]}, 'topK': {'value': 1, 'range': [1, 500]}, 'presencePenalty': {'value': 1, 'range': [0, 1]}, 'frequencyPenalty': {'value': 1, 'range': [0, 1]}, 'stopSequences': {'value': [], 'range': []}}, 'name': 'gpt-3.5-turbo'}, 'openai:text-ada-001': {'id': 'openai:text-ada-001', 'provider': 'openai', 'providerHumanName': 'OpenAI', 'makerHumanName': 'OpenAI', 'name': 'text-ada-001', 'parameters': {'temperature': {'value': 0.5, 'range': [0.1, 1]}, 'maximumLength': {'value': 200, 'range': [50, 1024]}, 'topP': {'value': 1, 'range': [0.1, 1]}, 'presencePenalty': {'value': 0, 'range': [0, 1]}, 'frequencyPenalty': {'value': 0, 'range': [0, 1]}, 'stopSequences': {'value': [], 'range': []}}}, 'openai:text-babbage-001': {'id': 'openai:text-babbage-001', 'provider': 'openai', 'providerHumanName': 'OpenAI', 'makerHumanName': 'OpenAI', 'name': 'text-babbage-001', 'parameters': {'temperature': {'value': 0.5, 'range': [0.1, 1]}, 'maximumLength': {'value': 200, 'range': [50, 1024]}, 'topP': {'value': 1, 'range': [0.1, 1]}, 'presencePenalty': {'value': 0, 'range': [0, 1]}, 'frequencyPenalty': {'value': 0, 'range': [0, 1]}, 'stopSequences': {'value': [], 'range': []}}}, 'openai:text-curie-001': {'id': 'openai:text-curie-001', 'provider': 'openai', 'providerHumanName': 'OpenAI', 'makerHumanName': 'OpenAI', 'name': 'text-curie-001', 'parameters': {'temperature': {'value': 0.5, 'range': [0.1, 1]}, 'maximumLength': {'value': 200, 'range': [50, 1024]}, 'topP': {'value': 1, 'range': [0.1, 1]}, 'presencePenalty': {'value': 0, 'range': [0, 1]}, 'frequencyPenalty': {'value': 0, 'range': [0, 1]}, 'stopSequences': {'value': [], 'range': []}}}, 'openai:text-davinci-002': {'id': 'openai:text-davinci-002', 'provider': 'openai', 'providerHumanName': 'OpenAI', 'makerHumanName': 'OpenAI', 'name': 'text-davinci-002', 'parameters': {'temperature': {'value': 0.5, 'range': [0.1, 1]}, 'maximumLength': {'value': 200, 'range': [50, 1024]}, 'topP': {'value': 1, 'range': [0.1, 1]}, 'presencePenalty': {'value': 0, 'range': [0, 1]}, 'frequencyPenalty': {'value': 0, 'range': [0, 1]}, 'stopSequences': {'value': [], 'range': []}}}, 'openai:text-davinci-003': {'id': 'openai:text-davinci-003', 'provider': 'openai', 'providerHumanName': 'OpenAI', 'makerHumanName': 'OpenAI', 'name': 'text-davinci-003', 'parameters': {'temperature': {'value': 0.5, 'range': [0.1, 1]}, 'maximumLength': {'value': 200, 'range': [50, 1024]}, 'topP': {'value': 1, 'range': [0.1, 1]}, 'presencePenalty': {'value': 0, 'range': [0, 1]}, 'frequencyPenalty': {'value': 0, 'range': [0, 1]}, 'stopSequences': {'value': [], 'range': []}}}}
-
-
-# based on https://github.com/ading2210/vercel-llm-api // modified
-class Client:
- def __init__(self):
- self.session = requests.Session()
- self.headers = {
- 'User-Agent': 'Mozilla/5.0 (Windows NT 10.0; Win64; x64) AppleWebKit/537.36 (KHTML, like Gecko) Chrome/110 Safari/537.36',
- 'Accept': 'text/html,application/xhtml+xml,application/xml;q=0.9,image/avif,image/webp,*/*;q=0.8',
- 'Accept-Encoding': 'gzip, deflate, br',
- 'Accept-Language': 'en-US,en;q=0.5',
- 'Te': 'trailers',
- 'Upgrade-Insecure-Requests': '1'
- }
- self.session.headers.update(self.headers)
-
- def get_token(self):
- b64 = self.session.get('https://sdk.vercel.ai/openai.jpeg').text
- data = json.loads(base64.b64decode(b64))
-
- code = 'const globalThis = {data: `sentinel`}; function token() {return (%s)(%s)}' % (
- data['c'], data['a'])
-
- token_string = json.dumps(separators=(',', ':'),
- obj={'r': execjs.compile(code).call('token'), 't': data['t']})
-
- return base64.b64encode(token_string.encode()).decode()
-
- def get_default_params(self, model_id):
- return {key: param['value'] for key, param in vercel_models[model_id]['parameters'].items()}
-
- def generate(self, model_id: str, prompt: str, params: dict = {}):
- if not ':' in model_id:
- model_id = models[model_id]
-
- defaults = self.get_default_params(model_id)
-
- payload = defaults | params | {
- 'prompt': prompt,
- 'model': model_id,
- }
-
- headers = self.headers | {
- 'Accept-Encoding': 'gzip, deflate, br',
- 'Custom-Encoding': self.get_token(),
- 'Host': 'sdk.vercel.ai',
- 'Origin': 'https://sdk.vercel.ai',
- 'Referrer': 'https://sdk.vercel.ai',
- 'Sec-Fetch-Dest': 'empty',
- 'Sec-Fetch-Mode': 'cors',
- 'Sec-Fetch-Site': 'same-origin',
- }
-
- chunks_queue = queue.Queue()
- error = None
- response = None
-
- def callback(data):
- chunks_queue.put(data.decode())
-
- def request_thread():
- nonlocal response, error
- for _ in range(3):
- try:
- response = self.session.post('https://sdk.vercel.ai/api/generate',
- json=payload, headers=headers, content_callback=callback)
- response.raise_for_status()
-
- except Exception as e:
- if _ == 2:
- error = e
-
- else:
- continue
-
- thread = threading.Thread(target=request_thread, daemon=True)
- thread.start()
-
- text = ''
- index = 0
- while True:
- try:
- chunk = chunks_queue.get(block=True, timeout=0.1)
-
- except queue.Empty:
- if error:
- raise error
-
- elif response:
- break
-
- else:
- continue
-
- text += chunk
- lines = text.split('\n')
-
- if len(lines) - 1 > index:
- new = lines[index:-1]
- for word in new:
- yield json.loads(word)
- index = len(lines) - 1
-
-def _create_completion(model: str, messages: list, stream: bool, **kwargs):
- yield 'Vercel is currently not working.'
- return
-
- conversation = 'This is a conversation between a human and a language model, respond to the last message accordingly, referring to the past history of messages if needed.\n'
-
- for message in messages:
- conversation += '%s: %s\n' % (message['role'], message['content'])
-
- conversation += 'assistant: '
-
- completion = Client().generate(model, conversation)
-
- for token in completion:
- yield token
-
-params = f'g4f.Providers.{os.path.basename(__file__)[:-3]} supports: ' + \
- '(%s)' % ', '.join([f"{name}: {get_type_hints(_create_completion)[name].__name__}" for name in _create_completion.__code__.co_varnames[:_create_completion.__code__.co_argcount]])
\ No newline at end of file
diff --git a/spaces/mshukor/UnIVAL/fairseq/examples/speech_synthesis/preprocessing/get_common_voice_audio_manifest.py b/spaces/mshukor/UnIVAL/fairseq/examples/speech_synthesis/preprocessing/get_common_voice_audio_manifest.py
deleted file mode 100644
index a30254604311a488a1d4959f941051890ed32b2e..0000000000000000000000000000000000000000
--- a/spaces/mshukor/UnIVAL/fairseq/examples/speech_synthesis/preprocessing/get_common_voice_audio_manifest.py
+++ /dev/null
@@ -1,140 +0,0 @@
-# Copyright (c) Facebook, Inc. and its affiliates.
-#
-# This source code is licensed under the MIT license found in the
-# LICENSE file in the root directory of this source tree.
-
-import argparse
-import logging
-from pathlib import Path
-from collections import defaultdict
-from typing import List, Dict, Tuple
-
-import pandas as pd
-import numpy as np
-import torchaudio
-from tqdm import tqdm
-
-from examples.speech_to_text.data_utils import load_df_from_tsv, save_df_to_tsv
-
-
-log = logging.getLogger(__name__)
-
-SPLITS = ["train", "dev", "test"]
-
-
-def get_top_n(
- root: Path, n_speakers: int = 10, min_n_tokens: int = 5
-) -> pd.DataFrame:
- df = load_df_from_tsv(root / "validated.tsv")
- df["n_tokens"] = [len(s.split()) for s in df["sentence"]]
- df = df[df["n_tokens"] >= min_n_tokens]
- df["n_frames"] = [
- torchaudio.info((root / "clips" / p).as_posix()).num_frames
- for p in tqdm(df["path"])
- ]
- df["id"] = [Path(p).stem for p in df["path"]]
- total_duration_ms = df.groupby("client_id")["n_frames"].agg(["sum"])
- total_duration_ms = total_duration_ms.sort_values("sum", ascending=False)
-
- top_n_total_duration_ms = total_duration_ms.head(n_speakers)
- top_n_client_ids = set(top_n_total_duration_ms.index.tolist())
- df_top_n = df[df["client_id"].isin(top_n_client_ids)]
- return df_top_n
-
-
-def get_splits(
- df, train_split_ratio=0.99, speaker_in_all_splits=False, rand_seed=0
-) -> Tuple[Dict[str, str], List[str]]:
- np.random.seed(rand_seed)
- dev_split_ratio = (1. - train_split_ratio) / 3
- grouped = list(df.groupby("client_id"))
- id_to_split = {}
- for _, cur_df in tqdm(grouped):
- cur_n_examples = len(cur_df)
- if speaker_in_all_splits and cur_n_examples < 3:
- continue
- cur_n_train = int(cur_n_examples * train_split_ratio)
- cur_n_dev = int(cur_n_examples * dev_split_ratio)
- cur_n_test = cur_n_examples - cur_n_dev - cur_n_train
- if speaker_in_all_splits and cur_n_dev * cur_n_test == 0:
- cur_n_dev, cur_n_test = 1, 1
- cur_n_train = cur_n_examples - cur_n_dev - cur_n_test
- cur_indices = cur_df.index.tolist()
- cur_shuffled_indices = np.random.permutation(cur_n_examples)
- cur_shuffled_indices = [cur_indices[i] for i in cur_shuffled_indices]
- cur_indices_by_split = {
- "train": cur_shuffled_indices[:cur_n_train],
- "dev": cur_shuffled_indices[cur_n_train: cur_n_train + cur_n_dev],
- "test": cur_shuffled_indices[cur_n_train + cur_n_dev:]
- }
- for split in SPLITS:
- for i in cur_indices_by_split[split]:
- id_ = df["id"].loc[i]
- id_to_split[id_] = split
- return id_to_split, sorted(df["client_id"].unique())
-
-
-def convert_to_wav(root: Path, filenames: List[str], target_sr=16_000):
- out_root = root / "wav"
- out_root.mkdir(exist_ok=True, parents=True)
- print("Converting to WAV...")
- for n in tqdm(filenames):
- in_path = (root / "clips" / n).as_posix()
- waveform, sr = torchaudio.load(in_path)
- converted, converted_sr = torchaudio.sox_effects.apply_effects_tensor(
- waveform, sr, [["rate", str(target_sr)], ["channels", "1"]]
- )
- out_path = (out_root / Path(n).with_suffix(".wav").name).as_posix()
- torchaudio.save(out_path, converted, converted_sr, encoding="PCM_S",
- bits_per_sample=16)
-
-
-def process(args):
- data_root = Path(args.data_root).absolute() / args.lang
-
- # Generate TSV manifest
- print("Generating manifest...")
-
- df_top_n = get_top_n(data_root)
- id_to_split, speakers = get_splits(df_top_n)
-
- if args.convert_to_wav:
- convert_to_wav(data_root, df_top_n["path"].tolist())
-
- manifest_by_split = {split: defaultdict(list) for split in SPLITS}
- for sample in tqdm(df_top_n.to_dict(orient="index").values()):
- sample_id = sample["id"]
- split = id_to_split[sample_id]
- manifest_by_split[split]["id"].append(sample_id)
- if args.convert_to_wav:
- audio_path = data_root / "wav" / f"{sample_id}.wav"
- else:
- audio_path = data_root / "clips" / f"{sample_id}.mp3"
- manifest_by_split[split]["audio"].append(audio_path.as_posix())
- manifest_by_split[split]["n_frames"].append(sample["n_frames"])
- manifest_by_split[split]["tgt_text"].append(sample["sentence"])
- manifest_by_split[split]["speaker"].append(sample["client_id"])
- manifest_by_split[split]["src_text"].append(sample["sentence"])
-
- output_root = Path(args.output_manifest_root).absolute()
- output_root.mkdir(parents=True, exist_ok=True)
- for split in SPLITS:
- save_df_to_tsv(
- pd.DataFrame.from_dict(manifest_by_split[split]),
- output_root / f"{split}.audio.tsv"
- )
-
-
-def main():
- parser = argparse.ArgumentParser()
- parser.add_argument("--data-root", "-d", required=True, type=str)
- parser.add_argument("--output-manifest-root", "-m", required=True, type=str)
- parser.add_argument("--lang", "-l", required=True, type=str)
- parser.add_argument("--convert-to-wav", action="store_true")
- args = parser.parse_args()
-
- process(args)
-
-
-if __name__ == "__main__":
- main()
diff --git a/spaces/mshukor/UnIVAL/fairseq/fairseq/models/roberta/model_xlmr.py b/spaces/mshukor/UnIVAL/fairseq/fairseq/models/roberta/model_xlmr.py
deleted file mode 100644
index cf6e354d53b918dd4c7c78bfcd38ac0d63cab3bd..0000000000000000000000000000000000000000
--- a/spaces/mshukor/UnIVAL/fairseq/fairseq/models/roberta/model_xlmr.py
+++ /dev/null
@@ -1,46 +0,0 @@
-# Copyright (c) Facebook, Inc. and its affiliates.
-#
-# This source code is licensed under the MIT license found in the
-# LICENSE file in the root directory of this source tree.
-"""
-Unsupervised Cross-lingual Representation Learning at Scale
-"""
-
-from fairseq.models import register_model
-
-from .hub_interface import RobertaHubInterface
-from .model import RobertaModel
-
-
-@register_model("xlmr")
-class XLMRModel(RobertaModel):
- @classmethod
- def hub_models(cls):
- return {
- "xlmr.base": "http://dl.fbaipublicfiles.com/fairseq/models/xlmr.base.tar.gz",
- "xlmr.large": "http://dl.fbaipublicfiles.com/fairseq/models/xlmr.large.tar.gz",
- "xlmr.xl": "http://dl.fbaipublicfiles.com/fairseq/models/xlmr/xlmr.xl.tar.gz",
- "xlmr.xxl": "http://dl.fbaipublicfiles.com/fairseq/models/xlmr/xlmr.xxl.tar.gz",
- }
-
- @classmethod
- def from_pretrained(
- cls,
- model_name_or_path,
- checkpoint_file="model.pt",
- data_name_or_path=".",
- bpe="sentencepiece",
- **kwargs
- ):
- from fairseq import hub_utils
-
- x = hub_utils.from_pretrained(
- model_name_or_path,
- checkpoint_file,
- data_name_or_path,
- archive_map=cls.hub_models(),
- bpe=bpe,
- load_checkpoint_heads=True,
- **kwargs,
- )
- return RobertaHubInterface(x["args"], x["task"], x["models"][0])
diff --git a/spaces/myrad01/Inpaint-Anything/third_party/segment-anything/demo/src/components/helpers/maskUtils.tsx b/spaces/myrad01/Inpaint-Anything/third_party/segment-anything/demo/src/components/helpers/maskUtils.tsx
deleted file mode 100644
index 709c77e28d2f3fbe457742dcfd2dccf28923e4a5..0000000000000000000000000000000000000000
--- a/spaces/myrad01/Inpaint-Anything/third_party/segment-anything/demo/src/components/helpers/maskUtils.tsx
+++ /dev/null
@@ -1,47 +0,0 @@
-// Copyright (c) Meta Platforms, Inc. and affiliates.
-// All rights reserved.
-
-// This source code is licensed under the license found in the
-// LICENSE file in the root directory of this source tree.
-
-// Convert the onnx model mask prediction to ImageData
-function arrayToImageData(input: any, width: number, height: number) {
- const [r, g, b, a] = [0, 114, 189, 255]; // the masks's blue color
- const arr = new Uint8ClampedArray(4 * width * height).fill(0);
- for (let i = 0; i < input.length; i++) {
-
- // Threshold the onnx model mask prediction at 0.0
- // This is equivalent to thresholding the mask using predictor.model.mask_threshold
- // in python
- if (input[i] > 0.0) {
- arr[4 * i + 0] = r;
- arr[4 * i + 1] = g;
- arr[4 * i + 2] = b;
- arr[4 * i + 3] = a;
- }
- }
- return new ImageData(arr, height, width);
-}
-
-// Use a Canvas element to produce an image from ImageData
-function imageDataToImage(imageData: ImageData) {
- const canvas = imageDataToCanvas(imageData);
- const image = new Image();
- image.src = canvas.toDataURL();
- return image;
-}
-
-// Canvas elements can be created from ImageData
-function imageDataToCanvas(imageData: ImageData) {
- const canvas = document.createElement("canvas");
- const ctx = canvas.getContext("2d");
- canvas.width = imageData.width;
- canvas.height = imageData.height;
- ctx?.putImageData(imageData, 0, 0);
- return canvas;
-}
-
-// Convert the onnx model mask output to an HTMLImageElement
-export function onnxMaskToImage(input: any, width: number, height: number) {
- return imageDataToImage(arrayToImageData(input, width, height));
-}
diff --git a/spaces/netiMophi/DreamlikeArt-Diffusion-1.0/ACDC The Complete Collection Collection 2012torrent.md b/spaces/netiMophi/DreamlikeArt-Diffusion-1.0/ACDC The Complete Collection Collection 2012torrent.md
deleted file mode 100644
index 46580d52cb7ae06d1078ef32b4777aacacff572a..0000000000000000000000000000000000000000
--- a/spaces/netiMophi/DreamlikeArt-Diffusion-1.0/ACDC The Complete Collection Collection 2012torrent.md
+++ /dev/null
@@ -1,14 +0,0 @@
-
-
AC/DC: The Complete Collection Review
-
AC/DC is one of the most iconic rock bands of all time, with a legacy of hard-hitting songs, electrifying performances and unforgettable albums. The Complete Collection is a 2012 box set that contains all 16 studio albums, four live albums and three EPs by the Australian legends, spanning from 1974 to 2008. It is a must-have for any fan of AC/DC or classic rock in general.
-
The Complete Collection showcases the evolution of AC/DC's sound and style, from the raw and bluesy early days with Bon Scott on vocals, to the more polished and powerful era with Brian Johnson. The box set includes some of the most influential and popular rock albums ever made, such as Highway to Hell, Back in Black, For Those About to Rock We Salute You and The Razor's Edge. It also features some of the band's lesser-known but equally impressive works, such as Powerage, Flick of the Switch and Stiff Upper Lip.
-
ACDC The Complete Collection Collection 2012torrent
The box set also contains some rare and live material that showcases AC/DC's incredible energy and charisma on stage. The Live at River Plate album captures the band's triumphant return to Argentina in 2009, where they played to over 200,000 fans. The Live from the Atlantic Studios album features a intimate performance from 1977, where the band played some of their classic songs in front of a small audience. The '74 Jailbreak EP contains some of the band's earliest recordings that were not released in the US until 1984.
-
The Complete Collection is a comprehensive and definitive collection of AC/DC's music, that celebrates their remarkable career and impact on rock history. It is a treasure trove of timeless tunes, riffs and lyrics that will never go out of style. It is a tribute to the band's enduring spirit and passion for rock 'n' roll.
The Complete Collection is not only a great way to enjoy AC/DC's music, but also a valuable piece of rock memorabilia. The box set comes in a sturdy and stylish black case, with the band's logo and name embossed on the front. Inside, each album is presented in a mini-LP replica sleeve, with the original artwork and liner notes. The box set also includes a 24-page booklet, with photos, credits and an introduction by David Fricke.
-
The Complete Collection is a must-have for any AC/DC fan or collector, as it offers the ultimate AC/DC experience. It is a testament to the band's longevity and relevance, as they continue to rock the world with their music. It is a perfect gift for yourself or someone you love, who appreciates the power and glory of AC/DC.
The Complete Collection is not only a great way to enjoy AC/DC's music, but also a valuable piece of rock memorabilia. The box set comes in a sturdy and stylish black case, with the band's logo and name embossed on the front. Inside, each album is presented in a mini-LP replica sleeve, with the original artwork and liner notes. The box set also includes a 24-page booklet, with photos, credits and an introduction by David Fricke.
-
The Complete Collection is a must-have for any AC/DC fan or collector, as it offers the ultimate AC/DC experience. It is a testament to the band's longevity and relevance, as they continue to rock the world with their music. It is a perfect gift for yourself or someone you love, who appreciates the power and glory of AC/DC.
-
If you are looking for a way to get your hands on this amazing box set, you can order it online from various retailers, such as Amazon, eBay or Discogs. You can also check your local record stores or music shops for availability. The price may vary depending on the seller and the condition of the box set, but it is worth every penny for the quality and quantity of music you get.
-
-
Don't miss this opportunity to own a piece of rock history, and enjoy the music of one of the greatest rock bands of all time. The Complete Collection by AC/DC is a box set that will make you feel like you are on a highway to hell, in a good way.
e93f5a0c3f
-
-
\ No newline at end of file
diff --git a/spaces/netiMophi/DreamlikeArt-Diffusion-1.0/Grigorigrabovoinumberspdf58 ((BETTER)).md b/spaces/netiMophi/DreamlikeArt-Diffusion-1.0/Grigorigrabovoinumberspdf58 ((BETTER)).md
deleted file mode 100644
index 8f1d1982fa9302b64484cf8ed5958aa4e05cf7ab..0000000000000000000000000000000000000000
--- a/spaces/netiMophi/DreamlikeArt-Diffusion-1.0/Grigorigrabovoinumberspdf58 ((BETTER)).md
+++ /dev/null
@@ -1,28 +0,0 @@
-
-
What are Grabovoi Numbers and How to Use Them for Manifestation?
-
Grabovoi numbers are special sequences of numbers that can help you manifest your desires into reality. They are based on the teachings of Grigori Grabovoi, a Russian scientist and healer who claimed to have the ability to heal people and restore matter using his mind and numerical codes. Grabovoi numbers are also known as "cheat codes to the universe" because they can help you bypass any obstacles or limitations that may be blocking your manifestation.
-
In this article, we will explain what Grabovoi numbers are, how they work, and how to use them effectively for manifestation. We will also provide you with a list of some common Grabovoi numbers that you can use for various purposes, such as money, love, health, and more. Finally, we will share with you a free PDF of 58 Grabovoi numbers that you can download and use anytime you want.
Grabovoi numbers are sequences of digits that have specific vibrational frequencies that correspond to different aspects of reality. By focusing on these numbers, you can align your own vibration with the vibration of your desired outcome and attract it into your life. According to Grabovoi, each number has a unique meaning and function, and by combining them in different ways, you can create complex codes that can address any situation or problem.
-
For example, the number 1 represents the beginning, unity, wholeness, and God. The number 2 represents duality, balance, harmony, and cooperation. The number 3 represents creativity, expression, communication, and growth. And so on. By using these numbers in specific combinations, you can create codes that can help you manifest anything you want.
-
How do Grabovoi Numbers Work?
-
Grabovoi numbers work by using the power of your mind and intention to influence reality. According to Grabovoi, everything in the universe is made of information and energy, and by changing the information and energy of something, you can change its physical manifestation. He also claimed that he could access the universal database of information and energy using his mind and numerical codes.
-
By using Grabovoi numbers, you can tap into this universal database and access the information and energy of your desired outcome. You can then use your intention and visualization to transfer this information and energy into your own reality and manifest it. The more you focus on the numbers and their meanings, the more you align your vibration with them and attract them into your life.
-
How to Use Grabovoi Numbers Effectively for Manifestation?
-
There are many ways to use Grabovoi numbers for manifestation, but here are some general guidelines that can help you get started:
-
-
Choose a Grabovoi number that matches your intention or goal. You can use one of the common numbers listed below or create your own code by combining different numbers.
-
Write down the number on a piece of paper or on your phone. You can also draw it on your skin, wear it on a bracelet or necklace, or place it under your pillow.
-
Focus on the number and its meaning for at least 5 minutes a day. You can repeat it out loud or in your mind, visualize it in front of you or in your third eye, or meditate on it.
-
While focusing on the number, imagine that you already have what you want. Feel the emotions of gratitude, joy, love, and satisfaction that come with having your desire. See yourself living your dream life as if it is already happening.
-
Let go of any doubts, fears, or worries that may arise. Trust that the universe is working in your favor and that everything is possible with Grabovoi numbers.
-
Be open to receive your manifestation in any way that it may come. Don't limit yourself to a specific time frame or method. Be flexible and adaptable to the signs and opportunities that may show up along the way.
-
-
List of Common Grabovoi Numbers
-
Here are some common Grabovoi numbers that you can use for different purposes:
-
-
Purpose
- cec2833e83
-
-
\ No newline at end of file
diff --git a/spaces/netiMophi/DreamlikeArt-Diffusion-1.0/NewAutoCADRevitLTSuite2008key.md b/spaces/netiMophi/DreamlikeArt-Diffusion-1.0/NewAutoCADRevitLTSuite2008key.md
deleted file mode 100644
index 462e0504fdb3e18d2f28b9287c9ed23d412f5974..0000000000000000000000000000000000000000
--- a/spaces/netiMophi/DreamlikeArt-Diffusion-1.0/NewAutoCADRevitLTSuite2008key.md
+++ /dev/null
@@ -1,30 +0,0 @@
-
-
New AutoCAD Revit LT Suite 2008 Key: How to Find and Activate It
-
If you are looking for a new product key for AutoCAD Revit LT Suite 2008, you may have some trouble finding it online. This is because Autodesk does not support product versions older than five years back, and AutoCAD Revit LT Suite 2008 is more than a decade old. However, there are still some ways to get a new product key and activate your software.
One way is to use the interactive lookup tool on Autodesk's website[^1^]. This tool allows you to find product keys for your Autodesk products by selecting the correct product name and year (version). You will need to enter your serial number and request code to get your activation code. If you get an error when you enter your product key, make sure you specified the correct product (or suite) and version in the lookup tool.
-
Another way is to contact Autodesk support or your reseller[^2^]. They may be able to provide you with a new product key or help you with the activation process. However, this may take some time and they may not be able to assist you with such an old product version. You may also need to provide proof of purchase or subscription.
-
A third way is to upgrade to a newer version of AutoCAD Revit LT Suite[^3^]. This will give you access to the latest features and updates, as well as a valid product key and activation code. You can purchase a subscription or a perpetual license from Autodesk's website or your reseller. You may also be eligible for a discount if you trade in your old license.
-
Whichever way you choose, make sure you do not use any illegal or pirated software or product keys. This may expose you to security risks, malware, errors, and legal consequences. Always use genuine Autodesk software and product keys from authorized sources.
-
-
AutoCAD Revit LT Suite is a software package that combines AutoCAD LT and Revit LT, two powerful tools for 2D and 3D design and documentation. AutoCAD LT allows you to create precise 2D drawings and edit them with ease. Revit LT allows you to create 3D models and generate high-quality renderings and animations. With AutoCAD Revit LT Suite, you can benefit from both workflows and collaborate with other professionals.
-
The latest version of AutoCAD Revit LT Suite is 2022, which was released in April 2021. This version offers several improvements and new features, such as:
-
-
-
Enhanced performance and stability for both AutoCAD LT and Revit LT.
-
New drawing history feature in AutoCAD LT that lets you compare past and present versions of your drawings and see the changes.
-
New generative design feature in Revit LT that lets you explore design options based on your goals and constraints.
-
New cloud collaboration feature in Revit LT that lets you share your models and work with others online.
-
New integration with Autodesk Docs that lets you access and manage your project data from anywhere.
-
-
If you want to upgrade to AutoCAD Revit LT Suite 2022, you have two options: subscription or perpetual license. A subscription gives you access to the latest software updates, technical support, cloud services, and more. A perpetual license gives you ownership of the software without expiration, but you will need to pay for maintenance plans to get updates and support. You can choose the option that suits your budget and needs.
-
If you have an old license of AutoCAD Revit LT Suite 2008 or any other Autodesk product, you may be able to trade it in for a discount on a new subscription or perpetual license. This is part of Autodesk's trade-in offer, which aims to help customers transition to the latest software versions. To qualify for the trade-in offer, you need to meet certain criteria, such as:
-
-
Your old license must be a perpetual license that is not on an active maintenance plan.
-
Your old license must be for a product that is 14 years old or less (2008 or later).
-
Your old license must be registered under your name or your company's name.
-
You must agree to discontinue using your old license after trading it in.
-
-
If you meet these criteria, you can contact Autodesk or your reseller to request a quote for the trade-in offer. You will need to provide your old serial number and product key. You can then choose the new product and license type that you want to purchase with the discount. The trade-in offer is valid until July 23, 2021, so act fast if you want to take advantage of it.
e93f5a0c3f
-
-
\ No newline at end of file
diff --git a/spaces/netiMophi/DreamlikeArt-Diffusion-1.0/Olai Chuvadi Tamil Book Free Download.md b/spaces/netiMophi/DreamlikeArt-Diffusion-1.0/Olai Chuvadi Tamil Book Free Download.md
deleted file mode 100644
index a05a584bf62ce3d56e081e9da5194ff93a5bdfc9..0000000000000000000000000000000000000000
--- a/spaces/netiMophi/DreamlikeArt-Diffusion-1.0/Olai Chuvadi Tamil Book Free Download.md
+++ /dev/null
@@ -1,26 +0,0 @@
-
-Here is a possible title and article for the keyword "Olai Chuvadi Tamil Book Free Download". I have used SEO optimization techniques such as using the keyword in the title, headings, and body text, as well as adding relevant links and images. I have also used HTML formatting to create a structured and appealing layout.
-
-```html
-
Olai Chuvadi Tamil Book Free Download: A Guide to the Ancient Palm Leaf Manuscripts
-
Olai Chuvadi is a Tamil term that means "palm leaf manuscript". These are ancient texts that were written on dried palm leaves using a stylus. They contain various forms of knowledge, such as astrology, medicine, history, literature, and spirituality. Olai Chuvadi is considered to be a treasure of Tamil culture and heritage.
-
-
If you are interested in learning more about Olai Chuvadi and reading some of the manuscripts yourself, you might be wondering where you can find them. Fortunately, there are some online sources that offer Olai Chuvadi Tamil book free download. In this article, we will introduce you to some of these websites and how you can access them.
Olai Chuvadi Tamil Book Free Download: Where to Find Them Online
-
There are several websites that offer Olai Chuvadi Tamil book free download. However, not all of them are reliable or authentic. Some of them may contain errors, incomplete texts, or low-quality scans. Therefore, it is important to be careful and selective when choosing a website to download Olai Chuvadi from.
-
Here are some of the websites that we recommend for Olai Chuvadi Tamil book free download:
-
-
-
Tamil Virtual Academy: This is an official website of the Government of Tamil Nadu that aims to preserve and promote Tamil language and culture. It has a large collection of Olai Chuvadi manuscripts on various topics, such as astrology, medicine, grammar, poetry, and religion. You can browse the manuscripts by category or search by title or author. You can also view the scanned images of the original palm leaves or download them as PDF files.
-
Scribd: This is a popular online platform that allows users to upload and share documents, books, magazines, and other types of content. It has a few Olai Chuvadi manuscripts that have been uploaded by users. You can read them online or download them as PDF files. However, you may need to create an account or pay a subscription fee to access some of the content.
-
Internet Archive: This is a non-profit organization that provides free access to millions of digital books, movies, music, and other media. It has a few Olai Chuvadi manuscripts that have been scanned and uploaded by users. You can view them online or download them as PDF files.
-
-
Olai Chuvadi Tamil Book Free Download: How to Read Them
-
Once you have downloaded the Olai Chuvadi manuscripts from one of the websites mentioned above, you might be wondering how to read them. After all, they are written in an ancient script and language that may not be familiar to modern readers.
-
Here are some tips on how to read Olai Chuvadi manuscripts:
-
-
Learn the basics of Tamil script and language: If you do not know how to read Tamil script or understand Tamil language, you may need to learn some basics before attempting to read Olai Chuvadi manuscripts. You can find some online resources that teach you how to read and write Tamil script here and how to speak and understand Tamil language here.
-
Use a dictionary or a translator: If you encounter words or phrases that you do not understand in the Olai Chuvadi manuscripts, you can use a dictionary or a translator to help you. 7196e7f11a
-
-
\ No newline at end of file
diff --git a/spaces/nomic-ai/kunishou_databricks-dolly-15k-ja/style.css b/spaces/nomic-ai/kunishou_databricks-dolly-15k-ja/style.css
deleted file mode 100644
index 114adf441e9032febb46bc056b2a8bb651075f0d..0000000000000000000000000000000000000000
--- a/spaces/nomic-ai/kunishou_databricks-dolly-15k-ja/style.css
+++ /dev/null
@@ -1,28 +0,0 @@
-body {
- padding: 2rem;
- font-family: -apple-system, BlinkMacSystemFont, "Arial", sans-serif;
-}
-
-h1 {
- font-size: 16px;
- margin-top: 0;
-}
-
-p {
- color: rgb(107, 114, 128);
- font-size: 15px;
- margin-bottom: 10px;
- margin-top: 5px;
-}
-
-.card {
- max-width: 620px;
- margin: 0 auto;
- padding: 16px;
- border: 1px solid lightgray;
- border-radius: 16px;
-}
-
-.card p:last-child {
- margin-bottom: 0;
-}
diff --git a/spaces/oguzakif/video-object-remover/FGT_codes/FGT/data/util/readers.py b/spaces/oguzakif/video-object-remover/FGT_codes/FGT/data/util/readers.py
deleted file mode 100644
index 71bb1cd3840ff390d4ca186b42d920c1e65494a0..0000000000000000000000000000000000000000
--- a/spaces/oguzakif/video-object-remover/FGT_codes/FGT/data/util/readers.py
+++ /dev/null
@@ -1,527 +0,0 @@
-import os
-import sys
-sys.path.append(os.path.dirname(os.path.dirname(os.path.abspath(__file__)))) # NOQA
-import argparse
-from math import ceil
-from glob import glob
-
-import numpy as np
-import cv2
-from PIL import Image, ImageDraw, ImageOps, ImageFont
-
-from utils.logging_config import logger
-from utils.util import make_dirs, bbox_offset
-
-
-DEFAULT_FPS = 6
-MAX_LENGTH = 60
-
-
-def parse_args():
- parser = argparse.ArgumentParser()
- parser.add_argument(
- '-fps', '--fps',
- type=int, default=DEFAULT_FPS,
- help="Output video FPS"
- )
- parser.add_argument(
- '-v', '--video_dir',
- type=str,
- help="Video directory name"
- )
- parser.add_argument(
- '-vs', '--video_dirs',
- nargs='+',
- type=str,
- help="Video directory names"
- )
- parser.add_argument(
- '-v2', '--video_dir2',
- type=str,
- help="Video directory name"
- )
- parser.add_argument(
- '-sd', '--segms_dir',
- type=str,
- help="Segmentation directory name"
- )
- parser.add_argument(
- '-fgd', '--fg_dir',
- type=str,
- help="Foreground directory name"
- )
- parser.add_argument(
- '-fgfd', '--fg_frames_dir',
- type=str,
- help="Foreground frames directory name"
- )
- parser.add_argument(
- '-fgsd', '--fg_segms_dir',
- type=str,
- help="Foreground segmentations directory name"
- )
- parser.add_argument(
- '-syfd', '--syn_frames_dir',
- type=str,
- help="Synthesized frames directory name"
- )
- parser.add_argument(
- '-bgfd', '--bg_frames_dir',
- type=str,
- help="Background frames directory name"
- )
- parser.add_argument(
- '-rt', '--reader_type',
- type=str,
- help="Type of reader"
- )
- parser.add_argument(
- '-od', '--output_dir',
- type=str,
- help="Output directory name"
- )
- parser.add_argument(
- '-o', '--output_filename',
- type=str, required=True,
- help="Output output filename"
- )
- args = parser.parse_args()
- return args
-
-
-class Reader:
- def __init__(self, dir_name, read=True, max_length=None, sample_period=1):
- self.dir_name = dir_name
- self.count = 0
- self.max_length = max_length
- self.filenames = []
- self.sample_period = sample_period
- if read:
- if os.path.exists(dir_name):
- # self.filenames = read_filenames_from_dir(dir_name, self.__class__.__name__)
- # ^^^^^ yield None when reading some videos of face forensics data
- # (related to 'Too many levels of symbolic links'?)
-
- self.filenames = sorted(glob(os.path.join(dir_name, '*')))
- self.filenames = [f for f in self.filenames if os.path.isfile(f)]
- self.filenames = self.filenames[::sample_period][:max_length]
- self.files = self.read_files(self.filenames)
- else:
- self.files = []
- logger.warning(f"Directory {dir_name} not exists!")
- else:
- self.files = []
- self.current_index = 0
-
- def append(self, file_):
- self.files.append(file_)
-
- def set_files(self, files):
- self.files = files
-
- def read_files(self, filenames):
- assert type(filenames) == list, f'filenames is not a list; dirname: {self.dir_name}'
- filenames.sort()
- frames = []
- for filename in filenames:
- file_ = self.read_file(filename)
- frames.append(file_)
- return frames
-
- def save_files(self, output_dir=None):
- make_dirs(output_dir)
- logger.info(f"Saving {self.__class__.__name__} files to {output_dir}")
- for i, file_ in enumerate(self.files):
- self._save_file(output_dir, i, file_)
-
- def _save_file(self, output_dir, i, file_):
- raise NotImplementedError("This is an abstract function")
-
- def read_file(self, filename):
- raise NotImplementedError("This is an abstract function")
-
- def __iter__(self):
- return self
-
- def __next__(self):
- if self.current_index < len(self.files):
- file_ = self.files[self.current_index]
- self.current_index += 1
- return file_
- else:
- self.current_index = 0
- raise StopIteration
-
- def __getitem__(self, key):
- return self.files[key]
-
- def __len__(self):
- return len(self.files)
-
-
-class FrameReader(Reader):
- def __init__(
- self, dir_name, resize=None, read=True, max_length=MAX_LENGTH,
- scale=1, sample_period=1
- ):
- self.resize = resize
- self.scale = scale
- self.sample_period = sample_period
- super().__init__(dir_name, read, max_length, sample_period)
-
- def read_file(self, filename):
- origin_frame = Image.open(filename)
- size = self.resize if self.resize is not None else origin_frame.size
- origin_frame_resized = origin_frame.resize(
- (int(size[0] * self.scale), int(size[1] * self.scale))
- )
- return origin_frame_resized
-
- def _save_file(self, output_dir, i, file_):
- if len(self.filenames) == len(self.files):
- name = sorted(self.filenames)[i].split('/')[-1]
- else:
- name = f"frame_{i:04}.png"
- filename = os.path.join(
- output_dir, name
- )
- file_.save(filename, "PNG")
-
- def write_files_to_video(self, output_filename, fps=DEFAULT_FPS, frame_num_when_repeat_list=[1]):
- logger.info(
- f"Writeing frames to video {output_filename} with FPS={fps}")
- video_writer = cv2.VideoWriter(
- output_filename,
- cv2.VideoWriter_fourcc(*"MJPG"),
- fps,
- self.files[0].size
- )
- for frame_num_when_repeat in frame_num_when_repeat_list:
- for frame in self.files:
- frame = frame.convert("RGB")
- frame_cv = np.array(frame)
- frame_cv = cv2.cvtColor(frame_cv, cv2.COLOR_RGB2BGR)
- for i in range(frame_num_when_repeat):
- video_writer.write(frame_cv)
- video_writer.release()
-
-
-class SynthesizedFrameReader(FrameReader):
- def __init__(
- self, bg_frames_dir, fg_frames_dir,
- fg_segms_dir, segm_bbox_mask_dir, fg_dir, dir_name,
- bboxes_list_dir,
- fg_scale=0.7, fg_location=(48, 27), mask_only=False
- ):
- self.bg_reader = FrameReader(bg_frames_dir)
- self.size = self.bg_reader[0].size
- # TODO: add different location and change scale to var
- self.fg_reader = ForegroundReader(
- fg_frames_dir, fg_segms_dir, fg_dir,
- resize=self.size,
- scale=fg_scale
- )
- self.fg_location = fg_location
- # self.masks = self.fg_reader.masks
- # self.bbox_masks = self.fg_reader.bbox_masks
- super().__init__(dir_name, read=False)
- self.files = self.synthesize_frames(
- self.bg_reader, self.fg_reader, mask_only)
- self.bbox_masks = MaskGenerator(
- segm_bbox_mask_dir, self.size, self.get_bboxeses()
- )
- self.bboxes_list_dir = bboxes_list_dir
- self.bboxes_list = self.get_bboxeses()
- self.save_bboxes()
-
- def save_bboxes(self):
- make_dirs(self.bboxes_list_dir)
- logger.info(f"Saving bboxes to {self.bboxes_list_dir}")
- for i, bboxes in enumerate(self.bboxes_list):
- save_path = os.path.join(self.bboxes_list_dir, f"bboxes_{i:04}.txt")
- if len(bboxes) > 0:
- np.savetxt(save_path, bboxes[0], fmt='%4u')
-
- def get_bboxeses(self):
- bboxeses = self.fg_reader.segms.bboxeses
- new_bboxeses = []
- for bboxes in bboxeses:
- new_bboxes = []
- for bbox in bboxes:
- offset_bbox = bbox_offset(bbox, self.fg_location)
- new_bboxes.append(offset_bbox)
- new_bboxeses.append(new_bboxes)
- return new_bboxeses
-
- def synthesize_frames(self, bg_reader, fg_reader, mask_only=False):
- logger.info(
- f"Synthesizing {bg_reader.dir_name} and {fg_reader.dir_name}"
- )
- synthesized_frames = []
- for i, bg in enumerate(bg_reader):
- if i == len(fg_reader):
- break
- fg = fg_reader[i]
- mask = fg_reader.get_mask(i)
- synthesized_frame = bg.copy()
- if mask_only:
- synthesized_frame.paste(mask, self.fg_location, mask)
- else:
- synthesized_frame.paste(fg, self.fg_location, mask)
- synthesized_frames.append(synthesized_frame)
- return synthesized_frames
-
-
-class WarpedFrameReader(FrameReader):
- def __init__(self, dir_name, i, ks):
- self.i = i
- self.ks = ks
- super().__init__(dir_name)
-
- def _save_file(self, output_dir, i, file_):
- filename = os.path.join(
- output_dir,
- f"warped_frame_{self.i:04}_k{self.ks[i]:02}.png"
- )
- file_.save(filename)
-
-
-class SegmentationReader(FrameReader):
- def __init__(
- self, dir_name,
- resize=None, scale=1
- ):
- super().__init__(
- dir_name, resize=resize, scale=scale
- )
-
- def read_file(self, filename):
- origin_frame = Image.open(filename)
- mask = ImageOps.invert(origin_frame.convert("L"))
- mask = mask.point(lambda x: 0 if x < 255 else 255, '1')
- size = self.resize if self.resize is not None else origin_frame.size
- mask_resized = mask.resize(
- (int(size[0] * self.scale), int(size[1] * self.scale))
- )
- return mask_resized
-
-
-class MaskReader(Reader):
- def __init__(self, dir_name, read=True):
- super().__init__(dir_name, read=read)
-
- def read_file(self, filename):
- mask = Image.open(filename)
- return mask
-
- def _save_file(self, output_dir, i, file_):
- filename = os.path.join(
- output_dir,
- f"mask_{i:04}.png"
- )
- file_.save(filename)
-
- def get_bboxes(self, i):
- # TODO: save bbox instead of looking for one
- mask = self.files[i]
- mask = ImageOps.invert(mask.convert("L")).convert("1")
- mask = np.array(mask)
- image, contours, hier = cv2.findContours(
- mask, cv2.RETR_EXTERNAL, cv2.CHAIN_APPROX_NONE)
- bboxes = []
- for c in contours:
- # get the bounding rect
- x, y, w, h = cv2.boundingRect(c)
- bbox = ((x, y), (x + w - 1, y + h - 1))
- bboxes.append(bbox)
- return bboxes
-
- def get_bbox(self, i):
- # TODO: save bbox instead of looking for one
- mask = self.files[i]
- mask = ImageOps.invert(mask.convert("L"))
- mask = np.array(mask)
- image, contours, hier = cv2.findContours(
- mask, cv2.RETR_EXTERNAL, cv2.CHAIN_APPROX_NONE)
- for c in contours:
- # get the bounding rect
- x, y, w, h = cv2.boundingRect(c)
- bbox = ((x, y), (x + w - 1, y + h - 1))
- return bbox
-
-
-class MaskGenerator(Reader):
- def __init__(
- self, mask_output_dir, size, bboxeses, save_masks=True
- ):
- self.bboxeses = bboxeses
- self.size = size
- super().__init__(mask_output_dir, read=False)
- self.files = self.generate_masks()
- if save_masks:
- make_dirs(mask_output_dir)
- self.save_files(mask_output_dir)
-
- def _save_file(self, output_dir, i, file_):
- filename = os.path.join(
- output_dir,
- f"mask_{i:04}.png"
- )
- file_.save(filename)
-
- def get_bboxes(self, i):
- return self.bboxeses[i]
-
- def generate_masks(self):
- masks = []
- for i in range(len(self.bboxeses)):
- mask = self.generate_mask(i)
- masks.append(mask)
- return masks
-
- def generate_mask(self, i):
- bboxes = self.bboxeses[i]
- mask = Image.new("1", self.size, 1)
- draw = ImageDraw.Draw(mask)
- for bbox in bboxes:
- draw.rectangle(
- bbox, fill=0
- )
- return mask
-
-
-class ForegroundReader(FrameReader):
- def __init__(
- self, frames_dir, segms_dir, dir_name,
- resize=None, scale=1
- ):
- self.frames_dir = frames_dir
- self.segms_dir = segms_dir
- self.frames = FrameReader(
- frames_dir,
- resize=resize, scale=scale
- )
- self.segms = SegmentationReader(
- segms_dir, resize=resize, scale=scale
- )
- super().__init__(dir_name, read=False)
- self.masks = self.segms.masks
- # self.bbox_masks = self.segms.bbox_masks
- self.files = self.generate_fg_frames(self.frames, self.segms)
-
- def get_mask(self, i):
- return self.masks[i]
-
- def generate_fg_frames(self, frames, segms):
- logger.info(
- f"Generating fg frames from {self.frames_dir} and {self.segms_dir}"
- )
- fg_frames = []
- for i, frame in enumerate(frames):
- mask = self.masks[i]
- fg_frame = Image.new("RGB", frame.size, (0, 0, 0))
- fg_frame.paste(
- frame, (0, 0),
- mask
- )
- fg_frames.append(fg_frame)
- return fg_frames
-
-
-class CompareFramesReader(FrameReader):
- def __init__(self, dir_names, col=2, names=[], mask_dir=None):
- self.videos = []
- for dir_name in dir_names:
- # If a method fails on this video, use None to indicate the situation
- try:
- self.videos.append(FrameReader(dir_name))
- except AssertionError:
- self.videos.append(None)
- if mask_dir is not None:
- self.masks = MaskReader(mask_dir)
- self.names = names
- self.files = self.combine_videos(self.videos, col)
-
- def combine_videos(self, videos, col=2, edge_offset=35, h_start_offset=35):
- combined_frames = []
- w, h = videos[0][0].size
- # Prevent the first method fails and have a "None" as its video
- i = 0
- while videos[i] is None:
- i += 1
- length = len(videos[i])
- video_num = len(videos)
- row = ceil(video_num / col)
- for frame_idx in range(length):
- width = col * w + (col - 1) * edge_offset
- height = row * h + (row - 1) * edge_offset + h_start_offset
- combined_frame = Image.new("RGBA", (width, height))
- draw = ImageDraw.Draw(combined_frame)
- for i, video in enumerate(videos):
- # Give the failed method a black output
- if video is None or frame_idx >= len(video):
- failed = True
- frame = Image.new("RGBA", (w, h))
- else:
- frame = video[frame_idx].convert("RGBA")
- failed = False
-
- f_x = (i % col) * (w + edge_offset)
- f_y = (i // col) * (h + edge_offset) + h_start_offset
- combined_frame.paste(frame, (f_x, f_y))
-
- # Draw name
- font = ImageFont.truetype("DejaVuSans.ttf", 12)
- # font = ImageFont.truetype("DejaVuSans-Bold.ttf", 13)
- # font = ImageFont.truetype("timesbd.ttf", 14)
- name = self.names[i] if not failed else f'{self.names[i]} (failed)'
- draw.text(
- (f_x + 10, f_y - 20),
- name, (255, 255, 255), font=font
- )
-
- combined_frames.append(combined_frame)
- return combined_frames
-
-
-class BoundingBoxesListReader(Reader):
- def __init__(
- self, dir_name, resize=None, read=True, max_length=MAX_LENGTH,
- scale=1
- ):
- self.resize = resize
- self.scale = scale
- super().__init__(dir_name, read, max_length)
-
- def read_file(self, filename):
- bboxes = np.loadtxt(filename, dtype=int)
- bboxes = [bboxes.tolist()]
- return bboxes
-
-
-def save_frames_to_dir(frames, dirname):
- reader = FrameReader(dirname, read=False)
- reader.set_files(frames)
- reader.save_files(dirname)
-
-
-if __name__ == "__main__":
- args = parse_args()
- if args.reader_type is None:
- reader = FrameReader(args.video_dir)
- elif args.reader_type == 'fg':
- reader = ForegroundReader(
- args.video_dir, args.segms_dir, args.fg_dir)
- elif args.reader_type == 'sy':
- reader = SynthesizedFrameReader(
- args.bg_frames_dir, args.fg_frames_dir,
- args.fg_segms_dir, args.fg_dir, args.syn_frames_dir
- )
- elif args.reader_type == 'com':
- reader = CompareFramesReader(
- args.video_dirs
- )
- reader.write_files_to_video(
- os.path.join(args.output_dir, args.output_filename),
- fps=args.fps
- )
diff --git a/spaces/oguzakif/video-object-remover/FGT_codes/LAFC/models/utils/fbConsistencyCheck.py b/spaces/oguzakif/video-object-remover/FGT_codes/LAFC/models/utils/fbConsistencyCheck.py
deleted file mode 100644
index 7d5b546c6124c8380e13f985199cf079350a8d2d..0000000000000000000000000000000000000000
--- a/spaces/oguzakif/video-object-remover/FGT_codes/LAFC/models/utils/fbConsistencyCheck.py
+++ /dev/null
@@ -1,127 +0,0 @@
-import torch
-import numpy as np
-from .sobel2 import SobelLayer, SeperateSobelLayer
-import torch.nn as nn
-import torch.nn.functional as F
-
-
-def image_warp(image, flow):
- '''
- image: 上一帧的图片,torch.Size([1, 3, 256, 256])
- flow: 光流, torch.Size([1, 2, 256, 256])
- final_grid: torch.Size([1, 2, 256, 256])
- '''
- b, c, h, w = image.size()
- device = image.device
- flow = torch.cat([flow[:, 0:1, :, :] / ((w - 1.0) / 2.0), flow[:, 1:2, :, :] / ((h - 1.0) / 2.0)],
- dim=1) # normalize to [-1~1](from upper left to lower right
- flow = flow.permute(0, 2, 3,
- 1) # if you wanna use grid_sample function, the channel(band) shape of show must be in the last dimension
- x = np.linspace(-1, 1, w)
- y = np.linspace(-1, 1, h)
- X, Y = np.meshgrid(x, y)
- grid = torch.cat((torch.from_numpy(X.astype('float32')).unsqueeze(0).unsqueeze(3),
- torch.from_numpy(Y.astype('float32')).unsqueeze(0).unsqueeze(3)), 3).to(device)
- output = torch.nn.functional.grid_sample(image, grid + flow, mode='bilinear', padding_mode='zeros')
- return output
-
-
-def length_sq(x):
- return torch.sum(torch.square(x), dim=1, keepdim=True)
-
-
-def fbConsistencyCheck(flow_fw, flow_bw, alpha1=0.01, alpha2=0.5):
- flow_bw_warped = image_warp(flow_bw, flow_fw) # wb(wf(x))
- flow_fw_warped = image_warp(flow_fw, flow_bw) # wf(wb(x))
- flow_diff_fw = flow_fw + flow_bw_warped # wf + wb(wf(x))
- flow_diff_bw = flow_bw + flow_fw_warped # wb + wf(wb(x))
-
- mag_sq_fw = length_sq(flow_fw) + length_sq(flow_bw_warped) # |wf| + |wb(wf(x))|
- mag_sq_bw = length_sq(flow_bw) + length_sq(flow_fw_warped) # |wb| + |wf(wb(x))|
- occ_thresh_fw = alpha1 * mag_sq_fw + alpha2
- occ_thresh_bw = alpha1 * mag_sq_bw + alpha2
-
- fb_occ_fw = (length_sq(flow_diff_fw) > occ_thresh_fw).float()
- fb_occ_bw = (length_sq(flow_diff_bw) > occ_thresh_bw).float()
-
- return fb_occ_fw, fb_occ_bw # fb_occ_fw -> frame2 area occluded by frame1, fb_occ_bw -> frame1 area occluded by frame2
-
-
-def rgb2gray(image):
- gray_image = image[:, 0] * 0.299 + image[:, 1] * 0.587 + 0.110 * image[:, 2]
- gray_image = gray_image.unsqueeze(1)
- return gray_image
-
-
-def ternary_transform(image, max_distance=1):
- device = image.device
- patch_size = 2 * max_distance + 1
- intensities = rgb2gray(image) * 255
- out_channels = patch_size * patch_size
- w = np.eye(out_channels).reshape(out_channels, 1, patch_size, patch_size)
- weights = torch.from_numpy(w).float().to(device)
- patches = F.conv2d(intensities, weights, stride=1, padding=1)
- transf = patches - intensities
- transf_norm = transf / torch.sqrt(0.81 + torch.square(transf))
- return transf_norm
-
-
-def hamming_distance(t1, t2):
- dist = torch.square(t1 - t2)
- dist_norm = dist / (0.1 + dist)
- dist_sum = torch.sum(dist_norm, dim=1, keepdim=True)
- return dist_sum
-
-
-def create_mask(mask, paddings):
- """
- padding: [[top, bottom], [left, right]]
- """
- shape = mask.shape
- inner_height = shape[2] - (paddings[0][0] + paddings[0][1])
- inner_width = shape[3] - (paddings[1][0] + paddings[1][1])
- inner = torch.ones([inner_height, inner_width])
-
- mask2d = F.pad(inner, pad=[paddings[1][0], paddings[1][1], paddings[0][0], paddings[0][1]]) # mask最外边一圈都pad成0了
- mask3d = mask2d.unsqueeze(0)
- mask4d = mask3d.unsqueeze(0).repeat(shape[0], 1, 1, 1)
- return mask4d.detach()
-
-
-def ternary_loss2(frame1, warp_frame21, confMask, masks, max_distance=1):
- """
-
- Args:
- frame1: torch tensor, with shape [b * t, c, h, w]
- warp_frame21: torch tensor, with shape [b * t, c, h, w]
- confMask: confidence mask, with shape [b * t, c, h, w]
- masks: torch tensor, with shape [b * t, c, h, w]
- max_distance: maximum distance.
-
- Returns: ternary loss
-
- """
- t1 = ternary_transform(frame1)
- t21 = ternary_transform(warp_frame21)
- dist = hamming_distance(t1, t21) # 近似求解,其实利用了mask区域和外界边缘交叉的那一部分像素
- loss = torch.mean(dist * confMask * masks) / torch.mean(masks)
- return loss
-
-
-def gradient_loss(frame1, frame2, confMask):
- device = frame1.device
- frame1_edge = SobelLayer(device)(frame1)
- frame2_edge = SobelLayer(device)(frame2)
- loss = torch.sum(torch.abs(frame1_edge * confMask - frame2_edge * confMask)) / (torch.sum(confMask) + 1) # escape divide 0
- return loss
-
-
-def seperate_gradient_loss(frame1, warp_frame21, confMask):
- device = frame1.device
- mask_x = create_mask(frame1, [[0, 0], [1, 1]]).to(device)
- mask_y = create_mask(frame1, [[1, 1], [0, 0]]).to(device)
- gradient_mask = torch.cat([mask_x, mask_y], dim=1).repeat(1, 3, 1, 1)
- frame1_edge = SeperateSobelLayer(device)(frame1)
- warp_frame21_edge = SeperateSobelLayer(device)(warp_frame21)
- loss = nn.L1Loss()(frame1_edge * confMask * gradient_mask, warp_frame21_edge * confMask * gradient_mask)
- return loss
diff --git a/spaces/omartine/prompt-generator/app.py b/spaces/omartine/prompt-generator/app.py
deleted file mode 100644
index a535880ec59270152e13461316b7ad0f06004eb9..0000000000000000000000000000000000000000
--- a/spaces/omartine/prompt-generator/app.py
+++ /dev/null
@@ -1,56 +0,0 @@
-from transformers import pipeline, set_seed
-import gradio as grad
-import random
-import re
-
-gpt2_pipe = pipeline('text-generation', model='succinctly/text2image-prompt-generator')
-
-with open("name.txt", "r") as f:
- line = f.readlines()
-
-
-def generate(starting_text):
- for count in range(6):
- seed = random.randint(100, 1000000)
- set_seed(seed)
-
- # If the text field is empty
- if starting_text == "":
- starting_text: str = line[random.randrange(0, len(line))].replace("\n", "").lower().capitalize()
- starting_text: str = re.sub(r"[,:\-–.!;?_]", '', starting_text)
- print(starting_text)
-
- response = gpt2_pipe(starting_text, max_length=random.randint(60, 90), num_return_sequences=8)
- response_list = []
- for x in response:
- resp = x['generated_text'].strip()
- if resp != starting_text and len(resp) > (len(starting_text) + 4) and resp.endswith((":", "-", "—")) is False:
- response_list.append(resp)
-
- response_end = "\n".join(response_list)
- response_end = re.sub('[^ ]+\.[^ ]+','', response_end)
- response_end = response_end.replace("<", "").replace(">", "")
- if response_end != "":
- return response_end
- if count == 5:
- return response_end
-
-
-txt = grad.Textbox(lines=1, label="English", placeholder="English Text here")
-out = grad.Textbox(lines=6, label="Generated Text")
-examples = [["mythology of the Slavs"], ["All-seeing eye monitors these world"], ["astronaut dog"],
- ["A monochrome forest of ebony trees"], ["sad view of worker in office,"],
- ["Headshot photo portrait of John Lennon"], ["wide field with thousands of blue nemophila,"]]
-title = "Midjourney Prompt Generator"
-description = "This is an unofficial demo for Midjourney Prompt Generator. To use it, simply send your text, or click one of the examples to load them. Read more at the links below. Model: https://huggingface.co/succinctly/text2image-prompt-generator Telegram bot: https://t.me/prompt_generator_bot [](https://twitter.com/DoEvent)"
-article = "
- This is an online demo of the
- GPT-2 output detector
- model, based on the 🤗/Transformers
- implementation of RoBERTa.
- Enter some text in the text box; the predicted probabilities will be displayed below.
- The results start to get reliable after around 50 tokens.
-
-
-
-
-
Real
-
-
Fake
-
-
-
-
-
-
-
-
-
-
-
diff --git a/spaces/openai/whisper/README.md b/spaces/openai/whisper/README.md
deleted file mode 100644
index 9908d27e54703e581dad9e88b7d14dc5a987ddae..0000000000000000000000000000000000000000
--- a/spaces/openai/whisper/README.md
+++ /dev/null
@@ -1,12 +0,0 @@
----
-title: Whisper
-emoji: 📉
-colorFrom: pink
-colorTo: yellow
-sdk: gradio
-sdk_version: 3.3.1
-app_file: app.py
-pinned: false
----
-
-Check out the configuration reference at https://huggingface.co/docs/hub/spaces-config-reference
diff --git a/spaces/perilli/tortoise-tts-v2/do_tts.py b/spaces/perilli/tortoise-tts-v2/do_tts.py
deleted file mode 100644
index fa0347e64c587786a90eeb053f7efb388f323bf9..0000000000000000000000000000000000000000
--- a/spaces/perilli/tortoise-tts-v2/do_tts.py
+++ /dev/null
@@ -1,34 +0,0 @@
-import argparse
-import os
-
-import torchaudio
-
-from api import TextToSpeech
-from utils.audio import load_audio, get_voices
-
-if __name__ == '__main__':
- parser = argparse.ArgumentParser()
- parser.add_argument('--text', type=str, help='Text to speak.', default="I am a language model that has learned to speak.")
- parser.add_argument('--voice', type=str, help='Selects the voice to use for generation. See options in voices/ directory (and add your own!) '
- 'Use the & character to join two voices together. Use a comma to perform inference on multiple voices.', default='pat')
- parser.add_argument('--preset', type=str, help='Which voice preset to use.', default='standard')
- parser.add_argument('--voice_diversity_intelligibility_slider', type=float,
- help='How to balance vocal diversity with the quality/intelligibility of the spoken text. 0 means highly diverse voice (not recommended), 1 means maximize intellibility',
- default=.5)
- parser.add_argument('--output_path', type=str, help='Where to store outputs.', default='results/')
- args = parser.parse_args()
- os.makedirs(args.output_path, exist_ok=True)
-
- tts = TextToSpeech()
-
- voices = get_voices()
- selected_voices = args.voice.split(',')
- for voice in selected_voices:
- cond_paths = voices[voice]
- conds = []
- for cond_path in cond_paths:
- c = load_audio(cond_path, 22050)
- conds.append(c)
- gen = tts.tts_with_preset(args.text, conds, preset=args.preset, clvp_cvvp_slider=args.voice_diversity_intelligibility_slider)
- torchaudio.save(os.path.join(args.output_path, f'{voice}.wav'), gen.squeeze(0).cpu(), 24000)
-
diff --git a/spaces/peteralexandercharles/wav2vec2-uk-demo/inference.py b/spaces/peteralexandercharles/wav2vec2-uk-demo/inference.py
deleted file mode 100644
index de9ed1de822a59a31d4232cc6ea55bdf929b2597..0000000000000000000000000000000000000000
--- a/spaces/peteralexandercharles/wav2vec2-uk-demo/inference.py
+++ /dev/null
@@ -1,68 +0,0 @@
-import argparse
-import torch
-import torchaudio
-from pathlib import Path
-from transformers import Wav2Vec2ProcessorWithLM, Wav2Vec2ForCTC
-
-
-def main(args):
- processor = Wav2Vec2ProcessorWithLM.from_pretrained(args.model_id)
- model = Wav2Vec2ForCTC.from_pretrained(args.model_id)
- model.to('cpu')
-
- files = args.path_files.split(',')
-
- for path_file in files:
- print('File:', path_file)
-
- wav_file_path = str(Path(path_file).absolute())
- waveform, sample_rate = torchaudio.load(wav_file_path)
-
- if sample_rate != 16000:
- resample = torchaudio.transforms.Resample(
- sample_rate, 16000, resampling_method='sinc_interpolation')
- speech_array = resample(waveform)
- sp = speech_array.squeeze().numpy()
- else:
- sp = waveform.squeeze().numpy()
-
- # stride_length_s is a tuple of the left and right stride length.
- # With only 1 number, both sides get the same stride, by default
- # the stride_length on one side is 1/6th of the chunk_length_s
- input_values = processor(sp,
- sample_rate=16000,
- chunk_length_s=args.chunk_length_s,
- stride_length_s=(args.stride_length_s_l, args.stride_length_s_r),
- return_tensors="pt").input_values
-
- with torch.no_grad():
- logits = model(input_values).logits
-
- prediction = processor.batch_decode(logits.numpy()).text
- print(prediction[0])
-
-
-if __name__ == "__main__":
- parser = argparse.ArgumentParser()
-
- parser.add_argument(
- "--path_files", type=str, required=True, help="WAV files to transcribe, separated by a comma"
- )
- parser.add_argument(
- "--model_id", type=str, required=True, help="Model identifier. Should be loadable with 🤗 Transformers"
- )
- parser.add_argument(
- "--chunk_length_s", type=float, default=None, help="Chunk length in seconds. Defaults to 5 seconds."
- )
- parser.add_argument(
- "--stride_length_s_l", type=int, default=None, help="Stride of the audio chunks, left value."
- )
- parser.add_argument(
- "--stride_length_s_r", type=int, default=None, help="Stride of the audio chunks, right value."
- )
- parser.add_argument(
- "--log_outputs", action="store_true", help="If defined, write outputs to log file for analysis."
- )
- args = parser.parse_args()
-
- main(args)
diff --git a/spaces/pix2pix-zero-library/pix2pix-zero-demo/utils/gradio_utils.py b/spaces/pix2pix-zero-library/pix2pix-zero-demo/utils/gradio_utils.py
deleted file mode 100644
index 1545d586bf46249c6d484ccd2f7abfee3c5ec02c..0000000000000000000000000000000000000000
--- a/spaces/pix2pix-zero-library/pix2pix-zero-demo/utils/gradio_utils.py
+++ /dev/null
@@ -1,616 +0,0 @@
-import gradio as gr
-
-def set_visible_true():
- return gr.update(visible=True)
-
-def set_visible_false():
- return gr.update(visible=False)
-
-
-# HTML_header = f"""
-#
-#
-# pix2pix-zero is a diffusion-based image-to-image approach that allows users to specify the edit direction on-the-fly
-# (e.g., cat to dog). Our method can directly use pre-trained text-to-image diffusion models, such as Stable Diffusion,
-# for editing real and synthetic images while preserving the input image's structure. Our method is training-free and prompt-free,
-# as it requires neither manual text prompting for each input image nor costly fine-tuning for each task.
-#
- This is a demo for pix2pix-zero, a diffusion-based image-to-image approach that allows users to
- specify the edit direction on-the-fly (e.g., cat to dog). Our method can directly use pre-trained text-to-image diffusion models, such as Stable Diffusion, for editing real and synthetic images while preserving the input image's structure. Our method is training-free and prompt-free, as it requires neither manual text prompting for each input image nor costly fine-tuning for each task.
-
- TL;DR: no finetuning required; no text input needed; input structure preserved.
-
-
-
-
-
-
-
-"""
-
-HTML_input_header = f"""
-
- Step 1: select a real input image.
-
-"""
-
-HTML_middle_header = f"""
-
- Step 2: select the editing options.
-
-"""
-
-
-HTML_output_header = f"""
-
- Step 3: translated image!
-
-"""
\ No newline at end of file
diff --git a/spaces/pknez/face-swap-docker/mynewshinyroop/Lib/site-packages/pip/_vendor/chardet/gb2312freq.py b/spaces/pknez/face-swap-docker/mynewshinyroop/Lib/site-packages/pip/_vendor/chardet/gb2312freq.py
deleted file mode 100644
index b32bfc74213d93d434f1f3a47cb5d7d0bf4863d3..0000000000000000000000000000000000000000
--- a/spaces/pknez/face-swap-docker/mynewshinyroop/Lib/site-packages/pip/_vendor/chardet/gb2312freq.py
+++ /dev/null
@@ -1,284 +0,0 @@
-######################## BEGIN LICENSE BLOCK ########################
-# The Original Code is Mozilla Communicator client code.
-#
-# The Initial Developer of the Original Code is
-# Netscape Communications Corporation.
-# Portions created by the Initial Developer are Copyright (C) 1998
-# the Initial Developer. All Rights Reserved.
-#
-# Contributor(s):
-# Mark Pilgrim - port to Python
-#
-# This library is free software; you can redistribute it and/or
-# modify it under the terms of the GNU Lesser General Public
-# License as published by the Free Software Foundation; either
-# version 2.1 of the License, or (at your option) any later version.
-#
-# This library is distributed in the hope that it will be useful,
-# but WITHOUT ANY WARRANTY; without even the implied warranty of
-# MERCHANTABILITY or FITNESS FOR A PARTICULAR PURPOSE. See the GNU
-# Lesser General Public License for more details.
-#
-# You should have received a copy of the GNU Lesser General Public
-# License along with this library; if not, write to the Free Software
-# Foundation, Inc., 51 Franklin St, Fifth Floor, Boston, MA
-# 02110-1301 USA
-######################### END LICENSE BLOCK #########################
-
-# GB2312 most frequently used character table
-#
-# Char to FreqOrder table , from hz6763
-
-# 512 --> 0.79 -- 0.79
-# 1024 --> 0.92 -- 0.13
-# 2048 --> 0.98 -- 0.06
-# 6768 --> 1.00 -- 0.02
-#
-# Ideal Distribution Ratio = 0.79135/(1-0.79135) = 3.79
-# Random Distribution Ration = 512 / (3755 - 512) = 0.157
-#
-# Typical Distribution Ratio about 25% of Ideal one, still much higher that RDR
-
-GB2312_TYPICAL_DISTRIBUTION_RATIO = 0.9
-
-GB2312_TABLE_SIZE = 3760
-
-# fmt: off
-GB2312_CHAR_TO_FREQ_ORDER = (
-1671, 749,1443,2364,3924,3807,2330,3921,1704,3463,2691,1511,1515, 572,3191,2205,
-2361, 224,2558, 479,1711, 963,3162, 440,4060,1905,2966,2947,3580,2647,3961,3842,
-2204, 869,4207, 970,2678,5626,2944,2956,1479,4048, 514,3595, 588,1346,2820,3409,
- 249,4088,1746,1873,2047,1774, 581,1813, 358,1174,3590,1014,1561,4844,2245, 670,
-1636,3112, 889,1286, 953, 556,2327,3060,1290,3141, 613, 185,3477,1367, 850,3820,
-1715,2428,2642,2303,2732,3041,2562,2648,3566,3946,1349, 388,3098,2091,1360,3585,
- 152,1687,1539, 738,1559, 59,1232,2925,2267,1388,1249,1741,1679,2960, 151,1566,
-1125,1352,4271, 924,4296, 385,3166,4459, 310,1245,2850, 70,3285,2729,3534,3575,
-2398,3298,3466,1960,2265, 217,3647, 864,1909,2084,4401,2773,1010,3269,5152, 853,
-3051,3121,1244,4251,1895, 364,1499,1540,2313,1180,3655,2268, 562, 715,2417,3061,
- 544, 336,3768,2380,1752,4075, 950, 280,2425,4382, 183,2759,3272, 333,4297,2155,
-1688,2356,1444,1039,4540, 736,1177,3349,2443,2368,2144,2225, 565, 196,1482,3406,
- 927,1335,4147, 692, 878,1311,1653,3911,3622,1378,4200,1840,2969,3149,2126,1816,
-2534,1546,2393,2760, 737,2494, 13, 447, 245,2747, 38,2765,2129,2589,1079, 606,
- 360, 471,3755,2890, 404, 848, 699,1785,1236, 370,2221,1023,3746,2074,2026,2023,
-2388,1581,2119, 812,1141,3091,2536,1519, 804,2053, 406,1596,1090, 784, 548,4414,
-1806,2264,2936,1100, 343,4114,5096, 622,3358, 743,3668,1510,1626,5020,3567,2513,
-3195,4115,5627,2489,2991, 24,2065,2697,1087,2719, 48,1634, 315, 68, 985,2052,
- 198,2239,1347,1107,1439, 597,2366,2172, 871,3307, 919,2487,2790,1867, 236,2570,
-1413,3794, 906,3365,3381,1701,1982,1818,1524,2924,1205, 616,2586,2072,2004, 575,
- 253,3099, 32,1365,1182, 197,1714,2454,1201, 554,3388,3224,2748, 756,2587, 250,
-2567,1507,1517,3529,1922,2761,2337,3416,1961,1677,2452,2238,3153, 615, 911,1506,
-1474,2495,1265,1906,2749,3756,3280,2161, 898,2714,1759,3450,2243,2444, 563, 26,
-3286,2266,3769,3344,2707,3677, 611,1402, 531,1028,2871,4548,1375, 261,2948, 835,
-1190,4134, 353, 840,2684,1900,3082,1435,2109,1207,1674, 329,1872,2781,4055,2686,
-2104, 608,3318,2423,2957,2768,1108,3739,3512,3271,3985,2203,1771,3520,1418,2054,
-1681,1153, 225,1627,2929, 162,2050,2511,3687,1954, 124,1859,2431,1684,3032,2894,
- 585,4805,3969,2869,2704,2088,2032,2095,3656,2635,4362,2209, 256, 518,2042,2105,
-3777,3657, 643,2298,1148,1779, 190, 989,3544, 414, 11,2135,2063,2979,1471, 403,
-3678, 126, 770,1563, 671,2499,3216,2877, 600,1179, 307,2805,4937,1268,1297,2694,
- 252,4032,1448,1494,1331,1394, 127,2256, 222,1647,1035,1481,3056,1915,1048, 873,
-3651, 210, 33,1608,2516, 200,1520, 415, 102, 0,3389,1287, 817, 91,3299,2940,
- 836,1814, 549,2197,1396,1669,2987,3582,2297,2848,4528,1070, 687, 20,1819, 121,
-1552,1364,1461,1968,2617,3540,2824,2083, 177, 948,4938,2291, 110,4549,2066, 648,
-3359,1755,2110,2114,4642,4845,1693,3937,3308,1257,1869,2123, 208,1804,3159,2992,
-2531,2549,3361,2418,1350,2347,2800,2568,1291,2036,2680, 72, 842,1990, 212,1233,
-1154,1586, 75,2027,3410,4900,1823,1337,2710,2676, 728,2810,1522,3026,4995, 157,
- 755,1050,4022, 710, 785,1936,2194,2085,1406,2777,2400, 150,1250,4049,1206, 807,
-1910, 534, 529,3309,1721,1660, 274, 39,2827, 661,2670,1578, 925,3248,3815,1094,
-4278,4901,4252, 41,1150,3747,2572,2227,4501,3658,4902,3813,3357,3617,2884,2258,
- 887, 538,4187,3199,1294,2439,3042,2329,2343,2497,1255, 107, 543,1527, 521,3478,
-3568, 194,5062, 15, 961,3870,1241,1192,2664, 66,5215,3260,2111,1295,1127,2152,
-3805,4135, 901,1164,1976, 398,1278, 530,1460, 748, 904,1054,1966,1426, 53,2909,
- 509, 523,2279,1534, 536,1019, 239,1685, 460,2353, 673,1065,2401,3600,4298,2272,
-1272,2363, 284,1753,3679,4064,1695, 81, 815,2677,2757,2731,1386, 859, 500,4221,
-2190,2566, 757,1006,2519,2068,1166,1455, 337,2654,3203,1863,1682,1914,3025,1252,
-1409,1366, 847, 714,2834,2038,3209, 964,2970,1901, 885,2553,1078,1756,3049, 301,
-1572,3326, 688,2130,1996,2429,1805,1648,2930,3421,2750,3652,3088, 262,1158,1254,
- 389,1641,1812, 526,1719, 923,2073,1073,1902, 468, 489,4625,1140, 857,2375,3070,
-3319,2863, 380, 116,1328,2693,1161,2244, 273,1212,1884,2769,3011,1775,1142, 461,
-3066,1200,2147,2212, 790, 702,2695,4222,1601,1058, 434,2338,5153,3640, 67,2360,
-4099,2502, 618,3472,1329, 416,1132, 830,2782,1807,2653,3211,3510,1662, 192,2124,
- 296,3979,1739,1611,3684, 23, 118, 324, 446,1239,1225, 293,2520,3814,3795,2535,
-3116, 17,1074, 467,2692,2201, 387,2922, 45,1326,3055,1645,3659,2817, 958, 243,
-1903,2320,1339,2825,1784,3289, 356, 576, 865,2315,2381,3377,3916,1088,3122,1713,
-1655, 935, 628,4689,1034,1327, 441, 800, 720, 894,1979,2183,1528,5289,2702,1071,
-4046,3572,2399,1571,3281, 79, 761,1103, 327, 134, 758,1899,1371,1615, 879, 442,
- 215,2605,2579, 173,2048,2485,1057,2975,3317,1097,2253,3801,4263,1403,1650,2946,
- 814,4968,3487,1548,2644,1567,1285, 2, 295,2636, 97, 946,3576, 832, 141,4257,
-3273, 760,3821,3521,3156,2607, 949,1024,1733,1516,1803,1920,2125,2283,2665,3180,
-1501,2064,3560,2171,1592, 803,3518,1416, 732,3897,4258,1363,1362,2458, 119,1427,
- 602,1525,2608,1605,1639,3175, 694,3064, 10, 465, 76,2000,4846,4208, 444,3781,
-1619,3353,2206,1273,3796, 740,2483, 320,1723,2377,3660,2619,1359,1137,1762,1724,
-2345,2842,1850,1862, 912, 821,1866, 612,2625,1735,2573,3369,1093, 844, 89, 937,
- 930,1424,3564,2413,2972,1004,3046,3019,2011, 711,3171,1452,4178, 428, 801,1943,
- 432, 445,2811, 206,4136,1472, 730, 349, 73, 397,2802,2547, 998,1637,1167, 789,
- 396,3217, 154,1218, 716,1120,1780,2819,4826,1931,3334,3762,2139,1215,2627, 552,
-3664,3628,3232,1405,2383,3111,1356,2652,3577,3320,3101,1703, 640,1045,1370,1246,
-4996, 371,1575,2436,1621,2210, 984,4033,1734,2638, 16,4529, 663,2755,3255,1451,
-3917,2257,1253,1955,2234,1263,2951, 214,1229, 617, 485, 359,1831,1969, 473,2310,
- 750,2058, 165, 80,2864,2419, 361,4344,2416,2479,1134, 796,3726,1266,2943, 860,
-2715, 938, 390,2734,1313,1384, 248, 202, 877,1064,2854, 522,3907, 279,1602, 297,
-2357, 395,3740, 137,2075, 944,4089,2584,1267,3802, 62,1533,2285, 178, 176, 780,
-2440, 201,3707, 590, 478,1560,4354,2117,1075, 30, 74,4643,4004,1635,1441,2745,
- 776,2596, 238,1077,1692,1912,2844, 605, 499,1742,3947, 241,3053, 980,1749, 936,
-2640,4511,2582, 515,1543,2162,5322,2892,2993, 890,2148,1924, 665,1827,3581,1032,
- 968,3163, 339,1044,1896, 270, 583,1791,1720,4367,1194,3488,3669, 43,2523,1657,
- 163,2167, 290,1209,1622,3378, 550, 634,2508,2510, 695,2634,2384,2512,1476,1414,
- 220,1469,2341,2138,2852,3183,2900,4939,2865,3502,1211,3680, 854,3227,1299,2976,
-3172, 186,2998,1459, 443,1067,3251,1495, 321,1932,3054, 909, 753,1410,1828, 436,
-2441,1119,1587,3164,2186,1258, 227, 231,1425,1890,3200,3942, 247, 959, 725,5254,
-2741, 577,2158,2079, 929, 120, 174, 838,2813, 591,1115, 417,2024, 40,3240,1536,
-1037, 291,4151,2354, 632,1298,2406,2500,3535,1825,1846,3451, 205,1171, 345,4238,
- 18,1163, 811, 685,2208,1217, 425,1312,1508,1175,4308,2552,1033, 587,1381,3059,
-2984,3482, 340,1316,4023,3972, 792,3176, 519, 777,4690, 918, 933,4130,2981,3741,
- 90,3360,2911,2200,5184,4550, 609,3079,2030, 272,3379,2736, 363,3881,1130,1447,
- 286, 779, 357,1169,3350,3137,1630,1220,2687,2391, 747,1277,3688,2618,2682,2601,
-1156,3196,5290,4034,3102,1689,3596,3128, 874, 219,2783, 798, 508,1843,2461, 269,
-1658,1776,1392,1913,2983,3287,2866,2159,2372, 829,4076, 46,4253,2873,1889,1894,
- 915,1834,1631,2181,2318, 298, 664,2818,3555,2735, 954,3228,3117, 527,3511,2173,
- 681,2712,3033,2247,2346,3467,1652, 155,2164,3382, 113,1994, 450, 899, 494, 994,
-1237,2958,1875,2336,1926,3727, 545,1577,1550, 633,3473, 204,1305,3072,2410,1956,
-2471, 707,2134, 841,2195,2196,2663,3843,1026,4940, 990,3252,4997, 368,1092, 437,
-3212,3258,1933,1829, 675,2977,2893, 412, 943,3723,4644,3294,3283,2230,2373,5154,
-2389,2241,2661,2323,1404,2524, 593, 787, 677,3008,1275,2059, 438,2709,2609,2240,
-2269,2246,1446, 36,1568,1373,3892,1574,2301,1456,3962, 693,2276,5216,2035,1143,
-2720,1919,1797,1811,2763,4137,2597,1830,1699,1488,1198,2090, 424,1694, 312,3634,
-3390,4179,3335,2252,1214, 561,1059,3243,2295,2561, 975,5155,2321,2751,3772, 472,
-1537,3282,3398,1047,2077,2348,2878,1323,3340,3076, 690,2906, 51, 369, 170,3541,
-1060,2187,2688,3670,2541,1083,1683, 928,3918, 459, 109,4427, 599,3744,4286, 143,
-2101,2730,2490, 82,1588,3036,2121, 281,1860, 477,4035,1238,2812,3020,2716,3312,
-1530,2188,2055,1317, 843, 636,1808,1173,3495, 649, 181,1002, 147,3641,1159,2414,
-3750,2289,2795, 813,3123,2610,1136,4368, 5,3391,4541,2174, 420, 429,1728, 754,
-1228,2115,2219, 347,2223,2733, 735,1518,3003,2355,3134,1764,3948,3329,1888,2424,
-1001,1234,1972,3321,3363,1672,1021,1450,1584, 226, 765, 655,2526,3404,3244,2302,
-3665, 731, 594,2184, 319,1576, 621, 658,2656,4299,2099,3864,1279,2071,2598,2739,
- 795,3086,3699,3908,1707,2352,2402,1382,3136,2475,1465,4847,3496,3865,1085,3004,
-2591,1084, 213,2287,1963,3565,2250, 822, 793,4574,3187,1772,1789,3050, 595,1484,
-1959,2770,1080,2650, 456, 422,2996, 940,3322,4328,4345,3092,2742, 965,2784, 739,
-4124, 952,1358,2498,2949,2565, 332,2698,2378, 660,2260,2473,4194,3856,2919, 535,
-1260,2651,1208,1428,1300,1949,1303,2942, 433,2455,2450,1251,1946, 614,1269, 641,
-1306,1810,2737,3078,2912, 564,2365,1419,1415,1497,4460,2367,2185,1379,3005,1307,
-3218,2175,1897,3063, 682,1157,4040,4005,1712,1160,1941,1399, 394, 402,2952,1573,
-1151,2986,2404, 862, 299,2033,1489,3006, 346, 171,2886,3401,1726,2932, 168,2533,
- 47,2507,1030,3735,1145,3370,1395,1318,1579,3609,4560,2857,4116,1457,2529,1965,
- 504,1036,2690,2988,2405, 745,5871, 849,2397,2056,3081, 863,2359,3857,2096, 99,
-1397,1769,2300,4428,1643,3455,1978,1757,3718,1440, 35,4879,3742,1296,4228,2280,
- 160,5063,1599,2013, 166, 520,3479,1646,3345,3012, 490,1937,1545,1264,2182,2505,
-1096,1188,1369,1436,2421,1667,2792,2460,1270,2122, 727,3167,2143, 806,1706,1012,
-1800,3037, 960,2218,1882, 805, 139,2456,1139,1521, 851,1052,3093,3089, 342,2039,
- 744,5097,1468,1502,1585,2087, 223, 939, 326,2140,2577, 892,2481,1623,4077, 982,
-3708, 135,2131, 87,2503,3114,2326,1106, 876,1616, 547,2997,2831,2093,3441,4530,
-4314, 9,3256,4229,4148, 659,1462,1986,1710,2046,2913,2231,4090,4880,5255,3392,
-3274,1368,3689,4645,1477, 705,3384,3635,1068,1529,2941,1458,3782,1509, 100,1656,
-2548, 718,2339, 408,1590,2780,3548,1838,4117,3719,1345,3530, 717,3442,2778,3220,
-2898,1892,4590,3614,3371,2043,1998,1224,3483, 891, 635, 584,2559,3355, 733,1766,
-1729,1172,3789,1891,2307, 781,2982,2271,1957,1580,5773,2633,2005,4195,3097,1535,
-3213,1189,1934,5693,3262, 586,3118,1324,1598, 517,1564,2217,1868,1893,4445,3728,
-2703,3139,1526,1787,1992,3882,2875,1549,1199,1056,2224,1904,2711,5098,4287, 338,
-1993,3129,3489,2689,1809,2815,1997, 957,1855,3898,2550,3275,3057,1105,1319, 627,
-1505,1911,1883,3526, 698,3629,3456,1833,1431, 746, 77,1261,2017,2296,1977,1885,
- 125,1334,1600, 525,1798,1109,2222,1470,1945, 559,2236,1186,3443,2476,1929,1411,
-2411,3135,1777,3372,2621,1841,1613,3229, 668,1430,1839,2643,2916, 195,1989,2671,
-2358,1387, 629,3205,2293,5256,4439, 123,1310, 888,1879,4300,3021,3605,1003,1162,
-3192,2910,2010, 140,2395,2859, 55,1082,2012,2901, 662, 419,2081,1438, 680,2774,
-4654,3912,1620,1731,1625,5035,4065,2328, 512,1344, 802,5443,2163,2311,2537, 524,
-3399, 98,1155,2103,1918,2606,3925,2816,1393,2465,1504,3773,2177,3963,1478,4346,
- 180,1113,4655,3461,2028,1698, 833,2696,1235,1322,1594,4408,3623,3013,3225,2040,
-3022, 541,2881, 607,3632,2029,1665,1219, 639,1385,1686,1099,2803,3231,1938,3188,
-2858, 427, 676,2772,1168,2025, 454,3253,2486,3556, 230,1950, 580, 791,1991,1280,
-1086,1974,2034, 630, 257,3338,2788,4903,1017, 86,4790, 966,2789,1995,1696,1131,
- 259,3095,4188,1308, 179,1463,5257, 289,4107,1248, 42,3413,1725,2288, 896,1947,
- 774,4474,4254, 604,3430,4264, 392,2514,2588, 452, 237,1408,3018, 988,4531,1970,
-3034,3310, 540,2370,1562,1288,2990, 502,4765,1147, 4,1853,2708, 207, 294,2814,
-4078,2902,2509, 684, 34,3105,3532,2551, 644, 709,2801,2344, 573,1727,3573,3557,
-2021,1081,3100,4315,2100,3681, 199,2263,1837,2385, 146,3484,1195,2776,3949, 997,
-1939,3973,1008,1091,1202,1962,1847,1149,4209,5444,1076, 493, 117,5400,2521, 972,
-1490,2934,1796,4542,2374,1512,2933,2657, 413,2888,1135,2762,2314,2156,1355,2369,
- 766,2007,2527,2170,3124,2491,2593,2632,4757,2437, 234,3125,3591,1898,1750,1376,
-1942,3468,3138, 570,2127,2145,3276,4131, 962, 132,1445,4196, 19, 941,3624,3480,
-3366,1973,1374,4461,3431,2629, 283,2415,2275, 808,2887,3620,2112,2563,1353,3610,
- 955,1089,3103,1053, 96, 88,4097, 823,3808,1583, 399, 292,4091,3313, 421,1128,
- 642,4006, 903,2539,1877,2082, 596, 29,4066,1790, 722,2157, 130, 995,1569, 769,
-1485, 464, 513,2213, 288,1923,1101,2453,4316, 133, 486,2445, 50, 625, 487,2207,
- 57, 423, 481,2962, 159,3729,1558, 491, 303, 482, 501, 240,2837, 112,3648,2392,
-1783, 362, 8,3433,3422, 610,2793,3277,1390,1284,1654, 21,3823, 734, 367, 623,
- 193, 287, 374,1009,1483, 816, 476, 313,2255,2340,1262,2150,2899,1146,2581, 782,
-2116,1659,2018,1880, 255,3586,3314,1110,2867,2137,2564, 986,2767,5185,2006, 650,
- 158, 926, 762, 881,3157,2717,2362,3587, 306,3690,3245,1542,3077,2427,1691,2478,
-2118,2985,3490,2438, 539,2305, 983, 129,1754, 355,4201,2386, 827,2923, 104,1773,
-2838,2771, 411,2905,3919, 376, 767, 122,1114, 828,2422,1817,3506, 266,3460,1007,
-1609,4998, 945,2612,4429,2274, 726,1247,1964,2914,2199,2070,4002,4108, 657,3323,
-1422, 579, 455,2764,4737,1222,2895,1670, 824,1223,1487,2525, 558, 861,3080, 598,
-2659,2515,1967, 752,2583,2376,2214,4180, 977, 704,2464,4999,2622,4109,1210,2961,
- 819,1541, 142,2284, 44, 418, 457,1126,3730,4347,4626,1644,1876,3671,1864, 302,
-1063,5694, 624, 723,1984,3745,1314,1676,2488,1610,1449,3558,3569,2166,2098, 409,
-1011,2325,3704,2306, 818,1732,1383,1824,1844,3757, 999,2705,3497,1216,1423,2683,
-2426,2954,2501,2726,2229,1475,2554,5064,1971,1794,1666,2014,1343, 783, 724, 191,
-2434,1354,2220,5065,1763,2752,2472,4152, 131, 175,2885,3434, 92,1466,4920,2616,
-3871,3872,3866, 128,1551,1632, 669,1854,3682,4691,4125,1230, 188,2973,3290,1302,
-1213, 560,3266, 917, 763,3909,3249,1760, 868,1958, 764,1782,2097, 145,2277,3774,
-4462, 64,1491,3062, 971,2132,3606,2442, 221,1226,1617, 218, 323,1185,3207,3147,
- 571, 619,1473,1005,1744,2281, 449,1887,2396,3685, 275, 375,3816,1743,3844,3731,
- 845,1983,2350,4210,1377, 773, 967,3499,3052,3743,2725,4007,1697,1022,3943,1464,
-3264,2855,2722,1952,1029,2839,2467, 84,4383,2215, 820,1391,2015,2448,3672, 377,
-1948,2168, 797,2545,3536,2578,2645, 94,2874,1678, 405,1259,3071, 771, 546,1315,
- 470,1243,3083, 895,2468, 981, 969,2037, 846,4181, 653,1276,2928, 14,2594, 557,
-3007,2474, 156, 902,1338,1740,2574, 537,2518, 973,2282,2216,2433,1928, 138,2903,
-1293,2631,1612, 646,3457, 839,2935, 111, 496,2191,2847, 589,3186, 149,3994,2060,
-4031,2641,4067,3145,1870, 37,3597,2136,1025,2051,3009,3383,3549,1121,1016,3261,
-1301, 251,2446,2599,2153, 872,3246, 637, 334,3705, 831, 884, 921,3065,3140,4092,
-2198,1944, 246,2964, 108,2045,1152,1921,2308,1031, 203,3173,4170,1907,3890, 810,
-1401,2003,1690, 506, 647,1242,2828,1761,1649,3208,2249,1589,3709,2931,5156,1708,
- 498, 666,2613, 834,3817,1231, 184,2851,1124, 883,3197,2261,3710,1765,1553,2658,
-1178,2639,2351, 93,1193, 942,2538,2141,4402, 235,1821, 870,1591,2192,1709,1871,
-3341,1618,4126,2595,2334, 603, 651, 69, 701, 268,2662,3411,2555,1380,1606, 503,
- 448, 254,2371,2646, 574,1187,2309,1770, 322,2235,1292,1801, 305, 566,1133, 229,
-2067,2057, 706, 167, 483,2002,2672,3295,1820,3561,3067, 316, 378,2746,3452,1112,
- 136,1981, 507,1651,2917,1117, 285,4591, 182,2580,3522,1304, 335,3303,1835,2504,
-1795,1792,2248, 674,1018,2106,2449,1857,2292,2845, 976,3047,1781,2600,2727,1389,
-1281, 52,3152, 153, 265,3950, 672,3485,3951,4463, 430,1183, 365, 278,2169, 27,
-1407,1336,2304, 209,1340,1730,2202,1852,2403,2883, 979,1737,1062, 631,2829,2542,
-3876,2592, 825,2086,2226,3048,3625, 352,1417,3724, 542, 991, 431,1351,3938,1861,
-2294, 826,1361,2927,3142,3503,1738, 463,2462,2723, 582,1916,1595,2808, 400,3845,
-3891,2868,3621,2254, 58,2492,1123, 910,2160,2614,1372,1603,1196,1072,3385,1700,
-3267,1980, 696, 480,2430, 920, 799,1570,2920,1951,2041,4047,2540,1321,4223,2469,
-3562,2228,1271,2602, 401,2833,3351,2575,5157, 907,2312,1256, 410, 263,3507,1582,
- 996, 678,1849,2316,1480, 908,3545,2237, 703,2322, 667,1826,2849,1531,2604,2999,
-2407,3146,2151,2630,1786,3711, 469,3542, 497,3899,2409, 858, 837,4446,3393,1274,
- 786, 620,1845,2001,3311, 484, 308,3367,1204,1815,3691,2332,1532,2557,1842,2020,
-2724,1927,2333,4440, 567, 22,1673,2728,4475,1987,1858,1144,1597, 101,1832,3601,
- 12, 974,3783,4391, 951,1412, 1,3720, 453,4608,4041, 528,1041,1027,3230,2628,
-1129, 875,1051,3291,1203,2262,1069,2860,2799,2149,2615,3278, 144,1758,3040, 31,
- 475,1680, 366,2685,3184, 311,1642,4008,2466,5036,1593,1493,2809, 216,1420,1668,
- 233, 304,2128,3284, 232,1429,1768,1040,2008,3407,2740,2967,2543, 242,2133, 778,
-1565,2022,2620, 505,2189,2756,1098,2273, 372,1614, 708, 553,2846,2094,2278, 169,
-3626,2835,4161, 228,2674,3165, 809,1454,1309, 466,1705,1095, 900,3423, 880,2667,
-3751,5258,2317,3109,2571,4317,2766,1503,1342, 866,4447,1118, 63,2076, 314,1881,
-1348,1061, 172, 978,3515,1747, 532, 511,3970, 6, 601, 905,2699,3300,1751, 276,
-1467,3725,2668, 65,4239,2544,2779,2556,1604, 578,2451,1802, 992,2331,2624,1320,
-3446, 713,1513,1013, 103,2786,2447,1661, 886,1702, 916, 654,3574,2031,1556, 751,
-2178,2821,2179,1498,1538,2176, 271, 914,2251,2080,1325, 638,1953,2937,3877,2432,
-2754, 95,3265,1716, 260,1227,4083, 775, 106,1357,3254, 426,1607, 555,2480, 772,
-1985, 244,2546, 474, 495,1046,2611,1851,2061, 71,2089,1675,2590, 742,3758,2843,
-3222,1433, 267,2180,2576,2826,2233,2092,3913,2435, 956,1745,3075, 856,2113,1116,
- 451, 3,1988,2896,1398, 993,2463,1878,2049,1341,2718,2721,2870,2108, 712,2904,
-4363,2753,2324, 277,2872,2349,2649, 384, 987, 435, 691,3000, 922, 164,3939, 652,
-1500,1184,4153,2482,3373,2165,4848,2335,3775,3508,3154,2806,2830,1554,2102,1664,
-2530,1434,2408, 893,1547,2623,3447,2832,2242,2532,3169,2856,3223,2078, 49,3770,
-3469, 462, 318, 656,2259,3250,3069, 679,1629,2758, 344,1138,1104,3120,1836,1283,
-3115,2154,1437,4448, 934, 759,1999, 794,2862,1038, 533,2560,1722,2342, 855,2626,
-1197,1663,4476,3127, 85,4240,2528, 25,1111,1181,3673, 407,3470,4561,2679,2713,
- 768,1925,2841,3986,1544,1165, 932, 373,1240,2146,1930,2673, 721,4766, 354,4333,
- 391,2963, 187, 61,3364,1442,1102, 330,1940,1767, 341,3809,4118, 393,2496,2062,
-2211, 105, 331, 300, 439, 913,1332, 626, 379,3304,1557, 328, 689,3952, 309,1555,
- 931, 317,2517,3027, 325, 569, 686,2107,3084, 60,1042,1333,2794, 264,3177,4014,
-1628, 258,3712, 7,4464,1176,1043,1778, 683, 114,1975, 78,1492, 383,1886, 510,
- 386, 645,5291,2891,2069,3305,4138,3867,2939,2603,2493,1935,1066,1848,3588,1015,
-1282,1289,4609, 697,1453,3044,2666,3611,1856,2412, 54, 719,1330, 568,3778,2459,
-1748, 788, 492, 551,1191,1000, 488,3394,3763, 282,1799, 348,2016,1523,3155,2390,
-1049, 382,2019,1788,1170, 729,2968,3523, 897,3926,2785,2938,3292, 350,2319,3238,
-1718,1717,2655,3453,3143,4465, 161,2889,2980,2009,1421, 56,1908,1640,2387,2232,
-1917,1874,2477,4921, 148, 83,3438, 592,4245,2882,1822,1055, 741, 115,1496,1624,
- 381,1638,4592,1020, 516,3214, 458, 947,4575,1432, 211,1514,2926,1865,2142, 189,
- 852,1221,1400,1486, 882,2299,4036, 351, 28,1122, 700,6479,6480,6481,6482,6483, #last 512
-)
-# fmt: on
diff --git a/spaces/profayle/TerrapinTalk/myenv/lib/python3.9/site-packages/gradio/ranged_response.py b/spaces/profayle/TerrapinTalk/myenv/lib/python3.9/site-packages/gradio/ranged_response.py
deleted file mode 100644
index f488776e6c7f3a58ce95375e043680b6c17257da..0000000000000000000000000000000000000000
--- a/spaces/profayle/TerrapinTalk/myenv/lib/python3.9/site-packages/gradio/ranged_response.py
+++ /dev/null
@@ -1,188 +0,0 @@
-# Taken from https://gist.github.com/kevinastone/a6a62db57577b3f24e8a6865ed311463
-# Context: https://github.com/encode/starlette/pull/1090
-from __future__ import annotations
-
-import os
-import re
-import stat
-from typing import NamedTuple
-from urllib.parse import quote
-
-import aiofiles
-from aiofiles.os import stat as aio_stat
-from starlette.datastructures import Headers
-from starlette.exceptions import HTTPException
-from starlette.responses import Response, guess_type
-from starlette.staticfiles import StaticFiles
-from starlette.types import Receive, Scope, Send
-
-RANGE_REGEX = re.compile(r"^bytes=(?P\d+)-(?P\d*)$")
-
-
-class ClosedRange(NamedTuple):
- start: int
- end: int
-
- def __len__(self) -> int:
- return self.end - self.start + 1
-
- def __bool__(self) -> bool:
- return len(self) > 0
-
-
-class OpenRange(NamedTuple):
- start: int
- end: int | None = None
-
- def clamp(self, start: int, end: int) -> ClosedRange:
- begin = max(self.start, start)
- end = min(x for x in (self.end, end) if x)
-
- begin = min(begin, end)
- end = max(begin, end)
-
- return ClosedRange(begin, end)
-
-
-class RangedFileResponse(Response):
- chunk_size = 4096
-
- def __init__(
- self,
- path: str | os.PathLike,
- range: OpenRange,
- headers: dict[str, str] | None = None,
- media_type: str | None = None,
- filename: str | None = None,
- stat_result: os.stat_result | None = None,
- method: str | None = None,
- ) -> None:
- if aiofiles is None:
- raise ModuleNotFoundError(
- "'aiofiles' must be installed to use FileResponse"
- )
- self.path = path
- self.range = range
- self.filename = filename
- self.background = None
- self.send_header_only = method is not None and method.upper() == "HEAD"
- if media_type is None:
- media_type = guess_type(filename or path)[0] or "text/plain"
- self.media_type = media_type
- self.init_headers(headers or {})
- if self.filename is not None:
- content_disposition_filename = quote(self.filename)
- if content_disposition_filename != self.filename:
- content_disposition = (
- f"attachment; filename*=utf-8''{content_disposition_filename}"
- )
- else:
- content_disposition = f'attachment; filename="{self.filename}"'
- self.headers.setdefault("content-disposition", content_disposition)
- self.stat_result = stat_result
-
- def set_range_headers(self, range: ClosedRange) -> None:
- assert self.stat_result
- total_length = self.stat_result.st_size
- content_length = len(range)
- self.headers[
- "content-range"
- ] = f"bytes {range.start}-{range.end}/{total_length}"
- self.headers["content-length"] = str(content_length)
- pass
-
- async def __call__(self, scope: Scope, receive: Receive, send: Send) -> None:
- if self.stat_result is None:
- try:
- stat_result = await aio_stat(self.path)
- self.stat_result = stat_result
- except FileNotFoundError as fnfe:
- raise RuntimeError(
- f"File at path {self.path} does not exist."
- ) from fnfe
- else:
- mode = stat_result.st_mode
- if not stat.S_ISREG(mode):
- raise RuntimeError(f"File at path {self.path} is not a file.")
-
- byte_range = self.range.clamp(0, self.stat_result.st_size)
- self.set_range_headers(byte_range)
-
- async with aiofiles.open(self.path, mode="rb") as file:
- await file.seek(byte_range.start)
- await send(
- {
- "type": "http.response.start",
- "status": 206,
- "headers": self.raw_headers,
- }
- )
- if self.send_header_only:
- await send(
- {"type": "http.response.body", "body": b"", "more_body": False}
- )
- else:
- remaining_bytes = len(byte_range)
-
- if not byte_range:
- await send(
- {"type": "http.response.body", "body": b"", "more_body": False}
- )
- return
-
- while remaining_bytes > 0:
- chunk_size = min(self.chunk_size, remaining_bytes)
- chunk = await file.read(chunk_size)
- remaining_bytes -= len(chunk)
- await send(
- {
- "type": "http.response.body",
- "body": chunk,
- "more_body": remaining_bytes > 0,
- }
- )
-
-
-class RangedStaticFiles(StaticFiles):
- def file_response(
- self,
- full_path: str | os.PathLike,
- stat_result: os.stat_result,
- scope: Scope,
- status_code: int = 200,
- ) -> Response:
- request_headers = Headers(scope=scope)
-
- if request_headers.get("range"):
- response = self.ranged_file_response(
- full_path, stat_result=stat_result, scope=scope
- )
- else:
- response = super().file_response(
- full_path, stat_result=stat_result, scope=scope, status_code=status_code
- )
- response.headers["accept-ranges"] = "bytes"
- return response
-
- def ranged_file_response(
- self,
- full_path: str | os.PathLike,
- stat_result: os.stat_result,
- scope: Scope,
- ) -> Response:
- method = scope["method"]
- request_headers = Headers(scope=scope)
-
- range_header = request_headers["range"]
-
- match = RANGE_REGEX.search(range_header)
- if not match:
- raise HTTPException(400)
-
- start, end = match.group("start"), match.group("end")
-
- range = OpenRange(int(start), int(end) if end else None)
-
- return RangedFileResponse(
- full_path, range, stat_result=stat_result, method=method
- )
diff --git a/spaces/profayle/TerrapinTalk/myenv/lib/python3.9/site-packages/pandas/core/apply.py b/spaces/profayle/TerrapinTalk/myenv/lib/python3.9/site-packages/pandas/core/apply.py
deleted file mode 100644
index e5683359c2fb95a99d17aea5d27963d48eb44136..0000000000000000000000000000000000000000
--- a/spaces/profayle/TerrapinTalk/myenv/lib/python3.9/site-packages/pandas/core/apply.py
+++ /dev/null
@@ -1,1833 +0,0 @@
-from __future__ import annotations
-
-import abc
-from collections import defaultdict
-from functools import partial
-import inspect
-from typing import (
- TYPE_CHECKING,
- Any,
- Callable,
- DefaultDict,
- Literal,
- cast,
-)
-import warnings
-
-import numpy as np
-
-from pandas._config import option_context
-
-from pandas._libs import lib
-from pandas._typing import (
- AggFuncType,
- AggFuncTypeBase,
- AggFuncTypeDict,
- AggObjType,
- Axis,
- AxisInt,
- NDFrameT,
- npt,
-)
-from pandas.errors import SpecificationError
-from pandas.util._decorators import cache_readonly
-from pandas.util._exceptions import find_stack_level
-
-from pandas.core.dtypes.cast import is_nested_object
-from pandas.core.dtypes.common import (
- is_dict_like,
- is_list_like,
- is_sequence,
-)
-from pandas.core.dtypes.dtypes import (
- CategoricalDtype,
- ExtensionDtype,
-)
-from pandas.core.dtypes.generic import (
- ABCDataFrame,
- ABCNDFrame,
- ABCSeries,
-)
-
-import pandas.core.common as com
-from pandas.core.construction import ensure_wrapped_if_datetimelike
-
-if TYPE_CHECKING:
- from collections.abc import (
- Hashable,
- Iterable,
- Iterator,
- Sequence,
- )
-
- from pandas import (
- DataFrame,
- Index,
- Series,
- )
- from pandas.core.groupby import GroupBy
- from pandas.core.resample import Resampler
- from pandas.core.window.rolling import BaseWindow
-
-
-ResType = dict[int, Any]
-
-
-def frame_apply(
- obj: DataFrame,
- func: AggFuncType,
- axis: Axis = 0,
- raw: bool = False,
- result_type: str | None = None,
- by_row: Literal[False, "compat"] = "compat",
- args=None,
- kwargs=None,
-) -> FrameApply:
- """construct and return a row or column based frame apply object"""
- axis = obj._get_axis_number(axis)
- klass: type[FrameApply]
- if axis == 0:
- klass = FrameRowApply
- elif axis == 1:
- klass = FrameColumnApply
-
- _, func, _, _ = reconstruct_func(func, **kwargs)
- assert func is not None
-
- return klass(
- obj,
- func,
- raw=raw,
- result_type=result_type,
- by_row=by_row,
- args=args,
- kwargs=kwargs,
- )
-
-
-class Apply(metaclass=abc.ABCMeta):
- axis: AxisInt
-
- def __init__(
- self,
- obj: AggObjType,
- func: AggFuncType,
- raw: bool,
- result_type: str | None,
- *,
- by_row: Literal[False, "compat", "_compat"] = "compat",
- args,
- kwargs,
- ) -> None:
- self.obj = obj
- self.raw = raw
-
- assert by_row is False or by_row in ["compat", "_compat"]
- self.by_row = by_row
-
- self.args = args or ()
- self.kwargs = kwargs or {}
-
- if result_type not in [None, "reduce", "broadcast", "expand"]:
- raise ValueError(
- "invalid value for result_type, must be one "
- "of {None, 'reduce', 'broadcast', 'expand'}"
- )
-
- self.result_type = result_type
-
- self.func = func
-
- @abc.abstractmethod
- def apply(self) -> DataFrame | Series:
- pass
-
- @abc.abstractmethod
- def agg_or_apply_list_like(
- self, op_name: Literal["agg", "apply"]
- ) -> DataFrame | Series:
- pass
-
- @abc.abstractmethod
- def agg_or_apply_dict_like(
- self, op_name: Literal["agg", "apply"]
- ) -> DataFrame | Series:
- pass
-
- def agg(self) -> DataFrame | Series | None:
- """
- Provide an implementation for the aggregators.
-
- Returns
- -------
- Result of aggregation, or None if agg cannot be performed by
- this method.
- """
- obj = self.obj
- func = self.func
- args = self.args
- kwargs = self.kwargs
-
- if isinstance(func, str):
- return self.apply_str()
-
- if is_dict_like(func):
- return self.agg_dict_like()
- elif is_list_like(func):
- # we require a list, but not a 'str'
- return self.agg_list_like()
-
- if callable(func):
- f = com.get_cython_func(func)
- if f and not args and not kwargs:
- warn_alias_replacement(obj, func, f)
- return getattr(obj, f)()
-
- # caller can react
- return None
-
- def transform(self) -> DataFrame | Series:
- """
- Transform a DataFrame or Series.
-
- Returns
- -------
- DataFrame or Series
- Result of applying ``func`` along the given axis of the
- Series or DataFrame.
-
- Raises
- ------
- ValueError
- If the transform function fails or does not transform.
- """
- obj = self.obj
- func = self.func
- axis = self.axis
- args = self.args
- kwargs = self.kwargs
-
- is_series = obj.ndim == 1
-
- if obj._get_axis_number(axis) == 1:
- assert not is_series
- return obj.T.transform(func, 0, *args, **kwargs).T
-
- if is_list_like(func) and not is_dict_like(func):
- func = cast(list[AggFuncTypeBase], func)
- # Convert func equivalent dict
- if is_series:
- func = {com.get_callable_name(v) or v: v for v in func}
- else:
- func = {col: func for col in obj}
-
- if is_dict_like(func):
- func = cast(AggFuncTypeDict, func)
- return self.transform_dict_like(func)
-
- # func is either str or callable
- func = cast(AggFuncTypeBase, func)
- try:
- result = self.transform_str_or_callable(func)
- except TypeError:
- raise
- except Exception as err:
- raise ValueError("Transform function failed") from err
-
- # Functions that transform may return empty Series/DataFrame
- # when the dtype is not appropriate
- if (
- isinstance(result, (ABCSeries, ABCDataFrame))
- and result.empty
- and not obj.empty
- ):
- raise ValueError("Transform function failed")
- # error: Argument 1 to "__get__" of "AxisProperty" has incompatible type
- # "Union[Series, DataFrame, GroupBy[Any], SeriesGroupBy,
- # DataFrameGroupBy, BaseWindow, Resampler]"; expected "Union[DataFrame,
- # Series]"
- if not isinstance(result, (ABCSeries, ABCDataFrame)) or not result.index.equals(
- obj.index # type: ignore[arg-type]
- ):
- raise ValueError("Function did not transform")
-
- return result
-
- def transform_dict_like(self, func):
- """
- Compute transform in the case of a dict-like func
- """
- from pandas.core.reshape.concat import concat
-
- obj = self.obj
- args = self.args
- kwargs = self.kwargs
-
- # transform is currently only for Series/DataFrame
- assert isinstance(obj, ABCNDFrame)
-
- if len(func) == 0:
- raise ValueError("No transform functions were provided")
-
- func = self.normalize_dictlike_arg("transform", obj, func)
-
- results: dict[Hashable, DataFrame | Series] = {}
- for name, how in func.items():
- colg = obj._gotitem(name, ndim=1)
- results[name] = colg.transform(how, 0, *args, **kwargs)
- return concat(results, axis=1)
-
- def transform_str_or_callable(self, func) -> DataFrame | Series:
- """
- Compute transform in the case of a string or callable func
- """
- obj = self.obj
- args = self.args
- kwargs = self.kwargs
-
- if isinstance(func, str):
- return self._apply_str(obj, func, *args, **kwargs)
-
- if not args and not kwargs:
- f = com.get_cython_func(func)
- if f:
- warn_alias_replacement(obj, func, f)
- return getattr(obj, f)()
-
- # Two possible ways to use a UDF - apply or call directly
- try:
- return obj.apply(func, args=args, **kwargs)
- except Exception:
- return func(obj, *args, **kwargs)
-
- def agg_list_like(self) -> DataFrame | Series:
- """
- Compute aggregation in the case of a list-like argument.
-
- Returns
- -------
- Result of aggregation.
- """
- return self.agg_or_apply_list_like(op_name="agg")
-
- def compute_list_like(
- self,
- op_name: Literal["agg", "apply"],
- selected_obj: Series | DataFrame,
- kwargs: dict[str, Any],
- ) -> tuple[list[Hashable], list[Any]]:
- """
- Compute agg/apply results for like-like input.
-
- Parameters
- ----------
- op_name : {"agg", "apply"}
- Operation being performed.
- selected_obj : Series or DataFrame
- Data to perform operation on.
- kwargs : dict
- Keyword arguments to pass to the functions.
-
- Returns
- -------
- keys : list[hashable]
- Index labels for result.
- results : list
- Data for result. When aggregating with a Series, this can contain any
- Python objects.
- """
- func = cast(list[AggFuncTypeBase], self.func)
- obj = self.obj
-
- results = []
- keys = []
-
- # degenerate case
- if selected_obj.ndim == 1:
- for a in func:
- colg = obj._gotitem(selected_obj.name, ndim=1, subset=selected_obj)
- args = (
- [self.axis, *self.args]
- if include_axis(op_name, colg)
- else self.args
- )
- new_res = getattr(colg, op_name)(a, *args, **kwargs)
- results.append(new_res)
-
- # make sure we find a good name
- name = com.get_callable_name(a) or a
- keys.append(name)
-
- else:
- indices = []
- for index, col in enumerate(selected_obj):
- colg = obj._gotitem(col, ndim=1, subset=selected_obj.iloc[:, index])
- args = (
- [self.axis, *self.args]
- if include_axis(op_name, colg)
- else self.args
- )
- new_res = getattr(colg, op_name)(func, *args, **kwargs)
- results.append(new_res)
- indices.append(index)
- keys = selected_obj.columns.take(indices)
-
- return keys, results
-
- def wrap_results_list_like(
- self, keys: list[Hashable], results: list[Series | DataFrame]
- ):
- from pandas.core.reshape.concat import concat
-
- obj = self.obj
-
- try:
- return concat(results, keys=keys, axis=1, sort=False)
- except TypeError as err:
- # we are concatting non-NDFrame objects,
- # e.g. a list of scalars
- from pandas import Series
-
- result = Series(results, index=keys, name=obj.name)
- if is_nested_object(result):
- raise ValueError(
- "cannot combine transform and aggregation operations"
- ) from err
- return result
-
- def agg_dict_like(self) -> DataFrame | Series:
- """
- Compute aggregation in the case of a dict-like argument.
-
- Returns
- -------
- Result of aggregation.
- """
- return self.agg_or_apply_dict_like(op_name="agg")
-
- def compute_dict_like(
- self,
- op_name: Literal["agg", "apply"],
- selected_obj: Series | DataFrame,
- selection: Hashable | Sequence[Hashable],
- kwargs: dict[str, Any],
- ) -> tuple[list[Hashable], list[Any]]:
- """
- Compute agg/apply results for dict-like input.
-
- Parameters
- ----------
- op_name : {"agg", "apply"}
- Operation being performed.
- selected_obj : Series or DataFrame
- Data to perform operation on.
- selection : hashable or sequence of hashables
- Used by GroupBy, Window, and Resample if selection is applied to the object.
- kwargs : dict
- Keyword arguments to pass to the functions.
-
- Returns
- -------
- keys : list[hashable]
- Index labels for result.
- results : list
- Data for result. When aggregating with a Series, this can contain any
- Python object.
- """
- from pandas.core.groupby.generic import (
- DataFrameGroupBy,
- SeriesGroupBy,
- )
-
- obj = self.obj
- is_groupby = isinstance(obj, (DataFrameGroupBy, SeriesGroupBy))
- func = cast(AggFuncTypeDict, self.func)
- func = self.normalize_dictlike_arg(op_name, selected_obj, func)
-
- is_non_unique_col = (
- selected_obj.ndim == 2
- and selected_obj.columns.nunique() < len(selected_obj.columns)
- )
-
- if selected_obj.ndim == 1:
- # key only used for output
- colg = obj._gotitem(selection, ndim=1)
- results = [getattr(colg, op_name)(how, **kwargs) for _, how in func.items()]
- keys = list(func.keys())
- elif not is_groupby and is_non_unique_col:
- # key used for column selection and output
- # GH#51099
- results = []
- keys = []
- for key, how in func.items():
- indices = selected_obj.columns.get_indexer_for([key])
- labels = selected_obj.columns.take(indices)
- label_to_indices = defaultdict(list)
- for index, label in zip(indices, labels):
- label_to_indices[label].append(index)
-
- key_data = [
- getattr(selected_obj._ixs(indice, axis=1), op_name)(how, **kwargs)
- for label, indices in label_to_indices.items()
- for indice in indices
- ]
-
- keys += [key] * len(key_data)
- results += key_data
- else:
- # key used for column selection and output
- results = [
- getattr(obj._gotitem(key, ndim=1), op_name)(how, **kwargs)
- for key, how in func.items()
- ]
- keys = list(func.keys())
-
- return keys, results
-
- def wrap_results_dict_like(
- self,
- selected_obj: Series | DataFrame,
- result_index: list[Hashable],
- result_data: list,
- ):
- from pandas import Index
- from pandas.core.reshape.concat import concat
-
- obj = self.obj
-
- # Avoid making two isinstance calls in all and any below
- is_ndframe = [isinstance(r, ABCNDFrame) for r in result_data]
-
- if all(is_ndframe):
- results = dict(zip(result_index, result_data))
- keys_to_use: Iterable[Hashable]
- keys_to_use = [k for k in result_index if not results[k].empty]
- # Have to check, if at least one DataFrame is not empty.
- keys_to_use = keys_to_use if keys_to_use != [] else result_index
- if selected_obj.ndim == 2:
- # keys are columns, so we can preserve names
- ktu = Index(keys_to_use)
- ktu._set_names(selected_obj.columns.names)
- keys_to_use = ktu
-
- axis: AxisInt = 0 if isinstance(obj, ABCSeries) else 1
- result = concat(
- {k: results[k] for k in keys_to_use},
- axis=axis,
- keys=keys_to_use,
- )
- elif any(is_ndframe):
- # There is a mix of NDFrames and scalars
- raise ValueError(
- "cannot perform both aggregation "
- "and transformation operations "
- "simultaneously"
- )
- else:
- from pandas import Series
-
- # we have a list of scalars
- # GH 36212 use name only if obj is a series
- if obj.ndim == 1:
- obj = cast("Series", obj)
- name = obj.name
- else:
- name = None
-
- result = Series(result_data, index=result_index, name=name)
-
- return result
-
- def apply_str(self) -> DataFrame | Series:
- """
- Compute apply in case of a string.
-
- Returns
- -------
- result: Series or DataFrame
- """
- # Caller is responsible for checking isinstance(self.f, str)
- func = cast(str, self.func)
-
- obj = self.obj
-
- from pandas.core.groupby.generic import (
- DataFrameGroupBy,
- SeriesGroupBy,
- )
-
- # Support for `frame.transform('method')`
- # Some methods (shift, etc.) require the axis argument, others
- # don't, so inspect and insert if necessary.
- method = getattr(obj, func, None)
- if callable(method):
- sig = inspect.getfullargspec(method)
- arg_names = (*sig.args, *sig.kwonlyargs)
- if self.axis != 0 and (
- "axis" not in arg_names or func in ("corrwith", "skew")
- ):
- raise ValueError(f"Operation {func} does not support axis=1")
- if "axis" in arg_names:
- if isinstance(obj, (SeriesGroupBy, DataFrameGroupBy)):
- # Try to avoid FutureWarning for deprecated axis keyword;
- # If self.axis matches the axis we would get by not passing
- # axis, we safely exclude the keyword.
-
- default_axis = 0
- if func in ["idxmax", "idxmin"]:
- # DataFrameGroupBy.idxmax, idxmin axis defaults to self.axis,
- # whereas other axis keywords default to 0
- default_axis = self.obj.axis
-
- if default_axis != self.axis:
- self.kwargs["axis"] = self.axis
- else:
- self.kwargs["axis"] = self.axis
- return self._apply_str(obj, func, *self.args, **self.kwargs)
-
- def apply_list_or_dict_like(self) -> DataFrame | Series:
- """
- Compute apply in case of a list-like or dict-like.
-
- Returns
- -------
- result: Series, DataFrame, or None
- Result when self.func is a list-like or dict-like, None otherwise.
- """
- if self.axis == 1 and isinstance(self.obj, ABCDataFrame):
- return self.obj.T.apply(self.func, 0, args=self.args, **self.kwargs).T
-
- func = self.func
- kwargs = self.kwargs
-
- if is_dict_like(func):
- result = self.agg_or_apply_dict_like(op_name="apply")
- else:
- result = self.agg_or_apply_list_like(op_name="apply")
-
- result = reconstruct_and_relabel_result(result, func, **kwargs)
-
- return result
-
- def normalize_dictlike_arg(
- self, how: str, obj: DataFrame | Series, func: AggFuncTypeDict
- ) -> AggFuncTypeDict:
- """
- Handler for dict-like argument.
-
- Ensures that necessary columns exist if obj is a DataFrame, and
- that a nested renamer is not passed. Also normalizes to all lists
- when values consists of a mix of list and non-lists.
- """
- assert how in ("apply", "agg", "transform")
-
- # Can't use func.values(); wouldn't work for a Series
- if (
- how == "agg"
- and isinstance(obj, ABCSeries)
- and any(is_list_like(v) for _, v in func.items())
- ) or (any(is_dict_like(v) for _, v in func.items())):
- # GH 15931 - deprecation of renaming keys
- raise SpecificationError("nested renamer is not supported")
-
- if obj.ndim != 1:
- # Check for missing columns on a frame
- from pandas import Index
-
- cols = Index(list(func.keys())).difference(obj.columns, sort=True)
- if len(cols) > 0:
- raise KeyError(f"Column(s) {list(cols)} do not exist")
-
- aggregator_types = (list, tuple, dict)
-
- # if we have a dict of any non-scalars
- # eg. {'A' : ['mean']}, normalize all to
- # be list-likes
- # Cannot use func.values() because arg may be a Series
- if any(isinstance(x, aggregator_types) for _, x in func.items()):
- new_func: AggFuncTypeDict = {}
- for k, v in func.items():
- if not isinstance(v, aggregator_types):
- new_func[k] = [v]
- else:
- new_func[k] = v
- func = new_func
- return func
-
- def _apply_str(self, obj, func: str, *args, **kwargs):
- """
- if arg is a string, then try to operate on it:
- - try to find a function (or attribute) on obj
- - try to find a numpy function
- - raise
- """
- assert isinstance(func, str)
-
- if hasattr(obj, func):
- f = getattr(obj, func)
- if callable(f):
- return f(*args, **kwargs)
-
- # people may aggregate on a non-callable attribute
- # but don't let them think they can pass args to it
- assert len(args) == 0
- assert len([kwarg for kwarg in kwargs if kwarg not in ["axis"]]) == 0
- return f
- elif hasattr(np, func) and hasattr(obj, "__array__"):
- # in particular exclude Window
- f = getattr(np, func)
- return f(obj, *args, **kwargs)
- else:
- msg = f"'{func}' is not a valid function for '{type(obj).__name__}' object"
- raise AttributeError(msg)
-
-
-class NDFrameApply(Apply):
- """
- Methods shared by FrameApply and SeriesApply but
- not GroupByApply or ResamplerWindowApply
- """
-
- obj: DataFrame | Series
-
- @property
- def index(self) -> Index:
- return self.obj.index
-
- @property
- def agg_axis(self) -> Index:
- return self.obj._get_agg_axis(self.axis)
-
- def agg_or_apply_list_like(
- self, op_name: Literal["agg", "apply"]
- ) -> DataFrame | Series:
- obj = self.obj
- kwargs = self.kwargs
-
- if op_name == "apply":
- if isinstance(self, FrameApply):
- by_row = self.by_row
-
- elif isinstance(self, SeriesApply):
- by_row = "_compat" if self.by_row else False
- else:
- by_row = False
- kwargs = {**kwargs, "by_row": by_row}
-
- if getattr(obj, "axis", 0) == 1:
- raise NotImplementedError("axis other than 0 is not supported")
-
- keys, results = self.compute_list_like(op_name, obj, kwargs)
- result = self.wrap_results_list_like(keys, results)
- return result
-
- def agg_or_apply_dict_like(
- self, op_name: Literal["agg", "apply"]
- ) -> DataFrame | Series:
- assert op_name in ["agg", "apply"]
- obj = self.obj
-
- kwargs = {}
- if op_name == "apply":
- by_row = "_compat" if self.by_row else False
- kwargs.update({"by_row": by_row})
-
- if getattr(obj, "axis", 0) == 1:
- raise NotImplementedError("axis other than 0 is not supported")
-
- selection = None
- result_index, result_data = self.compute_dict_like(
- op_name, obj, selection, kwargs
- )
- result = self.wrap_results_dict_like(obj, result_index, result_data)
- return result
-
-
-class FrameApply(NDFrameApply):
- obj: DataFrame
-
- def __init__(
- self,
- obj: AggObjType,
- func: AggFuncType,
- raw: bool,
- result_type: str | None,
- *,
- by_row: Literal[False, "compat"] = False,
- args,
- kwargs,
- ) -> None:
- if by_row is not False and by_row != "compat":
- raise ValueError(f"by_row={by_row} not allowed")
- super().__init__(
- obj, func, raw, result_type, by_row=by_row, args=args, kwargs=kwargs
- )
-
- # ---------------------------------------------------------------
- # Abstract Methods
-
- @property
- @abc.abstractmethod
- def result_index(self) -> Index:
- pass
-
- @property
- @abc.abstractmethod
- def result_columns(self) -> Index:
- pass
-
- @property
- @abc.abstractmethod
- def series_generator(self) -> Iterator[Series]:
- pass
-
- @abc.abstractmethod
- def wrap_results_for_axis(
- self, results: ResType, res_index: Index
- ) -> DataFrame | Series:
- pass
-
- # ---------------------------------------------------------------
-
- @property
- def res_columns(self) -> Index:
- return self.result_columns
-
- @property
- def columns(self) -> Index:
- return self.obj.columns
-
- @cache_readonly
- def values(self):
- return self.obj.values
-
- def apply(self) -> DataFrame | Series:
- """compute the results"""
- # dispatch to handle list-like or dict-like
- if is_list_like(self.func):
- return self.apply_list_or_dict_like()
-
- # all empty
- if len(self.columns) == 0 and len(self.index) == 0:
- return self.apply_empty_result()
-
- # string dispatch
- if isinstance(self.func, str):
- return self.apply_str()
-
- # ufunc
- elif isinstance(self.func, np.ufunc):
- with np.errstate(all="ignore"):
- results = self.obj._mgr.apply("apply", func=self.func)
- # _constructor will retain self.index and self.columns
- return self.obj._constructor_from_mgr(results, axes=results.axes)
-
- # broadcasting
- if self.result_type == "broadcast":
- return self.apply_broadcast(self.obj)
-
- # one axis empty
- elif not all(self.obj.shape):
- return self.apply_empty_result()
-
- # raw
- elif self.raw:
- return self.apply_raw()
-
- return self.apply_standard()
-
- def agg(self):
- obj = self.obj
- axis = self.axis
-
- # TODO: Avoid having to change state
- self.obj = self.obj if self.axis == 0 else self.obj.T
- self.axis = 0
-
- result = None
- try:
- result = super().agg()
- finally:
- self.obj = obj
- self.axis = axis
-
- if axis == 1:
- result = result.T if result is not None else result
-
- if result is None:
- result = self.obj.apply(self.func, axis, args=self.args, **self.kwargs)
-
- return result
-
- def apply_empty_result(self):
- """
- we have an empty result; at least 1 axis is 0
-
- we will try to apply the function to an empty
- series in order to see if this is a reduction function
- """
- assert callable(self.func)
-
- # we are not asked to reduce or infer reduction
- # so just return a copy of the existing object
- if self.result_type not in ["reduce", None]:
- return self.obj.copy()
-
- # we may need to infer
- should_reduce = self.result_type == "reduce"
-
- from pandas import Series
-
- if not should_reduce:
- try:
- if self.axis == 0:
- r = self.func(
- Series([], dtype=np.float64), *self.args, **self.kwargs
- )
- else:
- r = self.func(
- Series(index=self.columns, dtype=np.float64),
- *self.args,
- **self.kwargs,
- )
- except Exception:
- pass
- else:
- should_reduce = not isinstance(r, Series)
-
- if should_reduce:
- if len(self.agg_axis):
- r = self.func(Series([], dtype=np.float64), *self.args, **self.kwargs)
- else:
- r = np.nan
-
- return self.obj._constructor_sliced(r, index=self.agg_axis)
- else:
- return self.obj.copy()
-
- def apply_raw(self):
- """apply to the values as a numpy array"""
-
- def wrap_function(func):
- """
- Wrap user supplied function to work around numpy issue.
-
- see https://github.com/numpy/numpy/issues/8352
- """
-
- def wrapper(*args, **kwargs):
- result = func(*args, **kwargs)
- if isinstance(result, str):
- result = np.array(result, dtype=object)
- return result
-
- return wrapper
-
- result = np.apply_along_axis(wrap_function(self.func), self.axis, self.values)
-
- # TODO: mixed type case
- if result.ndim == 2:
- return self.obj._constructor(result, index=self.index, columns=self.columns)
- else:
- return self.obj._constructor_sliced(result, index=self.agg_axis)
-
- def apply_broadcast(self, target: DataFrame) -> DataFrame:
- assert callable(self.func)
-
- result_values = np.empty_like(target.values)
-
- # axis which we want to compare compliance
- result_compare = target.shape[0]
-
- for i, col in enumerate(target.columns):
- res = self.func(target[col], *self.args, **self.kwargs)
- ares = np.asarray(res).ndim
-
- # must be a scalar or 1d
- if ares > 1:
- raise ValueError("too many dims to broadcast")
- if ares == 1:
- # must match return dim
- if result_compare != len(res):
- raise ValueError("cannot broadcast result")
-
- result_values[:, i] = res
-
- # we *always* preserve the original index / columns
- result = self.obj._constructor(
- result_values, index=target.index, columns=target.columns
- )
- return result
-
- def apply_standard(self):
- results, res_index = self.apply_series_generator()
-
- # wrap results
- return self.wrap_results(results, res_index)
-
- def apply_series_generator(self) -> tuple[ResType, Index]:
- assert callable(self.func)
-
- series_gen = self.series_generator
- res_index = self.result_index
-
- results = {}
-
- with option_context("mode.chained_assignment", None):
- for i, v in enumerate(series_gen):
- # ignore SettingWithCopy here in case the user mutates
- results[i] = self.func(v, *self.args, **self.kwargs)
- if isinstance(results[i], ABCSeries):
- # If we have a view on v, we need to make a copy because
- # series_generator will swap out the underlying data
- results[i] = results[i].copy(deep=False)
-
- return results, res_index
-
- def wrap_results(self, results: ResType, res_index: Index) -> DataFrame | Series:
- from pandas import Series
-
- # see if we can infer the results
- if len(results) > 0 and 0 in results and is_sequence(results[0]):
- return self.wrap_results_for_axis(results, res_index)
-
- # dict of scalars
-
- # the default dtype of an empty Series is `object`, but this
- # code can be hit by df.mean() where the result should have dtype
- # float64 even if it's an empty Series.
- constructor_sliced = self.obj._constructor_sliced
- if len(results) == 0 and constructor_sliced is Series:
- result = constructor_sliced(results, dtype=np.float64)
- else:
- result = constructor_sliced(results)
- result.index = res_index
-
- return result
-
- def apply_str(self) -> DataFrame | Series:
- # Caller is responsible for checking isinstance(self.func, str)
- # TODO: GH#39993 - Avoid special-casing by replacing with lambda
- if self.func == "size":
- # Special-cased because DataFrame.size returns a single scalar
- obj = self.obj
- value = obj.shape[self.axis]
- return obj._constructor_sliced(value, index=self.agg_axis)
- return super().apply_str()
-
-
-class FrameRowApply(FrameApply):
- axis: AxisInt = 0
-
- @property
- def series_generator(self):
- return (self.obj._ixs(i, axis=1) for i in range(len(self.columns)))
-
- @property
- def result_index(self) -> Index:
- return self.columns
-
- @property
- def result_columns(self) -> Index:
- return self.index
-
- def wrap_results_for_axis(
- self, results: ResType, res_index: Index
- ) -> DataFrame | Series:
- """return the results for the rows"""
-
- if self.result_type == "reduce":
- # e.g. test_apply_dict GH#8735
- res = self.obj._constructor_sliced(results)
- res.index = res_index
- return res
-
- elif self.result_type is None and all(
- isinstance(x, dict) for x in results.values()
- ):
- # Our operation was a to_dict op e.g.
- # test_apply_dict GH#8735, test_apply_reduce_to_dict GH#25196 #37544
- res = self.obj._constructor_sliced(results)
- res.index = res_index
- return res
-
- try:
- result = self.obj._constructor(data=results)
- except ValueError as err:
- if "All arrays must be of the same length" in str(err):
- # e.g. result = [[2, 3], [1.5], ['foo', 'bar']]
- # see test_agg_listlike_result GH#29587
- res = self.obj._constructor_sliced(results)
- res.index = res_index
- return res
- else:
- raise
-
- if not isinstance(results[0], ABCSeries):
- if len(result.index) == len(self.res_columns):
- result.index = self.res_columns
-
- if len(result.columns) == len(res_index):
- result.columns = res_index
-
- return result
-
-
-class FrameColumnApply(FrameApply):
- axis: AxisInt = 1
-
- def apply_broadcast(self, target: DataFrame) -> DataFrame:
- result = super().apply_broadcast(target.T)
- return result.T
-
- @property
- def series_generator(self):
- values = self.values
- values = ensure_wrapped_if_datetimelike(values)
- assert len(values) > 0
-
- # We create one Series object, and will swap out the data inside
- # of it. Kids: don't do this at home.
- ser = self.obj._ixs(0, axis=0)
- mgr = ser._mgr
-
- if isinstance(ser.dtype, ExtensionDtype):
- # values will be incorrect for this block
- # TODO(EA2D): special case would be unnecessary with 2D EAs
- obj = self.obj
- for i in range(len(obj)):
- yield obj._ixs(i, axis=0)
-
- else:
- for arr, name in zip(values, self.index):
- # GH#35462 re-pin mgr in case setitem changed it
- ser._mgr = mgr
- mgr.set_values(arr)
- object.__setattr__(ser, "_name", name)
- yield ser
-
- @property
- def result_index(self) -> Index:
- return self.index
-
- @property
- def result_columns(self) -> Index:
- return self.columns
-
- def wrap_results_for_axis(
- self, results: ResType, res_index: Index
- ) -> DataFrame | Series:
- """return the results for the columns"""
- result: DataFrame | Series
-
- # we have requested to expand
- if self.result_type == "expand":
- result = self.infer_to_same_shape(results, res_index)
-
- # we have a non-series and don't want inference
- elif not isinstance(results[0], ABCSeries):
- result = self.obj._constructor_sliced(results)
- result.index = res_index
-
- # we may want to infer results
- else:
- result = self.infer_to_same_shape(results, res_index)
-
- return result
-
- def infer_to_same_shape(self, results: ResType, res_index: Index) -> DataFrame:
- """infer the results to the same shape as the input object"""
- result = self.obj._constructor(data=results)
- result = result.T
-
- # set the index
- result.index = res_index
-
- # infer dtypes
- result = result.infer_objects(copy=False)
-
- return result
-
-
-class SeriesApply(NDFrameApply):
- obj: Series
- axis: AxisInt = 0
- by_row: Literal[False, "compat", "_compat"] # only relevant for apply()
-
- def __init__(
- self,
- obj: Series,
- func: AggFuncType,
- *,
- convert_dtype: bool | lib.NoDefault = lib.no_default,
- by_row: Literal[False, "compat", "_compat"] = "compat",
- args,
- kwargs,
- ) -> None:
- if convert_dtype is lib.no_default:
- convert_dtype = True
- else:
- warnings.warn(
- "the convert_dtype parameter is deprecated and will be removed in a "
- "future version. Do ``ser.astype(object).apply()`` "
- "instead if you want ``convert_dtype=False``.",
- FutureWarning,
- stacklevel=find_stack_level(),
- )
- self.convert_dtype = convert_dtype
-
- super().__init__(
- obj,
- func,
- raw=False,
- result_type=None,
- by_row=by_row,
- args=args,
- kwargs=kwargs,
- )
-
- def apply(self) -> DataFrame | Series:
- obj = self.obj
-
- if len(obj) == 0:
- return self.apply_empty_result()
-
- # dispatch to handle list-like or dict-like
- if is_list_like(self.func):
- return self.apply_list_or_dict_like()
-
- if isinstance(self.func, str):
- # if we are a string, try to dispatch
- return self.apply_str()
-
- if self.by_row == "_compat":
- return self.apply_compat()
-
- # self.func is Callable
- return self.apply_standard()
-
- def agg(self):
- result = super().agg()
- if result is None:
- obj = self.obj
- func = self.func
- # string, list-like, and dict-like are entirely handled in super
- assert callable(func)
-
- # GH53325: The setup below is just to keep current behavior while emitting a
- # deprecation message. In the future this will all be replaced with a simple
- # `result = f(self.obj, *self.args, **self.kwargs)`.
- try:
- result = obj.apply(func, args=self.args, **self.kwargs)
- except (ValueError, AttributeError, TypeError):
- result = func(obj, *self.args, **self.kwargs)
- else:
- msg = (
- f"using {func} in {type(obj).__name__}.agg cannot aggregate and "
- f"has been deprecated. Use {type(obj).__name__}.transform to "
- f"keep behavior unchanged."
- )
- warnings.warn(msg, FutureWarning, stacklevel=find_stack_level())
-
- return result
-
- def apply_empty_result(self) -> Series:
- obj = self.obj
- return obj._constructor(dtype=obj.dtype, index=obj.index).__finalize__(
- obj, method="apply"
- )
-
- def apply_compat(self):
- """compat apply method for funcs in listlikes and dictlikes.
-
- Used for each callable when giving listlikes and dictlikes of callables to
- apply. Needed for compatibility with Pandas < v2.1.
-
- .. versionadded:: 2.1.0
- """
- obj = self.obj
- func = self.func
-
- if callable(func):
- f = com.get_cython_func(func)
- if f and not self.args and not self.kwargs:
- return obj.apply(func, by_row=False)
-
- try:
- result = obj.apply(func, by_row="compat")
- except (ValueError, AttributeError, TypeError):
- result = obj.apply(func, by_row=False)
- return result
-
- def apply_standard(self) -> DataFrame | Series:
- # caller is responsible for ensuring that f is Callable
- func = cast(Callable, self.func)
- obj = self.obj
-
- if isinstance(func, np.ufunc):
- with np.errstate(all="ignore"):
- return func(obj, *self.args, **self.kwargs)
- elif not self.by_row:
- return func(obj, *self.args, **self.kwargs)
-
- if self.args or self.kwargs:
- # _map_values does not support args/kwargs
- def curried(x):
- return func(x, *self.args, **self.kwargs)
-
- else:
- curried = func
-
- # row-wise access
- # apply doesn't have a `na_action` keyword and for backward compat reasons
- # we need to give `na_action="ignore"` for categorical data.
- # TODO: remove the `na_action="ignore"` when that default has been changed in
- # Categorical (GH51645).
- action = "ignore" if isinstance(obj.dtype, CategoricalDtype) else None
- mapped = obj._map_values(
- mapper=curried, na_action=action, convert=self.convert_dtype
- )
-
- if len(mapped) and isinstance(mapped[0], ABCSeries):
- # GH#43986 Need to do list(mapped) in order to get treated as nested
- # See also GH#25959 regarding EA support
- return obj._constructor_expanddim(list(mapped), index=obj.index)
- else:
- return obj._constructor(mapped, index=obj.index).__finalize__(
- obj, method="apply"
- )
-
-
-class GroupByApply(Apply):
- obj: GroupBy | Resampler | BaseWindow
-
- def __init__(
- self,
- obj: GroupBy[NDFrameT],
- func: AggFuncType,
- *,
- args,
- kwargs,
- ) -> None:
- kwargs = kwargs.copy()
- self.axis = obj.obj._get_axis_number(kwargs.get("axis", 0))
- super().__init__(
- obj,
- func,
- raw=False,
- result_type=None,
- args=args,
- kwargs=kwargs,
- )
-
- def apply(self):
- raise NotImplementedError
-
- def transform(self):
- raise NotImplementedError
-
- def agg_or_apply_list_like(
- self, op_name: Literal["agg", "apply"]
- ) -> DataFrame | Series:
- obj = self.obj
- kwargs = self.kwargs
- if op_name == "apply":
- kwargs = {**kwargs, "by_row": False}
-
- if getattr(obj, "axis", 0) == 1:
- raise NotImplementedError("axis other than 0 is not supported")
-
- if obj._selected_obj.ndim == 1:
- # For SeriesGroupBy this matches _obj_with_exclusions
- selected_obj = obj._selected_obj
- else:
- selected_obj = obj._obj_with_exclusions
-
- # Only set as_index=True on groupby objects, not Window or Resample
- # that inherit from this class.
- with com.temp_setattr(
- obj, "as_index", True, condition=hasattr(obj, "as_index")
- ):
- keys, results = self.compute_list_like(op_name, selected_obj, kwargs)
- result = self.wrap_results_list_like(keys, results)
- return result
-
- def agg_or_apply_dict_like(
- self, op_name: Literal["agg", "apply"]
- ) -> DataFrame | Series:
- from pandas.core.groupby.generic import (
- DataFrameGroupBy,
- SeriesGroupBy,
- )
-
- assert op_name in ["agg", "apply"]
-
- obj = self.obj
- kwargs = {}
- if op_name == "apply":
- by_row = "_compat" if self.by_row else False
- kwargs.update({"by_row": by_row})
-
- if getattr(obj, "axis", 0) == 1:
- raise NotImplementedError("axis other than 0 is not supported")
-
- selected_obj = obj._selected_obj
- selection = obj._selection
-
- is_groupby = isinstance(obj, (DataFrameGroupBy, SeriesGroupBy))
-
- # Numba Groupby engine/engine-kwargs passthrough
- if is_groupby:
- engine = self.kwargs.get("engine", None)
- engine_kwargs = self.kwargs.get("engine_kwargs", None)
- kwargs.update({"engine": engine, "engine_kwargs": engine_kwargs})
-
- with com.temp_setattr(
- obj, "as_index", True, condition=hasattr(obj, "as_index")
- ):
- result_index, result_data = self.compute_dict_like(
- op_name, selected_obj, selection, kwargs
- )
- result = self.wrap_results_dict_like(selected_obj, result_index, result_data)
- return result
-
-
-class ResamplerWindowApply(GroupByApply):
- axis: AxisInt = 0
- obj: Resampler | BaseWindow
-
- def __init__(
- self,
- obj: Resampler | BaseWindow,
- func: AggFuncType,
- *,
- args,
- kwargs,
- ) -> None:
- super(GroupByApply, self).__init__(
- obj,
- func,
- raw=False,
- result_type=None,
- args=args,
- kwargs=kwargs,
- )
-
- def apply(self):
- raise NotImplementedError
-
- def transform(self):
- raise NotImplementedError
-
-
-def reconstruct_func(
- func: AggFuncType | None, **kwargs
-) -> tuple[bool, AggFuncType, list[str] | None, npt.NDArray[np.intp] | None]:
- """
- This is the internal function to reconstruct func given if there is relabeling
- or not and also normalize the keyword to get new order of columns.
-
- If named aggregation is applied, `func` will be None, and kwargs contains the
- column and aggregation function information to be parsed;
- If named aggregation is not applied, `func` is either string (e.g. 'min') or
- Callable, or list of them (e.g. ['min', np.max]), or the dictionary of column name
- and str/Callable/list of them (e.g. {'A': 'min'}, or {'A': [np.min, lambda x: x]})
-
- If relabeling is True, will return relabeling, reconstructed func, column
- names, and the reconstructed order of columns.
- If relabeling is False, the columns and order will be None.
-
- Parameters
- ----------
- func: agg function (e.g. 'min' or Callable) or list of agg functions
- (e.g. ['min', np.max]) or dictionary (e.g. {'A': ['min', np.max]}).
- **kwargs: dict, kwargs used in is_multi_agg_with_relabel and
- normalize_keyword_aggregation function for relabelling
-
- Returns
- -------
- relabelling: bool, if there is relabelling or not
- func: normalized and mangled func
- columns: list of column names
- order: array of columns indices
-
- Examples
- --------
- >>> reconstruct_func(None, **{"foo": ("col", "min")})
- (True, defaultdict(, {'col': ['min']}), ('foo',), array([0]))
-
- >>> reconstruct_func("min")
- (False, 'min', None, None)
- """
- relabeling = func is None and is_multi_agg_with_relabel(**kwargs)
- columns: list[str] | None = None
- order: npt.NDArray[np.intp] | None = None
-
- if not relabeling:
- if isinstance(func, list) and len(func) > len(set(func)):
- # GH 28426 will raise error if duplicated function names are used and
- # there is no reassigned name
- raise SpecificationError(
- "Function names must be unique if there is no new column names "
- "assigned"
- )
- if func is None:
- # nicer error message
- raise TypeError("Must provide 'func' or tuples of '(column, aggfunc).")
-
- if relabeling:
- func, columns, order = normalize_keyword_aggregation(kwargs)
- assert func is not None
-
- return relabeling, func, columns, order
-
-
-def is_multi_agg_with_relabel(**kwargs) -> bool:
- """
- Check whether kwargs passed to .agg look like multi-agg with relabeling.
-
- Parameters
- ----------
- **kwargs : dict
-
- Returns
- -------
- bool
-
- Examples
- --------
- >>> is_multi_agg_with_relabel(a="max")
- False
- >>> is_multi_agg_with_relabel(a_max=("a", "max"), a_min=("a", "min"))
- True
- >>> is_multi_agg_with_relabel()
- False
- """
- return all(isinstance(v, tuple) and len(v) == 2 for v in kwargs.values()) and (
- len(kwargs) > 0
- )
-
-
-def normalize_keyword_aggregation(
- kwargs: dict,
-) -> tuple[dict, list[str], npt.NDArray[np.intp]]:
- """
- Normalize user-provided "named aggregation" kwargs.
- Transforms from the new ``Mapping[str, NamedAgg]`` style kwargs
- to the old Dict[str, List[scalar]]].
-
- Parameters
- ----------
- kwargs : dict
-
- Returns
- -------
- aggspec : dict
- The transformed kwargs.
- columns : List[str]
- The user-provided keys.
- col_idx_order : List[int]
- List of columns indices.
-
- Examples
- --------
- >>> normalize_keyword_aggregation({"output": ("input", "sum")})
- (defaultdict(, {'input': ['sum']}), ('output',), array([0]))
- """
- from pandas.core.indexes.base import Index
-
- # Normalize the aggregation functions as Mapping[column, List[func]],
- # process normally, then fixup the names.
- # TODO: aggspec type: typing.Dict[str, List[AggScalar]]
- # May be hitting https://github.com/python/mypy/issues/5958
- # saying it doesn't have an attribute __name__
- aggspec: DefaultDict = defaultdict(list)
- order = []
- columns, pairs = list(zip(*kwargs.items()))
-
- for column, aggfunc in pairs:
- aggspec[column].append(aggfunc)
- order.append((column, com.get_callable_name(aggfunc) or aggfunc))
-
- # uniquify aggfunc name if duplicated in order list
- uniquified_order = _make_unique_kwarg_list(order)
-
- # GH 25719, due to aggspec will change the order of assigned columns in aggregation
- # uniquified_aggspec will store uniquified order list and will compare it with order
- # based on index
- aggspec_order = [
- (column, com.get_callable_name(aggfunc) or aggfunc)
- for column, aggfuncs in aggspec.items()
- for aggfunc in aggfuncs
- ]
- uniquified_aggspec = _make_unique_kwarg_list(aggspec_order)
-
- # get the new index of columns by comparison
- col_idx_order = Index(uniquified_aggspec).get_indexer(uniquified_order)
- return aggspec, columns, col_idx_order
-
-
-def _make_unique_kwarg_list(
- seq: Sequence[tuple[Any, Any]]
-) -> Sequence[tuple[Any, Any]]:
- """
- Uniquify aggfunc name of the pairs in the order list
-
- Examples:
- --------
- >>> kwarg_list = [('a', ''), ('a', ''), ('b', '')]
- >>> _make_unique_kwarg_list(kwarg_list)
- [('a', '_0'), ('a', '_1'), ('b', '')]
- """
- return [
- (pair[0], f"{pair[1]}_{seq[:i].count(pair)}") if seq.count(pair) > 1 else pair
- for i, pair in enumerate(seq)
- ]
-
-
-def relabel_result(
- result: DataFrame | Series,
- func: dict[str, list[Callable | str]],
- columns: Iterable[Hashable],
- order: Iterable[int],
-) -> dict[Hashable, Series]:
- """
- Internal function to reorder result if relabelling is True for
- dataframe.agg, and return the reordered result in dict.
-
- Parameters:
- ----------
- result: Result from aggregation
- func: Dict of (column name, funcs)
- columns: New columns name for relabelling
- order: New order for relabelling
-
- Examples
- --------
- >>> from pandas.core.apply import relabel_result
- >>> result = pd.DataFrame(
- ... {"A": [np.nan, 2, np.nan], "C": [6, np.nan, np.nan], "B": [np.nan, 4, 2.5]},
- ... index=["max", "mean", "min"]
- ... )
- >>> funcs = {"A": ["max"], "C": ["max"], "B": ["mean", "min"]}
- >>> columns = ("foo", "aab", "bar", "dat")
- >>> order = [0, 1, 2, 3]
- >>> result_in_dict = relabel_result(result, funcs, columns, order)
- >>> pd.DataFrame(result_in_dict, index=columns)
- A C B
- foo 2.0 NaN NaN
- aab NaN 6.0 NaN
- bar NaN NaN 4.0
- dat NaN NaN 2.5
- """
- from pandas.core.indexes.base import Index
-
- reordered_indexes = [
- pair[0] for pair in sorted(zip(columns, order), key=lambda t: t[1])
- ]
- reordered_result_in_dict: dict[Hashable, Series] = {}
- idx = 0
-
- reorder_mask = not isinstance(result, ABCSeries) and len(result.columns) > 1
- for col, fun in func.items():
- s = result[col].dropna()
-
- # In the `_aggregate`, the callable names are obtained and used in `result`, and
- # these names are ordered alphabetically. e.g.
- # C2 C1
- # 1 NaN
- # amax NaN 4.0
- # max NaN 4.0
- # sum 18.0 6.0
- # Therefore, the order of functions for each column could be shuffled
- # accordingly so need to get the callable name if it is not parsed names, and
- # reorder the aggregated result for each column.
- # e.g. if df.agg(c1=("C2", sum), c2=("C2", lambda x: min(x))), correct order is
- # [sum, ], but in `result`, it will be [, sum], and we need to
- # reorder so that aggregated values map to their functions regarding the order.
-
- # However there is only one column being used for aggregation, not need to
- # reorder since the index is not sorted, and keep as is in `funcs`, e.g.
- # A
- # min 1.0
- # mean 1.5
- # mean 1.5
- if reorder_mask:
- fun = [
- com.get_callable_name(f) if not isinstance(f, str) else f for f in fun
- ]
- col_idx_order = Index(s.index).get_indexer(fun)
- s = s.iloc[col_idx_order]
-
- # assign the new user-provided "named aggregation" as index names, and reindex
- # it based on the whole user-provided names.
- s.index = reordered_indexes[idx : idx + len(fun)]
- reordered_result_in_dict[col] = s.reindex(columns, copy=False)
- idx = idx + len(fun)
- return reordered_result_in_dict
-
-
-def reconstruct_and_relabel_result(result, func, **kwargs) -> DataFrame | Series:
- from pandas import DataFrame
-
- relabeling, func, columns, order = reconstruct_func(func, **kwargs)
-
- if relabeling:
- # This is to keep the order to columns occurrence unchanged, and also
- # keep the order of new columns occurrence unchanged
-
- # For the return values of reconstruct_func, if relabeling is
- # False, columns and order will be None.
- assert columns is not None
- assert order is not None
-
- result_in_dict = relabel_result(result, func, columns, order)
- result = DataFrame(result_in_dict, index=columns)
-
- return result
-
-
-# TODO: Can't use, because mypy doesn't like us setting __name__
-# error: "partial[Any]" has no attribute "__name__"
-# the type is:
-# typing.Sequence[Callable[..., ScalarResult]]
-# -> typing.Sequence[Callable[..., ScalarResult]]:
-
-
-def _managle_lambda_list(aggfuncs: Sequence[Any]) -> Sequence[Any]:
- """
- Possibly mangle a list of aggfuncs.
-
- Parameters
- ----------
- aggfuncs : Sequence
-
- Returns
- -------
- mangled: list-like
- A new AggSpec sequence, where lambdas have been converted
- to have unique names.
-
- Notes
- -----
- If just one aggfunc is passed, the name will not be mangled.
- """
- if len(aggfuncs) <= 1:
- # don't mangle for .agg([lambda x: .])
- return aggfuncs
- i = 0
- mangled_aggfuncs = []
- for aggfunc in aggfuncs:
- if com.get_callable_name(aggfunc) == "":
- aggfunc = partial(aggfunc)
- aggfunc.__name__ = f""
- i += 1
- mangled_aggfuncs.append(aggfunc)
-
- return mangled_aggfuncs
-
-
-def maybe_mangle_lambdas(agg_spec: Any) -> Any:
- """
- Make new lambdas with unique names.
-
- Parameters
- ----------
- agg_spec : Any
- An argument to GroupBy.agg.
- Non-dict-like `agg_spec` are pass through as is.
- For dict-like `agg_spec` a new spec is returned
- with name-mangled lambdas.
-
- Returns
- -------
- mangled : Any
- Same type as the input.
-
- Examples
- --------
- >>> maybe_mangle_lambdas('sum')
- 'sum'
- >>> maybe_mangle_lambdas([lambda: 1, lambda: 2]) # doctest: +SKIP
- [,
- .f(*args, **kwargs)>]
- """
- is_dict = is_dict_like(agg_spec)
- if not (is_dict or is_list_like(agg_spec)):
- return agg_spec
- mangled_aggspec = type(agg_spec)() # dict or OrderedDict
-
- if is_dict:
- for key, aggfuncs in agg_spec.items():
- if is_list_like(aggfuncs) and not is_dict_like(aggfuncs):
- mangled_aggfuncs = _managle_lambda_list(aggfuncs)
- else:
- mangled_aggfuncs = aggfuncs
-
- mangled_aggspec[key] = mangled_aggfuncs
- else:
- mangled_aggspec = _managle_lambda_list(agg_spec)
-
- return mangled_aggspec
-
-
-def validate_func_kwargs(
- kwargs: dict,
-) -> tuple[list[str], list[str | Callable[..., Any]]]:
- """
- Validates types of user-provided "named aggregation" kwargs.
- `TypeError` is raised if aggfunc is not `str` or callable.
-
- Parameters
- ----------
- kwargs : dict
-
- Returns
- -------
- columns : List[str]
- List of user-provided keys.
- func : List[Union[str, callable[...,Any]]]
- List of user-provided aggfuncs
-
- Examples
- --------
- >>> validate_func_kwargs({'one': 'min', 'two': 'max'})
- (['one', 'two'], ['min', 'max'])
- """
- tuple_given_message = "func is expected but received {} in **kwargs."
- columns = list(kwargs)
- func = []
- for col_func in kwargs.values():
- if not (isinstance(col_func, str) or callable(col_func)):
- raise TypeError(tuple_given_message.format(type(col_func).__name__))
- func.append(col_func)
- if not columns:
- no_arg_message = "Must provide 'func' or named aggregation **kwargs."
- raise TypeError(no_arg_message)
- return columns, func
-
-
-def include_axis(op_name: Literal["agg", "apply"], colg: Series | DataFrame) -> bool:
- return isinstance(colg, ABCDataFrame) or (
- isinstance(colg, ABCSeries) and op_name == "agg"
- )
-
-
-def warn_alias_replacement(
- obj: AggObjType,
- func: Callable,
- alias: str,
-) -> None:
- if alias.startswith("np."):
- full_alias = alias
- else:
- full_alias = f"{type(obj).__name__}.{alias}"
- alias = f'"{alias}"'
- warnings.warn(
- f"The provided callable {func} is currently using "
- f"{full_alias}. In a future version of pandas, "
- f"the provided callable will be used directly. To keep current "
- f"behavior pass the string {alias} instead.",
- category=FutureWarning,
- stacklevel=find_stack_level(),
- )
diff --git a/spaces/profayle/TerrapinTalk/myenv/lib/python3.9/site-packages/pandas/tests/arrays/masked/test_function.py b/spaces/profayle/TerrapinTalk/myenv/lib/python3.9/site-packages/pandas/tests/arrays/masked/test_function.py
deleted file mode 100644
index 4c7bd6e293ef4a350dfe3b6aa7baa1513ee0946d..0000000000000000000000000000000000000000
--- a/spaces/profayle/TerrapinTalk/myenv/lib/python3.9/site-packages/pandas/tests/arrays/masked/test_function.py
+++ /dev/null
@@ -1,57 +0,0 @@
-import numpy as np
-import pytest
-
-from pandas.core.dtypes.common import is_integer_dtype
-
-import pandas as pd
-import pandas._testing as tm
-
-arrays = [pd.array([1, 2, 3, None], dtype=dtype) for dtype in tm.ALL_INT_EA_DTYPES]
-arrays += [
- pd.array([0.141, -0.268, 5.895, None], dtype=dtype) for dtype in tm.FLOAT_EA_DTYPES
-]
-
-
-@pytest.fixture(params=arrays, ids=[a.dtype.name for a in arrays])
-def data(request):
- """
- Fixture returning parametrized 'data' array with different integer and
- floating point types
- """
- return request.param
-
-
-@pytest.fixture()
-def numpy_dtype(data):
- """
- Fixture returning numpy dtype from 'data' input array.
- """
- # For integer dtype, the numpy conversion must be done to float
- if is_integer_dtype(data):
- numpy_dtype = float
- else:
- numpy_dtype = data.dtype.type
- return numpy_dtype
-
-
-def test_round(data, numpy_dtype):
- # No arguments
- result = data.round()
- expected = pd.array(
- np.round(data.to_numpy(dtype=numpy_dtype, na_value=None)), dtype=data.dtype
- )
- tm.assert_extension_array_equal(result, expected)
-
- # Decimals argument
- result = data.round(decimals=2)
- expected = pd.array(
- np.round(data.to_numpy(dtype=numpy_dtype, na_value=None), decimals=2),
- dtype=data.dtype,
- )
- tm.assert_extension_array_equal(result, expected)
-
-
-def test_tolist(data):
- result = data.tolist()
- expected = list(data)
- tm.assert_equal(result, expected)
diff --git a/spaces/profayle/TerrapinTalk/myenv/lib/python3.9/site-packages/pandas/tests/indexing/test_chaining_and_caching.py b/spaces/profayle/TerrapinTalk/myenv/lib/python3.9/site-packages/pandas/tests/indexing/test_chaining_and_caching.py
deleted file mode 100644
index f36fdf0d36ea94760baefb317729a7b6505490be..0000000000000000000000000000000000000000
--- a/spaces/profayle/TerrapinTalk/myenv/lib/python3.9/site-packages/pandas/tests/indexing/test_chaining_and_caching.py
+++ /dev/null
@@ -1,631 +0,0 @@
-from string import ascii_letters as letters
-
-import numpy as np
-import pytest
-
-from pandas.errors import (
- SettingWithCopyError,
- SettingWithCopyWarning,
-)
-import pandas.util._test_decorators as td
-
-import pandas as pd
-from pandas import (
- DataFrame,
- Series,
- Timestamp,
- date_range,
- option_context,
-)
-import pandas._testing as tm
-
-msg = "A value is trying to be set on a copy of a slice from a DataFrame"
-
-
-def random_text(nobs=100):
- # Construct a DataFrame where each row is a random slice from 'letters'
- idxs = np.random.default_rng(2).integers(len(letters), size=(nobs, 2))
- idxs.sort(axis=1)
- strings = [letters[x[0] : x[1]] for x in idxs]
-
- return DataFrame(strings, columns=["letters"])
-
-
-class TestCaching:
- def test_slice_consolidate_invalidate_item_cache(self, using_copy_on_write):
- # this is chained assignment, but will 'work'
- with option_context("chained_assignment", None):
- # #3970
- df = DataFrame({"aa": np.arange(5), "bb": [2.2] * 5})
-
- # Creates a second float block
- df["cc"] = 0.0
-
- # caches a reference to the 'bb' series
- df["bb"]
-
- # repr machinery triggers consolidation
- repr(df)
-
- # Assignment to wrong series
- if using_copy_on_write:
- with tm.raises_chained_assignment_error():
- df["bb"].iloc[0] = 0.17
- else:
- df["bb"].iloc[0] = 0.17
- df._clear_item_cache()
- if not using_copy_on_write:
- tm.assert_almost_equal(df["bb"][0], 0.17)
- else:
- # with ArrayManager, parent is not mutated with chained assignment
- tm.assert_almost_equal(df["bb"][0], 2.2)
-
- @pytest.mark.parametrize("do_ref", [True, False])
- def test_setitem_cache_updating(self, do_ref):
- # GH 5424
- cont = ["one", "two", "three", "four", "five", "six", "seven"]
-
- df = DataFrame({"a": cont, "b": cont[3:] + cont[:3], "c": np.arange(7)})
-
- # ref the cache
- if do_ref:
- df.loc[0, "c"]
-
- # set it
- df.loc[7, "c"] = 1
-
- assert df.loc[0, "c"] == 0.0
- assert df.loc[7, "c"] == 1.0
-
- def test_setitem_cache_updating_slices(self, using_copy_on_write):
- # GH 7084
- # not updating cache on series setting with slices
- expected = DataFrame(
- {"A": [600, 600, 600]}, index=date_range("5/7/2014", "5/9/2014")
- )
- out = DataFrame({"A": [0, 0, 0]}, index=date_range("5/7/2014", "5/9/2014"))
- df = DataFrame({"C": ["A", "A", "A"], "D": [100, 200, 300]})
-
- # loop through df to update out
- six = Timestamp("5/7/2014")
- eix = Timestamp("5/9/2014")
- for ix, row in df.iterrows():
- out.loc[six:eix, row["C"]] = out.loc[six:eix, row["C"]] + row["D"]
-
- tm.assert_frame_equal(out, expected)
- tm.assert_series_equal(out["A"], expected["A"])
-
- # try via a chain indexing
- # this actually works
- out = DataFrame({"A": [0, 0, 0]}, index=date_range("5/7/2014", "5/9/2014"))
- out_original = out.copy()
- for ix, row in df.iterrows():
- v = out[row["C"]][six:eix] + row["D"]
- if using_copy_on_write:
- with tm.raises_chained_assignment_error():
- out[row["C"]][six:eix] = v
- else:
- out[row["C"]][six:eix] = v
-
- if not using_copy_on_write:
- tm.assert_frame_equal(out, expected)
- tm.assert_series_equal(out["A"], expected["A"])
- else:
- tm.assert_frame_equal(out, out_original)
- tm.assert_series_equal(out["A"], out_original["A"])
-
- out = DataFrame({"A": [0, 0, 0]}, index=date_range("5/7/2014", "5/9/2014"))
- for ix, row in df.iterrows():
- out.loc[six:eix, row["C"]] += row["D"]
-
- tm.assert_frame_equal(out, expected)
- tm.assert_series_equal(out["A"], expected["A"])
-
- def test_altering_series_clears_parent_cache(self, using_copy_on_write):
- # GH #33675
- df = DataFrame([[1, 2], [3, 4]], index=["a", "b"], columns=["A", "B"])
- ser = df["A"]
-
- if using_copy_on_write:
- assert "A" not in df._item_cache
- else:
- assert "A" in df._item_cache
-
- # Adding a new entry to ser swaps in a new array, so "A" needs to
- # be removed from df._item_cache
- ser["c"] = 5
- assert len(ser) == 3
- assert "A" not in df._item_cache
- assert df["A"] is not ser
- assert len(df["A"]) == 2
-
-
-class TestChaining:
- def test_setitem_chained_setfault(self, using_copy_on_write):
- # GH6026
- data = ["right", "left", "left", "left", "right", "left", "timeout"]
- mdata = ["right", "left", "left", "left", "right", "left", "none"]
-
- df = DataFrame({"response": np.array(data)})
- mask = df.response == "timeout"
- if using_copy_on_write:
- with tm.raises_chained_assignment_error():
- df.response[mask] = "none"
- tm.assert_frame_equal(df, DataFrame({"response": data}))
- else:
- df.response[mask] = "none"
- tm.assert_frame_equal(df, DataFrame({"response": mdata}))
-
- recarray = np.rec.fromarrays([data], names=["response"])
- df = DataFrame(recarray)
- mask = df.response == "timeout"
- if using_copy_on_write:
- with tm.raises_chained_assignment_error():
- df.response[mask] = "none"
- tm.assert_frame_equal(df, DataFrame({"response": data}))
- else:
- df.response[mask] = "none"
- tm.assert_frame_equal(df, DataFrame({"response": mdata}))
-
- df = DataFrame({"response": data, "response1": data})
- df_original = df.copy()
- mask = df.response == "timeout"
- if using_copy_on_write:
- with tm.raises_chained_assignment_error():
- df.response[mask] = "none"
- tm.assert_frame_equal(df, df_original)
- else:
- df.response[mask] = "none"
- tm.assert_frame_equal(df, DataFrame({"response": mdata, "response1": data}))
-
- # GH 6056
- expected = DataFrame({"A": [np.nan, "bar", "bah", "foo", "bar"]})
- df = DataFrame({"A": np.array(["foo", "bar", "bah", "foo", "bar"])})
- if using_copy_on_write:
- with tm.raises_chained_assignment_error():
- df["A"].iloc[0] = np.nan
- expected = DataFrame({"A": ["foo", "bar", "bah", "foo", "bar"]})
- else:
- df["A"].iloc[0] = np.nan
- expected = DataFrame({"A": [np.nan, "bar", "bah", "foo", "bar"]})
- result = df.head()
- tm.assert_frame_equal(result, expected)
-
- df = DataFrame({"A": np.array(["foo", "bar", "bah", "foo", "bar"])})
- if using_copy_on_write:
- with tm.raises_chained_assignment_error():
- df.A.iloc[0] = np.nan
- else:
- df.A.iloc[0] = np.nan
- result = df.head()
- tm.assert_frame_equal(result, expected)
-
- @pytest.mark.arm_slow
- def test_detect_chained_assignment(self, using_copy_on_write):
- with option_context("chained_assignment", "raise"):
- # work with the chain
- expected = DataFrame([[-5, 1], [-6, 3]], columns=list("AB"))
- df = DataFrame(
- np.arange(4).reshape(2, 2), columns=list("AB"), dtype="int64"
- )
- df_original = df.copy()
- assert df._is_copy is None
-
- if using_copy_on_write:
- with tm.raises_chained_assignment_error():
- df["A"][0] = -5
- with tm.raises_chained_assignment_error():
- df["A"][1] = -6
- tm.assert_frame_equal(df, df_original)
- else:
- df["A"][0] = -5
- df["A"][1] = -6
- tm.assert_frame_equal(df, expected)
-
- @pytest.mark.arm_slow
- def test_detect_chained_assignment_raises(
- self, using_array_manager, using_copy_on_write
- ):
- # test with the chaining
- df = DataFrame(
- {
- "A": Series(range(2), dtype="int64"),
- "B": np.array(np.arange(2, 4), dtype=np.float64),
- }
- )
- df_original = df.copy()
- assert df._is_copy is None
-
- if using_copy_on_write:
- with tm.raises_chained_assignment_error():
- df["A"][0] = -5
- with tm.raises_chained_assignment_error():
- df["A"][1] = -6
- tm.assert_frame_equal(df, df_original)
- elif not using_array_manager:
- with pytest.raises(SettingWithCopyError, match=msg):
- df["A"][0] = -5
-
- with pytest.raises(SettingWithCopyError, match=msg):
- df["A"][1] = np.nan
-
- assert df["A"]._is_copy is None
- else:
- # INFO(ArrayManager) for ArrayManager it doesn't matter that it's
- # a mixed dataframe
- df["A"][0] = -5
- df["A"][1] = -6
- expected = DataFrame([[-5, 2], [-6, 3]], columns=list("AB"))
- expected["B"] = expected["B"].astype("float64")
- tm.assert_frame_equal(df, expected)
-
- @pytest.mark.arm_slow
- def test_detect_chained_assignment_fails(self, using_copy_on_write):
- # Using a copy (the chain), fails
- df = DataFrame(
- {
- "A": Series(range(2), dtype="int64"),
- "B": np.array(np.arange(2, 4), dtype=np.float64),
- }
- )
-
- if using_copy_on_write:
- with tm.raises_chained_assignment_error():
- df.loc[0]["A"] = -5
- else:
- with pytest.raises(SettingWithCopyError, match=msg):
- df.loc[0]["A"] = -5
-
- @pytest.mark.arm_slow
- def test_detect_chained_assignment_doc_example(self, using_copy_on_write):
- # Doc example
- df = DataFrame(
- {
- "a": ["one", "one", "two", "three", "two", "one", "six"],
- "c": Series(range(7), dtype="int64"),
- }
- )
- assert df._is_copy is None
-
- if using_copy_on_write:
- indexer = df.a.str.startswith("o")
- with tm.raises_chained_assignment_error():
- df[indexer]["c"] = 42
- else:
- with pytest.raises(SettingWithCopyError, match=msg):
- indexer = df.a.str.startswith("o")
- df[indexer]["c"] = 42
-
- @pytest.mark.arm_slow
- def test_detect_chained_assignment_object_dtype(
- self, using_array_manager, using_copy_on_write
- ):
- expected = DataFrame({"A": [111, "bbb", "ccc"], "B": [1, 2, 3]})
- df = DataFrame({"A": ["aaa", "bbb", "ccc"], "B": [1, 2, 3]})
- df_original = df.copy()
-
- if not using_copy_on_write:
- with pytest.raises(SettingWithCopyError, match=msg):
- df.loc[0]["A"] = 111
-
- if using_copy_on_write:
- with tm.raises_chained_assignment_error():
- df["A"][0] = 111
- tm.assert_frame_equal(df, df_original)
- elif not using_array_manager:
- with pytest.raises(SettingWithCopyError, match=msg):
- df["A"][0] = 111
-
- df.loc[0, "A"] = 111
- tm.assert_frame_equal(df, expected)
- else:
- # INFO(ArrayManager) for ArrayManager it doesn't matter that it's
- # a mixed dataframe
- df["A"][0] = 111
- tm.assert_frame_equal(df, expected)
-
- @pytest.mark.arm_slow
- def test_detect_chained_assignment_is_copy_pickle(self):
- # gh-5475: Make sure that is_copy is picked up reconstruction
- df = DataFrame({"A": [1, 2]})
- assert df._is_copy is None
-
- with tm.ensure_clean("__tmp__pickle") as path:
- df.to_pickle(path)
- df2 = pd.read_pickle(path)
- df2["B"] = df2["A"]
- df2["B"] = df2["A"]
-
- @pytest.mark.arm_slow
- def test_detect_chained_assignment_setting_entire_column(self):
- # gh-5597: a spurious raise as we are setting the entire column here
-
- df = random_text(100000)
-
- # Always a copy
- x = df.iloc[[0, 1, 2]]
- assert x._is_copy is not None
-
- x = df.iloc[[0, 1, 2, 4]]
- assert x._is_copy is not None
-
- # Explicitly copy
- indexer = df.letters.apply(lambda x: len(x) > 10)
- df = df.loc[indexer].copy()
-
- assert df._is_copy is None
- df["letters"] = df["letters"].apply(str.lower)
-
- @pytest.mark.arm_slow
- def test_detect_chained_assignment_implicit_take(self):
- # Implicitly take
- df = random_text(100000)
- indexer = df.letters.apply(lambda x: len(x) > 10)
- df = df.loc[indexer]
-
- assert df._is_copy is not None
- df["letters"] = df["letters"].apply(str.lower)
-
- @pytest.mark.arm_slow
- def test_detect_chained_assignment_implicit_take2(self, using_copy_on_write):
- if using_copy_on_write:
- pytest.skip("_is_copy is not always set for CoW")
- # Implicitly take 2
- df = random_text(100000)
- indexer = df.letters.apply(lambda x: len(x) > 10)
-
- df = df.loc[indexer]
- assert df._is_copy is not None
- df.loc[:, "letters"] = df["letters"].apply(str.lower)
-
- # with the enforcement of #45333 in 2.0, the .loc[:, letters] setting
- # is inplace, so df._is_copy remains non-None.
- assert df._is_copy is not None
-
- df["letters"] = df["letters"].apply(str.lower)
- assert df._is_copy is None
-
- @pytest.mark.arm_slow
- def test_detect_chained_assignment_str(self):
- df = random_text(100000)
- indexer = df.letters.apply(lambda x: len(x) > 10)
- df.loc[indexer, "letters"] = df.loc[indexer, "letters"].apply(str.lower)
-
- @pytest.mark.arm_slow
- def test_detect_chained_assignment_is_copy(self):
- # an identical take, so no copy
- df = DataFrame({"a": [1]}).dropna()
- assert df._is_copy is None
- df["a"] += 1
-
- @pytest.mark.arm_slow
- def test_detect_chained_assignment_sorting(self):
- df = DataFrame(np.random.default_rng(2).standard_normal((10, 4)))
- ser = df.iloc[:, 0].sort_values()
-
- tm.assert_series_equal(ser, df.iloc[:, 0].sort_values())
- tm.assert_series_equal(ser, df[0].sort_values())
-
- @pytest.mark.arm_slow
- def test_detect_chained_assignment_false_positives(self):
- # see gh-6025: false positives
- df = DataFrame({"column1": ["a", "a", "a"], "column2": [4, 8, 9]})
- str(df)
-
- df["column1"] = df["column1"] + "b"
- str(df)
-
- df = df[df["column2"] != 8]
- str(df)
-
- df["column1"] = df["column1"] + "c"
- str(df)
-
- @pytest.mark.arm_slow
- def test_detect_chained_assignment_undefined_column(self, using_copy_on_write):
- # from SO:
- # https://stackoverflow.com/questions/24054495/potential-bug-setting-value-for-undefined-column-using-iloc
- df = DataFrame(np.arange(0, 9), columns=["count"])
- df["group"] = "b"
- df_original = df.copy()
-
- if using_copy_on_write:
- with tm.raises_chained_assignment_error():
- df.iloc[0:5]["group"] = "a"
- tm.assert_frame_equal(df, df_original)
- else:
- with pytest.raises(SettingWithCopyError, match=msg):
- df.iloc[0:5]["group"] = "a"
-
- @pytest.mark.arm_slow
- def test_detect_chained_assignment_changing_dtype(
- self, using_array_manager, using_copy_on_write
- ):
- # Mixed type setting but same dtype & changing dtype
- df = DataFrame(
- {
- "A": date_range("20130101", periods=5),
- "B": np.random.default_rng(2).standard_normal(5),
- "C": np.arange(5, dtype="int64"),
- "D": ["a", "b", "c", "d", "e"],
- }
- )
- df_original = df.copy()
-
- if using_copy_on_write:
- with tm.raises_chained_assignment_error():
- df.loc[2]["D"] = "foo"
- with tm.raises_chained_assignment_error():
- df.loc[2]["C"] = "foo"
- with tm.raises_chained_assignment_error(extra_warnings=(FutureWarning,)):
- df["C"][2] = "foo"
- tm.assert_frame_equal(df, df_original)
-
- if not using_copy_on_write:
- with pytest.raises(SettingWithCopyError, match=msg):
- df.loc[2]["D"] = "foo"
-
- with pytest.raises(SettingWithCopyError, match=msg):
- df.loc[2]["C"] = "foo"
-
- if not using_array_manager:
- with pytest.raises(SettingWithCopyError, match=msg):
- df["C"][2] = "foo"
- else:
- # INFO(ArrayManager) for ArrayManager it doesn't matter if it's
- # changing the dtype or not
- df["C"][2] = "foo"
- assert df.loc[2, "C"] == "foo"
-
- def test_setting_with_copy_bug(self, using_copy_on_write):
- # operating on a copy
- df = DataFrame(
- {"a": list(range(4)), "b": list("ab.."), "c": ["a", "b", np.nan, "d"]}
- )
- df_original = df.copy()
- mask = pd.isna(df.c)
-
- if using_copy_on_write:
- with tm.raises_chained_assignment_error():
- df[["c"]][mask] = df[["b"]][mask]
- tm.assert_frame_equal(df, df_original)
- else:
- with pytest.raises(SettingWithCopyError, match=msg):
- df[["c"]][mask] = df[["b"]][mask]
-
- def test_setting_with_copy_bug_no_warning(self):
- # invalid warning as we are returning a new object
- # GH 8730
- df1 = DataFrame({"x": Series(["a", "b", "c"]), "y": Series(["d", "e", "f"])})
- df2 = df1[["x"]]
-
- # this should not raise
- df2["y"] = ["g", "h", "i"]
-
- def test_detect_chained_assignment_warnings_errors(self, using_copy_on_write):
- df = DataFrame({"A": ["aaa", "bbb", "ccc"], "B": [1, 2, 3]})
- if using_copy_on_write:
- with tm.raises_chained_assignment_error():
- df.loc[0]["A"] = 111
- return
-
- with option_context("chained_assignment", "warn"):
- with tm.assert_produces_warning(SettingWithCopyWarning):
- df.loc[0]["A"] = 111
-
- with option_context("chained_assignment", "raise"):
- with pytest.raises(SettingWithCopyError, match=msg):
- df.loc[0]["A"] = 111
-
- @pytest.mark.parametrize("rhs", [3, DataFrame({0: [1, 2, 3, 4]})])
- def test_detect_chained_assignment_warning_stacklevel(
- self, rhs, using_copy_on_write
- ):
- # GH#42570
- df = DataFrame(np.arange(25).reshape(5, 5))
- df_original = df.copy()
- chained = df.loc[:3]
- with option_context("chained_assignment", "warn"):
- if not using_copy_on_write:
- with tm.assert_produces_warning(SettingWithCopyWarning) as t:
- chained[2] = rhs
- assert t[0].filename == __file__
- else:
- # INFO(CoW) no warning, and original dataframe not changed
- with tm.assert_produces_warning(None):
- chained[2] = rhs
- tm.assert_frame_equal(df, df_original)
-
- # TODO(ArrayManager) fast_xs with array-like scalars is not yet working
- @td.skip_array_manager_not_yet_implemented
- def test_chained_getitem_with_lists(self):
- # GH6394
- # Regression in chained getitem indexing with embedded list-like from
- # 0.12
-
- df = DataFrame({"A": 5 * [np.zeros(3)], "B": 5 * [np.ones(3)]})
- expected = df["A"].iloc[2]
- result = df.loc[2, "A"]
- tm.assert_numpy_array_equal(result, expected)
- result2 = df.iloc[2]["A"]
- tm.assert_numpy_array_equal(result2, expected)
- result3 = df["A"].loc[2]
- tm.assert_numpy_array_equal(result3, expected)
- result4 = df["A"].iloc[2]
- tm.assert_numpy_array_equal(result4, expected)
-
- def test_cache_updating(self):
- # GH 4939, make sure to update the cache on setitem
-
- df = tm.makeDataFrame()
- df["A"] # cache series
- df.loc["Hello Friend"] = df.iloc[0]
- assert "Hello Friend" in df["A"].index
- assert "Hello Friend" in df["B"].index
-
- def test_cache_updating2(self, using_copy_on_write):
- # 10264
- df = DataFrame(
- np.zeros((5, 5), dtype="int64"),
- columns=["a", "b", "c", "d", "e"],
- index=range(5),
- )
- df["f"] = 0
- df_orig = df.copy()
- if using_copy_on_write:
- with pytest.raises(ValueError, match="read-only"):
- df.f.values[3] = 1
- tm.assert_frame_equal(df, df_orig)
- return
-
- df.f.values[3] = 1
-
- df.f.values[3] = 2
- expected = DataFrame(
- np.zeros((5, 6), dtype="int64"),
- columns=["a", "b", "c", "d", "e", "f"],
- index=range(5),
- )
- expected.at[3, "f"] = 2
- tm.assert_frame_equal(df, expected)
- expected = Series([0, 0, 0, 2, 0], name="f")
- tm.assert_series_equal(df.f, expected)
-
- def test_iloc_setitem_chained_assignment(self, using_copy_on_write):
- # GH#3970
- with option_context("chained_assignment", None):
- df = DataFrame({"aa": range(5), "bb": [2.2] * 5})
- df["cc"] = 0.0
-
- ck = [True] * len(df)
-
- if using_copy_on_write:
- with tm.raises_chained_assignment_error():
- df["bb"].iloc[0] = 0.13
- else:
- df["bb"].iloc[0] = 0.13
-
- # GH#3970 this lookup used to break the chained setting to 0.15
- df.iloc[ck]
-
- if using_copy_on_write:
- with tm.raises_chained_assignment_error():
- df["bb"].iloc[0] = 0.15
- else:
- df["bb"].iloc[0] = 0.15
-
- if not using_copy_on_write:
- assert df["bb"].iloc[0] == 0.15
- else:
- assert df["bb"].iloc[0] == 2.2
-
- def test_getitem_loc_assignment_slice_state(self, using_copy_on_write):
- # GH 13569
- df = DataFrame({"a": [10, 20, 30]})
- if using_copy_on_write:
- with tm.raises_chained_assignment_error():
- df["a"].loc[4] = 40
- else:
- df["a"].loc[4] = 40
- tm.assert_frame_equal(df, DataFrame({"a": [10, 20, 30]}))
- tm.assert_series_equal(df["a"], Series([10, 20, 30], name="a"))
diff --git a/spaces/profayle/TerrapinTalk/myenv/lib/python3.9/site-packages/pandas/tests/series/indexing/__init__.py b/spaces/profayle/TerrapinTalk/myenv/lib/python3.9/site-packages/pandas/tests/series/indexing/__init__.py
deleted file mode 100644
index e69de29bb2d1d6434b8b29ae775ad8c2e48c5391..0000000000000000000000000000000000000000
diff --git a/spaces/profayle/TerrapinTalk/myenv/lib/python3.9/site-packages/pandas/tests/series/methods/test_explode.py b/spaces/profayle/TerrapinTalk/myenv/lib/python3.9/site-packages/pandas/tests/series/methods/test_explode.py
deleted file mode 100644
index c8a9eb6f89fdef5fb16fe5f2a1d0490c02477ab8..0000000000000000000000000000000000000000
--- a/spaces/profayle/TerrapinTalk/myenv/lib/python3.9/site-packages/pandas/tests/series/methods/test_explode.py
+++ /dev/null
@@ -1,165 +0,0 @@
-import numpy as np
-import pytest
-
-import pandas as pd
-import pandas._testing as tm
-
-
-def test_basic():
- s = pd.Series([[0, 1, 2], np.nan, [], (3, 4)], index=list("abcd"), name="foo")
- result = s.explode()
- expected = pd.Series(
- [0, 1, 2, np.nan, np.nan, 3, 4], index=list("aaabcdd"), dtype=object, name="foo"
- )
- tm.assert_series_equal(result, expected)
-
-
-def test_mixed_type():
- s = pd.Series(
- [[0, 1, 2], np.nan, None, np.array([]), pd.Series(["a", "b"])], name="foo"
- )
- result = s.explode()
- expected = pd.Series(
- [0, 1, 2, np.nan, None, np.nan, "a", "b"],
- index=[0, 0, 0, 1, 2, 3, 4, 4],
- dtype=object,
- name="foo",
- )
- tm.assert_series_equal(result, expected)
-
-
-def test_empty():
- s = pd.Series(dtype=object)
- result = s.explode()
- expected = s.copy()
- tm.assert_series_equal(result, expected)
-
-
-def test_nested_lists():
- s = pd.Series([[[1, 2, 3]], [1, 2], 1])
- result = s.explode()
- expected = pd.Series([[1, 2, 3], 1, 2, 1], index=[0, 1, 1, 2])
- tm.assert_series_equal(result, expected)
-
-
-def test_multi_index():
- s = pd.Series(
- [[0, 1, 2], np.nan, [], (3, 4)],
- name="foo",
- index=pd.MultiIndex.from_product([list("ab"), range(2)], names=["foo", "bar"]),
- )
- result = s.explode()
- index = pd.MultiIndex.from_tuples(
- [("a", 0), ("a", 0), ("a", 0), ("a", 1), ("b", 0), ("b", 1), ("b", 1)],
- names=["foo", "bar"],
- )
- expected = pd.Series(
- [0, 1, 2, np.nan, np.nan, 3, 4], index=index, dtype=object, name="foo"
- )
- tm.assert_series_equal(result, expected)
-
-
-def test_large():
- s = pd.Series([range(256)]).explode()
- result = s.explode()
- tm.assert_series_equal(result, s)
-
-
-def test_invert_array():
- df = pd.DataFrame({"a": pd.date_range("20190101", periods=3, tz="UTC")})
-
- listify = df.apply(lambda x: x.array, axis=1)
- result = listify.explode()
- tm.assert_series_equal(result, df["a"].rename())
-
-
-@pytest.mark.parametrize(
- "s", [pd.Series([1, 2, 3]), pd.Series(pd.date_range("2019", periods=3, tz="UTC"))]
-)
-def test_non_object_dtype(s):
- result = s.explode()
- tm.assert_series_equal(result, s)
-
-
-def test_typical_usecase():
- df = pd.DataFrame(
- [{"var1": "a,b,c", "var2": 1}, {"var1": "d,e,f", "var2": 2}],
- columns=["var1", "var2"],
- )
- exploded = df.var1.str.split(",").explode()
- result = df[["var2"]].join(exploded)
- expected = pd.DataFrame(
- {"var2": [1, 1, 1, 2, 2, 2], "var1": list("abcdef")},
- columns=["var2", "var1"],
- index=[0, 0, 0, 1, 1, 1],
- )
- tm.assert_frame_equal(result, expected)
-
-
-def test_nested_EA():
- # a nested EA array
- s = pd.Series(
- [
- pd.date_range("20170101", periods=3, tz="UTC"),
- pd.date_range("20170104", periods=3, tz="UTC"),
- ]
- )
- result = s.explode()
- expected = pd.Series(
- pd.date_range("20170101", periods=6, tz="UTC"), index=[0, 0, 0, 1, 1, 1]
- )
- tm.assert_series_equal(result, expected)
-
-
-def test_duplicate_index():
- # GH 28005
- s = pd.Series([[1, 2], [3, 4]], index=[0, 0])
- result = s.explode()
- expected = pd.Series([1, 2, 3, 4], index=[0, 0, 0, 0], dtype=object)
- tm.assert_series_equal(result, expected)
-
-
-def test_ignore_index():
- # GH 34932
- s = pd.Series([[1, 2], [3, 4]])
- result = s.explode(ignore_index=True)
- expected = pd.Series([1, 2, 3, 4], index=[0, 1, 2, 3], dtype=object)
- tm.assert_series_equal(result, expected)
-
-
-def test_explode_sets():
- # https://github.com/pandas-dev/pandas/issues/35614
- s = pd.Series([{"a", "b", "c"}], index=[1])
- result = s.explode().sort_values()
- expected = pd.Series(["a", "b", "c"], index=[1, 1, 1])
- tm.assert_series_equal(result, expected)
-
-
-def test_explode_scalars_can_ignore_index():
- # https://github.com/pandas-dev/pandas/issues/40487
- s = pd.Series([1, 2, 3], index=["a", "b", "c"])
- result = s.explode(ignore_index=True)
- expected = pd.Series([1, 2, 3])
- tm.assert_series_equal(result, expected)
-
-
-@pytest.mark.parametrize("ignore_index", [True, False])
-def test_explode_pyarrow_list_type(ignore_index):
- # GH 53602
- pa = pytest.importorskip("pyarrow")
-
- data = [
- [None, None],
- [1],
- [],
- [2, 3],
- None,
- ]
- ser = pd.Series(data, dtype=pd.ArrowDtype(pa.list_(pa.int64())))
- result = ser.explode(ignore_index=ignore_index)
- expected = pd.Series(
- data=[None, None, 1, None, 2, 3, None],
- index=None if ignore_index else [0, 0, 1, 2, 3, 3, 4],
- dtype=pd.ArrowDtype(pa.int64()),
- )
- tm.assert_series_equal(result, expected)
diff --git a/spaces/profayle/TerrapinTalk/myenv/lib/python3.9/site-packages/pandas/tests/series/test_cumulative.py b/spaces/profayle/TerrapinTalk/myenv/lib/python3.9/site-packages/pandas/tests/series/test_cumulative.py
deleted file mode 100644
index e6f7b2a5e69e0a97e2f898c6a665372ba3ec2a6b..0000000000000000000000000000000000000000
--- a/spaces/profayle/TerrapinTalk/myenv/lib/python3.9/site-packages/pandas/tests/series/test_cumulative.py
+++ /dev/null
@@ -1,157 +0,0 @@
-"""
-Tests for Series cumulative operations.
-
-See also
---------
-tests.frame.test_cumulative
-"""
-
-import numpy as np
-import pytest
-
-import pandas as pd
-import pandas._testing as tm
-
-methods = {
- "cumsum": np.cumsum,
- "cumprod": np.cumprod,
- "cummin": np.minimum.accumulate,
- "cummax": np.maximum.accumulate,
-}
-
-
-class TestSeriesCumulativeOps:
- @pytest.mark.parametrize("func", [np.cumsum, np.cumprod])
- def test_datetime_series(self, datetime_series, func):
- tm.assert_numpy_array_equal(
- func(datetime_series).values,
- func(np.array(datetime_series)),
- check_dtype=True,
- )
-
- # with missing values
- ts = datetime_series.copy()
- ts[::2] = np.nan
-
- result = func(ts)[1::2]
- expected = func(np.array(ts.dropna()))
-
- tm.assert_numpy_array_equal(result.values, expected, check_dtype=False)
-
- @pytest.mark.parametrize("method", ["cummin", "cummax"])
- def test_cummin_cummax(self, datetime_series, method):
- ufunc = methods[method]
-
- result = getattr(datetime_series, method)().values
- expected = ufunc(np.array(datetime_series))
-
- tm.assert_numpy_array_equal(result, expected)
- ts = datetime_series.copy()
- ts[::2] = np.nan
- result = getattr(ts, method)()[1::2]
- expected = ufunc(ts.dropna())
-
- result.index = result.index._with_freq(None)
- tm.assert_series_equal(result, expected)
-
- @pytest.mark.parametrize(
- "ts",
- [
- pd.Timedelta(0),
- pd.Timestamp("1999-12-31"),
- pd.Timestamp("1999-12-31").tz_localize("US/Pacific"),
- ],
- )
- @pytest.mark.parametrize(
- "method, skipna, exp_tdi",
- [
- ["cummax", True, ["NaT", "2 days", "NaT", "2 days", "NaT", "3 days"]],
- ["cummin", True, ["NaT", "2 days", "NaT", "1 days", "NaT", "1 days"]],
- [
- "cummax",
- False,
- ["NaT", "NaT", "NaT", "NaT", "NaT", "NaT"],
- ],
- [
- "cummin",
- False,
- ["NaT", "NaT", "NaT", "NaT", "NaT", "NaT"],
- ],
- ],
- )
- def test_cummin_cummax_datetimelike(self, ts, method, skipna, exp_tdi):
- # with ts==pd.Timedelta(0), we are testing td64; with naive Timestamp
- # we are testing datetime64[ns]; with Timestamp[US/Pacific]
- # we are testing dt64tz
- tdi = pd.to_timedelta(["NaT", "2 days", "NaT", "1 days", "NaT", "3 days"])
- ser = pd.Series(tdi + ts)
-
- exp_tdi = pd.to_timedelta(exp_tdi)
- expected = pd.Series(exp_tdi + ts)
- result = getattr(ser, method)(skipna=skipna)
- tm.assert_series_equal(expected, result)
-
- @pytest.mark.parametrize(
- "func, exp",
- [
- ("cummin", pd.Period("2012-1-1", freq="D")),
- ("cummax", pd.Period("2012-1-2", freq="D")),
- ],
- )
- def test_cummin_cummax_period(self, func, exp):
- # GH#28385
- ser = pd.Series(
- [pd.Period("2012-1-1", freq="D"), pd.NaT, pd.Period("2012-1-2", freq="D")]
- )
- result = getattr(ser, func)(skipna=False)
- expected = pd.Series([pd.Period("2012-1-1", freq="D"), pd.NaT, pd.NaT])
- tm.assert_series_equal(result, expected)
-
- result = getattr(ser, func)(skipna=True)
- expected = pd.Series([pd.Period("2012-1-1", freq="D"), pd.NaT, exp])
- tm.assert_series_equal(result, expected)
-
- @pytest.mark.parametrize(
- "arg",
- [
- [False, False, False, True, True, False, False],
- [False, False, False, False, False, False, False],
- ],
- )
- @pytest.mark.parametrize(
- "func", [lambda x: x, lambda x: ~x], ids=["identity", "inverse"]
- )
- @pytest.mark.parametrize("method", methods.keys())
- def test_cummethods_bool(self, arg, func, method):
- # GH#6270
- # checking Series method vs the ufunc applied to the values
-
- ser = func(pd.Series(arg))
- ufunc = methods[method]
-
- exp_vals = ufunc(ser.values)
- expected = pd.Series(exp_vals)
-
- result = getattr(ser, method)()
-
- tm.assert_series_equal(result, expected)
-
- @pytest.mark.parametrize(
- "method, expected",
- [
- ["cumsum", pd.Series([0, 1, np.nan, 1], dtype=object)],
- ["cumprod", pd.Series([False, 0, np.nan, 0])],
- ["cummin", pd.Series([False, False, np.nan, False])],
- ["cummax", pd.Series([False, True, np.nan, True])],
- ],
- )
- def test_cummethods_bool_in_object_dtype(self, method, expected):
- ser = pd.Series([False, True, np.nan, False])
- result = getattr(ser, method)()
- tm.assert_series_equal(result, expected)
-
- def test_cumprod_timedelta(self):
- # GH#48111
- ser = pd.Series([pd.Timedelta(days=1), pd.Timedelta(days=3)])
- with pytest.raises(TypeError, match="cumprod not supported for Timedelta"):
- ser.cumprod()
diff --git a/spaces/profayle/TerrapinTalk/myenv/lib/python3.9/site-packages/pip/_vendor/html5lib/treebuilders/etree.py b/spaces/profayle/TerrapinTalk/myenv/lib/python3.9/site-packages/pip/_vendor/html5lib/treebuilders/etree.py
deleted file mode 100644
index ea92dc301fe3fcf2ec9839c39c7844ae9f5df614..0000000000000000000000000000000000000000
--- a/spaces/profayle/TerrapinTalk/myenv/lib/python3.9/site-packages/pip/_vendor/html5lib/treebuilders/etree.py
+++ /dev/null
@@ -1,343 +0,0 @@
-from __future__ import absolute_import, division, unicode_literals
-# pylint:disable=protected-access
-
-from pip._vendor.six import text_type
-
-import re
-
-from copy import copy
-
-from . import base
-from .. import _ihatexml
-from .. import constants
-from ..constants import namespaces
-from .._utils import moduleFactoryFactory
-
-tag_regexp = re.compile("{([^}]*)}(.*)")
-
-
-def getETreeBuilder(ElementTreeImplementation, fullTree=False):
- ElementTree = ElementTreeImplementation
- ElementTreeCommentType = ElementTree.Comment("asd").tag
-
- class Element(base.Node):
- def __init__(self, name, namespace=None):
- self._name = name
- self._namespace = namespace
- self._element = ElementTree.Element(self._getETreeTag(name,
- namespace))
- if namespace is None:
- self.nameTuple = namespaces["html"], self._name
- else:
- self.nameTuple = self._namespace, self._name
- self.parent = None
- self._childNodes = []
- self._flags = []
-
- def _getETreeTag(self, name, namespace):
- if namespace is None:
- etree_tag = name
- else:
- etree_tag = "{%s}%s" % (namespace, name)
- return etree_tag
-
- def _setName(self, name):
- self._name = name
- self._element.tag = self._getETreeTag(self._name, self._namespace)
-
- def _getName(self):
- return self._name
-
- name = property(_getName, _setName)
-
- def _setNamespace(self, namespace):
- self._namespace = namespace
- self._element.tag = self._getETreeTag(self._name, self._namespace)
-
- def _getNamespace(self):
- return self._namespace
-
- namespace = property(_getNamespace, _setNamespace)
-
- def _getAttributes(self):
- return self._element.attrib
-
- def _setAttributes(self, attributes):
- el_attrib = self._element.attrib
- el_attrib.clear()
- if attributes:
- # calling .items _always_ allocates, and the above truthy check is cheaper than the
- # allocation on average
- for key, value in attributes.items():
- if isinstance(key, tuple):
- name = "{%s}%s" % (key[2], key[1])
- else:
- name = key
- el_attrib[name] = value
-
- attributes = property(_getAttributes, _setAttributes)
-
- def _getChildNodes(self):
- return self._childNodes
-
- def _setChildNodes(self, value):
- del self._element[:]
- self._childNodes = []
- for element in value:
- self.insertChild(element)
-
- childNodes = property(_getChildNodes, _setChildNodes)
-
- def hasContent(self):
- """Return true if the node has children or text"""
- return bool(self._element.text or len(self._element))
-
- def appendChild(self, node):
- self._childNodes.append(node)
- self._element.append(node._element)
- node.parent = self
-
- def insertBefore(self, node, refNode):
- index = list(self._element).index(refNode._element)
- self._element.insert(index, node._element)
- node.parent = self
-
- def removeChild(self, node):
- self._childNodes.remove(node)
- self._element.remove(node._element)
- node.parent = None
-
- def insertText(self, data, insertBefore=None):
- if not(len(self._element)):
- if not self._element.text:
- self._element.text = ""
- self._element.text += data
- elif insertBefore is None:
- # Insert the text as the tail of the last child element
- if not self._element[-1].tail:
- self._element[-1].tail = ""
- self._element[-1].tail += data
- else:
- # Insert the text before the specified node
- children = list(self._element)
- index = children.index(insertBefore._element)
- if index > 0:
- if not self._element[index - 1].tail:
- self._element[index - 1].tail = ""
- self._element[index - 1].tail += data
- else:
- if not self._element.text:
- self._element.text = ""
- self._element.text += data
-
- def cloneNode(self):
- element = type(self)(self.name, self.namespace)
- if self._element.attrib:
- element._element.attrib = copy(self._element.attrib)
- return element
-
- def reparentChildren(self, newParent):
- if newParent.childNodes:
- newParent.childNodes[-1]._element.tail += self._element.text
- else:
- if not newParent._element.text:
- newParent._element.text = ""
- if self._element.text is not None:
- newParent._element.text += self._element.text
- self._element.text = ""
- base.Node.reparentChildren(self, newParent)
-
- class Comment(Element):
- def __init__(self, data):
- # Use the superclass constructor to set all properties on the
- # wrapper element
- self._element = ElementTree.Comment(data)
- self.parent = None
- self._childNodes = []
- self._flags = []
-
- def _getData(self):
- return self._element.text
-
- def _setData(self, value):
- self._element.text = value
-
- data = property(_getData, _setData)
-
- class DocumentType(Element):
- def __init__(self, name, publicId, systemId):
- Element.__init__(self, "")
- self._element.text = name
- self.publicId = publicId
- self.systemId = systemId
-
- def _getPublicId(self):
- return self._element.get("publicId", "")
-
- def _setPublicId(self, value):
- if value is not None:
- self._element.set("publicId", value)
-
- publicId = property(_getPublicId, _setPublicId)
-
- def _getSystemId(self):
- return self._element.get("systemId", "")
-
- def _setSystemId(self, value):
- if value is not None:
- self._element.set("systemId", value)
-
- systemId = property(_getSystemId, _setSystemId)
-
- class Document(Element):
- def __init__(self):
- Element.__init__(self, "DOCUMENT_ROOT")
-
- class DocumentFragment(Element):
- def __init__(self):
- Element.__init__(self, "DOCUMENT_FRAGMENT")
-
- def testSerializer(element):
- rv = []
-
- def serializeElement(element, indent=0):
- if not(hasattr(element, "tag")):
- element = element.getroot()
- if element.tag == "":
- if element.get("publicId") or element.get("systemId"):
- publicId = element.get("publicId") or ""
- systemId = element.get("systemId") or ""
- rv.append("""""" %
- (element.text, publicId, systemId))
- else:
- rv.append("" % (element.text,))
- elif element.tag == "DOCUMENT_ROOT":
- rv.append("#document")
- if element.text is not None:
- rv.append("|%s\"%s\"" % (' ' * (indent + 2), element.text))
- if element.tail is not None:
- raise TypeError("Document node cannot have tail")
- if hasattr(element, "attrib") and len(element.attrib):
- raise TypeError("Document node cannot have attributes")
- elif element.tag == ElementTreeCommentType:
- rv.append("|%s" % (' ' * indent, element.text))
- else:
- assert isinstance(element.tag, text_type), \
- "Expected unicode, got %s, %s" % (type(element.tag), element.tag)
- nsmatch = tag_regexp.match(element.tag)
-
- if nsmatch is None:
- name = element.tag
- else:
- ns, name = nsmatch.groups()
- prefix = constants.prefixes[ns]
- name = "%s %s" % (prefix, name)
- rv.append("|%s<%s>" % (' ' * indent, name))
-
- if hasattr(element, "attrib"):
- attributes = []
- for name, value in element.attrib.items():
- nsmatch = tag_regexp.match(name)
- if nsmatch is not None:
- ns, name = nsmatch.groups()
- prefix = constants.prefixes[ns]
- attr_string = "%s %s" % (prefix, name)
- else:
- attr_string = name
- attributes.append((attr_string, value))
-
- for name, value in sorted(attributes):
- rv.append('|%s%s="%s"' % (' ' * (indent + 2), name, value))
- if element.text:
- rv.append("|%s\"%s\"" % (' ' * (indent + 2), element.text))
- indent += 2
- for child in element:
- serializeElement(child, indent)
- if element.tail:
- rv.append("|%s\"%s\"" % (' ' * (indent - 2), element.tail))
- serializeElement(element, 0)
-
- return "\n".join(rv)
-
- def tostring(element): # pylint:disable=unused-variable
- """Serialize an element and its child nodes to a string"""
- rv = []
- filter = _ihatexml.InfosetFilter()
-
- def serializeElement(element):
- if isinstance(element, ElementTree.ElementTree):
- element = element.getroot()
-
- if element.tag == "":
- if element.get("publicId") or element.get("systemId"):
- publicId = element.get("publicId") or ""
- systemId = element.get("systemId") or ""
- rv.append("""""" %
- (element.text, publicId, systemId))
- else:
- rv.append("" % (element.text,))
- elif element.tag == "DOCUMENT_ROOT":
- if element.text is not None:
- rv.append(element.text)
- if element.tail is not None:
- raise TypeError("Document node cannot have tail")
- if hasattr(element, "attrib") and len(element.attrib):
- raise TypeError("Document node cannot have attributes")
-
- for child in element:
- serializeElement(child)
-
- elif element.tag == ElementTreeCommentType:
- rv.append("" % (element.text,))
- else:
- # This is assumed to be an ordinary element
- if not element.attrib:
- rv.append("<%s>" % (filter.fromXmlName(element.tag),))
- else:
- attr = " ".join(["%s=\"%s\"" % (
- filter.fromXmlName(name), value)
- for name, value in element.attrib.items()])
- rv.append("<%s %s>" % (element.tag, attr))
- if element.text:
- rv.append(element.text)
-
- for child in element:
- serializeElement(child)
-
- rv.append("%s>" % (element.tag,))
-
- if element.tail:
- rv.append(element.tail)
-
- serializeElement(element)
-
- return "".join(rv)
-
- class TreeBuilder(base.TreeBuilder): # pylint:disable=unused-variable
- documentClass = Document
- doctypeClass = DocumentType
- elementClass = Element
- commentClass = Comment
- fragmentClass = DocumentFragment
- implementation = ElementTreeImplementation
-
- def testSerializer(self, element):
- return testSerializer(element)
-
- def getDocument(self):
- if fullTree:
- return self.document._element
- else:
- if self.defaultNamespace is not None:
- return self.document._element.find(
- "{%s}html" % self.defaultNamespace)
- else:
- return self.document._element.find("html")
-
- def getFragment(self):
- return base.TreeBuilder.getFragment(self)._element
-
- return locals()
-
-
-getETreeModule = moduleFactoryFactory(getETreeBuilder)
diff --git a/spaces/profayle/TerrapinTalk/myenv/lib/python3.9/site-packages/pip/_vendor/pygments/lexer.py b/spaces/profayle/TerrapinTalk/myenv/lib/python3.9/site-packages/pip/_vendor/pygments/lexer.py
deleted file mode 100644
index b6d4b238845b15a3cfed958607ec41d35c9f425e..0000000000000000000000000000000000000000
--- a/spaces/profayle/TerrapinTalk/myenv/lib/python3.9/site-packages/pip/_vendor/pygments/lexer.py
+++ /dev/null
@@ -1,879 +0,0 @@
-"""
- pygments.lexer
- ~~~~~~~~~~~~~~
-
- Base lexer classes.
-
- :copyright: Copyright 2006-2021 by the Pygments team, see AUTHORS.
- :license: BSD, see LICENSE for details.
-"""
-
-import re
-import sys
-import time
-
-from pip._vendor.pygments.filter import apply_filters, Filter
-from pip._vendor.pygments.filters import get_filter_by_name
-from pip._vendor.pygments.token import Error, Text, Other, _TokenType
-from pip._vendor.pygments.util import get_bool_opt, get_int_opt, get_list_opt, \
- make_analysator, Future, guess_decode
-from pip._vendor.pygments.regexopt import regex_opt
-
-__all__ = ['Lexer', 'RegexLexer', 'ExtendedRegexLexer', 'DelegatingLexer',
- 'LexerContext', 'include', 'inherit', 'bygroups', 'using', 'this',
- 'default', 'words']
-
-
-_encoding_map = [(b'\xef\xbb\xbf', 'utf-8'),
- (b'\xff\xfe\0\0', 'utf-32'),
- (b'\0\0\xfe\xff', 'utf-32be'),
- (b'\xff\xfe', 'utf-16'),
- (b'\xfe\xff', 'utf-16be')]
-
-_default_analyse = staticmethod(lambda x: 0.0)
-
-
-class LexerMeta(type):
- """
- This metaclass automagically converts ``analyse_text`` methods into
- static methods which always return float values.
- """
-
- def __new__(mcs, name, bases, d):
- if 'analyse_text' in d:
- d['analyse_text'] = make_analysator(d['analyse_text'])
- return type.__new__(mcs, name, bases, d)
-
-
-class Lexer(metaclass=LexerMeta):
- """
- Lexer for a specific language.
-
- Basic options recognized:
- ``stripnl``
- Strip leading and trailing newlines from the input (default: True).
- ``stripall``
- Strip all leading and trailing whitespace from the input
- (default: False).
- ``ensurenl``
- Make sure that the input ends with a newline (default: True). This
- is required for some lexers that consume input linewise.
-
- .. versionadded:: 1.3
-
- ``tabsize``
- If given and greater than 0, expand tabs in the input (default: 0).
- ``encoding``
- If given, must be an encoding name. This encoding will be used to
- convert the input string to Unicode, if it is not already a Unicode
- string (default: ``'guess'``, which uses a simple UTF-8 / Locale /
- Latin1 detection. Can also be ``'chardet'`` to use the chardet
- library, if it is installed.
- ``inencoding``
- Overrides the ``encoding`` if given.
- """
-
- #: Name of the lexer
- name = None
-
- #: Shortcuts for the lexer
- aliases = []
-
- #: File name globs
- filenames = []
-
- #: Secondary file name globs
- alias_filenames = []
-
- #: MIME types
- mimetypes = []
-
- #: Priority, should multiple lexers match and no content is provided
- priority = 0
-
- def __init__(self, **options):
- self.options = options
- self.stripnl = get_bool_opt(options, 'stripnl', True)
- self.stripall = get_bool_opt(options, 'stripall', False)
- self.ensurenl = get_bool_opt(options, 'ensurenl', True)
- self.tabsize = get_int_opt(options, 'tabsize', 0)
- self.encoding = options.get('encoding', 'guess')
- self.encoding = options.get('inencoding') or self.encoding
- self.filters = []
- for filter_ in get_list_opt(options, 'filters', ()):
- self.add_filter(filter_)
-
- def __repr__(self):
- if self.options:
- return '' % (self.__class__.__name__,
- self.options)
- else:
- return '' % self.__class__.__name__
-
- def add_filter(self, filter_, **options):
- """
- Add a new stream filter to this lexer.
- """
- if not isinstance(filter_, Filter):
- filter_ = get_filter_by_name(filter_, **options)
- self.filters.append(filter_)
-
- def analyse_text(text):
- """
- Has to return a float between ``0`` and ``1`` that indicates
- if a lexer wants to highlight this text. Used by ``guess_lexer``.
- If this method returns ``0`` it won't highlight it in any case, if
- it returns ``1`` highlighting with this lexer is guaranteed.
-
- The `LexerMeta` metaclass automatically wraps this function so
- that it works like a static method (no ``self`` or ``cls``
- parameter) and the return value is automatically converted to
- `float`. If the return value is an object that is boolean `False`
- it's the same as if the return values was ``0.0``.
- """
-
- def get_tokens(self, text, unfiltered=False):
- """
- Return an iterable of (tokentype, value) pairs generated from
- `text`. If `unfiltered` is set to `True`, the filtering mechanism
- is bypassed even if filters are defined.
-
- Also preprocess the text, i.e. expand tabs and strip it if
- wanted and applies registered filters.
- """
- if not isinstance(text, str):
- if self.encoding == 'guess':
- text, _ = guess_decode(text)
- elif self.encoding == 'chardet':
- try:
- from pip._vendor import chardet
- except ImportError as e:
- raise ImportError('To enable chardet encoding guessing, '
- 'please install the chardet library '
- 'from http://chardet.feedparser.org/') from e
- # check for BOM first
- decoded = None
- for bom, encoding in _encoding_map:
- if text.startswith(bom):
- decoded = text[len(bom):].decode(encoding, 'replace')
- break
- # no BOM found, so use chardet
- if decoded is None:
- enc = chardet.detect(text[:1024]) # Guess using first 1KB
- decoded = text.decode(enc.get('encoding') or 'utf-8',
- 'replace')
- text = decoded
- else:
- text = text.decode(self.encoding)
- if text.startswith('\ufeff'):
- text = text[len('\ufeff'):]
- else:
- if text.startswith('\ufeff'):
- text = text[len('\ufeff'):]
-
- # text now *is* a unicode string
- text = text.replace('\r\n', '\n')
- text = text.replace('\r', '\n')
- if self.stripall:
- text = text.strip()
- elif self.stripnl:
- text = text.strip('\n')
- if self.tabsize > 0:
- text = text.expandtabs(self.tabsize)
- if self.ensurenl and not text.endswith('\n'):
- text += '\n'
-
- def streamer():
- for _, t, v in self.get_tokens_unprocessed(text):
- yield t, v
- stream = streamer()
- if not unfiltered:
- stream = apply_filters(stream, self.filters, self)
- return stream
-
- def get_tokens_unprocessed(self, text):
- """
- Return an iterable of (index, tokentype, value) pairs where "index"
- is the starting position of the token within the input text.
-
- In subclasses, implement this method as a generator to
- maximize effectiveness.
- """
- raise NotImplementedError
-
-
-class DelegatingLexer(Lexer):
- """
- This lexer takes two lexer as arguments. A root lexer and
- a language lexer. First everything is scanned using the language
- lexer, afterwards all ``Other`` tokens are lexed using the root
- lexer.
-
- The lexers from the ``template`` lexer package use this base lexer.
- """
-
- def __init__(self, _root_lexer, _language_lexer, _needle=Other, **options):
- self.root_lexer = _root_lexer(**options)
- self.language_lexer = _language_lexer(**options)
- self.needle = _needle
- Lexer.__init__(self, **options)
-
- def get_tokens_unprocessed(self, text):
- buffered = ''
- insertions = []
- lng_buffer = []
- for i, t, v in self.language_lexer.get_tokens_unprocessed(text):
- if t is self.needle:
- if lng_buffer:
- insertions.append((len(buffered), lng_buffer))
- lng_buffer = []
- buffered += v
- else:
- lng_buffer.append((i, t, v))
- if lng_buffer:
- insertions.append((len(buffered), lng_buffer))
- return do_insertions(insertions,
- self.root_lexer.get_tokens_unprocessed(buffered))
-
-
-# ------------------------------------------------------------------------------
-# RegexLexer and ExtendedRegexLexer
-#
-
-
-class include(str): # pylint: disable=invalid-name
- """
- Indicates that a state should include rules from another state.
- """
- pass
-
-
-class _inherit:
- """
- Indicates the a state should inherit from its superclass.
- """
- def __repr__(self):
- return 'inherit'
-
-inherit = _inherit() # pylint: disable=invalid-name
-
-
-class combined(tuple): # pylint: disable=invalid-name
- """
- Indicates a state combined from multiple states.
- """
-
- def __new__(cls, *args):
- return tuple.__new__(cls, args)
-
- def __init__(self, *args):
- # tuple.__init__ doesn't do anything
- pass
-
-
-class _PseudoMatch:
- """
- A pseudo match object constructed from a string.
- """
-
- def __init__(self, start, text):
- self._text = text
- self._start = start
-
- def start(self, arg=None):
- return self._start
-
- def end(self, arg=None):
- return self._start + len(self._text)
-
- def group(self, arg=None):
- if arg:
- raise IndexError('No such group')
- return self._text
-
- def groups(self):
- return (self._text,)
-
- def groupdict(self):
- return {}
-
-
-def bygroups(*args):
- """
- Callback that yields multiple actions for each group in the match.
- """
- def callback(lexer, match, ctx=None):
- for i, action in enumerate(args):
- if action is None:
- continue
- elif type(action) is _TokenType:
- data = match.group(i + 1)
- if data:
- yield match.start(i + 1), action, data
- else:
- data = match.group(i + 1)
- if data is not None:
- if ctx:
- ctx.pos = match.start(i + 1)
- for item in action(lexer,
- _PseudoMatch(match.start(i + 1), data), ctx):
- if item:
- yield item
- if ctx:
- ctx.pos = match.end()
- return callback
-
-
-class _This:
- """
- Special singleton used for indicating the caller class.
- Used by ``using``.
- """
-
-this = _This()
-
-
-def using(_other, **kwargs):
- """
- Callback that processes the match with a different lexer.
-
- The keyword arguments are forwarded to the lexer, except `state` which
- is handled separately.
-
- `state` specifies the state that the new lexer will start in, and can
- be an enumerable such as ('root', 'inline', 'string') or a simple
- string which is assumed to be on top of the root state.
-
- Note: For that to work, `_other` must not be an `ExtendedRegexLexer`.
- """
- gt_kwargs = {}
- if 'state' in kwargs:
- s = kwargs.pop('state')
- if isinstance(s, (list, tuple)):
- gt_kwargs['stack'] = s
- else:
- gt_kwargs['stack'] = ('root', s)
-
- if _other is this:
- def callback(lexer, match, ctx=None):
- # if keyword arguments are given the callback
- # function has to create a new lexer instance
- if kwargs:
- # XXX: cache that somehow
- kwargs.update(lexer.options)
- lx = lexer.__class__(**kwargs)
- else:
- lx = lexer
- s = match.start()
- for i, t, v in lx.get_tokens_unprocessed(match.group(), **gt_kwargs):
- yield i + s, t, v
- if ctx:
- ctx.pos = match.end()
- else:
- def callback(lexer, match, ctx=None):
- # XXX: cache that somehow
- kwargs.update(lexer.options)
- lx = _other(**kwargs)
-
- s = match.start()
- for i, t, v in lx.get_tokens_unprocessed(match.group(), **gt_kwargs):
- yield i + s, t, v
- if ctx:
- ctx.pos = match.end()
- return callback
-
-
-class default:
- """
- Indicates a state or state action (e.g. #pop) to apply.
- For example default('#pop') is equivalent to ('', Token, '#pop')
- Note that state tuples may be used as well.
-
- .. versionadded:: 2.0
- """
- def __init__(self, state):
- self.state = state
-
-
-class words(Future):
- """
- Indicates a list of literal words that is transformed into an optimized
- regex that matches any of the words.
-
- .. versionadded:: 2.0
- """
- def __init__(self, words, prefix='', suffix=''):
- self.words = words
- self.prefix = prefix
- self.suffix = suffix
-
- def get(self):
- return regex_opt(self.words, prefix=self.prefix, suffix=self.suffix)
-
-
-class RegexLexerMeta(LexerMeta):
- """
- Metaclass for RegexLexer, creates the self._tokens attribute from
- self.tokens on the first instantiation.
- """
-
- def _process_regex(cls, regex, rflags, state):
- """Preprocess the regular expression component of a token definition."""
- if isinstance(regex, Future):
- regex = regex.get()
- return re.compile(regex, rflags).match
-
- def _process_token(cls, token):
- """Preprocess the token component of a token definition."""
- assert type(token) is _TokenType or callable(token), \
- 'token type must be simple type or callable, not %r' % (token,)
- return token
-
- def _process_new_state(cls, new_state, unprocessed, processed):
- """Preprocess the state transition action of a token definition."""
- if isinstance(new_state, str):
- # an existing state
- if new_state == '#pop':
- return -1
- elif new_state in unprocessed:
- return (new_state,)
- elif new_state == '#push':
- return new_state
- elif new_state[:5] == '#pop:':
- return -int(new_state[5:])
- else:
- assert False, 'unknown new state %r' % new_state
- elif isinstance(new_state, combined):
- # combine a new state from existing ones
- tmp_state = '_tmp_%d' % cls._tmpname
- cls._tmpname += 1
- itokens = []
- for istate in new_state:
- assert istate != new_state, 'circular state ref %r' % istate
- itokens.extend(cls._process_state(unprocessed,
- processed, istate))
- processed[tmp_state] = itokens
- return (tmp_state,)
- elif isinstance(new_state, tuple):
- # push more than one state
- for istate in new_state:
- assert (istate in unprocessed or
- istate in ('#pop', '#push')), \
- 'unknown new state ' + istate
- return new_state
- else:
- assert False, 'unknown new state def %r' % new_state
-
- def _process_state(cls, unprocessed, processed, state):
- """Preprocess a single state definition."""
- assert type(state) is str, "wrong state name %r" % state
- assert state[0] != '#', "invalid state name %r" % state
- if state in processed:
- return processed[state]
- tokens = processed[state] = []
- rflags = cls.flags
- for tdef in unprocessed[state]:
- if isinstance(tdef, include):
- # it's a state reference
- assert tdef != state, "circular state reference %r" % state
- tokens.extend(cls._process_state(unprocessed, processed,
- str(tdef)))
- continue
- if isinstance(tdef, _inherit):
- # should be processed already, but may not in the case of:
- # 1. the state has no counterpart in any parent
- # 2. the state includes more than one 'inherit'
- continue
- if isinstance(tdef, default):
- new_state = cls._process_new_state(tdef.state, unprocessed, processed)
- tokens.append((re.compile('').match, None, new_state))
- continue
-
- assert type(tdef) is tuple, "wrong rule def %r" % tdef
-
- try:
- rex = cls._process_regex(tdef[0], rflags, state)
- except Exception as err:
- raise ValueError("uncompilable regex %r in state %r of %r: %s" %
- (tdef[0], state, cls, err)) from err
-
- token = cls._process_token(tdef[1])
-
- if len(tdef) == 2:
- new_state = None
- else:
- new_state = cls._process_new_state(tdef[2],
- unprocessed, processed)
-
- tokens.append((rex, token, new_state))
- return tokens
-
- def process_tokendef(cls, name, tokendefs=None):
- """Preprocess a dictionary of token definitions."""
- processed = cls._all_tokens[name] = {}
- tokendefs = tokendefs or cls.tokens[name]
- for state in list(tokendefs):
- cls._process_state(tokendefs, processed, state)
- return processed
-
- def get_tokendefs(cls):
- """
- Merge tokens from superclasses in MRO order, returning a single tokendef
- dictionary.
-
- Any state that is not defined by a subclass will be inherited
- automatically. States that *are* defined by subclasses will, by
- default, override that state in the superclass. If a subclass wishes to
- inherit definitions from a superclass, it can use the special value
- "inherit", which will cause the superclass' state definition to be
- included at that point in the state.
- """
- tokens = {}
- inheritable = {}
- for c in cls.__mro__:
- toks = c.__dict__.get('tokens', {})
-
- for state, items in toks.items():
- curitems = tokens.get(state)
- if curitems is None:
- # N.b. because this is assigned by reference, sufficiently
- # deep hierarchies are processed incrementally (e.g. for
- # A(B), B(C), C(RegexLexer), B will be premodified so X(B)
- # will not see any inherits in B).
- tokens[state] = items
- try:
- inherit_ndx = items.index(inherit)
- except ValueError:
- continue
- inheritable[state] = inherit_ndx
- continue
-
- inherit_ndx = inheritable.pop(state, None)
- if inherit_ndx is None:
- continue
-
- # Replace the "inherit" value with the items
- curitems[inherit_ndx:inherit_ndx+1] = items
- try:
- # N.b. this is the index in items (that is, the superclass
- # copy), so offset required when storing below.
- new_inh_ndx = items.index(inherit)
- except ValueError:
- pass
- else:
- inheritable[state] = inherit_ndx + new_inh_ndx
-
- return tokens
-
- def __call__(cls, *args, **kwds):
- """Instantiate cls after preprocessing its token definitions."""
- if '_tokens' not in cls.__dict__:
- cls._all_tokens = {}
- cls._tmpname = 0
- if hasattr(cls, 'token_variants') and cls.token_variants:
- # don't process yet
- pass
- else:
- cls._tokens = cls.process_tokendef('', cls.get_tokendefs())
-
- return type.__call__(cls, *args, **kwds)
-
-
-class RegexLexer(Lexer, metaclass=RegexLexerMeta):
- """
- Base for simple stateful regular expression-based lexers.
- Simplifies the lexing process so that you need only
- provide a list of states and regular expressions.
- """
-
- #: Flags for compiling the regular expressions.
- #: Defaults to MULTILINE.
- flags = re.MULTILINE
-
- #: At all time there is a stack of states. Initially, the stack contains
- #: a single state 'root'. The top of the stack is called "the current state".
- #:
- #: Dict of ``{'state': [(regex, tokentype, new_state), ...], ...}``
- #:
- #: ``new_state`` can be omitted to signify no state transition.
- #: If ``new_state`` is a string, it is pushed on the stack. This ensure
- #: the new current state is ``new_state``.
- #: If ``new_state`` is a tuple of strings, all of those strings are pushed
- #: on the stack and the current state will be the last element of the list.
- #: ``new_state`` can also be ``combined('state1', 'state2', ...)``
- #: to signify a new, anonymous state combined from the rules of two
- #: or more existing ones.
- #: Furthermore, it can be '#pop' to signify going back one step in
- #: the state stack, or '#push' to push the current state on the stack
- #: again. Note that if you push while in a combined state, the combined
- #: state itself is pushed, and not only the state in which the rule is
- #: defined.
- #:
- #: The tuple can also be replaced with ``include('state')``, in which
- #: case the rules from the state named by the string are included in the
- #: current one.
- tokens = {}
-
- def get_tokens_unprocessed(self, text, stack=('root',)):
- """
- Split ``text`` into (tokentype, text) pairs.
-
- ``stack`` is the inital stack (default: ``['root']``)
- """
- pos = 0
- tokendefs = self._tokens
- statestack = list(stack)
- statetokens = tokendefs[statestack[-1]]
- while 1:
- for rexmatch, action, new_state in statetokens:
- m = rexmatch(text, pos)
- if m:
- if action is not None:
- if type(action) is _TokenType:
- yield pos, action, m.group()
- else:
- yield from action(self, m)
- pos = m.end()
- if new_state is not None:
- # state transition
- if isinstance(new_state, tuple):
- for state in new_state:
- if state == '#pop':
- if len(statestack) > 1:
- statestack.pop()
- elif state == '#push':
- statestack.append(statestack[-1])
- else:
- statestack.append(state)
- elif isinstance(new_state, int):
- # pop, but keep at least one state on the stack
- # (random code leading to unexpected pops should
- # not allow exceptions)
- if abs(new_state) >= len(statestack):
- del statestack[1:]
- else:
- del statestack[new_state:]
- elif new_state == '#push':
- statestack.append(statestack[-1])
- else:
- assert False, "wrong state def: %r" % new_state
- statetokens = tokendefs[statestack[-1]]
- break
- else:
- # We are here only if all state tokens have been considered
- # and there was not a match on any of them.
- try:
- if text[pos] == '\n':
- # at EOL, reset state to "root"
- statestack = ['root']
- statetokens = tokendefs['root']
- yield pos, Text, '\n'
- pos += 1
- continue
- yield pos, Error, text[pos]
- pos += 1
- except IndexError:
- break
-
-
-class LexerContext:
- """
- A helper object that holds lexer position data.
- """
-
- def __init__(self, text, pos, stack=None, end=None):
- self.text = text
- self.pos = pos
- self.end = end or len(text) # end=0 not supported ;-)
- self.stack = stack or ['root']
-
- def __repr__(self):
- return 'LexerContext(%r, %r, %r)' % (
- self.text, self.pos, self.stack)
-
-
-class ExtendedRegexLexer(RegexLexer):
- """
- A RegexLexer that uses a context object to store its state.
- """
-
- def get_tokens_unprocessed(self, text=None, context=None):
- """
- Split ``text`` into (tokentype, text) pairs.
- If ``context`` is given, use this lexer context instead.
- """
- tokendefs = self._tokens
- if not context:
- ctx = LexerContext(text, 0)
- statetokens = tokendefs['root']
- else:
- ctx = context
- statetokens = tokendefs[ctx.stack[-1]]
- text = ctx.text
- while 1:
- for rexmatch, action, new_state in statetokens:
- m = rexmatch(text, ctx.pos, ctx.end)
- if m:
- if action is not None:
- if type(action) is _TokenType:
- yield ctx.pos, action, m.group()
- ctx.pos = m.end()
- else:
- yield from action(self, m, ctx)
- if not new_state:
- # altered the state stack?
- statetokens = tokendefs[ctx.stack[-1]]
- # CAUTION: callback must set ctx.pos!
- if new_state is not None:
- # state transition
- if isinstance(new_state, tuple):
- for state in new_state:
- if state == '#pop':
- if len(ctx.stack) > 1:
- ctx.stack.pop()
- elif state == '#push':
- ctx.stack.append(ctx.stack[-1])
- else:
- ctx.stack.append(state)
- elif isinstance(new_state, int):
- # see RegexLexer for why this check is made
- if abs(new_state) >= len(ctx.stack):
- del ctx.state[1:]
- else:
- del ctx.stack[new_state:]
- elif new_state == '#push':
- ctx.stack.append(ctx.stack[-1])
- else:
- assert False, "wrong state def: %r" % new_state
- statetokens = tokendefs[ctx.stack[-1]]
- break
- else:
- try:
- if ctx.pos >= ctx.end:
- break
- if text[ctx.pos] == '\n':
- # at EOL, reset state to "root"
- ctx.stack = ['root']
- statetokens = tokendefs['root']
- yield ctx.pos, Text, '\n'
- ctx.pos += 1
- continue
- yield ctx.pos, Error, text[ctx.pos]
- ctx.pos += 1
- except IndexError:
- break
-
-
-def do_insertions(insertions, tokens):
- """
- Helper for lexers which must combine the results of several
- sublexers.
-
- ``insertions`` is a list of ``(index, itokens)`` pairs.
- Each ``itokens`` iterable should be inserted at position
- ``index`` into the token stream given by the ``tokens``
- argument.
-
- The result is a combined token stream.
-
- TODO: clean up the code here.
- """
- insertions = iter(insertions)
- try:
- index, itokens = next(insertions)
- except StopIteration:
- # no insertions
- yield from tokens
- return
-
- realpos = None
- insleft = True
-
- # iterate over the token stream where we want to insert
- # the tokens from the insertion list.
- for i, t, v in tokens:
- # first iteration. store the postition of first item
- if realpos is None:
- realpos = i
- oldi = 0
- while insleft and i + len(v) >= index:
- tmpval = v[oldi:index - i]
- if tmpval:
- yield realpos, t, tmpval
- realpos += len(tmpval)
- for it_index, it_token, it_value in itokens:
- yield realpos, it_token, it_value
- realpos += len(it_value)
- oldi = index - i
- try:
- index, itokens = next(insertions)
- except StopIteration:
- insleft = False
- break # not strictly necessary
- if oldi < len(v):
- yield realpos, t, v[oldi:]
- realpos += len(v) - oldi
-
- # leftover tokens
- while insleft:
- # no normal tokens, set realpos to zero
- realpos = realpos or 0
- for p, t, v in itokens:
- yield realpos, t, v
- realpos += len(v)
- try:
- index, itokens = next(insertions)
- except StopIteration:
- insleft = False
- break # not strictly necessary
-
-
-class ProfilingRegexLexerMeta(RegexLexerMeta):
- """Metaclass for ProfilingRegexLexer, collects regex timing info."""
-
- def _process_regex(cls, regex, rflags, state):
- if isinstance(regex, words):
- rex = regex_opt(regex.words, prefix=regex.prefix,
- suffix=regex.suffix)
- else:
- rex = regex
- compiled = re.compile(rex, rflags)
-
- def match_func(text, pos, endpos=sys.maxsize):
- info = cls._prof_data[-1].setdefault((state, rex), [0, 0.0])
- t0 = time.time()
- res = compiled.match(text, pos, endpos)
- t1 = time.time()
- info[0] += 1
- info[1] += t1 - t0
- return res
- return match_func
-
-
-class ProfilingRegexLexer(RegexLexer, metaclass=ProfilingRegexLexerMeta):
- """Drop-in replacement for RegexLexer that does profiling of its regexes."""
-
- _prof_data = []
- _prof_sort_index = 4 # defaults to time per call
-
- def get_tokens_unprocessed(self, text, stack=('root',)):
- # this needs to be a stack, since using(this) will produce nested calls
- self.__class__._prof_data.append({})
- yield from RegexLexer.get_tokens_unprocessed(self, text, stack)
- rawdata = self.__class__._prof_data.pop()
- data = sorted(((s, repr(r).strip('u\'').replace('\\\\', '\\')[:65],
- n, 1000 * t, 1000 * t / n)
- for ((s, r), (n, t)) in rawdata.items()),
- key=lambda x: x[self._prof_sort_index],
- reverse=True)
- sum_total = sum(x[3] for x in data)
-
- print()
- print('Profiling result for %s lexing %d chars in %.3f ms' %
- (self.__class__.__name__, len(text), sum_total))
- print('=' * 110)
- print('%-20s %-64s ncalls tottime percall' % ('state', 'regex'))
- print('-' * 110)
- for d in data:
- print('%-20s %-65s %5d %8.4f %8.4f' % d)
- print('=' * 110)
diff --git a/spaces/profayle/TerrapinTalk/myenv/lib/python3.9/site-packages/pip/_vendor/tomli/_re.py b/spaces/profayle/TerrapinTalk/myenv/lib/python3.9/site-packages/pip/_vendor/tomli/_re.py
deleted file mode 100644
index 3883fdd9c9069c3655321da77d16499506b49958..0000000000000000000000000000000000000000
--- a/spaces/profayle/TerrapinTalk/myenv/lib/python3.9/site-packages/pip/_vendor/tomli/_re.py
+++ /dev/null
@@ -1,83 +0,0 @@
-from datetime import date, datetime, time, timedelta, timezone, tzinfo
-import re
-from typing import TYPE_CHECKING, Any, Optional, Union
-
-if TYPE_CHECKING:
- from re import Match
-
- from pip._vendor.tomli._parser import ParseFloat
-
-# E.g.
-# - 00:32:00.999999
-# - 00:32:00
-_TIME_RE_STR = r"([01][0-9]|2[0-3]):([0-5][0-9]):([0-5][0-9])(\.[0-9]+)?"
-
-RE_HEX = re.compile(r"[0-9A-Fa-f](?:_?[0-9A-Fa-f])*")
-RE_BIN = re.compile(r"[01](?:_?[01])*")
-RE_OCT = re.compile(r"[0-7](?:_?[0-7])*")
-RE_NUMBER = re.compile(
- r"[+-]?(?:0|[1-9](?:_?[0-9])*)" # integer
- + r"(?:\.[0-9](?:_?[0-9])*)?" # optional fractional part
- + r"(?:[eE][+-]?[0-9](?:_?[0-9])*)?" # optional exponent part
-)
-RE_LOCALTIME = re.compile(_TIME_RE_STR)
-RE_DATETIME = re.compile(
- r"([0-9]{4})-(0[1-9]|1[0-2])-(0[1-9]|1[0-9]|2[0-9]|3[01])" # date, e.g. 1988-10-27
- + r"(?:"
- + r"[T ]"
- + _TIME_RE_STR
- + r"(?:(Z)|([+-])([01][0-9]|2[0-3]):([0-5][0-9]))?" # time offset
- + r")?"
-)
-
-
-def match_to_datetime(match: "Match") -> Union[datetime, date]:
- """Convert a `RE_DATETIME` match to `datetime.datetime` or `datetime.date`.
-
- Raises ValueError if the match does not correspond to a valid date
- or datetime.
- """
- (
- year_str,
- month_str,
- day_str,
- hour_str,
- minute_str,
- sec_str,
- micros_str,
- zulu_time,
- offset_dir_str,
- offset_hour_str,
- offset_minute_str,
- ) = match.groups()
- year, month, day = int(year_str), int(month_str), int(day_str)
- if hour_str is None:
- return date(year, month, day)
- hour, minute, sec = int(hour_str), int(minute_str), int(sec_str)
- micros = int(micros_str[1:].ljust(6, "0")[:6]) if micros_str else 0
- if offset_dir_str:
- offset_dir = 1 if offset_dir_str == "+" else -1
- tz: Optional[tzinfo] = timezone(
- timedelta(
- hours=offset_dir * int(offset_hour_str),
- minutes=offset_dir * int(offset_minute_str),
- )
- )
- elif zulu_time:
- tz = timezone.utc
- else: # local date-time
- tz = None
- return datetime(year, month, day, hour, minute, sec, micros, tzinfo=tz)
-
-
-def match_to_localtime(match: "Match") -> time:
- hour_str, minute_str, sec_str, micros_str = match.groups()
- micros = int(micros_str[1:].ljust(6, "0")[:6]) if micros_str else 0
- return time(int(hour_str), int(minute_str), int(sec_str), micros)
-
-
-def match_to_number(match: "Match", parse_float: "ParseFloat") -> Any:
- match_str = match.group()
- if "." in match_str or "e" in match_str or "E" in match_str:
- return parse_float(match_str)
- return int(match_str)
diff --git a/spaces/profayle/TerrapinTalk/myenv/lib/python3.9/site-packages/tomlkit/_types.py b/spaces/profayle/TerrapinTalk/myenv/lib/python3.9/site-packages/tomlkit/_types.py
deleted file mode 100644
index cc1847b5e69447bb934076be14b66766aedb22c1..0000000000000000000000000000000000000000
--- a/spaces/profayle/TerrapinTalk/myenv/lib/python3.9/site-packages/tomlkit/_types.py
+++ /dev/null
@@ -1,65 +0,0 @@
-from __future__ import annotations
-
-from typing import TYPE_CHECKING
-from typing import Any
-from typing import TypeVar
-
-
-WT = TypeVar("WT", bound="WrapperType")
-
-if TYPE_CHECKING: # pragma: no cover
- # Define _CustomList and _CustomDict as a workaround for:
- # https://github.com/python/mypy/issues/11427
- #
- # According to this issue, the typeshed contains a "lie"
- # (it adds MutableSequence to the ancestry of list and MutableMapping to
- # the ancestry of dict) which completely messes with the type inference for
- # Table, InlineTable, Array and Container.
- #
- # Importing from builtins is preferred over simple assignment, see issues:
- # https://github.com/python/mypy/issues/8715
- # https://github.com/python/mypy/issues/10068
- from builtins import dict as _CustomDict # noqa: N812
- from builtins import float as _CustomFloat # noqa: N812
- from builtins import int as _CustomInt # noqa: N812
- from builtins import list as _CustomList # noqa: N812
- from typing import Callable
- from typing import Concatenate
- from typing import ParamSpec
- from typing import Protocol
-
- P = ParamSpec("P")
-
- class WrapperType(Protocol):
- def _new(self: WT, value: Any) -> WT:
- ...
-
-else:
- from collections.abc import MutableMapping
- from collections.abc import MutableSequence
- from numbers import Integral
- from numbers import Real
-
- class _CustomList(MutableSequence, list):
- """Adds MutableSequence mixin while pretending to be a builtin list"""
-
- class _CustomDict(MutableMapping, dict):
- """Adds MutableMapping mixin while pretending to be a builtin dict"""
-
- class _CustomInt(Integral, int):
- """Adds Integral mixin while pretending to be a builtin int"""
-
- class _CustomFloat(Real, float):
- """Adds Real mixin while pretending to be a builtin float"""
-
-
-def wrap_method(
- original_method: Callable[Concatenate[WT, P], Any]
-) -> Callable[Concatenate[WT, P], Any]:
- def wrapper(self: WT, *args: P.args, **kwargs: P.kwargs) -> Any:
- result = original_method(self, *args, **kwargs)
- if result is NotImplemented:
- return result
- return self._new(result)
-
- return wrapper
diff --git a/spaces/pycoming/bingo/src/components/ui/select.tsx b/spaces/pycoming/bingo/src/components/ui/select.tsx
deleted file mode 100644
index 77f12c2996f541b97663de4c9e20ab34d4ec2fac..0000000000000000000000000000000000000000
--- a/spaces/pycoming/bingo/src/components/ui/select.tsx
+++ /dev/null
@@ -1,123 +0,0 @@
-'use client'
-
-import * as React from 'react'
-import * as SelectPrimitive from '@radix-ui/react-select'
-
-import { cn } from '@/lib/utils'
-import {
- IconArrowDown,
- IconCheck,
- IconChevronUpDown
-} from '@/components/ui/icons'
-
-const Select = SelectPrimitive.Root
-
-const SelectGroup = SelectPrimitive.Group
-
-const SelectValue = SelectPrimitive.Value
-
-const SelectTrigger = React.forwardRef<
- React.ElementRef