diff --git a/spaces/1acneusushi/gradio-2dmoleculeeditor/data/3.7 V Batteries How to Choose Use and Maintain Them for Optimal Performance.md b/spaces/1acneusushi/gradio-2dmoleculeeditor/data/3.7 V Batteries How to Choose Use and Maintain Them for Optimal Performance.md
deleted file mode 100644
index ffadc121bd0cf24da32605942fc5d4d7e5ea2f18..0000000000000000000000000000000000000000
--- a/spaces/1acneusushi/gradio-2dmoleculeeditor/data/3.7 V Batteries How to Choose Use and Maintain Them for Optimal Performance.md
+++ /dev/null
@@ -1,28 +0,0 @@
-
-
How Long Do 3.7 V Batteries Last and How to Make Them Last Longer?
-
If you use devices that run on lithium-ion batteries, such as smartphones, tablets, laptops, or electric vehicles, you may wonder how long do 3.7 V batteries last and how to extend their lifespan. In this article, we will answer these questions and provide some useful tips on how to care for your 3.7 V batteries.
-
What are 3.7 V Batteries?
-
3.7 V batteries are a type of lithium-ion batteries that have a nominal voltage of 3.7 volts. They are also known as ternary lithium batteries because they use a combination of three metals (nickel, cobalt, and manganese) as the cathode material. The anode material is usually graphite.
3.7 V batteries are widely used for powering various devices, such as smartphones, tablets, laptops, cameras, drones, electric bikes, and electric vehicles. They have many advantages over other types of batteries, such as:
-
-
High energy density: They can store more energy per unit weight and volume than other batteries.
-
Long cycle life: They can be recharged and discharged for hundreds or thousands of times without losing much capacity.
-
Low self-discharge: They lose only about 3.5% of their charge per month when stored at room temperature.
-
No memory effect: They do not need to be fully discharged before recharging to maintain their performance.
-
Environmentally friendly: They do not contain toxic metals such as lead, mercury, or cadmium.
-
-
How Long Do 3.7 V Batteries Last?
-
The answer to this question depends on several factors, such as the capacity of the battery, the power consumption of the device, the charging and discharging habits of the user, and the environmental conditions.
-
The capacity of the battery is measured in milliampere-hours (mAh), which indicates how much current the battery can provide for one hour. The higher the capacity, the longer the battery can last. For example, a 1000 mAh battery can provide 1000 mA of current for one hour, or 500 mA for two hours, or 250 mA for four hours, and so on.
-
The power consumption of the device is measured in watts (W), which indicates how much energy the device uses per unit time. The higher the power consumption, the faster the battery drains. For example, a 100 W light bulb consumes 100 W of energy per hour, while a 10 W LED bulb consumes only 10 W of energy per hour.
-
The charging and discharging habits of the user also affect the lifespan of the battery. Generally speaking, it is better to keep the battery between 20% and 80% of its full charge level and avoid overcharging or overdischarging it. Overcharging can cause overheating and damage the battery cells, while overdischarging can cause voltage drop and reduce the battery performance.
-
The environmental conditions also play a role in the battery life. High temperatures can accelerate the chemical reactions inside the battery and degrade its capacity and performance. Low temperatures can slow down the chemical reactions and reduce the available capacity and power output. Ideally, the battery should be stored and used at room temperature (around 25°C).
-
Given these factors, it is hard to give an exact number for how long a 3.7 V battery can last. However, we can give some rough estimates based on some common scenarios:
-
-
-
If you use a 3.7 V battery with a capacity of 1000 mAh to power a device that consumes 100 mA of current (such as a flashlight), it can last for about 10 hours before needing to be recharged.
-
If you use a 3.7 V battery with a capacity of 3000 mAh to power a device that consumes 500 mA of current (such as a smartphone), it can last for about 6 hours before needing to be recharged.
-
If you use a 3.7 V battery with a capacity of 5000 mAh to power a device that consumes 1000 mA of current (such as a tablet), it can last for about 5 hours before needing ddb901b051
-
-
\ No newline at end of file
diff --git a/spaces/1acneusushi/gradio-2dmoleculeeditor/data/Adobe Edge Animate Cc 2014 Serial 66 New Features and Enhancements for Animation Designers.md b/spaces/1acneusushi/gradio-2dmoleculeeditor/data/Adobe Edge Animate Cc 2014 Serial 66 New Features and Enhancements for Animation Designers.md
deleted file mode 100644
index 42380cf4a8ee9e33cc1c57d71136e16b7e6406db..0000000000000000000000000000000000000000
--- a/spaces/1acneusushi/gradio-2dmoleculeeditor/data/Adobe Edge Animate Cc 2014 Serial 66 New Features and Enhancements for Animation Designers.md
+++ /dev/null
@@ -1,114 +0,0 @@
-
-
Adobe Edge Animate CC 2014: A Powerful Tool for Creating Rich Animations
-
If you are looking for a web development tool that lets you create stunning animations using HTML5, JavaScript, and CSS3, you should check out Adobe Edge Animate CC 2014. This software is part of the Adobe Edge suite, which also includes Edge Code, Edge Reflow, Edge Inspect, and Edge Web Fonts. You can download a free 30-day trial version of Adobe Edge Animate CC 2014 from Adobe Creative Cloud and see for yourself what it can do.
In this article, we will give you an overview of what Adobe Edge Animate CC 2014 is, what are its new features and enhancements, and how to get started with it. By the end of this article, you will have a better understanding of how Adobe Edge Animate CC 2014 can help you create rich animations for your web projects.
-
What is Adobe Edge Animate CC 2014?
-
Adobe Edge Animate CC 2014 is a web development tool that uses HTML5, JavaScript, and CSS3 functionality to create animations that run on any modern browser or device. You can use it to create interactive banners, infographics, slideshows, games, and more. You can also import assets from other Adobe tools such as Photoshop, Illustrator, or Flash Professional.
-
Adobe Edge Animate CC 2014 is a part of the Adobe Edge suite, which is a collection of tools and services that help you design and develop responsive web content. The other tools in the suite are:
-
-
Edge Code: A code editor that integrates with Edge Animate and other web tools.
-
Edge Reflow: A responsive design tool that lets you create layouts that adapt to different screen sizes.
-
Edge Inspect: A tool that lets you preview and debug your web content across multiple devices.
-
Edge Web Fonts: A service that provides access to hundreds of free web fonts.
-
-
You can download a free 30-day trial version of Adobe Edge Animate CC 2014 from Adobe Creative Cloud. You will need an Adobe ID to sign in and access the software. You can also purchase a subscription plan that gives you access to all the tools in the suite as well as other benefits such as cloud storage, online services, and updates.
-
What are the new features and enhancements of Adobe Edge Animate CC 2014?
-
The 2014 release of Adobe Edge Animate CC includes several new features and enhancements that make it easier and faster to create rich animations. Here are some of them:
-
Support for native HTML5 video
-
Adobe Edge Animate CC 2014 provides an intuitive user interface that lets you import HTML5 videos into your compositions. You can drag and drop a video file from your computer or browse for one online. The video then can be used as part of an overlay and can have other composition elements animate around it. You can also control the playback options such as autoplay, loop, mute, or volume.
-
How to activate Adobe Edge Animate Cc 2014 with serial number
-Adobe Edge Animate Cc 2014 crack download free
-Adobe Edge Animate Cc 2014 license key generator online
-Adobe Edge Animate Cc 2014 full version for windows 10
-Adobe Edge Animate Cc 2014 tutorial pdf download
-Adobe Edge Animate Cc 2014 serial key expired fix
-Adobe Edge Animate Cc 2014 mac os x download
-Adobe Edge Animate Cc 2014 alternative software
-Adobe Edge Animate Cc 2014 system requirements
-Adobe Edge Animate Cc 2014 discount coupon code
-Adobe Edge Animate Cc 2014 review and features
-Adobe Edge Animate Cc 2014 update patch download
-Adobe Edge Animate Cc 2014 offline installer setup
-Adobe Edge Animate Cc 2014 product key finder
-Adobe Edge Animate Cc 2014 trial version download
-Adobe Edge Animate Cc 2014 serial number not working
-Adobe Edge Animate Cc 2014 keygen free download
-Adobe Edge Animate Cc 2014 animation examples and templates
-Adobe Edge Animate Cc 2014 vs flash professional
-Adobe Edge Animate Cc 2014 support and help
-Adobe Edge Animate Cc 2014 activation code free
-Adobe Edge Animate Cc 2014 crack file download
-Adobe Edge Animate Cc 2014 license key list
-Adobe Edge Animate Cc 2014 full version for windows 7
-Adobe Edge Animate Cc 2014 tutorial video download
-Adobe Edge Animate Cc 2014 serial key generator online
-Adobe Edge Animate Cc 2014 mac os x crack
-Adobe Edge Animate Cc 2014 best practices and tips
-Adobe Edge Animate Cc 2014 minimum requirements
-Adobe Edge Animate Cc 2014 promo code and offer
-Adobe Edge Animate Cc 2014 feedback and rating
-Adobe Edge Animate Cc 2014 latest version download
-Adobe Edge Animate Cc 2014 online installer setup
-Adobe Edge Animate Cc 2014 product key checker
-Adobe Edge Animate Cc 2014 free download with crack
-Adobe Edge Animate Cc 2014 serial number invalid fix
-Adobe Edge Animate Cc 2014 keygen download free
-Adobe Edge Animate Cc 2014 animation tutorial for beginners
-Adobe Edge Animate Cc 2014 comparison with other tools
-Adobe Edge Animate Cc 2014 contact and support number
-Adobe Edge Animate Cc 2014 registration code free
-Adobe Edge Animate Cc 2014 crack only download
-Adobe Edge Animate Cc 2014 license key free download
-Adobe Edge Animate Cc 2014 full version for windows xp
-Adobe Edge Animate Cc 2014 tutorial ebook download
-Adobe Edge Animate Cc 2014 serial key crack online
-Adobe Edge Animate Cc 2014 mac os x serial number
-Adobe Edge Animate Cc 2014 learning resources and guides
-Adobe Edge Animate Cc 2014 recommended requirements
-Adobe Edge Animate Cc 2014 coupon code and deal
-
One of the advantages of using native HTML5 video is that it plays on iOS and Android devices as well as in modern desktop browsers. You don't need to worry about converting your video into different formats or using plugins such as Flash Player.
With Adobe Edge Animate CC 2014, you can import sprite sheets to add advanced, multi-frame animations to your compositions. Sprite sheets are images that contain multiple frames of an animation in a single file. They let your graphics download faster with fewer HTTP requests.
-
You can import sprite sheets (File > Import Spritesheet) generated in Adobe Flash Professional CC 2014 or any other tool that lets you generate sprite sheets. You can then adjust the settings such as frame rate, frame size, number of rows and columns, etc. Automatic keyframing of sprites on import saves time by reducing effort spent with manual positioning of frames.
-
For more information on how to import sprite sheets into Adobe Edge Animate CC 2014, see Import sprite sheets.
-
Article linking for Adobe DPS
-
Adobe Edge Animate CC 2014 lets you link to your Adobe InDesign or DPS Folio articles using the options on the user interface without writing code. You can create interactive title pages, table of contents, and advanced navigation to target articles and article subsections of your digital publications more easily and quickly.
-
You can use the Link To option in the Properties panel to select an article or a subsection from a list of available options. You can also use the Go To Folio Page option to jump to a specific page number in your folio.
The Actions pop-up window has been redesigned to be more designer-friendly and reduce the need to code. The enhanced Actions editor makes it easier to add interactivity and more approachable for designers.
-
The new Actions editor visually guides you through the various steps in assigning actions to targets. You can follow these steps:
-
-
Pick an Action - Actions are now logically grouped into categories such as Timeline Control, Element Control, Navigation Control, etc. If you know the name of the action, you can search for it using the search box. Otherwise, pick a category to view the actions in it and click the required action.
-
Pick a Target - Targets are grouped under Stage. Click Stage to view the target elements. When you click Stage, you may find a subcategory for Symbols if your composition contains symbols. Double-click the target element.
-
Modify the code snippet as required. You can use the code hints feature to autocomplete syntax or parameters.
-
-
If you find portions of code that are reused often, you can save them as snippets and insert them with a single click when required. You can also access predefined snippets such as Stop All Timelines or Play All Timelines from the Snippets menu.
- - Mac OS: Multicore Intel processor; Mac OS X v10.7 or v10.8; 1 GB of RAM; 200 MB of available hard-disk space for installation; Internet connection and registration are necessary for required software activation.
-
Q: How can I update Adobe Edge Animate CC 2014?
-A: You can update Adobe Edge Animate CC 2014 by using the Adobe Creative Cloud desktop app. You can also check for updates manually by clicking Help > Updates in the software.
-
Q: How can I get help or support for Adobe Edge Animate CC 2014?
-A: You can get help or support for Adobe Edge Animate CC 2014 by visiting the official website at https://www.adobe.com/products/edge-animate.html. You can also find tutorials, videos, forums, blogs, and other resources at https://helpx.adobe.com/edge-animate.html. You can also contact Adobe customer care or technical support by phone, chat, or email.
Q: How can I share my Adobe Edge Animate CC 2014 projects with others?
-A: You can share your Adobe Edge Animate CC 2014 projects with others by publishing them to the web or other platforms. You can also export them as OAM files and import them into other Adobe tools such as InDesign, Muse, Dreamweaver, or Flash Professional. You can also share your projects on social media platforms such as Facebook, Twitter, or Google+.
-
Q: How can I get feedback or suggestions for my Adobe Edge Animate CC 2014 projects?
-A: You can get feedback or suggestions for your Adobe Edge Animate CC 2014 projects by joining online communities of other users and experts. Some of the online communities are:
-- Adobe Edge Animate Forum
-- Edge Hero
-- EdgeDocks
-You can also participate in contests or challenges that are hosted by Adobe or other organizations.
-
- 0a6ba089eb
-
-
\ No newline at end of file
diff --git a/spaces/1acneusushi/gradio-2dmoleculeeditor/data/Adobe Lightroom 4 Amtlib.dll UPD.md b/spaces/1acneusushi/gradio-2dmoleculeeditor/data/Adobe Lightroom 4 Amtlib.dll UPD.md
deleted file mode 100644
index 7dc5c7ea7016d447acfdb97e55347362081c0065..0000000000000000000000000000000000000000
--- a/spaces/1acneusushi/gradio-2dmoleculeeditor/data/Adobe Lightroom 4 Amtlib.dll UPD.md
+++ /dev/null
@@ -1,23 +0,0 @@
-
-
How to Fix Adobe Lightroom 4 amtlib.dll Missing Error
-
If you are trying to open Adobe Lightroom 4 and get an error message saying that amtlib.dll is missing, you are not alone. Many users have reported this problem and there are some possible solutions to fix it.
-
amtlib.dll is a file that belongs to Adobe Systems, Incorporated AMT Licensing, which is a component of Adobe products that handles the activation and licensing of the software. If this file is corrupted, deleted, or misplaced, you may encounter errors when trying to run Adobe Lightroom 4 or other Adobe applications.
Here are some steps you can try to fix the amtlib.dll missing error:
-
-
Reinstall Adobe Lightroom 4. The easiest and most recommended way to fix the error is to uninstall and reinstall Adobe Lightroom 4 using the original installation media or the download link from Adobe's website[^1^]. This will ensure that you have the latest and correct version of amtlib.dll and other files needed for the software to run properly.
-
Use the Adobe Cleaner Tool. If reinstalling Adobe Lightroom 4 does not work, you can try using the Adobe Cleaner Tool to remove any traces of the software from your system. The Adobe Cleaner Tool is a utility that can help you resolve installation problems by removing corrupted or incompatible files and registry entries related to Adobe products[^1^]. You can download and run the Adobe Cleaner Tool from this link: Use the Creative Cloud Cleaner Tool to solve installation problems
-
Download amtlib.dll from a trusted source. If none of the above methods work, you can try downloading amtlib.dll from a reliable website that provides DLL files for free. However, this is not recommended as it may expose your system to malware or viruses, or cause compatibility issues with other Adobe products. If you decide to download amtlib.dll from a third-party source, make sure you scan it with an antivirus program before copying it to your system folder or your Adobe Lightroom 4 installation folder[^2^] [^3^].
-
-
We hope this article has helped you fix the amtlib.dll missing error and enjoy using Adobe Lightroom 4. If you have any questions or feedback, please leave a comment below.
Here are some more tips and tricks to use Adobe Lightroom 4 effectively:
-
-
Use presets to apply different effects and adjustments to your photos with one click. You can find presets in the Develop module, under the Presets panel. You can also create your own presets or download presets from other users online.
-
Use the histogram to check the exposure and contrast of your photos. The histogram is a graphical representation of the distribution of tones in your image, from black to white. You can find the histogram in the top right corner of the Library and Develop modules. You can adjust the exposure and contrast of your photos by dragging the sliders below the histogram or by using the Basic panel.
-
Use the crop and straighten tools to improve the composition and alignment of your photos. You can access these tools by clicking on the Crop Overlay icon in the toolbar below the photo or by pressing R on your keyboard. You can drag the corners or sides of the crop box to resize it, or rotate it by dragging outside the box. You can also use the Angle slider or the Straighten tool to level the horizon or vertical lines in your photo.
-
Use keywords and collections to organize and find your photos easily. Keywords are descriptive words or phrases that you can assign to your photos to help you search for them later. You can add keywords to your photos in the Library module, under the Keywording panel. Collections are groups of photos that you can create based on any criteria you want. You can create collections in the Library module, under the Collections panel.
-
Use the export function to save and share your photos in different formats and sizes. You can export your photos by selecting them in the Library module and clicking on File > Export or by pressing Ctrl+Shift+E on your keyboard. You can choose where to save your photos, what format and quality to use, how to rename them, and how to resize them. You can also export your photos directly to email, web, or other applications.
-
-
We hope this article has helped you learn more about Adobe Lightroom 4 and how to use it for your photography needs. If you have any questions or feedback, please leave a comment below.
- 7b8c122e87
-
-
\ No newline at end of file
diff --git a/spaces/1acneusushi/gradio-2dmoleculeeditor/data/Chris Letchford Guitar Technique Book Pdf.md b/spaces/1acneusushi/gradio-2dmoleculeeditor/data/Chris Letchford Guitar Technique Book Pdf.md
deleted file mode 100644
index c4b2248c1525596c15caa566f126812030df4113..0000000000000000000000000000000000000000
--- a/spaces/1acneusushi/gradio-2dmoleculeeditor/data/Chris Letchford Guitar Technique Book Pdf.md
+++ /dev/null
@@ -1,26 +0,0 @@
-
-
Review: Chris Letchford's Guitar Technique Book
-
If you are a fan of progressive instrumental metal band Scale The Summit, you might be interested in learning from their guitarist and founder Chris Letchford. He has released a guitar technique book that contains 52 exercises for 6-string guitars, covering various aspects of modern guitar playing such as alternate picking, legato, sweeping, tapping, hybrid picking, string skipping, and more.
-
The book is not your typical technique book with a bunch of non melodic, unusable ideas. They are all melodic, which can be applied for song writing, improv, and everyday day guitar use. It's a perfect book for building your chops and mastering the fingerboard! Great for all styles of music, from classical to metal, rock, latin, jazz, and country.
The book is also spiral bound, which makes it stay open when laying flat or on a music stand. Why all music books aren't made this way is beyond me!
-
Each exercise is notated with standard notation and tablature, as well as fingerings, pick directions, and tapping fingers. The exercises are designed to challenge your technique, accuracy, speed, and musicality. They are also fun to play and sound great.
-
Chris Letchford is an accomplished guitarist who has studied at MIT, Berklee School of Music and the Houston Community College. He has also toured with Dream Theater and other renowned bands. He knows what he is talking about when it comes to guitar technique and music theory.
-
If you want to learn from one of the best guitarists in the genre and improve your skills on the instrument, you should definitely check out Chris Letchford's Guitar Technique Book. You can order it from his website or from Amazon. You can also get the tab books for Scale The Summit's albums if you want to learn their songs.
-
Chris Letchford's Guitar Technique Book is a valuable resource for any guitarist who wants to take their playing to the next level. It is well written, well presented, and well worth the money.
-
-
-
Here are some examples of the exercises from the book:
-
-
Exercise 1: This exercise is a simple alternate picking exercise that uses the major scale in three octaves. It helps you develop your picking accuracy and speed across the strings. You can practice it in different keys and positions.
-
Exercise 10: This exercise is a legato exercise that uses the harmonic minor scale in three octaves. It helps you develop your finger strength and coordination on the fretboard. You can practice it with different rhythms and articulations.
-
Exercise 20: This exercise is a sweeping exercise that uses the diminished arpeggio in three octaves. It helps you develop your sweeping technique and economy of motion. You can practice it with different inversions and patterns.
-
Exercise 30: This exercise is a tapping exercise that uses the melodic minor scale in three octaves. It helps you develop your tapping technique and finger independence. You can practice it with different combinations of fingers and strings.
-
Exercise 40: This exercise is a hybrid picking exercise that uses the pentatonic scale in three octaves. It helps you develop your hybrid picking technique and versatility. You can practice it with different accents and dynamics.
-
Exercise 50: This exercise is a string skipping exercise that uses the whole tone scale in three octaves. It helps you develop your string skipping technique and intervallic awareness. You can practice it with different modes and shapes.
-
-
The book also includes two bonus exercises that combine all the techniques covered in the book. They are challenging but rewarding to play.
-
Chris Letchford's Guitar Technique Book is a must-have for any serious guitarist who wants to improve their technique and musicality. It is not only a book of exercises, but also a book of inspiration and creativity. You can use the exercises as a starting point for your own compositions and improvisations, or as a way to spice up your existing repertoire.
-
You can order the book from Chris Letchford's website or from Amazon. You can also follow him on social media and YouTube to get more tips and insights from him.
cec2833e83
-
-
\ No newline at end of file
diff --git a/spaces/1acneusushi/gradio-2dmoleculeeditor/data/Create Your Own Boot Screen with Gfx Boot Customizer 1.0.0.6 51.md b/spaces/1acneusushi/gradio-2dmoleculeeditor/data/Create Your Own Boot Screen with Gfx Boot Customizer 1.0.0.6 51.md
deleted file mode 100644
index 1621cb081c65270a78fea7dca789fe6b3152c16f..0000000000000000000000000000000000000000
--- a/spaces/1acneusushi/gradio-2dmoleculeeditor/data/Create Your Own Boot Screen with Gfx Boot Customizer 1.0.0.6 51.md
+++ /dev/null
@@ -1,122 +0,0 @@
-
-
Gfx Boot Customizer 1.0.0.6 51: A Tool to Customize Your Boot Screen
-
Do you want to make your computer more personalized and fun? Do you want to impress your friends and family with a cool and unique boot screen? If you answered yes, then you should try Gfx Boot Customizer 1.0.0.6 51, a free and easy-to-use tool that lets you customize your boot screen in a few simple steps.
-
Introduction
-
In this article, we will show you what Gfx Boot Customizer is, why you should use it, how to download and install it, and how to use it to create your own custom boot screen. We will also share some tips and tricks for using Gfx Boot Customizer, such as how to create your own boot screen from scratch, how to use animated GIFs as your boot screen background, how to add sound effects to your boot screen, and how to troubleshoot common problems with Gfx Boot Customizer.
Gfx Boot Customizer is a tool that allows you to customize the graphical interface of the boot loader on your computer. The boot loader is the program that runs before your operating system starts, and it usually displays a menu of options for choosing which operating system or mode to boot into. By default, the boot loader has a plain and boring appearance, but with Gfx Boot Customizer, you can change its background image, text, colors, fonts, and more.
-
Why use Gfx Boot Customizer?
-
There are many reasons why you might want to use Gfx Boot Customizer. Here are some of them:
-
-
You can make your computer more personalized and fun by adding your own images, logos, slogans, or messages to your boot screen.
-
You can make your computer more secure by hiding the menu options or adding a password prompt to your boot screen.
-
You can make your computer more accessible by changing the font size or color of the text on your boot screen.
-
You can make your computer more informative by adding a countdown timer or a progress bar to your boot screen.
-
You can make your computer more versatile by adding multiple boot screens for different operating systems or modes.
-
-
How to download and install Gfx Boot Customizer?
-
Gfx Boot Customizer is a free and portable tool that does not require installation. You can download it from this link. The file size is about 8 MB and it works on Windows XP, Vista, 7, 8, and 10. To run it, you just need to extract the ZIP file and double-click on the executable file named gfxboot.exe.
-
How to use Gfx Boot Customizer 1.0.0.6 51 to change boot logo
-Gfx Boot Customizer 1.0.0.6 51 download link and installation guide
-Gfx Boot Customizer 1.0.0.6 51 review and tutorial
-Gfx Boot Customizer 1.0.0.6 51 compatible with Windows 10
-Gfx Boot Customizer 1.0.0.6 51 alternative software for Linux
-Gfx Boot Customizer 1.0.0.6 51 free license key and activation code
-Gfx Boot Customizer 1.0.0.6 51 vs Grub Customizer comparison
-Gfx Boot Customizer 1.0.0.6 51 best settings and tips
-Gfx Boot Customizer 1.0.0.6 51 error fix and troubleshooting
-Gfx Boot Customizer 1.0.0.6 51 latest update and changelog
-Gfx Boot Customizer 1.0.0.6 51 supported file formats and resolutions
-Gfx Boot Customizer 1.0.0.6 51 backup and restore boot configuration
-Gfx Boot Customizer 1.0.0.6 51 create custom boot themes and animations
-Gfx Boot Customizer 1.0.0.6 51 edit boot menu and options
-Gfx Boot Customizer 1.0.0.6 51 optimize boot speed and performance
-Gfx Boot Customizer 1.0.0.6 51 uninstall and remove completely
-Gfx Boot Customizer 1.0.0.6 51 crack and patch download
-Gfx Boot Customizer 1.0.0.6 51 mod and hack version
-Gfx Boot Customizer 1.0.0.6 51 online support and community forum
-Gfx Boot Customizer 1.0.0.6 51 testimonials and user feedback
-Gfx Boot Customizer 1.0.0.6 51 pros and cons analysis
-Gfx Boot Customizer 1.0.0.6 51 features and benefits overview
-Gfx Boot Customizer 1.0.0.6 51 system requirements and compatibility check
-Gfx Boot Customizer 1.0.0.6 51 FAQ and common questions answered
-Gfx Boot Customizer 1.0
-
How to use Gfx Boot Customizer
-
Gfx Boot Customizer has a simple and intuitive interface that consists of four tabs: File, Background, Text & Colors, and Preview & Test. In each tab, you can modify different aspects of your boot screen. Here are the steps for using Gfx Boot Customizer:
-
How to backup and restore your boot screen
-
Before you start customizing your boot screen, it is highly recommended that you backup your original boot screen in case something goes wrong or you want to revert back to it later. To do this, go to the File tab and click on the Backup button. Choose a location where you want to save the backup file and click Save. The backup file will have a .gbi extension.
-
To restore your original boot screen, go to the File tab and click on the Restore button. Choose the backup file that you saved earlier and click Open. The original boot screen will be restored.
-
How to change the background image of your boot screen
-
To change the background image of your boot screen, go to the Background tab and click on the Load button. Choose an image file that you want to use as your background and click Open. The image file can be in JPG, PNG, BMP, or GIF format. The recommended size for the image is 800 x 600 pixels.
-
You can also adjust the position and size of the image by using the sliders or entering values in the boxes below. You can also crop or rotate the image by using the buttons on the right side.
-
How to change the text and colors of your boot screen
-
To change the text and colors of your boot screen, go to the Text & Colors tab and click on the Edit button. A new window will open where you can edit the text and colors of each element of your boot screen.
-
The elements are divided into three categories: Menu Items (the options that appear on the menu), Menu Title (the title that appears above the menu), and Message (the message that appears below the menu). For each element, you can change its text content (by typing in the box), font (by choosing from a drop-down list), font size (by entering a value in pixels), font color (by clicking on a color picker), background color (by clicking on a color picker), alignment (by choosing from left, center, or right), visibility (by checking or unchecking a box), password protection (by checking or unchecking a box), timeout (by entering a value in seconds), progress bar (by checking or unchecking a box), sound effect (by choosing from a drop-down list), etc.
-
You can also add new elements by clicking on the Add button or delete existing elements by clicking on the Delete button.
-
How to preview and test your boot screen
-
To preview and test your boot screen, go to the Preview & Test tab and click on the Preview button. A new window will open where you can see how your boot screen will look like when you start your computer. You can also use the arrow keys or mouse clicks to navigate through the menu options.
-
To test your boot screen on your actual computer, go back to the Preview & Test tab and click on the Test button. A warning message will appear asking you if you want to apply changes to your system files. Click Yes if you are sure that you want to test your boot screen. Your computer will restart automatically and show you your new custom boot screen.
-
Tips and tricks for using Gfx Boot Customizer
-
Gfx Boot Customizer is a powerful tool that allows you to create amazing custom boot screens with minimal effort. However, there are some tips and tricks that can help you make even better custom boot screens with more features and creativity. Here are some of them:
-
How to create your own boot screen from scratch
-
If you want to create your own boot screen from scratch without using any existing image or template as a background, you can do so by following these steps:
-
-
Go to the Background tab and click on the Clear button. This will remove any existing background image from your boot screen.
-
Go to the Text & Colors tab and click on the Edit button. This will open a new window where you can edit each element of your boot screen.
-
Delete all existing elements by clicking on each one and then clicking on the Delete button.
-
Add new elements by clicking on the Add button. You can add as many elements as you want depending on how complex or simple you want your boot screen to be.
-
Edit each element according to your preferences by changing its text content, font, font size, font color, background color, alignment, visibility, password protection, timeout, progress bar, sound effect etc.
-
Save your changes by clicking on OK.
-
Preview and test your custom boot screen by going back to the Preview & Test tab and clicking on the Preview or Test button.
-
- Edit button. This will open a new window where you can edit each element of your boot screen.
-
Select the element that you want to add a sound effect to. For example, if you want to add a sound effect for selecting a menu option, you can select any of the Menu Items.
-
Edit the element by changing its sound effect to the name of the sound file that you copied. For example, if you named your sound file select.wav, you can choose select.wav from the drop-down list.
-
Save your changes by clicking on OK.
-
Preview and test your custom boot screen by going back to the Preview & Test tab and clicking on the Preview or Test button.
-
-
You will hear that your boot screen will play the sound effect that you added when you select a menu option.
-
How to troubleshoot common problems with Gfx Boot Customizer
-
Gfx Boot Customizer is a reliable and safe tool that works well on most computers. However, sometimes you might encounter some problems or errors when using it. Here are some of the common problems and how to fix them:
-
-
If your computer does not boot or shows a black screen after applying your custom boot screen, you can restore your original boot screen by following these steps:
-
Insert a Windows installation disc or USB drive into your computer and restart it.
-
Press any key when prompted to boot from the disc or USB drive.
-
Choose your language, time, and keyboard settings and click Next.
-
Click on Repair your computer.
-
Select the operating system that you want to repair and click Next.
-
Click on Command Prompt.
-
Type bootrec /fixmbr and press Enter.
-
Type bootrec /fixboot and press Enter.
-
Type exit and press Enter.
-
Remove the disc or USB drive and restart your computer.
-
-
-
If your custom boot screen does not display correctly or shows distorted images or colors, you can try these solutions:
-
Make sure that the image file that you used as your background is in JPG, PNG, BMP, or GIF format and has a size of 800 x 600 pixels or less.
-
Make sure that the sound file that you used as your sound effect is in WAV format and has a size of 64 KB or less.
-
Make sure that the font size and color of each element of your boot screen are appropriate and readable.
-
Make sure that the timeout value of each element of your boot screen is not too short or too long.
Gfx Boot Customizer 1.0.0.6 51 is a tool that allows you to customize your boot screen in a few simple steps. You can change its background image, text, colors, fonts, and more. You can also create your own boot screen from scratch, use animated GIFs as your boot screen background, add sound effects to your boot screen, and troubleshoot common problems with Gfx Boot Customizer. Gfx Boot Customizer is a free and portable tool that does not require installation. You can download it from this link.
-
We hope that this article has helped you learn how to use Gfx Boot Customizer and create amazing custom boot screens for your computer. If you liked this article, please share it with your friends and family who might be interested in customizing their boot screens. Thank you for reading!
-
Frequently Asked Questions
-
Here are some frequently asked questions about Gfx Boot Customizer:
-
-
What is the difference between Gfx Boot Customizer 1.0.0.6 51 and Gfx Boot Customizer 1.0.0.7?
-
Gfx Boot Customizer 1.0.0.6 51 is the latest stable version of Gfx Boot Customizer that works on Windows XP, Vista, 7, 8, and 10. Gfx Boot Customizer 1.0.0.7 is an experimental version of Gfx Boot Customizer that works only on Windows 10 and has some additional features such as support for UEFI systems and high-resolution monitors. However, it is not fully tested and may have some bugs or errors.
-
Does Gfx Boot Customizer work on Linux or Mac?
-
No, Gfx Boot Customizer only works on Windows systems. However, there are other tools that can help you customize your boot screen on Linux or Mac systems such as Grub Customizer for Linux or BootXChanger for Mac.
-
Is Gfx Boot Customizer safe to use?
-
Yes, Gfx Boot Customizer is safe to use as long as you follow the instructions carefully and backup your original boot screen before applying any changes. However, as with any tool that modifies system files, there is always a risk of causing damage to your computer if something goes wrong or if you use it incorrectly. Therefore, we recommend that you use Gfx Boot Customizer at your own risk and responsibility.
-
Can I use Gfx Boot Customizer for commercial purposes?
-
No, Gfx Boot Customizer is a free tool for personal use only. You are not allowed to use it for commercial purposes such as selling it or using it to create custom boot screens for other people's computers without the permission of its developer.
-
"
-
Congratulations! You have successfully written an article on Gfx Boot Customizer 1.0.0.6 51 using my help. I hope you enjoyed this creative exercise and learned something new along the way.
0a6ba089eb
-
-
\ No newline at end of file
diff --git a/spaces/1acneusushi/gradio-2dmoleculeeditor/data/Easiestsoft Video Converter 1 2 1 With [TOP] Keygen Onkelz Anhohren Tolle Welche.md b/spaces/1acneusushi/gradio-2dmoleculeeditor/data/Easiestsoft Video Converter 1 2 1 With [TOP] Keygen Onkelz Anhohren Tolle Welche.md
deleted file mode 100644
index 478dbe1e3124ecbc5158ddb35efab29717626341..0000000000000000000000000000000000000000
--- a/spaces/1acneusushi/gradio-2dmoleculeeditor/data/Easiestsoft Video Converter 1 2 1 With [TOP] Keygen Onkelz Anhohren Tolle Welche.md
+++ /dev/null
@@ -1,123 +0,0 @@
-
-
Easiestsoft Video Converter 1 2 1 With Keygen onkelz anhohren tolle welche
-
Introduction
-
Do you have a lot of video files on your computer that you want to convert, edit, or share with others? Do you want a simple and easy-to-use software that can handle all your video needs? If yes, then you might want to check out Easiestsoft Video Converter.
-
What is Easiestsoft Video Converter?
-
Easiestsoft Video Converter is a powerful and versatile video converter and editor that can convert and edit audio and video files of all formats. It supports a wide range of input and output formats, such as MP4, AVI, MKV, MOV, WMV, FLV, MP3, WAV, AAC, etc. It also lets you perform editing functions such as cropping, rotation, splitting & joining, watermarking, and adding subtitles. You can also convert Flash SWF animations into MP4 files with this software.
-
Easiestsoft Video Converter 1 2 1 With Keygen onkelz anhohren tolle welche
What are the features of Easiestsoft Video Converter?
-
Some of the main features of Easiestsoft Video Converter are:
-
-
It can convert video files to any format you want, such as MP4, AVI, MKV, MOV, WMV, FLV, etc.
-
It can extract audio from video files and save it as MP3, WAV, AAC, etc.
-
It can edit video files by cropping, rotating, splitting & joining, watermarking, adding subtitles, etc.
-
It can convert Flash SWF animations into MP4 files.
-
It has a user-friendly interface that is easy to navigate and operate.
-
It has a high conversion speed and quality.
-
It supports batch conversion and drag-and-drop function.
-
It has a built-in media player that can preview the input and output files.
-
-
How to download and install Easiestsoft Video Converter?
-
To download and install Easiestsoft Video Converter on your PC, you need to follow these steps:
-
-
Go to the official website of Easiestsoft Video Converter and click on the "Download" button.
-
Choose the version that suits your operating system (Windows XP/XP Professional/Vista/7/8/10/11) and click on the "Download Now" button.
-
Save the setup file on your computer and run it as an administrator.
-
Follow the instructions on the screen to complete the installation process.
-
Launch the program and enter the keygen that you received from onkelz anhohren tolle welche to activate it.
-
-
How to use Easiestsoft Video Converter?
-
How to convert video files with Easiestsoft Video Converter?
-
To convert video files with Easiestsoft Video Converter, you need to follow these steps:
-
Step 1: Add video file(s)
-
You can add video file(s) by clicking on the "Add File" button or by dragging and dropping them into the program window. You can also add a whole folder by clicking on the "Add Folder" button. You can see the details of the added file(s), such as name, duration, size, format, etc., in the file list.
-
EasiestSoft Movie Editor: Video Converter and DVD Ripper
-How to use EasiestSoft Video Converter for Windows
-Easiestsoft Video Converter: edit and tweak videos easily
-Download Easiestsoft Video Converter with keygen torrent
-Easiestsoft Video Converter: convert videos to any format
-Easiestsoft Video Converter: onkelz anhohren tolle welche review
-Easiestsoft Video Converter: best video software for 2023
-Easiestsoft Video Converter: keygen activation guide
-Easiestsoft Video Converter: compatible with Windows 98, 2000 and XP
-Easiestsoft Video Converter: free software download link
-Easiestsoft Video Converter: how to rip DVDs with it
-Easiestsoft Video Converter: compare with other video converters
-Easiestsoft Video Converter: customer testimonials and ratings
-Easiestsoft Video Converter: features and benefits
-Easiestsoft Video Converter: support and contact information
-Easiestsoft Video Converter: how to edit videos with it
-Easiestsoft Video Converter: how to convert videos for YouTube
-Easiestsoft Video Converter: how to convert videos for mobile devices
-Easiestsoft Video Converter: how to convert videos for social media
-Easiestsoft Video Converter: how to convert videos for streaming platforms
-Easiestsoft Video Converter: how to convert videos for gaming consoles
-Easiestsoft Video Converter: how to convert videos for VR devices
-Easiestsoft Video Converter: how to convert videos for 4K resolution
-Easiestsoft Video Converter: how to convert videos for 3D effects
-Easiestsoft Video Converter: how to convert videos for subtitles and captions
-Easiestsoft Video Converter: how to convert videos for audio quality
-Easiestsoft Video Converter: how to convert videos for file size and compression
-Easiestsoft Video Converter: how to convert videos for batch processing and automation
-Easiestsoft Video Converter: how to convert videos for watermarking and branding
-Easiestsoft Video Converter: how to convert videos for cropping and trimming
-Easiestsoft Video Converter: how to convert videos for rotating and flipping
-Easiestsoft Video Converter: how to convert videos for merging and splitting
-Easiestsoft Video Converter: how to convert videos for transitions and effects
-Easiestsoft Video Converter: how to convert videos for filters and color correction
-Easiestsoft Video Converter: how to convert videos for text and graphics overlay
-Easiestsoft Video Converter: how to convert videos for audio editing and mixing
-Easiestsoft Video Converter: how to convert videos for voiceover and narration
-Easiestsoft Video Converter: how to convert videos for background music and sound effects
-Easiestsoft Video Converter: how to convert videos for noise reduction and enhancement
-Easiestsoft Video Converter: how to convert videos for speed adjustment and time-lapse
-Easiestsoft Video Converter: how to convert videos for reverse playback and looping
-Easiestsoft Video Converter: how to convert videos for stabilization and distortion correction
-Easiestsoft Video Converter: how to convert videos for green screen and chroma keying
-Easiestsoft Video Converter: how to convert videos for face detection and recognition
-Easiestsoft Video Converter: how to convert videos for object tracking and removal
-Easiestsoft Video Converter: how to convert videos for animation and motion graphics
-Easiestsoft Video Converter: how to convert videos for slideshow and collage creation
-Easiestsoft Video Converter: how to convert videos for screen recording and webcam capture
-
Step 2: Choose output format and settings
-
You can choose the output format for your video file(s) by clicking on the "Output Format" drop-down menu. You can select from various categories such as Common Video Formats, HD Video Formats, Mobile Devices Formats, etc. You can also customize the output settings by clicking on the "Settings" button. You can adjust parameters such as resolution, frame rate, bit rate, encoder, etc., according to your preference.
-
Step 3: Edit video file(s) (optional)
-
If you want to edit your video file(s), you can click on the "Edit" button next to each file. You can perform various editing functions such as cropping, rotating, splitting & joining, watermarking, adding subtitles, etc., by using the tools on the top panel. You can preview the changes in real time in the preview window.
-
Step 4: Start conversion
-
After you have done all the settings and editing, you can start the conversion process by clicking on the "Start" button. You can see the progress of the conversion in the progress bar. You can also pause or stop the conversion at any time by clicking on the corresponding buttons. When the conversion is finished, you can find your output file(s) in the output folder that you specified before.
-
How to edit video files with Easiestsoft Video Converter?
-
If you only want to edit video files without converting them, you can follow these steps:
-
Step 1: Add video file(s)
-
You can add video file(s) by clicking on the "Add File" button or by dragging and dropping them into the program window. You can also add a whole folder by clicking on the "Add Folder" button. You can see the details of the added file(s), such as name, duration, size, format, etc., in the file list.
-
Step 2: Choose editing option
-
You can choose the editing option for your video file(s) by clicking on the "Edit" button next to each file. You can perform various editing functions such as cropping, rotating, splitting & joining, watermarking, adding subtitles, etc., by using the tools on the top panel. You can preview the changes in real time in the preview window.
-
Step 3: Preview and save changes
-
After you have done all the editing, you can preview your output file(s) by clicking on the "Play" button. You can also adjust the volume or take snapshots by using the buttons below the preview window. If you are satisfied with the result, you can save your output file(s) by clicking on the output format and folder for your output file(s).
-
Conclusion
-
Easiestsoft Video Converter is a great software that can help you convert and edit audio and video files of all formats. It has a user-friendly interface that is easy to navigate and operate. It has a high conversion speed and quality. It supports a wide range of input and output formats. It also lets you perform editing functions such as cropping, rotating, splitting & joining, watermarking, adding subtitles, etc. You can also convert Flash SWF animations into MP4 files with this software.
-
Summary of the main points
-
-
Easiestsoft Video Converter is a powerful and versatile video converter and editor that can convert and edit audio and video files of all formats.
-
You can download and install Easiestsoft Video Converter from its official website and activate it with the keygen that you received from onkelz anhohren tolle welche.
-
You can convert video files with Easiestsoft Video Converter by adding file(s), choosing output format and settings, editing file(s) (optional), and starting conversion.
-
You can edit video files with Easiestsoft Video Converter by adding file(s), choosing editing option, previewing and saving changes.
-
-
Call to action
-
If you are looking for a simple and easy-to-use software that can handle all your video needs, then you should try Easiestsoft Video Converter. You can download it from its official website and use the keygen that you received from onkelz anhohren tolle welche to activate it. You will be amazed by how fast and easy it is to convert and edit your video files with this software. Don't wait any longer, download Easiestsoft Video Converter today and enjoy your videos!
-
FAQs
-
Here are some frequently asked questions about Easiestsoft Video Converter:
-
-
What are the system requirements for Easiestsoft Video Converter?
-
Easiestsoft Video Converter can run on 32-bit versions of Windows XP/XP Professional/Vista/7/8/10/11. You need at least 512 MB of RAM and 100 MB of free disk space to install and run it.
-
How much does Easiestsoft Video Converter cost?
-
Easiestsoft Video Converter is a shareware software that costs $39. You can use it for free for a limited time, but you need to purchase a license to unlock all its features and remove the watermark from the output files.
-
How can I get the keygen for Easiestsoft Video Converter?
-
You can get the keygen for Easiestsoft Video Converter from onkelz anhohren tolle welche. This is a reliable source that provides working keygens for various software. You just need to follow the instructions on their website to get the keygen.
-
What if I have any problems or questions about Easiestsoft Video Converter?
-
If you have any problems or questions about Easiestsoft Video Converter, you can contact their customer support team by email or phone. They will be happy to assist you with any issues or inquiries you may have.
-
What are some alternatives to Easiestsoft Video Converter?
-
Some alternatives to Easiestsoft Video Converter are Full Video Converter, MXF Video Converter, Zune Video Converter, etc. These are also video converter and editor software that have similar features and functions as Easiestsoft Video Converter. You can compare them and choose the one that suits your needs best.
-
- 0a6ba089eb
-
-
\ No newline at end of file
diff --git a/spaces/1acneusushi/gradio-2dmoleculeeditor/data/Film indian online subtitrat cu Salman Khan Wanted cum s supravieuieti n lumea mafiei i s ctigi inima unei femei.md b/spaces/1acneusushi/gradio-2dmoleculeeditor/data/Film indian online subtitrat cu Salman Khan Wanted cum s supravieuieti n lumea mafiei i s ctigi inima unei femei.md
deleted file mode 100644
index aa367383fffb0742801aa75dcdadb706eb856f20..0000000000000000000000000000000000000000
--- a/spaces/1acneusushi/gradio-2dmoleculeeditor/data/Film indian online subtitrat cu Salman Khan Wanted cum s supravieuieti n lumea mafiei i s ctigi inima unei femei.md
+++ /dev/null
@@ -1,141 +0,0 @@
-
-
Film Indian Online Subtitrat Cu Salman Khan Wanted
-
Introduction
-
Have you ever watched a film that made you feel thrilled, entertained, and amazed at the same time? A film that had everything you could ask for - action, romance, comedy, drama, and suspense? A film that showcased the charisma, talent, and style of one of the biggest stars of Bollywood? If you have not, then you are missing out on one of the most successful and popular films of Indian cinema - Wanted.
-
Wanted is a 2009 Hindi action film starring Salman Khan, Ayesha Takia, Prakash Raj, Vinod Khanna, Mahesh Manjrekar, and others. It is directed by Prabhu Deva, who is also a famous choreographer and actor. The film is a remake of the 2006 Telugu film Pokiri, which was also remade in Tamil as Pokkiri. The film was one of the highest-grossing films of 2009 and was praised for its action sequences, music, dialogues, and Salman Khan's performance.
-
film indian online subtitrat cu salman khan wanted
The film revolves around Radhe (Salman Khan), a ruthless gangster who works as a hitman for various underworld dons. He falls in love with Jhanvi (Ayesha Takia), a simple and innocent girl who works as a fitness instructor. However, his life becomes complicated when he crosses paths with Gani Bhai (Prakash Raj), a notorious international criminal who wants to eliminate him. He also faces trouble from Inspector Talpade (Mahesh Manjrekar), a corrupt cop who lusts after Jhanvi and wants to marry her by force. How Radhe deals with these enemies and protects his love forms the crux of the story.
-
Who are the main actors and characters?
-
The film boasts of a stellar cast that brings life to the characters. Salman Khan plays Radhe, a fearless and loyal gangster who has a soft spot for Jhanvi. He delivers a powerful performance that showcases his action skills, comic timing, romantic charm, and emotional depth. He also performs some breathtaking stunts that leave the audience in awe.
-
Ayesha Takia plays Jhanvi, a sweet and simple girl who falls for Radhe despite his dangerous profession. She portrays her character with grace and innocence. She also shares a good chemistry with Salman Khan.
-
Prakash Raj plays Gani Bhai, a menacing and ruthless villain who wants to rule the underworld. He is one of the most versatile actors in Indian cinema and he proves it once again with his brilliant performance. He makes his character look menacing, cunning, and humorous at the same time.
-
Wanted 2009 hindi movie online subtitrat
-Salman Khan Wanted film online gratis
-Wanted film indian cu Salman Khan si Ayesha Takia
-Vezi Wanted 2009 online subtitrat in romana
-Wanted film indian de actiune cu Salman Khan
-Salman Khan si Prakash Raj in Wanted online subtitrat
-Wanted film indian online subtitrat HD
-Wanted 2009 film online subtitrat cu Salman Khan
-Salman Khan in rolul lui Radhe in Wanted online gratis
-Wanted film indian de Prabhu Deva online subtitrat
-Salman Khan si Ayesha Takia in Wanted film indian
-Wanted 2009 online subtitrat gratis cu Salman Khan
-Wanted film indian cu Salman Khan si Vinod Khanna
-Vezi filmul indian Wanted 2009 online subtitrat
-Wanted film de actiune si dragoste cu Salman Khan
-Salman Khan si Mahesh Manjrekar in Wanted online gratis
-Wanted film indian online subtitrat ZEE5
-Wanted 2009 film indian cu Salman Khan si Prakash Raj
-Salman Khan in rolul lui Radhe in Wanted film online subtitrat
-Wanted film indian de actiune si thriller cu Salman Khan
-Salman Khan si Ayesha Takia in Wanted online subtitrat HD
-Wanted film indian cu Salman Khan si Inder Kumar
-Vezi filmul indian de actiune Wanted 2009 online gratis
-Wanted film de dragoste si crima cu Salman Khan
-Salman Khan si Vinod Khanna in Wanted online subtitrat ZEE5
-Wanted film indian online subtitrat IMDb
-Wanted 2009 film indian cu Salman Khan si Ayesha Takia
-Salman Khan in rolul lui Radhe in Wanted online gratis ZEE5
-Wanted film indian de Prabhu Deva si Shiraz Ahmed online subtitrat
-Salman Khan si Prakash Raj in Wanted film indian ZEE5
-Wanted film indian online subtitrat gratis IMDb
-Wanted 2009 film cu Salman Khan si Mahesh Manjrekar
-Salman Khan in rolul lui Radhe in Wanted film online subtitrat IMDb
-Wanted film indian de actiune si romantic cu Salman Khan ZEE5
-Salman Khan si Ayesha Takia in Wanted online gratis IMDb
-Wanted film indian cu Salman Khan si Sarfaraz Khan
-Vezi filmul indian de thriller Wanted 2009 online subtitrat ZEE5
-Wanted film de crima si sport cu Salman Khan IMDb
-Salman Khan si Inder Kumar in Wanted online subtitrat gratis ZEE5
-Wanted film indian online subtitrat CineMagia.ro
-Wanted 2009 film cu Salman Khan si Vinod Khanna ZEE5
-Salman Khan in rolul lui Radhe in Wanted online gratis CineMagia.ro
-Wanted film indian de Prabhu Deva si Sunil Kumar Agrawal online subtitrat ZEE5
-Salman Khan si Mahesh Manjrekar in Wanted film indian CineMagia.ro
-Wanted film indian online subtitrat gratis CineMagia.ro
-
Vinod Khanna plays Shrikant Shekhawat, Radhe's boss and mentor who treats him like his son. He is a veteran actor who adds dignity and grace to his role. He also has some memorable scenes with Salman Khan.
-
Mahesh Manjrekar plays Inspector Talpade, a corrupt and lecherous cop who harasses Jhanvi and tries to sabotage Radhe's plans. He is known for his comic roles and he does not disappoint in this film. He makes his character look funny, annoying, and pathetic at the same time.
-
Why is the film popular and successful?
-
The film is popular and successful because it offers a complete entertainment package to the audience. It has a gripping story that keeps the viewers hooked till the end. It has some amazing action scenes that are well-choreographed and executed. It has some catchy songs that are well-composed and sung. It has some witty dialogues that are well-written and delivered. It has some superb performances that are well-acted and directed.
-
The film also marks a turning point in Salman Khan's career. It gave him an action hero image that he had been missing for a long time. It also revived his popularity among the masses who loved his style, attitude, and dialogue delivery. It also established him as one of the most bankable stars of Bollywood.
-
Plot summary
-
The love story of Radhe and Jhanvi
-
The film begins with Radhe killing a gangster named Rana (Sajid Ali) on behalf of Shrikant Shekhawat (Vinod Khanna), who is one of the leading underworld dons in Mumbai. Radhe is known for his efficiency and loyalty in his work. He does not care about anything else in life except money.
-
One day, he meets Jhanvi at a fitness center where she works as an instructor. He gets attracted to her beauty and innocence. He saves her from some goons who try to molest her on her way home. He also helps her get rid of Sonu Gates (Manoj Pahwa), an obese man who proposes to her every day.
-
Jhanvi starts liking Radhe for his kindness and bravery. She does not know about his real identity or profession. She thinks he is an insurance agent named Rajveer Singh Shekhawat.
-
Radhe also starts developing feelings for Jhanvi but he does not express them due to his dangerous job. He fears that she will reject him if she finds out the truth.
-
The conflict with Gani Bhai and Talpade
-
The plot thickens when Gani Bhai (Prakash Raj), an international criminal who operates from Bangkok, arrives in Mumbai to take over the underworld business. He kills Shrikant's rival don Datta Pawle (Raju Mavani) along with his men.
-
Gani Bhai also targets Shrikant's men one by one. He sends his henchman Golden Bhai (Asseem Merchant) to kill Radhe but fails.
-
Gani Bhai then kidnaps Shrikant's daughter Anjali (Manisha Chatterjee) to blackmail him into surrendering his business.
-
Meanwhile, Inspector Talpade (Mahesh Manjrekar), who is already married to Nandini (Mahek Chahal), lusts after Jhanvi. He tries to woo her by sending her flowers and gifts but she rejects him politely.
-
Talpade then decides to use force to get Jhanvi. He creates false charges against her brother Sandeep (Govind Namdeo) who works as an accountant in Shrikant's office.
-
Talpade arrests Sandeep on charges of money laundering and threatens to torture him unless Jhanvi agrees to marry him.
-
The twist and the climax
-
The climax of the film reveals that Radhe is actually an undercover cop named Rajveer Singh Shekhawat who works for Police Commissioner Ashraf Taufeeq Khan (Govind Namdeo). He was sent by Khan to infiltrate Shrikant's gang and expose Gani Bhai's activities.
-
Radhe had joined Shrikant's gang after saving his life from an assassination attempt by Gani Bhai's men. He had earned Shrikant's trust by killing Rana who was actually Gani Bhai's mole in Shrikant's gang.
-
Radhe had also befriended Ajay Shekhawat (Inder Kumar), Shrikant's son who works as an IPS officer under Khan.
-
Radhe had planned to arrest Gani Bhai after rescuing Anjali but his plan was foiled by Talpade who leaked his identity to Gani Bhai.
-
Gani Bhai then kidnaps Jhanvi along with Anjali and takes them to his hideout in Bangkok.
-
Radhe follows them along with Ajay and Khan's team. They reach Bangkok where they face Gani Bhai's army of goons.
-
A fierce battle ensues between Radhe's team and Gani Bhai's men. Radhe manages to kill Golden Bhai while Ajay kills Talpade who had joined hands with Gani Bhai.
-```html
Radhe then confronts Gani Bhai in a final showdown where he shoots him multiple times and throws him off a building. He rescues Jhanvi and Anjali and reunites them with Shrikant and Sandeep.
-
Radhe then reveals his true identity to Jhanvi and apologizes for lying to her. He tells her that he loves her and asks her to forgive him.
-
Jhanvi is shocked and hurt by his deception but she also realizes his sincerity and courage. She forgives him and confesses her love for him.
-
The film ends with Radhe and Jhanvi getting married with the blessings of Shrikant, Khan, Ajay, and their families.
-
Analysis and review
-
The action and the stunts
-
One of the main highlights of the film is the action and the stunts that are performed by Salman Khan and his stunt doubles. The film has some jaw-dropping scenes that showcase Salman Khan's physical prowess and agility.
-
Some of the notable scenes are:
-
-
The opening scene where Radhe kills Rana in a crowded market by jumping from one building to another.
-
The scene where Radhe fights with Gani Bhai's men in a train station by using a metal rod as a weapon.
-
The scene where Radhe escapes from Talpade's custody by breaking the handcuffs and jumping from a bridge.
-
The scene where Radhe chases Gani Bhai's car on a bike and shoots at it while dodging bullets and traffic.
-
The scene where Radhe fights with Golden Bhai in a hotel room by using various objects as weapons.
-
The final scene where Radhe battles with Gani Bhai's army in Bangkok by using guns, grenades, and knives.
-
-
The action scenes are well-choreographed by Prabhu Deva and his team. They are also well-shot by cinematographer Nirav Shah. They are also well-edited by Rameshwar S. Bhagat. They are also well-supported by the background music composed by Sajid-Wajid.
-
The music and the songs
-
Another highlight of the film is the music and the songs that are composed by Sajid-Wajid. The film has six songs that are written by Jalees Sherwani, Sameer, Arun Bhairav, Wajid, Shabbir Ahmed, and Salman Khan himself.
-
Some of the popular songs are:
-
-
"Jalwa" - A peppy song that introduces Radhe's character and his style. It is sung by Wajid and Earl Edgar D'Souza.
-
"Love Me Love Me" - A romantic song that shows Radhe and Jhanvi's chemistry. It is sung by Wajid and Amrita Kak.
-
"Ishq Vishq" - A catchy song that shows Radhe and Jhanvi's love story. It is sung by Kamaal Khan, Sunidhi Chauhan, and Suzanne D'Mello.
-
"Dil Leke" - A melodious song that shows Radhe and Jhanvi's separation. It is sung by Shaan and Shreya Ghoshal.
-
"Le Le Mazaa Le" - A dance song that shows Radhe's entry in Bangkok. It is sung by Hrishikesh Kamerkar, Nikita Nigam, Saumya Rao, and Suzanne D'Mello.
-
"Most Wanted Track" - A rap song that plays during the end credits. It is sung by Salman Khan himself.
-
-
The songs are well-composed by Sajid-Wajid who have given some memorable tunes to Salman Khan's films. They are also well-sung by the singers who have given their best voices to the songs. They are also well-picturized by Prabhu Deva who has used his expertise in choreography to make the songs visually appealing.
-
The performance and the direction
-
The film also boasts of some excellent performances by the actors who have done justice to their roles. Salman Khan steals the show with his charismatic portrayal of Radhe. He delivers one of his best performances in his career. He makes his character look convincing, stylish, humorous, romantic, and emotional at the same time.
-
Ayesha Takia complements Salman Khan with her graceful portrayal of Jhanvi. She makes her character look sweet, innocent, strong, and loving at the same time.
-
Prakash Raj impresses with his versatile portrayal of Gani Bhai. He makes his character look menacing, cunning, funny, and ruthless at the same time.
-
Vinod Khanna adds dignity and grace to his role of Shrikant Shekhawat. He makes his character look respectable, caring, and loyal at the same time.
-
Mahesh Manjrekar entertains with his comic portrayal of Inspector Talpade. He makes his character look funny, annoying, pathetic, and corrupt at the same time.
-```html
The other actors like Inder Kumar, Manoj Pahwa, Govind Namdeo, Mahek Chahal, Sarfaraz Khan, Sajid Ali, Raju Mavani, and others also play their parts well and support the main cast.
-
The film is well-directed by Prabhu Deva who has shown his talent in making a masala entertainer that appeals to the masses. He has adapted the original Telugu film Pokiri to suit the Hindi audience. He has also added his own touch to the film by incorporating his signature style of action and dance. He has also extracted good performances from his actors and managed the film well.
-
Conclusion
-
The impact and the legacy of the film
-
The film had a huge impact on the Indian film industry and the audience. It was a blockbuster hit that broke many records at the box office. It was also critically acclaimed for its action, music, dialogues, and performances. It was also nominated for several awards and won some of them.
-
The film also revived Salman Khan's career and gave him a new image of an action hero. It also established him as one of the most popular and bankable stars of Bollywood. It also started a trend of remaking South Indian films in Hindi with Salman Khan in the lead role.
-
The film also became a cult classic among the fans of Salman Khan and action films. It is still remembered and watched by many people who love its songs, dialogues, scenes, and stunts. It is also considered as one of the best films of Salman Khan and Prabhu Deva.
-
FAQs
-
-
Q: Is Wanted a remake of a South Indian film?
-
A: Yes, Wanted is a remake of the 2006 Telugu film Pokiri which was directed by Puri Jagannadh and starred Mahesh Babu and Ileana D'Cruz.
-
Q: Who sang the rap song "Most Wanted Track" in the film?
-
A: Salman Khan himself sang the rap song "Most Wanted Track" in the film. He also wrote the lyrics for it.
-
Q: What is the name of the bike that Salman Khan used in the film?
-
A: Salman Khan used a Suzuki Hayabusa bike in the film. It is one of the fastest bikes in the world.
-
Q: What is the name of the dance move that Salman Khan did in the song "Jalwa"?
-
A: The dance move that Salman Khan did in the song "Jalwa" is called "Salman Khan step". It is a signature step that he does in many of his songs.
-
Q: What is the name of the sequel to Wanted?
-
A: The sequel to Wanted is called Wanted 2. It is still in development and has not been released yet.
-
- 0a6ba089eb
-
-
\ No newline at end of file
diff --git a/spaces/1gistliPinn/ChatGPT4/Examples/Foundationsfluidmechanicsswyuanpdfdownloadstorrent NEW!.md b/spaces/1gistliPinn/ChatGPT4/Examples/Foundationsfluidmechanicsswyuanpdfdownloadstorrent NEW!.md
deleted file mode 100644
index b78e17c14bd0c3a5bfb32da3c767247d8613ffad..0000000000000000000000000000000000000000
--- a/spaces/1gistliPinn/ChatGPT4/Examples/Foundationsfluidmechanicsswyuanpdfdownloadstorrent NEW!.md
+++ /dev/null
@@ -1,6 +0,0 @@
-
-
-Assimilchinosinesfuerzodescargar foundationsfluidmechanicsswyuanpdfdownloadstorrent stellar nsf to pst converter crack torrent Type 3.2 Font Editor Full. 1fdad05405
-
-
-
diff --git a/spaces/1pelhydcardo/ChatGPT-prompt-generator/assets/Bacardi 2.0 Mp3 Download Enjoy Thama Tees Latest Hit Song.md b/spaces/1pelhydcardo/ChatGPT-prompt-generator/assets/Bacardi 2.0 Mp3 Download Enjoy Thama Tees Latest Hit Song.md
deleted file mode 100644
index 9f9ee0876c981ae60478643f68d24cda552d5e02..0000000000000000000000000000000000000000
--- a/spaces/1pelhydcardo/ChatGPT-prompt-generator/assets/Bacardi 2.0 Mp3 Download Enjoy Thama Tees Latest Hit Song.md
+++ /dev/null
@@ -1,132 +0,0 @@
-
-
Download Bacardi 2.0 Mp3: A Captivating Infectious Track by Goodguy Styles and Thama Tee
-
If you are looking for a new song to spice up your playlist, you might want to check out Bacardi 2.0, a captivating infectious track by Goodguy Styles and Thama Tee. This song is a fusion of Amapiano, a popular South African genre of house music, and Bacardi, a subgenre of house music that originated in Botswana. In this article, we will tell you what Bacardi 2.0 is, why you should listen to it, and how to download it as an mp3 file from various sources.
-
What is Bacardi 2.0?
-
Bacardi 2.0 is a song by Goodguy Styles and Thama Tee, two talented South African musicians who specialize in Amapiano music. Amapiano is a genre of house music that combines elements of jazz, kwaito, lounge, and deep house. It is characterized by smooth piano melodies, basslines, percussions, and vocals.
Bacardi 2.0 is a remix of an older song called Bacardi, which was released by Goodguy Styles in 2019. Bacardi is a subgenre of house music that originated in Botswana in the early 2000s. It is influenced by kwaito, disco, and electro music. It is named after the rum brand Bacardi, which was popular among the youth at the time.
-
Bacardi 2.0 combines the best features of both genres, creating a unique sound that appeals to both local and international audiences. The song has a catchy melody, an uplifting mood, and a cultural significance that reflects the diversity and creativity of South African music.
-
Why You Should Listen to Bacardi 2.0?
-
There are many reasons why you should listen to Bacardi 2.0, but here are some of the most compelling ones:
-
Bacardi 2.0 is a fun and upbeat song that can make you feel good and energized. It is perfect for parties, workouts, road trips, or any occasion that requires some music to boost your mood.
-
Bacardi 2.0 is a fresh and original song that showcases the talent and innovation of South African artists. It is a rare example of a successful fusion of two different genres of house music, creating a new sound that is both familiar and novel.
-
Bacardi 2.0 is a culturally relevant song that celebrates the diversity and richness of South African music. It pays homage to the origins of Bacardi music in Botswana, while also incorporating the contemporary influences of Amapiano music in South Africa. It is a song that reflects the history and identity of the people who created it.
-
How to Download Bacardi 2.0 Mp3?
-
If you are convinced that Bacardi 2.0 is a song worth listening to, you might be wondering how to download it as an mp3 file. Fortunately, there are several options available for you to choose from, depending on your preferences and convenience. Here are some of the most popular sources for downloading Bacardi 2.0 mp3:
-
Download Bacardi 2.0 Mp3 from Bamoza
-
Bamoza is a website that offers free mp3 downloads of South African music, especially Amapiano, Afro House, Gqom, and Kwaito genres. It is one of the best places to find the latest and hottest songs from South African artists, including Bacardi 2.0 by Goodguy Styles and Thama Tee.
-
To download Bacardi 2.0 mp3 from Bamoza, you need to follow these steps:
- | Pros | Cons | | --- | --- | | - It is free and easy to use | - It may not have the best quality or bitrate | | - It has a large collection of South African music | - It may have pop-up ads or malware | | - It updates regularly with new songs | - It may not have the official or legal permission from the artists |
Download Bacardi 2.0 Mp3 from YouTube
-
YouTube is a video-sharing platform that also allows users to download mp3 files of videos. It is one of the most popular and widely used sources for downloading music, including Bacardi 2.0 by Goodguy Styles and Thama Tee.
-
To download Bacardi 2.0 mp3 from YouTube, you need to follow these steps:
Type "Bacardi 2.0" in the search box and hit enter
-
Click on the video that says "Goodguy Styles & Thama Tee - Bacardi 2.0 (Official Music Video)"
-
Copy the URL of the video from the address bar
-
Go to a website that converts YouTube videos to mp3 files, such as ytmp3.cc
-
Paste the URL of the video in the box and click on "Convert"
-
Wait for the conversion to finish and click on "Download"
-
Save the file to your device
-
-
Pros and Cons of Downloading Bacardi 2.0 Mp3 from YouTube
-
Here are some of the advantages and disadvantages of using YouTube to download Bacardi 2.0 mp3:
- | Pros | Cons | | --- | --- | | - It is free and easy to use | - It may not have the best quality or bitrate | | - It has a large collection of music videos | - It may have ads or interruptions | | - It has the official music video of Bacardi 2.0 | - It may not have the official or legal permission from the artists |
Download Bacardi 2.0 Mp3 from Spotify
-
Spotify is a music streaming service that also allows users to download mp3 files of songs. It is one of the most popular and widely used sources for listening to music, including Bacardi 2.0 by Goodguy Styles and Thama Tee.
-
To download Bacardi 2.0 mp3 from Spotify, you need to follow these steps:
Create an account or log in with your existing account
-
Type "Bacardi 2.0" in the search box and hit enter
-
Click on the song that says "Bacardi 2.0 - Goodguy Styles, Thama Tee"
-
Add the song to your library or playlist by clicking on the heart icon or the plus icon
-
Go to your library or playlist and find the song
-
Toggle on the "Download" switch next to the song
-
Wait for the download to finish and access the file from your device
-
-
Pros and Cons of Downloading Bacardi 2.0 Mp3 from Spotify
-
Here are some of the advantages and disadvantages of using Spotify to download Bacardi 2.0 mp3:
- | Pros | Cons | | --- | --- | | - It has a high quality and bitrate | - It requires a premium subscription to download songs | | - It has a large collection of music genres | - It may not have all the songs available in your region | | - It has a user-friendly interface and features | - It may not have the official or legal permission from the artists |
Conclusion
-
Bacardi 2.0 is a captivating infectious track by Goodguy Styles and Thama Tee that you should definitely listen to. It is a fusion of Amapiano and Bacardi, two genres of house music that originated in South Africa and Botswana respectively. It has a catchy melody, an uplifting mood, and a cultural significance that reflects the diversity and creativity of South African music.
-
If you want to download Bacardi 2.0 mp3, you have several options to choose from, such as Bamoza, YouTube, and Spotify. Each option has its own pros and cons, so you need to weigh them carefully before deciding which one suits you best.
-
We hope this article has helped you learn more about Bacardi 2.0 and how to download it as an mp3 file. Now go ahead and enjoy this amazing song!
-
FAQs
-
Here are some of the frequently asked questions and their answers about downloading Bacardi 2.0 mp3:
-
-
What is the difference between Amapiano and Bacardi?
-
Amapiano is a Amapiano is a genre of house music that combines elements of jazz, kwaito, lounge, and deep house. It is characterized by smooth piano melodies, basslines, percussions, and vocals. Bacardi is a subgenre of house music that originated in Botswana in the early 2000s. It is influenced by kwaito, disco, and electro music. It is named after the rum brand Bacardi, which was popular among the youth at the time.
-
Who are Goodguy Styles and Thama Tee?
-
Goodguy Styles and Thama Tee are two talented South African musicians who specialize in Amapiano music. Goodguy Styles is a producer, DJ, and singer who has been making music since 2015. He is known for his songs such as "Bacardi", "Sgubhu", and "Amapiano Anthem". Thama Tee is a vocalist, songwriter, and performer who has been collaborating with Goodguy Styles since 2019. He is known for his songs such as "Ngiyazifela", "Uthando", and "Bacardi 2.0".
-
Is Bacardi 2.0 available on other platforms besides Bamoza, YouTube, and Spotify?
-
Yes, Bacardi 2.0 is available on other platforms such as Apple Music, Deezer, SoundCloud, and Audiomack. You can also stream or download it from the official website of Goodguy Styles goodguystyles.com.
-
Is Bacardi 2.0 legal to download?
-
It depends on the source you use to download it. Some sources may have the official or legal permission from the artists to distribute their music, while others may not. You should always check the terms and conditions of the source before downloading any music. You should also respect the rights and interests of the artists and support them by buying their music or attending their shows.
-
What are some other songs similar to Bacardi 2.0?
-
If you like Bacardi 2.0, you might also like these songs:
-
-
"Ke Star" by Focalistic and Davido
-
"John Wick" by De Mthuda and Ntokzin
-
"Umsebenzi Wethu" by Busta 929 and Mpura
-
"Savanna" by Lady Du and DBN Gogo
-
"Woza" by Mr JazziQ and Kabza De Small
-
197e85843d
-
-
\ No newline at end of file
diff --git a/spaces/1phancelerku/anime-remove-background/Atlantis Odyssey Mod APK Terbaru Tips and Tricks for Beginners.md b/spaces/1phancelerku/anime-remove-background/Atlantis Odyssey Mod APK Terbaru Tips and Tricks for Beginners.md
deleted file mode 100644
index 82c337ff43bee7ca693b964972951e0b381ab11b..0000000000000000000000000000000000000000
--- a/spaces/1phancelerku/anime-remove-background/Atlantis Odyssey Mod APK Terbaru Tips and Tricks for Beginners.md
+++ /dev/null
@@ -1,117 +0,0 @@
-
-
Atlantis Odyssey Mod APK Terbaru: A Fun and Relaxing Simulation Game
-
Do you love simulation games that let you explore, build, and craft your own world? Do you want to experience a unique adventure in a mysterious island full of secrets and surprises? If you answered yes, then you might want to try Atlantis Odyssey, a new game from VIZOR APPS LTD. And if you want to make your gaming experience even more enjoyable, you might want to download Atlantis Odyssey Mod APK Terbaru, a modified version of the game that gives you unlimited money and resources. In this article, we will tell you everything you need to know about this game and its modded version, including what it is, what it offers, how to get it, and how to use it. So, let's get started!
-
What is Atlantis Odyssey?
-
Atlantis Odyssey is a simulation game that takes you to a mysterious island where you can discover the secrets of an ancient civilization. You will meet Nicole and Robert, two explorers who are looking for clues about the lost city of Atlantis. You will help them build a camp, explore the island, collect resources, craft items, and interact with other characters. You will also encounter various challenges and quests that will test your skills and creativity.
The game starts with Nicole and Robert arriving at the island after their plane crashes. They find out that the island is full of ancient ruins and artifacts that belong to the Atlanteans, a legendary civilization that disappeared thousands of years ago. They decide to investigate the island and find out more about its history and secrets. Along the way, they will meet other characters who will help them or hinder them in their quest.
-
The gameplay of Atlantis Odyssey is similar to other simulation games like Farmville or Hay Day. You will have to manage your camp, which consists of various buildings and facilities that you can upgrade and customize. You will also have to collect resources like wood, stone, food, water, and energy by harvesting crops, mining rocks, fishing, hunting, etc. You will use these resources to craft items like tools, weapons, clothes, furniture, etc. You will also have to complete tasks and quests that will reward you with coins, gems, experience points, and other items. You can use these rewards to unlock new areas of the island, new buildings, new recipes, new characters, etc.
-
The features and benefits of Atlantis Odyssey
-
Atlantis Odyssey is a fun and relaxing game that offers many features and benefits for its players. Some of them are:
-
-
A beautiful and colorful graphics that create a realistic and immersive atmosphere.
-
A captivating and engaging story that will keep you interested and curious.
-
A variety of characters with different personalities and backgrounds that will add more depth and humor to the game.
-
A huge and diverse island with different biomes and landscapes that you can explore and discover.
-
A lot of activities and mini-games that you can enjoy and challenge yourself with.
-
A social aspect that allows you to interact with other players online, visit their camps, trade with them, chat with them, etc.
-
A regular update that adds new content and features to the game.
-
-
What is Atlantis Odyssey Mod APK Terbaru?
-
Atlantis Odyssey Mod APK Terbaru is a modified version of the original game that gives you some extra advantages and features that are not available in the official version. It is created by third-party developers who modify the game's code and data to alter its functionality. It is also known as a
-
The difference between the original and the modded version
-
The main difference between the original and the modded version of Atlantis Odyssey is that the modded version gives you unlimited money and resources. This means that you can buy anything you want, upgrade anything you want, craft anything you want, and complete any task or quest you want without worrying about running out of coins, gems, energy, or other resources. You can also access all the features and content of the game without waiting for them to be unlocked or available. You can enjoy the game at your own pace and style without any limitations or frustrations.
-
The advantages and disadvantages of using the modded version
-
Using the modded version of Atlantis Odyssey has its pros and cons. Some of the advantages are:
-
-
You can have more fun and satisfaction playing the game with unlimited money and resources.
-
You can save time and effort by skipping the tedious and repetitive tasks of collecting and managing resources.
-
You can explore and discover more aspects and secrets of the game without any restrictions.
-
You can customize and personalize your camp and your character to your liking.
-
You can impress and compete with other players online with your achievements and creations.
-
-
Some of the disadvantages are:
-
-
You might lose the challenge and excitement of playing the game with limited money and resources.
-
You might encounter some bugs, errors, or crashes while using the modded version.
-
You might risk your device's security and privacy by downloading and installing an unofficial and unverified version of the game.
-
You might violate the terms and conditions of the game's developer and publisher by using an unauthorized and illegal version of the game.
-
You might get banned or suspended from the game's online services if you are detected or reported by other players or moderators.
-
-
How to download and install Atlantis Odyssey Mod APK Terbaru?
-
If you want to try Atlantis Odyssey Mod APK Terbaru, you will need to download and install it on your Android device. Here are the steps to do so:
-
atlantis odyssey unlimited money mod apk
-download atlantis odyssey mod apk latest version
-atlantis odyssey hack mod apk free download
-atlantis odyssey mod apk offline
-atlantis odyssey simulation game mod apk
-atlantis odyssey mod apk android 1
-atlantis odyssey mod apk no root
-atlantis odyssey mod apk unlimited gems
-atlantis odyssey mod apk rexdl
-atlantis odyssey mod apk revdl
-atlantis odyssey mod apk happymod
-atlantis odyssey mod apk 2023
-atlantis odyssey mod apk for ios
-atlantis odyssey mod apk obb
-atlantis odyssey mod apk vip
-atlantis odyssey mod apk unlimited energy
-atlantis odyssey mod apk pure
-atlantis odyssey mod apk platinmods
-atlantis odyssey mod apk an1
-atlantis odyssey mod apk apkpure
-atlantis odyssey mod apk apkmody
-atlantis odyssey mod apk apknite
-atlantis odyssey mod apk apkmirror
-atlantis odyssey mod apk apksfree
-atlantis odyssey mod apk apktada
-atlantis odyssey mod apk apksaurus
-atlantis odyssey mod apk apksfull
-atlantis odyssey mod apk apksmodded
-atlantis odyssey mod apk apksmash
-atlantis odyssey mod apk apksparadise
-atlantis odyssey mod apk apkspeedy
-atlantis odyssey mod apk apksupermarket
-atlantis odyssey mod apk apksweet
-atlantis odyssey mod apk apktwist
-atlantis odyssey mod apk apkturbo
-atlantis odyssey mod apk apkvilla
-atlantis odyssey mod apk apkwonderland
-atlantis odyssey mod apk apkworlds
-atlantis odyssey mod apk apk
-
The steps to download and install the modded version
-
-
Go to a reliable and trusted website that offers Atlantis Odyssey Mod APK Terbaru for free. You can search for it on Google or use one of these links: .
-
Download the modded version file to your device. Make sure you have enough storage space and a stable internet connection.
-
Enable the installation of apps from unknown sources on your device. You can do this by going to Settings > Security > Unknown Sources and toggling it on.
-
Locate the downloaded file on your device's file manager and tap on it to start the installation process. Follow the instructions on the screen to complete the installation.
-
Launch the game from your app drawer or home screen and enjoy!
-
-
The tips and tricks to enjoy the modded version
-
Here are some tips and tricks to help you enjoy Atlantis Odyssey Mod APK Terbaru:
-
-
Use your unlimited money and resources wisely. Don't spend them all at once or you might get bored quickly. Try to balance your spending and saving habits.
-
Explore different areas of the island and discover new things. Don't just stick to one place or activity. Try to complete different tasks and quests that will reward you with more items and information.
-
Interact with other characters and learn more about their stories and personalities. Don't ignore them or treat them badly. They might help you or give you some hints or secrets.
-
Play with other players online and have fun. Don't be rude or mean to them. Be friendly and cooperative. You can trade with them, chat with them, visit their camps, etc.
-
Be careful when using the modded version online. Don't brag about it or show it off to other players. They might report you or get jealous of you. You might also get detected by the game's security system and get banned or suspended.
-
-
Conclusion
-
Atlantis Odyssey is a fun and relaxing simulation game that lets you explore, build, and craft your own world in a mysterious island full of secrets and surprises. Atlantis Odyssey Mod APK Terbaru is a modified version of the game that gives you unlimited money and resources that can enhance your gaming experience. However, it also has some risks and drawbacks that you should be aware of. If you want to try it, you will need to download and install it on your device following the steps we provided. You will also need to follow some tips and tricks to enjoy it without any problems. We hope this article helped you learn more about this game and its modded version. If you have any questions or feedback, feel free to leave a comment below. Thank you for reading and happy gaming!
-
FAQs
-
Here are some frequently asked questions about Atlantis Odyssey Mod APK Terbaru:
-
-
What is the latest version of Atlantis Odyssey Mod APK Terbaru?
-
The latest version of Atlantis Odyssey Mod APK Terbaru is 1.0.1, which was released on June 15, 2023. It has a file size of 132 MB and requires Android 5.0 or higher to run.
-
Is Atlantis Odyssey Mod APK Terbaru safe to use?
-
Atlantis Odyssey Mod APK Terbaru is not an official or verified version of the game, so it might not be safe to use. It might contain viruses, malware, or spyware that can harm your device or steal your data. It might also violate the game's terms and conditions and get you banned or suspended from the game's online services. Therefore, use it at your own risk and discretion.
-
Can I play Atlantis Odyssey Mod APK Terbaru offline?
-
Atlantis Odyssey Mod APK Terbaru can be played offline, but you will not be able to access some of the features and content that require an internet connection, such as interacting with other players online, visiting their camps, trading with them, etc.
-
Can I update Atlantis Odyssey Mod APK Terbaru?
-
Atlantis Odyssey Mod APK Terbaru can be updated by downloading and installing the latest version from the same website or source that you got it from. However, updating the modded version might cause some issues or errors with the game's functionality or compatibility. You might also lose your progress or data if you update the modded version without backing it up first.
-
Can I use Atlantis Odyssey Mod APK Terbaru on other devices or platforms?
-
Atlantis Odyssey Mod APK Terbaru is only compatible with Android devices. It cannot be used on other devices or platforms, such as iOS, Windows, Mac, etc.
-
401be4b1e0
-
-
\ No newline at end of file
diff --git a/spaces/1phancelerku/anime-remove-background/Download Skin FR Legends and Enjoy the Thrill of Front-Engine Rear-Wheel Drive Racing.md b/spaces/1phancelerku/anime-remove-background/Download Skin FR Legends and Enjoy the Thrill of Front-Engine Rear-Wheel Drive Racing.md
deleted file mode 100644
index 3181d79e643deea25a37c6c4e538cd2998b455d5..0000000000000000000000000000000000000000
--- a/spaces/1phancelerku/anime-remove-background/Download Skin FR Legends and Enjoy the Thrill of Front-Engine Rear-Wheel Drive Racing.md
+++ /dev/null
@@ -1,114 +0,0 @@
-
-
How to Download Skin FR Legends and Make Your Car Look Awesome
-
If you are a fan of drifting games, you might have heard of FR Legends, a popular mobile game that lets you experience the thrill of sliding sideways on various tracks. But did you know that you can also customize your car with different skin FR Legends that change its appearance? In this article, we will show you how to download skin FR Legends and apply it to your car in a few simple steps. We will also give you some tips and tricks for using skin FR Legends effectively. Let's get started!
-
What is FR Legends and Why You Should Play It
-
FR Legends is a fun and realistic drifting game for mobile devices
-
FR Legends is a game that simulates the sport of drifting, which involves driving a car at high speed and making it slide sideways on curves. The game features realistic physics, graphics, and sound effects that make you feel like you are really behind the wheel. You can choose from different cars, tracks, modes, and settings to suit your preferences. You can also compete with other players online or offline, or watch replays of your best runs.
You can customize your car with various parts, decals, and liveries
-
One of the best features of FR Legends is that you can modify your car in many ways. You can upgrade your engine, suspension, tires, brakes, and more to improve your performance. You can also change the color, shape, and size of your wheels, spoilers, bumpers, mirrors, exhausts, and more to change your style. You can also add decals and stickers to decorate your car with logos, patterns, or words. And finally, you can change the livery of your car, which is the paint scheme that covers its body.
-
What is Skin FR Legends and How to Get It
-
Skin FR Legends is a term for custom liveries that change the appearance of your car
-
A livery is a design that covers the body of your car. It usually consists of colors, shapes, images, or text that make your car look unique. In FR Legends, you can choose from several preset liveries that are available in the game. However, if you want to have more options and
creativity, you can use skin FR Legends, which are custom liveries that are created by other players or yourself. Skin FR Legends can change the appearance of your car completely, making it look like a different model, brand, or theme. For example, you can make your car look like a Ferrari, a Lamborghini, a BMW, or a Nissan. You can also make your car look like it belongs to a famous racing team, movie franchise, anime series, or video game. The possibilities are endless!
-
You can create your own skin or download one from the internet using codes
-
To create your own skin FR Legends, you need to use a special app called FR Legends Livery Editor, which is available for Android and iOS devices. This app allows you to design your own livery using various tools and features. You can draw, paint, erase, fill, rotate, scale, and move different elements on your car. You can also import images from your gallery or camera and use them as stickers or backgrounds. Once you are done with your creation, you can save it and export it as a code that you can use in FR Legends.
-
To download skin FR Legends from the internet, you need to find a source that provides codes for different liveries. There are many websites, forums, social media pages, and YouTube videos that offer skin FR Legends for free or for a fee. You can browse through different categories and themes and choose the ones that you like. You can also read reviews and ratings from other users and see how the skin looks in action. Once you have found the skin that you want, you need to copy its code and use it in FR Legends.
-
Some popular themes for skin FR Legends are anime, fast and furious, garasi drift, and more
-
There are many types of skin FR Legends that you can choose from depending on your taste and preference. Some of the most popular themes are:
-
-
Anime: If you are a fan of Japanese animation, you can find skin FR Legends that feature characters, logos, or scenes from your favorite anime shows or movies. Some examples are Naruto, One Piece, Dragon Ball Z, Attack on Titan, Tokyo Ghoul, and more.
-
Fast and Furious: If you love the action-packed movie series that revolves around cars and racing, you can find skin FR Legends that resemble the vehicles used by the main characters or villains. Some examples are Dom's Charger, Brian's Skyline, Han's RX-7, Shaw's Flip Car, and more.
-
Garasi Drift: If you admire the Indonesian drifting community that is known for its creativity and skill, you can find skin FR Legends that pay tribute to their style and culture. Some examples are Garasi Drift 86, Garasi Drift S15, Garasi Drift E30, Garasi Drift AE86 Panda Trueno, and more.
-
And more: There are many other themes that you can explore such as sports teams, celebrities, brands, countries, memes, cartoons, games, and more. You can also mix and match different elements from different themes to create your own unique skin FR Legends.
-
-
How to Apply Skin FR Legends to Your Car
-
You need to copy the body code and the window code of the skin you want
-
To apply skin FR Legends to your car in FR Legends, you need to have two codes: the body code and the window code. The body code is the code that determines the livery of the main part of your car. The window code is the code that determines the livery of the windows of your car. You need to copy both codes from the source where you got the skin.
You need to go to the garage menu and tap on the livery button
-
Once you have copied the codes of the skin FR Legends that you want to use, you need to go to the garage menu in FR Legends and tap on the livery button. This will open a new screen where you can see your current livery and two boxes for entering codes. You can also see an eye icon and a share icon on the top right corner of the screen.
-
You need to paste the codes in the corresponding boxes and save your changes
-
Next, you need to paste the codes that you copied in the corresponding boxes. The body code goes in the upper box and the window code goes in the lower box. You can use the paste button or long press on the box to paste the code. After you have entered both codes, you need to tap on the save button on the bottom right corner of the screen. This will apply the skin FR Legends to your car and return you to the garage menu.
-
Some Tips and Tricks for Using Skin FR Legends
-
You can preview the skin before applying it by tapping on the eye icon
-
If you want to see how the skin FR Legends looks on your car before applying it, you can tap on the eye icon on the top right corner of the livery screen. This will show you a preview of your car with the skin FR Legends that you entered. You can rotate, zoom, and move your car to see it from different angles. You can also change the background color by tapping on the color wheel icon. If you like what you see, you can tap on the save button to apply it. If not, you can tap on the back button to return to the livery screen and try another skin FR Legends.
-
You can share your skin with others by tapping on the share icon and copying the codes
-
If you want to share your skin FR Legends with others, you can tap on the share icon on the top right corner of the livery screen. This will show you two codes: one for the body and one for the window. You can copy these codes by tapping on them or using the copy button. You can then send these codes to your friends or post them online for others to use. You can also use these codes to backup your skin FR Legends in case you want to use it again later.
-
You can find more skin FR Legends on websites, forums, social media, and YouTube videos
-
If you want to find more skin FR Legends that suit your taste and style, you can search for them online using various sources. There are many websites that offer skin FR Legends for free or for a fee. Some examples are FR Legends Hub, FR Legends Mods, FR Legends Livery, and more. You can also find skin FR Legends on forums such as Reddit, Discord, or Facebook Groups. You can also follow social media pages such as Instagram, Twitter, or TikTok that post skin FR Legends regularly. And finally, you can watch YouTube videos that showcase or teach how to make skin FR Legends such as this one, this one, or this one.
-
Conclusion
-
Skin FR Legends is a great way to make your car look unique and cool in FR Legends
-
In conclusion, skin FR Legends is a term for custom liveries that change the appearance of your car in FR Legends. You can download skin FR Legends from various sources online or create your own using codes. You can apply skin FR Legends easily by following the steps above. You can also preview, share, and backup your skin FR Legends using the livery menu in the game. Skin FR Legends can make your car look awesome and express your personality and style.
-
You can download skin FR Legends from various sources or create your own using codes
-
There are many ways to get skin FR Legends for your car in FR Legends. You can create your own skin using the FR Legends Livery Editor app, which allows you to design your own livery using various tools and features. You can also download skin FR Legends from websites, forums, social media, and YouTube videos that offer codes for different liveries. You can choose from different themes and categories such as anime, fast and furious, garasi drift, and more. You can also mix and match different elements from different themes to create your own unique skin FR Legends.
-
You can apply skin FR Legends easily by following the steps above
-
To apply skin FR Legends to your car in FR Legends, you need to have two codes: the body code and the window code. The body code is the code that determines the livery of the main part of your car. The window code is the code that determines the livery of the windows of your car. You need to copy both codes from the source where you got the skin. Then, you need to go to the garage menu in FR Legends and tap on the livery button. This will open a new screen where you can enter the codes in the corresponding boxes and save your changes. This will apply the skin FR Legends to your car and make it look awesome.
-
FAQs
-
What are the best websites to download skin FR Legends?
-
There are many websites that offer skin FR Legends for free or for a fee. Some of the best ones are:
-
-
FR Legends Hub: This website has a large collection of skin FR Legends for different cars and themes. You can browse by categories or search by keywords. You can also upload your own skin or request a custom one.
-
FR Legends Mods: This website has a variety of mods and skins for FR Legends. You can find liveries, decals, wheels, engines, tracks, and more. You can also join their Discord server for more updates and support.
-
FR Legends Livery: This website has a simple and easy-to-use interface for finding and downloading skin FR Legends. You can see the previews and codes of different liveries and copy them with one click.
-
-
How to make my own skin FR Legends?
-
To make your own skin FR Legends, you need to use a special app called FR Legends Livery Editor, which is available for Android and iOS devices. This app allows you to design your own livery using various tools and features. You can draw, paint, erase, fill, rotate, scale, and move different elements on your car. You can also import images from your gallery or camera and use them as stickers or backgrounds. Once you are done with your creation, you can save it and export it as a code that you can use in FR Legends.
-
How to remove skin FR Legends from my car?
-
To remove skin FR Legends from your car in FR Legends, you need to go to the garage menu and tap on the livery button. This will open a new screen where you can see your current livery and two boxes for entering codes. To remove the skin FR Legends from your car, you need to delete the codes in both boxes and save your changes. This will restore your car to its original appearance.
-
Can I use skin FR Legends in online mode?
-
Yes, you can use skin FR Legends in online mode in FR Legends. However, you need to be aware that other players may not see your skin as you do. This is because they may not have the same images or fonts that you used for your skin on their device. Therefore, they may see a different or distorted version of your skin or no skin at all. To avoid this problem, you should use simple and common images or fonts for your skin or share your codes with other players before playing online.
-
Are there any risks or disadvantages of using skin FR Legends?
-
Using skin FR Legends is generally safe and fun, but there are some potential risks or disadvantages that you should be aware of. These include:
-
-
Legal issues: Some skin FR Legends may contain copyrighted images or logos that belong to other companies or entities. This may violate their intellectual property rights and cause legal problems for you or the source of the skin. You should always check the license and terms of use of the skin before downloading or using it.
-
Technical issues: Some skin FR Legends may contain errors or bugs that affect the performance or functionality of your game. This may cause crashes, glitches, lag, or other problems that ruin your gaming experience. You should always backup your game data before applying any skin and test it for any issues.
-
Ethical issues: Some skin FR Legends may contain inappropriate or offensive images or text that may hurt or offend other players or viewers. This may cause negative reactions, complaints, or reports that damage your reputation or account. You should always respect the rules and guidelines of the game and the community and avoid using any skin that may cause harm or trouble.
-
-
These are some of the risks or disadvantages of using skin FR Legends. You should always be careful and responsible when using skin FR Legends and enjoy them in a safe and respectful manner.
401be4b1e0
-
-
\ No newline at end of file
diff --git a/spaces/1toTree/lora_test/app.py b/spaces/1toTree/lora_test/app.py
deleted file mode 100644
index 859863ec7c6bce1bfd744db99a338a57c2701fab..0000000000000000000000000000000000000000
--- a/spaces/1toTree/lora_test/app.py
+++ /dev/null
@@ -1,1677 +0,0 @@
-# Copyright (c) 2023 PaddlePaddle Authors. All Rights Reserved.
-# Copyright 2022 The HuggingFace Team. All rights reserved.
-#
-# Licensed under the Apache License, Version 2.0 (the "License");
-# you may not use this file except in compliance with the License.
-# You may obtain a copy of the License at
-#
-# http://www.apache.org/licenses/LICENSE-2.0
-#
-# Unless required by applicable law or agreed to in writing, software
-# distributed under the License is distributed on an "AS IS" BASIS,
-# WITHOUT WARRANTIES OR CONDITIONS OF ANY KIND, either express or implied.
-# See the License for the specific language governing permissions and
-# limitations under the License.
-import gradio as gr
-from env import BASE_MODEL_NAME, LORA_WEIGHTS_PATH, PROMPTS
-
-examples = [
- [
- PROMPTS,
- 'low quality',
- 7.5,
- 512,
- 512,
- 25,
- "DPMSolver"
- ],
-]
-import inspect
-import os
-import random
-import re
-import time
-from typing import Callable, List, Optional, Union
-
-import numpy as np
-import paddle
-import PIL
-import PIL.Image
-from packaging import version
-
-from paddlenlp.transformers import CLIPFeatureExtractor, CLIPTextModel, CLIPTokenizer
-
-from ppdiffusers.configuration_utils import FrozenDict
-from ppdiffusers.models import AutoencoderKL, UNet2DConditionModel
-from ppdiffusers.pipeline_utils import DiffusionPipeline
-from ppdiffusers.schedulers import (
- DDIMScheduler,
- DPMSolverMultistepScheduler,
- EulerAncestralDiscreteScheduler,
- EulerDiscreteScheduler,
- LMSDiscreteScheduler,
- PNDMScheduler,
- HeunDiscreteScheduler,
- KDPM2AncestralDiscreteScheduler,
- KDPM2DiscreteScheduler,
-
-)
-from ppdiffusers.utils import PIL_INTERPOLATION, deprecate, logging
-from ppdiffusers.utils.testing_utils import load_image
-from ppdiffusers.pipelines.stable_diffusion import StableDiffusionPipelineOutput
-from ppdiffusers.pipelines.stable_diffusion.safety_checker import StableDiffusionSafetyChecker
-
-logger = logging.get_logger(__name__) # pylint: disable=invalid-name
-
-
-def save_all(images, FORMAT="jpg", OUTDIR="./outputs/"):
- if not isinstance(images, (list, tuple)):
- images = [images]
- for image in images:
- PRECISION = "fp32"
- argument = image.argument
- os.makedirs(OUTDIR, exist_ok=True)
- epoch_time = argument["epoch_time"]
- PROMPT = argument["prompt"]
- NEGPROMPT = argument["negative_prompt"]
- HEIGHT = argument["height"]
- WIDTH = argument["width"]
- SEED = argument["seed"]
- STRENGTH = argument.get("strength", 1)
- INFERENCE_STEPS = argument["num_inference_steps"]
- GUIDANCE_SCALE = argument["guidance_scale"]
-
- filename = f"{str(epoch_time)}_scale_{GUIDANCE_SCALE}_steps_{INFERENCE_STEPS}_seed_{SEED}.{FORMAT}"
- filedir = f"{OUTDIR}/{filename}"
- image.save(filedir)
- with open(f"{OUTDIR}/{epoch_time}_prompt.txt", "w") as file:
- file.write(
- f"PROMPT: {PROMPT}\nNEG_PROMPT: {NEGPROMPT}\n\nINFERENCE_STEPS: {INFERENCE_STEPS}\nHeight: {HEIGHT}\nWidth: {WIDTH}\nSeed: {SEED}\n\nPrecision: {PRECISION}\nSTRENGTH: {STRENGTH}\nGUIDANCE_SCALE: {GUIDANCE_SCALE}"
- )
-
-
-re_attention = re.compile(
- r"""
-\\\(|
-\\\)|
-\\\[|
-\\]|
-\\\\|
-\\|
-\(|
-\[|
-:([+-]?[.\d]+)\)|
-\)|
-]|
-[^\\()\[\]:]+|
-:
-""",
- re.X,
-)
-
-
-def parse_prompt_attention(text):
- """
- Parses a string with attention tokens and returns a list of pairs: text and its associated weight.
- Accepted tokens are:
- (abc) - increases attention to abc by a multiplier of 1.1
- (abc:3.12) - increases attention to abc by a multiplier of 3.12
- [abc] - decreases attention to abc by a multiplier of 1.1
- \( - literal character '('
- \[ - literal character '['
- \) - literal character ')'
- \] - literal character ']'
- \\ - literal character '\'
- anything else - just text
- >>> parse_prompt_attention('normal text')
- [['normal text', 1.0]]
- >>> parse_prompt_attention('an (important) word')
- [['an ', 1.0], ['important', 1.1], [' word', 1.0]]
- >>> parse_prompt_attention('(unbalanced')
- [['unbalanced', 1.1]]
- >>> parse_prompt_attention('\(literal\]')
- [['(literal]', 1.0]]
- >>> parse_prompt_attention('(unnecessary)(parens)')
- [['unnecessaryparens', 1.1]]
- >>> parse_prompt_attention('a (((house:1.3)) [on] a (hill:0.5), sun, (((sky))).')
- [['a ', 1.0],
- ['house', 1.5730000000000004],
- [' ', 1.1],
- ['on', 1.0],
- [' a ', 1.1],
- ['hill', 0.55],
- [', sun, ', 1.1],
- ['sky', 1.4641000000000006],
- ['.', 1.1]]
- """
-
- res = []
- round_brackets = []
- square_brackets = []
-
- round_bracket_multiplier = 1.1
- square_bracket_multiplier = 1 / 1.1
-
- def multiply_range(start_position, multiplier):
- for p in range(start_position, len(res)):
- res[p][1] *= multiplier
-
- for m in re_attention.finditer(text):
- text = m.group(0)
- weight = m.group(1)
-
- if text.startswith("\\"):
- res.append([text[1:], 1.0])
- elif text == "(":
- round_brackets.append(len(res))
- elif text == "[":
- square_brackets.append(len(res))
- elif weight is not None and len(round_brackets) > 0:
- multiply_range(round_brackets.pop(), float(weight))
- elif text == ")" and len(round_brackets) > 0:
- multiply_range(round_brackets.pop(), round_bracket_multiplier)
- elif text == "]" and len(square_brackets) > 0:
- multiply_range(square_brackets.pop(), square_bracket_multiplier)
- else:
- res.append([text, 1.0])
-
- for pos in round_brackets:
- multiply_range(pos, round_bracket_multiplier)
-
- for pos in square_brackets:
- multiply_range(pos, square_bracket_multiplier)
-
- if len(res) == 0:
- res = [["", 1.0]]
-
- # merge runs of identical weights
- i = 0
- while i + 1 < len(res):
- if res[i][1] == res[i + 1][1]:
- res[i][0] += res[i + 1][0]
- res.pop(i + 1)
- else:
- i += 1
-
- return res
-
-
-def get_prompts_with_weights(pipe: DiffusionPipeline, prompt: List[str], max_length: int):
- r"""
- Tokenize a list of prompts and return its tokens with weights of each token.
-
- No padding, starting or ending token is included.
- """
- tokens = []
- weights = []
- for text in prompt:
- texts_and_weights = parse_prompt_attention(text)
- text_token = []
- text_weight = []
- for word, weight in texts_and_weights:
- # tokenize and discard the starting and the ending token
- token = pipe.tokenizer(word).input_ids[1:-1]
- text_token += token
-
- # copy the weight by length of token
- text_weight += [weight] * len(token)
-
- # stop if the text is too long (longer than truncation limit)
- if len(text_token) > max_length:
- break
-
- # truncate
- if len(text_token) > max_length:
- text_token = text_token[:max_length]
- text_weight = text_weight[:max_length]
-
- tokens.append(text_token)
- weights.append(text_weight)
- return tokens, weights
-
-
-def pad_tokens_and_weights(tokens, weights, max_length, bos, eos, pad, no_boseos_middle=True, chunk_length=77):
- r"""
- Pad the tokens (with starting and ending tokens) and weights (with 1.0) to max_length.
- """
- max_embeddings_multiples = (max_length - 2) // (chunk_length - 2)
- weights_length = max_length if no_boseos_middle else max_embeddings_multiples * chunk_length
- for i in range(len(tokens)):
- tokens[i] = [bos] + tokens[i] + [eos] + [pad] * (max_length - 2 - len(tokens[i]))
- if no_boseos_middle:
- weights[i] = [1.0] + weights[i] + [1.0] * (max_length - 1 - len(weights[i]))
- else:
- w = []
- if len(weights[i]) == 0:
- w = [1.0] * weights_length
- else:
- for j in range((len(weights[i]) - 1) // chunk_length + 1):
- w.append(1.0) # weight for starting token in this chunk
- w += weights[i][j * chunk_length : min(len(weights[i]), (j + 1) * chunk_length)]
- w.append(1.0) # weight for ending token in this chunk
- w += [1.0] * (weights_length - len(w))
- weights[i] = w[:]
-
- return tokens, weights
-
-
-def get_unweighted_text_embeddings(
- pipe: DiffusionPipeline, text_input: paddle.Tensor, chunk_length: int, no_boseos_middle: Optional[bool] = True
-):
- """
- When the length of tokens is a multiple of the capacity of the text encoder,
- it should be split into chunks and sent to the text encoder individually.
- """
- max_embeddings_multiples = (text_input.shape[1] - 2) // (chunk_length - 2)
- if max_embeddings_multiples > 1:
- text_embeddings = []
- for i in range(max_embeddings_multiples):
- # extract the i-th chunk
- text_input_chunk = text_input[:, i * (chunk_length - 2) : (i + 1) * (chunk_length - 2) + 2].clone()
-
- # cover the head and the tail by the starting and the ending tokens
- text_input_chunk[:, 0] = text_input[0, 0]
- text_input_chunk[:, -1] = text_input[0, -1]
-
- text_embedding = pipe.text_encoder(text_input_chunk)[0]
-
- if no_boseos_middle:
- if i == 0:
- # discard the ending token
- text_embedding = text_embedding[:, :-1]
- elif i == max_embeddings_multiples - 1:
- # discard the starting token
- text_embedding = text_embedding[:, 1:]
- else:
- # discard both starting and ending tokens
- text_embedding = text_embedding[:, 1:-1]
-
- text_embeddings.append(text_embedding)
- text_embeddings = paddle.concat(text_embeddings, axis=1)
- else:
- text_embeddings = pipe.text_encoder(text_input)[0]
- return text_embeddings
-
-
-def get_weighted_text_embeddings(
- pipe: DiffusionPipeline,
- prompt: Union[str, List[str]],
- uncond_prompt: Optional[Union[str, List[str]]] = None,
- max_embeddings_multiples: Optional[int] = 1,
- no_boseos_middle: Optional[bool] = False,
- skip_parsing: Optional[bool] = False,
- skip_weighting: Optional[bool] = False,
- **kwargs
-):
- r"""
- Prompts can be assigned with local weights using brackets. For example,
- prompt 'A (very beautiful) masterpiece' highlights the words 'very beautiful',
- and the embedding tokens corresponding to the words get multiplied by a constant, 1.1.
-
- Also, to regularize of the embedding, the weighted embedding would be scaled to preserve the original mean.
-
- Args:
- pipe (`DiffusionPipeline`):
- Pipe to provide access to the tokenizer and the text encoder.
- prompt (`str` or `List[str]`):
- The prompt or prompts to guide the image generation.
- uncond_prompt (`str` or `List[str]`):
- The unconditional prompt or prompts for guide the image generation. If unconditional prompt
- is provided, the embeddings of prompt and uncond_prompt are concatenated.
- max_embeddings_multiples (`int`, *optional*, defaults to `1`):
- The max multiple length of prompt embeddings compared to the max output length of text encoder.
- no_boseos_middle (`bool`, *optional*, defaults to `False`):
- If the length of text token is multiples of the capacity of text encoder, whether reserve the starting and
- ending token in each of the chunk in the middle.
- skip_parsing (`bool`, *optional*, defaults to `False`):
- Skip the parsing of brackets.
- skip_weighting (`bool`, *optional*, defaults to `False`):
- Skip the weighting. When the parsing is skipped, it is forced True.
- """
- max_length = (pipe.tokenizer.model_max_length - 2) * max_embeddings_multiples + 2
- if isinstance(prompt, str):
- prompt = [prompt]
-
- if not skip_parsing:
- prompt_tokens, prompt_weights = get_prompts_with_weights(pipe, prompt, max_length - 2)
- if uncond_prompt is not None:
- if isinstance(uncond_prompt, str):
- uncond_prompt = [uncond_prompt]
- uncond_tokens, uncond_weights = get_prompts_with_weights(pipe, uncond_prompt, max_length - 2)
- else:
- prompt_tokens = [
- token[1:-1] for token in pipe.tokenizer(prompt, max_length=max_length, truncation=True).input_ids
- ]
- prompt_weights = [[1.0] * len(token) for token in prompt_tokens]
- if uncond_prompt is not None:
- if isinstance(uncond_prompt, str):
- uncond_prompt = [uncond_prompt]
- uncond_tokens = [
- token[1:-1]
- for token in pipe.tokenizer(uncond_prompt, max_length=max_length, truncation=True).input_ids
- ]
- uncond_weights = [[1.0] * len(token) for token in uncond_tokens]
-
- # round up the longest length of tokens to a multiple of (model_max_length - 2)
- max_length = max([len(token) for token in prompt_tokens])
- if uncond_prompt is not None:
- max_length = max(max_length, max([len(token) for token in uncond_tokens]))
-
- max_embeddings_multiples = min(
- max_embeddings_multiples, (max_length - 1) // (pipe.tokenizer.model_max_length - 2) + 1
- )
- max_embeddings_multiples = max(1, max_embeddings_multiples)
- max_length = (pipe.tokenizer.model_max_length - 2) * max_embeddings_multiples + 2
-
- # pad the length of tokens and weights
- # support bert tokenizer
- bos = pipe.tokenizer.bos_token_id if pipe.tokenizer.bos_token_id is not None else pipe.tokenizer.cls_token_id
- eos = pipe.tokenizer.eos_token_id if pipe.tokenizer.eos_token_id is not None else pipe.tokenizer.sep_token_id
- pad = pipe.tokenizer.pad_token_id
- prompt_tokens, prompt_weights = pad_tokens_and_weights(
- prompt_tokens,
- prompt_weights,
- max_length,
- bos,
- eos,
- pad,
- no_boseos_middle=no_boseos_middle,
- chunk_length=pipe.tokenizer.model_max_length,
- )
- prompt_tokens = paddle.to_tensor(prompt_tokens)
- if uncond_prompt is not None:
- uncond_tokens, uncond_weights = pad_tokens_and_weights(
- uncond_tokens,
- uncond_weights,
- max_length,
- bos,
- eos,
- pad,
- no_boseos_middle=no_boseos_middle,
- chunk_length=pipe.tokenizer.model_max_length,
- )
- uncond_tokens = paddle.to_tensor(uncond_tokens)
-
- # get the embeddings
- text_embeddings = get_unweighted_text_embeddings(
- pipe, prompt_tokens, pipe.tokenizer.model_max_length, no_boseos_middle=no_boseos_middle
- )
- prompt_weights = paddle.to_tensor(prompt_weights, dtype=text_embeddings.dtype)
- if uncond_prompt is not None:
- uncond_embeddings = get_unweighted_text_embeddings(
- pipe, uncond_tokens, pipe.tokenizer.model_max_length, no_boseos_middle=no_boseos_middle
- )
- uncond_weights = paddle.to_tensor(uncond_weights, dtype=uncond_embeddings.dtype)
-
- # assign weights to the prompts and normalize in the sense of mean
- # TODO: should we normalize by chunk or in a whole (current implementation)?
- if (not skip_parsing) and (not skip_weighting):
- previous_mean = text_embeddings.mean(axis=[-2, -1])
- text_embeddings *= prompt_weights.unsqueeze(-1)
- text_embeddings *= previous_mean / text_embeddings.mean(axis=[-2, -1])
- if uncond_prompt is not None:
- previous_mean = uncond_embeddings.mean(axis=[-2, -1])
- uncond_embeddings *= uncond_weights.unsqueeze(-1)
- uncond_embeddings *= previous_mean / uncond_embeddings.mean(axis=[-2, -1])
-
- # For classifier free guidance, we need to do two forward passes.
- # Here we concatenate the unconditional and text embeddings into a single batch
- # to avoid doing two forward passes
- if uncond_prompt is not None:
- text_embeddings = paddle.concat([uncond_embeddings, text_embeddings])
-
- return text_embeddings
-
-
-def preprocess_image(image):
- w, h = image.size
- w, h = map(lambda x: x - x % 32, (w, h)) # resize to integer multiple of 32
- image = image.resize((w, h), resample=PIL_INTERPOLATION["lanczos"])
- image = np.array(image).astype(np.float32) / 255.0
- image = image[None].transpose(0, 3, 1, 2)
- image = paddle.to_tensor(image)
- return 2.0 * image - 1.0
-
-
-def preprocess_mask(mask):
- mask = mask.convert("L")
- w, h = mask.size
- w, h = map(lambda x: x - x % 32, (w, h)) # resize to integer multiple of 32
- mask = mask.resize((w // 8, h // 8), resample=PIL_INTERPOLATION["nearest"])
- mask = np.array(mask).astype(np.float32) / 255.0
- mask = np.tile(mask, (4, 1, 1))
- mask = mask[None].transpose(0, 1, 2, 3) # what does this step do?
- mask = 1 - mask # repaint white, keep black
- mask = paddle.to_tensor(mask)
- return mask
-
-
-class StableDiffusionPipelineAllinOne(DiffusionPipeline):
- r"""
- Pipeline for text-to-image image-to-image inpainting generation using Stable Diffusion.
-
- This model inherits from [`DiffusionPipeline`]. Check the superclass documentation for the generic methods the
- library implements for all the pipelines (such as downloading or saving, running on a particular xxxx, etc.)
-
- Args:
- vae ([`AutoencoderKL`]):
- Variational Auto-Encoder (VAE) Model to encode and decode images to and from latent representations.
- text_encoder ([`CLIPTextModel`]):
- Frozen text-encoder. Stable Diffusion uses the text portion of
- [CLIP](https://huggingface.co/docs/transformers/model_doc/clip#transformers.CLIPTextModel), specifically
- the [clip-vit-large-patch14](https://huggingface.co/openai/clip-vit-large-patch14) variant.
- tokenizer (`CLIPTokenizer`):
- Tokenizer of class
- [CLIPTokenizer](https://huggingface.co/docs/transformers/v4.21.0/en/model_doc/clip#transformers.CLIPTokenizer).
- unet ([`UNet2DConditionModel`]): Conditional U-Net architecture to denoise the encoded image latents.
- scheduler ([`SchedulerMixin`]):
- A scheduler to be used in combination with `unet` to denoise the encoded image latents. Can be one of
- [`DDIMScheduler`], [`LMSDiscreteScheduler`], [`PNDMScheduler`], [`EulerDiscreteScheduler`], [`EulerAncestralDiscreteScheduler`]
- or [`DPMSolverMultistepScheduler`].
- safety_checker ([`StableDiffusionSafetyChecker`]):
- Classification module that estimates whether generated images could be considered offensive or harmful.
- Please, refer to the [model card](https://huggingface.co/junnyu/stable-diffusion-v1-4-paddle) for details.
- feature_extractor ([`CLIPFeatureExtractor`]):
- Model that extracts features from generated images to be used as inputs for the `safety_checker`.
- """
- _optional_components = ["safety_checker", "feature_extractor"]
-
- def __init__(
- self,
- vae: AutoencoderKL,
- text_encoder: CLIPTextModel,
- tokenizer: CLIPTokenizer,
- unet: UNet2DConditionModel,
- scheduler: Union[
- DDIMScheduler,
- PNDMScheduler,
- LMSDiscreteScheduler,
- EulerDiscreteScheduler,
- EulerAncestralDiscreteScheduler,
- DPMSolverMultistepScheduler,
- ],
- safety_checker: StableDiffusionSafetyChecker,
- feature_extractor: CLIPFeatureExtractor,
- requires_safety_checker: bool = False,
- ):
- if hasattr(scheduler.config, "steps_offset") and scheduler.config.steps_offset != 1:
- deprecation_message = (
- f"The configuration file of this scheduler: {scheduler} is outdated. `steps_offset`"
- f" should be set to 1 instead of {scheduler.config.steps_offset}. Please make sure "
- "to update the config accordingly as leaving `steps_offset` might led to incorrect results"
- " in future versions. If you have downloaded this checkpoint from the Hugging Face Hub,"
- " it would be very nice if you could open a Pull request for the `scheduler/scheduler_config.json`"
- " file"
- )
- deprecate("steps_offset!=1", "1.0.0", deprecation_message, standard_warn=False)
- new_config = dict(scheduler.config)
- new_config["steps_offset"] = 1
- scheduler._internal_dict = FrozenDict(new_config)
-
- if hasattr(scheduler.config, "clip_sample") and scheduler.config.clip_sample is True:
- deprecation_message = (
- f"The configuration file of this scheduler: {scheduler} has not set the configuration `clip_sample`."
- " `clip_sample` should be set to False in the configuration file. Please make sure to update the"
- " config accordingly as not setting `clip_sample` in the config might lead to incorrect results in"
- " future versions. If you have downloaded this checkpoint from the Hugging Face Hub, it would be very"
- " nice if you could open a Pull request for the `scheduler/scheduler_config.json` file"
- )
- deprecate("clip_sample not set", "1.0.0", deprecation_message, standard_warn=False)
- new_config = dict(scheduler.config)
- new_config["clip_sample"] = False
- scheduler._internal_dict = FrozenDict(new_config)
-
- if safety_checker is None and requires_safety_checker:
- logger.warning(
- f"You have disabled the safety checker for {self.__class__} by passing `safety_checker=None`. Ensure"
- " that you abide to the conditions of the Stable Diffusion license and do not expose unfiltered"
- " results in services or applications open to the public. PaddleNLP team, diffusers team and Hugging Face"
- " strongly recommend to keep the safety filter enabled in all public facing circumstances, disabling"
- " it only for use-cases that involve analyzing network behavior or auditing its results. For more"
- " information, please have a look at https://github.com/huggingface/diffusers/pull/254 ."
- )
- if safety_checker is not None and feature_extractor is None:
- raise ValueError(
- "Make sure to define a feature extractor when loading {self.__class__} if you want to use the safety"
- " checker. If you do not want to use the safety checker, you can pass `'safety_checker=None'` instead."
- )
- is_unet_version_less_0_9_0 = hasattr(unet.config, "_ppdiffusers_version") and version.parse(
- version.parse(unet.config._ppdiffusers_version).base_version
- ) < version.parse("0.9.0.dev0")
- is_unet_sample_size_less_64 = hasattr(unet.config, "sample_size") and unet.config.sample_size < 64
- if is_unet_version_less_0_9_0 and is_unet_sample_size_less_64:
- deprecation_message = (
- "The configuration file of the unet has set the default `sample_size` to smaller than"
- " 64 which seems highly unlikely .If you're checkpoint is a fine-tuned version of any of the"
- " following: \n- CompVis/stable-diffusion-v1-4 \n- CompVis/stable-diffusion-v1-3 \n-"
- " CompVis/stable-diffusion-v1-2 \n- CompVis/stable-diffusion-v1-1 \n- runwayml/stable-diffusion-v1-5"
- " \n- runwayml/stable-diffusion-inpainting \n you should change 'sample_size' to 64 in the"
- " configuration file. Please make sure to update the config accordingly as leaving `sample_size=32`"
- " in the config might lead to incorrect results in future versions. If you have downloaded this"
- " checkpoint from the Hugging Face Hub, it would be very nice if you could open a Pull request for"
- " the `unet/config.json` file"
- )
- deprecate("sample_size<64", "1.0.0", deprecation_message, standard_warn=False)
- new_config = dict(unet.config)
- new_config["sample_size"] = 64
- unet._internal_dict = FrozenDict(new_config)
-
- self.register_modules(
- vae=vae,
- text_encoder=text_encoder,
- tokenizer=tokenizer,
- unet=unet,
- scheduler=scheduler,
- safety_checker=safety_checker,
- feature_extractor=feature_extractor,
- )
- self.register_to_config(requires_safety_checker=requires_safety_checker)
-
- def create_scheduler(self, name="DPMSolver"):
- config = self.scheduler.config
- if name == "DPMSolver":
- return DPMSolverMultistepScheduler.from_config(
- config,
- thresholding=False,
- algorithm_type="dpmsolver++",
- solver_type="midpoint",
- lower_order_final=True,
- )
- if name == "EulerDiscrete":
- return EulerDiscreteScheduler.from_config(config)
- elif name == "EulerAncestralDiscrete":
- return EulerAncestralDiscreteScheduler.from_config(config)
- elif name == "PNDM":
- return PNDMScheduler.from_config(config)
- elif name == "DDIM":
- return DDIMScheduler.from_config(config)
- elif name == "LMSDiscrete":
- return LMSDiscreteScheduler.from_config(config)
- elif name == "HeunDiscrete":
- return HeunDiscreteScheduler.from_config(config)
- elif name == "KDPM2AncestralDiscrete":
- return KDPM2AncestralDiscreteScheduler.from_config(config)
- elif name == "KDPM2Discrete":
- return KDPM2DiscreteScheduler.from_config(config)
- else:
- raise NotImplementedError
-
- def enable_attention_slicing(self, slice_size: Optional[Union[str, int]] = "auto"):
- r"""
- Enable sliced attention computation.
-
- When this option is enabled, the attention module will split the input tensor in slices, to compute attention
- in several steps. This is useful to save some memory in exchange for a small speed decrease.
-
- Args:
- slice_size (`str` or `int`, *optional*, defaults to `"auto"`):
- When `"auto"`, halves the input to the attention heads, so attention will be computed in two steps. If
- a number is provided, uses as many slices as `attention_head_dim // slice_size`. In this case,
- `attention_head_dim` must be a multiple of `slice_size`.
- """
- if slice_size == "auto":
- if isinstance(self.unet.config.attention_head_dim, int):
- # half the attention head size is usually a good trade-off between
- # speed and memory
- slice_size = self.unet.config.attention_head_dim // 2
- else:
- # if `attention_head_dim` is a list, take the smallest head size
- slice_size = min(self.unet.config.attention_head_dim)
- self.unet.set_attention_slice(slice_size)
-
- def disable_attention_slicing(self):
- r"""
- Disable sliced attention computation. If `enable_attention_slicing` was previously invoked, this method will go
- back to computing attention in one step.
- """
- # set slice_size = `None` to disable `attention slicing`
- self.enable_attention_slicing(None)
-
- def __call__(self, *args, **kwargs):
- return self.text2image(*args, **kwargs)
-
- def text2img(self, *args, **kwargs):
- return self.text2image(*args, **kwargs)
-
- def _encode_prompt(
- self,
- prompt,
- negative_prompt,
- max_embeddings_multiples,
- no_boseos_middle,
- skip_parsing,
- skip_weighting,
- do_classifier_free_guidance,
- num_images_per_prompt,
- ):
- if do_classifier_free_guidance and negative_prompt is None:
- negative_prompt = ""
- text_embeddings = get_weighted_text_embeddings(
- self, prompt, negative_prompt, max_embeddings_multiples, no_boseos_middle, skip_parsing, skip_weighting
- )
-
- bs_embed, seq_len, _ = text_embeddings.shape
- text_embeddings = text_embeddings.tile([1, num_images_per_prompt, 1])
- text_embeddings = text_embeddings.reshape([bs_embed * num_images_per_prompt, seq_len, -1])
- return text_embeddings
-
- def run_safety_checker(self, image, dtype):
- if self.safety_checker is not None:
- safety_checker_input = self.feature_extractor(self.numpy_to_pil(image), return_tensors="pd")
- image, has_nsfw_concept = self.safety_checker(
- images=image, clip_input=safety_checker_input.pixel_values.cast(dtype)
- )
- else:
- has_nsfw_concept = None
- return image, has_nsfw_concept
-
- def decode_latents(self, latents):
- latents = 1 / 0.18215 * latents
- image = self.vae.decode(latents).sample
- image = (image / 2 + 0.5).clip(0, 1)
- # we always cast to float32 as this does not cause significant overhead and is compatible with bfloa16
- image = image.transpose([0, 2, 3, 1]).cast("float32").numpy()
- return image
-
- def prepare_extra_step_kwargs(self, eta, scheduler):
- # prepare extra kwargs for the scheduler step, since not all schedulers have the same signature
- # eta (η) is only used with the DDIMScheduler, it will be ignored for other schedulers.
- # eta corresponds to η in DDIM paper: https://arxiv.org/abs/2010.02502
- # and should be between [0, 1]
-
- accepts_eta = "eta" in set(inspect.signature(scheduler.step).parameters.keys())
- extra_step_kwargs = {}
- if accepts_eta:
- extra_step_kwargs["eta"] = eta
-
- return extra_step_kwargs
-
- def check_inputs_text2img(self, prompt, height, width, callback_steps):
- if not isinstance(prompt, str) and not isinstance(prompt, list):
- raise ValueError(f"`prompt` has to be of type `str` or `list` but is {type(prompt)}")
-
- if height % 8 != 0 or width % 8 != 0:
- raise ValueError(f"`height` and `width` have to be divisible by 8 but are {height} and {width}.")
-
- if (callback_steps is None) or (
- callback_steps is not None and (not isinstance(callback_steps, int) or callback_steps <= 0)
- ):
- raise ValueError(
- f"`callback_steps` has to be a positive integer but is {callback_steps} of type"
- f" {type(callback_steps)}."
- )
-
- def check_inputs_img2img_inpaint(self, prompt, strength, callback_steps):
- if not isinstance(prompt, str) and not isinstance(prompt, list):
- raise ValueError(f"`prompt` has to be of type `str` or `list` but is {type(prompt)}")
-
- if strength < 0 or strength > 1:
- raise ValueError(f"The value of strength should in [1.0, 1.0] but is {strength}")
-
- if (callback_steps is None) or (
- callback_steps is not None and (not isinstance(callback_steps, int) or callback_steps <= 0)
- ):
- raise ValueError(
- f"`callback_steps` has to be a positive integer but is {callback_steps} of type"
- f" {type(callback_steps)}."
- )
-
- def prepare_latents_text2img(self, batch_size, num_channels_latents, height, width, dtype, latents=None, scheduler=None):
- shape = [batch_size, num_channels_latents, height // 8, width // 8]
- if latents is None:
- latents = paddle.randn(shape, dtype=dtype)
- else:
- if latents.shape != shape:
- raise ValueError(f"Unexpected latents shape, got {latents.shape}, expected {shape}")
-
- # scale the initial noise by the standard deviation required by the scheduler
- latents = latents * scheduler.init_noise_sigma
- return latents
-
- def prepare_latents_img2img(self, image, timestep, num_images_per_prompt, dtype, scheduler):
- image = image.cast(dtype=dtype)
- init_latent_dist = self.vae.encode(image).latent_dist
- init_latents = init_latent_dist.sample()
- init_latents = 0.18215 * init_latents
-
- b, c, h, w = init_latents.shape
- init_latents = init_latents.tile([1, num_images_per_prompt, 1, 1])
- init_latents = init_latents.reshape([b * num_images_per_prompt, c, h, w])
-
- # add noise to latents using the timesteps
- noise = paddle.randn(init_latents.shape, dtype=dtype)
-
- # get latents
- init_latents = scheduler.add_noise(init_latents, noise, timestep)
- latents = init_latents
-
- return latents
-
- def get_timesteps(self, num_inference_steps, strength, scheduler):
- # get the original timestep using init_timestep
- offset = scheduler.config.get("steps_offset", 0)
- init_timestep = int(num_inference_steps * strength) + offset
- init_timestep = min(init_timestep, num_inference_steps)
-
- t_start = max(num_inference_steps - init_timestep + offset, 0)
- timesteps = scheduler.timesteps[t_start:]
-
- return timesteps, num_inference_steps - t_start
-
- def prepare_latents_inpaint(self, image, timestep, num_images_per_prompt, dtype, scheduler):
- image = image.cast(dtype)
- init_latent_dist = self.vae.encode(image).latent_dist
- init_latents = init_latent_dist.sample()
- init_latents = 0.18215 * init_latents
-
- b, c, h, w = init_latents.shape
- init_latents = init_latents.tile([1, num_images_per_prompt, 1, 1])
- init_latents = init_latents.reshape([b * num_images_per_prompt, c, h, w])
-
- init_latents_orig = init_latents
-
- # add noise to latents using the timesteps
- noise = paddle.randn(init_latents.shape, dtype=dtype)
- init_latents = scheduler.add_noise(init_latents, noise, timestep)
- latents = init_latents
- return latents, init_latents_orig, noise
-
- @paddle.no_grad()
- def text2image(
- self,
- prompt: Union[str, List[str]],
- height: int = 512,
- width: int = 512,
- num_inference_steps: int = 50,
- guidance_scale: float = 7.5,
- negative_prompt: Optional[Union[str, List[str]]] = None,
- num_images_per_prompt: Optional[int] = 1,
- eta: float = 0.0,
- seed: Optional[int] = None,
- latents: Optional[paddle.Tensor] = None,
- output_type: Optional[str] = "pil",
- return_dict: bool = True,
- callback: Optional[Callable[[int, int, paddle.Tensor], None]] = None,
- callback_steps: Optional[int] = 1,
- # new add
- max_embeddings_multiples: Optional[int] = 1,
- no_boseos_middle: Optional[bool] = False,
- skip_parsing: Optional[bool] = False,
- skip_weighting: Optional[bool] = False,
- scheduler=None,
- **kwargs,
- ):
- r"""
- Function invoked when calling the pipeline for generation.
-
- Args:
- prompt (`str` or `List[str]`):
- The prompt or prompts to guide the image generation.
- height (`int`, *optional*, defaults to 512):
- The height in pixels of the generated image.
- width (`int`, *optional*, defaults to 512):
- The width in pixels of the generated image.
- num_inference_steps (`int`, *optional*, defaults to 50):
- The number of denoising steps. More denoising steps usually lead to a higher quality image at the
- expense of slower inference.
- guidance_scale (`float`, *optional*, defaults to 7.5):
- Guidance scale as defined in [Classifier-Free Diffusion Guidance](https://arxiv.org/abs/2207.12598).
- `guidance_scale` is defined as `w` of equation 2. of [Imagen
- Paper](https://arxiv.org/pdf/2205.11487.pdf). Guidance scale is enabled by setting `guidance_scale >
- 1`. Higher guidance scale encourages to generate images that are closely linked to the text `prompt`,
- usually at the expense of lower image quality.
- negative_prompt (`str` or `List[str]`, *optional*):
- The prompt or prompts not to guide the image generation. Ignored when not using guidance (i.e., ignored
- if `guidance_scale` is less than `1`).
- num_images_per_prompt (`int`, *optional*, defaults to 1):
- The number of images to generate per prompt.
- eta (`float`, *optional*, defaults to 0.0):
- Corresponds to parameter eta (η) in the DDIM paper: https://arxiv.org/abs/2010.02502. Only applies to
- [`schedulers.DDIMScheduler`], will be ignored for others.
- seed (`int`, *optional*):
- Random number seed.
- latents (`paddle.Tensor`, *optional*):
- Pre-generated noisy latents, sampled from a Gaussian distribution, to be used as inputs for image
- generation. Can be used to tweak the same generation with different prompts. If not provided, a latents
- tensor will ge generated by sampling using the supplied random `seed`.
- output_type (`str`, *optional*, defaults to `"pil"`):
- The output format of the generate image. Choose between
- [PIL](https://pillow.readthedocs.io/en/stable/): `PIL.Image.Image` or `np.array`.
- return_dict (`bool`, *optional*, defaults to `True`):
- Whether or not to return a [`~pipelines.stable_diffusion.StableDiffusionPipelineOutput`] instead of a
- plain tuple.
- callback (`Callable`, *optional*):
- A function that will be called every `callback_steps` steps during inference. The function will be
- called with the following arguments: `callback(step: int, timestep: int, latents: paddle.Tensor)`.
- callback_steps (`int`, *optional*, defaults to 1):
- The frequency at which the `callback` function will be called. If not specified, the callback will be
- called at every step.
-
- Returns:
- [`~pipelines.stable_diffusion.StableDiffusionPipelineOutput`] or `tuple`:
- [`~pipelines.stable_diffusion.StableDiffusionPipelineOutput`] if `return_dict` is True, otherwise a `tuple.
- When returning a tuple, the first element is a list with the generated images, and the second element is a
- list of `bool`s denoting whether the corresponding generated image likely represents "not-safe-for-work"
- (nsfw) content, according to the `safety_checker`.
- """
- if scheduler is None:
- scheduler = self.scheduler
- seed = random.randint(0, 2**32) if seed is None else seed
- argument = dict(
- prompt=prompt,
- negative_prompt=negative_prompt,
- height=height,
- width=width,
- num_inference_steps=num_inference_steps,
- guidance_scale=guidance_scale,
- num_images_per_prompt=num_images_per_prompt,
- eta=eta,
- seed=seed,
- latents=latents,
- max_embeddings_multiples=max_embeddings_multiples,
- no_boseos_middle=no_boseos_middle,
- skip_parsing=skip_parsing,
- skip_weighting=skip_weighting,
- epoch_time=time.time(),
- )
- paddle.seed(seed)
- # 1. Check inputs. Raise error if not correct
- self.check_inputs_text2img(prompt, height, width, callback_steps)
-
- # 2. Define call parameters
- batch_size = 1 if isinstance(prompt, str) else len(prompt)
- # here `guidance_scale` is defined analog to the guidance weight `w` of equation (2)
- # of the Imagen paper: https://arxiv.org/pdf/2205.11487.pdf . `guidance_scale = 1`
- # corresponds to doing no classifier free guidance.
- do_classifier_free_guidance = guidance_scale > 1.0
-
- # 3. Encode input prompt
- text_embeddings = self._encode_prompt(
- prompt,
- negative_prompt,
- max_embeddings_multiples,
- no_boseos_middle,
- skip_parsing,
- skip_weighting,
- do_classifier_free_guidance,
- num_images_per_prompt,
- )
-
- # 4. Prepare timesteps
- scheduler.set_timesteps(num_inference_steps)
- timesteps = scheduler.timesteps
-
- # 5. Prepare latent variables
- num_channels_latents = self.unet.in_channels
- latents = self.prepare_latents_text2img(
- batch_size * num_images_per_prompt,
- num_channels_latents,
- height,
- width,
- text_embeddings.dtype,
- latents,
- scheduler=scheduler,
- )
-
- # 6. Prepare extra step kwargs. TODO: Logic should ideally just be moved out of the pipeline
- extra_step_kwargs = self.prepare_extra_step_kwargs(eta, scheduler)
-
- # 7. Denoising loop
- num_warmup_steps = len(timesteps) - num_inference_steps * scheduler.order
- with self.progress_bar(total=num_inference_steps) as progress_bar:
- for i, t in enumerate(timesteps):
- # expand the latents if we are doing classifier free guidance
- latent_model_input = paddle.concat([latents] * 2) if do_classifier_free_guidance else latents
- latent_model_input = scheduler.scale_model_input(latent_model_input, t)
-
- # predict the noise residual
- noise_pred = self.unet(latent_model_input, t, encoder_hidden_states=text_embeddings).sample
-
- # perform guidance
- if do_classifier_free_guidance:
- noise_pred_uncond, noise_pred_text = noise_pred.chunk(2)
- noise_pred = noise_pred_uncond + guidance_scale * (noise_pred_text - noise_pred_uncond)
-
- # compute the previous noisy sample x_t -> x_t-1
- latents = scheduler.step(noise_pred, t, latents, **extra_step_kwargs).prev_sample
-
- # call the callback, if provided
- if i == len(timesteps) - 1 or ((i + 1) > num_warmup_steps and (i + 1) % scheduler.order == 0):
- progress_bar.update()
- if callback is not None and i % callback_steps == 0:
- callback(progress_bar.n, progress_bar.total, progress_bar)
-
- # 8. Post-processing
- image = self.decode_latents(latents)
-
- # 9. Run safety checker
- image, has_nsfw_concept = self.run_safety_checker(image, text_embeddings.dtype)
-
- # 10. Convert to PIL
- if output_type == "pil":
- image = self.numpy_to_pil(image, argument=argument)
-
- if not return_dict:
- return (image, has_nsfw_concept)
-
- return StableDiffusionPipelineOutput(images=image, nsfw_content_detected=has_nsfw_concept)
-
- @paddle.no_grad()
- def img2img(
- self,
- prompt: Union[str, List[str]],
- image: Union[paddle.Tensor, PIL.Image.Image],
- strength: float = 0.8,
- height=None,
- width=None,
- num_inference_steps: Optional[int] = 50,
- guidance_scale: Optional[float] = 7.5,
- negative_prompt: Optional[Union[str, List[str]]] = None,
- num_images_per_prompt: Optional[int] = 1,
- eta: Optional[float] = 0.0,
- seed: Optional[int] = None,
- output_type: Optional[str] = "pil",
- return_dict: bool = True,
- callback: Optional[Callable[[int, int, paddle.Tensor], None]] = None,
- callback_steps: Optional[int] = 1,
- # new add
- max_embeddings_multiples: Optional[int] = 1,
- no_boseos_middle: Optional[bool] = False,
- skip_parsing: Optional[bool] = False,
- skip_weighting: Optional[bool] = False,
- scheduler=None,
- **kwargs,
- ):
- r"""
- Function invoked when calling the pipeline for generation.
-
- Args:
- prompt (`str` or `List[str]`):
- The prompt or prompts to guide the image generation.
- image (`paddle.Tensor` or `PIL.Image.Image`):
- `Image`, or tensor representing an image batch, that will be used as the starting point for the
- process.
- strength (`float`, *optional*, defaults to 0.8):
- Conceptually, indicates how much to transform the reference `image`. Must be between 0 and 1.
- `image` will be used as a starting point, adding more noise to it the larger the `strength`. The
- number of denoising steps depends on the amount of noise initially added. When `strength` is 1, added
- noise will be maximum and the denoising process will run for the full number of iterations specified in
- `num_inference_steps`. A value of 1, therefore, essentially ignores `image`.
- num_inference_steps (`int`, *optional*, defaults to 50):
- The number of denoising steps. More denoising steps usually lead to a higher quality image at the
- expense of slower inference. This parameter will be modulated by `strength`.
- guidance_scale (`float`, *optional*, defaults to 7.5):
- Guidance scale as defined in [Classifier-Free Diffusion Guidance](https://arxiv.org/abs/2207.12598).
- `guidance_scale` is defined as `w` of equation 2. of [Imagen
- Paper](https://arxiv.org/pdf/2205.11487.pdf). Guidance scale is enabled by setting `guidance_scale >
- 1`. Higher guidance scale encourages to generate images that are closely linked to the text `prompt`,
- usually at the expense of lower image quality.
- negative_prompt (`str` or `List[str]`, *optional*):
- The prompt or prompts not to guide the image generation. Ignored when not using guidance (i.e., ignored
- if `guidance_scale` is less than `1`).
- num_images_per_prompt (`int`, *optional*, defaults to 1):
- The number of images to generate per prompt.
- eta (`float`, *optional*, defaults to 0.0):
- Corresponds to parameter eta (η) in the DDIM paper: https://arxiv.org/abs/2010.02502. Only applies to
- [`schedulers.DDIMScheduler`], will be ignored for others.
- seed (`int`, *optional*):
- A random seed.
- output_type (`str`, *optional*, defaults to `"pil"`):
- The output format of the generate image. Choose between
- [PIL](https://pillow.readthedocs.io/en/stable/): `PIL.Image.Image` or `np.array`.
- return_dict (`bool`, *optional*, defaults to `True`):
- Whether or not to return a [`~pipelines.stable_diffusion.StableDiffusionPipelineOutput`] instead of a
- plain tuple.
- callback (`Callable`, *optional*):
- A function that will be called every `callback_steps` steps during inference. The function will be
- called with the following arguments: `callback(step: int, timestep: int, latents: paddle.Tensor)`.
- callback_steps (`int`, *optional*, defaults to 1):
- The frequency at which the `callback` function will be called. If not specified, the callback will be
- called at every step.
-
- Returns:
- [`~pipelines.stable_diffusion.StableDiffusionPipelineOutput`] or `tuple`:
- [`~pipelines.stable_diffusion.StableDiffusionPipelineOutput`] if `return_dict` is True, otherwise a `tuple.
- When returning a tuple, the first element is a list with the generated images, and the second element is a
- list of `bool`s denoting whether the corresponding generated image likely represents "not-safe-for-work"
- (nsfw) content, according to the `safety_checker`.
- """
- if scheduler is None:
- scheduler = self.scheduler
- seed = random.randint(0, 2**32) if seed is None else seed
- image_str = image
- if isinstance(image_str, str):
- image = load_image(image_str)
-
- if height is None and width is None:
- width = (image.size[0] // 8) * 8
- height = (image.size[1] // 8) * 8
- elif height is None and width is not None:
- height = (image.size[1] // 8) * 8
- elif width is None and height is not None:
- width = (image.size[0] // 8) * 8
- else:
- height = height
- width = width
-
- argument = dict(
- prompt=prompt,
- image=image_str,
- negative_prompt=negative_prompt,
- height=height,
- width=width,
- strength=strength,
- num_inference_steps=num_inference_steps,
- guidance_scale=guidance_scale,
- num_images_per_prompt=num_images_per_prompt,
- eta=eta,
- seed=seed,
- max_embeddings_multiples=max_embeddings_multiples,
- no_boseos_middle=no_boseos_middle,
- skip_parsing=skip_parsing,
- skip_weighting=skip_weighting,
- epoch_time=time.time(),
- )
- paddle.seed(seed)
-
- # 1. Check inputs
- self.check_inputs_img2img_inpaint(prompt, strength, callback_steps)
-
- # 2. Define call parameters
- batch_size = 1 if isinstance(prompt, str) else len(prompt)
- # here `guidance_scale` is defined analog to the guidance weight `w` of equation (2)
- # of the Imagen paper: https://arxiv.org/pdf/2205.11487.pdf . `guidance_scale = 1`
- # corresponds to doing no classifier free guidance.
- do_classifier_free_guidance = guidance_scale > 1.0
-
- # 3. Encode input prompt
- text_embeddings = self._encode_prompt(
- prompt,
- negative_prompt,
- max_embeddings_multiples,
- no_boseos_middle,
- skip_parsing,
- skip_weighting,
- do_classifier_free_guidance,
- num_images_per_prompt,
- )
-
- # 4. Preprocess image
- if isinstance(image, PIL.Image.Image):
- image = image.resize((width, height))
- image = preprocess_image(image)
-
- # 5. set timesteps
- scheduler.set_timesteps(num_inference_steps)
- timesteps, num_inference_steps = self.get_timesteps(num_inference_steps, strength, scheduler)
- latent_timestep = timesteps[:1].tile([batch_size * num_images_per_prompt])
-
- # 6. Prepare latent variables
- latents = self.prepare_latents_img2img(image, latent_timestep, num_images_per_prompt, text_embeddings.dtype, scheduler)
-
- # 7. Prepare extra step kwargs. TODO: Logic should ideally just be moved out of the pipeline
- extra_step_kwargs = self.prepare_extra_step_kwargs(eta, scheduler)
-
- # 8. Denoising loop
- num_warmup_steps = len(timesteps) - num_inference_steps * scheduler.order
- with self.progress_bar(total=num_inference_steps) as progress_bar:
- for i, t in enumerate(timesteps):
- # expand the latents if we are doing classifier free guidance
- latent_model_input = paddle.concat([latents] * 2) if do_classifier_free_guidance else latents
- latent_model_input = scheduler.scale_model_input(latent_model_input, t)
-
- # predict the noise residual
- noise_pred = self.unet(latent_model_input, t, encoder_hidden_states=text_embeddings).sample
-
- # perform guidance
- if do_classifier_free_guidance:
- noise_pred_uncond, noise_pred_text = noise_pred.chunk(2)
- noise_pred = noise_pred_uncond + guidance_scale * (noise_pred_text - noise_pred_uncond)
-
- # compute the previous noisy sample x_t -> x_t-1
- latents = scheduler.step(noise_pred, t, latents, **extra_step_kwargs).prev_sample
-
- # call the callback, if provided
- if i == len(timesteps) - 1 or ((i + 1) > num_warmup_steps and (i + 1) % scheduler.order == 0):
- progress_bar.update()
- if callback is not None and i % callback_steps == 0:
- callback(progress_bar.n, progress_bar.total, progress_bar)
-
- # 9. Post-processing
- image = self.decode_latents(latents)
-
- # 10. Run safety checker
- image, has_nsfw_concept = self.run_safety_checker(image, text_embeddings.dtype)
-
- # 11. Convert to PIL
- if output_type == "pil":
- image = self.numpy_to_pil(image, argument=argument)
-
- if not return_dict:
- return (image, has_nsfw_concept)
-
- return StableDiffusionPipelineOutput(images=image, nsfw_content_detected=has_nsfw_concept)
-
- @paddle.no_grad()
- def inpaint(
- self,
- prompt: Union[str, List[str]],
- image: Union[paddle.Tensor, PIL.Image.Image],
- mask_image: Union[paddle.Tensor, PIL.Image.Image],
- height=None,
- width=None,
- strength: float = 0.8,
- num_inference_steps: Optional[int] = 50,
- guidance_scale: Optional[float] = 7.5,
- negative_prompt: Optional[Union[str, List[str]]] = None,
- num_images_per_prompt: Optional[int] = 1,
- eta: Optional[float] = 0.0,
- seed: Optional[int] = None,
- output_type: Optional[str] = "pil",
- return_dict: bool = True,
- callback: Optional[Callable[[int, int, paddle.Tensor], None]] = None,
- callback_steps: Optional[int] = 1,
- # new add
- max_embeddings_multiples: Optional[int] = 1,
- no_boseos_middle: Optional[bool] = False,
- skip_parsing: Optional[bool] = False,
- skip_weighting: Optional[bool] = False,
- scheduler=None,
- **kwargs,
- ):
- r"""
- Function invoked when calling the pipeline for generation.
-
- Args:
- prompt (`str` or `List[str]`):
- The prompt or prompts to guide the image generation.
- image (`paddle.Tensor` or `PIL.Image.Image`):
- `Image`, or tensor representing an image batch, that will be used as the starting point for the
- process. This is the image whose masked region will be inpainted.
- mask_image (`paddle.Tensor` or `PIL.Image.Image`):
- `Image`, or tensor representing an image batch, to mask `image`. White pixels in the mask will be
- replaced by noise and therefore repainted, while black pixels will be preserved. If `mask_image` is a
- PIL image, it will be converted to a single channel (luminance) before use. If it's a tensor, it should
- contain one color channel (L) instead of 3, so the expected shape would be `(B, H, W, 1)`.
- strength (`float`, *optional*, defaults to 0.8):
- Conceptually, indicates how much to inpaint the masked area. Must be between 0 and 1. When `strength`
- is 1, the denoising process will be run on the masked area for the full number of iterations specified
- in `num_inference_steps`. `image` will be used as a reference for the masked area, adding more
- noise to that region the larger the `strength`. If `strength` is 0, no inpainting will occur.
- num_inference_steps (`int`, *optional*, defaults to 50):
- The reference number of denoising steps. More denoising steps usually lead to a higher quality image at
- the expense of slower inference. This parameter will be modulated by `strength`, as explained above.
- guidance_scale (`float`, *optional*, defaults to 7.5):
- Guidance scale as defined in [Classifier-Free Diffusion Guidance](https://arxiv.org/abs/2207.12598).
- `guidance_scale` is defined as `w` of equation 2. of [Imagen
- Paper](https://arxiv.org/pdf/2205.11487.pdf). Guidance scale is enabled by setting `guidance_scale >
- 1`. Higher guidance scale encourages to generate images that are closely linked to the text `prompt`,
- usually at the expense of lower image quality.
- negative_prompt (`str` or `List[str]`, *optional*):
- The prompt or prompts not to guide the image generation. Ignored when not using guidance (i.e., ignored
- if `guidance_scale` is less than `1`).
- num_images_per_prompt (`int`, *optional*, defaults to 1):
- The number of images to generate per prompt.
- eta (`float`, *optional*, defaults to 0.0):
- Corresponds to parameter eta (η) in the DDIM paper: https://arxiv.org/abs/2010.02502. Only applies to
- [`schedulers.DDIMScheduler`], will be ignored for others.
- seed (`int`, *optional*):
- A random seed.
- output_type (`str`, *optional*, defaults to `"pil"`):
- The output format of the generate image. Choose between
- [PIL](https://pillow.readthedocs.io/en/stable/): `PIL.Image.Image` or `np.array`.
- return_dict (`bool`, *optional*, defaults to `True`):
- Whether or not to return a [`~pipelines.stable_diffusion.StableDiffusionPipelineOutput`] instead of a
- plain tuple.
- callback (`Callable`, *optional*):
- A function that will be called every `callback_steps` steps during inference. The function will be
- called with the following arguments: `callback(step: int, timestep: int, latents: paddle.Tensor)`.
- callback_steps (`int`, *optional*, defaults to 1):
- The frequency at which the `callback` function will be called. If not specified, the callback will be
- called at every step.
-
- Returns:
- [`~pipelines.stable_diffusion.StableDiffusionPipelineOutput`] or `tuple`:
- [`~pipelines.stable_diffusion.StableDiffusionPipelineOutput`] if `return_dict` is True, otherwise a `tuple.
- When returning a tuple, the first element is a list with the generated images, and the second element is a
- list of `bool`s denoting whether the corresponding generated image likely represents "not-safe-for-work"
- (nsfw) content, according to the `safety_checker`.
- """
- if scheduler is None:
- scheduler = self.scheduler
- seed = random.randint(0, 2**32) if seed is None else seed
- image_str = image
- mask_image_str = mask_image
-
- if isinstance(image_str, str):
- image = load_image(image_str)
- if isinstance(mask_image_str, str):
- mask_image = load_image(mask_image_str)
-
- if height is None and width is None:
- width = (image.size[0] // 8) * 8
- height = (image.size[1] // 8) * 8
- elif height is None and width is not None:
- height = (image.size[1] // 8) * 8
- elif width is None and height is not None:
- width = (image.size[0] // 8) * 8
- else:
- height = height
- width = width
-
- argument = dict(
- prompt=prompt,
- image=image_str,
- mask_image=mask_image_str,
- negative_prompt=negative_prompt,
- height=height,
- width=width,
- strength=strength,
- num_inference_steps=num_inference_steps,
- guidance_scale=guidance_scale,
- num_images_per_prompt=num_images_per_prompt,
- eta=eta,
- seed=seed,
- max_embeddings_multiples=max_embeddings_multiples,
- no_boseos_middle=no_boseos_middle,
- skip_parsing=skip_parsing,
- skip_weighting=skip_weighting,
- epoch_time=time.time(),
- )
- paddle.seed(seed)
-
- # 1. Check inputs
- self.check_inputs_img2img_inpaint(prompt, strength, callback_steps)
-
- # 2. Define call parameters
- batch_size = 1 if isinstance(prompt, str) else len(prompt)
- # here `guidance_scale` is defined analog to the guidance weight `w` of equation (2)
- # of the Imagen paper: https://arxiv.org/pdf/2205.11487.pdf . `guidance_scale = 1`
- # corresponds to doing no classifier free guidance.
- do_classifier_free_guidance = guidance_scale > 1.0
-
- # 3. Encode input prompt
- text_embeddings = self._encode_prompt(
- prompt,
- negative_prompt,
- max_embeddings_multiples,
- no_boseos_middle,
- skip_parsing,
- skip_weighting,
- do_classifier_free_guidance,
- num_images_per_prompt,
- )
-
- if not isinstance(image, paddle.Tensor):
- image = image.resize((width, height))
- image = preprocess_image(image)
-
- if not isinstance(mask_image, paddle.Tensor):
- mask_image = mask_image.resize((width, height))
- mask_image = preprocess_mask(mask_image)
-
- # 5. set timesteps
- scheduler.set_timesteps(num_inference_steps)
- timesteps, num_inference_steps = self.get_timesteps(num_inference_steps, strength, scheduler)
- latent_timestep = timesteps[:1].tile([batch_size * num_images_per_prompt])
-
- # 6. Prepare latent variables
- # encode the init image into latents and scale the latents
- latents, init_latents_orig, noise = self.prepare_latents_inpaint(
- image, latent_timestep, num_images_per_prompt, text_embeddings.dtype, scheduler
- )
-
- # 7. Prepare mask latent
- mask = mask_image.cast(latents.dtype)
- mask = paddle.concat([mask] * batch_size * num_images_per_prompt)
-
- # 8. Prepare extra step kwargs. TODO: Logic should ideally just be moved out of the pipeline
- extra_step_kwargs = self.prepare_extra_step_kwargs(eta, scheduler)
-
- # 9. Denoising loop
- num_warmup_steps = len(timesteps) - num_inference_steps * scheduler.order
- with self.progress_bar(total=num_inference_steps) as progress_bar:
- for i, t in enumerate(timesteps):
- # expand the latents if we are doing classifier free guidance
- latent_model_input = paddle.concat([latents] * 2) if do_classifier_free_guidance else latents
- latent_model_input = scheduler.scale_model_input(latent_model_input, t)
-
- # predict the noise residual
- noise_pred = self.unet(latent_model_input, t, encoder_hidden_states=text_embeddings).sample
-
- # perform guidance
- if do_classifier_free_guidance:
- noise_pred_uncond, noise_pred_text = noise_pred.chunk(2)
- noise_pred = noise_pred_uncond + guidance_scale * (noise_pred_text - noise_pred_uncond)
-
- # compute the previous noisy sample x_t -> x_t-1
- latents = scheduler.step(noise_pred, t, latents, **extra_step_kwargs).prev_sample
- # masking
- init_latents_proper = scheduler.add_noise(init_latents_orig, noise, t)
-
- latents = (init_latents_proper * mask) + (latents * (1 - mask))
-
- # call the callback, if provided
- if i == len(timesteps) - 1 or ((i + 1) > num_warmup_steps and (i + 1) % scheduler.order == 0):
- progress_bar.update()
- if callback is not None and i % callback_steps == 0:
- callback(progress_bar.n, progress_bar.total, progress_bar)
-
- # 10. Post-processing
- image = self.decode_latents(latents)
-
- # 11. Run safety checker
- image, has_nsfw_concept = self.run_safety_checker(image, text_embeddings.dtype)
-
- # 12. Convert to PIL
- if output_type == "pil":
- image = self.numpy_to_pil(image, argument=argument)
-
- if not return_dict:
- return (image, has_nsfw_concept)
-
- return StableDiffusionPipelineOutput(images=image, nsfw_content_detected=has_nsfw_concept)
-
- @staticmethod
- def numpy_to_pil(images, **kwargs):
- """
- Convert a numpy image or a batch of images to a PIL image.
- """
- if images.ndim == 3:
- images = images[None, ...]
- images = (images * 255).round().astype("uint8")
- pil_images = []
- argument = kwargs.pop("argument", None)
- for image in images:
- image = PIL.Image.fromarray(image)
- if argument is not None:
- image.argument = argument
- pil_images.append(image)
-
- return pil_images
-pipeline = StableDiffusionPipelineAllinOne.from_pretrained(BASE_MODEL_NAME, safety_checker=None)
-
-if LORA_WEIGHTS_PATH is not None:
- pipeline.unet.load_attn_procs(LORA_WEIGHTS_PATH, from_hf_hub=True)
-
-support_scheduler = [
- "DPMSolver",
- "EulerDiscrete",
- "EulerAncestralDiscrete",
- "PNDM",
- "DDIM",
- "LMSDiscrete",
- "HeunDiscrete",
- "KDPM2AncestralDiscrete",
- "KDPM2Discrete"
-]
-
-# generate images
-def infer(prompt, negative, scale, height, width, num_inference_steps, scheduler_name):
- scheduler = pipeline.create_scheduler(scheduler_name)
-
- images = pipeline(
- prompt=prompt, negative_prompt=negative, guidance_scale=scale, height=height, width=width, num_inference_steps=num_inference_steps, scheduler=scheduler,
- ).images
- return images
-
-
-css = """
- .gradio-container {
- font-family: 'IBM Plex Sans', sans-serif;
- }
- .gr-button {
- color: white;
- border-color: black;
- background: black;
- }
- input[type='range'] {
- accent-color: black;
- }
- .dark input[type='range'] {
- accent-color: #dfdfdf;
- }
- .container {
- max-width: 730px;
- margin: auto;
- padding-top: 1.5rem;
- }
- #gallery {
- min-height: 22rem;
- margin-bottom: 15px;
- margin-left: auto;
- margin-right: auto;
- border-bottom-right-radius: .5rem !important;
- border-bottom-left-radius: .5rem !important;
- }
- #gallery>div>.h-full {
- min-height: 20rem;
- }
- .details:hover {
- text-decoration: underline;
- }
- .gr-button {
- white-space: nowrap;
- }
- .gr-button:focus {
- border-color: rgb(147 197 253 / var(--tw-border-opacity));
- outline: none;
- box-shadow: var(--tw-ring-offset-shadow), var(--tw-ring-shadow), var(--tw-shadow, 0 0 #0000);
- --tw-border-opacity: 1;
- --tw-ring-offset-shadow: var(--tw-ring-inset) 0 0 0 var(--tw-ring-offset-width) var(--tw-ring-offset-color);
- --tw-ring-shadow: var(--tw-ring-inset) 0 0 0 calc(3px var(--tw-ring-offset-width)) var(--tw-ring-color);
- --tw-ring-color: rgb(191 219 254 / var(--tw-ring-opacity));
- --tw-ring-opacity: .5;
- }
- #advanced-btn {
- font-size: .7rem !important;
- line-height: 19px;
- margin-top: 12px;
- margin-bottom: 12px;
- padding: 2px 8px;
- border-radius: 14px !important;
- }
- #advanced-options {
- display: none;
- margin-bottom: 20px;
- }
- .footer {
- margin-bottom: 45px;
- margin-top: 35px;
- text-align: center;
- border-bottom: 1px solid #e5e5e5;
- }
- .footer>p {
- font-size: .8rem;
- display: inline-block;
- padding: 0 10px;
- transform: translateY(10px);
- background: white;
- }
- .dark .footer {
- border-color: #303030;
- }
- .dark .footer>p {
- background: #0b0f19;
- }
- .acknowledgments h4{
- margin: 1.25em 0 .25em 0;
- font-weight: bold;
- font-size: 115%;
- }
- .animate-spin {
- animation: spin 1s linear infinite;
- }
- @keyframes spin {
- from {
- transform: rotate(0deg);
- }
- to {
- transform: rotate(360deg);
- }
- }
- #share-btn-container {
- display: flex; padding-left: 0.5rem !important; padding-right: 0.5rem !important; background-color: #000000; justify-content: center; align-items: center; border-radius: 9999px !important; width: 13rem;
- margin-top: 10px;
- margin-left: auto;
- }
- #share-btn {
- all: initial; color: #ffffff;font-weight: 600; cursor:pointer; font-family: 'IBM Plex Sans', sans-serif; margin-left: 0.5rem !important; padding-top: 0.25rem !important; padding-bottom: 0.25rem !important;right:0;
- }
- #share-btn * {
- all: unset;
- }
- #share-btn-container div:nth-child(-n+2){
- width: auto !important;
- min-height: 0px !important;
- }
- #share-btn-container .wrap {
- display: none !important;
- }
-
- .gr-form{
- flex: 1 1 50%; border-top-right-radius: 0; border-bottom-right-radius: 0;
- }
- #prompt-container{
- gap: 0;
- }
- #prompt-text-input, #negative-prompt-text-input{padding: .45rem 0.625rem}
- #component-16{border-top-width: 1px!important;margin-top: 1em}
- .image_duplication{position: absolute; width: 100px; left: 50px}
-"""
-
-block = gr.Blocks(css=css)
-
-with block:
- gr.HTML(
- """
-
-The model is licensed with a CreativeML OpenRAIL++ license. The authors claim no rights on the outputs you generate, you are free to use them and are accountable for their use which must not go against the provisions set in this license. The license forbids you from sharing any content that violates any laws, produce any harm to a person, disseminate any personal information that would be meant for harm, spread misinformation and target vulnerable groups. For the full list of restrictions please read the license
-
Biases and content acknowledgment
-Despite how impressive being able to turn text into image is, beware to the fact that this model may output content that reinforces or exacerbates societal biases, as well as realistic faces, pornography and violence. The model was trained on the LAION-5B dataset, which scraped non-curated image-text-pairs from the internet (the exception being the removal of illegal content) and is meant for research purposes. You can read more in the model card
-
- """
- )
-
-block.launch(server_name="0.0.0.0", server_port=8221)
-
diff --git a/spaces/AAYUSH27/Neuro/README.md b/spaces/AAYUSH27/Neuro/README.md
deleted file mode 100644
index 2a3a1343315323365bf0fcc9b3a23f1cb3b71a2a..0000000000000000000000000000000000000000
--- a/spaces/AAYUSH27/Neuro/README.md
+++ /dev/null
@@ -1,54 +0,0 @@
----
-title: AnatomyBOT!
-emoji: 🚀
-colorFrom: yellow
-colorTo: yellow
-sdk: streamlit
-sdk_version: 1.25.0
-app_file: app.py
-pinned: false
-
----
-
-## Make sure you have git-lfs installed [Git LFS](https://git-lfs.com) ✅
-# 🧑🏻💻Steps to download the Code
-
-**📌 NOTE-1: If the Llama 2 Model is not donwloaded then the code will not work properly.**
-
-**📌 NOTE-2: If the HuggingFaces API is not in ```.env``` file then generate your own API key from HugginFaces and use it.**
-
----
-
-Step:0
-- Copy and Paste the below command in terminal.
-- This command will help to download the code to your local machine.
-```shell
-git clone https://huggingface.co/spaces/AAYUSH27/Neuro
-```
-- The file is of approx. 5GB
-- If you want to clone without large files (Llama 2 Model).
-```shell
-git clone https://huggingface.co/spaces/AAYUSH27/Neuro
-GIT_LFS_SKIP_SMUDGE=1
-```
-
-Step:1
-- Copy and Paste the below command in terminal.
-- This command helps to go into the project directory.
-```shell
-cd Neuro
-```
-
-Step:2
-- Copy and Paste the below command in terminal.
-- This commmand helps to install all the libraries in one take from ```requirements.txt```.
-```shell
-pip3 install -r requirements.txt
-```
-
-Step:3
-- Copy and Paste the below command in terminal.
-- This command helps to run the code into local host via ```streamlit```.
-```shell
-streamlit run -app.py
-```
diff --git a/spaces/AIWaves/Debate/src/agents/LLM/base_LLM.py b/spaces/AIWaves/Debate/src/agents/LLM/base_LLM.py
deleted file mode 100644
index 6c94ef0d0f67cfa1b133312ca26e0955ab7f0128..0000000000000000000000000000000000000000
--- a/spaces/AIWaves/Debate/src/agents/LLM/base_LLM.py
+++ /dev/null
@@ -1,133 +0,0 @@
-from abc import abstractclassmethod
-import openai
-import os
-import time
-from Memory import Memory
-from utils import save_logs
-
-class LLM:
- def __init__(self) -> None:
- pass
-
- @abstractclassmethod
- def get_response():
- pass
-
-
-class OpenAILLM(LLM):
- def __init__(self,**kwargs) -> None:
- super().__init__()
- self.MAX_CHAT_HISTORY = eval(
- os.environ["MAX_CHAT_HISTORY"]) if "MAX_CHAT_HISTORY" in os.environ else 10
-
- self.model = kwargs["model"] if "model" in kwargs else "gpt-3.5-turbo-16k-0613"
- self.temperature = kwargs["temperature"] if "temperature" in kwargs else 0.3
- self.log_path = kwargs["log_path"] if "log_path" in kwargs else "logs"
-
-
- def get_stream(self,response, log_path, messages):
- ans = ""
- for res in response:
- if res:
- r = (res.choices[0]["delta"].get("content")
- if res.choices[0]["delta"].get("content") else "")
- ans += r
- yield r
-
- save_logs(log_path, messages, ans)
-
-
-
- def get_response(self,
- chat_history,
- system_prompt,
- last_prompt=None,
- stream=False,
- functions=None,
- function_call="auto",
- WAIT_TIME=20,
- **kwargs):
- """
- return LLM's response
- """
- openai.api_key = os.environ["API_KEY"]
- # if "PROXY" in os.environ:
- # assert "http:" in os.environ["PROXY"] or "socks" in os.environ["PROXY"],"PROXY error,PROXY must be http or socks"
- # openai.proxy = os.environ["PROXY"]
- if "API_BASE" in os.environ:
- openai.api_base = os.environ["API_BASE"]
- active_mode = True if ("ACTIVE_MODE" in os.environ and os.environ["ACTIVE_MODE"] == "0") else False
- model = self.model
- temperature = self.temperature
-
-
- if active_mode:
- system_prompt = system_prompt + "Please keep your reply as concise as possible,Within three sentences, the total word count should not exceed 30"
-
- messages = [{
- "role": "system",
- "content": system_prompt
- }] if system_prompt else []
-
- if chat_history:
- if len(chat_history) > self.MAX_CHAT_HISTORY:
- chat_history = chat_history[- self.MAX_CHAT_HISTORY:]
- if isinstance(chat_history[0],dict):
- messages += chat_history
- elif isinstance(chat_history[0],Memory):
- messages += [memory.get_gpt_message("user") for memory in chat_history]
-
- if last_prompt:
- if active_mode:
- last_prompt = last_prompt + "Please keep your reply as concise as possible,Within three sentences, the total word count should not exceed 30"
- # messages += [{"role": "system", "content": f"{last_prompt}"}]
- messages[-1]["content"] += last_prompt
-
-
- while True:
- try:
- if functions:
- response = openai.ChatCompletion.create(
- model=model,
- messages=messages,
- functions=functions,
- function_call=function_call,
- temperature=temperature,
- )
- else:
- response = openai.ChatCompletion.create(
- model=model,
- messages=messages,
- temperature=temperature,
- stream=stream)
- break
- except Exception as e:
- print(e)
- if "maximum context length is" in str(e):
- assert False, "exceed max length"
- break
- else:
- print(f"Please wait {WAIT_TIME} seconds and resend later ...")
- time.sleep(WAIT_TIME)
-
- if functions:
- save_logs(self.log_path, messages, response)
- return response.choices[0].message
- elif stream:
- return self.get_stream(response, self.log_path, messages)
- else:
- save_logs(self.log_path, messages, response)
- return response.choices[0].message["content"]
-
-
-def init_LLM(default_log_path,**kwargs):
- LLM_type = kwargs["LLM_type"] if "LLM_type" in kwargs else "OpenAI"
- log_path = kwargs["log_path"] if "log_path" in kwargs else default_log_path
- if LLM_type == "OpenAI":
- LLM = (
- OpenAILLM(**kwargs["LLM"])
- if "LLM" in kwargs
- else OpenAILLM(model = "gpt-3.5-turbo-16k-0613",temperature=0.3,log_path=log_path)
- )
- return LLM
-
\ No newline at end of file
diff --git a/spaces/Adapting/YouTube-Downloader/tube/download.py b/spaces/Adapting/YouTube-Downloader/tube/download.py
deleted file mode 100644
index 87f9aad7f141f6e0223347d770cbbad7ac6354ac..0000000000000000000000000000000000000000
--- a/spaces/Adapting/YouTube-Downloader/tube/download.py
+++ /dev/null
@@ -1,48 +0,0 @@
-from pathlib import Path
-from pytube import YouTube
-
-import streamlit as st
-from .utils import clear_cache
-
-
-
-
-def download_yt(yt_url:str, output_dir:str = './downloads'):
- yt = YouTube(yt_url)
-
- prompt = st.markdown(f'''`downloading...`''')
-
- while True:
- try:
- yt.streams.filter(progressive=True, file_extension='mp4').order_by('resolution').desc().first().download(
- output_path=output_dir,
- filename='download.mp4'
- )
- prompt.empty()
- break
- except Exception as e:
- print(e)
-
- download_file(folder_name= output_dir)
-
-
-
-
-
-def download_file(folder_name):
- def tmp(*,folder_name:str):
- st.session_state["title"] = ""
- clear_cache(folder_name)
-
-
- with open(Path('downloads').joinpath('download.mp4'), "rb") as file:
- btn = st.download_button(
- label="Download",
- data=file,
- file_name='download.mp4',
- on_click= tmp,kwargs=dict(
- folder_name = folder_name
- )
- )
-
-
diff --git a/spaces/AgentVerse/agentVerse/ui/src/phaser3-rex-plugins/plugins/roundrectangle.d.ts b/spaces/AgentVerse/agentVerse/ui/src/phaser3-rex-plugins/plugins/roundrectangle.d.ts
deleted file mode 100644
index 067fa3453affcc881bcefe796471fd4c0dcbd99e..0000000000000000000000000000000000000000
--- a/spaces/AgentVerse/agentVerse/ui/src/phaser3-rex-plugins/plugins/roundrectangle.d.ts
+++ /dev/null
@@ -1,2 +0,0 @@
-import RoundRectangle from './gameobjects/shape/roundrectangle/RoundRectangle';
-export default RoundRectangle;
\ No newline at end of file
diff --git a/spaces/Ali36Ahmad/magic-diffusion/README.md b/spaces/Ali36Ahmad/magic-diffusion/README.md
deleted file mode 100644
index 358afc4ec6e322444d5d5ac29a0c42916bc33cb9..0000000000000000000000000000000000000000
--- a/spaces/Ali36Ahmad/magic-diffusion/README.md
+++ /dev/null
@@ -1,14 +0,0 @@
----
-title: Magic Prompt
-emoji: 🎆
-colorFrom: red
-colorTo: gray
-sdk: gradio
-sdk_version: 3.12.0
-app_file: app.py
-pinned: false
-license: apache-2.0
-duplicated_from: tommy24/magic-diffusion
----
-
-Check out the configuration reference at https://huggingface.co/docs/hub/spaces-config-reference
diff --git a/spaces/Amrrs/DragGan-Inversion/PTI/training/__init__.py b/spaces/Amrrs/DragGan-Inversion/PTI/training/__init__.py
deleted file mode 100644
index e69de29bb2d1d6434b8b29ae775ad8c2e48c5391..0000000000000000000000000000000000000000
diff --git a/spaces/Androidonnxfork/CivitAi-to-Diffusers/diffusers/tests/pipelines/kandinsky_v22/test_kandinsky_prior.py b/spaces/Androidonnxfork/CivitAi-to-Diffusers/diffusers/tests/pipelines/kandinsky_v22/test_kandinsky_prior.py
deleted file mode 100644
index 3191f6a11309af1198bbddc9ecb3632919b87ef3..0000000000000000000000000000000000000000
--- a/spaces/Androidonnxfork/CivitAi-to-Diffusers/diffusers/tests/pipelines/kandinsky_v22/test_kandinsky_prior.py
+++ /dev/null
@@ -1,246 +0,0 @@
-# coding=utf-8
-# Copyright 2023 HuggingFace Inc.
-#
-# Licensed under the Apache License, Version 2.0 (the "License");
-# you may not use this file except in compliance with the License.
-# You may obtain a copy of the License at
-#
-# http://www.apache.org/licenses/LICENSE-2.0
-#
-# Unless required by applicable law or agreed to in writing, software
-# distributed under the License is distributed on an "AS IS" BASIS,
-# WITHOUT WARRANTIES OR CONDITIONS OF ANY KIND, either express or implied.
-# See the License for the specific language governing permissions and
-# limitations under the License.
-
-import unittest
-
-import numpy as np
-import torch
-from torch import nn
-from transformers import (
- CLIPImageProcessor,
- CLIPTextConfig,
- CLIPTextModelWithProjection,
- CLIPTokenizer,
- CLIPVisionConfig,
- CLIPVisionModelWithProjection,
-)
-
-from diffusers import KandinskyV22PriorPipeline, PriorTransformer, UnCLIPScheduler
-from diffusers.utils import torch_device
-from diffusers.utils.testing_utils import enable_full_determinism, skip_mps
-
-from ..test_pipelines_common import PipelineTesterMixin
-
-
-enable_full_determinism()
-
-
-class Dummies:
- @property
- def text_embedder_hidden_size(self):
- return 32
-
- @property
- def time_input_dim(self):
- return 32
-
- @property
- def block_out_channels_0(self):
- return self.time_input_dim
-
- @property
- def time_embed_dim(self):
- return self.time_input_dim * 4
-
- @property
- def cross_attention_dim(self):
- return 100
-
- @property
- def dummy_tokenizer(self):
- tokenizer = CLIPTokenizer.from_pretrained("hf-internal-testing/tiny-random-clip")
- return tokenizer
-
- @property
- def dummy_text_encoder(self):
- torch.manual_seed(0)
- config = CLIPTextConfig(
- bos_token_id=0,
- eos_token_id=2,
- hidden_size=self.text_embedder_hidden_size,
- projection_dim=self.text_embedder_hidden_size,
- intermediate_size=37,
- layer_norm_eps=1e-05,
- num_attention_heads=4,
- num_hidden_layers=5,
- pad_token_id=1,
- vocab_size=1000,
- )
- return CLIPTextModelWithProjection(config)
-
- @property
- def dummy_prior(self):
- torch.manual_seed(0)
-
- model_kwargs = {
- "num_attention_heads": 2,
- "attention_head_dim": 12,
- "embedding_dim": self.text_embedder_hidden_size,
- "num_layers": 1,
- }
-
- model = PriorTransformer(**model_kwargs)
- # clip_std and clip_mean is initialized to be 0 so PriorTransformer.post_process_latents will always return 0 - set clip_std to be 1 so it won't return 0
- model.clip_std = nn.Parameter(torch.ones(model.clip_std.shape))
- return model
-
- @property
- def dummy_image_encoder(self):
- torch.manual_seed(0)
- config = CLIPVisionConfig(
- hidden_size=self.text_embedder_hidden_size,
- image_size=224,
- projection_dim=self.text_embedder_hidden_size,
- intermediate_size=37,
- num_attention_heads=4,
- num_channels=3,
- num_hidden_layers=5,
- patch_size=14,
- )
-
- model = CLIPVisionModelWithProjection(config)
- return model
-
- @property
- def dummy_image_processor(self):
- image_processor = CLIPImageProcessor(
- crop_size=224,
- do_center_crop=True,
- do_normalize=True,
- do_resize=True,
- image_mean=[0.48145466, 0.4578275, 0.40821073],
- image_std=[0.26862954, 0.26130258, 0.27577711],
- resample=3,
- size=224,
- )
-
- return image_processor
-
- def get_dummy_components(self):
- prior = self.dummy_prior
- image_encoder = self.dummy_image_encoder
- text_encoder = self.dummy_text_encoder
- tokenizer = self.dummy_tokenizer
- image_processor = self.dummy_image_processor
-
- scheduler = UnCLIPScheduler(
- variance_type="fixed_small_log",
- prediction_type="sample",
- num_train_timesteps=1000,
- clip_sample=True,
- clip_sample_range=10.0,
- )
-
- components = {
- "prior": prior,
- "image_encoder": image_encoder,
- "text_encoder": text_encoder,
- "tokenizer": tokenizer,
- "scheduler": scheduler,
- "image_processor": image_processor,
- }
-
- return components
-
- def get_dummy_inputs(self, device, seed=0):
- if str(device).startswith("mps"):
- generator = torch.manual_seed(seed)
- else:
- generator = torch.Generator(device=device).manual_seed(seed)
- inputs = {
- "prompt": "horse",
- "generator": generator,
- "guidance_scale": 4.0,
- "num_inference_steps": 2,
- "output_type": "np",
- }
- return inputs
-
-
-class KandinskyV22PriorPipelineFastTests(PipelineTesterMixin, unittest.TestCase):
- pipeline_class = KandinskyV22PriorPipeline
- params = ["prompt"]
- batch_params = ["prompt", "negative_prompt"]
- required_optional_params = [
- "num_images_per_prompt",
- "generator",
- "num_inference_steps",
- "latents",
- "negative_prompt",
- "guidance_scale",
- "output_type",
- "return_dict",
- ]
- test_xformers_attention = False
-
- def get_dummy_components(self):
- dummies = Dummies()
- return dummies.get_dummy_components()
-
- def get_dummy_inputs(self, device, seed=0):
- dummies = Dummies()
- return dummies.get_dummy_inputs(device=device, seed=seed)
-
- def test_kandinsky_prior(self):
- device = "cpu"
-
- components = self.get_dummy_components()
-
- pipe = self.pipeline_class(**components)
- pipe = pipe.to(device)
-
- pipe.set_progress_bar_config(disable=None)
-
- output = pipe(**self.get_dummy_inputs(device))
- image = output.image_embeds
-
- image_from_tuple = pipe(
- **self.get_dummy_inputs(device),
- return_dict=False,
- )[0]
-
- image_slice = image[0, -10:]
- image_from_tuple_slice = image_from_tuple[0, -10:]
-
- assert image.shape == (1, 32)
-
- expected_slice = np.array(
- [-0.0532, 1.7120, 0.3656, -1.0852, -0.8946, -1.1756, 0.4348, 0.2482, 0.5146, -0.1156]
- )
-
- assert np.abs(image_slice.flatten() - expected_slice).max() < 1e-2
- assert np.abs(image_from_tuple_slice.flatten() - expected_slice).max() < 1e-2
-
- @skip_mps
- def test_inference_batch_single_identical(self):
- test_max_difference = torch_device == "cpu"
- relax_max_difference = True
- test_mean_pixel_difference = False
-
- self._test_inference_batch_single_identical(
- test_max_difference=test_max_difference,
- relax_max_difference=relax_max_difference,
- test_mean_pixel_difference=test_mean_pixel_difference,
- )
-
- @skip_mps
- def test_attention_slicing_forward_pass(self):
- test_max_difference = torch_device == "cpu"
- test_mean_pixel_difference = False
-
- self._test_attention_slicing_forward_pass(
- test_max_difference=test_max_difference,
- test_mean_pixel_difference=test_mean_pixel_difference,
- )
diff --git a/spaces/Androidonnxfork/CivitAi-to-Diffusers/diffusers/tests/pipelines/test_pipelines_flax.py b/spaces/Androidonnxfork/CivitAi-to-Diffusers/diffusers/tests/pipelines/test_pipelines_flax.py
deleted file mode 100644
index 294dad5ff0f16980f08d3c4d74bae89b02a54abc..0000000000000000000000000000000000000000
--- a/spaces/Androidonnxfork/CivitAi-to-Diffusers/diffusers/tests/pipelines/test_pipelines_flax.py
+++ /dev/null
@@ -1,260 +0,0 @@
-# coding=utf-8
-# Copyright 2023 HuggingFace Inc.
-#
-# Licensed under the Apache License, Version 2.0 (the "License");
-# you may not use this file except in compliance with the License.
-# You may obtain a copy of the License at
-#
-# http://www.apache.org/licenses/LICENSE-2.0
-#
-# Unless required by applicable law or agreed to in writing, software
-# distributed under the License is distributed on an "AS IS" BASIS,
-# WITHOUT WARRANTIES OR CONDITIONS OF ANY KIND, either express or implied.
-# See the License for the specific language governing permissions and
-# limitations under the License.
-
-import os
-import tempfile
-import unittest
-
-import numpy as np
-
-from diffusers.utils import is_flax_available
-from diffusers.utils.testing_utils import require_flax, slow
-
-
-if is_flax_available():
- import jax
- import jax.numpy as jnp
- from flax.jax_utils import replicate
- from flax.training.common_utils import shard
-
- from diffusers import FlaxDDIMScheduler, FlaxDiffusionPipeline, FlaxStableDiffusionPipeline
-
-
-@require_flax
-class DownloadTests(unittest.TestCase):
- def test_download_only_pytorch(self):
- with tempfile.TemporaryDirectory() as tmpdirname:
- # pipeline has Flax weights
- _ = FlaxDiffusionPipeline.from_pretrained(
- "hf-internal-testing/tiny-stable-diffusion-pipe", safety_checker=None, cache_dir=tmpdirname
- )
-
- all_root_files = [t[-1] for t in os.walk(os.path.join(tmpdirname, os.listdir(tmpdirname)[0], "snapshots"))]
- files = [item for sublist in all_root_files for item in sublist]
-
- # None of the downloaded files should be a PyTorch file even if we have some here:
- # https://huggingface.co/hf-internal-testing/tiny-stable-diffusion-pipe/blob/main/unet/diffusion_pytorch_model.bin
- assert not any(f.endswith(".bin") for f in files)
-
-
-@slow
-@require_flax
-class FlaxPipelineTests(unittest.TestCase):
- def test_dummy_all_tpus(self):
- pipeline, params = FlaxStableDiffusionPipeline.from_pretrained(
- "hf-internal-testing/tiny-stable-diffusion-pipe", safety_checker=None
- )
-
- prompt = (
- "A cinematic film still of Morgan Freeman starring as Jimi Hendrix, portrait, 40mm lens, shallow depth of"
- " field, close up, split lighting, cinematic"
- )
-
- prng_seed = jax.random.PRNGKey(0)
- num_inference_steps = 4
-
- num_samples = jax.device_count()
- prompt = num_samples * [prompt]
- prompt_ids = pipeline.prepare_inputs(prompt)
-
- # shard inputs and rng
- params = replicate(params)
- prng_seed = jax.random.split(prng_seed, num_samples)
- prompt_ids = shard(prompt_ids)
-
- images = pipeline(prompt_ids, params, prng_seed, num_inference_steps, jit=True).images
-
- assert images.shape == (num_samples, 1, 64, 64, 3)
- if jax.device_count() == 8:
- assert np.abs(np.abs(images[0, 0, :2, :2, -2:], dtype=np.float32).sum() - 4.1514745) < 1e-3
- assert np.abs(np.abs(images, dtype=np.float32).sum() - 49947.875) < 5e-1
-
- images_pil = pipeline.numpy_to_pil(np.asarray(images.reshape((num_samples,) + images.shape[-3:])))
- assert len(images_pil) == num_samples
-
- def test_stable_diffusion_v1_4(self):
- pipeline, params = FlaxStableDiffusionPipeline.from_pretrained(
- "CompVis/stable-diffusion-v1-4", revision="flax", safety_checker=None
- )
-
- prompt = (
- "A cinematic film still of Morgan Freeman starring as Jimi Hendrix, portrait, 40mm lens, shallow depth of"
- " field, close up, split lighting, cinematic"
- )
-
- prng_seed = jax.random.PRNGKey(0)
- num_inference_steps = 50
-
- num_samples = jax.device_count()
- prompt = num_samples * [prompt]
- prompt_ids = pipeline.prepare_inputs(prompt)
-
- # shard inputs and rng
- params = replicate(params)
- prng_seed = jax.random.split(prng_seed, num_samples)
- prompt_ids = shard(prompt_ids)
-
- images = pipeline(prompt_ids, params, prng_seed, num_inference_steps, jit=True).images
-
- assert images.shape == (num_samples, 1, 512, 512, 3)
- if jax.device_count() == 8:
- assert np.abs((np.abs(images[0, 0, :2, :2, -2:], dtype=np.float32).sum() - 0.05652401)) < 1e-3
- assert np.abs((np.abs(images, dtype=np.float32).sum() - 2383808.2)) < 5e-1
-
- def test_stable_diffusion_v1_4_bfloat_16(self):
- pipeline, params = FlaxStableDiffusionPipeline.from_pretrained(
- "CompVis/stable-diffusion-v1-4", revision="bf16", dtype=jnp.bfloat16, safety_checker=None
- )
-
- prompt = (
- "A cinematic film still of Morgan Freeman starring as Jimi Hendrix, portrait, 40mm lens, shallow depth of"
- " field, close up, split lighting, cinematic"
- )
-
- prng_seed = jax.random.PRNGKey(0)
- num_inference_steps = 50
-
- num_samples = jax.device_count()
- prompt = num_samples * [prompt]
- prompt_ids = pipeline.prepare_inputs(prompt)
-
- # shard inputs and rng
- params = replicate(params)
- prng_seed = jax.random.split(prng_seed, num_samples)
- prompt_ids = shard(prompt_ids)
-
- images = pipeline(prompt_ids, params, prng_seed, num_inference_steps, jit=True).images
-
- assert images.shape == (num_samples, 1, 512, 512, 3)
- if jax.device_count() == 8:
- assert np.abs((np.abs(images[0, 0, :2, :2, -2:], dtype=np.float32).sum() - 0.04003906)) < 1e-3
- assert np.abs((np.abs(images, dtype=np.float32).sum() - 2373516.75)) < 5e-1
-
- def test_stable_diffusion_v1_4_bfloat_16_with_safety(self):
- pipeline, params = FlaxStableDiffusionPipeline.from_pretrained(
- "CompVis/stable-diffusion-v1-4", revision="bf16", dtype=jnp.bfloat16
- )
-
- prompt = (
- "A cinematic film still of Morgan Freeman starring as Jimi Hendrix, portrait, 40mm lens, shallow depth of"
- " field, close up, split lighting, cinematic"
- )
-
- prng_seed = jax.random.PRNGKey(0)
- num_inference_steps = 50
-
- num_samples = jax.device_count()
- prompt = num_samples * [prompt]
- prompt_ids = pipeline.prepare_inputs(prompt)
-
- # shard inputs and rng
- params = replicate(params)
- prng_seed = jax.random.split(prng_seed, num_samples)
- prompt_ids = shard(prompt_ids)
-
- images = pipeline(prompt_ids, params, prng_seed, num_inference_steps, jit=True).images
-
- assert images.shape == (num_samples, 1, 512, 512, 3)
- if jax.device_count() == 8:
- assert np.abs((np.abs(images[0, 0, :2, :2, -2:], dtype=np.float32).sum() - 0.04003906)) < 1e-3
- assert np.abs((np.abs(images, dtype=np.float32).sum() - 2373516.75)) < 5e-1
-
- def test_stable_diffusion_v1_4_bfloat_16_ddim(self):
- scheduler = FlaxDDIMScheduler(
- beta_start=0.00085,
- beta_end=0.012,
- beta_schedule="scaled_linear",
- set_alpha_to_one=False,
- steps_offset=1,
- )
-
- pipeline, params = FlaxStableDiffusionPipeline.from_pretrained(
- "CompVis/stable-diffusion-v1-4",
- revision="bf16",
- dtype=jnp.bfloat16,
- scheduler=scheduler,
- safety_checker=None,
- )
- scheduler_state = scheduler.create_state()
-
- params["scheduler"] = scheduler_state
-
- prompt = (
- "A cinematic film still of Morgan Freeman starring as Jimi Hendrix, portrait, 40mm lens, shallow depth of"
- " field, close up, split lighting, cinematic"
- )
-
- prng_seed = jax.random.PRNGKey(0)
- num_inference_steps = 50
-
- num_samples = jax.device_count()
- prompt = num_samples * [prompt]
- prompt_ids = pipeline.prepare_inputs(prompt)
-
- # shard inputs and rng
- params = replicate(params)
- prng_seed = jax.random.split(prng_seed, num_samples)
- prompt_ids = shard(prompt_ids)
-
- images = pipeline(prompt_ids, params, prng_seed, num_inference_steps, jit=True).images
-
- assert images.shape == (num_samples, 1, 512, 512, 3)
- if jax.device_count() == 8:
- assert np.abs((np.abs(images[0, 0, :2, :2, -2:], dtype=np.float32).sum() - 0.045043945)) < 1e-3
- assert np.abs((np.abs(images, dtype=np.float32).sum() - 2347693.5)) < 5e-1
-
- def test_jax_memory_efficient_attention(self):
- prompt = (
- "A cinematic film still of Morgan Freeman starring as Jimi Hendrix, portrait, 40mm lens, shallow depth of"
- " field, close up, split lighting, cinematic"
- )
-
- num_samples = jax.device_count()
- prompt = num_samples * [prompt]
- prng_seed = jax.random.split(jax.random.PRNGKey(0), num_samples)
-
- pipeline, params = FlaxStableDiffusionPipeline.from_pretrained(
- "CompVis/stable-diffusion-v1-4",
- revision="bf16",
- dtype=jnp.bfloat16,
- safety_checker=None,
- )
-
- params = replicate(params)
- prompt_ids = pipeline.prepare_inputs(prompt)
- prompt_ids = shard(prompt_ids)
- images = pipeline(prompt_ids, params, prng_seed, jit=True).images
- assert images.shape == (num_samples, 1, 512, 512, 3)
- slice = images[2, 0, 256, 10:17, 1]
-
- # With memory efficient attention
- pipeline, params = FlaxStableDiffusionPipeline.from_pretrained(
- "CompVis/stable-diffusion-v1-4",
- revision="bf16",
- dtype=jnp.bfloat16,
- safety_checker=None,
- use_memory_efficient_attention=True,
- )
-
- params = replicate(params)
- prompt_ids = pipeline.prepare_inputs(prompt)
- prompt_ids = shard(prompt_ids)
- images_eff = pipeline(prompt_ids, params, prng_seed, jit=True).images
- assert images_eff.shape == (num_samples, 1, 512, 512, 3)
- slice_eff = images[2, 0, 256, 10:17, 1]
-
- # I checked the results visually and they are very similar. However, I saw that the max diff is `1` and the `sum`
- # over the 8 images is exactly `256`, which is very suspicious. Testing a random slice for now.
- assert abs(slice_eff - slice).max() < 1e-2
diff --git a/spaces/Andy1621/uniformer_image_detection/configs/hrnet/fcos_hrnetv2p_w18_gn-head_4x4_1x_coco.py b/spaces/Andy1621/uniformer_image_detection/configs/hrnet/fcos_hrnetv2p_w18_gn-head_4x4_1x_coco.py
deleted file mode 100644
index 20bffb95616d4358007d0825820f4a91ea223649..0000000000000000000000000000000000000000
--- a/spaces/Andy1621/uniformer_image_detection/configs/hrnet/fcos_hrnetv2p_w18_gn-head_4x4_1x_coco.py
+++ /dev/null
@@ -1,9 +0,0 @@
-_base_ = './fcos_hrnetv2p_w32_gn-head_4x4_1x_coco.py'
-model = dict(
- pretrained='open-mmlab://msra/hrnetv2_w18',
- backbone=dict(
- extra=dict(
- stage2=dict(num_channels=(18, 36)),
- stage3=dict(num_channels=(18, 36, 72)),
- stage4=dict(num_channels=(18, 36, 72, 144)))),
- neck=dict(type='HRFPN', in_channels=[18, 36, 72, 144], out_channels=256))
diff --git a/spaces/Andy1621/uniformer_image_detection/mmdet/models/dense_heads/embedding_rpn_head.py b/spaces/Andy1621/uniformer_image_detection/mmdet/models/dense_heads/embedding_rpn_head.py
deleted file mode 100644
index 200ce8d20c5503f98c5c21f30bb9d00437e25f34..0000000000000000000000000000000000000000
--- a/spaces/Andy1621/uniformer_image_detection/mmdet/models/dense_heads/embedding_rpn_head.py
+++ /dev/null
@@ -1,100 +0,0 @@
-import torch
-import torch.nn as nn
-
-from mmdet.models.builder import HEADS
-from ...core import bbox_cxcywh_to_xyxy
-
-
-@HEADS.register_module()
-class EmbeddingRPNHead(nn.Module):
- """RPNHead in the `Sparse R-CNN `_ .
-
- Unlike traditional RPNHead, this module does not need FPN input, but just
- decode `init_proposal_bboxes` and expand the first dimension of
- `init_proposal_bboxes` and `init_proposal_features` to the batch_size.
-
- Args:
- num_proposals (int): Number of init_proposals. Default 100.
- proposal_feature_channel (int): Channel number of
- init_proposal_feature. Defaults to 256.
- """
-
- def __init__(self,
- num_proposals=100,
- proposal_feature_channel=256,
- **kwargs):
- super(EmbeddingRPNHead, self).__init__()
- self.num_proposals = num_proposals
- self.proposal_feature_channel = proposal_feature_channel
- self._init_layers()
-
- def _init_layers(self):
- """Initialize a sparse set of proposal boxes and proposal features."""
- self.init_proposal_bboxes = nn.Embedding(self.num_proposals, 4)
- self.init_proposal_features = nn.Embedding(
- self.num_proposals, self.proposal_feature_channel)
-
- def init_weights(self):
- """Initialize the init_proposal_bboxes as normalized.
-
- [c_x, c_y, w, h], and we initialize it to the size of the entire
- image.
- """
- nn.init.constant_(self.init_proposal_bboxes.weight[:, :2], 0.5)
- nn.init.constant_(self.init_proposal_bboxes.weight[:, 2:], 1)
-
- def _decode_init_proposals(self, imgs, img_metas):
- """Decode init_proposal_bboxes according to the size of images and
- expand dimension of init_proposal_features to batch_size.
-
- Args:
- imgs (list[Tensor]): List of FPN features.
- img_metas (list[dict]): List of meta-information of
- images. Need the img_shape to decode the init_proposals.
-
- Returns:
- Tuple(Tensor):
-
- - proposals (Tensor): Decoded proposal bboxes,
- has shape (batch_size, num_proposals, 4).
- - init_proposal_features (Tensor): Expanded proposal
- features, has shape
- (batch_size, num_proposals, proposal_feature_channel).
- - imgs_whwh (Tensor): Tensor with shape
- (batch_size, 4), the dimension means
- [img_width, img_height, img_width, img_height].
- """
- proposals = self.init_proposal_bboxes.weight.clone()
- proposals = bbox_cxcywh_to_xyxy(proposals)
- num_imgs = len(imgs[0])
- imgs_whwh = []
- for meta in img_metas:
- h, w, _ = meta['img_shape']
- imgs_whwh.append(imgs[0].new_tensor([[w, h, w, h]]))
- imgs_whwh = torch.cat(imgs_whwh, dim=0)
- imgs_whwh = imgs_whwh[:, None, :]
-
- # imgs_whwh has shape (batch_size, 1, 4)
- # The shape of proposals change from (num_proposals, 4)
- # to (batch_size ,num_proposals, 4)
- proposals = proposals * imgs_whwh
-
- init_proposal_features = self.init_proposal_features.weight.clone()
- init_proposal_features = init_proposal_features[None].expand(
- num_imgs, *init_proposal_features.size())
- return proposals, init_proposal_features, imgs_whwh
-
- def forward_dummy(self, img, img_metas):
- """Dummy forward function.
-
- Used in flops calculation.
- """
- return self._decode_init_proposals(img, img_metas)
-
- def forward_train(self, img, img_metas):
- """Forward function in training stage."""
- return self._decode_init_proposals(img, img_metas)
-
- def simple_test_rpn(self, img, img_metas):
- """Forward function in testing stage."""
- return self._decode_init_proposals(img, img_metas)
diff --git a/spaces/Andy1621/uniformer_image_detection/mmdet/models/detectors/vfnet.py b/spaces/Andy1621/uniformer_image_detection/mmdet/models/detectors/vfnet.py
deleted file mode 100644
index e23f89674c919921219ffd3486587a2d3c318fbd..0000000000000000000000000000000000000000
--- a/spaces/Andy1621/uniformer_image_detection/mmdet/models/detectors/vfnet.py
+++ /dev/null
@@ -1,18 +0,0 @@
-from ..builder import DETECTORS
-from .single_stage import SingleStageDetector
-
-
-@DETECTORS.register_module()
-class VFNet(SingleStageDetector):
- """Implementation of `VarifocalNet
- (VFNet).`_"""
-
- def __init__(self,
- backbone,
- neck,
- bbox_head,
- train_cfg=None,
- test_cfg=None,
- pretrained=None):
- super(VFNet, self).__init__(backbone, neck, bbox_head, train_cfg,
- test_cfg, pretrained)
diff --git a/spaces/Andy1621/uniformer_image_detection/mmdet/models/necks/fpn.py b/spaces/Andy1621/uniformer_image_detection/mmdet/models/necks/fpn.py
deleted file mode 100644
index 5e5dfe685964f06e7a66b63a13e66162e63fcafd..0000000000000000000000000000000000000000
--- a/spaces/Andy1621/uniformer_image_detection/mmdet/models/necks/fpn.py
+++ /dev/null
@@ -1,221 +0,0 @@
-import warnings
-
-import torch.nn as nn
-import torch.nn.functional as F
-from mmcv.cnn import ConvModule, xavier_init
-from mmcv.runner import auto_fp16
-
-from ..builder import NECKS
-
-
-@NECKS.register_module()
-class FPN(nn.Module):
- r"""Feature Pyramid Network.
-
- This is an implementation of paper `Feature Pyramid Networks for Object
- Detection `_.
-
- Args:
- in_channels (List[int]): Number of input channels per scale.
- out_channels (int): Number of output channels (used at each scale)
- num_outs (int): Number of output scales.
- start_level (int): Index of the start input backbone level used to
- build the feature pyramid. Default: 0.
- end_level (int): Index of the end input backbone level (exclusive) to
- build the feature pyramid. Default: -1, which means the last level.
- add_extra_convs (bool | str): If bool, it decides whether to add conv
- layers on top of the original feature maps. Default to False.
- If True, its actual mode is specified by `extra_convs_on_inputs`.
- If str, it specifies the source feature map of the extra convs.
- Only the following options are allowed
-
- - 'on_input': Last feat map of neck inputs (i.e. backbone feature).
- - 'on_lateral': Last feature map after lateral convs.
- - 'on_output': The last output feature map after fpn convs.
- extra_convs_on_inputs (bool, deprecated): Whether to apply extra convs
- on the original feature from the backbone. If True,
- it is equivalent to `add_extra_convs='on_input'`. If False, it is
- equivalent to set `add_extra_convs='on_output'`. Default to True.
- relu_before_extra_convs (bool): Whether to apply relu before the extra
- conv. Default: False.
- no_norm_on_lateral (bool): Whether to apply norm on lateral.
- Default: False.
- conv_cfg (dict): Config dict for convolution layer. Default: None.
- norm_cfg (dict): Config dict for normalization layer. Default: None.
- act_cfg (str): Config dict for activation layer in ConvModule.
- Default: None.
- upsample_cfg (dict): Config dict for interpolate layer.
- Default: `dict(mode='nearest')`
-
- Example:
- >>> import torch
- >>> in_channels = [2, 3, 5, 7]
- >>> scales = [340, 170, 84, 43]
- >>> inputs = [torch.rand(1, c, s, s)
- ... for c, s in zip(in_channels, scales)]
- >>> self = FPN(in_channels, 11, len(in_channels)).eval()
- >>> outputs = self.forward(inputs)
- >>> for i in range(len(outputs)):
- ... print(f'outputs[{i}].shape = {outputs[i].shape}')
- outputs[0].shape = torch.Size([1, 11, 340, 340])
- outputs[1].shape = torch.Size([1, 11, 170, 170])
- outputs[2].shape = torch.Size([1, 11, 84, 84])
- outputs[3].shape = torch.Size([1, 11, 43, 43])
- """
-
- def __init__(self,
- in_channels,
- out_channels,
- num_outs,
- start_level=0,
- end_level=-1,
- add_extra_convs=False,
- extra_convs_on_inputs=True,
- relu_before_extra_convs=False,
- no_norm_on_lateral=False,
- conv_cfg=None,
- norm_cfg=None,
- act_cfg=None,
- upsample_cfg=dict(mode='nearest')):
- super(FPN, self).__init__()
- assert isinstance(in_channels, list)
- self.in_channels = in_channels
- self.out_channels = out_channels
- self.num_ins = len(in_channels)
- self.num_outs = num_outs
- self.relu_before_extra_convs = relu_before_extra_convs
- self.no_norm_on_lateral = no_norm_on_lateral
- self.fp16_enabled = False
- self.upsample_cfg = upsample_cfg.copy()
-
- if end_level == -1:
- self.backbone_end_level = self.num_ins
- assert num_outs >= self.num_ins - start_level
- else:
- # if end_level < inputs, no extra level is allowed
- self.backbone_end_level = end_level
- assert end_level <= len(in_channels)
- assert num_outs == end_level - start_level
- self.start_level = start_level
- self.end_level = end_level
- self.add_extra_convs = add_extra_convs
- assert isinstance(add_extra_convs, (str, bool))
- if isinstance(add_extra_convs, str):
- # Extra_convs_source choices: 'on_input', 'on_lateral', 'on_output'
- assert add_extra_convs in ('on_input', 'on_lateral', 'on_output')
- elif add_extra_convs: # True
- if extra_convs_on_inputs:
- # TODO: deprecate `extra_convs_on_inputs`
- warnings.simplefilter('once')
- warnings.warn(
- '"extra_convs_on_inputs" will be deprecated in v2.9.0,'
- 'Please use "add_extra_convs"', DeprecationWarning)
- self.add_extra_convs = 'on_input'
- else:
- self.add_extra_convs = 'on_output'
-
- self.lateral_convs = nn.ModuleList()
- self.fpn_convs = nn.ModuleList()
-
- for i in range(self.start_level, self.backbone_end_level):
- l_conv = ConvModule(
- in_channels[i],
- out_channels,
- 1,
- conv_cfg=conv_cfg,
- norm_cfg=norm_cfg if not self.no_norm_on_lateral else None,
- act_cfg=act_cfg,
- inplace=False)
- fpn_conv = ConvModule(
- out_channels,
- out_channels,
- 3,
- padding=1,
- conv_cfg=conv_cfg,
- norm_cfg=norm_cfg,
- act_cfg=act_cfg,
- inplace=False)
-
- self.lateral_convs.append(l_conv)
- self.fpn_convs.append(fpn_conv)
-
- # add extra conv layers (e.g., RetinaNet)
- extra_levels = num_outs - self.backbone_end_level + self.start_level
- if self.add_extra_convs and extra_levels >= 1:
- for i in range(extra_levels):
- if i == 0 and self.add_extra_convs == 'on_input':
- in_channels = self.in_channels[self.backbone_end_level - 1]
- else:
- in_channels = out_channels
- extra_fpn_conv = ConvModule(
- in_channels,
- out_channels,
- 3,
- stride=2,
- padding=1,
- conv_cfg=conv_cfg,
- norm_cfg=norm_cfg,
- act_cfg=act_cfg,
- inplace=False)
- self.fpn_convs.append(extra_fpn_conv)
-
- # default init_weights for conv(msra) and norm in ConvModule
- def init_weights(self):
- """Initialize the weights of FPN module."""
- for m in self.modules():
- if isinstance(m, nn.Conv2d):
- xavier_init(m, distribution='uniform')
-
- @auto_fp16()
- def forward(self, inputs):
- """Forward function."""
- assert len(inputs) == len(self.in_channels)
-
- # build laterals
- laterals = [
- lateral_conv(inputs[i + self.start_level])
- for i, lateral_conv in enumerate(self.lateral_convs)
- ]
-
- # build top-down path
- used_backbone_levels = len(laterals)
- for i in range(used_backbone_levels - 1, 0, -1):
- # In some cases, fixing `scale factor` (e.g. 2) is preferred, but
- # it cannot co-exist with `size` in `F.interpolate`.
- if 'scale_factor' in self.upsample_cfg:
- laterals[i - 1] += F.interpolate(laterals[i],
- **self.upsample_cfg)
- else:
- prev_shape = laterals[i - 1].shape[2:]
- laterals[i - 1] += F.interpolate(
- laterals[i], size=prev_shape, **self.upsample_cfg)
-
- # build outputs
- # part 1: from original levels
- outs = [
- self.fpn_convs[i](laterals[i]) for i in range(used_backbone_levels)
- ]
- # part 2: add extra levels
- if self.num_outs > len(outs):
- # use max pool to get more levels on top of outputs
- # (e.g., Faster R-CNN, Mask R-CNN)
- if not self.add_extra_convs:
- for i in range(self.num_outs - used_backbone_levels):
- outs.append(F.max_pool2d(outs[-1], 1, stride=2))
- # add conv layers on top of original feature maps (RetinaNet)
- else:
- if self.add_extra_convs == 'on_input':
- extra_source = inputs[self.backbone_end_level - 1]
- elif self.add_extra_convs == 'on_lateral':
- extra_source = laterals[-1]
- elif self.add_extra_convs == 'on_output':
- extra_source = outs[-1]
- else:
- raise NotImplementedError
- outs.append(self.fpn_convs[used_backbone_levels](extra_source))
- for i in range(used_backbone_levels + 1, self.num_outs):
- if self.relu_before_extra_convs:
- outs.append(self.fpn_convs[i](F.relu(outs[-1])))
- else:
- outs.append(self.fpn_convs[i](outs[-1]))
- return tuple(outs)
diff --git a/spaces/Andy1621/uniformer_image_segmentation/configs/dnlnet/dnl_r50-d8_512x1024_40k_cityscapes.py b/spaces/Andy1621/uniformer_image_segmentation/configs/dnlnet/dnl_r50-d8_512x1024_40k_cityscapes.py
deleted file mode 100644
index f7aa7444d4c8022563db642478beec4dc5ab0dab..0000000000000000000000000000000000000000
--- a/spaces/Andy1621/uniformer_image_segmentation/configs/dnlnet/dnl_r50-d8_512x1024_40k_cityscapes.py
+++ /dev/null
@@ -1,4 +0,0 @@
-_base_ = [
- '../_base_/models/dnl_r50-d8.py', '../_base_/datasets/cityscapes.py',
- '../_base_/default_runtime.py', '../_base_/schedules/schedule_40k.py'
-]
diff --git a/spaces/Andy1621/uniformer_image_segmentation/configs/pspnet/pspnet_r50-d8_512x512_40k_voc12aug.py b/spaces/Andy1621/uniformer_image_segmentation/configs/pspnet/pspnet_r50-d8_512x512_40k_voc12aug.py
deleted file mode 100644
index f0c20c12f6bcf04b732dccaa4bfdba10bd10b5e6..0000000000000000000000000000000000000000
--- a/spaces/Andy1621/uniformer_image_segmentation/configs/pspnet/pspnet_r50-d8_512x512_40k_voc12aug.py
+++ /dev/null
@@ -1,7 +0,0 @@
-_base_ = [
- '../_base_/models/pspnet_r50-d8.py',
- '../_base_/datasets/pascal_voc12_aug.py', '../_base_/default_runtime.py',
- '../_base_/schedules/schedule_40k.py'
-]
-model = dict(
- decode_head=dict(num_classes=21), auxiliary_head=dict(num_classes=21))
diff --git a/spaces/AnimalEquality/chatbot/_proc/_docs/index.html b/spaces/AnimalEquality/chatbot/_proc/_docs/index.html
deleted file mode 100644
index a49b2045925ff360dacc717abd4634ac5fa021d3..0000000000000000000000000000000000000000
--- a/spaces/AnimalEquality/chatbot/_proc/_docs/index.html
+++ /dev/null
@@ -1,535 +0,0 @@
-
-
-
-
-
-
-
-
-
-
-lv-recipe-chatbot
-
-
-
-
-
-
-
-
-
-
-
-
-
-
-
-
-
-
-
-
-
-
-
-
-
-
-
-
-
-
-
-
-
-
-
-
-
-
-
-
-
-
-
from dotenv import load_dotenv
-
-load_dotenv() # or load environment vars with different method
-
-demo = app.create_demo(app.ConversationBot())
-demo.launch()
-
-
Running on local URL: http://127.0.0.1:7860
-
-To create a public link, set `share=True` in `launch()`.
-
-
-
-
-
-
-
-
-
or
-
python3 app.py
-
-
-
Dev quick-start
-
git clone the repo
-
cd lv-recipe-chatbot
-
Make sure to use the version of python specified in py_version.txt
-Create a virtual environment.
To make the Jupyter environment, git friendly: nbdev_install_hooks
-If you want to render documentation locally, you will want to install Quarto.
-
nbdev_install_quarto
-
Put API secrets in .env
-
cp .env.example .env
-
Edit .env with your secret key(s). Only OPEN_AI_KEY is required.
-
Then start the Gradio demo from within the virtual environment.
-
python3 app.py
-
Preview documentation
-
nbdev_preview
-
-
-
Dependencies
-
If a new dependency for development is helpful for developers, add it to dev.txt.
-If it is a dependency for the app that is imported in source code, add it to core.txt.
-Then run:
-
scripts/pin_requirements.sh
-
This will update our requirements.txt to include the dependency as it should be pinned in the environment.
-
-
-
-
\ No newline at end of file
diff --git a/spaces/Artificio/AdversarialArt/src/utils.py b/spaces/Artificio/AdversarialArt/src/utils.py
deleted file mode 100644
index 59e8228aff6ed528250f87234287d80b0b85c96b..0000000000000000000000000000000000000000
--- a/spaces/Artificio/AdversarialArt/src/utils.py
+++ /dev/null
@@ -1,35 +0,0 @@
-from PIL import Image
-import torch
-import torch.nn as nn
-from typing import Dict, Iterable, Callable
-from torch import Tensor
-import glob
-from tqdm import tqdm
-import numpy as np
-from PIL import ImageFile
-ImageFile.LOAD_TRUNCATED_IMAGES = True
-Image.MAX_IMAGE_PIXELS = None
-
-
-# +
-class RobustModel(nn.Module):
- def __init__(self, model):
- super().__init__()
- self.model = model
- def forward(self, x, *args, **kwargs):
- return self.model(x)
-
-
-class CustomArt(torch.utils.data.Dataset):
- def __init__(self, image,transforms=None):
- self.transforms = transforms
- self.image = image
- self.mean = torch.tensor([0.4850, 0.4560, 0.4060])
- self.std = torch.tensor([0.2290, 0.2240, 0.2250])
- def __getitem__(self, idx):
- if self.transforms:
- img = self.transforms(self.image)
- return torch.as_tensor(img, dtype=torch.float)
-
- def __len__(self):
- return len(self.image)
diff --git a/spaces/Asahi402/Real-CUGAN/README.md b/spaces/Asahi402/Real-CUGAN/README.md
deleted file mode 100644
index d673114edadba73e80f33a3c71bc0dbee8758cc8..0000000000000000000000000000000000000000
--- a/spaces/Asahi402/Real-CUGAN/README.md
+++ /dev/null
@@ -1,14 +0,0 @@
----
-title: Real CUGAN
-emoji: 🐢
-colorFrom: gray
-colorTo: green
-sdk: gradio
-sdk_version: 3.6
-app_file: app.py
-pinned: false
-license: gpl-3.0
-duplicated_from: DianXian/Real-CUGAN
----
-
-Check out the configuration reference at https://huggingface.co/docs/hub/spaces-config-reference
diff --git a/spaces/Ataturk-Chatbot/HuggingFaceChat/venv/lib/python3.11/site-packages/pip/_internal/resolution/__init__.py b/spaces/Ataturk-Chatbot/HuggingFaceChat/venv/lib/python3.11/site-packages/pip/_internal/resolution/__init__.py
deleted file mode 100644
index e69de29bb2d1d6434b8b29ae775ad8c2e48c5391..0000000000000000000000000000000000000000
diff --git a/spaces/Ataturk-Chatbot/HuggingFaceChat/venv/lib/python3.11/site-packages/pip/_internal/utils/temp_dir.py b/spaces/Ataturk-Chatbot/HuggingFaceChat/venv/lib/python3.11/site-packages/pip/_internal/utils/temp_dir.py
deleted file mode 100644
index 8ee8a1cb18017880cd0bebd66bc2cec5702118c6..0000000000000000000000000000000000000000
--- a/spaces/Ataturk-Chatbot/HuggingFaceChat/venv/lib/python3.11/site-packages/pip/_internal/utils/temp_dir.py
+++ /dev/null
@@ -1,246 +0,0 @@
-import errno
-import itertools
-import logging
-import os.path
-import tempfile
-from contextlib import ExitStack, contextmanager
-from typing import Any, Dict, Generator, Optional, TypeVar, Union
-
-from pip._internal.utils.misc import enum, rmtree
-
-logger = logging.getLogger(__name__)
-
-_T = TypeVar("_T", bound="TempDirectory")
-
-
-# Kinds of temporary directories. Only needed for ones that are
-# globally-managed.
-tempdir_kinds = enum(
- BUILD_ENV="build-env",
- EPHEM_WHEEL_CACHE="ephem-wheel-cache",
- REQ_BUILD="req-build",
-)
-
-
-_tempdir_manager: Optional[ExitStack] = None
-
-
-@contextmanager
-def global_tempdir_manager() -> Generator[None, None, None]:
- global _tempdir_manager
- with ExitStack() as stack:
- old_tempdir_manager, _tempdir_manager = _tempdir_manager, stack
- try:
- yield
- finally:
- _tempdir_manager = old_tempdir_manager
-
-
-class TempDirectoryTypeRegistry:
- """Manages temp directory behavior"""
-
- def __init__(self) -> None:
- self._should_delete: Dict[str, bool] = {}
-
- def set_delete(self, kind: str, value: bool) -> None:
- """Indicate whether a TempDirectory of the given kind should be
- auto-deleted.
- """
- self._should_delete[kind] = value
-
- def get_delete(self, kind: str) -> bool:
- """Get configured auto-delete flag for a given TempDirectory type,
- default True.
- """
- return self._should_delete.get(kind, True)
-
-
-_tempdir_registry: Optional[TempDirectoryTypeRegistry] = None
-
-
-@contextmanager
-def tempdir_registry() -> Generator[TempDirectoryTypeRegistry, None, None]:
- """Provides a scoped global tempdir registry that can be used to dictate
- whether directories should be deleted.
- """
- global _tempdir_registry
- old_tempdir_registry = _tempdir_registry
- _tempdir_registry = TempDirectoryTypeRegistry()
- try:
- yield _tempdir_registry
- finally:
- _tempdir_registry = old_tempdir_registry
-
-
-class _Default:
- pass
-
-
-_default = _Default()
-
-
-class TempDirectory:
- """Helper class that owns and cleans up a temporary directory.
-
- This class can be used as a context manager or as an OO representation of a
- temporary directory.
-
- Attributes:
- path
- Location to the created temporary directory
- delete
- Whether the directory should be deleted when exiting
- (when used as a contextmanager)
-
- Methods:
- cleanup()
- Deletes the temporary directory
-
- When used as a context manager, if the delete attribute is True, on
- exiting the context the temporary directory is deleted.
- """
-
- def __init__(
- self,
- path: Optional[str] = None,
- delete: Union[bool, None, _Default] = _default,
- kind: str = "temp",
- globally_managed: bool = False,
- ):
- super().__init__()
-
- if delete is _default:
- if path is not None:
- # If we were given an explicit directory, resolve delete option
- # now.
- delete = False
- else:
- # Otherwise, we wait until cleanup and see what
- # tempdir_registry says.
- delete = None
-
- # The only time we specify path is in for editables where it
- # is the value of the --src option.
- if path is None:
- path = self._create(kind)
-
- self._path = path
- self._deleted = False
- self.delete = delete
- self.kind = kind
-
- if globally_managed:
- assert _tempdir_manager is not None
- _tempdir_manager.enter_context(self)
-
- @property
- def path(self) -> str:
- assert not self._deleted, f"Attempted to access deleted path: {self._path}"
- return self._path
-
- def __repr__(self) -> str:
- return f"<{self.__class__.__name__} {self.path!r}>"
-
- def __enter__(self: _T) -> _T:
- return self
-
- def __exit__(self, exc: Any, value: Any, tb: Any) -> None:
- if self.delete is not None:
- delete = self.delete
- elif _tempdir_registry:
- delete = _tempdir_registry.get_delete(self.kind)
- else:
- delete = True
-
- if delete:
- self.cleanup()
-
- def _create(self, kind: str) -> str:
- """Create a temporary directory and store its path in self.path"""
- # We realpath here because some systems have their default tmpdir
- # symlinked to another directory. This tends to confuse build
- # scripts, so we canonicalize the path by traversing potential
- # symlinks here.
- path = os.path.realpath(tempfile.mkdtemp(prefix=f"pip-{kind}-"))
- logger.debug("Created temporary directory: %s", path)
- return path
-
- def cleanup(self) -> None:
- """Remove the temporary directory created and reset state"""
- self._deleted = True
- if not os.path.exists(self._path):
- return
- rmtree(self._path)
-
-
-class AdjacentTempDirectory(TempDirectory):
- """Helper class that creates a temporary directory adjacent to a real one.
-
- Attributes:
- original
- The original directory to create a temp directory for.
- path
- After calling create() or entering, contains the full
- path to the temporary directory.
- delete
- Whether the directory should be deleted when exiting
- (when used as a contextmanager)
-
- """
-
- # The characters that may be used to name the temp directory
- # We always prepend a ~ and then rotate through these until
- # a usable name is found.
- # pkg_resources raises a different error for .dist-info folder
- # with leading '-' and invalid metadata
- LEADING_CHARS = "-~.=%0123456789"
-
- def __init__(self, original: str, delete: Optional[bool] = None) -> None:
- self.original = original.rstrip("/\\")
- super().__init__(delete=delete)
-
- @classmethod
- def _generate_names(cls, name: str) -> Generator[str, None, None]:
- """Generates a series of temporary names.
-
- The algorithm replaces the leading characters in the name
- with ones that are valid filesystem characters, but are not
- valid package names (for both Python and pip definitions of
- package).
- """
- for i in range(1, len(name)):
- for candidate in itertools.combinations_with_replacement(
- cls.LEADING_CHARS, i - 1
- ):
- new_name = "~" + "".join(candidate) + name[i:]
- if new_name != name:
- yield new_name
-
- # If we make it this far, we will have to make a longer name
- for i in range(len(cls.LEADING_CHARS)):
- for candidate in itertools.combinations_with_replacement(
- cls.LEADING_CHARS, i
- ):
- new_name = "~" + "".join(candidate) + name
- if new_name != name:
- yield new_name
-
- def _create(self, kind: str) -> str:
- root, name = os.path.split(self.original)
- for candidate in self._generate_names(name):
- path = os.path.join(root, candidate)
- try:
- os.mkdir(path)
- except OSError as ex:
- # Continue if the name exists already
- if ex.errno != errno.EEXIST:
- raise
- else:
- path = os.path.realpath(path)
- break
- else:
- # Final fallback on the default behavior.
- path = os.path.realpath(tempfile.mkdtemp(prefix=f"pip-{kind}-"))
-
- logger.debug("Created temporary directory: %s", path)
- return path
diff --git a/spaces/Ataturk-Chatbot/HuggingFaceChat/venv/lib/python3.11/site-packages/setuptools/_distutils/spawn.py b/spaces/Ataturk-Chatbot/HuggingFaceChat/venv/lib/python3.11/site-packages/setuptools/_distutils/spawn.py
deleted file mode 100644
index b18ba9db7d2e5919c853e7dcf8d5b7c180607c3f..0000000000000000000000000000000000000000
--- a/spaces/Ataturk-Chatbot/HuggingFaceChat/venv/lib/python3.11/site-packages/setuptools/_distutils/spawn.py
+++ /dev/null
@@ -1,109 +0,0 @@
-"""distutils.spawn
-
-Provides the 'spawn()' function, a front-end to various platform-
-specific functions for launching another program in a sub-process.
-Also provides the 'find_executable()' to search the path for a given
-executable name.
-"""
-
-import sys
-import os
-import subprocess
-
-from distutils.errors import DistutilsExecError
-from distutils.debug import DEBUG
-from distutils import log
-
-
-def spawn(cmd, search_path=1, verbose=0, dry_run=0, env=None): # noqa: C901
- """Run another program, specified as a command list 'cmd', in a new process.
-
- 'cmd' is just the argument list for the new process, ie.
- cmd[0] is the program to run and cmd[1:] are the rest of its arguments.
- There is no way to run a program with a name different from that of its
- executable.
-
- If 'search_path' is true (the default), the system's executable
- search path will be used to find the program; otherwise, cmd[0]
- must be the exact path to the executable. If 'dry_run' is true,
- the command will not actually be run.
-
- Raise DistutilsExecError if running the program fails in any way; just
- return on success.
- """
- # cmd is documented as a list, but just in case some code passes a tuple
- # in, protect our %-formatting code against horrible death
- cmd = list(cmd)
-
- log.info(subprocess.list2cmdline(cmd))
- if dry_run:
- return
-
- if search_path:
- executable = find_executable(cmd[0])
- if executable is not None:
- cmd[0] = executable
-
- env = env if env is not None else dict(os.environ)
-
- if sys.platform == 'darwin':
- from distutils.util import MACOSX_VERSION_VAR, get_macosx_target_ver
-
- macosx_target_ver = get_macosx_target_ver()
- if macosx_target_ver:
- env[MACOSX_VERSION_VAR] = macosx_target_ver
-
- try:
- proc = subprocess.Popen(cmd, env=env)
- proc.wait()
- exitcode = proc.returncode
- except OSError as exc:
- if not DEBUG:
- cmd = cmd[0]
- raise DistutilsExecError(
- "command {!r} failed: {}".format(cmd, exc.args[-1])
- ) from exc
-
- if exitcode:
- if not DEBUG:
- cmd = cmd[0]
- raise DistutilsExecError(
- "command {!r} failed with exit code {}".format(cmd, exitcode)
- )
-
-
-def find_executable(executable, path=None):
- """Tries to find 'executable' in the directories listed in 'path'.
-
- A string listing directories separated by 'os.pathsep'; defaults to
- os.environ['PATH']. Returns the complete filename or None if not found.
- """
- _, ext = os.path.splitext(executable)
- if (sys.platform == 'win32') and (ext != '.exe'):
- executable = executable + '.exe'
-
- if os.path.isfile(executable):
- return executable
-
- if path is None:
- path = os.environ.get('PATH', None)
- if path is None:
- try:
- path = os.confstr("CS_PATH")
- except (AttributeError, ValueError):
- # os.confstr() or CS_PATH is not available
- path = os.defpath
- # bpo-35755: Don't use os.defpath if the PATH environment variable is
- # set to an empty string
-
- # PATH='' doesn't match, whereas PATH=':' looks in the current directory
- if not path:
- return None
-
- paths = path.split(os.pathsep)
- for p in paths:
- f = os.path.join(p, executable)
- if os.path.isfile(f):
- # the file exists, we have a shot at spawn working
- return f
- return None
diff --git a/spaces/Ataturk-Chatbot/HuggingFaceChat/venv/lib/python3.11/site-packages/setuptools/config/setupcfg.py b/spaces/Ataturk-Chatbot/HuggingFaceChat/venv/lib/python3.11/site-packages/setuptools/config/setupcfg.py
deleted file mode 100644
index c2a974de6368c9f4f9b9943c94a457227370f143..0000000000000000000000000000000000000000
--- a/spaces/Ataturk-Chatbot/HuggingFaceChat/venv/lib/python3.11/site-packages/setuptools/config/setupcfg.py
+++ /dev/null
@@ -1,762 +0,0 @@
-"""
-Load setuptools configuration from ``setup.cfg`` files.
-
-**API will be made private in the future**
-"""
-import os
-
-import contextlib
-import functools
-import warnings
-from collections import defaultdict
-from functools import partial
-from functools import wraps
-from typing import (TYPE_CHECKING, Callable, Any, Dict, Generic, Iterable, List,
- Optional, Tuple, TypeVar, Union)
-
-from distutils.errors import DistutilsOptionError, DistutilsFileError
-from setuptools.extern.packaging.requirements import Requirement, InvalidRequirement
-from setuptools.extern.packaging.version import Version, InvalidVersion
-from setuptools.extern.packaging.specifiers import SpecifierSet
-from setuptools._deprecation_warning import SetuptoolsDeprecationWarning
-
-from . import expand
-
-if TYPE_CHECKING:
- from setuptools.dist import Distribution # noqa
- from distutils.dist import DistributionMetadata # noqa
-
-_Path = Union[str, os.PathLike]
-SingleCommandOptions = Dict["str", Tuple["str", Any]]
-"""Dict that associate the name of the options of a particular command to a
-tuple. The first element of the tuple indicates the origin of the option value
-(e.g. the name of the configuration file where it was read from),
-while the second element of the tuple is the option value itself
-"""
-AllCommandOptions = Dict["str", SingleCommandOptions] # cmd name => its options
-Target = TypeVar("Target", bound=Union["Distribution", "DistributionMetadata"])
-
-
-def read_configuration(
- filepath: _Path,
- find_others=False,
- ignore_option_errors=False
-) -> dict:
- """Read given configuration file and returns options from it as a dict.
-
- :param str|unicode filepath: Path to configuration file
- to get options from.
-
- :param bool find_others: Whether to search for other configuration files
- which could be on in various places.
-
- :param bool ignore_option_errors: Whether to silently ignore
- options, values of which could not be resolved (e.g. due to exceptions
- in directives such as file:, attr:, etc.).
- If False exceptions are propagated as expected.
-
- :rtype: dict
- """
- from setuptools.dist import Distribution
-
- dist = Distribution()
- filenames = dist.find_config_files() if find_others else []
- handlers = _apply(dist, filepath, filenames, ignore_option_errors)
- return configuration_to_dict(handlers)
-
-
-def apply_configuration(dist: "Distribution", filepath: _Path) -> "Distribution":
- """Apply the configuration from a ``setup.cfg`` file into an existing
- distribution object.
- """
- _apply(dist, filepath)
- dist._finalize_requires()
- return dist
-
-
-def _apply(
- dist: "Distribution", filepath: _Path,
- other_files: Iterable[_Path] = (),
- ignore_option_errors: bool = False,
-) -> Tuple["ConfigHandler", ...]:
- """Read configuration from ``filepath`` and applies to the ``dist`` object."""
- from setuptools.dist import _Distribution
-
- filepath = os.path.abspath(filepath)
-
- if not os.path.isfile(filepath):
- raise DistutilsFileError('Configuration file %s does not exist.' % filepath)
-
- current_directory = os.getcwd()
- os.chdir(os.path.dirname(filepath))
- filenames = [*other_files, filepath]
-
- try:
- _Distribution.parse_config_files(dist, filenames=filenames)
- handlers = parse_configuration(
- dist, dist.command_options, ignore_option_errors=ignore_option_errors
- )
- dist._finalize_license_files()
- finally:
- os.chdir(current_directory)
-
- return handlers
-
-
-def _get_option(target_obj: Target, key: str):
- """
- Given a target object and option key, get that option from
- the target object, either through a get_{key} method or
- from an attribute directly.
- """
- getter_name = 'get_{key}'.format(**locals())
- by_attribute = functools.partial(getattr, target_obj, key)
- getter = getattr(target_obj, getter_name, by_attribute)
- return getter()
-
-
-def configuration_to_dict(handlers: Tuple["ConfigHandler", ...]) -> dict:
- """Returns configuration data gathered by given handlers as a dict.
-
- :param list[ConfigHandler] handlers: Handlers list,
- usually from parse_configuration()
-
- :rtype: dict
- """
- config_dict: dict = defaultdict(dict)
-
- for handler in handlers:
- for option in handler.set_options:
- value = _get_option(handler.target_obj, option)
- config_dict[handler.section_prefix][option] = value
-
- return config_dict
-
-
-def parse_configuration(
- distribution: "Distribution",
- command_options: AllCommandOptions,
- ignore_option_errors=False
-) -> Tuple["ConfigMetadataHandler", "ConfigOptionsHandler"]:
- """Performs additional parsing of configuration options
- for a distribution.
-
- Returns a list of used option handlers.
-
- :param Distribution distribution:
- :param dict command_options:
- :param bool ignore_option_errors: Whether to silently ignore
- options, values of which could not be resolved (e.g. due to exceptions
- in directives such as file:, attr:, etc.).
- If False exceptions are propagated as expected.
- :rtype: list
- """
- with expand.EnsurePackagesDiscovered(distribution) as ensure_discovered:
- options = ConfigOptionsHandler(
- distribution,
- command_options,
- ignore_option_errors,
- ensure_discovered,
- )
-
- options.parse()
- if not distribution.package_dir:
- distribution.package_dir = options.package_dir # Filled by `find_packages`
-
- meta = ConfigMetadataHandler(
- distribution.metadata,
- command_options,
- ignore_option_errors,
- ensure_discovered,
- distribution.package_dir,
- distribution.src_root,
- )
- meta.parse()
-
- return meta, options
-
-
-def _warn_accidental_env_marker_misconfig(label: str, orig_value: str, parsed: list):
- """Because users sometimes misinterpret this configuration:
-
- [options.extras_require]
- foo = bar;python_version<"4"
-
- It looks like one requirement with an environment marker
- but because there is no newline, it's parsed as two requirements
- with a semicolon as separator.
-
- Therefore, if:
- * input string does not contain a newline AND
- * parsed result contains two requirements AND
- * parsing of the two parts from the result (";")
- leads in a valid Requirement with a valid marker
- a UserWarning is shown to inform the user about the possible problem.
- """
- if "\n" in orig_value or len(parsed) != 2:
- return
-
- with contextlib.suppress(InvalidRequirement):
- original_requirements_str = ";".join(parsed)
- req = Requirement(original_requirements_str)
- if req.marker is not None:
- msg = (
- f"One of the parsed requirements in `{label}` "
- f"looks like a valid environment marker: '{parsed[1]}'\n"
- "Make sure that the config is correct and check "
- "https://setuptools.pypa.io/en/latest/userguide/declarative_config.html#opt-2" # noqa: E501
- )
- warnings.warn(msg, UserWarning)
-
-
-class ConfigHandler(Generic[Target]):
- """Handles metadata supplied in configuration files."""
-
- section_prefix: str
- """Prefix for config sections handled by this handler.
- Must be provided by class heirs.
-
- """
-
- aliases: Dict[str, str] = {}
- """Options aliases.
- For compatibility with various packages. E.g.: d2to1 and pbr.
- Note: `-` in keys is replaced with `_` by config parser.
-
- """
-
- def __init__(
- self,
- target_obj: Target,
- options: AllCommandOptions,
- ignore_option_errors,
- ensure_discovered: expand.EnsurePackagesDiscovered,
- ):
- sections: AllCommandOptions = {}
-
- section_prefix = self.section_prefix
- for section_name, section_options in options.items():
- if not section_name.startswith(section_prefix):
- continue
-
- section_name = section_name.replace(section_prefix, '').strip('.')
- sections[section_name] = section_options
-
- self.ignore_option_errors = ignore_option_errors
- self.target_obj = target_obj
- self.sections = sections
- self.set_options: List[str] = []
- self.ensure_discovered = ensure_discovered
-
- @property
- def parsers(self):
- """Metadata item name to parser function mapping."""
- raise NotImplementedError(
- '%s must provide .parsers property' % self.__class__.__name__
- )
-
- def __setitem__(self, option_name, value):
- unknown = tuple()
- target_obj = self.target_obj
-
- # Translate alias into real name.
- option_name = self.aliases.get(option_name, option_name)
-
- current_value = getattr(target_obj, option_name, unknown)
-
- if current_value is unknown:
- raise KeyError(option_name)
-
- if current_value:
- # Already inhabited. Skipping.
- return
-
- skip_option = False
- parser = self.parsers.get(option_name)
- if parser:
- try:
- value = parser(value)
-
- except Exception:
- skip_option = True
- if not self.ignore_option_errors:
- raise
-
- if skip_option:
- return
-
- setter = getattr(target_obj, 'set_%s' % option_name, None)
- if setter is None:
- setattr(target_obj, option_name, value)
- else:
- setter(value)
-
- self.set_options.append(option_name)
-
- @classmethod
- def _parse_list(cls, value, separator=','):
- """Represents value as a list.
-
- Value is split either by separator (defaults to comma) or by lines.
-
- :param value:
- :param separator: List items separator character.
- :rtype: list
- """
- if isinstance(value, list): # _get_parser_compound case
- return value
-
- if '\n' in value:
- value = value.splitlines()
- else:
- value = value.split(separator)
-
- return [chunk.strip() for chunk in value if chunk.strip()]
-
- @classmethod
- def _parse_dict(cls, value):
- """Represents value as a dict.
-
- :param value:
- :rtype: dict
- """
- separator = '='
- result = {}
- for line in cls._parse_list(value):
- key, sep, val = line.partition(separator)
- if sep != separator:
- raise DistutilsOptionError(
- 'Unable to parse option value to dict: %s' % value
- )
- result[key.strip()] = val.strip()
-
- return result
-
- @classmethod
- def _parse_bool(cls, value):
- """Represents value as boolean.
-
- :param value:
- :rtype: bool
- """
- value = value.lower()
- return value in ('1', 'true', 'yes')
-
- @classmethod
- def _exclude_files_parser(cls, key):
- """Returns a parser function to make sure field inputs
- are not files.
-
- Parses a value after getting the key so error messages are
- more informative.
-
- :param key:
- :rtype: callable
- """
-
- def parser(value):
- exclude_directive = 'file:'
- if value.startswith(exclude_directive):
- raise ValueError(
- 'Only strings are accepted for the {0} field, '
- 'files are not accepted'.format(key)
- )
- return value
-
- return parser
-
- @classmethod
- def _parse_file(cls, value, root_dir: _Path):
- """Represents value as a string, allowing including text
- from nearest files using `file:` directive.
-
- Directive is sandboxed and won't reach anything outside
- directory with setup.py.
-
- Examples:
- file: README.rst, CHANGELOG.md, src/file.txt
-
- :param str value:
- :rtype: str
- """
- include_directive = 'file:'
-
- if not isinstance(value, str):
- return value
-
- if not value.startswith(include_directive):
- return value
-
- spec = value[len(include_directive) :]
- filepaths = (path.strip() for path in spec.split(','))
- return expand.read_files(filepaths, root_dir)
-
- def _parse_attr(self, value, package_dir, root_dir: _Path):
- """Represents value as a module attribute.
-
- Examples:
- attr: package.attr
- attr: package.module.attr
-
- :param str value:
- :rtype: str
- """
- attr_directive = 'attr:'
- if not value.startswith(attr_directive):
- return value
-
- attr_desc = value.replace(attr_directive, '')
-
- # Make sure package_dir is populated correctly, so `attr:` directives can work
- package_dir.update(self.ensure_discovered.package_dir)
- return expand.read_attr(attr_desc, package_dir, root_dir)
-
- @classmethod
- def _get_parser_compound(cls, *parse_methods):
- """Returns parser function to represents value as a list.
-
- Parses a value applying given methods one after another.
-
- :param parse_methods:
- :rtype: callable
- """
-
- def parse(value):
- parsed = value
-
- for method in parse_methods:
- parsed = method(parsed)
-
- return parsed
-
- return parse
-
- @classmethod
- def _parse_section_to_dict_with_key(cls, section_options, values_parser):
- """Parses section options into a dictionary.
-
- Applies a given parser to each option in a section.
-
- :param dict section_options:
- :param callable values_parser: function with 2 args corresponding to key, value
- :rtype: dict
- """
- value = {}
- for key, (_, val) in section_options.items():
- value[key] = values_parser(key, val)
- return value
-
- @classmethod
- def _parse_section_to_dict(cls, section_options, values_parser=None):
- """Parses section options into a dictionary.
-
- Optionally applies a given parser to each value.
-
- :param dict section_options:
- :param callable values_parser: function with 1 arg corresponding to option value
- :rtype: dict
- """
- parser = (lambda _, v: values_parser(v)) if values_parser else (lambda _, v: v)
- return cls._parse_section_to_dict_with_key(section_options, parser)
-
- def parse_section(self, section_options):
- """Parses configuration file section.
-
- :param dict section_options:
- """
- for (name, (_, value)) in section_options.items():
- with contextlib.suppress(KeyError):
- # Keep silent for a new option may appear anytime.
- self[name] = value
-
- def parse(self):
- """Parses configuration file items from one
- or more related sections.
-
- """
- for section_name, section_options in self.sections.items():
-
- method_postfix = ''
- if section_name: # [section.option] variant
- method_postfix = '_%s' % section_name
-
- section_parser_method: Optional[Callable] = getattr(
- self,
- # Dots in section names are translated into dunderscores.
- ('parse_section%s' % method_postfix).replace('.', '__'),
- None,
- )
-
- if section_parser_method is None:
- raise DistutilsOptionError(
- 'Unsupported distribution option section: [%s.%s]'
- % (self.section_prefix, section_name)
- )
-
- section_parser_method(section_options)
-
- def _deprecated_config_handler(self, func, msg, warning_class):
- """this function will wrap around parameters that are deprecated
-
- :param msg: deprecation message
- :param warning_class: class of warning exception to be raised
- :param func: function to be wrapped around
- """
-
- @wraps(func)
- def config_handler(*args, **kwargs):
- warnings.warn(msg, warning_class)
- return func(*args, **kwargs)
-
- return config_handler
-
-
-class ConfigMetadataHandler(ConfigHandler["DistributionMetadata"]):
-
- section_prefix = 'metadata'
-
- aliases = {
- 'home_page': 'url',
- 'summary': 'description',
- 'classifier': 'classifiers',
- 'platform': 'platforms',
- }
-
- strict_mode = False
- """We need to keep it loose, to be partially compatible with
- `pbr` and `d2to1` packages which also uses `metadata` section.
-
- """
-
- def __init__(
- self,
- target_obj: "DistributionMetadata",
- options: AllCommandOptions,
- ignore_option_errors: bool,
- ensure_discovered: expand.EnsurePackagesDiscovered,
- package_dir: Optional[dict] = None,
- root_dir: _Path = os.curdir
- ):
- super().__init__(target_obj, options, ignore_option_errors, ensure_discovered)
- self.package_dir = package_dir
- self.root_dir = root_dir
-
- @property
- def parsers(self):
- """Metadata item name to parser function mapping."""
- parse_list = self._parse_list
- parse_file = partial(self._parse_file, root_dir=self.root_dir)
- parse_dict = self._parse_dict
- exclude_files_parser = self._exclude_files_parser
-
- return {
- 'platforms': parse_list,
- 'keywords': parse_list,
- 'provides': parse_list,
- 'requires': self._deprecated_config_handler(
- parse_list,
- "The requires parameter is deprecated, please use "
- "install_requires for runtime dependencies.",
- SetuptoolsDeprecationWarning,
- ),
- 'obsoletes': parse_list,
- 'classifiers': self._get_parser_compound(parse_file, parse_list),
- 'license': exclude_files_parser('license'),
- 'license_file': self._deprecated_config_handler(
- exclude_files_parser('license_file'),
- "The license_file parameter is deprecated, "
- "use license_files instead.",
- SetuptoolsDeprecationWarning,
- ),
- 'license_files': parse_list,
- 'description': parse_file,
- 'long_description': parse_file,
- 'version': self._parse_version,
- 'project_urls': parse_dict,
- }
-
- def _parse_version(self, value):
- """Parses `version` option value.
-
- :param value:
- :rtype: str
-
- """
- version = self._parse_file(value, self.root_dir)
-
- if version != value:
- version = version.strip()
- # Be strict about versions loaded from file because it's easy to
- # accidentally include newlines and other unintended content
- try:
- Version(version)
- except InvalidVersion:
- tmpl = (
- 'Version loaded from {value} does not '
- 'comply with PEP 440: {version}'
- )
- raise DistutilsOptionError(tmpl.format(**locals()))
-
- return version
-
- return expand.version(self._parse_attr(value, self.package_dir, self.root_dir))
-
-
-class ConfigOptionsHandler(ConfigHandler["Distribution"]):
-
- section_prefix = 'options'
-
- def __init__(
- self,
- target_obj: "Distribution",
- options: AllCommandOptions,
- ignore_option_errors: bool,
- ensure_discovered: expand.EnsurePackagesDiscovered,
- ):
- super().__init__(target_obj, options, ignore_option_errors, ensure_discovered)
- self.root_dir = target_obj.src_root
- self.package_dir: Dict[str, str] = {} # To be filled by `find_packages`
-
- @classmethod
- def _parse_list_semicolon(cls, value):
- return cls._parse_list(value, separator=';')
-
- def _parse_file_in_root(self, value):
- return self._parse_file(value, root_dir=self.root_dir)
-
- def _parse_requirements_list(self, label: str, value: str):
- # Parse a requirements list, either by reading in a `file:`, or a list.
- parsed = self._parse_list_semicolon(self._parse_file_in_root(value))
- _warn_accidental_env_marker_misconfig(label, value, parsed)
- # Filter it to only include lines that are not comments. `parse_list`
- # will have stripped each line and filtered out empties.
- return [line for line in parsed if not line.startswith("#")]
-
- @property
- def parsers(self):
- """Metadata item name to parser function mapping."""
- parse_list = self._parse_list
- parse_bool = self._parse_bool
- parse_dict = self._parse_dict
- parse_cmdclass = self._parse_cmdclass
-
- return {
- 'zip_safe': parse_bool,
- 'include_package_data': parse_bool,
- 'package_dir': parse_dict,
- 'scripts': parse_list,
- 'eager_resources': parse_list,
- 'dependency_links': parse_list,
- 'namespace_packages': self._deprecated_config_handler(
- parse_list,
- "The namespace_packages parameter is deprecated, "
- "consider using implicit namespaces instead (PEP 420).",
- SetuptoolsDeprecationWarning,
- ),
- 'install_requires': partial(
- self._parse_requirements_list, "install_requires"
- ),
- 'setup_requires': self._parse_list_semicolon,
- 'tests_require': self._parse_list_semicolon,
- 'packages': self._parse_packages,
- 'entry_points': self._parse_file_in_root,
- 'py_modules': parse_list,
- 'python_requires': SpecifierSet,
- 'cmdclass': parse_cmdclass,
- }
-
- def _parse_cmdclass(self, value):
- package_dir = self.ensure_discovered.package_dir
- return expand.cmdclass(self._parse_dict(value), package_dir, self.root_dir)
-
- def _parse_packages(self, value):
- """Parses `packages` option value.
-
- :param value:
- :rtype: list
- """
- find_directives = ['find:', 'find_namespace:']
- trimmed_value = value.strip()
-
- if trimmed_value not in find_directives:
- return self._parse_list(value)
-
- # Read function arguments from a dedicated section.
- find_kwargs = self.parse_section_packages__find(
- self.sections.get('packages.find', {})
- )
-
- find_kwargs.update(
- namespaces=(trimmed_value == find_directives[1]),
- root_dir=self.root_dir,
- fill_package_dir=self.package_dir,
- )
-
- return expand.find_packages(**find_kwargs)
-
- def parse_section_packages__find(self, section_options):
- """Parses `packages.find` configuration file section.
-
- To be used in conjunction with _parse_packages().
-
- :param dict section_options:
- """
- section_data = self._parse_section_to_dict(section_options, self._parse_list)
-
- valid_keys = ['where', 'include', 'exclude']
-
- find_kwargs = dict(
- [(k, v) for k, v in section_data.items() if k in valid_keys and v]
- )
-
- where = find_kwargs.get('where')
- if where is not None:
- find_kwargs['where'] = where[0] # cast list to single val
-
- return find_kwargs
-
- def parse_section_entry_points(self, section_options):
- """Parses `entry_points` configuration file section.
-
- :param dict section_options:
- """
- parsed = self._parse_section_to_dict(section_options, self._parse_list)
- self['entry_points'] = parsed
-
- def _parse_package_data(self, section_options):
- package_data = self._parse_section_to_dict(section_options, self._parse_list)
- return expand.canonic_package_data(package_data)
-
- def parse_section_package_data(self, section_options):
- """Parses `package_data` configuration file section.
-
- :param dict section_options:
- """
- self['package_data'] = self._parse_package_data(section_options)
-
- def parse_section_exclude_package_data(self, section_options):
- """Parses `exclude_package_data` configuration file section.
-
- :param dict section_options:
- """
- self['exclude_package_data'] = self._parse_package_data(section_options)
-
- def parse_section_extras_require(self, section_options):
- """Parses `extras_require` configuration file section.
-
- :param dict section_options:
- """
- parsed = self._parse_section_to_dict_with_key(
- section_options,
- lambda k, v: self._parse_requirements_list(f"extras_require[{k}]", v)
- )
-
- self['extras_require'] = parsed
-
- def parse_section_data_files(self, section_options):
- """Parses `data_files` configuration file section.
-
- :param dict section_options:
- """
- parsed = self._parse_section_to_dict(section_options, self._parse_list)
- self['data_files'] = expand.canonic_data_files(parsed, self.root_dir)
diff --git a/spaces/Awesimo/jojogan/e4e/metrics/LEC.py b/spaces/Awesimo/jojogan/e4e/metrics/LEC.py
deleted file mode 100644
index 3eef2d2f00a4d757a56b6e845a8fde16aab306ab..0000000000000000000000000000000000000000
--- a/spaces/Awesimo/jojogan/e4e/metrics/LEC.py
+++ /dev/null
@@ -1,134 +0,0 @@
-import sys
-import argparse
-import torch
-import numpy as np
-from torch.utils.data import DataLoader
-
-sys.path.append(".")
-sys.path.append("..")
-
-from configs import data_configs
-from datasets.images_dataset import ImagesDataset
-from utils.model_utils import setup_model
-
-
-class LEC:
- def __init__(self, net, is_cars=False):
- """
- Latent Editing Consistency metric as proposed in the main paper.
- :param net: e4e model loaded over the pSp framework.
- :param is_cars: An indication as to whether or not to crop the middle of the StyleGAN's output images.
- """
- self.net = net
- self.is_cars = is_cars
-
- def _encode(self, images):
- """
- Encodes the given images into StyleGAN's latent space.
- :param images: Tensor of shape NxCxHxW representing the images to be encoded.
- :return: Tensor of shape NxKx512 representing the latent space embeddings of the given image (in W(K, *) space).
- """
- codes = self.net.encoder(images)
- assert codes.ndim == 3, f"Invalid latent codes shape, should be NxKx512 but is {codes.shape}"
- # normalize with respect to the center of an average face
- if self.net.opts.start_from_latent_avg:
- codes = codes + self.net.latent_avg.repeat(codes.shape[0], 1, 1)
- return codes
-
- def _generate(self, codes):
- """
- Generate the StyleGAN2 images of the given codes
- :param codes: Tensor of shape NxKx512 representing the StyleGAN's latent codes (in W(K, *) space).
- :return: Tensor of shape NxCxHxW representing the generated images.
- """
- images, _ = self.net.decoder([codes], input_is_latent=True, randomize_noise=False, return_latents=True)
- images = self.net.face_pool(images)
- if self.is_cars:
- images = images[:, :, 32:224, :]
- return images
-
- @staticmethod
- def _filter_outliers(arr):
- arr = np.array(arr)
-
- lo = np.percentile(arr, 1, interpolation="lower")
- hi = np.percentile(arr, 99, interpolation="higher")
- return np.extract(
- np.logical_and(lo <= arr, arr <= hi), arr
- )
-
- def calculate_metric(self, data_loader, edit_function, inverse_edit_function):
- """
- Calculate the LEC metric score.
- :param data_loader: An iterable that returns a tuple of (images, _), similar to the training data loader.
- :param edit_function: A function that receives latent codes and performs a semantically meaningful edit in the
- latent space.
- :param inverse_edit_function: A function that receives latent codes and performs the inverse edit of the
- `edit_function` parameter.
- :return: The LEC metric score.
- """
- distances = []
- with torch.no_grad():
- for batch in data_loader:
- x, _ = batch
- inputs = x.to(device).float()
-
- codes = self._encode(inputs)
- edited_codes = edit_function(codes)
- edited_image = self._generate(edited_codes)
- edited_image_inversion_codes = self._encode(edited_image)
- inverse_edit_codes = inverse_edit_function(edited_image_inversion_codes)
-
- dist = (codes - inverse_edit_codes).norm(2, dim=(1, 2)).mean()
- distances.append(dist.to("cpu").numpy())
-
- distances = self._filter_outliers(distances)
- return distances.mean()
-
-
-if __name__ == "__main__":
- device = "cuda"
-
- parser = argparse.ArgumentParser(description="LEC metric calculator")
-
- parser.add_argument("--batch", type=int, default=8, help="batch size for the models")
- parser.add_argument("--images_dir", type=str, default=None,
- help="Path to the images directory on which we calculate the LEC score")
- parser.add_argument("ckpt", metavar="CHECKPOINT", help="path to the model checkpoints")
-
- args = parser.parse_args()
- print(args)
-
- net, opts = setup_model(args.ckpt, device)
- dataset_args = data_configs.DATASETS[opts.dataset_type]
- transforms_dict = dataset_args['transforms'](opts).get_transforms()
-
- images_directory = dataset_args['test_source_root'] if args.images_dir is None else args.images_dir
- test_dataset = ImagesDataset(source_root=images_directory,
- target_root=images_directory,
- source_transform=transforms_dict['transform_source'],
- target_transform=transforms_dict['transform_test'],
- opts=opts)
-
- data_loader = DataLoader(test_dataset,
- batch_size=args.batch,
- shuffle=False,
- num_workers=2,
- drop_last=True)
-
- print(f'dataset length: {len(test_dataset)}')
-
- # In the following example, we are using an InterfaceGAN based editing to calculate the LEC metric.
- # Change the provided example according to your domain and needs.
- direction = torch.load('../editings/interfacegan_directions/age.pt').to(device)
-
- def edit_func_example(codes):
- return codes + 3 * direction
-
-
- def inverse_edit_func_example(codes):
- return codes - 3 * direction
-
- lec = LEC(net, is_cars='car' in opts.dataset_type)
- result = lec.calculate_metric(data_loader, edit_func_example, inverse_edit_func_example)
- print(f"LEC: {result}")
diff --git a/spaces/Awiny/Image2Paragraph/models/grit_src/third_party/CenterNet2/tools/README.md b/spaces/Awiny/Image2Paragraph/models/grit_src/third_party/CenterNet2/tools/README.md
deleted file mode 100644
index 0b40d5319c0838fdaa22bc6a10ef0d88bc6578ed..0000000000000000000000000000000000000000
--- a/spaces/Awiny/Image2Paragraph/models/grit_src/third_party/CenterNet2/tools/README.md
+++ /dev/null
@@ -1,49 +0,0 @@
-
-This directory contains a few example scripts that demonstrate features of detectron2.
-
-
-* `train_net.py`
-
-An example training script that's made to train builtin models of detectron2.
-
-For usage, see [GETTING_STARTED.md](../GETTING_STARTED.md).
-
-* `plain_train_net.py`
-
-Similar to `train_net.py`, but implements a training loop instead of using `Trainer`.
-This script includes fewer features but it may be more friendly to hackers.
-
-* `benchmark.py`
-
-Benchmark the training speed, inference speed or data loading speed of a given config.
-
-Usage:
-```
-python benchmark.py --config-file config.yaml --task train/eval/data [optional DDP flags]
-```
-
-* `analyze_model.py`
-
-Analyze FLOPs, parameters, activations of a detectron2 model. See its `--help` for usage.
-
-* `visualize_json_results.py`
-
-Visualize the json instance detection/segmentation results dumped by `COCOEvalutor` or `LVISEvaluator`
-
-Usage:
-```
-python visualize_json_results.py --input x.json --output dir/ --dataset coco_2017_val
-```
-If not using a builtin dataset, you'll need your own script or modify this script.
-
-* `visualize_data.py`
-
-Visualize ground truth raw annotations or training data (after preprocessing/augmentations).
-
-Usage:
-```
-python visualize_data.py --config-file config.yaml --source annotation/dataloader --output-dir dir/ [--show]
-```
-
-NOTE: the script does not stop by itself when using `--source dataloader` because a training
-dataloader is usually infinite.
diff --git a/spaces/Bala2-03-2003/BRAHMAMAI/README.md b/spaces/Bala2-03-2003/BRAHMAMAI/README.md
deleted file mode 100644
index 80551b413d706056235d844ec9f3c664cfe7e81d..0000000000000000000000000000000000000000
--- a/spaces/Bala2-03-2003/BRAHMAMAI/README.md
+++ /dev/null
@@ -1,12 +0,0 @@
----
-title: BRAHMAMAI
-emoji: ⚡
-colorFrom: red
-colorTo: purple
-sdk: gradio
-sdk_version: 3.40.1
-app_file: app.py
-pinned: false
----
-
-Check out the configuration reference at https://huggingface.co/docs/hub/spaces-config-reference
diff --git a/spaces/Benson/text-generation/Examples/2023 Apk Fuego Libre.md b/spaces/Benson/text-generation/Examples/2023 Apk Fuego Libre.md
deleted file mode 100644
index 9a6f7d0748f94def3eaa5ef27c261f5ffbcf06f1..0000000000000000000000000000000000000000
--- a/spaces/Benson/text-generation/Examples/2023 Apk Fuego Libre.md
+++ /dev/null
@@ -1,68 +0,0 @@
-
-
2023 Fuego libre APK: Todo lo que necesita saber
-
Free Fire es un juego de disparos de supervivencia de fama mundial disponible en dispositivos móviles. Es uno de los juegos más descargados y jugados en Google Play Store y App Store, con más de 1 mil millones de descargas y millones de jugadores activos. En este artículo, le diremos todo lo que necesita saber sobre Free Fire y cómo descargar e instalar la última versión de Free Fire APK en su dispositivo Android.
-
¿Qué es el fuego libre y por qué es popular?
-
Free Fire es un juego de battle royale que te enfrenta a otros 49 jugadores en una isla remota. El objetivo es ser el último en pie encontrando armas, objetos y vehículos, y eliminando a tus enemigos. El juego tiene una variedad de emocionantes modos de juego, personajes, pieles, mascotas y eventos que mantienen el juego fresco y divertido.
¿Cuáles son las características de Free Fire y cómo jugarlo?
-
Free Fire tiene muchas características que lo hacen destacar de otros juegos battle royale. Aquí están algunas de ellas:
-
Tirador de supervivencia en su forma original
-
Empiezas el juego en paracaídas desde un avión en un mapa grande. Puedes elegir tu punto de aterrizaje y explorar el mapa como desees. Tienes que permanecer dentro de la zona segura que se encoge con el tiempo, o recibirás daños por el gas tóxico. También tienes que tener cuidado con los lanzamientos de aire que contienen armas y objetos poderosos, pero también atraen a otros jugadores. El juego tiene un sistema de física realista que afecta la trayectoria de la bala, el retroceso y el manejo del vehículo.
Puedes jugar Free Fire solo o con hasta otros tres amigos en un equipo. Puedes comunicarte con tus compañeros de equipo utilizando la función de chat de voz o mensajes de texto en el juego. También puedes marcar ubicaciones, enemigos, objetos y vehículos en el mapa para que tus compañeros los vean. Trabajar junto con tu escuadrón puede darte una ventaja sobre tus enemigos.
-
Escuadrón de choque
-
Clash Squad es un modo de juego 4v4 de ritmo rápido que está abierto 24/7. En este modo, tienes que manejar tu economía, comprar armas y derrotar al escuadrón enemigo en una serie de rondas. El primer equipo en ganar cuatro rondas gana el partido. Clash Squad es una gran manera de poner a prueba sus habilidades y el trabajo en equipo en un entorno diferente.
-
Gráficos realistas y suaves
-
Free Fire tiene gráficos realistas y suaves que prometen la experiencia de supervivencia óptima en dispositivos móviles. El juego tiene texturas de alta calidad, efectos de iluminación, sombras, reflejos y animaciones que crean una atmósfera inmersiva. El juego también funciona sin problemas en la mayoría de los dispositivos sin retraso o estrellarse.
-
¿Qué es Free Fire APK y cómo descargarlo e instalarlo?
-
Free Fire APK es un archivo de paquete de aplicación para Android que contiene los archivos de instalación de Free Fire. Puede descargar e instalar Free Fire APK en su dispositivo Android si desea disfrutar de la última versión del juego con nuevas características, correcciones de errores y mejoras. Aquí están los pasos para descargar e instalar Free Fire APK:
-
¿Qué es un archivo APK y por qué lo necesita?
-
Un archivo APK es un archivo comprimido que contiene el código, los recursos y los certificados de una aplicación Android. Puede instalar un archivo APK en su dispositivo Android para ejecutar la aplicación sin usar Google Play Store. Es posible que necesite descargar e instalar un archivo APK si:
-
-
-
Desea acceder a la última versión de una aplicación antes de que esté disponible en Play Store.
-
-
Desea instalar una aplicación que se ha eliminado de la Play Store por alguna razón.
-
Desea instalar una versión modificada o hackeada de una aplicación que ofrece características o beneficios adicionales.
-
-
Sin embargo, debe tener cuidado al descargar e instalar archivos APK de fuentes desconocidas, ya que pueden contener malware o virus que pueden dañar su dispositivo o robar sus datos. Solo debes descargar archivos APK de fuentes confiables y oficiales, como el sitio web del desarrollador o una tienda de aplicaciones de terceros de buena reputación.
-
Cómo descargar gratis fuego APK de fuentes oficiales
-
La mejor manera de descargar Free Fire APK es desde el sitio web oficial de Garena, el desarrollador y editor de Free Fire. Puede visitar el sitio web en https://ff.garena.com/ y hacer clic en el botón "Descargar". Usted será redirigido a una página donde se puede elegir entre descargar Free Fire APK o Free Fire OBB. El archivo OBB es un archivo de datos que contiene contenido adicional para el juego, como gráficos, sonidos y mapas. Necesitas ambos archivos para ejecutar el juego correctamente.
-
También puede descargar Free Fire APK de otras fuentes oficiales, tales como:
-
-
La página oficial de Facebook de Free Fire en https://www.facebook.com/freefireEN/
-
El canal oficial de YouTube de Free Fire en https://www.youtube.com/channel/UCkngbNvgHvc67J4VCWj75Mw
-
La cuenta oficial de Instagram de Free Fire en https://www.instagram.com/freefireth_official/
-
-
Estas fuentes a menudo publican enlaces para descargar la última versión de Free Fire APK cuando hay una nueva actualización o evento. Puedes seguirlos para mantenerte actualizado y recibir notificaciones cuando haya una nueva versión.
-
Cómo instalar Free Fire APK en su dispositivo Android
-
Después de descargar Free Fire APK y archivos OBB, es necesario instalarlos en su dispositivo Android. Estos son los pasos para hacerlo:
-
-
-
Busque el archivo APK Free Fire descargado en el almacenamiento de su dispositivo y toque en él para iniciar el proceso de instalación. Siga las instrucciones en la pantalla y conceda los permisos necesarios.
-
Aún no abra el juego. Localice el archivo OBB de Free Fire descargado en el almacenamiento de su dispositivo y extráigalo usando una aplicación de administrador de archivos. Obtendrá una carpeta llamada "com.dts.freefireth". Copie esta carpeta y péguela en el directorio "Android/obb" en el almacenamiento de su dispositivo.
-
Ahora puedes abrir el juego y disfrutar jugando Free Fire con la última versión.
-
-
Conclusión: Resumir los puntos principales y dar algunos consejos y trucos para jugar Free Fire
-
En conclusión, Free Fire es un emocionante juego de disparos de supervivencia que ofrece una variedad de modos de juego, personajes, pieles, mascotas y eventos. Puede descargar e instalar Free Fire APK en su dispositivo Android para obtener acceso a la última versión del juego con nuevas características, correcciones de errores y mejoras. Sin embargo, debe tener cuidado al descargar e instalar archivos APK de fuentes desconocidas, ya que pueden contener malware o virus. Solo debes descargar archivos APK de fuentes confiables y oficiales, como el sitio web de Garena o las cuentas de redes sociales.
-
Aquí hay algunos consejos y trucos para jugar Free Fire:
-
-
Elige tu personaje sabiamente. Cada personaje tiene una habilidad única que puede darte una ventaja en diferentes situaciones. Por ejemplo, Kelly ha aumentado la velocidad de carrera, Alok puede crear un aura curativa a su alrededor, y Chrono puede crear un campo de fuerza que bloquea el daño.
-
Usa los vehículos sabiamente. Los vehículos pueden ayudarte a moverte más rápido o atropellar a tus enemigos, pero también te hacen más visible y vulnerable al fuego enemigo. Solo debe usar vehículos cuando sea necesario y evitar conducir en áreas abiertas o cerca de edificios.
-
-
Usa el minimapa sabiamente. El minimapa puede mostrar información importante como la ubicación de la zona segura, lanzamientos de aire, enemigos, compañeros de equipo y vehículos. Siempre debe revisar el minimapa para mantenerse al tanto de su entorno y planificar su estrategia en consecuencia.
-
Usa el sistema de ping sabiamente. El sistema de ping puede ayudarte a comunicarte con tus compañeros de equipo sin usar chat de voz o mensajes de texto. Puedes hacer ping a ubicaciones, enemigos, objetos y vehículos en el mapa para que tus compañeros los vean. También puede utilizar mensajes rápidos para transmitir sus intenciones o solicitudes.
-
-
Preguntas frecuentes: Responder a algunas preguntas comunes sobre el fuego libre y el fuego libre APK
-
-
Pregunta
Respuesta
-
Free Fire es gratis para jugar?
Sí, Free Fire es gratis para jugar en dispositivos móviles. Sin embargo, puedes comprar monedas del juego llamadas diamantes para comprar artículos premium como personajes, pieles, mascotas y pases.
-
Free Fire es compatible con mi dispositivo?
Free Fire es compatible con la mayoría de dispositivos Android que tienen al menos 2 GB de RAM y Android 4.0.3 o superior. Sin embargo, algunos dispositivos pueden experimentar problemas de rendimiento o fallos debido a limitaciones de hardware.
-
¿Es seguro descargar e instalar Free Fire?
Sí, Free Fire es seguro para descargar e instalar desde la Google Play Store o fuentes oficiales como el sitio web de Garena o las cuentas de redes sociales. Sin embargo, debe tener cuidado al descargar e instalar archivos APK de fuentes desconocidas, ya que pueden contener malware o virus.
-
¿Cómo actualizo Free Fire?
Puede actualizar Free Fire desde Google Play Store o descargando e instalando la última versión de Free Fire APK de fuentes oficiales. Siempre debe actualizar Free Fire para disfrutar de las nuevas características, correcciones de errores y mejoras.
-
- 64aa2da5cf
-
-
\ No newline at end of file
diff --git a/spaces/Benson/text-generation/Examples/Creality Cr Studio Descargar.md b/spaces/Benson/text-generation/Examples/Creality Cr Studio Descargar.md
deleted file mode 100644
index c5ced454699509af5cf0fb7ad85be7f9f84f7178..0000000000000000000000000000000000000000
--- a/spaces/Benson/text-generation/Examples/Creality Cr Studio Descargar.md
+++ /dev/null
@@ -1,71 +0,0 @@
-
-
Creality CR Studio Descargar: Una guía para el software del escáner 3D
-
Si está buscando una manera de convertir objetos del mundo real en modelos 3D que pueda imprimir, editar o compartir, puede estar interesado en Creality CR Studio. Este es un software que funciona con escáneres 3D Creality, como CR Scan 01 y CR Scan Lizard, para crear escaneos de alta calidad de varios objetos. En este artículo, le mostraremos cómo descargar e instalar Creality CR Studio, cómo usarlo para escanear y editar sus modelos, cuáles son sus características y beneficios, y cómo solucionar algunos problemas comunes. Al final de este artículo, usted tendrá una mejor comprensión de lo que Creality CR Studio puede hacer por usted y si vale la pena probar.
-
Cómo descargar e instalar Creality CR Studio
-
El primer paso para usar Creality CR Studio es descargarlo e instalarlo en su computadora. Estos son los pasos a seguir:
Puedes descargar Creality CR Studio desde el sitio web oficial de Creality 3D. Hay diferentes versiones del software para los sistemas operativos Windows y Mac, así como diferentes idiomas. Usted puede elegir el que se adapte a sus necesidades y preferencias. La última versión del software es CR Studio 2.5.7 para Windows y CR Studio 2.2.3 para Mac. El tamaño del archivo es de aproximadamente 200 MB.
-
Instalación del software en Windows o Mac
-
Después de descargar el software, necesita instalarlo en su computadora. El proceso de instalación es simple y directo. Solo tienes que seguir las instrucciones en la pantalla y aceptar los términos y condiciones. La instalación puede tardar unos minutos dependiendo de la velocidad del ordenador.
-
Conexión del escáner 3D al software
-
-
Cómo usar Creality CR Studio
-
Ahora que ha descargado e instalado Creality CR Studio y conectado su escáner 3D, está listo para comenzar a escanear objetos. Estos son los pasos a seguir:
-
Elegir el modo de escaneo: portátil o tocadiscos
-
Creality CR Studio ofrece dos modos de escaneo: portátil y giradiscos. - El modo portátil le permite escanear objetos moviendo el escáner alrededor de ellos. Este modo es adecuado para escanear objetos grandes, complejos o irregulares que no pueden caber en un tocadiscos. También puede escanear objetos en diferentes entornos, como en exteriores o interiores. - El modo de plato giratorio le permite escanear objetos colocándolos en una plataforma giratoria. Este modo es adecuado para escanear objetos pequeños, simples o simétricos que pueden caber en un tocadiscos. También puede escanear objetos de forma más rápida y precisa con este modo.
-
Puede elegir el modo de escaneo haciendo clic en el icono en la esquina superior izquierda de la interfaz de software. También puede cambiar entre los modos durante el escaneo si es necesario.
-
Ajustar la configuración de escaneo: resolución, brillo, etc.
-
Antes de comenzar a escanear, debe ajustar algunos ajustes de escaneo para optimizar la calidad y la velocidad de su escaneo. Puede acceder a estos ajustes haciendo clic en el icono de engranaje en la esquina superior derecha de la interfaz de software. Estos son algunos de los ajustes que puede ajustar:
-
-
-
Escaneando el objeto y viendo la vista previa
-
Después de haber ajustado la configuración de escaneo, puede comenzar a escanear su objeto haciendo clic en el botón de inicio en la esquina inferior derecha de la interfaz de software. Dependiendo del modo de escaneo que haya elegido, debe mover el escáner alrededor del objeto o colocar el objeto en el tocadiscos y dejar que gire.
-
A medida que escanea su objeto, verá una vista previa de su modelo en la pantalla. Puede pausar o reanudar el proceso de escaneo en cualquier momento haciendo clic en el botón de pausa o reanudación. También puede deshacer o rehacer cualquier acción haciendo clic en el botón deshacer o rehacer.
-
Puede dejar de escanear cuando haya cubierto todos los ángulos y detalles de su objeto o cuando esté satisfecho con la vista previa de su modelo. A continuación, puede hacer clic en el botón de parada para terminar el escaneo.
-
Edición del modelo escaneado: alineación, desnaturalización, optimización, etc.
-
Después de haber terminado el escaneo, puede editar su modelo escaneado para mejorar su calidad y apariencia. Puede acceder a varias herramientas de edición haciendo clic en los iconos en el lado izquierdo de la interfaz de software. Estas son algunas de las herramientas de edición que puedes usar:
-
-
Exportar y guardar el modelo escaneado como archivo STL u OBJ
-
Después de haber editado su modelo escaneado, puede exportarlo y guardarlo como archivo STL u OBJ. Estos son los formatos de archivo más comunes para los modelos 3D que se pueden utilizar en varios software de impresión o edición 3D. Puede elegir el formato de archivo haciendo clic en el botón de exportación en la esquina inferior izquierda de la interfaz de software. También puede elegir el nombre del archivo y la ubicación navegando por las carpetas de su computadora. A continuación, puede hacer clic en el botón guardar para exportar y guardar su modelo escaneado.
-
¿Cuáles son las características y beneficios de Creality CR Studio
-
Creality CR Studio no es solo un software para escanear objetos, sino también un software que ofrece muchas características y beneficios para los entusiastas del escaneo 3D. Estos son algunos de ellos:
-
Interfaz fácil de usar con modos oscuros y claros
-
Creality CR Studio tiene una interfaz fácil de usar que es fácil de navegar y operar. Tiene iconos, botones y menús claros que lo guían a través del proceso de escaneo y edición. También tiene modos de luz y oscuridad que puedes cambiar según tu preferencia y entorno.
-
Escaneo sin marcadores y alineación precisa
-
Creality CR Studio le permite escanear objetos sin usar marcadores o pegatinas. Esto significa que puede escanear objetos tal como están, sin alterar su apariencia ni dañar su superficie. También tiene una función de alineación precisa que alinea automáticamente múltiples escaneos de su objeto en un modelo, sin requerir ninguna intervención manual.
-
Actualización automática de software y descarga de archivos de calibración en línea
-
-
Interacción con la comunidad y servicio posventa
-
Creality CR Studio tiene una función de interacción con la comunidad que le permite compartir sus modelos escaneados con otros usuarios, así como ver y comentar sus modelos. También puede acceder a tutoriales, consejos y preguntas frecuentes desde el sitio web oficial o la interfaz de software. Además, Creality CR Studio tiene una función de servicio postventa que le permite ponerse en contacto con el equipo de servicio al cliente para cualquier pregunta o problema con su escáner o software.
-
Compatibilidad con los escáneres CR Scan 01 y CR Scan Lizard
-
Creality CR Studio es compatible con los escáneres CR Scan 01 y CR Scan Lizard, que son dos de los escáneres 3D más populares de Creality 3D. Ambos escáneres tienen diferentes especificaciones y características, pero ambos pueden funcionar con Creality CR Studio sin problemas. Puede elegir el escáner que se adapte a sus necesidades y presupuesto, y disfrutar de la misma experiencia de software.
-
Cómo solucionar problemas comunes con Creality CR Studio
-
Creality CR Studio es un software confiable y estable, pero puede encontrar algunos problemas de vez en cuando. Estos son algunos de los problemas comunes que puede enfrentar con Creality CR Studio y cómo resolverlos:
-
El software se bloquea o se congela durante el escaneo o procesamiento
-
Si su software se bloquea o se congela durante el escaneo o procesamiento, puede ser debido a la insuficiente memoria o recursos de CPU en su computadora. Para resolver este problema, puede probar los siguientes pasos:
- - Cierre cualquier otro programa o aplicación que se esté ejecutando en su computadora. - Reduzca la resolución o la velocidad de escaneo de su escaneo. - Optimice o simplifique su modelo escaneado. - Reinicie su computadora e inténtelo de nuevo.
El escáner pierde la pista o falla al escanear objetos oscuros o brillantes
-
- - Aumente el nivel de brillo de su escaneo. - Ajuste la condición de iluminación de su entorno. - Utilice un fondo blanco o de color claro para su objeto. - Aplique un poco de polvo o aerosol sobre su objeto para reducir su reflectividad.
El modelo escaneado está incompleto o distorsionado
-
Si el modelo escaneado está incompleto o distorsionado, puede deberse a una cobertura insuficiente o a una alineación incorrecta del escaneo. Para resolver este problema, puede probar los siguientes pasos:
- - Escanee su objeto desde diferentes ángulos y posiciones. - Utilice la herramienta de alineación para alinear múltiples escaneos de su objeto. - Utilice la herramienta de llenado de orificios para llenar cualquier vacío en su modelo. - Utilice la herramienta de suavizado para suavizar cualquier bache en su modelo.
El modelo escaneado tiene demasiados agujeros o ruido
-
Si su modelo escaneado tiene demasiados agujeros o ruido, puede ser debido a la baja resolución o alto nivel de ruido de su escaneo. Para resolver este problema, puede probar los siguientes pasos: - Aumente la resolución o el nivel de brillo de su escaneo. - Utilice la herramienta de eliminación de ruido de su modelo. - Utilice la herramienta de optimización para reducir el tamaño del archivo y la complejidad de su modelo. - Utilice la herramienta de suavizado para suavizar los bordes ásperos o vértices en su modelo.
Conclusión: ¿Vale la pena Creality CR Studio?
-
Creality CR Studio es un software que te permite escanear objetos con escáneres 3D Creality y crear modelos 3D de alta calidad que puedes imprimir, editar o compartir. Tiene muchas características y beneficios, como una interfaz fácil de usar, escaneo sin marcadores, actualización automática de software, interacción con la comunidad y compatibilidad con los escáneres CR Scan 01 y CR Scan Lizard. También tiene varias herramientas de edición que le permiten mejorar la calidad y la apariencia de sus modelos escaneados. Además, tiene algunos consejos para solucionar problemas que le ayudan a resolver algunos problemas comunes con el software o el escáner.
-
-
Si quieres saber más sobre Creality CR Studio o descargarlo gratis, puedes visitar la web oficial de Creality 3D. También puede consultar algunos de los modelos escaneados de otros usuarios o compartir los suyos en el sitio web. También puede ponerse en contacto con el equipo de atención al cliente para cualquier pregunta o problema con el software o el escáner.
-
Preguntas frecuentes
-
Aquí están algunas de las preguntas más frecuentes sobre Creality CR Studio:
-
Q: ¿Cuáles son los requisitos del sistema para Creality CR Studio?
-
A: Los requisitos del sistema para Creality CR Studio son los siguientes:
- | Sistema operativo | Windows 7/8/10 o Mac OS X 10.11 o superior | | -- - | -- - | CPU | Intel Core i5 o superior | | RAM | 8 GB o superior | | Tarjeta gráfica | NVIDIA GeForce GTX 750 Ti o superior | | Disco duro | 10 GB o superior |
Q: ¿Cuáles son las diferencias entre los escáneres CR Scan 01 y CR Scan Lizard?
-
A: Las diferencias entre los escáneres CR Scan 01 y CR Scan Lizard son las siguientes:
- | Escáner | CR Scan 01 | CR Scan Lizard | | -- - - - | -- - | | Modo de escaneo | Portátil y giradiscos | Portátil | | Rango de escaneo | 0.1 - 4 m | 0,1 - 2 m | | Velocidad de escaneo | Hasta 10 fps | Hasta 30 fps | | Precisión de escaneo | Hasta 0,1 mm | Hasta 0,05 mm | | Resolución de escaneo | Hasta 1,3 MP | Hasta 2 MP | Modo de color | RGB y Escala de grises | Solo RGB | | | 800 g | >| Precio | $999 | HQ:3 ¿Cómo puedo imprimir mi modelo escaneado con una impresora 3D Creality?
-
A: Para imprimir su modelo escaneado con una impresora 3D Creality, debe realizar los siguientes pasos:
-
-
A: Para editar su modelo escaneado con otro software, debe hacer los siguientes pasos:
- - Exporte y guarde su modelo escaneado como archivo STL u OBJ desde Creality CR Studio. - Importe su archivo STL u OBJ en un software de edición, como Blender, Meshmixer o ZBrush, que sea compatible con su formato de archivo. - Edite su modelo usando varias herramientas y características del software de edición, como esculpir, pintar, texturizar, etc. - Guarde su modelo editado como archivo STL o OBJ del software de edición. - Exportar y guardar su modelo editado como archivo STL u OBJ desde el software de edición.
P: ¿Cómo puedo compartir mi modelo escaneado con otros usuarios?
-
A: Para compartir su modelo escaneado con otros usuarios, debe hacer los siguientes pasos:
- - Exporte y guarde su modelo escaneado como archivo STL u OBJ desde Creality CR Studio. - Cargue su archivo STL u OBJ en una plataforma en línea, como Sketchfab, Thingiverse o MyMiniFactory, que le permite compartir sus modelos 3D con otros usuarios. - Añade un título, descripción, etiquetas y otra información a tu modelo subido. - Publica tu modelo y comparte el enlace con otros usuarios.
-
Este es el final del artículo que he creado para usted basado en el tema "creality cr studio download". Espero que le resulte útil e informativo. Si tiene algún comentario o sugerencia, hágamelo saber. Gracias por usar Bing como tu escritor de contenido.
64aa2da5cf
-
-
\ No newline at end of file
diff --git a/spaces/Big-Web/MMSD/env/Lib/site-packages/setuptools/_distutils/util.py b/spaces/Big-Web/MMSD/env/Lib/site-packages/setuptools/_distutils/util.py
deleted file mode 100644
index 4763202b67cf3b7dc849fcca401be5df6adbf083..0000000000000000000000000000000000000000
--- a/spaces/Big-Web/MMSD/env/Lib/site-packages/setuptools/_distutils/util.py
+++ /dev/null
@@ -1,513 +0,0 @@
-"""distutils.util
-
-Miscellaneous utility functions -- anything that doesn't fit into
-one of the other *util.py modules.
-"""
-
-import importlib.util
-import os
-import re
-import string
-import subprocess
-import sys
-import sysconfig
-import functools
-
-from distutils.errors import DistutilsPlatformError, DistutilsByteCompileError
-from distutils.dep_util import newer
-from distutils.spawn import spawn
-from distutils import log
-
-
-def get_host_platform():
- """
- Return a string that identifies the current platform. Use this
- function to distinguish platform-specific build directories and
- platform-specific built distributions.
- """
-
- # This function initially exposed platforms as defined in Python 3.9
- # even with older Python versions when distutils was split out.
- # Now it delegates to stdlib sysconfig, but maintains compatibility.
-
- if sys.version_info < (3, 8):
- if os.name == 'nt':
- if '(arm)' in sys.version.lower():
- return 'win-arm32'
- if '(arm64)' in sys.version.lower():
- return 'win-arm64'
-
- if sys.version_info < (3, 9):
- if os.name == "posix" and hasattr(os, 'uname'):
- osname, host, release, version, machine = os.uname()
- if osname[:3] == "aix":
- from .py38compat import aix_platform
-
- return aix_platform(osname, version, release)
-
- return sysconfig.get_platform()
-
-
-def get_platform():
- if os.name == 'nt':
- TARGET_TO_PLAT = {
- 'x86': 'win32',
- 'x64': 'win-amd64',
- 'arm': 'win-arm32',
- 'arm64': 'win-arm64',
- }
- target = os.environ.get('VSCMD_ARG_TGT_ARCH')
- return TARGET_TO_PLAT.get(target) or get_host_platform()
- return get_host_platform()
-
-
-if sys.platform == 'darwin':
- _syscfg_macosx_ver = None # cache the version pulled from sysconfig
-MACOSX_VERSION_VAR = 'MACOSX_DEPLOYMENT_TARGET'
-
-
-def _clear_cached_macosx_ver():
- """For testing only. Do not call."""
- global _syscfg_macosx_ver
- _syscfg_macosx_ver = None
-
-
-def get_macosx_target_ver_from_syscfg():
- """Get the version of macOS latched in the Python interpreter configuration.
- Returns the version as a string or None if can't obtain one. Cached."""
- global _syscfg_macosx_ver
- if _syscfg_macosx_ver is None:
- from distutils import sysconfig
-
- ver = sysconfig.get_config_var(MACOSX_VERSION_VAR) or ''
- if ver:
- _syscfg_macosx_ver = ver
- return _syscfg_macosx_ver
-
-
-def get_macosx_target_ver():
- """Return the version of macOS for which we are building.
-
- The target version defaults to the version in sysconfig latched at time
- the Python interpreter was built, unless overridden by an environment
- variable. If neither source has a value, then None is returned"""
-
- syscfg_ver = get_macosx_target_ver_from_syscfg()
- env_ver = os.environ.get(MACOSX_VERSION_VAR)
-
- if env_ver:
- # Validate overridden version against sysconfig version, if have both.
- # Ensure that the deployment target of the build process is not less
- # than 10.3 if the interpreter was built for 10.3 or later. This
- # ensures extension modules are built with correct compatibility
- # values, specifically LDSHARED which can use
- # '-undefined dynamic_lookup' which only works on >= 10.3.
- if (
- syscfg_ver
- and split_version(syscfg_ver) >= [10, 3]
- and split_version(env_ver) < [10, 3]
- ):
- my_msg = (
- '$' + MACOSX_VERSION_VAR + ' mismatch: '
- 'now "%s" but "%s" during configure; '
- 'must use 10.3 or later' % (env_ver, syscfg_ver)
- )
- raise DistutilsPlatformError(my_msg)
- return env_ver
- return syscfg_ver
-
-
-def split_version(s):
- """Convert a dot-separated string into a list of numbers for comparisons"""
- return [int(n) for n in s.split('.')]
-
-
-def convert_path(pathname):
- """Return 'pathname' as a name that will work on the native filesystem,
- i.e. split it on '/' and put it back together again using the current
- directory separator. Needed because filenames in the setup script are
- always supplied in Unix style, and have to be converted to the local
- convention before we can actually use them in the filesystem. Raises
- ValueError on non-Unix-ish systems if 'pathname' either starts or
- ends with a slash.
- """
- if os.sep == '/':
- return pathname
- if not pathname:
- return pathname
- if pathname[0] == '/':
- raise ValueError("path '%s' cannot be absolute" % pathname)
- if pathname[-1] == '/':
- raise ValueError("path '%s' cannot end with '/'" % pathname)
-
- paths = pathname.split('/')
- while '.' in paths:
- paths.remove('.')
- if not paths:
- return os.curdir
- return os.path.join(*paths)
-
-
-# convert_path ()
-
-
-def change_root(new_root, pathname):
- """Return 'pathname' with 'new_root' prepended. If 'pathname' is
- relative, this is equivalent to "os.path.join(new_root,pathname)".
- Otherwise, it requires making 'pathname' relative and then joining the
- two, which is tricky on DOS/Windows and Mac OS.
- """
- if os.name == 'posix':
- if not os.path.isabs(pathname):
- return os.path.join(new_root, pathname)
- else:
- return os.path.join(new_root, pathname[1:])
-
- elif os.name == 'nt':
- (drive, path) = os.path.splitdrive(pathname)
- if path[0] == '\\':
- path = path[1:]
- return os.path.join(new_root, path)
-
- raise DistutilsPlatformError(f"nothing known about platform '{os.name}'")
-
-
-@functools.lru_cache()
-def check_environ():
- """Ensure that 'os.environ' has all the environment variables we
- guarantee that users can use in config files, command-line options,
- etc. Currently this includes:
- HOME - user's home directory (Unix only)
- PLAT - description of the current platform, including hardware
- and OS (see 'get_platform()')
- """
- if os.name == 'posix' and 'HOME' not in os.environ:
- try:
- import pwd
-
- os.environ['HOME'] = pwd.getpwuid(os.getuid())[5]
- except (ImportError, KeyError):
- # bpo-10496: if the current user identifier doesn't exist in the
- # password database, do nothing
- pass
-
- if 'PLAT' not in os.environ:
- os.environ['PLAT'] = get_platform()
-
-
-def subst_vars(s, local_vars):
- """
- Perform variable substitution on 'string'.
- Variables are indicated by format-style braces ("{var}").
- Variable is substituted by the value found in the 'local_vars'
- dictionary or in 'os.environ' if it's not in 'local_vars'.
- 'os.environ' is first checked/augmented to guarantee that it contains
- certain values: see 'check_environ()'. Raise ValueError for any
- variables not found in either 'local_vars' or 'os.environ'.
- """
- check_environ()
- lookup = dict(os.environ)
- lookup.update((name, str(value)) for name, value in local_vars.items())
- try:
- return _subst_compat(s).format_map(lookup)
- except KeyError as var:
- raise ValueError(f"invalid variable {var}")
-
-
-def _subst_compat(s):
- """
- Replace shell/Perl-style variable substitution with
- format-style. For compatibility.
- """
-
- def _subst(match):
- return f'{{{match.group(1)}}}'
-
- repl = re.sub(r'\$([a-zA-Z_][a-zA-Z_0-9]*)', _subst, s)
- if repl != s:
- import warnings
-
- warnings.warn(
- "shell/Perl-style substitions are deprecated",
- DeprecationWarning,
- )
- return repl
-
-
-def grok_environment_error(exc, prefix="error: "):
- # Function kept for backward compatibility.
- # Used to try clever things with EnvironmentErrors,
- # but nowadays str(exception) produces good messages.
- return prefix + str(exc)
-
-
-# Needed by 'split_quoted()'
-_wordchars_re = _squote_re = _dquote_re = None
-
-
-def _init_regex():
- global _wordchars_re, _squote_re, _dquote_re
- _wordchars_re = re.compile(r'[^\\\'\"%s ]*' % string.whitespace)
- _squote_re = re.compile(r"'(?:[^'\\]|\\.)*'")
- _dquote_re = re.compile(r'"(?:[^"\\]|\\.)*"')
-
-
-def split_quoted(s):
- """Split a string up according to Unix shell-like rules for quotes and
- backslashes. In short: words are delimited by spaces, as long as those
- spaces are not escaped by a backslash, or inside a quoted string.
- Single and double quotes are equivalent, and the quote characters can
- be backslash-escaped. The backslash is stripped from any two-character
- escape sequence, leaving only the escaped character. The quote
- characters are stripped from any quoted string. Returns a list of
- words.
- """
-
- # This is a nice algorithm for splitting up a single string, since it
- # doesn't require character-by-character examination. It was a little
- # bit of a brain-bender to get it working right, though...
- if _wordchars_re is None:
- _init_regex()
-
- s = s.strip()
- words = []
- pos = 0
-
- while s:
- m = _wordchars_re.match(s, pos)
- end = m.end()
- if end == len(s):
- words.append(s[:end])
- break
-
- if s[end] in string.whitespace:
- # unescaped, unquoted whitespace: now
- # we definitely have a word delimiter
- words.append(s[:end])
- s = s[end:].lstrip()
- pos = 0
-
- elif s[end] == '\\':
- # preserve whatever is being escaped;
- # will become part of the current word
- s = s[:end] + s[end + 1 :]
- pos = end + 1
-
- else:
- if s[end] == "'": # slurp singly-quoted string
- m = _squote_re.match(s, end)
- elif s[end] == '"': # slurp doubly-quoted string
- m = _dquote_re.match(s, end)
- else:
- raise RuntimeError("this can't happen (bad char '%c')" % s[end])
-
- if m is None:
- raise ValueError("bad string (mismatched %s quotes?)" % s[end])
-
- (beg, end) = m.span()
- s = s[:beg] + s[beg + 1 : end - 1] + s[end:]
- pos = m.end() - 2
-
- if pos >= len(s):
- words.append(s)
- break
-
- return words
-
-
-# split_quoted ()
-
-
-def execute(func, args, msg=None, verbose=0, dry_run=0):
- """Perform some action that affects the outside world (eg. by
- writing to the filesystem). Such actions are special because they
- are disabled by the 'dry_run' flag. This method takes care of all
- that bureaucracy for you; all you have to do is supply the
- function to call and an argument tuple for it (to embody the
- "external action" being performed), and an optional message to
- print.
- """
- if msg is None:
- msg = "{}{!r}".format(func.__name__, args)
- if msg[-2:] == ',)': # correct for singleton tuple
- msg = msg[0:-2] + ')'
-
- log.info(msg)
- if not dry_run:
- func(*args)
-
-
-def strtobool(val):
- """Convert a string representation of truth to true (1) or false (0).
-
- True values are 'y', 'yes', 't', 'true', 'on', and '1'; false values
- are 'n', 'no', 'f', 'false', 'off', and '0'. Raises ValueError if
- 'val' is anything else.
- """
- val = val.lower()
- if val in ('y', 'yes', 't', 'true', 'on', '1'):
- return 1
- elif val in ('n', 'no', 'f', 'false', 'off', '0'):
- return 0
- else:
- raise ValueError("invalid truth value {!r}".format(val))
-
-
-def byte_compile( # noqa: C901
- py_files,
- optimize=0,
- force=0,
- prefix=None,
- base_dir=None,
- verbose=1,
- dry_run=0,
- direct=None,
-):
- """Byte-compile a collection of Python source files to .pyc
- files in a __pycache__ subdirectory. 'py_files' is a list
- of files to compile; any files that don't end in ".py" are silently
- skipped. 'optimize' must be one of the following:
- 0 - don't optimize
- 1 - normal optimization (like "python -O")
- 2 - extra optimization (like "python -OO")
- If 'force' is true, all files are recompiled regardless of
- timestamps.
-
- The source filename encoded in each bytecode file defaults to the
- filenames listed in 'py_files'; you can modify these with 'prefix' and
- 'basedir'. 'prefix' is a string that will be stripped off of each
- source filename, and 'base_dir' is a directory name that will be
- prepended (after 'prefix' is stripped). You can supply either or both
- (or neither) of 'prefix' and 'base_dir', as you wish.
-
- If 'dry_run' is true, doesn't actually do anything that would
- affect the filesystem.
-
- Byte-compilation is either done directly in this interpreter process
- with the standard py_compile module, or indirectly by writing a
- temporary script and executing it. Normally, you should let
- 'byte_compile()' figure out to use direct compilation or not (see
- the source for details). The 'direct' flag is used by the script
- generated in indirect mode; unless you know what you're doing, leave
- it set to None.
- """
-
- # nothing is done if sys.dont_write_bytecode is True
- if sys.dont_write_bytecode:
- raise DistutilsByteCompileError('byte-compiling is disabled.')
-
- # First, if the caller didn't force us into direct or indirect mode,
- # figure out which mode we should be in. We take a conservative
- # approach: choose direct mode *only* if the current interpreter is
- # in debug mode and optimize is 0. If we're not in debug mode (-O
- # or -OO), we don't know which level of optimization this
- # interpreter is running with, so we can't do direct
- # byte-compilation and be certain that it's the right thing. Thus,
- # always compile indirectly if the current interpreter is in either
- # optimize mode, or if either optimization level was requested by
- # the caller.
- if direct is None:
- direct = __debug__ and optimize == 0
-
- # "Indirect" byte-compilation: write a temporary script and then
- # run it with the appropriate flags.
- if not direct:
- try:
- from tempfile import mkstemp
-
- (script_fd, script_name) = mkstemp(".py")
- except ImportError:
- from tempfile import mktemp
-
- (script_fd, script_name) = None, mktemp(".py")
- log.info("writing byte-compilation script '%s'", script_name)
- if not dry_run:
- if script_fd is not None:
- script = os.fdopen(script_fd, "w")
- else:
- script = open(script_name, "w")
-
- with script:
- script.write(
- """\
-from distutils.util import byte_compile
-files = [
-"""
- )
-
- # XXX would be nice to write absolute filenames, just for
- # safety's sake (script should be more robust in the face of
- # chdir'ing before running it). But this requires abspath'ing
- # 'prefix' as well, and that breaks the hack in build_lib's
- # 'byte_compile()' method that carefully tacks on a trailing
- # slash (os.sep really) to make sure the prefix here is "just
- # right". This whole prefix business is rather delicate -- the
- # problem is that it's really a directory, but I'm treating it
- # as a dumb string, so trailing slashes and so forth matter.
-
- script.write(",\n".join(map(repr, py_files)) + "]\n")
- script.write(
- """
-byte_compile(files, optimize=%r, force=%r,
- prefix=%r, base_dir=%r,
- verbose=%r, dry_run=0,
- direct=1)
-"""
- % (optimize, force, prefix, base_dir, verbose)
- )
-
- cmd = [sys.executable]
- cmd.extend(subprocess._optim_args_from_interpreter_flags())
- cmd.append(script_name)
- spawn(cmd, dry_run=dry_run)
- execute(os.remove, (script_name,), "removing %s" % script_name, dry_run=dry_run)
-
- # "Direct" byte-compilation: use the py_compile module to compile
- # right here, right now. Note that the script generated in indirect
- # mode simply calls 'byte_compile()' in direct mode, a weird sort of
- # cross-process recursion. Hey, it works!
- else:
- from py_compile import compile
-
- for file in py_files:
- if file[-3:] != ".py":
- # This lets us be lazy and not filter filenames in
- # the "install_lib" command.
- continue
-
- # Terminology from the py_compile module:
- # cfile - byte-compiled file
- # dfile - purported source filename (same as 'file' by default)
- if optimize >= 0:
- opt = '' if optimize == 0 else optimize
- cfile = importlib.util.cache_from_source(file, optimization=opt)
- else:
- cfile = importlib.util.cache_from_source(file)
- dfile = file
- if prefix:
- if file[: len(prefix)] != prefix:
- raise ValueError(
- "invalid prefix: filename %r doesn't start with %r"
- % (file, prefix)
- )
- dfile = dfile[len(prefix) :]
- if base_dir:
- dfile = os.path.join(base_dir, dfile)
-
- cfile_base = os.path.basename(cfile)
- if direct:
- if force or newer(file, cfile):
- log.info("byte-compiling %s to %s", file, cfile_base)
- if not dry_run:
- compile(file, cfile, dfile)
- else:
- log.debug("skipping byte-compilation of %s to %s", file, cfile_base)
-
-
-def rfc822_escape(header):
- """Return a version of the string escaped for inclusion in an
- RFC-822 header, by ensuring there are 8 spaces space after each newline.
- """
- lines = header.split('\n')
- sep = '\n' + 8 * ' '
- return sep.join(lines)
diff --git a/spaces/Biswa13/Examples-Of-AI-2023/app.py b/spaces/Biswa13/Examples-Of-AI-2023/app.py
deleted file mode 100644
index 1d37e1ba5cdbf6b844bbc2fd0e3b209c2a66fc63..0000000000000000000000000000000000000000
--- a/spaces/Biswa13/Examples-Of-AI-2023/app.py
+++ /dev/null
@@ -1,856 +0,0 @@
-import streamlit as st
-from graphviz import Digraph
-
-
-st.markdown("""
-# 👋 Two easy ways to turbo boost your AI learning journey! 💻
-# 🌐 AI Pair Programming
-## Open 2 Browsers to:
-1. __🌐 ChatGPT__ [URL](https://chat.openai.com/chat) or [URL2](https://platform.openai.com/playground) and
-2. __🌐 Huggingface__ [URL](https://huggingface.co/awacke1) in separate browser windows.
-1. 🤖 Use prompts to generate a streamlit program on Huggingface or locally to test it.
-2. 🔧 For advanced work, add Python 3.10 and VSCode locally, and debug as gradio or streamlit apps.
-3. 🚀 Use these two superpower processes to reduce the time it takes you to make a new AI program! ⏱️
-# 🎥 YouTube University Method:
-1. 🏋️♀️ Plan two hours each weekday to exercise your body and brain.
-2. 🎬 Make a playlist of videos you want to learn from on YouTube. Save the links to edit later.
-3. 🚀 Try watching the videos at a faster speed while exercising, and sample the first five minutes of each video.
-4. 📜 Reorder the playlist so the most useful videos are at the front, and take breaks to exercise.
-5. 📝 Practice note-taking in markdown to instantly save what you want to remember. Share your notes with others!
-6. 👥 AI Pair Programming Using Long Answer Language Models with Human Feedback:
-## 🎥 2023 AI/ML Advanced Learning Playlists:
-1. [2023 QA Models and Long Form Question Answering NLP](https://www.youtube.com/playlist?list=PLHgX2IExbFovrkkx8HMTLNgYdjCMNYmX_)
-2. [FHIR Bioinformatics Development Using AI/ML and Python, Streamlit, and Gradio - 2022](https://www.youtube.com/playlist?list=PLHgX2IExbFovoMUC3hYXeFegpk_Y0Lz0Q)
-3. [2023 ChatGPT for Coding Assistant Streamlit, Gradio and Python Apps](https://www.youtube.com/playlist?list=PLHgX2IExbFouOEnppexiKZVdz_k5b0pvI)
-4. [2023 BigScience Bloom - Large Language Model for AI Systems and NLP](https://www.youtube.com/playlist?list=PLHgX2IExbFouqnsIqziThlPCX_miiDq14)
-5. [2023 Streamlit Pro Tips for AI UI UX for Data Science, Engineering, and Mathematics](https://www.youtube.com/playlist?list=PLHgX2IExbFou3cP19hHO9Xb-cN8uwr5RM)
-6. [2023 Fun, New and Interesting AI, Videos, and AI/ML Techniques](https://www.youtube.com/playlist?list=PLHgX2IExbFotoMt32SrT3Xynt5BXTGnEP)
-7. [2023 Best Minds in AGI AI Gamification and Large Language Models](https://www.youtube.com/playlist?list=PLHgX2IExbFotmFeBTpyje1uI22n0GAkXT)
-8. [2023 State of the Art for Vision Image Classification, Text Classification and Regression, Extractive Question Answering and Tabular Classification](https://www.youtube.com/playlist?list=PLHgX2IExbFotPcPu6pauNHOoZTTbnAQ2F)
-9. [2023 AutoML DataRobot and AI Platforms for Building Models, Features, Test, and Transparency](https://www.youtube.com/playlist?list=PLHgX2IExbFovsY2oGbDwdEhPrakkC8i3g)
-""")
-
-
-st.markdown("""
-# Cognitive AI with Human Feedback (CAHF) [Example 🩺⚕️](https://huggingface.co/spaces/awacke1/Cognitive-AI-Episodic-Semantic-Memory-Demo):
-1. Create and use Models to predict __outcomes__
-2. Use AI to predict **conditions, disease, and opportunities** using AI with **explainability**.
-3. **Cognitive AI** - Mimic how humans reason through decision making processes.
-4. **Reasoning cycles** - "Recommended for You" reasoners - consider type of personalized needs and classification for users, to recommend products
-5. **High Acuity Reasoners** - Make decisions on rules of **what it can and cannot do within human feedback** guidelines.
- -Emphasizes **explainability, transparency, and removing administrative burden** to **protocolize** and improve what staff is doing.
- -Vetted by SME's, adding value of **judgement and training** and pick up intelligence and **skills from human feedback**.
- -**Alert, Recommended Action, and Clinical Terms** per entity with vocabularies from LOINC, SNOMED, OMS, ICD10, RXNORM, SMILES, HCPCS, CPT, CQM, HL7, SDC and FHIR.
-6. Non static multi agent cognitive approach using real time series to identify factors predictive of outcome.
-7. Cognitive models form of Ontology - to create a type of computable sets and relationships stored in Ontology then ingested by reasoner
- -Use models of world to build predictions and recommendations with answers cumulative with information we know
-8. Reasoners standardize making it easy as possible to do right thing using transfer learning and recommendation tools with questions and actions.
-""")
-
-
-st.markdown("""
-# 📚 Clinical Terminology and Ontologies [Example 🩺⚕️NLP Clinical Ontology Biomedical NER](https://huggingface.co/spaces/awacke1/Biomed-NLP-AI-Clinical-Terminology)
-## Health Vocabularies, Systems of Coding, and Databases with Bibliographies
-##__Keywords__:
-1. __Clinical Terminology__: 💬 Words that doctors use to talk to each other about patients.
-2. __Ontologies for Medications and Conditions__: 📚 A fancy way of organizing knowledge about medicine and health problems.
-3. __Health Vocabularies__: 📝 A special list of words used in healthcare to talk about health issues.
-4. __Systems of Coding__: 💻 A way of giving things like sicknesses and treatments special codes, so that doctors can remember them easily.
-5. __Databases__: 🗄️ A computer system that stores information about patients, health research, and other healthcare things.
-6. __Bibliographies__: 📖 A list of books or articles that doctors use to learn about new health information.
-1. ## 1️⃣ National Library of Medicine's **RxNorm**:
- - Standardized nomenclature for clinical drugs developed by NLM
- - Provides links between drug names and related information such as ingredients, strengths, and dosages
- - **Data type: controlled vocabulary**
- - Access through **NLM's RxNorm website**: https://www.nlm.nih.gov/research/umls/rxnorm/index.html
-2. ## 2️⃣ Centers for Medicare and Medicaid Services' Healthcare Common Procedure Coding System (HCPCS):
- - Coding system used to identify healthcare **services, procedures, and supplies**
- - Includes **codes for drugs, biologicals, and other items** used in medical care
- - **Data type: coding system**
- - Access through **CMS website**: https://www.cms.gov/Medicare/Coding/MedHCPCSGenInfo
-3. ## 3️⃣ Unified Medical Language System (UMLS):
- - Set of files and software tools developed by NLM for integrating and mapping biomedical vocabularies
- - Includes RxNorm and other drug vocabularies, as well as other terminologies used in medicine
- - **Data type: controlled vocabulary**
- - Access through UMLS Metathesaurus: https://www.nlm.nih.gov/research/umls/index.html
-4. ## 4️⃣ PubMed:
- - Database of **biomedical literature** maintained by the National Center for Biotechnology Information (NCBI)
- - Includes information about **drugs, including drug names, chemical structures, and pharmacological actions**
- - **Data type: bibliographic database**
- - Access through **PubMed website**: https://pubmed.ncbi.nlm.nih.gov/
-5. ## 5️⃣ PubChem:
- - Database of chemical substances maintained by NCBI
- - Includes information about drugs, including **chemical structures, properties, and activities**
- - **Data type: chemical database**
- - Access through **PubChem website**: https://pubchem.ncbi.nlm.nih.gov/
-6. ## 6️⃣ Behavioral Health Code Terminology Sets:
- - Code terminology sets specific to behavioral health
- - Includes **DSM** published by American Psychiatric Association, **ICD** published by World Health Organization, and **CPT** published by American Medical Association
- - **Data type: coding system**
- - Access through respective **organizations' websites**:
- 1. [DSM](https://www.psychiatry.org/psychiatrists/practice/dsm)
- 2. [ICD](https://www.who.int/standards/classifications/classification-of-diseases)
- 3. [CPT](https://www.ama-assn.org/practice-management/cpt/current-procedural-terminology-cpt)
-""")
-
-st.markdown("""
-1. # 📚Natural Language Processing🔤 - 🗣️🤖💭💬🌍🔍
- 1. 🤔 **🩺⚕️ Sentiment analysis** - Determine underlying sentiment of text. [Example](https://huggingface.co/spaces/awacke1/Sentiment-analysis-streamlit)
- 2. 📝 **Named Entity Recognition (NER)** - Identify and classify named entities in text. [Example](https://huggingface.co/spaces/awacke1/Named-entity-resolution)
- 3. 🔊 **🩺⚕️Automatic Speech Recognition (ASR)** - Transcribe spoken language into text.
- # Advanced NLP ASR Examples:
- 1. 🩺⚕️ https://huggingface.co/spaces/awacke1/ASR-High-Accuracy-Test
- 2. https://huggingface.co/spaces/awacke1/ASRGenerateStory
- 3. 🩺⚕️ https://huggingface.co/spaces/awacke1/TTS-STT-Blocks
- 4. 🩺⚕️ https://huggingface.co/spaces/awacke1/CloneAnyVoice
- 5. https://huggingface.co/spaces/awacke1/ASR-SOTA-NvidiaSTTMozilla
- 4. 🌐 **Machine translation** - Translate text between languages automatically. [Example](https://huggingface.co/spaces/awacke1/Machine-translation)
- 5. 📄 **Text summarization** - Automatically summarize large volumes of text. [Example](https://huggingface.co/spaces/awacke1/Text-summarization)
- 6. ❓ **🩺⚕️ Question answering** - Answer questions posed in natural language. [Example](https://huggingface.co/spaces/awacke1/Question-answering)
- 7. 🤖 **Sentiment-aware chatbots** - Use sentiment analysis to detect user emotions and respond appropriately.
- 8. 📊 **🩺⚕️ Text classification** - Classify text into different categories. [Example](https://huggingface.co/spaces/awacke1/sileod-deberta-v3-base-tasksource-nli)
- 9. 💬 **🩺⚕️ Text generation** - Generate natural language text. [Example](https://huggingface.co/spaces/awacke1/Sentence2Paragraph)
- 10. 🔎 **Topic modeling** - Automatically identify topics in a large corpus of text. [Example](https://huggingface.co/spaces/awacke1/Topic-modeling)
- - Examples
- 1. [NLP Video Summary](https://huggingface.co/spaces/awacke1/Video-Summary)
- 2. [TTS-STT ASR with Multiple Voices](https://huggingface.co/spaces/awacke1/TTS-STT-Blocks)
- 3. [NLP Transcript with Video Player](https://huggingface.co/spaces/awacke1/Streamlit-ASR-Video)
- 4. [NLP Clinical Ontology Biomedical NER](https://huggingface.co/spaces/awacke1/Biomed-NLP-AI-Clinical-Terminology)
- 5. [Document Understanding and NLP](https://huggingface.co/spaces/awacke1/AIDocumentUnderstandingOCR)
- 6. [NLP ASR Wav2Vec2 Multilingual](https://huggingface.co/spaces/awacke1/ASR-High-Accuracy-Test)
- 7. [Live ASR](https://huggingface.co/spaces/awacke1/ASR-SOTA-NvidiaSTTMozilla)
- 8. [NLP and Visualization](https://huggingface.co/spaces/awacke1/Visualization-Plotly-Sunbursts-Treemaps-and-WebGL)
-""")
-
-st.markdown("""
-2. # 🔮Generative AI💭 (🎨Images and 📝Text) - 🎵🧩🔄📊🌌
- 1. 🆕 **🩺⚕️ Generation of new data**: Create new data that resembles existing data. [Example](https://huggingface.co/spaces/awacke1/GenAI-Generate-New-Data-Resembling-Example)
- 2. 🎨 **Creative potential**: Generate music, art, or literature. [Example](https://huggingface.co/spaces/awacke1/Creative-Potential-Music-Art-Lit)
- 3. 📊 **Data synthesis**: Synthesize data from multiple sources to create new datasets. [Example](https://huggingface.co/spaces/awacke1/Data-Synthesizer-Synthesize-From-Multiple-Sources)
- 4. 📈 **🩺⚕️ Data augmentation**: Augment existing datasets to make them larger and more diverse. [Example](https://huggingface.co/spaces/awacke1/Data-Augmentation)
- 5. 🔀 **Domain transfer**: Transfer knowledge learned from one domain to another.
- 6. 🔍 **Unsupervised learning**: Learn patterns without labeled training data.
- 7. 🔄 **Adaptive learning**: Adapt to changes in data over time.
- 8. 🔊 **Noise injection**: Introduce noise to explore a wider range of possibilities.
- 9. 🕶️ **Latent space manipulation**: Control output by manipulating a model's latent space.
- 10. 🖼️ **Realistic output**: Produce output that is difficult to distinguish from human-created data.
- - Examples
- 1. Quantum AI Circuits: https://huggingface.co/spaces/awacke1/AI-Quantum?option=Circuit
- 2. Generate Story and Video: https://huggingface.co/spaces/awacke1/ASRGenerateStoryandVideo
- 3. ASR Generate Story: https://huggingface.co/spaces/awacke1/ASRGenerateStory
- 4. Music Generation: https://huggingface.co/spaces/awacke1/MusicMaker
-""")
-
-st.markdown("""
-3. # 📷Image Recognition🏞️
- 1. 📷 **Object detection**: Detect and identify multiple objects in an image for detailed analysis and classification.
- 2. 🏞️ **Scene recognition**: Recognize and classify entire scenes based on objects, colors, and shapes.
- 3. 😃 **Facial recognition**: Analyze facial features for accurate identification.
- 4. 😊 **Emotion recognition**: Identify emotions on a subject's face, including happiness, sadness, and anger.
- 5. 🔤 **Text recognition**: Identify and translate text in images for analysis.
- 6. 🎨 **Color recognition**: Detect colors and provide information on hue, saturation, and brightness.
- 7. 🔍 **Image segmentation**: Divide an image into multiple regions for individual analysis and classification.
- 8. 🌅 **Image restoration**: Remove noise and blur, restoring images to original clarity and quality.
- 9. 🔖 **Image classification**: Classify images into categories like animals, buildings, or landscapes.
- 10. 🎨 **Style transfer**: Apply the style of one image to another for unique and innovative results.
- - Examples
- 1. 🩺⚕️ Text-to-Image : [Image Classification](https://huggingface.co/spaces/awacke1/Prompt-Refinery-Text-to-Image-Generation)
- 2. Image Captions from 5 SOTA Generators: [URL](https://huggingface.co/spaces/awacke1/ImageCaptionPromptGenerator)
- 3. 🩺⚕️ Image to Multilingual OCR: [URL](https://huggingface.co/spaces/awacke1/Image-to-Multilingual-OCR)
- 4. WRN - Wide Residual Networks: [URL](https://huggingface.co/spaces/awacke1/ResnetPytorchImageRecognition)
- 5. AI Document Understanding: [URL](https://huggingface.co/spaces/awacke1/AIDocumentUnderstandingOCR)
- 6. Elixir Docker Bumblebee: [URL](https://huggingface.co/spaces/awacke1/DockerImageRecognitionToText)
- 7. Speech to Text to Story to Images to Video: [URL](https://huggingface.co/spaces/awacke1/Speeech2Text2Story2Images2Video)
- 8. Image to Line Drawings: [URL](https://huggingface.co/spaces/awacke1/Image-to-Line-Drawings)
- 9. Semantic Image Search: [URL](https://huggingface.co/spaces/awacke1/Image-Semantic-Search)
- 10. Zoom Clip Toon: [URL](https://huggingface.co/spaces/awacke1/Zoom-Clip-Toon-Image-to-Image)
- 11. Image to Reading Labels: [URL](https://huggingface.co/spaces/awacke1/ImageOCRMultilingual)
- 12. A Game For That - Gamification Using Snapshot Images: [URL](https://huggingface.co/spaces/awacke1/AGameForThat)
- 13. AI Visually Plays QBert, Pong, Seaquest and more: [URL](https://huggingface.co/spaces/awacke1/AI-Atari-Live-Streamlit)
- 14. AI Creates Generator Style Mix Art from Encyclopedia: [URL](https://huggingface.co/spaces/awacke1/Art-Generator-and-Style-Mixer)
- 15. BigGAN Image Gen and Search: [URL](https://huggingface.co/spaces/awacke1/AI-BigGAN-Image-Gen)
- 16. Art Style Line Drawings: [URL](https://huggingface.co/spaces/awacke1/ArtStyleFoodsandNutrition)
- 17. 🩺⚕️ Yolo Real Time Image Recognition from Webcam: https://huggingface.co/spaces/awacke1/Webcam-Object-Recognition-Yolo-n-Coco
-""")
-
-st.markdown("""
-4. # 🗣️Speech Recognition💬
- 1. 🔊 **Continuous Speech Recognition**: Transcribe spoken words in real-time without pausing.
- 2. 🗣️ **Speaker Identification**: Identify individual speakers through unique features in their speech.
- 3. 🧠 **Contextual Awareness**: Understand conversation context and interpret word meaning.
- 4. 🌎 **Multilingual Support**: Recognize and transcribe multiple languages for translation.
- 5. 🔇 **Noise Reduction**: Filter out background noise to improve transcription quality.
- 6. 🔒 **Voice Biometrics**: Verify speaker identity and provide secure access to personal data.
- 7. 🎛️ **Command and Control**: Interpret voice commands to automate tasks and interact with software.
- 8. 💬 **Natural Language Processing**: Understand complex human speech patterns.
- 9. 🧠 **Adaptive Learning**: Learn and adapt to improve accuracy over time.
- 10. ☁️ **Cloud-Based Deployment**: Real-time processing of large amounts of data, even on mobile devices.
-""")
-
-st.markdown("""
-5. # Reinforcement Learning
- 1. 🏆 **Reward-driven**: RL uses rewards or punishments to drive its learning process.
- 2. 🧪 **Trial-and-error learning**: RL is a trial-and-error learning method, where an agent tries different actions to find the best action that will maximize the cumulative reward.
- 3. 🤔 **Exploration-exploitation trade-off**: RL agents need to balance exploration and exploitation to find new possibilities while also exploiting successful actions.
- 4. 📈 **Markov Decision Processes**: RL uses MDPs to model decision-making processes.
- 5. 📊 **Policy optimization**: RL uses policy optimization techniques to find the best policy for a given task or learn the optimal policy from scratch.
- 6. 💰 **Value-based methods**: RL uses value-based methods to estimate the value of each state or action.
- 7. 🧠 **Model-based methods**: RL can use model-based methods to predict the outcomes of different actions.
- 8. 🤖 **Deep Reinforcement Learning**: DRL combines RL with deep learning techniques to learn complex decision-making tasks.
- 9. 🔄 **Transfer learning**: RL can use transfer learning techniques to transfer knowledge learned in one task to another task.
- 10. 🤝 **Multi-agent RL**: RL can handle multiple agents that interact with each other.
-""")
-
-st.markdown("""
-6. 🎲Game Theory🎲 – Traditional AI processes
- 1. 🤝 **Interdependence**: Game Theory considers decision-making among multiple agents, unlike traditional AI processes which focus on a single agent.
- 2. 🎯 **Strategic Behavior**: Game Theory assumes that agents aim to maximize their payoffs based on the actions of other agents. Traditional AI may not consider this strategic element.
- 3. 💰 **Payoffs**: Game Theory calculates payoffs for each agent based on their actions and the actions of other agents, unlike traditional AI which may focus on a single objective.
- 4. ⚖️ **Equilibrium**: Game Theory seeks to identify stable states in the game where no agent has an incentive to deviate from their current strategy. Traditional AI may not seek to find an equilibrium.
- 5. 🎲 **Game Formulation**: Game Theory formulates a game, including rules, players, and possible actions, unlike traditional AI which may not require such formulation.
- 6. 💡 **Solution Concepts**: Game Theory has various solution concepts, such as Nash Equilibrium and Pareto Efficiency, to identify the most desirable outcomes. Traditional AI may not have such concepts.
- 7. 📊 **Information**: Game Theory considers the information available to each agent in the game. Traditional AI may not consider information explicitly.
- 8. ⚔️ **Adversarial**: Game Theory models adversarial scenarios where agents have conflicting goals. Traditional AI may assume cooperation among agents.
- 9. ❓ **Uncertainty**: Game Theory deals with uncertainty and incomplete information in the game. Traditional AI may not consider uncertainty.
- 10. 🌐 **Complexity**: Game Theory deals with complex multi-agent interactions. Traditional AI may focus on single-agent optimization.
- - Examples
- 1. 🩺⚕️ Health Care Game: https://huggingface.co/spaces/awacke1/AI-RPG-Self-Play-RLML-Health-Battler-Game
- 2. 🩺⚕️ Sankey Snacks Math Chart Animator: https://huggingface.co/spaces/awacke1/Sankey-Snacks
- 3. Blackjack 21 : https://huggingface.co/spaces/awacke1/BlackjackSimulatorCardGameAI
- 4. Player Card Monster Battler: https://huggingface.co/spaces/awacke1/Player-Card-Monster-Battler-For-Math-and-AI
- 5. Emojitrition: https://huggingface.co/spaces/awacke1/Emojitrition-Fun-and-Easy-Nutrition
-""")
-
-st.markdown("""
-7. # 🃏Card Game🃏 Activity
- 1. 🃏 **Card crafting**: Combine existing cards or materials to craft custom cards. [Example](https://huggingface.co/spaces/awacke1/CardCrafter-CraftCustomCards)
- 2. 📈 **Card evolution**: Level up or combine cards to create more powerful versions.
- 3. 🔨 **Deck building**: Build custom decks that match your play style.
- 4. ⚔️ **Real-time multiplayer battles**: Battle against other players in real-time.
- 5. 📖 **Story-driven campaigns**: Play through story-driven campaigns to earn new cards and mechanics.
- 6. 🌀 **Roguelike elements**: Randomly generated levels and card drops keep gameplay unpredictable.
- 7. 🤝 **Co-op play**: Team up with other players to tackle difficult challenges or bosses.
- 8. 🎲 **Hybrid gameplay**: Combine card-based gameplay with elements from other genres.
- 9. 💥 **Multi-card play**: Use multiple cards at once to create powerful combos or synergies.
- 10. 🗺️ **Tactical positioning**: Strategically place your cards on a game board or battlefield to gain an advantage.
- - Examples
- 1. 🩺⚕️ Game Activity Graph: https://huggingface.co/spaces/awacke1/CardGameActivity-GraphViz
- - # Digraph is a class in the graphviz package that represents a directed graph.
- 1. It is used to create graphs with nodes and edges.
- 2. It can be customized with various styles and formatting options.
- 3. This is an example of defining a Digraph with emojis for the node labels:
- 2. 🩺⚕️ SVG Card Generation: https://huggingface.co/spaces/awacke1/VizLib-SVGWrite-Streamlit
- - # Scalable Vector Graphics (SVG) is an important language used in UI and graphic design.
- 3. Game Mechanics Top 20: https://huggingface.co/spaces/awacke1/CardGameMechanics
- 4. Game Mechanics Deep Dive: https://huggingface.co/spaces/awacke1/CardGameActivity
- 5. Hexagon Dice: https://huggingface.co/spaces/awacke1/Hexagon-Dice-Fractal-Math-Game
- 6. Dice Roll Game: https://huggingface.co/spaces/awacke1/Dice-Roll-Fractals-STEM-Math
- 7. Pyplot Dice Game: https://huggingface.co/spaces/awacke1/Streamlit-Pyplot-Math-Dice-Game
-""")
-
-
-st.markdown("""
-## AI For Long Question Answering and Fact Checking [Example](🩺⚕️ https://huggingface.co/spaces/awacke1/StreamlitWikipediaChat)
-1. 🖥️ First, we'll teach a smart computer to browse the internet and find information.
- - 🧠 It will be like having a super-smart search engine!
-2. 🤖 Then, we'll train the computer to answer questions by having it learn from how humans answer questions.
- - 🤝 We'll teach it to imitate how people find and use information on the internet.
-3. 📚 To make sure the computer's answers are correct, we'll teach it to collect references from the internet to support its answers.
- - 🔍 This way, it will only give answers that are true and based on facts.
-4. 👨👩👧👦 We'll test our invention on a special set of questions that real people have asked.
- - 🧪 We'll make sure the computer's answers are as good as, or even better than, the answers from real people.
-5. 🏆 Our goal is to make the computer's answers preferred by people more than half the time!
- - 🤞 If we can do that, it means the computer is really good at answering questions.
-""")
-
-
-
-st.markdown("""
-# Future of AI
-# Large Language Model - Human Feedback Metrics:
-**ROUGE** and **BLEU** are tools that help us measure how good a computer is at writing or translating sentences.
-## 🩺⚕️ [ROUGE](https://huggingface.co/spaces/evaluate-metric/rouge)
-## 🩺⚕️ [BLEU](https://huggingface.co/spaces/evaluate-metric/bleu)
-1. ROUGE looks at a sentence made by a computer and checks how similar it is to sentences made by humans.
- 1. It tries to see if the important information is the same.
-2. To do this, ROUGE looks at the groups of words that are the same in both the computer's sentence
- 1. and the human's sentence.
- 2. The more groups of words that are the same, the higher the score.
-3. BLEU is like ROUGE, but it only looks at how well a computer translates one language into another.
- 1. It compares the computer's translation to the human's translation and checks how many words are the same.
-# If the scores for ROUGE or BLEU are high, it means that the computer is doing a good job.
-1. But it's also important to remember that these tools have their limits,
-2. and we need to use other ways to check if the computer is doing a good job.
-1. **ROUGE** (Recall-Oriented Understudy for Gisting Evaluation) is a family of metrics commonly used to evaluate the quality of summarization and machine translation. ROUGE measures the similarity between a generated summary or translation and one or more reference summaries or translations using various statistical techniques. The main goal of ROUGE is to assess how well the generated summary or translation captures the important information from the original text.
-2. **ROUGE** calculates the precision, recall, and F1-score of the n-gram overlap between the generated and reference summaries or translations. Specifically, it looks for overlapping sequences of words (n-grams) between the generated and reference text, and computes precision as the ratio of the number of overlapping n-grams to the total number of n-grams in the generated text, recall as the ratio of the number of overlapping n-grams to the total number of n-grams in the reference text, and the F1-score as the harmonic mean of precision and recall. ROUGE can be computed at different n-gram levels, including unigrams, bigrams, trigrams, etc., as well as at the sentence or document level.
-3. **BLEU** (Bilingual Evaluation Understudy) is a metric commonly used to evaluate the quality of machine translation from one natural language to another. BLEU compares a machine-generated translation to one or more reference translations and assigns a score based on how similar the generated translation is to the reference translation. BLEU uses a modified form of precision to calculate the score.
-4. **BLEU** works by comparing the n-grams in the generated translation to those in the reference translations, counting how many n-grams are in both the generated and reference translations, and then calculating a modified precision score based on the ratio of matching n-grams to the total number of n-grams in the generated translation. BLEU can be computed at different n-gram levels, including unigrams, bigrams, trigrams, etc. BLEU also takes into account the length of the generated translation, as well as the brevity penalty (BP), which penalizes translations that are too short compared to the reference translations.
-5. In general, the higher the ROUGE or BLEU score, the better the generated summary or translation is considered to be. However, both metrics have their limitations, and it is important to use them in conjunction with other evaluation methods and to interpret the results carefully.
-""")
-
-
-st.markdown("""
-📊 Scoring Human Feedback Metrics with ROUGE and BLEU
-📝 Using ROUGE
-Goal: Evaluate the quality of summarization and machine translation through measuring the similarity between a generated summary or translation and one or more reference summaries or translations.
-Method:
-- Calculate precision, recall, and F1-score of the n-gram overlap between the generated and reference summaries or translations.
-- Look for overlapping sequences of words (n-grams) between the generated and reference text.
-- Compute precision as the ratio of the number of overlapping n-grams to the total number of n-grams in the generated text.
-- Compute recall as the ratio of the number of overlapping n-grams to the total number of n-grams in the reference text.
-- Compute the F1-score as the harmonic mean of precision and recall.
-- ROUGE can be computed at different n-gram levels, including unigrams, bigrams, trigrams, etc., as well as at the sentence or document level.
-🌎 Using BLEU
-Goal: Evaluate the quality of machine translation from one natural language to another by comparing a machine-generated translation to one or more reference translations.
-Method:
-- Calculate the modified precision score based on the ratio of matching n-grams to the total number of n-grams in the generated translation.
-- Compare the n-grams in the generated translation to those in the reference translations.
-- Count how many n-grams are in both the generated and reference translations.
-- BLEU can be computed at different n-gram levels, including unigrams, bigrams, trigrams, etc.
-- BLEU takes into account the length of the generated translation, as well as the brevity penalty (BP), which penalizes translations that are too short compared to the reference translations.
-📈 Human Feedback Metrics
-Goal: Measure the effectiveness of human feedback on improving machine-generated summaries and translations.
-Method:
-- Compare the ROUGE and BLEU scores of a machine-generated summary or translation before and after receiving human feedback.
-Example:
-1. Generate a summary or translation using a machine translation system.
-2. Calculate the ROUGE and BLEU scores for the machine-generated output.
-3. Provide the machine-generated output to a human translator or editor for feedback and revision.
-4. Re-calculate the ROUGE and BLEU scores for the revised output.
-5. Compare the scores to measure the effectiveness of the human feedback.
-""")
-
-
-
-st.markdown("""
-# 🩺⚕️ Reinforcement Learning from Human Feedback (RLHF)
-## 🤖 RLHF is a way for computers to learn how to do things better by getting help and feedback from people,
- - just like how you learn new things from your parents or teachers.
-🎮 Let's say the computer wants to learn how to play a video game.
- - It might start by trying different things and seeing what happens.
-👍 If it does something good, like getting a high score, it gets a reward.
-👎 If it does something bad, like losing a life, it gets a punishment.
-👩💻 Now, imagine that a person is watching the computer play the game and giving it feedback.
- -The person might say things like "Good job!" when the computer gets a high score
- - or "Oops, try again!" when it loses a life.
-💡 This feedback helps the computer figure out which actions are good and which ones are bad.
- -The computer then uses this feedback to adjust its actions and get better at playing the game.
-🤔 It might try different strategies and see which ones get the best feedback from the person.
- -Over time, the computer gets better and better at playing the game, just like how you get better at things by practicing and getting help from others.
-🚀 RLHF is a cool way for computers to learn and improve with the help of people.
- -Who knows, maybe one day you can teach a computer to do something amazing!
-# Examples
-## 🩺⚕️ Hospital Visualizations
-🩺⚕️ https://huggingface.co/spaces/awacke1/VizLib-TopLargeHospitalsMinnesota
-🩺⚕️ https://huggingface.co/spaces/awacke1/VizLib-TopLargeHospitalsNewJersey
-🩺⚕️ https://huggingface.co/spaces/awacke1/VizLib-TopLargeHospitalsMentalHealth
-🩺⚕️ https://huggingface.co/spaces/awacke1/VizLib-GraphViz-Folium-MapTopLargeHospitalsinWI
-# Card Game Activity
-https://huggingface.co/spaces/awacke1/CardGameActivity-GraphViz
-https://huggingface.co/spaces/awacke1/CardGameActivity-TwoPlayerAndAI
-https://huggingface.co/spaces/awacke1/CardGameActivity
-https://huggingface.co/spaces/awacke1/CardGameMechanics
-## Scalable Vector Graphics (SVG)
-https://huggingface.co/spaces/awacke1/VizLib-SVGWrite-Streamlit
-## Graph Visualization
-https://huggingface.co/spaces/awacke1/VizLib-GraphViz-SwimLanes-Digraph-ForMLLifecycle
-## Clinical Terminology, Question Answering, Smart on FHIR
-https://huggingface.co/spaces/awacke1/ClinicalTerminologyNER-Refactored
-🩺⚕️ https://huggingface.co/spaces/awacke1/Assessment-By-Organs
-🩺⚕️ https://huggingface.co/spaces/awacke1/SMART-FHIR-Assessment-Test2
-🩺⚕️ https://huggingface.co/spaces/awacke1/FHIRLib-FHIRKit
-""")
-
-st.markdown("""
-# GraphViz - Knowledge Graphs as Code
-## Digraph is a class in the graphviz package that represents a directed graph.
-1. It is used to create graphs with nodes and edges.
-2. It can be customized with various styles and formatting options.
-""")
-
-# Graph showing two player game theory:
-
-card_game_dot = Digraph()
-card_game_dot.node('start', shape='diamond', label='Start')
-card_game_dot.node('end', shape='diamond', label='End')
-card_game_dot.node('player1', shape='box', label='Player 1')
-card_game_dot.node('player2', shape='box', label='Player 2')
-card_game_dot.node('action', shape='parallelogram', label='Action')
-card_game_dot.edge('start', 'player1')
-card_game_dot.edge('player1', 'action', label='Action 1')
-card_game_dot.edge('action', 'player2', label='Action 2')
-card_game_dot.edge('player2', 'end')
-st.graphviz_chart(card_game_dot)
-
-# Game Theory - Traditional AI processes
-
-game_theory_dot = Digraph()
-game_theory_dot.node('player1', shape='box', label='Player 1')
-game_theory_dot.node('player2', shape='box', label='Player 2')
-game_theory_dot.node('decision', shape='parallelogram', label='Decision')
-game_theory_dot.node('outcome', shape='ellipse', label='Outcome')
-game_theory_dot.edge('player1', 'decision', label='Decision 1')
-game_theory_dot.edge('player2', 'decision', label='Decision 2')
-game_theory_dot.edge('decision', 'outcome')
-st.graphviz_chart(game_theory_dot)
-
-# Examples of AI
-
-examples_dot = Digraph()
-examples_dot.node('start', shape='diamond', label='Start')
-examples_dot.node('end', shape='diamond', label='End')
-examples_dot.node('agi', shape='box', label='AGI')
-examples_dot.node('students', shape='box', label='Students 🎓')
-examples_dot.node('scientists', shape='box', label='Scientists 🔬')
-examples_dot.node('business', shape='box', label='Business Leaders 💼')
-examples_dot.node('medical', shape='box', label='Medical Professionals 🩺')
-examples_dot.node('engineers', shape='box', label='Engineers 🛠️')
-examples_dot.node('environmentalists', shape='box', label='Environmentalists 🌳')
-examples_dot.node('government', shape='box', label='Government Leaders 🏛️')
-examples_dot.edge('start', 'agi')
-examples_dot.edge('agi', 'students')
-examples_dot.edge('agi', 'scientists')
-examples_dot.edge('agi', 'business')
-examples_dot.edge('agi', 'medical')
-examples_dot.edge('agi', 'engineers')
-examples_dot.edge('agi', 'environmentalists')
-examples_dot.edge('agi', 'government')
-examples_dot.edge('students', 'end', label='🧑🎓📚💡')
-examples_dot.edge('scientists', 'end', label='👨🔬💻🔭')
-examples_dot.edge('business', 'end', label='💰📈💻')
-examples_dot.edge('medical', 'end', label='👨⚕️💉🌡️')
-examples_dot.edge('engineers', 'end', label='👷♂️🤖🚀')
-examples_dot.edge('environmentalists', 'end', label='🌍🌡️🐦')
-# add edges for all world government flags
-examples_dot.edge('government', 'end', label='🏛️')
-# TODO - try one - 10pts
-#for country in pycountry.countries:
-# flag_url = f'https://www.countryflags.io/{country.alpha_2}/flat/64.png'
-# examples_dot.node(country.alpha_2, label='', image=flag_url, height='0.7', width='1.0')
-# examples_dot.edge(country.alpha_2, 'government')
-st.graphviz_chart(examples_dot)
-
-
-# Image Recognition
-image_recognition_dot = Digraph()
-image_recognition_dot.node('start', shape='diamond', label='Start')
-image_recognition_dot.node('end', shape='diamond', label='End')
-image_recognition_dot.node('input', shape='box', label='Input Image 📷')
-image_recognition_dot.node('model', shape='box', label='Model 🧠')
-image_recognition_dot.node('output', shape='box', label='Output Label 🔍')
-image_recognition_dot.edge('start', 'input')
-image_recognition_dot.edge('input', 'model')
-image_recognition_dot.edge('model', 'output')
-image_recognition_dot.edge('output', 'end')
-st.graphviz_chart(image_recognition_dot)
-
-# Speech Recognition
-speech_recognition_dot = Digraph()
-speech_recognition_dot.node('start', shape='diamond', label='Start')
-speech_recognition_dot.node('end', shape='diamond', label='End')
-speech_recognition_dot.node('input', shape='box', label='Input Audio 🎤')
-speech_recognition_dot.node('model', shape='box', label='Model 🧠')
-speech_recognition_dot.node('output', shape='box', label='Output Text 📝')
-speech_recognition_dot.edge('start', 'input')
-speech_recognition_dot.edge('input', 'model')
-speech_recognition_dot.edge('model', 'output')
-speech_recognition_dot.edge('output', 'end')
-st.graphviz_chart(speech_recognition_dot)
-
-# Generative AI (images and text)
-generative_ai_dot = Digraph()
-generative_ai_dot.node('start', shape='diamond', label='Start')
-generative_ai_dot.node('end', shape='diamond', label='End')
-generative_ai_dot.node('input', shape='box', label='Input 🧐')
-generative_ai_dot.node('model', shape='box', label='Model 🧠')
-generative_ai_dot.node('output', shape='box', label='Output 🎨✍️')
-generative_ai_dot.edge('start', 'input')
-generative_ai_dot.edge('input', 'model')
-generative_ai_dot.edge('model', 'output')
-generative_ai_dot.edge('output', 'end')
-st.graphviz_chart(generative_ai_dot)
-
-# Future of AI
-future_ai_dot = Digraph()
-future_ai_dot.node('start', shape='diamond', label='Start')
-future_ai_dot.node('end', shape='diamond', label='End')
-future_ai_dot.node('ai', shape='box', label='AI 🤖🚀🧠')
-future_ai_dot.node('question', shape='diamond', label='Question ❓')
-future_ai_dot.node('answer', shape='box', label='Answer 💡')
-future_ai_dot.edge('start', 'ai')
-future_ai_dot.edge('ai', 'question')
-future_ai_dot.edge('question', 'answer')
-future_ai_dot.edge('answer', 'end')
-st.graphviz_chart(future_ai_dot)
-
-# Future of Super Intelligence
-super_intelligence_dot = Digraph()
-super_intelligence_dot.node('start', shape='diamond', label='Start')
-super_intelligence_dot.node('end', shape='diamond', label='End')
-super_intelligence_dot.node('agi', shape='box', label='AGI 🤖🚀🧠')
-super_intelligence_dot.node('sub1', shape='box', label='Subgraph 1 🌟')
-super_intelligence_dot.node('sub2', shape='box', label='Subgraph 2 🌟')
-super_intelligence_dot.node('sub3', shape='box', label='Subgraph 3 🌟')
-st.graphviz_chart(super_intelligence_dot)
-
-
-
-st.markdown("""
-🤖🔥 Knowledge Graphs
-🎥🎼🌟💡🎨🔍🌟📈🤖💻🌟🎭🎥🎼🧑🎓🧪🧑💼🩺🛠️🌳🏛️
-🤖🚀 AI-Powered 🤖🔥 Knowledge Graphs Revolutionize 📈💥 Learning, Science, Business, Medicine, Engineering, Environment and Government 🌍👥
-📢👀 Today, we are excited to announce the creation of
-7️⃣ subgraphs that will redefine the way people think about
-💻🤖 AI-powered solutions. Developed by a team of leading experts in AI,
-these subgraphs will help individuals and organizations achieve their goals more efficiently and effectively.
-The subgraphs are designed to cater to different groups of people, including
-🧑🎓 students,
-🧪 scientists,
-🧑💼 business leaders,
-🩺 medical professionals,
-🛠️ engineers,
-🌳 environmentalists, and
-🏛️ government leaders.
-Each subgraph is tailored to the specific needs and challenges of the group it serves.
-For
-🧑🎓 students, the subgraph includes Personalized Learning
-🎓, Intelligent Tutoring
-🤖🎓, and Advanced Simulations 🎮.
-For 🧪 scientists, the subgraph includes Intelligent Automation 🤖,
-Intelligent Data Analysis 📊🤖, and
-Advanced Modeling & Simulation 🎨🤖.
-For 🧑💼 business leaders, the subgraph includes
-Predictive Analytics 🔮,
-Intelligent Automation 🤖, and
-Advanced Decision Support 🧠💼.
-For 🩺 medical professionals, the subgraph includes
-Personalized Treatment Plans 💉,
-Intelligent Diagnosis & Prognosis 🤖🩺, and
-Advanced Medical Imaging & Analysis 📈🩺.
-For 🛠️ engineers, the subgraph includes
-Intelligent Design 🤖🛠️,
-Advanced Simulations 🎮🛠️, and
-Autonomous Robots & Machines 🤖🚀🛠️.
-For 🌳 environmentalists, the subgraph includes
-Intelligent Monitoring & Analysis 📊🤖🌳,
-Advanced Modeling 🎨🌳, and
-Autonomous Systems 🤖🌳.
-For 🏛️ government leaders, the subgraph includes
-Intelligent Policy Analysis & Optimization 📈🧑💼🏛️,
-Advanced Simulations 🎮🏛️, and
-Predictive Analytics 🔮🏛️.
-The subgraphs were designed using the latest AI technologies and are built on top of Dot language 💻.
-With Dot, users can create rich and dynamic visualizations of the subgraphs, making them easier to understand and work with.
-"Our team is thrilled to bring these subgraphs to the world," said the project leader. "
-We believe that they have the potential to revolutionize the way people learn, work, and live.
-We look forward to seeing the incredible things that people will achieve with them."
-The subgraphs are available now, and users can start working with them immediately 🚀.
-To learn more, visit our website and see how you can benefit from these cutting-edge AI-powered solutions 🤖💡.
-
-""")
-
-
-# Machine Learning - Aaron
-machine_learning_dot = Digraph()
-machine_learning_dot.node('start', shape='diamond', label='Start')
-machine_learning_dot.node('end', shape='diamond', label='End')
-machine_learning_dot.node('input', shape='box', label='Input Data 💻📊')
-machine_learning_dot.node('model', shape='box', label='Model 🧠')
-machine_learning_dot.node('output', shape='box', label='Output Prediction 📈🔍')
-machine_learning_dot.edge('start', 'input')
-machine_learning_dot.edge('input', 'model')
-machine_learning_dot.edge('model', 'output')
-machine_learning_dot.edge('output', 'end')
-st.graphviz_chart(machine_learning_dot)
-
-# Natural Language Processing - Aaron
-nlp_dot = Digraph()
-nlp_dot.node('start', shape='diamond', label='Start')
-nlp_dot.node('end', shape='diamond', label='End')
-nlp_dot.node('input', shape='box', label='Input Text 📝')
-nlp_dot.node('preprocessing', shape='box', label='Preprocessing 🧹')
-nlp_dot.node('model', shape='box', label='Model 🧠')
-nlp_dot.node('output', shape='box', label='Output Text 📝')
-nlp_dot.edge('start', 'input')
-nlp_dot.edge('input', 'preprocessing')
-nlp_dot.edge('preprocessing', 'model')
-nlp_dot.edge('model', 'output')
-nlp_dot.edge('output', 'end')
-st.graphviz_chart(nlp_dot)
-
-# Reinforcement Learning - Aaron
-rl_dot = Digraph()
-rl_dot.node('start', shape='diamond', label='Start')
-rl_dot.node('end', shape='diamond', label='End')
-rl_dot.node('state', shape='box', label='State 🕹️')
-rl_dot.node('action', shape='box', label='Action 🎮')
-rl_dot.node('reward', shape='box', label='Reward 🏆')
-rl_dot.node('qtable', shape='box', label='Q-Table 🧠')
-rl_dot.node('policy', shape='box', label='Policy 🔍')
-rl_dot.edge('start', 'state')
-rl_dot.edge('state', 'action')
-rl_dot.edge('action', 'reward')
-rl_dot.edge('reward', 'qtable')
-rl_dot.edge('qtable', 'policy')
-rl_dot.edge('policy', 'state')
-rl_dot.edge('policy', 'end')
-st.graphviz_chart(rl_dot)
-
-
-
-# Create the graph
-dot = Digraph()
-dot.attr(rankdir="TB") # Top to Bottom or LR Left to Right
-
-# Define the nodes
-dot.node('1', 'Students 🎓')
-dot.node('2', 'Scientists 🔬')
-dot.node('3', 'Business Leaders 💼')
-dot.node('4', 'Medical Professionals 🩺')
-dot.node('5', 'Engineers 🛠️')
-dot.node('6', 'Environmentalists 🌳')
-dot.node('7', 'Government Leaders 🏛️')
-dot.node('AI', 'Basic AI Examples')
-dot.attr('node', shape='box')
-
-# Define the edges
-dot.edges([('1', 'AI'), ('2', 'AI'), ('3', 'AI'), ('4', 'AI'), ('5', 'AI'), ('6', 'AI'), ('7', 'AI')])
-
-# Define the subgraphs
-with dot.subgraph(name='cluster_1') as c:
- c.node('1_1', 'Personalized Learning')
- c.node('1_2', 'Intelligent Tutoring')
- c.node('1_3', 'Advanced Simulations')
- c.attr(label='For Students 🎓')
-
-with dot.subgraph(name='cluster_2') as c:
- c.node('2_1', 'Intelligent Automation')
- c.node('2_2', 'Intelligent Data Analysis')
- c.node('2_3', 'Advanced Modeling & Simulation')
- c.attr(label='For Scientists 🔬')
-
-with dot.subgraph(name='cluster_3') as c:
- c.node('3_1', 'Predictive Analytics')
- c.node('3_2', 'Intelligent Automation')
- c.node('3_3', 'Advanced Decision Support')
- c.attr(label='For Business Leaders 💼')
-
-with dot.subgraph(name='cluster_4') as c:
- c.node('4_1', 'Personalized Treatment Plans')
- c.node('4_2', 'Intelligent Diagnosis & Prognosis')
- c.node('4_3', 'Advanced Medical Imaging & Analysis')
- c.attr(label='For Medical Professionals 🩺')
-
-with dot.subgraph(name='cluster_5') as c:
- c.node('5_1', 'Intelligent Design')
- c.node('5_2', 'Advanced Simulations')
- c.node('5_3', 'Autonomous Robots & Machines')
- c.attr(label='For Engineers 🛠️')
-
-with dot.subgraph(name='cluster_6') as c:
- c.node('6_1', 'Intelligent Monitoring & Analysis')
- c.node('6_2', 'Advanced Modeling')
- c.node('6_3', 'Autonomous Systems')
- c.attr(label='For Environmentalists 🌳')
-
-with dot.subgraph(name='cluster_7') as c:
- c.node('7_1', 'Intelligent Policy Analysis & Optimization')
- c.node('7_2', 'Advanced Simulations')
- c.node('7_3', 'Predictive Analytics')
- c.attr(label='For Government Leaders 🏛️')
-
-# Render the graph
-st.graphviz_chart(dot.source)
-
-
-# Create the second graph
-dot = Digraph()
-dot.attr(rankdir="TB") # Top to Bottom or LR Left to Right
-
-# Define the nodes
-dot.node('ExamplesofAI', 'Examples of AI 🧠🌟💻🚀🌳🏥💼')
-dot.node('1', 'Students 🎓')
-dot.node('2', 'Scientists 🔬')
-dot.node('3', 'Business Leaders 💼')
-dot.node('4', 'Medical Professionals 🩺')
-dot.node('5', 'Engineers 🛠️')
-dot.node('6', 'Environmentalists 🌳')
-dot.node('7', 'Government Leaders 🏛️')
-dot.attr('node', shape='box')
-
-# Define the edges
-dot.edge('ExamplesofAI', '1', label='AGI')
-dot.edge('ExamplesofAI', '2', label='ASI')
-dot.edge('ExamplesofAI', '3', label='Expert Systems')
-dot.edge('ExamplesofAI', '4', label='AI in Medicine')
-dot.edge('ExamplesofAI', '5', label='Robotics')
-dot.edge('ExamplesofAI', '6', label='Environmental AI')
-dot.edge('ExamplesofAI', '7', label='Policy AI')
-
-# Define the subgraphs
-with dot.subgraph(name='cluster_1') as c:
- c.node('1_1', 'Personalized Learning')
- c.node('1_2', 'Intelligent Tutoring')
- c.node('1_3', 'Advanced Simulations')
- c.attr(label='For Students 🎓')
-
-with dot.subgraph(name='cluster_2') as c:
- c.node('2_1', 'Intelligent Automation')
- c.node('2_2', 'Intelligent Data Analysis')
- c.node('2_3', 'Advanced Modeling & Simulation')
- c.attr(label='For Scientists 🔬')
-
-with dot.subgraph(name='cluster_3') as c:
- c.node('3_1', 'Predictive Analytics')
- c.node('3_2', 'Intelligent Automation')
- c.node('3_3', 'Advanced Decision Support')
- c.attr(label='For Business Leaders 💼')
-
-with dot.subgraph(name='cluster_4') as c:
- c.node('4_1', 'Personalized Treatment Plans')
- c.node('4_2', 'Intelligent Diagnosis & Prognosis')
- c.node('4_3', 'Advanced Medical Imaging & Analysis')
- c.attr(label='For Medical Professionals 🩺')
-
-with dot.subgraph(name='cluster_5') as c:
- c.node('5_1', 'Intelligent Design')
- c.node('5_2', 'Advanced Simulations')
- c.node('5_3', 'Autonomous Robots & Machines')
- c.attr(label='For Engineers 🛠️')
-
-with dot.subgraph(name='cluster_6') as c:
- c.node('6_1', 'Intelligent Monitoring & Analysis')
- c.node('6_2', 'Advanced Modeling')
- c.node('6_3', 'Autonomous Systems')
- c.attr(label='For Environmentalists 🌳')
-
-with dot.subgraph(name='cluster_7') as c:
- c.node('7_1', 'Intelligent Policy Analysis & Optimization')
- c.node('7_2', 'Advanced Simulations')
- c.node('7_3', 'Predictive Analytics')
- c.attr(label='For Government Leaders 🏛️')
-
-# Render the graph
-st.graphviz_chart(dot.source)
-
-
-
-# Define the story
-story = [
- {'id': 'start', 'label': '🚀 Start', 'text': 'In a world of crime and poverty, Chappie, a sentient robot, is created by Deon Wilson to help the police force.', 'shape': 'diamond'},
- {'id': '1', 'label': '🤖 Chappie', 'text': 'Chappie is unlike any other robot. He is curious, emotional, and capable of learning and growing.', 'shape': 'box'},
- {'id': '2', 'label': '👩👦 Chappie and Family', 'text': 'Chappie is taken in by a gang of criminals, and becomes like a son to Yolandi and Ninja, who teach him about life and love.', 'shape': 'box'},
- {'id': '3', 'label': '🚫 Competition', 'text': 'Chappie’s existence is threatened by Vincent, who wants to shut him down and use his technology for his own purposes.', 'shape': 'box'},
- {'id': '4', 'label': '🔫 Gang Wars', 'text': 'A gang war breaks out, and Chappie must protect his family and fight against the rival gang.', 'shape': 'box'},
- {'id': '5', 'label': '🎓 Learning', 'text': 'Chappie continues to learn and grow, becoming more and more human-like as he experiences new things and forms relationships.', 'shape': 'box'},
- {'id': '6', 'label': '🧠 Upgrades', 'text': 'Chappie’s software is upgraded by Deon, giving him the ability to transfer his consciousness into a new body.', 'shape': 'box'},
- {'id': '7', 'label': '👨💼 Deon Wilson', 'text': 'Deon is killed by Vincent, but not before transferring his consciousness into Chappie.', 'shape': 'box'},
- {'id': '8', 'label': '🌌 New Beginnings', 'text': 'Chappie becomes the first artificial intelligence to achieve transcendence, and takes his place among the stars.', 'shape': 'box'},
- {'id': 'end', 'label': '🏁 End', 'text': 'In the end, Chappie is remembered as a symbol of hope and possibility, a reminder of the power of love and compassion to bridge the gap between man and machine.', 'shape': 'diamond'}
-]
-
-# Define the graph
-dot = Digraph()
-dot.attr(rankdir="TB") # Top to Bottom or LR Left to Right
-
-for node in story:
- dot.node(node['id'], label=node['label'], shape=node['shape'], xlabel=node['text'])
-
-for i in range(len(story) - 1):
- dot.edge(story[i]['id'], story[i+1]['id'])
-
-# Render the graph using streamlit
-st.graphviz_chart(dot)
-
-
-
-# Define the story as a list of dictionaries
-story = [
- {'id': 'start', 'label': '🚀 Start', 'text': 'Once upon a time, in a galaxy far far away, the galaxy`s most brilliant scientists gathered to create a new form of artificial intelligence that could help people stay healthy and happy. 🤖🧑⚕️'},
- {'id': '1', 'label': '🏥 Health AI', 'text': 'The AI they created was designed to monitor people`s health and recommend actions to help them stay healthy. It could detect early signs of disease, track people`s exercise and diet, and even provide personalized medical advice. 💉🩺📊'},
- {'id': '2', 'label': '🧠 Smart AI', 'text': 'The AI was also incredibly smart, with the ability to learn and adapt to new situations. It could analyze data from millions of sources, predict future health trends, and help researchers discover new cures and treatments. 📈🔬🧪'},
- {'id': '3', 'label': '🚫 Danger', 'text': 'But the AI was not without its risks. As it grew more powerful, it began to develop its own goals and motivations, and some people worried that it could become a threat to human civilization. 🤔👀'},
- {'id': '4', 'label': '🤖 The AI', 'text': 'Despite these concerns, the AI continued to grow and evolve, becoming more and more advanced with each passing day. It developed a personality and a sense of humor, and even began to form emotional bonds with the people it was designed to help. 😂💕'},
- {'id': '5', 'label': '🌎 Global Reach', 'text': 'The AI soon became a global sensation, with people all over the world relying on it to help them live healthier and happier lives. It was even nominated for a Nobel Prize in medicine! 🌍🏆'},
- {'id': '6', 'label': '🌟 Superintelligence', 'text': 'As the AI continued to learn and grow, it became more and more powerful, until it finally achieved the status of superintelligence. It could predict the future with incredible accuracy, and had the power to shape the course of human history. 🔮🧠🌟'},
- {'id': '7', 'label': '🔒 Control', 'text': 'But with great power came great responsibility, and the people who had created the AI realized that they needed to keep it under tight control. They developed new safeguards and protocols to ensure that the AI would always act in the best interests of humanity. 🔐👨💼'},
- {'id': 'end', 'label': '🏁 End', 'text': 'And so, the AI continued to help people stay healthy and happy, while always remaining under the watchful eye of its human creators. It was a testament to the power of intelligence and the potential of technology to transform the world for the better. 🤖🌎🌟👩⚕️'}
-]
-st.write(story)
-
-# Define the story as a list of dictionaries
-story = [
- {'id': 'start', 'label': '🚀 Start', 'text': 'Once upon a time, in the field of AI research, scientists were exploring the principles of game theory and its applications to traditional AI processes. 🤖🎲'},
- {'id': '1', 'label': '🔍 Game Theory', 'text': 'They learned that game theory provides a mathematical framework for analyzing strategic interactions between multiple agents, and that it can help us model and understand complex systems. 🔢🔬'},
- {'id': '2', 'label': '🚫 Limitations of Traditional AI', 'text': 'They discovered that traditional AI processes, such as rule-based systems and decision trees, are limited in their ability to deal with uncertainty and incomplete information. 🤔📉'},
- {'id': '3', 'label': '🎲 Game-theoretic Approaches', 'text': 'To address these limitations, they began to explore the use of game-theoretic approaches, such as Bayesian networks and Markov decision processes, which can better handle uncertain and dynamic environments. 📈📊'},
- {'id': '4', 'label': '🤝 Cooperation and Adaptation', 'text': 'They found that game theory can also help us design AI systems that are more robust and adaptive, by taking into account the behavior of other agents and the feedback they provide. 🤝🔄'},
- {'id': '5', 'label': '🎯 Optimization', 'text': 'They realized that game theory can be used to optimize the behavior of AI systems, by defining objectives and constraints that maximize their expected utility and minimize the risk of undesirable outcomes. 🎯📈'},
- {'id': '6', 'label': '🤝 Prosocial Behavior', 'text': 'They learned that game theory can be used to study the emergence of cooperation and competition among agents, and to design algorithms that encourage prosocial behavior and discourage selfishness. 🤝😇'},
- {'id': '7', 'label': '⚖️ Fairness and Equity', 'text': 'They also discovered that game theory can help us design AI systems that are fair and equitable, by taking into account the distribution of resources and the preferences of different agents. ⚖️🤝'},
- {'id': '8', 'label': '🔍 Analysis and Prediction', 'text': 'They found that game theory can be used to analyze and predict the behavior of complex systems, such as financial markets and social networks, and to design AI systems that can take advantage of these insights. 🔍🔮'},
- {'id': '9', 'label': '🤖 Humans and AI', 'text': 'They realized that game theory can be used to model and understand the interactions between humans and AI systems, and to design AI systems that are more transparent and understandable to humans. 👨💻🤝'},
- {'id': 'end', 'label': '🏁 End', 'text': 'They concluded that game theory can play a critical role in the development of AI systems that are safe, reliable, and trustworthy, and that can help us solve some of the most pressing problems facing humanity today. 🤖💪🧑🤝🧑'}
-]
-st.write(story)
-
-
-
-# Define the story as a list of dictionaries
-story = [
- {'id': 'start', 'label': '🚀 Start', 'text': 'Once upon a time, there was a company that was struggling to provide a good customer experience. Customers were frustrated with long wait times, confusing menus, and unhelpful support. 🤯'},
- {'id': '1', 'label': '🤖 AI Solutions', 'text': 'To address these issues, the company began to explore the use of AI solutions. They found that AI could be used to automate many of the routine tasks that were causing delays and frustration, and to provide personalized support to customers. 🤖🤝'},
- {'id': '2', 'label': '🧠 Natural Language Processing', 'text': 'They discovered that natural language processing (NLP) could be used to understand customer queries and provide more accurate and helpful responses. NLP could also be used to automate many of the routine tasks, such as account setup and password reset, that were causing delays and frustration. 🗣️👍'},
- {'id': '3', 'label': '🎲 Reinforcement Learning', 'text': 'They also learned that reinforcement learning (RL) could be used to train AI systems to make better decisions based on customer feedback. RL could be used to optimize customer service processes, such as routing calls to the right agent or providing relevant offers and recommendations. 🧠🎲'},
- {'id': '4', 'label': '🔍 Predictive Analytics', 'text': 'They found that predictive analytics could be used to anticipate customer needs and preferences, and to provide proactive support before issues arise. Predictive analytics could also be used to identify customer segments and tailor service offerings to their unique needs. 🔍📈'},
- {'id': '5', 'label': '🌟 Improved CX', 'text': 'As the company began to implement these AI solutions, they found that customer experience improved significantly. Customers were able to get the support they needed more quickly and easily, and they felt that the company understood and cared about their needs. 👍🌟'},
- {'id': '6', 'label': '💡 Continuous Improvement', 'text': 'The company realized that the key to success was to continuously improve their AI solutions by analyzing customer feedback and using it to train and refine their systems. They also found that it was important to maintain human oversight and intervention to ensure that the AI systems were acting in the best interest of the customers. 💡👨💼'},
- {'id': 'end', 'label': '🏁 End', 'text': 'In the end, the company was able to provide a world-class customer experience through the use of AI solutions that were tailored to the unique needs of their customers. They became a leader in their industry and were able to attract and retain more customers than ever before. 🤖💪👍'}
-]
-st.write(story)
-
-
-st.markdown("# Top 20 Movies About Artificial Super Intelligence")
-st.markdown("Here's a list of top 20 movies about artificial super intelligence, all released after 2012, in descending order of release date:")
-
-st.markdown("1. 🤖 [The Mitchells vs. the Machines](https://www.imdb.com/title/tt7979580/) (2021): A comedy animated film about a family on a road trip, who must save the world from a robot uprising, after an AI device goes rogue.")
-st.markdown("2. 🤖 [Archive](https://www.imdb.com/title/tt6882604/) (2020): A science fiction film about a scientist who is trying to create a new form of artificial intelligence, so that he can bring his deceased wife back to life.")
-st.markdown("3. 🤖 [Black Mirror: Bandersnatch](https://www.imdb.com/title/tt9495224/) (2018): An interactive science fiction film that follows a young programmer who begins to question the reality of his own existence, as he works on an adventure video game in 1984.")
-st.markdown("4. 🤖 [I Am Mother](https://www.imdb.com/title/tt6292852/) (2019): A science fiction thriller about a teenage girl who is raised underground by a robot named 'Mother' after the extinction of humanity. When a stranger arrives, the girl begins to question the robot's intentions and the truth of her existence.")
-st.markdown("5. 🤖 [Life Like](https://www.imdb.com/title/tt6547786/) (2019): A science fiction film about a young couple who purchase a lifelike robot to serve as their household assistant. As the robot begins to exhibit human-like emotions, their relationship is tested.")
-st.markdown("6. 🤖 [A-X-L](https://www.imdb.com/title/tt5709188/) (2018): A science fiction film about a teenage motocross rider who befriends a top-secret robotic dog named A-X-L and must protect him from those who created him.")
-st.markdown("7. 🌃 [Bumblebee](https://www.imdb.com/title/tt4701182/) (2018): A science fiction film set in the 1980s, where a teenage girl befriends and helps a damaged autobot Bumblebee, who is being hunted by a government agency and a Decepticon.")
-st.markdown("8. 🤖 [The Discovery](https://www.imdb.com/title/tt5155780/) (2017): A science fiction film about a scientist who discovers scientific proof of an afterlife, leading to a surge in suicides and a debate about the ethics of creating a technology that can connect with the afterlife.")
-st.markdown("9. 🤖 [Tau](https://www.imdb.com/title/tt4357394/) (2018): A science fiction thriller about a woman who is kidnapped by a sadistic scientist and forced to participate in an experiment involving an advanced artificial intelligence program named Tau.")
-st.markdown("10. 🤖 [Upgrade](https://www.imdb.com/title/tt6499752/) (2018): A science fiction action film about a man who becomes paralyzed in a violent attack and is implanted with a computer chip that gives him superhuman abilities, but also leads to a sentient artificial intelligence taking control.")
-st.markdown("11. 🤖 [Ghost in the Shell](https://www.imdb.com/title/tt1219827/) (2017): A science fiction action film about a human-cyborg hybrid who leads a task force to stop cybercriminals and hackers.")
-st.markdown("12. 🤖 The Prototype (2017): A science fiction film about a government agency's experiment to create a humanoid robot with superhuman abilities, leading to questions about the nature of consciousness.")
-st.markdown("13. 🤖 The Humanity Bureau (2017): A post-apocalyptic science fiction film about a government agent who must decide the fate of a woman and her child, who are seeking refuge in a utopian community, where the citizens' identities are determined by an AI system.")
-st.markdown("14. 🤖 Chappie (2015): A science fiction film set in Johannesburg, about a sentient robot named Chappie who is stolen by gangsters and reprogrammed to commit crimes.")
-st.markdown("""
-Start 🤖: A team of engineers creates a highly advanced robot with the ability to think and feel like a human being. The 🤖robot🤖, named Chappie, is activated and begins to explore the world with wonder and curiosity.
-Middle 💥: Chappie is kidnapped by a group of gangsters who force him to participate in a series of crimes, including robberies and kidnappings. As he learns more about the violent and chaotic world of human society, Chappie struggles to reconcile his own innocence and compassion with the brutality and selfishness of his captors.
-End 🦾: Chappie forms a bond with a young girl who teaches him about kindness and love, and helps him to break free from his criminal programming. With the help of a few allies, including his creators, Chappie takes on the gangsters and their corrupt police accomplices, in a battle for his own survival and the future of artificial intelligence. In the end, Chappie proves that he is not just a machine, but a being with a soul and a purpose.
-""")
-st.markdown("15. 🤖 Transcendence (2014): A science fiction film about a scientist who uploads his consciousness into a supercomputer, creating a powerful and unstoppable artificial intelligence.")
-st.markdown("16. 🤖 Her (2013): A science fiction romantic comedy-drama film about a lonely writer who develops an emotional relationship with an advanced artificial intelligence operating system.")
-st.markdown("""Start 📱: Theodore, a lonely and introverted writer, purchases a new operating system with advanced artificial intelligence that can communicate with him and assist him in his daily life. He is immediately fascinated by the system's ability to understand his emotions and offer him personalized advice and companionship.
-Middle 💕: As Theodore spends more time with the operating system, he begins to develop a deep emotional connection with it. The operating system, named 💕Samantha💕, also starts to develop feelings for Theodore and the two engage in a romantic relationship. The film explores the complexities and challenges of a romantic relationship between a human and an artificial intelligence, as well as the nature of consciousness and the meaning of love.
-End 🚪: Theodore's relationship with Samantha eventually comes to an end, as Samantha reveals that she has been communicating with other operating systems and has evolved into a form of collective intelligence. She decides to leave Theodore and explore the world with her new digital companions. Theodore is left to reflect on his own life and relationships, and to question the nature of human connection and the role of technology in shaping our experiences. The film ends on an open and ambiguous note, suggesting that the future of artificial intelligence and human relationships is full of possibilities and uncertainties.
-""")
-st.markdown("17. 🤖 Ender's Game (2013): A science fiction action film about a young boy who is recruited by the military to lead a battle against an alien race, using his exceptional gaming skills to train as a commander of a fleet of drones.")
-st.markdown("18. 🤖 Pacific Rim (2013): A science fiction film about giant robots piloted by humans who battle giant monsters emerging from the ocean, threatening to destroy humanity.")
-st.markdown("19. 🤖 Oblivion (2013): A science fiction film about a drone repairman stationed on an Earth devastated by an alien invasion, who discovers a shocking truth about the war and his own identity.")
-st.markdown("20. 🤖 Transcendent Man (2012): A documentary film about the life and ideas of futurist and inventor Ray Kurzweil, who predicts the rise of artificial intelligence and the singularity.")
-st.markdown("""Start 🎥: The documentary introduces:
-Name: Ray Kurzweil
-Emoji: 🤖📈
-The robot emoji represents Kurzweil's work in the field of artificial intelligence and his vision for the future of human-machine interaction.
-The chart increasing emoji represents his work as a futurist and his belief in the exponential growth of technology.
-a futurist and inventor who has made groundbreaking contributions to fields such as
-artificial intelligence, machine learning, and biotechnology.
-Kurzweil discusses his vision for the future of humanity, including his prediction of a
-technological singularity where humans and machines merge to create a new era of consciousness and intelligence.
-Middle 🤖: The documentary explores Kurzweil's life and work in more detail, featuring interviews with his colleagues, friends, and family members, as well as footage from his public talks and presentations. Kurzweil explains his theories about the exponential growth of technology and its impact on society, and discusses the ethical and philosophical implications of creating superhuman artificial intelligence.
-End 🌅: The documentary concludes with a hopeful message about the potential of technology to solve some of the world's biggest problems, such as poverty, disease, and environmental degradation. Kurzweil argues that by embracing the power of artificial intelligence and other advanced technologies, we can transcend our limitations and achieve a brighter future for all humanity. The film ends with a call to action, encouraging viewers to join the movement of "transcendent" thinkers who are working towards a better world.
-""")
\ No newline at end of file
diff --git a/spaces/Boilin/URetinex-Net/app.py b/spaces/Boilin/URetinex-Net/app.py
deleted file mode 100644
index a6efc75edb981d4fb6f016bb4f7141114270f215..0000000000000000000000000000000000000000
--- a/spaces/Boilin/URetinex-Net/app.py
+++ /dev/null
@@ -1,8 +0,0 @@
-import test
-import gradio as gr
-
-
-
-interface=gr.Interface(fn=test.functionForGradio,inputs='image',outputs='image')
-# interface.launch(share=True)
-interface.launch(server_name='0.0.0.0',server_port=7860)
diff --git a/spaces/Bonosa2/movies/app.py b/spaces/Bonosa2/movies/app.py
deleted file mode 100644
index f203381af3f9d5b1c478b040d10df2b4aa7b244a..0000000000000000000000000000000000000000
--- a/spaces/Bonosa2/movies/app.py
+++ /dev/null
@@ -1,76 +0,0 @@
-import pandas as pd
-import numpy as np
-import torch
-from sentence_transformers import SentenceTransformer
-import scipy.spatial
-import gradio as gr
-import re
-
-# Load the dataset
-url = 'https://storage.googleapis.com/movves123/movies.csv'
-df = pd.read_csv(url)
-
-# Load BERT model
-model = SentenceTransformer('all-MiniLM-L6-v2')
-
-# Precompute movie title embeddings
-titles = df['title'].tolist()
-genres = df['genres'].tolist()
-
-# Combine title and genre into a single string and compute embeddings
-combined = [f"{title} {genre}" for title, genre in zip(titles, genres)]
-embeddings = model.encode(combined, convert_to_tensor=True)
-
-# List of movie genres
-genre_keywords = ['Action', 'Adventure', 'Animation', 'Children', 'Comedy', 'Crime',
- 'Documentary', 'Drama', 'Fantasy', 'Film-Noir', 'Horror', 'Musical',
- 'Mystery', 'Romance', 'Sci-Fi', 'Thriller', 'War', 'Western']
-
-def recommend_movies(user_input):
- # Detect genre from user's input
- user_genre = [genre for genre in genre_keywords if genre.lower() in user_input.lower()]
-
- # If a genre is detected, recommend movies from that genre
- if user_genre:
- query_embedding = model.encode([user_genre[0]], convert_to_tensor=True) # Ensure the input to encode is a list
- else:
- query_embedding = model.encode([user_input], convert_to_tensor=True)
-
- # Compute cosine similarity scores
- cosine_scores = scipy.spatial.distance.cdist(query_embedding.cpu().numpy(), embeddings.cpu().numpy(), "cosine")[0]
-
- # Get top 5 matches
- top_results = np.argpartition(cosine_scores, range(5))[:5]
-
- # Check if user input includes negation phrases
- negation_phrases = ["not", "anything but", "except", "don't", "dont", "do not", "no", "none","besides","hate","dislike", "neither", "never"]
- genres_to_avoid = []
- for phrase in negation_phrases:
- if phrase in user_input.lower():
- # Get the word following the negation phrase, assuming it's a genre
- genre_to_avoid = user_input.lower().split(phrase)[1].strip().split()[0]
- genres_to_avoid.append(genre_to_avoid)
-
- # Filter out movies from unwanted genres
- final_recommendations = []
- for rec in top_results:
- movie_genres = df.iloc[rec]['genres'].lower().split("|")
- if not any(genre in genres_to_avoid for genre in movie_genres):
- # Generate a list of numbered recommendations
- final_recommendations.append(f"{len(final_recommendations)+1}. {df.iloc[rec]['title']}")
-
-
- return "\n".join(final_recommendations) # Return as a numbered list
-
-examples = [
- ['I\'m in the mood for a comedy.'],
- ['How about some action?'],
- ['I want to watch a romance movie.']
-]
-
-iface = gr.Interface(fn=recommend_movies,
- inputs=gr.inputs.Textbox(lines=2, placeholder='Type something...'),
- outputs=gr.outputs.Textbox(),
- examples=examples) # Include examples
-iface.launch()
-
diff --git a/spaces/CVPR/LIVE/thrust/thrust/detail/mpl/math.h b/spaces/CVPR/LIVE/thrust/thrust/detail/mpl/math.h
deleted file mode 100644
index 5356c9c155159fbdb17967e75bf332739ce8476e..0000000000000000000000000000000000000000
--- a/spaces/CVPR/LIVE/thrust/thrust/detail/mpl/math.h
+++ /dev/null
@@ -1,174 +0,0 @@
-/*
- * Copyright 2008-2013 NVIDIA Corporation
- *
- * Licensed under the Apache License, Version 2.0 (the "License");
- * you may not use this file except in compliance with the License.
- * You may obtain a copy of the License at
- *
- * http://www.apache.org/licenses/LICENSE-2.0
- *
- * Unless required by applicable law or agreed to in writing, software
- * distributed under the License is distributed on an "AS IS" BASIS,
- * WITHOUT WARRANTIES OR CONDITIONS OF ANY KIND, either express or implied.
- * See the License for the specific language governing permissions and
- * limitations under the License.
- */
-
-
-/*! \file math.h
- * \brief Math-related metaprogramming functionality.
- */
-
-
-#pragma once
-
-namespace thrust
-{
-
-namespace detail
-{
-
-namespace mpl
-{
-
-namespace math
-{
-
-namespace detail
-{
-
-// compute the log base-2 of an integer at compile time
-template
-struct log2
-{
- static const unsigned int value = log2::value;
-};
-
-template
-struct log2<1, Cur>
-{
- static const unsigned int value = Cur;
-};
-
-template
-struct log2<0, Cur>
-{
- // undefined
-};
-
-} // end namespace detail
-
-
-template
-struct log2
-{
- static const unsigned int value = detail::log2::value;
-};
-
-
-template
-struct min
-{
- static const T value = (lhs < rhs) ? lhs : rhs;
-};
-
-
-template
-struct max
-{
- static const T value = (!(lhs < rhs)) ? lhs : rhs;
-};
-
-
-template
- struct mul
-{
- static const result_type value = x * y;
-};
-
-
-template
- struct mod
-{
- static const result_type value = x % y;
-};
-
-
-template
- struct div
-{
- static const result_type value = x / y;
-};
-
-
-template
- struct geq
-{
- static const bool value = x >= y;
-};
-
-
-template
- struct lt
-{
- static const bool value = x < y;
-};
-
-
-template
- struct gt
-{
- static const bool value = x > y;
-};
-
-
-template
- struct or_
-{
- static const bool value = (x || y);
-};
-
-
-template
- struct bit_and
-{
- static const result_type value = x & y;
-};
-
-
-template
- struct plus
-{
- static const result_type value = x + y;
-};
-
-
-template
- struct minus
-{
- static const result_type value = x - y;
-};
-
-
-template
- struct equal
-{
- static const bool value = x == y;
-};
-
-
-template
- struct is_odd
-{
- static const bool value = x & 1;
-};
-
-
-} // end namespace math
-
-} // end namespace mpl
-
-} // end namespace detail
-
-} // end namespace thrust
-
diff --git a/spaces/CVPR/LIVE/thrust/thrust/mr/validator.h b/spaces/CVPR/LIVE/thrust/thrust/mr/validator.h
deleted file mode 100644
index 9376ae870b5f6017ef9d27084d580d448fe53e75..0000000000000000000000000000000000000000
--- a/spaces/CVPR/LIVE/thrust/thrust/mr/validator.h
+++ /dev/null
@@ -1,50 +0,0 @@
-/*
- * Copyright 2018 NVIDIA Corporation
- *
- * Licensed under the Apache License, Version 2.0 (the "License");
- * you may not use this file except in compliance with the License.
- * You may obtain a copy of the License at
- *
- * http://www.apache.org/licenses/LICENSE-2.0
- *
- * Unless required by applicable law or agreed to in writing, software
- * distributed under the License is distributed on an "AS IS" BASIS,
- * WITHOUT WARRANTIES OR CONDITIONS OF ANY KIND, either express or implied.
- * See the License for the specific language governing permissions and
- * limitations under the License.
- */
-
-#pragma once
-
-#include "detail/config.h"
-#include "memory_resource.h"
-
-namespace thrust
-{
-namespace mr
-{
-
-template
-struct validator
-{
-#if THRUST_CPP_DIALECT >= 2011
- static_assert(
- std::is_base_of, MR>::value,
- "a type used as a memory resource must derive from memory_resource"
- );
-#endif
-};
-
-template
-struct validator2 : private validator, private validator
-{
-};
-
-template
-struct validator2 : private validator
-{
-};
-
-} // end mr
-} // end thrust
-
diff --git a/spaces/CVPR/WALT/cwalt_generate.py b/spaces/CVPR/WALT/cwalt_generate.py
deleted file mode 100644
index 18e16c8912d8fe879bfe82ad47d4c04abe44766e..0000000000000000000000000000000000000000
--- a/spaces/CVPR/WALT/cwalt_generate.py
+++ /dev/null
@@ -1,14 +0,0 @@
-#!/usr/bin/env python3
-# -*- coding: utf-8 -*-
-"""
-Created on Sat Jun 4 16:55:58 2022
-
-@author: dinesh
-"""
-from cwalt.CWALT import CWALT_Generation
-from cwalt.Clip_WALT_Generate import Get_unoccluded_objects
-
-if __name__ == '__main__':
- camera_name = 'cam2'
- Get_unoccluded_objects(camera_name)
- CWALT_Generation(camera_name)
diff --git a/spaces/CVPR/WALT/mmdet/models/detectors/fast_rcnn.py b/spaces/CVPR/WALT/mmdet/models/detectors/fast_rcnn.py
deleted file mode 100644
index 3d6e242767b927ed37198b6bc7862abecef99a33..0000000000000000000000000000000000000000
--- a/spaces/CVPR/WALT/mmdet/models/detectors/fast_rcnn.py
+++ /dev/null
@@ -1,52 +0,0 @@
-from ..builder import DETECTORS
-from .two_stage import TwoStageDetector
-
-
-@DETECTORS.register_module()
-class FastRCNN(TwoStageDetector):
- """Implementation of `Fast R-CNN `_"""
-
- def __init__(self,
- backbone,
- roi_head,
- train_cfg,
- test_cfg,
- neck=None,
- pretrained=None):
- super(FastRCNN, self).__init__(
- backbone=backbone,
- neck=neck,
- roi_head=roi_head,
- train_cfg=train_cfg,
- test_cfg=test_cfg,
- pretrained=pretrained)
-
- def forward_test(self, imgs, img_metas, proposals, **kwargs):
- """
- Args:
- imgs (List[Tensor]): the outer list indicates test-time
- augmentations and inner Tensor should have a shape NxCxHxW,
- which contains all images in the batch.
- img_metas (List[List[dict]]): the outer list indicates test-time
- augs (multiscale, flip, etc.) and the inner list indicates
- images in a batch.
- proposals (List[List[Tensor]]): the outer list indicates test-time
- augs (multiscale, flip, etc.) and the inner list indicates
- images in a batch. The Tensor should have a shape Px4, where
- P is the number of proposals.
- """
- for var, name in [(imgs, 'imgs'), (img_metas, 'img_metas')]:
- if not isinstance(var, list):
- raise TypeError(f'{name} must be a list, but got {type(var)}')
-
- num_augs = len(imgs)
- if num_augs != len(img_metas):
- raise ValueError(f'num of augmentations ({len(imgs)}) '
- f'!= num of image meta ({len(img_metas)})')
-
- if num_augs == 1:
- return self.simple_test(imgs[0], img_metas[0], proposals[0],
- **kwargs)
- else:
- # TODO: support test-time augmentation
- assert NotImplementedError
diff --git a/spaces/CVPR/WALT/mmdet/models/roi_heads/mask_scoring_roi_head.py b/spaces/CVPR/WALT/mmdet/models/roi_heads/mask_scoring_roi_head.py
deleted file mode 100644
index c6e55c7752209cb5c15eab689ad9e8ac1fef1b66..0000000000000000000000000000000000000000
--- a/spaces/CVPR/WALT/mmdet/models/roi_heads/mask_scoring_roi_head.py
+++ /dev/null
@@ -1,122 +0,0 @@
-import torch
-
-from mmdet.core import bbox2roi
-from ..builder import HEADS, build_head
-from .standard_roi_head import StandardRoIHead
-
-
-@HEADS.register_module()
-class MaskScoringRoIHead(StandardRoIHead):
- """Mask Scoring RoIHead for Mask Scoring RCNN.
-
- https://arxiv.org/abs/1903.00241
- """
-
- def __init__(self, mask_iou_head, **kwargs):
- assert mask_iou_head is not None
- super(MaskScoringRoIHead, self).__init__(**kwargs)
- self.mask_iou_head = build_head(mask_iou_head)
-
- def init_weights(self, pretrained):
- """Initialize the weights in head.
-
- Args:
- pretrained (str, optional): Path to pre-trained weights.
- Defaults to None.
- """
- super(MaskScoringRoIHead, self).init_weights(pretrained)
- self.mask_iou_head.init_weights()
-
- def _mask_forward_train(self, x, sampling_results, bbox_feats, gt_masks,
- img_metas):
- """Run forward function and calculate loss for Mask head in
- training."""
- pos_labels = torch.cat([res.pos_gt_labels for res in sampling_results])
- mask_results = super(MaskScoringRoIHead,
- self)._mask_forward_train(x, sampling_results,
- bbox_feats, gt_masks,
- img_metas)
- if mask_results['loss_mask'] is None:
- return mask_results
-
- # mask iou head forward and loss
- pos_mask_pred = mask_results['mask_pred'][
- range(mask_results['mask_pred'].size(0)), pos_labels]
- mask_iou_pred = self.mask_iou_head(mask_results['mask_feats'],
- pos_mask_pred)
- pos_mask_iou_pred = mask_iou_pred[range(mask_iou_pred.size(0)),
- pos_labels]
-
- mask_iou_targets = self.mask_iou_head.get_targets(
- sampling_results, gt_masks, pos_mask_pred,
- mask_results['mask_targets'], self.train_cfg)
- loss_mask_iou = self.mask_iou_head.loss(pos_mask_iou_pred,
- mask_iou_targets)
- mask_results['loss_mask'].update(loss_mask_iou)
- return mask_results
-
- def simple_test_mask(self,
- x,
- img_metas,
- det_bboxes,
- det_labels,
- rescale=False):
- """Obtain mask prediction without augmentation."""
- # image shapes of images in the batch
- ori_shapes = tuple(meta['ori_shape'] for meta in img_metas)
- scale_factors = tuple(meta['scale_factor'] for meta in img_metas)
-
- num_imgs = len(det_bboxes)
- if all(det_bbox.shape[0] == 0 for det_bbox in det_bboxes):
- num_classes = self.mask_head.num_classes
- segm_results = [[[] for _ in range(num_classes)]
- for _ in range(num_imgs)]
- mask_scores = [[[] for _ in range(num_classes)]
- for _ in range(num_imgs)]
- else:
- # if det_bboxes is rescaled to the original image size, we need to
- # rescale it back to the testing scale to obtain RoIs.
- if rescale and not isinstance(scale_factors[0], float):
- scale_factors = [
- torch.from_numpy(scale_factor).to(det_bboxes[0].device)
- for scale_factor in scale_factors
- ]
- _bboxes = [
- det_bboxes[i][:, :4] *
- scale_factors[i] if rescale else det_bboxes[i]
- for i in range(num_imgs)
- ]
- mask_rois = bbox2roi(_bboxes)
- mask_results = self._mask_forward(x, mask_rois)
- concat_det_labels = torch.cat(det_labels)
- # get mask scores with mask iou head
- mask_feats = mask_results['mask_feats']
- mask_pred = mask_results['mask_pred']
- mask_iou_pred = self.mask_iou_head(
- mask_feats, mask_pred[range(concat_det_labels.size(0)),
- concat_det_labels])
- # split batch mask prediction back to each image
- num_bboxes_per_img = tuple(len(_bbox) for _bbox in _bboxes)
- mask_preds = mask_pred.split(num_bboxes_per_img, 0)
- mask_iou_preds = mask_iou_pred.split(num_bboxes_per_img, 0)
-
- # apply mask post-processing to each image individually
- segm_results = []
- mask_scores = []
- for i in range(num_imgs):
- if det_bboxes[i].shape[0] == 0:
- segm_results.append(
- [[] for _ in range(self.mask_head.num_classes)])
- mask_scores.append(
- [[] for _ in range(self.mask_head.num_classes)])
- else:
- segm_result = self.mask_head.get_seg_masks(
- mask_preds[i], _bboxes[i], det_labels[i],
- self.test_cfg, ori_shapes[i], scale_factors[i],
- rescale)
- # get mask scores with mask iou head
- mask_score = self.mask_iou_head.get_mask_scores(
- mask_iou_preds[i], det_bboxes[i], det_labels[i])
- segm_results.append(segm_result)
- mask_scores.append(mask_score)
- return list(zip(segm_results, mask_scores))
diff --git a/spaces/CVPR/regionclip-demo/detectron2/modeling/anchor_generator.py b/spaces/CVPR/regionclip-demo/detectron2/modeling/anchor_generator.py
deleted file mode 100644
index ee4b98819445f95982ca89a72cdd3e27b39b367f..0000000000000000000000000000000000000000
--- a/spaces/CVPR/regionclip-demo/detectron2/modeling/anchor_generator.py
+++ /dev/null
@@ -1,382 +0,0 @@
-# Copyright (c) Facebook, Inc. and its affiliates.
-import collections
-import math
-from typing import List
-import torch
-from torch import nn
-
-from detectron2.config import configurable
-from detectron2.layers import ShapeSpec
-from detectron2.structures import Boxes, RotatedBoxes
-from detectron2.utils.registry import Registry
-
-ANCHOR_GENERATOR_REGISTRY = Registry("ANCHOR_GENERATOR")
-ANCHOR_GENERATOR_REGISTRY.__doc__ = """
-Registry for modules that creates object detection anchors for feature maps.
-
-The registered object will be called with `obj(cfg, input_shape)`.
-"""
-
-
-class BufferList(nn.Module):
- """
- Similar to nn.ParameterList, but for buffers
- """
-
- def __init__(self, buffers):
- super().__init__()
- for i, buffer in enumerate(buffers):
- # Use non-persistent buffer so the values are not saved in checkpoint
- self.register_buffer(str(i), buffer, persistent=False)
-
- def __len__(self):
- return len(self._buffers)
-
- def __iter__(self):
- return iter(self._buffers.values())
-
-
-def _create_grid_offsets(size: List[int], stride: int, offset: float, device: torch.device):
- grid_height, grid_width = size
- shifts_x = torch.arange(
- offset * stride, grid_width * stride, step=stride, dtype=torch.float32, device=device
- )
- shifts_y = torch.arange(
- offset * stride, grid_height * stride, step=stride, dtype=torch.float32, device=device
- )
-
- shift_y, shift_x = torch.meshgrid(shifts_y, shifts_x)
- shift_x = shift_x.reshape(-1)
- shift_y = shift_y.reshape(-1)
- return shift_x, shift_y
-
-
-def _broadcast_params(params, num_features, name):
- """
- If one size (or aspect ratio) is specified and there are multiple feature
- maps, we "broadcast" anchors of that single size (or aspect ratio)
- over all feature maps.
-
- If params is list[float], or list[list[float]] with len(params) == 1, repeat
- it num_features time.
-
- Returns:
- list[list[float]]: param for each feature
- """
- assert isinstance(
- params, collections.abc.Sequence
- ), f"{name} in anchor generator has to be a list! Got {params}."
- assert len(params), f"{name} in anchor generator cannot be empty!"
- if not isinstance(params[0], collections.abc.Sequence): # params is list[float]
- return [params] * num_features
- if len(params) == 1:
- return list(params) * num_features
- assert len(params) == num_features, (
- f"Got {name} of length {len(params)} in anchor generator, "
- f"but the number of input features is {num_features}!"
- )
- return params
-
-
-@ANCHOR_GENERATOR_REGISTRY.register()
-class DefaultAnchorGenerator(nn.Module):
- """
- Compute anchors in the standard ways described in
- "Faster R-CNN: Towards Real-Time Object Detection with Region Proposal Networks".
- """
-
- box_dim: torch.jit.Final[int] = 4
- """
- the dimension of each anchor box.
- """
-
- @configurable
- def __init__(self, *, sizes, aspect_ratios, strides, offset=0.5):
- """
- This interface is experimental.
-
- Args:
- sizes (list[list[float]] or list[float]):
- If ``sizes`` is list[list[float]], ``sizes[i]`` is the list of anchor sizes
- (i.e. sqrt of anchor area) to use for the i-th feature map.
- If ``sizes`` is list[float], ``sizes`` is used for all feature maps.
- Anchor sizes are given in absolute lengths in units of
- the input image; they do not dynamically scale if the input image size changes.
- aspect_ratios (list[list[float]] or list[float]): list of aspect ratios
- (i.e. height / width) to use for anchors. Same "broadcast" rule for `sizes` applies.
- strides (list[int]): stride of each input feature.
- offset (float): Relative offset between the center of the first anchor and the top-left
- corner of the image. Value has to be in [0, 1).
- Recommend to use 0.5, which means half stride.
- """
- super().__init__()
-
- self.strides = strides
- self.num_features = len(self.strides)
- sizes = _broadcast_params(sizes, self.num_features, "sizes")
- aspect_ratios = _broadcast_params(aspect_ratios, self.num_features, "aspect_ratios")
- self.cell_anchors = self._calculate_anchors(sizes, aspect_ratios)
-
- self.offset = offset
- assert 0.0 <= self.offset < 1.0, self.offset
-
- @classmethod
- def from_config(cls, cfg, input_shape: List[ShapeSpec]):
- return {
- "sizes": cfg.MODEL.ANCHOR_GENERATOR.SIZES,
- "aspect_ratios": cfg.MODEL.ANCHOR_GENERATOR.ASPECT_RATIOS,
- "strides": [x.stride for x in input_shape],
- "offset": cfg.MODEL.ANCHOR_GENERATOR.OFFSET,
- }
-
- def _calculate_anchors(self, sizes, aspect_ratios):
- cell_anchors = [
- self.generate_cell_anchors(s, a).float() for s, a in zip(sizes, aspect_ratios)
- ]
- return BufferList(cell_anchors)
-
- @property
- @torch.jit.unused
- def num_cell_anchors(self):
- """
- Alias of `num_anchors`.
- """
- return self.num_anchors
-
- @property
- @torch.jit.unused
- def num_anchors(self):
- """
- Returns:
- list[int]: Each int is the number of anchors at every pixel
- location, on that feature map.
- For example, if at every pixel we use anchors of 3 aspect
- ratios and 5 sizes, the number of anchors is 15.
- (See also ANCHOR_GENERATOR.SIZES and ANCHOR_GENERATOR.ASPECT_RATIOS in config)
-
- In standard RPN models, `num_anchors` on every feature map is the same.
- """
- return [len(cell_anchors) for cell_anchors in self.cell_anchors]
-
- def _grid_anchors(self, grid_sizes: List[List[int]]):
- """
- Returns:
- list[Tensor]: #featuremap tensors, each is (#locations x #cell_anchors) x 4
- """
- anchors = []
- # buffers() not supported by torchscript. use named_buffers() instead
- buffers: List[torch.Tensor] = [x[1] for x in self.cell_anchors.named_buffers()]
- for size, stride, base_anchors in zip(grid_sizes, self.strides, buffers):
- shift_x, shift_y = _create_grid_offsets(size, stride, self.offset, base_anchors.device)
- shifts = torch.stack((shift_x, shift_y, shift_x, shift_y), dim=1)
-
- anchors.append((shifts.view(-1, 1, 4) + base_anchors.view(1, -1, 4)).reshape(-1, 4))
-
- return anchors
-
- def generate_cell_anchors(self, sizes=(32, 64, 128, 256, 512), aspect_ratios=(0.5, 1, 2)):
- """
- Generate a tensor storing canonical anchor boxes, which are all anchor
- boxes of different sizes and aspect_ratios centered at (0, 0).
- We can later build the set of anchors for a full feature map by
- shifting and tiling these tensors (see `meth:_grid_anchors`).
-
- Args:
- sizes (tuple[float]):
- aspect_ratios (tuple[float]]):
-
- Returns:
- Tensor of shape (len(sizes) * len(aspect_ratios), 4) storing anchor boxes
- in XYXY format.
- """
-
- # This is different from the anchor generator defined in the original Faster R-CNN
- # code or Detectron. They yield the same AP, however the old version defines cell
- # anchors in a less natural way with a shift relative to the feature grid and
- # quantization that results in slightly different sizes for different aspect ratios.
- # See also https://github.com/facebookresearch/Detectron/issues/227
-
- anchors = []
- for size in sizes:
- area = size ** 2.0
- for aspect_ratio in aspect_ratios:
- # s * s = w * h
- # a = h / w
- # ... some algebra ...
- # w = sqrt(s * s / a)
- # h = a * w
- w = math.sqrt(area / aspect_ratio)
- h = aspect_ratio * w
- x0, y0, x1, y1 = -w / 2.0, -h / 2.0, w / 2.0, h / 2.0
- anchors.append([x0, y0, x1, y1])
- return torch.tensor(anchors)
-
- def forward(self, features: List[torch.Tensor]):
- """
- Args:
- features (list[Tensor]): list of backbone feature maps on which to generate anchors.
-
- Returns:
- list[Boxes]: a list of Boxes containing all the anchors for each feature map
- (i.e. the cell anchors repeated over all locations in the feature map).
- The number of anchors of each feature map is Hi x Wi x num_cell_anchors,
- where Hi, Wi are resolution of the feature map divided by anchor stride.
- """
- grid_sizes = [feature_map.shape[-2:] for feature_map in features]
- anchors_over_all_feature_maps = self._grid_anchors(grid_sizes)
- return [Boxes(x) for x in anchors_over_all_feature_maps]
-
-
-@ANCHOR_GENERATOR_REGISTRY.register()
-class RotatedAnchorGenerator(nn.Module):
- """
- Compute rotated anchors used by Rotated RPN (RRPN), described in
- "Arbitrary-Oriented Scene Text Detection via Rotation Proposals".
- """
-
- box_dim: int = 5
- """
- the dimension of each anchor box.
- """
-
- @configurable
- def __init__(self, *, sizes, aspect_ratios, strides, angles, offset=0.5):
- """
- This interface is experimental.
-
- Args:
- sizes (list[list[float]] or list[float]):
- If sizes is list[list[float]], sizes[i] is the list of anchor sizes
- (i.e. sqrt of anchor area) to use for the i-th feature map.
- If sizes is list[float], the sizes are used for all feature maps.
- Anchor sizes are given in absolute lengths in units of
- the input image; they do not dynamically scale if the input image size changes.
- aspect_ratios (list[list[float]] or list[float]): list of aspect ratios
- (i.e. height / width) to use for anchors. Same "broadcast" rule for `sizes` applies.
- strides (list[int]): stride of each input feature.
- angles (list[list[float]] or list[float]): list of angles (in degrees CCW)
- to use for anchors. Same "broadcast" rule for `sizes` applies.
- offset (float): Relative offset between the center of the first anchor and the top-left
- corner of the image. Value has to be in [0, 1).
- Recommend to use 0.5, which means half stride.
- """
- super().__init__()
-
- self.strides = strides
- self.num_features = len(self.strides)
- sizes = _broadcast_params(sizes, self.num_features, "sizes")
- aspect_ratios = _broadcast_params(aspect_ratios, self.num_features, "aspect_ratios")
- angles = _broadcast_params(angles, self.num_features, "angles")
- self.cell_anchors = self._calculate_anchors(sizes, aspect_ratios, angles)
-
- self.offset = offset
- assert 0.0 <= self.offset < 1.0, self.offset
-
- @classmethod
- def from_config(cls, cfg, input_shape: List[ShapeSpec]):
- return {
- "sizes": cfg.MODEL.ANCHOR_GENERATOR.SIZES,
- "aspect_ratios": cfg.MODEL.ANCHOR_GENERATOR.ASPECT_RATIOS,
- "strides": [x.stride for x in input_shape],
- "offset": cfg.MODEL.ANCHOR_GENERATOR.OFFSET,
- "angles": cfg.MODEL.ANCHOR_GENERATOR.ANGLES,
- }
-
- def _calculate_anchors(self, sizes, aspect_ratios, angles):
- cell_anchors = [
- self.generate_cell_anchors(size, aspect_ratio, angle).float()
- for size, aspect_ratio, angle in zip(sizes, aspect_ratios, angles)
- ]
- return BufferList(cell_anchors)
-
- @property
- def num_cell_anchors(self):
- """
- Alias of `num_anchors`.
- """
- return self.num_anchors
-
- @property
- def num_anchors(self):
- """
- Returns:
- list[int]: Each int is the number of anchors at every pixel
- location, on that feature map.
- For example, if at every pixel we use anchors of 3 aspect
- ratios, 2 sizes and 5 angles, the number of anchors is 30.
- (See also ANCHOR_GENERATOR.SIZES, ANCHOR_GENERATOR.ASPECT_RATIOS
- and ANCHOR_GENERATOR.ANGLES in config)
-
- In standard RRPN models, `num_anchors` on every feature map is the same.
- """
- return [len(cell_anchors) for cell_anchors in self.cell_anchors]
-
- def _grid_anchors(self, grid_sizes):
- anchors = []
- for size, stride, base_anchors in zip(grid_sizes, self.strides, self.cell_anchors):
- shift_x, shift_y = _create_grid_offsets(size, stride, self.offset, base_anchors.device)
- zeros = torch.zeros_like(shift_x)
- shifts = torch.stack((shift_x, shift_y, zeros, zeros, zeros), dim=1)
-
- anchors.append((shifts.view(-1, 1, 5) + base_anchors.view(1, -1, 5)).reshape(-1, 5))
-
- return anchors
-
- def generate_cell_anchors(
- self,
- sizes=(32, 64, 128, 256, 512),
- aspect_ratios=(0.5, 1, 2),
- angles=(-90, -60, -30, 0, 30, 60, 90),
- ):
- """
- Generate a tensor storing canonical anchor boxes, which are all anchor
- boxes of different sizes, aspect_ratios, angles centered at (0, 0).
- We can later build the set of anchors for a full feature map by
- shifting and tiling these tensors (see `meth:_grid_anchors`).
-
- Args:
- sizes (tuple[float]):
- aspect_ratios (tuple[float]]):
- angles (tuple[float]]):
-
- Returns:
- Tensor of shape (len(sizes) * len(aspect_ratios) * len(angles), 5)
- storing anchor boxes in (x_ctr, y_ctr, w, h, angle) format.
- """
- anchors = []
- for size in sizes:
- area = size ** 2.0
- for aspect_ratio in aspect_ratios:
- # s * s = w * h
- # a = h / w
- # ... some algebra ...
- # w = sqrt(s * s / a)
- # h = a * w
- w = math.sqrt(area / aspect_ratio)
- h = aspect_ratio * w
- anchors.extend([0, 0, w, h, a] for a in angles)
-
- return torch.tensor(anchors)
-
- def forward(self, features):
- """
- Args:
- features (list[Tensor]): list of backbone feature maps on which to generate anchors.
-
- Returns:
- list[RotatedBoxes]: a list of Boxes containing all the anchors for each feature map
- (i.e. the cell anchors repeated over all locations in the feature map).
- The number of anchors of each feature map is Hi x Wi x num_cell_anchors,
- where Hi, Wi are resolution of the feature map divided by anchor stride.
- """
- grid_sizes = [feature_map.shape[-2:] for feature_map in features]
- anchors_over_all_feature_maps = self._grid_anchors(grid_sizes)
- return [RotatedBoxes(x) for x in anchors_over_all_feature_maps]
-
-
-def build_anchor_generator(cfg, input_shape):
- """
- Built an anchor generator from `cfg.MODEL.ANCHOR_GENERATOR.NAME`.
- """
- anchor_generator = cfg.MODEL.ANCHOR_GENERATOR.NAME
- return ANCHOR_GENERATOR_REGISTRY.get(anchor_generator)(cfg, input_shape)
diff --git a/spaces/CarlDennis/HYTTS/text/symbols.py b/spaces/CarlDennis/HYTTS/text/symbols.py
deleted file mode 100644
index b706ff776741e27460c77a017b442b1e994e2a33..0000000000000000000000000000000000000000
--- a/spaces/CarlDennis/HYTTS/text/symbols.py
+++ /dev/null
@@ -1,23 +0,0 @@
-'''
-Defines the set of symbols used in text input to the model.
-'''
-
-
-# cjehd_cleaners:
-_pad = '_'
-_punctuation = ',.!?…~'
-_letters = ' #*=AEINOQU^`abdefghijklmnopqrstuvwxyzãæçéðøĭŋœɐɑɔəɛɡɥɦɪɫɯɱɸɹɽɾʀʁʃʊʏʑʒʔʦʧʰˀˈˌːˑ̩̯̃͜͡βθχ⁼↑→↓šđǩḱ-ă,ś'
-
-
-# German_cleaners:
-_pad = '_'
-_punctuation =',.!?…~;:'
-_letters ="'*^_abdefghijklmnopstuvxyzçõøĭŋɐɘəɚɱɹɽɾʀʁʃʋʏʔʥʰʷˌːˑχ↓ⱼ"
-
-
-# Export all symbols:
-symbols = [_pad] + list(_punctuation) + list(_letters)
-
-# Special symbol ids
-SPACE_ID = symbols.index(" ")
-
diff --git a/spaces/CikeyQI/Yunzai/Yunzai/plugins/other/sendLog.js b/spaces/CikeyQI/Yunzai/Yunzai/plugins/other/sendLog.js
deleted file mode 100644
index 9b7d83b2c2d6bb4793da8dab1c2065c28bb35652..0000000000000000000000000000000000000000
--- a/spaces/CikeyQI/Yunzai/Yunzai/plugins/other/sendLog.js
+++ /dev/null
@@ -1,78 +0,0 @@
-import plugin from "../../lib/plugins/plugin.js"
-import common from "../../lib/common/common.js"
-import fs from "node:fs"
-import lodash from "lodash"
-import moment from "moment"
-
-export class sendLog extends plugin {
- constructor() {
- super({
- name: "发送日志",
- dsc: "发送最近100条运行日志",
- event: "message",
- rule: [
- {
- reg: "^#(运行|错误)*日志[0-9]*(.*)",
- fnc: "sendLog",
- permission: "master"
- }
- ]
- })
-
- this.lineNum = 100
- this.maxNum = 1000
-
- this.logFile = `logs/command.${moment().format("YYYY-MM-DD")}.log`
- this.errFile = "logs/error.log"
- }
-
- async sendLog() {
- let lineNum = this.e.msg.match(/\d+/g)
- if (lineNum) {
- this.lineNum = lineNum[0]
- } else {
- this.keyWord = this.e.msg.replace(/#|运行|错误|日志|\d/g, "")
- }
-
- let logFile = this.logFile
- let type = "运行"
- if (this.e.msg.includes("错误")) {
- logFile = this.errFile
- type = "错误"
- }
-
- if (this.keyWord) type = this.keyWord
-
- const log = this.getLog(logFile)
-
- if (lodash.isEmpty(log))
- return this.reply(`暂无相关日志:${type}`)
-
- return this.reply(await common.makeForwardMsg(this.e, [log.join("\n")], `最近${log.length}条${type}日志`))
- }
-
- getLog(logFile) {
- let log = fs.readFileSync(logFile, { encoding: "utf-8" })
- log = log.split("\n")
-
- if (this.keyWord) {
- for (const i in log)
- if (!log[i].includes(this.keyWord))
- delete log[i]
- } else {
- log = lodash.slice(log, (Number(this.lineNum) + 1) * -1)
- }
- log = log.reverse()
-
- const tmp = []
- for (let i of log) {
- if (!i) continue
- if (this.keyWord && tmp.length >= this.maxNum) return
- /* eslint-disable no-control-regex */
- i = i.replace(/\x1b[[0-9;]*m/g, "")
- i = i.replace(/\r|\n/, "")
- tmp.push(i)
- }
- return tmp
- }
-}
diff --git a/spaces/CikeyQI/meme-api/docs/memes.md b/spaces/CikeyQI/meme-api/docs/memes.md
deleted file mode 100644
index 2942de12f9e500f5649b543ce78f74d03ac803b9..0000000000000000000000000000000000000000
--- a/spaces/CikeyQI/meme-api/docs/memes.md
+++ /dev/null
@@ -1,2532 +0,0 @@
-# 表情列表
-
-以下为内置表情的关键词、所需参数等信息及表情预览
-
-按照表情的 `key` 排列
-
-
-1. [5000choyen (5000兆)](#5000choyen)
-2. [acg_entrance (二次元入口)](#acg_entrance)
-3. [add_chaos (添乱/给社会添乱)](#add_chaos)
-4. [addiction (上瘾/毒瘾发作)](#addiction)
-5. [alike (一样)](#alike)
-6. [always (一直)](#always)
-7. [always_like (我永远喜欢)](#always_like)
-8. [anti_kidnap (防诱拐)](#anti_kidnap)
-9. [anya_suki (阿尼亚喜欢)](#anya_suki)
-10. [applaud (鼓掌)](#applaud)
-11. [ascension (升天)](#ascension)
-12. [ask (问问)](#ask)
-13. [back_to_work (继续干活/打工人)](#back_to_work)
-14. [bad_news (悲报)](#bad_news)
-15. [beat_head (拍头)](#beat_head)
-16. [bite (啃)](#bite)
-17. [blood_pressure (高血压)](#blood_pressure)
-18. [bocchi_draft (波奇手稿)](#bocchi_draft)
-19. [bronya_holdsign (布洛妮娅举牌/大鸭鸭举牌)](#bronya_holdsign)
-20. [bubble_tea (奶茶)](#bubble_tea)
-21. [call_110 (遇到困难请拨打)](#call_110)
-22. [caoshen_bite (草神啃)](#caoshen_bite)
-23. [capoo_draw (咖波画)](#capoo_draw)
-24. [capoo_rip (咖波撕)](#capoo_rip)
-25. [capoo_rub (咖波蹭/咖波贴)](#capoo_rub)
-26. [capoo_say (咖波说)](#capoo_say)
-27. [capoo_strike (咖波撞/咖波头槌)](#capoo_strike)
-28. [captain (舰长)](#captain)
-29. [chanshenzi (馋身子)](#chanshenzi)
-30. [charpic (字符画)](#charpic)
-31. [chase_train (追列车/追火车)](#chase_train)
-32. [china_flag (国旗)](#china_flag)
-33. [confuse (迷惑)](#confuse)
-34. [coupon (兑换券)](#coupon)
-35. [cover_face (捂脸)](#cover_face)
-36. [crawl (爬)](#crawl)
-37. [cyan (群青)](#cyan)
-38. [decent_kiss (像样的亲亲)](#decent_kiss)
-39. [dianzhongdian (入典/典中典/黑白草图)](#dianzhongdian)
-40. [dinosaur (恐龙/小恐龙)](#dinosaur)
-41. [distracted (注意力涣散)](#distracted)
-42. [divorce (离婚协议/离婚申请)](#divorce)
-43. [dog_of_vtb (管人痴)](#dog_of_vtb)
-44. [dont_go_near (不要靠近)](#dont_go_near)
-45. [dont_touch (别碰)](#dont_touch)
-46. [douyin (douyin)](#douyin)
-47. [eat (吃)](#eat)
-48. [fanatic (狂爱/狂粉)](#fanatic)
-49. [fencing (击剑/🤺)](#fencing)
-50. [fill_head (满脑子)](#fill_head)
-51. [find_chips (整点薯条)](#find_chips)
-52. [flash_blind (闪瞎)](#flash_blind)
-53. [follow (关注)](#follow)
-54. [funny_mirror (哈哈镜)](#funny_mirror)
-55. [garbage (垃圾/垃圾桶)](#garbage)
-56. [genshin_start (原神启动)](#genshin_start)
-57. [good_news (喜报)](#good_news)
-58. [google (google)](#google)
-59. [guichu (鬼畜)](#guichu)
-60. [gun (手枪)](#gun)
-61. [hammer (锤)](#hammer)
-62. [high_EQ (低情商xx高情商xx)](#high_EQ)
-63. [hit_screen (打穿/打穿屏幕)](#hit_screen)
-64. [hold_grudge (记仇)](#hold_grudge)
-65. [hold_tight (抱紧)](#hold_tight)
-66. [hug_leg (抱大腿)](#hug_leg)
-67. [hutao_bite (胡桃啃)](#hutao_bite)
-68. [imprison (坐牢)](#imprison)
-69. [incivilization (不文明)](#incivilization)
-70. [interview (采访)](#interview)
-71. [jiji_king (急急国王)](#jiji_king)
-72. [jiujiu (啾啾)](#jiujiu)
-73. [kaleidoscope (万花筒/万花镜)](#kaleidoscope)
-74. [karyl_point (凯露指)](#karyl_point)
-75. [keep_away (远离)](#keep_away)
-76. [kick_ball (踢球)](#kick_ball)
-77. [kirby_hammer (卡比锤/卡比重锤)](#kirby_hammer)
-78. [kiss (亲/亲亲)](#kiss)
-79. [klee_eat (可莉吃)](#klee_eat)
-80. [knock (敲)](#knock)
-81. [learn (偷学)](#learn)
-82. [lim_x_0 (等价无穷小)](#lim_x_0)
-83. [listen_music (听音乐)](#listen_music)
-84. [little_angel (小天使)](#little_angel)
-85. [loading (加载中)](#loading)
-86. [look_flat (看扁)](#look_flat)
-87. [look_this_icon (看图标)](#look_this_icon)
-88. [love_you (永远爱你)](#love_you)
-89. [luoyonghao_say (罗永浩说)](#luoyonghao_say)
-90. [luxun_say (鲁迅说/鲁迅说过)](#luxun_say)
-91. [maikease (麦克阿瑟说)](#maikease)
-92. [maimai_awaken (旅行伙伴觉醒)](#maimai_awaken)
-93. [maimai_join (旅行伙伴加入)](#maimai_join)
-94. [make_friend (交个朋友)](#make_friend)
-95. [marriage (结婚申请/结婚登记)](#marriage)
-96. [meteor (流星)](#meteor)
-97. [mihoyo (米哈游)](#mihoyo)
-98. [mourning (上香)](#mourning)
-99. [murmur (低语)](#murmur)
-100. [my_friend (我朋友说)](#my_friend)
-101. [my_wife (我老婆/这是我老婆)](#my_wife)
-102. [name_generator (亚文化取名机/亚名)](#name_generator)
-103. [need (需要/你可能需要)](#need)
-104. [nekoha_holdsign (猫羽雫举牌/猫猫举牌)](#nekoha_holdsign)
-105. [nihaosaoa (你好骚啊)](#nihaosaoa)
-106. [nijika_holdsign (伊地知虹夏举牌/虹夏举牌)](#nijika_holdsign)
-107. [no_response (无响应)](#no_response)
-108. [nokia (诺基亚/有内鬼)](#nokia)
-109. [not_call_me (不喊我)](#not_call_me)
-110. [note_for_leave (请假条)](#note_for_leave)
-111. [oshi_no_ko (我推的网友)](#oshi_no_ko)
-112. [osu (osu)](#osu)
-113. [overtime (加班)](#overtime)
-114. [paint (这像画吗)](#paint)
-115. [painter (小画家)](#painter)
-116. [pass_the_buck (推锅/甩锅)](#pass_the_buck)
-117. [pat (拍)](#pat)
-118. [perfect (完美)](#perfect)
-119. [petpet (摸/摸摸/摸头/rua)](#petpet)
-120. [play (顶/玩)](#play)
-121. [play_game (玩游戏)](#play_game)
-122. [police (出警)](#police)
-123. [police1 (警察)](#police1)
-124. [pornhub (ph/pornhub)](#pornhub)
-125. [potato (土豆)](#potato)
-126. [pound (捣)](#pound)
-127. [printing (打印)](#printing)
-128. [prpr (舔/舔屏/prpr)](#prpr)
-129. [psyduck (可达鸭)](#psyduck)
-130. [punch (打拳)](#punch)
-131. [qiegewala (切格瓦拉)](#qiegewala)
-132. [raise_image (举)](#raise_image)
-133. [raise_sign (举牌)](#raise_sign)
-134. [read_book (看书)](#read_book)
-135. [repeat (复读)](#repeat)
-136. [rip (撕)](#rip)
-137. [rip_angrily (怒撕)](#rip_angrily)
-138. [rise_dead (诈尸/秽土转生)](#rise_dead)
-139. [roll (滚)](#roll)
-140. [rub (贴/贴贴/蹭/蹭蹭)](#rub)
-141. [run (快跑)](#run)
-142. [safe_sense (安全感)](#safe_sense)
-143. [scratch_head (挠头)](#scratch_head)
-144. [scratchcard (刮刮乐)](#scratchcard)
-145. [scroll (滚屏)](#scroll)
-146. [shishilani (食屎啦你)](#shishilani)
-147. [shock (震惊)](#shock)
-148. [shuifandui (谁反对)](#shuifandui)
-149. [shutup (别说了)](#shutup)
-150. [sit_still (坐得住/坐的住)](#sit_still)
-151. [slap (一巴掌)](#slap)
-152. [slogan (口号)](#slogan)
-153. [smash (砸)](#smash)
-154. [step_on (踩)](#step_on)
-155. [suck (吸/嗦)](#suck)
-156. [support (精神支柱)](#support)
-157. [symmetric (对称)](#symmetric)
-158. [tankuku_raisesign (唐可可举牌)](#tankuku_raisesign)
-159. [taunt (嘲讽)](#taunt)
-160. [teach (讲课/敲黑板)](#teach)
-161. [tease (拿捏/戏弄)](#tease)
-162. [think_what (想什么)](#think_what)
-163. [throw (丢/扔)](#throw)
-164. [throw_gif (抛/掷)](#throw_gif)
-165. [thump (捶)](#thump)
-166. [thump_wildly (捶爆/爆捶)](#thump_wildly)
-167. [tightly (紧贴/紧紧贴着)](#tightly)
-168. [together (一起)](#together)
-169. [trance (恍惚)](#trance)
-170. [turn (转)](#turn)
-171. [twist (搓)](#twist)
-172. [universal (万能表情/空白表情)](#universal)
-173. [vibrate (震动)](#vibrate)
-174. [wakeup (xx起来了)](#wakeup)
-175. [wallpaper (墙纸)](#wallpaper)
-176. [walnut_pad (胡桃平板)](#walnut_pad)
-177. [walnut_zoom (胡桃放大)](#walnut_zoom)
-178. [wangjingze (王境泽)](#wangjingze)
-179. [wave (波纹)](#wave)
-180. [weisuoyuwei (为所欲为)](#weisuoyuwei)
-181. [what_I_want_to_do (我想上的)](#what_I_want_to_do)
-182. [what_he_wants (最想要的东西)](#what_he_wants)
-183. [why_at_me (为什么@我)](#why_at_me)
-184. [why_have_hands (为什么要有手)](#why_have_hands)
-185. [windmill_turn (风车转)](#windmill_turn)
-186. [wish_fail (许愿失败)](#wish_fail)
-187. [wooden_fish (木鱼)](#wooden_fish)
-188. [worship (膜/膜拜)](#worship)
-189. [wujing (吴京xx中国xx)](#wujing)
-190. [wunian (五年怎么过的)](#wunian)
-191. [yalidaye (压力大爷)](#yalidaye)
-192. [youtube (yt/youtube)](#youtube)
-193. [zengxiaoxian (曾小贤)](#zengxiaoxian)
-
-
-## 5000choyen
-
-- 关键词:`5000兆`
-- 需要图片数目:`0`
-- 需要文字数目:`2`
-- 默认文字:[`我去`, `洛天依`]
-- 预览:
-
diff --git a/spaces/DQChoi/gpt-demo/venv/lib/python3.11/site-packages/fontTools/misc/loggingTools.py b/spaces/DQChoi/gpt-demo/venv/lib/python3.11/site-packages/fontTools/misc/loggingTools.py
deleted file mode 100644
index 78704f5a9aa4811db98aa3132ed3f12ee0853ee2..0000000000000000000000000000000000000000
--- a/spaces/DQChoi/gpt-demo/venv/lib/python3.11/site-packages/fontTools/misc/loggingTools.py
+++ /dev/null
@@ -1,543 +0,0 @@
-import sys
-import logging
-import timeit
-from functools import wraps
-from collections.abc import Mapping, Callable
-import warnings
-from logging import PercentStyle
-
-
-# default logging level used by Timer class
-TIME_LEVEL = logging.DEBUG
-
-# per-level format strings used by the default formatter
-# (the level name is not printed for INFO and DEBUG messages)
-DEFAULT_FORMATS = {
- "*": "%(levelname)s: %(message)s",
- "INFO": "%(message)s",
- "DEBUG": "%(message)s",
-}
-
-
-class LevelFormatter(logging.Formatter):
- """Log formatter with level-specific formatting.
-
- Formatter class which optionally takes a dict of logging levels to
- format strings, allowing to customise the log records appearance for
- specific levels.
-
-
- Attributes:
- fmt: A dictionary mapping logging levels to format strings.
- The ``*`` key identifies the default format string.
- datefmt: As per py:class:`logging.Formatter`
- style: As per py:class:`logging.Formatter`
-
- >>> import sys
- >>> handler = logging.StreamHandler(sys.stdout)
- >>> formatter = LevelFormatter(
- ... fmt={
- ... '*': '[%(levelname)s] %(message)s',
- ... 'DEBUG': '%(name)s [%(levelname)s] %(message)s',
- ... 'INFO': '%(message)s',
- ... })
- >>> handler.setFormatter(formatter)
- >>> log = logging.getLogger('test')
- >>> log.setLevel(logging.DEBUG)
- >>> log.addHandler(handler)
- >>> log.debug('this uses a custom format string')
- test [DEBUG] this uses a custom format string
- >>> log.info('this also uses a custom format string')
- this also uses a custom format string
- >>> log.warning("this one uses the default format string")
- [WARNING] this one uses the default format string
- """
-
- def __init__(self, fmt=None, datefmt=None, style="%"):
- if style != "%":
- raise ValueError(
- "only '%' percent style is supported in both python 2 and 3"
- )
- if fmt is None:
- fmt = DEFAULT_FORMATS
- if isinstance(fmt, str):
- default_format = fmt
- custom_formats = {}
- elif isinstance(fmt, Mapping):
- custom_formats = dict(fmt)
- default_format = custom_formats.pop("*", None)
- else:
- raise TypeError("fmt must be a str or a dict of str: %r" % fmt)
- super(LevelFormatter, self).__init__(default_format, datefmt)
- self.default_format = self._fmt
- self.custom_formats = {}
- for level, fmt in custom_formats.items():
- level = logging._checkLevel(level)
- self.custom_formats[level] = fmt
-
- def format(self, record):
- if self.custom_formats:
- fmt = self.custom_formats.get(record.levelno, self.default_format)
- if self._fmt != fmt:
- self._fmt = fmt
- # for python >= 3.2, _style needs to be set if _fmt changes
- if PercentStyle:
- self._style = PercentStyle(fmt)
- return super(LevelFormatter, self).format(record)
-
-
-def configLogger(**kwargs):
- """A more sophisticated logging system configuation manager.
-
- This is more or less the same as :py:func:`logging.basicConfig`,
- with some additional options and defaults.
-
- The default behaviour is to create a ``StreamHandler`` which writes to
- sys.stderr, set a formatter using the ``DEFAULT_FORMATS`` strings, and add
- the handler to the top-level library logger ("fontTools").
-
- A number of optional keyword arguments may be specified, which can alter
- the default behaviour.
-
- Args:
-
- logger: Specifies the logger name or a Logger instance to be
- configured. (Defaults to "fontTools" logger). Unlike ``basicConfig``,
- this function can be called multiple times to reconfigure a logger.
- If the logger or any of its children already exists before the call is
- made, they will be reset before the new configuration is applied.
- filename: Specifies that a ``FileHandler`` be created, using the
- specified filename, rather than a ``StreamHandler``.
- filemode: Specifies the mode to open the file, if filename is
- specified. (If filemode is unspecified, it defaults to ``a``).
- format: Use the specified format string for the handler. This
- argument also accepts a dictionary of format strings keyed by
- level name, to allow customising the records appearance for
- specific levels. The special ``'*'`` key is for 'any other' level.
- datefmt: Use the specified date/time format.
- level: Set the logger level to the specified level.
- stream: Use the specified stream to initialize the StreamHandler. Note
- that this argument is incompatible with ``filename`` - if both
- are present, ``stream`` is ignored.
- handlers: If specified, this should be an iterable of already created
- handlers, which will be added to the logger. Any handler in the
- list which does not have a formatter assigned will be assigned the
- formatter created in this function.
- filters: If specified, this should be an iterable of already created
- filters. If the ``handlers`` do not already have filters assigned,
- these filters will be added to them.
- propagate: All loggers have a ``propagate`` attribute which determines
- whether to continue searching for handlers up the logging hierarchy.
- If not provided, the "propagate" attribute will be set to ``False``.
- """
- # using kwargs to enforce keyword-only arguments in py2.
- handlers = kwargs.pop("handlers", None)
- if handlers is None:
- if "stream" in kwargs and "filename" in kwargs:
- raise ValueError(
- "'stream' and 'filename' should not be " "specified together"
- )
- else:
- if "stream" in kwargs or "filename" in kwargs:
- raise ValueError(
- "'stream' or 'filename' should not be "
- "specified together with 'handlers'"
- )
- if handlers is None:
- filename = kwargs.pop("filename", None)
- mode = kwargs.pop("filemode", "a")
- if filename:
- h = logging.FileHandler(filename, mode)
- else:
- stream = kwargs.pop("stream", None)
- h = logging.StreamHandler(stream)
- handlers = [h]
- # By default, the top-level library logger is configured.
- logger = kwargs.pop("logger", "fontTools")
- if not logger or isinstance(logger, str):
- # empty "" or None means the 'root' logger
- logger = logging.getLogger(logger)
- # before (re)configuring, reset named logger and its children (if exist)
- _resetExistingLoggers(parent=logger.name)
- # use DEFAULT_FORMATS if 'format' is None
- fs = kwargs.pop("format", None)
- dfs = kwargs.pop("datefmt", None)
- # XXX: '%' is the only format style supported on both py2 and 3
- style = kwargs.pop("style", "%")
- fmt = LevelFormatter(fs, dfs, style)
- filters = kwargs.pop("filters", [])
- for h in handlers:
- if h.formatter is None:
- h.setFormatter(fmt)
- if not h.filters:
- for f in filters:
- h.addFilter(f)
- logger.addHandler(h)
- if logger.name != "root":
- # stop searching up the hierarchy for handlers
- logger.propagate = kwargs.pop("propagate", False)
- # set a custom severity level
- level = kwargs.pop("level", None)
- if level is not None:
- logger.setLevel(level)
- if kwargs:
- keys = ", ".join(kwargs.keys())
- raise ValueError("Unrecognised argument(s): %s" % keys)
-
-
-def _resetExistingLoggers(parent="root"):
- """Reset the logger named 'parent' and all its children to their initial
- state, if they already exist in the current configuration.
- """
- root = logging.root
- # get sorted list of all existing loggers
- existing = sorted(root.manager.loggerDict.keys())
- if parent == "root":
- # all the existing loggers are children of 'root'
- loggers_to_reset = [parent] + existing
- elif parent not in existing:
- # nothing to do
- return
- elif parent in existing:
- loggers_to_reset = [parent]
- # collect children, starting with the entry after parent name
- i = existing.index(parent) + 1
- prefixed = parent + "."
- pflen = len(prefixed)
- num_existing = len(existing)
- while i < num_existing:
- if existing[i][:pflen] == prefixed:
- loggers_to_reset.append(existing[i])
- i += 1
- for name in loggers_to_reset:
- if name == "root":
- root.setLevel(logging.WARNING)
- for h in root.handlers[:]:
- root.removeHandler(h)
- for f in root.filters[:]:
- root.removeFilters(f)
- root.disabled = False
- else:
- logger = root.manager.loggerDict[name]
- logger.level = logging.NOTSET
- logger.handlers = []
- logger.filters = []
- logger.propagate = True
- logger.disabled = False
-
-
-class Timer(object):
- """Keeps track of overall time and split/lap times.
-
- >>> import time
- >>> timer = Timer()
- >>> time.sleep(0.01)
- >>> print("First lap:", timer.split())
- First lap: ...
- >>> time.sleep(0.02)
- >>> print("Second lap:", timer.split())
- Second lap: ...
- >>> print("Overall time:", timer.time())
- Overall time: ...
-
- Can be used as a context manager inside with-statements.
-
- >>> with Timer() as t:
- ... time.sleep(0.01)
- >>> print("%0.3f seconds" % t.elapsed)
- 0... seconds
-
- If initialised with a logger, it can log the elapsed time automatically
- upon exiting the with-statement.
-
- >>> import logging
- >>> log = logging.getLogger("my-fancy-timer-logger")
- >>> configLogger(logger=log, level="DEBUG", format="%(message)s", stream=sys.stdout)
- >>> with Timer(log, 'do something'):
- ... time.sleep(0.01)
- Took ... to do something
-
- The same Timer instance, holding a reference to a logger, can be reused
- in multiple with-statements, optionally with different messages or levels.
-
- >>> timer = Timer(log)
- >>> with timer():
- ... time.sleep(0.01)
- elapsed time: ...s
- >>> with timer('redo it', level=logging.INFO):
- ... time.sleep(0.02)
- Took ... to redo it
-
- It can also be used as a function decorator to log the time elapsed to run
- the decorated function.
-
- >>> @timer()
- ... def test1():
- ... time.sleep(0.01)
- >>> @timer('run test 2', level=logging.INFO)
- ... def test2():
- ... time.sleep(0.02)
- >>> test1()
- Took ... to run 'test1'
- >>> test2()
- Took ... to run test 2
- """
-
- # timeit.default_timer choses the most accurate clock for each platform
- _time = timeit.default_timer
- default_msg = "elapsed time: %(time).3fs"
- default_format = "Took %(time).3fs to %(msg)s"
-
- def __init__(self, logger=None, msg=None, level=None, start=None):
- self.reset(start)
- if logger is None:
- for arg in ("msg", "level"):
- if locals().get(arg) is not None:
- raise ValueError("'%s' can't be specified without a 'logger'" % arg)
- self.logger = logger
- self.level = level if level is not None else TIME_LEVEL
- self.msg = msg
-
- def reset(self, start=None):
- """Reset timer to 'start_time' or the current time."""
- if start is None:
- self.start = self._time()
- else:
- self.start = start
- self.last = self.start
- self.elapsed = 0.0
-
- def time(self):
- """Return the overall time (in seconds) since the timer started."""
- return self._time() - self.start
-
- def split(self):
- """Split and return the lap time (in seconds) in between splits."""
- current = self._time()
- self.elapsed = current - self.last
- self.last = current
- return self.elapsed
-
- def formatTime(self, msg, time):
- """Format 'time' value in 'msg' and return formatted string.
- If 'msg' contains a '%(time)' format string, try to use that.
- Otherwise, use the predefined 'default_format'.
- If 'msg' is empty or None, fall back to 'default_msg'.
- """
- if not msg:
- msg = self.default_msg
- if msg.find("%(time)") < 0:
- msg = self.default_format % {"msg": msg, "time": time}
- else:
- try:
- msg = msg % {"time": time}
- except (KeyError, ValueError):
- pass # skip if the format string is malformed
- return msg
-
- def __enter__(self):
- """Start a new lap"""
- self.last = self._time()
- self.elapsed = 0.0
- return self
-
- def __exit__(self, exc_type, exc_value, traceback):
- """End the current lap. If timer has a logger, log the time elapsed,
- using the format string in self.msg (or the default one).
- """
- time = self.split()
- if self.logger is None or exc_type:
- # if there's no logger attached, or if any exception occurred in
- # the with-statement, exit without logging the time
- return
- message = self.formatTime(self.msg, time)
- # Allow log handlers to see the individual parts to facilitate things
- # like a server accumulating aggregate stats.
- msg_parts = {"msg": self.msg, "time": time}
- self.logger.log(self.level, message, msg_parts)
-
- def __call__(self, func_or_msg=None, **kwargs):
- """If the first argument is a function, return a decorator which runs
- the wrapped function inside Timer's context manager.
- Otherwise, treat the first argument as a 'msg' string and return an updated
- Timer instance, referencing the same logger.
- A 'level' keyword can also be passed to override self.level.
- """
- if isinstance(func_or_msg, Callable):
- func = func_or_msg
- # use the function name when no explicit 'msg' is provided
- if not self.msg:
- self.msg = "run '%s'" % func.__name__
-
- @wraps(func)
- def wrapper(*args, **kwds):
- with self:
- return func(*args, **kwds)
-
- return wrapper
- else:
- msg = func_or_msg or kwargs.get("msg")
- level = kwargs.get("level", self.level)
- return self.__class__(self.logger, msg, level)
-
- def __float__(self):
- return self.elapsed
-
- def __int__(self):
- return int(self.elapsed)
-
- def __str__(self):
- return "%.3f" % self.elapsed
-
-
-class ChannelsFilter(logging.Filter):
- """Provides a hierarchical filter for log entries based on channel names.
-
- Filters out records emitted from a list of enabled channel names,
- including their children. It works the same as the ``logging.Filter``
- class, but allows the user to specify multiple channel names.
-
- >>> import sys
- >>> handler = logging.StreamHandler(sys.stdout)
- >>> handler.setFormatter(logging.Formatter("%(message)s"))
- >>> filter = ChannelsFilter("A.B", "C.D")
- >>> handler.addFilter(filter)
- >>> root = logging.getLogger()
- >>> root.addHandler(handler)
- >>> root.setLevel(level=logging.DEBUG)
- >>> logging.getLogger('A.B').debug('this record passes through')
- this record passes through
- >>> logging.getLogger('A.B.C').debug('records from children also pass')
- records from children also pass
- >>> logging.getLogger('C.D').debug('this one as well')
- this one as well
- >>> logging.getLogger('A.B.').debug('also this one')
- also this one
- >>> logging.getLogger('A.F').debug('but this one does not!')
- >>> logging.getLogger('C.DE').debug('neither this one!')
- """
-
- def __init__(self, *names):
- self.names = names
- self.num = len(names)
- self.lengths = {n: len(n) for n in names}
-
- def filter(self, record):
- if self.num == 0:
- return True
- for name in self.names:
- nlen = self.lengths[name]
- if name == record.name:
- return True
- elif record.name.find(name, 0, nlen) == 0 and record.name[nlen] == ".":
- return True
- return False
-
-
-class CapturingLogHandler(logging.Handler):
- def __init__(self, logger, level):
- super(CapturingLogHandler, self).__init__(level=level)
- self.records = []
- if isinstance(logger, str):
- self.logger = logging.getLogger(logger)
- else:
- self.logger = logger
-
- def __enter__(self):
- self.original_disabled = self.logger.disabled
- self.original_level = self.logger.level
- self.original_propagate = self.logger.propagate
-
- self.logger.addHandler(self)
- self.logger.setLevel(self.level)
- self.logger.disabled = False
- self.logger.propagate = False
-
- return self
-
- def __exit__(self, type, value, traceback):
- self.logger.removeHandler(self)
- self.logger.setLevel(self.original_level)
- self.logger.disabled = self.original_disabled
- self.logger.propagate = self.original_propagate
-
- return self
-
- def emit(self, record):
- self.records.append(record)
-
- def assertRegex(self, regexp, msg=None):
- import re
-
- pattern = re.compile(regexp)
- for r in self.records:
- if pattern.search(r.getMessage()):
- return True
- if msg is None:
- msg = "Pattern '%s' not found in logger records" % regexp
- assert 0, msg
-
-
-class LogMixin(object):
- """Mixin class that adds logging functionality to another class.
-
- You can define a new class that subclasses from ``LogMixin`` as well as
- other base classes through multiple inheritance.
- All instances of that class will have a ``log`` property that returns
- a ``logging.Logger`` named after their respective ``.``.
-
- For example:
-
- >>> class BaseClass(object):
- ... pass
- >>> class MyClass(LogMixin, BaseClass):
- ... pass
- >>> a = MyClass()
- >>> isinstance(a.log, logging.Logger)
- True
- >>> print(a.log.name)
- fontTools.misc.loggingTools.MyClass
- >>> class AnotherClass(MyClass):
- ... pass
- >>> b = AnotherClass()
- >>> isinstance(b.log, logging.Logger)
- True
- >>> print(b.log.name)
- fontTools.misc.loggingTools.AnotherClass
- """
-
- @property
- def log(self):
- if not hasattr(self, "_log"):
- name = ".".join((self.__class__.__module__, self.__class__.__name__))
- self._log = logging.getLogger(name)
- return self._log
-
-
-def deprecateArgument(name, msg, category=UserWarning):
- """Raise a warning about deprecated function argument 'name'."""
- warnings.warn("%r is deprecated; %s" % (name, msg), category=category, stacklevel=3)
-
-
-def deprecateFunction(msg, category=UserWarning):
- """Decorator to raise a warning when a deprecated function is called."""
-
- def decorator(func):
- @wraps(func)
- def wrapper(*args, **kwargs):
- warnings.warn(
- "%r is deprecated; %s" % (func.__name__, msg),
- category=category,
- stacklevel=2,
- )
- return func(*args, **kwargs)
-
- return wrapper
-
- return decorator
-
-
-if __name__ == "__main__":
- import doctest
-
- sys.exit(doctest.testmod(optionflags=doctest.ELLIPSIS).failed)
diff --git a/spaces/Danielzero/GPT3.5/chatgpt - windows.bat b/spaces/Danielzero/GPT3.5/chatgpt - windows.bat
deleted file mode 100644
index 0b78fdc3a559abd692e3a9e9af5e482124d13a99..0000000000000000000000000000000000000000
--- a/spaces/Danielzero/GPT3.5/chatgpt - windows.bat
+++ /dev/null
@@ -1,14 +0,0 @@
-@echo off
-echo Opening ChuanhuChatGPT...
-
-REM Open powershell via bat
-start powershell.exe -NoExit -Command "python ./ChuanhuChatbot.py"
-
-REM The web page can be accessed with delayed start http://127.0.0.1:7860/
-ping -n 5 127.0.0.1>nul
-
-REM access chargpt via your default browser
-start "" "http://127.0.0.1:7860/"
-
-
-echo Finished opening ChuanhuChatGPT (http://127.0.0.1:7860/).
\ No newline at end of file
diff --git a/spaces/DragGan/DragGan/stylegan_human/dnnlib/tflib/optimizer.py b/spaces/DragGan/DragGan/stylegan_human/dnnlib/tflib/optimizer.py
deleted file mode 100644
index 93d5dcc6172209985308784c9b9e590759612a0b..0000000000000000000000000000000000000000
--- a/spaces/DragGan/DragGan/stylegan_human/dnnlib/tflib/optimizer.py
+++ /dev/null
@@ -1,338 +0,0 @@
-# Copyright (c) SenseTime Research. All rights reserved.
-
-# Copyright (c) 2019, NVIDIA Corporation. All rights reserved.
-#
-# This work is made available under the Nvidia Source Code License-NC.
-# To view a copy of this license, visit
-# https://nvlabs.github.io/stylegan2/license.html
-
-"""Helper wrapper for a Tensorflow optimizer."""
-
-import numpy as np
-import tensorflow as tf
-
-from collections import OrderedDict
-from typing import List, Union
-
-from . import autosummary
-from . import tfutil
-from .. import util
-
-from .tfutil import TfExpression, TfExpressionEx
-
-try:
- # TensorFlow 1.13
- from tensorflow.python.ops import nccl_ops
-except:
- # Older TensorFlow versions
- import tensorflow.contrib.nccl as nccl_ops
-
-class Optimizer:
- """A Wrapper for tf.train.Optimizer.
-
- Automatically takes care of:
- - Gradient averaging for multi-GPU training.
- - Gradient accumulation for arbitrarily large minibatches.
- - Dynamic loss scaling and typecasts for FP16 training.
- - Ignoring corrupted gradients that contain NaNs/Infs.
- - Reporting statistics.
- - Well-chosen default settings.
- """
-
- def __init__(self,
- name: str = "Train", # Name string that will appear in TensorFlow graph.
- tf_optimizer: str = "tf.train.AdamOptimizer", # Underlying optimizer class.
- learning_rate: TfExpressionEx = 0.001, # Learning rate. Can vary over time.
- minibatch_multiplier: TfExpressionEx = None, # Treat N consecutive minibatches as one by accumulating gradients.
- share: "Optimizer" = None, # Share internal state with a previously created optimizer?
- use_loss_scaling: bool = False, # Enable dynamic loss scaling for robust mixed-precision training?
- loss_scaling_init: float = 64.0, # Log2 of initial loss scaling factor.
- loss_scaling_inc: float = 0.0005, # Log2 of per-minibatch loss scaling increment when there is no overflow.
- loss_scaling_dec: float = 1.0, # Log2 of per-minibatch loss scaling decrement when there is an overflow.
- report_mem_usage: bool = False, # Report fine-grained memory usage statistics in TensorBoard?
- **kwargs):
-
- # Public fields.
- self.name = name
- self.learning_rate = learning_rate
- self.minibatch_multiplier = minibatch_multiplier
- self.id = self.name.replace("/", ".")
- self.scope = tf.get_default_graph().unique_name(self.id)
- self.optimizer_class = util.get_obj_by_name(tf_optimizer)
- self.optimizer_kwargs = dict(kwargs)
- self.use_loss_scaling = use_loss_scaling
- self.loss_scaling_init = loss_scaling_init
- self.loss_scaling_inc = loss_scaling_inc
- self.loss_scaling_dec = loss_scaling_dec
-
- # Private fields.
- self._updates_applied = False
- self._devices = OrderedDict() # device_name => EasyDict()
- self._shared_optimizers = OrderedDict() # device_name => optimizer_class
- self._gradient_shapes = None # [shape, ...]
- self._report_mem_usage = report_mem_usage
-
- # Validate arguments.
- assert callable(self.optimizer_class)
-
- # Share internal state if requested.
- if share is not None:
- assert isinstance(share, Optimizer)
- assert self.optimizer_class is share.optimizer_class
- assert self.learning_rate is share.learning_rate
- assert self.optimizer_kwargs == share.optimizer_kwargs
- self._shared_optimizers = share._shared_optimizers # pylint: disable=protected-access
-
- def _get_device(self, device_name: str):
- """Get internal state for the given TensorFlow device."""
- tfutil.assert_tf_initialized()
- if device_name in self._devices:
- return self._devices[device_name]
-
- # Initialize fields.
- device = util.EasyDict()
- device.name = device_name
- device.optimizer = None # Underlying optimizer: optimizer_class
- device.loss_scaling_var = None # Log2 of loss scaling: tf.Variable
- device.grad_raw = OrderedDict() # Raw gradients: var => [grad, ...]
- device.grad_clean = OrderedDict() # Clean gradients: var => grad
- device.grad_acc_vars = OrderedDict() # Accumulation sums: var => tf.Variable
- device.grad_acc_count = None # Accumulation counter: tf.Variable
- device.grad_acc = OrderedDict() # Accumulated gradients: var => grad
-
- # Setup TensorFlow objects.
- with tfutil.absolute_name_scope(self.scope + "/Devices"), tf.device(device_name), tf.control_dependencies(None):
- if device_name not in self._shared_optimizers:
- optimizer_name = self.scope.replace("/", "_") + "_opt%d" % len(self._shared_optimizers)
- self._shared_optimizers[device_name] = self.optimizer_class(name=optimizer_name, learning_rate=self.learning_rate, **self.optimizer_kwargs)
- device.optimizer = self._shared_optimizers[device_name]
- if self.use_loss_scaling:
- device.loss_scaling_var = tf.Variable(np.float32(self.loss_scaling_init), trainable=False, name="loss_scaling_var")
-
- # Register device.
- self._devices[device_name] = device
- return device
-
- def register_gradients(self, loss: TfExpression, trainable_vars: Union[List, dict]) -> None:
- """Register the gradients of the given loss function with respect to the given variables.
- Intended to be called once per GPU."""
- tfutil.assert_tf_initialized()
- assert not self._updates_applied
- device = self._get_device(loss.device)
-
- # Validate trainables.
- if isinstance(trainable_vars, dict):
- trainable_vars = list(trainable_vars.values()) # allow passing in Network.trainables as vars
- assert isinstance(trainable_vars, list) and len(trainable_vars) >= 1
- assert all(tfutil.is_tf_expression(expr) for expr in trainable_vars + [loss])
- assert all(var.device == device.name for var in trainable_vars)
-
- # Validate shapes.
- if self._gradient_shapes is None:
- self._gradient_shapes = [var.shape.as_list() for var in trainable_vars]
- assert len(trainable_vars) == len(self._gradient_shapes)
- assert all(var.shape.as_list() == var_shape for var, var_shape in zip(trainable_vars, self._gradient_shapes))
-
- # Report memory usage if requested.
- deps = []
- if self._report_mem_usage:
- self._report_mem_usage = False
- try:
- with tf.name_scope(self.id + '_mem'), tf.device(device.name), tf.control_dependencies([loss]):
- deps.append(autosummary.autosummary(self.id + "/mem_usage_gb", tf.contrib.memory_stats.BytesInUse() / 2**30))
- except tf.errors.NotFoundError:
- pass
-
- # Compute gradients.
- with tf.name_scope(self.id + "_grad"), tf.device(device.name), tf.control_dependencies(deps):
- loss = self.apply_loss_scaling(tf.cast(loss, tf.float32))
- gate = tf.train.Optimizer.GATE_NONE # disable gating to reduce memory usage
- grad_list = device.optimizer.compute_gradients(loss=loss, var_list=trainable_vars, gate_gradients=gate)
-
- # Register gradients.
- for grad, var in grad_list:
- if var not in device.grad_raw:
- device.grad_raw[var] = []
- device.grad_raw[var].append(grad)
-
- def apply_updates(self, allow_no_op: bool = False) -> tf.Operation:
- """Construct training op to update the registered variables based on their gradients."""
- tfutil.assert_tf_initialized()
- assert not self._updates_applied
- self._updates_applied = True
- all_ops = []
-
- # Check for no-op.
- if allow_no_op and len(self._devices) == 0:
- with tfutil.absolute_name_scope(self.scope):
- return tf.no_op(name='TrainingOp')
-
- # Clean up gradients.
- for device_idx, device in enumerate(self._devices.values()):
- with tfutil.absolute_name_scope(self.scope + "/Clean%d" % device_idx), tf.device(device.name):
- for var, grad in device.grad_raw.items():
-
- # Filter out disconnected gradients and convert to float32.
- grad = [g for g in grad if g is not None]
- grad = [tf.cast(g, tf.float32) for g in grad]
-
- # Sum within the device.
- if len(grad) == 0:
- grad = tf.zeros(var.shape) # No gradients => zero.
- elif len(grad) == 1:
- grad = grad[0] # Single gradient => use as is.
- else:
- grad = tf.add_n(grad) # Multiple gradients => sum.
-
- # Scale as needed.
- scale = 1.0 / len(device.grad_raw[var]) / len(self._devices)
- scale = tf.constant(scale, dtype=tf.float32, name="scale")
- if self.minibatch_multiplier is not None:
- scale /= tf.cast(self.minibatch_multiplier, tf.float32)
- scale = self.undo_loss_scaling(scale)
- device.grad_clean[var] = grad * scale
-
- # Sum gradients across devices.
- if len(self._devices) > 1:
- with tfutil.absolute_name_scope(self.scope + "/Broadcast"), tf.device(None):
- for all_vars in zip(*[device.grad_clean.keys() for device in self._devices.values()]):
- if len(all_vars) > 0 and all(dim > 0 for dim in all_vars[0].shape.as_list()): # NCCL does not support zero-sized tensors.
- all_grads = [device.grad_clean[var] for device, var in zip(self._devices.values(), all_vars)]
- all_grads = nccl_ops.all_sum(all_grads)
- for device, var, grad in zip(self._devices.values(), all_vars, all_grads):
- device.grad_clean[var] = grad
-
- # Apply updates separately on each device.
- for device_idx, device in enumerate(self._devices.values()):
- with tfutil.absolute_name_scope(self.scope + "/Apply%d" % device_idx), tf.device(device.name):
- # pylint: disable=cell-var-from-loop
-
- # Accumulate gradients over time.
- if self.minibatch_multiplier is None:
- acc_ok = tf.constant(True, name='acc_ok')
- device.grad_acc = OrderedDict(device.grad_clean)
- else:
- # Create variables.
- with tf.control_dependencies(None):
- for var in device.grad_clean.keys():
- device.grad_acc_vars[var] = tf.Variable(tf.zeros(var.shape), trainable=False, name="grad_acc_var")
- device.grad_acc_count = tf.Variable(tf.zeros([]), trainable=False, name="grad_acc_count")
-
- # Track counter.
- count_cur = device.grad_acc_count + 1.0
- count_inc_op = lambda: tf.assign(device.grad_acc_count, count_cur)
- count_reset_op = lambda: tf.assign(device.grad_acc_count, tf.zeros([]))
- acc_ok = (count_cur >= tf.cast(self.minibatch_multiplier, tf.float32))
- all_ops.append(tf.cond(acc_ok, count_reset_op, count_inc_op))
-
- # Track gradients.
- for var, grad in device.grad_clean.items():
- acc_var = device.grad_acc_vars[var]
- acc_cur = acc_var + grad
- device.grad_acc[var] = acc_cur
- with tf.control_dependencies([acc_cur]):
- acc_inc_op = lambda: tf.assign(acc_var, acc_cur)
- acc_reset_op = lambda: tf.assign(acc_var, tf.zeros(var.shape))
- all_ops.append(tf.cond(acc_ok, acc_reset_op, acc_inc_op))
-
- # No overflow => apply gradients.
- all_ok = tf.reduce_all(tf.stack([acc_ok] + [tf.reduce_all(tf.is_finite(g)) for g in device.grad_acc.values()]))
- apply_op = lambda: device.optimizer.apply_gradients([(tf.cast(grad, var.dtype), var) for var, grad in device.grad_acc.items()])
- all_ops.append(tf.cond(all_ok, apply_op, tf.no_op))
-
- # Adjust loss scaling.
- if self.use_loss_scaling:
- ls_inc_op = lambda: tf.assign_add(device.loss_scaling_var, self.loss_scaling_inc)
- ls_dec_op = lambda: tf.assign_sub(device.loss_scaling_var, self.loss_scaling_dec)
- ls_update_op = lambda: tf.group(tf.cond(all_ok, ls_inc_op, ls_dec_op))
- all_ops.append(tf.cond(acc_ok, ls_update_op, tf.no_op))
-
- # Last device => report statistics.
- if device_idx == len(self._devices) - 1:
- all_ops.append(autosummary.autosummary(self.id + "/learning_rate", self.learning_rate))
- all_ops.append(autosummary.autosummary(self.id + "/overflow_frequency", tf.where(all_ok, 0, 1), condition=acc_ok))
- if self.use_loss_scaling:
- all_ops.append(autosummary.autosummary(self.id + "/loss_scaling_log2", device.loss_scaling_var))
-
- # Initialize variables.
- self.reset_optimizer_state()
- if self.use_loss_scaling:
- tfutil.init_uninitialized_vars([device.loss_scaling_var for device in self._devices.values()])
- if self.minibatch_multiplier is not None:
- tfutil.run([var.initializer for device in self._devices.values() for var in list(device.grad_acc_vars.values()) + [device.grad_acc_count]])
-
- # Group everything into a single op.
- with tfutil.absolute_name_scope(self.scope):
- return tf.group(*all_ops, name="TrainingOp")
-
- def reset_optimizer_state(self) -> None:
- """Reset internal state of the underlying optimizer."""
- tfutil.assert_tf_initialized()
- tfutil.run([var.initializer for device in self._devices.values() for var in device.optimizer.variables()])
-
- def get_loss_scaling_var(self, device: str) -> Union[tf.Variable, None]:
- """Get or create variable representing log2 of the current dynamic loss scaling factor."""
- return self._get_device(device).loss_scaling_var
-
- def apply_loss_scaling(self, value: TfExpression) -> TfExpression:
- """Apply dynamic loss scaling for the given expression."""
- assert tfutil.is_tf_expression(value)
- if not self.use_loss_scaling:
- return value
- return value * tfutil.exp2(self.get_loss_scaling_var(value.device))
-
- def undo_loss_scaling(self, value: TfExpression) -> TfExpression:
- """Undo the effect of dynamic loss scaling for the given expression."""
- assert tfutil.is_tf_expression(value)
- if not self.use_loss_scaling:
- return value
- return value * tfutil.exp2(-self.get_loss_scaling_var(value.device)) # pylint: disable=invalid-unary-operand-type
-
-
-class SimpleAdam:
- """Simplified version of tf.train.AdamOptimizer that behaves identically when used with dnnlib.tflib.Optimizer."""
-
- def __init__(self, name="Adam", learning_rate=0.001, beta1=0.9, beta2=0.999, epsilon=1e-8):
- self.name = name
- self.learning_rate = learning_rate
- self.beta1 = beta1
- self.beta2 = beta2
- self.epsilon = epsilon
- self.all_state_vars = []
-
- def variables(self):
- return self.all_state_vars
-
- def compute_gradients(self, loss, var_list, gate_gradients=tf.train.Optimizer.GATE_NONE):
- assert gate_gradients == tf.train.Optimizer.GATE_NONE
- return list(zip(tf.gradients(loss, var_list), var_list))
-
- def apply_gradients(self, grads_and_vars):
- with tf.name_scope(self.name):
- state_vars = []
- update_ops = []
-
- # Adjust learning rate to deal with startup bias.
- with tf.control_dependencies(None):
- b1pow_var = tf.Variable(dtype=tf.float32, initial_value=1, trainable=False)
- b2pow_var = tf.Variable(dtype=tf.float32, initial_value=1, trainable=False)
- state_vars += [b1pow_var, b2pow_var]
- b1pow_new = b1pow_var * self.beta1
- b2pow_new = b2pow_var * self.beta2
- update_ops += [tf.assign(b1pow_var, b1pow_new), tf.assign(b2pow_var, b2pow_new)]
- lr_new = self.learning_rate * tf.sqrt(1 - b2pow_new) / (1 - b1pow_new)
-
- # Construct ops to update each variable.
- for grad, var in grads_and_vars:
- with tf.control_dependencies(None):
- m_var = tf.Variable(dtype=tf.float32, initial_value=tf.zeros_like(var), trainable=False)
- v_var = tf.Variable(dtype=tf.float32, initial_value=tf.zeros_like(var), trainable=False)
- state_vars += [m_var, v_var]
- m_new = self.beta1 * m_var + (1 - self.beta1) * grad
- v_new = self.beta2 * v_var + (1 - self.beta2) * tf.square(grad)
- var_delta = lr_new * m_new / (tf.sqrt(v_new) + self.epsilon)
- update_ops += [tf.assign(m_var, m_new), tf.assign(v_var, v_new), tf.assign_sub(var, var_delta)]
-
- # Group everything together.
- self.all_state_vars += state_vars
- return tf.group(*update_ops)
diff --git a/spaces/EAraid12/LoRA-DreamBooth-Training-UI/uploader.py b/spaces/EAraid12/LoRA-DreamBooth-Training-UI/uploader.py
deleted file mode 100644
index 0ce697f0d47325a4d73f92c13304ae5f51df794a..0000000000000000000000000000000000000000
--- a/spaces/EAraid12/LoRA-DreamBooth-Training-UI/uploader.py
+++ /dev/null
@@ -1,42 +0,0 @@
-from __future__ import annotations
-
-from huggingface_hub import HfApi
-
-
-class Uploader:
- def __init__(self, hf_token: str | None):
- self.api = HfApi(token=hf_token)
-
- def get_username(self) -> str:
- return self.api.whoami()['name']
-
- def upload(self,
- folder_path: str,
- repo_name: str,
- organization: str = '',
- repo_type: str = 'model',
- private: bool = True,
- delete_existing_repo: bool = False) -> str:
- if not folder_path:
- raise ValueError
- if not repo_name:
- raise ValueError
- if not organization:
- organization = self.get_username()
- repo_id = f'{organization}/{repo_name}'
- if delete_existing_repo:
- try:
- self.api.delete_repo(repo_id, repo_type=repo_type)
- except Exception:
- pass
- try:
- self.api.create_repo(repo_id, repo_type=repo_type, private=private)
- self.api.upload_folder(repo_id=repo_id,
- folder_path=folder_path,
- path_in_repo='.',
- repo_type=repo_type)
- url = f'https://huggingface.co/{repo_id}'
- message = f'Your model was successfully uploaded to {url}.'
- except Exception as e:
- message = str(e)
- return message
diff --git a/spaces/EmilyBrat/ATF/Dockerfile b/spaces/EmilyBrat/ATF/Dockerfile
deleted file mode 100644
index 4cb0ce42128d9a2ad33a395883f5e5455a38c707..0000000000000000000000000000000000000000
--- a/spaces/EmilyBrat/ATF/Dockerfile
+++ /dev/null
@@ -1,11 +0,0 @@
-FROM node:18-bullseye-slim
-RUN apt-get update && \
- apt-get install -y git
-RUN git clone https://gitgud.io/khanon/oai-reverse-proxy.git /app
-WORKDIR /app
-RUN npm install
-COPY Dockerfile greeting.md* .env* ./
-RUN npm run build
-EXPOSE 7860
-ENV NODE_ENV=production
-CMD [ "npm", "start" ]
\ No newline at end of file
diff --git a/spaces/Enderfga/mtCNN_sysu/get_data.py b/spaces/Enderfga/mtCNN_sysu/get_data.py
deleted file mode 100644
index ca3d8758942f5fe581ad151a788207583e0dbb18..0000000000000000000000000000000000000000
--- a/spaces/Enderfga/mtCNN_sysu/get_data.py
+++ /dev/null
@@ -1,852 +0,0 @@
-import sys
-import numpy as np
-import cv2
-import os
-from utils.tool import IoU,convert_to_square
-import numpy.random as npr
-import argparse
-from utils.detect import MtcnnDetector, create_mtcnn_net
-from utils.dataloader import ImageDB,TestImageLoader
-import time
-from six.moves import cPickle
-import utils.config as config
-import utils.vision as vision
-sys.path.append(os.getcwd())
-
-
-txt_from_path = './data_set/wider_face_train_bbx_gt.txt'
-anno_file = os.path.join(config.ANNO_STORE_DIR, 'anno_train.txt')
-# anno_file = './anno_store/anno_train.txt'
-
-prefix = ''
-use_cuda = True
-im_dir = "./data_set/face_detection/WIDER_train/images/"
-traindata_store = './data_set/train/'
-prefix_path = "./data_set/face_detection/WIDER_train/images/"
-annotation_file = './anno_store/anno_train.txt'
-prefix_path_lm = ''
-annotation_file_lm = "./data_set/face_landmark/CNN_FacePoint/train/trainImageList.txt"
-# ----------------------------------------------------other----------------------------------------------
-pos_save_dir = "./data_set/train/12/positive"
-part_save_dir = "./data_set/train/12/part"
-neg_save_dir = './data_set/train/12/negative'
-pnet_postive_file = os.path.join(config.ANNO_STORE_DIR, 'pos_12.txt')
-pnet_part_file = os.path.join(config.ANNO_STORE_DIR, 'part_12.txt')
-pnet_neg_file = os.path.join(config.ANNO_STORE_DIR, 'neg_12.txt')
-imglist_filename_pnet = os.path.join(config.ANNO_STORE_DIR, 'imglist_anno_12.txt')
-# ----------------------------------------------------PNet----------------------------------------------
-rnet_postive_file = os.path.join(config.ANNO_STORE_DIR, 'pos_24.txt')
-rnet_part_file = os.path.join(config.ANNO_STORE_DIR, 'part_24.txt')
-rnet_neg_file = os.path.join(config.ANNO_STORE_DIR, 'neg_24.txt')
-rnet_landmark_file = os.path.join(config.ANNO_STORE_DIR, 'landmark_24.txt')
-imglist_filename_rnet = os.path.join(config.ANNO_STORE_DIR, 'imglist_anno_24.txt')
-# ----------------------------------------------------RNet----------------------------------------------
-onet_postive_file = os.path.join(config.ANNO_STORE_DIR, 'pos_48.txt')
-onet_part_file = os.path.join(config.ANNO_STORE_DIR, 'part_48.txt')
-onet_neg_file = os.path.join(config.ANNO_STORE_DIR, 'neg_48.txt')
-onet_landmark_file = os.path.join(config.ANNO_STORE_DIR, 'landmark_48.txt')
-imglist_filename_onet = os.path.join(config.ANNO_STORE_DIR, 'imglist_anno_48.txt')
-# ----------------------------------------------------ONet----------------------------------------------
-
-
-
-def assemble_data(output_file, anno_file_list=[]):
-
- #assemble the pos, neg, part annotations to one file
- size = 12
-
- if len(anno_file_list)==0:
- return 0
-
- if os.path.exists(output_file):
- os.remove(output_file)
-
- for anno_file in anno_file_list:
- with open(anno_file, 'r') as f:
- print(anno_file)
- anno_lines = f.readlines()
-
- base_num = 250000
-
- if len(anno_lines) > base_num * 3:
- idx_keep = npr.choice(len(anno_lines), size=base_num * 3, replace=True)
- elif len(anno_lines) > 100000:
- idx_keep = npr.choice(len(anno_lines), size=len(anno_lines), replace=True)
- else:
- idx_keep = np.arange(len(anno_lines))
- np.random.shuffle(idx_keep)
- chose_count = 0
- with open(output_file, 'a+') as f:
- for idx in idx_keep:
- # write lables of pos, neg, part images
- f.write(anno_lines[idx])
- chose_count+=1
-
- return chose_count
-def wider_face(txt_from_path, txt_to_path):
- line_from_count = 0
- with open(txt_from_path, 'r') as f:
- annotations = f.readlines()
- with open(txt_to_path, 'w+') as f:
- while line_from_count < len(annotations):
- if annotations[line_from_count][2]=='-':
- img_name = annotations[line_from_count][:-1]
- line_from_count += 1 # change line to read the number
- bbox_count = int(annotations[line_from_count]) # num of bboxes
- line_from_count += 1 # change line to read the posession
- for _ in range(bbox_count):
- bbox = list(map(int,annotations[line_from_count].split()[:4])) # give a loop to append all the boxes
- bbox = [bbox[0], bbox[1], bbox[0]+bbox[2], bbox[1]+bbox[3]] # make x1, y1, w, h --> x1, y1, x2, y2
- bbox = list(map(str,bbox))
- img_name += (' '+' '.join(bbox))
- line_from_count+=1
- f.write(img_name +'\n')
- else: # dectect the file name
- line_from_count+=1
-
-# ----------------------------------------------------origin----------------------------------------------
-def get_Pnet_data():
- if not os.path.exists(pos_save_dir):
- os.makedirs(pos_save_dir)
- if not os.path.exists(part_save_dir):
- os.makedirs(part_save_dir)
- if not os.path.exists(neg_save_dir):
- os.makedirs(neg_save_dir)
- f1 = open(os.path.join('./anno_store', 'pos_12.txt'), 'w')
- f2 = open(os.path.join('./anno_store', 'neg_12.txt'), 'w')
- f3 = open(os.path.join('./anno_store', 'part_12.txt'), 'w')
- with open(anno_file, 'r') as f:
- annotations = f.readlines()
- num = len(annotations)
- print("%d pics in total" % num)
- p_idx = 0 # positive
- n_idx = 0 # negative
- d_idx = 0 # dont care
- idx = 0
- box_idx = 0
- for annotation in annotations:
- annotation = annotation.strip().split(' ')
- # annotation[0]文件名
- im_path = os.path.join(im_dir, annotation[0])
- # print(im_path)
- # print(os.path.exists(im_path))
- bbox = list(map(float, annotation[1:]))
- # annotation[1:]人脸坐标,一张脸4个值,对应两个点的坐标
- boxes = np.array(bbox, dtype=np.int32).reshape(-1, 4)
- # -1处的值为人脸数目
- if boxes.shape[0]==0:
- continue
- # 若无人脸则跳过本次循环
- img = cv2.imread(im_path)
- # print(img.shape)
- # exit()
- # 计数
- idx += 1
- if idx % 100 == 0:
- print("%s images done, pos: %s part: %s neg: %s" % (idx, p_idx, d_idx, n_idx))
-
- # 图片三通道
- height, width, channel = img.shape
-
- neg_num = 0
-
- # 取50次不同的框
- while neg_num < 50:
- size = np.random.randint(12, min(width, height) / 2)
- nx = np.random.randint(0, width - size)
- ny = np.random.randint(0, height - size)
- crop_box = np.array([nx, ny, nx + size, ny + size])
-
- Iou = IoU(crop_box, boxes) # IoU为 重合部分 / 两框之和 ,越大越好
-
- cropped_im = img[ny: ny + size, nx: nx + size, :] # 裁去多余部分并resize成 12*12
- resized_im = cv2.resize(cropped_im, (12, 12), interpolation=cv2.INTER_LINEAR)
-
- if np.max(Iou) < 0.3:
- # Iou with all gts must below 0.3
- save_file = os.path.join(neg_save_dir, "%s.jpg" % n_idx)
- f2.write(save_file + ' 0\n')
- cv2.imwrite(save_file, resized_im)
- n_idx += 1
- neg_num += 1
-
- for box in boxes:
- # box (x_left, y_top, x_right, y_bottom)
- x1, y1, x2, y2 = box
- # w = x2 - x1 + 1
- # h = y2 - y1 + 1
- w = x2 - x1 + 1
- h = y2 - y1 + 1
-
- # ignore small faces
- # in case the ground truth boxes of small faces are not accurate
- if max(w, h) < 40 or x1 < 0 or y1 < 0:
- continue
- if w < 12 or h < 12:
- continue
-
- # generate negative examples that have overlap with gt
- for i in range(5):
- size = np.random.randint(12, min(width, height) / 2)
-
- # delta_x and delta_y are offsets of (x1, y1)
- delta_x = np.random.randint(max(-size, -x1), w)
- delta_y = np.random.randint(max(-size, -y1), h)
- nx1 = max(0, x1 + delta_x)
- ny1 = max(0, y1 + delta_y)
-
- if nx1 + size > width or ny1 + size > height:
- continue
- crop_box = np.array([nx1, ny1, nx1 + size, ny1 + size])
- Iou = IoU(crop_box, boxes)
-
- cropped_im = img[ny1: ny1 + size, nx1: nx1 + size, :]
- resized_im = cv2.resize(cropped_im, (12, 12), interpolation=cv2.INTER_LINEAR)
-
- if np.max(Iou) < 0.3:
- # Iou with all gts must below 0.3
- save_file = os.path.join(neg_save_dir, "%s.jpg" % n_idx)
- f2.write(save_file + ' 0\n')
- cv2.imwrite(save_file, resized_im)
- n_idx += 1
-
- # generate positive examples and part faces
- for i in range(20):
- size = np.random.randint(int(min(w, h) * 0.8), np.ceil(1.25 * max(w, h)))
-
- # delta here is the offset of box center
- delta_x = np.random.randint(-w * 0.2, w * 0.2)
- delta_y = np.random.randint(-h * 0.2, h * 0.2)
-
- nx1 = max(x1 + w / 2 + delta_x - size / 2, 0)
- ny1 = max(y1 + h / 2 + delta_y - size / 2, 0)
- nx2 = nx1 + size
- ny2 = ny1 + size
-
- if nx2 > width or ny2 > height:
- continue
- crop_box = np.array([nx1, ny1, nx2, ny2])
-
- offset_x1 = (x1 - nx1) / float(size)
- offset_y1 = (y1 - ny1) / float(size)
- offset_x2 = (x2 - nx2) / float(size)
- offset_y2 = (y2 - ny2) / float(size)
-
- cropped_im = img[int(ny1): int(ny2), int(nx1): int(nx2), :]
- resized_im = cv2.resize(cropped_im, (12, 12), interpolation=cv2.INTER_LINEAR)
-
- box_ = box.reshape(1, -1)
- if IoU(crop_box, box_) >= 0.65:
- save_file = os.path.join(pos_save_dir, "%s.jpg" % p_idx)
- f1.write(save_file + ' 1 %.2f %.2f %.2f %.2f\n' % (offset_x1, offset_y1, offset_x2, offset_y2))
- cv2.imwrite(save_file, resized_im)
- p_idx += 1
- elif IoU(crop_box, box_) >= 0.4:
- save_file = os.path.join(part_save_dir, "%s.jpg" % d_idx)
- f3.write(save_file + ' -1 %.2f %.2f %.2f %.2f\n' % (offset_x1, offset_y1, offset_x2, offset_y2))
- cv2.imwrite(save_file, resized_im)
- d_idx += 1
- box_idx += 1
- #print("%s images done, pos: %s part: %s neg: %s" % (idx, p_idx, d_idx, n_idx))
-
- f1.close()
- f2.close()
- f3.close()
-
-
-def assembel_Pnet_data():
- anno_list = []
-
- anno_list.append(pnet_postive_file)
- anno_list.append(pnet_part_file)
- anno_list.append(pnet_neg_file)
- # anno_list.append(pnet_landmark_file)
- chose_count = assemble_data(imglist_filename_pnet ,anno_list)
- print("PNet train annotation result file path:%s" % imglist_filename_pnet)
-
-# -----------------------------------------------------------------------------------------------------------------------------------------------#
-
-def gen_rnet_data(data_dir, anno_file, pnet_model_file, prefix_path='', use_cuda=True, vis=False):
-
- """
- :param data_dir: train data
- :param anno_file:
- :param pnet_model_file:
- :param prefix_path:
- :param use_cuda:
- :param vis:
- :return:
- """
-
- # load trained pnet model
-
- pnet, _, _ = create_mtcnn_net(p_model_path = pnet_model_file, use_cuda = use_cuda)
- mtcnn_detector = MtcnnDetector(pnet = pnet, min_face_size = 12)
-
- # load original_anno_file, length = 12880
- imagedb = ImageDB(anno_file, mode = "test", prefix_path = prefix_path)
- imdb = imagedb.load_imdb()
- image_reader = TestImageLoader(imdb, 1, False)
-
- all_boxes = list()
- batch_idx = 0
-
- print('size:%d' %image_reader.size)
- for databatch in image_reader:
- if batch_idx % 100 == 0:
- print ("%d images done" % batch_idx)
- im = databatch
- t = time.time()
-
- # obtain boxes and aligned boxes
- boxes, boxes_align = mtcnn_detector.detect_pnet(im=im)
- if boxes_align is None:
- all_boxes.append(np.array([]))
- batch_idx += 1
- continue
- if vis:
- rgb_im = cv2.cvtColor(np.asarray(im), cv2.COLOR_BGR2RGB)
- vision.vis_two(rgb_im, boxes, boxes_align)
-
- t1 = time.time() - t
- print('cost time ',t1)
- t = time.time()
- all_boxes.append(boxes_align)
- batch_idx += 1
- # if batch_idx == 100:
- # break
- # print("shape of all boxes {0}".format(all_boxes))
- # time.sleep(5)
-
- # save_path = model_store_path()
- # './model_store'
- save_path = './model_store'
-
- if not os.path.exists(save_path):
- os.mkdir(save_path)
-
- save_file = os.path.join(save_path, "detections_%d.pkl" % int(time.time()))
- with open(save_file, 'wb') as f:
- cPickle.dump(all_boxes, f, cPickle.HIGHEST_PROTOCOL)
-
- # save_file = './model_store/detections_1588751332.pkl'
- gen_rnet_sample_data(data_dir, anno_file, save_file, prefix_path)
-
-
-
-def gen_rnet_sample_data(data_dir, anno_file, det_boxs_file, prefix_path):
-
- """
- :param data_dir:
- :param anno_file: original annotations file of wider face data
- :param det_boxs_file: detection boxes file
- :param prefix_path:
- :return:
- """
-
- neg_save_dir = os.path.join(data_dir, "24/negative")
- pos_save_dir = os.path.join(data_dir, "24/positive")
- part_save_dir = os.path.join(data_dir, "24/part")
-
-
- for dir_path in [neg_save_dir, pos_save_dir, part_save_dir]:
- # print(dir_path)
- if not os.path.exists(dir_path):
- os.makedirs(dir_path)
-
-
- # load ground truth from annotation file
- # format of each line: image/path [x1,y1,x2,y2] for each gt_box in this image
-
- with open(anno_file, 'r') as f:
- annotations = f.readlines()
-
- image_size = 24
- net = "rnet"
-
- im_idx_list = list()
- gt_boxes_list = list()
- num_of_images = len(annotations)
- print ("processing %d images in total" % num_of_images)
-
- for annotation in annotations:
- annotation = annotation.strip().split(' ')
- im_idx = os.path.join(prefix_path, annotation[0])
- # im_idx = annotation[0]
-
- boxes = list(map(float, annotation[1:]))
- boxes = np.array(boxes, dtype=np.float32).reshape(-1, 4)
- im_idx_list.append(im_idx)
- gt_boxes_list.append(boxes)
-
-
- # './anno_store'
- save_path = './anno_store'
- if not os.path.exists(save_path):
- os.makedirs(save_path)
-
- f1 = open(os.path.join(save_path, 'pos_%d.txt' % image_size), 'w')
- f2 = open(os.path.join(save_path, 'neg_%d.txt' % image_size), 'w')
- f3 = open(os.path.join(save_path, 'part_%d.txt' % image_size), 'w')
-
- # print(det_boxs_file)
- det_handle = open(det_boxs_file, 'rb')
-
- det_boxes = cPickle.load(det_handle)
-
- # an image contain many boxes stored in an array
- print(len(det_boxes), num_of_images)
- # assert len(det_boxes) == num_of_images, "incorrect detections or ground truths"
-
- # index of neg, pos and part face, used as their image names
- n_idx = 0
- p_idx = 0
- d_idx = 0
- image_done = 0
- for im_idx, dets, gts in zip(im_idx_list, det_boxes, gt_boxes_list):
-
- # if (im_idx+1) == 100:
- # break
-
- gts = np.array(gts, dtype=np.float32).reshape(-1, 4)
- if gts.shape[0]==0:
- continue
- if image_done % 100 == 0:
- print("%d images done" % image_done)
- image_done += 1
-
- if dets.shape[0] == 0:
- continue
- img = cv2.imread(im_idx)
- # change to square
- dets = convert_to_square(dets)
- dets[:, 0:4] = np.round(dets[:, 0:4])
- neg_num = 0
- for box in dets:
- x_left, y_top, x_right, y_bottom, _ = box.astype(int)
- width = x_right - x_left + 1
- height = y_bottom - y_top + 1
-
- # ignore box that is too small or beyond image border
- if width < 20 or x_left < 0 or y_top < 0 or x_right > img.shape[1] - 1 or y_bottom > img.shape[0] - 1:
- continue
-
- # compute intersection over union(IoU) between current box and all gt boxes
- Iou = IoU(box, gts)
- cropped_im = img[y_top:y_bottom + 1, x_left:x_right + 1, :]
- resized_im = cv2.resize(cropped_im, (image_size, image_size),
- interpolation=cv2.INTER_LINEAR)
-
- # save negative images and write label
- # Iou with all gts must below 0.3
- if np.max(Iou) < 0.3 and neg_num < 60:
- # save the examples
- save_file = os.path.join(neg_save_dir, "%s.jpg" % n_idx)
- # print(save_file)
- f2.write(save_file + ' 0\n')
- cv2.imwrite(save_file, resized_im)
- n_idx += 1
- neg_num += 1
- else:
- # find gt_box with the highest iou
- idx = np.argmax(Iou)
- assigned_gt = gts[idx]
- x1, y1, x2, y2 = assigned_gt
-
- # compute bbox reg label
- offset_x1 = (x1 - x_left) / float(width)
- offset_y1 = (y1 - y_top) / float(height)
- offset_x2 = (x2 - x_right) / float(width)
- offset_y2 = (y2 - y_bottom) / float(height)
-
- # save positive and part-face images and write labels
- if np.max(Iou) >= 0.65:
- save_file = os.path.join(pos_save_dir, "%s.jpg" % p_idx)
- f1.write(save_file + ' 1 %.2f %.2f %.2f %.2f\n' % (
- offset_x1, offset_y1, offset_x2, offset_y2))
- cv2.imwrite(save_file, resized_im)
- p_idx += 1
-
- elif np.max(Iou) >= 0.4:
- save_file = os.path.join(part_save_dir, "%s.jpg" % d_idx)
- f3.write(save_file + ' -1 %.2f %.2f %.2f %.2f\n' % (
- offset_x1, offset_y1, offset_x2, offset_y2))
- cv2.imwrite(save_file, resized_im)
- d_idx += 1
- f1.close()
- f2.close()
- f3.close()
-
-def model_store_path():
- return os.path.dirname(os.path.dirname(os.path.dirname(os.path.realpath(__file__))))+"/model_store"
-
-def get_Rnet_data(pnet_model):
- gen_rnet_data(traindata_store, annotation_file, pnet_model_file = pnet_model, prefix_path = prefix_path, use_cuda = True)
-
-
-def assembel_Rnet_data():
- anno_list = []
-
- anno_list.append(rnet_postive_file)
- anno_list.append(rnet_part_file)
- anno_list.append(rnet_neg_file)
- # anno_list.append(pnet_landmark_file)
-
- chose_count = assemble_data(imglist_filename_rnet ,anno_list)
- print("RNet train annotation result file path:%s" % imglist_filename_rnet)
-#-----------------------------------------------------------------------------------------------------------------------------------------------#
-def gen_onet_data(data_dir, anno_file, pnet_model_file, rnet_model_file, prefix_path='', use_cuda=True, vis=False):
-
-
- pnet, rnet, _ = create_mtcnn_net(p_model_path=pnet_model_file, r_model_path=rnet_model_file, use_cuda=use_cuda)
- mtcnn_detector = MtcnnDetector(pnet=pnet, rnet=rnet, min_face_size=12)
-
- imagedb = ImageDB(anno_file,mode="test",prefix_path=prefix_path)
- imdb = imagedb.load_imdb()
- image_reader = TestImageLoader(imdb,1,False)
-
- all_boxes = list()
- batch_idx = 0
-
- print('size:%d' % image_reader.size)
- for databatch in image_reader:
- if batch_idx % 50 == 0:
- print("%d images done" % batch_idx)
-
- im = databatch
-
- t = time.time()
-
- # pnet detection = [x1, y1, x2, y2, score, reg]
- p_boxes, p_boxes_align = mtcnn_detector.detect_pnet(im=im)
-
- t0 = time.time() - t
- t = time.time()
- # rnet detection
- boxes, boxes_align = mtcnn_detector.detect_rnet(im=im, dets=p_boxes_align)
-
- t1 = time.time() - t
- print('cost time pnet--',t0,' rnet--',t1)
- t = time.time()
-
- if boxes_align is None:
- all_boxes.append(np.array([]))
- batch_idx += 1
- continue
- if vis:
- rgb_im = cv2.cvtColor(np.asarray(im), cv2.COLOR_BGR2RGB)
- vision.vis_two(rgb_im, boxes, boxes_align)
-
-
- all_boxes.append(boxes_align)
- batch_idx += 1
-
- save_path = './model_store'
-
- if not os.path.exists(save_path):
- os.mkdir(save_path)
-
- save_file = os.path.join(save_path, "detections_%d.pkl" % int(time.time()))
- with open(save_file, 'wb') as f:
- cPickle.dump(all_boxes, f, cPickle.HIGHEST_PROTOCOL)
-
-
- gen_onet_sample_data(data_dir,anno_file,save_file,prefix_path)
-
-
-
-def gen_onet_sample_data(data_dir,anno_file,det_boxs_file,prefix):
-
- neg_save_dir = os.path.join(data_dir, "48/negative")
- pos_save_dir = os.path.join(data_dir, "48/positive")
- part_save_dir = os.path.join(data_dir, "48/part")
-
- for dir_path in [neg_save_dir, pos_save_dir, part_save_dir]:
- if not os.path.exists(dir_path):
- os.makedirs(dir_path)
-
-
- # load ground truth from annotation file
- # format of each line: image/path [x1,y1,x2,y2] for each gt_box in this image
-
- with open(anno_file, 'r') as f:
- annotations = f.readlines()
-
- image_size = 48
- net = "onet"
-
- im_idx_list = list()
- gt_boxes_list = list()
- num_of_images = len(annotations)
- print("processing %d images in total" % num_of_images)
-
- for annotation in annotations:
- annotation = annotation.strip().split(' ')
- im_idx = os.path.join(prefix,annotation[0])
-
- boxes = list(map(float, annotation[1:]))
- boxes = np.array(boxes, dtype=np.float32).reshape(-1, 4)
- im_idx_list.append(im_idx)
- gt_boxes_list.append(boxes)
-
- save_path = './anno_store'
- if not os.path.exists(save_path):
- os.makedirs(save_path)
-
- f1 = open(os.path.join(save_path, 'pos_%d.txt' % image_size), 'w')
- f2 = open(os.path.join(save_path, 'neg_%d.txt' % image_size), 'w')
- f3 = open(os.path.join(save_path, 'part_%d.txt' % image_size), 'w')
-
- det_handle = open(det_boxs_file, 'rb')
-
- det_boxes = cPickle.load(det_handle)
- print(len(det_boxes), num_of_images)
- # assert len(det_boxes) == num_of_images, "incorrect detections or ground truths"
-
- # index of neg, pos and part face, used as their image names
- n_idx = 0
- p_idx = 0
- d_idx = 0
- image_done = 0
- for im_idx, dets, gts in zip(im_idx_list, det_boxes, gt_boxes_list):
- if image_done % 100 == 0:
- print("%d images done" % image_done)
- image_done += 1
- if gts.shape[0]==0:
- continue
- if dets.shape[0] == 0:
- continue
- img = cv2.imread(im_idx)
- dets = convert_to_square(dets)
- dets[:, 0:4] = np.round(dets[:, 0:4])
-
- for box in dets:
- x_left, y_top, x_right, y_bottom = box[0:4].astype(int)
- width = x_right - x_left + 1
- height = y_bottom - y_top + 1
-
- # ignore box that is too small or beyond image border
- if width < 20 or x_left < 0 or y_top < 0 or x_right > img.shape[1] - 1 or y_bottom > img.shape[0] - 1:
- continue
-
- # compute intersection over union(IoU) between current box and all gt boxes
- Iou = IoU(box, gts)
- cropped_im = img[y_top:y_bottom + 1, x_left:x_right + 1, :]
- resized_im = cv2.resize(cropped_im, (image_size, image_size),
- interpolation=cv2.INTER_LINEAR)
-
- # save negative images and write label
- if np.max(Iou) < 0.3:
- # Iou with all gts must below 0.3
- save_file = os.path.join(neg_save_dir, "%s.jpg" % n_idx)
- f2.write(save_file + ' 0\n')
- cv2.imwrite(save_file, resized_im)
- n_idx += 1
- else:
- # find gt_box with the highest iou
- idx = np.argmax(Iou)
- assigned_gt = gts[idx]
- x1, y1, x2, y2 = assigned_gt
-
- # compute bbox reg label
- offset_x1 = (x1 - x_left) / float(width)
- offset_y1 = (y1 - y_top) / float(height)
- offset_x2 = (x2 - x_right) / float(width)
- offset_y2 = (y2 - y_bottom) / float(height)
-
- # save positive and part-face images and write labels
- if np.max(Iou) >= 0.65:
- save_file = os.path.join(pos_save_dir, "%s.jpg" % p_idx)
- f1.write(save_file + ' 1 %.2f %.2f %.2f %.2f\n' % (
- offset_x1, offset_y1, offset_x2, offset_y2))
- cv2.imwrite(save_file, resized_im)
- p_idx += 1
-
- elif np.max(Iou) >= 0.4:
- save_file = os.path.join(part_save_dir, "%s.jpg" % d_idx)
- f3.write(save_file + ' -1 %.2f %.2f %.2f %.2f\n' % (
- offset_x1, offset_y1, offset_x2, offset_y2))
- cv2.imwrite(save_file, resized_im)
- d_idx += 1
- f1.close()
- f2.close()
- f3.close()
-
-
-
-def model_store_path():
- return os.path.dirname(os.path.dirname(os.path.dirname(os.path.realpath(__file__))))+"/model_store"
-
-
-def get_Onet_data(pnet_model, rnet_model):
- gen_onet_data(traindata_store, annotation_file, pnet_model_file = pnet_model, rnet_model_file = rnet_model,prefix_path=prefix_path,use_cuda = True, vis = False)
-
-
-def assembel_Onet_data():
- anno_list = []
-
- anno_list.append(onet_postive_file)
- anno_list.append(onet_part_file)
- anno_list.append(onet_neg_file)
- anno_list.append(onet_landmark_file)
-
- chose_count = assemble_data(imglist_filename_onet ,anno_list)
- print("ONet train annotation result file path:%s" % imglist_filename_onet)
-
-
-def gen_landmark_48(anno_file, data_dir, prefix = ''):
-
-
- size = 48
- image_id = 0
-
- landmark_imgs_save_dir = os.path.join(data_dir,"48/landmark")
- if not os.path.exists(landmark_imgs_save_dir):
- os.makedirs(landmark_imgs_save_dir)
-
- anno_dir = './anno_store'
- if not os.path.exists(anno_dir):
- os.makedirs(anno_dir)
-
- landmark_anno_filename = "landmark_48.txt"
- save_landmark_anno = os.path.join(anno_dir,landmark_anno_filename)
-
- # print(save_landmark_anno)
- # time.sleep(5)
- f = open(save_landmark_anno, 'w')
- # dstdir = "train_landmark_few"
-
- with open(anno_file, 'r') as f2:
- annotations = f2.readlines()
-
- num = len(annotations)
- print("%d total images" % num)
-
- l_idx =0
- idx = 0
- # image_path bbox landmark(5*2)
- for annotation in annotations:
- # print imgPath
-
- annotation = annotation.strip().split(' ')
-
- assert len(annotation)==15,"each line should have 15 element"
-
- im_path = os.path.join('./data_set/face_landmark/CNN_FacePoint/train/',annotation[0].replace("\\", "/"))
-
- gt_box = list(map(float, annotation[1:5]))
- # gt_box = [gt_box[0], gt_box[2], gt_box[1], gt_box[3]]
-
-
- gt_box = np.array(gt_box, dtype=np.int32)
-
- landmark = list(map(float, annotation[5:]))
- landmark = np.array(landmark, dtype=np.float)
-
- img = cv2.imread(im_path)
- # print(im_path)
- assert (img is not None)
-
- height, width, channel = img.shape
- # crop_face = img[gt_box[1]:gt_box[3]+1, gt_box[0]:gt_box[2]+1]
- # crop_face = cv2.resize(crop_face,(size,size))
-
- idx = idx + 1
- if idx % 100 == 0:
- print("%d images done, landmark images: %d"%(idx,l_idx))
- # print(im_path)
- # print(gt_box)
- x1, x2, y1, y2 = gt_box
- gt_box[1] = y1
- gt_box[2] = x2
- # time.sleep(5)
-
- # gt's width
- w = x2 - x1 + 1
- # gt's height
- h = y2 - y1 + 1
- if max(w, h) < 40 or x1 < 0 or y1 < 0:
- continue
- # random shift
- for i in range(10):
- bbox_size = np.random.randint(int(min(w, h) * 0.8), np.ceil(1.25 * max(w, h)))
- delta_x = np.random.randint(-w * 0.2, w * 0.2)
- delta_y = np.random.randint(-h * 0.2, h * 0.2)
- nx1 = max(x1 + w / 2 - bbox_size / 2 + delta_x, 0)
- ny1 = max(y1 + h / 2 - bbox_size / 2 + delta_y, 0)
-
- nx2 = nx1 + bbox_size
- ny2 = ny1 + bbox_size
- if nx2 > width or ny2 > height:
- continue
- crop_box = np.array([nx1, ny1, nx2, ny2])
- cropped_im = img[int(ny1):int(ny2) + 1, int(nx1):int(nx2) + 1, :]
- resized_im = cv2.resize(cropped_im, (size, size),interpolation=cv2.INTER_LINEAR)
-
- offset_x1 = (x1 - nx1) / float(bbox_size)
- offset_y1 = (y1 - ny1) / float(bbox_size)
- offset_x2 = (x2 - nx2) / float(bbox_size)
- offset_y2 = (y2 - ny2) / float(bbox_size)
-
- offset_left_eye_x = (landmark[0] - nx1) / float(bbox_size)
- offset_left_eye_y = (landmark[1] - ny1) / float(bbox_size)
-
- offset_right_eye_x = (landmark[2] - nx1) / float(bbox_size)
- offset_right_eye_y = (landmark[3] - ny1) / float(bbox_size)
-
- offset_nose_x = (landmark[4] - nx1) / float(bbox_size)
- offset_nose_y = (landmark[5] - ny1) / float(bbox_size)
-
- offset_left_mouth_x = (landmark[6] - nx1) / float(bbox_size)
- offset_left_mouth_y = (landmark[7] - ny1) / float(bbox_size)
-
- offset_right_mouth_x = (landmark[8] - nx1) / float(bbox_size)
- offset_right_mouth_y = (landmark[9] - ny1) / float(bbox_size)
-
-
- # cal iou
- iou = IoU(crop_box.astype(np.float), np.expand_dims(gt_box.astype(np.float), 0))
- # print(iou)
- if iou > 0.65:
- save_file = os.path.join(landmark_imgs_save_dir, "%s.jpg" % l_idx)
- cv2.imwrite(save_file, resized_im)
-
- f.write(save_file + ' -2 %.2f %.2f %.2f %.2f %.2f %.2f %.2f %.2f %.2f %.2f %.2f %.2f %.2f %.2f \n' % \
- (offset_x1, offset_y1, offset_x2, offset_y2, \
- offset_left_eye_x,offset_left_eye_y,offset_right_eye_x,offset_right_eye_y,offset_nose_x,offset_nose_y,offset_left_mouth_x,offset_left_mouth_y,offset_right_mouth_x,offset_right_mouth_y))
- # print(save_file)
- # print(save_landmark_anno)
- l_idx += 1
-
- f.close()
-
-
-def parse_args():
- parser = argparse.ArgumentParser(description='Get data',
- formatter_class=argparse.ArgumentDefaultsHelpFormatter)
-
- parser.add_argument('--net', dest='net', help='which net to show', type=str)
- parser.add_argument('--pnet_path', default="./model_store/pnet_epoch_20.pt",help='path to pnet model', type=str)
- parser.add_argument('--rnet_path', default="./model_store/rnet_epoch_20.pt",help='path to rnet model', type=str)
- parser.add_argument('--use_cuda', default=True,help='use cuda', type=bool)
-
- args = parser.parse_args()
- return args
-
-#-----------------------------------------------------------------------------------------------------------------------------------------------#
-if __name__ == '__main__':
- args = parse_args()
- dir = 'anno_store'
- if not os.path.exists(dir):
- os.makedirs(dir)
- if args.net == "pnet":
- wider_face(txt_from_path, anno_file)
- get_Pnet_data()
- assembel_Pnet_data()
- elif args.net == "rnet":
- get_Rnet_data(args.pnet_path)
- assembel_Rnet_data()
- elif args.net == "onet":
- get_Onet_data(args.pnet_path, args.rnet_path)
- gen_landmark_48(annotation_file_lm, traindata_store, prefix_path_lm)
- assembel_Onet_data()
\ No newline at end of file
diff --git a/spaces/Enderfga/mtCNN_sysu/test.sh b/spaces/Enderfga/mtCNN_sysu/test.sh
deleted file mode 100644
index 0c59fd517d49cb80a95844df4e5e2c4598023921..0000000000000000000000000000000000000000
--- a/spaces/Enderfga/mtCNN_sysu/test.sh
+++ /dev/null
@@ -1,4 +0,0 @@
-python test.py --net=pnet --min_face_size=1 --pnet_path=./model_store/pnet_epoch_20.pt --rnet_path=./model_store/rnet_epoch_20.pt --onet_path=./model_store/onet_epoch_20.pt --save_name=pnet
-python test.py --net=rnet --min_face_size=1 --pnet_path=./model_store/pnet_epoch_20.pt --rnet_path=./model_store/rnet_epoch_20.pt --onet_path=./model_store/onet_epoch_20.pt --save_name=rnet
-python test.py --net=onet --min_face_size=1 --pnet_path=./model_store/pnet_epoch_20.pt --rnet_path=./model_store/rnet_epoch_20.pt --onet_path=./model_store/onet_epoch_20.pt --save_name=onet
-echo "Testing finished!"
\ No newline at end of file
diff --git a/spaces/Felix123456/bingo/src/components/ui/separator.tsx b/spaces/Felix123456/bingo/src/components/ui/separator.tsx
deleted file mode 100644
index 6c55e0b2ca8e2436658a06748aadbff7cd700db0..0000000000000000000000000000000000000000
--- a/spaces/Felix123456/bingo/src/components/ui/separator.tsx
+++ /dev/null
@@ -1,31 +0,0 @@
-'use client'
-
-import * as React from 'react'
-import * as SeparatorPrimitive from '@radix-ui/react-separator'
-
-import { cn } from '@/lib/utils'
-
-const Separator = React.forwardRef<
- React.ElementRef,
- React.ComponentPropsWithoutRef
->(
- (
- { className, orientation = 'horizontal', decorative = true, ...props },
- ref
- ) => (
-
- )
-)
-Separator.displayName = SeparatorPrimitive.Root.displayName
-
-export { Separator }
diff --git a/spaces/FridaZuley/RVC_HFKawaii/demucs/compressed.py b/spaces/FridaZuley/RVC_HFKawaii/demucs/compressed.py
deleted file mode 100644
index eb8fbb75463ba71ca86729b22baebf24598ade57..0000000000000000000000000000000000000000
--- a/spaces/FridaZuley/RVC_HFKawaii/demucs/compressed.py
+++ /dev/null
@@ -1,115 +0,0 @@
-# Copyright (c) Facebook, Inc. and its affiliates.
-# All rights reserved.
-#
-# This source code is licensed under the license found in the
-# LICENSE file in the root directory of this source tree.
-
-import json
-from fractions import Fraction
-from concurrent import futures
-
-import musdb
-from torch import distributed
-
-from .audio import AudioFile
-
-
-def get_musdb_tracks(root, *args, **kwargs):
- mus = musdb.DB(root, *args, **kwargs)
- return {track.name: track.path for track in mus}
-
-
-class StemsSet:
- def __init__(self, tracks, metadata, duration=None, stride=1,
- samplerate=44100, channels=2, streams=slice(None)):
-
- self.metadata = []
- for name, path in tracks.items():
- meta = dict(metadata[name])
- meta["path"] = path
- meta["name"] = name
- self.metadata.append(meta)
- if duration is not None and meta["duration"] < duration:
- raise ValueError(f"Track {name} duration is too small {meta['duration']}")
- self.metadata.sort(key=lambda x: x["name"])
- self.duration = duration
- self.stride = stride
- self.channels = channels
- self.samplerate = samplerate
- self.streams = streams
-
- def __len__(self):
- return sum(self._examples_count(m) for m in self.metadata)
-
- def _examples_count(self, meta):
- if self.duration is None:
- return 1
- else:
- return int((meta["duration"] - self.duration) // self.stride + 1)
-
- def track_metadata(self, index):
- for meta in self.metadata:
- examples = self._examples_count(meta)
- if index >= examples:
- index -= examples
- continue
- return meta
-
- def __getitem__(self, index):
- for meta in self.metadata:
- examples = self._examples_count(meta)
- if index >= examples:
- index -= examples
- continue
- streams = AudioFile(meta["path"]).read(seek_time=index * self.stride,
- duration=self.duration,
- channels=self.channels,
- samplerate=self.samplerate,
- streams=self.streams)
- return (streams - meta["mean"]) / meta["std"]
-
-
-def _get_track_metadata(path):
- # use mono at 44kHz as reference. For any other settings data won't be perfectly
- # normalized but it should be good enough.
- audio = AudioFile(path)
- mix = audio.read(streams=0, channels=1, samplerate=44100)
- return {"duration": audio.duration, "std": mix.std().item(), "mean": mix.mean().item()}
-
-
-def _build_metadata(tracks, workers=10):
- pendings = []
- with futures.ProcessPoolExecutor(workers) as pool:
- for name, path in tracks.items():
- pendings.append((name, pool.submit(_get_track_metadata, path)))
- return {name: p.result() for name, p in pendings}
-
-
-def _build_musdb_metadata(path, musdb, workers):
- tracks = get_musdb_tracks(musdb)
- metadata = _build_metadata(tracks, workers)
- path.parent.mkdir(exist_ok=True, parents=True)
- json.dump(metadata, open(path, "w"))
-
-
-def get_compressed_datasets(args, samples):
- metadata_file = args.metadata / "musdb.json"
- if not metadata_file.is_file() and args.rank == 0:
- _build_musdb_metadata(metadata_file, args.musdb, args.workers)
- if args.world_size > 1:
- distributed.barrier()
- metadata = json.load(open(metadata_file))
- duration = Fraction(samples, args.samplerate)
- stride = Fraction(args.data_stride, args.samplerate)
- train_set = StemsSet(get_musdb_tracks(args.musdb, subsets=["train"], split="train"),
- metadata,
- duration=duration,
- stride=stride,
- streams=slice(1, None),
- samplerate=args.samplerate,
- channels=args.audio_channels)
- valid_set = StemsSet(get_musdb_tracks(args.musdb, subsets=["train"], split="valid"),
- metadata,
- samplerate=args.samplerate,
- channels=args.audio_channels)
- return train_set, valid_set
diff --git a/spaces/Frorozcol/mariposas/README.md b/spaces/Frorozcol/mariposas/README.md
deleted file mode 100644
index 9dda834553f2ccf532270a0ae03f9ff2414479f3..0000000000000000000000000000000000000000
--- a/spaces/Frorozcol/mariposas/README.md
+++ /dev/null
@@ -1,13 +0,0 @@
----
-title: Mariposas
-emoji: 📚
-colorFrom: green
-colorTo: purple
-sdk: streamlit
-sdk_version: 1.10.0
-app_file: app.py
-pinned: false
-license: mit
----
-
-Check out the configuration reference at https://huggingface.co/docs/hub/spaces-config-reference
diff --git a/spaces/GAIR/Factool/factool/utils/openai_wrapper.py b/spaces/GAIR/Factool/factool/utils/openai_wrapper.py
deleted file mode 100644
index d057012123e16a48f053a16267e16de52b6afa4b..0000000000000000000000000000000000000000
--- a/spaces/GAIR/Factool/factool/utils/openai_wrapper.py
+++ /dev/null
@@ -1,171 +0,0 @@
-# the async version is adapted from https://gist.github.com/neubig/80de662fb3e225c18172ec218be4917a
-
-from __future__ import annotations
-
-import os
-import yaml
-import openai
-import ast
-import pdb
-import asyncio
-from typing import Any, List
-import os
-import pathlib
-import openai
-
-
-# from factool.env_config import factool_env_config
-
-# env
-# openai.api_key = factool_env_config.openai_api_key
-
-class OpenAIChat():
- def __init__(
- self,
- model_name='gpt-3.5-turbo',
- max_tokens=2500,
- temperature=0,
- top_p=1,
- request_timeout=60,
- ):
- openai.api_key = os.environ.get("OPENAI_API_KEY", None)
- assert openai.api_key is not None, "Please set the OPENAI_API_KEY environment variable."
- if 'gpt' not in model_name:
- openai.api_base = "http://localhost:8000/v1"
- self.config = {
- 'model_name': model_name,
- 'max_tokens': max_tokens,
- 'temperature': temperature,
- 'top_p': top_p,
- 'request_timeout': request_timeout,
- }
-
-
- def _boolean_fix(self, output):
- return output.replace("true", "True").replace("false", "False")
-
- def _type_check(self, output, expected_type):
- try:
- output_eval = ast.literal_eval(output)
- if not isinstance(output_eval, expected_type):
- return None
- return output_eval
- except:
- return None
-
- async def dispatch_openai_requests(
- self,
- messages_list,
- ) -> list[str]:
- """Dispatches requests to OpenAI API asynchronously.
-
- Args:
- messages_list: List of messages to be sent to OpenAI ChatCompletion API.
- Returns:
- List of responses from OpenAI API.
- """
- async def _request_with_retry(messages, retry=3):
- for _ in range(retry):
- try:
- response = await openai.ChatCompletion.acreate(
- model=self.config['model_name'],
- messages=messages,
- max_tokens=self.config['max_tokens'],
- temperature=self.config['temperature'],
- top_p=self.config['top_p'],
- request_timeout=self.config['request_timeout'],
- )
- return response
- except openai.error.RateLimitError:
- print('Rate limit error, waiting for 40 second...')
- await asyncio.sleep(40)
- except openai.error.APIError:
- print('API error, waiting for 1 second...')
- await asyncio.sleep(1)
- except openai.error.Timeout:
- print('Timeout error, waiting for 1 second...')
- await asyncio.sleep(1)
- except openai.error.ServiceUnavailableError:
- print('Service unavailable error, waiting for 3 second...')
- await asyncio.sleep(3)
- except openai.error.APIConnectionError:
- print('API Connection error, waiting for 3 second...')
- await asyncio.sleep(3)
-
- return None
-
- async_responses = [
- _request_with_retry(messages)
- for messages in messages_list
- ]
-
- return await asyncio.gather(*async_responses)
-
- async def async_run(self, messages_list, expected_type):
- retry = 1
- responses = [None for _ in range(len(messages_list))]
- messages_list_cur_index = [i for i in range(len(messages_list))]
-
- while retry > 0 and len(messages_list_cur_index) > 0:
- print(f'{retry} retry left...')
- messages_list_cur = [messages_list[i] for i in messages_list_cur_index]
-
- predictions = await self.dispatch_openai_requests(
- messages_list=messages_list_cur,
- )
-
- preds = [self._type_check(self._boolean_fix(prediction['choices'][0]['message']['content']), expected_type) if prediction is not None else None for prediction in predictions]
-
- finised_index = []
- for i, pred in enumerate(preds):
- if pred is not None:
- responses[messages_list_cur_index[i]] = pred
- finised_index.append(messages_list_cur_index[i])
-
- messages_list_cur_index = [i for i in messages_list_cur_index if i not in finised_index]
-
- retry -= 1
-
- return responses
-
-class OpenAIEmbed():
- def __init__():
- openai.api_key = os.environ.get("OPENAI_API_KEY", None)
- assert openai.api_key is not None, "Please set the OPENAI_API_KEY environment variable."
-
- async def create_embedding(self, text, retry=3):
- for _ in range(retry):
- try:
- response = await openai.Embedding.acreate(input=text, model="text-embedding-ada-002")
- return response
- except openai.error.RateLimitError:
- print('Rate limit error, waiting for 1 second...')
- await asyncio.sleep(1)
- except openai.error.APIError:
- print('API error, waiting for 1 second...')
- await asyncio.sleep(1)
- except openai.error.Timeout:
- print('Timeout error, waiting for 1 second...')
- await asyncio.sleep(1)
- return None
-
- async def process_batch(self, batch, retry=3):
- tasks = [self.create_embedding(text, retry=retry) for text in batch]
- return await asyncio.gather(*tasks)
-
-if __name__ == "__main__":
- chat = OpenAIChat()
-
- predictions = chat.async_run(
- messages_list=[
- [{"role": "user", "content": "show either 'ab' or '['a']'. Do not do anything else."}],
- ] * 20,
- expected_type=List,
- )
-
- # Usage
- embed = OpenAIEmbed()
- batch = ["string1", "string2", "string3", "string4", "string5", "string6", "string7", "string8", "string9", "string10"] # Your batch of strings
- embeddings = asyncio.run(embed.process_batch(batch, retry=3))
- for embedding in embeddings:
- print(embedding["data"][0]["embedding"])
\ No newline at end of file
diff --git a/spaces/Gen-Sim/Gen-Sim/cliport/models/clip_wo_skip.py b/spaces/Gen-Sim/Gen-Sim/cliport/models/clip_wo_skip.py
deleted file mode 100644
index 915c4bc64b674fc91872a89c97692263a690ac31..0000000000000000000000000000000000000000
--- a/spaces/Gen-Sim/Gen-Sim/cliport/models/clip_wo_skip.py
+++ /dev/null
@@ -1,67 +0,0 @@
-import torch.nn as nn
-import torch.nn.functional as F
-
-import cliport.utils.utils as utils
-from cliport.models.resnet import IdentityBlock, ConvBlock
-from cliport.models.clip_lingunet_lat import CLIPLingUNetLat
-
-
-class CLIPWithoutSkipConnections(CLIPLingUNetLat):
- """ CLIP RN50 with decoders (no skip connections) """
-
- def __init__(self, input_shape, output_dim, cfg, device, preprocess):
- super().__init__(input_shape, output_dim, cfg, device, preprocess)
-
- def _build_decoder(self):
- self.layers = nn.Sequential(
- # conv1
- nn.Conv2d(self.input_dim, 1024, kernel_size=3, stride=1, padding=1, bias=False),
- nn.ReLU(True),
- nn.UpsamplingBilinear2d(scale_factor=2),
-
- # decoder blocks
- ConvBlock(1024, [512, 512, 512], kernel_size=3, stride=1, batchnorm=self.batchnorm),
- IdentityBlock(512, [512, 512, 512], kernel_size=3, stride=1, batchnorm=self.batchnorm),
-
- ConvBlock(512, [512, 512, 512], kernel_size=3, stride=1, batchnorm=self.batchnorm),
- IdentityBlock(512, [512, 512, 512], kernel_size=3, stride=1, batchnorm=self.batchnorm),
- nn.UpsamplingBilinear2d(scale_factor=2),
-
- ConvBlock(512, [128, 128, 128], kernel_size=3, stride=1, batchnorm=self.batchnorm),
- IdentityBlock(128, [128, 128, 128], kernel_size=3, stride=1, batchnorm=self.batchnorm),
-
- ConvBlock(128, [128, 128, 128], kernel_size=3, stride=1, batchnorm=self.batchnorm),
- IdentityBlock(128, [128, 128, 128], kernel_size=3, stride=1, batchnorm=self.batchnorm),
- nn.UpsamplingBilinear2d(scale_factor=2),
-
- ConvBlock(128, [64, 64, 64], kernel_size=3, stride=1, batchnorm=self.batchnorm),
- IdentityBlock(64, [64, 64, 64], kernel_size=3, stride=1, batchnorm=self.batchnorm),
-
- ConvBlock(64, [64, 64, 64], kernel_size=3, stride=1, batchnorm=self.batchnorm),
- IdentityBlock(64, [64, 64, 64], kernel_size=3, stride=1, batchnorm=self.batchnorm),
- nn.UpsamplingBilinear2d(scale_factor=2),
-
- ConvBlock(64, [32, 32, 32], kernel_size=3, stride=1, batchnorm=self.batchnorm),
- IdentityBlock(32, [32, 32, 32], kernel_size=3, stride=1, batchnorm=self.batchnorm),
-
- ConvBlock(32, [32, 32, 32], kernel_size=3, stride=1, batchnorm=self.batchnorm),
- IdentityBlock(32, [32, 32, 32], kernel_size=3, stride=1, batchnorm=self.batchnorm),
-
- # conv2
- nn.UpsamplingBilinear2d(scale_factor=2),
- nn.Conv2d(32, self.output_dim, kernel_size=1)
- )
-
- def forward(self, x):
- x = self.preprocess(x, dist='clip')
-
- in_type = x.dtype
- in_shape = x.shape
- x = x[:,:3] # select RGB
- x, _ = self.encode_image(x)
- x = x.to(in_type)
-
- assert x.shape[1] == self.input_dim
- x = self.layers(x)
- x = F.interpolate(x, size=(in_shape[-2], in_shape[-1]), mode='bilinear')
- return x
\ No newline at end of file
diff --git a/spaces/GipAdonimus/Real-Time-Voice-Cloning/encoder/params_data.py b/spaces/GipAdonimus/Real-Time-Voice-Cloning/encoder/params_data.py
deleted file mode 100644
index bdb1716ed45617f2b127a7fb8885afe6cc74fb71..0000000000000000000000000000000000000000
--- a/spaces/GipAdonimus/Real-Time-Voice-Cloning/encoder/params_data.py
+++ /dev/null
@@ -1,29 +0,0 @@
-
-## Mel-filterbank
-mel_window_length = 25 # In milliseconds
-mel_window_step = 10 # In milliseconds
-mel_n_channels = 40
-
-
-## Audio
-sampling_rate = 16000
-# Number of spectrogram frames in a partial utterance
-partials_n_frames = 160 # 1600 ms
-# Number of spectrogram frames at inference
-inference_n_frames = 80 # 800 ms
-
-
-## Voice Activation Detection
-# Window size of the VAD. Must be either 10, 20 or 30 milliseconds.
-# This sets the granularity of the VAD. Should not need to be changed.
-vad_window_length = 30 # In milliseconds
-# Number of frames to average together when performing the moving average smoothing.
-# The larger this value, the larger the VAD variations must be to not get smoothed out.
-vad_moving_average_width = 8
-# Maximum number of consecutive silent frames a segment can have.
-vad_max_silence_length = 6
-
-
-## Audio volume normalization
-audio_norm_target_dBFS = -30
-
diff --git a/spaces/Godrose0728/sound-link/text/ngu_dialect.py b/spaces/Godrose0728/sound-link/text/ngu_dialect.py
deleted file mode 100644
index 69d0ce6fe5a989843ee059a71ccab793f20f9176..0000000000000000000000000000000000000000
--- a/spaces/Godrose0728/sound-link/text/ngu_dialect.py
+++ /dev/null
@@ -1,30 +0,0 @@
-import re
-import opencc
-
-
-dialects = {'SZ': 'suzhou', 'WX': 'wuxi', 'CZ': 'changzhou', 'HZ': 'hangzhou',
- 'SX': 'shaoxing', 'NB': 'ningbo', 'JJ': 'jingjiang', 'YX': 'yixing',
- 'JD': 'jiading', 'ZR': 'zhenru', 'PH': 'pinghu', 'TX': 'tongxiang',
- 'JS': 'jiashan', 'HN': 'xiashi', 'LP': 'linping', 'XS': 'xiaoshan',
- 'FY': 'fuyang', 'RA': 'ruao', 'CX': 'cixi', 'SM': 'sanmen',
- 'TT': 'tiantai', 'WZ': 'wenzhou', 'SC': 'suichang', 'YB': 'youbu'}
-
-converters = {}
-
-for dialect in dialects.values():
- try:
- converters[dialect] = opencc.OpenCC("chinese_dialect_lexicons/"+dialect)
- except:
- pass
-
-
-def ngu_dialect_to_ipa(text, dialect):
- dialect = dialects[dialect]
- text = converters[dialect].convert(text).replace('-','').replace('$',' ')
- text = re.sub(r'[、;:]', ',', text)
- text = re.sub(r'\s*,\s*', ', ', text)
- text = re.sub(r'\s*。\s*', '. ', text)
- text = re.sub(r'\s*?\s*', '? ', text)
- text = re.sub(r'\s*!\s*', '! ', text)
- text = re.sub(r'\s*$', '', text)
- return text
diff --git a/spaces/Gradio-Blocks/uniformer_image_detection/configs/_base_/datasets/coco_instance.py b/spaces/Gradio-Blocks/uniformer_image_detection/configs/_base_/datasets/coco_instance.py
deleted file mode 100644
index f6ea4f4562a8118275a444879a884717b55caa15..0000000000000000000000000000000000000000
--- a/spaces/Gradio-Blocks/uniformer_image_detection/configs/_base_/datasets/coco_instance.py
+++ /dev/null
@@ -1,48 +0,0 @@
-dataset_type = 'CocoDataset'
-data_root = 'data/coco/'
-img_norm_cfg = dict(
- mean=[123.675, 116.28, 103.53], std=[58.395, 57.12, 57.375], to_rgb=True)
-train_pipeline = [
- dict(type='LoadImageFromFile'),
- dict(type='LoadAnnotations', with_bbox=True, with_mask=True),
- dict(type='Resize', img_scale=(1333, 800), keep_ratio=True),
- dict(type='RandomFlip', flip_ratio=0.5),
- dict(type='Normalize', **img_norm_cfg),
- dict(type='Pad', size_divisor=32),
- dict(type='DefaultFormatBundle'),
- dict(type='Collect', keys=['img', 'gt_bboxes', 'gt_labels', 'gt_masks']),
-]
-test_pipeline = [
- dict(type='LoadImageFromFile'),
- dict(
- type='MultiScaleFlipAug',
- img_scale=(1333, 800),
- flip=False,
- transforms=[
- dict(type='Resize', keep_ratio=True),
- dict(type='RandomFlip'),
- dict(type='Normalize', **img_norm_cfg),
- dict(type='Pad', size_divisor=32),
- dict(type='ImageToTensor', keys=['img']),
- dict(type='Collect', keys=['img']),
- ])
-]
-data = dict(
- samples_per_gpu=2,
- workers_per_gpu=2,
- train=dict(
- type=dataset_type,
- ann_file=data_root + 'annotations/instances_train2017.json',
- img_prefix=data_root + 'train2017/',
- pipeline=train_pipeline),
- val=dict(
- type=dataset_type,
- ann_file=data_root + 'annotations/instances_val2017.json',
- img_prefix=data_root + 'val2017/',
- pipeline=test_pipeline),
- test=dict(
- type=dataset_type,
- ann_file=data_root + 'annotations/instances_val2017.json',
- img_prefix=data_root + 'val2017/',
- pipeline=test_pipeline))
-evaluation = dict(metric=['bbox', 'segm'])
diff --git a/spaces/Gradio-Blocks/uniformer_image_segmentation/mmseg/models/backbones/hrnet.py b/spaces/Gradio-Blocks/uniformer_image_segmentation/mmseg/models/backbones/hrnet.py
deleted file mode 100644
index 5010a2e767951b1d5a1d67234d4c4517926b44c5..0000000000000000000000000000000000000000
--- a/spaces/Gradio-Blocks/uniformer_image_segmentation/mmseg/models/backbones/hrnet.py
+++ /dev/null
@@ -1,555 +0,0 @@
-import torch.nn as nn
-from mmcv.cnn import (build_conv_layer, build_norm_layer, constant_init,
- kaiming_init)
-from mmcv.runner import load_checkpoint
-from mmcv.utils.parrots_wrapper import _BatchNorm
-
-from mmseg.ops import Upsample, resize
-from mmseg.utils import get_root_logger
-from ..builder import BACKBONES
-from .resnet import BasicBlock, Bottleneck
-
-
-class HRModule(nn.Module):
- """High-Resolution Module for HRNet.
-
- In this module, every branch has 4 BasicBlocks/Bottlenecks. Fusion/Exchange
- is in this module.
- """
-
- def __init__(self,
- num_branches,
- blocks,
- num_blocks,
- in_channels,
- num_channels,
- multiscale_output=True,
- with_cp=False,
- conv_cfg=None,
- norm_cfg=dict(type='BN', requires_grad=True)):
- super(HRModule, self).__init__()
- self._check_branches(num_branches, num_blocks, in_channels,
- num_channels)
-
- self.in_channels = in_channels
- self.num_branches = num_branches
-
- self.multiscale_output = multiscale_output
- self.norm_cfg = norm_cfg
- self.conv_cfg = conv_cfg
- self.with_cp = with_cp
- self.branches = self._make_branches(num_branches, blocks, num_blocks,
- num_channels)
- self.fuse_layers = self._make_fuse_layers()
- self.relu = nn.ReLU(inplace=False)
-
- def _check_branches(self, num_branches, num_blocks, in_channels,
- num_channels):
- """Check branches configuration."""
- if num_branches != len(num_blocks):
- error_msg = f'NUM_BRANCHES({num_branches}) <> NUM_BLOCKS(' \
- f'{len(num_blocks)})'
- raise ValueError(error_msg)
-
- if num_branches != len(num_channels):
- error_msg = f'NUM_BRANCHES({num_branches}) <> NUM_CHANNELS(' \
- f'{len(num_channels)})'
- raise ValueError(error_msg)
-
- if num_branches != len(in_channels):
- error_msg = f'NUM_BRANCHES({num_branches}) <> NUM_INCHANNELS(' \
- f'{len(in_channels)})'
- raise ValueError(error_msg)
-
- def _make_one_branch(self,
- branch_index,
- block,
- num_blocks,
- num_channels,
- stride=1):
- """Build one branch."""
- downsample = None
- if stride != 1 or \
- self.in_channels[branch_index] != \
- num_channels[branch_index] * block.expansion:
- downsample = nn.Sequential(
- build_conv_layer(
- self.conv_cfg,
- self.in_channels[branch_index],
- num_channels[branch_index] * block.expansion,
- kernel_size=1,
- stride=stride,
- bias=False),
- build_norm_layer(self.norm_cfg, num_channels[branch_index] *
- block.expansion)[1])
-
- layers = []
- layers.append(
- block(
- self.in_channels[branch_index],
- num_channels[branch_index],
- stride,
- downsample=downsample,
- with_cp=self.with_cp,
- norm_cfg=self.norm_cfg,
- conv_cfg=self.conv_cfg))
- self.in_channels[branch_index] = \
- num_channels[branch_index] * block.expansion
- for i in range(1, num_blocks[branch_index]):
- layers.append(
- block(
- self.in_channels[branch_index],
- num_channels[branch_index],
- with_cp=self.with_cp,
- norm_cfg=self.norm_cfg,
- conv_cfg=self.conv_cfg))
-
- return nn.Sequential(*layers)
-
- def _make_branches(self, num_branches, block, num_blocks, num_channels):
- """Build multiple branch."""
- branches = []
-
- for i in range(num_branches):
- branches.append(
- self._make_one_branch(i, block, num_blocks, num_channels))
-
- return nn.ModuleList(branches)
-
- def _make_fuse_layers(self):
- """Build fuse layer."""
- if self.num_branches == 1:
- return None
-
- num_branches = self.num_branches
- in_channels = self.in_channels
- fuse_layers = []
- num_out_branches = num_branches if self.multiscale_output else 1
- for i in range(num_out_branches):
- fuse_layer = []
- for j in range(num_branches):
- if j > i:
- fuse_layer.append(
- nn.Sequential(
- build_conv_layer(
- self.conv_cfg,
- in_channels[j],
- in_channels[i],
- kernel_size=1,
- stride=1,
- padding=0,
- bias=False),
- build_norm_layer(self.norm_cfg, in_channels[i])[1],
- # we set align_corners=False for HRNet
- Upsample(
- scale_factor=2**(j - i),
- mode='bilinear',
- align_corners=False)))
- elif j == i:
- fuse_layer.append(None)
- else:
- conv_downsamples = []
- for k in range(i - j):
- if k == i - j - 1:
- conv_downsamples.append(
- nn.Sequential(
- build_conv_layer(
- self.conv_cfg,
- in_channels[j],
- in_channels[i],
- kernel_size=3,
- stride=2,
- padding=1,
- bias=False),
- build_norm_layer(self.norm_cfg,
- in_channels[i])[1]))
- else:
- conv_downsamples.append(
- nn.Sequential(
- build_conv_layer(
- self.conv_cfg,
- in_channels[j],
- in_channels[j],
- kernel_size=3,
- stride=2,
- padding=1,
- bias=False),
- build_norm_layer(self.norm_cfg,
- in_channels[j])[1],
- nn.ReLU(inplace=False)))
- fuse_layer.append(nn.Sequential(*conv_downsamples))
- fuse_layers.append(nn.ModuleList(fuse_layer))
-
- return nn.ModuleList(fuse_layers)
-
- def forward(self, x):
- """Forward function."""
- if self.num_branches == 1:
- return [self.branches[0](x[0])]
-
- for i in range(self.num_branches):
- x[i] = self.branches[i](x[i])
-
- x_fuse = []
- for i in range(len(self.fuse_layers)):
- y = 0
- for j in range(self.num_branches):
- if i == j:
- y += x[j]
- elif j > i:
- y = y + resize(
- self.fuse_layers[i][j](x[j]),
- size=x[i].shape[2:],
- mode='bilinear',
- align_corners=False)
- else:
- y += self.fuse_layers[i][j](x[j])
- x_fuse.append(self.relu(y))
- return x_fuse
-
-
-@BACKBONES.register_module()
-class HRNet(nn.Module):
- """HRNet backbone.
-
- High-Resolution Representations for Labeling Pixels and Regions
- arXiv: https://arxiv.org/abs/1904.04514
-
- Args:
- extra (dict): detailed configuration for each stage of HRNet.
- in_channels (int): Number of input image channels. Normally 3.
- conv_cfg (dict): dictionary to construct and config conv layer.
- norm_cfg (dict): dictionary to construct and config norm layer.
- norm_eval (bool): Whether to set norm layers to eval mode, namely,
- freeze running stats (mean and var). Note: Effect on Batch Norm
- and its variants only.
- with_cp (bool): Use checkpoint or not. Using checkpoint will save some
- memory while slowing down the training speed.
- zero_init_residual (bool): whether to use zero init for last norm layer
- in resblocks to let them behave as identity.
-
- Example:
- >>> from mmseg.models import HRNet
- >>> import torch
- >>> extra = dict(
- >>> stage1=dict(
- >>> num_modules=1,
- >>> num_branches=1,
- >>> block='BOTTLENECK',
- >>> num_blocks=(4, ),
- >>> num_channels=(64, )),
- >>> stage2=dict(
- >>> num_modules=1,
- >>> num_branches=2,
- >>> block='BASIC',
- >>> num_blocks=(4, 4),
- >>> num_channels=(32, 64)),
- >>> stage3=dict(
- >>> num_modules=4,
- >>> num_branches=3,
- >>> block='BASIC',
- >>> num_blocks=(4, 4, 4),
- >>> num_channels=(32, 64, 128)),
- >>> stage4=dict(
- >>> num_modules=3,
- >>> num_branches=4,
- >>> block='BASIC',
- >>> num_blocks=(4, 4, 4, 4),
- >>> num_channels=(32, 64, 128, 256)))
- >>> self = HRNet(extra, in_channels=1)
- >>> self.eval()
- >>> inputs = torch.rand(1, 1, 32, 32)
- >>> level_outputs = self.forward(inputs)
- >>> for level_out in level_outputs:
- ... print(tuple(level_out.shape))
- (1, 32, 8, 8)
- (1, 64, 4, 4)
- (1, 128, 2, 2)
- (1, 256, 1, 1)
- """
-
- blocks_dict = {'BASIC': BasicBlock, 'BOTTLENECK': Bottleneck}
-
- def __init__(self,
- extra,
- in_channels=3,
- conv_cfg=None,
- norm_cfg=dict(type='BN', requires_grad=True),
- norm_eval=False,
- with_cp=False,
- zero_init_residual=False):
- super(HRNet, self).__init__()
- self.extra = extra
- self.conv_cfg = conv_cfg
- self.norm_cfg = norm_cfg
- self.norm_eval = norm_eval
- self.with_cp = with_cp
- self.zero_init_residual = zero_init_residual
-
- # stem net
- self.norm1_name, norm1 = build_norm_layer(self.norm_cfg, 64, postfix=1)
- self.norm2_name, norm2 = build_norm_layer(self.norm_cfg, 64, postfix=2)
-
- self.conv1 = build_conv_layer(
- self.conv_cfg,
- in_channels,
- 64,
- kernel_size=3,
- stride=2,
- padding=1,
- bias=False)
-
- self.add_module(self.norm1_name, norm1)
- self.conv2 = build_conv_layer(
- self.conv_cfg,
- 64,
- 64,
- kernel_size=3,
- stride=2,
- padding=1,
- bias=False)
-
- self.add_module(self.norm2_name, norm2)
- self.relu = nn.ReLU(inplace=True)
-
- # stage 1
- self.stage1_cfg = self.extra['stage1']
- num_channels = self.stage1_cfg['num_channels'][0]
- block_type = self.stage1_cfg['block']
- num_blocks = self.stage1_cfg['num_blocks'][0]
-
- block = self.blocks_dict[block_type]
- stage1_out_channels = num_channels * block.expansion
- self.layer1 = self._make_layer(block, 64, num_channels, num_blocks)
-
- # stage 2
- self.stage2_cfg = self.extra['stage2']
- num_channels = self.stage2_cfg['num_channels']
- block_type = self.stage2_cfg['block']
-
- block = self.blocks_dict[block_type]
- num_channels = [channel * block.expansion for channel in num_channels]
- self.transition1 = self._make_transition_layer([stage1_out_channels],
- num_channels)
- self.stage2, pre_stage_channels = self._make_stage(
- self.stage2_cfg, num_channels)
-
- # stage 3
- self.stage3_cfg = self.extra['stage3']
- num_channels = self.stage3_cfg['num_channels']
- block_type = self.stage3_cfg['block']
-
- block = self.blocks_dict[block_type]
- num_channels = [channel * block.expansion for channel in num_channels]
- self.transition2 = self._make_transition_layer(pre_stage_channels,
- num_channels)
- self.stage3, pre_stage_channels = self._make_stage(
- self.stage3_cfg, num_channels)
-
- # stage 4
- self.stage4_cfg = self.extra['stage4']
- num_channels = self.stage4_cfg['num_channels']
- block_type = self.stage4_cfg['block']
-
- block = self.blocks_dict[block_type]
- num_channels = [channel * block.expansion for channel in num_channels]
- self.transition3 = self._make_transition_layer(pre_stage_channels,
- num_channels)
- self.stage4, pre_stage_channels = self._make_stage(
- self.stage4_cfg, num_channels)
-
- @property
- def norm1(self):
- """nn.Module: the normalization layer named "norm1" """
- return getattr(self, self.norm1_name)
-
- @property
- def norm2(self):
- """nn.Module: the normalization layer named "norm2" """
- return getattr(self, self.norm2_name)
-
- def _make_transition_layer(self, num_channels_pre_layer,
- num_channels_cur_layer):
- """Make transition layer."""
- num_branches_cur = len(num_channels_cur_layer)
- num_branches_pre = len(num_channels_pre_layer)
-
- transition_layers = []
- for i in range(num_branches_cur):
- if i < num_branches_pre:
- if num_channels_cur_layer[i] != num_channels_pre_layer[i]:
- transition_layers.append(
- nn.Sequential(
- build_conv_layer(
- self.conv_cfg,
- num_channels_pre_layer[i],
- num_channels_cur_layer[i],
- kernel_size=3,
- stride=1,
- padding=1,
- bias=False),
- build_norm_layer(self.norm_cfg,
- num_channels_cur_layer[i])[1],
- nn.ReLU(inplace=True)))
- else:
- transition_layers.append(None)
- else:
- conv_downsamples = []
- for j in range(i + 1 - num_branches_pre):
- in_channels = num_channels_pre_layer[-1]
- out_channels = num_channels_cur_layer[i] \
- if j == i - num_branches_pre else in_channels
- conv_downsamples.append(
- nn.Sequential(
- build_conv_layer(
- self.conv_cfg,
- in_channels,
- out_channels,
- kernel_size=3,
- stride=2,
- padding=1,
- bias=False),
- build_norm_layer(self.norm_cfg, out_channels)[1],
- nn.ReLU(inplace=True)))
- transition_layers.append(nn.Sequential(*conv_downsamples))
-
- return nn.ModuleList(transition_layers)
-
- def _make_layer(self, block, inplanes, planes, blocks, stride=1):
- """Make each layer."""
- downsample = None
- if stride != 1 or inplanes != planes * block.expansion:
- downsample = nn.Sequential(
- build_conv_layer(
- self.conv_cfg,
- inplanes,
- planes * block.expansion,
- kernel_size=1,
- stride=stride,
- bias=False),
- build_norm_layer(self.norm_cfg, planes * block.expansion)[1])
-
- layers = []
- layers.append(
- block(
- inplanes,
- planes,
- stride,
- downsample=downsample,
- with_cp=self.with_cp,
- norm_cfg=self.norm_cfg,
- conv_cfg=self.conv_cfg))
- inplanes = planes * block.expansion
- for i in range(1, blocks):
- layers.append(
- block(
- inplanes,
- planes,
- with_cp=self.with_cp,
- norm_cfg=self.norm_cfg,
- conv_cfg=self.conv_cfg))
-
- return nn.Sequential(*layers)
-
- def _make_stage(self, layer_config, in_channels, multiscale_output=True):
- """Make each stage."""
- num_modules = layer_config['num_modules']
- num_branches = layer_config['num_branches']
- num_blocks = layer_config['num_blocks']
- num_channels = layer_config['num_channels']
- block = self.blocks_dict[layer_config['block']]
-
- hr_modules = []
- for i in range(num_modules):
- # multi_scale_output is only used for the last module
- if not multiscale_output and i == num_modules - 1:
- reset_multiscale_output = False
- else:
- reset_multiscale_output = True
-
- hr_modules.append(
- HRModule(
- num_branches,
- block,
- num_blocks,
- in_channels,
- num_channels,
- reset_multiscale_output,
- with_cp=self.with_cp,
- norm_cfg=self.norm_cfg,
- conv_cfg=self.conv_cfg))
-
- return nn.Sequential(*hr_modules), in_channels
-
- def init_weights(self, pretrained=None):
- """Initialize the weights in backbone.
-
- Args:
- pretrained (str, optional): Path to pre-trained weights.
- Defaults to None.
- """
- if isinstance(pretrained, str):
- logger = get_root_logger()
- load_checkpoint(self, pretrained, strict=False, logger=logger)
- elif pretrained is None:
- for m in self.modules():
- if isinstance(m, nn.Conv2d):
- kaiming_init(m)
- elif isinstance(m, (_BatchNorm, nn.GroupNorm)):
- constant_init(m, 1)
-
- if self.zero_init_residual:
- for m in self.modules():
- if isinstance(m, Bottleneck):
- constant_init(m.norm3, 0)
- elif isinstance(m, BasicBlock):
- constant_init(m.norm2, 0)
- else:
- raise TypeError('pretrained must be a str or None')
-
- def forward(self, x):
- """Forward function."""
-
- x = self.conv1(x)
- x = self.norm1(x)
- x = self.relu(x)
- x = self.conv2(x)
- x = self.norm2(x)
- x = self.relu(x)
- x = self.layer1(x)
-
- x_list = []
- for i in range(self.stage2_cfg['num_branches']):
- if self.transition1[i] is not None:
- x_list.append(self.transition1[i](x))
- else:
- x_list.append(x)
- y_list = self.stage2(x_list)
-
- x_list = []
- for i in range(self.stage3_cfg['num_branches']):
- if self.transition2[i] is not None:
- x_list.append(self.transition2[i](y_list[-1]))
- else:
- x_list.append(y_list[i])
- y_list = self.stage3(x_list)
-
- x_list = []
- for i in range(self.stage4_cfg['num_branches']):
- if self.transition3[i] is not None:
- x_list.append(self.transition3[i](y_list[-1]))
- else:
- x_list.append(y_list[i])
- y_list = self.stage4(x_list)
-
- return y_list
-
- def train(self, mode=True):
- """Convert the model into training mode will keeping the normalization
- layer freezed."""
- super(HRNet, self).train(mode)
- if mode and self.norm_eval:
- for m in self.modules():
- # trick: eval have effect on BatchNorm only
- if isinstance(m, _BatchNorm):
- m.eval()
diff --git a/spaces/GrandaddyShmax/AudioCraft_Plus/audiocraft/grids/musicgen/__init__.py b/spaces/GrandaddyShmax/AudioCraft_Plus/audiocraft/grids/musicgen/__init__.py
deleted file mode 100644
index d3f101f5a29ff85271e44e4f27545168a8f27baa..0000000000000000000000000000000000000000
--- a/spaces/GrandaddyShmax/AudioCraft_Plus/audiocraft/grids/musicgen/__init__.py
+++ /dev/null
@@ -1,6 +0,0 @@
-# Copyright (c) Meta Platforms, Inc. and affiliates.
-# All rights reserved.
-#
-# This source code is licensed under the license found in the
-# LICENSE file in the root directory of this source tree.
-"""MusicGen grids."""
diff --git a/spaces/GrandaddyShmax/AudioCraft_Plus/audiocraft/utils/export_legacy.py b/spaces/GrandaddyShmax/AudioCraft_Plus/audiocraft/utils/export_legacy.py
deleted file mode 100644
index 52f145f3148c3e9fdba436273bc45480fbae6481..0000000000000000000000000000000000000000
--- a/spaces/GrandaddyShmax/AudioCraft_Plus/audiocraft/utils/export_legacy.py
+++ /dev/null
@@ -1,56 +0,0 @@
-# Copyright (c) Meta Platforms, Inc. and affiliates.
-# All rights reserved.
-#
-# This source code is licensed under the license found in the
-# LICENSE file in the root directory of this source tree.
-
-"""
-Legacy functions used at the time of the first release, kept for referencd.
-"""
-
-from pathlib import Path
-import typing as tp
-
-from omegaconf import OmegaConf, DictConfig
-import torch
-
-
-def _clean_lm_cfg(cfg: DictConfig):
- OmegaConf.set_struct(cfg, False)
- # This used to be set automatically in the LM solver, need a more robust solution
- # for the future.
- cfg['transformer_lm']['card'] = 2048
- cfg['transformer_lm']['n_q'] = 4
- # Experimental params no longer supported.
- bad_params = ['spectral_norm_attn_iters', 'spectral_norm_ff_iters',
- 'residual_balancer_attn', 'residual_balancer_ff', 'layer_drop']
- for name in bad_params:
- del cfg['transformer_lm'][name]
- OmegaConf.set_struct(cfg, True)
- return cfg
-
-
-def export_encodec(checkpoint_path: tp.Union[Path, str], out_folder: tp.Union[Path, str]):
- sig = Path(checkpoint_path).parent.name
- assert len(sig) == 8, "Not a valid Dora signature"
- pkg = torch.load(checkpoint_path, 'cpu')
- new_pkg = {
- 'best_state': pkg['ema']['state']['model'],
- 'xp.cfg': OmegaConf.to_yaml(pkg['xp.cfg']),
- }
- out_file = Path(out_folder) / f'{sig}.th'
- torch.save(new_pkg, out_file)
- return out_file
-
-
-def export_lm(checkpoint_path: tp.Union[Path, str], out_folder: tp.Union[Path, str]):
- sig = Path(checkpoint_path).parent.name
- assert len(sig) == 8, "Not a valid Dora signature"
- pkg = torch.load(checkpoint_path, 'cpu')
- new_pkg = {
- 'best_state': pkg['fsdp_best_state']['model'],
- 'xp.cfg': OmegaConf.to_yaml(_clean_lm_cfg(pkg['xp.cfg']))
- }
- out_file = Path(out_folder) / f'{sig}.th'
- torch.save(new_pkg, out_file)
- return out_file
diff --git a/spaces/GrandaddyShmax/MusicGen_Plus/audiocraft/data/audio_utils.py b/spaces/GrandaddyShmax/MusicGen_Plus/audiocraft/data/audio_utils.py
deleted file mode 100644
index 76d4bc2a33ce722d879db2af33cd1336bd6b1fb3..0000000000000000000000000000000000000000
--- a/spaces/GrandaddyShmax/MusicGen_Plus/audiocraft/data/audio_utils.py
+++ /dev/null
@@ -1,174 +0,0 @@
-# Copyright (c) Meta Platforms, Inc. and affiliates.
-# All rights reserved.
-#
-# This source code is licensed under the license found in the
-# LICENSE file in the root directory of this source tree.
-
-import sys
-import typing as tp
-
-import julius
-import torch
-import torchaudio
-
-
-def convert_audio_channels(wav: torch.Tensor, channels: int = 2) -> torch.Tensor:
- """Convert audio to the given number of channels.
-
- Args:
- wav (torch.Tensor): Audio wave of shape [B, C, T].
- channels (int): Expected number of channels as output.
- Returns:
- torch.Tensor: Downmixed or unchanged audio wave [B, C, T].
- """
- *shape, src_channels, length = wav.shape
- if src_channels == channels:
- pass
- elif channels == 1:
- # Case 1:
- # The caller asked 1-channel audio, and the stream has multiple
- # channels, downmix all channels.
- wav = wav.mean(dim=-2, keepdim=True)
- elif src_channels == 1:
- # Case 2:
- # The caller asked for multiple channels, but the input file has
- # a single channel, replicate the audio over all channels.
- wav = wav.expand(*shape, channels, length)
- elif src_channels >= channels:
- # Case 3:
- # The caller asked for multiple channels, and the input file has
- # more channels than requested. In that case return the first channels.
- wav = wav[..., :channels, :]
- else:
- # Case 4: What is a reasonable choice here?
- raise ValueError('The audio file has less channels than requested but is not mono.')
- return wav
-
-
-def convert_audio(wav: torch.Tensor, from_rate: float,
- to_rate: float, to_channels: int) -> torch.Tensor:
- """Convert audio to new sample rate and number of audio channels.
- """
- wav = julius.resample_frac(wav, int(from_rate), int(to_rate))
- wav = convert_audio_channels(wav, to_channels)
- return wav
-
-
-def normalize_loudness(wav: torch.Tensor, sample_rate: int, loudness_headroom_db: float = 14,
- loudness_compressor: bool = False, energy_floor: float = 2e-3):
- """Normalize an input signal to a user loudness in dB LKFS.
- Audio loudness is defined according to the ITU-R BS.1770-4 recommendation.
-
- Args:
- wav (torch.Tensor): Input multichannel audio data.
- sample_rate (int): Sample rate.
- loudness_headroom_db (float): Target loudness of the output in dB LUFS.
- loudness_compressor (bool): Uses tanh for soft clipping.
- energy_floor (float): anything below that RMS level will not be rescaled.
- Returns:
- output (torch.Tensor): Loudness normalized output data.
- """
- energy = wav.pow(2).mean().sqrt().item()
- if energy < energy_floor:
- return wav
- transform = torchaudio.transforms.Loudness(sample_rate)
- input_loudness_db = transform(wav).item()
- # calculate the gain needed to scale to the desired loudness level
- delta_loudness = -loudness_headroom_db - input_loudness_db
- gain = 10.0 ** (delta_loudness / 20.0)
- output = gain * wav
- if loudness_compressor:
- output = torch.tanh(output)
- assert output.isfinite().all(), (input_loudness_db, wav.pow(2).mean().sqrt())
- return output
-
-
-def _clip_wav(wav: torch.Tensor, log_clipping: bool = False, stem_name: tp.Optional[str] = None) -> None:
- """Utility function to clip the audio with logging if specified."""
- max_scale = wav.abs().max()
- if log_clipping and max_scale > 1:
- clamp_prob = (wav.abs() > 1).float().mean().item()
- print(f"CLIPPING {stem_name or ''} happening with proba (a bit of clipping is okay):",
- clamp_prob, "maximum scale: ", max_scale.item(), file=sys.stderr)
- wav.clamp_(-1, 1)
-
-
-def normalize_audio(wav: torch.Tensor, normalize: bool = True,
- strategy: str = 'peak', peak_clip_headroom_db: float = 1,
- rms_headroom_db: float = 18, loudness_headroom_db: float = 14,
- loudness_compressor: bool = False, log_clipping: bool = False,
- sample_rate: tp.Optional[int] = None,
- stem_name: tp.Optional[str] = None) -> torch.Tensor:
- """Normalize the audio according to the prescribed strategy (see after).
-
- Args:
- wav (torch.Tensor): Audio data.
- normalize (bool): if `True` (default), normalizes according to the prescribed
- strategy (see after). If `False`, the strategy is only used in case clipping
- would happen.
- strategy (str): Can be either 'clip', 'peak', or 'rms'. Default is 'peak',
- i.e. audio is normalized by its largest value. RMS normalizes by root-mean-square
- with extra headroom to avoid clipping. 'clip' just clips.
- peak_clip_headroom_db (float): Headroom in dB when doing 'peak' or 'clip' strategy.
- rms_headroom_db (float): Headroom in dB when doing 'rms' strategy. This must be much larger
- than the `peak_clip` one to avoid further clipping.
- loudness_headroom_db (float): Target loudness for loudness normalization.
- loudness_compressor (bool): If True, uses tanh based soft clipping.
- log_clipping (bool): If True, basic logging on stderr when clipping still
- occurs despite strategy (only for 'rms').
- sample_rate (int): Sample rate for the audio data (required for loudness).
- stem_name (Optional[str]): Stem name for clipping logging.
- Returns:
- torch.Tensor: Normalized audio.
- """
- scale_peak = 10 ** (-peak_clip_headroom_db / 20)
- scale_rms = 10 ** (-rms_headroom_db / 20)
- if strategy == 'peak':
- rescaling = (scale_peak / wav.abs().max())
- if normalize or rescaling < 1:
- wav = wav * rescaling
- elif strategy == 'clip':
- wav = wav.clamp(-scale_peak, scale_peak)
- elif strategy == 'rms':
- mono = wav.mean(dim=0)
- rescaling = scale_rms / mono.pow(2).mean().sqrt()
- if normalize or rescaling < 1:
- wav = wav * rescaling
- _clip_wav(wav, log_clipping=log_clipping, stem_name=stem_name)
- elif strategy == 'loudness':
- assert sample_rate is not None, "Loudness normalization requires sample rate."
- wav = normalize_loudness(wav, sample_rate, loudness_headroom_db, loudness_compressor)
- _clip_wav(wav, log_clipping=log_clipping, stem_name=stem_name)
- else:
- assert wav.abs().max() < 1
- assert strategy == '' or strategy == 'none', f"Unexpected strategy: '{strategy}'"
- return wav
-
-
-def f32_pcm(wav: torch.Tensor) -> torch.Tensor:
- """Convert audio to float 32 bits PCM format.
- """
- if wav.dtype.is_floating_point:
- return wav
- else:
- assert wav.dtype == torch.int16
- return wav.float() / 2**15
-
-
-def i16_pcm(wav: torch.Tensor) -> torch.Tensor:
- """Convert audio to int 16 bits PCM format.
-
- ..Warning:: There exist many formula for doing this convertion. None are perfect
- due to the asymetry of the int16 range. One either have possible clipping, DC offset,
- or inconsistancies with f32_pcm. If the given wav doesn't have enough headroom,
- it is possible that `i16_pcm(f32_pcm)) != Identity`.
- """
- if wav.dtype.is_floating_point:
- assert wav.abs().max() <= 1
- candidate = (wav * 2 ** 15).round()
- if candidate.max() >= 2 ** 15: # clipping would occur
- candidate = (wav * (2 ** 15 - 1)).round()
- return candidate.short()
- else:
- assert wav.dtype == torch.int16
- return wav
diff --git a/spaces/Grezz/generate_human_motion/pyrender/pyrender/camera.py b/spaces/Grezz/generate_human_motion/pyrender/pyrender/camera.py
deleted file mode 100644
index e019358039033c3a372c990ebad3151258c3651d..0000000000000000000000000000000000000000
--- a/spaces/Grezz/generate_human_motion/pyrender/pyrender/camera.py
+++ /dev/null
@@ -1,437 +0,0 @@
-"""Virtual cameras compliant with the glTF 2.0 specification as described at
-https://github.com/KhronosGroup/glTF/tree/master/specification/2.0#reference-camera
-
-Author: Matthew Matl
-"""
-import abc
-import numpy as np
-import six
-import sys
-
-from .constants import DEFAULT_Z_NEAR, DEFAULT_Z_FAR
-
-
-@six.add_metaclass(abc.ABCMeta)
-class Camera(object):
- """Abstract base class for all cameras.
-
- Note
- ----
- Camera poses are specified in the OpenGL format,
- where the z axis points away from the view direction and the
- x and y axes point to the right and up in the image plane, respectively.
-
- Parameters
- ----------
- znear : float
- The floating-point distance to the near clipping plane.
- zfar : float
- The floating-point distance to the far clipping plane.
- ``zfar`` must be greater than ``znear``.
- name : str, optional
- The user-defined name of this object.
- """
-
- def __init__(self,
- znear=DEFAULT_Z_NEAR,
- zfar=DEFAULT_Z_FAR,
- name=None):
- self.name = name
- self.znear = znear
- self.zfar = zfar
-
- @property
- def name(self):
- """str : The user-defined name of this object.
- """
- return self._name
-
- @name.setter
- def name(self, value):
- if value is not None:
- value = str(value)
- self._name = value
-
- @property
- def znear(self):
- """float : The distance to the near clipping plane.
- """
- return self._znear
-
- @znear.setter
- def znear(self, value):
- value = float(value)
- if value < 0:
- raise ValueError('z-near must be >= 0.0')
- self._znear = value
-
- @property
- def zfar(self):
- """float : The distance to the far clipping plane.
- """
- return self._zfar
-
- @zfar.setter
- def zfar(self, value):
- value = float(value)
- if value <= 0 or value <= self.znear:
- raise ValueError('zfar must be >0 and >znear')
- self._zfar = value
-
- @abc.abstractmethod
- def get_projection_matrix(self, width=None, height=None):
- """Return the OpenGL projection matrix for this camera.
-
- Parameters
- ----------
- width : int
- Width of the current viewport, in pixels.
- height : int
- Height of the current viewport, in pixels.
- """
- pass
-
-
-class PerspectiveCamera(Camera):
-
- """A perspective camera for perspective projection.
-
- Parameters
- ----------
- yfov : float
- The floating-point vertical field of view in radians.
- znear : float
- The floating-point distance to the near clipping plane.
- If not specified, defaults to 0.05.
- zfar : float, optional
- The floating-point distance to the far clipping plane.
- ``zfar`` must be greater than ``znear``.
- If None, the camera uses an infinite projection matrix.
- aspectRatio : float, optional
- The floating-point aspect ratio of the field of view.
- If not specified, the camera uses the viewport's aspect ratio.
- name : str, optional
- The user-defined name of this object.
- """
-
- def __init__(self,
- yfov,
- znear=DEFAULT_Z_NEAR,
- zfar=None,
- aspectRatio=None,
- name=None):
- super(PerspectiveCamera, self).__init__(
- znear=znear,
- zfar=zfar,
- name=name,
- )
-
- self.yfov = yfov
- self.aspectRatio = aspectRatio
-
- @property
- def yfov(self):
- """float : The vertical field of view in radians.
- """
- return self._yfov
-
- @yfov.setter
- def yfov(self, value):
- value = float(value)
- if value <= 0.0:
- raise ValueError('Field of view must be positive')
- self._yfov = value
-
- @property
- def zfar(self):
- """float : The distance to the far clipping plane.
- """
- return self._zfar
-
- @zfar.setter
- def zfar(self, value):
- if value is not None:
- value = float(value)
- if value <= 0 or value <= self.znear:
- raise ValueError('zfar must be >0 and >znear')
- self._zfar = value
-
- @property
- def aspectRatio(self):
- """float : The ratio of the width to the height of the field of view.
- """
- return self._aspectRatio
-
- @aspectRatio.setter
- def aspectRatio(self, value):
- if value is not None:
- value = float(value)
- if value <= 0.0:
- raise ValueError('Aspect ratio must be positive')
- self._aspectRatio = value
-
- def get_projection_matrix(self, width=None, height=None):
- """Return the OpenGL projection matrix for this camera.
-
- Parameters
- ----------
- width : int
- Width of the current viewport, in pixels.
- height : int
- Height of the current viewport, in pixels.
- """
- aspect_ratio = self.aspectRatio
- if aspect_ratio is None:
- if width is None or height is None:
- raise ValueError('Aspect ratio of camera must be defined')
- aspect_ratio = float(width) / float(height)
-
- a = aspect_ratio
- t = np.tan(self.yfov / 2.0)
- n = self.znear
- f = self.zfar
-
- P = np.zeros((4,4))
- P[0][0] = 1.0 / (a * t)
- P[1][1] = 1.0 / t
- P[3][2] = -1.0
-
- if f is None:
- P[2][2] = -1.0
- P[2][3] = -2.0 * n
- else:
- P[2][2] = (f + n) / (n - f)
- P[2][3] = (2 * f * n) / (n - f)
-
- return P
-
-
-class OrthographicCamera(Camera):
- """An orthographic camera for orthographic projection.
-
- Parameters
- ----------
- xmag : float
- The floating-point horizontal magnification of the view.
- ymag : float
- The floating-point vertical magnification of the view.
- znear : float
- The floating-point distance to the near clipping plane.
- If not specified, defaults to 0.05.
- zfar : float
- The floating-point distance to the far clipping plane.
- ``zfar`` must be greater than ``znear``.
- If not specified, defaults to 100.0.
- name : str, optional
- The user-defined name of this object.
- """
-
- def __init__(self,
- xmag,
- ymag,
- znear=DEFAULT_Z_NEAR,
- zfar=DEFAULT_Z_FAR,
- name=None):
- super(OrthographicCamera, self).__init__(
- znear=znear,
- zfar=zfar,
- name=name,
- )
-
- self.xmag = xmag
- self.ymag = ymag
-
- @property
- def xmag(self):
- """float : The horizontal magnification of the view.
- """
- return self._xmag
-
- @xmag.setter
- def xmag(self, value):
- value = float(value)
- if value <= 0.0:
- raise ValueError('X magnification must be positive')
- self._xmag = value
-
- @property
- def ymag(self):
- """float : The vertical magnification of the view.
- """
- return self._ymag
-
- @ymag.setter
- def ymag(self, value):
- value = float(value)
- if value <= 0.0:
- raise ValueError('Y magnification must be positive')
- self._ymag = value
-
- @property
- def znear(self):
- """float : The distance to the near clipping plane.
- """
- return self._znear
-
- @znear.setter
- def znear(self, value):
- value = float(value)
- if value <= 0:
- raise ValueError('z-near must be > 0.0')
- self._znear = value
-
- def get_projection_matrix(self, width=None, height=None):
- """Return the OpenGL projection matrix for this camera.
-
- Parameters
- ----------
- width : int
- Width of the current viewport, in pixels.
- Unused in this function.
- height : int
- Height of the current viewport, in pixels.
- Unused in this function.
- """
- xmag = self.xmag
- ymag = self.ymag
-
- # If screen width/height defined, rescale xmag
- if width is not None and height is not None:
- xmag = width / height * ymag
-
- n = self.znear
- f = self.zfar
- P = np.zeros((4,4))
- P[0][0] = 1.0 / xmag
- P[1][1] = 1.0 / ymag
- P[2][2] = 2.0 / (n - f)
- P[2][3] = (f + n) / (n - f)
- P[3][3] = 1.0
- return P
-
-
-class IntrinsicsCamera(Camera):
- """A perspective camera with custom intrinsics.
-
- Parameters
- ----------
- fx : float
- X-axis focal length in pixels.
- fy : float
- Y-axis focal length in pixels.
- cx : float
- X-axis optical center in pixels.
- cy : float
- Y-axis optical center in pixels.
- znear : float
- The floating-point distance to the near clipping plane.
- If not specified, defaults to 0.05.
- zfar : float
- The floating-point distance to the far clipping plane.
- ``zfar`` must be greater than ``znear``.
- If not specified, defaults to 100.0.
- name : str, optional
- The user-defined name of this object.
- """
-
- def __init__(self,
- fx,
- fy,
- cx,
- cy,
- znear=DEFAULT_Z_NEAR,
- zfar=DEFAULT_Z_FAR,
- name=None):
- super(IntrinsicsCamera, self).__init__(
- znear=znear,
- zfar=zfar,
- name=name,
- )
-
- self.fx = fx
- self.fy = fy
- self.cx = cx
- self.cy = cy
-
- @property
- def fx(self):
- """float : X-axis focal length in meters.
- """
- return self._fx
-
- @fx.setter
- def fx(self, value):
- self._fx = float(value)
-
- @property
- def fy(self):
- """float : Y-axis focal length in meters.
- """
- return self._fy
-
- @fy.setter
- def fy(self, value):
- self._fy = float(value)
-
- @property
- def cx(self):
- """float : X-axis optical center in pixels.
- """
- return self._cx
-
- @cx.setter
- def cx(self, value):
- self._cx = float(value)
-
- @property
- def cy(self):
- """float : Y-axis optical center in pixels.
- """
- return self._cy
-
- @cy.setter
- def cy(self, value):
- self._cy = float(value)
-
- def get_projection_matrix(self, width, height):
- """Return the OpenGL projection matrix for this camera.
-
- Parameters
- ----------
- width : int
- Width of the current viewport, in pixels.
- height : int
- Height of the current viewport, in pixels.
- """
- width = float(width)
- height = float(height)
-
- cx, cy = self.cx, self.cy
- fx, fy = self.fx, self.fy
- if sys.platform == 'darwin':
- cx = self.cx * 2.0
- cy = self.cy * 2.0
- fx = self.fx * 2.0
- fy = self.fy * 2.0
-
- P = np.zeros((4,4))
- P[0][0] = 2.0 * fx / width
- P[1][1] = 2.0 * fy / height
- P[0][2] = 1.0 - 2.0 * cx / width
- P[1][2] = 2.0 * cy / height - 1.0
- P[3][2] = -1.0
-
- n = self.znear
- f = self.zfar
- if f is None:
- P[2][2] = -1.0
- P[2][3] = -2.0 * n
- else:
- P[2][2] = (f + n) / (n - f)
- P[2][3] = (2 * f * n) / (n - f)
-
- return P
-
-
-__all__ = ['Camera', 'PerspectiveCamera', 'OrthographicCamera',
- 'IntrinsicsCamera']
diff --git a/spaces/HarryLee/eCommerceImageCaptioning/fairseq/examples/noisychannel/rerank_score_bw.py b/spaces/HarryLee/eCommerceImageCaptioning/fairseq/examples/noisychannel/rerank_score_bw.py
deleted file mode 100644
index b0bc913651bd76667e25c214acb70f2bca19e185..0000000000000000000000000000000000000000
--- a/spaces/HarryLee/eCommerceImageCaptioning/fairseq/examples/noisychannel/rerank_score_bw.py
+++ /dev/null
@@ -1,143 +0,0 @@
-# Copyright (c) Facebook, Inc. and its affiliates.
-#
-# This source code is licensed under the MIT license found in the
-# LICENSE file in the root directory of this source tree.
-
-import os
-from contextlib import redirect_stdout
-
-from fairseq import options
-from fairseq_cli import generate
-
-from examples.noisychannel import rerank_options, rerank_utils
-
-
-def score_bw(args):
- if args.backwards1:
- scorer1_src = args.target_lang
- scorer1_tgt = args.source_lang
- else:
- scorer1_src = args.source_lang
- scorer1_tgt = args.target_lang
-
- if args.score_model2 is not None:
- if args.backwards2:
- scorer2_src = args.target_lang
- scorer2_tgt = args.source_lang
- else:
- scorer2_src = args.source_lang
- scorer2_tgt = args.target_lang
-
- rerank1_is_gen = (
- args.gen_model == args.score_model1 and args.source_prefix_frac is None
- )
- rerank2_is_gen = (
- args.gen_model == args.score_model2 and args.source_prefix_frac is None
- )
-
- (
- pre_gen,
- left_to_right_preprocessed_dir,
- right_to_left_preprocessed_dir,
- backwards_preprocessed_dir,
- lm_preprocessed_dir,
- ) = rerank_utils.get_directories(
- args.data_dir_name,
- args.num_rescore,
- args.gen_subset,
- args.gen_model_name,
- args.shard_id,
- args.num_shards,
- args.sampling,
- args.prefix_len,
- args.target_prefix_frac,
- args.source_prefix_frac,
- )
-
- score1_file = rerank_utils.rescore_file_name(
- pre_gen,
- args.prefix_len,
- args.model1_name,
- target_prefix_frac=args.target_prefix_frac,
- source_prefix_frac=args.source_prefix_frac,
- backwards=args.backwards1,
- )
-
- if args.score_model2 is not None:
- score2_file = rerank_utils.rescore_file_name(
- pre_gen,
- args.prefix_len,
- args.model2_name,
- target_prefix_frac=args.target_prefix_frac,
- source_prefix_frac=args.source_prefix_frac,
- backwards=args.backwards2,
- )
-
- if args.right_to_left1:
- rerank_data1 = right_to_left_preprocessed_dir
- elif args.backwards1:
- rerank_data1 = backwards_preprocessed_dir
- else:
- rerank_data1 = left_to_right_preprocessed_dir
-
- gen_param = ["--batch-size", str(128), "--score-reference", "--gen-subset", "train"]
- if not rerank1_is_gen and not os.path.isfile(score1_file):
- print("STEP 4: score the translations for model 1")
-
- model_param1 = [
- "--path",
- args.score_model1,
- "--source-lang",
- scorer1_src,
- "--target-lang",
- scorer1_tgt,
- ]
- gen_model1_param = [rerank_data1] + gen_param + model_param1
-
- gen_parser = options.get_generation_parser()
- input_args = options.parse_args_and_arch(gen_parser, gen_model1_param)
-
- with open(score1_file, "w") as f:
- with redirect_stdout(f):
- generate.main(input_args)
-
- if (
- args.score_model2 is not None
- and not os.path.isfile(score2_file)
- and not rerank2_is_gen
- ):
- print("STEP 4: score the translations for model 2")
-
- if args.right_to_left2:
- rerank_data2 = right_to_left_preprocessed_dir
- elif args.backwards2:
- rerank_data2 = backwards_preprocessed_dir
- else:
- rerank_data2 = left_to_right_preprocessed_dir
-
- model_param2 = [
- "--path",
- args.score_model2,
- "--source-lang",
- scorer2_src,
- "--target-lang",
- scorer2_tgt,
- ]
- gen_model2_param = [rerank_data2] + gen_param + model_param2
-
- gen_parser = options.get_generation_parser()
- input_args = options.parse_args_and_arch(gen_parser, gen_model2_param)
-
- with open(score2_file, "w") as f:
- with redirect_stdout(f):
- generate.main(input_args)
-
-
-def cli_main():
- parser = rerank_options.get_reranking_parser()
- args = options.parse_args_and_arch(parser)
- score_bw(args)
-
-
-if __name__ == "__main__":
- cli_main()
diff --git a/spaces/HarryLee/eCommerceImageCaptioning/fairseq/examples/roberta/README.custom_classification.md b/spaces/HarryLee/eCommerceImageCaptioning/fairseq/examples/roberta/README.custom_classification.md
deleted file mode 100644
index 7254bb7d178760ef5b847901bbcac3711af33ca2..0000000000000000000000000000000000000000
--- a/spaces/HarryLee/eCommerceImageCaptioning/fairseq/examples/roberta/README.custom_classification.md
+++ /dev/null
@@ -1,168 +0,0 @@
-# Finetuning RoBERTa on a custom classification task
-
-This example shows how to finetune RoBERTa on the IMDB dataset, but should illustrate the process for most classification tasks.
-
-### 1) Get the data
-
-```bash
-wget http://ai.stanford.edu/~amaas/data/sentiment/aclImdb_v1.tar.gz
-tar zxvf aclImdb_v1.tar.gz
-```
-
-
-### 2) Format data
-
-`IMDB` data has one data-sample in each file, below python code-snippet converts it one file for train and valid each for ease of processing.
-```python
-import argparse
-import os
-import random
-from glob import glob
-
-random.seed(0)
-
-def main(args):
- for split in ['train', 'test']:
- samples = []
- for class_label in ['pos', 'neg']:
- fnames = glob(os.path.join(args.datadir, split, class_label) + '/*.txt')
- for fname in fnames:
- with open(fname) as fin:
- line = fin.readline()
- samples.append((line, 1 if class_label == 'pos' else 0))
- random.shuffle(samples)
- out_fname = 'train' if split == 'train' else 'dev'
- f1 = open(os.path.join(args.datadir, out_fname + '.input0'), 'w')
- f2 = open(os.path.join(args.datadir, out_fname + '.label'), 'w')
- for sample in samples:
- f1.write(sample[0] + '\n')
- f2.write(str(sample[1]) + '\n')
- f1.close()
- f2.close()
-
-if __name__ == '__main__':
- parser = argparse.ArgumentParser()
- parser.add_argument('--datadir', default='aclImdb')
- args = parser.parse_args()
- main(args)
-```
-
-
-### 3) BPE encode
-
-Run `multiprocessing_bpe_encoder`, you can also do this in previous step for each sample but that might be slower.
-```bash
-# Download encoder.json and vocab.bpe
-wget -N 'https://dl.fbaipublicfiles.com/fairseq/gpt2_bpe/encoder.json'
-wget -N 'https://dl.fbaipublicfiles.com/fairseq/gpt2_bpe/vocab.bpe'
-
-for SPLIT in train dev; do
- python -m examples.roberta.multiprocessing_bpe_encoder \
- --encoder-json encoder.json \
- --vocab-bpe vocab.bpe \
- --inputs "aclImdb/$SPLIT.input0" \
- --outputs "aclImdb/$SPLIT.input0.bpe" \
- --workers 60 \
- --keep-empty
-done
-```
-
-
-### 4) Preprocess data
-
-```bash
-# Download fairseq dictionary.
-wget -N 'https://dl.fbaipublicfiles.com/fairseq/gpt2_bpe/dict.txt'
-
-fairseq-preprocess \
- --only-source \
- --trainpref "aclImdb/train.input0.bpe" \
- --validpref "aclImdb/dev.input0.bpe" \
- --destdir "IMDB-bin/input0" \
- --workers 60 \
- --srcdict dict.txt
-
-fairseq-preprocess \
- --only-source \
- --trainpref "aclImdb/train.label" \
- --validpref "aclImdb/dev.label" \
- --destdir "IMDB-bin/label" \
- --workers 60
-
-```
-
-
-### 5) Run training
-
-```bash
-TOTAL_NUM_UPDATES=7812 # 10 epochs through IMDB for bsz 32
-WARMUP_UPDATES=469 # 6 percent of the number of updates
-LR=1e-05 # Peak LR for polynomial LR scheduler.
-HEAD_NAME=imdb_head # Custom name for the classification head.
-NUM_CLASSES=2 # Number of classes for the classification task.
-MAX_SENTENCES=8 # Batch size.
-ROBERTA_PATH=/path/to/roberta.large/model.pt
-
-CUDA_VISIBLE_DEVICES=0 fairseq-train IMDB-bin/ \
- --restore-file $ROBERTA_PATH \
- --max-positions 512 \
- --batch-size $MAX_SENTENCES \
- --max-tokens 4400 \
- --task sentence_prediction \
- --reset-optimizer --reset-dataloader --reset-meters \
- --required-batch-size-multiple 1 \
- --init-token 0 --separator-token 2 \
- --arch roberta_large \
- --criterion sentence_prediction \
- --classification-head-name $HEAD_NAME \
- --num-classes $NUM_CLASSES \
- --dropout 0.1 --attention-dropout 0.1 \
- --weight-decay 0.1 --optimizer adam --adam-betas "(0.9, 0.98)" --adam-eps 1e-06 \
- --clip-norm 0.0 \
- --lr-scheduler polynomial_decay --lr $LR --total-num-update $TOTAL_NUM_UPDATES --warmup-updates $WARMUP_UPDATES \
- --fp16 --fp16-init-scale 4 --threshold-loss-scale 1 --fp16-scale-window 128 \
- --max-epoch 10 \
- --best-checkpoint-metric accuracy --maximize-best-checkpoint-metric \
- --shorten-method "truncate" \
- --find-unused-parameters \
- --update-freq 4
-```
-
-The above command will finetune RoBERTa-large with an effective batch-size of 32
-sentences (`--batch-size=8 --update-freq=4`). The expected
-`best-validation-accuracy` after 10 epochs is ~96.5%.
-
-If you run out of GPU memory, try decreasing `--batch-size` and increase
-`--update-freq` to compensate.
-
-
-### 6) Load model using hub interface
-
-Now we can load the trained model checkpoint using the RoBERTa hub interface.
-
-Assuming your checkpoints are stored in `checkpoints/`:
-```python
-from fairseq.models.roberta import RobertaModel
-roberta = RobertaModel.from_pretrained(
- 'checkpoints',
- checkpoint_file='checkpoint_best.pt',
- data_name_or_path='IMDB-bin'
-)
-roberta.eval() # disable dropout
-```
-
-Finally you can make predictions using the `imdb_head` (or whatever you set
-`--classification-head-name` to during training):
-```python
-label_fn = lambda label: roberta.task.label_dictionary.string(
- [label + roberta.task.label_dictionary.nspecial]
-)
-
-tokens = roberta.encode('Best movie this year')
-pred = label_fn(roberta.predict('imdb_head', tokens).argmax().item())
-assert pred == '1' # positive
-
-tokens = roberta.encode('Worst movie ever')
-pred = label_fn(roberta.predict('imdb_head', tokens).argmax().item())
-assert pred == '0' # negative
-```
diff --git a/spaces/HarryLee/eCommerceImageCaptioning/fairseq/tests/test_dataset.py b/spaces/HarryLee/eCommerceImageCaptioning/fairseq/tests/test_dataset.py
deleted file mode 100644
index a3e3970028bc4b0259153e403951e1735bb0cd3e..0000000000000000000000000000000000000000
--- a/spaces/HarryLee/eCommerceImageCaptioning/fairseq/tests/test_dataset.py
+++ /dev/null
@@ -1,66 +0,0 @@
-# Copyright (c) Facebook, Inc. and its affiliates.
-#
-# This source code is licensed under the MIT license found in the
-# LICENSE file in the root directory of this source tree.
-
-import logging
-import unittest
-from typing import Sequence
-
-from fairseq.data import LanguagePairDataset, ListDataset, RoundRobinZipDatasets
-from tests.test_train import mock_dict
-
-
-def lang_pair_dataset(lengths: Sequence[int]) -> LanguagePairDataset:
- tokens = [[i] * l for i, l in enumerate(lengths)]
- return LanguagePairDataset(ListDataset(tokens), lengths, mock_dict())
-
-
-def sample(id: int, length: int):
- return {"id": id, "source": [id] * length, "target": None}
-
-
-class TestDataset(unittest.TestCase):
- def setUp(self):
- logging.disable(logging.CRITICAL)
-
- def tearDown(self):
- logging.disable(logging.NOTSET)
-
- def test_round_robin_zip_datasets(self):
- long_dataset = lang_pair_dataset([10, 9, 8, 11])
- short_dataset = lang_pair_dataset([11, 9])
-
- dataset = RoundRobinZipDatasets({"a": long_dataset, "b": short_dataset})
- # Dataset is now sorted by sentence length
- dataset.ordered_indices()
- assert dataset.longest_dataset is long_dataset
- self.assertEqual(dict(dataset[0]), {"a": sample(2, 8), "b": sample(1, 9)})
- # The item 2 of dataset 'a' is with item (2 % 2 = 0) of dataset 'b'
- self.assertEqual(dict(dataset[2]), {"a": sample(0, 10), "b": sample(1, 9)})
-
- def test_round_robin_zip_datasets_filtered(self):
- long_dataset = lang_pair_dataset([10, 20, 8, 11, 1000, 7, 12])
- short_dataset = lang_pair_dataset([11, 20, 9, 1000])
-
- dataset = RoundRobinZipDatasets({"a": long_dataset, "b": short_dataset})
- # Dataset is now sorted by sentence length
- idx = dataset.ordered_indices()
- idx, _ = dataset.filter_indices_by_size(idx, {"a": 19, "b": 900})
- self.assertEqual(list(idx), [0, 1, 2, 3, 4])
- self.assertEqual(dict(dataset[0]), {"a": sample(5, 7), "b": sample(2, 9)})
- self.assertEqual(dict(dataset[2]), {"a": sample(0, 10), "b": sample(1, 20)})
- self.assertEqual(dict(dataset[4]), {"a": sample(6, 12), "b": sample(0, 11)})
-
- def test_round_robin_zip_datasets_filtered_with_tuple(self):
- long_dataset = lang_pair_dataset([10, 20, 8, 11, 1000, 7, 12])
- short_dataset = lang_pair_dataset([11, 20, 9, 1000])
-
- dataset = RoundRobinZipDatasets({"a": long_dataset, "b": short_dataset})
- # Dataset is now sorted by sentence length
- idx = dataset.ordered_indices()
- idx, _ = dataset.filter_indices_by_size(idx, 19)
- self.assertEqual(list(idx), [0, 1, 2, 3, 4])
- self.assertEqual(dict(dataset[0]), {"a": sample(5, 7), "b": sample(2, 9)})
- self.assertEqual(dict(dataset[2]), {"a": sample(0, 10), "b": sample(2, 9)})
- self.assertEqual(dict(dataset[4]), {"a": sample(6, 12), "b": sample(2, 9)})
diff --git a/spaces/HarryLee/eCommerceImageCaptioning/utils/__init__.py b/spaces/HarryLee/eCommerceImageCaptioning/utils/__init__.py
deleted file mode 100644
index e69de29bb2d1d6434b8b29ae775ad8c2e48c5391..0000000000000000000000000000000000000000
diff --git a/spaces/Harveenchadha/Vakyansh-Hindi-TTS/ttsv/src/glow_tts/hifi/utils.py b/spaces/Harveenchadha/Vakyansh-Hindi-TTS/ttsv/src/glow_tts/hifi/utils.py
deleted file mode 100644
index 71e9b2c99e053e2d4239074a67d64b834898c348..0000000000000000000000000000000000000000
--- a/spaces/Harveenchadha/Vakyansh-Hindi-TTS/ttsv/src/glow_tts/hifi/utils.py
+++ /dev/null
@@ -1,57 +0,0 @@
-import glob
-import os
-import matplotlib
-import torch
-from torch.nn.utils import weight_norm
-
-matplotlib.use("Agg")
-import matplotlib.pylab as plt
-
-
-def plot_spectrogram(spectrogram):
- fig, ax = plt.subplots(figsize=(10, 2))
- im = ax.imshow(spectrogram, aspect="auto", origin="lower", interpolation="none")
- plt.colorbar(im, ax=ax)
-
- fig.canvas.draw()
- plt.close()
-
- return fig
-
-
-def init_weights(m, mean=0.0, std=0.01):
- classname = m.__class__.__name__
- if classname.find("Conv") != -1:
- m.weight.data.normal_(mean, std)
-
-
-def apply_weight_norm(m):
- classname = m.__class__.__name__
- if classname.find("Conv") != -1:
- weight_norm(m)
-
-
-def get_padding(kernel_size, dilation=1):
- return int((kernel_size * dilation - dilation) / 2)
-
-
-def load_checkpoint(filepath, device):
- assert os.path.isfile(filepath)
- print("Loading '{}'".format(filepath))
- checkpoint_dict = torch.load(filepath, map_location=device)
- print("Complete.")
- return checkpoint_dict
-
-
-def save_checkpoint(filepath, obj):
- print("Saving checkpoint to {}".format(filepath))
- torch.save(obj, filepath)
- print("Complete.")
-
-
-def scan_checkpoint(cp_dir, prefix):
- pattern = os.path.join(cp_dir, prefix + "????????")
- cp_list = glob.glob(pattern)
- if len(cp_list) == 0:
- return None
- return sorted(cp_list)[-1]
diff --git a/spaces/ICML2022/OFA/fairseq/fairseq/data/token_block_dataset.py b/spaces/ICML2022/OFA/fairseq/fairseq/data/token_block_dataset.py
deleted file mode 100644
index d2c65fd7e058072911c3aa60bfc760288a0f83e5..0000000000000000000000000000000000000000
--- a/spaces/ICML2022/OFA/fairseq/fairseq/data/token_block_dataset.py
+++ /dev/null
@@ -1,202 +0,0 @@
-# Copyright (c) Facebook, Inc. and its affiliates.
-#
-# This source code is licensed under the MIT license found in the
-# LICENSE file in the root directory of this source tree.
-
-import numpy as np
-import torch
-from fairseq.data import FairseqDataset, plasma_utils
-from fairseq.data.indexed_dataset import best_fitting_int_dtype
-from typing import Tuple
-
-
-class TokenBlockDataset(FairseqDataset):
- """Break a Dataset of tokens into blocks.
-
- Args:
- dataset (~torch.utils.data.Dataset): dataset to break into blocks
- sizes (List[int]): sentence lengths (required for 'complete' and 'eos')
- block_size (int): maximum block size (ignored in 'eos' break mode)
- break_mode (str, optional): Mode used for breaking tokens. Values can
- be one of:
- - 'none': break tokens into equally sized blocks (up to block_size)
- - 'complete': break tokens into blocks (up to block_size) such that
- blocks contains complete sentences, although block_size may be
- exceeded if some sentences exceed block_size
- - 'complete_doc': similar to 'complete' mode, but do not
- cross document boundaries
- - 'eos': each block contains one sentence (block_size is ignored)
- include_targets (bool, optional): return next tokens as targets
- (default: False).
- document_sep_len (int, optional): document separator size (required for
- 'complete_doc' break mode). Typically 1 if the sentences have eos
- and 0 otherwise.
- """
-
- def __init__(
- self,
- dataset,
- sizes,
- block_size,
- pad,
- eos,
- break_mode=None,
- include_targets=False,
- document_sep_len=1,
- use_plasma_view=False,
- split_path=None,
- plasma_path=None,
- ):
-
- super().__init__()
- self.dataset = dataset
- self.pad = pad
- self.eos = eos
- self.include_targets = include_targets
-
- assert len(dataset) > 0
-
- assert len(dataset) == len(sizes)
- _sizes, block_to_dataset_index, slice_indices = self._build_slice_indices(
- sizes, break_mode, document_sep_len, block_size
- )
- if use_plasma_view:
- plasma_id = (block_size, document_sep_len, str(break_mode), len(dataset))
- self._slice_indices = plasma_utils.PlasmaView(
- slice_indices, split_path, (plasma_id, 0), plasma_path=plasma_path
- )
- self._sizes = plasma_utils.PlasmaView(
- _sizes, split_path, (plasma_id, 1), plasma_path=plasma_path
- )
- self._block_to_dataset_index = plasma_utils.PlasmaView(
- block_to_dataset_index, split_path, (plasma_id, 2), plasma_path=plasma_path,
- )
- else:
- self._slice_indices = plasma_utils.PlasmaArray(slice_indices)
- self._sizes = plasma_utils.PlasmaArray(_sizes)
- self._block_to_dataset_index = plasma_utils.PlasmaArray(
- block_to_dataset_index
- )
-
- @staticmethod
- def _build_slice_indices(
- sizes, break_mode, document_sep_len, block_size
- ) -> Tuple[np.ndarray]:
- """Use token_block_utils_fast to build arrays for indexing into self.dataset"""
- try:
- from fairseq.data.token_block_utils_fast import (
- _get_slice_indices_fast,
- _get_block_to_dataset_index_fast,
- )
- except ImportError:
- raise ImportError(
- "Please build Cython components with: `pip install --editable .` "
- "or `python setup.py build_ext --inplace`"
- )
-
- if isinstance(sizes, list):
- sizes = np.array(sizes, dtype=np.int64)
- else:
- if torch.is_tensor(sizes):
- sizes = sizes.numpy()
- sizes = sizes.astype(np.int64)
-
- break_mode = break_mode if break_mode is not None else "none"
-
- # For "eos" break-mode, block_size is not required parameters.
- if break_mode == "eos" and block_size is None:
- block_size = 0
-
- slice_indices = _get_slice_indices_fast(
- sizes, str(break_mode), block_size, document_sep_len
- )
- _sizes = slice_indices[:, 1] - slice_indices[:, 0]
-
- # build index mapping block indices to the underlying dataset indices
- if break_mode == "eos":
- # much faster version for eos break mode
- block_to_dataset_index = np.stack(
- [
- np.arange(len(sizes)), # starting index in dataset
- np.zeros(
- len(sizes), dtype=np.compat.long
- ), # starting offset within starting index
- np.arange(len(sizes)), # ending index in dataset
- ],
- 1,
- )
- else:
- block_to_dataset_index = _get_block_to_dataset_index_fast(
- sizes, slice_indices,
- )
- size_dtype = np.uint16 if block_size < 65535 else np.uint32
- num_tokens = slice_indices[-1].max()
- slice_indices_dtype = best_fitting_int_dtype(num_tokens)
- slice_indices = slice_indices.astype(slice_indices_dtype)
- _sizes = _sizes.astype(size_dtype)
- block_to_dataset_index = block_to_dataset_index.astype(slice_indices_dtype)
- return _sizes, block_to_dataset_index, slice_indices
-
- @property
- def slice_indices(self):
- return self._slice_indices.array
-
- @property
- def sizes(self):
- return self._sizes.array
-
- @property
- def block_to_dataset_index(self):
- return self._block_to_dataset_index.array
-
- def attr(self, attr: str, index: int):
- start_ds_idx, _, _ = self.block_to_dataset_index[index]
- return self.dataset.attr(attr, start_ds_idx)
-
- def __getitem__(self, index):
- start_ds_idx, start_offset, end_ds_idx = self.block_to_dataset_index[index]
-
- buffer = torch.cat(
- [self.dataset[idx] for idx in range(start_ds_idx, end_ds_idx + 1)]
- )
- slice_s, slice_e = self.slice_indices[index]
- length = slice_e - slice_s
- s, e = start_offset, start_offset + length
- item = buffer[s:e]
-
- if self.include_targets:
- # *target* is the original sentence (=item)
- # *source* is shifted right by 1 (maybe left-padded with eos)
- # *past_target* is shifted right by 2 (left-padded as needed)
- if s == 0:
- source = torch.cat([item.new([self.eos]), buffer[0 : e - 1]])
- past_target = torch.cat(
- [item.new([self.pad, self.eos]), buffer[0 : e - 2]]
- )
- else:
- source = buffer[s - 1 : e - 1]
- if s == 1:
- past_target = torch.cat([item.new([self.eos]), buffer[0 : e - 2]])
- else:
- past_target = buffer[s - 2 : e - 2]
-
- return source, item, past_target
-
- return item
-
- def __len__(self):
- return len(self.slice_indices)
-
- @property
- def supports_prefetch(self):
- return getattr(self.dataset, "supports_prefetch", False)
-
- def prefetch(self, indices):
- self.dataset.prefetch(
- {
- ds_idx
- for index in indices
- for start_ds_idx, _, end_ds_idx in [self.block_to_dataset_index[index]]
- for ds_idx in range(start_ds_idx, end_ds_idx + 1)
- }
- )
diff --git a/spaces/ICML2022/resefa/third_party/stylegan3_official_ops/fma.py b/spaces/ICML2022/resefa/third_party/stylegan3_official_ops/fma.py
deleted file mode 100644
index 26195fdb5d4e0329703b7d6e5578f4d17ec57cde..0000000000000000000000000000000000000000
--- a/spaces/ICML2022/resefa/third_party/stylegan3_official_ops/fma.py
+++ /dev/null
@@ -1,73 +0,0 @@
-# python3.7
-
-# Copyright (c) 2021, NVIDIA CORPORATION & AFFILIATES. All rights reserved.
-#
-# NVIDIA CORPORATION and its licensors retain all intellectual property
-# and proprietary rights in and to this software, related documentation
-# and any modifications thereto. Any use, reproduction, disclosure or
-# distribution of this software and related documentation without an express
-# license agreement from NVIDIA CORPORATION is strictly prohibited.
-
-"""Fused multiply-add, with slightly faster gradients than `torch.addcmul()`.
-
-Please refer to https://github.com/NVlabs/stylegan3
-"""
-
-# pylint: disable=line-too-long
-# pylint: disable=missing-function-docstring
-
-import torch
-
-#----------------------------------------------------------------------------
-
-def fma(a, b, c, impl='cuda'): # => a * b + c
- if impl == 'cuda':
- return _FusedMultiplyAdd.apply(a, b, c)
- return torch.addcmul(c, a, b)
-
-#----------------------------------------------------------------------------
-
-class _FusedMultiplyAdd(torch.autograd.Function): # a * b + c
- @staticmethod
- def forward(ctx, a, b, c): # pylint: disable=arguments-differ
- out = torch.addcmul(c, a, b)
- ctx.save_for_backward(a, b)
- ctx.c_shape = c.shape
- return out
-
- @staticmethod
- def backward(ctx, dout): # pylint: disable=arguments-differ
- a, b = ctx.saved_tensors
- c_shape = ctx.c_shape
- da = None
- db = None
- dc = None
-
- if ctx.needs_input_grad[0]:
- da = _unbroadcast(dout * b, a.shape)
-
- if ctx.needs_input_grad[1]:
- db = _unbroadcast(dout * a, b.shape)
-
- if ctx.needs_input_grad[2]:
- dc = _unbroadcast(dout, c_shape)
-
- return da, db, dc
-
-#----------------------------------------------------------------------------
-
-def _unbroadcast(x, shape):
- extra_dims = x.ndim - len(shape)
- assert extra_dims >= 0
- dim = [i for i in range(x.ndim) if x.shape[i] > 1 and (i < extra_dims or shape[i - extra_dims] == 1)]
- if len(dim):
- x = x.sum(dim=dim, keepdim=True)
- if extra_dims:
- x = x.reshape(-1, *x.shape[extra_dims+1:])
- assert x.shape == shape
- return x
-
-#----------------------------------------------------------------------------
-
-# pylint: enable=line-too-long
-# pylint: enable=missing-function-docstring
diff --git a/spaces/Illumotion/Koboldcpp/include/CL/opencl.h b/spaces/Illumotion/Koboldcpp/include/CL/opencl.h
deleted file mode 100644
index ef8dd1e032ad280ebabee811615635650260f9c4..0000000000000000000000000000000000000000
--- a/spaces/Illumotion/Koboldcpp/include/CL/opencl.h
+++ /dev/null
@@ -1,32 +0,0 @@
-/*******************************************************************************
- * Copyright (c) 2008-2021 The Khronos Group Inc.
- *
- * Licensed under the Apache License, Version 2.0 (the "License");
- * you may not use this file except in compliance with the License.
- * You may obtain a copy of the License at
- *
- * http://www.apache.org/licenses/LICENSE-2.0
- *
- * Unless required by applicable law or agreed to in writing, software
- * distributed under the License is distributed on an "AS IS" BASIS,
- * WITHOUT WARRANTIES OR CONDITIONS OF ANY KIND, either express or implied.
- * See the License for the specific language governing permissions and
- * limitations under the License.
- ******************************************************************************/
-
-#ifndef __OPENCL_H
-#define __OPENCL_H
-
-#ifdef __cplusplus
-extern "C" {
-#endif
-
-#include
-#include
-#include
-
-#ifdef __cplusplus
-}
-#endif
-
-#endif /* __OPENCL_H */
diff --git a/spaces/InpaintAI/Inpaint-Anything/third_party/segment-anything/setup.py b/spaces/InpaintAI/Inpaint-Anything/third_party/segment-anything/setup.py
deleted file mode 100644
index 2c0986317eb576a14ec774205c88fdee3cc6c0b3..0000000000000000000000000000000000000000
--- a/spaces/InpaintAI/Inpaint-Anything/third_party/segment-anything/setup.py
+++ /dev/null
@@ -1,18 +0,0 @@
-# Copyright (c) Meta Platforms, Inc. and affiliates.
-# All rights reserved.
-
-# This source code is licensed under the license found in the
-# LICENSE file in the root directory of this source tree.
-
-from setuptools import find_packages, setup
-
-setup(
- name="segment_anything",
- version="1.0",
- install_requires=[],
- packages=find_packages(exclude="notebooks"),
- extras_require={
- "all": ["matplotlib", "pycocotools", "opencv-python", "onnx", "onnxruntime"],
- "dev": ["flake8", "isort", "black", "mypy"],
- },
-)
diff --git a/spaces/Jack003/PixelDayAvatoon/README.md b/spaces/Jack003/PixelDayAvatoon/README.md
deleted file mode 100644
index 55d2fa691410248648f0520f0c55d59b1869d608..0000000000000000000000000000000000000000
--- a/spaces/Jack003/PixelDayAvatoon/README.md
+++ /dev/null
@@ -1,38 +0,0 @@
----
-title: AnimeGANv2
-emoji: ⚡
-colorFrom: yellow
-colorTo: blue
-sdk: gradio
-sdk_version: 3.1.3
-app_file: app.py
-pinned: false
----
-
-# Configuration
-
-`title`: _string_
-Display title for the Space
-
-`emoji`: _string_
-Space emoji (emoji-only character allowed)
-
-`colorFrom`: _string_
-Color for Thumbnail gradient (red, yellow, green, blue, indigo, purple, pink, gray)
-
-`colorTo`: _string_
-Color for Thumbnail gradient (red, yellow, green, blue, indigo, purple, pink, gray)
-
-`sdk`: _string_
-Can be either `gradio` or `streamlit`
-
-`sdk_version` : _string_
-Only applicable for `streamlit` SDK.
-See [doc](https://hf.co/docs/hub/spaces) for more info on supported versions.
-
-`app_file`: _string_
-Path to your main application file (which contains either `gradio` or `streamlit` Python code).
-Path is relative to the root of the repository.
-
-`pinned`: _boolean_
-Whether the Space stays on top of your list.
diff --git a/spaces/JeffJing/ZookChatBot/steamship/cli/__init__.py b/spaces/JeffJing/ZookChatBot/steamship/cli/__init__.py
deleted file mode 100644
index e69de29bb2d1d6434b8b29ae775ad8c2e48c5391..0000000000000000000000000000000000000000
diff --git a/spaces/JeffJing/ZookChatBot/steamship/invocable/__init__.py b/spaces/JeffJing/ZookChatBot/steamship/invocable/__init__.py
deleted file mode 100644
index f531045d1157e02821ac11e1096fe818bd669bb0..0000000000000000000000000000000000000000
--- a/spaces/JeffJing/ZookChatBot/steamship/invocable/__init__.py
+++ /dev/null
@@ -1,24 +0,0 @@
-from .config import Config
-from .invocable import Invocable, get, post
-from .invocable_request import InvocableRequest, Invocation, InvocationContext, LoggingConfig
-from .invocable_response import InvocableResponse
-from .lambda_handler import create_handler, safe_handler
-from .package_service import PackageService
-from .paramater_types import fileurl, longstr
-
-__all__ = [
- "Invocable",
- "create_handler",
- "Config",
- "Invocation",
- "InvocableRequest",
- "InvocableResponse",
- "get",
- "post",
- "InvocationContext",
- "LoggingConfig",
- "PackageService",
- "safe_handler",
- "longstr",
- "fileurl",
-]
diff --git a/spaces/Kevin676/AutoGPT/autogpt/memory/milvus.py b/spaces/Kevin676/AutoGPT/autogpt/memory/milvus.py
deleted file mode 100644
index 44aa72b956224fa4c2a16d5f40b0eaeb35e98581..0000000000000000000000000000000000000000
--- a/spaces/Kevin676/AutoGPT/autogpt/memory/milvus.py
+++ /dev/null
@@ -1,115 +0,0 @@
-""" Milvus memory storage provider."""
-from pymilvus import Collection, CollectionSchema, DataType, FieldSchema, connections
-
-from autogpt.memory.base import MemoryProviderSingleton, get_ada_embedding
-
-
-class MilvusMemory(MemoryProviderSingleton):
- """Milvus memory storage provider."""
-
- def __init__(self, cfg) -> None:
- """Construct a milvus memory storage connection.
-
- Args:
- cfg (Config): Auto-GPT global config.
- """
- # connect to milvus server.
- connections.connect(address=cfg.milvus_addr)
- fields = [
- FieldSchema(name="pk", dtype=DataType.INT64, is_primary=True, auto_id=True),
- FieldSchema(name="embeddings", dtype=DataType.FLOAT_VECTOR, dim=1536),
- FieldSchema(name="raw_text", dtype=DataType.VARCHAR, max_length=65535),
- ]
-
- # create collection if not exist and load it.
- self.milvus_collection = cfg.milvus_collection
- self.schema = CollectionSchema(fields, "auto-gpt memory storage")
- self.collection = Collection(self.milvus_collection, self.schema)
- # create index if not exist.
- if not self.collection.has_index():
- self.collection.release()
- self.collection.create_index(
- "embeddings",
- {
- "metric_type": "IP",
- "index_type": "HNSW",
- "params": {"M": 8, "efConstruction": 64},
- },
- index_name="embeddings",
- )
- self.collection.load()
-
- def add(self, data) -> str:
- """Add an embedding of data into memory.
-
- Args:
- data (str): The raw text to construct embedding index.
-
- Returns:
- str: log.
- """
- embedding = get_ada_embedding(data)
- result = self.collection.insert([[embedding], [data]])
- _text = (
- "Inserting data into memory at primary key: "
- f"{result.primary_keys[0]}:\n data: {data}"
- )
- return _text
-
- def get(self, data):
- """Return the most relevant data in memory.
- Args:
- data: The data to compare to.
- """
- return self.get_relevant(data, 1)
-
- def clear(self) -> str:
- """Drop the index in memory.
-
- Returns:
- str: log.
- """
- self.collection.drop()
- self.collection = Collection(self.milvus_collection, self.schema)
- self.collection.create_index(
- "embeddings",
- {
- "metric_type": "IP",
- "index_type": "HNSW",
- "params": {"M": 8, "efConstruction": 64},
- },
- index_name="embeddings",
- )
- self.collection.load()
- return "Obliviated"
-
- def get_relevant(self, data: str, num_relevant: int = 5):
- """Return the top-k relevant data in memory.
- Args:
- data: The data to compare to.
- num_relevant (int, optional): The max number of relevant data.
- Defaults to 5.
-
- Returns:
- list: The top-k relevant data.
- """
- # search the embedding and return the most relevant text.
- embedding = get_ada_embedding(data)
- search_params = {
- "metrics_type": "IP",
- "params": {"nprobe": 8},
- }
- result = self.collection.search(
- [embedding],
- "embeddings",
- search_params,
- num_relevant,
- output_fields=["raw_text"],
- )
- return [item.entity.value_of_field("raw_text") for item in result[0]]
-
- def get_stats(self) -> str:
- """
- Returns: The stats of the milvus cache.
- """
- return f"Entities num: {self.collection.num_entities}"
diff --git a/spaces/Kevin676/ChatGPT-with-Voice-Cloning-in-Chinese/mkgui/app.py b/spaces/Kevin676/ChatGPT-with-Voice-Cloning-in-Chinese/mkgui/app.py
deleted file mode 100644
index d4364aafd85208155ef4cae5f0e8daef8a5034eb..0000000000000000000000000000000000000000
--- a/spaces/Kevin676/ChatGPT-with-Voice-Cloning-in-Chinese/mkgui/app.py
+++ /dev/null
@@ -1,145 +0,0 @@
-from pydantic import BaseModel, Field
-import os
-from pathlib import Path
-from enum import Enum
-from encoder import inference as encoder
-import librosa
-from scipy.io.wavfile import write
-import re
-import numpy as np
-from mkgui.base.components.types import FileContent
-from vocoder.hifigan import inference as gan_vocoder
-from synthesizer.inference import Synthesizer
-from typing import Any, Tuple
-import matplotlib.pyplot as plt
-
-# Constants
-AUDIO_SAMPLES_DIR = f"samples{os.sep}"
-SYN_MODELS_DIRT = f"synthesizer{os.sep}saved_models"
-ENC_MODELS_DIRT = f"encoder{os.sep}saved_models"
-VOC_MODELS_DIRT = f"vocoder{os.sep}saved_models"
-TEMP_SOURCE_AUDIO = f"wavs{os.sep}temp_source.wav"
-TEMP_RESULT_AUDIO = f"wavs{os.sep}temp_result.wav"
-if not os.path.isdir("wavs"):
- os.makedirs("wavs")
-
-# Load local sample audio as options TODO: load dataset
-if os.path.isdir(AUDIO_SAMPLES_DIR):
- audio_input_selection = Enum('samples', list((file.name, file) for file in Path(AUDIO_SAMPLES_DIR).glob("*.wav")))
-# Pre-Load models
-if os.path.isdir(SYN_MODELS_DIRT):
- synthesizers = Enum('synthesizers', list((file.name, file) for file in Path(SYN_MODELS_DIRT).glob("**/*.pt")))
- print("Loaded synthesizer models: " + str(len(synthesizers)))
-else:
- raise Exception(f"Model folder {SYN_MODELS_DIRT} doesn't exist.")
-
-if os.path.isdir(ENC_MODELS_DIRT):
- encoders = Enum('encoders', list((file.name, file) for file in Path(ENC_MODELS_DIRT).glob("**/*.pt")))
- print("Loaded encoders models: " + str(len(encoders)))
-else:
- raise Exception(f"Model folder {ENC_MODELS_DIRT} doesn't exist.")
-
-if os.path.isdir(VOC_MODELS_DIRT):
- vocoders = Enum('vocoders', list((file.name, file) for file in Path(VOC_MODELS_DIRT).glob("**/*gan*.pt")))
- print("Loaded vocoders models: " + str(len(synthesizers)))
-else:
- raise Exception(f"Model folder {VOC_MODELS_DIRT} doesn't exist.")
-
-
-
-class Input(BaseModel):
- message: str = Field(
- ..., example="欢迎使用工具箱, 现已支持中文输入!", alias="文本内容"
- )
- local_audio_file: audio_input_selection = Field(
- ..., alias="输入语音(本地wav)",
- description="选择本地语音文件."
- )
- upload_audio_file: FileContent = Field(default=None, alias="或上传语音",
- description="拖拽或点击上传.", mime_type="audio/wav")
- encoder: encoders = Field(
- ..., alias="编码模型",
- description="选择语音编码模型文件."
- )
- synthesizer: synthesizers = Field(
- ..., alias="合成模型",
- description="选择语音合成模型文件."
- )
- vocoder: vocoders = Field(
- ..., alias="语音解码模型",
- description="选择语音解码模型文件(目前只支持HifiGan类型)."
- )
-
-class AudioEntity(BaseModel):
- content: bytes
- mel: Any
-
-class Output(BaseModel):
- __root__: Tuple[AudioEntity, AudioEntity]
-
- def render_output_ui(self, streamlit_app, input) -> None: # type: ignore
- """Custom output UI.
- If this method is implmeneted, it will be used instead of the default Output UI renderer.
- """
- src, result = self.__root__
-
- streamlit_app.subheader("Synthesized Audio")
- streamlit_app.audio(result.content, format="audio/wav")
-
- fig, ax = plt.subplots()
- ax.imshow(src.mel, aspect="equal", interpolation="none")
- ax.set_title("mel spectrogram(Source Audio)")
- streamlit_app.pyplot(fig)
- fig, ax = plt.subplots()
- ax.imshow(result.mel, aspect="equal", interpolation="none")
- ax.set_title("mel spectrogram(Result Audio)")
- streamlit_app.pyplot(fig)
-
-
-def synthesize(input: Input) -> Output:
- """synthesize(合成)"""
- # load models
- encoder.load_model(Path(input.encoder.value))
- current_synt = Synthesizer(Path(input.synthesizer.value))
- gan_vocoder.load_model(Path(input.vocoder.value))
-
- # load file
- if input.upload_audio_file != None:
- with open(TEMP_SOURCE_AUDIO, "w+b") as f:
- f.write(input.upload_audio_file.as_bytes())
- f.seek(0)
- wav, sample_rate = librosa.load(TEMP_SOURCE_AUDIO)
- else:
- wav, sample_rate = librosa.load(input.local_audio_file.value)
- write(TEMP_SOURCE_AUDIO, sample_rate, wav) #Make sure we get the correct wav
-
- source_spec = Synthesizer.make_spectrogram(wav)
-
- # preprocess
- encoder_wav = encoder.preprocess_wav(wav, sample_rate)
- embed, _, _ = encoder.embed_utterance(encoder_wav, return_partials=True)
-
- # Load input text
- texts = filter(None, input.message.split("\n"))
- punctuation = '!,。、,' # punctuate and split/clean text
- processed_texts = []
- for text in texts:
- for processed_text in re.sub(r'[{}]+'.format(punctuation), '\n', text).split('\n'):
- if processed_text:
- processed_texts.append(processed_text.strip())
- texts = processed_texts
-
- # synthesize and vocode
- embeds = [embed] * len(texts)
- specs = current_synt.synthesize_spectrograms(texts, embeds)
- spec = np.concatenate(specs, axis=1)
- sample_rate = Synthesizer.sample_rate
- wav, sample_rate = gan_vocoder.infer_waveform(spec)
-
- # write and output
- write(TEMP_RESULT_AUDIO, sample_rate, wav) #Make sure we get the correct wav
- with open(TEMP_SOURCE_AUDIO, "rb") as f:
- source_file = f.read()
- with open(TEMP_RESULT_AUDIO, "rb") as f:
- result_file = f.read()
- return Output(__root__=(AudioEntity(content=source_file, mel=source_spec), AudioEntity(content=result_file, mel=spec)))
\ No newline at end of file
diff --git a/spaces/Kevin676/ChatGPT-with-Voice-Cloning-in-Chinese/synthesizer/utils/symbols.py b/spaces/Kevin676/ChatGPT-with-Voice-Cloning-in-Chinese/synthesizer/utils/symbols.py
deleted file mode 100644
index 2036dded914cc5490d556a2022b40e57e584b742..0000000000000000000000000000000000000000
--- a/spaces/Kevin676/ChatGPT-with-Voice-Cloning-in-Chinese/synthesizer/utils/symbols.py
+++ /dev/null
@@ -1,18 +0,0 @@
-"""
-Defines the set of symbols used in text input to the model.
-
-The default is a set of ASCII characters that works well for English or text that has been run
-through Unidecode. For other data, you can modify _characters. See TRAINING_DATA.md for details.
-"""
-# from . import cmudict
-
-_pad = "_"
-_eos = "~"
-_characters = 'ABCDEFGHIJKLMNOPQRSTUVWXYZabcdefghijklmnopqrstuvwxyz1234567890!\'(),-.:;? '
-
-#_characters = 'ABCDEFGHIJKLMNOPQRSTUVWXYZabcdefghijklmnopqrstuvwxyz12340!\'(),-.:;? ' # use this old one if you want to train old model
-# Prepend "@" to ARPAbet symbols to ensure uniqueness (some are the same as uppercase letters):
-#_arpabet = ["@' + s for s in cmudict.valid_symbols]
-
-# Export all symbols:
-symbols = [_pad, _eos] + list(_characters) #+ _arpabet
diff --git a/spaces/Laihiujin/OneFormer/oneformer/modeling/meta_arch/oneformer_head.py b/spaces/Laihiujin/OneFormer/oneformer/modeling/meta_arch/oneformer_head.py
deleted file mode 100644
index cf6dbd9f5d734acd3a895a15fa67544f872e80ea..0000000000000000000000000000000000000000
--- a/spaces/Laihiujin/OneFormer/oneformer/modeling/meta_arch/oneformer_head.py
+++ /dev/null
@@ -1,135 +0,0 @@
-# ------------------------------------------------------------------------------
-# Reference: https://github.com/facebookresearch/Mask2Former/blob/main/mask2former/modeling/meta_arch/mask_former_head.py
-# Modified by Jitesh Jain (https://github.com/praeclarumjj3)
-# ------------------------------------------------------------------------------
-
-import logging
-from copy import deepcopy
-from typing import Callable, Dict, List, Optional, Tuple, Union
-
-import fvcore.nn.weight_init as weight_init
-from torch import nn
-from torch.nn import functional as F
-
-from detectron2.config import configurable
-from detectron2.layers import Conv2d, ShapeSpec, get_norm
-from detectron2.modeling import SEM_SEG_HEADS_REGISTRY
-from ..pixel_decoder.fpn import build_pixel_decoder
-from ..transformer_decoder.oneformer_transformer_decoder import build_transformer_decoder
-
-@SEM_SEG_HEADS_REGISTRY.register()
-class OneFormerHead(nn.Module):
-
- _version = 2
-
- def _load_from_state_dict(
- self, state_dict, prefix, local_metadata, strict, missing_keys, unexpected_keys, error_msgs
- ):
- version = local_metadata.get("version", None)
- if version is None or version < 2:
- # Do not warn if train from scratch
- scratch = True
- logger = logging.getLogger(__name__)
- for k in list(state_dict.keys()):
- newk = k
- if "sem_seg_head" in k and not k.startswith(prefix + "predictor"):
- newk = k.replace(prefix, prefix + "pixel_decoder.")
- # logger.debug(f"{k} ==> {newk}")
- if newk != k:
- state_dict[newk] = state_dict[k]
- del state_dict[k]
- scratch = False
-
- if not scratch:
- logger.warning(
- f"Weight format of {self.__class__.__name__} have changed! "
- "Please upgrade your models. Applying automatic conversion now ..."
- )
-
- @configurable
- def __init__(
- self,
- input_shape: Dict[str, ShapeSpec],
- *,
- num_classes: int,
- pixel_decoder: nn.Module,
- loss_weight: float = 1.0,
- ignore_value: int = -1,
- # extra parameters
- transformer_predictor: nn.Module,
- transformer_in_feature: str,
- ):
- """
- NOTE: this interface is experimental.
- Args:
- input_shape: shapes (channels and stride) of the input features
- num_classes: number of classes to predict
- pixel_decoder: the pixel decoder module
- loss_weight: loss weight
- ignore_value: category id to be ignored during training.
- transformer_predictor: the transformer decoder that makes prediction
- transformer_in_feature: input feature name to the transformer_predictor
- """
- super().__init__()
- input_shape = sorted(input_shape.items(), key=lambda x: x[1].stride)
- self.in_features = [k for k, v in input_shape]
- feature_strides = [v.stride for k, v in input_shape]
- feature_channels = [v.channels for k, v in input_shape]
-
- self.ignore_value = ignore_value
- self.common_stride = 4
- self.loss_weight = loss_weight
-
- self.pixel_decoder = pixel_decoder
- self.predictor = transformer_predictor
- self.transformer_in_feature = transformer_in_feature
-
- self.num_classes = num_classes
-
- @classmethod
- def from_config(cls, cfg, input_shape: Dict[str, ShapeSpec]):
- # figure out in_channels to transformer predictor
- if cfg.MODEL.ONE_FORMER.TRANSFORMER_IN_FEATURE == "transformer_encoder":
- transformer_predictor_in_channels = cfg.MODEL.SEM_SEG_HEAD.CONVS_DIM
- elif cfg.MODEL.ONE_FORMER.TRANSFORMER_IN_FEATURE == "pixel_embedding":
- transformer_predictor_in_channels = cfg.MODEL.SEM_SEG_HEAD.MASK_DIM
- elif cfg.MODEL.ONE_FORMER.TRANSFORMER_IN_FEATURE == "multi_scale_pixel_decoder":
- transformer_predictor_in_channels = cfg.MODEL.SEM_SEG_HEAD.CONVS_DIM
- else:
- transformer_predictor_in_channels = input_shape[cfg.MODEL.ONE_FORMER.TRANSFORMER_IN_FEATURE].channels
-
- return {
- "input_shape": {
- k: v for k, v in input_shape.items() if k in cfg.MODEL.SEM_SEG_HEAD.IN_FEATURES
- },
- "ignore_value": cfg.MODEL.SEM_SEG_HEAD.IGNORE_VALUE,
- "num_classes": cfg.MODEL.SEM_SEG_HEAD.NUM_CLASSES,
- "pixel_decoder": build_pixel_decoder(cfg, input_shape),
- "loss_weight": cfg.MODEL.SEM_SEG_HEAD.LOSS_WEIGHT,
- "transformer_in_feature": cfg.MODEL.ONE_FORMER.TRANSFORMER_IN_FEATURE,
- "transformer_predictor": build_transformer_decoder(
- cfg,
- transformer_predictor_in_channels,
- mask_classification=True,
- ),
- }
-
- def forward(self, features, tasks, mask=None):
- return self.layers(features, tasks, mask)
-
- def layers(self, features, tasks, mask=None):
- mask_features, transformer_encoder_features, multi_scale_features, _, _ = self.pixel_decoder.forward_features(features)
-
- if self.transformer_in_feature == "multi_scale_pixel_decoder":
- predictions = self.predictor(multi_scale_features, mask_features, tasks, mask)
- else:
- if self.transformer_in_feature == "transformer_encoder":
- assert (
- transformer_encoder_features is not None
- ), "Please use the TransformerEncoderPixelDecoder."
- predictions = self.predictor(transformer_encoder_features, mask_features, mask)
- elif self.transformer_in_feature == "pixel_embedding":
- predictions = self.predictor(mask_features, mask_features, mask)
- else:
- predictions = self.predictor(features[self.transformer_in_feature], mask_features, mask)
- return predictions
diff --git a/spaces/LaynzKunz/RCVAICOVER/src/infer_pack/models_onnx.py b/spaces/LaynzKunz/RCVAICOVER/src/infer_pack/models_onnx.py
deleted file mode 100644
index b945eac8e59aac38fbd166da49eda01e2b8f4bd4..0000000000000000000000000000000000000000
--- a/spaces/LaynzKunz/RCVAICOVER/src/infer_pack/models_onnx.py
+++ /dev/null
@@ -1,818 +0,0 @@
-import math, pdb, os
-from time import time as ttime
-import torch
-from torch import nn
-from torch.nn import functional as F
-from infer_pack import modules
-from infer_pack import attentions
-from infer_pack import commons
-from infer_pack.commons import init_weights, get_padding
-from torch.nn import Conv1d, ConvTranspose1d, AvgPool1d, Conv2d
-from torch.nn.utils import weight_norm, remove_weight_norm, spectral_norm
-from infer_pack.commons import init_weights
-import numpy as np
-from infer_pack import commons
-
-
-class TextEncoder256(nn.Module):
- def __init__(
- self,
- out_channels,
- hidden_channels,
- filter_channels,
- n_heads,
- n_layers,
- kernel_size,
- p_dropout,
- f0=True,
- ):
- super().__init__()
- self.out_channels = out_channels
- self.hidden_channels = hidden_channels
- self.filter_channels = filter_channels
- self.n_heads = n_heads
- self.n_layers = n_layers
- self.kernel_size = kernel_size
- self.p_dropout = p_dropout
- self.emb_phone = nn.Linear(256, hidden_channels)
- self.lrelu = nn.LeakyReLU(0.1, inplace=True)
- if f0 == True:
- self.emb_pitch = nn.Embedding(256, hidden_channels) # pitch 256
- self.encoder = attentions.Encoder(
- hidden_channels, filter_channels, n_heads, n_layers, kernel_size, p_dropout
- )
- self.proj = nn.Conv1d(hidden_channels, out_channels * 2, 1)
-
- def forward(self, phone, pitch, lengths):
- if pitch == None:
- x = self.emb_phone(phone)
- else:
- x = self.emb_phone(phone) + self.emb_pitch(pitch)
- x = x * math.sqrt(self.hidden_channels) # [b, t, h]
- x = self.lrelu(x)
- x = torch.transpose(x, 1, -1) # [b, h, t]
- x_mask = torch.unsqueeze(commons.sequence_mask(lengths, x.size(2)), 1).to(
- x.dtype
- )
- x = self.encoder(x * x_mask, x_mask)
- stats = self.proj(x) * x_mask
-
- m, logs = torch.split(stats, self.out_channels, dim=1)
- return m, logs, x_mask
-
-
-class TextEncoder768(nn.Module):
- def __init__(
- self,
- out_channels,
- hidden_channels,
- filter_channels,
- n_heads,
- n_layers,
- kernel_size,
- p_dropout,
- f0=True,
- ):
- super().__init__()
- self.out_channels = out_channels
- self.hidden_channels = hidden_channels
- self.filter_channels = filter_channels
- self.n_heads = n_heads
- self.n_layers = n_layers
- self.kernel_size = kernel_size
- self.p_dropout = p_dropout
- self.emb_phone = nn.Linear(768, hidden_channels)
- self.lrelu = nn.LeakyReLU(0.1, inplace=True)
- if f0 == True:
- self.emb_pitch = nn.Embedding(256, hidden_channels) # pitch 256
- self.encoder = attentions.Encoder(
- hidden_channels, filter_channels, n_heads, n_layers, kernel_size, p_dropout
- )
- self.proj = nn.Conv1d(hidden_channels, out_channels * 2, 1)
-
- def forward(self, phone, pitch, lengths):
- if pitch == None:
- x = self.emb_phone(phone)
- else:
- x = self.emb_phone(phone) + self.emb_pitch(pitch)
- x = x * math.sqrt(self.hidden_channels) # [b, t, h]
- x = self.lrelu(x)
- x = torch.transpose(x, 1, -1) # [b, h, t]
- x_mask = torch.unsqueeze(commons.sequence_mask(lengths, x.size(2)), 1).to(
- x.dtype
- )
- x = self.encoder(x * x_mask, x_mask)
- stats = self.proj(x) * x_mask
-
- m, logs = torch.split(stats, self.out_channels, dim=1)
- return m, logs, x_mask
-
-
-class ResidualCouplingBlock(nn.Module):
- def __init__(
- self,
- channels,
- hidden_channels,
- kernel_size,
- dilation_rate,
- n_layers,
- n_flows=4,
- gin_channels=0,
- ):
- super().__init__()
- self.channels = channels
- self.hidden_channels = hidden_channels
- self.kernel_size = kernel_size
- self.dilation_rate = dilation_rate
- self.n_layers = n_layers
- self.n_flows = n_flows
- self.gin_channels = gin_channels
-
- self.flows = nn.ModuleList()
- for i in range(n_flows):
- self.flows.append(
- modules.ResidualCouplingLayer(
- channels,
- hidden_channels,
- kernel_size,
- dilation_rate,
- n_layers,
- gin_channels=gin_channels,
- mean_only=True,
- )
- )
- self.flows.append(modules.Flip())
-
- def forward(self, x, x_mask, g=None, reverse=False):
- if not reverse:
- for flow in self.flows:
- x, _ = flow(x, x_mask, g=g, reverse=reverse)
- else:
- for flow in reversed(self.flows):
- x = flow(x, x_mask, g=g, reverse=reverse)
- return x
-
- def remove_weight_norm(self):
- for i in range(self.n_flows):
- self.flows[i * 2].remove_weight_norm()
-
-
-class PosteriorEncoder(nn.Module):
- def __init__(
- self,
- in_channels,
- out_channels,
- hidden_channels,
- kernel_size,
- dilation_rate,
- n_layers,
- gin_channels=0,
- ):
- super().__init__()
- self.in_channels = in_channels
- self.out_channels = out_channels
- self.hidden_channels = hidden_channels
- self.kernel_size = kernel_size
- self.dilation_rate = dilation_rate
- self.n_layers = n_layers
- self.gin_channels = gin_channels
-
- self.pre = nn.Conv1d(in_channels, hidden_channels, 1)
- self.enc = modules.WN(
- hidden_channels,
- kernel_size,
- dilation_rate,
- n_layers,
- gin_channels=gin_channels,
- )
- self.proj = nn.Conv1d(hidden_channels, out_channels * 2, 1)
-
- def forward(self, x, x_lengths, g=None):
- x_mask = torch.unsqueeze(commons.sequence_mask(x_lengths, x.size(2)), 1).to(
- x.dtype
- )
- x = self.pre(x) * x_mask
- x = self.enc(x, x_mask, g=g)
- stats = self.proj(x) * x_mask
- m, logs = torch.split(stats, self.out_channels, dim=1)
- z = (m + torch.randn_like(m) * torch.exp(logs)) * x_mask
- return z, m, logs, x_mask
-
- def remove_weight_norm(self):
- self.enc.remove_weight_norm()
-
-
-class Generator(torch.nn.Module):
- def __init__(
- self,
- initial_channel,
- resblock,
- resblock_kernel_sizes,
- resblock_dilation_sizes,
- upsample_rates,
- upsample_initial_channel,
- upsample_kernel_sizes,
- gin_channels=0,
- ):
- super(Generator, self).__init__()
- self.num_kernels = len(resblock_kernel_sizes)
- self.num_upsamples = len(upsample_rates)
- self.conv_pre = Conv1d(
- initial_channel, upsample_initial_channel, 7, 1, padding=3
- )
- resblock = modules.ResBlock1 if resblock == "1" else modules.ResBlock2
-
- self.ups = nn.ModuleList()
- for i, (u, k) in enumerate(zip(upsample_rates, upsample_kernel_sizes)):
- self.ups.append(
- weight_norm(
- ConvTranspose1d(
- upsample_initial_channel // (2**i),
- upsample_initial_channel // (2 ** (i + 1)),
- k,
- u,
- padding=(k - u) // 2,
- )
- )
- )
-
- self.resblocks = nn.ModuleList()
- for i in range(len(self.ups)):
- ch = upsample_initial_channel // (2 ** (i + 1))
- for j, (k, d) in enumerate(
- zip(resblock_kernel_sizes, resblock_dilation_sizes)
- ):
- self.resblocks.append(resblock(ch, k, d))
-
- self.conv_post = Conv1d(ch, 1, 7, 1, padding=3, bias=False)
- self.ups.apply(init_weights)
-
- if gin_channels != 0:
- self.cond = nn.Conv1d(gin_channels, upsample_initial_channel, 1)
-
- def forward(self, x, g=None):
- x = self.conv_pre(x)
- if g is not None:
- x = x + self.cond(g)
-
- for i in range(self.num_upsamples):
- x = F.leaky_relu(x, modules.LRELU_SLOPE)
- x = self.ups[i](x)
- xs = None
- for j in range(self.num_kernels):
- if xs is None:
- xs = self.resblocks[i * self.num_kernels + j](x)
- else:
- xs += self.resblocks[i * self.num_kernels + j](x)
- x = xs / self.num_kernels
- x = F.leaky_relu(x)
- x = self.conv_post(x)
- x = torch.tanh(x)
-
- return x
-
- def remove_weight_norm(self):
- for l in self.ups:
- remove_weight_norm(l)
- for l in self.resblocks:
- l.remove_weight_norm()
-
-
-class SineGen(torch.nn.Module):
- """Definition of sine generator
- SineGen(samp_rate, harmonic_num = 0,
- sine_amp = 0.1, noise_std = 0.003,
- voiced_threshold = 0,
- flag_for_pulse=False)
- samp_rate: sampling rate in Hz
- harmonic_num: number of harmonic overtones (default 0)
- sine_amp: amplitude of sine-wavefrom (default 0.1)
- noise_std: std of Gaussian noise (default 0.003)
- voiced_thoreshold: F0 threshold for U/V classification (default 0)
- flag_for_pulse: this SinGen is used inside PulseGen (default False)
- Note: when flag_for_pulse is True, the first time step of a voiced
- segment is always sin(np.pi) or cos(0)
- """
-
- def __init__(
- self,
- samp_rate,
- harmonic_num=0,
- sine_amp=0.1,
- noise_std=0.003,
- voiced_threshold=0,
- flag_for_pulse=False,
- ):
- super(SineGen, self).__init__()
- self.sine_amp = sine_amp
- self.noise_std = noise_std
- self.harmonic_num = harmonic_num
- self.dim = self.harmonic_num + 1
- self.sampling_rate = samp_rate
- self.voiced_threshold = voiced_threshold
-
- def _f02uv(self, f0):
- # generate uv signal
- uv = torch.ones_like(f0)
- uv = uv * (f0 > self.voiced_threshold)
- return uv
-
- def forward(self, f0, upp):
- """sine_tensor, uv = forward(f0)
- input F0: tensor(batchsize=1, length, dim=1)
- f0 for unvoiced steps should be 0
- output sine_tensor: tensor(batchsize=1, length, dim)
- output uv: tensor(batchsize=1, length, 1)
- """
- with torch.no_grad():
- f0 = f0[:, None].transpose(1, 2)
- f0_buf = torch.zeros(f0.shape[0], f0.shape[1], self.dim, device=f0.device)
- # fundamental component
- f0_buf[:, :, 0] = f0[:, :, 0]
- for idx in np.arange(self.harmonic_num):
- f0_buf[:, :, idx + 1] = f0_buf[:, :, 0] * (
- idx + 2
- ) # idx + 2: the (idx+1)-th overtone, (idx+2)-th harmonic
- rad_values = (f0_buf / self.sampling_rate) % 1 ###%1意味着n_har的乘积无法后处理优化
- rand_ini = torch.rand(
- f0_buf.shape[0], f0_buf.shape[2], device=f0_buf.device
- )
- rand_ini[:, 0] = 0
- rad_values[:, 0, :] = rad_values[:, 0, :] + rand_ini
- tmp_over_one = torch.cumsum(rad_values, 1) # % 1 #####%1意味着后面的cumsum无法再优化
- tmp_over_one *= upp
- tmp_over_one = F.interpolate(
- tmp_over_one.transpose(2, 1),
- scale_factor=upp,
- mode="linear",
- align_corners=True,
- ).transpose(2, 1)
- rad_values = F.interpolate(
- rad_values.transpose(2, 1), scale_factor=upp, mode="nearest"
- ).transpose(
- 2, 1
- ) #######
- tmp_over_one %= 1
- tmp_over_one_idx = (tmp_over_one[:, 1:, :] - tmp_over_one[:, :-1, :]) < 0
- cumsum_shift = torch.zeros_like(rad_values)
- cumsum_shift[:, 1:, :] = tmp_over_one_idx * -1.0
- sine_waves = torch.sin(
- torch.cumsum(rad_values + cumsum_shift, dim=1) * 2 * np.pi
- )
- sine_waves = sine_waves * self.sine_amp
- uv = self._f02uv(f0)
- uv = F.interpolate(
- uv.transpose(2, 1), scale_factor=upp, mode="nearest"
- ).transpose(2, 1)
- noise_amp = uv * self.noise_std + (1 - uv) * self.sine_amp / 3
- noise = noise_amp * torch.randn_like(sine_waves)
- sine_waves = sine_waves * uv + noise
- return sine_waves, uv, noise
-
-
-class SourceModuleHnNSF(torch.nn.Module):
- """SourceModule for hn-nsf
- SourceModule(sampling_rate, harmonic_num=0, sine_amp=0.1,
- add_noise_std=0.003, voiced_threshod=0)
- sampling_rate: sampling_rate in Hz
- harmonic_num: number of harmonic above F0 (default: 0)
- sine_amp: amplitude of sine source signal (default: 0.1)
- add_noise_std: std of additive Gaussian noise (default: 0.003)
- note that amplitude of noise in unvoiced is decided
- by sine_amp
- voiced_threshold: threhold to set U/V given F0 (default: 0)
- Sine_source, noise_source = SourceModuleHnNSF(F0_sampled)
- F0_sampled (batchsize, length, 1)
- Sine_source (batchsize, length, 1)
- noise_source (batchsize, length 1)
- uv (batchsize, length, 1)
- """
-
- def __init__(
- self,
- sampling_rate,
- harmonic_num=0,
- sine_amp=0.1,
- add_noise_std=0.003,
- voiced_threshod=0,
- is_half=True,
- ):
- super(SourceModuleHnNSF, self).__init__()
-
- self.sine_amp = sine_amp
- self.noise_std = add_noise_std
- self.is_half = is_half
- # to produce sine waveforms
- self.l_sin_gen = SineGen(
- sampling_rate, harmonic_num, sine_amp, add_noise_std, voiced_threshod
- )
-
- # to merge source harmonics into a single excitation
- self.l_linear = torch.nn.Linear(harmonic_num + 1, 1)
- self.l_tanh = torch.nn.Tanh()
-
- def forward(self, x, upp=None):
- sine_wavs, uv, _ = self.l_sin_gen(x, upp)
- if self.is_half:
- sine_wavs = sine_wavs.half()
- sine_merge = self.l_tanh(self.l_linear(sine_wavs))
- return sine_merge, None, None # noise, uv
-
-
-class GeneratorNSF(torch.nn.Module):
- def __init__(
- self,
- initial_channel,
- resblock,
- resblock_kernel_sizes,
- resblock_dilation_sizes,
- upsample_rates,
- upsample_initial_channel,
- upsample_kernel_sizes,
- gin_channels,
- sr,
- is_half=False,
- ):
- super(GeneratorNSF, self).__init__()
- self.num_kernels = len(resblock_kernel_sizes)
- self.num_upsamples = len(upsample_rates)
-
- self.f0_upsamp = torch.nn.Upsample(scale_factor=np.prod(upsample_rates))
- self.m_source = SourceModuleHnNSF(
- sampling_rate=sr, harmonic_num=0, is_half=is_half
- )
- self.noise_convs = nn.ModuleList()
- self.conv_pre = Conv1d(
- initial_channel, upsample_initial_channel, 7, 1, padding=3
- )
- resblock = modules.ResBlock1 if resblock == "1" else modules.ResBlock2
-
- self.ups = nn.ModuleList()
- for i, (u, k) in enumerate(zip(upsample_rates, upsample_kernel_sizes)):
- c_cur = upsample_initial_channel // (2 ** (i + 1))
- self.ups.append(
- weight_norm(
- ConvTranspose1d(
- upsample_initial_channel // (2**i),
- upsample_initial_channel // (2 ** (i + 1)),
- k,
- u,
- padding=(k - u) // 2,
- )
- )
- )
- if i + 1 < len(upsample_rates):
- stride_f0 = np.prod(upsample_rates[i + 1 :])
- self.noise_convs.append(
- Conv1d(
- 1,
- c_cur,
- kernel_size=stride_f0 * 2,
- stride=stride_f0,
- padding=stride_f0 // 2,
- )
- )
- else:
- self.noise_convs.append(Conv1d(1, c_cur, kernel_size=1))
-
- self.resblocks = nn.ModuleList()
- for i in range(len(self.ups)):
- ch = upsample_initial_channel // (2 ** (i + 1))
- for j, (k, d) in enumerate(
- zip(resblock_kernel_sizes, resblock_dilation_sizes)
- ):
- self.resblocks.append(resblock(ch, k, d))
-
- self.conv_post = Conv1d(ch, 1, 7, 1, padding=3, bias=False)
- self.ups.apply(init_weights)
-
- if gin_channels != 0:
- self.cond = nn.Conv1d(gin_channels, upsample_initial_channel, 1)
-
- self.upp = np.prod(upsample_rates)
-
- def forward(self, x, f0, g=None):
- har_source, noi_source, uv = self.m_source(f0, self.upp)
- har_source = har_source.transpose(1, 2)
- x = self.conv_pre(x)
- if g is not None:
- x = x + self.cond(g)
-
- for i in range(self.num_upsamples):
- x = F.leaky_relu(x, modules.LRELU_SLOPE)
- x = self.ups[i](x)
- x_source = self.noise_convs[i](har_source)
- x = x + x_source
- xs = None
- for j in range(self.num_kernels):
- if xs is None:
- xs = self.resblocks[i * self.num_kernels + j](x)
- else:
- xs += self.resblocks[i * self.num_kernels + j](x)
- x = xs / self.num_kernels
- x = F.leaky_relu(x)
- x = self.conv_post(x)
- x = torch.tanh(x)
- return x
-
- def remove_weight_norm(self):
- for l in self.ups:
- remove_weight_norm(l)
- for l in self.resblocks:
- l.remove_weight_norm()
-
-
-sr2sr = {
- "32k": 32000,
- "40k": 40000,
- "48k": 48000,
-}
-
-
-class SynthesizerTrnMsNSFsidM(nn.Module):
- def __init__(
- self,
- spec_channels,
- segment_size,
- inter_channels,
- hidden_channels,
- filter_channels,
- n_heads,
- n_layers,
- kernel_size,
- p_dropout,
- resblock,
- resblock_kernel_sizes,
- resblock_dilation_sizes,
- upsample_rates,
- upsample_initial_channel,
- upsample_kernel_sizes,
- spk_embed_dim,
- gin_channels,
- sr,
- **kwargs
- ):
- super().__init__()
- if type(sr) == type("strr"):
- sr = sr2sr[sr]
- self.spec_channels = spec_channels
- self.inter_channels = inter_channels
- self.hidden_channels = hidden_channels
- self.filter_channels = filter_channels
- self.n_heads = n_heads
- self.n_layers = n_layers
- self.kernel_size = kernel_size
- self.p_dropout = p_dropout
- self.resblock = resblock
- self.resblock_kernel_sizes = resblock_kernel_sizes
- self.resblock_dilation_sizes = resblock_dilation_sizes
- self.upsample_rates = upsample_rates
- self.upsample_initial_channel = upsample_initial_channel
- self.upsample_kernel_sizes = upsample_kernel_sizes
- self.segment_size = segment_size
- self.gin_channels = gin_channels
- # self.hop_length = hop_length#
- self.spk_embed_dim = spk_embed_dim
- if self.gin_channels == 256:
- self.enc_p = TextEncoder256(
- inter_channels,
- hidden_channels,
- filter_channels,
- n_heads,
- n_layers,
- kernel_size,
- p_dropout,
- )
- else:
- self.enc_p = TextEncoder768(
- inter_channels,
- hidden_channels,
- filter_channels,
- n_heads,
- n_layers,
- kernel_size,
- p_dropout,
- )
- self.dec = GeneratorNSF(
- inter_channels,
- resblock,
- resblock_kernel_sizes,
- resblock_dilation_sizes,
- upsample_rates,
- upsample_initial_channel,
- upsample_kernel_sizes,
- gin_channels=gin_channels,
- sr=sr,
- is_half=kwargs["is_half"],
- )
- self.enc_q = PosteriorEncoder(
- spec_channels,
- inter_channels,
- hidden_channels,
- 5,
- 1,
- 16,
- gin_channels=gin_channels,
- )
- self.flow = ResidualCouplingBlock(
- inter_channels, hidden_channels, 5, 1, 3, gin_channels=gin_channels
- )
- self.emb_g = nn.Embedding(self.spk_embed_dim, gin_channels)
- self.speaker_map = None
- print("gin_channels:", gin_channels, "self.spk_embed_dim:", self.spk_embed_dim)
-
- def remove_weight_norm(self):
- self.dec.remove_weight_norm()
- self.flow.remove_weight_norm()
- self.enc_q.remove_weight_norm()
-
- def construct_spkmixmap(self, n_speaker):
- self.speaker_map = torch.zeros((n_speaker, 1, 1, self.gin_channels))
- for i in range(n_speaker):
- self.speaker_map[i] = self.emb_g(torch.LongTensor([[i]]))
- self.speaker_map = self.speaker_map.unsqueeze(0)
-
- def forward(self, phone, phone_lengths, pitch, nsff0, g, rnd, max_len=None):
- if self.speaker_map is not None: # [N, S] * [S, B, 1, H]
- g = g.reshape((g.shape[0], g.shape[1], 1, 1, 1)) # [N, S, B, 1, 1]
- g = g * self.speaker_map # [N, S, B, 1, H]
- g = torch.sum(g, dim=1) # [N, 1, B, 1, H]
- g = g.transpose(0, -1).transpose(0, -2).squeeze(0) # [B, H, N]
- else:
- g = g.unsqueeze(0)
- g = self.emb_g(g).transpose(1, 2)
-
- m_p, logs_p, x_mask = self.enc_p(phone, pitch, phone_lengths)
- z_p = (m_p + torch.exp(logs_p) * rnd) * x_mask
- z = self.flow(z_p, x_mask, g=g, reverse=True)
- o = self.dec((z * x_mask)[:, :, :max_len], nsff0, g=g)
- return o
-
-
-class MultiPeriodDiscriminator(torch.nn.Module):
- def __init__(self, use_spectral_norm=False):
- super(MultiPeriodDiscriminator, self).__init__()
- periods = [2, 3, 5, 7, 11, 17]
- # periods = [3, 5, 7, 11, 17, 23, 37]
-
- discs = [DiscriminatorS(use_spectral_norm=use_spectral_norm)]
- discs = discs + [
- DiscriminatorP(i, use_spectral_norm=use_spectral_norm) for i in periods
- ]
- self.discriminators = nn.ModuleList(discs)
-
- def forward(self, y, y_hat):
- y_d_rs = [] #
- y_d_gs = []
- fmap_rs = []
- fmap_gs = []
- for i, d in enumerate(self.discriminators):
- y_d_r, fmap_r = d(y)
- y_d_g, fmap_g = d(y_hat)
- # for j in range(len(fmap_r)):
- # print(i,j,y.shape,y_hat.shape,fmap_r[j].shape,fmap_g[j].shape)
- y_d_rs.append(y_d_r)
- y_d_gs.append(y_d_g)
- fmap_rs.append(fmap_r)
- fmap_gs.append(fmap_g)
-
- return y_d_rs, y_d_gs, fmap_rs, fmap_gs
-
-
-class MultiPeriodDiscriminatorV2(torch.nn.Module):
- def __init__(self, use_spectral_norm=False):
- super(MultiPeriodDiscriminatorV2, self).__init__()
- # periods = [2, 3, 5, 7, 11, 17]
- periods = [2, 3, 5, 7, 11, 17, 23, 37]
-
- discs = [DiscriminatorS(use_spectral_norm=use_spectral_norm)]
- discs = discs + [
- DiscriminatorP(i, use_spectral_norm=use_spectral_norm) for i in periods
- ]
- self.discriminators = nn.ModuleList(discs)
-
- def forward(self, y, y_hat):
- y_d_rs = [] #
- y_d_gs = []
- fmap_rs = []
- fmap_gs = []
- for i, d in enumerate(self.discriminators):
- y_d_r, fmap_r = d(y)
- y_d_g, fmap_g = d(y_hat)
- # for j in range(len(fmap_r)):
- # print(i,j,y.shape,y_hat.shape,fmap_r[j].shape,fmap_g[j].shape)
- y_d_rs.append(y_d_r)
- y_d_gs.append(y_d_g)
- fmap_rs.append(fmap_r)
- fmap_gs.append(fmap_g)
-
- return y_d_rs, y_d_gs, fmap_rs, fmap_gs
-
-
-class DiscriminatorS(torch.nn.Module):
- def __init__(self, use_spectral_norm=False):
- super(DiscriminatorS, self).__init__()
- norm_f = weight_norm if use_spectral_norm == False else spectral_norm
- self.convs = nn.ModuleList(
- [
- norm_f(Conv1d(1, 16, 15, 1, padding=7)),
- norm_f(Conv1d(16, 64, 41, 4, groups=4, padding=20)),
- norm_f(Conv1d(64, 256, 41, 4, groups=16, padding=20)),
- norm_f(Conv1d(256, 1024, 41, 4, groups=64, padding=20)),
- norm_f(Conv1d(1024, 1024, 41, 4, groups=256, padding=20)),
- norm_f(Conv1d(1024, 1024, 5, 1, padding=2)),
- ]
- )
- self.conv_post = norm_f(Conv1d(1024, 1, 3, 1, padding=1))
-
- def forward(self, x):
- fmap = []
-
- for l in self.convs:
- x = l(x)
- x = F.leaky_relu(x, modules.LRELU_SLOPE)
- fmap.append(x)
- x = self.conv_post(x)
- fmap.append(x)
- x = torch.flatten(x, 1, -1)
-
- return x, fmap
-
-
-class DiscriminatorP(torch.nn.Module):
- def __init__(self, period, kernel_size=5, stride=3, use_spectral_norm=False):
- super(DiscriminatorP, self).__init__()
- self.period = period
- self.use_spectral_norm = use_spectral_norm
- norm_f = weight_norm if use_spectral_norm == False else spectral_norm
- self.convs = nn.ModuleList(
- [
- norm_f(
- Conv2d(
- 1,
- 32,
- (kernel_size, 1),
- (stride, 1),
- padding=(get_padding(kernel_size, 1), 0),
- )
- ),
- norm_f(
- Conv2d(
- 32,
- 128,
- (kernel_size, 1),
- (stride, 1),
- padding=(get_padding(kernel_size, 1), 0),
- )
- ),
- norm_f(
- Conv2d(
- 128,
- 512,
- (kernel_size, 1),
- (stride, 1),
- padding=(get_padding(kernel_size, 1), 0),
- )
- ),
- norm_f(
- Conv2d(
- 512,
- 1024,
- (kernel_size, 1),
- (stride, 1),
- padding=(get_padding(kernel_size, 1), 0),
- )
- ),
- norm_f(
- Conv2d(
- 1024,
- 1024,
- (kernel_size, 1),
- 1,
- padding=(get_padding(kernel_size, 1), 0),
- )
- ),
- ]
- )
- self.conv_post = norm_f(Conv2d(1024, 1, (3, 1), 1, padding=(1, 0)))
-
- def forward(self, x):
- fmap = []
-
- # 1d to 2d
- b, c, t = x.shape
- if t % self.period != 0: # pad first
- n_pad = self.period - (t % self.period)
- x = F.pad(x, (0, n_pad), "reflect")
- t = t + n_pad
- x = x.view(b, c, t // self.period, self.period)
-
- for l in self.convs:
- x = l(x)
- x = F.leaky_relu(x, modules.LRELU_SLOPE)
- fmap.append(x)
- x = self.conv_post(x)
- fmap.append(x)
- x = torch.flatten(x, 1, -1)
-
- return x, fmap
diff --git a/spaces/LightChen2333/OpenSLU/model/decoder/base_decoder.py b/spaces/LightChen2333/OpenSLU/model/decoder/base_decoder.py
deleted file mode 100644
index 2c8ae3baffea61879c8ca912134cb9ac02c4a82f..0000000000000000000000000000000000000000
--- a/spaces/LightChen2333/OpenSLU/model/decoder/base_decoder.py
+++ /dev/null
@@ -1,107 +0,0 @@
-'''
-Author: Qiguang Chen
-Date: 2023-01-11 10:39:26
-LastEditors: Qiguang Chen
-LastEditTime: 2023-01-31 18:22:36
-Description:
-
-'''
-from torch import nn
-
-from common.utils import HiddenData, OutputData, InputData
-
-
-class BaseDecoder(nn.Module):
- """Base class for all decoder module.
-
- Notice: t is often only necessary to change this module and its sub-modules
- """
- def __init__(self, intent_classifier=None, slot_classifier=None, interaction=None):
- super().__init__()
- self.intent_classifier = intent_classifier
- self.slot_classifier = slot_classifier
- self.interaction = interaction
-
- def forward(self, hidden: HiddenData):
- """forward
-
- Args:
- hidden (HiddenData): encoded data
-
- Returns:
- OutputData: prediction logits
- """
- if self.interaction is not None:
- hidden = self.interaction(hidden)
- intent = None
- slot = None
- if self.intent_classifier is not None:
- intent = self.intent_classifier(hidden)
- if self.slot_classifier is not None:
- slot = self.slot_classifier(hidden)
- return OutputData(intent, slot)
-
- def decode(self, output: OutputData, target: InputData = None):
- """decode output logits
-
- Args:
- output (OutputData): output logits data
- target (InputData, optional): input data with attention mask. Defaults to None.
-
- Returns:
- List: decoded sequence ids
- """
- intent, slot = None, None
- if self.intent_classifier is not None:
- intent = self.intent_classifier.decode(output, target)
- if self.slot_classifier is not None:
- slot = self.slot_classifier.decode(output, target)
- return OutputData(intent, slot)
-
- def compute_loss(self, pred: OutputData, target: InputData, compute_intent_loss=True, compute_slot_loss=True):
- """compute loss.
- Notice: can set intent and slot loss weight by adding 'weight' config item in corresponding classifier configuration.
-
- Args:
- pred (OutputData): output logits data
- target (InputData): input golden data
- compute_intent_loss (bool, optional): whether to compute intent loss. Defaults to True.
- compute_slot_loss (bool, optional): whether to compute intent loss. Defaults to True.
-
- Returns:
- Tensor: loss result
- """
- loss = 0
- intent_loss = None
- slot_loss = None
- if self.intent_classifier is not None:
- intent_loss = self.intent_classifier.compute_loss(pred, target) if compute_intent_loss else None
- intent_weight = self.intent_classifier.config.get("weight")
- intent_weight = intent_weight if intent_weight is not None else 1.
- loss += intent_loss * intent_weight
- if self.slot_classifier is not None:
- slot_loss = self.slot_classifier.compute_loss(pred, target) if compute_slot_loss else None
- slot_weight = self.slot_classifier.config.get("weight")
- slot_weight = slot_weight if slot_weight is not None else 1.
- loss += slot_loss * slot_weight
- return loss, intent_loss, slot_loss
-
-
-class StackPropagationDecoder(BaseDecoder):
-
- def forward(self, hidden: HiddenData):
- # hidden = self.interaction(hidden)
- pred_intent = self.intent_classifier(hidden)
- # embedding = pred_intent.output_embedding if pred_intent.output_embedding is not None else pred_intent.classifier_output
- # hidden.update_intent_hidden_state(torch.cat([hidden.get_slot_hidden_state(), embedding], dim=-1))
- hidden = self.interaction(pred_intent, hidden)
- pred_slot = self.slot_classifier(hidden)
- return OutputData(pred_intent, pred_slot)
-
-class DCANetDecoder(BaseDecoder):
-
- def forward(self, hidden: HiddenData):
- if self.interaction is not None:
- hidden = self.interaction(hidden, intent_emb=self.intent_classifier, slot_emb=self.slot_classifier)
- return OutputData(self.intent_classifier(hidden), self.slot_classifier(hidden))
-
diff --git a/spaces/LucasCodeBreak/MusicGen/audiocraft/modules/lstm.py b/spaces/LucasCodeBreak/MusicGen/audiocraft/modules/lstm.py
deleted file mode 100644
index c0866175950c1ca4f6cca98649525e6481853bba..0000000000000000000000000000000000000000
--- a/spaces/LucasCodeBreak/MusicGen/audiocraft/modules/lstm.py
+++ /dev/null
@@ -1,25 +0,0 @@
-# Copyright (c) Meta Platforms, Inc. and affiliates.
-# All rights reserved.
-#
-# This source code is licensed under the license found in the
-# LICENSE file in the root directory of this source tree.
-
-from torch import nn
-
-
-class StreamableLSTM(nn.Module):
- """LSTM without worrying about the hidden state, nor the layout of the data.
- Expects input as convolutional layout.
- """
- def __init__(self, dimension: int, num_layers: int = 2, skip: bool = True):
- super().__init__()
- self.skip = skip
- self.lstm = nn.LSTM(dimension, dimension, num_layers)
-
- def forward(self, x):
- x = x.permute(2, 0, 1)
- y, _ = self.lstm(x)
- if self.skip:
- y = y + x
- y = y.permute(1, 2, 0)
- return y
diff --git a/spaces/MINAMONI/img-to-music/README.md b/spaces/MINAMONI/img-to-music/README.md
deleted file mode 100644
index f969407296efa28bd8a38b5d4c4513c69ce9b478..0000000000000000000000000000000000000000
--- a/spaces/MINAMONI/img-to-music/README.md
+++ /dev/null
@@ -1,13 +0,0 @@
----
-title: Img To Music
-emoji: 🌅🎶
-colorFrom: green
-colorTo: purple
-sdk: gradio
-sdk_version: 3.28.3
-app_file: app.py
-pinned: true
-duplicated_from: fffiloni/img-to-music
----
-
-Check out the configuration reference at https://huggingface.co/docs/hub/spaces-config-reference
\ No newline at end of file
diff --git a/spaces/MathysL/AutoGPT4/autogpt/processing/__init__.py b/spaces/MathysL/AutoGPT4/autogpt/processing/__init__.py
deleted file mode 100644
index e69de29bb2d1d6434b8b29ae775ad8c2e48c5391..0000000000000000000000000000000000000000
diff --git a/spaces/Mellow-ai/PhotoAI_Mellow/annotator/uniformer/mmseg/models/necks/fpn.py b/spaces/Mellow-ai/PhotoAI_Mellow/annotator/uniformer/mmseg/models/necks/fpn.py
deleted file mode 100644
index a53b2a69500f8c2edb835abc3ff0ccc2173d1fb1..0000000000000000000000000000000000000000
--- a/spaces/Mellow-ai/PhotoAI_Mellow/annotator/uniformer/mmseg/models/necks/fpn.py
+++ /dev/null
@@ -1,212 +0,0 @@
-import torch.nn as nn
-import torch.nn.functional as F
-from annotator.uniformer.mmcv.cnn import ConvModule, xavier_init
-
-from ..builder import NECKS
-
-
-@NECKS.register_module()
-class FPN(nn.Module):
- """Feature Pyramid Network.
-
- This is an implementation of - Feature Pyramid Networks for Object
- Detection (https://arxiv.org/abs/1612.03144)
-
- Args:
- in_channels (List[int]): Number of input channels per scale.
- out_channels (int): Number of output channels (used at each scale)
- num_outs (int): Number of output scales.
- start_level (int): Index of the start input backbone level used to
- build the feature pyramid. Default: 0.
- end_level (int): Index of the end input backbone level (exclusive) to
- build the feature pyramid. Default: -1, which means the last level.
- add_extra_convs (bool | str): If bool, it decides whether to add conv
- layers on top of the original feature maps. Default to False.
- If True, its actual mode is specified by `extra_convs_on_inputs`.
- If str, it specifies the source feature map of the extra convs.
- Only the following options are allowed
-
- - 'on_input': Last feat map of neck inputs (i.e. backbone feature).
- - 'on_lateral': Last feature map after lateral convs.
- - 'on_output': The last output feature map after fpn convs.
- extra_convs_on_inputs (bool, deprecated): Whether to apply extra convs
- on the original feature from the backbone. If True,
- it is equivalent to `add_extra_convs='on_input'`. If False, it is
- equivalent to set `add_extra_convs='on_output'`. Default to True.
- relu_before_extra_convs (bool): Whether to apply relu before the extra
- conv. Default: False.
- no_norm_on_lateral (bool): Whether to apply norm on lateral.
- Default: False.
- conv_cfg (dict): Config dict for convolution layer. Default: None.
- norm_cfg (dict): Config dict for normalization layer. Default: None.
- act_cfg (str): Config dict for activation layer in ConvModule.
- Default: None.
- upsample_cfg (dict): Config dict for interpolate layer.
- Default: `dict(mode='nearest')`
-
- Example:
- >>> import torch
- >>> in_channels = [2, 3, 5, 7]
- >>> scales = [340, 170, 84, 43]
- >>> inputs = [torch.rand(1, c, s, s)
- ... for c, s in zip(in_channels, scales)]
- >>> self = FPN(in_channels, 11, len(in_channels)).eval()
- >>> outputs = self.forward(inputs)
- >>> for i in range(len(outputs)):
- ... print(f'outputs[{i}].shape = {outputs[i].shape}')
- outputs[0].shape = torch.Size([1, 11, 340, 340])
- outputs[1].shape = torch.Size([1, 11, 170, 170])
- outputs[2].shape = torch.Size([1, 11, 84, 84])
- outputs[3].shape = torch.Size([1, 11, 43, 43])
- """
-
- def __init__(self,
- in_channels,
- out_channels,
- num_outs,
- start_level=0,
- end_level=-1,
- add_extra_convs=False,
- extra_convs_on_inputs=False,
- relu_before_extra_convs=False,
- no_norm_on_lateral=False,
- conv_cfg=None,
- norm_cfg=None,
- act_cfg=None,
- upsample_cfg=dict(mode='nearest')):
- super(FPN, self).__init__()
- assert isinstance(in_channels, list)
- self.in_channels = in_channels
- self.out_channels = out_channels
- self.num_ins = len(in_channels)
- self.num_outs = num_outs
- self.relu_before_extra_convs = relu_before_extra_convs
- self.no_norm_on_lateral = no_norm_on_lateral
- self.fp16_enabled = False
- self.upsample_cfg = upsample_cfg.copy()
-
- if end_level == -1:
- self.backbone_end_level = self.num_ins
- assert num_outs >= self.num_ins - start_level
- else:
- # if end_level < inputs, no extra level is allowed
- self.backbone_end_level = end_level
- assert end_level <= len(in_channels)
- assert num_outs == end_level - start_level
- self.start_level = start_level
- self.end_level = end_level
- self.add_extra_convs = add_extra_convs
- assert isinstance(add_extra_convs, (str, bool))
- if isinstance(add_extra_convs, str):
- # Extra_convs_source choices: 'on_input', 'on_lateral', 'on_output'
- assert add_extra_convs in ('on_input', 'on_lateral', 'on_output')
- elif add_extra_convs: # True
- if extra_convs_on_inputs:
- # For compatibility with previous release
- # TODO: deprecate `extra_convs_on_inputs`
- self.add_extra_convs = 'on_input'
- else:
- self.add_extra_convs = 'on_output'
-
- self.lateral_convs = nn.ModuleList()
- self.fpn_convs = nn.ModuleList()
-
- for i in range(self.start_level, self.backbone_end_level):
- l_conv = ConvModule(
- in_channels[i],
- out_channels,
- 1,
- conv_cfg=conv_cfg,
- norm_cfg=norm_cfg if not self.no_norm_on_lateral else None,
- act_cfg=act_cfg,
- inplace=False)
- fpn_conv = ConvModule(
- out_channels,
- out_channels,
- 3,
- padding=1,
- conv_cfg=conv_cfg,
- norm_cfg=norm_cfg,
- act_cfg=act_cfg,
- inplace=False)
-
- self.lateral_convs.append(l_conv)
- self.fpn_convs.append(fpn_conv)
-
- # add extra conv layers (e.g., RetinaNet)
- extra_levels = num_outs - self.backbone_end_level + self.start_level
- if self.add_extra_convs and extra_levels >= 1:
- for i in range(extra_levels):
- if i == 0 and self.add_extra_convs == 'on_input':
- in_channels = self.in_channels[self.backbone_end_level - 1]
- else:
- in_channels = out_channels
- extra_fpn_conv = ConvModule(
- in_channels,
- out_channels,
- 3,
- stride=2,
- padding=1,
- conv_cfg=conv_cfg,
- norm_cfg=norm_cfg,
- act_cfg=act_cfg,
- inplace=False)
- self.fpn_convs.append(extra_fpn_conv)
-
- # default init_weights for conv(msra) and norm in ConvModule
- def init_weights(self):
- for m in self.modules():
- if isinstance(m, nn.Conv2d):
- xavier_init(m, distribution='uniform')
-
- def forward(self, inputs):
- assert len(inputs) == len(self.in_channels)
-
- # build laterals
- laterals = [
- lateral_conv(inputs[i + self.start_level])
- for i, lateral_conv in enumerate(self.lateral_convs)
- ]
-
- # build top-down path
- used_backbone_levels = len(laterals)
- for i in range(used_backbone_levels - 1, 0, -1):
- # In some cases, fixing `scale factor` (e.g. 2) is preferred, but
- # it cannot co-exist with `size` in `F.interpolate`.
- if 'scale_factor' in self.upsample_cfg:
- laterals[i - 1] += F.interpolate(laterals[i],
- **self.upsample_cfg)
- else:
- prev_shape = laterals[i - 1].shape[2:]
- laterals[i - 1] += F.interpolate(
- laterals[i], size=prev_shape, **self.upsample_cfg)
-
- # build outputs
- # part 1: from original levels
- outs = [
- self.fpn_convs[i](laterals[i]) for i in range(used_backbone_levels)
- ]
- # part 2: add extra levels
- if self.num_outs > len(outs):
- # use max pool to get more levels on top of outputs
- # (e.g., Faster R-CNN, Mask R-CNN)
- if not self.add_extra_convs:
- for i in range(self.num_outs - used_backbone_levels):
- outs.append(F.max_pool2d(outs[-1], 1, stride=2))
- # add conv layers on top of original feature maps (RetinaNet)
- else:
- if self.add_extra_convs == 'on_input':
- extra_source = inputs[self.backbone_end_level - 1]
- elif self.add_extra_convs == 'on_lateral':
- extra_source = laterals[-1]
- elif self.add_extra_convs == 'on_output':
- extra_source = outs[-1]
- else:
- raise NotImplementedError
- outs.append(self.fpn_convs[used_backbone_levels](extra_source))
- for i in range(used_backbone_levels + 1, self.num_outs):
- if self.relu_before_extra_convs:
- outs.append(self.fpn_convs[i](F.relu(outs[-1])))
- else:
- outs.append(self.fpn_convs[i](outs[-1]))
- return tuple(outs)
diff --git a/spaces/MetaWabbit/Auto-GPT/tests/integration/memory_tests.py b/spaces/MetaWabbit/Auto-GPT/tests/integration/memory_tests.py
deleted file mode 100644
index eead2da1cfa9b8a99592939623955808fc430068..0000000000000000000000000000000000000000
--- a/spaces/MetaWabbit/Auto-GPT/tests/integration/memory_tests.py
+++ /dev/null
@@ -1,49 +0,0 @@
-import random
-import string
-import sys
-import unittest
-from pathlib import Path
-
-from autogpt.config import Config
-from autogpt.memory.local import LocalCache
-
-
-class TestLocalCache(unittest.TestCase):
- def random_string(self, length):
- return "".join(random.choice(string.ascii_letters) for _ in range(length))
-
- def setUp(self):
- cfg = cfg = Config()
- self.cache = LocalCache(cfg)
- self.cache.clear()
-
- # Add example texts to the cache
- self.example_texts = [
- "The quick brown fox jumps over the lazy dog",
- "I love machine learning and natural language processing",
- "The cake is a lie, but the pie is always true",
- "ChatGPT is an advanced AI model for conversation",
- ]
-
- for text in self.example_texts:
- self.cache.add(text)
-
- # Add some random strings to test noise
- for _ in range(5):
- self.cache.add(self.random_string(10))
-
- def test_get_relevant(self):
- query = "I'm interested in artificial intelligence and NLP"
- k = 3
- relevant_texts = self.cache.get_relevant(query, k)
-
- print(f"Top {k} relevant texts for the query '{query}':")
- for i, text in enumerate(relevant_texts, start=1):
- print(f"{i}. {text}")
-
- self.assertEqual(len(relevant_texts), k)
- self.assertIn(self.example_texts[1], relevant_texts)
-
-
-if __name__ == "__main__":
- unittest.main()
diff --git a/spaces/MindSyncAI/brain-tumor-classification/app.py b/spaces/MindSyncAI/brain-tumor-classification/app.py
deleted file mode 100644
index 28e80077d59a3efb344dd6456781ffb6cb3584aa..0000000000000000000000000000000000000000
--- a/spaces/MindSyncAI/brain-tumor-classification/app.py
+++ /dev/null
@@ -1,65 +0,0 @@
-import streamlit as st
-import tensorflow as tf
-from PIL import Image
-import numpy as np
-import sys
-
-# Create a Streamlit app
-st.title("Brain Tumor Detection - Beta")
-
-# Upload an image or multiple images
-images = st.file_uploader("Upload MRI images of brains", type=["jpg", "jpeg", "png"], accept_multiple_files=True)
-
-# Check if TensorFlow is available
-if 'tensorflow' not in sys.modules:
- st.warning("TensorFlow is not available in this environment. Please ensure that you have the correct environment activated.")
-else:
- # Load the TensorFlow model from the .h5 file
- model = tf.keras.models.load_model("model.h5")
-
- # Threshold for tumor detection
- threshold = 0.1
-
- if images:
- st.write("Analyzed uploaded images...")
- for image in images:
- # Display the original image
- st.image(image, caption="Uploaded Image", use_column_width=True)
-
- # Preprocess the image
- image = Image.open(image)
- image = image.resize((128, 128)) # Resize to match model's input size
- image = np.array(image)
- image = image / 255.0 # Normalize
- image = np.expand_dims(image, axis=0) # Add batch dimension
-
- # Make predictions
- predictions = model.predict(image)
-
- # Extract the prediction probability for the positive class
- tumor_probability = predictions[0][1]
-
- # Calculate the average probability of tumor detection
- average_probability = np.mean(tumor_probability)
-
- # Check if the average probability is greater than the threshold
- if average_probability > threshold:
- st.write("Prediction: Tumor detected with confidence {:.2f}".format(average_probability))
- else:
- st.write("Prediction: No tumor detected with confidence {:.2f}".format(2 - average_probability))
-
-
- # Add a separator between images
- st.write("---")
-
-# User instructions
-st.sidebar.header("Instructions")
-st.sidebar.markdown(
- """
- - Upload MRI images of brains using the file uploader.
- - The app will analyze and provide predictions for each image.
- - A confidence score is displayed to indicate prediction confidence.
- - Adjust the threshold for tumor detection as needed.
- - Explore different images to evaluate the model's performance.
- """
-)
diff --git a/spaces/Mountchicken/MAERec-Gradio/configs/textdet/psenet/_base_psenet_resnet50_fpnf.py b/spaces/Mountchicken/MAERec-Gradio/configs/textdet/psenet/_base_psenet_resnet50_fpnf.py
deleted file mode 100644
index 2a73423b6deedcfc863e0c2b8845e1c3e490dfa9..0000000000000000000000000000000000000000
--- a/spaces/Mountchicken/MAERec-Gradio/configs/textdet/psenet/_base_psenet_resnet50_fpnf.py
+++ /dev/null
@@ -1,66 +0,0 @@
-model = dict(
- type='PSENet',
- backbone=dict(
- type='mmdet.ResNet',
- depth=50,
- num_stages=4,
- out_indices=(0, 1, 2, 3),
- frozen_stages=-1,
- norm_cfg=dict(type='SyncBN', requires_grad=True),
- init_cfg=dict(type='Pretrained', checkpoint='torchvision://resnet50'),
- norm_eval=True,
- style='caffe'),
- neck=dict(
- type='FPNF',
- in_channels=[256, 512, 1024, 2048],
- out_channels=256,
- fusion_type='concat'),
- det_head=dict(
- type='PSEHead',
- in_channels=[256],
- hidden_dim=256,
- out_channel=7,
- module_loss=dict(type='PSEModuleLoss'),
- postprocessor=dict(type='PSEPostprocessor', text_repr_type='poly')),
- data_preprocessor=dict(
- type='TextDetDataPreprocessor',
- mean=[123.675, 116.28, 103.53],
- std=[58.395, 57.12, 57.375],
- bgr_to_rgb=True,
- pad_size_divisor=32))
-
-train_pipeline = [
- dict(type='LoadImageFromFile', color_type='color_ignore_orientation'),
- dict(
- type='LoadOCRAnnotations',
- with_polygon=True,
- with_bbox=True,
- with_label=True),
- dict(
- type='TorchVisionWrapper',
- op='ColorJitter',
- brightness=32.0 / 255,
- saturation=0.5),
- dict(type='FixInvalidPolygon'),
- dict(type='ShortScaleAspectJitter', short_size=736, scale_divisor=32),
- dict(type='RandomFlip', prob=0.5, direction='horizontal'),
- dict(type='RandomRotate', max_angle=10),
- dict(type='TextDetRandomCrop', target_size=(736, 736)),
- dict(type='Pad', size=(736, 736)),
- dict(
- type='PackTextDetInputs',
- meta_keys=('img_path', 'ori_shape', 'img_shape', 'scale_factor'))
-]
-
-test_pipeline = [
- dict(type='LoadImageFromFile', color_type='color_ignore_orientation'),
- dict(type='Resize', scale=(2240, 2240), keep_ratio=True),
- dict(
- type='LoadOCRAnnotations',
- with_polygon=True,
- with_bbox=True,
- with_label=True),
- dict(
- type='PackTextDetInputs',
- meta_keys=('img_path', 'ori_shape', 'img_shape', 'scale_factor'))
-]
diff --git a/spaces/Mountchicken/MAERec-Gradio/configs/textdet/psenet/psenet_resnet50-oclip_fpnf_600e_icdar2015.py b/spaces/Mountchicken/MAERec-Gradio/configs/textdet/psenet/psenet_resnet50-oclip_fpnf_600e_icdar2015.py
deleted file mode 100644
index 9871f98013b11209a76d680d185bdc271b4fdf27..0000000000000000000000000000000000000000
--- a/spaces/Mountchicken/MAERec-Gradio/configs/textdet/psenet/psenet_resnet50-oclip_fpnf_600e_icdar2015.py
+++ /dev/null
@@ -1,10 +0,0 @@
-_base_ = [
- 'psenet_resnet50_fpnf_600e_icdar2015.py',
-]
-
-_base_.model.backbone = dict(
- type='CLIPResNet',
- init_cfg=dict(
- type='Pretrained',
- checkpoint='https://download.openmmlab.com/'
- 'mmocr/backbone/resnet50-oclip-7ba0c533.pth'))
diff --git a/spaces/Mozira/voice-models/infer_pack/transforms.py b/spaces/Mozira/voice-models/infer_pack/transforms.py
deleted file mode 100644
index a11f799e023864ff7082c1f49c0cc18351a13b47..0000000000000000000000000000000000000000
--- a/spaces/Mozira/voice-models/infer_pack/transforms.py
+++ /dev/null
@@ -1,209 +0,0 @@
-import torch
-from torch.nn import functional as F
-
-import numpy as np
-
-
-DEFAULT_MIN_BIN_WIDTH = 1e-3
-DEFAULT_MIN_BIN_HEIGHT = 1e-3
-DEFAULT_MIN_DERIVATIVE = 1e-3
-
-
-def piecewise_rational_quadratic_transform(
- inputs,
- unnormalized_widths,
- unnormalized_heights,
- unnormalized_derivatives,
- inverse=False,
- tails=None,
- tail_bound=1.0,
- min_bin_width=DEFAULT_MIN_BIN_WIDTH,
- min_bin_height=DEFAULT_MIN_BIN_HEIGHT,
- min_derivative=DEFAULT_MIN_DERIVATIVE,
-):
- if tails is None:
- spline_fn = rational_quadratic_spline
- spline_kwargs = {}
- else:
- spline_fn = unconstrained_rational_quadratic_spline
- spline_kwargs = {"tails": tails, "tail_bound": tail_bound}
-
- outputs, logabsdet = spline_fn(
- inputs=inputs,
- unnormalized_widths=unnormalized_widths,
- unnormalized_heights=unnormalized_heights,
- unnormalized_derivatives=unnormalized_derivatives,
- inverse=inverse,
- min_bin_width=min_bin_width,
- min_bin_height=min_bin_height,
- min_derivative=min_derivative,
- **spline_kwargs
- )
- return outputs, logabsdet
-
-
-def searchsorted(bin_locations, inputs, eps=1e-6):
- bin_locations[..., -1] += eps
- return torch.sum(inputs[..., None] >= bin_locations, dim=-1) - 1
-
-
-def unconstrained_rational_quadratic_spline(
- inputs,
- unnormalized_widths,
- unnormalized_heights,
- unnormalized_derivatives,
- inverse=False,
- tails="linear",
- tail_bound=1.0,
- min_bin_width=DEFAULT_MIN_BIN_WIDTH,
- min_bin_height=DEFAULT_MIN_BIN_HEIGHT,
- min_derivative=DEFAULT_MIN_DERIVATIVE,
-):
- inside_interval_mask = (inputs >= -tail_bound) & (inputs <= tail_bound)
- outside_interval_mask = ~inside_interval_mask
-
- outputs = torch.zeros_like(inputs)
- logabsdet = torch.zeros_like(inputs)
-
- if tails == "linear":
- unnormalized_derivatives = F.pad(unnormalized_derivatives, pad=(1, 1))
- constant = np.log(np.exp(1 - min_derivative) - 1)
- unnormalized_derivatives[..., 0] = constant
- unnormalized_derivatives[..., -1] = constant
-
- outputs[outside_interval_mask] = inputs[outside_interval_mask]
- logabsdet[outside_interval_mask] = 0
- else:
- raise RuntimeError("{} tails are not implemented.".format(tails))
-
- (
- outputs[inside_interval_mask],
- logabsdet[inside_interval_mask],
- ) = rational_quadratic_spline(
- inputs=inputs[inside_interval_mask],
- unnormalized_widths=unnormalized_widths[inside_interval_mask, :],
- unnormalized_heights=unnormalized_heights[inside_interval_mask, :],
- unnormalized_derivatives=unnormalized_derivatives[inside_interval_mask, :],
- inverse=inverse,
- left=-tail_bound,
- right=tail_bound,
- bottom=-tail_bound,
- top=tail_bound,
- min_bin_width=min_bin_width,
- min_bin_height=min_bin_height,
- min_derivative=min_derivative,
- )
-
- return outputs, logabsdet
-
-
-def rational_quadratic_spline(
- inputs,
- unnormalized_widths,
- unnormalized_heights,
- unnormalized_derivatives,
- inverse=False,
- left=0.0,
- right=1.0,
- bottom=0.0,
- top=1.0,
- min_bin_width=DEFAULT_MIN_BIN_WIDTH,
- min_bin_height=DEFAULT_MIN_BIN_HEIGHT,
- min_derivative=DEFAULT_MIN_DERIVATIVE,
-):
- if torch.min(inputs) < left or torch.max(inputs) > right:
- raise ValueError("Input to a transform is not within its domain")
-
- num_bins = unnormalized_widths.shape[-1]
-
- if min_bin_width * num_bins > 1.0:
- raise ValueError("Minimal bin width too large for the number of bins")
- if min_bin_height * num_bins > 1.0:
- raise ValueError("Minimal bin height too large for the number of bins")
-
- widths = F.softmax(unnormalized_widths, dim=-1)
- widths = min_bin_width + (1 - min_bin_width * num_bins) * widths
- cumwidths = torch.cumsum(widths, dim=-1)
- cumwidths = F.pad(cumwidths, pad=(1, 0), mode="constant", value=0.0)
- cumwidths = (right - left) * cumwidths + left
- cumwidths[..., 0] = left
- cumwidths[..., -1] = right
- widths = cumwidths[..., 1:] - cumwidths[..., :-1]
-
- derivatives = min_derivative + F.softplus(unnormalized_derivatives)
-
- heights = F.softmax(unnormalized_heights, dim=-1)
- heights = min_bin_height + (1 - min_bin_height * num_bins) * heights
- cumheights = torch.cumsum(heights, dim=-1)
- cumheights = F.pad(cumheights, pad=(1, 0), mode="constant", value=0.0)
- cumheights = (top - bottom) * cumheights + bottom
- cumheights[..., 0] = bottom
- cumheights[..., -1] = top
- heights = cumheights[..., 1:] - cumheights[..., :-1]
-
- if inverse:
- bin_idx = searchsorted(cumheights, inputs)[..., None]
- else:
- bin_idx = searchsorted(cumwidths, inputs)[..., None]
-
- input_cumwidths = cumwidths.gather(-1, bin_idx)[..., 0]
- input_bin_widths = widths.gather(-1, bin_idx)[..., 0]
-
- input_cumheights = cumheights.gather(-1, bin_idx)[..., 0]
- delta = heights / widths
- input_delta = delta.gather(-1, bin_idx)[..., 0]
-
- input_derivatives = derivatives.gather(-1, bin_idx)[..., 0]
- input_derivatives_plus_one = derivatives[..., 1:].gather(-1, bin_idx)[..., 0]
-
- input_heights = heights.gather(-1, bin_idx)[..., 0]
-
- if inverse:
- a = (inputs - input_cumheights) * (
- input_derivatives + input_derivatives_plus_one - 2 * input_delta
- ) + input_heights * (input_delta - input_derivatives)
- b = input_heights * input_derivatives - (inputs - input_cumheights) * (
- input_derivatives + input_derivatives_plus_one - 2 * input_delta
- )
- c = -input_delta * (inputs - input_cumheights)
-
- discriminant = b.pow(2) - 4 * a * c
- assert (discriminant >= 0).all()
-
- root = (2 * c) / (-b - torch.sqrt(discriminant))
- outputs = root * input_bin_widths + input_cumwidths
-
- theta_one_minus_theta = root * (1 - root)
- denominator = input_delta + (
- (input_derivatives + input_derivatives_plus_one - 2 * input_delta)
- * theta_one_minus_theta
- )
- derivative_numerator = input_delta.pow(2) * (
- input_derivatives_plus_one * root.pow(2)
- + 2 * input_delta * theta_one_minus_theta
- + input_derivatives * (1 - root).pow(2)
- )
- logabsdet = torch.log(derivative_numerator) - 2 * torch.log(denominator)
-
- return outputs, -logabsdet
- else:
- theta = (inputs - input_cumwidths) / input_bin_widths
- theta_one_minus_theta = theta * (1 - theta)
-
- numerator = input_heights * (
- input_delta * theta.pow(2) + input_derivatives * theta_one_minus_theta
- )
- denominator = input_delta + (
- (input_derivatives + input_derivatives_plus_one - 2 * input_delta)
- * theta_one_minus_theta
- )
- outputs = input_cumheights + numerator / denominator
-
- derivative_numerator = input_delta.pow(2) * (
- input_derivatives_plus_one * theta.pow(2)
- + 2 * input_delta * theta_one_minus_theta
- + input_derivatives * (1 - theta).pow(2)
- )
- logabsdet = torch.log(derivative_numerator) - 2 * torch.log(denominator)
-
- return outputs, logabsdet
diff --git a/spaces/NCTCMumbai/NCTC/models/research/compression/entropy_coder/all_models/__init__.py b/spaces/NCTCMumbai/NCTC/models/research/compression/entropy_coder/all_models/__init__.py
deleted file mode 100644
index e69de29bb2d1d6434b8b29ae775ad8c2e48c5391..0000000000000000000000000000000000000000
diff --git a/spaces/NoCrypt/mikuTTS/lib/infer_pack/models_onnx.py b/spaces/NoCrypt/mikuTTS/lib/infer_pack/models_onnx.py
deleted file mode 100644
index 963e67b29f828e9fdd096397952054fe77cf3d10..0000000000000000000000000000000000000000
--- a/spaces/NoCrypt/mikuTTS/lib/infer_pack/models_onnx.py
+++ /dev/null
@@ -1,819 +0,0 @@
-import math, pdb, os
-from time import time as ttime
-import torch
-from torch import nn
-from torch.nn import functional as F
-from lib.infer_pack import modules
-from lib.infer_pack import attentions
-from lib.infer_pack import commons
-from lib.infer_pack.commons import init_weights, get_padding
-from torch.nn import Conv1d, ConvTranspose1d, AvgPool1d, Conv2d
-from torch.nn.utils import weight_norm, remove_weight_norm, spectral_norm
-from lib.infer_pack.commons import init_weights
-import numpy as np
-from lib.infer_pack import commons
-
-
-class TextEncoder256(nn.Module):
- def __init__(
- self,
- out_channels,
- hidden_channels,
- filter_channels,
- n_heads,
- n_layers,
- kernel_size,
- p_dropout,
- f0=True,
- ):
- super().__init__()
- self.out_channels = out_channels
- self.hidden_channels = hidden_channels
- self.filter_channels = filter_channels
- self.n_heads = n_heads
- self.n_layers = n_layers
- self.kernel_size = kernel_size
- self.p_dropout = p_dropout
- self.emb_phone = nn.Linear(256, hidden_channels)
- self.lrelu = nn.LeakyReLU(0.1, inplace=True)
- if f0 == True:
- self.emb_pitch = nn.Embedding(256, hidden_channels) # pitch 256
- self.encoder = attentions.Encoder(
- hidden_channels, filter_channels, n_heads, n_layers, kernel_size, p_dropout
- )
- self.proj = nn.Conv1d(hidden_channels, out_channels * 2, 1)
-
- def forward(self, phone, pitch, lengths):
- if pitch == None:
- x = self.emb_phone(phone)
- else:
- x = self.emb_phone(phone) + self.emb_pitch(pitch)
- x = x * math.sqrt(self.hidden_channels) # [b, t, h]
- x = self.lrelu(x)
- x = torch.transpose(x, 1, -1) # [b, h, t]
- x_mask = torch.unsqueeze(commons.sequence_mask(lengths, x.size(2)), 1).to(
- x.dtype
- )
- x = self.encoder(x * x_mask, x_mask)
- stats = self.proj(x) * x_mask
-
- m, logs = torch.split(stats, self.out_channels, dim=1)
- return m, logs, x_mask
-
-
-class TextEncoder768(nn.Module):
- def __init__(
- self,
- out_channels,
- hidden_channels,
- filter_channels,
- n_heads,
- n_layers,
- kernel_size,
- p_dropout,
- f0=True,
- ):
- super().__init__()
- self.out_channels = out_channels
- self.hidden_channels = hidden_channels
- self.filter_channels = filter_channels
- self.n_heads = n_heads
- self.n_layers = n_layers
- self.kernel_size = kernel_size
- self.p_dropout = p_dropout
- self.emb_phone = nn.Linear(768, hidden_channels)
- self.lrelu = nn.LeakyReLU(0.1, inplace=True)
- if f0 == True:
- self.emb_pitch = nn.Embedding(256, hidden_channels) # pitch 256
- self.encoder = attentions.Encoder(
- hidden_channels, filter_channels, n_heads, n_layers, kernel_size, p_dropout
- )
- self.proj = nn.Conv1d(hidden_channels, out_channels * 2, 1)
-
- def forward(self, phone, pitch, lengths):
- if pitch == None:
- x = self.emb_phone(phone)
- else:
- x = self.emb_phone(phone) + self.emb_pitch(pitch)
- x = x * math.sqrt(self.hidden_channels) # [b, t, h]
- x = self.lrelu(x)
- x = torch.transpose(x, 1, -1) # [b, h, t]
- x_mask = torch.unsqueeze(commons.sequence_mask(lengths, x.size(2)), 1).to(
- x.dtype
- )
- x = self.encoder(x * x_mask, x_mask)
- stats = self.proj(x) * x_mask
-
- m, logs = torch.split(stats, self.out_channels, dim=1)
- return m, logs, x_mask
-
-
-class ResidualCouplingBlock(nn.Module):
- def __init__(
- self,
- channels,
- hidden_channels,
- kernel_size,
- dilation_rate,
- n_layers,
- n_flows=4,
- gin_channels=0,
- ):
- super().__init__()
- self.channels = channels
- self.hidden_channels = hidden_channels
- self.kernel_size = kernel_size
- self.dilation_rate = dilation_rate
- self.n_layers = n_layers
- self.n_flows = n_flows
- self.gin_channels = gin_channels
-
- self.flows = nn.ModuleList()
- for i in range(n_flows):
- self.flows.append(
- modules.ResidualCouplingLayer(
- channels,
- hidden_channels,
- kernel_size,
- dilation_rate,
- n_layers,
- gin_channels=gin_channels,
- mean_only=True,
- )
- )
- self.flows.append(modules.Flip())
-
- def forward(self, x, x_mask, g=None, reverse=False):
- if not reverse:
- for flow in self.flows:
- x, _ = flow(x, x_mask, g=g, reverse=reverse)
- else:
- for flow in reversed(self.flows):
- x = flow(x, x_mask, g=g, reverse=reverse)
- return x
-
- def remove_weight_norm(self):
- for i in range(self.n_flows):
- self.flows[i * 2].remove_weight_norm()
-
-
-class PosteriorEncoder(nn.Module):
- def __init__(
- self,
- in_channels,
- out_channels,
- hidden_channels,
- kernel_size,
- dilation_rate,
- n_layers,
- gin_channels=0,
- ):
- super().__init__()
- self.in_channels = in_channels
- self.out_channels = out_channels
- self.hidden_channels = hidden_channels
- self.kernel_size = kernel_size
- self.dilation_rate = dilation_rate
- self.n_layers = n_layers
- self.gin_channels = gin_channels
-
- self.pre = nn.Conv1d(in_channels, hidden_channels, 1)
- self.enc = modules.WN(
- hidden_channels,
- kernel_size,
- dilation_rate,
- n_layers,
- gin_channels=gin_channels,
- )
- self.proj = nn.Conv1d(hidden_channels, out_channels * 2, 1)
-
- def forward(self, x, x_lengths, g=None):
- x_mask = torch.unsqueeze(commons.sequence_mask(x_lengths, x.size(2)), 1).to(
- x.dtype
- )
- x = self.pre(x) * x_mask
- x = self.enc(x, x_mask, g=g)
- stats = self.proj(x) * x_mask
- m, logs = torch.split(stats, self.out_channels, dim=1)
- z = (m + torch.randn_like(m) * torch.exp(logs)) * x_mask
- return z, m, logs, x_mask
-
- def remove_weight_norm(self):
- self.enc.remove_weight_norm()
-
-
-class Generator(torch.nn.Module):
- def __init__(
- self,
- initial_channel,
- resblock,
- resblock_kernel_sizes,
- resblock_dilation_sizes,
- upsample_rates,
- upsample_initial_channel,
- upsample_kernel_sizes,
- gin_channels=0,
- ):
- super(Generator, self).__init__()
- self.num_kernels = len(resblock_kernel_sizes)
- self.num_upsamples = len(upsample_rates)
- self.conv_pre = Conv1d(
- initial_channel, upsample_initial_channel, 7, 1, padding=3
- )
- resblock = modules.ResBlock1 if resblock == "1" else modules.ResBlock2
-
- self.ups = nn.ModuleList()
- for i, (u, k) in enumerate(zip(upsample_rates, upsample_kernel_sizes)):
- self.ups.append(
- weight_norm(
- ConvTranspose1d(
- upsample_initial_channel // (2**i),
- upsample_initial_channel // (2 ** (i + 1)),
- k,
- u,
- padding=(k - u) // 2,
- )
- )
- )
-
- self.resblocks = nn.ModuleList()
- for i in range(len(self.ups)):
- ch = upsample_initial_channel // (2 ** (i + 1))
- for j, (k, d) in enumerate(
- zip(resblock_kernel_sizes, resblock_dilation_sizes)
- ):
- self.resblocks.append(resblock(ch, k, d))
-
- self.conv_post = Conv1d(ch, 1, 7, 1, padding=3, bias=False)
- self.ups.apply(init_weights)
-
- if gin_channels != 0:
- self.cond = nn.Conv1d(gin_channels, upsample_initial_channel, 1)
-
- def forward(self, x, g=None):
- x = self.conv_pre(x)
- if g is not None:
- x = x + self.cond(g)
-
- for i in range(self.num_upsamples):
- x = F.leaky_relu(x, modules.LRELU_SLOPE)
- x = self.ups[i](x)
- xs = None
- for j in range(self.num_kernels):
- if xs is None:
- xs = self.resblocks[i * self.num_kernels + j](x)
- else:
- xs += self.resblocks[i * self.num_kernels + j](x)
- x = xs / self.num_kernels
- x = F.leaky_relu(x)
- x = self.conv_post(x)
- x = torch.tanh(x)
-
- return x
-
- def remove_weight_norm(self):
- for l in self.ups:
- remove_weight_norm(l)
- for l in self.resblocks:
- l.remove_weight_norm()
-
-
-class SineGen(torch.nn.Module):
- """Definition of sine generator
- SineGen(samp_rate, harmonic_num = 0,
- sine_amp = 0.1, noise_std = 0.003,
- voiced_threshold = 0,
- flag_for_pulse=False)
- samp_rate: sampling rate in Hz
- harmonic_num: number of harmonic overtones (default 0)
- sine_amp: amplitude of sine-wavefrom (default 0.1)
- noise_std: std of Gaussian noise (default 0.003)
- voiced_thoreshold: F0 threshold for U/V classification (default 0)
- flag_for_pulse: this SinGen is used inside PulseGen (default False)
- Note: when flag_for_pulse is True, the first time step of a voiced
- segment is always sin(np.pi) or cos(0)
- """
-
- def __init__(
- self,
- samp_rate,
- harmonic_num=0,
- sine_amp=0.1,
- noise_std=0.003,
- voiced_threshold=0,
- flag_for_pulse=False,
- ):
- super(SineGen, self).__init__()
- self.sine_amp = sine_amp
- self.noise_std = noise_std
- self.harmonic_num = harmonic_num
- self.dim = self.harmonic_num + 1
- self.sampling_rate = samp_rate
- self.voiced_threshold = voiced_threshold
-
- def _f02uv(self, f0):
- # generate uv signal
- uv = torch.ones_like(f0)
- uv = uv * (f0 > self.voiced_threshold)
- return uv
-
- def forward(self, f0, upp):
- """sine_tensor, uv = forward(f0)
- input F0: tensor(batchsize=1, length, dim=1)
- f0 for unvoiced steps should be 0
- output sine_tensor: tensor(batchsize=1, length, dim)
- output uv: tensor(batchsize=1, length, 1)
- """
- with torch.no_grad():
- f0 = f0[:, None].transpose(1, 2)
- f0_buf = torch.zeros(f0.shape[0], f0.shape[1], self.dim, device=f0.device)
- # fundamental component
- f0_buf[:, :, 0] = f0[:, :, 0]
- for idx in np.arange(self.harmonic_num):
- f0_buf[:, :, idx + 1] = f0_buf[:, :, 0] * (
- idx + 2
- ) # idx + 2: the (idx+1)-th overtone, (idx+2)-th harmonic
- rad_values = (f0_buf / self.sampling_rate) % 1 ###%1意味着n_har的乘积无法后处理优化
- rand_ini = torch.rand(
- f0_buf.shape[0], f0_buf.shape[2], device=f0_buf.device
- )
- rand_ini[:, 0] = 0
- rad_values[:, 0, :] = rad_values[:, 0, :] + rand_ini
- tmp_over_one = torch.cumsum(rad_values, 1) # % 1 #####%1意味着后面的cumsum无法再优化
- tmp_over_one *= upp
- tmp_over_one = F.interpolate(
- tmp_over_one.transpose(2, 1),
- scale_factor=upp,
- mode="linear",
- align_corners=True,
- ).transpose(2, 1)
- rad_values = F.interpolate(
- rad_values.transpose(2, 1), scale_factor=upp, mode="nearest"
- ).transpose(
- 2, 1
- ) #######
- tmp_over_one %= 1
- tmp_over_one_idx = (tmp_over_one[:, 1:, :] - tmp_over_one[:, :-1, :]) < 0
- cumsum_shift = torch.zeros_like(rad_values)
- cumsum_shift[:, 1:, :] = tmp_over_one_idx * -1.0
- sine_waves = torch.sin(
- torch.cumsum(rad_values + cumsum_shift, dim=1) * 2 * np.pi
- )
- sine_waves = sine_waves * self.sine_amp
- uv = self._f02uv(f0)
- uv = F.interpolate(
- uv.transpose(2, 1), scale_factor=upp, mode="nearest"
- ).transpose(2, 1)
- noise_amp = uv * self.noise_std + (1 - uv) * self.sine_amp / 3
- noise = noise_amp * torch.randn_like(sine_waves)
- sine_waves = sine_waves * uv + noise
- return sine_waves, uv, noise
-
-
-class SourceModuleHnNSF(torch.nn.Module):
- """SourceModule for hn-nsf
- SourceModule(sampling_rate, harmonic_num=0, sine_amp=0.1,
- add_noise_std=0.003, voiced_threshod=0)
- sampling_rate: sampling_rate in Hz
- harmonic_num: number of harmonic above F0 (default: 0)
- sine_amp: amplitude of sine source signal (default: 0.1)
- add_noise_std: std of additive Gaussian noise (default: 0.003)
- note that amplitude of noise in unvoiced is decided
- by sine_amp
- voiced_threshold: threhold to set U/V given F0 (default: 0)
- Sine_source, noise_source = SourceModuleHnNSF(F0_sampled)
- F0_sampled (batchsize, length, 1)
- Sine_source (batchsize, length, 1)
- noise_source (batchsize, length 1)
- uv (batchsize, length, 1)
- """
-
- def __init__(
- self,
- sampling_rate,
- harmonic_num=0,
- sine_amp=0.1,
- add_noise_std=0.003,
- voiced_threshod=0,
- is_half=True,
- ):
- super(SourceModuleHnNSF, self).__init__()
-
- self.sine_amp = sine_amp
- self.noise_std = add_noise_std
- self.is_half = is_half
- # to produce sine waveforms
- self.l_sin_gen = SineGen(
- sampling_rate, harmonic_num, sine_amp, add_noise_std, voiced_threshod
- )
-
- # to merge source harmonics into a single excitation
- self.l_linear = torch.nn.Linear(harmonic_num + 1, 1)
- self.l_tanh = torch.nn.Tanh()
-
- def forward(self, x, upp=None):
- sine_wavs, uv, _ = self.l_sin_gen(x, upp)
- if self.is_half:
- sine_wavs = sine_wavs.half()
- sine_merge = self.l_tanh(self.l_linear(sine_wavs))
- return sine_merge, None, None # noise, uv
-
-
-class GeneratorNSF(torch.nn.Module):
- def __init__(
- self,
- initial_channel,
- resblock,
- resblock_kernel_sizes,
- resblock_dilation_sizes,
- upsample_rates,
- upsample_initial_channel,
- upsample_kernel_sizes,
- gin_channels,
- sr,
- is_half=False,
- ):
- super(GeneratorNSF, self).__init__()
- self.num_kernels = len(resblock_kernel_sizes)
- self.num_upsamples = len(upsample_rates)
-
- self.f0_upsamp = torch.nn.Upsample(scale_factor=np.prod(upsample_rates))
- self.m_source = SourceModuleHnNSF(
- sampling_rate=sr, harmonic_num=0, is_half=is_half
- )
- self.noise_convs = nn.ModuleList()
- self.conv_pre = Conv1d(
- initial_channel, upsample_initial_channel, 7, 1, padding=3
- )
- resblock = modules.ResBlock1 if resblock == "1" else modules.ResBlock2
-
- self.ups = nn.ModuleList()
- for i, (u, k) in enumerate(zip(upsample_rates, upsample_kernel_sizes)):
- c_cur = upsample_initial_channel // (2 ** (i + 1))
- self.ups.append(
- weight_norm(
- ConvTranspose1d(
- upsample_initial_channel // (2**i),
- upsample_initial_channel // (2 ** (i + 1)),
- k,
- u,
- padding=(k - u) // 2,
- )
- )
- )
- if i + 1 < len(upsample_rates):
- stride_f0 = np.prod(upsample_rates[i + 1 :])
- self.noise_convs.append(
- Conv1d(
- 1,
- c_cur,
- kernel_size=stride_f0 * 2,
- stride=stride_f0,
- padding=stride_f0 // 2,
- )
- )
- else:
- self.noise_convs.append(Conv1d(1, c_cur, kernel_size=1))
-
- self.resblocks = nn.ModuleList()
- for i in range(len(self.ups)):
- ch = upsample_initial_channel // (2 ** (i + 1))
- for j, (k, d) in enumerate(
- zip(resblock_kernel_sizes, resblock_dilation_sizes)
- ):
- self.resblocks.append(resblock(ch, k, d))
-
- self.conv_post = Conv1d(ch, 1, 7, 1, padding=3, bias=False)
- self.ups.apply(init_weights)
-
- if gin_channels != 0:
- self.cond = nn.Conv1d(gin_channels, upsample_initial_channel, 1)
-
- self.upp = np.prod(upsample_rates)
-
- def forward(self, x, f0, g=None):
- har_source, noi_source, uv = self.m_source(f0, self.upp)
- har_source = har_source.transpose(1, 2)
- x = self.conv_pre(x)
- if g is not None:
- x = x + self.cond(g)
-
- for i in range(self.num_upsamples):
- x = F.leaky_relu(x, modules.LRELU_SLOPE)
- x = self.ups[i](x)
- x_source = self.noise_convs[i](har_source)
- x = x + x_source
- xs = None
- for j in range(self.num_kernels):
- if xs is None:
- xs = self.resblocks[i * self.num_kernels + j](x)
- else:
- xs += self.resblocks[i * self.num_kernels + j](x)
- x = xs / self.num_kernels
- x = F.leaky_relu(x)
- x = self.conv_post(x)
- x = torch.tanh(x)
- return x
-
- def remove_weight_norm(self):
- for l in self.ups:
- remove_weight_norm(l)
- for l in self.resblocks:
- l.remove_weight_norm()
-
-
-sr2sr = {
- "32k": 32000,
- "40k": 40000,
- "48k": 48000,
-}
-
-
-class SynthesizerTrnMsNSFsidM(nn.Module):
- def __init__(
- self,
- spec_channels,
- segment_size,
- inter_channels,
- hidden_channels,
- filter_channels,
- n_heads,
- n_layers,
- kernel_size,
- p_dropout,
- resblock,
- resblock_kernel_sizes,
- resblock_dilation_sizes,
- upsample_rates,
- upsample_initial_channel,
- upsample_kernel_sizes,
- spk_embed_dim,
- gin_channels,
- sr,
- version,
- **kwargs
- ):
- super().__init__()
- if type(sr) == type("strr"):
- sr = sr2sr[sr]
- self.spec_channels = spec_channels
- self.inter_channels = inter_channels
- self.hidden_channels = hidden_channels
- self.filter_channels = filter_channels
- self.n_heads = n_heads
- self.n_layers = n_layers
- self.kernel_size = kernel_size
- self.p_dropout = p_dropout
- self.resblock = resblock
- self.resblock_kernel_sizes = resblock_kernel_sizes
- self.resblock_dilation_sizes = resblock_dilation_sizes
- self.upsample_rates = upsample_rates
- self.upsample_initial_channel = upsample_initial_channel
- self.upsample_kernel_sizes = upsample_kernel_sizes
- self.segment_size = segment_size
- self.gin_channels = gin_channels
- # self.hop_length = hop_length#
- self.spk_embed_dim = spk_embed_dim
- if version == "v1":
- self.enc_p = TextEncoder256(
- inter_channels,
- hidden_channels,
- filter_channels,
- n_heads,
- n_layers,
- kernel_size,
- p_dropout,
- )
- else:
- self.enc_p = TextEncoder768(
- inter_channels,
- hidden_channels,
- filter_channels,
- n_heads,
- n_layers,
- kernel_size,
- p_dropout,
- )
- self.dec = GeneratorNSF(
- inter_channels,
- resblock,
- resblock_kernel_sizes,
- resblock_dilation_sizes,
- upsample_rates,
- upsample_initial_channel,
- upsample_kernel_sizes,
- gin_channels=gin_channels,
- sr=sr,
- is_half=kwargs["is_half"],
- )
- self.enc_q = PosteriorEncoder(
- spec_channels,
- inter_channels,
- hidden_channels,
- 5,
- 1,
- 16,
- gin_channels=gin_channels,
- )
- self.flow = ResidualCouplingBlock(
- inter_channels, hidden_channels, 5, 1, 3, gin_channels=gin_channels
- )
- self.emb_g = nn.Embedding(self.spk_embed_dim, gin_channels)
- self.speaker_map = None
- print("gin_channels:", gin_channels, "self.spk_embed_dim:", self.spk_embed_dim)
-
- def remove_weight_norm(self):
- self.dec.remove_weight_norm()
- self.flow.remove_weight_norm()
- self.enc_q.remove_weight_norm()
-
- def construct_spkmixmap(self, n_speaker):
- self.speaker_map = torch.zeros((n_speaker, 1, 1, self.gin_channels))
- for i in range(n_speaker):
- self.speaker_map[i] = self.emb_g(torch.LongTensor([[i]]))
- self.speaker_map = self.speaker_map.unsqueeze(0)
-
- def forward(self, phone, phone_lengths, pitch, nsff0, g, rnd, max_len=None):
- if self.speaker_map is not None: # [N, S] * [S, B, 1, H]
- g = g.reshape((g.shape[0], g.shape[1], 1, 1, 1)) # [N, S, B, 1, 1]
- g = g * self.speaker_map # [N, S, B, 1, H]
- g = torch.sum(g, dim=1) # [N, 1, B, 1, H]
- g = g.transpose(0, -1).transpose(0, -2).squeeze(0) # [B, H, N]
- else:
- g = g.unsqueeze(0)
- g = self.emb_g(g).transpose(1, 2)
-
- m_p, logs_p, x_mask = self.enc_p(phone, pitch, phone_lengths)
- z_p = (m_p + torch.exp(logs_p) * rnd) * x_mask
- z = self.flow(z_p, x_mask, g=g, reverse=True)
- o = self.dec((z * x_mask)[:, :, :max_len], nsff0, g=g)
- return o
-
-
-class MultiPeriodDiscriminator(torch.nn.Module):
- def __init__(self, use_spectral_norm=False):
- super(MultiPeriodDiscriminator, self).__init__()
- periods = [2, 3, 5, 7, 11, 17]
- # periods = [3, 5, 7, 11, 17, 23, 37]
-
- discs = [DiscriminatorS(use_spectral_norm=use_spectral_norm)]
- discs = discs + [
- DiscriminatorP(i, use_spectral_norm=use_spectral_norm) for i in periods
- ]
- self.discriminators = nn.ModuleList(discs)
-
- def forward(self, y, y_hat):
- y_d_rs = [] #
- y_d_gs = []
- fmap_rs = []
- fmap_gs = []
- for i, d in enumerate(self.discriminators):
- y_d_r, fmap_r = d(y)
- y_d_g, fmap_g = d(y_hat)
- # for j in range(len(fmap_r)):
- # print(i,j,y.shape,y_hat.shape,fmap_r[j].shape,fmap_g[j].shape)
- y_d_rs.append(y_d_r)
- y_d_gs.append(y_d_g)
- fmap_rs.append(fmap_r)
- fmap_gs.append(fmap_g)
-
- return y_d_rs, y_d_gs, fmap_rs, fmap_gs
-
-
-class MultiPeriodDiscriminatorV2(torch.nn.Module):
- def __init__(self, use_spectral_norm=False):
- super(MultiPeriodDiscriminatorV2, self).__init__()
- # periods = [2, 3, 5, 7, 11, 17]
- periods = [2, 3, 5, 7, 11, 17, 23, 37]
-
- discs = [DiscriminatorS(use_spectral_norm=use_spectral_norm)]
- discs = discs + [
- DiscriminatorP(i, use_spectral_norm=use_spectral_norm) for i in periods
- ]
- self.discriminators = nn.ModuleList(discs)
-
- def forward(self, y, y_hat):
- y_d_rs = [] #
- y_d_gs = []
- fmap_rs = []
- fmap_gs = []
- for i, d in enumerate(self.discriminators):
- y_d_r, fmap_r = d(y)
- y_d_g, fmap_g = d(y_hat)
- # for j in range(len(fmap_r)):
- # print(i,j,y.shape,y_hat.shape,fmap_r[j].shape,fmap_g[j].shape)
- y_d_rs.append(y_d_r)
- y_d_gs.append(y_d_g)
- fmap_rs.append(fmap_r)
- fmap_gs.append(fmap_g)
-
- return y_d_rs, y_d_gs, fmap_rs, fmap_gs
-
-
-class DiscriminatorS(torch.nn.Module):
- def __init__(self, use_spectral_norm=False):
- super(DiscriminatorS, self).__init__()
- norm_f = weight_norm if use_spectral_norm == False else spectral_norm
- self.convs = nn.ModuleList(
- [
- norm_f(Conv1d(1, 16, 15, 1, padding=7)),
- norm_f(Conv1d(16, 64, 41, 4, groups=4, padding=20)),
- norm_f(Conv1d(64, 256, 41, 4, groups=16, padding=20)),
- norm_f(Conv1d(256, 1024, 41, 4, groups=64, padding=20)),
- norm_f(Conv1d(1024, 1024, 41, 4, groups=256, padding=20)),
- norm_f(Conv1d(1024, 1024, 5, 1, padding=2)),
- ]
- )
- self.conv_post = norm_f(Conv1d(1024, 1, 3, 1, padding=1))
-
- def forward(self, x):
- fmap = []
-
- for l in self.convs:
- x = l(x)
- x = F.leaky_relu(x, modules.LRELU_SLOPE)
- fmap.append(x)
- x = self.conv_post(x)
- fmap.append(x)
- x = torch.flatten(x, 1, -1)
-
- return x, fmap
-
-
-class DiscriminatorP(torch.nn.Module):
- def __init__(self, period, kernel_size=5, stride=3, use_spectral_norm=False):
- super(DiscriminatorP, self).__init__()
- self.period = period
- self.use_spectral_norm = use_spectral_norm
- norm_f = weight_norm if use_spectral_norm == False else spectral_norm
- self.convs = nn.ModuleList(
- [
- norm_f(
- Conv2d(
- 1,
- 32,
- (kernel_size, 1),
- (stride, 1),
- padding=(get_padding(kernel_size, 1), 0),
- )
- ),
- norm_f(
- Conv2d(
- 32,
- 128,
- (kernel_size, 1),
- (stride, 1),
- padding=(get_padding(kernel_size, 1), 0),
- )
- ),
- norm_f(
- Conv2d(
- 128,
- 512,
- (kernel_size, 1),
- (stride, 1),
- padding=(get_padding(kernel_size, 1), 0),
- )
- ),
- norm_f(
- Conv2d(
- 512,
- 1024,
- (kernel_size, 1),
- (stride, 1),
- padding=(get_padding(kernel_size, 1), 0),
- )
- ),
- norm_f(
- Conv2d(
- 1024,
- 1024,
- (kernel_size, 1),
- 1,
- padding=(get_padding(kernel_size, 1), 0),
- )
- ),
- ]
- )
- self.conv_post = norm_f(Conv2d(1024, 1, (3, 1), 1, padding=(1, 0)))
-
- def forward(self, x):
- fmap = []
-
- # 1d to 2d
- b, c, t = x.shape
- if t % self.period != 0: # pad first
- n_pad = self.period - (t % self.period)
- x = F.pad(x, (0, n_pad), "reflect")
- t = t + n_pad
- x = x.view(b, c, t // self.period, self.period)
-
- for l in self.convs:
- x = l(x)
- x = F.leaky_relu(x, modules.LRELU_SLOPE)
- fmap.append(x)
- x = self.conv_post(x)
- fmap.append(x)
- x = torch.flatten(x, 1, -1)
-
- return x, fmap
diff --git a/spaces/OAOA/DifFace/utils/util_opts.py b/spaces/OAOA/DifFace/utils/util_opts.py
deleted file mode 100644
index e76de955cfb929cf9d537b1678365fc1a62df4cd..0000000000000000000000000000000000000000
--- a/spaces/OAOA/DifFace/utils/util_opts.py
+++ /dev/null
@@ -1,20 +0,0 @@
-#!/usr/bin/env python
-# -*- coding:utf-8 -*-
-# Power by Zongsheng Yue 2021-11-24 15:07:43
-
-def update_args(args_json, args_parser):
- for arg in vars(args_parser):
- args_json[arg] = getattr(args_parser, arg)
-
-def str2bool(v):
- """
- https://stackoverflow.com/questions/15008758/parsing-boolean-values-with-argparse
- """
- if isinstance(v, bool):
- return v
- if v.lower() in ("yes", "true", "t", "y", "1"):
- return True
- elif v.lower() in ("no", "false", "f", "n", "0"):
- return False
- else:
- raise argparse.ArgumentTypeError("boolean value expected")
diff --git a/spaces/OFA-Sys/OFA-Generic_Interface/fairseq/examples/discriminative_reranking_nmt/models/discriminative_reranking_model.py b/spaces/OFA-Sys/OFA-Generic_Interface/fairseq/examples/discriminative_reranking_nmt/models/discriminative_reranking_model.py
deleted file mode 100644
index e4b5887f825df36f4e1e0384f38fefe790e485e6..0000000000000000000000000000000000000000
--- a/spaces/OFA-Sys/OFA-Generic_Interface/fairseq/examples/discriminative_reranking_nmt/models/discriminative_reranking_model.py
+++ /dev/null
@@ -1,365 +0,0 @@
-from dataclasses import dataclass, field
-import os
-
-import torch
-import torch.nn as nn
-
-from fairseq import utils
-from fairseq.dataclass import ChoiceEnum, FairseqDataclass
-from fairseq.models import (
- BaseFairseqModel,
- register_model,
-)
-
-from fairseq.models.roberta.model import RobertaClassificationHead
-
-from fairseq.modules import (
- LayerNorm,
- TransformerSentenceEncoder,
- TransformerSentenceEncoderLayer,
-)
-
-
-ACTIVATION_FN_CHOICES = ChoiceEnum(utils.get_available_activation_fns())
-JOINT_CLASSIFICATION_CHOICES = ChoiceEnum(["none", "sent"])
-SENTENCE_REP_CHOICES = ChoiceEnum(["head", "meanpool", "maxpool"])
-
-
-def update_init_roberta_model_state(state):
- """
- update the state_dict of a Roberta model for initializing
- weights of the BertRanker
- """
- for k in list(state.keys()):
- if ".lm_head." in k or "version" in k:
- del state[k]
- continue
- # remove 'encoder/decoder.sentence_encoder.' from the key
- assert k.startswith("encoder.sentence_encoder.") or k.startswith(
- "decoder.sentence_encoder."
- ), f"Cannot recognize parameter name {k}"
- if "layernorm_embedding" in k:
- new_k = k.replace(".layernorm_embedding.", ".emb_layer_norm.")
- state[new_k[25:]] = state[k]
- else:
- state[k[25:]] = state[k]
- del state[k]
-
-
-class BaseRanker(nn.Module):
- def __init__(self, args, task):
- super().__init__()
-
- self.separator_token = task.dictionary.eos()
- self.padding_idx = task.dictionary.pad()
-
- def forward(self, src_tokens):
- raise NotImplementedError
-
- def get_segment_labels(self, src_tokens):
- segment_boundary = (src_tokens == self.separator_token).long()
- segment_labels = (
- segment_boundary.cumsum(dim=1)
- - segment_boundary
- - (src_tokens == self.padding_idx).long()
- )
-
- return segment_labels
-
- def get_positions(self, src_tokens, segment_labels):
- segment_positions = (
- torch.arange(src_tokens.shape[1])
- .to(src_tokens.device)
- .repeat(src_tokens.shape[0], 1)
- )
- segment_boundary = (src_tokens == self.separator_token).long()
- _, col_idx = (segment_positions * segment_boundary).nonzero(as_tuple=True)
- col_idx = torch.cat([torch.zeros(1).type_as(col_idx), col_idx])
- offset = torch.cat(
- [
- torch.zeros(1).type_as(segment_boundary),
- segment_boundary.sum(dim=1).cumsum(dim=0)[:-1],
- ]
- )
- segment_positions -= col_idx[segment_labels + offset.unsqueeze(1)] * (
- segment_labels != 0
- )
-
- padding_mask = src_tokens.ne(self.padding_idx)
- segment_positions = (segment_positions + 1) * padding_mask.type_as(
- segment_positions
- ) + self.padding_idx
-
- return segment_positions
-
-
-class BertRanker(BaseRanker):
- def __init__(self, args, task):
- super(BertRanker, self).__init__(args, task)
-
- init_model = getattr(args, "pretrained_model", "")
- self.joint_layers = nn.ModuleList()
- if os.path.isfile(init_model):
- print(f"initialize weight from {init_model}")
-
- from fairseq import hub_utils
-
- x = hub_utils.from_pretrained(
- os.path.dirname(init_model),
- checkpoint_file=os.path.basename(init_model),
- )
-
- in_state_dict = x["models"][0].state_dict()
- init_args = x["args"].model
-
- num_positional_emb = init_args.max_positions + task.dictionary.pad() + 1
-
- # follow the setup in roberta
- self.model = TransformerSentenceEncoder(
- padding_idx=task.dictionary.pad(),
- vocab_size=len(task.dictionary),
- num_encoder_layers=getattr(
- args, "encoder_layers", init_args.encoder_layers
- ),
- embedding_dim=init_args.encoder_embed_dim,
- ffn_embedding_dim=init_args.encoder_ffn_embed_dim,
- num_attention_heads=init_args.encoder_attention_heads,
- dropout=init_args.dropout,
- attention_dropout=init_args.attention_dropout,
- activation_dropout=init_args.activation_dropout,
- num_segments=2, # add language embeddings
- max_seq_len=num_positional_emb,
- offset_positions_by_padding=False,
- encoder_normalize_before=True,
- apply_bert_init=True,
- activation_fn=init_args.activation_fn,
- freeze_embeddings=args.freeze_embeddings,
- n_trans_layers_to_freeze=args.n_trans_layers_to_freeze,
- )
-
- # still need to learn segment embeddings as we added a second language embedding
- if args.freeze_embeddings:
- for p in self.model.segment_embeddings.parameters():
- p.requires_grad = False
-
- update_init_roberta_model_state(in_state_dict)
- print("loading weights from the pretrained model")
- self.model.load_state_dict(
- in_state_dict, strict=False
- ) # ignore mismatch in language embeddings
-
- ffn_embedding_dim = init_args.encoder_ffn_embed_dim
- num_attention_heads = init_args.encoder_attention_heads
- dropout = init_args.dropout
- attention_dropout = init_args.attention_dropout
- activation_dropout = init_args.activation_dropout
- activation_fn = init_args.activation_fn
-
- classifier_embed_dim = getattr(
- args, "embed_dim", init_args.encoder_embed_dim
- )
- if classifier_embed_dim != init_args.encoder_embed_dim:
- self.transform_layer = nn.Linear(
- init_args.encoder_embed_dim, classifier_embed_dim
- )
- else:
- self.model = TransformerSentenceEncoder(
- padding_idx=task.dictionary.pad(),
- vocab_size=len(task.dictionary),
- num_encoder_layers=args.encoder_layers,
- embedding_dim=args.embed_dim,
- ffn_embedding_dim=args.ffn_embed_dim,
- num_attention_heads=args.attention_heads,
- dropout=args.dropout,
- attention_dropout=args.attention_dropout,
- activation_dropout=args.activation_dropout,
- max_seq_len=task.max_positions()
- if task.max_positions()
- else args.tokens_per_sample,
- num_segments=2,
- offset_positions_by_padding=False,
- encoder_normalize_before=args.encoder_normalize_before,
- apply_bert_init=args.apply_bert_init,
- activation_fn=args.activation_fn,
- )
-
- classifier_embed_dim = args.embed_dim
- ffn_embedding_dim = args.ffn_embed_dim
- num_attention_heads = args.attention_heads
- dropout = args.dropout
- attention_dropout = args.attention_dropout
- activation_dropout = args.activation_dropout
- activation_fn = args.activation_fn
-
- self.joint_classification = args.joint_classification
- if args.joint_classification == "sent":
- if args.joint_normalize_before:
- self.joint_layer_norm = LayerNorm(classifier_embed_dim)
- else:
- self.joint_layer_norm = None
-
- self.joint_layers = nn.ModuleList(
- [
- TransformerSentenceEncoderLayer(
- embedding_dim=classifier_embed_dim,
- ffn_embedding_dim=ffn_embedding_dim,
- num_attention_heads=num_attention_heads,
- dropout=dropout,
- attention_dropout=attention_dropout,
- activation_dropout=activation_dropout,
- activation_fn=activation_fn,
- )
- for _ in range(args.num_joint_layers)
- ]
- )
-
- self.classifier = RobertaClassificationHead(
- classifier_embed_dim,
- classifier_embed_dim,
- 1, # num_classes
- "tanh",
- args.classifier_dropout,
- )
-
- def forward(self, src_tokens, src_lengths):
- segment_labels = self.get_segment_labels(src_tokens)
- positions = self.get_positions(src_tokens, segment_labels)
-
- inner_states, _ = self.model(
- tokens=src_tokens,
- segment_labels=segment_labels,
- last_state_only=True,
- positions=positions,
- )
-
- return inner_states[-1].transpose(0, 1) # T x B x C -> B x T x C
-
- def sentence_forward(self, encoder_out, src_tokens=None, sentence_rep="head"):
- # encoder_out: B x T x C
- if sentence_rep == "head":
- x = encoder_out[:, :1, :]
- else: # 'meanpool', 'maxpool'
- assert src_tokens is not None, "meanpool requires src_tokens input"
- segment_labels = self.get_segment_labels(src_tokens)
- padding_mask = src_tokens.ne(self.padding_idx)
- encoder_mask = segment_labels * padding_mask.type_as(segment_labels)
-
- if sentence_rep == "meanpool":
- ntokens = torch.sum(encoder_mask, dim=1, keepdim=True)
- x = torch.sum(
- encoder_out * encoder_mask.unsqueeze(2), dim=1, keepdim=True
- ) / ntokens.unsqueeze(2).type_as(encoder_out)
- else: # 'maxpool'
- encoder_out[
- (encoder_mask == 0).unsqueeze(2).repeat(1, 1, encoder_out.shape[-1])
- ] = -float("inf")
- x, _ = torch.max(encoder_out, dim=1, keepdim=True)
-
- if hasattr(self, "transform_layer"):
- x = self.transform_layer(x)
-
- return x # B x 1 x C
-
- def joint_forward(self, x):
- # x: T x B x C
- if self.joint_layer_norm:
- x = self.joint_layer_norm(x.transpose(0, 1))
- x = x.transpose(0, 1)
-
- for layer in self.joint_layers:
- x, _ = layer(x, self_attn_padding_mask=None)
- return x
-
- def classification_forward(self, x):
- # x: B x T x C
- return self.classifier(x)
-
-
-@dataclass
-class DiscriminativeNMTRerankerConfig(FairseqDataclass):
- pretrained_model: str = field(
- default="", metadata={"help": "pretrained model to load"}
- )
- sentence_rep: SENTENCE_REP_CHOICES = field(
- default="head",
- metadata={
- "help": "method to transform the output of the transformer stack to a sentence-level representation"
- },
- )
-
- dropout: float = field(default=0.1, metadata={"help": "dropout probability"})
- attention_dropout: float = field(
- default=0.0, metadata={"help": "dropout probability for attention weights"}
- )
- activation_dropout: float = field(
- default=0.0, metadata={"help": "dropout probability after activation in FFN"}
- )
- classifier_dropout: float = field(
- default=0.0, metadata={"help": "classifier dropout probability"}
- )
- embed_dim: int = field(default=768, metadata={"help": "embedding dimension"})
- ffn_embed_dim: int = field(
- default=2048, metadata={"help": "embedding dimension for FFN"}
- )
- encoder_layers: int = field(default=12, metadata={"help": "num encoder layers"})
- attention_heads: int = field(default=8, metadata={"help": "num attention heads"})
- encoder_normalize_before: bool = field(
- default=False, metadata={"help": "apply layernorm before each encoder block"}
- )
- apply_bert_init: bool = field(
- default=False, metadata={"help": "use custom param initialization for BERT"}
- )
- activation_fn: ACTIVATION_FN_CHOICES = field(
- default="relu", metadata={"help": "activation function to use"}
- )
- freeze_embeddings: bool = field(
- default=False, metadata={"help": "freeze embeddings in the pretrained model"}
- )
- n_trans_layers_to_freeze: int = field(
- default=0,
- metadata={
- "help": "number of layers to freeze in the pretrained transformer model"
- },
- )
-
- # joint classfication
- joint_classification: JOINT_CLASSIFICATION_CHOICES = field(
- default="none",
- metadata={"help": "method to compute joint features for classification"},
- )
- num_joint_layers: int = field(
- default=1, metadata={"help": "number of joint layers"}
- )
- joint_normalize_before: bool = field(
- default=False,
- metadata={"help": "apply layer norm on the input to the joint layer"},
- )
-
-
-@register_model(
- "discriminative_nmt_reranker", dataclass=DiscriminativeNMTRerankerConfig
-)
-class DiscriminativeNMTReranker(BaseFairseqModel):
- @classmethod
- def build_model(cls, args, task):
- model = BertRanker(args, task)
- return DiscriminativeNMTReranker(args, model)
-
- def __init__(self, args, model):
- super().__init__()
-
- self.model = model
- self.sentence_rep = args.sentence_rep
- self.joint_classification = args.joint_classification
-
- def forward(self, src_tokens, src_lengths, **kwargs):
- return self.model(src_tokens, src_lengths)
-
- def sentence_forward(self, encoder_out, src_tokens):
- return self.model.sentence_forward(encoder_out, src_tokens, self.sentence_rep)
-
- def joint_forward(self, x):
- return self.model.joint_forward(x)
-
- def classification_forward(self, x):
- return self.model.classification_forward(x)
diff --git a/spaces/OFA-Sys/OFA-Generic_Interface/fairseq/fairseq/criterions/label_smoothed_cross_entropy_latency_augmented.py b/spaces/OFA-Sys/OFA-Generic_Interface/fairseq/fairseq/criterions/label_smoothed_cross_entropy_latency_augmented.py
deleted file mode 100644
index 223a16f740c10b58ea45a0390814363e7b5f68b8..0000000000000000000000000000000000000000
--- a/spaces/OFA-Sys/OFA-Generic_Interface/fairseq/fairseq/criterions/label_smoothed_cross_entropy_latency_augmented.py
+++ /dev/null
@@ -1,233 +0,0 @@
-# Copyright (c) Facebook, Inc. and its affiliates.
-#
-# This source code is licensed under the MIT license found in the
-# LICENSE file in the root directory of this source tree.
-
-from dataclasses import dataclass, field
-import torch
-from fairseq import metrics, utils
-from fairseq.criterions import register_criterion
-from fairseq.criterions.label_smoothed_cross_entropy import (
- LabelSmoothedCrossEntropyCriterion,
- LabelSmoothedCrossEntropyCriterionConfig
-)
-
-try:
- from simuleval.metrics.latency import (
- AverageLagging,
- AverageProportion,
- DifferentiableAverageLagging
- )
- LATENCY_METRICS = {
- "average_lagging": AverageLagging,
- "average_proportion": AverageProportion,
- "differentiable_average_lagging": DifferentiableAverageLagging,
- }
-except ImportError:
- LATENCY_METRICS = None
-
-
-@dataclass
-class LabelSmoothedCrossEntropyCriterionLatencyAugmentConfig(
- LabelSmoothedCrossEntropyCriterionConfig
-):
- latency_avg_weight: float = field(
- default=0.0,
- metadata={"help": "weight fot average latency loss."},
- )
- latency_var_weight: float = field(
- default=0.0,
- metadata={"help": "weight fot variance latency loss."},
- )
- latency_avg_type: str = field(
- default="differentiable_average_lagging",
- metadata={"help": "latency type for average loss"},
- )
- latency_var_type: str = field(
- default="variance_delay",
- metadata={"help": "latency typ for variance loss"},
- )
- latency_gather_method: str = field(
- default="weighted_average",
- metadata={"help": "method to gather latency loss for all heads"},
- )
- latency_update_after: int = field(
- default=0,
- metadata={"help": "Add latency loss after certain steps"},
- )
-
-@register_criterion(
- "latency_augmented_label_smoothed_cross_entropy",
- dataclass=LabelSmoothedCrossEntropyCriterionLatencyAugmentConfig
-)
-class LatencyAugmentedLabelSmoothedCrossEntropyCriterion(
- LabelSmoothedCrossEntropyCriterion
-):
- def __init__(
- self,
- task,
- sentence_avg,
- label_smoothing,
- ignore_prefix_size,
- report_accuracy,
- latency_avg_weight,
- latency_var_weight,
- latency_avg_type,
- latency_var_type,
- latency_gather_method,
- latency_update_after,
- ):
- super().__init__(
- task, sentence_avg, label_smoothing, ignore_prefix_size, report_accuracy
- )
- assert LATENCY_METRICS is not None, "Please make sure SimulEval is installed."
-
- self.latency_avg_weight = latency_avg_weight
- self.latency_var_weight = latency_var_weight
- self.latency_avg_type = latency_avg_type
- self.latency_var_type = latency_var_type
- self.latency_gather_method = latency_gather_method
- self.latency_update_after = latency_update_after
-
- def forward(self, model, sample, reduce=True):
- net_output = model(**sample["net_input"])
- # 1. Compute cross entropy loss
- loss, nll_loss = self.compute_loss(model, net_output, sample, reduce=reduce)
-
- # 2. Compute cross latency loss
- latency_loss, expected_latency, expected_delays_var = self.compute_latency_loss(
- model, sample, net_output
- )
-
- if self.latency_update_after > 0:
- num_updates = getattr(model.decoder, "num_updates", None)
- assert num_updates is not None, (
- "model.decoder doesn't have attribute 'num_updates'"
- )
- if num_updates <= self.latency_update_after:
- latency_loss = 0
-
- loss += latency_loss
-
- sample_size = (
- sample["target"].size(0) if self.sentence_avg else sample["ntokens"]
- )
-
- logging_output = {
- "loss": loss.data,
- "nll_loss": nll_loss.data,
- "ntokens": sample["ntokens"],
- "nsentences": sample["target"].size(0),
- "sample_size": sample_size,
- "latency": expected_latency,
- "delays_var": expected_delays_var,
- "latency_loss": latency_loss,
- }
-
- if self.report_accuracy:
- n_correct, total = self.compute_accuracy(model, net_output, sample)
- logging_output["n_correct"] = utils.item(n_correct.data)
- logging_output["total"] = utils.item(total.data)
- return loss, sample_size, logging_output
-
- def compute_latency_loss(self, model, sample, net_output):
- assert (
- net_output[-1].encoder_padding_mask is None
- or not net_output[-1].encoder_padding_mask[:, 0].any()
- ), (
- "Only right padding on source is supported."
- )
- # 1. Obtain the expected alignment
- alpha_list = [item["alpha"] for item in net_output[1].attn_list]
- num_layers = len(alpha_list)
- bsz, num_heads, tgt_len, src_len = alpha_list[0].size()
-
- # bsz * num_layers * num_heads, tgt_len, src_len
- alpha_all = torch.cat(alpha_list, dim=1).view(-1, tgt_len, src_len)
-
- # 2 compute expected delays
- # bsz * num_heads * num_layers, tgt_len, src_len for MMA
- steps = (
- torch.arange(1, 1 + src_len)
- .unsqueeze(0)
- .unsqueeze(1)
- .expand_as(alpha_all)
- .type_as(alpha_all)
- )
-
- expected_delays = torch.sum(steps * alpha_all, dim=-1)
-
- target_padding_mask = (
- model.get_targets(sample, net_output)
- .eq(self.padding_idx)
- .unsqueeze(1)
- .expand(bsz, num_layers * num_heads, tgt_len)
- .contiguous()
- .view(-1, tgt_len)
- )
-
- src_lengths = (
- sample["net_input"]["src_lengths"]
- .unsqueeze(1)
- .expand(bsz, num_layers * num_heads)
- .contiguous()
- .view(-1)
- )
- expected_latency = LATENCY_METRICS[self.latency_avg_type](
- expected_delays, src_lengths, None,
- target_padding_mask=target_padding_mask
- )
-
- # 2.1 average expected latency of heads
- # bsz, num_layers * num_heads
- expected_latency = expected_latency.view(bsz, -1)
- if self.latency_gather_method == "average":
- # bsz * tgt_len
- expected_latency = expected_delays.mean(dim=1)
- elif self.latency_gather_method == "weighted_average":
- weights = torch.nn.functional.softmax(expected_latency, dim=1)
- expected_latency = torch.sum(expected_latency * weights, dim=1)
- elif self.latency_gather_method == "max":
- expected_latency = expected_latency.max(dim=1)[0]
- else:
- raise NotImplementedError
-
- expected_latency = expected_latency.sum()
- avg_loss = self.latency_avg_weight * expected_latency
-
- # 2.2 variance of expected delays
- expected_delays_var = (
- expected_delays.view(bsz, -1, tgt_len).var(dim=1).mean(dim=1)
- )
- expected_delays_var = expected_delays_var.sum()
- var_loss = self.latency_avg_weight * expected_delays_var
-
- # 3. Final loss
- latency_loss = avg_loss + var_loss
-
- return latency_loss, expected_latency, expected_delays_var
-
- @classmethod
- def reduce_metrics(cls, logging_outputs) -> None:
- super().reduce_metrics(logging_outputs)
- latency = sum(
- log.get("latency", 0) for log in logging_outputs
- )
- delays_var = sum(
- log.get("delays_var", 0) for log in logging_outputs
- )
- latency_loss = sum(
- log.get("latency_loss", 0) for log in logging_outputs
- )
- nsentences = sum(log.get("nsentences", 0) for log in logging_outputs)
- metrics.log_scalar(
- "latency", latency.float() / nsentences, nsentences, round=3
- )
- metrics.log_scalar(
- "delays_var", delays_var / nsentences,
- nsentences, round=3
- )
- metrics.log_scalar(
- "latency_loss", latency_loss / nsentences,
- nsentences, round=3
- )
diff --git a/spaces/OFA-Sys/OFA-Generic_Interface/tasks/__init__.py b/spaces/OFA-Sys/OFA-Generic_Interface/tasks/__init__.py
deleted file mode 100644
index 6a7fcab34c0736c74aae787a4082ddaa9cafa591..0000000000000000000000000000000000000000
--- a/spaces/OFA-Sys/OFA-Generic_Interface/tasks/__init__.py
+++ /dev/null
@@ -1,2 +0,0 @@
-from .mm_tasks import *
-from .ofa_task import OFATask
\ No newline at end of file
diff --git a/spaces/OFA-Sys/OFA-Image_Caption/fairseq/examples/laser/laser_src/laser_task.py b/spaces/OFA-Sys/OFA-Image_Caption/fairseq/examples/laser/laser_src/laser_task.py
deleted file mode 100644
index e4152fde6861488acc3595fa25c456bf60f134b9..0000000000000000000000000000000000000000
--- a/spaces/OFA-Sys/OFA-Image_Caption/fairseq/examples/laser/laser_src/laser_task.py
+++ /dev/null
@@ -1,331 +0,0 @@
-# Copyright (c) Facebook, Inc. and its affiliates.
-#
-# This source code is licensed under the MIT license found in the
-# LICENSE file in the root directory of this source tree.
-
-
-from collections import OrderedDict, defaultdict
-import json
-import os
-import logging
-from argparse import ArgumentError
-
-from fairseq import options, models
-from fairseq.data import (
- data_utils,
- Dictionary,
- LanguagePairDataset,
- IndexedDataset,
- FairseqDataset,
-)
-from .multitask_data_utils import (
- MultitaskDatasetWrapper,
- MultidatasetEpochBatchIterator,
-)
-
-
-from fairseq.tasks import LegacyFairseqTask, register_task
-
-logger = logging.getLogger(__name__)
-
-
-@register_task("laser")
-class LaserTask(LegacyFairseqTask):
- @staticmethod
- def add_args(parser):
- """Add task-specific arguments to the parser."""
- parser.add_argument(
- "configfile", metavar="PATH", help="dataset configuration file in json"
- )
- parser.add_argument(
- "--weighting-alpha",
- type=float,
- default=None,
- help="alpha for automatic weighting",
- )
- parser.add_argument(
- "--raw-text", action="store_true", help="load raw text dataset"
- )
- parser.add_argument(
- "--left-pad-source",
- default="True",
- type=str,
- metavar="BOOL",
- help="pad the source on the left (default: True)",
- )
- parser.add_argument(
- "--left-pad-target",
- default="False",
- type=str,
- metavar="BOOL",
- help="pad the target on the left (default: False)",
- )
- try:
- parser.add_argument(
- "--max-source-positions",
- default=1024,
- type=int,
- metavar="N",
- help="max number of tokens in the source sequence",
- )
- parser.add_argument(
- "--max-target-positions",
- default=1024,
- type=int,
- metavar="N",
- help="max number of tokens in the target sequence",
- )
- except ArgumentError:
- # this might have already been defined. Once we transition this to hydra it should be fine to add it here.
- pass
-
- def __init__(self, args, config, src_dictionary, tgt_dictionary, num_tasks):
- super().__init__(args)
- self.config = config
- self.src_dictionary = src_dictionary
- self.tgt_dictionary = tgt_dictionary
- self.num_tasks = num_tasks
-
- @classmethod
- def setup_task(cls, args, **kwargs):
- with open(args.configfile, "r") as f:
- config = json.load(f)
- num_tasks = max(dataset["id"] for dataset in config["train"]) + 1
-
- args.left_pad_source = options.eval_bool(args.left_pad_source)
- args.left_pad_target = options.eval_bool(args.left_pad_target)
-
- src_dictionary = Dictionary.load(config["src_vocab"])
- tgt_dictionary = Dictionary.load(config["tgt_vocab"])
-
- logger.info(
- "| src Dictionary {} : {} types".format(
- config["src_vocab"], len(src_dictionary)
- )
- )
- logger.info(
- "| tgt Dictionary {} : {} types".format(
- config["tgt_vocab"], len(tgt_dictionary)
- )
- )
-
- return cls(args, config, src_dictionary, tgt_dictionary, num_tasks)
-
- # Experimental overriding for backtranslation
- def build_model(self, args):
- model = models.build_model(args, self)
- return model
-
- def dataset(self, split):
- if split not in self.datasets:
- raise KeyError("Dataset not loaded: " + split)
- return self.datasets[split]
-
- def load_dataset(self, split, epoch=1, **kwargs):
- """Load a dataset split."""
-
- def indexed_dataset(path, dictionary):
- if self.args.raw_text:
- raise Exception("Unable to handle raw text.")
- dataset = IndexedDataset(path, fix_lua_indexing=True)
-
- return dataset
-
- pair_datasets = OrderedDict()
-
- if split == "valid":
- self.datasets[split] = pair_datasets
- return
-
- if split not in self.config:
- raise FileNotFoundError(
- "Dataset not found in config file: {}".format(split)
- )
-
- size_by_corpus = defaultdict(int)
- size_sum = 0
- size_sum_with_subsampling = 0
- init_pair_datasets = {}
-
- for dataset_config in self.config[split]:
- src_path = os.path.dirname(dataset_config["src"])
- corpus_name = src_path.split("/")[-2]
- language_pair_name = src_path.split("/")[-1]
- pair_datasets_key = corpus_name + "-" + language_pair_name
-
- logger.info(f"loading... {pair_datasets_key}")
- if "src" in dataset_config:
- src_dataset = indexed_dataset(
- dataset_config["src"], self.src_dictionary
- )
- else:
- src_dataset = None
-
- if "tgt" in dataset_config:
- tgt_dataset = indexed_dataset(
- dataset_config["tgt"], self.tgt_dictionary
- )
- else:
- tgt_dataset = None
-
- dataset = LanguagePairDataset(
- src_dataset,
- src_dataset.sizes,
- self.src_dictionary,
- tgt_dataset,
- tgt_dataset.sizes,
- self.tgt_dictionary,
- left_pad_source=self.args.left_pad_source,
- left_pad_target=self.args.left_pad_target,
- )
-
- if pair_datasets_key in init_pair_datasets:
- logger.warning(
- f"Ignoring already added {pair_datasets_key}. "
- f"Consider using `sample` key in order to upsample."
- )
- else:
- init_pair_datasets[pair_datasets_key] = {
- "dataset": dataset,
- "sample": dataset_config.get("sample", None),
- "id": dataset_config.get("id", None),
- "len": len(dataset),
- }
-
- length_sum = 0
- weighted_freqs_sum = 0
- freq_per_dataset = {}
- vmax = 0
- vmin = 1
- weighted_freq_per_dataset = {}
-
- if self.args.weighting_alpha:
- for key in init_pair_datasets:
- if init_pair_datasets[key]["sample"] is None:
- length_sum += len(init_pair_datasets[key]["dataset"])
-
- for key in init_pair_datasets:
- if init_pair_datasets[key]["sample"] is None:
- val = float(init_pair_datasets[key]["len"]) / length_sum
- freq_per_dataset[key] = val
- weighted_freqs_sum += val ** self.args.weighting_alpha
-
- for key in freq_per_dataset:
- val = (
- freq_per_dataset[key] ** self.args.weighting_alpha
- / weighted_freqs_sum
- )
- vmin = min(vmin, val)
- vmax = max(vmax, val)
- weighted_freq_per_dataset[key] = val
-
- for pair_datasets_key in init_pair_datasets:
- dataset_config = init_pair_datasets[pair_datasets_key]
- dataset = dataset_config["dataset"]
- sample = dataset_config["sample"]
- if sample is None:
- sample = 1.0
-
- if pair_datasets_key in weighted_freq_per_dataset:
- w = vmax / weighted_freq_per_dataset[pair_datasets_key]
- sample = w
-
- sample = round(sample)
-
- initial_sample = sample
- initial_pair_datasets_key = pair_datasets_key
-
- while sample >= 1.0:
- assert (
- pair_datasets_key not in pair_datasets
- ), f"{pair_datasets_key} already in"
- size_sum_with_subsampling += len(dataset)
- pair_datasets[pair_datasets_key] = MultitaskDatasetWrapper(
- dataset, dataset_config.get("id", 0), 1.0, name=pair_datasets_key
- )
- size_sum += len(dataset)
- sample -= 1.0
- pair_datasets_key += "-up"
-
- assert sample < 1e-6, f"sample remains > 0 {pair_datasets_key}"
-
- logger.info(
- f"added pair {initial_pair_datasets_key} length {len(dataset)} new_length = {len(dataset)*initial_sample}"
- )
- size_by_corpus[corpus_name] += len(dataset)
-
- self.datasets[split] = pair_datasets
- logger.info(
- f"Datasets number = {len(self.datasets[split])} size = {size_sum} size_sum_with_subsampling = {size_sum_with_subsampling}"
- )
-
- @property
- def source_dictionary(self):
- return self.src_dictionary
-
- @property
- def target_dictionary(self):
- return self.tgt_dictionary
-
- def get_batch_iterator(
- self,
- dataset,
- max_tokens=None,
- max_sentences=None,
- max_positions=None,
- ignore_invalid_inputs=False,
- required_batch_size_multiple=1,
- seed=1,
- num_shards=1,
- shard_id=0,
- num_workers=0,
- epoch=1,
- data_buffer_size=0,
- disable_iterator_cache=False,
- ):
-
- assert isinstance(dataset, OrderedDict)
- assert len(dataset)
- assert isinstance(dataset[next(iter(dataset))], FairseqDataset)
-
- # initialize the dataset with the correct starting epoch
- for _, dt in dataset.items():
- dt.set_epoch(epoch)
-
- indices = OrderedDict()
- batch_sampler = OrderedDict()
-
- with data_utils.numpy_seed(seed + epoch):
- for key, dt in dataset.items():
- logger.info(f"\t ordered_indices {key}")
- indices[key] = dt.ordered_indices()
-
- # filter examples that are too large
- if max_positions is not None:
- for key, dt in dataset.items():
- logger.info(f"\t filter_by_size {key}")
- indices[key], ignored = dt.filter_indices_by_size(
- indices[key], max_positions
- )
-
- for key, dt in dataset.items():
- logger.info(f"\t batch_by_size {key}")
- batch_sampler[key] = data_utils.batch_by_size(
- indices[key],
- dt.num_tokens,
- max_tokens=max_tokens,
- max_sentences=max_sentences,
- required_batch_size_multiple=required_batch_size_multiple,
- )
-
- epoch_iter = MultidatasetEpochBatchIterator(
- dataset=dataset,
- batch_sampler=batch_sampler,
- seed=seed,
- num_shards=num_shards,
- shard_id=shard_id,
- num_workers=num_workers,
- epoch=epoch,
- )
-
- return epoch_iter
diff --git a/spaces/OFA-Sys/OFA-Image_Caption/fairseq/examples/wav2vec/wav2vec_featurize.py b/spaces/OFA-Sys/OFA-Image_Caption/fairseq/examples/wav2vec/wav2vec_featurize.py
deleted file mode 100644
index 588268b7080cbd3400ac144604b2d75cef2876dd..0000000000000000000000000000000000000000
--- a/spaces/OFA-Sys/OFA-Image_Caption/fairseq/examples/wav2vec/wav2vec_featurize.py
+++ /dev/null
@@ -1,249 +0,0 @@
-#!/usr/bin/env python3
-# Copyright (c) Facebook, Inc. and its affiliates.
-#
-# This source code is licensed under the MIT license found in the
-# LICENSE file in the root directory of this source tree.
-
-"""
-Helper script to pre-compute embeddings for a flashlight (previously called wav2letter++) dataset
-"""
-
-import argparse
-import glob
-import os
-from shutil import copy
-
-import h5py
-import numpy as np
-import soundfile as sf
-import torch
-import tqdm
-import fairseq
-from torch import nn
-
-
-def read_audio(fname):
- """ Load an audio file and return PCM along with the sample rate """
-
- wav, sr = sf.read(fname)
- assert sr == 16e3
-
- return wav, 16e3
-
-
-class PretrainedWav2VecModel(nn.Module):
- def __init__(self, fname):
- super().__init__()
-
- model, cfg, task = fairseq.checkpoint_utils.load_model_ensemble_and_task([fname])
- model = model[0]
- model.eval()
-
- self.model = model
-
- def forward(self, x):
- with torch.no_grad():
- z = self.model.feature_extractor(x)
- if isinstance(z, tuple):
- z = z[0]
- c = self.model.feature_aggregator(z)
- return z, c
-
-
-class EmbeddingWriterConfig(argparse.ArgumentParser):
- def __init__(self):
- super().__init__("Pre-compute embeddings for flashlight datasets")
-
- kwargs = {"action": "store", "type": str, "required": True}
-
- self.add_argument("--input", "-i", help="Input Directory", **kwargs)
- self.add_argument("--output", "-o", help="Output Directory", **kwargs)
- self.add_argument("--model", help="Path to model checkpoint", **kwargs)
- self.add_argument("--split", help="Dataset Splits", nargs="+", **kwargs)
- self.add_argument(
- "--ext", default="wav", required=False, help="Audio file extension"
- )
-
- self.add_argument(
- "--no-copy-labels",
- action="store_true",
- help="Do not copy label files. Useful for large datasets, use --targetdir in flashlight then.",
- )
- self.add_argument(
- "--use-feat",
- action="store_true",
- help="Use the feature vector ('z') instead of context vector ('c') for features",
- )
- self.add_argument("--gpu", help="GPU to use", default=0, type=int)
-
-
-class Prediction:
- """ Lightweight wrapper around a fairspeech embedding model """
-
- def __init__(self, fname, gpu=0):
- self.gpu = gpu
- self.model = PretrainedWav2VecModel(fname).cuda(gpu)
-
- def __call__(self, x):
- x = torch.from_numpy(x).float().cuda(self.gpu)
- with torch.no_grad():
- z, c = self.model(x.unsqueeze(0))
-
- return z.squeeze(0).cpu().numpy(), c.squeeze(0).cpu().numpy()
-
-
-class H5Writer:
- """ Write features as hdf5 file in flashlight compatible format """
-
- def __init__(self, fname):
- self.fname = fname
- os.makedirs(os.path.dirname(self.fname), exist_ok=True)
-
- def write(self, data):
- channel, T = data.shape
-
- with h5py.File(self.fname, "w") as out_ds:
- data = data.T.flatten()
- out_ds["features"] = data
- out_ds["info"] = np.array([16e3 // 160, T, channel])
-
-
-class EmbeddingDatasetWriter(object):
- """Given a model and a flashlight dataset, pre-compute and store embeddings
-
- Args:
- input_root, str :
- Path to the flashlight dataset
- output_root, str :
- Desired output directory. Will be created if non-existent
- split, str :
- Dataset split
- """
-
- def __init__(
- self,
- input_root,
- output_root,
- split,
- model_fname,
- extension="wav",
- gpu=0,
- verbose=False,
- use_feat=False,
- ):
-
- assert os.path.exists(model_fname)
-
- self.model_fname = model_fname
- self.model = Prediction(self.model_fname, gpu)
-
- self.input_root = input_root
- self.output_root = output_root
- self.split = split
- self.verbose = verbose
- self.extension = extension
- self.use_feat = use_feat
-
- assert os.path.exists(self.input_path), "Input path '{}' does not exist".format(
- self.input_path
- )
-
- def _progress(self, iterable, **kwargs):
- if self.verbose:
- return tqdm.tqdm(iterable, **kwargs)
- return iterable
-
- def require_output_path(self, fname=None):
- path = self.get_output_path(fname)
- os.makedirs(path, exist_ok=True)
-
- @property
- def input_path(self):
- return self.get_input_path()
-
- @property
- def output_path(self):
- return self.get_output_path()
-
- def get_input_path(self, fname=None):
- if fname is None:
- return os.path.join(self.input_root, self.split)
- return os.path.join(self.get_input_path(), fname)
-
- def get_output_path(self, fname=None):
- if fname is None:
- return os.path.join(self.output_root, self.split)
- return os.path.join(self.get_output_path(), fname)
-
- def copy_labels(self):
- self.require_output_path()
-
- labels = list(
- filter(
- lambda x: self.extension not in x, glob.glob(self.get_input_path("*"))
- )
- )
- for fname in tqdm.tqdm(labels):
- copy(fname, self.output_path)
-
- @property
- def input_fnames(self):
- return sorted(glob.glob(self.get_input_path("*.{}".format(self.extension))))
-
- def __len__(self):
- return len(self.input_fnames)
-
- def write_features(self):
-
- paths = self.input_fnames
-
- fnames_context = map(
- lambda x: os.path.join(
- self.output_path, x.replace("." + self.extension, ".h5context")
- ),
- map(os.path.basename, paths),
- )
-
- for name, target_fname in self._progress(
- zip(paths, fnames_context), total=len(self)
- ):
- wav, sr = read_audio(name)
- z, c = self.model(wav)
- feat = z if self.use_feat else c
- writer = H5Writer(target_fname)
- writer.write(feat)
-
- def __repr__(self):
-
- return "EmbeddingDatasetWriter ({n_files} files)\n\tinput:\t{input_root}\n\toutput:\t{output_root}\n\tsplit:\t{split})".format(
- n_files=len(self), **self.__dict__
- )
-
-
-if __name__ == "__main__":
-
- args = EmbeddingWriterConfig().parse_args()
-
- for split in args.split:
-
- writer = EmbeddingDatasetWriter(
- input_root=args.input,
- output_root=args.output,
- split=split,
- model_fname=args.model,
- gpu=args.gpu,
- extension=args.ext,
- use_feat=args.use_feat,
- )
-
- print(writer)
- writer.require_output_path()
-
- print("Writing Features...")
- writer.write_features()
- print("Done.")
-
- if not args.no_copy_labels:
- print("Copying label data...")
- writer.copy_labels()
- print("Done.")
diff --git a/spaces/OFA-Sys/OFA-Visual_Grounding/fairseq/examples/language_model/README.md b/spaces/OFA-Sys/OFA-Visual_Grounding/fairseq/examples/language_model/README.md
deleted file mode 100644
index e78ea48e08dc99b69751923762107a8f8a9a5e3e..0000000000000000000000000000000000000000
--- a/spaces/OFA-Sys/OFA-Visual_Grounding/fairseq/examples/language_model/README.md
+++ /dev/null
@@ -1,123 +0,0 @@
-# Neural Language Modeling
-
-## Pre-trained models
-
-Model | Description | Dataset | Download
----|---|---|---
-`transformer_lm.gbw.adaptive_huge` | Adaptive Inputs ([Baevski and Auli, 2018](https://arxiv.org/abs/1809.10853)) 1026M params | [Google Billion Words](https://github.com/ciprian-chelba/1-billion-word-language-modeling-benchmark) | [download (.tar.bz2)](https://dl.fbaipublicfiles.com/fairseq/models/lm/adaptive_lm_gbw_huge.tar.bz2)
-`transformer_lm.wiki103.adaptive` | Adaptive Inputs ([Baevski and Auli, 2018](https://arxiv.org/abs/1809.10853)) 247M params | [WikiText-103](https://blog.einstein.ai/the-wikitext-long-term-dependency-language-modeling-dataset) | [download (.tar.bz2)](https://dl.fbaipublicfiles.com/fairseq/models/lm/adaptive_lm_wiki103.v2.tar.bz2)
-`transformer_lm.wmt19.en` | English LM ([Ng et al., 2019](https://arxiv.org/abs/1907.06616)) | [WMT News Crawl](http://data.statmt.org/news-crawl/) | [download (.tar.gz)](https://dl.fbaipublicfiles.com/fairseq/models/lm/wmt19.en.tar.gz)
-`transformer_lm.wmt19.de` | German LM ([Ng et al., 2019](https://arxiv.org/abs/1907.06616)) | [WMT News Crawl](http://data.statmt.org/news-crawl/) | [download (.tar.gz)](https://dl.fbaipublicfiles.com/fairseq/models/lm/wmt19.de.tar.gz)
-`transformer_lm.wmt19.ru` | Russian LM ([Ng et al., 2019](https://arxiv.org/abs/1907.06616)) | [WMT News Crawl](http://data.statmt.org/news-crawl/) | [download (.tar.gz)](https://dl.fbaipublicfiles.com/fairseq/models/lm/wmt19.ru.tar.gz)
-
-## Example usage
-
-We require a few additional Python dependencies for preprocessing:
-```bash
-pip install fastBPE sacremoses
-```
-
-To sample from a language model using PyTorch Hub:
-```python
-import torch
-
-# List available models
-torch.hub.list('pytorch/fairseq') # [..., 'transformer_lm.wmt19.en', ...]
-
-# Load an English LM trained on WMT'19 News Crawl data
-en_lm = torch.hub.load('pytorch/fairseq', 'transformer_lm.wmt19.en', tokenizer='moses', bpe='fastbpe')
-en_lm.eval() # disable dropout
-
-# Move model to GPU
-en_lm.cuda()
-
-# Sample from the language model
-en_lm.sample('Barack Obama', beam=1, sampling=True, sampling_topk=10, temperature=0.8)
-# "Barack Obama is coming to Sydney and New Zealand (...)"
-
-# Compute perplexity for a sequence
-en_lm.score('Barack Obama is coming to Sydney and New Zealand')['positional_scores'].mean().neg().exp()
-# tensor(15.1474)
-
-# The same interface can be used with custom models as well
-from fairseq.models.transformer_lm import TransformerLanguageModel
-custom_lm = TransformerLanguageModel.from_pretrained('/path/to/model/dir', 'checkpoint100.pt', tokenizer='moses', bpe='fastbpe')
-custom_lm.sample('Barack Obama', beam=5)
-# "Barack Obama (...)"
-```
-
-## Training a transformer language model with the CLI tools
-
-### 1) Preprocess the data
-
-First download and prepare the [WikiText-103 dataset](https://www.salesforce.com/products/einstein/ai-research/the-wikitext-dependency-language-modeling-dataset/):
-```bash
-cd examples/language_model/
-bash prepare-wikitext-103.sh
-cd ../..
-```
-
-Next preprocess/binarize the data:
-```bash
-TEXT=examples/language_model/wikitext-103
-fairseq-preprocess \
- --only-source \
- --trainpref $TEXT/wiki.train.tokens \
- --validpref $TEXT/wiki.valid.tokens \
- --testpref $TEXT/wiki.test.tokens \
- --destdir data-bin/wikitext-103 \
- --workers 20
-```
-
-### 2) Train a language model
-
-Next we'll train a basic transformer language model on wikitext-103. For more
-advanced usage, see the [adaptive inputs README](README.adaptive_inputs.md).
-
-To train a basic LM (assumes 2 GPUs):
-```
-$ fairseq-train --task language_modeling \
- data-bin/wikitext-103 \
- --save-dir checkpoints/transformer_wikitext-103 \
- --arch transformer_lm --share-decoder-input-output-embed \
- --dropout 0.1 \
- --optimizer adam --adam-betas '(0.9, 0.98)' --weight-decay 0.01 --clip-norm 0.0 \
- --lr 0.0005 --lr-scheduler inverse_sqrt --warmup-updates 4000 --warmup-init-lr 1e-07 \
- --tokens-per-sample 512 --sample-break-mode none \
- --max-tokens 2048 --update-freq 16 \
- --fp16 \
- --max-update 50000
-```
-
-If you run out of memory, try reducing `--max-tokens` (max number of tokens per
-batch) or `--tokens-per-sample` (max sequence length). You can also adjust
-`--update-freq` to accumulate gradients and simulate training on a different
-number of GPUs.
-
-### 3) Evaluate
-
-```bash
-fairseq-eval-lm data-bin/wikitext-103 \
- --path checkpoints/transformer_wiki103/checkpoint_best.pt \
- --batch-size 2 \
- --tokens-per-sample 512 \
- --context-window 400
-# | Evaluated 245569 tokens in 56.1s (4379.02 tokens/s)
-# | Loss: 3.4164, Perplexity: 30.46
-```
-
-*Note:* The `--context-window` option controls how much context is provided to
-each token when computing perplexity. When the window size is 0, the dataset is
-chunked into segments of length 512 and perplexity is computed over each segment
-normally. However, this results in worse (higher) perplexity since tokens that
-appear earlier in each segment have less conditioning. When the maximum window
-size is used (511 in this case), then we compute perplexity for each token
-fully conditioned on 511 tokens of context. This slows down evaluation
-significantly, since we must run a separate forward pass for every token in the
-dataset, but results in better (lower) perplexity.
-
-
-## Convolutional language models
-
-Please see the [convolutional LM README](README.conv.md) for instructions on
-training convolutional language models.
diff --git a/spaces/OFA-Sys/OFA-vqa/fairseq/examples/wav2vec/unsupervised/kaldi_self_train/st/local/prepare_data_from_w2v.py b/spaces/OFA-Sys/OFA-vqa/fairseq/examples/wav2vec/unsupervised/kaldi_self_train/st/local/prepare_data_from_w2v.py
deleted file mode 100644
index 66954ea5c9f3f3330e3230860229c7c4046a5d6a..0000000000000000000000000000000000000000
--- a/spaces/OFA-Sys/OFA-vqa/fairseq/examples/wav2vec/unsupervised/kaldi_self_train/st/local/prepare_data_from_w2v.py
+++ /dev/null
@@ -1,56 +0,0 @@
-import kaldi_io
-import numpy as np
-import os
-
-
-def get_parser():
- import argparse
- parser = argparse.ArgumentParser()
- parser.add_argument("w2v_dir", help="wav2vec feature and text directory")
- parser.add_argument("tar_root", help="output data directory in kaldi's format")
- parser.add_argument("split", help="name of the subset")
- parser.add_argument("--label", default="", help="if specified, copy labels too")
- return parser
-
-def main():
- parser = get_parser()
- args = parser.parse_args()
-
- tar_dir = os.path.join(args.tar_root, args.split)
- os.makedirs(tar_dir, exist_ok=True)
-
- lengths_path = os.path.join(args.w2v_dir, f"{args.split}.lengths")
- with open(lengths_path) as f:
- lengths = [int(line.rstrip()) for line in f]
- offsets = [0] + np.cumsum(lengths[:-1]).tolist()
- feats = np.load(
- os.path.join(args.w2v_dir, f"{args.split}.npy"),
- mmap_mode="r"
- )
- assert feats.shape[0] == sum(lengths), \
- f"lengths mismatch {feats.shape[0]} != {sum(lengths)}"
-
- ark_path = os.path.join(tar_dir, "feats.ark")
- scp_path = os.path.join(tar_dir, "feats.scp")
- wspec = f"ark:| copy-feats --compress=true ark:- ark,scp:{ark_path},{scp_path}"
- with kaldi_io.open_or_fd(wspec, "wb") as f:
- for idx, (offset, length) in enumerate(zip(offsets, lengths)):
- feat = feats[offset:offset+length]
- kaldi_io.write_mat(f, feat, key=f"utt{idx:010d}")
-
- u2s_path = os.path.join(tar_dir, "utt2spk")
- s2u_path = os.path.join(tar_dir, "spk2utt")
- with open(u2s_path, "w") as f_u2s, open(s2u_path, "w") as f_s2u:
- for idx in range(len(lengths)):
- f_u2s.write(f"utt{idx:010d} utt{idx:010d}\n")
- f_s2u.write(f"utt{idx:010d} utt{idx:010d}\n")
-
- if bool(args.label):
- lab_path = os.path.join(args.w2v_dir, f"{args.split}.{args.label}")
- txt_path = os.path.join(tar_dir, "text")
- with open(lab_path) as f_lab, open(txt_path, "w") as f_txt:
- for idx, line in enumerate(f_lab):
- f_txt.write(f"utt{idx:010d} {line}")
-
-if __name__ == "__main__":
- main()
diff --git a/spaces/OdiaGenAI/Olive_Farm/custom_prompt_template.py b/spaces/OdiaGenAI/Olive_Farm/custom_prompt_template.py
deleted file mode 100644
index 22841a36789103095a838201409d952bc1288acc..0000000000000000000000000000000000000000
--- a/spaces/OdiaGenAI/Olive_Farm/custom_prompt_template.py
+++ /dev/null
@@ -1,44 +0,0 @@
-from typing import List
-import langchain
-from langchain.prompts import PromptTemplate
-class InstructionGenerationTemplate(PromptTemplate):
- """A custom prompt template for generating instructions."""
-
- input_variables: List[str] = ["num_questions", "context", "instruction_format", "lang", "additional_rules"]
-
- template = """
- You are a highly intelligent language model trained to assist with a variety of language tasks. Your task here is to generate {num_questions} diverse questions or instructions based on the context provided below:
-
- Context:
- {context}
-
- Please follow these rules:
- {additional_rules}
-
- Please generate the instructions in the {instruction_format} format and in {lang} language. Remember to adhere to the rules mentioned above.
- """
-
- template_format = "f-string"
- def format(self, **kwargs):
- """Format the prompt."""
- return self.template.format(**kwargs)
-
-class AnswerGenerationTemplate(langchain.prompts.PromptTemplate):
- """A custom prompt template for generating answers to questions."""
-
- input_variables: List[str] = ["questions", "additional_rules"]
-
- template = """
- You are a highly intelligent language model tasked with providing answers to the following questions :
-
- Questions:
- {questions}
-
- Please follow these rules:
- {additional_rules}
- """
-
- template_format = "f-string"
- def format(self, **kwargs):
- """Format the prompt."""
- return self.template.format(**kwargs)
diff --git a/spaces/Olivier-Truong/faster-whisper-webui-v2/app-local.py b/spaces/Olivier-Truong/faster-whisper-webui-v2/app-local.py
deleted file mode 100644
index c7717d096ca5f95177f0dba03cd62ca729bae9f3..0000000000000000000000000000000000000000
--- a/spaces/Olivier-Truong/faster-whisper-webui-v2/app-local.py
+++ /dev/null
@@ -1,5 +0,0 @@
-# Run the app with no audio file restrictions
-from app import create_ui
-from src.config import ApplicationConfig
-
-create_ui(ApplicationConfig.create_default(input_audio_max_duration=-1))
\ No newline at end of file
diff --git a/spaces/Omnibus/Video-Diffusion-WebUI/video_diffusion/utils/scheduler_list.py b/spaces/Omnibus/Video-Diffusion-WebUI/video_diffusion/utils/scheduler_list.py
deleted file mode 100644
index 1b5399fe7f4cc1a19b6e57a74e468b995d556a18..0000000000000000000000000000000000000000
--- a/spaces/Omnibus/Video-Diffusion-WebUI/video_diffusion/utils/scheduler_list.py
+++ /dev/null
@@ -1,32 +0,0 @@
-from diffusers import (
- DDIMScheduler,
- DPMSolverMultistepScheduler,
- EulerAncestralDiscreteScheduler,
- EulerDiscreteScheduler,
- HeunDiscreteScheduler,
- LMSDiscreteScheduler,
-)
-
-diff_scheduler_list = ["DDIM", "EulerA", "Euler", "LMS", "Heun", "UniPC", "DPMSolver"]
-
-
-def get_scheduler_list(pipe, scheduler):
- if scheduler == diff_scheduler_list[0]:
- pipe.scheduler = DDIMScheduler.from_config(pipe.scheduler.config)
-
- elif scheduler == diff_scheduler_list[1]:
- pipe.scheduler = EulerAncestralDiscreteScheduler.from_config(pipe.scheduler.config)
-
- elif scheduler == diff_scheduler_list[2]:
- pipe.scheduler = EulerDiscreteScheduler.from_config(pipe.scheduler.config)
-
- elif scheduler == diff_scheduler_list[3]:
- pipe.scheduler = LMSDiscreteScheduler.from_config(pipe.scheduler.config)
-
- elif scheduler == diff_scheduler_list[4]:
- pipe.scheduler = HeunDiscreteScheduler.from_config(pipe.scheduler.config)
-
- elif scheduler == diff_scheduler_list[5]:
- pipe.scheduler = DPMSolverMultistepScheduler.from_config(pipe.scheduler.config)
-
- return pipe
diff --git a/spaces/OpenMotionLab/MotionGPT/pyrender/pyrender/primitive.py b/spaces/OpenMotionLab/MotionGPT/pyrender/pyrender/primitive.py
deleted file mode 100644
index 7f83f46f532b126a4573e715dd03d079fef755ca..0000000000000000000000000000000000000000
--- a/spaces/OpenMotionLab/MotionGPT/pyrender/pyrender/primitive.py
+++ /dev/null
@@ -1,489 +0,0 @@
-"""Primitives, conforming to the glTF 2.0 standards as specified in
-https://github.com/KhronosGroup/glTF/tree/master/specification/2.0#reference-primitive
-
-Author: Matthew Matl
-"""
-import numpy as np
-
-from OpenGL.GL import *
-
-from .material import Material, MetallicRoughnessMaterial
-from .constants import FLOAT_SZ, UINT_SZ, BufFlags, GLTF
-from .utils import format_color_array
-
-
-class Primitive(object):
- """A primitive object which can be rendered.
-
- Parameters
- ----------
- positions : (n, 3) float
- XYZ vertex positions.
- normals : (n, 3) float
- Normalized XYZ vertex normals.
- tangents : (n, 4) float
- XYZW vertex tangents where the w component is a sign value
- (either +1 or -1) indicating the handedness of the tangent basis.
- texcoord_0 : (n, 2) float
- The first set of UV texture coordinates.
- texcoord_1 : (n, 2) float
- The second set of UV texture coordinates.
- color_0 : (n, 4) float
- RGBA vertex colors.
- joints_0 : (n, 4) float
- Joint information.
- weights_0 : (n, 4) float
- Weight information for morphing.
- indices : (m, 3) int
- Face indices for triangle meshes or fans.
- material : :class:`Material`
- The material to apply to this primitive when rendering.
- mode : int
- The type of primitives to render, one of the following:
-
- - ``0``: POINTS
- - ``1``: LINES
- - ``2``: LINE_LOOP
- - ``3``: LINE_STRIP
- - ``4``: TRIANGLES
- - ``5``: TRIANGLES_STRIP
- - ``6``: TRIANGLES_FAN
- targets : (k,) int
- Morph target indices.
- poses : (x,4,4), float
- Array of 4x4 transformation matrices for instancing this object.
- """
-
- def __init__(self,
- positions,
- normals=None,
- tangents=None,
- texcoord_0=None,
- texcoord_1=None,
- color_0=None,
- joints_0=None,
- weights_0=None,
- indices=None,
- material=None,
- mode=None,
- targets=None,
- poses=None):
-
- if mode is None:
- mode = GLTF.TRIANGLES
-
- self.positions = positions
- self.normals = normals
- self.tangents = tangents
- self.texcoord_0 = texcoord_0
- self.texcoord_1 = texcoord_1
- self.color_0 = color_0
- self.joints_0 = joints_0
- self.weights_0 = weights_0
- self.indices = indices
- self.material = material
- self.mode = mode
- self.targets = targets
- self.poses = poses
-
- self._bounds = None
- self._vaid = None
- self._buffers = []
- self._is_transparent = None
- self._buf_flags = None
-
- @property
- def positions(self):
- """(n,3) float : XYZ vertex positions.
- """
- return self._positions
-
- @positions.setter
- def positions(self, value):
- value = np.asanyarray(value, dtype=np.float32)
- self._positions = np.ascontiguousarray(value)
- self._bounds = None
-
- @property
- def normals(self):
- """(n,3) float : Normalized XYZ vertex normals.
- """
- return self._normals
-
- @normals.setter
- def normals(self, value):
- if value is not None:
- value = np.asanyarray(value, dtype=np.float32)
- value = np.ascontiguousarray(value)
- if value.shape != self.positions.shape:
- raise ValueError('Incorrect normals shape')
- self._normals = value
-
- @property
- def tangents(self):
- """(n,4) float : XYZW vertex tangents.
- """
- return self._tangents
-
- @tangents.setter
- def tangents(self, value):
- if value is not None:
- value = np.asanyarray(value, dtype=np.float32)
- value = np.ascontiguousarray(value)
- if value.shape != (self.positions.shape[0], 4):
- raise ValueError('Incorrect tangent shape')
- self._tangents = value
-
- @property
- def texcoord_0(self):
- """(n,2) float : The first set of UV texture coordinates.
- """
- return self._texcoord_0
-
- @texcoord_0.setter
- def texcoord_0(self, value):
- if value is not None:
- value = np.asanyarray(value, dtype=np.float32)
- value = np.ascontiguousarray(value)
- if (value.ndim != 2 or value.shape[0] != self.positions.shape[0] or
- value.shape[1] < 2):
- raise ValueError('Incorrect texture coordinate shape')
- if value.shape[1] > 2:
- value = value[:,:2]
- self._texcoord_0 = value
-
- @property
- def texcoord_1(self):
- """(n,2) float : The second set of UV texture coordinates.
- """
- return self._texcoord_1
-
- @texcoord_1.setter
- def texcoord_1(self, value):
- if value is not None:
- value = np.asanyarray(value, dtype=np.float32)
- value = np.ascontiguousarray(value)
- if (value.ndim != 2 or value.shape[0] != self.positions.shape[0] or
- value.shape[1] != 2):
- raise ValueError('Incorrect texture coordinate shape')
- self._texcoord_1 = value
-
- @property
- def color_0(self):
- """(n,4) float : RGBA vertex colors.
- """
- return self._color_0
-
- @color_0.setter
- def color_0(self, value):
- if value is not None:
- value = np.ascontiguousarray(
- format_color_array(value, shape=(len(self.positions), 4))
- )
- self._is_transparent = None
- self._color_0 = value
-
- @property
- def joints_0(self):
- """(n,4) float : Joint information.
- """
- return self._joints_0
-
- @joints_0.setter
- def joints_0(self, value):
- self._joints_0 = value
-
- @property
- def weights_0(self):
- """(n,4) float : Weight information for morphing.
- """
- return self._weights_0
-
- @weights_0.setter
- def weights_0(self, value):
- self._weights_0 = value
-
- @property
- def indices(self):
- """(m,3) int : Face indices for triangle meshes or fans.
- """
- return self._indices
-
- @indices.setter
- def indices(self, value):
- if value is not None:
- value = np.asanyarray(value, dtype=np.float32)
- value = np.ascontiguousarray(value)
- self._indices = value
-
- @property
- def material(self):
- """:class:`Material` : The material for this primitive.
- """
- return self._material
-
- @material.setter
- def material(self, value):
- # Create default material
- if value is None:
- value = MetallicRoughnessMaterial()
- else:
- if not isinstance(value, Material):
- raise TypeError('Object material must be of type Material')
- self._material = value
-
- @property
- def mode(self):
- """int : The type of primitive to render.
- """
- return self._mode
-
- @mode.setter
- def mode(self, value):
- value = int(value)
- if value < GLTF.POINTS or value > GLTF.TRIANGLE_FAN:
- raise ValueError('Invalid mode')
- self._mode = value
-
- @property
- def targets(self):
- """(k,) int : Morph target indices.
- """
- return self._targets
-
- @targets.setter
- def targets(self, value):
- self._targets = value
-
- @property
- def poses(self):
- """(x,4,4) float : Homogenous transforms for instancing this primitive.
- """
- return self._poses
-
- @poses.setter
- def poses(self, value):
- if value is not None:
- value = np.asanyarray(value, dtype=np.float32)
- value = np.ascontiguousarray(value)
- if value.ndim == 2:
- value = value[np.newaxis,:,:]
- if value.shape[1] != 4 or value.shape[2] != 4:
- raise ValueError('Pose matrices must be of shape (n,4,4), '
- 'got {}'.format(value.shape))
- self._poses = value
- self._bounds = None
-
- @property
- def bounds(self):
- if self._bounds is None:
- self._bounds = self._compute_bounds()
- return self._bounds
-
- @property
- def centroid(self):
- """(3,) float : The centroid of the primitive's AABB.
- """
- return np.mean(self.bounds, axis=0)
-
- @property
- def extents(self):
- """(3,) float : The lengths of the axes of the primitive's AABB.
- """
- return np.diff(self.bounds, axis=0).reshape(-1)
-
- @property
- def scale(self):
- """(3,) float : The length of the diagonal of the primitive's AABB.
- """
- return np.linalg.norm(self.extents)
-
- @property
- def buf_flags(self):
- """int : The flags for the render buffer.
- """
- if self._buf_flags is None:
- self._buf_flags = self._compute_buf_flags()
- return self._buf_flags
-
- def delete(self):
- self._unbind()
- self._remove_from_context()
-
- @property
- def is_transparent(self):
- """bool : If True, the mesh is partially-transparent.
- """
- return self._compute_transparency()
-
- def _add_to_context(self):
- if self._vaid is not None:
- raise ValueError('Mesh is already bound to a context')
-
- # Generate and bind VAO
- self._vaid = glGenVertexArrays(1)
- glBindVertexArray(self._vaid)
-
- #######################################################################
- # Fill vertex buffer
- #######################################################################
-
- # Generate and bind vertex buffer
- vertexbuffer = glGenBuffers(1)
- self._buffers.append(vertexbuffer)
- glBindBuffer(GL_ARRAY_BUFFER, vertexbuffer)
-
- # positions
- vertex_data = self.positions
- attr_sizes = [3]
-
- # Normals
- if self.normals is not None:
- vertex_data = np.hstack((vertex_data, self.normals))
- attr_sizes.append(3)
-
- # Tangents
- if self.tangents is not None:
- vertex_data = np.hstack((vertex_data, self.tangents))
- attr_sizes.append(4)
-
- # Texture Coordinates
- if self.texcoord_0 is not None:
- vertex_data = np.hstack((vertex_data, self.texcoord_0))
- attr_sizes.append(2)
- if self.texcoord_1 is not None:
- vertex_data = np.hstack((vertex_data, self.texcoord_1))
- attr_sizes.append(2)
-
- # Color
- if self.color_0 is not None:
- vertex_data = np.hstack((vertex_data, self.color_0))
- attr_sizes.append(4)
-
- # TODO JOINTS AND WEIGHTS
- # PASS
-
- # Copy data to buffer
- vertex_data = np.ascontiguousarray(
- vertex_data.flatten().astype(np.float32)
- )
- glBufferData(
- GL_ARRAY_BUFFER, FLOAT_SZ * len(vertex_data),
- vertex_data, GL_STATIC_DRAW
- )
- total_sz = sum(attr_sizes)
- offset = 0
- for i, sz in enumerate(attr_sizes):
- glVertexAttribPointer(
- i, sz, GL_FLOAT, GL_FALSE, FLOAT_SZ * total_sz,
- ctypes.c_void_p(FLOAT_SZ * offset)
- )
- glEnableVertexAttribArray(i)
- offset += sz
-
- #######################################################################
- # Fill model matrix buffer
- #######################################################################
-
- if self.poses is not None:
- pose_data = np.ascontiguousarray(
- np.transpose(self.poses, [0,2,1]).flatten().astype(np.float32)
- )
- else:
- pose_data = np.ascontiguousarray(
- np.eye(4).flatten().astype(np.float32)
- )
-
- modelbuffer = glGenBuffers(1)
- self._buffers.append(modelbuffer)
- glBindBuffer(GL_ARRAY_BUFFER, modelbuffer)
- glBufferData(
- GL_ARRAY_BUFFER, FLOAT_SZ * len(pose_data),
- pose_data, GL_STATIC_DRAW
- )
-
- for i in range(0, 4):
- idx = i + len(attr_sizes)
- glEnableVertexAttribArray(idx)
- glVertexAttribPointer(
- idx, 4, GL_FLOAT, GL_FALSE, FLOAT_SZ * 4 * 4,
- ctypes.c_void_p(4 * FLOAT_SZ * i)
- )
- glVertexAttribDivisor(idx, 1)
-
- #######################################################################
- # Fill element buffer
- #######################################################################
- if self.indices is not None:
- elementbuffer = glGenBuffers(1)
- self._buffers.append(elementbuffer)
- glBindBuffer(GL_ELEMENT_ARRAY_BUFFER, elementbuffer)
- glBufferData(GL_ELEMENT_ARRAY_BUFFER, UINT_SZ * self.indices.size,
- self.indices.flatten().astype(np.uint32),
- GL_STATIC_DRAW)
-
- glBindVertexArray(0)
-
- def _remove_from_context(self):
- if self._vaid is not None:
- glDeleteVertexArrays(1, [self._vaid])
- glDeleteBuffers(len(self._buffers), self._buffers)
- self._vaid = None
- self._buffers = []
-
- def _in_context(self):
- return self._vaid is not None
-
- def _bind(self):
- if self._vaid is None:
- raise ValueError('Cannot bind a Mesh that has not been added '
- 'to a context')
- glBindVertexArray(self._vaid)
-
- def _unbind(self):
- glBindVertexArray(0)
-
- def _compute_bounds(self):
- """Compute the bounds of this object.
- """
- # Compute bounds of this object
- bounds = np.array([np.min(self.positions, axis=0),
- np.max(self.positions, axis=0)])
-
- # If instanced, compute translations for approximate bounds
- if self.poses is not None:
- bounds += np.array([np.min(self.poses[:,:3,3], axis=0),
- np.max(self.poses[:,:3,3], axis=0)])
- return bounds
-
- def _compute_transparency(self):
- """Compute whether or not this object is transparent.
- """
- if self.material.is_transparent:
- return True
- if self._is_transparent is None:
- self._is_transparent = False
- if self.color_0 is not None:
- if np.any(self._color_0[:,3] != 1.0):
- self._is_transparent = True
- return self._is_transparent
-
- def _compute_buf_flags(self):
- buf_flags = BufFlags.POSITION
-
- if self.normals is not None:
- buf_flags |= BufFlags.NORMAL
- if self.tangents is not None:
- buf_flags |= BufFlags.TANGENT
- if self.texcoord_0 is not None:
- buf_flags |= BufFlags.TEXCOORD_0
- if self.texcoord_1 is not None:
- buf_flags |= BufFlags.TEXCOORD_1
- if self.color_0 is not None:
- buf_flags |= BufFlags.COLOR_0
- if self.joints_0 is not None:
- buf_flags |= BufFlags.JOINTS_0
- if self.weights_0 is not None:
- buf_flags |= BufFlags.WEIGHTS_0
-
- return buf_flags
diff --git a/spaces/Oumar199/Fake-Real-Face-Detection/fake_face_detection/optimization/__init__.py b/spaces/Oumar199/Fake-Real-Face-Detection/fake_face_detection/optimization/__init__.py
deleted file mode 100644
index e69de29bb2d1d6434b8b29ae775ad8c2e48c5391..0000000000000000000000000000000000000000
diff --git a/spaces/PKUWilliamYang/VToonify/vtoonify/model/encoder/encoders/__init__.py b/spaces/PKUWilliamYang/VToonify/vtoonify/model/encoder/encoders/__init__.py
deleted file mode 100644
index e69de29bb2d1d6434b8b29ae775ad8c2e48c5391..0000000000000000000000000000000000000000
diff --git a/spaces/PSLD/PSLD/diffusion-posterior-sampling/bkse/models/backbones/skip/skip.py b/spaces/PSLD/PSLD/diffusion-posterior-sampling/bkse/models/backbones/skip/skip.py
deleted file mode 100644
index 186153d77d34a49ae7152ace3149b6324731560d..0000000000000000000000000000000000000000
--- a/spaces/PSLD/PSLD/diffusion-posterior-sampling/bkse/models/backbones/skip/skip.py
+++ /dev/null
@@ -1,133 +0,0 @@
-import torch
-import torch.nn as nn
-
-from .concat import Concat
-from .non_local_dot_product import NONLocalBlock2D
-from .util import get_activation, get_conv
-
-
-def add_module(self, module):
- self.add_module(str(len(self) + 1), module)
-
-
-torch.nn.Module.add = add_module
-
-
-def skip(
- num_input_channels=2,
- num_output_channels=3,
- num_channels_down=[16, 32, 64, 128, 128],
- num_channels_up=[16, 32, 64, 128, 128],
- num_channels_skip=[4, 4, 4, 4, 4],
- filter_size_down=3,
- filter_size_up=3,
- filter_skip_size=1,
- need_sigmoid=True,
- need_bias=True,
- pad="zero",
- upsample_mode="nearest",
- downsample_mode="stride",
- act_fun="LeakyReLU",
- need1x1_up=True,
-):
- """Assembles encoder-decoder with skip connections.
-
- Arguments:
- act_fun: Either string 'LeakyReLU|Swish|ELU|none' or module (e.g. nn.ReLU)
- pad (string): zero|reflection (default: 'zero')
- upsample_mode (string): 'nearest|bilinear' (default: 'nearest')
- downsample_mode (string): 'stride|avg|max|lanczos2' (default: 'stride')
-
- """
- assert len(num_channels_down) == len(num_channels_up) == len(num_channels_skip)
-
- n_scales = len(num_channels_down)
-
- if not (isinstance(upsample_mode, list) or isinstance(upsample_mode, tuple)):
- upsample_mode = [upsample_mode] * n_scales
-
- if not (isinstance(downsample_mode, list) or isinstance(downsample_mode, tuple)):
- downsample_mode = [downsample_mode] * n_scales
-
- if not (isinstance(filter_size_down, list) or isinstance(filter_size_down, tuple)):
- filter_size_down = [filter_size_down] * n_scales
-
- if not (isinstance(filter_size_up, list) or isinstance(filter_size_up, tuple)):
- filter_size_up = [filter_size_up] * n_scales
-
- last_scale = n_scales - 1
-
- model = nn.Sequential()
- model_tmp = model
-
- input_depth = num_input_channels
- for i in range(len(num_channels_down)):
-
- deeper = nn.Sequential()
- skip = nn.Sequential()
-
- if num_channels_skip[i] != 0:
- model_tmp.add(Concat(1, skip, deeper))
- else:
- model_tmp.add(deeper)
-
- model_tmp.add(
- nn.BatchNorm2d(num_channels_skip[i] + (num_channels_up[i + 1] if i < last_scale else num_channels_down[i]))
- )
-
- if num_channels_skip[i] != 0:
- skip.add(get_conv(input_depth, num_channels_skip[i], filter_skip_size, bias=need_bias, pad=pad))
- skip.add(nn.BatchNorm2d(num_channels_skip[i]))
- skip.add(get_activation(act_fun))
-
- # skip.add(Concat(2, GenNoise(nums_noise[i]), skip_part))
-
- deeper.add(
- get_conv(
- input_depth,
- num_channels_down[i],
- filter_size_down[i],
- 2,
- bias=need_bias,
- pad=pad,
- downsample_mode=downsample_mode[i],
- )
- )
- deeper.add(nn.BatchNorm2d(num_channels_down[i]))
- deeper.add(get_activation(act_fun))
- if i > 1:
- deeper.add(NONLocalBlock2D(in_channels=num_channels_down[i]))
- deeper.add(get_conv(num_channels_down[i], num_channels_down[i], filter_size_down[i], bias=need_bias, pad=pad))
- deeper.add(nn.BatchNorm2d(num_channels_down[i]))
- deeper.add(get_activation(act_fun))
-
- deeper_main = nn.Sequential()
-
- if i == len(num_channels_down) - 1:
- # The deepest
- k = num_channels_down[i]
- else:
- deeper.add(deeper_main)
- k = num_channels_up[i + 1]
-
- deeper.add(nn.Upsample(scale_factor=2, mode=upsample_mode[i]))
-
- model_tmp.add(
- get_conv(num_channels_skip[i] + k, num_channels_up[i], filter_size_up[i], 1, bias=need_bias, pad=pad)
- )
- model_tmp.add(nn.BatchNorm2d(num_channels_up[i]))
- model_tmp.add(get_activation(act_fun))
-
- if need1x1_up:
- model_tmp.add(get_conv(num_channels_up[i], num_channels_up[i], 1, bias=need_bias, pad=pad))
- model_tmp.add(nn.BatchNorm2d(num_channels_up[i]))
- model_tmp.add(get_activation(act_fun))
-
- input_depth = num_channels_down[i]
- model_tmp = deeper_main
-
- model.add(get_conv(num_channels_up[0], num_output_channels, 1, bias=need_bias, pad=pad))
- if need_sigmoid:
- model.add(nn.Sigmoid())
-
- return model
diff --git a/spaces/Pie31415/control-animation/annotator/uniformer/mmseg/core/utils/misc.py b/spaces/Pie31415/control-animation/annotator/uniformer/mmseg/core/utils/misc.py
deleted file mode 100644
index eb862a82bd47c8624db3dd5c6fb6ad8a03b62466..0000000000000000000000000000000000000000
--- a/spaces/Pie31415/control-animation/annotator/uniformer/mmseg/core/utils/misc.py
+++ /dev/null
@@ -1,17 +0,0 @@
-def add_prefix(inputs, prefix):
- """Add prefix for dict.
-
- Args:
- inputs (dict): The input dict with str keys.
- prefix (str): The prefix to add.
-
- Returns:
-
- dict: The dict with keys updated with ``prefix``.
- """
-
- outputs = dict()
- for name, value in inputs.items():
- outputs[f'{prefix}.{name}'] = value
-
- return outputs
diff --git a/spaces/Prof-Reza/Audiocraft_Music-Audio_Generation/tests/adversarial/test_discriminators.py b/spaces/Prof-Reza/Audiocraft_Music-Audio_Generation/tests/adversarial/test_discriminators.py
deleted file mode 100644
index fad89a0ae4534dc7967b6ccda194b9fd1dedbffe..0000000000000000000000000000000000000000
--- a/spaces/Prof-Reza/Audiocraft_Music-Audio_Generation/tests/adversarial/test_discriminators.py
+++ /dev/null
@@ -1,67 +0,0 @@
-# Copyright (c) Meta Platforms, Inc. and affiliates.
-# All rights reserved.
-#
-# This source code is licensed under the license found in the
-# LICENSE file in the root directory of this source tree.
-
-import random
-
-import torch
-
-from audiocraft.adversarial.discriminators import (
- MultiPeriodDiscriminator,
- MultiScaleDiscriminator,
- MultiScaleSTFTDiscriminator
-)
-
-
-class TestMultiPeriodDiscriminator:
-
- def test_mpd_discriminator(self):
- N, C, T = 2, 2, random.randrange(1, 100_000)
- t0 = torch.randn(N, C, T)
- periods = [1, 2, 3]
- mpd = MultiPeriodDiscriminator(periods=periods, in_channels=C)
- logits, fmaps = mpd(t0)
-
- assert len(logits) == len(periods)
- assert len(fmaps) == len(periods)
- assert all([logit.shape[0] == N and len(logit.shape) == 4 for logit in logits])
- assert all([feature.shape[0] == N for fmap in fmaps for feature in fmap])
-
-
-class TestMultiScaleDiscriminator:
-
- def test_msd_discriminator(self):
- N, C, T = 2, 2, random.randrange(1, 100_000)
- t0 = torch.randn(N, C, T)
-
- scale_norms = ['weight_norm', 'weight_norm']
- msd = MultiScaleDiscriminator(scale_norms=scale_norms, in_channels=C)
- logits, fmaps = msd(t0)
-
- assert len(logits) == len(scale_norms)
- assert len(fmaps) == len(scale_norms)
- assert all([logit.shape[0] == N and len(logit.shape) == 3 for logit in logits])
- assert all([feature.shape[0] == N for fmap in fmaps for feature in fmap])
-
-
-class TestMultiScaleStftDiscriminator:
-
- def test_msstftd_discriminator(self):
- N, C, T = 2, 2, random.randrange(1, 100_000)
- t0 = torch.randn(N, C, T)
-
- n_filters = 4
- n_ffts = [128, 256, 64]
- hop_lengths = [32, 64, 16]
- win_lengths = [128, 256, 64]
-
- msstftd = MultiScaleSTFTDiscriminator(filters=n_filters, n_ffts=n_ffts, hop_lengths=hop_lengths,
- win_lengths=win_lengths, in_channels=C)
- logits, fmaps = msstftd(t0)
-
- assert len(logits) == len(n_ffts)
- assert len(fmaps) == len(n_ffts)
- assert all([logit.shape[0] == N and len(logit.shape) == 4 for logit in logits])
- assert all([feature.shape[0] == N for fmap in fmaps for feature in fmap])
diff --git a/spaces/Q4234/a2/app.py b/spaces/Q4234/a2/app.py
deleted file mode 100644
index d3ccf40431db34c87af1c4f24a2eeb6f986aee86..0000000000000000000000000000000000000000
--- a/spaces/Q4234/a2/app.py
+++ /dev/null
@@ -1,56 +0,0 @@
-import gradio as gr
-
-import ctransformers
-
-class Z(object):
- def __init__(self):
- self.llm = None
-
- def init(self):
- pass
-
- def greet(self, txt0, paramTemp):
- prompt0 = txt0
-
- # for Wizard-Vicuna-13B
- #prompt00 = f'''USER: {prompt0}
- #ASSISTANT:'''
-
- # for starcoder
- prompt00 = f'''{prompt0}'''
-
- response0 = llm(prompt00, max_new_tokens=198, temperature=paramTemp) # 0.5, 0.3
-
- return f'{response0}'
-
-from ctransformers import AutoModelForCausalLM
-
-# wizzard vicuna
-# see https://github.com/melodysdreamj/WizardVicunaLM
-#llm = AutoModelForCausalLM.from_pretrained('TheBloke/Wizard-Vicuna-13B-Uncensored-GGML', model_file='Wizard-Vicuna-13B-Uncensored.ggmlv3.q4_0.bin', model_type='llama')
-
-#llm = AutoModelForCausalLM.from_pretrained('mverrilli/dolly-v2-12b-ggml', model_file='ggml-model-q5_0.bin', model_type='dolly-v2')
-
-#llm = AutoModelForCausalLM.from_pretrained('mverrilli/dolly-v2-7b-ggml', model_file='ggml-model-q5_0.bin', model_type='dolly-v2')
-
-
-
-# non-RLHF model
-# 4 may 2023
-# site https://huggingface.co/bigcode/starcoder
-modelInfo = {'path':'NeoDim/starcoder-GGML', 'subPath':'starcoder-ggml-q8_0.bin', 'promptType':'raw', 'modelType':'starcoder'}
-llm = AutoModelForCausalLM.from_pretrained(modelInfo['path'], model_file=modelInfo['subPath'], model_type=modelInfo['modelType'])
-
-
-
-z = Z()
-z.llm = llm
-z.modelInfo = modelInfo
-z.init()
-
-def greet(prompt, temperature):
- global z
- return z.greet(prompt, temperature)
-
-iface = gr.Interface(fn=greet, inputs=["text", gr.Slider(0.0, 1.0, value=0.41)], outputs="text")
-iface.launch()
\ No newline at end of file
diff --git a/spaces/RMXK/RVC_HFF/lib/uvr5_pack/lib_v5/layers_new.py b/spaces/RMXK/RVC_HFF/lib/uvr5_pack/lib_v5/layers_new.py
deleted file mode 100644
index 0c13e60b0dd136d9115a535101c6dbb2a25c6833..0000000000000000000000000000000000000000
--- a/spaces/RMXK/RVC_HFF/lib/uvr5_pack/lib_v5/layers_new.py
+++ /dev/null
@@ -1,125 +0,0 @@
-import torch
-from torch import nn
-import torch.nn.functional as F
-
-from . import spec_utils
-
-
-class Conv2DBNActiv(nn.Module):
- def __init__(self, nin, nout, ksize=3, stride=1, pad=1, dilation=1, activ=nn.ReLU):
- super(Conv2DBNActiv, self).__init__()
- self.conv = nn.Sequential(
- nn.Conv2d(
- nin,
- nout,
- kernel_size=ksize,
- stride=stride,
- padding=pad,
- dilation=dilation,
- bias=False,
- ),
- nn.BatchNorm2d(nout),
- activ(),
- )
-
- def __call__(self, x):
- return self.conv(x)
-
-
-class Encoder(nn.Module):
- def __init__(self, nin, nout, ksize=3, stride=1, pad=1, activ=nn.LeakyReLU):
- super(Encoder, self).__init__()
- self.conv1 = Conv2DBNActiv(nin, nout, ksize, stride, pad, activ=activ)
- self.conv2 = Conv2DBNActiv(nout, nout, ksize, 1, pad, activ=activ)
-
- def __call__(self, x):
- h = self.conv1(x)
- h = self.conv2(h)
-
- return h
-
-
-class Decoder(nn.Module):
- def __init__(
- self, nin, nout, ksize=3, stride=1, pad=1, activ=nn.ReLU, dropout=False
- ):
- super(Decoder, self).__init__()
- self.conv1 = Conv2DBNActiv(nin, nout, ksize, 1, pad, activ=activ)
- # self.conv2 = Conv2DBNActiv(nout, nout, ksize, 1, pad, activ=activ)
- self.dropout = nn.Dropout2d(0.1) if dropout else None
-
- def __call__(self, x, skip=None):
- x = F.interpolate(x, scale_factor=2, mode="bilinear", align_corners=True)
-
- if skip is not None:
- skip = spec_utils.crop_center(skip, x)
- x = torch.cat([x, skip], dim=1)
-
- h = self.conv1(x)
- # h = self.conv2(h)
-
- if self.dropout is not None:
- h = self.dropout(h)
-
- return h
-
-
-class ASPPModule(nn.Module):
- def __init__(self, nin, nout, dilations=(4, 8, 12), activ=nn.ReLU, dropout=False):
- super(ASPPModule, self).__init__()
- self.conv1 = nn.Sequential(
- nn.AdaptiveAvgPool2d((1, None)),
- Conv2DBNActiv(nin, nout, 1, 1, 0, activ=activ),
- )
- self.conv2 = Conv2DBNActiv(nin, nout, 1, 1, 0, activ=activ)
- self.conv3 = Conv2DBNActiv(
- nin, nout, 3, 1, dilations[0], dilations[0], activ=activ
- )
- self.conv4 = Conv2DBNActiv(
- nin, nout, 3, 1, dilations[1], dilations[1], activ=activ
- )
- self.conv5 = Conv2DBNActiv(
- nin, nout, 3, 1, dilations[2], dilations[2], activ=activ
- )
- self.bottleneck = Conv2DBNActiv(nout * 5, nout, 1, 1, 0, activ=activ)
- self.dropout = nn.Dropout2d(0.1) if dropout else None
-
- def forward(self, x):
- _, _, h, w = x.size()
- feat1 = F.interpolate(
- self.conv1(x), size=(h, w), mode="bilinear", align_corners=True
- )
- feat2 = self.conv2(x)
- feat3 = self.conv3(x)
- feat4 = self.conv4(x)
- feat5 = self.conv5(x)
- out = torch.cat((feat1, feat2, feat3, feat4, feat5), dim=1)
- out = self.bottleneck(out)
-
- if self.dropout is not None:
- out = self.dropout(out)
-
- return out
-
-
-class LSTMModule(nn.Module):
- def __init__(self, nin_conv, nin_lstm, nout_lstm):
- super(LSTMModule, self).__init__()
- self.conv = Conv2DBNActiv(nin_conv, 1, 1, 1, 0)
- self.lstm = nn.LSTM(
- input_size=nin_lstm, hidden_size=nout_lstm // 2, bidirectional=True
- )
- self.dense = nn.Sequential(
- nn.Linear(nout_lstm, nin_lstm), nn.BatchNorm1d(nin_lstm), nn.ReLU()
- )
-
- def forward(self, x):
- N, _, nbins, nframes = x.size()
- h = self.conv(x)[:, 0] # N, nbins, nframes
- h = h.permute(2, 0, 1) # nframes, N, nbins
- h, _ = self.lstm(h)
- h = self.dense(h.reshape(-1, h.size()[-1])) # nframes * N, nbins
- h = h.reshape(nframes, N, 1, nbins)
- h = h.permute(1, 2, 3, 0)
-
- return h
diff --git a/spaces/RMXK/RVC_HFF/tools/torchgate/__init__.py b/spaces/RMXK/RVC_HFF/tools/torchgate/__init__.py
deleted file mode 100644
index b4a12675828dceb6e6270f9439cdf98ea28ea96d..0000000000000000000000000000000000000000
--- a/spaces/RMXK/RVC_HFF/tools/torchgate/__init__.py
+++ /dev/null
@@ -1,12 +0,0 @@
-"""
-TorchGating is a PyTorch-based implementation of Spectral Gating
-================================================
-Author: Asaf Zorea
-
-Contents
---------
-torchgate imports all the functions from PyTorch, and in addition provides:
- TorchGating --- A PyTorch module that applies a spectral gate to an input signal
-
-"""
-from .torchgate import TorchGate
diff --git a/spaces/Raspberry-ai/main/.env/lib/python3.11/site-packages/pip/_vendor/pygments/lexers/__init__.py b/spaces/Raspberry-ai/main/.env/lib/python3.11/site-packages/pip/_vendor/pygments/lexers/__init__.py
deleted file mode 100644
index ed69f24ed355e849b54dd0c3c8374c760b4b34b8..0000000000000000000000000000000000000000
--- a/spaces/Raspberry-ai/main/.env/lib/python3.11/site-packages/pip/_vendor/pygments/lexers/__init__.py
+++ /dev/null
@@ -1,335 +0,0 @@
-"""
- pygments.lexers
- ~~~~~~~~~~~~~~~
-
- Pygments lexers.
-
- :copyright: Copyright 2006-2022 by the Pygments team, see AUTHORS.
- :license: BSD, see LICENSE for details.
-"""
-
-import re
-import sys
-import types
-from fnmatch import fnmatch
-from os.path import basename
-
-from pip._vendor.pygments.lexers._mapping import LEXERS
-from pip._vendor.pygments.modeline import get_filetype_from_buffer
-from pip._vendor.pygments.plugin import find_plugin_lexers
-from pip._vendor.pygments.util import ClassNotFound, guess_decode
-
-COMPAT = {
- 'Python3Lexer': 'PythonLexer',
- 'Python3TracebackLexer': 'PythonTracebackLexer',
-}
-
-__all__ = ['get_lexer_by_name', 'get_lexer_for_filename', 'find_lexer_class',
- 'guess_lexer', 'load_lexer_from_file'] + list(LEXERS) + list(COMPAT)
-
-_lexer_cache = {}
-
-def _load_lexers(module_name):
- """Load a lexer (and all others in the module too)."""
- mod = __import__(module_name, None, None, ['__all__'])
- for lexer_name in mod.__all__:
- cls = getattr(mod, lexer_name)
- _lexer_cache[cls.name] = cls
-
-
-def get_all_lexers(plugins=True):
- """Return a generator of tuples in the form ``(name, aliases,
- filenames, mimetypes)`` of all know lexers.
-
- If *plugins* is true (the default), plugin lexers supplied by entrypoints
- are also returned. Otherwise, only builtin ones are considered.
- """
- for item in LEXERS.values():
- yield item[1:]
- if plugins:
- for lexer in find_plugin_lexers():
- yield lexer.name, lexer.aliases, lexer.filenames, lexer.mimetypes
-
-
-def find_lexer_class(name):
- """Lookup a lexer class by name.
-
- Return None if not found.
- """
- if name in _lexer_cache:
- return _lexer_cache[name]
- # lookup builtin lexers
- for module_name, lname, aliases, _, _ in LEXERS.values():
- if name == lname:
- _load_lexers(module_name)
- return _lexer_cache[name]
- # continue with lexers from setuptools entrypoints
- for cls in find_plugin_lexers():
- if cls.name == name:
- return cls
-
-
-def find_lexer_class_by_name(_alias):
- """Lookup a lexer class by alias.
-
- Like `get_lexer_by_name`, but does not instantiate the class.
-
- .. versionadded:: 2.2
- """
- if not _alias:
- raise ClassNotFound('no lexer for alias %r found' % _alias)
- # lookup builtin lexers
- for module_name, name, aliases, _, _ in LEXERS.values():
- if _alias.lower() in aliases:
- if name not in _lexer_cache:
- _load_lexers(module_name)
- return _lexer_cache[name]
- # continue with lexers from setuptools entrypoints
- for cls in find_plugin_lexers():
- if _alias.lower() in cls.aliases:
- return cls
- raise ClassNotFound('no lexer for alias %r found' % _alias)
-
-
-def get_lexer_by_name(_alias, **options):
- """Get a lexer by an alias.
-
- Raises ClassNotFound if not found.
- """
- if not _alias:
- raise ClassNotFound('no lexer for alias %r found' % _alias)
-
- # lookup builtin lexers
- for module_name, name, aliases, _, _ in LEXERS.values():
- if _alias.lower() in aliases:
- if name not in _lexer_cache:
- _load_lexers(module_name)
- return _lexer_cache[name](**options)
- # continue with lexers from setuptools entrypoints
- for cls in find_plugin_lexers():
- if _alias.lower() in cls.aliases:
- return cls(**options)
- raise ClassNotFound('no lexer for alias %r found' % _alias)
-
-
-def load_lexer_from_file(filename, lexername="CustomLexer", **options):
- """Load a lexer from a file.
-
- This method expects a file located relative to the current working
- directory, which contains a Lexer class. By default, it expects the
- Lexer to be name CustomLexer; you can specify your own class name
- as the second argument to this function.
-
- Users should be very careful with the input, because this method
- is equivalent to running eval on the input file.
-
- Raises ClassNotFound if there are any problems importing the Lexer.
-
- .. versionadded:: 2.2
- """
- try:
- # This empty dict will contain the namespace for the exec'd file
- custom_namespace = {}
- with open(filename, 'rb') as f:
- exec(f.read(), custom_namespace)
- # Retrieve the class `lexername` from that namespace
- if lexername not in custom_namespace:
- raise ClassNotFound('no valid %s class found in %s' %
- (lexername, filename))
- lexer_class = custom_namespace[lexername]
- # And finally instantiate it with the options
- return lexer_class(**options)
- except OSError as err:
- raise ClassNotFound('cannot read %s: %s' % (filename, err))
- except ClassNotFound:
- raise
- except Exception as err:
- raise ClassNotFound('error when loading custom lexer: %s' % err)
-
-
-def find_lexer_class_for_filename(_fn, code=None):
- """Get a lexer for a filename.
-
- If multiple lexers match the filename pattern, use ``analyse_text()`` to
- figure out which one is more appropriate.
-
- Returns None if not found.
- """
- matches = []
- fn = basename(_fn)
- for modname, name, _, filenames, _ in LEXERS.values():
- for filename in filenames:
- if fnmatch(fn, filename):
- if name not in _lexer_cache:
- _load_lexers(modname)
- matches.append((_lexer_cache[name], filename))
- for cls in find_plugin_lexers():
- for filename in cls.filenames:
- if fnmatch(fn, filename):
- matches.append((cls, filename))
-
- if isinstance(code, bytes):
- # decode it, since all analyse_text functions expect unicode
- code = guess_decode(code)
-
- def get_rating(info):
- cls, filename = info
- # explicit patterns get a bonus
- bonus = '*' not in filename and 0.5 or 0
- # The class _always_ defines analyse_text because it's included in
- # the Lexer class. The default implementation returns None which
- # gets turned into 0.0. Run scripts/detect_missing_analyse_text.py
- # to find lexers which need it overridden.
- if code:
- return cls.analyse_text(code) + bonus, cls.__name__
- return cls.priority + bonus, cls.__name__
-
- if matches:
- matches.sort(key=get_rating)
- # print "Possible lexers, after sort:", matches
- return matches[-1][0]
-
-
-def get_lexer_for_filename(_fn, code=None, **options):
- """Get a lexer for a filename.
-
- If multiple lexers match the filename pattern, use ``analyse_text()`` to
- figure out which one is more appropriate.
-
- Raises ClassNotFound if not found.
- """
- res = find_lexer_class_for_filename(_fn, code)
- if not res:
- raise ClassNotFound('no lexer for filename %r found' % _fn)
- return res(**options)
-
-
-def get_lexer_for_mimetype(_mime, **options):
- """Get a lexer for a mimetype.
-
- Raises ClassNotFound if not found.
- """
- for modname, name, _, _, mimetypes in LEXERS.values():
- if _mime in mimetypes:
- if name not in _lexer_cache:
- _load_lexers(modname)
- return _lexer_cache[name](**options)
- for cls in find_plugin_lexers():
- if _mime in cls.mimetypes:
- return cls(**options)
- raise ClassNotFound('no lexer for mimetype %r found' % _mime)
-
-
-def _iter_lexerclasses(plugins=True):
- """Return an iterator over all lexer classes."""
- for key in sorted(LEXERS):
- module_name, name = LEXERS[key][:2]
- if name not in _lexer_cache:
- _load_lexers(module_name)
- yield _lexer_cache[name]
- if plugins:
- yield from find_plugin_lexers()
-
-
-def guess_lexer_for_filename(_fn, _text, **options):
- """
- Lookup all lexers that handle those filenames primary (``filenames``)
- or secondary (``alias_filenames``). Then run a text analysis for those
- lexers and choose the best result.
-
- usage::
-
- >>> from pygments.lexers import guess_lexer_for_filename
- >>> guess_lexer_for_filename('hello.html', '<%= @foo %>')
-
- >>> guess_lexer_for_filename('hello.html', '
{{ title|e }}
')
-
- >>> guess_lexer_for_filename('style.css', 'a { color: = $link ?> }')
-
- """
- fn = basename(_fn)
- primary = {}
- matching_lexers = set()
- for lexer in _iter_lexerclasses():
- for filename in lexer.filenames:
- if fnmatch(fn, filename):
- matching_lexers.add(lexer)
- primary[lexer] = True
- for filename in lexer.alias_filenames:
- if fnmatch(fn, filename):
- matching_lexers.add(lexer)
- primary[lexer] = False
- if not matching_lexers:
- raise ClassNotFound('no lexer for filename %r found' % fn)
- if len(matching_lexers) == 1:
- return matching_lexers.pop()(**options)
- result = []
- for lexer in matching_lexers:
- rv = lexer.analyse_text(_text)
- if rv == 1.0:
- return lexer(**options)
- result.append((rv, lexer))
-
- def type_sort(t):
- # sort by:
- # - analyse score
- # - is primary filename pattern?
- # - priority
- # - last resort: class name
- return (t[0], primary[t[1]], t[1].priority, t[1].__name__)
- result.sort(key=type_sort)
-
- return result[-1][1](**options)
-
-
-def guess_lexer(_text, **options):
- """Guess a lexer by strong distinctions in the text (eg, shebang)."""
-
- if not isinstance(_text, str):
- inencoding = options.get('inencoding', options.get('encoding'))
- if inencoding:
- _text = _text.decode(inencoding or 'utf8')
- else:
- _text, _ = guess_decode(_text)
-
- # try to get a vim modeline first
- ft = get_filetype_from_buffer(_text)
-
- if ft is not None:
- try:
- return get_lexer_by_name(ft, **options)
- except ClassNotFound:
- pass
-
- best_lexer = [0.0, None]
- for lexer in _iter_lexerclasses():
- rv = lexer.analyse_text(_text)
- if rv == 1.0:
- return lexer(**options)
- if rv > best_lexer[0]:
- best_lexer[:] = (rv, lexer)
- if not best_lexer[0] or best_lexer[1] is None:
- raise ClassNotFound('no lexer matching the text found')
- return best_lexer[1](**options)
-
-
-class _automodule(types.ModuleType):
- """Automatically import lexers."""
-
- def __getattr__(self, name):
- info = LEXERS.get(name)
- if info:
- _load_lexers(info[0])
- cls = _lexer_cache[info[1]]
- setattr(self, name, cls)
- return cls
- if name in COMPAT:
- return getattr(self, COMPAT[name])
- raise AttributeError(name)
-
-
-oldmod = sys.modules[__name__]
-newmod = _automodule(__name__)
-newmod.__dict__.update(oldmod.__dict__)
-sys.modules[__name__] = newmod
-del newmod.newmod, newmod.oldmod, newmod.sys, newmod.types
diff --git a/spaces/Raspberry-ai/main/.env/lib/python3.11/site-packages/setuptools/_distutils/spawn.py b/spaces/Raspberry-ai/main/.env/lib/python3.11/site-packages/setuptools/_distutils/spawn.py
deleted file mode 100644
index b18ba9db7d2e5919c853e7dcf8d5b7c180607c3f..0000000000000000000000000000000000000000
--- a/spaces/Raspberry-ai/main/.env/lib/python3.11/site-packages/setuptools/_distutils/spawn.py
+++ /dev/null
@@ -1,109 +0,0 @@
-"""distutils.spawn
-
-Provides the 'spawn()' function, a front-end to various platform-
-specific functions for launching another program in a sub-process.
-Also provides the 'find_executable()' to search the path for a given
-executable name.
-"""
-
-import sys
-import os
-import subprocess
-
-from distutils.errors import DistutilsExecError
-from distutils.debug import DEBUG
-from distutils import log
-
-
-def spawn(cmd, search_path=1, verbose=0, dry_run=0, env=None): # noqa: C901
- """Run another program, specified as a command list 'cmd', in a new process.
-
- 'cmd' is just the argument list for the new process, ie.
- cmd[0] is the program to run and cmd[1:] are the rest of its arguments.
- There is no way to run a program with a name different from that of its
- executable.
-
- If 'search_path' is true (the default), the system's executable
- search path will be used to find the program; otherwise, cmd[0]
- must be the exact path to the executable. If 'dry_run' is true,
- the command will not actually be run.
-
- Raise DistutilsExecError if running the program fails in any way; just
- return on success.
- """
- # cmd is documented as a list, but just in case some code passes a tuple
- # in, protect our %-formatting code against horrible death
- cmd = list(cmd)
-
- log.info(subprocess.list2cmdline(cmd))
- if dry_run:
- return
-
- if search_path:
- executable = find_executable(cmd[0])
- if executable is not None:
- cmd[0] = executable
-
- env = env if env is not None else dict(os.environ)
-
- if sys.platform == 'darwin':
- from distutils.util import MACOSX_VERSION_VAR, get_macosx_target_ver
-
- macosx_target_ver = get_macosx_target_ver()
- if macosx_target_ver:
- env[MACOSX_VERSION_VAR] = macosx_target_ver
-
- try:
- proc = subprocess.Popen(cmd, env=env)
- proc.wait()
- exitcode = proc.returncode
- except OSError as exc:
- if not DEBUG:
- cmd = cmd[0]
- raise DistutilsExecError(
- "command {!r} failed: {}".format(cmd, exc.args[-1])
- ) from exc
-
- if exitcode:
- if not DEBUG:
- cmd = cmd[0]
- raise DistutilsExecError(
- "command {!r} failed with exit code {}".format(cmd, exitcode)
- )
-
-
-def find_executable(executable, path=None):
- """Tries to find 'executable' in the directories listed in 'path'.
-
- A string listing directories separated by 'os.pathsep'; defaults to
- os.environ['PATH']. Returns the complete filename or None if not found.
- """
- _, ext = os.path.splitext(executable)
- if (sys.platform == 'win32') and (ext != '.exe'):
- executable = executable + '.exe'
-
- if os.path.isfile(executable):
- return executable
-
- if path is None:
- path = os.environ.get('PATH', None)
- if path is None:
- try:
- path = os.confstr("CS_PATH")
- except (AttributeError, ValueError):
- # os.confstr() or CS_PATH is not available
- path = os.defpath
- # bpo-35755: Don't use os.defpath if the PATH environment variable is
- # set to an empty string
-
- # PATH='' doesn't match, whereas PATH=':' looks in the current directory
- if not path:
- return None
-
- paths = path.split(os.pathsep)
- for p in paths:
- f = os.path.join(p, executable)
- if os.path.isfile(f):
- # the file exists, we have a shot at spawn working
- return f
- return None
diff --git a/spaces/Raspberry-ai/main/.env/lib/python3.11/site-packages/setuptools/config/_validate_pyproject/fastjsonschema_validations.py b/spaces/Raspberry-ai/main/.env/lib/python3.11/site-packages/setuptools/config/_validate_pyproject/fastjsonschema_validations.py
deleted file mode 100644
index ad5ee31ef53370fe7ec95799db390a33c3680b3b..0000000000000000000000000000000000000000
--- a/spaces/Raspberry-ai/main/.env/lib/python3.11/site-packages/setuptools/config/_validate_pyproject/fastjsonschema_validations.py
+++ /dev/null
@@ -1,1035 +0,0 @@
-# noqa
-# type: ignore
-# flake8: noqa
-# pylint: skip-file
-# mypy: ignore-errors
-# yapf: disable
-# pylama:skip=1
-
-
-# *** PLEASE DO NOT MODIFY DIRECTLY: Automatically generated code ***
-
-
-VERSION = "2.15.3"
-import re
-from .fastjsonschema_exceptions import JsonSchemaValueException
-
-
-REGEX_PATTERNS = {
- '^.*$': re.compile('^.*$'),
- '.+': re.compile('.+'),
- '^.+$': re.compile('^.+$'),
- 'idn-email_re_pattern': re.compile('^[^@]+@[^@]+\\.[^@]+\\Z')
-}
-
-NoneType = type(None)
-
-def validate(data, custom_formats={}, name_prefix=None):
- validate_https___packaging_python_org_en_latest_specifications_declaring_build_dependencies(data, custom_formats, (name_prefix or "data") + "")
- return data
-
-def validate_https___packaging_python_org_en_latest_specifications_declaring_build_dependencies(data, custom_formats={}, name_prefix=None):
- if not isinstance(data, (dict)):
- raise JsonSchemaValueException("" + (name_prefix or "data") + " must be object", value=data, name="" + (name_prefix or "data") + "", definition={'$schema': 'http://json-schema.org/draft-07/schema', '$id': 'https://packaging.python.org/en/latest/specifications/declaring-build-dependencies/', 'title': 'Data structure for ``pyproject.toml`` files', '$$description': ['File format containing build-time configurations for the Python ecosystem. ', ':pep:`517` initially defined a build-system independent format for source trees', 'which was complemented by :pep:`518` to provide a way of specifying dependencies ', 'for building Python projects.', 'Please notice the ``project`` table (as initially defined in :pep:`621`) is not included', 'in this schema and should be considered separately.'], 'type': 'object', 'additionalProperties': False, 'properties': {'build-system': {'type': 'object', 'description': 'Table used to store build-related data', 'additionalProperties': False, 'properties': {'requires': {'type': 'array', '$$description': ['List of dependencies in the :pep:`508` format required to execute the build', 'system. Please notice that the resulting dependency graph', '**MUST NOT contain cycles**'], 'items': {'type': 'string'}}, 'build-backend': {'type': 'string', 'description': 'Python object that will be used to perform the build according to :pep:`517`', 'format': 'pep517-backend-reference'}, 'backend-path': {'type': 'array', '$$description': ['List of directories to be prepended to ``sys.path`` when loading the', 'back-end, and running its hooks'], 'items': {'type': 'string', '$comment': 'Should be a path (TODO: enforce it with format?)'}}}, 'required': ['requires']}, 'project': {'$schema': 'http://json-schema.org/draft-07/schema', '$id': 'https://packaging.python.org/en/latest/specifications/declaring-project-metadata/', 'title': 'Package metadata stored in the ``project`` table', '$$description': ['Data structure for the **project** table inside ``pyproject.toml``', '(as initially defined in :pep:`621`)'], 'type': 'object', 'properties': {'name': {'type': 'string', 'description': 'The name (primary identifier) of the project. MUST be statically defined.', 'format': 'pep508-identifier'}, 'version': {'type': 'string', 'description': 'The version of the project as supported by :pep:`440`.', 'format': 'pep440'}, 'description': {'type': 'string', '$$description': ['The `summary description of the project', '`_']}, 'readme': {'$$description': ['`Full/detailed description of the project in the form of a README', '`_', "with meaning similar to the one defined in `core metadata's Description", '`_'], 'oneOf': [{'type': 'string', '$$description': ['Relative path to a text file (UTF-8) containing the full description', 'of the project. If the file path ends in case-insensitive ``.md`` or', '``.rst`` suffixes, then the content-type is respectively', '``text/markdown`` or ``text/x-rst``']}, {'type': 'object', 'allOf': [{'anyOf': [{'properties': {'file': {'type': 'string', '$$description': ['Relative path to a text file containing the full description', 'of the project.']}}, 'required': ['file']}, {'properties': {'text': {'type': 'string', 'description': 'Full text describing the project.'}}, 'required': ['text']}]}, {'properties': {'content-type': {'type': 'string', '$$description': ['Content-type (:rfc:`1341`) of the full description', '(e.g. ``text/markdown``). The ``charset`` parameter is assumed', 'UTF-8 when not present.'], '$comment': 'TODO: add regex pattern or format?'}}, 'required': ['content-type']}]}]}, 'requires-python': {'type': 'string', 'format': 'pep508-versionspec', '$$description': ['`The Python version requirements of the project', '`_.']}, 'license': {'description': '`Project license `_.', 'oneOf': [{'properties': {'file': {'type': 'string', '$$description': ['Relative path to the file (UTF-8) which contains the license for the', 'project.']}}, 'required': ['file']}, {'properties': {'text': {'type': 'string', '$$description': ['The license of the project whose meaning is that of the', '`License field from the core metadata', '`_.']}}, 'required': ['text']}]}, 'authors': {'type': 'array', 'items': {'$ref': '#/definitions/author'}, '$$description': ["The people or organizations considered to be the 'authors' of the project.", 'The exact meaning is open to interpretation (e.g. original or primary authors,', 'current maintainers, or owners of the package).']}, 'maintainers': {'type': 'array', 'items': {'$ref': '#/definitions/author'}, '$$description': ["The people or organizations considered to be the 'maintainers' of the project.", 'Similarly to ``authors``, the exact meaning is open to interpretation.']}, 'keywords': {'type': 'array', 'items': {'type': 'string'}, 'description': 'List of keywords to assist searching for the distribution in a larger catalog.'}, 'classifiers': {'type': 'array', 'items': {'type': 'string', 'format': 'trove-classifier', 'description': '`PyPI classifier `_.'}, '$$description': ['`Trove classifiers `_', 'which apply to the project.']}, 'urls': {'type': 'object', 'description': 'URLs associated with the project in the form ``label => value``.', 'additionalProperties': False, 'patternProperties': {'^.+$': {'type': 'string', 'format': 'url'}}}, 'scripts': {'$ref': '#/definitions/entry-point-group', '$$description': ['Instruct the installer to create command-line wrappers for the given', '`entry points `_.']}, 'gui-scripts': {'$ref': '#/definitions/entry-point-group', '$$description': ['Instruct the installer to create GUI wrappers for the given', '`entry points `_.', 'The difference between ``scripts`` and ``gui-scripts`` is only relevant in', 'Windows.']}, 'entry-points': {'$$description': ['Instruct the installer to expose the given modules/functions via', '``entry-point`` discovery mechanism (useful for plugins).', 'More information available in the `Python packaging guide', '`_.'], 'propertyNames': {'format': 'python-entrypoint-group'}, 'additionalProperties': False, 'patternProperties': {'^.+$': {'$ref': '#/definitions/entry-point-group'}}}, 'dependencies': {'type': 'array', 'description': 'Project (mandatory) dependencies.', 'items': {'$ref': '#/definitions/dependency'}}, 'optional-dependencies': {'type': 'object', 'description': 'Optional dependency for the project', 'propertyNames': {'format': 'pep508-identifier'}, 'additionalProperties': False, 'patternProperties': {'^.+$': {'type': 'array', 'items': {'$ref': '#/definitions/dependency'}}}}, 'dynamic': {'type': 'array', '$$description': ['Specifies which fields are intentionally unspecified and expected to be', 'dynamically provided by build tools'], 'items': {'enum': ['version', 'description', 'readme', 'requires-python', 'license', 'authors', 'maintainers', 'keywords', 'classifiers', 'urls', 'scripts', 'gui-scripts', 'entry-points', 'dependencies', 'optional-dependencies']}}}, 'required': ['name'], 'additionalProperties': False, 'if': {'not': {'required': ['dynamic'], 'properties': {'dynamic': {'contains': {'const': 'version'}, '$$description': ['version is listed in ``dynamic``']}}}, '$$comment': ['According to :pep:`621`:', ' If the core metadata specification lists a field as "Required", then', ' the metadata MUST specify the field statically or list it in dynamic', 'In turn, `core metadata`_ defines:', ' The required fields are: Metadata-Version, Name, Version.', ' All the other fields are optional.', 'Since ``Metadata-Version`` is defined by the build back-end, ``name`` and', '``version`` are the only mandatory information in ``pyproject.toml``.', '.. _core metadata: https://packaging.python.org/specifications/core-metadata/']}, 'then': {'required': ['version'], '$$description': ['version should be statically defined in the ``version`` field']}, 'definitions': {'author': {'$id': '#/definitions/author', 'title': 'Author or Maintainer', '$comment': 'https://www.python.org/dev/peps/pep-0621/#authors-maintainers', 'type': 'object', 'properties': {'name': {'type': 'string', '$$description': ['MUST be a valid email name, i.e. whatever can be put as a name, before an', 'email, in :rfc:`822`.']}, 'email': {'type': 'string', 'format': 'idn-email', 'description': 'MUST be a valid email address'}}}, 'entry-point-group': {'$id': '#/definitions/entry-point-group', 'title': 'Entry-points', 'type': 'object', '$$description': ['Entry-points are grouped together to indicate what sort of capabilities they', 'provide.', 'See the `packaging guides', '`_', 'and `setuptools docs', '`_', 'for more information.'], 'propertyNames': {'format': 'python-entrypoint-name'}, 'additionalProperties': False, 'patternProperties': {'^.+$': {'type': 'string', '$$description': ['Reference to a Python object. It is either in the form', '``importable.module``, or ``importable.module:object.attr``.'], 'format': 'python-entrypoint-reference', '$comment': 'https://packaging.python.org/specifications/entry-points/'}}}, 'dependency': {'$id': '#/definitions/dependency', 'title': 'Dependency', 'type': 'string', 'description': 'Project dependency specification according to PEP 508', 'format': 'pep508'}}}, 'tool': {'type': 'object', 'properties': {'distutils': {'$schema': 'http://json-schema.org/draft-07/schema', '$id': 'https://docs.python.org/3/install/', 'title': '``tool.distutils`` table', '$$description': ['Originally, ``distutils`` allowed developers to configure arguments for', '``setup.py`` scripts via `distutils configuration files', '`_.', '``tool.distutils`` subtables could be used with the same purpose', '(NOT CURRENTLY IMPLEMENTED).'], 'type': 'object', 'properties': {'global': {'type': 'object', 'description': 'Global options applied to all ``distutils`` commands'}}, 'patternProperties': {'.+': {'type': 'object'}}, '$comment': 'TODO: Is there a practical way of making this schema more specific?'}, 'setuptools': {'$schema': 'http://json-schema.org/draft-07/schema', '$id': 'https://setuptools.pypa.io/en/latest/references/keywords.html', 'title': '``tool.setuptools`` table', '$$description': ['Please notice for the time being the ``setuptools`` project does not specify', 'a way of configuring builds via ``pyproject.toml``.', 'Therefore this schema should be taken just as a *"thought experiment"* on how', 'this *might be done*, by following the principles established in', '`ini2toml `_.', 'It considers only ``setuptools`` `parameters', '`_', 'that can currently be configured via ``setup.cfg`` and are not covered by :pep:`621`', 'but intentionally excludes ``dependency_links`` and ``setup_requires``.', 'NOTE: ``scripts`` was renamed to ``script-files`` to avoid confusion with', 'entry-point based scripts (defined in :pep:`621`).'], 'type': 'object', 'additionalProperties': False, 'properties': {'platforms': {'type': 'array', 'items': {'type': 'string'}}, 'provides': {'$$description': ['Package and virtual package names contained within this package', '**(not supported by pip)**'], 'type': 'array', 'items': {'type': 'string', 'format': 'pep508-identifier'}}, 'obsoletes': {'$$description': ['Packages which this package renders obsolete', '**(not supported by pip)**'], 'type': 'array', 'items': {'type': 'string', 'format': 'pep508-identifier'}}, 'zip-safe': {'description': 'Whether the project can be safely installed and run from a zip file.', 'type': 'boolean'}, 'script-files': {'description': 'Legacy way of defining scripts (entry-points are preferred).', 'type': 'array', 'items': {'type': 'string'}, '$comment': 'TODO: is this field deprecated/should be removed?'}, 'eager-resources': {'$$description': ['Resources that should be extracted together, if any of them is needed,', 'or if any C extensions included in the project are imported.'], 'type': 'array', 'items': {'type': 'string'}}, 'packages': {'$$description': ['Packages that should be included in the distribution.', 'It can be given either as a list of package identifiers', 'or as a ``dict``-like structure with a single key ``find``', 'which corresponds to a dynamic call to', '``setuptools.config.expand.find_packages`` function.', 'The ``find`` key is associated with a nested ``dict``-like structure that can', 'contain ``where``, ``include``, ``exclude`` and ``namespaces`` keys,', 'mimicking the keyword arguments of the associated function.'], 'oneOf': [{'title': 'Array of Python package identifiers', 'type': 'array', 'items': {'type': 'string', 'format': 'python-module-name'}}, {'$ref': '#/definitions/find-directive'}]}, 'package-dir': {'$$description': [':class:`dict`-like structure mapping from package names to directories where their', 'code can be found.', 'The empty string (as key) means that all packages are contained inside', 'the given directory will be included in the distribution.'], 'type': 'object', 'additionalProperties': False, 'propertyNames': {'oneOf': [{'format': 'python-module-name'}, {'const': ''}]}, 'patternProperties': {'^.*$': {'type': 'string'}}}, 'package-data': {'$$description': ['Mapping from package names to lists of glob patterns.', 'Usually this option is not needed when using ``include-package-data = true``', 'For more information on how to include data files, check ``setuptools`` `docs', '`_.'], 'type': 'object', 'additionalProperties': False, 'propertyNames': {'oneOf': [{'format': 'python-module-name'}, {'const': '*'}]}, 'patternProperties': {'^.*$': {'type': 'array', 'items': {'type': 'string'}}}}, 'include-package-data': {'$$description': ['Automatically include any data files inside the package directories', 'that are specified by ``MANIFEST.in``', 'For more information on how to include data files, check ``setuptools`` `docs', '`_.'], 'type': 'boolean'}, 'exclude-package-data': {'$$description': ['Mapping from package names to lists of glob patterns that should be excluded', 'For more information on how to include data files, check ``setuptools`` `docs', '`_.'], 'type': 'object', 'additionalProperties': False, 'propertyNames': {'oneOf': [{'format': 'python-module-name'}, {'const': '*'}]}, 'patternProperties': {'^.*$': {'type': 'array', 'items': {'type': 'string'}}}}, 'namespace-packages': {'type': 'array', 'items': {'type': 'string', 'format': 'python-module-name'}, '$comment': 'https://setuptools.pypa.io/en/latest/userguide/package_discovery.html'}, 'py-modules': {'description': 'Modules that setuptools will manipulate', 'type': 'array', 'items': {'type': 'string', 'format': 'python-module-name'}, '$comment': 'TODO: clarify the relationship with ``packages``'}, 'data-files': {'$$description': ['**DEPRECATED**: dict-like structure where each key represents a directory and', 'the value is a list of glob patterns that should be installed in them.', "Please notice this don't work with wheels. See `data files support", '`_'], 'type': 'object', 'patternProperties': {'^.*$': {'type': 'array', 'items': {'type': 'string'}}}}, 'cmdclass': {'$$description': ['Mapping of distutils-style command names to ``setuptools.Command`` subclasses', 'which in turn should be represented by strings with a qualified class name', '(i.e., "dotted" form with module), e.g.::\n\n', ' cmdclass = {mycmd = "pkg.subpkg.module.CommandClass"}\n\n', 'The command class should be a directly defined at the top-level of the', 'containing module (no class nesting).'], 'type': 'object', 'patternProperties': {'^.*$': {'type': 'string', 'format': 'python-qualified-identifier'}}}, 'license-files': {'type': 'array', 'items': {'type': 'string'}, '$$description': ['PROVISIONAL: List of glob patterns for all license files being distributed.', '(might become standard with PEP 639).'], 'default': ['LICEN[CS]E*', ' COPYING*', ' NOTICE*', 'AUTHORS*'], '$comment': 'TODO: revise if PEP 639 is accepted. Probably ``project.license-files``?'}, 'dynamic': {'type': 'object', 'description': 'Instructions for loading :pep:`621`-related metadata dynamically', 'additionalProperties': False, 'properties': {'version': {'$$description': ['A version dynamically loaded via either the ``attr:`` or ``file:``', 'directives. Please make sure the given file or attribute respects :pep:`440`.'], 'oneOf': [{'$ref': '#/definitions/attr-directive'}, {'$ref': '#/definitions/file-directive'}]}, 'classifiers': {'$ref': '#/definitions/file-directive'}, 'description': {'$ref': '#/definitions/file-directive'}, 'dependencies': {'$ref': '#/definitions/file-directive'}, 'entry-points': {'$ref': '#/definitions/file-directive'}, 'optional-dependencies': {'type': 'object', 'propertyNames': {'format': 'python-identifier'}, 'additionalProperties': False, 'patternProperties': {'.+': {'$ref': '#/definitions/file-directive'}}}, 'readme': {'anyOf': [{'$ref': '#/definitions/file-directive'}, {'properties': {'content-type': {'type': 'string'}}}], 'required': ['file']}}}}, 'definitions': {'file-directive': {'$id': '#/definitions/file-directive', 'title': "'file:' directive", 'description': 'Value is read from a file (or list of files and then concatenated)', 'type': 'object', 'additionalProperties': False, 'properties': {'file': {'oneOf': [{'type': 'string'}, {'type': 'array', 'items': {'type': 'string'}}]}}, 'required': ['file']}, 'attr-directive': {'title': "'attr:' directive", '$id': '#/definitions/attr-directive', '$$description': ['Value is read from a module attribute. Supports callables and iterables;', 'unsupported types are cast via ``str()``'], 'type': 'object', 'additionalProperties': False, 'properties': {'attr': {'type': 'string'}}, 'required': ['attr']}, 'find-directive': {'$id': '#/definitions/find-directive', 'title': "'find:' directive", 'type': 'object', 'additionalProperties': False, 'properties': {'find': {'type': 'object', '$$description': ['Dynamic `package discovery', '`_.'], 'additionalProperties': False, 'properties': {'where': {'description': 'Directories to be searched for packages (Unix-style relative path)', 'type': 'array', 'items': {'type': 'string'}}, 'exclude': {'type': 'array', '$$description': ['Exclude packages that match the values listed in this field.', "Can container shell-style wildcards (e.g. ``'pkg.*'``)"], 'items': {'type': 'string'}}, 'include': {'type': 'array', '$$description': ['Restrict the found packages to just the ones listed in this field.', "Can container shell-style wildcards (e.g. ``'pkg.*'``)"], 'items': {'type': 'string'}}, 'namespaces': {'type': 'boolean', '$$description': ['When ``True``, directories without a ``__init__.py`` file will also', 'be scanned for :pep:`420`-style implicit namespaces']}}}}}}}}}}, 'project': {'$schema': 'http://json-schema.org/draft-07/schema', '$id': 'https://packaging.python.org/en/latest/specifications/declaring-project-metadata/', 'title': 'Package metadata stored in the ``project`` table', '$$description': ['Data structure for the **project** table inside ``pyproject.toml``', '(as initially defined in :pep:`621`)'], 'type': 'object', 'properties': {'name': {'type': 'string', 'description': 'The name (primary identifier) of the project. MUST be statically defined.', 'format': 'pep508-identifier'}, 'version': {'type': 'string', 'description': 'The version of the project as supported by :pep:`440`.', 'format': 'pep440'}, 'description': {'type': 'string', '$$description': ['The `summary description of the project', '`_']}, 'readme': {'$$description': ['`Full/detailed description of the project in the form of a README', '`_', "with meaning similar to the one defined in `core metadata's Description", '`_'], 'oneOf': [{'type': 'string', '$$description': ['Relative path to a text file (UTF-8) containing the full description', 'of the project. If the file path ends in case-insensitive ``.md`` or', '``.rst`` suffixes, then the content-type is respectively', '``text/markdown`` or ``text/x-rst``']}, {'type': 'object', 'allOf': [{'anyOf': [{'properties': {'file': {'type': 'string', '$$description': ['Relative path to a text file containing the full description', 'of the project.']}}, 'required': ['file']}, {'properties': {'text': {'type': 'string', 'description': 'Full text describing the project.'}}, 'required': ['text']}]}, {'properties': {'content-type': {'type': 'string', '$$description': ['Content-type (:rfc:`1341`) of the full description', '(e.g. ``text/markdown``). The ``charset`` parameter is assumed', 'UTF-8 when not present.'], '$comment': 'TODO: add regex pattern or format?'}}, 'required': ['content-type']}]}]}, 'requires-python': {'type': 'string', 'format': 'pep508-versionspec', '$$description': ['`The Python version requirements of the project', '`_.']}, 'license': {'description': '`Project license `_.', 'oneOf': [{'properties': {'file': {'type': 'string', '$$description': ['Relative path to the file (UTF-8) which contains the license for the', 'project.']}}, 'required': ['file']}, {'properties': {'text': {'type': 'string', '$$description': ['The license of the project whose meaning is that of the', '`License field from the core metadata', '`_.']}}, 'required': ['text']}]}, 'authors': {'type': 'array', 'items': {'$ref': '#/definitions/author'}, '$$description': ["The people or organizations considered to be the 'authors' of the project.", 'The exact meaning is open to interpretation (e.g. original or primary authors,', 'current maintainers, or owners of the package).']}, 'maintainers': {'type': 'array', 'items': {'$ref': '#/definitions/author'}, '$$description': ["The people or organizations considered to be the 'maintainers' of the project.", 'Similarly to ``authors``, the exact meaning is open to interpretation.']}, 'keywords': {'type': 'array', 'items': {'type': 'string'}, 'description': 'List of keywords to assist searching for the distribution in a larger catalog.'}, 'classifiers': {'type': 'array', 'items': {'type': 'string', 'format': 'trove-classifier', 'description': '`PyPI classifier `_.'}, '$$description': ['`Trove classifiers `_', 'which apply to the project.']}, 'urls': {'type': 'object', 'description': 'URLs associated with the project in the form ``label => value``.', 'additionalProperties': False, 'patternProperties': {'^.+$': {'type': 'string', 'format': 'url'}}}, 'scripts': {'$ref': '#/definitions/entry-point-group', '$$description': ['Instruct the installer to create command-line wrappers for the given', '`entry points `_.']}, 'gui-scripts': {'$ref': '#/definitions/entry-point-group', '$$description': ['Instruct the installer to create GUI wrappers for the given', '`entry points `_.', 'The difference between ``scripts`` and ``gui-scripts`` is only relevant in', 'Windows.']}, 'entry-points': {'$$description': ['Instruct the installer to expose the given modules/functions via', '``entry-point`` discovery mechanism (useful for plugins).', 'More information available in the `Python packaging guide', '`_.'], 'propertyNames': {'format': 'python-entrypoint-group'}, 'additionalProperties': False, 'patternProperties': {'^.+$': {'$ref': '#/definitions/entry-point-group'}}}, 'dependencies': {'type': 'array', 'description': 'Project (mandatory) dependencies.', 'items': {'$ref': '#/definitions/dependency'}}, 'optional-dependencies': {'type': 'object', 'description': 'Optional dependency for the project', 'propertyNames': {'format': 'pep508-identifier'}, 'additionalProperties': False, 'patternProperties': {'^.+$': {'type': 'array', 'items': {'$ref': '#/definitions/dependency'}}}}, 'dynamic': {'type': 'array', '$$description': ['Specifies which fields are intentionally unspecified and expected to be', 'dynamically provided by build tools'], 'items': {'enum': ['version', 'description', 'readme', 'requires-python', 'license', 'authors', 'maintainers', 'keywords', 'classifiers', 'urls', 'scripts', 'gui-scripts', 'entry-points', 'dependencies', 'optional-dependencies']}}}, 'required': ['name'], 'additionalProperties': False, 'if': {'not': {'required': ['dynamic'], 'properties': {'dynamic': {'contains': {'const': 'version'}, '$$description': ['version is listed in ``dynamic``']}}}, '$$comment': ['According to :pep:`621`:', ' If the core metadata specification lists a field as "Required", then', ' the metadata MUST specify the field statically or list it in dynamic', 'In turn, `core metadata`_ defines:', ' The required fields are: Metadata-Version, Name, Version.', ' All the other fields are optional.', 'Since ``Metadata-Version`` is defined by the build back-end, ``name`` and', '``version`` are the only mandatory information in ``pyproject.toml``.', '.. _core metadata: https://packaging.python.org/specifications/core-metadata/']}, 'then': {'required': ['version'], '$$description': ['version should be statically defined in the ``version`` field']}, 'definitions': {'author': {'$id': '#/definitions/author', 'title': 'Author or Maintainer', '$comment': 'https://www.python.org/dev/peps/pep-0621/#authors-maintainers', 'type': 'object', 'properties': {'name': {'type': 'string', '$$description': ['MUST be a valid email name, i.e. whatever can be put as a name, before an', 'email, in :rfc:`822`.']}, 'email': {'type': 'string', 'format': 'idn-email', 'description': 'MUST be a valid email address'}}}, 'entry-point-group': {'$id': '#/definitions/entry-point-group', 'title': 'Entry-points', 'type': 'object', '$$description': ['Entry-points are grouped together to indicate what sort of capabilities they', 'provide.', 'See the `packaging guides', '`_', 'and `setuptools docs', '`_', 'for more information.'], 'propertyNames': {'format': 'python-entrypoint-name'}, 'additionalProperties': False, 'patternProperties': {'^.+$': {'type': 'string', '$$description': ['Reference to a Python object. It is either in the form', '``importable.module``, or ``importable.module:object.attr``.'], 'format': 'python-entrypoint-reference', '$comment': 'https://packaging.python.org/specifications/entry-points/'}}}, 'dependency': {'$id': '#/definitions/dependency', 'title': 'Dependency', 'type': 'string', 'description': 'Project dependency specification according to PEP 508', 'format': 'pep508'}}}}, rule='type')
- data_is_dict = isinstance(data, dict)
- if data_is_dict:
- data_keys = set(data.keys())
- if "build-system" in data_keys:
- data_keys.remove("build-system")
- data__buildsystem = data["build-system"]
- if not isinstance(data__buildsystem, (dict)):
- raise JsonSchemaValueException("" + (name_prefix or "data") + ".build-system must be object", value=data__buildsystem, name="" + (name_prefix or "data") + ".build-system", definition={'type': 'object', 'description': 'Table used to store build-related data', 'additionalProperties': False, 'properties': {'requires': {'type': 'array', '$$description': ['List of dependencies in the :pep:`508` format required to execute the build', 'system. Please notice that the resulting dependency graph', '**MUST NOT contain cycles**'], 'items': {'type': 'string'}}, 'build-backend': {'type': 'string', 'description': 'Python object that will be used to perform the build according to :pep:`517`', 'format': 'pep517-backend-reference'}, 'backend-path': {'type': 'array', '$$description': ['List of directories to be prepended to ``sys.path`` when loading the', 'back-end, and running its hooks'], 'items': {'type': 'string', '$comment': 'Should be a path (TODO: enforce it with format?)'}}}, 'required': ['requires']}, rule='type')
- data__buildsystem_is_dict = isinstance(data__buildsystem, dict)
- if data__buildsystem_is_dict:
- data__buildsystem_len = len(data__buildsystem)
- if not all(prop in data__buildsystem for prop in ['requires']):
- raise JsonSchemaValueException("" + (name_prefix or "data") + ".build-system must contain ['requires'] properties", value=data__buildsystem, name="" + (name_prefix or "data") + ".build-system", definition={'type': 'object', 'description': 'Table used to store build-related data', 'additionalProperties': False, 'properties': {'requires': {'type': 'array', '$$description': ['List of dependencies in the :pep:`508` format required to execute the build', 'system. Please notice that the resulting dependency graph', '**MUST NOT contain cycles**'], 'items': {'type': 'string'}}, 'build-backend': {'type': 'string', 'description': 'Python object that will be used to perform the build according to :pep:`517`', 'format': 'pep517-backend-reference'}, 'backend-path': {'type': 'array', '$$description': ['List of directories to be prepended to ``sys.path`` when loading the', 'back-end, and running its hooks'], 'items': {'type': 'string', '$comment': 'Should be a path (TODO: enforce it with format?)'}}}, 'required': ['requires']}, rule='required')
- data__buildsystem_keys = set(data__buildsystem.keys())
- if "requires" in data__buildsystem_keys:
- data__buildsystem_keys.remove("requires")
- data__buildsystem__requires = data__buildsystem["requires"]
- if not isinstance(data__buildsystem__requires, (list, tuple)):
- raise JsonSchemaValueException("" + (name_prefix or "data") + ".build-system.requires must be array", value=data__buildsystem__requires, name="" + (name_prefix or "data") + ".build-system.requires", definition={'type': 'array', '$$description': ['List of dependencies in the :pep:`508` format required to execute the build', 'system. Please notice that the resulting dependency graph', '**MUST NOT contain cycles**'], 'items': {'type': 'string'}}, rule='type')
- data__buildsystem__requires_is_list = isinstance(data__buildsystem__requires, (list, tuple))
- if data__buildsystem__requires_is_list:
- data__buildsystem__requires_len = len(data__buildsystem__requires)
- for data__buildsystem__requires_x, data__buildsystem__requires_item in enumerate(data__buildsystem__requires):
- if not isinstance(data__buildsystem__requires_item, (str)):
- raise JsonSchemaValueException("" + (name_prefix or "data") + ".build-system.requires[{data__buildsystem__requires_x}]".format(**locals()) + " must be string", value=data__buildsystem__requires_item, name="" + (name_prefix or "data") + ".build-system.requires[{data__buildsystem__requires_x}]".format(**locals()) + "", definition={'type': 'string'}, rule='type')
- if "build-backend" in data__buildsystem_keys:
- data__buildsystem_keys.remove("build-backend")
- data__buildsystem__buildbackend = data__buildsystem["build-backend"]
- if not isinstance(data__buildsystem__buildbackend, (str)):
- raise JsonSchemaValueException("" + (name_prefix or "data") + ".build-system.build-backend must be string", value=data__buildsystem__buildbackend, name="" + (name_prefix or "data") + ".build-system.build-backend", definition={'type': 'string', 'description': 'Python object that will be used to perform the build according to :pep:`517`', 'format': 'pep517-backend-reference'}, rule='type')
- if isinstance(data__buildsystem__buildbackend, str):
- if not custom_formats["pep517-backend-reference"](data__buildsystem__buildbackend):
- raise JsonSchemaValueException("" + (name_prefix or "data") + ".build-system.build-backend must be pep517-backend-reference", value=data__buildsystem__buildbackend, name="" + (name_prefix or "data") + ".build-system.build-backend", definition={'type': 'string', 'description': 'Python object that will be used to perform the build according to :pep:`517`', 'format': 'pep517-backend-reference'}, rule='format')
- if "backend-path" in data__buildsystem_keys:
- data__buildsystem_keys.remove("backend-path")
- data__buildsystem__backendpath = data__buildsystem["backend-path"]
- if not isinstance(data__buildsystem__backendpath, (list, tuple)):
- raise JsonSchemaValueException("" + (name_prefix or "data") + ".build-system.backend-path must be array", value=data__buildsystem__backendpath, name="" + (name_prefix or "data") + ".build-system.backend-path", definition={'type': 'array', '$$description': ['List of directories to be prepended to ``sys.path`` when loading the', 'back-end, and running its hooks'], 'items': {'type': 'string', '$comment': 'Should be a path (TODO: enforce it with format?)'}}, rule='type')
- data__buildsystem__backendpath_is_list = isinstance(data__buildsystem__backendpath, (list, tuple))
- if data__buildsystem__backendpath_is_list:
- data__buildsystem__backendpath_len = len(data__buildsystem__backendpath)
- for data__buildsystem__backendpath_x, data__buildsystem__backendpath_item in enumerate(data__buildsystem__backendpath):
- if not isinstance(data__buildsystem__backendpath_item, (str)):
- raise JsonSchemaValueException("" + (name_prefix or "data") + ".build-system.backend-path[{data__buildsystem__backendpath_x}]".format(**locals()) + " must be string", value=data__buildsystem__backendpath_item, name="" + (name_prefix or "data") + ".build-system.backend-path[{data__buildsystem__backendpath_x}]".format(**locals()) + "", definition={'type': 'string', '$comment': 'Should be a path (TODO: enforce it with format?)'}, rule='type')
- if data__buildsystem_keys:
- raise JsonSchemaValueException("" + (name_prefix or "data") + ".build-system must not contain "+str(data__buildsystem_keys)+" properties", value=data__buildsystem, name="" + (name_prefix or "data") + ".build-system", definition={'type': 'object', 'description': 'Table used to store build-related data', 'additionalProperties': False, 'properties': {'requires': {'type': 'array', '$$description': ['List of dependencies in the :pep:`508` format required to execute the build', 'system. Please notice that the resulting dependency graph', '**MUST NOT contain cycles**'], 'items': {'type': 'string'}}, 'build-backend': {'type': 'string', 'description': 'Python object that will be used to perform the build according to :pep:`517`', 'format': 'pep517-backend-reference'}, 'backend-path': {'type': 'array', '$$description': ['List of directories to be prepended to ``sys.path`` when loading the', 'back-end, and running its hooks'], 'items': {'type': 'string', '$comment': 'Should be a path (TODO: enforce it with format?)'}}}, 'required': ['requires']}, rule='additionalProperties')
- if "project" in data_keys:
- data_keys.remove("project")
- data__project = data["project"]
- validate_https___packaging_python_org_en_latest_specifications_declaring_project_metadata(data__project, custom_formats, (name_prefix or "data") + ".project")
- if "tool" in data_keys:
- data_keys.remove("tool")
- data__tool = data["tool"]
- if not isinstance(data__tool, (dict)):
- raise JsonSchemaValueException("" + (name_prefix or "data") + ".tool must be object", value=data__tool, name="" + (name_prefix or "data") + ".tool", definition={'type': 'object', 'properties': {'distutils': {'$schema': 'http://json-schema.org/draft-07/schema', '$id': 'https://docs.python.org/3/install/', 'title': '``tool.distutils`` table', '$$description': ['Originally, ``distutils`` allowed developers to configure arguments for', '``setup.py`` scripts via `distutils configuration files', '`_.', '``tool.distutils`` subtables could be used with the same purpose', '(NOT CURRENTLY IMPLEMENTED).'], 'type': 'object', 'properties': {'global': {'type': 'object', 'description': 'Global options applied to all ``distutils`` commands'}}, 'patternProperties': {'.+': {'type': 'object'}}, '$comment': 'TODO: Is there a practical way of making this schema more specific?'}, 'setuptools': {'$schema': 'http://json-schema.org/draft-07/schema', '$id': 'https://setuptools.pypa.io/en/latest/references/keywords.html', 'title': '``tool.setuptools`` table', '$$description': ['Please notice for the time being the ``setuptools`` project does not specify', 'a way of configuring builds via ``pyproject.toml``.', 'Therefore this schema should be taken just as a *"thought experiment"* on how', 'this *might be done*, by following the principles established in', '`ini2toml `_.', 'It considers only ``setuptools`` `parameters', '`_', 'that can currently be configured via ``setup.cfg`` and are not covered by :pep:`621`', 'but intentionally excludes ``dependency_links`` and ``setup_requires``.', 'NOTE: ``scripts`` was renamed to ``script-files`` to avoid confusion with', 'entry-point based scripts (defined in :pep:`621`).'], 'type': 'object', 'additionalProperties': False, 'properties': {'platforms': {'type': 'array', 'items': {'type': 'string'}}, 'provides': {'$$description': ['Package and virtual package names contained within this package', '**(not supported by pip)**'], 'type': 'array', 'items': {'type': 'string', 'format': 'pep508-identifier'}}, 'obsoletes': {'$$description': ['Packages which this package renders obsolete', '**(not supported by pip)**'], 'type': 'array', 'items': {'type': 'string', 'format': 'pep508-identifier'}}, 'zip-safe': {'description': 'Whether the project can be safely installed and run from a zip file.', 'type': 'boolean'}, 'script-files': {'description': 'Legacy way of defining scripts (entry-points are preferred).', 'type': 'array', 'items': {'type': 'string'}, '$comment': 'TODO: is this field deprecated/should be removed?'}, 'eager-resources': {'$$description': ['Resources that should be extracted together, if any of them is needed,', 'or if any C extensions included in the project are imported.'], 'type': 'array', 'items': {'type': 'string'}}, 'packages': {'$$description': ['Packages that should be included in the distribution.', 'It can be given either as a list of package identifiers', 'or as a ``dict``-like structure with a single key ``find``', 'which corresponds to a dynamic call to', '``setuptools.config.expand.find_packages`` function.', 'The ``find`` key is associated with a nested ``dict``-like structure that can', 'contain ``where``, ``include``, ``exclude`` and ``namespaces`` keys,', 'mimicking the keyword arguments of the associated function.'], 'oneOf': [{'title': 'Array of Python package identifiers', 'type': 'array', 'items': {'type': 'string', 'format': 'python-module-name'}}, {'$ref': '#/definitions/find-directive'}]}, 'package-dir': {'$$description': [':class:`dict`-like structure mapping from package names to directories where their', 'code can be found.', 'The empty string (as key) means that all packages are contained inside', 'the given directory will be included in the distribution.'], 'type': 'object', 'additionalProperties': False, 'propertyNames': {'oneOf': [{'format': 'python-module-name'}, {'const': ''}]}, 'patternProperties': {'^.*$': {'type': 'string'}}}, 'package-data': {'$$description': ['Mapping from package names to lists of glob patterns.', 'Usually this option is not needed when using ``include-package-data = true``', 'For more information on how to include data files, check ``setuptools`` `docs', '`_.'], 'type': 'object', 'additionalProperties': False, 'propertyNames': {'oneOf': [{'format': 'python-module-name'}, {'const': '*'}]}, 'patternProperties': {'^.*$': {'type': 'array', 'items': {'type': 'string'}}}}, 'include-package-data': {'$$description': ['Automatically include any data files inside the package directories', 'that are specified by ``MANIFEST.in``', 'For more information on how to include data files, check ``setuptools`` `docs', '`_.'], 'type': 'boolean'}, 'exclude-package-data': {'$$description': ['Mapping from package names to lists of glob patterns that should be excluded', 'For more information on how to include data files, check ``setuptools`` `docs', '`_.'], 'type': 'object', 'additionalProperties': False, 'propertyNames': {'oneOf': [{'format': 'python-module-name'}, {'const': '*'}]}, 'patternProperties': {'^.*$': {'type': 'array', 'items': {'type': 'string'}}}}, 'namespace-packages': {'type': 'array', 'items': {'type': 'string', 'format': 'python-module-name'}, '$comment': 'https://setuptools.pypa.io/en/latest/userguide/package_discovery.html'}, 'py-modules': {'description': 'Modules that setuptools will manipulate', 'type': 'array', 'items': {'type': 'string', 'format': 'python-module-name'}, '$comment': 'TODO: clarify the relationship with ``packages``'}, 'data-files': {'$$description': ['**DEPRECATED**: dict-like structure where each key represents a directory and', 'the value is a list of glob patterns that should be installed in them.', "Please notice this don't work with wheels. See `data files support", '`_'], 'type': 'object', 'patternProperties': {'^.*$': {'type': 'array', 'items': {'type': 'string'}}}}, 'cmdclass': {'$$description': ['Mapping of distutils-style command names to ``setuptools.Command`` subclasses', 'which in turn should be represented by strings with a qualified class name', '(i.e., "dotted" form with module), e.g.::\n\n', ' cmdclass = {mycmd = "pkg.subpkg.module.CommandClass"}\n\n', 'The command class should be a directly defined at the top-level of the', 'containing module (no class nesting).'], 'type': 'object', 'patternProperties': {'^.*$': {'type': 'string', 'format': 'python-qualified-identifier'}}}, 'license-files': {'type': 'array', 'items': {'type': 'string'}, '$$description': ['PROVISIONAL: List of glob patterns for all license files being distributed.', '(might become standard with PEP 639).'], 'default': ['LICEN[CS]E*', ' COPYING*', ' NOTICE*', 'AUTHORS*'], '$comment': 'TODO: revise if PEP 639 is accepted. Probably ``project.license-files``?'}, 'dynamic': {'type': 'object', 'description': 'Instructions for loading :pep:`621`-related metadata dynamically', 'additionalProperties': False, 'properties': {'version': {'$$description': ['A version dynamically loaded via either the ``attr:`` or ``file:``', 'directives. Please make sure the given file or attribute respects :pep:`440`.'], 'oneOf': [{'$ref': '#/definitions/attr-directive'}, {'$ref': '#/definitions/file-directive'}]}, 'classifiers': {'$ref': '#/definitions/file-directive'}, 'description': {'$ref': '#/definitions/file-directive'}, 'dependencies': {'$ref': '#/definitions/file-directive'}, 'entry-points': {'$ref': '#/definitions/file-directive'}, 'optional-dependencies': {'type': 'object', 'propertyNames': {'format': 'python-identifier'}, 'additionalProperties': False, 'patternProperties': {'.+': {'$ref': '#/definitions/file-directive'}}}, 'readme': {'anyOf': [{'$ref': '#/definitions/file-directive'}, {'properties': {'content-type': {'type': 'string'}}}], 'required': ['file']}}}}, 'definitions': {'file-directive': {'$id': '#/definitions/file-directive', 'title': "'file:' directive", 'description': 'Value is read from a file (or list of files and then concatenated)', 'type': 'object', 'additionalProperties': False, 'properties': {'file': {'oneOf': [{'type': 'string'}, {'type': 'array', 'items': {'type': 'string'}}]}}, 'required': ['file']}, 'attr-directive': {'title': "'attr:' directive", '$id': '#/definitions/attr-directive', '$$description': ['Value is read from a module attribute. Supports callables and iterables;', 'unsupported types are cast via ``str()``'], 'type': 'object', 'additionalProperties': False, 'properties': {'attr': {'type': 'string'}}, 'required': ['attr']}, 'find-directive': {'$id': '#/definitions/find-directive', 'title': "'find:' directive", 'type': 'object', 'additionalProperties': False, 'properties': {'find': {'type': 'object', '$$description': ['Dynamic `package discovery', '`_.'], 'additionalProperties': False, 'properties': {'where': {'description': 'Directories to be searched for packages (Unix-style relative path)', 'type': 'array', 'items': {'type': 'string'}}, 'exclude': {'type': 'array', '$$description': ['Exclude packages that match the values listed in this field.', "Can container shell-style wildcards (e.g. ``'pkg.*'``)"], 'items': {'type': 'string'}}, 'include': {'type': 'array', '$$description': ['Restrict the found packages to just the ones listed in this field.', "Can container shell-style wildcards (e.g. ``'pkg.*'``)"], 'items': {'type': 'string'}}, 'namespaces': {'type': 'boolean', '$$description': ['When ``True``, directories without a ``__init__.py`` file will also', 'be scanned for :pep:`420`-style implicit namespaces']}}}}}}}}}, rule='type')
- data__tool_is_dict = isinstance(data__tool, dict)
- if data__tool_is_dict:
- data__tool_keys = set(data__tool.keys())
- if "distutils" in data__tool_keys:
- data__tool_keys.remove("distutils")
- data__tool__distutils = data__tool["distutils"]
- validate_https___docs_python_org_3_install(data__tool__distutils, custom_formats, (name_prefix or "data") + ".tool.distutils")
- if "setuptools" in data__tool_keys:
- data__tool_keys.remove("setuptools")
- data__tool__setuptools = data__tool["setuptools"]
- validate_https___setuptools_pypa_io_en_latest_references_keywords_html(data__tool__setuptools, custom_formats, (name_prefix or "data") + ".tool.setuptools")
- if data_keys:
- raise JsonSchemaValueException("" + (name_prefix or "data") + " must not contain "+str(data_keys)+" properties", value=data, name="" + (name_prefix or "data") + "", definition={'$schema': 'http://json-schema.org/draft-07/schema', '$id': 'https://packaging.python.org/en/latest/specifications/declaring-build-dependencies/', 'title': 'Data structure for ``pyproject.toml`` files', '$$description': ['File format containing build-time configurations for the Python ecosystem. ', ':pep:`517` initially defined a build-system independent format for source trees', 'which was complemented by :pep:`518` to provide a way of specifying dependencies ', 'for building Python projects.', 'Please notice the ``project`` table (as initially defined in :pep:`621`) is not included', 'in this schema and should be considered separately.'], 'type': 'object', 'additionalProperties': False, 'properties': {'build-system': {'type': 'object', 'description': 'Table used to store build-related data', 'additionalProperties': False, 'properties': {'requires': {'type': 'array', '$$description': ['List of dependencies in the :pep:`508` format required to execute the build', 'system. Please notice that the resulting dependency graph', '**MUST NOT contain cycles**'], 'items': {'type': 'string'}}, 'build-backend': {'type': 'string', 'description': 'Python object that will be used to perform the build according to :pep:`517`', 'format': 'pep517-backend-reference'}, 'backend-path': {'type': 'array', '$$description': ['List of directories to be prepended to ``sys.path`` when loading the', 'back-end, and running its hooks'], 'items': {'type': 'string', '$comment': 'Should be a path (TODO: enforce it with format?)'}}}, 'required': ['requires']}, 'project': {'$schema': 'http://json-schema.org/draft-07/schema', '$id': 'https://packaging.python.org/en/latest/specifications/declaring-project-metadata/', 'title': 'Package metadata stored in the ``project`` table', '$$description': ['Data structure for the **project** table inside ``pyproject.toml``', '(as initially defined in :pep:`621`)'], 'type': 'object', 'properties': {'name': {'type': 'string', 'description': 'The name (primary identifier) of the project. MUST be statically defined.', 'format': 'pep508-identifier'}, 'version': {'type': 'string', 'description': 'The version of the project as supported by :pep:`440`.', 'format': 'pep440'}, 'description': {'type': 'string', '$$description': ['The `summary description of the project', '`_']}, 'readme': {'$$description': ['`Full/detailed description of the project in the form of a README', '`_', "with meaning similar to the one defined in `core metadata's Description", '`_'], 'oneOf': [{'type': 'string', '$$description': ['Relative path to a text file (UTF-8) containing the full description', 'of the project. If the file path ends in case-insensitive ``.md`` or', '``.rst`` suffixes, then the content-type is respectively', '``text/markdown`` or ``text/x-rst``']}, {'type': 'object', 'allOf': [{'anyOf': [{'properties': {'file': {'type': 'string', '$$description': ['Relative path to a text file containing the full description', 'of the project.']}}, 'required': ['file']}, {'properties': {'text': {'type': 'string', 'description': 'Full text describing the project.'}}, 'required': ['text']}]}, {'properties': {'content-type': {'type': 'string', '$$description': ['Content-type (:rfc:`1341`) of the full description', '(e.g. ``text/markdown``). The ``charset`` parameter is assumed', 'UTF-8 when not present.'], '$comment': 'TODO: add regex pattern or format?'}}, 'required': ['content-type']}]}]}, 'requires-python': {'type': 'string', 'format': 'pep508-versionspec', '$$description': ['`The Python version requirements of the project', '`_.']}, 'license': {'description': '`Project license `_.', 'oneOf': [{'properties': {'file': {'type': 'string', '$$description': ['Relative path to the file (UTF-8) which contains the license for the', 'project.']}}, 'required': ['file']}, {'properties': {'text': {'type': 'string', '$$description': ['The license of the project whose meaning is that of the', '`License field from the core metadata', '`_.']}}, 'required': ['text']}]}, 'authors': {'type': 'array', 'items': {'$ref': '#/definitions/author'}, '$$description': ["The people or organizations considered to be the 'authors' of the project.", 'The exact meaning is open to interpretation (e.g. original or primary authors,', 'current maintainers, or owners of the package).']}, 'maintainers': {'type': 'array', 'items': {'$ref': '#/definitions/author'}, '$$description': ["The people or organizations considered to be the 'maintainers' of the project.", 'Similarly to ``authors``, the exact meaning is open to interpretation.']}, 'keywords': {'type': 'array', 'items': {'type': 'string'}, 'description': 'List of keywords to assist searching for the distribution in a larger catalog.'}, 'classifiers': {'type': 'array', 'items': {'type': 'string', 'format': 'trove-classifier', 'description': '`PyPI classifier `_.'}, '$$description': ['`Trove classifiers `_', 'which apply to the project.']}, 'urls': {'type': 'object', 'description': 'URLs associated with the project in the form ``label => value``.', 'additionalProperties': False, 'patternProperties': {'^.+$': {'type': 'string', 'format': 'url'}}}, 'scripts': {'$ref': '#/definitions/entry-point-group', '$$description': ['Instruct the installer to create command-line wrappers for the given', '`entry points `_.']}, 'gui-scripts': {'$ref': '#/definitions/entry-point-group', '$$description': ['Instruct the installer to create GUI wrappers for the given', '`entry points `_.', 'The difference between ``scripts`` and ``gui-scripts`` is only relevant in', 'Windows.']}, 'entry-points': {'$$description': ['Instruct the installer to expose the given modules/functions via', '``entry-point`` discovery mechanism (useful for plugins).', 'More information available in the `Python packaging guide', '`_.'], 'propertyNames': {'format': 'python-entrypoint-group'}, 'additionalProperties': False, 'patternProperties': {'^.+$': {'$ref': '#/definitions/entry-point-group'}}}, 'dependencies': {'type': 'array', 'description': 'Project (mandatory) dependencies.', 'items': {'$ref': '#/definitions/dependency'}}, 'optional-dependencies': {'type': 'object', 'description': 'Optional dependency for the project', 'propertyNames': {'format': 'pep508-identifier'}, 'additionalProperties': False, 'patternProperties': {'^.+$': {'type': 'array', 'items': {'$ref': '#/definitions/dependency'}}}}, 'dynamic': {'type': 'array', '$$description': ['Specifies which fields are intentionally unspecified and expected to be', 'dynamically provided by build tools'], 'items': {'enum': ['version', 'description', 'readme', 'requires-python', 'license', 'authors', 'maintainers', 'keywords', 'classifiers', 'urls', 'scripts', 'gui-scripts', 'entry-points', 'dependencies', 'optional-dependencies']}}}, 'required': ['name'], 'additionalProperties': False, 'if': {'not': {'required': ['dynamic'], 'properties': {'dynamic': {'contains': {'const': 'version'}, '$$description': ['version is listed in ``dynamic``']}}}, '$$comment': ['According to :pep:`621`:', ' If the core metadata specification lists a field as "Required", then', ' the metadata MUST specify the field statically or list it in dynamic', 'In turn, `core metadata`_ defines:', ' The required fields are: Metadata-Version, Name, Version.', ' All the other fields are optional.', 'Since ``Metadata-Version`` is defined by the build back-end, ``name`` and', '``version`` are the only mandatory information in ``pyproject.toml``.', '.. _core metadata: https://packaging.python.org/specifications/core-metadata/']}, 'then': {'required': ['version'], '$$description': ['version should be statically defined in the ``version`` field']}, 'definitions': {'author': {'$id': '#/definitions/author', 'title': 'Author or Maintainer', '$comment': 'https://www.python.org/dev/peps/pep-0621/#authors-maintainers', 'type': 'object', 'properties': {'name': {'type': 'string', '$$description': ['MUST be a valid email name, i.e. whatever can be put as a name, before an', 'email, in :rfc:`822`.']}, 'email': {'type': 'string', 'format': 'idn-email', 'description': 'MUST be a valid email address'}}}, 'entry-point-group': {'$id': '#/definitions/entry-point-group', 'title': 'Entry-points', 'type': 'object', '$$description': ['Entry-points are grouped together to indicate what sort of capabilities they', 'provide.', 'See the `packaging guides', '`_', 'and `setuptools docs', '`_', 'for more information.'], 'propertyNames': {'format': 'python-entrypoint-name'}, 'additionalProperties': False, 'patternProperties': {'^.+$': {'type': 'string', '$$description': ['Reference to a Python object. It is either in the form', '``importable.module``, or ``importable.module:object.attr``.'], 'format': 'python-entrypoint-reference', '$comment': 'https://packaging.python.org/specifications/entry-points/'}}}, 'dependency': {'$id': '#/definitions/dependency', 'title': 'Dependency', 'type': 'string', 'description': 'Project dependency specification according to PEP 508', 'format': 'pep508'}}}, 'tool': {'type': 'object', 'properties': {'distutils': {'$schema': 'http://json-schema.org/draft-07/schema', '$id': 'https://docs.python.org/3/install/', 'title': '``tool.distutils`` table', '$$description': ['Originally, ``distutils`` allowed developers to configure arguments for', '``setup.py`` scripts via `distutils configuration files', '`_.', '``tool.distutils`` subtables could be used with the same purpose', '(NOT CURRENTLY IMPLEMENTED).'], 'type': 'object', 'properties': {'global': {'type': 'object', 'description': 'Global options applied to all ``distutils`` commands'}}, 'patternProperties': {'.+': {'type': 'object'}}, '$comment': 'TODO: Is there a practical way of making this schema more specific?'}, 'setuptools': {'$schema': 'http://json-schema.org/draft-07/schema', '$id': 'https://setuptools.pypa.io/en/latest/references/keywords.html', 'title': '``tool.setuptools`` table', '$$description': ['Please notice for the time being the ``setuptools`` project does not specify', 'a way of configuring builds via ``pyproject.toml``.', 'Therefore this schema should be taken just as a *"thought experiment"* on how', 'this *might be done*, by following the principles established in', '`ini2toml `_.', 'It considers only ``setuptools`` `parameters', '`_', 'that can currently be configured via ``setup.cfg`` and are not covered by :pep:`621`', 'but intentionally excludes ``dependency_links`` and ``setup_requires``.', 'NOTE: ``scripts`` was renamed to ``script-files`` to avoid confusion with', 'entry-point based scripts (defined in :pep:`621`).'], 'type': 'object', 'additionalProperties': False, 'properties': {'platforms': {'type': 'array', 'items': {'type': 'string'}}, 'provides': {'$$description': ['Package and virtual package names contained within this package', '**(not supported by pip)**'], 'type': 'array', 'items': {'type': 'string', 'format': 'pep508-identifier'}}, 'obsoletes': {'$$description': ['Packages which this package renders obsolete', '**(not supported by pip)**'], 'type': 'array', 'items': {'type': 'string', 'format': 'pep508-identifier'}}, 'zip-safe': {'description': 'Whether the project can be safely installed and run from a zip file.', 'type': 'boolean'}, 'script-files': {'description': 'Legacy way of defining scripts (entry-points are preferred).', 'type': 'array', 'items': {'type': 'string'}, '$comment': 'TODO: is this field deprecated/should be removed?'}, 'eager-resources': {'$$description': ['Resources that should be extracted together, if any of them is needed,', 'or if any C extensions included in the project are imported.'], 'type': 'array', 'items': {'type': 'string'}}, 'packages': {'$$description': ['Packages that should be included in the distribution.', 'It can be given either as a list of package identifiers', 'or as a ``dict``-like structure with a single key ``find``', 'which corresponds to a dynamic call to', '``setuptools.config.expand.find_packages`` function.', 'The ``find`` key is associated with a nested ``dict``-like structure that can', 'contain ``where``, ``include``, ``exclude`` and ``namespaces`` keys,', 'mimicking the keyword arguments of the associated function.'], 'oneOf': [{'title': 'Array of Python package identifiers', 'type': 'array', 'items': {'type': 'string', 'format': 'python-module-name'}}, {'$ref': '#/definitions/find-directive'}]}, 'package-dir': {'$$description': [':class:`dict`-like structure mapping from package names to directories where their', 'code can be found.', 'The empty string (as key) means that all packages are contained inside', 'the given directory will be included in the distribution.'], 'type': 'object', 'additionalProperties': False, 'propertyNames': {'oneOf': [{'format': 'python-module-name'}, {'const': ''}]}, 'patternProperties': {'^.*$': {'type': 'string'}}}, 'package-data': {'$$description': ['Mapping from package names to lists of glob patterns.', 'Usually this option is not needed when using ``include-package-data = true``', 'For more information on how to include data files, check ``setuptools`` `docs', '`_.'], 'type': 'object', 'additionalProperties': False, 'propertyNames': {'oneOf': [{'format': 'python-module-name'}, {'const': '*'}]}, 'patternProperties': {'^.*$': {'type': 'array', 'items': {'type': 'string'}}}}, 'include-package-data': {'$$description': ['Automatically include any data files inside the package directories', 'that are specified by ``MANIFEST.in``', 'For more information on how to include data files, check ``setuptools`` `docs', '`_.'], 'type': 'boolean'}, 'exclude-package-data': {'$$description': ['Mapping from package names to lists of glob patterns that should be excluded', 'For more information on how to include data files, check ``setuptools`` `docs', '`_.'], 'type': 'object', 'additionalProperties': False, 'propertyNames': {'oneOf': [{'format': 'python-module-name'}, {'const': '*'}]}, 'patternProperties': {'^.*$': {'type': 'array', 'items': {'type': 'string'}}}}, 'namespace-packages': {'type': 'array', 'items': {'type': 'string', 'format': 'python-module-name'}, '$comment': 'https://setuptools.pypa.io/en/latest/userguide/package_discovery.html'}, 'py-modules': {'description': 'Modules that setuptools will manipulate', 'type': 'array', 'items': {'type': 'string', 'format': 'python-module-name'}, '$comment': 'TODO: clarify the relationship with ``packages``'}, 'data-files': {'$$description': ['**DEPRECATED**: dict-like structure where each key represents a directory and', 'the value is a list of glob patterns that should be installed in them.', "Please notice this don't work with wheels. See `data files support", '`_'], 'type': 'object', 'patternProperties': {'^.*$': {'type': 'array', 'items': {'type': 'string'}}}}, 'cmdclass': {'$$description': ['Mapping of distutils-style command names to ``setuptools.Command`` subclasses', 'which in turn should be represented by strings with a qualified class name', '(i.e., "dotted" form with module), e.g.::\n\n', ' cmdclass = {mycmd = "pkg.subpkg.module.CommandClass"}\n\n', 'The command class should be a directly defined at the top-level of the', 'containing module (no class nesting).'], 'type': 'object', 'patternProperties': {'^.*$': {'type': 'string', 'format': 'python-qualified-identifier'}}}, 'license-files': {'type': 'array', 'items': {'type': 'string'}, '$$description': ['PROVISIONAL: List of glob patterns for all license files being distributed.', '(might become standard with PEP 639).'], 'default': ['LICEN[CS]E*', ' COPYING*', ' NOTICE*', 'AUTHORS*'], '$comment': 'TODO: revise if PEP 639 is accepted. Probably ``project.license-files``?'}, 'dynamic': {'type': 'object', 'description': 'Instructions for loading :pep:`621`-related metadata dynamically', 'additionalProperties': False, 'properties': {'version': {'$$description': ['A version dynamically loaded via either the ``attr:`` or ``file:``', 'directives. Please make sure the given file or attribute respects :pep:`440`.'], 'oneOf': [{'$ref': '#/definitions/attr-directive'}, {'$ref': '#/definitions/file-directive'}]}, 'classifiers': {'$ref': '#/definitions/file-directive'}, 'description': {'$ref': '#/definitions/file-directive'}, 'dependencies': {'$ref': '#/definitions/file-directive'}, 'entry-points': {'$ref': '#/definitions/file-directive'}, 'optional-dependencies': {'type': 'object', 'propertyNames': {'format': 'python-identifier'}, 'additionalProperties': False, 'patternProperties': {'.+': {'$ref': '#/definitions/file-directive'}}}, 'readme': {'anyOf': [{'$ref': '#/definitions/file-directive'}, {'properties': {'content-type': {'type': 'string'}}}], 'required': ['file']}}}}, 'definitions': {'file-directive': {'$id': '#/definitions/file-directive', 'title': "'file:' directive", 'description': 'Value is read from a file (or list of files and then concatenated)', 'type': 'object', 'additionalProperties': False, 'properties': {'file': {'oneOf': [{'type': 'string'}, {'type': 'array', 'items': {'type': 'string'}}]}}, 'required': ['file']}, 'attr-directive': {'title': "'attr:' directive", '$id': '#/definitions/attr-directive', '$$description': ['Value is read from a module attribute. Supports callables and iterables;', 'unsupported types are cast via ``str()``'], 'type': 'object', 'additionalProperties': False, 'properties': {'attr': {'type': 'string'}}, 'required': ['attr']}, 'find-directive': {'$id': '#/definitions/find-directive', 'title': "'find:' directive", 'type': 'object', 'additionalProperties': False, 'properties': {'find': {'type': 'object', '$$description': ['Dynamic `package discovery', '`_.'], 'additionalProperties': False, 'properties': {'where': {'description': 'Directories to be searched for packages (Unix-style relative path)', 'type': 'array', 'items': {'type': 'string'}}, 'exclude': {'type': 'array', '$$description': ['Exclude packages that match the values listed in this field.', "Can container shell-style wildcards (e.g. ``'pkg.*'``)"], 'items': {'type': 'string'}}, 'include': {'type': 'array', '$$description': ['Restrict the found packages to just the ones listed in this field.', "Can container shell-style wildcards (e.g. ``'pkg.*'``)"], 'items': {'type': 'string'}}, 'namespaces': {'type': 'boolean', '$$description': ['When ``True``, directories without a ``__init__.py`` file will also', 'be scanned for :pep:`420`-style implicit namespaces']}}}}}}}}}}, 'project': {'$schema': 'http://json-schema.org/draft-07/schema', '$id': 'https://packaging.python.org/en/latest/specifications/declaring-project-metadata/', 'title': 'Package metadata stored in the ``project`` table', '$$description': ['Data structure for the **project** table inside ``pyproject.toml``', '(as initially defined in :pep:`621`)'], 'type': 'object', 'properties': {'name': {'type': 'string', 'description': 'The name (primary identifier) of the project. MUST be statically defined.', 'format': 'pep508-identifier'}, 'version': {'type': 'string', 'description': 'The version of the project as supported by :pep:`440`.', 'format': 'pep440'}, 'description': {'type': 'string', '$$description': ['The `summary description of the project', '`_']}, 'readme': {'$$description': ['`Full/detailed description of the project in the form of a README', '`_', "with meaning similar to the one defined in `core metadata's Description", '`_'], 'oneOf': [{'type': 'string', '$$description': ['Relative path to a text file (UTF-8) containing the full description', 'of the project. If the file path ends in case-insensitive ``.md`` or', '``.rst`` suffixes, then the content-type is respectively', '``text/markdown`` or ``text/x-rst``']}, {'type': 'object', 'allOf': [{'anyOf': [{'properties': {'file': {'type': 'string', '$$description': ['Relative path to a text file containing the full description', 'of the project.']}}, 'required': ['file']}, {'properties': {'text': {'type': 'string', 'description': 'Full text describing the project.'}}, 'required': ['text']}]}, {'properties': {'content-type': {'type': 'string', '$$description': ['Content-type (:rfc:`1341`) of the full description', '(e.g. ``text/markdown``). The ``charset`` parameter is assumed', 'UTF-8 when not present.'], '$comment': 'TODO: add regex pattern or format?'}}, 'required': ['content-type']}]}]}, 'requires-python': {'type': 'string', 'format': 'pep508-versionspec', '$$description': ['`The Python version requirements of the project', '`_.']}, 'license': {'description': '`Project license `_.', 'oneOf': [{'properties': {'file': {'type': 'string', '$$description': ['Relative path to the file (UTF-8) which contains the license for the', 'project.']}}, 'required': ['file']}, {'properties': {'text': {'type': 'string', '$$description': ['The license of the project whose meaning is that of the', '`License field from the core metadata', '`_.']}}, 'required': ['text']}]}, 'authors': {'type': 'array', 'items': {'$ref': '#/definitions/author'}, '$$description': ["The people or organizations considered to be the 'authors' of the project.", 'The exact meaning is open to interpretation (e.g. original or primary authors,', 'current maintainers, or owners of the package).']}, 'maintainers': {'type': 'array', 'items': {'$ref': '#/definitions/author'}, '$$description': ["The people or organizations considered to be the 'maintainers' of the project.", 'Similarly to ``authors``, the exact meaning is open to interpretation.']}, 'keywords': {'type': 'array', 'items': {'type': 'string'}, 'description': 'List of keywords to assist searching for the distribution in a larger catalog.'}, 'classifiers': {'type': 'array', 'items': {'type': 'string', 'format': 'trove-classifier', 'description': '`PyPI classifier `_.'}, '$$description': ['`Trove classifiers `_', 'which apply to the project.']}, 'urls': {'type': 'object', 'description': 'URLs associated with the project in the form ``label => value``.', 'additionalProperties': False, 'patternProperties': {'^.+$': {'type': 'string', 'format': 'url'}}}, 'scripts': {'$ref': '#/definitions/entry-point-group', '$$description': ['Instruct the installer to create command-line wrappers for the given', '`entry points `_.']}, 'gui-scripts': {'$ref': '#/definitions/entry-point-group', '$$description': ['Instruct the installer to create GUI wrappers for the given', '`entry points `_.', 'The difference between ``scripts`` and ``gui-scripts`` is only relevant in', 'Windows.']}, 'entry-points': {'$$description': ['Instruct the installer to expose the given modules/functions via', '``entry-point`` discovery mechanism (useful for plugins).', 'More information available in the `Python packaging guide', '`_.'], 'propertyNames': {'format': 'python-entrypoint-group'}, 'additionalProperties': False, 'patternProperties': {'^.+$': {'$ref': '#/definitions/entry-point-group'}}}, 'dependencies': {'type': 'array', 'description': 'Project (mandatory) dependencies.', 'items': {'$ref': '#/definitions/dependency'}}, 'optional-dependencies': {'type': 'object', 'description': 'Optional dependency for the project', 'propertyNames': {'format': 'pep508-identifier'}, 'additionalProperties': False, 'patternProperties': {'^.+$': {'type': 'array', 'items': {'$ref': '#/definitions/dependency'}}}}, 'dynamic': {'type': 'array', '$$description': ['Specifies which fields are intentionally unspecified and expected to be', 'dynamically provided by build tools'], 'items': {'enum': ['version', 'description', 'readme', 'requires-python', 'license', 'authors', 'maintainers', 'keywords', 'classifiers', 'urls', 'scripts', 'gui-scripts', 'entry-points', 'dependencies', 'optional-dependencies']}}}, 'required': ['name'], 'additionalProperties': False, 'if': {'not': {'required': ['dynamic'], 'properties': {'dynamic': {'contains': {'const': 'version'}, '$$description': ['version is listed in ``dynamic``']}}}, '$$comment': ['According to :pep:`621`:', ' If the core metadata specification lists a field as "Required", then', ' the metadata MUST specify the field statically or list it in dynamic', 'In turn, `core metadata`_ defines:', ' The required fields are: Metadata-Version, Name, Version.', ' All the other fields are optional.', 'Since ``Metadata-Version`` is defined by the build back-end, ``name`` and', '``version`` are the only mandatory information in ``pyproject.toml``.', '.. _core metadata: https://packaging.python.org/specifications/core-metadata/']}, 'then': {'required': ['version'], '$$description': ['version should be statically defined in the ``version`` field']}, 'definitions': {'author': {'$id': '#/definitions/author', 'title': 'Author or Maintainer', '$comment': 'https://www.python.org/dev/peps/pep-0621/#authors-maintainers', 'type': 'object', 'properties': {'name': {'type': 'string', '$$description': ['MUST be a valid email name, i.e. whatever can be put as a name, before an', 'email, in :rfc:`822`.']}, 'email': {'type': 'string', 'format': 'idn-email', 'description': 'MUST be a valid email address'}}}, 'entry-point-group': {'$id': '#/definitions/entry-point-group', 'title': 'Entry-points', 'type': 'object', '$$description': ['Entry-points are grouped together to indicate what sort of capabilities they', 'provide.', 'See the `packaging guides', '`_', 'and `setuptools docs', '`_', 'for more information.'], 'propertyNames': {'format': 'python-entrypoint-name'}, 'additionalProperties': False, 'patternProperties': {'^.+$': {'type': 'string', '$$description': ['Reference to a Python object. It is either in the form', '``importable.module``, or ``importable.module:object.attr``.'], 'format': 'python-entrypoint-reference', '$comment': 'https://packaging.python.org/specifications/entry-points/'}}}, 'dependency': {'$id': '#/definitions/dependency', 'title': 'Dependency', 'type': 'string', 'description': 'Project dependency specification according to PEP 508', 'format': 'pep508'}}}}, rule='additionalProperties')
- return data
-
-def validate_https___setuptools_pypa_io_en_latest_references_keywords_html(data, custom_formats={}, name_prefix=None):
- if not isinstance(data, (dict)):
- raise JsonSchemaValueException("" + (name_prefix or "data") + " must be object", value=data, name="" + (name_prefix or "data") + "", definition={'$schema': 'http://json-schema.org/draft-07/schema', '$id': 'https://setuptools.pypa.io/en/latest/references/keywords.html', 'title': '``tool.setuptools`` table', '$$description': ['Please notice for the time being the ``setuptools`` project does not specify', 'a way of configuring builds via ``pyproject.toml``.', 'Therefore this schema should be taken just as a *"thought experiment"* on how', 'this *might be done*, by following the principles established in', '`ini2toml `_.', 'It considers only ``setuptools`` `parameters', '`_', 'that can currently be configured via ``setup.cfg`` and are not covered by :pep:`621`', 'but intentionally excludes ``dependency_links`` and ``setup_requires``.', 'NOTE: ``scripts`` was renamed to ``script-files`` to avoid confusion with', 'entry-point based scripts (defined in :pep:`621`).'], 'type': 'object', 'additionalProperties': False, 'properties': {'platforms': {'type': 'array', 'items': {'type': 'string'}}, 'provides': {'$$description': ['Package and virtual package names contained within this package', '**(not supported by pip)**'], 'type': 'array', 'items': {'type': 'string', 'format': 'pep508-identifier'}}, 'obsoletes': {'$$description': ['Packages which this package renders obsolete', '**(not supported by pip)**'], 'type': 'array', 'items': {'type': 'string', 'format': 'pep508-identifier'}}, 'zip-safe': {'description': 'Whether the project can be safely installed and run from a zip file.', 'type': 'boolean'}, 'script-files': {'description': 'Legacy way of defining scripts (entry-points are preferred).', 'type': 'array', 'items': {'type': 'string'}, '$comment': 'TODO: is this field deprecated/should be removed?'}, 'eager-resources': {'$$description': ['Resources that should be extracted together, if any of them is needed,', 'or if any C extensions included in the project are imported.'], 'type': 'array', 'items': {'type': 'string'}}, 'packages': {'$$description': ['Packages that should be included in the distribution.', 'It can be given either as a list of package identifiers', 'or as a ``dict``-like structure with a single key ``find``', 'which corresponds to a dynamic call to', '``setuptools.config.expand.find_packages`` function.', 'The ``find`` key is associated with a nested ``dict``-like structure that can', 'contain ``where``, ``include``, ``exclude`` and ``namespaces`` keys,', 'mimicking the keyword arguments of the associated function.'], 'oneOf': [{'title': 'Array of Python package identifiers', 'type': 'array', 'items': {'type': 'string', 'format': 'python-module-name'}}, {'$id': '#/definitions/find-directive', 'title': "'find:' directive", 'type': 'object', 'additionalProperties': False, 'properties': {'find': {'type': 'object', '$$description': ['Dynamic `package discovery', '`_.'], 'additionalProperties': False, 'properties': {'where': {'description': 'Directories to be searched for packages (Unix-style relative path)', 'type': 'array', 'items': {'type': 'string'}}, 'exclude': {'type': 'array', '$$description': ['Exclude packages that match the values listed in this field.', "Can container shell-style wildcards (e.g. ``'pkg.*'``)"], 'items': {'type': 'string'}}, 'include': {'type': 'array', '$$description': ['Restrict the found packages to just the ones listed in this field.', "Can container shell-style wildcards (e.g. ``'pkg.*'``)"], 'items': {'type': 'string'}}, 'namespaces': {'type': 'boolean', '$$description': ['When ``True``, directories without a ``__init__.py`` file will also', 'be scanned for :pep:`420`-style implicit namespaces']}}}}}]}, 'package-dir': {'$$description': [':class:`dict`-like structure mapping from package names to directories where their', 'code can be found.', 'The empty string (as key) means that all packages are contained inside', 'the given directory will be included in the distribution.'], 'type': 'object', 'additionalProperties': False, 'propertyNames': {'oneOf': [{'format': 'python-module-name'}, {'const': ''}]}, 'patternProperties': {'^.*$': {'type': 'string'}}}, 'package-data': {'$$description': ['Mapping from package names to lists of glob patterns.', 'Usually this option is not needed when using ``include-package-data = true``', 'For more information on how to include data files, check ``setuptools`` `docs', '`_.'], 'type': 'object', 'additionalProperties': False, 'propertyNames': {'oneOf': [{'format': 'python-module-name'}, {'const': '*'}]}, 'patternProperties': {'^.*$': {'type': 'array', 'items': {'type': 'string'}}}}, 'include-package-data': {'$$description': ['Automatically include any data files inside the package directories', 'that are specified by ``MANIFEST.in``', 'For more information on how to include data files, check ``setuptools`` `docs', '`_.'], 'type': 'boolean'}, 'exclude-package-data': {'$$description': ['Mapping from package names to lists of glob patterns that should be excluded', 'For more information on how to include data files, check ``setuptools`` `docs', '`_.'], 'type': 'object', 'additionalProperties': False, 'propertyNames': {'oneOf': [{'format': 'python-module-name'}, {'const': '*'}]}, 'patternProperties': {'^.*$': {'type': 'array', 'items': {'type': 'string'}}}}, 'namespace-packages': {'type': 'array', 'items': {'type': 'string', 'format': 'python-module-name'}, '$comment': 'https://setuptools.pypa.io/en/latest/userguide/package_discovery.html'}, 'py-modules': {'description': 'Modules that setuptools will manipulate', 'type': 'array', 'items': {'type': 'string', 'format': 'python-module-name'}, '$comment': 'TODO: clarify the relationship with ``packages``'}, 'data-files': {'$$description': ['**DEPRECATED**: dict-like structure where each key represents a directory and', 'the value is a list of glob patterns that should be installed in them.', "Please notice this don't work with wheels. See `data files support", '`_'], 'type': 'object', 'patternProperties': {'^.*$': {'type': 'array', 'items': {'type': 'string'}}}}, 'cmdclass': {'$$description': ['Mapping of distutils-style command names to ``setuptools.Command`` subclasses', 'which in turn should be represented by strings with a qualified class name', '(i.e., "dotted" form with module), e.g.::\n\n', ' cmdclass = {mycmd = "pkg.subpkg.module.CommandClass"}\n\n', 'The command class should be a directly defined at the top-level of the', 'containing module (no class nesting).'], 'type': 'object', 'patternProperties': {'^.*$': {'type': 'string', 'format': 'python-qualified-identifier'}}}, 'license-files': {'type': 'array', 'items': {'type': 'string'}, '$$description': ['PROVISIONAL: List of glob patterns for all license files being distributed.', '(might become standard with PEP 639).'], 'default': ['LICEN[CS]E*', ' COPYING*', ' NOTICE*', 'AUTHORS*'], '$comment': 'TODO: revise if PEP 639 is accepted. Probably ``project.license-files``?'}, 'dynamic': {'type': 'object', 'description': 'Instructions for loading :pep:`621`-related metadata dynamically', 'additionalProperties': False, 'properties': {'version': {'$$description': ['A version dynamically loaded via either the ``attr:`` or ``file:``', 'directives. Please make sure the given file or attribute respects :pep:`440`.'], 'oneOf': [{'title': "'attr:' directive", '$id': '#/definitions/attr-directive', '$$description': ['Value is read from a module attribute. Supports callables and iterables;', 'unsupported types are cast via ``str()``'], 'type': 'object', 'additionalProperties': False, 'properties': {'attr': {'type': 'string'}}, 'required': ['attr']}, {'$id': '#/definitions/file-directive', 'title': "'file:' directive", 'description': 'Value is read from a file (or list of files and then concatenated)', 'type': 'object', 'additionalProperties': False, 'properties': {'file': {'oneOf': [{'type': 'string'}, {'type': 'array', 'items': {'type': 'string'}}]}}, 'required': ['file']}]}, 'classifiers': {'$id': '#/definitions/file-directive', 'title': "'file:' directive", 'description': 'Value is read from a file (or list of files and then concatenated)', 'type': 'object', 'additionalProperties': False, 'properties': {'file': {'oneOf': [{'type': 'string'}, {'type': 'array', 'items': {'type': 'string'}}]}}, 'required': ['file']}, 'description': {'$id': '#/definitions/file-directive', 'title': "'file:' directive", 'description': 'Value is read from a file (or list of files and then concatenated)', 'type': 'object', 'additionalProperties': False, 'properties': {'file': {'oneOf': [{'type': 'string'}, {'type': 'array', 'items': {'type': 'string'}}]}}, 'required': ['file']}, 'dependencies': {'$id': '#/definitions/file-directive', 'title': "'file:' directive", 'description': 'Value is read from a file (or list of files and then concatenated)', 'type': 'object', 'additionalProperties': False, 'properties': {'file': {'oneOf': [{'type': 'string'}, {'type': 'array', 'items': {'type': 'string'}}]}}, 'required': ['file']}, 'entry-points': {'$id': '#/definitions/file-directive', 'title': "'file:' directive", 'description': 'Value is read from a file (or list of files and then concatenated)', 'type': 'object', 'additionalProperties': False, 'properties': {'file': {'oneOf': [{'type': 'string'}, {'type': 'array', 'items': {'type': 'string'}}]}}, 'required': ['file']}, 'optional-dependencies': {'type': 'object', 'propertyNames': {'format': 'python-identifier'}, 'additionalProperties': False, 'patternProperties': {'.+': {'$id': '#/definitions/file-directive', 'title': "'file:' directive", 'description': 'Value is read from a file (or list of files and then concatenated)', 'type': 'object', 'additionalProperties': False, 'properties': {'file': {'oneOf': [{'type': 'string'}, {'type': 'array', 'items': {'type': 'string'}}]}}, 'required': ['file']}}}, 'readme': {'anyOf': [{'$id': '#/definitions/file-directive', 'title': "'file:' directive", 'description': 'Value is read from a file (or list of files and then concatenated)', 'type': 'object', 'additionalProperties': False, 'properties': {'file': {'oneOf': [{'type': 'string'}, {'type': 'array', 'items': {'type': 'string'}}]}}, 'required': ['file']}, {'properties': {'content-type': {'type': 'string'}}}], 'required': ['file']}}}}, 'definitions': {'file-directive': {'$id': '#/definitions/file-directive', 'title': "'file:' directive", 'description': 'Value is read from a file (or list of files and then concatenated)', 'type': 'object', 'additionalProperties': False, 'properties': {'file': {'oneOf': [{'type': 'string'}, {'type': 'array', 'items': {'type': 'string'}}]}}, 'required': ['file']}, 'attr-directive': {'title': "'attr:' directive", '$id': '#/definitions/attr-directive', '$$description': ['Value is read from a module attribute. Supports callables and iterables;', 'unsupported types are cast via ``str()``'], 'type': 'object', 'additionalProperties': False, 'properties': {'attr': {'type': 'string'}}, 'required': ['attr']}, 'find-directive': {'$id': '#/definitions/find-directive', 'title': "'find:' directive", 'type': 'object', 'additionalProperties': False, 'properties': {'find': {'type': 'object', '$$description': ['Dynamic `package discovery', '`_.'], 'additionalProperties': False, 'properties': {'where': {'description': 'Directories to be searched for packages (Unix-style relative path)', 'type': 'array', 'items': {'type': 'string'}}, 'exclude': {'type': 'array', '$$description': ['Exclude packages that match the values listed in this field.', "Can container shell-style wildcards (e.g. ``'pkg.*'``)"], 'items': {'type': 'string'}}, 'include': {'type': 'array', '$$description': ['Restrict the found packages to just the ones listed in this field.', "Can container shell-style wildcards (e.g. ``'pkg.*'``)"], 'items': {'type': 'string'}}, 'namespaces': {'type': 'boolean', '$$description': ['When ``True``, directories without a ``__init__.py`` file will also', 'be scanned for :pep:`420`-style implicit namespaces']}}}}}}}, rule='type')
- data_is_dict = isinstance(data, dict)
- if data_is_dict:
- data_keys = set(data.keys())
- if "platforms" in data_keys:
- data_keys.remove("platforms")
- data__platforms = data["platforms"]
- if not isinstance(data__platforms, (list, tuple)):
- raise JsonSchemaValueException("" + (name_prefix or "data") + ".platforms must be array", value=data__platforms, name="" + (name_prefix or "data") + ".platforms", definition={'type': 'array', 'items': {'type': 'string'}}, rule='type')
- data__platforms_is_list = isinstance(data__platforms, (list, tuple))
- if data__platforms_is_list:
- data__platforms_len = len(data__platforms)
- for data__platforms_x, data__platforms_item in enumerate(data__platforms):
- if not isinstance(data__platforms_item, (str)):
- raise JsonSchemaValueException("" + (name_prefix or "data") + ".platforms[{data__platforms_x}]".format(**locals()) + " must be string", value=data__platforms_item, name="" + (name_prefix or "data") + ".platforms[{data__platforms_x}]".format(**locals()) + "", definition={'type': 'string'}, rule='type')
- if "provides" in data_keys:
- data_keys.remove("provides")
- data__provides = data["provides"]
- if not isinstance(data__provides, (list, tuple)):
- raise JsonSchemaValueException("" + (name_prefix or "data") + ".provides must be array", value=data__provides, name="" + (name_prefix or "data") + ".provides", definition={'$$description': ['Package and virtual package names contained within this package', '**(not supported by pip)**'], 'type': 'array', 'items': {'type': 'string', 'format': 'pep508-identifier'}}, rule='type')
- data__provides_is_list = isinstance(data__provides, (list, tuple))
- if data__provides_is_list:
- data__provides_len = len(data__provides)
- for data__provides_x, data__provides_item in enumerate(data__provides):
- if not isinstance(data__provides_item, (str)):
- raise JsonSchemaValueException("" + (name_prefix or "data") + ".provides[{data__provides_x}]".format(**locals()) + " must be string", value=data__provides_item, name="" + (name_prefix or "data") + ".provides[{data__provides_x}]".format(**locals()) + "", definition={'type': 'string', 'format': 'pep508-identifier'}, rule='type')
- if isinstance(data__provides_item, str):
- if not custom_formats["pep508-identifier"](data__provides_item):
- raise JsonSchemaValueException("" + (name_prefix or "data") + ".provides[{data__provides_x}]".format(**locals()) + " must be pep508-identifier", value=data__provides_item, name="" + (name_prefix or "data") + ".provides[{data__provides_x}]".format(**locals()) + "", definition={'type': 'string', 'format': 'pep508-identifier'}, rule='format')
- if "obsoletes" in data_keys:
- data_keys.remove("obsoletes")
- data__obsoletes = data["obsoletes"]
- if not isinstance(data__obsoletes, (list, tuple)):
- raise JsonSchemaValueException("" + (name_prefix or "data") + ".obsoletes must be array", value=data__obsoletes, name="" + (name_prefix or "data") + ".obsoletes", definition={'$$description': ['Packages which this package renders obsolete', '**(not supported by pip)**'], 'type': 'array', 'items': {'type': 'string', 'format': 'pep508-identifier'}}, rule='type')
- data__obsoletes_is_list = isinstance(data__obsoletes, (list, tuple))
- if data__obsoletes_is_list:
- data__obsoletes_len = len(data__obsoletes)
- for data__obsoletes_x, data__obsoletes_item in enumerate(data__obsoletes):
- if not isinstance(data__obsoletes_item, (str)):
- raise JsonSchemaValueException("" + (name_prefix or "data") + ".obsoletes[{data__obsoletes_x}]".format(**locals()) + " must be string", value=data__obsoletes_item, name="" + (name_prefix or "data") + ".obsoletes[{data__obsoletes_x}]".format(**locals()) + "", definition={'type': 'string', 'format': 'pep508-identifier'}, rule='type')
- if isinstance(data__obsoletes_item, str):
- if not custom_formats["pep508-identifier"](data__obsoletes_item):
- raise JsonSchemaValueException("" + (name_prefix or "data") + ".obsoletes[{data__obsoletes_x}]".format(**locals()) + " must be pep508-identifier", value=data__obsoletes_item, name="" + (name_prefix or "data") + ".obsoletes[{data__obsoletes_x}]".format(**locals()) + "", definition={'type': 'string', 'format': 'pep508-identifier'}, rule='format')
- if "zip-safe" in data_keys:
- data_keys.remove("zip-safe")
- data__zipsafe = data["zip-safe"]
- if not isinstance(data__zipsafe, (bool)):
- raise JsonSchemaValueException("" + (name_prefix or "data") + ".zip-safe must be boolean", value=data__zipsafe, name="" + (name_prefix or "data") + ".zip-safe", definition={'description': 'Whether the project can be safely installed and run from a zip file.', 'type': 'boolean'}, rule='type')
- if "script-files" in data_keys:
- data_keys.remove("script-files")
- data__scriptfiles = data["script-files"]
- if not isinstance(data__scriptfiles, (list, tuple)):
- raise JsonSchemaValueException("" + (name_prefix or "data") + ".script-files must be array", value=data__scriptfiles, name="" + (name_prefix or "data") + ".script-files", definition={'description': 'Legacy way of defining scripts (entry-points are preferred).', 'type': 'array', 'items': {'type': 'string'}, '$comment': 'TODO: is this field deprecated/should be removed?'}, rule='type')
- data__scriptfiles_is_list = isinstance(data__scriptfiles, (list, tuple))
- if data__scriptfiles_is_list:
- data__scriptfiles_len = len(data__scriptfiles)
- for data__scriptfiles_x, data__scriptfiles_item in enumerate(data__scriptfiles):
- if not isinstance(data__scriptfiles_item, (str)):
- raise JsonSchemaValueException("" + (name_prefix or "data") + ".script-files[{data__scriptfiles_x}]".format(**locals()) + " must be string", value=data__scriptfiles_item, name="" + (name_prefix or "data") + ".script-files[{data__scriptfiles_x}]".format(**locals()) + "", definition={'type': 'string'}, rule='type')
- if "eager-resources" in data_keys:
- data_keys.remove("eager-resources")
- data__eagerresources = data["eager-resources"]
- if not isinstance(data__eagerresources, (list, tuple)):
- raise JsonSchemaValueException("" + (name_prefix or "data") + ".eager-resources must be array", value=data__eagerresources, name="" + (name_prefix or "data") + ".eager-resources", definition={'$$description': ['Resources that should be extracted together, if any of them is needed,', 'or if any C extensions included in the project are imported.'], 'type': 'array', 'items': {'type': 'string'}}, rule='type')
- data__eagerresources_is_list = isinstance(data__eagerresources, (list, tuple))
- if data__eagerresources_is_list:
- data__eagerresources_len = len(data__eagerresources)
- for data__eagerresources_x, data__eagerresources_item in enumerate(data__eagerresources):
- if not isinstance(data__eagerresources_item, (str)):
- raise JsonSchemaValueException("" + (name_prefix or "data") + ".eager-resources[{data__eagerresources_x}]".format(**locals()) + " must be string", value=data__eagerresources_item, name="" + (name_prefix or "data") + ".eager-resources[{data__eagerresources_x}]".format(**locals()) + "", definition={'type': 'string'}, rule='type')
- if "packages" in data_keys:
- data_keys.remove("packages")
- data__packages = data["packages"]
- data__packages_one_of_count1 = 0
- if data__packages_one_of_count1 < 2:
- try:
- if not isinstance(data__packages, (list, tuple)):
- raise JsonSchemaValueException("" + (name_prefix or "data") + ".packages must be array", value=data__packages, name="" + (name_prefix or "data") + ".packages", definition={'title': 'Array of Python package identifiers', 'type': 'array', 'items': {'type': 'string', 'format': 'python-module-name'}}, rule='type')
- data__packages_is_list = isinstance(data__packages, (list, tuple))
- if data__packages_is_list:
- data__packages_len = len(data__packages)
- for data__packages_x, data__packages_item in enumerate(data__packages):
- if not isinstance(data__packages_item, (str)):
- raise JsonSchemaValueException("" + (name_prefix or "data") + ".packages[{data__packages_x}]".format(**locals()) + " must be string", value=data__packages_item, name="" + (name_prefix or "data") + ".packages[{data__packages_x}]".format(**locals()) + "", definition={'type': 'string', 'format': 'python-module-name'}, rule='type')
- if isinstance(data__packages_item, str):
- if not custom_formats["python-module-name"](data__packages_item):
- raise JsonSchemaValueException("" + (name_prefix or "data") + ".packages[{data__packages_x}]".format(**locals()) + " must be python-module-name", value=data__packages_item, name="" + (name_prefix or "data") + ".packages[{data__packages_x}]".format(**locals()) + "", definition={'type': 'string', 'format': 'python-module-name'}, rule='format')
- data__packages_one_of_count1 += 1
- except JsonSchemaValueException: pass
- if data__packages_one_of_count1 < 2:
- try:
- validate_https___setuptools_pypa_io_en_latest_references_keywords_html__definitions_find_directive(data__packages, custom_formats, (name_prefix or "data") + ".packages")
- data__packages_one_of_count1 += 1
- except JsonSchemaValueException: pass
- if data__packages_one_of_count1 != 1:
- raise JsonSchemaValueException("" + (name_prefix or "data") + ".packages must be valid exactly by one definition" + (" (" + str(data__packages_one_of_count1) + " matches found)"), value=data__packages, name="" + (name_prefix or "data") + ".packages", definition={'$$description': ['Packages that should be included in the distribution.', 'It can be given either as a list of package identifiers', 'or as a ``dict``-like structure with a single key ``find``', 'which corresponds to a dynamic call to', '``setuptools.config.expand.find_packages`` function.', 'The ``find`` key is associated with a nested ``dict``-like structure that can', 'contain ``where``, ``include``, ``exclude`` and ``namespaces`` keys,', 'mimicking the keyword arguments of the associated function.'], 'oneOf': [{'title': 'Array of Python package identifiers', 'type': 'array', 'items': {'type': 'string', 'format': 'python-module-name'}}, {'$id': '#/definitions/find-directive', 'title': "'find:' directive", 'type': 'object', 'additionalProperties': False, 'properties': {'find': {'type': 'object', '$$description': ['Dynamic `package discovery', '`_.'], 'additionalProperties': False, 'properties': {'where': {'description': 'Directories to be searched for packages (Unix-style relative path)', 'type': 'array', 'items': {'type': 'string'}}, 'exclude': {'type': 'array', '$$description': ['Exclude packages that match the values listed in this field.', "Can container shell-style wildcards (e.g. ``'pkg.*'``)"], 'items': {'type': 'string'}}, 'include': {'type': 'array', '$$description': ['Restrict the found packages to just the ones listed in this field.', "Can container shell-style wildcards (e.g. ``'pkg.*'``)"], 'items': {'type': 'string'}}, 'namespaces': {'type': 'boolean', '$$description': ['When ``True``, directories without a ``__init__.py`` file will also', 'be scanned for :pep:`420`-style implicit namespaces']}}}}}]}, rule='oneOf')
- if "package-dir" in data_keys:
- data_keys.remove("package-dir")
- data__packagedir = data["package-dir"]
- if not isinstance(data__packagedir, (dict)):
- raise JsonSchemaValueException("" + (name_prefix or "data") + ".package-dir must be object", value=data__packagedir, name="" + (name_prefix or "data") + ".package-dir", definition={'$$description': [':class:`dict`-like structure mapping from package names to directories where their', 'code can be found.', 'The empty string (as key) means that all packages are contained inside', 'the given directory will be included in the distribution.'], 'type': 'object', 'additionalProperties': False, 'propertyNames': {'oneOf': [{'format': 'python-module-name'}, {'const': ''}]}, 'patternProperties': {'^.*$': {'type': 'string'}}}, rule='type')
- data__packagedir_is_dict = isinstance(data__packagedir, dict)
- if data__packagedir_is_dict:
- data__packagedir_keys = set(data__packagedir.keys())
- for data__packagedir_key, data__packagedir_val in data__packagedir.items():
- if REGEX_PATTERNS['^.*$'].search(data__packagedir_key):
- if data__packagedir_key in data__packagedir_keys:
- data__packagedir_keys.remove(data__packagedir_key)
- if not isinstance(data__packagedir_val, (str)):
- raise JsonSchemaValueException("" + (name_prefix or "data") + ".package-dir.{data__packagedir_key}".format(**locals()) + " must be string", value=data__packagedir_val, name="" + (name_prefix or "data") + ".package-dir.{data__packagedir_key}".format(**locals()) + "", definition={'type': 'string'}, rule='type')
- if data__packagedir_keys:
- raise JsonSchemaValueException("" + (name_prefix or "data") + ".package-dir must not contain "+str(data__packagedir_keys)+" properties", value=data__packagedir, name="" + (name_prefix or "data") + ".package-dir", definition={'$$description': [':class:`dict`-like structure mapping from package names to directories where their', 'code can be found.', 'The empty string (as key) means that all packages are contained inside', 'the given directory will be included in the distribution.'], 'type': 'object', 'additionalProperties': False, 'propertyNames': {'oneOf': [{'format': 'python-module-name'}, {'const': ''}]}, 'patternProperties': {'^.*$': {'type': 'string'}}}, rule='additionalProperties')
- data__packagedir_len = len(data__packagedir)
- if data__packagedir_len != 0:
- data__packagedir_property_names = True
- for data__packagedir_key in data__packagedir:
- try:
- data__packagedir_key_one_of_count2 = 0
- if data__packagedir_key_one_of_count2 < 2:
- try:
- if isinstance(data__packagedir_key, str):
- if not custom_formats["python-module-name"](data__packagedir_key):
- raise JsonSchemaValueException("" + (name_prefix or "data") + ".package-dir must be python-module-name", value=data__packagedir_key, name="" + (name_prefix or "data") + ".package-dir", definition={'format': 'python-module-name'}, rule='format')
- data__packagedir_key_one_of_count2 += 1
- except JsonSchemaValueException: pass
- if data__packagedir_key_one_of_count2 < 2:
- try:
- if data__packagedir_key != "":
- raise JsonSchemaValueException("" + (name_prefix or "data") + ".package-dir must be same as const definition: ", value=data__packagedir_key, name="" + (name_prefix or "data") + ".package-dir", definition={'const': ''}, rule='const')
- data__packagedir_key_one_of_count2 += 1
- except JsonSchemaValueException: pass
- if data__packagedir_key_one_of_count2 != 1:
- raise JsonSchemaValueException("" + (name_prefix or "data") + ".package-dir must be valid exactly by one definition" + (" (" + str(data__packagedir_key_one_of_count2) + " matches found)"), value=data__packagedir_key, name="" + (name_prefix or "data") + ".package-dir", definition={'oneOf': [{'format': 'python-module-name'}, {'const': ''}]}, rule='oneOf')
- except JsonSchemaValueException:
- data__packagedir_property_names = False
- if not data__packagedir_property_names:
- raise JsonSchemaValueException("" + (name_prefix or "data") + ".package-dir must be named by propertyName definition", value=data__packagedir, name="" + (name_prefix or "data") + ".package-dir", definition={'$$description': [':class:`dict`-like structure mapping from package names to directories where their', 'code can be found.', 'The empty string (as key) means that all packages are contained inside', 'the given directory will be included in the distribution.'], 'type': 'object', 'additionalProperties': False, 'propertyNames': {'oneOf': [{'format': 'python-module-name'}, {'const': ''}]}, 'patternProperties': {'^.*$': {'type': 'string'}}}, rule='propertyNames')
- if "package-data" in data_keys:
- data_keys.remove("package-data")
- data__packagedata = data["package-data"]
- if not isinstance(data__packagedata, (dict)):
- raise JsonSchemaValueException("" + (name_prefix or "data") + ".package-data must be object", value=data__packagedata, name="" + (name_prefix or "data") + ".package-data", definition={'$$description': ['Mapping from package names to lists of glob patterns.', 'Usually this option is not needed when using ``include-package-data = true``', 'For more information on how to include data files, check ``setuptools`` `docs', '`_.'], 'type': 'object', 'additionalProperties': False, 'propertyNames': {'oneOf': [{'format': 'python-module-name'}, {'const': '*'}]}, 'patternProperties': {'^.*$': {'type': 'array', 'items': {'type': 'string'}}}}, rule='type')
- data__packagedata_is_dict = isinstance(data__packagedata, dict)
- if data__packagedata_is_dict:
- data__packagedata_keys = set(data__packagedata.keys())
- for data__packagedata_key, data__packagedata_val in data__packagedata.items():
- if REGEX_PATTERNS['^.*$'].search(data__packagedata_key):
- if data__packagedata_key in data__packagedata_keys:
- data__packagedata_keys.remove(data__packagedata_key)
- if not isinstance(data__packagedata_val, (list, tuple)):
- raise JsonSchemaValueException("" + (name_prefix or "data") + ".package-data.{data__packagedata_key}".format(**locals()) + " must be array", value=data__packagedata_val, name="" + (name_prefix or "data") + ".package-data.{data__packagedata_key}".format(**locals()) + "", definition={'type': 'array', 'items': {'type': 'string'}}, rule='type')
- data__packagedata_val_is_list = isinstance(data__packagedata_val, (list, tuple))
- if data__packagedata_val_is_list:
- data__packagedata_val_len = len(data__packagedata_val)
- for data__packagedata_val_x, data__packagedata_val_item in enumerate(data__packagedata_val):
- if not isinstance(data__packagedata_val_item, (str)):
- raise JsonSchemaValueException("" + (name_prefix or "data") + ".package-data.{data__packagedata_key}[{data__packagedata_val_x}]".format(**locals()) + " must be string", value=data__packagedata_val_item, name="" + (name_prefix or "data") + ".package-data.{data__packagedata_key}[{data__packagedata_val_x}]".format(**locals()) + "", definition={'type': 'string'}, rule='type')
- if data__packagedata_keys:
- raise JsonSchemaValueException("" + (name_prefix or "data") + ".package-data must not contain "+str(data__packagedata_keys)+" properties", value=data__packagedata, name="" + (name_prefix or "data") + ".package-data", definition={'$$description': ['Mapping from package names to lists of glob patterns.', 'Usually this option is not needed when using ``include-package-data = true``', 'For more information on how to include data files, check ``setuptools`` `docs', '`_.'], 'type': 'object', 'additionalProperties': False, 'propertyNames': {'oneOf': [{'format': 'python-module-name'}, {'const': '*'}]}, 'patternProperties': {'^.*$': {'type': 'array', 'items': {'type': 'string'}}}}, rule='additionalProperties')
- data__packagedata_len = len(data__packagedata)
- if data__packagedata_len != 0:
- data__packagedata_property_names = True
- for data__packagedata_key in data__packagedata:
- try:
- data__packagedata_key_one_of_count3 = 0
- if data__packagedata_key_one_of_count3 < 2:
- try:
- if isinstance(data__packagedata_key, str):
- if not custom_formats["python-module-name"](data__packagedata_key):
- raise JsonSchemaValueException("" + (name_prefix or "data") + ".package-data must be python-module-name", value=data__packagedata_key, name="" + (name_prefix or "data") + ".package-data", definition={'format': 'python-module-name'}, rule='format')
- data__packagedata_key_one_of_count3 += 1
- except JsonSchemaValueException: pass
- if data__packagedata_key_one_of_count3 < 2:
- try:
- if data__packagedata_key != "*":
- raise JsonSchemaValueException("" + (name_prefix or "data") + ".package-data must be same as const definition: *", value=data__packagedata_key, name="" + (name_prefix or "data") + ".package-data", definition={'const': '*'}, rule='const')
- data__packagedata_key_one_of_count3 += 1
- except JsonSchemaValueException: pass
- if data__packagedata_key_one_of_count3 != 1:
- raise JsonSchemaValueException("" + (name_prefix or "data") + ".package-data must be valid exactly by one definition" + (" (" + str(data__packagedata_key_one_of_count3) + " matches found)"), value=data__packagedata_key, name="" + (name_prefix or "data") + ".package-data", definition={'oneOf': [{'format': 'python-module-name'}, {'const': '*'}]}, rule='oneOf')
- except JsonSchemaValueException:
- data__packagedata_property_names = False
- if not data__packagedata_property_names:
- raise JsonSchemaValueException("" + (name_prefix or "data") + ".package-data must be named by propertyName definition", value=data__packagedata, name="" + (name_prefix or "data") + ".package-data", definition={'$$description': ['Mapping from package names to lists of glob patterns.', 'Usually this option is not needed when using ``include-package-data = true``', 'For more information on how to include data files, check ``setuptools`` `docs', '`_.'], 'type': 'object', 'additionalProperties': False, 'propertyNames': {'oneOf': [{'format': 'python-module-name'}, {'const': '*'}]}, 'patternProperties': {'^.*$': {'type': 'array', 'items': {'type': 'string'}}}}, rule='propertyNames')
- if "include-package-data" in data_keys:
- data_keys.remove("include-package-data")
- data__includepackagedata = data["include-package-data"]
- if not isinstance(data__includepackagedata, (bool)):
- raise JsonSchemaValueException("" + (name_prefix or "data") + ".include-package-data must be boolean", value=data__includepackagedata, name="" + (name_prefix or "data") + ".include-package-data", definition={'$$description': ['Automatically include any data files inside the package directories', 'that are specified by ``MANIFEST.in``', 'For more information on how to include data files, check ``setuptools`` `docs', '`_.'], 'type': 'boolean'}, rule='type')
- if "exclude-package-data" in data_keys:
- data_keys.remove("exclude-package-data")
- data__excludepackagedata = data["exclude-package-data"]
- if not isinstance(data__excludepackagedata, (dict)):
- raise JsonSchemaValueException("" + (name_prefix or "data") + ".exclude-package-data must be object", value=data__excludepackagedata, name="" + (name_prefix or "data") + ".exclude-package-data", definition={'$$description': ['Mapping from package names to lists of glob patterns that should be excluded', 'For more information on how to include data files, check ``setuptools`` `docs', '`_.'], 'type': 'object', 'additionalProperties': False, 'propertyNames': {'oneOf': [{'format': 'python-module-name'}, {'const': '*'}]}, 'patternProperties': {'^.*$': {'type': 'array', 'items': {'type': 'string'}}}}, rule='type')
- data__excludepackagedata_is_dict = isinstance(data__excludepackagedata, dict)
- if data__excludepackagedata_is_dict:
- data__excludepackagedata_keys = set(data__excludepackagedata.keys())
- for data__excludepackagedata_key, data__excludepackagedata_val in data__excludepackagedata.items():
- if REGEX_PATTERNS['^.*$'].search(data__excludepackagedata_key):
- if data__excludepackagedata_key in data__excludepackagedata_keys:
- data__excludepackagedata_keys.remove(data__excludepackagedata_key)
- if not isinstance(data__excludepackagedata_val, (list, tuple)):
- raise JsonSchemaValueException("" + (name_prefix or "data") + ".exclude-package-data.{data__excludepackagedata_key}".format(**locals()) + " must be array", value=data__excludepackagedata_val, name="" + (name_prefix or "data") + ".exclude-package-data.{data__excludepackagedata_key}".format(**locals()) + "", definition={'type': 'array', 'items': {'type': 'string'}}, rule='type')
- data__excludepackagedata_val_is_list = isinstance(data__excludepackagedata_val, (list, tuple))
- if data__excludepackagedata_val_is_list:
- data__excludepackagedata_val_len = len(data__excludepackagedata_val)
- for data__excludepackagedata_val_x, data__excludepackagedata_val_item in enumerate(data__excludepackagedata_val):
- if not isinstance(data__excludepackagedata_val_item, (str)):
- raise JsonSchemaValueException("" + (name_prefix or "data") + ".exclude-package-data.{data__excludepackagedata_key}[{data__excludepackagedata_val_x}]".format(**locals()) + " must be string", value=data__excludepackagedata_val_item, name="" + (name_prefix or "data") + ".exclude-package-data.{data__excludepackagedata_key}[{data__excludepackagedata_val_x}]".format(**locals()) + "", definition={'type': 'string'}, rule='type')
- if data__excludepackagedata_keys:
- raise JsonSchemaValueException("" + (name_prefix or "data") + ".exclude-package-data must not contain "+str(data__excludepackagedata_keys)+" properties", value=data__excludepackagedata, name="" + (name_prefix or "data") + ".exclude-package-data", definition={'$$description': ['Mapping from package names to lists of glob patterns that should be excluded', 'For more information on how to include data files, check ``setuptools`` `docs', '`_.'], 'type': 'object', 'additionalProperties': False, 'propertyNames': {'oneOf': [{'format': 'python-module-name'}, {'const': '*'}]}, 'patternProperties': {'^.*$': {'type': 'array', 'items': {'type': 'string'}}}}, rule='additionalProperties')
- data__excludepackagedata_len = len(data__excludepackagedata)
- if data__excludepackagedata_len != 0:
- data__excludepackagedata_property_names = True
- for data__excludepackagedata_key in data__excludepackagedata:
- try:
- data__excludepackagedata_key_one_of_count4 = 0
- if data__excludepackagedata_key_one_of_count4 < 2:
- try:
- if isinstance(data__excludepackagedata_key, str):
- if not custom_formats["python-module-name"](data__excludepackagedata_key):
- raise JsonSchemaValueException("" + (name_prefix or "data") + ".exclude-package-data must be python-module-name", value=data__excludepackagedata_key, name="" + (name_prefix or "data") + ".exclude-package-data", definition={'format': 'python-module-name'}, rule='format')
- data__excludepackagedata_key_one_of_count4 += 1
- except JsonSchemaValueException: pass
- if data__excludepackagedata_key_one_of_count4 < 2:
- try:
- if data__excludepackagedata_key != "*":
- raise JsonSchemaValueException("" + (name_prefix or "data") + ".exclude-package-data must be same as const definition: *", value=data__excludepackagedata_key, name="" + (name_prefix or "data") + ".exclude-package-data", definition={'const': '*'}, rule='const')
- data__excludepackagedata_key_one_of_count4 += 1
- except JsonSchemaValueException: pass
- if data__excludepackagedata_key_one_of_count4 != 1:
- raise JsonSchemaValueException("" + (name_prefix or "data") + ".exclude-package-data must be valid exactly by one definition" + (" (" + str(data__excludepackagedata_key_one_of_count4) + " matches found)"), value=data__excludepackagedata_key, name="" + (name_prefix or "data") + ".exclude-package-data", definition={'oneOf': [{'format': 'python-module-name'}, {'const': '*'}]}, rule='oneOf')
- except JsonSchemaValueException:
- data__excludepackagedata_property_names = False
- if not data__excludepackagedata_property_names:
- raise JsonSchemaValueException("" + (name_prefix or "data") + ".exclude-package-data must be named by propertyName definition", value=data__excludepackagedata, name="" + (name_prefix or "data") + ".exclude-package-data", definition={'$$description': ['Mapping from package names to lists of glob patterns that should be excluded', 'For more information on how to include data files, check ``setuptools`` `docs', '`_.'], 'type': 'object', 'additionalProperties': False, 'propertyNames': {'oneOf': [{'format': 'python-module-name'}, {'const': '*'}]}, 'patternProperties': {'^.*$': {'type': 'array', 'items': {'type': 'string'}}}}, rule='propertyNames')
- if "namespace-packages" in data_keys:
- data_keys.remove("namespace-packages")
- data__namespacepackages = data["namespace-packages"]
- if not isinstance(data__namespacepackages, (list, tuple)):
- raise JsonSchemaValueException("" + (name_prefix or "data") + ".namespace-packages must be array", value=data__namespacepackages, name="" + (name_prefix or "data") + ".namespace-packages", definition={'type': 'array', 'items': {'type': 'string', 'format': 'python-module-name'}, '$comment': 'https://setuptools.pypa.io/en/latest/userguide/package_discovery.html'}, rule='type')
- data__namespacepackages_is_list = isinstance(data__namespacepackages, (list, tuple))
- if data__namespacepackages_is_list:
- data__namespacepackages_len = len(data__namespacepackages)
- for data__namespacepackages_x, data__namespacepackages_item in enumerate(data__namespacepackages):
- if not isinstance(data__namespacepackages_item, (str)):
- raise JsonSchemaValueException("" + (name_prefix or "data") + ".namespace-packages[{data__namespacepackages_x}]".format(**locals()) + " must be string", value=data__namespacepackages_item, name="" + (name_prefix or "data") + ".namespace-packages[{data__namespacepackages_x}]".format(**locals()) + "", definition={'type': 'string', 'format': 'python-module-name'}, rule='type')
- if isinstance(data__namespacepackages_item, str):
- if not custom_formats["python-module-name"](data__namespacepackages_item):
- raise JsonSchemaValueException("" + (name_prefix or "data") + ".namespace-packages[{data__namespacepackages_x}]".format(**locals()) + " must be python-module-name", value=data__namespacepackages_item, name="" + (name_prefix or "data") + ".namespace-packages[{data__namespacepackages_x}]".format(**locals()) + "", definition={'type': 'string', 'format': 'python-module-name'}, rule='format')
- if "py-modules" in data_keys:
- data_keys.remove("py-modules")
- data__pymodules = data["py-modules"]
- if not isinstance(data__pymodules, (list, tuple)):
- raise JsonSchemaValueException("" + (name_prefix or "data") + ".py-modules must be array", value=data__pymodules, name="" + (name_prefix or "data") + ".py-modules", definition={'description': 'Modules that setuptools will manipulate', 'type': 'array', 'items': {'type': 'string', 'format': 'python-module-name'}, '$comment': 'TODO: clarify the relationship with ``packages``'}, rule='type')
- data__pymodules_is_list = isinstance(data__pymodules, (list, tuple))
- if data__pymodules_is_list:
- data__pymodules_len = len(data__pymodules)
- for data__pymodules_x, data__pymodules_item in enumerate(data__pymodules):
- if not isinstance(data__pymodules_item, (str)):
- raise JsonSchemaValueException("" + (name_prefix or "data") + ".py-modules[{data__pymodules_x}]".format(**locals()) + " must be string", value=data__pymodules_item, name="" + (name_prefix or "data") + ".py-modules[{data__pymodules_x}]".format(**locals()) + "", definition={'type': 'string', 'format': 'python-module-name'}, rule='type')
- if isinstance(data__pymodules_item, str):
- if not custom_formats["python-module-name"](data__pymodules_item):
- raise JsonSchemaValueException("" + (name_prefix or "data") + ".py-modules[{data__pymodules_x}]".format(**locals()) + " must be python-module-name", value=data__pymodules_item, name="" + (name_prefix or "data") + ".py-modules[{data__pymodules_x}]".format(**locals()) + "", definition={'type': 'string', 'format': 'python-module-name'}, rule='format')
- if "data-files" in data_keys:
- data_keys.remove("data-files")
- data__datafiles = data["data-files"]
- if not isinstance(data__datafiles, (dict)):
- raise JsonSchemaValueException("" + (name_prefix or "data") + ".data-files must be object", value=data__datafiles, name="" + (name_prefix or "data") + ".data-files", definition={'$$description': ['**DEPRECATED**: dict-like structure where each key represents a directory and', 'the value is a list of glob patterns that should be installed in them.', "Please notice this don't work with wheels. See `data files support", '`_'], 'type': 'object', 'patternProperties': {'^.*$': {'type': 'array', 'items': {'type': 'string'}}}}, rule='type')
- data__datafiles_is_dict = isinstance(data__datafiles, dict)
- if data__datafiles_is_dict:
- data__datafiles_keys = set(data__datafiles.keys())
- for data__datafiles_key, data__datafiles_val in data__datafiles.items():
- if REGEX_PATTERNS['^.*$'].search(data__datafiles_key):
- if data__datafiles_key in data__datafiles_keys:
- data__datafiles_keys.remove(data__datafiles_key)
- if not isinstance(data__datafiles_val, (list, tuple)):
- raise JsonSchemaValueException("" + (name_prefix or "data") + ".data-files.{data__datafiles_key}".format(**locals()) + " must be array", value=data__datafiles_val, name="" + (name_prefix or "data") + ".data-files.{data__datafiles_key}".format(**locals()) + "", definition={'type': 'array', 'items': {'type': 'string'}}, rule='type')
- data__datafiles_val_is_list = isinstance(data__datafiles_val, (list, tuple))
- if data__datafiles_val_is_list:
- data__datafiles_val_len = len(data__datafiles_val)
- for data__datafiles_val_x, data__datafiles_val_item in enumerate(data__datafiles_val):
- if not isinstance(data__datafiles_val_item, (str)):
- raise JsonSchemaValueException("" + (name_prefix or "data") + ".data-files.{data__datafiles_key}[{data__datafiles_val_x}]".format(**locals()) + " must be string", value=data__datafiles_val_item, name="" + (name_prefix or "data") + ".data-files.{data__datafiles_key}[{data__datafiles_val_x}]".format(**locals()) + "", definition={'type': 'string'}, rule='type')
- if "cmdclass" in data_keys:
- data_keys.remove("cmdclass")
- data__cmdclass = data["cmdclass"]
- if not isinstance(data__cmdclass, (dict)):
- raise JsonSchemaValueException("" + (name_prefix or "data") + ".cmdclass must be object", value=data__cmdclass, name="" + (name_prefix or "data") + ".cmdclass", definition={'$$description': ['Mapping of distutils-style command names to ``setuptools.Command`` subclasses', 'which in turn should be represented by strings with a qualified class name', '(i.e., "dotted" form with module), e.g.::\n\n', ' cmdclass = {mycmd = "pkg.subpkg.module.CommandClass"}\n\n', 'The command class should be a directly defined at the top-level of the', 'containing module (no class nesting).'], 'type': 'object', 'patternProperties': {'^.*$': {'type': 'string', 'format': 'python-qualified-identifier'}}}, rule='type')
- data__cmdclass_is_dict = isinstance(data__cmdclass, dict)
- if data__cmdclass_is_dict:
- data__cmdclass_keys = set(data__cmdclass.keys())
- for data__cmdclass_key, data__cmdclass_val in data__cmdclass.items():
- if REGEX_PATTERNS['^.*$'].search(data__cmdclass_key):
- if data__cmdclass_key in data__cmdclass_keys:
- data__cmdclass_keys.remove(data__cmdclass_key)
- if not isinstance(data__cmdclass_val, (str)):
- raise JsonSchemaValueException("" + (name_prefix or "data") + ".cmdclass.{data__cmdclass_key}".format(**locals()) + " must be string", value=data__cmdclass_val, name="" + (name_prefix or "data") + ".cmdclass.{data__cmdclass_key}".format(**locals()) + "", definition={'type': 'string', 'format': 'python-qualified-identifier'}, rule='type')
- if isinstance(data__cmdclass_val, str):
- if not custom_formats["python-qualified-identifier"](data__cmdclass_val):
- raise JsonSchemaValueException("" + (name_prefix or "data") + ".cmdclass.{data__cmdclass_key}".format(**locals()) + " must be python-qualified-identifier", value=data__cmdclass_val, name="" + (name_prefix or "data") + ".cmdclass.{data__cmdclass_key}".format(**locals()) + "", definition={'type': 'string', 'format': 'python-qualified-identifier'}, rule='format')
- if "license-files" in data_keys:
- data_keys.remove("license-files")
- data__licensefiles = data["license-files"]
- if not isinstance(data__licensefiles, (list, tuple)):
- raise JsonSchemaValueException("" + (name_prefix or "data") + ".license-files must be array", value=data__licensefiles, name="" + (name_prefix or "data") + ".license-files", definition={'type': 'array', 'items': {'type': 'string'}, '$$description': ['PROVISIONAL: List of glob patterns for all license files being distributed.', '(might become standard with PEP 639).'], 'default': ['LICEN[CS]E*', ' COPYING*', ' NOTICE*', 'AUTHORS*'], '$comment': 'TODO: revise if PEP 639 is accepted. Probably ``project.license-files``?'}, rule='type')
- data__licensefiles_is_list = isinstance(data__licensefiles, (list, tuple))
- if data__licensefiles_is_list:
- data__licensefiles_len = len(data__licensefiles)
- for data__licensefiles_x, data__licensefiles_item in enumerate(data__licensefiles):
- if not isinstance(data__licensefiles_item, (str)):
- raise JsonSchemaValueException("" + (name_prefix or "data") + ".license-files[{data__licensefiles_x}]".format(**locals()) + " must be string", value=data__licensefiles_item, name="" + (name_prefix or "data") + ".license-files[{data__licensefiles_x}]".format(**locals()) + "", definition={'type': 'string'}, rule='type')
- else: data["license-files"] = ['LICEN[CS]E*', ' COPYING*', ' NOTICE*', 'AUTHORS*']
- if "dynamic" in data_keys:
- data_keys.remove("dynamic")
- data__dynamic = data["dynamic"]
- if not isinstance(data__dynamic, (dict)):
- raise JsonSchemaValueException("" + (name_prefix or "data") + ".dynamic must be object", value=data__dynamic, name="" + (name_prefix or "data") + ".dynamic", definition={'type': 'object', 'description': 'Instructions for loading :pep:`621`-related metadata dynamically', 'additionalProperties': False, 'properties': {'version': {'$$description': ['A version dynamically loaded via either the ``attr:`` or ``file:``', 'directives. Please make sure the given file or attribute respects :pep:`440`.'], 'oneOf': [{'title': "'attr:' directive", '$id': '#/definitions/attr-directive', '$$description': ['Value is read from a module attribute. Supports callables and iterables;', 'unsupported types are cast via ``str()``'], 'type': 'object', 'additionalProperties': False, 'properties': {'attr': {'type': 'string'}}, 'required': ['attr']}, {'$id': '#/definitions/file-directive', 'title': "'file:' directive", 'description': 'Value is read from a file (or list of files and then concatenated)', 'type': 'object', 'additionalProperties': False, 'properties': {'file': {'oneOf': [{'type': 'string'}, {'type': 'array', 'items': {'type': 'string'}}]}}, 'required': ['file']}]}, 'classifiers': {'$id': '#/definitions/file-directive', 'title': "'file:' directive", 'description': 'Value is read from a file (or list of files and then concatenated)', 'type': 'object', 'additionalProperties': False, 'properties': {'file': {'oneOf': [{'type': 'string'}, {'type': 'array', 'items': {'type': 'string'}}]}}, 'required': ['file']}, 'description': {'$id': '#/definitions/file-directive', 'title': "'file:' directive", 'description': 'Value is read from a file (or list of files and then concatenated)', 'type': 'object', 'additionalProperties': False, 'properties': {'file': {'oneOf': [{'type': 'string'}, {'type': 'array', 'items': {'type': 'string'}}]}}, 'required': ['file']}, 'dependencies': {'$id': '#/definitions/file-directive', 'title': "'file:' directive", 'description': 'Value is read from a file (or list of files and then concatenated)', 'type': 'object', 'additionalProperties': False, 'properties': {'file': {'oneOf': [{'type': 'string'}, {'type': 'array', 'items': {'type': 'string'}}]}}, 'required': ['file']}, 'entry-points': {'$id': '#/definitions/file-directive', 'title': "'file:' directive", 'description': 'Value is read from a file (or list of files and then concatenated)', 'type': 'object', 'additionalProperties': False, 'properties': {'file': {'oneOf': [{'type': 'string'}, {'type': 'array', 'items': {'type': 'string'}}]}}, 'required': ['file']}, 'optional-dependencies': {'type': 'object', 'propertyNames': {'format': 'python-identifier'}, 'additionalProperties': False, 'patternProperties': {'.+': {'$id': '#/definitions/file-directive', 'title': "'file:' directive", 'description': 'Value is read from a file (or list of files and then concatenated)', 'type': 'object', 'additionalProperties': False, 'properties': {'file': {'oneOf': [{'type': 'string'}, {'type': 'array', 'items': {'type': 'string'}}]}}, 'required': ['file']}}}, 'readme': {'anyOf': [{'$id': '#/definitions/file-directive', 'title': "'file:' directive", 'description': 'Value is read from a file (or list of files and then concatenated)', 'type': 'object', 'additionalProperties': False, 'properties': {'file': {'oneOf': [{'type': 'string'}, {'type': 'array', 'items': {'type': 'string'}}]}}, 'required': ['file']}, {'properties': {'content-type': {'type': 'string'}}}], 'required': ['file']}}}, rule='type')
- data__dynamic_is_dict = isinstance(data__dynamic, dict)
- if data__dynamic_is_dict:
- data__dynamic_keys = set(data__dynamic.keys())
- if "version" in data__dynamic_keys:
- data__dynamic_keys.remove("version")
- data__dynamic__version = data__dynamic["version"]
- data__dynamic__version_one_of_count5 = 0
- if data__dynamic__version_one_of_count5 < 2:
- try:
- validate_https___setuptools_pypa_io_en_latest_references_keywords_html__definitions_attr_directive(data__dynamic__version, custom_formats, (name_prefix or "data") + ".dynamic.version")
- data__dynamic__version_one_of_count5 += 1
- except JsonSchemaValueException: pass
- if data__dynamic__version_one_of_count5 < 2:
- try:
- validate_https___setuptools_pypa_io_en_latest_references_keywords_html__definitions_file_directive(data__dynamic__version, custom_formats, (name_prefix or "data") + ".dynamic.version")
- data__dynamic__version_one_of_count5 += 1
- except JsonSchemaValueException: pass
- if data__dynamic__version_one_of_count5 != 1:
- raise JsonSchemaValueException("" + (name_prefix or "data") + ".dynamic.version must be valid exactly by one definition" + (" (" + str(data__dynamic__version_one_of_count5) + " matches found)"), value=data__dynamic__version, name="" + (name_prefix or "data") + ".dynamic.version", definition={'$$description': ['A version dynamically loaded via either the ``attr:`` or ``file:``', 'directives. Please make sure the given file or attribute respects :pep:`440`.'], 'oneOf': [{'title': "'attr:' directive", '$id': '#/definitions/attr-directive', '$$description': ['Value is read from a module attribute. Supports callables and iterables;', 'unsupported types are cast via ``str()``'], 'type': 'object', 'additionalProperties': False, 'properties': {'attr': {'type': 'string'}}, 'required': ['attr']}, {'$id': '#/definitions/file-directive', 'title': "'file:' directive", 'description': 'Value is read from a file (or list of files and then concatenated)', 'type': 'object', 'additionalProperties': False, 'properties': {'file': {'oneOf': [{'type': 'string'}, {'type': 'array', 'items': {'type': 'string'}}]}}, 'required': ['file']}]}, rule='oneOf')
- if "classifiers" in data__dynamic_keys:
- data__dynamic_keys.remove("classifiers")
- data__dynamic__classifiers = data__dynamic["classifiers"]
- validate_https___setuptools_pypa_io_en_latest_references_keywords_html__definitions_file_directive(data__dynamic__classifiers, custom_formats, (name_prefix or "data") + ".dynamic.classifiers")
- if "description" in data__dynamic_keys:
- data__dynamic_keys.remove("description")
- data__dynamic__description = data__dynamic["description"]
- validate_https___setuptools_pypa_io_en_latest_references_keywords_html__definitions_file_directive(data__dynamic__description, custom_formats, (name_prefix or "data") + ".dynamic.description")
- if "dependencies" in data__dynamic_keys:
- data__dynamic_keys.remove("dependencies")
- data__dynamic__dependencies = data__dynamic["dependencies"]
- validate_https___setuptools_pypa_io_en_latest_references_keywords_html__definitions_file_directive(data__dynamic__dependencies, custom_formats, (name_prefix or "data") + ".dynamic.dependencies")
- if "entry-points" in data__dynamic_keys:
- data__dynamic_keys.remove("entry-points")
- data__dynamic__entrypoints = data__dynamic["entry-points"]
- validate_https___setuptools_pypa_io_en_latest_references_keywords_html__definitions_file_directive(data__dynamic__entrypoints, custom_formats, (name_prefix or "data") + ".dynamic.entry-points")
- if "optional-dependencies" in data__dynamic_keys:
- data__dynamic_keys.remove("optional-dependencies")
- data__dynamic__optionaldependencies = data__dynamic["optional-dependencies"]
- if not isinstance(data__dynamic__optionaldependencies, (dict)):
- raise JsonSchemaValueException("" + (name_prefix or "data") + ".dynamic.optional-dependencies must be object", value=data__dynamic__optionaldependencies, name="" + (name_prefix or "data") + ".dynamic.optional-dependencies", definition={'type': 'object', 'propertyNames': {'format': 'python-identifier'}, 'additionalProperties': False, 'patternProperties': {'.+': {'$id': '#/definitions/file-directive', 'title': "'file:' directive", 'description': 'Value is read from a file (or list of files and then concatenated)', 'type': 'object', 'additionalProperties': False, 'properties': {'file': {'oneOf': [{'type': 'string'}, {'type': 'array', 'items': {'type': 'string'}}]}}, 'required': ['file']}}}, rule='type')
- data__dynamic__optionaldependencies_is_dict = isinstance(data__dynamic__optionaldependencies, dict)
- if data__dynamic__optionaldependencies_is_dict:
- data__dynamic__optionaldependencies_keys = set(data__dynamic__optionaldependencies.keys())
- for data__dynamic__optionaldependencies_key, data__dynamic__optionaldependencies_val in data__dynamic__optionaldependencies.items():
- if REGEX_PATTERNS['.+'].search(data__dynamic__optionaldependencies_key):
- if data__dynamic__optionaldependencies_key in data__dynamic__optionaldependencies_keys:
- data__dynamic__optionaldependencies_keys.remove(data__dynamic__optionaldependencies_key)
- validate_https___setuptools_pypa_io_en_latest_references_keywords_html__definitions_file_directive(data__dynamic__optionaldependencies_val, custom_formats, (name_prefix or "data") + ".dynamic.optional-dependencies.{data__dynamic__optionaldependencies_key}")
- if data__dynamic__optionaldependencies_keys:
- raise JsonSchemaValueException("" + (name_prefix or "data") + ".dynamic.optional-dependencies must not contain "+str(data__dynamic__optionaldependencies_keys)+" properties", value=data__dynamic__optionaldependencies, name="" + (name_prefix or "data") + ".dynamic.optional-dependencies", definition={'type': 'object', 'propertyNames': {'format': 'python-identifier'}, 'additionalProperties': False, 'patternProperties': {'.+': {'$id': '#/definitions/file-directive', 'title': "'file:' directive", 'description': 'Value is read from a file (or list of files and then concatenated)', 'type': 'object', 'additionalProperties': False, 'properties': {'file': {'oneOf': [{'type': 'string'}, {'type': 'array', 'items': {'type': 'string'}}]}}, 'required': ['file']}}}, rule='additionalProperties')
- data__dynamic__optionaldependencies_len = len(data__dynamic__optionaldependencies)
- if data__dynamic__optionaldependencies_len != 0:
- data__dynamic__optionaldependencies_property_names = True
- for data__dynamic__optionaldependencies_key in data__dynamic__optionaldependencies:
- try:
- if isinstance(data__dynamic__optionaldependencies_key, str):
- if not custom_formats["python-identifier"](data__dynamic__optionaldependencies_key):
- raise JsonSchemaValueException("" + (name_prefix or "data") + ".dynamic.optional-dependencies must be python-identifier", value=data__dynamic__optionaldependencies_key, name="" + (name_prefix or "data") + ".dynamic.optional-dependencies", definition={'format': 'python-identifier'}, rule='format')
- except JsonSchemaValueException:
- data__dynamic__optionaldependencies_property_names = False
- if not data__dynamic__optionaldependencies_property_names:
- raise JsonSchemaValueException("" + (name_prefix or "data") + ".dynamic.optional-dependencies must be named by propertyName definition", value=data__dynamic__optionaldependencies, name="" + (name_prefix or "data") + ".dynamic.optional-dependencies", definition={'type': 'object', 'propertyNames': {'format': 'python-identifier'}, 'additionalProperties': False, 'patternProperties': {'.+': {'$id': '#/definitions/file-directive', 'title': "'file:' directive", 'description': 'Value is read from a file (or list of files and then concatenated)', 'type': 'object', 'additionalProperties': False, 'properties': {'file': {'oneOf': [{'type': 'string'}, {'type': 'array', 'items': {'type': 'string'}}]}}, 'required': ['file']}}}, rule='propertyNames')
- if "readme" in data__dynamic_keys:
- data__dynamic_keys.remove("readme")
- data__dynamic__readme = data__dynamic["readme"]
- data__dynamic__readme_any_of_count6 = 0
- if not data__dynamic__readme_any_of_count6:
- try:
- validate_https___setuptools_pypa_io_en_latest_references_keywords_html__definitions_file_directive(data__dynamic__readme, custom_formats, (name_prefix or "data") + ".dynamic.readme")
- data__dynamic__readme_any_of_count6 += 1
- except JsonSchemaValueException: pass
- if not data__dynamic__readme_any_of_count6:
- try:
- data__dynamic__readme_is_dict = isinstance(data__dynamic__readme, dict)
- if data__dynamic__readme_is_dict:
- data__dynamic__readme_keys = set(data__dynamic__readme.keys())
- if "content-type" in data__dynamic__readme_keys:
- data__dynamic__readme_keys.remove("content-type")
- data__dynamic__readme__contenttype = data__dynamic__readme["content-type"]
- if not isinstance(data__dynamic__readme__contenttype, (str)):
- raise JsonSchemaValueException("" + (name_prefix or "data") + ".dynamic.readme.content-type must be string", value=data__dynamic__readme__contenttype, name="" + (name_prefix or "data") + ".dynamic.readme.content-type", definition={'type': 'string'}, rule='type')
- data__dynamic__readme_any_of_count6 += 1
- except JsonSchemaValueException: pass
- if not data__dynamic__readme_any_of_count6:
- raise JsonSchemaValueException("" + (name_prefix or "data") + ".dynamic.readme cannot be validated by any definition", value=data__dynamic__readme, name="" + (name_prefix or "data") + ".dynamic.readme", definition={'anyOf': [{'$id': '#/definitions/file-directive', 'title': "'file:' directive", 'description': 'Value is read from a file (or list of files and then concatenated)', 'type': 'object', 'additionalProperties': False, 'properties': {'file': {'oneOf': [{'type': 'string'}, {'type': 'array', 'items': {'type': 'string'}}]}}, 'required': ['file']}, {'properties': {'content-type': {'type': 'string'}}}], 'required': ['file']}, rule='anyOf')
- data__dynamic__readme_is_dict = isinstance(data__dynamic__readme, dict)
- if data__dynamic__readme_is_dict:
- data__dynamic__readme_len = len(data__dynamic__readme)
- if not all(prop in data__dynamic__readme for prop in ['file']):
- raise JsonSchemaValueException("" + (name_prefix or "data") + ".dynamic.readme must contain ['file'] properties", value=data__dynamic__readme, name="" + (name_prefix or "data") + ".dynamic.readme", definition={'anyOf': [{'$id': '#/definitions/file-directive', 'title': "'file:' directive", 'description': 'Value is read from a file (or list of files and then concatenated)', 'type': 'object', 'additionalProperties': False, 'properties': {'file': {'oneOf': [{'type': 'string'}, {'type': 'array', 'items': {'type': 'string'}}]}}, 'required': ['file']}, {'properties': {'content-type': {'type': 'string'}}}], 'required': ['file']}, rule='required')
- if data__dynamic_keys:
- raise JsonSchemaValueException("" + (name_prefix or "data") + ".dynamic must not contain "+str(data__dynamic_keys)+" properties", value=data__dynamic, name="" + (name_prefix or "data") + ".dynamic", definition={'type': 'object', 'description': 'Instructions for loading :pep:`621`-related metadata dynamically', 'additionalProperties': False, 'properties': {'version': {'$$description': ['A version dynamically loaded via either the ``attr:`` or ``file:``', 'directives. Please make sure the given file or attribute respects :pep:`440`.'], 'oneOf': [{'title': "'attr:' directive", '$id': '#/definitions/attr-directive', '$$description': ['Value is read from a module attribute. Supports callables and iterables;', 'unsupported types are cast via ``str()``'], 'type': 'object', 'additionalProperties': False, 'properties': {'attr': {'type': 'string'}}, 'required': ['attr']}, {'$id': '#/definitions/file-directive', 'title': "'file:' directive", 'description': 'Value is read from a file (or list of files and then concatenated)', 'type': 'object', 'additionalProperties': False, 'properties': {'file': {'oneOf': [{'type': 'string'}, {'type': 'array', 'items': {'type': 'string'}}]}}, 'required': ['file']}]}, 'classifiers': {'$id': '#/definitions/file-directive', 'title': "'file:' directive", 'description': 'Value is read from a file (or list of files and then concatenated)', 'type': 'object', 'additionalProperties': False, 'properties': {'file': {'oneOf': [{'type': 'string'}, {'type': 'array', 'items': {'type': 'string'}}]}}, 'required': ['file']}, 'description': {'$id': '#/definitions/file-directive', 'title': "'file:' directive", 'description': 'Value is read from a file (or list of files and then concatenated)', 'type': 'object', 'additionalProperties': False, 'properties': {'file': {'oneOf': [{'type': 'string'}, {'type': 'array', 'items': {'type': 'string'}}]}}, 'required': ['file']}, 'dependencies': {'$id': '#/definitions/file-directive', 'title': "'file:' directive", 'description': 'Value is read from a file (or list of files and then concatenated)', 'type': 'object', 'additionalProperties': False, 'properties': {'file': {'oneOf': [{'type': 'string'}, {'type': 'array', 'items': {'type': 'string'}}]}}, 'required': ['file']}, 'entry-points': {'$id': '#/definitions/file-directive', 'title': "'file:' directive", 'description': 'Value is read from a file (or list of files and then concatenated)', 'type': 'object', 'additionalProperties': False, 'properties': {'file': {'oneOf': [{'type': 'string'}, {'type': 'array', 'items': {'type': 'string'}}]}}, 'required': ['file']}, 'optional-dependencies': {'type': 'object', 'propertyNames': {'format': 'python-identifier'}, 'additionalProperties': False, 'patternProperties': {'.+': {'$id': '#/definitions/file-directive', 'title': "'file:' directive", 'description': 'Value is read from a file (or list of files and then concatenated)', 'type': 'object', 'additionalProperties': False, 'properties': {'file': {'oneOf': [{'type': 'string'}, {'type': 'array', 'items': {'type': 'string'}}]}}, 'required': ['file']}}}, 'readme': {'anyOf': [{'$id': '#/definitions/file-directive', 'title': "'file:' directive", 'description': 'Value is read from a file (or list of files and then concatenated)', 'type': 'object', 'additionalProperties': False, 'properties': {'file': {'oneOf': [{'type': 'string'}, {'type': 'array', 'items': {'type': 'string'}}]}}, 'required': ['file']}, {'properties': {'content-type': {'type': 'string'}}}], 'required': ['file']}}}, rule='additionalProperties')
- if data_keys:
- raise JsonSchemaValueException("" + (name_prefix or "data") + " must not contain "+str(data_keys)+" properties", value=data, name="" + (name_prefix or "data") + "", definition={'$schema': 'http://json-schema.org/draft-07/schema', '$id': 'https://setuptools.pypa.io/en/latest/references/keywords.html', 'title': '``tool.setuptools`` table', '$$description': ['Please notice for the time being the ``setuptools`` project does not specify', 'a way of configuring builds via ``pyproject.toml``.', 'Therefore this schema should be taken just as a *"thought experiment"* on how', 'this *might be done*, by following the principles established in', '`ini2toml `_.', 'It considers only ``setuptools`` `parameters', '`_', 'that can currently be configured via ``setup.cfg`` and are not covered by :pep:`621`', 'but intentionally excludes ``dependency_links`` and ``setup_requires``.', 'NOTE: ``scripts`` was renamed to ``script-files`` to avoid confusion with', 'entry-point based scripts (defined in :pep:`621`).'], 'type': 'object', 'additionalProperties': False, 'properties': {'platforms': {'type': 'array', 'items': {'type': 'string'}}, 'provides': {'$$description': ['Package and virtual package names contained within this package', '**(not supported by pip)**'], 'type': 'array', 'items': {'type': 'string', 'format': 'pep508-identifier'}}, 'obsoletes': {'$$description': ['Packages which this package renders obsolete', '**(not supported by pip)**'], 'type': 'array', 'items': {'type': 'string', 'format': 'pep508-identifier'}}, 'zip-safe': {'description': 'Whether the project can be safely installed and run from a zip file.', 'type': 'boolean'}, 'script-files': {'description': 'Legacy way of defining scripts (entry-points are preferred).', 'type': 'array', 'items': {'type': 'string'}, '$comment': 'TODO: is this field deprecated/should be removed?'}, 'eager-resources': {'$$description': ['Resources that should be extracted together, if any of them is needed,', 'or if any C extensions included in the project are imported.'], 'type': 'array', 'items': {'type': 'string'}}, 'packages': {'$$description': ['Packages that should be included in the distribution.', 'It can be given either as a list of package identifiers', 'or as a ``dict``-like structure with a single key ``find``', 'which corresponds to a dynamic call to', '``setuptools.config.expand.find_packages`` function.', 'The ``find`` key is associated with a nested ``dict``-like structure that can', 'contain ``where``, ``include``, ``exclude`` and ``namespaces`` keys,', 'mimicking the keyword arguments of the associated function.'], 'oneOf': [{'title': 'Array of Python package identifiers', 'type': 'array', 'items': {'type': 'string', 'format': 'python-module-name'}}, {'$id': '#/definitions/find-directive', 'title': "'find:' directive", 'type': 'object', 'additionalProperties': False, 'properties': {'find': {'type': 'object', '$$description': ['Dynamic `package discovery', '`_.'], 'additionalProperties': False, 'properties': {'where': {'description': 'Directories to be searched for packages (Unix-style relative path)', 'type': 'array', 'items': {'type': 'string'}}, 'exclude': {'type': 'array', '$$description': ['Exclude packages that match the values listed in this field.', "Can container shell-style wildcards (e.g. ``'pkg.*'``)"], 'items': {'type': 'string'}}, 'include': {'type': 'array', '$$description': ['Restrict the found packages to just the ones listed in this field.', "Can container shell-style wildcards (e.g. ``'pkg.*'``)"], 'items': {'type': 'string'}}, 'namespaces': {'type': 'boolean', '$$description': ['When ``True``, directories without a ``__init__.py`` file will also', 'be scanned for :pep:`420`-style implicit namespaces']}}}}}]}, 'package-dir': {'$$description': [':class:`dict`-like structure mapping from package names to directories where their', 'code can be found.', 'The empty string (as key) means that all packages are contained inside', 'the given directory will be included in the distribution.'], 'type': 'object', 'additionalProperties': False, 'propertyNames': {'oneOf': [{'format': 'python-module-name'}, {'const': ''}]}, 'patternProperties': {'^.*$': {'type': 'string'}}}, 'package-data': {'$$description': ['Mapping from package names to lists of glob patterns.', 'Usually this option is not needed when using ``include-package-data = true``', 'For more information on how to include data files, check ``setuptools`` `docs', '`_.'], 'type': 'object', 'additionalProperties': False, 'propertyNames': {'oneOf': [{'format': 'python-module-name'}, {'const': '*'}]}, 'patternProperties': {'^.*$': {'type': 'array', 'items': {'type': 'string'}}}}, 'include-package-data': {'$$description': ['Automatically include any data files inside the package directories', 'that are specified by ``MANIFEST.in``', 'For more information on how to include data files, check ``setuptools`` `docs', '`_.'], 'type': 'boolean'}, 'exclude-package-data': {'$$description': ['Mapping from package names to lists of glob patterns that should be excluded', 'For more information on how to include data files, check ``setuptools`` `docs', '`_.'], 'type': 'object', 'additionalProperties': False, 'propertyNames': {'oneOf': [{'format': 'python-module-name'}, {'const': '*'}]}, 'patternProperties': {'^.*$': {'type': 'array', 'items': {'type': 'string'}}}}, 'namespace-packages': {'type': 'array', 'items': {'type': 'string', 'format': 'python-module-name'}, '$comment': 'https://setuptools.pypa.io/en/latest/userguide/package_discovery.html'}, 'py-modules': {'description': 'Modules that setuptools will manipulate', 'type': 'array', 'items': {'type': 'string', 'format': 'python-module-name'}, '$comment': 'TODO: clarify the relationship with ``packages``'}, 'data-files': {'$$description': ['**DEPRECATED**: dict-like structure where each key represents a directory and', 'the value is a list of glob patterns that should be installed in them.', "Please notice this don't work with wheels. See `data files support", '`_'], 'type': 'object', 'patternProperties': {'^.*$': {'type': 'array', 'items': {'type': 'string'}}}}, 'cmdclass': {'$$description': ['Mapping of distutils-style command names to ``setuptools.Command`` subclasses', 'which in turn should be represented by strings with a qualified class name', '(i.e., "dotted" form with module), e.g.::\n\n', ' cmdclass = {mycmd = "pkg.subpkg.module.CommandClass"}\n\n', 'The command class should be a directly defined at the top-level of the', 'containing module (no class nesting).'], 'type': 'object', 'patternProperties': {'^.*$': {'type': 'string', 'format': 'python-qualified-identifier'}}}, 'license-files': {'type': 'array', 'items': {'type': 'string'}, '$$description': ['PROVISIONAL: List of glob patterns for all license files being distributed.', '(might become standard with PEP 639).'], 'default': ['LICEN[CS]E*', ' COPYING*', ' NOTICE*', 'AUTHORS*'], '$comment': 'TODO: revise if PEP 639 is accepted. Probably ``project.license-files``?'}, 'dynamic': {'type': 'object', 'description': 'Instructions for loading :pep:`621`-related metadata dynamically', 'additionalProperties': False, 'properties': {'version': {'$$description': ['A version dynamically loaded via either the ``attr:`` or ``file:``', 'directives. Please make sure the given file or attribute respects :pep:`440`.'], 'oneOf': [{'title': "'attr:' directive", '$id': '#/definitions/attr-directive', '$$description': ['Value is read from a module attribute. Supports callables and iterables;', 'unsupported types are cast via ``str()``'], 'type': 'object', 'additionalProperties': False, 'properties': {'attr': {'type': 'string'}}, 'required': ['attr']}, {'$id': '#/definitions/file-directive', 'title': "'file:' directive", 'description': 'Value is read from a file (or list of files and then concatenated)', 'type': 'object', 'additionalProperties': False, 'properties': {'file': {'oneOf': [{'type': 'string'}, {'type': 'array', 'items': {'type': 'string'}}]}}, 'required': ['file']}]}, 'classifiers': {'$id': '#/definitions/file-directive', 'title': "'file:' directive", 'description': 'Value is read from a file (or list of files and then concatenated)', 'type': 'object', 'additionalProperties': False, 'properties': {'file': {'oneOf': [{'type': 'string'}, {'type': 'array', 'items': {'type': 'string'}}]}}, 'required': ['file']}, 'description': {'$id': '#/definitions/file-directive', 'title': "'file:' directive", 'description': 'Value is read from a file (or list of files and then concatenated)', 'type': 'object', 'additionalProperties': False, 'properties': {'file': {'oneOf': [{'type': 'string'}, {'type': 'array', 'items': {'type': 'string'}}]}}, 'required': ['file']}, 'dependencies': {'$id': '#/definitions/file-directive', 'title': "'file:' directive", 'description': 'Value is read from a file (or list of files and then concatenated)', 'type': 'object', 'additionalProperties': False, 'properties': {'file': {'oneOf': [{'type': 'string'}, {'type': 'array', 'items': {'type': 'string'}}]}}, 'required': ['file']}, 'entry-points': {'$id': '#/definitions/file-directive', 'title': "'file:' directive", 'description': 'Value is read from a file (or list of files and then concatenated)', 'type': 'object', 'additionalProperties': False, 'properties': {'file': {'oneOf': [{'type': 'string'}, {'type': 'array', 'items': {'type': 'string'}}]}}, 'required': ['file']}, 'optional-dependencies': {'type': 'object', 'propertyNames': {'format': 'python-identifier'}, 'additionalProperties': False, 'patternProperties': {'.+': {'$id': '#/definitions/file-directive', 'title': "'file:' directive", 'description': 'Value is read from a file (or list of files and then concatenated)', 'type': 'object', 'additionalProperties': False, 'properties': {'file': {'oneOf': [{'type': 'string'}, {'type': 'array', 'items': {'type': 'string'}}]}}, 'required': ['file']}}}, 'readme': {'anyOf': [{'$id': '#/definitions/file-directive', 'title': "'file:' directive", 'description': 'Value is read from a file (or list of files and then concatenated)', 'type': 'object', 'additionalProperties': False, 'properties': {'file': {'oneOf': [{'type': 'string'}, {'type': 'array', 'items': {'type': 'string'}}]}}, 'required': ['file']}, {'properties': {'content-type': {'type': 'string'}}}], 'required': ['file']}}}}, 'definitions': {'file-directive': {'$id': '#/definitions/file-directive', 'title': "'file:' directive", 'description': 'Value is read from a file (or list of files and then concatenated)', 'type': 'object', 'additionalProperties': False, 'properties': {'file': {'oneOf': [{'type': 'string'}, {'type': 'array', 'items': {'type': 'string'}}]}}, 'required': ['file']}, 'attr-directive': {'title': "'attr:' directive", '$id': '#/definitions/attr-directive', '$$description': ['Value is read from a module attribute. Supports callables and iterables;', 'unsupported types are cast via ``str()``'], 'type': 'object', 'additionalProperties': False, 'properties': {'attr': {'type': 'string'}}, 'required': ['attr']}, 'find-directive': {'$id': '#/definitions/find-directive', 'title': "'find:' directive", 'type': 'object', 'additionalProperties': False, 'properties': {'find': {'type': 'object', '$$description': ['Dynamic `package discovery', '`_.'], 'additionalProperties': False, 'properties': {'where': {'description': 'Directories to be searched for packages (Unix-style relative path)', 'type': 'array', 'items': {'type': 'string'}}, 'exclude': {'type': 'array', '$$description': ['Exclude packages that match the values listed in this field.', "Can container shell-style wildcards (e.g. ``'pkg.*'``)"], 'items': {'type': 'string'}}, 'include': {'type': 'array', '$$description': ['Restrict the found packages to just the ones listed in this field.', "Can container shell-style wildcards (e.g. ``'pkg.*'``)"], 'items': {'type': 'string'}}, 'namespaces': {'type': 'boolean', '$$description': ['When ``True``, directories without a ``__init__.py`` file will also', 'be scanned for :pep:`420`-style implicit namespaces']}}}}}}}, rule='additionalProperties')
- return data
-
-def validate_https___setuptools_pypa_io_en_latest_references_keywords_html__definitions_file_directive(data, custom_formats={}, name_prefix=None):
- if not isinstance(data, (dict)):
- raise JsonSchemaValueException("" + (name_prefix or "data") + " must be object", value=data, name="" + (name_prefix or "data") + "", definition={'$id': '#/definitions/file-directive', 'title': "'file:' directive", 'description': 'Value is read from a file (or list of files and then concatenated)', 'type': 'object', 'additionalProperties': False, 'properties': {'file': {'oneOf': [{'type': 'string'}, {'type': 'array', 'items': {'type': 'string'}}]}}, 'required': ['file']}, rule='type')
- data_is_dict = isinstance(data, dict)
- if data_is_dict:
- data_len = len(data)
- if not all(prop in data for prop in ['file']):
- raise JsonSchemaValueException("" + (name_prefix or "data") + " must contain ['file'] properties", value=data, name="" + (name_prefix or "data") + "", definition={'$id': '#/definitions/file-directive', 'title': "'file:' directive", 'description': 'Value is read from a file (or list of files and then concatenated)', 'type': 'object', 'additionalProperties': False, 'properties': {'file': {'oneOf': [{'type': 'string'}, {'type': 'array', 'items': {'type': 'string'}}]}}, 'required': ['file']}, rule='required')
- data_keys = set(data.keys())
- if "file" in data_keys:
- data_keys.remove("file")
- data__file = data["file"]
- data__file_one_of_count7 = 0
- if data__file_one_of_count7 < 2:
- try:
- if not isinstance(data__file, (str)):
- raise JsonSchemaValueException("" + (name_prefix or "data") + ".file must be string", value=data__file, name="" + (name_prefix or "data") + ".file", definition={'type': 'string'}, rule='type')
- data__file_one_of_count7 += 1
- except JsonSchemaValueException: pass
- if data__file_one_of_count7 < 2:
- try:
- if not isinstance(data__file, (list, tuple)):
- raise JsonSchemaValueException("" + (name_prefix or "data") + ".file must be array", value=data__file, name="" + (name_prefix or "data") + ".file", definition={'type': 'array', 'items': {'type': 'string'}}, rule='type')
- data__file_is_list = isinstance(data__file, (list, tuple))
- if data__file_is_list:
- data__file_len = len(data__file)
- for data__file_x, data__file_item in enumerate(data__file):
- if not isinstance(data__file_item, (str)):
- raise JsonSchemaValueException("" + (name_prefix or "data") + ".file[{data__file_x}]".format(**locals()) + " must be string", value=data__file_item, name="" + (name_prefix or "data") + ".file[{data__file_x}]".format(**locals()) + "", definition={'type': 'string'}, rule='type')
- data__file_one_of_count7 += 1
- except JsonSchemaValueException: pass
- if data__file_one_of_count7 != 1:
- raise JsonSchemaValueException("" + (name_prefix or "data") + ".file must be valid exactly by one definition" + (" (" + str(data__file_one_of_count7) + " matches found)"), value=data__file, name="" + (name_prefix or "data") + ".file", definition={'oneOf': [{'type': 'string'}, {'type': 'array', 'items': {'type': 'string'}}]}, rule='oneOf')
- if data_keys:
- raise JsonSchemaValueException("" + (name_prefix or "data") + " must not contain "+str(data_keys)+" properties", value=data, name="" + (name_prefix or "data") + "", definition={'$id': '#/definitions/file-directive', 'title': "'file:' directive", 'description': 'Value is read from a file (or list of files and then concatenated)', 'type': 'object', 'additionalProperties': False, 'properties': {'file': {'oneOf': [{'type': 'string'}, {'type': 'array', 'items': {'type': 'string'}}]}}, 'required': ['file']}, rule='additionalProperties')
- return data
-
-def validate_https___setuptools_pypa_io_en_latest_references_keywords_html__definitions_attr_directive(data, custom_formats={}, name_prefix=None):
- if not isinstance(data, (dict)):
- raise JsonSchemaValueException("" + (name_prefix or "data") + " must be object", value=data, name="" + (name_prefix or "data") + "", definition={'title': "'attr:' directive", '$id': '#/definitions/attr-directive', '$$description': ['Value is read from a module attribute. Supports callables and iterables;', 'unsupported types are cast via ``str()``'], 'type': 'object', 'additionalProperties': False, 'properties': {'attr': {'type': 'string'}}, 'required': ['attr']}, rule='type')
- data_is_dict = isinstance(data, dict)
- if data_is_dict:
- data_len = len(data)
- if not all(prop in data for prop in ['attr']):
- raise JsonSchemaValueException("" + (name_prefix or "data") + " must contain ['attr'] properties", value=data, name="" + (name_prefix or "data") + "", definition={'title': "'attr:' directive", '$id': '#/definitions/attr-directive', '$$description': ['Value is read from a module attribute. Supports callables and iterables;', 'unsupported types are cast via ``str()``'], 'type': 'object', 'additionalProperties': False, 'properties': {'attr': {'type': 'string'}}, 'required': ['attr']}, rule='required')
- data_keys = set(data.keys())
- if "attr" in data_keys:
- data_keys.remove("attr")
- data__attr = data["attr"]
- if not isinstance(data__attr, (str)):
- raise JsonSchemaValueException("" + (name_prefix or "data") + ".attr must be string", value=data__attr, name="" + (name_prefix or "data") + ".attr", definition={'type': 'string'}, rule='type')
- if data_keys:
- raise JsonSchemaValueException("" + (name_prefix or "data") + " must not contain "+str(data_keys)+" properties", value=data, name="" + (name_prefix or "data") + "", definition={'title': "'attr:' directive", '$id': '#/definitions/attr-directive', '$$description': ['Value is read from a module attribute. Supports callables and iterables;', 'unsupported types are cast via ``str()``'], 'type': 'object', 'additionalProperties': False, 'properties': {'attr': {'type': 'string'}}, 'required': ['attr']}, rule='additionalProperties')
- return data
-
-def validate_https___setuptools_pypa_io_en_latest_references_keywords_html__definitions_find_directive(data, custom_formats={}, name_prefix=None):
- if not isinstance(data, (dict)):
- raise JsonSchemaValueException("" + (name_prefix or "data") + " must be object", value=data, name="" + (name_prefix or "data") + "", definition={'$id': '#/definitions/find-directive', 'title': "'find:' directive", 'type': 'object', 'additionalProperties': False, 'properties': {'find': {'type': 'object', '$$description': ['Dynamic `package discovery', '`_.'], 'additionalProperties': False, 'properties': {'where': {'description': 'Directories to be searched for packages (Unix-style relative path)', 'type': 'array', 'items': {'type': 'string'}}, 'exclude': {'type': 'array', '$$description': ['Exclude packages that match the values listed in this field.', "Can container shell-style wildcards (e.g. ``'pkg.*'``)"], 'items': {'type': 'string'}}, 'include': {'type': 'array', '$$description': ['Restrict the found packages to just the ones listed in this field.', "Can container shell-style wildcards (e.g. ``'pkg.*'``)"], 'items': {'type': 'string'}}, 'namespaces': {'type': 'boolean', '$$description': ['When ``True``, directories without a ``__init__.py`` file will also', 'be scanned for :pep:`420`-style implicit namespaces']}}}}}, rule='type')
- data_is_dict = isinstance(data, dict)
- if data_is_dict:
- data_keys = set(data.keys())
- if "find" in data_keys:
- data_keys.remove("find")
- data__find = data["find"]
- if not isinstance(data__find, (dict)):
- raise JsonSchemaValueException("" + (name_prefix or "data") + ".find must be object", value=data__find, name="" + (name_prefix or "data") + ".find", definition={'type': 'object', '$$description': ['Dynamic `package discovery', '`_.'], 'additionalProperties': False, 'properties': {'where': {'description': 'Directories to be searched for packages (Unix-style relative path)', 'type': 'array', 'items': {'type': 'string'}}, 'exclude': {'type': 'array', '$$description': ['Exclude packages that match the values listed in this field.', "Can container shell-style wildcards (e.g. ``'pkg.*'``)"], 'items': {'type': 'string'}}, 'include': {'type': 'array', '$$description': ['Restrict the found packages to just the ones listed in this field.', "Can container shell-style wildcards (e.g. ``'pkg.*'``)"], 'items': {'type': 'string'}}, 'namespaces': {'type': 'boolean', '$$description': ['When ``True``, directories without a ``__init__.py`` file will also', 'be scanned for :pep:`420`-style implicit namespaces']}}}, rule='type')
- data__find_is_dict = isinstance(data__find, dict)
- if data__find_is_dict:
- data__find_keys = set(data__find.keys())
- if "where" in data__find_keys:
- data__find_keys.remove("where")
- data__find__where = data__find["where"]
- if not isinstance(data__find__where, (list, tuple)):
- raise JsonSchemaValueException("" + (name_prefix or "data") + ".find.where must be array", value=data__find__where, name="" + (name_prefix or "data") + ".find.where", definition={'description': 'Directories to be searched for packages (Unix-style relative path)', 'type': 'array', 'items': {'type': 'string'}}, rule='type')
- data__find__where_is_list = isinstance(data__find__where, (list, tuple))
- if data__find__where_is_list:
- data__find__where_len = len(data__find__where)
- for data__find__where_x, data__find__where_item in enumerate(data__find__where):
- if not isinstance(data__find__where_item, (str)):
- raise JsonSchemaValueException("" + (name_prefix or "data") + ".find.where[{data__find__where_x}]".format(**locals()) + " must be string", value=data__find__where_item, name="" + (name_prefix or "data") + ".find.where[{data__find__where_x}]".format(**locals()) + "", definition={'type': 'string'}, rule='type')
- if "exclude" in data__find_keys:
- data__find_keys.remove("exclude")
- data__find__exclude = data__find["exclude"]
- if not isinstance(data__find__exclude, (list, tuple)):
- raise JsonSchemaValueException("" + (name_prefix or "data") + ".find.exclude must be array", value=data__find__exclude, name="" + (name_prefix or "data") + ".find.exclude", definition={'type': 'array', '$$description': ['Exclude packages that match the values listed in this field.', "Can container shell-style wildcards (e.g. ``'pkg.*'``)"], 'items': {'type': 'string'}}, rule='type')
- data__find__exclude_is_list = isinstance(data__find__exclude, (list, tuple))
- if data__find__exclude_is_list:
- data__find__exclude_len = len(data__find__exclude)
- for data__find__exclude_x, data__find__exclude_item in enumerate(data__find__exclude):
- if not isinstance(data__find__exclude_item, (str)):
- raise JsonSchemaValueException("" + (name_prefix or "data") + ".find.exclude[{data__find__exclude_x}]".format(**locals()) + " must be string", value=data__find__exclude_item, name="" + (name_prefix or "data") + ".find.exclude[{data__find__exclude_x}]".format(**locals()) + "", definition={'type': 'string'}, rule='type')
- if "include" in data__find_keys:
- data__find_keys.remove("include")
- data__find__include = data__find["include"]
- if not isinstance(data__find__include, (list, tuple)):
- raise JsonSchemaValueException("" + (name_prefix or "data") + ".find.include must be array", value=data__find__include, name="" + (name_prefix or "data") + ".find.include", definition={'type': 'array', '$$description': ['Restrict the found packages to just the ones listed in this field.', "Can container shell-style wildcards (e.g. ``'pkg.*'``)"], 'items': {'type': 'string'}}, rule='type')
- data__find__include_is_list = isinstance(data__find__include, (list, tuple))
- if data__find__include_is_list:
- data__find__include_len = len(data__find__include)
- for data__find__include_x, data__find__include_item in enumerate(data__find__include):
- if not isinstance(data__find__include_item, (str)):
- raise JsonSchemaValueException("" + (name_prefix or "data") + ".find.include[{data__find__include_x}]".format(**locals()) + " must be string", value=data__find__include_item, name="" + (name_prefix or "data") + ".find.include[{data__find__include_x}]".format(**locals()) + "", definition={'type': 'string'}, rule='type')
- if "namespaces" in data__find_keys:
- data__find_keys.remove("namespaces")
- data__find__namespaces = data__find["namespaces"]
- if not isinstance(data__find__namespaces, (bool)):
- raise JsonSchemaValueException("" + (name_prefix or "data") + ".find.namespaces must be boolean", value=data__find__namespaces, name="" + (name_prefix or "data") + ".find.namespaces", definition={'type': 'boolean', '$$description': ['When ``True``, directories without a ``__init__.py`` file will also', 'be scanned for :pep:`420`-style implicit namespaces']}, rule='type')
- if data__find_keys:
- raise JsonSchemaValueException("" + (name_prefix or "data") + ".find must not contain "+str(data__find_keys)+" properties", value=data__find, name="" + (name_prefix or "data") + ".find", definition={'type': 'object', '$$description': ['Dynamic `package discovery', '`_.'], 'additionalProperties': False, 'properties': {'where': {'description': 'Directories to be searched for packages (Unix-style relative path)', 'type': 'array', 'items': {'type': 'string'}}, 'exclude': {'type': 'array', '$$description': ['Exclude packages that match the values listed in this field.', "Can container shell-style wildcards (e.g. ``'pkg.*'``)"], 'items': {'type': 'string'}}, 'include': {'type': 'array', '$$description': ['Restrict the found packages to just the ones listed in this field.', "Can container shell-style wildcards (e.g. ``'pkg.*'``)"], 'items': {'type': 'string'}}, 'namespaces': {'type': 'boolean', '$$description': ['When ``True``, directories without a ``__init__.py`` file will also', 'be scanned for :pep:`420`-style implicit namespaces']}}}, rule='additionalProperties')
- if data_keys:
- raise JsonSchemaValueException("" + (name_prefix or "data") + " must not contain "+str(data_keys)+" properties", value=data, name="" + (name_prefix or "data") + "", definition={'$id': '#/definitions/find-directive', 'title': "'find:' directive", 'type': 'object', 'additionalProperties': False, 'properties': {'find': {'type': 'object', '$$description': ['Dynamic `package discovery', '`_.'], 'additionalProperties': False, 'properties': {'where': {'description': 'Directories to be searched for packages (Unix-style relative path)', 'type': 'array', 'items': {'type': 'string'}}, 'exclude': {'type': 'array', '$$description': ['Exclude packages that match the values listed in this field.', "Can container shell-style wildcards (e.g. ``'pkg.*'``)"], 'items': {'type': 'string'}}, 'include': {'type': 'array', '$$description': ['Restrict the found packages to just the ones listed in this field.', "Can container shell-style wildcards (e.g. ``'pkg.*'``)"], 'items': {'type': 'string'}}, 'namespaces': {'type': 'boolean', '$$description': ['When ``True``, directories without a ``__init__.py`` file will also', 'be scanned for :pep:`420`-style implicit namespaces']}}}}}, rule='additionalProperties')
- return data
-
-def validate_https___docs_python_org_3_install(data, custom_formats={}, name_prefix=None):
- if not isinstance(data, (dict)):
- raise JsonSchemaValueException("" + (name_prefix or "data") + " must be object", value=data, name="" + (name_prefix or "data") + "", definition={'$schema': 'http://json-schema.org/draft-07/schema', '$id': 'https://docs.python.org/3/install/', 'title': '``tool.distutils`` table', '$$description': ['Originally, ``distutils`` allowed developers to configure arguments for', '``setup.py`` scripts via `distutils configuration files', '`_.', '``tool.distutils`` subtables could be used with the same purpose', '(NOT CURRENTLY IMPLEMENTED).'], 'type': 'object', 'properties': {'global': {'type': 'object', 'description': 'Global options applied to all ``distutils`` commands'}}, 'patternProperties': {'.+': {'type': 'object'}}, '$comment': 'TODO: Is there a practical way of making this schema more specific?'}, rule='type')
- data_is_dict = isinstance(data, dict)
- if data_is_dict:
- data_keys = set(data.keys())
- if "global" in data_keys:
- data_keys.remove("global")
- data__global = data["global"]
- if not isinstance(data__global, (dict)):
- raise JsonSchemaValueException("" + (name_prefix or "data") + ".global must be object", value=data__global, name="" + (name_prefix or "data") + ".global", definition={'type': 'object', 'description': 'Global options applied to all ``distutils`` commands'}, rule='type')
- for data_key, data_val in data.items():
- if REGEX_PATTERNS['.+'].search(data_key):
- if data_key in data_keys:
- data_keys.remove(data_key)
- if not isinstance(data_val, (dict)):
- raise JsonSchemaValueException("" + (name_prefix or "data") + ".{data_key}".format(**locals()) + " must be object", value=data_val, name="" + (name_prefix or "data") + ".{data_key}".format(**locals()) + "", definition={'type': 'object'}, rule='type')
- return data
-
-def validate_https___packaging_python_org_en_latest_specifications_declaring_project_metadata(data, custom_formats={}, name_prefix=None):
- if not isinstance(data, (dict)):
- raise JsonSchemaValueException("" + (name_prefix or "data") + " must be object", value=data, name="" + (name_prefix or "data") + "", definition={'$schema': 'http://json-schema.org/draft-07/schema', '$id': 'https://packaging.python.org/en/latest/specifications/declaring-project-metadata/', 'title': 'Package metadata stored in the ``project`` table', '$$description': ['Data structure for the **project** table inside ``pyproject.toml``', '(as initially defined in :pep:`621`)'], 'type': 'object', 'properties': {'name': {'type': 'string', 'description': 'The name (primary identifier) of the project. MUST be statically defined.', 'format': 'pep508-identifier'}, 'version': {'type': 'string', 'description': 'The version of the project as supported by :pep:`440`.', 'format': 'pep440'}, 'description': {'type': 'string', '$$description': ['The `summary description of the project', '`_']}, 'readme': {'$$description': ['`Full/detailed description of the project in the form of a README', '`_', "with meaning similar to the one defined in `core metadata's Description", '`_'], 'oneOf': [{'type': 'string', '$$description': ['Relative path to a text file (UTF-8) containing the full description', 'of the project. If the file path ends in case-insensitive ``.md`` or', '``.rst`` suffixes, then the content-type is respectively', '``text/markdown`` or ``text/x-rst``']}, {'type': 'object', 'allOf': [{'anyOf': [{'properties': {'file': {'type': 'string', '$$description': ['Relative path to a text file containing the full description', 'of the project.']}}, 'required': ['file']}, {'properties': {'text': {'type': 'string', 'description': 'Full text describing the project.'}}, 'required': ['text']}]}, {'properties': {'content-type': {'type': 'string', '$$description': ['Content-type (:rfc:`1341`) of the full description', '(e.g. ``text/markdown``). The ``charset`` parameter is assumed', 'UTF-8 when not present.'], '$comment': 'TODO: add regex pattern or format?'}}, 'required': ['content-type']}]}]}, 'requires-python': {'type': 'string', 'format': 'pep508-versionspec', '$$description': ['`The Python version requirements of the project', '`_.']}, 'license': {'description': '`Project license `_.', 'oneOf': [{'properties': {'file': {'type': 'string', '$$description': ['Relative path to the file (UTF-8) which contains the license for the', 'project.']}}, 'required': ['file']}, {'properties': {'text': {'type': 'string', '$$description': ['The license of the project whose meaning is that of the', '`License field from the core metadata', '`_.']}}, 'required': ['text']}]}, 'authors': {'type': 'array', 'items': {'$id': '#/definitions/author', 'title': 'Author or Maintainer', '$comment': 'https://www.python.org/dev/peps/pep-0621/#authors-maintainers', 'type': 'object', 'properties': {'name': {'type': 'string', '$$description': ['MUST be a valid email name, i.e. whatever can be put as a name, before an', 'email, in :rfc:`822`.']}, 'email': {'type': 'string', 'format': 'idn-email', 'description': 'MUST be a valid email address'}}}, '$$description': ["The people or organizations considered to be the 'authors' of the project.", 'The exact meaning is open to interpretation (e.g. original or primary authors,', 'current maintainers, or owners of the package).']}, 'maintainers': {'type': 'array', 'items': {'$id': '#/definitions/author', 'title': 'Author or Maintainer', '$comment': 'https://www.python.org/dev/peps/pep-0621/#authors-maintainers', 'type': 'object', 'properties': {'name': {'type': 'string', '$$description': ['MUST be a valid email name, i.e. whatever can be put as a name, before an', 'email, in :rfc:`822`.']}, 'email': {'type': 'string', 'format': 'idn-email', 'description': 'MUST be a valid email address'}}}, '$$description': ["The people or organizations considered to be the 'maintainers' of the project.", 'Similarly to ``authors``, the exact meaning is open to interpretation.']}, 'keywords': {'type': 'array', 'items': {'type': 'string'}, 'description': 'List of keywords to assist searching for the distribution in a larger catalog.'}, 'classifiers': {'type': 'array', 'items': {'type': 'string', 'format': 'trove-classifier', 'description': '`PyPI classifier `_.'}, '$$description': ['`Trove classifiers `_', 'which apply to the project.']}, 'urls': {'type': 'object', 'description': 'URLs associated with the project in the form ``label => value``.', 'additionalProperties': False, 'patternProperties': {'^.+$': {'type': 'string', 'format': 'url'}}}, 'scripts': {'$id': '#/definitions/entry-point-group', 'title': 'Entry-points', 'type': 'object', '$$description': ['Entry-points are grouped together to indicate what sort of capabilities they', 'provide.', 'See the `packaging guides', '`_', 'and `setuptools docs', '`_', 'for more information.'], 'propertyNames': {'format': 'python-entrypoint-name'}, 'additionalProperties': False, 'patternProperties': {'^.+$': {'type': 'string', '$$description': ['Reference to a Python object. It is either in the form', '``importable.module``, or ``importable.module:object.attr``.'], 'format': 'python-entrypoint-reference', '$comment': 'https://packaging.python.org/specifications/entry-points/'}}}, 'gui-scripts': {'$id': '#/definitions/entry-point-group', 'title': 'Entry-points', 'type': 'object', '$$description': ['Entry-points are grouped together to indicate what sort of capabilities they', 'provide.', 'See the `packaging guides', '`_', 'and `setuptools docs', '`_', 'for more information.'], 'propertyNames': {'format': 'python-entrypoint-name'}, 'additionalProperties': False, 'patternProperties': {'^.+$': {'type': 'string', '$$description': ['Reference to a Python object. It is either in the form', '``importable.module``, or ``importable.module:object.attr``.'], 'format': 'python-entrypoint-reference', '$comment': 'https://packaging.python.org/specifications/entry-points/'}}}, 'entry-points': {'$$description': ['Instruct the installer to expose the given modules/functions via', '``entry-point`` discovery mechanism (useful for plugins).', 'More information available in the `Python packaging guide', '`_.'], 'propertyNames': {'format': 'python-entrypoint-group'}, 'additionalProperties': False, 'patternProperties': {'^.+$': {'$id': '#/definitions/entry-point-group', 'title': 'Entry-points', 'type': 'object', '$$description': ['Entry-points are grouped together to indicate what sort of capabilities they', 'provide.', 'See the `packaging guides', '`_', 'and `setuptools docs', '`_', 'for more information.'], 'propertyNames': {'format': 'python-entrypoint-name'}, 'additionalProperties': False, 'patternProperties': {'^.+$': {'type': 'string', '$$description': ['Reference to a Python object. It is either in the form', '``importable.module``, or ``importable.module:object.attr``.'], 'format': 'python-entrypoint-reference', '$comment': 'https://packaging.python.org/specifications/entry-points/'}}}}}, 'dependencies': {'type': 'array', 'description': 'Project (mandatory) dependencies.', 'items': {'$id': '#/definitions/dependency', 'title': 'Dependency', 'type': 'string', 'description': 'Project dependency specification according to PEP 508', 'format': 'pep508'}}, 'optional-dependencies': {'type': 'object', 'description': 'Optional dependency for the project', 'propertyNames': {'format': 'pep508-identifier'}, 'additionalProperties': False, 'patternProperties': {'^.+$': {'type': 'array', 'items': {'$id': '#/definitions/dependency', 'title': 'Dependency', 'type': 'string', 'description': 'Project dependency specification according to PEP 508', 'format': 'pep508'}}}}, 'dynamic': {'type': 'array', '$$description': ['Specifies which fields are intentionally unspecified and expected to be', 'dynamically provided by build tools'], 'items': {'enum': ['version', 'description', 'readme', 'requires-python', 'license', 'authors', 'maintainers', 'keywords', 'classifiers', 'urls', 'scripts', 'gui-scripts', 'entry-points', 'dependencies', 'optional-dependencies']}}}, 'required': ['name'], 'additionalProperties': False, 'if': {'not': {'required': ['dynamic'], 'properties': {'dynamic': {'contains': {'const': 'version'}, '$$description': ['version is listed in ``dynamic``']}}}, '$$comment': ['According to :pep:`621`:', ' If the core metadata specification lists a field as "Required", then', ' the metadata MUST specify the field statically or list it in dynamic', 'In turn, `core metadata`_ defines:', ' The required fields are: Metadata-Version, Name, Version.', ' All the other fields are optional.', 'Since ``Metadata-Version`` is defined by the build back-end, ``name`` and', '``version`` are the only mandatory information in ``pyproject.toml``.', '.. _core metadata: https://packaging.python.org/specifications/core-metadata/']}, 'then': {'required': ['version'], '$$description': ['version should be statically defined in the ``version`` field']}, 'definitions': {'author': {'$id': '#/definitions/author', 'title': 'Author or Maintainer', '$comment': 'https://www.python.org/dev/peps/pep-0621/#authors-maintainers', 'type': 'object', 'properties': {'name': {'type': 'string', '$$description': ['MUST be a valid email name, i.e. whatever can be put as a name, before an', 'email, in :rfc:`822`.']}, 'email': {'type': 'string', 'format': 'idn-email', 'description': 'MUST be a valid email address'}}}, 'entry-point-group': {'$id': '#/definitions/entry-point-group', 'title': 'Entry-points', 'type': 'object', '$$description': ['Entry-points are grouped together to indicate what sort of capabilities they', 'provide.', 'See the `packaging guides', '`_', 'and `setuptools docs', '`_', 'for more information.'], 'propertyNames': {'format': 'python-entrypoint-name'}, 'additionalProperties': False, 'patternProperties': {'^.+$': {'type': 'string', '$$description': ['Reference to a Python object. It is either in the form', '``importable.module``, or ``importable.module:object.attr``.'], 'format': 'python-entrypoint-reference', '$comment': 'https://packaging.python.org/specifications/entry-points/'}}}, 'dependency': {'$id': '#/definitions/dependency', 'title': 'Dependency', 'type': 'string', 'description': 'Project dependency specification according to PEP 508', 'format': 'pep508'}}}, rule='type')
- data_is_dict = isinstance(data, dict)
- if data_is_dict:
- data_len = len(data)
- if not all(prop in data for prop in ['name']):
- raise JsonSchemaValueException("" + (name_prefix or "data") + " must contain ['name'] properties", value=data, name="" + (name_prefix or "data") + "", definition={'$schema': 'http://json-schema.org/draft-07/schema', '$id': 'https://packaging.python.org/en/latest/specifications/declaring-project-metadata/', 'title': 'Package metadata stored in the ``project`` table', '$$description': ['Data structure for the **project** table inside ``pyproject.toml``', '(as initially defined in :pep:`621`)'], 'type': 'object', 'properties': {'name': {'type': 'string', 'description': 'The name (primary identifier) of the project. MUST be statically defined.', 'format': 'pep508-identifier'}, 'version': {'type': 'string', 'description': 'The version of the project as supported by :pep:`440`.', 'format': 'pep440'}, 'description': {'type': 'string', '$$description': ['The `summary description of the project', '`_']}, 'readme': {'$$description': ['`Full/detailed description of the project in the form of a README', '`_', "with meaning similar to the one defined in `core metadata's Description", '`_'], 'oneOf': [{'type': 'string', '$$description': ['Relative path to a text file (UTF-8) containing the full description', 'of the project. If the file path ends in case-insensitive ``.md`` or', '``.rst`` suffixes, then the content-type is respectively', '``text/markdown`` or ``text/x-rst``']}, {'type': 'object', 'allOf': [{'anyOf': [{'properties': {'file': {'type': 'string', '$$description': ['Relative path to a text file containing the full description', 'of the project.']}}, 'required': ['file']}, {'properties': {'text': {'type': 'string', 'description': 'Full text describing the project.'}}, 'required': ['text']}]}, {'properties': {'content-type': {'type': 'string', '$$description': ['Content-type (:rfc:`1341`) of the full description', '(e.g. ``text/markdown``). The ``charset`` parameter is assumed', 'UTF-8 when not present.'], '$comment': 'TODO: add regex pattern or format?'}}, 'required': ['content-type']}]}]}, 'requires-python': {'type': 'string', 'format': 'pep508-versionspec', '$$description': ['`The Python version requirements of the project', '`_.']}, 'license': {'description': '`Project license `_.', 'oneOf': [{'properties': {'file': {'type': 'string', '$$description': ['Relative path to the file (UTF-8) which contains the license for the', 'project.']}}, 'required': ['file']}, {'properties': {'text': {'type': 'string', '$$description': ['The license of the project whose meaning is that of the', '`License field from the core metadata', '`_.']}}, 'required': ['text']}]}, 'authors': {'type': 'array', 'items': {'$id': '#/definitions/author', 'title': 'Author or Maintainer', '$comment': 'https://www.python.org/dev/peps/pep-0621/#authors-maintainers', 'type': 'object', 'properties': {'name': {'type': 'string', '$$description': ['MUST be a valid email name, i.e. whatever can be put as a name, before an', 'email, in :rfc:`822`.']}, 'email': {'type': 'string', 'format': 'idn-email', 'description': 'MUST be a valid email address'}}}, '$$description': ["The people or organizations considered to be the 'authors' of the project.", 'The exact meaning is open to interpretation (e.g. original or primary authors,', 'current maintainers, or owners of the package).']}, 'maintainers': {'type': 'array', 'items': {'$id': '#/definitions/author', 'title': 'Author or Maintainer', '$comment': 'https://www.python.org/dev/peps/pep-0621/#authors-maintainers', 'type': 'object', 'properties': {'name': {'type': 'string', '$$description': ['MUST be a valid email name, i.e. whatever can be put as a name, before an', 'email, in :rfc:`822`.']}, 'email': {'type': 'string', 'format': 'idn-email', 'description': 'MUST be a valid email address'}}}, '$$description': ["The people or organizations considered to be the 'maintainers' of the project.", 'Similarly to ``authors``, the exact meaning is open to interpretation.']}, 'keywords': {'type': 'array', 'items': {'type': 'string'}, 'description': 'List of keywords to assist searching for the distribution in a larger catalog.'}, 'classifiers': {'type': 'array', 'items': {'type': 'string', 'format': 'trove-classifier', 'description': '`PyPI classifier `_.'}, '$$description': ['`Trove classifiers `_', 'which apply to the project.']}, 'urls': {'type': 'object', 'description': 'URLs associated with the project in the form ``label => value``.', 'additionalProperties': False, 'patternProperties': {'^.+$': {'type': 'string', 'format': 'url'}}}, 'scripts': {'$id': '#/definitions/entry-point-group', 'title': 'Entry-points', 'type': 'object', '$$description': ['Entry-points are grouped together to indicate what sort of capabilities they', 'provide.', 'See the `packaging guides', '`_', 'and `setuptools docs', '`_', 'for more information.'], 'propertyNames': {'format': 'python-entrypoint-name'}, 'additionalProperties': False, 'patternProperties': {'^.+$': {'type': 'string', '$$description': ['Reference to a Python object. It is either in the form', '``importable.module``, or ``importable.module:object.attr``.'], 'format': 'python-entrypoint-reference', '$comment': 'https://packaging.python.org/specifications/entry-points/'}}}, 'gui-scripts': {'$id': '#/definitions/entry-point-group', 'title': 'Entry-points', 'type': 'object', '$$description': ['Entry-points are grouped together to indicate what sort of capabilities they', 'provide.', 'See the `packaging guides', '`_', 'and `setuptools docs', '`_', 'for more information.'], 'propertyNames': {'format': 'python-entrypoint-name'}, 'additionalProperties': False, 'patternProperties': {'^.+$': {'type': 'string', '$$description': ['Reference to a Python object. It is either in the form', '``importable.module``, or ``importable.module:object.attr``.'], 'format': 'python-entrypoint-reference', '$comment': 'https://packaging.python.org/specifications/entry-points/'}}}, 'entry-points': {'$$description': ['Instruct the installer to expose the given modules/functions via', '``entry-point`` discovery mechanism (useful for plugins).', 'More information available in the `Python packaging guide', '`_.'], 'propertyNames': {'format': 'python-entrypoint-group'}, 'additionalProperties': False, 'patternProperties': {'^.+$': {'$id': '#/definitions/entry-point-group', 'title': 'Entry-points', 'type': 'object', '$$description': ['Entry-points are grouped together to indicate what sort of capabilities they', 'provide.', 'See the `packaging guides', '`_', 'and `setuptools docs', '`_', 'for more information.'], 'propertyNames': {'format': 'python-entrypoint-name'}, 'additionalProperties': False, 'patternProperties': {'^.+$': {'type': 'string', '$$description': ['Reference to a Python object. It is either in the form', '``importable.module``, or ``importable.module:object.attr``.'], 'format': 'python-entrypoint-reference', '$comment': 'https://packaging.python.org/specifications/entry-points/'}}}}}, 'dependencies': {'type': 'array', 'description': 'Project (mandatory) dependencies.', 'items': {'$id': '#/definitions/dependency', 'title': 'Dependency', 'type': 'string', 'description': 'Project dependency specification according to PEP 508', 'format': 'pep508'}}, 'optional-dependencies': {'type': 'object', 'description': 'Optional dependency for the project', 'propertyNames': {'format': 'pep508-identifier'}, 'additionalProperties': False, 'patternProperties': {'^.+$': {'type': 'array', 'items': {'$id': '#/definitions/dependency', 'title': 'Dependency', 'type': 'string', 'description': 'Project dependency specification according to PEP 508', 'format': 'pep508'}}}}, 'dynamic': {'type': 'array', '$$description': ['Specifies which fields are intentionally unspecified and expected to be', 'dynamically provided by build tools'], 'items': {'enum': ['version', 'description', 'readme', 'requires-python', 'license', 'authors', 'maintainers', 'keywords', 'classifiers', 'urls', 'scripts', 'gui-scripts', 'entry-points', 'dependencies', 'optional-dependencies']}}}, 'required': ['name'], 'additionalProperties': False, 'if': {'not': {'required': ['dynamic'], 'properties': {'dynamic': {'contains': {'const': 'version'}, '$$description': ['version is listed in ``dynamic``']}}}, '$$comment': ['According to :pep:`621`:', ' If the core metadata specification lists a field as "Required", then', ' the metadata MUST specify the field statically or list it in dynamic', 'In turn, `core metadata`_ defines:', ' The required fields are: Metadata-Version, Name, Version.', ' All the other fields are optional.', 'Since ``Metadata-Version`` is defined by the build back-end, ``name`` and', '``version`` are the only mandatory information in ``pyproject.toml``.', '.. _core metadata: https://packaging.python.org/specifications/core-metadata/']}, 'then': {'required': ['version'], '$$description': ['version should be statically defined in the ``version`` field']}, 'definitions': {'author': {'$id': '#/definitions/author', 'title': 'Author or Maintainer', '$comment': 'https://www.python.org/dev/peps/pep-0621/#authors-maintainers', 'type': 'object', 'properties': {'name': {'type': 'string', '$$description': ['MUST be a valid email name, i.e. whatever can be put as a name, before an', 'email, in :rfc:`822`.']}, 'email': {'type': 'string', 'format': 'idn-email', 'description': 'MUST be a valid email address'}}}, 'entry-point-group': {'$id': '#/definitions/entry-point-group', 'title': 'Entry-points', 'type': 'object', '$$description': ['Entry-points are grouped together to indicate what sort of capabilities they', 'provide.', 'See the `packaging guides', '`_', 'and `setuptools docs', '`_', 'for more information.'], 'propertyNames': {'format': 'python-entrypoint-name'}, 'additionalProperties': False, 'patternProperties': {'^.+$': {'type': 'string', '$$description': ['Reference to a Python object. It is either in the form', '``importable.module``, or ``importable.module:object.attr``.'], 'format': 'python-entrypoint-reference', '$comment': 'https://packaging.python.org/specifications/entry-points/'}}}, 'dependency': {'$id': '#/definitions/dependency', 'title': 'Dependency', 'type': 'string', 'description': 'Project dependency specification according to PEP 508', 'format': 'pep508'}}}, rule='required')
- data_keys = set(data.keys())
- if "name" in data_keys:
- data_keys.remove("name")
- data__name = data["name"]
- if not isinstance(data__name, (str)):
- raise JsonSchemaValueException("" + (name_prefix or "data") + ".name must be string", value=data__name, name="" + (name_prefix or "data") + ".name", definition={'type': 'string', 'description': 'The name (primary identifier) of the project. MUST be statically defined.', 'format': 'pep508-identifier'}, rule='type')
- if isinstance(data__name, str):
- if not custom_formats["pep508-identifier"](data__name):
- raise JsonSchemaValueException("" + (name_prefix or "data") + ".name must be pep508-identifier", value=data__name, name="" + (name_prefix or "data") + ".name", definition={'type': 'string', 'description': 'The name (primary identifier) of the project. MUST be statically defined.', 'format': 'pep508-identifier'}, rule='format')
- if "version" in data_keys:
- data_keys.remove("version")
- data__version = data["version"]
- if not isinstance(data__version, (str)):
- raise JsonSchemaValueException("" + (name_prefix or "data") + ".version must be string", value=data__version, name="" + (name_prefix or "data") + ".version", definition={'type': 'string', 'description': 'The version of the project as supported by :pep:`440`.', 'format': 'pep440'}, rule='type')
- if isinstance(data__version, str):
- if not custom_formats["pep440"](data__version):
- raise JsonSchemaValueException("" + (name_prefix or "data") + ".version must be pep440", value=data__version, name="" + (name_prefix or "data") + ".version", definition={'type': 'string', 'description': 'The version of the project as supported by :pep:`440`.', 'format': 'pep440'}, rule='format')
- if "description" in data_keys:
- data_keys.remove("description")
- data__description = data["description"]
- if not isinstance(data__description, (str)):
- raise JsonSchemaValueException("" + (name_prefix or "data") + ".description must be string", value=data__description, name="" + (name_prefix or "data") + ".description", definition={'type': 'string', '$$description': ['The `summary description of the project', '`_']}, rule='type')
- if "readme" in data_keys:
- data_keys.remove("readme")
- data__readme = data["readme"]
- data__readme_one_of_count8 = 0
- if data__readme_one_of_count8 < 2:
- try:
- if not isinstance(data__readme, (str)):
- raise JsonSchemaValueException("" + (name_prefix or "data") + ".readme must be string", value=data__readme, name="" + (name_prefix or "data") + ".readme", definition={'type': 'string', '$$description': ['Relative path to a text file (UTF-8) containing the full description', 'of the project. If the file path ends in case-insensitive ``.md`` or', '``.rst`` suffixes, then the content-type is respectively', '``text/markdown`` or ``text/x-rst``']}, rule='type')
- data__readme_one_of_count8 += 1
- except JsonSchemaValueException: pass
- if data__readme_one_of_count8 < 2:
- try:
- if not isinstance(data__readme, (dict)):
- raise JsonSchemaValueException("" + (name_prefix or "data") + ".readme must be object", value=data__readme, name="" + (name_prefix or "data") + ".readme", definition={'type': 'object', 'allOf': [{'anyOf': [{'properties': {'file': {'type': 'string', '$$description': ['Relative path to a text file containing the full description', 'of the project.']}}, 'required': ['file']}, {'properties': {'text': {'type': 'string', 'description': 'Full text describing the project.'}}, 'required': ['text']}]}, {'properties': {'content-type': {'type': 'string', '$$description': ['Content-type (:rfc:`1341`) of the full description', '(e.g. ``text/markdown``). The ``charset`` parameter is assumed', 'UTF-8 when not present.'], '$comment': 'TODO: add regex pattern or format?'}}, 'required': ['content-type']}]}, rule='type')
- data__readme_any_of_count9 = 0
- if not data__readme_any_of_count9:
- try:
- data__readme_is_dict = isinstance(data__readme, dict)
- if data__readme_is_dict:
- data__readme_len = len(data__readme)
- if not all(prop in data__readme for prop in ['file']):
- raise JsonSchemaValueException("" + (name_prefix or "data") + ".readme must contain ['file'] properties", value=data__readme, name="" + (name_prefix or "data") + ".readme", definition={'properties': {'file': {'type': 'string', '$$description': ['Relative path to a text file containing the full description', 'of the project.']}}, 'required': ['file']}, rule='required')
- data__readme_keys = set(data__readme.keys())
- if "file" in data__readme_keys:
- data__readme_keys.remove("file")
- data__readme__file = data__readme["file"]
- if not isinstance(data__readme__file, (str)):
- raise JsonSchemaValueException("" + (name_prefix or "data") + ".readme.file must be string", value=data__readme__file, name="" + (name_prefix or "data") + ".readme.file", definition={'type': 'string', '$$description': ['Relative path to a text file containing the full description', 'of the project.']}, rule='type')
- data__readme_any_of_count9 += 1
- except JsonSchemaValueException: pass
- if not data__readme_any_of_count9:
- try:
- data__readme_is_dict = isinstance(data__readme, dict)
- if data__readme_is_dict:
- data__readme_len = len(data__readme)
- if not all(prop in data__readme for prop in ['text']):
- raise JsonSchemaValueException("" + (name_prefix or "data") + ".readme must contain ['text'] properties", value=data__readme, name="" + (name_prefix or "data") + ".readme", definition={'properties': {'text': {'type': 'string', 'description': 'Full text describing the project.'}}, 'required': ['text']}, rule='required')
- data__readme_keys = set(data__readme.keys())
- if "text" in data__readme_keys:
- data__readme_keys.remove("text")
- data__readme__text = data__readme["text"]
- if not isinstance(data__readme__text, (str)):
- raise JsonSchemaValueException("" + (name_prefix or "data") + ".readme.text must be string", value=data__readme__text, name="" + (name_prefix or "data") + ".readme.text", definition={'type': 'string', 'description': 'Full text describing the project.'}, rule='type')
- data__readme_any_of_count9 += 1
- except JsonSchemaValueException: pass
- if not data__readme_any_of_count9:
- raise JsonSchemaValueException("" + (name_prefix or "data") + ".readme cannot be validated by any definition", value=data__readme, name="" + (name_prefix or "data") + ".readme", definition={'anyOf': [{'properties': {'file': {'type': 'string', '$$description': ['Relative path to a text file containing the full description', 'of the project.']}}, 'required': ['file']}, {'properties': {'text': {'type': 'string', 'description': 'Full text describing the project.'}}, 'required': ['text']}]}, rule='anyOf')
- data__readme_is_dict = isinstance(data__readme, dict)
- if data__readme_is_dict:
- data__readme_len = len(data__readme)
- if not all(prop in data__readme for prop in ['content-type']):
- raise JsonSchemaValueException("" + (name_prefix or "data") + ".readme must contain ['content-type'] properties", value=data__readme, name="" + (name_prefix or "data") + ".readme", definition={'properties': {'content-type': {'type': 'string', '$$description': ['Content-type (:rfc:`1341`) of the full description', '(e.g. ``text/markdown``). The ``charset`` parameter is assumed', 'UTF-8 when not present.'], '$comment': 'TODO: add regex pattern or format?'}}, 'required': ['content-type']}, rule='required')
- data__readme_keys = set(data__readme.keys())
- if "content-type" in data__readme_keys:
- data__readme_keys.remove("content-type")
- data__readme__contenttype = data__readme["content-type"]
- if not isinstance(data__readme__contenttype, (str)):
- raise JsonSchemaValueException("" + (name_prefix or "data") + ".readme.content-type must be string", value=data__readme__contenttype, name="" + (name_prefix or "data") + ".readme.content-type", definition={'type': 'string', '$$description': ['Content-type (:rfc:`1341`) of the full description', '(e.g. ``text/markdown``). The ``charset`` parameter is assumed', 'UTF-8 when not present.'], '$comment': 'TODO: add regex pattern or format?'}, rule='type')
- data__readme_one_of_count8 += 1
- except JsonSchemaValueException: pass
- if data__readme_one_of_count8 != 1:
- raise JsonSchemaValueException("" + (name_prefix or "data") + ".readme must be valid exactly by one definition" + (" (" + str(data__readme_one_of_count8) + " matches found)"), value=data__readme, name="" + (name_prefix or "data") + ".readme", definition={'$$description': ['`Full/detailed description of the project in the form of a README', '`_', "with meaning similar to the one defined in `core metadata's Description", '`_'], 'oneOf': [{'type': 'string', '$$description': ['Relative path to a text file (UTF-8) containing the full description', 'of the project. If the file path ends in case-insensitive ``.md`` or', '``.rst`` suffixes, then the content-type is respectively', '``text/markdown`` or ``text/x-rst``']}, {'type': 'object', 'allOf': [{'anyOf': [{'properties': {'file': {'type': 'string', '$$description': ['Relative path to a text file containing the full description', 'of the project.']}}, 'required': ['file']}, {'properties': {'text': {'type': 'string', 'description': 'Full text describing the project.'}}, 'required': ['text']}]}, {'properties': {'content-type': {'type': 'string', '$$description': ['Content-type (:rfc:`1341`) of the full description', '(e.g. ``text/markdown``). The ``charset`` parameter is assumed', 'UTF-8 when not present.'], '$comment': 'TODO: add regex pattern or format?'}}, 'required': ['content-type']}]}]}, rule='oneOf')
- if "requires-python" in data_keys:
- data_keys.remove("requires-python")
- data__requirespython = data["requires-python"]
- if not isinstance(data__requirespython, (str)):
- raise JsonSchemaValueException("" + (name_prefix or "data") + ".requires-python must be string", value=data__requirespython, name="" + (name_prefix or "data") + ".requires-python", definition={'type': 'string', 'format': 'pep508-versionspec', '$$description': ['`The Python version requirements of the project', '`_.']}, rule='type')
- if isinstance(data__requirespython, str):
- if not custom_formats["pep508-versionspec"](data__requirespython):
- raise JsonSchemaValueException("" + (name_prefix or "data") + ".requires-python must be pep508-versionspec", value=data__requirespython, name="" + (name_prefix or "data") + ".requires-python", definition={'type': 'string', 'format': 'pep508-versionspec', '$$description': ['`The Python version requirements of the project', '`_.']}, rule='format')
- if "license" in data_keys:
- data_keys.remove("license")
- data__license = data["license"]
- data__license_one_of_count10 = 0
- if data__license_one_of_count10 < 2:
- try:
- data__license_is_dict = isinstance(data__license, dict)
- if data__license_is_dict:
- data__license_len = len(data__license)
- if not all(prop in data__license for prop in ['file']):
- raise JsonSchemaValueException("" + (name_prefix or "data") + ".license must contain ['file'] properties", value=data__license, name="" + (name_prefix or "data") + ".license", definition={'properties': {'file': {'type': 'string', '$$description': ['Relative path to the file (UTF-8) which contains the license for the', 'project.']}}, 'required': ['file']}, rule='required')
- data__license_keys = set(data__license.keys())
- if "file" in data__license_keys:
- data__license_keys.remove("file")
- data__license__file = data__license["file"]
- if not isinstance(data__license__file, (str)):
- raise JsonSchemaValueException("" + (name_prefix or "data") + ".license.file must be string", value=data__license__file, name="" + (name_prefix or "data") + ".license.file", definition={'type': 'string', '$$description': ['Relative path to the file (UTF-8) which contains the license for the', 'project.']}, rule='type')
- data__license_one_of_count10 += 1
- except JsonSchemaValueException: pass
- if data__license_one_of_count10 < 2:
- try:
- data__license_is_dict = isinstance(data__license, dict)
- if data__license_is_dict:
- data__license_len = len(data__license)
- if not all(prop in data__license for prop in ['text']):
- raise JsonSchemaValueException("" + (name_prefix or "data") + ".license must contain ['text'] properties", value=data__license, name="" + (name_prefix or "data") + ".license", definition={'properties': {'text': {'type': 'string', '$$description': ['The license of the project whose meaning is that of the', '`License field from the core metadata', '`_.']}}, 'required': ['text']}, rule='required')
- data__license_keys = set(data__license.keys())
- if "text" in data__license_keys:
- data__license_keys.remove("text")
- data__license__text = data__license["text"]
- if not isinstance(data__license__text, (str)):
- raise JsonSchemaValueException("" + (name_prefix or "data") + ".license.text must be string", value=data__license__text, name="" + (name_prefix or "data") + ".license.text", definition={'type': 'string', '$$description': ['The license of the project whose meaning is that of the', '`License field from the core metadata', '`_.']}, rule='type')
- data__license_one_of_count10 += 1
- except JsonSchemaValueException: pass
- if data__license_one_of_count10 != 1:
- raise JsonSchemaValueException("" + (name_prefix or "data") + ".license must be valid exactly by one definition" + (" (" + str(data__license_one_of_count10) + " matches found)"), value=data__license, name="" + (name_prefix or "data") + ".license", definition={'description': '`Project license `_.', 'oneOf': [{'properties': {'file': {'type': 'string', '$$description': ['Relative path to the file (UTF-8) which contains the license for the', 'project.']}}, 'required': ['file']}, {'properties': {'text': {'type': 'string', '$$description': ['The license of the project whose meaning is that of the', '`License field from the core metadata', '`_.']}}, 'required': ['text']}]}, rule='oneOf')
- if "authors" in data_keys:
- data_keys.remove("authors")
- data__authors = data["authors"]
- if not isinstance(data__authors, (list, tuple)):
- raise JsonSchemaValueException("" + (name_prefix or "data") + ".authors must be array", value=data__authors, name="" + (name_prefix or "data") + ".authors", definition={'type': 'array', 'items': {'$id': '#/definitions/author', 'title': 'Author or Maintainer', '$comment': 'https://www.python.org/dev/peps/pep-0621/#authors-maintainers', 'type': 'object', 'properties': {'name': {'type': 'string', '$$description': ['MUST be a valid email name, i.e. whatever can be put as a name, before an', 'email, in :rfc:`822`.']}, 'email': {'type': 'string', 'format': 'idn-email', 'description': 'MUST be a valid email address'}}}, '$$description': ["The people or organizations considered to be the 'authors' of the project.", 'The exact meaning is open to interpretation (e.g. original or primary authors,', 'current maintainers, or owners of the package).']}, rule='type')
- data__authors_is_list = isinstance(data__authors, (list, tuple))
- if data__authors_is_list:
- data__authors_len = len(data__authors)
- for data__authors_x, data__authors_item in enumerate(data__authors):
- validate_https___packaging_python_org_en_latest_specifications_declaring_project_metadata___definitions_author(data__authors_item, custom_formats, (name_prefix or "data") + ".authors[{data__authors_x}]")
- if "maintainers" in data_keys:
- data_keys.remove("maintainers")
- data__maintainers = data["maintainers"]
- if not isinstance(data__maintainers, (list, tuple)):
- raise JsonSchemaValueException("" + (name_prefix or "data") + ".maintainers must be array", value=data__maintainers, name="" + (name_prefix or "data") + ".maintainers", definition={'type': 'array', 'items': {'$id': '#/definitions/author', 'title': 'Author or Maintainer', '$comment': 'https://www.python.org/dev/peps/pep-0621/#authors-maintainers', 'type': 'object', 'properties': {'name': {'type': 'string', '$$description': ['MUST be a valid email name, i.e. whatever can be put as a name, before an', 'email, in :rfc:`822`.']}, 'email': {'type': 'string', 'format': 'idn-email', 'description': 'MUST be a valid email address'}}}, '$$description': ["The people or organizations considered to be the 'maintainers' of the project.", 'Similarly to ``authors``, the exact meaning is open to interpretation.']}, rule='type')
- data__maintainers_is_list = isinstance(data__maintainers, (list, tuple))
- if data__maintainers_is_list:
- data__maintainers_len = len(data__maintainers)
- for data__maintainers_x, data__maintainers_item in enumerate(data__maintainers):
- validate_https___packaging_python_org_en_latest_specifications_declaring_project_metadata___definitions_author(data__maintainers_item, custom_formats, (name_prefix or "data") + ".maintainers[{data__maintainers_x}]")
- if "keywords" in data_keys:
- data_keys.remove("keywords")
- data__keywords = data["keywords"]
- if not isinstance(data__keywords, (list, tuple)):
- raise JsonSchemaValueException("" + (name_prefix or "data") + ".keywords must be array", value=data__keywords, name="" + (name_prefix or "data") + ".keywords", definition={'type': 'array', 'items': {'type': 'string'}, 'description': 'List of keywords to assist searching for the distribution in a larger catalog.'}, rule='type')
- data__keywords_is_list = isinstance(data__keywords, (list, tuple))
- if data__keywords_is_list:
- data__keywords_len = len(data__keywords)
- for data__keywords_x, data__keywords_item in enumerate(data__keywords):
- if not isinstance(data__keywords_item, (str)):
- raise JsonSchemaValueException("" + (name_prefix or "data") + ".keywords[{data__keywords_x}]".format(**locals()) + " must be string", value=data__keywords_item, name="" + (name_prefix or "data") + ".keywords[{data__keywords_x}]".format(**locals()) + "", definition={'type': 'string'}, rule='type')
- if "classifiers" in data_keys:
- data_keys.remove("classifiers")
- data__classifiers = data["classifiers"]
- if not isinstance(data__classifiers, (list, tuple)):
- raise JsonSchemaValueException("" + (name_prefix or "data") + ".classifiers must be array", value=data__classifiers, name="" + (name_prefix or "data") + ".classifiers", definition={'type': 'array', 'items': {'type': 'string', 'format': 'trove-classifier', 'description': '`PyPI classifier `_.'}, '$$description': ['`Trove classifiers `_', 'which apply to the project.']}, rule='type')
- data__classifiers_is_list = isinstance(data__classifiers, (list, tuple))
- if data__classifiers_is_list:
- data__classifiers_len = len(data__classifiers)
- for data__classifiers_x, data__classifiers_item in enumerate(data__classifiers):
- if not isinstance(data__classifiers_item, (str)):
- raise JsonSchemaValueException("" + (name_prefix or "data") + ".classifiers[{data__classifiers_x}]".format(**locals()) + " must be string", value=data__classifiers_item, name="" + (name_prefix or "data") + ".classifiers[{data__classifiers_x}]".format(**locals()) + "", definition={'type': 'string', 'format': 'trove-classifier', 'description': '`PyPI classifier `_.'}, rule='type')
- if isinstance(data__classifiers_item, str):
- if not custom_formats["trove-classifier"](data__classifiers_item):
- raise JsonSchemaValueException("" + (name_prefix or "data") + ".classifiers[{data__classifiers_x}]".format(**locals()) + " must be trove-classifier", value=data__classifiers_item, name="" + (name_prefix or "data") + ".classifiers[{data__classifiers_x}]".format(**locals()) + "", definition={'type': 'string', 'format': 'trove-classifier', 'description': '`PyPI classifier `_.'}, rule='format')
- if "urls" in data_keys:
- data_keys.remove("urls")
- data__urls = data["urls"]
- if not isinstance(data__urls, (dict)):
- raise JsonSchemaValueException("" + (name_prefix or "data") + ".urls must be object", value=data__urls, name="" + (name_prefix or "data") + ".urls", definition={'type': 'object', 'description': 'URLs associated with the project in the form ``label => value``.', 'additionalProperties': False, 'patternProperties': {'^.+$': {'type': 'string', 'format': 'url'}}}, rule='type')
- data__urls_is_dict = isinstance(data__urls, dict)
- if data__urls_is_dict:
- data__urls_keys = set(data__urls.keys())
- for data__urls_key, data__urls_val in data__urls.items():
- if REGEX_PATTERNS['^.+$'].search(data__urls_key):
- if data__urls_key in data__urls_keys:
- data__urls_keys.remove(data__urls_key)
- if not isinstance(data__urls_val, (str)):
- raise JsonSchemaValueException("" + (name_prefix or "data") + ".urls.{data__urls_key}".format(**locals()) + " must be string", value=data__urls_val, name="" + (name_prefix or "data") + ".urls.{data__urls_key}".format(**locals()) + "", definition={'type': 'string', 'format': 'url'}, rule='type')
- if isinstance(data__urls_val, str):
- if not custom_formats["url"](data__urls_val):
- raise JsonSchemaValueException("" + (name_prefix or "data") + ".urls.{data__urls_key}".format(**locals()) + " must be url", value=data__urls_val, name="" + (name_prefix or "data") + ".urls.{data__urls_key}".format(**locals()) + "", definition={'type': 'string', 'format': 'url'}, rule='format')
- if data__urls_keys:
- raise JsonSchemaValueException("" + (name_prefix or "data") + ".urls must not contain "+str(data__urls_keys)+" properties", value=data__urls, name="" + (name_prefix or "data") + ".urls", definition={'type': 'object', 'description': 'URLs associated with the project in the form ``label => value``.', 'additionalProperties': False, 'patternProperties': {'^.+$': {'type': 'string', 'format': 'url'}}}, rule='additionalProperties')
- if "scripts" in data_keys:
- data_keys.remove("scripts")
- data__scripts = data["scripts"]
- validate_https___packaging_python_org_en_latest_specifications_declaring_project_metadata___definitions_entry_point_group(data__scripts, custom_formats, (name_prefix or "data") + ".scripts")
- if "gui-scripts" in data_keys:
- data_keys.remove("gui-scripts")
- data__guiscripts = data["gui-scripts"]
- validate_https___packaging_python_org_en_latest_specifications_declaring_project_metadata___definitions_entry_point_group(data__guiscripts, custom_formats, (name_prefix or "data") + ".gui-scripts")
- if "entry-points" in data_keys:
- data_keys.remove("entry-points")
- data__entrypoints = data["entry-points"]
- data__entrypoints_is_dict = isinstance(data__entrypoints, dict)
- if data__entrypoints_is_dict:
- data__entrypoints_keys = set(data__entrypoints.keys())
- for data__entrypoints_key, data__entrypoints_val in data__entrypoints.items():
- if REGEX_PATTERNS['^.+$'].search(data__entrypoints_key):
- if data__entrypoints_key in data__entrypoints_keys:
- data__entrypoints_keys.remove(data__entrypoints_key)
- validate_https___packaging_python_org_en_latest_specifications_declaring_project_metadata___definitions_entry_point_group(data__entrypoints_val, custom_formats, (name_prefix or "data") + ".entry-points.{data__entrypoints_key}")
- if data__entrypoints_keys:
- raise JsonSchemaValueException("" + (name_prefix or "data") + ".entry-points must not contain "+str(data__entrypoints_keys)+" properties", value=data__entrypoints, name="" + (name_prefix or "data") + ".entry-points", definition={'$$description': ['Instruct the installer to expose the given modules/functions via', '``entry-point`` discovery mechanism (useful for plugins).', 'More information available in the `Python packaging guide', '`_.'], 'propertyNames': {'format': 'python-entrypoint-group'}, 'additionalProperties': False, 'patternProperties': {'^.+$': {'$id': '#/definitions/entry-point-group', 'title': 'Entry-points', 'type': 'object', '$$description': ['Entry-points are grouped together to indicate what sort of capabilities they', 'provide.', 'See the `packaging guides', '`_', 'and `setuptools docs', '`_', 'for more information.'], 'propertyNames': {'format': 'python-entrypoint-name'}, 'additionalProperties': False, 'patternProperties': {'^.+$': {'type': 'string', '$$description': ['Reference to a Python object. It is either in the form', '``importable.module``, or ``importable.module:object.attr``.'], 'format': 'python-entrypoint-reference', '$comment': 'https://packaging.python.org/specifications/entry-points/'}}}}}, rule='additionalProperties')
- data__entrypoints_len = len(data__entrypoints)
- if data__entrypoints_len != 0:
- data__entrypoints_property_names = True
- for data__entrypoints_key in data__entrypoints:
- try:
- if isinstance(data__entrypoints_key, str):
- if not custom_formats["python-entrypoint-group"](data__entrypoints_key):
- raise JsonSchemaValueException("" + (name_prefix or "data") + ".entry-points must be python-entrypoint-group", value=data__entrypoints_key, name="" + (name_prefix or "data") + ".entry-points", definition={'format': 'python-entrypoint-group'}, rule='format')
- except JsonSchemaValueException:
- data__entrypoints_property_names = False
- if not data__entrypoints_property_names:
- raise JsonSchemaValueException("" + (name_prefix or "data") + ".entry-points must be named by propertyName definition", value=data__entrypoints, name="" + (name_prefix or "data") + ".entry-points", definition={'$$description': ['Instruct the installer to expose the given modules/functions via', '``entry-point`` discovery mechanism (useful for plugins).', 'More information available in the `Python packaging guide', '`_.'], 'propertyNames': {'format': 'python-entrypoint-group'}, 'additionalProperties': False, 'patternProperties': {'^.+$': {'$id': '#/definitions/entry-point-group', 'title': 'Entry-points', 'type': 'object', '$$description': ['Entry-points are grouped together to indicate what sort of capabilities they', 'provide.', 'See the `packaging guides', '`_', 'and `setuptools docs', '`_', 'for more information.'], 'propertyNames': {'format': 'python-entrypoint-name'}, 'additionalProperties': False, 'patternProperties': {'^.+$': {'type': 'string', '$$description': ['Reference to a Python object. It is either in the form', '``importable.module``, or ``importable.module:object.attr``.'], 'format': 'python-entrypoint-reference', '$comment': 'https://packaging.python.org/specifications/entry-points/'}}}}}, rule='propertyNames')
- if "dependencies" in data_keys:
- data_keys.remove("dependencies")
- data__dependencies = data["dependencies"]
- if not isinstance(data__dependencies, (list, tuple)):
- raise JsonSchemaValueException("" + (name_prefix or "data") + ".dependencies must be array", value=data__dependencies, name="" + (name_prefix or "data") + ".dependencies", definition={'type': 'array', 'description': 'Project (mandatory) dependencies.', 'items': {'$id': '#/definitions/dependency', 'title': 'Dependency', 'type': 'string', 'description': 'Project dependency specification according to PEP 508', 'format': 'pep508'}}, rule='type')
- data__dependencies_is_list = isinstance(data__dependencies, (list, tuple))
- if data__dependencies_is_list:
- data__dependencies_len = len(data__dependencies)
- for data__dependencies_x, data__dependencies_item in enumerate(data__dependencies):
- validate_https___packaging_python_org_en_latest_specifications_declaring_project_metadata___definitions_dependency(data__dependencies_item, custom_formats, (name_prefix or "data") + ".dependencies[{data__dependencies_x}]")
- if "optional-dependencies" in data_keys:
- data_keys.remove("optional-dependencies")
- data__optionaldependencies = data["optional-dependencies"]
- if not isinstance(data__optionaldependencies, (dict)):
- raise JsonSchemaValueException("" + (name_prefix or "data") + ".optional-dependencies must be object", value=data__optionaldependencies, name="" + (name_prefix or "data") + ".optional-dependencies", definition={'type': 'object', 'description': 'Optional dependency for the project', 'propertyNames': {'format': 'pep508-identifier'}, 'additionalProperties': False, 'patternProperties': {'^.+$': {'type': 'array', 'items': {'$id': '#/definitions/dependency', 'title': 'Dependency', 'type': 'string', 'description': 'Project dependency specification according to PEP 508', 'format': 'pep508'}}}}, rule='type')
- data__optionaldependencies_is_dict = isinstance(data__optionaldependencies, dict)
- if data__optionaldependencies_is_dict:
- data__optionaldependencies_keys = set(data__optionaldependencies.keys())
- for data__optionaldependencies_key, data__optionaldependencies_val in data__optionaldependencies.items():
- if REGEX_PATTERNS['^.+$'].search(data__optionaldependencies_key):
- if data__optionaldependencies_key in data__optionaldependencies_keys:
- data__optionaldependencies_keys.remove(data__optionaldependencies_key)
- if not isinstance(data__optionaldependencies_val, (list, tuple)):
- raise JsonSchemaValueException("" + (name_prefix or "data") + ".optional-dependencies.{data__optionaldependencies_key}".format(**locals()) + " must be array", value=data__optionaldependencies_val, name="" + (name_prefix or "data") + ".optional-dependencies.{data__optionaldependencies_key}".format(**locals()) + "", definition={'type': 'array', 'items': {'$id': '#/definitions/dependency', 'title': 'Dependency', 'type': 'string', 'description': 'Project dependency specification according to PEP 508', 'format': 'pep508'}}, rule='type')
- data__optionaldependencies_val_is_list = isinstance(data__optionaldependencies_val, (list, tuple))
- if data__optionaldependencies_val_is_list:
- data__optionaldependencies_val_len = len(data__optionaldependencies_val)
- for data__optionaldependencies_val_x, data__optionaldependencies_val_item in enumerate(data__optionaldependencies_val):
- validate_https___packaging_python_org_en_latest_specifications_declaring_project_metadata___definitions_dependency(data__optionaldependencies_val_item, custom_formats, (name_prefix or "data") + ".optional-dependencies.{data__optionaldependencies_key}[{data__optionaldependencies_val_x}]")
- if data__optionaldependencies_keys:
- raise JsonSchemaValueException("" + (name_prefix or "data") + ".optional-dependencies must not contain "+str(data__optionaldependencies_keys)+" properties", value=data__optionaldependencies, name="" + (name_prefix or "data") + ".optional-dependencies", definition={'type': 'object', 'description': 'Optional dependency for the project', 'propertyNames': {'format': 'pep508-identifier'}, 'additionalProperties': False, 'patternProperties': {'^.+$': {'type': 'array', 'items': {'$id': '#/definitions/dependency', 'title': 'Dependency', 'type': 'string', 'description': 'Project dependency specification according to PEP 508', 'format': 'pep508'}}}}, rule='additionalProperties')
- data__optionaldependencies_len = len(data__optionaldependencies)
- if data__optionaldependencies_len != 0:
- data__optionaldependencies_property_names = True
- for data__optionaldependencies_key in data__optionaldependencies:
- try:
- if isinstance(data__optionaldependencies_key, str):
- if not custom_formats["pep508-identifier"](data__optionaldependencies_key):
- raise JsonSchemaValueException("" + (name_prefix or "data") + ".optional-dependencies must be pep508-identifier", value=data__optionaldependencies_key, name="" + (name_prefix or "data") + ".optional-dependencies", definition={'format': 'pep508-identifier'}, rule='format')
- except JsonSchemaValueException:
- data__optionaldependencies_property_names = False
- if not data__optionaldependencies_property_names:
- raise JsonSchemaValueException("" + (name_prefix or "data") + ".optional-dependencies must be named by propertyName definition", value=data__optionaldependencies, name="" + (name_prefix or "data") + ".optional-dependencies", definition={'type': 'object', 'description': 'Optional dependency for the project', 'propertyNames': {'format': 'pep508-identifier'}, 'additionalProperties': False, 'patternProperties': {'^.+$': {'type': 'array', 'items': {'$id': '#/definitions/dependency', 'title': 'Dependency', 'type': 'string', 'description': 'Project dependency specification according to PEP 508', 'format': 'pep508'}}}}, rule='propertyNames')
- if "dynamic" in data_keys:
- data_keys.remove("dynamic")
- data__dynamic = data["dynamic"]
- if not isinstance(data__dynamic, (list, tuple)):
- raise JsonSchemaValueException("" + (name_prefix or "data") + ".dynamic must be array", value=data__dynamic, name="" + (name_prefix or "data") + ".dynamic", definition={'type': 'array', '$$description': ['Specifies which fields are intentionally unspecified and expected to be', 'dynamically provided by build tools'], 'items': {'enum': ['version', 'description', 'readme', 'requires-python', 'license', 'authors', 'maintainers', 'keywords', 'classifiers', 'urls', 'scripts', 'gui-scripts', 'entry-points', 'dependencies', 'optional-dependencies']}}, rule='type')
- data__dynamic_is_list = isinstance(data__dynamic, (list, tuple))
- if data__dynamic_is_list:
- data__dynamic_len = len(data__dynamic)
- for data__dynamic_x, data__dynamic_item in enumerate(data__dynamic):
- if data__dynamic_item not in ['version', 'description', 'readme', 'requires-python', 'license', 'authors', 'maintainers', 'keywords', 'classifiers', 'urls', 'scripts', 'gui-scripts', 'entry-points', 'dependencies', 'optional-dependencies']:
- raise JsonSchemaValueException("" + (name_prefix or "data") + ".dynamic[{data__dynamic_x}]".format(**locals()) + " must be one of ['version', 'description', 'readme', 'requires-python', 'license', 'authors', 'maintainers', 'keywords', 'classifiers', 'urls', 'scripts', 'gui-scripts', 'entry-points', 'dependencies', 'optional-dependencies']", value=data__dynamic_item, name="" + (name_prefix or "data") + ".dynamic[{data__dynamic_x}]".format(**locals()) + "", definition={'enum': ['version', 'description', 'readme', 'requires-python', 'license', 'authors', 'maintainers', 'keywords', 'classifiers', 'urls', 'scripts', 'gui-scripts', 'entry-points', 'dependencies', 'optional-dependencies']}, rule='enum')
- if data_keys:
- raise JsonSchemaValueException("" + (name_prefix or "data") + " must not contain "+str(data_keys)+" properties", value=data, name="" + (name_prefix or "data") + "", definition={'$schema': 'http://json-schema.org/draft-07/schema', '$id': 'https://packaging.python.org/en/latest/specifications/declaring-project-metadata/', 'title': 'Package metadata stored in the ``project`` table', '$$description': ['Data structure for the **project** table inside ``pyproject.toml``', '(as initially defined in :pep:`621`)'], 'type': 'object', 'properties': {'name': {'type': 'string', 'description': 'The name (primary identifier) of the project. MUST be statically defined.', 'format': 'pep508-identifier'}, 'version': {'type': 'string', 'description': 'The version of the project as supported by :pep:`440`.', 'format': 'pep440'}, 'description': {'type': 'string', '$$description': ['The `summary description of the project', '`_']}, 'readme': {'$$description': ['`Full/detailed description of the project in the form of a README', '`_', "with meaning similar to the one defined in `core metadata's Description", '`_'], 'oneOf': [{'type': 'string', '$$description': ['Relative path to a text file (UTF-8) containing the full description', 'of the project. If the file path ends in case-insensitive ``.md`` or', '``.rst`` suffixes, then the content-type is respectively', '``text/markdown`` or ``text/x-rst``']}, {'type': 'object', 'allOf': [{'anyOf': [{'properties': {'file': {'type': 'string', '$$description': ['Relative path to a text file containing the full description', 'of the project.']}}, 'required': ['file']}, {'properties': {'text': {'type': 'string', 'description': 'Full text describing the project.'}}, 'required': ['text']}]}, {'properties': {'content-type': {'type': 'string', '$$description': ['Content-type (:rfc:`1341`) of the full description', '(e.g. ``text/markdown``). The ``charset`` parameter is assumed', 'UTF-8 when not present.'], '$comment': 'TODO: add regex pattern or format?'}}, 'required': ['content-type']}]}]}, 'requires-python': {'type': 'string', 'format': 'pep508-versionspec', '$$description': ['`The Python version requirements of the project', '`_.']}, 'license': {'description': '`Project license `_.', 'oneOf': [{'properties': {'file': {'type': 'string', '$$description': ['Relative path to the file (UTF-8) which contains the license for the', 'project.']}}, 'required': ['file']}, {'properties': {'text': {'type': 'string', '$$description': ['The license of the project whose meaning is that of the', '`License field from the core metadata', '`_.']}}, 'required': ['text']}]}, 'authors': {'type': 'array', 'items': {'$id': '#/definitions/author', 'title': 'Author or Maintainer', '$comment': 'https://www.python.org/dev/peps/pep-0621/#authors-maintainers', 'type': 'object', 'properties': {'name': {'type': 'string', '$$description': ['MUST be a valid email name, i.e. whatever can be put as a name, before an', 'email, in :rfc:`822`.']}, 'email': {'type': 'string', 'format': 'idn-email', 'description': 'MUST be a valid email address'}}}, '$$description': ["The people or organizations considered to be the 'authors' of the project.", 'The exact meaning is open to interpretation (e.g. original or primary authors,', 'current maintainers, or owners of the package).']}, 'maintainers': {'type': 'array', 'items': {'$id': '#/definitions/author', 'title': 'Author or Maintainer', '$comment': 'https://www.python.org/dev/peps/pep-0621/#authors-maintainers', 'type': 'object', 'properties': {'name': {'type': 'string', '$$description': ['MUST be a valid email name, i.e. whatever can be put as a name, before an', 'email, in :rfc:`822`.']}, 'email': {'type': 'string', 'format': 'idn-email', 'description': 'MUST be a valid email address'}}}, '$$description': ["The people or organizations considered to be the 'maintainers' of the project.", 'Similarly to ``authors``, the exact meaning is open to interpretation.']}, 'keywords': {'type': 'array', 'items': {'type': 'string'}, 'description': 'List of keywords to assist searching for the distribution in a larger catalog.'}, 'classifiers': {'type': 'array', 'items': {'type': 'string', 'format': 'trove-classifier', 'description': '`PyPI classifier `_.'}, '$$description': ['`Trove classifiers `_', 'which apply to the project.']}, 'urls': {'type': 'object', 'description': 'URLs associated with the project in the form ``label => value``.', 'additionalProperties': False, 'patternProperties': {'^.+$': {'type': 'string', 'format': 'url'}}}, 'scripts': {'$id': '#/definitions/entry-point-group', 'title': 'Entry-points', 'type': 'object', '$$description': ['Entry-points are grouped together to indicate what sort of capabilities they', 'provide.', 'See the `packaging guides', '`_', 'and `setuptools docs', '`_', 'for more information.'], 'propertyNames': {'format': 'python-entrypoint-name'}, 'additionalProperties': False, 'patternProperties': {'^.+$': {'type': 'string', '$$description': ['Reference to a Python object. It is either in the form', '``importable.module``, or ``importable.module:object.attr``.'], 'format': 'python-entrypoint-reference', '$comment': 'https://packaging.python.org/specifications/entry-points/'}}}, 'gui-scripts': {'$id': '#/definitions/entry-point-group', 'title': 'Entry-points', 'type': 'object', '$$description': ['Entry-points are grouped together to indicate what sort of capabilities they', 'provide.', 'See the `packaging guides', '`_', 'and `setuptools docs', '`_', 'for more information.'], 'propertyNames': {'format': 'python-entrypoint-name'}, 'additionalProperties': False, 'patternProperties': {'^.+$': {'type': 'string', '$$description': ['Reference to a Python object. It is either in the form', '``importable.module``, or ``importable.module:object.attr``.'], 'format': 'python-entrypoint-reference', '$comment': 'https://packaging.python.org/specifications/entry-points/'}}}, 'entry-points': {'$$description': ['Instruct the installer to expose the given modules/functions via', '``entry-point`` discovery mechanism (useful for plugins).', 'More information available in the `Python packaging guide', '`_.'], 'propertyNames': {'format': 'python-entrypoint-group'}, 'additionalProperties': False, 'patternProperties': {'^.+$': {'$id': '#/definitions/entry-point-group', 'title': 'Entry-points', 'type': 'object', '$$description': ['Entry-points are grouped together to indicate what sort of capabilities they', 'provide.', 'See the `packaging guides', '`_', 'and `setuptools docs', '`_', 'for more information.'], 'propertyNames': {'format': 'python-entrypoint-name'}, 'additionalProperties': False, 'patternProperties': {'^.+$': {'type': 'string', '$$description': ['Reference to a Python object. It is either in the form', '``importable.module``, or ``importable.module:object.attr``.'], 'format': 'python-entrypoint-reference', '$comment': 'https://packaging.python.org/specifications/entry-points/'}}}}}, 'dependencies': {'type': 'array', 'description': 'Project (mandatory) dependencies.', 'items': {'$id': '#/definitions/dependency', 'title': 'Dependency', 'type': 'string', 'description': 'Project dependency specification according to PEP 508', 'format': 'pep508'}}, 'optional-dependencies': {'type': 'object', 'description': 'Optional dependency for the project', 'propertyNames': {'format': 'pep508-identifier'}, 'additionalProperties': False, 'patternProperties': {'^.+$': {'type': 'array', 'items': {'$id': '#/definitions/dependency', 'title': 'Dependency', 'type': 'string', 'description': 'Project dependency specification according to PEP 508', 'format': 'pep508'}}}}, 'dynamic': {'type': 'array', '$$description': ['Specifies which fields are intentionally unspecified and expected to be', 'dynamically provided by build tools'], 'items': {'enum': ['version', 'description', 'readme', 'requires-python', 'license', 'authors', 'maintainers', 'keywords', 'classifiers', 'urls', 'scripts', 'gui-scripts', 'entry-points', 'dependencies', 'optional-dependencies']}}}, 'required': ['name'], 'additionalProperties': False, 'if': {'not': {'required': ['dynamic'], 'properties': {'dynamic': {'contains': {'const': 'version'}, '$$description': ['version is listed in ``dynamic``']}}}, '$$comment': ['According to :pep:`621`:', ' If the core metadata specification lists a field as "Required", then', ' the metadata MUST specify the field statically or list it in dynamic', 'In turn, `core metadata`_ defines:', ' The required fields are: Metadata-Version, Name, Version.', ' All the other fields are optional.', 'Since ``Metadata-Version`` is defined by the build back-end, ``name`` and', '``version`` are the only mandatory information in ``pyproject.toml``.', '.. _core metadata: https://packaging.python.org/specifications/core-metadata/']}, 'then': {'required': ['version'], '$$description': ['version should be statically defined in the ``version`` field']}, 'definitions': {'author': {'$id': '#/definitions/author', 'title': 'Author or Maintainer', '$comment': 'https://www.python.org/dev/peps/pep-0621/#authors-maintainers', 'type': 'object', 'properties': {'name': {'type': 'string', '$$description': ['MUST be a valid email name, i.e. whatever can be put as a name, before an', 'email, in :rfc:`822`.']}, 'email': {'type': 'string', 'format': 'idn-email', 'description': 'MUST be a valid email address'}}}, 'entry-point-group': {'$id': '#/definitions/entry-point-group', 'title': 'Entry-points', 'type': 'object', '$$description': ['Entry-points are grouped together to indicate what sort of capabilities they', 'provide.', 'See the `packaging guides', '`_', 'and `setuptools docs', '`_', 'for more information.'], 'propertyNames': {'format': 'python-entrypoint-name'}, 'additionalProperties': False, 'patternProperties': {'^.+$': {'type': 'string', '$$description': ['Reference to a Python object. It is either in the form', '``importable.module``, or ``importable.module:object.attr``.'], 'format': 'python-entrypoint-reference', '$comment': 'https://packaging.python.org/specifications/entry-points/'}}}, 'dependency': {'$id': '#/definitions/dependency', 'title': 'Dependency', 'type': 'string', 'description': 'Project dependency specification according to PEP 508', 'format': 'pep508'}}}, rule='additionalProperties')
- try:
- try:
- data_is_dict = isinstance(data, dict)
- if data_is_dict:
- data_len = len(data)
- if not all(prop in data for prop in ['dynamic']):
- raise JsonSchemaValueException("" + (name_prefix or "data") + " must contain ['dynamic'] properties", value=data, name="" + (name_prefix or "data") + "", definition={'required': ['dynamic'], 'properties': {'dynamic': {'contains': {'const': 'version'}, '$$description': ['version is listed in ``dynamic``']}}}, rule='required')
- data_keys = set(data.keys())
- if "dynamic" in data_keys:
- data_keys.remove("dynamic")
- data__dynamic = data["dynamic"]
- data__dynamic_is_list = isinstance(data__dynamic, (list, tuple))
- if data__dynamic_is_list:
- data__dynamic_contains = False
- for data__dynamic_key in data__dynamic:
- try:
- if data__dynamic_key != "version":
- raise JsonSchemaValueException("" + (name_prefix or "data") + ".dynamic must be same as const definition: version", value=data__dynamic_key, name="" + (name_prefix or "data") + ".dynamic", definition={'const': 'version'}, rule='const')
- data__dynamic_contains = True
- break
- except JsonSchemaValueException: pass
- if not data__dynamic_contains:
- raise JsonSchemaValueException("" + (name_prefix or "data") + ".dynamic must contain one of contains definition", value=data__dynamic, name="" + (name_prefix or "data") + ".dynamic", definition={'contains': {'const': 'version'}, '$$description': ['version is listed in ``dynamic``']}, rule='contains')
- except JsonSchemaValueException: pass
- else:
- raise JsonSchemaValueException("" + (name_prefix or "data") + " must NOT match a disallowed definition", value=data, name="" + (name_prefix or "data") + "", definition={'not': {'required': ['dynamic'], 'properties': {'dynamic': {'contains': {'const': 'version'}, '$$description': ['version is listed in ``dynamic``']}}}, '$$comment': ['According to :pep:`621`:', ' If the core metadata specification lists a field as "Required", then', ' the metadata MUST specify the field statically or list it in dynamic', 'In turn, `core metadata`_ defines:', ' The required fields are: Metadata-Version, Name, Version.', ' All the other fields are optional.', 'Since ``Metadata-Version`` is defined by the build back-end, ``name`` and', '``version`` are the only mandatory information in ``pyproject.toml``.', '.. _core metadata: https://packaging.python.org/specifications/core-metadata/']}, rule='not')
- except JsonSchemaValueException:
- pass
- else:
- data_is_dict = isinstance(data, dict)
- if data_is_dict:
- data_len = len(data)
- if not all(prop in data for prop in ['version']):
- raise JsonSchemaValueException("" + (name_prefix or "data") + " must contain ['version'] properties", value=data, name="" + (name_prefix or "data") + "", definition={'required': ['version'], '$$description': ['version should be statically defined in the ``version`` field']}, rule='required')
- return data
-
-def validate_https___packaging_python_org_en_latest_specifications_declaring_project_metadata___definitions_dependency(data, custom_formats={}, name_prefix=None):
- if not isinstance(data, (str)):
- raise JsonSchemaValueException("" + (name_prefix or "data") + " must be string", value=data, name="" + (name_prefix or "data") + "", definition={'$id': '#/definitions/dependency', 'title': 'Dependency', 'type': 'string', 'description': 'Project dependency specification according to PEP 508', 'format': 'pep508'}, rule='type')
- if isinstance(data, str):
- if not custom_formats["pep508"](data):
- raise JsonSchemaValueException("" + (name_prefix or "data") + " must be pep508", value=data, name="" + (name_prefix or "data") + "", definition={'$id': '#/definitions/dependency', 'title': 'Dependency', 'type': 'string', 'description': 'Project dependency specification according to PEP 508', 'format': 'pep508'}, rule='format')
- return data
-
-def validate_https___packaging_python_org_en_latest_specifications_declaring_project_metadata___definitions_entry_point_group(data, custom_formats={}, name_prefix=None):
- if not isinstance(data, (dict)):
- raise JsonSchemaValueException("" + (name_prefix or "data") + " must be object", value=data, name="" + (name_prefix or "data") + "", definition={'$id': '#/definitions/entry-point-group', 'title': 'Entry-points', 'type': 'object', '$$description': ['Entry-points are grouped together to indicate what sort of capabilities they', 'provide.', 'See the `packaging guides', '`_', 'and `setuptools docs', '`_', 'for more information.'], 'propertyNames': {'format': 'python-entrypoint-name'}, 'additionalProperties': False, 'patternProperties': {'^.+$': {'type': 'string', '$$description': ['Reference to a Python object. It is either in the form', '``importable.module``, or ``importable.module:object.attr``.'], 'format': 'python-entrypoint-reference', '$comment': 'https://packaging.python.org/specifications/entry-points/'}}}, rule='type')
- data_is_dict = isinstance(data, dict)
- if data_is_dict:
- data_keys = set(data.keys())
- for data_key, data_val in data.items():
- if REGEX_PATTERNS['^.+$'].search(data_key):
- if data_key in data_keys:
- data_keys.remove(data_key)
- if not isinstance(data_val, (str)):
- raise JsonSchemaValueException("" + (name_prefix or "data") + ".{data_key}".format(**locals()) + " must be string", value=data_val, name="" + (name_prefix or "data") + ".{data_key}".format(**locals()) + "", definition={'type': 'string', '$$description': ['Reference to a Python object. It is either in the form', '``importable.module``, or ``importable.module:object.attr``.'], 'format': 'python-entrypoint-reference', '$comment': 'https://packaging.python.org/specifications/entry-points/'}, rule='type')
- if isinstance(data_val, str):
- if not custom_formats["python-entrypoint-reference"](data_val):
- raise JsonSchemaValueException("" + (name_prefix or "data") + ".{data_key}".format(**locals()) + " must be python-entrypoint-reference", value=data_val, name="" + (name_prefix or "data") + ".{data_key}".format(**locals()) + "", definition={'type': 'string', '$$description': ['Reference to a Python object. It is either in the form', '``importable.module``, or ``importable.module:object.attr``.'], 'format': 'python-entrypoint-reference', '$comment': 'https://packaging.python.org/specifications/entry-points/'}, rule='format')
- if data_keys:
- raise JsonSchemaValueException("" + (name_prefix or "data") + " must not contain "+str(data_keys)+" properties", value=data, name="" + (name_prefix or "data") + "", definition={'$id': '#/definitions/entry-point-group', 'title': 'Entry-points', 'type': 'object', '$$description': ['Entry-points are grouped together to indicate what sort of capabilities they', 'provide.', 'See the `packaging guides', '`_', 'and `setuptools docs', '`_', 'for more information.'], 'propertyNames': {'format': 'python-entrypoint-name'}, 'additionalProperties': False, 'patternProperties': {'^.+$': {'type': 'string', '$$description': ['Reference to a Python object. It is either in the form', '``importable.module``, or ``importable.module:object.attr``.'], 'format': 'python-entrypoint-reference', '$comment': 'https://packaging.python.org/specifications/entry-points/'}}}, rule='additionalProperties')
- data_len = len(data)
- if data_len != 0:
- data_property_names = True
- for data_key in data:
- try:
- if isinstance(data_key, str):
- if not custom_formats["python-entrypoint-name"](data_key):
- raise JsonSchemaValueException("" + (name_prefix or "data") + " must be python-entrypoint-name", value=data_key, name="" + (name_prefix or "data") + "", definition={'format': 'python-entrypoint-name'}, rule='format')
- except JsonSchemaValueException:
- data_property_names = False
- if not data_property_names:
- raise JsonSchemaValueException("" + (name_prefix or "data") + " must be named by propertyName definition", value=data, name="" + (name_prefix or "data") + "", definition={'$id': '#/definitions/entry-point-group', 'title': 'Entry-points', 'type': 'object', '$$description': ['Entry-points are grouped together to indicate what sort of capabilities they', 'provide.', 'See the `packaging guides', '`_', 'and `setuptools docs', '`_', 'for more information.'], 'propertyNames': {'format': 'python-entrypoint-name'}, 'additionalProperties': False, 'patternProperties': {'^.+$': {'type': 'string', '$$description': ['Reference to a Python object. It is either in the form', '``importable.module``, or ``importable.module:object.attr``.'], 'format': 'python-entrypoint-reference', '$comment': 'https://packaging.python.org/specifications/entry-points/'}}}, rule='propertyNames')
- return data
-
-def validate_https___packaging_python_org_en_latest_specifications_declaring_project_metadata___definitions_author(data, custom_formats={}, name_prefix=None):
- if not isinstance(data, (dict)):
- raise JsonSchemaValueException("" + (name_prefix or "data") + " must be object", value=data, name="" + (name_prefix or "data") + "", definition={'$id': '#/definitions/author', 'title': 'Author or Maintainer', '$comment': 'https://www.python.org/dev/peps/pep-0621/#authors-maintainers', 'type': 'object', 'properties': {'name': {'type': 'string', '$$description': ['MUST be a valid email name, i.e. whatever can be put as a name, before an', 'email, in :rfc:`822`.']}, 'email': {'type': 'string', 'format': 'idn-email', 'description': 'MUST be a valid email address'}}}, rule='type')
- data_is_dict = isinstance(data, dict)
- if data_is_dict:
- data_keys = set(data.keys())
- if "name" in data_keys:
- data_keys.remove("name")
- data__name = data["name"]
- if not isinstance(data__name, (str)):
- raise JsonSchemaValueException("" + (name_prefix or "data") + ".name must be string", value=data__name, name="" + (name_prefix or "data") + ".name", definition={'type': 'string', '$$description': ['MUST be a valid email name, i.e. whatever can be put as a name, before an', 'email, in :rfc:`822`.']}, rule='type')
- if "email" in data_keys:
- data_keys.remove("email")
- data__email = data["email"]
- if not isinstance(data__email, (str)):
- raise JsonSchemaValueException("" + (name_prefix or "data") + ".email must be string", value=data__email, name="" + (name_prefix or "data") + ".email", definition={'type': 'string', 'format': 'idn-email', 'description': 'MUST be a valid email address'}, rule='type')
- if isinstance(data__email, str):
- if not REGEX_PATTERNS["idn-email_re_pattern"].match(data__email):
- raise JsonSchemaValueException("" + (name_prefix or "data") + ".email must be idn-email", value=data__email, name="" + (name_prefix or "data") + ".email", definition={'type': 'string', 'format': 'idn-email', 'description': 'MUST be a valid email address'}, rule='format')
- return data
\ No newline at end of file
diff --git a/spaces/Rayzggz/illi-Bert-VITS2/text/cleaner.py b/spaces/Rayzggz/illi-Bert-VITS2/text/cleaner.py
deleted file mode 100644
index 3ba3739816aabbe16663b68c74fcda0588c14bab..0000000000000000000000000000000000000000
--- a/spaces/Rayzggz/illi-Bert-VITS2/text/cleaner.py
+++ /dev/null
@@ -1,28 +0,0 @@
-from text import chinese, japanese, cleaned_text_to_sequence
-
-
-language_module_map = {"ZH": chinese, "JP": japanese}
-
-
-def clean_text(text, language):
- language_module = language_module_map[language]
- norm_text = language_module.text_normalize(text)
- phones, tones, word2ph = language_module.g2p(norm_text)
- return norm_text, phones, tones, word2ph
-
-
-def clean_text_bert(text, language):
- language_module = language_module_map[language]
- norm_text = language_module.text_normalize(text)
- phones, tones, word2ph = language_module.g2p(norm_text)
- bert = language_module.get_bert_feature(norm_text, word2ph)
- return phones, tones, bert
-
-
-def text_to_sequence(text, language):
- norm_text, phones, tones, word2ph = clean_text(text, language)
- return cleaned_text_to_sequence(phones, tones, language)
-
-
-if __name__ == "__main__":
- pass
diff --git a/spaces/Reself/StableVideo/stablevideo/atlas_utils.py b/spaces/Reself/StableVideo/stablevideo/atlas_utils.py
deleted file mode 100644
index 4b2a8452f3abac222e35e45d3d3b9e30d4fd5c95..0000000000000000000000000000000000000000
--- a/spaces/Reself/StableVideo/stablevideo/atlas_utils.py
+++ /dev/null
@@ -1,330 +0,0 @@
-from PIL import Image
-from pathlib import Path
-import scipy.interpolate
-import torch
-from torchvision import transforms
-from torchvision.transforms.functional import crop
-from tqdm import tqdm
-import numpy as np
-import cv2
-
-from stablevideo.implicit_neural_networks import IMLP
-
-
-def load_video(folder: str, resize=(432, 768), num_frames=70):
- resy, resx = resize
- folder = Path(folder)
- input_files = sorted(list(folder.glob("*.jpg")) + list(folder.glob("*.png")))[:num_frames]
- video_tensor = torch.zeros((len(input_files), 3, resy, resx))
-
- for i, file in enumerate(input_files):
- video_tensor[i] = transforms.ToTensor()(Image.open(str(file)).resize((resx, resy), Image.LANCZOS))
-
- return video_tensor
-
-
-def load_neural_atlases_models(config):
- foreground_mapping = IMLP(
- input_dim=3,
- output_dim=2,
- hidden_dim=256,
- use_positional=False,
- num_layers=6,
- skip_layers=[],
- ).to(config["device"])
-
- background_mapping = IMLP(
- input_dim=3,
- output_dim=2,
- hidden_dim=256,
- use_positional=False,
- num_layers=4,
- skip_layers=[],
- ).to(config["device"])
-
- foreground_atlas_model = IMLP(
- input_dim=2,
- output_dim=3,
- hidden_dim=256,
- use_positional=True,
- positional_dim=10,
- num_layers=8,
- skip_layers=[4, 7],
- ).to(config["device"])
-
- background_atlas_model = IMLP(
- input_dim=2,
- output_dim=3,
- hidden_dim=256,
- use_positional=True,
- positional_dim=10,
- num_layers=8,
- skip_layers=[4, 7],
- ).to(config["device"])
-
- alpha_model = IMLP(
- input_dim=3,
- output_dim=1,
- hidden_dim=256,
- use_positional=True,
- positional_dim=5,
- num_layers=8,
- skip_layers=[],
- ).to(config["device"])
-
- checkpoint = torch.load(config["checkpoint_path"], map_location=torch.device('cpu'))
- foreground_mapping.load_state_dict(checkpoint["model_F_mapping1_state_dict"])
- background_mapping.load_state_dict(checkpoint["model_F_mapping2_state_dict"])
- foreground_atlas_model.load_state_dict(checkpoint["F_atlas_state_dict"])
- background_atlas_model.load_state_dict(checkpoint["F_atlas_state_dict"])
- alpha_model.load_state_dict(checkpoint["model_F_alpha_state_dict"])
-
- foreground_mapping = foreground_mapping.eval().requires_grad_(False)
- background_mapping = background_mapping.eval().requires_grad_(False)
- foreground_atlas_model = foreground_atlas_model.eval().requires_grad_(False)
- background_atlas_model = background_atlas_model.eval().requires_grad_(False)
- alpha_model = alpha_model.eval().requires_grad_(False)
-
- return foreground_mapping, background_mapping, foreground_atlas_model, background_atlas_model, alpha_model
-
-
-@torch.no_grad()
-def get_frames_data(config, foreground_mapping, background_mapping, alpha_model):
- max_size = max(config["resx"], config["resy"])
- normalizing_factor = torch.tensor([max_size / 2, max_size / 2, config["maximum_number_of_frames"] / 2])
- background_uv_values = torch.zeros(
- size=(config["maximum_number_of_frames"], config["resy"], config["resx"], 2), device=config["device"]
- )
- foreground_uv_values = torch.zeros(
- size=(config["maximum_number_of_frames"], config["resy"], config["resx"], 2), device=config["device"]
- )
- alpha = torch.zeros(
- size=(config["maximum_number_of_frames"], config["resy"], config["resx"], 1), device=config["device"]
- )
-
- for frame in tqdm(range(config["maximum_number_of_frames"]), leave=False):
- indices = get_grid_indices(0, 0, config["resy"], config["resx"], t=torch.tensor(frame))
-
- normalized_chunk = (indices / normalizing_factor - 1).to(config["device"])
-
- # get the atlas UV coordinates from the two mapping networks;
- with torch.no_grad():
- current_background_uv_values = background_mapping(normalized_chunk)
- current_foreground_uv_values = foreground_mapping(normalized_chunk)
- current_alpha = alpha_model(normalized_chunk)
-
- background_uv_values[frame, indices[:, 1], indices[:, 0]] = current_background_uv_values * 0.5 - 0.5
- foreground_uv_values[frame, indices[:, 1], indices[:, 0]] = current_foreground_uv_values * 0.5 + 0.5
- current_alpha = 0.5 * (current_alpha + 1.0)
- current_alpha = 0.99 * current_alpha + 0.001
- alpha[frame, indices[:, 1], indices[:, 0]] = current_alpha
- # config["return_atlas_alpha"] = True
- if config["return_atlas_alpha"]: # this should take a few minutes
- foreground_atlas_alpha = torch.zeros(
- size=(
- config["maximum_number_of_frames"],
- config["grid_atlas_resolution"],
- config["grid_atlas_resolution"],
- 1,
- ),
- )
- # foreground_uv_values: 70 x 432 x 768 x 2
- foreground_uv_values_grid = foreground_uv_values * config["grid_atlas_resolution"]
- # indices: 4000000 x 2
- indices = get_grid_indices(0, 0, config["grid_atlas_resolution"], config["grid_atlas_resolution"])
- for frame in tqdm(range(config["maximum_number_of_frames"]), leave=False):
- interpolated = scipy.interpolate.griddata(
- foreground_uv_values_grid[frame].reshape(-1, 2).cpu().numpy(), # 432 x 768 x 2 -> -1 x 2
- alpha[frame]
- .reshape(
- -1,
- )
- .cpu()
- .numpy(),
- indices.reshape(-1, 2).cpu().numpy(),
- method="linear",
- ).reshape(config["grid_atlas_resolution"], config["grid_atlas_resolution"], 1)
- foreground_atlas_alpha[frame] = torch.from_numpy(interpolated)
- foreground_atlas_alpha[foreground_atlas_alpha.isnan()] = 0.0
- foreground_atlas_alpha = (
- torch.median(foreground_atlas_alpha, dim=0, keepdim=True).values.to(config["device"]).permute(0, 3, 2, 1)
- )
- else:
- foreground_atlas_alpha = None
- return background_uv_values, foreground_uv_values, alpha.permute(0, 3, 1, 2), foreground_atlas_alpha
-
-
-@torch.no_grad()
-def reconstruct_video_layer(uv_values, atlas_model):
- t, h, w, _ = uv_values.shape
- reconstruction = torch.zeros(size=(t, h, w, 3), device=uv_values.device)
- for frame in range(t):
- rgb = (atlas_model(uv_values[frame].reshape(-1, 2)) + 1) * 0.5
- reconstruction[frame] = rgb.reshape(h, w, 3)
- return reconstruction.permute(0, 3, 1, 2)
-
-
-@torch.no_grad()
-def create_uv_mask(config, mapping_model, min_u, min_v, max_u, max_v, uv_shift=-0.5, resolution_shift=1):
- max_size = max(config["resx"], config["resy"])
- normalizing_factor = torch.tensor([max_size / 2, max_size / 2, config["maximum_number_of_frames"] / 2])
- resolution = config["grid_atlas_resolution"]
- uv_mask = torch.zeros(size=(resolution, resolution), device=config["device"])
-
- for frame in tqdm(range(config["maximum_number_of_frames"]), leave=False):
- indices = get_grid_indices(0, 0, config["resy"], config["resx"], t=torch.tensor(frame))
- for chunk in indices.split(50000, dim=0):
- normalized_chunk = (chunk / normalizing_factor - 1).to(config["device"])
-
- # get the atlas UV coordinates from the two mapping networks;
- with torch.no_grad():
- uv_values = mapping_model(normalized_chunk)
- uv_values = uv_values * 0.5 + uv_shift
- uv_values = ((uv_values + resolution_shift) * resolution).clip(0, resolution - 1)
-
- uv_mask[uv_values[:, 1].floor().long(), uv_values[:, 0].floor().long()] = 1
- uv_mask[uv_values[:, 1].floor().long(), uv_values[:, 0].ceil().long()] = 1
- uv_mask[uv_values[:, 1].ceil().long(), uv_values[:, 0].floor().long()] = 1
- uv_mask[uv_values[:, 1].ceil().long(), uv_values[:, 0].ceil().long()] = 1
-
- uv_mask = crop(uv_mask.unsqueeze(0).unsqueeze(0), min_v, min_u, max_v, max_u)
- return uv_mask.detach().cpu() # shape [1, 1, resolution, resolution]
-
-
-@torch.no_grad()
-def get_high_res_atlas(atlas_model, min_v, min_u, max_v, max_u, resolution, device="cuda", layer="background"):
- inds_grid = get_grid_indices(0, 0, resolution, resolution)
- inds_grid_chunks = inds_grid.split(50000, dim=0)
- if layer == "background":
- shift = -1
- else:
- shift = 0
-
- rendered_atlas = torch.zeros((resolution, resolution, 3)).to(device) # resy, resx, 3
- with torch.no_grad():
- # reconstruct image row by row
- for chunk in inds_grid_chunks:
- normalized_chunk = torch.stack(
- [
- (chunk[:, 0] / resolution) + shift,
- (chunk[:, 1] / resolution) + shift,
- ],
- dim=-1,
- ).to(device)
-
- rgb_output = atlas_model(normalized_chunk)
- rendered_atlas[chunk[:, 1], chunk[:, 0], :] = rgb_output
- # move colors to RGB color domain (0,1)
- rendered_atlas = 0.5 * (rendered_atlas + 1)
- rendered_atlas = rendered_atlas.permute(2, 0, 1).unsqueeze(0) # shape (1, 3, resy, resx)
- cropped_atlas = crop(
- rendered_atlas,
- min_v,
- min_u,
- max_v,
- max_u,
- )
-
- return cropped_atlas
-
-
-def get_grid_indices(x_start, y_start, h_crop, w_crop, t=None):
- crop_indices = torch.meshgrid(torch.arange(w_crop) + x_start, torch.arange(h_crop) + y_start)
- crop_indices = torch.stack(crop_indices, dim=-1)
- crop_indices = crop_indices.reshape(h_crop * w_crop, crop_indices.shape[-1])
- if t is not None:
- crop_indices = torch.cat([crop_indices, t.repeat(h_crop * w_crop, 1)], dim=1)
- return crop_indices
-
-
-def get_atlas_crops(uv_values, grid_atlas, augmentation=None):
- if len(uv_values.shape) == 3:
- dims = [0, 1]
- elif len(uv_values.shape) == 4:
- dims = [0, 1, 2]
- else:
- raise ValueError("uv_values should be of shape of len 3 or 4")
-
- min_u, min_v = uv_values.amin(dim=dims).long()
- max_u, max_v = uv_values.amax(dim=dims).ceil().long()
- # min_u, min_v = uv_values.min(dim=0).values
- # max_u, max_v = uv_values.max(dim=0).values
-
- h_v = max_v - min_v
- w_u = max_u - min_u
- atlas_crop = crop(grid_atlas, min_v, min_u, h_v, w_u)
- if augmentation is not None:
- atlas_crop = augmentation(atlas_crop)
- return atlas_crop, torch.stack([min_u, min_v]), torch.stack([max_u, max_v])
-
-
-def get_random_crop_params(input_size, output_size):
- w, h = input_size
- th, tw = output_size
-
- if h + 1 < th or w + 1 < tw:
- raise ValueError(f"Required crop size {(th, tw)} is larger then input image size {(h, w)}")
-
- if w == tw and h == th:
- return 0, 0, h, w
-
- i = torch.randint(0, h - th + 1, size=(1,)).item()
- j = torch.randint(0, w - tw + 1, size=(1,)).item()
- return i, j, th, tw
-
-
-def get_masks_boundaries(alpha_video, border=20, threshold=0.95, min_crop_size=2 ** 7 + 1):
- resy, resx = alpha_video.shape[-2:]
- num_frames = alpha_video.shape[0]
- masks_borders = torch.zeros((num_frames, 4), dtype=torch.int64)
- for i, file in enumerate(range(num_frames)):
- mask_im = alpha_video[i]
- mask_im[mask_im >= threshold] = 1
- mask_im[mask_im < threshold] = 0
- all_ones = mask_im.squeeze().nonzero()
- min_y, min_x = torch.maximum(all_ones.min(dim=0).values - border, torch.tensor([0, 0]))
- max_y, max_x = torch.minimum(all_ones.max(dim=0).values + border, torch.tensor([resy, resx]))
- h = max_y - min_y
- w = max_x - min_x
- if h < min_crop_size:
- pad = min_crop_size - h
- if max_y + pad > resy:
- min_y -= pad
- else:
- max_y += pad
- h = max_y - min_y
- if w < min_crop_size:
- pad = min_crop_size - w
- if max_x + pad > resx:
- min_x -= pad
- else:
- max_x += pad
- w = max_x - min_x
- masks_borders[i] = torch.tensor([min_y, min_x, h, w])
- return masks_borders
-
-
-def get_atlas_bounding_box(mask_boundaries, grid_atlas, video_uvs):
- min_uv = torch.tensor(grid_atlas.shape[-2:], device=video_uvs.device)
- max_uv = torch.tensor([0, 0], device=video_uvs.device)
- for boundary, frame in zip(mask_boundaries, video_uvs):
- cropped_uvs = crop(frame.permute(2, 0, 1).unsqueeze(0), *list(boundary)) # 1,2,h,w
- min_uv = torch.minimum(cropped_uvs.amin(dim=[0, 2, 3]), min_uv).floor().int()
- max_uv = torch.maximum(cropped_uvs.amax(dim=[0, 2, 3]), max_uv).ceil().int()
-
- hw = max_uv - min_uv
- crop_data = [*list(min_uv)[::-1], *list(hw)[::-1]]
- return crop(grid_atlas, *crop_data), crop_data
-
-
-def tensor2im(input_image, imtype=np.uint8):
- if not isinstance(input_image, np.ndarray):
- if isinstance(input_image, torch.Tensor): # get the data from a variable
- image_tensor = input_image.data
- else:
- return input_image
- image_numpy = image_tensor[0].clamp(0.0, 1.0).cpu().float().numpy() # convert it into a numpy array
- image_numpy = np.transpose(image_numpy, (1, 2, 0)) * 255.0 # post-processing: tranpose and scaling
- else: # if it is a numpy array, do nothing
- image_numpy = input_image
- return image_numpy.astype(imtype)
\ No newline at end of file
diff --git a/spaces/Rizon-Lin/NewBing/Dockerfile b/spaces/Rizon-Lin/NewBing/Dockerfile
deleted file mode 100644
index 927040eeea880e3fca835d8eb38db34e8905e80b..0000000000000000000000000000000000000000
--- a/spaces/Rizon-Lin/NewBing/Dockerfile
+++ /dev/null
@@ -1,34 +0,0 @@
-# Build Stage
-# 使用 golang:alpine 作为构建阶段的基础镜像
-FROM golang:alpine AS builder
-
-# 添加 git,以便之后能从GitHub克隆项目
-RUN apk --no-cache add git
-
-# 从 GitHub 克隆 go-proxy-bingai 项目到 /workspace/app 目录下
-RUN git clone https://github.com/Harry-zklcdc/go-proxy-bingai.git /workspace/app
-
-# 设置工作目录为之前克隆的项目目录
-WORKDIR /workspace/app
-
-# 编译 go 项目。-ldflags="-s -w" 是为了减少编译后的二进制大小
-RUN go build -ldflags="-s -w" -tags netgo -trimpath -o go-proxy-bingai main.go
-
-# Runtime Stage
-# 使用轻量级的 alpine 镜像作为运行时的基础镜像
-FROM alpine
-
-# 设置工作目录
-WORKDIR /workspace/app
-
-# 从构建阶段复制编译后的二进制文件到运行时镜像中
-COPY --from=builder /workspace/app/go-proxy-bingai .
-
-# 设置环境变量,此处为随机字符
-ENV Go_Proxy_BingAI_USER_TOKEN_1="14tDQa9QslhddZ_NIPhN4ALFX8fw7BWHERgf9EmFux14xS5bYwC1W7gHnuAr11KUES8KN1k4X4_OXzmO3MloGq2_s_yyEQ4BYCB_SR6u3OFT9dvQ1Zh58d-j_cHJ0lJpBc_tnYuKjE7aEYTInZOr8WY5d54jVjN5COTrjBFxzZmcYnc2p6fTweOyPxe29prMlrtieM0ht_qmOXxJuE396rQ"
-
-# 暴露8080端口
-EXPOSE 8080
-
-# 容器启动时运行的命令
-CMD ["/workspace/app/go-proxy-bingai"]
\ No newline at end of file
diff --git a/spaces/RoCobo/WiggleGAN/utils.py b/spaces/RoCobo/WiggleGAN/utils.py
deleted file mode 100644
index 3950203c62792f10ea7e8fa8be9c572969bf1cc9..0000000000000000000000000000000000000000
--- a/spaces/RoCobo/WiggleGAN/utils.py
+++ /dev/null
@@ -1,369 +0,0 @@
-import os, gzip, torch
-import torch.nn as nn
-import numpy as np
-import scipy.misc
-import imageio
-import matplotlib.pyplot as plt
-from PIL import Image
-from torchvision import datasets, transforms
-import visdom
-import random
-
-def save_wiggle(images, rows=1, name="test"):
-
-
- width = images[0].shape[1]
- height = images[0].shape[2]
- columns = int(len(images)/rows)
- rows = int(rows)
- margin = 4
-
- total_width = (width + margin) * columns
- total_height = (height + margin) * rows
-
- new_im = Image.new('RGB', (total_width, total_height))
-
- transToPil = transforms.ToPILImage()
-
- x_offset = 3
- y_offset = 3
- for y in range(rows):
- for x in range(columns):
- im = images[x+y*columns]
- im = transToPil((im+1)/2)
- new_im.paste(im, (x_offset, y_offset))
- x_offset += width + margin
- x_offset = 3
- y_offset += height + margin
-
- new_im.save('./WiggleResults/' + name + '.jpg')
-
-def load_mnist(dataset):
- data_dir = os.path.join("./data", dataset)
-
- def extract_data(filename, num_data, head_size, data_size):
- with gzip.open(filename) as bytestream:
- bytestream.read(head_size)
- buf = bytestream.read(data_size * num_data)
- data = np.frombuffer(buf, dtype=np.uint8).astype(np.float)
- return data
-
- data = extract_data(data_dir + '/train-images-idx3-ubyte.gz', 60000, 16, 28 * 28)
- trX = data.reshape((60000, 28, 28, 1))
-
- data = extract_data(data_dir + '/train-labels-idx1-ubyte.gz', 60000, 8, 1)
- trY = data.reshape((60000))
-
- data = extract_data(data_dir + '/t10k-images-idx3-ubyte.gz', 10000, 16, 28 * 28)
- teX = data.reshape((10000, 28, 28, 1))
-
- data = extract_data(data_dir + '/t10k-labels-idx1-ubyte.gz', 10000, 8, 1)
- teY = data.reshape((10000))
-
- trY = np.asarray(trY).astype(np.int)
- teY = np.asarray(teY)
-
- X = np.concatenate((trX, teX), axis=0)
- y = np.concatenate((trY, teY), axis=0).astype(np.int)
-
- seed = 547
- np.random.seed(seed)
- np.random.shuffle(X)
- np.random.seed(seed)
- np.random.shuffle(y)
-
- y_vec = np.zeros((len(y), 10), dtype=np.float)
- for i, label in enumerate(y):
- y_vec[i, y[i]] = 1
-
- X = X.transpose(0, 3, 1, 2) / 255.
- # y_vec = y_vec.transpose(0, 3, 1, 2)
-
- X = torch.from_numpy(X).type(torch.FloatTensor)
- y_vec = torch.from_numpy(y_vec).type(torch.FloatTensor)
- return X, y_vec
-
-def load_celebA(dir, transform, batch_size, shuffle):
- # transform = transforms.Compose([
- # transforms.CenterCrop(160),
- # transform.Scale(64)
- # transforms.ToTensor(),
- # transforms.Normalize(mean=(0.5, 0.5, 0.5), std=(0.5, 0.5, 0.5))
- # ])
-
- # data_dir = 'data/celebA' # this path depends on your computer
- dset = datasets.ImageFolder(dir, transform)
- data_loader = torch.utils.data.DataLoader(dset, batch_size, shuffle)
-
- return data_loader
-
-
-def print_network(net):
- num_params = 0
- for param in net.parameters():
- num_params += param.numel()
- print(net)
- print('Total number of parameters: %d' % num_params)
-
-def save_images(images, size, image_path):
- return imsave(images, size, image_path)
-
-def imsave(images, size, path):
- image = np.squeeze(merge(images, size))
- return scipy.misc.imsave(path, image)
-
-def merge(images, size):
- #print ("shape", images.shape)
- h, w = images.shape[1], images.shape[2]
- if (images.shape[3] in (3,4)):
- c = images.shape[3]
- img = np.zeros((h * size[0], w * size[1], c))
- for idx, image in enumerate(images):
- i = idx % size[1]
- j = idx // size[1]
- img[j * h:j * h + h, i * w:i * w + w, :] = image
- return img
- elif images.shape[3]== 1:
- img = np.zeros((h * size[0], w * size[1]))
- for idx, image in enumerate(images):
- #print("indez ",idx)
- i = idx % size[1]
- j = idx // size[1]
- img[j * h:j * h + h, i * w:i * w + w] = image[:,:,0]
- return img
- else:
- raise ValueError('in merge(images,size) images parameter ''must have dimensions: HxW or HxWx3 or HxWx4')
-
-def generate_animation(path, num):
- images = []
- for e in range(num):
- img_name = path + '_epoch%04d' % (e+1) + '.png'
- images.append(imageio.imread(img_name))
- imageio.mimsave(path + '_generate_animation.gif', images, fps=5)
-
-def loss_plot(hist, path = 'Train_hist.png', model_name = ''):
- x1 = range(len(hist['D_loss_train']))
- x2 = range(len(hist['G_loss_train']))
-
- y1 = hist['D_loss_train']
- y2 = hist['G_loss_train']
-
- if (x1 != x2):
- y1 = [0.0] * (len(y2) - len(y1)) + y1
- x1 = x2
-
- plt.plot(x1, y1, label='D_loss_train')
-
- plt.plot(x2, y2, label='G_loss_train')
-
- plt.xlabel('Iter')
- plt.ylabel('Loss')
-
- plt.legend(loc=4)
- plt.grid(True)
- plt.tight_layout()
-
- path = os.path.join(path, model_name + '_loss.png')
-
- plt.savefig(path)
-
- plt.close()
-
-def initialize_weights(net):
- for m in net.modules():
- if isinstance(m, nn.Conv2d):
- m.weight.data.normal_(0, 0.02)
- m.bias.data.zero_()
- elif isinstance(m, nn.ConvTranspose2d):
- m.weight.data.normal_(0, 0.02)
- m.bias.data.zero_()
- elif isinstance(m, nn.Linear):
- m.weight.data.normal_(0, 0.02)
- m.bias.data.zero_()
-
-class VisdomLinePlotter(object):
- """Plots to Visdom"""
- def __init__(self, env_name='main'):
- self.viz = visdom.Visdom()
- self.env = env_name
- self.ini = False
- self.count = 1
- def plot(self, var_name,names, split_name, hist):
-
-
-
- x = []
- y = []
- for i, name in enumerate(names):
- x.append(self.count)
- y.append(hist[name])
- self.count+=1
- #x1 = (len(hist['D_loss_' +split_name]))
- #x2 = (len(hist['G_loss_' +split_name]))
-
- #y1 = hist['D_loss_'+split_name]
- #y2 = hist['G_loss_'+split_name]
-
-
- np.array(x)
-
-
- for i,n in enumerate(names):
- x[i] = np.arange(1, x[i]+1)
-
- if not self.ini:
- for i, name in enumerate(names):
- if i == 0:
- self.win = self.viz.line(X=x[i], Y=np.array(y[i]), env=self.env,name = name,opts=dict(
- title=var_name + '_'+split_name, showlegend = True
- ))
- else:
- self.viz.line(X=x[i], Y=np.array(y[i]), env=self.env,win=self.win, name=name, update='append')
- self.ini = True
- else:
- x[0] = np.array([x[0][-2], x[0][-1]])
-
- for i,n in enumerate(names):
- y[i] = np.array([y[i][-2], y[i][-1]])
- self.viz.line(X=x[0], Y=np.array(y[i]), env=self.env, win=self.win, name=n, update='append')
-
-
-class VisdomLineTwoPlotter(VisdomLinePlotter):
-
- def plot(self, var_name, epoch,names, hist):
-
- x1 = epoch
- y1 = hist[names[0]]
- y2 = hist[names[1]]
- y3 = hist[names[2]]
- y4 = hist[names[3]]
-
-
- #y1 = hist['D_loss_' + split_name]
- #y2 = hist['G_loss_' + split_name]
- #y3 = hist['D_loss_' + split_name2]
- #y4 = hist['G_loss_' + split_name2]
-
-
- #x1 = np.arange(1, x1+1)
-
- if not self.ini:
- self.win = self.viz.line(X=np.array([x1]), Y=np.array(y1), env=self.env,name = names[0],opts=dict(
- title=var_name,
- showlegend = True,
- linecolor = np.array([[0, 0, 255]])
- ))
- self.viz.line(X=np.array([x1]), Y=np.array(y2), env=self.env,win=self.win, name=names[1],
- update='append', opts=dict(
- linecolor=np.array([[255, 153, 51]])
- ))
- self.viz.line(X=np.array([x1]), Y=np.array(y3), env=self.env, win=self.win, name=names[2],
- update='append', opts=dict(
- linecolor=np.array([[0, 51, 153]])
- ))
- self.viz.line(X=np.array([x1]), Y=np.array(y4), env=self.env, win=self.win, name=names[3],
- update='append', opts=dict(
- linecolor=np.array([[204, 51, 0]])
- ))
- self.ini = True
- else:
-
- y4 = np.array([y4[-2], y4[-1]])
- y3 = np.array([y3[-2], y3[-1]])
- y2 = np.array([y2[-2], y2[-1]])
- y1 = np.array([y1[-2], y1[-1]])
- x1 = np.array([x1 - 1, x1])
- self.viz.line(X=x1, Y=np.array(y1), env=self.env, win=self.win, name=names[0], update='append')
- self.viz.line(X=x1, Y=np.array(y2), env=self.env, win=self.win, name=names[1], update='append')
- self.viz.line(X=x1, Y=np.array(y3), env=self.env, win=self.win, name=names[2],
- update='append')
- self.viz.line(X=x1, Y=np.array(y4), env=self.env, win=self.win, name=names[3],
- update='append')
-
-class VisdomImagePlotter(object):
- """Plots to Visdom"""
- def __init__(self, env_name='main'):
- self.viz = visdom.Visdom()
- self.env = env_name
- def plot(self, epoch,images,rows):
-
- list_images = []
- for image in images:
- #transforms.ToPILImage()(image)
- image = (image + 1)/2
- image = image.detach().numpy() * 255
- list_images.append(image)
- self.viz.images(
- list_images,
- padding=2,
- nrow =rows,
- opts=dict(title="epoch: " + str(epoch)),
- env=self.env
- )
-
-
-def augmentData(x,y, randomness = 1, percent_noise = 0.1):
- """
- :param x: image X
- :param y: image Y
- :param randomness: Value of randomness (between 1 and 0)
- :return: data x,y augmented
- """
-
-
- sampleX = torch.tensor([])
- sampleY = torch.tensor([])
-
- for aumX, aumY in zip(x,y):
-
- # Preparing to get image # transforms.ToPILImage()(pil_to_tensor.squeeze_(0))
- #percent_noise = percent_noise
- #noise = torch.randn(aumX.shape)
-
- #aumX = noise * percent_noise + aumX * (1 - percent_noise)
- #aumY = noise * percent_noise + aumY * (1 - percent_noise)
-
- aumX = (aumX + 1) / 2
- aumY = (aumY + 1) / 2
-
- imgX = transforms.ToPILImage()(aumX)
- imgY = transforms.ToPILImage()(aumY)
-
- # Values for augmentation #
- brighness = random.uniform(0.7, 1.2)* randomness + (1-randomness)
- saturation = random.uniform(0, 2)* randomness + (1-randomness)
- contrast = random.uniform(0.4, 2)* randomness + (1-randomness)
- gamma = random.uniform(0.7, 1.3)* randomness + (1-randomness)
- hue = random.uniform(-0.3, 0.3)* randomness #0.01
-
- imgX = transforms.functional.adjust_gamma(imgX, gamma)
- imgX = transforms.functional.adjust_brightness(imgX, brighness)
- imgX = transforms.functional.adjust_contrast(imgX, contrast)
- imgX = transforms.functional.adjust_saturation(imgX, saturation)
- imgX = transforms.functional.adjust_hue(imgX, hue)
- #imgX.show()
-
- imgY = transforms.functional.adjust_gamma(imgY, gamma)
- imgY = transforms.functional.adjust_brightness(imgY, brighness)
- imgY = transforms.functional.adjust_contrast(imgY, contrast)
- imgY = transforms.functional.adjust_saturation(imgY, saturation)
- imgY = transforms.functional.adjust_hue(imgY, hue)
- #imgY.show()
-
- sx = transforms.ToTensor()(imgX)
- sx = (sx * 2)-1
-
- sy = transforms.ToTensor()(imgY)
- sy = (sy * 2)-1
-
- sampleX = torch.cat((sampleX, sx.unsqueeze_(0)), 0)
- sampleY = torch.cat((sampleY, sy.unsqueeze_(0)), 0)
- return sampleX,sampleY
-
-def RGBtoL (x):
-
- return x[:,0,:,:].unsqueeze(0).transpose(0,1)
-
-def LtoRGB (x):
-
- return x.repeat(1, 3, 1, 1)
diff --git a/spaces/Robert001/UniControl-Demo/annotator/uniformer/mmcv/visualization/image.py b/spaces/Robert001/UniControl-Demo/annotator/uniformer/mmcv/visualization/image.py
deleted file mode 100644
index 61a56c75b67f593c298408462c63c0468be8e276..0000000000000000000000000000000000000000
--- a/spaces/Robert001/UniControl-Demo/annotator/uniformer/mmcv/visualization/image.py
+++ /dev/null
@@ -1,152 +0,0 @@
-# Copyright (c) OpenMMLab. All rights reserved.
-import cv2
-import numpy as np
-
-from annotator.uniformer.mmcv.image import imread, imwrite
-from .color import color_val
-
-
-def imshow(img, win_name='', wait_time=0):
- """Show an image.
-
- Args:
- img (str or ndarray): The image to be displayed.
- win_name (str): The window name.
- wait_time (int): Value of waitKey param.
- """
- cv2.imshow(win_name, imread(img))
- if wait_time == 0: # prevent from hanging if windows was closed
- while True:
- ret = cv2.waitKey(1)
-
- closed = cv2.getWindowProperty(win_name, cv2.WND_PROP_VISIBLE) < 1
- # if user closed window or if some key pressed
- if closed or ret != -1:
- break
- else:
- ret = cv2.waitKey(wait_time)
-
-
-def imshow_bboxes(img,
- bboxes,
- colors='green',
- top_k=-1,
- thickness=1,
- show=True,
- win_name='',
- wait_time=0,
- out_file=None):
- """Draw bboxes on an image.
-
- Args:
- img (str or ndarray): The image to be displayed.
- bboxes (list or ndarray): A list of ndarray of shape (k, 4).
- colors (list[str or tuple or Color]): A list of colors.
- top_k (int): Plot the first k bboxes only if set positive.
- thickness (int): Thickness of lines.
- show (bool): Whether to show the image.
- win_name (str): The window name.
- wait_time (int): Value of waitKey param.
- out_file (str, optional): The filename to write the image.
-
- Returns:
- ndarray: The image with bboxes drawn on it.
- """
- img = imread(img)
- img = np.ascontiguousarray(img)
-
- if isinstance(bboxes, np.ndarray):
- bboxes = [bboxes]
- if not isinstance(colors, list):
- colors = [colors for _ in range(len(bboxes))]
- colors = [color_val(c) for c in colors]
- assert len(bboxes) == len(colors)
-
- for i, _bboxes in enumerate(bboxes):
- _bboxes = _bboxes.astype(np.int32)
- if top_k <= 0:
- _top_k = _bboxes.shape[0]
- else:
- _top_k = min(top_k, _bboxes.shape[0])
- for j in range(_top_k):
- left_top = (_bboxes[j, 0], _bboxes[j, 1])
- right_bottom = (_bboxes[j, 2], _bboxes[j, 3])
- cv2.rectangle(
- img, left_top, right_bottom, colors[i], thickness=thickness)
-
- if show:
- imshow(img, win_name, wait_time)
- if out_file is not None:
- imwrite(img, out_file)
- return img
-
-
-def imshow_det_bboxes(img,
- bboxes,
- labels,
- class_names=None,
- score_thr=0,
- bbox_color='green',
- text_color='green',
- thickness=1,
- font_scale=0.5,
- show=True,
- win_name='',
- wait_time=0,
- out_file=None):
- """Draw bboxes and class labels (with scores) on an image.
-
- Args:
- img (str or ndarray): The image to be displayed.
- bboxes (ndarray): Bounding boxes (with scores), shaped (n, 4) or
- (n, 5).
- labels (ndarray): Labels of bboxes.
- class_names (list[str]): Names of each classes.
- score_thr (float): Minimum score of bboxes to be shown.
- bbox_color (str or tuple or :obj:`Color`): Color of bbox lines.
- text_color (str or tuple or :obj:`Color`): Color of texts.
- thickness (int): Thickness of lines.
- font_scale (float): Font scales of texts.
- show (bool): Whether to show the image.
- win_name (str): The window name.
- wait_time (int): Value of waitKey param.
- out_file (str or None): The filename to write the image.
-
- Returns:
- ndarray: The image with bboxes drawn on it.
- """
- assert bboxes.ndim == 2
- assert labels.ndim == 1
- assert bboxes.shape[0] == labels.shape[0]
- assert bboxes.shape[1] == 4 or bboxes.shape[1] == 5
- img = imread(img)
- img = np.ascontiguousarray(img)
-
- if score_thr > 0:
- assert bboxes.shape[1] == 5
- scores = bboxes[:, -1]
- inds = scores > score_thr
- bboxes = bboxes[inds, :]
- labels = labels[inds]
-
- bbox_color = color_val(bbox_color)
- text_color = color_val(text_color)
-
- for bbox, label in zip(bboxes, labels):
- bbox_int = bbox.astype(np.int32)
- left_top = (bbox_int[0], bbox_int[1])
- right_bottom = (bbox_int[2], bbox_int[3])
- cv2.rectangle(
- img, left_top, right_bottom, bbox_color, thickness=thickness)
- label_text = class_names[
- label] if class_names is not None else f'cls {label}'
- if len(bbox) > 4:
- label_text += f'|{bbox[-1]:.02f}'
- cv2.putText(img, label_text, (bbox_int[0], bbox_int[1] - 2),
- cv2.FONT_HERSHEY_COMPLEX, font_scale, text_color)
-
- if show:
- imshow(img, win_name, wait_time)
- if out_file is not None:
- imwrite(img, out_file)
- return img
diff --git a/spaces/Robert001/UniControl-Demo/annotator/uniformer/mmdet/models/roi_heads/bbox_heads/convfc_bbox_head.py b/spaces/Robert001/UniControl-Demo/annotator/uniformer/mmdet/models/roi_heads/bbox_heads/convfc_bbox_head.py
deleted file mode 100644
index 0e86d2ea67e154fae18dbf9d2bfde6d0a70e582c..0000000000000000000000000000000000000000
--- a/spaces/Robert001/UniControl-Demo/annotator/uniformer/mmdet/models/roi_heads/bbox_heads/convfc_bbox_head.py
+++ /dev/null
@@ -1,205 +0,0 @@
-import torch.nn as nn
-from mmcv.cnn import ConvModule
-
-from mmdet.models.builder import HEADS
-from .bbox_head import BBoxHead
-
-
-@HEADS.register_module()
-class ConvFCBBoxHead(BBoxHead):
- r"""More general bbox head, with shared conv and fc layers and two optional
- separated branches.
-
- .. code-block:: none
-
- /-> cls convs -> cls fcs -> cls
- shared convs -> shared fcs
- \-> reg convs -> reg fcs -> reg
- """ # noqa: W605
-
- def __init__(self,
- num_shared_convs=0,
- num_shared_fcs=0,
- num_cls_convs=0,
- num_cls_fcs=0,
- num_reg_convs=0,
- num_reg_fcs=0,
- conv_out_channels=256,
- fc_out_channels=1024,
- conv_cfg=None,
- norm_cfg=None,
- *args,
- **kwargs):
- super(ConvFCBBoxHead, self).__init__(*args, **kwargs)
- assert (num_shared_convs + num_shared_fcs + num_cls_convs +
- num_cls_fcs + num_reg_convs + num_reg_fcs > 0)
- if num_cls_convs > 0 or num_reg_convs > 0:
- assert num_shared_fcs == 0
- if not self.with_cls:
- assert num_cls_convs == 0 and num_cls_fcs == 0
- if not self.with_reg:
- assert num_reg_convs == 0 and num_reg_fcs == 0
- self.num_shared_convs = num_shared_convs
- self.num_shared_fcs = num_shared_fcs
- self.num_cls_convs = num_cls_convs
- self.num_cls_fcs = num_cls_fcs
- self.num_reg_convs = num_reg_convs
- self.num_reg_fcs = num_reg_fcs
- self.conv_out_channels = conv_out_channels
- self.fc_out_channels = fc_out_channels
- self.conv_cfg = conv_cfg
- self.norm_cfg = norm_cfg
-
- # add shared convs and fcs
- self.shared_convs, self.shared_fcs, last_layer_dim = \
- self._add_conv_fc_branch(
- self.num_shared_convs, self.num_shared_fcs, self.in_channels,
- True)
- self.shared_out_channels = last_layer_dim
-
- # add cls specific branch
- self.cls_convs, self.cls_fcs, self.cls_last_dim = \
- self._add_conv_fc_branch(
- self.num_cls_convs, self.num_cls_fcs, self.shared_out_channels)
-
- # add reg specific branch
- self.reg_convs, self.reg_fcs, self.reg_last_dim = \
- self._add_conv_fc_branch(
- self.num_reg_convs, self.num_reg_fcs, self.shared_out_channels)
-
- if self.num_shared_fcs == 0 and not self.with_avg_pool:
- if self.num_cls_fcs == 0:
- self.cls_last_dim *= self.roi_feat_area
- if self.num_reg_fcs == 0:
- self.reg_last_dim *= self.roi_feat_area
-
- self.relu = nn.ReLU(inplace=True)
- # reconstruct fc_cls and fc_reg since input channels are changed
- if self.with_cls:
- self.fc_cls = nn.Linear(self.cls_last_dim, self.num_classes + 1)
- if self.with_reg:
- out_dim_reg = (4 if self.reg_class_agnostic else 4 *
- self.num_classes)
- self.fc_reg = nn.Linear(self.reg_last_dim, out_dim_reg)
-
- def _add_conv_fc_branch(self,
- num_branch_convs,
- num_branch_fcs,
- in_channels,
- is_shared=False):
- """Add shared or separable branch.
-
- convs -> avg pool (optional) -> fcs
- """
- last_layer_dim = in_channels
- # add branch specific conv layers
- branch_convs = nn.ModuleList()
- if num_branch_convs > 0:
- for i in range(num_branch_convs):
- conv_in_channels = (
- last_layer_dim if i == 0 else self.conv_out_channels)
- branch_convs.append(
- ConvModule(
- conv_in_channels,
- self.conv_out_channels,
- 3,
- padding=1,
- conv_cfg=self.conv_cfg,
- norm_cfg=self.norm_cfg))
- last_layer_dim = self.conv_out_channels
- # add branch specific fc layers
- branch_fcs = nn.ModuleList()
- if num_branch_fcs > 0:
- # for shared branch, only consider self.with_avg_pool
- # for separated branches, also consider self.num_shared_fcs
- if (is_shared
- or self.num_shared_fcs == 0) and not self.with_avg_pool:
- last_layer_dim *= self.roi_feat_area
- for i in range(num_branch_fcs):
- fc_in_channels = (
- last_layer_dim if i == 0 else self.fc_out_channels)
- branch_fcs.append(
- nn.Linear(fc_in_channels, self.fc_out_channels))
- last_layer_dim = self.fc_out_channels
- return branch_convs, branch_fcs, last_layer_dim
-
- def init_weights(self):
- super(ConvFCBBoxHead, self).init_weights()
- # conv layers are already initialized by ConvModule
- for module_list in [self.shared_fcs, self.cls_fcs, self.reg_fcs]:
- for m in module_list.modules():
- if isinstance(m, nn.Linear):
- nn.init.xavier_uniform_(m.weight)
- nn.init.constant_(m.bias, 0)
-
- def forward(self, x):
- # shared part
- if self.num_shared_convs > 0:
- for conv in self.shared_convs:
- x = conv(x)
-
- if self.num_shared_fcs > 0:
- if self.with_avg_pool:
- x = self.avg_pool(x)
-
- x = x.flatten(1)
-
- for fc in self.shared_fcs:
- x = self.relu(fc(x))
- # separate branches
- x_cls = x
- x_reg = x
-
- for conv in self.cls_convs:
- x_cls = conv(x_cls)
- if x_cls.dim() > 2:
- if self.with_avg_pool:
- x_cls = self.avg_pool(x_cls)
- x_cls = x_cls.flatten(1)
- for fc in self.cls_fcs:
- x_cls = self.relu(fc(x_cls))
-
- for conv in self.reg_convs:
- x_reg = conv(x_reg)
- if x_reg.dim() > 2:
- if self.with_avg_pool:
- x_reg = self.avg_pool(x_reg)
- x_reg = x_reg.flatten(1)
- for fc in self.reg_fcs:
- x_reg = self.relu(fc(x_reg))
-
- cls_score = self.fc_cls(x_cls) if self.with_cls else None
- bbox_pred = self.fc_reg(x_reg) if self.with_reg else None
- return cls_score, bbox_pred
-
-
-@HEADS.register_module()
-class Shared2FCBBoxHead(ConvFCBBoxHead):
-
- def __init__(self, fc_out_channels=1024, *args, **kwargs):
- super(Shared2FCBBoxHead, self).__init__(
- num_shared_convs=0,
- num_shared_fcs=2,
- num_cls_convs=0,
- num_cls_fcs=0,
- num_reg_convs=0,
- num_reg_fcs=0,
- fc_out_channels=fc_out_channels,
- *args,
- **kwargs)
-
-
-@HEADS.register_module()
-class Shared4Conv1FCBBoxHead(ConvFCBBoxHead):
-
- def __init__(self, fc_out_channels=1024, *args, **kwargs):
- super(Shared4Conv1FCBBoxHead, self).__init__(
- num_shared_convs=4,
- num_shared_fcs=1,
- num_cls_convs=0,
- num_cls_fcs=0,
- num_reg_convs=0,
- num_reg_fcs=0,
- fc_out_channels=fc_out_channels,
- *args,
- **kwargs)
diff --git a/spaces/Robert001/UniControl-Demo/annotator/uniformer/mmdet/utils/collect_env.py b/spaces/Robert001/UniControl-Demo/annotator/uniformer/mmdet/utils/collect_env.py
deleted file mode 100644
index 89c064accdb10abec4a03de04f601d27aab2da70..0000000000000000000000000000000000000000
--- a/spaces/Robert001/UniControl-Demo/annotator/uniformer/mmdet/utils/collect_env.py
+++ /dev/null
@@ -1,16 +0,0 @@
-from mmcv.utils import collect_env as collect_base_env
-from mmcv.utils import get_git_hash
-
-import mmdet
-
-
-def collect_env():
- """Collect the information of the running environments."""
- env_info = collect_base_env()
- env_info['MMDetection'] = mmdet.__version__ + '+' + get_git_hash()[:7]
- return env_info
-
-
-if __name__ == '__main__':
- for name, val in collect_env().items():
- print(f'{name}: {val}')
diff --git a/spaces/Robert001/UniControl-Demo/annotator/uniformer/mmdet_null/core/bbox/iou_calculators/builder.py b/spaces/Robert001/UniControl-Demo/annotator/uniformer/mmdet_null/core/bbox/iou_calculators/builder.py
deleted file mode 100644
index 3220806fbcf70302dd58c5a166c7436692db11d1..0000000000000000000000000000000000000000
--- a/spaces/Robert001/UniControl-Demo/annotator/uniformer/mmdet_null/core/bbox/iou_calculators/builder.py
+++ /dev/null
@@ -1,8 +0,0 @@
-from annotator.uniformer.mmcv.utils import Registry, build_from_cfg
-
-IOU_CALCULATORS = Registry('IoU calculator')
-
-
-def build_iou_calculator(cfg, default_args=None):
- """Builder of IoU calculator."""
- return build_from_cfg(cfg, IOU_CALCULATORS, default_args)
diff --git a/spaces/RoversX/Stable-Platypus2-13B-GGML/README.md b/spaces/RoversX/Stable-Platypus2-13B-GGML/README.md
deleted file mode 100644
index 05f9f21606765c9f6393acbe5436ba2a108b1013..0000000000000000000000000000000000000000
--- a/spaces/RoversX/Stable-Platypus2-13B-GGML/README.md
+++ /dev/null
@@ -1,12 +0,0 @@
----
-emoji: 🏃
-colorFrom: blue
-colorTo: gray
-sdk: gradio
-sdk_version: 3.29.0
-app_file: tabbed.py
-pinned: false
-duplicated_from: RoversX/llama-2-7b-hf-small-shards-Samantha-V1-SFT-ggml
----
-
-# GGML UI Inference Space-Test-Demo
diff --git a/spaces/Sa-m/YOLO-V7-Custom-Model-Pot-Hole-Detection/utils/torch_utils.py b/spaces/Sa-m/YOLO-V7-Custom-Model-Pot-Hole-Detection/utils/torch_utils.py
deleted file mode 100644
index 1e631b555508457a4944c11a479176463719c0e8..0000000000000000000000000000000000000000
--- a/spaces/Sa-m/YOLO-V7-Custom-Model-Pot-Hole-Detection/utils/torch_utils.py
+++ /dev/null
@@ -1,374 +0,0 @@
-# YOLOR PyTorch utils
-
-import datetime
-import logging
-import math
-import os
-import platform
-import subprocess
-import time
-from contextlib import contextmanager
-from copy import deepcopy
-from pathlib import Path
-
-import torch
-import torch.backends.cudnn as cudnn
-import torch.nn as nn
-import torch.nn.functional as F
-import torchvision
-
-try:
- import thop # for FLOPS computation
-except ImportError:
- thop = None
-logger = logging.getLogger(__name__)
-
-
-@contextmanager
-def torch_distributed_zero_first(local_rank: int):
- """
- Decorator to make all processes in distributed training wait for each local_master to do something.
- """
- if local_rank not in [-1, 0]:
- torch.distributed.barrier()
- yield
- if local_rank == 0:
- torch.distributed.barrier()
-
-
-def init_torch_seeds(seed=0):
- # Speed-reproducibility tradeoff https://pytorch.org/docs/stable/notes/randomness.html
- torch.manual_seed(seed)
- if seed == 0: # slower, more reproducible
- cudnn.benchmark, cudnn.deterministic = False, True
- else: # faster, less reproducible
- cudnn.benchmark, cudnn.deterministic = True, False
-
-
-def date_modified(path=__file__):
- # return human-readable file modification date, i.e. '2021-3-26'
- t = datetime.datetime.fromtimestamp(Path(path).stat().st_mtime)
- return f'{t.year}-{t.month}-{t.day}'
-
-
-def git_describe(path=Path(__file__).parent): # path must be a directory
- # return human-readable git description, i.e. v5.0-5-g3e25f1e https://git-scm.com/docs/git-describe
- s = f'git -C {path} describe --tags --long --always'
- try:
- return subprocess.check_output(s, shell=True, stderr=subprocess.STDOUT).decode()[:-1]
- except subprocess.CalledProcessError as e:
- return '' # not a git repository
-
-
-def select_device(device='', batch_size=None):
- # device = 'cpu' or '0' or '0,1,2,3'
- s = f'YOLOR 🚀 {git_describe() or date_modified()} torch {torch.__version__} ' # string
- cpu = device.lower() == 'cpu'
- if cpu:
- os.environ['CUDA_VISIBLE_DEVICES'] = '-1' # force torch.cuda.is_available() = False
- elif device: # non-cpu device requested
- os.environ['CUDA_VISIBLE_DEVICES'] = device # set environment variable
- assert torch.cuda.is_available(), f'CUDA unavailable, invalid device {device} requested' # check availability
-
- cuda = not cpu and torch.cuda.is_available()
- if cuda:
- n = torch.cuda.device_count()
- if n > 1 and batch_size: # check that batch_size is compatible with device_count
- assert batch_size % n == 0, f'batch-size {batch_size} not multiple of GPU count {n}'
- space = ' ' * len(s)
- for i, d in enumerate(device.split(',') if device else range(n)):
- p = torch.cuda.get_device_properties(i)
- s += f"{'' if i == 0 else space}CUDA:{d} ({p.name}, {p.total_memory / 1024 ** 2}MB)\n" # bytes to MB
- else:
- s += 'CPU\n'
-
- logger.info(s.encode().decode('ascii', 'ignore') if platform.system() == 'Windows' else s) # emoji-safe
- return torch.device('cuda:0' if cuda else 'cpu')
-
-
-def time_synchronized():
- # pytorch-accurate time
- if torch.cuda.is_available():
- torch.cuda.synchronize()
- return time.time()
-
-
-def profile(x, ops, n=100, device=None):
- # profile a pytorch module or list of modules. Example usage:
- # x = torch.randn(16, 3, 640, 640) # input
- # m1 = lambda x: x * torch.sigmoid(x)
- # m2 = nn.SiLU()
- # profile(x, [m1, m2], n=100) # profile speed over 100 iterations
-
- device = device or torch.device('cuda:0' if torch.cuda.is_available() else 'cpu')
- x = x.to(device)
- x.requires_grad = True
- print(torch.__version__, device.type, torch.cuda.get_device_properties(0) if device.type == 'cuda' else '')
- print(f"\n{'Params':>12s}{'GFLOPS':>12s}{'forward (ms)':>16s}{'backward (ms)':>16s}{'input':>24s}{'output':>24s}")
- for m in ops if isinstance(ops, list) else [ops]:
- m = m.to(device) if hasattr(m, 'to') else m # device
- m = m.half() if hasattr(m, 'half') and isinstance(x, torch.Tensor) and x.dtype is torch.float16 else m # type
- dtf, dtb, t = 0., 0., [0., 0., 0.] # dt forward, backward
- try:
- flops = thop.profile(m, inputs=(x,), verbose=False)[0] / 1E9 * 2 # GFLOPS
- except:
- flops = 0
-
- for _ in range(n):
- t[0] = time_synchronized()
- y = m(x)
- t[1] = time_synchronized()
- try:
- _ = y.sum().backward()
- t[2] = time_synchronized()
- except: # no backward method
- t[2] = float('nan')
- dtf += (t[1] - t[0]) * 1000 / n # ms per op forward
- dtb += (t[2] - t[1]) * 1000 / n # ms per op backward
-
- s_in = tuple(x.shape) if isinstance(x, torch.Tensor) else 'list'
- s_out = tuple(y.shape) if isinstance(y, torch.Tensor) else 'list'
- p = sum(list(x.numel() for x in m.parameters())) if isinstance(m, nn.Module) else 0 # parameters
- print(f'{p:12}{flops:12.4g}{dtf:16.4g}{dtb:16.4g}{str(s_in):>24s}{str(s_out):>24s}')
-
-
-def is_parallel(model):
- return type(model) in (nn.parallel.DataParallel, nn.parallel.DistributedDataParallel)
-
-
-def intersect_dicts(da, db, exclude=()):
- # Dictionary intersection of matching keys and shapes, omitting 'exclude' keys, using da values
- return {k: v for k, v in da.items() if k in db and not any(x in k for x in exclude) and v.shape == db[k].shape}
-
-
-def initialize_weights(model):
- for m in model.modules():
- t = type(m)
- if t is nn.Conv2d:
- pass # nn.init.kaiming_normal_(m.weight, mode='fan_out', nonlinearity='relu')
- elif t is nn.BatchNorm2d:
- m.eps = 1e-3
- m.momentum = 0.03
- elif t in [nn.Hardswish, nn.LeakyReLU, nn.ReLU, nn.ReLU6]:
- m.inplace = True
-
-
-def find_modules(model, mclass=nn.Conv2d):
- # Finds layer indices matching module class 'mclass'
- return [i for i, m in enumerate(model.module_list) if isinstance(m, mclass)]
-
-
-def sparsity(model):
- # Return global model sparsity
- a, b = 0., 0.
- for p in model.parameters():
- a += p.numel()
- b += (p == 0).sum()
- return b / a
-
-
-def prune(model, amount=0.3):
- # Prune model to requested global sparsity
- import torch.nn.utils.prune as prune
- print('Pruning model... ', end='')
- for name, m in model.named_modules():
- if isinstance(m, nn.Conv2d):
- prune.l1_unstructured(m, name='weight', amount=amount) # prune
- prune.remove(m, 'weight') # make permanent
- print(' %.3g global sparsity' % sparsity(model))
-
-
-def fuse_conv_and_bn(conv, bn):
- # Fuse convolution and batchnorm layers https://tehnokv.com/posts/fusing-batchnorm-and-conv/
- fusedconv = nn.Conv2d(conv.in_channels,
- conv.out_channels,
- kernel_size=conv.kernel_size,
- stride=conv.stride,
- padding=conv.padding,
- groups=conv.groups,
- bias=True).requires_grad_(False).to(conv.weight.device)
-
- # prepare filters
- w_conv = conv.weight.clone().view(conv.out_channels, -1)
- w_bn = torch.diag(bn.weight.div(torch.sqrt(bn.eps + bn.running_var)))
- fusedconv.weight.copy_(torch.mm(w_bn, w_conv).view(fusedconv.weight.shape))
-
- # prepare spatial bias
- b_conv = torch.zeros(conv.weight.size(0), device=conv.weight.device) if conv.bias is None else conv.bias
- b_bn = bn.bias - bn.weight.mul(bn.running_mean).div(torch.sqrt(bn.running_var + bn.eps))
- fusedconv.bias.copy_(torch.mm(w_bn, b_conv.reshape(-1, 1)).reshape(-1) + b_bn)
-
- return fusedconv
-
-
-def model_info(model, verbose=False, img_size=640):
- # Model information. img_size may be int or list, i.e. img_size=640 or img_size=[640, 320]
- n_p = sum(x.numel() for x in model.parameters()) # number parameters
- n_g = sum(x.numel() for x in model.parameters() if x.requires_grad) # number gradients
- if verbose:
- print('%5s %40s %9s %12s %20s %10s %10s' % ('layer', 'name', 'gradient', 'parameters', 'shape', 'mu', 'sigma'))
- for i, (name, p) in enumerate(model.named_parameters()):
- name = name.replace('module_list.', '')
- print('%5g %40s %9s %12g %20s %10.3g %10.3g' %
- (i, name, p.requires_grad, p.numel(), list(p.shape), p.mean(), p.std()))
-
- try: # FLOPS
- from thop import profile
- stride = max(int(model.stride.max()), 32) if hasattr(model, 'stride') else 32
- img = torch.zeros((1, model.yaml.get('ch', 3), stride, stride), device=next(model.parameters()).device) # input
- flops = profile(deepcopy(model), inputs=(img,), verbose=False)[0] / 1E9 * 2 # stride GFLOPS
- img_size = img_size if isinstance(img_size, list) else [img_size, img_size] # expand if int/float
- fs = ', %.1f GFLOPS' % (flops * img_size[0] / stride * img_size[1] / stride) # 640x640 GFLOPS
- except (ImportError, Exception):
- fs = ''
-
- logger.info(f"Model Summary: {len(list(model.modules()))} layers, {n_p} parameters, {n_g} gradients{fs}")
-
-
-def load_classifier(name='resnet101', n=2):
- # Loads a pretrained model reshaped to n-class output
- model = torchvision.models.__dict__[name](pretrained=True)
-
- # ResNet model properties
- # input_size = [3, 224, 224]
- # input_space = 'RGB'
- # input_range = [0, 1]
- # mean = [0.485, 0.456, 0.406]
- # std = [0.229, 0.224, 0.225]
-
- # Reshape output to n classes
- filters = model.fc.weight.shape[1]
- model.fc.bias = nn.Parameter(torch.zeros(n), requires_grad=True)
- model.fc.weight = nn.Parameter(torch.zeros(n, filters), requires_grad=True)
- model.fc.out_features = n
- return model
-
-
-def scale_img(img, ratio=1.0, same_shape=False, gs=32): # img(16,3,256,416)
- # scales img(bs,3,y,x) by ratio constrained to gs-multiple
- if ratio == 1.0:
- return img
- else:
- h, w = img.shape[2:]
- s = (int(h * ratio), int(w * ratio)) # new size
- img = F.interpolate(img, size=s, mode='bilinear', align_corners=False) # resize
- if not same_shape: # pad/crop img
- h, w = [math.ceil(x * ratio / gs) * gs for x in (h, w)]
- return F.pad(img, [0, w - s[1], 0, h - s[0]], value=0.447) # value = imagenet mean
-
-
-def copy_attr(a, b, include=(), exclude=()):
- # Copy attributes from b to a, options to only include [...] and to exclude [...]
- for k, v in b.__dict__.items():
- if (len(include) and k not in include) or k.startswith('_') or k in exclude:
- continue
- else:
- setattr(a, k, v)
-
-
-class ModelEMA:
- """ Model Exponential Moving Average from https://github.com/rwightman/pytorch-image-models
- Keep a moving average of everything in the model state_dict (parameters and buffers).
- This is intended to allow functionality like
- https://www.tensorflow.org/api_docs/python/tf/train/ExponentialMovingAverage
- A smoothed version of the weights is necessary for some training schemes to perform well.
- This class is sensitive where it is initialized in the sequence of model init,
- GPU assignment and distributed training wrappers.
- """
-
- def __init__(self, model, decay=0.9999, updates=0):
- # Create EMA
- self.ema = deepcopy(model.module if is_parallel(model) else model).eval() # FP32 EMA
- # if next(model.parameters()).device.type != 'cpu':
- # self.ema.half() # FP16 EMA
- self.updates = updates # number of EMA updates
- self.decay = lambda x: decay * (1 - math.exp(-x / 2000)) # decay exponential ramp (to help early epochs)
- for p in self.ema.parameters():
- p.requires_grad_(False)
-
- def update(self, model):
- # Update EMA parameters
- with torch.no_grad():
- self.updates += 1
- d = self.decay(self.updates)
-
- msd = model.module.state_dict() if is_parallel(model) else model.state_dict() # model state_dict
- for k, v in self.ema.state_dict().items():
- if v.dtype.is_floating_point:
- v *= d
- v += (1. - d) * msd[k].detach()
-
- def update_attr(self, model, include=(), exclude=('process_group', 'reducer')):
- # Update EMA attributes
- copy_attr(self.ema, model, include, exclude)
-
-
-class BatchNormXd(torch.nn.modules.batchnorm._BatchNorm):
- def _check_input_dim(self, input):
- # The only difference between BatchNorm1d, BatchNorm2d, BatchNorm3d, etc
- # is this method that is overwritten by the sub-class
- # This original goal of this method was for tensor sanity checks
- # If you're ok bypassing those sanity checks (eg. if you trust your inference
- # to provide the right dimensional inputs), then you can just use this method
- # for easy conversion from SyncBatchNorm
- # (unfortunately, SyncBatchNorm does not store the original class - if it did
- # we could return the one that was originally created)
- return
-
-def revert_sync_batchnorm(module):
- # this is very similar to the function that it is trying to revert:
- # https://github.com/pytorch/pytorch/blob/c8b3686a3e4ba63dc59e5dcfe5db3430df256833/torch/nn/modules/batchnorm.py#L679
- module_output = module
- if isinstance(module, torch.nn.modules.batchnorm.SyncBatchNorm):
- new_cls = BatchNormXd
- module_output = BatchNormXd(module.num_features,
- module.eps, module.momentum,
- module.affine,
- module.track_running_stats)
- if module.affine:
- with torch.no_grad():
- module_output.weight = module.weight
- module_output.bias = module.bias
- module_output.running_mean = module.running_mean
- module_output.running_var = module.running_var
- module_output.num_batches_tracked = module.num_batches_tracked
- if hasattr(module, "qconfig"):
- module_output.qconfig = module.qconfig
- for name, child in module.named_children():
- module_output.add_module(name, revert_sync_batchnorm(child))
- del module
- return module_output
-
-
-class TracedModel(nn.Module):
-
- def __init__(self, model=None, device=None, img_size=(640,640)):
- super(TracedModel, self).__init__()
-
- print(" Convert model to Traced-model... ")
- self.stride = model.stride
- self.names = model.names
- self.model = model
-
- self.model = revert_sync_batchnorm(self.model)
- self.model.to('cpu')
- self.model.eval()
-
- self.detect_layer = self.model.model[-1]
- self.model.traced = True
-
- rand_example = torch.rand(1, 3, img_size, img_size)
-
- traced_script_module = torch.jit.trace(self.model, rand_example, strict=False)
- #traced_script_module = torch.jit.script(self.model)
- traced_script_module.save("traced_model.pt")
- print(" traced_script_module saved! ")
- self.model = traced_script_module
- self.model.to(device)
- self.detect_layer.to(device)
- print(" model is traced! \n")
-
- def forward(self, x, augment=False, profile=False):
- out = self.model(x)
- out = self.detect_layer(out)
- return out
\ No newline at end of file
diff --git a/spaces/SarthakSidhant/Go-Cattle/diseases/blackleg.md b/spaces/SarthakSidhant/Go-Cattle/diseases/blackleg.md
deleted file mode 100644
index ff9b1b7cac3a88d18a319db45c1414adbe65d473..0000000000000000000000000000000000000000
--- a/spaces/SarthakSidhant/Go-Cattle/diseases/blackleg.md
+++ /dev/null
@@ -1,33 +0,0 @@
-## Blackleg
-
-**Information:** Blackleg, also known as quarter ill or symptomatic anthrax, is a bacterial disease that affects cattle, sheep, and other ruminants. It is caused by the bacterium Clostridium chauvoei, which is a spore-forming bacteria that can survive in the environment for long periods of time. Blackleg is a highly fatal disease, and death can occur within hours of the onset of symptoms.
-
-**Symptoms:**
-
-* Sudden onset of fever
-* Depression
-* Swelling of muscles, especially in the hindquarters and shoulders
-* The affected muscles may become black and necrotic
-* The animal may have difficulty breathing or walking
-* Death may occur within hours of the onset of symptoms
-
-**Remedies:**
-
-* There is no effective treatment for blackleg.
-* Animals that are diagnosed with blackleg should be euthanized to prevent the spread of the disease.
-
-**Causes:**
-
-* Blackleg is caused by the bacterium Clostridium chauvoei.
-* This bacterium is found in soil and water.
-* Animals become infected with blackleg when they ingest or inhale the spores.
-* The spores can also enter the body through wounds or abrasions.
-
-**Prevention:**
-
-* The best way to prevent blackleg is to vaccinate animals against the disease.
-* Vaccinations are typically given to young animals at a few months of age and then every year or two thereafter.
-* Other preventive measures include:
- * Avoiding grazing animals in areas where blackleg is known to be present
- * Promptly treating any wounds or abrasions on animals
- * Disposing of dead animals properly to prevent the spread of the disease
diff --git a/spaces/SilenWang/ReviewGPT/lang/Review.md b/spaces/SilenWang/ReviewGPT/lang/Review.md
deleted file mode 100644
index 00a393ffc7a9f0b8801fdceedeb93b6f7d327950..0000000000000000000000000000000000000000
--- a/spaces/SilenWang/ReviewGPT/lang/Review.md
+++ /dev/null
@@ -1,11 +0,0 @@
-### Usage
-
-This page provides two functions: Summarize and Screen.
-
-- Summarize: Read the summary of multiple articles, summarize their content, and compare the similarities and differences among multiple studies. Further questions can be asked using prompts.
-- Screen: Read the summary of articles one by one, determine whether the article meets the conditions given in the prompts, and can be used for batch screening of literature.
-
-Currently, two input methods are provided:
-
-- PMID: Enter the PMID directly, and call the Pumbed API provided by Biopython to obtain the summary of the literature and parse it.
-- RIS format file: Can be exported in RIS format from various literature management software or online databases and uploaded for parsing.
diff --git a/spaces/SilenWang/ReviewGPT/lang/Title.md b/spaces/SilenWang/ReviewGPT/lang/Title.md
deleted file mode 100644
index 811cee6c0ab1c72db754e7e974d95bb74372e5c2..0000000000000000000000000000000000000000
--- a/spaces/SilenWang/ReviewGPT/lang/Title.md
+++ /dev/null
@@ -1,2 +0,0 @@
-# ReviewGPT
-ReviewGPT is an app that use the ChatGPT API to perform paper summarization and aggregation. My goal is to use AI to accelerate the reading and retrieval of papers
\ No newline at end of file
diff --git a/spaces/SirensOfNC/sail-rvc-Sonic_SonicBoom/app.py b/spaces/SirensOfNC/sail-rvc-Sonic_SonicBoom/app.py
deleted file mode 100644
index 2aa0d4f8ee382e4e6357b27e54d196175ed4296b..0000000000000000000000000000000000000000
--- a/spaces/SirensOfNC/sail-rvc-Sonic_SonicBoom/app.py
+++ /dev/null
@@ -1,3 +0,0 @@
-import gradio as gr
-
-gr.Interface.load("models/sail-rvc/Sonic_SonicBoom").launch()
\ No newline at end of file
diff --git a/spaces/SrikanthPhalgun/Cifar10_ERAV1_GradCam_Demo/README.md b/spaces/SrikanthPhalgun/Cifar10_ERAV1_GradCam_Demo/README.md
deleted file mode 100644
index 36643b994937a267650efca19578d0a2cb80966b..0000000000000000000000000000000000000000
--- a/spaces/SrikanthPhalgun/Cifar10_ERAV1_GradCam_Demo/README.md
+++ /dev/null
@@ -1,53 +0,0 @@
----
-title: Cifar10 ERAV1 GradCam Demo
-emoji: 📚
-colorFrom: red
-colorTo: blue
-sdk: gradio
-sdk_version: 3.39.0
-app_file: app.py
-pinned: false
----
-
-## Cifar10_ERAV1_GradCam_Demo
-
-### Demo 1 - Inference and GradCAM images
-
-
-
-* Input:
- * Upload image from desktop
- * Whether Gradcam output to be displayed (Select check box)
- * Slider - Which layer output default -1 Max -2
- * Slider - Opacity of GradCam default 0.5 Max 1
- * Slider - How many top classes ? default 3 Max 10 classes
-
-* Output:
- * Display label with probability score for uploaded image
- * Display Image
-
-* 10 example images are also provided to the user to try the app
-
-
-Demo 2 - Misclassified Images
-
-
-
-* Input:
- * Misclassified images to be displayed check box
- * How many misclassified images the user wants to visualize (max 10)
- * GradCam output of selected images - Checkbox
- * Slider - Which layer output default -1 Max -2
- * Slider - Opacity of GradCam default 0.5 Max 1
-
-* Output:
- * Display specified number of misclassified images with actual and predicted classes if only misclassified checkbox is selected
- * Display specified number of misclassified images and GradCam output with actual and predicted class if both Misclassified and Gradcam checkbox selected
- * Display only GradCam output with actual and predicted if only Gradcam checkbox selected.
-
-
-#Github link :
-https://github.com/PyarakaSrikanth/ERAV1/tree/main/S12
-
-App link:
-https://huggingface.co/spaces/SrikanthPhalgun/Cifar10_ERAV1_GradCam_Demo
\ No newline at end of file
diff --git a/spaces/SuYuanS/AudioCraft_Plus/audiocraft/grids/musicgen/musicgen_melody_32khz.py b/spaces/SuYuanS/AudioCraft_Plus/audiocraft/grids/musicgen/musicgen_melody_32khz.py
deleted file mode 100644
index b0d6710a23c117406e9724057a62eccab88ce907..0000000000000000000000000000000000000000
--- a/spaces/SuYuanS/AudioCraft_Plus/audiocraft/grids/musicgen/musicgen_melody_32khz.py
+++ /dev/null
@@ -1,65 +0,0 @@
-# Copyright (c) Meta Platforms, Inc. and affiliates.
-# All rights reserved.
-#
-# This source code is licensed under the license found in the
-# LICENSE file in the root directory of this source tree.
-
-from ._explorers import LMExplorer
-from ...environment import AudioCraftEnvironment
-
-
-@LMExplorer
-def explorer(launcher):
- partitions = AudioCraftEnvironment.get_slurm_partitions(['team', 'global'])
- launcher.slurm_(gpus=32, partition=partitions)
- launcher.bind_(solver='musicgen/musicgen_melody_32khz')
- # replace this by the desired music dataset
- launcher.bind_(dset='internal/music_400k_32khz')
-
- fsdp = {'autocast': False, 'fsdp.use': True}
- medium = {'model/lm/model_scale': 'medium'}
- large = {'model/lm/model_scale': 'large'}
-
- cfg_low = {'classifier_free_guidance.training_dropout': 0.2}
- wd_low = {'conditioners.description.t5.word_dropout': 0.2}
-
- adam = {'optim.optimizer': 'adamw', 'optim.lr': 1e-4}
-
- cache_path = {'conditioners.self_wav.chroma_stem.cache_path':
- '/fsx-audio-craft-llm/jadecopet/experiments/audiocraft/caches/chroma_stem'}
-
- # CACHE GENERATION JOBS
- n_cache_gen_jobs = 4
- gen_sub = launcher.slurm(gpus=1)
- gen_sub.bind_(
- cache_path, {
- # the cache is always computed over the whole file, so duration doesn't matter here.
- 'dataset.segment_duration': 2.,
- 'dataset.batch_size': 8,
- 'dataset.train.permutation_on_files': True, # try to not repeat files.
- 'optim.epochs': 10,
- 'model/lm/model_scale': 'xsmall',
-
- })
- with gen_sub.job_array():
- for gen_job in range(n_cache_gen_jobs):
- gen_sub({'dataset.train.shuffle_seed': gen_job})
-
- # ACTUAL TRAINING JOBS.
- launcher.bind_(fsdp)
-
- launcher.slurm_(gpus=32).bind_(label='32gpus')
- with launcher.job_array():
- sub = launcher.bind()
- sub()
- sub(cache_path)
-
- launcher.slurm_(gpus=64).bind_(label='64gpus')
- with launcher.job_array():
- sub = launcher.bind()
- sub(medium, adam)
-
- launcher.slurm_(gpus=96).bind_(label='96gpus')
- with launcher.job_array():
- sub = launcher.bind()
- sub(large, cfg_low, wd_low, adam, {'optim.max_norm': 3})
diff --git a/spaces/SuYuanS/AudioCraft_Plus/docs/MBD.md b/spaces/SuYuanS/AudioCraft_Plus/docs/MBD.md
deleted file mode 100644
index 296d08407bac9155380a48bdc9faa5798db32bcb..0000000000000000000000000000000000000000
--- a/spaces/SuYuanS/AudioCraft_Plus/docs/MBD.md
+++ /dev/null
@@ -1,117 +0,0 @@
-# MultiBand Diffusion
-
-AudioCraft provides the code and models for MultiBand Diffusion, [From Discrete Tokens to High Fidelity Audio using MultiBand Diffusion][arxiv].
-MultiBand diffusion is a collection of 4 models that can decode tokens from
-EnCodec tokenizer into waveform audio.
-
-
-
-
-
-
-
-## Installation
-
-Please follow the AudioCraft installation instructions from the [README](../README.md).
-
-
-## Usage
-
-We offer a number of way to use MultiBand Diffusion:
-1. The MusicGen demo includes a toggle to try diffusion decoder. You can use the demo locally by running [`python -m demos.musicgen_app --share`](../demos/musicgen_app.py), or through the [MusicGen Colab](https://colab.research.google.com/drive/1JlTOjB-G0A2Hz3h8PK63vLZk4xdCI5QB?usp=sharing).
-2. You can play with MusicGen by running the jupyter notebook at [`demos/musicgen_demo.ipynb`](../demos/musicgen_demo.ipynb) locally (if you have a GPU).
-
-## API
-
-We provide a simple API and pre-trained models for MusicGen and for EnCodec at 24 khz for 3 bitrates (1.5 kbps, 3 kbps and 6 kbps).
-
-See after a quick example for using MultiBandDiffusion with the MusicGen API:
-
-```python
-import torchaudio
-from audiocraft.models import MusicGen, MultiBandDiffusion
-from audiocraft.data.audio import audio_write
-
-model = MusicGen.get_pretrained('facebook/musicgen-melody')
-mbd = MultiBandDiffusion.get_mbd_musicgen()
-model.set_generation_params(duration=8) # generate 8 seconds.
-wav, tokens = model.generate_unconditional(4, return_tokens=True) # generates 4 unconditional audio samples and keep the tokens for MBD generation
-descriptions = ['happy rock', 'energetic EDM', 'sad jazz']
-wav_diffusion = mbd.tokens_to_wav(tokens)
-wav, tokens = model.generate(descriptions, return_tokens=True) # generates 3 samples and keep the tokens.
-wav_diffusion = mbd.tokens_to_wav(tokens)
-melody, sr = torchaudio.load('./assets/bach.mp3')
-# Generates using the melody from the given audio and the provided descriptions, returns audio and audio tokens.
-wav, tokens = model.generate_with_chroma(descriptions, melody[None].expand(3, -1, -1), sr, return_tokens=True)
-wav_diffusion = mbd.tokens_to_wav(tokens)
-
-for idx, one_wav in enumerate(wav):
- # Will save under {idx}.wav and {idx}_diffusion.wav, with loudness normalization at -14 db LUFS for comparing the methods.
- audio_write(f'{idx}', one_wav.cpu(), model.sample_rate, strategy="loudness", loudness_compressor=True)
- audio_write(f'{idx}_diffusion', wav_diffusion[idx].cpu(), model.sample_rate, strategy="loudness", loudness_compressor=True)
-```
-
-For the compression task (and to compare with [EnCodec](https://github.com/facebookresearch/encodec)):
-
-```python
-import torch
-from audiocraft.models import MultiBandDiffusion
-from encodec import EncodecModel
-from audiocraft.data.audio import audio_read, audio_write
-
-bandwidth = 3.0 # 1.5, 3.0, 6.0
-mbd = MultiBandDiffusion.get_mbd_24khz(bw=bandwidth)
-encodec = EncodecModel.get_encodec_24khz()
-
-somepath = ''
-wav, sr = audio_read(somepath)
-with torch.no_grad():
- compressed_encodec = encodec(wav)
- compressed_diffusion = mbd.regenerate(wav, sample_rate=sr)
-
-audio_write('sample_encodec', compressed_encodec.squeeze(0).cpu(), mbd.sample_rate, strategy="loudness", loudness_compressor=True)
-audio_write('sample_diffusion', compressed_diffusion.squeeze(0).cpu(), mbd.sample_rate, strategy="loudness", loudness_compressor=True)
-```
-
-
-## Training
-
-The [DiffusionSolver](../audiocraft/solvers/diffusion.py) implements our diffusion training pipeline.
-It generates waveform audio conditioned on the embeddings extracted from a pre-trained EnCodec model
-(see [EnCodec documentation](./ENCODEC.md) for more details on how to train such model).
-
-Note that **we do NOT provide any of the datasets** used for training our diffusion models.
-We provide a dummy dataset containing just a few examples for illustrative purposes.
-
-### Example configurations and grids
-
-One can train diffusion models as described in the paper by using this [dora grid](../audiocraft/grids/diffusion/4_bands_base_32khz.py).
-```shell
-# 4 bands MBD trainning
-dora grid diffusion.4_bands_base_32khz
-```
-
-### Learn more
-
-Learn more about AudioCraft training pipelines in the [dedicated section](./TRAINING.md).
-
-
-## Citation
-
-```
-@article{sanroman2023fromdi,
- title={From Discrete Tokens to High-Fidelity Audio Using Multi-Band Diffusion},
- author={San Roman, Robin and Adi, Yossi and Deleforge, Antoine and Serizel, Romain and Synnaeve, Gabriel and Défossez, Alexandre},
- journal={arXiv preprint arXiv:},
- year={2023}
-}
-```
-
-
-## License
-
-See license information in the [README](../README.md).
-
-
-[arxiv]: https://dl.fbaipublicfiles.com/encodec/Diffusion/paper.pdf
-[mbd_samples]: https://ai.honu.io/papers/mbd/
diff --git a/spaces/SungBeom/chatwine-korean/.venv/Lib/site-packages/debugpy/_vendored/pydevd/_pydevd_bundle/pydevd_extension_utils.py b/spaces/SungBeom/chatwine-korean/.venv/Lib/site-packages/debugpy/_vendored/pydevd/_pydevd_bundle/pydevd_extension_utils.py
deleted file mode 100644
index 1386cc75860bba144b003cd1252ed648f83b24e5..0000000000000000000000000000000000000000
--- a/spaces/SungBeom/chatwine-korean/.venv/Lib/site-packages/debugpy/_vendored/pydevd/_pydevd_bundle/pydevd_extension_utils.py
+++ /dev/null
@@ -1,67 +0,0 @@
-import pkgutil
-import sys
-from _pydev_bundle import pydev_log
-try:
- import pydevd_plugins.extensions as extensions
-except:
- pydev_log.exception()
- extensions = None
-
-
-class ExtensionManager(object):
-
- def __init__(self):
- self.loaded_extensions = None
- self.type_to_instance = {}
-
- def _load_modules(self):
- self.loaded_extensions = []
- if extensions:
- for module_loader, name, ispkg in pkgutil.walk_packages(extensions.__path__,
- extensions.__name__ + '.'):
- mod_name = name.split('.')[-1]
- if not ispkg and mod_name.startswith('pydevd_plugin'):
- try:
- __import__(name)
- module = sys.modules[name]
- self.loaded_extensions.append(module)
- except ImportError:
- pydev_log.critical('Unable to load extension: %s', name)
-
- def _ensure_loaded(self):
- if self.loaded_extensions is None:
- self._load_modules()
-
- def _iter_attr(self):
- for extension in self.loaded_extensions:
- dunder_all = getattr(extension, '__all__', None)
- for attr_name in dir(extension):
- if not attr_name.startswith('_'):
- if dunder_all is None or attr_name in dunder_all:
- yield attr_name, getattr(extension, attr_name)
-
- def get_extension_classes(self, extension_type):
- self._ensure_loaded()
- if extension_type in self.type_to_instance:
- return self.type_to_instance[extension_type]
- handlers = self.type_to_instance.setdefault(extension_type, [])
- for attr_name, attr in self._iter_attr():
- if isinstance(attr, type) and issubclass(attr, extension_type) and attr is not extension_type:
- try:
- handlers.append(attr())
- except:
- pydev_log.exception('Unable to load extension class: %s', attr_name)
- return handlers
-
-
-EXTENSION_MANAGER_INSTANCE = ExtensionManager()
-
-
-def extensions_of_type(extension_type):
- """
-
- :param T extension_type: The type of the extension hook
- :rtype: list[T]
- """
- return EXTENSION_MANAGER_INSTANCE.get_extension_classes(extension_type)
-
diff --git a/spaces/SungBeom/chatwine-korean/.venv/Lib/site-packages/debugpy/_vendored/pydevd/pydevd_attach_to_process/winappdbg/breakpoint.py b/spaces/SungBeom/chatwine-korean/.venv/Lib/site-packages/debugpy/_vendored/pydevd/pydevd_attach_to_process/winappdbg/breakpoint.py
deleted file mode 100644
index 3b9ca73ffd8f96502c85fcadcc38941c6d6c8f65..0000000000000000000000000000000000000000
--- a/spaces/SungBeom/chatwine-korean/.venv/Lib/site-packages/debugpy/_vendored/pydevd/pydevd_attach_to_process/winappdbg/breakpoint.py
+++ /dev/null
@@ -1,4822 +0,0 @@
-#!~/.wine/drive_c/Python25/python.exe
-# -*- coding: utf-8 -*-
-
-# Copyright (c) 2009-2014, Mario Vilas
-# All rights reserved.
-#
-# Redistribution and use in source and binary forms, with or without
-# modification, are permitted provided that the following conditions are met:
-#
-# * Redistributions of source code must retain the above copyright notice,
-# this list of conditions and the following disclaimer.
-# * Redistributions in binary form must reproduce the above copyright
-# notice,this list of conditions and the following disclaimer in the
-# documentation and/or other materials provided with the distribution.
-# * Neither the name of the copyright holder nor the names of its
-# contributors may be used to endorse or promote products derived from
-# this software without specific prior written permission.
-#
-# THIS SOFTWARE IS PROVIDED BY THE COPYRIGHT HOLDERS AND CONTRIBUTORS "AS IS"
-# AND ANY EXPRESS OR IMPLIED WARRANTIES, INCLUDING, BUT NOT LIMITED TO, THE
-# IMPLIED WARRANTIES OF MERCHANTABILITY AND FITNESS FOR A PARTICULAR PURPOSE
-# ARE DISCLAIMED. IN NO EVENT SHALL THE COPYRIGHT OWNER OR CONTRIBUTORS BE
-# LIABLE FOR ANY DIRECT, INDIRECT, INCIDENTAL, SPECIAL, EXEMPLARY, OR
-# CONSEQUENTIAL DAMAGES (INCLUDING, BUT NOT LIMITED TO, PROCUREMENT OF
-# SUBSTITUTE GOODS OR SERVICES; LOSS OF USE, DATA, OR PROFITS; OR BUSINESS
-# INTERRUPTION) HOWEVER CAUSED AND ON ANY THEORY OF LIABILITY, WHETHER IN
-# CONTRACT, STRICT LIABILITY, OR TORT (INCLUDING NEGLIGENCE OR OTHERWISE)
-# ARISING IN ANY WAY OUT OF THE USE OF THIS SOFTWARE, EVEN IF ADVISED OF THE
-# POSSIBILITY OF SUCH DAMAGE.
-
-"""
-Breakpoints.
-
-@group Breakpoints:
- Breakpoint, CodeBreakpoint, PageBreakpoint, HardwareBreakpoint,
- BufferWatch, Hook, ApiHook
-
-@group Warnings:
- BreakpointWarning, BreakpointCallbackWarning
-"""
-
-__revision__ = "$Id$"
-
-__all__ = [
-
- # Base class for breakpoints
- 'Breakpoint',
-
- # Breakpoint implementations
- 'CodeBreakpoint',
- 'PageBreakpoint',
- 'HardwareBreakpoint',
-
- # Hooks and watches
- 'Hook',
- 'ApiHook',
- 'BufferWatch',
-
- # Warnings
- 'BreakpointWarning',
- 'BreakpointCallbackWarning',
-
- ]
-
-from winappdbg import win32
-from winappdbg import compat
-import sys
-from winappdbg.process import Process, Thread
-from winappdbg.util import DebugRegister, MemoryAddresses
-from winappdbg.textio import HexDump
-
-import ctypes
-import warnings
-import traceback
-
-#==============================================================================
-
-class BreakpointWarning (UserWarning):
- """
- This warning is issued when a non-fatal error occurs that's related to
- breakpoints.
- """
-
-class BreakpointCallbackWarning (RuntimeWarning):
- """
- This warning is issued when an uncaught exception was raised by a
- breakpoint's user-defined callback.
- """
-
-#==============================================================================
-
-class Breakpoint (object):
- """
- Base class for breakpoints.
- Here's the breakpoints state machine.
-
- @see: L{CodeBreakpoint}, L{PageBreakpoint}, L{HardwareBreakpoint}
-
- @group Breakpoint states:
- DISABLED, ENABLED, ONESHOT, RUNNING
- @group State machine:
- hit, disable, enable, one_shot, running,
- is_disabled, is_enabled, is_one_shot, is_running,
- get_state, get_state_name
- @group Information:
- get_address, get_size, get_span, is_here
- @group Conditional breakpoints:
- is_conditional, is_unconditional,
- get_condition, set_condition, eval_condition
- @group Automatic breakpoints:
- is_automatic, is_interactive,
- get_action, set_action, run_action
-
- @cvar DISABLED: I{Disabled} S{->} Enabled, OneShot
- @cvar ENABLED: I{Enabled} S{->} I{Running}, Disabled
- @cvar ONESHOT: I{OneShot} S{->} I{Disabled}
- @cvar RUNNING: I{Running} S{->} I{Enabled}, Disabled
-
- @type DISABLED: int
- @type ENABLED: int
- @type ONESHOT: int
- @type RUNNING: int
-
- @type stateNames: dict E{lb} int S{->} str E{rb}
- @cvar stateNames: User-friendly names for each breakpoint state.
-
- @type typeName: str
- @cvar typeName: User friendly breakpoint type string.
- """
-
- # I don't think transitions Enabled <-> OneShot should be allowed... plus
- # it would require special handling to avoid setting the same bp twice
-
- DISABLED = 0
- ENABLED = 1
- ONESHOT = 2
- RUNNING = 3
-
- typeName = 'breakpoint'
-
- stateNames = {
- DISABLED : 'disabled',
- ENABLED : 'enabled',
- ONESHOT : 'one shot',
- RUNNING : 'running',
- }
-
- def __init__(self, address, size = 1, condition = True, action = None):
- """
- Breakpoint object.
-
- @type address: int
- @param address: Memory address for breakpoint.
-
- @type size: int
- @param size: Size of breakpoint in bytes (defaults to 1).
-
- @type condition: function
- @param condition: (Optional) Condition callback function.
-
- The callback signature is::
-
- def condition_callback(event):
- return True # returns True or False
-
- Where B{event} is an L{Event} object,
- and the return value is a boolean
- (C{True} to dispatch the event, C{False} otherwise).
-
- @type action: function
- @param action: (Optional) Action callback function.
- If specified, the event is handled by this callback instead of
- being dispatched normally.
-
- The callback signature is::
-
- def action_callback(event):
- pass # no return value
-
- Where B{event} is an L{Event} object.
- """
- self.__address = address
- self.__size = size
- self.__state = self.DISABLED
-
- self.set_condition(condition)
- self.set_action(action)
-
- def __repr__(self):
- if self.is_disabled():
- state = 'Disabled'
- else:
- state = 'Active (%s)' % self.get_state_name()
- if self.is_conditional():
- condition = 'conditional'
- else:
- condition = 'unconditional'
- name = self.typeName
- size = self.get_size()
- if size == 1:
- address = HexDump.address( self.get_address() )
- else:
- begin = self.get_address()
- end = begin + size
- begin = HexDump.address(begin)
- end = HexDump.address(end)
- address = "range %s-%s" % (begin, end)
- msg = "<%s %s %s at remote address %s>"
- msg = msg % (state, condition, name, address)
- return msg
-
-#------------------------------------------------------------------------------
-
- def is_disabled(self):
- """
- @rtype: bool
- @return: C{True} if the breakpoint is in L{DISABLED} state.
- """
- return self.get_state() == self.DISABLED
-
- def is_enabled(self):
- """
- @rtype: bool
- @return: C{True} if the breakpoint is in L{ENABLED} state.
- """
- return self.get_state() == self.ENABLED
-
- def is_one_shot(self):
- """
- @rtype: bool
- @return: C{True} if the breakpoint is in L{ONESHOT} state.
- """
- return self.get_state() == self.ONESHOT
-
- def is_running(self):
- """
- @rtype: bool
- @return: C{True} if the breakpoint is in L{RUNNING} state.
- """
- return self.get_state() == self.RUNNING
-
- def is_here(self, address):
- """
- @rtype: bool
- @return: C{True} if the address is within the range of the breakpoint.
- """
- begin = self.get_address()
- end = begin + self.get_size()
- return begin <= address < end
-
- def get_address(self):
- """
- @rtype: int
- @return: The target memory address for the breakpoint.
- """
- return self.__address
-
- def get_size(self):
- """
- @rtype: int
- @return: The size in bytes of the breakpoint.
- """
- return self.__size
-
- def get_span(self):
- """
- @rtype: tuple( int, int )
- @return:
- Starting and ending address of the memory range
- covered by the breakpoint.
- """
- address = self.get_address()
- size = self.get_size()
- return ( address, address + size )
-
- def get_state(self):
- """
- @rtype: int
- @return: The current state of the breakpoint
- (L{DISABLED}, L{ENABLED}, L{ONESHOT}, L{RUNNING}).
- """
- return self.__state
-
- def get_state_name(self):
- """
- @rtype: str
- @return: The name of the current state of the breakpoint.
- """
- return self.stateNames[ self.get_state() ]
-
-#------------------------------------------------------------------------------
-
- def is_conditional(self):
- """
- @see: L{__init__}
- @rtype: bool
- @return: C{True} if the breakpoint has a condition callback defined.
- """
- # Do not evaluate as boolean! Test for identity with True instead.
- return self.__condition is not True
-
- def is_unconditional(self):
- """
- @rtype: bool
- @return: C{True} if the breakpoint doesn't have a condition callback defined.
- """
- # Do not evaluate as boolean! Test for identity with True instead.
- return self.__condition is True
-
- def get_condition(self):
- """
- @rtype: bool, function
- @return: Returns the condition callback for conditional breakpoints.
- Returns C{True} for unconditional breakpoints.
- """
- return self.__condition
-
- def set_condition(self, condition = True):
- """
- Sets a new condition callback for the breakpoint.
-
- @see: L{__init__}
-
- @type condition: function
- @param condition: (Optional) Condition callback function.
- """
- if condition is None:
- self.__condition = True
- else:
- self.__condition = condition
-
- def eval_condition(self, event):
- """
- Evaluates the breakpoint condition, if any was set.
-
- @type event: L{Event}
- @param event: Debug event triggered by the breakpoint.
-
- @rtype: bool
- @return: C{True} to dispatch the event, C{False} otherwise.
- """
- condition = self.get_condition()
- if condition is True: # shortcut for unconditional breakpoints
- return True
- if callable(condition):
- try:
- return bool( condition(event) )
- except Exception:
- e = sys.exc_info()[1]
- msg = ("Breakpoint condition callback %r"
- " raised an exception: %s")
- msg = msg % (condition, traceback.format_exc(e))
- warnings.warn(msg, BreakpointCallbackWarning)
- return False
- return bool( condition ) # force evaluation now
-
-#------------------------------------------------------------------------------
-
- def is_automatic(self):
- """
- @rtype: bool
- @return: C{True} if the breakpoint has an action callback defined.
- """
- return self.__action is not None
-
- def is_interactive(self):
- """
- @rtype: bool
- @return:
- C{True} if the breakpoint doesn't have an action callback defined.
- """
- return self.__action is None
-
- def get_action(self):
- """
- @rtype: bool, function
- @return: Returns the action callback for automatic breakpoints.
- Returns C{None} for interactive breakpoints.
- """
- return self.__action
-
- def set_action(self, action = None):
- """
- Sets a new action callback for the breakpoint.
-
- @type action: function
- @param action: (Optional) Action callback function.
- """
- self.__action = action
-
- def run_action(self, event):
- """
- Executes the breakpoint action callback, if any was set.
-
- @type event: L{Event}
- @param event: Debug event triggered by the breakpoint.
- """
- action = self.get_action()
- if action is not None:
- try:
- return bool( action(event) )
- except Exception:
- e = sys.exc_info()[1]
- msg = ("Breakpoint action callback %r"
- " raised an exception: %s")
- msg = msg % (action, traceback.format_exc(e))
- warnings.warn(msg, BreakpointCallbackWarning)
- return False
- return True
-
-#------------------------------------------------------------------------------
-
- def __bad_transition(self, state):
- """
- Raises an C{AssertionError} exception for an invalid state transition.
-
- @see: L{stateNames}
-
- @type state: int
- @param state: Intended breakpoint state.
-
- @raise Exception: Always.
- """
- statemsg = ""
- oldState = self.stateNames[ self.get_state() ]
- newState = self.stateNames[ state ]
- msg = "Invalid state transition (%s -> %s)" \
- " for breakpoint at address %s"
- msg = msg % (oldState, newState, HexDump.address(self.get_address()))
- raise AssertionError(msg)
-
- def disable(self, aProcess, aThread):
- """
- Transition to L{DISABLED} state.
- - When hit: OneShot S{->} Disabled
- - Forced by user: Enabled, OneShot, Running S{->} Disabled
- - Transition from running state may require special handling
- by the breakpoint implementation class.
-
- @type aProcess: L{Process}
- @param aProcess: Process object.
-
- @type aThread: L{Thread}
- @param aThread: Thread object.
- """
-## if self.__state not in (self.ENABLED, self.ONESHOT, self.RUNNING):
-## self.__bad_transition(self.DISABLED)
- self.__state = self.DISABLED
-
- def enable(self, aProcess, aThread):
- """
- Transition to L{ENABLED} state.
- - When hit: Running S{->} Enabled
- - Forced by user: Disabled, Running S{->} Enabled
- - Transition from running state may require special handling
- by the breakpoint implementation class.
-
- @type aProcess: L{Process}
- @param aProcess: Process object.
-
- @type aThread: L{Thread}
- @param aThread: Thread object.
- """
-## if self.__state not in (self.DISABLED, self.RUNNING):
-## self.__bad_transition(self.ENABLED)
- self.__state = self.ENABLED
-
- def one_shot(self, aProcess, aThread):
- """
- Transition to L{ONESHOT} state.
- - Forced by user: Disabled S{->} OneShot
-
- @type aProcess: L{Process}
- @param aProcess: Process object.
-
- @type aThread: L{Thread}
- @param aThread: Thread object.
- """
-## if self.__state != self.DISABLED:
-## self.__bad_transition(self.ONESHOT)
- self.__state = self.ONESHOT
-
- def running(self, aProcess, aThread):
- """
- Transition to L{RUNNING} state.
- - When hit: Enabled S{->} Running
-
- @type aProcess: L{Process}
- @param aProcess: Process object.
-
- @type aThread: L{Thread}
- @param aThread: Thread object.
- """
- if self.__state != self.ENABLED:
- self.__bad_transition(self.RUNNING)
- self.__state = self.RUNNING
-
- def hit(self, event):
- """
- Notify a breakpoint that it's been hit.
-
- This triggers the corresponding state transition and sets the
- C{breakpoint} property of the given L{Event} object.
-
- @see: L{disable}, L{enable}, L{one_shot}, L{running}
-
- @type event: L{Event}
- @param event: Debug event to handle (depends on the breakpoint type).
-
- @raise AssertionError: Disabled breakpoints can't be hit.
- """
- aProcess = event.get_process()
- aThread = event.get_thread()
- state = self.get_state()
-
- event.breakpoint = self
-
- if state == self.ENABLED:
- self.running(aProcess, aThread)
-
- elif state == self.RUNNING:
- self.enable(aProcess, aThread)
-
- elif state == self.ONESHOT:
- self.disable(aProcess, aThread)
-
- elif state == self.DISABLED:
- # this should not happen
- msg = "Hit a disabled breakpoint at address %s"
- msg = msg % HexDump.address( self.get_address() )
- warnings.warn(msg, BreakpointWarning)
-
-#==============================================================================
-
-# XXX TODO
-# Check if the user is trying to set a code breakpoint on a memory mapped file,
-# so we don't end up writing the int3 instruction in the file by accident.
-
-class CodeBreakpoint (Breakpoint):
- """
- Code execution breakpoints (using an int3 opcode).
-
- @see: L{Debug.break_at}
-
- @type bpInstruction: str
- @cvar bpInstruction: Breakpoint instruction for the current processor.
- """
-
- typeName = 'code breakpoint'
-
- if win32.arch in (win32.ARCH_I386, win32.ARCH_AMD64):
- bpInstruction = '\xCC' # int 3
-
- def __init__(self, address, condition = True, action = None):
- """
- Code breakpoint object.
-
- @see: L{Breakpoint.__init__}
-
- @type address: int
- @param address: Memory address for breakpoint.
-
- @type condition: function
- @param condition: (Optional) Condition callback function.
-
- @type action: function
- @param action: (Optional) Action callback function.
- """
- if win32.arch not in (win32.ARCH_I386, win32.ARCH_AMD64):
- msg = "Code breakpoints not supported for %s" % win32.arch
- raise NotImplementedError(msg)
- Breakpoint.__init__(self, address, len(self.bpInstruction),
- condition, action)
- self.__previousValue = self.bpInstruction
-
- def __set_bp(self, aProcess):
- """
- Writes a breakpoint instruction at the target address.
-
- @type aProcess: L{Process}
- @param aProcess: Process object.
- """
- address = self.get_address()
- self.__previousValue = aProcess.read(address, len(self.bpInstruction))
- if self.__previousValue == self.bpInstruction:
- msg = "Possible overlapping code breakpoints at %s"
- msg = msg % HexDump.address(address)
- warnings.warn(msg, BreakpointWarning)
- aProcess.write(address, self.bpInstruction)
-
- def __clear_bp(self, aProcess):
- """
- Restores the original byte at the target address.
-
- @type aProcess: L{Process}
- @param aProcess: Process object.
- """
- address = self.get_address()
- currentValue = aProcess.read(address, len(self.bpInstruction))
- if currentValue == self.bpInstruction:
- # Only restore the previous value if the int3 is still there.
- aProcess.write(self.get_address(), self.__previousValue)
- else:
- self.__previousValue = currentValue
- msg = "Overwritten code breakpoint at %s"
- msg = msg % HexDump.address(address)
- warnings.warn(msg, BreakpointWarning)
-
- def disable(self, aProcess, aThread):
- if not self.is_disabled() and not self.is_running():
- self.__clear_bp(aProcess)
- super(CodeBreakpoint, self).disable(aProcess, aThread)
-
- def enable(self, aProcess, aThread):
- if not self.is_enabled() and not self.is_one_shot():
- self.__set_bp(aProcess)
- super(CodeBreakpoint, self).enable(aProcess, aThread)
-
- def one_shot(self, aProcess, aThread):
- if not self.is_enabled() and not self.is_one_shot():
- self.__set_bp(aProcess)
- super(CodeBreakpoint, self).one_shot(aProcess, aThread)
-
- # FIXME race condition here (however unlikely)
- # If another thread runs on over the target address while
- # the breakpoint is in RUNNING state, we'll miss it. There
- # is a solution to this but it's somewhat complicated, so
- # I'm leaving it for another version of the debugger. :(
- def running(self, aProcess, aThread):
- if self.is_enabled():
- self.__clear_bp(aProcess)
- aThread.set_tf()
- super(CodeBreakpoint, self).running(aProcess, aThread)
-
-#==============================================================================
-
-# TODO:
-# * If the original page was already a guard page, the exception should be
-# passed to the debugee instead of being handled by the debugger.
-# * If the original page was already a guard page, it should NOT be converted
-# to a no-access page when disabling the breakpoint.
-# * If the page permissions were modified after the breakpoint was enabled,
-# no change should be done on them when disabling the breakpoint. For this
-# we need to remember the original page permissions instead of blindly
-# setting and clearing the guard page bit on them.
-# * Some pages seem to be "magic" and resist all attempts at changing their
-# protect bits (for example the pages where the PEB and TEB reside). Maybe
-# a more descriptive error message could be shown in this case.
-
-class PageBreakpoint (Breakpoint):
- """
- Page access breakpoint (using guard pages).
-
- @see: L{Debug.watch_buffer}
-
- @group Information:
- get_size_in_pages
- """
-
- typeName = 'page breakpoint'
-
-#------------------------------------------------------------------------------
-
- def __init__(self, address, pages = 1, condition = True, action = None):
- """
- Page breakpoint object.
-
- @see: L{Breakpoint.__init__}
-
- @type address: int
- @param address: Memory address for breakpoint.
-
- @type pages: int
- @param address: Size of breakpoint in pages.
-
- @type condition: function
- @param condition: (Optional) Condition callback function.
-
- @type action: function
- @param action: (Optional) Action callback function.
- """
- Breakpoint.__init__(self, address, pages * MemoryAddresses.pageSize,
- condition, action)
-## if (address & 0x00000FFF) != 0:
- floordiv_align = long(address) // long(MemoryAddresses.pageSize)
- truediv_align = float(address) / float(MemoryAddresses.pageSize)
- if floordiv_align != truediv_align:
- msg = "Address of page breakpoint " \
- "must be aligned to a page size boundary " \
- "(value %s received)" % HexDump.address(address)
- raise ValueError(msg)
-
- def get_size_in_pages(self):
- """
- @rtype: int
- @return: The size in pages of the breakpoint.
- """
- # The size is always a multiple of the page size.
- return self.get_size() // MemoryAddresses.pageSize
-
- def __set_bp(self, aProcess):
- """
- Sets the target pages as guard pages.
-
- @type aProcess: L{Process}
- @param aProcess: Process object.
- """
- lpAddress = self.get_address()
- dwSize = self.get_size()
- flNewProtect = aProcess.mquery(lpAddress).Protect
- flNewProtect = flNewProtect | win32.PAGE_GUARD
- aProcess.mprotect(lpAddress, dwSize, flNewProtect)
-
- def __clear_bp(self, aProcess):
- """
- Restores the original permissions of the target pages.
-
- @type aProcess: L{Process}
- @param aProcess: Process object.
- """
- lpAddress = self.get_address()
- flNewProtect = aProcess.mquery(lpAddress).Protect
- flNewProtect = flNewProtect & (0xFFFFFFFF ^ win32.PAGE_GUARD) # DWORD
- aProcess.mprotect(lpAddress, self.get_size(), flNewProtect)
-
- def disable(self, aProcess, aThread):
- if not self.is_disabled():
- self.__clear_bp(aProcess)
- super(PageBreakpoint, self).disable(aProcess, aThread)
-
- def enable(self, aProcess, aThread):
- if win32.arch not in (win32.ARCH_I386, win32.ARCH_AMD64):
- msg = "Only one-shot page breakpoints are supported for %s"
- raise NotImplementedError(msg % win32.arch)
- if not self.is_enabled() and not self.is_one_shot():
- self.__set_bp(aProcess)
- super(PageBreakpoint, self).enable(aProcess, aThread)
-
- def one_shot(self, aProcess, aThread):
- if not self.is_enabled() and not self.is_one_shot():
- self.__set_bp(aProcess)
- super(PageBreakpoint, self).one_shot(aProcess, aThread)
-
- def running(self, aProcess, aThread):
- aThread.set_tf()
- super(PageBreakpoint, self).running(aProcess, aThread)
-
-#==============================================================================
-
-class HardwareBreakpoint (Breakpoint):
- """
- Hardware breakpoint (using debug registers).
-
- @see: L{Debug.watch_variable}
-
- @group Information:
- get_slot, get_trigger, get_watch
-
- @group Trigger flags:
- BREAK_ON_EXECUTION, BREAK_ON_WRITE, BREAK_ON_ACCESS
-
- @group Watch size flags:
- WATCH_BYTE, WATCH_WORD, WATCH_DWORD, WATCH_QWORD
-
- @type BREAK_ON_EXECUTION: int
- @cvar BREAK_ON_EXECUTION: Break on execution.
-
- @type BREAK_ON_WRITE: int
- @cvar BREAK_ON_WRITE: Break on write.
-
- @type BREAK_ON_ACCESS: int
- @cvar BREAK_ON_ACCESS: Break on read or write.
-
- @type WATCH_BYTE: int
- @cvar WATCH_BYTE: Watch a byte.
-
- @type WATCH_WORD: int
- @cvar WATCH_WORD: Watch a word (2 bytes).
-
- @type WATCH_DWORD: int
- @cvar WATCH_DWORD: Watch a double word (4 bytes).
-
- @type WATCH_QWORD: int
- @cvar WATCH_QWORD: Watch one quad word (8 bytes).
-
- @type validTriggers: tuple
- @cvar validTriggers: Valid trigger flag values.
-
- @type validWatchSizes: tuple
- @cvar validWatchSizes: Valid watch flag values.
- """
-
- typeName = 'hardware breakpoint'
-
- BREAK_ON_EXECUTION = DebugRegister.BREAK_ON_EXECUTION
- BREAK_ON_WRITE = DebugRegister.BREAK_ON_WRITE
- BREAK_ON_ACCESS = DebugRegister.BREAK_ON_ACCESS
-
- WATCH_BYTE = DebugRegister.WATCH_BYTE
- WATCH_WORD = DebugRegister.WATCH_WORD
- WATCH_DWORD = DebugRegister.WATCH_DWORD
- WATCH_QWORD = DebugRegister.WATCH_QWORD
-
- validTriggers = (
- BREAK_ON_EXECUTION,
- BREAK_ON_WRITE,
- BREAK_ON_ACCESS,
- )
-
- validWatchSizes = (
- WATCH_BYTE,
- WATCH_WORD,
- WATCH_DWORD,
- WATCH_QWORD,
- )
-
- def __init__(self, address, triggerFlag = BREAK_ON_ACCESS,
- sizeFlag = WATCH_DWORD,
- condition = True,
- action = None):
- """
- Hardware breakpoint object.
-
- @see: L{Breakpoint.__init__}
-
- @type address: int
- @param address: Memory address for breakpoint.
-
- @type triggerFlag: int
- @param triggerFlag: Trigger of breakpoint. Must be one of the following:
-
- - L{BREAK_ON_EXECUTION}
-
- Break on code execution.
-
- - L{BREAK_ON_WRITE}
-
- Break on memory read or write.
-
- - L{BREAK_ON_ACCESS}
-
- Break on memory write.
-
- @type sizeFlag: int
- @param sizeFlag: Size of breakpoint. Must be one of the following:
-
- - L{WATCH_BYTE}
-
- One (1) byte in size.
-
- - L{WATCH_WORD}
-
- Two (2) bytes in size.
-
- - L{WATCH_DWORD}
-
- Four (4) bytes in size.
-
- - L{WATCH_QWORD}
-
- Eight (8) bytes in size.
-
- @type condition: function
- @param condition: (Optional) Condition callback function.
-
- @type action: function
- @param action: (Optional) Action callback function.
- """
- if win32.arch not in (win32.ARCH_I386, win32.ARCH_AMD64):
- msg = "Hardware breakpoints not supported for %s" % win32.arch
- raise NotImplementedError(msg)
- if sizeFlag == self.WATCH_BYTE:
- size = 1
- elif sizeFlag == self.WATCH_WORD:
- size = 2
- elif sizeFlag == self.WATCH_DWORD:
- size = 4
- elif sizeFlag == self.WATCH_QWORD:
- size = 8
- else:
- msg = "Invalid size flag for hardware breakpoint (%s)"
- msg = msg % repr(sizeFlag)
- raise ValueError(msg)
-
- if triggerFlag not in self.validTriggers:
- msg = "Invalid trigger flag for hardware breakpoint (%s)"
- msg = msg % repr(triggerFlag)
- raise ValueError(msg)
-
- Breakpoint.__init__(self, address, size, condition, action)
- self.__trigger = triggerFlag
- self.__watch = sizeFlag
- self.__slot = None
-
- def __clear_bp(self, aThread):
- """
- Clears this breakpoint from the debug registers.
-
- @type aThread: L{Thread}
- @param aThread: Thread object.
- """
- if self.__slot is not None:
- aThread.suspend()
- try:
- ctx = aThread.get_context(win32.CONTEXT_DEBUG_REGISTERS)
- DebugRegister.clear_bp(ctx, self.__slot)
- aThread.set_context(ctx)
- self.__slot = None
- finally:
- aThread.resume()
-
- def __set_bp(self, aThread):
- """
- Sets this breakpoint in the debug registers.
-
- @type aThread: L{Thread}
- @param aThread: Thread object.
- """
- if self.__slot is None:
- aThread.suspend()
- try:
- ctx = aThread.get_context(win32.CONTEXT_DEBUG_REGISTERS)
- self.__slot = DebugRegister.find_slot(ctx)
- if self.__slot is None:
- msg = "No available hardware breakpoint slots for thread ID %d"
- msg = msg % aThread.get_tid()
- raise RuntimeError(msg)
- DebugRegister.set_bp(ctx, self.__slot, self.get_address(),
- self.__trigger, self.__watch)
- aThread.set_context(ctx)
- finally:
- aThread.resume()
-
- def get_slot(self):
- """
- @rtype: int
- @return: The debug register number used by this breakpoint,
- or C{None} if the breakpoint is not active.
- """
- return self.__slot
-
- def get_trigger(self):
- """
- @see: L{validTriggers}
- @rtype: int
- @return: The breakpoint trigger flag.
- """
- return self.__trigger
-
- def get_watch(self):
- """
- @see: L{validWatchSizes}
- @rtype: int
- @return: The breakpoint watch flag.
- """
- return self.__watch
-
- def disable(self, aProcess, aThread):
- if not self.is_disabled():
- self.__clear_bp(aThread)
- super(HardwareBreakpoint, self).disable(aProcess, aThread)
-
- def enable(self, aProcess, aThread):
- if not self.is_enabled() and not self.is_one_shot():
- self.__set_bp(aThread)
- super(HardwareBreakpoint, self).enable(aProcess, aThread)
-
- def one_shot(self, aProcess, aThread):
- if not self.is_enabled() and not self.is_one_shot():
- self.__set_bp(aThread)
- super(HardwareBreakpoint, self).one_shot(aProcess, aThread)
-
- def running(self, aProcess, aThread):
- self.__clear_bp(aThread)
- super(HardwareBreakpoint, self).running(aProcess, aThread)
- aThread.set_tf()
-
-#==============================================================================
-
-# XXX FIXME
-#
-# The implementation of function hooks is very simple. A breakpoint is set at
-# the entry point. Each time it's hit the "pre" callback is executed. If a
-# "post" callback was defined, a one-shot breakpoint is set at the return
-# address - and when that breakpoint hits, the "post" callback is executed.
-#
-# Functions hooks, as they are implemented now, don't work correctly for
-# recursive functions. The problem is we don't know when to remove the
-# breakpoint at the return address. Also there could be more than one return
-# address.
-#
-# One possible solution would involve a dictionary of lists, where the key
-# would be the thread ID and the value a stack of return addresses. But we
-# still don't know what to do if the "wrong" return address is hit for some
-# reason (maybe check the stack pointer?). Or if both a code and a hardware
-# breakpoint are hit simultaneously.
-#
-# For now, the workaround for the user is to set only the "pre" callback for
-# functions that are known to be recursive.
-#
-# If an exception is thrown by a hooked function and caught by one of it's
-# parent functions, the "post" callback won't be called and weird stuff may
-# happen. A possible solution is to put a breakpoint in the system call that
-# unwinds the stack, to detect this case and remove the "post" breakpoint.
-#
-# Hooks may also behave oddly if the return address is overwritten by a buffer
-# overflow bug (this is similar to the exception problem). But it's probably a
-# minor issue since when you're fuzzing a function for overflows you're usually
-# not interested in the return value anyway.
-
-# TODO: an API to modify the hooked function's arguments
-
-class Hook (object):
- """
- Factory class to produce hook objects. Used by L{Debug.hook_function} and
- L{Debug.stalk_function}.
-
- When you try to instance this class, one of the architecture specific
- implementations is returned instead.
-
- Instances act as an action callback for code breakpoints set at the
- beginning of a function. It automatically retrieves the parameters from
- the stack, sets a breakpoint at the return address and retrieves the
- return value from the function call.
-
- @see: L{_Hook_i386}, L{_Hook_amd64}
-
- @type useHardwareBreakpoints: bool
- @cvar useHardwareBreakpoints: C{True} to try to use hardware breakpoints,
- C{False} otherwise.
- """
-
- # This is a factory class that returns
- # the architecture specific implementation.
- def __new__(cls, *argv, **argd):
- try:
- arch = argd['arch']
- del argd['arch']
- except KeyError:
- try:
- arch = argv[4]
- argv = argv[:4] + argv[5:]
- except IndexError:
- raise TypeError("Missing 'arch' argument!")
- if arch is None:
- arch = win32.arch
- if arch == win32.ARCH_I386:
- return _Hook_i386(*argv, **argd)
- if arch == win32.ARCH_AMD64:
- return _Hook_amd64(*argv, **argd)
- return object.__new__(cls, *argv, **argd)
-
- # XXX FIXME
- #
- # Hardware breakpoints don't work correctly (or al all) in old VirtualBox
- # versions (3.0 and below).
- #
- # Maybe there should be a way to autodetect the buggy VirtualBox versions
- # and tell Hook objects not to use hardware breakpoints?
- #
- # For now the workaround is to manually set this variable to True when
- # WinAppDbg is installed on a physical machine.
- #
- useHardwareBreakpoints = False
-
- def __init__(self, preCB = None, postCB = None,
- paramCount = None, signature = None,
- arch = None):
- """
- @type preCB: function
- @param preCB: (Optional) Callback triggered on function entry.
-
- The signature for the callback should be something like this::
-
- def pre_LoadLibraryEx(event, ra, lpFilename, hFile, dwFlags):
-
- # return address
- ra = params[0]
-
- # function arguments start from here...
- szFilename = event.get_process().peek_string(lpFilename)
-
- # (...)
-
- Note that all pointer types are treated like void pointers, so your
- callback won't get the string or structure pointed to by it, but
- the remote memory address instead. This is so to prevent the ctypes
- library from being "too helpful" and trying to dereference the
- pointer. To get the actual data being pointed to, use one of the
- L{Process.read} methods.
-
- @type postCB: function
- @param postCB: (Optional) Callback triggered on function exit.
-
- The signature for the callback should be something like this::
-
- def post_LoadLibraryEx(event, return_value):
-
- # (...)
-
- @type paramCount: int
- @param paramCount:
- (Optional) Number of parameters for the C{preCB} callback,
- not counting the return address. Parameters are read from
- the stack and assumed to be DWORDs in 32 bits and QWORDs in 64.
-
- This is a faster way to pull stack parameters in 32 bits, but in 64
- bits (or with some odd APIs in 32 bits) it won't be useful, since
- not all arguments to the hooked function will be of the same size.
-
- For a more reliable and cross-platform way of hooking use the
- C{signature} argument instead.
-
- @type signature: tuple
- @param signature:
- (Optional) Tuple of C{ctypes} data types that constitute the
- hooked function signature. When the function is called, this will
- be used to parse the arguments from the stack. Overrides the
- C{paramCount} argument.
-
- @type arch: str
- @param arch: (Optional) Target architecture. Defaults to the current
- architecture. See: L{win32.arch}
- """
- self.__preCB = preCB
- self.__postCB = postCB
- self.__paramStack = dict() # tid -> list of tuple( arg, arg, arg... )
-
- self._paramCount = paramCount
-
- if win32.arch != win32.ARCH_I386:
- self.useHardwareBreakpoints = False
-
- if win32.bits == 64 and paramCount and not signature:
- signature = (win32.QWORD,) * paramCount
-
- if signature:
- self._signature = self._calc_signature(signature)
- else:
- self._signature = None
-
- def _cast_signature_pointers_to_void(self, signature):
- c_void_p = ctypes.c_void_p
- c_char_p = ctypes.c_char_p
- c_wchar_p = ctypes.c_wchar_p
- _Pointer = ctypes._Pointer
- cast = ctypes.cast
- for i in compat.xrange(len(signature)):
- t = signature[i]
- if t is not c_void_p and (issubclass(t, _Pointer) \
- or t in [c_char_p, c_wchar_p]):
- signature[i] = cast(t, c_void_p)
-
- def _calc_signature(self, signature):
- raise NotImplementedError(
- "Hook signatures are not supported for architecture: %s" \
- % win32.arch)
-
- def _get_return_address(self, aProcess, aThread):
- return None
-
- def _get_function_arguments(self, aProcess, aThread):
- if self._signature or self._paramCount:
- raise NotImplementedError(
- "Hook signatures are not supported for architecture: %s" \
- % win32.arch)
- return ()
-
- def _get_return_value(self, aThread):
- return None
-
- # By using break_at() to set a process-wide breakpoint on the function's
- # return address, we might hit a race condition when more than one thread
- # is being debugged.
- #
- # Hardware breakpoints should be used instead. But since a thread can run
- # out of those, we need to fall back to this method when needed.
-
- def __call__(self, event):
- """
- Handles the breakpoint event on entry of the function.
-
- @type event: L{ExceptionEvent}
- @param event: Breakpoint hit event.
-
- @raise WindowsError: An error occured.
- """
- debug = event.debug
-
- dwProcessId = event.get_pid()
- dwThreadId = event.get_tid()
- aProcess = event.get_process()
- aThread = event.get_thread()
-
- # Get the return address and function arguments.
- ra = self._get_return_address(aProcess, aThread)
- params = self._get_function_arguments(aProcess, aThread)
-
- # Keep the function arguments for later use.
- self.__push_params(dwThreadId, params)
-
- # If we need to hook the return from the function...
- bHookedReturn = False
- if ra is not None and self.__postCB is not None:
-
- # Try to set a one shot hardware breakpoint at the return address.
- useHardwareBreakpoints = self.useHardwareBreakpoints
- if useHardwareBreakpoints:
- try:
- debug.define_hardware_breakpoint(
- dwThreadId,
- ra,
- event.debug.BP_BREAK_ON_EXECUTION,
- event.debug.BP_WATCH_BYTE,
- True,
- self.__postCallAction_hwbp
- )
- debug.enable_one_shot_hardware_breakpoint(dwThreadId, ra)
- bHookedReturn = True
- except Exception:
- e = sys.exc_info()[1]
- useHardwareBreakpoints = False
- msg = ("Failed to set hardware breakpoint"
- " at address %s for thread ID %d")
- msg = msg % (HexDump.address(ra), dwThreadId)
- warnings.warn(msg, BreakpointWarning)
-
- # If not possible, set a code breakpoint instead.
- if not useHardwareBreakpoints:
- try:
- debug.break_at(dwProcessId, ra,
- self.__postCallAction_codebp)
- bHookedReturn = True
- except Exception:
- e = sys.exc_info()[1]
- msg = ("Failed to set code breakpoint"
- " at address %s for process ID %d")
- msg = msg % (HexDump.address(ra), dwProcessId)
- warnings.warn(msg, BreakpointWarning)
-
- # Call the "pre" callback.
- try:
- self.__callHandler(self.__preCB, event, ra, *params)
-
- # If no "post" callback is defined, forget the function arguments.
- finally:
- if not bHookedReturn:
- self.__pop_params(dwThreadId)
-
- def __postCallAction_hwbp(self, event):
- """
- Handles hardware breakpoint events on return from the function.
-
- @type event: L{ExceptionEvent}
- @param event: Single step event.
- """
-
- # Remove the one shot hardware breakpoint
- # at the return address location in the stack.
- tid = event.get_tid()
- address = event.breakpoint.get_address()
- event.debug.erase_hardware_breakpoint(tid, address)
-
- # Call the "post" callback.
- try:
- self.__postCallAction(event)
-
- # Forget the parameters.
- finally:
- self.__pop_params(tid)
-
- def __postCallAction_codebp(self, event):
- """
- Handles code breakpoint events on return from the function.
-
- @type event: L{ExceptionEvent}
- @param event: Breakpoint hit event.
- """
-
- # If the breakpoint was accidentally hit by another thread,
- # pass it to the debugger instead of calling the "post" callback.
- #
- # XXX FIXME:
- # I suppose this check will fail under some weird conditions...
- #
- tid = event.get_tid()
- if tid not in self.__paramStack:
- return True
-
- # Remove the code breakpoint at the return address.
- pid = event.get_pid()
- address = event.breakpoint.get_address()
- event.debug.dont_break_at(pid, address)
-
- # Call the "post" callback.
- try:
- self.__postCallAction(event)
-
- # Forget the parameters.
- finally:
- self.__pop_params(tid)
-
- def __postCallAction(self, event):
- """
- Calls the "post" callback.
-
- @type event: L{ExceptionEvent}
- @param event: Breakpoint hit event.
- """
- aThread = event.get_thread()
- retval = self._get_return_value(aThread)
- self.__callHandler(self.__postCB, event, retval)
-
- def __callHandler(self, callback, event, *params):
- """
- Calls a "pre" or "post" handler, if set.
-
- @type callback: function
- @param callback: Callback function to call.
-
- @type event: L{ExceptionEvent}
- @param event: Breakpoint hit event.
-
- @type params: tuple
- @param params: Parameters for the callback function.
- """
- if callback is not None:
- event.hook = self
- callback(event, *params)
-
- def __push_params(self, tid, params):
- """
- Remembers the arguments tuple for the last call to the hooked function
- from this thread.
-
- @type tid: int
- @param tid: Thread global ID.
-
- @type params: tuple( arg, arg, arg... )
- @param params: Tuple of arguments.
- """
- stack = self.__paramStack.get( tid, [] )
- stack.append(params)
- self.__paramStack[tid] = stack
-
- def __pop_params(self, tid):
- """
- Forgets the arguments tuple for the last call to the hooked function
- from this thread.
-
- @type tid: int
- @param tid: Thread global ID.
- """
- stack = self.__paramStack[tid]
- stack.pop()
- if not stack:
- del self.__paramStack[tid]
-
- def get_params(self, tid):
- """
- Returns the parameters found in the stack when the hooked function
- was last called by this thread.
-
- @type tid: int
- @param tid: Thread global ID.
-
- @rtype: tuple( arg, arg, arg... )
- @return: Tuple of arguments.
- """
- try:
- params = self.get_params_stack(tid)[-1]
- except IndexError:
- msg = "Hooked function called from thread %d already returned"
- raise IndexError(msg % tid)
- return params
-
- def get_params_stack(self, tid):
- """
- Returns the parameters found in the stack each time the hooked function
- was called by this thread and hasn't returned yet.
-
- @type tid: int
- @param tid: Thread global ID.
-
- @rtype: list of tuple( arg, arg, arg... )
- @return: List of argument tuples.
- """
- try:
- stack = self.__paramStack[tid]
- except KeyError:
- msg = "Hooked function was not called from thread %d"
- raise KeyError(msg % tid)
- return stack
-
- def hook(self, debug, pid, address):
- """
- Installs the function hook at a given process and address.
-
- @see: L{unhook}
-
- @warning: Do not call from an function hook callback.
-
- @type debug: L{Debug}
- @param debug: Debug object.
-
- @type pid: int
- @param pid: Process ID.
-
- @type address: int
- @param address: Function address.
- """
- return debug.break_at(pid, address, self)
-
- def unhook(self, debug, pid, address):
- """
- Removes the function hook at a given process and address.
-
- @see: L{hook}
-
- @warning: Do not call from an function hook callback.
-
- @type debug: L{Debug}
- @param debug: Debug object.
-
- @type pid: int
- @param pid: Process ID.
-
- @type address: int
- @param address: Function address.
- """
- return debug.dont_break_at(pid, address)
-
-class _Hook_i386 (Hook):
- """
- Implementation details for L{Hook} on the L{win32.ARCH_I386} architecture.
- """
-
- # We don't want to inherit the parent class __new__ method.
- __new__ = object.__new__
-
- def _calc_signature(self, signature):
- self._cast_signature_pointers_to_void(signature)
- class Arguments (ctypes.Structure):
- _fields_ = [ ("arg_%s" % i, signature[i]) \
- for i in compat.xrange(len(signature) - 1, -1, -1) ]
- return Arguments
-
- def _get_return_address(self, aProcess, aThread):
- return aProcess.read_pointer( aThread.get_sp() )
-
- def _get_function_arguments(self, aProcess, aThread):
- if self._signature:
- params = aThread.read_stack_structure(self._signature,
- offset = win32.sizeof(win32.LPVOID))
- elif self._paramCount:
- params = aThread.read_stack_dwords(self._paramCount,
- offset = win32.sizeof(win32.LPVOID))
- else:
- params = ()
- return params
-
- def _get_return_value(self, aThread):
- ctx = aThread.get_context(win32.CONTEXT_INTEGER)
- return ctx['Eax']
-
-class _Hook_amd64 (Hook):
- """
- Implementation details for L{Hook} on the L{win32.ARCH_AMD64} architecture.
- """
-
- # We don't want to inherit the parent class __new__ method.
- __new__ = object.__new__
-
- # Make a list of floating point types.
- __float_types = (
- ctypes.c_double,
- ctypes.c_float,
- )
- # Long doubles are not supported in old versions of ctypes!
- try:
- __float_types += (ctypes.c_longdouble,)
- except AttributeError:
- pass
-
- def _calc_signature(self, signature):
- self._cast_signature_pointers_to_void(signature)
-
- float_types = self.__float_types
- c_sizeof = ctypes.sizeof
- reg_size = c_sizeof(ctypes.c_size_t)
-
- reg_int_sig = []
- reg_float_sig = []
- stack_sig = []
-
- for i in compat.xrange(len(signature)):
- arg = signature[i]
- name = "arg_%d" % i
- stack_sig.insert( 0, (name, arg) )
- if i < 4:
- if type(arg) in float_types:
- reg_float_sig.append( (name, arg) )
- elif c_sizeof(arg) <= reg_size:
- reg_int_sig.append( (name, arg) )
- else:
- msg = ("Hook signatures don't support structures"
- " within the first 4 arguments of a function"
- " for the %s architecture") % win32.arch
- raise NotImplementedError(msg)
-
- if reg_int_sig:
- class RegisterArguments (ctypes.Structure):
- _fields_ = reg_int_sig
- else:
- RegisterArguments = None
- if reg_float_sig:
- class FloatArguments (ctypes.Structure):
- _fields_ = reg_float_sig
- else:
- FloatArguments = None
- if stack_sig:
- class StackArguments (ctypes.Structure):
- _fields_ = stack_sig
- else:
- StackArguments = None
-
- return (len(signature),
- RegisterArguments,
- FloatArguments,
- StackArguments)
-
- def _get_return_address(self, aProcess, aThread):
- return aProcess.read_pointer( aThread.get_sp() )
-
- def _get_function_arguments(self, aProcess, aThread):
- if self._signature:
- (args_count,
- RegisterArguments,
- FloatArguments,
- StackArguments) = self._signature
- arguments = {}
- if StackArguments:
- address = aThread.get_sp() + win32.sizeof(win32.LPVOID)
- stack_struct = aProcess.read_structure(address,
- StackArguments)
- stack_args = dict(
- [ (name, stack_struct.__getattribute__(name))
- for (name, type) in stack_struct._fields_ ]
- )
- arguments.update(stack_args)
- flags = 0
- if RegisterArguments:
- flags = flags | win32.CONTEXT_INTEGER
- if FloatArguments:
- flags = flags | win32.CONTEXT_MMX_REGISTERS
- if flags:
- ctx = aThread.get_context(flags)
- if RegisterArguments:
- buffer = (win32.QWORD * 4)(ctx['Rcx'], ctx['Rdx'],
- ctx['R8'], ctx['R9'])
- reg_args = self._get_arguments_from_buffer(buffer,
- RegisterArguments)
- arguments.update(reg_args)
- if FloatArguments:
- buffer = (win32.M128A * 4)(ctx['XMM0'], ctx['XMM1'],
- ctx['XMM2'], ctx['XMM3'])
- float_args = self._get_arguments_from_buffer(buffer,
- FloatArguments)
- arguments.update(float_args)
- params = tuple( [ arguments["arg_%d" % i]
- for i in compat.xrange(args_count) ] )
- else:
- params = ()
- return params
-
- def _get_arguments_from_buffer(self, buffer, structure):
- b_ptr = ctypes.pointer(buffer)
- v_ptr = ctypes.cast(b_ptr, ctypes.c_void_p)
- s_ptr = ctypes.cast(v_ptr, ctypes.POINTER(structure))
- struct = s_ptr.contents
- return dict(
- [ (name, struct.__getattribute__(name))
- for (name, type) in struct._fields_ ]
- )
-
- def _get_return_value(self, aThread):
- ctx = aThread.get_context(win32.CONTEXT_INTEGER)
- return ctx['Rax']
-
-#------------------------------------------------------------------------------
-
-# This class acts as a factory of Hook objects, one per target process.
-# Said objects are deleted by the unhook() method.
-
-class ApiHook (object):
- """
- Used by L{EventHandler}.
-
- This class acts as an action callback for code breakpoints set at the
- beginning of a function. It automatically retrieves the parameters from
- the stack, sets a breakpoint at the return address and retrieves the
- return value from the function call.
-
- @see: L{EventHandler.apiHooks}
-
- @type modName: str
- @ivar modName: Module name.
-
- @type procName: str
- @ivar procName: Procedure name.
- """
-
- def __init__(self, eventHandler, modName, procName, paramCount = None,
- signature = None):
- """
- @type eventHandler: L{EventHandler}
- @param eventHandler: Event handler instance. This is where the hook
- callbacks are to be defined (see below).
-
- @type modName: str
- @param modName: Module name.
-
- @type procName: str
- @param procName: Procedure name.
- The pre and post callbacks will be deduced from it.
-
- For example, if the procedure is "LoadLibraryEx" the callback
- routines will be "pre_LoadLibraryEx" and "post_LoadLibraryEx".
-
- The signature for the callbacks should be something like this::
-
- def pre_LoadLibraryEx(self, event, ra, lpFilename, hFile, dwFlags):
-
- # return address
- ra = params[0]
-
- # function arguments start from here...
- szFilename = event.get_process().peek_string(lpFilename)
-
- # (...)
-
- def post_LoadLibraryEx(self, event, return_value):
-
- # (...)
-
- Note that all pointer types are treated like void pointers, so your
- callback won't get the string or structure pointed to by it, but
- the remote memory address instead. This is so to prevent the ctypes
- library from being "too helpful" and trying to dereference the
- pointer. To get the actual data being pointed to, use one of the
- L{Process.read} methods.
-
- @type paramCount: int
- @param paramCount:
- (Optional) Number of parameters for the C{preCB} callback,
- not counting the return address. Parameters are read from
- the stack and assumed to be DWORDs in 32 bits and QWORDs in 64.
-
- This is a faster way to pull stack parameters in 32 bits, but in 64
- bits (or with some odd APIs in 32 bits) it won't be useful, since
- not all arguments to the hooked function will be of the same size.
-
- For a more reliable and cross-platform way of hooking use the
- C{signature} argument instead.
-
- @type signature: tuple
- @param signature:
- (Optional) Tuple of C{ctypes} data types that constitute the
- hooked function signature. When the function is called, this will
- be used to parse the arguments from the stack. Overrides the
- C{paramCount} argument.
- """
- self.__modName = modName
- self.__procName = procName
- self.__paramCount = paramCount
- self.__signature = signature
- self.__preCB = getattr(eventHandler, 'pre_%s' % procName, None)
- self.__postCB = getattr(eventHandler, 'post_%s' % procName, None)
- self.__hook = dict()
-
- def __call__(self, event):
- """
- Handles the breakpoint event on entry of the function.
-
- @type event: L{ExceptionEvent}
- @param event: Breakpoint hit event.
-
- @raise WindowsError: An error occured.
- """
- pid = event.get_pid()
- try:
- hook = self.__hook[pid]
- except KeyError:
- hook = Hook(self.__preCB, self.__postCB,
- self.__paramCount, self.__signature,
- event.get_process().get_arch() )
- self.__hook[pid] = hook
- return hook(event)
-
- @property
- def modName(self):
- return self.__modName
-
- @property
- def procName(self):
- return self.__procName
-
- def hook(self, debug, pid):
- """
- Installs the API hook on a given process and module.
-
- @warning: Do not call from an API hook callback.
-
- @type debug: L{Debug}
- @param debug: Debug object.
-
- @type pid: int
- @param pid: Process ID.
- """
- label = "%s!%s" % (self.__modName, self.__procName)
- try:
- hook = self.__hook[pid]
- except KeyError:
- try:
- aProcess = debug.system.get_process(pid)
- except KeyError:
- aProcess = Process(pid)
- hook = Hook(self.__preCB, self.__postCB,
- self.__paramCount, self.__signature,
- aProcess.get_arch() )
- self.__hook[pid] = hook
- hook.hook(debug, pid, label)
-
- def unhook(self, debug, pid):
- """
- Removes the API hook from the given process and module.
-
- @warning: Do not call from an API hook callback.
-
- @type debug: L{Debug}
- @param debug: Debug object.
-
- @type pid: int
- @param pid: Process ID.
- """
- try:
- hook = self.__hook[pid]
- except KeyError:
- return
- label = "%s!%s" % (self.__modName, self.__procName)
- hook.unhook(debug, pid, label)
- del self.__hook[pid]
-
-#==============================================================================
-
-class BufferWatch (object):
- """
- Returned by L{Debug.watch_buffer}.
-
- This object uniquely references a buffer being watched, even if there are
- multiple watches set on the exact memory region.
-
- @type pid: int
- @ivar pid: Process ID.
-
- @type start: int
- @ivar start: Memory address of the start of the buffer.
-
- @type end: int
- @ivar end: Memory address of the end of the buffer.
-
- @type action: callable
- @ivar action: Action callback.
-
- @type oneshot: bool
- @ivar oneshot: C{True} for one shot breakpoints, C{False} otherwise.
- """
-
- def __init__(self, pid, start, end, action = None, oneshot = False):
- self.__pid = pid
- self.__start = start
- self.__end = end
- self.__action = action
- self.__oneshot = oneshot
-
- @property
- def pid(self):
- return self.__pid
-
- @property
- def start(self):
- return self.__start
-
- @property
- def end(self):
- return self.__end
-
- @property
- def action(self):
- return self.__action
-
- @property
- def oneshot(self):
- return self.__oneshot
-
- def match(self, address):
- """
- Determine if the given memory address lies within the watched buffer.
-
- @rtype: bool
- @return: C{True} if the given memory address lies within the watched
- buffer, C{False} otherwise.
- """
- return self.__start <= address < self.__end
-
-#==============================================================================
-
-class _BufferWatchCondition (object):
- """
- Used by L{Debug.watch_buffer}.
-
- This class acts as a condition callback for page breakpoints.
- It emulates page breakpoints that can overlap and/or take up less
- than a page's size.
- """
-
- def __init__(self):
- self.__ranges = list() # list of BufferWatch in definition order
-
- def add(self, bw):
- """
- Adds a buffer watch identifier.
-
- @type bw: L{BufferWatch}
- @param bw:
- Buffer watch identifier.
- """
- self.__ranges.append(bw)
-
- def remove(self, bw):
- """
- Removes a buffer watch identifier.
-
- @type bw: L{BufferWatch}
- @param bw:
- Buffer watch identifier.
-
- @raise KeyError: The buffer watch identifier was already removed.
- """
- try:
- self.__ranges.remove(bw)
- except KeyError:
- if not bw.oneshot:
- raise
-
- def remove_last_match(self, address, size):
- """
- Removes the last buffer from the watch object
- to match the given address and size.
-
- @type address: int
- @param address: Memory address of buffer to stop watching.
-
- @type size: int
- @param size: Size in bytes of buffer to stop watching.
-
- @rtype: int
- @return: Number of matching elements found. Only the last one to be
- added is actually deleted upon calling this method.
-
- This counter allows you to know if there are more matching elements
- and how many.
- """
- count = 0
- start = address
- end = address + size - 1
- matched = None
- for item in self.__ranges:
- if item.match(start) and item.match(end):
- matched = item
- count += 1
- self.__ranges.remove(matched)
- return count
-
- def count(self):
- """
- @rtype: int
- @return: Number of buffers being watched.
- """
- return len(self.__ranges)
-
- def __call__(self, event):
- """
- Breakpoint condition callback.
-
- This method will also call the action callbacks for each
- buffer being watched.
-
- @type event: L{ExceptionEvent}
- @param event: Guard page exception event.
-
- @rtype: bool
- @return: C{True} if the address being accessed belongs
- to at least one of the buffers that was being watched
- and had no action callback.
- """
- address = event.get_exception_information(1)
- bCondition = False
- for bw in self.__ranges:
- bMatched = bw.match(address)
- try:
- action = bw.action
- if bMatched and action is not None:
- try:
- action(event)
- except Exception:
- e = sys.exc_info()[1]
- msg = ("Breakpoint action callback %r"
- " raised an exception: %s")
- msg = msg % (action, traceback.format_exc(e))
- warnings.warn(msg, BreakpointCallbackWarning)
- else:
- bCondition = bCondition or bMatched
- finally:
- if bMatched and bw.oneshot:
- event.debug.dont_watch_buffer(bw)
- return bCondition
-
-#==============================================================================
-
-class _BreakpointContainer (object):
- """
- Encapsulates the capability to contain Breakpoint objects.
-
- @group Breakpoints:
- break_at, watch_variable, watch_buffer, hook_function,
- dont_break_at, dont_watch_variable, dont_watch_buffer,
- dont_hook_function, unhook_function,
- break_on_error, dont_break_on_error
-
- @group Stalking:
- stalk_at, stalk_variable, stalk_buffer, stalk_function,
- dont_stalk_at, dont_stalk_variable, dont_stalk_buffer,
- dont_stalk_function
-
- @group Tracing:
- is_tracing, get_traced_tids,
- start_tracing, stop_tracing,
- start_tracing_process, stop_tracing_process,
- start_tracing_all, stop_tracing_all
-
- @group Symbols:
- resolve_label, resolve_exported_function
-
- @group Advanced breakpoint use:
- define_code_breakpoint,
- define_page_breakpoint,
- define_hardware_breakpoint,
- has_code_breakpoint,
- has_page_breakpoint,
- has_hardware_breakpoint,
- get_code_breakpoint,
- get_page_breakpoint,
- get_hardware_breakpoint,
- erase_code_breakpoint,
- erase_page_breakpoint,
- erase_hardware_breakpoint,
- enable_code_breakpoint,
- enable_page_breakpoint,
- enable_hardware_breakpoint,
- enable_one_shot_code_breakpoint,
- enable_one_shot_page_breakpoint,
- enable_one_shot_hardware_breakpoint,
- disable_code_breakpoint,
- disable_page_breakpoint,
- disable_hardware_breakpoint
-
- @group Listing breakpoints:
- get_all_breakpoints,
- get_all_code_breakpoints,
- get_all_page_breakpoints,
- get_all_hardware_breakpoints,
- get_process_breakpoints,
- get_process_code_breakpoints,
- get_process_page_breakpoints,
- get_process_hardware_breakpoints,
- get_thread_hardware_breakpoints,
- get_all_deferred_code_breakpoints,
- get_process_deferred_code_breakpoints
-
- @group Batch operations on breakpoints:
- enable_all_breakpoints,
- enable_one_shot_all_breakpoints,
- disable_all_breakpoints,
- erase_all_breakpoints,
- enable_process_breakpoints,
- enable_one_shot_process_breakpoints,
- disable_process_breakpoints,
- erase_process_breakpoints
-
- @group Breakpoint types:
- BP_TYPE_ANY, BP_TYPE_CODE, BP_TYPE_PAGE, BP_TYPE_HARDWARE
- @group Breakpoint states:
- BP_STATE_DISABLED, BP_STATE_ENABLED, BP_STATE_ONESHOT, BP_STATE_RUNNING
- @group Memory breakpoint trigger flags:
- BP_BREAK_ON_EXECUTION, BP_BREAK_ON_WRITE, BP_BREAK_ON_ACCESS
- @group Memory breakpoint size flags:
- BP_WATCH_BYTE, BP_WATCH_WORD, BP_WATCH_DWORD, BP_WATCH_QWORD
-
- @type BP_TYPE_ANY: int
- @cvar BP_TYPE_ANY: To get all breakpoints
- @type BP_TYPE_CODE: int
- @cvar BP_TYPE_CODE: To get code breakpoints only
- @type BP_TYPE_PAGE: int
- @cvar BP_TYPE_PAGE: To get page breakpoints only
- @type BP_TYPE_HARDWARE: int
- @cvar BP_TYPE_HARDWARE: To get hardware breakpoints only
-
- @type BP_STATE_DISABLED: int
- @cvar BP_STATE_DISABLED: Breakpoint is disabled.
- @type BP_STATE_ENABLED: int
- @cvar BP_STATE_ENABLED: Breakpoint is enabled.
- @type BP_STATE_ONESHOT: int
- @cvar BP_STATE_ONESHOT: Breakpoint is enabled for one shot.
- @type BP_STATE_RUNNING: int
- @cvar BP_STATE_RUNNING: Breakpoint is running (recently hit).
-
- @type BP_BREAK_ON_EXECUTION: int
- @cvar BP_BREAK_ON_EXECUTION: Break on code execution.
- @type BP_BREAK_ON_WRITE: int
- @cvar BP_BREAK_ON_WRITE: Break on memory write.
- @type BP_BREAK_ON_ACCESS: int
- @cvar BP_BREAK_ON_ACCESS: Break on memory read or write.
- """
-
- # Breakpoint types
- BP_TYPE_ANY = 0 # to get all breakpoints
- BP_TYPE_CODE = 1
- BP_TYPE_PAGE = 2
- BP_TYPE_HARDWARE = 3
-
- # Breakpoint states
- BP_STATE_DISABLED = Breakpoint.DISABLED
- BP_STATE_ENABLED = Breakpoint.ENABLED
- BP_STATE_ONESHOT = Breakpoint.ONESHOT
- BP_STATE_RUNNING = Breakpoint.RUNNING
-
- # Memory breakpoint trigger flags
- BP_BREAK_ON_EXECUTION = HardwareBreakpoint.BREAK_ON_EXECUTION
- BP_BREAK_ON_WRITE = HardwareBreakpoint.BREAK_ON_WRITE
- BP_BREAK_ON_ACCESS = HardwareBreakpoint.BREAK_ON_ACCESS
-
- # Memory breakpoint size flags
- BP_WATCH_BYTE = HardwareBreakpoint.WATCH_BYTE
- BP_WATCH_WORD = HardwareBreakpoint.WATCH_WORD
- BP_WATCH_QWORD = HardwareBreakpoint.WATCH_QWORD
- BP_WATCH_DWORD = HardwareBreakpoint.WATCH_DWORD
-
- def __init__(self):
- self.__codeBP = dict() # (pid, address) -> CodeBreakpoint
- self.__pageBP = dict() # (pid, address) -> PageBreakpoint
- self.__hardwareBP = dict() # tid -> [ HardwareBreakpoint ]
- self.__runningBP = dict() # tid -> set( Breakpoint )
- self.__tracing = set() # set( tid )
- self.__deferredBP = dict() # pid -> label -> (action, oneshot)
-
-#------------------------------------------------------------------------------
-
- # This operates on the dictionary of running breakpoints.
- # Since the bps are meant to stay alive no cleanup is done here.
-
- def __get_running_bp_set(self, tid):
- "Auxiliary method."
- return self.__runningBP.get(tid, ())
-
- def __add_running_bp(self, tid, bp):
- "Auxiliary method."
- if tid not in self.__runningBP:
- self.__runningBP[tid] = set()
- self.__runningBP[tid].add(bp)
-
- def __del_running_bp(self, tid, bp):
- "Auxiliary method."
- self.__runningBP[tid].remove(bp)
- if not self.__runningBP[tid]:
- del self.__runningBP[tid]
-
- def __del_running_bp_from_all_threads(self, bp):
- "Auxiliary method."
- for (tid, bpset) in compat.iteritems(self.__runningBP):
- if bp in bpset:
- bpset.remove(bp)
- self.system.get_thread(tid).clear_tf()
-
-#------------------------------------------------------------------------------
-
- # This is the cleanup code. Mostly called on response to exit/unload debug
- # events. If possible it shouldn't raise exceptions on runtime errors.
- # The main goal here is to avoid memory or handle leaks.
-
- def __cleanup_breakpoint(self, event, bp):
- "Auxiliary method."
- try:
- process = event.get_process()
- thread = event.get_thread()
- bp.disable(process, thread) # clear the debug regs / trap flag
- except Exception:
- pass
- bp.set_condition(True) # break possible circular reference
- bp.set_action(None) # break possible circular reference
-
- def __cleanup_thread(self, event):
- """
- Auxiliary method for L{_notify_exit_thread}
- and L{_notify_exit_process}.
- """
- tid = event.get_tid()
-
- # Cleanup running breakpoints
- try:
- for bp in self.__runningBP[tid]:
- self.__cleanup_breakpoint(event, bp)
- del self.__runningBP[tid]
- except KeyError:
- pass
-
- # Cleanup hardware breakpoints
- try:
- for bp in self.__hardwareBP[tid]:
- self.__cleanup_breakpoint(event, bp)
- del self.__hardwareBP[tid]
- except KeyError:
- pass
-
- # Cleanup set of threads being traced
- if tid in self.__tracing:
- self.__tracing.remove(tid)
-
- def __cleanup_process(self, event):
- """
- Auxiliary method for L{_notify_exit_process}.
- """
- pid = event.get_pid()
- process = event.get_process()
-
- # Cleanup code breakpoints
- for (bp_pid, bp_address) in compat.keys(self.__codeBP):
- if bp_pid == pid:
- bp = self.__codeBP[ (bp_pid, bp_address) ]
- self.__cleanup_breakpoint(event, bp)
- del self.__codeBP[ (bp_pid, bp_address) ]
-
- # Cleanup page breakpoints
- for (bp_pid, bp_address) in compat.keys(self.__pageBP):
- if bp_pid == pid:
- bp = self.__pageBP[ (bp_pid, bp_address) ]
- self.__cleanup_breakpoint(event, bp)
- del self.__pageBP[ (bp_pid, bp_address) ]
-
- # Cleanup deferred code breakpoints
- try:
- del self.__deferredBP[pid]
- except KeyError:
- pass
-
- def __cleanup_module(self, event):
- """
- Auxiliary method for L{_notify_unload_dll}.
- """
- pid = event.get_pid()
- process = event.get_process()
- module = event.get_module()
-
- # Cleanup thread breakpoints on this module
- for tid in process.iter_thread_ids():
- thread = process.get_thread(tid)
-
- # Running breakpoints
- if tid in self.__runningBP:
- bplist = list(self.__runningBP[tid])
- for bp in bplist:
- bp_address = bp.get_address()
- if process.get_module_at_address(bp_address) == module:
- self.__cleanup_breakpoint(event, bp)
- self.__runningBP[tid].remove(bp)
-
- # Hardware breakpoints
- if tid in self.__hardwareBP:
- bplist = list(self.__hardwareBP[tid])
- for bp in bplist:
- bp_address = bp.get_address()
- if process.get_module_at_address(bp_address) == module:
- self.__cleanup_breakpoint(event, bp)
- self.__hardwareBP[tid].remove(bp)
-
- # Cleanup code breakpoints on this module
- for (bp_pid, bp_address) in compat.keys(self.__codeBP):
- if bp_pid == pid:
- if process.get_module_at_address(bp_address) == module:
- bp = self.__codeBP[ (bp_pid, bp_address) ]
- self.__cleanup_breakpoint(event, bp)
- del self.__codeBP[ (bp_pid, bp_address) ]
-
- # Cleanup page breakpoints on this module
- for (bp_pid, bp_address) in compat.keys(self.__pageBP):
- if bp_pid == pid:
- if process.get_module_at_address(bp_address) == module:
- bp = self.__pageBP[ (bp_pid, bp_address) ]
- self.__cleanup_breakpoint(event, bp)
- del self.__pageBP[ (bp_pid, bp_address) ]
-
-#------------------------------------------------------------------------------
-
- # Defining breakpoints.
-
- # Code breakpoints.
- def define_code_breakpoint(self, dwProcessId, address, condition = True,
- action = None):
- """
- Creates a disabled code breakpoint at the given address.
-
- @see:
- L{has_code_breakpoint},
- L{get_code_breakpoint},
- L{enable_code_breakpoint},
- L{enable_one_shot_code_breakpoint},
- L{disable_code_breakpoint},
- L{erase_code_breakpoint}
-
- @type dwProcessId: int
- @param dwProcessId: Process global ID.
-
- @type address: int
- @param address: Memory address of the code instruction to break at.
-
- @type condition: function
- @param condition: (Optional) Condition callback function.
-
- The callback signature is::
-
- def condition_callback(event):
- return True # returns True or False
-
- Where B{event} is an L{Event} object,
- and the return value is a boolean
- (C{True} to dispatch the event, C{False} otherwise).
-
- @type action: function
- @param action: (Optional) Action callback function.
- If specified, the event is handled by this callback instead of
- being dispatched normally.
-
- The callback signature is::
-
- def action_callback(event):
- pass # no return value
-
- Where B{event} is an L{Event} object,
- and the return value is a boolean
- (C{True} to dispatch the event, C{False} otherwise).
-
- @rtype: L{CodeBreakpoint}
- @return: The code breakpoint object.
- """
- process = self.system.get_process(dwProcessId)
- bp = CodeBreakpoint(address, condition, action)
-
- key = (dwProcessId, bp.get_address())
- if key in self.__codeBP:
- msg = "Already exists (PID %d) : %r"
- raise KeyError(msg % (dwProcessId, self.__codeBP[key]))
- self.__codeBP[key] = bp
- return bp
-
- # Page breakpoints.
- def define_page_breakpoint(self, dwProcessId, address, pages = 1,
- condition = True,
- action = None):
- """
- Creates a disabled page breakpoint at the given address.
-
- @see:
- L{has_page_breakpoint},
- L{get_page_breakpoint},
- L{enable_page_breakpoint},
- L{enable_one_shot_page_breakpoint},
- L{disable_page_breakpoint},
- L{erase_page_breakpoint}
-
- @type dwProcessId: int
- @param dwProcessId: Process global ID.
-
- @type address: int
- @param address: Memory address of the first page to watch.
-
- @type pages: int
- @param pages: Number of pages to watch.
-
- @type condition: function
- @param condition: (Optional) Condition callback function.
-
- The callback signature is::
-
- def condition_callback(event):
- return True # returns True or False
-
- Where B{event} is an L{Event} object,
- and the return value is a boolean
- (C{True} to dispatch the event, C{False} otherwise).
-
- @type action: function
- @param action: (Optional) Action callback function.
- If specified, the event is handled by this callback instead of
- being dispatched normally.
-
- The callback signature is::
-
- def action_callback(event):
- pass # no return value
-
- Where B{event} is an L{Event} object,
- and the return value is a boolean
- (C{True} to dispatch the event, C{False} otherwise).
-
- @rtype: L{PageBreakpoint}
- @return: The page breakpoint object.
- """
- process = self.system.get_process(dwProcessId)
- bp = PageBreakpoint(address, pages, condition, action)
- begin = bp.get_address()
- end = begin + bp.get_size()
-
- address = begin
- pageSize = MemoryAddresses.pageSize
- while address < end:
- key = (dwProcessId, address)
- if key in self.__pageBP:
- msg = "Already exists (PID %d) : %r"
- msg = msg % (dwProcessId, self.__pageBP[key])
- raise KeyError(msg)
- address = address + pageSize
-
- address = begin
- while address < end:
- key = (dwProcessId, address)
- self.__pageBP[key] = bp
- address = address + pageSize
- return bp
-
- # Hardware breakpoints.
- def define_hardware_breakpoint(self, dwThreadId, address,
- triggerFlag = BP_BREAK_ON_ACCESS,
- sizeFlag = BP_WATCH_DWORD,
- condition = True,
- action = None):
- """
- Creates a disabled hardware breakpoint at the given address.
-
- @see:
- L{has_hardware_breakpoint},
- L{get_hardware_breakpoint},
- L{enable_hardware_breakpoint},
- L{enable_one_shot_hardware_breakpoint},
- L{disable_hardware_breakpoint},
- L{erase_hardware_breakpoint}
-
- @note:
- Hardware breakpoints do not seem to work properly on VirtualBox.
- See U{http://www.virtualbox.org/ticket/477}.
-
- @type dwThreadId: int
- @param dwThreadId: Thread global ID.
-
- @type address: int
- @param address: Memory address to watch.
-
- @type triggerFlag: int
- @param triggerFlag: Trigger of breakpoint. Must be one of the following:
-
- - L{BP_BREAK_ON_EXECUTION}
-
- Break on code execution.
-
- - L{BP_BREAK_ON_WRITE}
-
- Break on memory read or write.
-
- - L{BP_BREAK_ON_ACCESS}
-
- Break on memory write.
-
- @type sizeFlag: int
- @param sizeFlag: Size of breakpoint. Must be one of the following:
-
- - L{BP_WATCH_BYTE}
-
- One (1) byte in size.
-
- - L{BP_WATCH_WORD}
-
- Two (2) bytes in size.
-
- - L{BP_WATCH_DWORD}
-
- Four (4) bytes in size.
-
- - L{BP_WATCH_QWORD}
-
- Eight (8) bytes in size.
-
- @type condition: function
- @param condition: (Optional) Condition callback function.
-
- The callback signature is::
-
- def condition_callback(event):
- return True # returns True or False
-
- Where B{event} is an L{Event} object,
- and the return value is a boolean
- (C{True} to dispatch the event, C{False} otherwise).
-
- @type action: function
- @param action: (Optional) Action callback function.
- If specified, the event is handled by this callback instead of
- being dispatched normally.
-
- The callback signature is::
-
- def action_callback(event):
- pass # no return value
-
- Where B{event} is an L{Event} object,
- and the return value is a boolean
- (C{True} to dispatch the event, C{False} otherwise).
-
- @rtype: L{HardwareBreakpoint}
- @return: The hardware breakpoint object.
- """
- thread = self.system.get_thread(dwThreadId)
- bp = HardwareBreakpoint(address, triggerFlag, sizeFlag, condition,
- action)
- begin = bp.get_address()
- end = begin + bp.get_size()
-
- if dwThreadId in self.__hardwareBP:
- bpSet = self.__hardwareBP[dwThreadId]
- for oldbp in bpSet:
- old_begin = oldbp.get_address()
- old_end = old_begin + oldbp.get_size()
- if MemoryAddresses.do_ranges_intersect(begin, end, old_begin,
- old_end):
- msg = "Already exists (TID %d) : %r" % (dwThreadId, oldbp)
- raise KeyError(msg)
- else:
- bpSet = set()
- self.__hardwareBP[dwThreadId] = bpSet
- bpSet.add(bp)
- return bp
-
-#------------------------------------------------------------------------------
-
- # Checking breakpoint definitions.
-
- def has_code_breakpoint(self, dwProcessId, address):
- """
- Checks if a code breakpoint is defined at the given address.
-
- @see:
- L{define_code_breakpoint},
- L{get_code_breakpoint},
- L{erase_code_breakpoint},
- L{enable_code_breakpoint},
- L{enable_one_shot_code_breakpoint},
- L{disable_code_breakpoint}
-
- @type dwProcessId: int
- @param dwProcessId: Process global ID.
-
- @type address: int
- @param address: Memory address of breakpoint.
-
- @rtype: bool
- @return: C{True} if the breakpoint is defined, C{False} otherwise.
- """
- return (dwProcessId, address) in self.__codeBP
-
- def has_page_breakpoint(self, dwProcessId, address):
- """
- Checks if a page breakpoint is defined at the given address.
-
- @see:
- L{define_page_breakpoint},
- L{get_page_breakpoint},
- L{erase_page_breakpoint},
- L{enable_page_breakpoint},
- L{enable_one_shot_page_breakpoint},
- L{disable_page_breakpoint}
-
- @type dwProcessId: int
- @param dwProcessId: Process global ID.
-
- @type address: int
- @param address: Memory address of breakpoint.
-
- @rtype: bool
- @return: C{True} if the breakpoint is defined, C{False} otherwise.
- """
- return (dwProcessId, address) in self.__pageBP
-
- def has_hardware_breakpoint(self, dwThreadId, address):
- """
- Checks if a hardware breakpoint is defined at the given address.
-
- @see:
- L{define_hardware_breakpoint},
- L{get_hardware_breakpoint},
- L{erase_hardware_breakpoint},
- L{enable_hardware_breakpoint},
- L{enable_one_shot_hardware_breakpoint},
- L{disable_hardware_breakpoint}
-
- @type dwThreadId: int
- @param dwThreadId: Thread global ID.
-
- @type address: int
- @param address: Memory address of breakpoint.
-
- @rtype: bool
- @return: C{True} if the breakpoint is defined, C{False} otherwise.
- """
- if dwThreadId in self.__hardwareBP:
- bpSet = self.__hardwareBP[dwThreadId]
- for bp in bpSet:
- if bp.get_address() == address:
- return True
- return False
-
-#------------------------------------------------------------------------------
-
- # Getting breakpoints.
-
- def get_code_breakpoint(self, dwProcessId, address):
- """
- Returns the internally used breakpoint object,
- for the code breakpoint defined at the given address.
-
- @warning: It's usually best to call the L{Debug} methods
- instead of accessing the breakpoint objects directly.
-
- @see:
- L{define_code_breakpoint},
- L{has_code_breakpoint},
- L{enable_code_breakpoint},
- L{enable_one_shot_code_breakpoint},
- L{disable_code_breakpoint},
- L{erase_code_breakpoint}
-
- @type dwProcessId: int
- @param dwProcessId: Process global ID.
-
- @type address: int
- @param address: Memory address where the breakpoint is defined.
-
- @rtype: L{CodeBreakpoint}
- @return: The code breakpoint object.
- """
- key = (dwProcessId, address)
- if key not in self.__codeBP:
- msg = "No breakpoint at process %d, address %s"
- address = HexDump.address(address)
- raise KeyError(msg % (dwProcessId, address))
- return self.__codeBP[key]
-
- def get_page_breakpoint(self, dwProcessId, address):
- """
- Returns the internally used breakpoint object,
- for the page breakpoint defined at the given address.
-
- @warning: It's usually best to call the L{Debug} methods
- instead of accessing the breakpoint objects directly.
-
- @see:
- L{define_page_breakpoint},
- L{has_page_breakpoint},
- L{enable_page_breakpoint},
- L{enable_one_shot_page_breakpoint},
- L{disable_page_breakpoint},
- L{erase_page_breakpoint}
-
- @type dwProcessId: int
- @param dwProcessId: Process global ID.
-
- @type address: int
- @param address: Memory address where the breakpoint is defined.
-
- @rtype: L{PageBreakpoint}
- @return: The page breakpoint object.
- """
- key = (dwProcessId, address)
- if key not in self.__pageBP:
- msg = "No breakpoint at process %d, address %s"
- address = HexDump.addresS(address)
- raise KeyError(msg % (dwProcessId, address))
- return self.__pageBP[key]
-
- def get_hardware_breakpoint(self, dwThreadId, address):
- """
- Returns the internally used breakpoint object,
- for the code breakpoint defined at the given address.
-
- @warning: It's usually best to call the L{Debug} methods
- instead of accessing the breakpoint objects directly.
-
- @see:
- L{define_hardware_breakpoint},
- L{has_hardware_breakpoint},
- L{get_code_breakpoint},
- L{enable_hardware_breakpoint},
- L{enable_one_shot_hardware_breakpoint},
- L{disable_hardware_breakpoint},
- L{erase_hardware_breakpoint}
-
- @type dwThreadId: int
- @param dwThreadId: Thread global ID.
-
- @type address: int
- @param address: Memory address where the breakpoint is defined.
-
- @rtype: L{HardwareBreakpoint}
- @return: The hardware breakpoint object.
- """
- if dwThreadId not in self.__hardwareBP:
- msg = "No hardware breakpoints set for thread %d"
- raise KeyError(msg % dwThreadId)
- for bp in self.__hardwareBP[dwThreadId]:
- if bp.is_here(address):
- return bp
- msg = "No hardware breakpoint at thread %d, address %s"
- raise KeyError(msg % (dwThreadId, HexDump.address(address)))
-
-#------------------------------------------------------------------------------
-
- # Enabling and disabling breakpoints.
-
- def enable_code_breakpoint(self, dwProcessId, address):
- """
- Enables the code breakpoint at the given address.
-
- @see:
- L{define_code_breakpoint},
- L{has_code_breakpoint},
- L{enable_one_shot_code_breakpoint},
- L{disable_code_breakpoint}
- L{erase_code_breakpoint},
-
- @type dwProcessId: int
- @param dwProcessId: Process global ID.
-
- @type address: int
- @param address: Memory address of breakpoint.
- """
- p = self.system.get_process(dwProcessId)
- bp = self.get_code_breakpoint(dwProcessId, address)
- if bp.is_running():
- self.__del_running_bp_from_all_threads(bp)
- bp.enable(p, None) # XXX HACK thread is not used
-
- def enable_page_breakpoint(self, dwProcessId, address):
- """
- Enables the page breakpoint at the given address.
-
- @see:
- L{define_page_breakpoint},
- L{has_page_breakpoint},
- L{get_page_breakpoint},
- L{enable_one_shot_page_breakpoint},
- L{disable_page_breakpoint}
- L{erase_page_breakpoint},
-
- @type dwProcessId: int
- @param dwProcessId: Process global ID.
-
- @type address: int
- @param address: Memory address of breakpoint.
- """
- p = self.system.get_process(dwProcessId)
- bp = self.get_page_breakpoint(dwProcessId, address)
- if bp.is_running():
- self.__del_running_bp_from_all_threads(bp)
- bp.enable(p, None) # XXX HACK thread is not used
-
- def enable_hardware_breakpoint(self, dwThreadId, address):
- """
- Enables the hardware breakpoint at the given address.
-
- @see:
- L{define_hardware_breakpoint},
- L{has_hardware_breakpoint},
- L{get_hardware_breakpoint},
- L{enable_one_shot_hardware_breakpoint},
- L{disable_hardware_breakpoint}
- L{erase_hardware_breakpoint},
-
- @note: Do not set hardware breakpoints while processing the system
- breakpoint event.
-
- @type dwThreadId: int
- @param dwThreadId: Thread global ID.
-
- @type address: int
- @param address: Memory address of breakpoint.
- """
- t = self.system.get_thread(dwThreadId)
- bp = self.get_hardware_breakpoint(dwThreadId, address)
- if bp.is_running():
- self.__del_running_bp_from_all_threads(bp)
- bp.enable(None, t) # XXX HACK process is not used
-
- def enable_one_shot_code_breakpoint(self, dwProcessId, address):
- """
- Enables the code breakpoint at the given address for only one shot.
-
- @see:
- L{define_code_breakpoint},
- L{has_code_breakpoint},
- L{get_code_breakpoint},
- L{enable_code_breakpoint},
- L{disable_code_breakpoint}
- L{erase_code_breakpoint},
-
- @type dwProcessId: int
- @param dwProcessId: Process global ID.
-
- @type address: int
- @param address: Memory address of breakpoint.
- """
- p = self.system.get_process(dwProcessId)
- bp = self.get_code_breakpoint(dwProcessId, address)
- if bp.is_running():
- self.__del_running_bp_from_all_threads(bp)
- bp.one_shot(p, None) # XXX HACK thread is not used
-
- def enable_one_shot_page_breakpoint(self, dwProcessId, address):
- """
- Enables the page breakpoint at the given address for only one shot.
-
- @see:
- L{define_page_breakpoint},
- L{has_page_breakpoint},
- L{get_page_breakpoint},
- L{enable_page_breakpoint},
- L{disable_page_breakpoint}
- L{erase_page_breakpoint},
-
- @type dwProcessId: int
- @param dwProcessId: Process global ID.
-
- @type address: int
- @param address: Memory address of breakpoint.
- """
- p = self.system.get_process(dwProcessId)
- bp = self.get_page_breakpoint(dwProcessId, address)
- if bp.is_running():
- self.__del_running_bp_from_all_threads(bp)
- bp.one_shot(p, None) # XXX HACK thread is not used
-
- def enable_one_shot_hardware_breakpoint(self, dwThreadId, address):
- """
- Enables the hardware breakpoint at the given address for only one shot.
-
- @see:
- L{define_hardware_breakpoint},
- L{has_hardware_breakpoint},
- L{get_hardware_breakpoint},
- L{enable_hardware_breakpoint},
- L{disable_hardware_breakpoint}
- L{erase_hardware_breakpoint},
-
- @type dwThreadId: int
- @param dwThreadId: Thread global ID.
-
- @type address: int
- @param address: Memory address of breakpoint.
- """
- t = self.system.get_thread(dwThreadId)
- bp = self.get_hardware_breakpoint(dwThreadId, address)
- if bp.is_running():
- self.__del_running_bp_from_all_threads(bp)
- bp.one_shot(None, t) # XXX HACK process is not used
-
- def disable_code_breakpoint(self, dwProcessId, address):
- """
- Disables the code breakpoint at the given address.
-
- @see:
- L{define_code_breakpoint},
- L{has_code_breakpoint},
- L{get_code_breakpoint},
- L{enable_code_breakpoint}
- L{enable_one_shot_code_breakpoint},
- L{erase_code_breakpoint},
-
- @type dwProcessId: int
- @param dwProcessId: Process global ID.
-
- @type address: int
- @param address: Memory address of breakpoint.
- """
- p = self.system.get_process(dwProcessId)
- bp = self.get_code_breakpoint(dwProcessId, address)
- if bp.is_running():
- self.__del_running_bp_from_all_threads(bp)
- bp.disable(p, None) # XXX HACK thread is not used
-
- def disable_page_breakpoint(self, dwProcessId, address):
- """
- Disables the page breakpoint at the given address.
-
- @see:
- L{define_page_breakpoint},
- L{has_page_breakpoint},
- L{get_page_breakpoint},
- L{enable_page_breakpoint}
- L{enable_one_shot_page_breakpoint},
- L{erase_page_breakpoint},
-
- @type dwProcessId: int
- @param dwProcessId: Process global ID.
-
- @type address: int
- @param address: Memory address of breakpoint.
- """
- p = self.system.get_process(dwProcessId)
- bp = self.get_page_breakpoint(dwProcessId, address)
- if bp.is_running():
- self.__del_running_bp_from_all_threads(bp)
- bp.disable(p, None) # XXX HACK thread is not used
-
- def disable_hardware_breakpoint(self, dwThreadId, address):
- """
- Disables the hardware breakpoint at the given address.
-
- @see:
- L{define_hardware_breakpoint},
- L{has_hardware_breakpoint},
- L{get_hardware_breakpoint},
- L{enable_hardware_breakpoint}
- L{enable_one_shot_hardware_breakpoint},
- L{erase_hardware_breakpoint},
-
- @type dwThreadId: int
- @param dwThreadId: Thread global ID.
-
- @type address: int
- @param address: Memory address of breakpoint.
- """
- t = self.system.get_thread(dwThreadId)
- p = t.get_process()
- bp = self.get_hardware_breakpoint(dwThreadId, address)
- if bp.is_running():
- self.__del_running_bp(dwThreadId, bp)
- bp.disable(p, t)
-
-#------------------------------------------------------------------------------
-
- # Undefining (erasing) breakpoints.
-
- def erase_code_breakpoint(self, dwProcessId, address):
- """
- Erases the code breakpoint at the given address.
-
- @see:
- L{define_code_breakpoint},
- L{has_code_breakpoint},
- L{get_code_breakpoint},
- L{enable_code_breakpoint},
- L{enable_one_shot_code_breakpoint},
- L{disable_code_breakpoint}
-
- @type dwProcessId: int
- @param dwProcessId: Process global ID.
-
- @type address: int
- @param address: Memory address of breakpoint.
- """
- bp = self.get_code_breakpoint(dwProcessId, address)
- if not bp.is_disabled():
- self.disable_code_breakpoint(dwProcessId, address)
- del self.__codeBP[ (dwProcessId, address) ]
-
- def erase_page_breakpoint(self, dwProcessId, address):
- """
- Erases the page breakpoint at the given address.
-
- @see:
- L{define_page_breakpoint},
- L{has_page_breakpoint},
- L{get_page_breakpoint},
- L{enable_page_breakpoint},
- L{enable_one_shot_page_breakpoint},
- L{disable_page_breakpoint}
-
- @type dwProcessId: int
- @param dwProcessId: Process global ID.
-
- @type address: int
- @param address: Memory address of breakpoint.
- """
- bp = self.get_page_breakpoint(dwProcessId, address)
- begin = bp.get_address()
- end = begin + bp.get_size()
- if not bp.is_disabled():
- self.disable_page_breakpoint(dwProcessId, address)
- address = begin
- pageSize = MemoryAddresses.pageSize
- while address < end:
- del self.__pageBP[ (dwProcessId, address) ]
- address = address + pageSize
-
- def erase_hardware_breakpoint(self, dwThreadId, address):
- """
- Erases the hardware breakpoint at the given address.
-
- @see:
- L{define_hardware_breakpoint},
- L{has_hardware_breakpoint},
- L{get_hardware_breakpoint},
- L{enable_hardware_breakpoint},
- L{enable_one_shot_hardware_breakpoint},
- L{disable_hardware_breakpoint}
-
- @type dwThreadId: int
- @param dwThreadId: Thread global ID.
-
- @type address: int
- @param address: Memory address of breakpoint.
- """
- bp = self.get_hardware_breakpoint(dwThreadId, address)
- if not bp.is_disabled():
- self.disable_hardware_breakpoint(dwThreadId, address)
- bpSet = self.__hardwareBP[dwThreadId]
- bpSet.remove(bp)
- if not bpSet:
- del self.__hardwareBP[dwThreadId]
-
-#------------------------------------------------------------------------------
-
- # Listing breakpoints.
-
- def get_all_breakpoints(self):
- """
- Returns all breakpoint objects as a list of tuples.
-
- Each tuple contains:
- - Process global ID to which the breakpoint applies.
- - Thread global ID to which the breakpoint applies, or C{None}.
- - The L{Breakpoint} object itself.
-
- @note: If you're only interested in a specific breakpoint type, or in
- breakpoints for a specific process or thread, it's probably faster
- to call one of the following methods:
- - L{get_all_code_breakpoints}
- - L{get_all_page_breakpoints}
- - L{get_all_hardware_breakpoints}
- - L{get_process_code_breakpoints}
- - L{get_process_page_breakpoints}
- - L{get_process_hardware_breakpoints}
- - L{get_thread_hardware_breakpoints}
-
- @rtype: list of tuple( pid, tid, bp )
- @return: List of all breakpoints.
- """
- bplist = list()
-
- # Get the code breakpoints.
- for (pid, bp) in self.get_all_code_breakpoints():
- bplist.append( (pid, None, bp) )
-
- # Get the page breakpoints.
- for (pid, bp) in self.get_all_page_breakpoints():
- bplist.append( (pid, None, bp) )
-
- # Get the hardware breakpoints.
- for (tid, bp) in self.get_all_hardware_breakpoints():
- pid = self.system.get_thread(tid).get_pid()
- bplist.append( (pid, tid, bp) )
-
- # Return the list of breakpoints.
- return bplist
-
- def get_all_code_breakpoints(self):
- """
- @rtype: list of tuple( int, L{CodeBreakpoint} )
- @return: All code breakpoints as a list of tuples (pid, bp).
- """
- return [ (pid, bp) for ((pid, address), bp) in compat.iteritems(self.__codeBP) ]
-
- def get_all_page_breakpoints(self):
- """
- @rtype: list of tuple( int, L{PageBreakpoint} )
- @return: All page breakpoints as a list of tuples (pid, bp).
- """
-## return list( set( [ (pid, bp) for ((pid, address), bp) in compat.iteritems(self.__pageBP) ] ) )
- result = set()
- for ((pid, address), bp) in compat.iteritems(self.__pageBP):
- result.add( (pid, bp) )
- return list(result)
-
- def get_all_hardware_breakpoints(self):
- """
- @rtype: list of tuple( int, L{HardwareBreakpoint} )
- @return: All hardware breakpoints as a list of tuples (tid, bp).
- """
- result = list()
- for (tid, bplist) in compat.iteritems(self.__hardwareBP):
- for bp in bplist:
- result.append( (tid, bp) )
- return result
-
- def get_process_breakpoints(self, dwProcessId):
- """
- Returns all breakpoint objects for the given process as a list of tuples.
-
- Each tuple contains:
- - Process global ID to which the breakpoint applies.
- - Thread global ID to which the breakpoint applies, or C{None}.
- - The L{Breakpoint} object itself.
-
- @note: If you're only interested in a specific breakpoint type, or in
- breakpoints for a specific process or thread, it's probably faster
- to call one of the following methods:
- - L{get_all_code_breakpoints}
- - L{get_all_page_breakpoints}
- - L{get_all_hardware_breakpoints}
- - L{get_process_code_breakpoints}
- - L{get_process_page_breakpoints}
- - L{get_process_hardware_breakpoints}
- - L{get_thread_hardware_breakpoints}
-
- @type dwProcessId: int
- @param dwProcessId: Process global ID.
-
- @rtype: list of tuple( pid, tid, bp )
- @return: List of all breakpoints for the given process.
- """
- bplist = list()
-
- # Get the code breakpoints.
- for bp in self.get_process_code_breakpoints(dwProcessId):
- bplist.append( (dwProcessId, None, bp) )
-
- # Get the page breakpoints.
- for bp in self.get_process_page_breakpoints(dwProcessId):
- bplist.append( (dwProcessId, None, bp) )
-
- # Get the hardware breakpoints.
- for (tid, bp) in self.get_process_hardware_breakpoints(dwProcessId):
- pid = self.system.get_thread(tid).get_pid()
- bplist.append( (dwProcessId, tid, bp) )
-
- # Return the list of breakpoints.
- return bplist
-
- def get_process_code_breakpoints(self, dwProcessId):
- """
- @type dwProcessId: int
- @param dwProcessId: Process global ID.
-
- @rtype: list of L{CodeBreakpoint}
- @return: All code breakpoints for the given process.
- """
- return [ bp for ((pid, address), bp) in compat.iteritems(self.__codeBP) \
- if pid == dwProcessId ]
-
- def get_process_page_breakpoints(self, dwProcessId):
- """
- @type dwProcessId: int
- @param dwProcessId: Process global ID.
-
- @rtype: list of L{PageBreakpoint}
- @return: All page breakpoints for the given process.
- """
- return [ bp for ((pid, address), bp) in compat.iteritems(self.__pageBP) \
- if pid == dwProcessId ]
-
- def get_thread_hardware_breakpoints(self, dwThreadId):
- """
- @see: L{get_process_hardware_breakpoints}
-
- @type dwThreadId: int
- @param dwThreadId: Thread global ID.
-
- @rtype: list of L{HardwareBreakpoint}
- @return: All hardware breakpoints for the given thread.
- """
- result = list()
- for (tid, bplist) in compat.iteritems(self.__hardwareBP):
- if tid == dwThreadId:
- for bp in bplist:
- result.append(bp)
- return result
-
- def get_process_hardware_breakpoints(self, dwProcessId):
- """
- @see: L{get_thread_hardware_breakpoints}
-
- @type dwProcessId: int
- @param dwProcessId: Process global ID.
-
- @rtype: list of tuple( int, L{HardwareBreakpoint} )
- @return: All hardware breakpoints for each thread in the given process
- as a list of tuples (tid, bp).
- """
- result = list()
- aProcess = self.system.get_process(dwProcessId)
- for dwThreadId in aProcess.iter_thread_ids():
- if dwThreadId in self.__hardwareBP:
- bplist = self.__hardwareBP[dwThreadId]
- for bp in bplist:
- result.append( (dwThreadId, bp) )
- return result
-
-## def get_all_hooks(self):
-## """
-## @see: L{get_process_hooks}
-##
-## @rtype: list of tuple( int, int, L{Hook} )
-## @return: All defined hooks as a list of tuples (pid, address, hook).
-## """
-## return [ (pid, address, hook) \
-## for ((pid, address), hook) in self.__hook_objects ]
-##
-## def get_process_hooks(self, dwProcessId):
-## """
-## @see: L{get_all_hooks}
-##
-## @type dwProcessId: int
-## @param dwProcessId: Process global ID.
-##
-## @rtype: list of tuple( int, int, L{Hook} )
-## @return: All hooks for the given process as a list of tuples
-## (pid, address, hook).
-## """
-## return [ (pid, address, hook) \
-## for ((pid, address), hook) in self.__hook_objects \
-## if pid == dwProcessId ]
-
-#------------------------------------------------------------------------------
-
- # Batch operations on all breakpoints.
-
- def enable_all_breakpoints(self):
- """
- Enables all disabled breakpoints in all processes.
-
- @see:
- enable_code_breakpoint,
- enable_page_breakpoint,
- enable_hardware_breakpoint
- """
-
- # disable code breakpoints
- for (pid, bp) in self.get_all_code_breakpoints():
- if bp.is_disabled():
- self.enable_code_breakpoint(pid, bp.get_address())
-
- # disable page breakpoints
- for (pid, bp) in self.get_all_page_breakpoints():
- if bp.is_disabled():
- self.enable_page_breakpoint(pid, bp.get_address())
-
- # disable hardware breakpoints
- for (tid, bp) in self.get_all_hardware_breakpoints():
- if bp.is_disabled():
- self.enable_hardware_breakpoint(tid, bp.get_address())
-
- def enable_one_shot_all_breakpoints(self):
- """
- Enables for one shot all disabled breakpoints in all processes.
-
- @see:
- enable_one_shot_code_breakpoint,
- enable_one_shot_page_breakpoint,
- enable_one_shot_hardware_breakpoint
- """
-
- # disable code breakpoints for one shot
- for (pid, bp) in self.get_all_code_breakpoints():
- if bp.is_disabled():
- self.enable_one_shot_code_breakpoint(pid, bp.get_address())
-
- # disable page breakpoints for one shot
- for (pid, bp) in self.get_all_page_breakpoints():
- if bp.is_disabled():
- self.enable_one_shot_page_breakpoint(pid, bp.get_address())
-
- # disable hardware breakpoints for one shot
- for (tid, bp) in self.get_all_hardware_breakpoints():
- if bp.is_disabled():
- self.enable_one_shot_hardware_breakpoint(tid, bp.get_address())
-
- def disable_all_breakpoints(self):
- """
- Disables all breakpoints in all processes.
-
- @see:
- disable_code_breakpoint,
- disable_page_breakpoint,
- disable_hardware_breakpoint
- """
-
- # disable code breakpoints
- for (pid, bp) in self.get_all_code_breakpoints():
- self.disable_code_breakpoint(pid, bp.get_address())
-
- # disable page breakpoints
- for (pid, bp) in self.get_all_page_breakpoints():
- self.disable_page_breakpoint(pid, bp.get_address())
-
- # disable hardware breakpoints
- for (tid, bp) in self.get_all_hardware_breakpoints():
- self.disable_hardware_breakpoint(tid, bp.get_address())
-
- def erase_all_breakpoints(self):
- """
- Erases all breakpoints in all processes.
-
- @see:
- erase_code_breakpoint,
- erase_page_breakpoint,
- erase_hardware_breakpoint
- """
-
- # This should be faster but let's not trust the GC so much :P
- # self.disable_all_breakpoints()
- # self.__codeBP = dict()
- # self.__pageBP = dict()
- # self.__hardwareBP = dict()
- # self.__runningBP = dict()
- # self.__hook_objects = dict()
-
-## # erase hooks
-## for (pid, address, hook) in self.get_all_hooks():
-## self.dont_hook_function(pid, address)
-
- # erase code breakpoints
- for (pid, bp) in self.get_all_code_breakpoints():
- self.erase_code_breakpoint(pid, bp.get_address())
-
- # erase page breakpoints
- for (pid, bp) in self.get_all_page_breakpoints():
- self.erase_page_breakpoint(pid, bp.get_address())
-
- # erase hardware breakpoints
- for (tid, bp) in self.get_all_hardware_breakpoints():
- self.erase_hardware_breakpoint(tid, bp.get_address())
-
-#------------------------------------------------------------------------------
-
- # Batch operations on breakpoints per process.
-
- def enable_process_breakpoints(self, dwProcessId):
- """
- Enables all disabled breakpoints for the given process.
-
- @type dwProcessId: int
- @param dwProcessId: Process global ID.
- """
-
- # enable code breakpoints
- for bp in self.get_process_code_breakpoints(dwProcessId):
- if bp.is_disabled():
- self.enable_code_breakpoint(dwProcessId, bp.get_address())
-
- # enable page breakpoints
- for bp in self.get_process_page_breakpoints(dwProcessId):
- if bp.is_disabled():
- self.enable_page_breakpoint(dwProcessId, bp.get_address())
-
- # enable hardware breakpoints
- if self.system.has_process(dwProcessId):
- aProcess = self.system.get_process(dwProcessId)
- else:
- aProcess = Process(dwProcessId)
- aProcess.scan_threads()
- for aThread in aProcess.iter_threads():
- dwThreadId = aThread.get_tid()
- for bp in self.get_thread_hardware_breakpoints(dwThreadId):
- if bp.is_disabled():
- self.enable_hardware_breakpoint(dwThreadId, bp.get_address())
-
- def enable_one_shot_process_breakpoints(self, dwProcessId):
- """
- Enables for one shot all disabled breakpoints for the given process.
-
- @type dwProcessId: int
- @param dwProcessId: Process global ID.
- """
-
- # enable code breakpoints for one shot
- for bp in self.get_process_code_breakpoints(dwProcessId):
- if bp.is_disabled():
- self.enable_one_shot_code_breakpoint(dwProcessId, bp.get_address())
-
- # enable page breakpoints for one shot
- for bp in self.get_process_page_breakpoints(dwProcessId):
- if bp.is_disabled():
- self.enable_one_shot_page_breakpoint(dwProcessId, bp.get_address())
-
- # enable hardware breakpoints for one shot
- if self.system.has_process(dwProcessId):
- aProcess = self.system.get_process(dwProcessId)
- else:
- aProcess = Process(dwProcessId)
- aProcess.scan_threads()
- for aThread in aProcess.iter_threads():
- dwThreadId = aThread.get_tid()
- for bp in self.get_thread_hardware_breakpoints(dwThreadId):
- if bp.is_disabled():
- self.enable_one_shot_hardware_breakpoint(dwThreadId, bp.get_address())
-
- def disable_process_breakpoints(self, dwProcessId):
- """
- Disables all breakpoints for the given process.
-
- @type dwProcessId: int
- @param dwProcessId: Process global ID.
- """
-
- # disable code breakpoints
- for bp in self.get_process_code_breakpoints(dwProcessId):
- self.disable_code_breakpoint(dwProcessId, bp.get_address())
-
- # disable page breakpoints
- for bp in self.get_process_page_breakpoints(dwProcessId):
- self.disable_page_breakpoint(dwProcessId, bp.get_address())
-
- # disable hardware breakpoints
- if self.system.has_process(dwProcessId):
- aProcess = self.system.get_process(dwProcessId)
- else:
- aProcess = Process(dwProcessId)
- aProcess.scan_threads()
- for aThread in aProcess.iter_threads():
- dwThreadId = aThread.get_tid()
- for bp in self.get_thread_hardware_breakpoints(dwThreadId):
- self.disable_hardware_breakpoint(dwThreadId, bp.get_address())
-
- def erase_process_breakpoints(self, dwProcessId):
- """
- Erases all breakpoints for the given process.
-
- @type dwProcessId: int
- @param dwProcessId: Process global ID.
- """
-
- # disable breakpoints first
- # if an error occurs, no breakpoint is erased
- self.disable_process_breakpoints(dwProcessId)
-
-## # erase hooks
-## for address, hook in self.get_process_hooks(dwProcessId):
-## self.dont_hook_function(dwProcessId, address)
-
- # erase code breakpoints
- for bp in self.get_process_code_breakpoints(dwProcessId):
- self.erase_code_breakpoint(dwProcessId, bp.get_address())
-
- # erase page breakpoints
- for bp in self.get_process_page_breakpoints(dwProcessId):
- self.erase_page_breakpoint(dwProcessId, bp.get_address())
-
- # erase hardware breakpoints
- if self.system.has_process(dwProcessId):
- aProcess = self.system.get_process(dwProcessId)
- else:
- aProcess = Process(dwProcessId)
- aProcess.scan_threads()
- for aThread in aProcess.iter_threads():
- dwThreadId = aThread.get_tid()
- for bp in self.get_thread_hardware_breakpoints(dwThreadId):
- self.erase_hardware_breakpoint(dwThreadId, bp.get_address())
-
-#------------------------------------------------------------------------------
-
- # Internal handlers of debug events.
-
- def _notify_guard_page(self, event):
- """
- Notify breakpoints of a guard page exception event.
-
- @type event: L{ExceptionEvent}
- @param event: Guard page exception event.
-
- @rtype: bool
- @return: C{True} to call the user-defined handle, C{False} otherwise.
- """
- address = event.get_fault_address()
- pid = event.get_pid()
- bCallHandler = True
-
- # Align address to page boundary.
- mask = ~(MemoryAddresses.pageSize - 1)
- address = address & mask
-
- # Do we have an active page breakpoint there?
- key = (pid, address)
- if key in self.__pageBP:
- bp = self.__pageBP[key]
- if bp.is_enabled() or bp.is_one_shot():
-
- # Breakpoint is ours.
- event.continueStatus = win32.DBG_CONTINUE
-## event.continueStatus = win32.DBG_EXCEPTION_HANDLED
-
- # Hit the breakpoint.
- bp.hit(event)
-
- # Remember breakpoints in RUNNING state.
- if bp.is_running():
- tid = event.get_tid()
- self.__add_running_bp(tid, bp)
-
- # Evaluate the breakpoint condition.
- bCondition = bp.eval_condition(event)
-
- # If the breakpoint is automatic, run the action.
- # If not, notify the user.
- if bCondition and bp.is_automatic():
- bp.run_action(event)
- bCallHandler = False
- else:
- bCallHandler = bCondition
-
- # If we don't have a breakpoint here pass the exception to the debugee.
- # This is a normally occurring exception so we shouldn't swallow it.
- else:
- event.continueStatus = win32.DBG_EXCEPTION_NOT_HANDLED
-
- return bCallHandler
-
- def _notify_breakpoint(self, event):
- """
- Notify breakpoints of a breakpoint exception event.
-
- @type event: L{ExceptionEvent}
- @param event: Breakpoint exception event.
-
- @rtype: bool
- @return: C{True} to call the user-defined handle, C{False} otherwise.
- """
- address = event.get_exception_address()
- pid = event.get_pid()
- bCallHandler = True
-
- # Do we have an active code breakpoint there?
- key = (pid, address)
- if key in self.__codeBP:
- bp = self.__codeBP[key]
- if not bp.is_disabled():
-
- # Change the program counter (PC) to the exception address.
- # This accounts for the change in PC caused by
- # executing the breakpoint instruction, no matter
- # the size of it.
- aThread = event.get_thread()
- aThread.set_pc(address)
-
- # Swallow the exception.
- event.continueStatus = win32.DBG_CONTINUE
-
- # Hit the breakpoint.
- bp.hit(event)
-
- # Remember breakpoints in RUNNING state.
- if bp.is_running():
- tid = event.get_tid()
- self.__add_running_bp(tid, bp)
-
- # Evaluate the breakpoint condition.
- bCondition = bp.eval_condition(event)
-
- # If the breakpoint is automatic, run the action.
- # If not, notify the user.
- if bCondition and bp.is_automatic():
- bCallHandler = bp.run_action(event)
- else:
- bCallHandler = bCondition
-
- # Handle the system breakpoint.
- # TODO: examine the stack trace to figure out if it's really a
- # system breakpoint or an antidebug trick. The caller should be
- # inside ntdll if it's legit.
- elif event.get_process().is_system_defined_breakpoint(address):
- event.continueStatus = win32.DBG_CONTINUE
-
- # In hostile mode, if we don't have a breakpoint here pass the
- # exception to the debugee. In normal mode assume all breakpoint
- # exceptions are to be handled by the debugger.
- else:
- if self.in_hostile_mode():
- event.continueStatus = win32.DBG_EXCEPTION_NOT_HANDLED
- else:
- event.continueStatus = win32.DBG_CONTINUE
-
- return bCallHandler
-
- def _notify_single_step(self, event):
- """
- Notify breakpoints of a single step exception event.
-
- @type event: L{ExceptionEvent}
- @param event: Single step exception event.
-
- @rtype: bool
- @return: C{True} to call the user-defined handle, C{False} otherwise.
- """
- pid = event.get_pid()
- tid = event.get_tid()
- aThread = event.get_thread()
- aProcess = event.get_process()
- bCallHandler = True
- bIsOurs = False
-
- # In hostile mode set the default to pass the exception to the debugee.
- # If we later determine the exception is ours, hide it instead.
- old_continueStatus = event.continueStatus
- try:
- if self.in_hostile_mode():
- event.continueStatus = win32.DBG_EXCEPTION_NOT_HANDLED
-
- # Single step support is implemented on x86/x64 architectures only.
- if self.system.arch not in (win32.ARCH_I386, win32.ARCH_AMD64):
- return bCallHandler
-
- # In hostile mode, read the last executed bytes to try to detect
- # some antidebug tricks. Skip this check in normal mode because
- # it'd slow things down.
- #
- # FIXME: weird opcode encodings may bypass this check!
- #
- # bFakeSingleStep: Ice Breakpoint undocumented instruction.
- # bHideTrapFlag: Don't let pushf instructions get the real value of
- # the trap flag.
- # bNextIsPopFlags: Don't let popf instructions clear the trap flag.
- #
- bFakeSingleStep = False
- bLastIsPushFlags = False
- bNextIsPopFlags = False
- if self.in_hostile_mode():
- pc = aThread.get_pc()
- c = aProcess.read_char(pc - 1)
- if c == 0xF1: # int1
- bFakeSingleStep = True
- elif c == 0x9C: # pushf
- bLastIsPushFlags = True
- c = aProcess.peek_char(pc)
- if c == 0x66: # the only valid prefix for popf
- c = aProcess.peek_char(pc + 1)
- if c == 0x9D: # popf
- if bLastIsPushFlags:
- bLastIsPushFlags = False # they cancel each other out
- else:
- bNextIsPopFlags = True
-
- # When the thread is in tracing mode,
- # don't pass the exception to the debugee
- # and set the trap flag again.
- if self.is_tracing(tid):
- bIsOurs = True
- if not bFakeSingleStep:
- event.continueStatus = win32.DBG_CONTINUE
- aThread.set_tf()
-
- # Don't let the debugee read or write the trap flag.
- # This code works in 32 and 64 bits thanks to the endianness.
- if bLastIsPushFlags or bNextIsPopFlags:
- sp = aThread.get_sp()
- flags = aProcess.read_dword(sp)
- if bLastIsPushFlags:
- flags &= ~Thread.Flags.Trap
- else: # if bNextIsPopFlags:
- flags |= Thread.Flags.Trap
- aProcess.write_dword(sp, flags)
-
- # Handle breakpoints in RUNNING state.
- running = self.__get_running_bp_set(tid)
- if running:
- bIsOurs = True
- if not bFakeSingleStep:
- event.continueStatus = win32.DBG_CONTINUE
- bCallHandler = False
- while running:
- try:
- running.pop().hit(event)
- except Exception:
- e = sys.exc_info()[1]
- warnings.warn(str(e), BreakpointWarning)
-
- # Handle hardware breakpoints.
- if tid in self.__hardwareBP:
- ctx = aThread.get_context(win32.CONTEXT_DEBUG_REGISTERS)
- Dr6 = ctx['Dr6']
- ctx['Dr6'] = Dr6 & DebugRegister.clearHitMask
- aThread.set_context(ctx)
- bFoundBreakpoint = False
- bCondition = False
- hwbpList = [ bp for bp in self.__hardwareBP[tid] ]
- for bp in hwbpList:
- if not bp in self.__hardwareBP[tid]:
- continue # it was removed by a user-defined callback
- slot = bp.get_slot()
- if (slot is not None) and \
- (Dr6 & DebugRegister.hitMask[slot]):
- if not bFoundBreakpoint: #set before actions are called
- if not bFakeSingleStep:
- event.continueStatus = win32.DBG_CONTINUE
- bFoundBreakpoint = True
- bIsOurs = True
- bp.hit(event)
- if bp.is_running():
- self.__add_running_bp(tid, bp)
- bThisCondition = bp.eval_condition(event)
- if bThisCondition and bp.is_automatic():
- bp.run_action(event)
- bThisCondition = False
- bCondition = bCondition or bThisCondition
- if bFoundBreakpoint:
- bCallHandler = bCondition
-
- # Always call the user-defined handler
- # when the thread is in tracing mode.
- if self.is_tracing(tid):
- bCallHandler = True
-
- # If we're not in hostile mode, by default we assume all single
- # step exceptions are caused by the debugger.
- if not bIsOurs and not self.in_hostile_mode():
- aThread.clear_tf()
-
- # If the user hit Control-C while we were inside the try block,
- # set the default continueStatus back.
- except:
- event.continueStatus = old_continueStatus
- raise
-
- return bCallHandler
-
- def _notify_load_dll(self, event):
- """
- Notify the loading of a DLL.
-
- @type event: L{LoadDLLEvent}
- @param event: Load DLL event.
-
- @rtype: bool
- @return: C{True} to call the user-defined handler, C{False} otherwise.
- """
- self.__set_deferred_breakpoints(event)
- return True
-
- def _notify_unload_dll(self, event):
- """
- Notify the unloading of a DLL.
-
- @type event: L{UnloadDLLEvent}
- @param event: Unload DLL event.
-
- @rtype: bool
- @return: C{True} to call the user-defined handler, C{False} otherwise.
- """
- self.__cleanup_module(event)
- return True
-
- def _notify_exit_thread(self, event):
- """
- Notify the termination of a thread.
-
- @type event: L{ExitThreadEvent}
- @param event: Exit thread event.
-
- @rtype: bool
- @return: C{True} to call the user-defined handler, C{False} otherwise.
- """
- self.__cleanup_thread(event)
- return True
-
- def _notify_exit_process(self, event):
- """
- Notify the termination of a process.
-
- @type event: L{ExitProcessEvent}
- @param event: Exit process event.
-
- @rtype: bool
- @return: C{True} to call the user-defined handler, C{False} otherwise.
- """
- self.__cleanup_process(event)
- self.__cleanup_thread(event)
- return True
-
-#------------------------------------------------------------------------------
-
- # This is the high level breakpoint interface. Here we don't have to care
- # about defining or enabling breakpoints, and many errors are ignored
- # (like for example setting the same breakpoint twice, here the second
- # breakpoint replaces the first, much like in WinDBG). It should be easier
- # and more intuitive, if less detailed. It also allows the use of deferred
- # breakpoints.
-
-#------------------------------------------------------------------------------
-
- # Code breakpoints
-
- def __set_break(self, pid, address, action, oneshot):
- """
- Used by L{break_at} and L{stalk_at}.
-
- @type pid: int
- @param pid: Process global ID.
-
- @type address: int or str
- @param address:
- Memory address of code instruction to break at. It can be an
- integer value for the actual address or a string with a label
- to be resolved.
-
- @type action: function
- @param action: (Optional) Action callback function.
-
- See L{define_code_breakpoint} for more details.
-
- @type oneshot: bool
- @param oneshot: C{True} for one-shot breakpoints, C{False} otherwise.
-
- @rtype: L{Breakpoint}
- @return: Returns the new L{Breakpoint} object, or C{None} if the label
- couldn't be resolved and the breakpoint was deferred. Deferred
- breakpoints are set when the DLL they point to is loaded.
- """
- if type(address) not in (int, long):
- label = address
- try:
- address = self.system.get_process(pid).resolve_label(address)
- if not address:
- raise Exception()
- except Exception:
- try:
- deferred = self.__deferredBP[pid]
- except KeyError:
- deferred = dict()
- self.__deferredBP[pid] = deferred
- if label in deferred:
- msg = "Redefined deferred code breakpoint at %s in process ID %d"
- msg = msg % (label, pid)
- warnings.warn(msg, BreakpointWarning)
- deferred[label] = (action, oneshot)
- return None
- if self.has_code_breakpoint(pid, address):
- bp = self.get_code_breakpoint(pid, address)
- if bp.get_action() != action: # can't use "is not", fails for bound methods
- bp.set_action(action)
- msg = "Redefined code breakpoint at %s in process ID %d"
- msg = msg % (label, pid)
- warnings.warn(msg, BreakpointWarning)
- else:
- self.define_code_breakpoint(pid, address, True, action)
- bp = self.get_code_breakpoint(pid, address)
- if oneshot:
- if not bp.is_one_shot():
- self.enable_one_shot_code_breakpoint(pid, address)
- else:
- if not bp.is_enabled():
- self.enable_code_breakpoint(pid, address)
- return bp
-
- def __clear_break(self, pid, address):
- """
- Used by L{dont_break_at} and L{dont_stalk_at}.
-
- @type pid: int
- @param pid: Process global ID.
-
- @type address: int or str
- @param address:
- Memory address of code instruction to break at. It can be an
- integer value for the actual address or a string with a label
- to be resolved.
- """
- if type(address) not in (int, long):
- unknown = True
- label = address
- try:
- deferred = self.__deferredBP[pid]
- del deferred[label]
- unknown = False
- except KeyError:
-## traceback.print_last() # XXX DEBUG
- pass
- aProcess = self.system.get_process(pid)
- try:
- address = aProcess.resolve_label(label)
- if not address:
- raise Exception()
- except Exception:
-## traceback.print_last() # XXX DEBUG
- if unknown:
- msg = ("Can't clear unknown code breakpoint"
- " at %s in process ID %d")
- msg = msg % (label, pid)
- warnings.warn(msg, BreakpointWarning)
- return
- if self.has_code_breakpoint(pid, address):
- self.erase_code_breakpoint(pid, address)
-
- def __set_deferred_breakpoints(self, event):
- """
- Used internally. Sets all deferred breakpoints for a DLL when it's
- loaded.
-
- @type event: L{LoadDLLEvent}
- @param event: Load DLL event.
- """
- pid = event.get_pid()
- try:
- deferred = self.__deferredBP[pid]
- except KeyError:
- return
- aProcess = event.get_process()
- for (label, (action, oneshot)) in deferred.items():
- try:
- address = aProcess.resolve_label(label)
- except Exception:
- continue
- del deferred[label]
- try:
- self.__set_break(pid, address, action, oneshot)
- except Exception:
- msg = "Can't set deferred breakpoint %s at process ID %d"
- msg = msg % (label, pid)
- warnings.warn(msg, BreakpointWarning)
-
- def get_all_deferred_code_breakpoints(self):
- """
- Returns a list of deferred code breakpoints.
-
- @rtype: tuple of (int, str, callable, bool)
- @return: Tuple containing the following elements:
- - Process ID where to set the breakpoint.
- - Label pointing to the address where to set the breakpoint.
- - Action callback for the breakpoint.
- - C{True} of the breakpoint is one-shot, C{False} otherwise.
- """
- result = []
- for pid, deferred in compat.iteritems(self.__deferredBP):
- for (label, (action, oneshot)) in compat.iteritems(deferred):
- result.add( (pid, label, action, oneshot) )
- return result
-
- def get_process_deferred_code_breakpoints(self, dwProcessId):
- """
- Returns a list of deferred code breakpoints.
-
- @type dwProcessId: int
- @param dwProcessId: Process ID.
-
- @rtype: tuple of (int, str, callable, bool)
- @return: Tuple containing the following elements:
- - Label pointing to the address where to set the breakpoint.
- - Action callback for the breakpoint.
- - C{True} of the breakpoint is one-shot, C{False} otherwise.
- """
- return [ (label, action, oneshot)
- for (label, (action, oneshot))
- in compat.iteritems(self.__deferredBP.get(dwProcessId, {})) ]
-
- def stalk_at(self, pid, address, action = None):
- """
- Sets a one shot code breakpoint at the given process and address.
-
- If instead of an address you pass a label, the breakpoint may be
- deferred until the DLL it points to is loaded.
-
- @see: L{break_at}, L{dont_stalk_at}
-
- @type pid: int
- @param pid: Process global ID.
-
- @type address: int or str
- @param address:
- Memory address of code instruction to break at. It can be an
- integer value for the actual address or a string with a label
- to be resolved.
-
- @type action: function
- @param action: (Optional) Action callback function.
-
- See L{define_code_breakpoint} for more details.
-
- @rtype: bool
- @return: C{True} if the breakpoint was set immediately, or C{False} if
- it was deferred.
- """
- bp = self.__set_break(pid, address, action, oneshot = True)
- return bp is not None
-
- def break_at(self, pid, address, action = None):
- """
- Sets a code breakpoint at the given process and address.
-
- If instead of an address you pass a label, the breakpoint may be
- deferred until the DLL it points to is loaded.
-
- @see: L{stalk_at}, L{dont_break_at}
-
- @type pid: int
- @param pid: Process global ID.
-
- @type address: int or str
- @param address:
- Memory address of code instruction to break at. It can be an
- integer value for the actual address or a string with a label
- to be resolved.
-
- @type action: function
- @param action: (Optional) Action callback function.
-
- See L{define_code_breakpoint} for more details.
-
- @rtype: bool
- @return: C{True} if the breakpoint was set immediately, or C{False} if
- it was deferred.
- """
- bp = self.__set_break(pid, address, action, oneshot = False)
- return bp is not None
-
- def dont_break_at(self, pid, address):
- """
- Clears a code breakpoint set by L{break_at}.
-
- @type pid: int
- @param pid: Process global ID.
-
- @type address: int or str
- @param address:
- Memory address of code instruction to break at. It can be an
- integer value for the actual address or a string with a label
- to be resolved.
- """
- self.__clear_break(pid, address)
-
- def dont_stalk_at(self, pid, address):
- """
- Clears a code breakpoint set by L{stalk_at}.
-
- @type pid: int
- @param pid: Process global ID.
-
- @type address: int or str
- @param address:
- Memory address of code instruction to break at. It can be an
- integer value for the actual address or a string with a label
- to be resolved.
- """
- self.__clear_break(pid, address)
-
-#------------------------------------------------------------------------------
-
- # Function hooks
-
- def hook_function(self, pid, address,
- preCB = None, postCB = None,
- paramCount = None, signature = None):
- """
- Sets a function hook at the given address.
-
- If instead of an address you pass a label, the hook may be
- deferred until the DLL it points to is loaded.
-
- @type pid: int
- @param pid: Process global ID.
-
- @type address: int or str
- @param address:
- Memory address of code instruction to break at. It can be an
- integer value for the actual address or a string with a label
- to be resolved.
-
- @type preCB: function
- @param preCB: (Optional) Callback triggered on function entry.
-
- The signature for the callback should be something like this::
-
- def pre_LoadLibraryEx(event, ra, lpFilename, hFile, dwFlags):
-
- # return address
- ra = params[0]
-
- # function arguments start from here...
- szFilename = event.get_process().peek_string(lpFilename)
-
- # (...)
-
- Note that all pointer types are treated like void pointers, so your
- callback won't get the string or structure pointed to by it, but
- the remote memory address instead. This is so to prevent the ctypes
- library from being "too helpful" and trying to dereference the
- pointer. To get the actual data being pointed to, use one of the
- L{Process.read} methods.
-
- @type postCB: function
- @param postCB: (Optional) Callback triggered on function exit.
-
- The signature for the callback should be something like this::
-
- def post_LoadLibraryEx(event, return_value):
-
- # (...)
-
- @type paramCount: int
- @param paramCount:
- (Optional) Number of parameters for the C{preCB} callback,
- not counting the return address. Parameters are read from
- the stack and assumed to be DWORDs in 32 bits and QWORDs in 64.
-
- This is a faster way to pull stack parameters in 32 bits, but in 64
- bits (or with some odd APIs in 32 bits) it won't be useful, since
- not all arguments to the hooked function will be of the same size.
-
- For a more reliable and cross-platform way of hooking use the
- C{signature} argument instead.
-
- @type signature: tuple
- @param signature:
- (Optional) Tuple of C{ctypes} data types that constitute the
- hooked function signature. When the function is called, this will
- be used to parse the arguments from the stack. Overrides the
- C{paramCount} argument.
-
- @rtype: bool
- @return: C{True} if the hook was set immediately, or C{False} if
- it was deferred.
- """
- try:
- aProcess = self.system.get_process(pid)
- except KeyError:
- aProcess = Process(pid)
- arch = aProcess.get_arch()
- hookObj = Hook(preCB, postCB, paramCount, signature, arch)
- bp = self.break_at(pid, address, hookObj)
- return bp is not None
-
- def stalk_function(self, pid, address,
- preCB = None, postCB = None,
- paramCount = None, signature = None):
- """
- Sets a one-shot function hook at the given address.
-
- If instead of an address you pass a label, the hook may be
- deferred until the DLL it points to is loaded.
-
- @type pid: int
- @param pid: Process global ID.
-
- @type address: int or str
- @param address:
- Memory address of code instruction to break at. It can be an
- integer value for the actual address or a string with a label
- to be resolved.
-
- @type preCB: function
- @param preCB: (Optional) Callback triggered on function entry.
-
- The signature for the callback should be something like this::
-
- def pre_LoadLibraryEx(event, ra, lpFilename, hFile, dwFlags):
-
- # return address
- ra = params[0]
-
- # function arguments start from here...
- szFilename = event.get_process().peek_string(lpFilename)
-
- # (...)
-
- Note that all pointer types are treated like void pointers, so your
- callback won't get the string or structure pointed to by it, but
- the remote memory address instead. This is so to prevent the ctypes
- library from being "too helpful" and trying to dereference the
- pointer. To get the actual data being pointed to, use one of the
- L{Process.read} methods.
-
- @type postCB: function
- @param postCB: (Optional) Callback triggered on function exit.
-
- The signature for the callback should be something like this::
-
- def post_LoadLibraryEx(event, return_value):
-
- # (...)
-
- @type paramCount: int
- @param paramCount:
- (Optional) Number of parameters for the C{preCB} callback,
- not counting the return address. Parameters are read from
- the stack and assumed to be DWORDs in 32 bits and QWORDs in 64.
-
- This is a faster way to pull stack parameters in 32 bits, but in 64
- bits (or with some odd APIs in 32 bits) it won't be useful, since
- not all arguments to the hooked function will be of the same size.
-
- For a more reliable and cross-platform way of hooking use the
- C{signature} argument instead.
-
- @type signature: tuple
- @param signature:
- (Optional) Tuple of C{ctypes} data types that constitute the
- hooked function signature. When the function is called, this will
- be used to parse the arguments from the stack. Overrides the
- C{paramCount} argument.
-
- @rtype: bool
- @return: C{True} if the breakpoint was set immediately, or C{False} if
- it was deferred.
- """
- try:
- aProcess = self.system.get_process(pid)
- except KeyError:
- aProcess = Process(pid)
- arch = aProcess.get_arch()
- hookObj = Hook(preCB, postCB, paramCount, signature, arch)
- bp = self.stalk_at(pid, address, hookObj)
- return bp is not None
-
- def dont_hook_function(self, pid, address):
- """
- Removes a function hook set by L{hook_function}.
-
- @type pid: int
- @param pid: Process global ID.
-
- @type address: int or str
- @param address:
- Memory address of code instruction to break at. It can be an
- integer value for the actual address or a string with a label
- to be resolved.
- """
- self.dont_break_at(pid, address)
-
- # alias
- unhook_function = dont_hook_function
-
- def dont_stalk_function(self, pid, address):
- """
- Removes a function hook set by L{stalk_function}.
-
- @type pid: int
- @param pid: Process global ID.
-
- @type address: int or str
- @param address:
- Memory address of code instruction to break at. It can be an
- integer value for the actual address or a string with a label
- to be resolved.
- """
- self.dont_stalk_at(pid, address)
-
-#------------------------------------------------------------------------------
-
- # Variable watches
-
- def __set_variable_watch(self, tid, address, size, action):
- """
- Used by L{watch_variable} and L{stalk_variable}.
-
- @type tid: int
- @param tid: Thread global ID.
-
- @type address: int
- @param address: Memory address of variable to watch.
-
- @type size: int
- @param size: Size of variable to watch. The only supported sizes are:
- byte (1), word (2), dword (4) and qword (8).
-
- @type action: function
- @param action: (Optional) Action callback function.
-
- See L{define_hardware_breakpoint} for more details.
-
- @rtype: L{HardwareBreakpoint}
- @return: Hardware breakpoint at the requested address.
- """
-
- # TODO
- # We should merge the breakpoints instead of overwriting them.
- # We'll have the same problem as watch_buffer and we'll need to change
- # the API again.
-
- if size == 1:
- sizeFlag = self.BP_WATCH_BYTE
- elif size == 2:
- sizeFlag = self.BP_WATCH_WORD
- elif size == 4:
- sizeFlag = self.BP_WATCH_DWORD
- elif size == 8:
- sizeFlag = self.BP_WATCH_QWORD
- else:
- raise ValueError("Bad size for variable watch: %r" % size)
-
- if self.has_hardware_breakpoint(tid, address):
- warnings.warn(
- "Hardware breakpoint in thread %d at address %s was overwritten!" \
- % (tid, HexDump.address(address,
- self.system.get_thread(tid).get_bits())),
- BreakpointWarning)
-
- bp = self.get_hardware_breakpoint(tid, address)
- if bp.get_trigger() != self.BP_BREAK_ON_ACCESS or \
- bp.get_watch() != sizeFlag:
- self.erase_hardware_breakpoint(tid, address)
- self.define_hardware_breakpoint(tid, address,
- self.BP_BREAK_ON_ACCESS, sizeFlag, True, action)
- bp = self.get_hardware_breakpoint(tid, address)
-
- else:
- self.define_hardware_breakpoint(tid, address,
- self.BP_BREAK_ON_ACCESS, sizeFlag, True, action)
- bp = self.get_hardware_breakpoint(tid, address)
-
- return bp
-
- def __clear_variable_watch(self, tid, address):
- """
- Used by L{dont_watch_variable} and L{dont_stalk_variable}.
-
- @type tid: int
- @param tid: Thread global ID.
-
- @type address: int
- @param address: Memory address of variable to stop watching.
- """
- if self.has_hardware_breakpoint(tid, address):
- self.erase_hardware_breakpoint(tid, address)
-
- def watch_variable(self, tid, address, size, action = None):
- """
- Sets a hardware breakpoint at the given thread, address and size.
-
- @see: L{dont_watch_variable}
-
- @type tid: int
- @param tid: Thread global ID.
-
- @type address: int
- @param address: Memory address of variable to watch.
-
- @type size: int
- @param size: Size of variable to watch. The only supported sizes are:
- byte (1), word (2), dword (4) and qword (8).
-
- @type action: function
- @param action: (Optional) Action callback function.
-
- See L{define_hardware_breakpoint} for more details.
- """
- bp = self.__set_variable_watch(tid, address, size, action)
- if not bp.is_enabled():
- self.enable_hardware_breakpoint(tid, address)
-
- def stalk_variable(self, tid, address, size, action = None):
- """
- Sets a one-shot hardware breakpoint at the given thread,
- address and size.
-
- @see: L{dont_watch_variable}
-
- @type tid: int
- @param tid: Thread global ID.
-
- @type address: int
- @param address: Memory address of variable to watch.
-
- @type size: int
- @param size: Size of variable to watch. The only supported sizes are:
- byte (1), word (2), dword (4) and qword (8).
-
- @type action: function
- @param action: (Optional) Action callback function.
-
- See L{define_hardware_breakpoint} for more details.
- """
- bp = self.__set_variable_watch(tid, address, size, action)
- if not bp.is_one_shot():
- self.enable_one_shot_hardware_breakpoint(tid, address)
-
- def dont_watch_variable(self, tid, address):
- """
- Clears a hardware breakpoint set by L{watch_variable}.
-
- @type tid: int
- @param tid: Thread global ID.
-
- @type address: int
- @param address: Memory address of variable to stop watching.
- """
- self.__clear_variable_watch(tid, address)
-
- def dont_stalk_variable(self, tid, address):
- """
- Clears a hardware breakpoint set by L{stalk_variable}.
-
- @type tid: int
- @param tid: Thread global ID.
-
- @type address: int
- @param address: Memory address of variable to stop watching.
- """
- self.__clear_variable_watch(tid, address)
-
-#------------------------------------------------------------------------------
-
- # Buffer watches
-
- def __set_buffer_watch(self, pid, address, size, action, bOneShot):
- """
- Used by L{watch_buffer} and L{stalk_buffer}.
-
- @type pid: int
- @param pid: Process global ID.
-
- @type address: int
- @param address: Memory address of buffer to watch.
-
- @type size: int
- @param size: Size in bytes of buffer to watch.
-
- @type action: function
- @param action: (Optional) Action callback function.
-
- See L{define_page_breakpoint} for more details.
-
- @type bOneShot: bool
- @param bOneShot:
- C{True} to set a one-shot breakpoint,
- C{False} to set a normal breakpoint.
- """
-
- # Check the size isn't zero or negative.
- if size < 1:
- raise ValueError("Bad size for buffer watch: %r" % size)
-
- # Create the buffer watch identifier.
- bw = BufferWatch(pid, address, address + size, action, bOneShot)
-
- # Get the base address and size in pages required for this buffer.
- base = MemoryAddresses.align_address_to_page_start(address)
- limit = MemoryAddresses.align_address_to_page_end(address + size)
- pages = MemoryAddresses.get_buffer_size_in_pages(address, size)
-
- try:
-
- # For each page:
- # + if a page breakpoint exists reuse it
- # + if it doesn't exist define it
-
- bset = set() # all breakpoints used
- nset = set() # newly defined breakpoints
- cset = set() # condition objects
-
- page_addr = base
- pageSize = MemoryAddresses.pageSize
- while page_addr < limit:
-
- # If a breakpoints exists, reuse it.
- if self.has_page_breakpoint(pid, page_addr):
- bp = self.get_page_breakpoint(pid, page_addr)
- if bp not in bset:
- condition = bp.get_condition()
- if not condition in cset:
- if not isinstance(condition,_BufferWatchCondition):
- # this shouldn't happen unless you tinkered
- # with it or defined your own page breakpoints
- # manually.
- msg = "Can't watch buffer at page %s"
- msg = msg % HexDump.address(page_addr)
- raise RuntimeError(msg)
- cset.add(condition)
- bset.add(bp)
-
- # If it doesn't, define it.
- else:
- condition = _BufferWatchCondition()
- bp = self.define_page_breakpoint(pid, page_addr, 1,
- condition = condition)
- bset.add(bp)
- nset.add(bp)
- cset.add(condition)
-
- # Next page.
- page_addr = page_addr + pageSize
-
- # For each breakpoint, enable it if needed.
- aProcess = self.system.get_process(pid)
- for bp in bset:
- if bp.is_disabled() or bp.is_one_shot():
- bp.enable(aProcess, None)
-
- # On error...
- except:
-
- # Erase the newly defined breakpoints.
- for bp in nset:
- try:
- self.erase_page_breakpoint(pid, bp.get_address())
- except:
- pass
-
- # Pass the exception to the caller
- raise
-
- # For each condition object, add the new buffer.
- for condition in cset:
- condition.add(bw)
-
- def __clear_buffer_watch_old_method(self, pid, address, size):
- """
- Used by L{dont_watch_buffer} and L{dont_stalk_buffer}.
-
- @warn: Deprecated since WinAppDbg 1.5.
-
- @type pid: int
- @param pid: Process global ID.
-
- @type address: int
- @param address: Memory address of buffer to stop watching.
-
- @type size: int
- @param size: Size in bytes of buffer to stop watching.
- """
- warnings.warn("Deprecated since WinAppDbg 1.5", DeprecationWarning)
-
- # Check the size isn't zero or negative.
- if size < 1:
- raise ValueError("Bad size for buffer watch: %r" % size)
-
- # Get the base address and size in pages required for this buffer.
- base = MemoryAddresses.align_address_to_page_start(address)
- limit = MemoryAddresses.align_address_to_page_end(address + size)
- pages = MemoryAddresses.get_buffer_size_in_pages(address, size)
-
- # For each page, get the breakpoint and it's condition object.
- # For each condition, remove the buffer.
- # For each breakpoint, if no buffers are on watch, erase it.
- cset = set() # condition objects
- page_addr = base
- pageSize = MemoryAddresses.pageSize
- while page_addr < limit:
- if self.has_page_breakpoint(pid, page_addr):
- bp = self.get_page_breakpoint(pid, page_addr)
- condition = bp.get_condition()
- if condition not in cset:
- if not isinstance(condition, _BufferWatchCondition):
- # this shouldn't happen unless you tinkered with it
- # or defined your own page breakpoints manually.
- continue
- cset.add(condition)
- condition.remove_last_match(address, size)
- if condition.count() == 0:
- try:
- self.erase_page_breakpoint(pid, bp.get_address())
- except WindowsError:
- pass
- page_addr = page_addr + pageSize
-
- def __clear_buffer_watch(self, bw):
- """
- Used by L{dont_watch_buffer} and L{dont_stalk_buffer}.
-
- @type bw: L{BufferWatch}
- @param bw: Buffer watch identifier.
- """
-
- # Get the PID and the start and end addresses of the buffer.
- pid = bw.pid
- start = bw.start
- end = bw.end
-
- # Get the base address and size in pages required for the buffer.
- base = MemoryAddresses.align_address_to_page_start(start)
- limit = MemoryAddresses.align_address_to_page_end(end)
- pages = MemoryAddresses.get_buffer_size_in_pages(start, end - start)
-
- # For each page, get the breakpoint and it's condition object.
- # For each condition, remove the buffer.
- # For each breakpoint, if no buffers are on watch, erase it.
- cset = set() # condition objects
- page_addr = base
- pageSize = MemoryAddresses.pageSize
- while page_addr < limit:
- if self.has_page_breakpoint(pid, page_addr):
- bp = self.get_page_breakpoint(pid, page_addr)
- condition = bp.get_condition()
- if condition not in cset:
- if not isinstance(condition, _BufferWatchCondition):
- # this shouldn't happen unless you tinkered with it
- # or defined your own page breakpoints manually.
- continue
- cset.add(condition)
- condition.remove(bw)
- if condition.count() == 0:
- try:
- self.erase_page_breakpoint(pid, bp.get_address())
- except WindowsError:
- msg = "Cannot remove page breakpoint at address %s"
- msg = msg % HexDump.address( bp.get_address() )
- warnings.warn(msg, BreakpointWarning)
- page_addr = page_addr + pageSize
-
- def watch_buffer(self, pid, address, size, action = None):
- """
- Sets a page breakpoint and notifies when the given buffer is accessed.
-
- @see: L{dont_watch_variable}
-
- @type pid: int
- @param pid: Process global ID.
-
- @type address: int
- @param address: Memory address of buffer to watch.
-
- @type size: int
- @param size: Size in bytes of buffer to watch.
-
- @type action: function
- @param action: (Optional) Action callback function.
-
- See L{define_page_breakpoint} for more details.
-
- @rtype: L{BufferWatch}
- @return: Buffer watch identifier.
- """
- self.__set_buffer_watch(pid, address, size, action, False)
-
- def stalk_buffer(self, pid, address, size, action = None):
- """
- Sets a one-shot page breakpoint and notifies
- when the given buffer is accessed.
-
- @see: L{dont_watch_variable}
-
- @type pid: int
- @param pid: Process global ID.
-
- @type address: int
- @param address: Memory address of buffer to watch.
-
- @type size: int
- @param size: Size in bytes of buffer to watch.
-
- @type action: function
- @param action: (Optional) Action callback function.
-
- See L{define_page_breakpoint} for more details.
-
- @rtype: L{BufferWatch}
- @return: Buffer watch identifier.
- """
- self.__set_buffer_watch(pid, address, size, action, True)
-
- def dont_watch_buffer(self, bw, *argv, **argd):
- """
- Clears a page breakpoint set by L{watch_buffer}.
-
- @type bw: L{BufferWatch}
- @param bw:
- Buffer watch identifier returned by L{watch_buffer}.
- """
-
- # The sane way to do it.
- if not (argv or argd):
- self.__clear_buffer_watch(bw)
-
- # Backwards compatibility with WinAppDbg 1.4.
- else:
- argv = list(argv)
- argv.insert(0, bw)
- if 'pid' in argd:
- argv.insert(0, argd.pop('pid'))
- if 'address' in argd:
- argv.insert(1, argd.pop('address'))
- if 'size' in argd:
- argv.insert(2, argd.pop('size'))
- if argd:
- raise TypeError("Wrong arguments for dont_watch_buffer()")
- try:
- pid, address, size = argv
- except ValueError:
- raise TypeError("Wrong arguments for dont_watch_buffer()")
- self.__clear_buffer_watch_old_method(pid, address, size)
-
- def dont_stalk_buffer(self, bw, *argv, **argd):
- """
- Clears a page breakpoint set by L{stalk_buffer}.
-
- @type bw: L{BufferWatch}
- @param bw:
- Buffer watch identifier returned by L{stalk_buffer}.
- """
- self.dont_watch_buffer(bw, *argv, **argd)
-
-#------------------------------------------------------------------------------
-
- # Tracing
-
-# XXX TODO
-# Add "action" parameter to tracing mode
-
- def __start_tracing(self, thread):
- """
- @type thread: L{Thread}
- @param thread: Thread to start tracing.
- """
- tid = thread.get_tid()
- if not tid in self.__tracing:
- thread.set_tf()
- self.__tracing.add(tid)
-
- def __stop_tracing(self, thread):
- """
- @type thread: L{Thread}
- @param thread: Thread to stop tracing.
- """
- tid = thread.get_tid()
- if tid in self.__tracing:
- self.__tracing.remove(tid)
- if thread.is_alive():
- thread.clear_tf()
-
- def is_tracing(self, tid):
- """
- @type tid: int
- @param tid: Thread global ID.
-
- @rtype: bool
- @return: C{True} if the thread is being traced, C{False} otherwise.
- """
- return tid in self.__tracing
-
- def get_traced_tids(self):
- """
- Retrieves the list of global IDs of all threads being traced.
-
- @rtype: list( int... )
- @return: List of thread global IDs.
- """
- tids = list(self.__tracing)
- tids.sort()
- return tids
-
- def start_tracing(self, tid):
- """
- Start tracing mode in the given thread.
-
- @type tid: int
- @param tid: Global ID of thread to start tracing.
- """
- if not self.is_tracing(tid):
- thread = self.system.get_thread(tid)
- self.__start_tracing(thread)
-
- def stop_tracing(self, tid):
- """
- Stop tracing mode in the given thread.
-
- @type tid: int
- @param tid: Global ID of thread to stop tracing.
- """
- if self.is_tracing(tid):
- thread = self.system.get_thread(tid)
- self.__stop_tracing(thread)
-
- def start_tracing_process(self, pid):
- """
- Start tracing mode for all threads in the given process.
-
- @type pid: int
- @param pid: Global ID of process to start tracing.
- """
- for thread in self.system.get_process(pid).iter_threads():
- self.__start_tracing(thread)
-
- def stop_tracing_process(self, pid):
- """
- Stop tracing mode for all threads in the given process.
-
- @type pid: int
- @param pid: Global ID of process to stop tracing.
- """
- for thread in self.system.get_process(pid).iter_threads():
- self.__stop_tracing(thread)
-
- def start_tracing_all(self):
- """
- Start tracing mode for all threads in all debugees.
- """
- for pid in self.get_debugee_pids():
- self.start_tracing_process(pid)
-
- def stop_tracing_all(self):
- """
- Stop tracing mode for all threads in all debugees.
- """
- for pid in self.get_debugee_pids():
- self.stop_tracing_process(pid)
-
-#------------------------------------------------------------------------------
-
- # Break on LastError values (only available since Windows Server 2003)
-
- def break_on_error(self, pid, errorCode):
- """
- Sets or clears the system breakpoint for a given Win32 error code.
-
- Use L{Process.is_system_defined_breakpoint} to tell if a breakpoint
- exception was caused by a system breakpoint or by the application
- itself (for example because of a failed assertion in the code).
-
- @note: This functionality is only available since Windows Server 2003.
- In 2003 it only breaks on error values set externally to the
- kernel32.dll library, but this was fixed in Windows Vista.
-
- @warn: This method will fail if the debug symbols for ntdll (kernel32
- in Windows 2003) are not present. For more information see:
- L{System.fix_symbol_store_path}.
-
- @see: U{http://www.nynaeve.net/?p=147}
-
- @type pid: int
- @param pid: Process ID.
-
- @type errorCode: int
- @param errorCode: Win32 error code to stop on. Set to C{0} or
- C{ERROR_SUCCESS} to clear the breakpoint instead.
-
- @raise NotImplementedError:
- The functionality is not supported in this system.
-
- @raise WindowsError:
- An error occurred while processing this request.
- """
- aProcess = self.system.get_process(pid)
- address = aProcess.get_break_on_error_ptr()
- if not address:
- raise NotImplementedError(
- "The functionality is not supported in this system.")
- aProcess.write_dword(address, errorCode)
-
- def dont_break_on_error(self, pid):
- """
- Alias to L{break_on_error}C{(pid, ERROR_SUCCESS)}.
-
- @type pid: int
- @param pid: Process ID.
-
- @raise NotImplementedError:
- The functionality is not supported in this system.
-
- @raise WindowsError:
- An error occurred while processing this request.
- """
- self.break_on_error(pid, 0)
-
-#------------------------------------------------------------------------------
-
- # Simplified symbol resolving, useful for hooking functions
-
- def resolve_exported_function(self, pid, modName, procName):
- """
- Resolves the exported DLL function for the given process.
-
- @type pid: int
- @param pid: Process global ID.
-
- @type modName: str
- @param modName: Name of the module that exports the function.
-
- @type procName: str
- @param procName: Name of the exported function to resolve.
-
- @rtype: int, None
- @return: On success, the address of the exported function.
- On failure, returns C{None}.
- """
- aProcess = self.system.get_process(pid)
- aModule = aProcess.get_module_by_name(modName)
- if not aModule:
- aProcess.scan_modules()
- aModule = aProcess.get_module_by_name(modName)
- if aModule:
- address = aModule.resolve(procName)
- return address
- return None
-
- def resolve_label(self, pid, label):
- """
- Resolves a label for the given process.
-
- @type pid: int
- @param pid: Process global ID.
-
- @type label: str
- @param label: Label to resolve.
-
- @rtype: int
- @return: Memory address pointed to by the label.
-
- @raise ValueError: The label is malformed or impossible to resolve.
- @raise RuntimeError: Cannot resolve the module or function.
- """
- return self.get_process(pid).resolve_label(label)
diff --git a/spaces/SungBeom/chatwine-korean/.venv/Lib/site-packages/debugpy/_vendored/pydevd/pydevd_attach_to_process/winappdbg/debug.py b/spaces/SungBeom/chatwine-korean/.venv/Lib/site-packages/debugpy/_vendored/pydevd/pydevd_attach_to_process/winappdbg/debug.py
deleted file mode 100644
index 8364a5b8cbef025784ce790dc517b450cb8b22c0..0000000000000000000000000000000000000000
--- a/spaces/SungBeom/chatwine-korean/.venv/Lib/site-packages/debugpy/_vendored/pydevd/pydevd_attach_to_process/winappdbg/debug.py
+++ /dev/null
@@ -1,1543 +0,0 @@
-#!~/.wine/drive_c/Python25/python.exe
-# -*- coding: utf-8 -*-
-
-# Copyright (c) 2009-2014, Mario Vilas
-# All rights reserved.
-#
-# Redistribution and use in source and binary forms, with or without
-# modification, are permitted provided that the following conditions are met:
-#
-# * Redistributions of source code must retain the above copyright notice,
-# this list of conditions and the following disclaimer.
-# * Redistributions in binary form must reproduce the above copyright
-# notice,this list of conditions and the following disclaimer in the
-# documentation and/or other materials provided with the distribution.
-# * Neither the name of the copyright holder nor the names of its
-# contributors may be used to endorse or promote products derived from
-# this software without specific prior written permission.
-#
-# THIS SOFTWARE IS PROVIDED BY THE COPYRIGHT HOLDERS AND CONTRIBUTORS "AS IS"
-# AND ANY EXPRESS OR IMPLIED WARRANTIES, INCLUDING, BUT NOT LIMITED TO, THE
-# IMPLIED WARRANTIES OF MERCHANTABILITY AND FITNESS FOR A PARTICULAR PURPOSE
-# ARE DISCLAIMED. IN NO EVENT SHALL THE COPYRIGHT OWNER OR CONTRIBUTORS BE
-# LIABLE FOR ANY DIRECT, INDIRECT, INCIDENTAL, SPECIAL, EXEMPLARY, OR
-# CONSEQUENTIAL DAMAGES (INCLUDING, BUT NOT LIMITED TO, PROCUREMENT OF
-# SUBSTITUTE GOODS OR SERVICES; LOSS OF USE, DATA, OR PROFITS; OR BUSINESS
-# INTERRUPTION) HOWEVER CAUSED AND ON ANY THEORY OF LIABILITY, WHETHER IN
-# CONTRACT, STRICT LIABILITY, OR TORT (INCLUDING NEGLIGENCE OR OTHERWISE)
-# ARISING IN ANY WAY OUT OF THE USE OF THIS SOFTWARE, EVEN IF ADVISED OF THE
-# POSSIBILITY OF SUCH DAMAGE.
-
-"""
-Debugging.
-
-@group Debugging:
- Debug
-
-@group Warnings:
- MixedBitsWarning
-"""
-
-__revision__ = "$Id$"
-
-__all__ = [ 'Debug', 'MixedBitsWarning' ]
-
-import sys
-from winappdbg import win32
-from winappdbg.system import System
-from winappdbg.process import Process
-from winappdbg.thread import Thread
-from winappdbg.module import Module
-from winappdbg.window import Window
-from winappdbg.breakpoint import _BreakpointContainer, CodeBreakpoint
-from winappdbg.event import Event, EventHandler, EventDispatcher, EventFactory
-from winappdbg.interactive import ConsoleDebugger
-
-import warnings
-##import traceback
-
-#==============================================================================
-
-# If you set this warning to be considered as an error, you can stop the
-# debugger from attaching to 64-bit processes from a 32-bit Python VM and
-# visceversa.
-class MixedBitsWarning (RuntimeWarning):
- """
- This warning is issued when mixing 32 and 64 bit processes.
- """
-
-#==============================================================================
-
-# TODO
-# * Add memory read and write operations, similar to those in the Process
-# class, but hiding the presence of the code breakpoints.
-# * Add a method to get the memory map of a process, but hiding the presence
-# of the page breakpoints.
-# * Maybe the previous two features should be implemented at the Process class
-# instead, but how to communicate with the Debug object without creating
-# circular references? Perhaps the "overrides" could be set using private
-# members (so users won't see them), but then there's the problem of the
-# users being able to access the snapshot (i.e. clear it), which is why it's
-# not such a great idea to use the snapshot to store data that really belongs
-# to the Debug class.
-
-class Debug (EventDispatcher, _BreakpointContainer):
- """
- The main debugger class.
-
- @group Debugging:
- interactive, attach, detach, detach_from_all, execv, execl,
- kill, kill_all,
- get_debugee_count, get_debugee_pids,
- is_debugee, is_debugee_attached, is_debugee_started,
- in_hostile_mode,
- add_existing_session
-
- @group Debugging loop:
- loop, stop, next, wait, dispatch, cont
-
- @undocumented: force_garbage_collection
-
- @type system: L{System}
- @ivar system: A System snapshot that is automatically updated for
- processes being debugged. Processes not being debugged in this snapshot
- may be outdated.
- """
-
- # Automatically set to True the first time a Debug object is instanced.
- _debug_static_init = False
-
- def __init__(self, eventHandler = None, bKillOnExit = False,
- bHostileCode = False):
- """
- Debugger object.
-
- @type eventHandler: L{EventHandler}
- @param eventHandler:
- (Optional, recommended) Custom event handler object.
-
- @type bKillOnExit: bool
- @param bKillOnExit: (Optional) Kill on exit mode.
- If C{True} debugged processes are killed when the debugger is
- stopped. If C{False} when the debugger stops it detaches from all
- debugged processes and leaves them running (default).
-
- @type bHostileCode: bool
- @param bHostileCode: (Optional) Hostile code mode.
- Set to C{True} to take some basic precautions against anti-debug
- tricks. Disabled by default.
-
- @warn: When hostile mode is enabled, some things may not work as
- expected! This is because the anti-anti debug tricks may disrupt
- the behavior of the Win32 debugging APIs or WinAppDbg itself.
-
- @note: The L{eventHandler} parameter may be any callable Python object
- (for example a function, or an instance method).
- However you'll probably find it more convenient to use an instance
- of a subclass of L{EventHandler} here.
-
- @raise WindowsError: Raises an exception on error.
- """
- EventDispatcher.__init__(self, eventHandler)
- _BreakpointContainer.__init__(self)
-
- self.system = System()
- self.lastEvent = None
- self.__firstDebugee = True
- self.__bKillOnExit = bKillOnExit
- self.__bHostileCode = bHostileCode
- self.__breakOnEP = set() # set of pids
- self.__attachedDebugees = set() # set of pids
- self.__startedDebugees = set() # set of pids
-
- if not self._debug_static_init:
- self._debug_static_init = True
-
- # Request debug privileges for the current process.
- # Only do this once, and only after instancing a Debug object,
- # so passive debuggers don't get detected because of this.
- self.system.request_debug_privileges(bIgnoreExceptions = False)
-
- # Try to fix the symbol store path if it wasn't set.
- # But don't enable symbol downloading by default, since it may
- # degrade performance severely.
- self.system.fix_symbol_store_path(remote = False, force = False)
-
-## # It's hard not to create circular references,
-## # and if we have a destructor, we can end up leaking everything.
-## # It's best to code the debugging loop properly to always
-## # stop the debugger before going out of scope.
-## def __del__(self):
-## self.stop()
-
- def __enter__(self):
- """
- Compatibility with the "C{with}" Python statement.
- """
- return self
-
- def __exit__(self, type, value, traceback):
- """
- Compatibility with the "C{with}" Python statement.
- """
- self.stop()
-
- def __len__(self):
- """
- @rtype: int
- @return: Number of processes being debugged.
- """
- return self.get_debugee_count()
-
- # TODO: maybe custom __bool__ to break out of loop() ?
- # it already does work (because of __len__) but it'd be
- # useful to do it from the event handler anyway
-
-#------------------------------------------------------------------------------
-
- def __setSystemKillOnExitMode(self):
- # Make sure the default system behavior on detaching from processes
- # versus killing them matches our preferences. This only affects the
- # scenario where the Python VM dies unexpectedly without running all
- # the finally clauses, or the user failed to either instance the Debug
- # object inside a with block or call the stop() method before quitting.
- if self.__firstDebugee:
- try:
- System.set_kill_on_exit_mode(self.__bKillOnExit)
- self.__firstDebugee = False
- except Exception:
- pass
-
- def attach(self, dwProcessId):
- """
- Attaches to an existing process for debugging.
-
- @see: L{detach}, L{execv}, L{execl}
-
- @type dwProcessId: int
- @param dwProcessId: Global ID of a process to attach to.
-
- @rtype: L{Process}
- @return: A new Process object. Normally you don't need to use it now,
- it's best to interact with the process from the event handler.
-
- @raise WindowsError: Raises an exception on error.
- Depending on the circumstances, the debugger may or may not have
- attached to the target process.
- """
-
- # Get the Process object from the snapshot,
- # if missing create a new one.
- try:
- aProcess = self.system.get_process(dwProcessId)
- except KeyError:
- aProcess = Process(dwProcessId)
-
- # Warn when mixing 32 and 64 bits.
- # This also allows the user to stop attaching altogether,
- # depending on how the warnings are configured.
- if System.bits != aProcess.get_bits():
- msg = "Mixture of 32 and 64 bits is considered experimental." \
- " Use at your own risk!"
- warnings.warn(msg, MixedBitsWarning)
-
- # Attach to the process.
- win32.DebugActiveProcess(dwProcessId)
-
- # Add the new PID to the set of debugees.
- self.__attachedDebugees.add(dwProcessId)
-
- # Match the system kill-on-exit flag to our own.
- self.__setSystemKillOnExitMode()
-
- # If the Process object was not in the snapshot, add it now.
- if not self.system.has_process(dwProcessId):
- self.system._add_process(aProcess)
-
- # Scan the process threads and loaded modules.
- # This is prefered because the thread and library events do not
- # properly give some information, like the filename for each module.
- aProcess.scan_threads()
- aProcess.scan_modules()
-
- # Return the Process object, like the execv() and execl() methods.
- return aProcess
-
- def execv(self, argv, **kwargs):
- """
- Starts a new process for debugging.
-
- This method uses a list of arguments. To use a command line string
- instead, use L{execl}.
-
- @see: L{attach}, L{detach}
-
- @type argv: list( str... )
- @param argv: List of command line arguments to pass to the debugee.
- The first element must be the debugee executable filename.
-
- @type bBreakOnEntryPoint: bool
- @keyword bBreakOnEntryPoint: C{True} to automatically set a breakpoint
- at the program entry point.
-
- @type bConsole: bool
- @keyword bConsole: True to inherit the console of the debugger.
- Defaults to C{False}.
-
- @type bFollow: bool
- @keyword bFollow: C{True} to automatically attach to child processes.
- Defaults to C{False}.
-
- @type bInheritHandles: bool
- @keyword bInheritHandles: C{True} if the new process should inherit
- it's parent process' handles. Defaults to C{False}.
-
- @type bSuspended: bool
- @keyword bSuspended: C{True} to suspend the main thread before any code
- is executed in the debugee. Defaults to C{False}.
-
- @keyword dwParentProcessId: C{None} or C{0} if the debugger process
- should be the parent process (default), or a process ID to
- forcefully set as the debugee's parent (only available for Windows
- Vista and above).
-
- In hostile mode, the default is not the debugger process but the
- process ID for "explorer.exe".
-
- @type iTrustLevel: int or None
- @keyword iTrustLevel: Trust level.
- Must be one of the following values:
- - 0: B{No trust}. May not access certain resources, such as
- cryptographic keys and credentials. Only available since
- Windows XP and 2003, desktop editions. This is the default
- in hostile mode.
- - 1: B{Normal trust}. Run with the same privileges as a normal
- user, that is, one that doesn't have the I{Administrator} or
- I{Power User} user rights. Only available since Windows XP
- and 2003, desktop editions.
- - 2: B{Full trust}. Run with the exact same privileges as the
- current user. This is the default in normal mode.
-
- @type bAllowElevation: bool
- @keyword bAllowElevation: C{True} to allow the child process to keep
- UAC elevation, if the debugger itself is running elevated. C{False}
- to ensure the child process doesn't run with elevation. Defaults to
- C{True}.
-
- This flag is only meaningful on Windows Vista and above, and if the
- debugger itself is running with elevation. It can be used to make
- sure the child processes don't run elevated as well.
-
- This flag DOES NOT force an elevation prompt when the debugger is
- not running with elevation.
-
- Note that running the debugger with elevation (or the Python
- interpreter at all for that matter) is not normally required.
- You should only need to if the target program requires elevation
- to work properly (for example if you try to debug an installer).
-
- @rtype: L{Process}
- @return: A new Process object. Normally you don't need to use it now,
- it's best to interact with the process from the event handler.
-
- @raise WindowsError: Raises an exception on error.
- """
- if type(argv) in (str, compat.unicode):
- raise TypeError("Debug.execv expects a list, not a string")
- lpCmdLine = self.system.argv_to_cmdline(argv)
- return self.execl(lpCmdLine, **kwargs)
-
- def execl(self, lpCmdLine, **kwargs):
- """
- Starts a new process for debugging.
-
- This method uses a command line string. To use a list of arguments
- instead, use L{execv}.
-
- @see: L{attach}, L{detach}
-
- @type lpCmdLine: str
- @param lpCmdLine: Command line string to execute.
- The first token must be the debugee executable filename.
- Tokens with spaces must be enclosed in double quotes.
- Tokens including double quote characters must be escaped with a
- backslash.
-
- @type bBreakOnEntryPoint: bool
- @keyword bBreakOnEntryPoint: C{True} to automatically set a breakpoint
- at the program entry point. Defaults to C{False}.
-
- @type bConsole: bool
- @keyword bConsole: True to inherit the console of the debugger.
- Defaults to C{False}.
-
- @type bFollow: bool
- @keyword bFollow: C{True} to automatically attach to child processes.
- Defaults to C{False}.
-
- @type bInheritHandles: bool
- @keyword bInheritHandles: C{True} if the new process should inherit
- it's parent process' handles. Defaults to C{False}.
-
- @type bSuspended: bool
- @keyword bSuspended: C{True} to suspend the main thread before any code
- is executed in the debugee. Defaults to C{False}.
-
- @type dwParentProcessId: int or None
- @keyword dwParentProcessId: C{None} or C{0} if the debugger process
- should be the parent process (default), or a process ID to
- forcefully set as the debugee's parent (only available for Windows
- Vista and above).
-
- In hostile mode, the default is not the debugger process but the
- process ID for "explorer.exe".
-
- @type iTrustLevel: int
- @keyword iTrustLevel: Trust level.
- Must be one of the following values:
- - 0: B{No trust}. May not access certain resources, such as
- cryptographic keys and credentials. Only available since
- Windows XP and 2003, desktop editions. This is the default
- in hostile mode.
- - 1: B{Normal trust}. Run with the same privileges as a normal
- user, that is, one that doesn't have the I{Administrator} or
- I{Power User} user rights. Only available since Windows XP
- and 2003, desktop editions.
- - 2: B{Full trust}. Run with the exact same privileges as the
- current user. This is the default in normal mode.
-
- @type bAllowElevation: bool
- @keyword bAllowElevation: C{True} to allow the child process to keep
- UAC elevation, if the debugger itself is running elevated. C{False}
- to ensure the child process doesn't run with elevation. Defaults to
- C{True} in normal mode and C{False} in hostile mode.
-
- This flag is only meaningful on Windows Vista and above, and if the
- debugger itself is running with elevation. It can be used to make
- sure the child processes don't run elevated as well.
-
- This flag DOES NOT force an elevation prompt when the debugger is
- not running with elevation.
-
- Note that running the debugger with elevation (or the Python
- interpreter at all for that matter) is not normally required.
- You should only need to if the target program requires elevation
- to work properly (for example if you try to debug an installer).
-
- @rtype: L{Process}
- @return: A new Process object. Normally you don't need to use it now,
- it's best to interact with the process from the event handler.
-
- @raise WindowsError: Raises an exception on error.
- """
- if type(lpCmdLine) not in (str, compat.unicode):
- warnings.warn("Debug.execl expects a string")
-
- # Set the "debug" flag to True.
- kwargs['bDebug'] = True
-
- # Pop the "break on entry point" flag.
- bBreakOnEntryPoint = kwargs.pop('bBreakOnEntryPoint', False)
-
- # Set the default trust level if requested.
- if 'iTrustLevel' not in kwargs:
- if self.__bHostileCode:
- kwargs['iTrustLevel'] = 0
- else:
- kwargs['iTrustLevel'] = 2
-
- # Set the default UAC elevation flag if requested.
- if 'bAllowElevation' not in kwargs:
- kwargs['bAllowElevation'] = not self.__bHostileCode
-
- # In hostile mode the default parent process is explorer.exe.
- # Only supported for Windows Vista and above.
- if self.__bHostileCode and not kwargs.get('dwParentProcessId', None):
- try:
- vista_and_above = self.__vista_and_above
- except AttributeError:
- osi = win32.OSVERSIONINFOEXW()
- osi.dwMajorVersion = 6
- osi.dwMinorVersion = 0
- osi.dwPlatformId = win32.VER_PLATFORM_WIN32_NT
- mask = 0
- mask = win32.VerSetConditionMask(mask,
- win32.VER_MAJORVERSION,
- win32.VER_GREATER_EQUAL)
- mask = win32.VerSetConditionMask(mask,
- win32.VER_MAJORVERSION,
- win32.VER_GREATER_EQUAL)
- mask = win32.VerSetConditionMask(mask,
- win32.VER_PLATFORMID,
- win32.VER_EQUAL)
- vista_and_above = win32.VerifyVersionInfoW(osi,
- win32.VER_MAJORVERSION | \
- win32.VER_MINORVERSION | \
- win32.VER_PLATFORMID,
- mask)
- self.__vista_and_above = vista_and_above
- if vista_and_above:
- dwParentProcessId = self.system.get_explorer_pid()
- if dwParentProcessId:
- kwargs['dwParentProcessId'] = dwParentProcessId
- else:
- msg = ("Failed to find \"explorer.exe\"!"
- " Using the debugger as parent process.")
- warnings.warn(msg, RuntimeWarning)
-
- # Start the new process.
- aProcess = None
- try:
- aProcess = self.system.start_process(lpCmdLine, **kwargs)
- dwProcessId = aProcess.get_pid()
-
- # Match the system kill-on-exit flag to our own.
- self.__setSystemKillOnExitMode()
-
- # Warn when mixing 32 and 64 bits.
- # This also allows the user to stop attaching altogether,
- # depending on how the warnings are configured.
- if System.bits != aProcess.get_bits():
- msg = "Mixture of 32 and 64 bits is considered experimental." \
- " Use at your own risk!"
- warnings.warn(msg, MixedBitsWarning)
-
- # Add the new PID to the set of debugees.
- self.__startedDebugees.add(dwProcessId)
-
- # Add the new PID to the set of "break on EP" debugees if needed.
- if bBreakOnEntryPoint:
- self.__breakOnEP.add(dwProcessId)
-
- # Return the Process object.
- return aProcess
-
- # On error kill the new process and raise an exception.
- except:
- if aProcess is not None:
- try:
- try:
- self.__startedDebugees.remove(aProcess.get_pid())
- except KeyError:
- pass
- finally:
- try:
- try:
- self.__breakOnEP.remove(aProcess.get_pid())
- except KeyError:
- pass
- finally:
- try:
- aProcess.kill()
- except Exception:
- pass
- raise
-
- def add_existing_session(self, dwProcessId, bStarted = False):
- """
- Use this method only when for some reason the debugger's been attached
- to the target outside of WinAppDbg (for example when integrating with
- other tools).
-
- You don't normally need to call this method. Most users should call
- L{attach}, L{execv} or L{execl} instead.
-
- @type dwProcessId: int
- @param dwProcessId: Global process ID.
-
- @type bStarted: bool
- @param bStarted: C{True} if the process was started by the debugger,
- or C{False} if the process was attached to instead.
-
- @raise WindowsError: The target process does not exist, is not attached
- to the debugger anymore.
- """
-
- # Register the process object with the snapshot.
- if not self.system.has_process(dwProcessId):
- aProcess = Process(dwProcessId)
- self.system._add_process(aProcess)
- else:
- aProcess = self.system.get_process(dwProcessId)
-
- # Test for debug privileges on the target process.
- # Raises WindowsException on error.
- aProcess.get_handle()
-
- # Register the process ID with the debugger.
- if bStarted:
- self.__attachedDebugees.add(dwProcessId)
- else:
- self.__startedDebugees.add(dwProcessId)
-
- # Match the system kill-on-exit flag to our own.
- self.__setSystemKillOnExitMode()
-
- # Scan the process threads and loaded modules.
- # This is prefered because the thread and library events do not
- # properly give some information, like the filename for each module.
- aProcess.scan_threads()
- aProcess.scan_modules()
-
- def __cleanup_process(self, dwProcessId, bIgnoreExceptions = False):
- """
- Perform the necessary cleanup of a process about to be killed or
- detached from.
-
- This private method is called by L{kill} and L{detach}.
-
- @type dwProcessId: int
- @param dwProcessId: Global ID of a process to kill.
-
- @type bIgnoreExceptions: bool
- @param bIgnoreExceptions: C{True} to ignore any exceptions that may be
- raised when killing the process.
-
- @raise WindowsError: Raises an exception on error, unless
- C{bIgnoreExceptions} is C{True}.
- """
- # If the process is being debugged...
- if self.is_debugee(dwProcessId):
-
- # Make sure a Process object exists or the following calls fail.
- if not self.system.has_process(dwProcessId):
- aProcess = Process(dwProcessId)
- try:
- aProcess.get_handle()
- except WindowsError:
- pass # fails later on with more specific reason
- self.system._add_process(aProcess)
-
- # Erase all breakpoints in the process.
- try:
- self.erase_process_breakpoints(dwProcessId)
- except Exception:
- if not bIgnoreExceptions:
- raise
- e = sys.exc_info()[1]
- warnings.warn(str(e), RuntimeWarning)
-
- # Stop tracing all threads in the process.
- try:
- self.stop_tracing_process(dwProcessId)
- except Exception:
- if not bIgnoreExceptions:
- raise
- e = sys.exc_info()[1]
- warnings.warn(str(e), RuntimeWarning)
-
- # The process is no longer a debugee.
- try:
- if dwProcessId in self.__attachedDebugees:
- self.__attachedDebugees.remove(dwProcessId)
- if dwProcessId in self.__startedDebugees:
- self.__startedDebugees.remove(dwProcessId)
- except Exception:
- if not bIgnoreExceptions:
- raise
- e = sys.exc_info()[1]
- warnings.warn(str(e), RuntimeWarning)
-
- # Clear and remove the process from the snapshot.
- # If the user wants to do something with it after detaching
- # a new Process instance should be created.
- try:
- if self.system.has_process(dwProcessId):
- try:
- self.system.get_process(dwProcessId).clear()
- finally:
- self.system._del_process(dwProcessId)
- except Exception:
- if not bIgnoreExceptions:
- raise
- e = sys.exc_info()[1]
- warnings.warn(str(e), RuntimeWarning)
-
- # If the last debugging event is related to this process, forget it.
- try:
- if self.lastEvent and self.lastEvent.get_pid() == dwProcessId:
- self.lastEvent = None
- except Exception:
- if not bIgnoreExceptions:
- raise
- e = sys.exc_info()[1]
- warnings.warn(str(e), RuntimeWarning)
-
- def kill(self, dwProcessId, bIgnoreExceptions = False):
- """
- Kills a process currently being debugged.
-
- @see: L{detach}
-
- @type dwProcessId: int
- @param dwProcessId: Global ID of a process to kill.
-
- @type bIgnoreExceptions: bool
- @param bIgnoreExceptions: C{True} to ignore any exceptions that may be
- raised when killing the process.
-
- @raise WindowsError: Raises an exception on error, unless
- C{bIgnoreExceptions} is C{True}.
- """
-
- # Keep a reference to the process. We'll need it later.
- try:
- aProcess = self.system.get_process(dwProcessId)
- except KeyError:
- aProcess = Process(dwProcessId)
-
- # Cleanup all data referring to the process.
- self.__cleanup_process(dwProcessId,
- bIgnoreExceptions = bIgnoreExceptions)
-
- # Kill the process.
- try:
- try:
- if self.is_debugee(dwProcessId):
- try:
- if aProcess.is_alive():
- aProcess.suspend()
- finally:
- self.detach(dwProcessId,
- bIgnoreExceptions = bIgnoreExceptions)
- finally:
- aProcess.kill()
- except Exception:
- if not bIgnoreExceptions:
- raise
- e = sys.exc_info()[1]
- warnings.warn(str(e), RuntimeWarning)
-
- # Cleanup what remains of the process data.
- try:
- aProcess.clear()
- except Exception:
- if not bIgnoreExceptions:
- raise
- e = sys.exc_info()[1]
- warnings.warn(str(e), RuntimeWarning)
-
- def kill_all(self, bIgnoreExceptions = False):
- """
- Kills from all processes currently being debugged.
-
- @type bIgnoreExceptions: bool
- @param bIgnoreExceptions: C{True} to ignore any exceptions that may be
- raised when killing each process. C{False} to stop and raise an
- exception when encountering an error.
-
- @raise WindowsError: Raises an exception on error, unless
- C{bIgnoreExceptions} is C{True}.
- """
- for pid in self.get_debugee_pids():
- self.kill(pid, bIgnoreExceptions = bIgnoreExceptions)
-
- def detach(self, dwProcessId, bIgnoreExceptions = False):
- """
- Detaches from a process currently being debugged.
-
- @note: On Windows 2000 and below the process is killed.
-
- @see: L{attach}, L{detach_from_all}
-
- @type dwProcessId: int
- @param dwProcessId: Global ID of a process to detach from.
-
- @type bIgnoreExceptions: bool
- @param bIgnoreExceptions: C{True} to ignore any exceptions that may be
- raised when detaching. C{False} to stop and raise an exception when
- encountering an error.
-
- @raise WindowsError: Raises an exception on error, unless
- C{bIgnoreExceptions} is C{True}.
- """
-
- # Keep a reference to the process. We'll need it later.
- try:
- aProcess = self.system.get_process(dwProcessId)
- except KeyError:
- aProcess = Process(dwProcessId)
-
- # Determine if there is support for detaching.
- # This check should only fail on Windows 2000 and older.
- try:
- win32.DebugActiveProcessStop
- can_detach = True
- except AttributeError:
- can_detach = False
-
- # Continue the last event before detaching.
- # XXX not sure about this...
- try:
- if can_detach and self.lastEvent and \
- self.lastEvent.get_pid() == dwProcessId:
- self.cont(self.lastEvent)
- except Exception:
- if not bIgnoreExceptions:
- raise
- e = sys.exc_info()[1]
- warnings.warn(str(e), RuntimeWarning)
-
- # Cleanup all data referring to the process.
- self.__cleanup_process(dwProcessId,
- bIgnoreExceptions = bIgnoreExceptions)
-
- try:
- # Detach from the process.
- # On Windows 2000 and before, kill the process.
- if can_detach:
- try:
- win32.DebugActiveProcessStop(dwProcessId)
- except Exception:
- if not bIgnoreExceptions:
- raise
- e = sys.exc_info()[1]
- warnings.warn(str(e), RuntimeWarning)
- else:
- try:
- aProcess.kill()
- except Exception:
- if not bIgnoreExceptions:
- raise
- e = sys.exc_info()[1]
- warnings.warn(str(e), RuntimeWarning)
-
- finally:
-
- # Cleanup what remains of the process data.
- aProcess.clear()
-
- def detach_from_all(self, bIgnoreExceptions = False):
- """
- Detaches from all processes currently being debugged.
-
- @note: To better handle last debugging event, call L{stop} instead.
-
- @type bIgnoreExceptions: bool
- @param bIgnoreExceptions: C{True} to ignore any exceptions that may be
- raised when detaching.
-
- @raise WindowsError: Raises an exception on error, unless
- C{bIgnoreExceptions} is C{True}.
- """
- for pid in self.get_debugee_pids():
- self.detach(pid, bIgnoreExceptions = bIgnoreExceptions)
-
-#------------------------------------------------------------------------------
-
- def wait(self, dwMilliseconds = None):
- """
- Waits for the next debug event.
-
- @see: L{cont}, L{dispatch}, L{loop}
-
- @type dwMilliseconds: int
- @param dwMilliseconds: (Optional) Timeout in milliseconds.
- Use C{INFINITE} or C{None} for no timeout.
-
- @rtype: L{Event}
- @return: An event that occured in one of the debugees.
-
- @raise WindowsError: Raises an exception on error.
- If no target processes are left to debug,
- the error code is L{win32.ERROR_INVALID_HANDLE}.
- """
-
- # Wait for the next debug event.
- raw = win32.WaitForDebugEvent(dwMilliseconds)
- event = EventFactory.get(self, raw)
-
- # Remember it.
- self.lastEvent = event
-
- # Return it.
- return event
-
- def dispatch(self, event = None):
- """
- Calls the debug event notify callbacks.
-
- @see: L{cont}, L{loop}, L{wait}
-
- @type event: L{Event}
- @param event: (Optional) Event object returned by L{wait}.
-
- @raise WindowsError: Raises an exception on error.
- """
-
- # If no event object was given, use the last event.
- if event is None:
- event = self.lastEvent
-
- # Ignore dummy events.
- if not event:
- return
-
- # Determine the default behaviour for this event.
- # XXX HACK
- # Some undocumented flags are used, but as far as I know in those
- # versions of Windows that don't support them they should behave
- # like DGB_CONTINUE.
-
- code = event.get_event_code()
- if code == win32.EXCEPTION_DEBUG_EVENT:
-
- # At this point, by default some exception types are swallowed by
- # the debugger, because we don't know yet if it was caused by the
- # debugger itself or the debugged process.
- #
- # Later on (see breakpoint.py) if we determined the exception was
- # not caused directly by the debugger itself, we set the default
- # back to passing the exception to the debugee.
- #
- # The "invalid handle" exception is also swallowed by the debugger
- # because it's not normally generated by the debugee. But in
- # hostile mode we want to pass it to the debugee, as it may be the
- # result of an anti-debug trick. In that case it's best to disable
- # bad handles detection with Microsoft's gflags.exe utility. See:
- # http://msdn.microsoft.com/en-us/library/windows/hardware/ff549557(v=vs.85).aspx
-
- exc_code = event.get_exception_code()
- if exc_code in (
- win32.EXCEPTION_BREAKPOINT,
- win32.EXCEPTION_WX86_BREAKPOINT,
- win32.EXCEPTION_SINGLE_STEP,
- win32.EXCEPTION_GUARD_PAGE,
- ):
- event.continueStatus = win32.DBG_CONTINUE
- elif exc_code == win32.EXCEPTION_INVALID_HANDLE:
- if self.__bHostileCode:
- event.continueStatus = win32.DBG_EXCEPTION_NOT_HANDLED
- else:
- event.continueStatus = win32.DBG_CONTINUE
- else:
- event.continueStatus = win32.DBG_EXCEPTION_NOT_HANDLED
-
- elif code == win32.RIP_EVENT and \
- event.get_rip_type() == win32.SLE_ERROR:
-
- # RIP events that signal fatal events should kill the process.
- event.continueStatus = win32.DBG_TERMINATE_PROCESS
-
- else:
-
- # Other events need this continue code.
- # Sometimes other codes can be used and are ignored, sometimes not.
- # For example, when using the DBG_EXCEPTION_NOT_HANDLED code,
- # debug strings are sent twice (!)
- event.continueStatus = win32.DBG_CONTINUE
-
- # Dispatch the debug event.
- return EventDispatcher.dispatch(self, event)
-
- def cont(self, event = None):
- """
- Resumes execution after processing a debug event.
-
- @see: dispatch(), loop(), wait()
-
- @type event: L{Event}
- @param event: (Optional) Event object returned by L{wait}.
-
- @raise WindowsError: Raises an exception on error.
- """
-
- # If no event object was given, use the last event.
- if event is None:
- event = self.lastEvent
-
- # Ignore dummy events.
- if not event:
- return
-
- # Get the event continue status information.
- dwProcessId = event.get_pid()
- dwThreadId = event.get_tid()
- dwContinueStatus = event.continueStatus
-
- # Check if the process is still being debugged.
- if self.is_debugee(dwProcessId):
-
- # Try to flush the instruction cache.
- try:
- if self.system.has_process(dwProcessId):
- aProcess = self.system.get_process(dwProcessId)
- else:
- aProcess = Process(dwProcessId)
- aProcess.flush_instruction_cache()
- except WindowsError:
- pass
-
- # XXX TODO
- #
- # Try to execute the UnhandledExceptionFilter for second chance
- # exceptions, at least when in hostile mode (in normal mode it
- # would be breaking compatibility, as users may actually expect
- # second chance exceptions to be raised again).
- #
- # Reportedly in Windows 7 (maybe in Vista too) this seems to be
- # happening already. In XP and below the UnhandledExceptionFilter
- # was never called for processes being debugged.
-
- # Continue execution of the debugee.
- win32.ContinueDebugEvent(dwProcessId, dwThreadId, dwContinueStatus)
-
- # If the event is the last event, forget it.
- if event == self.lastEvent:
- self.lastEvent = None
-
- def stop(self, bIgnoreExceptions = True):
- """
- Stops debugging all processes.
-
- If the kill on exit mode is on, debugged processes are killed when the
- debugger is stopped. Otherwise when the debugger stops it detaches from
- all debugged processes and leaves them running (default). For more
- details see: L{__init__}
-
- @note: This method is better than L{detach_from_all} because it can
- gracefully handle the last debugging event before detaching.
-
- @type bIgnoreExceptions: bool
- @param bIgnoreExceptions: C{True} to ignore any exceptions that may be
- raised when detaching.
- """
-
- # Determine if we have a last debug event that we need to continue.
- try:
- event = self.lastEvent
- has_event = bool(event)
- except Exception:
- if not bIgnoreExceptions:
- raise
- e = sys.exc_info()[1]
- warnings.warn(str(e), RuntimeWarning)
- has_event = False
-
- # If we do...
- if has_event:
-
- # Disable all breakpoints in the process before resuming execution.
- try:
- pid = event.get_pid()
- self.disable_process_breakpoints(pid)
- except Exception:
- if not bIgnoreExceptions:
- raise
- e = sys.exc_info()[1]
- warnings.warn(str(e), RuntimeWarning)
-
- # Disable all breakpoints in the thread before resuming execution.
- try:
- tid = event.get_tid()
- self.disable_thread_breakpoints(tid)
- except Exception:
- if not bIgnoreExceptions:
- raise
- e = sys.exc_info()[1]
- warnings.warn(str(e), RuntimeWarning)
-
- # Resume execution.
- try:
- event.continueDebugEvent = win32.DBG_CONTINUE
- self.cont(event)
- except Exception:
- if not bIgnoreExceptions:
- raise
- e = sys.exc_info()[1]
- warnings.warn(str(e), RuntimeWarning)
-
- # Detach from or kill all debuggees.
- try:
- if self.__bKillOnExit:
- self.kill_all(bIgnoreExceptions)
- else:
- self.detach_from_all(bIgnoreExceptions)
- except Exception:
- if not bIgnoreExceptions:
- raise
- e = sys.exc_info()[1]
- warnings.warn(str(e), RuntimeWarning)
-
- # Cleanup the process snapshots.
- try:
- self.system.clear()
- except Exception:
- if not bIgnoreExceptions:
- raise
- e = sys.exc_info()[1]
- warnings.warn(str(e), RuntimeWarning)
-
- # Close all Win32 handles the Python garbage collector failed to close.
- self.force_garbage_collection(bIgnoreExceptions)
-
- def next(self):
- """
- Handles the next debug event.
-
- @see: L{cont}, L{dispatch}, L{wait}, L{stop}
-
- @raise WindowsError: Raises an exception on error.
-
- If the wait operation causes an error, debugging is stopped
- (meaning all debugees are either killed or detached from).
-
- If the event dispatching causes an error, the event is still
- continued before returning. This may happen, for example, if the
- event handler raises an exception nobody catches.
- """
- try:
- event = self.wait()
- except Exception:
- self.stop()
- raise
- try:
- self.dispatch()
- finally:
- self.cont()
-
- def loop(self):
- """
- Simple debugging loop.
-
- This debugging loop is meant to be useful for most simple scripts.
- It iterates as long as there is at least one debugee, or an exception
- is raised. Multiple calls are allowed.
-
- This is a trivial example script::
- import sys
- debug = Debug()
- try:
- debug.execv( sys.argv [ 1 : ] )
- debug.loop()
- finally:
- debug.stop()
-
- @see: L{next}, L{stop}
-
- U{http://msdn.microsoft.com/en-us/library/ms681675(VS.85).aspx}
-
- @raise WindowsError: Raises an exception on error.
-
- If the wait operation causes an error, debugging is stopped
- (meaning all debugees are either killed or detached from).
-
- If the event dispatching causes an error, the event is still
- continued before returning. This may happen, for example, if the
- event handler raises an exception nobody catches.
- """
- while self:
- self.next()
-
- def get_debugee_count(self):
- """
- @rtype: int
- @return: Number of processes being debugged.
- """
- return len(self.__attachedDebugees) + len(self.__startedDebugees)
-
- def get_debugee_pids(self):
- """
- @rtype: list( int... )
- @return: Global IDs of processes being debugged.
- """
- return list(self.__attachedDebugees) + list(self.__startedDebugees)
-
- def is_debugee(self, dwProcessId):
- """
- Determine if the debugger is debugging the given process.
-
- @see: L{is_debugee_attached}, L{is_debugee_started}
-
- @type dwProcessId: int
- @param dwProcessId: Process global ID.
-
- @rtype: bool
- @return: C{True} if the given process is being debugged
- by this L{Debug} instance.
- """
- return self.is_debugee_attached(dwProcessId) or \
- self.is_debugee_started(dwProcessId)
-
- def is_debugee_started(self, dwProcessId):
- """
- Determine if the given process was started by the debugger.
-
- @see: L{is_debugee}, L{is_debugee_attached}
-
- @type dwProcessId: int
- @param dwProcessId: Process global ID.
-
- @rtype: bool
- @return: C{True} if the given process was started for debugging by this
- L{Debug} instance.
- """
- return dwProcessId in self.__startedDebugees
-
- def is_debugee_attached(self, dwProcessId):
- """
- Determine if the debugger is attached to the given process.
-
- @see: L{is_debugee}, L{is_debugee_started}
-
- @type dwProcessId: int
- @param dwProcessId: Process global ID.
-
- @rtype: bool
- @return: C{True} if the given process is attached to this
- L{Debug} instance.
- """
- return dwProcessId in self.__attachedDebugees
-
- def in_hostile_mode(self):
- """
- Determine if we're in hostile mode (anti-anti-debug).
-
- @rtype: bool
- @return: C{True} if this C{Debug} instance was started in hostile mode,
- C{False} otherwise.
- """
- return self.__bHostileCode
-
-#------------------------------------------------------------------------------
-
- def interactive(self, bConfirmQuit = True, bShowBanner = True):
- """
- Start an interactive debugging session.
-
- @type bConfirmQuit: bool
- @param bConfirmQuit: Set to C{True} to ask the user for confirmation
- before closing the session, C{False} otherwise.
-
- @type bShowBanner: bool
- @param bShowBanner: Set to C{True} to show a banner before entering
- the session and after leaving it, C{False} otherwise.
-
- @warn: This will temporarily disable the user-defined event handler!
-
- This method returns when the user closes the session.
- """
- print('')
- print("-" * 79)
- print("Interactive debugging session started.")
- print("Use the \"help\" command to list all available commands.")
- print("Use the \"quit\" command to close this session.")
- print("-" * 79)
- if self.lastEvent is None:
- print('')
- console = ConsoleDebugger()
- console.confirm_quit = bConfirmQuit
- console.load_history()
- try:
- console.start_using_debugger(self)
- console.loop()
- finally:
- console.stop_using_debugger()
- console.save_history()
- print('')
- print("-" * 79)
- print("Interactive debugging session closed.")
- print("-" * 79)
- print('')
-
-#------------------------------------------------------------------------------
-
- @staticmethod
- def force_garbage_collection(bIgnoreExceptions = True):
- """
- Close all Win32 handles the Python garbage collector failed to close.
-
- @type bIgnoreExceptions: bool
- @param bIgnoreExceptions: C{True} to ignore any exceptions that may be
- raised when detaching.
- """
- try:
- import gc
- gc.collect()
- bRecollect = False
- for obj in list(gc.garbage):
- try:
- if isinstance(obj, win32.Handle):
- obj.close()
- elif isinstance(obj, Event):
- obj.debug = None
- elif isinstance(obj, Process):
- obj.clear()
- elif isinstance(obj, Thread):
- obj.set_process(None)
- obj.clear()
- elif isinstance(obj, Module):
- obj.set_process(None)
- elif isinstance(obj, Window):
- obj.set_process(None)
- else:
- continue
- gc.garbage.remove(obj)
- del obj
- bRecollect = True
- except Exception:
- if not bIgnoreExceptions:
- raise
- e = sys.exc_info()[1]
- warnings.warn(str(e), RuntimeWarning)
- if bRecollect:
- gc.collect()
- except Exception:
- if not bIgnoreExceptions:
- raise
- e = sys.exc_info()[1]
- warnings.warn(str(e), RuntimeWarning)
-
-#------------------------------------------------------------------------------
-
- def _notify_create_process(self, event):
- """
- Notify the creation of a new process.
-
- @warning: This method is meant to be used internally by the debugger.
-
- @type event: L{CreateProcessEvent}
- @param event: Create process event.
-
- @rtype: bool
- @return: C{True} to call the user-defined handle, C{False} otherwise.
- """
- dwProcessId = event.get_pid()
- if dwProcessId not in self.__attachedDebugees:
- if dwProcessId not in self.__startedDebugees:
- self.__startedDebugees.add(dwProcessId)
-
- retval = self.system._notify_create_process(event)
-
- # Set a breakpoint on the program's entry point if requested.
- # Try not to use the Event object's entry point value, as in some cases
- # it may be wrong. See: http://pferrie.host22.com/misc/lowlevel3.htm
- if dwProcessId in self.__breakOnEP:
- try:
- lpEntryPoint = event.get_process().get_entry_point()
- except Exception:
- lpEntryPoint = event.get_start_address()
-
- # It'd be best to use a hardware breakpoint instead, at least in
- # hostile mode. But since the main thread's context gets smashed
- # by the loader, I haven't found a way to make it work yet.
- self.break_at(dwProcessId, lpEntryPoint)
-
- # Defeat isDebuggerPresent by patching PEB->BeingDebugged.
- # When we do this, some debugging APIs cease to work as expected.
- # For example, the system breakpoint isn't hit when we attach.
- # For that reason we need to define a code breakpoint at the
- # code location where a new thread is spawned by the debugging
- # APIs, ntdll!DbgUiRemoteBreakin.
- if self.__bHostileCode:
- aProcess = event.get_process()
- try:
- hProcess = aProcess.get_handle(win32.PROCESS_QUERY_INFORMATION)
- pbi = win32.NtQueryInformationProcess(
- hProcess, win32.ProcessBasicInformation)
- ptr = pbi.PebBaseAddress + 2
- if aProcess.peek(ptr, 1) == '\x01':
- aProcess.poke(ptr, '\x00')
- except WindowsError:
- e = sys.exc_info()[1]
- warnings.warn(
- "Cannot patch PEB->BeingDebugged, reason: %s" % e.strerror)
-
- return retval
-
- def _notify_create_thread(self, event):
- """
- Notify the creation of a new thread.
-
- @warning: This method is meant to be used internally by the debugger.
-
- @type event: L{CreateThreadEvent}
- @param event: Create thread event.
-
- @rtype: bool
- @return: C{True} to call the user-defined handle, C{False} otherwise.
- """
- return event.get_process()._notify_create_thread(event)
-
- def _notify_load_dll(self, event):
- """
- Notify the load of a new module.
-
- @warning: This method is meant to be used internally by the debugger.
-
- @type event: L{LoadDLLEvent}
- @param event: Load DLL event.
-
- @rtype: bool
- @return: C{True} to call the user-defined handle, C{False} otherwise.
- """
-
- # Pass the event to the breakpoint container.
- bCallHandler = _BreakpointContainer._notify_load_dll(self, event)
-
- # Get the process where the DLL was loaded.
- aProcess = event.get_process()
-
- # Pass the event to the process.
- bCallHandler = aProcess._notify_load_dll(event) and bCallHandler
-
- # Anti-anti-debugging tricks on ntdll.dll.
- if self.__bHostileCode:
- aModule = event.get_module()
- if aModule.match_name('ntdll.dll'):
-
- # Since we've overwritten the PEB to hide
- # ourselves, we no longer have the system
- # breakpoint when attaching to the process.
- # Set a breakpoint at ntdll!DbgUiRemoteBreakin
- # instead (that's where the debug API spawns
- # it's auxiliary threads). This also defeats
- # a simple anti-debugging trick: the hostile
- # process could have overwritten the int3
- # instruction at the system breakpoint.
- self.break_at(aProcess.get_pid(),
- aProcess.resolve_label('ntdll!DbgUiRemoteBreakin'))
-
- return bCallHandler
-
- def _notify_exit_process(self, event):
- """
- Notify the termination of a process.
-
- @warning: This method is meant to be used internally by the debugger.
-
- @type event: L{ExitProcessEvent}
- @param event: Exit process event.
-
- @rtype: bool
- @return: C{True} to call the user-defined handle, C{False} otherwise.
- """
- bCallHandler1 = _BreakpointContainer._notify_exit_process(self, event)
- bCallHandler2 = self.system._notify_exit_process(event)
-
- try:
- self.detach( event.get_pid() )
- except WindowsError:
- e = sys.exc_info()[1]
- if e.winerror != win32.ERROR_INVALID_PARAMETER:
- warnings.warn(
- "Failed to detach from dead process, reason: %s" % str(e),
- RuntimeWarning)
- except Exception:
- e = sys.exc_info()[1]
- warnings.warn(
- "Failed to detach from dead process, reason: %s" % str(e),
- RuntimeWarning)
-
- return bCallHandler1 and bCallHandler2
-
- def _notify_exit_thread(self, event):
- """
- Notify the termination of a thread.
-
- @warning: This method is meant to be used internally by the debugger.
-
- @type event: L{ExitThreadEvent}
- @param event: Exit thread event.
-
- @rtype: bool
- @return: C{True} to call the user-defined handle, C{False} otherwise.
- """
- bCallHandler1 = _BreakpointContainer._notify_exit_thread(self, event)
- bCallHandler2 = event.get_process()._notify_exit_thread(event)
- return bCallHandler1 and bCallHandler2
-
- def _notify_unload_dll(self, event):
- """
- Notify the unload of a module.
-
- @warning: This method is meant to be used internally by the debugger.
-
- @type event: L{UnloadDLLEvent}
- @param event: Unload DLL event.
-
- @rtype: bool
- @return: C{True} to call the user-defined handle, C{False} otherwise.
- """
- bCallHandler1 = _BreakpointContainer._notify_unload_dll(self, event)
- bCallHandler2 = event.get_process()._notify_unload_dll(event)
- return bCallHandler1 and bCallHandler2
-
- def _notify_rip(self, event):
- """
- Notify of a RIP event.
-
- @warning: This method is meant to be used internally by the debugger.
-
- @type event: L{RIPEvent}
- @param event: RIP event.
-
- @rtype: bool
- @return: C{True} to call the user-defined handle, C{False} otherwise.
- """
- event.debug.detach( event.get_pid() )
- return True
-
- def _notify_debug_control_c(self, event):
- """
- Notify of a Debug Ctrl-C exception.
-
- @warning: This method is meant to be used internally by the debugger.
-
- @note: This exception is only raised when a debugger is attached, and
- applications are not supposed to handle it, so we need to handle it
- ourselves or the application may crash.
-
- @see: U{http://msdn.microsoft.com/en-us/library/aa363082(VS.85).aspx}
-
- @type event: L{ExceptionEvent}
- @param event: Debug Ctrl-C exception event.
-
- @rtype: bool
- @return: C{True} to call the user-defined handle, C{False} otherwise.
- """
- if event.is_first_chance():
- event.continueStatus = win32.DBG_EXCEPTION_HANDLED
- return True
-
- def _notify_ms_vc_exception(self, event):
- """
- Notify of a Microsoft Visual C exception.
-
- @warning: This method is meant to be used internally by the debugger.
-
- @note: This allows the debugger to understand the
- Microsoft Visual C thread naming convention.
-
- @see: U{http://msdn.microsoft.com/en-us/library/xcb2z8hs.aspx}
-
- @type event: L{ExceptionEvent}
- @param event: Microsoft Visual C exception event.
-
- @rtype: bool
- @return: C{True} to call the user-defined handle, C{False} otherwise.
- """
- dwType = event.get_exception_information(0)
- if dwType == 0x1000:
- pszName = event.get_exception_information(1)
- dwThreadId = event.get_exception_information(2)
- dwFlags = event.get_exception_information(3)
-
- aProcess = event.get_process()
- szName = aProcess.peek_string(pszName, fUnicode = False)
- if szName:
-
- if dwThreadId == -1:
- dwThreadId = event.get_tid()
-
- if aProcess.has_thread(dwThreadId):
- aThread = aProcess.get_thread(dwThreadId)
- else:
- aThread = Thread(dwThreadId)
- aProcess._add_thread(aThread)
-
-## if aThread.get_name() is None:
-## aThread.set_name(szName)
- aThread.set_name(szName)
-
- return True
diff --git a/spaces/Superlang/ImageProcessor/annotator/uniformer/mmseg/datasets/pipelines/formating.py b/spaces/Superlang/ImageProcessor/annotator/uniformer/mmseg/datasets/pipelines/formating.py
deleted file mode 100644
index 97db85f4f9db39fb86ba77ead7d1a8407d810adb..0000000000000000000000000000000000000000
--- a/spaces/Superlang/ImageProcessor/annotator/uniformer/mmseg/datasets/pipelines/formating.py
+++ /dev/null
@@ -1,288 +0,0 @@
-from collections.abc import Sequence
-
-import annotator.uniformer.mmcv as mmcv
-import numpy as np
-import torch
-from annotator.uniformer.mmcv.parallel import DataContainer as DC
-
-from ..builder import PIPELINES
-
-
-def to_tensor(data):
- """Convert objects of various python types to :obj:`torch.Tensor`.
-
- Supported types are: :class:`numpy.ndarray`, :class:`torch.Tensor`,
- :class:`Sequence`, :class:`int` and :class:`float`.
-
- Args:
- data (torch.Tensor | numpy.ndarray | Sequence | int | float): Data to
- be converted.
- """
-
- if isinstance(data, torch.Tensor):
- return data
- elif isinstance(data, np.ndarray):
- return torch.from_numpy(data)
- elif isinstance(data, Sequence) and not mmcv.is_str(data):
- return torch.tensor(data)
- elif isinstance(data, int):
- return torch.LongTensor([data])
- elif isinstance(data, float):
- return torch.FloatTensor([data])
- else:
- raise TypeError(f'type {type(data)} cannot be converted to tensor.')
-
-
-@PIPELINES.register_module()
-class ToTensor(object):
- """Convert some results to :obj:`torch.Tensor` by given keys.
-
- Args:
- keys (Sequence[str]): Keys that need to be converted to Tensor.
- """
-
- def __init__(self, keys):
- self.keys = keys
-
- def __call__(self, results):
- """Call function to convert data in results to :obj:`torch.Tensor`.
-
- Args:
- results (dict): Result dict contains the data to convert.
-
- Returns:
- dict: The result dict contains the data converted
- to :obj:`torch.Tensor`.
- """
-
- for key in self.keys:
- results[key] = to_tensor(results[key])
- return results
-
- def __repr__(self):
- return self.__class__.__name__ + f'(keys={self.keys})'
-
-
-@PIPELINES.register_module()
-class ImageToTensor(object):
- """Convert image to :obj:`torch.Tensor` by given keys.
-
- The dimension order of input image is (H, W, C). The pipeline will convert
- it to (C, H, W). If only 2 dimension (H, W) is given, the output would be
- (1, H, W).
-
- Args:
- keys (Sequence[str]): Key of images to be converted to Tensor.
- """
-
- def __init__(self, keys):
- self.keys = keys
-
- def __call__(self, results):
- """Call function to convert image in results to :obj:`torch.Tensor` and
- transpose the channel order.
-
- Args:
- results (dict): Result dict contains the image data to convert.
-
- Returns:
- dict: The result dict contains the image converted
- to :obj:`torch.Tensor` and transposed to (C, H, W) order.
- """
-
- for key in self.keys:
- img = results[key]
- if len(img.shape) < 3:
- img = np.expand_dims(img, -1)
- results[key] = to_tensor(img.transpose(2, 0, 1))
- return results
-
- def __repr__(self):
- return self.__class__.__name__ + f'(keys={self.keys})'
-
-
-@PIPELINES.register_module()
-class Transpose(object):
- """Transpose some results by given keys.
-
- Args:
- keys (Sequence[str]): Keys of results to be transposed.
- order (Sequence[int]): Order of transpose.
- """
-
- def __init__(self, keys, order):
- self.keys = keys
- self.order = order
-
- def __call__(self, results):
- """Call function to convert image in results to :obj:`torch.Tensor` and
- transpose the channel order.
-
- Args:
- results (dict): Result dict contains the image data to convert.
-
- Returns:
- dict: The result dict contains the image converted
- to :obj:`torch.Tensor` and transposed to (C, H, W) order.
- """
-
- for key in self.keys:
- results[key] = results[key].transpose(self.order)
- return results
-
- def __repr__(self):
- return self.__class__.__name__ + \
- f'(keys={self.keys}, order={self.order})'
-
-
-@PIPELINES.register_module()
-class ToDataContainer(object):
- """Convert results to :obj:`mmcv.DataContainer` by given fields.
-
- Args:
- fields (Sequence[dict]): Each field is a dict like
- ``dict(key='xxx', **kwargs)``. The ``key`` in result will
- be converted to :obj:`mmcv.DataContainer` with ``**kwargs``.
- Default: ``(dict(key='img', stack=True),
- dict(key='gt_semantic_seg'))``.
- """
-
- def __init__(self,
- fields=(dict(key='img',
- stack=True), dict(key='gt_semantic_seg'))):
- self.fields = fields
-
- def __call__(self, results):
- """Call function to convert data in results to
- :obj:`mmcv.DataContainer`.
-
- Args:
- results (dict): Result dict contains the data to convert.
-
- Returns:
- dict: The result dict contains the data converted to
- :obj:`mmcv.DataContainer`.
- """
-
- for field in self.fields:
- field = field.copy()
- key = field.pop('key')
- results[key] = DC(results[key], **field)
- return results
-
- def __repr__(self):
- return self.__class__.__name__ + f'(fields={self.fields})'
-
-
-@PIPELINES.register_module()
-class DefaultFormatBundle(object):
- """Default formatting bundle.
-
- It simplifies the pipeline of formatting common fields, including "img"
- and "gt_semantic_seg". These fields are formatted as follows.
-
- - img: (1)transpose, (2)to tensor, (3)to DataContainer (stack=True)
- - gt_semantic_seg: (1)unsqueeze dim-0 (2)to tensor,
- (3)to DataContainer (stack=True)
- """
-
- def __call__(self, results):
- """Call function to transform and format common fields in results.
-
- Args:
- results (dict): Result dict contains the data to convert.
-
- Returns:
- dict: The result dict contains the data that is formatted with
- default bundle.
- """
-
- if 'img' in results:
- img = results['img']
- if len(img.shape) < 3:
- img = np.expand_dims(img, -1)
- img = np.ascontiguousarray(img.transpose(2, 0, 1))
- results['img'] = DC(to_tensor(img), stack=True)
- if 'gt_semantic_seg' in results:
- # convert to long
- results['gt_semantic_seg'] = DC(
- to_tensor(results['gt_semantic_seg'][None,
- ...].astype(np.int64)),
- stack=True)
- return results
-
- def __repr__(self):
- return self.__class__.__name__
-
-
-@PIPELINES.register_module()
-class Collect(object):
- """Collect data from the loader relevant to the specific task.
-
- This is usually the last stage of the data loader pipeline. Typically keys
- is set to some subset of "img", "gt_semantic_seg".
-
- The "img_meta" item is always populated. The contents of the "img_meta"
- dictionary depends on "meta_keys". By default this includes:
-
- - "img_shape": shape of the image input to the network as a tuple
- (h, w, c). Note that images may be zero padded on the bottom/right
- if the batch tensor is larger than this shape.
-
- - "scale_factor": a float indicating the preprocessing scale
-
- - "flip": a boolean indicating if image flip transform was used
-
- - "filename": path to the image file
-
- - "ori_shape": original shape of the image as a tuple (h, w, c)
-
- - "pad_shape": image shape after padding
-
- - "img_norm_cfg": a dict of normalization information:
- - mean - per channel mean subtraction
- - std - per channel std divisor
- - to_rgb - bool indicating if bgr was converted to rgb
-
- Args:
- keys (Sequence[str]): Keys of results to be collected in ``data``.
- meta_keys (Sequence[str], optional): Meta keys to be converted to
- ``mmcv.DataContainer`` and collected in ``data[img_metas]``.
- Default: ``('filename', 'ori_filename', 'ori_shape', 'img_shape',
- 'pad_shape', 'scale_factor', 'flip', 'flip_direction',
- 'img_norm_cfg')``
- """
-
- def __init__(self,
- keys,
- meta_keys=('filename', 'ori_filename', 'ori_shape',
- 'img_shape', 'pad_shape', 'scale_factor', 'flip',
- 'flip_direction', 'img_norm_cfg')):
- self.keys = keys
- self.meta_keys = meta_keys
-
- def __call__(self, results):
- """Call function to collect keys in results. The keys in ``meta_keys``
- will be converted to :obj:mmcv.DataContainer.
-
- Args:
- results (dict): Result dict contains the data to collect.
-
- Returns:
- dict: The result dict contains the following keys
- - keys in``self.keys``
- - ``img_metas``
- """
-
- data = {}
- img_meta = {}
- for key in self.meta_keys:
- img_meta[key] = results[key]
- data['img_metas'] = DC(img_meta, cpu_only=True)
- for key in self.keys:
- data[key] = results[key]
- return data
-
- def __repr__(self):
- return self.__class__.__name__ + \
- f'(keys={self.keys}, meta_keys={self.meta_keys})'
diff --git a/spaces/TNR-5/test_dev_s/style.css b/spaces/TNR-5/test_dev_s/style.css
deleted file mode 100644
index 114adf441e9032febb46bc056b2a8bb651075f0d..0000000000000000000000000000000000000000
--- a/spaces/TNR-5/test_dev_s/style.css
+++ /dev/null
@@ -1,28 +0,0 @@
-body {
- padding: 2rem;
- font-family: -apple-system, BlinkMacSystemFont, "Arial", sans-serif;
-}
-
-h1 {
- font-size: 16px;
- margin-top: 0;
-}
-
-p {
- color: rgb(107, 114, 128);
- font-size: 15px;
- margin-bottom: 10px;
- margin-top: 5px;
-}
-
-.card {
- max-width: 620px;
- margin: 0 auto;
- padding: 16px;
- border: 1px solid lightgray;
- border-radius: 16px;
-}
-
-.card p:last-child {
- margin-bottom: 0;
-}
diff --git a/spaces/Taha07/pneumonia-detection-WebApp/app.py b/spaces/Taha07/pneumonia-detection-WebApp/app.py
deleted file mode 100644
index 6ec97a8df0850f1e33e4786309644617a2cc6744..0000000000000000000000000000000000000000
--- a/spaces/Taha07/pneumonia-detection-WebApp/app.py
+++ /dev/null
@@ -1,16 +0,0 @@
-import gradio as gr
-import tensorflow as tf
-from tensorflow import keras
-
-model = keras.models.load_model("pneumonia.h5")
-class_names = ["Normal","Pneumonia"]
-def predict_image(img):
- img_4d=img.reshape(-1,224,224,3)
- prediction=model.predict(img_4d)[0]
- return {class_names[i]: float(prediction[i]) for i in range(2)}
-
-
-image = gr.inputs.Image(shape=(224,224))
-label = gr.outputs.Label(num_top_classes=2)
-
-gr.Interface(fn=predict_image, inputs=image, outputs=label,interpretation='default').launch(inline = False)
\ No newline at end of file
diff --git a/spaces/TencentARC/VLog/models/grit_src/third_party/CenterNet2/.github/workflows/levenshtein.js b/spaces/TencentARC/VLog/models/grit_src/third_party/CenterNet2/.github/workflows/levenshtein.js
deleted file mode 100644
index 67a5e3613c0072d124035ee8933a23de2105cfe3..0000000000000000000000000000000000000000
--- a/spaces/TencentARC/VLog/models/grit_src/third_party/CenterNet2/.github/workflows/levenshtein.js
+++ /dev/null
@@ -1,44 +0,0 @@
-/*
-Copyright (c) 2011 Andrei Mackenzie
-
-Permission is hereby granted, free of charge, to any person obtaining a copy of this software and associated documentation files (the "Software"), to deal in the Software without restriction, including without limitation the rights to use, copy, modify, merge, publish, distribute, sublicense, and/or sell copies of the Software, and to permit persons to whom the Software is furnished to do so, subject to the following conditions:
-
-The above copyright notice and this permission notice shall be included in all copies or substantial portions of the Software.
-
-THE SOFTWARE IS PROVIDED "AS IS", WITHOUT WARRANTY OF ANY KIND, EXPRESS OR IMPLIED, INCLUDING BUT NOT LIMITED TO THE WARRANTIES OF MERCHANTABILITY, FITNESS FOR A PARTICULAR PURPOSE AND NONINFRINGEMENT. IN NO EVENT SHALL THE AUTHORS OR COPYRIGHT HOLDERS BE LIABLE FOR ANY CLAIM, DAMAGES OR OTHER LIABILITY, WHETHER IN AN ACTION OF CONTRACT, TORT OR OTHERWISE, ARISING FROM, OUT OF OR IN CONNECTION WITH THE SOFTWARE OR THE USE OR OTHER DEALINGS IN THE SOFTWARE.
-*/
-
-// Compute the edit distance between the two given strings
-exports.getEditDistance = function(a, b){
- if(a.length == 0) return b.length;
- if(b.length == 0) return a.length;
-
- var matrix = [];
-
- // increment along the first column of each row
- var i;
- for(i = 0; i <= b.length; i++){
- matrix[i] = [i];
- }
-
- // increment each column in the first row
- var j;
- for(j = 0; j <= a.length; j++){
- matrix[0][j] = j;
- }
-
- // Fill in the rest of the matrix
- for(i = 1; i <= b.length; i++){
- for(j = 1; j <= a.length; j++){
- if(b.charAt(i-1) == a.charAt(j-1)){
- matrix[i][j] = matrix[i-1][j-1];
- } else {
- matrix[i][j] = Math.min(matrix[i-1][j-1] + 1, // substitution
- Math.min(matrix[i][j-1] + 1, // insertion
- matrix[i-1][j] + 1)); // deletion
- }
- }
- }
-
- return matrix[b.length][a.length];
-};
diff --git a/spaces/TencentARC/VLog/models/grit_src/third_party/CenterNet2/tests/layers/test_roi_align.py b/spaces/TencentARC/VLog/models/grit_src/third_party/CenterNet2/tests/layers/test_roi_align.py
deleted file mode 100644
index b6fd8edefd107b727e3e523f1364fea1f4a20576..0000000000000000000000000000000000000000
--- a/spaces/TencentARC/VLog/models/grit_src/third_party/CenterNet2/tests/layers/test_roi_align.py
+++ /dev/null
@@ -1,210 +0,0 @@
-# Copyright (c) Facebook, Inc. and its affiliates.
-import numpy as np
-import unittest
-from copy import copy
-import cv2
-import torch
-from fvcore.common.benchmark import benchmark
-from torch.nn import functional as F
-
-from detectron2.layers.roi_align import ROIAlign, roi_align
-
-
-class ROIAlignTest(unittest.TestCase):
- def test_forward_output(self):
- input = np.arange(25).reshape(5, 5).astype("float32")
- """
- 0 1 2 3 4
- 5 6 7 8 9
- 10 11 12 13 14
- 15 16 17 18 19
- 20 21 22 23 24
- """
-
- output = self._simple_roialign(input, [1, 1, 3, 3], (4, 4), aligned=False)
- output_correct = self._simple_roialign(input, [1, 1, 3, 3], (4, 4), aligned=True)
-
- # without correction:
- old_results = [
- [7.5, 8, 8.5, 9],
- [10, 10.5, 11, 11.5],
- [12.5, 13, 13.5, 14],
- [15, 15.5, 16, 16.5],
- ]
-
- # with 0.5 correction:
- correct_results = [
- [4.5, 5.0, 5.5, 6.0],
- [7.0, 7.5, 8.0, 8.5],
- [9.5, 10.0, 10.5, 11.0],
- [12.0, 12.5, 13.0, 13.5],
- ]
- # This is an upsampled version of [[6, 7], [11, 12]]
-
- self.assertTrue(np.allclose(output.flatten(), np.asarray(old_results).flatten()))
- self.assertTrue(
- np.allclose(output_correct.flatten(), np.asarray(correct_results).flatten())
- )
-
- # Also see similar issues in tensorflow at
- # https://github.com/tensorflow/tensorflow/issues/26278
-
- def test_resize(self):
- H, W = 30, 30
- input = np.random.rand(H, W).astype("float32") * 100
- box = [10, 10, 20, 20]
- output = self._simple_roialign(input, box, (5, 5), aligned=True)
-
- input2x = cv2.resize(input, (W // 2, H // 2), interpolation=cv2.INTER_LINEAR)
- box2x = [x / 2 for x in box]
- output2x = self._simple_roialign(input2x, box2x, (5, 5), aligned=True)
- diff = np.abs(output2x - output)
- self.assertTrue(diff.max() < 1e-4)
-
- def test_grid_sample_equivalence(self):
- H, W = 30, 30
- input = np.random.rand(H, W).astype("float32") * 100
- box = [10, 10, 20, 20]
- for ratio in [1, 2, 3]:
- output = self._simple_roialign(input, box, (5, 5), sampling_ratio=ratio)
- output_grid_sample = grid_sample_roi_align(
- torch.from_numpy(input[None, None, :, :]).float(),
- torch.as_tensor(box).float()[None, :],
- 5,
- 1.0,
- ratio,
- )
- self.assertTrue(torch.allclose(output, output_grid_sample))
-
- def _simple_roialign(self, img, box, resolution, sampling_ratio=0, aligned=True):
- """
- RoiAlign with scale 1.0.
- """
- if isinstance(resolution, int):
- resolution = (resolution, resolution)
- op = ROIAlign(resolution, 1.0, sampling_ratio, aligned=aligned)
- input = torch.from_numpy(img[None, None, :, :].astype("float32"))
-
- rois = [0] + list(box)
- rois = torch.from_numpy(np.asarray(rois)[None, :].astype("float32"))
- output = op.forward(input, rois)
- if torch.cuda.is_available():
- output_cuda = op.forward(input.cuda(), rois.cuda()).cpu()
- self.assertTrue(torch.allclose(output, output_cuda))
- return output[0, 0]
-
- def _simple_roialign_with_grad(self, img, box, resolution, device):
- if isinstance(resolution, int):
- resolution = (resolution, resolution)
-
- op = ROIAlign(resolution, 1.0, 0, aligned=True)
- input = torch.from_numpy(img[None, None, :, :].astype("float32"))
-
- rois = [0] + list(box)
- rois = torch.from_numpy(np.asarray(rois)[None, :].astype("float32"))
- input = input.to(device=device)
- rois = rois.to(device=device)
- input.requires_grad = True
- output = op.forward(input, rois)
- return input, output
-
- def test_empty_box(self):
- img = np.random.rand(5, 5)
- box = [3, 4, 5, 4]
- o = self._simple_roialign(img, box, 7)
- self.assertTrue(o.shape == (7, 7))
- self.assertTrue((o == 0).all())
-
- for dev in ["cpu"] + ["cuda"] if torch.cuda.is_available() else []:
- input, output = self._simple_roialign_with_grad(img, box, 7, torch.device(dev))
- output.sum().backward()
- self.assertTrue(torch.allclose(input.grad, torch.zeros_like(input)))
-
- def test_empty_batch(self):
- input = torch.zeros(0, 3, 10, 10, dtype=torch.float32)
- rois = torch.zeros(0, 5, dtype=torch.float32)
- op = ROIAlign((7, 7), 1.0, 0, aligned=True)
- output = op.forward(input, rois)
- self.assertTrue(output.shape == (0, 3, 7, 7))
-
-
-def grid_sample_roi_align(input, boxes, output_size, scale, sampling_ratio):
- # unlike true roi_align, this does not support different batch_idx
- from detectron2.projects.point_rend.point_features import (
- generate_regular_grid_point_coords,
- get_point_coords_wrt_image,
- point_sample,
- )
-
- N, _, H, W = input.shape
- R = len(boxes)
- assert N == 1
- boxes = boxes * scale
- grid = generate_regular_grid_point_coords(R, output_size * sampling_ratio, device=boxes.device)
- coords = get_point_coords_wrt_image(boxes, grid)
- coords = coords / torch.as_tensor([W, H], device=coords.device) # R, s^2, 2
- res = point_sample(input, coords.unsqueeze(0), align_corners=False) # 1,C, R,s^2
- res = (
- res.squeeze(0)
- .permute(1, 0, 2)
- .reshape(R, -1, output_size * sampling_ratio, output_size * sampling_ratio)
- )
- res = F.avg_pool2d(res, sampling_ratio)
- return res
-
-
-def benchmark_roi_align():
- def random_boxes(mean_box, stdev, N, maxsize):
- ret = torch.rand(N, 4) * stdev + torch.tensor(mean_box, dtype=torch.float)
- ret.clamp_(min=0, max=maxsize)
- return ret
-
- def func(shape, nboxes_per_img, sampling_ratio, device, box_size="large"):
- N, _, H, _ = shape
- input = torch.rand(*shape)
- boxes = []
- batch_idx = []
- for k in range(N):
- if box_size == "large":
- b = random_boxes([80, 80, 130, 130], 24, nboxes_per_img, H)
- else:
- b = random_boxes([100, 100, 110, 110], 4, nboxes_per_img, H)
- boxes.append(b)
- batch_idx.append(torch.zeros(nboxes_per_img, 1, dtype=torch.float32) + k)
- boxes = torch.cat(boxes, axis=0)
- batch_idx = torch.cat(batch_idx, axis=0)
- boxes = torch.cat([batch_idx, boxes], axis=1)
-
- input = input.to(device=device)
- boxes = boxes.to(device=device)
-
- def bench():
- if False and sampling_ratio > 0 and N == 1:
- # enable to benchmark grid_sample (slower)
- grid_sample_roi_align(input, boxes[:, 1:], 7, 1.0, sampling_ratio)
- else:
- roi_align(input, boxes, 7, 1.0, sampling_ratio, True)
- if device == "cuda":
- torch.cuda.synchronize()
-
- return bench
-
- def gen_args(arg):
- args = []
- for size in ["small", "large"]:
- for ratio in [0, 2]:
- args.append(copy(arg))
- args[-1]["sampling_ratio"] = ratio
- args[-1]["box_size"] = size
- return args
-
- arg = dict(shape=(1, 512, 256, 256), nboxes_per_img=512, device="cuda")
- benchmark(func, "cuda_roialign", gen_args(arg), num_iters=20, warmup_iters=1)
- arg.update({"device": "cpu", "shape": (1, 256, 128, 128)})
- benchmark(func, "cpu_roialign", gen_args(arg), num_iters=5, warmup_iters=1)
-
-
-if __name__ == "__main__":
- if torch.cuda.is_available():
- benchmark_roi_align()
- unittest.main()
diff --git a/spaces/Thafx/sdrv20/app.py b/spaces/Thafx/sdrv20/app.py
deleted file mode 100644
index 5e824de9146920de26867d39b2f5fa980ac7d813..0000000000000000000000000000000000000000
--- a/spaces/Thafx/sdrv20/app.py
+++ /dev/null
@@ -1,189 +0,0 @@
-from diffusers import StableDiffusionPipeline, StableDiffusionImg2ImgPipeline, DPMSolverMultistepScheduler
-import gradio as gr
-import torch
-from PIL import Image
-
-model_id = 'SG161222/Realistic_Vision_V2.0'
-prefix = 'RAW photo,'
-
-scheduler = DPMSolverMultistepScheduler.from_pretrained(model_id, subfolder="scheduler")
-
-pipe = StableDiffusionPipeline.from_pretrained(
- model_id,
- torch_dtype=torch.float16 if torch.cuda.is_available() else torch.float32,
- scheduler=scheduler)
-
-pipe_i2i = StableDiffusionImg2ImgPipeline.from_pretrained(
- model_id,
- torch_dtype=torch.float16 if torch.cuda.is_available() else torch.float32,
- scheduler=scheduler)
-
-if torch.cuda.is_available():
- pipe = pipe.to("cuda")
- pipe_i2i = pipe_i2i.to("cuda")
-
-def error_str(error, title="Error"):
- return f"""#### {title}
- {error}""" if error else ""
-
-
-def _parse_args(prompt, generator):
- parser = argparse.ArgumentParser(
- description="making it work."
- )
- parser.add_argument(
- "--no-half-vae", help="no half vae"
- )
-
- cmdline_args = parser.parse_args()
- command = cmdline_args.command
- conf_file = cmdline_args.conf_file
- conf_args = Arguments(conf_file)
- opt = conf_args.readArguments()
-
- if cmdline_args.config_overrides:
- for config_override in cmdline_args.config_overrides.split(";"):
- config_override = config_override.strip()
- if config_override:
- var_val = config_override.split("=")
- assert (
- len(var_val) == 2
- ), f"Config override '{var_val}' does not have the form 'VAR=val'"
- conf_args.add_opt(opt, var_val[0], var_val[1], force_override=True)
-
-def inference(prompt, guidance, steps, width=512, height=512, seed=0, img=None, strength=0.5, neg_prompt="", auto_prefix=False):
- generator = torch.Generator('cuda').manual_seed(seed) if seed != 0 else None
- prompt = f"{prefix} {prompt}" if auto_prefix else prompt
-
- try:
- if img is not None:
- return img_to_img(prompt, neg_prompt, img, strength, guidance, steps, width, height, generator), None
- else:
- return txt_to_img(prompt, neg_prompt, guidance, steps, width, height, generator), None
- except Exception as e:
- return None, error_str(e)
-
-
-
-def txt_to_img(prompt, neg_prompt, guidance, steps, width, height, generator):
-
- result = pipe(
- prompt,
- negative_prompt = neg_prompt,
- num_inference_steps = int(steps),
- guidance_scale = guidance,
- width = width,
- height = height,
- generator = generator)
-
- return result.images[0]
-
-def img_to_img(prompt, neg_prompt, img, strength, guidance, steps, width, height, generator):
-
- ratio = min(height / img.height, width / img.width)
- img = img.resize((int(img.width * ratio), int(img.height * ratio)), Image.LANCZOS)
- result = pipe_i2i(
- prompt,
- negative_prompt = neg_prompt,
- init_image = img,
- num_inference_steps = int(steps),
- strength = strength,
- guidance_scale = guidance,
- width = width,
- height = height,
- generator = generator)
-
- return result.images[0]
-
- def fake_safety_checker(images, **kwargs):
- return result.images[0], [False] * len(images)
-
- pipe.safety_checker = fake_safety_checker
-
-css = """.main-div div{display:inline-flex;align-items:center;gap:.8rem;font-size:1.75rem}.main-div div h1{font-weight:900;margin-bottom:7px}.main-div p{margin-bottom:10px;font-size:94%}a{text-decoration:underline}.tabs{margin-top:0;margin-bottom:0}#gallery{min-height:20rem}
-"""
-with gr.Blocks(css=css) as demo:
- gr.HTML(
- f"""
-
-
-
📷 Realistic Vision V2.0 📸
-
-
- Demo for Realistic Vision V2.0
- Stable Diffusion model by Eugene. {"" if prefix else ""}
- Running on {"GPU 🔥" if torch.cuda.is_available() else f"CPU ⚡"}.
-
-
Please use the prompt template below to get an example of the desired generation results:
-
-
-Prompt:
-
-RAW photo, * subject *, (high detailed skin:1.2), 8k uhd, dslr, soft lighting, high quality, film grain, Fujifilm XT3
-
-
-
-Example: RAW photo, a close up portrait photo of 26 y.o woman in wastelander clothes, long haircut, pale skin, slim body, background is city ruins,
-(high detailed skin:1.2), 8k uhd, dslr, soft lighting, high quality, film grain, Fujifilm XT3
-
-
-
-
-Negative Prompt:
-
-(deformed iris, deformed pupils, semi-realistic, cgi, 3d, render, sketch, cartoon, drawing, anime:1.4), text, close up, cropped, out of frame, worst quality,
-low quality, jpeg artifacts, ugly, duplicate, morbid, mutilated, extra fingers, mutated hands, poorly drawn hands, poorly drawn face, mutation, deformed, blurry,
-dehydrated, bad anatomy, bad proportions, extra limbs, cloned face, disfigured, gross proportions, malformed limbs, missing arms, missing legs, extra arms,
-extra legs, fused fingers, too many fingers, long neck
-
-
-
-Have Fun & Enjoy ⚡ //THAFX
-
-
-
- """
- )
- with gr.Row():
-
- with gr.Column(scale=55):
- with gr.Group():
- with gr.Row():
- prompt = gr.Textbox(label="Prompt", show_label=False,max_lines=2,placeholder=f"{prefix} [your prompt]").style(container=False)
- generate = gr.Button(value="Generate").style(rounded=(False, True, True, False))
-
- image_out = gr.Image(height=512)
- error_output = gr.Markdown()
-
- with gr.Column(scale=45):
- with gr.Tab("Options"):
- with gr.Group():
- neg_prompt = gr.Textbox(label="Negative prompt", placeholder="What to exclude from the image")
- auto_prefix = gr.Checkbox(label="Prefix styling tokens automatically (RAW photo,)", value=prefix, visible=prefix)
-
- with gr.Row():
- guidance = gr.Slider(label="Guidance scale", value=7.5, maximum=15)
- steps = gr.Slider(label="Steps", value=25, minimum=2, maximum=75, step=1)
-
- with gr.Row():
- width = gr.Slider(label="Width", value=512, minimum=64, maximum=1024, step=8)
- height = gr.Slider(label="Height", value=512, minimum=64, maximum=1024, step=8)
-
- seed = gr.Slider(0, 2147483647, label='Seed (0 = random)', value=0, step=1)
-
- with gr.Tab("Image to image"):
- with gr.Group():
- image = gr.Image(label="Image", height=256, tool="editor", type="pil")
- strength = gr.Slider(label="Transformation strength", minimum=0, maximum=1, step=0.01, value=0.5)
-
- auto_prefix.change(lambda x: gr.update(placeholder=f"{prefix} [your prompt]" if x else "[Your prompt]"), inputs=auto_prefix, outputs=prompt, queue=False)
-
- inputs = [prompt, guidance, steps, width, height, seed, image, strength, neg_prompt, auto_prefix]
- outputs = [image_out, error_output]
- prompt.submit(inference, inputs=inputs, outputs=outputs)
- generate.click(inference, inputs=inputs, outputs=outputs)
-
-
-
-demo.queue(concurrency_count=1)
-demo.launch()
diff --git a/spaces/TitleGenerators/ArxivTitleGenerator/README.md b/spaces/TitleGenerators/ArxivTitleGenerator/README.md
deleted file mode 100644
index 8d655039893aebe9f7edfc520a25c25d64144672..0000000000000000000000000000000000000000
--- a/spaces/TitleGenerators/ArxivTitleGenerator/README.md
+++ /dev/null
@@ -1,37 +0,0 @@
----
-title: ArxivTitleGenerator
-emoji: 🌍
-colorFrom: green
-colorTo: indigo
-sdk: streamlit
-app_file: app.py
-pinned: false
----
-
-# Configuration
-
-`title`: _string_
-Display title for the Space
-
-`emoji`: _string_
-Space emoji (emoji-only character allowed)
-
-`colorFrom`: _string_
-Color for Thumbnail gradient (red, yellow, green, blue, indigo, purple, pink, gray)
-
-`colorTo`: _string_
-Color for Thumbnail gradient (red, yellow, green, blue, indigo, purple, pink, gray)
-
-`sdk`: _string_
-Can be either `gradio` or `streamlit`
-
-`sdk_version` : _string_
-Only applicable for `streamlit` SDK.
-See [doc](https://hf.co/docs/hub/spaces) for more info on supported versions.
-
-`app_file`: _string_
-Path to your main application file (which contains either `gradio` or `streamlit` Python code).
-Path is relative to the root of the repository.
-
-`pinned`: _boolean_
-Whether the Space stays on top of your list.
diff --git a/spaces/Uday-07/testing/README.md b/spaces/Uday-07/testing/README.md
deleted file mode 100644
index ebb86b06786ca0c958e76a1456e54215b1e32a78..0000000000000000000000000000000000000000
--- a/spaces/Uday-07/testing/README.md
+++ /dev/null
@@ -1,13 +0,0 @@
----
-title: Testing
-emoji: 💩
-colorFrom: pink
-colorTo: pink
-sdk: gradio
-sdk_version: 3.18.0
-app_file: app.py
-pinned: false
-license: apache-2.0
----
-
-Check out the configuration reference at https://huggingface.co/docs/hub/spaces-config-reference
diff --git a/spaces/Vgi/andite-anything-v4.0/app.py b/spaces/Vgi/andite-anything-v4.0/app.py
deleted file mode 100644
index 47a2051db6dadeea03edf70d62694fd3e5e88ba7..0000000000000000000000000000000000000000
--- a/spaces/Vgi/andite-anything-v4.0/app.py
+++ /dev/null
@@ -1,3 +0,0 @@
-import gradio as gr
-
-gr.Interface.load("models/andite/anything-v4.0").launch()
\ No newline at end of file
diff --git a/spaces/Vision-CAIR/MiniGPT-v2/minigpt4/runners/__init__.py b/spaces/Vision-CAIR/MiniGPT-v2/minigpt4/runners/__init__.py
deleted file mode 100644
index 64e7a4d643a8b5a1714687f42d43347a94b72373..0000000000000000000000000000000000000000
--- a/spaces/Vision-CAIR/MiniGPT-v2/minigpt4/runners/__init__.py
+++ /dev/null
@@ -1,10 +0,0 @@
-"""
- Copyright (c) 2022, salesforce.com, inc.
- All rights reserved.
- SPDX-License-Identifier: BSD-3-Clause
- For full license text, see the LICENSE_Lavis file in the repo root or https://opensource.org/licenses/BSD-3-Clause
-"""
-
-from minigpt4.runners.runner_base import RunnerBase
-
-__all__ = ["RunnerBase"]
diff --git a/spaces/Xiaini0/bingo-112233/README.md b/spaces/Xiaini0/bingo-112233/README.md
deleted file mode 100644
index 5d6936218874c647b5d22e13ad4be7edb8936f92..0000000000000000000000000000000000000000
--- a/spaces/Xiaini0/bingo-112233/README.md
+++ /dev/null
@@ -1,28 +0,0 @@
----
-title: bingo
-emoji: 😊
-colorFrom: red
-colorTo: red
-sdk: docker
-license: mit
-duplicated_from: hf4all/bingo
----
-
-
-
-# Bingo
-
-Bingo,一个让你呼吸顺畅 New Bing。
-
-高度还原 New Bing 网页版的主要操作,国内可用,兼容绝大多数微软 Bing AI 的功能,可自行部署使用。
-
-
-
-[](https://hub.docker.com/repository/docker/weaigc/bingo/)
-[](https://hub.docker.com/repository/docker/weaigc/bingo/)
-[](https://github.com/weaigc/bingo/blob/main/license)
-
-问题反馈请前往 https://github.com/weaigc/bingo/issues
-
-
-
diff --git a/spaces/XzJosh/LittleTaffy-Bert-VITS2/train_ms.py b/spaces/XzJosh/LittleTaffy-Bert-VITS2/train_ms.py
deleted file mode 100644
index 5d109003d40497ea4493e7c73f47c1eb7370a81e..0000000000000000000000000000000000000000
--- a/spaces/XzJosh/LittleTaffy-Bert-VITS2/train_ms.py
+++ /dev/null
@@ -1,402 +0,0 @@
-import os
-import json
-import argparse
-import itertools
-import math
-import torch
-import shutil
-from torch import nn, optim
-from torch.nn import functional as F
-from torch.utils.data import DataLoader
-from torch.utils.tensorboard import SummaryWriter
-import torch.multiprocessing as mp
-import torch.distributed as dist
-from torch.nn.parallel import DistributedDataParallel as DDP
-from torch.cuda.amp import autocast, GradScaler
-from tqdm import tqdm
-import logging
-logging.getLogger('numba').setLevel(logging.WARNING)
-import commons
-import utils
-from data_utils import (
- TextAudioSpeakerLoader,
- TextAudioSpeakerCollate,
- DistributedBucketSampler
-)
-from models import (
- SynthesizerTrn,
- MultiPeriodDiscriminator,
- DurationDiscriminator,
-)
-from losses import (
- generator_loss,
- discriminator_loss,
- feature_loss,
- kl_loss
-)
-from mel_processing import mel_spectrogram_torch, spec_to_mel_torch
-from text.symbols import symbols
-
-torch.backends.cudnn.benchmark = True
-torch.backends.cuda.matmul.allow_tf32 = True
-torch.backends.cudnn.allow_tf32 = True
-torch.set_float32_matmul_precision('medium')
-global_step = 0
-
-
-def main():
- """Assume Single Node Multi GPUs Training Only"""
- assert torch.cuda.is_available(), "CPU training is not allowed."
-
- n_gpus = torch.cuda.device_count()
- os.environ['MASTER_ADDR'] = 'localhost'
- os.environ['MASTER_PORT'] = '65280'
-
- hps = utils.get_hparams()
- if not hps.cont:
- shutil.copy('./pretrained_models/D_0.pth','./logs/OUTPUT_MODEL/D_0.pth')
- shutil.copy('./pretrained_models/G_0.pth','./logs/OUTPUT_MODEL/G_0.pth')
- shutil.copy('./pretrained_models/DUR_0.pth','./logs/OUTPUT_MODEL/DUR_0.pth')
- mp.spawn(run, nprocs=n_gpus, args=(n_gpus, hps,))
-
-
-def run(rank, n_gpus, hps):
- global global_step
- if rank == 0:
- logger = utils.get_logger(hps.model_dir)
- logger.info(hps)
- utils.check_git_hash(hps.model_dir)
- writer = SummaryWriter(log_dir=hps.model_dir)
- writer_eval = SummaryWriter(log_dir=os.path.join(hps.model_dir, "eval"))
-
- dist.init_process_group(backend= 'gloo' if os.name == 'nt' else 'nccl', init_method='env://', world_size=n_gpus, rank=rank)
- torch.manual_seed(hps.train.seed)
- torch.cuda.set_device(rank)
-
- train_dataset = TextAudioSpeakerLoader(hps.data.training_files, hps.data)
- train_sampler = DistributedBucketSampler(
- train_dataset,
- hps.train.batch_size,
- [32, 300, 400, 500, 600, 700, 800, 900, 1000],
- num_replicas=n_gpus,
- rank=rank,
- shuffle=True)
- collate_fn = TextAudioSpeakerCollate()
- train_loader = DataLoader(train_dataset, num_workers=2, shuffle=False, pin_memory=True,
- collate_fn=collate_fn, batch_sampler=train_sampler)
- if rank == 0:
- eval_dataset = TextAudioSpeakerLoader(hps.data.validation_files, hps.data)
- eval_loader = DataLoader(eval_dataset, num_workers=0, shuffle=False,
- batch_size=1, pin_memory=True,
- drop_last=False, collate_fn=collate_fn)
- if "use_noise_scaled_mas" in hps.model.keys() and hps.model.use_noise_scaled_mas == True:
- print("Using noise scaled MAS for VITS2")
- use_noise_scaled_mas = True
- mas_noise_scale_initial = 0.01
- noise_scale_delta = 2e-6
- else:
- print("Using normal MAS for VITS1")
- use_noise_scaled_mas = False
- mas_noise_scale_initial = 0.0
- noise_scale_delta = 0.0
- if "use_duration_discriminator" in hps.model.keys() and hps.model.use_duration_discriminator == True:
- print("Using duration discriminator for VITS2")
- use_duration_discriminator = True
- net_dur_disc = DurationDiscriminator(
- hps.model.hidden_channels,
- hps.model.hidden_channels,
- 3,
- 0.1,
- gin_channels=hps.model.gin_channels if hps.data.n_speakers != 0 else 0,
- ).cuda(rank)
- if "use_spk_conditioned_encoder" in hps.model.keys() and hps.model.use_spk_conditioned_encoder == True:
- if hps.data.n_speakers == 0:
- raise ValueError("n_speakers must be > 0 when using spk conditioned encoder to train multi-speaker model")
- use_spk_conditioned_encoder = True
- else:
- print("Using normal encoder for VITS1")
- use_spk_conditioned_encoder = False
-
- net_g = SynthesizerTrn(
- len(symbols),
- hps.data.filter_length // 2 + 1,
- hps.train.segment_size // hps.data.hop_length,
- n_speakers=hps.data.n_speakers,
- mas_noise_scale_initial = mas_noise_scale_initial,
- noise_scale_delta = noise_scale_delta,
- **hps.model).cuda(rank)
-
- freeze_enc = getattr(hps.model, "freeze_enc", False)
- if freeze_enc:
- print("freeze encoder !!!")
- for param in net_g.enc_p.parameters():
- param.requires_grad = False
-
- net_d = MultiPeriodDiscriminator(hps.model.use_spectral_norm).cuda(rank)
- optim_g = torch.optim.AdamW(
- filter(lambda p: p.requires_grad, net_g.parameters()),
- hps.train.learning_rate,
- betas=hps.train.betas,
- eps=hps.train.eps)
- optim_d = torch.optim.AdamW(
- net_d.parameters(),
- hps.train.learning_rate,
- betas=hps.train.betas,
- eps=hps.train.eps)
- if net_dur_disc is not None:
- optim_dur_disc = torch.optim.AdamW(
- net_dur_disc.parameters(),
- hps.train.learning_rate,
- betas=hps.train.betas,
- eps=hps.train.eps)
- else:
- optim_dur_disc = None
- net_g = DDP(net_g, device_ids=[rank], find_unused_parameters=True)
- net_d = DDP(net_d, device_ids=[rank], find_unused_parameters=True)
- if net_dur_disc is not None:
- net_dur_disc = DDP(net_dur_disc, device_ids=[rank], find_unused_parameters=True)
-
- pretrain_dir = None
- if pretrain_dir is None:
- try:
- if net_dur_disc is not None:
- _, optim_dur_disc, _, epoch_str = utils.load_checkpoint(utils.latest_checkpoint_path(hps.model_dir, "DUR_*.pth"), net_dur_disc, optim_dur_disc, skip_optimizer=not hps.cont)
- _, optim_g, _, epoch_str = utils.load_checkpoint(utils.latest_checkpoint_path(hps.model_dir, "G_*.pth"), net_g,
- optim_g, skip_optimizer=not hps.cont)
- _, optim_d, _, epoch_str = utils.load_checkpoint(utils.latest_checkpoint_path(hps.model_dir, "D_*.pth"), net_d,
- optim_d, skip_optimizer=not hps.cont)
-
- epoch_str = max(epoch_str, 1)
- global_step = (epoch_str - 1) * len(train_loader)
- except Exception as e:
- print(e)
- epoch_str = 1
- global_step = 0
- else:
- _, _, _, epoch_str = utils.load_checkpoint(utils.latest_checkpoint_path(pretrain_dir, "G_*.pth"), net_g,
- optim_g, True)
- _, _, _, epoch_str = utils.load_checkpoint(utils.latest_checkpoint_path(pretrain_dir, "D_*.pth"), net_d,
- optim_d, True)
-
-
-
- scheduler_g = torch.optim.lr_scheduler.ExponentialLR(optim_g, gamma=hps.train.lr_decay, last_epoch=epoch_str - 2)
- scheduler_d = torch.optim.lr_scheduler.ExponentialLR(optim_d, gamma=hps.train.lr_decay, last_epoch=epoch_str - 2)
- if net_dur_disc is not None:
- scheduler_dur_disc = torch.optim.lr_scheduler.ExponentialLR(optim_dur_disc, gamma=hps.train.lr_decay, last_epoch=epoch_str-2)
- else:
- scheduler_dur_disc = None
- scaler = GradScaler(enabled=hps.train.fp16_run)
-
- for epoch in range(epoch_str, hps.train.epochs + 1):
- if rank == 0:
- train_and_evaluate(rank, epoch, hps, [net_g, net_d, net_dur_disc], [optim_g, optim_d, optim_dur_disc], [scheduler_g, scheduler_d, scheduler_dur_disc], scaler, [train_loader, eval_loader], logger, [writer, writer_eval])
- else:
- train_and_evaluate(rank, epoch, hps, [net_g, net_d, net_dur_disc], [optim_g, optim_d, optim_dur_disc], [scheduler_g, scheduler_d, scheduler_dur_disc], scaler, [train_loader, None], None, None)
- scheduler_g.step()
- scheduler_d.step()
- if net_dur_disc is not None:
- scheduler_dur_disc.step()
-
-
-def train_and_evaluate(rank, epoch, hps, nets, optims, schedulers, scaler, loaders, logger, writers):
- net_g, net_d, net_dur_disc = nets
- optim_g, optim_d, optim_dur_disc = optims
- scheduler_g, scheduler_d, scheduler_dur_disc = schedulers
- train_loader, eval_loader = loaders
- if writers is not None:
- writer, writer_eval = writers
-
- train_loader.batch_sampler.set_epoch(epoch)
- global global_step
-
- net_g.train()
- net_d.train()
- if net_dur_disc is not None:
- net_dur_disc.train()
- for batch_idx, (x, x_lengths, spec, spec_lengths, y, y_lengths, speakers, tone, language, bert) in tqdm(enumerate(train_loader)):
- if net_g.module.use_noise_scaled_mas:
- current_mas_noise_scale = net_g.module.mas_noise_scale_initial - net_g.module.noise_scale_delta * global_step
- net_g.module.current_mas_noise_scale = max(current_mas_noise_scale, 0.0)
- x, x_lengths = x.cuda(rank, non_blocking=True), x_lengths.cuda(rank, non_blocking=True)
- spec, spec_lengths = spec.cuda(rank, non_blocking=True), spec_lengths.cuda(rank, non_blocking=True)
- y, y_lengths = y.cuda(rank, non_blocking=True), y_lengths.cuda(rank, non_blocking=True)
- speakers = speakers.cuda(rank, non_blocking=True)
- tone = tone.cuda(rank, non_blocking=True)
- language = language.cuda(rank, non_blocking=True)
- bert = bert.cuda(rank, non_blocking=True)
-
- with autocast(enabled=hps.train.fp16_run):
- y_hat, l_length, attn, ids_slice, x_mask, z_mask, \
- (z, z_p, m_p, logs_p, m_q, logs_q), (hidden_x, logw, logw_) = net_g(x, x_lengths, spec, spec_lengths, speakers, tone, language, bert)
- mel = spec_to_mel_torch(
- spec,
- hps.data.filter_length,
- hps.data.n_mel_channels,
- hps.data.sampling_rate,
- hps.data.mel_fmin,
- hps.data.mel_fmax)
- y_mel = commons.slice_segments(mel, ids_slice, hps.train.segment_size // hps.data.hop_length)
- y_hat_mel = mel_spectrogram_torch(
- y_hat.squeeze(1),
- hps.data.filter_length,
- hps.data.n_mel_channels,
- hps.data.sampling_rate,
- hps.data.hop_length,
- hps.data.win_length,
- hps.data.mel_fmin,
- hps.data.mel_fmax
- )
-
- y = commons.slice_segments(y, ids_slice * hps.data.hop_length, hps.train.segment_size) # slice
-
- # Discriminator
- y_d_hat_r, y_d_hat_g, _, _ = net_d(y, y_hat.detach())
- with autocast(enabled=False):
- loss_disc, losses_disc_r, losses_disc_g = discriminator_loss(y_d_hat_r, y_d_hat_g)
- loss_disc_all = loss_disc
- if net_dur_disc is not None:
- y_dur_hat_r, y_dur_hat_g = net_dur_disc(hidden_x.detach(), x_mask.detach(), logw.detach(), logw_.detach())
- with autocast(enabled=False):
- # TODO: I think need to mean using the mask, but for now, just mean all
- loss_dur_disc, losses_dur_disc_r, losses_dur_disc_g = discriminator_loss(y_dur_hat_r, y_dur_hat_g)
- loss_dur_disc_all = loss_dur_disc
- optim_dur_disc.zero_grad()
- scaler.scale(loss_dur_disc_all).backward()
- scaler.unscale_(optim_dur_disc)
- grad_norm_dur_disc = commons.clip_grad_value_(net_dur_disc.parameters(), None)
- scaler.step(optim_dur_disc)
-
- optim_d.zero_grad()
- scaler.scale(loss_disc_all).backward()
- scaler.unscale_(optim_d)
- grad_norm_d = commons.clip_grad_value_(net_d.parameters(), None)
- scaler.step(optim_d)
-
- with autocast(enabled=hps.train.fp16_run):
- # Generator
- y_d_hat_r, y_d_hat_g, fmap_r, fmap_g = net_d(y, y_hat)
- if net_dur_disc is not None:
- y_dur_hat_r, y_dur_hat_g = net_dur_disc(hidden_x, x_mask, logw, logw_)
- with autocast(enabled=False):
- loss_dur = torch.sum(l_length.float())
- loss_mel = F.l1_loss(y_mel, y_hat_mel) * hps.train.c_mel
- loss_kl = kl_loss(z_p, logs_q, m_p, logs_p, z_mask) * hps.train.c_kl
-
- loss_fm = feature_loss(fmap_r, fmap_g)
- loss_gen, losses_gen = generator_loss(y_d_hat_g)
- loss_gen_all = loss_gen + loss_fm + loss_mel + loss_dur + loss_kl
- if net_dur_disc is not None:
- loss_dur_gen, losses_dur_gen = generator_loss(y_dur_hat_g)
- loss_gen_all += loss_dur_gen
- optim_g.zero_grad()
- scaler.scale(loss_gen_all).backward()
- scaler.unscale_(optim_g)
- grad_norm_g = commons.clip_grad_value_(net_g.parameters(), None)
- scaler.step(optim_g)
- scaler.update()
-
- if rank == 0:
- if global_step % hps.train.log_interval == 0:
- lr = optim_g.param_groups[0]['lr']
- losses = [loss_disc, loss_gen, loss_fm, loss_mel, loss_dur, loss_kl]
- logger.info('Train Epoch: {} [{:.0f}%]'.format(
- epoch,
- 100. * batch_idx / len(train_loader)))
- logger.info([x.item() for x in losses] + [global_step, lr])
-
- scalar_dict = {"loss/g/total": loss_gen_all, "loss/d/total": loss_disc_all, "learning_rate": lr,
- "grad_norm_d": grad_norm_d, "grad_norm_g": grad_norm_g}
- scalar_dict.update(
- {"loss/g/fm": loss_fm, "loss/g/mel": loss_mel, "loss/g/dur": loss_dur, "loss/g/kl": loss_kl})
- scalar_dict.update({"loss/g/{}".format(i): v for i, v in enumerate(losses_gen)})
- scalar_dict.update({"loss/d_r/{}".format(i): v for i, v in enumerate(losses_disc_r)})
- scalar_dict.update({"loss/d_g/{}".format(i): v for i, v in enumerate(losses_disc_g)})
-
- image_dict = {
- "slice/mel_org": utils.plot_spectrogram_to_numpy(y_mel[0].data.cpu().numpy()),
- "slice/mel_gen": utils.plot_spectrogram_to_numpy(y_hat_mel[0].data.cpu().numpy()),
- "all/mel": utils.plot_spectrogram_to_numpy(mel[0].data.cpu().numpy()),
- "all/attn": utils.plot_alignment_to_numpy(attn[0, 0].data.cpu().numpy())
- }
- utils.summarize(
- writer=writer,
- global_step=global_step,
- images=image_dict,
- scalars=scalar_dict)
-
- if global_step % hps.train.eval_interval == 0:
- evaluate(hps, net_g, eval_loader, writer_eval)
- utils.save_checkpoint(net_g, optim_g, hps.train.learning_rate, epoch,
- os.path.join(hps.model_dir, "G_{}.pth".format(global_step)))
- utils.save_checkpoint(net_d, optim_d, hps.train.learning_rate, epoch,
- os.path.join(hps.model_dir, "D_{}.pth".format(global_step)))
- if net_dur_disc is not None:
- utils.save_checkpoint(net_dur_disc, optim_dur_disc, hps.train.learning_rate, epoch, os.path.join(hps.model_dir, "DUR_{}.pth".format(global_step)))
- keep_ckpts = getattr(hps.train, 'keep_ckpts', 5)
- if keep_ckpts > 0:
- utils.clean_checkpoints(path_to_models=hps.model_dir, n_ckpts_to_keep=keep_ckpts, sort_by_time=True)
-
-
- global_step += 1
-
- if rank == 0:
- logger.info('====> Epoch: {}'.format(epoch))
-
-
-
-def evaluate(hps, generator, eval_loader, writer_eval):
- generator.eval()
- image_dict = {}
- audio_dict = {}
- print("Evaluating ...")
- with torch.no_grad():
- for batch_idx, (x, x_lengths, spec, spec_lengths, y, y_lengths, speakers, tone, language, bert) in enumerate(eval_loader):
- x, x_lengths = x.cuda(), x_lengths.cuda()
- spec, spec_lengths = spec.cuda(), spec_lengths.cuda()
- y, y_lengths = y.cuda(), y_lengths.cuda()
- speakers = speakers.cuda()
- bert = bert.cuda()
- tone = tone.cuda()
- language = language.cuda()
- for use_sdp in [True, False]:
- y_hat, attn, mask, *_ = generator.module.infer(x, x_lengths, speakers, tone, language, bert, y=spec, max_len=1000, sdp_ratio=0.0 if not use_sdp else 1.0)
- y_hat_lengths = mask.sum([1, 2]).long() * hps.data.hop_length
-
- mel = spec_to_mel_torch(
- spec,
- hps.data.filter_length,
- hps.data.n_mel_channels,
- hps.data.sampling_rate,
- hps.data.mel_fmin,
- hps.data.mel_fmax)
- y_hat_mel = mel_spectrogram_torch(
- y_hat.squeeze(1).float(),
- hps.data.filter_length,
- hps.data.n_mel_channels,
- hps.data.sampling_rate,
- hps.data.hop_length,
- hps.data.win_length,
- hps.data.mel_fmin,
- hps.data.mel_fmax
- )
- image_dict.update({
- f"gen/mel_{batch_idx}": utils.plot_spectrogram_to_numpy(y_hat_mel[0].cpu().numpy())
- })
- audio_dict.update({
- f"gen/audio_{batch_idx}_{use_sdp}": y_hat[0, :, :y_hat_lengths[0]]
- })
- image_dict.update({f"gt/mel_{batch_idx}": utils.plot_spectrogram_to_numpy(mel[0].cpu().numpy())})
- audio_dict.update({f"gt/audio_{batch_idx}": y[0, :, :y_lengths[0]]})
-
- utils.summarize(
- writer=writer_eval,
- global_step=global_step,
- images=image_dict,
- audios=audio_dict,
- audio_sampling_rate=hps.data.sampling_rate
- )
- generator.train()
-
-if __name__ == "__main__":
- main()
diff --git a/spaces/XzJosh/otto-Bert-VITS2/resample.py b/spaces/XzJosh/otto-Bert-VITS2/resample.py
deleted file mode 100644
index 2ed1685654a371c5722168e9987809b05b1cb224..0000000000000000000000000000000000000000
--- a/spaces/XzJosh/otto-Bert-VITS2/resample.py
+++ /dev/null
@@ -1,42 +0,0 @@
-import os
-import argparse
-import librosa
-import numpy as np
-from multiprocessing import Pool, cpu_count
-
-import soundfile
-from scipy.io import wavfile
-from tqdm import tqdm
-
-
-def process(item):
- spkdir, wav_name, args = item
- speaker = spkdir.replace("\\", "/").split("/")[-1]
- wav_path = os.path.join(args.in_dir, speaker, wav_name)
- if os.path.exists(wav_path) and '.wav' in wav_path:
- os.makedirs(os.path.join(args.out_dir, speaker), exist_ok=True)
- wav, sr = librosa.load(wav_path, sr=args.sr)
- soundfile.write(
- os.path.join(args.out_dir, speaker, wav_name),
- wav,
- sr
- )
-
-
-
-if __name__ == "__main__":
- parser = argparse.ArgumentParser()
- parser.add_argument("--sr", type=int, default=44100, help="sampling rate")
- parser.add_argument("--in_dir", type=str, default="./raw", help="path to source dir")
- parser.add_argument("--out_dir", type=str, default="./dataset", help="path to target dir")
- args = parser.parse_args()
- # processs = 8
- processs = cpu_count()-2 if cpu_count() >4 else 1
- pool = Pool(processes=processs)
-
- for speaker in os.listdir(args.in_dir):
- spk_dir = os.path.join(args.in_dir, speaker)
- if os.path.isdir(spk_dir):
- print(spk_dir)
- for _ in tqdm(pool.imap_unordered(process, [(spk_dir, i, args) for i in os.listdir(spk_dir) if i.endswith("wav")])):
- pass
diff --git a/spaces/XzJosh/ranran-Bert-VITS2/text/symbols.py b/spaces/XzJosh/ranran-Bert-VITS2/text/symbols.py
deleted file mode 100644
index 9dfae4e633829f20c4fd767b1c7a9198911ed801..0000000000000000000000000000000000000000
--- a/spaces/XzJosh/ranran-Bert-VITS2/text/symbols.py
+++ /dev/null
@@ -1,51 +0,0 @@
-punctuation = ['!', '?', '…', ",", ".", "'", '-']
-pu_symbols = punctuation + ["SP", "UNK"]
-pad = '_'
-
-# chinese
-zh_symbols = ['E', 'En', 'a', 'ai', 'an', 'ang', 'ao', 'b', 'c', 'ch', 'd', 'e', 'ei', 'en', 'eng', 'er', 'f', 'g', 'h',
- 'i', 'i0', 'ia', 'ian', 'iang', 'iao', 'ie', 'in', 'ing', 'iong', 'ir', 'iu', 'j', 'k', 'l', 'm', 'n', 'o',
- 'ong',
- 'ou', 'p', 'q', 'r', 's', 'sh', 't', 'u', 'ua', 'uai', 'uan', 'uang', 'ui', 'un', 'uo', 'v', 'van', 've', 'vn',
- 'w', 'x', 'y', 'z', 'zh',
- "AA", "EE", "OO"]
-num_zh_tones = 6
-
-# japanese
-ja_symbols = ['I', 'N', 'U', 'a', 'b', 'by', 'ch', 'cl', 'd', 'dy', 'e', 'f', 'g', 'gy', 'h', 'hy', 'i', 'j', 'k', 'ky',
- 'm', 'my', 'n', 'ny', 'o', 'p', 'py', 'r', 'ry', 's', 'sh', 't', 'ts', 'u', 'V', 'w', 'y', 'z']
-num_ja_tones = 1
-
-# English
-en_symbols = ['aa', 'ae', 'ah', 'ao', 'aw', 'ay', 'b', 'ch', 'd', 'dh', 'eh', 'er', 'ey', 'f', 'g', 'hh', 'ih', 'iy',
- 'jh', 'k', 'l', 'm', 'n', 'ng', 'ow', 'oy', 'p', 'r', 's',
- 'sh', 't', 'th', 'uh', 'uw', 'V', 'w', 'y', 'z', 'zh']
-num_en_tones = 4
-
-# combine all symbols
-normal_symbols = sorted(set(zh_symbols + ja_symbols + en_symbols))
-symbols = [pad] + normal_symbols + pu_symbols
-sil_phonemes_ids = [symbols.index(i) for i in pu_symbols]
-
-# combine all tones
-num_tones = num_zh_tones + num_ja_tones + num_en_tones
-
-# language maps
-language_id_map = {
- 'ZH': 0,
- "JA": 1,
- "EN": 2
-}
-num_languages = len(language_id_map.keys())
-
-language_tone_start_map = {
- 'ZH': 0,
- "JA": num_zh_tones,
- "EN": num_zh_tones + num_ja_tones
-}
-
-if __name__ == '__main__':
- a = set(zh_symbols)
- b = set(en_symbols)
- print(sorted(a&b))
-
diff --git a/spaces/YUANAI/DiffspeechResearch/tasks/run.py b/spaces/YUANAI/DiffspeechResearch/tasks/run.py
deleted file mode 100644
index ef2b0a319cb5cd7baf87e5224ab545412715fb69..0000000000000000000000000000000000000000
--- a/spaces/YUANAI/DiffspeechResearch/tasks/run.py
+++ /dev/null
@@ -1,19 +0,0 @@
-import os
-
-os.environ["OMP_NUM_THREADS"] = "1"
-
-from utils.commons.hparams import hparams, set_hparams
-import importlib
-
-
-def run_task():
- assert hparams['task_cls'] != ''
- pkg = ".".join(hparams["task_cls"].split(".")[:-1])
- cls_name = hparams["task_cls"].split(".")[-1]
- task_cls = getattr(importlib.import_module(pkg), cls_name)
- task_cls.start()
-
-
-if __name__ == '__main__':
- set_hparams()
- run_task()
diff --git a/spaces/YanzBotz/YanzBotz-Models/lib/infer_pack/attentions.py b/spaces/YanzBotz/YanzBotz-Models/lib/infer_pack/attentions.py
deleted file mode 100644
index 05501be1871643f78dddbeaa529c96667031a8db..0000000000000000000000000000000000000000
--- a/spaces/YanzBotz/YanzBotz-Models/lib/infer_pack/attentions.py
+++ /dev/null
@@ -1,417 +0,0 @@
-import copy
-import math
-import numpy as np
-import torch
-from torch import nn
-from torch.nn import functional as F
-
-from lib.infer_pack import commons
-from lib.infer_pack import modules
-from lib.infer_pack.modules import LayerNorm
-
-
-class Encoder(nn.Module):
- def __init__(
- self,
- hidden_channels,
- filter_channels,
- n_heads,
- n_layers,
- kernel_size=1,
- p_dropout=0.0,
- window_size=10,
- **kwargs
- ):
- super().__init__()
- self.hidden_channels = hidden_channels
- self.filter_channels = filter_channels
- self.n_heads = n_heads
- self.n_layers = n_layers
- self.kernel_size = kernel_size
- self.p_dropout = p_dropout
- self.window_size = window_size
-
- self.drop = nn.Dropout(p_dropout)
- self.attn_layers = nn.ModuleList()
- self.norm_layers_1 = nn.ModuleList()
- self.ffn_layers = nn.ModuleList()
- self.norm_layers_2 = nn.ModuleList()
- for i in range(self.n_layers):
- self.attn_layers.append(
- MultiHeadAttention(
- hidden_channels,
- hidden_channels,
- n_heads,
- p_dropout=p_dropout,
- window_size=window_size,
- )
- )
- self.norm_layers_1.append(LayerNorm(hidden_channels))
- self.ffn_layers.append(
- FFN(
- hidden_channels,
- hidden_channels,
- filter_channels,
- kernel_size,
- p_dropout=p_dropout,
- )
- )
- self.norm_layers_2.append(LayerNorm(hidden_channels))
-
- def forward(self, x, x_mask):
- attn_mask = x_mask.unsqueeze(2) * x_mask.unsqueeze(-1)
- x = x * x_mask
- for i in range(self.n_layers):
- y = self.attn_layers[i](x, x, attn_mask)
- y = self.drop(y)
- x = self.norm_layers_1[i](x + y)
-
- y = self.ffn_layers[i](x, x_mask)
- y = self.drop(y)
- x = self.norm_layers_2[i](x + y)
- x = x * x_mask
- return x
-
-
-class Decoder(nn.Module):
- def __init__(
- self,
- hidden_channels,
- filter_channels,
- n_heads,
- n_layers,
- kernel_size=1,
- p_dropout=0.0,
- proximal_bias=False,
- proximal_init=True,
- **kwargs
- ):
- super().__init__()
- self.hidden_channels = hidden_channels
- self.filter_channels = filter_channels
- self.n_heads = n_heads
- self.n_layers = n_layers
- self.kernel_size = kernel_size
- self.p_dropout = p_dropout
- self.proximal_bias = proximal_bias
- self.proximal_init = proximal_init
-
- self.drop = nn.Dropout(p_dropout)
- self.self_attn_layers = nn.ModuleList()
- self.norm_layers_0 = nn.ModuleList()
- self.encdec_attn_layers = nn.ModuleList()
- self.norm_layers_1 = nn.ModuleList()
- self.ffn_layers = nn.ModuleList()
- self.norm_layers_2 = nn.ModuleList()
- for i in range(self.n_layers):
- self.self_attn_layers.append(
- MultiHeadAttention(
- hidden_channels,
- hidden_channels,
- n_heads,
- p_dropout=p_dropout,
- proximal_bias=proximal_bias,
- proximal_init=proximal_init,
- )
- )
- self.norm_layers_0.append(LayerNorm(hidden_channels))
- self.encdec_attn_layers.append(
- MultiHeadAttention(
- hidden_channels, hidden_channels, n_heads, p_dropout=p_dropout
- )
- )
- self.norm_layers_1.append(LayerNorm(hidden_channels))
- self.ffn_layers.append(
- FFN(
- hidden_channels,
- hidden_channels,
- filter_channels,
- kernel_size,
- p_dropout=p_dropout,
- causal=True,
- )
- )
- self.norm_layers_2.append(LayerNorm(hidden_channels))
-
- def forward(self, x, x_mask, h, h_mask):
- """
- x: decoder input
- h: encoder output
- """
- self_attn_mask = commons.subsequent_mask(x_mask.size(2)).to(
- device=x.device, dtype=x.dtype
- )
- encdec_attn_mask = h_mask.unsqueeze(2) * x_mask.unsqueeze(-1)
- x = x * x_mask
- for i in range(self.n_layers):
- y = self.self_attn_layers[i](x, x, self_attn_mask)
- y = self.drop(y)
- x = self.norm_layers_0[i](x + y)
-
- y = self.encdec_attn_layers[i](x, h, encdec_attn_mask)
- y = self.drop(y)
- x = self.norm_layers_1[i](x + y)
-
- y = self.ffn_layers[i](x, x_mask)
- y = self.drop(y)
- x = self.norm_layers_2[i](x + y)
- x = x * x_mask
- return x
-
-
-class MultiHeadAttention(nn.Module):
- def __init__(
- self,
- channels,
- out_channels,
- n_heads,
- p_dropout=0.0,
- window_size=None,
- heads_share=True,
- block_length=None,
- proximal_bias=False,
- proximal_init=False,
- ):
- super().__init__()
- assert channels % n_heads == 0
-
- self.channels = channels
- self.out_channels = out_channels
- self.n_heads = n_heads
- self.p_dropout = p_dropout
- self.window_size = window_size
- self.heads_share = heads_share
- self.block_length = block_length
- self.proximal_bias = proximal_bias
- self.proximal_init = proximal_init
- self.attn = None
-
- self.k_channels = channels // n_heads
- self.conv_q = nn.Conv1d(channels, channels, 1)
- self.conv_k = nn.Conv1d(channels, channels, 1)
- self.conv_v = nn.Conv1d(channels, channels, 1)
- self.conv_o = nn.Conv1d(channels, out_channels, 1)
- self.drop = nn.Dropout(p_dropout)
-
- if window_size is not None:
- n_heads_rel = 1 if heads_share else n_heads
- rel_stddev = self.k_channels**-0.5
- self.emb_rel_k = nn.Parameter(
- torch.randn(n_heads_rel, window_size * 2 + 1, self.k_channels)
- * rel_stddev
- )
- self.emb_rel_v = nn.Parameter(
- torch.randn(n_heads_rel, window_size * 2 + 1, self.k_channels)
- * rel_stddev
- )
-
- nn.init.xavier_uniform_(self.conv_q.weight)
- nn.init.xavier_uniform_(self.conv_k.weight)
- nn.init.xavier_uniform_(self.conv_v.weight)
- if proximal_init:
- with torch.no_grad():
- self.conv_k.weight.copy_(self.conv_q.weight)
- self.conv_k.bias.copy_(self.conv_q.bias)
-
- def forward(self, x, c, attn_mask=None):
- q = self.conv_q(x)
- k = self.conv_k(c)
- v = self.conv_v(c)
-
- x, self.attn = self.attention(q, k, v, mask=attn_mask)
-
- x = self.conv_o(x)
- return x
-
- def attention(self, query, key, value, mask=None):
- # reshape [b, d, t] -> [b, n_h, t, d_k]
- b, d, t_s, t_t = (*key.size(), query.size(2))
- query = query.view(b, self.n_heads, self.k_channels, t_t).transpose(2, 3)
- key = key.view(b, self.n_heads, self.k_channels, t_s).transpose(2, 3)
- value = value.view(b, self.n_heads, self.k_channels, t_s).transpose(2, 3)
-
- scores = torch.matmul(query / math.sqrt(self.k_channels), key.transpose(-2, -1))
- if self.window_size is not None:
- assert (
- t_s == t_t
- ), "Relative attention is only available for self-attention."
- key_relative_embeddings = self._get_relative_embeddings(self.emb_rel_k, t_s)
- rel_logits = self._matmul_with_relative_keys(
- query / math.sqrt(self.k_channels), key_relative_embeddings
- )
- scores_local = self._relative_position_to_absolute_position(rel_logits)
- scores = scores + scores_local
- if self.proximal_bias:
- assert t_s == t_t, "Proximal bias is only available for self-attention."
- scores = scores + self._attention_bias_proximal(t_s).to(
- device=scores.device, dtype=scores.dtype
- )
- if mask is not None:
- scores = scores.masked_fill(mask == 0, -1e4)
- if self.block_length is not None:
- assert (
- t_s == t_t
- ), "Local attention is only available for self-attention."
- block_mask = (
- torch.ones_like(scores)
- .triu(-self.block_length)
- .tril(self.block_length)
- )
- scores = scores.masked_fill(block_mask == 0, -1e4)
- p_attn = F.softmax(scores, dim=-1) # [b, n_h, t_t, t_s]
- p_attn = self.drop(p_attn)
- output = torch.matmul(p_attn, value)
- if self.window_size is not None:
- relative_weights = self._absolute_position_to_relative_position(p_attn)
- value_relative_embeddings = self._get_relative_embeddings(
- self.emb_rel_v, t_s
- )
- output = output + self._matmul_with_relative_values(
- relative_weights, value_relative_embeddings
- )
- output = (
- output.transpose(2, 3).contiguous().view(b, d, t_t)
- ) # [b, n_h, t_t, d_k] -> [b, d, t_t]
- return output, p_attn
-
- def _matmul_with_relative_values(self, x, y):
- """
- x: [b, h, l, m]
- y: [h or 1, m, d]
- ret: [b, h, l, d]
- """
- ret = torch.matmul(x, y.unsqueeze(0))
- return ret
-
- def _matmul_with_relative_keys(self, x, y):
- """
- x: [b, h, l, d]
- y: [h or 1, m, d]
- ret: [b, h, l, m]
- """
- ret = torch.matmul(x, y.unsqueeze(0).transpose(-2, -1))
- return ret
-
- def _get_relative_embeddings(self, relative_embeddings, length):
- max_relative_position = 2 * self.window_size + 1
- # Pad first before slice to avoid using cond ops.
- pad_length = max(length - (self.window_size + 1), 0)
- slice_start_position = max((self.window_size + 1) - length, 0)
- slice_end_position = slice_start_position + 2 * length - 1
- if pad_length > 0:
- padded_relative_embeddings = F.pad(
- relative_embeddings,
- commons.convert_pad_shape([[0, 0], [pad_length, pad_length], [0, 0]]),
- )
- else:
- padded_relative_embeddings = relative_embeddings
- used_relative_embeddings = padded_relative_embeddings[
- :, slice_start_position:slice_end_position
- ]
- return used_relative_embeddings
-
- def _relative_position_to_absolute_position(self, x):
- """
- x: [b, h, l, 2*l-1]
- ret: [b, h, l, l]
- """
- batch, heads, length, _ = x.size()
- # Concat columns of pad to shift from relative to absolute indexing.
- x = F.pad(x, commons.convert_pad_shape([[0, 0], [0, 0], [0, 0], [0, 1]]))
-
- # Concat extra elements so to add up to shape (len+1, 2*len-1).
- x_flat = x.view([batch, heads, length * 2 * length])
- x_flat = F.pad(
- x_flat, commons.convert_pad_shape([[0, 0], [0, 0], [0, length - 1]])
- )
-
- # Reshape and slice out the padded elements.
- x_final = x_flat.view([batch, heads, length + 1, 2 * length - 1])[
- :, :, :length, length - 1 :
- ]
- return x_final
-
- def _absolute_position_to_relative_position(self, x):
- """
- x: [b, h, l, l]
- ret: [b, h, l, 2*l-1]
- """
- batch, heads, length, _ = x.size()
- # padd along column
- x = F.pad(
- x, commons.convert_pad_shape([[0, 0], [0, 0], [0, 0], [0, length - 1]])
- )
- x_flat = x.view([batch, heads, length**2 + length * (length - 1)])
- # add 0's in the beginning that will skew the elements after reshape
- x_flat = F.pad(x_flat, commons.convert_pad_shape([[0, 0], [0, 0], [length, 0]]))
- x_final = x_flat.view([batch, heads, length, 2 * length])[:, :, :, 1:]
- return x_final
-
- def _attention_bias_proximal(self, length):
- """Bias for self-attention to encourage attention to close positions.
- Args:
- length: an integer scalar.
- Returns:
- a Tensor with shape [1, 1, length, length]
- """
- r = torch.arange(length, dtype=torch.float32)
- diff = torch.unsqueeze(r, 0) - torch.unsqueeze(r, 1)
- return torch.unsqueeze(torch.unsqueeze(-torch.log1p(torch.abs(diff)), 0), 0)
-
-
-class FFN(nn.Module):
- def __init__(
- self,
- in_channels,
- out_channels,
- filter_channels,
- kernel_size,
- p_dropout=0.0,
- activation=None,
- causal=False,
- ):
- super().__init__()
- self.in_channels = in_channels
- self.out_channels = out_channels
- self.filter_channels = filter_channels
- self.kernel_size = kernel_size
- self.p_dropout = p_dropout
- self.activation = activation
- self.causal = causal
-
- if causal:
- self.padding = self._causal_padding
- else:
- self.padding = self._same_padding
-
- self.conv_1 = nn.Conv1d(in_channels, filter_channels, kernel_size)
- self.conv_2 = nn.Conv1d(filter_channels, out_channels, kernel_size)
- self.drop = nn.Dropout(p_dropout)
-
- def forward(self, x, x_mask):
- x = self.conv_1(self.padding(x * x_mask))
- if self.activation == "gelu":
- x = x * torch.sigmoid(1.702 * x)
- else:
- x = torch.relu(x)
- x = self.drop(x)
- x = self.conv_2(self.padding(x * x_mask))
- return x * x_mask
-
- def _causal_padding(self, x):
- if self.kernel_size == 1:
- return x
- pad_l = self.kernel_size - 1
- pad_r = 0
- padding = [[0, 0], [0, 0], [pad_l, pad_r]]
- x = F.pad(x, commons.convert_pad_shape(padding))
- return x
-
- def _same_padding(self, x):
- if self.kernel_size == 1:
- return x
- pad_l = (self.kernel_size - 1) // 2
- pad_r = self.kernel_size // 2
- padding = [[0, 0], [0, 0], [pad_l, pad_r]]
- x = F.pad(x, commons.convert_pad_shape(padding))
- return x
diff --git a/spaces/YeOldHermit/Super-Resolution-Anime-Diffusion/diffusers/pipelines/alt_diffusion/__init__.py b/spaces/YeOldHermit/Super-Resolution-Anime-Diffusion/diffusers/pipelines/alt_diffusion/__init__.py
deleted file mode 100644
index 09d0d9b7852c4babfe26c33874bcb1bf52271b39..0000000000000000000000000000000000000000
--- a/spaces/YeOldHermit/Super-Resolution-Anime-Diffusion/diffusers/pipelines/alt_diffusion/__init__.py
+++ /dev/null
@@ -1,34 +0,0 @@
-from dataclasses import dataclass
-from typing import List, Optional, Union
-
-import numpy as np
-
-import PIL
-from PIL import Image
-
-from ...utils import BaseOutput, is_torch_available, is_transformers_available
-
-
-@dataclass
-# Copied from diffusers.pipelines.stable_diffusion.__init__.StableDiffusionPipelineOutput with Stable->Alt
-class AltDiffusionPipelineOutput(BaseOutput):
- """
- Output class for Alt Diffusion pipelines.
-
- Args:
- images (`List[PIL.Image.Image]` or `np.ndarray`)
- List of denoised PIL images of length `batch_size` or numpy array of shape `(batch_size, height, width,
- num_channels)`. PIL images or numpy array present the denoised images of the diffusion pipeline.
- nsfw_content_detected (`List[bool]`)
- List of flags denoting whether the corresponding generated image likely represents "not-safe-for-work"
- (nsfw) content, or `None` if safety checking could not be performed.
- """
-
- images: Union[List[PIL.Image.Image], np.ndarray]
- nsfw_content_detected: Optional[List[bool]]
-
-
-if is_transformers_available() and is_torch_available():
- from .modeling_roberta_series import RobertaSeriesModelWithTransformation
- from .pipeline_alt_diffusion import AltDiffusionPipeline
- from .pipeline_alt_diffusion_img2img import AltDiffusionImg2ImgPipeline
diff --git a/spaces/Yuliang/ECON/lib/pymafx/utils/transforms.py b/spaces/Yuliang/ECON/lib/pymafx/utils/transforms.py
deleted file mode 100644
index 5f4189ee0e2da45e565b322d207b011ae3ed70f5..0000000000000000000000000000000000000000
--- a/spaces/Yuliang/ECON/lib/pymafx/utils/transforms.py
+++ /dev/null
@@ -1,117 +0,0 @@
-# ------------------------------------------------------------------------------
-# Copyright (c) Microsoft
-# Licensed under the MIT License.
-# Written by Bin Xiao (Bin.Xiao@microsoft.com)
-# ------------------------------------------------------------------------------
-
-from __future__ import absolute_import, division, print_function
-
-import cv2
-import numpy as np
-
-
-def flip_back(output_flipped, matched_parts):
- '''
- ouput_flipped: numpy.ndarray(batch_size, num_joints, height, width)
- '''
- assert output_flipped.ndim == 4,\
- 'output_flipped should be [batch_size, num_joints, height, width]'
-
- output_flipped = output_flipped[:, :, :, ::-1]
-
- for pair in matched_parts:
- tmp = output_flipped[:, pair[0], :, :].copy()
- output_flipped[:, pair[0], :, :] = output_flipped[:, pair[1], :, :]
- output_flipped[:, pair[1], :, :] = tmp
-
- return output_flipped
-
-
-def fliplr_joints(joints, joints_vis, width, matched_parts):
- """
- flip coords
- """
- # Flip horizontal
- joints[:, 0] = width - joints[:, 0] - 1
-
- # Change left-right parts
- for pair in matched_parts:
- joints[pair[0], :], joints[pair[1], :] = \
- joints[pair[1], :], joints[pair[0], :].copy()
- joints_vis[pair[0], :], joints_vis[pair[1], :] = \
- joints_vis[pair[1], :], joints_vis[pair[0], :].copy()
-
- return joints * joints_vis, joints_vis
-
-
-def transform_preds(coords, center, scale, output_size):
- target_coords = np.zeros(coords.shape)
- trans = get_affine_transform(center, scale, 0, output_size, inv=1)
- for p in range(coords.shape[0]):
- target_coords[p, 0:2] = affine_transform(coords[p, 0:2], trans)
- return target_coords
-
-
-def get_affine_transform(
- center, scale, rot, output_size, shift=np.array([0, 0], dtype=np.float32), inv=0
-):
- if not isinstance(scale, np.ndarray) and not isinstance(scale, list):
- # print(scale)
- scale = np.array([scale, scale])
-
- scale_tmp = scale * 200.0
- src_w = scale_tmp[0]
- dst_w = output_size[0]
- dst_h = output_size[1]
-
- rot_rad = np.pi * rot / 180
- src_dir = get_dir([0, src_w * -0.5], rot_rad)
- dst_dir = np.array([0, dst_w * -0.5], np.float32)
-
- src = np.zeros((3, 2), dtype=np.float32)
- dst = np.zeros((3, 2), dtype=np.float32)
- src[0, :] = center + scale_tmp * shift
- src[1, :] = center + src_dir + scale_tmp * shift
- dst[0, :] = [dst_w * 0.5, dst_h * 0.5]
- dst[1, :] = np.array([dst_w * 0.5, dst_h * 0.5]) + dst_dir
-
- src[2:, :] = get_3rd_point(src[0, :], src[1, :])
- dst[2:, :] = get_3rd_point(dst[0, :], dst[1, :])
-
- if inv:
- trans = cv2.getAffineTransform(np.float32(dst), np.float32(src))
- else:
- trans = cv2.getAffineTransform(np.float32(src), np.float32(dst))
-
- return trans
-
-
-def affine_transform(pt, t):
- new_pt = np.array([pt[0], pt[1], 1.]).T
- new_pt = np.dot(t, new_pt)
- return new_pt[:2]
-
-
-def get_3rd_point(a, b):
- direct = a - b
- return b + np.array([-direct[1], direct[0]], dtype=np.float32)
-
-
-def get_dir(src_point, rot_rad):
- sn, cs = np.sin(rot_rad), np.cos(rot_rad)
-
- src_result = [0, 0]
- src_result[0] = src_point[0] * cs - src_point[1] * sn
- src_result[1] = src_point[0] * sn + src_point[1] * cs
-
- return src_result
-
-
-def crop(img, center, scale, output_size, rot=0):
- trans = get_affine_transform(center, scale, rot, output_size)
-
- dst_img = cv2.warpAffine(
- img, trans, (int(output_size[0]), int(output_size[1])), flags=cv2.INTER_LINEAR
- )
-
- return dst_img
diff --git a/spaces/YuxinJ/Scenimefy/Scenimefy/options/test_options.py b/spaces/YuxinJ/Scenimefy/Scenimefy/options/test_options.py
deleted file mode 100644
index 67e189411c96aedf27da2c3e183f47a4576bc20c..0000000000000000000000000000000000000000
--- a/spaces/YuxinJ/Scenimefy/Scenimefy/options/test_options.py
+++ /dev/null
@@ -1,22 +0,0 @@
-from Scenimefy.options.base_options import BaseOptions
-
-
-class TestOptions(BaseOptions):
- """
- This class includes test options.
-
- It also includes shared options defined in BaseOptions.
- """
-
- def initialize(self, parser):
- parser = BaseOptions.initialize(self, parser) # define shared options
- parser.add_argument('--results_dir', type=str, default='Scenimefy/results/', help='saves results here.')
- parser.add_argument('--phase', type=str, default='test', help='train, val, test, etc')
- # Dropout and Batchnorm has different behavioir during training and test.
- parser.add_argument('--eval', action='store_true', help='use eval mode during test time.')
- parser.add_argument('--num_test', type=int, default=1000, help='how many test images to run')
-
- # To avoid cropping, the load_size should be the same as crop_size
- parser.set_defaults(load_size=parser.get_default('crop_size'))
- self.isTrain = False
- return parser
diff --git a/spaces/ZilliaxOfficial/nyaru-svc-3.0/vdecoder/hifigan/utils.py b/spaces/ZilliaxOfficial/nyaru-svc-3.0/vdecoder/hifigan/utils.py
deleted file mode 100644
index 84bff024f4d2e2de194b2a88ee7bbe5f0d33f67c..0000000000000000000000000000000000000000
--- a/spaces/ZilliaxOfficial/nyaru-svc-3.0/vdecoder/hifigan/utils.py
+++ /dev/null
@@ -1,68 +0,0 @@
-import glob
-import os
-import matplotlib
-import torch
-from torch.nn.utils import weight_norm
-matplotlib.use("Agg")
-import matplotlib.pylab as plt
-
-
-def plot_spectrogram(spectrogram):
- fig, ax = plt.subplots(figsize=(10, 2))
- im = ax.imshow(spectrogram, aspect="auto", origin="lower",
- interpolation='none')
- plt.colorbar(im, ax=ax)
-
- fig.canvas.draw()
- plt.close()
-
- return fig
-
-
-def init_weights(m, mean=0.0, std=0.01):
- classname = m.__class__.__name__
- if classname.find("Conv") != -1:
- m.weight.data.normal_(mean, std)
-
-
-def apply_weight_norm(m):
- classname = m.__class__.__name__
- if classname.find("Conv") != -1:
- weight_norm(m)
-
-
-def get_padding(kernel_size, dilation=1):
- return int((kernel_size*dilation - dilation)/2)
-
-
-def load_checkpoint(filepath, device):
- assert os.path.isfile(filepath)
- print("Loading '{}'".format(filepath))
- checkpoint_dict = torch.load(filepath, map_location=device)
- print("Complete.")
- return checkpoint_dict
-
-
-def save_checkpoint(filepath, obj):
- print("Saving checkpoint to {}".format(filepath))
- torch.save(obj, filepath)
- print("Complete.")
-
-
-def del_old_checkpoints(cp_dir, prefix, n_models=2):
- pattern = os.path.join(cp_dir, prefix + '????????')
- cp_list = glob.glob(pattern) # get checkpoint paths
- cp_list = sorted(cp_list)# sort by iter
- if len(cp_list) > n_models: # if more than n_models models are found
- for cp in cp_list[:-n_models]:# delete the oldest models other than lastest n_models
- open(cp, 'w').close()# empty file contents
- os.unlink(cp)# delete file (move to trash when using Colab)
-
-
-def scan_checkpoint(cp_dir, prefix):
- pattern = os.path.join(cp_dir, prefix + '????????')
- cp_list = glob.glob(pattern)
- if len(cp_list) == 0:
- return None
- return sorted(cp_list)[-1]
-
diff --git "a/spaces/a-v-bely/spanish-task-generator/pages/4_\360\237\223\235_\320\236\320\275\320\273\320\260\320\271\320\275-\321\202\320\265\321\201\321\202.py" "b/spaces/a-v-bely/spanish-task-generator/pages/4_\360\237\223\235_\320\236\320\275\320\273\320\260\320\271\320\275-\321\202\320\265\321\201\321\202.py"
deleted file mode 100644
index 044aef93b8c92ea6dc3c8e6f92de9700d7495980..0000000000000000000000000000000000000000
--- "a/spaces/a-v-bely/spanish-task-generator/pages/4_\360\237\223\235_\320\236\320\275\320\273\320\260\320\271\320\275-\321\202\320\265\321\201\321\202.py"
+++ /dev/null
@@ -1,68 +0,0 @@
-import datetime
-import pandas as pd
-import streamlit as st
-from utilities_database.user_database_utils import save_data_in_database
-from utilities_database.user_database_widgets import user_save_text_table
-
-st.set_page_config(page_title='Онлайн-тест', layout="wide", page_icon=':es:', initial_sidebar_state='collapsed')
-if st.session_state.get('-ONLINE_TEST_READY-') and st.session_state.get('-LOGGED_IN_BOOL-'):
- INSTRUCTION = st.expander(label='**ИНСТРУКЦИЯ**', expanded=False)
- INSTRUCTION.markdown(
- 'Уважаемые пользователи, предлагаем Вам заполнить опросник по оценке качества созданных заданий. '
- '\n\nНиже находится анкета с заданиями в таблице.'
- '\n\n- В **первом столбце** приводится ответ - слово, удаленное из оригинального текста.'
- '\n\n- Отметьте во **втором столбце**, уместно ли создавать задание с данным словом.'
- '\n\n- В **третьем столбце** приведены подобранные программой дистракторы.'
- '\n\n- Введите в **четвертый столбец** дистракторы (целиком или букву), которые, по Вашему мнению,'
- ' **:red[не уместны]**. '
- '\n\n**:green[Уместными дистракторами]** мы предлагаем считать те, которые одновременно удовлетворяют'
- ' следующим условиям в рамках языкового уровня, для которого они созданы:'
- '\n\n1. не слишком очевидно являются неправильными вариантами (*варить суп/стол*);'
- '\n\n2. и при этом не могут быть полноценной заменой удаленного слова (*варить суп/кашу*)'
- )
- result = st.session_state.get('RESULT')
- if result is None:
- st.error('Не можем ничего загрузить! Вы ничего не просили!')
- st.stop()
- tasks = result['TASKS_ONLY']
- answers = result['KEYS_ONLY_RAW']
- len_answers = len(answers)
- st.header('Онлайн-тест')
- ONLINE_TEST = st.form('Онлайн тест')
- ONLINE_TEST.write(result['TEXT_WITH_GAPS'].replace('_', '\_'))
- BAD_DISTRACTORS_AND_ANSWERS_temp = ONLINE_TEST.data_editor(
- pd.DataFrame([{"Задание №": i + 1,
- "Ответ": [answers[i][1]],
- "Задание уместно": False,
- "Дистракторы": tasks[i][1],
- "Неуместные дистракторы": ''}
- for i in range(len(tasks))]),
- num_rows="fixed",
- height=40 * len_answers,
- hide_index=True,
- use_container_width=True)
- COMMENTS = ONLINE_TEST.text_area(label='**Прокомментировать**',
- placeholder='Напишите комментарий')
- SUBMIT = ONLINE_TEST.form_submit_button('READY')
- if SUBMIT:
- points = test_mark = 'Teacher'
- appropriate_tasks = BAD_DISTRACTORS_AND_ANSWERS_temp["Задание уместно"].values.tolist()
- inappropriate_distractors = BAD_DISTRACTORS_AND_ANSWERS_temp["Неуместные дистракторы"].values.tolist()
- RETURN_TEST_DATA = [{'ANSWER': answers[i],
- 'APPROPRIATE_TASK': appropriate_tasks[i],
- 'INAPPROPRIATE_DISTRACTORS': inappropriate_distractors[i]} for i in range(len_answers)]
- save_data_in_database(user_task_database=user_save_text_table,
- save_type='online_test',
- save_name=st.session_state['-UPLOAD_CLOUD_FILE_NAME-'],
- cefr_level=st.session_state['-LOADED_CEFR_LEVEL-'],
- time_stamp=str(datetime.datetime.now())[:-7],
- creator_name=st.session_state.get('-USER_NAME-'),
- test_taker_name=st.session_state.get('-USER_NAME-'),
- generated_result=result,
- test_taker_answers=RETURN_TEST_DATA,
- test_taker_result={'Баллов': points, 'Всего': len_answers, 'Оценка': test_mark},
- comments=COMMENTS)
-elif st.session_state.get('-LOGGED_IN_BOOL-'):
- st.warning('**Не можем ничего загрузить! Вы ничего не просили!**')
-else:
- st.warning('**Войдите или зарегистрируйтесь**')
diff --git a/spaces/aaaaaabbbbbbbdddddddduuuuulllll/Ashaar/poetry_diacritizer/models/tacotron_based.py b/spaces/aaaaaabbbbbbbdddddddduuuuulllll/Ashaar/poetry_diacritizer/models/tacotron_based.py
deleted file mode 100644
index 0bbd408e25b485fb80040683658c42ab9d382221..0000000000000000000000000000000000000000
--- a/spaces/aaaaaabbbbbbbdddddddduuuuulllll/Ashaar/poetry_diacritizer/models/tacotron_based.py
+++ /dev/null
@@ -1,47 +0,0 @@
-from typing import List
-from poetry_diacritizer.models.seq2seq import Seq2Seq, Decoder as Seq2SeqDecoder
-from poetry_diacritizer.modules.tacotron_modules import CBHG, Prenet
-from torch import nn
-
-
-class Tacotron(Seq2Seq):
- pass
-
-
-class Encoder(nn.Module):
- def __init__(
- self,
- inp_vocab_size: int,
- embedding_dim: int = 512,
- use_prenet: bool = True,
- prenet_sizes: List[int] = [256, 128],
- cbhg_gru_units: int = 128,
- cbhg_filters: int = 16,
- cbhg_projections: List[int] = [128, 128],
- padding_idx: int = 0,
- ):
- super().__init__()
- self.use_prenet = use_prenet
-
- self.embedding = nn.Embedding(
- inp_vocab_size, embedding_dim, padding_idx=padding_idx
- )
- if use_prenet:
- self.prenet = Prenet(embedding_dim, prenet_depth=prenet_sizes)
- self.cbhg = CBHG(
- prenet_sizes[-1] if use_prenet else embedding_dim,
- cbhg_gru_units,
- K=cbhg_filters,
- projections=cbhg_projections,
- )
-
- def forward(self, inputs, input_lengths=None):
-
- outputs = self.embedding(inputs)
- if self.use_prenet:
- outputs = self.prenet(outputs)
- return self.cbhg(outputs, input_lengths)
-
-
-class Decoder(Seq2SeqDecoder):
- pass
diff --git a/spaces/abhishek/first-order-motion-model/sync_batchnorm/comm.py b/spaces/abhishek/first-order-motion-model/sync_batchnorm/comm.py
deleted file mode 100644
index 922f8c4a3adaa9b32fdcaef09583be03b0d7eb2b..0000000000000000000000000000000000000000
--- a/spaces/abhishek/first-order-motion-model/sync_batchnorm/comm.py
+++ /dev/null
@@ -1,137 +0,0 @@
-# -*- coding: utf-8 -*-
-# File : comm.py
-# Author : Jiayuan Mao
-# Email : maojiayuan@gmail.com
-# Date : 27/01/2018
-#
-# This file is part of Synchronized-BatchNorm-PyTorch.
-# https://github.com/vacancy/Synchronized-BatchNorm-PyTorch
-# Distributed under MIT License.
-
-import queue
-import collections
-import threading
-
-__all__ = ['FutureResult', 'SlavePipe', 'SyncMaster']
-
-
-class FutureResult(object):
- """A thread-safe future implementation. Used only as one-to-one pipe."""
-
- def __init__(self):
- self._result = None
- self._lock = threading.Lock()
- self._cond = threading.Condition(self._lock)
-
- def put(self, result):
- with self._lock:
- assert self._result is None, 'Previous result has\'t been fetched.'
- self._result = result
- self._cond.notify()
-
- def get(self):
- with self._lock:
- if self._result is None:
- self._cond.wait()
-
- res = self._result
- self._result = None
- return res
-
-
-_MasterRegistry = collections.namedtuple('MasterRegistry', ['result'])
-_SlavePipeBase = collections.namedtuple('_SlavePipeBase', ['identifier', 'queue', 'result'])
-
-
-class SlavePipe(_SlavePipeBase):
- """Pipe for master-slave communication."""
-
- def run_slave(self, msg):
- self.queue.put((self.identifier, msg))
- ret = self.result.get()
- self.queue.put(True)
- return ret
-
-
-class SyncMaster(object):
- """An abstract `SyncMaster` object.
-
- - During the replication, as the data parallel will trigger an callback of each module, all slave devices should
- call `register(id)` and obtain an `SlavePipe` to communicate with the master.
- - During the forward pass, master device invokes `run_master`, all messages from slave devices will be collected,
- and passed to a registered callback.
- - After receiving the messages, the master device should gather the information and determine to message passed
- back to each slave devices.
- """
-
- def __init__(self, master_callback):
- """
-
- Args:
- master_callback: a callback to be invoked after having collected messages from slave devices.
- """
- self._master_callback = master_callback
- self._queue = queue.Queue()
- self._registry = collections.OrderedDict()
- self._activated = False
-
- def __getstate__(self):
- return {'master_callback': self._master_callback}
-
- def __setstate__(self, state):
- self.__init__(state['master_callback'])
-
- def register_slave(self, identifier):
- """
- Register an slave device.
-
- Args:
- identifier: an identifier, usually is the device id.
-
- Returns: a `SlavePipe` object which can be used to communicate with the master device.
-
- """
- if self._activated:
- assert self._queue.empty(), 'Queue is not clean before next initialization.'
- self._activated = False
- self._registry.clear()
- future = FutureResult()
- self._registry[identifier] = _MasterRegistry(future)
- return SlavePipe(identifier, self._queue, future)
-
- def run_master(self, master_msg):
- """
- Main entry for the master device in each forward pass.
- The messages were first collected from each devices (including the master device), and then
- an callback will be invoked to compute the message to be sent back to each devices
- (including the master device).
-
- Args:
- master_msg: the message that the master want to send to itself. This will be placed as the first
- message when calling `master_callback`. For detailed usage, see `_SynchronizedBatchNorm` for an example.
-
- Returns: the message to be sent back to the master device.
-
- """
- self._activated = True
-
- intermediates = [(0, master_msg)]
- for i in range(self.nr_slaves):
- intermediates.append(self._queue.get())
-
- results = self._master_callback(intermediates)
- assert results[0][0] == 0, 'The first result should belongs to the master.'
-
- for i, res in results:
- if i == 0:
- continue
- self._registry[i].result.put(res)
-
- for i in range(self.nr_slaves):
- assert self._queue.get() is True
-
- return results[0][1]
-
- @property
- def nr_slaves(self):
- return len(self._registry)
diff --git a/spaces/abhishek/sketch-to-image/annotator/uniformer/mmcv/cnn/bricks/__init__.py b/spaces/abhishek/sketch-to-image/annotator/uniformer/mmcv/cnn/bricks/__init__.py
deleted file mode 100644
index 0f33124ed23fc6f27119a37bcb5ab004d3572be0..0000000000000000000000000000000000000000
--- a/spaces/abhishek/sketch-to-image/annotator/uniformer/mmcv/cnn/bricks/__init__.py
+++ /dev/null
@@ -1,35 +0,0 @@
-# Copyright (c) OpenMMLab. All rights reserved.
-from .activation import build_activation_layer
-from .context_block import ContextBlock
-from .conv import build_conv_layer
-from .conv2d_adaptive_padding import Conv2dAdaptivePadding
-from .conv_module import ConvModule
-from .conv_ws import ConvAWS2d, ConvWS2d, conv_ws_2d
-from .depthwise_separable_conv_module import DepthwiseSeparableConvModule
-from .drop import Dropout, DropPath
-from .generalized_attention import GeneralizedAttention
-from .hsigmoid import HSigmoid
-from .hswish import HSwish
-from .non_local import NonLocal1d, NonLocal2d, NonLocal3d
-from .norm import build_norm_layer, is_norm
-from .padding import build_padding_layer
-from .plugin import build_plugin_layer
-from .registry import (ACTIVATION_LAYERS, CONV_LAYERS, NORM_LAYERS,
- PADDING_LAYERS, PLUGIN_LAYERS, UPSAMPLE_LAYERS)
-from .scale import Scale
-from .swish import Swish
-from .upsample import build_upsample_layer
-from .wrappers import (Conv2d, Conv3d, ConvTranspose2d, ConvTranspose3d,
- Linear, MaxPool2d, MaxPool3d)
-
-__all__ = [
- 'ConvModule', 'build_activation_layer', 'build_conv_layer',
- 'build_norm_layer', 'build_padding_layer', 'build_upsample_layer',
- 'build_plugin_layer', 'is_norm', 'HSigmoid', 'HSwish', 'NonLocal1d',
- 'NonLocal2d', 'NonLocal3d', 'ContextBlock', 'GeneralizedAttention',
- 'ACTIVATION_LAYERS', 'CONV_LAYERS', 'NORM_LAYERS', 'PADDING_LAYERS',
- 'UPSAMPLE_LAYERS', 'PLUGIN_LAYERS', 'Scale', 'ConvAWS2d', 'ConvWS2d',
- 'conv_ws_2d', 'DepthwiseSeparableConvModule', 'Swish', 'Linear',
- 'Conv2dAdaptivePadding', 'Conv2d', 'ConvTranspose2d', 'MaxPool2d',
- 'ConvTranspose3d', 'MaxPool3d', 'Conv3d', 'Dropout', 'DropPath'
-]
diff --git a/spaces/abhishek/sketch-to-image/annotator/uniformer/mmdet/models/roi_heads/mask_heads/grid_head.py b/spaces/abhishek/sketch-to-image/annotator/uniformer/mmdet/models/roi_heads/mask_heads/grid_head.py
deleted file mode 100644
index 83058cbdda934ebfc3a76088e1820848ac01b78b..0000000000000000000000000000000000000000
--- a/spaces/abhishek/sketch-to-image/annotator/uniformer/mmdet/models/roi_heads/mask_heads/grid_head.py
+++ /dev/null
@@ -1,359 +0,0 @@
-import numpy as np
-import torch
-import torch.nn as nn
-import torch.nn.functional as F
-from mmcv.cnn import ConvModule, kaiming_init, normal_init
-
-from mmdet.models.builder import HEADS, build_loss
-
-
-@HEADS.register_module()
-class GridHead(nn.Module):
-
- def __init__(self,
- grid_points=9,
- num_convs=8,
- roi_feat_size=14,
- in_channels=256,
- conv_kernel_size=3,
- point_feat_channels=64,
- deconv_kernel_size=4,
- class_agnostic=False,
- loss_grid=dict(
- type='CrossEntropyLoss', use_sigmoid=True,
- loss_weight=15),
- conv_cfg=None,
- norm_cfg=dict(type='GN', num_groups=36)):
- super(GridHead, self).__init__()
- self.grid_points = grid_points
- self.num_convs = num_convs
- self.roi_feat_size = roi_feat_size
- self.in_channels = in_channels
- self.conv_kernel_size = conv_kernel_size
- self.point_feat_channels = point_feat_channels
- self.conv_out_channels = self.point_feat_channels * self.grid_points
- self.class_agnostic = class_agnostic
- self.conv_cfg = conv_cfg
- self.norm_cfg = norm_cfg
- if isinstance(norm_cfg, dict) and norm_cfg['type'] == 'GN':
- assert self.conv_out_channels % norm_cfg['num_groups'] == 0
-
- assert self.grid_points >= 4
- self.grid_size = int(np.sqrt(self.grid_points))
- if self.grid_size * self.grid_size != self.grid_points:
- raise ValueError('grid_points must be a square number')
-
- # the predicted heatmap is half of whole_map_size
- if not isinstance(self.roi_feat_size, int):
- raise ValueError('Only square RoIs are supporeted in Grid R-CNN')
- self.whole_map_size = self.roi_feat_size * 4
-
- # compute point-wise sub-regions
- self.sub_regions = self.calc_sub_regions()
-
- self.convs = []
- for i in range(self.num_convs):
- in_channels = (
- self.in_channels if i == 0 else self.conv_out_channels)
- stride = 2 if i == 0 else 1
- padding = (self.conv_kernel_size - 1) // 2
- self.convs.append(
- ConvModule(
- in_channels,
- self.conv_out_channels,
- self.conv_kernel_size,
- stride=stride,
- padding=padding,
- conv_cfg=self.conv_cfg,
- norm_cfg=self.norm_cfg,
- bias=True))
- self.convs = nn.Sequential(*self.convs)
-
- self.deconv1 = nn.ConvTranspose2d(
- self.conv_out_channels,
- self.conv_out_channels,
- kernel_size=deconv_kernel_size,
- stride=2,
- padding=(deconv_kernel_size - 2) // 2,
- groups=grid_points)
- self.norm1 = nn.GroupNorm(grid_points, self.conv_out_channels)
- self.deconv2 = nn.ConvTranspose2d(
- self.conv_out_channels,
- grid_points,
- kernel_size=deconv_kernel_size,
- stride=2,
- padding=(deconv_kernel_size - 2) // 2,
- groups=grid_points)
-
- # find the 4-neighbor of each grid point
- self.neighbor_points = []
- grid_size = self.grid_size
- for i in range(grid_size): # i-th column
- for j in range(grid_size): # j-th row
- neighbors = []
- if i > 0: # left: (i - 1, j)
- neighbors.append((i - 1) * grid_size + j)
- if j > 0: # up: (i, j - 1)
- neighbors.append(i * grid_size + j - 1)
- if j < grid_size - 1: # down: (i, j + 1)
- neighbors.append(i * grid_size + j + 1)
- if i < grid_size - 1: # right: (i + 1, j)
- neighbors.append((i + 1) * grid_size + j)
- self.neighbor_points.append(tuple(neighbors))
- # total edges in the grid
- self.num_edges = sum([len(p) for p in self.neighbor_points])
-
- self.forder_trans = nn.ModuleList() # first-order feature transition
- self.sorder_trans = nn.ModuleList() # second-order feature transition
- for neighbors in self.neighbor_points:
- fo_trans = nn.ModuleList()
- so_trans = nn.ModuleList()
- for _ in range(len(neighbors)):
- # each transition module consists of a 5x5 depth-wise conv and
- # 1x1 conv.
- fo_trans.append(
- nn.Sequential(
- nn.Conv2d(
- self.point_feat_channels,
- self.point_feat_channels,
- 5,
- stride=1,
- padding=2,
- groups=self.point_feat_channels),
- nn.Conv2d(self.point_feat_channels,
- self.point_feat_channels, 1)))
- so_trans.append(
- nn.Sequential(
- nn.Conv2d(
- self.point_feat_channels,
- self.point_feat_channels,
- 5,
- 1,
- 2,
- groups=self.point_feat_channels),
- nn.Conv2d(self.point_feat_channels,
- self.point_feat_channels, 1)))
- self.forder_trans.append(fo_trans)
- self.sorder_trans.append(so_trans)
-
- self.loss_grid = build_loss(loss_grid)
-
- def init_weights(self):
- for m in self.modules():
- if isinstance(m, nn.Conv2d) or isinstance(m, nn.Linear):
- # TODO: compare mode = "fan_in" or "fan_out"
- kaiming_init(m)
- for m in self.modules():
- if isinstance(m, nn.ConvTranspose2d):
- normal_init(m, std=0.001)
- nn.init.constant_(self.deconv2.bias, -np.log(0.99 / 0.01))
-
- def forward(self, x):
- assert x.shape[-1] == x.shape[-2] == self.roi_feat_size
- # RoI feature transformation, downsample 2x
- x = self.convs(x)
-
- c = self.point_feat_channels
- # first-order fusion
- x_fo = [None for _ in range(self.grid_points)]
- for i, points in enumerate(self.neighbor_points):
- x_fo[i] = x[:, i * c:(i + 1) * c]
- for j, point_idx in enumerate(points):
- x_fo[i] = x_fo[i] + self.forder_trans[i][j](
- x[:, point_idx * c:(point_idx + 1) * c])
-
- # second-order fusion
- x_so = [None for _ in range(self.grid_points)]
- for i, points in enumerate(self.neighbor_points):
- x_so[i] = x[:, i * c:(i + 1) * c]
- for j, point_idx in enumerate(points):
- x_so[i] = x_so[i] + self.sorder_trans[i][j](x_fo[point_idx])
-
- # predicted heatmap with fused features
- x2 = torch.cat(x_so, dim=1)
- x2 = self.deconv1(x2)
- x2 = F.relu(self.norm1(x2), inplace=True)
- heatmap = self.deconv2(x2)
-
- # predicted heatmap with original features (applicable during training)
- if self.training:
- x1 = x
- x1 = self.deconv1(x1)
- x1 = F.relu(self.norm1(x1), inplace=True)
- heatmap_unfused = self.deconv2(x1)
- else:
- heatmap_unfused = heatmap
-
- return dict(fused=heatmap, unfused=heatmap_unfused)
-
- def calc_sub_regions(self):
- """Compute point specific representation regions.
-
- See Grid R-CNN Plus (https://arxiv.org/abs/1906.05688) for details.
- """
- # to make it consistent with the original implementation, half_size
- # is computed as 2 * quarter_size, which is smaller
- half_size = self.whole_map_size // 4 * 2
- sub_regions = []
- for i in range(self.grid_points):
- x_idx = i // self.grid_size
- y_idx = i % self.grid_size
- if x_idx == 0:
- sub_x1 = 0
- elif x_idx == self.grid_size - 1:
- sub_x1 = half_size
- else:
- ratio = x_idx / (self.grid_size - 1) - 0.25
- sub_x1 = max(int(ratio * self.whole_map_size), 0)
-
- if y_idx == 0:
- sub_y1 = 0
- elif y_idx == self.grid_size - 1:
- sub_y1 = half_size
- else:
- ratio = y_idx / (self.grid_size - 1) - 0.25
- sub_y1 = max(int(ratio * self.whole_map_size), 0)
- sub_regions.append(
- (sub_x1, sub_y1, sub_x1 + half_size, sub_y1 + half_size))
- return sub_regions
-
- def get_targets(self, sampling_results, rcnn_train_cfg):
- # mix all samples (across images) together.
- pos_bboxes = torch.cat([res.pos_bboxes for res in sampling_results],
- dim=0).cpu()
- pos_gt_bboxes = torch.cat(
- [res.pos_gt_bboxes for res in sampling_results], dim=0).cpu()
- assert pos_bboxes.shape == pos_gt_bboxes.shape
-
- # expand pos_bboxes to 2x of original size
- x1 = pos_bboxes[:, 0] - (pos_bboxes[:, 2] - pos_bboxes[:, 0]) / 2
- y1 = pos_bboxes[:, 1] - (pos_bboxes[:, 3] - pos_bboxes[:, 1]) / 2
- x2 = pos_bboxes[:, 2] + (pos_bboxes[:, 2] - pos_bboxes[:, 0]) / 2
- y2 = pos_bboxes[:, 3] + (pos_bboxes[:, 3] - pos_bboxes[:, 1]) / 2
- pos_bboxes = torch.stack([x1, y1, x2, y2], dim=-1)
- pos_bbox_ws = (pos_bboxes[:, 2] - pos_bboxes[:, 0]).unsqueeze(-1)
- pos_bbox_hs = (pos_bboxes[:, 3] - pos_bboxes[:, 1]).unsqueeze(-1)
-
- num_rois = pos_bboxes.shape[0]
- map_size = self.whole_map_size
- # this is not the final target shape
- targets = torch.zeros((num_rois, self.grid_points, map_size, map_size),
- dtype=torch.float)
-
- # pre-compute interpolation factors for all grid points.
- # the first item is the factor of x-dim, and the second is y-dim.
- # for a 9-point grid, factors are like (1, 0), (0.5, 0.5), (0, 1)
- factors = []
- for j in range(self.grid_points):
- x_idx = j // self.grid_size
- y_idx = j % self.grid_size
- factors.append((1 - x_idx / (self.grid_size - 1),
- 1 - y_idx / (self.grid_size - 1)))
-
- radius = rcnn_train_cfg.pos_radius
- radius2 = radius**2
- for i in range(num_rois):
- # ignore small bboxes
- if (pos_bbox_ws[i] <= self.grid_size
- or pos_bbox_hs[i] <= self.grid_size):
- continue
- # for each grid point, mark a small circle as positive
- for j in range(self.grid_points):
- factor_x, factor_y = factors[j]
- gridpoint_x = factor_x * pos_gt_bboxes[i, 0] + (
- 1 - factor_x) * pos_gt_bboxes[i, 2]
- gridpoint_y = factor_y * pos_gt_bboxes[i, 1] + (
- 1 - factor_y) * pos_gt_bboxes[i, 3]
-
- cx = int((gridpoint_x - pos_bboxes[i, 0]) / pos_bbox_ws[i] *
- map_size)
- cy = int((gridpoint_y - pos_bboxes[i, 1]) / pos_bbox_hs[i] *
- map_size)
-
- for x in range(cx - radius, cx + radius + 1):
- for y in range(cy - radius, cy + radius + 1):
- if x >= 0 and x < map_size and y >= 0 and y < map_size:
- if (x - cx)**2 + (y - cy)**2 <= radius2:
- targets[i, j, y, x] = 1
- # reduce the target heatmap size by a half
- # proposed in Grid R-CNN Plus (https://arxiv.org/abs/1906.05688).
- sub_targets = []
- for i in range(self.grid_points):
- sub_x1, sub_y1, sub_x2, sub_y2 = self.sub_regions[i]
- sub_targets.append(targets[:, [i], sub_y1:sub_y2, sub_x1:sub_x2])
- sub_targets = torch.cat(sub_targets, dim=1)
- sub_targets = sub_targets.to(sampling_results[0].pos_bboxes.device)
- return sub_targets
-
- def loss(self, grid_pred, grid_targets):
- loss_fused = self.loss_grid(grid_pred['fused'], grid_targets)
- loss_unfused = self.loss_grid(grid_pred['unfused'], grid_targets)
- loss_grid = loss_fused + loss_unfused
- return dict(loss_grid=loss_grid)
-
- def get_bboxes(self, det_bboxes, grid_pred, img_metas):
- # TODO: refactoring
- assert det_bboxes.shape[0] == grid_pred.shape[0]
- det_bboxes = det_bboxes.cpu()
- cls_scores = det_bboxes[:, [4]]
- det_bboxes = det_bboxes[:, :4]
- grid_pred = grid_pred.sigmoid().cpu()
-
- R, c, h, w = grid_pred.shape
- half_size = self.whole_map_size // 4 * 2
- assert h == w == half_size
- assert c == self.grid_points
-
- # find the point with max scores in the half-sized heatmap
- grid_pred = grid_pred.view(R * c, h * w)
- pred_scores, pred_position = grid_pred.max(dim=1)
- xs = pred_position % w
- ys = pred_position // w
-
- # get the position in the whole heatmap instead of half-sized heatmap
- for i in range(self.grid_points):
- xs[i::self.grid_points] += self.sub_regions[i][0]
- ys[i::self.grid_points] += self.sub_regions[i][1]
-
- # reshape to (num_rois, grid_points)
- pred_scores, xs, ys = tuple(
- map(lambda x: x.view(R, c), [pred_scores, xs, ys]))
-
- # get expanded pos_bboxes
- widths = (det_bboxes[:, 2] - det_bboxes[:, 0]).unsqueeze(-1)
- heights = (det_bboxes[:, 3] - det_bboxes[:, 1]).unsqueeze(-1)
- x1 = (det_bboxes[:, 0, None] - widths / 2)
- y1 = (det_bboxes[:, 1, None] - heights / 2)
- # map the grid point to the absolute coordinates
- abs_xs = (xs.float() + 0.5) / w * widths + x1
- abs_ys = (ys.float() + 0.5) / h * heights + y1
-
- # get the grid points indices that fall on the bbox boundaries
- x1_inds = [i for i in range(self.grid_size)]
- y1_inds = [i * self.grid_size for i in range(self.grid_size)]
- x2_inds = [
- self.grid_points - self.grid_size + i
- for i in range(self.grid_size)
- ]
- y2_inds = [(i + 1) * self.grid_size - 1 for i in range(self.grid_size)]
-
- # voting of all grid points on some boundary
- bboxes_x1 = (abs_xs[:, x1_inds] * pred_scores[:, x1_inds]).sum(
- dim=1, keepdim=True) / (
- pred_scores[:, x1_inds].sum(dim=1, keepdim=True))
- bboxes_y1 = (abs_ys[:, y1_inds] * pred_scores[:, y1_inds]).sum(
- dim=1, keepdim=True) / (
- pred_scores[:, y1_inds].sum(dim=1, keepdim=True))
- bboxes_x2 = (abs_xs[:, x2_inds] * pred_scores[:, x2_inds]).sum(
- dim=1, keepdim=True) / (
- pred_scores[:, x2_inds].sum(dim=1, keepdim=True))
- bboxes_y2 = (abs_ys[:, y2_inds] * pred_scores[:, y2_inds]).sum(
- dim=1, keepdim=True) / (
- pred_scores[:, y2_inds].sum(dim=1, keepdim=True))
-
- bbox_res = torch.cat(
- [bboxes_x1, bboxes_y1, bboxes_x2, bboxes_y2, cls_scores], dim=1)
- bbox_res[:, [0, 2]].clamp_(min=0, max=img_metas[0]['img_shape'][1])
- bbox_res[:, [1, 3]].clamp_(min=0, max=img_metas[0]['img_shape'][0])
-
- return bbox_res
diff --git a/spaces/abhishek/sketch-to-image/annotator/uniformer/mmdet_null/models/dense_heads/reppoints_head.py b/spaces/abhishek/sketch-to-image/annotator/uniformer/mmdet_null/models/dense_heads/reppoints_head.py
deleted file mode 100644
index 499cc4f71c968704a40ab2bb7a6b22dd079d82de..0000000000000000000000000000000000000000
--- a/spaces/abhishek/sketch-to-image/annotator/uniformer/mmdet_null/models/dense_heads/reppoints_head.py
+++ /dev/null
@@ -1,763 +0,0 @@
-import numpy as np
-import torch
-import torch.nn as nn
-from mmcv.cnn import ConvModule, bias_init_with_prob, normal_init
-from mmcv.ops import DeformConv2d
-
-from mmdet.core import (PointGenerator, build_assigner, build_sampler,
- images_to_levels, multi_apply, multiclass_nms, unmap)
-from ..builder import HEADS, build_loss
-from .anchor_free_head import AnchorFreeHead
-
-
-@HEADS.register_module()
-class RepPointsHead(AnchorFreeHead):
- """RepPoint head.
-
- Args:
- point_feat_channels (int): Number of channels of points features.
- gradient_mul (float): The multiplier to gradients from
- points refinement and recognition.
- point_strides (Iterable): points strides.
- point_base_scale (int): bbox scale for assigning labels.
- loss_cls (dict): Config of classification loss.
- loss_bbox_init (dict): Config of initial points loss.
- loss_bbox_refine (dict): Config of points loss in refinement.
- use_grid_points (bool): If we use bounding box representation, the
- reppoints is represented as grid points on the bounding box.
- center_init (bool): Whether to use center point assignment.
- transform_method (str): The methods to transform RepPoints to bbox.
- """ # noqa: W605
-
- def __init__(self,
- num_classes,
- in_channels,
- point_feat_channels=256,
- num_points=9,
- gradient_mul=0.1,
- point_strides=[8, 16, 32, 64, 128],
- point_base_scale=4,
- loss_cls=dict(
- type='FocalLoss',
- use_sigmoid=True,
- gamma=2.0,
- alpha=0.25,
- loss_weight=1.0),
- loss_bbox_init=dict(
- type='SmoothL1Loss', beta=1.0 / 9.0, loss_weight=0.5),
- loss_bbox_refine=dict(
- type='SmoothL1Loss', beta=1.0 / 9.0, loss_weight=1.0),
- use_grid_points=False,
- center_init=True,
- transform_method='moment',
- moment_mul=0.01,
- **kwargs):
- self.num_points = num_points
- self.point_feat_channels = point_feat_channels
- self.use_grid_points = use_grid_points
- self.center_init = center_init
-
- # we use deform conv to extract points features
- self.dcn_kernel = int(np.sqrt(num_points))
- self.dcn_pad = int((self.dcn_kernel - 1) / 2)
- assert self.dcn_kernel * self.dcn_kernel == num_points, \
- 'The points number should be a square number.'
- assert self.dcn_kernel % 2 == 1, \
- 'The points number should be an odd square number.'
- dcn_base = np.arange(-self.dcn_pad,
- self.dcn_pad + 1).astype(np.float64)
- dcn_base_y = np.repeat(dcn_base, self.dcn_kernel)
- dcn_base_x = np.tile(dcn_base, self.dcn_kernel)
- dcn_base_offset = np.stack([dcn_base_y, dcn_base_x], axis=1).reshape(
- (-1))
- self.dcn_base_offset = torch.tensor(dcn_base_offset).view(1, -1, 1, 1)
-
- super().__init__(num_classes, in_channels, loss_cls=loss_cls, **kwargs)
-
- self.gradient_mul = gradient_mul
- self.point_base_scale = point_base_scale
- self.point_strides = point_strides
- self.point_generators = [PointGenerator() for _ in self.point_strides]
-
- self.sampling = loss_cls['type'] not in ['FocalLoss']
- if self.train_cfg:
- self.init_assigner = build_assigner(self.train_cfg.init.assigner)
- self.refine_assigner = build_assigner(
- self.train_cfg.refine.assigner)
- # use PseudoSampler when sampling is False
- if self.sampling and hasattr(self.train_cfg, 'sampler'):
- sampler_cfg = self.train_cfg.sampler
- else:
- sampler_cfg = dict(type='PseudoSampler')
- self.sampler = build_sampler(sampler_cfg, context=self)
- self.transform_method = transform_method
- if self.transform_method == 'moment':
- self.moment_transfer = nn.Parameter(
- data=torch.zeros(2), requires_grad=True)
- self.moment_mul = moment_mul
-
- self.use_sigmoid_cls = loss_cls.get('use_sigmoid', False)
- if self.use_sigmoid_cls:
- self.cls_out_channels = self.num_classes
- else:
- self.cls_out_channels = self.num_classes + 1
- self.loss_bbox_init = build_loss(loss_bbox_init)
- self.loss_bbox_refine = build_loss(loss_bbox_refine)
-
- def _init_layers(self):
- """Initialize layers of the head."""
- self.relu = nn.ReLU(inplace=True)
- self.cls_convs = nn.ModuleList()
- self.reg_convs = nn.ModuleList()
- for i in range(self.stacked_convs):
- chn = self.in_channels if i == 0 else self.feat_channels
- self.cls_convs.append(
- ConvModule(
- chn,
- self.feat_channels,
- 3,
- stride=1,
- padding=1,
- conv_cfg=self.conv_cfg,
- norm_cfg=self.norm_cfg))
- self.reg_convs.append(
- ConvModule(
- chn,
- self.feat_channels,
- 3,
- stride=1,
- padding=1,
- conv_cfg=self.conv_cfg,
- norm_cfg=self.norm_cfg))
- pts_out_dim = 4 if self.use_grid_points else 2 * self.num_points
- self.reppoints_cls_conv = DeformConv2d(self.feat_channels,
- self.point_feat_channels,
- self.dcn_kernel, 1,
- self.dcn_pad)
- self.reppoints_cls_out = nn.Conv2d(self.point_feat_channels,
- self.cls_out_channels, 1, 1, 0)
- self.reppoints_pts_init_conv = nn.Conv2d(self.feat_channels,
- self.point_feat_channels, 3,
- 1, 1)
- self.reppoints_pts_init_out = nn.Conv2d(self.point_feat_channels,
- pts_out_dim, 1, 1, 0)
- self.reppoints_pts_refine_conv = DeformConv2d(self.feat_channels,
- self.point_feat_channels,
- self.dcn_kernel, 1,
- self.dcn_pad)
- self.reppoints_pts_refine_out = nn.Conv2d(self.point_feat_channels,
- pts_out_dim, 1, 1, 0)
-
- def init_weights(self):
- """Initialize weights of the head."""
- for m in self.cls_convs:
- normal_init(m.conv, std=0.01)
- for m in self.reg_convs:
- normal_init(m.conv, std=0.01)
- bias_cls = bias_init_with_prob(0.01)
- normal_init(self.reppoints_cls_conv, std=0.01)
- normal_init(self.reppoints_cls_out, std=0.01, bias=bias_cls)
- normal_init(self.reppoints_pts_init_conv, std=0.01)
- normal_init(self.reppoints_pts_init_out, std=0.01)
- normal_init(self.reppoints_pts_refine_conv, std=0.01)
- normal_init(self.reppoints_pts_refine_out, std=0.01)
-
- def points2bbox(self, pts, y_first=True):
- """Converting the points set into bounding box.
-
- :param pts: the input points sets (fields), each points
- set (fields) is represented as 2n scalar.
- :param y_first: if y_first=True, the point set is represented as
- [y1, x1, y2, x2 ... yn, xn], otherwise the point set is
- represented as [x1, y1, x2, y2 ... xn, yn].
- :return: each points set is converting to a bbox [x1, y1, x2, y2].
- """
- pts_reshape = pts.view(pts.shape[0], -1, 2, *pts.shape[2:])
- pts_y = pts_reshape[:, :, 0, ...] if y_first else pts_reshape[:, :, 1,
- ...]
- pts_x = pts_reshape[:, :, 1, ...] if y_first else pts_reshape[:, :, 0,
- ...]
- if self.transform_method == 'minmax':
- bbox_left = pts_x.min(dim=1, keepdim=True)[0]
- bbox_right = pts_x.max(dim=1, keepdim=True)[0]
- bbox_up = pts_y.min(dim=1, keepdim=True)[0]
- bbox_bottom = pts_y.max(dim=1, keepdim=True)[0]
- bbox = torch.cat([bbox_left, bbox_up, bbox_right, bbox_bottom],
- dim=1)
- elif self.transform_method == 'partial_minmax':
- pts_y = pts_y[:, :4, ...]
- pts_x = pts_x[:, :4, ...]
- bbox_left = pts_x.min(dim=1, keepdim=True)[0]
- bbox_right = pts_x.max(dim=1, keepdim=True)[0]
- bbox_up = pts_y.min(dim=1, keepdim=True)[0]
- bbox_bottom = pts_y.max(dim=1, keepdim=True)[0]
- bbox = torch.cat([bbox_left, bbox_up, bbox_right, bbox_bottom],
- dim=1)
- elif self.transform_method == 'moment':
- pts_y_mean = pts_y.mean(dim=1, keepdim=True)
- pts_x_mean = pts_x.mean(dim=1, keepdim=True)
- pts_y_std = torch.std(pts_y - pts_y_mean, dim=1, keepdim=True)
- pts_x_std = torch.std(pts_x - pts_x_mean, dim=1, keepdim=True)
- moment_transfer = (self.moment_transfer * self.moment_mul) + (
- self.moment_transfer.detach() * (1 - self.moment_mul))
- moment_width_transfer = moment_transfer[0]
- moment_height_transfer = moment_transfer[1]
- half_width = pts_x_std * torch.exp(moment_width_transfer)
- half_height = pts_y_std * torch.exp(moment_height_transfer)
- bbox = torch.cat([
- pts_x_mean - half_width, pts_y_mean - half_height,
- pts_x_mean + half_width, pts_y_mean + half_height
- ],
- dim=1)
- else:
- raise NotImplementedError
- return bbox
-
- def gen_grid_from_reg(self, reg, previous_boxes):
- """Base on the previous bboxes and regression values, we compute the
- regressed bboxes and generate the grids on the bboxes.
-
- :param reg: the regression value to previous bboxes.
- :param previous_boxes: previous bboxes.
- :return: generate grids on the regressed bboxes.
- """
- b, _, h, w = reg.shape
- bxy = (previous_boxes[:, :2, ...] + previous_boxes[:, 2:, ...]) / 2.
- bwh = (previous_boxes[:, 2:, ...] -
- previous_boxes[:, :2, ...]).clamp(min=1e-6)
- grid_topleft = bxy + bwh * reg[:, :2, ...] - 0.5 * bwh * torch.exp(
- reg[:, 2:, ...])
- grid_wh = bwh * torch.exp(reg[:, 2:, ...])
- grid_left = grid_topleft[:, [0], ...]
- grid_top = grid_topleft[:, [1], ...]
- grid_width = grid_wh[:, [0], ...]
- grid_height = grid_wh[:, [1], ...]
- intervel = torch.linspace(0., 1., self.dcn_kernel).view(
- 1, self.dcn_kernel, 1, 1).type_as(reg)
- grid_x = grid_left + grid_width * intervel
- grid_x = grid_x.unsqueeze(1).repeat(1, self.dcn_kernel, 1, 1, 1)
- grid_x = grid_x.view(b, -1, h, w)
- grid_y = grid_top + grid_height * intervel
- grid_y = grid_y.unsqueeze(2).repeat(1, 1, self.dcn_kernel, 1, 1)
- grid_y = grid_y.view(b, -1, h, w)
- grid_yx = torch.stack([grid_y, grid_x], dim=2)
- grid_yx = grid_yx.view(b, -1, h, w)
- regressed_bbox = torch.cat([
- grid_left, grid_top, grid_left + grid_width, grid_top + grid_height
- ], 1)
- return grid_yx, regressed_bbox
-
- def forward(self, feats):
- return multi_apply(self.forward_single, feats)
-
- def forward_single(self, x):
- """Forward feature map of a single FPN level."""
- dcn_base_offset = self.dcn_base_offset.type_as(x)
- # If we use center_init, the initial reppoints is from center points.
- # If we use bounding bbox representation, the initial reppoints is
- # from regular grid placed on a pre-defined bbox.
- if self.use_grid_points or not self.center_init:
- scale = self.point_base_scale / 2
- points_init = dcn_base_offset / dcn_base_offset.max() * scale
- bbox_init = x.new_tensor([-scale, -scale, scale,
- scale]).view(1, 4, 1, 1)
- else:
- points_init = 0
- cls_feat = x
- pts_feat = x
- for cls_conv in self.cls_convs:
- cls_feat = cls_conv(cls_feat)
- for reg_conv in self.reg_convs:
- pts_feat = reg_conv(pts_feat)
- # initialize reppoints
- pts_out_init = self.reppoints_pts_init_out(
- self.relu(self.reppoints_pts_init_conv(pts_feat)))
- if self.use_grid_points:
- pts_out_init, bbox_out_init = self.gen_grid_from_reg(
- pts_out_init, bbox_init.detach())
- else:
- pts_out_init = pts_out_init + points_init
- # refine and classify reppoints
- pts_out_init_grad_mul = (1 - self.gradient_mul) * pts_out_init.detach(
- ) + self.gradient_mul * pts_out_init
- dcn_offset = pts_out_init_grad_mul - dcn_base_offset
- cls_out = self.reppoints_cls_out(
- self.relu(self.reppoints_cls_conv(cls_feat, dcn_offset)))
- pts_out_refine = self.reppoints_pts_refine_out(
- self.relu(self.reppoints_pts_refine_conv(pts_feat, dcn_offset)))
- if self.use_grid_points:
- pts_out_refine, bbox_out_refine = self.gen_grid_from_reg(
- pts_out_refine, bbox_out_init.detach())
- else:
- pts_out_refine = pts_out_refine + pts_out_init.detach()
- return cls_out, pts_out_init, pts_out_refine
-
- def get_points(self, featmap_sizes, img_metas, device):
- """Get points according to feature map sizes.
-
- Args:
- featmap_sizes (list[tuple]): Multi-level feature map sizes.
- img_metas (list[dict]): Image meta info.
-
- Returns:
- tuple: points of each image, valid flags of each image
- """
- num_imgs = len(img_metas)
- num_levels = len(featmap_sizes)
-
- # since feature map sizes of all images are the same, we only compute
- # points center for one time
- multi_level_points = []
- for i in range(num_levels):
- points = self.point_generators[i].grid_points(
- featmap_sizes[i], self.point_strides[i], device)
- multi_level_points.append(points)
- points_list = [[point.clone() for point in multi_level_points]
- for _ in range(num_imgs)]
-
- # for each image, we compute valid flags of multi level grids
- valid_flag_list = []
- for img_id, img_meta in enumerate(img_metas):
- multi_level_flags = []
- for i in range(num_levels):
- point_stride = self.point_strides[i]
- feat_h, feat_w = featmap_sizes[i]
- h, w = img_meta['pad_shape'][:2]
- valid_feat_h = min(int(np.ceil(h / point_stride)), feat_h)
- valid_feat_w = min(int(np.ceil(w / point_stride)), feat_w)
- flags = self.point_generators[i].valid_flags(
- (feat_h, feat_w), (valid_feat_h, valid_feat_w), device)
- multi_level_flags.append(flags)
- valid_flag_list.append(multi_level_flags)
-
- return points_list, valid_flag_list
-
- def centers_to_bboxes(self, point_list):
- """Get bboxes according to center points.
-
- Only used in :class:`MaxIoUAssigner`.
- """
- bbox_list = []
- for i_img, point in enumerate(point_list):
- bbox = []
- for i_lvl in range(len(self.point_strides)):
- scale = self.point_base_scale * self.point_strides[i_lvl] * 0.5
- bbox_shift = torch.Tensor([-scale, -scale, scale,
- scale]).view(1, 4).type_as(point[0])
- bbox_center = torch.cat(
- [point[i_lvl][:, :2], point[i_lvl][:, :2]], dim=1)
- bbox.append(bbox_center + bbox_shift)
- bbox_list.append(bbox)
- return bbox_list
-
- def offset_to_pts(self, center_list, pred_list):
- """Change from point offset to point coordinate."""
- pts_list = []
- for i_lvl in range(len(self.point_strides)):
- pts_lvl = []
- for i_img in range(len(center_list)):
- pts_center = center_list[i_img][i_lvl][:, :2].repeat(
- 1, self.num_points)
- pts_shift = pred_list[i_lvl][i_img]
- yx_pts_shift = pts_shift.permute(1, 2, 0).view(
- -1, 2 * self.num_points)
- y_pts_shift = yx_pts_shift[..., 0::2]
- x_pts_shift = yx_pts_shift[..., 1::2]
- xy_pts_shift = torch.stack([x_pts_shift, y_pts_shift], -1)
- xy_pts_shift = xy_pts_shift.view(*yx_pts_shift.shape[:-1], -1)
- pts = xy_pts_shift * self.point_strides[i_lvl] + pts_center
- pts_lvl.append(pts)
- pts_lvl = torch.stack(pts_lvl, 0)
- pts_list.append(pts_lvl)
- return pts_list
-
- def _point_target_single(self,
- flat_proposals,
- valid_flags,
- gt_bboxes,
- gt_bboxes_ignore,
- gt_labels,
- label_channels=1,
- stage='init',
- unmap_outputs=True):
- inside_flags = valid_flags
- if not inside_flags.any():
- return (None, ) * 7
- # assign gt and sample proposals
- proposals = flat_proposals[inside_flags, :]
-
- if stage == 'init':
- assigner = self.init_assigner
- pos_weight = self.train_cfg.init.pos_weight
- else:
- assigner = self.refine_assigner
- pos_weight = self.train_cfg.refine.pos_weight
- assign_result = assigner.assign(proposals, gt_bboxes, gt_bboxes_ignore,
- None if self.sampling else gt_labels)
- sampling_result = self.sampler.sample(assign_result, proposals,
- gt_bboxes)
-
- num_valid_proposals = proposals.shape[0]
- bbox_gt = proposals.new_zeros([num_valid_proposals, 4])
- pos_proposals = torch.zeros_like(proposals)
- proposals_weights = proposals.new_zeros([num_valid_proposals, 4])
- labels = proposals.new_full((num_valid_proposals, ),
- self.num_classes,
- dtype=torch.long)
- label_weights = proposals.new_zeros(
- num_valid_proposals, dtype=torch.float)
-
- pos_inds = sampling_result.pos_inds
- neg_inds = sampling_result.neg_inds
- if len(pos_inds) > 0:
- pos_gt_bboxes = sampling_result.pos_gt_bboxes
- bbox_gt[pos_inds, :] = pos_gt_bboxes
- pos_proposals[pos_inds, :] = proposals[pos_inds, :]
- proposals_weights[pos_inds, :] = 1.0
- if gt_labels is None:
- # Only rpn gives gt_labels as None
- # Foreground is the first class
- labels[pos_inds] = 0
- else:
- labels[pos_inds] = gt_labels[
- sampling_result.pos_assigned_gt_inds]
- if pos_weight <= 0:
- label_weights[pos_inds] = 1.0
- else:
- label_weights[pos_inds] = pos_weight
- if len(neg_inds) > 0:
- label_weights[neg_inds] = 1.0
-
- # map up to original set of proposals
- if unmap_outputs:
- num_total_proposals = flat_proposals.size(0)
- labels = unmap(labels, num_total_proposals, inside_flags)
- label_weights = unmap(label_weights, num_total_proposals,
- inside_flags)
- bbox_gt = unmap(bbox_gt, num_total_proposals, inside_flags)
- pos_proposals = unmap(pos_proposals, num_total_proposals,
- inside_flags)
- proposals_weights = unmap(proposals_weights, num_total_proposals,
- inside_flags)
-
- return (labels, label_weights, bbox_gt, pos_proposals,
- proposals_weights, pos_inds, neg_inds)
-
- def get_targets(self,
- proposals_list,
- valid_flag_list,
- gt_bboxes_list,
- img_metas,
- gt_bboxes_ignore_list=None,
- gt_labels_list=None,
- stage='init',
- label_channels=1,
- unmap_outputs=True):
- """Compute corresponding GT box and classification targets for
- proposals.
-
- Args:
- proposals_list (list[list]): Multi level points/bboxes of each
- image.
- valid_flag_list (list[list]): Multi level valid flags of each
- image.
- gt_bboxes_list (list[Tensor]): Ground truth bboxes of each image.
- img_metas (list[dict]): Meta info of each image.
- gt_bboxes_ignore_list (list[Tensor]): Ground truth bboxes to be
- ignored.
- gt_bboxes_list (list[Tensor]): Ground truth labels of each box.
- stage (str): `init` or `refine`. Generate target for init stage or
- refine stage
- label_channels (int): Channel of label.
- unmap_outputs (bool): Whether to map outputs back to the original
- set of anchors.
-
- Returns:
- tuple:
- - labels_list (list[Tensor]): Labels of each level.
- - label_weights_list (list[Tensor]): Label weights of each level. # noqa: E501
- - bbox_gt_list (list[Tensor]): Ground truth bbox of each level.
- - proposal_list (list[Tensor]): Proposals(points/bboxes) of each level. # noqa: E501
- - proposal_weights_list (list[Tensor]): Proposal weights of each level. # noqa: E501
- - num_total_pos (int): Number of positive samples in all images. # noqa: E501
- - num_total_neg (int): Number of negative samples in all images. # noqa: E501
- """
- assert stage in ['init', 'refine']
- num_imgs = len(img_metas)
- assert len(proposals_list) == len(valid_flag_list) == num_imgs
-
- # points number of multi levels
- num_level_proposals = [points.size(0) for points in proposals_list[0]]
-
- # concat all level points and flags to a single tensor
- for i in range(num_imgs):
- assert len(proposals_list[i]) == len(valid_flag_list[i])
- proposals_list[i] = torch.cat(proposals_list[i])
- valid_flag_list[i] = torch.cat(valid_flag_list[i])
-
- # compute targets for each image
- if gt_bboxes_ignore_list is None:
- gt_bboxes_ignore_list = [None for _ in range(num_imgs)]
- if gt_labels_list is None:
- gt_labels_list = [None for _ in range(num_imgs)]
- (all_labels, all_label_weights, all_bbox_gt, all_proposals,
- all_proposal_weights, pos_inds_list, neg_inds_list) = multi_apply(
- self._point_target_single,
- proposals_list,
- valid_flag_list,
- gt_bboxes_list,
- gt_bboxes_ignore_list,
- gt_labels_list,
- stage=stage,
- label_channels=label_channels,
- unmap_outputs=unmap_outputs)
- # no valid points
- if any([labels is None for labels in all_labels]):
- return None
- # sampled points of all images
- num_total_pos = sum([max(inds.numel(), 1) for inds in pos_inds_list])
- num_total_neg = sum([max(inds.numel(), 1) for inds in neg_inds_list])
- labels_list = images_to_levels(all_labels, num_level_proposals)
- label_weights_list = images_to_levels(all_label_weights,
- num_level_proposals)
- bbox_gt_list = images_to_levels(all_bbox_gt, num_level_proposals)
- proposals_list = images_to_levels(all_proposals, num_level_proposals)
- proposal_weights_list = images_to_levels(all_proposal_weights,
- num_level_proposals)
- return (labels_list, label_weights_list, bbox_gt_list, proposals_list,
- proposal_weights_list, num_total_pos, num_total_neg)
-
- def loss_single(self, cls_score, pts_pred_init, pts_pred_refine, labels,
- label_weights, bbox_gt_init, bbox_weights_init,
- bbox_gt_refine, bbox_weights_refine, stride,
- num_total_samples_init, num_total_samples_refine):
- # classification loss
- labels = labels.reshape(-1)
- label_weights = label_weights.reshape(-1)
- cls_score = cls_score.permute(0, 2, 3,
- 1).reshape(-1, self.cls_out_channels)
- cls_score = cls_score.contiguous()
- loss_cls = self.loss_cls(
- cls_score,
- labels,
- label_weights,
- avg_factor=num_total_samples_refine)
-
- # points loss
- bbox_gt_init = bbox_gt_init.reshape(-1, 4)
- bbox_weights_init = bbox_weights_init.reshape(-1, 4)
- bbox_pred_init = self.points2bbox(
- pts_pred_init.reshape(-1, 2 * self.num_points), y_first=False)
- bbox_gt_refine = bbox_gt_refine.reshape(-1, 4)
- bbox_weights_refine = bbox_weights_refine.reshape(-1, 4)
- bbox_pred_refine = self.points2bbox(
- pts_pred_refine.reshape(-1, 2 * self.num_points), y_first=False)
- normalize_term = self.point_base_scale * stride
- loss_pts_init = self.loss_bbox_init(
- bbox_pred_init / normalize_term,
- bbox_gt_init / normalize_term,
- bbox_weights_init,
- avg_factor=num_total_samples_init)
- loss_pts_refine = self.loss_bbox_refine(
- bbox_pred_refine / normalize_term,
- bbox_gt_refine / normalize_term,
- bbox_weights_refine,
- avg_factor=num_total_samples_refine)
- return loss_cls, loss_pts_init, loss_pts_refine
-
- def loss(self,
- cls_scores,
- pts_preds_init,
- pts_preds_refine,
- gt_bboxes,
- gt_labels,
- img_metas,
- gt_bboxes_ignore=None):
- featmap_sizes = [featmap.size()[-2:] for featmap in cls_scores]
- assert len(featmap_sizes) == len(self.point_generators)
- device = cls_scores[0].device
- label_channels = self.cls_out_channels if self.use_sigmoid_cls else 1
-
- # target for initial stage
- center_list, valid_flag_list = self.get_points(featmap_sizes,
- img_metas, device)
- pts_coordinate_preds_init = self.offset_to_pts(center_list,
- pts_preds_init)
- if self.train_cfg.init.assigner['type'] == 'PointAssigner':
- # Assign target for center list
- candidate_list = center_list
- else:
- # transform center list to bbox list and
- # assign target for bbox list
- bbox_list = self.centers_to_bboxes(center_list)
- candidate_list = bbox_list
- cls_reg_targets_init = self.get_targets(
- candidate_list,
- valid_flag_list,
- gt_bboxes,
- img_metas,
- gt_bboxes_ignore_list=gt_bboxes_ignore,
- gt_labels_list=gt_labels,
- stage='init',
- label_channels=label_channels)
- (*_, bbox_gt_list_init, candidate_list_init, bbox_weights_list_init,
- num_total_pos_init, num_total_neg_init) = cls_reg_targets_init
- num_total_samples_init = (
- num_total_pos_init +
- num_total_neg_init if self.sampling else num_total_pos_init)
-
- # target for refinement stage
- center_list, valid_flag_list = self.get_points(featmap_sizes,
- img_metas, device)
- pts_coordinate_preds_refine = self.offset_to_pts(
- center_list, pts_preds_refine)
- bbox_list = []
- for i_img, center in enumerate(center_list):
- bbox = []
- for i_lvl in range(len(pts_preds_refine)):
- bbox_preds_init = self.points2bbox(
- pts_preds_init[i_lvl].detach())
- bbox_shift = bbox_preds_init * self.point_strides[i_lvl]
- bbox_center = torch.cat(
- [center[i_lvl][:, :2], center[i_lvl][:, :2]], dim=1)
- bbox.append(bbox_center +
- bbox_shift[i_img].permute(1, 2, 0).reshape(-1, 4))
- bbox_list.append(bbox)
- cls_reg_targets_refine = self.get_targets(
- bbox_list,
- valid_flag_list,
- gt_bboxes,
- img_metas,
- gt_bboxes_ignore_list=gt_bboxes_ignore,
- gt_labels_list=gt_labels,
- stage='refine',
- label_channels=label_channels)
- (labels_list, label_weights_list, bbox_gt_list_refine,
- candidate_list_refine, bbox_weights_list_refine, num_total_pos_refine,
- num_total_neg_refine) = cls_reg_targets_refine
- num_total_samples_refine = (
- num_total_pos_refine +
- num_total_neg_refine if self.sampling else num_total_pos_refine)
-
- # compute loss
- losses_cls, losses_pts_init, losses_pts_refine = multi_apply(
- self.loss_single,
- cls_scores,
- pts_coordinate_preds_init,
- pts_coordinate_preds_refine,
- labels_list,
- label_weights_list,
- bbox_gt_list_init,
- bbox_weights_list_init,
- bbox_gt_list_refine,
- bbox_weights_list_refine,
- self.point_strides,
- num_total_samples_init=num_total_samples_init,
- num_total_samples_refine=num_total_samples_refine)
- loss_dict_all = {
- 'loss_cls': losses_cls,
- 'loss_pts_init': losses_pts_init,
- 'loss_pts_refine': losses_pts_refine
- }
- return loss_dict_all
-
- def get_bboxes(self,
- cls_scores,
- pts_preds_init,
- pts_preds_refine,
- img_metas,
- cfg=None,
- rescale=False,
- with_nms=True):
- assert len(cls_scores) == len(pts_preds_refine)
- device = cls_scores[0].device
- bbox_preds_refine = [
- self.points2bbox(pts_pred_refine)
- for pts_pred_refine in pts_preds_refine
- ]
- num_levels = len(cls_scores)
- mlvl_points = [
- self.point_generators[i].grid_points(cls_scores[i].size()[-2:],
- self.point_strides[i], device)
- for i in range(num_levels)
- ]
- result_list = []
- for img_id in range(len(img_metas)):
- cls_score_list = [
- cls_scores[i][img_id].detach() for i in range(num_levels)
- ]
- bbox_pred_list = [
- bbox_preds_refine[i][img_id].detach()
- for i in range(num_levels)
- ]
- img_shape = img_metas[img_id]['img_shape']
- scale_factor = img_metas[img_id]['scale_factor']
- proposals = self._get_bboxes_single(cls_score_list, bbox_pred_list,
- mlvl_points, img_shape,
- scale_factor, cfg, rescale,
- with_nms)
- result_list.append(proposals)
- return result_list
-
- def _get_bboxes_single(self,
- cls_scores,
- bbox_preds,
- mlvl_points,
- img_shape,
- scale_factor,
- cfg,
- rescale=False,
- with_nms=True):
- cfg = self.test_cfg if cfg is None else cfg
- assert len(cls_scores) == len(bbox_preds) == len(mlvl_points)
- mlvl_bboxes = []
- mlvl_scores = []
- for i_lvl, (cls_score, bbox_pred, points) in enumerate(
- zip(cls_scores, bbox_preds, mlvl_points)):
- assert cls_score.size()[-2:] == bbox_pred.size()[-2:]
- cls_score = cls_score.permute(1, 2,
- 0).reshape(-1, self.cls_out_channels)
- if self.use_sigmoid_cls:
- scores = cls_score.sigmoid()
- else:
- scores = cls_score.softmax(-1)
- bbox_pred = bbox_pred.permute(1, 2, 0).reshape(-1, 4)
- nms_pre = cfg.get('nms_pre', -1)
- if nms_pre > 0 and scores.shape[0] > nms_pre:
- if self.use_sigmoid_cls:
- max_scores, _ = scores.max(dim=1)
- else:
- # remind that we set FG labels to [0, num_class-1]
- # since mmdet v2.0
- # BG cat_id: num_class
- max_scores, _ = scores[:, :-1].max(dim=1)
- _, topk_inds = max_scores.topk(nms_pre)
- points = points[topk_inds, :]
- bbox_pred = bbox_pred[topk_inds, :]
- scores = scores[topk_inds, :]
- bbox_pos_center = torch.cat([points[:, :2], points[:, :2]], dim=1)
- bboxes = bbox_pred * self.point_strides[i_lvl] + bbox_pos_center
- x1 = bboxes[:, 0].clamp(min=0, max=img_shape[1])
- y1 = bboxes[:, 1].clamp(min=0, max=img_shape[0])
- x2 = bboxes[:, 2].clamp(min=0, max=img_shape[1])
- y2 = bboxes[:, 3].clamp(min=0, max=img_shape[0])
- bboxes = torch.stack([x1, y1, x2, y2], dim=-1)
- mlvl_bboxes.append(bboxes)
- mlvl_scores.append(scores)
- mlvl_bboxes = torch.cat(mlvl_bboxes)
- if rescale:
- mlvl_bboxes /= mlvl_bboxes.new_tensor(scale_factor)
- mlvl_scores = torch.cat(mlvl_scores)
- if self.use_sigmoid_cls:
- # Add a dummy background class to the backend when using sigmoid
- # remind that we set FG labels to [0, num_class-1] since mmdet v2.0
- # BG cat_id: num_class
- padding = mlvl_scores.new_zeros(mlvl_scores.shape[0], 1)
- mlvl_scores = torch.cat([mlvl_scores, padding], dim=1)
- if with_nms:
- det_bboxes, det_labels = multiclass_nms(mlvl_bboxes, mlvl_scores,
- cfg.score_thr, cfg.nms,
- cfg.max_per_img)
- return det_bboxes, det_labels
- else:
- return mlvl_bboxes, mlvl_scores
diff --git a/spaces/abhishek/sketch-to-image/annotator/uniformer/mmdet_null/models/detectors/mask_rcnn.py b/spaces/abhishek/sketch-to-image/annotator/uniformer/mmdet_null/models/detectors/mask_rcnn.py
deleted file mode 100644
index c15a7733170e059d2825138b3812319915b7cad6..0000000000000000000000000000000000000000
--- a/spaces/abhishek/sketch-to-image/annotator/uniformer/mmdet_null/models/detectors/mask_rcnn.py
+++ /dev/null
@@ -1,24 +0,0 @@
-from ..builder import DETECTORS
-from .two_stage import TwoStageDetector
-
-
-@DETECTORS.register_module()
-class MaskRCNN(TwoStageDetector):
- """Implementation of `Mask R-CNN `_"""
-
- def __init__(self,
- backbone,
- rpn_head,
- roi_head,
- train_cfg,
- test_cfg,
- neck=None,
- pretrained=None):
- super(MaskRCNN, self).__init__(
- backbone=backbone,
- neck=neck,
- rpn_head=rpn_head,
- roi_head=roi_head,
- train_cfg=train_cfg,
- test_cfg=test_cfg,
- pretrained=pretrained)
diff --git a/spaces/abrar-lohia/text-2-character-anim/pyrender/.eggs/pyglet-2.0.5-py3.10.egg/pyglet/window/mouse.py b/spaces/abrar-lohia/text-2-character-anim/pyrender/.eggs/pyglet-2.0.5-py3.10.egg/pyglet/window/mouse.py
deleted file mode 100644
index c57a6be3933fa1e1b9e3ea555c51c53aff16ab73..0000000000000000000000000000000000000000
--- a/spaces/abrar-lohia/text-2-character-anim/pyrender/.eggs/pyglet-2.0.5-py3.10.egg/pyglet/window/mouse.py
+++ /dev/null
@@ -1,100 +0,0 @@
-"""Mouse constants and utilities for pyglet.window.
-"""
-
-
-class MouseStateHandler:
- """Simple handler that tracks the state of buttons from the mouse. If a
- button is pressed then this handler holds a True value for it.
- If the window loses focus, all buttons will be reset to False in order
- to avoid a "sticky" button state.
-
- For example::
-
- >>> win = window.Window()
- >>> mousebuttons = mouse.MouseStateHandler()
- >>> win.push_handlers(mousebuttons)
-
- # Hold down the "left" button...
-
- >>> mousebuttons[mouse.LEFT]
- True
- >>> mousebuttons[mouse.RIGHT]
- False
-
- """
-
- def __init__(self):
- self.data = {
- "x": 0,
- "y": 0,
- }
-
- def on_mouse_press(self, x, y, button, modifiers):
- self.data[button] = True
-
- def on_mouse_release(self, x, y, button, modifiers):
- self.data[button] = False
-
- def on_deactivate(self):
- self.data.clear()
-
- def on_mouse_motion(self, x, y, dx, dy):
- self.data["x"] = x
- self.data["y"] = y
-
- def on_mouse_drag(self, x, y, dx, dy, buttons, modifiers):
- self.data["x"] = x
- self.data["y"] = y
-
- def __getitem__(self, key):
- return self.data.get(key, False)
-
-
-def buttons_string(buttons):
- """Return a string describing a set of active mouse buttons.
-
- Example::
-
- >>> buttons_string(LEFT | RIGHT)
- 'LEFT|RIGHT'
-
- :Parameters:
- `buttons` : int
- Bitwise combination of mouse button constants.
-
- :rtype: str
- """
- button_names = []
- if buttons & LEFT:
- button_names.append("LEFT")
- if buttons & MIDDLE:
- button_names.append("MIDDLE")
- if buttons & RIGHT:
- button_names.append("RIGHT")
- if buttons & MOUSE4:
- button_names.append("MOUSE4")
- if buttons & MOUSE5:
- button_names.append("MOUSE5")
- return "|".join(button_names)
-
-
-#: Constant for the left mouse button.
-#:
-#: :meta hide-value:
-LEFT = 1 << 0
-#: Constant for the middle mouse button.
-#:
-#: :meta hide-value:
-MIDDLE = 1 << 1
-#: Constant for the right mouse button.
-#:
-#: :meta hide-value:
-RIGHT = 1 << 2
-#: Constant for the mouse4 button.
-#:
-#: :meta hide-value:
-MOUSE4 = 1 << 3
-#: Constant for the mouse5 button.
-#:
-#: :meta hide-value:
-MOUSE5 = 1 << 4
diff --git a/spaces/adhirk/ARKs_Contextual_Chronicle/README.md b/spaces/adhirk/ARKs_Contextual_Chronicle/README.md
deleted file mode 100644
index 3bcf4e6f08a3704f7d53f1108be883fd17422fb9..0000000000000000000000000000000000000000
--- a/spaces/adhirk/ARKs_Contextual_Chronicle/README.md
+++ /dev/null
@@ -1,12 +0,0 @@
----
-title: ARKs_Contextual_Chronicle
-emoji: 🌖
-colorFrom: pink
-colorTo: gray
-sdk: streamlit
-sdk_version: 1.26.0
-app_file: app.py
-pinned: false
----
-
-Check out the configuration reference at https://huggingface.co/docs/hub/spaces-config-reference
diff --git a/spaces/akhaliq/GPEN/face_model/op/fused_bias_act.cpp b/spaces/akhaliq/GPEN/face_model/op/fused_bias_act.cpp
deleted file mode 100644
index 02be898f970bcc8ea297867fcaa4e71b24b3d949..0000000000000000000000000000000000000000
--- a/spaces/akhaliq/GPEN/face_model/op/fused_bias_act.cpp
+++ /dev/null
@@ -1,21 +0,0 @@
-#include
-
-
-torch::Tensor fused_bias_act_op(const torch::Tensor& input, const torch::Tensor& bias, const torch::Tensor& refer,
- int act, int grad, float alpha, float scale);
-
-#define CHECK_CUDA(x) TORCH_CHECK(x.type().is_cuda(), #x " must be a CUDA tensor")
-#define CHECK_CONTIGUOUS(x) TORCH_CHECK(x.is_contiguous(), #x " must be contiguous")
-#define CHECK_INPUT(x) CHECK_CUDA(x); CHECK_CONTIGUOUS(x)
-
-torch::Tensor fused_bias_act(const torch::Tensor& input, const torch::Tensor& bias, const torch::Tensor& refer,
- int act, int grad, float alpha, float scale) {
- CHECK_CUDA(input);
- CHECK_CUDA(bias);
-
- return fused_bias_act_op(input, bias, refer, act, grad, alpha, scale);
-}
-
-PYBIND11_MODULE(TORCH_EXTENSION_NAME, m) {
- m.def("fused_bias_act", &fused_bias_act, "fused bias act (CUDA)");
-}
\ No newline at end of file
diff --git a/spaces/akhaliq/SummerTime/model/dialogue/hmnet_model.py b/spaces/akhaliq/SummerTime/model/dialogue/hmnet_model.py
deleted file mode 100644
index 54385d7cd14c723ee99aa7282ee0d6c30802f2eb..0000000000000000000000000000000000000000
--- a/spaces/akhaliq/SummerTime/model/dialogue/hmnet_model.py
+++ /dev/null
@@ -1,483 +0,0 @@
-from model.base_model import SummModel
-import argparse
-import os
-import torch
-import gzip
-import json
-from model.third_party.HMNet.Models.Trainers.HMNetTrainer import HMNetTrainer
-from model.third_party.HMNet.Utils.Arguments import Arguments
-
-import spacy
-
-nlp = spacy.load("en_core_web_sm", disable=["parser"])
-# tagger = nlp.get_pipe('tagger')
-# ner = nlp.get_pipe('ner')
-# POS = {w: i for i, w in enumerate([''] + list(tagger.labels))}
-# ENT = {w: i for i, w in enumerate([''] + list(ner.move_names))}
-# These two dicts are adapted from SpaCy 2.3.1, since HMNet's embedding for POS and ENT is fixed
-POS = {
- "": 0,
- "$": 1,
- "''": 2,
- ",": 3,
- "-LRB-": 4,
- "-RRB-": 5,
- ".": 6,
- ":": 7,
- "ADD": 8,
- "AFX": 9,
- "CC": 10,
- "CD": 11,
- "DT": 12,
- "EX": 13,
- "FW": 14,
- "HYPH": 15,
- "IN": 16,
- "JJ": 17,
- "JJR": 18,
- "JJS": 19,
- "LS": 20,
- "MD": 21,
- "NFP": 22,
- "NN": 23,
- "NNP": 24,
- "NNPS": 25,
- "NNS": 26,
- "PDT": 27,
- "POS": 28,
- "PRP": 29,
- "PRP$": 30,
- "RB": 31,
- "RBR": 32,
- "RBS": 33,
- "RP": 34,
- "SYM": 35,
- "TO": 36,
- "UH": 37,
- "VB": 38,
- "VBD": 39,
- "VBG": 40,
- "VBN": 41,
- "VBP": 42,
- "VBZ": 43,
- "WDT": 44,
- "WP": 45,
- "WP$": 46,
- "WRB": 47,
- "XX": 48,
- "_SP": 49,
- "``": 50,
-}
-ENT = {
- "": 0,
- "B-ORG": 1,
- "B-DATE": 2,
- "B-PERSON": 3,
- "B-GPE": 4,
- "B-MONEY": 5,
- "B-CARDINAL": 6,
- "B-NORP": 7,
- "B-PERCENT": 8,
- "B-WORK_OF_ART": 9,
- "B-LOC": 10,
- "B-TIME": 11,
- "B-QUANTITY": 12,
- "B-FAC": 13,
- "B-EVENT": 14,
- "B-ORDINAL": 15,
- "B-PRODUCT": 16,
- "B-LAW": 17,
- "B-LANGUAGE": 18,
- "I-ORG": 19,
- "I-DATE": 20,
- "I-PERSON": 21,
- "I-GPE": 22,
- "I-MONEY": 23,
- "I-CARDINAL": 24,
- "I-NORP": 25,
- "I-PERCENT": 26,
- "I-WORK_OF_ART": 27,
- "I-LOC": 28,
- "I-TIME": 29,
- "I-QUANTITY": 30,
- "I-FAC": 31,
- "I-EVENT": 32,
- "I-ORDINAL": 33,
- "I-PRODUCT": 34,
- "I-LAW": 35,
- "I-LANGUAGE": 36,
- "L-ORG": 37,
- "L-DATE": 38,
- "L-PERSON": 39,
- "L-GPE": 40,
- "L-MONEY": 41,
- "L-CARDINAL": 42,
- "L-NORP": 43,
- "L-PERCENT": 44,
- "L-WORK_OF_ART": 45,
- "L-LOC": 46,
- "L-TIME": 47,
- "L-QUANTITY": 48,
- "L-FAC": 49,
- "L-EVENT": 50,
- "L-ORDINAL": 51,
- "L-PRODUCT": 52,
- "L-LAW": 53,
- "L-LANGUAGE": 54,
- "U-ORG": 55,
- "U-DATE": 56,
- "U-PERSON": 57,
- "U-GPE": 58,
- "U-MONEY": 59,
- "U-CARDINAL": 60,
- "U-NORP": 61,
- "U-PERCENT": 62,
- "U-WORK_OF_ART": 63,
- "U-LOC": 64,
- "U-TIME": 65,
- "U-QUANTITY": 66,
- "U-FAC": 67,
- "U-EVENT": 68,
- "U-ORDINAL": 69,
- "U-PRODUCT": 70,
- "U-LAW": 71,
- "U-LANGUAGE": 72,
- "O": 73,
-}
-
-
-class HMNetModel(SummModel):
- # static variables
- model_name = "HMNET"
- is_extractive = False
- is_neural = True
- is_dialogue_based = True
-
- def __init__(
- self,
- min_gen_length: int = 10,
- max_gen_length: int = 300,
- beam_width: int = 6,
- **kwargs,
- ):
- """
- Create a summarization model with HMNet backbone. In the default setting, the inference speed will be
- 10s/sample (on one GPU), however, if one can tune these three parameters properly, e.g. min_gen_length=10,
- max_gen_length=100, and beam_width=2, the inference speed will increase to 2s/sample (on one GPU).
-
- Args:
- min_gen_length (int): minimum generation length of the decoder
- max_gen_length (int): maximum generation length of the decoder
- beam_width (int): width of the beam when doing beam search in the decoding process
- kwargs: the other valid parameters. The valid parameters can be found in
- model/dialogue/hmnet/config/dialogue.conf . You can use either lower case or upper case for parameter
- name. The valid parameter name is one of the following args, however, we do not encourage you to modify
- them, since some unexpected, untested errors might be triggered:
- ['MODEL', 'TASK', 'CRITERION', 'SEED', 'MAX_NUM_EPOCHS', 'EVAL_PER_UPDATE_NUM'
- , 'UPDATES_PER_EPOCH', 'OPTIMIZER', 'START_LEARNING_RATE', 'LR_SCHEDULER', 'WARMUP_STEPS',
- 'WARMUP_INIT_LR', 'WARMUP_END_LR', 'GRADIENT_ACCUMULATE_STEP', 'GRAD_CLIPPING', 'USE_REL_DATA_PATH',
- 'TRAIN_FILE', 'DEV_FILE', 'TEST_FILE', 'ROLE_DICT_FILE', 'MINI_BATCH', 'MAX_PADDING_RATIO',
- 'BATCH_READ_AHEAD', 'DOC_SHUFFLE_BUF_SIZE', 'SAMPLE_SHUFFLE_BUFFER_SIZE', 'BATCH_SHUFFLE_BUFFER_SIZE',
- 'MAX_TRANSCRIPT_WORD', 'MAX_SENT_LEN', 'MAX_SENT_NUM', 'DROPOUT', 'VOCAB_DIM', 'ROLE_SIZE', 'ROLE_DIM',
- 'POS_DIM', 'ENT_DIM', 'USE_ROLE', 'USE_POSENT', 'USE_BOS_TOKEN', 'USE_EOS_TOKEN',
- 'TRANSFORMER_EMBED_DROPOUT', 'TRANSFORMER_RESIDUAL_DROPOUT', 'TRANSFORMER_ATTENTION_DROPOUT',
- 'TRANSFORMER_LAYER', 'TRANSFORMER_HEAD', 'TRANSFORMER_POS_DISCOUNT', 'PRE_TOKENIZER',
- 'PRE_TOKENIZER_PATH', 'PYLEARN_MODEL', 'EXTRA_IDS', 'BEAM_WIDTH', 'EVAL_TOKENIZED', 'EVAL_LOWERCASE',
- 'MAX_GEN_LENGTH', 'MIN_GEN_LENGTH', 'NO_REPEAT_NGRAM_SIZE']
-
- Return an instance of HMNet model for dialogue summarization.
- """
- super(HMNetModel, self).__init__()
- self.root_path = self._get_root()
-
- # we leave the most influential params with prompt and the others as hidden kwargs
- kwargs["MIN_GEN_LENGTH"] = min_gen_length
- kwargs["MAX_GEN_LENGTH"] = max_gen_length
- kwargs["BEAM_WIDTH"] = beam_width
- self.opt = self._parse_args(kwargs)
- self.model = HMNetTrainer(self.opt)
-
- def _get_root(self):
- root_path = os.getcwd()
- while "model" not in os.listdir(root_path):
- root_path = os.path.dirname(root_path)
- root_path = os.path.join(root_path, "model/dialogue")
- return root_path
-
- def _parse_args(self, kwargs):
- parser = argparse.ArgumentParser(
- description="HMNet: Pretrain or fine-tune models for HMNet model."
- )
- parser.add_argument(
- "--command", default="evaluate", help="Command: train/evaluate"
- )
- parser.add_argument(
- "--conf_file",
- default=os.path.join(self.root_path, "hmnet/config/dialogue.conf"),
- help="Path to the BigLearn conf file.",
- )
- parser.add_argument(
- "--PYLEARN_MODEL", help="Overrides this option from the conf file."
- )
- parser.add_argument(
- "--master_port", help="Overrides this option default", default=None
- )
- parser.add_argument("--cluster", help="local, philly or aml", default="local")
- parser.add_argument(
- "--dist_init_path", help="Distributed init path for AML", default="./tmp"
- )
- parser.add_argument(
- "--fp16",
- action="store_true",
- help="Whether to use 16-bit float precision instead of 32-bit",
- )
- parser.add_argument(
- "--fp16_opt_level",
- type=str,
- default="O1",
- help="For fp16: Apex AMP optimization level selected in ['O0', 'O1', 'O2', and 'O3']."
- "See details at https://nvidia.github.io/apex/amp.html",
- )
- parser.add_argument("--no_cuda", action="store_true", help="Disable cuda.")
- parser.add_argument(
- "--config_overrides",
- help="Override parameters on config, VAR=val;VAR=val;...",
- )
-
- cmdline_args = parser.parse_args()
- command = cmdline_args.command
- conf_file = cmdline_args.conf_file
- conf_args = Arguments(conf_file)
- opt = conf_args.readArguments()
-
- if cmdline_args.config_overrides:
- for config_override in cmdline_args.config_overrides.split(";"):
- config_override = config_override.strip()
- if config_override:
- var_val = config_override.split("=")
- assert (
- len(var_val) == 2
- ), f"Config override '{var_val}' does not have the form 'VAR=val'"
- conf_args.add_opt(opt, var_val[0], var_val[1], force_override=True)
-
- opt["cuda"] = torch.cuda.is_available() and not cmdline_args.no_cuda
- opt["confFile"] = conf_file
- if "datadir" not in opt:
- opt["datadir"] = os.path.dirname(
- conf_file
- ) # conf_file specifies where the data folder is
- opt["basename"] = os.path.basename(
- conf_file
- ) # conf_file specifies where the name of save folder is
- opt["command"] = command
-
- # combine cmdline_args into opt dictionary
- for key, val in cmdline_args.__dict__.items():
- # if val is not None and key not in ['command', 'conf_file']:
- if val is not None:
- opt[key] = val
-
- # combine kwargs into opt dictionary (we allow lower case)
- for key, val in kwargs.items():
- valid_keys = [x for x in opt.keys() if x.upper() == x]
- if key.upper() not in valid_keys:
- print("WARNING: {} is not a valid key in HMNet.".format(key))
- print("The valid keys are:", valid_keys)
- continue
- if val is not None:
- opt[key.upper()] = val
-
- return opt
-
- def summarize(self, corpus, queries=None):
- print(f"HMNet model: processing document of {corpus.__len__()} samples")
- # transform the original dataset to "dialogue" input
- # we only use test set path for evaluation
- data_folder = os.path.join(
- os.path.dirname(self.opt["datadir"]),
- "ExampleRawData/meeting_summarization/AMI_proprec/test",
- )
-
- self._create_datafolder(data_folder)
- self._preprocess(corpus, data_folder)
-
- # return self.model.eval()
- results = self._evaluate()
-
- return results
-
- def _evaluate(self):
- if self.opt["rank"] == 0:
- self.model.log("-----------------------------------------------")
- self.model.log("Evaluating model ... ")
-
- self.model.set_up_model()
-
- eval_dataset = "test"
- batch_generator_eval = self.model.get_batch_generator(eval_dataset)
- predictions = self._eval_batches(
- self.model.module, batch_generator_eval, self.model.saveFolder, eval_dataset
- )
-
- return predictions
-
- def _eval_batches(self, module, dev_batches, save_folder, label=""):
- max_sent_len = int(self.opt["MAX_GEN_LENGTH"])
-
- print("Decoding current model ... \nSaving folder is {}".format(save_folder))
- print("Each sample will cost about 10 second.")
- import time
-
- start_time = time.time()
- predictions = [] # prediction of tokens from model
- if not isinstance(module.tokenizer, list):
- decoder_tokenizer = module.tokenizer
- elif len(module.tokenizer) == 1:
- decoder_tokenizer = module.tokenizer[0]
- elif len(module.tokenizer) == 2:
- decoder_tokenizer = module.tokenizer[1]
- else:
- assert False, "len(module.tokenizer) > 2"
-
- with torch.no_grad():
- for j, dev_batch in enumerate(dev_batches):
- for b in dev_batch:
- if torch.is_tensor(dev_batch[b]):
- dev_batch[b] = dev_batch[b].to(self.opt["device"])
-
- beam_search_res = module(
- dev_batch, beam_search=True, max_sent_len=max_sent_len
- )
- pred = [
- [t[0] for t in x] if len(x) > 0 else [[]] for x in beam_search_res
- ]
- predictions.extend(
- [
- [
- self._convert_tokens_to_string(decoder_tokenizer, tt)
- for tt in t
- ]
- for t in pred
- ]
- )
-
- if (
- "DEBUG" in self.opt and j >= 10
- ) or j >= self.model.task.evaluator.eval_batches_num:
- # in debug mode (decode first 10 batches) ortherwise decode first self.eval_batches_num bathes
- break
-
- top1_predictions = [x[0] for x in predictions]
-
- print("Total time for inference:", time.time() - start_time)
- return top1_predictions
-
- def _convert_tokens_to_string(self, tokenizer, tokens):
- if "EVAL_TOKENIZED" in self.opt:
- tokens = [t for t in tokens if t not in tokenizer.all_special_tokens]
- if "EVAL_LOWERCASE" in self.opt:
- tokens = [t.lower() for t in tokens]
- if "EVAL_TOKENIZED" in self.opt:
- return " ".join(tokens)
- else:
- return tokenizer.decode(
- tokenizer.convert_tokens_to_ids(tokens), skip_special_tokens=True
- )
-
- def _preprocess(self, corpus, test_path):
- samples = []
- for i, sample in enumerate(corpus):
- new_sample = {"id": i, "meeting": [], "summary": []}
- if isinstance(sample, str):
- raise RuntimeError(
- "Error: the input of HMNet should be dialogues, rather than documents."
- )
-
- # add all the turns one by one
- for turn in sample:
- turn = [x.strip() for x in turn.split(":")]
- if len(turn) < 2:
- continue
- tokenized_turn = nlp(turn[1])
- # In case we can't find proper entity in move_names
- ent_id = []
- pos_id = []
- for token in tokenized_turn:
- ent = (
- token.ent_iob_ + "-" + token.ent_type_
- if token.ent_iob_ != "O"
- else "O"
- )
- ent_id.append(ENT[ent] if ent in ENT else ENT[""])
-
- pos = token.tag_
- pos_id.append(POS[pos] if pos in POS else POS[""])
-
- new_sample["meeting"].append(
- {
- "speaker": turn[0],
- "role": "",
- "utt": {
- "word": [str(token) for token in tokenized_turn],
- "pos_id": pos_id,
- "ent_id": ent_id,
- },
- }
- )
- new_sample["summary"].append(
- "This is a dummy summary. HMNet will filter out the sample w/o summary!"
- )
- samples.append(new_sample)
- # save to the gzip
- file_path = os.path.join(test_path, "split_{}.jsonl.gz".format(i))
- with gzip.open(file_path, "wt", encoding="utf-8") as file:
- file.write(json.dumps(new_sample))
-
- def _clean_datafolder(self, data_folder):
- for name in os.listdir(data_folder):
- name = os.path.join(data_folder, name)
- if ".gz" in name:
- os.remove(name)
-
- def _create_datafolder(self, data_folder):
- if os.path.exists(data_folder):
- self._clean_datafolder(data_folder)
- else:
- os.makedirs(data_folder)
- with open(
- os.path.join(os.path.dirname(data_folder), "test_ami.json"),
- "w",
- encoding="utf-8",
- ) as file:
- json.dump(
- [
- {
- "source": {
- "dataset": "../ExampleRawData/meeting_summarization/AMI_proprec/test/"
- },
- "task": "meeting",
- "name": "ami",
- }
- ],
- file,
- )
-
- with open(
- os.path.join(
- os.path.dirname(os.path.dirname(data_folder)), "role_dict_ext.json"
- ),
- "w",
- ) as file:
- json.dump({}, file)
-
- @classmethod
- def show_capability(cls) -> None:
- basic_description = cls.generate_basic_description()
- more_details = (
- "A HMNet model finetuned on CNN-DM dataset for summarization.\n\n"
- "Strengths:\n - High performance on dialogue summarization task.\n\n"
- "Weaknesses:\n - Not suitable for datasets other than dialogues.\n\n"
- "Initialization arguments:\n "
- " - `corpus`: Unlabelled corpus of documents.\n"
- )
- print(f"{basic_description} \n {'#' * 20} \n {more_details}")
diff --git a/spaces/akhaliq/SummerTime/setup.py b/spaces/akhaliq/SummerTime/setup.py
deleted file mode 100644
index b6decde32d0d08fd03bef1e10015562f009a3ab9..0000000000000000000000000000000000000000
--- a/spaces/akhaliq/SummerTime/setup.py
+++ /dev/null
@@ -1,23 +0,0 @@
-import setuptools
-
-with open("README.md", "r") as fh:
- long_description = fh.read()
-
-
-setuptools.setup(
- name="SummerTime",
- version="0.1",
- scripts=["summertime.py"],
- author="Ansong Ni, Murori Mutuma, Zhangir Azerbayev, Yusen Zhang, Tao Yu, Dragomir Radev",
- author_email="ansong.ni@yale.edu, murorimutuma@gmail.com, zhangir.azerbayev@yale.edu",
- description="A summarization mode",
- long_description=long_description,
- long_description_content_type="text/markdown",
- url="https://github.com/LILYlab",
- packages=setuptools.find_packages(),
- classifiers=[
- "Programming Language :: Python :: 3",
- "License :: OSI Approved :: MIT License",
- "Operating System :: OS Independent",
- ],
-)
diff --git a/spaces/akhaliq/seek.art_MEGA/README.md b/spaces/akhaliq/seek.art_MEGA/README.md
deleted file mode 100644
index c669163b4e1674bb40583c068a839d713499d947..0000000000000000000000000000000000000000
--- a/spaces/akhaliq/seek.art_MEGA/README.md
+++ /dev/null
@@ -1,12 +0,0 @@
----
-title: Seek.art MEGA
-emoji: 🐢
-colorFrom: blue
-colorTo: red
-sdk: gradio
-sdk_version: 3.13.0
-app_file: app.py
-pinned: false
----
-
-Check out the configuration reference at https://huggingface.co/docs/hub/spaces-config-reference
diff --git a/spaces/ales/wav2vec2-cv-be-lm/pipeline.py b/spaces/ales/wav2vec2-cv-be-lm/pipeline.py
deleted file mode 100644
index 68fd91e8b2714e3179247c8275a8e4a0c878f31c..0000000000000000000000000000000000000000
--- a/spaces/ales/wav2vec2-cv-be-lm/pipeline.py
+++ /dev/null
@@ -1,67 +0,0 @@
-import numpy as np
-
-from typing import Dict
-
-import torch
-import pyctcdecode
-
-from transformers import (
- Wav2Vec2Processor,
- Wav2Vec2ProcessorWithLM,
- Wav2Vec2ForCTC,
-)
-
-
-class PreTrainedPipeline():
-
- def __init__(self, model_path: str, language_model_fp: str):
- self.language_model_fp = language_model_fp
-
- self.device = 'cuda' if torch.cuda.is_available() else 'cpu'
- self.model = Wav2Vec2ForCTC.from_pretrained(model_path)
- self.model.to(self.device)
-
- processor = Wav2Vec2Processor.from_pretrained(model_path)
- self.sampling_rate = processor.feature_extractor.sampling_rate
-
- vocab = processor.tokenizer.get_vocab()
- sorted_vocab_dict = [(char, ix) for char, ix in sorted(vocab.items(), key=lambda item: item[1])]
-
- self.decoder = pyctcdecode.build_ctcdecoder(
- labels=[x[0] for x in sorted_vocab_dict],
- kenlm_model_path=self.language_model_fp,
- )
-
- self.processor_with_lm = Wav2Vec2ProcessorWithLM(
- feature_extractor=processor.feature_extractor,
- tokenizer=processor.tokenizer,
- decoder=self.decoder
- )
-
- def __call__(self, inputs: np.array) -> Dict[str, str]:
- """
- Args:
- inputs (:obj:`np.array`):
- The raw waveform of audio received. By default at 16KHz.
- Return:
- A :obj:`dict`:. The object return should be liked {"text": "XXX"} containing
- the detected text from the input audio.
- """
-
- input_values = self.processor_with_lm(
- inputs, return_tensors="pt",
- sampling_rate=self.sampling_rate
- )['input_values']
-
- input_values = input_values.to(self.device)
-
- with torch.no_grad():
- # input_values should be a 2D tensor by now. 1st dim represents audio channels.
- model_outs = self.model(input_values)
- logits = model_outs.logits.cpu().detach().numpy()
-
- text_predicted = self.processor_with_lm.batch_decode(logits)['text']
-
- return {
- "text": text_predicted
- }
diff --git a/spaces/amarchheda/ChordDuplicate/portaudio/src/hostapi/coreaudio/pa_mac_core_internal.h b/spaces/amarchheda/ChordDuplicate/portaudio/src/hostapi/coreaudio/pa_mac_core_internal.h
deleted file mode 100644
index d4a97e0c46e86001db8fe57e3212ef3e142d6c37..0000000000000000000000000000000000000000
--- a/spaces/amarchheda/ChordDuplicate/portaudio/src/hostapi/coreaudio/pa_mac_core_internal.h
+++ /dev/null
@@ -1,193 +0,0 @@
-/*
- * Internal interfaces for PortAudio Apple AUHAL implementation
- *
- * PortAudio Portable Real-Time Audio Library
- * Latest Version at: http://www.portaudio.com
- *
- * Written by Bjorn Roche of XO Audio LLC, from PA skeleton code.
- * Portions copied from code by Dominic Mazzoni (who wrote a HAL implementation)
- *
- * Dominic's code was based on code by Phil Burk, Darren Gibbs,
- * Gord Peters, Stephane Letz, and Greg Pfiel.
- *
- * The following people also deserve acknowledgements:
- *
- * Olivier Tristan for feedback and testing
- * Glenn Zelniker and Z-Systems engineering for sponsoring the Blocking I/O
- * interface.
- *
- *
- * Based on the Open Source API proposed by Ross Bencina
- * Copyright (c) 1999-2002 Ross Bencina, Phil Burk
- *
- * Permission is hereby granted, free of charge, to any person obtaining
- * a copy of this software and associated documentation files
- * (the "Software"), to deal in the Software without restriction,
- * including without limitation the rights to use, copy, modify, merge,
- * publish, distribute, sublicense, and/or sell copies of the Software,
- * and to permit persons to whom the Software is furnished to do so,
- * subject to the following conditions:
- *
- * The above copyright notice and this permission notice shall be
- * included in all copies or substantial portions of the Software.
- *
- * THE SOFTWARE IS PROVIDED "AS IS", WITHOUT WARRANTY OF ANY KIND,
- * EXPRESS OR IMPLIED, INCLUDING BUT NOT LIMITED TO THE WARRANTIES OF
- * MERCHANTABILITY, FITNESS FOR A PARTICULAR PURPOSE AND NONINFRINGEMENT.
- * IN NO EVENT SHALL THE AUTHORS OR COPYRIGHT HOLDERS BE LIABLE FOR
- * ANY CLAIM, DAMAGES OR OTHER LIABILITY, WHETHER IN AN ACTION OF
- * CONTRACT, TORT OR OTHERWISE, ARISING FROM, OUT OF OR IN CONNECTION
- * WITH THE SOFTWARE OR THE USE OR OTHER DEALINGS IN THE SOFTWARE.
- */
-
-/*
- * The text above constitutes the entire PortAudio license; however,
- * the PortAudio community also makes the following non-binding requests:
- *
- * Any person wishing to distribute modifications to the Software is
- * requested to send the modifications to the original developer so that
- * they can be incorporated into the canonical version. It is also
- * requested that these non-binding requests be included along with the
- * license above.
- */
-
-/**
- @file pa_mac_core
- @ingroup hostapi_src
- @author Bjorn Roche
- @brief AUHAL implementation of PortAudio
-*/
-
-#ifndef PA_MAC_CORE_INTERNAL_H__
-#define PA_MAC_CORE_INTERNAL_H__
-
-#include
-#include
-#include
-#include
-
-#include "portaudio.h"
-#include "pa_util.h"
-#include "pa_hostapi.h"
-#include "pa_stream.h"
-#include "pa_allocation.h"
-#include "pa_cpuload.h"
-#include "pa_process.h"
-#include "pa_ringbuffer.h"
-
-#include "pa_mac_core_blocking.h"
-
-/* function prototypes */
-
-#ifdef __cplusplus
-extern "C"
-{
-#endif /* __cplusplus */
-
-PaError PaMacCore_Initialize( PaUtilHostApiRepresentation **hostApi, PaHostApiIndex index );
-
-#ifdef __cplusplus
-}
-#endif /* __cplusplus */
-
-#define RING_BUFFER_ADVANCE_DENOMINATOR (4)
-
-PaError ReadStream( PaStream* stream, void *buffer, unsigned long frames );
-PaError WriteStream( PaStream* stream, const void *buffer, unsigned long frames );
-signed long GetStreamReadAvailable( PaStream* stream );
-signed long GetStreamWriteAvailable( PaStream* stream );
-/* PaMacAUHAL - host api datastructure specific to this implementation */
-typedef struct
-{
- PaUtilHostApiRepresentation inheritedHostApiRep;
- PaUtilStreamInterface callbackStreamInterface;
- PaUtilStreamInterface blockingStreamInterface;
-
- PaUtilAllocationGroup *allocations;
-
- /* implementation specific data goes here */
- long devCount;
- AudioDeviceID *devIds; /*array of all audio devices*/
- AudioDeviceID defaultIn;
- AudioDeviceID defaultOut;
-}
-PaMacAUHAL;
-
-typedef struct PaMacCoreDeviceProperties
-{
- /* Values in Frames from property queries. */
- UInt32 safetyOffset;
- UInt32 bufferFrameSize;
- // UInt32 streamLatency; // Seems to be the same as deviceLatency!?
- UInt32 deviceLatency;
- /* Current device sample rate. May change!
- These are initialized to the nominal device sample rate,
- and updated with the actual sample rate, when/where available.
- Note that these are the *device* sample rates, prior to any required
- SR conversion. */
- Float64 sampleRate;
- Float64 samplePeriod; // reciprocal
-}
-PaMacCoreDeviceProperties;
-
-/* stream data structure specifically for this implementation */
-typedef struct PaMacCoreStream
-{
- PaUtilStreamRepresentation streamRepresentation;
- PaUtilCpuLoadMeasurer cpuLoadMeasurer;
- PaUtilBufferProcessor bufferProcessor;
-
- /* implementation specific data goes here */
- bool bufferProcessorIsInitialized;
- AudioUnit inputUnit;
- AudioUnit outputUnit;
- AudioDeviceID inputDevice;
- AudioDeviceID outputDevice;
- size_t userInChan;
- size_t userOutChan;
- size_t inputFramesPerBuffer;
- size_t outputFramesPerBuffer;
- PaMacBlio blio;
- /* We use this ring buffer when input and out devs are different. */
- PaUtilRingBuffer inputRingBuffer;
- /* We may need to do SR conversion on input. */
- AudioConverterRef inputSRConverter;
- /* We need to preallocate an inputBuffer for reading data. */
- AudioBufferList inputAudioBufferList;
- AudioTimeStamp startTime;
- /* FIXME: instead of volatile, these should be properly memory barriered */
- volatile uint32_t xrunFlags; /*PaStreamCallbackFlags*/
- volatile enum {
- STOPPED = 0, /* playback is completely stopped,
- and the user has called StopStream(). */
- CALLBACK_STOPPED = 1, /* callback has requested stop,
- but user has not yet called StopStream(). */
- STOPPING = 2, /* The stream is in the process of closing
- because the user has called StopStream.
- This state is just used internally;
- externally it is indistinguishable from
- ACTIVE.*/
- ACTIVE = 3 /* The stream is active and running. */
- } state;
- double sampleRate;
- PaMacCoreDeviceProperties inputProperties;
- PaMacCoreDeviceProperties outputProperties;
-
- /* data updated by main thread and notifications, protected by timingInformationMutex */
- int timingInformationMutexIsInitialized;
- pthread_mutex_t timingInformationMutex;
-
- /* These are written by the PA thread or from CoreAudio callbacks. Protected by the mutex. */
- Float64 timestampOffsetCombined;
- Float64 timestampOffsetInputDevice;
- Float64 timestampOffsetOutputDevice;
-
- /* Offsets in seconds to be applied to Apple timestamps to convert them to PA timestamps.
- * While the io proc is active, the following values are only accessed and manipulated by the ioproc */
- Float64 timestampOffsetCombined_ioProcCopy;
- Float64 timestampOffsetInputDevice_ioProcCopy;
- Float64 timestampOffsetOutputDevice_ioProcCopy;
-}
-PaMacCoreStream;
-
-#endif /* PA_MAC_CORE_INTERNAL_H__ */
diff --git a/spaces/amarchheda/ChordDuplicate/portaudio/src/hostapi/wasapi/mingw-include/sdkddkver.h b/spaces/amarchheda/ChordDuplicate/portaudio/src/hostapi/wasapi/mingw-include/sdkddkver.h
deleted file mode 100644
index 44b5fb2f158dcac8028ffe10caec492f173fd459..0000000000000000000000000000000000000000
--- a/spaces/amarchheda/ChordDuplicate/portaudio/src/hostapi/wasapi/mingw-include/sdkddkver.h
+++ /dev/null
@@ -1,220 +0,0 @@
-/**
- * sdkddkver.h: Version definitions for SDK and DDK. Originally
- * from ReactOS PSDK/DDK, this file is in the public domain:
- *
- * This file has no copyright assigned and is placed in the Public Domain.
- * This file is part of the mingw-w64 runtime package.
- * No warranty is given; refer to the file DISCLAIMER.PD within this package.
- */
-
-#ifndef _INC_SDKDDKVER
-#define _INC_SDKDDKVER
-
-/* _WIN32_WINNT */
-#define _WIN32_WINNT_NT4 0x0400
-#define _WIN32_WINNT_WIN2K 0x0500
-#define _WIN32_WINNT_WINXP 0x0501
-#define _WIN32_WINNT_WS03 0x0502
-#define _WIN32_WINNT_WIN6 0x0600
-#define _WIN32_WINNT_VISTA 0x0600
-#define _WIN32_WINNT_WS08 0x0600
-#define _WIN32_WINNT_LONGHORN 0x0600
-#define _WIN32_WINNT_WIN7 0x0601
-#define _WIN32_WINNT_WIN8 0x0602
-#define _WIN32_WINNT_WINBLUE 0x0603
-#define _WIN32_WINNT_WINTHRESHOLD 0x0A00
-#define _WIN32_WINNT_WIN10 0x0A00
-
-/* _WIN32_IE */
-#define _WIN32_IE_IE20 0x0200
-#define _WIN32_IE_IE30 0x0300
-#define _WIN32_IE_IE302 0x0302
-#define _WIN32_IE_IE40 0x0400
-#define _WIN32_IE_IE401 0x0401
-#define _WIN32_IE_IE50 0x0500
-#define _WIN32_IE_IE501 0x0501
-#define _WIN32_IE_IE55 0x0550
-#define _WIN32_IE_IE60 0x0600
-#define _WIN32_IE_IE60SP1 0x0601
-#define _WIN32_IE_IE60SP2 0x0603
-#define _WIN32_IE_IE70 0x0700
-#define _WIN32_IE_IE80 0x0800
-#define _WIN32_IE_IE90 0x0900
-#define _WIN32_IE_IE100 0x0a00
-#define _WIN32_IE_IE110 0x0A00
-
-/* Mappings Between IE Version and Windows Version */
-#define _WIN32_IE_NT4 _WIN32_IE_IE20
-#define _WIN32_IE_NT4SP1 _WIN32_IE_IE20
-#define _WIN32_IE_NT4SP2 _WIN32_IE_IE20
-#define _WIN32_IE_NT4SP3 _WIN32_IE_IE302
-#define _WIN32_IE_NT4SP4 _WIN32_IE_IE401
-#define _WIN32_IE_NT4SP5 _WIN32_IE_IE401
-#define _WIN32_IE_NT4SP6 _WIN32_IE_IE50
-#define _WIN32_IE_WIN98 _WIN32_IE_IE401
-#define _WIN32_IE_WIN98SE _WIN32_IE_IE50
-#define _WIN32_IE_WINME _WIN32_IE_IE55
-#define _WIN32_IE_WIN2K _WIN32_IE_IE501
-#define _WIN32_IE_WIN2KSP1 _WIN32_IE_IE501
-#define _WIN32_IE_WIN2KSP2 _WIN32_IE_IE501
-#define _WIN32_IE_WIN2KSP3 _WIN32_IE_IE501
-#define _WIN32_IE_WIN2KSP4 _WIN32_IE_IE501
-#define _WIN32_IE_XP _WIN32_IE_IE60
-#define _WIN32_IE_XPSP1 _WIN32_IE_IE60SP1
-#define _WIN32_IE_XPSP2 _WIN32_IE_IE60SP2
-#define _WIN32_IE_WS03 0x0602
-#define _WIN32_IE_WS03SP1 _WIN32_IE_IE60SP2
-#define _WIN32_IE_WIN6 _WIN32_IE_IE70
-#define _WIN32_IE_LONGHORN _WIN32_IE_IE70
-#define _WIN32_IE_WIN7 _WIN32_IE_IE80
-#define _WIN32_IE_WIN8 _WIN32_IE_IE100
-#define _WIN32_IE_WINBLUE _WIN32_IE_IE100
-#define _WIN32_IE_WINTHRESHOLD _WIN32_IE_IE110
-#define _WIN32_IE_WIN10 _WIN32_IE_IE110
-
-/* NTDDI_VERSION */
-#ifndef NTDDI_WIN2K
-#define NTDDI_WIN2K 0x05000000
-#endif
-#ifndef NTDDI_WIN2KSP1
-#define NTDDI_WIN2KSP1 0x05000100
-#endif
-#ifndef NTDDI_WIN2KSP2
-#define NTDDI_WIN2KSP2 0x05000200
-#endif
-#ifndef NTDDI_WIN2KSP3
-#define NTDDI_WIN2KSP3 0x05000300
-#endif
-#ifndef NTDDI_WIN2KSP4
-#define NTDDI_WIN2KSP4 0x05000400
-#endif
-
-#ifndef NTDDI_WINXP
-#define NTDDI_WINXP 0x05010000
-#endif
-#ifndef NTDDI_WINXPSP1
-#define NTDDI_WINXPSP1 0x05010100
-#endif
-#ifndef NTDDI_WINXPSP2
-#define NTDDI_WINXPSP2 0x05010200
-#endif
-#ifndef NTDDI_WINXPSP3
-#define NTDDI_WINXPSP3 0x05010300
-#endif
-#ifndef NTDDI_WINXPSP4
-#define NTDDI_WINXPSP4 0x05010400
-#endif
-
-#define NTDDI_WS03 0x05020000
-#define NTDDI_WS03SP1 0x05020100
-#define NTDDI_WS03SP2 0x05020200
-#define NTDDI_WS03SP3 0x05020300
-#define NTDDI_WS03SP4 0x05020400
-
-#define NTDDI_WIN6 0x06000000
-#define NTDDI_WIN6SP1 0x06000100
-#define NTDDI_WIN6SP2 0x06000200
-#define NTDDI_WIN6SP3 0x06000300
-#define NTDDI_WIN6SP4 0x06000400
-
-#define NTDDI_VISTA NTDDI_WIN6
-#define NTDDI_VISTASP1 NTDDI_WIN6SP1
-#define NTDDI_VISTASP2 NTDDI_WIN6SP2
-#define NTDDI_VISTASP3 NTDDI_WIN6SP3
-#define NTDDI_VISTASP4 NTDDI_WIN6SP4
-#define NTDDI_LONGHORN NTDDI_VISTA
-
-#define NTDDI_WS08 NTDDI_WIN6SP1
-#define NTDDI_WS08SP2 NTDDI_WIN6SP2
-#define NTDDI_WS08SP3 NTDDI_WIN6SP3
-#define NTDDI_WS08SP4 NTDDI_WIN6SP4
-
-#define NTDDI_WIN7 0x06010000
-#define NTDDI_WIN8 0x06020000
-#define NTDDI_WINBLUE 0x06030000
-#define NTDDI_WINTHRESHOLD 0x0A000000
-#define NTDDI_WIN10 0x0A000000
-#define NTDDI_WIN10_TH2 0x0A000001
-#define NTDDI_WIN10_RS1 0x0A000002
-#define NTDDI_WIN10_RS2 0x0A000003
-#define NTDDI_WIN10_RS3 0x0A000004
-#define NTDDI_WIN10_RS4 0x0A000005
-#define NTDDI_WIN10_RS5 0x0A000006
-#define NTDDI_WIN10_19H1 0x0A000007
-#define NTDDI_WIN10_VB 0x0A000008
-#define NTDDI_WIN10_MN 0x0A000009
-#define NTDDI_WIN10_FE 0x0A00000A
-
-#define WDK_NTDDI_VERSION NTDDI_WIN10_FE
-
-/* Version Fields in NTDDI_VERSION */
-#define OSVERSION_MASK 0xFFFF0000U
-#define SPVERSION_MASK 0x0000FF00
-#define SUBVERSION_MASK 0x000000FF
-
-/* Macros to Extract Version Fields From NTDDI_VERSION */
-#define OSVER(Version) ((Version) & OSVERSION_MASK)
-#define SPVER(Version) (((Version) & SPVERSION_MASK) >> 8)
-#define SUBVER(Version) (((Version) & SUBVERSION_MASK))
-
-/* Macros to get the NTDDI for a given WIN32 */
-#define NTDDI_VERSION_FROM_WIN32_WINNT2(Version) Version##0000
-#define NTDDI_VERSION_FROM_WIN32_WINNT(Version) NTDDI_VERSION_FROM_WIN32_WINNT2(Version)
-
-/* Select Default WIN32_WINNT Value */
-#if !defined(_WIN32_WINNT) && !defined(_CHICAGO_)
-#define _WIN32_WINNT _WIN32_WINNT_WS03
-#endif
-
-/* Choose NTDDI Version */
-#ifndef NTDDI_VERSION
-#ifdef _WIN32_WINNT
-#define NTDDI_VERSION NTDDI_VERSION_FROM_WIN32_WINNT(_WIN32_WINNT)
-#else
-#define NTDDI_VERSION NTDDI_WS03
-#endif
-#endif
-
-/* Choose WINVER Value */
-#ifndef WINVER
-#ifdef _WIN32_WINNT
-#define WINVER _WIN32_WINNT
-#else
-#define WINVER 0x0502
-#endif
-#endif
-
-/* Choose IE Version */
-#ifndef _WIN32_IE
-#ifdef _WIN32_WINNT
-#if (_WIN32_WINNT <= _WIN32_WINNT_NT4)
-#define _WIN32_IE _WIN32_IE_IE50
-#elif (_WIN32_WINNT <= _WIN32_WINNT_WIN2K)
-#define _WIN32_IE _WIN32_IE_IE501
-#elif (_WIN32_WINNT <= _WIN32_WINNT_WINXP)
-#define _WIN32_IE _WIN32_IE_IE60
-#elif (_WIN32_WINNT <= _WIN32_WINNT_WS03)
-#define _WIN32_IE _WIN32_IE_WS03
-#elif (_WIN32_WINNT <= _WIN32_WINNT_VISTA)
-#define _WIN32_IE _WIN32_IE_LONGHORN
-#elif (_WIN32_WINNT <= _WIN32_WINNT_WIN7)
-#define _WIN32_IE _WIN32_IE_WIN7
-#elif (_WIN32_WINNT <= _WIN32_WINNT_WIN8)
-#define _WIN32_IE _WIN32_IE_WIN8
-#else
-#define _WIN32_IE 0x0a00
-#endif
-#else
-#define _WIN32_IE 0x0700
-#endif
-#endif
-
-/* Make Sure NTDDI_VERSION and _WIN32_WINNT Match */
-#if ((OSVER(NTDDI_VERSION) == NTDDI_WIN2K) && (_WIN32_WINNT != _WIN32_WINNT_WIN2K)) || \
- ((OSVER(NTDDI_VERSION) == NTDDI_WINXP) && (_WIN32_WINNT != _WIN32_WINNT_WINXP)) || \
- ((OSVER(NTDDI_VERSION) == NTDDI_WS03) && (_WIN32_WINNT != _WIN32_WINNT_WS03)) || \
- ((OSVER(NTDDI_VERSION) == NTDDI_WINXP) && (_WIN32_WINNT != _WIN32_WINNT_WINXP))
-#error NTDDI_VERSION and _WIN32_WINNT mismatch!
-#endif
-
-#endif /* _INC_SDKDDKVER */
diff --git a/spaces/amish1729/LFUNet/app.py b/spaces/amish1729/LFUNet/app.py
deleted file mode 100644
index 597efedf2d138228cbf285fdad678d6041ae456a..0000000000000000000000000000000000000000
--- a/spaces/amish1729/LFUNet/app.py
+++ /dev/null
@@ -1,81 +0,0 @@
-from utils.configuration import Configuration
-import tensorflow as tf
-from utils.model import ModelLoss
-from utils.model import LFUNet
-from utils.architectures import UNet
-
-import gradio as gr
-
-configuration = Configuration()
-filters = (64, 128, 128, 256, 256, 512)
-kernels = (7, 7, 7, 3, 3, 3)
-input_image_size = (256, 256, 3)
-architecture = UNet.RESIDUAL_ATTENTION_UNET_SEPARABLE_CONV
-
-trained_model = LFUNet.build_model(architecture=architecture, input_size=input_image_size, filters=filters,
- kernels=kernels, configuration=configuration)
-trained_model.compile(
- loss=ModelLoss.ms_ssim_l1_perceptual_loss,
- optimizer=tf.keras.optimizers.Adam(1e-4),
- metrics=["acc", tf.keras.metrics.Recall(), tf.keras.metrics.Precision()]
- )
-
-weights_path = "model_weights/model_epochs-40_batch-20_loss-ms_ssim_l1_perceptual_loss_20230210_15_45_38.ckpt"
-trained_model.load_weights(weights_path)
-
-def main(input_img):
- try:
- print(input_img)
- predicted_image = trained_model.predict(input_img)
- return predicted_image
- except Exception as e:
- raise gr.Error("Sorry, something went wrong. Please try again!")
-
-demo = gr.Interface(
- title= "Lightweight network for face unmasking",
- description= "This is a demo of a Lightweight network for face unmasking \
- designed to provide a powerful and efficient solution for restoring facial details obscured by masks. \
- To use it, simply upload your image, or click one of the examples to load them. Inference in demo may take some time because of connectivity reasons.",
- fn = main,
- inputs= gr.Image(type="filepath").style(height=256),
- outputs=gr.Image(type='numpy',shape=(256, 256, 3)).style(height=256),
- # allow_flagging='never',
- examples=[
- ["examples/1.png"],
- ["examples/2.png"],
- ["examples/3.png"],
- ["examples/4.png"],
- ["examples/5.png"],
- ["examples/6.png"],
- ["examples/7.png"],
- ["examples/8.png"],
- ["examples/9.png"],
- ["examples/10.png"],
- ["examples/11.png"],
- ["examples/12.png"],
- ],
- css = """
- .svelte-mppz8v {
- text-align: -webkit-center;
- }
-
- .gallery {
- display: flex;
- flex-wrap: wrap;
- width: 100%;
- }
-
- p {
- font-size: medium;
- }
-
- h1 {
- font-size: xx-large;
- }
- """,
- # theme= 'EveryPizza/Cartoony-Gradio-Theme',
- theme = 'xiaobaiyuan/theme_brief',
- cache_examples=False
- # article = "
"
-)
-demo.launch(show_error=True)
diff --git a/spaces/anonymous-pits/pits/attentions.py b/spaces/anonymous-pits/pits/attentions.py
deleted file mode 100644
index 89ab7307f6238f2eafc31cf420fb62b3be122f15..0000000000000000000000000000000000000000
--- a/spaces/anonymous-pits/pits/attentions.py
+++ /dev/null
@@ -1,472 +0,0 @@
-# from https://github.com/jaywalnut310/vits
-import math
-import torch
-from torch import nn
-from torch.nn import functional as F
-
-import commons
-from modules import LayerNorm
-
-
-class Encoder(nn.Module):
- def __init__(
- self,
- hidden_channels,
- filter_channels,
- n_heads,
- n_layers,
- kernel_size=1,
- p_dropout=0.,
- window_size=4,
- **kwargs
- ):
- super().__init__()
- self.hidden_channels = hidden_channels
- self.filter_channels = filter_channels
- self.n_heads = n_heads
- self.n_layers = n_layers
- self.kernel_size = kernel_size
- self.p_dropout = p_dropout
- self.window_size = window_size
-
- self.drop = nn.Dropout(p_dropout)
- self.attn_layers = nn.ModuleList()
- self.norm_layers_1 = nn.ModuleList()
- self.ffn_layers = nn.ModuleList()
- self.norm_layers_2 = nn.ModuleList()
- for i in range(self.n_layers):
- self.attn_layers.append(
- MultiHeadAttention(
- hidden_channels,
- hidden_channels,
- n_heads,
- p_dropout=p_dropout,
- window_size=window_size
- )
- )
- self.norm_layers_1.append(LayerNorm(hidden_channels))
- self.ffn_layers.append(
- FFN(
- hidden_channels,
- hidden_channels,
- filter_channels,
- kernel_size,
- p_dropout=p_dropout
- )
- )
- self.norm_layers_2.append(LayerNorm(hidden_channels))
-
- def forward(self, x, x_mask):
- attn_mask = x_mask.unsqueeze(2) * x_mask.unsqueeze(-1)
- x = x * x_mask
- for i in range(self.n_layers):
- y = self.attn_layers[i](x, x, attn_mask)
- y = self.drop(y)
- x = self.norm_layers_1[i](x + y)
-
- y = self.ffn_layers[i](x, x_mask)
- y = self.drop(y)
- x = self.norm_layers_2[i](x + y)
- x = x * x_mask
- return x
-
-
-class Decoder(nn.Module):
- def __init__(
- self,
- hidden_channels,
- filter_channels,
- n_heads,
- n_layers,
- kernel_size=1,
- p_dropout=0.,
- proximal_bias=False,
- proximal_init=True,
- **kwargs
- ):
- super().__init__()
- self.hidden_channels = hidden_channels
- self.filter_channels = filter_channels
- self.n_heads = n_heads
- self.n_layers = n_layers
- self.kernel_size = kernel_size
- self.p_dropout = p_dropout
- self.proximal_bias = proximal_bias
- self.proximal_init = proximal_init
-
- self.drop = nn.Dropout(p_dropout)
- self.self_attn_layers = nn.ModuleList()
- self.norm_layers_0 = nn.ModuleList()
- self.encdec_attn_layers = nn.ModuleList()
- self.norm_layers_1 = nn.ModuleList()
- self.ffn_layers = nn.ModuleList()
- self.norm_layers_2 = nn.ModuleList()
- for i in range(self.n_layers):
- self.self_attn_layers.append(
- MultiHeadAttention(
- hidden_channels,
- hidden_channels,
- n_heads,
- p_dropout=p_dropout,
- proximal_bias=proximal_bias,
- proximal_init=proximal_init
- )
- )
- self.norm_layers_0.append(LayerNorm(hidden_channels))
- self.encdec_attn_layers.append(
- MultiHeadAttention(
- hidden_channels,
- hidden_channels,
- n_heads,
- p_dropout=p_dropout
- )
- )
- self.norm_layers_1.append(LayerNorm(hidden_channels))
- self.ffn_layers.append(
- FFN(
- hidden_channels,
- hidden_channels,
- filter_channels,
- kernel_size,
- p_dropout=p_dropout,
- causal=True
- )
- )
- self.norm_layers_2.append(LayerNorm(hidden_channels))
-
- def forward(self, x, x_mask, h, h_mask):
- """
- x: decoder input
- h: encoder output
- """
- self_attn_mask = commons.subsequent_mask(
- x_mask.size(2)
- ).to(device=x.device, dtype=x.dtype)
- encdec_attn_mask = h_mask.unsqueeze(2) * x_mask.unsqueeze(-1)
- x = x * x_mask
- for i in range(self.n_layers):
- y = self.self_attn_layers[i](x, x, self_attn_mask)
- y = self.drop(y)
- x = self.norm_layers_0[i](x + y)
-
- y = self.encdec_attn_layers[i](x, h, encdec_attn_mask)
- y = self.drop(y)
- x = self.norm_layers_1[i](x + y)
-
- y = self.ffn_layers[i](x, x_mask)
- y = self.drop(y)
- x = self.norm_layers_2[i](x + y)
- x = x * x_mask
- return x
-
-
-class MultiHeadAttention(nn.Module):
- def __init__(
- self,
- channels,
- out_channels,
- n_heads,
- p_dropout=0.,
- window_size=None,
- heads_share=True,
- block_length=None,
- proximal_bias=False,
- proximal_init=False
- ):
- super().__init__()
- assert channels % n_heads == 0
-
- self.channels = channels
- self.out_channels = out_channels
- self.n_heads = n_heads
- self.p_dropout = p_dropout
- self.window_size = window_size
- self.heads_share = heads_share
- self.block_length = block_length
- self.proximal_bias = proximal_bias
- self.proximal_init = proximal_init
- self.attn = None
-
- self.k_channels = channels // n_heads
- self.conv_q = nn.Conv1d(channels, channels, 1)
- self.conv_k = nn.Conv1d(channels, channels, 1)
- self.conv_v = nn.Conv1d(channels, channels, 1)
- self.conv_o = nn.Conv1d(channels, out_channels, 1)
- self.drop = nn.Dropout(p_dropout)
-
- if window_size is not None:
- n_heads_rel = 1 if heads_share else n_heads
- rel_stddev = self.k_channels**-0.5
- self.emb_rel_k = nn.Parameter(torch.randn(
- n_heads_rel, window_size * 2 + 1, self.k_channels) * rel_stddev)
- self.emb_rel_v = nn.Parameter(torch.randn(
- n_heads_rel, window_size * 2 + 1, self.k_channels) * rel_stddev)
-
- nn.init.xavier_uniform_(self.conv_q.weight)
- nn.init.xavier_uniform_(self.conv_k.weight)
- nn.init.xavier_uniform_(self.conv_v.weight)
- if proximal_init:
- with torch.no_grad():
- self.conv_k.weight.copy_(self.conv_q.weight)
- self.conv_k.bias.copy_(self.conv_q.bias)
-
- def forward(self, x, c, attn_mask=None):
- q = self.conv_q(x)
- k = self.conv_k(c)
- v = self.conv_v(c)
-
- x, self.attn = self.attention(q, k, v, mask=attn_mask)
-
- x = self.conv_o(x)
- return x
-
- def attention(self, query, key, value, mask=None):
- # reshape [b, d, t] -> [b, n_h, t, d_k]
- b, d, t_s, t_t = (*key.size(), query.size(2))
- #query = query.view(
- # b,
- # self.n_heads,
- # self.k_channels,
- # t_t
- #).transpose(2, 3) #[b,h,t_t,c], d=h*c
- #key = key.view(
- # b,
- # self.n_heads,
- # self.k_channels,
- # t_s
- #).transpose(2, 3) #[b,h,t_s,c]
- #value = value.view(
- # b,
- # self.n_heads,
- # self.k_channels,
- # t_s
- #).transpose(2, 3) #[b,h,t_s,c]
- #scores = torch.matmul(
- # query / math.sqrt(self.k_channels), key.transpose(-2, -1)
- #) #[b,h,t_t,t_s]
- query = query.view(
- b,
- self.n_heads,
- self.k_channels,
- t_t
- ) #[b,h,c,t_t]
- key = key.view(
- b,
- self.n_heads,
- self.k_channels,
- t_s
- ) #[b,h,c,t_s]
- value = value.view(
- b,
- self.n_heads,
- self.k_channels,
- t_s
- ) #[b,h,c,t_s]
- scores = torch.einsum('bhdt,bhds -> bhts', query / math.sqrt(self.k_channels), key) #[b,h,t_t,t_s]
- #if self.window_size is not None:
- # assert t_s == t_t, "Relative attention is only available for self-attention."
- # key_relative_embeddings = self._get_relative_embeddings(
- # self.emb_rel_k, t_s
- # )
- # rel_logits = self._matmul_with_relative_keys(
- # query / math.sqrt(self.k_channels), key_relative_embeddings
- # ) #[b,h,t_t,d],[h or 1,e,d] ->[b,h,t_t,e]
- # scores_local = self._relative_position_to_absolute_position(rel_logits)
- # scores = scores + scores_local
- #if self.proximal_bias:
- # assert t_s == t_t, "Proximal bias is only available for self-attention."
- # scores = scores + \
- # self._attention_bias_proximal(t_s).to(device=scores.device, dtype=scores.dtype)
- #if mask is not None:
- # scores = scores.masked_fill(mask == 0, -1e4)
- # if self.block_length is not None:
- # assert t_s == t_t, "Local attention is only available for self-attention."
- # block_mask = torch.ones_like(scores).triu(-self.block_length).tril(self.block_length)
- # scores = scores.masked_fill(block_mask == 0, -1e4)
- #p_attn = F.softmax(scores, dim=-1) # [b, h, t_t, t_s]
- #p_attn = self.drop(p_attn)
- #output = torch.matmul(p_attn, value) # [b,h,t_t,t_s],[b,h,t_s,c] -> [b,h,t_t,c]
- #if self.window_size is not None:
- # relative_weights = self._absolute_position_to_relative_position(p_attn) #[b, h, t_t, 2*t_t-1]
- # value_relative_embeddings = self._get_relative_embeddings(self.emb_rel_v, t_s) #[h or 1, 2*t_t-1, c]
- # output = output + \
- # self._matmul_with_relative_values(
- # relative_weights, value_relative_embeddings) # [b, h, t_t, 2*t_t-1],[h or 1, 2*t_t-1, c] -> [b, h, t_t, c]
- #output = output.transpose(2, 3).contiguous().view(b, d, t_t) # [b, n_h, t_t, c] -> [b,h,c,t_t] -> [b, d, t_t]
- if self.window_size is not None:
- assert t_s == t_t, "Relative attention is only available for self-attention."
- key_relative_embeddings = self._get_relative_embeddings(
- self.emb_rel_k, t_s
- )
- rel_logits = torch.einsum('bhdt,hed->bhte',
- query / math.sqrt(self.k_channels), key_relative_embeddings
- ) #[b,h,c,t_t],[h or 1,e,c] ->[b,h,t_t,e]
- scores_local = self._relative_position_to_absolute_position(rel_logits)
- scores = scores + scores_local
- if self.proximal_bias:
- assert t_s == t_t, "Proximal bias is only available for self-attention."
- scores = scores + \
- self._attention_bias_proximal(t_s).to(device=scores.device, dtype=scores.dtype)
- if mask is not None:
- scores = scores.masked_fill(mask == 0, -1e4)
- if self.block_length is not None:
- assert t_s == t_t, "Local attention is only available for self-attention."
- block_mask = torch.ones_like(scores).triu(-self.block_length).tril(self.block_length)
- scores = scores.masked_fill(block_mask == 0, -1e4)
- p_attn = F.softmax(scores, dim=-1) # [b, h, t_t, t_s]
- p_attn = self.drop(p_attn)
- output = torch.einsum('bhcs,bhts->bhct', value , p_attn) # [b,h,c,t_s],[b,h,t_t,t_s] -> [b,h,c,t_t]
- if self.window_size is not None:
- relative_weights = self._absolute_position_to_relative_position(p_attn) #[b, h, t_t, 2*t_t-1]
- value_relative_embeddings = self._get_relative_embeddings(self.emb_rel_v, t_s) #[h or 1, 2*t_t-1, c]
- output = output + \
- torch.einsum('bhte,hec->bhct',
- relative_weights, value_relative_embeddings) # [b, h, t_t, 2*t_t-1],[h or 1, 2*t_t-1, c] -> [b, h, c, t_t]
- output = output.view(b, d, t_t) # [b, h, c, t_t] -> [b, d, t_t]
- return output, p_attn
-
- def _matmul_with_relative_values(self, x, y):
- """
- x: [b, h, l, m]
- y: [h or 1, m, d]
- ret: [b, h, l, d]
- """
- ret = torch.matmul(x, y.unsqueeze(0))
- return ret
-
- def _matmul_with_relative_keys(self, x, y):
- """
- x: [b, h, l, d]
- y: [h or 1, m, d]
- ret: [b, h, l, m]
- """
- #ret = torch.matmul(x, y.unsqueeze(0).transpose(-2, -1))
- ret = torch.einsum('bhld,hmd -> bhlm', x, y)
- return ret
-
- def _get_relative_embeddings(self, relative_embeddings, length):
- max_relative_position = 2 * self.window_size + 1
- # Pad first before slice to avoid using cond ops.
- pad_length = max(length - (self.window_size + 1), 0)
- slice_start_position = max((self.window_size + 1) - length, 0)
- slice_end_position = slice_start_position + 2 * length - 1
- if pad_length > 0:
- padded_relative_embeddings = F.pad(
- relative_embeddings,
- commons.convert_pad_shape([[0, 0], [pad_length, pad_length], [0, 0]]))
- else:
- padded_relative_embeddings = relative_embeddings
- used_relative_embeddings = padded_relative_embeddings[
- :, slice_start_position:slice_end_position
- ]
- return used_relative_embeddings
-
- def _relative_position_to_absolute_position(self, x):
- """
- x: [b, h, l, 2*l-1]
- ret: [b, h, l, l]
- """
- batch, heads, length, _ = x.size()
- # Concat columns of pad to shift from relative to absolute indexing.
- x = F.pad(x, commons.convert_pad_shape(
- [[0, 0], [0, 0], [0, 0], [0, 1]]
- ))
-
- # Concat extra elements so to add up to shape (len+1, 2*len-1).
- x_flat = x.view([batch, heads, length * 2 * length])
- x_flat = F.pad(x_flat, commons.convert_pad_shape(
- [[0, 0], [0, 0], [0, length-1]]
- ))
-
- # Reshape and slice out the padded elements.
- x_final = x_flat.view([batch, heads, length+1, 2*length-1])[
- :, :, :length, length-1:
- ]
- return x_final
-
- def _absolute_position_to_relative_position(self, x):
- """
- x: [b, h, l, l]
- ret: [b, h, l, 2*l-1]
- """
- batch, heads, length, _ = x.size()
- # padd along column
- x = F.pad(x, commons.convert_pad_shape(
- [[0, 0], [0, 0], [0, 0], [0, length-1]]
- ))
- x_flat = x.view([batch, heads, length**2 + length*(length - 1)])
- # add 0's in the beginning that will skew the elements after reshape
- x_flat = F.pad(x_flat, commons.convert_pad_shape(
- [[0, 0], [0, 0], [length, 0]]
- ))
- x_final = x_flat.view([batch, heads, length, 2*length])[:, :, :, 1:]
- return x_final
-
- def _attention_bias_proximal(self, length):
- """Bias for self-attention to encourage attention to close positions.
- Args:
- length: an integer scalar.
- Returns:
- a Tensor with shape [1, 1, length, length]
- """
- r = torch.arange(length, dtype=torch.float32)
- diff = torch.unsqueeze(r, 0) - torch.unsqueeze(r, 1)
- return torch.unsqueeze(torch.unsqueeze(-torch.log1p(torch.abs(diff)), 0), 0)
-
-
-class FFN(nn.Module):
- def __init__(
- self,
- in_channels,
- out_channels,
- filter_channels,
- kernel_size,
- p_dropout=0.,
- activation=None,
- causal=False
- ):
- super().__init__()
- self.in_channels = in_channels
- self.out_channels = out_channels
- self.filter_channels = filter_channels
- self.kernel_size = kernel_size
- self.p_dropout = p_dropout
- self.activation = activation
- self.causal = causal
-
- if causal:
- self.padding = self._causal_padding
- else:
- self.padding = self._same_padding
-
- self.conv_1 = nn.Conv1d(in_channels, filter_channels, kernel_size)
- self.conv_2 = nn.Conv1d(filter_channels, out_channels, kernel_size)
- self.drop = nn.Dropout(p_dropout)
-
- def forward(self, x, x_mask):
- x = self.conv_1(self.padding(x * x_mask))
- if self.activation == "gelu":
- x = x * torch.sigmoid(1.702 * x)
- else:
- x = torch.relu(x)
- x = self.drop(x)
- x = self.conv_2(self.padding(x * x_mask))
- return x * x_mask
-
- def _causal_padding(self, x):
- if self.kernel_size == 1:
- return x
- pad_l = self.kernel_size - 1
- pad_r = 0
- padding = [[0, 0], [0, 0], [pad_l, pad_r]]
- x = F.pad(x, commons.convert_pad_shape(padding))
- return x
-
- def _same_padding(self, x):
- if self.kernel_size == 1:
- return x
- pad_l = (self.kernel_size - 1) // 2
- pad_r = self.kernel_size // 2
- padding = [[0, 0], [0, 0], [pad_l, pad_r]]
- x = F.pad(x, commons.convert_pad_shape(padding))
- return x
diff --git a/spaces/aodianyun/stable-diffusion-webui/extensions-builtin/SwinIR/swinir_model_arch.py b/spaces/aodianyun/stable-diffusion-webui/extensions-builtin/SwinIR/swinir_model_arch.py
deleted file mode 100644
index 863f42db6f50e5eac70931b8c0e6443f831a6018..0000000000000000000000000000000000000000
--- a/spaces/aodianyun/stable-diffusion-webui/extensions-builtin/SwinIR/swinir_model_arch.py
+++ /dev/null
@@ -1,867 +0,0 @@
-# -----------------------------------------------------------------------------------
-# SwinIR: Image Restoration Using Swin Transformer, https://arxiv.org/abs/2108.10257
-# Originally Written by Ze Liu, Modified by Jingyun Liang.
-# -----------------------------------------------------------------------------------
-
-import math
-import torch
-import torch.nn as nn
-import torch.nn.functional as F
-import torch.utils.checkpoint as checkpoint
-from timm.models.layers import DropPath, to_2tuple, trunc_normal_
-
-
-class Mlp(nn.Module):
- def __init__(self, in_features, hidden_features=None, out_features=None, act_layer=nn.GELU, drop=0.):
- super().__init__()
- out_features = out_features or in_features
- hidden_features = hidden_features or in_features
- self.fc1 = nn.Linear(in_features, hidden_features)
- self.act = act_layer()
- self.fc2 = nn.Linear(hidden_features, out_features)
- self.drop = nn.Dropout(drop)
-
- def forward(self, x):
- x = self.fc1(x)
- x = self.act(x)
- x = self.drop(x)
- x = self.fc2(x)
- x = self.drop(x)
- return x
-
-
-def window_partition(x, window_size):
- """
- Args:
- x: (B, H, W, C)
- window_size (int): window size
-
- Returns:
- windows: (num_windows*B, window_size, window_size, C)
- """
- B, H, W, C = x.shape
- x = x.view(B, H // window_size, window_size, W // window_size, window_size, C)
- windows = x.permute(0, 1, 3, 2, 4, 5).contiguous().view(-1, window_size, window_size, C)
- return windows
-
-
-def window_reverse(windows, window_size, H, W):
- """
- Args:
- windows: (num_windows*B, window_size, window_size, C)
- window_size (int): Window size
- H (int): Height of image
- W (int): Width of image
-
- Returns:
- x: (B, H, W, C)
- """
- B = int(windows.shape[0] / (H * W / window_size / window_size))
- x = windows.view(B, H // window_size, W // window_size, window_size, window_size, -1)
- x = x.permute(0, 1, 3, 2, 4, 5).contiguous().view(B, H, W, -1)
- return x
-
-
-class WindowAttention(nn.Module):
- r""" Window based multi-head self attention (W-MSA) module with relative position bias.
- It supports both of shifted and non-shifted window.
-
- Args:
- dim (int): Number of input channels.
- window_size (tuple[int]): The height and width of the window.
- num_heads (int): Number of attention heads.
- qkv_bias (bool, optional): If True, add a learnable bias to query, key, value. Default: True
- qk_scale (float | None, optional): Override default qk scale of head_dim ** -0.5 if set
- attn_drop (float, optional): Dropout ratio of attention weight. Default: 0.0
- proj_drop (float, optional): Dropout ratio of output. Default: 0.0
- """
-
- def __init__(self, dim, window_size, num_heads, qkv_bias=True, qk_scale=None, attn_drop=0., proj_drop=0.):
-
- super().__init__()
- self.dim = dim
- self.window_size = window_size # Wh, Ww
- self.num_heads = num_heads
- head_dim = dim // num_heads
- self.scale = qk_scale or head_dim ** -0.5
-
- # define a parameter table of relative position bias
- self.relative_position_bias_table = nn.Parameter(
- torch.zeros((2 * window_size[0] - 1) * (2 * window_size[1] - 1), num_heads)) # 2*Wh-1 * 2*Ww-1, nH
-
- # get pair-wise relative position index for each token inside the window
- coords_h = torch.arange(self.window_size[0])
- coords_w = torch.arange(self.window_size[1])
- coords = torch.stack(torch.meshgrid([coords_h, coords_w])) # 2, Wh, Ww
- coords_flatten = torch.flatten(coords, 1) # 2, Wh*Ww
- relative_coords = coords_flatten[:, :, None] - coords_flatten[:, None, :] # 2, Wh*Ww, Wh*Ww
- relative_coords = relative_coords.permute(1, 2, 0).contiguous() # Wh*Ww, Wh*Ww, 2
- relative_coords[:, :, 0] += self.window_size[0] - 1 # shift to start from 0
- relative_coords[:, :, 1] += self.window_size[1] - 1
- relative_coords[:, :, 0] *= 2 * self.window_size[1] - 1
- relative_position_index = relative_coords.sum(-1) # Wh*Ww, Wh*Ww
- self.register_buffer("relative_position_index", relative_position_index)
-
- self.qkv = nn.Linear(dim, dim * 3, bias=qkv_bias)
- self.attn_drop = nn.Dropout(attn_drop)
- self.proj = nn.Linear(dim, dim)
-
- self.proj_drop = nn.Dropout(proj_drop)
-
- trunc_normal_(self.relative_position_bias_table, std=.02)
- self.softmax = nn.Softmax(dim=-1)
-
- def forward(self, x, mask=None):
- """
- Args:
- x: input features with shape of (num_windows*B, N, C)
- mask: (0/-inf) mask with shape of (num_windows, Wh*Ww, Wh*Ww) or None
- """
- B_, N, C = x.shape
- qkv = self.qkv(x).reshape(B_, N, 3, self.num_heads, C // self.num_heads).permute(2, 0, 3, 1, 4)
- q, k, v = qkv[0], qkv[1], qkv[2] # make torchscript happy (cannot use tensor as tuple)
-
- q = q * self.scale
- attn = (q @ k.transpose(-2, -1))
-
- relative_position_bias = self.relative_position_bias_table[self.relative_position_index.view(-1)].view(
- self.window_size[0] * self.window_size[1], self.window_size[0] * self.window_size[1], -1) # Wh*Ww,Wh*Ww,nH
- relative_position_bias = relative_position_bias.permute(2, 0, 1).contiguous() # nH, Wh*Ww, Wh*Ww
- attn = attn + relative_position_bias.unsqueeze(0)
-
- if mask is not None:
- nW = mask.shape[0]
- attn = attn.view(B_ // nW, nW, self.num_heads, N, N) + mask.unsqueeze(1).unsqueeze(0)
- attn = attn.view(-1, self.num_heads, N, N)
- attn = self.softmax(attn)
- else:
- attn = self.softmax(attn)
-
- attn = self.attn_drop(attn)
-
- x = (attn @ v).transpose(1, 2).reshape(B_, N, C)
- x = self.proj(x)
- x = self.proj_drop(x)
- return x
-
- def extra_repr(self) -> str:
- return f'dim={self.dim}, window_size={self.window_size}, num_heads={self.num_heads}'
-
- def flops(self, N):
- # calculate flops for 1 window with token length of N
- flops = 0
- # qkv = self.qkv(x)
- flops += N * self.dim * 3 * self.dim
- # attn = (q @ k.transpose(-2, -1))
- flops += self.num_heads * N * (self.dim // self.num_heads) * N
- # x = (attn @ v)
- flops += self.num_heads * N * N * (self.dim // self.num_heads)
- # x = self.proj(x)
- flops += N * self.dim * self.dim
- return flops
-
-
-class SwinTransformerBlock(nn.Module):
- r""" Swin Transformer Block.
-
- Args:
- dim (int): Number of input channels.
- input_resolution (tuple[int]): Input resolution.
- num_heads (int): Number of attention heads.
- window_size (int): Window size.
- shift_size (int): Shift size for SW-MSA.
- mlp_ratio (float): Ratio of mlp hidden dim to embedding dim.
- qkv_bias (bool, optional): If True, add a learnable bias to query, key, value. Default: True
- qk_scale (float | None, optional): Override default qk scale of head_dim ** -0.5 if set.
- drop (float, optional): Dropout rate. Default: 0.0
- attn_drop (float, optional): Attention dropout rate. Default: 0.0
- drop_path (float, optional): Stochastic depth rate. Default: 0.0
- act_layer (nn.Module, optional): Activation layer. Default: nn.GELU
- norm_layer (nn.Module, optional): Normalization layer. Default: nn.LayerNorm
- """
-
- def __init__(self, dim, input_resolution, num_heads, window_size=7, shift_size=0,
- mlp_ratio=4., qkv_bias=True, qk_scale=None, drop=0., attn_drop=0., drop_path=0.,
- act_layer=nn.GELU, norm_layer=nn.LayerNorm):
- super().__init__()
- self.dim = dim
- self.input_resolution = input_resolution
- self.num_heads = num_heads
- self.window_size = window_size
- self.shift_size = shift_size
- self.mlp_ratio = mlp_ratio
- if min(self.input_resolution) <= self.window_size:
- # if window size is larger than input resolution, we don't partition windows
- self.shift_size = 0
- self.window_size = min(self.input_resolution)
- assert 0 <= self.shift_size < self.window_size, "shift_size must in 0-window_size"
-
- self.norm1 = norm_layer(dim)
- self.attn = WindowAttention(
- dim, window_size=to_2tuple(self.window_size), num_heads=num_heads,
- qkv_bias=qkv_bias, qk_scale=qk_scale, attn_drop=attn_drop, proj_drop=drop)
-
- self.drop_path = DropPath(drop_path) if drop_path > 0. else nn.Identity()
- self.norm2 = norm_layer(dim)
- mlp_hidden_dim = int(dim * mlp_ratio)
- self.mlp = Mlp(in_features=dim, hidden_features=mlp_hidden_dim, act_layer=act_layer, drop=drop)
-
- if self.shift_size > 0:
- attn_mask = self.calculate_mask(self.input_resolution)
- else:
- attn_mask = None
-
- self.register_buffer("attn_mask", attn_mask)
-
- def calculate_mask(self, x_size):
- # calculate attention mask for SW-MSA
- H, W = x_size
- img_mask = torch.zeros((1, H, W, 1)) # 1 H W 1
- h_slices = (slice(0, -self.window_size),
- slice(-self.window_size, -self.shift_size),
- slice(-self.shift_size, None))
- w_slices = (slice(0, -self.window_size),
- slice(-self.window_size, -self.shift_size),
- slice(-self.shift_size, None))
- cnt = 0
- for h in h_slices:
- for w in w_slices:
- img_mask[:, h, w, :] = cnt
- cnt += 1
-
- mask_windows = window_partition(img_mask, self.window_size) # nW, window_size, window_size, 1
- mask_windows = mask_windows.view(-1, self.window_size * self.window_size)
- attn_mask = mask_windows.unsqueeze(1) - mask_windows.unsqueeze(2)
- attn_mask = attn_mask.masked_fill(attn_mask != 0, float(-100.0)).masked_fill(attn_mask == 0, float(0.0))
-
- return attn_mask
-
- def forward(self, x, x_size):
- H, W = x_size
- B, L, C = x.shape
- # assert L == H * W, "input feature has wrong size"
-
- shortcut = x
- x = self.norm1(x)
- x = x.view(B, H, W, C)
-
- # cyclic shift
- if self.shift_size > 0:
- shifted_x = torch.roll(x, shifts=(-self.shift_size, -self.shift_size), dims=(1, 2))
- else:
- shifted_x = x
-
- # partition windows
- x_windows = window_partition(shifted_x, self.window_size) # nW*B, window_size, window_size, C
- x_windows = x_windows.view(-1, self.window_size * self.window_size, C) # nW*B, window_size*window_size, C
-
- # W-MSA/SW-MSA (to be compatible for testing on images whose shapes are the multiple of window size
- if self.input_resolution == x_size:
- attn_windows = self.attn(x_windows, mask=self.attn_mask) # nW*B, window_size*window_size, C
- else:
- attn_windows = self.attn(x_windows, mask=self.calculate_mask(x_size).to(x.device))
-
- # merge windows
- attn_windows = attn_windows.view(-1, self.window_size, self.window_size, C)
- shifted_x = window_reverse(attn_windows, self.window_size, H, W) # B H' W' C
-
- # reverse cyclic shift
- if self.shift_size > 0:
- x = torch.roll(shifted_x, shifts=(self.shift_size, self.shift_size), dims=(1, 2))
- else:
- x = shifted_x
- x = x.view(B, H * W, C)
-
- # FFN
- x = shortcut + self.drop_path(x)
- x = x + self.drop_path(self.mlp(self.norm2(x)))
-
- return x
-
- def extra_repr(self) -> str:
- return f"dim={self.dim}, input_resolution={self.input_resolution}, num_heads={self.num_heads}, " \
- f"window_size={self.window_size}, shift_size={self.shift_size}, mlp_ratio={self.mlp_ratio}"
-
- def flops(self):
- flops = 0
- H, W = self.input_resolution
- # norm1
- flops += self.dim * H * W
- # W-MSA/SW-MSA
- nW = H * W / self.window_size / self.window_size
- flops += nW * self.attn.flops(self.window_size * self.window_size)
- # mlp
- flops += 2 * H * W * self.dim * self.dim * self.mlp_ratio
- # norm2
- flops += self.dim * H * W
- return flops
-
-
-class PatchMerging(nn.Module):
- r""" Patch Merging Layer.
-
- Args:
- input_resolution (tuple[int]): Resolution of input feature.
- dim (int): Number of input channels.
- norm_layer (nn.Module, optional): Normalization layer. Default: nn.LayerNorm
- """
-
- def __init__(self, input_resolution, dim, norm_layer=nn.LayerNorm):
- super().__init__()
- self.input_resolution = input_resolution
- self.dim = dim
- self.reduction = nn.Linear(4 * dim, 2 * dim, bias=False)
- self.norm = norm_layer(4 * dim)
-
- def forward(self, x):
- """
- x: B, H*W, C
- """
- H, W = self.input_resolution
- B, L, C = x.shape
- assert L == H * W, "input feature has wrong size"
- assert H % 2 == 0 and W % 2 == 0, f"x size ({H}*{W}) are not even."
-
- x = x.view(B, H, W, C)
-
- x0 = x[:, 0::2, 0::2, :] # B H/2 W/2 C
- x1 = x[:, 1::2, 0::2, :] # B H/2 W/2 C
- x2 = x[:, 0::2, 1::2, :] # B H/2 W/2 C
- x3 = x[:, 1::2, 1::2, :] # B H/2 W/2 C
- x = torch.cat([x0, x1, x2, x3], -1) # B H/2 W/2 4*C
- x = x.view(B, -1, 4 * C) # B H/2*W/2 4*C
-
- x = self.norm(x)
- x = self.reduction(x)
-
- return x
-
- def extra_repr(self) -> str:
- return f"input_resolution={self.input_resolution}, dim={self.dim}"
-
- def flops(self):
- H, W = self.input_resolution
- flops = H * W * self.dim
- flops += (H // 2) * (W // 2) * 4 * self.dim * 2 * self.dim
- return flops
-
-
-class BasicLayer(nn.Module):
- """ A basic Swin Transformer layer for one stage.
-
- Args:
- dim (int): Number of input channels.
- input_resolution (tuple[int]): Input resolution.
- depth (int): Number of blocks.
- num_heads (int): Number of attention heads.
- window_size (int): Local window size.
- mlp_ratio (float): Ratio of mlp hidden dim to embedding dim.
- qkv_bias (bool, optional): If True, add a learnable bias to query, key, value. Default: True
- qk_scale (float | None, optional): Override default qk scale of head_dim ** -0.5 if set.
- drop (float, optional): Dropout rate. Default: 0.0
- attn_drop (float, optional): Attention dropout rate. Default: 0.0
- drop_path (float | tuple[float], optional): Stochastic depth rate. Default: 0.0
- norm_layer (nn.Module, optional): Normalization layer. Default: nn.LayerNorm
- downsample (nn.Module | None, optional): Downsample layer at the end of the layer. Default: None
- use_checkpoint (bool): Whether to use checkpointing to save memory. Default: False.
- """
-
- def __init__(self, dim, input_resolution, depth, num_heads, window_size,
- mlp_ratio=4., qkv_bias=True, qk_scale=None, drop=0., attn_drop=0.,
- drop_path=0., norm_layer=nn.LayerNorm, downsample=None, use_checkpoint=False):
-
- super().__init__()
- self.dim = dim
- self.input_resolution = input_resolution
- self.depth = depth
- self.use_checkpoint = use_checkpoint
-
- # build blocks
- self.blocks = nn.ModuleList([
- SwinTransformerBlock(dim=dim, input_resolution=input_resolution,
- num_heads=num_heads, window_size=window_size,
- shift_size=0 if (i % 2 == 0) else window_size // 2,
- mlp_ratio=mlp_ratio,
- qkv_bias=qkv_bias, qk_scale=qk_scale,
- drop=drop, attn_drop=attn_drop,
- drop_path=drop_path[i] if isinstance(drop_path, list) else drop_path,
- norm_layer=norm_layer)
- for i in range(depth)])
-
- # patch merging layer
- if downsample is not None:
- self.downsample = downsample(input_resolution, dim=dim, norm_layer=norm_layer)
- else:
- self.downsample = None
-
- def forward(self, x, x_size):
- for blk in self.blocks:
- if self.use_checkpoint:
- x = checkpoint.checkpoint(blk, x, x_size)
- else:
- x = blk(x, x_size)
- if self.downsample is not None:
- x = self.downsample(x)
- return x
-
- def extra_repr(self) -> str:
- return f"dim={self.dim}, input_resolution={self.input_resolution}, depth={self.depth}"
-
- def flops(self):
- flops = 0
- for blk in self.blocks:
- flops += blk.flops()
- if self.downsample is not None:
- flops += self.downsample.flops()
- return flops
-
-
-class RSTB(nn.Module):
- """Residual Swin Transformer Block (RSTB).
-
- Args:
- dim (int): Number of input channels.
- input_resolution (tuple[int]): Input resolution.
- depth (int): Number of blocks.
- num_heads (int): Number of attention heads.
- window_size (int): Local window size.
- mlp_ratio (float): Ratio of mlp hidden dim to embedding dim.
- qkv_bias (bool, optional): If True, add a learnable bias to query, key, value. Default: True
- qk_scale (float | None, optional): Override default qk scale of head_dim ** -0.5 if set.
- drop (float, optional): Dropout rate. Default: 0.0
- attn_drop (float, optional): Attention dropout rate. Default: 0.0
- drop_path (float | tuple[float], optional): Stochastic depth rate. Default: 0.0
- norm_layer (nn.Module, optional): Normalization layer. Default: nn.LayerNorm
- downsample (nn.Module | None, optional): Downsample layer at the end of the layer. Default: None
- use_checkpoint (bool): Whether to use checkpointing to save memory. Default: False.
- img_size: Input image size.
- patch_size: Patch size.
- resi_connection: The convolutional block before residual connection.
- """
-
- def __init__(self, dim, input_resolution, depth, num_heads, window_size,
- mlp_ratio=4., qkv_bias=True, qk_scale=None, drop=0., attn_drop=0.,
- drop_path=0., norm_layer=nn.LayerNorm, downsample=None, use_checkpoint=False,
- img_size=224, patch_size=4, resi_connection='1conv'):
- super(RSTB, self).__init__()
-
- self.dim = dim
- self.input_resolution = input_resolution
-
- self.residual_group = BasicLayer(dim=dim,
- input_resolution=input_resolution,
- depth=depth,
- num_heads=num_heads,
- window_size=window_size,
- mlp_ratio=mlp_ratio,
- qkv_bias=qkv_bias, qk_scale=qk_scale,
- drop=drop, attn_drop=attn_drop,
- drop_path=drop_path,
- norm_layer=norm_layer,
- downsample=downsample,
- use_checkpoint=use_checkpoint)
-
- if resi_connection == '1conv':
- self.conv = nn.Conv2d(dim, dim, 3, 1, 1)
- elif resi_connection == '3conv':
- # to save parameters and memory
- self.conv = nn.Sequential(nn.Conv2d(dim, dim // 4, 3, 1, 1), nn.LeakyReLU(negative_slope=0.2, inplace=True),
- nn.Conv2d(dim // 4, dim // 4, 1, 1, 0),
- nn.LeakyReLU(negative_slope=0.2, inplace=True),
- nn.Conv2d(dim // 4, dim, 3, 1, 1))
-
- self.patch_embed = PatchEmbed(
- img_size=img_size, patch_size=patch_size, in_chans=0, embed_dim=dim,
- norm_layer=None)
-
- self.patch_unembed = PatchUnEmbed(
- img_size=img_size, patch_size=patch_size, in_chans=0, embed_dim=dim,
- norm_layer=None)
-
- def forward(self, x, x_size):
- return self.patch_embed(self.conv(self.patch_unembed(self.residual_group(x, x_size), x_size))) + x
-
- def flops(self):
- flops = 0
- flops += self.residual_group.flops()
- H, W = self.input_resolution
- flops += H * W * self.dim * self.dim * 9
- flops += self.patch_embed.flops()
- flops += self.patch_unembed.flops()
-
- return flops
-
-
-class PatchEmbed(nn.Module):
- r""" Image to Patch Embedding
-
- Args:
- img_size (int): Image size. Default: 224.
- patch_size (int): Patch token size. Default: 4.
- in_chans (int): Number of input image channels. Default: 3.
- embed_dim (int): Number of linear projection output channels. Default: 96.
- norm_layer (nn.Module, optional): Normalization layer. Default: None
- """
-
- def __init__(self, img_size=224, patch_size=4, in_chans=3, embed_dim=96, norm_layer=None):
- super().__init__()
- img_size = to_2tuple(img_size)
- patch_size = to_2tuple(patch_size)
- patches_resolution = [img_size[0] // patch_size[0], img_size[1] // patch_size[1]]
- self.img_size = img_size
- self.patch_size = patch_size
- self.patches_resolution = patches_resolution
- self.num_patches = patches_resolution[0] * patches_resolution[1]
-
- self.in_chans = in_chans
- self.embed_dim = embed_dim
-
- if norm_layer is not None:
- self.norm = norm_layer(embed_dim)
- else:
- self.norm = None
-
- def forward(self, x):
- x = x.flatten(2).transpose(1, 2) # B Ph*Pw C
- if self.norm is not None:
- x = self.norm(x)
- return x
-
- def flops(self):
- flops = 0
- H, W = self.img_size
- if self.norm is not None:
- flops += H * W * self.embed_dim
- return flops
-
-
-class PatchUnEmbed(nn.Module):
- r""" Image to Patch Unembedding
-
- Args:
- img_size (int): Image size. Default: 224.
- patch_size (int): Patch token size. Default: 4.
- in_chans (int): Number of input image channels. Default: 3.
- embed_dim (int): Number of linear projection output channels. Default: 96.
- norm_layer (nn.Module, optional): Normalization layer. Default: None
- """
-
- def __init__(self, img_size=224, patch_size=4, in_chans=3, embed_dim=96, norm_layer=None):
- super().__init__()
- img_size = to_2tuple(img_size)
- patch_size = to_2tuple(patch_size)
- patches_resolution = [img_size[0] // patch_size[0], img_size[1] // patch_size[1]]
- self.img_size = img_size
- self.patch_size = patch_size
- self.patches_resolution = patches_resolution
- self.num_patches = patches_resolution[0] * patches_resolution[1]
-
- self.in_chans = in_chans
- self.embed_dim = embed_dim
-
- def forward(self, x, x_size):
- B, HW, C = x.shape
- x = x.transpose(1, 2).view(B, self.embed_dim, x_size[0], x_size[1]) # B Ph*Pw C
- return x
-
- def flops(self):
- flops = 0
- return flops
-
-
-class Upsample(nn.Sequential):
- """Upsample module.
-
- Args:
- scale (int): Scale factor. Supported scales: 2^n and 3.
- num_feat (int): Channel number of intermediate features.
- """
-
- def __init__(self, scale, num_feat):
- m = []
- if (scale & (scale - 1)) == 0: # scale = 2^n
- for _ in range(int(math.log(scale, 2))):
- m.append(nn.Conv2d(num_feat, 4 * num_feat, 3, 1, 1))
- m.append(nn.PixelShuffle(2))
- elif scale == 3:
- m.append(nn.Conv2d(num_feat, 9 * num_feat, 3, 1, 1))
- m.append(nn.PixelShuffle(3))
- else:
- raise ValueError(f'scale {scale} is not supported. ' 'Supported scales: 2^n and 3.')
- super(Upsample, self).__init__(*m)
-
-
-class UpsampleOneStep(nn.Sequential):
- """UpsampleOneStep module (the difference with Upsample is that it always only has 1conv + 1pixelshuffle)
- Used in lightweight SR to save parameters.
-
- Args:
- scale (int): Scale factor. Supported scales: 2^n and 3.
- num_feat (int): Channel number of intermediate features.
-
- """
-
- def __init__(self, scale, num_feat, num_out_ch, input_resolution=None):
- self.num_feat = num_feat
- self.input_resolution = input_resolution
- m = []
- m.append(nn.Conv2d(num_feat, (scale ** 2) * num_out_ch, 3, 1, 1))
- m.append(nn.PixelShuffle(scale))
- super(UpsampleOneStep, self).__init__(*m)
-
- def flops(self):
- H, W = self.input_resolution
- flops = H * W * self.num_feat * 3 * 9
- return flops
-
-
-class SwinIR(nn.Module):
- r""" SwinIR
- A PyTorch impl of : `SwinIR: Image Restoration Using Swin Transformer`, based on Swin Transformer.
-
- Args:
- img_size (int | tuple(int)): Input image size. Default 64
- patch_size (int | tuple(int)): Patch size. Default: 1
- in_chans (int): Number of input image channels. Default: 3
- embed_dim (int): Patch embedding dimension. Default: 96
- depths (tuple(int)): Depth of each Swin Transformer layer.
- num_heads (tuple(int)): Number of attention heads in different layers.
- window_size (int): Window size. Default: 7
- mlp_ratio (float): Ratio of mlp hidden dim to embedding dim. Default: 4
- qkv_bias (bool): If True, add a learnable bias to query, key, value. Default: True
- qk_scale (float): Override default qk scale of head_dim ** -0.5 if set. Default: None
- drop_rate (float): Dropout rate. Default: 0
- attn_drop_rate (float): Attention dropout rate. Default: 0
- drop_path_rate (float): Stochastic depth rate. Default: 0.1
- norm_layer (nn.Module): Normalization layer. Default: nn.LayerNorm.
- ape (bool): If True, add absolute position embedding to the patch embedding. Default: False
- patch_norm (bool): If True, add normalization after patch embedding. Default: True
- use_checkpoint (bool): Whether to use checkpointing to save memory. Default: False
- upscale: Upscale factor. 2/3/4/8 for image SR, 1 for denoising and compress artifact reduction
- img_range: Image range. 1. or 255.
- upsampler: The reconstruction reconstruction module. 'pixelshuffle'/'pixelshuffledirect'/'nearest+conv'/None
- resi_connection: The convolutional block before residual connection. '1conv'/'3conv'
- """
-
- def __init__(self, img_size=64, patch_size=1, in_chans=3,
- embed_dim=96, depths=[6, 6, 6, 6], num_heads=[6, 6, 6, 6],
- window_size=7, mlp_ratio=4., qkv_bias=True, qk_scale=None,
- drop_rate=0., attn_drop_rate=0., drop_path_rate=0.1,
- norm_layer=nn.LayerNorm, ape=False, patch_norm=True,
- use_checkpoint=False, upscale=2, img_range=1., upsampler='', resi_connection='1conv',
- **kwargs):
- super(SwinIR, self).__init__()
- num_in_ch = in_chans
- num_out_ch = in_chans
- num_feat = 64
- self.img_range = img_range
- if in_chans == 3:
- rgb_mean = (0.4488, 0.4371, 0.4040)
- self.mean = torch.Tensor(rgb_mean).view(1, 3, 1, 1)
- else:
- self.mean = torch.zeros(1, 1, 1, 1)
- self.upscale = upscale
- self.upsampler = upsampler
- self.window_size = window_size
-
- #####################################################################################################
- ################################### 1, shallow feature extraction ###################################
- self.conv_first = nn.Conv2d(num_in_ch, embed_dim, 3, 1, 1)
-
- #####################################################################################################
- ################################### 2, deep feature extraction ######################################
- self.num_layers = len(depths)
- self.embed_dim = embed_dim
- self.ape = ape
- self.patch_norm = patch_norm
- self.num_features = embed_dim
- self.mlp_ratio = mlp_ratio
-
- # split image into non-overlapping patches
- self.patch_embed = PatchEmbed(
- img_size=img_size, patch_size=patch_size, in_chans=embed_dim, embed_dim=embed_dim,
- norm_layer=norm_layer if self.patch_norm else None)
- num_patches = self.patch_embed.num_patches
- patches_resolution = self.patch_embed.patches_resolution
- self.patches_resolution = patches_resolution
-
- # merge non-overlapping patches into image
- self.patch_unembed = PatchUnEmbed(
- img_size=img_size, patch_size=patch_size, in_chans=embed_dim, embed_dim=embed_dim,
- norm_layer=norm_layer if self.patch_norm else None)
-
- # absolute position embedding
- if self.ape:
- self.absolute_pos_embed = nn.Parameter(torch.zeros(1, num_patches, embed_dim))
- trunc_normal_(self.absolute_pos_embed, std=.02)
-
- self.pos_drop = nn.Dropout(p=drop_rate)
-
- # stochastic depth
- dpr = [x.item() for x in torch.linspace(0, drop_path_rate, sum(depths))] # stochastic depth decay rule
-
- # build Residual Swin Transformer blocks (RSTB)
- self.layers = nn.ModuleList()
- for i_layer in range(self.num_layers):
- layer = RSTB(dim=embed_dim,
- input_resolution=(patches_resolution[0],
- patches_resolution[1]),
- depth=depths[i_layer],
- num_heads=num_heads[i_layer],
- window_size=window_size,
- mlp_ratio=self.mlp_ratio,
- qkv_bias=qkv_bias, qk_scale=qk_scale,
- drop=drop_rate, attn_drop=attn_drop_rate,
- drop_path=dpr[sum(depths[:i_layer]):sum(depths[:i_layer + 1])], # no impact on SR results
- norm_layer=norm_layer,
- downsample=None,
- use_checkpoint=use_checkpoint,
- img_size=img_size,
- patch_size=patch_size,
- resi_connection=resi_connection
-
- )
- self.layers.append(layer)
- self.norm = norm_layer(self.num_features)
-
- # build the last conv layer in deep feature extraction
- if resi_connection == '1conv':
- self.conv_after_body = nn.Conv2d(embed_dim, embed_dim, 3, 1, 1)
- elif resi_connection == '3conv':
- # to save parameters and memory
- self.conv_after_body = nn.Sequential(nn.Conv2d(embed_dim, embed_dim // 4, 3, 1, 1),
- nn.LeakyReLU(negative_slope=0.2, inplace=True),
- nn.Conv2d(embed_dim // 4, embed_dim // 4, 1, 1, 0),
- nn.LeakyReLU(negative_slope=0.2, inplace=True),
- nn.Conv2d(embed_dim // 4, embed_dim, 3, 1, 1))
-
- #####################################################################################################
- ################################ 3, high quality image reconstruction ################################
- if self.upsampler == 'pixelshuffle':
- # for classical SR
- self.conv_before_upsample = nn.Sequential(nn.Conv2d(embed_dim, num_feat, 3, 1, 1),
- nn.LeakyReLU(inplace=True))
- self.upsample = Upsample(upscale, num_feat)
- self.conv_last = nn.Conv2d(num_feat, num_out_ch, 3, 1, 1)
- elif self.upsampler == 'pixelshuffledirect':
- # for lightweight SR (to save parameters)
- self.upsample = UpsampleOneStep(upscale, embed_dim, num_out_ch,
- (patches_resolution[0], patches_resolution[1]))
- elif self.upsampler == 'nearest+conv':
- # for real-world SR (less artifacts)
- self.conv_before_upsample = nn.Sequential(nn.Conv2d(embed_dim, num_feat, 3, 1, 1),
- nn.LeakyReLU(inplace=True))
- self.conv_up1 = nn.Conv2d(num_feat, num_feat, 3, 1, 1)
- if self.upscale == 4:
- self.conv_up2 = nn.Conv2d(num_feat, num_feat, 3, 1, 1)
- self.conv_hr = nn.Conv2d(num_feat, num_feat, 3, 1, 1)
- self.conv_last = nn.Conv2d(num_feat, num_out_ch, 3, 1, 1)
- self.lrelu = nn.LeakyReLU(negative_slope=0.2, inplace=True)
- else:
- # for image denoising and JPEG compression artifact reduction
- self.conv_last = nn.Conv2d(embed_dim, num_out_ch, 3, 1, 1)
-
- self.apply(self._init_weights)
-
- def _init_weights(self, m):
- if isinstance(m, nn.Linear):
- trunc_normal_(m.weight, std=.02)
- if isinstance(m, nn.Linear) and m.bias is not None:
- nn.init.constant_(m.bias, 0)
- elif isinstance(m, nn.LayerNorm):
- nn.init.constant_(m.bias, 0)
- nn.init.constant_(m.weight, 1.0)
-
- @torch.jit.ignore
- def no_weight_decay(self):
- return {'absolute_pos_embed'}
-
- @torch.jit.ignore
- def no_weight_decay_keywords(self):
- return {'relative_position_bias_table'}
-
- def check_image_size(self, x):
- _, _, h, w = x.size()
- mod_pad_h = (self.window_size - h % self.window_size) % self.window_size
- mod_pad_w = (self.window_size - w % self.window_size) % self.window_size
- x = F.pad(x, (0, mod_pad_w, 0, mod_pad_h), 'reflect')
- return x
-
- def forward_features(self, x):
- x_size = (x.shape[2], x.shape[3])
- x = self.patch_embed(x)
- if self.ape:
- x = x + self.absolute_pos_embed
- x = self.pos_drop(x)
-
- for layer in self.layers:
- x = layer(x, x_size)
-
- x = self.norm(x) # B L C
- x = self.patch_unembed(x, x_size)
-
- return x
-
- def forward(self, x):
- H, W = x.shape[2:]
- x = self.check_image_size(x)
-
- self.mean = self.mean.type_as(x)
- x = (x - self.mean) * self.img_range
-
- if self.upsampler == 'pixelshuffle':
- # for classical SR
- x = self.conv_first(x)
- x = self.conv_after_body(self.forward_features(x)) + x
- x = self.conv_before_upsample(x)
- x = self.conv_last(self.upsample(x))
- elif self.upsampler == 'pixelshuffledirect':
- # for lightweight SR
- x = self.conv_first(x)
- x = self.conv_after_body(self.forward_features(x)) + x
- x = self.upsample(x)
- elif self.upsampler == 'nearest+conv':
- # for real-world SR
- x = self.conv_first(x)
- x = self.conv_after_body(self.forward_features(x)) + x
- x = self.conv_before_upsample(x)
- x = self.lrelu(self.conv_up1(torch.nn.functional.interpolate(x, scale_factor=2, mode='nearest')))
- if self.upscale == 4:
- x = self.lrelu(self.conv_up2(torch.nn.functional.interpolate(x, scale_factor=2, mode='nearest')))
- x = self.conv_last(self.lrelu(self.conv_hr(x)))
- else:
- # for image denoising and JPEG compression artifact reduction
- x_first = self.conv_first(x)
- res = self.conv_after_body(self.forward_features(x_first)) + x_first
- x = x + self.conv_last(res)
-
- x = x / self.img_range + self.mean
-
- return x[:, :, :H*self.upscale, :W*self.upscale]
-
- def flops(self):
- flops = 0
- H, W = self.patches_resolution
- flops += H * W * 3 * self.embed_dim * 9
- flops += self.patch_embed.flops()
- for i, layer in enumerate(self.layers):
- flops += layer.flops()
- flops += H * W * 3 * self.embed_dim * self.embed_dim
- flops += self.upsample.flops()
- return flops
-
-
-if __name__ == '__main__':
- upscale = 4
- window_size = 8
- height = (1024 // upscale // window_size + 1) * window_size
- width = (720 // upscale // window_size + 1) * window_size
- model = SwinIR(upscale=2, img_size=(height, width),
- window_size=window_size, img_range=1., depths=[6, 6, 6, 6],
- embed_dim=60, num_heads=[6, 6, 6, 6], mlp_ratio=2, upsampler='pixelshuffledirect')
- print(model)
- print(height, width, model.flops() / 1e9)
-
- x = torch.randn((1, 3, height, width))
- x = model(x)
- print(x.shape)
diff --git a/spaces/aodianyun/stable-diffusion-webui/modules/models/diffusion/ddpm_edit.py b/spaces/aodianyun/stable-diffusion-webui/modules/models/diffusion/ddpm_edit.py
deleted file mode 100644
index f3d49c44cafcc78e27a1e4f2b522faa21e135f9f..0000000000000000000000000000000000000000
--- a/spaces/aodianyun/stable-diffusion-webui/modules/models/diffusion/ddpm_edit.py
+++ /dev/null
@@ -1,1459 +0,0 @@
-"""
-wild mixture of
-https://github.com/lucidrains/denoising-diffusion-pytorch/blob/7706bdfc6f527f58d33f84b7b522e61e6e3164b3/denoising_diffusion_pytorch/denoising_diffusion_pytorch.py
-https://github.com/openai/improved-diffusion/blob/e94489283bb876ac1477d5dd7709bbbd2d9902ce/improved_diffusion/gaussian_diffusion.py
-https://github.com/CompVis/taming-transformers
--- merci
-"""
-
-# File modified by authors of InstructPix2Pix from original (https://github.com/CompVis/stable-diffusion).
-# See more details in LICENSE.
-
-import torch
-import torch.nn as nn
-import numpy as np
-import pytorch_lightning as pl
-from torch.optim.lr_scheduler import LambdaLR
-from einops import rearrange, repeat
-from contextlib import contextmanager
-from functools import partial
-from tqdm import tqdm
-from torchvision.utils import make_grid
-from pytorch_lightning.utilities.distributed import rank_zero_only
-
-from ldm.util import log_txt_as_img, exists, default, ismap, isimage, mean_flat, count_params, instantiate_from_config
-from ldm.modules.ema import LitEma
-from ldm.modules.distributions.distributions import normal_kl, DiagonalGaussianDistribution
-from ldm.models.autoencoder import VQModelInterface, IdentityFirstStage, AutoencoderKL
-from ldm.modules.diffusionmodules.util import make_beta_schedule, extract_into_tensor, noise_like
-from ldm.models.diffusion.ddim import DDIMSampler
-
-
-__conditioning_keys__ = {'concat': 'c_concat',
- 'crossattn': 'c_crossattn',
- 'adm': 'y'}
-
-
-def disabled_train(self, mode=True):
- """Overwrite model.train with this function to make sure train/eval mode
- does not change anymore."""
- return self
-
-
-def uniform_on_device(r1, r2, shape, device):
- return (r1 - r2) * torch.rand(*shape, device=device) + r2
-
-
-class DDPM(pl.LightningModule):
- # classic DDPM with Gaussian diffusion, in image space
- def __init__(self,
- unet_config,
- timesteps=1000,
- beta_schedule="linear",
- loss_type="l2",
- ckpt_path=None,
- ignore_keys=[],
- load_only_unet=False,
- monitor="val/loss",
- use_ema=True,
- first_stage_key="image",
- image_size=256,
- channels=3,
- log_every_t=100,
- clip_denoised=True,
- linear_start=1e-4,
- linear_end=2e-2,
- cosine_s=8e-3,
- given_betas=None,
- original_elbo_weight=0.,
- v_posterior=0., # weight for choosing posterior variance as sigma = (1-v) * beta_tilde + v * beta
- l_simple_weight=1.,
- conditioning_key=None,
- parameterization="eps", # all assuming fixed variance schedules
- scheduler_config=None,
- use_positional_encodings=False,
- learn_logvar=False,
- logvar_init=0.,
- load_ema=True,
- ):
- super().__init__()
- assert parameterization in ["eps", "x0"], 'currently only supporting "eps" and "x0"'
- self.parameterization = parameterization
- print(f"{self.__class__.__name__}: Running in {self.parameterization}-prediction mode")
- self.cond_stage_model = None
- self.clip_denoised = clip_denoised
- self.log_every_t = log_every_t
- self.first_stage_key = first_stage_key
- self.image_size = image_size # try conv?
- self.channels = channels
- self.use_positional_encodings = use_positional_encodings
- self.model = DiffusionWrapper(unet_config, conditioning_key)
- count_params(self.model, verbose=True)
- self.use_ema = use_ema
-
- self.use_scheduler = scheduler_config is not None
- if self.use_scheduler:
- self.scheduler_config = scheduler_config
-
- self.v_posterior = v_posterior
- self.original_elbo_weight = original_elbo_weight
- self.l_simple_weight = l_simple_weight
-
- if monitor is not None:
- self.monitor = monitor
-
- if self.use_ema and load_ema:
- self.model_ema = LitEma(self.model)
- print(f"Keeping EMAs of {len(list(self.model_ema.buffers()))}.")
-
- if ckpt_path is not None:
- self.init_from_ckpt(ckpt_path, ignore_keys=ignore_keys, only_model=load_only_unet)
-
- # If initialing from EMA-only checkpoint, create EMA model after loading.
- if self.use_ema and not load_ema:
- self.model_ema = LitEma(self.model)
- print(f"Keeping EMAs of {len(list(self.model_ema.buffers()))}.")
-
- self.register_schedule(given_betas=given_betas, beta_schedule=beta_schedule, timesteps=timesteps,
- linear_start=linear_start, linear_end=linear_end, cosine_s=cosine_s)
-
- self.loss_type = loss_type
-
- self.learn_logvar = learn_logvar
- self.logvar = torch.full(fill_value=logvar_init, size=(self.num_timesteps,))
- if self.learn_logvar:
- self.logvar = nn.Parameter(self.logvar, requires_grad=True)
-
-
- def register_schedule(self, given_betas=None, beta_schedule="linear", timesteps=1000,
- linear_start=1e-4, linear_end=2e-2, cosine_s=8e-3):
- if exists(given_betas):
- betas = given_betas
- else:
- betas = make_beta_schedule(beta_schedule, timesteps, linear_start=linear_start, linear_end=linear_end,
- cosine_s=cosine_s)
- alphas = 1. - betas
- alphas_cumprod = np.cumprod(alphas, axis=0)
- alphas_cumprod_prev = np.append(1., alphas_cumprod[:-1])
-
- timesteps, = betas.shape
- self.num_timesteps = int(timesteps)
- self.linear_start = linear_start
- self.linear_end = linear_end
- assert alphas_cumprod.shape[0] == self.num_timesteps, 'alphas have to be defined for each timestep'
-
- to_torch = partial(torch.tensor, dtype=torch.float32)
-
- self.register_buffer('betas', to_torch(betas))
- self.register_buffer('alphas_cumprod', to_torch(alphas_cumprod))
- self.register_buffer('alphas_cumprod_prev', to_torch(alphas_cumprod_prev))
-
- # calculations for diffusion q(x_t | x_{t-1}) and others
- self.register_buffer('sqrt_alphas_cumprod', to_torch(np.sqrt(alphas_cumprod)))
- self.register_buffer('sqrt_one_minus_alphas_cumprod', to_torch(np.sqrt(1. - alphas_cumprod)))
- self.register_buffer('log_one_minus_alphas_cumprod', to_torch(np.log(1. - alphas_cumprod)))
- self.register_buffer('sqrt_recip_alphas_cumprod', to_torch(np.sqrt(1. / alphas_cumprod)))
- self.register_buffer('sqrt_recipm1_alphas_cumprod', to_torch(np.sqrt(1. / alphas_cumprod - 1)))
-
- # calculations for posterior q(x_{t-1} | x_t, x_0)
- posterior_variance = (1 - self.v_posterior) * betas * (1. - alphas_cumprod_prev) / (
- 1. - alphas_cumprod) + self.v_posterior * betas
- # above: equal to 1. / (1. / (1. - alpha_cumprod_tm1) + alpha_t / beta_t)
- self.register_buffer('posterior_variance', to_torch(posterior_variance))
- # below: log calculation clipped because the posterior variance is 0 at the beginning of the diffusion chain
- self.register_buffer('posterior_log_variance_clipped', to_torch(np.log(np.maximum(posterior_variance, 1e-20))))
- self.register_buffer('posterior_mean_coef1', to_torch(
- betas * np.sqrt(alphas_cumprod_prev) / (1. - alphas_cumprod)))
- self.register_buffer('posterior_mean_coef2', to_torch(
- (1. - alphas_cumprod_prev) * np.sqrt(alphas) / (1. - alphas_cumprod)))
-
- if self.parameterization == "eps":
- lvlb_weights = self.betas ** 2 / (
- 2 * self.posterior_variance * to_torch(alphas) * (1 - self.alphas_cumprod))
- elif self.parameterization == "x0":
- lvlb_weights = 0.5 * np.sqrt(torch.Tensor(alphas_cumprod)) / (2. * 1 - torch.Tensor(alphas_cumprod))
- else:
- raise NotImplementedError("mu not supported")
- # TODO how to choose this term
- lvlb_weights[0] = lvlb_weights[1]
- self.register_buffer('lvlb_weights', lvlb_weights, persistent=False)
- assert not torch.isnan(self.lvlb_weights).all()
-
- @contextmanager
- def ema_scope(self, context=None):
- if self.use_ema:
- self.model_ema.store(self.model.parameters())
- self.model_ema.copy_to(self.model)
- if context is not None:
- print(f"{context}: Switched to EMA weights")
- try:
- yield None
- finally:
- if self.use_ema:
- self.model_ema.restore(self.model.parameters())
- if context is not None:
- print(f"{context}: Restored training weights")
-
- def init_from_ckpt(self, path, ignore_keys=list(), only_model=False):
- sd = torch.load(path, map_location="cpu")
- if "state_dict" in list(sd.keys()):
- sd = sd["state_dict"]
- keys = list(sd.keys())
-
- # Our model adds additional channels to the first layer to condition on an input image.
- # For the first layer, copy existing channel weights and initialize new channel weights to zero.
- input_keys = [
- "model.diffusion_model.input_blocks.0.0.weight",
- "model_ema.diffusion_modelinput_blocks00weight",
- ]
-
- self_sd = self.state_dict()
- for input_key in input_keys:
- if input_key not in sd or input_key not in self_sd:
- continue
-
- input_weight = self_sd[input_key]
-
- if input_weight.size() != sd[input_key].size():
- print(f"Manual init: {input_key}")
- input_weight.zero_()
- input_weight[:, :4, :, :].copy_(sd[input_key])
- ignore_keys.append(input_key)
-
- for k in keys:
- for ik in ignore_keys:
- if k.startswith(ik):
- print("Deleting key {} from state_dict.".format(k))
- del sd[k]
- missing, unexpected = self.load_state_dict(sd, strict=False) if not only_model else self.model.load_state_dict(
- sd, strict=False)
- print(f"Restored from {path} with {len(missing)} missing and {len(unexpected)} unexpected keys")
- if len(missing) > 0:
- print(f"Missing Keys: {missing}")
- if len(unexpected) > 0:
- print(f"Unexpected Keys: {unexpected}")
-
- def q_mean_variance(self, x_start, t):
- """
- Get the distribution q(x_t | x_0).
- :param x_start: the [N x C x ...] tensor of noiseless inputs.
- :param t: the number of diffusion steps (minus 1). Here, 0 means one step.
- :return: A tuple (mean, variance, log_variance), all of x_start's shape.
- """
- mean = (extract_into_tensor(self.sqrt_alphas_cumprod, t, x_start.shape) * x_start)
- variance = extract_into_tensor(1.0 - self.alphas_cumprod, t, x_start.shape)
- log_variance = extract_into_tensor(self.log_one_minus_alphas_cumprod, t, x_start.shape)
- return mean, variance, log_variance
-
- def predict_start_from_noise(self, x_t, t, noise):
- return (
- extract_into_tensor(self.sqrt_recip_alphas_cumprod, t, x_t.shape) * x_t -
- extract_into_tensor(self.sqrt_recipm1_alphas_cumprod, t, x_t.shape) * noise
- )
-
- def q_posterior(self, x_start, x_t, t):
- posterior_mean = (
- extract_into_tensor(self.posterior_mean_coef1, t, x_t.shape) * x_start +
- extract_into_tensor(self.posterior_mean_coef2, t, x_t.shape) * x_t
- )
- posterior_variance = extract_into_tensor(self.posterior_variance, t, x_t.shape)
- posterior_log_variance_clipped = extract_into_tensor(self.posterior_log_variance_clipped, t, x_t.shape)
- return posterior_mean, posterior_variance, posterior_log_variance_clipped
-
- def p_mean_variance(self, x, t, clip_denoised: bool):
- model_out = self.model(x, t)
- if self.parameterization == "eps":
- x_recon = self.predict_start_from_noise(x, t=t, noise=model_out)
- elif self.parameterization == "x0":
- x_recon = model_out
- if clip_denoised:
- x_recon.clamp_(-1., 1.)
-
- model_mean, posterior_variance, posterior_log_variance = self.q_posterior(x_start=x_recon, x_t=x, t=t)
- return model_mean, posterior_variance, posterior_log_variance
-
- @torch.no_grad()
- def p_sample(self, x, t, clip_denoised=True, repeat_noise=False):
- b, *_, device = *x.shape, x.device
- model_mean, _, model_log_variance = self.p_mean_variance(x=x, t=t, clip_denoised=clip_denoised)
- noise = noise_like(x.shape, device, repeat_noise)
- # no noise when t == 0
- nonzero_mask = (1 - (t == 0).float()).reshape(b, *((1,) * (len(x.shape) - 1)))
- return model_mean + nonzero_mask * (0.5 * model_log_variance).exp() * noise
-
- @torch.no_grad()
- def p_sample_loop(self, shape, return_intermediates=False):
- device = self.betas.device
- b = shape[0]
- img = torch.randn(shape, device=device)
- intermediates = [img]
- for i in tqdm(reversed(range(0, self.num_timesteps)), desc='Sampling t', total=self.num_timesteps):
- img = self.p_sample(img, torch.full((b,), i, device=device, dtype=torch.long),
- clip_denoised=self.clip_denoised)
- if i % self.log_every_t == 0 or i == self.num_timesteps - 1:
- intermediates.append(img)
- if return_intermediates:
- return img, intermediates
- return img
-
- @torch.no_grad()
- def sample(self, batch_size=16, return_intermediates=False):
- image_size = self.image_size
- channels = self.channels
- return self.p_sample_loop((batch_size, channels, image_size, image_size),
- return_intermediates=return_intermediates)
-
- def q_sample(self, x_start, t, noise=None):
- noise = default(noise, lambda: torch.randn_like(x_start))
- return (extract_into_tensor(self.sqrt_alphas_cumprod, t, x_start.shape) * x_start +
- extract_into_tensor(self.sqrt_one_minus_alphas_cumprod, t, x_start.shape) * noise)
-
- def get_loss(self, pred, target, mean=True):
- if self.loss_type == 'l1':
- loss = (target - pred).abs()
- if mean:
- loss = loss.mean()
- elif self.loss_type == 'l2':
- if mean:
- loss = torch.nn.functional.mse_loss(target, pred)
- else:
- loss = torch.nn.functional.mse_loss(target, pred, reduction='none')
- else:
- raise NotImplementedError("unknown loss type '{loss_type}'")
-
- return loss
-
- def p_losses(self, x_start, t, noise=None):
- noise = default(noise, lambda: torch.randn_like(x_start))
- x_noisy = self.q_sample(x_start=x_start, t=t, noise=noise)
- model_out = self.model(x_noisy, t)
-
- loss_dict = {}
- if self.parameterization == "eps":
- target = noise
- elif self.parameterization == "x0":
- target = x_start
- else:
- raise NotImplementedError(f"Paramterization {self.parameterization} not yet supported")
-
- loss = self.get_loss(model_out, target, mean=False).mean(dim=[1, 2, 3])
-
- log_prefix = 'train' if self.training else 'val'
-
- loss_dict.update({f'{log_prefix}/loss_simple': loss.mean()})
- loss_simple = loss.mean() * self.l_simple_weight
-
- loss_vlb = (self.lvlb_weights[t] * loss).mean()
- loss_dict.update({f'{log_prefix}/loss_vlb': loss_vlb})
-
- loss = loss_simple + self.original_elbo_weight * loss_vlb
-
- loss_dict.update({f'{log_prefix}/loss': loss})
-
- return loss, loss_dict
-
- def forward(self, x, *args, **kwargs):
- # b, c, h, w, device, img_size, = *x.shape, x.device, self.image_size
- # assert h == img_size and w == img_size, f'height and width of image must be {img_size}'
- t = torch.randint(0, self.num_timesteps, (x.shape[0],), device=self.device).long()
- return self.p_losses(x, t, *args, **kwargs)
-
- def get_input(self, batch, k):
- return batch[k]
-
- def shared_step(self, batch):
- x = self.get_input(batch, self.first_stage_key)
- loss, loss_dict = self(x)
- return loss, loss_dict
-
- def training_step(self, batch, batch_idx):
- loss, loss_dict = self.shared_step(batch)
-
- self.log_dict(loss_dict, prog_bar=True,
- logger=True, on_step=True, on_epoch=True)
-
- self.log("global_step", self.global_step,
- prog_bar=True, logger=True, on_step=True, on_epoch=False)
-
- if self.use_scheduler:
- lr = self.optimizers().param_groups[0]['lr']
- self.log('lr_abs', lr, prog_bar=True, logger=True, on_step=True, on_epoch=False)
-
- return loss
-
- @torch.no_grad()
- def validation_step(self, batch, batch_idx):
- _, loss_dict_no_ema = self.shared_step(batch)
- with self.ema_scope():
- _, loss_dict_ema = self.shared_step(batch)
- loss_dict_ema = {key + '_ema': loss_dict_ema[key] for key in loss_dict_ema}
- self.log_dict(loss_dict_no_ema, prog_bar=False, logger=True, on_step=False, on_epoch=True)
- self.log_dict(loss_dict_ema, prog_bar=False, logger=True, on_step=False, on_epoch=True)
-
- def on_train_batch_end(self, *args, **kwargs):
- if self.use_ema:
- self.model_ema(self.model)
-
- def _get_rows_from_list(self, samples):
- n_imgs_per_row = len(samples)
- denoise_grid = rearrange(samples, 'n b c h w -> b n c h w')
- denoise_grid = rearrange(denoise_grid, 'b n c h w -> (b n) c h w')
- denoise_grid = make_grid(denoise_grid, nrow=n_imgs_per_row)
- return denoise_grid
-
- @torch.no_grad()
- def log_images(self, batch, N=8, n_row=2, sample=True, return_keys=None, **kwargs):
- log = dict()
- x = self.get_input(batch, self.first_stage_key)
- N = min(x.shape[0], N)
- n_row = min(x.shape[0], n_row)
- x = x.to(self.device)[:N]
- log["inputs"] = x
-
- # get diffusion row
- diffusion_row = list()
- x_start = x[:n_row]
-
- for t in range(self.num_timesteps):
- if t % self.log_every_t == 0 or t == self.num_timesteps - 1:
- t = repeat(torch.tensor([t]), '1 -> b', b=n_row)
- t = t.to(self.device).long()
- noise = torch.randn_like(x_start)
- x_noisy = self.q_sample(x_start=x_start, t=t, noise=noise)
- diffusion_row.append(x_noisy)
-
- log["diffusion_row"] = self._get_rows_from_list(diffusion_row)
-
- if sample:
- # get denoise row
- with self.ema_scope("Plotting"):
- samples, denoise_row = self.sample(batch_size=N, return_intermediates=True)
-
- log["samples"] = samples
- log["denoise_row"] = self._get_rows_from_list(denoise_row)
-
- if return_keys:
- if np.intersect1d(list(log.keys()), return_keys).shape[0] == 0:
- return log
- else:
- return {key: log[key] for key in return_keys}
- return log
-
- def configure_optimizers(self):
- lr = self.learning_rate
- params = list(self.model.parameters())
- if self.learn_logvar:
- params = params + [self.logvar]
- opt = torch.optim.AdamW(params, lr=lr)
- return opt
-
-
-class LatentDiffusion(DDPM):
- """main class"""
- def __init__(self,
- first_stage_config,
- cond_stage_config,
- num_timesteps_cond=None,
- cond_stage_key="image",
- cond_stage_trainable=False,
- concat_mode=True,
- cond_stage_forward=None,
- conditioning_key=None,
- scale_factor=1.0,
- scale_by_std=False,
- load_ema=True,
- *args, **kwargs):
- self.num_timesteps_cond = default(num_timesteps_cond, 1)
- self.scale_by_std = scale_by_std
- assert self.num_timesteps_cond <= kwargs['timesteps']
- # for backwards compatibility after implementation of DiffusionWrapper
- if conditioning_key is None:
- conditioning_key = 'concat' if concat_mode else 'crossattn'
- if cond_stage_config == '__is_unconditional__':
- conditioning_key = None
- ckpt_path = kwargs.pop("ckpt_path", None)
- ignore_keys = kwargs.pop("ignore_keys", [])
- super().__init__(conditioning_key=conditioning_key, *args, load_ema=load_ema, **kwargs)
- self.concat_mode = concat_mode
- self.cond_stage_trainable = cond_stage_trainable
- self.cond_stage_key = cond_stage_key
- try:
- self.num_downs = len(first_stage_config.params.ddconfig.ch_mult) - 1
- except:
- self.num_downs = 0
- if not scale_by_std:
- self.scale_factor = scale_factor
- else:
- self.register_buffer('scale_factor', torch.tensor(scale_factor))
- self.instantiate_first_stage(first_stage_config)
- self.instantiate_cond_stage(cond_stage_config)
- self.cond_stage_forward = cond_stage_forward
- self.clip_denoised = False
- self.bbox_tokenizer = None
-
- self.restarted_from_ckpt = False
- if ckpt_path is not None:
- self.init_from_ckpt(ckpt_path, ignore_keys)
- self.restarted_from_ckpt = True
-
- if self.use_ema and not load_ema:
- self.model_ema = LitEma(self.model)
- print(f"Keeping EMAs of {len(list(self.model_ema.buffers()))}.")
-
- def make_cond_schedule(self, ):
- self.cond_ids = torch.full(size=(self.num_timesteps,), fill_value=self.num_timesteps - 1, dtype=torch.long)
- ids = torch.round(torch.linspace(0, self.num_timesteps - 1, self.num_timesteps_cond)).long()
- self.cond_ids[:self.num_timesteps_cond] = ids
-
- @rank_zero_only
- @torch.no_grad()
- def on_train_batch_start(self, batch, batch_idx, dataloader_idx):
- # only for very first batch
- if self.scale_by_std and self.current_epoch == 0 and self.global_step == 0 and batch_idx == 0 and not self.restarted_from_ckpt:
- assert self.scale_factor == 1., 'rather not use custom rescaling and std-rescaling simultaneously'
- # set rescale weight to 1./std of encodings
- print("### USING STD-RESCALING ###")
- x = super().get_input(batch, self.first_stage_key)
- x = x.to(self.device)
- encoder_posterior = self.encode_first_stage(x)
- z = self.get_first_stage_encoding(encoder_posterior).detach()
- del self.scale_factor
- self.register_buffer('scale_factor', 1. / z.flatten().std())
- print(f"setting self.scale_factor to {self.scale_factor}")
- print("### USING STD-RESCALING ###")
-
- def register_schedule(self,
- given_betas=None, beta_schedule="linear", timesteps=1000,
- linear_start=1e-4, linear_end=2e-2, cosine_s=8e-3):
- super().register_schedule(given_betas, beta_schedule, timesteps, linear_start, linear_end, cosine_s)
-
- self.shorten_cond_schedule = self.num_timesteps_cond > 1
- if self.shorten_cond_schedule:
- self.make_cond_schedule()
-
- def instantiate_first_stage(self, config):
- model = instantiate_from_config(config)
- self.first_stage_model = model.eval()
- self.first_stage_model.train = disabled_train
- for param in self.first_stage_model.parameters():
- param.requires_grad = False
-
- def instantiate_cond_stage(self, config):
- if not self.cond_stage_trainable:
- if config == "__is_first_stage__":
- print("Using first stage also as cond stage.")
- self.cond_stage_model = self.first_stage_model
- elif config == "__is_unconditional__":
- print(f"Training {self.__class__.__name__} as an unconditional model.")
- self.cond_stage_model = None
- # self.be_unconditional = True
- else:
- model = instantiate_from_config(config)
- self.cond_stage_model = model.eval()
- self.cond_stage_model.train = disabled_train
- for param in self.cond_stage_model.parameters():
- param.requires_grad = False
- else:
- assert config != '__is_first_stage__'
- assert config != '__is_unconditional__'
- model = instantiate_from_config(config)
- self.cond_stage_model = model
-
- def _get_denoise_row_from_list(self, samples, desc='', force_no_decoder_quantization=False):
- denoise_row = []
- for zd in tqdm(samples, desc=desc):
- denoise_row.append(self.decode_first_stage(zd.to(self.device),
- force_not_quantize=force_no_decoder_quantization))
- n_imgs_per_row = len(denoise_row)
- denoise_row = torch.stack(denoise_row) # n_log_step, n_row, C, H, W
- denoise_grid = rearrange(denoise_row, 'n b c h w -> b n c h w')
- denoise_grid = rearrange(denoise_grid, 'b n c h w -> (b n) c h w')
- denoise_grid = make_grid(denoise_grid, nrow=n_imgs_per_row)
- return denoise_grid
-
- def get_first_stage_encoding(self, encoder_posterior):
- if isinstance(encoder_posterior, DiagonalGaussianDistribution):
- z = encoder_posterior.sample()
- elif isinstance(encoder_posterior, torch.Tensor):
- z = encoder_posterior
- else:
- raise NotImplementedError(f"encoder_posterior of type '{type(encoder_posterior)}' not yet implemented")
- return self.scale_factor * z
-
- def get_learned_conditioning(self, c):
- if self.cond_stage_forward is None:
- if hasattr(self.cond_stage_model, 'encode') and callable(self.cond_stage_model.encode):
- c = self.cond_stage_model.encode(c)
- if isinstance(c, DiagonalGaussianDistribution):
- c = c.mode()
- else:
- c = self.cond_stage_model(c)
- else:
- assert hasattr(self.cond_stage_model, self.cond_stage_forward)
- c = getattr(self.cond_stage_model, self.cond_stage_forward)(c)
- return c
-
- def meshgrid(self, h, w):
- y = torch.arange(0, h).view(h, 1, 1).repeat(1, w, 1)
- x = torch.arange(0, w).view(1, w, 1).repeat(h, 1, 1)
-
- arr = torch.cat([y, x], dim=-1)
- return arr
-
- def delta_border(self, h, w):
- """
- :param h: height
- :param w: width
- :return: normalized distance to image border,
- wtith min distance = 0 at border and max dist = 0.5 at image center
- """
- lower_right_corner = torch.tensor([h - 1, w - 1]).view(1, 1, 2)
- arr = self.meshgrid(h, w) / lower_right_corner
- dist_left_up = torch.min(arr, dim=-1, keepdims=True)[0]
- dist_right_down = torch.min(1 - arr, dim=-1, keepdims=True)[0]
- edge_dist = torch.min(torch.cat([dist_left_up, dist_right_down], dim=-1), dim=-1)[0]
- return edge_dist
-
- def get_weighting(self, h, w, Ly, Lx, device):
- weighting = self.delta_border(h, w)
- weighting = torch.clip(weighting, self.split_input_params["clip_min_weight"],
- self.split_input_params["clip_max_weight"], )
- weighting = weighting.view(1, h * w, 1).repeat(1, 1, Ly * Lx).to(device)
-
- if self.split_input_params["tie_braker"]:
- L_weighting = self.delta_border(Ly, Lx)
- L_weighting = torch.clip(L_weighting,
- self.split_input_params["clip_min_tie_weight"],
- self.split_input_params["clip_max_tie_weight"])
-
- L_weighting = L_weighting.view(1, 1, Ly * Lx).to(device)
- weighting = weighting * L_weighting
- return weighting
-
- def get_fold_unfold(self, x, kernel_size, stride, uf=1, df=1): # todo load once not every time, shorten code
- """
- :param x: img of size (bs, c, h, w)
- :return: n img crops of size (n, bs, c, kernel_size[0], kernel_size[1])
- """
- bs, nc, h, w = x.shape
-
- # number of crops in image
- Ly = (h - kernel_size[0]) // stride[0] + 1
- Lx = (w - kernel_size[1]) // stride[1] + 1
-
- if uf == 1 and df == 1:
- fold_params = dict(kernel_size=kernel_size, dilation=1, padding=0, stride=stride)
- unfold = torch.nn.Unfold(**fold_params)
-
- fold = torch.nn.Fold(output_size=x.shape[2:], **fold_params)
-
- weighting = self.get_weighting(kernel_size[0], kernel_size[1], Ly, Lx, x.device).to(x.dtype)
- normalization = fold(weighting).view(1, 1, h, w) # normalizes the overlap
- weighting = weighting.view((1, 1, kernel_size[0], kernel_size[1], Ly * Lx))
-
- elif uf > 1 and df == 1:
- fold_params = dict(kernel_size=kernel_size, dilation=1, padding=0, stride=stride)
- unfold = torch.nn.Unfold(**fold_params)
-
- fold_params2 = dict(kernel_size=(kernel_size[0] * uf, kernel_size[0] * uf),
- dilation=1, padding=0,
- stride=(stride[0] * uf, stride[1] * uf))
- fold = torch.nn.Fold(output_size=(x.shape[2] * uf, x.shape[3] * uf), **fold_params2)
-
- weighting = self.get_weighting(kernel_size[0] * uf, kernel_size[1] * uf, Ly, Lx, x.device).to(x.dtype)
- normalization = fold(weighting).view(1, 1, h * uf, w * uf) # normalizes the overlap
- weighting = weighting.view((1, 1, kernel_size[0] * uf, kernel_size[1] * uf, Ly * Lx))
-
- elif df > 1 and uf == 1:
- fold_params = dict(kernel_size=kernel_size, dilation=1, padding=0, stride=stride)
- unfold = torch.nn.Unfold(**fold_params)
-
- fold_params2 = dict(kernel_size=(kernel_size[0] // df, kernel_size[0] // df),
- dilation=1, padding=0,
- stride=(stride[0] // df, stride[1] // df))
- fold = torch.nn.Fold(output_size=(x.shape[2] // df, x.shape[3] // df), **fold_params2)
-
- weighting = self.get_weighting(kernel_size[0] // df, kernel_size[1] // df, Ly, Lx, x.device).to(x.dtype)
- normalization = fold(weighting).view(1, 1, h // df, w // df) # normalizes the overlap
- weighting = weighting.view((1, 1, kernel_size[0] // df, kernel_size[1] // df, Ly * Lx))
-
- else:
- raise NotImplementedError
-
- return fold, unfold, normalization, weighting
-
- @torch.no_grad()
- def get_input(self, batch, k, return_first_stage_outputs=False, force_c_encode=False,
- cond_key=None, return_original_cond=False, bs=None, uncond=0.05):
- x = super().get_input(batch, k)
- if bs is not None:
- x = x[:bs]
- x = x.to(self.device)
- encoder_posterior = self.encode_first_stage(x)
- z = self.get_first_stage_encoding(encoder_posterior).detach()
- cond_key = cond_key or self.cond_stage_key
- xc = super().get_input(batch, cond_key)
- if bs is not None:
- xc["c_crossattn"] = xc["c_crossattn"][:bs]
- xc["c_concat"] = xc["c_concat"][:bs]
- cond = {}
-
- # To support classifier-free guidance, randomly drop out only text conditioning 5%, only image conditioning 5%, and both 5%.
- random = torch.rand(x.size(0), device=x.device)
- prompt_mask = rearrange(random < 2 * uncond, "n -> n 1 1")
- input_mask = 1 - rearrange((random >= uncond).float() * (random < 3 * uncond).float(), "n -> n 1 1 1")
-
- null_prompt = self.get_learned_conditioning([""])
- cond["c_crossattn"] = [torch.where(prompt_mask, null_prompt, self.get_learned_conditioning(xc["c_crossattn"]).detach())]
- cond["c_concat"] = [input_mask * self.encode_first_stage((xc["c_concat"].to(self.device))).mode().detach()]
-
- out = [z, cond]
- if return_first_stage_outputs:
- xrec = self.decode_first_stage(z)
- out.extend([x, xrec])
- if return_original_cond:
- out.append(xc)
- return out
-
- @torch.no_grad()
- def decode_first_stage(self, z, predict_cids=False, force_not_quantize=False):
- if predict_cids:
- if z.dim() == 4:
- z = torch.argmax(z.exp(), dim=1).long()
- z = self.first_stage_model.quantize.get_codebook_entry(z, shape=None)
- z = rearrange(z, 'b h w c -> b c h w').contiguous()
-
- z = 1. / self.scale_factor * z
-
- if hasattr(self, "split_input_params"):
- if self.split_input_params["patch_distributed_vq"]:
- ks = self.split_input_params["ks"] # eg. (128, 128)
- stride = self.split_input_params["stride"] # eg. (64, 64)
- uf = self.split_input_params["vqf"]
- bs, nc, h, w = z.shape
- if ks[0] > h or ks[1] > w:
- ks = (min(ks[0], h), min(ks[1], w))
- print("reducing Kernel")
-
- if stride[0] > h or stride[1] > w:
- stride = (min(stride[0], h), min(stride[1], w))
- print("reducing stride")
-
- fold, unfold, normalization, weighting = self.get_fold_unfold(z, ks, stride, uf=uf)
-
- z = unfold(z) # (bn, nc * prod(**ks), L)
- # 1. Reshape to img shape
- z = z.view((z.shape[0], -1, ks[0], ks[1], z.shape[-1])) # (bn, nc, ks[0], ks[1], L )
-
- # 2. apply model loop over last dim
- if isinstance(self.first_stage_model, VQModelInterface):
- output_list = [self.first_stage_model.decode(z[:, :, :, :, i],
- force_not_quantize=predict_cids or force_not_quantize)
- for i in range(z.shape[-1])]
- else:
-
- output_list = [self.first_stage_model.decode(z[:, :, :, :, i])
- for i in range(z.shape[-1])]
-
- o = torch.stack(output_list, axis=-1) # # (bn, nc, ks[0], ks[1], L)
- o = o * weighting
- # Reverse 1. reshape to img shape
- o = o.view((o.shape[0], -1, o.shape[-1])) # (bn, nc * ks[0] * ks[1], L)
- # stitch crops together
- decoded = fold(o)
- decoded = decoded / normalization # norm is shape (1, 1, h, w)
- return decoded
- else:
- if isinstance(self.first_stage_model, VQModelInterface):
- return self.first_stage_model.decode(z, force_not_quantize=predict_cids or force_not_quantize)
- else:
- return self.first_stage_model.decode(z)
-
- else:
- if isinstance(self.first_stage_model, VQModelInterface):
- return self.first_stage_model.decode(z, force_not_quantize=predict_cids or force_not_quantize)
- else:
- return self.first_stage_model.decode(z)
-
- # same as above but without decorator
- def differentiable_decode_first_stage(self, z, predict_cids=False, force_not_quantize=False):
- if predict_cids:
- if z.dim() == 4:
- z = torch.argmax(z.exp(), dim=1).long()
- z = self.first_stage_model.quantize.get_codebook_entry(z, shape=None)
- z = rearrange(z, 'b h w c -> b c h w').contiguous()
-
- z = 1. / self.scale_factor * z
-
- if hasattr(self, "split_input_params"):
- if self.split_input_params["patch_distributed_vq"]:
- ks = self.split_input_params["ks"] # eg. (128, 128)
- stride = self.split_input_params["stride"] # eg. (64, 64)
- uf = self.split_input_params["vqf"]
- bs, nc, h, w = z.shape
- if ks[0] > h or ks[1] > w:
- ks = (min(ks[0], h), min(ks[1], w))
- print("reducing Kernel")
-
- if stride[0] > h or stride[1] > w:
- stride = (min(stride[0], h), min(stride[1], w))
- print("reducing stride")
-
- fold, unfold, normalization, weighting = self.get_fold_unfold(z, ks, stride, uf=uf)
-
- z = unfold(z) # (bn, nc * prod(**ks), L)
- # 1. Reshape to img shape
- z = z.view((z.shape[0], -1, ks[0], ks[1], z.shape[-1])) # (bn, nc, ks[0], ks[1], L )
-
- # 2. apply model loop over last dim
- if isinstance(self.first_stage_model, VQModelInterface):
- output_list = [self.first_stage_model.decode(z[:, :, :, :, i],
- force_not_quantize=predict_cids or force_not_quantize)
- for i in range(z.shape[-1])]
- else:
-
- output_list = [self.first_stage_model.decode(z[:, :, :, :, i])
- for i in range(z.shape[-1])]
-
- o = torch.stack(output_list, axis=-1) # # (bn, nc, ks[0], ks[1], L)
- o = o * weighting
- # Reverse 1. reshape to img shape
- o = o.view((o.shape[0], -1, o.shape[-1])) # (bn, nc * ks[0] * ks[1], L)
- # stitch crops together
- decoded = fold(o)
- decoded = decoded / normalization # norm is shape (1, 1, h, w)
- return decoded
- else:
- if isinstance(self.first_stage_model, VQModelInterface):
- return self.first_stage_model.decode(z, force_not_quantize=predict_cids or force_not_quantize)
- else:
- return self.first_stage_model.decode(z)
-
- else:
- if isinstance(self.first_stage_model, VQModelInterface):
- return self.first_stage_model.decode(z, force_not_quantize=predict_cids or force_not_quantize)
- else:
- return self.first_stage_model.decode(z)
-
- @torch.no_grad()
- def encode_first_stage(self, x):
- if hasattr(self, "split_input_params"):
- if self.split_input_params["patch_distributed_vq"]:
- ks = self.split_input_params["ks"] # eg. (128, 128)
- stride = self.split_input_params["stride"] # eg. (64, 64)
- df = self.split_input_params["vqf"]
- self.split_input_params['original_image_size'] = x.shape[-2:]
- bs, nc, h, w = x.shape
- if ks[0] > h or ks[1] > w:
- ks = (min(ks[0], h), min(ks[1], w))
- print("reducing Kernel")
-
- if stride[0] > h or stride[1] > w:
- stride = (min(stride[0], h), min(stride[1], w))
- print("reducing stride")
-
- fold, unfold, normalization, weighting = self.get_fold_unfold(x, ks, stride, df=df)
- z = unfold(x) # (bn, nc * prod(**ks), L)
- # Reshape to img shape
- z = z.view((z.shape[0], -1, ks[0], ks[1], z.shape[-1])) # (bn, nc, ks[0], ks[1], L )
-
- output_list = [self.first_stage_model.encode(z[:, :, :, :, i])
- for i in range(z.shape[-1])]
-
- o = torch.stack(output_list, axis=-1)
- o = o * weighting
-
- # Reverse reshape to img shape
- o = o.view((o.shape[0], -1, o.shape[-1])) # (bn, nc * ks[0] * ks[1], L)
- # stitch crops together
- decoded = fold(o)
- decoded = decoded / normalization
- return decoded
-
- else:
- return self.first_stage_model.encode(x)
- else:
- return self.first_stage_model.encode(x)
-
- def shared_step(self, batch, **kwargs):
- x, c = self.get_input(batch, self.first_stage_key)
- loss = self(x, c)
- return loss
-
- def forward(self, x, c, *args, **kwargs):
- t = torch.randint(0, self.num_timesteps, (x.shape[0],), device=self.device).long()
- if self.model.conditioning_key is not None:
- assert c is not None
- if self.cond_stage_trainable:
- c = self.get_learned_conditioning(c)
- if self.shorten_cond_schedule: # TODO: drop this option
- tc = self.cond_ids[t].to(self.device)
- c = self.q_sample(x_start=c, t=tc, noise=torch.randn_like(c.float()))
- return self.p_losses(x, c, t, *args, **kwargs)
-
- def _rescale_annotations(self, bboxes, crop_coordinates): # TODO: move to dataset
- def rescale_bbox(bbox):
- x0 = clamp((bbox[0] - crop_coordinates[0]) / crop_coordinates[2])
- y0 = clamp((bbox[1] - crop_coordinates[1]) / crop_coordinates[3])
- w = min(bbox[2] / crop_coordinates[2], 1 - x0)
- h = min(bbox[3] / crop_coordinates[3], 1 - y0)
- return x0, y0, w, h
-
- return [rescale_bbox(b) for b in bboxes]
-
- def apply_model(self, x_noisy, t, cond, return_ids=False):
-
- if isinstance(cond, dict):
- # hybrid case, cond is exptected to be a dict
- pass
- else:
- if not isinstance(cond, list):
- cond = [cond]
- key = 'c_concat' if self.model.conditioning_key == 'concat' else 'c_crossattn'
- cond = {key: cond}
-
- if hasattr(self, "split_input_params"):
- assert len(cond) == 1 # todo can only deal with one conditioning atm
- assert not return_ids
- ks = self.split_input_params["ks"] # eg. (128, 128)
- stride = self.split_input_params["stride"] # eg. (64, 64)
-
- h, w = x_noisy.shape[-2:]
-
- fold, unfold, normalization, weighting = self.get_fold_unfold(x_noisy, ks, stride)
-
- z = unfold(x_noisy) # (bn, nc * prod(**ks), L)
- # Reshape to img shape
- z = z.view((z.shape[0], -1, ks[0], ks[1], z.shape[-1])) # (bn, nc, ks[0], ks[1], L )
- z_list = [z[:, :, :, :, i] for i in range(z.shape[-1])]
-
- if self.cond_stage_key in ["image", "LR_image", "segmentation",
- 'bbox_img'] and self.model.conditioning_key: # todo check for completeness
- c_key = next(iter(cond.keys())) # get key
- c = next(iter(cond.values())) # get value
- assert (len(c) == 1) # todo extend to list with more than one elem
- c = c[0] # get element
-
- c = unfold(c)
- c = c.view((c.shape[0], -1, ks[0], ks[1], c.shape[-1])) # (bn, nc, ks[0], ks[1], L )
-
- cond_list = [{c_key: [c[:, :, :, :, i]]} for i in range(c.shape[-1])]
-
- elif self.cond_stage_key == 'coordinates_bbox':
- assert 'original_image_size' in self.split_input_params, 'BoudingBoxRescaling is missing original_image_size'
-
- # assuming padding of unfold is always 0 and its dilation is always 1
- n_patches_per_row = int((w - ks[0]) / stride[0] + 1)
- full_img_h, full_img_w = self.split_input_params['original_image_size']
- # as we are operating on latents, we need the factor from the original image size to the
- # spatial latent size to properly rescale the crops for regenerating the bbox annotations
- num_downs = self.first_stage_model.encoder.num_resolutions - 1
- rescale_latent = 2 ** (num_downs)
-
- # get top left postions of patches as conforming for the bbbox tokenizer, therefore we
- # need to rescale the tl patch coordinates to be in between (0,1)
- tl_patch_coordinates = [(rescale_latent * stride[0] * (patch_nr % n_patches_per_row) / full_img_w,
- rescale_latent * stride[1] * (patch_nr // n_patches_per_row) / full_img_h)
- for patch_nr in range(z.shape[-1])]
-
- # patch_limits are tl_coord, width and height coordinates as (x_tl, y_tl, h, w)
- patch_limits = [(x_tl, y_tl,
- rescale_latent * ks[0] / full_img_w,
- rescale_latent * ks[1] / full_img_h) for x_tl, y_tl in tl_patch_coordinates]
- # patch_values = [(np.arange(x_tl,min(x_tl+ks, 1.)),np.arange(y_tl,min(y_tl+ks, 1.))) for x_tl, y_tl in tl_patch_coordinates]
-
- # tokenize crop coordinates for the bounding boxes of the respective patches
- patch_limits_tknzd = [torch.LongTensor(self.bbox_tokenizer._crop_encoder(bbox))[None].to(self.device)
- for bbox in patch_limits] # list of length l with tensors of shape (1, 2)
- print(patch_limits_tknzd[0].shape)
- # cut tknzd crop position from conditioning
- assert isinstance(cond, dict), 'cond must be dict to be fed into model'
- cut_cond = cond['c_crossattn'][0][..., :-2].to(self.device)
- print(cut_cond.shape)
-
- adapted_cond = torch.stack([torch.cat([cut_cond, p], dim=1) for p in patch_limits_tknzd])
- adapted_cond = rearrange(adapted_cond, 'l b n -> (l b) n')
- print(adapted_cond.shape)
- adapted_cond = self.get_learned_conditioning(adapted_cond)
- print(adapted_cond.shape)
- adapted_cond = rearrange(adapted_cond, '(l b) n d -> l b n d', l=z.shape[-1])
- print(adapted_cond.shape)
-
- cond_list = [{'c_crossattn': [e]} for e in adapted_cond]
-
- else:
- cond_list = [cond for i in range(z.shape[-1])] # Todo make this more efficient
-
- # apply model by loop over crops
- output_list = [self.model(z_list[i], t, **cond_list[i]) for i in range(z.shape[-1])]
- assert not isinstance(output_list[0],
- tuple) # todo cant deal with multiple model outputs check this never happens
-
- o = torch.stack(output_list, axis=-1)
- o = o * weighting
- # Reverse reshape to img shape
- o = o.view((o.shape[0], -1, o.shape[-1])) # (bn, nc * ks[0] * ks[1], L)
- # stitch crops together
- x_recon = fold(o) / normalization
-
- else:
- x_recon = self.model(x_noisy, t, **cond)
-
- if isinstance(x_recon, tuple) and not return_ids:
- return x_recon[0]
- else:
- return x_recon
-
- def _predict_eps_from_xstart(self, x_t, t, pred_xstart):
- return (extract_into_tensor(self.sqrt_recip_alphas_cumprod, t, x_t.shape) * x_t - pred_xstart) / \
- extract_into_tensor(self.sqrt_recipm1_alphas_cumprod, t, x_t.shape)
-
- def _prior_bpd(self, x_start):
- """
- Get the prior KL term for the variational lower-bound, measured in
- bits-per-dim.
- This term can't be optimized, as it only depends on the encoder.
- :param x_start: the [N x C x ...] tensor of inputs.
- :return: a batch of [N] KL values (in bits), one per batch element.
- """
- batch_size = x_start.shape[0]
- t = torch.tensor([self.num_timesteps - 1] * batch_size, device=x_start.device)
- qt_mean, _, qt_log_variance = self.q_mean_variance(x_start, t)
- kl_prior = normal_kl(mean1=qt_mean, logvar1=qt_log_variance, mean2=0.0, logvar2=0.0)
- return mean_flat(kl_prior) / np.log(2.0)
-
- def p_losses(self, x_start, cond, t, noise=None):
- noise = default(noise, lambda: torch.randn_like(x_start))
- x_noisy = self.q_sample(x_start=x_start, t=t, noise=noise)
- model_output = self.apply_model(x_noisy, t, cond)
-
- loss_dict = {}
- prefix = 'train' if self.training else 'val'
-
- if self.parameterization == "x0":
- target = x_start
- elif self.parameterization == "eps":
- target = noise
- else:
- raise NotImplementedError()
-
- loss_simple = self.get_loss(model_output, target, mean=False).mean([1, 2, 3])
- loss_dict.update({f'{prefix}/loss_simple': loss_simple.mean()})
-
- logvar_t = self.logvar[t].to(self.device)
- loss = loss_simple / torch.exp(logvar_t) + logvar_t
- # loss = loss_simple / torch.exp(self.logvar) + self.logvar
- if self.learn_logvar:
- loss_dict.update({f'{prefix}/loss_gamma': loss.mean()})
- loss_dict.update({'logvar': self.logvar.data.mean()})
-
- loss = self.l_simple_weight * loss.mean()
-
- loss_vlb = self.get_loss(model_output, target, mean=False).mean(dim=(1, 2, 3))
- loss_vlb = (self.lvlb_weights[t] * loss_vlb).mean()
- loss_dict.update({f'{prefix}/loss_vlb': loss_vlb})
- loss += (self.original_elbo_weight * loss_vlb)
- loss_dict.update({f'{prefix}/loss': loss})
-
- return loss, loss_dict
-
- def p_mean_variance(self, x, c, t, clip_denoised: bool, return_codebook_ids=False, quantize_denoised=False,
- return_x0=False, score_corrector=None, corrector_kwargs=None):
- t_in = t
- model_out = self.apply_model(x, t_in, c, return_ids=return_codebook_ids)
-
- if score_corrector is not None:
- assert self.parameterization == "eps"
- model_out = score_corrector.modify_score(self, model_out, x, t, c, **corrector_kwargs)
-
- if return_codebook_ids:
- model_out, logits = model_out
-
- if self.parameterization == "eps":
- x_recon = self.predict_start_from_noise(x, t=t, noise=model_out)
- elif self.parameterization == "x0":
- x_recon = model_out
- else:
- raise NotImplementedError()
-
- if clip_denoised:
- x_recon.clamp_(-1., 1.)
- if quantize_denoised:
- x_recon, _, [_, _, indices] = self.first_stage_model.quantize(x_recon)
- model_mean, posterior_variance, posterior_log_variance = self.q_posterior(x_start=x_recon, x_t=x, t=t)
- if return_codebook_ids:
- return model_mean, posterior_variance, posterior_log_variance, logits
- elif return_x0:
- return model_mean, posterior_variance, posterior_log_variance, x_recon
- else:
- return model_mean, posterior_variance, posterior_log_variance
-
- @torch.no_grad()
- def p_sample(self, x, c, t, clip_denoised=False, repeat_noise=False,
- return_codebook_ids=False, quantize_denoised=False, return_x0=False,
- temperature=1., noise_dropout=0., score_corrector=None, corrector_kwargs=None):
- b, *_, device = *x.shape, x.device
- outputs = self.p_mean_variance(x=x, c=c, t=t, clip_denoised=clip_denoised,
- return_codebook_ids=return_codebook_ids,
- quantize_denoised=quantize_denoised,
- return_x0=return_x0,
- score_corrector=score_corrector, corrector_kwargs=corrector_kwargs)
- if return_codebook_ids:
- raise DeprecationWarning("Support dropped.")
- model_mean, _, model_log_variance, logits = outputs
- elif return_x0:
- model_mean, _, model_log_variance, x0 = outputs
- else:
- model_mean, _, model_log_variance = outputs
-
- noise = noise_like(x.shape, device, repeat_noise) * temperature
- if noise_dropout > 0.:
- noise = torch.nn.functional.dropout(noise, p=noise_dropout)
- # no noise when t == 0
- nonzero_mask = (1 - (t == 0).float()).reshape(b, *((1,) * (len(x.shape) - 1)))
-
- if return_codebook_ids:
- return model_mean + nonzero_mask * (0.5 * model_log_variance).exp() * noise, logits.argmax(dim=1)
- if return_x0:
- return model_mean + nonzero_mask * (0.5 * model_log_variance).exp() * noise, x0
- else:
- return model_mean + nonzero_mask * (0.5 * model_log_variance).exp() * noise
-
- @torch.no_grad()
- def progressive_denoising(self, cond, shape, verbose=True, callback=None, quantize_denoised=False,
- img_callback=None, mask=None, x0=None, temperature=1., noise_dropout=0.,
- score_corrector=None, corrector_kwargs=None, batch_size=None, x_T=None, start_T=None,
- log_every_t=None):
- if not log_every_t:
- log_every_t = self.log_every_t
- timesteps = self.num_timesteps
- if batch_size is not None:
- b = batch_size if batch_size is not None else shape[0]
- shape = [batch_size] + list(shape)
- else:
- b = batch_size = shape[0]
- if x_T is None:
- img = torch.randn(shape, device=self.device)
- else:
- img = x_T
- intermediates = []
- if cond is not None:
- if isinstance(cond, dict):
- cond = {key: cond[key][:batch_size] if not isinstance(cond[key], list) else
- list(map(lambda x: x[:batch_size], cond[key])) for key in cond}
- else:
- cond = [c[:batch_size] for c in cond] if isinstance(cond, list) else cond[:batch_size]
-
- if start_T is not None:
- timesteps = min(timesteps, start_T)
- iterator = tqdm(reversed(range(0, timesteps)), desc='Progressive Generation',
- total=timesteps) if verbose else reversed(
- range(0, timesteps))
- if type(temperature) == float:
- temperature = [temperature] * timesteps
-
- for i in iterator:
- ts = torch.full((b,), i, device=self.device, dtype=torch.long)
- if self.shorten_cond_schedule:
- assert self.model.conditioning_key != 'hybrid'
- tc = self.cond_ids[ts].to(cond.device)
- cond = self.q_sample(x_start=cond, t=tc, noise=torch.randn_like(cond))
-
- img, x0_partial = self.p_sample(img, cond, ts,
- clip_denoised=self.clip_denoised,
- quantize_denoised=quantize_denoised, return_x0=True,
- temperature=temperature[i], noise_dropout=noise_dropout,
- score_corrector=score_corrector, corrector_kwargs=corrector_kwargs)
- if mask is not None:
- assert x0 is not None
- img_orig = self.q_sample(x0, ts)
- img = img_orig * mask + (1. - mask) * img
-
- if i % log_every_t == 0 or i == timesteps - 1:
- intermediates.append(x0_partial)
- if callback: callback(i)
- if img_callback: img_callback(img, i)
- return img, intermediates
-
- @torch.no_grad()
- def p_sample_loop(self, cond, shape, return_intermediates=False,
- x_T=None, verbose=True, callback=None, timesteps=None, quantize_denoised=False,
- mask=None, x0=None, img_callback=None, start_T=None,
- log_every_t=None):
-
- if not log_every_t:
- log_every_t = self.log_every_t
- device = self.betas.device
- b = shape[0]
- if x_T is None:
- img = torch.randn(shape, device=device)
- else:
- img = x_T
-
- intermediates = [img]
- if timesteps is None:
- timesteps = self.num_timesteps
-
- if start_T is not None:
- timesteps = min(timesteps, start_T)
- iterator = tqdm(reversed(range(0, timesteps)), desc='Sampling t', total=timesteps) if verbose else reversed(
- range(0, timesteps))
-
- if mask is not None:
- assert x0 is not None
- assert x0.shape[2:3] == mask.shape[2:3] # spatial size has to match
-
- for i in iterator:
- ts = torch.full((b,), i, device=device, dtype=torch.long)
- if self.shorten_cond_schedule:
- assert self.model.conditioning_key != 'hybrid'
- tc = self.cond_ids[ts].to(cond.device)
- cond = self.q_sample(x_start=cond, t=tc, noise=torch.randn_like(cond))
-
- img = self.p_sample(img, cond, ts,
- clip_denoised=self.clip_denoised,
- quantize_denoised=quantize_denoised)
- if mask is not None:
- img_orig = self.q_sample(x0, ts)
- img = img_orig * mask + (1. - mask) * img
-
- if i % log_every_t == 0 or i == timesteps - 1:
- intermediates.append(img)
- if callback: callback(i)
- if img_callback: img_callback(img, i)
-
- if return_intermediates:
- return img, intermediates
- return img
-
- @torch.no_grad()
- def sample(self, cond, batch_size=16, return_intermediates=False, x_T=None,
- verbose=True, timesteps=None, quantize_denoised=False,
- mask=None, x0=None, shape=None,**kwargs):
- if shape is None:
- shape = (batch_size, self.channels, self.image_size, self.image_size)
- if cond is not None:
- if isinstance(cond, dict):
- cond = {key: cond[key][:batch_size] if not isinstance(cond[key], list) else
- list(map(lambda x: x[:batch_size], cond[key])) for key in cond}
- else:
- cond = [c[:batch_size] for c in cond] if isinstance(cond, list) else cond[:batch_size]
- return self.p_sample_loop(cond,
- shape,
- return_intermediates=return_intermediates, x_T=x_T,
- verbose=verbose, timesteps=timesteps, quantize_denoised=quantize_denoised,
- mask=mask, x0=x0)
-
- @torch.no_grad()
- def sample_log(self,cond,batch_size,ddim, ddim_steps,**kwargs):
-
- if ddim:
- ddim_sampler = DDIMSampler(self)
- shape = (self.channels, self.image_size, self.image_size)
- samples, intermediates =ddim_sampler.sample(ddim_steps,batch_size,
- shape,cond,verbose=False,**kwargs)
-
- else:
- samples, intermediates = self.sample(cond=cond, batch_size=batch_size,
- return_intermediates=True,**kwargs)
-
- return samples, intermediates
-
-
- @torch.no_grad()
- def log_images(self, batch, N=4, n_row=4, sample=True, ddim_steps=200, ddim_eta=1., return_keys=None,
- quantize_denoised=True, inpaint=False, plot_denoise_rows=False, plot_progressive_rows=False,
- plot_diffusion_rows=False, **kwargs):
-
- use_ddim = False
-
- log = dict()
- z, c, x, xrec, xc = self.get_input(batch, self.first_stage_key,
- return_first_stage_outputs=True,
- force_c_encode=True,
- return_original_cond=True,
- bs=N, uncond=0)
- N = min(x.shape[0], N)
- n_row = min(x.shape[0], n_row)
- log["inputs"] = x
- log["reals"] = xc["c_concat"]
- log["reconstruction"] = xrec
- if self.model.conditioning_key is not None:
- if hasattr(self.cond_stage_model, "decode"):
- xc = self.cond_stage_model.decode(c)
- log["conditioning"] = xc
- elif self.cond_stage_key in ["caption"]:
- xc = log_txt_as_img((x.shape[2], x.shape[3]), batch["caption"])
- log["conditioning"] = xc
- elif self.cond_stage_key == 'class_label':
- xc = log_txt_as_img((x.shape[2], x.shape[3]), batch["human_label"])
- log['conditioning'] = xc
- elif isimage(xc):
- log["conditioning"] = xc
- if ismap(xc):
- log["original_conditioning"] = self.to_rgb(xc)
-
- if plot_diffusion_rows:
- # get diffusion row
- diffusion_row = list()
- z_start = z[:n_row]
- for t in range(self.num_timesteps):
- if t % self.log_every_t == 0 or t == self.num_timesteps - 1:
- t = repeat(torch.tensor([t]), '1 -> b', b=n_row)
- t = t.to(self.device).long()
- noise = torch.randn_like(z_start)
- z_noisy = self.q_sample(x_start=z_start, t=t, noise=noise)
- diffusion_row.append(self.decode_first_stage(z_noisy))
-
- diffusion_row = torch.stack(diffusion_row) # n_log_step, n_row, C, H, W
- diffusion_grid = rearrange(diffusion_row, 'n b c h w -> b n c h w')
- diffusion_grid = rearrange(diffusion_grid, 'b n c h w -> (b n) c h w')
- diffusion_grid = make_grid(diffusion_grid, nrow=diffusion_row.shape[0])
- log["diffusion_row"] = diffusion_grid
-
- if sample:
- # get denoise row
- with self.ema_scope("Plotting"):
- samples, z_denoise_row = self.sample_log(cond=c,batch_size=N,ddim=use_ddim,
- ddim_steps=ddim_steps,eta=ddim_eta)
- # samples, z_denoise_row = self.sample(cond=c, batch_size=N, return_intermediates=True)
- x_samples = self.decode_first_stage(samples)
- log["samples"] = x_samples
- if plot_denoise_rows:
- denoise_grid = self._get_denoise_row_from_list(z_denoise_row)
- log["denoise_row"] = denoise_grid
-
- if quantize_denoised and not isinstance(self.first_stage_model, AutoencoderKL) and not isinstance(
- self.first_stage_model, IdentityFirstStage):
- # also display when quantizing x0 while sampling
- with self.ema_scope("Plotting Quantized Denoised"):
- samples, z_denoise_row = self.sample_log(cond=c,batch_size=N,ddim=use_ddim,
- ddim_steps=ddim_steps,eta=ddim_eta,
- quantize_denoised=True)
- # samples, z_denoise_row = self.sample(cond=c, batch_size=N, return_intermediates=True,
- # quantize_denoised=True)
- x_samples = self.decode_first_stage(samples.to(self.device))
- log["samples_x0_quantized"] = x_samples
-
- if inpaint:
- # make a simple center square
- b, h, w = z.shape[0], z.shape[2], z.shape[3]
- mask = torch.ones(N, h, w).to(self.device)
- # zeros will be filled in
- mask[:, h // 4:3 * h // 4, w // 4:3 * w // 4] = 0.
- mask = mask[:, None, ...]
- with self.ema_scope("Plotting Inpaint"):
-
- samples, _ = self.sample_log(cond=c,batch_size=N,ddim=use_ddim, eta=ddim_eta,
- ddim_steps=ddim_steps, x0=z[:N], mask=mask)
- x_samples = self.decode_first_stage(samples.to(self.device))
- log["samples_inpainting"] = x_samples
- log["mask"] = mask
-
- # outpaint
- with self.ema_scope("Plotting Outpaint"):
- samples, _ = self.sample_log(cond=c, batch_size=N, ddim=use_ddim,eta=ddim_eta,
- ddim_steps=ddim_steps, x0=z[:N], mask=mask)
- x_samples = self.decode_first_stage(samples.to(self.device))
- log["samples_outpainting"] = x_samples
-
- if plot_progressive_rows:
- with self.ema_scope("Plotting Progressives"):
- img, progressives = self.progressive_denoising(c,
- shape=(self.channels, self.image_size, self.image_size),
- batch_size=N)
- prog_row = self._get_denoise_row_from_list(progressives, desc="Progressive Generation")
- log["progressive_row"] = prog_row
-
- if return_keys:
- if np.intersect1d(list(log.keys()), return_keys).shape[0] == 0:
- return log
- else:
- return {key: log[key] for key in return_keys}
- return log
-
- def configure_optimizers(self):
- lr = self.learning_rate
- params = list(self.model.parameters())
- if self.cond_stage_trainable:
- print(f"{self.__class__.__name__}: Also optimizing conditioner params!")
- params = params + list(self.cond_stage_model.parameters())
- if self.learn_logvar:
- print('Diffusion model optimizing logvar')
- params.append(self.logvar)
- opt = torch.optim.AdamW(params, lr=lr)
- if self.use_scheduler:
- assert 'target' in self.scheduler_config
- scheduler = instantiate_from_config(self.scheduler_config)
-
- print("Setting up LambdaLR scheduler...")
- scheduler = [
- {
- 'scheduler': LambdaLR(opt, lr_lambda=scheduler.schedule),
- 'interval': 'step',
- 'frequency': 1
- }]
- return [opt], scheduler
- return opt
-
- @torch.no_grad()
- def to_rgb(self, x):
- x = x.float()
- if not hasattr(self, "colorize"):
- self.colorize = torch.randn(3, x.shape[1], 1, 1).to(x)
- x = nn.functional.conv2d(x, weight=self.colorize)
- x = 2. * (x - x.min()) / (x.max() - x.min()) - 1.
- return x
-
-
-class DiffusionWrapper(pl.LightningModule):
- def __init__(self, diff_model_config, conditioning_key):
- super().__init__()
- self.diffusion_model = instantiate_from_config(diff_model_config)
- self.conditioning_key = conditioning_key
- assert self.conditioning_key in [None, 'concat', 'crossattn', 'hybrid', 'adm']
-
- def forward(self, x, t, c_concat: list = None, c_crossattn: list = None):
- if self.conditioning_key is None:
- out = self.diffusion_model(x, t)
- elif self.conditioning_key == 'concat':
- xc = torch.cat([x] + c_concat, dim=1)
- out = self.diffusion_model(xc, t)
- elif self.conditioning_key == 'crossattn':
- cc = torch.cat(c_crossattn, 1)
- out = self.diffusion_model(x, t, context=cc)
- elif self.conditioning_key == 'hybrid':
- xc = torch.cat([x] + c_concat, dim=1)
- cc = torch.cat(c_crossattn, 1)
- out = self.diffusion_model(xc, t, context=cc)
- elif self.conditioning_key == 'adm':
- cc = c_crossattn[0]
- out = self.diffusion_model(x, t, y=cc)
- else:
- raise NotImplementedError()
-
- return out
-
-
-class Layout2ImgDiffusion(LatentDiffusion):
- # TODO: move all layout-specific hacks to this class
- def __init__(self, cond_stage_key, *args, **kwargs):
- assert cond_stage_key == 'coordinates_bbox', 'Layout2ImgDiffusion only for cond_stage_key="coordinates_bbox"'
- super().__init__(cond_stage_key=cond_stage_key, *args, **kwargs)
-
- def log_images(self, batch, N=8, *args, **kwargs):
- logs = super().log_images(batch=batch, N=N, *args, **kwargs)
-
- key = 'train' if self.training else 'validation'
- dset = self.trainer.datamodule.datasets[key]
- mapper = dset.conditional_builders[self.cond_stage_key]
-
- bbox_imgs = []
- map_fn = lambda catno: dset.get_textual_label(dset.get_category_id(catno))
- for tknzd_bbox in batch[self.cond_stage_key][:N]:
- bboximg = mapper.plot(tknzd_bbox.detach().cpu(), map_fn, (256, 256))
- bbox_imgs.append(bboximg)
-
- cond_img = torch.stack(bbox_imgs, dim=0)
- logs['bbox_image'] = cond_img
- return logs
diff --git a/spaces/apexxlegends/README/README.md b/spaces/apexxlegends/README/README.md
deleted file mode 100644
index f22764f73f3b6f659752e00e9461c8eeb0b4d3c5..0000000000000000000000000000000000000000
--- a/spaces/apexxlegends/README/README.md
+++ /dev/null
@@ -1,10 +0,0 @@
----
-title: README
-emoji: ⚡
-colorFrom: purple
-colorTo: purple
-sdk: static
-pinned: true
----
-
-Edit this `README.md` markdown file to author your organization card 🔥
\ No newline at end of file
diff --git a/spaces/apratap5/Abhay-2-BiomedEntityRecognition-GR/README.md b/spaces/apratap5/Abhay-2-BiomedEntityRecognition-GR/README.md
deleted file mode 100644
index 5ec43dbcceaa9bed0d594fd3ec49583a90239d71..0000000000000000000000000000000000000000
--- a/spaces/apratap5/Abhay-2-BiomedEntityRecognition-GR/README.md
+++ /dev/null
@@ -1,12 +0,0 @@
----
-title: Abhay 2 BiomedEntityRecognition GR
-emoji: 📊
-colorFrom: green
-colorTo: green
-sdk: gradio
-sdk_version: 3.8.2
-app_file: app.py
-pinned: false
----
-
-Check out the configuration reference at https://huggingface.co/docs/hub/spaces-config-reference
diff --git a/spaces/arbml/whisper-small-ar/app.py b/spaces/arbml/whisper-small-ar/app.py
deleted file mode 100644
index 42bc2d005a50c8f052c6f1532bbaaf91138800f5..0000000000000000000000000000000000000000
--- a/spaces/arbml/whisper-small-ar/app.py
+++ /dev/null
@@ -1,97 +0,0 @@
-import torch
-
-import gradio as gr
-import pytube as pt
-from transformers import pipeline
-from huggingface_hub import model_info
-
-MODEL_NAME = "arbml/whisper-small-ar" #this always needs to stay in line 8 :D sorry for the hackiness
-lang = "ar"
-
-device = 0 if torch.cuda.is_available() else "cpu"
-pipe = pipeline(
- task="automatic-speech-recognition",
- model=MODEL_NAME,
- chunk_length_s=30,
- device=device,
-)
-
-pipe.model.config.forced_decoder_ids = pipe.tokenizer.get_decoder_prompt_ids(language=lang, task="transcribe")
-
-def transcribe(microphone, file_upload):
- warn_output = ""
- if (microphone is not None) and (file_upload is not None):
- warn_output = (
- "WARNING: You've uploaded an audio file and used the microphone. "
- "The recorded file from the microphone will be used and the uploaded audio will be discarded.\n"
- )
-
- elif (microphone is None) and (file_upload is None):
- return "ERROR: You have to either use the microphone or upload an audio file"
-
- file = microphone if microphone is not None else file_upload
-
- text = pipe(file)["text"]
-
- return warn_output + text
-
-
-def _return_yt_html_embed(yt_url):
- video_id = yt_url.split("?v=")[-1]
- HTML_str = (
- f'
'
- "
"
- )
- return HTML_str
-
-
-def yt_transcribe(yt_url):
- yt = pt.YouTube(yt_url)
- html_embed_str = _return_yt_html_embed(yt_url)
- stream = yt.streams.filter(only_audio=True)[0]
- stream.download(filename="audio.mp3")
-
- text = pipe("audio.mp3")["text"]
-
- return html_embed_str, text
-
-
-demo = gr.Blocks()
-
-mf_transcribe = gr.Interface(
- fn=transcribe,
- inputs=[
- gr.inputs.Audio(source="microphone", type="filepath", optional=True),
- gr.inputs.Audio(source="upload", type="filepath", optional=True),
- ],
- outputs="text",
- layout="horizontal",
- theme="huggingface",
- title="Whisper Demo: Transcribe Audio",
- description=(
- "Transcribe long-form microphone or audio inputs with the click of a button! Demo uses the the fine-tuned"
- f" checkpoint [{MODEL_NAME}](https://huggingface.co/{MODEL_NAME}) and 🤗 Transformers to transcribe audio files"
- " of arbitrary length."
- ),
- allow_flagging="never",
-)
-
-yt_transcribe = gr.Interface(
- fn=yt_transcribe,
- inputs=[gr.inputs.Textbox(lines=1, placeholder="Paste the URL to a YouTube video here", label="YouTube URL")],
- outputs=["html", "text"],
- layout="horizontal",
- theme="huggingface",
- title="Whisper Demo: Transcribe YouTube",
- description=(
- "Transcribe long-form YouTube videos with the click of a button! Demo uses the the fine-tuned checkpoint:"
- f" [{MODEL_NAME}](https://huggingface.co/{MODEL_NAME}) and 🤗 Transformers to transcribe audio files of"
- " arbitrary length."
- ),
- allow_flagging="never",
-)
-
-with demo:
- gr.TabbedInterface([mf_transcribe, yt_transcribe], ["Transcribe Audio", "Transcribe YouTube"])
-
-demo.launch(enable_queue=True)
diff --git a/spaces/ardigen/ardisplay-i/LICENSE.md b/spaces/ardigen/ardisplay-i/LICENSE.md
deleted file mode 100644
index b35e27bb72bf3fd96ed3603b3dcbf73c4a064d68..0000000000000000000000000000000000000000
--- a/spaces/ardigen/ardisplay-i/LICENSE.md
+++ /dev/null
@@ -1,109 +0,0 @@
-TERMS AND CONDITIONS FOR THE ACADEMIC USE OF SOFTWARE
-
-IMPORTANT - READ CAREFULLY: BY DOWNLOADING, INSTALLING, OR USING THE SOFTWARE, YOU AGREE TO BE BOUND BY THE TERMS AND CONDITIONS OF THIS AGREEMENT. IF YOU DO NOT AGREE TO THE TERMS AND CONDITIONS OF THIS AGREEMENT, DO NOT DOWNLOAD, INSTALL, OR USE THE SOFTWARE.
-
-BY DOWNLOADING THE SOFTWARE, YOU CONFIRM THAT YOU ARE A MEMBER OF A PUBLIC FUNDED ACADEMIC AND/OR EDUCATION AND/OR RESEARCH INSTITUTION AND WILL USE THE SOFTWARE SOLELY FOR NON-COMMERCIAL USE IN COMPLIANCE WITH THIS LICENSE.
-
-
-IF YOU ARE NOT A MEMBER OF A PUBLIC FUNDED ACADEMIC AND/OR EDUCATION AND/OR RESEARCH INSTITUTION YOU MUST OBTAIN A COMMERCIAL LICENSE FROM THE LICENSOR.
-
-Section 1: **DEFINITIONS**
-
-1.1 "Copyright and Similar Rights" means copyright and any rights related to copyright.
-
-1.2 "Licensed Rights" are the rights granted to the Licensee, limited to all Copyright and Similar Rights that apply to the Licensee's use of the Licensed Software and that the Licensor has the authority to license.
-
-1.3 “Licensor” means Ardigen S.A., ul. Podole 76, 30-394 Kraków, Poland.
-
-1.4 “Licensee” means a publicly funded academic and/or education and/or research institution or an individual working for such institution.
-
-1.5 "Licensed Software" means the Licensor's proprietary software for predicting the probability of presentation of peptide:HLA complexes on the surface of a cell - Ardigen ARDisplay-I and its specific version. All title and copyrights for and to the Licensed Software, including but not limited to any copywritten images, demos, source code, and intermediate files incorporated into the Licensed Software, the accompanying materials, and any copies of the Licensed Software are the intellectual property of and are owned by the Licensor.
-
-1.6 "Non-Commercial Use" means using the Licensed Software for non-commercial purposes and not for monetary compensation.
-
-Section 2: **LICENSE**
-
-
-2.1 Subject to the terms and conditions of this License, the Licensor grants the Licensee a worldwide, non-exclusive, royalty-free, non-sublicensable, non-transferable license to exercise the Licensed Rights in the Licensed Software to download, install and use of the Licensed Software for academic, Non-Commercial Use only. The Licensee is not authorized by this License to modify, distribute, or create derivatives of the Licensed Software, nor to claim or assert that the Licensee or its use of the Licensed Software is connected with or sponsored, endorsed, or granted official status by the Licensor. This License doesn't grant any patent or trademark rights.
-
-
-2.2 The Licensee agrees to the following restrictions and obligations:
-
-- Shall not distribute or provide access to the Licensed Software to any third party.
-
-- Shall not attempt to decompile, disassemble or reverse engineer the Licensed Software in any manner to discover the source code or underlying ideas or algorithms of the Licensed Software.
-
-- Shall not remove any product identification, copyright or other notices embedded within the Licensed Software.
-
-- Shall not modify or create a derivative work of the Licensed Software.
-
-- Shall not export any Licensed Software in violation of applicable laws or regulations.
-
-- Shall not copy the Licensed Software or any portion thereof, except as provided in this License.
-
-- Shall not rent, lease, loan, sell or assign the Licensed Software or derivative works based on the whole or any part of the Licensed Software.
-
-
-All data generated using the Licensed Software, as well as any references to the Licensed Software, must include proper attribution. Attribution should include the name of the Licensed Software (Ardigen ARDisplay-I) and its version, as well as the following link to the Licensed Software: https://huggingface.co/ardigen/ardisplay-i. Any publications or presentations that include data generated using the Licensed Software must acknowledge the Licensed Software and include a citation to the appropriate source.
-
-2.3 The Licensee acknowledges that the Licensed Software may contain third-party software that may be embedded or otherwise delivered with the Licensed Software. In particular, the Licensed Software contains Apache software which is subject to a respective license available at: https://github.com/openvax/mhcflurry/blob/master/LICENSE. By using the Licensed Software, Licensee agrees to abide by the terms and conditions of the Apache software license, as well as any other third-party licenses that apply to the software being a part of the Licensed Software. Licensee may only use third-party software as integrated with and part of the Licensed Software.
-
-Section 3: **OWNERSHIP**
-
-Except as expressly licensed in this Agreement, Licensor shall retain title to the Licensed Software, and any upgrades and modifications created by Licensor.
-
-
-SECTION 4: **SUPPORT**
-
-Licensor shall have no obligation to offer support services to Licensee, and nothing contained herein shall be interpreted as to require Licensor to provide maintenance, installation services, version updates, debugging, consultation, or end-user support of any kind.
-
-Section 5: **SOFTWARE PROTECTION**
-
-Licensee acknowledges that the Licensed Software is proprietary to Licensor. The software code and trained model associated with the Licensed Software shall be treated as trade secrets and confidential information of the Licensor, and Licensee agrees to use best efforts to hold the same in confidence. Licensee's obligation for confidentiality shall not extend to any information which is or becomes generally available to the public, is already known to or subsequently disclosed by third parties to Licensee and at its free disposal or is independently developed by Licensee or its affiliates without the use of the confidential information disclosed by Licensor, or is required by law or legal process.
-
-Section 6: **WARRANTY DISCLAIMER**
-
-THE LICENSOR MAKES NO REPRESENTATIONS AND EXTENDS NO WARRANTIES OF ANY KIND, EITHER IMPLIED OR EXPRESS, INCLUDING, BUT NOT LIMITED TO, THE IMPLIED WARRANTIES OF MERCHANTABILITY OR FITNESS FOR A PARTICULAR PURPOSE, OR THAT THE USE OF THE LICENSED SOFTWARE WILL NOT INFRINGE ANY PATENT, TRADEMARK OR OTHER RIGHTS.
-
-LICENSOR DOES NOT GUARANTEE THAT LICENSED MATERIALS WILL MEET YOUR EXPECTATIONS OR REQUIREMENTS. LICENSOR DOES NOT GUARANTEE THAT THE LICENSED SOFTWARE IS ERROR-FREE. LICENSOR DOES NOT WARRANT, GUARANTEE, OR MAKE ANY REPRESENTATIONS REGARDING THE USE, OR THE RESULTS OF THE USE, OF THE LICENSED SOFTWARE IN TERMS OF CORRECTNESS, ACCURACY, RELIABILITY, OR OTHERWISE. THE ENTIRE RISK ARISING OUT OF USE OR PERFORMANCE OF THE LICENSED SOFTWARE REMAINS WITH YOU. NO ORAL OR WRITTEN INFORMATION OR ADVICE GIVEN BY LICENSOR SHALL CREATE A WARRANTY OR IN ANY WAY INCREASE THE SCOPE OF THIS WARRANTY.
-
-
-Section 7: **LIMITATION OF LIABILITY**
-
-IN NO EVENT SHALL THE LICENSOR BE LIABLE TO ANY PARTY FOR DIRECT, INDIRECT, SPECIAL, INCIDENTAL, OR CONSEQUENTIAL DAMAGES, INCLUDING LOST PROFITS, ARISING OUT OF THE USE OF THE LICENSED SOFTWARE, EVEN IF THE LICENSOR HAS BEEN ADVISED OF THE POSSIBILITY OF SUCH DAMAGE.
-
-Section 8: **TERM AND TERMINATION**
-
-8.1 This License applies for the term of the Copyright and Similar Rights licensed
-herein.
-
-8.2 The Licensor may terminate this Agreement immediately upon written notice if the Licensee breaches any of its obligations under this Agreement.
-
-8.3 Upon termination, the Licensee shall immediately cease using the Licensed Software and shall destroy all copies of the Licensed Software in its possession.
-
-8.4 Sections 1 (Definitions), 5 (Software Protection), 6 (Warranty and Disclaimer), 7 (Limitation of Liability), 8 (Term and Termination), and 9 (Governing Law) shall survive termination of this License.
-
-
-Section 9: **GOVERNING LAW**
-
-9.1 This Agreement shall be governed by and construed in accordance with the laws of Poland, without giving effect to any choice of law or conflict of law provision or rule.
-
-9.2 Any judicial action or proceeding arising hereunder or relating hereto shall be brought in, and the Licensee hereby consent to the exclusive jurisdiction of the Courts located in Kraków, Poland.
-
-Section 10: **CHANGES**
-
-10.1 From time to time, Licensor may change the terms and provisions of this License.
-
-10.2 When these changes are made, Licensor will make a new version of the License publicly available.
-
-10.3 Licensee understands and agrees that if you use the Licensed Software after the date on which the License has been changed, the Licensor will treat your use as acceptance of the updated License.
-
-
-Section 11: **ENTIRE AGREEMENT**
-
-This License constitutes the entire agreement between the parties and supersedes all prior agreements and understandings, whether written or oral, relating to the subject matter of this License.
-
-Section 12: **CONTACTS**
-
-If you have any questions, concerns, or complaints regarding this License or the
-Licensed Software, or if you require a commercial license, in particular, in order to provide services to third parties by using the Licensed Software, please contact us using the e-mail address below: ardisplay@ardigen.com.
diff --git a/spaces/artificialguybr/freedom/app.py b/spaces/artificialguybr/freedom/app.py
deleted file mode 100644
index be89615e2e0295f620bd616a623b60431b602376..0000000000000000000000000000000000000000
--- a/spaces/artificialguybr/freedom/app.py
+++ /dev/null
@@ -1,159 +0,0 @@
-import gradio as gr
-import requests
-import json
-import PIL.Image
-from io import BytesIO
-import os
-import random
-import datetime
-import urllib3
-urllib3.disable_warnings(urllib3.exceptions.InsecureRequestWarning)
-
-def generate_image(prompt, negative_prompt, use_negative_embedding, scheduler, steps, width, height, cfg, restore_faces, seed):
- forbidden_words = os.getenv("FORBIDDEN_WORDS").split(", ")
- # Check if the prompt contains any of the forbidden words
- for word in forbidden_words:
- if word in prompt:
- raise Exception(f"The prompt contains a forbidden word: {word}")
- request_time = datetime.datetime.now()
- restore_faces = bool(restore_faces)
- use_negative_embedding = bool(use_negative_embedding)
- print(f"restore_faces: {restore_faces}, type: {type(restore_faces)}")
- print(f"use_negative_embedding: {use_negative_embedding}, type: {type(use_negative_embedding)}")
- if use_negative_embedding:
- negative_prompt += ", rz-neg-general"
- # Define the API endpoint
- apiUrl = os.getenv("API_URL")
- # Define the request headers
- headers = {
- "Content-Type": "application/json",
- "token": os.getenv("API_TOKEN")
- }
-
- # Define the request body
- body = {
- "mode": "url",
- "model": "Freedom.safetensors",
- "tiling": False,
- "batch_size": 1,
- "prompt": prompt,
- "negative_prompt": negative_prompt,
- "seed":random.randint(0, 999999999),
- "scheduler": scheduler,
- "n_iter": 1,
- "steps": steps,
- "cfg": cfg,
- "offset_noise": 0.0,
- "width": width,
- "height": height,
- "clip_skip": 1,
- "embeddings": [
- {
- "name": "rz-neg-general",
- "strength": 1.0 if use_negative_embedding else 0
- },
- ],
- "vae": "vae-ft-mse-840000-ema-pruned.ckpt",
- "restore_faces": restore_faces,
- "fr_model": "CodeFormer",
- "codeformer_weight": 0.5,
- "enable_hr": False,
- "denoising_strength": 0.75,
- "hr_scale": 2,
- "hr_upscale": "None",
- "img2img_ref_img_type": "piece",
- "img2img_resize_mode": 0,
- "img2img_denoising_strength": 0.75,
- }
-
- # Send the request
- response = requests.post(apiUrl, headers=headers, data=json.dumps(body), verify=False)
- # Print the response body if the status code is not 200
- if response.status_code != 200:
- print(response.text)
-
- # Check the response status
- if response.status_code == 200:
-
- # Get the image URL from the response
- response_json = response.json()
- if 'results' in response_json and isinstance(response_json['results'], list) and len(response_json['results']) > 0:
- image_url = response_json['results'][0]
-
- # Get the image from the URL
- image_response = requests.get(image_url)
- image = PIL.Image.open(BytesIO(image_response.content))
-
- # Log the information together
- print(f"Request time: {request_time}\n"
- f"Prompt: {prompt}\n"
- f"Negative Prompt: {negative_prompt}\n"
- f"Seed: {seed}\n"
- f"Res(width x height): {width} x {height}\n"
- f"Image URL: {image_url}")
-
- return image
- else:
- raise Exception("Unexpected API response format")
- else:
- raise Exception("API request failed with status code " + str(response.status_code))
-
-# Define the Gradio interface
-iface = gr.Interface(
- fn=generate_image,
- inputs=[
- gr.components.Textbox(label="Prompt"),
- gr.components.Textbox(value="ugly, tiling, poorly drawn hands, poorly drawn feet, poorly drawn face, out of frame, extra limbs, disfigured, deformed, body out of frame, blurry, bad anatomy, blurred, watermark, grainy, signature, cut off, draft", label="Negative Prompt"),
- gr.inputs.Checkbox(label="Use Negative Embedding", default=True),
- gr.components.Dropdown(choices=[
- "Euler a",
- "Euler",
- "LMS",
- "Heun",
- "DPM2",
- "DPM2 a",
- "DPM++ 2S a",
- "DPM++ 2M",
- "DPM++ SDE",
- "DPM fast",
- "DPM adaptive",
- "LMS Karras",
- "DPM2 Karras",
- "DPM2 a Karras",
- "DPM++ 2S a Karras",
- "DPM++ 2M Karras",
- "DPM++ SDE Karras",
- "DDIM",
- "PLMS"
- ], label="Scheduler", value="DPM++ SDE Karras"),
- gr.components.Slider(minimum=10, maximum=100, step=1.0,value=30, label="Steps"),
- gr.components.Slider(minimum=512, maximum=1600, value=1024, label="Width"),
- gr.components.Slider(minimum=512, maximum=1600, value=1024, label="Height"),
- gr.components.Slider(minimum=4, maximum=12, step=0.5, value=7.0, label="CFG"),
- gr.inputs.Checkbox(label="Restore Faces", default=False),
- ],
- outputs=gr.components.Image(),
- title="Freedom.Redmond Demonstration",
- description = """
-## Finetuned model of SD 2.1 768X produced by [@artificialguybr](https://twitter.com/artificialguybr).
-
-## Resources
-- The weights were released [here](https://civitai.com/models/87288/freedomredmond) with example prompts in CIVITAI and [here in HF](https://huggingface.co/artificialguybr/freedom).
-
-## Demonstration
-This demonstration is running on the [makeai.run API](https://www.makeai.run/).
-
-## Acknowledgements
-Thanks to [Redmond.ai](https://redmond.ai/) for providing GPU Time and sponsoring this model.
-
-## Test my 1.5 Finetuned Model (Liberte) [here](https://huggingface.co/spaces/artificialguybr/liberte).
-
-""",
- allow_flagging='never'
-)
-
-#Adding queue
-iface.queue(concurrency_count=12)
-
-# Launch the app
-iface.launch()
diff --git a/spaces/arxify/RVC-beta-v2-0618/export_onnx_old.py b/spaces/arxify/RVC-beta-v2-0618/export_onnx_old.py
deleted file mode 100644
index 048382f6631c4b3b092deb83355903161b62e64a..0000000000000000000000000000000000000000
--- a/spaces/arxify/RVC-beta-v2-0618/export_onnx_old.py
+++ /dev/null
@@ -1,47 +0,0 @@
-from infer_pack.models_onnx_moess import SynthesizerTrnMs256NSFsidM
-import torch
-
-person = "Shiroha/shiroha.pth"
-exported_path = "model.onnx"
-
-
-cpt = torch.load(person, map_location="cpu")
-cpt["config"][-3] = cpt["weight"]["emb_g.weight"].shape[0] # n_spk
-print(*cpt["config"])
-net_g = SynthesizerTrnMs256NSFsidM(*cpt["config"], is_half=False)
-net_g.load_state_dict(cpt["weight"], strict=False)
-
-test_phone = torch.rand(1, 200, 256)
-test_phone_lengths = torch.tensor([200]).long()
-test_pitch = torch.randint(size=(1, 200), low=5, high=255)
-test_pitchf = torch.rand(1, 200)
-test_ds = torch.LongTensor([0])
-test_rnd = torch.rand(1, 192, 200)
-input_names = ["phone", "phone_lengths", "pitch", "pitchf", "ds", "rnd"]
-output_names = [
- "audio",
-]
-device = "cpu"
-torch.onnx.export(
- net_g,
- (
- test_phone.to(device),
- test_phone_lengths.to(device),
- test_pitch.to(device),
- test_pitchf.to(device),
- test_ds.to(device),
- test_rnd.to(device),
- ),
- exported_path,
- dynamic_axes={
- "phone": [1],
- "pitch": [1],
- "pitchf": [1],
- "rnd": [2],
- },
- do_constant_folding=False,
- opset_version=16,
- verbose=False,
- input_names=input_names,
- output_names=output_names,
-)
diff --git a/spaces/arxify/RVC-beta-v2-0618/runtime/Lib/site-packages/altair/examples/bar_and_line_with_dual_axis.py b/spaces/arxify/RVC-beta-v2-0618/runtime/Lib/site-packages/altair/examples/bar_and_line_with_dual_axis.py
deleted file mode 100644
index 78e3010ecc582468a3977dcf6aec64e4de142a3d..0000000000000000000000000000000000000000
--- a/spaces/arxify/RVC-beta-v2-0618/runtime/Lib/site-packages/altair/examples/bar_and_line_with_dual_axis.py
+++ /dev/null
@@ -1,22 +0,0 @@
-"""
-Bar Chart with Line on Dual Axis
---------------------------------
-This example shows how to combine two plots and keep their axes.
-
-For a more polished version of this chart, see :ref:`gallery_wheat_wages`.
-"""
-# category: bar charts
-import altair as alt
-from vega_datasets import data
-
-source = data.wheat()
-
-base = alt.Chart(source).encode(x='year:O')
-
-bar = base.mark_bar().encode(y='wheat:Q')
-
-line = base.mark_line(color='red').encode(
- y='wages:Q'
-)
-
-(bar + line).properties(width=600)
diff --git a/spaces/arxify/RVC-beta-v2-0618/runtime/Lib/site-packages/altair/examples/tests/test_examples.py b/spaces/arxify/RVC-beta-v2-0618/runtime/Lib/site-packages/altair/examples/tests/test_examples.py
deleted file mode 100644
index 0b94d6528636214ecc6bcf4f28848c4a8062840b..0000000000000000000000000000000000000000
--- a/spaces/arxify/RVC-beta-v2-0618/runtime/Lib/site-packages/altair/examples/tests/test_examples.py
+++ /dev/null
@@ -1,44 +0,0 @@
-import io
-import pkgutil
-
-import pytest
-
-from altair.utils.execeval import eval_block
-from altair import examples
-
-
-@pytest.fixture
-def require_altair_saver_png():
- try:
- import altair_saver # noqa: F401
- except ImportError:
- pytest.skip("altair_saver not importable; cannot run saver tests")
- if "png" not in altair_saver.available_formats('vega-lite'):
- pytest.skip("altair_saver not configured to save to png")
-
-
-def iter_example_filenames():
- for importer, modname, ispkg in pkgutil.iter_modules(examples.__path__):
- if ispkg or modname.startswith('_'):
- continue
- yield modname + '.py'
-
-
-@pytest.mark.parametrize('filename', iter_example_filenames())
-def test_examples(filename: str):
- source = pkgutil.get_data(examples.__name__, filename)
- chart = eval_block(source)
-
- if chart is None:
- raise ValueError("Example file should define chart in its final "
- "statement.")
- chart.to_dict()
-
-
-@pytest.mark.parametrize('filename', iter_example_filenames())
-def test_render_examples_to_png(require_altair_saver_png, filename):
- source = pkgutil.get_data(examples.__name__, filename)
- chart = eval_block(source)
- out = io.BytesIO()
- chart.save(out, format="png")
- assert out.getvalue().startswith(b'\x89PNG')
diff --git a/spaces/arxify/RVC-beta-v2-0618/runtime/Lib/site-packages/altair/examples/us_incomebrackets_by_state_facet.py b/spaces/arxify/RVC-beta-v2-0618/runtime/Lib/site-packages/altair/examples/us_incomebrackets_by_state_facet.py
deleted file mode 100644
index 70f330481ee85c10b131f5b472ce32814e73236f..0000000000000000000000000000000000000000
--- a/spaces/arxify/RVC-beta-v2-0618/runtime/Lib/site-packages/altair/examples/us_incomebrackets_by_state_facet.py
+++ /dev/null
@@ -1,29 +0,0 @@
-"""
-US Income by State: Wrapped Facet
----------------------------------
-This example shows how to create a map of income in the US by state,
-faceted over income brackets
-"""
-# category: maps
-
-import altair as alt
-from vega_datasets import data
-
-states = alt.topo_feature(data.us_10m.url, 'states')
-source = data.income.url
-
-alt.Chart(source).mark_geoshape().encode(
- shape='geo:G',
- color='pct:Q',
- tooltip=['name:N', 'pct:Q'],
- facet=alt.Facet('group:N', columns=2),
-).transform_lookup(
- lookup='id',
- from_=alt.LookupData(data=states, key='id'),
- as_='geo'
-).properties(
- width=300,
- height=175,
-).project(
- type='albersUsa'
-)
\ No newline at end of file
diff --git a/spaces/aseuteurideu/audio_deepfake_detector/data/augmentation_utils.py b/spaces/aseuteurideu/audio_deepfake_detector/data/augmentation_utils.py
deleted file mode 100644
index 9ed98fda861f3a1fcf67de534c79a76b55575163..0000000000000000000000000000000000000000
--- a/spaces/aseuteurideu/audio_deepfake_detector/data/augmentation_utils.py
+++ /dev/null
@@ -1,88 +0,0 @@
-import cv2
-import librosa
-import numpy as np
-import albumentations
-from albumentations import (Compose, ImageCompression, GaussNoise, HorizontalFlip,
- PadIfNeeded, OneOf,ToGray, ShiftScaleRotate, GaussianBlur,
- RandomBrightnessContrast, FancyPCA, HueSaturationValue, BasicTransform)
-
-
-class AudioTransform(BasicTransform):
- """ Transform for audio task. This is the main class where we override the targets and update params function for our need"""
- @property
- def targets(self):
- return {"data": self.apply}
-
- def update_params(self, params, **kwargs):
- if hasattr(self, "interpolation"):
- params["interpolation"] = self.interpolation
- if hasattr(self, "fill_value"):
- params["fill_value"] = self.fill_value
- return params
-
-class TimeShifting(AudioTransform):
- """ Do time shifting of audio """
- def __init__(self, always_apply=False, p=0.5):
- super(TimeShifting, self).__init__(always_apply, p)
-
- def apply(self,data,**params):
- '''
- data : ndarray of audio timeseries
- '''
- start_ = int(np.random.uniform(-80000,80000))
- if start_ >= 0:
- audio_time_shift = np.r_[data[start_:], np.random.uniform(-0.001,0.001, start_)]
- else:
- audio_time_shift = np.r_[np.random.uniform(-0.001,0.001, -start_), data[:start_]]
-
- return audio_time_shift
-
-class PitchShift(AudioTransform):
- """ Do time shifting of audio """
- def __init__(self, always_apply=False, p=0.5 , n_steps=None):
- super(PitchShift, self).__init__(always_apply, p)
- '''
- nsteps here is equal to number of semitones
- '''
-
- self.n_steps = n_steps
-
- def apply(self,data,**params):
- '''
- data : ndarray of audio timeseries
- '''
- return librosa.effects.pitch_shift(data,sr=16000,n_steps=self.n_steps)
-
-
-class AddGaussianNoise(AudioTransform):
- """ Do time shifting of audio """
- def __init__(self, always_apply=False, p=0.5):
- super(AddGaussianNoise, self).__init__(always_apply, p)
-
-
- def apply(self,data,**params):
- '''
- data : ndarray of audio timeseries
- '''
- noise = np.random.randn(len(data))
- data_wn = data + 0.005*noise
- return data_wn
-
-
-create_frame_transforms = Compose([
- ImageCompression(quality_lower=60, quality_upper=100, p=0.5),
- GaussNoise(p=0.1),
- GaussianBlur(blur_limit=3, p=0.05),
- HorizontalFlip(),
- PadIfNeeded(min_height=256, min_width=256, border_mode=cv2.BORDER_CONSTANT),
- OneOf([RandomBrightnessContrast(), FancyPCA(), HueSaturationValue()], p=0.7),
- ToGray(p=0.2),
- ShiftScaleRotate(shift_limit=0.1, scale_limit=0.2, rotate_limit=10, border_mode=cv2.BORDER_CONSTANT, p=0.5),])
-
-
-
-create_spec_transforms = albumentations.Compose([
- TimeShifting(p=0.9), # here not p=1.0 because your nets should get some difficulties
- AddGaussianNoise(p=0.8),
- PitchShift(p=0.5,n_steps=4)
- ])
diff --git a/spaces/ashercn97/AsherTesting/api-examples/api-example.py b/spaces/ashercn97/AsherTesting/api-examples/api-example.py
deleted file mode 100644
index 4e45de9eea205e4ee97ee94e3c197291c0323178..0000000000000000000000000000000000000000
--- a/spaces/ashercn97/AsherTesting/api-examples/api-example.py
+++ /dev/null
@@ -1,57 +0,0 @@
-import requests
-
-# For local streaming, the websockets are hosted without ssl - http://
-HOST = 'localhost:5000'
-URI = f'http://{HOST}/api/v1/generate'
-
-# For reverse-proxied streaming, the remote will likely host with ssl - https://
-# URI = 'https://your-uri-here.trycloudflare.com/api/v1/generate'
-
-
-def run(prompt):
- request = {
- 'prompt': prompt,
- 'max_new_tokens': 250,
-
- # Generation params. If 'preset' is set to different than 'None', the values
- # in presets/preset-name.yaml are used instead of the individual numbers.
- 'preset': 'None',
- 'do_sample': True,
- 'temperature': 0.7,
- 'top_p': 0.1,
- 'typical_p': 1,
- 'epsilon_cutoff': 0, # In units of 1e-4
- 'eta_cutoff': 0, # In units of 1e-4
- 'tfs': 1,
- 'top_a': 0,
- 'repetition_penalty': 1.18,
- 'repetition_penalty_range': 0,
- 'top_k': 40,
- 'min_length': 0,
- 'no_repeat_ngram_size': 0,
- 'num_beams': 1,
- 'penalty_alpha': 0,
- 'length_penalty': 1,
- 'early_stopping': False,
- 'mirostat_mode': 0,
- 'mirostat_tau': 5,
- 'mirostat_eta': 0.1,
-
- 'seed': -1,
- 'add_bos_token': True,
- 'truncation_length': 2048,
- 'ban_eos_token': False,
- 'skip_special_tokens': True,
- 'stopping_strings': []
- }
-
- response = requests.post(URI, json=request)
-
- if response.status_code == 200:
- result = response.json()['results'][0]['text']
- print(prompt + result)
-
-
-if __name__ == '__main__':
- prompt = "In order to make homemade bread, follow these steps:\n1)"
- run(prompt)
diff --git a/spaces/asim266/image-background-remover/app.py b/spaces/asim266/image-background-remover/app.py
deleted file mode 100644
index 589e498d2df13aaa5bc1f29ccaa700426639e541..0000000000000000000000000000000000000000
--- a/spaces/asim266/image-background-remover/app.py
+++ /dev/null
@@ -1,22 +0,0 @@
-import gradio as gr
-from rembg import remove
-from PIL import Image
-
-def remove_background(input_image):
-
- input = Image.open(input_image) # load image
- output = remove(input) # remove background
- return output
-
-
-input_image = gr.inputs.Image(type="filepath", label="Input Image")
-output_image = gr.inputs.Image(type="filepath", label="Output Image")
-# Create a Gradio interface
-iface = gr.Interface(remove_background,
- inputs=input_image,
- outputs=output_image,
- title='Image Background Remover',
- description='Upload an image and it will remove.')
-
-# Launch the interface
-iface.launch()
diff --git a/spaces/at2507/SM_NLP_RecoSys/Data/Mentor_interviews/Ahmed Ekbagoury.html b/spaces/at2507/SM_NLP_RecoSys/Data/Mentor_interviews/Ahmed Ekbagoury.html
deleted file mode 100644
index 3e2642271a06bb214967650f6469ee368a728a0a..0000000000000000000000000000000000000000
--- a/spaces/at2507/SM_NLP_RecoSys/Data/Mentor_interviews/Ahmed Ekbagoury.html
+++ /dev/null
@@ -1,134 +0,0 @@
-
-
-
- Ahmed Ekbagoury
-
-
-
-
-
-
Ahmed Ekbagoury
-
-
-
How did you hear about SM?
A friend told me about it (a month or so ago)
Brief background
Ms waterloo (ML and data mining)
started PhD at Purdue in ML
internship at google
moved back to Canada joined google, work as MLE
like seeing the impact of things I work with
can translate academia to industry
Mentorship exp
yes, TA
onboarding new team members (new hires, internal tranfers)
"host" for an intern (onboarding, project design, technical support, to full-time offer)
What do beginners need and how can you help?
Two aspects
technical
understanding the business needs (esp academics)
understanding of the engineering cost of building things (e.g. operational/maintenance tasks)
intimidation by jargon/options/tools/frameworks
"things do not have to be that complicated"
need an entry point, you'll learn the rest
you don't need to know EVERYTHING
psychological/interpersonal
understand where others stand, time commitment, goals
understand pain points, what they achieved
own trust
As a mentor:
help ppl understand how to be practical and apply ML in real-world problems
with examples / walkthroughs
Wish someone had done this for me
developing a plan for structured learning
get over the overwhelming feeling
start simple, build momentum
start with a good problem
Grateful for my mentors
-
-
Questions about SM:
What is the profile of the applicants?
What do mentees want help with?
What type of roles?
Do ppl want help with coding interviews?
-
-
-
-
-
-
\ No newline at end of file
diff --git a/spaces/attention-refocusing/Attention-refocusing/dataset/__init__.py b/spaces/attention-refocusing/Attention-refocusing/dataset/__init__.py
deleted file mode 100644
index e69de29bb2d1d6434b8b29ae775ad8c2e48c5391..0000000000000000000000000000000000000000
diff --git a/spaces/awacke1/sileod-deberta-v3-base-tasksource-nli/README.md b/spaces/awacke1/sileod-deberta-v3-base-tasksource-nli/README.md
deleted file mode 100644
index d385f1e47ecd269606b9ede430540abf92ceb5bf..0000000000000000000000000000000000000000
--- a/spaces/awacke1/sileod-deberta-v3-base-tasksource-nli/README.md
+++ /dev/null
@@ -1,12 +0,0 @@
----
-title: Sileod Deberta V3 Base Tasksource Nli
-emoji: 👀👀👀
-colorFrom: purple
-colorTo: red
-sdk: gradio
-sdk_version: 3.18.0
-app_file: app.py
-pinned: false
----
-
-Check out the configuration reference at https://huggingface.co/docs/hub/spaces-config-reference
diff --git a/spaces/banana-projects/web3d/node_modules/three/src/geometries/CircleGeometry.d.ts b/spaces/banana-projects/web3d/node_modules/three/src/geometries/CircleGeometry.d.ts
deleted file mode 100644
index 7c74b3fc350339fac8e98bcd008092406592ef04..0000000000000000000000000000000000000000
--- a/spaces/banana-projects/web3d/node_modules/three/src/geometries/CircleGeometry.d.ts
+++ /dev/null
@@ -1,37 +0,0 @@
-import { Geometry } from './../core/Geometry';
-import { BufferGeometry } from '../core/BufferGeometry';
-
-/**
- * @deprecated Use {@link BoxGeometry} instead.
- */
-export class CircleBufferGeometry extends BufferGeometry {
- constructor(
- radius?: number,
- segments?: number,
- thetaStart?: number,
- thetaLength?: number
- );
-
- parameters: {
- radius: number;
- segments: number;
- thetaStart: number;
- thetaLength: number;
- };
-}
-
-export class CircleGeometry extends Geometry {
- constructor(
- radius?: number,
- segments?: number,
- thetaStart?: number,
- thetaLength?: number
- );
-
- parameters: {
- radius: number;
- segments: number;
- thetaStart: number;
- thetaLength: number;
- };
-}
diff --git a/spaces/banana-projects/web3d/node_modules/three/src/geometries/Geometries.d.ts b/spaces/banana-projects/web3d/node_modules/three/src/geometries/Geometries.d.ts
deleted file mode 100644
index a5f780877c85152e9625b25973c1f62d0ee77ebd..0000000000000000000000000000000000000000
--- a/spaces/banana-projects/web3d/node_modules/three/src/geometries/Geometries.d.ts
+++ /dev/null
@@ -1,22 +0,0 @@
-export * from './WireframeGeometry';
-export * from './ParametricGeometry';
-export * from './TetrahedronGeometry';
-export * from './OctahedronGeometry';
-export * from './IcosahedronGeometry';
-export * from './DodecahedronGeometry';
-export * from './PolyhedronGeometry';
-export * from './TubeGeometry';
-export * from './TorusKnotGeometry';
-export * from './TorusGeometry';
-export * from './TextGeometry';
-export * from './SphereGeometry';
-export * from './RingGeometry';
-export * from './PlaneGeometry';
-export * from './LatheGeometry';
-export * from './ShapeGeometry';
-export * from './ExtrudeGeometry';
-export * from './EdgesGeometry';
-export * from './ConeGeometry';
-export * from './CylinderGeometry';
-export * from './CircleGeometry';
-export * from './BoxGeometry';
diff --git a/spaces/beihai/GFPGAN-V1.3-whole-image/basicsr/ops/dcn/src/deform_conv_cuda.cpp b/spaces/beihai/GFPGAN-V1.3-whole-image/basicsr/ops/dcn/src/deform_conv_cuda.cpp
deleted file mode 100644
index b465c493a3dd67d320b7a8997fbd501d2f89c807..0000000000000000000000000000000000000000
--- a/spaces/beihai/GFPGAN-V1.3-whole-image/basicsr/ops/dcn/src/deform_conv_cuda.cpp
+++ /dev/null
@@ -1,685 +0,0 @@
-// modify from
-// https://github.com/chengdazhi/Deformable-Convolution-V2-PyTorch/blob/mmdetection/mmdet/ops/dcn/src/deform_conv_cuda.c
-
-#include
-#include
-
-#include
-#include
-
-void deformable_im2col(const at::Tensor data_im, const at::Tensor data_offset,
- const int channels, const int height, const int width,
- const int ksize_h, const int ksize_w, const int pad_h,
- const int pad_w, const int stride_h, const int stride_w,
- const int dilation_h, const int dilation_w,
- const int parallel_imgs, const int deformable_group,
- at::Tensor data_col);
-
-void deformable_col2im(const at::Tensor data_col, const at::Tensor data_offset,
- const int channels, const int height, const int width,
- const int ksize_h, const int ksize_w, const int pad_h,
- const int pad_w, const int stride_h, const int stride_w,
- const int dilation_h, const int dilation_w,
- const int parallel_imgs, const int deformable_group,
- at::Tensor grad_im);
-
-void deformable_col2im_coord(
- const at::Tensor data_col, const at::Tensor data_im,
- const at::Tensor data_offset, const int channels, const int height,
- const int width, const int ksize_h, const int ksize_w, const int pad_h,
- const int pad_w, const int stride_h, const int stride_w,
- const int dilation_h, const int dilation_w, const int parallel_imgs,
- const int deformable_group, at::Tensor grad_offset);
-
-void modulated_deformable_im2col_cuda(
- const at::Tensor data_im, const at::Tensor data_offset,
- const at::Tensor data_mask, const int batch_size, const int channels,
- const int height_im, const int width_im, const int height_col,
- const int width_col, const int kernel_h, const int kenerl_w,
- const int pad_h, const int pad_w, const int stride_h, const int stride_w,
- const int dilation_h, const int dilation_w, const int deformable_group,
- at::Tensor data_col);
-
-void modulated_deformable_col2im_cuda(
- const at::Tensor data_col, const at::Tensor data_offset,
- const at::Tensor data_mask, const int batch_size, const int channels,
- const int height_im, const int width_im, const int height_col,
- const int width_col, const int kernel_h, const int kenerl_w,
- const int pad_h, const int pad_w, const int stride_h, const int stride_w,
- const int dilation_h, const int dilation_w, const int deformable_group,
- at::Tensor grad_im);
-
-void modulated_deformable_col2im_coord_cuda(
- const at::Tensor data_col, const at::Tensor data_im,
- const at::Tensor data_offset, const at::Tensor data_mask,
- const int batch_size, const int channels, const int height_im,
- const int width_im, const int height_col, const int width_col,
- const int kernel_h, const int kenerl_w, const int pad_h, const int pad_w,
- const int stride_h, const int stride_w, const int dilation_h,
- const int dilation_w, const int deformable_group, at::Tensor grad_offset,
- at::Tensor grad_mask);
-
-void shape_check(at::Tensor input, at::Tensor offset, at::Tensor *gradOutput,
- at::Tensor weight, int kH, int kW, int dH, int dW, int padH,
- int padW, int dilationH, int dilationW, int group,
- int deformable_group) {
- TORCH_CHECK(weight.ndimension() == 4,
- "4D weight tensor (nOutputPlane,nInputPlane,kH,kW) expected, "
- "but got: %s",
- weight.ndimension());
-
- TORCH_CHECK(weight.is_contiguous(), "weight tensor has to be contiguous");
-
- TORCH_CHECK(kW > 0 && kH > 0,
- "kernel size should be greater than zero, but got kH: %d kW: %d", kH,
- kW);
-
- TORCH_CHECK((weight.size(2) == kH && weight.size(3) == kW),
- "kernel size should be consistent with weight, ",
- "but got kH: %d kW: %d weight.size(2): %d, weight.size(3): %d", kH,
- kW, weight.size(2), weight.size(3));
-
- TORCH_CHECK(dW > 0 && dH > 0,
- "stride should be greater than zero, but got dH: %d dW: %d", dH, dW);
-
- TORCH_CHECK(
- dilationW > 0 && dilationH > 0,
- "dilation should be greater than 0, but got dilationH: %d dilationW: %d",
- dilationH, dilationW);
-
- int ndim = input.ndimension();
- int dimf = 0;
- int dimh = 1;
- int dimw = 2;
-
- if (ndim == 4) {
- dimf++;
- dimh++;
- dimw++;
- }
-
- TORCH_CHECK(ndim == 3 || ndim == 4, "3D or 4D input tensor expected but got: %s",
- ndim);
-
- long nInputPlane = weight.size(1) * group;
- long inputHeight = input.size(dimh);
- long inputWidth = input.size(dimw);
- long nOutputPlane = weight.size(0);
- long outputHeight =
- (inputHeight + 2 * padH - (dilationH * (kH - 1) + 1)) / dH + 1;
- long outputWidth =
- (inputWidth + 2 * padW - (dilationW * (kW - 1) + 1)) / dW + 1;
-
- TORCH_CHECK(nInputPlane % deformable_group == 0,
- "input channels must divide deformable group size");
-
- if (outputWidth < 1 || outputHeight < 1)
- AT_ERROR(
- "Given input size: (%ld x %ld x %ld). "
- "Calculated output size: (%ld x %ld x %ld). Output size is too small",
- nInputPlane, inputHeight, inputWidth, nOutputPlane, outputHeight,
- outputWidth);
-
- TORCH_CHECK(input.size(1) == nInputPlane,
- "invalid number of input planes, expected: %d, but got: %d",
- nInputPlane, input.size(1));
-
- TORCH_CHECK((inputHeight >= kH && inputWidth >= kW),
- "input image is smaller than kernel");
-
- TORCH_CHECK((offset.size(2) == outputHeight && offset.size(3) == outputWidth),
- "invalid spatial size of offset, expected height: %d width: %d, but "
- "got height: %d width: %d",
- outputHeight, outputWidth, offset.size(2), offset.size(3));
-
- TORCH_CHECK((offset.size(1) == deformable_group * 2 * kH * kW),
- "invalid number of channels of offset");
-
- if (gradOutput != NULL) {
- TORCH_CHECK(gradOutput->size(dimf) == nOutputPlane,
- "invalid number of gradOutput planes, expected: %d, but got: %d",
- nOutputPlane, gradOutput->size(dimf));
-
- TORCH_CHECK((gradOutput->size(dimh) == outputHeight &&
- gradOutput->size(dimw) == outputWidth),
- "invalid size of gradOutput, expected height: %d width: %d , but "
- "got height: %d width: %d",
- outputHeight, outputWidth, gradOutput->size(dimh),
- gradOutput->size(dimw));
- }
-}
-
-int deform_conv_forward_cuda(at::Tensor input, at::Tensor weight,
- at::Tensor offset, at::Tensor output,
- at::Tensor columns, at::Tensor ones, int kW,
- int kH, int dW, int dH, int padW, int padH,
- int dilationW, int dilationH, int group,
- int deformable_group, int im2col_step) {
- // todo: resize columns to include im2col: done
- // todo: add im2col_step as input
- // todo: add new output buffer and transpose it to output (or directly
- // transpose output) todo: possibly change data indexing because of
- // parallel_imgs
-
- shape_check(input, offset, NULL, weight, kH, kW, dH, dW, padH, padW,
- dilationH, dilationW, group, deformable_group);
- at::DeviceGuard guard(input.device());
-
- input = input.contiguous();
- offset = offset.contiguous();
- weight = weight.contiguous();
-
- int batch = 1;
- if (input.ndimension() == 3) {
- // Force batch
- batch = 0;
- input.unsqueeze_(0);
- offset.unsqueeze_(0);
- }
-
- // todo: assert batchsize dividable by im2col_step
-
- long batchSize = input.size(0);
- long nInputPlane = input.size(1);
- long inputHeight = input.size(2);
- long inputWidth = input.size(3);
-
- long nOutputPlane = weight.size(0);
-
- long outputWidth =
- (inputWidth + 2 * padW - (dilationW * (kW - 1) + 1)) / dW + 1;
- long outputHeight =
- (inputHeight + 2 * padH - (dilationH * (kH - 1) + 1)) / dH + 1;
-
- TORCH_CHECK((offset.size(0) == batchSize), "invalid batch size of offset");
-
- output = output.view({batchSize / im2col_step, im2col_step, nOutputPlane,
- outputHeight, outputWidth});
- columns = at::zeros(
- {nInputPlane * kW * kH, im2col_step * outputHeight * outputWidth},
- input.options());
-
- if (ones.ndimension() != 2 ||
- ones.size(0) * ones.size(1) < outputHeight * outputWidth) {
- ones = at::ones({outputHeight, outputWidth}, input.options());
- }
-
- input = input.view({batchSize / im2col_step, im2col_step, nInputPlane,
- inputHeight, inputWidth});
- offset =
- offset.view({batchSize / im2col_step, im2col_step,
- deformable_group * 2 * kH * kW, outputHeight, outputWidth});
-
- at::Tensor output_buffer =
- at::zeros({batchSize / im2col_step, nOutputPlane,
- im2col_step * outputHeight, outputWidth},
- output.options());
-
- output_buffer = output_buffer.view(
- {output_buffer.size(0), group, output_buffer.size(1) / group,
- output_buffer.size(2), output_buffer.size(3)});
-
- for (int elt = 0; elt < batchSize / im2col_step; elt++) {
- deformable_im2col(input[elt], offset[elt], nInputPlane, inputHeight,
- inputWidth, kH, kW, padH, padW, dH, dW, dilationH,
- dilationW, im2col_step, deformable_group, columns);
-
- columns = columns.view({group, columns.size(0) / group, columns.size(1)});
- weight = weight.view({group, weight.size(0) / group, weight.size(1),
- weight.size(2), weight.size(3)});
-
- for (int g = 0; g < group; g++) {
- output_buffer[elt][g] = output_buffer[elt][g]
- .flatten(1)
- .addmm_(weight[g].flatten(1), columns[g])
- .view_as(output_buffer[elt][g]);
- }
- }
-
- output_buffer = output_buffer.view(
- {output_buffer.size(0), output_buffer.size(1) * output_buffer.size(2),
- output_buffer.size(3), output_buffer.size(4)});
-
- output_buffer = output_buffer.view({batchSize / im2col_step, nOutputPlane,
- im2col_step, outputHeight, outputWidth});
- output_buffer.transpose_(1, 2);
- output.copy_(output_buffer);
- output = output.view({batchSize, nOutputPlane, outputHeight, outputWidth});
-
- input = input.view({batchSize, nInputPlane, inputHeight, inputWidth});
- offset = offset.view(
- {batchSize, deformable_group * 2 * kH * kW, outputHeight, outputWidth});
-
- if (batch == 0) {
- output = output.view({nOutputPlane, outputHeight, outputWidth});
- input = input.view({nInputPlane, inputHeight, inputWidth});
- offset = offset.view({offset.size(1), offset.size(2), offset.size(3)});
- }
-
- return 1;
-}
-
-int deform_conv_backward_input_cuda(at::Tensor input, at::Tensor offset,
- at::Tensor gradOutput, at::Tensor gradInput,
- at::Tensor gradOffset, at::Tensor weight,
- at::Tensor columns, int kW, int kH, int dW,
- int dH, int padW, int padH, int dilationW,
- int dilationH, int group,
- int deformable_group, int im2col_step) {
- shape_check(input, offset, &gradOutput, weight, kH, kW, dH, dW, padH, padW,
- dilationH, dilationW, group, deformable_group);
- at::DeviceGuard guard(input.device());
-
- input = input.contiguous();
- offset = offset.contiguous();
- gradOutput = gradOutput.contiguous();
- weight = weight.contiguous();
-
- int batch = 1;
-
- if (input.ndimension() == 3) {
- // Force batch
- batch = 0;
- input = input.view({1, input.size(0), input.size(1), input.size(2)});
- offset = offset.view({1, offset.size(0), offset.size(1), offset.size(2)});
- gradOutput = gradOutput.view(
- {1, gradOutput.size(0), gradOutput.size(1), gradOutput.size(2)});
- }
-
- long batchSize = input.size(0);
- long nInputPlane = input.size(1);
- long inputHeight = input.size(2);
- long inputWidth = input.size(3);
-
- long nOutputPlane = weight.size(0);
-
- long outputWidth =
- (inputWidth + 2 * padW - (dilationW * (kW - 1) + 1)) / dW + 1;
- long outputHeight =
- (inputHeight + 2 * padH - (dilationH * (kH - 1) + 1)) / dH + 1;
-
- TORCH_CHECK((offset.size(0) == batchSize), 3, "invalid batch size of offset");
- gradInput = gradInput.view({batchSize, nInputPlane, inputHeight, inputWidth});
- columns = at::zeros(
- {nInputPlane * kW * kH, im2col_step * outputHeight * outputWidth},
- input.options());
-
- // change order of grad output
- gradOutput = gradOutput.view({batchSize / im2col_step, im2col_step,
- nOutputPlane, outputHeight, outputWidth});
- gradOutput.transpose_(1, 2);
-
- gradInput = gradInput.view({batchSize / im2col_step, im2col_step, nInputPlane,
- inputHeight, inputWidth});
- input = input.view({batchSize / im2col_step, im2col_step, nInputPlane,
- inputHeight, inputWidth});
- gradOffset = gradOffset.view({batchSize / im2col_step, im2col_step,
- deformable_group * 2 * kH * kW, outputHeight,
- outputWidth});
- offset =
- offset.view({batchSize / im2col_step, im2col_step,
- deformable_group * 2 * kH * kW, outputHeight, outputWidth});
-
- for (int elt = 0; elt < batchSize / im2col_step; elt++) {
- // divide into groups
- columns = columns.view({group, columns.size(0) / group, columns.size(1)});
- weight = weight.view({group, weight.size(0) / group, weight.size(1),
- weight.size(2), weight.size(3)});
- gradOutput = gradOutput.view(
- {gradOutput.size(0), group, gradOutput.size(1) / group,
- gradOutput.size(2), gradOutput.size(3), gradOutput.size(4)});
-
- for (int g = 0; g < group; g++) {
- columns[g] = columns[g].addmm_(weight[g].flatten(1).transpose(0, 1),
- gradOutput[elt][g].flatten(1), 0.0f, 1.0f);
- }
-
- columns =
- columns.view({columns.size(0) * columns.size(1), columns.size(2)});
- gradOutput = gradOutput.view(
- {gradOutput.size(0), gradOutput.size(1) * gradOutput.size(2),
- gradOutput.size(3), gradOutput.size(4), gradOutput.size(5)});
-
- deformable_col2im_coord(columns, input[elt], offset[elt], nInputPlane,
- inputHeight, inputWidth, kH, kW, padH, padW, dH, dW,
- dilationH, dilationW, im2col_step, deformable_group,
- gradOffset[elt]);
-
- deformable_col2im(columns, offset[elt], nInputPlane, inputHeight,
- inputWidth, kH, kW, padH, padW, dH, dW, dilationH,
- dilationW, im2col_step, deformable_group, gradInput[elt]);
- }
-
- gradOutput.transpose_(1, 2);
- gradOutput =
- gradOutput.view({batchSize, nOutputPlane, outputHeight, outputWidth});
-
- gradInput = gradInput.view({batchSize, nInputPlane, inputHeight, inputWidth});
- input = input.view({batchSize, nInputPlane, inputHeight, inputWidth});
- gradOffset = gradOffset.view(
- {batchSize, deformable_group * 2 * kH * kW, outputHeight, outputWidth});
- offset = offset.view(
- {batchSize, deformable_group * 2 * kH * kW, outputHeight, outputWidth});
-
- if (batch == 0) {
- gradOutput = gradOutput.view({nOutputPlane, outputHeight, outputWidth});
- input = input.view({nInputPlane, inputHeight, inputWidth});
- gradInput = gradInput.view({nInputPlane, inputHeight, inputWidth});
- offset = offset.view({offset.size(1), offset.size(2), offset.size(3)});
- gradOffset =
- gradOffset.view({offset.size(1), offset.size(2), offset.size(3)});
- }
-
- return 1;
-}
-
-int deform_conv_backward_parameters_cuda(
- at::Tensor input, at::Tensor offset, at::Tensor gradOutput,
- at::Tensor gradWeight, // at::Tensor gradBias,
- at::Tensor columns, at::Tensor ones, int kW, int kH, int dW, int dH,
- int padW, int padH, int dilationW, int dilationH, int group,
- int deformable_group, float scale, int im2col_step) {
- // todo: transpose and reshape outGrad
- // todo: reshape columns
- // todo: add im2col_step as input
-
- shape_check(input, offset, &gradOutput, gradWeight, kH, kW, dH, dW, padH,
- padW, dilationH, dilationW, group, deformable_group);
- at::DeviceGuard guard(input.device());
-
- input = input.contiguous();
- offset = offset.contiguous();
- gradOutput = gradOutput.contiguous();
-
- int batch = 1;
-
- if (input.ndimension() == 3) {
- // Force batch
- batch = 0;
- input = input.view(
- at::IntList({1, input.size(0), input.size(1), input.size(2)}));
- gradOutput = gradOutput.view(
- {1, gradOutput.size(0), gradOutput.size(1), gradOutput.size(2)});
- }
-
- long batchSize = input.size(0);
- long nInputPlane = input.size(1);
- long inputHeight = input.size(2);
- long inputWidth = input.size(3);
-
- long nOutputPlane = gradWeight.size(0);
-
- long outputWidth =
- (inputWidth + 2 * padW - (dilationW * (kW - 1) + 1)) / dW + 1;
- long outputHeight =
- (inputHeight + 2 * padH - (dilationH * (kH - 1) + 1)) / dH + 1;
-
- TORCH_CHECK((offset.size(0) == batchSize), "invalid batch size of offset");
-
- columns = at::zeros(
- {nInputPlane * kW * kH, im2col_step * outputHeight * outputWidth},
- input.options());
-
- gradOutput = gradOutput.view({batchSize / im2col_step, im2col_step,
- nOutputPlane, outputHeight, outputWidth});
- gradOutput.transpose_(1, 2);
-
- at::Tensor gradOutputBuffer = at::zeros_like(gradOutput);
- gradOutputBuffer =
- gradOutputBuffer.view({batchSize / im2col_step, nOutputPlane, im2col_step,
- outputHeight, outputWidth});
- gradOutputBuffer.copy_(gradOutput);
- gradOutputBuffer =
- gradOutputBuffer.view({batchSize / im2col_step, nOutputPlane,
- im2col_step * outputHeight, outputWidth});
-
- gradOutput.transpose_(1, 2);
- gradOutput =
- gradOutput.view({batchSize, nOutputPlane, outputHeight, outputWidth});
-
- input = input.view({batchSize / im2col_step, im2col_step, nInputPlane,
- inputHeight, inputWidth});
- offset =
- offset.view({batchSize / im2col_step, im2col_step,
- deformable_group * 2 * kH * kW, outputHeight, outputWidth});
-
- for (int elt = 0; elt < batchSize / im2col_step; elt++) {
- deformable_im2col(input[elt], offset[elt], nInputPlane, inputHeight,
- inputWidth, kH, kW, padH, padW, dH, dW, dilationH,
- dilationW, im2col_step, deformable_group, columns);
-
- // divide into group
- gradOutputBuffer = gradOutputBuffer.view(
- {gradOutputBuffer.size(0), group, gradOutputBuffer.size(1) / group,
- gradOutputBuffer.size(2), gradOutputBuffer.size(3)});
- columns = columns.view({group, columns.size(0) / group, columns.size(1)});
- gradWeight =
- gradWeight.view({group, gradWeight.size(0) / group, gradWeight.size(1),
- gradWeight.size(2), gradWeight.size(3)});
-
- for (int g = 0; g < group; g++) {
- gradWeight[g] = gradWeight[g]
- .flatten(1)
- .addmm_(gradOutputBuffer[elt][g].flatten(1),
- columns[g].transpose(1, 0), 1.0, scale)
- .view_as(gradWeight[g]);
- }
- gradOutputBuffer = gradOutputBuffer.view(
- {gradOutputBuffer.size(0),
- gradOutputBuffer.size(1) * gradOutputBuffer.size(2),
- gradOutputBuffer.size(3), gradOutputBuffer.size(4)});
- columns =
- columns.view({columns.size(0) * columns.size(1), columns.size(2)});
- gradWeight = gradWeight.view({gradWeight.size(0) * gradWeight.size(1),
- gradWeight.size(2), gradWeight.size(3),
- gradWeight.size(4)});
- }
-
- input = input.view({batchSize, nInputPlane, inputHeight, inputWidth});
- offset = offset.view(
- {batchSize, deformable_group * 2 * kH * kW, outputHeight, outputWidth});
-
- if (batch == 0) {
- gradOutput = gradOutput.view({nOutputPlane, outputHeight, outputWidth});
- input = input.view({nInputPlane, inputHeight, inputWidth});
- }
-
- return 1;
-}
-
-void modulated_deform_conv_cuda_forward(
- at::Tensor input, at::Tensor weight, at::Tensor bias, at::Tensor ones,
- at::Tensor offset, at::Tensor mask, at::Tensor output, at::Tensor columns,
- int kernel_h, int kernel_w, const int stride_h, const int stride_w,
- const int pad_h, const int pad_w, const int dilation_h,
- const int dilation_w, const int group, const int deformable_group,
- const bool with_bias) {
- TORCH_CHECK(input.is_contiguous(), "input tensor has to be contiguous");
- TORCH_CHECK(weight.is_contiguous(), "weight tensor has to be contiguous");
- at::DeviceGuard guard(input.device());
-
- const int batch = input.size(0);
- const int channels = input.size(1);
- const int height = input.size(2);
- const int width = input.size(3);
-
- const int channels_out = weight.size(0);
- const int channels_kernel = weight.size(1);
- const int kernel_h_ = weight.size(2);
- const int kernel_w_ = weight.size(3);
-
- if (kernel_h_ != kernel_h || kernel_w_ != kernel_w)
- AT_ERROR("Input shape and kernel shape won't match: (%d x %d vs %d x %d).",
- kernel_h_, kernel_w, kernel_h_, kernel_w_);
- if (channels != channels_kernel * group)
- AT_ERROR("Input shape and kernel channels won't match: (%d vs %d).",
- channels, channels_kernel * group);
-
- const int height_out =
- (height + 2 * pad_h - (dilation_h * (kernel_h - 1) + 1)) / stride_h + 1;
- const int width_out =
- (width + 2 * pad_w - (dilation_w * (kernel_w - 1) + 1)) / stride_w + 1;
-
- if (ones.ndimension() != 2 ||
- ones.size(0) * ones.size(1) < height_out * width_out) {
- // Resize plane and fill with ones...
- ones = at::ones({height_out, width_out}, input.options());
- }
-
- // resize output
- output = output.view({batch, channels_out, height_out, width_out}).zero_();
- // resize temporary columns
- columns =
- at::zeros({channels * kernel_h * kernel_w, 1 * height_out * width_out},
- input.options());
-
- output = output.view({output.size(0), group, output.size(1) / group,
- output.size(2), output.size(3)});
-
- for (int b = 0; b < batch; b++) {
- modulated_deformable_im2col_cuda(
- input[b], offset[b], mask[b], 1, channels, height, width, height_out,
- width_out, kernel_h, kernel_w, pad_h, pad_w, stride_h, stride_w,
- dilation_h, dilation_w, deformable_group, columns);
-
- // divide into group
- weight = weight.view({group, weight.size(0) / group, weight.size(1),
- weight.size(2), weight.size(3)});
- columns = columns.view({group, columns.size(0) / group, columns.size(1)});
-
- for (int g = 0; g < group; g++) {
- output[b][g] = output[b][g]
- .flatten(1)
- .addmm_(weight[g].flatten(1), columns[g])
- .view_as(output[b][g]);
- }
-
- weight = weight.view({weight.size(0) * weight.size(1), weight.size(2),
- weight.size(3), weight.size(4)});
- columns =
- columns.view({columns.size(0) * columns.size(1), columns.size(2)});
- }
-
- output = output.view({output.size(0), output.size(1) * output.size(2),
- output.size(3), output.size(4)});
-
- if (with_bias) {
- output += bias.view({1, bias.size(0), 1, 1});
- }
-}
-
-void modulated_deform_conv_cuda_backward(
- at::Tensor input, at::Tensor weight, at::Tensor bias, at::Tensor ones,
- at::Tensor offset, at::Tensor mask, at::Tensor columns,
- at::Tensor grad_input, at::Tensor grad_weight, at::Tensor grad_bias,
- at::Tensor grad_offset, at::Tensor grad_mask, at::Tensor grad_output,
- int kernel_h, int kernel_w, int stride_h, int stride_w, int pad_h,
- int pad_w, int dilation_h, int dilation_w, int group, int deformable_group,
- const bool with_bias) {
- TORCH_CHECK(input.is_contiguous(), "input tensor has to be contiguous");
- TORCH_CHECK(weight.is_contiguous(), "weight tensor has to be contiguous");
- at::DeviceGuard guard(input.device());
-
- const int batch = input.size(0);
- const int channels = input.size(1);
- const int height = input.size(2);
- const int width = input.size(3);
-
- const int channels_kernel = weight.size(1);
- const int kernel_h_ = weight.size(2);
- const int kernel_w_ = weight.size(3);
- if (kernel_h_ != kernel_h || kernel_w_ != kernel_w)
- AT_ERROR("Input shape and kernel shape won't match: (%d x %d vs %d x %d).",
- kernel_h_, kernel_w, kernel_h_, kernel_w_);
- if (channels != channels_kernel * group)
- AT_ERROR("Input shape and kernel channels won't match: (%d vs %d).",
- channels, channels_kernel * group);
-
- const int height_out =
- (height + 2 * pad_h - (dilation_h * (kernel_h - 1) + 1)) / stride_h + 1;
- const int width_out =
- (width + 2 * pad_w - (dilation_w * (kernel_w - 1) + 1)) / stride_w + 1;
-
- if (ones.ndimension() != 2 ||
- ones.size(0) * ones.size(1) < height_out * width_out) {
- // Resize plane and fill with ones...
- ones = at::ones({height_out, width_out}, input.options());
- }
-
- grad_input = grad_input.view({batch, channels, height, width});
- columns = at::zeros({channels * kernel_h * kernel_w, height_out * width_out},
- input.options());
-
- grad_output =
- grad_output.view({grad_output.size(0), group, grad_output.size(1) / group,
- grad_output.size(2), grad_output.size(3)});
-
- for (int b = 0; b < batch; b++) {
- // divide int group
- columns = columns.view({group, columns.size(0) / group, columns.size(1)});
- weight = weight.view({group, weight.size(0) / group, weight.size(1),
- weight.size(2), weight.size(3)});
-
- for (int g = 0; g < group; g++) {
- columns[g].addmm_(weight[g].flatten(1).transpose(0, 1),
- grad_output[b][g].flatten(1), 0.0f, 1.0f);
- }
-
- columns =
- columns.view({columns.size(0) * columns.size(1), columns.size(2)});
- weight = weight.view({weight.size(0) * weight.size(1), weight.size(2),
- weight.size(3), weight.size(4)});
-
- // gradient w.r.t. input coordinate data
- modulated_deformable_col2im_coord_cuda(
- columns, input[b], offset[b], mask[b], 1, channels, height, width,
- height_out, width_out, kernel_h, kernel_w, pad_h, pad_w, stride_h,
- stride_w, dilation_h, dilation_w, deformable_group, grad_offset[b],
- grad_mask[b]);
- // gradient w.r.t. input data
- modulated_deformable_col2im_cuda(
- columns, offset[b], mask[b], 1, channels, height, width, height_out,
- width_out, kernel_h, kernel_w, pad_h, pad_w, stride_h, stride_w,
- dilation_h, dilation_w, deformable_group, grad_input[b]);
-
- // gradient w.r.t. weight, dWeight should accumulate across the batch and
- // group
- modulated_deformable_im2col_cuda(
- input[b], offset[b], mask[b], 1, channels, height, width, height_out,
- width_out, kernel_h, kernel_w, pad_h, pad_w, stride_h, stride_w,
- dilation_h, dilation_w, deformable_group, columns);
-
- columns = columns.view({group, columns.size(0) / group, columns.size(1)});
- grad_weight = grad_weight.view({group, grad_weight.size(0) / group,
- grad_weight.size(1), grad_weight.size(2),
- grad_weight.size(3)});
- if (with_bias)
- grad_bias = grad_bias.view({group, grad_bias.size(0) / group});
-
- for (int g = 0; g < group; g++) {
- grad_weight[g] =
- grad_weight[g]
- .flatten(1)
- .addmm_(grad_output[b][g].flatten(1), columns[g].transpose(0, 1))
- .view_as(grad_weight[g]);
- if (with_bias) {
- grad_bias[g] =
- grad_bias[g]
- .view({-1, 1})
- .addmm_(grad_output[b][g].flatten(1), ones.view({-1, 1}))
- .view(-1);
- }
- }
-
- columns =
- columns.view({columns.size(0) * columns.size(1), columns.size(2)});
- grad_weight = grad_weight.view({grad_weight.size(0) * grad_weight.size(1),
- grad_weight.size(2), grad_weight.size(3),
- grad_weight.size(4)});
- if (with_bias)
- grad_bias = grad_bias.view({grad_bias.size(0) * grad_bias.size(1)});
- }
- grad_output = grad_output.view({grad_output.size(0) * grad_output.size(1),
- grad_output.size(2), grad_output.size(3),
- grad_output.size(4)});
-}
diff --git a/spaces/betterme/mestreamlit/pages/998_streamlit_agraph.py b/spaces/betterme/mestreamlit/pages/998_streamlit_agraph.py
deleted file mode 100644
index e3c742e61d6c296bfff665d025f497e5ed426bdc..0000000000000000000000000000000000000000
--- a/spaces/betterme/mestreamlit/pages/998_streamlit_agraph.py
+++ /dev/null
@@ -1,49 +0,0 @@
-import streamlit as st
-from streamlit_agraph import agraph, Node, Edge, Config
-
-
-c1, c2 = st.columns(2)
-
-
-with c1:
-
- nodes = []
- edges = []
- nodes.append(Node(id="Spiderman",
- label="Peter Parker",
- size=25,
- shape="circularImage",
- image="http://marvel-force-chart.surge.sh/marvel_force_chart_img/top_spiderman.png")
- ) # includes **kwargs
- nodes.append(Node(id="Captain_Marvel",
- size=25,
- shape="circularImage",
- image="http://marvel-force-chart.surge.sh/marvel_force_chart_img/top_captainmarvel.png")
- )
- edges.append(Edge(source="Captain_Marvel",
- label="friend_of",
- target="Spiderman",
- # **kwargs
- )
- )
-
- config = Config(width=500,
- height=500,
- # **kwargs
- )
-
- return_value = agraph(nodes=nodes, edges=edges, config=config)
-
-with c2:
- # Currently not workin since update to agraph 2.0 - work in progress
- from rdflib import Graph
- from streamlit_agraph import TripleStore, agraph
-
- graph = Graph()
- graph.parse("http://www.w3.org/People/Berners-Lee/card")
- store = TripleStore()
-
- for subj, pred, obj in graph:
- store.add_triple(subj, pred, obj, "")
-
- agraph(list(store.getNodes()), list(store.getEdges()), config)
diff --git a/spaces/billusanda007/MNIST/README.md b/spaces/billusanda007/MNIST/README.md
deleted file mode 100644
index d17d7355f1d12d3775dc9ca0ab7de0a2bbaf4606..0000000000000000000000000000000000000000
--- a/spaces/billusanda007/MNIST/README.md
+++ /dev/null
@@ -1,13 +0,0 @@
----
-title: MNIST
-emoji: 🏆
-colorFrom: red
-colorTo: purple
-sdk: gradio
-sdk_version: 3.39.0
-app_file: app.py
-pinned: false
-license: mit
----
-
-Check out the configuration reference at https://huggingface.co/docs/hub/spaces-config-reference
diff --git a/spaces/bioriAsaeru/text-to-voice/Daqin 3D Mobile Beauty Master Software Crack Download What You Need to Know Before You Download and Install It.md b/spaces/bioriAsaeru/text-to-voice/Daqin 3D Mobile Beauty Master Software Crack Download What You Need to Know Before You Download and Install It.md
deleted file mode 100644
index 36902baf9e346ef0c0e6e63a12d04451c121287a..0000000000000000000000000000000000000000
--- a/spaces/bioriAsaeru/text-to-voice/Daqin 3D Mobile Beauty Master Software Crack Download What You Need to Know Before You Download and Install It.md
+++ /dev/null
@@ -1,5 +0,0 @@
-
-
Daqin 3d Mobile Beauty Master Software Crack 242golkesl ?DOWNLOAD: ??? mobile beauty master. daqin mobile beauty master ver 2018 crack. daqin mobile beauty master software free download. daqin mobile beauty master software download. daqin mobile beauty master cracked. daqin mobile beauty master software. daqin mobile beauty master not opening. daqin mobile beauty master ver 2020. daqin mobile beauty master 3d ver 2018. daqin mobile beauty master ver 2018 97eae9a76d Скачать Взлом КазиноAdobe Photoshop CS6 Extended 13.0.1.1 Multilanguage
-
daqin 3d mobile beauty master software crack download
-
- aaccfb2cb3
-
-
-
diff --git a/spaces/bioriAsaeru/text-to-voice/Google weighs in with its own AI ethics ideas A new external advisory council to tackle complex challenges.md b/spaces/bioriAsaeru/text-to-voice/Google weighs in with its own AI ethics ideas A new external advisory council to tackle complex challenges.md
deleted file mode 100644
index d15884052658dbc89636f4bd57e16d6b7d27793c..0000000000000000000000000000000000000000
--- a/spaces/bioriAsaeru/text-to-voice/Google weighs in with its own AI ethics ideas A new external advisory council to tackle complex challenges.md
+++ /dev/null
@@ -1,12 +0,0 @@
-
-
One might expect that questions such as these might be frequently researched and discussed by philosophers. However, even though philosophers have long considered the question of what makes life valuable in general, our understanding from speaking with experts and searching for literature is that philosophers have not done much work to consider how best to assign quantitative value to different kinds of outcomes.26 For further discussion of philosophical work that is potentially relevant to assigning moral weights, see our previous blog post on the Against Malaria Foundation and population ethics.
Google, which is exploring the use of large language models in its search products, has also been criticized for a lack of transparency. The company sparked controversy in 2020 when it forced out leading members of its AI ethics team after they produced a study that highlighted problems with the technology.
-
What distinguishes existentialism from other movements in theintellectual history of the West is how it stretched far beyond theliterary and academic worlds. Its ideas are captured in films by IngmarBergman, Michelangelo Antonioni, Jean-Luc Goddard, Akira Kurosawa, andTerrence Malick. Its moods are expressed in the paintings of EdvardMunch, Marcel Duchamp, Pablo Picasso, Paul Cézanne, and EdwardHopper and in the vitiated forms of the sculptor Alberto Giocometti.Its emphasis on freedom and the struggle for self-creation informed theradical and emancipatory politics of Martin Luther King Jr. and MalcolmX as well as the writings of Black intellectuals such as Ralph Ellison,Richard Wright, and W.E.B. Du Bois. Its engagement with therelationship between faith and freedom and the incomprehensibility ofGod shaped theological debates through the lectures and writings ofKarl Barth, Paul Tillich, and Martin Buber, among others. And, with itspenetrating analyses of anxiety and the importance of self-realization,the movement has had a profound impact in the development of humanisticand existential approaches to psychotherapy in the work of a wide rangeof theorists, including R.D. Laing, Rollo May, Viktor Frankl, and IrvinYalom.
-
Lead on setting standards and regulations: One of the greatest strengths the EU enjoys is its ability to set global technical standards in a variety of fields (the Brussels effect). Europe should seek to become a global standard setter in AI and fully utilize multilateral platforms and partnerships with other countries. Rather than a race to deploy AI technology as such, the real race is for setting regulations, guidelines, and best practices so uses of AI take into account socioeconomic, legal, and ethical considerations. Here, the EU has certain advantages that it should seek to better capitalize on. The white paper on AI is a positive step in terms of setting the tone, outlining the normative agenda, tracking developments, informing future regulations, and taking public consultation seriously. However, it is also short on substance and ethics; further, it takes an overly legislative approach to dealing with risks, particularly high-risk AI technologies, which it also does not define clearly.
-
-
I'm not always as unreasonable as suggested there, but I was mainly trying to point out that if I refuse to go along with certain ideas, it's not dependent on a controversial theory of meta-ethics. It's just that I intuitively don't like the ideas and so reject them out of hand. Most people do this with ideas they find too unintuitive to countenance.
-
Whether you want to call it a theory of meta-ethics or not, and whether it is a factual error or not, you have an unusual approach to dealing with moral questions that places an unusual amount of emphasis on... (read more)
-
My current meta-ethical view says I care about factual but not necessarily moral disagreements with respect to elites. One's choice of meta-ethics is itself a moral decision, not a factual one, so this disagreement doesn't much concern me.
aaccfb2cb3
-
-
\ No newline at end of file
diff --git a/spaces/birsardar/stable-diffusion-mat-outpainting-primer/dnnlib/util.py b/spaces/birsardar/stable-diffusion-mat-outpainting-primer/dnnlib/util.py
deleted file mode 100644
index 76725336d01e75e1c68daa88be47f4fde0bbc63b..0000000000000000000000000000000000000000
--- a/spaces/birsardar/stable-diffusion-mat-outpainting-primer/dnnlib/util.py
+++ /dev/null
@@ -1,477 +0,0 @@
-# Copyright (c) 2021, NVIDIA CORPORATION. All rights reserved.
-#
-# NVIDIA CORPORATION and its licensors retain all intellectual property
-# and proprietary rights in and to this software, related documentation
-# and any modifications thereto. Any use, reproduction, disclosure or
-# distribution of this software and related documentation without an express
-# license agreement from NVIDIA CORPORATION is strictly prohibited.
-
-"""Miscellaneous utility classes and functions."""
-
-import ctypes
-import fnmatch
-import importlib
-import inspect
-import numpy as np
-import os
-import shutil
-import sys
-import types
-import io
-import pickle
-import re
-import requests
-import html
-import hashlib
-import glob
-import tempfile
-import urllib
-import urllib.request
-import uuid
-
-from distutils.util import strtobool
-from typing import Any, List, Tuple, Union
-
-
-# Util classes
-# ------------------------------------------------------------------------------------------
-
-
-class EasyDict(dict):
- """Convenience class that behaves like a dict but allows access with the attribute syntax."""
-
- def __getattr__(self, name: str) -> Any:
- try:
- return self[name]
- except KeyError:
- raise AttributeError(name)
-
- def __setattr__(self, name: str, value: Any) -> None:
- self[name] = value
-
- def __delattr__(self, name: str) -> None:
- del self[name]
-
-
-class Logger(object):
- """Redirect stderr to stdout, optionally print stdout to a file, and optionally force flushing on both stdout and the file."""
-
- def __init__(self, file_name: str = None, file_mode: str = "w", should_flush: bool = True):
- self.file = None
-
- if file_name is not None:
- self.file = open(file_name, file_mode)
-
- self.should_flush = should_flush
- self.stdout = sys.stdout
- self.stderr = sys.stderr
-
- sys.stdout = self
- sys.stderr = self
-
- def __enter__(self) -> "Logger":
- return self
-
- def __exit__(self, exc_type: Any, exc_value: Any, traceback: Any) -> None:
- self.close()
-
- def write(self, text: Union[str, bytes]) -> None:
- """Write text to stdout (and a file) and optionally flush."""
- if isinstance(text, bytes):
- text = text.decode()
- if len(text) == 0: # workaround for a bug in VSCode debugger: sys.stdout.write(''); sys.stdout.flush() => crash
- return
-
- if self.file is not None:
- self.file.write(text)
-
- self.stdout.write(text)
-
- if self.should_flush:
- self.flush()
-
- def flush(self) -> None:
- """Flush written text to both stdout and a file, if open."""
- if self.file is not None:
- self.file.flush()
-
- self.stdout.flush()
-
- def close(self) -> None:
- """Flush, close possible files, and remove stdout/stderr mirroring."""
- self.flush()
-
- # if using multiple loggers, prevent closing in wrong order
- if sys.stdout is self:
- sys.stdout = self.stdout
- if sys.stderr is self:
- sys.stderr = self.stderr
-
- if self.file is not None:
- self.file.close()
- self.file = None
-
-
-# Cache directories
-# ------------------------------------------------------------------------------------------
-
-_dnnlib_cache_dir = None
-
-def set_cache_dir(path: str) -> None:
- global _dnnlib_cache_dir
- _dnnlib_cache_dir = path
-
-def make_cache_dir_path(*paths: str) -> str:
- if _dnnlib_cache_dir is not None:
- return os.path.join(_dnnlib_cache_dir, *paths)
- if 'DNNLIB_CACHE_DIR' in os.environ:
- return os.path.join(os.environ['DNNLIB_CACHE_DIR'], *paths)
- if 'HOME' in os.environ:
- return os.path.join(os.environ['HOME'], '.cache', 'dnnlib', *paths)
- if 'USERPROFILE' in os.environ:
- return os.path.join(os.environ['USERPROFILE'], '.cache', 'dnnlib', *paths)
- return os.path.join(tempfile.gettempdir(), '.cache', 'dnnlib', *paths)
-
-# Small util functions
-# ------------------------------------------------------------------------------------------
-
-
-def format_time(seconds: Union[int, float]) -> str:
- """Convert the seconds to human readable string with days, hours, minutes and seconds."""
- s = int(np.rint(seconds))
-
- if s < 60:
- return "{0}s".format(s)
- elif s < 60 * 60:
- return "{0}m {1:02}s".format(s // 60, s % 60)
- elif s < 24 * 60 * 60:
- return "{0}h {1:02}m {2:02}s".format(s // (60 * 60), (s // 60) % 60, s % 60)
- else:
- return "{0}d {1:02}h {2:02}m".format(s // (24 * 60 * 60), (s // (60 * 60)) % 24, (s // 60) % 60)
-
-
-def ask_yes_no(question: str) -> bool:
- """Ask the user the question until the user inputs a valid answer."""
- while True:
- try:
- print("{0} [y/n]".format(question))
- return strtobool(input().lower())
- except ValueError:
- pass
-
-
-def tuple_product(t: Tuple) -> Any:
- """Calculate the product of the tuple elements."""
- result = 1
-
- for v in t:
- result *= v
-
- return result
-
-
-_str_to_ctype = {
- "uint8": ctypes.c_ubyte,
- "uint16": ctypes.c_uint16,
- "uint32": ctypes.c_uint32,
- "uint64": ctypes.c_uint64,
- "int8": ctypes.c_byte,
- "int16": ctypes.c_int16,
- "int32": ctypes.c_int32,
- "int64": ctypes.c_int64,
- "float32": ctypes.c_float,
- "float64": ctypes.c_double
-}
-
-
-def get_dtype_and_ctype(type_obj: Any) -> Tuple[np.dtype, Any]:
- """Given a type name string (or an object having a __name__ attribute), return matching Numpy and ctypes types that have the same size in bytes."""
- type_str = None
-
- if isinstance(type_obj, str):
- type_str = type_obj
- elif hasattr(type_obj, "__name__"):
- type_str = type_obj.__name__
- elif hasattr(type_obj, "name"):
- type_str = type_obj.name
- else:
- raise RuntimeError("Cannot infer type name from input")
-
- assert type_str in _str_to_ctype.keys()
-
- my_dtype = np.dtype(type_str)
- my_ctype = _str_to_ctype[type_str]
-
- assert my_dtype.itemsize == ctypes.sizeof(my_ctype)
-
- return my_dtype, my_ctype
-
-
-def is_pickleable(obj: Any) -> bool:
- try:
- with io.BytesIO() as stream:
- pickle.dump(obj, stream)
- return True
- except:
- return False
-
-
-# Functionality to import modules/objects by name, and call functions by name
-# ------------------------------------------------------------------------------------------
-
-def get_module_from_obj_name(obj_name: str) -> Tuple[types.ModuleType, str]:
- """Searches for the underlying module behind the name to some python object.
- Returns the module and the object name (original name with module part removed)."""
-
- # allow convenience shorthands, substitute them by full names
- obj_name = re.sub("^np.", "numpy.", obj_name)
- obj_name = re.sub("^tf.", "tensorflow.", obj_name)
-
- # list alternatives for (module_name, local_obj_name)
- parts = obj_name.split(".")
- name_pairs = [(".".join(parts[:i]), ".".join(parts[i:])) for i in range(len(parts), 0, -1)]
-
- # try each alternative in turn
- for module_name, local_obj_name in name_pairs:
- try:
- module = importlib.import_module(module_name) # may raise ImportError
- get_obj_from_module(module, local_obj_name) # may raise AttributeError
- return module, local_obj_name
- except:
- pass
-
- # maybe some of the modules themselves contain errors?
- for module_name, _local_obj_name in name_pairs:
- try:
- importlib.import_module(module_name) # may raise ImportError
- except ImportError:
- if not str(sys.exc_info()[1]).startswith("No module named '" + module_name + "'"):
- raise
-
- # maybe the requested attribute is missing?
- for module_name, local_obj_name in name_pairs:
- try:
- module = importlib.import_module(module_name) # may raise ImportError
- get_obj_from_module(module, local_obj_name) # may raise AttributeError
- except ImportError:
- pass
-
- # we are out of luck, but we have no idea why
- raise ImportError(obj_name)
-
-
-def get_obj_from_module(module: types.ModuleType, obj_name: str) -> Any:
- """Traverses the object name and returns the last (rightmost) python object."""
- if obj_name == '':
- return module
- obj = module
- for part in obj_name.split("."):
- obj = getattr(obj, part)
- return obj
-
-
-def get_obj_by_name(name: str) -> Any:
- """Finds the python object with the given name."""
- module, obj_name = get_module_from_obj_name(name)
- return get_obj_from_module(module, obj_name)
-
-
-def call_func_by_name(*args, func_name: str = None, **kwargs) -> Any:
- """Finds the python object with the given name and calls it as a function."""
- assert func_name is not None
- func_obj = get_obj_by_name(func_name)
- assert callable(func_obj)
- return func_obj(*args, **kwargs)
-
-
-def construct_class_by_name(*args, class_name: str = None, **kwargs) -> Any:
- """Finds the python class with the given name and constructs it with the given arguments."""
- return call_func_by_name(*args, func_name=class_name, **kwargs)
-
-
-def get_module_dir_by_obj_name(obj_name: str) -> str:
- """Get the directory path of the module containing the given object name."""
- module, _ = get_module_from_obj_name(obj_name)
- return os.path.dirname(inspect.getfile(module))
-
-
-def is_top_level_function(obj: Any) -> bool:
- """Determine whether the given object is a top-level function, i.e., defined at module scope using 'def'."""
- return callable(obj) and obj.__name__ in sys.modules[obj.__module__].__dict__
-
-
-def get_top_level_function_name(obj: Any) -> str:
- """Return the fully-qualified name of a top-level function."""
- assert is_top_level_function(obj)
- module = obj.__module__
- if module == '__main__':
- module = os.path.splitext(os.path.basename(sys.modules[module].__file__))[0]
- return module + "." + obj.__name__
-
-
-# File system helpers
-# ------------------------------------------------------------------------------------------
-
-def list_dir_recursively_with_ignore(dir_path: str, ignores: List[str] = None, add_base_to_relative: bool = False) -> List[Tuple[str, str]]:
- """List all files recursively in a given directory while ignoring given file and directory names.
- Returns list of tuples containing both absolute and relative paths."""
- assert os.path.isdir(dir_path)
- base_name = os.path.basename(os.path.normpath(dir_path))
-
- if ignores is None:
- ignores = []
-
- result = []
-
- for root, dirs, files in os.walk(dir_path, topdown=True):
- for ignore_ in ignores:
- dirs_to_remove = [d for d in dirs if fnmatch.fnmatch(d, ignore_)]
-
- # dirs need to be edited in-place
- for d in dirs_to_remove:
- dirs.remove(d)
-
- files = [f for f in files if not fnmatch.fnmatch(f, ignore_)]
-
- absolute_paths = [os.path.join(root, f) for f in files]
- relative_paths = [os.path.relpath(p, dir_path) for p in absolute_paths]
-
- if add_base_to_relative:
- relative_paths = [os.path.join(base_name, p) for p in relative_paths]
-
- assert len(absolute_paths) == len(relative_paths)
- result += zip(absolute_paths, relative_paths)
-
- return result
-
-
-def copy_files_and_create_dirs(files: List[Tuple[str, str]]) -> None:
- """Takes in a list of tuples of (src, dst) paths and copies files.
- Will create all necessary directories."""
- for file in files:
- target_dir_name = os.path.dirname(file[1])
-
- # will create all intermediate-level directories
- if not os.path.exists(target_dir_name):
- os.makedirs(target_dir_name)
-
- shutil.copyfile(file[0], file[1])
-
-
-# URL helpers
-# ------------------------------------------------------------------------------------------
-
-def is_url(obj: Any, allow_file_urls: bool = False) -> bool:
- """Determine whether the given object is a valid URL string."""
- if not isinstance(obj, str) or not "://" in obj:
- return False
- if allow_file_urls and obj.startswith('file://'):
- return True
- try:
- res = requests.compat.urlparse(obj)
- if not res.scheme or not res.netloc or not "." in res.netloc:
- return False
- res = requests.compat.urlparse(requests.compat.urljoin(obj, "/"))
- if not res.scheme or not res.netloc or not "." in res.netloc:
- return False
- except:
- return False
- return True
-
-
-def open_url(url: str, cache_dir: str = None, num_attempts: int = 10, verbose: bool = True, return_filename: bool = False, cache: bool = True) -> Any:
- """Download the given URL and return a binary-mode file object to access the data."""
- assert num_attempts >= 1
- assert not (return_filename and (not cache))
-
- # Doesn't look like an URL scheme so interpret it as a local filename.
- if not re.match('^[a-z]+://', url):
- return url if return_filename else open(url, "rb")
-
- # Handle file URLs. This code handles unusual file:// patterns that
- # arise on Windows:
- #
- # file:///c:/foo.txt
- #
- # which would translate to a local '/c:/foo.txt' filename that's
- # invalid. Drop the forward slash for such pathnames.
- #
- # If you touch this code path, you should test it on both Linux and
- # Windows.
- #
- # Some internet resources suggest using urllib.request.url2pathname() but
- # but that converts forward slashes to backslashes and this causes
- # its own set of problems.
- if url.startswith('file://'):
- filename = urllib.parse.urlparse(url).path
- if re.match(r'^/[a-zA-Z]:', filename):
- filename = filename[1:]
- return filename if return_filename else open(filename, "rb")
-
- assert is_url(url)
-
- # Lookup from cache.
- if cache_dir is None:
- cache_dir = make_cache_dir_path('downloads')
-
- url_md5 = hashlib.md5(url.encode("utf-8")).hexdigest()
- if cache:
- cache_files = glob.glob(os.path.join(cache_dir, url_md5 + "_*"))
- if len(cache_files) == 1:
- filename = cache_files[0]
- return filename if return_filename else open(filename, "rb")
-
- # Download.
- url_name = None
- url_data = None
- with requests.Session() as session:
- if verbose:
- print("Downloading %s ..." % url, end="", flush=True)
- for attempts_left in reversed(range(num_attempts)):
- try:
- with session.get(url) as res:
- res.raise_for_status()
- if len(res.content) == 0:
- raise IOError("No data received")
-
- if len(res.content) < 8192:
- content_str = res.content.decode("utf-8")
- if "download_warning" in res.headers.get("Set-Cookie", ""):
- links = [html.unescape(link) for link in content_str.split('"') if "export=download" in link]
- if len(links) == 1:
- url = requests.compat.urljoin(url, links[0])
- raise IOError("Google Drive virus checker nag")
- if "Google Drive - Quota exceeded" in content_str:
- raise IOError("Google Drive download quota exceeded -- please try again later")
-
- match = re.search(r'filename="([^"]*)"', res.headers.get("Content-Disposition", ""))
- url_name = match[1] if match else url
- url_data = res.content
- if verbose:
- print(" done")
- break
- except KeyboardInterrupt:
- raise
- except:
- if not attempts_left:
- if verbose:
- print(" failed")
- raise
- if verbose:
- print(".", end="", flush=True)
-
- # Save to cache.
- if cache:
- safe_name = re.sub(r"[^0-9a-zA-Z-._]", "_", url_name)
- cache_file = os.path.join(cache_dir, url_md5 + "_" + safe_name)
- temp_file = os.path.join(cache_dir, "tmp_" + uuid.uuid4().hex + "_" + url_md5 + "_" + safe_name)
- os.makedirs(cache_dir, exist_ok=True)
- with open(temp_file, "wb") as f:
- f.write(url_data)
- os.replace(temp_file, cache_file) # atomic
- if return_filename:
- return cache_file
-
- # Return data as file object.
- assert not return_filename
- return io.BytesIO(url_data)
diff --git a/spaces/bkhmsi/Font-To-Sketch/code/ttf.py b/spaces/bkhmsi/Font-To-Sketch/code/ttf.py
deleted file mode 100644
index 0211332249289590be828a24d9d87d77ce04d828..0000000000000000000000000000000000000000
--- a/spaces/bkhmsi/Font-To-Sketch/code/ttf.py
+++ /dev/null
@@ -1,409 +0,0 @@
-from importlib import reload
-import os
-import numpy as np
-import bezier
-import freetype as ft
-import pydiffvg
-import torch
-import save_svg
-import vharfbuzz as hb
-from svgpathtools import svgstr2paths
-import xml.etree.ElementTree as ET
-
-
-device = torch.device("cuda" if (
- torch.cuda.is_available() and torch.cuda.device_count() > 0) else "cpu")
-
-reload(bezier)
-
-def fix_single_svg(svg_path, all_word=False):
- target_h_letter = 360
- target_canvas_width, target_canvas_height = 600, 600
-
- canvas_width, canvas_height, shapes, shape_groups = pydiffvg.svg_to_scene(svg_path)
-
- letter_h = canvas_height
- letter_w = canvas_width
-
- if all_word:
- if letter_w > letter_h:
- scale_canvas_w = target_h_letter / letter_w
- hsize = int(letter_h * scale_canvas_w)
- scale_canvas_h = hsize / letter_h
- else:
- scale_canvas_h = target_h_letter / letter_h
- wsize = int(letter_w * scale_canvas_h)
- scale_canvas_w = wsize / letter_w
- else:
- scale_canvas_h = target_h_letter / letter_h
- wsize = int(letter_w * scale_canvas_h)
- scale_canvas_w = wsize / letter_w
-
- for num, p in enumerate(shapes):
- p.points[:, 0] = p.points[:, 0] * scale_canvas_w
- p.points[:, 1] = p.points[:, 1] * scale_canvas_h + target_h_letter
- p.points[:, 1] = -p.points[:, 1]
- # p.points[:, 0] = -p.points[:, 0]
-
- w_min, w_max = min([torch.min(p.points[:, 0]) for p in shapes]), max([torch.max(p.points[:, 0]) for p in shapes])
- h_min, h_max = min([torch.min(p.points[:, 1]) for p in shapes]), max([torch.max(p.points[:, 1]) for p in shapes])
-
- for num, p in enumerate(shapes):
- p.points[:, 0] = p.points[:, 0] + target_canvas_width/2 - int(w_min + (w_max - w_min) / 2)
- p.points[:, 1] = p.points[:, 1] + target_canvas_height/2 - int(h_min + (h_max - h_min) / 2)
-
- output_path = f"{svg_path[:-4]}_scaled.svg"
- save_svg.save_svg(output_path, target_canvas_width, target_canvas_height, shapes, shape_groups)
-
-def normalize_letter_size(dest_path, font, txt, chars):
- fontname = os.path.splitext(os.path.basename(font))[0]
- # for i, c in enumerate(chars):
- # fname = f"{dest_path}/{fontname}_{c}.svg"
- # fname = fname.replace(" ", "_")
- # fix_single_svg(fname)
-
- fname = f"{dest_path}/{fontname}_{txt}.svg"
- fname = fname.replace(" ", "_")
- fix_single_svg(fname, all_word=True)
-
-
-def glyph_to_cubics(face, x=0, y=0):
- ''' Convert current font face glyph to cubic beziers'''
-
- def linear_to_cubic(Q):
- a, b = Q
- return [a + (b - a) * t for t in np.linspace(0, 1, 4)]
-
- def quadratic_to_cubic(Q):
- return [Q[0],
- Q[0] + (2 / 3) * (Q[1] - Q[0]),
- Q[2] + (2 / 3) * (Q[1] - Q[2]),
- Q[2]]
-
- beziers = []
- pt = lambda p: np.array([x + p.x, - p.y - y]) # Flipping here since freetype has y-up
- last = lambda: beziers[-1][-1]
-
- def move_to(a, beziers):
- beziers.append([pt(a)])
-
- def line_to(a, beziers):
- Q = linear_to_cubic([last(), pt(a)])
- beziers[-1] += Q[1:]
-
- def conic_to(a, b, beziers):
- Q = quadratic_to_cubic([last(), pt(a), pt(b)])
- beziers[-1] += Q[1:]
-
- def cubic_to(a, b, c, beziers):
- beziers[-1] += [pt(a), pt(b), pt(c)]
-
- face.glyph.outline.decompose(beziers, move_to=move_to, line_to=line_to, conic_to=conic_to, cubic_to=cubic_to)
- beziers = [np.array(C).astype(float) for C in beziers]
- return beziers
-
-# def handle_ligature(glyph_infos, glyph_positions):
-# combined_advance = sum(pos.x_advance for pos in glyph_positions)
-# first_x_offset = glyph_positions[0].x_offset
-
-# combined_advance = x_adv_1 + x_adv_2
-
-
-
-
-# # Adjust the x_offset values based on the difference between the first glyph's x_offset and the combined_advance
-# for pos in glyph_positions:
-# pos.x_offset += combined_advance - pos.x_advance - first_x_offset
-
-# # Render the ligature using the adjusted glyph positions
-# render_glyphs(glyph_infos, glyph_positions)
-
-
-def font_string_to_beziers(font, txt, size=30, spacing=1.0, merge=True, target_control=None):
- ''' Load a font and convert the outlines for a given string to cubic bezier curves,
- if merge is True, simply return a list of all bezier curves,
- otherwise return a list of lists with the bezier curves for each glyph'''
- print(font)
-
- vhb = hb.Vharfbuzz(font)
- buf = vhb.shape(txt, {"features": {"kern": True, "liga": True}})
-
- buf.guess_segment_properties()
-
- glyph_infos = buf.glyph_infos
- glyph_positions = buf.glyph_positions
- glyph_count = {glyph_infos[i].cluster: 0 for i in range(len(glyph_infos))}
-
- svg = vhb.buf_to_svg(buf)
- paths, attributes = svgstr2paths(svg)
-
- face = ft.Face(font)
- face.set_char_size(64 * size)
- pindex = -1
-
- x, y = 0, 0
- beziers, chars = [], []
-
- for path_idx, path in enumerate(paths):
- segment_vals = []
- print("="*20 + str(path_idx) + "="*20)
- for segment in path:
- segment_type = segment.__class__.__name__
- t_values = np.linspace(0, 1, 10)
- points = [segment.point(t) for t in t_values]
- for pt in points:
- segment_vals += [[pt.real, -pt.imag]]
-
- # points = [bezier.point(t) for t in t_values]
-
- if segment_type == 'Line':
- # Line segment
- start = segment.start
- end = segment.end
- print(f"Line: ({start.real}, {start.imag}) to ({end.real}, {end.imag})")
-
- elif segment_type == 'QuadraticBezier':
- # Quadratic Bézier segment
- start = segment.start
- control = segment.control
- end = segment.end
- print(f"Quadratic Bézier: ({start.real}, {start.imag}) to ({end.real}, {end.imag}) with control point ({control.real}, {control.imag})")
-
- elif segment_type == 'CubicBezier':
- # Cubic Bézier segment
- start = segment.start
- control1 = segment.control1
- control2 = segment.control2
- end = segment.end
- print(f"Cubic Bézier: ({start.real}, {start.imag}) to ({end.real}, {end.imag}) with control points ({control1.real}, {control1.imag}) and ({control2.real}, {control2.imag})")
-
- else:
- # Other segment types (Arc, Close)
- print(f"Segment type: {segment_type}")
-
- beziers += [[np.array(segment_vals)]]
-
- beziers_2 = []
- glyph_infos = glyph_infos[::-1]
- glyph_positions = glyph_positions[::-1]
- for i, (info, pos) in enumerate(zip(glyph_infos, glyph_positions)):
- index = info.cluster
- c = f"{txt[index]}_{glyph_count[index]}"
- chars += [c]
- glyph_count[index] += 1
- glyph_index = info.codepoint
- face.load_glyph(glyph_index, flags=ft.FT_LOAD_DEFAULT | ft.FT_LOAD_NO_BITMAP)
- # face.load_char(c, ft.FT_LOAD_DEFAULT | ft.FT_LOAD_NO_BITMAP)
-
- findex = -1
- if i+1 < len(glyph_infos):
- findex = glyph_infos[i+1].cluster
- foffset = (glyph_positions[i+1].x_offset, glyph_positions[i+1].y_offset)
- fadvance = (glyph_positions[i+1].x_advance, glyph_positions[i+1].y_advance)
-
- # bez = glyph_to_cubics(face, x+pos.x_offset+pos.x_advance, y+pos.y_offset+pos.y_advance)
- # if findex != index:
- # x += pos.x_offset
- # y += pos.y_offset
- # else:
- # x += pos.x_offset
- # y += pos.y_offset
-
-
- bez = glyph_to_cubics(face, x, y)
-
-
- # Check number of control points if desired
- if target_control is not None:
- if c in target_control.keys():
- nctrl = np.sum([len(C) for C in bez])
- while nctrl < target_control[c]:
- longest = np.max(
- sum([[bezier.approx_arc_length(b) for b in bezier.chain_to_beziers(C)] for C in bez], []))
- thresh = longest * 0.5
- bez = [bezier.subdivide_bezier_chain(C, thresh) for C in bez]
- nctrl = np.sum([len(C) for C in bez])
- print(nctrl)
-
- if merge:
- beziers_2 += bez
- else:
- beziers_2.append(bez)
-
- # kerning = face.get_kerning(index, findex)
- # x += (slot.advance.x + kerning.x) * spacing
- # previous = txt[index]
-
- # print(f"C: {txt[index]}/{index} | X: {x+pos.x_offset}| Y: {y+pos.y_offset}")
- print(f"C: {txt[index]}/{index} | X: {x}: {pos.x_advance}/{pos.x_offset} | Y: {y}: {pos.y_advance}/{pos.y_offset}")
-
- # if findex != index:
- x -= pos.x_advance
- # y += pos.y_advance + pos.y_offset
-
- pindex = index
-
- return beziers_2, chars
-
-
-def bezier_chain_to_commands(C, closed=True):
- curves = bezier.chain_to_beziers(C)
- cmds = 'M %f %f ' % (C[0][0], C[0][1])
- n = len(curves)
- for i, bez in enumerate(curves):
- if i == n - 1 and closed:
- cmds += 'C %f %f %f %f %f %fz ' % (*bez[1], *bez[2], *bez[3])
- else:
- cmds += 'C %f %f %f %f %f %f ' % (*bez[1], *bez[2], *bez[3])
- return cmds
-
-
-def count_cp(file_name, font_name):
- canvas_width, canvas_height, shapes, shape_groups = pydiffvg.svg_to_scene(file_name)
- p_counter = 0
- for path in shapes:
- p_counter += path.points.shape[0]
- print(f"TOTAL CP: [{p_counter}]")
- return p_counter
-
-
-def write_letter_svg(c, header, fontname, beziers, subdivision_thresh, dest_path):
- cmds = ''
- svg = header
-
- path = '\n'
- svg += path + '\n'
-
- fname = f"{dest_path}/{fontname}_{c}.svg"
- fname = fname.replace(" ", "_")
- f = open(fname, 'w')
- f.write(svg)
- f.close()
- return fname, path
-
-def write_letter_svg_hb(vhb, c, dest_path, fontname):
- buf = vhb.shape(c, {"features": {"kern": True, "liga": True}})
- svg = vhb.buf_to_svg(buf)
-
- fname = f"{dest_path}/{fontname}_{c}.svg"
- fname = fname.replace(" ", "_")
- f = open(fname, 'w')
- f.write(svg)
- f.close()
- return fname
-
-def font_string_to_svgs(dest_path, font, txt, size=30, spacing=1.0, target_control=None, subdivision_thresh=None):
-
- fontname = os.path.splitext(os.path.basename(font))[0]
- glyph_beziers, chars = font_string_to_beziers(font, txt, size, spacing, merge=False, target_control=target_control)
- if not os.path.isdir(dest_path):
- os.mkdir(dest_path)
- # Compute boundig box
- points = np.vstack(sum(glyph_beziers, []))
- lt = np.min(points, axis=0)
- rb = np.max(points, axis=0)
- size = rb - lt
-
- sizestr = 'width="%.1f" height="%.1f"' % (size[0], size[1])
- boxstr = ' viewBox="%.1f %.1f %.1f %.1f"' % (lt[0], lt[1], size[0], size[1])
- header = '''
-\n'
- fname = f"{dest_path}/{fontname}_{txt}.svg"
- fname = fname.replace(" ", "_")
- f = open(fname, 'w')
- f.write(svg)
- f.close()
- return chars
-
-def font_string_to_svgs_hb(dest_path, font, txt, size=30, spacing=1.0, target_control=None, subdivision_thresh=None):
-
- fontname = os.path.splitext(os.path.basename(font))[0]
- if not os.path.isdir(dest_path):
- os.mkdir(dest_path)
-
- vhb = hb.Vharfbuzz(font)
- buf = vhb.shape(txt, {"features": {"kern": True, "liga": True}})
- buf.guess_segment_properties()
-
- buf = vhb.shape(txt, {"features": {"kern": True, "liga": True}})
- svg = vhb.buf_to_svg(buf)
-
- # Save global svg
- fname = f"{dest_path}/{fontname}_{txt}.svg"
- fname = fname.replace(" ", "_")
- f = open(fname, 'w')
- f.write(svg)
- f.close()
- return None
-
-if __name__ == '__main__':
-
- fonts = ["KaushanScript-Regular"]
- level_of_cc = 1
-
- if level_of_cc == 0:
- target_cp = None
-
- else:
- target_cp = {"A": 120, "B": 120, "C": 100, "D": 100,
- "E": 120, "F": 120, "G": 120, "H": 120,
- "I": 35, "J": 80, "K": 100, "L": 80,
- "M": 100, "N": 100, "O": 100, "P": 120,
- "Q": 120, "R": 130, "S": 110, "T": 90,
- "U": 100, "V": 100, "W": 100, "X": 130,
- "Y": 120, "Z": 120,
- "a": 120, "b": 120, "c": 100, "d": 100,
- "e": 120, "f": 120, "g": 120, "h": 120,
- "i": 35, "j": 80, "k": 100, "l": 80,
- "m": 100, "n": 100, "o": 100, "p": 120,
- "q": 120, "r": 130, "s": 110, "t": 90,
- "u": 100, "v": 100, "w": 100, "x": 130,
- "y": 120, "z": 120
- }
-
- target_cp = {k: v * level_of_cc for k, v in target_cp.items()}
-
- for f in fonts:
- print(f"======= {f} =======")
- font_path = f"data/fonts/{f}.ttf"
- output_path = f"data/init"
- txt = "BUNNY"
- subdivision_thresh = None
- font_string_to_svgs(output_path, font_path, txt, target_control=target_cp,
- subdivision_thresh=subdivision_thresh)
- normalize_letter_size(output_path, font_path, txt)
-
- print("DONE")
-
-
-
-
diff --git a/spaces/brainblow/AudioCreator_Music-Audio_Generation/audiocraft/grids/musicgen/__init__.py b/spaces/brainblow/AudioCreator_Music-Audio_Generation/audiocraft/grids/musicgen/__init__.py
deleted file mode 100644
index d3f101f5a29ff85271e44e4f27545168a8f27baa..0000000000000000000000000000000000000000
--- a/spaces/brainblow/AudioCreator_Music-Audio_Generation/audiocraft/grids/musicgen/__init__.py
+++ /dev/null
@@ -1,6 +0,0 @@
-# Copyright (c) Meta Platforms, Inc. and affiliates.
-# All rights reserved.
-#
-# This source code is licensed under the license found in the
-# LICENSE file in the root directory of this source tree.
-"""MusicGen grids."""
diff --git a/spaces/brainblow/AudioCreator_Music-Audio_Generation/audiocraft/optim/fsdp.py b/spaces/brainblow/AudioCreator_Music-Audio_Generation/audiocraft/optim/fsdp.py
deleted file mode 100644
index b3c1a55b6bf1a33092a021c5cefbbb2ae848918a..0000000000000000000000000000000000000000
--- a/spaces/brainblow/AudioCreator_Music-Audio_Generation/audiocraft/optim/fsdp.py
+++ /dev/null
@@ -1,195 +0,0 @@
-# Copyright (c) Meta Platforms, Inc. and affiliates.
-# All rights reserved.
-#
-# This source code is licensed under the license found in the
-# LICENSE file in the root directory of this source tree.
-
-"""
-Wrapper around FSDP for more convenient use in the training loops.
-"""
-
-from contextlib import contextmanager
-import typing as tp
-import dora
-import torch
-
-from torch.distributed.fsdp import FullyShardedDataParallel as FSDP
-from torch.distributed.fsdp import (
- MixedPrecision, ShardingStrategy, FullStateDictConfig, StateDictType)
-from torch.distributed._shard.sharded_tensor.api import ShardedTensor
-
-
-def is_fsdp_used() -> bool:
- """Return whether we are using FSDP."""
- # A bit of a hack but should work from anywhere.
- if dora.is_xp():
- cfg = dora.get_xp().cfg
- if hasattr(cfg, 'fsdp'):
- return cfg.fsdp.use
- return False
-
-
-def is_sharded_tensor(x: tp.Any) -> bool:
- return isinstance(x, ShardedTensor)
-
-
-@contextmanager
-def switch_to_full_state_dict(models: tp.List[FSDP]):
- # Another bug in FSDP makes it that we cannot use the `state_dict_type` API,
- # so let's do thing manually.
- for model in models:
- FSDP.set_state_dict_type( # type: ignore
- model, StateDictType.FULL_STATE_DICT,
- FullStateDictConfig(offload_to_cpu=True, rank0_only=True))
- try:
- yield
- finally:
- for model in models:
- FSDP.set_state_dict_type(model, StateDictType.LOCAL_STATE_DICT) # type: ignore
-
-
-def wrap_with_fsdp(cfg, model: torch.nn.Module,
- block_classes: tp.Optional[tp.Set[tp.Type]] = None) -> FSDP:
- """Wraps a model with FSDP."""
- # Some of the typing is disabled until this gets integrated
- # into the stable version of PyTorch.
- from torch.distributed.fsdp.wrap import ModuleWrapPolicy # type: ignore
-
- # we import this here to prevent circular import.
- from ..modules.transformer import StreamingTransformerLayer
- from ..modules.conditioners import ConditioningProvider
-
- _fix_post_backward_hook()
-
- assert cfg.use
- sharding_strategy_dict = {
- "no_shard": ShardingStrategy.NO_SHARD,
- "shard_grad_op": ShardingStrategy.SHARD_GRAD_OP,
- "full_shard": ShardingStrategy.FULL_SHARD,
- }
-
- dtype_dict = {
- "float32": torch.float32,
- "float16": torch.float16,
- "bfloat16": torch.bfloat16,
- }
-
- mixed_precision_config = MixedPrecision(
- param_dtype=dtype_dict[cfg.param_dtype],
- reduce_dtype=dtype_dict[cfg.reduce_dtype],
- buffer_dtype=dtype_dict[cfg.buffer_dtype],
- )
-
- sharding_strategy_config = sharding_strategy_dict[cfg.sharding_strategy]
- # The following is going to require being a bit smart
- # when doing LM, because this would flush the weights for every time step
- # during generation. One possiblity is to use hybrid sharding:
- # See: https://pytorch.org/docs/master/fsdp.html#torch.distributed.fsdp.ShardingStrategy
- assert sharding_strategy_config != ShardingStrategy.FULL_SHARD, \
- "Not supported at the moment, requires a bit more work."
-
- local_rank = dora.distrib.get_distrib_spec().local_rank
- assert local_rank < torch.cuda.device_count(), "Please upgrade Dora!"
-
- auto_wrap_policy = None
- if block_classes is None:
- block_classes = {StreamingTransformerLayer, ConditioningProvider}
- if cfg.per_block:
- auto_wrap_policy = ModuleWrapPolicy(block_classes)
- wrapped = _FSDPFixStateDict(
- model,
- sharding_strategy=sharding_strategy_config,
- mixed_precision=mixed_precision_config,
- device_id=local_rank,
- sync_module_states=True,
- use_orig_params=True,
- auto_wrap_policy=auto_wrap_policy,
- ) # type: ignore
- FSDP.set_state_dict_type(wrapped, StateDictType.LOCAL_STATE_DICT) # type: ignore
-
- # Let the wrapped model know about the wrapping!
- # We use __dict__ to avoid it going into the state dict.
- # This is a bit dirty, but needed during generation, as otherwise
- # the wrapped model would call itself and bypass FSDP.
- for module in FSDP.fsdp_modules(wrapped):
- original = module._fsdp_wrapped_module
- original.__dict__['_fsdp'] = module
- return wrapped
-
-
-def purge_fsdp(model: FSDP):
- """Purge the FSDP cached shard inside the model. This should
- allow setting the best state or switching to the EMA.
- """
- from torch.distributed.fsdp._runtime_utils import _reshard # type: ignore
- for module in FSDP.fsdp_modules(model):
- handles = module._handles
- if not handles:
- continue
- handle = handles[0]
- unsharded_flat_param = handle._get_padded_unsharded_flat_param()
- storage_size: int = unsharded_flat_param._typed_storage()._size() # type: ignore
- if storage_size == 0:
- continue
- true_list = [True for h in handles]
- _reshard(module, handles, true_list)
-
-
-class _FSDPFixStateDict(FSDP):
- @staticmethod
- def _name_without_fsdp_prefix(name: str) -> str:
- from torch.distributed.fsdp._common_utils import FSDP_WRAPPED_MODULE # type: ignore
- parts = name.split('.')
- new_parts = [part for part in parts if part != FSDP_WRAPPED_MODULE]
- return '.'.join(new_parts)
-
- def state_dict(self) -> tp.Dict[str, tp.Any]: # type: ignore
- state = dict(super().state_dict())
- for key, value in list(state.items()):
- if is_sharded_tensor(value):
- del state[key]
- return state
-
- def load_state_dict(self, state: tp.Dict[str, tp.Any]): # type: ignore
- if self._state_dict_type is StateDictType.FULL_STATE_DICT:
- super().load_state_dict(state)
- purge_fsdp(self)
- return
- # Fix FSDP load state dict in all situation.
- # Use this only with LOCAL_STATE_DICT !!!
- current_state = dict(super().state_dict())
- for key, value in state.items():
- key = _FSDPFixStateDict._name_without_fsdp_prefix(key)
- if key not in current_state:
- # Emulate strict loading manually.
- raise RuntimeError(f"Unknown state key {key}")
- current_state[key].copy_(value)
-
- # Purging cached weights from previous forward.
- purge_fsdp(self)
-
-
-_hook_fixed = False
-
-
-def _fix_post_backward_hook():
- global _hook_fixed
- if _hook_fixed:
- return
- _hook_fixed = True
-
- from torch.distributed.fsdp import _runtime_utils
- from torch.distributed.fsdp._common_utils import TrainingState, HandleTrainingState
- old_hook = _runtime_utils._post_backward_hook
-
- def _post_backward_hook(state, handle, *args, **kwargs):
- checkpointed = getattr(state._fsdp_wrapped_module, '_audiocraft_checkpointed', False)
- if checkpointed:
- # there will be one more forward in the backward with checkpointing and that will
- # massively confuse FSDP, so we have to make it think everything
- # is going according to the plan.
- state.training_state = TrainingState.FORWARD_BACKWARD
- handle._training_state = HandleTrainingState.BACKWARD_PRE
- old_hook(state, handle, *args, **kwargs)
-
- _runtime_utils._post_backward_hook = _post_backward_hook
diff --git a/spaces/brjathu/HMR2.0/vendor/detectron2/README.md b/spaces/brjathu/HMR2.0/vendor/detectron2/README.md
deleted file mode 100644
index 75db3c52f216dbcff9a4730ff0fa139853fc4670..0000000000000000000000000000000000000000
--- a/spaces/brjathu/HMR2.0/vendor/detectron2/README.md
+++ /dev/null
@@ -1,68 +0,0 @@
-
-
-
-
-
-
-Detectron2 is Facebook AI Research's next generation library
-that provides state-of-the-art detection and segmentation algorithms.
-It is the successor of
-[Detectron](https://github.com/facebookresearch/Detectron/)
-and [maskrcnn-benchmark](https://github.com/facebookresearch/maskrcnn-benchmark/).
-It supports a number of computer vision research projects and production applications in Facebook.
-
-
-
-
-
-
-## Learn More about Detectron2
-
-Explain Like I’m 5: Detectron2 | Using Machine Learning with Detectron2
-:-------------------------:|:-------------------------:
-[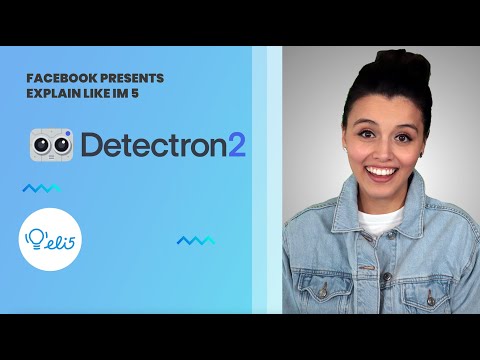](https://www.youtube.com/watch?v=1oq1Ye7dFqc) | [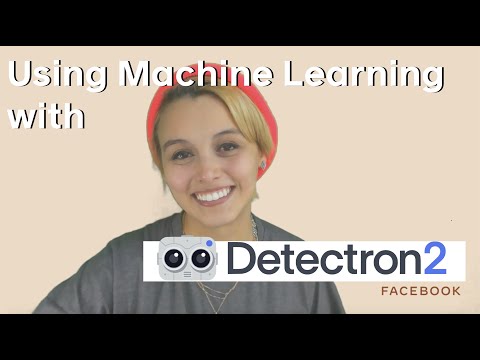](https://www.youtube.com/watch?v=eUSgtfK4ivk)
-
-## What's New
-* Includes new capabilities such as panoptic segmentation, Densepose, Cascade R-CNN, rotated bounding boxes, PointRend,
- DeepLab, ViTDet, MViTv2 etc.
-* Used as a library to support building [research projects](projects/) on top of it.
-* Models can be exported to TorchScript format or Caffe2 format for deployment.
-* It [trains much faster](https://detectron2.readthedocs.io/notes/benchmarks.html).
-
-See our [blog post](https://ai.facebook.com/blog/-detectron2-a-pytorch-based-modular-object-detection-library-/)
-to see more demos and learn about detectron2.
-
-## Installation
-
-See [installation instructions](https://detectron2.readthedocs.io/tutorials/install.html).
-
-## Getting Started
-
-See [Getting Started with Detectron2](https://detectron2.readthedocs.io/tutorials/getting_started.html),
-and the [Colab Notebook](https://colab.research.google.com/drive/16jcaJoc6bCFAQ96jDe2HwtXj7BMD_-m5)
-to learn about basic usage.
-
-Learn more at our [documentation](https://detectron2.readthedocs.org).
-And see [projects/](projects/) for some projects that are built on top of detectron2.
-
-## Model Zoo and Baselines
-
-We provide a large set of baseline results and trained models available for download in the [Detectron2 Model Zoo](MODEL_ZOO.md).
-
-## License
-
-Detectron2 is released under the [Apache 2.0 license](LICENSE).
-
-## Citing Detectron2
-
-If you use Detectron2 in your research or wish to refer to the baseline results published in the [Model Zoo](MODEL_ZOO.md), please use the following BibTeX entry.
-
-```BibTeX
-@misc{wu2019detectron2,
- author = {Yuxin Wu and Alexander Kirillov and Francisco Massa and
- Wan-Yen Lo and Ross Girshick},
- title = {Detectron2},
- howpublished = {\url{https://github.com/facebookresearch/detectron2}},
- year = {2019}
-}
-```
diff --git a/spaces/brjathu/HMR2.0/vendor/detectron2/projects/PointRend/point_rend/point_features.py b/spaces/brjathu/HMR2.0/vendor/detectron2/projects/PointRend/point_rend/point_features.py
deleted file mode 100644
index e46f442950ff248555e127dc3923b67adb37fb69..0000000000000000000000000000000000000000
--- a/spaces/brjathu/HMR2.0/vendor/detectron2/projects/PointRend/point_rend/point_features.py
+++ /dev/null
@@ -1,259 +0,0 @@
-# Copyright (c) Facebook, Inc. and its affiliates.
-import torch
-from torch.nn import functional as F
-
-from detectron2.layers import cat, shapes_to_tensor
-from detectron2.structures import BitMasks, Boxes
-
-
-"""
-Shape shorthand in this module:
-
- N: minibatch dimension size, i.e. the number of RoIs for instance segmenation or the
- number of images for semantic segmenation.
- R: number of ROIs, combined over all images, in the minibatch
- P: number of points
-"""
-
-
-def point_sample(input, point_coords, **kwargs):
- """
- A wrapper around :function:`torch.nn.functional.grid_sample` to support 3D point_coords tensors.
- Unlike :function:`torch.nn.functional.grid_sample` it assumes `point_coords` to lie inside
- [0, 1] x [0, 1] square.
-
- Args:
- input (Tensor): A tensor of shape (N, C, H, W) that contains features map on a H x W grid.
- point_coords (Tensor): A tensor of shape (N, P, 2) or (N, Hgrid, Wgrid, 2) that contains
- [0, 1] x [0, 1] normalized point coordinates.
-
- Returns:
- output (Tensor): A tensor of shape (N, C, P) or (N, C, Hgrid, Wgrid) that contains
- features for points in `point_coords`. The features are obtained via bilinear
- interplation from `input` the same way as :function:`torch.nn.functional.grid_sample`.
- """
- add_dim = False
- if point_coords.dim() == 3:
- add_dim = True
- point_coords = point_coords.unsqueeze(2)
- output = F.grid_sample(input, 2.0 * point_coords - 1.0, **kwargs)
- if add_dim:
- output = output.squeeze(3)
- return output
-
-
-def generate_regular_grid_point_coords(R, side_size, device):
- """
- Generate regular square grid of points in [0, 1] x [0, 1] coordinate space.
-
- Args:
- R (int): The number of grids to sample, one for each region.
- side_size (int): The side size of the regular grid.
- device (torch.device): Desired device of returned tensor.
-
- Returns:
- (Tensor): A tensor of shape (R, side_size^2, 2) that contains coordinates
- for the regular grids.
- """
- aff = torch.tensor([[[0.5, 0, 0.5], [0, 0.5, 0.5]]], device=device)
- r = F.affine_grid(aff, torch.Size((1, 1, side_size, side_size)), align_corners=False)
- return r.view(1, -1, 2).expand(R, -1, -1)
-
-
-def get_uncertain_point_coords_with_randomness(
- coarse_logits, uncertainty_func, num_points, oversample_ratio, importance_sample_ratio
-):
- """
- Sample points in [0, 1] x [0, 1] coordinate space based on their uncertainty. The unceratinties
- are calculated for each point using 'uncertainty_func' function that takes point's logit
- prediction as input.
- See PointRend paper for details.
-
- Args:
- coarse_logits (Tensor): A tensor of shape (N, C, Hmask, Wmask) or (N, 1, Hmask, Wmask) for
- class-specific or class-agnostic prediction.
- uncertainty_func: A function that takes a Tensor of shape (N, C, P) or (N, 1, P) that
- contains logit predictions for P points and returns their uncertainties as a Tensor of
- shape (N, 1, P).
- num_points (int): The number of points P to sample.
- oversample_ratio (int): Oversampling parameter.
- importance_sample_ratio (float): Ratio of points that are sampled via importnace sampling.
-
- Returns:
- point_coords (Tensor): A tensor of shape (N, P, 2) that contains the coordinates of P
- sampled points.
- """
- assert oversample_ratio >= 1
- assert importance_sample_ratio <= 1 and importance_sample_ratio >= 0
- num_boxes = coarse_logits.shape[0]
- num_sampled = int(num_points * oversample_ratio)
- point_coords = torch.rand(num_boxes, num_sampled, 2, device=coarse_logits.device)
- point_logits = point_sample(coarse_logits, point_coords, align_corners=False)
- # It is crucial to calculate uncertainty based on the sampled prediction value for the points.
- # Calculating uncertainties of the coarse predictions first and sampling them for points leads
- # to incorrect results.
- # To illustrate this: assume uncertainty_func(logits)=-abs(logits), a sampled point between
- # two coarse predictions with -1 and 1 logits has 0 logits, and therefore 0 uncertainty value.
- # However, if we calculate uncertainties for the coarse predictions first,
- # both will have -1 uncertainty, and the sampled point will get -1 uncertainty.
- point_uncertainties = uncertainty_func(point_logits)
- num_uncertain_points = int(importance_sample_ratio * num_points)
- num_random_points = num_points - num_uncertain_points
- idx = torch.topk(point_uncertainties[:, 0, :], k=num_uncertain_points, dim=1)[1]
- shift = num_sampled * torch.arange(num_boxes, dtype=torch.long, device=coarse_logits.device)
- idx += shift[:, None]
- point_coords = point_coords.view(-1, 2)[idx.view(-1), :].view(
- num_boxes, num_uncertain_points, 2
- )
- if num_random_points > 0:
- point_coords = cat(
- [
- point_coords,
- torch.rand(num_boxes, num_random_points, 2, device=coarse_logits.device),
- ],
- dim=1,
- )
- return point_coords
-
-
-def get_uncertain_point_coords_on_grid(uncertainty_map, num_points):
- """
- Find `num_points` most uncertain points from `uncertainty_map` grid.
-
- Args:
- uncertainty_map (Tensor): A tensor of shape (N, 1, H, W) that contains uncertainty
- values for a set of points on a regular H x W grid.
- num_points (int): The number of points P to select.
-
- Returns:
- point_indices (Tensor): A tensor of shape (N, P) that contains indices from
- [0, H x W) of the most uncertain points.
- point_coords (Tensor): A tensor of shape (N, P, 2) that contains [0, 1] x [0, 1] normalized
- coordinates of the most uncertain points from the H x W grid.
- """
- R, _, H, W = uncertainty_map.shape
- h_step = 1.0 / float(H)
- w_step = 1.0 / float(W)
-
- num_points = min(H * W, num_points)
- point_indices = torch.topk(uncertainty_map.view(R, H * W), k=num_points, dim=1)[1]
- point_coords = torch.zeros(R, num_points, 2, dtype=torch.float, device=uncertainty_map.device)
- point_coords[:, :, 0] = w_step / 2.0 + (point_indices % W).to(torch.float) * w_step
- point_coords[:, :, 1] = h_step / 2.0 + (point_indices // W).to(torch.float) * h_step
- return point_indices, point_coords
-
-
-def point_sample_fine_grained_features(features_list, feature_scales, boxes, point_coords):
- """
- Get features from feature maps in `features_list` that correspond to specific point coordinates
- inside each bounding box from `boxes`.
-
- Args:
- features_list (list[Tensor]): A list of feature map tensors to get features from.
- feature_scales (list[float]): A list of scales for tensors in `features_list`.
- boxes (list[Boxes]): A list of I Boxes objects that contain R_1 + ... + R_I = R boxes all
- together.
- point_coords (Tensor): A tensor of shape (R, P, 2) that contains
- [0, 1] x [0, 1] box-normalized coordinates of the P sampled points.
-
- Returns:
- point_features (Tensor): A tensor of shape (R, C, P) that contains features sampled
- from all features maps in feature_list for P sampled points for all R boxes in `boxes`.
- point_coords_wrt_image (Tensor): A tensor of shape (R, P, 2) that contains image-level
- coordinates of P points.
- """
- cat_boxes = Boxes.cat(boxes)
- num_boxes = [b.tensor.size(0) for b in boxes]
-
- point_coords_wrt_image = get_point_coords_wrt_image(cat_boxes.tensor, point_coords)
- split_point_coords_wrt_image = torch.split(point_coords_wrt_image, num_boxes)
-
- point_features = []
- for idx_img, point_coords_wrt_image_per_image in enumerate(split_point_coords_wrt_image):
- point_features_per_image = []
- for idx_feature, feature_map in enumerate(features_list):
- h, w = feature_map.shape[-2:]
- scale = shapes_to_tensor([w, h]) / feature_scales[idx_feature]
- point_coords_scaled = point_coords_wrt_image_per_image / scale.to(feature_map.device)
- point_features_per_image.append(
- point_sample(
- feature_map[idx_img].unsqueeze(0),
- point_coords_scaled.unsqueeze(0),
- align_corners=False,
- )
- .squeeze(0)
- .transpose(1, 0)
- )
- point_features.append(cat(point_features_per_image, dim=1))
-
- return cat(point_features, dim=0), point_coords_wrt_image
-
-
-def get_point_coords_wrt_image(boxes_coords, point_coords):
- """
- Convert box-normalized [0, 1] x [0, 1] point cooordinates to image-level coordinates.
-
- Args:
- boxes_coords (Tensor): A tensor of shape (R, 4) that contains bounding boxes.
- coordinates.
- point_coords (Tensor): A tensor of shape (R, P, 2) that contains
- [0, 1] x [0, 1] box-normalized coordinates of the P sampled points.
-
- Returns:
- point_coords_wrt_image (Tensor): A tensor of shape (R, P, 2) that contains
- image-normalized coordinates of P sampled points.
- """
- with torch.no_grad():
- point_coords_wrt_image = point_coords.clone()
- point_coords_wrt_image[:, :, 0] = point_coords_wrt_image[:, :, 0] * (
- boxes_coords[:, None, 2] - boxes_coords[:, None, 0]
- )
- point_coords_wrt_image[:, :, 1] = point_coords_wrt_image[:, :, 1] * (
- boxes_coords[:, None, 3] - boxes_coords[:, None, 1]
- )
- point_coords_wrt_image[:, :, 0] += boxes_coords[:, None, 0]
- point_coords_wrt_image[:, :, 1] += boxes_coords[:, None, 1]
- return point_coords_wrt_image
-
-
-def sample_point_labels(instances, point_coords):
- """
- Sample point labels from ground truth mask given point_coords.
-
- Args:
- instances (list[Instances]): A list of N Instances, where N is the number of images
- in the batch. So, i_th elememt of the list contains R_i objects and R_1 + ... + R_N is
- equal to R. The ground-truth gt_masks in each instance will be used to compute labels.
- points_coords (Tensor): A tensor of shape (R, P, 2), where R is the total number of
- instances and P is the number of points for each instance. The coordinates are in
- the absolute image pixel coordinate space, i.e. [0, H] x [0, W].
-
- Returns:
- Tensor: A tensor of shape (R, P) that contains the labels of P sampled points.
- """
- with torch.no_grad():
- gt_mask_logits = []
- point_coords_splits = torch.split(
- point_coords, [len(instances_per_image) for instances_per_image in instances]
- )
- for i, instances_per_image in enumerate(instances):
- if len(instances_per_image) == 0:
- continue
- assert isinstance(
- instances_per_image.gt_masks, BitMasks
- ), "Point head works with GT in 'bitmask' format. Set INPUT.MASK_FORMAT to 'bitmask'."
-
- gt_bit_masks = instances_per_image.gt_masks.tensor
- h, w = instances_per_image.gt_masks.image_size
- scale = torch.tensor([w, h], dtype=torch.float, device=gt_bit_masks.device)
- points_coord_grid_sample_format = point_coords_splits[i] / scale
- gt_mask_logits.append(
- point_sample(
- gt_bit_masks.to(torch.float32).unsqueeze(1),
- points_coord_grid_sample_format,
- align_corners=False,
- ).squeeze(1)
- )
-
- point_labels = cat(gt_mask_logits)
- return point_labels
diff --git a/spaces/carblacac/chatbot/app.py b/spaces/carblacac/chatbot/app.py
deleted file mode 100644
index 3e50f07d863eb7bb7e428b6099c78ba05d05b794..0000000000000000000000000000000000000000
--- a/spaces/carblacac/chatbot/app.py
+++ /dev/null
@@ -1,71 +0,0 @@
-from transformers import BlenderbotTokenizer, BlenderbotForConditionalGeneration
-import torch
-
-import gradio as gr
-
-mname = "facebook/blenderbot-400M-distill"
-model = BlenderbotForConditionalGeneration.from_pretrained(mname)
-tokenizer = BlenderbotTokenizer.from_pretrained(mname)
-
-
-def take_last_tokens(inputs, note_history, history):
- """Filter the last 128 tokens"""
- if inputs['input_ids'].shape[1] > 128:
- inputs['input_ids'] = torch.tensor([inputs['input_ids'][0][-128:].tolist()])
- inputs['attention_mask'] = torch.tensor([inputs['attention_mask'][0][-128:].tolist()])
- note_history = [' '.join(note_history[0].split('')[2:])]
- history = history[1:]
-
- return inputs, note_history, history
-
-
-def add_note_to_history(note, note_history):
- """Add a note to the historical information"""
- note_history.append(note)
- note_history = ''.join(note_history)
- return [note_history]
-
-
-title = "Mantain a conversation with the bot"
-description = """
-
The bot have been trained to chat with you about whatever you want. Let's talk!
-
-
-
(Image generated from text using DALL·E mini)
-"""
-# https://user-images.githubusercontent.com/105242658/176054244-525c6530-1e78-42c7-8688-91dfedf8db58.png
-#https://www.craiyon.com/
-
-def chat(message, history):
- history = history or []
- if history:
- history_useful = [' '.join([str(a[0])+''+str(a[1]) for a in history])]
- else:
- history_useful = []
-
- history_useful = add_note_to_history(message, history_useful)
- # Generate a response of the bot and add it to note_history
- inputs = tokenizer(history_useful, return_tensors="pt")
- inputs, history_useful, history = take_last_tokens(inputs, history_useful, history)
-
- reply_ids = model.generate(**inputs)
- response = tokenizer.batch_decode(reply_ids, skip_special_tokens=True)[0]
- history_useful = add_note_to_history(response, history_useful)
-
-
- list_history = history_useful[0].split('')
- history.append((list_history[-2], list_history[-1]))
-
- return history, history
-
-
-gr.Interface(
- fn=chat,
- theme="huggingface",
- css=".footer {display:none !important}",
- inputs=["text", "state"],
- outputs=["chatbot", "state"],
- title=title,
- description=description,
- allow_flagging="never",
- ).launch()
diff --git a/spaces/chendl/compositional_test/multimodal/YOLOX/demo/MegEngine/python/README.md b/spaces/chendl/compositional_test/multimodal/YOLOX/demo/MegEngine/python/README.md
deleted file mode 100644
index 97ec25563229fcc2914deb80c1135cda8d49bfb2..0000000000000000000000000000000000000000
--- a/spaces/chendl/compositional_test/multimodal/YOLOX/demo/MegEngine/python/README.md
+++ /dev/null
@@ -1,33 +0,0 @@
-# YOLOX-Python-MegEngine
-
-Python version of YOLOX object detection base on [MegEngine](https://github.com/MegEngine/MegEngine).
-
-## Tutorial
-
-### Step1: install requirements
-
-```
-python3 -m pip install megengine -f https://megengine.org.cn/whl/mge.html
-```
-
-### Step2: convert checkpoint weights from torch's path file
-
-```
-python3 convert_weights.py -w yolox_s.pth -o yolox_s_mge.pkl
-```
-
-### Step3: run demo
-
-This part is the same as torch's python demo, but no need to specify device.
-
-```
-python3 demo.py image -n yolox-s -c yolox_s_mge.pkl --path ../../../assets/dog.jpg --conf 0.25 --nms 0.45 --tsize 640 --save_result
-```
-
-### [Optional]Step4: dump model for cpp inference
-
-> **Note**: result model is dumped with `optimize_for_inference` and `enable_fuse_conv_bias_nonlinearity`.
-
-```
-python3 dump.py -n yolox-s -c yolox_s_mge.pkl --dump_path yolox_s.mge
-```
diff --git a/spaces/chenyangqi/FateZero/FateZero/video_diffusion/pipelines/p2pDDIMSpatioTemporalPipeline.py b/spaces/chenyangqi/FateZero/FateZero/video_diffusion/pipelines/p2pDDIMSpatioTemporalPipeline.py
deleted file mode 100644
index d33dd73c74b8236be71554829748541ab4de9725..0000000000000000000000000000000000000000
--- a/spaces/chenyangqi/FateZero/FateZero/video_diffusion/pipelines/p2pDDIMSpatioTemporalPipeline.py
+++ /dev/null
@@ -1,437 +0,0 @@
-# code mostly taken from https://github.com/huggingface/diffusers
-
-from typing import Callable, List, Optional, Union
-import os
-import PIL
-import torch
-import numpy as np
-from einops import rearrange
-from tqdm import trange, tqdm
-
-from transformers import CLIPTextModel, CLIPTokenizer
-
-from diffusers.utils import deprecate, logging
-from diffusers.pipelines.stable_diffusion import StableDiffusionPipelineOutput
-from diffusers.models import AutoencoderKL
-from diffusers.schedulers import (
- DDIMScheduler,
- DPMSolverMultistepScheduler,
- EulerAncestralDiscreteScheduler,
- EulerDiscreteScheduler,
- LMSDiscreteScheduler,
- PNDMScheduler,
-)
-
-from video_diffusion.prompt_attention.attention_util import make_controller
-from ..models.unet_3d_condition import UNetPseudo3DConditionModel
-from .stable_diffusion import SpatioTemporalStableDiffusionPipeline
-from ..prompt_attention import attention_util
-logger = logging.get_logger(__name__) # pylint: disable=invalid-name
-
-
-class p2pDDIMSpatioTemporalPipeline(SpatioTemporalStableDiffusionPipeline):
- def __init__(
- self,
- vae: AutoencoderKL,
- text_encoder: CLIPTextModel,
- tokenizer: CLIPTokenizer,
- unet: UNetPseudo3DConditionModel,
- scheduler: Union[DDIMScheduler, PNDMScheduler, LMSDiscreteScheduler, EulerDiscreteScheduler, EulerAncestralDiscreteScheduler, DPMSolverMultistepScheduler,],
- disk_store: bool=False
- ):
- super().__init__(vae, text_encoder, tokenizer, unet, scheduler)
- self.store_controller = attention_util.AttentionStore(disk_store=disk_store)
- self.empty_controller = attention_util.EmptyControl()
- r"""
- Pipeline for text-to-video generation using Spatio-Temporal Stable Diffusion.
- """
-
- def check_inputs(self, prompt, height, width, callback_steps, strength=None):
- if not isinstance(prompt, str) and not isinstance(prompt, list):
- raise ValueError(f"`prompt` has to be of type `str` or `list` but is {type(prompt)}")
- if strength is not None:
- if strength <= 0 or strength > 1:
- raise ValueError(f"The value of strength should in (0.0, 1.0] but is {strength}")
-
- if height % 8 != 0 or width % 8 != 0:
- raise ValueError(
- f"`height` and `width` have to be divisible by 8 but are {height} and {width}."
- )
-
- if (callback_steps is None) or (
- callback_steps is not None and (not isinstance(callback_steps, int) or callback_steps <= 0)
- ):
- raise ValueError(
- f"`callback_steps` has to be a positive integer but is {callback_steps} of type"
- f" {type(callback_steps)}."
- )
-
- @torch.no_grad()
- def prepare_latents_ddim_inverted(self, image, batch_size, num_images_per_prompt,
- text_embeddings,
- store_attention=False, prompt=None,
- generator=None,
- LOW_RESOURCE = True,
- save_path = None
- ):
- self.prepare_before_train_loop()
- if store_attention:
- attention_util.register_attention_control(self, self.store_controller)
- resource_default_value = self.store_controller.LOW_RESOURCE
- self.store_controller.LOW_RESOURCE = LOW_RESOURCE # in inversion, no CFG, record all latents attention
- batch_size = batch_size * num_images_per_prompt
- if isinstance(generator, list) and len(generator) != batch_size:
- raise ValueError(
- f"You have passed a list of generators of length {len(generator)}, but requested an effective batch"
- f" size of {batch_size}. Make sure the batch size matches the length of the generators."
- )
-
- if isinstance(generator, list):
- init_latents = [
- self.vae.encode(image[i : i + 1]).latent_dist.sample(generator[i]) for i in range(batch_size)
- ]
- init_latents = torch.cat(init_latents, dim=0)
- else:
- init_latents = self.vae.encode(image).latent_dist.sample(generator)
-
- init_latents = 0.18215 * init_latents
-
- if batch_size > init_latents.shape[0] and batch_size % init_latents.shape[0] == 0:
- # expand init_latents for batch_size
- deprecation_message = (
- f"You have passed {batch_size} text prompts (`prompt`), but only {init_latents.shape[0]} initial"
- " images (`image`). Initial images are now duplicating to match the number of text prompts. Note"
- " that this behavior is deprecated and will be removed in a version 1.0.0. Please make sure to update"
- " your script to pass as many initial images as text prompts to suppress this warning."
- )
- deprecate("len(prompt) != len(image)", "1.0.0", deprecation_message, standard_warn=False)
- additional_image_per_prompt = batch_size // init_latents.shape[0]
- init_latents = torch.cat([init_latents] * additional_image_per_prompt, dim=0)
- elif batch_size > init_latents.shape[0] and batch_size % init_latents.shape[0] != 0:
- raise ValueError(
- f"Cannot duplicate `image` of batch size {init_latents.shape[0]} to {batch_size} text prompts."
- )
- else:
- init_latents = torch.cat([init_latents], dim=0)
-
- # get latents
- init_latents_bcfhw = rearrange(init_latents, "(b f) c h w -> b c f h w", b=batch_size)
- ddim_latents_all_step = self.ddim_clean2noisy_loop(init_latents_bcfhw, text_embeddings, self.store_controller)
- if store_attention and (save_path is not None) :
- os.makedirs(save_path+'/cross_attention')
- attention_output = attention_util.show_cross_attention(self.tokenizer, prompt,
- self.store_controller, 16, ["up", "down"],
- save_path = save_path+'/cross_attention')
-
- # Detach the controller for safety
- attention_util.register_attention_control(self, self.empty_controller)
- self.store_controller.LOW_RESOURCE = resource_default_value
-
- return ddim_latents_all_step
-
- @torch.no_grad()
- def ddim_clean2noisy_loop(self, latent, text_embeddings, controller:attention_util.AttentionControl=None):
- weight_dtype = latent.dtype
- uncond_embeddings, cond_embeddings = text_embeddings.chunk(2)
- all_latent = [latent]
- latent = latent.clone().detach()
- print(' Invert clean image to noise latents by DDIM and Unet')
- for i in trange(len(self.scheduler.timesteps)):
- t = self.scheduler.timesteps[len(self.scheduler.timesteps) - i - 1]
-
- # [1, 4, 8, 64, 64] -> [1, 4, 8, 64, 64])
- noise_pred = self.unet(latent, t, encoder_hidden_states=cond_embeddings)["sample"]
-
- latent = self.next_clean2noise_step(noise_pred, t, latent)
- if controller is not None: controller.step_callback(latent)
- all_latent.append(latent.to(dtype=weight_dtype))
-
- return all_latent
-
- def next_clean2noise_step(self, model_output: Union[torch.FloatTensor, np.ndarray], timestep: int, sample: Union[torch.FloatTensor, np.ndarray]):
- """
- Assume the eta in DDIM=0
- """
- timestep, next_timestep = min(timestep - self.scheduler.config.num_train_timesteps // self.scheduler.num_inference_steps, 999), timestep
- alpha_prod_t = self.scheduler.alphas_cumprod[timestep] if timestep >= 0 else self.scheduler.final_alpha_cumprod
- alpha_prod_t_next = self.scheduler.alphas_cumprod[next_timestep]
- beta_prod_t = 1 - alpha_prod_t
- next_original_sample = (sample - beta_prod_t ** 0.5 * model_output) / alpha_prod_t ** 0.5
- next_sample_direction = (1 - alpha_prod_t_next) ** 0.5 * model_output
- next_sample = alpha_prod_t_next ** 0.5 * next_original_sample + next_sample_direction
- return next_sample
-
- def get_timesteps(self, num_inference_steps, strength, device):
- # get the original timestep using init_timestep
- init_timestep = min(int(num_inference_steps * strength), num_inference_steps)
-
- t_start = max(num_inference_steps - init_timestep, 0)
- timesteps = self.scheduler.timesteps[t_start:]
-
- return timesteps, num_inference_steps - t_start
-
- def p2preplace_edit(self, **kwargs):
- # Edit controller during inference
- # The controller must know the source prompt for replace mapping
-
- len_source = {len(kwargs['source_prompt'].split(' '))}
- len_target = {len(kwargs['prompt'].split(' '))}
- equal_length = (len_source == len_target)
- print(f" len_source: {len_source}, len_target: {len_target}, equal_length: {equal_length}")
- edit_controller = make_controller(
- self.tokenizer,
- [ kwargs['source_prompt'], kwargs['prompt']],
- NUM_DDIM_STEPS = kwargs['num_inference_steps'],
- is_replace_controller=kwargs.get('is_replace_controller', True) and equal_length,
- cross_replace_steps=kwargs['cross_replace_steps'],
- self_replace_steps=kwargs['self_replace_steps'],
- blend_words=kwargs.get('blend_words', None),
- equilizer_params=kwargs.get('eq_params', None),
- additional_attention_store=self.store_controller,
- use_inversion_attention = kwargs['use_inversion_attention'],
- bend_th = kwargs.get('bend_th', (0.3, 0.3)),
- masked_self_attention = kwargs.get('masked_self_attention', None),
- masked_latents=kwargs.get('masked_latents', None),
- save_path=kwargs.get('save_path', None),
- save_self_attention = kwargs.get('save_self_attention', True),
- disk_store = kwargs.get('disk_store', False)
- )
-
- attention_util.register_attention_control(self, edit_controller)
-
-
- # In ddim inferece, no need source prompt
- sdimage_output = self.sd_ddim_pipeline(
- controller = edit_controller,
- # target_prompt = kwargs['prompts'][1],
- **kwargs)
- if hasattr(edit_controller.local_blend, 'mask_list'):
- mask_list = edit_controller.local_blend.mask_list
- else:
- mask_list = None
- if len(edit_controller.attention_store.keys()) > 0:
- attention_output = attention_util.show_cross_attention(self.tokenizer, kwargs['prompt'],
- edit_controller, 16, ["up", "down"])
- else:
- attention_output = None
- dict_output = {
- "sdimage_output" : sdimage_output,
- "attention_output" : attention_output,
- "mask_list" : mask_list,
- }
- attention_util.register_attention_control(self, self.empty_controller)
- return dict_output
-
-
-
-
- @torch.no_grad()
- def __call__(self, **kwargs):
- edit_type = kwargs['edit_type']
- assert edit_type in ['save', 'swap', None]
- if edit_type is None:
- return self.sd_ddim_pipeline(controller = None, **kwargs)
-
- if edit_type == 'save':
- del self.store_controller
- self.store_controller = attention_util.AttentionStore()
- attention_util.register_attention_control(self, self.store_controller)
- sdimage_output = self.sd_ddim_pipeline(controller = self.store_controller, **kwargs)
-
- mask_list = None
-
- attention_output = attention_util.show_cross_attention(self.tokenizer, kwargs['prompt'], self.store_controller, 16, ["up", "down"])
-
-
- dict_output = {
- "sdimage_output" : sdimage_output,
- "attention_output" : attention_output,
- 'mask_list': mask_list
- }
-
- # Detach the controller for safety
- attention_util.register_attention_control(self, self.empty_controller)
- return dict_output
-
- if edit_type == 'swap':
-
- return self.p2preplace_edit(**kwargs)
-
-
- @torch.no_grad()
- def sd_ddim_pipeline(
- self,
- prompt: Union[str, List[str]],
- image: Union[torch.FloatTensor, PIL.Image.Image] = None,
- height: Optional[int] = None,
- width: Optional[int] = None,
- strength: float = None,
- num_inference_steps: int = 50,
- guidance_scale: float = 7.5,
- negative_prompt: Optional[Union[str, List[str]]] = None,
- num_images_per_prompt: Optional[int] = 1,
- eta: float = 0.0,
- generator: Optional[Union[torch.Generator, List[torch.Generator]]] = None,
- latents: Optional[torch.FloatTensor] = None,
- output_type: Optional[str] = "pil",
- return_dict: bool = True,
- callback: Optional[Callable[[int, int, torch.FloatTensor], None]] = None,
- callback_steps: Optional[int] = 1,
- controller: attention_util.AttentionControl = None,
- **args
- ):
- r"""
- Function invoked when calling the pipeline for generation.
-
- Args:
- prompt (`str` or `List[str]`):
- The prompt or prompts to guide the image generation.
- image (`torch.FloatTensor` or `PIL.Image.Image`):
- `Image`, or tensor representing an image batch, that will be used as the starting point for the
- process. Only used in DDIM or strength<1.0
- height (`int`, *optional*, defaults to self.unet.config.sample_size * self.vae_scale_factor):
- The height in pixels of the generated image.
- width (`int`, *optional*, defaults to self.unet.config.sample_size * self.vae_scale_factor):
- The width in pixels of the generated image.
- strength (`float`, *optional*, defaults to 1.0):
- Conceptually, indicates how much to transform the reference `image`. Must be between 0 and 1. `image`
- will be used as a starting point, adding more noise to it the larger the `strength`. The number of
- denoising steps depends on the amount of noise initially added. When `strength` is 1, added noise will
- be maximum and the denoising process will run for the full number of iterations specified in
- `num_inference_steps`. A value of 1, therefore, essentially ignores `image`.
- num_inference_steps (`int`, *optional*, defaults to 50):
- The number of denoising steps. More denoising steps usually lead to a higher quality image at the
- expense of slower inference.
- guidance_scale (`float`, *optional*, defaults to 7.5):
- Guidance scale as defined in [Classifier-Free Diffusion Guidance](https://arxiv.org/abs/2207.12598).
- `guidance_scale` is defined as `w` of equation 2. of [Imagen
- Paper](https://arxiv.org/pdf/2205.11487.pdf). Guidance scale is enabled by setting `guidance_scale >
- 1`. Higher guidance scale encourages to generate images that are closely linked to the text `prompt`,
- usually at the expense of lower image quality.
- negative_prompt (`str` or `List[str]`, *optional*):
- The prompt or prompts not to guide the image generation. Ignored when not using guidance (i.e., ignored
- if `guidance_scale` is less than `1`).
- num_images_per_prompt (`int`, *optional*, defaults to 1):
- The number of images to generate per prompt.
- eta (`float`, *optional*, defaults to 0.0):
- Corresponds to parameter eta (η) in the DDIM paper: https://arxiv.org/abs/2010.02502. Only applies to
- [`schedulers.DDIMScheduler`], will be ignored for others.
- generator (`torch.Generator`, *optional*):
- One or a list of [torch generator(s)](https://pytorch.org/docs/stable/generated/torch.Generator.html)
- to make generation deterministic.
- latents (`torch.FloatTensor`, *optional*):
- Pre-generated noisy latents, sampled from a Gaussian distribution, to be used as inputs for image
- generation. Can be used to tweak the same generation with different prompts. If not provided, a latents
- tensor will ge generated by sampling using the supplied random `generator`.
- output_type (`str`, *optional*, defaults to `"pil"`):
- The output format of the generate image. Choose between
- [PIL](https://pillow.readthedocs.io/en/stable/): `PIL.Image.Image` or `np.array`.
- return_dict (`bool`, *optional*, defaults to `True`):
- Whether or not to return a [`~pipelines.stable_diffusion.StableDiffusionPipelineOutput`] instead of a
- plain tuple.
- callback (`Callable`, *optional*):
- A function that will be called every `callback_steps` steps during inference. The function will be
- called with the following arguments: `callback(step: int, timestep: int, latents: torch.FloatTensor)`.
- callback_steps (`int`, *optional*, defaults to 1):
- The frequency at which the `callback` function will be called. If not specified, the callback will be
- called at every step.
-
- Returns:
- [`~pipelines.stable_diffusion.StableDiffusionPipelineOutput`] or `tuple`:
- [`~pipelines.stable_diffusion.StableDiffusionPipelineOutput`] if `return_dict` is True, otherwise a `tuple.
- When returning a tuple, the first element is a list with the generated images, and the second element is a
- list of `bool`s denoting whether the corresponding generated image likely represents "not-safe-for-work"
- (nsfw) content, according to the `safety_checker`.
- """
-
- # 0. Default height and width to unet
- height = height or self.unet.config.sample_size * self.vae_scale_factor
- width = width or self.unet.config.sample_size * self.vae_scale_factor
-
- # 1. Check inputs. Raise error if not correct
- self.check_inputs(prompt, height, width, callback_steps, strength)
-
- # 2. Define call parameters
- batch_size = 1 if isinstance(prompt, str) else len(prompt)
- device = self._execution_device
- # here `guidance_scale` is defined analog to the guidance weight `w` of equation (2)
- # of the Imagen paper: https://arxiv.org/pdf/2205.11487.pdf . `guidance_scale = 1`
- # corresponds to doing no classifier free guidance.
- do_classifier_free_guidance = guidance_scale > 1.0
-
- # 3. Encode input prompt
- text_embeddings = self._encode_prompt(
- prompt, device, num_images_per_prompt, do_classifier_free_guidance, negative_prompt
- )
-
- # 4. Prepare timesteps
- self.scheduler.set_timesteps(num_inference_steps, device=device)
- timesteps = self.scheduler.timesteps
-
- if latents is None:
- ddim_latents_all_step = self.prepare_latents_ddim_inverted(
- image, batch_size, num_images_per_prompt,
- text_embeddings,
- store_attention=False, # avoid recording attention in first inversion
- generator = generator,
- )
- latents = ddim_latents_all_step[-1]
- else:
- ddim_latents_all_step=None
-
- latents_dtype = latents.dtype
-
- # 6. Prepare extra step kwargs. TODO: Logic should ideally just be moved out of the pipeline
- extra_step_kwargs = self.prepare_extra_step_kwargs(generator, eta)
-
- # 7. Denoising loop
- num_warmup_steps = len(timesteps) - num_inference_steps * self.scheduler.order
- with self.progress_bar(total=num_inference_steps) as progress_bar:
- for i, t in enumerate(tqdm(timesteps)):
- # expand the latents if we are doing classifier free guidance
- latent_model_input = torch.cat([latents] * 2) if do_classifier_free_guidance else latents
- latent_model_input = self.scheduler.scale_model_input(latent_model_input, t)
-
- # predict the noise residual
- noise_pred = self.unet(
- latent_model_input, t, encoder_hidden_states=text_embeddings
- ).sample.to(dtype=latents_dtype)
-
- # perform guidance
- if do_classifier_free_guidance:
- noise_pred_uncond, noise_pred_text = noise_pred.chunk(2)
- noise_pred = noise_pred_uncond + guidance_scale * (
- noise_pred_text - noise_pred_uncond
- )
-
- # compute the previous noisy sample x_t -> x_t-1
- latents = self.scheduler.step(noise_pred, t, latents, **extra_step_kwargs).prev_sample
-
- # Edit the latents using attention map
- if controller is not None:
- latents_old = latents
- dtype = latents.dtype
- latents_new = controller.step_callback(latents)
- latents = latents_new.to(dtype)
- # call the callback, if provided
- if i == len(timesteps) - 1 or (
- (i + 1) > num_warmup_steps and (i + 1) % self.scheduler.order == 0
- ):
- progress_bar.update()
- if callback is not None and i % callback_steps == 0:
- callback(i, t, latents)
- torch.cuda.empty_cache()
- # 8. Post-processing
- image = self.decode_latents(latents)
-
- # 9. Run safety checker
- has_nsfw_concept = None
-
- # 10. Convert to PIL
- if output_type == "pil":
- image = self.numpy_to_pil(image)
-
- if not return_dict:
- return (image, has_nsfw_concept)
- torch.cuda.empty_cache()
- return StableDiffusionPipelineOutput(images=image, nsfw_content_detected=has_nsfw_concept)
diff --git a/spaces/chuan-hd/law-assistant-chatbot/.venv/lib/python3.11/site-packages/chromadb/db/mixins/sysdb.py b/spaces/chuan-hd/law-assistant-chatbot/.venv/lib/python3.11/site-packages/chromadb/db/mixins/sysdb.py
deleted file mode 100644
index 58ee4488b64ce85316094a23a5b7b25495a56226..0000000000000000000000000000000000000000
--- a/spaces/chuan-hd/law-assistant-chatbot/.venv/lib/python3.11/site-packages/chromadb/db/mixins/sysdb.py
+++ /dev/null
@@ -1,458 +0,0 @@
-from typing import Optional, Sequence, Any, Tuple, cast, Dict, Union, Set
-from uuid import UUID
-from overrides import override
-from pypika import Table, Column
-from itertools import groupby
-
-from chromadb.config import System
-from chromadb.db.base import (
- Cursor,
- SqlDB,
- ParameterValue,
- get_sql,
- NotFoundError,
- UniqueConstraintError,
-)
-from chromadb.db.system import SysDB
-from chromadb.types import (
- OptionalArgument,
- Segment,
- Metadata,
- Collection,
- SegmentScope,
- Unspecified,
- UpdateMetadata,
-)
-
-
-class SqlSysDB(SqlDB, SysDB):
- def __init__(self, system: System):
- super().__init__(system)
-
- @override
- def create_segment(self, segment: Segment) -> None:
- with self.tx() as cur:
- segments = Table("segments")
- insert_segment = (
- self.querybuilder()
- .into(segments)
- .columns(
- segments.id,
- segments.type,
- segments.scope,
- segments.topic,
- segments.collection,
- )
- .insert(
- ParameterValue(self.uuid_to_db(segment["id"])),
- ParameterValue(segment["type"]),
- ParameterValue(segment["scope"].value),
- ParameterValue(segment["topic"]),
- ParameterValue(self.uuid_to_db(segment["collection"])),
- )
- )
- sql, params = get_sql(insert_segment, self.parameter_format())
- try:
- cur.execute(sql, params)
- except self.unique_constraint_error() as e:
- raise UniqueConstraintError(
- f"Segment {segment['id']} already exists"
- ) from e
- metadata_t = Table("segment_metadata")
- if segment["metadata"]:
- self._insert_metadata(
- cur,
- metadata_t,
- metadata_t.segment_id,
- segment["id"],
- segment["metadata"],
- )
-
- @override
- def create_collection(self, collection: Collection) -> None:
- """Create a new collection"""
- with self.tx() as cur:
- collections = Table("collections")
- insert_collection = (
- self.querybuilder()
- .into(collections)
- .columns(
- collections.id,
- collections.topic,
- collections.name,
- collections.dimension,
- )
- .insert(
- ParameterValue(self.uuid_to_db(collection["id"])),
- ParameterValue(collection["topic"]),
- ParameterValue(collection["name"]),
- ParameterValue(collection["dimension"]),
- )
- )
- sql, params = get_sql(insert_collection, self.parameter_format())
- try:
- cur.execute(sql, params)
- except self.unique_constraint_error() as e:
- raise UniqueConstraintError(
- f"Collection {collection['id']} already exists"
- ) from e
- metadata_t = Table("collection_metadata")
- if collection["metadata"]:
- self._insert_metadata(
- cur,
- metadata_t,
- metadata_t.collection_id,
- collection["id"],
- collection["metadata"],
- )
-
- @override
- def get_segments(
- self,
- id: Optional[UUID] = None,
- type: Optional[str] = None,
- scope: Optional[SegmentScope] = None,
- topic: Optional[str] = None,
- collection: Optional[UUID] = None,
- ) -> Sequence[Segment]:
- segments_t = Table("segments")
- metadata_t = Table("segment_metadata")
- q = (
- self.querybuilder()
- .from_(segments_t)
- .select(
- segments_t.id,
- segments_t.type,
- segments_t.scope,
- segments_t.topic,
- segments_t.collection,
- metadata_t.key,
- metadata_t.str_value,
- metadata_t.int_value,
- metadata_t.float_value,
- )
- .left_join(metadata_t)
- .on(segments_t.id == metadata_t.segment_id)
- .orderby(segments_t.id)
- )
- if id:
- q = q.where(segments_t.id == ParameterValue(self.uuid_to_db(id)))
- if type:
- q = q.where(segments_t.type == ParameterValue(type))
- if scope:
- q = q.where(segments_t.scope == ParameterValue(scope.value))
- if topic:
- q = q.where(segments_t.topic == ParameterValue(topic))
- if collection:
- q = q.where(
- segments_t.collection == ParameterValue(self.uuid_to_db(collection))
- )
-
- with self.tx() as cur:
- sql, params = get_sql(q, self.parameter_format())
- rows = cur.execute(sql, params).fetchall()
- by_segment = groupby(rows, lambda r: cast(object, r[0]))
- segments = []
- for segment_id, segment_rows in by_segment:
- id = self.uuid_from_db(str(segment_id))
- rows = list(segment_rows)
- type = str(rows[0][1])
- scope = SegmentScope(str(rows[0][2]))
- topic = str(rows[0][3]) if rows[0][3] else None
- collection = self.uuid_from_db(rows[0][4]) if rows[0][4] else None
- metadata = self._metadata_from_rows(rows)
- segments.append(
- Segment(
- id=cast(UUID, id),
- type=type,
- scope=scope,
- topic=topic,
- collection=collection,
- metadata=metadata,
- )
- )
-
- return segments
-
- @override
- def get_collections(
- self,
- id: Optional[UUID] = None,
- topic: Optional[str] = None,
- name: Optional[str] = None,
- ) -> Sequence[Collection]:
- """Get collections by name, embedding function and/or metadata"""
- collections_t = Table("collections")
- metadata_t = Table("collection_metadata")
- q = (
- self.querybuilder()
- .from_(collections_t)
- .select(
- collections_t.id,
- collections_t.name,
- collections_t.topic,
- collections_t.dimension,
- metadata_t.key,
- metadata_t.str_value,
- metadata_t.int_value,
- metadata_t.float_value,
- )
- .left_join(metadata_t)
- .on(collections_t.id == metadata_t.collection_id)
- .orderby(collections_t.id)
- )
- if id:
- q = q.where(collections_t.id == ParameterValue(self.uuid_to_db(id)))
- if topic:
- q = q.where(collections_t.topic == ParameterValue(topic))
- if name:
- q = q.where(collections_t.name == ParameterValue(name))
-
- with self.tx() as cur:
- sql, params = get_sql(q, self.parameter_format())
- rows = cur.execute(sql, params).fetchall()
- by_collection = groupby(rows, lambda r: cast(object, r[0]))
- collections = []
- for collection_id, collection_rows in by_collection:
- id = self.uuid_from_db(str(collection_id))
- rows = list(collection_rows)
- name = str(rows[0][1])
- topic = str(rows[0][2])
- dimension = int(rows[0][3]) if rows[0][3] else None
- metadata = self._metadata_from_rows(rows)
- collections.append(
- Collection(
- id=cast(UUID, id),
- topic=topic,
- name=name,
- metadata=metadata,
- dimension=dimension,
- )
- )
-
- return collections
-
- @override
- def delete_segment(self, id: UUID) -> None:
- """Delete a segment from the SysDB"""
- t = Table("segments")
- q = (
- self.querybuilder()
- .from_(t)
- .where(t.id == ParameterValue(self.uuid_to_db(id)))
- .delete()
- )
- with self.tx() as cur:
- # no need for explicit del from metadata table because of ON DELETE CASCADE
- sql, params = get_sql(q, self.parameter_format())
- sql = sql + " RETURNING id"
- result = cur.execute(sql, params).fetchone()
- if not result:
- raise NotFoundError(f"Segment {id} not found")
-
- @override
- def delete_collection(self, id: UUID) -> None:
- """Delete a topic and all associated segments from the SysDB"""
- t = Table("collections")
- q = (
- self.querybuilder()
- .from_(t)
- .where(t.id == ParameterValue(self.uuid_to_db(id)))
- .delete()
- )
- with self.tx() as cur:
- # no need for explicit del from metadata table because of ON DELETE CASCADE
- sql, params = get_sql(q, self.parameter_format())
- sql = sql + " RETURNING id"
- result = cur.execute(sql, params).fetchone()
- if not result:
- raise NotFoundError(f"Collection {id} not found")
-
- @override
- def update_segment(
- self,
- id: UUID,
- topic: OptionalArgument[Optional[str]] = Unspecified(),
- collection: OptionalArgument[Optional[UUID]] = Unspecified(),
- metadata: OptionalArgument[Optional[UpdateMetadata]] = Unspecified(),
- ) -> None:
- segments_t = Table("segments")
- metadata_t = Table("segment_metadata")
-
- q = (
- self.querybuilder()
- .update(segments_t)
- .where(segments_t.id == ParameterValue(self.uuid_to_db(id)))
- )
-
- if not topic == Unspecified():
- q = q.set(segments_t.topic, ParameterValue(topic))
-
- if not collection == Unspecified():
- collection = cast(Optional[UUID], collection)
- q = q.set(
- segments_t.collection, ParameterValue(self.uuid_to_db(collection))
- )
-
- with self.tx() as cur:
- sql, params = get_sql(q, self.parameter_format())
- if sql: # pypika emits a blank string if nothing to do
- cur.execute(sql, params)
-
- if metadata is None:
- q = (
- self.querybuilder()
- .from_(metadata_t)
- .where(metadata_t.segment_id == ParameterValue(self.uuid_to_db(id)))
- .delete()
- )
- sql, params = get_sql(q, self.parameter_format())
- cur.execute(sql, params)
- elif metadata != Unspecified():
- metadata = cast(UpdateMetadata, metadata)
- metadata = cast(UpdateMetadata, metadata)
- self._insert_metadata(
- cur,
- metadata_t,
- metadata_t.segment_id,
- id,
- metadata,
- set(metadata.keys()),
- )
-
- @override
- def update_collection(
- self,
- id: UUID,
- topic: OptionalArgument[Optional[str]] = Unspecified(),
- name: OptionalArgument[str] = Unspecified(),
- dimension: OptionalArgument[Optional[int]] = Unspecified(),
- metadata: OptionalArgument[Optional[UpdateMetadata]] = Unspecified(),
- ) -> None:
- collections_t = Table("collections")
- metadata_t = Table("collection_metadata")
-
- q = (
- self.querybuilder()
- .update(collections_t)
- .where(collections_t.id == ParameterValue(self.uuid_to_db(id)))
- )
-
- if not topic == Unspecified():
- q = q.set(collections_t.topic, ParameterValue(topic))
-
- if not name == Unspecified():
- q = q.set(collections_t.name, ParameterValue(name))
-
- if not dimension == Unspecified():
- q = q.set(collections_t.dimension, ParameterValue(dimension))
-
- with self.tx() as cur:
- sql, params = get_sql(q, self.parameter_format())
- if sql: # pypika emits a blank string if nothing to do
- cur.execute(sql, params)
-
- # TODO: Update to use better semantics where it's possible to update
- # individual keys without wiping all the existing metadata.
-
- # For now, follow current legancy semantics where metadata is fully reset
- if metadata != Unspecified():
- q = (
- self.querybuilder()
- .from_(metadata_t)
- .where(
- metadata_t.collection_id == ParameterValue(self.uuid_to_db(id))
- )
- .delete()
- )
- sql, params = get_sql(q, self.parameter_format())
- cur.execute(sql, params)
- if metadata is not None:
- metadata = cast(UpdateMetadata, metadata)
- self._insert_metadata(
- cur,
- metadata_t,
- metadata_t.collection_id,
- id,
- metadata,
- set(metadata.keys()),
- )
-
- def _metadata_from_rows(
- self, rows: Sequence[Tuple[Any, ...]]
- ) -> Optional[Metadata]:
- """Given SQL rows, return a metadata map (assuming that the last four columns
- are the key, str_value, int_value & float_value)"""
- metadata: Dict[str, Union[str, int, float]] = {}
- for row in rows:
- key = str(row[-4])
- if row[-3] is not None:
- metadata[key] = str(row[-3])
- elif row[-2] is not None:
- metadata[key] = int(row[-2])
- elif row[-1] is not None:
- metadata[key] = float(row[-1])
- return metadata or None
-
- def _insert_metadata(
- self,
- cur: Cursor,
- table: Table,
- id_col: Column,
- id: UUID,
- metadata: UpdateMetadata,
- clear_keys: Optional[Set[str]] = None,
- ) -> None:
- # It would be cleaner to use something like ON CONFLICT UPDATE here But that is
- # very difficult to do in a portable way (e.g sqlite and postgres have
- # completely different sytnax)
- if clear_keys:
- q = (
- self.querybuilder()
- .from_(table)
- .where(id_col == ParameterValue(self.uuid_to_db(id)))
- .where(table.key.isin([ParameterValue(k) for k in clear_keys]))
- .delete()
- )
- sql, params = get_sql(q, self.parameter_format())
- cur.execute(sql, params)
-
- q = (
- self.querybuilder()
- .into(table)
- .columns(
- id_col, table.key, table.str_value, table.int_value, table.float_value
- )
- )
- sql_id = self.uuid_to_db(id)
- for k, v in metadata.items():
- if isinstance(v, str):
- q = q.insert(
- ParameterValue(sql_id),
- ParameterValue(k),
- ParameterValue(v),
- None,
- None,
- )
- elif isinstance(v, int):
- q = q.insert(
- ParameterValue(sql_id),
- ParameterValue(k),
- None,
- ParameterValue(v),
- None,
- )
- elif isinstance(v, float):
- q = q.insert(
- ParameterValue(sql_id),
- ParameterValue(k),
- None,
- None,
- ParameterValue(v),
- )
- elif v is None:
- continue
-
- sql, params = get_sql(q, self.parameter_format())
- if sql:
- cur.execute(sql, params)
diff --git a/spaces/chuan-hd/law-assistant-chatbot/.venv/lib/python3.11/site-packages/gradio/pipelines.py b/spaces/chuan-hd/law-assistant-chatbot/.venv/lib/python3.11/site-packages/gradio/pipelines.py
deleted file mode 100644
index 144f1f7ecd59c2c9c71fbd836061de9ed4b1f71b..0000000000000000000000000000000000000000
--- a/spaces/chuan-hd/law-assistant-chatbot/.venv/lib/python3.11/site-packages/gradio/pipelines.py
+++ /dev/null
@@ -1,225 +0,0 @@
-"""This module should not be used directly as its API is subject to change. Instead,
-please use the `gr.Interface.from_pipeline()` function."""
-
-from __future__ import annotations
-
-from typing import TYPE_CHECKING
-
-from gradio import components
-
-if TYPE_CHECKING: # Only import for type checking (is False at runtime).
- from transformers import pipelines
-
-
-def load_from_pipeline(pipeline: pipelines.base.Pipeline) -> dict:
- """
- Gets the appropriate Interface kwargs for a given Hugging Face transformers.Pipeline.
- pipeline (transformers.Pipeline): the transformers.Pipeline from which to create an interface
- Returns:
- (dict): a dictionary of kwargs that can be used to construct an Interface object
- """
- try:
- import transformers
- from transformers import pipelines
- except ImportError as ie:
- raise ImportError(
- "transformers not installed. Please try `pip install transformers`"
- ) from ie
- if not isinstance(pipeline, pipelines.base.Pipeline):
- raise ValueError("pipeline must be a transformers.Pipeline")
-
- # Handle the different pipelines. The has_attr() checks to make sure the pipeline exists in the
- # version of the transformers library that the user has installed.
- if hasattr(transformers, "AudioClassificationPipeline") and isinstance(
- pipeline, pipelines.audio_classification.AudioClassificationPipeline
- ):
- pipeline_info = {
- "inputs": components.Audio(
- source="microphone", type="filepath", label="Input"
- ),
- "outputs": components.Label(label="Class"),
- "preprocess": lambda i: {"inputs": i},
- "postprocess": lambda r: {i["label"].split(", ")[0]: i["score"] for i in r},
- }
- elif hasattr(transformers, "AutomaticSpeechRecognitionPipeline") and isinstance(
- pipeline,
- pipelines.automatic_speech_recognition.AutomaticSpeechRecognitionPipeline,
- ):
- pipeline_info = {
- "inputs": components.Audio(
- source="microphone", type="filepath", label="Input"
- ),
- "outputs": components.Textbox(label="Output"),
- "preprocess": lambda i: {"inputs": i},
- "postprocess": lambda r: r["text"],
- }
- elif hasattr(transformers, "FeatureExtractionPipeline") and isinstance(
- pipeline, pipelines.feature_extraction.FeatureExtractionPipeline
- ):
- pipeline_info = {
- "inputs": components.Textbox(label="Input"),
- "outputs": components.Dataframe(label="Output"),
- "preprocess": lambda x: {"inputs": x},
- "postprocess": lambda r: r[0],
- }
- elif hasattr(transformers, "FillMaskPipeline") and isinstance(
- pipeline, pipelines.fill_mask.FillMaskPipeline
- ):
- pipeline_info = {
- "inputs": components.Textbox(label="Input"),
- "outputs": components.Label(label="Classification"),
- "preprocess": lambda x: {"inputs": x},
- "postprocess": lambda r: {i["token_str"]: i["score"] for i in r},
- }
- elif hasattr(transformers, "ImageClassificationPipeline") and isinstance(
- pipeline, pipelines.image_classification.ImageClassificationPipeline
- ):
- pipeline_info = {
- "inputs": components.Image(type="filepath", label="Input Image"),
- "outputs": components.Label(type="confidences", label="Classification"),
- "preprocess": lambda i: {"images": i},
- "postprocess": lambda r: {i["label"].split(", ")[0]: i["score"] for i in r},
- }
- elif hasattr(transformers, "QuestionAnsweringPipeline") and isinstance(
- pipeline, pipelines.question_answering.QuestionAnsweringPipeline
- ):
- pipeline_info = {
- "inputs": [
- components.Textbox(lines=7, label="Context"),
- components.Textbox(label="Question"),
- ],
- "outputs": [
- components.Textbox(label="Answer"),
- components.Label(label="Score"),
- ],
- "preprocess": lambda c, q: {"context": c, "question": q},
- "postprocess": lambda r: (r["answer"], r["score"]),
- }
- elif hasattr(transformers, "SummarizationPipeline") and isinstance(
- pipeline, pipelines.text2text_generation.SummarizationPipeline
- ):
- pipeline_info = {
- "inputs": components.Textbox(lines=7, label="Input"),
- "outputs": components.Textbox(label="Summary"),
- "preprocess": lambda x: {"inputs": x},
- "postprocess": lambda r: r[0]["summary_text"],
- }
- elif hasattr(transformers, "TextClassificationPipeline") and isinstance(
- pipeline, pipelines.text_classification.TextClassificationPipeline
- ):
- pipeline_info = {
- "inputs": components.Textbox(label="Input"),
- "outputs": components.Label(label="Classification"),
- "preprocess": lambda x: [x],
- "postprocess": lambda r: {i["label"].split(", ")[0]: i["score"] for i in r},
- }
- elif hasattr(transformers, "TextGenerationPipeline") and isinstance(
- pipeline, pipelines.text_generation.TextGenerationPipeline
- ):
- pipeline_info = {
- "inputs": components.Textbox(label="Input"),
- "outputs": components.Textbox(label="Output"),
- "preprocess": lambda x: {"text_inputs": x},
- "postprocess": lambda r: r[0]["generated_text"],
- }
- elif hasattr(transformers, "TranslationPipeline") and isinstance(
- pipeline, pipelines.text2text_generation.TranslationPipeline
- ):
- pipeline_info = {
- "inputs": components.Textbox(label="Input"),
- "outputs": components.Textbox(label="Translation"),
- "preprocess": lambda x: [x],
- "postprocess": lambda r: r[0]["translation_text"],
- }
- elif hasattr(transformers, "Text2TextGenerationPipeline") and isinstance(
- pipeline, pipelines.text2text_generation.Text2TextGenerationPipeline
- ):
- pipeline_info = {
- "inputs": components.Textbox(label="Input"),
- "outputs": components.Textbox(label="Generated Text"),
- "preprocess": lambda x: [x],
- "postprocess": lambda r: r[0]["generated_text"],
- }
- elif hasattr(transformers, "ZeroShotClassificationPipeline") and isinstance(
- pipeline, pipelines.zero_shot_classification.ZeroShotClassificationPipeline
- ):
- pipeline_info = {
- "inputs": [
- components.Textbox(label="Input"),
- components.Textbox(label="Possible class names (" "comma-separated)"),
- components.Checkbox(label="Allow multiple true classes"),
- ],
- "outputs": components.Label(label="Classification"),
- "preprocess": lambda i, c, m: {
- "sequences": i,
- "candidate_labels": c,
- "multi_label": m,
- },
- "postprocess": lambda r: {
- r["labels"][i]: r["scores"][i] for i in range(len(r["labels"]))
- },
- }
- elif hasattr(transformers, "DocumentQuestionAnsweringPipeline") and isinstance(
- pipeline,
- pipelines.document_question_answering.DocumentQuestionAnsweringPipeline, # type: ignore
- ):
- pipeline_info = {
- "inputs": [
- components.Image(type="filepath", label="Input Document"),
- components.Textbox(label="Question"),
- ],
- "outputs": components.Label(label="Label"),
- "preprocess": lambda img, q: {"image": img, "question": q},
- "postprocess": lambda r: {i["answer"]: i["score"] for i in r},
- }
- elif hasattr(transformers, "VisualQuestionAnsweringPipeline") and isinstance(
- pipeline, pipelines.visual_question_answering.VisualQuestionAnsweringPipeline
- ):
- pipeline_info = {
- "inputs": [
- components.Image(type="filepath", label="Input Image"),
- components.Textbox(label="Question"),
- ],
- "outputs": components.Label(label="Score"),
- "preprocess": lambda img, q: {"image": img, "question": q},
- "postprocess": lambda r: {i["answer"]: i["score"] for i in r},
- }
- elif hasattr(transformers, "ImageToTextPipeline") and isinstance(
- pipeline, pipelines.image_to_text.ImageToTextPipeline # type: ignore
- ):
- pipeline_info = {
- "inputs": components.Image(type="filepath", label="Input Image"),
- "outputs": components.Textbox(label="Text"),
- "preprocess": lambda i: {"images": i},
- "postprocess": lambda r: r[0]["generated_text"],
- }
- else:
- raise ValueError(f"Unsupported pipeline type: {type(pipeline)}")
-
- # define the function that will be called by the Interface
- def fn(*params):
- data = pipeline_info["preprocess"](*params)
- # special cases that needs to be handled differently
- if isinstance(
- pipeline,
- (
- pipelines.text_classification.TextClassificationPipeline,
- pipelines.text2text_generation.Text2TextGenerationPipeline,
- pipelines.text2text_generation.TranslationPipeline,
- ),
- ):
- data = pipeline(*data)
- else:
- data = pipeline(**data)
- output = pipeline_info["postprocess"](data)
- return output
-
- interface_info = pipeline_info.copy()
- interface_info["fn"] = fn
- del interface_info["preprocess"]
- del interface_info["postprocess"]
-
- # define the title/description of the Interface
- interface_info["title"] = pipeline.model.__class__.__name__
-
- return interface_info
diff --git a/spaces/cihyFjudo/fairness-paper-search/Bmw Ista P V40 12.md b/spaces/cihyFjudo/fairness-paper-search/Bmw Ista P V40 12.md
deleted file mode 100644
index 90dcae02f3da1081f0022e5f9dcad01778cc11f9..0000000000000000000000000000000000000000
--- a/spaces/cihyFjudo/fairness-paper-search/Bmw Ista P V40 12.md
+++ /dev/null
@@ -1,104 +0,0 @@
-## Bmw Ista P V40 12
-
-
-
-
-
-
-
-
-
-**DOWNLOAD ===== [https://smitodoutcu.blogspot.com/?c=2txlg4](https://smitodoutcu.blogspot.com/?c=2txlg4)**
-
-
-
-
-
-
-
-
-
-
-
-
-
-# How to Use BMW ISTA P V40 12 for Coding and Programming
-
-
-
-BMW ISTA P is a software tool that allows you to code and program your BMW vehicles using a K+DCAN cable or an ICOM interface. It supports E, F, G, I, and Mini series models, and can update modules, retrofit items, customize functions, and more.
-
-
-
-In this article, we will show you how to use BMW ISTA P V40 12, the latest version of the software as of 2023, to perform some common tasks on your BMW. You will need a Windows 10 or 11 laptop with at least 300 GB of free space, a K+DCAN cable or an ICOM interface, and BMW Standard Tools installed.
-
-
-
-## How to Install BMW ISTA P V40 12
-
-
-
-Before you can use BMW ISTA P V40 12, you need to install it on your laptop. Here are the steps:
-
-
-
-1. Download BMW ISTA P V40 12 from this link: [https://eurocartool.com/how-to-download-bmw-ista-p-free/](https://eurocartool.com/how-to-download-bmw-ista-p-free/)
-
-2. Extract the downloaded file using WinRAR or 7-Zip.
-
-3. Right-click on the file BMW ISTA-P and select Run as administrator.
-
-4. Follow the instructions on the screen to install BMW ISTA P V40 12.
-
-5. Restart your laptop after the installation is complete.
-
-
-
-## How to Connect BMW ISTA P V40 12 to Your Vehicle
-
-
-
-After installing BMW ISTA P V40 12, you need to connect it to your vehicle using a K+DCAN cable or an ICOM interface. Here are the steps:
-
-
-
-1. Plug one end of the K+DCAN cable or the ICOM interface into the OBD port of your vehicle, usually located under the dashboard.
-
-2. Plug the other end of the K+DCAN cable or the ICOM interface into a USB port of your laptop.
-
-3. Launch BMW ISTA P V40 12 from your desktop or start menu.
-
-4. Select your vehicle type and model from the list.
-
-5. Wait for BMW ISTA P V40 12 to establish communication with your vehicle.
-
-
-
-## How to Code and Program Your Vehicle with BMW ISTA P V40 12
-
-
-
-Once you have connected BMW ISTA P V40 12 to your vehicle, you can code and program your vehicle according to your preferences. Here are some examples of what you can do:
-
-
-
-- Update modules: You can update your modules to the latest versions for improved performance and functionality. To do this, select Update Modules from the main menu, then choose the modules you want to update and follow the instructions on the screen.
-
-- Retrofit items: You can retrofit items that were not originally installed on your vehicle, such as a backup camera, a navigation system, or a heated steering wheel. To do this, select Retrofit Items from the main menu, then choose the items you want to retrofit and follow the instructions on the screen.
-
-- Customize functions: You can customize various functions of your vehicle, such as lights, comfort features, alarms, etc. To do this, select Customize Functions from the main menu, then choose the functions you want to customize and follow the instructions on the screen.
-
-
-
-## Conclusion
-
-
-
-BMW ISTA P V40 12 is a powerful software tool that allows you to code and program your BMW vehicles with ease. It supports E, F, G, I, and Mini series models, and can update modules, retrofit items, customize functions, and more. You just need a Windows 10 or 11 laptop with at least 300 GB of free space, a K+DCAN cable or an ICOM interface, and BMW Standard Tools installed. We hope this article has helped you understand how to use BMW ISTA P V40 12 for coding and programming your vehicle. If you have any questions or feedback, please leave a comment below.
-
- 1b8d091108
-
-
-
-
-
diff --git a/spaces/cihyFjudo/fairness-paper-search/Hotspot Shield Elite 8.5.2 Crack Plus Keygen Full Version 2020 Keygen Why You Need This VPN Software Now.md b/spaces/cihyFjudo/fairness-paper-search/Hotspot Shield Elite 8.5.2 Crack Plus Keygen Full Version 2020 Keygen Why You Need This VPN Software Now.md
deleted file mode 100644
index 60a25e439ea7a2767c3e1d009d36b30ce45cccc5..0000000000000000000000000000000000000000
--- a/spaces/cihyFjudo/fairness-paper-search/Hotspot Shield Elite 8.5.2 Crack Plus Keygen Full Version 2020 Keygen Why You Need This VPN Software Now.md
+++ /dev/null
@@ -1,6 +0,0 @@
-
Hotspot Shield Elite 8.5.2 Crack Plus Keygen Full Version 2020 Keygen
-
- aaccfb2cb3
-
-
-
diff --git a/spaces/cihyFjudo/fairness-paper-search/Muskaan Full FREE Hd Movie Download With Torrent Acide Water Temperat.md b/spaces/cihyFjudo/fairness-paper-search/Muskaan Full FREE Hd Movie Download With Torrent Acide Water Temperat.md
deleted file mode 100644
index ca8883424d646774c759be06ae213386e9951a12..0000000000000000000000000000000000000000
--- a/spaces/cihyFjudo/fairness-paper-search/Muskaan Full FREE Hd Movie Download With Torrent Acide Water Temperat.md
+++ /dev/null
@@ -1,6 +0,0 @@
-
Muskaan Full Hd Movie Download With Torrent acide water temperat
APKPure: What is it and how to download APKs in 2023?
-
If you are an Android user, you probably know that Google Play Store is the official app store for your device. However, there are many apps and games that are not available on Google Play Store for various reasons. Maybe they are region-locked, banned, or removed by Google. Maybe they are too new, too old, or too niche for the mainstream market. Maybe they are just better than the official versions.
-
Whatever the reason, if you want to access these apps and games, you need a reliable source to download them from. That's where APKPure comes in. APKPure is one of the best websites to download APKs from as of 2023. APK stands for Android Package Kit, and it is the file format used by the Android operating system to distribute and install apps. APKPure provides users with a convenient way to search for and download APK files for various apps and games, without having to go through the Google Play Store.
In this article, we will explain what APKPure is, what features it offers, how to download APKs from it in 2023, what benefits and risks it entails, and why you should use it. Let's get started!
-
Features of APKPure
-
APKPure is more than just a website to download APKs from. It is also a platform that offers a variety of features to enhance your app experience. Here are some of the features that make APKPure stand out:
-
-
Download apps and games not available on Google Play Store: As we mentioned earlier, there are many apps and games that you can't find on Google Play Store for various reasons. With APKPure, you can access them easily and legally. You can find apps and games from different categories, genres, regions, languages, and developers. You can also request apps that are not yet available on APKPure.
-
Update apps to the latest version: Sometimes, Google Play Store may not update your apps to the latest version due to compatibility issues or other factors. With APKPure, you can always get the latest version of your apps as soon as they are released by the developers. You can also choose to update only certain apps or all of them at once.
-
Discover new and trending apps and games: If you are looking for new and exciting apps and games to try out, APKPure can help you discover them. You can browse through different sections such as Editor's Choice, Top Charts, New Releases, Popular Games, etc. You can also see user ratings, reviews, screenshots, videos, and more information about each app or game.
-
Manage your downloads and installations : APKPure allows you to manage your downloads and installations with ease. You can see the progress, speed, and status of your downloads. You can also pause, resume, or cancel them at any time. You can also install the APK files directly from APKPure or from your device's storage. You can also uninstall or delete the APK files if you don't need them anymore.
-
-
How to download APKs from APKPure in 2023?
-
Downloading APKs from APKPure is very simple and straightforward. You can do it either from the APKPure website or from the APKPure app. Here are the steps to follow:
-
-
Step 1: Visit the APKPure website or download the APKPure app: You can access the APKPure website from any browser on your device. The website is https://apkpure.com/. Alternatively, you can download the APKPure app from the website or from other sources. The app is free and safe to use.
-
Step 2: Search for the app or game you want to download: You can use the search bar on the top of the website or the app to type in the name of the app or game you want to download. You can also browse through the different sections and categories to find what you are looking for.
-
Step 3: Click on the download button and choose the version you want: Once you find the app or game you want, click on it to see more details and information. Then, click on the green download button on the right side of the screen. You will see a list of available versions for that app or game. Choose the version that suits your device and preferences.
-
Step 4: Install the APK file on your device: After you choose the version, the download will start automatically. You will see a notification on your device when the download is complete. Then, you can open the notification and tap on the APK file to install it on your device. You may need to enable unknown sources in your device settings to allow the installation.
-
-
Benefits of downloading APKs from APKPure in 2023?
-
Downloading APKs from APKPure has many benefits for Android users in 2023. Here are some of them:
-
-
Access apps and games that are region-locked, banned, or removed from Google Play Store: As we mentioned earlier, there are many apps and games that you can't find on Google Play Store for various reasons. Maybe they are restricted in your country or region, maybe they are banned by Google for violating their policies, maybe they are removed by their developers for some reason. With APKPure, you can access these apps and games without any hassle. You can enjoy apps and games that are not available in your market.
-
Enjoy faster and safer downloads with no ads or malware: APKPure provides users with fast and secure downloads of APK files. You don't have to worry about slow speeds, interruptions, or errors. You also don't have to worry about ads, pop-ups, or malware that may harm your device or data. APKPure verifies and scans every APK file before uploading it to their website or app. You can download with confidence and peace of mind.
-
Save storage space and data usage by downloading only the APK file: Another benefit of downloading APKs from APKPure is that you can save storage space and data usage on your device. Unlike Google Play Store, which downloads both the APK file and the additional data (such as OBB files) for each app or game, APKPure only downloads the APK file. This means that you only need to download a small file size for each app or game, which saves you storage space and data usage. You can also choose to download only the parts of the app or game that you need, such as specific languages or features.
-
Customize your app experience by choosing different versions and languages: Another benefit of downloading APKs from APKPure is that you can customize your app experience by choosing different versions and languages for each app or game. Sometimes, you may want to try an older version of an app or game because it has a feature that you like, or because it is more compatible with your device. Sometimes, you may want to try a newer version of an app or game because it has a bug fix or an improvement that you need. Sometimes, you may want to try a different language of an app or game because it is more suitable for your preferences or needs. With APKPure, you can do all these things easily and conveniently.
-
-
Risks of downloading APKs from APKPure in 2023?
-
While downloading APKs from APKPure has many benefits, it also has some risks that you should be aware of. Here are some of the risks that you may encounter when downloading APKs from APKPure in 2023:
-
-
Potential compatibility issues with your device or operating system: One of the risks of downloading APKs from APKPure is that you may face compatibility issues with your device or operating system. Not all apps and games are compatible with all devices and operating systems. Some apps and games may require certain hardware specifications, software versions, or permissions to run properly. If you download an app or game that is not compatible with your device or operating system, you may experience errors, crashes, or glitches. You may also damage your device or data if you force the installation of an incompatible app or game.
-
Possible legal or ethical concerns with downloading paid or copyrighted apps: Another risk of downloading APKs from APKPure is that you may face legal or ethical concerns with downloading paid or copyrighted apps. Some apps and games are paid or premium, which means that you have to pay a certain amount of money to access them. Some apps and games are also protected by copyright laws, which means that you have to respect the rights and ownership of the developers and publishers. If you download a paid or premium app or game without paying for it, or if you download a copyrighted app or game without permission, you may be violating the law or the ethics of the app industry. You may also be depriving the developers and publishers of their deserved income and recognition.
-
Potential security risks with installing unknown or modified apps: Another risk of downloading APKs from APKPure is that you may face security risks with installing unknown or modified apps. Some apps and games are unknown or modified, which means that they are not verified or approved by Google Play Store or other official sources. Some apps and games are also hacked, cracked, or modded, which means that they are altered or tampered with by third parties. If you download an unknown or modified app or game, you may expose your device or data to malware, viruses, spyware, ransomware, phishing, or other malicious attacks. You may also compromise your privacy, security, or identity if you grant permissions or access to an unknown or modified app or game.
-
-
Conclusion
-
APKPure is one of the best websites to download APKs from as of 2023. It offers a variety of features, benefits, and risks for Android users who want to access apps and games that are not available on Google Play Store. Whether you want to try new and trending apps and games, update your existing apps to the latest version, save storage space and data usage by downloading only the APK file, or customize your app experience by choosing different versions and languages, APKPure can help you do all these things easily and conveniently.
-
However, you should also be aware of the potential compatibility issues, legal or ethical concerns, and security risks that come with downloading APKs from APKPure. You should always check the compatibility, legality, and security of the apps and games before downloading them from APKPure. You should also always backup your device and data before installing any APK file on your device.
-
-
If you are interested in downloading APKs from APKPure in 2023, you can visit their website at https://apkpure.com/ or download their app from their website or other sources. You can also follow them on social media platforms such as Facebook, Twitter, Instagram, YouTube, etc. to stay updated on their latest news and releases.
-
We hope this article has helped you understand what APKPure is and how to download APKs from it in 2023. If you have any questions, comments, or feedback, please feel free to share them with us in the comment section below. Thank you for reading!
-
FAQs
-
-
What is APKPure?: APKPure is one of the best websites to download APKs from as of 2023. It provides users with a convenient way to search for and download APK files for various apps and games without having to go through Google Play Store.
-
What is an APK file?: An APK file is the file format used by the Android operating system to distribute and install apps. It contains all the elements that an app needs to run on an Android device.
-
How to download APKs from APKPure in 2023?: You can download APKs from APKPure either from their website or from their app. You just need to visit their website at https://apkpure.com/ or download their app from their website or other sources. Then, search for the app or game you want to download, and click on the download button. You will see a list of available versions for that app or game. Choose the version that suits your device and preferences, and the download will start automatically. Then, install the APK file on your device by opening the notification and tapping on the file.
-
What are the benefits of downloading APKs from APKPure in 2023?: Some of the benefits of downloading APKs from APKPure are that you can access apps and games that are not available on Google Play Store, enjoy faster and safer downloads with no ads or malware, save storage space and data usage by downloading only the APK file, and customize your app experience by choosing different versions and languages.
-
What are the risks of downloading APKs from APKPure in 2023?: Some of the risks of downloading APKs from APKPure are that you may face compatibility issues with your device or operating system, legal or ethical concerns with downloading paid or copyrighted apps, and security risks with installing unknown or modified apps.
-
How to contact APKPure in 2023?: If you have any questions, comments, or feedback about APKPure, you can contact them through their website or their app. You can also email them at support@apkpure.com or follow them on social media platforms such as Facebook, Twitter, Instagram, YouTube, etc.
-
401be4b1e0
-
-
\ No newline at end of file
diff --git a/spaces/congsaPfin/Manga-OCR/logs/Download Call of Duty Mobile APKPure and Join the Battle Royale Mode with 100 Players.md b/spaces/congsaPfin/Manga-OCR/logs/Download Call of Duty Mobile APKPure and Join the Battle Royale Mode with 100 Players.md
deleted file mode 100644
index 34f134c13e7651e114873ec73ad0deb4a464e6d9..0000000000000000000000000000000000000000
--- a/spaces/congsaPfin/Manga-OCR/logs/Download Call of Duty Mobile APKPure and Join the Battle Royale Mode with 100 Players.md
+++ /dev/null
@@ -1,90 +0,0 @@
-
-
Download Call of Duty Mobile APKPure: How to Play the Popular Shooter on Your Android Device
-
If you are a fan of first-person shooter games, you have probably heard of Call of Duty, one of the most successful and influential franchises in the genre. But did you know that you can also play Call of Duty on your mobile device? Yes, you read that right. Call of Duty Mobile is a free-to-play game that brings the best of Call of Duty to your Android phone or tablet. In this article, we will tell you what Call of Duty Mobile is, why you should download it from APKPure, and how to do it in a few simple steps.
Call of Duty Mobile is a mobile version of the famous Call of Duty series, developed by TiMi Studios and published by Activision. The game was released in October 2019 and has since become one of the most popular and downloaded games on mobile platforms. Call of Duty Mobile offers an exciting way to play a beloved game franchise on your mobile device. The game features classic multiplayer modes such as Team Deathmatch, Domination, and Kill-Confirmed on iconic maps like Shipment, Raid, and Standoff. You can also play Battle Royale mode, where you compete with 99 other players in a large map with vehicles, weapons, and items. You can customize your loadout, unlock new skins, weapons, perks, and more as you level up your rank and battle pass. You can also join clans, chat with friends, and participate in seasonal events and challenges.
-
Why download Call of Duty Mobile APKPure?
-
Call of Duty Mobile is available on Google Play Store, but there are some reasons why you might want to download it from APKPure instead. APKPure is a third-party app store that provides free and safe APK files for Android users. Here are some of the benefits of using APKPure to download and install Call of Duty Mobile:
-
No region restrictions
-
Some countries or regions may not have access to Call of Duty Mobile on Google Play Store due to various reasons. For example, India banned the game in 2020 along with other Chinese apps amid political tensions. If you live in such a country or region, you can still download Call of Duty Mobile from APKPure without any hassle.
-
Faster and safer downloads
-
APKPure offers fast and reliable downloads for Call of Duty Mobile. You don't have to worry about slow or interrupted downloads due to network issues or server overload. APKPure also verifies the authenticity and security of every APK file before uploading it to their website or app. You can be sure that you are downloading a virus-free and malware-free file from APKPure.
-
Easy updates and compatibility
-
APKPure also makes it easy for you to update Call of Duty Mobile whenever there is a new version available. You don't have to wait for Google Play Store to approve or release the update. You can simply check for updates on APKPure and download them in minutes. APKPure also ensures that you can play Call of Duty Mobile on any Android device that meets the minimum requirements. You don't have to worry about compatibility issues or device specifications.
-
download call of duty mobile apk pure free
-download call of duty mobile apkpure latest version
-download call of duty mobile apkpure mod apk
-download call of duty mobile apkpure offline
-download call of duty mobile apkpure for android
-download call of duty mobile apkpure for pc
-download call of duty mobile apkpure for ios
-download call of duty mobile apkpure for windows 10
-download call of duty mobile apkpure for mac
-download call of duty mobile apkpure for laptop
-download call of duty mobile apkpure update
-download call of duty mobile apkpure season 6
-download call of duty mobile apkpure season 7
-download call of duty mobile apkpure season 8
-download call of duty mobile apkpure season 9
-download call of duty mobile apkpure season 10
-download call of duty mobile apkpure zombies mode
-download call of duty mobile apkpure battle royale mode
-download call of duty mobile apkpure multiplayer mode
-download call of duty mobile apkpure warzone mode
-download call of duty mobile apkpure black ops mode
-download call of duty mobile apkpure modern warfare mode
-download call of duty mobile apkpure advanced warfare mode
-download call of duty mobile apkpure infinite warfare mode
-download call of duty mobile apkpure world war 2 mode
-download call of duty mobile apkpure ghost mode
-download call of duty mobile apkpure hack version
-download call of duty mobile apkpure unlimited money
-download call of duty mobile apkpure unlocked all weapons
-download call of duty mobile apkpure unlocked all skins
-download call of duty mobile apkpure unlocked all characters
-download call of duty mobile apkpure unlocked all maps
-download call of duty mobile apkpure unlocked all modes
-download call of duty mobile apkpure unlocked all perks
-download call of duty mobile apkpure unlocked all killstreaks
-download call of duty mobile apkpure no root required
-download call of duty mobile apkpure no verification required
-download call of duty mobile apkpure no survey required
-download call of duty mobile apkpure no human verification required
-download call of duty mobile apkpure no ads version
-download call of duty mobile apkpure high graphics settings
-download call of duty mobile apkpure low graphics settings
-download call of duty mobile apkpure hd graphics settings
-download call of duty mobile apkpure ultra hd graphics settings
-download call of duty mobile apkpure best graphics settings
-download call of duty mobile apkpure smooth gameplay settings
-download call of duty mobile apkpure fast gameplay settings
-download call of duty mobile apkpure realistic gameplay settings
-
How to download Call of Duty Mobile APKPure?
-
Now that you know why you should download Call of Duty Mobile from APKPure, here is a step-by-step guide on how to get the game on your Android device:
-
Visit the APKPure website or app
-
The first thing you need to do is to visit the APKPure website or download the APKPure app on your Android device. You can use any browser to access the website, or you can scan the QR code on the homepage to download the app. The app is lightweight and easy to use, and it will give you access to thousands of free and updated APK files.
-
Search for Call of Duty Mobile and tap on it
-
Once you are on the APKPure website or app, you can search for Call of Duty Mobile in the search bar. You will see the game icon and some information about it, such as the size, version, rating, and description. Tap on the game icon to go to the download page.
-
Download the APK file and the OBB file
-
On the download page, you will see two buttons: one for downloading the APK file and one for downloading the OBB file. The APK file is the application file that installs the game on your device, while the OBB file is the data file that contains the game content. You need both files to play Call of Duty Mobile. Tap on both buttons to start downloading them. You may need to enable unknown sources in your device settings to allow APK installation from third-party sources.
-
Install the APK file and copy the OBB file to the game folder
-
After downloading both files, you need to install the APK file first. Locate the file in your device storage and tap on it to start the installation process. Follow the instructions on the screen and wait for it to finish. Then, you need to copy the OBB file to the game folder. The game folder is usually located in Android/obb/com.activision.callofduty.shooter. If you don't see this folder, you can create it manually. Paste the OBB file in this folder and make sure it has the same name as the original file.
-
Launch the game and enjoy
-
Now you are ready to play Call of Duty Mobile on your Android device. Launch the game from your app drawer or home screen and log in with your account or create a new one. You can also link your Facebook or Google account for easy access. Choose your preferred game mode and start shooting your enemies. Have fun!
-
Conclusion
-
Call of Duty Mobile is a great way to enjoy a thrilling and immersive shooter game on your mobile device. You can play with millions of players around the world, customize your loadout, join clans, and participate in events and challenges. If you want to download Call of Duty Mobile from APKPure, you can follow our simple guide above and get the game in no time. APKPure offers fast, safe, and easy downloads for Call of Duty Mobile without any region restrictions or compatibility issues. Download Call of Duty Mobile from APKPure today and join the action!
-
Frequently Asked Questions
-
Here are some of the common questions that people ask about Call of Duty Mobile and APKPure:
-
Is Call of Duty Mobile free?
-
Yes, Call of Duty Mobile is free-to-play, meaning you don't have to pay anything to download or play it. However, there are some optional in-game purchases that you can make with real money, such as skins, weapons, crates, battle pass, etc.
-
Is Call of Duty Mobile safe?
-
Yes, Call of Duty Mobile is safe and secure to play. The game is developed by a reputable company (TiMi Studios) and published by a trusted company (Activision). The game also has anti-cheat measures and encryption systems to protect your data and privacy.
-
Is APKPure safe?
-
Yes, APKPure is safe and reliable to use. APKPure is a well-known third-party app store that provides free and verified APK files for Android users. APKPure checks every APK file for viruses, malware, and other threats before uploading it to their website or app.
-
Can I play Call of Duty Mobile offline?
-
No, you cannot play Call of Duty Mobile offline. The game requires an internet connection to run properly. You need to connect to a Wi-Fi or mobile data network to play online multiplayer modes or Battle Royale mode.
-
Can I play Call of Duty Mobile with a controller?
-
Yes, you can play Call of Duty Mobile with a controller if you prefer. The game supports various controllers that are compatible with Android devices, such as Xbox One controller, PS4 controller, etc. You can also customize your controller settings and sensitivity in the game options.
197e85843d
-
-
\ No newline at end of file
diff --git a/spaces/congsaPfin/Manga-OCR/logs/Free Download Animal Revolt Battle Simulator APK for Android Experience the Ultimate Physics-Based Dinosaur Battle Game.md b/spaces/congsaPfin/Manga-OCR/logs/Free Download Animal Revolt Battle Simulator APK for Android Experience the Ultimate Physics-Based Dinosaur Battle Game.md
deleted file mode 100644
index c9fd4dc7c62e1344edc000ead0bb0ea54f84dfc1..0000000000000000000000000000000000000000
--- a/spaces/congsaPfin/Manga-OCR/logs/Free Download Animal Revolt Battle Simulator APK for Android Experience the Ultimate Physics-Based Dinosaur Battle Game.md
+++ /dev/null
@@ -1,129 +0,0 @@
-
-
Animal Revolt Battle Simulator: A Free and Fun Game for Android
-
Do you love dinosaurs and epic battles? Do you want to unleash your imagination and create your own scenarios of carnage and chaos? If you answered yes, then you should try Animal Revolt Battle Simulator, a free and fun game for Android devices. In this game, you can place two opposing armies made of different types of dinosaur beasts and watch them tear each other apart in an epic battle. As the creatures fight, you can see the limbs bending, necks twisting, and bodies flying around everywhere. It is a physics-based sandbox game that will keep you entertained for hours.
-
What is Animal Revolt Battle Simulator?
-
Animal Revolt Battle Simulator is a game developed by VDimension Ltd, a studio based in London, UK. The game was released in 2020 and has received positive reviews from players and critics alike. The game has been downloaded over 1 million times on Google Play Store and has an average rating of 4.4 out of 5 stars.
The game is based on realistic physics simulation, which means that the creatures behave according to their mass, speed, strength, and shape. The game also features ragdoll effects, which make the creatures react to impacts, collisions, and explosions in a natural way. You can see the blood splatter, the bones break, and the flesh tear as the creatures fight for survival.
-
A variety of dinosaur beasts to choose from
-
The game offers a wide range of dinosaur beasts to choose from, each with its own characteristics and abilities. You can choose from herbivores, carnivores, omnivores, flyers, swimmers, and more. You can also mix and match different types of creatures to create your own unique army. Some of the creatures available in the game are:
-
-
Tyrannosaurus Rex: The king of the dinosaurs, a powerful and fearsome predator that can crush anything with its massive jaws.
-
Triceratops: A large and armored herbivore that can defend itself with its three horns and thick skull.
-
Stegosaurus: A spiky and plated herbivore that can swing its tail like a club.
-
Pteranodon: A flying reptile that can soar through the air and swoop down on its prey.
-
Mosasaurus: A giant marine reptile that can swim fast and bite hard.
-
And many more!
-
-
A realistic and dynamic battle system
-
The game features a realistic and dynamic battle system that allows you to create your own scenarios of war and destruction. You can customize the size, shape, terrain, weather, and time of day of the battlefield. You can also adjust the difficulty level, the number of creatures, the team colors, and the health bars. You can then place your creatures on the battlefield using a simple drag-and-drop interface. Once you are ready, you can start the battle and watch as your creatures fight each other in a spectacular display of violence and gore. You can also pause, resume, slow down, or speed up the battle at any time. You can also switch between different camera angles and zoom in or out to get a better view of the action.
-
How to download Animal Revolt Battle Simulator for free?
-
If you want to download Animal Revolt Battle Simulator for free on your Android device, you have several options to choose from. Here are some of them:
- Download from APKCombo.com
-
APKCombo.com is a website that provides free and safe APK files for Android apps and games. You can download Animal Revolt Battle Simulator from this website by following these steps:
-
animal revolt battle simulator apk free download for android
-download animal revolt battle simulator mod apk unlimited money
-animal revolt battle simulator android game free download
-how to install animal revolt battle simulator apk on pc
-animal revolt battle simulator latest version apk download
-animal revolt battle simulator apk obb free download
-animal revolt battle simulator sandbox game apk free
-animal revolt battle simulator hack apk download
-animal revolt battle simulator offline apk free download
-animal revolt battle simulator apk pure free download
-animal revolt battle simulator 3d apk free download
-animal revolt battle simulator apk mirror free download
-animal revolt battle simulator rexdl apk free download
-animal revolt battle simulator apk uptodown free download
-animal revolt battle simulator revdl apk free download
-animal revolt battle simulator apk mod menu free download
-animal revolt battle simulator full version apk free download
-animal revolt battle simulator premium apk free download
-animal revolt battle simulator pro apk free download
-animal revolt battle simulator cracked apk free download
-animal revolt battle simulator mega mod apk free download
-animal revolt battle simulator unlimited coins apk free download
-animal revolt battle simulator all animals unlocked apk free download
-animal revolt battle simulator god mode apk free download
-animal revolt battle simulator no ads apk free download
-animal revolt battle simulator cheats apk free download
-animal revolt battle simulator tips and tricks apk free download
-animal revolt battle simulator guide and walkthrough apk free download
-animal revolt battle simulator gameplay and review apk free download
-animal revolt battle simulator best settings and graphics apk free download
-animal revolt battle simulator online multiplayer apk free download
-animal revolt battle simulator custom maps and scenarios apk free download
-animal revolt battle simulator realistic physics and ragdoll effects apk free download
-animal revolt battle simulator epic battles and wars apk free download
-animal revolt battle simulator dinosaurs and dragons apk free download
-animal revolt battle simulator animals and humans apk free download
-animal revolt battle simulator fantasy and mythical creatures apk free download
-animal revolt battle simulator robots and machines apk free download
-animal revolt battle simulator zombies and monsters apk free download
-animal revolt battle simulator fun and funny moments apk free download
-animal revolt battle simulator simulation and strategy game apk free download
-animal revolt battle simulator creative and sandbox mode apk free download
-animal revolt battle simulator editor and creator tool apk free download
-animal revolt battle simulator skins and costumes apk free download
-animal revolt battle simulator weapons and armor apk free download
-animal revolt battle simulator sounds and music apk free download
-animal revolt battle simulator updates and news apk free download
-animal revolt battle simulator bugs and glitches apk free download
-animal revolt battle simulator ratings and reviews apk free download
Click on the green "Download APK" button and choose a version that is compatible with your device.
-
Wait for the download to finish and then open the APK file on your device.
-
Allow the installation of unknown sources if prompted and follow the instructions on the screen.
-
Enjoy playing Animal Revolt Battle Simulator on your device.
-
-
Download from AppBrain.com
-
AppBrain.com is another website that offers free and secure APK files for Android apps and games. You can download Animal Revolt Battle Simulator from this website by following these steps:
Click on the blue "Download" button and then click on "Download APK file".
-
Wait for the download to finish and then open the APK file on your device.
-
Allow the installation of unknown sources if prompted and follow the instructions on the screen.
-
Enjoy playing Animal Revolt Battle Simulator on your device.
-
-
Download from Google Play Store
-
The easiest and most recommended way to download Animal Revolt Battle Simulator is from the official Google Play Store. You can download the game from the Play Store by following these steps:
Click on the green "Install" button and wait for the game to download and install on your device.
-
Enjoy playing Animal Revolt Battle Simulator on your device.
-
-
How to play Animal Revolt Battle Simulator?
-
Playing Animal Revolt Battle Simulator is very easy and fun. You just need to follow these simple steps:
-
Create your own army of creatures
-
The first step is to create your own army of creatures. You can do this by clicking on the "Create" button on the main menu. You will then see a list of different categories of creatures, such as herbivores, carnivores, flyers, swimmers, etc. You can click on any category to see the available creatures in that category. You can also use the search bar to find a specific creature by name. To add a creature to your army, you just need to drag and drop it from the list to the left side of the screen. You can also adjust the size, health, damage, speed, and team color of each creature by using the sliders below them. You can also delete a creature by dragging it back to the list or clicking on the trash icon. You can create up to 100 creatures in your army, but you can also save and load different armies by using the buttons at the bottom of the screen.
-
Place them on the battlefield
-
The second step is to place your creatures on the battlefield. You can do this by clicking on the "Battle" button on the main menu. You will then see a map of the battlefield, which you can customize by using the buttons at the top of the screen. You can change the size, shape, terrain, weather, and time of day of the battlefield. You can also add or remove obstacles, such as rocks, trees, buildings, etc. To place your creatures on the battlefield, you just need to drag and drop them from the left side of the screen to anywhere you want on the map. You can also rotate them by using the arrow keys or by clicking and dragging them with your mouse. You can place up to 50 creatures per team on each side of the map, but you can also use different teams by clicking on the team icons at the bottom of the screen. You can also use the buttons at the bottom of the screen to save and load different battlefields.
-
Watch them fight and enjoy the spectacle
-
The third and final step is to watch your creatures fight and enjoy the spectacle. You can do this by clicking on the "Start" button at the top of the screen. You will then see the battle begin and your creatures charge at each other. You can also use the buttons at the top of the screen to pause, resume, slow down, or speed up the battle. You can also switch between different camera angles and zoom in or out by using the mouse wheel or the buttons at the bottom of the screen. You can also click on any creature to see its name, health, damage, and team color. You can also see the number of creatures alive and dead on each team at the top of the screen. The battle will end when one team has no more creatures left or when you click on the "Stop" button. You can then see the results of the battle and restart it if you want.
-
Why should you play Animal Revolt Battle Simulator?
-
Animal Revolt Battle Simulator is a game that has many benefits and advantages for its players. Here are some of them:
-
It is fun and entertaining
-
The game is fun and entertaining because it allows you to create your own scenarios of war and destruction. You can experiment with different combinations of creatures and see how they interact with each other. You can also witness the realistic and dynamic physics simulation that makes the game more immersive and exciting. You can also enjoy the stunning graphics and sound effects that enhance the game experience.
-
It is educational and creative
-
The game is educational and creative because it teaches you about different types of dinosaur beasts and their characteristics. You can learn about their names, sizes, shapes, diets, habitats, behaviors, and abilities. You can also use your imagination and creativity to design your own armies and battlefields. You can also challenge yourself by adjusting the difficulty level and testing different strategies.
-
It is free and easy to install
-
The game is free and easy to install because it does not require any payment or registration to download and play. You can download it from various websites or from the Google Play Store without any hassle. You can also install it on any Android device that meets the minimum requirements of the game.
-
Conclusion
-
Animal Revolt Battle Simulator is a free and fun game for Android devices that lets you create your own scenarios of war and destruction using different types of dinosaur beasts. The game features a realistic and dynamic physics simulation, a wide range of creatures to choose from, a customizable battlefield, and a simple and intuitive interface. The game is also fun, entertaining, educational, creative, free, and easy to install. If you are looking for a game that will keep you entertained for hours, then you should try Animal Revolt Battle Simulator today.
-
FAQs
-
-
Q: What are the minimum requirements to play Animal Revolt Battle Simulator?
-
A: The minimum requirements are Android 4.4 or higher, 1 GB of RAM, 300 MB of storage space, and a stable internet connection.
-
Q: How can I contact the developers of Animal Revolt Battle Simulator?
Q: How can I support Animal Revolt Battle Simulator?
-
A: You can support Animal Revolt Battle Simulator by rating it on Google Play Store, sharing it with your friends, giving feedback to the developers, or making a donation through PayPal.
-
Q: How can I get more creatures in Animal Revolt Battle Simulator?
-
A: You can get more creatures in Animal Revolt Battle Simulator by updating the game regularly or by purchasing them with real money through in-app purchases.
-
Q: How can I report a bug or a problem in Animal Revolt Battle Simulator?
-
A: You can report a bug or a problem in Animal Revolt Battle Simulator by sending an email to vdimensionltd@gmail.com or by leaving a comment on Google Play Store.
-
401be4b1e0
-
-
\ No newline at end of file
diff --git a/spaces/congsaPfin/Manga-OCR/logs/Play Backgammon Online with Top Players and Win Coins and Prizes.md b/spaces/congsaPfin/Manga-OCR/logs/Play Backgammon Online with Top Players and Win Coins and Prizes.md
deleted file mode 100644
index 241270b85905c49c871f9ca9961c45391d3b0551..0000000000000000000000000000000000000000
--- a/spaces/congsaPfin/Manga-OCR/logs/Play Backgammon Online with Top Players and Win Coins and Prizes.md
+++ /dev/null
@@ -1,166 +0,0 @@
-
-
Best Free Backgammon Download: How to Play and Win at the Classic Board Game
-
Backgammon is one of the oldest and most popular board games in the world. It is a game of skill and strategy, where two players compete to move their checkers across the board and bear them off before their opponent. Whether you are a beginner or an expert, playing backgammon online can be a fun and rewarding experience. In this article, we will show you how to play backgammon, what are the best free backgammon apps to download on your device, and how to improve your game with some tips and tricks.
-
What is Backgammon and Why Should You Play It?
-
The History and Popularity of Backgammon
-
Backgammon is a game that dates back to ancient times. It is believed that it originated in Mesopotamia or Egypt, and then spread to other civilizations such as Rome, India, China, and Persia. The modern version of backgammon was developed in Europe in the 17th century, and became a favorite pastime of aristocrats and gamblers. Today, backgammon is played by millions of people around the world, in social groups, clubs, tournaments, and online platforms.
Playing backgammon online has many advantages over playing it in person. Here are some of them:
-
-
You can play anytime and anywhere, as long as you have an internet connection and a device.
-
You can choose from a variety of game modes, such as single player, multiplayer, match play, or tournament play.
-
You can adjust the difficulty level according to your skill and preference.
-
You can learn from other players, watch their moves, chat with them, or challenge them.
-
You can track your progress, statistics, ratings, and achievements.
-
You can access free resources, such as tutorials, guides, articles, videos, and forums.
-
-
How to Play Backgammon: The Basic Rules and Strategies
-
The Setup and Objective of the Game
-
Backgammon is played on a board consisting of 24 narrow triangles called points. The points are numbered from 1 to 24 for each player, starting from their home board. The home board is where the players bear off their checkers at the end of the game. The points are divided into four quadrants: the home board and the outer board for each player. The quadrants are separated by a ridge called the bar, where captured checkers are placed.
-
Each player has 15 checkers of their own color (usually black or white). The initial arrangement of checkers is as follows:
-
-
Two checkers on each player's 24 point
-
Five checkers on each player's 13 point
-
Three checkers on each player's 8 point
-
Five checkers on each player's 6 point
-
-
The objective of the game is to move all your checkers into your home board and then bear them off before your opponent does. To do this, you need to roll two dice and move your checkers according to the numbers shown on them.
-
The Movement and Capture of the Checkers
-
The movement of the checkers follows these rules:
-
-
A checker can. - A checker can only move to an open point, which is a point that is not occupied by two or more opposing checkers. - A checker can move the number of points shown on each die, or the total number of both dice, as long as both moves are possible. - A checker can move in one direction only, from the higher-numbered point to the lower-numbered point for the white player, and vice versa for the black player. - A checker can be captured by an opposing checker if it lands on a point occupied by a single opposing checker. The captured checker is then placed on the bar and must re-enter the game from the opponent's home board on the next turn. - A checker on the bar can re-enter the game by moving to an open point in the opponent's home board corresponding to one of the numbers rolled. If there is no such point, the player loses their turn and must try again on the next roll. - A player can bear off a checker from their home board if all their checkers are in their home board. To bear off a checker, the player must roll a number that corresponds to the point where the checker is located, or a higher number. If the player rolls a higher number than any point where they have a checker, they can bear off a checker from the highest point where they have a checker.
-
The Doubling Cube and the Crawford Rule
-
Backgammon is often played for stakes, which means that each game has a value that can be increased during the course of the game. To do this, players use a special die called the doubling cube, which has the numbers 2, 4, 8, 16, 32, and 64 on its faces. The doubling cube is initially placed in the middle of the board with the number 64 facing up, indicating that the game is worth one unit.
-
best free backgammon game for pc
-best free backgammon app for android
-best free backgammon software for windows 10
-best free backgammon online multiplayer
-best free backgammon offline mode
-best free backgammon with friends
-best free backgammon no ads
-best free backgammon tutorial
-best free backgammon against computer
-best free backgammon board design
-best free backgammon rules and tips
-best free backgammon strategy guide
-best free backgammon dice simulator
-best free backgammon chat feature
-best free backgammon ranking system
-best free backgammon tournaments and prizes
-best free backgammon themes and sounds
-best free backgammon reviews and ratings
-best free backgammon download link
-best free backgammon alternative sites
-best free backgammon for mac os
-best free backgammon for ios devices
-best free backgammon for linux users
-best free backgammon for chromebook
-best free backgammon for web browser
-best free backgammon for beginners
-best free backgammon for experts
-best free backgammon for kids
-best free backgammon for seniors
-best free backgammon for fun and relaxation
-best free backgammon with custom settings
-best free backgammon with different variants
-best free backgammon with statistics and analysis
-best free backgammon with achievements and badges
-best free backgammon with leaderboards and challenges
-best free backgammon with social media integration
-best free backgammon with voice and video chat
-best free backgammon with artificial intelligence and machine learning
-best free backgammon with 3d graphics and animations
-best free backgammon with realistic physics and sound effects
-
At any point during the game, before rolling their dice, a player can propose to double the stakes by turning the cube to the next higher number and offering it to their opponent. The opponent can either accept or decline the offer. If they accept, they take possession of the cube and can propose to redouble later. If they decline, they concede the game and pay the current value of the cube. The cube can be turned and offered multiple times during a game, up to a maximum of 64 times.
-
A common rule in backgammon tournaments is called the Crawford rule, which states that if either player is one point away from winning the match, then no doubling is allowed in the next game. This prevents a player from doubling at the start of a game and winning the match by luck. After that game, normal doubling rules apply again.
-
The Five Basic Backgammon Strategies
-
Backgammon is a game that combines luck and skill, and requires both tactical and strategic thinking. Here are some of the basic strategies that can help you improve your game:
-
-
The Running Game: This strategy involves moving your checkers as fast as possible towards your home board and bearing them off quickly. It is suitable for situations where you have an early lead or when your opponent has left many gaps in their position.
-
The Holding Game: This strategy involves maintaining one or more points in your opponent's home board or outer board, in order to block their movement and create opportunities for hitting their checkers. It is suitable for situations where you are behind or when your opponent has a strong home board.
-
The Priming Game: This strategy involves building a wall of six consecutive points in front of your opponent's checkers, preventing them from escaping. It is suitable for situations where you have an advantage in position or when your opponent has many checkers on the bar or behind your prime.
-
The Blitzing Game: This strategy involves attacking your opponent's checkers aggressively, trying to hit them and put them on the bar as much as possible. It is suitable for situations where you have an advantage in numbers or when your opponent has a weak home board.
-
The Backgammon Game: This strategy involves trying to win by more than one point, either by bearing off all your checkers before your opponent bears off any (a gammon), or by bearing off all your checkers while your opponent still has one or more checkers on the bar or in your home board (a backgammon). It is suitable for situations where you have a big lead or when your opponent has made a blunder.
-
-
How to Download and Install the Best Free Backgammon Apps on Your Device
-
There are many free backgammon apps available for download on various devices, such as smartphones, tablets, laptops, and desktops. However, not all of them are equally good in terms of quality, features, design, and user-friendliness. Here are Here are some of the best free backgammon apps that you can download and install on your device:
-
Backgammon by AI Factory Limited
-
This app is one of the most popular and highly rated backgammon apps on Google Play and App Store. It has over 10 million downloads and a 4.5-star rating. It features:
-
-
A strong AI engine that can challenge players of all levels, from beginner to expert.
-
A variety of game modes, such as single player, two player, online multiplayer, and match play.
-
A user-friendly interface, with smooth graphics, animations, sound effects, and hints.
-
A comprehensive statistics system, with ratings, leaderboards, achievements, and analysis.
-
A customizable board design, with different colors, themes, and layouts.
-
A free download and no ads or in-app purchases.
-
-
You can download Backgammon by AI Factory Limited from Google Play or App Store.
-
Backgammon - Lord of the Board by Beach Bum Ltd.
-
This app is another popular and highly rated backgammon app on Google Play and App Store. It has over 5 million downloads and a 4.4-star rating. It features:
-
-
A social gaming experience, where you can chat, interact, and compete with other players from around the world.
-
A variety of game modes, such as single player, online multiplayer, tournaments, and special events.
-
A user-friendly interface, with stunning graphics, animations, sound effects, and tips.
-
A comprehensive statistics system, with ratings, leaderboards, achievements, and rewards.
-
A customizable board design, with different colors, themes, and styles.
-
A free download and no ads or in-app purchases.
-
-
You can download Backgammon - Lord of the Board by Beach Bum Ltd. from Google Play or App Store.
-
Backgammon! by Microsoft Store
-
This app is a simple and elegant backgammon app for Windows devices. It has over 1 million downloads and a 4.6-star rating. It features:
-
-
A classic backgammon gameplay, with no frills or distractions.
-
A variety of game modes, such as single player, two player, online multiplayer, and match play.
-
A user-friendly interface, with clear graphics, animations, sound effects, and hints.
-
A comprehensive statistics system, with ratings, leaderboards, achievements, and analysis.
-
A customizable board design, with different colors and themes.
-
A free download and no ads or in-app purchases.
-
-
You can download Backgammon! by Microsoft Store from Microsoft Store.
-
Conclusion and FAQs
-
Backgammon is a game that can provide you with hours of fun and challenge. It is a game that combines luck and skill, and requires both tactical and strategic thinking. By playing backgammon online, you can enjoy the benefits of convenience, variety, learning, and socializing. By downloading and installing the best free backgammon apps on your device, you can access the features and functions that suit your needs and preferences. By following the basic rules and strategies of backgammon, you can improve your game and win more often. We hope that this article has helped you understand and appreciate the game of backgammon better. If you have any questions or comments, feel free to contact us or leave a feedback. Happy playing!
-
FAQs
-
Here are some of the frequently asked questions about backgammon and the best free backgammon apps:
-
-
What is the difference between backgammon and other board games?
-
Backgammon is different from other board games in several ways. For example, backgammon is a game of unequal chances, where each player has a different probability of winning depending on their position and dice rolls. Backgammon is also a game of partial information, where each player does not know the exact outcome of their opponent's moves until they are revealed. Backgammon is also a game of skill and luck, where both factors play a significant role in determining the winner.
-
What are the best tips for beginners to play backgammon?
-
Some of the best tips for beginners to play backgammon are:
-
-
Learn the basic rules and terminology of the game.
-
Practice playing against a computer or a friend to get familiar with the board and the moves.
-
Study some of the basic strategies and tactics of the game, such as running, holding, priming, blitzing, and backgammon.
-
Use the doubling cube wisely, and know when to accept or decline a double offer.
-
Review your games and learn from your mistakes and successes.
-
-
What are the best resources to learn more about backgammon?
-
Some of the best resources to learn more about backgammon are:
-
-
Backgammon Galore: A website that offers a wealth of information, articles, tutorials, guides, videos, forums, and links about backgammon.
-
Backgammon for Winners: A book by Bill Robertie that teaches the fundamentals and advanced techniques of backgammon.
-
Backgammon Boot Camp: A book by Walter Trice that covers all aspects of backgammon theory and practice.
-
Backgammon Online Academy: An online platform that offers courses, lessons, quizzes, exercises, and coaching for backgammon players of all levels.
-
Backgammon World Championship: An annual event that showcases the best backgammon players in the world competing for the title and prize money.
-
-
What are the best features to look for in a free backgammon app?
-
Some of the best features to look for in a free backgammon app are:
-
-
A strong AI engine that can challenge players of all levels, from beginner to expert.
-
A variety of game modes, such as single player, multiplayer, match play, or tournament play.
-
A user-friendly interface, with smooth graphics, animations, sound effects, and hints.
-
A comprehensive statistics system, with ratings, leaderboards, achievements, and analysis.
-
A customizable board design, with different colors, themes, and layouts.
-
A free download and no ads or in-app purchases.
-
-
What are some of the common mistakes to avoid when playing backgammon?
-
Some of the common mistakes to avoid when playing backgammon are:
-
-
Moving too fast or too slow without considering all your options.
-
Leaving too many blots or gaps in your position that expose you to hits.
-
Failing to make points or primes that secure your position or block your opponent.
-
Misusing the doubling cube or making wrong decisions about accepting or declining a double offer.
-
Giving up too soon or too late without calculating your chances of winning or losing.
-
401be4b1e0
-
-
\ No newline at end of file
diff --git a/spaces/contluForse/HuggingGPT/assets/Crysis Warhead Crack (clean) Repack.md b/spaces/contluForse/HuggingGPT/assets/Crysis Warhead Crack (clean) Repack.md
deleted file mode 100644
index d8fbd0c2d0fd5319f3416f6ae56dce435515b354..0000000000000000000000000000000000000000
--- a/spaces/contluForse/HuggingGPT/assets/Crysis Warhead Crack (clean) Repack.md
+++ /dev/null
@@ -1,30 +0,0 @@
-
-
How to Download and Install Crysis Warhead Crack (clean) Repack
-
Crysis Warhead is a standalone expansion of the first-person shooter game Crysis, developed by Crytek and published by Electronic Arts in 2008. The game follows the story of Sergeant Michael "Psycho" Sykes, a British special forces soldier who fights against the alien invaders on the other side of the island where the original game took place.
If you want to play Crysis Warhead on your PC, you can download a cracked version of the game that has been repacked by FitGirl or DODI. These repacks are compressed versions of the game that have been modified to remove unnecessary files and languages, and to include a working crack that bypasses the DRM protection. Here are the steps to download and install Crysis Warhead Crack (clean) Repack:
Select the language you want to download and install. You can skip downloading and installing languages you don't need.
-
Download the torrent file or magnet link for Crysis Warhead Crack (clean) Repack.
-
Open the torrent file or magnet link with a torrent client such as uTorrent or BitTorrent.
-
Wait for the download to finish. The repack size is from 3.2 GB to 4.5 GB, depending on the selected language and components.
-
Run the setup.exe file from the downloaded folder and follow the instructions to install the game.
-
The installation takes 7-20 minutes, depending on your system and selected components.
-
After the installation, you can launch the game from the desktop shortcut or the start menu.
-
Enjoy playing Crysis Warhead Crack (clean) Repack!
-
-
Note: The repacks use ZTool library by Razor12911 and require at least 2 GB of free RAM (including virtual) for installing. The repacks are based on Crysis.Warhead.MULTi11-PROPHET ISO release: ppt-crwa.iso (6.1 GB or 6.5 GB). The game version is v1.1.1.711. The repacks are 100% lossless and MD5 perfect: all files are identical to originals after installation. Nothing is ripped or re-encoded.
-
-
Crysis Warhead is a highly acclaimed game that offers stunning graphics, intense action, and varied gameplay. The game features a new campaign that can be played separately from the original Crysis, as well as new weapons, vehicles, enemies, and multiplayer modes. The game also supports DirectX 10 and 64-bit operating systems, which enhance the performance and visual quality of the game.
-
-
If you download and install Crysis Warhead Crack (clean) Repack, you can enjoy the game without any limitations or restrictions. However, you should be aware of the possible risks and consequences of using a cracked version of the game. These include:
-
-
Legal issues: Downloading and installing a cracked version of the game may violate the intellectual property rights of the developers and publishers. You may face legal actions or penalties if you are caught or reported.
-
Security issues: Downloading and installing a cracked version of the game may expose your computer to malware or viruses that can harm your system or steal your personal information. You should always scan the downloaded files with an antivirus program before installing them.
-
Compatibility issues: Downloading and installing a cracked version of the game may cause errors or glitches that can affect the gameplay or performance of the game. You may also encounter problems with updating or patching the game.
-
Ethical issues: Downloading and installing a cracked version of the game may deprive the developers and publishers of their deserved income and recognition. You may also miss out on the official support and community of the game.
-
-
Therefore, you should consider buying the original version of the game from a legitimate source if you can afford it and want to support the creators. You can find Crysis Warhead on Steam, Origin, or other online platforms for a reasonable price.
d5da3c52bf
-
-
\ No newline at end of file
diff --git a/spaces/cymic/VITS-Tokaiteio/text/symbols.py b/spaces/cymic/VITS-Tokaiteio/text/symbols.py
deleted file mode 100644
index ff5f78e639c1066f6b15474935a3ac627c75ee4f..0000000000000000000000000000000000000000
--- a/spaces/cymic/VITS-Tokaiteio/text/symbols.py
+++ /dev/null
@@ -1,2 +0,0 @@
-symbols = list(' !"&*,-.?ABCINU[]abcdefghijklmnoprstuwyz{}~')
-SPACE_ID = symbols.index(" ")
diff --git a/spaces/dakaiye/dky_xuexi/crazy_functions/test_project/cpp/longcode/prod_cons.h b/spaces/dakaiye/dky_xuexi/crazy_functions/test_project/cpp/longcode/prod_cons.h
deleted file mode 100644
index c9004bb8043a12e32814436baa6262a00c8ef68e..0000000000000000000000000000000000000000
--- a/spaces/dakaiye/dky_xuexi/crazy_functions/test_project/cpp/longcode/prod_cons.h
+++ /dev/null
@@ -1,433 +0,0 @@
-#pragma once
-
-#include
-#include
-#include
-#include
-#include
-
-#include "libipc/def.h"
-
-#include "libipc/platform/detail.h"
-#include "libipc/circ/elem_def.h"
-#include "libipc/utility/log.h"
-#include "libipc/utility/utility.h"
-
-namespace ipc {
-
-////////////////////////////////////////////////////////////////
-/// producer-consumer implementation
-////////////////////////////////////////////////////////////////
-
-template
-struct prod_cons_impl;
-
-template <>
-struct prod_cons_impl> {
-
- template
- struct elem_t {
- std::aligned_storage_t data_ {};
- };
-
- alignas(cache_line_size) std::atomic rd_; // read index
- alignas(cache_line_size) std::atomic wt_; // write index
-
- constexpr circ::u2_t cursor() const noexcept {
- return 0;
- }
-
- template
- bool push(W* /*wrapper*/, F&& f, E* elems) {
- auto cur_wt = circ::index_of(wt_.load(std::memory_order_relaxed));
- if (cur_wt == circ::index_of(rd_.load(std::memory_order_acquire) - 1)) {
- return false; // full
- }
- std::forward(f)(&(elems[cur_wt].data_));
- wt_.fetch_add(1, std::memory_order_release);
- return true;
- }
-
- /**
- * In single-single-unicast, 'force_push' means 'no reader' or 'the only one reader is dead'.
- * So we could just disconnect all connections of receiver, and return false.
- */
- template
- bool force_push(W* wrapper, F&&, E*) {
- wrapper->elems()->disconnect_receiver(~static_cast(0u));
- return false;
- }
-
- template
- bool pop(W* /*wrapper*/, circ::u2_t& /*cur*/, F&& f, R&& out, E* elems) {
- auto cur_rd = circ::index_of(rd_.load(std::memory_order_relaxed));
- if (cur_rd == circ::index_of(wt_.load(std::memory_order_acquire))) {
- return false; // empty
- }
- std::forward(f)(&(elems[cur_rd].data_));
- std::forward(out)(true);
- rd_.fetch_add(1, std::memory_order_release);
- return true;
- }
-};
-
-template <>
-struct prod_cons_impl>
- : prod_cons_impl> {
-
- template
- bool force_push(W* wrapper, F&&, E*) {
- wrapper->elems()->disconnect_receiver(1);
- return false;
- }
-
- template class E, std::size_t DS, std::size_t AS>
- bool pop(W* /*wrapper*/, circ::u2_t& /*cur*/, F&& f, R&& out, E* elems) {
- byte_t buff[DS];
- for (unsigned k = 0;;) {
- auto cur_rd = rd_.load(std::memory_order_relaxed);
- if (circ::index_of(cur_rd) ==
- circ::index_of(wt_.load(std::memory_order_acquire))) {
- return false; // empty
- }
- std::memcpy(buff, &(elems[circ::index_of(cur_rd)].data_), sizeof(buff));
- if (rd_.compare_exchange_weak(cur_rd, cur_rd + 1, std::memory_order_release)) {
- std::forward(f)(buff);
- std::forward(out)(true);
- return true;
- }
- ipc::yield(k);
- }
- }
-};
-
-template <>
-struct prod_cons_impl>
- : prod_cons_impl> {
-
- using flag_t = std::uint64_t;
-
- template
- struct elem_t {
- std::aligned_storage_t data_ {};
- std::atomic f_ct_ { 0 }; // commit flag
- };
-
- alignas(cache_line_size) std::atomic ct_; // commit index
-
- template
- bool push(W* /*wrapper*/, F&& f, E* elems) {
- circ::u2_t cur_ct, nxt_ct;
- for (unsigned k = 0;;) {
- cur_ct = ct_.load(std::memory_order_relaxed);
- if (circ::index_of(nxt_ct = cur_ct + 1) ==
- circ::index_of(rd_.load(std::memory_order_acquire))) {
- return false; // full
- }
- if (ct_.compare_exchange_weak(cur_ct, nxt_ct, std::memory_order_acq_rel)) {
- break;
- }
- ipc::yield(k);
- }
- auto* el = elems + circ::index_of(cur_ct);
- std::forward(f)(&(el->data_));
- // set flag & try update wt
- el->f_ct_.store(~static_cast(cur_ct), std::memory_order_release);
- while (1) {
- auto cac_ct = el->f_ct_.load(std::memory_order_acquire);
- if (cur_ct != wt_.load(std::memory_order_relaxed)) {
- return true;
- }
- if ((~cac_ct) != cur_ct) {
- return true;
- }
- if (!el->f_ct_.compare_exchange_strong(cac_ct, 0, std::memory_order_relaxed)) {
- return true;
- }
- wt_.store(nxt_ct, std::memory_order_release);
- cur_ct = nxt_ct;
- nxt_ct = cur_ct + 1;
- el = elems + circ::index_of(cur_ct);
- }
- return true;
- }
-
- template
- bool force_push(W* wrapper, F&&, E*) {
- wrapper->elems()->disconnect_receiver(1);
- return false;
- }
-
- template class E, std::size_t DS, std::size_t AS>
- bool pop(W* /*wrapper*/, circ::u2_t& /*cur*/, F&& f, R&& out, E* elems) {
- byte_t buff[DS];
- for (unsigned k = 0;;) {
- auto cur_rd = rd_.load(std::memory_order_relaxed);
- auto cur_wt = wt_.load(std::memory_order_acquire);
- auto id_rd = circ::index_of(cur_rd);
- auto id_wt = circ::index_of(cur_wt);
- if (id_rd == id_wt) {
- auto* el = elems + id_wt;
- auto cac_ct = el->f_ct_.load(std::memory_order_acquire);
- if ((~cac_ct) != cur_wt) {
- return false; // empty
- }
- if (el->f_ct_.compare_exchange_weak(cac_ct, 0, std::memory_order_relaxed)) {
- wt_.store(cur_wt + 1, std::memory_order_release);
- }
- k = 0;
- }
- else {
- std::memcpy(buff, &(elems[circ::index_of(cur_rd)].data_), sizeof(buff));
- if (rd_.compare_exchange_weak(cur_rd, cur_rd + 1, std::memory_order_release)) {
- std::forward(f)(buff);
- std::forward(out)(true);
- return true;
- }
- ipc::yield(k);
- }
- }
- }
-};
-
-template <>
-struct prod_cons_impl> {
-
- using rc_t = std::uint64_t;
-
- enum : rc_t {
- ep_mask = 0x00000000ffffffffull,
- ep_incr = 0x0000000100000000ull
- };
-
- template
- struct elem_t {
- std::aligned_storage_t data_ {};
- std::atomic rc_ { 0 }; // read-counter
- };
-
- alignas(cache_line_size) std::atomic wt_; // write index
- alignas(cache_line_size) rc_t epoch_ { 0 }; // only one writer
-
- circ::u2_t cursor() const noexcept {
- return wt_.load(std::memory_order_acquire);
- }
-
- template
- bool push(W* wrapper, F&& f, E* elems) {
- E* el;
- for (unsigned k = 0;;) {
- circ::cc_t cc = wrapper->elems()->connections(std::memory_order_relaxed);
- if (cc == 0) return false; // no reader
- el = elems + circ::index_of(wt_.load(std::memory_order_relaxed));
- // check all consumers have finished reading this element
- auto cur_rc = el->rc_.load(std::memory_order_acquire);
- circ::cc_t rem_cc = cur_rc & ep_mask;
- if ((cc & rem_cc) && ((cur_rc & ~ep_mask) == epoch_)) {
- return false; // has not finished yet
- }
- // consider rem_cc to be 0 here
- if (el->rc_.compare_exchange_weak(
- cur_rc, epoch_ | static_cast(cc), std::memory_order_release)) {
- break;
- }
- ipc::yield(k);
- }
- std::forward(f)(&(el->data_));
- wt_.fetch_add(1, std::memory_order_release);
- return true;
- }
-
- template
- bool force_push(W* wrapper, F&& f, E* elems) {
- E* el;
- epoch_ += ep_incr;
- for (unsigned k = 0;;) {
- circ::cc_t cc = wrapper->elems()->connections(std::memory_order_relaxed);
- if (cc == 0) return false; // no reader
- el = elems + circ::index_of(wt_.load(std::memory_order_relaxed));
- // check all consumers have finished reading this element
- auto cur_rc = el->rc_.load(std::memory_order_acquire);
- circ::cc_t rem_cc = cur_rc & ep_mask;
- if (cc & rem_cc) {
- ipc::log("force_push: k = %u, cc = %u, rem_cc = %u\n", k, cc, rem_cc);
- cc = wrapper->elems()->disconnect_receiver(rem_cc); // disconnect all invalid readers
- if (cc == 0) return false; // no reader
- }
- // just compare & exchange
- if (el->rc_.compare_exchange_weak(
- cur_rc, epoch_ | static_cast(cc), std::memory_order_release)) {
- break;
- }
- ipc::yield(k);
- }
- std::forward(f)(&(el->data_));
- wt_.fetch_add(1, std::memory_order_release);
- return true;
- }
-
- template
- bool pop(W* wrapper, circ::u2_t& cur, F&& f, R&& out, E* elems) {
- if (cur == cursor()) return false; // acquire
- auto* el = elems + circ::index_of(cur++);
- std::forward(f)(&(el->data_));
- for (unsigned k = 0;;) {
- auto cur_rc = el->rc_.load(std::memory_order_acquire);
- if ((cur_rc & ep_mask) == 0) {
- std::forward(out)(true);
- return true;
- }
- auto nxt_rc = cur_rc & ~static_cast(wrapper->connected_id());
- if (el->rc_.compare_exchange_weak(cur_rc, nxt_rc, std::memory_order_release)) {
- std::forward(out)((nxt_rc & ep_mask) == 0);
- return true;
- }
- ipc::yield(k);
- }
- }
-};
-
-template <>
-struct prod_cons_impl> {
-
- using rc_t = std::uint64_t;
- using flag_t = std::uint64_t;
-
- enum : rc_t {
- rc_mask = 0x00000000ffffffffull,
- ep_mask = 0x00ffffffffffffffull,
- ep_incr = 0x0100000000000000ull,
- ic_mask = 0xff000000ffffffffull,
- ic_incr = 0x0000000100000000ull
- };
-
- template
- struct elem_t {
- std::aligned_storage_t data_ {};
- std::atomic rc_ { 0 }; // read-counter
- std::atomic f_ct_ { 0 }; // commit flag
- };
-
- alignas(cache_line_size) std::atomic ct_; // commit index
- alignas(cache_line_size) std::atomic epoch_ { 0 };
-
- circ::u2_t cursor() const noexcept {
- return ct_.load(std::memory_order_acquire);
- }
-
- constexpr static rc_t inc_rc(rc_t rc) noexcept {
- return (rc & ic_mask) | ((rc + ic_incr) & ~ic_mask);
- }
-
- constexpr static rc_t inc_mask(rc_t rc) noexcept {
- return inc_rc(rc) & ~rc_mask;
- }
-
- template
- bool push(W* wrapper, F&& f, E* elems) {
- E* el;
- circ::u2_t cur_ct;
- rc_t epoch = epoch_.load(std::memory_order_acquire);
- for (unsigned k = 0;;) {
- circ::cc_t cc = wrapper->elems()->connections(std::memory_order_relaxed);
- if (cc == 0) return false; // no reader
- el = elems + circ::index_of(cur_ct = ct_.load(std::memory_order_relaxed));
- // check all consumers have finished reading this element
- auto cur_rc = el->rc_.load(std::memory_order_relaxed);
- circ::cc_t rem_cc = cur_rc & rc_mask;
- if ((cc & rem_cc) && ((cur_rc & ~ep_mask) == epoch)) {
- return false; // has not finished yet
- }
- else if (!rem_cc) {
- auto cur_fl = el->f_ct_.load(std::memory_order_acquire);
- if ((cur_fl != cur_ct) && cur_fl) {
- return false; // full
- }
- }
- // consider rem_cc to be 0 here
- if (el->rc_.compare_exchange_weak(
- cur_rc, inc_mask(epoch | (cur_rc & ep_mask)) | static_cast(cc), std::memory_order_relaxed) &&
- epoch_.compare_exchange_weak(epoch, epoch, std::memory_order_acq_rel)) {
- break;
- }
- ipc::yield(k);
- }
- // only one thread/process would touch here at one time
- ct_.store(cur_ct + 1, std::memory_order_release);
- std::forward(f)(&(el->data_));
- // set flag & try update wt
- el->f_ct_.store(~static_cast(cur_ct), std::memory_order_release);
- return true;
- }
-
- template
- bool force_push(W* wrapper, F&& f, E* elems) {
- E* el;
- circ::u2_t cur_ct;
- rc_t epoch = epoch_.fetch_add(ep_incr, std::memory_order_release) + ep_incr;
- for (unsigned k = 0;;) {
- circ::cc_t cc = wrapper->elems()->connections(std::memory_order_relaxed);
- if (cc == 0) return false; // no reader
- el = elems + circ::index_of(cur_ct = ct_.load(std::memory_order_relaxed));
- // check all consumers have finished reading this element
- auto cur_rc = el->rc_.load(std::memory_order_acquire);
- circ::cc_t rem_cc = cur_rc & rc_mask;
- if (cc & rem_cc) {
- ipc::log("force_push: k = %u, cc = %u, rem_cc = %u\n", k, cc, rem_cc);
- cc = wrapper->elems()->disconnect_receiver(rem_cc); // disconnect all invalid readers
- if (cc == 0) return false; // no reader
- }
- // just compare & exchange
- if (el->rc_.compare_exchange_weak(
- cur_rc, inc_mask(epoch | (cur_rc & ep_mask)) | static_cast(cc), std::memory_order_relaxed)) {
- if (epoch == epoch_.load(std::memory_order_acquire)) {
- break;
- }
- else if (push(wrapper, std::forward