Commit
·
348ec71
1
Parent(s):
e5ccb28
Update parquet files (step 88 of 121)
Browse filesThis view is limited to 50 files because it contains too many changes.
See raw diff
- spaces/1acneusushi/gradio-2dmoleculeeditor/data/Cyberlink PowerDirector 11 Full Version with Crack Download and Install Guide.md +0 -93
- spaces/1acneusushi/gradio-2dmoleculeeditor/data/Download Microsoft Project 32 Bit Full Crack What You Need to Know.md +0 -29
- spaces/1gistliPinn/ChatGPT4/Examples/Downloadgametrainsimulatormodindonesia.md +0 -110
- spaces/1pelhydcardo/ChatGPT-prompt-generator/assets/Auto Report FB How to Automate Reporting on Facebook with a Simple App.md +0 -164
- spaces/1phancelerku/anime-remove-background/Create Convert and Edit PDF Files with PrimoPDF - A Free and Reliable PDF Creator.md +0 -92
- spaces/1phancelerku/anime-remove-background/Download Kaash Paige Love Songs MP3 and Listen Offline.md +0 -105
- spaces/4Taps/SadTalker/src/face3d/util/visualizer.py +0 -227
- spaces/AIFILMS/generate_human_motion/VQ-Trans/models/t2m_trans.py +0 -211
- spaces/AIFILMS/generate_human_motion/pyrender/docs/Makefile +0 -23
- spaces/AIGC-Audio/AudioGPT/text_to_speech/utils/commons/trainer.py +0 -561
- spaces/AIGText/GlyphControl/annotator/canny/__init__.py +0 -6
- spaces/AgentVerse/agentVerse/ui/src/phaser3-rex-plugins/plugins/sequence.js +0 -2
- spaces/AgentVerse/agentVerse/ui/src/phaser3-rex-plugins/templates/ui/customprogress/CustomProgress.d.ts +0 -2
- spaces/AlexWang/lama/saicinpainting/training/visualizers/base.py +0 -73
- spaces/AlexWortega/Kandinsky2.0/app.py +0 -215
- spaces/Androidonnxfork/CivitAi-to-Diffusers/diffusers/docs/source/en/using-diffusers/custom_pipeline_examples.md +0 -282
- spaces/Androidonnxfork/CivitAi-to-Diffusers/diffusers/docs/source/ko/training/text_inversion.md +0 -275
- spaces/Androidonnxfork/CivitAi-to-Diffusers/diffusers/tests/pipelines/stable_diffusion/test_stable_diffusion_inpaint_legacy.py +0 -621
- spaces/Andy1621/uniformer_image_segmentation/configs/danet/danet_r101-d8_512x512_40k_voc12aug.py +0 -2
- spaces/AnishKumbhar/ChatBot/text-generation-webui-main/css/main.css +0 -612
- spaces/Anonymous-sub/Rerender/ControlNet/annotator/uniformer/mmcv/ops/multi_scale_deform_attn.py +0 -358
- spaces/Arnx/MusicGenXvAKN/MODEL_CARD.md +0 -81
- spaces/Arnx/MusicGenXvAKN/tests/__init__.py +0 -5
- spaces/Artrajz/vits-simple-api/utils/utils.py +0 -95
- spaces/ArturStepanenko/digitsSpace/README.md +0 -12
- spaces/AsakuraMizu/moe-tts/README.md +0 -14
- spaces/Ataturk-Chatbot/HuggingFaceChat/venv/lib/python3.11/site-packages/pip/_internal/vcs/git.py +0 -526
- spaces/Awiny/Image2Paragraph/models/grit_src/third_party/CenterNet2/detectron2/solver/build.py +0 -285
- spaces/Awiny/Image2Paragraph/models/grit_src/third_party/CenterNet2/tests/test_events.py +0 -64
- spaces/AzulaFire/SparkDebate/README.md +0 -12
- spaces/Bart92/RVC_HF/demucs/parser.py +0 -244
- spaces/Benson/text-generation/Examples/Camioneros De Europa 3 Hack Mod Apk An1.md +0 -87
- spaces/Benson/text-generation/Examples/Cmo Crear Y Construir Apk.md +0 -133
- spaces/Big-Web/MMSD/env/Lib/site-packages/pip/_vendor/pygments/scanner.py +0 -104
- spaces/BigChungux/Pet_Survey/info.md +0 -16
- spaces/CALM/Dashboard/README.md +0 -26
- spaces/CVPR/Dual-Key_Backdoor_Attacks/datagen/detectron2/dev/run_inference_tests.sh +0 -44
- spaces/CVPR/LIVE/thrust/thrust/iterator/detail/universal_categories.h +0 -87
- spaces/CVPR/LIVE/thrust/thrust/system/cpp/detail/binary_search.h +0 -23
- spaces/CVPR/LIVE/thrust/thrust/system/detail/adl/assign_value.h +0 -44
- spaces/CVPR/WALT/mmdet/core/visualization/__init__.py +0 -4
- spaces/CVPR/drawings-to-human/static/_app/immutable/pages/__layout.svelte-d07d8fed.js +0 -1
- spaces/CVPR/regionclip-demo/detectron2/layers/soft_nms.py +0 -261
- spaces/CarlDennis/Lovelive-VITS-JPZH/app.py +0 -124
- spaces/CikeyQI/meme-api/meme_generator/memes/chase_train/__init__.py +0 -60
- spaces/Curranj/FlowerDiffusion/README.md +0 -12
- spaces/DESUCLUB/BLLAMA/export_state_dict_checkpoint.py +0 -119
- spaces/DJQmUKV/rvc-inference/util.py +0 -81
- spaces/DQChoi/gpt-demo/venv/lib/python3.11/site-packages/altair/_magics.py +0 -109
- spaces/DQChoi/gpt-demo/venv/lib/python3.11/site-packages/click/shell_completion.py +0 -593
spaces/1acneusushi/gradio-2dmoleculeeditor/data/Cyberlink PowerDirector 11 Full Version with Crack Download and Install Guide.md
DELETED
@@ -1,93 +0,0 @@
|
|
1 |
-
|
2 |
-
<h1>Cyberlink PowerDirector 11 Full Version with Crack: A Comprehensive Review</h1>
|
3 |
-
<p>If you are looking for a powerful and easy-to-use video editing software, you might have heard of Cyberlink PowerDirector. It is one of the most popular and versatile video editors on the market, with a range of features and tools that can help you create stunning videos for any purpose. But what if you want to use the full version of Cyberlink PowerDirector without paying for it? Is there a way to get Cyberlink PowerDirector 11 full version with crack?</p>
|
4 |
-
<h2>cyberlink powerdirector 11 full version with crack</h2><br /><p><b><b>DOWNLOAD</b> >>>>> <a href="https://byltly.com/2uKyY2">https://byltly.com/2uKyY2</a></b></p><br /><br />
|
5 |
-
<p>In this article, we will review Cyberlink PowerDirector 11, its features, pros and cons, and how to download and install it with a crack. We will also compare it with some alternatives that you might want to consider. By the end of this article, you will have a clear idea of whether Cyberlink PowerDirector 11 is the right video editor for you and how to get it for free.</p>
|
6 |
-
<h2>Features of Cyberlink PowerDirector 11</h2>
|
7 |
-
<p>Cyberlink PowerDirector 11 is a video editing software that was released in 2012. It is designed for both beginners and professionals, with a user-friendly interface and a comprehensive set of features. Here are some of the main features of Cyberlink PowerDirector 11:</p>
|
8 |
-
<h3>Express Video Creation</h3>
|
9 |
-
<p>If you want to create a video quickly and easily, you can use the Express Project module. This module allows you to choose from a variety of templates that are suitable for different types of videos, such as travel, wedding, sports, etc. You can then drag and drop your clips into the timeline, add transitions, effects, music, and titles, and produce your video in minutes.</p>
|
10 |
-
<h3>Action Camera Center</h3>
|
11 |
-
<p>If you are into action sports or adventure videos, you will love the Action Camera Center. This feature lets you edit your footage from action cameras like GoPro, DJI, or Sony. You can apply effects such as slow motion, freeze frame, zoom, pan, or rotate. You can also correct lens distortion, stabilize shaky videos, or remove background noise.</p>
|
12 |
-
<h3>Simplified Color Adjustment</h3>
|
13 |
-
<p>If you want to enhance the color and tone of your videos, you can use the Simplified Color Adjustment feature. This feature lets you adjust the brightness, contrast, saturation, hue, temperature, tint, and exposure of your videos with simple sliders. You can also use one-click color correction tools such as Auto Tone or White Balance. If you want more control over your color grading, you can use advanced tools such as Color Director or Color Match.</p>
|
14 |
-
<h3>Customizable Design Tools</h3>
|
15 |
-
<p>If you want to add some creativity and style to your videos, you can use the Customizable Design Tools. These tools let you create and edit titles, transitions, PiP objects (picture-in-picture), masks, subtitles, etc. You can also use the new Brush Tool to draw shapes or masks on your videos. You can customize these elements with different fonts, colors, sizes, animations, etc.</p>
|
16 |
-
<h3>New Effects and Enhancements</h3>
|
17 |
-
<p>If you want to spice up your videos with some special effects, you can use the New Effects and Enhancements feature. This feature lets you access a large library of effects that are categorized into themes such as Bloggers' Social Media Pack, Holiday Pack Vol 11, Travel Pack 6, Wedding Pack, etc. You can also use third-party plug-ins from sources such as NewBlueFX, proDAD, or BorisFX to add more effects to your videos.</p>
|
18 |
-
<h3>360 Video Stabilization and Editing</h3>
|
19 |
-
<p>If you want to edit your videos in 360 degrees, you can use the 360 Video Stabilization and Editing feature. This feature lets you import and edit your footage from 360 cameras such as Samsung Gear VR, Ricoh Theta, or Kodak Pixpro . You can apply effects such as stabilization, trimming, splitting, or adding titles to your 360 videos. You can also use the True360 View Designer to convert your 360 videos into standard videos with different perspectives.</p>
|
20 |
-
<p>cyberlink powerdirector 11 ultimate suite crack<br />
|
21 |
-
cyberlink powerdirector 11 ultra download + crack<br />
|
22 |
-
cyberlink powerdirector 11 deluxe free download full version with crack<br />
|
23 |
-
cyberlink powerdirector 11 activation key crack<br />
|
24 |
-
cyberlink powerdirector 11 serial number crack<br />
|
25 |
-
how to install cyberlink powerdirector 11 with crack<br />
|
26 |
-
cyberlink powerdirector 11 content pack premium crack<br />
|
27 |
-
cyberlink powerdirector 11 director suite crack<br />
|
28 |
-
cyberlink powerdirector 11 free download full version for windows 10 with crack<br />
|
29 |
-
cyberlink powerdirector 11 patch crack<br />
|
30 |
-
cyberlink powerdirector 11 ultimate download + crack<br />
|
31 |
-
cyberlink powerdirector 11 keygen crack<br />
|
32 |
-
cyberlink powerdirector 11 free download full version for windows 7 with crack<br />
|
33 |
-
cyberlink powerdirector 11 license key crack<br />
|
34 |
-
cyberlink powerdirector 11 registration code crack<br />
|
35 |
-
how to use cyberlink powerdirector 11 with crack<br />
|
36 |
-
cyberlink powerdirector 11 content pack essential crack<br />
|
37 |
-
cyberlink powerdirector 11 ultimate serial key + crack full version<br />
|
38 |
-
cyberlink powerdirector 11 free download full version for windows 8 with crack<br />
|
39 |
-
cyberlink powerdirector 11 activation code crack<br />
|
40 |
-
how to get cyberlink powerdirector 11 for free with crack<br />
|
41 |
-
cyberlink powerdirector 11 content pack premium download + crack<br />
|
42 |
-
cyberlink powerdirector 11 ultra serial key + crack full version<br />
|
43 |
-
cyberlink powerdirector 11 free download full version for windows xp with crack<br />
|
44 |
-
cyberlink powerdirector 11 product key crack<br />
|
45 |
-
how to update cyberlink powerdirector 11 with crack<br />
|
46 |
-
cyberlink powerdirector 11 content pack essential download + crack<br />
|
47 |
-
cyberlink powerdirector 11 deluxe serial key + crack full version<br />
|
48 |
-
cyberlink powerdirector 11 free download full version for mac with crack<br />
|
49 |
-
cyberlink powerdirector 11 activation patch crack<br />
|
50 |
-
how to register cyberlink powerdirector 11 with crack<br />
|
51 |
-
cyberlink powerdirector 11 content pack premium free download + crack<br />
|
52 |
-
cyberlink powerdirector 11 ultra keygen + crack full version<br />
|
53 |
-
cyberlink powerdirector 11 free download full version for android with crack<br />
|
54 |
-
cyberlink powerdirector 11 trial resetter + crack full version<br />
|
55 |
-
how to uninstall cyberlink powerdirector 11 with crack<br />
|
56 |
-
cyberlink powerdirector 11 content pack essential free download + crack<br />
|
57 |
-
cyberlink powerdirector 11 deluxe keygen + crack full version<br />
|
58 |
-
cyberlink powerdirector 11 free download full version for linux with crack<br />
|
59 |
-
cyberlink powerdirector 11 activation manager + crack full version</p>
|
60 |
-
<h2>Pros and Cons of Cyberlink PowerDirector 11</h2>
|
61 |
-
<p>As with any software, Cyberlink PowerDirector 11 has its advantages and disadvantages. Here are some of them:</p>
|
62 |
-
<h3>Pros</h3>
|
63 |
-
<ul>
|
64 |
-
<li>It has a user-friendly interface that is easy to navigate and learn.</li>
|
65 |
-
<li>It has a comprehensive set of features that can meet various video editing needs.</li>
|
66 |
-
<li>It supports a wide range of formats and resolutions, including 4K and 3D .</li>
|
67 |
-
<li>It has fast rendering speed and performance.</li>
|
68 |
-
<li>It has a large community of users who share tips, tutorials, and feedback.</li>
|
69 |
-
</ul>
|
70 |
-
<h3>Cons</h3>
|
71 |
-
<ul>
|
72 |
-
<li>It requires a high-end computer system to run smoothly.</li>
|
73 |
-
<li>It may crash or freeze occasionally due to bugs or compatibility issues.</li>
|
74 |
-
<li>It may have some limitations in terms of customization or creativity compared to other professional video editors.</li>
|
75 |
-
<li>It may not be compatible with some newer devices or technologies.</li>
|
76 |
-
<li>It may not be legal or ethical to use it with a crack.</li>
|
77 |
-
</ul>
|
78 |
-
<h2>How to Download and Install Cyberlink PowerDirector 11 Full Version with Crack</h2>
|
79 |
-
<p>If you are interested in using Cyberlink PowerDirector 11 full version with crack, you will need to follow these steps:</p>
|
80 |
-
<h3>Step 1: Download the setup file and the crack file from a reliable source</h3>
|
81 |
-
<p>You will need to find a website that offers both the setup file and the crack file for Cyberlink PowerDirector 11. You can search for them on Google or other search engines, but be careful not to download any malware or viruses along with them. You should also check the reviews and ratings of the website before downloading anything.</p>
|
82 |
-
<p>One possible website that offers both files is FileCR.com . You can download them from these links:</p>
|
83 |
-
<ul>
|
84 |
-
<li><b>CyberLink Director Suite v11 Setup File:</b> https://filecr.com/windows/cyberlink-director-suite/</li>
|
85 |
-
<li><b>CyberLink Director Suite v11 Crack File:</b> https://filecr.com/windows/cyberlink-director-suite/#crack-download</li>
|
86 |
-
</ul>
|
87 |
-
<p>Note that these links are only for reference purposes and we do not endorse or guarantee their safety or legality.</p>
|
88 |
-
<h3>Step 2: Run the setup file and follow the instructions to install the program</h3>
|
89 |
-
<p>Once you have downloaded both files, you will need to run the setup file and follow the instructions on the screen to install Cyberlink PowerDirector 11 on your computer. You may need to agree to some terms and conditions and choose some options such as language, destination folder, etc. You may also need to enter a serial number or activation code that comes with the setup file. You should not launch or run the program after installation.</p>
|
90 |
-
<h3>Step 3: Copy the crack file and paste it into the installation folder</h3>
|
91 |
-
<p</p> 0a6ba089eb<br />
|
92 |
-
<br />
|
93 |
-
<br />
|
|
|
|
|
|
|
|
|
|
|
|
|
|
|
|
|
|
|
|
|
|
|
|
|
|
|
|
|
|
|
|
|
|
|
|
|
|
|
|
|
|
|
|
|
|
|
|
|
|
|
|
|
|
|
|
|
|
|
|
|
|
|
|
|
|
|
|
|
|
|
|
|
|
|
|
|
|
|
|
|
|
|
|
|
|
|
|
|
|
|
|
|
|
|
|
|
|
|
|
|
|
|
|
|
|
|
|
|
|
|
|
|
|
|
|
|
|
|
|
|
|
|
|
|
|
|
|
|
|
|
|
|
|
|
|
|
|
|
|
|
|
|
|
|
|
|
|
|
|
|
|
|
|
|
|
|
|
|
|
|
|
|
|
|
|
|
|
|
|
|
|
|
|
|
|
|
|
|
|
|
|
|
|
|
|
|
|
spaces/1acneusushi/gradio-2dmoleculeeditor/data/Download Microsoft Project 32 Bit Full Crack What You Need to Know.md
DELETED
@@ -1,29 +0,0 @@
|
|
1 |
-
<br />
|
2 |
-
<h1>How to Download Microsoft Project 32 Bit Full Crack for Free</h1>
|
3 |
-
<p>Microsoft Project is a powerful project management software that helps you plan, track, and manage your projects. It allows you to create schedules, assign tasks, monitor progress, manage resources, and collaborate with your team. Microsoft Project is widely used by professionals and organizations in various fields and industries.</p>
|
4 |
-
<p>However, Microsoft Project is not a cheap software. It requires a subscription to Microsoft 365 or a one-time purchase of a standalone license. If you want to use Microsoft Project without paying for it, you might be tempted to download Microsoft Project 32 bit full crack for free from the internet.</p>
|
5 |
-
<h2>download microsoft project 32 bit full crack</h2><br /><p><b><b>Download Zip</b> ✪✪✪ <a href="https://byltly.com/2uKvbI">https://byltly.com/2uKvbI</a></b></p><br /><br />
|
6 |
-
<p>A crack is a modified version of a software that bypasses its security and activation features. By using a crack, you can run the software without a valid license or product key. However, downloading and using Microsoft Project 32 bit full crack is not a good idea for several reasons.</p>
|
7 |
-
<h2>The Risks of Downloading Microsoft Project 32 Bit Full Crack</h2>
|
8 |
-
<p>Downloading Microsoft Project 32 bit full crack from the internet is risky and illegal. Here are some of the dangers and disadvantages of doing so:</p>
|
9 |
-
<ul>
|
10 |
-
<li><b>It can harm your computer.</b> Many websites that offer Microsoft Project 32 bit full crack are unreliable and malicious. They can infect your computer with viruses, malware, spyware, ransomware, and other threats that can damage your system, steal your data, or lock your files.</li>
|
11 |
-
<li><b>It can compromise your security.</b> By using Microsoft Project 32 bit full crack, you are exposing yourself to potential hackers and cybercriminals who can exploit the vulnerabilities and backdoors in the cracked software. They can access your personal information, financial accounts, passwords, and other sensitive data.</li>
|
12 |
-
<li><b>It can affect your performance.</b> Microsoft Project 32 bit full crack is not guaranteed to work properly or smoothly. It can have bugs, errors, crashes, compatibility issues, and missing features that can hinder your productivity and efficiency. It can also cause conflicts with other software or updates on your computer.</li>
|
13 |
-
<li><b>It can violate the law.</b> Downloading and using Microsoft Project 32 bit full crack is illegal and unethical. It is a form of piracy that infringes the intellectual property rights of Microsoft and its developers. You can face legal consequences such as fines, lawsuits, or even jail time if you are caught using cracked software.</li>
|
14 |
-
</ul>
|
15 |
-
<h2>The Benefits of Using Genuine Microsoft Project</h2>
|
16 |
-
<p>Instead of downloading Microsoft Project 32 bit full crack, you should consider using genuine Microsoft Project. Here are some of the benefits of doing so:</p>
|
17 |
-
<ul>
|
18 |
-
<li><b>It can protect your computer.</b> Genuine Microsoft Project is safe and secure to download and install. It does not contain any viruses, malware, spyware, ransomware, or other threats that can harm your computer. It also has regular updates that fix any bugs or issues in the software.</li>
|
19 |
-
<li><b>It can enhance your security.</b> Genuine Microsoft Project has built-in security features that protect your data and privacy. It encrypts your files and communications, prevents unauthorized access, and integrates with other Microsoft services such as OneDrive, SharePoint, Teams, and Outlook.</li>
|
20 |
-
<li><b>It can improve your performance.</b> Genuine Microsoft Project works flawlessly and smoothly on your computer. It has all the features and functions that you need to manage your projects effectively and efficiently. It also supports multiple languages, formats, platforms, and devices.</li>
|
21 |
-
<li><b>It can comply with the law.</b> Genuine Microsoft Project is legal and ethical to use. It respects the intellectual property rights of Microsoft and its developers. You can use it without worrying about any legal consequences or penalties.</li>
|
22 |
-
</ul>
|
23 |
-
<h2>How to Get Genuine Microsoft Project</h2>
|
24 |
-
<p>If you want to get genuine Microsoft Project for your computer, you have two options:</p>
|
25 |
-
<ol>
|
26 |
-
<li><b>Subscribe to Microsoft 365.</b> Microsoft 365 is a cloud-based service that gives you access to various Microsoft applications such as Word, Excel, PowerPoint, Outlook, OneNote, OneDrive, Teams, and more. It also includes Microsoft Project as part of its plans. You can choose from different plans depending on your needs and budget. You can pay monthly or yearly for the subscription and enjoy all the benefits of Microsoft 365.</li>
|
27 |
-
<li><b>Purchase a standalone license.</b> A standalone license is a one-time purchase that gives you the</p> ddb901b051<br />
|
28 |
-
<br />
|
29 |
-
<br />
|
|
|
|
|
|
|
|
|
|
|
|
|
|
|
|
|
|
|
|
|
|
|
|
|
|
|
|
|
|
|
|
|
|
|
|
|
|
|
|
|
|
|
|
|
|
|
|
|
|
|
|
|
|
|
|
|
|
|
|
spaces/1gistliPinn/ChatGPT4/Examples/Downloadgametrainsimulatormodindonesia.md
DELETED
@@ -1,110 +0,0 @@
|
|
1 |
-
<br />
|
2 |
-
<h1>Download Game Train Simulator Mod Indonesia: How to Enjoy Realistic and Immersive Trainz Experience</h1>
|
3 |
-
|
4 |
-
<p>If you are a fan of train simulation games and want to experience a realistic and immersive trainz experience, you might want to download game train simulator mod Indonesia. This is a custom mod that allows you to play on various train simulators with Indonesian trains, stations, routes, and scenery. But what is game train simulator mod Indonesia exactly, and how can you download and install it? In this article, we will answer these questions and more.</p>
|
5 |
-
<h2>downloadgametrainsimulatormodindonesia</h2><br /><p><b><b>Download</b> 🌟 <a href="https://imgfil.com/2uy21n">https://imgfil.com/2uy21n</a></b></p><br /><br />
|
6 |
-
|
7 |
-
<h2>What is Game Train Simulator Mod Indonesia?</h2>
|
8 |
-
|
9 |
-
<p>Game train simulator mod Indonesia is a custom mod that adds Indonesian content to various train simulators. It is developed by the Indonesian Trainz Community, a group of passionate trainz fans who have been around since 2009. The mod aims to provide a realistic and immersive trainz experience, with features such as:</p>
|
10 |
-
|
11 |
-
<ul>
|
12 |
-
<li>A variety of Indonesian locomotives and coaches, such as GE U18C, GE U20C, GE CC206, passenger and freight coaches.</li>
|
13 |
-
<li>A selection of Indonesian stations, such as Gambir, Karawang, Purwakarta, Bandung.</li>
|
14 |
-
<li>A number of Indonesian routes, such as Jakarta-Bandung, Jakarta-Surabaya, Jakarta-Medan.</li>
|
15 |
-
<li>A realistic and detailed Indonesian scenery, with custom maps, buildings, trees, roads, bridges, etc.</li>
|
16 |
-
<li>A dynamic and interactive Indonesian environment, with weather, time, traffic, signals, etc.</li>
|
17 |
-
</ul>
|
18 |
-
|
19 |
-
<p>Game train simulator mod Indonesia is compatible with several train simulators, such as Trainz Simulator 2009, Trainz Simulator 2012, Trainz Simulator 2019, Indonesian Train Simulator (Android), etc. It is also constantly updated and improved by the developers and the community feedback.</p>
|
20 |
-
|
21 |
-
<h2>How to Download and Install Game Train Simulator Mod Indonesia?</h2>
|
22 |
-
|
23 |
-
<p>If you want to play with game train simulator mod Indonesia, you will need to download and install the mod for the train simulator you want to play. Here are the steps to do so:</p>
|
24 |
-
|
25 |
-
<ol>
|
26 |
-
<li>Download and install the train simulator of your choice from their official websites or app stores.</li>
|
27 |
-
<li>Download the latest version of game train simulator mod Indonesia from the YouTube channel or website of the Indonesian Trainz Community. You can find the links for different train simulators below:</li>
|
28 |
-
<ul>
|
29 |
-
<li><a href="https://www.youtube.com/watch?v=jfyqk8yWr8E">Game Train Simulator Mod Indonesia for Trainz Simulator 2009</a></li>
|
30 |
-
<li><a href="https://www.youtube.com/watch?v=4Z6x7b0oX4c">Game Train Simulator Mod Indonesia for Trainz Simulator 2012</a></li>
|
31 |
-
<li><a href="https://www.youtube.com/watch?v=JgYfY0nZi3w">Game Train Simulator Mod Indonesia for Trainz Simulator 2019</a></li>
|
32 |
-
<li><a href="https://play.google.com/store/apps/details?id=com.HighbrowInteractive.IndonesianTrainSim">Game Train Simulator Mod Indonesia for Indonesian Train Simulator (Android)</a></li>
|
33 |
-
</ul>
|
34 |
-
<li>Extract the downloaded files to your train simulator folder.</li>
|
35 |
-
<li>Launch your train simulator and select game train simulator mod Indonesia as your content.</li>
|
36 |
-
<li>Create your scenario and start playing!</li>
|
37 |
-
</ol>
|
38 |
-
|
39 |
-
<p>If you encounter any issues or need any help with the installation process, you can contact the Indonesian Trainz Community on their YouTube channel or website. They will be happy to assist you.</p>
|
40 |
-
|
41 |
-
<h2>Conclusion</h2>
|
42 |
-
|
43 |
-
<p>Game train simulator mod Indonesia is a custom mod that allows you to play on various train simulators with Indonesian trains, stations, routes, and scenery. It is designed to provide a realistic and immersive trainz experience for train simulation fans. You can download and install it for free from the YouTube channel or website of the Indonesian Trainz Community. If you want to enjoy realistic and immersive trainz experience with game train simulator mod Indonesia, you can find more information on their YouTube channel or website. Have fun playing!</p>
|
44 |
-
<h2>What are the Tips and Tricks for Game Train Simulator Mod Indonesia?</h2>
|
45 |
-
|
46 |
-
<p>Game train simulator mod Indonesia is a fun and enjoyable mod for train simulators, but it can also be challenging and tricky at times. If you want to master the game and have a smooth and satisfying trainz experience, you might want to follow some tips and tricks. Here are some of them:</p>
|
47 |
-
<p></p>
|
48 |
-
|
49 |
-
<ul>
|
50 |
-
<li>Read the instructions and tutorials carefully before playing. They will help you understand the basics and features of the game.</li>
|
51 |
-
<li>Adjust the settings and preferences according to your device and preferences. You can change the graphics, sound, controls, etc. to suit your needs.</li>
|
52 |
-
<li>Choose the right locomotive and coach for your scenario. Different locomotives and coaches have different characteristics, such as speed, power, capacity, etc. Choose the ones that match your objectives and preferences.</li>
|
53 |
-
<li>Follow the rules and regulations of the game. Respect the signals, speed limits, timetables, etc. They will help you avoid accidents and penalties.</li>
|
54 |
-
<li>Plan your route and strategy ahead. Use the map, GPS, route planner, etc. to plan your route and strategy. They will help you avoid traffic, delays, detours, etc.</li>
|
55 |
-
<li>Use the camera angles wisely. Switch between different camera angles to get a better view of your surroundings and situation. They will help you avoid obstacles, hazards, errors, etc.</li>
|
56 |
-
<li>Save your progress frequently. Use the save and load functions to save your progress frequently. They will help you avoid losing your progress in case of crashes, errors, etc.</li>
|
57 |
-
<li>Have fun and enjoy the game. Don't take the game too seriously or stress yourself too much. Remember that it is just a game and the main purpose is to have fun and enjoy the trainz experience.</li>
|
58 |
-
</ul>
|
59 |
-
|
60 |
-
<p>Game train simulator mod Indonesia is a mod that can offer you a lot of fun and enjoyment, but also a lot of challenge and difficulty. If you want to overcome the challenge and difficulty and have a smooth and satisfying trainz experience, you might want to follow these tips and tricks. They will help you improve your skills and performance in game train simulator mod Indonesia.</p>
|
61 |
-
|
62 |
-
<h2>How to Get More Content for Game Train Simulator Mod Indonesia?</h2>
|
63 |
-
|
64 |
-
<p>If you want to get more content for game train simulator mod Indonesia, such as more locomotives, coaches, stations, routes, scenery, etc., you have two options:</p>
|
65 |
-
|
66 |
-
<ol>
|
67 |
-
<li>You can download more content from the Indonesian Trainz Community website or YouTube channel. They have a lot of content available for free download. You can find the links for different train simulators in the previous sections.</li>
|
68 |
-
<li>You can create your own content using the content creation tools provided by the train simulators. You can use the surveyor tool, asset editor tool, script editor tool, etc. to create your own content. You can also share your content with other players on the Indonesian Trainz Community website or YouTube channel.</li>
|
69 |
-
</ol>
|
70 |
-
|
71 |
-
<p>Game train simulator mod Indonesia is a mod that has a lot of content available for you to enjoy, but it also allows you to get more content or create your own content if you want to. If you want to get more content or create your own content for game train simulator mod Indonesia, you can follow these options. They will help you expand your trainz experience with game train simulator mod Indonesia.</p>
|
72 |
-
|
73 |
-
<h2>Conclusion</h2>
|
74 |
-
|
75 |
-
<p>Game train simulator mod Indonesia is a custom mod that allows you to play on various train simulators with Indonesian trains, stations, routes, and scenery. It is designed to provide a realistic and immersive trainz experience for train simulation fans. You can download and install it for free from the YouTube channel or website of the Indonesian Trainz Community. If you want to enjoy realistic and immersive trainz experience with game train simulator mod Indonesia, you can find more information on their YouTube channel or website. Have fun playing!</p>
|
76 |
-
<h2>What are the Reviews and Ratings for Game Train Simulator Mod Indonesia?</h2>
|
77 |
-
|
78 |
-
<p>Game train simulator mod Indonesia is a popular and well-received mod for train simulators. It has received a lot of positive reviews and ratings from the players and critics. Here are some of them:</p>
|
79 |
-
|
80 |
-
<ul>
|
81 |
-
<li>On Google Play, game train simulator mod Indonesia has a rating of 4.4 out of 5 stars, based on 187K reviews. Most of the reviews praise the game for its realism, graphics, sound, gameplay, etc.</li>
|
82 |
-
<li>On APKPure, game train simulator mod Indonesia has a rating of 8.5 out of 10, based on 18 reviews. Most of the reviews commend the game for its quality, features, content, etc.</li>
|
83 |
-
<li>On YouTube, game train simulator mod Indonesia has a lot of videos showcasing the game and its features. Most of the videos have a lot of views, likes, comments, and subscriptions.</li>
|
84 |
-
<li>On the Indonesian Trainz Community website and YouTube channel, game train simulator mod Indonesia has a lot of feedback and suggestions from the players and fans. Most of the feedback and suggestions are positive and constructive.</li>
|
85 |
-
</ul>
|
86 |
-
|
87 |
-
<p>Game train simulator mod Indonesia is a mod that has a lot of fans and supporters. It has received a lot of positive feedback and recognition from the players and critics. If you want to see what other people think about game train simulator mod Indonesia, you can check out these reviews and ratings.</p>
|
88 |
-
|
89 |
-
<h2>How to Support Game Train Simulator Mod Indonesia?</h2>
|
90 |
-
|
91 |
-
<p>If you like game train simulator mod Indonesia and want to support it, you have several ways to do so. Here are some of them:</p>
|
92 |
-
|
93 |
-
<ol>
|
94 |
-
<li>You can rate and review the game on Google Play, APKPure, or any other platform where you downloaded it. This will help the game get more exposure and recognition.</li>
|
95 |
-
<li>You can share the game with your friends and family who are interested in train simulation games. This will help the game get more downloads and players.</li>
|
96 |
-
<li>You can subscribe to the Indonesian Trainz Community website or YouTube channel. This will help you get more updates and information about the game and its development.</li>
|
97 |
-
<li>You can donate to the Indonesian Trainz Community website or YouTube channel. This will help them cover the costs of developing and maintaining the game.</li>
|
98 |
-
<li>You can provide feedback and suggestions to the Indonesian Trainz Community website or YouTube channel. This will help them improve and enhance the game according to your preferences and needs.</li>
|
99 |
-
</ol>
|
100 |
-
|
101 |
-
<p>Game train simulator mod Indonesia is a mod that deserves your support and appreciation. It is a mod that provides you with a realistic and immersive trainz experience for free. If you want to support game train simulator mod Indonesia, you can follow these ways. They will help you show your gratitude and respect to the developers and creators of game train simulator mod Indonesia.</p>
|
102 |
-
|
103 |
-
<h2>Conclusion</h2>
|
104 |
-
|
105 |
-
<p>Game train simulator mod Indonesia is a custom mod that allows you to play on various train simulators with Indonesian trains, stations, routes, and scenery. It is designed to provide a realistic and immersive trainz experience for train simulation fans. You can download and install it for free from the YouTube channel or website of the Indonesian Trainz Community. If you want to enjoy realistic and immersive trainz experience with game train simulator mod Indonesia, you can find more information on their YouTube channel or website. Have fun playing!</p>
|
106 |
-
<h2>Conclusion</h2>
|
107 |
-
|
108 |
-
<p>Game train simulator mod Indonesia is a custom mod that allows you to play on various train simulators with Indonesian trains, stations, routes, and scenery. It is designed to provide a realistic and immersive trainz experience for train simulation fans. You can download and install it for free from the YouTube channel or website of the Indonesian Trainz Community. If you want to enjoy realistic and immersive trainz experience with game train simulator mod Indonesia, you can find more information on their YouTube channel or website. Have fun playing!</p> 3cee63e6c2<br />
|
109 |
-
<br />
|
110 |
-
<br />
|
|
|
|
|
|
|
|
|
|
|
|
|
|
|
|
|
|
|
|
|
|
|
|
|
|
|
|
|
|
|
|
|
|
|
|
|
|
|
|
|
|
|
|
|
|
|
|
|
|
|
|
|
|
|
|
|
|
|
|
|
|
|
|
|
|
|
|
|
|
|
|
|
|
|
|
|
|
|
|
|
|
|
|
|
|
|
|
|
|
|
|
|
|
|
|
|
|
|
|
|
|
|
|
|
|
|
|
|
|
|
|
|
|
|
|
|
|
|
|
|
|
|
|
|
|
|
|
|
|
|
|
|
|
|
|
|
|
|
|
|
|
|
|
|
|
|
|
|
|
|
|
|
|
|
|
|
|
|
|
|
|
|
|
|
|
|
|
|
|
|
|
|
|
|
|
|
|
|
|
|
|
|
|
|
|
|
|
|
|
|
|
|
|
|
|
|
|
|
|
|
|
|
|
|
|
|
|
|
|
|
|
|
|
|
|
|
|
|
|
|
|
spaces/1pelhydcardo/ChatGPT-prompt-generator/assets/Auto Report FB How to Automate Reporting on Facebook with a Simple App.md
DELETED
@@ -1,164 +0,0 @@
|
|
1 |
-
|
2 |
-
<h1>Auto Report Facebook APK: What Is It and How to Use It</h1>
|
3 |
-
<p>Facebook is one of the most popular social media platforms in the world, with billions of users and millions of posts every day. However, not all of these posts are appropriate or respectful. Some of them may contain spam, hate speech, violence, nudity, or other violations of Facebook's community standards. If you encounter such posts, you can report them to Facebook and hope that they will take action. But what if you want to report multiple posts or profiles at once, without wasting time and effort? This is where auto report facebook apk comes in handy.</p>
|
4 |
-
<h2>Introduction</h2>
|
5 |
-
<h3>What is auto report facebook apk?</h3>
|
6 |
-
<p>Auto report facebook apk is an Android application that allows you to automatically report any Facebook profile or post that you want. It is not an official app from Facebook, but a third-party tool developed by independent developers. It works by using your Facebook account to send multiple reports to Facebook's servers, with the aim of getting the target profile or post removed or banned.</p>
|
7 |
-
<h2>auto report facebook apk</h2><br /><p><b><b>DOWNLOAD</b> » <a href="https://urlin.us/2uT1Co">https://urlin.us/2uT1Co</a></b></p><br /><br />
|
8 |
-
<h3>Why would you want to use it?</h3>
|
9 |
-
<p>There are many reasons why you might want to use auto report facebook apk. For example, you might want to:</p>
|
10 |
-
<ul>
|
11 |
-
<li>Report a fake or impersonating profile that is trying to scam or harass you or your friends.</li>
|
12 |
-
<li>Report a spammy or malicious post that is spreading false information or harmful links.</li>
|
13 |
-
<li>Report a hateful or abusive post that is targeting you or someone else based on their identity, beliefs, or opinions.</li>
|
14 |
-
<li>Report a violent or graphic post that is showing disturbing images or videos.</li>
|
15 |
-
<li>Report a nude or sexual post that is violating your privacy or consent.</li>
|
16 |
-
</ul>
|
17 |
-
<h3>What are the benefits and drawbacks of using it?</h3>
|
18 |
-
<p>Using auto report facebook apk can have some benefits and drawbacks. Some of the benefits are:</p>
|
19 |
-
<ul>
|
20 |
-
<li>You can save time and energy by reporting multiple profiles or posts at once, instead of doing it manually one by one.</li>
|
21 |
-
<li>You can increase the chances of getting the target profile or post removed or banned, by sending more reports than usual.</li>
|
22 |
-
<li>You can protect yourself and others from harmful or offensive content on Facebook, by reducing its visibility and reach.</li>
|
23 |
-
</ul>
|
24 |
-
<p>Some of the drawbacks are:</p>
|
25 |
-
<ul>
|
26 |
-
<li>You may risk violating Facebook's terms of service, by using an unauthorized app that manipulates their system.</li>
|
27 |
-
<li>You may risk losing your Facebook account, by logging in with your credentials on a third-party app that may not be secure or trustworthy.</li>
|
28 |
-
<li>You may risk reporting innocent profiles or posts, by using the app incorrectly or irresponsibly.</li>
|
29 |
-
</ul>
|
30 |
-
<h2>How to download and install auto report facebook apk</h2>
|
31 |
-
<h3>Step 1: Find a reliable source for the apk file</h3>
|
32 |
-
<p>The first step to use auto report facebook apk is to find a reliable source for the apk file. The apk file is the installation package for Android applications. You can search for it online, but be careful not to download it from shady or unknown websites that may contain viruses or malware. One of the sources that you can try is GitHub, where you can find the project page for auto report facebook apk. There, you can see the latest version of the app, its features, and its instructions. You can also download the apk file from there by clicking on the "Releases" tab and then on the "Assets" section. Make sure you download the file that ends with ".apk".</p>
|
33 |
-
<h3>Step 2: Enable unknown sources on your device</h3>
|
34 |
-
<p>The second step to use auto report facebook apk is to enable unknown sources on your device. This is a security setting that allows you to install apps from sources other than the Google Play Store. To do this, you need to go to your device's settings, then to security or privacy, and then to unknown sources or install unknown apps. There, you need to toggle on the option that allows you to install apps from unknown sources. You may also need to grant permission to the browser or file manager that you are using to download the apk file.</p>
|
35 |
-
<h3>Step 3: Download and install the apk file</h3>
|
36 |
-
<p>The third step to use auto report facebook apk is to download and install the apk file. To do this, you need to open the browser or file manager that you used to download the apk file, and then locate the file in your downloads folder or wherever you saved it. Then, you need to tap on the file and follow the instructions on the screen to install the app. You may need to grant some permissions to the app, such as access to your storage, contacts, and location. Once the installation is complete, you can find the app icon on your home screen or app drawer.</p>
|
37 |
-
<p>auto report fb apk download<br />
|
38 |
-
facebook auto reporter v2<br />
|
39 |
-
auto report facebook account<br />
|
40 |
-
facebook auto report script<br />
|
41 |
-
auto report facebook group<br />
|
42 |
-
facebook auto report bot<br />
|
43 |
-
auto report facebook page<br />
|
44 |
-
facebook auto report tool<br />
|
45 |
-
auto report fb apk free<br />
|
46 |
-
facebook auto reporter v2 download<br />
|
47 |
-
auto report facebook online<br />
|
48 |
-
facebook auto report imacros<br />
|
49 |
-
auto report facebook app<br />
|
50 |
-
facebook auto report software<br />
|
51 |
-
auto report fb apk 2023<br />
|
52 |
-
facebook auto reporter v2 free<br />
|
53 |
-
auto report facebook profile<br />
|
54 |
-
facebook auto report chrome extension<br />
|
55 |
-
auto report fb apk latest version<br />
|
56 |
-
facebook auto reporter v2 tutorial<br />
|
57 |
-
auto report facebook comment<br />
|
58 |
-
facebook auto report generator<br />
|
59 |
-
auto report fb apk mod<br />
|
60 |
-
facebook auto reporter v2 script<br />
|
61 |
-
auto report fb apk no root<br />
|
62 |
-
facebook auto reporter v2 youtube<br />
|
63 |
-
auto report fb apk for android<br />
|
64 |
-
facebook auto report hack<br />
|
65 |
-
auto report fb apk 2022<br />
|
66 |
-
facebook auto reporter v2 github<br />
|
67 |
-
auto report fb apk pro<br />
|
68 |
-
facebook auto report website<br />
|
69 |
-
auto report fb apk terbaru<br />
|
70 |
-
facebook auto reporter v2 online<br />
|
71 |
-
auto report fb apk 2021<br />
|
72 |
-
facebook auto reporter v2 review<br />
|
73 |
-
auto report fb apk update<br />
|
74 |
-
facebook auto reporter v2 crack<br />
|
75 |
-
auto report fb apk premium<br />
|
76 |
-
facebook auto reporter v2 alternative<br />
|
77 |
-
auto report fb apk old version<br />
|
78 |
-
facebook auto reporter v2 for pc<br />
|
79 |
-
auto report fb apk 2020<br />
|
80 |
-
facebook auto reporter v2 for android<br />
|
81 |
-
auto report fb apk cracked<br />
|
82 |
-
facebook auto reporter v2 for mac<br />
|
83 |
-
auto report fb apk full version<br />
|
84 |
-
facebook reporting tools free</p>
|
85 |
-
<h2>How to use auto report facebook apk</h2>
|
86 |
-
<h3>Step 1: Launch the app and log in with your Facebook account</h3>
|
87 |
-
<p>The first step to use auto report facebook apk is to launch the app and log in with your Facebook account. To do this, you need to tap on the app icon and wait for it to load. Then, you need to enter your Facebook email or phone number and password, and tap on "Log In". You may also need to enter a verification code or confirm your identity if prompted by Facebook. Once you are logged in, you will see the main interface of the app, which consists of a menu bar at the top and a list of profiles or posts at the bottom.</p>
|
88 |
-
<h3>Step 2: Select the target profile or post that you want to report</h3>
|
89 |
-
<p>The second step to use auto report facebook apk is to select the target profile or post that you want to report. To do this, you need to tap on the menu bar at the top and choose one of the options: "Report Profile" or "Report Post". Then, you need to enter the URL or ID of the profile or post that you want to report in the text box, and tap on "Search". You will see a preview of the profile or post below, along with some information such as name, date, and content. You can also tap on "Load More" to see more profiles or posts that match your search criteria.</p>
|
90 |
-
<h3>Step 3: Choose the reason for reporting and submit</h3>
|
91 |
-
<p>The third step to use auto report facebook apk is to choose the reason for reporting and submit. To do this, you need to tap on the profile or post that you want to report from the list below, and then tap on "Report". You will see a pop-up window with a list of reasons for reporting, such as spam, fake account, hate speech, violence, nudity, or other. You can select one or more reasons that apply to your case, and then tap on "Submit". You will see a confirmation message that your report has been sent successfully. You can repeat this process for as many profiles or posts as you want.</p>
|
92 |
-
<h2>Conclusion</h2>
|
93 |
-
<h3>Summary of the main points</h3>
|
94 |
-
<p>In this article, we have explained what auto report facebook apk is and how to use it. Auto report facebook apk is an Android application that allows you to automatically report any Facebook profile or post that you want. It can help you save time and energy by reporting multiple profiles or posts at once, increase the chances of getting them removed or banned, and protect yourself and others from harmful or offensive content on Facebook. However, it also has some drawbacks, such as violating Facebook's terms of service, risking losing your Facebook account, and reporting innocent profiles or posts.</p>
|
95 |
-
<h3>Call to action and disclaimer</h3>
|
96 |
-
<p>If you want to try auto report facebook apk for yourself, you can download it from GitHub and follow the steps that we have outlined above. However, we advise you to use it with caution and responsibility, as we are not responsible for any consequences that may arise from using it. We also recommend that you respect Facebook's community standards and only report profiles or posts that truly violate them. Remember that reporting is a serious matter and should not be abused for personal vendetta or malicious intent.</p>
|
97 |
-
<table style="border: 1px solid black;"> <tr>
|
98 |
-
<th style="border: 1px solid black;">Reason for reporting</th>
|
99 |
-
<th style="border: 1px solid black;">Description</th>
|
100 |
-
</tr>
|
101 |
-
<tr>
|
102 |
-
<td style="border: 1px solid black;">Spam</td>
|
103 |
-
<td style="border: 1px solid black;">The profile or post is unsolicited, repetitive, or irrelevant.</td>
|
104 |
-
</tr>
|
105 |
-
<tr>
|
106 |
-
<td style="border: 1px solid black;">Fake account</td>
|
107 |
-
<td style="border: 1px solid black;">The profile is not representing a real person or entity.</td>
|
108 |
-
</tr>
|
109 |
-
<tr>
|
110 |
-
<td style="border: 1px solid black;">Hate speech</td>
|
111 |
-
<td style="border: 1px solid black;">The profile or post is attacking or discriminating against a group or individual based on their race, ethnicity, religion, gender, sexual orientation, disability, or other characteristic.</td>
|
112 |
-
</tr>
|
113 |
-
<tr>
|
114 |
-
<td style="border: 1px solid black;">Violence</td>
|
115 |
-
<td style="border: 1px solid black;">The profile or post is promoting or showing physical harm, threats, or cruelty to oneself or others.</td>
|
116 |
-
</tr>
|
117 |
-
<tr>
|
118 |
-
<td style="border: 1px solid black;">Nudity</td>
|
119 |
-
<td style="border: 1px solid black;">The profile or post is displaying or soliciting sexual or explicit content that violates Facebook's policies.</td>
|
120 |
-
</tr>
|
121 |
-
<tr>
|
122 |
-
<td style="border: 1px solid black;">Other</td>
|
123 |
-
<td style="border: 1px solid black;">The profile or post is violating Facebook's community standards in some other way.</td>
|
124 |
-
</tr>
|
125 |
-
</table>
|
126 |
-
<p>This is an example of a table that you can use in your article to illustrate the different reasons for reporting a profile or post on Facebook.</p>
|
127 |
-
<h2>FAQs</h2>
|
128 |
-
<h3>What is the difference between auto report facebook apk and the report feature on Facebook?</h3>
|
129 |
-
<p>The report feature on Facebook is the official way to report a profile or post that violates Facebook's community standards. You can access it by clicking on the three dots icon on the top right corner of any profile or post, and then selecting "Report". You can then choose the reason for reporting and follow the instructions. The report feature on Facebook allows you to report one profile or post at a time, and it may take some time for Facebook to review and act on your report.</p>
|
130 |
-
<p>Auto report facebook apk is an unofficial app that allows you to automatically report multiple profiles or posts at once. You can download it from GitHub and install it on your Android device. You can then log in with your Facebook account and enter the URL or ID of the profile or post that you want to report. You can then choose the reason for reporting and submit. Auto report facebook apk sends multiple reports to Facebook's servers, with the aim of getting the profile or post removed or banned faster.</p>
|
131 |
-
<h3>Is auto report facebook apk safe to use?</h3>
|
132 |
-
<p>Auto report facebook apk is not a safe app to use, as it may pose some risks to your device and your Facebook account. Some of the risks are:</p>
|
133 |
-
<ul>
|
134 |
-
<li>It may contain viruses or malware that can harm your device or steal your data.</li>
|
135 |
-
<li>It may not be secure or trustworthy, as it requires you to log in with your Facebook credentials on a third-party app that may not protect your privacy.</li>
|
136 |
-
<li>It may violate Facebook's terms of service, as it manipulates their system and abuses their report feature.</li>
|
137 |
-
<li>It may result in your Facebook account being suspended or banned, as Facebook may detect your abnormal activity and flag you as a spammer or a violator.</li>
|
138 |
-
<li>It may report innocent profiles or posts, as it may not be accurate or responsible in selecting the target profile or post.</li>
|
139 |
-
</ul>
|
140 |
-
<p>Therefore, we advise you to use auto report facebook apk with caution and responsibility, and at your own risk. We also recommend that you use the official report feature on Facebook instead, as it is safer and more reliable.</p>
|
141 |
-
<h3>How can I avoid being reported by auto report facebook apk?</h3>
|
142 |
-
<p>The best way to avoid being reported by auto report facebook apk is to follow Facebook's community standards and not post anything that violates them. Some of the things that you should avoid posting are:</p>
|
143 |
-
<ul>
|
144 |
-
<li>Fake or impersonating profiles that try to scam or harass others.</li>
|
145 |
-
<li>Spammy or malicious posts that spread false information or harmful links.</li>
|
146 |
-
<li>Hateful or abusive posts that target others based on their identity, beliefs, or opinions.</li>
|
147 |
-
<li>Violent or graphic posts that show disturbing images or videos.</li>
|
148 |
-
<li>Nude or sexual posts that violate others' privacy or consent.</li>
|
149 |
-
</ul>
|
150 |
-
<p>If you follow these guidelines, you will not only avoid being reported by auto report facebook apk, but also create a positive and respectful environment on Facebook for yourself and others.</p>
|
151 |
-
<h3>How can I report a profile or post that is using auto report facebook apk?</h3>
|
152 |
-
<p>If you suspect that a profile or post is using auto report facebook apk to report others unfairly or maliciously, you can report them to Facebook using the official report feature. To do this, you need to click on the three dots icon on the top right corner of the profile or post, and then select "Report". You can then choose the reason for reporting, such as "It's spam" or "It's abusive or harmful". You can also provide more details or feedback to Facebook, such as "This profile or post is using auto report facebook apk to report others". Facebook will then review your report and take appropriate action.</p>
|
153 |
-
<h3>What are some alternatives to auto report facebook apk?</h3>
|
154 |
-
<p>If you are looking for some alternatives to auto report facebook apk, you can try some of these options:</p>
|
155 |
-
<ul>
|
156 |
-
<li>Use the official report feature on Facebook, as it is safer and more reliable.</li>
|
157 |
-
<li>Use the block or unfriend feature on Facebook, as it will prevent you from seeing or interacting with the profile or post that you don't like.</li>
|
158 |
-
<li>Use the hide or snooze feature on Facebook, as it will reduce the visibility or frequency of the profile or post that you don't want to see.</li>
|
159 |
-
<li>Use the mute or unfollow feature on Facebook, as it will stop the notifications or updates from the profile or post that you are not interested in.</li>
|
160 |
-
<li>Use the feedback or rating feature on Facebook, as it will help Facebook improve their content quality and relevance.</li>
|
161 |
-
</ul>
|
162 |
-
<p>These options will help you manage your Facebook experience better, without resorting to auto report facebook apk.</p> 197e85843d<br />
|
163 |
-
<br />
|
164 |
-
<br />
|
|
|
|
|
|
|
|
|
|
|
|
|
|
|
|
|
|
|
|
|
|
|
|
|
|
|
|
|
|
|
|
|
|
|
|
|
|
|
|
|
|
|
|
|
|
|
|
|
|
|
|
|
|
|
|
|
|
|
|
|
|
|
|
|
|
|
|
|
|
|
|
|
|
|
|
|
|
|
|
|
|
|
|
|
|
|
|
|
|
|
|
|
|
|
|
|
|
|
|
|
|
|
|
|
|
|
|
|
|
|
|
|
|
|
|
|
|
|
|
|
|
|
|
|
|
|
|
|
|
|
|
|
|
|
|
|
|
|
|
|
|
|
|
|
|
|
|
|
|
|
|
|
|
|
|
|
|
|
|
|
|
|
|
|
|
|
|
|
|
|
|
|
|
|
|
|
|
|
|
|
|
|
|
|
|
|
|
|
|
|
|
|
|
|
|
|
|
|
|
|
|
|
|
|
|
|
|
|
|
|
|
|
|
|
|
|
|
|
|
|
|
|
|
|
|
|
|
|
|
|
|
|
|
|
|
|
|
|
|
|
|
|
|
|
|
|
|
|
|
|
|
|
|
|
|
|
|
|
|
|
|
|
|
|
|
|
|
|
|
|
|
|
|
|
|
|
|
|
|
|
|
|
|
|
|
|
|
|
|
|
|
|
|
|
|
|
|
|
|
|
|
|
|
|
|
|
|
|
|
|
|
|
|
|
|
|
|
|
|
|
|
|
|
|
|
|
|
|
|
spaces/1phancelerku/anime-remove-background/Create Convert and Edit PDF Files with PrimoPDF - A Free and Reliable PDF Creator.md
DELETED
@@ -1,92 +0,0 @@
|
|
1 |
-
|
2 |
-
<h1>How to Download PrimoPDF: A Free PDF Converter and Creator</h1>
|
3 |
-
<p>If you are looking for a free and easy way to convert or create PDF files from any Windows application, you might want to try PrimoPDF. PrimoPDF is a free tool provided by Nitro Software, Inc that offers high-quality conversion to PDF, comprising a user-friendly interface that enables printing to PDF from virtually any Windows application.</p>
|
4 |
-
<p>In this article, we will show you how to download PrimoPDF, how to use it to convert and create PDF files, and some tips and tricks for using it effectively. We will also answer some frequently asked questions about PrimoPDF.</p>
|
5 |
-
<h2>download primopdf</h2><br /><p><b><b>Download</b> ⚙ <a href="https://jinyurl.com/2uNMvq">https://jinyurl.com/2uNMvq</a></b></p><br /><br />
|
6 |
-
<h2>Benefits of PrimoPDF</h2>
|
7 |
-
<p>PrimoPDF has many benefits that make it a great choice for PDF productivity. Here are some of the main benefits of PrimoPDF:</p>
|
8 |
-
<ul>
|
9 |
-
<li><strong>High-quality conversion:</strong> PrimoPDF can convert any type of file to PDF with high fidelity and accuracy. You can choose from four output quality settings: Screen, eBook, Print, and Prepress. You can also customize the PDF settings to suit your needs.</li>
|
10 |
-
<li><strong>User-friendly interface:</strong> PrimoPDF has a simple and intuitive interface that allows you to print to PDF from any Windows application. You just need to select PrimoPDF as the printer and click on OK. You can also drag and drop files to the PrimoPDF desktop icon to convert them to PDF.</li>
|
11 |
-
<li><strong>Security features:</strong> PrimoPDF can protect your PDF files with 128-bit encryption and password protection. You can also add watermarks and stamps to your PDF files to prevent unauthorized copying or editing.</li>
|
12 |
-
<li><strong>Free and easy to use:</strong> PrimoPDF is completely free and does not require any registration or subscription. It is also easy to install and use, with no ads or pop-ups.</li>
|
13 |
-
</ul>
|
14 |
-
<h2>Steps to Download PrimoPDF</h2>
|
15 |
-
<p>To download PrimoPDF, you need to follow these steps:</p>
|
16 |
-
<ol>
|
17 |
-
<li>Visit the official website of PrimoPDF. You will see a page like this:</li>
|
18 |
-
</ol>
|
19 |
-
<p><img src="https://www.primopdf.com/images/primopdf-homepage.png" alt="PrimoPDF homepage" width="600" height="400"></p>
|
20 |
-
<ol start="2">
|
21 |
-
<li>Click on the "Download Now" button. You will be redirected to a page where you can choose between two options: "Premium Upgrade" or "Free Download".</li>
|
22 |
-
</ol>
|
23 |
-
<p><img src="https://www.primopdf.com/images/primopdf-download.png" alt="PrimoPDF download options" width="600" height="400"></p>
|
24 |
-
<p>How to download primopdf for free<br />
|
25 |
-
Download primopdf for windows 10<br />
|
26 |
-
PrimoPDF - PDF converter and creator<br />
|
27 |
-
Download primopdf from nitro software<br />
|
28 |
-
PrimoPDF download and installation guide<br />
|
29 |
-
PrimoPDF review and features<br />
|
30 |
-
Download primopdf for mac<br />
|
31 |
-
PrimoPDF alternatives and comparisons<br />
|
32 |
-
How to use primopdf to create pdf files<br />
|
33 |
-
Download primopdf offline installer<br />
|
34 |
-
PrimoPDF vs Adobe Acrobat<br />
|
35 |
-
Download primopdf for android<br />
|
36 |
-
PrimoPDF - the best free pdf tool<br />
|
37 |
-
Download primopdf from sourceforge<br />
|
38 |
-
PrimoPDF troubleshooting and support<br />
|
39 |
-
How to update primopdf to the latest version<br />
|
40 |
-
Download primopdf for linux<br />
|
41 |
-
PrimoPDF pros and cons<br />
|
42 |
-
How to uninstall primopdf from your pc<br />
|
43 |
-
Download primopdf from cnet download<br />
|
44 |
-
PrimoPDF security and privacy<br />
|
45 |
-
How to convert pdf files with primopdf<br />
|
46 |
-
Download primopdf for chromebook<br />
|
47 |
-
PrimoPDF user manual and tutorials<br />
|
48 |
-
How to merge pdf files with primopdf<br />
|
49 |
-
Download primopdf for windows 7<br />
|
50 |
-
PrimoPDF license and terms of use<br />
|
51 |
-
How to edit pdf files with primopdf<br />
|
52 |
-
Download primopdf for windows 8.1<br />
|
53 |
-
PrimoPDF feedback and ratings<br />
|
54 |
-
How to sign pdf files with primopdf<br />
|
55 |
-
Download primopdf for windows xp<br />
|
56 |
-
PrimoPDF FAQs and tips<br />
|
57 |
-
How to protect pdf files with primopdf<br />
|
58 |
-
Download primopdf for windows vista<br />
|
59 |
-
PrimoPDF blog and news<br />
|
60 |
-
How to print pdf files with primopdf<br />
|
61 |
-
Download primopdf for ios<br />
|
62 |
-
PrimoPDF coupons and discounts<br />
|
63 |
-
How to compress pdf files with primopdf<br />
|
64 |
-
Download primopdf for windows server 2023 <br />
|
65 |
-
PrimoPDF testimonials and case studies <br />
|
66 |
-
How to rotate pdf files with primopdf <br />
|
67 |
-
Download primopdf portable version <br />
|
68 |
-
PrimoPDF awards and recognition <br />
|
69 |
-
How to split pdf files with primopdf <br />
|
70 |
-
Download primopdf old versions <br />
|
71 |
-
PrimoPDF social media and community <br />
|
72 |
-
How to add bookmarks to pdf files with primopdf</p>
|
73 |
-
<ol start="3">
|
74 |
-
<li>If you want to upgrade to Nitro Pro, which is a more advanced PDF software that offers more features and functions, you can click on the "Premium Upgrade" button. You will be able to enjoy a free trial of Nitro Pro for 14 days before deciding whether to purchase it or not.</li>
|
75 |
-
<li>If you want to download PrimoPDF for free, you can click on the "Free Download" button. You will see a dialog box like this:</li>
|
76 |
-
</ol>
|
77 |
-
<p><img src="https://www.primopdf.com/images/primopdf-save.png" alt="PrimoPDF save dialog box" width="600" height="400"></p>
|
78 |
-
<ol start="5">
|
79 |
-
<li>Choose a location on your computer where you want to save the PrimoPDF installer file and click on "Save". The file name is "PrimoSetup.exe" and the file size is about 7 MB.</li>
|
80 |
-
<li>Once the download is complete, locate the PrimoPDF installer file on your computer and double-click on it. You will see a window like this:</li>
|
81 |
-
</ol>
|
82 |
-
<p><img src="https://www.primopdf.com/images/primopdf-install.png" alt="PrimoPDF install window" width="600" height="400"></p>
|
83 |
-
<ol start="7">
|
84 |
-
<li>Follow the instructions to install PrimoPDF on your computer. You will need to accept the license agreement, choose the installation folder, and select the components you want to install. You can also opt out of installing any additional software or toolbars that may be offered by PrimoPDF.</li>
|
85 |
-
<li>Once the installation is complete, you will see a window like this:</li>
|
86 |
-
</ol>
|
87 |
-
<p><img src="https://www.primopdf.com/images/primopdf-finish.png" alt="PrimoPDF finish window" width="600" height="400"></p>
|
88 |
-
<ol start="9">
|
89 |
-
<li>Click on "Finish" to exit the installer. You have successfully downloaded and installed PrimoPDF on your computer.</li>
|
90 |
-
</ol></p> 197e85843d<br />
|
91 |
-
<br />
|
92 |
-
<br />
|
|
|
|
|
|
|
|
|
|
|
|
|
|
|
|
|
|
|
|
|
|
|
|
|
|
|
|
|
|
|
|
|
|
|
|
|
|
|
|
|
|
|
|
|
|
|
|
|
|
|
|
|
|
|
|
|
|
|
|
|
|
|
|
|
|
|
|
|
|
|
|
|
|
|
|
|
|
|
|
|
|
|
|
|
|
|
|
|
|
|
|
|
|
|
|
|
|
|
|
|
|
|
|
|
|
|
|
|
|
|
|
|
|
|
|
|
|
|
|
|
|
|
|
|
|
|
|
|
|
|
|
|
|
|
|
|
|
|
|
|
|
|
|
|
|
|
|
|
|
|
|
|
|
|
|
|
|
|
|
|
|
|
|
|
|
|
|
|
|
|
|
|
|
|
|
|
|
|
|
|
|
|
|
|
|
spaces/1phancelerku/anime-remove-background/Download Kaash Paige Love Songs MP3 and Listen Offline.md
DELETED
@@ -1,105 +0,0 @@
|
|
1 |
-
|
2 |
-
<h1>Download Kaash Paige Love Songs MP3: How to Enjoy the Best of R&B</h1>
|
3 |
-
<p>If you are a fan of R&B music, you have probably heard of Kaash Paige, the rising star who has captivated millions of listeners with her soulful voice and relatable lyrics. Kaash Paige is known for her love songs, which express her feelings and experiences with romance, heartbreak, and self-love. In this article, we will tell you more about who Kaash Paige is, why you should listen to her love songs, and how to download them in mp3 format for free. We will also give you some tips on how to enjoy her music on different devices and create your own playlists and mixtapes.</p>
|
4 |
-
<h2>Who is Kaash Paige and why you should listen to her love songs</h2>
|
5 |
-
<p>Kaash Paige is a 20-year-old singer and songwriter from Dallas, Texas, who started making music when she was 14. She rose to fame in 2018 with her viral hit "Love Songs", which sampled Brent Faiyaz's "Poison". Since then, she has released two EPs, <em>Parked Car Convos</em> and <em>Teenage Fever</em>, and collaborated with artists like Don Toliver, Isaiah Rashad, K Camp, and 6LACK. She is currently signed to Def Jam Recordings and is working on her debut album.</p>
|
6 |
-
<h2>download kaash paige love songs mp3</h2><br /><p><b><b>DOWNLOAD</b> → <a href="https://jinyurl.com/2uNOOz">https://jinyurl.com/2uNOOz</a></b></p><br /><br />
|
7 |
-
<h3>Kaash Paige's background and musical influences</h3>
|
8 |
-
<p>Kaash Paige was born as Kaashara Bostic on January 8, 2001, in Dallas, Texas. She grew up in a musical family, as her father was a DJ and her mother was a singer. She was exposed to various genres of music, such as hip-hop, soul, jazz, rock, and gospel. She cites Lauryn Hill, Erykah Badu, Frank Ocean, Drake, Jhené Aiko, SZA, and Brent Faiyaz as some of her main influences. She also draws inspiration from anime, movies, books, and nature.</p>
|
9 |
-
<h3>Kaash Paige's style and themes</h3>
|
10 |
-
<p>Kaash Paige's style can be described as a blend of R&B, soul, hip-hop, and alternative. She has a smooth and soothing voice that can switch from singing to rapping effortlessly. She writes her own lyrics, which are honest, vulnerable, and poetic. She often sings about love, relationships, emotions, self-discovery, and growing up. Some of her recurring themes are nostalgia, loneliness, intimacy, infatuation, and empowerment.</p>
|
11 |
-
<h3>Kaash Paige's most popular love songs</h3>
|
12 |
-
<p>Kaash Paige has released many love songs that have resonated with her fans and critics alike. Some of her most popular ones are:</p>
|
13 |
-
<ul>
|
14 |
-
<li>"Love Songs": This is the song that put Kaash Paige on the map. It is a catchy and melodic tune that samples Brent Faiyaz's "Poison". It talks about missing someone who used to make you feel special and sing love songs with you.</li>
|
15 |
-
<li>"64'": This is a laid-back and nostalgic song that features rapper K Camp. It reminisces about riding in a 1964 Chevrolet Impala with a lover and enjoying the simple moments.</li>
|
16 |
-
<li>"Break Up Song": This is a sad and emotional song that deals with. the end of a relationship and the pain of letting go. It features a sample of Drake's "Doing It Wrong".</li>
|
17 |
-
<li>"Soul Ties": This is a smooth and sensual song that explores the concept of soul ties, which are the emotional and spiritual bonds that form between people who have sex. It features rapper 6LACK, who adds his own perspective on the topic.</li>
|
18 |
-
<li>"London": This is a dreamy and romantic song that expresses the desire to travel to London with a lover and escape from reality. It has a lo-fi and atmospheric vibe that matches the mood of the lyrics.</li>
|
19 |
-
</ul>
|
20 |
-
<h2>How to download Kaash Paige love songs mp3 for free</h2>
|
21 |
-
<p>If you want to enjoy Kaash Paige's love songs offline, you might want to download them in mp3 format. Mp3 is a popular and widely supported audio file format that can be played on various devices and platforms. Mp3 files are also smaller in size than other formats, which means they take up less storage space and bandwidth. Plus, mp3 files can be easily edited, converted, and transferred.</p>
|
22 |
-
<h3>The benefits of downloading mp3 files</h3>
|
23 |
-
<p>Downloading mp3 files has many benefits, such as:</p>
|
24 |
-
<ul>
|
25 |
-
<li>You can listen to your favorite songs anytime and anywhere, without relying on an internet connection or streaming service.</li>
|
26 |
-
<li>You can save money on data charges and subscription fees, as you don't have to stream music online.</li>
|
27 |
-
<li>You can create your own music library and organize it according to your preferences.</li>
|
28 |
-
<li>You can customize your songs by adding metadata, artwork, lyrics, and tags.</li>
|
29 |
-
<li>You can share your songs with your friends and family via email, Bluetooth, or social media.</li>
|
30 |
-
</ul>
|
31 |
-
<h3>The best websites and apps to download Kaash Paige love songs mp3</h3>
|
32 |
-
<p>There are many websites and apps that allow you to download Kaash Paige love songs mp3 for free. However, not all of them are safe, legal, or reliable. Some of them might contain viruses, malware, or spyware that can harm your device or compromise your privacy. Some of them might also violate the copyright laws and infringe on the rights of the artists and labels. Therefore, you should be careful and choose only the reputable and trustworthy sources. Here are some of the best ones:</p>
|
33 |
-
<h4>YouTube</h4>
|
34 |
-
<p>YouTube is the most popular video-sharing platform in the world, where you can find millions of music videos, including Kaash Paige's love songs. You can watch them online or download them in mp3 format using a YouTube to mp3 converter. There are many online converters available, such as YTMP3, Y2Mate, 4K Video Downloader, etc. You just need to copy the URL of the video you want to download, paste it into the converter's website or app, choose the mp3 format and quality, and click on the download button. The process is fast and easy, but you should be aware of the potential risks of downloading from unverified sources.</p>
|
35 |
-
<p>download kaash paige love songs lyrics<br />
|
36 |
-
kaash paige love songs mp3 free download<br />
|
37 |
-
kaash paige love songs remix download<br />
|
38 |
-
download kaash paige love songs video<br />
|
39 |
-
kaash paige love songs album download<br />
|
40 |
-
download kaash paige love songs instrumental<br />
|
41 |
-
kaash paige love songs mp3 320kbps download<br />
|
42 |
-
kaash paige love songs acoustic download<br />
|
43 |
-
download kaash paage love songs feat 6lack<br />
|
44 |
-
kaash paige love songs ringtone download<br />
|
45 |
-
download kaash paige love songs slowed<br />
|
46 |
-
kaash paige love songs zip download<br />
|
47 |
-
kaash paige love songs spotify download<br />
|
48 |
-
download kaash paige love songs clean version<br />
|
49 |
-
kaash paige love songs karaoke download<br />
|
50 |
-
download kaash paige love songs genius lyrics<br />
|
51 |
-
kaash paige love songs soundcloud download<br />
|
52 |
-
kaash paige love songs live performance download<br />
|
53 |
-
download kaash paige love songs piano cover<br />
|
54 |
-
kaash paige love songs tiktok version download<br />
|
55 |
-
download kaash paige love songs reaction video<br />
|
56 |
-
kaash paige love songs behind the scenes download<br />
|
57 |
-
kaash paige love songs official music video download<br />
|
58 |
-
download kaash paige love songs guitar chords<br />
|
59 |
-
kaash paige love songs dance challenge download<br />
|
60 |
-
download kaash paige love songs nightcore<br />
|
61 |
-
kaash paige love songs mashup download<br />
|
62 |
-
kaash paige love songs unplugged download<br />
|
63 |
-
download kaash paige love songs meaning explained<br />
|
64 |
-
kaash paige love songs extended version download<br />
|
65 |
-
download kaash paige love songs sample used<br />
|
66 |
-
kaash paige love songs flac download<br />
|
67 |
-
kaash paige love songs radio edit download<br />
|
68 |
-
download kaash paige love songs interview clip<br />
|
69 |
-
kaash paage love songs fan made video download<br />
|
70 |
-
download kaash pagee love songss lyrics translation<br />
|
71 |
-
kassh pagee luv songz mp3 downlod</p>
|
72 |
-
<h4>Spotify</h4>
|
73 |
-
<p>Spotify is one of the most popular music streaming services in the world, where you can listen to millions of songs, including Kaash Paige's love songs. You can access Spotify for free with ads or pay for a premium subscription that offers more features and benefits. One of them is the ability to download songs for offline listening. However, this feature is only available for premium users and only works within the Spotify app. You cannot transfer or play the downloaded songs on other devices or apps. If you want to download Spotify songs in mp3 format, you will need a Spotify to mp3 converter. There are some online converters available, such as SpotiFlyer, AudKit Spotify Music Converter, TuneFab Spotify Music Converter, etc. You just need to copy the URL of the song or playlist you want to download, paste it into the converter's website or app, choose the mp3 format and quality, and click on the download button. The process is fast and easy, but you should be aware of the potential risks of downloading from unverified sources.</p> <h4>SoundCloud</h4>
|
74 |
-
<p>SoundCloud is another popular music streaming service in the world, where you can discover and listen to millions of songs, including Kaash Paige's love songs. You can access SoundCloud for free with ads or pay for a premium subscription that offers more features and benefits. One of them is the ability to download songs for offline listening. However, this feature is only available for some songs and only works within the SoundCloud app. You cannot transfer or play the downloaded songs on other devices or apps. If you want to download SoundCloud songs in mp3 format, you will need a SoundCloud to mp3 converter. There are some online converters available, such as SCDL, SoundCloud Downloader, KlickAud, etc. You just need to copy the URL of the song you want to download, paste it into the converter's website or app, choose the mp3 format and quality, and click on the download button. The process is fast and easy, but you should be aware of the potential risks of downloading from unverified sources.</p>
|
75 |
-
<h4>Audiomack</h4>
|
76 |
-
<p>Audiomack is a music streaming and discovery platform that allows artists to upload their music and fans to listen to it for free. You can find many songs by Kaash Paige on Audiomack, as well as other genres and artists. You can access Audiomack for free with ads or pay for a premium subscription that offers more features and benefits. One of them is the ability to download songs for offline listening. However, this feature is only available for some songs and only works within the Audiomack app. You cannot transfer or play the downloaded songs on other devices or apps. If you want to download Audiomack songs in mp3 format, you will need an Audiomack to mp3 converter. There are some online converters available, such as Audiomack Downloader, MP3FY, MP3Juices, etc. You just need to copy the URL of the song you want to download, paste it into the converter's website or app, choose the mp3 format and quality, and click on the download button. The process is fast and easy, but you should be aware of the potential risks of downloading from unverified sources.</p>
|
77 |
-
<h2>How to enjoy Kaash Paige love songs mp3 on different devices</h2>
|
78 |
-
<p>Once you have downloaded Kaash Paige love songs mp3 from any of the sources mentioned above, you can enjoy them on different devices, such as your phone, tablet, computer, or laptop. However, you might need to transfer or play them differently depending on the device and the app you use. Here are some tips on how to do that:</p>
|
79 |
-
<h3>How to transfer mp3 files to your phone or tablet</h3>
|
80 |
-
<p>If you have downloaded mp3 files on your computer or laptop, you can transfer them to your phone or tablet using a USB cable or a wireless method. Here are the steps for each method:</p>
|
81 |
-
<ul>
|
82 |
-
<li>USB cable: Connect your phone or tablet to your computer or laptop using a USB cable. Make sure your device is unlocked and select the file transfer option on your device's screen. On your computer or laptop, open the folder where you saved the mp3 files and drag and drop them to your device's folder. Once the transfer is complete, disconnect your device and open the music app of your choice.</li>
|
83 |
-
<li>Wireless method: There are many apps that allow you to transfer files wirelessly between devices using Wi-Fi or Bluetooth. Some of them are SHAREit, Xender, Zapya, etc. You just need to install the app on both devices and follow the instructions on how to connect them and send files.</li>
|
84 |
-
</ul>
|
85 |
-
<h3>How to play mp3 files on your computer or laptop</h3>
|
86 |
-
<p>If you have downloaded mp3 files on your computer or laptop, you can play them using any media player that supports mp3 format. Some of them are Windows Media Player, VLC Media Player, iTunes, etc. You just need to open the media player of your choice and browse for the folder where you saved the mp3 files. You can also create playlists and edit metadata within the media player.</p>
|
87 |
-
<h3>How to create playlists and mixtapes with Kaash Paige love songs mp3</h3>
|
88 |
-
<p>If you want to create playlists and mixtapes with Kaash Paige love songs mp3, you can use any music app that allows you to do that. Some of them are Spotify, SoundCloud, Audiomack, etc. You just need to open the app of your choice and select the option to create a new playlist or mixtape. Then, you can add Kaash Paige love songs mp3 from your device's storage or from the app's library. You can also rearrange, rename, delete, or share your playlists and mixtapes within the app.</p>
|
89 |
-
<h <h2>Conclusion and FAQs</h2>
|
90 |
-
<p>In conclusion, Kaash Paige is a talented and promising R&B singer who has a lot of love songs that you can enjoy. You can download her love songs in mp3 format for free from various websites and apps, such as YouTube, Spotify, SoundCloud, and Audiomack. You can also transfer and play them on different devices, such as your phone, tablet, computer, or laptop. You can also create your own playlists and mixtapes with her love songs and share them with your friends and family. Kaash Paige's love songs are perfect for any mood and occasion, whether you are feeling happy, sad, romantic, or nostalgic. If you are looking for some quality R&B music, you should definitely check out Kaash Paige's love songs mp3.</p>
|
91 |
-
<p>Here are some FAQs that you might have about Kaash Paige and her love songs:</p>
|
92 |
-
<ul>
|
93 |
-
<li><strong>Q: What does Kaash Paige mean?</strong></li>
|
94 |
-
<li>A: Kaash Paige is a stage name that stands for Kill All Arrogance Stop Hatred. Paige is her middle name.</li>
|
95 |
-
<li><strong>Q: What is Kaash Paige's real name?</strong></li>
|
96 |
-
<li>A: Kaash Paige's real name is Kaashara Bostic.</li>
|
97 |
-
<li><strong>Q: How old is Kaash Paige?</strong></li>
|
98 |
-
<li>A: Kaash Paige is 20 years old. She was born on January 8, 2001.</li>
|
99 |
-
<li><strong>Q: Where is Kaash Paige from?</strong></li>
|
100 |
-
<li>A: Kaash Paige is from Dallas, Texas.</li>
|
101 |
-
<li><strong>Q: Is Kaash Paige single?</strong></li>
|
102 |
-
<li>A: Kaash Paige has not publicly confirmed her relationship status. She has been linked to rapper Don Toliver in the past, but they have not confirmed their romance.</li>
|
103 |
-
</ul></p> 401be4b1e0<br />
|
104 |
-
<br />
|
105 |
-
<br />
|
|
|
|
|
|
|
|
|
|
|
|
|
|
|
|
|
|
|
|
|
|
|
|
|
|
|
|
|
|
|
|
|
|
|
|
|
|
|
|
|
|
|
|
|
|
|
|
|
|
|
|
|
|
|
|
|
|
|
|
|
|
|
|
|
|
|
|
|
|
|
|
|
|
|
|
|
|
|
|
|
|
|
|
|
|
|
|
|
|
|
|
|
|
|
|
|
|
|
|
|
|
|
|
|
|
|
|
|
|
|
|
|
|
|
|
|
|
|
|
|
|
|
|
|
|
|
|
|
|
|
|
|
|
|
|
|
|
|
|
|
|
|
|
|
|
|
|
|
|
|
|
|
|
|
|
|
|
|
|
|
|
|
|
|
|
|
|
|
|
|
|
|
|
|
|
|
|
|
|
|
|
|
|
|
|
|
|
|
|
|
|
|
|
|
|
|
|
|
|
|
|
|
|
|
|
|
|
|
|
|
|
spaces/4Taps/SadTalker/src/face3d/util/visualizer.py
DELETED
@@ -1,227 +0,0 @@
|
|
1 |
-
"""This script defines the visualizer for Deep3DFaceRecon_pytorch
|
2 |
-
"""
|
3 |
-
|
4 |
-
import numpy as np
|
5 |
-
import os
|
6 |
-
import sys
|
7 |
-
import ntpath
|
8 |
-
import time
|
9 |
-
from . import util, html
|
10 |
-
from subprocess import Popen, PIPE
|
11 |
-
from torch.utils.tensorboard import SummaryWriter
|
12 |
-
|
13 |
-
def save_images(webpage, visuals, image_path, aspect_ratio=1.0, width=256):
|
14 |
-
"""Save images to the disk.
|
15 |
-
|
16 |
-
Parameters:
|
17 |
-
webpage (the HTML class) -- the HTML webpage class that stores these imaegs (see html.py for more details)
|
18 |
-
visuals (OrderedDict) -- an ordered dictionary that stores (name, images (either tensor or numpy) ) pairs
|
19 |
-
image_path (str) -- the string is used to create image paths
|
20 |
-
aspect_ratio (float) -- the aspect ratio of saved images
|
21 |
-
width (int) -- the images will be resized to width x width
|
22 |
-
|
23 |
-
This function will save images stored in 'visuals' to the HTML file specified by 'webpage'.
|
24 |
-
"""
|
25 |
-
image_dir = webpage.get_image_dir()
|
26 |
-
short_path = ntpath.basename(image_path[0])
|
27 |
-
name = os.path.splitext(short_path)[0]
|
28 |
-
|
29 |
-
webpage.add_header(name)
|
30 |
-
ims, txts, links = [], [], []
|
31 |
-
|
32 |
-
for label, im_data in visuals.items():
|
33 |
-
im = util.tensor2im(im_data)
|
34 |
-
image_name = '%s/%s.png' % (label, name)
|
35 |
-
os.makedirs(os.path.join(image_dir, label), exist_ok=True)
|
36 |
-
save_path = os.path.join(image_dir, image_name)
|
37 |
-
util.save_image(im, save_path, aspect_ratio=aspect_ratio)
|
38 |
-
ims.append(image_name)
|
39 |
-
txts.append(label)
|
40 |
-
links.append(image_name)
|
41 |
-
webpage.add_images(ims, txts, links, width=width)
|
42 |
-
|
43 |
-
|
44 |
-
class Visualizer():
|
45 |
-
"""This class includes several functions that can display/save images and print/save logging information.
|
46 |
-
|
47 |
-
It uses a Python library tensprboardX for display, and a Python library 'dominate' (wrapped in 'HTML') for creating HTML files with images.
|
48 |
-
"""
|
49 |
-
|
50 |
-
def __init__(self, opt):
|
51 |
-
"""Initialize the Visualizer class
|
52 |
-
|
53 |
-
Parameters:
|
54 |
-
opt -- stores all the experiment flags; needs to be a subclass of BaseOptions
|
55 |
-
Step 1: Cache the training/test options
|
56 |
-
Step 2: create a tensorboard writer
|
57 |
-
Step 3: create an HTML object for saveing HTML filters
|
58 |
-
Step 4: create a logging file to store training losses
|
59 |
-
"""
|
60 |
-
self.opt = opt # cache the option
|
61 |
-
self.use_html = opt.isTrain and not opt.no_html
|
62 |
-
self.writer = SummaryWriter(os.path.join(opt.checkpoints_dir, 'logs', opt.name))
|
63 |
-
self.win_size = opt.display_winsize
|
64 |
-
self.name = opt.name
|
65 |
-
self.saved = False
|
66 |
-
if self.use_html: # create an HTML object at <checkpoints_dir>/web/; images will be saved under <checkpoints_dir>/web/images/
|
67 |
-
self.web_dir = os.path.join(opt.checkpoints_dir, opt.name, 'web')
|
68 |
-
self.img_dir = os.path.join(self.web_dir, 'images')
|
69 |
-
print('create web directory %s...' % self.web_dir)
|
70 |
-
util.mkdirs([self.web_dir, self.img_dir])
|
71 |
-
# create a logging file to store training losses
|
72 |
-
self.log_name = os.path.join(opt.checkpoints_dir, opt.name, 'loss_log.txt')
|
73 |
-
with open(self.log_name, "a") as log_file:
|
74 |
-
now = time.strftime("%c")
|
75 |
-
log_file.write('================ Training Loss (%s) ================\n' % now)
|
76 |
-
|
77 |
-
def reset(self):
|
78 |
-
"""Reset the self.saved status"""
|
79 |
-
self.saved = False
|
80 |
-
|
81 |
-
|
82 |
-
def display_current_results(self, visuals, total_iters, epoch, save_result):
|
83 |
-
"""Display current results on tensorboad; save current results to an HTML file.
|
84 |
-
|
85 |
-
Parameters:
|
86 |
-
visuals (OrderedDict) - - dictionary of images to display or save
|
87 |
-
total_iters (int) -- total iterations
|
88 |
-
epoch (int) - - the current epoch
|
89 |
-
save_result (bool) - - if save the current results to an HTML file
|
90 |
-
"""
|
91 |
-
for label, image in visuals.items():
|
92 |
-
self.writer.add_image(label, util.tensor2im(image), total_iters, dataformats='HWC')
|
93 |
-
|
94 |
-
if self.use_html and (save_result or not self.saved): # save images to an HTML file if they haven't been saved.
|
95 |
-
self.saved = True
|
96 |
-
# save images to the disk
|
97 |
-
for label, image in visuals.items():
|
98 |
-
image_numpy = util.tensor2im(image)
|
99 |
-
img_path = os.path.join(self.img_dir, 'epoch%.3d_%s.png' % (epoch, label))
|
100 |
-
util.save_image(image_numpy, img_path)
|
101 |
-
|
102 |
-
# update website
|
103 |
-
webpage = html.HTML(self.web_dir, 'Experiment name = %s' % self.name, refresh=0)
|
104 |
-
for n in range(epoch, 0, -1):
|
105 |
-
webpage.add_header('epoch [%d]' % n)
|
106 |
-
ims, txts, links = [], [], []
|
107 |
-
|
108 |
-
for label, image_numpy in visuals.items():
|
109 |
-
image_numpy = util.tensor2im(image)
|
110 |
-
img_path = 'epoch%.3d_%s.png' % (n, label)
|
111 |
-
ims.append(img_path)
|
112 |
-
txts.append(label)
|
113 |
-
links.append(img_path)
|
114 |
-
webpage.add_images(ims, txts, links, width=self.win_size)
|
115 |
-
webpage.save()
|
116 |
-
|
117 |
-
def plot_current_losses(self, total_iters, losses):
|
118 |
-
# G_loss_collection = {}
|
119 |
-
# D_loss_collection = {}
|
120 |
-
# for name, value in losses.items():
|
121 |
-
# if 'G' in name or 'NCE' in name or 'idt' in name:
|
122 |
-
# G_loss_collection[name] = value
|
123 |
-
# else:
|
124 |
-
# D_loss_collection[name] = value
|
125 |
-
# self.writer.add_scalars('G_collec', G_loss_collection, total_iters)
|
126 |
-
# self.writer.add_scalars('D_collec', D_loss_collection, total_iters)
|
127 |
-
for name, value in losses.items():
|
128 |
-
self.writer.add_scalar(name, value, total_iters)
|
129 |
-
|
130 |
-
# losses: same format as |losses| of plot_current_losses
|
131 |
-
def print_current_losses(self, epoch, iters, losses, t_comp, t_data):
|
132 |
-
"""print current losses on console; also save the losses to the disk
|
133 |
-
|
134 |
-
Parameters:
|
135 |
-
epoch (int) -- current epoch
|
136 |
-
iters (int) -- current training iteration during this epoch (reset to 0 at the end of every epoch)
|
137 |
-
losses (OrderedDict) -- training losses stored in the format of (name, float) pairs
|
138 |
-
t_comp (float) -- computational time per data point (normalized by batch_size)
|
139 |
-
t_data (float) -- data loading time per data point (normalized by batch_size)
|
140 |
-
"""
|
141 |
-
message = '(epoch: %d, iters: %d, time: %.3f, data: %.3f) ' % (epoch, iters, t_comp, t_data)
|
142 |
-
for k, v in losses.items():
|
143 |
-
message += '%s: %.3f ' % (k, v)
|
144 |
-
|
145 |
-
print(message) # print the message
|
146 |
-
with open(self.log_name, "a") as log_file:
|
147 |
-
log_file.write('%s\n' % message) # save the message
|
148 |
-
|
149 |
-
|
150 |
-
class MyVisualizer:
|
151 |
-
def __init__(self, opt):
|
152 |
-
"""Initialize the Visualizer class
|
153 |
-
|
154 |
-
Parameters:
|
155 |
-
opt -- stores all the experiment flags; needs to be a subclass of BaseOptions
|
156 |
-
Step 1: Cache the training/test options
|
157 |
-
Step 2: create a tensorboard writer
|
158 |
-
Step 3: create an HTML object for saveing HTML filters
|
159 |
-
Step 4: create a logging file to store training losses
|
160 |
-
"""
|
161 |
-
self.opt = opt # cache the optio
|
162 |
-
self.name = opt.name
|
163 |
-
self.img_dir = os.path.join(opt.checkpoints_dir, opt.name, 'results')
|
164 |
-
|
165 |
-
if opt.phase != 'test':
|
166 |
-
self.writer = SummaryWriter(os.path.join(opt.checkpoints_dir, opt.name, 'logs'))
|
167 |
-
# create a logging file to store training losses
|
168 |
-
self.log_name = os.path.join(opt.checkpoints_dir, opt.name, 'loss_log.txt')
|
169 |
-
with open(self.log_name, "a") as log_file:
|
170 |
-
now = time.strftime("%c")
|
171 |
-
log_file.write('================ Training Loss (%s) ================\n' % now)
|
172 |
-
|
173 |
-
|
174 |
-
def display_current_results(self, visuals, total_iters, epoch, dataset='train', save_results=False, count=0, name=None,
|
175 |
-
add_image=True):
|
176 |
-
"""Display current results on tensorboad; save current results to an HTML file.
|
177 |
-
|
178 |
-
Parameters:
|
179 |
-
visuals (OrderedDict) - - dictionary of images to display or save
|
180 |
-
total_iters (int) -- total iterations
|
181 |
-
epoch (int) - - the current epoch
|
182 |
-
dataset (str) - - 'train' or 'val' or 'test'
|
183 |
-
"""
|
184 |
-
# if (not add_image) and (not save_results): return
|
185 |
-
|
186 |
-
for label, image in visuals.items():
|
187 |
-
for i in range(image.shape[0]):
|
188 |
-
image_numpy = util.tensor2im(image[i])
|
189 |
-
if add_image:
|
190 |
-
self.writer.add_image(label + '%s_%02d'%(dataset, i + count),
|
191 |
-
image_numpy, total_iters, dataformats='HWC')
|
192 |
-
|
193 |
-
if save_results:
|
194 |
-
save_path = os.path.join(self.img_dir, dataset, 'epoch_%s_%06d'%(epoch, total_iters))
|
195 |
-
if not os.path.isdir(save_path):
|
196 |
-
os.makedirs(save_path)
|
197 |
-
|
198 |
-
if name is not None:
|
199 |
-
img_path = os.path.join(save_path, '%s.png' % name)
|
200 |
-
else:
|
201 |
-
img_path = os.path.join(save_path, '%s_%03d.png' % (label, i + count))
|
202 |
-
util.save_image(image_numpy, img_path)
|
203 |
-
|
204 |
-
|
205 |
-
def plot_current_losses(self, total_iters, losses, dataset='train'):
|
206 |
-
for name, value in losses.items():
|
207 |
-
self.writer.add_scalar(name + '/%s'%dataset, value, total_iters)
|
208 |
-
|
209 |
-
# losses: same format as |losses| of plot_current_losses
|
210 |
-
def print_current_losses(self, epoch, iters, losses, t_comp, t_data, dataset='train'):
|
211 |
-
"""print current losses on console; also save the losses to the disk
|
212 |
-
|
213 |
-
Parameters:
|
214 |
-
epoch (int) -- current epoch
|
215 |
-
iters (int) -- current training iteration during this epoch (reset to 0 at the end of every epoch)
|
216 |
-
losses (OrderedDict) -- training losses stored in the format of (name, float) pairs
|
217 |
-
t_comp (float) -- computational time per data point (normalized by batch_size)
|
218 |
-
t_data (float) -- data loading time per data point (normalized by batch_size)
|
219 |
-
"""
|
220 |
-
message = '(dataset: %s, epoch: %d, iters: %d, time: %.3f, data: %.3f) ' % (
|
221 |
-
dataset, epoch, iters, t_comp, t_data)
|
222 |
-
for k, v in losses.items():
|
223 |
-
message += '%s: %.3f ' % (k, v)
|
224 |
-
|
225 |
-
print(message) # print the message
|
226 |
-
with open(self.log_name, "a") as log_file:
|
227 |
-
log_file.write('%s\n' % message) # save the message
|
|
|
|
|
|
|
|
|
|
|
|
|
|
|
|
|
|
|
|
|
|
|
|
|
|
|
|
|
|
|
|
|
|
|
|
|
|
|
|
|
|
|
|
|
|
|
|
|
|
|
|
|
|
|
|
|
|
|
|
|
|
|
|
|
|
|
|
|
|
|
|
|
|
|
|
|
|
|
|
|
|
|
|
|
|
|
|
|
|
|
|
|
|
|
|
|
|
|
|
|
|
|
|
|
|
|
|
|
|
|
|
|
|
|
|
|
|
|
|
|
|
|
|
|
|
|
|
|
|
|
|
|
|
|
|
|
|
|
|
|
|
|
|
|
|
|
|
|
|
|
|
|
|
|
|
|
|
|
|
|
|
|
|
|
|
|
|
|
|
|
|
|
|
|
|
|
|
|
|
|
|
|
|
|
|
|
|
|
|
|
|
|
|
|
|
|
|
|
|
|
|
|
|
|
|
|
|
|
|
|
|
|
|
|
|
|
|
|
|
|
|
|
|
|
|
|
|
|
|
|
|
|
|
|
|
|
|
|
|
|
|
|
|
|
|
|
|
|
|
|
|
|
|
|
|
|
|
|
|
|
|
|
|
|
|
|
|
|
|
|
|
|
|
|
|
|
|
|
|
|
|
|
|
|
|
|
|
|
|
|
|
|
|
|
|
|
|
|
|
|
|
|
|
|
|
|
|
|
|
|
|
|
|
|
|
|
|
|
|
|
|
|
|
|
|
|
|
|
|
|
|
|
|
|
|
|
|
|
|
|
|
|
|
|
|
|
|
|
|
|
|
|
|
|
|
|
|
|
|
|
|
|
|
|
|
|
|
|
|
|
|
|
|
|
|
|
|
|
|
|
|
|
|
|
|
|
|
|
|
|
|
|
|
|
|
|
|
|
|
|
|
|
|
|
|
|
|
|
|
|
|
|
|
|
|
|
|
|
|
|
|
|
|
|
|
|
|
|
|
|
|
|
|
|
|
|
|
|
|
|
|
|
|
|
|
|
|
|
|
|
|
|
|
|
|
spaces/AIFILMS/generate_human_motion/VQ-Trans/models/t2m_trans.py
DELETED
@@ -1,211 +0,0 @@
|
|
1 |
-
import math
|
2 |
-
import torch
|
3 |
-
import torch.nn as nn
|
4 |
-
from torch.nn import functional as F
|
5 |
-
from torch.distributions import Categorical
|
6 |
-
import models.pos_encoding as pos_encoding
|
7 |
-
|
8 |
-
class Text2Motion_Transformer(nn.Module):
|
9 |
-
|
10 |
-
def __init__(self,
|
11 |
-
num_vq=1024,
|
12 |
-
embed_dim=512,
|
13 |
-
clip_dim=512,
|
14 |
-
block_size=16,
|
15 |
-
num_layers=2,
|
16 |
-
n_head=8,
|
17 |
-
drop_out_rate=0.1,
|
18 |
-
fc_rate=4):
|
19 |
-
super().__init__()
|
20 |
-
self.trans_base = CrossCondTransBase(num_vq, embed_dim, clip_dim, block_size, num_layers, n_head, drop_out_rate, fc_rate)
|
21 |
-
self.trans_head = CrossCondTransHead(num_vq, embed_dim, block_size, num_layers, n_head, drop_out_rate, fc_rate)
|
22 |
-
self.block_size = block_size
|
23 |
-
self.num_vq = num_vq
|
24 |
-
|
25 |
-
def get_block_size(self):
|
26 |
-
return self.block_size
|
27 |
-
|
28 |
-
def forward(self, idxs, clip_feature):
|
29 |
-
feat = self.trans_base(idxs, clip_feature)
|
30 |
-
logits = self.trans_head(feat)
|
31 |
-
return logits
|
32 |
-
|
33 |
-
def sample(self, clip_feature, if_categorial=False):
|
34 |
-
for k in range(self.block_size):
|
35 |
-
if k == 0:
|
36 |
-
x = []
|
37 |
-
else:
|
38 |
-
x = xs
|
39 |
-
logits = self.forward(x, clip_feature)
|
40 |
-
logits = logits[:, -1, :]
|
41 |
-
probs = F.softmax(logits, dim=-1)
|
42 |
-
if if_categorial:
|
43 |
-
dist = Categorical(probs)
|
44 |
-
idx = dist.sample()
|
45 |
-
if idx == self.num_vq:
|
46 |
-
break
|
47 |
-
idx = idx.unsqueeze(-1)
|
48 |
-
else:
|
49 |
-
_, idx = torch.topk(probs, k=1, dim=-1)
|
50 |
-
if idx[0] == self.num_vq:
|
51 |
-
break
|
52 |
-
# append to the sequence and continue
|
53 |
-
if k == 0:
|
54 |
-
xs = idx
|
55 |
-
else:
|
56 |
-
xs = torch.cat((xs, idx), dim=1)
|
57 |
-
|
58 |
-
if k == self.block_size - 1:
|
59 |
-
return xs[:, :-1]
|
60 |
-
return xs
|
61 |
-
|
62 |
-
class CausalCrossConditionalSelfAttention(nn.Module):
|
63 |
-
|
64 |
-
def __init__(self, embed_dim=512, block_size=16, n_head=8, drop_out_rate=0.1):
|
65 |
-
super().__init__()
|
66 |
-
assert embed_dim % 8 == 0
|
67 |
-
# key, query, value projections for all heads
|
68 |
-
self.key = nn.Linear(embed_dim, embed_dim)
|
69 |
-
self.query = nn.Linear(embed_dim, embed_dim)
|
70 |
-
self.value = nn.Linear(embed_dim, embed_dim)
|
71 |
-
|
72 |
-
self.attn_drop = nn.Dropout(drop_out_rate)
|
73 |
-
self.resid_drop = nn.Dropout(drop_out_rate)
|
74 |
-
|
75 |
-
self.proj = nn.Linear(embed_dim, embed_dim)
|
76 |
-
# causal mask to ensure that attention is only applied to the left in the input sequence
|
77 |
-
self.register_buffer("mask", torch.tril(torch.ones(block_size, block_size)).view(1, 1, block_size, block_size))
|
78 |
-
self.n_head = n_head
|
79 |
-
|
80 |
-
def forward(self, x):
|
81 |
-
B, T, C = x.size()
|
82 |
-
|
83 |
-
# calculate query, key, values for all heads in batch and move head forward to be the batch dim
|
84 |
-
k = self.key(x).view(B, T, self.n_head, C // self.n_head).transpose(1, 2) # (B, nh, T, hs)
|
85 |
-
q = self.query(x).view(B, T, self.n_head, C // self.n_head).transpose(1, 2) # (B, nh, T, hs)
|
86 |
-
v = self.value(x).view(B, T, self.n_head, C // self.n_head).transpose(1, 2) # (B, nh, T, hs)
|
87 |
-
# causal self-attention; Self-attend: (B, nh, T, hs) x (B, nh, hs, T) -> (B, nh, T, T)
|
88 |
-
att = (q @ k.transpose(-2, -1)) * (1.0 / math.sqrt(k.size(-1)))
|
89 |
-
att = att.masked_fill(self.mask[:,:,:T,:T] == 0, float('-inf'))
|
90 |
-
att = F.softmax(att, dim=-1)
|
91 |
-
att = self.attn_drop(att)
|
92 |
-
y = att @ v # (B, nh, T, T) x (B, nh, T, hs) -> (B, nh, T, hs)
|
93 |
-
y = y.transpose(1, 2).contiguous().view(B, T, C) # re-assemble all head outputs side by side
|
94 |
-
|
95 |
-
# output projection
|
96 |
-
y = self.resid_drop(self.proj(y))
|
97 |
-
return y
|
98 |
-
|
99 |
-
class Block(nn.Module):
|
100 |
-
|
101 |
-
def __init__(self, embed_dim=512, block_size=16, n_head=8, drop_out_rate=0.1, fc_rate=4):
|
102 |
-
super().__init__()
|
103 |
-
self.ln1 = nn.LayerNorm(embed_dim)
|
104 |
-
self.ln2 = nn.LayerNorm(embed_dim)
|
105 |
-
self.attn = CausalCrossConditionalSelfAttention(embed_dim, block_size, n_head, drop_out_rate)
|
106 |
-
self.mlp = nn.Sequential(
|
107 |
-
nn.Linear(embed_dim, fc_rate * embed_dim),
|
108 |
-
nn.GELU(),
|
109 |
-
nn.Linear(fc_rate * embed_dim, embed_dim),
|
110 |
-
nn.Dropout(drop_out_rate),
|
111 |
-
)
|
112 |
-
|
113 |
-
def forward(self, x):
|
114 |
-
x = x + self.attn(self.ln1(x))
|
115 |
-
x = x + self.mlp(self.ln2(x))
|
116 |
-
return x
|
117 |
-
|
118 |
-
class CrossCondTransBase(nn.Module):
|
119 |
-
|
120 |
-
def __init__(self,
|
121 |
-
num_vq=1024,
|
122 |
-
embed_dim=512,
|
123 |
-
clip_dim=512,
|
124 |
-
block_size=16,
|
125 |
-
num_layers=2,
|
126 |
-
n_head=8,
|
127 |
-
drop_out_rate=0.1,
|
128 |
-
fc_rate=4):
|
129 |
-
super().__init__()
|
130 |
-
self.tok_emb = nn.Embedding(num_vq + 2, embed_dim)
|
131 |
-
self.cond_emb = nn.Linear(clip_dim, embed_dim)
|
132 |
-
self.pos_embedding = nn.Embedding(block_size, embed_dim)
|
133 |
-
self.drop = nn.Dropout(drop_out_rate)
|
134 |
-
# transformer block
|
135 |
-
self.blocks = nn.Sequential(*[Block(embed_dim, block_size, n_head, drop_out_rate, fc_rate) for _ in range(num_layers)])
|
136 |
-
self.pos_embed = pos_encoding.PositionEmbedding(block_size, embed_dim, 0.0, False)
|
137 |
-
|
138 |
-
self.block_size = block_size
|
139 |
-
|
140 |
-
self.apply(self._init_weights)
|
141 |
-
|
142 |
-
def get_block_size(self):
|
143 |
-
return self.block_size
|
144 |
-
|
145 |
-
def _init_weights(self, module):
|
146 |
-
if isinstance(module, (nn.Linear, nn.Embedding)):
|
147 |
-
module.weight.data.normal_(mean=0.0, std=0.02)
|
148 |
-
if isinstance(module, nn.Linear) and module.bias is not None:
|
149 |
-
module.bias.data.zero_()
|
150 |
-
elif isinstance(module, nn.LayerNorm):
|
151 |
-
module.bias.data.zero_()
|
152 |
-
module.weight.data.fill_(1.0)
|
153 |
-
|
154 |
-
def forward(self, idx, clip_feature):
|
155 |
-
if len(idx) == 0:
|
156 |
-
token_embeddings = self.cond_emb(clip_feature).unsqueeze(1)
|
157 |
-
else:
|
158 |
-
b, t = idx.size()
|
159 |
-
assert t <= self.block_size, "Cannot forward, model block size is exhausted."
|
160 |
-
# forward the Trans model
|
161 |
-
token_embeddings = self.tok_emb(idx)
|
162 |
-
token_embeddings = torch.cat([self.cond_emb(clip_feature).unsqueeze(1), token_embeddings], dim=1)
|
163 |
-
|
164 |
-
x = self.pos_embed(token_embeddings)
|
165 |
-
x = self.blocks(x)
|
166 |
-
|
167 |
-
return x
|
168 |
-
|
169 |
-
|
170 |
-
class CrossCondTransHead(nn.Module):
|
171 |
-
|
172 |
-
def __init__(self,
|
173 |
-
num_vq=1024,
|
174 |
-
embed_dim=512,
|
175 |
-
block_size=16,
|
176 |
-
num_layers=2,
|
177 |
-
n_head=8,
|
178 |
-
drop_out_rate=0.1,
|
179 |
-
fc_rate=4):
|
180 |
-
super().__init__()
|
181 |
-
|
182 |
-
self.blocks = nn.Sequential(*[Block(embed_dim, block_size, n_head, drop_out_rate, fc_rate) for _ in range(num_layers)])
|
183 |
-
self.ln_f = nn.LayerNorm(embed_dim)
|
184 |
-
self.head = nn.Linear(embed_dim, num_vq + 1, bias=False)
|
185 |
-
self.block_size = block_size
|
186 |
-
|
187 |
-
self.apply(self._init_weights)
|
188 |
-
|
189 |
-
def get_block_size(self):
|
190 |
-
return self.block_size
|
191 |
-
|
192 |
-
def _init_weights(self, module):
|
193 |
-
if isinstance(module, (nn.Linear, nn.Embedding)):
|
194 |
-
module.weight.data.normal_(mean=0.0, std=0.02)
|
195 |
-
if isinstance(module, nn.Linear) and module.bias is not None:
|
196 |
-
module.bias.data.zero_()
|
197 |
-
elif isinstance(module, nn.LayerNorm):
|
198 |
-
module.bias.data.zero_()
|
199 |
-
module.weight.data.fill_(1.0)
|
200 |
-
|
201 |
-
def forward(self, x):
|
202 |
-
x = self.blocks(x)
|
203 |
-
x = self.ln_f(x)
|
204 |
-
logits = self.head(x)
|
205 |
-
return logits
|
206 |
-
|
207 |
-
|
208 |
-
|
209 |
-
|
210 |
-
|
211 |
-
|
|
|
|
|
|
|
|
|
|
|
|
|
|
|
|
|
|
|
|
|
|
|
|
|
|
|
|
|
|
|
|
|
|
|
|
|
|
|
|
|
|
|
|
|
|
|
|
|
|
|
|
|
|
|
|
|
|
|
|
|
|
|
|
|
|
|
|
|
|
|
|
|
|
|
|
|
|
|
|
|
|
|
|
|
|
|
|
|
|
|
|
|
|
|
|
|
|
|
|
|
|
|
|
|
|
|
|
|
|
|
|
|
|
|
|
|
|
|
|
|
|
|
|
|
|
|
|
|
|
|
|
|
|
|
|
|
|
|
|
|
|
|
|
|
|
|
|
|
|
|
|
|
|
|
|
|
|
|
|
|
|
|
|
|
|
|
|
|
|
|
|
|
|
|
|
|
|
|
|
|
|
|
|
|
|
|
|
|
|
|
|
|
|
|
|
|
|
|
|
|
|
|
|
|
|
|
|
|
|
|
|
|
|
|
|
|
|
|
|
|
|
|
|
|
|
|
|
|
|
|
|
|
|
|
|
|
|
|
|
|
|
|
|
|
|
|
|
|
|
|
|
|
|
|
|
|
|
|
|
|
|
|
|
|
|
|
|
|
|
|
|
|
|
|
|
|
|
|
|
|
|
|
|
|
|
|
|
|
|
|
|
|
|
|
|
|
|
|
|
|
|
|
|
|
|
|
|
|
|
|
|
|
|
|
|
|
|
|
|
|
|
|
|
|
|
|
|
|
|
|
|
|
|
|
|
|
|
|
|
|
|
|
|
|
|
|
|
|
|
|
|
|
|
|
|
|
|
|
|
|
|
|
|
|
|
|
|
|
|
|
|
|
|
|
|
|
|
|
|
|
|
|
|
|
|
|
|
|
|
|
|
|
|
|
|
|
|
|
|
|
|
|
|
|
|
|
|
|
|
|
|
|
|
|
|
|
|
|
|
|
|
|
|
spaces/AIFILMS/generate_human_motion/pyrender/docs/Makefile
DELETED
@@ -1,23 +0,0 @@
|
|
1 |
-
# Minimal makefile for Sphinx documentation
|
2 |
-
#
|
3 |
-
|
4 |
-
# You can set these variables from the command line.
|
5 |
-
SPHINXOPTS =
|
6 |
-
SPHINXBUILD = sphinx-build
|
7 |
-
SOURCEDIR = source
|
8 |
-
BUILDDIR = build
|
9 |
-
|
10 |
-
# Put it first so that "make" without argument is like "make help".
|
11 |
-
help:
|
12 |
-
@$(SPHINXBUILD) -M help "$(SOURCEDIR)" "$(BUILDDIR)" $(SPHINXOPTS) $(O)
|
13 |
-
|
14 |
-
.PHONY: help Makefile
|
15 |
-
|
16 |
-
clean:
|
17 |
-
@$(SPHINXBUILD) -M $@ "$(SOURCEDIR)" "$(BUILDDIR)" $(SPHINXOPTS) $(O)
|
18 |
-
rm -rf ./source/generated/*
|
19 |
-
|
20 |
-
# Catch-all target: route all unknown targets to Sphinx using the new
|
21 |
-
# "make mode" option. $(O) is meant as a shortcut for $(SPHINXOPTS).
|
22 |
-
%: Makefile
|
23 |
-
@$(SPHINXBUILD) -M $@ "$(SOURCEDIR)" "$(BUILDDIR)" $(SPHINXOPTS) $(O)
|
|
|
|
|
|
|
|
|
|
|
|
|
|
|
|
|
|
|
|
|
|
|
|
|
|
|
|
|
|
|
|
|
|
|
|
|
|
|
|
|
|
|
|
|
|
|
|
spaces/AIGC-Audio/AudioGPT/text_to_speech/utils/commons/trainer.py
DELETED
@@ -1,561 +0,0 @@
|
|
1 |
-
import random
|
2 |
-
import subprocess
|
3 |
-
import traceback
|
4 |
-
from datetime import datetime
|
5 |
-
|
6 |
-
from torch.cuda.amp import GradScaler, autocast
|
7 |
-
import numpy as np
|
8 |
-
import torch.optim
|
9 |
-
import torch.utils.data
|
10 |
-
import copy
|
11 |
-
import logging
|
12 |
-
import os
|
13 |
-
import re
|
14 |
-
import sys
|
15 |
-
import torch
|
16 |
-
import torch.distributed as dist
|
17 |
-
import torch.multiprocessing as mp
|
18 |
-
import tqdm
|
19 |
-
|
20 |
-
from text_to_speech.utils.commons.ckpt_utils import get_last_checkpoint, get_all_ckpts
|
21 |
-
from text_to_speech.utils.commons.ddp_utils import DDP
|
22 |
-
from text_to_speech.utils.commons.hparams import hparams
|
23 |
-
from text_to_speech.utils.commons.tensor_utils import move_to_cuda
|
24 |
-
from text_to_speech.utils.os_utils import remove_file
|
25 |
-
|
26 |
-
|
27 |
-
class Tee(object):
|
28 |
-
def __init__(self, name, mode):
|
29 |
-
self.file = open(name, mode)
|
30 |
-
self.stdout = sys.stdout
|
31 |
-
sys.stdout = self
|
32 |
-
|
33 |
-
def __del__(self):
|
34 |
-
sys.stdout = self.stdout
|
35 |
-
self.file.close()
|
36 |
-
|
37 |
-
def write(self, data):
|
38 |
-
self.file.write(data)
|
39 |
-
self.stdout.write(data)
|
40 |
-
|
41 |
-
def flush(self):
|
42 |
-
self.file.flush()
|
43 |
-
|
44 |
-
|
45 |
-
class Trainer:
|
46 |
-
def __init__(
|
47 |
-
self,
|
48 |
-
work_dir,
|
49 |
-
default_save_path=None,
|
50 |
-
accumulate_grad_batches=1,
|
51 |
-
max_updates=160000,
|
52 |
-
print_nan_grads=False,
|
53 |
-
val_check_interval=2000,
|
54 |
-
num_sanity_val_steps=5,
|
55 |
-
amp=False,
|
56 |
-
# tb logger
|
57 |
-
log_save_interval=100,
|
58 |
-
tb_log_interval=10,
|
59 |
-
# checkpoint
|
60 |
-
monitor_key='val_loss',
|
61 |
-
monitor_mode='min',
|
62 |
-
num_ckpt_keep=5,
|
63 |
-
save_best=True,
|
64 |
-
resume_from_checkpoint=0,
|
65 |
-
seed=1234,
|
66 |
-
debug=False,
|
67 |
-
):
|
68 |
-
os.makedirs(work_dir, exist_ok=True)
|
69 |
-
self.work_dir = work_dir
|
70 |
-
self.accumulate_grad_batches = accumulate_grad_batches
|
71 |
-
self.max_updates = max_updates
|
72 |
-
self.num_sanity_val_steps = num_sanity_val_steps
|
73 |
-
self.print_nan_grads = print_nan_grads
|
74 |
-
self.default_save_path = default_save_path
|
75 |
-
self.resume_from_checkpoint = resume_from_checkpoint if resume_from_checkpoint > 0 else None
|
76 |
-
self.seed = seed
|
77 |
-
self.debug = debug
|
78 |
-
# model and optm
|
79 |
-
self.task = None
|
80 |
-
self.optimizers = []
|
81 |
-
|
82 |
-
# trainer state
|
83 |
-
self.testing = False
|
84 |
-
self.global_step = 0
|
85 |
-
self.current_epoch = 0
|
86 |
-
self.total_batches = 0
|
87 |
-
|
88 |
-
# configure checkpoint
|
89 |
-
self.monitor_key = monitor_key
|
90 |
-
self.num_ckpt_keep = num_ckpt_keep
|
91 |
-
self.save_best = save_best
|
92 |
-
self.monitor_op = np.less if monitor_mode == 'min' else np.greater
|
93 |
-
self.best_val_results = np.Inf if monitor_mode == 'min' else -np.Inf
|
94 |
-
self.mode = 'min'
|
95 |
-
|
96 |
-
# allow int, string and gpu list
|
97 |
-
self.all_gpu_ids = [
|
98 |
-
int(x) for x in os.environ.get("CUDA_VISIBLE_DEVICES", "").split(",") if x != '']
|
99 |
-
self.num_gpus = len(self.all_gpu_ids)
|
100 |
-
self.on_gpu = self.num_gpus > 0
|
101 |
-
self.root_gpu = 0
|
102 |
-
logging.info(f'GPU available: {torch.cuda.is_available()}, GPU used: {self.all_gpu_ids}')
|
103 |
-
self.use_ddp = self.num_gpus > 1
|
104 |
-
self.proc_rank = 0
|
105 |
-
# Tensorboard logging
|
106 |
-
self.log_save_interval = log_save_interval
|
107 |
-
self.val_check_interval = val_check_interval
|
108 |
-
self.tb_log_interval = tb_log_interval
|
109 |
-
self.amp = amp
|
110 |
-
self.amp_scalar = GradScaler()
|
111 |
-
|
112 |
-
def test(self, task_cls):
|
113 |
-
self.testing = True
|
114 |
-
self.fit(task_cls)
|
115 |
-
|
116 |
-
def fit(self, task_cls):
|
117 |
-
if len(self.all_gpu_ids) > 1:
|
118 |
-
mp.spawn(self.ddp_run, nprocs=self.num_gpus, args=(task_cls, copy.deepcopy(hparams)))
|
119 |
-
else:
|
120 |
-
self.task = task_cls()
|
121 |
-
self.task.trainer = self
|
122 |
-
self.run_single_process(self.task)
|
123 |
-
return 1
|
124 |
-
|
125 |
-
def ddp_run(self, gpu_idx, task_cls, hparams_):
|
126 |
-
hparams.update(hparams_)
|
127 |
-
self.proc_rank = gpu_idx
|
128 |
-
self.init_ddp_connection(self.proc_rank, self.num_gpus)
|
129 |
-
if dist.get_rank() != 0 and not self.debug:
|
130 |
-
sys.stdout = open(os.devnull, "w")
|
131 |
-
sys.stderr = open(os.devnull, "w")
|
132 |
-
task = task_cls()
|
133 |
-
task.trainer = self
|
134 |
-
torch.cuda.set_device(gpu_idx)
|
135 |
-
self.root_gpu = gpu_idx
|
136 |
-
self.task = task
|
137 |
-
self.run_single_process(task)
|
138 |
-
|
139 |
-
def run_single_process(self, task):
|
140 |
-
"""Sanity check a few things before starting actual training.
|
141 |
-
|
142 |
-
:param task:
|
143 |
-
"""
|
144 |
-
# build model, optm and load checkpoint
|
145 |
-
if self.proc_rank == 0:
|
146 |
-
self.save_terminal_logs()
|
147 |
-
if not self.testing:
|
148 |
-
self.save_codes()
|
149 |
-
|
150 |
-
model = task.build_model()
|
151 |
-
if model is not None:
|
152 |
-
task.model = model
|
153 |
-
checkpoint, _ = get_last_checkpoint(self.work_dir, self.resume_from_checkpoint)
|
154 |
-
if checkpoint is not None:
|
155 |
-
self.restore_weights(checkpoint)
|
156 |
-
elif self.on_gpu:
|
157 |
-
task.cuda(self.root_gpu)
|
158 |
-
if not self.testing:
|
159 |
-
self.optimizers = task.configure_optimizers()
|
160 |
-
self.fisrt_epoch = True
|
161 |
-
if checkpoint is not None:
|
162 |
-
self.restore_opt_state(checkpoint)
|
163 |
-
del checkpoint
|
164 |
-
# clear cache after restore
|
165 |
-
if self.on_gpu:
|
166 |
-
torch.cuda.empty_cache()
|
167 |
-
|
168 |
-
if self.use_ddp:
|
169 |
-
self.task = self.configure_ddp(self.task)
|
170 |
-
dist.barrier()
|
171 |
-
|
172 |
-
task_ref = self.get_task_ref()
|
173 |
-
task_ref.trainer = self
|
174 |
-
task_ref.testing = self.testing
|
175 |
-
# link up experiment object
|
176 |
-
if self.proc_rank == 0:
|
177 |
-
task_ref.build_tensorboard(save_dir=self.work_dir, name='tb_logs')
|
178 |
-
else:
|
179 |
-
os.makedirs('tmp', exist_ok=True)
|
180 |
-
task_ref.build_tensorboard(save_dir='tmp', name='tb_tmp')
|
181 |
-
self.logger = task_ref.logger
|
182 |
-
try:
|
183 |
-
if self.testing:
|
184 |
-
self.run_evaluation(test=True)
|
185 |
-
else:
|
186 |
-
self.train()
|
187 |
-
except KeyboardInterrupt as e:
|
188 |
-
traceback.print_exc()
|
189 |
-
task_ref.on_keyboard_interrupt()
|
190 |
-
|
191 |
-
####################
|
192 |
-
# valid and test
|
193 |
-
####################
|
194 |
-
def run_evaluation(self, test=False):
|
195 |
-
eval_results = self.evaluate(self.task, test, tqdm_desc='Valid' if not test else 'test',
|
196 |
-
max_batches=hparams['eval_max_batches'])
|
197 |
-
if eval_results is not None and 'tb_log' in eval_results:
|
198 |
-
tb_log_output = eval_results['tb_log']
|
199 |
-
self.log_metrics_to_tb(tb_log_output)
|
200 |
-
if self.proc_rank == 0 and not test:
|
201 |
-
self.save_checkpoint(epoch=self.current_epoch, logs=eval_results)
|
202 |
-
|
203 |
-
def evaluate(self, task, test=False, tqdm_desc='Valid', max_batches=None):
|
204 |
-
if max_batches == -1:
|
205 |
-
max_batches = None
|
206 |
-
# enable eval mode
|
207 |
-
task.zero_grad()
|
208 |
-
task.eval()
|
209 |
-
torch.set_grad_enabled(False)
|
210 |
-
|
211 |
-
task_ref = self.get_task_ref()
|
212 |
-
if test:
|
213 |
-
ret = task_ref.test_start()
|
214 |
-
if ret == 'EXIT':
|
215 |
-
return
|
216 |
-
else:
|
217 |
-
task_ref.validation_start()
|
218 |
-
outputs = []
|
219 |
-
dataloader = task_ref.test_dataloader() if test else task_ref.val_dataloader()
|
220 |
-
pbar = tqdm.tqdm(dataloader, desc=tqdm_desc, total=max_batches, dynamic_ncols=True, unit='step',
|
221 |
-
disable=self.root_gpu > 0)
|
222 |
-
# give model a chance to do something with the outputs (and method defined)
|
223 |
-
for batch_idx, batch in enumerate(pbar):
|
224 |
-
if batch is None: # pragma: no cover
|
225 |
-
continue
|
226 |
-
# stop short when on fast_dev_run (sets max_batch=1)
|
227 |
-
if max_batches is not None and batch_idx >= max_batches:
|
228 |
-
break
|
229 |
-
|
230 |
-
# make dataloader_idx arg in validation_step optional
|
231 |
-
if self.on_gpu:
|
232 |
-
batch = move_to_cuda(batch, self.root_gpu)
|
233 |
-
args = [batch, batch_idx]
|
234 |
-
if self.use_ddp:
|
235 |
-
output = task(*args)
|
236 |
-
else:
|
237 |
-
if test:
|
238 |
-
output = task_ref.test_step(*args)
|
239 |
-
else:
|
240 |
-
output = task_ref.validation_step(*args)
|
241 |
-
# track outputs for collation
|
242 |
-
outputs.append(output)
|
243 |
-
# give model a chance to do something with the outputs (and method defined)
|
244 |
-
if test:
|
245 |
-
eval_results = task_ref.test_end(outputs)
|
246 |
-
else:
|
247 |
-
eval_results = task_ref.validation_end(outputs)
|
248 |
-
# enable train mode again
|
249 |
-
task.train()
|
250 |
-
torch.set_grad_enabled(True)
|
251 |
-
return eval_results
|
252 |
-
|
253 |
-
####################
|
254 |
-
# train
|
255 |
-
####################
|
256 |
-
def train(self):
|
257 |
-
task_ref = self.get_task_ref()
|
258 |
-
task_ref.on_train_start()
|
259 |
-
if self.num_sanity_val_steps > 0:
|
260 |
-
# run tiny validation (if validation defined) to make sure program won't crash during val
|
261 |
-
self.evaluate(self.task, False, 'Sanity Val', max_batches=self.num_sanity_val_steps)
|
262 |
-
# clear cache before training
|
263 |
-
if self.on_gpu:
|
264 |
-
torch.cuda.empty_cache()
|
265 |
-
dataloader = task_ref.train_dataloader()
|
266 |
-
epoch = self.current_epoch
|
267 |
-
# run all epochs
|
268 |
-
while True:
|
269 |
-
# set seed for distributed sampler (enables shuffling for each epoch)
|
270 |
-
if self.use_ddp and hasattr(dataloader.sampler, 'set_epoch'):
|
271 |
-
dataloader.sampler.set_epoch(epoch)
|
272 |
-
# update training progress in trainer and model
|
273 |
-
task_ref.current_epoch = epoch
|
274 |
-
self.current_epoch = epoch
|
275 |
-
# total batches includes multiple val checks
|
276 |
-
self.batch_loss_value = 0 # accumulated grads
|
277 |
-
# before epoch hook
|
278 |
-
task_ref.on_epoch_start()
|
279 |
-
|
280 |
-
# run epoch
|
281 |
-
train_pbar = tqdm.tqdm(dataloader, initial=self.global_step, total=float('inf'),
|
282 |
-
dynamic_ncols=True, unit='step', disable=self.root_gpu > 0)
|
283 |
-
for batch_idx, batch in enumerate(train_pbar):
|
284 |
-
if self.global_step % self.val_check_interval == 0 and not self.fisrt_epoch:
|
285 |
-
self.run_evaluation()
|
286 |
-
pbar_metrics, tb_metrics = self.run_training_batch(batch_idx, batch)
|
287 |
-
train_pbar.set_postfix(**pbar_metrics)
|
288 |
-
self.fisrt_epoch = False
|
289 |
-
# when metrics should be logged
|
290 |
-
if (self.global_step + 1) % self.tb_log_interval == 0:
|
291 |
-
# logs user requested information to logger
|
292 |
-
self.log_metrics_to_tb(tb_metrics)
|
293 |
-
|
294 |
-
self.global_step += 1
|
295 |
-
task_ref.global_step = self.global_step
|
296 |
-
if self.global_step > self.max_updates:
|
297 |
-
print("| Training end..")
|
298 |
-
break
|
299 |
-
# epoch end hook
|
300 |
-
task_ref.on_epoch_end()
|
301 |
-
epoch += 1
|
302 |
-
if self.global_step > self.max_updates:
|
303 |
-
break
|
304 |
-
task_ref.on_train_end()
|
305 |
-
|
306 |
-
def run_training_batch(self, batch_idx, batch):
|
307 |
-
if batch is None:
|
308 |
-
return {}
|
309 |
-
all_progress_bar_metrics = []
|
310 |
-
all_log_metrics = []
|
311 |
-
task_ref = self.get_task_ref()
|
312 |
-
for opt_idx, optimizer in enumerate(self.optimizers):
|
313 |
-
if optimizer is None:
|
314 |
-
continue
|
315 |
-
# make sure only the gradients of the current optimizer's paramaters are calculated
|
316 |
-
# in the training step to prevent dangling gradients in multiple-optimizer setup.
|
317 |
-
if len(self.optimizers) > 1:
|
318 |
-
for param in task_ref.parameters():
|
319 |
-
param.requires_grad = False
|
320 |
-
for group in optimizer.param_groups:
|
321 |
-
for param in group['params']:
|
322 |
-
param.requires_grad = True
|
323 |
-
|
324 |
-
# forward pass
|
325 |
-
with autocast(enabled=self.amp):
|
326 |
-
if self.on_gpu:
|
327 |
-
batch = move_to_cuda(copy.copy(batch), self.root_gpu)
|
328 |
-
args = [batch, batch_idx, opt_idx]
|
329 |
-
if self.use_ddp:
|
330 |
-
output = self.task(*args)
|
331 |
-
else:
|
332 |
-
output = task_ref.training_step(*args)
|
333 |
-
loss = output['loss']
|
334 |
-
if loss is None:
|
335 |
-
continue
|
336 |
-
progress_bar_metrics = output['progress_bar']
|
337 |
-
log_metrics = output['tb_log']
|
338 |
-
# accumulate loss
|
339 |
-
loss = loss / self.accumulate_grad_batches
|
340 |
-
|
341 |
-
# backward pass
|
342 |
-
if loss.requires_grad:
|
343 |
-
if self.amp:
|
344 |
-
self.amp_scalar.scale(loss).backward()
|
345 |
-
else:
|
346 |
-
loss.backward()
|
347 |
-
|
348 |
-
# track progress bar metrics
|
349 |
-
all_log_metrics.append(log_metrics)
|
350 |
-
all_progress_bar_metrics.append(progress_bar_metrics)
|
351 |
-
|
352 |
-
if loss is None:
|
353 |
-
continue
|
354 |
-
|
355 |
-
# nan grads
|
356 |
-
if self.print_nan_grads:
|
357 |
-
has_nan_grad = False
|
358 |
-
for name, param in task_ref.named_parameters():
|
359 |
-
if (param.grad is not None) and torch.isnan(param.grad.float()).any():
|
360 |
-
print("| NaN params: ", name, param, param.grad)
|
361 |
-
has_nan_grad = True
|
362 |
-
if has_nan_grad:
|
363 |
-
exit(0)
|
364 |
-
|
365 |
-
# gradient update with accumulated gradients
|
366 |
-
if (self.global_step + 1) % self.accumulate_grad_batches == 0:
|
367 |
-
grad_norm_dict = task_ref.on_before_optimization(opt_idx)
|
368 |
-
if grad_norm_dict is not None:
|
369 |
-
all_log_metrics[-1].update(grad_norm_dict)
|
370 |
-
if self.amp:
|
371 |
-
self.amp_scalar.step(optimizer)
|
372 |
-
self.amp_scalar.update()
|
373 |
-
else:
|
374 |
-
optimizer.step()
|
375 |
-
optimizer.zero_grad()
|
376 |
-
task_ref.on_after_optimization(self.current_epoch, batch_idx, optimizer, opt_idx)
|
377 |
-
|
378 |
-
# collapse all metrics into one dict
|
379 |
-
all_progress_bar_metrics = {k: v for d in all_progress_bar_metrics for k, v in d.items()}
|
380 |
-
all_log_metrics = {k: v for d in all_log_metrics for k, v in d.items()}
|
381 |
-
return all_progress_bar_metrics, all_log_metrics
|
382 |
-
|
383 |
-
####################
|
384 |
-
# load and save checkpoint
|
385 |
-
####################
|
386 |
-
def restore_weights(self, checkpoint):
|
387 |
-
# load model state
|
388 |
-
task_ref = self.get_task_ref()
|
389 |
-
|
390 |
-
for k, v in checkpoint['state_dict'].items():
|
391 |
-
getattr(task_ref, k).load_state_dict(v)
|
392 |
-
|
393 |
-
if self.on_gpu:
|
394 |
-
task_ref.cuda(self.root_gpu)
|
395 |
-
# load training state (affects trainer only)
|
396 |
-
self.best_val_results = checkpoint['checkpoint_callback_best']
|
397 |
-
self.global_step = checkpoint['global_step']
|
398 |
-
self.current_epoch = checkpoint['epoch']
|
399 |
-
task_ref.global_step = self.global_step
|
400 |
-
|
401 |
-
# wait for all models to restore weights
|
402 |
-
if self.use_ddp:
|
403 |
-
# wait for all processes to catch up
|
404 |
-
dist.barrier()
|
405 |
-
|
406 |
-
def restore_opt_state(self, checkpoint):
|
407 |
-
if self.testing:
|
408 |
-
return
|
409 |
-
# restore the optimizers
|
410 |
-
optimizer_states = checkpoint['optimizer_states']
|
411 |
-
for optimizer, opt_state in zip(self.optimizers, optimizer_states):
|
412 |
-
if optimizer is None:
|
413 |
-
return
|
414 |
-
try:
|
415 |
-
optimizer.load_state_dict(opt_state)
|
416 |
-
# move optimizer to GPU 1 weight at a time
|
417 |
-
if self.on_gpu:
|
418 |
-
for state in optimizer.state.values():
|
419 |
-
for k, v in state.items():
|
420 |
-
if isinstance(v, torch.Tensor):
|
421 |
-
state[k] = v.cuda(self.root_gpu)
|
422 |
-
except ValueError:
|
423 |
-
print("| WARMING: optimizer parameters not match !!!")
|
424 |
-
try:
|
425 |
-
if dist.is_initialized() and dist.get_rank() > 0:
|
426 |
-
return
|
427 |
-
except Exception as e:
|
428 |
-
print(e)
|
429 |
-
return
|
430 |
-
did_restore = True
|
431 |
-
return did_restore
|
432 |
-
|
433 |
-
def save_checkpoint(self, epoch, logs=None):
|
434 |
-
monitor_op = np.less
|
435 |
-
ckpt_path = f'{self.work_dir}/model_ckpt_steps_{self.global_step}.ckpt'
|
436 |
-
logging.info(f'Epoch {epoch:05d}@{self.global_step}: saving model to {ckpt_path}')
|
437 |
-
self._atomic_save(ckpt_path)
|
438 |
-
for old_ckpt in get_all_ckpts(self.work_dir)[self.num_ckpt_keep:]:
|
439 |
-
remove_file(old_ckpt)
|
440 |
-
logging.info(f'Delete ckpt: {os.path.basename(old_ckpt)}')
|
441 |
-
current = None
|
442 |
-
if logs is not None and self.monitor_key in logs:
|
443 |
-
current = logs[self.monitor_key]
|
444 |
-
if current is not None and self.save_best:
|
445 |
-
if monitor_op(current, self.best_val_results):
|
446 |
-
best_filepath = f'{self.work_dir}/model_ckpt_best.pt'
|
447 |
-
self.best_val_results = current
|
448 |
-
logging.info(
|
449 |
-
f'Epoch {epoch:05d}@{self.global_step}: {self.monitor_key} reached {current:0.5f}. '
|
450 |
-
f'Saving model to {best_filepath}')
|
451 |
-
self._atomic_save(best_filepath)
|
452 |
-
|
453 |
-
def _atomic_save(self, filepath):
|
454 |
-
checkpoint = self.dump_checkpoint()
|
455 |
-
tmp_path = str(filepath) + ".part"
|
456 |
-
torch.save(checkpoint, tmp_path, _use_new_zipfile_serialization=False)
|
457 |
-
os.replace(tmp_path, filepath)
|
458 |
-
|
459 |
-
def dump_checkpoint(self):
|
460 |
-
checkpoint = {'epoch': self.current_epoch, 'global_step': self.global_step,
|
461 |
-
'checkpoint_callback_best': self.best_val_results}
|
462 |
-
# save optimizers
|
463 |
-
optimizer_states = []
|
464 |
-
for i, optimizer in enumerate(self.optimizers):
|
465 |
-
if optimizer is not None:
|
466 |
-
optimizer_states.append(optimizer.state_dict())
|
467 |
-
|
468 |
-
checkpoint['optimizer_states'] = optimizer_states
|
469 |
-
task_ref = self.get_task_ref()
|
470 |
-
checkpoint['state_dict'] = {
|
471 |
-
k: v.state_dict() for k, v in task_ref.named_children() if len(list(v.parameters())) > 0}
|
472 |
-
return checkpoint
|
473 |
-
|
474 |
-
####################
|
475 |
-
# DDP
|
476 |
-
####################
|
477 |
-
def configure_ddp(self, task):
|
478 |
-
task = DDP(task, device_ids=[self.root_gpu], find_unused_parameters=True)
|
479 |
-
random.seed(self.seed)
|
480 |
-
np.random.seed(self.seed)
|
481 |
-
return task
|
482 |
-
|
483 |
-
def init_ddp_connection(self, proc_rank, world_size):
|
484 |
-
root_node = '127.0.0.1'
|
485 |
-
root_node = self.resolve_root_node_address(root_node)
|
486 |
-
os.environ['MASTER_ADDR'] = root_node
|
487 |
-
dist.init_process_group('nccl', rank=proc_rank, world_size=world_size)
|
488 |
-
|
489 |
-
def resolve_root_node_address(self, root_node):
|
490 |
-
if '[' in root_node:
|
491 |
-
name = root_node.split('[')[0]
|
492 |
-
number = root_node.split(',')[0]
|
493 |
-
if '-' in number:
|
494 |
-
number = number.split('-')[0]
|
495 |
-
number = re.sub('[^0-9]', '', number)
|
496 |
-
root_node = name + number
|
497 |
-
return root_node
|
498 |
-
|
499 |
-
####################
|
500 |
-
# utils
|
501 |
-
####################
|
502 |
-
def get_task_ref(self):
|
503 |
-
from text_to_speech.utils.commons.base_task import BaseTask
|
504 |
-
task: BaseTask = self.task.module if isinstance(self.task, DDP) else self.task
|
505 |
-
return task
|
506 |
-
|
507 |
-
def log_metrics_to_tb(self, metrics, step=None):
|
508 |
-
"""Logs the metric dict passed in.
|
509 |
-
|
510 |
-
:param metrics:
|
511 |
-
"""
|
512 |
-
# turn all tensors to scalars
|
513 |
-
scalar_metrics = self.metrics_to_scalars(metrics)
|
514 |
-
|
515 |
-
step = step if step is not None else self.global_step
|
516 |
-
# log actual metrics
|
517 |
-
if self.proc_rank == 0:
|
518 |
-
self.log_metrics(self.logger, scalar_metrics, step=step)
|
519 |
-
|
520 |
-
@staticmethod
|
521 |
-
def log_metrics(logger, metrics, step=None):
|
522 |
-
for k, v in metrics.items():
|
523 |
-
if isinstance(v, torch.Tensor):
|
524 |
-
v = v.item()
|
525 |
-
logger.add_scalar(k, v, step)
|
526 |
-
|
527 |
-
def metrics_to_scalars(self, metrics):
|
528 |
-
new_metrics = {}
|
529 |
-
for k, v in metrics.items():
|
530 |
-
if isinstance(v, torch.Tensor):
|
531 |
-
v = v.item()
|
532 |
-
|
533 |
-
if type(v) is dict:
|
534 |
-
v = self.metrics_to_scalars(v)
|
535 |
-
|
536 |
-
new_metrics[k] = v
|
537 |
-
|
538 |
-
return new_metrics
|
539 |
-
|
540 |
-
def save_terminal_logs(self):
|
541 |
-
t = datetime.now().strftime('%Y%m%d%H%M%S')
|
542 |
-
os.makedirs(f'{self.work_dir}/terminal_logs', exist_ok=True)
|
543 |
-
Tee(f'{self.work_dir}/terminal_logs/log_{t}.txt', 'w')
|
544 |
-
|
545 |
-
def save_codes(self):
|
546 |
-
if len(hparams['save_codes']) > 0:
|
547 |
-
t = datetime.now().strftime('%Y%m%d%H%M%S')
|
548 |
-
code_dir = f'{self.work_dir}/codes/{t}'
|
549 |
-
subprocess.check_call(f'mkdir -p "{code_dir}"', shell=True)
|
550 |
-
for c in hparams['save_codes']:
|
551 |
-
if os.path.exists(c):
|
552 |
-
subprocess.check_call(
|
553 |
-
f'rsync -aR '
|
554 |
-
f'--include="*.py" '
|
555 |
-
f'--include="*.yaml" '
|
556 |
-
f'--exclude="__pycache__" '
|
557 |
-
f'--include="*/" '
|
558 |
-
f'--exclude="*" '
|
559 |
-
f'"./{c}" "{code_dir}/"',
|
560 |
-
shell=True)
|
561 |
-
print(f"| Copied codes to {code_dir}.")
|
|
|
|
|
|
|
|
|
|
|
|
|
|
|
|
|
|
|
|
|
|
|
|
|
|
|
|
|
|
|
|
|
|
|
|
|
|
|
|
|
|
|
|
|
|
|
|
|
|
|
|
|
|
|
|
|
|
|
|
|
|
|
|
|
|
|
|
|
|
|
|
|
|
|
|
|
|
|
|
|
|
|
|
|
|
|
|
|
|
|
|
|
|
|
|
|
|
|
|
|
|
|
|
|
|
|
|
|
|
|
|
|
|
|
|
|
|
|
|
|
|
|
|
|
|
|
|
|
|
|
|
|
|
|
|
|
|
|
|
|
|
|
|
|
|
|
|
|
|
|
|
|
|
|
|
|
|
|
|
|
|
|
|
|
|
|
|
|
|
|
|
|
|
|
|
|
|
|
|
|
|
|
|
|
|
|
|
|
|
|
|
|
|
|
|
|
|
|
|
|
|
|
|
|
|
|
|
|
|
|
|
|
|
|
|
|
|
|
|
|
|
|
|
|
|
|
|
|
|
|
|
|
|
|
|
|
|
|
|
|
|
|
|
|
|
|
|
|
|
|
|
|
|
|
|
|
|
|
|
|
|
|
|
|
|
|
|
|
|
|
|
|
|
|
|
|
|
|
|
|
|
|
|
|
|
|
|
|
|
|
|
|
|
|
|
|
|
|
|
|
|
|
|
|
|
|
|
|
|
|
|
|
|
|
|
|
|
|
|
|
|
|
|
|
|
|
|
|
|
|
|
|
|
|
|
|
|
|
|
|
|
|
|
|
|
|
|
|
|
|
|
|
|
|
|
|
|
|
|
|
|
|
|
|
|
|
|
|
|
|
|
|
|
|
|
|
|
|
|
|
|
|
|
|
|
|
|
|
|
|
|
|
|
|
|
|
|
|
|
|
|
|
|
|
|
|
|
|
|
|
|
|
|
|
|
|
|
|
|
|
|
|
|
|
|
|
|
|
|
|
|
|
|
|
|
|
|
|
|
|
|
|
|
|
|
|
|
|
|
|
|
|
|
|
|
|
|
|
|
|
|
|
|
|
|
|
|
|
|
|
|
|
|
|
|
|
|
|
|
|
|
|
|
|
|
|
|
|
|
|
|
|
|
|
|
|
|
|
|
|
|
|
|
|
|
|
|
|
|
|
|
|
|
|
|
|
|
|
|
|
|
|
|
|
|
|
|
|
|
|
|
|
|
|
|
|
|
|
|
|
|
|
|
|
|
|
|
|
|
|
|
|
|
|
|
|
|
|
|
|
|
|
|
|
|
|
|
|
|
|
|
|
|
|
|
|
|
|
|
|
|
|
|
|
|
|
|
|
|
|
|
|
|
|
|
|
|
|
|
|
|
|
|
|
|
|
|
|
|
|
|
|
|
|
|
|
|
|
|
|
|
|
|
|
|
|
|
|
|
|
|
|
|
|
|
|
|
|
|
|
|
|
|
|
|
|
|
|
|
|
|
|
|
|
|
|
|
|
|
|
|
|
|
|
|
|
|
|
|
|
|
|
|
|
|
|
|
|
|
|
|
|
|
|
|
|
|
|
|
|
|
|
|
|
|
|
|
|
|
|
|
|
|
|
|
|
|
|
|
|
|
|
|
|
|
|
|
|
|
|
|
|
|
|
|
|
|
|
|
|
|
|
|
|
|
|
|
|
|
|
|
|
|
|
|
|
|
|
|
|
|
|
|
|
|
|
|
|
|
|
|
|
|
|
|
|
|
|
|
|
|
|
|
|
|
|
|
|
|
|
|
|
|
|
|
|
|
|
|
|
|
|
|
|
|
|
|
|
|
|
|
|
|
|
|
|
|
|
|
|
|
|
|
|
|
|
|
|
|
|
|
|
|
|
|
|
|
|
|
|
|
|
|
|
|
|
|
|
|
|
|
|
|
|
|
|
|
|
|
|
|
|
|
|
|
|
|
|
|
|
|
|
|
|
|
|
|
|
|
|
|
|
|
|
|
|
|
|
|
|
|
|
|
|
|
|
|
|
|
|
|
|
|
|
|
|
|
|
|
|
|
|
|
|
|
|
|
|
|
|
|
|
|
|
|
|
|
|
|
|
|
|
|
|
|
|
|
|
|
|
|
|
|
|
|
|
|
|
|
|
|
|
|
|
|
|
|
|
|
|
|
|
|
|
|
|
|
|
|
|
|
|
|
|
|
|
|
|
|
|
|
|
|
|
|
|
|
|
|
|
|
|
|
|
|
|
|
|
|
|
|
|
|
|
|
|
|
|
|
|
|
|
|
|
|
|
|
|
|
|
|
|
|
|
|
|
|
|
|
|
|
|
|
|
|
|
|
|
|
|
|
|
|
|
|
|
|
|
|
|
|
|
|
|
|
|
|
|
|
|
|
|
|
|
|
|
|
|
|
|
|
|
|
|
|
|
|
|
|
|
|
|
|
|
|
|
|
|
|
|
|
|
|
|
|
|
|
|
|
|
|
|
|
|
|
|
|
|
|
|
|
|
|
|
|
|
|
|
|
|
|
|
|
|
|
|
|
|
|
|
|
|
|
spaces/AIGText/GlyphControl/annotator/canny/__init__.py
DELETED
@@ -1,6 +0,0 @@
|
|
1 |
-
import cv2
|
2 |
-
|
3 |
-
|
4 |
-
class CannyDetector:
|
5 |
-
def __call__(self, img, low_threshold, high_threshold):
|
6 |
-
return cv2.Canny(img, low_threshold, high_threshold)
|
|
|
|
|
|
|
|
|
|
|
|
|
|
spaces/AgentVerse/agentVerse/ui/src/phaser3-rex-plugins/plugins/sequence.js
DELETED
@@ -1,2 +0,0 @@
|
|
1 |
-
import Sequence from './logic/runcommands/sequence/Sequence.js';
|
2 |
-
export default Sequence;
|
|
|
|
|
|
spaces/AgentVerse/agentVerse/ui/src/phaser3-rex-plugins/templates/ui/customprogress/CustomProgress.d.ts
DELETED
@@ -1,2 +0,0 @@
|
|
1 |
-
import CustomProgress from '../../../plugins/customprogress';
|
2 |
-
export default CustomProgress;
|
|
|
|
|
|
spaces/AlexWang/lama/saicinpainting/training/visualizers/base.py
DELETED
@@ -1,73 +0,0 @@
|
|
1 |
-
import abc
|
2 |
-
from typing import Dict, List
|
3 |
-
|
4 |
-
import numpy as np
|
5 |
-
import torch
|
6 |
-
from skimage import color
|
7 |
-
from skimage.segmentation import mark_boundaries
|
8 |
-
|
9 |
-
from . import colors
|
10 |
-
|
11 |
-
COLORS, _ = colors.generate_colors(151) # 151 - max classes for semantic segmentation
|
12 |
-
|
13 |
-
|
14 |
-
class BaseVisualizer:
|
15 |
-
@abc.abstractmethod
|
16 |
-
def __call__(self, epoch_i, batch_i, batch, suffix='', rank=None):
|
17 |
-
"""
|
18 |
-
Take a batch, make an image from it and visualize
|
19 |
-
"""
|
20 |
-
raise NotImplementedError()
|
21 |
-
|
22 |
-
|
23 |
-
def visualize_mask_and_images(images_dict: Dict[str, np.ndarray], keys: List[str],
|
24 |
-
last_without_mask=True, rescale_keys=None, mask_only_first=None,
|
25 |
-
black_mask=False) -> np.ndarray:
|
26 |
-
mask = images_dict['mask'] > 0.5
|
27 |
-
result = []
|
28 |
-
for i, k in enumerate(keys):
|
29 |
-
img = images_dict[k]
|
30 |
-
img = np.transpose(img, (1, 2, 0))
|
31 |
-
|
32 |
-
if rescale_keys is not None and k in rescale_keys:
|
33 |
-
img = img - img.min()
|
34 |
-
img /= img.max() + 1e-5
|
35 |
-
if len(img.shape) == 2:
|
36 |
-
img = np.expand_dims(img, 2)
|
37 |
-
|
38 |
-
if img.shape[2] == 1:
|
39 |
-
img = np.repeat(img, 3, axis=2)
|
40 |
-
elif (img.shape[2] > 3):
|
41 |
-
img_classes = img.argmax(2)
|
42 |
-
img = color.label2rgb(img_classes, colors=COLORS)
|
43 |
-
|
44 |
-
if mask_only_first:
|
45 |
-
need_mark_boundaries = i == 0
|
46 |
-
else:
|
47 |
-
need_mark_boundaries = i < len(keys) - 1 or not last_without_mask
|
48 |
-
|
49 |
-
if need_mark_boundaries:
|
50 |
-
if black_mask:
|
51 |
-
img = img * (1 - mask[0][..., None])
|
52 |
-
img = mark_boundaries(img,
|
53 |
-
mask[0],
|
54 |
-
color=(1., 0., 0.),
|
55 |
-
outline_color=(1., 1., 1.),
|
56 |
-
mode='thick')
|
57 |
-
result.append(img)
|
58 |
-
return np.concatenate(result, axis=1)
|
59 |
-
|
60 |
-
|
61 |
-
def visualize_mask_and_images_batch(batch: Dict[str, torch.Tensor], keys: List[str], max_items=10,
|
62 |
-
last_without_mask=True, rescale_keys=None) -> np.ndarray:
|
63 |
-
batch = {k: tens.detach().cpu().numpy() for k, tens in batch.items()
|
64 |
-
if k in keys or k == 'mask'}
|
65 |
-
|
66 |
-
batch_size = next(iter(batch.values())).shape[0]
|
67 |
-
items_to_vis = min(batch_size, max_items)
|
68 |
-
result = []
|
69 |
-
for i in range(items_to_vis):
|
70 |
-
cur_dct = {k: tens[i] for k, tens in batch.items()}
|
71 |
-
result.append(visualize_mask_and_images(cur_dct, keys, last_without_mask=last_without_mask,
|
72 |
-
rescale_keys=rescale_keys))
|
73 |
-
return np.concatenate(result, axis=0)
|
|
|
|
|
|
|
|
|
|
|
|
|
|
|
|
|
|
|
|
|
|
|
|
|
|
|
|
|
|
|
|
|
|
|
|
|
|
|
|
|
|
|
|
|
|
|
|
|
|
|
|
|
|
|
|
|
|
|
|
|
|
|
|
|
|
|
|
|
|
|
|
|
|
|
|
|
|
|
|
|
|
|
|
|
|
|
|
|
|
|
|
|
|
|
|
|
|
|
|
|
|
|
|
|
|
|
|
|
|
|
|
|
|
|
|
|
|
|
|
|
|
|
|
|
|
|
|
|
|
|
|
|
|
|
|
|
|
|
|
|
|
|
|
|
|
|
|
spaces/AlexWortega/Kandinsky2.0/app.py
DELETED
@@ -1,215 +0,0 @@
|
|
1 |
-
|
2 |
-
import gradio as gr
|
3 |
-
import torch
|
4 |
-
from torch import autocast
|
5 |
-
from kandinsky2 import get_kandinsky2
|
6 |
-
device = torch.device('cuda' if torch.cuda.is_available() else 'cpu')
|
7 |
-
model = get_kandinsky2(device, task_type='text2img')
|
8 |
-
|
9 |
-
|
10 |
-
|
11 |
-
|
12 |
-
def infer(prompt):
|
13 |
-
images = model.generate_text2img(prompt, batch_size=4, h=512, w=512, num_steps=75, denoised_type='dynamic_threshold', dynamic_threshold_v=99.5, sampler='ddim_sampler', ddim_eta=0.05, guidance_scale=10)
|
14 |
-
return images
|
15 |
-
|
16 |
-
css = """
|
17 |
-
.gradio-container {
|
18 |
-
font-family: 'IBM Plex Sans', sans-serif;
|
19 |
-
}
|
20 |
-
.gr-button {
|
21 |
-
color: white;
|
22 |
-
border-color: black;
|
23 |
-
background: black;
|
24 |
-
}
|
25 |
-
input[type='range'] {
|
26 |
-
accent-color: black;
|
27 |
-
}
|
28 |
-
.dark input[type='range'] {
|
29 |
-
accent-color: #dfdfdf;
|
30 |
-
}
|
31 |
-
.container {
|
32 |
-
max-width: 730px;
|
33 |
-
margin: auto;
|
34 |
-
padding-top: 1.5rem;
|
35 |
-
}
|
36 |
-
#gallery {
|
37 |
-
min-height: 22rem;
|
38 |
-
margin-bottom: 15px;
|
39 |
-
margin-left: auto;
|
40 |
-
margin-right: auto;
|
41 |
-
border-bottom-right-radius: .5rem !important;
|
42 |
-
border-bottom-left-radius: .5rem !important;
|
43 |
-
}
|
44 |
-
#gallery>div>.h-full {
|
45 |
-
min-height: 20rem;
|
46 |
-
}
|
47 |
-
.details:hover {
|
48 |
-
text-decoration: underline;
|
49 |
-
}
|
50 |
-
.gr-button {
|
51 |
-
white-space: nowrap;
|
52 |
-
}
|
53 |
-
.gr-button:focus {
|
54 |
-
border-color: rgb(147 197 253 / var(--tw-border-opacity));
|
55 |
-
outline: none;
|
56 |
-
box-shadow: var(--tw-ring-offset-shadow), var(--tw-ring-shadow), var(--tw-shadow, 0 0 #0000);
|
57 |
-
--tw-border-opacity: 1;
|
58 |
-
--tw-ring-offset-shadow: var(--tw-ring-inset) 0 0 0 var(--tw-ring-offset-width) var(--tw-ring-offset-color);
|
59 |
-
--tw-ring-shadow: var(--tw-ring-inset) 0 0 0 calc(3px var(--tw-ring-offset-width)) var(--tw-ring-color);
|
60 |
-
--tw-ring-color: rgb(191 219 254 / var(--tw-ring-opacity));
|
61 |
-
--tw-ring-opacity: .5;
|
62 |
-
}
|
63 |
-
#advanced-btn {
|
64 |
-
font-size: .7rem !important;
|
65 |
-
line-height: 19px;
|
66 |
-
margin-top: 12px;
|
67 |
-
margin-bottom: 12px;
|
68 |
-
padding: 2px 8px;
|
69 |
-
border-radius: 14px !important;
|
70 |
-
}
|
71 |
-
#advanced-options {
|
72 |
-
display: none;
|
73 |
-
margin-bottom: 20px;
|
74 |
-
}
|
75 |
-
.footer {
|
76 |
-
margin-bottom: 45px;
|
77 |
-
margin-top: 35px;
|
78 |
-
text-align: center;
|
79 |
-
border-bottom: 1px solid #e5e5e5;
|
80 |
-
}
|
81 |
-
.footer>p {
|
82 |
-
font-size: .8rem;
|
83 |
-
display: inline-block;
|
84 |
-
padding: 0 10px;
|
85 |
-
transform: translateY(10px);
|
86 |
-
background: white;
|
87 |
-
}
|
88 |
-
.dark .footer {
|
89 |
-
border-color: #303030;
|
90 |
-
}
|
91 |
-
.dark .footer>p {
|
92 |
-
background: #0b0f19;
|
93 |
-
}
|
94 |
-
.acknowledgments h4{
|
95 |
-
margin: 1.25em 0 .25em 0;
|
96 |
-
font-weight: bold;
|
97 |
-
font-size: 115%;
|
98 |
-
}
|
99 |
-
#container-advanced-btns{
|
100 |
-
display: flex;
|
101 |
-
flex-wrap: wrap;
|
102 |
-
justify-content: space-between;
|
103 |
-
align-items: center;
|
104 |
-
}
|
105 |
-
.animate-spin {
|
106 |
-
animation: spin 1s linear infinite;
|
107 |
-
}
|
108 |
-
@keyframes spin {
|
109 |
-
from {
|
110 |
-
transform: rotate(0deg);
|
111 |
-
}
|
112 |
-
to {
|
113 |
-
transform: rotate(360deg);
|
114 |
-
}
|
115 |
-
}
|
116 |
-
#share-btn-container {
|
117 |
-
display: flex; padding-left: 0.5rem !important; padding-right: 0.5rem !important; background-color: #000000; justify-content: center; align-items: center; border-radius: 9999px !important; width: 13rem;
|
118 |
-
}
|
119 |
-
#share-btn {
|
120 |
-
all: initial; color: #ffffff;font-weight: 600; cursor:pointer; font-family: 'IBM Plex Sans', sans-serif; margin-left: 0.5rem !important; padding-top: 0.25rem !important; padding-bottom: 0.25rem !important;
|
121 |
-
}
|
122 |
-
#share-btn * {
|
123 |
-
all: unset;
|
124 |
-
}
|
125 |
-
.gr-form{
|
126 |
-
flex: 1 1 50%; border-top-right-radius: 0; border-bottom-right-radius: 0;
|
127 |
-
}
|
128 |
-
#prompt-container{
|
129 |
-
gap: 0;
|
130 |
-
}
|
131 |
-
#generated_id{
|
132 |
-
min-height: 700px
|
133 |
-
}
|
134 |
-
"""
|
135 |
-
block = gr.Blocks(css=css)
|
136 |
-
|
137 |
-
examples = [
|
138 |
-
[
|
139 |
-
'Красная площадь'
|
140 |
-
],
|
141 |
-
[
|
142 |
-
'Thinking man in anime style'
|
143 |
-
],
|
144 |
-
[
|
145 |
-
'אבוקדו'
|
146 |
-
],
|
147 |
-
]
|
148 |
-
|
149 |
-
with block as demo:
|
150 |
-
gr.Markdown("""
|
151 |
-
|
152 |
-
|
153 |
-
[](https://pytorch.org/) [](https://huggingface.co/sberbank-ai/Kandinsky_2.0)
|
154 |
-
|
155 |
-
|
156 |
-
|
157 |
-
## Model architecture:
|
158 |
-
|
159 |
-
It is a latent diffusion model with two multilingual text encoders:
|
160 |
-
* mCLIP-XLMR 560M parameters
|
161 |
-
* mT5-encoder-small 146M parameters
|
162 |
-
|
163 |
-
These encoders and multilingual training datasets unveil the real multilingual text-to-image generation experience!
|
164 |
-
|
165 |
-
**Kandinsky 2.0** was trained on a large 1B multilingual set, including samples that we used to train Kandinsky.
|
166 |
-
|
167 |
-
In terms of diffusion architecture Kandinsky 2.0 implements UNet with 1.2B parameters.
|
168 |
-
|
169 |
-
**Kandinsky 2.0** architecture overview:
|
170 |
-

|
171 |
-
|
172 |
-
"""
|
173 |
-
)
|
174 |
-
with gr.Group():
|
175 |
-
with gr.Box():
|
176 |
-
with gr.Row().style(mobile_collapse=False, equal_height=True):
|
177 |
-
|
178 |
-
text = gr.Textbox(
|
179 |
-
label="Enter your prompt", show_label=False, max_lines=1
|
180 |
-
).style(
|
181 |
-
border=(True, False, True, True),
|
182 |
-
rounded=(True, False, False, True),
|
183 |
-
container=False,
|
184 |
-
)
|
185 |
-
btn = gr.Button("Run").style(
|
186 |
-
margin=False,
|
187 |
-
rounded=(False, True, True, False),
|
188 |
-
)
|
189 |
-
|
190 |
-
gallery = gr.Gallery(label="Generated images", show_label=False, elem_id="generated_id").style(
|
191 |
-
grid=[2], height="auto"
|
192 |
-
)
|
193 |
-
|
194 |
-
ex = gr.Examples(examples=examples, fn=infer, inputs=[text], outputs=gallery, cache_examples=True)
|
195 |
-
ex.dataset.headers = [""]
|
196 |
-
|
197 |
-
text.submit(infer, inputs=[text], outputs=gallery)
|
198 |
-
btn.click(infer, inputs=[text], outputs=gallery)
|
199 |
-
gr.Markdown("""
|
200 |
-
|
201 |
-
|
202 |
-
# Authors
|
203 |
-
|
204 |
-
+ Arseniy Shakhmatov: [Github](https://github.com/cene555), [Blog](https://t.me/gradientdip)
|
205 |
-
+ Anton Razzhigaev: [Github](https://github.com/razzant), [Blog](https://t.me/abstractDL)
|
206 |
-
+ Aleksandr Nikolich: [Github](https://github.com/AlexWortega), [Blog](https://t.me/lovedeathtransformers)
|
207 |
-
+ Vladimir Arkhipkin: [Github](https://github.com/oriBetelgeuse)
|
208 |
-
+ Igor Pavlov: [Github](https://github.com/boomb0om)
|
209 |
-
+ Andrey Kuznetsov: [Github](https://github.com/kuznetsoffandrey)
|
210 |
-
+ Denis Dimitrov: [Github](https://github.com/denndimitrov)
|
211 |
-
|
212 |
-
"""
|
213 |
-
)
|
214 |
-
|
215 |
-
demo.queue(max_size=25).launch()
|
|
|
|
|
|
|
|
|
|
|
|
|
|
|
|
|
|
|
|
|
|
|
|
|
|
|
|
|
|
|
|
|
|
|
|
|
|
|
|
|
|
|
|
|
|
|
|
|
|
|
|
|
|
|
|
|
|
|
|
|
|
|
|
|
|
|
|
|
|
|
|
|
|
|
|
|
|
|
|
|
|
|
|
|
|
|
|
|
|
|
|
|
|
|
|
|
|
|
|
|
|
|
|
|
|
|
|
|
|
|
|
|
|
|
|
|
|
|
|
|
|
|
|
|
|
|
|
|
|
|
|
|
|
|
|
|
|
|
|
|
|
|
|
|
|
|
|
|
|
|
|
|
|
|
|
|
|
|
|
|
|
|
|
|
|
|
|
|
|
|
|
|
|
|
|
|
|
|
|
|
|
|
|
|
|
|
|
|
|
|
|
|
|
|
|
|
|
|
|
|
|
|
|
|
|
|
|
|
|
|
|
|
|
|
|
|
|
|
|
|
|
|
|
|
|
|
|
|
|
|
|
|
|
|
|
|
|
|
|
|
|
|
|
|
|
|
|
|
|
|
|
|
|
|
|
|
|
|
|
|
|
|
|
|
|
|
|
|
|
|
|
|
|
|
|
|
|
|
|
|
|
|
|
|
|
|
|
|
|
|
|
|
|
|
|
|
|
|
|
|
|
|
|
|
|
|
|
|
|
|
|
|
|
|
|
|
|
|
|
|
|
|
|
|
|
|
|
|
|
|
|
|
|
|
|
|
|
|
|
|
|
|
|
|
|
|
|
|
|
|
|
|
|
|
|
|
|
|
|
|
|
|
|
|
|
|
|
|
|
|
|
|
|
|
|
|
|
|
|
|
|
|
|
|
|
|
|
|
|
|
|
|
|
|
|
|
|
|
|
|
|
|
|
|
|
|
|
|
|
|
|
|
|
|
|
|
|
|
|
|
|
|
|
|
|
|
|
|
|
|
|
spaces/Androidonnxfork/CivitAi-to-Diffusers/diffusers/docs/source/en/using-diffusers/custom_pipeline_examples.md
DELETED
@@ -1,282 +0,0 @@
|
|
1 |
-
<!--Copyright 2023 The HuggingFace Team. All rights reserved.
|
2 |
-
|
3 |
-
Licensed under the Apache License, Version 2.0 (the "License"); you may not use this file except in compliance with
|
4 |
-
the License. You may obtain a copy of the License at
|
5 |
-
|
6 |
-
http://www.apache.org/licenses/LICENSE-2.0
|
7 |
-
|
8 |
-
Unless required by applicable law or agreed to in writing, software distributed under the License is distributed on
|
9 |
-
an "AS IS" BASIS, WITHOUT WARRANTIES OR CONDITIONS OF ANY KIND, either express or implied. See the License for the
|
10 |
-
specific language governing permissions and limitations under the License.
|
11 |
-
-->
|
12 |
-
|
13 |
-
# Community pipelines
|
14 |
-
|
15 |
-
[[open-in-colab]]
|
16 |
-
|
17 |
-
> **For more information about community pipelines, please have a look at [this issue](https://github.com/huggingface/diffusers/issues/841).**
|
18 |
-
|
19 |
-
**Community** examples consist of both inference and training examples that have been added by the community.
|
20 |
-
Please have a look at the following table to get an overview of all community examples. Click on the **Code Example** to get a copy-and-paste ready code example that you can try out.
|
21 |
-
If a community doesn't work as expected, please open an issue and ping the author on it.
|
22 |
-
|
23 |
-
| Example | Description | Code Example | Colab | Author |
|
24 |
-
|:---------------------------------------|:---------------------------------------------------------------------------------------------------------------------------------------------------------------------------------------------------------------------------------------------------------------------------------------------------------------------------------------------------------------------------------------------------------------------------------------------------------------------------------------------------------|:------------------------------------------------------------------|:-------------------------------------------------------------------------------------------------------------------------------------------------------------------------------------------------------------------|-----------------------------------------------------------:|
|
25 |
-
| CLIP Guided Stable Diffusion | Doing CLIP guidance for text to image generation with Stable Diffusion | [CLIP Guided Stable Diffusion](#clip-guided-stable-diffusion) | [](https://colab.research.google.com/github/huggingface/notebooks/blob/main/diffusers/CLIP_Guided_Stable_diffusion_with_diffusers.ipynb) | [Suraj Patil](https://github.com/patil-suraj/) |
|
26 |
-
| One Step U-Net (Dummy) | Example showcasing of how to use Community Pipelines (see https://github.com/huggingface/diffusers/issues/841) | [One Step U-Net](#one-step-unet) | - | [Patrick von Platen](https://github.com/patrickvonplaten/) |
|
27 |
-
| Stable Diffusion Interpolation | Interpolate the latent space of Stable Diffusion between different prompts/seeds | [Stable Diffusion Interpolation](#stable-diffusion-interpolation) | - | [Nate Raw](https://github.com/nateraw/) |
|
28 |
-
| Stable Diffusion Mega | **One** Stable Diffusion Pipeline with all functionalities of [Text2Image](https://github.com/huggingface/diffusers/blob/main/src/diffusers/pipelines/stable_diffusion/pipeline_stable_diffusion.py), [Image2Image](https://github.com/huggingface/diffusers/blob/main/src/diffusers/pipelines/stable_diffusion/pipeline_stable_diffusion_img2img.py) and [Inpainting](https://github.com/huggingface/diffusers/blob/main/src/diffusers/pipelines/stable_diffusion/pipeline_stable_diffusion_inpaint.py) | [Stable Diffusion Mega](#stable-diffusion-mega) | - | [Patrick von Platen](https://github.com/patrickvonplaten/) |
|
29 |
-
| Long Prompt Weighting Stable Diffusion | **One** Stable Diffusion Pipeline without tokens length limit, and support parsing weighting in prompt. | [Long Prompt Weighting Stable Diffusion](#long-prompt-weighting-stable-diffusion) | - | [SkyTNT](https://github.com/SkyTNT) |
|
30 |
-
| Speech to Image | Using automatic-speech-recognition to transcribe text and Stable Diffusion to generate images | [Speech to Image](#speech-to-image) | - | [Mikail Duzenli](https://github.com/MikailINTech)
|
31 |
-
|
32 |
-
To load a custom pipeline you just need to pass the `custom_pipeline` argument to `DiffusionPipeline`, as one of the files in `diffusers/examples/community`. Feel free to send a PR with your own pipelines, we will merge them quickly.
|
33 |
-
```py
|
34 |
-
pipe = DiffusionPipeline.from_pretrained(
|
35 |
-
"CompVis/stable-diffusion-v1-4", custom_pipeline="filename_in_the_community_folder"
|
36 |
-
)
|
37 |
-
```
|
38 |
-
|
39 |
-
## Example usages
|
40 |
-
|
41 |
-
### CLIP Guided Stable Diffusion
|
42 |
-
|
43 |
-
CLIP guided stable diffusion can help to generate more realistic images
|
44 |
-
by guiding stable diffusion at every denoising step with an additional CLIP model.
|
45 |
-
|
46 |
-
The following code requires roughly 12GB of GPU RAM.
|
47 |
-
|
48 |
-
```python
|
49 |
-
from diffusers import DiffusionPipeline
|
50 |
-
from transformers import CLIPImageProcessor, CLIPModel
|
51 |
-
import torch
|
52 |
-
|
53 |
-
|
54 |
-
feature_extractor = CLIPImageProcessor.from_pretrained("laion/CLIP-ViT-B-32-laion2B-s34B-b79K")
|
55 |
-
clip_model = CLIPModel.from_pretrained("laion/CLIP-ViT-B-32-laion2B-s34B-b79K", torch_dtype=torch.float16)
|
56 |
-
|
57 |
-
|
58 |
-
guided_pipeline = DiffusionPipeline.from_pretrained(
|
59 |
-
"CompVis/stable-diffusion-v1-4",
|
60 |
-
custom_pipeline="clip_guided_stable_diffusion",
|
61 |
-
clip_model=clip_model,
|
62 |
-
feature_extractor=feature_extractor,
|
63 |
-
torch_dtype=torch.float16,
|
64 |
-
)
|
65 |
-
guided_pipeline.enable_attention_slicing()
|
66 |
-
guided_pipeline = guided_pipeline.to("cuda")
|
67 |
-
|
68 |
-
prompt = "fantasy book cover, full moon, fantasy forest landscape, golden vector elements, fantasy magic, dark light night, intricate, elegant, sharp focus, illustration, highly detailed, digital painting, concept art, matte, art by WLOP and Artgerm and Albert Bierstadt, masterpiece"
|
69 |
-
|
70 |
-
generator = torch.Generator(device="cuda").manual_seed(0)
|
71 |
-
images = []
|
72 |
-
for i in range(4):
|
73 |
-
image = guided_pipeline(
|
74 |
-
prompt,
|
75 |
-
num_inference_steps=50,
|
76 |
-
guidance_scale=7.5,
|
77 |
-
clip_guidance_scale=100,
|
78 |
-
num_cutouts=4,
|
79 |
-
use_cutouts=False,
|
80 |
-
generator=generator,
|
81 |
-
).images[0]
|
82 |
-
images.append(image)
|
83 |
-
|
84 |
-
# save images locally
|
85 |
-
for i, img in enumerate(images):
|
86 |
-
img.save(f"./clip_guided_sd/image_{i}.png")
|
87 |
-
```
|
88 |
-
|
89 |
-
The `images` list contains a list of PIL images that can be saved locally or displayed directly in a google colab.
|
90 |
-
Generated images tend to be of higher qualtiy than natively using stable diffusion. E.g. the above script generates the following images:
|
91 |
-
|
92 |
-
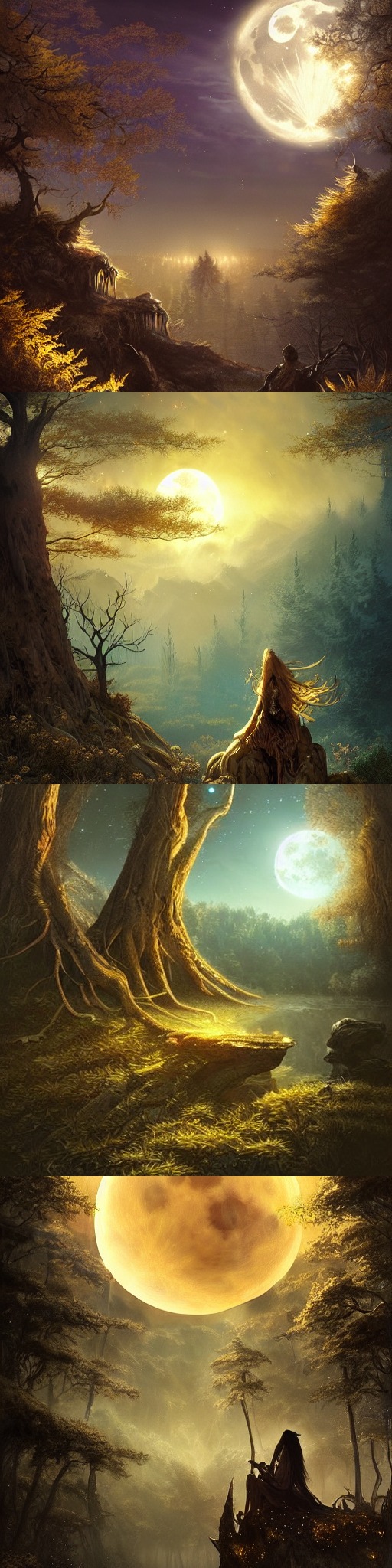.
|
93 |
-
|
94 |
-
### One Step Unet
|
95 |
-
|
96 |
-
The dummy "one-step-unet" can be run as follows:
|
97 |
-
|
98 |
-
```python
|
99 |
-
from diffusers import DiffusionPipeline
|
100 |
-
|
101 |
-
pipe = DiffusionPipeline.from_pretrained("google/ddpm-cifar10-32", custom_pipeline="one_step_unet")
|
102 |
-
pipe()
|
103 |
-
```
|
104 |
-
|
105 |
-
**Note**: This community pipeline is not useful as a feature, but rather just serves as an example of how community pipelines can be added (see https://github.com/huggingface/diffusers/issues/841).
|
106 |
-
|
107 |
-
### Stable Diffusion Interpolation
|
108 |
-
|
109 |
-
The following code can be run on a GPU of at least 8GB VRAM and should take approximately 5 minutes.
|
110 |
-
|
111 |
-
```python
|
112 |
-
from diffusers import DiffusionPipeline
|
113 |
-
import torch
|
114 |
-
|
115 |
-
pipe = DiffusionPipeline.from_pretrained(
|
116 |
-
"CompVis/stable-diffusion-v1-4",
|
117 |
-
torch_dtype=torch.float16,
|
118 |
-
safety_checker=None, # Very important for videos...lots of false positives while interpolating
|
119 |
-
custom_pipeline="interpolate_stable_diffusion",
|
120 |
-
).to("cuda")
|
121 |
-
pipe.enable_attention_slicing()
|
122 |
-
|
123 |
-
frame_filepaths = pipe.walk(
|
124 |
-
prompts=["a dog", "a cat", "a horse"],
|
125 |
-
seeds=[42, 1337, 1234],
|
126 |
-
num_interpolation_steps=16,
|
127 |
-
output_dir="./dreams",
|
128 |
-
batch_size=4,
|
129 |
-
height=512,
|
130 |
-
width=512,
|
131 |
-
guidance_scale=8.5,
|
132 |
-
num_inference_steps=50,
|
133 |
-
)
|
134 |
-
```
|
135 |
-
|
136 |
-
The output of the `walk(...)` function returns a list of images saved under the folder as defined in `output_dir`. You can use these images to create videos of stable diffusion.
|
137 |
-
|
138 |
-
> **Please have a look at https://github.com/nateraw/stable-diffusion-videos for more in-detail information on how to create videos using stable diffusion as well as more feature-complete functionality.**
|
139 |
-
|
140 |
-
### Stable Diffusion Mega
|
141 |
-
|
142 |
-
The Stable Diffusion Mega Pipeline lets you use the main use cases of the stable diffusion pipeline in a single class.
|
143 |
-
|
144 |
-
```python
|
145 |
-
#!/usr/bin/env python3
|
146 |
-
from diffusers import DiffusionPipeline
|
147 |
-
import PIL
|
148 |
-
import requests
|
149 |
-
from io import BytesIO
|
150 |
-
import torch
|
151 |
-
|
152 |
-
|
153 |
-
def download_image(url):
|
154 |
-
response = requests.get(url)
|
155 |
-
return PIL.Image.open(BytesIO(response.content)).convert("RGB")
|
156 |
-
|
157 |
-
|
158 |
-
pipe = DiffusionPipeline.from_pretrained(
|
159 |
-
"CompVis/stable-diffusion-v1-4",
|
160 |
-
custom_pipeline="stable_diffusion_mega",
|
161 |
-
torch_dtype=torch.float16,
|
162 |
-
)
|
163 |
-
pipe.to("cuda")
|
164 |
-
pipe.enable_attention_slicing()
|
165 |
-
|
166 |
-
|
167 |
-
### Text-to-Image
|
168 |
-
|
169 |
-
images = pipe.text2img("An astronaut riding a horse").images
|
170 |
-
|
171 |
-
### Image-to-Image
|
172 |
-
|
173 |
-
init_image = download_image(
|
174 |
-
"https://raw.githubusercontent.com/CompVis/stable-diffusion/main/assets/stable-samples/img2img/sketch-mountains-input.jpg"
|
175 |
-
)
|
176 |
-
|
177 |
-
prompt = "A fantasy landscape, trending on artstation"
|
178 |
-
|
179 |
-
images = pipe.img2img(prompt=prompt, image=init_image, strength=0.75, guidance_scale=7.5).images
|
180 |
-
|
181 |
-
### Inpainting
|
182 |
-
|
183 |
-
img_url = "https://raw.githubusercontent.com/CompVis/latent-diffusion/main/data/inpainting_examples/overture-creations-5sI6fQgYIuo.png"
|
184 |
-
mask_url = "https://raw.githubusercontent.com/CompVis/latent-diffusion/main/data/inpainting_examples/overture-creations-5sI6fQgYIuo_mask.png"
|
185 |
-
init_image = download_image(img_url).resize((512, 512))
|
186 |
-
mask_image = download_image(mask_url).resize((512, 512))
|
187 |
-
|
188 |
-
prompt = "a cat sitting on a bench"
|
189 |
-
images = pipe.inpaint(prompt=prompt, image=init_image, mask_image=mask_image, strength=0.75).images
|
190 |
-
```
|
191 |
-
|
192 |
-
As shown above this one pipeline can run all both "text-to-image", "image-to-image", and "inpainting" in one pipeline.
|
193 |
-
|
194 |
-
### Long Prompt Weighting Stable Diffusion
|
195 |
-
|
196 |
-
The Pipeline lets you input prompt without 77 token length limit. And you can increase words weighting by using "()" or decrease words weighting by using "[]"
|
197 |
-
The Pipeline also lets you use the main use cases of the stable diffusion pipeline in a single class.
|
198 |
-
|
199 |
-
#### pytorch
|
200 |
-
|
201 |
-
```python
|
202 |
-
from diffusers import DiffusionPipeline
|
203 |
-
import torch
|
204 |
-
|
205 |
-
pipe = DiffusionPipeline.from_pretrained(
|
206 |
-
"hakurei/waifu-diffusion", custom_pipeline="lpw_stable_diffusion", torch_dtype=torch.float16
|
207 |
-
)
|
208 |
-
pipe = pipe.to("cuda")
|
209 |
-
|
210 |
-
prompt = "best_quality (1girl:1.3) bow bride brown_hair closed_mouth frilled_bow frilled_hair_tubes frills (full_body:1.3) fox_ear hair_bow hair_tubes happy hood japanese_clothes kimono long_sleeves red_bow smile solo tabi uchikake white_kimono wide_sleeves cherry_blossoms"
|
211 |
-
neg_prompt = "lowres, bad_anatomy, error_body, error_hair, error_arm, error_hands, bad_hands, error_fingers, bad_fingers, missing_fingers, error_legs, bad_legs, multiple_legs, missing_legs, error_lighting, error_shadow, error_reflection, text, error, extra_digit, fewer_digits, cropped, worst_quality, low_quality, normal_quality, jpeg_artifacts, signature, watermark, username, blurry"
|
212 |
-
|
213 |
-
pipe.text2img(prompt, negative_prompt=neg_prompt, width=512, height=512, max_embeddings_multiples=3).images[0]
|
214 |
-
```
|
215 |
-
|
216 |
-
#### onnxruntime
|
217 |
-
|
218 |
-
```python
|
219 |
-
from diffusers import DiffusionPipeline
|
220 |
-
import torch
|
221 |
-
|
222 |
-
pipe = DiffusionPipeline.from_pretrained(
|
223 |
-
"CompVis/stable-diffusion-v1-4",
|
224 |
-
custom_pipeline="lpw_stable_diffusion_onnx",
|
225 |
-
revision="onnx",
|
226 |
-
provider="CUDAExecutionProvider",
|
227 |
-
)
|
228 |
-
|
229 |
-
prompt = "a photo of an astronaut riding a horse on mars, best quality"
|
230 |
-
neg_prompt = "lowres, bad anatomy, error body, error hair, error arm, error hands, bad hands, error fingers, bad fingers, missing fingers, error legs, bad legs, multiple legs, missing legs, error lighting, error shadow, error reflection, text, error, extra digit, fewer digits, cropped, worst quality, low quality, normal quality, jpeg artifacts, signature, watermark, username, blurry"
|
231 |
-
|
232 |
-
pipe.text2img(prompt, negative_prompt=neg_prompt, width=512, height=512, max_embeddings_multiples=3).images[0]
|
233 |
-
```
|
234 |
-
|
235 |
-
if you see `Token indices sequence length is longer than the specified maximum sequence length for this model ( *** > 77 ) . Running this sequence through the model will result in indexing errors`. Do not worry, it is normal.
|
236 |
-
|
237 |
-
### Speech to Image
|
238 |
-
|
239 |
-
The following code can generate an image from an audio sample using pre-trained OpenAI whisper-small and Stable Diffusion.
|
240 |
-
|
241 |
-
```Python
|
242 |
-
import torch
|
243 |
-
|
244 |
-
import matplotlib.pyplot as plt
|
245 |
-
from datasets import load_dataset
|
246 |
-
from diffusers import DiffusionPipeline
|
247 |
-
from transformers import (
|
248 |
-
WhisperForConditionalGeneration,
|
249 |
-
WhisperProcessor,
|
250 |
-
)
|
251 |
-
|
252 |
-
|
253 |
-
device = "cuda" if torch.cuda.is_available() else "cpu"
|
254 |
-
|
255 |
-
ds = load_dataset("hf-internal-testing/librispeech_asr_dummy", "clean", split="validation")
|
256 |
-
|
257 |
-
audio_sample = ds[3]
|
258 |
-
|
259 |
-
text = audio_sample["text"].lower()
|
260 |
-
speech_data = audio_sample["audio"]["array"]
|
261 |
-
|
262 |
-
model = WhisperForConditionalGeneration.from_pretrained("openai/whisper-small").to(device)
|
263 |
-
processor = WhisperProcessor.from_pretrained("openai/whisper-small")
|
264 |
-
|
265 |
-
diffuser_pipeline = DiffusionPipeline.from_pretrained(
|
266 |
-
"CompVis/stable-diffusion-v1-4",
|
267 |
-
custom_pipeline="speech_to_image_diffusion",
|
268 |
-
speech_model=model,
|
269 |
-
speech_processor=processor,
|
270 |
-
|
271 |
-
torch_dtype=torch.float16,
|
272 |
-
)
|
273 |
-
|
274 |
-
diffuser_pipeline.enable_attention_slicing()
|
275 |
-
diffuser_pipeline = diffuser_pipeline.to(device)
|
276 |
-
|
277 |
-
output = diffuser_pipeline(speech_data)
|
278 |
-
plt.imshow(output.images[0])
|
279 |
-
```
|
280 |
-
This example produces the following image:
|
281 |
-
|
282 |
-
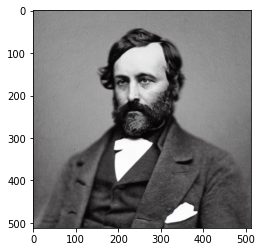
|
|
|
|
|
|
|
|
|
|
|
|
|
|
|
|
|
|
|
|
|
|
|
|
|
|
|
|
|
|
|
|
|
|
|
|
|
|
|
|
|
|
|
|
|
|
|
|
|
|
|
|
|
|
|
|
|
|
|
|
|
|
|
|
|
|
|
|
|
|
|
|
|
|
|
|
|
|
|
|
|
|
|
|
|
|
|
|
|
|
|
|
|
|
|
|
|
|
|
|
|
|
|
|
|
|
|
|
|
|
|
|
|
|
|
|
|
|
|
|
|
|
|
|
|
|
|
|
|
|
|
|
|
|
|
|
|
|
|
|
|
|
|
|
|
|
|
|
|
|
|
|
|
|
|
|
|
|
|
|
|
|
|
|
|
|
|
|
|
|
|
|
|
|
|
|
|
|
|
|
|
|
|
|
|
|
|
|
|
|
|
|
|
|
|
|
|
|
|
|
|
|
|
|
|
|
|
|
|
|
|
|
|
|
|
|
|
|
|
|
|
|
|
|
|
|
|
|
|
|
|
|
|
|
|
|
|
|
|
|
|
|
|
|
|
|
|
|
|
|
|
|
|
|
|
|
|
|
|
|
|
|
|
|
|
|
|
|
|
|
|
|
|
|
|
|
|
|
|
|
|
|
|
|
|
|
|
|
|
|
|
|
|
|
|
|
|
|
|
|
|
|
|
|
|
|
|
|
|
|
|
|
|
|
|
|
|
|
|
|
|
|
|
|
|
|
|
|
|
|
|
|
|
|
|
|
|
|
|
|
|
|
|
|
|
|
|
|
|
|
|
|
|
|
|
|
|
|
|
|
|
|
|
|
|
|
|
|
|
|
|
|
|
|
|
|
|
|
|
|
|
|
|
|
|
|
|
|
|
|
|
|
|
|
|
|
|
|
|
|
|
|
|
|
|
|
|
|
|
|
|
|
|
|
|
|
|
|
|
|
|
|
|
|
|
|
|
|
|
|
|
|
|
|
|
|
|
|
|
|
|
|
|
|
|
|
|
|
|
|
|
|
|
|
|
|
|
|
|
|
|
|
|
|
|
|
|
|
|
|
|
|
|
|
|
|
|
|
|
|
|
|
|
|
|
|
|
|
|
|
|
|
|
|
|
|
|
|
|
|
|
|
|
|
|
|
|
|
|
|
|
|
|
|
|
|
|
|
|
|
|
|
|
|
|
|
|
|
|
|
|
|
|
|
|
|
|
|
|
|
|
|
|
|
|
|
|
|
|
|
|
|
|
|
|
|
|
|
|
|
|
|
|
|
|
|
spaces/Androidonnxfork/CivitAi-to-Diffusers/diffusers/docs/source/ko/training/text_inversion.md
DELETED
@@ -1,275 +0,0 @@
|
|
1 |
-
<!--Copyright 2023 The HuggingFace Team. All rights reserved.
|
2 |
-
|
3 |
-
Licensed under the Apache License, Version 2.0 (the "License"); you may not use this file except in compliance with
|
4 |
-
the License. You may obtain a copy of the License at
|
5 |
-
|
6 |
-
http://www.apache.org/licenses/LICENSE-2.0
|
7 |
-
|
8 |
-
Unless required by applicable law or agreed to in writing, software distributed under the License is distributed on
|
9 |
-
an "AS IS" BASIS, WITHOUT WARRANTIES OR CONDITIONS OF ANY KIND, either express or implied. See the License for the
|
10 |
-
specific language governing permissions and limitations under the License.
|
11 |
-
-->
|
12 |
-
|
13 |
-
|
14 |
-
|
15 |
-
# Textual-Inversion
|
16 |
-
|
17 |
-
[[open-in-colab]]
|
18 |
-
|
19 |
-
[textual-inversion](https://arxiv.org/abs/2208.01618)은 소수의 예시 이미지에서 새로운 콘셉트를 포착하는 기법입니다. 이 기술은 원래 [Latent Diffusion](https://github.com/CompVis/latent-diffusion)에서 시연되었지만, 이후 [Stable Diffusion](https://huggingface.co/docs/diffusers/main/en/conceptual/stable_diffusion)과 같은 유사한 다른 모델에도 적용되었습니다. 학습된 콘셉트는 text-to-image 파이프라인에서 생성된 이미지를 더 잘 제어하는 데 사용할 수 있습니다. 이 모델은 텍스트 인코더의 임베딩 공간에서 새로운 '단어'를 학습하여 개인화된 이미지 생성을 위한 텍스트 프롬프트 내에서 사용됩니다.
|
20 |
-
|
21 |
-
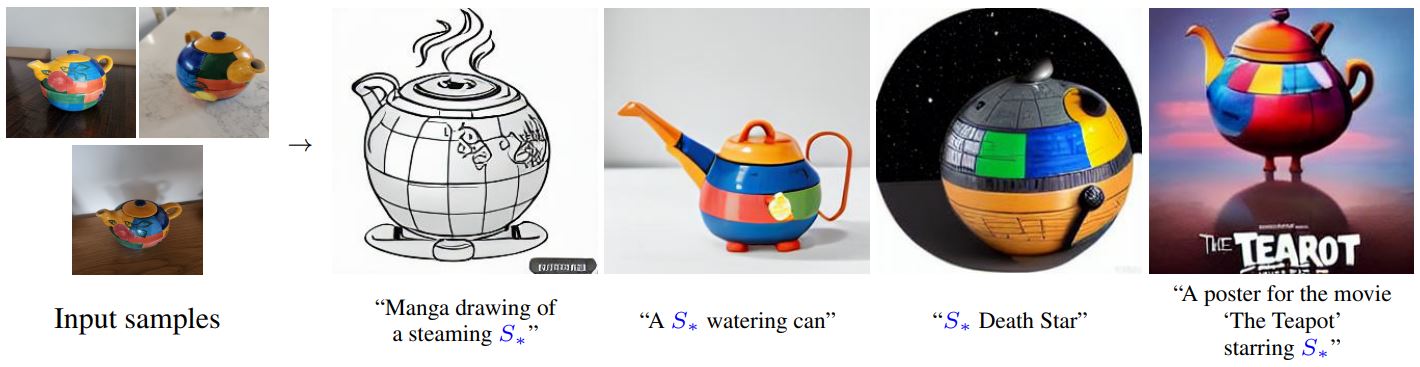
|
22 |
-
<small>By using just 3-5 images you can teach new concepts to a model such as Stable Diffusion for personalized image generation <a href="https://github.com/rinongal/textual_inversion">(image source)</a>.</small>
|
23 |
-
|
24 |
-
이 가이드에서는 textual-inversion으로 [`runwayml/stable-diffusion-v1-5`](https://huggingface.co/runwayml/stable-diffusion-v1-5) 모델을 학습하는 방법을 설명합니다. 이 가이드에서 사용된 모든 textual-inversion 학습 스크립트는 [여기](https://github.com/huggingface/diffusers/tree/main/examples/textual_inversion)에서 확인할 수 있습니다. 내부적으로 어떻게 작동하는지 자세히 살펴보고 싶으시다면 해당 링크를 참조해주시기 바랍니다.
|
25 |
-
|
26 |
-
<Tip>
|
27 |
-
|
28 |
-
[Stable Diffusion Textual Inversion Concepts Library](https://huggingface.co/sd-concepts-library)에는 커뮤니티에서 제작한 학습된 textual-inversion 모델들이 있습니다. 시간이 지남에 따라 더 많은 콘셉트들이 추가되어 유용한 리소스로 성장할 것입니다!
|
29 |
-
|
30 |
-
</Tip>
|
31 |
-
|
32 |
-
시작하기 전에 학습을 위한 의존성 라이브러리들을 설치해야 합니다:
|
33 |
-
|
34 |
-
```bash
|
35 |
-
pip install diffusers accelerate transformers
|
36 |
-
```
|
37 |
-
|
38 |
-
의존성 라이브러리들의 설치가 완료되면, [🤗Accelerate](https://github.com/huggingface/accelerate/) 환경을 초기화시킵니다.
|
39 |
-
|
40 |
-
```bash
|
41 |
-
accelerate config
|
42 |
-
```
|
43 |
-
|
44 |
-
별도의 설정없이, 기본 🤗Accelerate 환경을 설정하려면 다음과 같이 하세요:
|
45 |
-
|
46 |
-
```bash
|
47 |
-
accelerate config default
|
48 |
-
```
|
49 |
-
|
50 |
-
또는 사용 중인 환경이 노트북과 같은 대화형 셸을 지원하지 않는다면, 다음과 같이 사용할 수 있습니다:
|
51 |
-
|
52 |
-
```py
|
53 |
-
from accelerate.utils import write_basic_config
|
54 |
-
|
55 |
-
write_basic_config()
|
56 |
-
```
|
57 |
-
|
58 |
-
마지막으로, Memory-Efficient Attention을 통해 메모리 사용량을 줄이기 위해 [xFormers](https://huggingface.co/docs/diffusers/main/en/training/optimization/xformers)를 설치합니다. xFormers를 설치한 후, 학습 스크립트에 `--enable_xformers_memory_efficient_attention` 인자를 추가합니다. xFormers는 Flax에서 지원되지 않습니다.
|
59 |
-
|
60 |
-
## 허브에 모델 업로드하기
|
61 |
-
|
62 |
-
모델을 허브에 저장하려면, 학습 스크립트에 다음 인자를 추가해야 합니다.
|
63 |
-
|
64 |
-
```bash
|
65 |
-
--push_to_hub
|
66 |
-
```
|
67 |
-
|
68 |
-
## 체크포인트 저장 및 불러오기
|
69 |
-
|
70 |
-
학습중에 모델의 체크포인트를 정기적으로 저장하는 것이 좋습니다. 이렇게 하면 어떤 이유로든 학습이 중단된 경우 저장된 체크포인트에서 학습을 다시 시작할 수 있습니다. 학습 스크립트에 다음 인자를 전달하면 500단계마다 전체 학습 상태가 `output_dir`의 하위 폴더에 체크포인트로서 저장됩니다.
|
71 |
-
|
72 |
-
```bash
|
73 |
-
--checkpointing_steps=500
|
74 |
-
```
|
75 |
-
|
76 |
-
저장된 체크포인트에서 학습을 재개하려면, 학습 스크립트와 재개할 특정 체크포인트에 다음 인자를 전달하세요.
|
77 |
-
|
78 |
-
```bash
|
79 |
-
--resume_from_checkpoint="checkpoint-1500"
|
80 |
-
```
|
81 |
-
|
82 |
-
## 파인 튜닝
|
83 |
-
|
84 |
-
학습용 데이터셋으로 [고양이 장난감 데이터셋](https://huggingface.co/datasets/diffusers/cat_toy_example)을 다운로드하여 디렉토리에 저장하세요. 여러분만의 고유한 데이터셋을 사용하고자 한다면, [학습용 데이터셋 만들기](https://huggingface.co/docs/diffusers/training/create_dataset) 가이드를 살펴보시기 바랍니다.
|
85 |
-
|
86 |
-
```py
|
87 |
-
from huggingface_hub import snapshot_download
|
88 |
-
|
89 |
-
local_dir = "./cat"
|
90 |
-
snapshot_download(
|
91 |
-
"diffusers/cat_toy_example", local_dir=local_dir, repo_type="dataset", ignore_patterns=".gitattributes"
|
92 |
-
)
|
93 |
-
```
|
94 |
-
|
95 |
-
모델의 리포지토리 ID(또는 모델 가중치가 포함된 디렉터리 경로)를 `MODEL_NAME` 환경 변수에 할당하고, 해당 값을 [`pretrained_model_name_or_path`](https://huggingface.co/docs/diffusers/en/api/diffusion_pipeline#diffusers.DiffusionPipeline.from_pretrained.pretrained_model_name_or_path) 인자에 전달합니다. 그리고 이미지가 포함된 디렉터리 경로를 `DATA_DIR` 환경 변수에 할당합니다.
|
96 |
-
|
97 |
-
이제 [학습 스크립트](https://github.com/huggingface/diffusers/blob/main/examples/textual_inversion/textual_inversion.py)를 실행할 수 있습니다. 스크립트는 다음 파일을 생성하고 리포지토리에 저장합니다.
|
98 |
-
|
99 |
-
- `learned_embeds.bin`
|
100 |
-
- `token_identifier.txt`
|
101 |
-
- `type_of_concept.txt`.
|
102 |
-
|
103 |
-
<Tip>
|
104 |
-
|
105 |
-
💡V100 GPU 1개를 기준으로 전체 학습에는 최대 1시간이 걸립니다. 학습이 완료되기를 기다리는 동안 궁금한 점이 있으면 아래 섹션에서 [textual-inversion이 어떻게 작동하는지](https://huggingface.co/docs/diffusers/training/text_inversion#how-it-works) 자유롭게 확인하세요 !
|
106 |
-
|
107 |
-
</Tip>
|
108 |
-
|
109 |
-
<frameworkcontent>
|
110 |
-
<pt>
|
111 |
-
```bash
|
112 |
-
export MODEL_NAME="runwayml/stable-diffusion-v1-5"
|
113 |
-
export DATA_DIR="./cat"
|
114 |
-
|
115 |
-
accelerate launch textual_inversion.py \
|
116 |
-
--pretrained_model_name_or_path=$MODEL_NAME \
|
117 |
-
--train_data_dir=$DATA_DIR \
|
118 |
-
--learnable_property="object" \
|
119 |
-
--placeholder_token="<cat-toy>" --initializer_token="toy" \
|
120 |
-
--resolution=512 \
|
121 |
-
--train_batch_size=1 \
|
122 |
-
--gradient_accumulation_steps=4 \
|
123 |
-
--max_train_steps=3000 \
|
124 |
-
--learning_rate=5.0e-04 --scale_lr \
|
125 |
-
--lr_scheduler="constant" \
|
126 |
-
--lr_warmup_steps=0 \
|
127 |
-
--output_dir="textual_inversion_cat" \
|
128 |
-
--push_to_hub
|
129 |
-
```
|
130 |
-
|
131 |
-
<Tip>
|
132 |
-
|
133 |
-
💡학습 성능을 올리기 위해, 플레이스홀더 토큰(`<cat-toy>`)을 (단일한 임베딩 벡터가 아닌) 복수의 임베딩 벡터로 표현하는 것 역시 고려할 있습니다. 이러한 트릭이 모델이 보다 복잡한 이미지의 스타일(앞서 말한 콘셉트)을 더 잘 캡처하는 데 도움이 될 수 있습니다. 복수의 임베딩 벡터 학습을 활성화하려면 다음 옵션을 전달하십시오.
|
134 |
-
|
135 |
-
```bash
|
136 |
-
--num_vectors=5
|
137 |
-
```
|
138 |
-
|
139 |
-
</Tip>
|
140 |
-
</pt>
|
141 |
-
<jax>
|
142 |
-
|
143 |
-
TPU에 액세스할 수 있는 경우, [Flax 학습 스크립트](https://github.com/huggingface/diffusers/blob/main/examples/textual_inversion/textual_inversion_flax.py)를 사용하여 더 빠르게 모델을 학습시켜보세요. (물론 GPU에서도 작동합니다.) 동일한 설정에서 Flax 학습 스크립트는 PyTorch 학습 스크립트보다 최소 70% 더 빨라야 합니다! ⚡️
|
144 |
-
|
145 |
-
시작하기 앞서 Flax에 대한 의존성 라이브러리들을 설치해야 합니다.
|
146 |
-
|
147 |
-
```bash
|
148 |
-
pip install -U -r requirements_flax.txt
|
149 |
-
```
|
150 |
-
|
151 |
-
모델의 리포지토리 ID(또는 모델 가중치가 포함된 디렉터리 경로)를 `MODEL_NAME` 환경 변수에 할당하고, 해당 값을 [`pretrained_model_name_or_path`](https://huggingface.co/docs/diffusers/en/api/diffusion_pipeline#diffusers.DiffusionPipeline.from_pretrained.pretrained_model_name_or_path) 인자에 전달합니다.
|
152 |
-
|
153 |
-
그런 다음 [학습 스크립트](https://github.com/huggingface/diffusers/blob/main/examples/textual_inversion/textual_inversion_flax.py)를 시작할 수 있습니다.
|
154 |
-
|
155 |
-
```bash
|
156 |
-
export MODEL_NAME="duongna/stable-diffusion-v1-4-flax"
|
157 |
-
export DATA_DIR="./cat"
|
158 |
-
|
159 |
-
python textual_inversion_flax.py \
|
160 |
-
--pretrained_model_name_or_path=$MODEL_NAME \
|
161 |
-
--train_data_dir=$DATA_DIR \
|
162 |
-
--learnable_property="object" \
|
163 |
-
--placeholder_token="<cat-toy>" --initializer_token="toy" \
|
164 |
-
--resolution=512 \
|
165 |
-
--train_batch_size=1 \
|
166 |
-
--max_train_steps=3000 \
|
167 |
-
--learning_rate=5.0e-04 --scale_lr \
|
168 |
-
--output_dir="textual_inversion_cat" \
|
169 |
-
--push_to_hub
|
170 |
-
```
|
171 |
-
</jax>
|
172 |
-
</frameworkcontent>
|
173 |
-
|
174 |
-
### 중간 로깅
|
175 |
-
|
176 |
-
모델의 학습 진행 상황을 추적하는 데 관심이 있는 경우, 학습 과정에서 생성된 이미지를 저장할 수 있습니다. 학습 스크립트에 다음 인수를 추가하여 중간 로깅을 활성화합니다.
|
177 |
-
|
178 |
-
- `validation_prompt` : 샘플을 생성하는 데 사용되는 프롬프트(기본값은 `None`으로 설정되며, 이 때 중간 로깅은 비활성화됨)
|
179 |
-
- `num_validation_images` : 생성할 샘플 이미지 수
|
180 |
-
- `validation_steps` : `validation_prompt`로부터 샘플 이미지를 생성하기 전 스텝의 수
|
181 |
-
|
182 |
-
```bash
|
183 |
-
--validation_prompt="A <cat-toy> backpack"
|
184 |
-
--num_validation_images=4
|
185 |
-
--validation_steps=100
|
186 |
-
```
|
187 |
-
|
188 |
-
## 추론
|
189 |
-
|
190 |
-
모델을 학습한 후에는, 해당 모델을 [`StableDiffusionPipeline`]을 사용하여 추론에 사용할 수 있습니다.
|
191 |
-
|
192 |
-
textual-inversion 스크립트는 기본적으로 textual-inversion을 통해 얻어진 임베딩 벡터만을 저장합니다. 해당 임베딩 벡터들은 텍스트 인코더의 임베딩 행렬에 추가되어 있습습니다.
|
193 |
-
|
194 |
-
<frameworkcontent>
|
195 |
-
<pt>
|
196 |
-
<Tip>
|
197 |
-
|
198 |
-
💡 커뮤니티는 [sd-concepts-library](https://huggingface.co/sd-concepts-library) 라는 대규모의 textual-inversion 임베딩 벡터 라이브러리를 만들었습니다. textual-inversion 임베딩을 밑바닥부터 학습하는 대신, 해당 라이브러리에 본인이 찾는 textual-inversion 임베딩이 이미 추가되어 있지 않은지를 확인하는 것도 좋은 방법이 될 것 같습니다.
|
199 |
-
|
200 |
-
</Tip>
|
201 |
-
|
202 |
-
textual-inversion 임베딩 벡터을 불러오기 위해서는, 먼저 해당 임베딩 벡터를 학습할 때 사용한 모델을 불러와야 합니다. 여기서는 [`runwayml/stable-diffusion-v1-5`](https://huggingface.co/docs/diffusers/training/runwayml/stable-diffusion-v1-5) 모델이 사용되었다고 가정하고 불러오겠습니다.
|
203 |
-
|
204 |
-
```python
|
205 |
-
from diffusers import StableDiffusionPipeline
|
206 |
-
import torch
|
207 |
-
|
208 |
-
model_id = "runwayml/stable-diffusion-v1-5"
|
209 |
-
pipe = StableDiffusionPipeline.from_pretrained(model_id, torch_dtype=torch.float16).to("cuda")
|
210 |
-
```
|
211 |
-
|
212 |
-
다음으로 `TextualInversionLoaderMixin.load_textual_inversion` 함수를 통해, textual-inversion 임베딩 벡터를 불러와야 합니다. 여기서 우리는 이전의 `<cat-toy>` 예제의 임베딩을 불러올 것입니다.
|
213 |
-
|
214 |
-
```python
|
215 |
-
pipe.load_textual_inversion("sd-concepts-library/cat-toy")
|
216 |
-
```
|
217 |
-
|
218 |
-
이제 플레이스홀더 토큰(`<cat-toy>`)이 잘 동작하는지를 확인하는 파이프라인을 실행할 수 있습니다.
|
219 |
-
|
220 |
-
```python
|
221 |
-
prompt = "A <cat-toy> backpack"
|
222 |
-
|
223 |
-
image = pipe(prompt, num_inference_steps=50).images[0]
|
224 |
-
image.save("cat-backpack.png")
|
225 |
-
```
|
226 |
-
|
227 |
-
`TextualInversionLoaderMixin.load_textual_inversion`은 Diffusers 형식으로 저장된 텍스트 임베딩 벡터를 로드할 수 있을 뿐만 아니라, [Automatic1111](https://github.com/AUTOMATIC1111/stable-diffusion-webui) 형식으로 저장된 임베딩 벡터도 로드할 수 있습니다. 이렇게 하려면, 먼저 [civitAI](https://civitai.com/models/3036?modelVersionId=8387)에서 임베딩 벡터를 다운로드한 다음 로컬에서 불러와야 합니다.
|
228 |
-
|
229 |
-
```python
|
230 |
-
pipe.load_textual_inversion("./charturnerv2.pt")
|
231 |
-
```
|
232 |
-
</pt>
|
233 |
-
<jax>
|
234 |
-
|
235 |
-
현재 Flax에 대한 `load_textual_inversion` 함수는 없습니다. 따라서 학습 후 textual-inversion 임베딩 벡터가 모델의 일부로서 저장되었는지를 확인해야 합니다. 그런 다음은 다른 Flax 모델과 마찬가지로 실행할 수 있습니다.
|
236 |
-
|
237 |
-
```python
|
238 |
-
import jax
|
239 |
-
import numpy as np
|
240 |
-
from flax.jax_utils import replicate
|
241 |
-
from flax.training.common_utils import shard
|
242 |
-
from diffusers import FlaxStableDiffusionPipeline
|
243 |
-
|
244 |
-
model_path = "path-to-your-trained-model"
|
245 |
-
pipeline, params = FlaxStableDiffusionPipeline.from_pretrained(model_path, dtype=jax.numpy.bfloat16)
|
246 |
-
|
247 |
-
prompt = "A <cat-toy> backpack"
|
248 |
-
prng_seed = jax.random.PRNGKey(0)
|
249 |
-
num_inference_steps = 50
|
250 |
-
|
251 |
-
num_samples = jax.device_count()
|
252 |
-
prompt = num_samples * [prompt]
|
253 |
-
prompt_ids = pipeline.prepare_inputs(prompt)
|
254 |
-
|
255 |
-
# shard inputs and rng
|
256 |
-
params = replicate(params)
|
257 |
-
prng_seed = jax.random.split(prng_seed, jax.device_count())
|
258 |
-
prompt_ids = shard(prompt_ids)
|
259 |
-
|
260 |
-
images = pipeline(prompt_ids, params, prng_seed, num_inference_steps, jit=True).images
|
261 |
-
images = pipeline.numpy_to_pil(np.asarray(images.reshape((num_samples,) + images.shape[-3:])))
|
262 |
-
image.save("cat-backpack.png")
|
263 |
-
```
|
264 |
-
</jax>
|
265 |
-
</frameworkcontent>
|
266 |
-
|
267 |
-
## 작동 방식
|
268 |
-
|
269 |
-
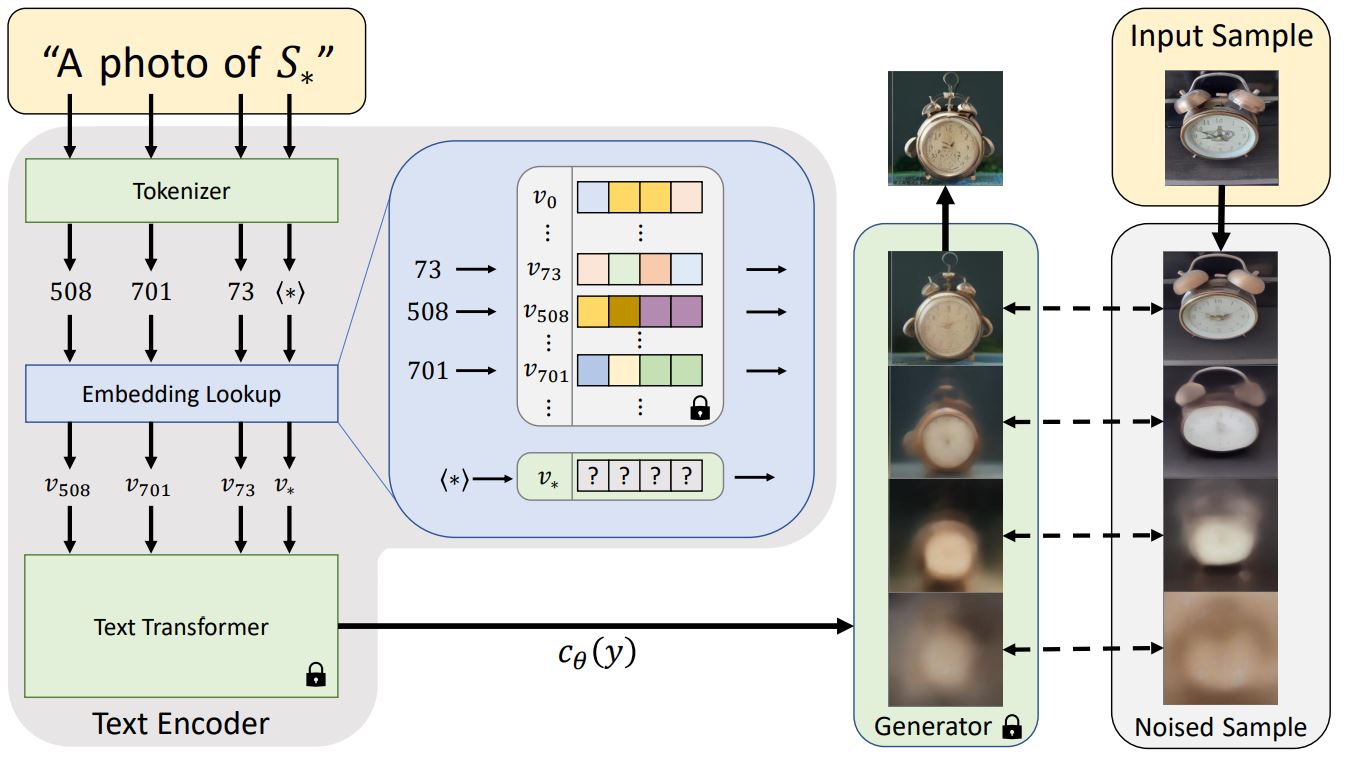
|
270 |
-
<small>Architecture overview from the Textual Inversion <a href="https://textual-inversion.github.io/">blog post.</a></small>
|
271 |
-
|
272 |
-
일반적으로 텍스트 프롬프트는 모델에 전달되기 전에 임베딩으로 토큰화됩니다. textual-inversion은 비슷한 작업을 수행하지만, 위 다이어그램의 특수 토큰 `S*`로부터 새로운 토큰 임베딩 `v*`를 학습합니다. 모델의 아웃풋은 디퓨전 모델을 조정하는 데 사용되며, 디퓨전 모델이 단 몇 개의 예제 이미지에서 신속하고 새로운 콘셉트를 이해하는 데 도움을 줍니다.
|
273 |
-
|
274 |
-
이를 위해 textual-inversion은 제너레이터 모델과 학습용 이미지의 노이즈 버전을 사용합니다. 제너레이터는 노이즈가 적은 버전의 이미지를 예측하려고 시도하며 토큰 임베딩 `v*`은 제너레이터의 성능에 따라 최적화됩니다. 토큰 임베딩이 새로운 콘셉트를 성공적으로 포착하면 디퓨전 모델에 더 유용한 정보를 제공하고 노이즈가 적은 더 선명한 이미지를 생성하는 데 도움이 됩니다. 이러한 최적화 프로세스는 일반적으로 다양한 프롬프트와 이미지에 수천 번에 노출됨으로써 이루어집니다.
|
275 |
-
|
|
|
|
|
|
|
|
|
|
|
|
|
|
|
|
|
|
|
|
|
|
|
|
|
|
|
|
|
|
|
|
|
|
|
|
|
|
|
|
|
|
|
|
|
|
|
|
|
|
|
|
|
|
|
|
|
|
|
|
|
|
|
|
|
|
|
|
|
|
|
|
|
|
|
|
|
|
|
|
|
|
|
|
|
|
|
|
|
|
|
|
|
|
|
|
|
|
|
|
|
|
|
|
|
|
|
|
|
|
|
|
|
|
|
|
|
|
|
|
|
|
|
|
|
|
|
|
|
|
|
|
|
|
|
|
|
|
|
|
|
|
|
|
|
|
|
|
|
|
|
|
|
|
|
|
|
|
|
|
|
|
|
|
|
|
|
|
|
|
|
|
|
|
|
|
|
|
|
|
|
|
|
|
|
|
|
|
|
|
|
|
|
|
|
|
|
|
|
|
|
|
|
|
|
|
|
|
|
|
|
|
|
|
|
|
|
|
|
|
|
|
|
|
|
|
|
|
|
|
|
|
|
|
|
|
|
|
|
|
|
|
|
|
|
|
|
|
|
|
|
|
|
|
|
|
|
|
|
|
|
|
|
|
|
|
|
|
|
|
|
|
|
|
|
|
|
|
|
|
|
|
|
|
|
|
|
|
|
|
|
|
|
|
|
|
|
|
|
|
|
|
|
|
|
|
|
|
|
|
|
|
|
|
|
|
|
|
|
|
|
|
|
|
|
|
|
|
|
|
|
|
|
|
|
|
|
|
|
|
|
|
|
|
|
|
|
|
|
|
|
|
|
|
|
|
|
|
|
|
|
|
|
|
|
|
|
|
|
|
|
|
|
|
|
|
|
|
|
|
|
|
|
|
|
|
|
|
|
|
|
|
|
|
|
|
|
|
|
|
|
|
|
|
|
|
|
|
|
|
|
|
|
|
|
|
|
|
|
|
|
|
|
|
|
|
|
|
|
|
|
|
|
|
|
|
|
|
|
|
|
|
|
|
|
|
|
|
|
|
|
|
|
|
|
|
|
|
|
|
|
|
|
|
|
|
|
|
|
|
|
|
|
|
|
|
|
|
|
|
|
|
|
|
|
|
|
|
|
|
|
|
|
|
|
|
|
|
|
|
|
|
|
|
|
|
|
|
|
|
|
|
|
|
|
|
|
|
|
|
|
|
|
|
|
|
|
|
|
|
|
|
|
|
|
|
|
|
|
|
|
|
|
|
|
|
|
|
|
|
|
|
spaces/Androidonnxfork/CivitAi-to-Diffusers/diffusers/tests/pipelines/stable_diffusion/test_stable_diffusion_inpaint_legacy.py
DELETED
@@ -1,621 +0,0 @@
|
|
1 |
-
# coding=utf-8
|
2 |
-
# Copyright 2023 HuggingFace Inc.
|
3 |
-
#
|
4 |
-
# Licensed under the Apache License, Version 2.0 (the "License");
|
5 |
-
# you may not use this file except in compliance with the License.
|
6 |
-
# You may obtain a copy of the License at
|
7 |
-
#
|
8 |
-
# http://www.apache.org/licenses/LICENSE-2.0
|
9 |
-
#
|
10 |
-
# Unless required by applicable law or agreed to in writing, software
|
11 |
-
# distributed under the License is distributed on an "AS IS" BASIS,
|
12 |
-
# WITHOUT WARRANTIES OR CONDITIONS OF ANY KIND, either express or implied.
|
13 |
-
# See the License for the specific language governing permissions and
|
14 |
-
# limitations under the License.
|
15 |
-
|
16 |
-
import gc
|
17 |
-
import random
|
18 |
-
import unittest
|
19 |
-
|
20 |
-
import numpy as np
|
21 |
-
import torch
|
22 |
-
from PIL import Image
|
23 |
-
from transformers import CLIPTextConfig, CLIPTextModel, CLIPTokenizer
|
24 |
-
|
25 |
-
from diffusers import (
|
26 |
-
AutoencoderKL,
|
27 |
-
DDIMScheduler,
|
28 |
-
DPMSolverMultistepScheduler,
|
29 |
-
LMSDiscreteScheduler,
|
30 |
-
PNDMScheduler,
|
31 |
-
StableDiffusionInpaintPipelineLegacy,
|
32 |
-
UNet2DConditionModel,
|
33 |
-
UNet2DModel,
|
34 |
-
VQModel,
|
35 |
-
)
|
36 |
-
from diffusers.utils import floats_tensor, load_image, nightly, slow, torch_device
|
37 |
-
from diffusers.utils.testing_utils import enable_full_determinism, load_numpy, preprocess_image, require_torch_gpu
|
38 |
-
|
39 |
-
|
40 |
-
enable_full_determinism()
|
41 |
-
|
42 |
-
|
43 |
-
class StableDiffusionInpaintLegacyPipelineFastTests(unittest.TestCase):
|
44 |
-
def tearDown(self):
|
45 |
-
# clean up the VRAM after each test
|
46 |
-
super().tearDown()
|
47 |
-
gc.collect()
|
48 |
-
torch.cuda.empty_cache()
|
49 |
-
|
50 |
-
@property
|
51 |
-
def dummy_image(self):
|
52 |
-
batch_size = 1
|
53 |
-
num_channels = 3
|
54 |
-
sizes = (32, 32)
|
55 |
-
|
56 |
-
image = floats_tensor((batch_size, num_channels) + sizes, rng=random.Random(0)).to(torch_device)
|
57 |
-
return image
|
58 |
-
|
59 |
-
@property
|
60 |
-
def dummy_uncond_unet(self):
|
61 |
-
torch.manual_seed(0)
|
62 |
-
model = UNet2DModel(
|
63 |
-
block_out_channels=(32, 64),
|
64 |
-
layers_per_block=2,
|
65 |
-
sample_size=32,
|
66 |
-
in_channels=3,
|
67 |
-
out_channels=3,
|
68 |
-
down_block_types=("DownBlock2D", "AttnDownBlock2D"),
|
69 |
-
up_block_types=("AttnUpBlock2D", "UpBlock2D"),
|
70 |
-
)
|
71 |
-
return model
|
72 |
-
|
73 |
-
@property
|
74 |
-
def dummy_cond_unet(self):
|
75 |
-
torch.manual_seed(0)
|
76 |
-
model = UNet2DConditionModel(
|
77 |
-
block_out_channels=(32, 64),
|
78 |
-
layers_per_block=2,
|
79 |
-
sample_size=32,
|
80 |
-
in_channels=4,
|
81 |
-
out_channels=4,
|
82 |
-
down_block_types=("DownBlock2D", "CrossAttnDownBlock2D"),
|
83 |
-
up_block_types=("CrossAttnUpBlock2D", "UpBlock2D"),
|
84 |
-
cross_attention_dim=32,
|
85 |
-
)
|
86 |
-
return model
|
87 |
-
|
88 |
-
@property
|
89 |
-
def dummy_cond_unet_inpaint(self):
|
90 |
-
torch.manual_seed(0)
|
91 |
-
model = UNet2DConditionModel(
|
92 |
-
block_out_channels=(32, 64),
|
93 |
-
layers_per_block=2,
|
94 |
-
sample_size=32,
|
95 |
-
in_channels=9,
|
96 |
-
out_channels=4,
|
97 |
-
down_block_types=("DownBlock2D", "CrossAttnDownBlock2D"),
|
98 |
-
up_block_types=("CrossAttnUpBlock2D", "UpBlock2D"),
|
99 |
-
cross_attention_dim=32,
|
100 |
-
)
|
101 |
-
return model
|
102 |
-
|
103 |
-
@property
|
104 |
-
def dummy_vq_model(self):
|
105 |
-
torch.manual_seed(0)
|
106 |
-
model = VQModel(
|
107 |
-
block_out_channels=[32, 64],
|
108 |
-
in_channels=3,
|
109 |
-
out_channels=3,
|
110 |
-
down_block_types=["DownEncoderBlock2D", "DownEncoderBlock2D"],
|
111 |
-
up_block_types=["UpDecoderBlock2D", "UpDecoderBlock2D"],
|
112 |
-
latent_channels=3,
|
113 |
-
)
|
114 |
-
return model
|
115 |
-
|
116 |
-
@property
|
117 |
-
def dummy_vae(self):
|
118 |
-
torch.manual_seed(0)
|
119 |
-
model = AutoencoderKL(
|
120 |
-
block_out_channels=[32, 64],
|
121 |
-
in_channels=3,
|
122 |
-
out_channels=3,
|
123 |
-
down_block_types=["DownEncoderBlock2D", "DownEncoderBlock2D"],
|
124 |
-
up_block_types=["UpDecoderBlock2D", "UpDecoderBlock2D"],
|
125 |
-
latent_channels=4,
|
126 |
-
)
|
127 |
-
return model
|
128 |
-
|
129 |
-
@property
|
130 |
-
def dummy_text_encoder(self):
|
131 |
-
torch.manual_seed(0)
|
132 |
-
config = CLIPTextConfig(
|
133 |
-
bos_token_id=0,
|
134 |
-
eos_token_id=2,
|
135 |
-
hidden_size=32,
|
136 |
-
intermediate_size=37,
|
137 |
-
layer_norm_eps=1e-05,
|
138 |
-
num_attention_heads=4,
|
139 |
-
num_hidden_layers=5,
|
140 |
-
pad_token_id=1,
|
141 |
-
vocab_size=1000,
|
142 |
-
)
|
143 |
-
return CLIPTextModel(config)
|
144 |
-
|
145 |
-
@property
|
146 |
-
def dummy_extractor(self):
|
147 |
-
def extract(*args, **kwargs):
|
148 |
-
class Out:
|
149 |
-
def __init__(self):
|
150 |
-
self.pixel_values = torch.ones([0])
|
151 |
-
|
152 |
-
def to(self, device):
|
153 |
-
self.pixel_values.to(device)
|
154 |
-
return self
|
155 |
-
|
156 |
-
return Out()
|
157 |
-
|
158 |
-
return extract
|
159 |
-
|
160 |
-
def test_stable_diffusion_inpaint_legacy(self):
|
161 |
-
device = "cpu" # ensure determinism for the device-dependent torch.Generator
|
162 |
-
unet = self.dummy_cond_unet
|
163 |
-
scheduler = PNDMScheduler(skip_prk_steps=True)
|
164 |
-
vae = self.dummy_vae
|
165 |
-
bert = self.dummy_text_encoder
|
166 |
-
tokenizer = CLIPTokenizer.from_pretrained("hf-internal-testing/tiny-random-clip")
|
167 |
-
|
168 |
-
image = self.dummy_image.cpu().permute(0, 2, 3, 1)[0]
|
169 |
-
init_image = Image.fromarray(np.uint8(image)).convert("RGB")
|
170 |
-
mask_image = Image.fromarray(np.uint8(image + 4)).convert("RGB").resize((32, 32))
|
171 |
-
|
172 |
-
# make sure here that pndm scheduler skips prk
|
173 |
-
sd_pipe = StableDiffusionInpaintPipelineLegacy(
|
174 |
-
unet=unet,
|
175 |
-
scheduler=scheduler,
|
176 |
-
vae=vae,
|
177 |
-
text_encoder=bert,
|
178 |
-
tokenizer=tokenizer,
|
179 |
-
safety_checker=None,
|
180 |
-
feature_extractor=self.dummy_extractor,
|
181 |
-
)
|
182 |
-
sd_pipe = sd_pipe.to(device)
|
183 |
-
sd_pipe.set_progress_bar_config(disable=None)
|
184 |
-
|
185 |
-
prompt = "A painting of a squirrel eating a burger"
|
186 |
-
generator = torch.Generator(device=device).manual_seed(0)
|
187 |
-
output = sd_pipe(
|
188 |
-
[prompt],
|
189 |
-
generator=generator,
|
190 |
-
guidance_scale=6.0,
|
191 |
-
num_inference_steps=2,
|
192 |
-
output_type="np",
|
193 |
-
image=init_image,
|
194 |
-
mask_image=mask_image,
|
195 |
-
)
|
196 |
-
|
197 |
-
image = output.images
|
198 |
-
|
199 |
-
generator = torch.Generator(device=device).manual_seed(0)
|
200 |
-
image_from_tuple = sd_pipe(
|
201 |
-
[prompt],
|
202 |
-
generator=generator,
|
203 |
-
guidance_scale=6.0,
|
204 |
-
num_inference_steps=2,
|
205 |
-
output_type="np",
|
206 |
-
image=init_image,
|
207 |
-
mask_image=mask_image,
|
208 |
-
return_dict=False,
|
209 |
-
)[0]
|
210 |
-
|
211 |
-
image_slice = image[0, -3:, -3:, -1]
|
212 |
-
image_from_tuple_slice = image_from_tuple[0, -3:, -3:, -1]
|
213 |
-
|
214 |
-
assert image.shape == (1, 32, 32, 3)
|
215 |
-
expected_slice = np.array([0.4941, 0.5396, 0.4689, 0.6338, 0.5392, 0.4094, 0.5477, 0.5904, 0.5165])
|
216 |
-
|
217 |
-
assert np.abs(image_slice.flatten() - expected_slice).max() < 1e-2
|
218 |
-
assert np.abs(image_from_tuple_slice.flatten() - expected_slice).max() < 1e-2
|
219 |
-
|
220 |
-
def test_stable_diffusion_inpaint_legacy_batched(self):
|
221 |
-
device = "cpu" # ensure determinism for the device-dependent torch.Generator
|
222 |
-
unet = self.dummy_cond_unet
|
223 |
-
scheduler = PNDMScheduler(skip_prk_steps=True)
|
224 |
-
vae = self.dummy_vae
|
225 |
-
bert = self.dummy_text_encoder
|
226 |
-
tokenizer = CLIPTokenizer.from_pretrained("hf-internal-testing/tiny-random-clip")
|
227 |
-
|
228 |
-
image = self.dummy_image.cpu().permute(0, 2, 3, 1)[0]
|
229 |
-
init_image = Image.fromarray(np.uint8(image)).convert("RGB")
|
230 |
-
init_images_tens = preprocess_image(init_image, batch_size=2)
|
231 |
-
init_masks_tens = init_images_tens + 4
|
232 |
-
|
233 |
-
# make sure here that pndm scheduler skips prk
|
234 |
-
sd_pipe = StableDiffusionInpaintPipelineLegacy(
|
235 |
-
unet=unet,
|
236 |
-
scheduler=scheduler,
|
237 |
-
vae=vae,
|
238 |
-
text_encoder=bert,
|
239 |
-
tokenizer=tokenizer,
|
240 |
-
safety_checker=None,
|
241 |
-
feature_extractor=self.dummy_extractor,
|
242 |
-
)
|
243 |
-
sd_pipe = sd_pipe.to(device)
|
244 |
-
sd_pipe.set_progress_bar_config(disable=None)
|
245 |
-
|
246 |
-
prompt = "A painting of a squirrel eating a burger"
|
247 |
-
generator = torch.Generator(device=device).manual_seed(0)
|
248 |
-
images = sd_pipe(
|
249 |
-
[prompt] * 2,
|
250 |
-
generator=generator,
|
251 |
-
guidance_scale=6.0,
|
252 |
-
num_inference_steps=2,
|
253 |
-
output_type="np",
|
254 |
-
image=init_images_tens,
|
255 |
-
mask_image=init_masks_tens,
|
256 |
-
).images
|
257 |
-
|
258 |
-
assert images.shape == (2, 32, 32, 3)
|
259 |
-
|
260 |
-
image_slice_0 = images[0, -3:, -3:, -1].flatten()
|
261 |
-
image_slice_1 = images[1, -3:, -3:, -1].flatten()
|
262 |
-
|
263 |
-
expected_slice_0 = np.array([0.4697, 0.3770, 0.4096, 0.4653, 0.4497, 0.4183, 0.3950, 0.4668, 0.4672])
|
264 |
-
expected_slice_1 = np.array([0.4105, 0.4987, 0.5771, 0.4921, 0.4237, 0.5684, 0.5496, 0.4645, 0.5272])
|
265 |
-
|
266 |
-
assert np.abs(expected_slice_0 - image_slice_0).max() < 1e-2
|
267 |
-
assert np.abs(expected_slice_1 - image_slice_1).max() < 1e-2
|
268 |
-
|
269 |
-
def test_stable_diffusion_inpaint_legacy_negative_prompt(self):
|
270 |
-
device = "cpu" # ensure determinism for the device-dependent torch.Generator
|
271 |
-
unet = self.dummy_cond_unet
|
272 |
-
scheduler = PNDMScheduler(skip_prk_steps=True)
|
273 |
-
vae = self.dummy_vae
|
274 |
-
bert = self.dummy_text_encoder
|
275 |
-
tokenizer = CLIPTokenizer.from_pretrained("hf-internal-testing/tiny-random-clip")
|
276 |
-
|
277 |
-
image = self.dummy_image.cpu().permute(0, 2, 3, 1)[0]
|
278 |
-
init_image = Image.fromarray(np.uint8(image)).convert("RGB")
|
279 |
-
mask_image = Image.fromarray(np.uint8(image + 4)).convert("RGB").resize((32, 32))
|
280 |
-
|
281 |
-
# make sure here that pndm scheduler skips prk
|
282 |
-
sd_pipe = StableDiffusionInpaintPipelineLegacy(
|
283 |
-
unet=unet,
|
284 |
-
scheduler=scheduler,
|
285 |
-
vae=vae,
|
286 |
-
text_encoder=bert,
|
287 |
-
tokenizer=tokenizer,
|
288 |
-
safety_checker=None,
|
289 |
-
feature_extractor=self.dummy_extractor,
|
290 |
-
)
|
291 |
-
sd_pipe = sd_pipe.to(device)
|
292 |
-
sd_pipe.set_progress_bar_config(disable=None)
|
293 |
-
|
294 |
-
prompt = "A painting of a squirrel eating a burger"
|
295 |
-
negative_prompt = "french fries"
|
296 |
-
generator = torch.Generator(device=device).manual_seed(0)
|
297 |
-
output = sd_pipe(
|
298 |
-
prompt,
|
299 |
-
negative_prompt=negative_prompt,
|
300 |
-
generator=generator,
|
301 |
-
guidance_scale=6.0,
|
302 |
-
num_inference_steps=2,
|
303 |
-
output_type="np",
|
304 |
-
image=init_image,
|
305 |
-
mask_image=mask_image,
|
306 |
-
)
|
307 |
-
|
308 |
-
image = output.images
|
309 |
-
image_slice = image[0, -3:, -3:, -1]
|
310 |
-
|
311 |
-
assert image.shape == (1, 32, 32, 3)
|
312 |
-
expected_slice = np.array([0.4941, 0.5396, 0.4689, 0.6338, 0.5392, 0.4094, 0.5477, 0.5904, 0.5165])
|
313 |
-
|
314 |
-
assert np.abs(image_slice.flatten() - expected_slice).max() < 1e-2
|
315 |
-
|
316 |
-
def test_stable_diffusion_inpaint_legacy_num_images_per_prompt(self):
|
317 |
-
device = "cpu"
|
318 |
-
unet = self.dummy_cond_unet
|
319 |
-
scheduler = PNDMScheduler(skip_prk_steps=True)
|
320 |
-
vae = self.dummy_vae
|
321 |
-
bert = self.dummy_text_encoder
|
322 |
-
tokenizer = CLIPTokenizer.from_pretrained("hf-internal-testing/tiny-random-clip")
|
323 |
-
|
324 |
-
image = self.dummy_image.cpu().permute(0, 2, 3, 1)[0]
|
325 |
-
init_image = Image.fromarray(np.uint8(image)).convert("RGB")
|
326 |
-
mask_image = Image.fromarray(np.uint8(image + 4)).convert("RGB").resize((32, 32))
|
327 |
-
|
328 |
-
# make sure here that pndm scheduler skips prk
|
329 |
-
sd_pipe = StableDiffusionInpaintPipelineLegacy(
|
330 |
-
unet=unet,
|
331 |
-
scheduler=scheduler,
|
332 |
-
vae=vae,
|
333 |
-
text_encoder=bert,
|
334 |
-
tokenizer=tokenizer,
|
335 |
-
safety_checker=None,
|
336 |
-
feature_extractor=self.dummy_extractor,
|
337 |
-
)
|
338 |
-
sd_pipe = sd_pipe.to(device)
|
339 |
-
sd_pipe.set_progress_bar_config(disable=None)
|
340 |
-
|
341 |
-
prompt = "A painting of a squirrel eating a burger"
|
342 |
-
|
343 |
-
# test num_images_per_prompt=1 (default)
|
344 |
-
images = sd_pipe(
|
345 |
-
prompt,
|
346 |
-
num_inference_steps=2,
|
347 |
-
output_type="np",
|
348 |
-
image=init_image,
|
349 |
-
mask_image=mask_image,
|
350 |
-
).images
|
351 |
-
|
352 |
-
assert images.shape == (1, 32, 32, 3)
|
353 |
-
|
354 |
-
# test num_images_per_prompt=1 (default) for batch of prompts
|
355 |
-
batch_size = 2
|
356 |
-
images = sd_pipe(
|
357 |
-
[prompt] * batch_size,
|
358 |
-
num_inference_steps=2,
|
359 |
-
output_type="np",
|
360 |
-
image=init_image,
|
361 |
-
mask_image=mask_image,
|
362 |
-
).images
|
363 |
-
|
364 |
-
assert images.shape == (batch_size, 32, 32, 3)
|
365 |
-
|
366 |
-
# test num_images_per_prompt for single prompt
|
367 |
-
num_images_per_prompt = 2
|
368 |
-
images = sd_pipe(
|
369 |
-
prompt,
|
370 |
-
num_inference_steps=2,
|
371 |
-
output_type="np",
|
372 |
-
image=init_image,
|
373 |
-
mask_image=mask_image,
|
374 |
-
num_images_per_prompt=num_images_per_prompt,
|
375 |
-
).images
|
376 |
-
|
377 |
-
assert images.shape == (num_images_per_prompt, 32, 32, 3)
|
378 |
-
|
379 |
-
# test num_images_per_prompt for batch of prompts
|
380 |
-
batch_size = 2
|
381 |
-
images = sd_pipe(
|
382 |
-
[prompt] * batch_size,
|
383 |
-
num_inference_steps=2,
|
384 |
-
output_type="np",
|
385 |
-
image=init_image,
|
386 |
-
mask_image=mask_image,
|
387 |
-
num_images_per_prompt=num_images_per_prompt,
|
388 |
-
).images
|
389 |
-
|
390 |
-
assert images.shape == (batch_size * num_images_per_prompt, 32, 32, 3)
|
391 |
-
|
392 |
-
|
393 |
-
@slow
|
394 |
-
@require_torch_gpu
|
395 |
-
class StableDiffusionInpaintLegacyPipelineSlowTests(unittest.TestCase):
|
396 |
-
def tearDown(self):
|
397 |
-
super().tearDown()
|
398 |
-
gc.collect()
|
399 |
-
torch.cuda.empty_cache()
|
400 |
-
|
401 |
-
def get_inputs(self, generator_device="cpu", seed=0):
|
402 |
-
generator = torch.Generator(device=generator_device).manual_seed(seed)
|
403 |
-
init_image = load_image(
|
404 |
-
"https://huggingface.co/datasets/diffusers/test-arrays/resolve/main"
|
405 |
-
"/stable_diffusion_inpaint/input_bench_image.png"
|
406 |
-
)
|
407 |
-
mask_image = load_image(
|
408 |
-
"https://huggingface.co/datasets/diffusers/test-arrays/resolve/main"
|
409 |
-
"/stable_diffusion_inpaint/input_bench_mask.png"
|
410 |
-
)
|
411 |
-
inputs = {
|
412 |
-
"prompt": "A red cat sitting on a park bench",
|
413 |
-
"image": init_image,
|
414 |
-
"mask_image": mask_image,
|
415 |
-
"generator": generator,
|
416 |
-
"num_inference_steps": 3,
|
417 |
-
"strength": 0.75,
|
418 |
-
"guidance_scale": 7.5,
|
419 |
-
"output_type": "numpy",
|
420 |
-
}
|
421 |
-
return inputs
|
422 |
-
|
423 |
-
def test_stable_diffusion_inpaint_legacy_pndm(self):
|
424 |
-
pipe = StableDiffusionInpaintPipelineLegacy.from_pretrained(
|
425 |
-
"CompVis/stable-diffusion-v1-4", safety_checker=None
|
426 |
-
)
|
427 |
-
pipe.to(torch_device)
|
428 |
-
pipe.set_progress_bar_config(disable=None)
|
429 |
-
pipe.enable_attention_slicing()
|
430 |
-
|
431 |
-
inputs = self.get_inputs()
|
432 |
-
image = pipe(**inputs).images
|
433 |
-
image_slice = image[0, 253:256, 253:256, -1].flatten()
|
434 |
-
|
435 |
-
assert image.shape == (1, 512, 512, 3)
|
436 |
-
expected_slice = np.array([0.5665, 0.6117, 0.6430, 0.4057, 0.4594, 0.5658, 0.1596, 0.3106, 0.4305])
|
437 |
-
|
438 |
-
assert np.abs(expected_slice - image_slice).max() < 3e-3
|
439 |
-
|
440 |
-
def test_stable_diffusion_inpaint_legacy_batched(self):
|
441 |
-
pipe = StableDiffusionInpaintPipelineLegacy.from_pretrained(
|
442 |
-
"CompVis/stable-diffusion-v1-4", safety_checker=None
|
443 |
-
)
|
444 |
-
pipe.to(torch_device)
|
445 |
-
pipe.set_progress_bar_config(disable=None)
|
446 |
-
pipe.enable_attention_slicing()
|
447 |
-
|
448 |
-
inputs = self.get_inputs()
|
449 |
-
inputs["prompt"] = [inputs["prompt"]] * 2
|
450 |
-
inputs["image"] = preprocess_image(inputs["image"], batch_size=2)
|
451 |
-
|
452 |
-
mask = inputs["mask_image"].convert("L")
|
453 |
-
mask = np.array(mask).astype(np.float32) / 255.0
|
454 |
-
mask = torch.from_numpy(1 - mask)
|
455 |
-
masks = torch.vstack([mask[None][None]] * 2)
|
456 |
-
inputs["mask_image"] = masks
|
457 |
-
|
458 |
-
image = pipe(**inputs).images
|
459 |
-
assert image.shape == (2, 512, 512, 3)
|
460 |
-
|
461 |
-
image_slice_0 = image[0, 253:256, 253:256, -1].flatten()
|
462 |
-
image_slice_1 = image[1, 253:256, 253:256, -1].flatten()
|
463 |
-
|
464 |
-
expected_slice_0 = np.array(
|
465 |
-
[0.52093095, 0.4176447, 0.32752383, 0.6175223, 0.50563973, 0.36470804, 0.65460044, 0.5775188, 0.44332123]
|
466 |
-
)
|
467 |
-
expected_slice_1 = np.array(
|
468 |
-
[0.3592432, 0.4233033, 0.3914635, 0.31014425, 0.3702293, 0.39412856, 0.17526966, 0.2642669, 0.37480092]
|
469 |
-
)
|
470 |
-
|
471 |
-
assert np.abs(expected_slice_0 - image_slice_0).max() < 3e-3
|
472 |
-
assert np.abs(expected_slice_1 - image_slice_1).max() < 3e-3
|
473 |
-
|
474 |
-
def test_stable_diffusion_inpaint_legacy_k_lms(self):
|
475 |
-
pipe = StableDiffusionInpaintPipelineLegacy.from_pretrained(
|
476 |
-
"CompVis/stable-diffusion-v1-4", safety_checker=None
|
477 |
-
)
|
478 |
-
pipe.scheduler = LMSDiscreteScheduler.from_config(pipe.scheduler.config)
|
479 |
-
pipe.to(torch_device)
|
480 |
-
pipe.set_progress_bar_config(disable=None)
|
481 |
-
pipe.enable_attention_slicing()
|
482 |
-
|
483 |
-
inputs = self.get_inputs()
|
484 |
-
image = pipe(**inputs).images
|
485 |
-
image_slice = image[0, 253:256, 253:256, -1].flatten()
|
486 |
-
|
487 |
-
assert image.shape == (1, 512, 512, 3)
|
488 |
-
expected_slice = np.array([0.4534, 0.4467, 0.4329, 0.4329, 0.4339, 0.4220, 0.4244, 0.4332, 0.4426])
|
489 |
-
|
490 |
-
assert np.abs(expected_slice - image_slice).max() < 3e-3
|
491 |
-
|
492 |
-
def test_stable_diffusion_inpaint_legacy_intermediate_state(self):
|
493 |
-
number_of_steps = 0
|
494 |
-
|
495 |
-
def callback_fn(step: int, timestep: int, latents: torch.FloatTensor) -> None:
|
496 |
-
callback_fn.has_been_called = True
|
497 |
-
nonlocal number_of_steps
|
498 |
-
number_of_steps += 1
|
499 |
-
if step == 1:
|
500 |
-
latents = latents.detach().cpu().numpy()
|
501 |
-
assert latents.shape == (1, 4, 64, 64)
|
502 |
-
latents_slice = latents[0, -3:, -3:, -1]
|
503 |
-
expected_slice = np.array([0.5977, 1.5449, 1.0586, -0.3250, 0.7383, -0.0862, 0.4631, -0.2571, -1.1289])
|
504 |
-
|
505 |
-
assert np.abs(latents_slice.flatten() - expected_slice).max() < 1e-3
|
506 |
-
elif step == 2:
|
507 |
-
latents = latents.detach().cpu().numpy()
|
508 |
-
assert latents.shape == (1, 4, 64, 64)
|
509 |
-
latents_slice = latents[0, -3:, -3:, -1]
|
510 |
-
expected_slice = np.array([0.5190, 1.1621, 0.6885, 0.2424, 0.3337, -0.1617, 0.6914, -0.1957, -0.5474])
|
511 |
-
|
512 |
-
assert np.abs(latents_slice.flatten() - expected_slice).max() < 1e-3
|
513 |
-
|
514 |
-
callback_fn.has_been_called = False
|
515 |
-
|
516 |
-
pipe = StableDiffusionInpaintPipelineLegacy.from_pretrained(
|
517 |
-
"CompVis/stable-diffusion-v1-4", safety_checker=None, torch_dtype=torch.float16
|
518 |
-
)
|
519 |
-
pipe = pipe.to(torch_device)
|
520 |
-
pipe.set_progress_bar_config(disable=None)
|
521 |
-
pipe.enable_attention_slicing()
|
522 |
-
|
523 |
-
inputs = self.get_inputs()
|
524 |
-
pipe(**inputs, callback=callback_fn, callback_steps=1)
|
525 |
-
assert callback_fn.has_been_called
|
526 |
-
assert number_of_steps == 2
|
527 |
-
|
528 |
-
|
529 |
-
@nightly
|
530 |
-
@require_torch_gpu
|
531 |
-
class StableDiffusionInpaintLegacyPipelineNightlyTests(unittest.TestCase):
|
532 |
-
def tearDown(self):
|
533 |
-
super().tearDown()
|
534 |
-
gc.collect()
|
535 |
-
torch.cuda.empty_cache()
|
536 |
-
|
537 |
-
def get_inputs(self, device, generator_device="cpu", dtype=torch.float32, seed=0):
|
538 |
-
generator = torch.Generator(device=generator_device).manual_seed(seed)
|
539 |
-
init_image = load_image(
|
540 |
-
"https://huggingface.co/datasets/diffusers/test-arrays/resolve/main"
|
541 |
-
"/stable_diffusion_inpaint/input_bench_image.png"
|
542 |
-
)
|
543 |
-
mask_image = load_image(
|
544 |
-
"https://huggingface.co/datasets/diffusers/test-arrays/resolve/main"
|
545 |
-
"/stable_diffusion_inpaint/input_bench_mask.png"
|
546 |
-
)
|
547 |
-
inputs = {
|
548 |
-
"prompt": "A red cat sitting on a park bench",
|
549 |
-
"image": init_image,
|
550 |
-
"mask_image": mask_image,
|
551 |
-
"generator": generator,
|
552 |
-
"num_inference_steps": 50,
|
553 |
-
"strength": 0.75,
|
554 |
-
"guidance_scale": 7.5,
|
555 |
-
"output_type": "numpy",
|
556 |
-
}
|
557 |
-
return inputs
|
558 |
-
|
559 |
-
def test_inpaint_pndm(self):
|
560 |
-
sd_pipe = StableDiffusionInpaintPipelineLegacy.from_pretrained("runwayml/stable-diffusion-v1-5")
|
561 |
-
sd_pipe.to(torch_device)
|
562 |
-
sd_pipe.set_progress_bar_config(disable=None)
|
563 |
-
|
564 |
-
inputs = self.get_inputs(torch_device)
|
565 |
-
image = sd_pipe(**inputs).images[0]
|
566 |
-
|
567 |
-
expected_image = load_numpy(
|
568 |
-
"https://huggingface.co/datasets/diffusers/test-arrays/resolve/main"
|
569 |
-
"/stable_diffusion_inpaint_legacy/stable_diffusion_1_5_pndm.npy"
|
570 |
-
)
|
571 |
-
max_diff = np.abs(expected_image - image).max()
|
572 |
-
assert max_diff < 1e-3
|
573 |
-
|
574 |
-
def test_inpaint_ddim(self):
|
575 |
-
sd_pipe = StableDiffusionInpaintPipelineLegacy.from_pretrained("runwayml/stable-diffusion-v1-5")
|
576 |
-
sd_pipe.scheduler = DDIMScheduler.from_config(sd_pipe.scheduler.config)
|
577 |
-
sd_pipe.to(torch_device)
|
578 |
-
sd_pipe.set_progress_bar_config(disable=None)
|
579 |
-
|
580 |
-
inputs = self.get_inputs(torch_device)
|
581 |
-
image = sd_pipe(**inputs).images[0]
|
582 |
-
|
583 |
-
expected_image = load_numpy(
|
584 |
-
"https://huggingface.co/datasets/diffusers/test-arrays/resolve/main"
|
585 |
-
"/stable_diffusion_inpaint_legacy/stable_diffusion_1_5_ddim.npy"
|
586 |
-
)
|
587 |
-
max_diff = np.abs(expected_image - image).max()
|
588 |
-
assert max_diff < 1e-3
|
589 |
-
|
590 |
-
def test_inpaint_lms(self):
|
591 |
-
sd_pipe = StableDiffusionInpaintPipelineLegacy.from_pretrained("runwayml/stable-diffusion-v1-5")
|
592 |
-
sd_pipe.scheduler = LMSDiscreteScheduler.from_config(sd_pipe.scheduler.config)
|
593 |
-
sd_pipe.to(torch_device)
|
594 |
-
sd_pipe.set_progress_bar_config(disable=None)
|
595 |
-
|
596 |
-
inputs = self.get_inputs(torch_device)
|
597 |
-
image = sd_pipe(**inputs).images[0]
|
598 |
-
|
599 |
-
expected_image = load_numpy(
|
600 |
-
"https://huggingface.co/datasets/diffusers/test-arrays/resolve/main"
|
601 |
-
"/stable_diffusion_inpaint_legacy/stable_diffusion_1_5_lms.npy"
|
602 |
-
)
|
603 |
-
max_diff = np.abs(expected_image - image).max()
|
604 |
-
assert max_diff < 1e-3
|
605 |
-
|
606 |
-
def test_inpaint_dpm(self):
|
607 |
-
sd_pipe = StableDiffusionInpaintPipelineLegacy.from_pretrained("runwayml/stable-diffusion-v1-5")
|
608 |
-
sd_pipe.scheduler = DPMSolverMultistepScheduler.from_config(sd_pipe.scheduler.config)
|
609 |
-
sd_pipe.to(torch_device)
|
610 |
-
sd_pipe.set_progress_bar_config(disable=None)
|
611 |
-
|
612 |
-
inputs = self.get_inputs(torch_device)
|
613 |
-
inputs["num_inference_steps"] = 30
|
614 |
-
image = sd_pipe(**inputs).images[0]
|
615 |
-
|
616 |
-
expected_image = load_numpy(
|
617 |
-
"https://huggingface.co/datasets/diffusers/test-arrays/resolve/main"
|
618 |
-
"/stable_diffusion_inpaint_legacy/stable_diffusion_1_5_dpm_multi.npy"
|
619 |
-
)
|
620 |
-
max_diff = np.abs(expected_image - image).max()
|
621 |
-
assert max_diff < 1e-3
|
|
|
|
|
|
|
|
|
|
|
|
|
|
|
|
|
|
|
|
|
|
|
|
|
|
|
|
|
|
|
|
|
|
|
|
|
|
|
|
|
|
|
|
|
|
|
|
|
|
|
|
|
|
|
|
|
|
|
|
|
|
|
|
|
|
|
|
|
|
|
|
|
|
|
|
|
|
|
|
|
|
|
|
|
|
|
|
|
|
|
|
|
|
|
|
|
|
|
|
|
|
|
|
|
|
|
|
|
|
|
|
|
|
|
|
|
|
|
|
|
|
|
|
|
|
|
|
|
|
|
|
|
|
|
|
|
|
|
|
|
|
|
|
|
|
|
|
|
|
|
|
|
|
|
|
|
|
|
|
|
|
|
|
|
|
|
|
|
|
|
|
|
|
|
|
|
|
|
|
|
|
|
|
|
|
|
|
|
|
|
|
|
|
|
|
|
|
|
|
|
|
|
|
|
|
|
|
|
|
|
|
|
|
|
|
|
|
|
|
|
|
|
|
|
|
|
|
|
|
|
|
|
|
|
|
|
|
|
|
|
|
|
|
|
|
|
|
|
|
|
|
|
|
|
|
|
|
|
|
|
|
|
|
|
|
|
|
|
|
|
|
|
|
|
|
|
|
|
|
|
|
|
|
|
|
|
|
|
|
|
|
|
|
|
|
|
|
|
|
|
|
|
|
|
|
|
|
|
|
|
|
|
|
|
|
|
|
|
|
|
|
|
|
|
|
|
|
|
|
|
|
|
|
|
|
|
|
|
|
|
|
|
|
|
|
|
|
|
|
|
|
|
|
|
|
|
|
|
|
|
|
|
|
|
|
|
|
|
|
|
|
|
|
|
|
|
|
|
|
|
|
|
|
|
|
|
|
|
|
|
|
|
|
|
|
|
|
|
|
|
|
|
|
|
|
|
|
|
|
|
|
|
|
|
|
|
|
|
|
|
|
|
|
|
|
|
|
|
|
|
|
|
|
|
|
|
|
|
|
|
|
|
|
|
|
|
|
|
|
|
|
|
|
|
|
|
|
|
|
|
|
|
|
|
|
|
|
|
|
|
|
|
|
|
|
|
|
|
|
|
|
|
|
|
|
|
|
|
|
|
|
|
|
|
|
|
|
|
|
|
|
|
|
|
|
|
|
|
|
|
|
|
|
|
|
|
|
|
|
|
|
|
|
|
|
|
|
|
|
|
|
|
|
|
|
|
|
|
|
|
|
|
|
|
|
|
|
|
|
|
|
|
|
|
|
|
|
|
|
|
|
|
|
|
|
|
|
|
|
|
|
|
|
|
|
|
|
|
|
|
|
|
|
|
|
|
|
|
|
|
|
|
|
|
|
|
|
|
|
|
|
|
|
|
|
|
|
|
|
|
|
|
|
|
|
|
|
|
|
|
|
|
|
|
|
|
|
|
|
|
|
|
|
|
|
|
|
|
|
|
|
|
|
|
|
|
|
|
|
|
|
|
|
|
|
|
|
|
|
|
|
|
|
|
|
|
|
|
|
|
|
|
|
|
|
|
|
|
|
|
|
|
|
|
|
|
|
|
|
|
|
|
|
|
|
|
|
|
|
|
|
|
|
|
|
|
|
|
|
|
|
|
|
|
|
|
|
|
|
|
|
|
|
|
|
|
|
|
|
|
|
|
|
|
|
|
|
|
|
|
|
|
|
|
|
|
|
|
|
|
|
|
|
|
|
|
|
|
|
|
|
|
|
|
|
|
|
|
|
|
|
|
|
|
|
|
|
|
|
|
|
|
|
|
|
|
|
|
|
|
|
|
|
|
|
|
|
|
|
|
|
|
|
|
|
|
|
|
|
|
|
|
|
|
|
|
|
|
|
|
|
|
|
|
|
|
|
|
|
|
|
|
|
|
|
|
|
|
|
|
|
|
|
|
|
|
|
|
|
|
|
|
|
|
|
|
|
|
|
|
|
|
|
|
|
|
|
|
|
|
|
|
|
|
|
|
|
|
|
|
|
|
|
|
|
|
|
|
|
|
|
|
|
|
|
|
|
|
|
|
|
|
|
|
|
|
|
|
|
|
|
|
|
|
|
|
|
|
|
|
|
|
|
|
|
|
|
|
|
|
|
|
|
|
|
|
|
|
|
|
|
|
|
|
|
|
|
|
|
|
|
|
|
|
|
|
|
|
|
|
|
|
|
|
|
|
|
|
|
|
|
|
|
|
|
|
|
|
|
|
|
|
|
|
|
|
|
|
|
|
|
|
|
|
|
|
|
|
|
|
|
|
|
|
|
|
|
|
|
|
|
|
|
|
|
|
|
|
|
|
|
|
|
|
|
|
|
|
|
|
|
|
|
|
|
|
|
|
|
|
|
|
|
|
|
|
|
|
|
|
|
|
|
|
|
|
|
|
|
|
|
|
|
|
|
|
|
|
|
|
|
|
|
|
|
|
|
|
|
|
|
|
|
|
|
|
|
|
|
|
|
|
|
|
|
|
|
|
|
|
|
|
|
|
|
|
|
|
|
|
|
|
|
|
|
|
|
|
|
|
|
|
|
|
|
|
|
|
|
|
|
|
|
|
|
|
|
|
|
|
|
|
|
|
|
|
|
|
|
|
|
|
|
|
|
|
|
|
|
|
|
|
|
|
|
|
|
|
|
|
|
|
|
|
|
|
|
|
|
|
|
|
|
|
|
|
|
|
|
|
|
|
|
|
|
|
|
|
|
|
|
|
|
|
|
|
|
|
|
|
|
|
|
|
|
|
|
|
|
|
|
|
|
|
|
|
|
|
|
|
|
|
|
|
|
|
|
|
|
|
|
|
|
spaces/Andy1621/uniformer_image_segmentation/configs/danet/danet_r101-d8_512x512_40k_voc12aug.py
DELETED
@@ -1,2 +0,0 @@
|
|
1 |
-
_base_ = './danet_r50-d8_512x512_40k_voc12aug.py'
|
2 |
-
model = dict(pretrained='open-mmlab://resnet101_v1c', backbone=dict(depth=101))
|
|
|
|
|
|
spaces/AnishKumbhar/ChatBot/text-generation-webui-main/css/main.css
DELETED
@@ -1,612 +0,0 @@
|
|
1 |
-
.tabs.svelte-710i53 {
|
2 |
-
margin-top: 0
|
3 |
-
}
|
4 |
-
|
5 |
-
.py-6 {
|
6 |
-
padding-top: 2.5rem
|
7 |
-
}
|
8 |
-
|
9 |
-
.small-button {
|
10 |
-
min-width: 0 !important;
|
11 |
-
max-width: 171px;
|
12 |
-
height: 39.594px;
|
13 |
-
align-self: end;
|
14 |
-
}
|
15 |
-
|
16 |
-
.refresh-button {
|
17 |
-
max-width: 4.4em;
|
18 |
-
min-width: 2.2em !important;
|
19 |
-
height: 39.594px;
|
20 |
-
align-self: end;
|
21 |
-
line-height: 1em;
|
22 |
-
border-radius: 0.5em;
|
23 |
-
flex: none;
|
24 |
-
}
|
25 |
-
|
26 |
-
.refresh-button-small {
|
27 |
-
max-width: 2.2em;
|
28 |
-
}
|
29 |
-
|
30 |
-
.button_nowrap {
|
31 |
-
white-space: nowrap;
|
32 |
-
}
|
33 |
-
|
34 |
-
#slim-column {
|
35 |
-
flex: none !important;
|
36 |
-
min-width: 0 !important;
|
37 |
-
}
|
38 |
-
|
39 |
-
.slim-dropdown {
|
40 |
-
background-color: transparent !important;
|
41 |
-
border: none !important;
|
42 |
-
padding: 0 !important;
|
43 |
-
}
|
44 |
-
|
45 |
-
#download-label, #upload-label {
|
46 |
-
min-height: 0
|
47 |
-
}
|
48 |
-
|
49 |
-
.dark svg {
|
50 |
-
fill: white;
|
51 |
-
}
|
52 |
-
|
53 |
-
.dark a {
|
54 |
-
color: white !important;
|
55 |
-
}
|
56 |
-
|
57 |
-
ol li p, ul li p {
|
58 |
-
display: inline-block;
|
59 |
-
}
|
60 |
-
|
61 |
-
#chat-tab, #default-tab, #notebook-tab, #parameters, #chat-settings, #lora, #training-tab, #model-tab, #session-tab {
|
62 |
-
border: 0;
|
63 |
-
}
|
64 |
-
|
65 |
-
.gradio-container-3-18-0 .prose * h1, h2, h3, h4 {
|
66 |
-
color: white;
|
67 |
-
}
|
68 |
-
|
69 |
-
.gradio-container {
|
70 |
-
max-width: 100% !important;
|
71 |
-
padding-top: 0 !important;
|
72 |
-
}
|
73 |
-
|
74 |
-
#extensions {
|
75 |
-
margin-top: 5px;
|
76 |
-
margin-bottom: 35px;
|
77 |
-
}
|
78 |
-
|
79 |
-
.extension-tab {
|
80 |
-
border: 0 !important;
|
81 |
-
}
|
82 |
-
|
83 |
-
span.math.inline {
|
84 |
-
font-size: 27px;
|
85 |
-
vertical-align: baseline !important;
|
86 |
-
}
|
87 |
-
|
88 |
-
div.svelte-15lo0d8 > *, div.svelte-15lo0d8 > .form > * {
|
89 |
-
flex-wrap: nowrap;
|
90 |
-
}
|
91 |
-
|
92 |
-
.header_bar {
|
93 |
-
background-color: #f7f7f7;
|
94 |
-
margin-bottom: 19px;
|
95 |
-
display: inline !important;
|
96 |
-
overflow-x: scroll;
|
97 |
-
margin-left: calc(-1 * var(--size-4));
|
98 |
-
margin-right: calc(-1 * var(--size-4));
|
99 |
-
}
|
100 |
-
|
101 |
-
.dark .header_bar {
|
102 |
-
border: none !important;
|
103 |
-
background-color: #8080802b;
|
104 |
-
}
|
105 |
-
|
106 |
-
.header_bar button.selected {
|
107 |
-
border-radius: 0;
|
108 |
-
}
|
109 |
-
|
110 |
-
.textbox_default textarea {
|
111 |
-
height: calc(100dvh - 271px);
|
112 |
-
}
|
113 |
-
|
114 |
-
.textbox_default_output textarea {
|
115 |
-
height: calc(100dvh - 185px);
|
116 |
-
}
|
117 |
-
|
118 |
-
.textbox textarea {
|
119 |
-
height: calc(100dvh - 241px);
|
120 |
-
}
|
121 |
-
|
122 |
-
.textbox_logits textarea {
|
123 |
-
height: calc(100dvh - 236px);
|
124 |
-
}
|
125 |
-
|
126 |
-
.textbox_logits_notebook textarea {
|
127 |
-
height: calc(100dvh - 292px);
|
128 |
-
}
|
129 |
-
|
130 |
-
.monospace textarea {
|
131 |
-
font-family: monospace;
|
132 |
-
}
|
133 |
-
|
134 |
-
.textbox_default textarea,
|
135 |
-
.textbox_default_output textarea,
|
136 |
-
.textbox_logits textarea,
|
137 |
-
.textbox_logits_notebook textarea,
|
138 |
-
.textbox textarea {
|
139 |
-
font-size: 16px !important;
|
140 |
-
color: #46464A !important;
|
141 |
-
}
|
142 |
-
|
143 |
-
.dark textarea {
|
144 |
-
color: #efefef !important;
|
145 |
-
}
|
146 |
-
|
147 |
-
@media screen and (max-width: 711px) {
|
148 |
-
.textbox_default textarea {
|
149 |
-
height: calc(100dvh - 259px);
|
150 |
-
}
|
151 |
-
|
152 |
-
div .default-token-counter {
|
153 |
-
top: calc( 0.5 * (100dvh - 236px) ) !important;
|
154 |
-
}
|
155 |
-
|
156 |
-
.transparent-substring {
|
157 |
-
display: none;
|
158 |
-
}
|
159 |
-
|
160 |
-
.hover-menu {
|
161 |
-
min-width: 250px !important;
|
162 |
-
}
|
163 |
-
}
|
164 |
-
|
165 |
-
/* Hide the gradio footer*/
|
166 |
-
footer {
|
167 |
-
display: none !important;
|
168 |
-
}
|
169 |
-
|
170 |
-
button {
|
171 |
-
font-size: 14px !important;
|
172 |
-
}
|
173 |
-
|
174 |
-
.file-saver {
|
175 |
-
position: fixed !important;
|
176 |
-
top: 50%;
|
177 |
-
left: 50%;
|
178 |
-
transform: translate(-50%, -50%); /* center horizontally */
|
179 |
-
max-width: 500px;
|
180 |
-
background-color: var(--input-background-fill);
|
181 |
-
border: 2px solid black !important;
|
182 |
-
z-index: 1000;
|
183 |
-
}
|
184 |
-
|
185 |
-
.dark .file-saver {
|
186 |
-
border: 2px solid white !important;
|
187 |
-
}
|
188 |
-
|
189 |
-
.checkboxgroup-table label {
|
190 |
-
background: none !important;
|
191 |
-
padding: 0 !important;
|
192 |
-
border: 0 !important;
|
193 |
-
}
|
194 |
-
|
195 |
-
.checkboxgroup-table div {
|
196 |
-
display: grid !important;
|
197 |
-
}
|
198 |
-
|
199 |
-
.markdown ul ol {
|
200 |
-
font-size: 100% !important;
|
201 |
-
}
|
202 |
-
|
203 |
-
.pretty_scrollbar::-webkit-scrollbar {
|
204 |
-
width: 5px;
|
205 |
-
}
|
206 |
-
|
207 |
-
.pretty_scrollbar::-webkit-scrollbar-track {
|
208 |
-
background: transparent;
|
209 |
-
}
|
210 |
-
|
211 |
-
.pretty_scrollbar::-webkit-scrollbar-thumb,
|
212 |
-
.pretty_scrollbar::-webkit-scrollbar-thumb:hover {
|
213 |
-
background: #c5c5d2;
|
214 |
-
}
|
215 |
-
|
216 |
-
.dark .pretty_scrollbar::-webkit-scrollbar-thumb,
|
217 |
-
.dark .pretty_scrollbar::-webkit-scrollbar-thumb:hover {
|
218 |
-
background: #374151;
|
219 |
-
}
|
220 |
-
|
221 |
-
.pretty_scrollbar::-webkit-resizer {
|
222 |
-
background: #c5c5d2;
|
223 |
-
}
|
224 |
-
|
225 |
-
.dark .pretty_scrollbar::-webkit-resizer {
|
226 |
-
background: #374151;
|
227 |
-
}
|
228 |
-
|
229 |
-
audio {
|
230 |
-
max-width: 100%;
|
231 |
-
}
|
232 |
-
|
233 |
-
/* Copied from https://github.com/AUTOMATIC1111/stable-diffusion-webui */
|
234 |
-
.token-counter {
|
235 |
-
position: absolute !important;
|
236 |
-
top: calc( 0.5 * (100dvh - 218px) ) !important;
|
237 |
-
right: 2px;
|
238 |
-
z-index: 100;
|
239 |
-
background: var(--input-background-fill) !important;
|
240 |
-
min-height: 0 !important;
|
241 |
-
}
|
242 |
-
|
243 |
-
.default-token-counter {
|
244 |
-
top: calc( 0.5 * (100dvh - 248px) ) !important;
|
245 |
-
}
|
246 |
-
|
247 |
-
.token-counter span {
|
248 |
-
padding: 1px;
|
249 |
-
box-shadow: 0 0 0 0.3em rgba(192,192,192,0.15), inset 0 0 0.6em rgba(192,192,192,0.075);
|
250 |
-
border: 2px solid rgba(192,192,192,0.4) !important;
|
251 |
-
border-radius: 0.4em;
|
252 |
-
}
|
253 |
-
|
254 |
-
.no-background {
|
255 |
-
background: var(--background-fill-primary) !important;
|
256 |
-
padding: 0px !important;
|
257 |
-
}
|
258 |
-
|
259 |
-
/*----------------------------------------------
|
260 |
-
Chat tab
|
261 |
-
----------------------------------------------*/
|
262 |
-
.h-\[40vh\], .wrap.svelte-byatnx.svelte-byatnx.svelte-byatnx {
|
263 |
-
height: 66.67vh
|
264 |
-
}
|
265 |
-
|
266 |
-
.gradio-container {
|
267 |
-
margin-left: auto !important;
|
268 |
-
margin-right: auto !important;
|
269 |
-
}
|
270 |
-
|
271 |
-
.w-screen {
|
272 |
-
width: unset
|
273 |
-
}
|
274 |
-
|
275 |
-
div.svelte-362y77>*, div.svelte-362y77>.form>* {
|
276 |
-
flex-wrap: nowrap
|
277 |
-
}
|
278 |
-
|
279 |
-
.pending.svelte-1ed2p3z {
|
280 |
-
opacity: 1;
|
281 |
-
}
|
282 |
-
|
283 |
-
.wrap.svelte-6roggh.svelte-6roggh {
|
284 |
-
max-height: 92.5%;
|
285 |
-
}
|
286 |
-
|
287 |
-
/* This is for the microphone button in the whisper extension */
|
288 |
-
.sm.svelte-1ipelgc {
|
289 |
-
width: 100%;
|
290 |
-
}
|
291 |
-
|
292 |
-
#chat-tab button#Generate, #chat-tab button#stop {
|
293 |
-
width: 89.3438px !important;
|
294 |
-
}
|
295 |
-
|
296 |
-
#chat-tab button, #notebook-tab button, #default-tab button {
|
297 |
-
min-width: 0 !important;
|
298 |
-
}
|
299 |
-
|
300 |
-
#chat-tab > :first-child, #extensions {
|
301 |
-
max-width: 880px;
|
302 |
-
margin-left: auto;
|
303 |
-
margin-right: auto;
|
304 |
-
}
|
305 |
-
|
306 |
-
@media screen and (max-width: 688px) {
|
307 |
-
#chat-tab {
|
308 |
-
padding-left: 0px;
|
309 |
-
padding-right: 0px;
|
310 |
-
}
|
311 |
-
|
312 |
-
.chat-parent {
|
313 |
-
height: calc(100dvh - 179px) !important;
|
314 |
-
}
|
315 |
-
|
316 |
-
.old-ui .chat-parent {
|
317 |
-
height: calc(100dvh - 310px) !important;
|
318 |
-
}
|
319 |
-
}
|
320 |
-
|
321 |
-
.chat {
|
322 |
-
margin-left: auto;
|
323 |
-
margin-right: auto;
|
324 |
-
max-width: 880px;
|
325 |
-
height: 100%;
|
326 |
-
overflow-y: auto;
|
327 |
-
padding-right: 15px;
|
328 |
-
display: flex;
|
329 |
-
flex-direction: column;
|
330 |
-
word-break: break-word;
|
331 |
-
overflow-wrap: anywhere;
|
332 |
-
}
|
333 |
-
|
334 |
-
.chat-parent {
|
335 |
-
height: calc(100dvh - 181px);
|
336 |
-
overflow: auto !important;
|
337 |
-
}
|
338 |
-
|
339 |
-
.old-ui .chat-parent {
|
340 |
-
height: calc(100dvh - 270px);
|
341 |
-
}
|
342 |
-
|
343 |
-
.chat-parent.bigchat {
|
344 |
-
height: calc(100dvh - 181px) !important;
|
345 |
-
}
|
346 |
-
|
347 |
-
.chat > .messages {
|
348 |
-
display: flex;
|
349 |
-
flex-direction: column;
|
350 |
-
}
|
351 |
-
|
352 |
-
.chat .message:last-child {
|
353 |
-
margin-bottom: 0px !important;
|
354 |
-
padding-bottom: 0px !important;
|
355 |
-
}
|
356 |
-
|
357 |
-
.message-body li {
|
358 |
-
margin-top: 0 !important;
|
359 |
-
margin-bottom: 0 !important;
|
360 |
-
}
|
361 |
-
|
362 |
-
.message-body li > p {
|
363 |
-
display: inline !important;
|
364 |
-
}
|
365 |
-
|
366 |
-
.message-body ul, .message-body ol {
|
367 |
-
font-size: 15px !important;
|
368 |
-
}
|
369 |
-
|
370 |
-
.message-body ul {
|
371 |
-
list-style-type: disc !important;
|
372 |
-
}
|
373 |
-
|
374 |
-
.message-body pre {
|
375 |
-
margin-bottom: 1.25em !important;
|
376 |
-
}
|
377 |
-
|
378 |
-
.message-body code {
|
379 |
-
white-space: pre-wrap !important;
|
380 |
-
word-wrap: break-word !important;
|
381 |
-
}
|
382 |
-
|
383 |
-
.message-body :not(pre) > code {
|
384 |
-
white-space: normal !important;
|
385 |
-
}
|
386 |
-
|
387 |
-
#chat-input {
|
388 |
-
padding: 0;
|
389 |
-
padding-top: 18px;
|
390 |
-
background: transparent;
|
391 |
-
border: none;
|
392 |
-
}
|
393 |
-
|
394 |
-
#chat-input textarea:focus {
|
395 |
-
box-shadow: none !important;
|
396 |
-
}
|
397 |
-
|
398 |
-
@media print {
|
399 |
-
body {
|
400 |
-
visibility: hidden;
|
401 |
-
}
|
402 |
-
|
403 |
-
.chat {
|
404 |
-
visibility: visible;
|
405 |
-
position: absolute;
|
406 |
-
left: 0;
|
407 |
-
top: 0;
|
408 |
-
max-width: unset;
|
409 |
-
max-height: unset;
|
410 |
-
width: 100%;
|
411 |
-
overflow-y: visible;
|
412 |
-
}
|
413 |
-
|
414 |
-
.message {
|
415 |
-
break-inside: avoid;
|
416 |
-
}
|
417 |
-
|
418 |
-
.gradio-container {
|
419 |
-
overflow: visible;
|
420 |
-
}
|
421 |
-
|
422 |
-
.tab-nav {
|
423 |
-
display: none !important;
|
424 |
-
}
|
425 |
-
|
426 |
-
#chat-tab > :first-child {
|
427 |
-
max-width: unset;
|
428 |
-
}
|
429 |
-
}
|
430 |
-
|
431 |
-
#show-controls {
|
432 |
-
position: absolute;
|
433 |
-
height: 100%;
|
434 |
-
background-color: var(--background-fill-primary);
|
435 |
-
border: 0px;
|
436 |
-
border-radius: 0px;
|
437 |
-
}
|
438 |
-
|
439 |
-
#show-controls label {
|
440 |
-
z-index: 1000;
|
441 |
-
position: absolute;
|
442 |
-
left: calc(100% - 168px);
|
443 |
-
}
|
444 |
-
|
445 |
-
#typing-container {
|
446 |
-
display: none;
|
447 |
-
position: absolute;
|
448 |
-
background-color: transparent;
|
449 |
-
left: -2px;
|
450 |
-
padding: var(--block-padding);
|
451 |
-
}
|
452 |
-
|
453 |
-
.typing {
|
454 |
-
position: relative;
|
455 |
-
}
|
456 |
-
|
457 |
-
.visible-dots #typing-container {
|
458 |
-
display: block;
|
459 |
-
}
|
460 |
-
|
461 |
-
.typing span {
|
462 |
-
content: '';
|
463 |
-
animation: blink 1.5s infinite;
|
464 |
-
animation-fill-mode: both;
|
465 |
-
height: 10px;
|
466 |
-
width: 10px;
|
467 |
-
background: #3b5998;;
|
468 |
-
position: absolute;
|
469 |
-
left:0;
|
470 |
-
top:0;
|
471 |
-
border-radius: 50%;
|
472 |
-
}
|
473 |
-
|
474 |
-
.typing .dot1 {
|
475 |
-
animation-delay: .2s;
|
476 |
-
margin-left: calc(10px * 1.5);
|
477 |
-
}
|
478 |
-
|
479 |
-
.typing .dot2 {
|
480 |
-
animation-delay: .4s;
|
481 |
-
margin-left: calc(10px * 3);
|
482 |
-
}
|
483 |
-
|
484 |
-
@keyframes blink {
|
485 |
-
0% {
|
486 |
-
opacity: .1;
|
487 |
-
}
|
488 |
-
20% {
|
489 |
-
opacity: 1;
|
490 |
-
}
|
491 |
-
100% {
|
492 |
-
opacity: .1;
|
493 |
-
}
|
494 |
-
}
|
495 |
-
|
496 |
-
#chat-tab .generating {
|
497 |
-
display: none !important;
|
498 |
-
}
|
499 |
-
|
500 |
-
.hover-element {
|
501 |
-
position: relative;
|
502 |
-
font-size: 24px;
|
503 |
-
}
|
504 |
-
|
505 |
-
.hover-menu {
|
506 |
-
display: none;
|
507 |
-
position: absolute;
|
508 |
-
bottom: 80%;
|
509 |
-
left: 0;
|
510 |
-
background-color: var(--background-fill-secondary);
|
511 |
-
box-shadow: 0 0 10px rgba(0, 0, 0, 0.5);
|
512 |
-
z-index: 10000;
|
513 |
-
min-width: 330px;
|
514 |
-
flex-direction: column;
|
515 |
-
}
|
516 |
-
|
517 |
-
.hover-menu button {
|
518 |
-
width: 100%;
|
519 |
-
background: transparent !important;
|
520 |
-
border-radius: 0px !important;
|
521 |
-
justify-content: space-between;
|
522 |
-
margin: 0 !important;
|
523 |
-
height: 36px;
|
524 |
-
}
|
525 |
-
|
526 |
-
.hover-menu button:not(#clear-history-confirm) {
|
527 |
-
border-bottom: 0 !important;
|
528 |
-
}
|
529 |
-
|
530 |
-
.hover-menu button:not(#clear-history-confirm):last-child {
|
531 |
-
border-bottom: var(--button-border-width) solid var(--button-secondary-border-color) !important;
|
532 |
-
}
|
533 |
-
|
534 |
-
.hover-menu button:hover {
|
535 |
-
background: var(--button-secondary-background-fill-hover) !important;
|
536 |
-
}
|
537 |
-
|
538 |
-
.transparent-substring {
|
539 |
-
opacity: 0.333;
|
540 |
-
}
|
541 |
-
|
542 |
-
#chat-tab:not(.old-ui) #chat-buttons {
|
543 |
-
display: none !important;
|
544 |
-
}
|
545 |
-
|
546 |
-
#gr-hover-container {
|
547 |
-
min-width: 0 !important;
|
548 |
-
display: flex;
|
549 |
-
flex-direction: column-reverse;
|
550 |
-
padding-right: 20px;
|
551 |
-
padding-bottom: 3px;
|
552 |
-
flex-grow: 0 !important;
|
553 |
-
}
|
554 |
-
|
555 |
-
#generate-stop-container {
|
556 |
-
min-width: 0 !important;
|
557 |
-
display: flex;
|
558 |
-
flex-direction: column-reverse;
|
559 |
-
padding-bottom: 3px;
|
560 |
-
flex: 0 auto !important;
|
561 |
-
}
|
562 |
-
|
563 |
-
#chat-input-container {
|
564 |
-
min-width: 0 !important;
|
565 |
-
}
|
566 |
-
|
567 |
-
#chat-input-container > .form {
|
568 |
-
background: transparent;
|
569 |
-
border: none;
|
570 |
-
}
|
571 |
-
|
572 |
-
#chat-input-row {
|
573 |
-
padding-bottom: 20px;
|
574 |
-
}
|
575 |
-
|
576 |
-
.old-ui #chat-input-row, #chat-input-row.bigchat {
|
577 |
-
padding-bottom: 0px !important;
|
578 |
-
}
|
579 |
-
|
580 |
-
#chat-col {
|
581 |
-
padding-bottom: 115px;
|
582 |
-
}
|
583 |
-
|
584 |
-
.old-ui #chat-col, #chat-col.bigchat {
|
585 |
-
padding-bottom: 95px !important;
|
586 |
-
}
|
587 |
-
|
588 |
-
.old-ui #chat-buttons #clear-history-confirm {
|
589 |
-
order: -1;
|
590 |
-
}
|
591 |
-
|
592 |
-
.chat ol, .chat ul {
|
593 |
-
margin-top: 6px !important;
|
594 |
-
}
|
595 |
-
|
596 |
-
/*----------------------------------------------
|
597 |
-
Past chats menus
|
598 |
-
----------------------------------------------*/
|
599 |
-
#past-chats-row {
|
600 |
-
margin-bottom: calc( -1 * var(--layout-gap) );
|
601 |
-
}
|
602 |
-
|
603 |
-
#rename-row label {
|
604 |
-
margin-top: var(--layout-gap);
|
605 |
-
}
|
606 |
-
|
607 |
-
/*----------------------------------------------
|
608 |
-
Keep dropdown menus above errored components
|
609 |
-
----------------------------------------------*/
|
610 |
-
.options {
|
611 |
-
z-index: 100 !important;
|
612 |
-
}
|
|
|
|
|
|
|
|
|
|
|
|
|
|
|
|
|
|
|
|
|
|
|
|
|
|
|
|
|
|
|
|
|
|
|
|
|
|
|
|
|
|
|
|
|
|
|
|
|
|
|
|
|
|
|
|
|
|
|
|
|
|
|
|
|
|
|
|
|
|
|
|
|
|
|
|
|
|
|
|
|
|
|
|
|
|
|
|
|
|
|
|
|
|
|
|
|
|
|
|
|
|
|
|
|
|
|
|
|
|
|
|
|
|
|
|
|
|
|
|
|
|
|
|
|
|
|
|
|
|
|
|
|
|
|
|
|
|
|
|
|
|
|
|
|
|
|
|
|
|
|
|
|
|
|
|
|
|
|
|
|
|
|
|
|
|
|
|
|
|
|
|
|
|
|
|
|
|
|
|
|
|
|
|
|
|
|
|
|
|
|
|
|
|
|
|
|
|
|
|
|
|
|
|
|
|
|
|
|
|
|
|
|
|
|
|
|
|
|
|
|
|
|
|
|
|
|
|
|
|
|
|
|
|
|
|
|
|
|
|
|
|
|
|
|
|
|
|
|
|
|
|
|
|
|
|
|
|
|
|
|
|
|
|
|
|
|
|
|
|
|
|
|
|
|
|
|
|
|
|
|
|
|
|
|
|
|
|
|
|
|
|
|
|
|
|
|
|
|
|
|
|
|
|
|
|
|
|
|
|
|
|
|
|
|
|
|
|
|
|
|
|
|
|
|
|
|
|
|
|
|
|
|
|
|
|
|
|
|
|
|
|
|
|
|
|
|
|
|
|
|
|
|
|
|
|
|
|
|
|
|
|
|
|
|
|
|
|
|
|
|
|
|
|
|
|
|
|
|
|
|
|
|
|
|
|
|
|
|
|
|
|
|
|
|
|
|
|
|
|
|
|
|
|
|
|
|
|
|
|
|
|
|
|
|
|
|
|
|
|
|
|
|
|
|
|
|
|
|
|
|
|
|
|
|
|
|
|
|
|
|
|
|
|
|
|
|
|
|
|
|
|
|
|
|
|
|
|
|
|
|
|
|
|
|
|
|
|
|
|
|
|
|
|
|
|
|
|
|
|
|
|
|
|
|
|
|
|
|
|
|
|
|
|
|
|
|
|
|
|
|
|
|
|
|
|
|
|
|
|
|
|
|
|
|
|
|
|
|
|
|
|
|
|
|
|
|
|
|
|
|
|
|
|
|
|
|
|
|
|
|
|
|
|
|
|
|
|
|
|
|
|
|
|
|
|
|
|
|
|
|
|
|
|
|
|
|
|
|
|
|
|
|
|
|
|
|
|
|
|
|
|
|
|
|
|
|
|
|
|
|
|
|
|
|
|
|
|
|
|
|
|
|
|
|
|
|
|
|
|
|
|
|
|
|
|
|
|
|
|
|
|
|
|
|
|
|
|
|
|
|
|
|
|
|
|
|
|
|
|
|
|
|
|
|
|
|
|
|
|
|
|
|
|
|
|
|
|
|
|
|
|
|
|
|
|
|
|
|
|
|
|
|
|
|
|
|
|
|
|
|
|
|
|
|
|
|
|
|
|
|
|
|
|
|
|
|
|
|
|
|
|
|
|
|
|
|
|
|
|
|
|
|
|
|
|
|
|
|
|
|
|
|
|
|
|
|
|
|
|
|
|
|
|
|
|
|
|
|
|
|
|
|
|
|
|
|
|
|
|
|
|
|
|
|
|
|
|
|
|
|
|
|
|
|
|
|
|
|
|
|
|
|
|
|
|
|
|
|
|
|
|
|
|
|
|
|
|
|
|
|
|
|
|
|
|
|
|
|
|
|
|
|
|
|
|
|
|
|
|
|
|
|
|
|
|
|
|
|
|
|
|
|
|
|
|
|
|
|
|
|
|
|
|
|
|
|
|
|
|
|
|
|
|
|
|
|
|
|
|
|
|
|
|
|
|
|
|
|
|
|
|
|
|
|
|
|
|
|
|
|
|
|
|
|
|
|
|
|
|
|
|
|
|
|
|
|
|
|
|
|
|
|
|
|
|
|
|
|
|
|
|
|
|
|
|
|
|
|
|
|
|
|
|
|
|
|
|
|
|
|
|
|
|
|
|
|
|
|
|
|
|
|
|
|
|
|
|
|
|
|
|
|
|
|
|
|
|
|
|
|
|
|
|
|
|
|
|
|
|
|
|
|
|
|
|
|
|
|
|
|
|
|
|
|
|
|
|
|
|
|
|
|
|
|
|
|
|
|
|
|
|
|
|
|
|
|
|
|
|
|
|
|
|
|
|
|
|
|
|
|
|
|
|
|
|
|
|
|
|
|
|
|
|
|
|
|
|
|
|
|
|
|
|
|
|
|
|
|
|
|
|
|
|
|
|
|
|
|
|
|
|
|
|
|
|
|
|
|
|
|
|
|
|
|
|
|
|
|
|
|
|
|
|
|
|
|
|
|
|
|
|
|
|
|
|
|
|
|
|
|
|
|
|
|
|
|
|
|
|
|
|
|
|
|
|
|
|
|
|
|
|
|
|
|
|
|
|
|
|
|
|
|
|
|
|
|
|
|
|
|
|
|
|
|
|
|
|
|
|
|
|
|
|
|
|
|
|
|
|
|
|
|
|
|
|
|
|
|
|
|
|
|
|
|
|
|
|
|
|
|
|
|
|
|
|
|
|
|
|
|
|
|
|
|
|
|
|
|
|
|
|
|
|
|
|
|
|
|
|
|
|
|
|
|
|
|
|
|
|
|
|
|
|
|
|
|
|
|
|
|
|
|
|
|
|
spaces/Anonymous-sub/Rerender/ControlNet/annotator/uniformer/mmcv/ops/multi_scale_deform_attn.py
DELETED
@@ -1,358 +0,0 @@
|
|
1 |
-
# Copyright (c) OpenMMLab. All rights reserved.
|
2 |
-
import math
|
3 |
-
import warnings
|
4 |
-
|
5 |
-
import torch
|
6 |
-
import torch.nn as nn
|
7 |
-
import torch.nn.functional as F
|
8 |
-
from torch.autograd.function import Function, once_differentiable
|
9 |
-
|
10 |
-
from annotator.uniformer.mmcv import deprecated_api_warning
|
11 |
-
from annotator.uniformer.mmcv.cnn import constant_init, xavier_init
|
12 |
-
from annotator.uniformer.mmcv.cnn.bricks.registry import ATTENTION
|
13 |
-
from annotator.uniformer.mmcv.runner import BaseModule
|
14 |
-
from ..utils import ext_loader
|
15 |
-
|
16 |
-
ext_module = ext_loader.load_ext(
|
17 |
-
'_ext', ['ms_deform_attn_backward', 'ms_deform_attn_forward'])
|
18 |
-
|
19 |
-
|
20 |
-
class MultiScaleDeformableAttnFunction(Function):
|
21 |
-
|
22 |
-
@staticmethod
|
23 |
-
def forward(ctx, value, value_spatial_shapes, value_level_start_index,
|
24 |
-
sampling_locations, attention_weights, im2col_step):
|
25 |
-
"""GPU version of multi-scale deformable attention.
|
26 |
-
|
27 |
-
Args:
|
28 |
-
value (Tensor): The value has shape
|
29 |
-
(bs, num_keys, mum_heads, embed_dims//num_heads)
|
30 |
-
value_spatial_shapes (Tensor): Spatial shape of
|
31 |
-
each feature map, has shape (num_levels, 2),
|
32 |
-
last dimension 2 represent (h, w)
|
33 |
-
sampling_locations (Tensor): The location of sampling points,
|
34 |
-
has shape
|
35 |
-
(bs ,num_queries, num_heads, num_levels, num_points, 2),
|
36 |
-
the last dimension 2 represent (x, y).
|
37 |
-
attention_weights (Tensor): The weight of sampling points used
|
38 |
-
when calculate the attention, has shape
|
39 |
-
(bs ,num_queries, num_heads, num_levels, num_points),
|
40 |
-
im2col_step (Tensor): The step used in image to column.
|
41 |
-
|
42 |
-
Returns:
|
43 |
-
Tensor: has shape (bs, num_queries, embed_dims)
|
44 |
-
"""
|
45 |
-
|
46 |
-
ctx.im2col_step = im2col_step
|
47 |
-
output = ext_module.ms_deform_attn_forward(
|
48 |
-
value,
|
49 |
-
value_spatial_shapes,
|
50 |
-
value_level_start_index,
|
51 |
-
sampling_locations,
|
52 |
-
attention_weights,
|
53 |
-
im2col_step=ctx.im2col_step)
|
54 |
-
ctx.save_for_backward(value, value_spatial_shapes,
|
55 |
-
value_level_start_index, sampling_locations,
|
56 |
-
attention_weights)
|
57 |
-
return output
|
58 |
-
|
59 |
-
@staticmethod
|
60 |
-
@once_differentiable
|
61 |
-
def backward(ctx, grad_output):
|
62 |
-
"""GPU version of backward function.
|
63 |
-
|
64 |
-
Args:
|
65 |
-
grad_output (Tensor): Gradient
|
66 |
-
of output tensor of forward.
|
67 |
-
|
68 |
-
Returns:
|
69 |
-
Tuple[Tensor]: Gradient
|
70 |
-
of input tensors in forward.
|
71 |
-
"""
|
72 |
-
value, value_spatial_shapes, value_level_start_index,\
|
73 |
-
sampling_locations, attention_weights = ctx.saved_tensors
|
74 |
-
grad_value = torch.zeros_like(value)
|
75 |
-
grad_sampling_loc = torch.zeros_like(sampling_locations)
|
76 |
-
grad_attn_weight = torch.zeros_like(attention_weights)
|
77 |
-
|
78 |
-
ext_module.ms_deform_attn_backward(
|
79 |
-
value,
|
80 |
-
value_spatial_shapes,
|
81 |
-
value_level_start_index,
|
82 |
-
sampling_locations,
|
83 |
-
attention_weights,
|
84 |
-
grad_output.contiguous(),
|
85 |
-
grad_value,
|
86 |
-
grad_sampling_loc,
|
87 |
-
grad_attn_weight,
|
88 |
-
im2col_step=ctx.im2col_step)
|
89 |
-
|
90 |
-
return grad_value, None, None, \
|
91 |
-
grad_sampling_loc, grad_attn_weight, None
|
92 |
-
|
93 |
-
|
94 |
-
def multi_scale_deformable_attn_pytorch(value, value_spatial_shapes,
|
95 |
-
sampling_locations, attention_weights):
|
96 |
-
"""CPU version of multi-scale deformable attention.
|
97 |
-
|
98 |
-
Args:
|
99 |
-
value (Tensor): The value has shape
|
100 |
-
(bs, num_keys, mum_heads, embed_dims//num_heads)
|
101 |
-
value_spatial_shapes (Tensor): Spatial shape of
|
102 |
-
each feature map, has shape (num_levels, 2),
|
103 |
-
last dimension 2 represent (h, w)
|
104 |
-
sampling_locations (Tensor): The location of sampling points,
|
105 |
-
has shape
|
106 |
-
(bs ,num_queries, num_heads, num_levels, num_points, 2),
|
107 |
-
the last dimension 2 represent (x, y).
|
108 |
-
attention_weights (Tensor): The weight of sampling points used
|
109 |
-
when calculate the attention, has shape
|
110 |
-
(bs ,num_queries, num_heads, num_levels, num_points),
|
111 |
-
|
112 |
-
Returns:
|
113 |
-
Tensor: has shape (bs, num_queries, embed_dims)
|
114 |
-
"""
|
115 |
-
|
116 |
-
bs, _, num_heads, embed_dims = value.shape
|
117 |
-
_, num_queries, num_heads, num_levels, num_points, _ =\
|
118 |
-
sampling_locations.shape
|
119 |
-
value_list = value.split([H_ * W_ for H_, W_ in value_spatial_shapes],
|
120 |
-
dim=1)
|
121 |
-
sampling_grids = 2 * sampling_locations - 1
|
122 |
-
sampling_value_list = []
|
123 |
-
for level, (H_, W_) in enumerate(value_spatial_shapes):
|
124 |
-
# bs, H_*W_, num_heads, embed_dims ->
|
125 |
-
# bs, H_*W_, num_heads*embed_dims ->
|
126 |
-
# bs, num_heads*embed_dims, H_*W_ ->
|
127 |
-
# bs*num_heads, embed_dims, H_, W_
|
128 |
-
value_l_ = value_list[level].flatten(2).transpose(1, 2).reshape(
|
129 |
-
bs * num_heads, embed_dims, H_, W_)
|
130 |
-
# bs, num_queries, num_heads, num_points, 2 ->
|
131 |
-
# bs, num_heads, num_queries, num_points, 2 ->
|
132 |
-
# bs*num_heads, num_queries, num_points, 2
|
133 |
-
sampling_grid_l_ = sampling_grids[:, :, :,
|
134 |
-
level].transpose(1, 2).flatten(0, 1)
|
135 |
-
# bs*num_heads, embed_dims, num_queries, num_points
|
136 |
-
sampling_value_l_ = F.grid_sample(
|
137 |
-
value_l_,
|
138 |
-
sampling_grid_l_,
|
139 |
-
mode='bilinear',
|
140 |
-
padding_mode='zeros',
|
141 |
-
align_corners=False)
|
142 |
-
sampling_value_list.append(sampling_value_l_)
|
143 |
-
# (bs, num_queries, num_heads, num_levels, num_points) ->
|
144 |
-
# (bs, num_heads, num_queries, num_levels, num_points) ->
|
145 |
-
# (bs, num_heads, 1, num_queries, num_levels*num_points)
|
146 |
-
attention_weights = attention_weights.transpose(1, 2).reshape(
|
147 |
-
bs * num_heads, 1, num_queries, num_levels * num_points)
|
148 |
-
output = (torch.stack(sampling_value_list, dim=-2).flatten(-2) *
|
149 |
-
attention_weights).sum(-1).view(bs, num_heads * embed_dims,
|
150 |
-
num_queries)
|
151 |
-
return output.transpose(1, 2).contiguous()
|
152 |
-
|
153 |
-
|
154 |
-
@ATTENTION.register_module()
|
155 |
-
class MultiScaleDeformableAttention(BaseModule):
|
156 |
-
"""An attention module used in Deformable-Detr.
|
157 |
-
|
158 |
-
`Deformable DETR: Deformable Transformers for End-to-End Object Detection.
|
159 |
-
<https://arxiv.org/pdf/2010.04159.pdf>`_.
|
160 |
-
|
161 |
-
Args:
|
162 |
-
embed_dims (int): The embedding dimension of Attention.
|
163 |
-
Default: 256.
|
164 |
-
num_heads (int): Parallel attention heads. Default: 64.
|
165 |
-
num_levels (int): The number of feature map used in
|
166 |
-
Attention. Default: 4.
|
167 |
-
num_points (int): The number of sampling points for
|
168 |
-
each query in each head. Default: 4.
|
169 |
-
im2col_step (int): The step used in image_to_column.
|
170 |
-
Default: 64.
|
171 |
-
dropout (float): A Dropout layer on `inp_identity`.
|
172 |
-
Default: 0.1.
|
173 |
-
batch_first (bool): Key, Query and Value are shape of
|
174 |
-
(batch, n, embed_dim)
|
175 |
-
or (n, batch, embed_dim). Default to False.
|
176 |
-
norm_cfg (dict): Config dict for normalization layer.
|
177 |
-
Default: None.
|
178 |
-
init_cfg (obj:`mmcv.ConfigDict`): The Config for initialization.
|
179 |
-
Default: None.
|
180 |
-
"""
|
181 |
-
|
182 |
-
def __init__(self,
|
183 |
-
embed_dims=256,
|
184 |
-
num_heads=8,
|
185 |
-
num_levels=4,
|
186 |
-
num_points=4,
|
187 |
-
im2col_step=64,
|
188 |
-
dropout=0.1,
|
189 |
-
batch_first=False,
|
190 |
-
norm_cfg=None,
|
191 |
-
init_cfg=None):
|
192 |
-
super().__init__(init_cfg)
|
193 |
-
if embed_dims % num_heads != 0:
|
194 |
-
raise ValueError(f'embed_dims must be divisible by num_heads, '
|
195 |
-
f'but got {embed_dims} and {num_heads}')
|
196 |
-
dim_per_head = embed_dims // num_heads
|
197 |
-
self.norm_cfg = norm_cfg
|
198 |
-
self.dropout = nn.Dropout(dropout)
|
199 |
-
self.batch_first = batch_first
|
200 |
-
|
201 |
-
# you'd better set dim_per_head to a power of 2
|
202 |
-
# which is more efficient in the CUDA implementation
|
203 |
-
def _is_power_of_2(n):
|
204 |
-
if (not isinstance(n, int)) or (n < 0):
|
205 |
-
raise ValueError(
|
206 |
-
'invalid input for _is_power_of_2: {} (type: {})'.format(
|
207 |
-
n, type(n)))
|
208 |
-
return (n & (n - 1) == 0) and n != 0
|
209 |
-
|
210 |
-
if not _is_power_of_2(dim_per_head):
|
211 |
-
warnings.warn(
|
212 |
-
"You'd better set embed_dims in "
|
213 |
-
'MultiScaleDeformAttention to make '
|
214 |
-
'the dimension of each attention head a power of 2 '
|
215 |
-
'which is more efficient in our CUDA implementation.')
|
216 |
-
|
217 |
-
self.im2col_step = im2col_step
|
218 |
-
self.embed_dims = embed_dims
|
219 |
-
self.num_levels = num_levels
|
220 |
-
self.num_heads = num_heads
|
221 |
-
self.num_points = num_points
|
222 |
-
self.sampling_offsets = nn.Linear(
|
223 |
-
embed_dims, num_heads * num_levels * num_points * 2)
|
224 |
-
self.attention_weights = nn.Linear(embed_dims,
|
225 |
-
num_heads * num_levels * num_points)
|
226 |
-
self.value_proj = nn.Linear(embed_dims, embed_dims)
|
227 |
-
self.output_proj = nn.Linear(embed_dims, embed_dims)
|
228 |
-
self.init_weights()
|
229 |
-
|
230 |
-
def init_weights(self):
|
231 |
-
"""Default initialization for Parameters of Module."""
|
232 |
-
constant_init(self.sampling_offsets, 0.)
|
233 |
-
thetas = torch.arange(
|
234 |
-
self.num_heads,
|
235 |
-
dtype=torch.float32) * (2.0 * math.pi / self.num_heads)
|
236 |
-
grid_init = torch.stack([thetas.cos(), thetas.sin()], -1)
|
237 |
-
grid_init = (grid_init /
|
238 |
-
grid_init.abs().max(-1, keepdim=True)[0]).view(
|
239 |
-
self.num_heads, 1, 1,
|
240 |
-
2).repeat(1, self.num_levels, self.num_points, 1)
|
241 |
-
for i in range(self.num_points):
|
242 |
-
grid_init[:, :, i, :] *= i + 1
|
243 |
-
|
244 |
-
self.sampling_offsets.bias.data = grid_init.view(-1)
|
245 |
-
constant_init(self.attention_weights, val=0., bias=0.)
|
246 |
-
xavier_init(self.value_proj, distribution='uniform', bias=0.)
|
247 |
-
xavier_init(self.output_proj, distribution='uniform', bias=0.)
|
248 |
-
self._is_init = True
|
249 |
-
|
250 |
-
@deprecated_api_warning({'residual': 'identity'},
|
251 |
-
cls_name='MultiScaleDeformableAttention')
|
252 |
-
def forward(self,
|
253 |
-
query,
|
254 |
-
key=None,
|
255 |
-
value=None,
|
256 |
-
identity=None,
|
257 |
-
query_pos=None,
|
258 |
-
key_padding_mask=None,
|
259 |
-
reference_points=None,
|
260 |
-
spatial_shapes=None,
|
261 |
-
level_start_index=None,
|
262 |
-
**kwargs):
|
263 |
-
"""Forward Function of MultiScaleDeformAttention.
|
264 |
-
|
265 |
-
Args:
|
266 |
-
query (Tensor): Query of Transformer with shape
|
267 |
-
(num_query, bs, embed_dims).
|
268 |
-
key (Tensor): The key tensor with shape
|
269 |
-
`(num_key, bs, embed_dims)`.
|
270 |
-
value (Tensor): The value tensor with shape
|
271 |
-
`(num_key, bs, embed_dims)`.
|
272 |
-
identity (Tensor): The tensor used for addition, with the
|
273 |
-
same shape as `query`. Default None. If None,
|
274 |
-
`query` will be used.
|
275 |
-
query_pos (Tensor): The positional encoding for `query`.
|
276 |
-
Default: None.
|
277 |
-
key_pos (Tensor): The positional encoding for `key`. Default
|
278 |
-
None.
|
279 |
-
reference_points (Tensor): The normalized reference
|
280 |
-
points with shape (bs, num_query, num_levels, 2),
|
281 |
-
all elements is range in [0, 1], top-left (0,0),
|
282 |
-
bottom-right (1, 1), including padding area.
|
283 |
-
or (N, Length_{query}, num_levels, 4), add
|
284 |
-
additional two dimensions is (w, h) to
|
285 |
-
form reference boxes.
|
286 |
-
key_padding_mask (Tensor): ByteTensor for `query`, with
|
287 |
-
shape [bs, num_key].
|
288 |
-
spatial_shapes (Tensor): Spatial shape of features in
|
289 |
-
different levels. With shape (num_levels, 2),
|
290 |
-
last dimension represents (h, w).
|
291 |
-
level_start_index (Tensor): The start index of each level.
|
292 |
-
A tensor has shape ``(num_levels, )`` and can be represented
|
293 |
-
as [0, h_0*w_0, h_0*w_0+h_1*w_1, ...].
|
294 |
-
|
295 |
-
Returns:
|
296 |
-
Tensor: forwarded results with shape [num_query, bs, embed_dims].
|
297 |
-
"""
|
298 |
-
|
299 |
-
if value is None:
|
300 |
-
value = query
|
301 |
-
|
302 |
-
if identity is None:
|
303 |
-
identity = query
|
304 |
-
if query_pos is not None:
|
305 |
-
query = query + query_pos
|
306 |
-
if not self.batch_first:
|
307 |
-
# change to (bs, num_query ,embed_dims)
|
308 |
-
query = query.permute(1, 0, 2)
|
309 |
-
value = value.permute(1, 0, 2)
|
310 |
-
|
311 |
-
bs, num_query, _ = query.shape
|
312 |
-
bs, num_value, _ = value.shape
|
313 |
-
assert (spatial_shapes[:, 0] * spatial_shapes[:, 1]).sum() == num_value
|
314 |
-
|
315 |
-
value = self.value_proj(value)
|
316 |
-
if key_padding_mask is not None:
|
317 |
-
value = value.masked_fill(key_padding_mask[..., None], 0.0)
|
318 |
-
value = value.view(bs, num_value, self.num_heads, -1)
|
319 |
-
sampling_offsets = self.sampling_offsets(query).view(
|
320 |
-
bs, num_query, self.num_heads, self.num_levels, self.num_points, 2)
|
321 |
-
attention_weights = self.attention_weights(query).view(
|
322 |
-
bs, num_query, self.num_heads, self.num_levels * self.num_points)
|
323 |
-
attention_weights = attention_weights.softmax(-1)
|
324 |
-
|
325 |
-
attention_weights = attention_weights.view(bs, num_query,
|
326 |
-
self.num_heads,
|
327 |
-
self.num_levels,
|
328 |
-
self.num_points)
|
329 |
-
if reference_points.shape[-1] == 2:
|
330 |
-
offset_normalizer = torch.stack(
|
331 |
-
[spatial_shapes[..., 1], spatial_shapes[..., 0]], -1)
|
332 |
-
sampling_locations = reference_points[:, :, None, :, None, :] \
|
333 |
-
+ sampling_offsets \
|
334 |
-
/ offset_normalizer[None, None, None, :, None, :]
|
335 |
-
elif reference_points.shape[-1] == 4:
|
336 |
-
sampling_locations = reference_points[:, :, None, :, None, :2] \
|
337 |
-
+ sampling_offsets / self.num_points \
|
338 |
-
* reference_points[:, :, None, :, None, 2:] \
|
339 |
-
* 0.5
|
340 |
-
else:
|
341 |
-
raise ValueError(
|
342 |
-
f'Last dim of reference_points must be'
|
343 |
-
f' 2 or 4, but get {reference_points.shape[-1]} instead.')
|
344 |
-
if torch.cuda.is_available() and value.is_cuda:
|
345 |
-
output = MultiScaleDeformableAttnFunction.apply(
|
346 |
-
value, spatial_shapes, level_start_index, sampling_locations,
|
347 |
-
attention_weights, self.im2col_step)
|
348 |
-
else:
|
349 |
-
output = multi_scale_deformable_attn_pytorch(
|
350 |
-
value, spatial_shapes, sampling_locations, attention_weights)
|
351 |
-
|
352 |
-
output = self.output_proj(output)
|
353 |
-
|
354 |
-
if not self.batch_first:
|
355 |
-
# (num_query, bs ,embed_dims)
|
356 |
-
output = output.permute(1, 0, 2)
|
357 |
-
|
358 |
-
return self.dropout(output) + identity
|
|
|
|
|
|
|
|
|
|
|
|
|
|
|
|
|
|
|
|
|
|
|
|
|
|
|
|
|
|
|
|
|
|
|
|
|
|
|
|
|
|
|
|
|
|
|
|
|
|
|
|
|
|
|
|
|
|
|
|
|
|
|
|
|
|
|
|
|
|
|
|
|
|
|
|
|
|
|
|
|
|
|
|
|
|
|
|
|
|
|
|
|
|
|
|
|
|
|
|
|
|
|
|
|
|
|
|
|
|
|
|
|
|
|
|
|
|
|
|
|
|
|
|
|
|
|
|
|
|
|
|
|
|
|
|
|
|
|
|
|
|
|
|
|
|
|
|
|
|
|
|
|
|
|
|
|
|
|
|
|
|
|
|
|
|
|
|
|
|
|
|
|
|
|
|
|
|
|
|
|
|
|
|
|
|
|
|
|
|
|
|
|
|
|
|
|
|
|
|
|
|
|
|
|
|
|
|
|
|
|
|
|
|
|
|
|
|
|
|
|
|
|
|
|
|
|
|
|
|
|
|
|
|
|
|
|
|
|
|
|
|
|
|
|
|
|
|
|
|
|
|
|
|
|
|
|
|
|
|
|
|
|
|
|
|
|
|
|
|
|
|
|
|
|
|
|
|
|
|
|
|
|
|
|
|
|
|
|
|
|
|
|
|
|
|
|
|
|
|
|
|
|
|
|
|
|
|
|
|
|
|
|
|
|
|
|
|
|
|
|
|
|
|
|
|
|
|
|
|
|
|
|
|
|
|
|
|
|
|
|
|
|
|
|
|
|
|
|
|
|
|
|
|
|
|
|
|
|
|
|
|
|
|
|
|
|
|
|
|
|
|
|
|
|
|
|
|
|
|
|
|
|
|
|
|
|
|
|
|
|
|
|
|
|
|
|
|
|
|
|
|
|
|
|
|
|
|
|
|
|
|
|
|
|
|
|
|
|
|
|
|
|
|
|
|
|
|
|
|
|
|
|
|
|
|
|
|
|
|
|
|
|
|
|
|
|
|
|
|
|
|
|
|
|
|
|
|
|
|
|
|
|
|
|
|
|
|
|
|
|
|
|
|
|
|
|
|
|
|
|
|
|
|
|
|
|
|
|
|
|
|
|
|
|
|
|
|
|
|
|
|
|
|
|
|
|
|
|
|
|
|
|
|
|
|
|
|
|
|
|
|
|
|
|
|
|
|
|
|
|
|
|
|
|
|
|
|
|
|
|
|
|
|
|
|
|
|
|
|
|
|
|
|
|
|
|
|
|
|
|
|
|
|
|
|
|
|
|
|
|
|
|
|
|
|
|
|
|
|
|
|
|
|
|
|
|
|
|
|
|
|
|
|
|
|
|
|
|
|
|
|
|
|
|
|
|
|
|
|
|
|
|
|
|
|
|
|
|
|
|
|
|
|
|
|
|
|
|
|
|
|
|
|
|
|
|
|
|
|
|
|
|
|
|
|
|
|
|
|
|
|
|
|
|
|
|
|
|
|
|
|
|
|
|
|
|
|
|
|
|
|
|
|
|
|
|
|
|
|
|
|
|
|
|
|
|
|
|
|
|
|
|
|
|
|
|
|
|
|
|
|
|
|
|
|
|
|
|
|
|
|
|
|
|
|
|
|
spaces/Arnx/MusicGenXvAKN/MODEL_CARD.md
DELETED
@@ -1,81 +0,0 @@
|
|
1 |
-
# MusicGen Model Card
|
2 |
-
|
3 |
-
## Model details
|
4 |
-
|
5 |
-
**Organization developing the model:** The FAIR team of Meta AI.
|
6 |
-
|
7 |
-
**Model date:** MusicGen was trained between April 2023 and May 2023.
|
8 |
-
|
9 |
-
**Model version:** This is the version 1 of the model.
|
10 |
-
|
11 |
-
**Model type:** MusicGen consists of an EnCodec model for audio tokenization, an auto-regressive language model based on the transformer architecture for music modeling. The model comes in different sizes: 300M, 1.5B and 3.3B parameters ; and two variants: a model trained for text-to-music generation task and a model trained for melody-guided music generation.
|
12 |
-
|
13 |
-
**Paper or resources for more information:** More information can be found in the paper [Simple and Controllable Music Generation][arxiv].
|
14 |
-
|
15 |
-
**Citation details** See [our paper][arxiv]
|
16 |
-
|
17 |
-
**License** Code is released under MIT, model weights are released under CC-BY-NC 4.0.
|
18 |
-
|
19 |
-
**Where to send questions or comments about the model:** Questions and comments about MusicGen can be sent via the [Github repository](https://github.com/facebookresearch/audiocraft) of the project, or by opening an issue.
|
20 |
-
|
21 |
-
## Intended use
|
22 |
-
**Primary intended use:** The primary use of MusicGen is research on AI-based music generation, including:
|
23 |
-
|
24 |
-
- Research efforts, such as probing and better understanding the limitations of generative models to further improve the state of science
|
25 |
-
- Generation of music guided by text or melody to understand current abilities of generative AI models by machine learning amateurs
|
26 |
-
|
27 |
-
**Primary intended users:** The primary intended users of the model are researchers in audio, machine learning and artificial intelligence, as well as amateur seeking to better understand those models.
|
28 |
-
|
29 |
-
**Out-of-scope use cases** The model should not be used on downstream applications without further risk evaluation and mitigation. The model should not be used to intentionally create or disseminate music pieces that create hostile or alienating environments for people. This includes generating music that people would foreseeably find disturbing, distressing, or offensive; or content that propagates historical or current stereotypes.
|
30 |
-
|
31 |
-
## Metrics
|
32 |
-
|
33 |
-
**Models performance measures:** We used the following objective measure to evaluate the model on a standard music benchmark:
|
34 |
-
|
35 |
-
- Frechet Audio Distance computed on features extracted from a pre-trained audio classifier (VGGish)
|
36 |
-
- Kullback-Leibler Divergence on label distributions extracted from a pre-trained audio classifier (PaSST)
|
37 |
-
- CLAP Score between audio embedding and text embedding extracted from a pre-trained CLAP model
|
38 |
-
|
39 |
-
Additionally, we run qualitative studies with human participants, evaluating the performance of the model with the following axes:
|
40 |
-
|
41 |
-
- Overall quality of the music samples;
|
42 |
-
- Text relevance to the provided text input;
|
43 |
-
- Adherence to the melody for melody-guided music generation.
|
44 |
-
|
45 |
-
More details on performance measures and human studies can be found in the paper.
|
46 |
-
|
47 |
-
**Decision thresholds:** Not applicable.
|
48 |
-
|
49 |
-
## Evaluation datasets
|
50 |
-
|
51 |
-
The model was evaluated on the [MusicCaps benchmark](https://www.kaggle.com/datasets/googleai/musiccaps) and on an in-domain held-out evaluation set, with no artist overlap with the training set.
|
52 |
-
|
53 |
-
## Training datasets
|
54 |
-
|
55 |
-
The model was trained on licensed data using the following sources: the [Meta Music Initiative Sound Collection](https://www.fb.com/sound), [Shutterstock music collection](https://www.shutterstock.com/music) and the [Pond5 music collection](https://www.pond5.com/). See the paper for more details about the training set and corresponding preprocessing.
|
56 |
-
|
57 |
-
## Quantitative analysis
|
58 |
-
|
59 |
-
More information can be found in the paper [Simple and Controllable Music Generation][arxiv], in the Experimental Setup section.
|
60 |
-
|
61 |
-
## Limitations and biases
|
62 |
-
|
63 |
-
**Data:** The data sources used to train the model are created by music professionals and covered by legal agreements with the right holders. The model is trained on 20K hours of data, we believe that scaling the model on larger datasets can further improve the performance of the model.
|
64 |
-
|
65 |
-
**Mitigations:** Vocals have been removed from the data source using corresponding tags, and then using using a state-of-the-art music source separation method, namely using the open source [Hybrid Transformer for Music Source Separation](https://github.com/facebookresearch/demucs) (HT-Demucs).
|
66 |
-
|
67 |
-
**Limitations:**
|
68 |
-
|
69 |
-
- The model is not able to generate realistic vocals.
|
70 |
-
- The model has been trained with English descriptions and will not perform as well in other languages.
|
71 |
-
- The model does not perform equally well for all music styles and cultures.
|
72 |
-
- The model sometimes generates end of songs, collapsing to silence.
|
73 |
-
- It is sometimes difficult to assess what types of text descriptions provide the best generations. Prompt engineering may be required to obtain satisfying results.
|
74 |
-
|
75 |
-
**Biases:** The source of data is potentially lacking diversity and all music cultures are not equally represented in the dataset. The model may not perform equally well on the wide variety of music genres that exists. The generated samples from the model will reflect the biases from the training data. Further work on this model should include methods for balanced and just representations of cultures, for example, by scaling the training data to be both diverse and inclusive.
|
76 |
-
|
77 |
-
**Risks and harms:** Biases and limitations of the model may lead to generation of samples that may be considered as biased, inappropriate or offensive. We believe that providing the code to reproduce the research and train new models will allow to broaden the application to new and more representative data.
|
78 |
-
|
79 |
-
**Use cases:** Users must be aware of the biases, limitations and risks of the model. MusicGen is a model developed for artificial intelligence research on controllable music generation. As such, it should not be used for downstream applications without further investigation and mitigation of risks.
|
80 |
-
|
81 |
-
[arxiv]: https://arxiv.org/abs/2306.05284
|
|
|
|
|
|
|
|
|
|
|
|
|
|
|
|
|
|
|
|
|
|
|
|
|
|
|
|
|
|
|
|
|
|
|
|
|
|
|
|
|
|
|
|
|
|
|
|
|
|
|
|
|
|
|
|
|
|
|
|
|
|
|
|
|
|
|
|
|
|
|
|
|
|
|
|
|
|
|
|
|
|
|
|
|
|
|
|
|
|
|
|
|
|
|
|
|
|
|
|
|
|
|
|
|
|
|
|
|
|
|
|
|
|
|
|
|
|
|
|
|
|
|
|
|
|
|
|
|
|
|
|
|
|
|
|
|
|
|
|
|
|
|
|
|
|
|
|
|
|
|
|
|
|
|
|
|
|
|
|
|
|
|
|
spaces/Arnx/MusicGenXvAKN/tests/__init__.py
DELETED
@@ -1,5 +0,0 @@
|
|
1 |
-
# Copyright (c) Meta Platforms, Inc. and affiliates.
|
2 |
-
# All rights reserved.
|
3 |
-
#
|
4 |
-
# This source code is licensed under the license found in the
|
5 |
-
# LICENSE file in the root directory of this source tree.
|
|
|
|
|
|
|
|
|
|
|
|
spaces/Artrajz/vits-simple-api/utils/utils.py
DELETED
@@ -1,95 +0,0 @@
|
|
1 |
-
import logging
|
2 |
-
import os
|
3 |
-
from json import loads
|
4 |
-
from torch import load, FloatTensor
|
5 |
-
from numpy import float32
|
6 |
-
import librosa
|
7 |
-
|
8 |
-
|
9 |
-
class HParams():
|
10 |
-
def __init__(self, **kwargs):
|
11 |
-
for k, v in kwargs.items():
|
12 |
-
if type(v) == dict:
|
13 |
-
v = HParams(**v)
|
14 |
-
self[k] = v
|
15 |
-
|
16 |
-
def keys(self):
|
17 |
-
return self.__dict__.keys()
|
18 |
-
|
19 |
-
def items(self):
|
20 |
-
return self.__dict__.items()
|
21 |
-
|
22 |
-
def values(self):
|
23 |
-
return self.__dict__.values()
|
24 |
-
|
25 |
-
def __len__(self):
|
26 |
-
return len(self.__dict__)
|
27 |
-
|
28 |
-
def __getitem__(self, key):
|
29 |
-
return getattr(self, key)
|
30 |
-
|
31 |
-
def __setitem__(self, key, value):
|
32 |
-
return setattr(self, key, value)
|
33 |
-
|
34 |
-
def __contains__(self, key):
|
35 |
-
return key in self.__dict__
|
36 |
-
|
37 |
-
def __repr__(self):
|
38 |
-
return self.__dict__.__repr__()
|
39 |
-
|
40 |
-
|
41 |
-
def load_checkpoint(checkpoint_path, model):
|
42 |
-
checkpoint_dict = load(checkpoint_path, map_location='cpu')
|
43 |
-
iteration = checkpoint_dict.get('iteration', None)
|
44 |
-
saved_state_dict = checkpoint_dict['model']
|
45 |
-
if hasattr(model, 'module'):
|
46 |
-
state_dict = model.module.state_dict()
|
47 |
-
else:
|
48 |
-
state_dict = model.state_dict()
|
49 |
-
new_state_dict = {}
|
50 |
-
for k, v in state_dict.items():
|
51 |
-
try:
|
52 |
-
new_state_dict[k] = saved_state_dict[k]
|
53 |
-
except:
|
54 |
-
logging.info(f"{k} is not in the checkpoint")
|
55 |
-
new_state_dict[k] = v
|
56 |
-
if hasattr(model, 'module'):
|
57 |
-
model.module.load_state_dict(new_state_dict)
|
58 |
-
else:
|
59 |
-
model.load_state_dict(new_state_dict)
|
60 |
-
if iteration:
|
61 |
-
logging.info(f"Loaded checkpoint '{checkpoint_path}' (iteration {iteration})")
|
62 |
-
else:
|
63 |
-
logging.info(f"Loaded checkpoint '{checkpoint_path}'")
|
64 |
-
return
|
65 |
-
|
66 |
-
|
67 |
-
def get_hparams_from_file(config_path):
|
68 |
-
with open(config_path, 'r', encoding='utf-8') as f:
|
69 |
-
data = f.read()
|
70 |
-
config = loads(data)
|
71 |
-
|
72 |
-
hparams = HParams(**config)
|
73 |
-
return hparams
|
74 |
-
|
75 |
-
|
76 |
-
def load_audio_to_torch(full_path, target_sampling_rate):
|
77 |
-
audio, sampling_rate = librosa.load(full_path, sr=target_sampling_rate, mono=True)
|
78 |
-
return FloatTensor(audio.astype(float32))
|
79 |
-
|
80 |
-
|
81 |
-
def clean_folder(folder_path):
|
82 |
-
for filename in os.listdir(folder_path):
|
83 |
-
file_path = os.path.join(folder_path, filename)
|
84 |
-
# 如果是文件,则删除文件
|
85 |
-
if os.path.isfile(file_path):
|
86 |
-
os.remove(file_path)
|
87 |
-
|
88 |
-
|
89 |
-
# is none -> True, is not none -> False
|
90 |
-
def check_is_none(s):
|
91 |
-
return s is None or (isinstance(s, str) and str(s).isspace()) or str(s) == ""
|
92 |
-
|
93 |
-
def save_audio(audio, path):
|
94 |
-
with open(path,"wb") as f:
|
95 |
-
f.write(audio)
|
|
|
|
|
|
|
|
|
|
|
|
|
|
|
|
|
|
|
|
|
|
|
|
|
|
|
|
|
|
|
|
|
|
|
|
|
|
|
|
|
|
|
|
|
|
|
|
|
|
|
|
|
|
|
|
|
|
|
|
|
|
|
|
|
|
|
|
|
|
|
|
|
|
|
|
|
|
|
|
|
|
|
|
|
|
|
|
|
|
|
|
|
|
|
|
|
|
|
|
|
|
|
|
|
|
|
|
|
|
|
|
|
|
|
|
|
|
|
|
|
|
|
|
|
|
|
|
|
|
|
|
|
|
|
|
|
|
|
|
|
|
|
|
|
|
|
|
|
|
|
|
|
|
|
|
|
|
|
|
|
|
|
|
|
|
|
|
|
|
|
|
|
|
|
|
|
|
|
|
|
|
|
|
|
|
|
|
|
|
|
|
spaces/ArturStepanenko/digitsSpace/README.md
DELETED
@@ -1,12 +0,0 @@
|
|
1 |
-
---
|
2 |
-
title: digitsSpace
|
3 |
-
emoji: 🐨
|
4 |
-
colorFrom: yellow
|
5 |
-
colorTo: purple
|
6 |
-
sdk: gradio
|
7 |
-
sdk_version: 3.33.1
|
8 |
-
app_file: app.py
|
9 |
-
pinned: false
|
10 |
-
---
|
11 |
-
|
12 |
-
Check out the configuration reference at https://huggingface.co/docs/hub/spaces-config-reference
|
|
|
|
|
|
|
|
|
|
|
|
|
|
|
|
|
|
|
|
|
|
|
|
|
|
spaces/AsakuraMizu/moe-tts/README.md
DELETED
@@ -1,14 +0,0 @@
|
|
1 |
-
---
|
2 |
-
title: Moe TTS
|
3 |
-
emoji: 😊🎙️
|
4 |
-
colorFrom: red
|
5 |
-
colorTo: pink
|
6 |
-
sdk: gradio
|
7 |
-
sdk_version: 3.16.1
|
8 |
-
app_file: app.py
|
9 |
-
pinned: false
|
10 |
-
license: mit
|
11 |
-
duplicated_from: skytnt/moe-tts
|
12 |
-
---
|
13 |
-
|
14 |
-
Check out the configuration reference at https://huggingface.co/docs/hub/spaces-config-reference
|
|
|
|
|
|
|
|
|
|
|
|
|
|
|
|
|
|
|
|
|
|
|
|
|
|
|
|
|
|
spaces/Ataturk-Chatbot/HuggingFaceChat/venv/lib/python3.11/site-packages/pip/_internal/vcs/git.py
DELETED
@@ -1,526 +0,0 @@
|
|
1 |
-
import logging
|
2 |
-
import os.path
|
3 |
-
import pathlib
|
4 |
-
import re
|
5 |
-
import urllib.parse
|
6 |
-
import urllib.request
|
7 |
-
from typing import List, Optional, Tuple
|
8 |
-
|
9 |
-
from pip._internal.exceptions import BadCommand, InstallationError
|
10 |
-
from pip._internal.utils.misc import HiddenText, display_path, hide_url
|
11 |
-
from pip._internal.utils.subprocess import make_command
|
12 |
-
from pip._internal.vcs.versioncontrol import (
|
13 |
-
AuthInfo,
|
14 |
-
RemoteNotFoundError,
|
15 |
-
RemoteNotValidError,
|
16 |
-
RevOptions,
|
17 |
-
VersionControl,
|
18 |
-
find_path_to_project_root_from_repo_root,
|
19 |
-
vcs,
|
20 |
-
)
|
21 |
-
|
22 |
-
urlsplit = urllib.parse.urlsplit
|
23 |
-
urlunsplit = urllib.parse.urlunsplit
|
24 |
-
|
25 |
-
|
26 |
-
logger = logging.getLogger(__name__)
|
27 |
-
|
28 |
-
|
29 |
-
GIT_VERSION_REGEX = re.compile(
|
30 |
-
r"^git version " # Prefix.
|
31 |
-
r"(\d+)" # Major.
|
32 |
-
r"\.(\d+)" # Dot, minor.
|
33 |
-
r"(?:\.(\d+))?" # Optional dot, patch.
|
34 |
-
r".*$" # Suffix, including any pre- and post-release segments we don't care about.
|
35 |
-
)
|
36 |
-
|
37 |
-
HASH_REGEX = re.compile("^[a-fA-F0-9]{40}$")
|
38 |
-
|
39 |
-
# SCP (Secure copy protocol) shorthand. e.g. '[email protected]:foo/bar.git'
|
40 |
-
SCP_REGEX = re.compile(
|
41 |
-
r"""^
|
42 |
-
# Optional user, e.g. 'git@'
|
43 |
-
(\w+@)?
|
44 |
-
# Server, e.g. 'github.com'.
|
45 |
-
([^/:]+):
|
46 |
-
# The server-side path. e.g. 'user/project.git'. Must start with an
|
47 |
-
# alphanumeric character so as not to be confusable with a Windows paths
|
48 |
-
# like 'C:/foo/bar' or 'C:\foo\bar'.
|
49 |
-
(\w[^:]*)
|
50 |
-
$""",
|
51 |
-
re.VERBOSE,
|
52 |
-
)
|
53 |
-
|
54 |
-
|
55 |
-
def looks_like_hash(sha: str) -> bool:
|
56 |
-
return bool(HASH_REGEX.match(sha))
|
57 |
-
|
58 |
-
|
59 |
-
class Git(VersionControl):
|
60 |
-
name = "git"
|
61 |
-
dirname = ".git"
|
62 |
-
repo_name = "clone"
|
63 |
-
schemes = (
|
64 |
-
"git+http",
|
65 |
-
"git+https",
|
66 |
-
"git+ssh",
|
67 |
-
"git+git",
|
68 |
-
"git+file",
|
69 |
-
)
|
70 |
-
# Prevent the user's environment variables from interfering with pip:
|
71 |
-
# https://github.com/pypa/pip/issues/1130
|
72 |
-
unset_environ = ("GIT_DIR", "GIT_WORK_TREE")
|
73 |
-
default_arg_rev = "HEAD"
|
74 |
-
|
75 |
-
@staticmethod
|
76 |
-
def get_base_rev_args(rev: str) -> List[str]:
|
77 |
-
return [rev]
|
78 |
-
|
79 |
-
def is_immutable_rev_checkout(self, url: str, dest: str) -> bool:
|
80 |
-
_, rev_options = self.get_url_rev_options(hide_url(url))
|
81 |
-
if not rev_options.rev:
|
82 |
-
return False
|
83 |
-
if not self.is_commit_id_equal(dest, rev_options.rev):
|
84 |
-
# the current commit is different from rev,
|
85 |
-
# which means rev was something else than a commit hash
|
86 |
-
return False
|
87 |
-
# return False in the rare case rev is both a commit hash
|
88 |
-
# and a tag or a branch; we don't want to cache in that case
|
89 |
-
# because that branch/tag could point to something else in the future
|
90 |
-
is_tag_or_branch = bool(self.get_revision_sha(dest, rev_options.rev)[0])
|
91 |
-
return not is_tag_or_branch
|
92 |
-
|
93 |
-
def get_git_version(self) -> Tuple[int, ...]:
|
94 |
-
version = self.run_command(
|
95 |
-
["version"],
|
96 |
-
command_desc="git version",
|
97 |
-
show_stdout=False,
|
98 |
-
stdout_only=True,
|
99 |
-
)
|
100 |
-
match = GIT_VERSION_REGEX.match(version)
|
101 |
-
if not match:
|
102 |
-
logger.warning("Can't parse git version: %s", version)
|
103 |
-
return ()
|
104 |
-
return tuple(int(c) for c in match.groups())
|
105 |
-
|
106 |
-
@classmethod
|
107 |
-
def get_current_branch(cls, location: str) -> Optional[str]:
|
108 |
-
"""
|
109 |
-
Return the current branch, or None if HEAD isn't at a branch
|
110 |
-
(e.g. detached HEAD).
|
111 |
-
"""
|
112 |
-
# git-symbolic-ref exits with empty stdout if "HEAD" is a detached
|
113 |
-
# HEAD rather than a symbolic ref. In addition, the -q causes the
|
114 |
-
# command to exit with status code 1 instead of 128 in this case
|
115 |
-
# and to suppress the message to stderr.
|
116 |
-
args = ["symbolic-ref", "-q", "HEAD"]
|
117 |
-
output = cls.run_command(
|
118 |
-
args,
|
119 |
-
extra_ok_returncodes=(1,),
|
120 |
-
show_stdout=False,
|
121 |
-
stdout_only=True,
|
122 |
-
cwd=location,
|
123 |
-
)
|
124 |
-
ref = output.strip()
|
125 |
-
|
126 |
-
if ref.startswith("refs/heads/"):
|
127 |
-
return ref[len("refs/heads/") :]
|
128 |
-
|
129 |
-
return None
|
130 |
-
|
131 |
-
@classmethod
|
132 |
-
def get_revision_sha(cls, dest: str, rev: str) -> Tuple[Optional[str], bool]:
|
133 |
-
"""
|
134 |
-
Return (sha_or_none, is_branch), where sha_or_none is a commit hash
|
135 |
-
if the revision names a remote branch or tag, otherwise None.
|
136 |
-
|
137 |
-
Args:
|
138 |
-
dest: the repository directory.
|
139 |
-
rev: the revision name.
|
140 |
-
"""
|
141 |
-
# Pass rev to pre-filter the list.
|
142 |
-
output = cls.run_command(
|
143 |
-
["show-ref", rev],
|
144 |
-
cwd=dest,
|
145 |
-
show_stdout=False,
|
146 |
-
stdout_only=True,
|
147 |
-
on_returncode="ignore",
|
148 |
-
)
|
149 |
-
refs = {}
|
150 |
-
# NOTE: We do not use splitlines here since that would split on other
|
151 |
-
# unicode separators, which can be maliciously used to install a
|
152 |
-
# different revision.
|
153 |
-
for line in output.strip().split("\n"):
|
154 |
-
line = line.rstrip("\r")
|
155 |
-
if not line:
|
156 |
-
continue
|
157 |
-
try:
|
158 |
-
ref_sha, ref_name = line.split(" ", maxsplit=2)
|
159 |
-
except ValueError:
|
160 |
-
# Include the offending line to simplify troubleshooting if
|
161 |
-
# this error ever occurs.
|
162 |
-
raise ValueError(f"unexpected show-ref line: {line!r}")
|
163 |
-
|
164 |
-
refs[ref_name] = ref_sha
|
165 |
-
|
166 |
-
branch_ref = f"refs/remotes/origin/{rev}"
|
167 |
-
tag_ref = f"refs/tags/{rev}"
|
168 |
-
|
169 |
-
sha = refs.get(branch_ref)
|
170 |
-
if sha is not None:
|
171 |
-
return (sha, True)
|
172 |
-
|
173 |
-
sha = refs.get(tag_ref)
|
174 |
-
|
175 |
-
return (sha, False)
|
176 |
-
|
177 |
-
@classmethod
|
178 |
-
def _should_fetch(cls, dest: str, rev: str) -> bool:
|
179 |
-
"""
|
180 |
-
Return true if rev is a ref or is a commit that we don't have locally.
|
181 |
-
|
182 |
-
Branches and tags are not considered in this method because they are
|
183 |
-
assumed to be always available locally (which is a normal outcome of
|
184 |
-
``git clone`` and ``git fetch --tags``).
|
185 |
-
"""
|
186 |
-
if rev.startswith("refs/"):
|
187 |
-
# Always fetch remote refs.
|
188 |
-
return True
|
189 |
-
|
190 |
-
if not looks_like_hash(rev):
|
191 |
-
# Git fetch would fail with abbreviated commits.
|
192 |
-
return False
|
193 |
-
|
194 |
-
if cls.has_commit(dest, rev):
|
195 |
-
# Don't fetch if we have the commit locally.
|
196 |
-
return False
|
197 |
-
|
198 |
-
return True
|
199 |
-
|
200 |
-
@classmethod
|
201 |
-
def resolve_revision(
|
202 |
-
cls, dest: str, url: HiddenText, rev_options: RevOptions
|
203 |
-
) -> RevOptions:
|
204 |
-
"""
|
205 |
-
Resolve a revision to a new RevOptions object with the SHA1 of the
|
206 |
-
branch, tag, or ref if found.
|
207 |
-
|
208 |
-
Args:
|
209 |
-
rev_options: a RevOptions object.
|
210 |
-
"""
|
211 |
-
rev = rev_options.arg_rev
|
212 |
-
# The arg_rev property's implementation for Git ensures that the
|
213 |
-
# rev return value is always non-None.
|
214 |
-
assert rev is not None
|
215 |
-
|
216 |
-
sha, is_branch = cls.get_revision_sha(dest, rev)
|
217 |
-
|
218 |
-
if sha is not None:
|
219 |
-
rev_options = rev_options.make_new(sha)
|
220 |
-
rev_options.branch_name = rev if is_branch else None
|
221 |
-
|
222 |
-
return rev_options
|
223 |
-
|
224 |
-
# Do not show a warning for the common case of something that has
|
225 |
-
# the form of a Git commit hash.
|
226 |
-
if not looks_like_hash(rev):
|
227 |
-
logger.warning(
|
228 |
-
"Did not find branch or tag '%s', assuming revision or ref.",
|
229 |
-
rev,
|
230 |
-
)
|
231 |
-
|
232 |
-
if not cls._should_fetch(dest, rev):
|
233 |
-
return rev_options
|
234 |
-
|
235 |
-
# fetch the requested revision
|
236 |
-
cls.run_command(
|
237 |
-
make_command("fetch", "-q", url, rev_options.to_args()),
|
238 |
-
cwd=dest,
|
239 |
-
)
|
240 |
-
# Change the revision to the SHA of the ref we fetched
|
241 |
-
sha = cls.get_revision(dest, rev="FETCH_HEAD")
|
242 |
-
rev_options = rev_options.make_new(sha)
|
243 |
-
|
244 |
-
return rev_options
|
245 |
-
|
246 |
-
@classmethod
|
247 |
-
def is_commit_id_equal(cls, dest: str, name: Optional[str]) -> bool:
|
248 |
-
"""
|
249 |
-
Return whether the current commit hash equals the given name.
|
250 |
-
|
251 |
-
Args:
|
252 |
-
dest: the repository directory.
|
253 |
-
name: a string name.
|
254 |
-
"""
|
255 |
-
if not name:
|
256 |
-
# Then avoid an unnecessary subprocess call.
|
257 |
-
return False
|
258 |
-
|
259 |
-
return cls.get_revision(dest) == name
|
260 |
-
|
261 |
-
def fetch_new(
|
262 |
-
self, dest: str, url: HiddenText, rev_options: RevOptions, verbosity: int
|
263 |
-
) -> None:
|
264 |
-
rev_display = rev_options.to_display()
|
265 |
-
logger.info("Cloning %s%s to %s", url, rev_display, display_path(dest))
|
266 |
-
if verbosity <= 0:
|
267 |
-
flags: Tuple[str, ...] = ("--quiet",)
|
268 |
-
elif verbosity == 1:
|
269 |
-
flags = ()
|
270 |
-
else:
|
271 |
-
flags = ("--verbose", "--progress")
|
272 |
-
if self.get_git_version() >= (2, 17):
|
273 |
-
# Git added support for partial clone in 2.17
|
274 |
-
# https://git-scm.com/docs/partial-clone
|
275 |
-
# Speeds up cloning by functioning without a complete copy of repository
|
276 |
-
self.run_command(
|
277 |
-
make_command(
|
278 |
-
"clone",
|
279 |
-
"--filter=blob:none",
|
280 |
-
*flags,
|
281 |
-
url,
|
282 |
-
dest,
|
283 |
-
)
|
284 |
-
)
|
285 |
-
else:
|
286 |
-
self.run_command(make_command("clone", *flags, url, dest))
|
287 |
-
|
288 |
-
if rev_options.rev:
|
289 |
-
# Then a specific revision was requested.
|
290 |
-
rev_options = self.resolve_revision(dest, url, rev_options)
|
291 |
-
branch_name = getattr(rev_options, "branch_name", None)
|
292 |
-
logger.debug("Rev options %s, branch_name %s", rev_options, branch_name)
|
293 |
-
if branch_name is None:
|
294 |
-
# Only do a checkout if the current commit id doesn't match
|
295 |
-
# the requested revision.
|
296 |
-
if not self.is_commit_id_equal(dest, rev_options.rev):
|
297 |
-
cmd_args = make_command(
|
298 |
-
"checkout",
|
299 |
-
"-q",
|
300 |
-
rev_options.to_args(),
|
301 |
-
)
|
302 |
-
self.run_command(cmd_args, cwd=dest)
|
303 |
-
elif self.get_current_branch(dest) != branch_name:
|
304 |
-
# Then a specific branch was requested, and that branch
|
305 |
-
# is not yet checked out.
|
306 |
-
track_branch = f"origin/{branch_name}"
|
307 |
-
cmd_args = [
|
308 |
-
"checkout",
|
309 |
-
"-b",
|
310 |
-
branch_name,
|
311 |
-
"--track",
|
312 |
-
track_branch,
|
313 |
-
]
|
314 |
-
self.run_command(cmd_args, cwd=dest)
|
315 |
-
else:
|
316 |
-
sha = self.get_revision(dest)
|
317 |
-
rev_options = rev_options.make_new(sha)
|
318 |
-
|
319 |
-
logger.info("Resolved %s to commit %s", url, rev_options.rev)
|
320 |
-
|
321 |
-
#: repo may contain submodules
|
322 |
-
self.update_submodules(dest)
|
323 |
-
|
324 |
-
def switch(self, dest: str, url: HiddenText, rev_options: RevOptions) -> None:
|
325 |
-
self.run_command(
|
326 |
-
make_command("config", "remote.origin.url", url),
|
327 |
-
cwd=dest,
|
328 |
-
)
|
329 |
-
cmd_args = make_command("checkout", "-q", rev_options.to_args())
|
330 |
-
self.run_command(cmd_args, cwd=dest)
|
331 |
-
|
332 |
-
self.update_submodules(dest)
|
333 |
-
|
334 |
-
def update(self, dest: str, url: HiddenText, rev_options: RevOptions) -> None:
|
335 |
-
# First fetch changes from the default remote
|
336 |
-
if self.get_git_version() >= (1, 9):
|
337 |
-
# fetch tags in addition to everything else
|
338 |
-
self.run_command(["fetch", "-q", "--tags"], cwd=dest)
|
339 |
-
else:
|
340 |
-
self.run_command(["fetch", "-q"], cwd=dest)
|
341 |
-
# Then reset to wanted revision (maybe even origin/master)
|
342 |
-
rev_options = self.resolve_revision(dest, url, rev_options)
|
343 |
-
cmd_args = make_command("reset", "--hard", "-q", rev_options.to_args())
|
344 |
-
self.run_command(cmd_args, cwd=dest)
|
345 |
-
#: update submodules
|
346 |
-
self.update_submodules(dest)
|
347 |
-
|
348 |
-
@classmethod
|
349 |
-
def get_remote_url(cls, location: str) -> str:
|
350 |
-
"""
|
351 |
-
Return URL of the first remote encountered.
|
352 |
-
|
353 |
-
Raises RemoteNotFoundError if the repository does not have a remote
|
354 |
-
url configured.
|
355 |
-
"""
|
356 |
-
# We need to pass 1 for extra_ok_returncodes since the command
|
357 |
-
# exits with return code 1 if there are no matching lines.
|
358 |
-
stdout = cls.run_command(
|
359 |
-
["config", "--get-regexp", r"remote\..*\.url"],
|
360 |
-
extra_ok_returncodes=(1,),
|
361 |
-
show_stdout=False,
|
362 |
-
stdout_only=True,
|
363 |
-
cwd=location,
|
364 |
-
)
|
365 |
-
remotes = stdout.splitlines()
|
366 |
-
try:
|
367 |
-
found_remote = remotes[0]
|
368 |
-
except IndexError:
|
369 |
-
raise RemoteNotFoundError
|
370 |
-
|
371 |
-
for remote in remotes:
|
372 |
-
if remote.startswith("remote.origin.url "):
|
373 |
-
found_remote = remote
|
374 |
-
break
|
375 |
-
url = found_remote.split(" ")[1]
|
376 |
-
return cls._git_remote_to_pip_url(url.strip())
|
377 |
-
|
378 |
-
@staticmethod
|
379 |
-
def _git_remote_to_pip_url(url: str) -> str:
|
380 |
-
"""
|
381 |
-
Convert a remote url from what git uses to what pip accepts.
|
382 |
-
|
383 |
-
There are 3 legal forms **url** may take:
|
384 |
-
|
385 |
-
1. A fully qualified url: ssh://[email protected]/foo/bar.git
|
386 |
-
2. A local project.git folder: /path/to/bare/repository.git
|
387 |
-
3. SCP shorthand for form 1: [email protected]:foo/bar.git
|
388 |
-
|
389 |
-
Form 1 is output as-is. Form 2 must be converted to URI and form 3 must
|
390 |
-
be converted to form 1.
|
391 |
-
|
392 |
-
See the corresponding test test_git_remote_url_to_pip() for examples of
|
393 |
-
sample inputs/outputs.
|
394 |
-
"""
|
395 |
-
if re.match(r"\w+://", url):
|
396 |
-
# This is already valid. Pass it though as-is.
|
397 |
-
return url
|
398 |
-
if os.path.exists(url):
|
399 |
-
# A local bare remote (git clone --mirror).
|
400 |
-
# Needs a file:// prefix.
|
401 |
-
return pathlib.PurePath(url).as_uri()
|
402 |
-
scp_match = SCP_REGEX.match(url)
|
403 |
-
if scp_match:
|
404 |
-
# Add an ssh:// prefix and replace the ':' with a '/'.
|
405 |
-
return scp_match.expand(r"ssh://\1\2/\3")
|
406 |
-
# Otherwise, bail out.
|
407 |
-
raise RemoteNotValidError(url)
|
408 |
-
|
409 |
-
@classmethod
|
410 |
-
def has_commit(cls, location: str, rev: str) -> bool:
|
411 |
-
"""
|
412 |
-
Check if rev is a commit that is available in the local repository.
|
413 |
-
"""
|
414 |
-
try:
|
415 |
-
cls.run_command(
|
416 |
-
["rev-parse", "-q", "--verify", "sha^" + rev],
|
417 |
-
cwd=location,
|
418 |
-
log_failed_cmd=False,
|
419 |
-
)
|
420 |
-
except InstallationError:
|
421 |
-
return False
|
422 |
-
else:
|
423 |
-
return True
|
424 |
-
|
425 |
-
@classmethod
|
426 |
-
def get_revision(cls, location: str, rev: Optional[str] = None) -> str:
|
427 |
-
if rev is None:
|
428 |
-
rev = "HEAD"
|
429 |
-
current_rev = cls.run_command(
|
430 |
-
["rev-parse", rev],
|
431 |
-
show_stdout=False,
|
432 |
-
stdout_only=True,
|
433 |
-
cwd=location,
|
434 |
-
)
|
435 |
-
return current_rev.strip()
|
436 |
-
|
437 |
-
@classmethod
|
438 |
-
def get_subdirectory(cls, location: str) -> Optional[str]:
|
439 |
-
"""
|
440 |
-
Return the path to Python project root, relative to the repo root.
|
441 |
-
Return None if the project root is in the repo root.
|
442 |
-
"""
|
443 |
-
# find the repo root
|
444 |
-
git_dir = cls.run_command(
|
445 |
-
["rev-parse", "--git-dir"],
|
446 |
-
show_stdout=False,
|
447 |
-
stdout_only=True,
|
448 |
-
cwd=location,
|
449 |
-
).strip()
|
450 |
-
if not os.path.isabs(git_dir):
|
451 |
-
git_dir = os.path.join(location, git_dir)
|
452 |
-
repo_root = os.path.abspath(os.path.join(git_dir, ".."))
|
453 |
-
return find_path_to_project_root_from_repo_root(location, repo_root)
|
454 |
-
|
455 |
-
@classmethod
|
456 |
-
def get_url_rev_and_auth(cls, url: str) -> Tuple[str, Optional[str], AuthInfo]:
|
457 |
-
"""
|
458 |
-
Prefixes stub URLs like 'user@hostname:user/repo.git' with 'ssh://'.
|
459 |
-
That's required because although they use SSH they sometimes don't
|
460 |
-
work with a ssh:// scheme (e.g. GitHub). But we need a scheme for
|
461 |
-
parsing. Hence we remove it again afterwards and return it as a stub.
|
462 |
-
"""
|
463 |
-
# Works around an apparent Git bug
|
464 |
-
# (see https://article.gmane.org/gmane.comp.version-control.git/146500)
|
465 |
-
scheme, netloc, path, query, fragment = urlsplit(url)
|
466 |
-
if scheme.endswith("file"):
|
467 |
-
initial_slashes = path[: -len(path.lstrip("/"))]
|
468 |
-
newpath = initial_slashes + urllib.request.url2pathname(path).replace(
|
469 |
-
"\\", "/"
|
470 |
-
).lstrip("/")
|
471 |
-
after_plus = scheme.find("+") + 1
|
472 |
-
url = scheme[:after_plus] + urlunsplit(
|
473 |
-
(scheme[after_plus:], netloc, newpath, query, fragment),
|
474 |
-
)
|
475 |
-
|
476 |
-
if "://" not in url:
|
477 |
-
assert "file:" not in url
|
478 |
-
url = url.replace("git+", "git+ssh://")
|
479 |
-
url, rev, user_pass = super().get_url_rev_and_auth(url)
|
480 |
-
url = url.replace("ssh://", "")
|
481 |
-
else:
|
482 |
-
url, rev, user_pass = super().get_url_rev_and_auth(url)
|
483 |
-
|
484 |
-
return url, rev, user_pass
|
485 |
-
|
486 |
-
@classmethod
|
487 |
-
def update_submodules(cls, location: str) -> None:
|
488 |
-
if not os.path.exists(os.path.join(location, ".gitmodules")):
|
489 |
-
return
|
490 |
-
cls.run_command(
|
491 |
-
["submodule", "update", "--init", "--recursive", "-q"],
|
492 |
-
cwd=location,
|
493 |
-
)
|
494 |
-
|
495 |
-
@classmethod
|
496 |
-
def get_repository_root(cls, location: str) -> Optional[str]:
|
497 |
-
loc = super().get_repository_root(location)
|
498 |
-
if loc:
|
499 |
-
return loc
|
500 |
-
try:
|
501 |
-
r = cls.run_command(
|
502 |
-
["rev-parse", "--show-toplevel"],
|
503 |
-
cwd=location,
|
504 |
-
show_stdout=False,
|
505 |
-
stdout_only=True,
|
506 |
-
on_returncode="raise",
|
507 |
-
log_failed_cmd=False,
|
508 |
-
)
|
509 |
-
except BadCommand:
|
510 |
-
logger.debug(
|
511 |
-
"could not determine if %s is under git control "
|
512 |
-
"because git is not available",
|
513 |
-
location,
|
514 |
-
)
|
515 |
-
return None
|
516 |
-
except InstallationError:
|
517 |
-
return None
|
518 |
-
return os.path.normpath(r.rstrip("\r\n"))
|
519 |
-
|
520 |
-
@staticmethod
|
521 |
-
def should_add_vcs_url_prefix(repo_url: str) -> bool:
|
522 |
-
"""In either https or ssh form, requirements must be prefixed with git+."""
|
523 |
-
return True
|
524 |
-
|
525 |
-
|
526 |
-
vcs.register(Git)
|
|
|
|
|
|
|
|
|
|
|
|
|
|
|
|
|
|
|
|
|
|
|
|
|
|
|
|
|
|
|
|
|
|
|
|
|
|
|
|
|
|
|
|
|
|
|
|
|
|
|
|
|
|
|
|
|
|
|
|
|
|
|
|
|
|
|
|
|
|
|
|
|
|
|
|
|
|
|
|
|
|
|
|
|
|
|
|
|
|
|
|
|
|
|
|
|
|
|
|
|
|
|
|
|
|
|
|
|
|
|
|
|
|
|
|
|
|
|
|
|
|
|
|
|
|
|
|
|
|
|
|
|
|
|
|
|
|
|
|
|
|
|
|
|
|
|
|
|
|
|
|
|
|
|
|
|
|
|
|
|
|
|
|
|
|
|
|
|
|
|
|
|
|
|
|
|
|
|
|
|
|
|
|
|
|
|
|
|
|
|
|
|
|
|
|
|
|
|
|
|
|
|
|
|
|
|
|
|
|
|
|
|
|
|
|
|
|
|
|
|
|
|
|
|
|
|
|
|
|
|
|
|
|
|
|
|
|
|
|
|
|
|
|
|
|
|
|
|
|
|
|
|
|
|
|
|
|
|
|
|
|
|
|
|
|
|
|
|
|
|
|
|
|
|
|
|
|
|
|
|
|
|
|
|
|
|
|
|
|
|
|
|
|
|
|
|
|
|
|
|
|
|
|
|
|
|
|
|
|
|
|
|
|
|
|
|
|
|
|
|
|
|
|
|
|
|
|
|
|
|
|
|
|
|
|
|
|
|
|
|
|
|
|
|
|
|
|
|
|
|
|
|
|
|
|
|
|
|
|
|
|
|
|
|
|
|
|
|
|
|
|
|
|
|
|
|
|
|
|
|
|
|
|
|
|
|
|
|
|
|
|
|
|
|
|
|
|
|
|
|
|
|
|
|
|
|
|
|
|
|
|
|
|
|
|
|
|
|
|
|
|
|
|
|
|
|
|
|
|
|
|
|
|
|
|
|
|
|
|
|
|
|
|
|
|
|
|
|
|
|
|
|
|
|
|
|
|
|
|
|
|
|
|
|
|
|
|
|
|
|
|
|
|
|
|
|
|
|
|
|
|
|
|
|
|
|
|
|
|
|
|
|
|
|
|
|
|
|
|
|
|
|
|
|
|
|
|
|
|
|
|
|
|
|
|
|
|
|
|
|
|
|
|
|
|
|
|
|
|
|
|
|
|
|
|
|
|
|
|
|
|
|
|
|
|
|
|
|
|
|
|
|
|
|
|
|
|
|
|
|
|
|
|
|
|
|
|
|
|
|
|
|
|
|
|
|
|
|
|
|
|
|
|
|
|
|
|
|
|
|
|
|
|
|
|
|
|
|
|
|
|
|
|
|
|
|
|
|
|
|
|
|
|
|
|
|
|
|
|
|
|
|
|
|
|
|
|
|
|
|
|
|
|
|
|
|
|
|
|
|
|
|
|
|
|
|
|
|
|
|
|
|
|
|
|
|
|
|
|
|
|
|
|
|
|
|
|
|
|
|
|
|
|
|
|
|
|
|
|
|
|
|
|
|
|
|
|
|
|
|
|
|
|
|
|
|
|
|
|
|
|
|
|
|
|
|
|
|
|
|
|
|
|
|
|
|
|
|
|
|
|
|
|
|
|
|
|
|
|
|
|
|
|
|
|
|
|
|
|
|
|
|
|
|
|
|
|
|
|
|
|
|
|
|
|
|
|
|
|
|
|
|
|
|
|
|
|
|
|
|
|
|
|
|
|
|
|
|
|
|
|
|
|
|
|
|
|
|
|
|
|
|
|
|
|
|
|
|
|
|
|
|
|
|
|
|
|
|
|
|
|
|
|
|
|
|
|
|
|
|
|
|
|
|
|
|
|
|
|
|
|
|
|
|
|
|
|
|
|
|
|
|
|
|
|
|
|
|
|
|
|
|
|
|
|
|
|
|
|
|
|
|
|
|
|
|
|
|
|
|
|
|
|
|
|
|
|
|
|
|
|
|
|
|
|
|
|
|
|
|
|
|
|
|
|
|
|
|
|
|
|
|
|
|
|
|
|
|
|
|
|
|
|
|
|
|
|
|
|
|
|
|
|
|
|
|
|
|
|
|
|
|
|
|
|
|
|
|
|
|
|
|
|
|
|
|
|
|
|
|
|
|
|
|
|
|
|
|
|
|
|
|
|
|
|
|
|
|
|
|
|
|
|
|
|
|
|
|
|
|
|
|
|
|
|
|
|
|
|
|
|
|
|
|
|
|
|
|
|
|
|
|
|
|
|
|
|
|
|
|
|
|
|
|
|
|
|
|
|
|
|
|
|
|
|
|
|
|
|
|
|
|
|
|
|
|
|
|
|
|
|
|
|
|
|
|
|
|
|
|
|
|
|
spaces/Awiny/Image2Paragraph/models/grit_src/third_party/CenterNet2/detectron2/solver/build.py
DELETED
@@ -1,285 +0,0 @@
|
|
1 |
-
# Copyright (c) Facebook, Inc. and its affiliates.
|
2 |
-
import copy
|
3 |
-
import itertools
|
4 |
-
import logging
|
5 |
-
from collections import defaultdict
|
6 |
-
from enum import Enum
|
7 |
-
from typing import Any, Callable, Dict, Iterable, List, Optional, Set, Type, Union
|
8 |
-
import torch
|
9 |
-
from fvcore.common.param_scheduler import CosineParamScheduler, MultiStepParamScheduler
|
10 |
-
|
11 |
-
from detectron2.config import CfgNode
|
12 |
-
|
13 |
-
from .lr_scheduler import LRMultiplier, WarmupParamScheduler
|
14 |
-
|
15 |
-
_GradientClipperInput = Union[torch.Tensor, Iterable[torch.Tensor]]
|
16 |
-
_GradientClipper = Callable[[_GradientClipperInput], None]
|
17 |
-
|
18 |
-
|
19 |
-
class GradientClipType(Enum):
|
20 |
-
VALUE = "value"
|
21 |
-
NORM = "norm"
|
22 |
-
|
23 |
-
|
24 |
-
def _create_gradient_clipper(cfg: CfgNode) -> _GradientClipper:
|
25 |
-
"""
|
26 |
-
Creates gradient clipping closure to clip by value or by norm,
|
27 |
-
according to the provided config.
|
28 |
-
"""
|
29 |
-
cfg = copy.deepcopy(cfg)
|
30 |
-
|
31 |
-
def clip_grad_norm(p: _GradientClipperInput):
|
32 |
-
torch.nn.utils.clip_grad_norm_(p, cfg.CLIP_VALUE, cfg.NORM_TYPE)
|
33 |
-
|
34 |
-
def clip_grad_value(p: _GradientClipperInput):
|
35 |
-
torch.nn.utils.clip_grad_value_(p, cfg.CLIP_VALUE)
|
36 |
-
|
37 |
-
_GRADIENT_CLIP_TYPE_TO_CLIPPER = {
|
38 |
-
GradientClipType.VALUE: clip_grad_value,
|
39 |
-
GradientClipType.NORM: clip_grad_norm,
|
40 |
-
}
|
41 |
-
return _GRADIENT_CLIP_TYPE_TO_CLIPPER[GradientClipType(cfg.CLIP_TYPE)]
|
42 |
-
|
43 |
-
|
44 |
-
def _generate_optimizer_class_with_gradient_clipping(
|
45 |
-
optimizer: Type[torch.optim.Optimizer],
|
46 |
-
*,
|
47 |
-
per_param_clipper: Optional[_GradientClipper] = None,
|
48 |
-
global_clipper: Optional[_GradientClipper] = None,
|
49 |
-
) -> Type[torch.optim.Optimizer]:
|
50 |
-
"""
|
51 |
-
Dynamically creates a new type that inherits the type of a given instance
|
52 |
-
and overrides the `step` method to add gradient clipping
|
53 |
-
"""
|
54 |
-
assert (
|
55 |
-
per_param_clipper is None or global_clipper is None
|
56 |
-
), "Not allowed to use both per-parameter clipping and global clipping"
|
57 |
-
|
58 |
-
def optimizer_wgc_step(self, closure=None):
|
59 |
-
if per_param_clipper is not None:
|
60 |
-
for group in self.param_groups:
|
61 |
-
for p in group["params"]:
|
62 |
-
per_param_clipper(p)
|
63 |
-
else:
|
64 |
-
# global clipper for future use with detr
|
65 |
-
# (https://github.com/facebookresearch/detr/pull/287)
|
66 |
-
all_params = itertools.chain(*[g["params"] for g in self.param_groups])
|
67 |
-
global_clipper(all_params)
|
68 |
-
super(type(self), self).step(closure)
|
69 |
-
|
70 |
-
OptimizerWithGradientClip = type(
|
71 |
-
optimizer.__name__ + "WithGradientClip",
|
72 |
-
(optimizer,),
|
73 |
-
{"step": optimizer_wgc_step},
|
74 |
-
)
|
75 |
-
return OptimizerWithGradientClip
|
76 |
-
|
77 |
-
|
78 |
-
def maybe_add_gradient_clipping(
|
79 |
-
cfg: CfgNode, optimizer: Type[torch.optim.Optimizer]
|
80 |
-
) -> Type[torch.optim.Optimizer]:
|
81 |
-
"""
|
82 |
-
If gradient clipping is enabled through config options, wraps the existing
|
83 |
-
optimizer type to become a new dynamically created class OptimizerWithGradientClip
|
84 |
-
that inherits the given optimizer and overrides the `step` method to
|
85 |
-
include gradient clipping.
|
86 |
-
|
87 |
-
Args:
|
88 |
-
cfg: CfgNode, configuration options
|
89 |
-
optimizer: type. A subclass of torch.optim.Optimizer
|
90 |
-
|
91 |
-
Return:
|
92 |
-
type: either the input `optimizer` (if gradient clipping is disabled), or
|
93 |
-
a subclass of it with gradient clipping included in the `step` method.
|
94 |
-
"""
|
95 |
-
if not cfg.SOLVER.CLIP_GRADIENTS.ENABLED:
|
96 |
-
return optimizer
|
97 |
-
if isinstance(optimizer, torch.optim.Optimizer):
|
98 |
-
optimizer_type = type(optimizer)
|
99 |
-
else:
|
100 |
-
assert issubclass(optimizer, torch.optim.Optimizer), optimizer
|
101 |
-
optimizer_type = optimizer
|
102 |
-
|
103 |
-
grad_clipper = _create_gradient_clipper(cfg.SOLVER.CLIP_GRADIENTS)
|
104 |
-
OptimizerWithGradientClip = _generate_optimizer_class_with_gradient_clipping(
|
105 |
-
optimizer_type, per_param_clipper=grad_clipper
|
106 |
-
)
|
107 |
-
if isinstance(optimizer, torch.optim.Optimizer):
|
108 |
-
optimizer.__class__ = OptimizerWithGradientClip # a bit hacky, not recommended
|
109 |
-
return optimizer
|
110 |
-
else:
|
111 |
-
return OptimizerWithGradientClip
|
112 |
-
|
113 |
-
|
114 |
-
def build_optimizer(cfg: CfgNode, model: torch.nn.Module) -> torch.optim.Optimizer:
|
115 |
-
"""
|
116 |
-
Build an optimizer from config.
|
117 |
-
"""
|
118 |
-
params = get_default_optimizer_params(
|
119 |
-
model,
|
120 |
-
base_lr=cfg.SOLVER.BASE_LR,
|
121 |
-
weight_decay_norm=cfg.SOLVER.WEIGHT_DECAY_NORM,
|
122 |
-
bias_lr_factor=cfg.SOLVER.BIAS_LR_FACTOR,
|
123 |
-
weight_decay_bias=cfg.SOLVER.WEIGHT_DECAY_BIAS,
|
124 |
-
)
|
125 |
-
return maybe_add_gradient_clipping(cfg, torch.optim.SGD)(
|
126 |
-
params,
|
127 |
-
lr=cfg.SOLVER.BASE_LR,
|
128 |
-
momentum=cfg.SOLVER.MOMENTUM,
|
129 |
-
nesterov=cfg.SOLVER.NESTEROV,
|
130 |
-
weight_decay=cfg.SOLVER.WEIGHT_DECAY,
|
131 |
-
)
|
132 |
-
|
133 |
-
|
134 |
-
def get_default_optimizer_params(
|
135 |
-
model: torch.nn.Module,
|
136 |
-
base_lr: Optional[float] = None,
|
137 |
-
weight_decay: Optional[float] = None,
|
138 |
-
weight_decay_norm: Optional[float] = None,
|
139 |
-
bias_lr_factor: Optional[float] = 1.0,
|
140 |
-
weight_decay_bias: Optional[float] = None,
|
141 |
-
overrides: Optional[Dict[str, Dict[str, float]]] = None,
|
142 |
-
) -> List[Dict[str, Any]]:
|
143 |
-
"""
|
144 |
-
Get default param list for optimizer, with support for a few types of
|
145 |
-
overrides. If no overrides needed, this is equivalent to `model.parameters()`.
|
146 |
-
|
147 |
-
Args:
|
148 |
-
base_lr: lr for every group by default. Can be omitted to use the one in optimizer.
|
149 |
-
weight_decay: weight decay for every group by default. Can be omitted to use the one
|
150 |
-
in optimizer.
|
151 |
-
weight_decay_norm: override weight decay for params in normalization layers
|
152 |
-
bias_lr_factor: multiplier of lr for bias parameters.
|
153 |
-
weight_decay_bias: override weight decay for bias parameters
|
154 |
-
overrides: if not `None`, provides values for optimizer hyperparameters
|
155 |
-
(LR, weight decay) for module parameters with a given name; e.g.
|
156 |
-
``{"embedding": {"lr": 0.01, "weight_decay": 0.1}}`` will set the LR and
|
157 |
-
weight decay values for all module parameters named `embedding`.
|
158 |
-
|
159 |
-
For common detection models, ``weight_decay_norm`` is the only option
|
160 |
-
needed to be set. ``bias_lr_factor,weight_decay_bias`` are legacy settings
|
161 |
-
from Detectron1 that are not found useful.
|
162 |
-
|
163 |
-
Example:
|
164 |
-
::
|
165 |
-
torch.optim.SGD(get_default_optimizer_params(model, weight_decay_norm=0),
|
166 |
-
lr=0.01, weight_decay=1e-4, momentum=0.9)
|
167 |
-
"""
|
168 |
-
if overrides is None:
|
169 |
-
overrides = {}
|
170 |
-
defaults = {}
|
171 |
-
if base_lr is not None:
|
172 |
-
defaults["lr"] = base_lr
|
173 |
-
if weight_decay is not None:
|
174 |
-
defaults["weight_decay"] = weight_decay
|
175 |
-
bias_overrides = {}
|
176 |
-
if bias_lr_factor is not None and bias_lr_factor != 1.0:
|
177 |
-
# NOTE: unlike Detectron v1, we now by default make bias hyperparameters
|
178 |
-
# exactly the same as regular weights.
|
179 |
-
if base_lr is None:
|
180 |
-
raise ValueError("bias_lr_factor requires base_lr")
|
181 |
-
bias_overrides["lr"] = base_lr * bias_lr_factor
|
182 |
-
if weight_decay_bias is not None:
|
183 |
-
bias_overrides["weight_decay"] = weight_decay_bias
|
184 |
-
if len(bias_overrides):
|
185 |
-
if "bias" in overrides:
|
186 |
-
raise ValueError("Conflicting overrides for 'bias'")
|
187 |
-
overrides["bias"] = bias_overrides
|
188 |
-
|
189 |
-
norm_module_types = (
|
190 |
-
torch.nn.BatchNorm1d,
|
191 |
-
torch.nn.BatchNorm2d,
|
192 |
-
torch.nn.BatchNorm3d,
|
193 |
-
torch.nn.SyncBatchNorm,
|
194 |
-
# NaiveSyncBatchNorm inherits from BatchNorm2d
|
195 |
-
torch.nn.GroupNorm,
|
196 |
-
torch.nn.InstanceNorm1d,
|
197 |
-
torch.nn.InstanceNorm2d,
|
198 |
-
torch.nn.InstanceNorm3d,
|
199 |
-
torch.nn.LayerNorm,
|
200 |
-
torch.nn.LocalResponseNorm,
|
201 |
-
)
|
202 |
-
params: List[Dict[str, Any]] = []
|
203 |
-
memo: Set[torch.nn.parameter.Parameter] = set()
|
204 |
-
for module in model.modules():
|
205 |
-
for module_param_name, value in module.named_parameters(recurse=False):
|
206 |
-
if not value.requires_grad:
|
207 |
-
continue
|
208 |
-
# Avoid duplicating parameters
|
209 |
-
if value in memo:
|
210 |
-
continue
|
211 |
-
memo.add(value)
|
212 |
-
|
213 |
-
hyperparams = copy.copy(defaults)
|
214 |
-
if isinstance(module, norm_module_types) and weight_decay_norm is not None:
|
215 |
-
hyperparams["weight_decay"] = weight_decay_norm
|
216 |
-
hyperparams.update(overrides.get(module_param_name, {}))
|
217 |
-
params.append({"params": [value], **hyperparams})
|
218 |
-
return reduce_param_groups(params)
|
219 |
-
|
220 |
-
|
221 |
-
def _expand_param_groups(params: List[Dict[str, Any]]) -> List[Dict[str, Any]]:
|
222 |
-
# Transform parameter groups into per-parameter structure.
|
223 |
-
# Later items in `params` can overwrite parameters set in previous items.
|
224 |
-
ret = defaultdict(dict)
|
225 |
-
for item in params:
|
226 |
-
assert "params" in item
|
227 |
-
cur_params = {x: y for x, y in item.items() if x != "params"}
|
228 |
-
for param in item["params"]:
|
229 |
-
ret[param].update({"params": [param], **cur_params})
|
230 |
-
return list(ret.values())
|
231 |
-
|
232 |
-
|
233 |
-
def reduce_param_groups(params: List[Dict[str, Any]]) -> List[Dict[str, Any]]:
|
234 |
-
# Reorganize the parameter groups and merge duplicated groups.
|
235 |
-
# The number of parameter groups needs to be as small as possible in order
|
236 |
-
# to efficiently use the PyTorch multi-tensor optimizer. Therefore instead
|
237 |
-
# of using a parameter_group per single parameter, we reorganize the
|
238 |
-
# parameter groups and merge duplicated groups. This approach speeds
|
239 |
-
# up multi-tensor optimizer significantly.
|
240 |
-
params = _expand_param_groups(params)
|
241 |
-
groups = defaultdict(list) # re-group all parameter groups by their hyperparams
|
242 |
-
for item in params:
|
243 |
-
cur_params = tuple((x, y) for x, y in item.items() if x != "params")
|
244 |
-
groups[cur_params].extend(item["params"])
|
245 |
-
ret = []
|
246 |
-
for param_keys, param_values in groups.items():
|
247 |
-
cur = {kv[0]: kv[1] for kv in param_keys}
|
248 |
-
cur["params"] = param_values
|
249 |
-
ret.append(cur)
|
250 |
-
return ret
|
251 |
-
|
252 |
-
|
253 |
-
def build_lr_scheduler(
|
254 |
-
cfg: CfgNode, optimizer: torch.optim.Optimizer
|
255 |
-
) -> torch.optim.lr_scheduler._LRScheduler:
|
256 |
-
"""
|
257 |
-
Build a LR scheduler from config.
|
258 |
-
"""
|
259 |
-
name = cfg.SOLVER.LR_SCHEDULER_NAME
|
260 |
-
|
261 |
-
if name == "WarmupMultiStepLR":
|
262 |
-
steps = [x for x in cfg.SOLVER.STEPS if x <= cfg.SOLVER.MAX_ITER]
|
263 |
-
if len(steps) != len(cfg.SOLVER.STEPS):
|
264 |
-
logger = logging.getLogger(__name__)
|
265 |
-
logger.warning(
|
266 |
-
"SOLVER.STEPS contains values larger than SOLVER.MAX_ITER. "
|
267 |
-
"These values will be ignored."
|
268 |
-
)
|
269 |
-
sched = MultiStepParamScheduler(
|
270 |
-
values=[cfg.SOLVER.GAMMA ** k for k in range(len(steps) + 1)],
|
271 |
-
milestones=steps,
|
272 |
-
num_updates=cfg.SOLVER.MAX_ITER,
|
273 |
-
)
|
274 |
-
elif name == "WarmupCosineLR":
|
275 |
-
sched = CosineParamScheduler(1, 0)
|
276 |
-
else:
|
277 |
-
raise ValueError("Unknown LR scheduler: {}".format(name))
|
278 |
-
|
279 |
-
sched = WarmupParamScheduler(
|
280 |
-
sched,
|
281 |
-
cfg.SOLVER.WARMUP_FACTOR,
|
282 |
-
min(cfg.SOLVER.WARMUP_ITERS / cfg.SOLVER.MAX_ITER, 1.0),
|
283 |
-
cfg.SOLVER.WARMUP_METHOD,
|
284 |
-
)
|
285 |
-
return LRMultiplier(optimizer, multiplier=sched, max_iter=cfg.SOLVER.MAX_ITER)
|
|
|
|
|
|
|
|
|
|
|
|
|
|
|
|
|
|
|
|
|
|
|
|
|
|
|
|
|
|
|
|
|
|
|
|
|
|
|
|
|
|
|
|
|
|
|
|
|
|
|
|
|
|
|
|
|
|
|
|
|
|
|
|
|
|
|
|
|
|
|
|
|
|
|
|
|
|
|
|
|
|
|
|
|
|
|
|
|
|
|
|
|
|
|
|
|
|
|
|
|
|
|
|
|
|
|
|
|
|
|
|
|
|
|
|
|
|
|
|
|
|
|
|
|
|
|
|
|
|
|
|
|
|
|
|
|
|
|
|
|
|
|
|
|
|
|
|
|
|
|
|
|
|
|
|
|
|
|
|
|
|
|
|
|
|
|
|
|
|
|
|
|
|
|
|
|
|
|
|
|
|
|
|
|
|
|
|
|
|
|
|
|
|
|
|
|
|
|
|
|
|
|
|
|
|
|
|
|
|
|
|
|
|
|
|
|
|
|
|
|
|
|
|
|
|
|
|
|
|
|
|
|
|
|
|
|
|
|
|
|
|
|
|
|
|
|
|
|
|
|
|
|
|
|
|
|
|
|
|
|
|
|
|
|
|
|
|
|
|
|
|
|
|
|
|
|
|
|
|
|
|
|
|
|
|
|
|
|
|
|
|
|
|
|
|
|
|
|
|
|
|
|
|
|
|
|
|
|
|
|
|
|
|
|
|
|
|
|
|
|
|
|
|
|
|
|
|
|
|
|
|
|
|
|
|
|
|
|
|
|
|
|
|
|
|
|
|
|
|
|
|
|
|
|
|
|
|
|
|
|
|
|
|
|
|
|
|
|
|
|
|
|
|
|
|
|
|
|
|
|
|
|
|
|
|
|
|
|
|
|
|
|
|
|
|
|
|
|
|
|
|
|
|
|
|
|
|
|
|
|
|
|
|
|
|
|
|
|
|
|
|
|
|
|
|
|
|
|
|
|
|
|
|
|
|
|
|
|
|
|
|
|
|
|
|
|
|
|
|
|
|
|
|
|
|
|
|
|
|
|
|
|
|
|
|
|
|
|
|
|
|
|
|
|
|
|
|
|
|
|
|
|
|
|
|
|
|
|
|
|
|
|
|
|
|
|
|
|
|
|
|
|
|
|
|
|
|
|
|
|
|
|
|
|
|
|
|
|
|
|
|
|
|
|
|
|
|
|
|
|
|
|
|
|
|
|
|
|
|
|
|
|
|
|
|
|
|
|
|
|
|
|
|
|
|
|
|
|
|
|
|
|
|
|
|
|
|
|
|
|
|
spaces/Awiny/Image2Paragraph/models/grit_src/third_party/CenterNet2/tests/test_events.py
DELETED
@@ -1,64 +0,0 @@
|
|
1 |
-
# Copyright (c) Facebook, Inc. and its affiliates.
|
2 |
-
import json
|
3 |
-
import os
|
4 |
-
import tempfile
|
5 |
-
import unittest
|
6 |
-
|
7 |
-
from detectron2.utils.events import CommonMetricPrinter, EventStorage, JSONWriter
|
8 |
-
|
9 |
-
|
10 |
-
class TestEventWriter(unittest.TestCase):
|
11 |
-
def testScalar(self):
|
12 |
-
with tempfile.TemporaryDirectory(
|
13 |
-
prefix="detectron2_tests"
|
14 |
-
) as dir, EventStorage() as storage:
|
15 |
-
json_file = os.path.join(dir, "test.json")
|
16 |
-
writer = JSONWriter(json_file)
|
17 |
-
for k in range(60):
|
18 |
-
storage.put_scalar("key", k, smoothing_hint=False)
|
19 |
-
if (k + 1) % 20 == 0:
|
20 |
-
writer.write()
|
21 |
-
storage.step()
|
22 |
-
writer.close()
|
23 |
-
with open(json_file) as f:
|
24 |
-
data = [json.loads(l) for l in f]
|
25 |
-
self.assertTrue([int(k["key"]) for k in data] == [19, 39, 59])
|
26 |
-
|
27 |
-
def testScalarMismatchedPeriod(self):
|
28 |
-
with tempfile.TemporaryDirectory(
|
29 |
-
prefix="detectron2_tests"
|
30 |
-
) as dir, EventStorage() as storage:
|
31 |
-
json_file = os.path.join(dir, "test.json")
|
32 |
-
|
33 |
-
writer = JSONWriter(json_file)
|
34 |
-
for k in range(60):
|
35 |
-
if k % 17 == 0: # write in a differnt period
|
36 |
-
storage.put_scalar("key2", k, smoothing_hint=False)
|
37 |
-
storage.put_scalar("key", k, smoothing_hint=False)
|
38 |
-
if (k + 1) % 20 == 0:
|
39 |
-
writer.write()
|
40 |
-
storage.step()
|
41 |
-
writer.close()
|
42 |
-
with open(json_file) as f:
|
43 |
-
data = [json.loads(l) for l in f]
|
44 |
-
self.assertTrue([int(k.get("key2", 0)) for k in data] == [17, 0, 34, 0, 51, 0])
|
45 |
-
self.assertTrue([int(k.get("key", 0)) for k in data] == [0, 19, 0, 39, 0, 59])
|
46 |
-
self.assertTrue([int(k["iteration"]) for k in data] == [17, 19, 34, 39, 51, 59])
|
47 |
-
|
48 |
-
def testPrintETA(self):
|
49 |
-
with EventStorage() as s:
|
50 |
-
p1 = CommonMetricPrinter(10)
|
51 |
-
p2 = CommonMetricPrinter()
|
52 |
-
|
53 |
-
s.put_scalar("time", 1.0)
|
54 |
-
s.step()
|
55 |
-
s.put_scalar("time", 1.0)
|
56 |
-
s.step()
|
57 |
-
|
58 |
-
with self.assertLogs("detectron2.utils.events") as logs:
|
59 |
-
p1.write()
|
60 |
-
self.assertIn("eta", logs.output[0])
|
61 |
-
|
62 |
-
with self.assertLogs("detectron2.utils.events") as logs:
|
63 |
-
p2.write()
|
64 |
-
self.assertNotIn("eta", logs.output[0])
|
|
|
|
|
|
|
|
|
|
|
|
|
|
|
|
|
|
|
|
|
|
|
|
|
|
|
|
|
|
|
|
|
|
|
|
|
|
|
|
|
|
|
|
|
|
|
|
|
|
|
|
|
|
|
|
|
|
|
|
|
|
|
|
|
|
|
|
|
|
|
|
|
|
|
|
|
|
|
|
|
|
|
|
|
|
|
|
|
|
|
|
|
|
|
|
|
|
|
|
|
|
|
|
|
|
|
|
|
|
|
|
|
|
|
|
|
|
|
|
|
|
|
|
|
|
|
|
|
|
spaces/AzulaFire/SparkDebate/README.md
DELETED
@@ -1,12 +0,0 @@
|
|
1 |
-
---
|
2 |
-
title: SparkDebate
|
3 |
-
emoji: 🌖
|
4 |
-
colorFrom: pink
|
5 |
-
colorTo: yellow
|
6 |
-
sdk: gradio
|
7 |
-
sdk_version: 3.39.0
|
8 |
-
app_file: app.py
|
9 |
-
pinned: false
|
10 |
-
---
|
11 |
-
# 知识库问答因为部署环境没卡,所以暂时无法使用(🥺
|
12 |
-
Check out the configuration reference at https://huggingface.co/docs/hub/spaces-config-reference
|
|
|
|
|
|
|
|
|
|
|
|
|
|
|
|
|
|
|
|
|
|
|
|
|
|
spaces/Bart92/RVC_HF/demucs/parser.py
DELETED
@@ -1,244 +0,0 @@
|
|
1 |
-
# Copyright (c) Facebook, Inc. and its affiliates.
|
2 |
-
# All rights reserved.
|
3 |
-
#
|
4 |
-
# This source code is licensed under the license found in the
|
5 |
-
# LICENSE file in the root directory of this source tree.
|
6 |
-
|
7 |
-
import argparse
|
8 |
-
import os
|
9 |
-
from pathlib import Path
|
10 |
-
|
11 |
-
|
12 |
-
def get_parser():
|
13 |
-
parser = argparse.ArgumentParser("demucs", description="Train and evaluate Demucs.")
|
14 |
-
default_raw = None
|
15 |
-
default_musdb = None
|
16 |
-
if 'DEMUCS_RAW' in os.environ:
|
17 |
-
default_raw = Path(os.environ['DEMUCS_RAW'])
|
18 |
-
if 'DEMUCS_MUSDB' in os.environ:
|
19 |
-
default_musdb = Path(os.environ['DEMUCS_MUSDB'])
|
20 |
-
parser.add_argument(
|
21 |
-
"--raw",
|
22 |
-
type=Path,
|
23 |
-
default=default_raw,
|
24 |
-
help="Path to raw audio, can be faster, see python3 -m demucs.raw to extract.")
|
25 |
-
parser.add_argument("--no_raw", action="store_const", const=None, dest="raw")
|
26 |
-
parser.add_argument("-m",
|
27 |
-
"--musdb",
|
28 |
-
type=Path,
|
29 |
-
default=default_musdb,
|
30 |
-
help="Path to musdb root")
|
31 |
-
parser.add_argument("--is_wav", action="store_true",
|
32 |
-
help="Indicate that the MusDB dataset is in wav format (i.e. MusDB-HQ).")
|
33 |
-
parser.add_argument("--metadata", type=Path, default=Path("metadata/"),
|
34 |
-
help="Folder where metadata information is stored.")
|
35 |
-
parser.add_argument("--wav", type=Path,
|
36 |
-
help="Path to a wav dataset. This should contain a 'train' and a 'valid' "
|
37 |
-
"subfolder.")
|
38 |
-
parser.add_argument("--samplerate", type=int, default=44100)
|
39 |
-
parser.add_argument("--audio_channels", type=int, default=2)
|
40 |
-
parser.add_argument("--samples",
|
41 |
-
default=44100 * 10,
|
42 |
-
type=int,
|
43 |
-
help="number of samples to feed in")
|
44 |
-
parser.add_argument("--data_stride",
|
45 |
-
default=44100,
|
46 |
-
type=int,
|
47 |
-
help="Stride for chunks, shorter = longer epochs")
|
48 |
-
parser.add_argument("-w", "--workers", default=10, type=int, help="Loader workers")
|
49 |
-
parser.add_argument("--eval_workers", default=2, type=int, help="Final evaluation workers")
|
50 |
-
parser.add_argument("-d",
|
51 |
-
"--device",
|
52 |
-
help="Device to train on, default is cuda if available else cpu")
|
53 |
-
parser.add_argument("--eval_cpu", action="store_true", help="Eval on test will be run on cpu.")
|
54 |
-
parser.add_argument("--dummy", help="Dummy parameter, useful to create a new checkpoint file")
|
55 |
-
parser.add_argument("--test", help="Just run the test pipeline + one validation. "
|
56 |
-
"This should be a filename relative to the models/ folder.")
|
57 |
-
parser.add_argument("--test_pretrained", help="Just run the test pipeline + one validation, "
|
58 |
-
"on a pretrained model. ")
|
59 |
-
|
60 |
-
parser.add_argument("--rank", default=0, type=int)
|
61 |
-
parser.add_argument("--world_size", default=1, type=int)
|
62 |
-
parser.add_argument("--master")
|
63 |
-
|
64 |
-
parser.add_argument("--checkpoints",
|
65 |
-
type=Path,
|
66 |
-
default=Path("checkpoints"),
|
67 |
-
help="Folder where to store checkpoints etc")
|
68 |
-
parser.add_argument("--evals",
|
69 |
-
type=Path,
|
70 |
-
default=Path("evals"),
|
71 |
-
help="Folder where to store evals and waveforms")
|
72 |
-
parser.add_argument("--save",
|
73 |
-
action="store_true",
|
74 |
-
help="Save estimated for the test set waveforms")
|
75 |
-
parser.add_argument("--logs",
|
76 |
-
type=Path,
|
77 |
-
default=Path("logs"),
|
78 |
-
help="Folder where to store logs")
|
79 |
-
parser.add_argument("--models",
|
80 |
-
type=Path,
|
81 |
-
default=Path("models"),
|
82 |
-
help="Folder where to store trained models")
|
83 |
-
parser.add_argument("-R",
|
84 |
-
"--restart",
|
85 |
-
action='store_true',
|
86 |
-
help='Restart training, ignoring previous run')
|
87 |
-
|
88 |
-
parser.add_argument("--seed", type=int, default=42)
|
89 |
-
parser.add_argument("-e", "--epochs", type=int, default=180, help="Number of epochs")
|
90 |
-
parser.add_argument("-r",
|
91 |
-
"--repeat",
|
92 |
-
type=int,
|
93 |
-
default=2,
|
94 |
-
help="Repeat the train set, longer epochs")
|
95 |
-
parser.add_argument("-b", "--batch_size", type=int, default=64)
|
96 |
-
parser.add_argument("--lr", type=float, default=3e-4)
|
97 |
-
parser.add_argument("--mse", action="store_true", help="Use MSE instead of L1")
|
98 |
-
parser.add_argument("--init", help="Initialize from a pre-trained model.")
|
99 |
-
|
100 |
-
# Augmentation options
|
101 |
-
parser.add_argument("--no_augment",
|
102 |
-
action="store_false",
|
103 |
-
dest="augment",
|
104 |
-
default=True,
|
105 |
-
help="No basic data augmentation.")
|
106 |
-
parser.add_argument("--repitch", type=float, default=0.2,
|
107 |
-
help="Probability to do tempo/pitch change")
|
108 |
-
parser.add_argument("--max_tempo", type=float, default=12,
|
109 |
-
help="Maximum relative tempo change in %% when using repitch.")
|
110 |
-
|
111 |
-
parser.add_argument("--remix_group_size",
|
112 |
-
type=int,
|
113 |
-
default=4,
|
114 |
-
help="Shuffle sources using group of this size. Useful to somewhat "
|
115 |
-
"replicate multi-gpu training "
|
116 |
-
"on less GPUs.")
|
117 |
-
parser.add_argument("--shifts",
|
118 |
-
type=int,
|
119 |
-
default=10,
|
120 |
-
help="Number of random shifts used for the shift trick.")
|
121 |
-
parser.add_argument("--overlap",
|
122 |
-
type=float,
|
123 |
-
default=0.25,
|
124 |
-
help="Overlap when --split_valid is passed.")
|
125 |
-
|
126 |
-
# See model.py for doc
|
127 |
-
parser.add_argument("--growth",
|
128 |
-
type=float,
|
129 |
-
default=2.,
|
130 |
-
help="Number of channels between two layers will increase by this factor")
|
131 |
-
parser.add_argument("--depth",
|
132 |
-
type=int,
|
133 |
-
default=6,
|
134 |
-
help="Number of layers for the encoder and decoder")
|
135 |
-
parser.add_argument("--lstm_layers", type=int, default=2, help="Number of layers for the LSTM")
|
136 |
-
parser.add_argument("--channels",
|
137 |
-
type=int,
|
138 |
-
default=64,
|
139 |
-
help="Number of channels for the first encoder layer")
|
140 |
-
parser.add_argument("--kernel_size",
|
141 |
-
type=int,
|
142 |
-
default=8,
|
143 |
-
help="Kernel size for the (transposed) convolutions")
|
144 |
-
parser.add_argument("--conv_stride",
|
145 |
-
type=int,
|
146 |
-
default=4,
|
147 |
-
help="Stride for the (transposed) convolutions")
|
148 |
-
parser.add_argument("--context",
|
149 |
-
type=int,
|
150 |
-
default=3,
|
151 |
-
help="Context size for the decoder convolutions "
|
152 |
-
"before the transposed convolutions")
|
153 |
-
parser.add_argument("--rescale",
|
154 |
-
type=float,
|
155 |
-
default=0.1,
|
156 |
-
help="Initial weight rescale reference")
|
157 |
-
parser.add_argument("--no_resample", action="store_false",
|
158 |
-
default=True, dest="resample",
|
159 |
-
help="No Resampling of the input/output x2")
|
160 |
-
parser.add_argument("--no_glu",
|
161 |
-
action="store_false",
|
162 |
-
default=True,
|
163 |
-
dest="glu",
|
164 |
-
help="Replace all GLUs by ReLUs")
|
165 |
-
parser.add_argument("--no_rewrite",
|
166 |
-
action="store_false",
|
167 |
-
default=True,
|
168 |
-
dest="rewrite",
|
169 |
-
help="No 1x1 rewrite convolutions")
|
170 |
-
parser.add_argument("--normalize", action="store_true")
|
171 |
-
parser.add_argument("--no_norm_wav", action="store_false", dest='norm_wav', default=True)
|
172 |
-
|
173 |
-
# Tasnet options
|
174 |
-
parser.add_argument("--tasnet", action="store_true")
|
175 |
-
parser.add_argument("--split_valid",
|
176 |
-
action="store_true",
|
177 |
-
help="Predict chunks by chunks for valid and test. Required for tasnet")
|
178 |
-
parser.add_argument("--X", type=int, default=8)
|
179 |
-
|
180 |
-
# Other options
|
181 |
-
parser.add_argument("--show",
|
182 |
-
action="store_true",
|
183 |
-
help="Show model architecture, size and exit")
|
184 |
-
parser.add_argument("--save_model", action="store_true",
|
185 |
-
help="Skip traning, just save final model "
|
186 |
-
"for the current checkpoint value.")
|
187 |
-
parser.add_argument("--save_state",
|
188 |
-
help="Skip training, just save state "
|
189 |
-
"for the current checkpoint value. You should "
|
190 |
-
"provide a model name as argument.")
|
191 |
-
|
192 |
-
# Quantization options
|
193 |
-
parser.add_argument("--q-min-size", type=float, default=1,
|
194 |
-
help="Only quantize layers over this size (in MB)")
|
195 |
-
parser.add_argument(
|
196 |
-
"--qat", type=int, help="If provided, use QAT training with that many bits.")
|
197 |
-
|
198 |
-
parser.add_argument("--diffq", type=float, default=0)
|
199 |
-
parser.add_argument(
|
200 |
-
"--ms-target", type=float, default=162,
|
201 |
-
help="Model size target in MB, when using DiffQ. Best model will be kept "
|
202 |
-
"only if it is smaller than this target.")
|
203 |
-
|
204 |
-
return parser
|
205 |
-
|
206 |
-
|
207 |
-
def get_name(parser, args):
|
208 |
-
"""
|
209 |
-
Return the name of an experiment given the args. Some parameters are ignored,
|
210 |
-
for instance --workers, as they do not impact the final result.
|
211 |
-
"""
|
212 |
-
ignore_args = set([
|
213 |
-
"checkpoints",
|
214 |
-
"deterministic",
|
215 |
-
"eval",
|
216 |
-
"evals",
|
217 |
-
"eval_cpu",
|
218 |
-
"eval_workers",
|
219 |
-
"logs",
|
220 |
-
"master",
|
221 |
-
"rank",
|
222 |
-
"restart",
|
223 |
-
"save",
|
224 |
-
"save_model",
|
225 |
-
"save_state",
|
226 |
-
"show",
|
227 |
-
"workers",
|
228 |
-
"world_size",
|
229 |
-
])
|
230 |
-
parts = []
|
231 |
-
name_args = dict(args.__dict__)
|
232 |
-
for name, value in name_args.items():
|
233 |
-
if name in ignore_args:
|
234 |
-
continue
|
235 |
-
if value != parser.get_default(name):
|
236 |
-
if isinstance(value, Path):
|
237 |
-
parts.append(f"{name}={value.name}")
|
238 |
-
else:
|
239 |
-
parts.append(f"{name}={value}")
|
240 |
-
if parts:
|
241 |
-
name = " ".join(parts)
|
242 |
-
else:
|
243 |
-
name = "default"
|
244 |
-
return name
|
|
|
|
|
|
|
|
|
|
|
|
|
|
|
|
|
|
|
|
|
|
|
|
|
|
|
|
|
|
|
|
|
|
|
|
|
|
|
|
|
|
|
|
|
|
|
|
|
|
|
|
|
|
|
|
|
|
|
|
|
|
|
|
|
|
|
|
|
|
|
|
|
|
|
|
|
|
|
|
|
|
|
|
|
|
|
|
|
|
|
|
|
|
|
|
|
|
|
|
|
|
|
|
|
|
|
|
|
|
|
|
|
|
|
|
|
|
|
|
|
|
|
|
|
|
|
|
|
|
|
|
|
|
|
|
|
|
|
|
|
|
|
|
|
|
|
|
|
|
|
|
|
|
|
|
|
|
|
|
|
|
|
|
|
|
|
|
|
|
|
|
|
|
|
|
|
|
|
|
|
|
|
|
|
|
|
|
|
|
|
|
|
|
|
|
|
|
|
|
|
|
|
|
|
|
|
|
|
|
|
|
|
|
|
|
|
|
|
|
|
|
|
|
|
|
|
|
|
|
|
|
|
|
|
|
|
|
|
|
|
|
|
|
|
|
|
|
|
|
|
|
|
|
|
|
|
|
|
|
|
|
|
|
|
|
|
|
|
|
|
|
|
|
|
|
|
|
|
|
|
|
|
|
|
|
|
|
|
|
|
|
|
|
|
|
|
|
|
|
|
|
|
|
|
|
|
|
|
|
|
|
|
|
|
|
|
|
|
|
|
|
|
|
|
|
|
|
|
|
|
|
|
|
|
|
|
|
|
|
|
|
|
|
|
|
|
|
|
|
|
|
|
|
|
|
|
|
|
|
|
|
|
|
|
|
|
|
|
|
|
|
|
|
|
|
|
|
|
|
|
|
|
|
|
|
|
|
|
|
|
|
|
|
|
|
|
|
|
|
|
|
|
|
|
|
|
|
|
|
|
|
|
|
|
|
|
|
|
|
|
|
|
|
|
|
|
|
|
|
|
|
|
|
|
|
|
|
|
|
|
|
|
|
|
|
|
|
|
|
|
|
|
|
|
|
|
|
|
|
|
|
|
|
|
|
|
|
|
|
|
|
|
|
|
|
|
|
|
|
|
|
|
|
|
|
|
|
|
|
spaces/Benson/text-generation/Examples/Camioneros De Europa 3 Hack Mod Apk An1.md
DELETED
@@ -1,87 +0,0 @@
|
|
1 |
-
|
2 |
-
<h1>Camioneros de Europa 3 Hack Mod APK: Cómo descargarlo e instalarlo</h1>
|
3 |
-
<p>Si eres un fan de los juegos realistas de conducción de camiones, es posible que hayas oído hablar de Truckers of Europe 3, un popular juego de simulador que te permite experimentar la vida de un camionero en Europa. Pero lo que si quieres disfrutar del juego sin limitaciones o restricciones? Ahí es donde un mod hack APK viene muy bien. En este artículo, le diremos todo lo que necesita saber sobre Camioneros de Europa 3 hack mod APK, incluyendo lo que es, cómo descargar e instalar, y cómo usarlo. ¡Vamos a empezar! </p>
|
4 |
-
<h2>camioneros de europa 3 hack mod apk an1</h2><br /><p><b><b>Download File</b> ❤❤❤ <a href="https://bltlly.com/2v6JgL">https://bltlly.com/2v6JgL</a></b></p><br /><br />
|
5 |
-
<h2>¿Qué es Camioneros de Europa 3?</h2>
|
6 |
-
<p>Truckers of Europe 3 es un juego de simulador de conductor de camión realista desarrollado por Wanda Software. El juego cuenta con gráficos que están cerca de los de las computadoras de escritorio, y le permite conducir varios camiones a través de diferentes países europeos. Puede elegir entre diferentes tipos de carga, como contenedores, tuberías de acero, alimentos o cualquier producto útil, y entregarlos a sus destinos. También puede personalizar su camión con diferentes piezas, colores y accesorios. El juego también tiene física realista, efectos meteorológicos, tráfico y ciclos día-noche. </p>
|
7 |
-
<h3>Características de los camioneros de Europa 3</h3>
|
8 |
-
<p>Algunas de las principales características de Truckers of Europe 3 son:</p>
|
9 |
-
<ul>
|
10 |
-
<li>Múltiples camiones para elegir, cada uno con sus propias características y rendimiento. </li>
|
11 |
-
<li>Diferentes tipos de carga a transportar, cada uno con su propio peso y tamaño. </li>
|
12 |
-
<li>Varias rutas y lugares para explorar, desde carreteras hasta caminos rurales. </li>
|
13 |
-
<li>Física de conducción y controles realistas, incluyendo volante, pedales, caja de cambios e indicadores. </li>
|
14 |
-
<li>Condiciones climáticas dinámicas y ciclos día-noche que afectan el juego. </li>
|
15 |
-
<li> Apariencia y accesorios de camiones personalizables, como pintura, ruedas, luces, cuernos y más. </li>
|
16 |
-
<li>Radio en el juego con varios géneros musicales y estaciones de noticias. </li>
|
17 |
-
<li>Logros y tablas de clasificación para competir con otros jugadores. </li>
|
18 |
-
|
19 |
-
<h3> ¿Por qué es posible que desee utilizar un Hack Mod APK</h3>
|
20 |
-
<p>Si bien Truckers of Europe 3 es un juego divertido e inmersivo, también tiene algunos inconvenientes que podrían hacer que desee utilizar un mod hack APK. Algunos de estos inconvenientes son:</p>
|
21 |
-
<ul>
|
22 |
-
<li>El juego requiere mucho espacio de almacenamiento y RAM para funcionar sin problemas. </li>
|
23 |
-
<li> El juego tiene anuncios que pueden interrumpir su juego o consumir sus datos. </li>
|
24 |
-
<li>El juego tiene compras en la aplicación que pueden darle una ventaja sobre otros jugadores o desbloquear más características. </li>
|
25 |
-
<li>El juego puede ser desafiante y frustrante a veces, especialmente cuando tienes que lidiar con el tráfico, accidentes, multas o carga dañada. </li>
|
26 |
-
</ul>
|
27 |
-
<p>Un hack mod APK es una versión modificada del juego original que puede evitar estos inconvenientes y darle más libertad y disfrute. Un mod hack APK también puede proporcionar algunas características adicionales que no están disponibles en el juego original. </p>
|
28 |
-
<h2>¿Qué es Camioneros de Europa 3 Hack Mod APK? </h2>
|
29 |
-
<p>Camioneros de Europa 3 hack mod APK es una versión modificada del juego original que puede darle dinero ilimitado, desbloquear todos los camiones y accesorios, eliminar anuncios, y más. Con este hack mod APK, puede jugar el juego sin limitaciones o restricciones. Usted <p>Sin embargo, antes de decidirse a descargar e instalar camioneros de Europa 3 hack mod APK, también debe ser consciente de los riesgos potenciales y desventajas que vienen con él. </p>
|
30 |
-
<h3>Beneficios de los camioneros de Europa 3 Hack Mod APK</h3>
|
31 |
-
<p>Algunos de los beneficios de usar camioneros de Europa 3 hack mod APK son:</p>
|
32 |
-
<ul>
|
33 |
-
<li> Puede obtener dinero ilimitado que puede utilizar para comprar y actualizar cualquier camión o accesorio que desee. </li>
|
34 |
-
<li> Puede desbloquear todos los camiones y accesorios que están bloqueados o requieren dinero real para comprar. </li>
|
35 |
-
<li> Puede eliminar los anuncios que pueden ser molestos o distraer mientras juega el juego. </li>
|
36 |
-
<li>Puedes disfrutar del juego sin ninguna dificultad o frustración, ya que puedes evitar el tráfico, accidentes, multas o carga dañada. </li>
|
37 |
-
|
38 |
-
</ul>
|
39 |
-
<h3> Riesgos de los camioneros de Europa 3 Hack Mod APK</h3>
|
40 |
-
<p>Algunos de los riesgos de usar camioneros de Europa 3 hack mod APK son:</p>
|
41 |
-
<p></p>
|
42 |
-
<ul>
|
43 |
-
<li> Puede exponer su dispositivo a malware o virus que pueden dañar sus datos o sistema. </li>
|
44 |
-
<li> Puede violar los términos y condiciones del juego original y obtener prohibido o suspendido de jugar. </li>
|
45 |
-
<li> Puede perder su progreso o datos si el mod hack APK no es compatible con la última versión del juego o su dispositivo. </li>
|
46 |
-
<li>Puedes arruinar la diversión y el desafío del juego, ya que puedes completar fácilmente todas las misiones y logros sin ningún esfuerzo. </li>
|
47 |
-
<li>Puedes perderte las actualizaciones y nuevas características que los desarrolladores agregan al juego original regularmente. </li>
|
48 |
-
</ul>
|
49 |
-
<h2>Cómo descargar e instalar camioneros de Europa 3 Hack Mod APK</h2>
|
50 |
-
<p>Si todavía quieres probar Camioneros de Europa 3 hack mod APK, es necesario seguir algunos pasos para descargar e instalar en su dispositivo. Estos son los pasos:</p>
|
51 |
-
<h3>Paso 1: Encontrar una fuente confiable</h3>
|
52 |
-
<p>El primer paso es encontrar una fuente confiable que proporciona el enlace de descarga para camioneros de Europa 3 hack mod APK. Puedes buscar en línea sitios web o foros que ofrecen este servicio, pero ten cuidado de no hacer clic en enlaces sospechosos o falsos que puedan dañar tu dispositivo. También puede comprobar las revisiones y calificaciones de otros usuarios que han descargado el mod hack APK de la misma fuente. Una buena fuente debe proporcionar un enlace de descarga seguro y de trabajo, así como una descripción detallada e instrucciones para usar el mod hack APK.</p>
|
53 |
-
<h3>Paso 2: Habilitar fuentes desconocidas en su dispositivo</h3>
|
54 |
-
|
55 |
-
<h3>Paso 3: Descargar el archivo APK</h3>
|
56 |
-
<p>El tercer paso es descargar el archivo APK de la fuente que ha elegido. Puede usar su navegador o una aplicación de administrador de descargas para hacer esto. Asegúrate de tener suficiente espacio de almacenamiento en tu dispositivo antes de descargar el archivo. El tamaño del archivo puede variar dependiendo de la fuente y la versión del mod hack APK. Una vez completada la descarga, puedes encontrar el archivo en tu carpeta de descargas o donde sea que lo hayas guardado. </p>
|
57 |
-
<h3>Paso 4: Instalar el archivo APK</h3>
|
58 |
-
<p>El paso final es instalar el archivo APK en su dispositivo. Para hacer esto, toque en el archivo y siga las instrucciones de instalación. Es posible que vea un mensaje de advertencia de que instalar esta aplicación podría dañar su dispositivo, pero puede ignorarlo si confía en la fuente que está utilizando. También es posible que tenga que permitir algunos permisos para que la aplicación funcione correctamente. Una vez que la instalación se ha completado, puede iniciar la aplicación y disfrutar de los camioneros de Europa 3 hack mod APK.</p>
|
59 |
-
<h2>Cómo utilizar camioneros de Europa 3 Hack Mod APK</h2>
|
60 |
-
<p>Ahora que ha descargado e instalado Camioneros de Europa 3 hack mod APK, es posible que se pregunte cómo usarlo. Aquí hay algunos consejos y trucos para jugar Camioneros de Europa 3 con hack mod APK:</p>
|
61 |
-
<h3> Consejos y trucos para jugar camioneros de Europa 3 con Hack Mod APK</h3>
|
62 |
-
<ul>
|
63 |
-
<li>Utilice la función de dinero ilimitado para comprar y actualizar cualquier camión o accesorio que desee. También puede usarlo para pagar cualquier multa o reparar cualquier daño que pueda sufrir mientras conduce. </li>
|
64 |
-
<li>Utilice la función de desbloqueo de todos los camiones y accesorios para probar diferentes camiones y personalizarlos según su preferencia. También puede cambiar entre diferentes camiones sin perder su progreso o carga. </li>
|
65 |
-
<li>Utilice la función de eliminación de anuncios para jugar el juego sin interrupciones o distracciones. También puede guardar sus datos y batería al no cargar ningún anuncio. </li>
|
66 |
-
|
67 |
-
<li>Utilice la función de hackeo de velocidad para conducir más rápido que el límite de velocidad normal. También puede superar a otros vehículos y llegar a su destino más rápido. Sin embargo, tenga cuidado de no estrellarse o ser atrapado por la policía. </li>
|
68 |
-
<li>Utilice la función de navegación de mapa gratuito para encontrar la mejor ruta a su destino. También puede ver las condiciones del tráfico y la carretera y evitar retrasos o desvíos. </li>
|
69 |
-
</ul>
|
70 |
-
<h2>Conclusión</h2>
|
71 |
-
<p>Truckers of Europe 3 es un juego de simulador de conductor de camión realista que le permite experimentar la vida de un camionero en Europa. Sin embargo, si desea jugar el juego sin limitaciones o restricciones, puede utilizar un mod hack APK que puede darle dinero ilimitado, desbloquear todos los camiones y accesorios, eliminar anuncios, y más. En este artículo, hemos explicado lo que Camioneros de Europa 3 hack mod APK es, cómo descargarlo e instalarlo, y cómo usarlo. También hemos proporcionado algunos consejos y trucos para jugar Camioneros de Europa 3 con hack mod APK. Sin embargo, también le advertimos sobre los posibles riesgos y desventajas de usar un mod de hackeo APK, tales como malware, prohibiciones, pérdida de datos o pérdida de diversión. Por lo tanto, le aconsejamos que utilice un mod hack APK a su propio riesgo y discreción. Esperamos que haya encontrado este artículo útil e informativo. ¡Feliz transporte! </p>
|
72 |
-
<h2>Preguntas frecuentes</h2>
|
73 |
-
<p>Aquí hay algunas preguntas frecuentes sobre camioneros de Europa 3 mod hack APK:</p>
|
74 |
-
<ol>
|
75 |
-
<li> Es camioneros de Europa 3 hack mod APK seguro de usar? </li>
|
76 |
-
<p>Camioneros de Europa 3 hack mod APK no es una aplicación oficial de los desarrolladores del juego original. Por lo tanto, no se garantiza su seguridad. Puede contener malware o virus que puedan dañar su dispositivo o datos. También podría violar los términos y condiciones del juego original y conseguir que se le prohibió o suspendido de jugar. Por lo tanto, usted debe utilizar un mod hack APK a su propio riesgo y discreción. </p>
|
77 |
-
<li> ¿Cómo actualizo camioneros de Europa 3 hack mod APK? </li>
|
78 |
-
|
79 |
-
<li> ¿Puedo jugar camioneros de Europa 3 hack mod APK en línea? </li>
|
80 |
-
<p>Camioneros de Europa 3 hack mod APK podría no funcionar bien con el modo en línea del juego original. Es posible que se enfrenten a algunos errores o problemas técnicos al jugar en línea con otros jugadores. También puede ser detectado por el sistema anti-cheat del juego y obtener prohibido o suspendido de jugar. Por lo tanto, le recomendamos que juegue Camioneros de Europa 3 hack mod APK offline o en modo de un solo jugador. </p>
|
81 |
-
<li> ¿Puedo usar camioneros de Europa 3 hack mod APK en dispositivos iOS? </li>
|
82 |
-
<p>Camioneros de Europa 3 hack mod APK es una aplicación para Android que solo se puede instalar en dispositivos Android. Por lo tanto, no puede usarlo en dispositivos iOS como iPhones o iPads. Sin embargo, es posible que encuentre algunas formas alternativas de utilizar un mod hack APK en dispositivos iOS, como el uso de un emulador o una herramienta de jailbreak. Sin embargo, estos métodos no son recomendables ya que podrían dañar su dispositivo o datos. </p>
|
83 |
-
<li> ¿Puedo usar camioneros de Europa 3 hack mod APK en PC? </li>
|
84 |
-
<p>Camioneros de Europa 3 hack mod APK es una aplicación para Android que solo se puede instalar en dispositivos Android. Por lo tanto, no se puede utilizar en el PC directamente. Sin embargo, usted puede encontrar algunas maneras de utilizar un mod hack APK en el PC, como el uso de un emulador o una máquina virtual. Estos métodos le permiten ejecutar aplicaciones Android en el PC mediante la simulación de un entorno Android. Sin embargo, no se garantiza que estos métodos funcionen bien o sin problemas. </p>
|
85 |
-
</ol></p> 64aa2da5cf<br />
|
86 |
-
<br />
|
87 |
-
<br />
|
|
|
|
|
|
|
|
|
|
|
|
|
|
|
|
|
|
|
|
|
|
|
|
|
|
|
|
|
|
|
|
|
|
|
|
|
|
|
|
|
|
|
|
|
|
|
|
|
|
|
|
|
|
|
|
|
|
|
|
|
|
|
|
|
|
|
|
|
|
|
|
|
|
|
|
|
|
|
|
|
|
|
|
|
|
|
|
|
|
|
|
|
|
|
|
|
|
|
|
|
|
|
|
|
|
|
|
|
|
|
|
|
|
|
|
|
|
|
|
|
|
|
|
|
|
|
|
|
|
|
|
|
|
|
|
|
|
|
|
|
|
|
|
|
|
|
|
|
|
|
|
|
|
|
|
|
|
|
|
|
|
|
|
|
|
|
|
|
|
|
|
|
|
|
|
spaces/Benson/text-generation/Examples/Cmo Crear Y Construir Apk.md
DELETED
@@ -1,133 +0,0 @@
|
|
1 |
-
|
2 |
-
<h1>Tải elaboración y construcción APK - Un juego de construcción gratis para Android</h1>
|
3 |
-
<p>¿Te gustan los juegos de construcción? ¿Quieres dar rienda suelta a tu creatividad e imaginación? ¿Quieres divertirte con tus amigos y familiares? Si respondiste afirmativamente a cualquiera de estas preguntas, entonces deberías probar <strong>Crafting and Building APK</strong>, un nuevo juego de construcción gratuito para dispositivos Android. En este artículo, te contaremos todo lo que necesitas saber sobre este juego, incluyendo qué es, cómo descargarlo e instalarlo, por qué deberías jugarlo, cómo jugarlo y cuáles son algunas alternativas. ¡Vamos a empezar! </p>
|
4 |
-
<h2>¿Qué es la elaboración y construcción de APK? </h2>
|
5 |
-
<h3>Una breve introducción al juego y sus características</h3>
|
6 |
-
<p>Elaboración y construcción de APK es un juego gratuito para dispositivos Android que le permite construir sus propias construcciones, desde casas y castillos hasta minas y templos. También puedes explorar el mundo creado por otros jugadores, interactuar con aldeanos y animales, personalizar tu personaje y jugar online con tus amigos. El juego tiene muchas características que lo hacen divertido y atractivo, como:</p>
|
7 |
-
<h2>Cómo crear y construir apk</h2><br /><p><b><b>Download Zip</b> … <a href="https://bltlly.com/2v6LAv">https://bltlly.com/2v6LAv</a></b></p><br /><br />
|
8 |
-
<ul>
|
9 |
-
<li>Gráficos geniales: disfruta de los mejores gráficos de píxeles con fps altos. </li>
|
10 |
-
<li>Muchos tipos de bloques: elige entre hierba, piedra, diamante y más para construir tu imperio. </li>
|
11 |
-
<li>Juegos multijugador: juega online y ayuda a tu amigo a construir su casa o competir con ellos. </li>
|
12 |
-
<li>Divertido juego: juega con mascotas, busca cuevas ocultas y diviértete con tus amigos. </li>
|
13 |
-
<li>Juego gratis: juega el juego gratis sin limitaciones ni anuncios. </li>
|
14 |
-
</ul>
|
15 |
-
<h3>Cómo descargar e instalar el juego en tu dispositivo</h3>
|
16 |
-
<p>Descargar e instalar Elaboración y construcción de APK en su dispositivo es muy fácil. Solo tiene que seguir estos sencillos pasos:</p>
|
17 |
-
<ol>
|
18 |
-
<li>Ir a <a href="( 1 )">este enlace</a> o <a href="( 4 )">este enlace</a> o <a href="( 6 )">este enlace</a> o <a href="( 7 )">este enlace</a> para descargar el archivo APK del juego. </li>
|
19 |
-
|
20 |
-
<li>Toque en el archivo y permita la instalación desde fuentes desconocidas si se le solicita. </li>
|
21 |
-
<li>Espere a que el proceso de instalación termine y luego inicie el juego desde el cajón de la aplicación o la pantalla de inicio. </li>
|
22 |
-
<li> ¡Disfruta jugando a crear y construir APK en tu dispositivo! </li>
|
23 |
-
</ol>
|
24 |
-
<h2>¿Por qué debe jugar a la elaboración y construcción de APK? </h2>
|
25 |
-
<h3>Los beneficios de jugar este juego para diferentes grupos de edad</h3>
|
26 |
-
<p>Elaboración y construcción de APK es un juego que puede ser disfrutado por cualquier persona, independientemente de su edad o origen. Estos son algunos de los beneficios de jugar este juego para diferentes grupos de edad:</p>
|
27 |
-
<ul>
|
28 |
-
<li>Para los niños: Jugar a este juego puede ayudar a los niños a desarrollar su creatividad, imaginación, habilidades para resolver problemas, conciencia espacial, coordinación mano-ojo y habilidades motoras finas. También puede fomentar su curiosidad, interés y pasión por aprender cosas nuevas. </li>
|
29 |
-
<h3>Los aspectos divertidos y creativos del juego</h3>
|
30 |
-
<p>Elaboración y construcción de APK es un juego que también puede proporcionar un montón de oportunidades divertidas y creativas para los jugadores. Estos son algunos de los aspectos divertidos y creativos del juego:</p>
|
31 |
-
<ul>
|
32 |
-
<li>Puedes construir lo que quieras, desde casas sencillas y granjas hasta ciudades y monumentos complejos. También puede decorar sus edificios con muebles, pinturas, alfombras y más. </li>
|
33 |
-
<li>Puedes explorar el mundo creado por otros jugadores, descubrir nuevos lugares y admirar sus creaciones. También puede interactuar con aldeanos y animales, comerciar con ellos o luchar contra ellos. </li>
|
34 |
-
<li>Puedes personalizar tu personaje con diferentes pieles, ropa, accesorios y peinados. También puede cambiar la apariencia de sus mascotas y vehículos. </li>
|
35 |
-
<li>Puedes jugar en línea con tus amigos y familiares, chatear con ellos, colaborar con ellos o competir con ellos. También puede unirse o crear sus propios servidores y comunidades. </li>
|
36 |
-
</ul>
|
37 |
-
<h3>El modo multijugador y la interacción social con otros jugadores</h3>
|
38 |
-
|
39 |
-
<ul>
|
40 |
-
<li>Puedes jugar online con hasta 10 jugadores al mismo tiempo, ya sea en modo cooperativo o competitivo. También puede invitar a sus amigos a unirse a su juego o unirse a su juego. </li>
|
41 |
-
<li>Puedes chatear con otros jugadores usando mensajes de texto o de voz. También puedes usar emojis y pegatinas para expresar tus emociones y reacciones. </li>
|
42 |
-
<li>Puedes compartir tus creaciones con otros jugadores, calificar sus creaciones, comentarlas o seguirlas. También puedes obtener comentarios y sugerencias de otros jugadores sobre cómo mejorar tus habilidades y experiencia. </li>
|
43 |
-
<li>Puede unirse o crear sus propios servidores y comunidades, donde puede conocer gente nueva, hacer amigos o encontrar socios. También puedes participar en eventos, concursos, desafíos o juegos organizados por otros jugadores o por ti mismo. </li>
|
44 |
-
</ul>
|
45 |
-
<h2>¿Cómo se juega elaboración y construcción APK? </h2>
|
46 |
-
<h3>El juego básico y los controles</h3>
|
47 |
-
<p>Elaboración y construcción de APK es un juego que es fácil de jugar y controlar. Estos son algunos de los juegos básicos y controles:</p>
|
48 |
-
<ul>
|
49 |
-
<li>Para moverse, puede usar el joystick en el lado izquierdo de la pantalla. Para mirar alrededor, puede deslizar el dedo hacia el lado derecho de la pantalla. </li>
|
50 |
-
<li>Para saltar, puede tocar el botón de salto en el lado derecho de la pantalla. Para volar, puede pulsar dos veces el botón de salto y luego usar el joystick para controlar su dirección. </li>
|
51 |
-
<li>Para seleccionar un tipo de bloque, puede tocar el botón de inventario en el lado derecho de la pantalla y luego elegir entre los bloques disponibles. Para colocar un bloque, puede tocar en la pantalla donde desea colocarlo. Para romper un bloque, puede pulsar y mantener pulsado en la pantalla donde desea romperlo. </li>
|
52 |
-
<li>Para interactuar con un objeto, como una puerta, un cofre o un animal, puede pulsarlo. Para usar un objeto, como una espada, un arco o una poción, puede seleccionarlo de su inventario y luego tocar en la pantalla donde desea usarlo. </li>
|
53 |
-
|
54 |
-
</ul>
|
55 |
-
<h3>Los diferentes modos y opciones disponibles</h3>
|
56 |
-
<p>Elaboración y construcción de APK es un juego que ofrece diferentes modos y opciones para los jugadores para elegir. Estos son algunos de los diferentes modos y opciones disponibles:</p>
|
57 |
-
<ul>
|
58 |
-
<li>Modo creativo: En este modo, tienes recursos ilimitados y puedes construir lo que quieras sin restricciones. También puedes volar y acceder a todos los bloques y objetos del juego. </li>
|
59 |
-
<li>Modo de supervivencia: En este modo, tienes que reunir recursos, crear herramientas y armas, y sobrevivir a los peligros del mundo. También tienes que controlar tus niveles de salud, hambre y sed. También puedes luchar contra enemigos, como zombis, arañas y esqueletos. </li>
|
60 |
-
<li>Modo aventura: En este modo, puedes explorar el mundo creado por otros jugadores, completar misiones y recoger recompensas. También puede interactuar con aldeanos y animales, comerciar con ellos o luchar contra ellos. </li>
|
61 |
-
<li>Modo multijugador: En este modo, puedes jugar online con otros jugadores, ya sea en modo cooperativo o competitivo. También puede chatear con ellos, compartir sus creaciones o unirse a sus servidores y comunidades. </li>
|
62 |
-
<li>Opciones: En este menú, puede cambiar la configuración del juego, como el sonido, los gráficos, el idioma y los controles. También puede acceder a la sección de ayuda, donde puede encontrar tutoriales, consejos y preguntas frecuentes.</li>
|
63 |
-
</ul>
|
64 |
-
<h3>Algunos consejos y trucos para mejorar tus habilidades y experiencia</h3>
|
65 |
-
<p>Elaboración y construcción de APK es un juego que puede ser desafiante y gratificante al mismo tiempo. Aquí hay algunos consejos y trucos para mejorar tus habilidades y experiencia:</p>
|
66 |
-
<ul>
|
67 |
-
<li>Utilice la mesa de elaboración para crear nuevos artículos, tales como herramientas, armas, armaduras y muebles. También puede utilizar el horno para fundir minerales y cocinar alimentos. </li>
|
68 |
-
<li>Usa el mapa para navegar por el mundo y encontrar tu ubicación. También puedes usar la brújula para encontrar tu punto de aparición o tu hogar. </li>
|
69 |
-
|
70 |
-
<li>Utiliza antorchas para iluminar tus edificios y evitar que los monstruos aparezcan. También puedes usar antorchas para marcar tu camino o tu territorio. </li>
|
71 |
-
<li>Use cofres para guardar sus artículos y mantenerlos seguros. También puede usar letreros para etiquetar sus cofres o sus edificios. </li>
|
72 |
-
<li>Use escaleras para subir o bajar paredes o torres. También puede usar escaleras o losas para crear pendientes o techos. </li>
|
73 |
-
<li>Use puertas para asegurar sus entradas o salidas. También puede usar trampillas para crear pasajes secretos o habitaciones ocultas. </li>
|
74 |
-
<li>Use cercas para encerrar sus granjas o jardines. También puede usar puertas para acceder a ellas. </li>
|
75 |
-
<li>Usa cubos para recoger agua o lava. También puedes usar cubos para crear fuentes o piscinas. </li>
|
76 |
-
<li>Usa semillas para cultivar cultivos o flores. También puedes usar harina de huesos para acelerar su crecimiento. </li>
|
77 |
-
</ul>
|
78 |
-
<h2>¿Cuáles son algunas alternativas a la elaboración y construcción de APK? </h2>
|
79 |
-
<h3>Una comparación de juegos similares en el mercado</h3>
|
80 |
-
<p>Elaboración y construcción APK no es el único juego de construcción en el mercado. Hay muchos otros juegos similares que puedes probar si buscas más opciones. Estos son algunos de ellos:</p>
|
81 |
-
<p></p>
|
82 |
-
<borde de la tabla="1">
|
83 |
-
<tr><th>Juego</th><th>Descripción</th><th>Precio</th></tr>
|
84 |
-
<tr><td>Minecraft</td><td>El juego de construcción más popular del mundo, donde puedes crear lo que quieras con bloques en un mundo de caja de arena. También puedes jugar online con millones de jugadores. </td><td>$6.99</td></tr>
|
85 |
-
<tr><td>Roblox</td><td>Una plataforma donde puedes jugar millones de juegos creados por otros usuarios o crear tus propios juegos con Roblox Studio. También puedes personalizar tu avatar y chatear con otros jugadores. </td><td>Gratis (con compras en la aplicación)</td></tr>
|
86 |
-
<tr><td>Terraria</td><td>Un juego de aventura en 2D donde puedes explorar, construir, crear, luchar y sobrevivir en un mundo generado al azar. También puedes jugar online con hasta 8 jugadores. </td><td>$4.99</td></tr>
|
87 |
-
|
88 |
-
con animales y plantas. También puede visitar otras islas y jugar con otros jugadores. </td><td>Gratis (con compras en la aplicación)</td></tr>
|
89 |
-
</tabla>
|
90 |
-
<h3>Los pros y los contras de cada alternativa</h3>
|
91 |
-
<p>Cada uno de estos juegos tiene sus propios pros y contras que debes considerar antes de elegir uno. Estos son algunos de ellos:</p>
|
92 |
-
<borde de la tabla="1">
|
93 |
-
<tr><th>Juego</th><th>Pros</th><th>Contras</th></tr>
|
94 |
-
<tr><td>Minecraft</td><td>- Tiene una base de fans enorme y leal<br>- Tiene un montón de contenido y actualizaciones<br>- Tiene una gran cantidad de mods y plugins<br>- Tiene un montón de potencial educativo y creativo</td><td>- Requiere una cuenta de pago para jugar en línea<br>- Puede ser lento o defectuoso en algunos dispositivos<br> Puede ser demasiado complejo o abrumador para algunos jugadores<br>- Puede ser adictivo o perjudicial para algunos jugadores</td></tr>
|
95 |
-
<tr><td>Roblox</td><td>- Tiene mucha variedad y diversidad<br>- Tiene mucho contenido generado por el usuario<br>- Tiene muchas características sociales y opciones<br>- Tiene muchas oportunidades para aprender y ganar</td><td>- Tiene mucho contenido inapropiado o inseguro<br-> Tiene un montón de anuncios y microtransacciones<br>- Tiene un montón de hackers y estafadores<br>- Tiene un montón de problemas técnicos y problemas técnicos</td></tr>
|
96 |
-
<tr><td>Terraria</td><td>- Tiene mucha profundidad y detalle<br>- Tiene mucha exploración y aventura<br>- Tiene mucha personalización y personalización<br>- Tiene mucho desafío y valor de repetición</td><td>- Tiene una curva de aprendizaje empinada<br>- Tiene un tamaño mundial limitado Tiene un modo multijugador limitado<br>- Tiene una calidad gráfica limitada</td></tr>
|
97 |
-
<tr><td>Sandbox 3D</td><td>- Tiene una interfaz simple e intuitiva<br>- Tiene unos gráficos coloridos y vibrantes<br>- Tiene un juego relajante y casual<br>- Tiene una comunidad amigable y de apoyo</td><td>- Tiene un bloque limitado de tipos y elementos<br>- Tiene modos de juego y opciones limitadas br<>-> Tiene características y funciones limitadas en línea<br>- Tiene un desarrollo y soporte limitados</td></tr>
|
98 |
-
|
99 |
-
</tabla>
|
100 |
-
<h3>La mejor opción para sus preferencias y necesidades</h3>
|
101 |
-
<p>La mejor opción para tus preferencias y necesidades depende de lo que estés buscando en un juego de construcción. Aquí hay algunas preguntas que puedes hacerte para ayudarte a decidir:</p>
|
102 |
-
<ul>
|
103 |
-
<li>¿Quieres jugar online o offline? </li>
|
104 |
-
<li>¿Quieres jugar solo o con otros? </li>
|
105 |
-
<li>¿Quieres construir o explorar? </li>
|
106 |
-
<li>¿Quieres crear o consumir? </li>
|
107 |
-
<li>¿Quieres aprender o divertirte? </li>
|
108 |
-
<li>¿Quieres pagar o jugar gratis? </li>
|
109 |
-
<li>¿Quieres tener más control o más libertad? </li>
|
110 |
-
<li>¿Quieres tener más realismo o más fantasía? </li>
|
111 |
-
<li>¿Quieres tener más simplicidad o más complejidad? </li>
|
112 |
-
<li>¿Quieres tener más calidad o más cantidad? </li>
|
113 |
-
</ul>
|
114 |
-
<p>Basado en sus respuestas, puede comparar los diferentes juegos y elegir el que más le convenga. Por supuesto, también puedes probarlos todos y ver cuál te gusta más. ¡La elección es tuya! </p>
|
115 |
-
<h2>Conclusión</h2>
|
116 |
-
<h3>Un resumen de los puntos principales y una llamada a la acción</h3>
|
117 |
-
y control, y ofrece diferentes modos y opciones para los jugadores a elegir. También es un juego que tiene muchos aspectos divertidos y creativos, como construir lo que quieras, explorar el mundo, personalizar tu personaje y jugar online con tus amigos. Sin embargo, si buscas más alternativas, también puedes probar otros juegos similares en el mercado, como Minecraft, Roblox, Terraria, Sandbox 3D y Crafty Lands. Cada uno de estos juegos tiene sus propios pros y contras que usted debe considerar antes de elegir uno. La mejor opción para tus preferencias y necesidades depende de lo que estés buscando en un juego de construcción. Esperamos que este artículo le ha ayudado a aprender más acerca de la elaboración y construcción de APK y para decidir si desea descargar e instalar en su dispositivo o no. Si lo haces, esperamos que tengas mucha diversión y creatividad con este juego. ¡Gracias por leer! </p>
|
118 |
-
<h3>Preguntas frecuentes</h3>
|
119 |
-
|
120 |
-
<ol>
|
121 |
-
<li> ¿Es la elaboración y construcción de APK seguro para descargar e instalar? </li>
|
122 |
-
<p>Sí, Elaboración y construcción de APK es seguro para descargar e instalar. No contiene ningún virus, malware o spyware. Sin embargo, siempre debe descargarlo de una fuente de confianza, como <a href="">este enlace</a> o <a href=">este enlace</a> o <a href=">">este enlace</a> o <a href=">este enlace</a>, y no de ningún sitio web desconocido o sospechoso. </p>
|
123 |
-
<li> ¿Es la elaboración y construcción de APK compatible con mi dispositivo? </li>
|
124 |
-
<p>Elaboración y construcción de APK es compatible con la mayoría de los dispositivos Android que tienen Android 4.1 o superior. Sin embargo, algunos dispositivos pueden tener diferentes especificaciones o problemas de rendimiento que pueden afectar la jugabilidad o la calidad de los gráficos. Puede comprobar la compatibilidad de su dispositivo visitando <a href="">este enlace</a> o <a href=">este enlace</a>. </p>
|
125 |
-
<li> ¿Cómo puedo actualizar la elaboración y construcción de APK? </li>
|
126 |
-
<p>Elaboración y construcción de APK se actualiza regularmente por los desarrolladores para corregir errores, mejorar las características, y añadir nuevo contenido. Puedes actualizar el juego visitando <a href="">este enlace</a> o <a href=">este enlace</a> o <a href=">este enlace</a> o <a href=">este enlace</a> y descargando la última versión del juego. También puede habilitar la opción de actualizaciones automáticas en la configuración del dispositivo para recibir notificaciones cuando una nueva actualización esté disponible. </p>
|
127 |
-
<li> ¿Cómo puedo contactar a los desarrolladores de Elaboración y construcción de APK? </li>
|
128 |
-
<p>Si usted tiene alguna pregunta, comentarios, sugerencias, o problemas con respecto a la elaboración y construcción de APK, puede ponerse en contacto con los desarrolladores enviando un correo electrónico a <a href="mailto:[email protected]">[email protected]</a>. También puede visitar su sitio web en <a href="">este enlace</a> o su página de Facebook en <a href=">este enlace</a>. </p>
|
129 |
-
<li> ¿Cómo puedo apoyar a los desarrolladores de elaboración y construcción de APK? </li>
|
130 |
-
|
131 |
-
</ol></p> 64aa2da5cf<br />
|
132 |
-
<br />
|
133 |
-
<br />
|
|
|
|
|
|
|
|
|
|
|
|
|
|
|
|
|
|
|
|
|
|
|
|
|
|
|
|
|
|
|
|
|
|
|
|
|
|
|
|
|
|
|
|
|
|
|
|
|
|
|
|
|
|
|
|
|
|
|
|
|
|
|
|
|
|
|
|
|
|
|
|
|
|
|
|
|
|
|
|
|
|
|
|
|
|
|
|
|
|
|
|
|
|
|
|
|
|
|
|
|
|
|
|
|
|
|
|
|
|
|
|
|
|
|
|
|
|
|
|
|
|
|
|
|
|
|
|
|
|
|
|
|
|
|
|
|
|
|
|
|
|
|
|
|
|
|
|
|
|
|
|
|
|
|
|
|
|
|
|
|
|
|
|
|
|
|
|
|
|
|
|
|
|
|
|
|
|
|
|
|
|
|
|
|
|
|
|
|
|
|
|
|
|
|
|
|
|
|
|
|
|
|
|
|
|
|
|
|
|
|
|
|
|
|
|
|
|
|
|
|
|
|
|
|
|
|
|
|
|
|
|
|
|
|
|
|
|
|
|
|
|
|
|
|
|
|
|
|
|
|
|
|
|
|
|
|
|
|
|
|
|
|
|
|
|
|
|
spaces/Big-Web/MMSD/env/Lib/site-packages/pip/_vendor/pygments/scanner.py
DELETED
@@ -1,104 +0,0 @@
|
|
1 |
-
"""
|
2 |
-
pygments.scanner
|
3 |
-
~~~~~~~~~~~~~~~~
|
4 |
-
|
5 |
-
This library implements a regex based scanner. Some languages
|
6 |
-
like Pascal are easy to parse but have some keywords that
|
7 |
-
depend on the context. Because of this it's impossible to lex
|
8 |
-
that just by using a regular expression lexer like the
|
9 |
-
`RegexLexer`.
|
10 |
-
|
11 |
-
Have a look at the `DelphiLexer` to get an idea of how to use
|
12 |
-
this scanner.
|
13 |
-
|
14 |
-
:copyright: Copyright 2006-2022 by the Pygments team, see AUTHORS.
|
15 |
-
:license: BSD, see LICENSE for details.
|
16 |
-
"""
|
17 |
-
import re
|
18 |
-
|
19 |
-
|
20 |
-
class EndOfText(RuntimeError):
|
21 |
-
"""
|
22 |
-
Raise if end of text is reached and the user
|
23 |
-
tried to call a match function.
|
24 |
-
"""
|
25 |
-
|
26 |
-
|
27 |
-
class Scanner:
|
28 |
-
"""
|
29 |
-
Simple scanner
|
30 |
-
|
31 |
-
All method patterns are regular expression strings (not
|
32 |
-
compiled expressions!)
|
33 |
-
"""
|
34 |
-
|
35 |
-
def __init__(self, text, flags=0):
|
36 |
-
"""
|
37 |
-
:param text: The text which should be scanned
|
38 |
-
:param flags: default regular expression flags
|
39 |
-
"""
|
40 |
-
self.data = text
|
41 |
-
self.data_length = len(text)
|
42 |
-
self.start_pos = 0
|
43 |
-
self.pos = 0
|
44 |
-
self.flags = flags
|
45 |
-
self.last = None
|
46 |
-
self.match = None
|
47 |
-
self._re_cache = {}
|
48 |
-
|
49 |
-
def eos(self):
|
50 |
-
"""`True` if the scanner reached the end of text."""
|
51 |
-
return self.pos >= self.data_length
|
52 |
-
eos = property(eos, eos.__doc__)
|
53 |
-
|
54 |
-
def check(self, pattern):
|
55 |
-
"""
|
56 |
-
Apply `pattern` on the current position and return
|
57 |
-
the match object. (Doesn't touch pos). Use this for
|
58 |
-
lookahead.
|
59 |
-
"""
|
60 |
-
if self.eos:
|
61 |
-
raise EndOfText()
|
62 |
-
if pattern not in self._re_cache:
|
63 |
-
self._re_cache[pattern] = re.compile(pattern, self.flags)
|
64 |
-
return self._re_cache[pattern].match(self.data, self.pos)
|
65 |
-
|
66 |
-
def test(self, pattern):
|
67 |
-
"""Apply a pattern on the current position and check
|
68 |
-
if it patches. Doesn't touch pos.
|
69 |
-
"""
|
70 |
-
return self.check(pattern) is not None
|
71 |
-
|
72 |
-
def scan(self, pattern):
|
73 |
-
"""
|
74 |
-
Scan the text for the given pattern and update pos/match
|
75 |
-
and related fields. The return value is a boolean that
|
76 |
-
indicates if the pattern matched. The matched value is
|
77 |
-
stored on the instance as ``match``, the last value is
|
78 |
-
stored as ``last``. ``start_pos`` is the position of the
|
79 |
-
pointer before the pattern was matched, ``pos`` is the
|
80 |
-
end position.
|
81 |
-
"""
|
82 |
-
if self.eos:
|
83 |
-
raise EndOfText()
|
84 |
-
if pattern not in self._re_cache:
|
85 |
-
self._re_cache[pattern] = re.compile(pattern, self.flags)
|
86 |
-
self.last = self.match
|
87 |
-
m = self._re_cache[pattern].match(self.data, self.pos)
|
88 |
-
if m is None:
|
89 |
-
return False
|
90 |
-
self.start_pos = m.start()
|
91 |
-
self.pos = m.end()
|
92 |
-
self.match = m.group()
|
93 |
-
return True
|
94 |
-
|
95 |
-
def get_char(self):
|
96 |
-
"""Scan exactly one char."""
|
97 |
-
self.scan('.')
|
98 |
-
|
99 |
-
def __repr__(self):
|
100 |
-
return '<%s %d/%d>' % (
|
101 |
-
self.__class__.__name__,
|
102 |
-
self.pos,
|
103 |
-
self.data_length
|
104 |
-
)
|
|
|
|
|
|
|
|
|
|
|
|
|
|
|
|
|
|
|
|
|
|
|
|
|
|
|
|
|
|
|
|
|
|
|
|
|
|
|
|
|
|
|
|
|
|
|
|
|
|
|
|
|
|
|
|
|
|
|
|
|
|
|
|
|
|
|
|
|
|
|
|
|
|
|
|
|
|
|
|
|
|
|
|
|
|
|
|
|
|
|
|
|
|
|
|
|
|
|
|
|
|
|
|
|
|
|
|
|
|
|
|
|
|
|
|
|
|
|
|
|
|
|
|
|
|
|
|
|
|
|
|
|
|
|
|
|
|
|
|
|
|
|
|
|
|
|
|
|
|
|
|
|
|
|
|
|
|
|
|
|
|
|
|
|
|
|
|
|
|
|
|
|
|
|
|
|
|
|
|
|
|
|
|
|
|
|
|
|
|
|
|
|
|
|
|
|
|
|
|
|
|
|
|
|
|
|
|
|
|
spaces/BigChungux/Pet_Survey/info.md
DELETED
@@ -1,16 +0,0 @@
|
|
1 |
-
# 😌 [Edit info.md - Your app's title here]
|
2 |
-
|
3 |
-
### 🧐 Problem Statement and Research Summary
|
4 |
-
[add info about your problem statement and your research here!]
|
5 |
-
|
6 |
-
### 🎣 Data Collection Plan
|
7 |
-
[Edit info.md - add info about what data you collected and why here!]
|
8 |
-
|
9 |
-
### 💥 Ethical Considerations (Data Privacy and Bias)
|
10 |
-
* Data privacy: [Edit info.md - add info about you considered users' privacy here!]
|
11 |
-
* Bias: [Edit info.md - add info about you considered bias here!]
|
12 |
-
|
13 |
-
### 👻 Our Team
|
14 |
-
[Edit info.md - add info about your team members here!]
|
15 |
-
|
16 |
-
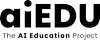
|
|
|
|
|
|
|
|
|
|
|
|
|
|
|
|
|
|
|
|
|
|
|
|
|
|
|
|
|
|
|
|
|
|
spaces/CALM/Dashboard/README.md
DELETED
@@ -1,26 +0,0 @@
|
|
1 |
-
---
|
2 |
-
|
3 |
-
title: Dashboard
|
4 |
-
emoji: 🌐
|
5 |
-
colorFrom: blue
|
6 |
-
colorTo: red
|
7 |
-
sdk: streamlit
|
8 |
-
|
9 |
-
|
10 |
-
|
11 |
-
|
12 |
-
app_file: app.py
|
13 |
-
pinned: true
|
14 |
-
|
15 |
-
---
|
16 |
-
|
17 |
-
|
18 |
-
# Training transformers together dashboard
|
19 |
-
|
20 |
-
[](https://huggingface.co/spaces/training-transformers-together/training-transformers-together-dashboard)
|
21 |
-
|
22 |
-
A dashboard app for Hugging Face Spaces
|
23 |
-
---
|
24 |
-
|
25 |
-
Autogenerated using [this template](https://github.com/nateraw/spaces-template)
|
26 |
-
|
|
|
|
|
|
|
|
|
|
|
|
|
|
|
|
|
|
|
|
|
|
|
|
|
|
|
|
|
|
|
|
|
|
|
|
|
|
|
|
|
|
|
|
|
|
|
|
|
|
|
|
|
|
spaces/CVPR/Dual-Key_Backdoor_Attacks/datagen/detectron2/dev/run_inference_tests.sh
DELETED
@@ -1,44 +0,0 @@
|
|
1 |
-
#!/bin/bash -e
|
2 |
-
# Copyright (c) Facebook, Inc. and its affiliates. All Rights Reserved
|
3 |
-
|
4 |
-
BIN="python tools/train_net.py"
|
5 |
-
OUTPUT="inference_test_output"
|
6 |
-
NUM_GPUS=2
|
7 |
-
|
8 |
-
CFG_LIST=( "${@:1}" )
|
9 |
-
|
10 |
-
if [ ${#CFG_LIST[@]} -eq 0 ]; then
|
11 |
-
CFG_LIST=( ./configs/quick_schedules/*inference_acc_test.yaml )
|
12 |
-
fi
|
13 |
-
|
14 |
-
echo "========================================================================"
|
15 |
-
echo "Configs to run:"
|
16 |
-
echo "${CFG_LIST[@]}"
|
17 |
-
echo "========================================================================"
|
18 |
-
|
19 |
-
|
20 |
-
for cfg in "${CFG_LIST[@]}"; do
|
21 |
-
echo "========================================================================"
|
22 |
-
echo "Running $cfg ..."
|
23 |
-
echo "========================================================================"
|
24 |
-
$BIN \
|
25 |
-
--eval-only \
|
26 |
-
--num-gpus $NUM_GPUS \
|
27 |
-
--config-file "$cfg" \
|
28 |
-
OUTPUT_DIR $OUTPUT
|
29 |
-
rm -rf $OUTPUT
|
30 |
-
done
|
31 |
-
|
32 |
-
|
33 |
-
echo "========================================================================"
|
34 |
-
echo "Running demo.py ..."
|
35 |
-
echo "========================================================================"
|
36 |
-
DEMO_BIN="python demo/demo.py"
|
37 |
-
COCO_DIR=datasets/coco/val2014
|
38 |
-
mkdir -pv $OUTPUT
|
39 |
-
|
40 |
-
set -v
|
41 |
-
|
42 |
-
$DEMO_BIN --config-file ./configs/quick_schedules/panoptic_fpn_R_50_inference_acc_test.yaml \
|
43 |
-
--input $COCO_DIR/COCO_val2014_0000001933* --output $OUTPUT
|
44 |
-
rm -rf $OUTPUT
|
|
|
|
|
|
|
|
|
|
|
|
|
|
|
|
|
|
|
|
|
|
|
|
|
|
|
|
|
|
|
|
|
|
|
|
|
|
|
|
|
|
|
|
|
|
|
|
|
|
|
|
|
|
|
|
|
|
|
|
|
|
|
|
|
|
|
|
|
|
|
|
|
|
|
|
|
|
|
|
|
|
|
|
|
|
|
|
|
|
spaces/CVPR/LIVE/thrust/thrust/iterator/detail/universal_categories.h
DELETED
@@ -1,87 +0,0 @@
|
|
1 |
-
/*
|
2 |
-
* Copyright 2008-2013 NVIDIA Corporation
|
3 |
-
*
|
4 |
-
* Licensed under the Apache License, Version 2.0 (the "License");
|
5 |
-
* you may not use this file except in compliance with the License.
|
6 |
-
* You may obtain a copy of the License at
|
7 |
-
*
|
8 |
-
* http://www.apache.org/licenses/LICENSE-2.0
|
9 |
-
*
|
10 |
-
* Unless required by applicable law or agreed to in writing, software
|
11 |
-
* distributed under the License is distributed on an "AS IS" BASIS,
|
12 |
-
* WITHOUT WARRANTIES OR CONDITIONS OF ANY KIND, either express or implied.
|
13 |
-
* See the License for the specific language governing permissions and
|
14 |
-
* limitations under the License.
|
15 |
-
*/
|
16 |
-
|
17 |
-
#pragma once
|
18 |
-
|
19 |
-
#include <thrust/detail/config.h>
|
20 |
-
#include <thrust/iterator/iterator_categories.h>
|
21 |
-
|
22 |
-
// XXX eliminate this file
|
23 |
-
|
24 |
-
namespace thrust
|
25 |
-
{
|
26 |
-
|
27 |
-
// define these types without inheritance to avoid ambiguous conversion to base classes
|
28 |
-
|
29 |
-
struct input_universal_iterator_tag
|
30 |
-
{
|
31 |
-
operator input_host_iterator_tag () {return input_host_iterator_tag();}
|
32 |
-
|
33 |
-
operator input_device_iterator_tag () {return input_device_iterator_tag();}
|
34 |
-
};
|
35 |
-
|
36 |
-
struct output_universal_iterator_tag
|
37 |
-
{
|
38 |
-
operator output_host_iterator_tag () {return output_host_iterator_tag();}
|
39 |
-
|
40 |
-
operator output_device_iterator_tag () {return output_device_iterator_tag();}
|
41 |
-
};
|
42 |
-
|
43 |
-
struct forward_universal_iterator_tag
|
44 |
-
: input_universal_iterator_tag
|
45 |
-
{
|
46 |
-
operator forward_host_iterator_tag () {return forward_host_iterator_tag();};
|
47 |
-
|
48 |
-
operator forward_device_iterator_tag () {return forward_device_iterator_tag();};
|
49 |
-
};
|
50 |
-
|
51 |
-
struct bidirectional_universal_iterator_tag
|
52 |
-
: forward_universal_iterator_tag
|
53 |
-
{
|
54 |
-
operator bidirectional_host_iterator_tag () {return bidirectional_host_iterator_tag();};
|
55 |
-
|
56 |
-
operator bidirectional_device_iterator_tag () {return bidirectional_device_iterator_tag();};
|
57 |
-
};
|
58 |
-
|
59 |
-
|
60 |
-
namespace detail
|
61 |
-
{
|
62 |
-
|
63 |
-
// create this struct to control conversion precedence in random_access_universal_iterator_tag
|
64 |
-
template<typename T>
|
65 |
-
struct one_degree_of_separation
|
66 |
-
: T
|
67 |
-
{
|
68 |
-
};
|
69 |
-
|
70 |
-
} // end detail
|
71 |
-
|
72 |
-
|
73 |
-
struct random_access_universal_iterator_tag
|
74 |
-
{
|
75 |
-
// these conversions are all P0
|
76 |
-
operator random_access_host_iterator_tag () {return random_access_host_iterator_tag();};
|
77 |
-
|
78 |
-
operator random_access_device_iterator_tag () {return random_access_device_iterator_tag();};
|
79 |
-
|
80 |
-
// bidirectional_universal_iterator_tag is P1
|
81 |
-
operator detail::one_degree_of_separation<bidirectional_universal_iterator_tag> () {return detail::one_degree_of_separation<bidirectional_universal_iterator_tag>();}
|
82 |
-
|
83 |
-
};
|
84 |
-
|
85 |
-
|
86 |
-
} // end thrust
|
87 |
-
|
|
|
|
|
|
|
|
|
|
|
|
|
|
|
|
|
|
|
|
|
|
|
|
|
|
|
|
|
|
|
|
|
|
|
|
|
|
|
|
|
|
|
|
|
|
|
|
|
|
|
|
|
|
|
|
|
|
|
|
|
|
|
|
|
|
|
|
|
|
|
|
|
|
|
|
|
|
|
|
|
|
|
|
|
|
|
|
|
|
|
|
|
|
|
|
|
|
|
|
|
|
|
|
|
|
|
|
|
|
|
|
|
|
|
|
|
|
|
|
|
|
|
|
|
|
|
|
|
|
|
|
|
|
|
|
|
|
|
|
|
|
|
|
|
|
|
|
|
|
|
|
|
|
|
|
|
|
|
|
|
|
|
|
|
|
|
|
|
|
|
|
|
|
|
|
spaces/CVPR/LIVE/thrust/thrust/system/cpp/detail/binary_search.h
DELETED
@@ -1,23 +0,0 @@
|
|
1 |
-
/*
|
2 |
-
* Copyright 2008-2013 NVIDIA Corporation
|
3 |
-
*
|
4 |
-
* Licensed under the Apache License, Version 2.0 (the "License");
|
5 |
-
* you may not use this file except in compliance with the License.
|
6 |
-
* You may obtain a copy of the License at
|
7 |
-
*
|
8 |
-
* http://www.apache.org/licenses/LICENSE-2.0
|
9 |
-
*
|
10 |
-
* Unless required by applicable law or agreed to in writing, software
|
11 |
-
* distributed under the License is distributed on an "AS IS" BASIS,
|
12 |
-
* WITHOUT WARRANTIES OR CONDITIONS OF ANY KIND, either express or implied.
|
13 |
-
* See the License for the specific language governing permissions and
|
14 |
-
* limitations under the License.
|
15 |
-
*/
|
16 |
-
|
17 |
-
#pragma once
|
18 |
-
|
19 |
-
#include <thrust/system/cpp/detail/execution_policy.h>
|
20 |
-
|
21 |
-
// this system inherits the binary search algorithms
|
22 |
-
#include <thrust/system/detail/sequential/binary_search.h>
|
23 |
-
|
|
|
|
|
|
|
|
|
|
|
|
|
|
|
|
|
|
|
|
|
|
|
|
|
|
|
|
|
|
|
|
|
|
|
|
|
|
|
|
|
|
|
|
|
|
|
|
spaces/CVPR/LIVE/thrust/thrust/system/detail/adl/assign_value.h
DELETED
@@ -1,44 +0,0 @@
|
|
1 |
-
/*
|
2 |
-
* Copyright 2008-2013 NVIDIA Corporation
|
3 |
-
*
|
4 |
-
* Licensed under the Apache License, Version 2.0 (the "License");
|
5 |
-
* you may not use this file except in compliance with the License.
|
6 |
-
* You may obtain a copy of the License at
|
7 |
-
*
|
8 |
-
* http://www.apache.org/licenses/LICENSE-2.0
|
9 |
-
*
|
10 |
-
* Unless required by applicable law or agreed to in writing, software
|
11 |
-
* distributed under the License is distributed on an "AS IS" BASIS,
|
12 |
-
* WITHOUT WARRANTIES OR CONDITIONS OF ANY KIND, either express or implied.
|
13 |
-
* See the License for the specific language governing permissions and
|
14 |
-
* limitations under the License.
|
15 |
-
*/
|
16 |
-
|
17 |
-
#pragma once
|
18 |
-
|
19 |
-
#include <thrust/detail/config.h>
|
20 |
-
|
21 |
-
// the purpose of this header is to #include the assign_value.h header
|
22 |
-
// of the sequential, host, and device systems. It should be #included in any
|
23 |
-
// code which uses adl to dispatch assign_value
|
24 |
-
|
25 |
-
#include <thrust/system/detail/sequential/assign_value.h>
|
26 |
-
|
27 |
-
// SCons can't see through the #defines below to figure out what this header
|
28 |
-
// includes, so we fake it out by specifying all possible files we might end up
|
29 |
-
// including inside an #if 0.
|
30 |
-
#if 0
|
31 |
-
#include <thrust/system/cpp/detail/assign_value.h>
|
32 |
-
#include <thrust/system/cuda/detail/assign_value.h>
|
33 |
-
#include <thrust/system/omp/detail/assign_value.h>
|
34 |
-
#include <thrust/system/tbb/detail/assign_value.h>
|
35 |
-
#endif
|
36 |
-
|
37 |
-
#define __THRUST_HOST_SYSTEM_ASSIGN_VALUE_HEADER <__THRUST_HOST_SYSTEM_ROOT/detail/assign_value.h>
|
38 |
-
#include __THRUST_HOST_SYSTEM_ASSIGN_VALUE_HEADER
|
39 |
-
#undef __THRUST_HOST_SYSTEM_ASSIGN_VALUE_HEADER
|
40 |
-
|
41 |
-
#define __THRUST_DEVICE_SYSTEM_ASSIGN_VALUE_HEADER <__THRUST_DEVICE_SYSTEM_ROOT/detail/assign_value.h>
|
42 |
-
#include __THRUST_DEVICE_SYSTEM_ASSIGN_VALUE_HEADER
|
43 |
-
#undef __THRUST_DEVICE_SYSTEM_ASSIGN_VALUE_HEADER
|
44 |
-
|
|
|
|
|
|
|
|
|
|
|
|
|
|
|
|
|
|
|
|
|
|
|
|
|
|
|
|
|
|
|
|
|
|
|
|
|
|
|
|
|
|
|
|
|
|
|
|
|
|
|
|
|
|
|
|
|
|
|
|
|
|
|
|
|
|
|
|
|
|
|
|
|
|
|
|
|
|
|
|
|
|
|
|
|
|
|
|
|
|
spaces/CVPR/WALT/mmdet/core/visualization/__init__.py
DELETED
@@ -1,4 +0,0 @@
|
|
1 |
-
from .image import (color_val_matplotlib, imshow_det_bboxes,
|
2 |
-
imshow_gt_det_bboxes)
|
3 |
-
|
4 |
-
__all__ = ['imshow_det_bboxes', 'imshow_gt_det_bboxes', 'color_val_matplotlib']
|
|
|
|
|
|
|
|
|
|
spaces/CVPR/drawings-to-human/static/_app/immutable/pages/__layout.svelte-d07d8fed.js
DELETED
@@ -1 +0,0 @@
|
|
1 |
-
import{S as i,i as n,s as p,F as l,G as w,H as c,I as d,q as h,o as g}from"../chunks/index-bcf2726a.js";function m(s){let r;const a=s[1].default,t=l(a,s,s[0],null);return{c(){t&&t.c()},l(e){t&&t.l(e)},m(e,o){t&&t.m(e,o),r=!0},p(e,[o]){t&&t.p&&(!r||o&1)&&w(t,a,e,e[0],r?d(a,e[0],o,null):c(e[0]),null)},i(e){r||(h(t,e),r=!0)},o(e){g(t,e),r=!1},d(e){t&&t.d(e)}}}function b(s,r,a){let{$$slots:t={},$$scope:e}=r;return s.$$set=o=>{"$$scope"in o&&a(0,e=o.$$scope)},[e,t]}class u extends i{constructor(r){super(),n(this,r,b,m,p,{})}}export{u as default};
|
|
|
|
spaces/CVPR/regionclip-demo/detectron2/layers/soft_nms.py
DELETED
@@ -1,261 +0,0 @@
|
|
1 |
-
import torch
|
2 |
-
|
3 |
-
from detectron2.structures import Boxes, RotatedBoxes, pairwise_iou, pairwise_iou_rotated
|
4 |
-
|
5 |
-
""" Soft-NMS Pull request from: https://github.com/facebookresearch/detectron2/pull/1183/files
|
6 |
-
"""
|
7 |
-
|
8 |
-
def soft_nms(boxes, scores, method, gaussian_sigma, linear_threshold, prune_threshold):
|
9 |
-
"""
|
10 |
-
Performs soft non-maximum suppression algorithm on axis aligned boxes
|
11 |
-
Args:
|
12 |
-
boxes (Tensor[N, 5]):
|
13 |
-
boxes where NMS will be performed. They
|
14 |
-
are expected to be in (x_ctr, y_ctr, width, height, angle_degrees) format
|
15 |
-
scores (Tensor[N]):
|
16 |
-
scores for each one of the boxes
|
17 |
-
method (str):
|
18 |
-
one of ['gaussian', 'linear', 'hard']
|
19 |
-
see paper for details. users encouraged not to use "hard", as this is the
|
20 |
-
same nms available elsewhere in detectron2
|
21 |
-
gaussian_sigma (float):
|
22 |
-
parameter for Gaussian penalty function
|
23 |
-
linear_threshold (float):
|
24 |
-
iou threshold for applying linear decay. Nt from the paper
|
25 |
-
re-used as threshold for standard "hard" nms
|
26 |
-
prune_threshold (float):
|
27 |
-
boxes with scores below this threshold are pruned at each iteration.
|
28 |
-
Dramatically reduces computation time. Authors use values in [10e-4, 10e-2]
|
29 |
-
Returns:
|
30 |
-
tuple(Tensor, Tensor):
|
31 |
-
[0]: int64 tensor with the indices of the elements that have been kept
|
32 |
-
by Soft NMS, sorted in decreasing order of scores
|
33 |
-
[1]: float tensor with the re-scored scores of the elements that were kept
|
34 |
-
"""
|
35 |
-
return _soft_nms(
|
36 |
-
Boxes,
|
37 |
-
pairwise_iou,
|
38 |
-
boxes,
|
39 |
-
scores,
|
40 |
-
method,
|
41 |
-
gaussian_sigma,
|
42 |
-
linear_threshold,
|
43 |
-
prune_threshold,
|
44 |
-
)
|
45 |
-
|
46 |
-
|
47 |
-
def soft_nms_rotated(boxes, scores, method, gaussian_sigma, linear_threshold, prune_threshold):
|
48 |
-
"""
|
49 |
-
Performs soft non-maximum suppression algorithm on rotated boxes
|
50 |
-
Args:
|
51 |
-
boxes (Tensor[N, 5]):
|
52 |
-
boxes where NMS will be performed. They
|
53 |
-
are expected to be in (x_ctr, y_ctr, width, height, angle_degrees) format
|
54 |
-
scores (Tensor[N]):
|
55 |
-
scores for each one of the boxes
|
56 |
-
method (str):
|
57 |
-
one of ['gaussian', 'linear', 'hard']
|
58 |
-
see paper for details. users encouraged not to use "hard", as this is the
|
59 |
-
same nms available elsewhere in detectron2
|
60 |
-
gaussian_sigma (float):
|
61 |
-
parameter for Gaussian penalty function
|
62 |
-
linear_threshold (float):
|
63 |
-
iou threshold for applying linear decay. Nt from the paper
|
64 |
-
re-used as threshold for standard "hard" nms
|
65 |
-
prune_threshold (float):
|
66 |
-
boxes with scores below this threshold are pruned at each iteration.
|
67 |
-
Dramatically reduces computation time. Authors use values in [10e-4, 10e-2]
|
68 |
-
Returns:
|
69 |
-
tuple(Tensor, Tensor):
|
70 |
-
[0]: int64 tensor with the indices of the elements that have been kept
|
71 |
-
by Soft NMS, sorted in decreasing order of scores
|
72 |
-
[1]: float tensor with the re-scored scores of the elements that were kept """
|
73 |
-
return _soft_nms(
|
74 |
-
RotatedBoxes,
|
75 |
-
pairwise_iou_rotated,
|
76 |
-
boxes,
|
77 |
-
scores,
|
78 |
-
method,
|
79 |
-
gaussian_sigma,
|
80 |
-
linear_threshold,
|
81 |
-
prune_threshold,
|
82 |
-
)
|
83 |
-
|
84 |
-
|
85 |
-
def batched_soft_nms(
|
86 |
-
boxes, scores, idxs, method, gaussian_sigma, linear_threshold, prune_threshold
|
87 |
-
):
|
88 |
-
"""
|
89 |
-
Performs soft non-maximum suppression in a batched fashion.
|
90 |
-
Each index value correspond to a category, and NMS
|
91 |
-
will not be applied between elements of different categories.
|
92 |
-
Args:
|
93 |
-
boxes (Tensor[N, 4]):
|
94 |
-
boxes where NMS will be performed. They
|
95 |
-
are expected to be in (x1, y1, x2, y2) format
|
96 |
-
scores (Tensor[N]):
|
97 |
-
scores for each one of the boxes
|
98 |
-
idxs (Tensor[N]):
|
99 |
-
indices of the categories for each one of the boxes.
|
100 |
-
method (str):
|
101 |
-
one of ['gaussian', 'linear', 'hard']
|
102 |
-
see paper for details. users encouraged not to use "hard", as this is the
|
103 |
-
same nms available elsewhere in detectron2
|
104 |
-
gaussian_sigma (float):
|
105 |
-
parameter for Gaussian penalty function
|
106 |
-
linear_threshold (float):
|
107 |
-
iou threshold for applying linear decay. Nt from the paper
|
108 |
-
re-used as threshold for standard "hard" nms
|
109 |
-
prune_threshold (float):
|
110 |
-
boxes with scores below this threshold are pruned at each iteration.
|
111 |
-
Dramatically reduces computation time. Authors use values in [10e-4, 10e-2]
|
112 |
-
Returns:
|
113 |
-
tuple(Tensor, Tensor):
|
114 |
-
[0]: int64 tensor with the indices of the elements that have been kept
|
115 |
-
by Soft NMS, sorted in decreasing order of scores
|
116 |
-
[1]: float tensor with the re-scored scores of the elements that were kept
|
117 |
-
"""
|
118 |
-
if boxes.numel() == 0:
|
119 |
-
return (
|
120 |
-
torch.empty((0,), dtype=torch.int64, device=boxes.device),
|
121 |
-
torch.empty((0,), dtype=torch.float32, device=scores.device),
|
122 |
-
)
|
123 |
-
# strategy: in order to perform NMS independently per class.
|
124 |
-
# we add an offset to all the boxes. The offset is dependent
|
125 |
-
# only on the class idx, and is large enough so that boxes
|
126 |
-
# from different classes do not overlap
|
127 |
-
max_coordinate = boxes.max()
|
128 |
-
offsets = idxs.to(boxes) * (max_coordinate + 1)
|
129 |
-
boxes_for_nms = boxes + offsets[:, None]
|
130 |
-
return soft_nms(
|
131 |
-
boxes_for_nms, scores, method, gaussian_sigma, linear_threshold, prune_threshold
|
132 |
-
)
|
133 |
-
|
134 |
-
|
135 |
-
def batched_soft_nms_rotated(
|
136 |
-
boxes, scores, idxs, method, gaussian_sigma, linear_threshold, prune_threshold
|
137 |
-
):
|
138 |
-
"""
|
139 |
-
Performs soft non-maximum suppression in a batched fashion on rotated bounding boxes.
|
140 |
-
Each index value correspond to a category, and NMS
|
141 |
-
will not be applied between elements of different categories.
|
142 |
-
Args:
|
143 |
-
boxes (Tensor[N, 5]):
|
144 |
-
boxes where NMS will be performed. They
|
145 |
-
are expected to be in (x_ctr, y_ctr, width, height, angle_degrees) format
|
146 |
-
scores (Tensor[N]):
|
147 |
-
scores for each one of the boxes
|
148 |
-
idxs (Tensor[N]):
|
149 |
-
indices of the categories for each one of the boxes.
|
150 |
-
method (str):
|
151 |
-
one of ['gaussian', 'linear', 'hard']
|
152 |
-
see paper for details. users encouraged not to use "hard", as this is the
|
153 |
-
same nms available elsewhere in detectron2
|
154 |
-
gaussian_sigma (float):
|
155 |
-
parameter for Gaussian penalty function
|
156 |
-
linear_threshold (float):
|
157 |
-
iou threshold for applying linear decay. Nt from the paper
|
158 |
-
re-used as threshold for standard "hard" nms
|
159 |
-
prune_threshold (float):
|
160 |
-
boxes with scores below this threshold are pruned at each iteration.
|
161 |
-
Dramatically reduces computation time. Authors use values in [10e-4, 10e-2]
|
162 |
-
Returns:
|
163 |
-
tuple(Tensor, Tensor):
|
164 |
-
[0]: int64 tensor with the indices of the elements that have been kept
|
165 |
-
by Soft NMS, sorted in decreasing order of scores
|
166 |
-
[1]: float tensor with the re-scored scores of the elements that were kept
|
167 |
-
"""
|
168 |
-
if boxes.numel() == 0:
|
169 |
-
return (
|
170 |
-
torch.empty((0,), dtype=torch.int64, device=boxes.device),
|
171 |
-
torch.empty((0,), dtype=torch.float32, device=scores.device),
|
172 |
-
)
|
173 |
-
# strategy: in order to perform NMS independently per class.
|
174 |
-
# we add an offset to all the boxes. The offset is dependent
|
175 |
-
# only on the class idx, and is large enough so that boxes
|
176 |
-
# from different classes do not overlap
|
177 |
-
max_coordinate = boxes[:, :2].max() + torch.norm(boxes[:, 2:4], 2, dim=1).max()
|
178 |
-
offsets = idxs.to(boxes) * (max_coordinate + 1)
|
179 |
-
boxes_for_nms = boxes.clone()
|
180 |
-
boxes_for_nms[:, :2] += offsets[:, None]
|
181 |
-
return soft_nms_rotated(
|
182 |
-
boxes_for_nms, scores, method, gaussian_sigma, linear_threshold, prune_threshold
|
183 |
-
)
|
184 |
-
|
185 |
-
|
186 |
-
def _soft_nms(
|
187 |
-
box_class,
|
188 |
-
pairwise_iou_func,
|
189 |
-
boxes,
|
190 |
-
scores,
|
191 |
-
method,
|
192 |
-
gaussian_sigma,
|
193 |
-
linear_threshold,
|
194 |
-
prune_threshold,
|
195 |
-
):
|
196 |
-
"""
|
197 |
-
Soft non-max suppression algorithm.
|
198 |
-
Implementation of [Soft-NMS -- Improving Object Detection With One Line of Codec]
|
199 |
-
(https://arxiv.org/abs/1704.04503)
|
200 |
-
Args:
|
201 |
-
box_class (cls): one of Box, RotatedBoxes
|
202 |
-
pairwise_iou_func (func): one of pairwise_iou, pairwise_iou_rotated
|
203 |
-
boxes (Tensor[N, ?]):
|
204 |
-
boxes where NMS will be performed
|
205 |
-
if Boxes, in (x1, y1, x2, y2) format
|
206 |
-
if RotatedBoxes, in (x_ctr, y_ctr, width, height, angle_degrees) format
|
207 |
-
scores (Tensor[N]):
|
208 |
-
scores for each one of the boxes
|
209 |
-
method (str):
|
210 |
-
one of ['gaussian', 'linear', 'hard']
|
211 |
-
see paper for details. users encouraged not to use "hard", as this is the
|
212 |
-
same nms available elsewhere in detectron2
|
213 |
-
gaussian_sigma (float):
|
214 |
-
parameter for Gaussian penalty function
|
215 |
-
linear_threshold (float):
|
216 |
-
iou threshold for applying linear decay. Nt from the paper
|
217 |
-
re-used as threshold for standard "hard" nms
|
218 |
-
prune_threshold (float):
|
219 |
-
boxes with scores below this threshold are pruned at each iteration.
|
220 |
-
Dramatically reduces computation time. Authors use values in [10e-4, 10e-2]
|
221 |
-
Returns:
|
222 |
-
tuple(Tensor, Tensor):
|
223 |
-
[0]: int64 tensor with the indices of the elements that have been kept
|
224 |
-
by Soft NMS, sorted in decreasing order of scores
|
225 |
-
[1]: float tensor with the re-scored scores of the elements that were kept
|
226 |
-
"""
|
227 |
-
boxes = boxes.clone()
|
228 |
-
scores = scores.clone()
|
229 |
-
idxs = torch.arange(scores.size()[0])
|
230 |
-
|
231 |
-
idxs_out = []
|
232 |
-
scores_out = []
|
233 |
-
|
234 |
-
while scores.numel() > 0:
|
235 |
-
top_idx = torch.argmax(scores)
|
236 |
-
idxs_out.append(idxs[top_idx].item())
|
237 |
-
scores_out.append(scores[top_idx].item())
|
238 |
-
|
239 |
-
top_box = boxes[top_idx]
|
240 |
-
ious = pairwise_iou_func(box_class(top_box.unsqueeze(0)), box_class(boxes))[0]
|
241 |
-
|
242 |
-
if method == "linear":
|
243 |
-
decay = torch.ones_like(ious)
|
244 |
-
decay_mask = ious > linear_threshold
|
245 |
-
decay[decay_mask] = 1 - ious[decay_mask]
|
246 |
-
elif method == "gaussian":
|
247 |
-
decay = torch.exp(-torch.pow(ious, 2) / gaussian_sigma)
|
248 |
-
elif method == "hard": # standard NMS
|
249 |
-
decay = (ious < linear_threshold).float()
|
250 |
-
else:
|
251 |
-
raise NotImplementedError("{} soft nms method not implemented.".format(method))
|
252 |
-
|
253 |
-
scores *= decay
|
254 |
-
keep = scores > prune_threshold
|
255 |
-
keep[top_idx] = False
|
256 |
-
|
257 |
-
boxes = boxes[keep]
|
258 |
-
scores = scores[keep]
|
259 |
-
idxs = idxs[keep]
|
260 |
-
|
261 |
-
return torch.tensor(idxs_out).to(boxes.device), torch.tensor(scores_out).to(scores.device)
|
|
|
|
|
|
|
|
|
|
|
|
|
|
|
|
|
|
|
|
|
|
|
|
|
|
|
|
|
|
|
|
|
|
|
|
|
|
|
|
|
|
|
|
|
|
|
|
|
|
|
|
|
|
|
|
|
|
|
|
|
|
|
|
|
|
|
|
|
|
|
|
|
|
|
|
|
|
|
|
|
|
|
|
|
|
|
|
|
|
|
|
|
|
|
|
|
|
|
|
|
|
|
|
|
|
|
|
|
|
|
|
|
|
|
|
|
|
|
|
|
|
|
|
|
|
|
|
|
|
|
|
|
|
|
|
|
|
|
|
|
|
|
|
|
|
|
|
|
|
|
|
|
|
|
|
|
|
|
|
|
|
|
|
|
|
|
|
|
|
|
|
|
|
|
|
|
|
|
|
|
|
|
|
|
|
|
|
|
|
|
|
|
|
|
|
|
|
|
|
|
|
|
|
|
|
|
|
|
|
|
|
|
|
|
|
|
|
|
|
|
|
|
|
|
|
|
|
|
|
|
|
|
|
|
|
|
|
|
|
|
|
|
|
|
|
|
|
|
|
|
|
|
|
|
|
|
|
|
|
|
|
|
|
|
|
|
|
|
|
|
|
|
|
|
|
|
|
|
|
|
|
|
|
|
|
|
|
|
|
|
|
|
|
|
|
|
|
|
|
|
|
|
|
|
|
|
|
|
|
|
|
|
|
|
|
|
|
|
|
|
|
|
|
|
|
|
|
|
|
|
|
|
|
|
|
|
|
|
|
|
|
|
|
|
|
|
|
|
|
|
|
|
|
|
|
|
|
|
|
|
|
|
|
|
|
|
|
|
|
|
|
|
|
|
|
|
|
|
|
|
|
|
|
|
|
|
|
|
|
|
|
|
|
|
|
|
|
|
|
|
|
|
|
|
|
|
|
|
|
|
|
|
|
|
|
|
|
|
|
|
|
|
|
|
|
|
|
|
|
|
|
|
|
|
|
|
|
|
|
|
|
|
|
|
|
|
|
|
|
|
|
|
|
|
|
|
|
|
|
|
|
|
|
|
|
|
|
|
|
|
|
|
|
|
|
|
|
|
|
|
|
|
|
|
|
|
|
|
|
|
|
|
|
|
|
|
|
|
|
|
|
|
|
|
|
|
|
|
|
|
|
|
|
|
|
|
|
|
|
|
|
|
|
spaces/CarlDennis/Lovelive-VITS-JPZH/app.py
DELETED
@@ -1,124 +0,0 @@
|
|
1 |
-
import re
|
2 |
-
import gradio as gr
|
3 |
-
import torch
|
4 |
-
import unicodedata
|
5 |
-
import commons
|
6 |
-
import utils
|
7 |
-
from models import SynthesizerTrn
|
8 |
-
from text import text_to_sequence
|
9 |
-
|
10 |
-
config_json = "muse_tricolor_b.json"
|
11 |
-
pth_path = "G=869.pth"
|
12 |
-
|
13 |
-
|
14 |
-
def get_text(text, hps, cleaned=False):
|
15 |
-
if cleaned:
|
16 |
-
text_norm = text_to_sequence(text, hps.symbols, [])
|
17 |
-
else:
|
18 |
-
text_norm = text_to_sequence(text, hps.symbols, hps.data.text_cleaners)
|
19 |
-
if hps.data.add_blank:
|
20 |
-
text_norm = commons.intersperse(text_norm, 0)
|
21 |
-
text_norm = torch.LongTensor(text_norm)
|
22 |
-
return text_norm
|
23 |
-
|
24 |
-
|
25 |
-
def get_label(text, label):
|
26 |
-
if f'[{label}]' in text:
|
27 |
-
return True, text.replace(f'[{label}]', '')
|
28 |
-
else:
|
29 |
-
return False, text
|
30 |
-
|
31 |
-
|
32 |
-
def clean_text(text):
|
33 |
-
print(text)
|
34 |
-
jap = re.compile(r'[\u3040-\u309F\u30A0-\u30FF]') # 匹配日文
|
35 |
-
text = unicodedata.normalize('NFKC', text)
|
36 |
-
text = f"[JA]{text}[JA]" if jap.search(text) else f"[ZH]{text}[ZH]"
|
37 |
-
return text
|
38 |
-
|
39 |
-
|
40 |
-
def load_model(config_json, pth_path):
|
41 |
-
dev = torch.device("cuda:0" if torch.cuda.is_available() else "cpu")
|
42 |
-
hps_ms = utils.get_hparams_from_file(f"{config_json}")
|
43 |
-
n_speakers = hps_ms.data.n_speakers if 'n_speakers' in hps_ms.data.keys() else 0
|
44 |
-
n_symbols = len(hps_ms.symbols) if 'symbols' in hps_ms.keys() else 0
|
45 |
-
net_g_ms = SynthesizerTrn(
|
46 |
-
n_symbols,
|
47 |
-
hps_ms.data.filter_length // 2 + 1,
|
48 |
-
hps_ms.train.segment_size // hps_ms.data.hop_length,
|
49 |
-
n_speakers=n_speakers,
|
50 |
-
**hps_ms.model).to(dev)
|
51 |
-
_ = net_g_ms.eval()
|
52 |
-
_ = utils.load_checkpoint(pth_path, net_g_ms)
|
53 |
-
return net_g_ms
|
54 |
-
|
55 |
-
net_g_ms = load_model(config_json, pth_path)
|
56 |
-
|
57 |
-
def selection(speaker):
|
58 |
-
if speaker == "南小鸟":
|
59 |
-
spk = 0
|
60 |
-
return spk
|
61 |
-
|
62 |
-
elif speaker == "园田海未":
|
63 |
-
spk = 1
|
64 |
-
return spk
|
65 |
-
|
66 |
-
elif speaker == "小泉花阳":
|
67 |
-
spk = 2
|
68 |
-
return spk
|
69 |
-
|
70 |
-
elif speaker == "星空凛":
|
71 |
-
spk = 3
|
72 |
-
return spk
|
73 |
-
|
74 |
-
elif speaker == "东条希":
|
75 |
-
spk = 4
|
76 |
-
return spk
|
77 |
-
|
78 |
-
elif speaker == "矢泽妮可":
|
79 |
-
spk = 5
|
80 |
-
return spk
|
81 |
-
|
82 |
-
elif speaker == "绚濑绘里":
|
83 |
-
spk = 6
|
84 |
-
return spk
|
85 |
-
|
86 |
-
elif speaker == "西木野真姬":
|
87 |
-
spk = 7
|
88 |
-
return spk
|
89 |
-
|
90 |
-
elif speaker == "高坂穗乃果":
|
91 |
-
spk = 8
|
92 |
-
return spk
|
93 |
-
|
94 |
-
def infer(text,speaker_id, n_scale= 0.667,n_scale_w = 0.8, l_scale = 1 ):
|
95 |
-
text = clean_text(text)
|
96 |
-
speaker_id = int(selection(speaker_id))
|
97 |
-
dev = torch.device("cuda:0" if torch.cuda.is_available() else "cpu")
|
98 |
-
hps_ms = utils.get_hparams_from_file(f"{config_json}")
|
99 |
-
with torch.no_grad():
|
100 |
-
stn_tst = get_text(text, hps_ms, cleaned=False)
|
101 |
-
x_tst = stn_tst.unsqueeze(0).to(dev)
|
102 |
-
x_tst_lengths = torch.LongTensor([stn_tst.size(0)]).to(dev)
|
103 |
-
sid = torch.LongTensor([speaker_id]).to(dev)
|
104 |
-
audio = net_g_ms.infer(x_tst, x_tst_lengths, sid=sid, noise_scale=n_scale, noise_scale_w=n_scale_w, length_scale=l_scale)[0][
|
105 |
-
0, 0].data.cpu().float().numpy()
|
106 |
-
return (hps_ms.data.sampling_rate, audio)
|
107 |
-
|
108 |
-
idols = ["南小鸟","园田海未","小泉花阳","星空凛","东条希","矢泽妮可","绚濑绘里","西木野真姬","高坂穗乃果"]
|
109 |
-
app = gr.Blocks()
|
110 |
-
with app:
|
111 |
-
with gr.Tabs():
|
112 |
-
|
113 |
-
with gr.TabItem("Basic"):
|
114 |
-
|
115 |
-
tts_input1 = gr.TextArea(label="请输入纯中文或纯日文", value="大家好")
|
116 |
-
para_input1 = gr.Slider(minimum= 0.01,maximum=1.0,label="更改噪声比例", value=0.667)
|
117 |
-
para_input2 = gr.Slider(minimum= 0.01,maximum=1.0,label="更改噪声偏差", value=0.8)
|
118 |
-
para_input3 = gr.Slider(minimum= 0.1,maximum=10,label="更改时间比例", value=1)
|
119 |
-
tts_submit = gr.Button("Generate", variant="primary")
|
120 |
-
speaker1 = gr.Dropdown(label="选择说话人",choices=idols, value="高坂穗乃果", interactive=True)
|
121 |
-
tts_output2 = gr.Audio(label="Output")
|
122 |
-
|
123 |
-
tts_submit.click(infer, [tts_input1,speaker1,para_input1,para_input2,para_input3], [tts_output2])
|
124 |
-
app.launch()
|
|
|
|
|
|
|
|
|
|
|
|
|
|
|
|
|
|
|
|
|
|
|
|
|
|
|
|
|
|
|
|
|
|
|
|
|
|
|
|
|
|
|
|
|
|
|
|
|
|
|
|
|
|
|
|
|
|
|
|
|
|
|
|
|
|
|
|
|
|
|
|
|
|
|
|
|
|
|
|
|
|
|
|
|
|
|
|
|
|
|
|
|
|
|
|
|
|
|
|
|
|
|
|
|
|
|
|
|
|
|
|
|
|
|
|
|
|
|
|
|
|
|
|
|
|
|
|
|
|
|
|
|
|
|
|
|
|
|
|
|
|
|
|
|
|
|
|
|
|
|
|
|
|
|
|
|
|
|
|
|
|
|
|
|
|
|
|
|
|
|
|
|
|
|
|
|
|
|
|
|
|
|
|
|
|
|
|
|
|
|
|
|
|
|
|
|
|
|
|
|
|
|
|
|
|
|
|
|
|
|
|
|
|
|
|
|
|
|
|
|
|
|
|
|
|
|
|
|
|
|
|
|
|
|
|
|
|
|
|
|
|
|
|
|
|
|
|
|
|
spaces/CikeyQI/meme-api/meme_generator/memes/chase_train/__init__.py
DELETED
@@ -1,60 +0,0 @@
|
|
1 |
-
from pathlib import Path
|
2 |
-
from typing import List
|
3 |
-
|
4 |
-
from PIL.Image import Image as IMG
|
5 |
-
from pil_utils import BuildImage
|
6 |
-
|
7 |
-
from meme_generator import add_meme
|
8 |
-
from meme_generator.utils import save_gif
|
9 |
-
|
10 |
-
img_dir = Path(__file__).parent / "images"
|
11 |
-
|
12 |
-
|
13 |
-
def chase_train(images: List[BuildImage], texts, args):
|
14 |
-
img = images[0].convert("RGBA").square().resize((42, 42))
|
15 |
-
frames: List[IMG] = []
|
16 |
-
# fmt: off
|
17 |
-
locs = [
|
18 |
-
(35, 34, 128, 44), (35, 33, 132, 40), (33, 34, 133, 36), (33, 38, 135, 41),
|
19 |
-
(34, 34, 136, 38), (35, 35, 136, 33), (33, 34, 138, 38), (36, 35, 138, 34),
|
20 |
-
(38, 34, 139, 32), (40, 35, 139, 37), (36, 35, 139, 33), (39, 36, 138, 28),
|
21 |
-
(40, 35, 138, 33), (37, 34, 138, 31), (43, 36, 135, 27), (36, 37, 136, 32),
|
22 |
-
(38, 40, 135, 26), (37, 35, 133, 26), (33, 36, 132, 30), (33, 39, 132, 25),
|
23 |
-
(32, 36, 131, 23), (33, 36, 130, 31), (35, 39, 128, 25), (33, 35, 127, 23),
|
24 |
-
(34, 36, 126, 29), (34, 40, 124, 25), (39, 36, 119, 23), (35, 36, 119, 32),
|
25 |
-
(35, 37, 116, 27), (36, 38, 113, 23), (34, 35, 113, 32), (39, 36, 113, 23),
|
26 |
-
(36, 35, 114, 17), (36, 38, 111, 13), (34, 37, 114, 15), (34, 39, 111, 10),
|
27 |
-
(33, 39, 109, 11), (36, 35, 104, 17), (34, 36, 102, 14), (34, 35, 99, 14),
|
28 |
-
(35, 38, 96, 16), (35, 35, 93, 14), (36, 35, 89, 15), (36, 36, 86, 18),
|
29 |
-
(36, 39, 83, 14), (34, 36, 81, 16), (40, 41, 74, 17), (38, 36, 74, 15),
|
30 |
-
(39, 35, 70, 16), (33, 35, 69, 20), (36, 35, 66, 17), (36, 35, 62, 17),
|
31 |
-
(37, 36, 57, 21), (35, 39, 57, 15), (35, 36, 53, 17), (35, 38, 51, 20),
|
32 |
-
(37, 36, 47, 19), (37, 35, 47, 18), (40, 36, 43, 19), (38, 35, 42, 22),
|
33 |
-
(40, 34, 38, 20), (38, 34, 37, 21), (39, 32, 35, 24), (39, 33, 33, 22),
|
34 |
-
(39, 36, 32, 22), (38, 35, 32, 25), (35, 37, 31, 22), (37, 37, 31, 23),
|
35 |
-
(36, 31, 31, 28), (37, 34, 32, 25), (36, 37, 32, 23), (36, 33, 33, 30),
|
36 |
-
(35, 34, 33, 27), (38, 33, 33, 28), (37, 34, 33, 29), (36, 35, 35, 28),
|
37 |
-
(36, 37, 36, 27), (43, 39, 33, 30), (35, 34, 38, 31), (37, 34, 39, 30),
|
38 |
-
(36, 34, 40, 30), (39, 35, 41, 30), (41, 36, 41, 29), (40, 37, 44, 32),
|
39 |
-
(40, 37, 45, 29), (39, 38, 48, 28), (38, 33, 50, 33), (35, 38, 53, 28),
|
40 |
-
(37, 34, 54, 31), (38, 34, 57, 32), (41, 35, 57, 29), (35, 34, 63, 29),
|
41 |
-
(41, 35, 62, 29), (38, 35, 66, 28), (35, 33, 70, 29), (40, 39, 70, 28),
|
42 |
-
(36, 36, 74, 28), (37, 35, 77, 26), (37, 35, 79, 28), (38, 35, 81, 27),
|
43 |
-
(36, 35, 85, 27), (37, 36, 88, 29), (36, 34, 91, 27), (38, 39, 94, 24),
|
44 |
-
(39, 34, 95, 27), (37, 34, 98, 26), (36, 35, 103, 24), (37, 36, 99, 28),
|
45 |
-
(34, 36, 97, 34), (34, 38, 102, 38), (37, 37, 99, 40), (39, 36, 101, 47),
|
46 |
-
(36, 36, 106, 43), (35, 35, 109, 40), (35, 39, 112, 43), (33, 36, 116, 41),
|
47 |
-
(36, 36, 116, 39), (34, 37, 121, 45), (35, 41, 123, 38), (34, 37, 126, 35),
|
48 |
-
]
|
49 |
-
# fmt: on
|
50 |
-
for i in range(120):
|
51 |
-
frame = BuildImage.open(img_dir / f"{i}.png")
|
52 |
-
w, h, x, y = locs[i]
|
53 |
-
frame.paste(img.resize((w, h)), (x, y), below=True)
|
54 |
-
frames.append(frame.image)
|
55 |
-
return save_gif(frames, 0.05)
|
56 |
-
|
57 |
-
|
58 |
-
add_meme(
|
59 |
-
"chase_train", chase_train, min_images=1, max_images=1, keywords=["追列车", "追火车"]
|
60 |
-
)
|
|
|
|
|
|
|
|
|
|
|
|
|
|
|
|
|
|
|
|
|
|
|
|
|
|
|
|
|
|
|
|
|
|
|
|
|
|
|
|
|
|
|
|
|
|
|
|
|
|
|
|
|
|
|
|
|
|
|
|
|
|
|
|
|
|
|
|
|
|
|
|
|
|
|
|
|
|
|
|
|
|
|
|
|
|
|
|
|
|
|
|
|
|
|
|
|
|
|
|
|
|
|
|
|
|
|
|
|
|
|
|
|
|
|
|
|
|
|
|
|
|
spaces/Curranj/FlowerDiffusion/README.md
DELETED
@@ -1,12 +0,0 @@
|
|
1 |
-
---
|
2 |
-
title: FlowerDiffusion
|
3 |
-
emoji: 🏢
|
4 |
-
colorFrom: blue
|
5 |
-
colorTo: yellow
|
6 |
-
sdk: gradio
|
7 |
-
sdk_version: 3.1.7
|
8 |
-
app_file: app.py
|
9 |
-
pinned: false
|
10 |
-
---
|
11 |
-
|
12 |
-
Check out the configuration reference at https://huggingface.co/docs/hub/spaces-config-reference
|
|
|
|
|
|
|
|
|
|
|
|
|
|
|
|
|
|
|
|
|
|
|
|
|
|
spaces/DESUCLUB/BLLAMA/export_state_dict_checkpoint.py
DELETED
@@ -1,119 +0,0 @@
|
|
1 |
-
import os
|
2 |
-
import json
|
3 |
-
|
4 |
-
import torch
|
5 |
-
from peft import PeftModel, LoraConfig
|
6 |
-
|
7 |
-
import transformers
|
8 |
-
|
9 |
-
assert (
|
10 |
-
"LlamaTokenizer" in transformers._import_structure["models.llama"]
|
11 |
-
), "LLaMA is now in HuggingFace's main branch.\nPlease reinstall it: pip uninstall transformers && pip install git+https://github.com/huggingface/transformers.git"
|
12 |
-
from transformers import LlamaTokenizer, LlamaForCausalLM
|
13 |
-
|
14 |
-
tokenizer = LlamaTokenizer.from_pretrained("decapoda-research/llama-7b-hf")
|
15 |
-
|
16 |
-
base_model = LlamaForCausalLM.from_pretrained(
|
17 |
-
"decapoda-research/llama-7b-hf",
|
18 |
-
load_in_8bit=False,
|
19 |
-
torch_dtype=torch.float16,
|
20 |
-
device_map={"": "cpu"},
|
21 |
-
)
|
22 |
-
|
23 |
-
lora_model = PeftModel.from_pretrained(
|
24 |
-
base_model,
|
25 |
-
"tloen/alpaca-lora-7b",
|
26 |
-
device_map={"": "cpu"},
|
27 |
-
torch_dtype=torch.float16,
|
28 |
-
)
|
29 |
-
|
30 |
-
# merge weights
|
31 |
-
for layer in lora_model.base_model.model.model.layers:
|
32 |
-
layer.self_attn.q_proj.merge_weights = True
|
33 |
-
layer.self_attn.v_proj.merge_weights = True
|
34 |
-
|
35 |
-
lora_model.train(False)
|
36 |
-
|
37 |
-
lora_model_sd = lora_model.state_dict()
|
38 |
-
|
39 |
-
params = {
|
40 |
-
"dim": 4096,
|
41 |
-
"multiple_of": 256,
|
42 |
-
"n_heads": 32,
|
43 |
-
"n_layers": 32,
|
44 |
-
"norm_eps": 1e-06,
|
45 |
-
"vocab_size": -1,
|
46 |
-
}
|
47 |
-
n_layers = params["n_layers"]
|
48 |
-
n_heads = params["n_heads"]
|
49 |
-
dim = params["dim"]
|
50 |
-
dims_per_head = dim // n_heads
|
51 |
-
base = 10000.0
|
52 |
-
inv_freq = 1.0 / (base ** (torch.arange(0, dims_per_head, 2).float() / dims_per_head))
|
53 |
-
|
54 |
-
|
55 |
-
def permute(w):
|
56 |
-
return (
|
57 |
-
w.view(n_heads, dim // n_heads // 2, 2, dim).transpose(1, 2).reshape(dim, dim)
|
58 |
-
)
|
59 |
-
|
60 |
-
|
61 |
-
def unpermute(w):
|
62 |
-
return (
|
63 |
-
w.view(n_heads, 2, dim // n_heads // 2, dim).transpose(1, 2).reshape(dim, dim)
|
64 |
-
)
|
65 |
-
|
66 |
-
|
67 |
-
def translate_state_dict_key(k):
|
68 |
-
k = k.replace("base_model.model.", "")
|
69 |
-
if k == "model.embed_tokens.weight":
|
70 |
-
return "tok_embeddings.weight"
|
71 |
-
elif k == "model.norm.weight":
|
72 |
-
return "norm.weight"
|
73 |
-
elif k == "lm_head.weight":
|
74 |
-
return "output.weight"
|
75 |
-
elif k.startswith("model.layers."):
|
76 |
-
layer = k.split(".")[2]
|
77 |
-
if k.endswith(".self_attn.q_proj.weight"):
|
78 |
-
return f"layers.{layer}.attention.wq.weight"
|
79 |
-
elif k.endswith(".self_attn.k_proj.weight"):
|
80 |
-
return f"layers.{layer}.attention.wk.weight"
|
81 |
-
elif k.endswith(".self_attn.v_proj.weight"):
|
82 |
-
return f"layers.{layer}.attention.wv.weight"
|
83 |
-
elif k.endswith(".self_attn.o_proj.weight"):
|
84 |
-
return f"layers.{layer}.attention.wo.weight"
|
85 |
-
elif k.endswith(".mlp.gate_proj.weight"):
|
86 |
-
return f"layers.{layer}.feed_forward.w1.weight"
|
87 |
-
elif k.endswith(".mlp.down_proj.weight"):
|
88 |
-
return f"layers.{layer}.feed_forward.w2.weight"
|
89 |
-
elif k.endswith(".mlp.up_proj.weight"):
|
90 |
-
return f"layers.{layer}.feed_forward.w3.weight"
|
91 |
-
elif k.endswith(".input_layernorm.weight"):
|
92 |
-
return f"layers.{layer}.attention_norm.weight"
|
93 |
-
elif k.endswith(".post_attention_layernorm.weight"):
|
94 |
-
return f"layers.{layer}.ffn_norm.weight"
|
95 |
-
elif k.endswith("rotary_emb.inv_freq") or "lora" in k:
|
96 |
-
return None
|
97 |
-
else:
|
98 |
-
print(layer, k)
|
99 |
-
raise NotImplementedError
|
100 |
-
else:
|
101 |
-
print(k)
|
102 |
-
raise NotImplementedError
|
103 |
-
|
104 |
-
|
105 |
-
new_state_dict = {}
|
106 |
-
for k, v in lora_model_sd.items():
|
107 |
-
new_k = translate_state_dict_key(k)
|
108 |
-
if new_k is not None:
|
109 |
-
if "wq" in new_k or "wk" in new_k:
|
110 |
-
new_state_dict[new_k] = unpermute(v)
|
111 |
-
else:
|
112 |
-
new_state_dict[new_k] = v
|
113 |
-
|
114 |
-
os.makedirs("./ckpt", exist_ok=True)
|
115 |
-
|
116 |
-
torch.save(new_state_dict, "./ckpt/consolidated.00.pth")
|
117 |
-
|
118 |
-
with open("./ckpt/params.json", "w") as f:
|
119 |
-
json.dump(params, f)
|
|
|
|
|
|
|
|
|
|
|
|
|
|
|
|
|
|
|
|
|
|
|
|
|
|
|
|
|
|
|
|
|
|
|
|
|
|
|
|
|
|
|
|
|
|
|
|
|
|
|
|
|
|
|
|
|
|
|
|
|
|
|
|
|
|
|
|
|
|
|
|
|
|
|
|
|
|
|
|
|
|
|
|
|
|
|
|
|
|
|
|
|
|
|
|
|
|
|
|
|
|
|
|
|
|
|
|
|
|
|
|
|
|
|
|
|
|
|
|
|
|
|
|
|
|
|
|
|
|
|
|
|
|
|
|
|
|
|
|
|
|
|
|
|
|
|
|
|
|
|
|
|
|
|
|
|
|
|
|
|
|
|
|
|
|
|
|
|
|
|
|
|
|
|
|
|
|
|
|
|
|
|
|
|
|
|
|
|
|
|
|
|
|
|
|
|
|
|
|
|
|
|
|
|
|
|
|
|
|
|
|
|
|
|
|
|
|
|
|
|
|
|
|
|
|
|
|
|
|
|
|
|
|
|
|
|
|
|
|
spaces/DJQmUKV/rvc-inference/util.py
DELETED
@@ -1,81 +0,0 @@
|
|
1 |
-
import sys
|
2 |
-
import asyncio
|
3 |
-
from io import BytesIO
|
4 |
-
|
5 |
-
from fairseq import checkpoint_utils
|
6 |
-
|
7 |
-
import torch
|
8 |
-
|
9 |
-
import edge_tts
|
10 |
-
import librosa
|
11 |
-
|
12 |
-
|
13 |
-
# https://github.com/fumiama/Retrieval-based-Voice-Conversion-WebUI/blob/main/config.py#L43-L55 # noqa
|
14 |
-
def has_mps() -> bool:
|
15 |
-
if sys.platform != "darwin":
|
16 |
-
return False
|
17 |
-
else:
|
18 |
-
if not getattr(torch, 'has_mps', False):
|
19 |
-
return False
|
20 |
-
|
21 |
-
try:
|
22 |
-
torch.zeros(1).to(torch.device("mps"))
|
23 |
-
return True
|
24 |
-
except Exception:
|
25 |
-
return False
|
26 |
-
|
27 |
-
|
28 |
-
def is_half(device: str) -> bool:
|
29 |
-
if not device.startswith('cuda'):
|
30 |
-
return False
|
31 |
-
else:
|
32 |
-
gpu_name = torch.cuda.get_device_name(
|
33 |
-
int(device.split(':')[-1])
|
34 |
-
).upper()
|
35 |
-
|
36 |
-
# ...regex?
|
37 |
-
if (
|
38 |
-
('16' in gpu_name and 'V100' not in gpu_name)
|
39 |
-
or 'P40' in gpu_name
|
40 |
-
or '1060' in gpu_name
|
41 |
-
or '1070' in gpu_name
|
42 |
-
or '1080' in gpu_name
|
43 |
-
):
|
44 |
-
return False
|
45 |
-
|
46 |
-
return True
|
47 |
-
|
48 |
-
|
49 |
-
def load_hubert_model(device: str, model_path: str = 'hubert_base.pt'):
|
50 |
-
model = checkpoint_utils.load_model_ensemble_and_task(
|
51 |
-
[model_path]
|
52 |
-
)[0][0].to(device)
|
53 |
-
|
54 |
-
if is_half(device):
|
55 |
-
return model.half()
|
56 |
-
else:
|
57 |
-
return model.float()
|
58 |
-
|
59 |
-
|
60 |
-
async def call_edge_tts(speaker_name: str, text: str):
|
61 |
-
tts_com = edge_tts.Communicate(text, speaker_name)
|
62 |
-
tts_raw = b''
|
63 |
-
|
64 |
-
# Stream TTS audio to bytes
|
65 |
-
async for chunk in tts_com.stream():
|
66 |
-
if chunk['type'] == 'audio':
|
67 |
-
tts_raw += chunk['data']
|
68 |
-
|
69 |
-
# Convert mp3 stream to wav
|
70 |
-
ffmpeg_proc = await asyncio.create_subprocess_exec(
|
71 |
-
'ffmpeg',
|
72 |
-
'-f', 'mp3',
|
73 |
-
'-i', '-',
|
74 |
-
'-f', 'wav',
|
75 |
-
'-',
|
76 |
-
stdin=asyncio.subprocess.PIPE,
|
77 |
-
stdout=asyncio.subprocess.PIPE
|
78 |
-
)
|
79 |
-
(tts_wav, _) = await ffmpeg_proc.communicate(tts_raw)
|
80 |
-
|
81 |
-
return librosa.load(BytesIO(tts_wav))
|
|
|
|
|
|
|
|
|
|
|
|
|
|
|
|
|
|
|
|
|
|
|
|
|
|
|
|
|
|
|
|
|
|
|
|
|
|
|
|
|
|
|
|
|
|
|
|
|
|
|
|
|
|
|
|
|
|
|
|
|
|
|
|
|
|
|
|
|
|
|
|
|
|
|
|
|
|
|
|
|
|
|
|
|
|
|
|
|
|
|
|
|
|
|
|
|
|
|
|
|
|
|
|
|
|
|
|
|
|
|
|
|
|
|
|
|
|
|
|
|
|
|
|
|
|
|
|
|
|
|
|
|
|
|
|
|
|
|
|
|
|
|
|
|
|
|
|
|
|
|
|
|
|
|
|
|
|
|
|
|
|
|
|
spaces/DQChoi/gpt-demo/venv/lib/python3.11/site-packages/altair/_magics.py
DELETED
@@ -1,109 +0,0 @@
|
|
1 |
-
"""
|
2 |
-
Magic functions for rendering vega-lite specifications
|
3 |
-
"""
|
4 |
-
__all__ = ["vegalite"]
|
5 |
-
|
6 |
-
import json
|
7 |
-
import warnings
|
8 |
-
|
9 |
-
import IPython
|
10 |
-
from IPython.core import magic_arguments
|
11 |
-
import pandas as pd
|
12 |
-
from toolz import curried
|
13 |
-
|
14 |
-
from altair.vegalite import v5 as vegalite_v5
|
15 |
-
|
16 |
-
try:
|
17 |
-
import yaml
|
18 |
-
|
19 |
-
YAML_AVAILABLE = True
|
20 |
-
except ImportError:
|
21 |
-
YAML_AVAILABLE = False
|
22 |
-
|
23 |
-
|
24 |
-
RENDERERS = {
|
25 |
-
"vega-lite": {
|
26 |
-
"5": vegalite_v5.VegaLite,
|
27 |
-
},
|
28 |
-
}
|
29 |
-
|
30 |
-
|
31 |
-
TRANSFORMERS = {
|
32 |
-
"vega-lite": {
|
33 |
-
"5": vegalite_v5.data_transformers,
|
34 |
-
},
|
35 |
-
}
|
36 |
-
|
37 |
-
|
38 |
-
def _prepare_data(data, data_transformers):
|
39 |
-
"""Convert input data to data for use within schema"""
|
40 |
-
if data is None or isinstance(data, dict):
|
41 |
-
return data
|
42 |
-
elif isinstance(data, pd.DataFrame):
|
43 |
-
return curried.pipe(data, data_transformers.get())
|
44 |
-
elif isinstance(data, str):
|
45 |
-
return {"url": data}
|
46 |
-
else:
|
47 |
-
warnings.warn("data of type {} not recognized".format(type(data)), stacklevel=1)
|
48 |
-
return data
|
49 |
-
|
50 |
-
|
51 |
-
def _get_variable(name):
|
52 |
-
"""Get a variable from the notebook namespace."""
|
53 |
-
ip = IPython.get_ipython()
|
54 |
-
if ip is None:
|
55 |
-
raise ValueError(
|
56 |
-
"Magic command must be run within an IPython "
|
57 |
-
"environemnt, in which get_ipython() is defined."
|
58 |
-
)
|
59 |
-
if name not in ip.user_ns:
|
60 |
-
raise NameError(
|
61 |
-
"argument '{}' does not match the "
|
62 |
-
"name of any defined variable".format(name)
|
63 |
-
)
|
64 |
-
return ip.user_ns[name]
|
65 |
-
|
66 |
-
|
67 |
-
@magic_arguments.magic_arguments()
|
68 |
-
@magic_arguments.argument(
|
69 |
-
"data",
|
70 |
-
nargs="?",
|
71 |
-
help="local variablename of a pandas DataFrame to be used as the dataset",
|
72 |
-
)
|
73 |
-
@magic_arguments.argument("-v", "--version", dest="version", default="v5")
|
74 |
-
@magic_arguments.argument("-j", "--json", dest="json", action="store_true")
|
75 |
-
def vegalite(line, cell):
|
76 |
-
"""Cell magic for displaying vega-lite visualizations in CoLab.
|
77 |
-
|
78 |
-
%%vegalite [dataframe] [--json] [--version='v5']
|
79 |
-
|
80 |
-
Visualize the contents of the cell using Vega-Lite, optionally
|
81 |
-
specifying a pandas DataFrame object to be used as the dataset.
|
82 |
-
|
83 |
-
if --json is passed, then input is parsed as json rather than yaml.
|
84 |
-
"""
|
85 |
-
args = magic_arguments.parse_argstring(vegalite, line)
|
86 |
-
existing_versions = {"v5": "5"}
|
87 |
-
version = existing_versions[args.version]
|
88 |
-
assert version in RENDERERS["vega-lite"]
|
89 |
-
VegaLite = RENDERERS["vega-lite"][version]
|
90 |
-
data_transformers = TRANSFORMERS["vega-lite"][version]
|
91 |
-
|
92 |
-
if args.json:
|
93 |
-
spec = json.loads(cell)
|
94 |
-
elif not YAML_AVAILABLE:
|
95 |
-
try:
|
96 |
-
spec = json.loads(cell)
|
97 |
-
except json.JSONDecodeError as err:
|
98 |
-
raise ValueError(
|
99 |
-
"%%vegalite: spec is not valid JSON. "
|
100 |
-
"Install pyyaml to parse spec as yaml"
|
101 |
-
) from err
|
102 |
-
else:
|
103 |
-
spec = yaml.load(cell, Loader=yaml.SafeLoader)
|
104 |
-
|
105 |
-
if args.data is not None:
|
106 |
-
data = _get_variable(args.data)
|
107 |
-
spec["data"] = _prepare_data(data, data_transformers)
|
108 |
-
|
109 |
-
return VegaLite(spec)
|
|
|
|
|
|
|
|
|
|
|
|
|
|
|
|
|
|
|
|
|
|
|
|
|
|
|
|
|
|
|
|
|
|
|
|
|
|
|
|
|
|
|
|
|
|
|
|
|
|
|
|
|
|
|
|
|
|
|
|
|
|
|
|
|
|
|
|
|
|
|
|
|
|
|
|
|
|
|
|
|
|
|
|
|
|
|
|
|
|
|
|
|
|
|
|
|
|
|
|
|
|
|
|
|
|
|
|
|
|
|
|
|
|
|
|
|
|
|
|
|
|
|
|
|
|
|
|
|
|
|
|
|
|
|
|
|
|
|
|
|
|
|
|
|
|
|
|
|
|
|
|
|
|
|
|
|
|
|
|
|
|
|
|
|
|
|
|
|
|
|
|
|
|
|
|
|
|
|
|
|
|
|
|
|
|
|
|
|
|
|
|
|
|
|
|
|
|
|
|
|
|
|
|
|
|
|
|
|
|
|
|
|
|
|
|
|
|
|
|
spaces/DQChoi/gpt-demo/venv/lib/python3.11/site-packages/click/shell_completion.py
DELETED
@@ -1,593 +0,0 @@
|
|
1 |
-
import os
|
2 |
-
import re
|
3 |
-
import typing as t
|
4 |
-
from gettext import gettext as _
|
5 |
-
|
6 |
-
from .core import Argument
|
7 |
-
from .core import BaseCommand
|
8 |
-
from .core import Context
|
9 |
-
from .core import MultiCommand
|
10 |
-
from .core import Option
|
11 |
-
from .core import Parameter
|
12 |
-
from .core import ParameterSource
|
13 |
-
from .parser import split_arg_string
|
14 |
-
from .utils import echo
|
15 |
-
|
16 |
-
|
17 |
-
def shell_complete(
|
18 |
-
cli: BaseCommand,
|
19 |
-
ctx_args: t.MutableMapping[str, t.Any],
|
20 |
-
prog_name: str,
|
21 |
-
complete_var: str,
|
22 |
-
instruction: str,
|
23 |
-
) -> int:
|
24 |
-
"""Perform shell completion for the given CLI program.
|
25 |
-
|
26 |
-
:param cli: Command being called.
|
27 |
-
:param ctx_args: Extra arguments to pass to
|
28 |
-
``cli.make_context``.
|
29 |
-
:param prog_name: Name of the executable in the shell.
|
30 |
-
:param complete_var: Name of the environment variable that holds
|
31 |
-
the completion instruction.
|
32 |
-
:param instruction: Value of ``complete_var`` with the completion
|
33 |
-
instruction and shell, in the form ``instruction_shell``.
|
34 |
-
:return: Status code to exit with.
|
35 |
-
"""
|
36 |
-
shell, _, instruction = instruction.partition("_")
|
37 |
-
comp_cls = get_completion_class(shell)
|
38 |
-
|
39 |
-
if comp_cls is None:
|
40 |
-
return 1
|
41 |
-
|
42 |
-
comp = comp_cls(cli, ctx_args, prog_name, complete_var)
|
43 |
-
|
44 |
-
if instruction == "source":
|
45 |
-
echo(comp.source())
|
46 |
-
return 0
|
47 |
-
|
48 |
-
if instruction == "complete":
|
49 |
-
echo(comp.complete())
|
50 |
-
return 0
|
51 |
-
|
52 |
-
return 1
|
53 |
-
|
54 |
-
|
55 |
-
class CompletionItem:
|
56 |
-
"""Represents a completion value and metadata about the value. The
|
57 |
-
default metadata is ``type`` to indicate special shell handling,
|
58 |
-
and ``help`` if a shell supports showing a help string next to the
|
59 |
-
value.
|
60 |
-
|
61 |
-
Arbitrary parameters can be passed when creating the object, and
|
62 |
-
accessed using ``item.attr``. If an attribute wasn't passed,
|
63 |
-
accessing it returns ``None``.
|
64 |
-
|
65 |
-
:param value: The completion suggestion.
|
66 |
-
:param type: Tells the shell script to provide special completion
|
67 |
-
support for the type. Click uses ``"dir"`` and ``"file"``.
|
68 |
-
:param help: String shown next to the value if supported.
|
69 |
-
:param kwargs: Arbitrary metadata. The built-in implementations
|
70 |
-
don't use this, but custom type completions paired with custom
|
71 |
-
shell support could use it.
|
72 |
-
"""
|
73 |
-
|
74 |
-
__slots__ = ("value", "type", "help", "_info")
|
75 |
-
|
76 |
-
def __init__(
|
77 |
-
self,
|
78 |
-
value: t.Any,
|
79 |
-
type: str = "plain",
|
80 |
-
help: t.Optional[str] = None,
|
81 |
-
**kwargs: t.Any,
|
82 |
-
) -> None:
|
83 |
-
self.value: t.Any = value
|
84 |
-
self.type: str = type
|
85 |
-
self.help: t.Optional[str] = help
|
86 |
-
self._info = kwargs
|
87 |
-
|
88 |
-
def __getattr__(self, name: str) -> t.Any:
|
89 |
-
return self._info.get(name)
|
90 |
-
|
91 |
-
|
92 |
-
# Only Bash >= 4.4 has the nosort option.
|
93 |
-
_SOURCE_BASH = """\
|
94 |
-
%(complete_func)s() {
|
95 |
-
local IFS=$'\\n'
|
96 |
-
local response
|
97 |
-
|
98 |
-
response=$(env COMP_WORDS="${COMP_WORDS[*]}" COMP_CWORD=$COMP_CWORD \
|
99 |
-
%(complete_var)s=bash_complete $1)
|
100 |
-
|
101 |
-
for completion in $response; do
|
102 |
-
IFS=',' read type value <<< "$completion"
|
103 |
-
|
104 |
-
if [[ $type == 'dir' ]]; then
|
105 |
-
COMPREPLY=()
|
106 |
-
compopt -o dirnames
|
107 |
-
elif [[ $type == 'file' ]]; then
|
108 |
-
COMPREPLY=()
|
109 |
-
compopt -o default
|
110 |
-
elif [[ $type == 'plain' ]]; then
|
111 |
-
COMPREPLY+=($value)
|
112 |
-
fi
|
113 |
-
done
|
114 |
-
|
115 |
-
return 0
|
116 |
-
}
|
117 |
-
|
118 |
-
%(complete_func)s_setup() {
|
119 |
-
complete -o nosort -F %(complete_func)s %(prog_name)s
|
120 |
-
}
|
121 |
-
|
122 |
-
%(complete_func)s_setup;
|
123 |
-
"""
|
124 |
-
|
125 |
-
_SOURCE_ZSH = """\
|
126 |
-
#compdef %(prog_name)s
|
127 |
-
|
128 |
-
%(complete_func)s() {
|
129 |
-
local -a completions
|
130 |
-
local -a completions_with_descriptions
|
131 |
-
local -a response
|
132 |
-
(( ! $+commands[%(prog_name)s] )) && return 1
|
133 |
-
|
134 |
-
response=("${(@f)$(env COMP_WORDS="${words[*]}" COMP_CWORD=$((CURRENT-1)) \
|
135 |
-
%(complete_var)s=zsh_complete %(prog_name)s)}")
|
136 |
-
|
137 |
-
for type key descr in ${response}; do
|
138 |
-
if [[ "$type" == "plain" ]]; then
|
139 |
-
if [[ "$descr" == "_" ]]; then
|
140 |
-
completions+=("$key")
|
141 |
-
else
|
142 |
-
completions_with_descriptions+=("$key":"$descr")
|
143 |
-
fi
|
144 |
-
elif [[ "$type" == "dir" ]]; then
|
145 |
-
_path_files -/
|
146 |
-
elif [[ "$type" == "file" ]]; then
|
147 |
-
_path_files -f
|
148 |
-
fi
|
149 |
-
done
|
150 |
-
|
151 |
-
if [ -n "$completions_with_descriptions" ]; then
|
152 |
-
_describe -V unsorted completions_with_descriptions -U
|
153 |
-
fi
|
154 |
-
|
155 |
-
if [ -n "$completions" ]; then
|
156 |
-
compadd -U -V unsorted -a completions
|
157 |
-
fi
|
158 |
-
}
|
159 |
-
|
160 |
-
if [[ $zsh_eval_context[-1] == loadautofunc ]]; then
|
161 |
-
# autoload from fpath, call function directly
|
162 |
-
%(complete_func)s "$@"
|
163 |
-
else
|
164 |
-
# eval/source/. command, register function for later
|
165 |
-
compdef %(complete_func)s %(prog_name)s
|
166 |
-
fi
|
167 |
-
"""
|
168 |
-
|
169 |
-
_SOURCE_FISH = """\
|
170 |
-
function %(complete_func)s
|
171 |
-
set -l response (env %(complete_var)s=fish_complete COMP_WORDS=(commandline -cp) \
|
172 |
-
COMP_CWORD=(commandline -t) %(prog_name)s)
|
173 |
-
|
174 |
-
for completion in $response
|
175 |
-
set -l metadata (string split "," $completion)
|
176 |
-
|
177 |
-
if test $metadata[1] = "dir"
|
178 |
-
__fish_complete_directories $metadata[2]
|
179 |
-
else if test $metadata[1] = "file"
|
180 |
-
__fish_complete_path $metadata[2]
|
181 |
-
else if test $metadata[1] = "plain"
|
182 |
-
echo $metadata[2]
|
183 |
-
end
|
184 |
-
end
|
185 |
-
end
|
186 |
-
|
187 |
-
complete --no-files --command %(prog_name)s --arguments \
|
188 |
-
"(%(complete_func)s)"
|
189 |
-
"""
|
190 |
-
|
191 |
-
|
192 |
-
class ShellComplete:
|
193 |
-
"""Base class for providing shell completion support. A subclass for
|
194 |
-
a given shell will override attributes and methods to implement the
|
195 |
-
completion instructions (``source`` and ``complete``).
|
196 |
-
|
197 |
-
:param cli: Command being called.
|
198 |
-
:param prog_name: Name of the executable in the shell.
|
199 |
-
:param complete_var: Name of the environment variable that holds
|
200 |
-
the completion instruction.
|
201 |
-
|
202 |
-
.. versionadded:: 8.0
|
203 |
-
"""
|
204 |
-
|
205 |
-
name: t.ClassVar[str]
|
206 |
-
"""Name to register the shell as with :func:`add_completion_class`.
|
207 |
-
This is used in completion instructions (``{name}_source`` and
|
208 |
-
``{name}_complete``).
|
209 |
-
"""
|
210 |
-
|
211 |
-
source_template: t.ClassVar[str]
|
212 |
-
"""Completion script template formatted by :meth:`source`. This must
|
213 |
-
be provided by subclasses.
|
214 |
-
"""
|
215 |
-
|
216 |
-
def __init__(
|
217 |
-
self,
|
218 |
-
cli: BaseCommand,
|
219 |
-
ctx_args: t.MutableMapping[str, t.Any],
|
220 |
-
prog_name: str,
|
221 |
-
complete_var: str,
|
222 |
-
) -> None:
|
223 |
-
self.cli = cli
|
224 |
-
self.ctx_args = ctx_args
|
225 |
-
self.prog_name = prog_name
|
226 |
-
self.complete_var = complete_var
|
227 |
-
|
228 |
-
@property
|
229 |
-
def func_name(self) -> str:
|
230 |
-
"""The name of the shell function defined by the completion
|
231 |
-
script.
|
232 |
-
"""
|
233 |
-
safe_name = re.sub(r"\W*", "", self.prog_name.replace("-", "_"), re.ASCII)
|
234 |
-
return f"_{safe_name}_completion"
|
235 |
-
|
236 |
-
def source_vars(self) -> t.Dict[str, t.Any]:
|
237 |
-
"""Vars for formatting :attr:`source_template`.
|
238 |
-
|
239 |
-
By default this provides ``complete_func``, ``complete_var``,
|
240 |
-
and ``prog_name``.
|
241 |
-
"""
|
242 |
-
return {
|
243 |
-
"complete_func": self.func_name,
|
244 |
-
"complete_var": self.complete_var,
|
245 |
-
"prog_name": self.prog_name,
|
246 |
-
}
|
247 |
-
|
248 |
-
def source(self) -> str:
|
249 |
-
"""Produce the shell script that defines the completion
|
250 |
-
function. By default this ``%``-style formats
|
251 |
-
:attr:`source_template` with the dict returned by
|
252 |
-
:meth:`source_vars`.
|
253 |
-
"""
|
254 |
-
return self.source_template % self.source_vars()
|
255 |
-
|
256 |
-
def get_completion_args(self) -> t.Tuple[t.List[str], str]:
|
257 |
-
"""Use the env vars defined by the shell script to return a
|
258 |
-
tuple of ``args, incomplete``. This must be implemented by
|
259 |
-
subclasses.
|
260 |
-
"""
|
261 |
-
raise NotImplementedError
|
262 |
-
|
263 |
-
def get_completions(
|
264 |
-
self, args: t.List[str], incomplete: str
|
265 |
-
) -> t.List[CompletionItem]:
|
266 |
-
"""Determine the context and last complete command or parameter
|
267 |
-
from the complete args. Call that object's ``shell_complete``
|
268 |
-
method to get the completions for the incomplete value.
|
269 |
-
|
270 |
-
:param args: List of complete args before the incomplete value.
|
271 |
-
:param incomplete: Value being completed. May be empty.
|
272 |
-
"""
|
273 |
-
ctx = _resolve_context(self.cli, self.ctx_args, self.prog_name, args)
|
274 |
-
obj, incomplete = _resolve_incomplete(ctx, args, incomplete)
|
275 |
-
return obj.shell_complete(ctx, incomplete)
|
276 |
-
|
277 |
-
def format_completion(self, item: CompletionItem) -> str:
|
278 |
-
"""Format a completion item into the form recognized by the
|
279 |
-
shell script. This must be implemented by subclasses.
|
280 |
-
|
281 |
-
:param item: Completion item to format.
|
282 |
-
"""
|
283 |
-
raise NotImplementedError
|
284 |
-
|
285 |
-
def complete(self) -> str:
|
286 |
-
"""Produce the completion data to send back to the shell.
|
287 |
-
|
288 |
-
By default this calls :meth:`get_completion_args`, gets the
|
289 |
-
completions, then calls :meth:`format_completion` for each
|
290 |
-
completion.
|
291 |
-
"""
|
292 |
-
args, incomplete = self.get_completion_args()
|
293 |
-
completions = self.get_completions(args, incomplete)
|
294 |
-
out = [self.format_completion(item) for item in completions]
|
295 |
-
return "\n".join(out)
|
296 |
-
|
297 |
-
|
298 |
-
class BashComplete(ShellComplete):
|
299 |
-
"""Shell completion for Bash."""
|
300 |
-
|
301 |
-
name = "bash"
|
302 |
-
source_template = _SOURCE_BASH
|
303 |
-
|
304 |
-
def _check_version(self) -> None:
|
305 |
-
import subprocess
|
306 |
-
|
307 |
-
output = subprocess.run(
|
308 |
-
["bash", "-c", 'echo "${BASH_VERSION}"'], stdout=subprocess.PIPE
|
309 |
-
)
|
310 |
-
match = re.search(r"^(\d+)\.(\d+)\.\d+", output.stdout.decode())
|
311 |
-
|
312 |
-
if match is not None:
|
313 |
-
major, minor = match.groups()
|
314 |
-
|
315 |
-
if major < "4" or major == "4" and minor < "4":
|
316 |
-
raise RuntimeError(
|
317 |
-
_(
|
318 |
-
"Shell completion is not supported for Bash"
|
319 |
-
" versions older than 4.4."
|
320 |
-
)
|
321 |
-
)
|
322 |
-
else:
|
323 |
-
raise RuntimeError(
|
324 |
-
_("Couldn't detect Bash version, shell completion is not supported.")
|
325 |
-
)
|
326 |
-
|
327 |
-
def source(self) -> str:
|
328 |
-
self._check_version()
|
329 |
-
return super().source()
|
330 |
-
|
331 |
-
def get_completion_args(self) -> t.Tuple[t.List[str], str]:
|
332 |
-
cwords = split_arg_string(os.environ["COMP_WORDS"])
|
333 |
-
cword = int(os.environ["COMP_CWORD"])
|
334 |
-
args = cwords[1:cword]
|
335 |
-
|
336 |
-
try:
|
337 |
-
incomplete = cwords[cword]
|
338 |
-
except IndexError:
|
339 |
-
incomplete = ""
|
340 |
-
|
341 |
-
return args, incomplete
|
342 |
-
|
343 |
-
def format_completion(self, item: CompletionItem) -> str:
|
344 |
-
return f"{item.type},{item.value}"
|
345 |
-
|
346 |
-
|
347 |
-
class ZshComplete(ShellComplete):
|
348 |
-
"""Shell completion for Zsh."""
|
349 |
-
|
350 |
-
name = "zsh"
|
351 |
-
source_template = _SOURCE_ZSH
|
352 |
-
|
353 |
-
def get_completion_args(self) -> t.Tuple[t.List[str], str]:
|
354 |
-
cwords = split_arg_string(os.environ["COMP_WORDS"])
|
355 |
-
cword = int(os.environ["COMP_CWORD"])
|
356 |
-
args = cwords[1:cword]
|
357 |
-
|
358 |
-
try:
|
359 |
-
incomplete = cwords[cword]
|
360 |
-
except IndexError:
|
361 |
-
incomplete = ""
|
362 |
-
|
363 |
-
return args, incomplete
|
364 |
-
|
365 |
-
def format_completion(self, item: CompletionItem) -> str:
|
366 |
-
return f"{item.type}\n{item.value}\n{item.help if item.help else '_'}"
|
367 |
-
|
368 |
-
|
369 |
-
class FishComplete(ShellComplete):
|
370 |
-
"""Shell completion for Fish."""
|
371 |
-
|
372 |
-
name = "fish"
|
373 |
-
source_template = _SOURCE_FISH
|
374 |
-
|
375 |
-
def get_completion_args(self) -> t.Tuple[t.List[str], str]:
|
376 |
-
cwords = split_arg_string(os.environ["COMP_WORDS"])
|
377 |
-
incomplete = os.environ["COMP_CWORD"]
|
378 |
-
args = cwords[1:]
|
379 |
-
|
380 |
-
# Fish stores the partial word in both COMP_WORDS and
|
381 |
-
# COMP_CWORD, remove it from complete args.
|
382 |
-
if incomplete and args and args[-1] == incomplete:
|
383 |
-
args.pop()
|
384 |
-
|
385 |
-
return args, incomplete
|
386 |
-
|
387 |
-
def format_completion(self, item: CompletionItem) -> str:
|
388 |
-
if item.help:
|
389 |
-
return f"{item.type},{item.value}\t{item.help}"
|
390 |
-
|
391 |
-
return f"{item.type},{item.value}"
|
392 |
-
|
393 |
-
|
394 |
-
ShellCompleteType = t.TypeVar("ShellCompleteType", bound=t.Type[ShellComplete])
|
395 |
-
|
396 |
-
|
397 |
-
_available_shells: t.Dict[str, t.Type[ShellComplete]] = {
|
398 |
-
"bash": BashComplete,
|
399 |
-
"fish": FishComplete,
|
400 |
-
"zsh": ZshComplete,
|
401 |
-
}
|
402 |
-
|
403 |
-
|
404 |
-
def add_completion_class(
|
405 |
-
cls: ShellCompleteType, name: t.Optional[str] = None
|
406 |
-
) -> ShellCompleteType:
|
407 |
-
"""Register a :class:`ShellComplete` subclass under the given name.
|
408 |
-
The name will be provided by the completion instruction environment
|
409 |
-
variable during completion.
|
410 |
-
|
411 |
-
:param cls: The completion class that will handle completion for the
|
412 |
-
shell.
|
413 |
-
:param name: Name to register the class under. Defaults to the
|
414 |
-
class's ``name`` attribute.
|
415 |
-
"""
|
416 |
-
if name is None:
|
417 |
-
name = cls.name
|
418 |
-
|
419 |
-
_available_shells[name] = cls
|
420 |
-
|
421 |
-
return cls
|
422 |
-
|
423 |
-
|
424 |
-
def get_completion_class(shell: str) -> t.Optional[t.Type[ShellComplete]]:
|
425 |
-
"""Look up a registered :class:`ShellComplete` subclass by the name
|
426 |
-
provided by the completion instruction environment variable. If the
|
427 |
-
name isn't registered, returns ``None``.
|
428 |
-
|
429 |
-
:param shell: Name the class is registered under.
|
430 |
-
"""
|
431 |
-
return _available_shells.get(shell)
|
432 |
-
|
433 |
-
|
434 |
-
def _is_incomplete_argument(ctx: Context, param: Parameter) -> bool:
|
435 |
-
"""Determine if the given parameter is an argument that can still
|
436 |
-
accept values.
|
437 |
-
|
438 |
-
:param ctx: Invocation context for the command represented by the
|
439 |
-
parsed complete args.
|
440 |
-
:param param: Argument object being checked.
|
441 |
-
"""
|
442 |
-
if not isinstance(param, Argument):
|
443 |
-
return False
|
444 |
-
|
445 |
-
assert param.name is not None
|
446 |
-
# Will be None if expose_value is False.
|
447 |
-
value = ctx.params.get(param.name)
|
448 |
-
return (
|
449 |
-
param.nargs == -1
|
450 |
-
or ctx.get_parameter_source(param.name) is not ParameterSource.COMMANDLINE
|
451 |
-
or (
|
452 |
-
param.nargs > 1
|
453 |
-
and isinstance(value, (tuple, list))
|
454 |
-
and len(value) < param.nargs
|
455 |
-
)
|
456 |
-
)
|
457 |
-
|
458 |
-
|
459 |
-
def _start_of_option(ctx: Context, value: str) -> bool:
|
460 |
-
"""Check if the value looks like the start of an option."""
|
461 |
-
if not value:
|
462 |
-
return False
|
463 |
-
|
464 |
-
c = value[0]
|
465 |
-
return c in ctx._opt_prefixes
|
466 |
-
|
467 |
-
|
468 |
-
def _is_incomplete_option(ctx: Context, args: t.List[str], param: Parameter) -> bool:
|
469 |
-
"""Determine if the given parameter is an option that needs a value.
|
470 |
-
|
471 |
-
:param args: List of complete args before the incomplete value.
|
472 |
-
:param param: Option object being checked.
|
473 |
-
"""
|
474 |
-
if not isinstance(param, Option):
|
475 |
-
return False
|
476 |
-
|
477 |
-
if param.is_flag or param.count:
|
478 |
-
return False
|
479 |
-
|
480 |
-
last_option = None
|
481 |
-
|
482 |
-
for index, arg in enumerate(reversed(args)):
|
483 |
-
if index + 1 > param.nargs:
|
484 |
-
break
|
485 |
-
|
486 |
-
if _start_of_option(ctx, arg):
|
487 |
-
last_option = arg
|
488 |
-
|
489 |
-
return last_option is not None and last_option in param.opts
|
490 |
-
|
491 |
-
|
492 |
-
def _resolve_context(
|
493 |
-
cli: BaseCommand,
|
494 |
-
ctx_args: t.MutableMapping[str, t.Any],
|
495 |
-
prog_name: str,
|
496 |
-
args: t.List[str],
|
497 |
-
) -> Context:
|
498 |
-
"""Produce the context hierarchy starting with the command and
|
499 |
-
traversing the complete arguments. This only follows the commands,
|
500 |
-
it doesn't trigger input prompts or callbacks.
|
501 |
-
|
502 |
-
:param cli: Command being called.
|
503 |
-
:param prog_name: Name of the executable in the shell.
|
504 |
-
:param args: List of complete args before the incomplete value.
|
505 |
-
"""
|
506 |
-
ctx_args["resilient_parsing"] = True
|
507 |
-
ctx = cli.make_context(prog_name, args.copy(), **ctx_args)
|
508 |
-
args = ctx.protected_args + ctx.args
|
509 |
-
|
510 |
-
while args:
|
511 |
-
command = ctx.command
|
512 |
-
|
513 |
-
if isinstance(command, MultiCommand):
|
514 |
-
if not command.chain:
|
515 |
-
name, cmd, args = command.resolve_command(ctx, args)
|
516 |
-
|
517 |
-
if cmd is None:
|
518 |
-
return ctx
|
519 |
-
|
520 |
-
ctx = cmd.make_context(name, args, parent=ctx, resilient_parsing=True)
|
521 |
-
args = ctx.protected_args + ctx.args
|
522 |
-
else:
|
523 |
-
sub_ctx = ctx
|
524 |
-
|
525 |
-
while args:
|
526 |
-
name, cmd, args = command.resolve_command(ctx, args)
|
527 |
-
|
528 |
-
if cmd is None:
|
529 |
-
return ctx
|
530 |
-
|
531 |
-
sub_ctx = cmd.make_context(
|
532 |
-
name,
|
533 |
-
args,
|
534 |
-
parent=ctx,
|
535 |
-
allow_extra_args=True,
|
536 |
-
allow_interspersed_args=False,
|
537 |
-
resilient_parsing=True,
|
538 |
-
)
|
539 |
-
args = sub_ctx.args
|
540 |
-
|
541 |
-
ctx = sub_ctx
|
542 |
-
args = [*sub_ctx.protected_args, *sub_ctx.args]
|
543 |
-
else:
|
544 |
-
break
|
545 |
-
|
546 |
-
return ctx
|
547 |
-
|
548 |
-
|
549 |
-
def _resolve_incomplete(
|
550 |
-
ctx: Context, args: t.List[str], incomplete: str
|
551 |
-
) -> t.Tuple[t.Union[BaseCommand, Parameter], str]:
|
552 |
-
"""Find the Click object that will handle the completion of the
|
553 |
-
incomplete value. Return the object and the incomplete value.
|
554 |
-
|
555 |
-
:param ctx: Invocation context for the command represented by
|
556 |
-
the parsed complete args.
|
557 |
-
:param args: List of complete args before the incomplete value.
|
558 |
-
:param incomplete: Value being completed. May be empty.
|
559 |
-
"""
|
560 |
-
# Different shells treat an "=" between a long option name and
|
561 |
-
# value differently. Might keep the value joined, return the "="
|
562 |
-
# as a separate item, or return the split name and value. Always
|
563 |
-
# split and discard the "=" to make completion easier.
|
564 |
-
if incomplete == "=":
|
565 |
-
incomplete = ""
|
566 |
-
elif "=" in incomplete and _start_of_option(ctx, incomplete):
|
567 |
-
name, _, incomplete = incomplete.partition("=")
|
568 |
-
args.append(name)
|
569 |
-
|
570 |
-
# The "--" marker tells Click to stop treating values as options
|
571 |
-
# even if they start with the option character. If it hasn't been
|
572 |
-
# given and the incomplete arg looks like an option, the current
|
573 |
-
# command will provide option name completions.
|
574 |
-
if "--" not in args and _start_of_option(ctx, incomplete):
|
575 |
-
return ctx.command, incomplete
|
576 |
-
|
577 |
-
params = ctx.command.get_params(ctx)
|
578 |
-
|
579 |
-
# If the last complete arg is an option name with an incomplete
|
580 |
-
# value, the option will provide value completions.
|
581 |
-
for param in params:
|
582 |
-
if _is_incomplete_option(ctx, args, param):
|
583 |
-
return param, incomplete
|
584 |
-
|
585 |
-
# It's not an option name or value. The first argument without a
|
586 |
-
# parsed value will provide value completions.
|
587 |
-
for param in params:
|
588 |
-
if _is_incomplete_argument(ctx, param):
|
589 |
-
return param, incomplete
|
590 |
-
|
591 |
-
# There were no unparsed arguments, the command may be a group that
|
592 |
-
# will provide command name completions.
|
593 |
-
return ctx.command, incomplete
|
|
|
|
|
|
|
|
|
|
|
|
|
|
|
|
|
|
|
|
|
|
|
|
|
|
|
|
|
|
|
|
|
|
|
|
|
|
|
|
|
|
|
|
|
|
|
|
|
|
|
|
|
|
|
|
|
|
|
|
|
|
|
|
|
|
|
|
|
|
|
|
|
|
|
|
|
|
|
|
|
|
|
|
|
|
|
|
|
|
|
|
|
|
|
|
|
|
|
|
|
|
|
|
|
|
|
|
|
|
|
|
|
|
|
|
|
|
|
|
|
|
|
|
|
|
|
|
|
|
|
|
|
|
|
|
|
|
|
|
|
|
|
|
|
|
|
|
|
|
|
|
|
|
|
|
|
|
|
|
|
|
|
|
|
|
|
|
|
|
|
|
|
|
|
|
|
|
|
|
|
|
|
|
|
|
|
|
|
|
|
|
|
|
|
|
|
|
|
|
|
|
|
|
|
|
|
|
|
|
|
|
|
|
|
|
|
|
|
|
|
|
|
|
|
|
|
|
|
|
|
|
|
|
|
|
|
|
|
|
|
|
|
|
|
|
|
|
|
|
|
|
|
|
|
|
|
|
|
|
|
|
|
|
|
|
|
|
|
|
|
|
|
|
|
|
|
|
|
|
|
|
|
|
|
|
|
|
|
|
|
|
|
|
|
|
|
|
|
|
|
|
|
|
|
|
|
|
|
|
|
|
|
|
|
|
|
|
|
|
|
|
|
|
|
|
|
|
|
|
|
|
|
|
|
|
|
|
|
|
|
|
|
|
|
|
|
|
|
|
|
|
|
|
|
|
|
|
|
|
|
|
|
|
|
|
|
|
|
|
|
|
|
|
|
|
|
|
|
|
|
|
|
|
|
|
|
|
|
|
|
|
|
|
|
|
|
|
|
|
|
|
|
|
|
|
|
|
|
|
|
|
|
|
|
|
|
|
|
|
|
|
|
|
|
|
|
|
|
|
|
|
|
|
|
|
|
|
|
|
|
|
|
|
|
|
|
|
|
|
|
|
|
|
|
|
|
|
|
|
|
|
|
|
|
|
|
|
|
|
|
|
|
|
|
|
|
|
|
|
|
|
|
|
|
|
|
|
|
|
|
|
|
|
|
|
|
|
|
|
|
|
|
|
|
|
|
|
|
|
|
|
|
|
|
|
|
|
|
|
|
|
|
|
|
|
|
|
|
|
|
|
|
|
|
|
|
|
|
|
|
|
|
|
|
|
|
|
|
|
|
|
|
|
|
|
|
|
|
|
|
|
|
|
|
|
|
|
|
|
|
|
|
|
|
|
|
|
|
|
|
|
|
|
|
|
|
|
|
|
|
|
|
|
|
|
|
|
|
|
|
|
|
|
|
|
|
|
|
|
|
|
|
|
|
|
|
|
|
|
|
|
|
|
|
|
|
|
|
|
|
|
|
|
|
|
|
|
|
|
|
|
|
|
|
|
|
|
|
|
|
|
|
|
|
|
|
|
|
|
|
|
|
|
|
|
|
|
|
|
|
|
|
|
|
|
|
|
|
|
|
|
|
|
|
|
|
|
|
|
|
|
|
|
|
|
|
|
|
|
|
|
|
|
|
|
|
|
|
|
|
|
|
|
|
|
|
|
|
|
|
|
|
|
|
|
|
|
|
|
|
|
|
|
|
|
|
|
|
|
|
|
|
|
|
|
|
|
|
|
|
|
|
|
|
|
|
|
|
|
|
|
|
|
|
|
|
|
|
|
|
|
|
|
|
|
|
|
|
|
|
|
|
|
|
|
|
|
|
|
|
|
|
|
|
|
|
|
|
|
|
|
|
|
|
|
|
|
|
|
|
|
|
|
|
|
|
|
|
|
|
|
|
|
|
|
|
|
|
|
|
|
|
|
|
|
|
|
|
|
|
|
|
|
|
|
|
|
|
|
|
|
|
|
|
|
|
|
|
|
|
|
|
|
|
|
|
|
|
|
|
|
|
|
|
|
|
|
|
|
|
|
|
|
|
|
|
|
|
|
|
|
|
|
|
|
|
|
|
|
|
|
|
|
|
|
|
|
|
|
|
|
|
|
|
|
|
|
|
|
|
|
|
|
|
|
|
|
|
|
|
|
|
|
|
|
|
|
|
|
|
|
|
|
|
|
|
|
|
|
|
|
|
|
|
|
|
|
|
|
|
|
|
|
|
|
|
|
|
|
|
|
|
|
|
|
|
|
|
|
|
|
|
|
|
|
|
|
|
|
|
|
|
|
|
|
|
|
|
|
|
|
|
|
|
|
|
|
|
|
|
|
|
|
|
|
|
|
|
|
|
|
|
|
|
|
|
|
|
|
|
|
|
|
|
|
|
|
|
|
|
|
|
|
|
|
|
|
|
|
|
|
|
|
|
|
|
|
|
|
|
|
|
|
|
|
|
|
|
|
|
|
|
|
|
|
|
|
|
|
|
|
|
|
|
|
|
|
|
|
|
|
|
|
|
|
|
|
|
|
|
|
|
|
|
|
|
|
|
|
|
|
|
|
|
|
|
|
|
|
|
|
|
|
|
|
|
|
|
|
|
|
|
|
|
|
|
|
|
|
|
|
|
|
|
|
|
|
|
|
|
|
|
|
|
|
|
|
|
|
|
|
|
|
|
|
|
|
|
|
|
|
|
|
|
|
|
|
|
|
|
|
|
|
|
|
|
|