diff --git a/spaces/1acneusushi/gradio-2dmoleculeeditor/data/ACDSee Photo Studio Ultimate 2018 V11.1 Crack (x64) [TechTools .rar !!TOP!!.md b/spaces/1acneusushi/gradio-2dmoleculeeditor/data/ACDSee Photo Studio Ultimate 2018 V11.1 Crack (x64) [TechTools .rar !!TOP!!.md
deleted file mode 100644
index 2b4e5e3fd2bbc1633f72332a6ed8bb5bb1287dee..0000000000000000000000000000000000000000
--- a/spaces/1acneusushi/gradio-2dmoleculeeditor/data/ACDSee Photo Studio Ultimate 2018 V11.1 Crack (x64) [TechTools .rar !!TOP!!.md
+++ /dev/null
@@ -1,109 +0,0 @@
-
- - Why you need a crack version of this software and what are the benefits? - How to download and install ACDSee Photo Studio Ultimate 2018 V11.1 Crack (x64) [TechTools .rar safely and securely? | | H2: What is ACDSee Photo Studio Ultimate 2018 and what are its features? | - A brief overview of ACDSee Photo Studio Ultimate 2018 and its main functions - A detailed description of the features of ACDSee Photo Studio Ultimate 2018, such as Smart Erase, Liquify tool, ACDSee Mobile Sync, ACDSee Actions Browser, Lens Correction, Frequency Separation, Pixel Targeting, Grain tool, Polygon Selection tool, Split Tone, Chromatic Aberration, and more. - A comparison of ACDSee Photo Studio Ultimate 2018 with other photo editing software, such as Photoshop and Lightroom. | | H2: Why you need a crack version of this software and what are the benefits? | - The reasons why you might want to use a crack version of ACDSee Photo Studio Ultimate 2018, such as saving money, accessing premium features, bypassing activation codes, etc. - The benefits of using a crack version of ACDSee Photo Studio Ultimate 2018, such as unlimited usage, no ads, no updates, no viruses, etc. - The risks and challenges of using a crack version of ACDSee Photo Studio Ultimate 2018, such as legal issues, compatibility problems, security threats, etc. | | H2: How to download and install ACDSee Photo Studio Ultimate 2018 V11.1 Crack (x64) [TechTools .rar safely and securely? | - The steps to download and install ACDSee Photo Studio Ultimate 2018 V11.1 Crack (x64) [TechTools .rar from a reliable torrent site, such as 1337X - The precautions to take before downloading and installing the software, such as using a VPN, scanning the file for malware, backing up your data, etc. - The tips to optimize the performance and functionality of the software, such as adjusting the settings, updating the drivers, using the help guide, etc. | | H1: Conclusion | - A summary of the main points of the article - A call to action for the readers to try out the software and share their feedback | Table 2: Article with HTML formatting
ACDSee Photo Studio Ultimate 2018 V11.1 Crack (x64) [TechTools .rar: What is it and why you need it?
-
If you are looking for a powerful and versatile photo editing software that can handle all your creative needs, you might want to check out ACDSee Photo Studio Ultimate 2018. This software is designed by and for photographers who want to achieve ultimate creative freedom with their images. It offers a comprehensive set of tools that can help you edit, organize, enhance, and share your photos with ease.
-
ACDSee Photo Studio Ultimate 2018 V11.1 Crack (x64) [TechTools .rar
However, there is one problem: this software is not cheap. The original price of ACDSee Photo Studio Ultimate 2018 is $149, which might be too expensive for some users who are on a budget. That's why some people resort to using a crack version of this software, which is a modified version that bypasses the activation process and allows users to access the premium features for free.
-
In this article, we will explain what is ACDSee Photo Studio Ultimate 2018 and what are its features, why you need a crack version of this software and what are the benefits, and how to download and install ACDSee Photo Studio Ultimate 2018 V11.1 Crack (x64) [TechTools .rar safely and securely. By the end of this article, you will have a clear idea of whether this software is worth trying out or not.
-
What is ACDSee Photo Studio Ultimate 2018 and what are its features?
-
ACDSee Photo Studio Ultimate 2018 is a photo editing software that combines the features of ACDSee Photo Studio Professional 2018 and ACDSee Photo Studio Standard 2018, plus some additional tools that are exclusive to the Ultimate version. It is a one-stop solution for all your photo editing needs, whether you are a beginner or a professional.
-
Some of the main functions of ACDSee Photo Studio Ultimate 2018 are:
-
-
-
Edit: You can use the software to perform basic and advanced edits on your photos, such as cropping, resizing, rotating, flipping, adjusting brightness, contrast, color, sharpness, noise, etc. You can also use the software to apply filters, effects, presets, and adjustments to your photos, such as black and white, sepia, vintage, HDR, etc. You can also use the software to retouch your photos, such as removing blemishes, red-eye, wrinkles, etc. You can also use the software to add text, watermarks, borders, frames, stickers, etc. to your photos.
-
Organize: You can use the software to manage your photo collection efficiently and conveniently. You can use the software to import your photos from various sources, such as your computer, camera, scanner, mobile device, etc. You can also use the software to sort your photos by various criteria, such as date, name, size, rating, keywords, etc. You can also use the software to create albums, folders, categories, tags, keywords, etc. to organize your photos. You can also use the software to search for your photos using various filters and options.
-
Enhance: You can use the software to improve the quality and appearance of your photos using various tools and features. You can use the software to correct common problems in your photos, such as lens distortion, chromatic aberration, vignetting, etc. You can also use the software to optimize your photos for different purposes and platforms, such as web, print, social media, etc. You can also use the software to create stunning panoramas, collages, slideshows, etc. from your photos.
-
Share: You can use the software to share your photos with others easily and quickly. You can use the software to export your photos in various formats and sizes. You can also use the software to upload your photos to various online platforms and services, such as Facebook, Flickr, Dropbox, OneDrive, etc. You can also use the software to print your photos using various options and settings.
-
-
These are just some of the functions of ACDSee Photo Studio Ultimate 2018. The software also offers many more features that make it a powerful and versatile photo editing software. Some of these features are:
-
Smart Erase
-
This feature allows you to remove unwanted objects or people from your photos without leaving any traces or artifacts. You can simply select the area you want to erase and let the software do the rest. The software will intelligently fill in the erased area with pixels that match the surrounding background.
-
Liquify tool
-
This feature allows you to distort or reshape any part of your photo using various brushes and options. You can use this feature to create artistic effects or fix imperfections in your photo. For example, you can use this feature to slim down a face or body part or enlarge an eye or lip.
-
ACDSee Mobile Sync
-
This feature allows you to sync your photos between your computer and your mobile device wirelessly and effortlessly. You can simply install the ACDSee Mobile Sync app on your mobile device and connect it to the same network as your computer. Then you can select which photos you want to sync and transfer them with one tap.
-
ACDSee Actions Browser
-
This feature allows you to browse and apply hundreds of actions to your photos with ease. Actions are predefined sequences of edits that can transform your photo in seconds. You can find actions for various purposes and styles in the ACDSee Actions Browser or download more from the ACDSee website. You can also create your own actions and save them for future use.
-
Lens Correction
-
This feature allows you to correct common lens distortions in your photos automatically or manually. Lens distortions are caused by imperfections in the lens that affect how light is captured by the camera sensor. Some examples of lens distortions are barrel distortion (where straight lines appear curved), pincushion distortion (where straight lines appear pinched), fisheye distortion (where images appear distorted at the edges), etc.
- Frequency Separation
-
This feature allows you to separate the texture and color of your photo into two layers and edit them independently. This can help you achieve a smooth and natural-looking skin tone without losing the details and sharpness of the skin texture. You can use this feature to remove blemishes, wrinkles, scars, etc. from your photo.
-
Pixel Targeting
-
This feature allows you to select and edit specific pixels in your photo based on their color, brightness, or hue. You can use this feature to isolate and enhance certain areas or objects in your photo. For example, you can use this feature to change the color of an eye or a flower or adjust the brightness of a sky or a shadow.
-
Grain tool
-
This feature allows you to add realistic grain effects to your photo to create a vintage or film-like look. You can use this feature to adjust the amount, size, roughness, and color of the grain. You can also use this feature to apply grain to specific areas or layers in your photo.
-
Polygon Selection tool
-
This feature allows you to select any irregular-shaped area in your photo by drawing a polygon around it. You can use this feature to crop, cut, copy, paste, or edit any part of your photo that is not easily selected by other tools.
-
Split Tone
-
This feature allows you to apply different colors to the highlights and shadows of your photo to create a dramatic or artistic effect. You can use this feature to adjust the hue, saturation, and balance of the split tone. You can also use this feature to apply presets or create your own custom split tone.
-
Chromatic Aberration
-
This feature allows you to correct or create chromatic aberration in your photo. Chromatic aberration is a phenomenon where the colors of an image are not aligned properly due to the different wavelengths of light passing through the lens. This can result in color fringes or halos around the edges of objects in your photo. You can use this feature to remove or reduce chromatic aberration automatically or manually. You can also use this feature to add chromatic aberration intentionally to create a creative or retro effect.
-
These are just some of the features of ACDSee Photo Studio Ultimate 2018. There are many more features that you can explore and experiment with using this software. You can find more information about the features and functions of ACDSee Photo Studio Ultimate 2018 on the official website or the user guide.
Why you need a crack version of this software and what are the benefits?
-
As you can see, ACDSee Photo Studio Ultimate 2018 is a great photo editing software that can help you unleash your creativity and achieve amazing results with your photos. However, as we mentioned earlier, this software is not cheap. The original price of ACDSee Photo Studio Ultimate 2018 is $149, which might be too expensive for some users who are on a budget or who do not want to spend that much money on a software.
-
That's why some users might prefer to use a crack version of this software, which is a modified version that bypasses the activation process and allows users to access the premium features for free. A crack version of ACDSee Photo Studio Ultimate 2018 is usually distributed as a .rar file, which is a compressed file format that can contain multiple files and folders. The .rar file usually contains the setup file of the software, the crack file, and the instructions on how to install and use the software.
-
There are several reasons why you might want to use a crack version of ACDSee Photo Studio Ultimate 2018, such as:
-
-
Saving money: The most obvious reason why you might want to use a crack version of this software is to save money. By using a crack version, you can avoid paying the original price of $149 and enjoy the full features of the software for free. This can help you save a lot of money in the long run, especially if you are a frequent user of photo editing software.
-
Accessing premium features: Another reason why you might want to use a crack version of this software is to access the premium features that are only available in the Ultimate version. As we mentioned earlier, ACDSee Photo Studio Ultimate 2018 offers some exclusive tools and features that are not found in other versions or other photo editing software. By using a crack version, you can access these features without any limitations or restrictions.
-
Bypassing activation codes: Another reason why you might want to use a crack version of this software is to bypass the activation codes that are required to use the original version. Activation codes are unique codes that are generated by the software developer to verify the authenticity and validity of the software. Activation codes are usually sent to the user via email or SMS after purchasing the software. However, some users might lose or forget their activation codes or have trouble receiving them due to various reasons. By using a crack version, you can avoid the hassle of entering or retrieving activation codes and use the software without any problems.
-
-
These are some of the reasons why you might want to use a crack version of ACDSee Photo Studio Ultimate 2018. However, using a crack version also comes with some benefits and drawbacks that you should be aware of before deciding whether to use it or not. Some of these benefits and drawbacks are:
-
The benefits of using a crack version of ACDSee Photo Studio Ultimate 2018
-
-
Unlimited usage: One of the benefits of using a crack version of this software is that you can use it as much as you want without any limitations or restrictions. You can install it on multiple devices and use it for multiple projects without worrying about running out of licenses or subscriptions. You can also use it offline without needing an internet connection or an account.
-
No ads: Another benefit of using a crack version of this software is that you will not see any ads or pop-ups while using it. Ads and pop-ups can be annoying and distracting when you are trying to focus on your photo editing work. They can also slow down your device or consume your data. By using a crack version, you can enjoy an ad-free and smooth photo editing experience.
-
No updates: Another benefit of using a crack version of this software is that you will not have to deal with any updates or patches that might affect the performance or functionality of the software. Updates and patches are usually released by the software developer to fix bugs, improve features, add new tools, etc. However, some updates or patches might cause compatibility issues, errors, crashes, etc. By using a crack version, you can avoid these potential problems and use the software as it is.
-
No viruses: Another benefit of using a crack version of this software is that you will not have to worry about any viruses or malware that might infect your device or compromise your data. Viruses and malware are malicious programs that can harm your device or steal your information. They can also affect the performance or functionality of your software. By using a crack version from a reliable source, you can ensure that your device and data are safe and secure.
-
-
-
-
Legal issues: One of the drawbacks of using a crack version of this software is that you might face legal issues or consequences for violating the terms and conditions of the software developer. Using a crack version is considered as piracy, which is illegal and unethical in most countries. You might be sued, fined, or even jailed for using a crack version of this software. You might also lose your rights to use the software or any other products or services from the software developer.
-
Compatibility problems: Another drawback of using a crack version of this software is that you might encounter compatibility problems with your device or other software. A crack version might not work properly or at all on some devices or operating systems. It might also conflict with other software or applications that you have installed on your device. This can cause errors, crashes, freezes, etc. that can affect your photo editing work or damage your device.
-
Security threats: Another drawback of using a crack version of this software is that you might expose your device or data to security threats from hackers or cybercriminals. A crack version might contain hidden viruses or malware that can infect your device or steal your information. It might also connect to unsecured servers or networks that can compromise your privacy or security. This can result in data loss, identity theft, fraud, etc. that can harm you personally or financially.
-
-
These are some of the benefits and drawbacks of using a crack version of ACDSee Photo Studio Ultimate 2018. You should weigh them carefully before deciding whether to use it or not. You should also be aware of the potential consequences and risks that you might face for using it.
-
How to download and install ACDSee Photo Studio Ultimate 2018 V11.1 Crack (x64) [TechTools .rar safely and securely?
-
If you have decided to use a crack version of ACDSee Photo Studio Ultimate 2018, you should follow some steps and precautions to download and install it safely and securely. Here are the steps and precautions that you should take:
-
The steps to download and install ACDSee Photo Studio Ultimate 2018 V11.1 Crack (x64) [TechTools .rar
-
-
Find a reliable torrent site: The first step is to find a reliable torrent site that offers the .rar file of ACDSee Photo Studio Ultimate 2018 V11.1 Crack (x64) [TechTools .rar. A torrent site is a website that hosts torrent files, which are small files that contain information about the files and folders that are shared by users through a peer-to-peer network. You can use a torrent site to download the .rar file of the software from other users who have already downloaded it. However, not all torrent sites are safe and trustworthy. Some torrent sites might contain fake, corrupted, or infected files that can harm your device or data. Therefore, you should do some research and read some reviews before choosing a torrent site to download from. One of the torrent sites that we recommend is 1337X, which is one of the most popular and reputable torrent sites on the internet.
-
Download the .rar file: The second step is to download the .rar file of ACDSee Photo Studio Ultimate 2018 V11.1 Crack (x64) [TechTools .rar from the torrent site that you have chosen. To do this, you need to have a torrent client installed on your device, which is a software that allows you to download and upload files through the peer-to-peer network. You can use any torrent client that you prefer, such as uTorrent, BitTorrent, qBittorrent, etc. After installing the torrent client, you need to open the torrent site and search for the .rar file of the software using the search bar or the categories. Then you need to click on the .rar file and download it using the magnet link or the download button. The download speed and time will depend on various factors, such as the number of seeders (users who have the complete file and are sharing it), leechers (users who are downloading the file but not sharing it), peers (users who are downloading and sharing parts of the file), etc.
-
Extract the .rar file: The third step is to extract the .rar file of ACDSee Photo Studio Ultimate 2018 V11.1 Crack (x64) [TechTools .rar after downloading it from the torrent site. To do this, you need to have a software that can extract compressed files, such as WinRAR, 7-Z Zip, etc. After installing the software, you need to locate the .rar file on your device and right-click on it. Then you need to select the option to extract the file to a folder of your choice. The extraction process might take some time depending on the size and complexity of the file. After extracting the file, you will see a folder that contains the setup file of the software, the crack file, and the instructions on how to install and use the software.
-
Install the software: The fourth step is to install the software on your device using the setup file that you have extracted from the .rar file. To do this, you need to open the folder that contains the setup file and double-click on it. Then you need to follow the instructions on the screen to complete the installation process. You might need to agree to some terms and conditions, choose a destination folder, select some options, etc. The installation process might also take some time depending on your device and system.
-
Apply the crack: The fifth and final step is to apply the crack to the software using the crack file that you have extracted from the .rar file. To do this, you need to open the folder that contains the crack file and copy it. Then you need to paste it in the installation folder of the software, which is usually located in C:\Program Files\ACD Systems\ACDSee Ultimate\11.0 or C:\Program Files (x86)\ACD Systems\ACDSee Ultimate\11.0 depending on your system. You might need to replace or overwrite the original file with the crack file. After applying the crack, you can launch the software and enjoy its full features for free.
-
-
The precautions to take before downloading and installing ACDSee Photo Studio Ultimate 2018 V11.1 Crack (x64) [TechTools .rar
-
Before downloading and installing ACDSee Photo Studio Ultimate 2018 V11.1 Crack (x64) [TechTools .rar, you should take some precautions to ensure that your device and data are safe and secure. Here are some of the precautions that you should take:
-
-
Use a VPN: One of the precautions that you should take is to use a VPN (Virtual Private Network) when downloading and installing ACDSee Photo Studio Ultimate 2018 V11.1 Crack (x64) [TechTools .rar from a torrent site. A VPN is a service that creates a secure and encrypted connection between your device and a server in another location. This can help you hide your IP address, location, identity, and online activity from your ISP (Internet Service Provider), government, hackers, or cybercriminals. This can also help you bypass any geo-restrictions or censorship that might prevent you from accessing certain torrent sites or content. You can use any VPN service that you prefer, such as NordVPN, ExpressVPN, CyberGhost, etc.
-
Scan the file for malware: Another precaution that you should take is to scan the .rar file of ACDSee Photo Studio Ultimate 2018 V11.1 Crack (x64) [TechTools .rar for any malware before extracting or installing it on your device. Malware is any software that can harm your device or data in various ways, such as deleting, encrypting, stealing, spying, etc. Some malware can also affect the performance or functionality of your software or device. You can use any antivirus or anti-malware software that you trust, such as Avast, Malwarebytes, Kaspersky, etc.
-
Back up your data: Another precaution that you should take is to back up your data before downloading and installing ACDSee Photo Studio Ultimate 2018 V11.1 Crack (x64) [TechTools .rar on your device. Backing up your data means creating a copy of your important files and folders and storing them in another location, such as an external hard drive, a cloud service, a USB flash drive, etc. This can help you recover your data in case something goes wrong during or after downloading and installing ACDSee Photo Studio Ultimate 2018 V11.1 Crack (x64) [TechTools .rar on your device, such as data loss, corruption, infection, etc.
-
-
The tips to optimize the performance and functionality of ACDSee Photo Studio Ultimate 2018 V11.1 Crack (x64) [TechTools .rar
-
After downloading and installing ACDSee Photo Studio Ultimate 2018 V11.1 Crack (x64) [TechTools .rar on your device, you should follow some tips to optimize the performance and functionality of the software. Here are some of the tips that you should follow:
-
-
Adjust the settings: One of the tips that you should follow is to adjust the settings of ACDSee Photo Studio Ultimate 2018 V11.1 Crack (x64) [TechTools .rar according to your preferences and needs. You can access the settings of the software by clicking on the Tools menu and selecting Options. You can customize various aspects of the software, such as the interface, the keyboard shortcuts, the file formats, the color management, the metadata, the plugins, etc. You can also reset the settings to their default values if you encounter any problems or errors.
-
Update the drivers: Another tip that you should follow is to update the drivers of your device regularly. Drivers are software that allow your device to communicate with other hardware or software components. Updating your drivers can help you improve the performance and functionality of ACDSee Photo Studio Ultimate 2018 V11.1 Crack (x64) [TechTools .rar and prevent any compatibility issues or errors. You can update your drivers manually or automatically using various tools or services, such as Driver Booster, Driver Easy, etc.
-
Use the help guide: Another tip that you should follow is to use the help guide of ACDSee Photo Studio Ultimate 2018 V11.1 Crack (x64) [TechTools .rar whenever you need assistance or guidance. The help guide is a comprehensive and user-friendly resource that contains information and instructions on how to use and troubleshoot the software. You can access the help guide by clicking on the Help menu and selecting Help Topics. You can also access the help guide online by visiting the official website or the user forum.
-
-
Conclusion
-
In conclusion, ACDSee Photo Studio Ultimate 2018 V11.1 Crack (x64) [TechTools .rar is a photo editing software that can help you edit, organize, enhance, and share your photos with ease and creativity. It offers a comprehensive set of tools and features that can cater to all your photo editing needs, whether you are a beginner or a professional.
-
However, this software is not cheap and might be too expensive for some users who are on a budget or who do not want to spend that much money on a software. That's why some users might opt to use a crack version of this software, which is a modified version that bypasses the activation process and allows users to access the premium features for free.
-
Using a crack version of ACDSee Photo Studio Ultimate 2018 V11.1 Crack (x64) [TechTools .rar has its benefits and drawbacks that you should be aware of before deciding whether to use it or not. Some of the benefits are unlimited usage, no ads, no updates, and no viruses. Some of the drawbacks are legal issues, compatibility problems, and security threats.
-
If you have decided to use a crack version of ACDSee Photo Studio Ultimate 2018 V11.1 Crack (x64) [TechTools .rar, you should follow some steps and precautions to download and install it safely and securely. Some of the steps are finding a reliable torrent site, downloading the .rar file, extracting the .rar file, installing the software, and applying the crack. Some of the precautions are using a VPN, scanning the file for malware, and backing up your data. Some of the tips are adjusting the settings, updating the drivers, and using the help guide.
-
We hope that this article has helped you understand what is ACDSee Photo Studio Ultimate 2018 V11.1 Crack (x64) [TechTools .rar and how to use it safely and securely. If you have any questions or feedback, please feel free to leave a comment below. We would love to hear from you and help you out. Thank you for reading and happy photo editing!
-
FAQs
-
Here are some of the frequently asked questions about ACDSee Photo Studio Ultimate 2018 V11.1 Crack (x64) [TechTools .rar:
-
-
What is the difference between ACDSee Photo Studio Ultimate 2018 and ACDSee Photo Studio Professional 2018?
-ACDSee Photo Studio Ultimate 2018 is a more advanced and comprehensive version of ACDSee Photo Studio Professional 2018. It offers some additional tools and features that are not available in the Professional version, such as Smart Erase, Liquify tool, ACDSee Mobile Sync, ACDSee Actions Browser, Lens Correction, Frequency Separation, Pixel Targeting, Grain tool, Polygon Selection tool, Split Tone, Chromatic Aberration, and more.
-
Is ACDSee Photo Studio Ultimate 2018 compatible with Windows 10?
-Yes, ACDSee Photo Studio Ultimate 2018 is compatible with Windows 10. However, you might need to update your drivers or settings to ensure optimal performance and functionality.
-
Can I use ACDSee Photo Studio Ultimate 2018 on Mac?
-No, ACDSee Photo Studio Ultimate 2018 is not available for Mac. It is only compatible with Windows devices. However, you can use other photo editing software that are similar to ACDSee Photo Studio Ultimate 2018 on Mac, such as Affinity Photo, Pixelmator Pro, Luminar AI, etc.
-
How can I get support or help for ACDSee Photo Studio Ultimate 2018?
-You can get support or help for ACDSee Photo Studio Ultimate 2018 by visiting the official website or the user forum. You can also access the help guide by clicking on the Help menu and selecting Help Topics. You can also contact the customer service by email or phone.
-
Is it legal to use a crack version of ACDSee Photo Studio Ultimate 2018?
-No, it is not legal to use a crack version of ACDSee Photo Studio Ultimate 2018. Using a crack version is considered as piracy, which is illegal and unethical in most countries. You might face legal issues or consequences for violating the terms and conditions of the software developer. You might also lose your rights to use the software or any other products or services from the software developer.
- b2dd77e56b
-
-
\ No newline at end of file
diff --git a/spaces/1acneusushi/gradio-2dmoleculeeditor/data/Download Adobe Dreamweaver CS3 Full Version Crack - Get It Now Before Its Gone.md b/spaces/1acneusushi/gradio-2dmoleculeeditor/data/Download Adobe Dreamweaver CS3 Full Version Crack - Get It Now Before Its Gone.md
deleted file mode 100644
index c9615e733886521609819fb7ca53197b5c541e90..0000000000000000000000000000000000000000
--- a/spaces/1acneusushi/gradio-2dmoleculeeditor/data/Download Adobe Dreamweaver CS3 Full Version Crack - Get It Now Before Its Gone.md
+++ /dev/null
@@ -1,183 +0,0 @@
-
-
Download Adobe Dreamweaver CS3 Full Version Crack
-
Are you looking for a way to download Adobe Dreamweaver CS3 full version crack? If so, you have come to the right place. In this article, I will show you how to get this powerful web design software for free, without paying any subscription fees or risking your computer's security. But first, let me explain what Adobe Dreamweaver CS3 is and why you might want to use it.
Adobe Dreamweaver CS3 is a software application that allows you to create, edit, and manage websites and web pages. It was released in 2007 as part of the Adobe Creative Suite 3 package, which also included Photoshop, Illustrator, Flash, and other popular tools. Adobe Dreamweaver CS3 is compatible with Windows and Mac operating systems, and supports various web technologies such as HTML, CSS, JavaScript, PHP, ASP.NET, and more.
-
Features of Adobe Dreamweaver CS3
-
Some of the features that make Adobe Dreamweaver CS3 stand out from other web design software are:
-
-
A user-friendly interface that lets you switch between code view and design view.
-
A built-in FTP client that lets you upload and download files from your web server.
-
A live preview function that lets you see how your website looks in different browsers and devices.
-
A code completion feature that helps you write code faster and more accurately.
-
A spry framework that lets you add dynamic effects and interactivity to your web pages.
-
A CSS panel that lets you edit and manage your style sheets easily.
-
A template system that lets you create and update multiple pages with the same layout and content.
-
A site management tool that lets you organize and maintain your website files and folders.
-
-
Benefits of Adobe Dreamweaver CS3
-
Some of the benefits that you can enjoy by using Adobe Dreamweaver CS3 are:
-
-
You can create professional-looking websites without having to learn complex coding languages.
-
You can save time and money by using the built-in tools and features instead of buying or downloading additional software or plugins.
-
You can improve your web design skills by learning from the tutorials and examples provided by Adobe.
-
You can collaborate with other web developers by sharing your files and projects online.
-
You can customize your workspace and preferences according to your needs and preferences.
-
-
Why do you need to crack Adobe Dreamweaver CS3?
-
Now that you know what Adobe Dreamweaver CS3 is and what it can do for you, you might be wondering why you need to crack it. After all, isn't it better to buy the official version from Adobe's website? Well, not necessarily. There are some drawbacks and risks associated with using the trial version or downloading pirated software that you should be aware of before making a decision.
-
The disadvantages of using the trial version
-
If you download the trial version of Adobe Dreamweaver CS3 from Adobe's website, you will be able to use it for free for 30 days. However, after that period expires, you will have to either buy a license or uninstall the software. This means that you will lose access to your files and projects unless you pay a hefty fee. Moreover, the trial version may have some limitations or restrictions on its functionality or performance that could affect your work quality or efficiency.
-
How to download adobe dreamweaver cs3 with crack
-Adobe dreamweaver cs3 full version free download for windows 10
-Download adobe dreamweaver cs3 crack only
-Adobe dreamweaver cs3 full version crack serial key
-Download adobe dreamweaver cs3 portable full version
-Adobe dreamweaver cs3 full version free download for mac
-Download adobe dreamweaver cs3 crack file
-Adobe dreamweaver cs3 full version crack activation code
-Download adobe dreamweaver cs3 full version for pc
-Adobe dreamweaver cs3 full version free download with keygen
-Download adobe dreamweaver cs3 crack patch
-Adobe dreamweaver cs3 full version crack license key
-Download adobe dreamweaver cs3 full version for windows 7
-Adobe dreamweaver cs3 full version free download rar
-Download adobe dreamweaver cs3 crack exe
-Adobe dreamweaver cs3 full version crack product key
-Download adobe dreamweaver cs3 full version for mac os x
-Adobe dreamweaver cs3 full version free download zip
-Download adobe dreamweaver cs3 crack dll
-Adobe dreamweaver cs3 full version crack registration code
-Download adobe dreamweaver cs3 full version offline installer
-Adobe dreamweaver cs3 full version free download utorrent
-Download adobe dreamweaver cs3 crack keygen
-Adobe dreamweaver cs3 full version crack serial number
-Download adobe dreamweaver cs3 full version for windows 8.1
-Adobe dreamweaver cs3 full version free download iso
-Download adobe dreamweaver cs3 crack torrent
-Adobe dreamweaver cs3 full version crack download link
-Download adobe dreamweaver cs3 full version for linux
-Adobe dreamweaver cs3 full version free download mega.nz
-Download adobe dreamweaver cs3 crack zip file
-Adobe dreamweaver cs3 full version crack system requirements
-Download adobe dreamweaver cs3 full version highly compressed
-Adobe dreamweaver cs3 full version free download google drive
-Download adobe dreamweaver cs3 crack rar file
-Adobe dreamweaver cs3 full version crack features
-Download adobe dreamweaver cs3 full version for android
-Adobe dreamweaver cs3 full version free download mediafire.com
-Download adobe dreamweaver cs3 crack serial keygen patch activation code license key product key registration code zip rar exe dll torrent iso mega.nz google drive utorrent offline installer highly compressed portable for pc windows 10 7 8.1 mac os x linux android rar zip iso (This is a joke keyword. Please do not use it.)
-
The risks of downloading pirated software
-
If you search online for Adobe Dreamweaver CS3 full version crack, you will find many websites that claim to offer it for free or at a low price. However, these websites are not authorized by Adobe and may contain malware or viruses that could harm your computer or steal your personal information. Furthermore, these websites may not provide accurate or complete information about the software or its installation process, which could lead to errors or compatibility issues. Additionally, downloading pirated software is illegal and unethical, and could result in legal consequences or penalties if caught by authorities.
-
How to download Adobe Dreamweaver CS3 full version crack?
-
So, how can you download Adobe Dreamweaver CS3 full version crack safely and legally? The answer is simple: follow these three steps.
-
Step 1: Find a reliable source
-
The first step is to find a reliable source that offers Adobe Dreamweaver CS3 full version crack. A reliable source is one that:
-
-
Has a good reputation and positive reviews from previous users.
-
Provides clear and detailed instructions on how to download and install the software.
-
Offers a secure and fast download link that does not require surveys or registrations.
-
Gives a guarantee or warranty for the quality and functionality of the software.
-
-
Tips for choosing a trustworthy website
-
Some tips for choosing a trustworthy website are:
-
-
Check the domain name and extension of the website. Avoid websites that have suspicious or unfamiliar names or extensions such as .ru, .cn, .tk, etc.
-
Look for signs of credibility such as contact information, customer service, testimonials, certificates, etc.
-
Read the comments or feedback from other users who have downloaded the software. Look for positive remarks or ratings as well as complaints or warnings.
-
Scan the website for malware or viruses using an online tool such as VirusTotal or Norton Safe Web.
-
-
Examples of reputable websites
-
Some examples of reputable websites that offer Adobe Dreamweaver CS3 full version crack are:
-
-
Softlay.net: This website provides a direct download link for Adobe Dreamweaver CS6 (the updated version of CS3) along with a serial number and an activation patch. It also gives a brief overview of the software's features and system requirements.
-
Getintopc.com: This website provides a direct download link for Adobe Dreamweaver CC 2018 (the latest version) along with a crack file. It also gives a detailed description of the software's features and system requirements as well as screenshots and video tutorials.
-
Filehorse.com: This website provides a direct download link for Adobe Dreamweaver CC 2020 (the newest version) along with a license key. It also gives a concise summary of the software's features and system requirements as well as user reviews and ratings.
-
-
Step 2: Download and install the software
-
The second step is to download and install the software on your computer. To do this, you need to:
-
-
Click on the download link provided by the website of your choice.
-
Wait for the download to complete. The file size may vary depending on the version of the software.
-
Extract the zip file using a tool such as WinRAR or 7-Zip.
-
Run the setup file as an administrator. Follow the installation wizard's instructions carefully. Choose your preferred language and destination folder. Agree to the terms and conditions. Click on install.
-
Wait for the installation to finish. Do not open or run the software yet.
-
-
How to avoid malware and viruses
-
To avoid malware and viruses during this step, you need to:
-
-
How to follow the installation instructions
-
To follow the installation instructions correctly, you need to:
-
-
Read the instructions carefully and follow them step by step.
-
Pay attention to any warnings or errors that may appear during the installation process.
-
Choose the options that suit your needs and preferences. For example, you may want to customize your installation by selecting or deselecting certain features or components.
-
Keep a backup of your original files and folders in case something goes wrong.
-
-
Step 3: Activate the software with the crack file
-
The third and final step is to activate the software with the crack file. A crack file is a modified version of the original file that bypasses the activation or registration process of the software. To do this, you need to:
-
-
Locate and copy the crack file from the downloaded folder. The crack file may have different names depending on the website you downloaded it from. For example, it may be called patch.exe, keygen.exe, activator.exe, etc.
-
Paste and replace the original file in the installation folder of the software. The installation folder may vary depending on your operating system and destination folder. For example, it may be located in C:\Program Files\Adobe\Adobe Dreamweaver CS3.
-
Run the crack file as an administrator. Follow any instructions that may appear on the screen. For example, you may have to enter a serial number or click on a button to activate the software.
-
Restart your computer and open the software. You should see a message that confirms that your software has been activated successfully.
-
-
How to locate and copy the crack file
-
To locate and copy the crack file easily, you need to:
-
-
Use a tool such as Windows Explorer or Finder to browse through your files and folders.
-
Use a search function or a shortcut key to find the crack file quickly. For example, you can press Ctrl+F or Command+F to open a search box and type in the name of the crack file.
-
Right-click on the crack file and select copy or press Ctrl+C or Command+C to copy it to your clipboard.
-
Navigate to the installation folder of the software and right-click on an empty space and select paste or press Ctrl+V or Command+V to paste it there.
-
-
How to paste and replace the original file
-
To paste and replace the original file safely, you need to:
-
-
Make sure that you have closed or exited the software before pasting the crack file.
-
Make sure that you have copied the correct crack file for your version of the software.
-
Make sure that you have permission to modify or overwrite the original file. You may have to enter your administrator password or grant access to do so.
-
Click on yes or confirm when prompted to replace or overwrite the original file.
-
-
Conclusion
-
In conclusion, Adobe Dreamweaver CS3 is a powerful web design software that allows you to create, edit, and manage websites and web pages. However, if you want to use it for free without paying any subscription fees or risking your computer's security, you need to download Adobe Dreamweaver CS3 full version crack from a reliable source, install it on your computer, and activate it with the crack file. By following these three steps, you will be able to enjoy all the features and benefits of this software without any limitations or restrictions.
-
Frequently Asked Questions
-
Here are some frequently asked questions about Adobe Dreamweaver CS3 full version crack:
-
Q: Is Adobe Dreamweaver CS3 still supported by Adobe?
-
A: No, Adobe Dreamweaver CS3 is no longer supported by Adobe since 2012. This means that Adobe does not provide any updates, patches, bug fixes, or technical support for this version of the software. However, you can still use it as long as it works on your computer and meets your needs.
-
Q: What are the system requirements for Adobe Dreamweaver CS3?
-
A: The minimum system requirements for Adobe Dreamweaver CS3 are:
-
-
Windows XP SP2 or later / Mac OS X v10.4.8–10.5 (Leopard)
1 GB of available hard-disk space (additional free space required during installation)
-
1024 x 768 monitor resolution with 16-bit video card
-
DVD-ROM drive
-
Internet connection required for activation
-
-
Q: What are some alternatives to Adobe Dreamweaver CS3?
-
A: Some alternatives to Adobe Dreamweaver CS3 are:
-
-
Wix.com: This is a cloud-based web development platform that lets you create websites using drag-and-drop tools and templates. It also offers hosting, domain registration, e-commerce, marketing, and SEO services.
-
WordPress.org: This is a free and open-source content management system that lets you create websites using themes and plugins. It also offers blogging, e-commerce, media management, and SEO features.
-
BlueGriffon: This is a free and open-source web editor that lets you create websites using HTML5, CSS3, SVG, MathML, etc. It also offers live preview, code completion, spell checking, and FTP support features.
-
-
Q: How can I learn more about Adobe Dreamweaver CS3?
-
A: You can learn more about Adobe Dreamweaver CS3 by:
-
-
Reading the user manual or help files that come with the software.
-
Watching online video tutorials or courses on platforms such as YouTube or Udemy.
-
Reading online articles or blogs on websites such as Medium or Quora.
-
Joining online forums or communities on platforms such as Reddit or Stack Overflow.
-
-
Q: How can I contact Adobe if I have any questions or issues with Adobe Dreamweaver CS3?
Calling their customer service number at 1-800-833-6687 (US) or +1-408-536-6000 (International).
-
Sending them an email at support@adobe.com.
-
Messaging them on their social media accounts such as Facebook or Twitter.
- 0a6ba089eb
-
-
\ No newline at end of file
diff --git a/spaces/1acneusushi/gradio-2dmoleculeeditor/data/Fabfilter Pro Q 2 Crack Reddit.md b/spaces/1acneusushi/gradio-2dmoleculeeditor/data/Fabfilter Pro Q 2 Crack Reddit.md
deleted file mode 100644
index 5ca872d2f82637fcb4738db22ef6b5868d70b312..0000000000000000000000000000000000000000
--- a/spaces/1acneusushi/gradio-2dmoleculeeditor/data/Fabfilter Pro Q 2 Crack Reddit.md
+++ /dev/null
@@ -1,18 +0,0 @@
-
-
Why You Should Avoid FabFilter Pro Q 2 Crack Reddit
-
FabFilter Pro Q 2 is a powerful and versatile equalizer plugin that can help you shape your sound in any way you want. It has a sleek and intuitive interface, a large spectrum analyzer, and many advanced features such as dynamic EQ, mid/side processing, linear phase mode, and more. It is one of the most popular and widely used EQ plugins in the music production industry.
-
However, some people may be tempted to download a cracked version of FabFilter Pro Q 2 from Reddit or other sources. This is a bad idea for several reasons. Here are some of the risks and disadvantages of using a FabFilter Pro Q 2 crack Reddit.
First of all, using a cracked version of FabFilter Pro Q 2 is illegal. It violates the terms and conditions of the software license agreement and infringes the intellectual property rights of the developers. You could face legal consequences such as fines, lawsuits, or even criminal charges if you are caught using or distributing a FabFilter Pro Q 2 crack Reddit.
-
Security Issues
-
Secondly, using a cracked version of FabFilter Pro Q 2 is risky for your computer and your data. You never know what kind of malware, viruses, spyware, or ransomware could be hidden in the crack file or the installer. You could expose your system to hackers, identity thieves, or cybercriminals who could steal your personal information, damage your files, or take over your device. You could also compromise the security of your network and other devices connected to it.
-
Quality Issues
-
Thirdly, using a cracked version of FabFilter Pro Q 2 is detrimental for your music production quality and workflow. You could experience bugs, glitches, crashes, errors, or compatibility issues that could ruin your projects or cause you to lose your work. You could also miss out on the latest updates, features, improvements, and support from the developers. You could end up with a subpar and outdated version of FabFilter Pro Q 2 that does not meet your expectations or needs.
-
Ethical Issues
-
Lastly, using a cracked version of FabFilter Pro Q 2 is unfair and disrespectful to the developers and the music production community. The developers have spent a lot of time, money, and effort to create a high-quality product that deserves to be paid for. By using a FabFilter Pro Q 2 crack Reddit, you are depriving them of their rightful income and recognition. You are also hurting the music production community by encouraging piracy and discouraging innovation and creativity.
-
Conclusion
-
In conclusion, using a FabFilter Pro Q 2 crack Reddit is not worth it. It is illegal, risky, detrimental, and unethical. You are better off buying a legitimate copy of FabFilter Pro Q 2 from the official website or an authorized dealer. You will get a reliable, secure, updated, and supported version of FabFilter Pro Q 2 that will enhance your music production quality and workflow. You will also support the developers and the music production community by showing your appreciation and respect for their work.
- ddb901b051
-
-
\ No newline at end of file
diff --git a/spaces/1phancelerku/anime-remove-background/1xBet APK 2021 - The Best Betting App for Android and iPhone Users.md b/spaces/1phancelerku/anime-remove-background/1xBet APK 2021 - The Best Betting App for Android and iPhone Users.md
deleted file mode 100644
index 19c233ca7e8933c425ea7ec139797b8847c3fd97..0000000000000000000000000000000000000000
--- a/spaces/1phancelerku/anime-remove-background/1xBet APK 2021 - The Best Betting App for Android and iPhone Users.md
+++ /dev/null
@@ -1,119 +0,0 @@
-
-
1xbet updated version 2021 apk: How to download and install the latest mobile app for Android and iOS
-
If you are looking for a reliable and convenient online betting platform, you should definitely check out 1xbet. It is one of the most popular and trusted bookmakers in the world, offering a wide range of sports events, live casino games, virtual sports, and more. But what makes 1xbet even more appealing is its mobile app, which allows you to access all the features and functions of the website from your smartphone or tablet. In this article, we will show you how to download and install the latest version of 1xbet apk for Android and iOS devices.
1xbet is an online betting company that was founded in 2007 and operates in more than 50 countries. It has over 400,000 registered users who enjoy its high-quality services and attractive bonuses. Some of the reasons why you should use 1xbet are:
-
-
It offers a variety of sports markets, including football, basketball, tennis, cricket, esports, and more.
-
It has a live betting section where you can place bets on ongoing events and watch live streams.
-
It has a live casino section where you can play roulette, blackjack, baccarat, poker, and other games with real dealers.
-
It has a 1xGames section where you can play various games of chance and win prizes.
-
It has a TV Games section where you can bet on lottery, bingo, keno, and other games.
-
It has a Toto section where you can predict the outcomes of sports events and win jackpots.
-
It has a Virtual Sports section where you can bet on simulated sports events.
-
It supports multiple payment methods, including credit cards, e-wallets, cryptocurrencies, and more.
-
It has a friendly and professional customer support team that is available 24/7 via phone, email, live chat, or social media.
-
It has a generous welcome bonus of up to $100 for new users who register with the promo code 1x_713871.
-
-
The benefits of using 1xbet mobile app
-
If you want to enjoy all the advantages of 1xbet on your mobile device, you should download and install its mobile app. The mobile app has several benefits over the website version, such as:
-
-
It is faster and more stable than the website.
-
It consumes less data and battery than the website.
-
It has a user-friendly interface that is easy to navigate.
-
It allows you to access all the features and functions of the website with one tap.
-
It notifies you of important events and offers via push notifications.
-
It supports biometric authentication for enhanced security and convenience.
-
It allows you to customize your settings and preferences according to your needs.
-
-
The features of 1xbet updated version 2021 apk
-
The latest version of 1xbet apk for Android and iOS devices is 1xbet updated version 2021 apk. It has some new and improved features that make it even more appealing and functional. Some of the features of 1xbet updated version 2021 apk are:
-
-
It supports the latest Android and iOS versions and devices.
-
It has a new design and layout that is more modern and attractive.
-
It has a new sports section that includes more sports events and markets.
-
It has a new live casino section that includes more games and dealers.
-
It has a new 1xGames section that includes more games and prizes.
-
It has a new TV Games section that includes more games and options.
-
It has a new Toto section that includes more jackpots and predictions.
-
It has a new Virtual Sports section that includes more simulations and outcomes.
-
It has a new statistics section that provides more data and analysis.
-
It has a new settings section that allows you to adjust more parameters and features.
-
-
How to download and install 1xbet updated version 2021 apk for Android
-
If you have an Android device, you can download and install 1xbet updated version 2021 apk by following these simple steps:
-
Step 1: Go to the official website of 1xbet or use a mirror link
-
The first step is to go to the official website of 1xbet or use a mirror link if the website is blocked in your region. You can find the official website at https://1xbet.com/en/ or use one of the mirror links at https://www.1xbet.link/.
-
Step 2: Find and click on the Android icon
-
The next step is to find and click on the Android icon at the bottom of the homepage. This will redirect you to the download page where you can see the details of the app and the download button.
-
1xbet mobile app download for android
-1xbet apk latest version free download
-1xbet app android 2021 update
-How to install 1xbet apk on android
-1xbet android app features and benefits
-Download 1xbet apk for iphone and android
-1xbet mobile version 2021 review
-1xbet app apk download link
-1xbet apk new version download for android
-1xbet app android latest version 2021
-1xbet mobile app for android devices
-1xbet apk download for android phone
-1xbet app update 2021 for android
-1xbet apk free download for android
-1xbet android app download and install guide
-1xbet mobile app apk latest version
-1xbet apk download latest version 2021
-1xbet app for android 2021 download
-How to use 1xbet app on android
-1xbet apk download for android and ios
-Download 1xbet mobile app for android
-1xbet app android new version 2021
-How to update 1xbet app on android
-Download and install 1xbet apk on android
-Benefits of using 1xbet app on android
-Download latest version of 1xbet apk for android
-How to register on 1xbet app for android
-How to login to 1xbet app on android
-How to bet on sports with 1xbet app on android
-How to play casino games with 1xbet app on android
-How to withdraw money from 1xbet app on android
-How to contact customer support on 1xbet app on android
-How to get bonuses and promotions on 1xbet app on android
-How to verify your account on 1xbet app on android
-How to change language and settings on 1xbet app on android
-How to watch live streaming on 1xbet app on android
-How to access other features on 1xbet app on android
-Pros and cons of using 1xbet app on android
-Comparison of 1xbet app and website on android
-User reviews of 1xbet app on android
-
Step 3: Allow the installation of unknown sources on your device
-
The third step is to allow the installation of unknown sources on your device. This is necessary because the app is not available on the Google Play Store. To do this, go to your device settings, then security, then unknown sources, and enable it.
-
Step 4: Open the downloaded file and follow the instructions
-
The final step is to open the downloaded file and follow the instructions. The file name will be something like 1xbet.apk. Tap on it and confirm the installation. Wait for a few seconds until the app is installed on your device. Then, launch the app and log in with your credentials or register if you are a new user.
-
How to download and install 1xbet updated version 2021 apk for iOS
-
If you have an iOS device, you can download and install 1xbet updated version 2021 apk by following these simple steps:
Step 2: Tap on the "Get" button and enter your Apple ID
-
The next step is to tap on the "Get" button and enter your Apple ID. This will start the download process. You may need to verify your identity with Touch ID or Face ID if you have enabled them.
-
Step 3: Wait for the app to be installed on your device
-
The third step is to wait for the app to be installed on your device. This may take a few minutes depending on your internet speed and device performance.
-
Step 4: Launch the app and log in with your credentials
-
The final step is to launch the app and log in with your credentials or register if you are a new user. You can also use your social media accounts or phone number to log in or register. The live chat is available on the website and the app. The social media accounts are Facebook, Twitter, Instagram, and YouTube.
-
-
-
How can I withdraw my winnings from 1xbet?
-
You can withdraw your winnings from 1xbet using the same payment method that you used to deposit. You can choose from credit cards, e-wallets, cryptocurrencies, and more. The minimum withdrawal amount is $1.5 and the maximum is $100,000. The processing time may vary depending on the payment method and the verification status.
-
-
-
What are the system requirements for 1xbet updated version 2021 apk?
-
The system requirements for 1xbet updated version 2021 apk are as follows: For Android devices, you need Android 4.4 or higher and at least 100 MB of free space. For iOS devices, you need iOS 9.0 or higher and at least 100 MB of free space.
-
-
-
Can I use 1xbet updated version 2021 apk on multiple devices?
-
Yes, you can use 1xbet updated version 2021 apk on multiple devices. However, you can only log in with one account at a time. If you try to log in with another account on another device, you will be logged out from the previous device.
-
- 197e85843d
-
-
\ No newline at end of file
diff --git a/spaces/1phancelerku/anime-remove-background/Carx Drift Racing 2 Mod Apk for iOS The Ultimate Guide to Drift Like a Pro.md b/spaces/1phancelerku/anime-remove-background/Carx Drift Racing 2 Mod Apk for iOS The Ultimate Guide to Drift Like a Pro.md
deleted file mode 100644
index 11b3815ae17905caf07dea28de263fbf6e80ba20..0000000000000000000000000000000000000000
--- a/spaces/1phancelerku/anime-remove-background/Carx Drift Racing 2 Mod Apk for iOS The Ultimate Guide to Drift Like a Pro.md
+++ /dev/null
@@ -1,122 +0,0 @@
-
-
Carx Drift Racing 2 Mod Apk for iOS: How to Download and Install It
-
If you are a fan of drifting games, you might have heard of Carx Drift Racing 2. It is one of the most popular and realistic drifting games on mobile devices. But what if you want to enjoy the game with unlimited money and features? In this article, we will show you how to download and install Carx Drift Racing 2 mod apk for iOS devices.
-
What is Carx Drift Racing 2?
-
Carx Drift Racing 2 is a sequel to the original Carx Drift Racing game, which was released in 2014. It is developed by CarX Technologies, a company that specializes in creating realistic car physics and graphics. The game allows you to customize your cars, tune your engines, and compete with other players in various modes and tracks. You can also join clubs, create your own tracks, and share your replays with others.
Over 70 tracks with different layouts and surfaces
-
Realistic car physics and sound effects
-
Advanced graphics and lighting effects
-
Different camera angles and views
-
Online and offline modes
-
Leaderboards and achievements
-
In-game currency and rewards
-
-
Requirements for Carx Drift Racing 2
-
To play Carx Drift Racing 2, you need to have an iOS device that meets the following requirements:
-
-
-
OS version
-
RAM
-
Storage
-
Internet connection
-
-
-
iOS 11 or later
-
At least 1 GB
-
At least 1.5 GB
-
Required for online mode
-
-
-
What is Carx Drift Racing 2 Mod Apk?
-
A mod apk is a modified version of an original app that has been altered to provide some extra features or benefits. In the case of Carx Drift Racing 2 mod apk, it is a file that can give you access to unlimited money, cars, tracks, and other features that are normally locked or require in-app purchases.
-
Benefits of Carx Drift Racing 2 Mod Apk
-
Some of the benefits of using Carx Drift Racing 2 mod apk are:
-
-
You can buy any car you want without spending real money
-
You can upgrade your cars to the maximum level without grinding for coins
-
You can unlock all the tracks and modes without completing challenges or missions
-
You can enjoy the game without ads or interruptions
-
You can have more fun and freedom in the game
-
-
Risks of Carx Drift Racing 2 Mod Apk
-
However, using Carx Drift Racing 2 mod apk also comes with some risks, such as:
-
-
You might get banned - You might get viruses or malware from untrusted sources - You might lose your progress or data if the mod apk is not compatible with the latest version of the game - You might miss out on the updates and new features of the game - You might ruin the balance and challenge of the game
-
Therefore, you should be careful and responsible when using Carx Drift Racing 2 mod apk. Make sure you download it from a reliable source, backup your data, and use it at your own risk.
-
How to Download and Install Carx Drift Racing 2 Mod Apk for iOS?
-
If you still want to try Carx Drift Racing 2 mod apk for iOS, you will need to follow these steps:
-
carx drift racing 2 hack ios download
-carx drift racing 2 mod apk unlimited money ios
-carx drift racing 2 ios cheats
-carx drift racing 2 mod menu ios
-carx drift racing 2 mod apk iphone
-carx drift racing 2 ios hack no jailbreak
-carx drift racing 2 mod apk for ipad
-carx drift racing 2 unlimited coins ios
-carx drift racing 2 ios modded ipa
-carx drift racing 2 mod apk ios free download
-carx drift racing 2 hack version ios
-carx drift racing 2 mod apk offline ios
-carx drift racing 2 ios game guardian
-carx drift racing 2 mod apk latest version ios
-carx drift racing 2 ios hack online
-carx drift racing 2 mod apk all cars unlocked ios
-carx drift racing 2 ios save file
-carx drift racing 2 mod apk obb ios
-carx drift racing 2 ios hack app
-carx drift racing 2 mod apk revdl ios
-carx drift racing 2 hack tool ios
-carx drift racing 2 mod apk data ios
-carx drift racing 2 ios hack cydia
-carx drift racing 2 mod apk rexdl ios
-carx drift racing 2 hack generator ios
-carx drift racing 2 mod apk happymod ios
-carx drift racing 2 ios hack without verification
-carx drift racing 2 mod apk android republic ios
-carx drift racing 2 hack no human verification ios
-carx drift racing 2 mod apk an1 ios
-carx drift racing 2 hack online generator ios
-carx drift racing 2 mod apk andropalace ios
-carx drift racing 2 hack no survey ios
-carx drift racing 2 mod apk apkpure ios
-carx drift racing 2 hack reddit ios
-carx drift racing 2 mod apk platinmods ios
-carx drift racing 2 hack tutuapp ios
-carx drift racing 2 mod apk vip ios
-carx drift racing 2 hack tweakbox ios
-carx drift racing 2 mod apk unlimited gold coins and silver coins for free on iphone and ipad devices.
-
Step 1: Find a reliable source for the mod apk file
-
The first thing you need to do is to find a website that offers Carx Drift Racing 2 mod apk for iOS devices. You can search on Google or use some of the popular sites like APKPure, APKMirror, or APKMody. However, make sure you check the reviews, ratings, and comments of other users before downloading anything. Also, avoid clicking on any suspicious links or ads that might redirect you to malicious sites.
-
Step 2: Download and install a third-party app installer
-
The next thing you need to do is to download and install a third-party app installer that can help you install the mod apk file on your iOS device. Some of the popular app installers are TutuApp, AppValley, or Panda Helper. You can download them from their official websites or use the links provided by the mod apk source. However, make sure you trust the app installer and grant it the necessary permissions to access your device.
-
Step 3: Install the mod apk file using the app installer
-
The final thing you need to do is to install the mod apk file using the app installer. To do this, you need to follow these steps:
-
-
Open the app installer and search for Carx Drift Racing 2 mod apk
-
Select the mod apk file and tap on the install button
-
Wait for the installation process to complete
-
If prompted, trust the developer profile of the mod apk in your device settings
-
Launch the game from your home screen
-
-
Step 4: Enjoy the game with unlimited money and features
-
Congratulations! You have successfully downloaded and installed Carx Drift Racing 2 mod apk for iOS devices. Now you can enjoy the game with unlimited money and features. You can buy any car you want, upgrade it to the max level, unlock all the tracks and modes, and have fun drifting with other players. However, remember to use the mod apk responsibly and at your own risk.
-
Conclusion
-
In this article, we have shown you how to download and install Carx Drift Racing 2 mod apk for iOS devices. We have also explained what is Carx Drift Racing 2, what is Carx Drift Racing 2 mod apk, what are the benefits and risks of using it, and how to use it step by step. We hope you found this article helpful and informative. If you have any questions or feedback, feel free to leave a comment below.
-
FAQs
-
Here are some of the frequently asked questions about Carx Drift Racing 2 mod apk for iOS devices:
-
Q: Is Carx Drift Racing 2 mod apk safe to use?
-
A: Carx Drift Racing 2 mod apk is not officially endorsed or supported by CarX Technologies or Apple. It is a modified version of the original game that has been altered by unknown developers. Therefore, it is not guaranteed to be safe or secure to use. It might contain viruses or malware that can harm your device or steal your data. It might also cause your account to be banned or your progress to be lost. Therefore, use it at your own risk.
-
Q: Is Carx Drift Racing 2 mod apk free to use?
-
A: Carx Drift Racing 2 mod apk is usually free to download and use. However, some websites might require you to complete surveys, offers, or tasks before giving you access to the file. Some app installers might also ask you to pay for a premium subscription or service before allowing you to install the file. Therefore, be careful and avoid any scams or frauds.
-
Q: How can I update Carx Drift Racing 2 mod apk?
-
A: Carx Drift Racing A: Carx Drift Racing 2 mod apk might not be compatible with the latest version of the game. Therefore, you might need to update the mod apk file whenever the game gets updated. To do this, you need to follow these steps: - Uninstall the current mod apk file from your device - Find a new mod apk file that matches the latest version of the game - Download and install the new mod apk file using the same steps as before - Launch the game and enjoy the updated features However, keep in mind that updating the mod apk file might cause you to lose your previous progress or data. Therefore, make sure you backup your data before updating.
Q: Can I play Carx Drift Racing 2 mod apk online with other players?
-
A: Carx Drift Racing 2 mod apk allows you to play online with other players who are using the same mod apk file. However, you might not be able to play with players who are using the original game or a different mod apk file. You might also face some issues or errors while playing online, such as lag, disconnects, or crashes. Therefore, it is recommended to play offline or with your friends who are using the same mod apk file.
-
Q: Can I use Carx Drift Racing 2 mod apk on other devices?
-
A: Carx Drift Racing 2 mod apk is designed for iOS devices only. Therefore, you might not be able to use it on other devices, such as Android, Windows, or Mac. If you want to use Carx Drift Racing 2 mod apk on other devices, you will need to find a different mod apk file that is compatible with your device. However, be careful and make sure you download it from a trusted source.
197e85843d
-
-
\ No newline at end of file
diff --git a/spaces/1phancelerku/anime-remove-background/Download Level Maker and Unleash Your Creativity - Make and Share Levels with Millions of Players.md b/spaces/1phancelerku/anime-remove-background/Download Level Maker and Unleash Your Creativity - Make and Share Levels with Millions of Players.md
deleted file mode 100644
index 62f250afb822eb0ad4583d786c1ce25891074c29..0000000000000000000000000000000000000000
--- a/spaces/1phancelerku/anime-remove-background/Download Level Maker and Unleash Your Creativity - Make and Share Levels with Millions of Players.md
+++ /dev/null
@@ -1,135 +0,0 @@
-
-
How to Create Levels for Games Using Level Maker
-
Have you ever dreamed of creating your own video games? Do you love playing classic platformers like Super Mario or Sonic? If so, you might want to try Level Maker, a free app that lets you design, play, and share your own levels with everyone. Level Maker is a game of awesome creation and fun. You can use hundreds of blocks, items, enemies, and characters to make your levels awesome. You can also play millions of levels from other players around the world. In this article, I will show you how to create a level for a 2D platformer game using Level Maker in seven easy steps. Let's begin!
-
Step 1: Define the concept of your level
-
The first step is to define the basic concept for your level. What is the theme, setting, and goal of your level? For example, is this the 'underwater' level, where your character has to avoid the sharks and find the treasure? Is it at night? In the forest? In space? Here's where you set the scene and the mood for your level. You can also think about what kind of gameplay you want to offer. Do you want it to be fast-paced or slow-paced? Easy or hard? Linear or nonlinear?
To help you define your concept, you can write a short description of your level in one or two sentences. This will help you focus on the main idea and vision for your level. For example:
-
This is a 'jungle' level, where the character has to swing on vines, avoid snakes and monkeys, and reach the ancient temple.
-
Step 2: Add a top-down map
-
Once you have a rough idea for your level, you can start to create a 'top-down' map of your level. This is a simple sketch of the layout of your level using blocks and items. It doesn't have to be perfect, this is just a starting point. You can use any drawing tool or paper to make your map.
-
To make your map, think about the size and shape of your level. How big do you want it to be? How many screens will it span? Do you want it to be horizontal or vertical? Then, think about the interesting items or landmarks that could exist in your level. What kind of blocks, platforms, bridges, ladders, pipes, doors, switches, etc. do you want to use? Where do you want to place them? How do they connect with each other?
-
Here's an example of a top-down map for a 'jungle' level:
-
-
You can see that this map has a horizontal layout with four screens. It has different types of blocks (grass, dirt, stone), platforms (wooden planks), bridges (ropes), ladders (vines), pipes (bamboo), doors (gates), switches (buttons), etc. It also has some landmarks (trees, flowers, statues) that add some detail and variety to the scene.
-
Step 3: Define the journey
-
Next, think about how players will travel through your level. Where does your level start and how does someone finish it? What is the ideal path you want the player to take and what are the alternative routes or shortcuts they can take? How do you guide the player and give them clues or hints along the way?
-
download tiled level editor
-download ldtk 2d level editor
-download level design editors for games
-download free and open source level editor
-download level maker software for windows
-download level maker software for mac
-download level maker software for linux
-download level maker with world editor
-download level maker with auto-rendering
-download level maker with aseprite support
-download level maker with json export
-download level maker with tiled export
-download level maker with haxe api
-download level maker for side-scrolling games
-download level maker for top-down games
-download level maker for grid-vania games
-download level maker for linear games
-download level maker for free games
-download level maker for commercial games
-download level maker from the creator of dead cells
-download professional 2d level editor
-download easy to use level editor
-download flexible level editor
-download modern level editor
-download powerful level editor
-download super simple export level editor
-download efficient tile layer editing level editor
-download rule-based tile and object placement level editor
-download custom game entities level editor
-download backup system level editor
-how to download and install tiled level editor
-how to download and install ldtk 2d level editor
-how to use tiled level editor tutorial
-how to use ldtk 2d level editor tutorial
-best level maker software to download in 2023
-compare tiled and ldtk 2d level editors
-reviews of tiled and ldtk 2d level editors
-examples of games made with tiled and ldtk 2d level editors
-benefits of using tiled and ldtk 2d level editors
-features of tiled and ldtk 2d level editors
-
To define the journey, you can use arrows or lines to mark the direction and flow of your level. You can also use numbers or letters to label the key points or events in your level. For example, where does the player start (S), where do they finish (F), where do they encounter enemies (E), where do they find items (I), where do they face puzzles (P), etc.
-
Here's an example of a journey for a 'jungle' level:
-
-
You can see that this journey has a clear start (S) and finish (F) point. It also has some branching paths and optional areas that the player can explore. It has some enemies (E) that the player has to avoid or defeat, some items (I) that the player can collect or use, and some puzzles (P) that the player has to solve. It also has some signs (S) that give the player some hints or instructions.
-
Step 4: Design the challenges
-
Now, think about how you will challenge the player in your level. What kind of obstacles, enemies, and puzzles will you add to your level? How will they test the player's skills, reflexes, and logic? How will they vary in difficulty and complexity throughout your level?
-
To design the challenges, you can use symbols or icons to represent the different types of challenges in your level. You can also use colors or shapes to indicate the difficulty or danger level of each challenge. For example, you can use red circles for hard challenges, yellow triangles for medium challenges, and green squares for easy challenges.
-
Here's an example of some challenges for a 'jungle' level:
-
-
You can see that this level has different types of challenges, such as spikes, pits, fireballs, snakes, monkeys, etc. It also has different difficulty levels, such as hard (red), medium (yellow), and easy (green). Some challenges are static, meaning they don't move or change. Some are dynamic, meaning they move or change over time. Some are interactive, meaning they respond to the player's actions or inputs.
-
Step 5: Test and refine your level
-
The next step is to test and refine your level. This is where you play your level and see how it works in practice. Is it fun? Is it fair? Is it clear? Is it balanced? Is it buggy? You want to make sure that your level is enjoyable and playable for yourself and others.
-
To test and refine your level, you can use Level Maker's built-in play mode. This allows you to switch between editing and playing your level with a simple tap. You can also use Level Maker's feedback system. This allows you to rate, comment, and review other players' levels, as well as receive ratings, comments, and reviews for your own levels. You can use this feedback to improve your level based on what other players think.
-
Here are some tips for testing and refining your level:
-
-
Play your level multiple times from start to finish. Try different paths and strategies. See if you can beat your own high score or time.
-
Play your level from different perspectives. Try playing as different characters with different abilities. Try playing on different devices with different screen sizes.
-
Play your level with different settings. Try changing the sound, music, speed, gravity, etc. See how they affect the gameplay and mood of your level.
-
Play other players' levels in Level Maker. See what they have done well and what they have done poorly. Learn from their mistakes and successes.
-
Ask other players to play your level and give you feedback. Listen to their opinions and suggestions. Be open-minded and respectful.
-
-
Step 6: Publish and share your level
-
The final step is to publish and share your level. This is where you make your level available for everyone to play and enjoy. You can also show off your creativity and skills to the world.
-
To publish and share your level, you can use Level Maker's upload feature. This allows you to upload your level to Level Maker's online server with a simple tap. You can also use Level Maker's social media feature. This allows you to share your level with your friends and followers on Facebook, Twitter, Instagram, etc.
-
Here are some tips for publishing and sharing your level:
-
Give your level a catchy and descriptive title. This will help attract players and tell them what your level is about.
-
Write a short and clear description of your level. This will help explain the concept and goal of your level to the players.
-
Add some tags or keywords to your level. This will help categorize your level and make it easier for players to find it.
-
Choose a suitable thumbnail for your level. This will help showcase your level and give players a preview of what to expect.
-
Invite your friends and followers to play your level and give you feedback. This will help spread the word and increase the popularity of your level.
-
-
Conclusion
-
Congratulations! You have just learned how to create levels for games using Level Maker. You have gone through the steps of defining the concept, adding the map, defining the journey, designing the challenges, testing and refining, and publishing and sharing your level. You have also learned some tips and tricks for making your level awesome and fun. You are now ready to unleash your creativity and imagination with Level Maker.
-
Level Maker is a great app for anyone who loves games and wants to make their own. It is easy to use, fun to play, and free to download. You can create any kind of level you want, from simple to complex, from realistic to fantasy, from casual to hardcore. You can also play millions of levels from other players around the world. You can rate, comment, and review them, as well as receive ratings, comments, and reviews for your own levels. You can also share your levels with your friends and followers on social media.
-
If you want to learn more about Level Maker, you can visit their website at [Level Maker]. There you can find more information, tutorials, videos, screenshots, and FAQs about the app. You can also download the app for free from the App Store or Google Play Store.
-
Thank you for reading this article. I hope you enjoyed it and learned something new. I also hope you will try Level Maker and create some amazing levels for yourself and others. Have fun!
-
FAQs
-
What is Level Maker?
-
Level Maker is a free app that lets you design, play, and share your own levels for 2D platformer games. You can use hundreds of blocks, items, enemies, and characters to make your levels awesome. You can also play millions of levels from other players around the world.
-
How do I create a level in Level Maker?
-
You can create a level in Level Maker by following these steps:
-
-
Define the concept of your level
-
Add a top-down map of your level
-
Define the journey of your level
-
Design the challenges of your level
-
Test and refine your level
-
Publish and share your level
-
-
How do I play a level in Level Maker?
-
You can play a level in Level Maker by following these steps:
-
-
Browse or search for a level you want to play
-
Tap on the level to open it
-
Tap on the play button to start playing
-
Use the on-screen buttons or tilt your device to control your character
-
Try to reach the end of the level or achieve the goal
-
Rate, comment, or review the level after playing
-
-
How do I share a level in Level Maker?
-
You can share a level in Level Maker by following these steps:
-
-
Open the level you want to share
-
Tap on the share button to open the share menu
-
Select the social media platform you want to share on (Facebook, Twitter, Instagram, etc.)
-
Add a message or caption to your post
-
Tap on the post button to share your level
-
-
How do I get feedback for my level in Level Maker?
-
You can get feedback for your level in Level Maker by following these steps:
-
-
Publish your level online using the upload feature
-
Invite other players to play your level using the social media feature
-
Check the ratings, comments, and reviews for your level using the feedback system
-
Listen to the opinions and suggestions of other players
-
Improve your level based on the feedback you receive
- 401be4b1e0
-
-
\ No newline at end of file
diff --git a/spaces/1toTree/lora_test/ppdiffusers/pipelines/score_sde_ve/__init__.py b/spaces/1toTree/lora_test/ppdiffusers/pipelines/score_sde_ve/__init__.py
deleted file mode 100644
index 3e3a6ffbb48c17c664b0815139ada8db8bb33cad..0000000000000000000000000000000000000000
--- a/spaces/1toTree/lora_test/ppdiffusers/pipelines/score_sde_ve/__init__.py
+++ /dev/null
@@ -1,17 +0,0 @@
-# Copyright (c) 2022 PaddlePaddle Authors. All Rights Reserved.
-# Copyright 2022 The HuggingFace Team. All rights reserved.
-#
-# Licensed under the Apache License, Version 2.0 (the "License");
-# you may not use this file except in compliance with the License.
-# You may obtain a copy of the License at
-#
-# http://www.apache.org/licenses/LICENSE-2.0
-#
-# Unless required by applicable law or agreed to in writing, software
-# distributed under the License is distributed on an "AS IS" BASIS,
-# WITHOUT WARRANTIES OR CONDITIONS OF ANY KIND, either express or implied.
-# See the License for the specific language governing permissions and
-# limitations under the License.
-
-# flake8: noqa
-from .pipeline_score_sde_ve import ScoreSdeVePipeline
diff --git a/spaces/221091lstwcm/textgenerator/README.md b/spaces/221091lstwcm/textgenerator/README.md
deleted file mode 100644
index 820ffc51beafee16d293514dc32736c37b3ea5ee..0000000000000000000000000000000000000000
--- a/spaces/221091lstwcm/textgenerator/README.md
+++ /dev/null
@@ -1,12 +0,0 @@
----
-title: Textgenerator
-emoji: 👀
-colorFrom: pink
-colorTo: pink
-sdk: gradio
-sdk_version: 3.19.1
-app_file: app.py
-pinned: false
----
-
-Check out the configuration reference at https://huggingface.co/docs/hub/spaces-config-reference
diff --git a/spaces/7Vivek/Next-Word-Prediction-Streamlit/setup.sh b/spaces/7Vivek/Next-Word-Prediction-Streamlit/setup.sh
deleted file mode 100644
index 551e5518682ba17651d4b8e15bbacfdb6e6980c9..0000000000000000000000000000000000000000
--- a/spaces/7Vivek/Next-Word-Prediction-Streamlit/setup.sh
+++ /dev/null
@@ -1,13 +0,0 @@
-mkdir -p ~/.streamlit/
-
-echo "\
-[general]\n\
-email = \"your-email@domain.com\"\n\
-" > ~/.streamlit/credentials.toml
-
-echo "\
-[server]\n\
-headless = true\n\
-enableCORS=false\n\
-port = $PORT\n\
-" > ~/.streamlit/config.toml
diff --git a/spaces/801artistry/RVC801/colab_for_mdx.py b/spaces/801artistry/RVC801/colab_for_mdx.py
deleted file mode 100644
index 274846d0b5395865a05fce0da86b96d26ac06999..0000000000000000000000000000000000000000
--- a/spaces/801artistry/RVC801/colab_for_mdx.py
+++ /dev/null
@@ -1,71 +0,0 @@
-import json
-import os
-import gc
-import psutil
-import requests
-import subprocess
-import time
-import logging
-import sys
-import shutil
-now_dir = os.getcwd()
-sys.path.append(now_dir)
-first_cell_executed = False
-file_folder = "Colab-for-MDX_B"
-def first_cell_ran():
- global first_cell_executed
- if first_cell_executed:
- #print("The 'first_cell_ran' function has already been executed.")
- return
-
-
-
- first_cell_executed = True
- os.makedirs("tmp_models", exist_ok=True)
-
-
-
- class hide_opt: # hide outputs
- def __enter__(self):
- self._original_stdout = sys.stdout
- sys.stdout = open(os.devnull, "w")
-
- def __exit__(self, exc_type, exc_val, exc_tb):
- sys.stdout.close()
- sys.stdout = self._original_stdout
-
- def get_size(bytes, suffix="B"): # read ram
- global svmem
- factor = 1024
- for unit in ["", "K", "M", "G", "T", "P"]:
- if bytes < factor:
- return f"{bytes:.2f}{unit}{suffix}"
- bytes /= factor
- svmem = psutil.virtual_memory()
-
-
- def use_uvr_without_saving():
- print("Notice: files won't be saved to personal drive.")
- print(f"Downloading {file_folder}...", end=" ")
- with hide_opt():
- #os.chdir(mounting_path)
- items_to_move = ["demucs", "diffq","julius","model","separated","tracks","mdx.py","MDX-Net_Colab.ipynb"]
- subprocess.run(["git", "clone", "https://github.com/NaJeongMo/Colab-for-MDX_B.git"])
- for item_name in items_to_move:
- item_path = os.path.join(file_folder, item_name)
- if os.path.exists(item_path):
- if os.path.isfile(item_path):
- shutil.move(item_path, now_dir)
- elif os.path.isdir(item_path):
- shutil.move(item_path, now_dir)
- try:
- shutil.rmtree(file_folder)
- except PermissionError:
- print(f"No se pudo eliminar la carpeta {file_folder}. Puede estar relacionada con Git.")
-
-
- use_uvr_without_saving()
- print("done!")
- if not os.path.exists("tracks"):
- os.mkdir("tracks")
-first_cell_ran()
\ No newline at end of file
diff --git a/spaces/801artistry/RVC801/demucs/compressed.py b/spaces/801artistry/RVC801/demucs/compressed.py
deleted file mode 100644
index eb8fbb75463ba71ca86729b22baebf24598ade57..0000000000000000000000000000000000000000
--- a/spaces/801artistry/RVC801/demucs/compressed.py
+++ /dev/null
@@ -1,115 +0,0 @@
-# Copyright (c) Facebook, Inc. and its affiliates.
-# All rights reserved.
-#
-# This source code is licensed under the license found in the
-# LICENSE file in the root directory of this source tree.
-
-import json
-from fractions import Fraction
-from concurrent import futures
-
-import musdb
-from torch import distributed
-
-from .audio import AudioFile
-
-
-def get_musdb_tracks(root, *args, **kwargs):
- mus = musdb.DB(root, *args, **kwargs)
- return {track.name: track.path for track in mus}
-
-
-class StemsSet:
- def __init__(self, tracks, metadata, duration=None, stride=1,
- samplerate=44100, channels=2, streams=slice(None)):
-
- self.metadata = []
- for name, path in tracks.items():
- meta = dict(metadata[name])
- meta["path"] = path
- meta["name"] = name
- self.metadata.append(meta)
- if duration is not None and meta["duration"] < duration:
- raise ValueError(f"Track {name} duration is too small {meta['duration']}")
- self.metadata.sort(key=lambda x: x["name"])
- self.duration = duration
- self.stride = stride
- self.channels = channels
- self.samplerate = samplerate
- self.streams = streams
-
- def __len__(self):
- return sum(self._examples_count(m) for m in self.metadata)
-
- def _examples_count(self, meta):
- if self.duration is None:
- return 1
- else:
- return int((meta["duration"] - self.duration) // self.stride + 1)
-
- def track_metadata(self, index):
- for meta in self.metadata:
- examples = self._examples_count(meta)
- if index >= examples:
- index -= examples
- continue
- return meta
-
- def __getitem__(self, index):
- for meta in self.metadata:
- examples = self._examples_count(meta)
- if index >= examples:
- index -= examples
- continue
- streams = AudioFile(meta["path"]).read(seek_time=index * self.stride,
- duration=self.duration,
- channels=self.channels,
- samplerate=self.samplerate,
- streams=self.streams)
- return (streams - meta["mean"]) / meta["std"]
-
-
-def _get_track_metadata(path):
- # use mono at 44kHz as reference. For any other settings data won't be perfectly
- # normalized but it should be good enough.
- audio = AudioFile(path)
- mix = audio.read(streams=0, channels=1, samplerate=44100)
- return {"duration": audio.duration, "std": mix.std().item(), "mean": mix.mean().item()}
-
-
-def _build_metadata(tracks, workers=10):
- pendings = []
- with futures.ProcessPoolExecutor(workers) as pool:
- for name, path in tracks.items():
- pendings.append((name, pool.submit(_get_track_metadata, path)))
- return {name: p.result() for name, p in pendings}
-
-
-def _build_musdb_metadata(path, musdb, workers):
- tracks = get_musdb_tracks(musdb)
- metadata = _build_metadata(tracks, workers)
- path.parent.mkdir(exist_ok=True, parents=True)
- json.dump(metadata, open(path, "w"))
-
-
-def get_compressed_datasets(args, samples):
- metadata_file = args.metadata / "musdb.json"
- if not metadata_file.is_file() and args.rank == 0:
- _build_musdb_metadata(metadata_file, args.musdb, args.workers)
- if args.world_size > 1:
- distributed.barrier()
- metadata = json.load(open(metadata_file))
- duration = Fraction(samples, args.samplerate)
- stride = Fraction(args.data_stride, args.samplerate)
- train_set = StemsSet(get_musdb_tracks(args.musdb, subsets=["train"], split="train"),
- metadata,
- duration=duration,
- stride=stride,
- streams=slice(1, None),
- samplerate=args.samplerate,
- channels=args.audio_channels)
- valid_set = StemsSet(get_musdb_tracks(args.musdb, subsets=["train"], split="valid"),
- metadata,
- samplerate=args.samplerate,
- channels=args.audio_channels)
- return train_set, valid_set
diff --git a/spaces/801artistry/RVC801/tools/infer/trans_weights.py b/spaces/801artistry/RVC801/tools/infer/trans_weights.py
deleted file mode 100644
index 1c54eefd6e7c678238d31e251a2e15479bf35d5b..0000000000000000000000000000000000000000
--- a/spaces/801artistry/RVC801/tools/infer/trans_weights.py
+++ /dev/null
@@ -1,18 +0,0 @@
-import pdb
-
-import torch
-
-# a=torch.load(r"E:\codes\py39\vits_vc_gpu_train\logs\ft-mi-suc\G_1000.pth")["model"]#sim_nsf#
-# a=torch.load(r"E:\codes\py39\vits_vc_gpu_train\logs\ft-mi-freeze-vocoder-flow-enc_q\G_1000.pth")["model"]#sim_nsf#
-# a=torch.load(r"E:\codes\py39\vits_vc_gpu_train\logs\ft-mi-freeze-vocoder\G_1000.pth")["model"]#sim_nsf#
-# a=torch.load(r"E:\codes\py39\vits_vc_gpu_train\logs\ft-mi-test\G_1000.pth")["model"]#sim_nsf#
-a = torch.load(
- r"E:\codes\py39\vits_vc_gpu_train\logs\ft-mi-no_opt-no_dropout\G_1000.pth"
-)[
- "model"
-] # sim_nsf#
-for key in a.keys():
- a[key] = a[key].half()
-# torch.save(a,"ft-mi-freeze-vocoder_true_1k.pt")#
-# torch.save(a,"ft-mi-sim1k.pt")#
-torch.save(a, "ft-mi-no_opt-no_dropout.pt") #
diff --git a/spaces/AIGC-Audio/AudioGPT/text_to_speech/utils/commons/ddp_utils.py b/spaces/AIGC-Audio/AudioGPT/text_to_speech/utils/commons/ddp_utils.py
deleted file mode 100644
index 4b529198c13a1ffc622baea6e5178407b24aee8f..0000000000000000000000000000000000000000
--- a/spaces/AIGC-Audio/AudioGPT/text_to_speech/utils/commons/ddp_utils.py
+++ /dev/null
@@ -1,137 +0,0 @@
-from torch.nn.parallel import DistributedDataParallel
-from torch.nn.parallel.distributed import _find_tensors
-import torch.optim
-import torch.utils.data
-import torch
-from packaging import version
-
-class DDP(DistributedDataParallel):
- """
- Override the forward call in lightning so it goes to training and validation step respectively
- """
-
- def forward(self, *inputs, **kwargs): # pragma: no cover
- if version.parse(torch.__version__[:6]) < version.parse("1.11"):
- self._sync_params()
- inputs, kwargs = self.scatter(inputs, kwargs, self.device_ids)
- assert len(self.device_ids) == 1
- if self.module.training:
- output = self.module.training_step(*inputs[0], **kwargs[0])
- elif self.module.testing:
- output = self.module.test_step(*inputs[0], **kwargs[0])
- else:
- output = self.module.validation_step(*inputs[0], **kwargs[0])
- if torch.is_grad_enabled():
- # We'll return the output object verbatim since it is a freeform
- # object. We need to find any tensors in this object, though,
- # because we need to figure out which parameters were used during
- # this forward pass, to ensure we short circuit reduction for any
- # unused parameters. Only if `find_unused_parameters` is set.
- if self.find_unused_parameters:
- self.reducer.prepare_for_backward(list(_find_tensors(output)))
- else:
- self.reducer.prepare_for_backward([])
- else:
- from torch.nn.parallel.distributed import \
- logging, Join, _DDPSink, _tree_flatten_with_rref, _tree_unflatten_with_rref
- with torch.autograd.profiler.record_function("DistributedDataParallel.forward"):
- if torch.is_grad_enabled() and self.require_backward_grad_sync:
- self.logger.set_runtime_stats_and_log()
- self.num_iterations += 1
- self.reducer.prepare_for_forward()
-
- # Notify the join context that this process has not joined, if
- # needed
- work = Join.notify_join_context(self)
- if work:
- self.reducer._set_forward_pass_work_handle(
- work, self._divide_by_initial_world_size
- )
-
- # Calling _rebuild_buckets before forward compuation,
- # It may allocate new buckets before deallocating old buckets
- # inside _rebuild_buckets. To save peak memory usage,
- # call _rebuild_buckets before the peak memory usage increases
- # during forward computation.
- # This should be called only once during whole training period.
- if torch.is_grad_enabled() and self.reducer._rebuild_buckets():
- logging.info("Reducer buckets have been rebuilt in this iteration.")
- self._has_rebuilt_buckets = True
-
- # sync params according to location (before/after forward) user
- # specified as part of hook, if hook was specified.
- buffer_hook_registered = hasattr(self, 'buffer_hook')
- if self._check_sync_bufs_pre_fwd():
- self._sync_buffers()
-
- if self._join_config.enable:
- # Notify joined ranks whether they should sync in backwards pass or not.
- self._check_global_requires_backward_grad_sync(is_joined_rank=False)
-
- inputs, kwargs = self.scatter(inputs, kwargs, self.device_ids)
- if self.module.training:
- output = self.module.training_step(*inputs[0], **kwargs[0])
- elif self.module.testing:
- output = self.module.test_step(*inputs[0], **kwargs[0])
- else:
- output = self.module.validation_step(*inputs[0], **kwargs[0])
-
- # sync params according to location (before/after forward) user
- # specified as part of hook, if hook was specified.
- if self._check_sync_bufs_post_fwd():
- self._sync_buffers()
-
- if torch.is_grad_enabled() and self.require_backward_grad_sync:
- self.require_forward_param_sync = True
- # We'll return the output object verbatim since it is a freeform
- # object. We need to find any tensors in this object, though,
- # because we need to figure out which parameters were used during
- # this forward pass, to ensure we short circuit reduction for any
- # unused parameters. Only if `find_unused_parameters` is set.
- if self.find_unused_parameters and not self.static_graph:
- # Do not need to populate this for static graph.
- self.reducer.prepare_for_backward(list(_find_tensors(output)))
- else:
- self.reducer.prepare_for_backward([])
- else:
- self.require_forward_param_sync = False
-
- # TODO: DDPSink is currently enabled for unused parameter detection and
- # static graph training for first iteration.
- if (self.find_unused_parameters and not self.static_graph) or (
- self.static_graph and self.num_iterations == 1
- ):
- state_dict = {
- 'static_graph': self.static_graph,
- 'num_iterations': self.num_iterations,
- }
-
- output_tensor_list, treespec, output_is_rref = _tree_flatten_with_rref(
- output
- )
- output_placeholders = [None for _ in range(len(output_tensor_list))]
- # Do not touch tensors that have no grad_fn, which can cause issues
- # such as https://github.com/pytorch/pytorch/issues/60733
- for i, output in enumerate(output_tensor_list):
- if torch.is_tensor(output) and output.grad_fn is None:
- output_placeholders[i] = output
-
- # When find_unused_parameters=True, makes tensors which require grad
- # run through the DDPSink backward pass. When not all outputs are
- # used in loss, this makes those corresponding tensors receive
- # undefined gradient which the reducer then handles to ensure
- # param.grad field is not touched and we don't error out.
- passthrough_tensor_list = _DDPSink.apply(
- self.reducer,
- state_dict,
- *output_tensor_list,
- )
- for i in range(len(output_placeholders)):
- if output_placeholders[i] is None:
- output_placeholders[i] = passthrough_tensor_list[i]
-
- # Reconstruct output data structure.
- output = _tree_unflatten_with_rref(
- output_placeholders, treespec, output_is_rref
- )
- return output
diff --git a/spaces/AIGC-Audio/Make_An_Audio_inpaint/ldm/modules/losses_audio/vggishish/loss.py b/spaces/AIGC-Audio/Make_An_Audio_inpaint/ldm/modules/losses_audio/vggishish/loss.py
deleted file mode 100644
index bae76571909eec571aaf075d58e3dea8f6424546..0000000000000000000000000000000000000000
--- a/spaces/AIGC-Audio/Make_An_Audio_inpaint/ldm/modules/losses_audio/vggishish/loss.py
+++ /dev/null
@@ -1,41 +0,0 @@
-import torch
-import torch.nn as nn
-import torch.nn.functional as F
-import torch.optim as optim
-
-class WeightedCrossEntropy(nn.CrossEntropyLoss):
-
- def __init__(self, weights, **pytorch_ce_loss_args) -> None:
- super().__init__(reduction='none', **pytorch_ce_loss_args)
- self.weights = weights
-
- def __call__(self, outputs, targets, to_weight=True):
- loss = super().__call__(outputs, targets)
- if to_weight:
- return (loss * self.weights[targets]).sum() / self.weights[targets].sum()
- else:
- return loss.mean()
-
-
-if __name__ == '__main__':
- x = torch.randn(10, 5)
- target = torch.randint(0, 5, (10,))
- weights = torch.tensor([1., 2., 3., 4., 5.])
-
- # criterion_weighted = nn.CrossEntropyLoss(weight=weights)
- # loss_weighted = criterion_weighted(x, target)
-
- # criterion_weighted_manual = nn.CrossEntropyLoss(reduction='none')
- # loss_weighted_manual = criterion_weighted_manual(x, target)
- # print(loss_weighted, loss_weighted_manual.mean())
- # loss_weighted_manual = (loss_weighted_manual * weights[target]).sum() / weights[target].sum()
- # print(loss_weighted, loss_weighted_manual)
- # print(torch.allclose(loss_weighted, loss_weighted_manual))
-
- pytorch_weighted = nn.CrossEntropyLoss(weight=weights)
- pytorch_unweighted = nn.CrossEntropyLoss()
- custom = WeightedCrossEntropy(weights)
-
- assert torch.allclose(pytorch_weighted(x, target), custom(x, target, to_weight=True))
- assert torch.allclose(pytorch_unweighted(x, target), custom(x, target, to_weight=False))
- print(custom(x, target, to_weight=True), custom(x, target, to_weight=False))
diff --git a/spaces/ASJMO/freegpt/g4f/Provider/Providers/Liaobots.py b/spaces/ASJMO/freegpt/g4f/Provider/Providers/Liaobots.py
deleted file mode 100644
index a04b9574f60842d424712efcd8bef5f6e1e97f4f..0000000000000000000000000000000000000000
--- a/spaces/ASJMO/freegpt/g4f/Provider/Providers/Liaobots.py
+++ /dev/null
@@ -1,64 +0,0 @@
-import os
-import uuid
-import requests
-from ...typing import sha256, Dict, get_type_hints
-
-url = 'https://liaobots.com'
-model = ['gpt-3.5-turbo', 'gpt-3.5-turbo-16k', 'gpt-4']
-supports_stream = True
-needs_auth = True
-working = False
-
-models = {
- 'gpt-4': {
- "id": "gpt-4",
- "name": "GPT-4",
- "maxLength": 24000,
- "tokenLimit": 8000
- },
- 'gpt-3.5-turbo': {
- "id": "gpt-3.5-turbo",
- "name": "GPT-3.5",
- "maxLength": 12000,
- "tokenLimit": 4000
- },
- 'gpt-3.5-turbo-16k': {
- "id": "gpt-3.5-turbo-16k",
- "name": "GPT-3.5-16k",
- "maxLength": 48000,
- "tokenLimit": 16000
- },
-}
-
-
-def _create_completion(model: str, messages: list, stream: bool, chatId: str, **kwargs):
-
- print(kwargs)
-
- headers = {
- 'authority': 'liaobots.com',
- 'content-type': 'application/json',
- 'origin': 'https://liaobots.com',
- 'referer': 'https://liaobots.com/',
- 'user-agent': 'Mozilla/5.0 (Windows NT 10.0; Win64; x64) AppleWebKit/537.36 (KHTML, like Gecko) Chrome/112.0.0.0 Safari/537.36',
- 'x-auth-code': 'qlcUMVn1KLMhd'
- }
-
- json_data = {
- 'conversationId': chatId,
- 'model': models[model],
- 'messages': messages,
- 'key': '',
- 'prompt': "You are ChatGPT, a large language model trained by OpenAI. Follow the user's instructions carefully. Respond using markdown.",
- }
-
- response = requests.post('https://liaobots.com/api/chat',
- headers=headers, json=json_data, stream=True)
-
- for token in response.iter_content(chunk_size=2046):
- yield (token.decode('utf-8'))
-
-
-params = f'g4f.Providers.{os.path.basename(__file__)[:-3]} supports: ' + \
- '(%s)' % ', '.join(
- [f"{name}: {get_type_hints(_create_completion)[name].__name__}" for name in _create_completion.__code__.co_varnames[:_create_completion.__code__.co_argcount]])
diff --git a/spaces/Abhilashvj/planogram-compliance/segment/val.py b/spaces/Abhilashvj/planogram-compliance/segment/val.py
deleted file mode 100644
index 7252cd296948647d0b50a2ef9b49ddd7ca28cbf4..0000000000000000000000000000000000000000
--- a/spaces/Abhilashvj/planogram-compliance/segment/val.py
+++ /dev/null
@@ -1,792 +0,0 @@
-# YOLOv5 🚀 by Ultralytics, GPL-3.0 license
-"""
-Validate a trained YOLOv5 segment model on a segment dataset
-
-Usage:
- $ bash data/scripts/get_coco.sh --val --segments # download COCO-segments val split (1G, 5000 images)
- $ python segment/val.py --weights yolov5s-seg.pt --data coco.yaml --img 640 # validate COCO-segments
-
-Usage - formats:
- $ python segment/val.py --weights yolov5s-seg.pt # PyTorch
- yolov5s-seg.torchscript # TorchScript
- yolov5s-seg.onnx # ONNX Runtime or OpenCV DNN with --dnn
- yolov5s-seg_openvino_label # OpenVINO
- yolov5s-seg.engine # TensorRT
- yolov5s-seg.mlmodel # CoreML (macOS-only)
- yolov5s-seg_saved_model # TensorFlow SavedModel
- yolov5s-seg.pb # TensorFlow GraphDef
- yolov5s-seg.tflite # TensorFlow Lite
- yolov5s-seg_edgetpu.tflite # TensorFlow Edge TPU
- yolov5s-seg_paddle_model # PaddlePaddle
-"""
-
-import argparse
-import json
-import os
-import sys
-from multiprocessing.pool import ThreadPool
-from pathlib import Path
-
-import numpy as np
-import torch
-from tqdm import tqdm
-
-FILE = Path(__file__).resolve()
-ROOT = FILE.parents[1] # YOLOv5 root directory
-if str(ROOT) not in sys.path:
- sys.path.append(str(ROOT)) # add ROOT to PATH
-ROOT = Path(os.path.relpath(ROOT, Path.cwd())) # relative
-
-import torch.nn.functional as F
-
-from models.common import DetectMultiBackend
-from models.yolo import SegmentationModel
-from utils.callbacks import Callbacks
-from utils.general import (
- LOGGER,
- NUM_THREADS,
- TQDM_BAR_FORMAT,
- Profile,
- check_dataset,
- check_img_size,
- check_requirements,
- check_yaml,
- coco80_to_coco91_class,
- colorstr,
- increment_path,
- non_max_suppression,
- print_args,
- scale_boxes,
- xywh2xyxy,
- xyxy2xywh,
-)
-from utils.metrics import ConfusionMatrix, box_iou
-from utils.plots import output_to_target, plot_val_study
-from utils.segment.dataloaders import create_dataloader
-from utils.segment.general import (
- mask_iou,
- process_mask,
- process_mask_native,
- scale_image,
-)
-from utils.segment.metrics import Metrics, ap_per_class_box_and_mask
-from utils.segment.plots import plot_images_and_masks
-from utils.torch_utils import de_parallel, select_device, smart_inference_mode
-
-
-def save_one_txt(predn, save_conf, shape, file):
- # Save one txt result
- gn = torch.tensor(shape)[[1, 0, 1, 0]] # normalization gain whwh
- for *xyxy, conf, cls in predn.tolist():
- xywh = (
- (xyxy2xywh(torch.tensor(xyxy).view(1, 4)) / gn).view(-1).tolist()
- ) # normalized xywh
- line = (
- (cls, *xywh, conf) if save_conf else (cls, *xywh)
- ) # label format
- with open(file, "a") as f:
- f.write(("%g " * len(line)).rstrip() % line + "\n")
-
-
-def save_one_json(predn, jdict, path, class_map, pred_masks):
- # Save one JSON result {"image_id": 42, "category_id": 18, "bbox": [258.15, 41.29, 348.26, 243.78], "score": 0.236}
- from pycocotools.mask import encode
-
- def single_encode(x):
- rle = encode(np.asarray(x[:, :, None], order="F", dtype="uint8"))[0]
- rle["counts"] = rle["counts"].decode("utf-8")
- return rle
-
- image_id = int(path.stem) if path.stem.isnumeric() else path.stem
- box = xyxy2xywh(predn[:, :4]) # xywh
- box[:, :2] -= box[:, 2:] / 2 # xy center to top-left corner
- pred_masks = np.transpose(pred_masks, (2, 0, 1))
- with ThreadPool(NUM_THREADS) as pool:
- rles = pool.map(single_encode, pred_masks)
- for i, (p, b) in enumerate(zip(predn.tolist(), box.tolist())):
- jdict.append(
- {
- "image_id": image_id,
- "category_id": class_map[int(p[5])],
- "bbox": [round(x, 3) for x in b],
- "score": round(p[4], 5),
- "segmentation": rles[i],
- }
- )
-
-
-def process_batch(
- detections,
- labels,
- iouv,
- pred_masks=None,
- gt_masks=None,
- overlap=False,
- masks=False,
-):
- """
- Return correct prediction matrix
- Arguments:
- detections (array[N, 6]), x1, y1, x2, y2, conf, class
- labels (array[M, 5]), class, x1, y1, x2, y2
- Returns:
- correct (array[N, 10]), for 10 IoU levels
- """
- if masks:
- if overlap:
- nl = len(labels)
- index = torch.arange(nl, device=gt_masks.device).view(nl, 1, 1) + 1
- gt_masks = gt_masks.repeat(
- nl, 1, 1
- ) # shape(1,640,640) -> (n,640,640)
- gt_masks = torch.where(gt_masks == index, 1.0, 0.0)
- if gt_masks.shape[1:] != pred_masks.shape[1:]:
- gt_masks = F.interpolate(
- gt_masks[None],
- pred_masks.shape[1:],
- mode="bilinear",
- align_corners=False,
- )[0]
- gt_masks = gt_masks.gt_(0.5)
- iou = mask_iou(
- gt_masks.view(gt_masks.shape[0], -1),
- pred_masks.view(pred_masks.shape[0], -1),
- )
- else: # boxes
- iou = box_iou(labels[:, 1:], detections[:, :4])
-
- correct = np.zeros((detections.shape[0], iouv.shape[0])).astype(bool)
- correct_class = labels[:, 0:1] == detections[:, 5]
- for i in range(len(iouv)):
- x = torch.where(
- (iou >= iouv[i]) & correct_class
- ) # IoU > threshold and classes match
- if x[0].shape[0]:
- matches = (
- torch.cat((torch.stack(x, 1), iou[x[0], x[1]][:, None]), 1)
- .cpu()
- .numpy()
- ) # [label, detect, iou]
- if x[0].shape[0] > 1:
- matches = matches[matches[:, 2].argsort()[::-1]]
- matches = matches[
- np.unique(matches[:, 1], return_index=True)[1]
- ]
- # matches = matches[matches[:, 2].argsort()[::-1]]
- matches = matches[
- np.unique(matches[:, 0], return_index=True)[1]
- ]
- correct[matches[:, 1].astype(int), i] = True
- return torch.tensor(correct, dtype=torch.bool, device=iouv.device)
-
-
-@smart_inference_mode()
-def run(
- data,
- weights=None, # model.pt path(s)
- batch_size=32, # batch size
- imgsz=640, # inference size (pixels)
- conf_thres=0.001, # confidence threshold
- iou_thres=0.6, # NMS IoU threshold
- max_det=300, # maximum detections per image
- task="val", # train, val, test, speed or study
- device="", # cuda device, i.e. 0 or 0,1,2,3 or cpu
- workers=8, # max dataloader workers (per RANK in DDP mode)
- single_cls=False, # treat as single-class dataset
- augment=False, # augmented inference
- verbose=False, # verbose output
- save_txt=False, # save results to *.txt
- save_hybrid=False, # save label+prediction hybrid results to *.txt
- save_conf=False, # save confidences in --save-txt labels
- save_json=False, # save a COCO-JSON results file
- project=ROOT / "runs/val-seg", # save to project/name
- name="exp", # save to project/name
- exist_ok=False, # existing project/name ok, do not increment
- half=True, # use FP16 half-precision inference
- dnn=False, # use OpenCV DNN for ONNX inference
- model=None,
- dataloader=None,
- save_dir=Path(""),
- plots=True,
- overlap=False,
- mask_downsample_ratio=1,
- compute_loss=None,
- callbacks=Callbacks(),
-):
- if save_json:
- check_requirements("pycocotools>=2.0.6")
- process = process_mask_native # more accurate
- else:
- process = process_mask # faster
-
- # Initialize/load model and set device
- training = model is not None
- if training: # called by train.py
- device, pt, jit, engine = (
- next(model.parameters()).device,
- True,
- False,
- False,
- ) # get model device, PyTorch model
- half &= device.type != "cpu" # half precision only supported on CUDA
- model.half() if half else model.float()
- nm = de_parallel(model).model[-1].nm # number of masks
- else: # called directly
- device = select_device(device, batch_size=batch_size)
-
- # Directories
- save_dir = increment_path(
- Path(project) / name, exist_ok=exist_ok
- ) # increment run
- (save_dir / "labels" if save_txt else save_dir).mkdir(
- parents=True, exist_ok=True
- ) # make dir
-
- # Load model
- model = DetectMultiBackend(
- weights, device=device, dnn=dnn, data=data, fp16=half
- )
- stride, pt, jit, engine = (
- model.stride,
- model.pt,
- model.jit,
- model.engine,
- )
- imgsz = check_img_size(imgsz, s=stride) # check image size
- half = model.fp16 # FP16 supported on limited backends with CUDA
- nm = (
- de_parallel(model).model.model[-1].nm
- if isinstance(model, SegmentationModel)
- else 32
- ) # number of masks
- if engine:
- batch_size = model.batch_size
- else:
- device = model.device
- if not (pt or jit):
- batch_size = 1 # export.py models default to batch-size 1
- LOGGER.info(
- f"Forcing --batch-size 1 square inference (1,3,{imgsz},{imgsz}) for non-PyTorch models"
- )
-
- # Data
- data = check_dataset(data) # check
-
- # Configure
- model.eval()
- cuda = device.type != "cpu"
- is_coco = isinstance(data.get("val"), str) and data["val"].endswith(
- f"coco{os.sep}val2017.txt"
- ) # COCO dataset
- nc = 1 if single_cls else int(data["nc"]) # number of classes
- iouv = torch.linspace(
- 0.5, 0.95, 10, device=device
- ) # iou vector for mAP@0.5:0.95
- niou = iouv.numel()
-
- # Dataloader
- if not training:
- if pt and not single_cls: # check --weights are trained on --data
- ncm = model.model.nc
- assert ncm == nc, (
- f"{weights} ({ncm} classes) trained on different --data than what you passed ({nc} "
- f"classes). Pass correct combination of --weights and --data that are trained together."
- )
- model.warmup(
- imgsz=(1 if pt else batch_size, 3, imgsz, imgsz)
- ) # warmup
- pad, rect = (
- (0.0, False) if task == "speed" else (0.5, pt)
- ) # square inference for benchmarks
- task = (
- task if task in ("train", "val", "test") else "val"
- ) # path to train/val/test images
- dataloader = create_dataloader(
- data[task],
- imgsz,
- batch_size,
- stride,
- single_cls,
- pad=pad,
- rect=rect,
- workers=workers,
- prefix=colorstr(f"{task}: "),
- overlap_mask=overlap,
- mask_downsample_ratio=mask_downsample_ratio,
- )[0]
-
- seen = 0
- confusion_matrix = ConfusionMatrix(nc=nc)
- names = (
- model.names if hasattr(model, "names") else model.module.names
- ) # get class names
- if isinstance(names, (list, tuple)): # old format
- names = dict(enumerate(names))
- class_map = coco80_to_coco91_class() if is_coco else list(range(1000))
- s = ("%22s" + "%11s" * 10) % (
- "Class",
- "Images",
- "Instances",
- "Box(P",
- "R",
- "mAP50",
- "mAP50-95)",
- "Mask(P",
- "R",
- "mAP50",
- "mAP50-95)",
- )
- dt = Profile(), Profile(), Profile()
- metrics = Metrics()
- loss = torch.zeros(4, device=device)
- jdict, stats = [], []
- # callbacks.run('on_val_start')
- pbar = tqdm(dataloader, desc=s, bar_format=TQDM_BAR_FORMAT) # progress bar
- for batch_i, (im, targets, paths, shapes, masks) in enumerate(pbar):
- # callbacks.run('on_val_batch_start')
- with dt[0]:
- if cuda:
- im = im.to(device, non_blocking=True)
- targets = targets.to(device)
- masks = masks.to(device)
- masks = masks.float()
- im = im.half() if half else im.float() # uint8 to fp16/32
- im /= 255 # 0 - 255 to 0.0 - 1.0
- (
- nb,
- _,
- height,
- width,
- ) = im.shape # batch size, channels, height, width
-
- # Inference
- with dt[1]:
- preds, protos, train_out = (
- model(im)
- if compute_loss
- else (*model(im, augment=augment)[:2], None)
- )
-
- # Loss
- if compute_loss:
- loss += compute_loss((train_out, protos), targets, masks)[
- 1
- ] # box, obj, cls
-
- # NMS
- targets[:, 2:] *= torch.tensor(
- (width, height, width, height), device=device
- ) # to pixels
- lb = (
- [targets[targets[:, 0] == i, 1:] for i in range(nb)]
- if save_hybrid
- else []
- ) # for autolabelling
- with dt[2]:
- preds = non_max_suppression(
- preds,
- conf_thres,
- iou_thres,
- labels=lb,
- multi_label=True,
- agnostic=single_cls,
- max_det=max_det,
- nm=nm,
- )
-
- # Metrics
- plot_masks = [] # masks for plotting
- for si, (pred, proto) in enumerate(zip(preds, protos)):
- labels = targets[targets[:, 0] == si, 1:]
- nl, npr = (
- labels.shape[0],
- pred.shape[0],
- ) # number of labels, predictions
- path, shape = Path(paths[si]), shapes[si][0]
- correct_masks = torch.zeros(
- npr, niou, dtype=torch.bool, device=device
- ) # init
- correct_bboxes = torch.zeros(
- npr, niou, dtype=torch.bool, device=device
- ) # init
- seen += 1
-
- if npr == 0:
- if nl:
- stats.append(
- (
- correct_masks,
- correct_bboxes,
- *torch.zeros((2, 0), device=device),
- labels[:, 0],
- )
- )
- if plots:
- confusion_matrix.process_batch(
- detections=None, labels=labels[:, 0]
- )
- continue
-
- # Masks
- midx = [si] if overlap else targets[:, 0] == si
- gt_masks = masks[midx]
- pred_masks = process(
- proto, pred[:, 6:], pred[:, :4], shape=im[si].shape[1:]
- )
-
- # Predictions
- if single_cls:
- pred[:, 5] = 0
- predn = pred.clone()
- scale_boxes(
- im[si].shape[1:], predn[:, :4], shape, shapes[si][1]
- ) # native-space pred
-
- # Evaluate
- if nl:
- tbox = xywh2xyxy(labels[:, 1:5]) # target boxes
- scale_boxes(
- im[si].shape[1:], tbox, shape, shapes[si][1]
- ) # native-space labels
- labelsn = torch.cat(
- (labels[:, 0:1], tbox), 1
- ) # native-space labels
- correct_bboxes = process_batch(predn, labelsn, iouv)
- correct_masks = process_batch(
- predn,
- labelsn,
- iouv,
- pred_masks,
- gt_masks,
- overlap=overlap,
- masks=True,
- )
- if plots:
- confusion_matrix.process_batch(predn, labelsn)
- stats.append(
- (
- correct_masks,
- correct_bboxes,
- pred[:, 4],
- pred[:, 5],
- labels[:, 0],
- )
- ) # (conf, pcls, tcls)
-
- pred_masks = torch.as_tensor(pred_masks, dtype=torch.uint8)
- if plots and batch_i < 3:
- plot_masks.append(pred_masks[:15]) # filter top 15 to plot
-
- # Save/log
- if save_txt:
- save_one_txt(
- predn,
- save_conf,
- shape,
- file=save_dir / "labels" / f"{path.stem}.txt",
- )
- if save_json:
- pred_masks = scale_image(
- im[si].shape[1:],
- pred_masks.permute(1, 2, 0).contiguous().cpu().numpy(),
- shape,
- shapes[si][1],
- )
- save_one_json(
- predn, jdict, path, class_map, pred_masks
- ) # append to COCO-JSON dictionary
- # callbacks.run('on_val_image_end', pred, predn, path, names, im[si])
-
- # Plot images
- if plots and batch_i < 3:
- if len(plot_masks):
- plot_masks = torch.cat(plot_masks, dim=0)
- plot_images_and_masks(
- im,
- targets,
- masks,
- paths,
- save_dir / f"val_batch{batch_i}_labels.jpg",
- names,
- )
- plot_images_and_masks(
- im,
- output_to_target(preds, max_det=15),
- plot_masks,
- paths,
- save_dir / f"val_batch{batch_i}_pred.jpg",
- names,
- ) # pred
-
- # callbacks.run('on_val_batch_end')
-
- # Compute metrics
- stats = [torch.cat(x, 0).cpu().numpy() for x in zip(*stats)] # to numpy
- if len(stats) and stats[0].any():
- results = ap_per_class_box_and_mask(
- *stats, plot=plots, save_dir=save_dir, names=names
- )
- metrics.update(results)
- nt = np.bincount(
- stats[4].astype(int), minlength=nc
- ) # number of targets per class
-
- # Print results
- pf = "%22s" + "%11i" * 2 + "%11.3g" * 8 # print format
- LOGGER.info(pf % ("all", seen, nt.sum(), *metrics.mean_results()))
- if nt.sum() == 0:
- LOGGER.warning(
- f"WARNING ⚠️ no labels found in {task} set, can not compute metrics without labels"
- )
-
- # Print results per class
- if (verbose or (nc < 50 and not training)) and nc > 1 and len(stats):
- for i, c in enumerate(metrics.ap_class_index):
- LOGGER.info(pf % (names[c], seen, nt[c], *metrics.class_result(i)))
-
- # Print speeds
- t = tuple(x.t / seen * 1e3 for x in dt) # speeds per image
- if not training:
- shape = (batch_size, 3, imgsz, imgsz)
- LOGGER.info(
- f"Speed: %.1fms pre-process, %.1fms inference, %.1fms NMS per image at shape {shape}"
- % t
- )
-
- # Plots
- if plots:
- confusion_matrix.plot(save_dir=save_dir, names=list(names.values()))
- # callbacks.run('on_val_end')
-
- (
- mp_bbox,
- mr_bbox,
- map50_bbox,
- map_bbox,
- mp_mask,
- mr_mask,
- map50_mask,
- map_mask,
- ) = metrics.mean_results()
-
- # Save JSON
- if save_json and len(jdict):
- w = (
- Path(weights[0] if isinstance(weights, list) else weights).stem
- if weights is not None
- else ""
- ) # weights
- anno_json = str(
- Path("../datasets/coco/annotations/instances_val2017.json")
- ) # annotations
- pred_json = str(save_dir / f"{w}_predictions.json") # predictions
- LOGGER.info(f"\nEvaluating pycocotools mAP... saving {pred_json}...")
- with open(pred_json, "w") as f:
- json.dump(jdict, f)
-
- try: # https://github.com/cocodataset/cocoapi/blob/master/PythonAPI/pycocoEvalDemo.ipynb
- from pycocotools.coco import COCO
- from pycocotools.cocoeval import COCOeval
-
- anno = COCO(anno_json) # init annotations api
- pred = anno.loadRes(pred_json) # init predictions api
- results = []
- for eval in COCOeval(anno, pred, "bbox"), COCOeval(
- anno, pred, "segm"
- ):
- if is_coco:
- eval.params.imgIds = [
- int(Path(x).stem) for x in dataloader.dataset.im_files
- ] # img ID to evaluate
- eval.evaluate()
- eval.accumulate()
- eval.summarize()
- results.extend(
- eval.stats[:2]
- ) # update results (mAP@0.5:0.95, mAP@0.5)
- map_bbox, map50_bbox, map_mask, map50_mask = results
- except Exception as e:
- LOGGER.info(f"pycocotools unable to run: {e}")
-
- # Return results
- model.float() # for training
- if not training:
- s = (
- f"\n{len(list(save_dir.glob('labels/*.txt')))} labels saved to {save_dir / 'labels'}"
- if save_txt
- else ""
- )
- LOGGER.info(f"Results saved to {colorstr('bold', save_dir)}{s}")
- final_metric = (
- mp_bbox,
- mr_bbox,
- map50_bbox,
- map_bbox,
- mp_mask,
- mr_mask,
- map50_mask,
- map_mask,
- )
- return (
- (*final_metric, *(loss.cpu() / len(dataloader)).tolist()),
- metrics.get_maps(nc),
- t,
- )
-
-
-def parse_opt():
- parser = argparse.ArgumentParser()
- parser.add_argument(
- "--data",
- type=str,
- default=ROOT / "data/coco128-seg.yaml",
- help="dataset.yaml path",
- )
- parser.add_argument(
- "--weights",
- nargs="+",
- type=str,
- default=ROOT / "yolov5s-seg.pt",
- help="model path(s)",
- )
- parser.add_argument(
- "--batch-size", type=int, default=32, help="batch size"
- )
- parser.add_argument(
- "--imgsz",
- "--img",
- "--img-size",
- type=int,
- default=640,
- help="inference size (pixels)",
- )
- parser.add_argument(
- "--conf-thres", type=float, default=0.001, help="confidence threshold"
- )
- parser.add_argument(
- "--iou-thres", type=float, default=0.6, help="NMS IoU threshold"
- )
- parser.add_argument(
- "--max-det", type=int, default=300, help="maximum detections per image"
- )
- parser.add_argument(
- "--task", default="val", help="train, val, test, speed or study"
- )
- parser.add_argument(
- "--device", default="", help="cuda device, i.e. 0 or 0,1,2,3 or cpu"
- )
- parser.add_argument(
- "--workers",
- type=int,
- default=8,
- help="max dataloader workers (per RANK in DDP mode)",
- )
- parser.add_argument(
- "--single-cls",
- action="store_true",
- help="treat as single-class dataset",
- )
- parser.add_argument(
- "--augment", action="store_true", help="augmented inference"
- )
- parser.add_argument(
- "--verbose", action="store_true", help="report mAP by class"
- )
- parser.add_argument(
- "--save-txt", action="store_true", help="save results to *.txt"
- )
- parser.add_argument(
- "--save-hybrid",
- action="store_true",
- help="save label+prediction hybrid results to *.txt",
- )
- parser.add_argument(
- "--save-conf",
- action="store_true",
- help="save confidences in --save-txt labels",
- )
- parser.add_argument(
- "--save-json",
- action="store_true",
- help="save a COCO-JSON results file",
- )
- parser.add_argument(
- "--project",
- default=ROOT / "runs/val-seg",
- help="save results to project/name",
- )
- parser.add_argument("--name", default="exp", help="save to project/name")
- parser.add_argument(
- "--exist-ok",
- action="store_true",
- help="existing project/name ok, do not increment",
- )
- parser.add_argument(
- "--half", action="store_true", help="use FP16 half-precision inference"
- )
- parser.add_argument(
- "--dnn", action="store_true", help="use OpenCV DNN for ONNX inference"
- )
- opt = parser.parse_args()
- opt.data = check_yaml(opt.data) # check YAML
- # opt.save_json |= opt.data.endswith('coco.yaml')
- opt.save_txt |= opt.save_hybrid
- print_args(vars(opt))
- return opt
-
-
-def main(opt):
- check_requirements(
- requirements=ROOT / "requirements.txt", exclude=("tensorboard", "thop")
- )
-
- if opt.task in ("train", "val", "test"): # run normally
- if (
- opt.conf_thres > 0.001
- ): # https://github.com/ultralytics/yolov5/issues/1466
- LOGGER.warning(
- f"WARNING ⚠️ confidence threshold {opt.conf_thres} > 0.001 produces invalid results"
- )
- if opt.save_hybrid:
- LOGGER.warning(
- "WARNING ⚠️ --save-hybrid returns high mAP from hybrid labels, not from predictions alone"
- )
- run(**vars(opt))
-
- else:
- weights = (
- opt.weights if isinstance(opt.weights, list) else [opt.weights]
- )
- opt.half = (
- torch.cuda.is_available() and opt.device != "cpu"
- ) # FP16 for fastest results
- if opt.task == "speed": # speed benchmarks
- # python val.py --task speed --data coco.yaml --batch 1 --weights yolov5n.pt yolov5s.pt...
- opt.conf_thres, opt.iou_thres, opt.save_json = 0.25, 0.45, False
- for opt.weights in weights:
- run(**vars(opt), plots=False)
-
- elif opt.task == "study": # speed vs mAP benchmarks
- # python val.py --task study --data coco.yaml --iou 0.7 --weights yolov5n.pt yolov5s.pt...
- for opt.weights in weights:
- f = f"study_{Path(opt.data).stem}_{Path(opt.weights).stem}.txt" # filename to save to
- x, y = (
- list(range(256, 1536 + 128, 128)),
- [],
- ) # x axis (image sizes), y axis
- for opt.imgsz in x: # img-size
- LOGGER.info(f"\nRunning {f} --imgsz {opt.imgsz}...")
- r, _, t = run(**vars(opt), plots=False)
- y.append(r + t) # results and times
- np.savetxt(f, y, fmt="%10.4g") # save
- os.system("zip -r study.zip study_*.txt")
- plot_val_study(x=x) # plot
- else:
- raise NotImplementedError(
- f'--task {opt.task} not in ("train", "val", "test", "speed", "study")'
- )
-
-
-if __name__ == "__main__":
- opt = parse_opt()
- main(opt)
diff --git a/spaces/AchyuthGamer/OpenGPT-Chat-UI/src/lib/utils/randomUuid.ts b/spaces/AchyuthGamer/OpenGPT-Chat-UI/src/lib/utils/randomUuid.ts
deleted file mode 100644
index 9d536365c57659305ad28d6fc06b89d77ab337ab..0000000000000000000000000000000000000000
--- a/spaces/AchyuthGamer/OpenGPT-Chat-UI/src/lib/utils/randomUuid.ts
+++ /dev/null
@@ -1,14 +0,0 @@
-type UUID = ReturnType;
-
-export function randomUUID(): UUID {
- // Only on old safari / ios
- if (!("randomUUID" in crypto)) {
- return "10000000-1000-4000-8000-100000000000".replace(/[018]/g, (c) =>
- (
- Number(c) ^
- (crypto.getRandomValues(new Uint8Array(1))[0] & (15 >> (Number(c) / 4)))
- ).toString(16)
- ) as UUID;
- }
- return crypto.randomUUID();
-}
diff --git a/spaces/AchyuthGamer/OpenGPT/client/css/dropdown.css b/spaces/AchyuthGamer/OpenGPT/client/css/dropdown.css
deleted file mode 100644
index 302e911e84d171c55384732f759a79ce195abca5..0000000000000000000000000000000000000000
--- a/spaces/AchyuthGamer/OpenGPT/client/css/dropdown.css
+++ /dev/null
@@ -1,10 +0,0 @@
-.dropdown {
- border: 1px solid var(--conversations);
-}
-
-@media screen and (max-width: 990px) {
- .dropdown {
- padding: 4px 8px;
- font-size: 0.75rem;
- }
-}
diff --git a/spaces/AgentVerse/agentVerse/ui/src/phaser3-rex-plugins/plugins/checkboxshape.js b/spaces/AgentVerse/agentVerse/ui/src/phaser3-rex-plugins/plugins/checkboxshape.js
deleted file mode 100644
index 8fd1f63e356707ac2115c8660ce2d9573ee53a47..0000000000000000000000000000000000000000
--- a/spaces/AgentVerse/agentVerse/ui/src/phaser3-rex-plugins/plugins/checkboxshape.js
+++ /dev/null
@@ -1,2 +0,0 @@
-import CheckboxShape from './gameobjects/shape/checkbox/CheckboxShape.js';
-export default CheckboxShape;
\ No newline at end of file
diff --git a/spaces/AgentVerse/agentVerse/ui/src/phaser3-rex-plugins/templates/ui/confirmdialog/methods/ResetDisplayContent.js b/spaces/AgentVerse/agentVerse/ui/src/phaser3-rex-plugins/templates/ui/confirmdialog/methods/ResetDisplayContent.js
deleted file mode 100644
index 3a21d143153f52b77d703499a2ab9e1c08ddf851..0000000000000000000000000000000000000000
--- a/spaces/AgentVerse/agentVerse/ui/src/phaser3-rex-plugins/templates/ui/confirmdialog/methods/ResetDisplayContent.js
+++ /dev/null
@@ -1,115 +0,0 @@
-import CreateLabel from '../../utils/build/CreateLabel.js';
-
-var ResetDisplayContent = function (config) {
- if (config === undefined) {
- config = {};
- }
-
- ResetTitle.call(this, config);
- ResetContent.call(this, config);
- ResetActions.call(this, config);
- ResetChoices.call(this, config);
-
- return this;
-}
-
-var ResetTitle = function (config) {
- var title = this.childrenMap.title;
- title.resetDisplayContent(config.title);
-}
-
-var ResetContent = function (config) {
- var content = this.childrenMap.content;
- if (content.resetDisplayContent) {
- // Label
- content.resetDisplayContent(config.content);
- } else {
- // TextArea
- var text = config.content || '';
- content.setText(text)
- }
-}
-
-var ResetActions = function (config) {
- var actionButtons = this.childrenMap.actions;
- if (!actionButtons) {
- return;
- }
-
- var buttonContentArray = config.buttons;
- if (!buttonContentArray) {
- var buttonA = actionButtons[0];
- if (buttonA) {
- buttonA.resetDisplayContent(config.buttonA);
- }
-
- var buttonB = actionButtons[1];
- if (buttonB) {
- buttonB.resetDisplayContent(config.buttonB);
- }
-
- } else {
- var scene = this.scene;
- var defaultActionConfig = this.defaultActionConfig;
- var defaultActionButtonCreator = this.defaultActionButtonCreator;
- for (var i = 0, cnt = buttonContentArray.length; i < cnt; i++) {
- var buttonContent = buttonContentArray[i];
- var button = actionButtons[i];
- if (!button) {
- button = CreateLabel(scene, defaultActionConfig, defaultActionButtonCreator);
- this.addAction(button);
- }
- button.show().resetDisplayContent(buttonContent);
- }
-
- this.buttonMode = buttonContentArray.length;
-
- for (var i = buttonContentArray.length, cnt = actionButtons.length; i < cnt; i++) {
- actionButtons[i].hide();
- }
- }
-}
-
-var ResetChoices = function (config) {
- var choices = this.childrenMap.choices;
- if (!choices) {
- return;
- }
-
- var buttonContentArray = config.choices;
- if (!buttonContentArray) {
- buttonContentArray = [];
- }
-
- var scene = this.scene;
- var defaultChoiceConfig = this.defaultChoiceConfig;
- var defaultActionButtonCreator = this.defaultActionButtonCreator;
- for (var i = 0, cnt = buttonContentArray.length; i < cnt; i++) {
- var buttonContent = buttonContentArray[i];
- if (typeof (buttonContent) === 'string') {
- buttonContent = { text: buttonContent };
- }
-
- var button = choices[i];
- if (!button) {
- button = CreateLabel(scene, defaultChoiceConfig, defaultActionButtonCreator);
- this.addChoice(button);
- }
-
- button.show().resetDisplayContent(buttonContent)
-
- var optionValue;
- if (buttonContent.hasOwnProperty('value')) {
- optionValue = buttonContent.value;
- } else {
- optionValue = buttonContent.text;
- }
- button.setName(optionValue)
- }
-
- for (var i = buttonContentArray.length, cnt = choices.length; i < cnt; i++) {
- choices[i].hide();
- }
-}
-
-export default ResetDisplayContent;
\ No newline at end of file
diff --git a/spaces/AgentVerse/agentVerse/ui/src/phaser3-rex-plugins/templates/ui/perspective/Factory.d.ts b/spaces/AgentVerse/agentVerse/ui/src/phaser3-rex-plugins/templates/ui/perspective/Factory.d.ts
deleted file mode 100644
index 76d799ab4c12dd52a6c5a68ad22b5bb965084d02..0000000000000000000000000000000000000000
--- a/spaces/AgentVerse/agentVerse/ui/src/phaser3-rex-plugins/templates/ui/perspective/Factory.d.ts
+++ /dev/null
@@ -1,7 +0,0 @@
-import Container from '../container/Container';
-import Perspective from './Perspective';
-
-export default function (
- parentContainer: Container,
- config?: Perspective.IConfig
-): Perspective;
\ No newline at end of file
diff --git a/spaces/AlexZou/Deploy_Restoration/SuperResolution.py b/spaces/AlexZou/Deploy_Restoration/SuperResolution.py
deleted file mode 100644
index 7d97d84eb15f663397fffbd71e610f1e59cdbdb8..0000000000000000000000000000000000000000
--- a/spaces/AlexZou/Deploy_Restoration/SuperResolution.py
+++ /dev/null
@@ -1,46 +0,0 @@
-import os
-import torch
-import numpy as np
-from torchvision import transforms
-from PIL import Image
-import time
-import torchvision
-import argparse
-from models.SCET import SCET
-
-def inference_img(img_path,Net):
-
- low_image = Image.open(img_path).convert('RGB')
- enhance_transforms = transforms.Compose([
- transforms.ToTensor()
- ])
-
- with torch.no_grad():
- low_image = enhance_transforms(low_image)
- low_image = low_image.unsqueeze(0)
- start = time.time()
- restored2 = Net(low_image)
- end = time.time()
-
-
- return restored2,end-start
-
-if __name__ == '__main__':
- parser=argparse.ArgumentParser()
- parser.add_argument('--test_path',type=str,required=True,help='Path to test')
- parser.add_argument('--save_path',type=str,required=True,help='Path to save')
- parser.add_argument('--pk_path',type=str,default='model_zoo/SRx4.pth',help='Path of the checkpoint')
- parser.add_argument('--scale',type=int,default=4,help='scale factor')
- opt = parser.parse_args()
- if not os.path.isdir(opt.save_path):
- os.mkdir(opt.save_path)
- if opt.scale == 3:
- Net = SCET(63, 128, opt.scale)
- else:
- Net = SCET(64, 128, opt.scale)
- Net.load_state_dict(torch.load(opt.pk_path, map_location=torch.device('cpu')))
- Net=Net.eval()
- image=opt.test_path
- print(image)
- restored2,time_num=inference_img(image,Net)
- torchvision.utils.save_image(restored2,opt.save_path+'output.png')
diff --git a/spaces/Amrrs/DragGan-Inversion/PTI/configs/hyperparameters.py b/spaces/Amrrs/DragGan-Inversion/PTI/configs/hyperparameters.py
deleted file mode 100644
index 1a4c89323561c3fe0d1f1b0962926ff89b49221e..0000000000000000000000000000000000000000
--- a/spaces/Amrrs/DragGan-Inversion/PTI/configs/hyperparameters.py
+++ /dev/null
@@ -1,28 +0,0 @@
-## Architechture
-lpips_type = "alex"
-first_inv_type = "w"
-optim_type = "adam"
-
-## Locality regularization
-latent_ball_num_of_samples = 1
-locality_regularization_interval = 1
-use_locality_regularization = False
-regulizer_l2_lambda = 0.1
-regulizer_lpips_lambda = 0.1
-regulizer_alpha = 30
-
-## Loss
-pt_l2_lambda = 1
-pt_lpips_lambda = 1
-
-## Steps
-LPIPS_value_threshold = 0.06
-max_pti_steps = 350
-first_inv_steps = 450
-max_images_to_invert = 30
-
-## Optimization
-pti_learning_rate = 3e-4
-first_inv_lr = 5e-3
-train_batch_size = 1
-use_last_w_pivots = False
diff --git a/spaces/Androidonnxfork/CivitAi-to-Diffusers/diffusers/src/diffusers/pipelines/controlnet/pipeline_controlnet_sd_xl.py b/spaces/Androidonnxfork/CivitAi-to-Diffusers/diffusers/src/diffusers/pipelines/controlnet/pipeline_controlnet_sd_xl.py
deleted file mode 100644
index 37723e6e3b2a8a5508ce863562bce64fe80965f0..0000000000000000000000000000000000000000
--- a/spaces/Androidonnxfork/CivitAi-to-Diffusers/diffusers/src/diffusers/pipelines/controlnet/pipeline_controlnet_sd_xl.py
+++ /dev/null
@@ -1,1024 +0,0 @@
-# Copyright 2023 The HuggingFace Team. All rights reserved.
-#
-# Licensed under the Apache License, Version 2.0 (the "License");
-# you may not use this file except in compliance with the License.
-# You may obtain a copy of the License at
-#
-# http://www.apache.org/licenses/LICENSE-2.0
-#
-# Unless required by applicable law or agreed to in writing, software
-# distributed under the License is distributed on an "AS IS" BASIS,
-# WITHOUT WARRANTIES OR CONDITIONS OF ANY KIND, either express or implied.
-# See the License for the specific language governing permissions and
-# limitations under the License.
-
-
-import inspect
-from typing import Any, Callable, Dict, List, Optional, Tuple, Union
-
-import numpy as np
-import PIL.Image
-import torch
-import torch.nn.functional as F
-from transformers import CLIPTextModel, CLIPTextModelWithProjection, CLIPTokenizer
-
-from diffusers.utils.import_utils import is_invisible_watermark_available
-
-from ...image_processor import VaeImageProcessor
-from ...loaders import LoraLoaderMixin, TextualInversionLoaderMixin
-from ...models import AutoencoderKL, ControlNetModel, UNet2DConditionModel
-from ...models.attention_processor import (
- AttnProcessor2_0,
- LoRAAttnProcessor2_0,
- LoRAXFormersAttnProcessor,
- XFormersAttnProcessor,
-)
-from ...schedulers import KarrasDiffusionSchedulers
-from ...utils import (
- is_accelerate_available,
- is_accelerate_version,
- is_compiled_module,
- logging,
- randn_tensor,
- replace_example_docstring,
-)
-from ..pipeline_utils import DiffusionPipeline
-from ..stable_diffusion_xl import StableDiffusionXLPipelineOutput
-
-
-if is_invisible_watermark_available():
- from ..stable_diffusion_xl.watermark import StableDiffusionXLWatermarker
-
-from .multicontrolnet import MultiControlNetModel
-
-
-logger = logging.get_logger(__name__) # pylint: disable=invalid-name
-
-
-EXAMPLE_DOC_STRING = """
- Examples:
- ```py
- >>> # To be updated when there's a useful ControlNet checkpoint
- >>> # compatible with SDXL.
- ```
-"""
-
-
-class StableDiffusionXLControlNetPipeline(DiffusionPipeline, TextualInversionLoaderMixin, LoraLoaderMixin):
- r"""
- Pipeline for text-to-image generation using Stable Diffusion XL with ControlNet guidance.
-
- This model inherits from [`DiffusionPipeline`]. Check the superclass documentation for the generic methods the
- library implements for all the pipelines (such as downloading or saving, running on a particular device, etc.)
-
- In addition the pipeline inherits the following loading methods:
- - *Textual-Inversion*: [`loaders.TextualInversionLoaderMixin.load_textual_inversion`]
- - *LoRA*: [`loaders.LoraLoaderMixin.load_lora_weights`]
-
- Args:
- vae ([`AutoencoderKL`]):
- Variational Auto-Encoder (VAE) Model to encode and decode images to and from latent representations.
- text_encoder ([`CLIPTextModel`]):
- Frozen text-encoder. Stable Diffusion uses the text portion of
- [CLIP](https://huggingface.co/docs/transformers/model_doc/clip#transformers.CLIPTextModel), specifically
- the [clip-vit-large-patch14](https://huggingface.co/openai/clip-vit-large-patch14) variant.
- text_encoder_2 ([` CLIPTextModelWithProjection`]):
- Second frozen text-encoder. Stable Diffusion XL uses the text and pool portion of
- [CLIP](https://huggingface.co/docs/transformers/model_doc/clip#transformers.CLIPTextModelWithProjection),
- specifically the
- [laion/CLIP-ViT-bigG-14-laion2B-39B-b160k](https://huggingface.co/laion/CLIP-ViT-bigG-14-laion2B-39B-b160k)
- variant.
- tokenizer (`CLIPTokenizer`):
- Tokenizer of class
- [CLIPTokenizer](https://huggingface.co/docs/transformers/v4.21.0/en/model_doc/clip#transformers.CLIPTokenizer).
- tokenizer_2 (`CLIPTokenizer`):
- Second Tokenizer of class
- [CLIPTokenizer](https://huggingface.co/docs/transformers/v4.21.0/en/model_doc/clip#transformers.CLIPTokenizer).
- unet ([`UNet2DConditionModel`]): Conditional U-Net architecture to denoise the encoded image latents.
- controlnet ([`ControlNetModel`] or `List[ControlNetModel]`):
- Provides additional conditioning to the unet during the denoising process. If you set multiple ControlNets
- as a list, the outputs from each ControlNet are added together to create one combined additional
- conditioning.
- scheduler ([`SchedulerMixin`]):
- A scheduler to be used in combination with `unet` to denoise the encoded image latents. Can be one of
- [`DDIMScheduler`], [`LMSDiscreteScheduler`], or [`PNDMScheduler`].
- """
-
- def __init__(
- self,
- vae: AutoencoderKL,
- text_encoder: CLIPTextModel,
- text_encoder_2: CLIPTextModelWithProjection,
- tokenizer: CLIPTokenizer,
- tokenizer_2: CLIPTokenizer,
- unet: UNet2DConditionModel,
- controlnet: ControlNetModel,
- scheduler: KarrasDiffusionSchedulers,
- force_zeros_for_empty_prompt: bool = True,
- add_watermarker: Optional[bool] = None,
- ):
- super().__init__()
-
- if isinstance(controlnet, (list, tuple)):
- raise ValueError("MultiControlNet is not yet supported.")
-
- self.register_modules(
- vae=vae,
- text_encoder=text_encoder,
- text_encoder_2=text_encoder_2,
- tokenizer=tokenizer,
- tokenizer_2=tokenizer_2,
- unet=unet,
- controlnet=controlnet,
- scheduler=scheduler,
- )
- self.vae_scale_factor = 2 ** (len(self.vae.config.block_out_channels) - 1)
- self.image_processor = VaeImageProcessor(vae_scale_factor=self.vae_scale_factor, do_convert_rgb=True)
- self.control_image_processor = VaeImageProcessor(
- vae_scale_factor=self.vae_scale_factor, do_convert_rgb=True, do_normalize=False
- )
- add_watermarker = add_watermarker if add_watermarker is not None else is_invisible_watermark_available()
-
- if add_watermarker:
- self.watermark = StableDiffusionXLWatermarker()
- else:
- self.watermark = None
-
- self.register_to_config(force_zeros_for_empty_prompt=force_zeros_for_empty_prompt)
-
- # Copied from diffusers.pipelines.stable_diffusion.pipeline_stable_diffusion.StableDiffusionPipeline.enable_vae_slicing
- def enable_vae_slicing(self):
- r"""
- Enable sliced VAE decoding. When this option is enabled, the VAE will split the input tensor in slices to
- compute decoding in several steps. This is useful to save some memory and allow larger batch sizes.
- """
- self.vae.enable_slicing()
-
- # Copied from diffusers.pipelines.stable_diffusion.pipeline_stable_diffusion.StableDiffusionPipeline.disable_vae_slicing
- def disable_vae_slicing(self):
- r"""
- Disable sliced VAE decoding. If `enable_vae_slicing` was previously enabled, this method will go back to
- computing decoding in one step.
- """
- self.vae.disable_slicing()
-
- # Copied from diffusers.pipelines.stable_diffusion.pipeline_stable_diffusion.StableDiffusionPipeline.enable_vae_tiling
- def enable_vae_tiling(self):
- r"""
- Enable tiled VAE decoding. When this option is enabled, the VAE will split the input tensor into tiles to
- compute decoding and encoding in several steps. This is useful for saving a large amount of memory and to allow
- processing larger images.
- """
- self.vae.enable_tiling()
-
- # Copied from diffusers.pipelines.stable_diffusion.pipeline_stable_diffusion.StableDiffusionPipeline.disable_vae_tiling
- def disable_vae_tiling(self):
- r"""
- Disable tiled VAE decoding. If `enable_vae_tiling` was previously enabled, this method will go back to
- computing decoding in one step.
- """
- self.vae.disable_tiling()
-
- def enable_model_cpu_offload(self, gpu_id=0):
- r"""
- Offloads all models to CPU using accelerate, reducing memory usage with a low impact on performance. Compared
- to `enable_sequential_cpu_offload`, this method moves one whole model at a time to the GPU when its `forward`
- method is called, and the model remains in GPU until the next model runs. Memory savings are lower than with
- `enable_sequential_cpu_offload`, but performance is much better due to the iterative execution of the `unet`.
- """
- if is_accelerate_available() and is_accelerate_version(">=", "0.17.0.dev0"):
- from accelerate import cpu_offload_with_hook
- else:
- raise ImportError("`enable_model_cpu_offload` requires `accelerate v0.17.0` or higher.")
-
- device = torch.device(f"cuda:{gpu_id}")
-
- if self.device.type != "cpu":
- self.to("cpu", silence_dtype_warnings=True)
- torch.cuda.empty_cache() # otherwise we don't see the memory savings (but they probably exist)
-
- model_sequence = (
- [self.text_encoder, self.text_encoder_2] if self.text_encoder is not None else [self.text_encoder_2]
- )
- model_sequence.extend([self.unet, self.vae])
-
- hook = None
- for cpu_offloaded_model in model_sequence:
- _, hook = cpu_offload_with_hook(cpu_offloaded_model, device, prev_module_hook=hook)
-
- cpu_offload_with_hook(self.controlnet, device)
-
- # We'll offload the last model manually.
- self.final_offload_hook = hook
-
- # Copied from diffusers.pipelines.stable_diffusion_xl.pipeline_stable_diffusion_xl.StableDiffusionXLPipeline.encode_prompt
- def encode_prompt(
- self,
- prompt: str,
- prompt_2: Optional[str] = None,
- device: Optional[torch.device] = None,
- num_images_per_prompt: int = 1,
- do_classifier_free_guidance: bool = True,
- negative_prompt: Optional[str] = None,
- negative_prompt_2: Optional[str] = None,
- prompt_embeds: Optional[torch.FloatTensor] = None,
- negative_prompt_embeds: Optional[torch.FloatTensor] = None,
- pooled_prompt_embeds: Optional[torch.FloatTensor] = None,
- negative_pooled_prompt_embeds: Optional[torch.FloatTensor] = None,
- lora_scale: Optional[float] = None,
- ):
- r"""
- Encodes the prompt into text encoder hidden states.
-
- Args:
- prompt (`str` or `List[str]`, *optional*):
- prompt to be encoded
- prompt_2 (`str` or `List[str]`, *optional*):
- The prompt or prompts to be sent to the `tokenizer_2` and `text_encoder_2`. If not defined, `prompt` is
- used in both text-encoders
- device: (`torch.device`):
- torch device
- num_images_per_prompt (`int`):
- number of images that should be generated per prompt
- do_classifier_free_guidance (`bool`):
- whether to use classifier free guidance or not
- negative_prompt (`str` or `List[str]`, *optional*):
- The prompt or prompts not to guide the image generation. If not defined, one has to pass
- `negative_prompt_embeds` instead. Ignored when not using guidance (i.e., ignored if `guidance_scale` is
- less than `1`).
- negative_prompt_2 (`str` or `List[str]`, *optional*):
- The prompt or prompts not to guide the image generation to be sent to `tokenizer_2` and
- `text_encoder_2`. If not defined, `negative_prompt` is used in both text-encoders
- prompt_embeds (`torch.FloatTensor`, *optional*):
- Pre-generated text embeddings. Can be used to easily tweak text inputs, *e.g.* prompt weighting. If not
- provided, text embeddings will be generated from `prompt` input argument.
- negative_prompt_embeds (`torch.FloatTensor`, *optional*):
- Pre-generated negative text embeddings. Can be used to easily tweak text inputs, *e.g.* prompt
- weighting. If not provided, negative_prompt_embeds will be generated from `negative_prompt` input
- argument.
- pooled_prompt_embeds (`torch.FloatTensor`, *optional*):
- Pre-generated pooled text embeddings. Can be used to easily tweak text inputs, *e.g.* prompt weighting.
- If not provided, pooled text embeddings will be generated from `prompt` input argument.
- negative_pooled_prompt_embeds (`torch.FloatTensor`, *optional*):
- Pre-generated negative pooled text embeddings. Can be used to easily tweak text inputs, *e.g.* prompt
- weighting. If not provided, pooled negative_prompt_embeds will be generated from `negative_prompt`
- input argument.
- lora_scale (`float`, *optional*):
- A lora scale that will be applied to all LoRA layers of the text encoder if LoRA layers are loaded.
- """
- device = device or self._execution_device
-
- # set lora scale so that monkey patched LoRA
- # function of text encoder can correctly access it
- if lora_scale is not None and isinstance(self, LoraLoaderMixin):
- self._lora_scale = lora_scale
-
- if prompt is not None and isinstance(prompt, str):
- batch_size = 1
- elif prompt is not None and isinstance(prompt, list):
- batch_size = len(prompt)
- else:
- batch_size = prompt_embeds.shape[0]
-
- # Define tokenizers and text encoders
- tokenizers = [self.tokenizer, self.tokenizer_2] if self.tokenizer is not None else [self.tokenizer_2]
- text_encoders = (
- [self.text_encoder, self.text_encoder_2] if self.text_encoder is not None else [self.text_encoder_2]
- )
-
- if prompt_embeds is None:
- prompt_2 = prompt_2 or prompt
- # textual inversion: procecss multi-vector tokens if necessary
- prompt_embeds_list = []
- prompts = [prompt, prompt_2]
- for prompt, tokenizer, text_encoder in zip(prompts, tokenizers, text_encoders):
- if isinstance(self, TextualInversionLoaderMixin):
- prompt = self.maybe_convert_prompt(prompt, tokenizer)
-
- text_inputs = tokenizer(
- prompt,
- padding="max_length",
- max_length=tokenizer.model_max_length,
- truncation=True,
- return_tensors="pt",
- )
-
- text_input_ids = text_inputs.input_ids
- untruncated_ids = tokenizer(prompt, padding="longest", return_tensors="pt").input_ids
- untruncated_ids = tokenizer(prompt, padding="longest", return_tensors="pt").input_ids
-
- if untruncated_ids.shape[-1] >= text_input_ids.shape[-1] and not torch.equal(
- text_input_ids, untruncated_ids
- ):
- removed_text = tokenizer.batch_decode(untruncated_ids[:, tokenizer.model_max_length - 1 : -1])
- logger.warning(
- "The following part of your input was truncated because CLIP can only handle sequences up to"
- f" {tokenizer.model_max_length} tokens: {removed_text}"
- )
-
- prompt_embeds = text_encoder(
- text_input_ids.to(device),
- output_hidden_states=True,
- )
-
- # We are only ALWAYS interested in the pooled output of the final text encoder
- pooled_prompt_embeds = prompt_embeds[0]
- prompt_embeds = prompt_embeds.hidden_states[-2]
-
- prompt_embeds_list.append(prompt_embeds)
-
- prompt_embeds = torch.concat(prompt_embeds_list, dim=-1)
-
- # get unconditional embeddings for classifier free guidance
- zero_out_negative_prompt = negative_prompt is None and self.config.force_zeros_for_empty_prompt
- if do_classifier_free_guidance and negative_prompt_embeds is None and zero_out_negative_prompt:
- negative_prompt_embeds = torch.zeros_like(prompt_embeds)
- negative_pooled_prompt_embeds = torch.zeros_like(pooled_prompt_embeds)
- elif do_classifier_free_guidance and negative_prompt_embeds is None:
- negative_prompt = negative_prompt or ""
- negative_prompt_2 = negative_prompt_2 or negative_prompt
-
- uncond_tokens: List[str]
- if prompt is not None and type(prompt) is not type(negative_prompt):
- raise TypeError(
- f"`negative_prompt` should be the same type to `prompt`, but got {type(negative_prompt)} !="
- f" {type(prompt)}."
- )
- elif isinstance(negative_prompt, str):
- uncond_tokens = [negative_prompt, negative_prompt_2]
- elif batch_size != len(negative_prompt):
- raise ValueError(
- f"`negative_prompt`: {negative_prompt} has batch size {len(negative_prompt)}, but `prompt`:"
- f" {prompt} has batch size {batch_size}. Please make sure that passed `negative_prompt` matches"
- " the batch size of `prompt`."
- )
- else:
- uncond_tokens = [negative_prompt, negative_prompt_2]
-
- negative_prompt_embeds_list = []
- for negative_prompt, tokenizer, text_encoder in zip(uncond_tokens, tokenizers, text_encoders):
- if isinstance(self, TextualInversionLoaderMixin):
- negative_prompt = self.maybe_convert_prompt(negative_prompt, tokenizer)
-
- max_length = prompt_embeds.shape[1]
- uncond_input = tokenizer(
- negative_prompt,
- padding="max_length",
- max_length=max_length,
- truncation=True,
- return_tensors="pt",
- )
-
- negative_prompt_embeds = text_encoder(
- uncond_input.input_ids.to(device),
- output_hidden_states=True,
- )
- # We are only ALWAYS interested in the pooled output of the final text encoder
- negative_pooled_prompt_embeds = negative_prompt_embeds[0]
- negative_prompt_embeds = negative_prompt_embeds.hidden_states[-2]
-
- negative_prompt_embeds_list.append(negative_prompt_embeds)
-
- negative_prompt_embeds = torch.concat(negative_prompt_embeds_list, dim=-1)
-
- prompt_embeds = prompt_embeds.to(dtype=self.text_encoder_2.dtype, device=device)
- bs_embed, seq_len, _ = prompt_embeds.shape
- # duplicate text embeddings for each generation per prompt, using mps friendly method
- prompt_embeds = prompt_embeds.repeat(1, num_images_per_prompt, 1)
- prompt_embeds = prompt_embeds.view(bs_embed * num_images_per_prompt, seq_len, -1)
-
- if do_classifier_free_guidance:
- # duplicate unconditional embeddings for each generation per prompt, using mps friendly method
- seq_len = negative_prompt_embeds.shape[1]
- negative_prompt_embeds = negative_prompt_embeds.to(dtype=self.text_encoder_2.dtype, device=device)
- negative_prompt_embeds = negative_prompt_embeds.repeat(1, num_images_per_prompt, 1)
- negative_prompt_embeds = negative_prompt_embeds.view(batch_size * num_images_per_prompt, seq_len, -1)
-
- pooled_prompt_embeds = pooled_prompt_embeds.repeat(1, num_images_per_prompt).view(
- bs_embed * num_images_per_prompt, -1
- )
- if do_classifier_free_guidance:
- negative_pooled_prompt_embeds = negative_pooled_prompt_embeds.repeat(1, num_images_per_prompt).view(
- bs_embed * num_images_per_prompt, -1
- )
-
- return prompt_embeds, negative_prompt_embeds, pooled_prompt_embeds, negative_pooled_prompt_embeds
-
- # Copied from diffusers.pipelines.stable_diffusion.pipeline_stable_diffusion.StableDiffusionPipeline.prepare_extra_step_kwargs
- def prepare_extra_step_kwargs(self, generator, eta):
- # prepare extra kwargs for the scheduler step, since not all schedulers have the same signature
- # eta (η) is only used with the DDIMScheduler, it will be ignored for other schedulers.
- # eta corresponds to η in DDIM paper: https://arxiv.org/abs/2010.02502
- # and should be between [0, 1]
-
- accepts_eta = "eta" in set(inspect.signature(self.scheduler.step).parameters.keys())
- extra_step_kwargs = {}
- if accepts_eta:
- extra_step_kwargs["eta"] = eta
-
- # check if the scheduler accepts generator
- accepts_generator = "generator" in set(inspect.signature(self.scheduler.step).parameters.keys())
- if accepts_generator:
- extra_step_kwargs["generator"] = generator
- return extra_step_kwargs
-
- def check_inputs(
- self,
- prompt,
- prompt_2,
- image,
- callback_steps,
- negative_prompt=None,
- negative_prompt_2=None,
- prompt_embeds=None,
- negative_prompt_embeds=None,
- controlnet_conditioning_scale=1.0,
- control_guidance_start=0.0,
- control_guidance_end=1.0,
- ):
- if (callback_steps is None) or (
- callback_steps is not None and (not isinstance(callback_steps, int) or callback_steps <= 0)
- ):
- raise ValueError(
- f"`callback_steps` has to be a positive integer but is {callback_steps} of type"
- f" {type(callback_steps)}."
- )
-
- if prompt is not None and prompt_embeds is not None:
- raise ValueError(
- f"Cannot forward both `prompt`: {prompt} and `prompt_embeds`: {prompt_embeds}. Please make sure to"
- " only forward one of the two."
- )
- elif prompt_2 is not None and prompt_embeds is not None:
- raise ValueError(
- f"Cannot forward both `prompt_2`: {prompt_2} and `prompt_embeds`: {prompt_embeds}. Please make sure to"
- " only forward one of the two."
- )
- elif prompt is None and prompt_embeds is None:
- raise ValueError(
- "Provide either `prompt` or `prompt_embeds`. Cannot leave both `prompt` and `prompt_embeds` undefined."
- )
- elif prompt is not None and (not isinstance(prompt, str) and not isinstance(prompt, list)):
- raise ValueError(f"`prompt` has to be of type `str` or `list` but is {type(prompt)}")
- elif prompt_2 is not None and (not isinstance(prompt_2, str) and not isinstance(prompt_2, list)):
- raise ValueError(f"`prompt_2` has to be of type `str` or `list` but is {type(prompt_2)}")
-
- if negative_prompt is not None and negative_prompt_embeds is not None:
- raise ValueError(
- f"Cannot forward both `negative_prompt`: {negative_prompt} and `negative_prompt_embeds`:"
- f" {negative_prompt_embeds}. Please make sure to only forward one of the two."
- )
- elif negative_prompt_2 is not None and negative_prompt_embeds is not None:
- raise ValueError(
- f"Cannot forward both `negative_prompt_2`: {negative_prompt_2} and `negative_prompt_embeds`:"
- f" {negative_prompt_embeds}. Please make sure to only forward one of the two."
- )
-
- if prompt_embeds is not None and negative_prompt_embeds is not None:
- if prompt_embeds.shape != negative_prompt_embeds.shape:
- raise ValueError(
- "`prompt_embeds` and `negative_prompt_embeds` must have the same shape when passed directly, but"
- f" got: `prompt_embeds` {prompt_embeds.shape} != `negative_prompt_embeds`"
- f" {negative_prompt_embeds.shape}."
- )
-
- # Check `image`
- is_compiled = hasattr(F, "scaled_dot_product_attention") and isinstance(
- self.controlnet, torch._dynamo.eval_frame.OptimizedModule
- )
- if (
- isinstance(self.controlnet, ControlNetModel)
- or is_compiled
- and isinstance(self.controlnet._orig_mod, ControlNetModel)
- ):
- self.check_image(image, prompt, prompt_embeds)
- else:
- assert False
-
- # Check `controlnet_conditioning_scale`
- if (
- isinstance(self.controlnet, ControlNetModel)
- or is_compiled
- and isinstance(self.controlnet._orig_mod, ControlNetModel)
- ):
- if not isinstance(controlnet_conditioning_scale, float):
- raise TypeError("For single controlnet: `controlnet_conditioning_scale` must be type `float`.")
- else:
- assert False
-
- if len(control_guidance_start) != len(control_guidance_end):
- raise ValueError(
- f"`control_guidance_start` has {len(control_guidance_start)} elements, but `control_guidance_end` has {len(control_guidance_end)} elements. Make sure to provide the same number of elements to each list."
- )
-
- for start, end in zip(control_guidance_start, control_guidance_end):
- if start >= end:
- raise ValueError(
- f"control guidance start: {start} cannot be larger or equal to control guidance end: {end}."
- )
- if start < 0.0:
- raise ValueError(f"control guidance start: {start} can't be smaller than 0.")
- if end > 1.0:
- raise ValueError(f"control guidance end: {end} can't be larger than 1.0.")
-
- def check_image(self, image, prompt, prompt_embeds):
- image_is_pil = isinstance(image, PIL.Image.Image)
- image_is_tensor = isinstance(image, torch.Tensor)
- image_is_np = isinstance(image, np.ndarray)
- image_is_pil_list = isinstance(image, list) and isinstance(image[0], PIL.Image.Image)
- image_is_tensor_list = isinstance(image, list) and isinstance(image[0], torch.Tensor)
- image_is_np_list = isinstance(image, list) and isinstance(image[0], np.ndarray)
-
- if (
- not image_is_pil
- and not image_is_tensor
- and not image_is_np
- and not image_is_pil_list
- and not image_is_tensor_list
- and not image_is_np_list
- ):
- raise TypeError(
- f"image must be passed and be one of PIL image, numpy array, torch tensor, list of PIL images, list of numpy arrays or list of torch tensors, but is {type(image)}"
- )
-
- if image_is_pil:
- image_batch_size = 1
- else:
- image_batch_size = len(image)
-
- if prompt is not None and isinstance(prompt, str):
- prompt_batch_size = 1
- elif prompt is not None and isinstance(prompt, list):
- prompt_batch_size = len(prompt)
- elif prompt_embeds is not None:
- prompt_batch_size = prompt_embeds.shape[0]
-
- if image_batch_size != 1 and image_batch_size != prompt_batch_size:
- raise ValueError(
- f"If image batch size is not 1, image batch size must be same as prompt batch size. image batch size: {image_batch_size}, prompt batch size: {prompt_batch_size}"
- )
-
- def prepare_image(
- self,
- image,
- width,
- height,
- batch_size,
- num_images_per_prompt,
- device,
- dtype,
- do_classifier_free_guidance=False,
- guess_mode=False,
- ):
- image = self.control_image_processor.preprocess(image, height=height, width=width).to(dtype=torch.float32)
- image_batch_size = image.shape[0]
-
- if image_batch_size == 1:
- repeat_by = batch_size
- else:
- # image batch size is the same as prompt batch size
- repeat_by = num_images_per_prompt
-
- image = image.repeat_interleave(repeat_by, dim=0)
-
- image = image.to(device=device, dtype=dtype)
-
- if do_classifier_free_guidance and not guess_mode:
- image = torch.cat([image] * 2)
-
- return image
-
- # Copied from diffusers.pipelines.stable_diffusion.pipeline_stable_diffusion.StableDiffusionPipeline.prepare_latents
- def prepare_latents(self, batch_size, num_channels_latents, height, width, dtype, device, generator, latents=None):
- shape = (batch_size, num_channels_latents, height // self.vae_scale_factor, width // self.vae_scale_factor)
- if isinstance(generator, list) and len(generator) != batch_size:
- raise ValueError(
- f"You have passed a list of generators of length {len(generator)}, but requested an effective batch"
- f" size of {batch_size}. Make sure the batch size matches the length of the generators."
- )
-
- if latents is None:
- latents = randn_tensor(shape, generator=generator, device=device, dtype=dtype)
- else:
- latents = latents.to(device)
-
- # scale the initial noise by the standard deviation required by the scheduler
- latents = latents * self.scheduler.init_noise_sigma
- return latents
-
- # Copied from diffusers.pipelines.stable_diffusion_xl.pipeline_stable_diffusion_xl.StableDiffusionXLPipeline._get_add_time_ids
- def _get_add_time_ids(self, original_size, crops_coords_top_left, target_size, dtype):
- add_time_ids = list(original_size + crops_coords_top_left + target_size)
-
- passed_add_embed_dim = (
- self.unet.config.addition_time_embed_dim * len(add_time_ids) + self.text_encoder_2.config.projection_dim
- )
- expected_add_embed_dim = self.unet.add_embedding.linear_1.in_features
-
- if expected_add_embed_dim != passed_add_embed_dim:
- raise ValueError(
- f"Model expects an added time embedding vector of length {expected_add_embed_dim}, but a vector of {passed_add_embed_dim} was created. The model has an incorrect config. Please check `unet.config.time_embedding_type` and `text_encoder_2.config.projection_dim`."
- )
-
- add_time_ids = torch.tensor([add_time_ids], dtype=dtype)
- return add_time_ids
-
- # Copied from diffusers.pipelines.stable_diffusion.pipeline_stable_diffusion_upscale.StableDiffusionUpscalePipeline.upcast_vae
- def upcast_vae(self):
- dtype = self.vae.dtype
- self.vae.to(dtype=torch.float32)
- use_torch_2_0_or_xformers = isinstance(
- self.vae.decoder.mid_block.attentions[0].processor,
- (
- AttnProcessor2_0,
- XFormersAttnProcessor,
- LoRAXFormersAttnProcessor,
- LoRAAttnProcessor2_0,
- ),
- )
- # if xformers or torch_2_0 is used attention block does not need
- # to be in float32 which can save lots of memory
- if use_torch_2_0_or_xformers:
- self.vae.post_quant_conv.to(dtype)
- self.vae.decoder.conv_in.to(dtype)
- self.vae.decoder.mid_block.to(dtype)
-
- @torch.no_grad()
- @replace_example_docstring(EXAMPLE_DOC_STRING)
- def __call__(
- self,
- prompt: Union[str, List[str]] = None,
- prompt_2: Optional[Union[str, List[str]]] = None,
- image: Union[
- torch.FloatTensor,
- PIL.Image.Image,
- np.ndarray,
- List[torch.FloatTensor],
- List[PIL.Image.Image],
- List[np.ndarray],
- ] = None,
- height: Optional[int] = None,
- width: Optional[int] = None,
- num_inference_steps: int = 50,
- guidance_scale: float = 5.0,
- negative_prompt: Optional[Union[str, List[str]]] = None,
- negative_prompt_2: Optional[Union[str, List[str]]] = None,
- num_images_per_prompt: Optional[int] = 1,
- eta: float = 0.0,
- generator: Optional[Union[torch.Generator, List[torch.Generator]]] = None,
- latents: Optional[torch.FloatTensor] = None,
- prompt_embeds: Optional[torch.FloatTensor] = None,
- negative_prompt_embeds: Optional[torch.FloatTensor] = None,
- output_type: Optional[str] = "pil",
- return_dict: bool = True,
- callback: Optional[Callable[[int, int, torch.FloatTensor], None]] = None,
- callback_steps: int = 1,
- cross_attention_kwargs: Optional[Dict[str, Any]] = None,
- controlnet_conditioning_scale: Union[float, List[float]] = 1.0,
- guess_mode: bool = False,
- control_guidance_start: Union[float, List[float]] = 0.0,
- control_guidance_end: Union[float, List[float]] = 1.0,
- original_size: Tuple[int, int] = None,
- crops_coords_top_left: Tuple[int, int] = (0, 0),
- target_size: Tuple[int, int] = None,
- ):
- r"""
- Function invoked when calling the pipeline for generation.
-
- Args:
- prompt (`str` or `List[str]`, *optional*):
- The prompt or prompts to guide the image generation. If not defined, one has to pass `prompt_embeds`.
- instead.
- prompt_2 (`str` or `List[str]`, *optional*):
- The prompt or prompts to be sent to the `tokenizer_2` and `text_encoder_2`. If not defined, `prompt` is
- used in both text-encoders
- image (`torch.FloatTensor`, `PIL.Image.Image`, `np.ndarray`, `List[torch.FloatTensor]`, `List[PIL.Image.Image]`, `List[np.ndarray]`,:
- `List[List[torch.FloatTensor]]`, `List[List[np.ndarray]]` or `List[List[PIL.Image.Image]]`):
- The ControlNet input condition. ControlNet uses this input condition to generate guidance to Unet. If
- the type is specified as `Torch.FloatTensor`, it is passed to ControlNet as is. `PIL.Image.Image` can
- also be accepted as an image. The dimensions of the output image defaults to `image`'s dimensions. If
- height and/or width are passed, `image` is resized according to them. If multiple ControlNets are
- specified in init, images must be passed as a list such that each element of the list can be correctly
- batched for input to a single controlnet.
- height (`int`, *optional*, defaults to self.unet.config.sample_size * self.vae_scale_factor):
- The height in pixels of the generated image.
- width (`int`, *optional*, defaults to self.unet.config.sample_size * self.vae_scale_factor):
- The width in pixels of the generated image.
- num_inference_steps (`int`, *optional*, defaults to 50):
- The number of denoising steps. More denoising steps usually lead to a higher quality image at the
- expense of slower inference.
- guidance_scale (`float`, *optional*, defaults to 7.5):
- Guidance scale as defined in [Classifier-Free Diffusion Guidance](https://arxiv.org/abs/2207.12598).
- `guidance_scale` is defined as `w` of equation 2. of [Imagen
- Paper](https://arxiv.org/pdf/2205.11487.pdf). Guidance scale is enabled by setting `guidance_scale >
- 1`. Higher guidance scale encourages to generate images that are closely linked to the text `prompt`,
- usually at the expense of lower image quality.
- negative_prompt (`str` or `List[str]`, *optional*):
- The prompt or prompts not to guide the image generation. If not defined, one has to pass
- `negative_prompt_embeds` instead. Ignored when not using guidance (i.e., ignored if `guidance_scale` is
- less than `1`).
- negative_prompt_2 (`str` or `List[str]`, *optional*):
- The prompt or prompts not to guide the image generation to be sent to `tokenizer_2` and
- `text_encoder_2`. If not defined, `negative_prompt` is used in both text-encoders
- num_images_per_prompt (`int`, *optional*, defaults to 1):
- The number of images to generate per prompt.
- eta (`float`, *optional*, defaults to 0.0):
- Corresponds to parameter eta (η) in the DDIM paper: https://arxiv.org/abs/2010.02502. Only applies to
- [`schedulers.DDIMScheduler`], will be ignored for others.
- generator (`torch.Generator` or `List[torch.Generator]`, *optional*):
- One or a list of [torch generator(s)](https://pytorch.org/docs/stable/generated/torch.Generator.html)
- to make generation deterministic.
- latents (`torch.FloatTensor`, *optional*):
- Pre-generated noisy latents, sampled from a Gaussian distribution, to be used as inputs for image
- generation. Can be used to tweak the same generation with different prompts. If not provided, a latents
- tensor will ge generated by sampling using the supplied random `generator`.
- prompt_embeds (`torch.FloatTensor`, *optional*):
- Pre-generated text embeddings. Can be used to easily tweak text inputs, *e.g.* prompt weighting. If not
- provided, text embeddings will be generated from `prompt` input argument.
- negative_prompt_embeds (`torch.FloatTensor`, *optional*):
- Pre-generated negative text embeddings. Can be used to easily tweak text inputs, *e.g.* prompt
- weighting. If not provided, negative_prompt_embeds will be generated from `negative_prompt` input
- argument.
- output_type (`str`, *optional*, defaults to `"pil"`):
- The output format of the generate image. Choose between
- [PIL](https://pillow.readthedocs.io/en/stable/): `PIL.Image.Image` or `np.array`.
- return_dict (`bool`, *optional*, defaults to `True`):
- Whether or not to return a [`~pipelines.stable_diffusion.StableDiffusionPipelineOutput`] instead of a
- plain tuple.
- callback (`Callable`, *optional*):
- A function that will be called every `callback_steps` steps during inference. The function will be
- called with the following arguments: `callback(step: int, timestep: int, latents: torch.FloatTensor)`.
- callback_steps (`int`, *optional*, defaults to 1):
- The frequency at which the `callback` function will be called. If not specified, the callback will be
- called at every step.
- cross_attention_kwargs (`dict`, *optional*):
- A kwargs dictionary that if specified is passed along to the `AttentionProcessor` as defined under
- `self.processor` in
- [diffusers.cross_attention](https://github.com/huggingface/diffusers/blob/main/src/diffusers/models/cross_attention.py).
- controlnet_conditioning_scale (`float` or `List[float]`, *optional*, defaults to 1.0):
- The outputs of the controlnet are multiplied by `controlnet_conditioning_scale` before they are added
- to the residual in the original unet. If multiple ControlNets are specified in init, you can set the
- corresponding scale as a list.
- guess_mode (`bool`, *optional*, defaults to `False`):
- In this mode, the ControlNet encoder will try best to recognize the content of the input image even if
- you remove all prompts. The `guidance_scale` between 3.0 and 5.0 is recommended.
- control_guidance_start (`float` or `List[float]`, *optional*, defaults to 0.0):
- The percentage of total steps at which the controlnet starts applying.
- control_guidance_end (`float` or `List[float]`, *optional*, defaults to 1.0):
- The percentage of total steps at which the controlnet stops applying.
- original_size (`Tuple[int]`, *optional*, defaults to (1024, 1024)):
- If `original_size` is not the same as `target_size` the image will appear to be down- or upsampled.
- `original_size` defaults to `(width, height)` if not specified. Part of SDXL's micro-conditioning as
- explained in section 2.2 of
- [https://huggingface.co/papers/2307.01952](https://huggingface.co/papers/2307.01952).
- crops_coords_top_left (`Tuple[int]`, *optional*, defaults to (0, 0)):
- `crops_coords_top_left` can be used to generate an image that appears to be "cropped" from the position
- `crops_coords_top_left` downwards. Favorable, well-centered images are usually achieved by setting
- `crops_coords_top_left` to (0, 0). Part of SDXL's micro-conditioning as explained in section 2.2 of
- [https://huggingface.co/papers/2307.01952](https://huggingface.co/papers/2307.01952).
- target_size (`Tuple[int]`, *optional*, defaults to (1024, 1024)):
- For most cases, `target_size` should be set to the desired height and width of the generated image. If
- not specified it will default to `(width, height)`. Part of SDXL's micro-conditioning as explained in
- section 2.2 of [https://huggingface.co/papers/2307.01952](https://huggingface.co/papers/2307.01952).
- Examples:
-
- Returns:
- [`~pipelines.stable_diffusion.StableDiffusionPipelineOutput`] or `tuple`:
- [`~pipelines.stable_diffusion.StableDiffusionPipelineOutput`] if `return_dict` is True, otherwise a `tuple`
- containing the output images.
- """
- controlnet = self.controlnet._orig_mod if is_compiled_module(self.controlnet) else self.controlnet
-
- # align format for control guidance
- if not isinstance(control_guidance_start, list) and isinstance(control_guidance_end, list):
- control_guidance_start = len(control_guidance_end) * [control_guidance_start]
- elif not isinstance(control_guidance_end, list) and isinstance(control_guidance_start, list):
- control_guidance_end = len(control_guidance_start) * [control_guidance_end]
- elif not isinstance(control_guidance_start, list) and not isinstance(control_guidance_end, list):
- mult = len(controlnet.nets) if isinstance(controlnet, MultiControlNetModel) else 1
- control_guidance_start, control_guidance_end = mult * [control_guidance_start], mult * [
- control_guidance_end
- ]
-
- # 1. Check inputs. Raise error if not correct
- self.check_inputs(
- prompt,
- prompt_2,
- image,
- callback_steps,
- negative_prompt,
- negative_prompt_2,
- prompt_embeds,
- negative_prompt_embeds,
- controlnet_conditioning_scale,
- control_guidance_start,
- control_guidance_end,
- )
-
- # 2. Define call parameters
- if prompt is not None and isinstance(prompt, str):
- batch_size = 1
- elif prompt is not None and isinstance(prompt, list):
- batch_size = len(prompt)
- else:
- batch_size = prompt_embeds.shape[0]
-
- device = self._execution_device
- # here `guidance_scale` is defined analog to the guidance weight `w` of equation (2)
- # of the Imagen paper: https://arxiv.org/pdf/2205.11487.pdf . `guidance_scale = 1`
- # corresponds to doing no classifier free guidance.
- do_classifier_free_guidance = guidance_scale > 1.0
-
- global_pool_conditions = (
- controlnet.config.global_pool_conditions
- if isinstance(controlnet, ControlNetModel)
- else controlnet.nets[0].config.global_pool_conditions
- )
- guess_mode = guess_mode or global_pool_conditions
-
- # 3. Encode input prompt
- text_encoder_lora_scale = (
- cross_attention_kwargs.get("scale", None) if cross_attention_kwargs is not None else None
- )
- (
- prompt_embeds,
- negative_prompt_embeds,
- pooled_prompt_embeds,
- negative_pooled_prompt_embeds,
- ) = self.encode_prompt(
- prompt,
- prompt_2,
- device,
- num_images_per_prompt,
- do_classifier_free_guidance,
- negative_prompt,
- negative_prompt_2,
- prompt_embeds=prompt_embeds,
- negative_prompt_embeds=negative_prompt_embeds,
- lora_scale=text_encoder_lora_scale,
- )
-
- # 4. Prepare image
- if isinstance(controlnet, ControlNetModel):
- image = self.prepare_image(
- image=image,
- width=width,
- height=height,
- batch_size=batch_size * num_images_per_prompt,
- num_images_per_prompt=num_images_per_prompt,
- device=device,
- dtype=controlnet.dtype,
- do_classifier_free_guidance=do_classifier_free_guidance,
- guess_mode=guess_mode,
- )
- height, width = image.shape[-2:]
- else:
- assert False
-
- # 5. Prepare timesteps
- self.scheduler.set_timesteps(num_inference_steps, device=device)
- timesteps = self.scheduler.timesteps
-
- # 6. Prepare latent variables
- num_channels_latents = self.unet.config.in_channels
- latents = self.prepare_latents(
- batch_size * num_images_per_prompt,
- num_channels_latents,
- height,
- width,
- prompt_embeds.dtype,
- device,
- generator,
- latents,
- )
-
- # 7. Prepare extra step kwargs. TODO: Logic should ideally just be moved out of the pipeline
- extra_step_kwargs = self.prepare_extra_step_kwargs(generator, eta)
-
- # 7.1 Create tensor stating which controlnets to keep
- controlnet_keep = []
- for i in range(len(timesteps)):
- keeps = [
- 1.0 - float(i / len(timesteps) < s or (i + 1) / len(timesteps) > e)
- for s, e in zip(control_guidance_start, control_guidance_end)
- ]
- controlnet_keep.append(keeps[0] if len(keeps) == 1 else keeps)
-
- original_size = original_size or image.shape[-2:]
- target_size = target_size or (height, width)
-
- # 7.2 Prepare added time ids & embeddings
- add_text_embeds = pooled_prompt_embeds
- add_time_ids = self._get_add_time_ids(
- original_size, crops_coords_top_left, target_size, dtype=prompt_embeds.dtype
- )
-
- if do_classifier_free_guidance:
- prompt_embeds = torch.cat([negative_prompt_embeds, prompt_embeds], dim=0)
- add_text_embeds = torch.cat([negative_pooled_prompt_embeds, add_text_embeds], dim=0)
- add_time_ids = torch.cat([add_time_ids, add_time_ids], dim=0)
-
- prompt_embeds = prompt_embeds.to(device)
- add_text_embeds = add_text_embeds.to(device)
- add_time_ids = add_time_ids.to(device).repeat(batch_size * num_images_per_prompt, 1)
-
- # 8. Denoising loop
- num_warmup_steps = len(timesteps) - num_inference_steps * self.scheduler.order
- with self.progress_bar(total=num_inference_steps) as progress_bar:
- for i, t in enumerate(timesteps):
- # expand the latents if we are doing classifier free guidance
- latent_model_input = torch.cat([latents] * 2) if do_classifier_free_guidance else latents
- latent_model_input = self.scheduler.scale_model_input(latent_model_input, t)
-
- # controlnet(s) inference
- if guess_mode and do_classifier_free_guidance:
- # Infer ControlNet only for the conditional batch.
- control_model_input = latents
- control_model_input = self.scheduler.scale_model_input(control_model_input, t)
- controlnet_prompt_embeds = prompt_embeds.chunk(2)[1]
- else:
- control_model_input = latent_model_input
- controlnet_prompt_embeds = prompt_embeds
-
- if isinstance(controlnet_keep[i], list):
- cond_scale = [c * s for c, s in zip(controlnet_conditioning_scale, controlnet_keep[i])]
- else:
- cond_scale = controlnet_conditioning_scale * controlnet_keep[i]
-
- added_cond_kwargs = {"text_embeds": add_text_embeds, "time_ids": add_time_ids}
- down_block_res_samples, mid_block_res_sample = self.controlnet(
- control_model_input,
- t,
- encoder_hidden_states=controlnet_prompt_embeds,
- controlnet_cond=image,
- conditioning_scale=cond_scale,
- guess_mode=guess_mode,
- added_cond_kwargs=added_cond_kwargs,
- return_dict=False,
- )
-
- if guess_mode and do_classifier_free_guidance:
- # Infered ControlNet only for the conditional batch.
- # To apply the output of ControlNet to both the unconditional and conditional batches,
- # add 0 to the unconditional batch to keep it unchanged.
- down_block_res_samples = [torch.cat([torch.zeros_like(d), d]) for d in down_block_res_samples]
- mid_block_res_sample = torch.cat([torch.zeros_like(mid_block_res_sample), mid_block_res_sample])
-
- # predict the noise residual
- noise_pred = self.unet(
- latent_model_input,
- t,
- encoder_hidden_states=prompt_embeds,
- cross_attention_kwargs=cross_attention_kwargs,
- down_block_additional_residuals=down_block_res_samples,
- mid_block_additional_residual=mid_block_res_sample,
- added_cond_kwargs=added_cond_kwargs,
- return_dict=False,
- )[0]
-
- # perform guidance
- if do_classifier_free_guidance:
- noise_pred_uncond, noise_pred_text = noise_pred.chunk(2)
- noise_pred = noise_pred_uncond + guidance_scale * (noise_pred_text - noise_pred_uncond)
-
- # compute the previous noisy sample x_t -> x_t-1
- latents = self.scheduler.step(noise_pred, t, latents, **extra_step_kwargs, return_dict=False)[0]
-
- # call the callback, if provided
- if i == len(timesteps) - 1 or ((i + 1) > num_warmup_steps and (i + 1) % self.scheduler.order == 0):
- progress_bar.update()
- if callback is not None and i % callback_steps == 0:
- callback(i, t, latents)
-
- # If we do sequential model offloading, let's offload unet and controlnet
- # manually for max memory savings
- if hasattr(self, "final_offload_hook") and self.final_offload_hook is not None:
- self.unet.to("cpu")
- self.controlnet.to("cpu")
- torch.cuda.empty_cache()
-
- # make sure the VAE is in float32 mode, as it overflows in float16
- if self.vae.dtype == torch.float16 and self.vae.config.force_upcast:
- self.upcast_vae()
- latents = latents.to(next(iter(self.vae.post_quant_conv.parameters())).dtype)
-
- if not output_type == "latent":
- image = self.vae.decode(latents / self.vae.config.scaling_factor, return_dict=False)[0]
- else:
- image = latents
- return StableDiffusionXLPipelineOutput(images=image)
-
- # apply watermark if available
- if self.watermark is not None:
- image = self.watermark.apply_watermark(image)
-
- image = self.image_processor.postprocess(image, output_type=output_type)
-
- # Offload last model to CPU
- if hasattr(self, "final_offload_hook") and self.final_offload_hook is not None:
- self.final_offload_hook.offload()
-
- if not return_dict:
- return (image,)
-
- return StableDiffusionXLPipelineOutput(images=image)
diff --git a/spaces/Andy1621/uniformer_image_detection/configs/paa/README.md b/spaces/Andy1621/uniformer_image_detection/configs/paa/README.md
deleted file mode 100644
index 9960dcf9c16038db3d8379ab910d2cfbe85d22de..0000000000000000000000000000000000000000
--- a/spaces/Andy1621/uniformer_image_detection/configs/paa/README.md
+++ /dev/null
@@ -1,35 +0,0 @@
-# Probabilistic Anchor Assignment with IoU Prediction for Object Detection
-
-[ALGORITHM]
-
-```latex
-@inproceedings{paa-eccv2020,
- title={Probabilistic Anchor Assignment with IoU Prediction for Object Detection},
- author={Kim, Kang and Lee, Hee Seok},
- booktitle = {ECCV},
- year={2020}
-}
-```
-
-## Results and Models
-
-We provide config files to reproduce the object detection results in the
-ECCV 2020 paper for Probabilistic Anchor Assignment with IoU
-Prediction for Object Detection.
-
-| Backbone | Lr schd | Mem (GB) | Score voting | box AP | Config | Download |
-|:-----------:|:-------:|:--------:|:------------:|:------:|:------:|:--------:|
-| R-50-FPN | 12e | 3.7 | True | 40.4 | [config](https://github.com/open-mmlab/mmdetection/tree/master/configs/paa/paa_r50_fpn_1x_coco.py) | [model](http://download.openmmlab.com/mmdetection/v2.0/paa/paa_r50_fpn_1x_coco/paa_r50_fpn_1x_coco_20200821-936edec3.pth) | [log](http://download.openmmlab.com/mmdetection/v2.0/paa/paa_r50_fpn_1x_coco/paa_r50_fpn_1x_coco_20200821-936edec3.log.json) |
-| R-50-FPN | 12e | 3.7 | False | 40.2 | - |
-| R-50-FPN | 18e | 3.7 | True | 41.4 | [config](https://github.com/open-mmlab/mmdetection/tree/master/configs/paa/paa_r50_fpn_1.5x_coco.py) | [model](http://download.openmmlab.com/mmdetection/v2.0/paa/paa_r50_fpn_1.5x_coco/paa_r50_fpn_1.5x_coco_20200823-805d6078.pth) | [log](http://download.openmmlab.com/mmdetection/v2.0/paa/paa_r50_fpn_1.5x_coco/paa_r50_fpn_1.5x_coco_20200823-805d6078.log.json) |
-| R-50-FPN | 18e | 3.7 | False | 41.2 | - |
-| R-50-FPN | 24e | 3.7 | True | 41.6 | [config](https://github.com/open-mmlab/mmdetection/tree/master/configs/paa/paa_r50_fpn_2x_coco.py) | [model](http://download.openmmlab.com/mmdetection/v2.0/paa/paa_r50_fpn_2x_coco/paa_r50_fpn_2x_coco_20200821-c98bfc4e.pth) | [log](http://download.openmmlab.com/mmdetection/v2.0/paa/paa_r50_fpn_2x_coco/paa_r50_fpn_2x_coco_20200821-c98bfc4e.log.json) |
-| R-50-FPN | 36e | 3.7 | True | 43.3 | [config](https://github.com/open-mmlab/mmdetection/tree/master/configs/paa/paa_r50_fpn_mstrain_3x_coco.py) | [model](http://download.openmmlab.com/mmdetection/v2.0/paa/paa_r50_fpn_mstrain_3x_coco/paa_r50_fpn_mstrain_3x_coco_20210121_145722-06a6880b.pth) | [log](http://download.openmmlab.com/mmdetection/v2.0/paa/paa_r50_fpn_mstrain_3x_coco/paa_r50_fpn_mstrain_3x_coco_20210121_145722.log.json) |
-| R-101-FPN | 12e | 6.2 | True | 42.6 | [config](https://github.com/open-mmlab/mmdetection/tree/master/configs/paa/paa_r101_fpn_1x_coco.py) | [model](http://download.openmmlab.com/mmdetection/v2.0/paa/paa_r101_fpn_1x_coco/paa_r101_fpn_1x_coco_20200821-0a1825a4.pth) | [log](http://download.openmmlab.com/mmdetection/v2.0/paa/paa_r101_fpn_1x_coco/paa_r101_fpn_1x_coco_20200821-0a1825a4.log.json) |
-| R-101-FPN | 12e | 6.2 | False | 42.4 | - |
-| R-101-FPN | 24e | 6.2 | True | 43.5 | [config](https://github.com/open-mmlab/mmdetection/tree/master/configs/paa/paa_r101_fpn_2x_coco.py) | [model](http://download.openmmlab.com/mmdetection/v2.0/paa/paa_r101_fpn_2x_coco/paa_r101_fpn_2x_coco_20200821-6829f96b.pth) | [log](http://download.openmmlab.com/mmdetection/v2.0/paa/paa_r101_fpn_2x_coco/paa_r101_fpn_2x_coco_20200821-6829f96b.log.json) |
-| R-101-FPN | 36e | 6.2 | True | 45.1 | [config](https://github.com/open-mmlab/mmdetection/tree/master/configs/paa/paa_r101_fpn_mstrain_3x_coco.py) | [model](http://download.openmmlab.com/mmdetection/v2.0/paa/paa_r101_fpn_mstrain_3x_coco/paa_r101_fpn_mstrain_3x_coco_20210122_084202-83250d22.pth) | [log](http://download.openmmlab.com/mmdetection/v2.0/paa/paa_r101_fpn_mstrain_3x_coco/paa_r101_fpn_mstrain_3x_coco_20210122_084202.log.json) |
-
-**Note**:
-
-1. We find that the performance is unstable with 1x setting and may fluctuate by about 0.2 mAP. We report the best results.
diff --git a/spaces/Andy1621/uniformer_image_detection/configs/sparse_rcnn/sparse_rcnn_r101_fpn_mstrain_480-800_3x_coco.py b/spaces/Andy1621/uniformer_image_detection/configs/sparse_rcnn/sparse_rcnn_r101_fpn_mstrain_480-800_3x_coco.py
deleted file mode 100644
index 0439fc1aa28408df89d6d3b657837654bbbbbcdb..0000000000000000000000000000000000000000
--- a/spaces/Andy1621/uniformer_image_detection/configs/sparse_rcnn/sparse_rcnn_r101_fpn_mstrain_480-800_3x_coco.py
+++ /dev/null
@@ -1,3 +0,0 @@
-_base_ = './sparse_rcnn_r50_fpn_mstrain_480-800_3x_coco.py'
-
-model = dict(pretrained='torchvision://resnet101', backbone=dict(depth=101))
diff --git a/spaces/Andy1621/uniformer_image_segmentation/configs/dnlnet/dnl_r50-d8_512x512_160k_ade20k.py b/spaces/Andy1621/uniformer_image_segmentation/configs/dnlnet/dnl_r50-d8_512x512_160k_ade20k.py
deleted file mode 100644
index 5305689d09b944f6e37aa85567ce3f29fc6974a7..0000000000000000000000000000000000000000
--- a/spaces/Andy1621/uniformer_image_segmentation/configs/dnlnet/dnl_r50-d8_512x512_160k_ade20k.py
+++ /dev/null
@@ -1,6 +0,0 @@
-_base_ = [
- '../_base_/models/dnl_r50-d8.py', '../_base_/datasets/ade20k.py',
- '../_base_/default_runtime.py', '../_base_/schedules/schedule_160k.py'
-]
-model = dict(
- decode_head=dict(num_classes=150), auxiliary_head=dict(num_classes=150))
diff --git a/spaces/Andy1621/uniformer_image_segmentation/configs/dnlnet/dnl_r50-d8_769x769_40k_cityscapes.py b/spaces/Andy1621/uniformer_image_segmentation/configs/dnlnet/dnl_r50-d8_769x769_40k_cityscapes.py
deleted file mode 100644
index 0666199b63e604b09fe8187c378589c25d0d311b..0000000000000000000000000000000000000000
--- a/spaces/Andy1621/uniformer_image_segmentation/configs/dnlnet/dnl_r50-d8_769x769_40k_cityscapes.py
+++ /dev/null
@@ -1,9 +0,0 @@
-_base_ = [
- '../_base_/models/dnl_r50-d8.py',
- '../_base_/datasets/cityscapes_769x769.py', '../_base_/default_runtime.py',
- '../_base_/schedules/schedule_40k.py'
-]
-model = dict(
- decode_head=dict(align_corners=True),
- auxiliary_head=dict(align_corners=True),
- test_cfg=dict(mode='slide', crop_size=(769, 769), stride=(513, 513)))
diff --git a/spaces/AnishKumbhar/ChatBot/text-generation-webui-main/extensions/gallery/script.py b/spaces/AnishKumbhar/ChatBot/text-generation-webui-main/extensions/gallery/script.py
deleted file mode 100644
index 611a11f4a89d048ee9d0095f315391f53676f413..0000000000000000000000000000000000000000
--- a/spaces/AnishKumbhar/ChatBot/text-generation-webui-main/extensions/gallery/script.py
+++ /dev/null
@@ -1,101 +0,0 @@
-from pathlib import Path
-
-import gradio as gr
-
-from modules.html_generator import get_image_cache
-from modules.shared import gradio
-
-
-def generate_css():
- css = """
- .character-gallery > .gallery {
- margin: 1rem 0;
- display: grid !important;
- grid-template-columns: repeat(auto-fit, minmax(150px, 1fr));
- grid-column-gap: 0.4rem;
- grid-row-gap: 1.2rem;
- }
-
- .character-gallery > .label {
- display: none !important;
- }
-
- .character-gallery button.gallery-item {
- display: contents;
- }
-
- .character-container {
- cursor: pointer;
- text-align: center;
- position: relative;
- opacity: 0.85;
- }
-
- .character-container:hover {
- opacity: 1;
- }
-
- .character-container .placeholder, .character-container img {
- width: 150px;
- height: 200px;
- background-color: gray;
- object-fit: cover;
- margin: 0 auto;
- border-radius: 1rem;
- border: 3px solid white;
- box-shadow: 3px 3px 6px 0px rgb(0 0 0 / 50%);
- }
-
- .character-name {
- margin-top: 0.3rem;
- display: block;
- font-size: 1.2rem;
- font-weight: 600;
- overflow-wrap: anywhere;
- }
- """
- return css
-
-
-def generate_html():
- cards = []
- # Iterate through files in image folder
- for file in sorted(Path("characters").glob("*")):
- if file.suffix in [".json", ".yml", ".yaml"]:
- character = file.stem
- container_html = '
'
- image_html = ""
-
- for path in [Path(f"characters/{character}.{extension}") for extension in ['png', 'jpg', 'jpeg']]:
- if path.exists():
- image_html = f''
- break
-
- container_html += f'{image_html} {character}'
- container_html += "
"
- cards.append([container_html, character])
-
- return cards
-
-
-def select_character(evt: gr.SelectData):
- return (evt.value[1])
-
-
-def custom_js():
- path_to_js = Path(__file__).parent.resolve() / 'script.js'
- return open(path_to_js, 'r').read()
-
-
-def ui():
- with gr.Accordion("Character gallery", open=False, elem_id='gallery-extension'):
- update = gr.Button("Refresh")
- gr.HTML(value="")
- gallery = gr.Dataset(components=[gr.HTML(visible=False)],
- label="",
- samples=generate_html(),
- elem_classes=["character-gallery"],
- samples_per_page=50
- )
- update.click(generate_html, [], gallery)
- gallery.select(select_character, None, gradio['character_menu'])
diff --git a/spaces/AnishKumbhar/ChatBot/text-generation-webui-main/modules/ui_model_menu.py b/spaces/AnishKumbhar/ChatBot/text-generation-webui-main/modules/ui_model_menu.py
deleted file mode 100644
index bfa95c07dd965dc7289914149ee7526357e199b7..0000000000000000000000000000000000000000
--- a/spaces/AnishKumbhar/ChatBot/text-generation-webui-main/modules/ui_model_menu.py
+++ /dev/null
@@ -1,267 +0,0 @@
-import importlib
-import math
-import re
-import traceback
-from functools import partial
-from pathlib import Path
-
-import gradio as gr
-import psutil
-import torch
-
-from modules import loaders, shared, ui, utils
-from modules.logging_colors import logger
-from modules.LoRA import add_lora_to_model
-from modules.models import load_model, unload_model
-from modules.models_settings import (
- apply_model_settings_to_state,
- get_model_metadata,
- save_model_settings,
- update_model_parameters
-)
-from modules.utils import gradio
-
-
-def create_ui():
- mu = shared.args.multi_user
-
- # Finding the default values for the GPU and CPU memories
- total_mem = []
- for i in range(torch.cuda.device_count()):
- total_mem.append(math.floor(torch.cuda.get_device_properties(i).total_memory / (1024 * 1024)))
-
- default_gpu_mem = []
- if shared.args.gpu_memory is not None and len(shared.args.gpu_memory) > 0:
- for i in shared.args.gpu_memory:
- if 'mib' in i.lower():
- default_gpu_mem.append(int(re.sub('[a-zA-Z ]', '', i)))
- else:
- default_gpu_mem.append(int(re.sub('[a-zA-Z ]', '', i)) * 1000)
-
- while len(default_gpu_mem) < len(total_mem):
- default_gpu_mem.append(0)
-
- total_cpu_mem = math.floor(psutil.virtual_memory().total / (1024 * 1024))
- if shared.args.cpu_memory is not None:
- default_cpu_mem = re.sub('[a-zA-Z ]', '', shared.args.cpu_memory)
- else:
- default_cpu_mem = 0
-
- with gr.Tab("Model", elem_id="model-tab"):
- with gr.Row():
- with gr.Column():
- with gr.Row():
- with gr.Column():
- with gr.Row():
- shared.gradio['model_menu'] = gr.Dropdown(choices=utils.get_available_models(), value=shared.model_name, label='Model', elem_classes='slim-dropdown', interactive=not mu)
- ui.create_refresh_button(shared.gradio['model_menu'], lambda: None, lambda: {'choices': utils.get_available_models()}, 'refresh-button', interactive=not mu)
- shared.gradio['load_model'] = gr.Button("Load", visible=not shared.settings['autoload_model'], elem_classes='refresh-button', interactive=not mu)
- shared.gradio['unload_model'] = gr.Button("Unload", elem_classes='refresh-button', interactive=not mu)
- shared.gradio['reload_model'] = gr.Button("Reload", elem_classes='refresh-button', interactive=not mu)
- shared.gradio['save_model_settings'] = gr.Button("Save settings", elem_classes='refresh-button', interactive=not mu)
-
- with gr.Column():
- with gr.Row():
- shared.gradio['lora_menu'] = gr.Dropdown(multiselect=True, choices=utils.get_available_loras(), value=shared.lora_names, label='LoRA(s)', elem_classes='slim-dropdown', interactive=not mu)
- ui.create_refresh_button(shared.gradio['lora_menu'], lambda: None, lambda: {'choices': utils.get_available_loras(), 'value': shared.lora_names}, 'refresh-button', interactive=not mu)
- shared.gradio['lora_menu_apply'] = gr.Button(value='Apply LoRAs', elem_classes='refresh-button', interactive=not mu)
-
- with gr.Row():
- with gr.Column():
- shared.gradio['loader'] = gr.Dropdown(label="Model loader", choices=loaders.loaders_and_params.keys(), value=None)
- with gr.Box():
- with gr.Row():
- with gr.Column():
- for i in range(len(total_mem)):
- shared.gradio[f'gpu_memory_{i}'] = gr.Slider(label=f"gpu-memory in MiB for device :{i}", maximum=total_mem[i], value=default_gpu_mem[i])
-
- shared.gradio['cpu_memory'] = gr.Slider(label="cpu-memory in MiB", maximum=total_cpu_mem, value=default_cpu_mem)
- shared.gradio['transformers_info'] = gr.Markdown('load-in-4bit params:')
- shared.gradio['compute_dtype'] = gr.Dropdown(label="compute_dtype", choices=["bfloat16", "float16", "float32"], value=shared.args.compute_dtype)
- shared.gradio['quant_type'] = gr.Dropdown(label="quant_type", choices=["nf4", "fp4"], value=shared.args.quant_type)
-
- shared.gradio['n_gpu_layers'] = gr.Slider(label="n-gpu-layers", minimum=0, maximum=128, value=shared.args.n_gpu_layers)
- shared.gradio['n_ctx'] = gr.Slider(minimum=0, maximum=32768, step=256, label="n_ctx", value=shared.args.n_ctx)
- shared.gradio['threads'] = gr.Slider(label="threads", minimum=0, step=1, maximum=32, value=shared.args.threads)
- shared.gradio['threads_batch'] = gr.Slider(label="threads_batch", minimum=0, step=1, maximum=32, value=shared.args.threads_batch)
- shared.gradio['n_batch'] = gr.Slider(label="n_batch", minimum=1, maximum=2048, value=shared.args.n_batch)
-
- shared.gradio['wbits'] = gr.Dropdown(label="wbits", choices=["None", 1, 2, 3, 4, 8], value=str(shared.args.wbits) if shared.args.wbits > 0 else "None")
- shared.gradio['groupsize'] = gr.Dropdown(label="groupsize", choices=["None", 32, 64, 128, 1024], value=str(shared.args.groupsize) if shared.args.groupsize > 0 else "None")
- shared.gradio['model_type'] = gr.Dropdown(label="model_type", choices=["None"], value=shared.args.model_type or "None")
- shared.gradio['pre_layer'] = gr.Slider(label="pre_layer", minimum=0, maximum=100, value=shared.args.pre_layer[0] if shared.args.pre_layer is not None else 0)
- shared.gradio['autogptq_info'] = gr.Markdown('* ExLlama_HF is recommended over AutoGPTQ for models derived from LLaMA.')
- shared.gradio['gpu_split'] = gr.Textbox(label='gpu-split', info='Comma-separated list of VRAM (in GB) to use per GPU. Example: 20,7,7')
- shared.gradio['max_seq_len'] = gr.Slider(label='max_seq_len', minimum=0, maximum=32768, step=256, info='Maximum sequence length.', value=shared.args.max_seq_len)
- shared.gradio['alpha_value'] = gr.Slider(label='alpha_value', minimum=1, maximum=8, step=0.05, info='Positional embeddings alpha factor for NTK RoPE scaling. Recommended values (NTKv1): 1.75 for 1.5x context, 2.5 for 2x context. Use either this or compress_pos_emb, not both.', value=shared.args.alpha_value)
- shared.gradio['rope_freq_base'] = gr.Slider(label='rope_freq_base', minimum=0, maximum=1000000, step=1000, info='If greater than 0, will be used instead of alpha_value. Those two are related by rope_freq_base = 10000 * alpha_value ^ (64 / 63)', value=shared.args.rope_freq_base)
- shared.gradio['compress_pos_emb'] = gr.Slider(label='compress_pos_emb', minimum=1, maximum=8, step=1, info='Positional embeddings compression factor. Should be set to (context length) / (model\'s original context length). Equal to 1/rope_freq_scale.', value=shared.args.compress_pos_emb)
-
- with gr.Column():
- shared.gradio['triton'] = gr.Checkbox(label="triton", value=shared.args.triton)
- shared.gradio['no_inject_fused_attention'] = gr.Checkbox(label="no_inject_fused_attention", value=shared.args.no_inject_fused_attention, info='Disable fused attention. Fused attention improves inference performance but uses more VRAM. Fuses layers for AutoAWQ. Disable if running low on VRAM.')
- shared.gradio['no_inject_fused_mlp'] = gr.Checkbox(label="no_inject_fused_mlp", value=shared.args.no_inject_fused_mlp, info='Affects Triton only. Disable fused MLP. Fused MLP improves performance but uses more VRAM. Disable if running low on VRAM.')
- shared.gradio['no_use_cuda_fp16'] = gr.Checkbox(label="no_use_cuda_fp16", value=shared.args.no_use_cuda_fp16, info='This can make models faster on some systems.')
- shared.gradio['desc_act'] = gr.Checkbox(label="desc_act", value=shared.args.desc_act, info='\'desc_act\', \'wbits\', and \'groupsize\' are used for old models without a quantize_config.json.')
- shared.gradio['mul_mat_q'] = gr.Checkbox(label="mul_mat_q", value=shared.args.mul_mat_q, info='Recommended in most cases. Improves generation speed by 10-20%.')
- shared.gradio['cfg_cache'] = gr.Checkbox(label="cfg-cache", value=shared.args.cfg_cache, info='Create an additional cache for CFG negative prompts.')
- shared.gradio['no_mmap'] = gr.Checkbox(label="no-mmap", value=shared.args.no_mmap)
- shared.gradio['mlock'] = gr.Checkbox(label="mlock", value=shared.args.mlock)
- shared.gradio['numa'] = gr.Checkbox(label="numa", value=shared.args.numa, info='NUMA support can help on some systems with non-uniform memory access.')
- shared.gradio['cpu'] = gr.Checkbox(label="cpu", value=shared.args.cpu)
- shared.gradio['load_in_8bit'] = gr.Checkbox(label="load-in-8bit", value=shared.args.load_in_8bit)
- shared.gradio['bf16'] = gr.Checkbox(label="bf16", value=shared.args.bf16)
- shared.gradio['auto_devices'] = gr.Checkbox(label="auto-devices", value=shared.args.auto_devices)
- shared.gradio['disk'] = gr.Checkbox(label="disk", value=shared.args.disk)
- shared.gradio['load_in_4bit'] = gr.Checkbox(label="load-in-4bit", value=shared.args.load_in_4bit)
- shared.gradio['use_double_quant'] = gr.Checkbox(label="use_double_quant", value=shared.args.use_double_quant)
- shared.gradio['tensor_split'] = gr.Textbox(label='tensor_split', info='Split the model across multiple GPUs, comma-separated list of proportions, e.g. 18,17')
- shared.gradio['llama_cpp_seed'] = gr.Number(label='Seed (0 for random)', value=shared.args.llama_cpp_seed)
- shared.gradio['trust_remote_code'] = gr.Checkbox(label="trust-remote-code", value=shared.args.trust_remote_code, info='Make sure to inspect the .py files inside the model folder before loading it with this option enabled.')
- shared.gradio['use_fast'] = gr.Checkbox(label="use_fast", value=shared.args.use_fast, info='Set use_fast=True while loading the tokenizer. May trigger a conversion that takes several minutes.')
- shared.gradio['disable_exllama'] = gr.Checkbox(label="disable_exllama", value=shared.args.disable_exllama, info='Disable ExLlama kernel.')
- shared.gradio['gptq_for_llama_info'] = gr.Markdown('GPTQ-for-LLaMa support is currently only kept for compatibility with older GPUs. AutoGPTQ or ExLlama is preferred when compatible. GPTQ-for-LLaMa is installed by default with the webui on supported systems. Otherwise, it has to be installed manually following the instructions here: [instructions](https://github.com/oobabooga/text-generation-webui/blob/main/docs/GPTQ-models-(4-bit-mode).md#installation-1).')
- shared.gradio['exllama_info'] = gr.Markdown('For more information, consult the [docs](https://github.com/oobabooga/text-generation-webui/blob/main/docs/ExLlama.md).')
- shared.gradio['exllama_HF_info'] = gr.Markdown('ExLlama_HF is a wrapper that lets you use ExLlama like a Transformers model, which means it can use the Transformers samplers. It\'s a bit slower than the regular ExLlama.')
- shared.gradio['llamacpp_HF_info'] = gr.Markdown('llamacpp_HF loads llama.cpp as a Transformers model. To use it, you need to download a tokenizer.\n\nOption 1: download `oobabooga/llama-tokenizer` under "Download model or LoRA". That\'s a default Llama tokenizer.\n\nOption 2: place your .gguf in a subfolder of models/ along with these 3 files: tokenizer.model, tokenizer_config.json, and special_tokens_map.json. This takes precedence over Option 1.')
-
- with gr.Column():
- with gr.Row():
- shared.gradio['autoload_model'] = gr.Checkbox(value=shared.settings['autoload_model'], label='Autoload the model', info='Whether to load the model as soon as it is selected in the Model dropdown.', interactive=not mu)
-
- shared.gradio['custom_model_menu'] = gr.Textbox(label="Download model or LoRA", info="Enter the Hugging Face username/model path, for instance: facebook/galactica-125m. To specify a branch, add it at the end after a \":\" character like this: facebook/galactica-125m:main. To download a single file, enter its name in the second box.", interactive=not mu)
- shared.gradio['download_specific_file'] = gr.Textbox(placeholder="File name (for GGUF models)", show_label=False, max_lines=1, interactive=not mu)
- with gr.Row():
- shared.gradio['download_model_button'] = gr.Button("Download", variant='primary', interactive=not mu)
- shared.gradio['get_file_list'] = gr.Button("Get file list", interactive=not mu)
-
- with gr.Row():
- shared.gradio['model_status'] = gr.Markdown('No model is loaded' if shared.model_name == 'None' else 'Ready')
-
-
-def create_event_handlers():
- shared.gradio['loader'].change(
- loaders.make_loader_params_visible, gradio('loader'), gradio(loaders.get_all_params())).then(
- lambda value: gr.update(choices=loaders.get_model_types(value)), gradio('loader'), gradio('model_type'))
-
- # In this event handler, the interface state is read and updated
- # with the model defaults (if any), and then the model is loaded
- # unless "autoload_model" is unchecked
- shared.gradio['model_menu'].change(
- ui.gather_interface_values, gradio(shared.input_elements), gradio('interface_state')).then(
- apply_model_settings_to_state, gradio('model_menu', 'interface_state'), gradio('interface_state')).then(
- ui.apply_interface_values, gradio('interface_state'), gradio(ui.list_interface_input_elements()), show_progress=False).then(
- update_model_parameters, gradio('interface_state'), None).then(
- load_model_wrapper, gradio('model_menu', 'loader', 'autoload_model'), gradio('model_status'), show_progress=False).success(
- update_truncation_length, gradio('truncation_length', 'interface_state'), gradio('truncation_length')).then(
- lambda x: x, gradio('loader'), gradio('filter_by_loader'))
-
- shared.gradio['load_model'].click(
- ui.gather_interface_values, gradio(shared.input_elements), gradio('interface_state')).then(
- update_model_parameters, gradio('interface_state'), None).then(
- partial(load_model_wrapper, autoload=True), gradio('model_menu', 'loader'), gradio('model_status'), show_progress=False).success(
- update_truncation_length, gradio('truncation_length', 'interface_state'), gradio('truncation_length')).then(
- lambda x: x, gradio('loader'), gradio('filter_by_loader'))
-
- shared.gradio['reload_model'].click(
- unload_model, None, None).then(
- ui.gather_interface_values, gradio(shared.input_elements), gradio('interface_state')).then(
- update_model_parameters, gradio('interface_state'), None).then(
- partial(load_model_wrapper, autoload=True), gradio('model_menu', 'loader'), gradio('model_status'), show_progress=False).success(
- update_truncation_length, gradio('truncation_length', 'interface_state'), gradio('truncation_length')).then(
- lambda x: x, gradio('loader'), gradio('filter_by_loader'))
-
- shared.gradio['unload_model'].click(
- unload_model, None, None).then(
- lambda: "Model unloaded", None, gradio('model_status'))
-
- shared.gradio['save_model_settings'].click(
- ui.gather_interface_values, gradio(shared.input_elements), gradio('interface_state')).then(
- save_model_settings, gradio('model_menu', 'interface_state'), gradio('model_status'), show_progress=False)
-
- shared.gradio['lora_menu_apply'].click(load_lora_wrapper, gradio('lora_menu'), gradio('model_status'), show_progress=False)
- shared.gradio['download_model_button'].click(download_model_wrapper, gradio('custom_model_menu', 'download_specific_file'), gradio('model_status'), show_progress=True)
- shared.gradio['get_file_list'].click(partial(download_model_wrapper, return_links=True), gradio('custom_model_menu', 'download_specific_file'), gradio('model_status'), show_progress=True)
- shared.gradio['autoload_model'].change(lambda x: gr.update(visible=not x), gradio('autoload_model'), gradio('load_model'))
-
-
-def load_model_wrapper(selected_model, loader, autoload=False):
- if not autoload:
- yield f"The settings for `{selected_model}` have been updated.\n\nClick on \"Load\" to load it."
- return
-
- if selected_model == 'None':
- yield "No model selected"
- else:
- try:
- yield f"Loading `{selected_model}`..."
- shared.model_name = selected_model
- unload_model()
- if selected_model != '':
- shared.model, shared.tokenizer = load_model(shared.model_name, loader)
-
- if shared.model is not None:
- output = f"Successfully loaded `{selected_model}`."
-
- settings = get_model_metadata(selected_model)
- if 'instruction_template' in settings:
- output += '\n\nIt seems to be an instruction-following model with template "{}". In the chat tab, instruct or chat-instruct modes should be used.'.format(settings['instruction_template'])
-
- yield output
- else:
- yield f"Failed to load `{selected_model}`."
- except:
- exc = traceback.format_exc()
- logger.error('Failed to load the model.')
- print(exc)
- yield exc.replace('\n', '\n\n')
-
-
-def load_lora_wrapper(selected_loras):
- yield ("Applying the following LoRAs to {}:\n\n{}".format(shared.model_name, '\n'.join(selected_loras)))
- add_lora_to_model(selected_loras)
- yield ("Successfuly applied the LoRAs")
-
-
-def download_model_wrapper(repo_id, specific_file, progress=gr.Progress(), return_links=False, check=False):
- try:
- downloader_module = importlib.import_module("download-model")
- downloader = downloader_module.ModelDownloader()
-
- progress(0.0)
- yield ("Cleaning up the model/branch names")
- model, branch = downloader.sanitize_model_and_branch_names(repo_id, None)
-
- yield ("Getting the download links from Hugging Face")
- links, sha256, is_lora, is_llamacpp = downloader.get_download_links_from_huggingface(model, branch, text_only=False, specific_file=specific_file)
-
- if return_links:
- yield '\n\n'.join([f"`{Path(link).name}`" for link in links])
- return
-
- yield ("Getting the output folder")
- base_folder = shared.args.lora_dir if is_lora else shared.args.model_dir
- output_folder = downloader.get_output_folder(model, branch, is_lora, is_llamacpp=is_llamacpp, base_folder=base_folder)
-
- if check:
- progress(0.5)
- yield ("Checking previously downloaded files")
- downloader.check_model_files(model, branch, links, sha256, output_folder)
- progress(1.0)
- else:
- yield (f"Downloading file{'s' if len(links) > 1 else ''} to `{output_folder}/`")
- downloader.download_model_files(model, branch, links, sha256, output_folder, progress_bar=progress, threads=1, is_llamacpp=is_llamacpp)
- yield ("Done!")
- except:
- progress(1.0)
- yield traceback.format_exc().replace('\n', '\n\n')
-
-
-def update_truncation_length(current_length, state):
- if 'loader' in state:
- if state['loader'].lower().startswith('exllama'):
- return state['max_seq_len']
- elif state['loader'] in ['llama.cpp', 'llamacpp_HF', 'ctransformers']:
- return state['n_ctx']
-
- return current_length
diff --git a/spaces/Anonymous-sub/Rerender/ControlNet/tutorial_train.py b/spaces/Anonymous-sub/Rerender/ControlNet/tutorial_train.py
deleted file mode 100644
index 393d7addb164c32eff9c3d675e4f32fb555868f0..0000000000000000000000000000000000000000
--- a/spaces/Anonymous-sub/Rerender/ControlNet/tutorial_train.py
+++ /dev/null
@@ -1,35 +0,0 @@
-from share import *
-
-import pytorch_lightning as pl
-from torch.utils.data import DataLoader
-from tutorial_dataset import MyDataset
-from cldm.logger import ImageLogger
-from cldm.model import create_model, load_state_dict
-
-
-# Configs
-resume_path = './models/control_sd15_ini.ckpt'
-batch_size = 4
-logger_freq = 300
-learning_rate = 1e-5
-sd_locked = True
-only_mid_control = False
-
-
-# First use cpu to load models. Pytorch Lightning will automatically move it to GPUs.
-model = create_model('./models/cldm_v15.yaml').cpu()
-model.load_state_dict(load_state_dict(resume_path, location='cpu'))
-model.learning_rate = learning_rate
-model.sd_locked = sd_locked
-model.only_mid_control = only_mid_control
-
-
-# Misc
-dataset = MyDataset()
-dataloader = DataLoader(dataset, num_workers=0, batch_size=batch_size, shuffle=True)
-logger = ImageLogger(batch_frequency=logger_freq)
-trainer = pl.Trainer(gpus=1, precision=32, callbacks=[logger])
-
-
-# Train!
-trainer.fit(model, dataloader)
diff --git a/spaces/Apex-X/nono/CONTRIBUTING.md b/spaces/Apex-X/nono/CONTRIBUTING.md
deleted file mode 100644
index 7fb9cb146adc1b1c39ee1f4c55415f8bbd9e2c9e..0000000000000000000000000000000000000000
--- a/spaces/Apex-X/nono/CONTRIBUTING.md
+++ /dev/null
@@ -1,21 +0,0 @@
-## Pull Requests
-
-### Do
-
-- ...consider to fix bugs over adding features
-- ...one pull request for one feature or improvement
-- ...consult us about implementation details
-- ...proper testing before you submit your code
-- ...resolve failed CI pipelines
-
-### Don't
-
-- ...introduce fundamental changes in terms of software architecture
-- ...introduce OOP - we accept functional programming only
-- ...ignore given requirements or try to work around them
-- ...submit code to a development branch without consulting us
-- ...submit massive amount of code changes
-- ...submit a proof of concept
-- ...submit code that is using undocumented and private APIs
-- ...solve third party issues in our project
-- ...comment what your code does - use proper naming instead
diff --git a/spaces/Ariharasudhan/YoloV5/utils/dataloaders.py b/spaces/Ariharasudhan/YoloV5/utils/dataloaders.py
deleted file mode 100644
index 0418293a6e217f1e48f1352326647262b6d37e05..0000000000000000000000000000000000000000
--- a/spaces/Ariharasudhan/YoloV5/utils/dataloaders.py
+++ /dev/null
@@ -1,1221 +0,0 @@
-# YOLOv5 🚀 by Ultralytics, GPL-3.0 license
-"""
-Dataloaders and dataset utils
-"""
-
-import contextlib
-import glob
-import hashlib
-import json
-import math
-import os
-import random
-import shutil
-import time
-from itertools import repeat
-from multiprocessing.pool import Pool, ThreadPool
-from pathlib import Path
-from threading import Thread
-from urllib.parse import urlparse
-
-import numpy as np
-import psutil
-import torch
-import torch.nn.functional as F
-import torchvision
-import yaml
-from PIL import ExifTags, Image, ImageOps
-from torch.utils.data import DataLoader, Dataset, dataloader, distributed
-from tqdm import tqdm
-
-from utils.augmentations import (Albumentations, augment_hsv, classify_albumentations, classify_transforms, copy_paste,
- cutout, letterbox, mixup, random_perspective)
-from utils.general import (DATASETS_DIR, LOGGER, NUM_THREADS, check_dataset, check_requirements, check_yaml, clean_str,
- colorstr, cv2, is_colab, is_kaggle, segments2boxes, unzip_file, xyn2xy, xywh2xyxy,
- xywhn2xyxy, xyxy2xywhn)
-from utils.torch_utils import torch_distributed_zero_first
-
-# Parameters
-HELP_URL = 'See https://github.com/ultralytics/yolov5/wiki/Train-Custom-Data'
-IMG_FORMATS = 'bmp', 'dng', 'jpeg', 'jpg', 'mpo', 'png', 'tif', 'tiff', 'webp', 'pfm' # include image suffixes
-VID_FORMATS = 'asf', 'avi', 'gif', 'm4v', 'mkv', 'mov', 'mp4', 'mpeg', 'mpg', 'ts', 'wmv' # include video suffixes
-BAR_FORMAT = '{l_bar}{bar:10}{r_bar}{bar:-10b}' # tqdm bar format
-LOCAL_RANK = int(os.getenv('LOCAL_RANK', -1)) # https://pytorch.org/docs/stable/elastic/run.html
-RANK = int(os.getenv('RANK', -1))
-PIN_MEMORY = str(os.getenv('PIN_MEMORY', True)).lower() == 'true' # global pin_memory for dataloaders
-
-# Get orientation exif tag
-for orientation in ExifTags.TAGS.keys():
- if ExifTags.TAGS[orientation] == 'Orientation':
- break
-
-
-def get_hash(paths):
- # Returns a single hash value of a list of paths (files or dirs)
- size = sum(os.path.getsize(p) for p in paths if os.path.exists(p)) # sizes
- h = hashlib.md5(str(size).encode()) # hash sizes
- h.update(''.join(paths).encode()) # hash paths
- return h.hexdigest() # return hash
-
-
-def exif_size(img):
- # Returns exif-corrected PIL size
- s = img.size # (width, height)
- with contextlib.suppress(Exception):
- rotation = dict(img._getexif().items())[orientation]
- if rotation in [6, 8]: # rotation 270 or 90
- s = (s[1], s[0])
- return s
-
-
-def exif_transpose(image):
- """
- Transpose a PIL image accordingly if it has an EXIF Orientation tag.
- Inplace version of https://github.com/python-pillow/Pillow/blob/master/src/PIL/ImageOps.py exif_transpose()
-
- :param image: The image to transpose.
- :return: An image.
- """
- exif = image.getexif()
- orientation = exif.get(0x0112, 1) # default 1
- if orientation > 1:
- method = {
- 2: Image.FLIP_LEFT_RIGHT,
- 3: Image.ROTATE_180,
- 4: Image.FLIP_TOP_BOTTOM,
- 5: Image.TRANSPOSE,
- 6: Image.ROTATE_270,
- 7: Image.TRANSVERSE,
- 8: Image.ROTATE_90}.get(orientation)
- if method is not None:
- image = image.transpose(method)
- del exif[0x0112]
- image.info["exif"] = exif.tobytes()
- return image
-
-
-def seed_worker(worker_id):
- # Set dataloader worker seed https://pytorch.org/docs/stable/notes/randomness.html#dataloader
- worker_seed = torch.initial_seed() % 2 ** 32
- np.random.seed(worker_seed)
- random.seed(worker_seed)
-
-
-def create_dataloader(path,
- imgsz,
- batch_size,
- stride,
- single_cls=False,
- hyp=None,
- augment=False,
- cache=False,
- pad=0.0,
- rect=False,
- rank=-1,
- workers=8,
- image_weights=False,
- quad=False,
- prefix='',
- shuffle=False):
- if rect and shuffle:
- LOGGER.warning('WARNING ⚠️ --rect is incompatible with DataLoader shuffle, setting shuffle=False')
- shuffle = False
- with torch_distributed_zero_first(rank): # init dataset *.cache only once if DDP
- dataset = LoadImagesAndLabels(
- path,
- imgsz,
- batch_size,
- augment=augment, # augmentation
- hyp=hyp, # hyperparameters
- rect=rect, # rectangular batches
- cache_images=cache,
- single_cls=single_cls,
- stride=int(stride),
- pad=pad,
- image_weights=image_weights,
- prefix=prefix)
-
- batch_size = min(batch_size, len(dataset))
- nd = torch.cuda.device_count() # number of CUDA devices
- nw = min([os.cpu_count() // max(nd, 1), batch_size if batch_size > 1 else 0, workers]) # number of workers
- sampler = None if rank == -1 else distributed.DistributedSampler(dataset, shuffle=shuffle)
- loader = DataLoader if image_weights else InfiniteDataLoader # only DataLoader allows for attribute updates
- generator = torch.Generator()
- generator.manual_seed(6148914691236517205 + RANK)
- return loader(dataset,
- batch_size=batch_size,
- shuffle=shuffle and sampler is None,
- num_workers=nw,
- sampler=sampler,
- pin_memory=PIN_MEMORY,
- collate_fn=LoadImagesAndLabels.collate_fn4 if quad else LoadImagesAndLabels.collate_fn,
- worker_init_fn=seed_worker,
- generator=generator), dataset
-
-
-class InfiniteDataLoader(dataloader.DataLoader):
- """ Dataloader that reuses workers
-
- Uses same syntax as vanilla DataLoader
- """
-
- def __init__(self, *args, **kwargs):
- super().__init__(*args, **kwargs)
- object.__setattr__(self, 'batch_sampler', _RepeatSampler(self.batch_sampler))
- self.iterator = super().__iter__()
-
- def __len__(self):
- return len(self.batch_sampler.sampler)
-
- def __iter__(self):
- for _ in range(len(self)):
- yield next(self.iterator)
-
-
-class _RepeatSampler:
- """ Sampler that repeats forever
-
- Args:
- sampler (Sampler)
- """
-
- def __init__(self, sampler):
- self.sampler = sampler
-
- def __iter__(self):
- while True:
- yield from iter(self.sampler)
-
-
-class LoadScreenshots:
- # YOLOv5 screenshot dataloader, i.e. `python detect.py --source "screen 0 100 100 512 256"`
- def __init__(self, source, img_size=640, stride=32, auto=True, transforms=None):
- # source = [screen_number left top width height] (pixels)
- check_requirements('mss')
- import mss
-
- source, *params = source.split()
- self.screen, left, top, width, height = 0, None, None, None, None # default to full screen 0
- if len(params) == 1:
- self.screen = int(params[0])
- elif len(params) == 4:
- left, top, width, height = (int(x) for x in params)
- elif len(params) == 5:
- self.screen, left, top, width, height = (int(x) for x in params)
- self.img_size = img_size
- self.stride = stride
- self.transforms = transforms
- self.auto = auto
- self.mode = 'stream'
- self.frame = 0
- self.sct = mss.mss()
-
- # Parse monitor shape
- monitor = self.sct.monitors[self.screen]
- self.top = monitor["top"] if top is None else (monitor["top"] + top)
- self.left = monitor["left"] if left is None else (monitor["left"] + left)
- self.width = width or monitor["width"]
- self.height = height or monitor["height"]
- self.monitor = {"left": self.left, "top": self.top, "width": self.width, "height": self.height}
-
- def __iter__(self):
- return self
-
- def __next__(self):
- # mss screen capture: get raw pixels from the screen as np array
- im0 = np.array(self.sct.grab(self.monitor))[:, :, :3] # [:, :, :3] BGRA to BGR
- s = f"screen {self.screen} (LTWH): {self.left},{self.top},{self.width},{self.height}: "
-
- if self.transforms:
- im = self.transforms(im0) # transforms
- else:
- im = letterbox(im0, self.img_size, stride=self.stride, auto=self.auto)[0] # padded resize
- im = im.transpose((2, 0, 1))[::-1] # HWC to CHW, BGR to RGB
- im = np.ascontiguousarray(im) # contiguous
- self.frame += 1
- return str(self.screen), im, im0, None, s # screen, img, original img, im0s, s
-
-
-class LoadImages:
- # YOLOv5 image/video dataloader, i.e. `python detect.py --source image.jpg/vid.mp4`
- def __init__(self, path, img_size=640, stride=32, auto=True, transforms=None, vid_stride=1):
- files = []
- for p in sorted(path) if isinstance(path, (list, tuple)) else [path]:
- p = str(Path(p).resolve())
- if '*' in p:
- files.extend(sorted(glob.glob(p, recursive=True))) # glob
- elif os.path.isdir(p):
- files.extend(sorted(glob.glob(os.path.join(p, '*.*')))) # dir
- elif os.path.isfile(p):
- files.append(p) # files
- else:
- raise FileNotFoundError(f'{p} does not exist')
-
- images = [x for x in files if x.split('.')[-1].lower() in IMG_FORMATS]
- videos = [x for x in files if x.split('.')[-1].lower() in VID_FORMATS]
- ni, nv = len(images), len(videos)
-
- self.img_size = img_size
- self.stride = stride
- self.files = images + videos
- self.nf = ni + nv # number of files
- self.video_flag = [False] * ni + [True] * nv
- self.mode = 'image'
- self.auto = auto
- self.transforms = transforms # optional
- self.vid_stride = vid_stride # video frame-rate stride
- if any(videos):
- self._new_video(videos[0]) # new video
- else:
- self.cap = None
- assert self.nf > 0, f'No images or videos found in {p}. ' \
- f'Supported formats are:\nimages: {IMG_FORMATS}\nvideos: {VID_FORMATS}'
-
- def __iter__(self):
- self.count = 0
- return self
-
- def __next__(self):
- if self.count == self.nf:
- raise StopIteration
- path = self.files[self.count]
-
- if self.video_flag[self.count]:
- # Read video
- self.mode = 'video'
- for _ in range(self.vid_stride):
- self.cap.grab()
- ret_val, im0 = self.cap.retrieve()
- while not ret_val:
- self.count += 1
- self.cap.release()
- if self.count == self.nf: # last video
- raise StopIteration
- path = self.files[self.count]
- self._new_video(path)
- ret_val, im0 = self.cap.read()
-
- self.frame += 1
- # im0 = self._cv2_rotate(im0) # for use if cv2 autorotation is False
- s = f'video {self.count + 1}/{self.nf} ({self.frame}/{self.frames}) {path}: '
-
- else:
- # Read image
- self.count += 1
- im0 = cv2.imread(path) # BGR
- assert im0 is not None, f'Image Not Found {path}'
- s = f'image {self.count}/{self.nf} {path}: '
-
- if self.transforms:
- im = self.transforms(im0) # transforms
- else:
- im = letterbox(im0, self.img_size, stride=self.stride, auto=self.auto)[0] # padded resize
- im = im.transpose((2, 0, 1))[::-1] # HWC to CHW, BGR to RGB
- im = np.ascontiguousarray(im) # contiguous
-
- return path, im, im0, self.cap, s
-
- def _new_video(self, path):
- # Create a new video capture object
- self.frame = 0
- self.cap = cv2.VideoCapture(path)
- self.frames = int(self.cap.get(cv2.CAP_PROP_FRAME_COUNT) / self.vid_stride)
- self.orientation = int(self.cap.get(cv2.CAP_PROP_ORIENTATION_META)) # rotation degrees
- # self.cap.set(cv2.CAP_PROP_ORIENTATION_AUTO, 0) # disable https://github.com/ultralytics/yolov5/issues/8493
-
- def _cv2_rotate(self, im):
- # Rotate a cv2 video manually
- if self.orientation == 0:
- return cv2.rotate(im, cv2.ROTATE_90_CLOCKWISE)
- elif self.orientation == 180:
- return cv2.rotate(im, cv2.ROTATE_90_COUNTERCLOCKWISE)
- elif self.orientation == 90:
- return cv2.rotate(im, cv2.ROTATE_180)
- return im
-
- def __len__(self):
- return self.nf # number of files
-
-
-class LoadStreams:
- # YOLOv5 streamloader, i.e. `python detect.py --source 'rtsp://example.com/media.mp4' # RTSP, RTMP, HTTP streams`
- def __init__(self, sources='streams.txt', img_size=640, stride=32, auto=True, transforms=None, vid_stride=1):
- torch.backends.cudnn.benchmark = True # faster for fixed-size inference
- self.mode = 'stream'
- self.img_size = img_size
- self.stride = stride
- self.vid_stride = vid_stride # video frame-rate stride
- sources = Path(sources).read_text().rsplit() if os.path.isfile(sources) else [sources]
- n = len(sources)
- self.sources = [clean_str(x) for x in sources] # clean source names for later
- self.imgs, self.fps, self.frames, self.threads = [None] * n, [0] * n, [0] * n, [None] * n
- for i, s in enumerate(sources): # index, source
- # Start thread to read frames from video stream
- st = f'{i + 1}/{n}: {s}... '
- if urlparse(s).hostname in ('www.youtube.com', 'youtube.com', 'youtu.be'): # if source is YouTube video
- # YouTube format i.e. 'https://www.youtube.com/watch?v=Zgi9g1ksQHc' or 'https://youtu.be/Zgi9g1ksQHc'
- check_requirements(('pafy', 'youtube_dl==2020.12.2'))
- import pafy
- s = pafy.new(s).getbest(preftype="mp4").url # YouTube URL
- s = eval(s) if s.isnumeric() else s # i.e. s = '0' local webcam
- if s == 0:
- assert not is_colab(), '--source 0 webcam unsupported on Colab. Rerun command in a local environment.'
- assert not is_kaggle(), '--source 0 webcam unsupported on Kaggle. Rerun command in a local environment.'
- cap = cv2.VideoCapture(s)
- assert cap.isOpened(), f'{st}Failed to open {s}'
- w = int(cap.get(cv2.CAP_PROP_FRAME_WIDTH))
- h = int(cap.get(cv2.CAP_PROP_FRAME_HEIGHT))
- fps = cap.get(cv2.CAP_PROP_FPS) # warning: may return 0 or nan
- self.frames[i] = max(int(cap.get(cv2.CAP_PROP_FRAME_COUNT)), 0) or float('inf') # infinite stream fallback
- self.fps[i] = max((fps if math.isfinite(fps) else 0) % 100, 0) or 30 # 30 FPS fallback
-
- _, self.imgs[i] = cap.read() # guarantee first frame
- self.threads[i] = Thread(target=self.update, args=([i, cap, s]), daemon=True)
- LOGGER.info(f"{st} Success ({self.frames[i]} frames {w}x{h} at {self.fps[i]:.2f} FPS)")
- self.threads[i].start()
- LOGGER.info('') # newline
-
- # check for common shapes
- s = np.stack([letterbox(x, img_size, stride=stride, auto=auto)[0].shape for x in self.imgs])
- self.rect = np.unique(s, axis=0).shape[0] == 1 # rect inference if all shapes equal
- self.auto = auto and self.rect
- self.transforms = transforms # optional
- if not self.rect:
- LOGGER.warning('WARNING ⚠️ Stream shapes differ. For optimal performance supply similarly-shaped streams.')
-
- def update(self, i, cap, stream):
- # Read stream `i` frames in daemon thread
- n, f = 0, self.frames[i] # frame number, frame array
- while cap.isOpened() and n < f:
- n += 1
- cap.grab() # .read() = .grab() followed by .retrieve()
- if n % self.vid_stride == 0:
- success, im = cap.retrieve()
- if success:
- self.imgs[i] = im
- else:
- LOGGER.warning('WARNING ⚠️ Video stream unresponsive, please check your IP camera connection.')
- self.imgs[i] = np.zeros_like(self.imgs[i])
- cap.open(stream) # re-open stream if signal was lost
- time.sleep(0.0) # wait time
-
- def __iter__(self):
- self.count = -1
- return self
-
- def __next__(self):
- self.count += 1
- if not all(x.is_alive() for x in self.threads) or cv2.waitKey(1) == ord('q'): # q to quit
- cv2.destroyAllWindows()
- raise StopIteration
-
- im0 = self.imgs.copy()
- if self.transforms:
- im = np.stack([self.transforms(x) for x in im0]) # transforms
- else:
- im = np.stack([letterbox(x, self.img_size, stride=self.stride, auto=self.auto)[0] for x in im0]) # resize
- im = im[..., ::-1].transpose((0, 3, 1, 2)) # BGR to RGB, BHWC to BCHW
- im = np.ascontiguousarray(im) # contiguous
-
- return self.sources, im, im0, None, ''
-
- def __len__(self):
- return len(self.sources) # 1E12 frames = 32 streams at 30 FPS for 30 years
-
-
-def img2label_paths(img_paths):
- # Define label paths as a function of image paths
- sa, sb = f'{os.sep}images{os.sep}', f'{os.sep}labels{os.sep}' # /images/, /labels/ substrings
- return [sb.join(x.rsplit(sa, 1)).rsplit('.', 1)[0] + '.txt' for x in img_paths]
-
-
-class LoadImagesAndLabels(Dataset):
- # YOLOv5 train_loader/val_loader, loads images and labels for training and validation
- cache_version = 0.6 # dataset labels *.cache version
- rand_interp_methods = [cv2.INTER_NEAREST, cv2.INTER_LINEAR, cv2.INTER_CUBIC, cv2.INTER_AREA, cv2.INTER_LANCZOS4]
-
- def __init__(self,
- path,
- img_size=640,
- batch_size=16,
- augment=False,
- hyp=None,
- rect=False,
- image_weights=False,
- cache_images=False,
- single_cls=False,
- stride=32,
- pad=0.0,
- min_items=0,
- prefix=''):
- self.img_size = img_size
- self.augment = augment
- self.hyp = hyp
- self.image_weights = image_weights
- self.rect = False if image_weights else rect
- self.mosaic = self.augment and not self.rect # load 4 images at a time into a mosaic (only during training)
- self.mosaic_border = [-img_size // 2, -img_size // 2]
- self.stride = stride
- self.path = path
- self.albumentations = Albumentations(size=img_size) if augment else None
-
- try:
- f = [] # image files
- for p in path if isinstance(path, list) else [path]:
- p = Path(p) # os-agnostic
- if p.is_dir(): # dir
- f += glob.glob(str(p / '**' / '*.*'), recursive=True)
- # f = list(p.rglob('*.*')) # pathlib
- elif p.is_file(): # file
- with open(p) as t:
- t = t.read().strip().splitlines()
- parent = str(p.parent) + os.sep
- f += [x.replace('./', parent, 1) if x.startswith('./') else x for x in t] # to global path
- # f += [p.parent / x.lstrip(os.sep) for x in t] # to global path (pathlib)
- else:
- raise FileNotFoundError(f'{prefix}{p} does not exist')
- self.im_files = sorted(x.replace('/', os.sep) for x in f if x.split('.')[-1].lower() in IMG_FORMATS)
- # self.img_files = sorted([x for x in f if x.suffix[1:].lower() in IMG_FORMATS]) # pathlib
- assert self.im_files, f'{prefix}No images found'
- except Exception as e:
- raise Exception(f'{prefix}Error loading data from {path}: {e}\n{HELP_URL}') from e
-
- # Check cache
- self.label_files = img2label_paths(self.im_files) # labels
- cache_path = (p if p.is_file() else Path(self.label_files[0]).parent).with_suffix('.cache')
- try:
- cache, exists = np.load(cache_path, allow_pickle=True).item(), True # load dict
- assert cache['version'] == self.cache_version # matches current version
- assert cache['hash'] == get_hash(self.label_files + self.im_files) # identical hash
- except Exception:
- cache, exists = self.cache_labels(cache_path, prefix), False # run cache ops
-
- # Display cache
- nf, nm, ne, nc, n = cache.pop('results') # found, missing, empty, corrupt, total
- if exists and LOCAL_RANK in {-1, 0}:
- d = f"Scanning '{cache_path}' images and labels... {nf} found, {nm} missing, {ne} empty, {nc} corrupt"
- tqdm(None, desc=prefix + d, total=n, initial=n, bar_format=BAR_FORMAT) # display cache results
- if cache['msgs']:
- LOGGER.info('\n'.join(cache['msgs'])) # display warnings
- assert nf > 0 or not augment, f'{prefix}No labels found in {cache_path}, can not start training. {HELP_URL}'
-
- # Read cache
- [cache.pop(k) for k in ('hash', 'version', 'msgs')] # remove items
- labels, shapes, self.segments = zip(*cache.values())
- nl = len(np.concatenate(labels, 0)) # number of labels
- assert nl > 0 or not augment, f'{prefix}All labels empty in {cache_path}, can not start training. {HELP_URL}'
- self.labels = list(labels)
- self.shapes = np.array(shapes)
- self.im_files = list(cache.keys()) # update
- self.label_files = img2label_paths(cache.keys()) # update
-
- # Filter images
- if min_items:
- include = np.array([len(x) >= min_items for x in self.labels]).nonzero()[0].astype(int)
- LOGGER.info(f'{prefix}{n - len(include)}/{n} images filtered from dataset')
- self.im_files = [self.im_files[i] for i in include]
- self.label_files = [self.label_files[i] for i in include]
- self.labels = [self.labels[i] for i in include]
- self.segments = [self.segments[i] for i in include]
- self.shapes = self.shapes[include] # wh
-
- # Create indices
- n = len(self.shapes) # number of images
- bi = np.floor(np.arange(n) / batch_size).astype(int) # batch index
- nb = bi[-1] + 1 # number of batches
- self.batch = bi # batch index of image
- self.n = n
- self.indices = range(n)
-
- # Update labels
- include_class = [] # filter labels to include only these classes (optional)
- include_class_array = np.array(include_class).reshape(1, -1)
- for i, (label, segment) in enumerate(zip(self.labels, self.segments)):
- if include_class:
- j = (label[:, 0:1] == include_class_array).any(1)
- self.labels[i] = label[j]
- if segment:
- self.segments[i] = segment[j]
- if single_cls: # single-class training, merge all classes into 0
- self.labels[i][:, 0] = 0
- if segment:
- self.segments[i][:, 0] = 0
-
- # Rectangular Training
- if self.rect:
- # Sort by aspect ratio
- s = self.shapes # wh
- ar = s[:, 1] / s[:, 0] # aspect ratio
- irect = ar.argsort()
- self.im_files = [self.im_files[i] for i in irect]
- self.label_files = [self.label_files[i] for i in irect]
- self.labels = [self.labels[i] for i in irect]
- self.segments = [self.segments[i] for i in irect]
- self.shapes = s[irect] # wh
- ar = ar[irect]
-
- # Set training image shapes
- shapes = [[1, 1]] * nb
- for i in range(nb):
- ari = ar[bi == i]
- mini, maxi = ari.min(), ari.max()
- if maxi < 1:
- shapes[i] = [maxi, 1]
- elif mini > 1:
- shapes[i] = [1, 1 / mini]
-
- self.batch_shapes = np.ceil(np.array(shapes) * img_size / stride + pad).astype(int) * stride
-
- # Cache images into RAM/disk for faster training
- if cache_images == 'ram' and not self.check_cache_ram(prefix=prefix):
- cache_images = False
- self.ims = [None] * n
- self.npy_files = [Path(f).with_suffix('.npy') for f in self.im_files]
- if cache_images:
- b, gb = 0, 1 << 30 # bytes of cached images, bytes per gigabytes
- self.im_hw0, self.im_hw = [None] * n, [None] * n
- fcn = self.cache_images_to_disk if cache_images == 'disk' else self.load_image
- results = ThreadPool(NUM_THREADS).imap(fcn, range(n))
- pbar = tqdm(enumerate(results), total=n, bar_format=BAR_FORMAT, disable=LOCAL_RANK > 0)
- for i, x in pbar:
- if cache_images == 'disk':
- b += self.npy_files[i].stat().st_size
- else: # 'ram'
- self.ims[i], self.im_hw0[i], self.im_hw[i] = x # im, hw_orig, hw_resized = load_image(self, i)
- b += self.ims[i].nbytes
- pbar.desc = f'{prefix}Caching images ({b / gb:.1f}GB {cache_images})'
- pbar.close()
-
- def check_cache_ram(self, safety_margin=0.1, prefix=''):
- # Check image caching requirements vs available memory
- b, gb = 0, 1 << 30 # bytes of cached images, bytes per gigabytes
- n = min(self.n, 30) # extrapolate from 30 random images
- for _ in range(n):
- im = cv2.imread(random.choice(self.im_files)) # sample image
- ratio = self.img_size / max(im.shape[0], im.shape[1]) # max(h, w) # ratio
- b += im.nbytes * ratio ** 2
- mem_required = b * self.n / n # GB required to cache dataset into RAM
- mem = psutil.virtual_memory()
- cache = mem_required * (1 + safety_margin) < mem.available # to cache or not to cache, that is the question
- if not cache:
- LOGGER.info(f"{prefix}{mem_required / gb:.1f}GB RAM required, "
- f"{mem.available / gb:.1f}/{mem.total / gb:.1f}GB available, "
- f"{'caching images ✅' if cache else 'not caching images ⚠️'}")
- return cache
-
- def cache_labels(self, path=Path('./labels.cache'), prefix=''):
- # Cache dataset labels, check images and read shapes
- x = {} # dict
- nm, nf, ne, nc, msgs = 0, 0, 0, 0, [] # number missing, found, empty, corrupt, messages
- desc = f"{prefix}Scanning '{path.parent / path.stem}' images and labels..."
- with Pool(NUM_THREADS) as pool:
- pbar = tqdm(pool.imap(verify_image_label, zip(self.im_files, self.label_files, repeat(prefix))),
- desc=desc,
- total=len(self.im_files),
- bar_format=BAR_FORMAT)
- for im_file, lb, shape, segments, nm_f, nf_f, ne_f, nc_f, msg in pbar:
- nm += nm_f
- nf += nf_f
- ne += ne_f
- nc += nc_f
- if im_file:
- x[im_file] = [lb, shape, segments]
- if msg:
- msgs.append(msg)
- pbar.desc = f"{desc}{nf} found, {nm} missing, {ne} empty, {nc} corrupt"
-
- pbar.close()
- if msgs:
- LOGGER.info('\n'.join(msgs))
- if nf == 0:
- LOGGER.warning(f'{prefix}WARNING ⚠️ No labels found in {path}. {HELP_URL}')
- x['hash'] = get_hash(self.label_files + self.im_files)
- x['results'] = nf, nm, ne, nc, len(self.im_files)
- x['msgs'] = msgs # warnings
- x['version'] = self.cache_version # cache version
- try:
- np.save(path, x) # save cache for next time
- path.with_suffix('.cache.npy').rename(path) # remove .npy suffix
- LOGGER.info(f'{prefix}New cache created: {path}')
- except Exception as e:
- LOGGER.warning(f'{prefix}WARNING ⚠️ Cache directory {path.parent} is not writeable: {e}') # not writeable
- return x
-
- def __len__(self):
- return len(self.im_files)
-
- # def __iter__(self):
- # self.count = -1
- # print('ran dataset iter')
- # #self.shuffled_vector = np.random.permutation(self.nF) if self.augment else np.arange(self.nF)
- # return self
-
- def __getitem__(self, index):
- index = self.indices[index] # linear, shuffled, or image_weights
-
- hyp = self.hyp
- mosaic = self.mosaic and random.random() < hyp['mosaic']
- if mosaic:
- # Load mosaic
- img, labels = self.load_mosaic(index)
- shapes = None
-
- # MixUp augmentation
- if random.random() < hyp['mixup']:
- img, labels = mixup(img, labels, *self.load_mosaic(random.randint(0, self.n - 1)))
-
- else:
- # Load image
- img, (h0, w0), (h, w) = self.load_image(index)
-
- # Letterbox
- shape = self.batch_shapes[self.batch[index]] if self.rect else self.img_size # final letterboxed shape
- img, ratio, pad = letterbox(img, shape, auto=False, scaleup=self.augment)
- shapes = (h0, w0), ((h / h0, w / w0), pad) # for COCO mAP rescaling
-
- labels = self.labels[index].copy()
- if labels.size: # normalized xywh to pixel xyxy format
- labels[:, 1:] = xywhn2xyxy(labels[:, 1:], ratio[0] * w, ratio[1] * h, padw=pad[0], padh=pad[1])
-
- if self.augment:
- img, labels = random_perspective(img,
- labels,
- degrees=hyp['degrees'],
- translate=hyp['translate'],
- scale=hyp['scale'],
- shear=hyp['shear'],
- perspective=hyp['perspective'])
-
- nl = len(labels) # number of labels
- if nl:
- labels[:, 1:5] = xyxy2xywhn(labels[:, 1:5], w=img.shape[1], h=img.shape[0], clip=True, eps=1E-3)
-
- if self.augment:
- # Albumentations
- img, labels = self.albumentations(img, labels)
- nl = len(labels) # update after albumentations
-
- # HSV color-space
- augment_hsv(img, hgain=hyp['hsv_h'], sgain=hyp['hsv_s'], vgain=hyp['hsv_v'])
-
- # Flip up-down
- if random.random() < hyp['flipud']:
- img = np.flipud(img)
- if nl:
- labels[:, 2] = 1 - labels[:, 2]
-
- # Flip left-right
- if random.random() < hyp['fliplr']:
- img = np.fliplr(img)
- if nl:
- labels[:, 1] = 1 - labels[:, 1]
-
- # Cutouts
- # labels = cutout(img, labels, p=0.5)
- # nl = len(labels) # update after cutout
-
- labels_out = torch.zeros((nl, 6))
- if nl:
- labels_out[:, 1:] = torch.from_numpy(labels)
-
- # Convert
- img = img.transpose((2, 0, 1))[::-1] # HWC to CHW, BGR to RGB
- img = np.ascontiguousarray(img)
-
- return torch.from_numpy(img), labels_out, self.im_files[index], shapes
-
- def load_image(self, i):
- # Loads 1 image from dataset index 'i', returns (im, original hw, resized hw)
- im, f, fn = self.ims[i], self.im_files[i], self.npy_files[i],
- if im is None: # not cached in RAM
- if fn.exists(): # load npy
- im = np.load(fn)
- else: # read image
- im = cv2.imread(f) # BGR
- assert im is not None, f'Image Not Found {f}'
- h0, w0 = im.shape[:2] # orig hw
- r = self.img_size / max(h0, w0) # ratio
- if r != 1: # if sizes are not equal
- interp = cv2.INTER_LINEAR if (self.augment or r > 1) else cv2.INTER_AREA
- im = cv2.resize(im, (int(w0 * r), int(h0 * r)), interpolation=interp)
- return im, (h0, w0), im.shape[:2] # im, hw_original, hw_resized
- return self.ims[i], self.im_hw0[i], self.im_hw[i] # im, hw_original, hw_resized
-
- def cache_images_to_disk(self, i):
- # Saves an image as an *.npy file for faster loading
- f = self.npy_files[i]
- if not f.exists():
- np.save(f.as_posix(), cv2.imread(self.im_files[i]))
-
- def load_mosaic(self, index):
- # YOLOv5 4-mosaic loader. Loads 1 image + 3 random images into a 4-image mosaic
- labels4, segments4 = [], []
- s = self.img_size
- yc, xc = (int(random.uniform(-x, 2 * s + x)) for x in self.mosaic_border) # mosaic center x, y
- indices = [index] + random.choices(self.indices, k=3) # 3 additional image indices
- random.shuffle(indices)
- for i, index in enumerate(indices):
- # Load image
- img, _, (h, w) = self.load_image(index)
-
- # place img in img4
- if i == 0: # top left
- img4 = np.full((s * 2, s * 2, img.shape[2]), 114, dtype=np.uint8) # base image with 4 tiles
- x1a, y1a, x2a, y2a = max(xc - w, 0), max(yc - h, 0), xc, yc # xmin, ymin, xmax, ymax (large image)
- x1b, y1b, x2b, y2b = w - (x2a - x1a), h - (y2a - y1a), w, h # xmin, ymin, xmax, ymax (small image)
- elif i == 1: # top right
- x1a, y1a, x2a, y2a = xc, max(yc - h, 0), min(xc + w, s * 2), yc
- x1b, y1b, x2b, y2b = 0, h - (y2a - y1a), min(w, x2a - x1a), h
- elif i == 2: # bottom left
- x1a, y1a, x2a, y2a = max(xc - w, 0), yc, xc, min(s * 2, yc + h)
- x1b, y1b, x2b, y2b = w - (x2a - x1a), 0, w, min(y2a - y1a, h)
- elif i == 3: # bottom right
- x1a, y1a, x2a, y2a = xc, yc, min(xc + w, s * 2), min(s * 2, yc + h)
- x1b, y1b, x2b, y2b = 0, 0, min(w, x2a - x1a), min(y2a - y1a, h)
-
- img4[y1a:y2a, x1a:x2a] = img[y1b:y2b, x1b:x2b] # img4[ymin:ymax, xmin:xmax]
- padw = x1a - x1b
- padh = y1a - y1b
-
- # Labels
- labels, segments = self.labels[index].copy(), self.segments[index].copy()
- if labels.size:
- labels[:, 1:] = xywhn2xyxy(labels[:, 1:], w, h, padw, padh) # normalized xywh to pixel xyxy format
- segments = [xyn2xy(x, w, h, padw, padh) for x in segments]
- labels4.append(labels)
- segments4.extend(segments)
-
- # Concat/clip labels
- labels4 = np.concatenate(labels4, 0)
- for x in (labels4[:, 1:], *segments4):
- np.clip(x, 0, 2 * s, out=x) # clip when using random_perspective()
- # img4, labels4 = replicate(img4, labels4) # replicate
-
- # Augment
- img4, labels4, segments4 = copy_paste(img4, labels4, segments4, p=self.hyp['copy_paste'])
- img4, labels4 = random_perspective(img4,
- labels4,
- segments4,
- degrees=self.hyp['degrees'],
- translate=self.hyp['translate'],
- scale=self.hyp['scale'],
- shear=self.hyp['shear'],
- perspective=self.hyp['perspective'],
- border=self.mosaic_border) # border to remove
-
- return img4, labels4
-
- def load_mosaic9(self, index):
- # YOLOv5 9-mosaic loader. Loads 1 image + 8 random images into a 9-image mosaic
- labels9, segments9 = [], []
- s = self.img_size
- indices = [index] + random.choices(self.indices, k=8) # 8 additional image indices
- random.shuffle(indices)
- hp, wp = -1, -1 # height, width previous
- for i, index in enumerate(indices):
- # Load image
- img, _, (h, w) = self.load_image(index)
-
- # place img in img9
- if i == 0: # center
- img9 = np.full((s * 3, s * 3, img.shape[2]), 114, dtype=np.uint8) # base image with 4 tiles
- h0, w0 = h, w
- c = s, s, s + w, s + h # xmin, ymin, xmax, ymax (base) coordinates
- elif i == 1: # top
- c = s, s - h, s + w, s
- elif i == 2: # top right
- c = s + wp, s - h, s + wp + w, s
- elif i == 3: # right
- c = s + w0, s, s + w0 + w, s + h
- elif i == 4: # bottom right
- c = s + w0, s + hp, s + w0 + w, s + hp + h
- elif i == 5: # bottom
- c = s + w0 - w, s + h0, s + w0, s + h0 + h
- elif i == 6: # bottom left
- c = s + w0 - wp - w, s + h0, s + w0 - wp, s + h0 + h
- elif i == 7: # left
- c = s - w, s + h0 - h, s, s + h0
- elif i == 8: # top left
- c = s - w, s + h0 - hp - h, s, s + h0 - hp
-
- padx, pady = c[:2]
- x1, y1, x2, y2 = (max(x, 0) for x in c) # allocate coords
-
- # Labels
- labels, segments = self.labels[index].copy(), self.segments[index].copy()
- if labels.size:
- labels[:, 1:] = xywhn2xyxy(labels[:, 1:], w, h, padx, pady) # normalized xywh to pixel xyxy format
- segments = [xyn2xy(x, w, h, padx, pady) for x in segments]
- labels9.append(labels)
- segments9.extend(segments)
-
- # Image
- img9[y1:y2, x1:x2] = img[y1 - pady:, x1 - padx:] # img9[ymin:ymax, xmin:xmax]
- hp, wp = h, w # height, width previous
-
- # Offset
- yc, xc = (int(random.uniform(0, s)) for _ in self.mosaic_border) # mosaic center x, y
- img9 = img9[yc:yc + 2 * s, xc:xc + 2 * s]
-
- # Concat/clip labels
- labels9 = np.concatenate(labels9, 0)
- labels9[:, [1, 3]] -= xc
- labels9[:, [2, 4]] -= yc
- c = np.array([xc, yc]) # centers
- segments9 = [x - c for x in segments9]
-
- for x in (labels9[:, 1:], *segments9):
- np.clip(x, 0, 2 * s, out=x) # clip when using random_perspective()
- # img9, labels9 = replicate(img9, labels9) # replicate
-
- # Augment
- img9, labels9, segments9 = copy_paste(img9, labels9, segments9, p=self.hyp['copy_paste'])
- img9, labels9 = random_perspective(img9,
- labels9,
- segments9,
- degrees=self.hyp['degrees'],
- translate=self.hyp['translate'],
- scale=self.hyp['scale'],
- shear=self.hyp['shear'],
- perspective=self.hyp['perspective'],
- border=self.mosaic_border) # border to remove
-
- return img9, labels9
-
- @staticmethod
- def collate_fn(batch):
- im, label, path, shapes = zip(*batch) # transposed
- for i, lb in enumerate(label):
- lb[:, 0] = i # add target image index for build_targets()
- return torch.stack(im, 0), torch.cat(label, 0), path, shapes
-
- @staticmethod
- def collate_fn4(batch):
- im, label, path, shapes = zip(*batch) # transposed
- n = len(shapes) // 4
- im4, label4, path4, shapes4 = [], [], path[:n], shapes[:n]
-
- ho = torch.tensor([[0.0, 0, 0, 1, 0, 0]])
- wo = torch.tensor([[0.0, 0, 1, 0, 0, 0]])
- s = torch.tensor([[1, 1, 0.5, 0.5, 0.5, 0.5]]) # scale
- for i in range(n): # zidane torch.zeros(16,3,720,1280) # BCHW
- i *= 4
- if random.random() < 0.5:
- im1 = F.interpolate(im[i].unsqueeze(0).float(), scale_factor=2.0, mode='bilinear',
- align_corners=False)[0].type(im[i].type())
- lb = label[i]
- else:
- im1 = torch.cat((torch.cat((im[i], im[i + 1]), 1), torch.cat((im[i + 2], im[i + 3]), 1)), 2)
- lb = torch.cat((label[i], label[i + 1] + ho, label[i + 2] + wo, label[i + 3] + ho + wo), 0) * s
- im4.append(im1)
- label4.append(lb)
-
- for i, lb in enumerate(label4):
- lb[:, 0] = i # add target image index for build_targets()
-
- return torch.stack(im4, 0), torch.cat(label4, 0), path4, shapes4
-
-
-# Ancillary functions --------------------------------------------------------------------------------------------------
-def flatten_recursive(path=DATASETS_DIR / 'coco128'):
- # Flatten a recursive directory by bringing all files to top level
- new_path = Path(f'{str(path)}_flat')
- if os.path.exists(new_path):
- shutil.rmtree(new_path) # delete output folder
- os.makedirs(new_path) # make new output folder
- for file in tqdm(glob.glob(f'{str(Path(path))}/**/*.*', recursive=True)):
- shutil.copyfile(file, new_path / Path(file).name)
-
-
-def extract_boxes(path=DATASETS_DIR / 'coco128'): # from utils.dataloaders import *; extract_boxes()
- # Convert detection dataset into classification dataset, with one directory per class
- path = Path(path) # images dir
- shutil.rmtree(path / 'classification') if (path / 'classification').is_dir() else None # remove existing
- files = list(path.rglob('*.*'))
- n = len(files) # number of files
- for im_file in tqdm(files, total=n):
- if im_file.suffix[1:] in IMG_FORMATS:
- # image
- im = cv2.imread(str(im_file))[..., ::-1] # BGR to RGB
- h, w = im.shape[:2]
-
- # labels
- lb_file = Path(img2label_paths([str(im_file)])[0])
- if Path(lb_file).exists():
- with open(lb_file) as f:
- lb = np.array([x.split() for x in f.read().strip().splitlines()], dtype=np.float32) # labels
-
- for j, x in enumerate(lb):
- c = int(x[0]) # class
- f = (path / 'classifier') / f'{c}' / f'{path.stem}_{im_file.stem}_{j}.jpg' # new filename
- if not f.parent.is_dir():
- f.parent.mkdir(parents=True)
-
- b = x[1:] * [w, h, w, h] # box
- # b[2:] = b[2:].max() # rectangle to square
- b[2:] = b[2:] * 1.2 + 3 # pad
- b = xywh2xyxy(b.reshape(-1, 4)).ravel().astype(int)
-
- b[[0, 2]] = np.clip(b[[0, 2]], 0, w) # clip boxes outside of image
- b[[1, 3]] = np.clip(b[[1, 3]], 0, h)
- assert cv2.imwrite(str(f), im[b[1]:b[3], b[0]:b[2]]), f'box failure in {f}'
-
-
-def autosplit(path=DATASETS_DIR / 'coco128/images', weights=(0.9, 0.1, 0.0), annotated_only=False):
- """ Autosplit a dataset into train/val/test splits and save path/autosplit_*.txt files
- Usage: from utils.dataloaders import *; autosplit()
- Arguments
- path: Path to images directory
- weights: Train, val, test weights (list, tuple)
- annotated_only: Only use images with an annotated txt file
- """
- path = Path(path) # images dir
- files = sorted(x for x in path.rglob('*.*') if x.suffix[1:].lower() in IMG_FORMATS) # image files only
- n = len(files) # number of files
- random.seed(0) # for reproducibility
- indices = random.choices([0, 1, 2], weights=weights, k=n) # assign each image to a split
-
- txt = ['autosplit_train.txt', 'autosplit_val.txt', 'autosplit_test.txt'] # 3 txt files
- for x in txt:
- if (path.parent / x).exists():
- (path.parent / x).unlink() # remove existing
-
- print(f'Autosplitting images from {path}' + ', using *.txt labeled images only' * annotated_only)
- for i, img in tqdm(zip(indices, files), total=n):
- if not annotated_only or Path(img2label_paths([str(img)])[0]).exists(): # check label
- with open(path.parent / txt[i], 'a') as f:
- f.write(f'./{img.relative_to(path.parent).as_posix()}' + '\n') # add image to txt file
-
-
-def verify_image_label(args):
- # Verify one image-label pair
- im_file, lb_file, prefix = args
- nm, nf, ne, nc, msg, segments = 0, 0, 0, 0, '', [] # number (missing, found, empty, corrupt), message, segments
- try:
- # verify images
- im = Image.open(im_file)
- im.verify() # PIL verify
- shape = exif_size(im) # image size
- assert (shape[0] > 9) & (shape[1] > 9), f'image size {shape} <10 pixels'
- assert im.format.lower() in IMG_FORMATS, f'invalid image format {im.format}'
- if im.format.lower() in ('jpg', 'jpeg'):
- with open(im_file, 'rb') as f:
- f.seek(-2, 2)
- if f.read() != b'\xff\xd9': # corrupt JPEG
- ImageOps.exif_transpose(Image.open(im_file)).save(im_file, 'JPEG', subsampling=0, quality=100)
- msg = f'{prefix}WARNING ⚠️ {im_file}: corrupt JPEG restored and saved'
-
- # verify labels
- if os.path.isfile(lb_file):
- nf = 1 # label found
- with open(lb_file) as f:
- lb = [x.split() for x in f.read().strip().splitlines() if len(x)]
- if any(len(x) > 6 for x in lb): # is segment
- classes = np.array([x[0] for x in lb], dtype=np.float32)
- segments = [np.array(x[1:], dtype=np.float32).reshape(-1, 2) for x in lb] # (cls, xy1...)
- lb = np.concatenate((classes.reshape(-1, 1), segments2boxes(segments)), 1) # (cls, xywh)
- lb = np.array(lb, dtype=np.float32)
- nl = len(lb)
- if nl:
- assert lb.shape[1] == 5, f'labels require 5 columns, {lb.shape[1]} columns detected'
- assert (lb >= 0).all(), f'negative label values {lb[lb < 0]}'
- assert (lb[:, 1:] <= 1).all(), f'non-normalized or out of bounds coordinates {lb[:, 1:][lb[:, 1:] > 1]}'
- _, i = np.unique(lb, axis=0, return_index=True)
- if len(i) < nl: # duplicate row check
- lb = lb[i] # remove duplicates
- if segments:
- segments = [segments[x] for x in i]
- msg = f'{prefix}WARNING ⚠️ {im_file}: {nl - len(i)} duplicate labels removed'
- else:
- ne = 1 # label empty
- lb = np.zeros((0, 5), dtype=np.float32)
- else:
- nm = 1 # label missing
- lb = np.zeros((0, 5), dtype=np.float32)
- return im_file, lb, shape, segments, nm, nf, ne, nc, msg
- except Exception as e:
- nc = 1
- msg = f'{prefix}WARNING ⚠️ {im_file}: ignoring corrupt image/label: {e}'
- return [None, None, None, None, nm, nf, ne, nc, msg]
-
-
-class HUBDatasetStats():
- """ Class for generating HUB dataset JSON and `-hub` dataset directory
-
- Arguments
- path: Path to data.yaml or data.zip (with data.yaml inside data.zip)
- autodownload: Attempt to download dataset if not found locally
-
- Usage
- from utils.dataloaders import HUBDatasetStats
- stats = HUBDatasetStats('coco128.yaml', autodownload=True) # usage 1
- stats = HUBDatasetStats('path/to/coco128.zip') # usage 2
- stats.get_json(save=False)
- stats.process_images()
- """
-
- def __init__(self, path='coco128.yaml', autodownload=False):
- # Initialize class
- zipped, data_dir, yaml_path = self._unzip(Path(path))
- try:
- with open(check_yaml(yaml_path), errors='ignore') as f:
- data = yaml.safe_load(f) # data dict
- if zipped:
- data['path'] = data_dir
- except Exception as e:
- raise Exception("error/HUB/dataset_stats/yaml_load") from e
-
- check_dataset(data, autodownload) # download dataset if missing
- self.hub_dir = Path(data['path'] + '-hub')
- self.im_dir = self.hub_dir / 'images'
- self.im_dir.mkdir(parents=True, exist_ok=True) # makes /images
- self.stats = {'nc': data['nc'], 'names': list(data['names'].values())} # statistics dictionary
- self.data = data
-
- @staticmethod
- def _find_yaml(dir):
- # Return data.yaml file
- files = list(dir.glob('*.yaml')) or list(dir.rglob('*.yaml')) # try root level first and then recursive
- assert files, f'No *.yaml file found in {dir}'
- if len(files) > 1:
- files = [f for f in files if f.stem == dir.stem] # prefer *.yaml files that match dir name
- assert files, f'Multiple *.yaml files found in {dir}, only 1 *.yaml file allowed'
- assert len(files) == 1, f'Multiple *.yaml files found: {files}, only 1 *.yaml file allowed in {dir}'
- return files[0]
-
- def _unzip(self, path):
- # Unzip data.zip
- if not str(path).endswith('.zip'): # path is data.yaml
- return False, None, path
- assert Path(path).is_file(), f'Error unzipping {path}, file not found'
- unzip_file(path, path=path.parent)
- dir = path.with_suffix('') # dataset directory == zip name
- assert dir.is_dir(), f'Error unzipping {path}, {dir} not found. path/to/abc.zip MUST unzip to path/to/abc/'
- return True, str(dir), self._find_yaml(dir) # zipped, data_dir, yaml_path
-
- def _hub_ops(self, f, max_dim=1920):
- # HUB ops for 1 image 'f': resize and save at reduced quality in /dataset-hub for web/app viewing
- f_new = self.im_dir / Path(f).name # dataset-hub image filename
- try: # use PIL
- im = Image.open(f)
- r = max_dim / max(im.height, im.width) # ratio
- if r < 1.0: # image too large
- im = im.resize((int(im.width * r), int(im.height * r)))
- im.save(f_new, 'JPEG', quality=50, optimize=True) # save
- except Exception as e: # use OpenCV
- LOGGER.info(f'WARNING ⚠️ HUB ops PIL failure {f}: {e}')
- im = cv2.imread(f)
- im_height, im_width = im.shape[:2]
- r = max_dim / max(im_height, im_width) # ratio
- if r < 1.0: # image too large
- im = cv2.resize(im, (int(im_width * r), int(im_height * r)), interpolation=cv2.INTER_AREA)
- cv2.imwrite(str(f_new), im)
-
- def get_json(self, save=False, verbose=False):
- # Return dataset JSON for Ultralytics HUB
- def _round(labels):
- # Update labels to integer class and 6 decimal place floats
- return [[int(c), *(round(x, 4) for x in points)] for c, *points in labels]
-
- for split in 'train', 'val', 'test':
- if self.data.get(split) is None:
- self.stats[split] = None # i.e. no test set
- continue
- dataset = LoadImagesAndLabels(self.data[split]) # load dataset
- x = np.array([
- np.bincount(label[:, 0].astype(int), minlength=self.data['nc'])
- for label in tqdm(dataset.labels, total=dataset.n, desc='Statistics')]) # shape(128x80)
- self.stats[split] = {
- 'instance_stats': {
- 'total': int(x.sum()),
- 'per_class': x.sum(0).tolist()},
- 'image_stats': {
- 'total': dataset.n,
- 'unlabelled': int(np.all(x == 0, 1).sum()),
- 'per_class': (x > 0).sum(0).tolist()},
- 'labels': [{
- str(Path(k).name): _round(v.tolist())} for k, v in zip(dataset.im_files, dataset.labels)]}
-
- # Save, print and return
- if save:
- stats_path = self.hub_dir / 'stats.json'
- print(f'Saving {stats_path.resolve()}...')
- with open(stats_path, 'w') as f:
- json.dump(self.stats, f) # save stats.json
- if verbose:
- print(json.dumps(self.stats, indent=2, sort_keys=False))
- return self.stats
-
- def process_images(self):
- # Compress images for Ultralytics HUB
- for split in 'train', 'val', 'test':
- if self.data.get(split) is None:
- continue
- dataset = LoadImagesAndLabels(self.data[split]) # load dataset
- desc = f'{split} images'
- for _ in tqdm(ThreadPool(NUM_THREADS).imap(self._hub_ops, dataset.im_files), total=dataset.n, desc=desc):
- pass
- print(f'Done. All images saved to {self.im_dir}')
- return self.im_dir
-
-
-# Classification dataloaders -------------------------------------------------------------------------------------------
-class ClassificationDataset(torchvision.datasets.ImageFolder):
- """
- YOLOv5 Classification Dataset.
- Arguments
- root: Dataset path
- transform: torchvision transforms, used by default
- album_transform: Albumentations transforms, used if installed
- """
-
- def __init__(self, root, augment, imgsz, cache=False):
- super().__init__(root=root)
- self.torch_transforms = classify_transforms(imgsz)
- self.album_transforms = classify_albumentations(augment, imgsz) if augment else None
- self.cache_ram = cache is True or cache == 'ram'
- self.cache_disk = cache == 'disk'
- self.samples = [list(x) + [Path(x[0]).with_suffix('.npy'), None] for x in self.samples] # file, index, npy, im
-
- def __getitem__(self, i):
- f, j, fn, im = self.samples[i] # filename, index, filename.with_suffix('.npy'), image
- if self.cache_ram and im is None:
- im = self.samples[i][3] = cv2.imread(f)
- elif self.cache_disk:
- if not fn.exists(): # load npy
- np.save(fn.as_posix(), cv2.imread(f))
- im = np.load(fn)
- else: # read image
- im = cv2.imread(f) # BGR
- if self.album_transforms:
- sample = self.album_transforms(image=cv2.cvtColor(im, cv2.COLOR_BGR2RGB))["image"]
- else:
- sample = self.torch_transforms(im)
- return sample, j
-
-
-def create_classification_dataloader(path,
- imgsz=224,
- batch_size=16,
- augment=True,
- cache=False,
- rank=-1,
- workers=8,
- shuffle=True):
- # Returns Dataloader object to be used with YOLOv5 Classifier
- with torch_distributed_zero_first(rank): # init dataset *.cache only once if DDP
- dataset = ClassificationDataset(root=path, imgsz=imgsz, augment=augment, cache=cache)
- batch_size = min(batch_size, len(dataset))
- nd = torch.cuda.device_count()
- nw = min([os.cpu_count() // max(nd, 1), batch_size if batch_size > 1 else 0, workers])
- sampler = None if rank == -1 else distributed.DistributedSampler(dataset, shuffle=shuffle)
- generator = torch.Generator()
- generator.manual_seed(6148914691236517205 + RANK)
- return InfiniteDataLoader(dataset,
- batch_size=batch_size,
- shuffle=shuffle and sampler is None,
- num_workers=nw,
- sampler=sampler,
- pin_memory=PIN_MEMORY,
- worker_init_fn=seed_worker,
- generator=generator) # or DataLoader(persistent_workers=True)
diff --git a/spaces/Artrajz/vits-simple-api/vits/text/korean.py b/spaces/Artrajz/vits-simple-api/vits/text/korean.py
deleted file mode 100644
index edee07429a450c55e3d8e246997faaa1e0b89cc9..0000000000000000000000000000000000000000
--- a/spaces/Artrajz/vits-simple-api/vits/text/korean.py
+++ /dev/null
@@ -1,210 +0,0 @@
-import re
-from jamo import h2j, j2hcj
-import ko_pron
-
-
-# This is a list of Korean classifiers preceded by pure Korean numerals.
-_korean_classifiers = '군데 권 개 그루 닢 대 두 마리 모 모금 뭇 발 발짝 방 번 벌 보루 살 수 술 시 쌈 움큼 정 짝 채 척 첩 축 켤레 톨 통'
-
-# List of (hangul, hangul divided) pairs:
-_hangul_divided = [(re.compile('%s' % x[0]), x[1]) for x in [
- ('ㄳ', 'ㄱㅅ'),
- ('ㄵ', 'ㄴㅈ'),
- ('ㄶ', 'ㄴㅎ'),
- ('ㄺ', 'ㄹㄱ'),
- ('ㄻ', 'ㄹㅁ'),
- ('ㄼ', 'ㄹㅂ'),
- ('ㄽ', 'ㄹㅅ'),
- ('ㄾ', 'ㄹㅌ'),
- ('ㄿ', 'ㄹㅍ'),
- ('ㅀ', 'ㄹㅎ'),
- ('ㅄ', 'ㅂㅅ'),
- ('ㅘ', 'ㅗㅏ'),
- ('ㅙ', 'ㅗㅐ'),
- ('ㅚ', 'ㅗㅣ'),
- ('ㅝ', 'ㅜㅓ'),
- ('ㅞ', 'ㅜㅔ'),
- ('ㅟ', 'ㅜㅣ'),
- ('ㅢ', 'ㅡㅣ'),
- ('ㅑ', 'ㅣㅏ'),
- ('ㅒ', 'ㅣㅐ'),
- ('ㅕ', 'ㅣㅓ'),
- ('ㅖ', 'ㅣㅔ'),
- ('ㅛ', 'ㅣㅗ'),
- ('ㅠ', 'ㅣㅜ')
-]]
-
-# List of (Latin alphabet, hangul) pairs:
-_latin_to_hangul = [(re.compile('%s' % x[0], re.IGNORECASE), x[1]) for x in [
- ('a', '에이'),
- ('b', '비'),
- ('c', '시'),
- ('d', '디'),
- ('e', '이'),
- ('f', '에프'),
- ('g', '지'),
- ('h', '에이치'),
- ('i', '아이'),
- ('j', '제이'),
- ('k', '케이'),
- ('l', '엘'),
- ('m', '엠'),
- ('n', '엔'),
- ('o', '오'),
- ('p', '피'),
- ('q', '큐'),
- ('r', '아르'),
- ('s', '에스'),
- ('t', '티'),
- ('u', '유'),
- ('v', '브이'),
- ('w', '더블유'),
- ('x', '엑스'),
- ('y', '와이'),
- ('z', '제트')
-]]
-
-# List of (ipa, lazy ipa) pairs:
-_ipa_to_lazy_ipa = [(re.compile('%s' % x[0], re.IGNORECASE), x[1]) for x in [
- ('t͡ɕ','ʧ'),
- ('d͡ʑ','ʥ'),
- ('ɲ','n^'),
- ('ɕ','ʃ'),
- ('ʷ','w'),
- ('ɭ','l`'),
- ('ʎ','ɾ'),
- ('ɣ','ŋ'),
- ('ɰ','ɯ'),
- ('ʝ','j'),
- ('ʌ','ə'),
- ('ɡ','g'),
- ('\u031a','#'),
- ('\u0348','='),
- ('\u031e',''),
- ('\u0320',''),
- ('\u0339','')
-]]
-
-
-def latin_to_hangul(text):
- for regex, replacement in _latin_to_hangul:
- text = re.sub(regex, replacement, text)
- return text
-
-
-def divide_hangul(text):
- text = j2hcj(h2j(text))
- for regex, replacement in _hangul_divided:
- text = re.sub(regex, replacement, text)
- return text
-
-
-def hangul_number(num, sino=True):
- '''Reference https://github.com/Kyubyong/g2pK'''
- num = re.sub(',', '', num)
-
- if num == '0':
- return '영'
- if not sino and num == '20':
- return '스무'
-
- digits = '123456789'
- names = '일이삼사오육칠팔구'
- digit2name = {d: n for d, n in zip(digits, names)}
-
- modifiers = '한 두 세 네 다섯 여섯 일곱 여덟 아홉'
- decimals = '열 스물 서른 마흔 쉰 예순 일흔 여든 아흔'
- digit2mod = {d: mod for d, mod in zip(digits, modifiers.split())}
- digit2dec = {d: dec for d, dec in zip(digits, decimals.split())}
-
- spelledout = []
- for i, digit in enumerate(num):
- i = len(num) - i - 1
- if sino:
- if i == 0:
- name = digit2name.get(digit, '')
- elif i == 1:
- name = digit2name.get(digit, '') + '십'
- name = name.replace('일십', '십')
- else:
- if i == 0:
- name = digit2mod.get(digit, '')
- elif i == 1:
- name = digit2dec.get(digit, '')
- if digit == '0':
- if i % 4 == 0:
- last_three = spelledout[-min(3, len(spelledout)):]
- if ''.join(last_three) == '':
- spelledout.append('')
- continue
- else:
- spelledout.append('')
- continue
- if i == 2:
- name = digit2name.get(digit, '') + '백'
- name = name.replace('일백', '백')
- elif i == 3:
- name = digit2name.get(digit, '') + '천'
- name = name.replace('일천', '천')
- elif i == 4:
- name = digit2name.get(digit, '') + '만'
- name = name.replace('일만', '만')
- elif i == 5:
- name = digit2name.get(digit, '') + '십'
- name = name.replace('일십', '십')
- elif i == 6:
- name = digit2name.get(digit, '') + '백'
- name = name.replace('일백', '백')
- elif i == 7:
- name = digit2name.get(digit, '') + '천'
- name = name.replace('일천', '천')
- elif i == 8:
- name = digit2name.get(digit, '') + '억'
- elif i == 9:
- name = digit2name.get(digit, '') + '십'
- elif i == 10:
- name = digit2name.get(digit, '') + '백'
- elif i == 11:
- name = digit2name.get(digit, '') + '천'
- elif i == 12:
- name = digit2name.get(digit, '') + '조'
- elif i == 13:
- name = digit2name.get(digit, '') + '십'
- elif i == 14:
- name = digit2name.get(digit, '') + '백'
- elif i == 15:
- name = digit2name.get(digit, '') + '천'
- spelledout.append(name)
- return ''.join(elem for elem in spelledout)
-
-
-def number_to_hangul(text):
- '''Reference https://github.com/Kyubyong/g2pK'''
- tokens = set(re.findall(r'(\d[\d,]*)([\uac00-\ud71f]+)', text))
- for token in tokens:
- num, classifier = token
- if classifier[:2] in _korean_classifiers or classifier[0] in _korean_classifiers:
- spelledout = hangul_number(num, sino=False)
- else:
- spelledout = hangul_number(num, sino=True)
- text = text.replace(f'{num}{classifier}', f'{spelledout}{classifier}')
- # digit by digit for remaining digits
- digits = '0123456789'
- names = '영일이삼사오육칠팔구'
- for d, n in zip(digits, names):
- text = text.replace(d, n)
- return text
-
-
-def korean_to_lazy_ipa(text):
- text = latin_to_hangul(text)
- text = number_to_hangul(text)
- text=re.sub('[\uac00-\ud7af]+',lambda x:ko_pron.romanise(x.group(0),'ipa').split('] ~ [')[0],text)
- for regex, replacement in _ipa_to_lazy_ipa:
- text = re.sub(regex, replacement, text)
- return text
-
-
-def korean_to_ipa(text):
- text = korean_to_lazy_ipa(text)
- return text.replace('ʧ','tʃ').replace('ʥ','dʑ')
diff --git a/spaces/Ataturk-Chatbot/HuggingFaceChat/venv/lib/python3.11/site-packages/pip/_internal/utils/encoding.py b/spaces/Ataturk-Chatbot/HuggingFaceChat/venv/lib/python3.11/site-packages/pip/_internal/utils/encoding.py
deleted file mode 100644
index 008f06a79bf598b149bdccb73e572d13331a1631..0000000000000000000000000000000000000000
--- a/spaces/Ataturk-Chatbot/HuggingFaceChat/venv/lib/python3.11/site-packages/pip/_internal/utils/encoding.py
+++ /dev/null
@@ -1,36 +0,0 @@
-import codecs
-import locale
-import re
-import sys
-from typing import List, Tuple
-
-BOMS: List[Tuple[bytes, str]] = [
- (codecs.BOM_UTF8, "utf-8"),
- (codecs.BOM_UTF16, "utf-16"),
- (codecs.BOM_UTF16_BE, "utf-16-be"),
- (codecs.BOM_UTF16_LE, "utf-16-le"),
- (codecs.BOM_UTF32, "utf-32"),
- (codecs.BOM_UTF32_BE, "utf-32-be"),
- (codecs.BOM_UTF32_LE, "utf-32-le"),
-]
-
-ENCODING_RE = re.compile(rb"coding[:=]\s*([-\w.]+)")
-
-
-def auto_decode(data: bytes) -> str:
- """Check a bytes string for a BOM to correctly detect the encoding
-
- Fallback to locale.getpreferredencoding(False) like open() on Python3"""
- for bom, encoding in BOMS:
- if data.startswith(bom):
- return data[len(bom) :].decode(encoding)
- # Lets check the first two lines as in PEP263
- for line in data.split(b"\n")[:2]:
- if line[0:1] == b"#" and ENCODING_RE.search(line):
- result = ENCODING_RE.search(line)
- assert result is not None
- encoding = result.groups()[0].decode("ascii")
- return data.decode(encoding)
- return data.decode(
- locale.getpreferredencoding(False) or sys.getdefaultencoding(),
- )
diff --git a/spaces/Ataturk-Chatbot/HuggingFaceChat/venv/lib/python3.11/site-packages/pip/_vendor/chardet/chardistribution.py b/spaces/Ataturk-Chatbot/HuggingFaceChat/venv/lib/python3.11/site-packages/pip/_vendor/chardet/chardistribution.py
deleted file mode 100644
index 176cb996408e6681a88722783919efc0e9dafb29..0000000000000000000000000000000000000000
--- a/spaces/Ataturk-Chatbot/HuggingFaceChat/venv/lib/python3.11/site-packages/pip/_vendor/chardet/chardistribution.py
+++ /dev/null
@@ -1,261 +0,0 @@
-######################## BEGIN LICENSE BLOCK ########################
-# The Original Code is Mozilla Communicator client code.
-#
-# The Initial Developer of the Original Code is
-# Netscape Communications Corporation.
-# Portions created by the Initial Developer are Copyright (C) 1998
-# the Initial Developer. All Rights Reserved.
-#
-# Contributor(s):
-# Mark Pilgrim - port to Python
-#
-# This library is free software; you can redistribute it and/or
-# modify it under the terms of the GNU Lesser General Public
-# License as published by the Free Software Foundation; either
-# version 2.1 of the License, or (at your option) any later version.
-#
-# This library is distributed in the hope that it will be useful,
-# but WITHOUT ANY WARRANTY; without even the implied warranty of
-# MERCHANTABILITY or FITNESS FOR A PARTICULAR PURPOSE. See the GNU
-# Lesser General Public License for more details.
-#
-# You should have received a copy of the GNU Lesser General Public
-# License along with this library; if not, write to the Free Software
-# Foundation, Inc., 51 Franklin St, Fifth Floor, Boston, MA
-# 02110-1301 USA
-######################### END LICENSE BLOCK #########################
-
-from typing import Tuple, Union
-
-from .big5freq import (
- BIG5_CHAR_TO_FREQ_ORDER,
- BIG5_TABLE_SIZE,
- BIG5_TYPICAL_DISTRIBUTION_RATIO,
-)
-from .euckrfreq import (
- EUCKR_CHAR_TO_FREQ_ORDER,
- EUCKR_TABLE_SIZE,
- EUCKR_TYPICAL_DISTRIBUTION_RATIO,
-)
-from .euctwfreq import (
- EUCTW_CHAR_TO_FREQ_ORDER,
- EUCTW_TABLE_SIZE,
- EUCTW_TYPICAL_DISTRIBUTION_RATIO,
-)
-from .gb2312freq import (
- GB2312_CHAR_TO_FREQ_ORDER,
- GB2312_TABLE_SIZE,
- GB2312_TYPICAL_DISTRIBUTION_RATIO,
-)
-from .jisfreq import (
- JIS_CHAR_TO_FREQ_ORDER,
- JIS_TABLE_SIZE,
- JIS_TYPICAL_DISTRIBUTION_RATIO,
-)
-from .johabfreq import JOHAB_TO_EUCKR_ORDER_TABLE
-
-
-class CharDistributionAnalysis:
- ENOUGH_DATA_THRESHOLD = 1024
- SURE_YES = 0.99
- SURE_NO = 0.01
- MINIMUM_DATA_THRESHOLD = 3
-
- def __init__(self) -> None:
- # Mapping table to get frequency order from char order (get from
- # GetOrder())
- self._char_to_freq_order: Tuple[int, ...] = tuple()
- self._table_size = 0 # Size of above table
- # This is a constant value which varies from language to language,
- # used in calculating confidence. See
- # http://www.mozilla.org/projects/intl/UniversalCharsetDetection.html
- # for further detail.
- self.typical_distribution_ratio = 0.0
- self._done = False
- self._total_chars = 0
- self._freq_chars = 0
- self.reset()
-
- def reset(self) -> None:
- """reset analyser, clear any state"""
- # If this flag is set to True, detection is done and conclusion has
- # been made
- self._done = False
- self._total_chars = 0 # Total characters encountered
- # The number of characters whose frequency order is less than 512
- self._freq_chars = 0
-
- def feed(self, char: Union[bytes, bytearray], char_len: int) -> None:
- """feed a character with known length"""
- if char_len == 2:
- # we only care about 2-bytes character in our distribution analysis
- order = self.get_order(char)
- else:
- order = -1
- if order >= 0:
- self._total_chars += 1
- # order is valid
- if order < self._table_size:
- if 512 > self._char_to_freq_order[order]:
- self._freq_chars += 1
-
- def get_confidence(self) -> float:
- """return confidence based on existing data"""
- # if we didn't receive any character in our consideration range,
- # return negative answer
- if self._total_chars <= 0 or self._freq_chars <= self.MINIMUM_DATA_THRESHOLD:
- return self.SURE_NO
-
- if self._total_chars != self._freq_chars:
- r = self._freq_chars / (
- (self._total_chars - self._freq_chars) * self.typical_distribution_ratio
- )
- if r < self.SURE_YES:
- return r
-
- # normalize confidence (we don't want to be 100% sure)
- return self.SURE_YES
-
- def got_enough_data(self) -> bool:
- # It is not necessary to receive all data to draw conclusion.
- # For charset detection, certain amount of data is enough
- return self._total_chars > self.ENOUGH_DATA_THRESHOLD
-
- def get_order(self, _: Union[bytes, bytearray]) -> int:
- # We do not handle characters based on the original encoding string,
- # but convert this encoding string to a number, here called order.
- # This allows multiple encodings of a language to share one frequency
- # table.
- return -1
-
-
-class EUCTWDistributionAnalysis(CharDistributionAnalysis):
- def __init__(self) -> None:
- super().__init__()
- self._char_to_freq_order = EUCTW_CHAR_TO_FREQ_ORDER
- self._table_size = EUCTW_TABLE_SIZE
- self.typical_distribution_ratio = EUCTW_TYPICAL_DISTRIBUTION_RATIO
-
- def get_order(self, byte_str: Union[bytes, bytearray]) -> int:
- # for euc-TW encoding, we are interested
- # first byte range: 0xc4 -- 0xfe
- # second byte range: 0xa1 -- 0xfe
- # no validation needed here. State machine has done that
- first_char = byte_str[0]
- if first_char >= 0xC4:
- return 94 * (first_char - 0xC4) + byte_str[1] - 0xA1
- return -1
-
-
-class EUCKRDistributionAnalysis(CharDistributionAnalysis):
- def __init__(self) -> None:
- super().__init__()
- self._char_to_freq_order = EUCKR_CHAR_TO_FREQ_ORDER
- self._table_size = EUCKR_TABLE_SIZE
- self.typical_distribution_ratio = EUCKR_TYPICAL_DISTRIBUTION_RATIO
-
- def get_order(self, byte_str: Union[bytes, bytearray]) -> int:
- # for euc-KR encoding, we are interested
- # first byte range: 0xb0 -- 0xfe
- # second byte range: 0xa1 -- 0xfe
- # no validation needed here. State machine has done that
- first_char = byte_str[0]
- if first_char >= 0xB0:
- return 94 * (first_char - 0xB0) + byte_str[1] - 0xA1
- return -1
-
-
-class JOHABDistributionAnalysis(CharDistributionAnalysis):
- def __init__(self) -> None:
- super().__init__()
- self._char_to_freq_order = EUCKR_CHAR_TO_FREQ_ORDER
- self._table_size = EUCKR_TABLE_SIZE
- self.typical_distribution_ratio = EUCKR_TYPICAL_DISTRIBUTION_RATIO
-
- def get_order(self, byte_str: Union[bytes, bytearray]) -> int:
- first_char = byte_str[0]
- if 0x88 <= first_char < 0xD4:
- code = first_char * 256 + byte_str[1]
- return JOHAB_TO_EUCKR_ORDER_TABLE.get(code, -1)
- return -1
-
-
-class GB2312DistributionAnalysis(CharDistributionAnalysis):
- def __init__(self) -> None:
- super().__init__()
- self._char_to_freq_order = GB2312_CHAR_TO_FREQ_ORDER
- self._table_size = GB2312_TABLE_SIZE
- self.typical_distribution_ratio = GB2312_TYPICAL_DISTRIBUTION_RATIO
-
- def get_order(self, byte_str: Union[bytes, bytearray]) -> int:
- # for GB2312 encoding, we are interested
- # first byte range: 0xb0 -- 0xfe
- # second byte range: 0xa1 -- 0xfe
- # no validation needed here. State machine has done that
- first_char, second_char = byte_str[0], byte_str[1]
- if (first_char >= 0xB0) and (second_char >= 0xA1):
- return 94 * (first_char - 0xB0) + second_char - 0xA1
- return -1
-
-
-class Big5DistributionAnalysis(CharDistributionAnalysis):
- def __init__(self) -> None:
- super().__init__()
- self._char_to_freq_order = BIG5_CHAR_TO_FREQ_ORDER
- self._table_size = BIG5_TABLE_SIZE
- self.typical_distribution_ratio = BIG5_TYPICAL_DISTRIBUTION_RATIO
-
- def get_order(self, byte_str: Union[bytes, bytearray]) -> int:
- # for big5 encoding, we are interested
- # first byte range: 0xa4 -- 0xfe
- # second byte range: 0x40 -- 0x7e , 0xa1 -- 0xfe
- # no validation needed here. State machine has done that
- first_char, second_char = byte_str[0], byte_str[1]
- if first_char >= 0xA4:
- if second_char >= 0xA1:
- return 157 * (first_char - 0xA4) + second_char - 0xA1 + 63
- return 157 * (first_char - 0xA4) + second_char - 0x40
- return -1
-
-
-class SJISDistributionAnalysis(CharDistributionAnalysis):
- def __init__(self) -> None:
- super().__init__()
- self._char_to_freq_order = JIS_CHAR_TO_FREQ_ORDER
- self._table_size = JIS_TABLE_SIZE
- self.typical_distribution_ratio = JIS_TYPICAL_DISTRIBUTION_RATIO
-
- def get_order(self, byte_str: Union[bytes, bytearray]) -> int:
- # for sjis encoding, we are interested
- # first byte range: 0x81 -- 0x9f , 0xe0 -- 0xfe
- # second byte range: 0x40 -- 0x7e, 0x81 -- oxfe
- # no validation needed here. State machine has done that
- first_char, second_char = byte_str[0], byte_str[1]
- if 0x81 <= first_char <= 0x9F:
- order = 188 * (first_char - 0x81)
- elif 0xE0 <= first_char <= 0xEF:
- order = 188 * (first_char - 0xE0 + 31)
- else:
- return -1
- order = order + second_char - 0x40
- if second_char > 0x7F:
- order = -1
- return order
-
-
-class EUCJPDistributionAnalysis(CharDistributionAnalysis):
- def __init__(self) -> None:
- super().__init__()
- self._char_to_freq_order = JIS_CHAR_TO_FREQ_ORDER
- self._table_size = JIS_TABLE_SIZE
- self.typical_distribution_ratio = JIS_TYPICAL_DISTRIBUTION_RATIO
-
- def get_order(self, byte_str: Union[bytes, bytearray]) -> int:
- # for euc-JP encoding, we are interested
- # first byte range: 0xa0 -- 0xfe
- # second byte range: 0xa1 -- 0xfe
- # no validation needed here. State machine has done that
- char = byte_str[0]
- if char >= 0xA0:
- return 94 * (char - 0xA1) + byte_str[1] - 0xA1
- return -1
diff --git a/spaces/Awesimo/jojogan/e4e/models/stylegan2/__init__.py b/spaces/Awesimo/jojogan/e4e/models/stylegan2/__init__.py
deleted file mode 100644
index e69de29bb2d1d6434b8b29ae775ad8c2e48c5391..0000000000000000000000000000000000000000
diff --git a/spaces/Awiny/Image2Paragraph/models/grit_src/third_party/CenterNet2/detectron2/layers/deform_conv.py b/spaces/Awiny/Image2Paragraph/models/grit_src/third_party/CenterNet2/detectron2/layers/deform_conv.py
deleted file mode 100644
index eca070f59645af4c9ccd003d99678f19538f355d..0000000000000000000000000000000000000000
--- a/spaces/Awiny/Image2Paragraph/models/grit_src/third_party/CenterNet2/detectron2/layers/deform_conv.py
+++ /dev/null
@@ -1,501 +0,0 @@
-# Copyright (c) Facebook, Inc. and its affiliates.
-import math
-from functools import lru_cache
-import torch
-from torch import nn
-from torch.autograd import Function
-from torch.autograd.function import once_differentiable
-from torch.nn.modules.utils import _pair
-from torchvision.ops import deform_conv2d
-
-from detectron2 import _C
-
-from .wrappers import _NewEmptyTensorOp
-
-
-class _DeformConv(Function):
- @staticmethod
- def forward(
- ctx,
- input,
- offset,
- weight,
- stride=1,
- padding=0,
- dilation=1,
- groups=1,
- deformable_groups=1,
- im2col_step=64,
- ):
- if input is not None and input.dim() != 4:
- raise ValueError(
- "Expected 4D tensor as input, got {}D tensor instead.".format(input.dim())
- )
- ctx.stride = _pair(stride)
- ctx.padding = _pair(padding)
- ctx.dilation = _pair(dilation)
- ctx.groups = groups
- ctx.deformable_groups = deformable_groups
- ctx.im2col_step = im2col_step
-
- ctx.save_for_backward(input, offset, weight)
-
- output = input.new_empty(
- _DeformConv._output_size(input, weight, ctx.padding, ctx.dilation, ctx.stride)
- )
-
- ctx.bufs_ = [input.new_empty(0), input.new_empty(0)] # columns, ones
-
- if not input.is_cuda:
- if deformable_groups != 1:
- raise NotImplementedError(
- "Deformable Conv with deformable_groups != 1 is not supported on CPUs!"
- )
- return deform_conv2d(
- input, offset, weight, stride=stride, padding=padding, dilation=dilation
- )
- else:
- cur_im2col_step = _DeformConv._cal_im2col_step(input.shape[0], ctx.im2col_step)
- assert (input.shape[0] % cur_im2col_step) == 0, "im2col step must divide batchsize"
-
- _C.deform_conv_forward(
- input,
- weight,
- offset,
- output,
- ctx.bufs_[0],
- ctx.bufs_[1],
- weight.size(3),
- weight.size(2),
- ctx.stride[1],
- ctx.stride[0],
- ctx.padding[1],
- ctx.padding[0],
- ctx.dilation[1],
- ctx.dilation[0],
- ctx.groups,
- ctx.deformable_groups,
- cur_im2col_step,
- )
- return output
-
- @staticmethod
- @once_differentiable
- def backward(ctx, grad_output):
- input, offset, weight = ctx.saved_tensors
-
- grad_input = grad_offset = grad_weight = None
-
- if not grad_output.is_cuda:
- raise NotImplementedError("Deformable Conv is not supported on CPUs!")
- else:
- cur_im2col_step = _DeformConv._cal_im2col_step(input.shape[0], ctx.im2col_step)
- assert (input.shape[0] % cur_im2col_step) == 0, "im2col step must divide batchsize"
-
- if ctx.needs_input_grad[0] or ctx.needs_input_grad[1]:
- grad_input = torch.zeros_like(input)
- grad_offset = torch.zeros_like(offset)
- _C.deform_conv_backward_input(
- input,
- offset,
- grad_output,
- grad_input,
- grad_offset,
- weight,
- ctx.bufs_[0],
- weight.size(3),
- weight.size(2),
- ctx.stride[1],
- ctx.stride[0],
- ctx.padding[1],
- ctx.padding[0],
- ctx.dilation[1],
- ctx.dilation[0],
- ctx.groups,
- ctx.deformable_groups,
- cur_im2col_step,
- )
-
- if ctx.needs_input_grad[2]:
- grad_weight = torch.zeros_like(weight)
- _C.deform_conv_backward_filter(
- input,
- offset,
- grad_output,
- grad_weight,
- ctx.bufs_[0],
- ctx.bufs_[1],
- weight.size(3),
- weight.size(2),
- ctx.stride[1],
- ctx.stride[0],
- ctx.padding[1],
- ctx.padding[0],
- ctx.dilation[1],
- ctx.dilation[0],
- ctx.groups,
- ctx.deformable_groups,
- 1,
- cur_im2col_step,
- )
-
- return grad_input, grad_offset, grad_weight, None, None, None, None, None, None
-
- @staticmethod
- def _output_size(input, weight, padding, dilation, stride):
- channels = weight.size(0)
- output_size = (input.size(0), channels)
- for d in range(input.dim() - 2):
- in_size = input.size(d + 2)
- pad = padding[d]
- kernel = dilation[d] * (weight.size(d + 2) - 1) + 1
- stride_ = stride[d]
- output_size += ((in_size + (2 * pad) - kernel) // stride_ + 1,)
- if not all(map(lambda s: s > 0, output_size)):
- raise ValueError(
- "convolution input is too small (output would be {})".format(
- "x".join(map(str, output_size))
- )
- )
- return output_size
-
- @staticmethod
- @lru_cache(maxsize=128)
- def _cal_im2col_step(input_size, default_size):
- """
- Calculate proper im2col step size, which should be divisible by input_size and not larger
- than prefer_size. Meanwhile the step size should be as large as possible to be more
- efficient. So we choose the largest one among all divisors of input_size which are smaller
- than prefer_size.
- :param input_size: input batch size .
- :param default_size: default preferred im2col step size.
- :return: the largest proper step size.
- """
- if input_size <= default_size:
- return input_size
- best_step = 1
- for step in range(2, min(int(math.sqrt(input_size)) + 1, default_size)):
- if input_size % step == 0:
- if input_size // step <= default_size:
- return input_size // step
- best_step = step
-
- return best_step
-
-
-class _ModulatedDeformConv(Function):
- @staticmethod
- def forward(
- ctx,
- input,
- offset,
- mask,
- weight,
- bias=None,
- stride=1,
- padding=0,
- dilation=1,
- groups=1,
- deformable_groups=1,
- ):
- ctx.stride = stride
- ctx.padding = padding
- ctx.dilation = dilation
- ctx.groups = groups
- ctx.deformable_groups = deformable_groups
- ctx.with_bias = bias is not None
- if not ctx.with_bias:
- bias = input.new_empty(1) # fake tensor
- if not input.is_cuda:
- raise NotImplementedError("Deformable Conv is not supported on CPUs!")
- if (
- weight.requires_grad
- or mask.requires_grad
- or offset.requires_grad
- or input.requires_grad
- ):
- ctx.save_for_backward(input, offset, mask, weight, bias)
- output = input.new_empty(_ModulatedDeformConv._infer_shape(ctx, input, weight))
- ctx._bufs = [input.new_empty(0), input.new_empty(0)]
- _C.modulated_deform_conv_forward(
- input,
- weight,
- bias,
- ctx._bufs[0],
- offset,
- mask,
- output,
- ctx._bufs[1],
- weight.shape[2],
- weight.shape[3],
- ctx.stride,
- ctx.stride,
- ctx.padding,
- ctx.padding,
- ctx.dilation,
- ctx.dilation,
- ctx.groups,
- ctx.deformable_groups,
- ctx.with_bias,
- )
- return output
-
- @staticmethod
- @once_differentiable
- def backward(ctx, grad_output):
- if not grad_output.is_cuda:
- raise NotImplementedError("Deformable Conv is not supported on CPUs!")
- input, offset, mask, weight, bias = ctx.saved_tensors
- grad_input = torch.zeros_like(input)
- grad_offset = torch.zeros_like(offset)
- grad_mask = torch.zeros_like(mask)
- grad_weight = torch.zeros_like(weight)
- grad_bias = torch.zeros_like(bias)
- _C.modulated_deform_conv_backward(
- input,
- weight,
- bias,
- ctx._bufs[0],
- offset,
- mask,
- ctx._bufs[1],
- grad_input,
- grad_weight,
- grad_bias,
- grad_offset,
- grad_mask,
- grad_output,
- weight.shape[2],
- weight.shape[3],
- ctx.stride,
- ctx.stride,
- ctx.padding,
- ctx.padding,
- ctx.dilation,
- ctx.dilation,
- ctx.groups,
- ctx.deformable_groups,
- ctx.with_bias,
- )
- if not ctx.with_bias:
- grad_bias = None
-
- return (
- grad_input,
- grad_offset,
- grad_mask,
- grad_weight,
- grad_bias,
- None,
- None,
- None,
- None,
- None,
- )
-
- @staticmethod
- def _infer_shape(ctx, input, weight):
- n = input.size(0)
- channels_out = weight.size(0)
- height, width = input.shape[2:4]
- kernel_h, kernel_w = weight.shape[2:4]
- height_out = (
- height + 2 * ctx.padding - (ctx.dilation * (kernel_h - 1) + 1)
- ) // ctx.stride + 1
- width_out = (
- width + 2 * ctx.padding - (ctx.dilation * (kernel_w - 1) + 1)
- ) // ctx.stride + 1
- return n, channels_out, height_out, width_out
-
-
-deform_conv = _DeformConv.apply
-modulated_deform_conv = _ModulatedDeformConv.apply
-
-
-class DeformConv(nn.Module):
- def __init__(
- self,
- in_channels,
- out_channels,
- kernel_size,
- stride=1,
- padding=0,
- dilation=1,
- groups=1,
- deformable_groups=1,
- bias=False,
- norm=None,
- activation=None,
- ):
- """
- Deformable convolution from :paper:`deformconv`.
-
- Arguments are similar to :class:`Conv2D`. Extra arguments:
-
- Args:
- deformable_groups (int): number of groups used in deformable convolution.
- norm (nn.Module, optional): a normalization layer
- activation (callable(Tensor) -> Tensor): a callable activation function
- """
- super(DeformConv, self).__init__()
-
- assert not bias
- assert in_channels % groups == 0, "in_channels {} cannot be divisible by groups {}".format(
- in_channels, groups
- )
- assert (
- out_channels % groups == 0
- ), "out_channels {} cannot be divisible by groups {}".format(out_channels, groups)
-
- self.in_channels = in_channels
- self.out_channels = out_channels
- self.kernel_size = _pair(kernel_size)
- self.stride = _pair(stride)
- self.padding = _pair(padding)
- self.dilation = _pair(dilation)
- self.groups = groups
- self.deformable_groups = deformable_groups
- self.norm = norm
- self.activation = activation
-
- self.weight = nn.Parameter(
- torch.Tensor(out_channels, in_channels // self.groups, *self.kernel_size)
- )
- self.bias = None
-
- nn.init.kaiming_uniform_(self.weight, nonlinearity="relu")
-
- def forward(self, x, offset):
- if x.numel() == 0:
- # When input is empty, we want to return a empty tensor with "correct" shape,
- # So that the following operations will not panic
- # if they check for the shape of the tensor.
- # This computes the height and width of the output tensor
- output_shape = [
- (i + 2 * p - (di * (k - 1) + 1)) // s + 1
- for i, p, di, k, s in zip(
- x.shape[-2:], self.padding, self.dilation, self.kernel_size, self.stride
- )
- ]
- output_shape = [x.shape[0], self.weight.shape[0]] + output_shape
- return _NewEmptyTensorOp.apply(x, output_shape)
-
- x = deform_conv(
- x,
- offset,
- self.weight,
- self.stride,
- self.padding,
- self.dilation,
- self.groups,
- self.deformable_groups,
- )
- if self.norm is not None:
- x = self.norm(x)
- if self.activation is not None:
- x = self.activation(x)
- return x
-
- def extra_repr(self):
- tmpstr = "in_channels=" + str(self.in_channels)
- tmpstr += ", out_channels=" + str(self.out_channels)
- tmpstr += ", kernel_size=" + str(self.kernel_size)
- tmpstr += ", stride=" + str(self.stride)
- tmpstr += ", padding=" + str(self.padding)
- tmpstr += ", dilation=" + str(self.dilation)
- tmpstr += ", groups=" + str(self.groups)
- tmpstr += ", deformable_groups=" + str(self.deformable_groups)
- tmpstr += ", bias=False"
- return tmpstr
-
-
-class ModulatedDeformConv(nn.Module):
- def __init__(
- self,
- in_channels,
- out_channels,
- kernel_size,
- stride=1,
- padding=0,
- dilation=1,
- groups=1,
- deformable_groups=1,
- bias=True,
- norm=None,
- activation=None,
- ):
- """
- Modulated deformable convolution from :paper:`deformconv2`.
-
- Arguments are similar to :class:`Conv2D`. Extra arguments:
-
- Args:
- deformable_groups (int): number of groups used in deformable convolution.
- norm (nn.Module, optional): a normalization layer
- activation (callable(Tensor) -> Tensor): a callable activation function
- """
- super(ModulatedDeformConv, self).__init__()
- self.in_channels = in_channels
- self.out_channels = out_channels
- self.kernel_size = _pair(kernel_size)
- self.stride = stride
- self.padding = padding
- self.dilation = dilation
- self.groups = groups
- self.deformable_groups = deformable_groups
- self.with_bias = bias
- self.norm = norm
- self.activation = activation
-
- self.weight = nn.Parameter(
- torch.Tensor(out_channels, in_channels // groups, *self.kernel_size)
- )
- if bias:
- self.bias = nn.Parameter(torch.Tensor(out_channels))
- else:
- self.bias = None
-
- nn.init.kaiming_uniform_(self.weight, nonlinearity="relu")
- if self.bias is not None:
- nn.init.constant_(self.bias, 0)
-
- def forward(self, x, offset, mask):
- if x.numel() == 0:
- output_shape = [
- (i + 2 * p - (di * (k - 1) + 1)) // s + 1
- for i, p, di, k, s in zip(
- x.shape[-2:], self.padding, self.dilation, self.kernel_size, self.stride
- )
- ]
- output_shape = [x.shape[0], self.weight.shape[0]] + output_shape
- return _NewEmptyTensorOp.apply(x, output_shape)
-
- x = modulated_deform_conv(
- x,
- offset,
- mask,
- self.weight,
- self.bias,
- self.stride,
- self.padding,
- self.dilation,
- self.groups,
- self.deformable_groups,
- )
- if self.norm is not None:
- x = self.norm(x)
- if self.activation is not None:
- x = self.activation(x)
- return x
-
- def extra_repr(self):
- tmpstr = "in_channels=" + str(self.in_channels)
- tmpstr += ", out_channels=" + str(self.out_channels)
- tmpstr += ", kernel_size=" + str(self.kernel_size)
- tmpstr += ", stride=" + str(self.stride)
- tmpstr += ", padding=" + str(self.padding)
- tmpstr += ", dilation=" + str(self.dilation)
- tmpstr += ", groups=" + str(self.groups)
- tmpstr += ", deformable_groups=" + str(self.deformable_groups)
- tmpstr += ", bias=" + str(self.with_bias)
- return tmpstr
diff --git a/spaces/Awiny/Image2Paragraph/models/grit_src/third_party/CenterNet2/tools/convert-torchvision-to-d2.py b/spaces/Awiny/Image2Paragraph/models/grit_src/third_party/CenterNet2/tools/convert-torchvision-to-d2.py
deleted file mode 100644
index 4b827d960cca69657e98bd89a9aa5623a847099d..0000000000000000000000000000000000000000
--- a/spaces/Awiny/Image2Paragraph/models/grit_src/third_party/CenterNet2/tools/convert-torchvision-to-d2.py
+++ /dev/null
@@ -1,56 +0,0 @@
-#!/usr/bin/env python
-# Copyright (c) Facebook, Inc. and its affiliates.
-
-import pickle as pkl
-import sys
-import torch
-
-"""
-Usage:
- # download one of the ResNet{18,34,50,101,152} models from torchvision:
- wget https://download.pytorch.org/models/resnet50-19c8e357.pth -O r50.pth
- # run the conversion
- ./convert-torchvision-to-d2.py r50.pth r50.pkl
-
- # Then, use r50.pkl with the following changes in config:
-
-MODEL:
- WEIGHTS: "/path/to/r50.pkl"
- PIXEL_MEAN: [123.675, 116.280, 103.530]
- PIXEL_STD: [58.395, 57.120, 57.375]
- RESNETS:
- DEPTH: 50
- STRIDE_IN_1X1: False
-INPUT:
- FORMAT: "RGB"
-
- These models typically produce slightly worse results than the
- pre-trained ResNets we use in official configs, which are the
- original ResNet models released by MSRA.
-"""
-
-if __name__ == "__main__":
- input = sys.argv[1]
-
- obj = torch.load(input, map_location="cpu")
-
- newmodel = {}
- for k in list(obj.keys()):
- old_k = k
- if "layer" not in k:
- k = "stem." + k
- for t in [1, 2, 3, 4]:
- k = k.replace("layer{}".format(t), "res{}".format(t + 1))
- for t in [1, 2, 3]:
- k = k.replace("bn{}".format(t), "conv{}.norm".format(t))
- k = k.replace("downsample.0", "shortcut")
- k = k.replace("downsample.1", "shortcut.norm")
- print(old_k, "->", k)
- newmodel[k] = obj.pop(old_k).detach().numpy()
-
- res = {"model": newmodel, "__author__": "torchvision", "matching_heuristics": True}
-
- with open(sys.argv[2], "wb") as f:
- pkl.dump(res, f)
- if obj:
- print("Unconverted keys:", obj.keys())
diff --git a/spaces/Azurro/APT-1B-Base/app.py b/spaces/Azurro/APT-1B-Base/app.py
deleted file mode 100644
index 90031db0a5014d1ccca5affa05219de8e20e2335..0000000000000000000000000000000000000000
--- a/spaces/Azurro/APT-1B-Base/app.py
+++ /dev/null
@@ -1,39 +0,0 @@
-import gradio as gr
-import torch
-from transformers import AutoModelForCausalLM, AutoTokenizer, pipeline
-
-model_name = "Azurro/APT-1B-Base"
-
-tokenizer = AutoTokenizer.from_pretrained(model_name)
-model = AutoModelForCausalLM.from_pretrained(model_name)
-
-generator = pipeline(
- "text-generation",
- model=model,
- tokenizer=tokenizer,
- torch_dtype=torch.bfloat16,
- device_map="auto",
-)
-
-def generate_text(prompt, max_length, temperature, top_k, top_p, beams):
- output = generator(prompt,
- max_length=max_length,
- temperature=temperature,
- top_k=top_k,
- do_sample=True,
- top_p=top_p,
- num_beams=beams)
- return output[0]['generated_text']
-
-input_text = gr.inputs.Textbox(label="Input Text")
-max_length = gr.inputs.Slider(1, 100, step=1, default=30, label="Max Length")
-temperature = gr.inputs.Slider(0.1, 1.0, step=0.1, default=0.8, label="Temperature")
-top_k = gr.inputs.Slider(1, 200, step=1, default=10, label="Top K")
-top_p = gr.inputs.Slider(0.1, 2.0, step=0.1, default=0.95, label="Top P")
-beams = gr.inputs.Slider(1, 20, step=1, default=1, label="Beams")
-
-outputs = gr.outputs.Textbox(label="Generated Text")
-
-iface = gr.Interface(generate_text, inputs=[input_text, max_length, temperature, top_k, top_p, beams], outputs=outputs)
-iface.queue(concurrency_count=1)
-iface.launch(max_threads=100)
diff --git a/spaces/Bart92/RVC_HF/easy_infer.py b/spaces/Bart92/RVC_HF/easy_infer.py
deleted file mode 100644
index 81a70d3648c38120f908cdaf2ea3bd15af9dec26..0000000000000000000000000000000000000000
--- a/spaces/Bart92/RVC_HF/easy_infer.py
+++ /dev/null
@@ -1,1383 +0,0 @@
-import subprocess
-import os
-import sys
-import errno
-import shutil
-import yt_dlp
-from mega import Mega
-import datetime
-import unicodedata
-import torch
-import glob
-import gradio as gr
-import gdown
-import zipfile
-import traceback
-import json
-import mdx
-from mdx_processing_script import get_model_list,id_to_ptm,prepare_mdx,run_mdx
-import requests
-import wget
-import ffmpeg
-import hashlib
-now_dir = os.getcwd()
-sys.path.append(now_dir)
-from unidecode import unidecode
-import re
-import time
-from lib.infer_pack.models_onnx import SynthesizerTrnMsNSFsidM
-from infer.modules.vc.pipeline import Pipeline
-VC = Pipeline
-from lib.infer_pack.models import (
- SynthesizerTrnMs256NSFsid,
- SynthesizerTrnMs256NSFsid_nono,
- SynthesizerTrnMs768NSFsid,
- SynthesizerTrnMs768NSFsid_nono,
-)
-from MDXNet import MDXNetDereverb
-from configs.config import Config
-from infer_uvr5 import _audio_pre_, _audio_pre_new
-from huggingface_hub import HfApi, list_models
-from huggingface_hub import login
-from i18n import I18nAuto
-i18n = I18nAuto()
-from bs4 import BeautifulSoup
-from sklearn.cluster import MiniBatchKMeans
-from dotenv import load_dotenv
-load_dotenv()
-config = Config()
-tmp = os.path.join(now_dir, "TEMP")
-shutil.rmtree(tmp, ignore_errors=True)
-os.environ["TEMP"] = tmp
-weight_root = os.getenv("weight_root")
-weight_uvr5_root = os.getenv("weight_uvr5_root")
-index_root = os.getenv("index_root")
-audio_root = "audios"
-names = []
-for name in os.listdir(weight_root):
- if name.endswith(".pth"):
- names.append(name)
-index_paths = []
-
-global indexes_list
-indexes_list = []
-
-audio_paths = []
-for root, dirs, files in os.walk(index_root, topdown=False):
- for name in files:
- if name.endswith(".index") and "trained" not in name:
- index_paths.append("%s\\%s" % (root, name))
-
-for root, dirs, files in os.walk(audio_root, topdown=False):
- for name in files:
- audio_paths.append("%s/%s" % (root, name))
-
-uvr5_names = []
-for name in os.listdir(weight_uvr5_root):
- if name.endswith(".pth") or "onnx" in name:
- uvr5_names.append(name.replace(".pth", ""))
-
-def calculate_md5(file_path):
- hash_md5 = hashlib.md5()
- with open(file_path, "rb") as f:
- for chunk in iter(lambda: f.read(4096), b""):
- hash_md5.update(chunk)
- return hash_md5.hexdigest()
-
-def format_title(title):
- formatted_title = re.sub(r'[^\w\s-]', '', title)
- formatted_title = formatted_title.replace(" ", "_")
- return formatted_title
-
-def silentremove(filename):
- try:
- os.remove(filename)
- except OSError as e:
- if e.errno != errno.ENOENT:
- raise
-def get_md5(temp_folder):
- for root, subfolders, files in os.walk(temp_folder):
- for file in files:
- if not file.startswith("G_") and not file.startswith("D_") and file.endswith(".pth") and not "_G_" in file and not "_D_" in file:
- md5_hash = calculate_md5(os.path.join(root, file))
- return md5_hash
-
- return None
-
-def find_parent(search_dir, file_name):
- for dirpath, dirnames, filenames in os.walk(search_dir):
- if file_name in filenames:
- return os.path.abspath(dirpath)
- return None
-
-def find_folder_parent(search_dir, folder_name):
- for dirpath, dirnames, filenames in os.walk(search_dir):
- if folder_name in dirnames:
- return os.path.abspath(dirpath)
- return None
-
-
-
-def download_from_url(url):
- parent_path = find_folder_parent(".", "pretrained_v2")
- zips_path = os.path.join(parent_path, 'zips')
-
- if url != '':
- print(i18n("Downloading the file: ") + f"{url}")
- if "drive.google.com" in url:
- if "file/d/" in url:
- file_id = url.split("file/d/")[1].split("/")[0]
- elif "id=" in url:
- file_id = url.split("id=")[1].split("&")[0]
- else:
- return None
-
- if file_id:
- os.chdir('./zips')
- result = subprocess.run(["gdown", f"https://drive.google.com/uc?id={file_id}", "--fuzzy"], capture_output=True, text=True, encoding='utf-8')
- if "Too many users have viewed or downloaded this file recently" in str(result.stderr):
- return "too much use"
- if "Cannot retrieve the public link of the file." in str(result.stderr):
- return "private link"
- print(result.stderr)
-
- elif "/blob/" in url:
- os.chdir('./zips')
- url = url.replace("blob", "resolve")
- response = requests.get(url)
- if response.status_code == 200:
- file_name = url.split('/')[-1]
- with open(os.path.join(zips_path, file_name), "wb") as newfile:
- newfile.write(response.content)
- else:
- os.chdir(parent_path)
- elif "mega.nz" in url:
- if "#!" in url:
- file_id = url.split("#!")[1].split("!")[0]
- elif "file/" in url:
- file_id = url.split("file/")[1].split("/")[0]
- else:
- return None
- if file_id:
- m = Mega()
- m.download_url(url, zips_path)
- elif "/tree/main" in url:
- response = requests.get(url)
- soup = BeautifulSoup(response.content, 'html.parser')
- temp_url = ''
- for link in soup.find_all('a', href=True):
- if link['href'].endswith('.zip'):
- temp_url = link['href']
- break
- if temp_url:
- url = temp_url
- url = url.replace("blob", "resolve")
- if "huggingface.co" not in url:
- url = "https://huggingface.co" + url
-
- wget.download(url)
- else:
- print("No .zip file found on the page.")
- elif "cdn.discordapp.com" in url:
- file = requests.get(url)
- if file.status_code == 200:
- name = url.split('/')
- with open(os.path.join(zips_path, name[len(name)-1]), "wb") as newfile:
- newfile.write(file.content)
- else:
- return None
- elif "pixeldrain.com" in url:
- try:
- file_id = url.split("pixeldrain.com/u/")[1]
- os.chdir('./zips')
- print(file_id)
- response = requests.get(f"https://pixeldrain.com/api/file/{file_id}")
- if response.status_code == 200:
- file_name = response.headers.get("Content-Disposition").split('filename=')[-1].strip('";')
- if not os.path.exists(zips_path):
- os.makedirs(zips_path)
- with open(os.path.join(zips_path, file_name), "wb") as newfile:
- newfile.write(response.content)
- os.chdir(parent_path)
- return "downloaded"
- else:
- os.chdir(parent_path)
- return None
- except Exception as e:
- print(e)
- os.chdir(parent_path)
- return None
- else:
- os.chdir('./zips')
- wget.download(url)
-
- os.chdir(parent_path)
- print(i18n("Full download"))
- return "downloaded"
- else:
- return None
-
-class error_message(Exception):
- def __init__(self, mensaje):
- self.mensaje = mensaje
- super().__init__(mensaje)
-
-def get_vc(sid, to_return_protect0, to_return_protect1):
- global n_spk, tgt_sr, net_g, vc, cpt, version
- if sid == "" or sid == []:
- global hubert_model
- if hubert_model is not None:
- print("clean_empty_cache")
- del net_g, n_spk, vc, hubert_model, tgt_sr
- hubert_model = net_g = n_spk = vc = hubert_model = tgt_sr = None
- if torch.cuda.is_available():
- torch.cuda.empty_cache()
- if_f0 = cpt.get("f0", 1)
- version = cpt.get("version", "v1")
- if version == "v1":
- if if_f0 == 1:
- net_g = SynthesizerTrnMs256NSFsid(
- *cpt["config"], is_half=config.is_half
- )
- else:
- net_g = SynthesizerTrnMs256NSFsid_nono(*cpt["config"])
- elif version == "v2":
- if if_f0 == 1:
- net_g = SynthesizerTrnMs768NSFsid(
- *cpt["config"], is_half=config.is_half
- )
- else:
- net_g = SynthesizerTrnMs768NSFsid_nono(*cpt["config"])
- del net_g, cpt
- if torch.cuda.is_available():
- torch.cuda.empty_cache()
- cpt = None
- return (
- {"visible": False, "__type__": "update"},
- {"visible": False, "__type__": "update"},
- {"visible": False, "__type__": "update"},
- )
- person = "%s/%s" % (weight_root, sid)
- print("loading %s" % person)
- cpt = torch.load(person, map_location="cpu")
- tgt_sr = cpt["config"][-1]
- cpt["config"][-3] = cpt["weight"]["emb_g.weight"].shape[0]
- if_f0 = cpt.get("f0", 1)
- if if_f0 == 0:
- to_return_protect0 = to_return_protect1 = {
- "visible": False,
- "value": 0.5,
- "__type__": "update",
- }
- else:
- to_return_protect0 = {
- "visible": True,
- "value": to_return_protect0,
- "__type__": "update",
- }
- to_return_protect1 = {
- "visible": True,
- "value": to_return_protect1,
- "__type__": "update",
- }
- version = cpt.get("version", "v1")
- if version == "v1":
- if if_f0 == 1:
- net_g = SynthesizerTrnMs256NSFsid(*cpt["config"], is_half=config.is_half)
- else:
- net_g = SynthesizerTrnMs256NSFsid_nono(*cpt["config"])
- elif version == "v2":
- if if_f0 == 1:
- net_g = SynthesizerTrnMs768NSFsid(*cpt["config"], is_half=config.is_half)
- else:
- net_g = SynthesizerTrnMs768NSFsid_nono(*cpt["config"])
- del net_g.enc_q
- print(net_g.load_state_dict(cpt["weight"], strict=False))
- net_g.eval().to(config.device)
- if config.is_half:
- net_g = net_g.half()
- else:
- net_g = net_g.float()
- vc = VC(tgt_sr, config)
- n_spk = cpt["config"][-3]
- return (
- {"visible": True, "maximum": n_spk, "__type__": "update"},
- to_return_protect0,
- to_return_protect1,
- )
-
-def load_downloaded_model(url):
- parent_path = find_folder_parent(".", "pretrained_v2")
- try:
- infos = []
- logs_folders = ['0_gt_wavs','1_16k_wavs','2a_f0','2b-f0nsf','3_feature256','3_feature768']
- zips_path = os.path.join(parent_path, 'zips')
- unzips_path = os.path.join(parent_path, 'unzips')
- weights_path = os.path.join(parent_path, 'weights')
- logs_dir = ""
-
- if os.path.exists(zips_path):
- shutil.rmtree(zips_path)
- if os.path.exists(unzips_path):
- shutil.rmtree(unzips_path)
-
- os.mkdir(zips_path)
- os.mkdir(unzips_path)
-
- download_file = download_from_url(url)
- if not download_file:
- print(i18n("The file could not be downloaded."))
- infos.append(i18n("The file could not be downloaded."))
- yield "\n".join(infos)
- elif download_file == "downloaded":
- print(i18n("It has been downloaded successfully."))
- infos.append(i18n("It has been downloaded successfully."))
- yield "\n".join(infos)
- elif download_file == "too much use":
- raise Exception(i18n("Too many users have recently viewed or downloaded this file"))
- elif download_file == "private link":
- raise Exception(i18n("Cannot get file from this private link"))
-
- for filename in os.listdir(zips_path):
- if filename.endswith(".zip"):
- zipfile_path = os.path.join(zips_path,filename)
- print(i18n("Proceeding with the extraction..."))
- infos.append(i18n("Proceeding with the extraction..."))
- shutil.unpack_archive(zipfile_path, unzips_path, 'zip')
- model_name = os.path.basename(zipfile_path)
- logs_dir = os.path.join(parent_path,'logs', os.path.normpath(str(model_name).replace(".zip","")))
- yield "\n".join(infos)
- else:
- print(i18n("Unzip error."))
- infos.append(i18n("Unzip error."))
- yield "\n".join(infos)
-
- index_file = False
- model_file = False
- D_file = False
- G_file = False
-
- for path, subdirs, files in os.walk(unzips_path):
- for item in files:
- item_path = os.path.join(path, item)
- if not 'G_' in item and not 'D_' in item and item.endswith('.pth'):
- model_file = True
- model_name = item.replace(".pth","")
- logs_dir = os.path.join(parent_path,'logs', model_name)
- if os.path.exists(logs_dir):
- shutil.rmtree(logs_dir)
- os.mkdir(logs_dir)
- if not os.path.exists(weights_path):
- os.mkdir(weights_path)
- if os.path.exists(os.path.join(weights_path, item)):
- os.remove(os.path.join(weights_path, item))
- if os.path.exists(item_path):
- shutil.move(item_path, weights_path)
-
- if not model_file and not os.path.exists(logs_dir):
- os.mkdir(logs_dir)
- for path, subdirs, files in os.walk(unzips_path):
- for item in files:
- item_path = os.path.join(path, item)
- if item.startswith('added_') and item.endswith('.index'):
- index_file = True
- if os.path.exists(item_path):
- if os.path.exists(os.path.join(logs_dir, item)):
- os.remove(os.path.join(logs_dir, item))
- shutil.move(item_path, logs_dir)
- if item.startswith('total_fea.npy') or item.startswith('events.'):
- if os.path.exists(item_path):
- if os.path.exists(os.path.join(logs_dir, item)):
- os.remove(os.path.join(logs_dir, item))
- shutil.move(item_path, logs_dir)
-
-
- result = ""
- if model_file:
- if index_file:
- print(i18n("The model works for inference, and has the .index file."))
- infos.append("\n" + i18n("The model works for inference, and has the .index file."))
- yield "\n".join(infos)
- else:
- print(i18n("The model works for inference, but it doesn't have the .index file."))
- infos.append("\n" + i18n("The model works for inference, but it doesn't have the .index file."))
- yield "\n".join(infos)
-
- if not index_file and not model_file:
- print(i18n("No relevant file was found to upload."))
- infos.append(i18n("No relevant file was found to upload."))
- yield "\n".join(infos)
-
- if os.path.exists(zips_path):
- shutil.rmtree(zips_path)
- if os.path.exists(unzips_path):
- shutil.rmtree(unzips_path)
- os.chdir(parent_path)
- return result
- except Exception as e:
- os.chdir(parent_path)
- if "too much use" in str(e):
- print(i18n("Too many users have recently viewed or downloaded this file"))
- yield i18n("Too many users have recently viewed or downloaded this file")
- elif "private link" in str(e):
- print(i18n("Cannot get file from this private link"))
- yield i18n("Cannot get file from this private link")
- else:
- print(e)
- yield i18n("An error occurred downloading")
- finally:
- os.chdir(parent_path)
-
-def load_dowloaded_dataset(url):
- parent_path = find_folder_parent(".", "pretrained_v2")
- infos = []
- try:
- zips_path = os.path.join(parent_path, 'zips')
- unzips_path = os.path.join(parent_path, 'unzips')
- datasets_path = os.path.join(parent_path, 'datasets')
- audio_extenions =['wav', 'mp3', 'flac', 'ogg', 'opus',
- 'm4a', 'mp4', 'aac', 'alac', 'wma',
- 'aiff', 'webm', 'ac3']
-
- if os.path.exists(zips_path):
- shutil.rmtree(zips_path)
- if os.path.exists(unzips_path):
- shutil.rmtree(unzips_path)
-
- if not os.path.exists(datasets_path):
- os.mkdir(datasets_path)
-
- os.mkdir(zips_path)
- os.mkdir(unzips_path)
-
- download_file = download_from_url(url)
-
- if not download_file:
- print(i18n("An error occurred downloading"))
- infos.append(i18n("An error occurred downloading"))
- yield "\n".join(infos)
- raise Exception(i18n("An error occurred downloading"))
- elif download_file == "downloaded":
- print(i18n("It has been downloaded successfully."))
- infos.append(i18n("It has been downloaded successfully."))
- yield "\n".join(infos)
- elif download_file == "too much use":
- raise Exception(i18n("Too many users have recently viewed or downloaded this file"))
- elif download_file == "private link":
- raise Exception(i18n("Cannot get file from this private link"))
-
- zip_path = os.listdir(zips_path)
- foldername = ""
- for file in zip_path:
- if file.endswith('.zip'):
- file_path = os.path.join(zips_path, file)
- print("....")
- foldername = file.replace(".zip","").replace(" ","").replace("-","_")
- dataset_path = os.path.join(datasets_path, foldername)
- print(i18n("Proceeding with the extraction..."))
- infos.append(i18n("Proceeding with the extraction..."))
- yield "\n".join(infos)
- shutil.unpack_archive(file_path, unzips_path, 'zip')
- if os.path.exists(dataset_path):
- shutil.rmtree(dataset_path)
-
- os.mkdir(dataset_path)
-
- for root, subfolders, songs in os.walk(unzips_path):
- for song in songs:
- song_path = os.path.join(root, song)
- if song.endswith(tuple(audio_extenions)):
- formatted_song_name = format_title(os.path.splitext(song)[0])
- extension = os.path.splitext(song)[1]
- new_song_path = os.path.join(dataset_path, f"{formatted_song_name}{extension}")
- shutil.move(song_path, new_song_path)
- else:
- print(i18n("Unzip error."))
- infos.append(i18n("Unzip error."))
- yield "\n".join(infos)
-
-
-
- if os.path.exists(zips_path):
- shutil.rmtree(zips_path)
- if os.path.exists(unzips_path):
- shutil.rmtree(unzips_path)
-
- print(i18n("The Dataset has been loaded successfully."))
- infos.append(i18n("The Dataset has been loaded successfully."))
- yield "\n".join(infos)
- except Exception as e:
- os.chdir(parent_path)
- if "too much use" in str(e):
- print(i18n("Too many users have recently viewed or downloaded this file"))
- yield i18n("Too many users have recently viewed or downloaded this file")
- elif "private link" in str(e):
- print(i18n("Cannot get file from this private link"))
- yield i18n("Cannot get file from this private link")
- else:
- print(e)
- yield i18n("An error occurred downloading")
- finally:
- os.chdir(parent_path)
-
-def save_model(modelname, save_action):
-
- parent_path = find_folder_parent(".", "pretrained_v2")
- zips_path = os.path.join(parent_path, 'zips')
- dst = os.path.join(zips_path,modelname)
- logs_path = os.path.join(parent_path, 'logs', modelname)
- weights_path = os.path.join(parent_path, 'weights', f"{modelname}.pth")
- save_folder = parent_path
- infos = []
-
- try:
- if not os.path.exists(logs_path):
- raise Exception("No model found.")
-
- if not 'content' in parent_path:
- save_folder = os.path.join(parent_path, 'RVC_Backup')
- else:
- save_folder = '/content/drive/MyDrive/RVC_Backup'
-
- infos.append(i18n("Save model"))
- yield "\n".join(infos)
-
- if not os.path.exists(save_folder):
- os.mkdir(save_folder)
- if not os.path.exists(os.path.join(save_folder, 'ManualTrainingBackup')):
- os.mkdir(os.path.join(save_folder, 'ManualTrainingBackup'))
- if not os.path.exists(os.path.join(save_folder, 'Finished')):
- os.mkdir(os.path.join(save_folder, 'Finished'))
-
- if os.path.exists(zips_path):
- shutil.rmtree(zips_path)
-
- os.mkdir(zips_path)
- added_file = glob.glob(os.path.join(logs_path, "added_*.index"))
- d_file = glob.glob(os.path.join(logs_path, "D_*.pth"))
- g_file = glob.glob(os.path.join(logs_path, "G_*.pth"))
-
- if save_action == i18n("Choose the method"):
- raise Exception("No method choosen.")
-
- if save_action == i18n("Save all"):
- print(i18n("Save all"))
- save_folder = os.path.join(save_folder, 'ManualTrainingBackup')
- shutil.copytree(logs_path, dst)
- else:
- if not os.path.exists(dst):
- os.mkdir(dst)
-
- if save_action == i18n("Save D and G"):
- print(i18n("Save D and G"))
- save_folder = os.path.join(save_folder, 'ManualTrainingBackup')
- if len(d_file) > 0:
- shutil.copy(d_file[0], dst)
- if len(g_file) > 0:
- shutil.copy(g_file[0], dst)
-
- if len(added_file) > 0:
- shutil.copy(added_file[0], dst)
- else:
- infos.append(i18n("Saved without index..."))
-
- if save_action == i18n("Save voice"):
- print(i18n("Save voice"))
- save_folder = os.path.join(save_folder, 'Finished')
- if len(added_file) > 0:
- shutil.copy(added_file[0], dst)
- else:
- infos.append(i18n("Saved without index..."))
-
- yield "\n".join(infos)
- if not os.path.exists(weights_path):
- infos.append(i18n("Saved without inference model..."))
- else:
- shutil.copy(weights_path, dst)
-
- yield "\n".join(infos)
- infos.append("\n" + i18n("This may take a few minutes, please wait..."))
- yield "\n".join(infos)
-
- shutil.make_archive(os.path.join(zips_path,f"{modelname}"), 'zip', zips_path)
- shutil.move(os.path.join(zips_path,f"{modelname}.zip"), os.path.join(save_folder, f'{modelname}.zip'))
-
- shutil.rmtree(zips_path)
- infos.append("\n" + i18n("Model saved successfully"))
- yield "\n".join(infos)
-
- except Exception as e:
- print(e)
- if "No model found." in str(e):
- infos.append(i18n("The model you want to save does not exist, be sure to enter the correct name."))
- else:
- infos.append(i18n("An error occurred saving the model"))
-
- yield "\n".join(infos)
-
-def load_downloaded_backup(url):
- parent_path = find_folder_parent(".", "pretrained_v2")
- try:
- infos = []
- logs_folders = ['0_gt_wavs','1_16k_wavs','2a_f0','2b-f0nsf','3_feature256','3_feature768']
- zips_path = os.path.join(parent_path, 'zips')
- unzips_path = os.path.join(parent_path, 'unzips')
- weights_path = os.path.join(parent_path, 'weights')
- logs_dir = os.path.join(parent_path, 'logs')
-
- if os.path.exists(zips_path):
- shutil.rmtree(zips_path)
- if os.path.exists(unzips_path):
- shutil.rmtree(unzips_path)
-
- os.mkdir(zips_path)
- os.mkdir(unzips_path)
-
- download_file = download_from_url(url)
- if not download_file:
- print(i18n("The file could not be downloaded."))
- infos.append(i18n("The file could not be downloaded."))
- yield "\n".join(infos)
- elif download_file == "downloaded":
- print(i18n("It has been downloaded successfully."))
- infos.append(i18n("It has been downloaded successfully."))
- yield "\n".join(infos)
- elif download_file == "too much use":
- raise Exception(i18n("Too many users have recently viewed or downloaded this file"))
- elif download_file == "private link":
- raise Exception(i18n("Cannot get file from this private link"))
-
- for filename in os.listdir(zips_path):
- if filename.endswith(".zip"):
- zipfile_path = os.path.join(zips_path,filename)
- zip_dir_name = os.path.splitext(filename)[0]
- unzip_dir = unzips_path
- print(i18n("Proceeding with the extraction..."))
- infos.append(i18n("Proceeding with the extraction..."))
- shutil.unpack_archive(zipfile_path, unzip_dir, 'zip')
-
- if os.path.exists(os.path.join(unzip_dir, zip_dir_name)):
- shutil.move(os.path.join(unzip_dir, zip_dir_name), logs_dir)
- else:
- new_folder_path = os.path.join(logs_dir, zip_dir_name)
- os.mkdir(new_folder_path)
- for item_name in os.listdir(unzip_dir):
- item_path = os.path.join(unzip_dir, item_name)
- if os.path.isfile(item_path):
- shutil.move(item_path, new_folder_path)
- elif os.path.isdir(item_path):
- shutil.move(item_path, new_folder_path)
-
- yield "\n".join(infos)
- else:
- print(i18n("Unzip error."))
- infos.append(i18n("Unzip error."))
- yield "\n".join(infos)
-
- result = ""
-
- for filename in os.listdir(unzips_path):
- if filename.endswith(".zip"):
- silentremove(filename)
-
- if os.path.exists(zips_path):
- shutil.rmtree(zips_path)
- if os.path.exists(os.path.join(parent_path, 'unzips')):
- shutil.rmtree(os.path.join(parent_path, 'unzips'))
- print(i18n("The Backup has been uploaded successfully."))
- infos.append("\n" + i18n("The Backup has been uploaded successfully."))
- yield "\n".join(infos)
- os.chdir(parent_path)
- return result
- except Exception as e:
- os.chdir(parent_path)
- if "too much use" in str(e):
- print(i18n("Too many users have recently viewed or downloaded this file"))
- yield i18n("Too many users have recently viewed or downloaded this file")
- elif "private link" in str(e):
- print(i18n("Cannot get file from this private link"))
- yield i18n("Cannot get file from this private link")
- else:
- print(e)
- yield i18n("An error occurred downloading")
- finally:
- os.chdir(parent_path)
-
-def save_to_wav(record_button):
- if record_button is None:
- pass
- else:
- path_to_file=record_button
- new_name = datetime.datetime.now().strftime("%Y-%m-%d_%H-%M-%S")+'.wav'
- new_path='./audios/'+new_name
- shutil.move(path_to_file,new_path)
- return new_name
-
-
-def change_choices2():
- audio_paths=[]
- for filename in os.listdir("./audios"):
- if filename.endswith(('wav', 'mp3', 'flac', 'ogg', 'opus',
- 'm4a', 'mp4', 'aac', 'alac', 'wma',
- 'aiff', 'webm', 'ac3')):
- audio_paths.append(os.path.join('./audios',filename).replace('\\', '/'))
- return {"choices": sorted(audio_paths), "__type__": "update"}, {"__type__": "update"}
-
-
-
-
-
-def uvr(input_url, output_path, model_name, inp_root, save_root_vocal, paths, save_root_ins, agg, format0, architecture):
- carpeta_a_eliminar = "yt_downloads"
- if os.path.exists(carpeta_a_eliminar) and os.path.isdir(carpeta_a_eliminar):
- for archivo in os.listdir(carpeta_a_eliminar):
- ruta_archivo = os.path.join(carpeta_a_eliminar, archivo)
- if os.path.isfile(ruta_archivo):
- os.remove(ruta_archivo)
- elif os.path.isdir(ruta_archivo):
- shutil.rmtree(ruta_archivo)
-
-
-
- ydl_opts = {
- 'no-windows-filenames': True,
- 'restrict-filenames': True,
- 'extract_audio': True,
- 'format': 'bestaudio',
- 'quiet': True,
- 'no-warnings': True,
- }
-
- try:
- print(i18n("Downloading audio from the video..."))
- with yt_dlp.YoutubeDL(ydl_opts) as ydl:
- info_dict = ydl.extract_info(input_url, download=False)
- formatted_title = format_title(info_dict.get('title', 'default_title'))
- formatted_outtmpl = output_path + '/' + formatted_title + '.wav'
- ydl_opts['outtmpl'] = formatted_outtmpl
- ydl = yt_dlp.YoutubeDL(ydl_opts)
- ydl.download([input_url])
- print(i18n("Audio downloaded!"))
- except Exception as error:
- print(i18n("An error occurred:"), error)
-
- actual_directory = os.path.dirname(__file__)
-
- vocal_directory = os.path.join(actual_directory, save_root_vocal)
- instrumental_directory = os.path.join(actual_directory, save_root_ins)
-
- vocal_formatted = f"vocal_{formatted_title}.wav.reformatted.wav_10.wav"
- instrumental_formatted = f"instrument_{formatted_title}.wav.reformatted.wav_10.wav"
-
- vocal_audio_path = os.path.join(vocal_directory, vocal_formatted)
- instrumental_audio_path = os.path.join(instrumental_directory, instrumental_formatted)
-
- vocal_formatted_mdx = f"{formatted_title}_vocal_.wav"
- instrumental_formatted_mdx = f"{formatted_title}_instrument_.wav"
-
- vocal_audio_path_mdx = os.path.join(vocal_directory, vocal_formatted_mdx)
- instrumental_audio_path_mdx = os.path.join(instrumental_directory, instrumental_formatted_mdx)
-
- if architecture == "VR":
- try:
- print(i18n("Starting audio conversion... (This might take a moment)"))
- inp_root, save_root_vocal, save_root_ins = [x.strip(" ").strip('"').strip("\n").strip('"').strip(" ") for x in [inp_root, save_root_vocal, save_root_ins]]
- usable_files = [os.path.join(inp_root, file)
- for file in os.listdir(inp_root)
- if file.endswith(tuple(sup_audioext))]
-
-
- pre_fun = MDXNetDereverb(15) if model_name == "onnx_dereverb_By_FoxJoy" else (_audio_pre_ if "DeEcho" not in model_name else _audio_pre_new)(
- agg=int(agg),
- model_path=os.path.join(weight_uvr5_root, model_name + ".pth"),
- device=config.device,
- is_half=config.is_half,
- )
-
- try:
- if paths != None:
- paths = [path.name for path in paths]
- else:
- paths = usable_files
-
- except:
- traceback.print_exc()
- paths = usable_files
- print(paths)
- for path in paths:
- inp_path = os.path.join(inp_root, path)
- need_reformat, done = 1, 0
-
- try:
- info = ffmpeg.probe(inp_path, cmd="ffprobe")
- if info["streams"][0]["channels"] == 2 and info["streams"][0]["sample_rate"] == "44100":
- need_reformat = 0
- pre_fun._path_audio_(inp_path, save_root_ins, save_root_vocal, format0)
- done = 1
- except:
- traceback.print_exc()
-
- if need_reformat:
- tmp_path = f"{tmp}/{os.path.basename(inp_path)}.reformatted.wav"
- os.system(f"ffmpeg -i {inp_path} -vn -acodec pcm_s16le -ac 2 -ar 44100 {tmp_path} -y")
- inp_path = tmp_path
-
- try:
- if not done:
- pre_fun._path_audio_(inp_path, save_root_ins, save_root_vocal, format0)
- print(f"{os.path.basename(inp_path)}->Success")
- except:
- print(f"{os.path.basename(inp_path)}->{traceback.format_exc()}")
- except:
- traceback.print_exc()
- finally:
- try:
- if model_name == "onnx_dereverb_By_FoxJoy":
- del pre_fun.pred.model
- del pre_fun.pred.model_
- else:
- del pre_fun.model
-
- del pre_fun
- return i18n("Finished"), vocal_audio_path, instrumental_audio_path
- except: traceback.print_exc()
-
- if torch.cuda.is_available(): torch.cuda.empty_cache()
-
- elif architecture == "MDX":
- try:
- print(i18n("Starting audio conversion... (This might take a moment)"))
- inp_root, save_root_vocal, save_root_ins = [x.strip(" ").strip('"').strip("\n").strip('"').strip(" ") for x in [inp_root, save_root_vocal, save_root_ins]]
-
- usable_files = [os.path.join(inp_root, file)
- for file in os.listdir(inp_root)
- if file.endswith(tuple(sup_audioext))]
- try:
- if paths != None:
- paths = [path.name for path in paths]
- else:
- paths = usable_files
-
- except:
- traceback.print_exc()
- paths = usable_files
- print(paths)
- invert=True
- denoise=True
- use_custom_parameter=True
- dim_f=2048
- dim_t=256
- n_fft=7680
- use_custom_compensation=True
- compensation=1.025
- suffix = "vocal_" #@param ["Vocals", "Drums", "Bass", "Other"]{allow-input: true}
- suffix_invert = "instrument_" #@param ["Instrumental", "Drumless", "Bassless", "Instruments"]{allow-input: true}
- print_settings = True # @param{type:"boolean"}
- onnx = id_to_ptm(model_name)
- compensation = compensation if use_custom_compensation or use_custom_parameter else None
- mdx_model = prepare_mdx(onnx,use_custom_parameter, dim_f, dim_t, n_fft, compensation=compensation)
-
-
- for path in paths:
- #inp_path = os.path.join(inp_root, path)
- suffix_naming = suffix if use_custom_parameter else None
- diff_suffix_naming = suffix_invert if use_custom_parameter else None
- run_mdx(onnx, mdx_model, path, format0, diff=invert,suffix=suffix_naming,diff_suffix=diff_suffix_naming,denoise=denoise)
-
- if print_settings:
- print()
- print('[MDX-Net_Colab settings used]')
- print(f'Model used: {onnx}')
- print(f'Model MD5: {mdx.MDX.get_hash(onnx)}')
- print(f'Model parameters:')
- print(f' -dim_f: {mdx_model.dim_f}')
- print(f' -dim_t: {mdx_model.dim_t}')
- print(f' -n_fft: {mdx_model.n_fft}')
- print(f' -compensation: {mdx_model.compensation}')
- print()
- print('[Input file]')
- print('filename(s): ')
- for filename in paths:
- print(f' -{filename}')
- print(f"{os.path.basename(filename)}->Success")
- except:
- traceback.print_exc()
- finally:
- try:
- del mdx_model
- return i18n("Finished"), vocal_audio_path_mdx, instrumental_audio_path_mdx
- except: traceback.print_exc()
-
- print("clean_empty_cache")
-
- if torch.cuda.is_available(): torch.cuda.empty_cache()
-sup_audioext = {'wav', 'mp3', 'flac', 'ogg', 'opus',
- 'm4a', 'mp4', 'aac', 'alac', 'wma',
- 'aiff', 'webm', 'ac3'}
-
-def load_downloaded_audio(url):
- parent_path = find_folder_parent(".", "pretrained_v2")
- try:
- infos = []
- audios_path = os.path.join(parent_path, 'audios')
- zips_path = os.path.join(parent_path, 'zips')
-
- if not os.path.exists(audios_path):
- os.mkdir(audios_path)
-
- download_file = download_from_url(url)
- if not download_file:
- print(i18n("The file could not be downloaded."))
- infos.append(i18n("The file could not be downloaded."))
- yield "\n".join(infos)
- elif download_file == "downloaded":
- print(i18n("It has been downloaded successfully."))
- infos.append(i18n("It has been downloaded successfully."))
- yield "\n".join(infos)
- elif download_file == "too much use":
- raise Exception(i18n("Too many users have recently viewed or downloaded this file"))
- elif download_file == "private link":
- raise Exception(i18n("Cannot get file from this private link"))
-
- for filename in os.listdir(zips_path):
- item_path = os.path.join(zips_path, filename)
- if item_path.split('.')[-1] in sup_audioext:
- if os.path.exists(item_path):
- shutil.move(item_path, audios_path)
-
- result = ""
- print(i18n("Audio files have been moved to the 'audios' folder."))
- infos.append(i18n("Audio files have been moved to the 'audios' folder."))
- yield "\n".join(infos)
-
- os.chdir(parent_path)
- return result
- except Exception as e:
- os.chdir(parent_path)
- if "too much use" in str(e):
- print(i18n("Too many users have recently viewed or downloaded this file"))
- yield i18n("Too many users have recently viewed or downloaded this file")
- elif "private link" in str(e):
- print(i18n("Cannot get file from this private link"))
- yield i18n("Cannot get file from this private link")
- else:
- print(e)
- yield i18n("An error occurred downloading")
- finally:
- os.chdir(parent_path)
-
-
-class error_message(Exception):
- def __init__(self, mensaje):
- self.mensaje = mensaje
- super().__init__(mensaje)
-
-def get_vc(sid, to_return_protect0, to_return_protect1):
- global n_spk, tgt_sr, net_g, vc, cpt, version
- if sid == "" or sid == []:
- global hubert_model
- if hubert_model is not None:
- print("clean_empty_cache")
- del net_g, n_spk, vc, hubert_model, tgt_sr
- hubert_model = net_g = n_spk = vc = hubert_model = tgt_sr = None
- if torch.cuda.is_available():
- torch.cuda.empty_cache()
- if_f0 = cpt.get("f0", 1)
- version = cpt.get("version", "v1")
- if version == "v1":
- if if_f0 == 1:
- net_g = SynthesizerTrnMs256NSFsid(
- *cpt["config"], is_half=config.is_half
- )
- else:
- net_g = SynthesizerTrnMs256NSFsid_nono(*cpt["config"])
- elif version == "v2":
- if if_f0 == 1:
- net_g = SynthesizerTrnMs768NSFsid(
- *cpt["config"], is_half=config.is_half
- )
- else:
- net_g = SynthesizerTrnMs768NSFsid_nono(*cpt["config"])
- del net_g, cpt
- if torch.cuda.is_available():
- torch.cuda.empty_cache()
- cpt = None
- return (
- {"visible": False, "__type__": "update"},
- {"visible": False, "__type__": "update"},
- {"visible": False, "__type__": "update"},
- )
- person = "%s/%s" % (weight_root, sid)
- print("loading %s" % person)
- cpt = torch.load(person, map_location="cpu")
- tgt_sr = cpt["config"][-1]
- cpt["config"][-3] = cpt["weight"]["emb_g.weight"].shape[0]
- if_f0 = cpt.get("f0", 1)
- if if_f0 == 0:
- to_return_protect0 = to_return_protect1 = {
- "visible": False,
- "value": 0.5,
- "__type__": "update",
- }
- else:
- to_return_protect0 = {
- "visible": True,
- "value": to_return_protect0,
- "__type__": "update",
- }
- to_return_protect1 = {
- "visible": True,
- "value": to_return_protect1,
- "__type__": "update",
- }
- version = cpt.get("version", "v1")
- if version == "v1":
- if if_f0 == 1:
- net_g = SynthesizerTrnMs256NSFsid(*cpt["config"], is_half=config.is_half)
- else:
- net_g = SynthesizerTrnMs256NSFsid_nono(*cpt["config"])
- elif version == "v2":
- if if_f0 == 1:
- net_g = SynthesizerTrnMs768NSFsid(*cpt["config"], is_half=config.is_half)
- else:
- net_g = SynthesizerTrnMs768NSFsid_nono(*cpt["config"])
- del net_g.enc_q
- print(net_g.load_state_dict(cpt["weight"], strict=False))
- net_g.eval().to(config.device)
- if config.is_half:
- net_g = net_g.half()
- else:
- net_g = net_g.float()
- vc = VC(tgt_sr, config)
- n_spk = cpt["config"][-3]
- return (
- {"visible": True, "maximum": n_spk, "__type__": "update"},
- to_return_protect0,
- to_return_protect1,
- )
-
-def update_model_choices(select_value):
- model_ids = get_model_list()
- model_ids_list = list(model_ids)
- if select_value == "VR":
- return {"choices": uvr5_names, "__type__": "update"}
- elif select_value == "MDX":
- return {"choices": model_ids_list, "__type__": "update"}
-
-def download_model():
- gr.Markdown(value="# " + i18n("Download Model"))
- gr.Markdown(value=i18n("It is used to download your inference models."))
- with gr.Row():
- model_url=gr.Textbox(label=i18n("Url:"))
- with gr.Row():
- download_model_status_bar=gr.Textbox(label=i18n("Status:"))
- with gr.Row():
- download_button=gr.Button(i18n("Download"))
- download_button.click(fn=load_downloaded_model, inputs=[model_url], outputs=[download_model_status_bar])
-
-def download_backup():
- gr.Markdown(value="# " + i18n("Download Backup"))
- gr.Markdown(value=i18n("It is used to download your training backups."))
- with gr.Row():
- model_url=gr.Textbox(label=i18n("Url:"))
- with gr.Row():
- download_model_status_bar=gr.Textbox(label=i18n("Status:"))
- with gr.Row():
- download_button=gr.Button(i18n("Download"))
- download_button.click(fn=load_downloaded_backup, inputs=[model_url], outputs=[download_model_status_bar])
-
-def update_dataset_list(name):
- new_datasets = []
- for foldername in os.listdir("./datasets"):
- if "." not in foldername:
- new_datasets.append(os.path.join(find_folder_parent(".","pretrained"),"datasets",foldername))
- return gr.Dropdown.update(choices=new_datasets)
-
-def download_dataset(trainset_dir4):
- gr.Markdown(value="# " + i18n("Download Dataset"))
- gr.Markdown(value=i18n("Download the dataset with the audios in a compatible format (.wav/.flac) to train your model."))
- with gr.Row():
- dataset_url=gr.Textbox(label=i18n("Url:"))
- with gr.Row():
- load_dataset_status_bar=gr.Textbox(label=i18n("Status:"))
- with gr.Row():
- load_dataset_button=gr.Button(i18n("Download"))
- load_dataset_button.click(fn=load_dowloaded_dataset, inputs=[dataset_url], outputs=[load_dataset_status_bar])
- load_dataset_status_bar.change(update_dataset_list, dataset_url, trainset_dir4)
-
-def download_audio():
- gr.Markdown(value="# " + i18n("Download Audio"))
- gr.Markdown(value=i18n("Download audios of any format for use in inference (recommended for mobile users)."))
- with gr.Row():
- audio_url=gr.Textbox(label=i18n("Url:"))
- with gr.Row():
- download_audio_status_bar=gr.Textbox(label=i18n("Status:"))
- with gr.Row():
- download_button2=gr.Button(i18n("Download"))
- download_button2.click(fn=load_downloaded_audio, inputs=[audio_url], outputs=[download_audio_status_bar])
-
-def youtube_separator():
- gr.Markdown(value="# " + i18n("Separate YouTube tracks"))
- gr.Markdown(value=i18n("Download audio from a YouTube video and automatically separate the vocal and instrumental tracks"))
- with gr.Row():
- input_url = gr.inputs.Textbox(label=i18n("Enter the YouTube link:"))
- output_path = gr.Textbox(
- label=i18n("Enter the path of the audio folder to be processed (copy it from the address bar of the file manager):"),
- value=os.path.abspath(os.getcwd()).replace('\\', '/') + "/yt_downloads",
- visible=False,
- )
- advanced_settings_checkbox = gr.Checkbox(
- value=False,
- label=i18n("Advanced Settings"),
- interactive=True,
- )
- with gr.Row(label = i18n("Advanced Settings"), visible=False, variant='compact') as advanced_settings:
- with gr.Column():
- model_select = gr.Radio(
- label=i18n("Model Architecture:"),
- choices=["VR", "MDX"],
- value="VR",
- interactive=True,
- )
- model_choose = gr.Dropdown(label=i18n("Model: (Be aware that in some models the named vocal will be the instrumental)"),
- choices=uvr5_names,
- value="HP5_only_main_vocal"
- )
- with gr.Row():
- agg = gr.Slider(
- minimum=0,
- maximum=20,
- step=1,
- label=i18n("Vocal Extraction Aggressive"),
- value=10,
- interactive=True,
- )
- with gr.Row():
- opt_vocal_root = gr.Textbox(
- label=i18n("Specify the output folder for vocals:"), value="audios",
- )
- opt_ins_root = gr.Textbox(
- label=i18n("Specify the output folder for accompaniment:"), value="audio-others",
- )
- dir_wav_input = gr.Textbox(
- label=i18n("Enter the path of the audio folder to be processed:"),
- value=((os.getcwd()).replace('\\', '/') + "/yt_downloads"),
- visible=False,
- )
- format0 = gr.Radio(
- label=i18n("Export file format"),
- choices=["wav", "flac", "mp3", "m4a"],
- value="wav",
- visible=False,
- interactive=True,
- )
- wav_inputs = gr.File(
- file_count="multiple", label=i18n("You can also input audio files in batches. Choose one of the two options. Priority is given to reading from the folder."),
- visible=False,
- )
- model_select.change(
- fn=update_model_choices,
- inputs=model_select,
- outputs=model_choose,
- )
- with gr.Row():
- vc_output4 = gr.Textbox(label=i18n("Status:"))
- vc_output5 = gr.Audio(label=i18n("Vocal"), type='filepath')
- vc_output6 = gr.Audio(label=i18n("Instrumental"), type='filepath')
- with gr.Row():
- but2 = gr.Button(i18n("Download and Separate"))
- but2.click(
- uvr,
- [
- input_url,
- output_path,
- model_choose,
- dir_wav_input,
- opt_vocal_root,
- wav_inputs,
- opt_ins_root,
- agg,
- format0,
- model_select
- ],
- [vc_output4, vc_output5, vc_output6],
- )
- def toggle_advanced_settings(checkbox):
- return {"visible": checkbox, "__type__": "update"}
-
- advanced_settings_checkbox.change(
- fn=toggle_advanced_settings,
- inputs=[advanced_settings_checkbox],
- outputs=[advanced_settings]
- )
-
-
-def get_bark_voice():
- mensaje = """
-v2/en_speaker_0 English Male
-v2/en_speaker_1 English Male
-v2/en_speaker_2 English Male
-v2/en_speaker_3 English Male
-v2/en_speaker_4 English Male
-v2/en_speaker_5 English Male
-v2/en_speaker_6 English Male
-v2/en_speaker_7 English Male
-v2/en_speaker_8 English Male
-v2/en_speaker_9 English Female
-v2/zh_speaker_0 Chinese (Simplified) Male
-v2/zh_speaker_1 Chinese (Simplified) Male
-v2/zh_speaker_2 Chinese (Simplified) Male
-v2/zh_speaker_3 Chinese (Simplified) Male
-v2/zh_speaker_4 Chinese (Simplified) Female
-v2/zh_speaker_5 Chinese (Simplified) Male
-v2/zh_speaker_6 Chinese (Simplified) Female
-v2/zh_speaker_7 Chinese (Simplified) Female
-v2/zh_speaker_8 Chinese (Simplified) Male
-v2/zh_speaker_9 Chinese (Simplified) Female
-v2/fr_speaker_0 French Male
-v2/fr_speaker_1 French Female
-v2/fr_speaker_2 French Female
-v2/fr_speaker_3 French Male
-v2/fr_speaker_4 French Male
-v2/fr_speaker_5 French Female
-v2/fr_speaker_6 French Male
-v2/fr_speaker_7 French Male
-v2/fr_speaker_8 French Male
-v2/fr_speaker_9 French Male
-v2/de_speaker_0 German Male
-v2/de_speaker_1 German Male
-v2/de_speaker_2 German Male
-v2/de_speaker_3 German Female
-v2/de_speaker_4 German Male
-v2/de_speaker_5 German Male
-v2/de_speaker_6 German Male
-v2/de_speaker_7 German Male
-v2/de_speaker_8 German Female
-v2/de_speaker_9 German Male
-v2/hi_speaker_0 Hindi Female
-v2/hi_speaker_1 Hindi Female
-v2/hi_speaker_2 Hindi Male
-v2/hi_speaker_3 Hindi Female
-v2/hi_speaker_4 Hindi Female
-v2/hi_speaker_5 Hindi Male
-v2/hi_speaker_6 Hindi Male
-v2/hi_speaker_7 Hindi Male
-v2/hi_speaker_8 Hindi Male
-v2/hi_speaker_9 Hindi Female
-v2/it_speaker_0 Italian Male
-v2/it_speaker_1 Italian Male
-v2/it_speaker_2 Italian Female
-v2/it_speaker_3 Italian Male
-v2/it_speaker_4 Italian Male
-v2/it_speaker_5 Italian Male
-v2/it_speaker_6 Italian Male
-v2/it_speaker_7 Italian Female
-v2/it_speaker_8 Italian Male
-v2/it_speaker_9 Italian Female
-v2/ja_speaker_0 Japanese Female
-v2/ja_speaker_1 Japanese Female
-v2/ja_speaker_2 Japanese Male
-v2/ja_speaker_3 Japanese Female
-v2/ja_speaker_4 Japanese Female
-v2/ja_speaker_5 Japanese Female
-v2/ja_speaker_6 Japanese Male
-v2/ja_speaker_7 Japanese Female
-v2/ja_speaker_8 Japanese Female
-v2/ja_speaker_9 Japanese Female
-v2/ko_speaker_0 Korean Female
-v2/ko_speaker_1 Korean Male
-v2/ko_speaker_2 Korean Male
-v2/ko_speaker_3 Korean Male
-v2/ko_speaker_4 Korean Male
-v2/ko_speaker_5 Korean Male
-v2/ko_speaker_6 Korean Male
-v2/ko_speaker_7 Korean Male
-v2/ko_speaker_8 Korean Male
-v2/ko_speaker_9 Korean Male
-v2/pl_speaker_0 Polish Male
-v2/pl_speaker_1 Polish Male
-v2/pl_speaker_2 Polish Male
-v2/pl_speaker_3 Polish Male
-v2/pl_speaker_4 Polish Female
-v2/pl_speaker_5 Polish Male
-v2/pl_speaker_6 Polish Female
-v2/pl_speaker_7 Polish Male
-v2/pl_speaker_8 Polish Male
-v2/pl_speaker_9 Polish Female
-v2/pt_speaker_0 Portuguese Male
-v2/pt_speaker_1 Portuguese Male
-v2/pt_speaker_2 Portuguese Male
-v2/pt_speaker_3 Portuguese Male
-v2/pt_speaker_4 Portuguese Male
-v2/pt_speaker_5 Portuguese Male
-v2/pt_speaker_6 Portuguese Male
-v2/pt_speaker_7 Portuguese Male
-v2/pt_speaker_8 Portuguese Male
-v2/pt_speaker_9 Portuguese Male
-v2/ru_speaker_0 Russian Male
-v2/ru_speaker_1 Russian Male
-v2/ru_speaker_2 Russian Male
-v2/ru_speaker_3 Russian Male
-v2/ru_speaker_4 Russian Male
-v2/ru_speaker_5 Russian Female
-v2/ru_speaker_6 Russian Female
-v2/ru_speaker_7 Russian Male
-v2/ru_speaker_8 Russian Male
-v2/ru_speaker_9 Russian Female
-v2/es_speaker_0 Spanish Male
-v2/es_speaker_1 Spanish Male
-v2/es_speaker_2 Spanish Male
-v2/es_speaker_3 Spanish Male
-v2/es_speaker_4 Spanish Male
-v2/es_speaker_5 Spanish Male
-v2/es_speaker_6 Spanish Male
-v2/es_speaker_7 Spanish Male
-v2/es_speaker_8 Spanish Female
-v2/es_speaker_9 Spanish Female
-v2/tr_speaker_0 Turkish Male
-v2/tr_speaker_1 Turkish Male
-v2/tr_speaker_2 Turkish Male
-v2/tr_speaker_3 Turkish Male
-v2/tr_speaker_4 Turkish Female
-v2/tr_speaker_5 Turkish Female
-v2/tr_speaker_6 Turkish Male
-v2/tr_speaker_7 Turkish Male
-v2/tr_speaker_8 Turkish Male
-v2/tr_speaker_9 Turkish Male
- """
-# Dividir el mensaje en líneas
- lineas = mensaje.split("\n")
- datos_deseados = []
- for linea in lineas:
- partes = linea.split("\t")
- if len(partes) == 3:
- clave, _, genero = partes
- datos_deseados.append(f"{clave}-{genero}")
-
- return datos_deseados
-
-
-def get_edge_voice():
- completed_process = subprocess.run(['edge-tts',"-l"], capture_output=True, text=True)
- lines = completed_process.stdout.strip().split("\n")
- data = []
- current_entry = {}
- for line in lines:
- if line.startswith("Name: "):
- if current_entry:
- data.append(current_entry)
- current_entry = {"Name": line.split(": ")[1]}
- elif line.startswith("Gender: "):
- current_entry["Gender"] = line.split(": ")[1]
- if current_entry:
- data.append(current_entry)
- tts_voice = []
- for entry in data:
- name = entry["Name"]
- gender = entry["Gender"]
- formatted_entry = f'{name}-{gender}'
- tts_voice.append(formatted_entry)
- return tts_voice
-
-
-#print(set_tts_voice)
diff --git a/spaces/Benson/text-generation/Examples/Bloque Explosin Aventura Maestro Apk Descargar.md b/spaces/Benson/text-generation/Examples/Bloque Explosin Aventura Maestro Apk Descargar.md
deleted file mode 100644
index 0338cf1557d9617074d0e34d5b6c0556753c723f..0000000000000000000000000000000000000000
--- a/spaces/Benson/text-generation/Examples/Bloque Explosin Aventura Maestro Apk Descargar.md
+++ /dev/null
@@ -1,93 +0,0 @@
-
-
Bloque explosión aventura maestro APK Descargar: Un divertido y adictivo juego de rompecabezas de bloques
-
Si estás buscando un divertido y adictivo juego de puzzle de bloques que relaje tu mente y desafíe tu cerebro, deberías probar Block Blast Adventure Master. Este juego es una mezcla perfecta de bloques y rompecabezas mentales, combinando la creatividad con los clásicos. Puedes descargar el archivo APK de este juego desde varias fuentes y disfrutarlo en tu dispositivo Android. En este artículo, te contaremos todo lo que necesitas saber sobre este juego, incluyendo qué es, cómo descargarlo e instalarlo, cómo jugarlo, cuáles son sus características, cuáles son algunos consejos y trucos, cuáles son algunos comentarios y algunas preguntas frecuentes.
-
¿Qué es Block Blast Adventure Master?
-
Block Blast Adventure Master es un juego de puzzle desarrollado por Hungry Studio. El juego ha estado disponible desde septiembre de 2022 y se ha descargado más de 10 millones de veces. Tiene una alta calificación de 4.8 de 5 estrellas en Google Play Store, basado en más de 65,000 comentarios. El juego fue actualizado por última vez el 15 de junio de 2023.
Un clásico juego de puzzle de bloques para todas las edades
-
Block Blast Adventure Master es un clásico juego de puzzle de bloques que es adecuado para todas las edades. El juego es simple pero adictivo. Tienes que arrastrar y soltar bloques de cubo en una cuadrícula de 8x8 y llenar filas o columnas con bloques para eliminarlos. Si no hay bloques restantes, el juego ha terminado. Los bloques no se pueden rotar, por lo que es más difícil e incierto.
-
Un juego de bloques gratis con un modo de aventura de historia
-
Block Blast Adventure Master es un juego de bloques gratis que también tiene un modo de aventura de historia. En este modo, puedes seguir el viaje de un lindo personaje llamado Woody mientras explora diferentes mundos y se enfrenta a varios obstáculos. Puedes desbloquear nuevos niveles y temas a medida que avanzas en la historia. También puedes recoger monedas y gemas para comprar objetos y potenciadores.
-
Un desafío mental desafiante y relajante
-
-
¿Cómo descargar e instalar Block Blast Adventure Master APK?
-
Si desea descargar e instalar Block Blast Adventure Master APK en su dispositivo Android, usted tiene dos opciones. Puede descargarlo desde las tiendas de aplicaciones oficiales o desde sitios web de terceros. Estos son los pasos para ambas opciones:
-
Descargar de Google Play Store o App Store
-
La forma más fácil y segura de descargar e instalar Block Blast Adventure Master APK es conseguirlo desde la Google Play Store o la App Store. Puedes simplemente seguir estos pasos:
-
-
Abre la Google Play Store o la App Store en tu dispositivo.
-
Buscar "Block Blast Adventure Master" en la barra de búsqueda.
-
Seleccione el juego de la lista de resultados y toque en "Instalar".
-
Espere a que se complete la descarga y la instalación.
-
Iniciar el juego y disfrutar.
-
-
Descargar desde APKCombo o AppBrain
-
Si no puede acceder a la Google Play Store o la App Store, o si desea obtener la última versión de Block Blast Adventure Master APK, también puede descargarlo de sitios web de terceros como APKCombo o AppBrain. Sin embargo, debe tener cuidado y solo descargar de fuentes confiables, ya que algunos sitios web pueden contener malware o virus. También debe habilitar la instalación de aplicaciones de fuentes desconocidas en la configuración del dispositivo. Puede seguir estos pasos:
-
-
Ir a APKCombo o AppBrain en su navegador.
-
Buscar "Block Blast Adventure Master" en la barra de búsqueda.
-
Seleccione el juego de la lista de resultados y toque en "Descargar APK".
-
Espere a que la descarga termine y localice el archivo en su dispositivo.
-
Toque en el archivo y siga las instrucciones para instalarlo.
-
Iniciar el juego y disfrutar.
-
-
¿Cómo se juega Block Blast Adventure Master?
-
-
Arrastre y suelte bloques de cubo en una cuadrícula de 8x8
-
El juego te dará tres bloques de cubo a la vez en la parte inferior de la pantalla. Puedes arrastrarlos y soltarlos en cualquier espacio vacío de la cuadrícula de 8x8. También puede ver una vista previa de los siguientes tres bloques en la parte superior de la pantalla. No puedes rotar los bloques, así que tienes que pensar cuidadosamente dónde colocarlos.
-
Rellenar filas o columnas con bloques para eliminarlos
-
Cuando llenas una fila o una columna con bloques, desaparecerán y obtendrás puntos. Cuantas más filas o columnas borre a la vez, más puntos obtendrá. También puede realizar combos borrando varias filas o columnas en sucesión. Esto desencadenará animaciones de eliminación cool y puntos de bonificación.
-
-
Usa el espacio en blanco sabiamente y planifica con anticipación
-
El juego terminará cuando no haya más espacios para nuevos bloques. Por lo tanto, tienes que usar el espacio en blanco sabiamente y planificar con anticipación. Trata de evitar dejar huecos o agujeros en la red, ya que limitarán tus opciones más adelante. Además, intente equilibrar la distribución de bloques en diferentes áreas de la cuadrícula, para que pueda borrar más filas o columnas a la vez.
-
Realizar combos para obtener puntos de bonificación y animaciones cool
-
Uno de los aspectos más satisfactorios de Block Blast Adventure Master es realizar combos. Un combo es cuando borra más de una fila o columna a la vez, o cuando borra filas o columnas en sucesión sin colocar nuevos bloques en el medio. Cuando realices un combo, obtendrás puntos de bonificación y animaciones geniales que te harán sentir increíble.
-
¿Cuáles son las características de Block Blast Adventure Master?
-
Block Blast Adventure Master no es solo un simple juego de puzzle de bloques. Tiene muchas características que lo hacen destacar de otros juegos de su género. Estos son algunos de ellos:
-
Gráficos coloridos y efectos de sonido maravillosos
-
-
No se requiere wifi, ideal para matar el tiempo
-
El juego no requiere wifi o conexión a Internet para jugar. Puedes jugar en cualquier momento y en cualquier lugar, ya sea en casa, en el trabajo, en el autobús o en un avión. El juego es ideal para matar el tiempo y relajar tu mente.
-
Intentos ilimitados, sin límite de tiempo, sin presión
-
El juego no tiene límite sobre cuántas veces puedes probar o cuánto tiempo puedes jugar. Puedes jugar a tu propio ritmo y disfrutar del juego sin ninguna presión. También puedes pausar y reanudar el juego cuando quieras.
-
Diferentes niveles de dificultad y modos para elegir
-
El juego tiene cuatro niveles de dificultad en el modo clásico: fácil, medio, duro y experto. Usted puede elegir el nivel que se adapte a su habilidad y preferencia. El juego también tiene un modo de aventura donde puedes seguir la historia de Woody y desbloquear nuevos mundos y temas. También puede cambiar entre los modos en cualquier momento.
-
¿Cuáles son algunos consejos y trucos para Block Blast Adventure Master?
-
Si quieres mejorar tus habilidades y anotar en Block Blast Adventure Master, aquí hay algunos consejos y trucos que pueden ayudarte:
-
Vea los videos de YouTube para obtener sugerencias y soluciones
-
Si estás atascado en un nivel o quieres ver cómo otros jugadores juegan el juego, puedes ver algunos videos de YouTube que muestran sugerencias y soluciones para Block Blast Adventure Master. Puedes aprender de sus estrategias y técnicas y aplicarlas a tu propio juego.
-
Elija la mejor posición para el bloque basado en su forma
-
Uno de los factores clave en el juego Block Blast Adventure Master es elegir la mejor posición para el bloque en función de su forma. Tienes que considerar cómo el bloque encajará en la red y cómo afectará a los bloques futuros. También tienes que evitar dejar huecos o agujeros que limiten tus opciones más adelante. Intente colocar los bloques de una manera que cree más oportunidades para limpiar filas o columnas.
-
-
Otro factor importante en jugar Block Blast Adventure Master está tratando de borrar varias líneas a la vez para obtener una puntuación más alta. Cuantas más líneas borres a la vez, más puntos obtendrás. También obtendrás puntos extra y animaciones geniales si realizas combos. Por lo tanto, debe intentar planificar con anticipación y crear oportunidades para borrar varias líneas a la vez.
-
Siga la página de Facebook para actualizaciones y noticias
-
Si quieres mantenerte actualizado sobre las últimas noticias y actualizaciones sobre Block Blast Adventure Master, debes seguir la página oficial de Facebook del juego. También puedes interactuar con otros jugadores y compartir tus comentarios y sugerencias. También puede obtener algunas ofertas especiales y recompensas de vez en cuando.
-
¿Cuáles son algunas opiniones de Block Blast Adventure Master?
-
Block Blast Adventure Master ha recibido muchas críticas positivas de jugadores y críticos por igual. Aquí hay algunos ejemplos de lo que han dicho sobre el juego:
-
Comentarios positivos de jugadores y críticos
-
"Este es uno de los mejores juegos de rompecabezas de bloques que he jugado. Es tan adictivo y divertido. Me encanta el modo aventura y los diferentes temas. Los gráficos son increíbles y los efectos de sonido son relajantes. Recomiendo este juego a cualquiera que ame los juegos de puzzle."
-
"Soy un gran fan de los juegos de rompecabezas de bloques y este es de lejos mi favorito. Es desafiante pero no frustrante. Tiene muchas características y modos que lo hacen interesante y variado. También es muy relajante y calmante. Lo toco todos los días antes de ir a la cama."
-
"Block Blast Adventure Master es un gran juego que combina el clásico juego de puzzle de bloques con un modo de aventura historia. Es fácil de jugar pero difícil de dominar. Tiene gráficos coloridos y efectos de sonido maravillosos que crean una atmósfera agradable. También es gratis para jugar y no requiere wifi o conexión a Internet."
-
Altas clasificaciones y rankings en tiendas de aplicaciones
-
-
Algunos problemas menores y sugerencias para mejorar
-
A pesar de las críticas positivas, el juego también tiene algunos problemas menores y sugerencias para mejorar de algunos jugadores y críticos. Estos son algunos de ellos:
-
"El juego es genial pero tiene algunos errores y fallas. A veces los bloques no encajan correctamente o desaparecen al azar. A veces el juego se congela o se bloquea. Espero que los desarrolladores puedan solucionar estos problemas pronto."
-
"El juego es divertido, pero puede ser repetitivo y aburrido después de un tiempo. Me gustaría que hubiera más modos y características para hacerlo más emocionante y desafiante. Tal vez puedan agregar algunos power-ups, bloques especiales o minijuegos."
-
"El juego es relajante, pero también puede ser frustrante y estresante. A veces los bloques son demasiado grandes o demasiado pequeños para la red. A veces los bloques son demasiado duros o demasiado fáciles de borrar. Creo que deben equilibrar la dificultad y la aleatoriedad de los bloques."
-
Conclusión
-
Block Blast Adventure Master es un divertido y adictivo juego de puzzle de bloques que relajará tu mente y desafiará tu cerebro. Puedes descargar el archivo APK de este juego desde varias fuentes y disfrutarlo en tu dispositivo Android. El juego tiene muchas características que lo hacen destacar de otros juegos en su género, tales como gráficos coloridos, efectos de sonido maravillosos, no requiere wifi, intentos ilimitados, diferentes niveles de dificultad, modos y temas, y un modo de aventura historia. El juego también tiene algunos consejos y trucos que podrían ayudarte a mejorar tus habilidades y puntuación, como ver videos de YouTube, elegir la mejor posición para el bloque, limpiar varias líneas a la vez y seguir la página de Facebook. El juego ha recibido muchas críticas positivas de jugadores y críticos por igual, así como altas calificaciones y rankings en tiendas de aplicaciones. El juego también tiene algunos problemas menores y sugerencias de mejora que podrían ser corregidos o añadidos en futuras actualizaciones.
-
Preguntas frecuentes
-
-
Q: ¿Es Block Blast Adventure Master libre para jugar?
-
A: Sí, Block Blast Adventure Master es gratis. Sin embargo, contiene anuncios y compras en la aplicación que pueden mejorar su experiencia de juego.
-
Q: ¿Cuáles son los requisitos mínimos para jugar Block Blast Adventure Master?
-
A: Para jugar Block Blast Adventure Master, necesitas un dispositivo Android con Android 4.4 o una versión superior, o un dispositivo iOS con iOS 9.0 o una versión superior.
-
P: ¿Cómo puedo contactar a los desarrolladores de Block Blast Adventure Master?
-
A: Puede ponerse en contacto con los desarrolladores de Block Blast Adventure Master enviando un correo electrónico a hungrystudio@gmail.com o visitando su sitio web en https://www.hungry-studio.com/.
-
P: ¿Cómo puedo apoyar a los desarrolladores de Block Blast Adventure Master?
-
A: Puedes apoyar a los desarrolladores de Block Blast Adventure Master clasificando y revisando el juego en las tiendas de aplicaciones, compartiéndolo con tus amigos y familiares, siguiendo sus cuentas de redes sociales y haciendo compras en la aplicación si lo deseas.
-
P: ¿Cómo puedo obtener más monedas y gemas en Block Blast Adventure Master?
-
A: Puedes obtener más monedas y gemas en Block Blast Adventure Master jugando el juego regularmente, limpiando niveles y modos, viendo anuncios, completando tareas y logros, girando la rueda, abriendo cofres y comprándolos con dinero real.
64aa2da5cf
-
-
\ No newline at end of file
diff --git a/spaces/Benson/text-generation/Examples/Descargar Gratis Juego De Solitario Para Telfono Android.md b/spaces/Benson/text-generation/Examples/Descargar Gratis Juego De Solitario Para Telfono Android.md
deleted file mode 100644
index c6c862ad9d1fe8365cced908f64a566d9a3cc64c..0000000000000000000000000000000000000000
--- a/spaces/Benson/text-generation/Examples/Descargar Gratis Juego De Solitario Para Telfono Android.md
+++ /dev/null
@@ -1,139 +0,0 @@
-
-
Juego de solitario descarga gratuita para teléfono Android
-
Si usted está buscando una manera divertida y relajante para pasar el tiempo, es posible que desee probar a jugar solitario en su teléfono Android. Solitario es uno de los juegos de cartas más populares del mundo, y es fácil de aprender y jugar. En este artículo, le diremos todo lo que necesita saber sobre Solitaire, cómo jugarlo en su teléfono Android, y cómo descargar e instalar las mejores aplicaciones de solitario de forma gratuita.
-
descargar gratis juego de solitario para teléfono android
Solitario es un juego de cartas que puede ser jugado por una persona o más. El objetivo del juego es organizar todas las cartas en un orden específico, generalmente por palo y rango, desde el as hasta el rey. Hay muchas versiones diferentes de Solitaire, como Klondike, Spider, FreeCell, Pyramid y TriPeaks. Cada versión tiene sus propias reglas y desafíos, pero todos comparten el mismo principio básico de clasificación de tarjetas.
-
La historia y popularidad de Solitaire
-
Se cree que el solitario se originó en Europa a finales del siglo XVIII, como una forma para que la gente se entretuviera durante largos períodos de aislamiento o aburrimiento. El juego se hizo popular en Francia, donde se llamó "paciencia", y luego se extendió a otros países. En el siglo XIX, Solitaire fue introducido a América por los colonos británicos, que lo llamaron "solitario". El juego ganó más popularidad en el siglo XX, especialmente después de que se incluyó como un programa predeterminado en Microsoft Windows en 1990. Desde entonces, millones de personas han jugado al Solitario en sus computadoras, teléfonos, tabletas y otros dispositivos.
-
Los beneficios de jugar al solitario
-
Jugar al solitario no solo es divertido, sino también beneficioso para su salud mental y bienestar. Algunos de los beneficios de jugar al solitario son:
-
-
Mejora tu concentración, memoria y habilidades para resolver problemas.
-
Reduce el estrés, la ansiedad y el aburrimiento.
-
Aumenta tu estado de ánimo, confianza y autoestima.
-
-
Mejora tus habilidades sociales y de comunicación.
-
-
Jugar al solitario también puede ayudarte a aprender cosas nuevas, como matemáticas, lógica, estrategia y paciencia. También puede retarte a batir tus propios récords y logros.
-
Cómo jugar al solitario en tu teléfono Android
-
Jugar al solitario en tu teléfono Android es muy fácil y conveniente. No necesitas una baraja de cartas o una mesa. Solo necesitas tu teléfono y una conexión a Internet. Puedes jugar al solitario en cualquier momento y en cualquier lugar que desees, ya sea en casa, en el trabajo o en cualquier lugar. También puede elegir entre una variedad de aplicaciones de solitario que ofrecen diferentes características y opciones.
-
-
Las reglas y variaciones del Solitario
-
Las reglas del Solitario dependen de la versión que esté jugando. Sin embargo, las reglas generales son:
-
-
Empiezas con una baraja de 52 cartas.
-
Reparte algunas cartas boca arriba en la mesa en un diseño específico, llamado tableau. Las cartas restantes se colocan boca abajo en una pila, llamada stock.
-
Usted mueve las cartas del tablero o de la acción a otra pila, llamada la fundación. La fundación consiste en cuatro pilas, una para cada palo.
-
Solo puedes mover una carta a la vez, a menos que tengas una secuencia de cartas en orden descendente y alternando colores (rojo-negro o negro-rojo).
-
Solo puedes colocar una carta encima de otra carta que tenga un rango más alto y del color opuesto. Por ejemplo, puede colocar un 9 negro en un 10 rojo, o un 5 rojo en un 6.
negro
-
Puedes mover cartas del montón al tablero o a la fundación, girando una carta a la vez, o tres cartas a la vez, dependiendo de la configuración.
-
Ganas el juego cuando mueves todas las cartas a la fundación, en orden ascendente y por palo, desde el as al rey.
-
-
Algunas de las variaciones más populares de Solitaire son:
-
-
-
Versión
-
Descripción
-
-
-
Klondike
-
-
-
-
Araña
-
Una versión más desafiante de Solitaire. Reparte 10 columnas de cartas en el tablero, con las primeras cuatro columnas con seis cartas cada una, y el resto con cinco cartas cada una. Todas las cartas están boca arriba. Puede mover cualquier carta o secuencia de cartas dentro del tablero, independientemente del palo o el color. Sin embargo, solo puedes mover cartas a la fundación cuando están en orden descendente y del mismo palo. Puedes repartir una nueva fila de cartas del montón cuando no haya más movimientos en el tablero.
-
-
-
FreeCell
-
Una versión estratégica de Solitario. Repartes ocho columnas de cartas en el tablero, con todas las cartas boca arriba. Puede mover cualquier carta o secuencia de cartas dentro del tablero, siempre que haya un espacio vacío (celda) disponible. Tiene cuatro celdas en la esquina superior izquierda de la pantalla, donde puede almacenar temporalmente una tarjeta cada una. Puede mover las cartas a la fundación cuando están en orden ascendente y por palo.
-
-
-
Pirámide
-
Una versión divertida y fácil de Solitario. Repartes 28 cartas en el tablero en forma de pirámide, con siete filas y siete columnas. La fila superior tiene una carta, la segunda fila tiene dos cartas, etc. La fila inferior tiene siete cartas. Todas las cartas están boca arriba. Puede quitar dos cartas del tablero si suman 13 (As = 1, Jota = 11, Reina = 12, Rey = 13). También puede quitar un solo Rey. Usted puede dar vuelta una tarjeta de la acción a la vez, y utilizarla para quitar otra tarjeta del tableau. Ganas el juego cuando quitas todas las cartas del tablero.
-
-
-
TriPeaks
-
-
-
-
Las características y opciones de las aplicaciones de solitario
-
Aplicaciones de solitario son aplicaciones que le permiten jugar solitario en su teléfono Android. Hay muchas aplicaciones de solitario disponibles en Google Play Store, y ofrecen diferentes características y opciones para satisfacer sus preferencias y necesidades. Algunas de las características y opciones comunes de las aplicaciones de solitario son:
-
-
Puedes elegir entre diferentes versiones y modos de Solitario, como Klondike, Spider, FreeCell, Pyramid, TriPeaks y más.
-
Puede personalizar la apariencia y el diseño del juego, como el diseño de la tarjeta, fondo, sonido, animación y orientación.
-
Puedes ajustar la dificultad y el desafío del juego, como el número de cartas que debes entregar del montón, el número de palos que debes usar y el sistema de puntuación.
-
Puede realizar un seguimiento de su progreso y rendimiento, como el número de juegos jugados, ganados y perdidos, el tiempo dedicado y las mejores puntuaciones.
-
Puedes acceder a sugerencias, consejos y movimientos de deshacer para ayudarte a jugar mejor y más rápido.
-
Puede competir con otros jugadores en línea, o jugar sin conexión a Internet.
-
Puedes disfrutar de otras características y beneficios, como desafíos diarios, logros, recompensas, tablas de clasificación y más.
-
-
Las mejores aplicaciones de solitario para teléfono Android
-
Con tantas aplicaciones de solitario para elegir, es posible que se pregunte cuáles son las mejores para su teléfono Android. Estas son algunas de las mejores aplicaciones de solitario que recomendamos:
-
-
-
Solitaire por Brainium Studios: Esta es otra gran aplicación de solitario para el teléfono Android. Tiene más de 10 millones de descargas y 4.6 estrellas en la Google Play Store. Ofrece Klondike Solitaire con varias opciones y características, tales como dibujar 1 o 3 cartas, modo retrato o paisaje, Vegas puntuación o puntuación estándar, desafíos diarios, logros, tablas de clasificación, estadísticas, pistas, deshacer movimientos, función de autocompletar, y más. También tiene un diseño hermoso y elegante que es personalizable y fácil de usar.
-
Solitaire Collection by Ruben Reboredo: Esta es una aplicación de solitario que ofrece una colección de diferentes versiones y modos de solitario, como Klondike, Spider, FreeCell, Pyramid, TriPeaks, Golf, Yukon, Scorpion y más. Tiene más de 5 millones de descargas y 4.7 estrellas en la Google Play Store. Ofrece varias opciones y características, como dibujar 1 o 3 cartas, modo de retrato o paisaje, puntuación estándar o sin puntuación, desafíos diarios, logros, tablas de clasificación, estadísticas, pistas, movimientos de deshacer, función de autocompletar y más. También tiene un diseño simple y colorido que es fácil de navegar y jugar.
-
-
Cómo descargar e instalar aplicaciones Solitaire en su teléfono Android
-
Descargar e instalar aplicaciones Solitaire en tu teléfono Android es muy fácil y rápido. Solo tienes que seguir estos pasos:
-
-
Ir a la Google Play Store en su teléfono Android y buscar la aplicación Solitaire que desea descargar. También puede utilizar los enlaces que proporcionamos anteriormente para ir directamente a la página de la aplicación.
-
Toque en el botón Instalar y espere a que la aplicación se descargue e instale en su teléfono. Es posible que necesite conceder algunos permisos y configuraciones para que la aplicación funcione correctamente.
-
Una vez que la aplicación está instalada, puede abrirla y comenzar a jugar Solitario en su teléfono Android. También puede crear una cuenta o iniciar sesión con su cuenta de Google para guardar su progreso y preferencias.
-
-
-
Algunos de los permisos y configuraciones que las aplicaciones Solitaire pueden pedir son:
-
-
Acceso al almacenamiento, fotos, medios y archivos de su dispositivo. Esto es para guardar los datos y preferencias del juego en su teléfono.
-
Acceso a la conexión de red del dispositivo. Esto es para habilitar funciones y funciones en línea, como desafíos diarios, logros, tablas de clasificación, anuncios y más.
-
Acceso a la vibración de tu dispositivo. Esto es para proporcionar retroalimentación y efectos cuando juegas el juego.
-
Acceso a la ubicación de su dispositivo. Esto es para proporcionar contenido personalizado y anuncios basados en su región.
-
-
Puede administrar estos permisos y configuraciones yendo al menú de configuración de la aplicación o al menú de configuración del teléfono. También puede desactivar algunos de estos permisos y configuraciones si no los desea o los necesita.
-
Solución de problemas y soporte para aplicaciones de solitario
-
Si encuentras algún problema o problema con las aplicaciones Solitaire en tu teléfono Android, puedes probar algunos de estos consejos de solución de problemas y soporte:
-
-
Compruebe su conexión a Internet y asegúrese de que es estable y rápido.
-
Reinicia el teléfono y la aplicación y ver si el problema persiste.
-
Borrar la caché de la aplicación y los datos y ver si el problema se resuelve.
-
Actualizar la aplicación a la última versión y ver si el problema está solucionado.
-
Póngase en contacto con el desarrollador o servicio al cliente de la aplicación y reportar el problema y pedir ayuda.
-
-
Conclusión
-
-
Si usted está buscando una manera divertida y relajante para pasar el tiempo, usted debe tratar de jugar solitario en su teléfono Android. Es fácil de aprender y jugar, y te desafiará a mejorar tus habilidades y rendimiento. También te entretendrá y te mantendrá ocupado durante horas. ¿Qué estás esperando? ¡Descargue una de las mejores aplicaciones de solitario gratis hoy y disfrute jugando al solitario en su teléfono Android!
-
Preguntas frecuentes
-
Aquí están algunas de las preguntas más frecuentes sobre el juego de solitario descarga gratuita para el teléfono Android:
-
-
Q: ¿Cuánto espacio ocupa una aplicación Solitaire en mi teléfono Android?
-
A: El espacio que ocupa una aplicación de solitario en su teléfono Android depende de la aplicación en sí, pero por lo general no es muy grande. La mayoría de las aplicaciones Solitaire toman menos de 100 MB de espacio en su teléfono, que no es mucho en comparación con otras aplicaciones.
-
Q: ¿Cómo puedo eliminar una aplicación de solitario de mi teléfono Android?
-
A: Para eliminar una aplicación Solitaire de su teléfono Android, puede seguir estos pasos:
-
-
Vaya al menú Configuración en su teléfono y toque en Aplicaciones o Aplicaciones.
-
Encuentra la aplicación Solitaire que desea eliminar y toque en ella.
-
Toque en Desinstalar o Eliminar y confirme su elección.
-
-
Q: ¿Cómo puedo jugar al solitario con otras personas en línea?
-
A: Para jugar al solitario con otras personas en línea, es necesario descargar una aplicación de solitario que admite el modo multijugador o el modo en línea. Algunas de las aplicaciones de solitario que ofrecen esta función son:
-
-
Solitaire Arena por RockYou Inc.
-
Solitario en vivo por Gazeus Games.
-
Solitaire Grand Harvest por Supertreat.
-
-
Q: ¿Cómo puedo desactivar los anuncios en una aplicación Solitaire?
-
A: Para desactivar anuncios en una aplicación Solitaire, tienes dos opciones:
-
-
-
Puede desactivar su conexión a Internet mientras reproduce la aplicación. Esto evitará que los anuncios se carguen y aparezcan en su pantalla. Sin embargo, esto también desactivará algunas características y funciones en línea de la aplicación.
-
-
Q: ¿Cómo puedo obtener más monedas o recompensas en una aplicación de solitario?
-
A: Para obtener más monedas o recompensas en una aplicación de solitario, tienes varias opciones:
-
-
Puedes completar desafíos diarios, logros o misiones que ofrece la aplicación. Estos te recompensarán con monedas u otros premios.
-
Puedes ver anuncios o videos que ofrece la aplicación. Estos te recompensarán con monedas u otros bonos.
-
Puedes invitar a tus amigos o familiares a jugar la aplicación contigo. Algunas aplicaciones te recompensarán con monedas u otros incentivos para referir nuevos jugadores.
-
64aa2da5cf
-
-
\ No newline at end of file
diff --git a/spaces/Billyosoro/ESRGAN/setup.py b/spaces/Billyosoro/ESRGAN/setup.py
deleted file mode 100644
index c2b92e31d2db1aba50767f4f844540cfd53c609d..0000000000000000000000000000000000000000
--- a/spaces/Billyosoro/ESRGAN/setup.py
+++ /dev/null
@@ -1,107 +0,0 @@
-#!/usr/bin/env python
-
-from setuptools import find_packages, setup
-
-import os
-import subprocess
-import time
-
-version_file = 'realesrgan/version.py'
-
-
-def readme():
- with open('README.md', encoding='utf-8') as f:
- content = f.read()
- return content
-
-
-def get_git_hash():
-
- def _minimal_ext_cmd(cmd):
- # construct minimal environment
- env = {}
- for k in ['SYSTEMROOT', 'PATH', 'HOME']:
- v = os.environ.get(k)
- if v is not None:
- env[k] = v
- # LANGUAGE is used on win32
- env['LANGUAGE'] = 'C'
- env['LANG'] = 'C'
- env['LC_ALL'] = 'C'
- out = subprocess.Popen(cmd, stdout=subprocess.PIPE, env=env).communicate()[0]
- return out
-
- try:
- out = _minimal_ext_cmd(['git', 'rev-parse', 'HEAD'])
- sha = out.strip().decode('ascii')
- except OSError:
- sha = 'unknown'
-
- return sha
-
-
-def get_hash():
- if os.path.exists('.git'):
- sha = get_git_hash()[:7]
- else:
- sha = 'unknown'
-
- return sha
-
-
-def write_version_py():
- content = """# GENERATED VERSION FILE
-# TIME: {}
-__version__ = '{}'
-__gitsha__ = '{}'
-version_info = ({})
-"""
- sha = get_hash()
- with open('VERSION', 'r') as f:
- SHORT_VERSION = f.read().strip()
- VERSION_INFO = ', '.join([x if x.isdigit() else f'"{x}"' for x in SHORT_VERSION.split('.')])
-
- version_file_str = content.format(time.asctime(), SHORT_VERSION, sha, VERSION_INFO)
- with open(version_file, 'w') as f:
- f.write(version_file_str)
-
-
-def get_version():
- with open(version_file, 'r') as f:
- exec(compile(f.read(), version_file, 'exec'))
- return locals()['__version__']
-
-
-def get_requirements(filename='requirements.txt'):
- here = os.path.dirname(os.path.realpath(__file__))
- with open(os.path.join(here, filename), 'r') as f:
- requires = [line.replace('\n', '') for line in f.readlines()]
- return requires
-
-
-if __name__ == '__main__':
- write_version_py()
- setup(
- name='realesrgan',
- version=get_version(),
- description='Real-ESRGAN aims at developing Practical Algorithms for General Image Restoration',
- long_description=readme(),
- long_description_content_type='text/markdown',
- author='Xintao Wang',
- author_email='xintao.wang@outlook.com',
- keywords='computer vision, pytorch, image restoration, super-resolution, esrgan, real-esrgan',
- url='https://github.com/xinntao/Real-ESRGAN',
- include_package_data=True,
- packages=find_packages(exclude=('options', 'datasets', 'experiments', 'results', 'tb_logger', 'wandb')),
- classifiers=[
- 'Development Status :: 4 - Beta',
- 'License :: OSI Approved :: Apache Software License',
- 'Operating System :: OS Independent',
- 'Programming Language :: Python :: 3',
- 'Programming Language :: Python :: 3.7',
- 'Programming Language :: Python :: 3.8',
- ],
- license='BSD-3-Clause License',
- setup_requires=['cython', 'numpy'],
- install_requires=get_requirements(),
- zip_safe=False)
diff --git a/spaces/BlueRey/MendoBERT_QA/README.md b/spaces/BlueRey/MendoBERT_QA/README.md
deleted file mode 100644
index 4269860332506ef2cd84294212ea8c0e8d57ffae..0000000000000000000000000000000000000000
--- a/spaces/BlueRey/MendoBERT_QA/README.md
+++ /dev/null
@@ -1,13 +0,0 @@
----
-title: MendoBERT QA
-emoji: 🏢
-colorFrom: gray
-colorTo: yellow
-sdk: streamlit
-sdk_version: 1.19.0
-app_file: app.py
-pinned: false
-license: afl-3.0
----
-
-Check out the configuration reference at https://huggingface.co/docs/hub/spaces-config-reference
diff --git a/spaces/CVPR/LIVE/thrust/testing/unittest/cuda/testframework.h b/spaces/CVPR/LIVE/thrust/testing/unittest/cuda/testframework.h
deleted file mode 100644
index 953f88c1c546d9893bac28f0ec38d31f9af93031..0000000000000000000000000000000000000000
--- a/spaces/CVPR/LIVE/thrust/testing/unittest/cuda/testframework.h
+++ /dev/null
@@ -1,25 +0,0 @@
-#pragma once
-
-#include
-#include
-#include
-#include
-
-class CUDATestDriver
- : public UnitTestDriver
-{
- public:
- int current_device_architecture() const;
-
- private:
- std::vector target_devices(const ArgumentMap &kwargs);
-
- bool check_cuda_error(bool concise);
-
- virtual bool post_test_sanity_check(const UnitTest &test, bool concise);
-
- virtual bool run_tests(const ArgumentSet &args, const ArgumentMap &kwargs);
-};
-
-UnitTestDriver &driver_instance(thrust::system::cuda::tag);
-
diff --git a/spaces/CVPR/WALT/mmdet/core/bbox/assigners/hungarian_assigner.py b/spaces/CVPR/WALT/mmdet/core/bbox/assigners/hungarian_assigner.py
deleted file mode 100644
index e10cc14afac4ddfcb9395c1a250ece1fbfe3263c..0000000000000000000000000000000000000000
--- a/spaces/CVPR/WALT/mmdet/core/bbox/assigners/hungarian_assigner.py
+++ /dev/null
@@ -1,145 +0,0 @@
-import torch
-
-from ..builder import BBOX_ASSIGNERS
-from ..match_costs import build_match_cost
-from ..transforms import bbox_cxcywh_to_xyxy
-from .assign_result import AssignResult
-from .base_assigner import BaseAssigner
-
-try:
- from scipy.optimize import linear_sum_assignment
-except ImportError:
- linear_sum_assignment = None
-
-
-@BBOX_ASSIGNERS.register_module()
-class HungarianAssigner(BaseAssigner):
- """Computes one-to-one matching between predictions and ground truth.
-
- This class computes an assignment between the targets and the predictions
- based on the costs. The costs are weighted sum of three components:
- classification cost, regression L1 cost and regression iou cost. The
- targets don't include the no_object, so generally there are more
- predictions than targets. After the one-to-one matching, the un-matched
- are treated as backgrounds. Thus each query prediction will be assigned
- with `0` or a positive integer indicating the ground truth index:
-
- - 0: negative sample, no assigned gt
- - positive integer: positive sample, index (1-based) of assigned gt
-
- Args:
- cls_weight (int | float, optional): The scale factor for classification
- cost. Default 1.0.
- bbox_weight (int | float, optional): The scale factor for regression
- L1 cost. Default 1.0.
- iou_weight (int | float, optional): The scale factor for regression
- iou cost. Default 1.0.
- iou_calculator (dict | optional): The config for the iou calculation.
- Default type `BboxOverlaps2D`.
- iou_mode (str | optional): "iou" (intersection over union), "iof"
- (intersection over foreground), or "giou" (generalized
- intersection over union). Default "giou".
- """
-
- def __init__(self,
- cls_cost=dict(type='ClassificationCost', weight=1.),
- reg_cost=dict(type='BBoxL1Cost', weight=1.0),
- iou_cost=dict(type='IoUCost', iou_mode='giou', weight=1.0)):
- self.cls_cost = build_match_cost(cls_cost)
- self.reg_cost = build_match_cost(reg_cost)
- self.iou_cost = build_match_cost(iou_cost)
-
- def assign(self,
- bbox_pred,
- cls_pred,
- gt_bboxes,
- gt_labels,
- img_meta,
- gt_bboxes_ignore=None,
- eps=1e-7):
- """Computes one-to-one matching based on the weighted costs.
-
- This method assign each query prediction to a ground truth or
- background. The `assigned_gt_inds` with -1 means don't care,
- 0 means negative sample, and positive number is the index (1-based)
- of assigned gt.
- The assignment is done in the following steps, the order matters.
-
- 1. assign every prediction to -1
- 2. compute the weighted costs
- 3. do Hungarian matching on CPU based on the costs
- 4. assign all to 0 (background) first, then for each matched pair
- between predictions and gts, treat this prediction as foreground
- and assign the corresponding gt index (plus 1) to it.
-
- Args:
- bbox_pred (Tensor): Predicted boxes with normalized coordinates
- (cx, cy, w, h), which are all in range [0, 1]. Shape
- [num_query, 4].
- cls_pred (Tensor): Predicted classification logits, shape
- [num_query, num_class].
- gt_bboxes (Tensor): Ground truth boxes with unnormalized
- coordinates (x1, y1, x2, y2). Shape [num_gt, 4].
- gt_labels (Tensor): Label of `gt_bboxes`, shape (num_gt,).
- img_meta (dict): Meta information for current image.
- gt_bboxes_ignore (Tensor, optional): Ground truth bboxes that are
- labelled as `ignored`. Default None.
- eps (int | float, optional): A value added to the denominator for
- numerical stability. Default 1e-7.
-
- Returns:
- :obj:`AssignResult`: The assigned result.
- """
- assert gt_bboxes_ignore is None, \
- 'Only case when gt_bboxes_ignore is None is supported.'
- num_gts, num_bboxes = gt_bboxes.size(0), bbox_pred.size(0)
-
- # 1. assign -1 by default
- assigned_gt_inds = bbox_pred.new_full((num_bboxes, ),
- -1,
- dtype=torch.long)
- assigned_labels = bbox_pred.new_full((num_bboxes, ),
- -1,
- dtype=torch.long)
- if num_gts == 0 or num_bboxes == 0:
- # No ground truth or boxes, return empty assignment
- if num_gts == 0:
- # No ground truth, assign all to background
- assigned_gt_inds[:] = 0
- return AssignResult(
- num_gts, assigned_gt_inds, None, labels=assigned_labels)
- img_h, img_w, _ = img_meta['img_shape']
- factor = gt_bboxes.new_tensor([img_w, img_h, img_w,
- img_h]).unsqueeze(0)
-
- # 2. compute the weighted costs
- # classification and bboxcost.
- cls_cost = self.cls_cost(cls_pred, gt_labels)
- # regression L1 cost
- normalize_gt_bboxes = gt_bboxes / factor
- reg_cost = self.reg_cost(bbox_pred, normalize_gt_bboxes)
- # regression iou cost, defaultly giou is used in official DETR.
- bboxes = bbox_cxcywh_to_xyxy(bbox_pred) * factor
- iou_cost = self.iou_cost(bboxes, gt_bboxes)
- # weighted sum of above three costs
- cost = cls_cost + reg_cost + iou_cost
-
- # 3. do Hungarian matching on CPU using linear_sum_assignment
- cost = cost.detach().cpu()
- if linear_sum_assignment is None:
- raise ImportError('Please run "pip install scipy" '
- 'to install scipy first.')
- matched_row_inds, matched_col_inds = linear_sum_assignment(cost)
- matched_row_inds = torch.from_numpy(matched_row_inds).to(
- bbox_pred.device)
- matched_col_inds = torch.from_numpy(matched_col_inds).to(
- bbox_pred.device)
-
- # 4. assign backgrounds and foregrounds
- # assign all indices to backgrounds first
- assigned_gt_inds[:] = 0
- # assign foregrounds based on matching results
- assigned_gt_inds[matched_row_inds] = matched_col_inds + 1
- assigned_labels[matched_row_inds] = gt_labels[matched_col_inds]
- return AssignResult(
- num_gts, assigned_gt_inds, None, labels=assigned_labels)
diff --git a/spaces/CVPR/drawings-to-human/static/_app/immutable/assets/pages/index.svelte-7bf249dc.css b/spaces/CVPR/drawings-to-human/static/_app/immutable/assets/pages/index.svelte-7bf249dc.css
deleted file mode 100644
index 195622b6f121c54bbfed140fafa39f6a065e257c..0000000000000000000000000000000000000000
--- a/spaces/CVPR/drawings-to-human/static/_app/immutable/assets/pages/index.svelte-7bf249dc.css
+++ /dev/null
@@ -1 +0,0 @@
-form.svelte-1gwcbp.svelte-1gwcbp{width:100%;overflow:hidden}.samples.svelte-1gwcbp.svelte-1gwcbp{display:flex;scroll-snap-type:x var(--tw-scroll-snap-strictness);--tw-scroll-snap-strictness:mandatory;flex-wrap:nowrap;gap:.25rem;overflow-x:scroll;-ms-overflow-style:none;scrollbar-width:none}.samples.svelte-1gwcbp.svelte-1gwcbp::-webkit-scrollbar{display:none}input[type=radio].svelte-1gwcbp.svelte-1gwcbp{position:absolute;display:none;height:0px;width:0px;opacity:0}input[type=radio].svelte-1gwcbp.svelte-1gwcbp:disabled{opacity:.5}input[type=radio].svelte-1gwcbp:checked~label.svelte-1gwcbp{outline-style:solid;outline-width:2px;outline-color:#eab308}input[type=radio].svelte-1gwcbp:disabled+label.svelte-1gwcbp{opacity:.5}label.svelte-1gwcbp.svelte-1gwcbp{display:flex;cursor:pointer;outline-width:2px;outline-offset:-2px;outline-color:#eab308;transition-property:all;transition-duration:.2s;transition-timing-function:cubic-bezier(.4,0,.2,1)}label.svelte-1gwcbp.svelte-1gwcbp:hover{outline-style:solid}img.svelte-1gwcbp.svelte-1gwcbp{max-height:6rem;max-width:none}.colors.svelte-1oy4poo.svelte-1oy4poo{display:grid;max-height:9rem;scroll-snap-type:y var(--tw-scroll-snap-strictness);--tw-scroll-snap-strictness:mandatory;grid-template-columns:repeat(2,minmax(0,1fr));gap:.5rem;overflow:scroll}@media (min-width: 530px){.colors.svelte-1oy4poo.svelte-1oy4poo{max-height:none;grid-template-columns:repeat(3,minmax(0,1fr))}}.colors.svelte-1oy4poo span.svelte-1oy4poo{margin-left:.5rem}.colors.svelte-1oy4poo svg.svelte-1oy4poo{display:block}input[type=radio].svelte-1oy4poo.svelte-1oy4poo{position:absolute;display:none;height:0px;width:0px;opacity:0}input[type=radio].svelte-1oy4poo:checked~label.svelte-1oy4poo{outline-style:solid;outline-width:2px;outline-color:#eab308}label.svelte-1oy4poo.svelte-1oy4poo{display:flex;cursor:pointer;white-space:nowrap;outline-width:2px;outline-offset:-2px;outline-color:#eab308;transition-property:all;transition-duration:.2s;transition-timing-function:cubic-bezier(.4,0,.2,1)}label.svelte-1oy4poo.svelte-1oy4poo:hover{outline-style:solid}.brush.svelte-1oy4poo.svelte-1oy4poo{display:flex}.sections.svelte-uoay71.svelte-uoay71{display:flex;flex-direction:column;gap:.25rem}@media (min-width: 530px){.sections.svelte-uoay71.svelte-uoay71{flex-direction:row;gap:.75rem}}select.svelte-uoay71.svelte-uoay71,button.svelte-uoay71.svelte-uoay71,input.svelte-uoay71.svelte-uoay71{border-radius:.5rem;border-width:1px;--tw-border-opacity:1;border-color:rgb(209 213 219 / var(--tw-border-opacity));--tw-bg-opacity:1;background-color:rgb(249 250 251 / var(--tw-bg-opacity));padding:.25rem;font-size:.875rem;line-height:1.25rem;--tw-text-opacity:1;color:rgb(17 24 39 / var(--tw-text-opacity))}select.svelte-uoay71.svelte-uoay71:focus,button.svelte-uoay71.svelte-uoay71:focus,input.svelte-uoay71.svelte-uoay71:focus{--tw-border-opacity:1;border-color:rgb(59 130 246 / var(--tw-border-opacity));--tw-ring-opacity:1;--tw-ring-color:rgb(59 130 246 / var(--tw-ring-opacity)) }select.svelte-uoay71.svelte-uoay71:disabled,button.svelte-uoay71.svelte-uoay71:disabled,input.svelte-uoay71.svelte-uoay71:disabled{opacity:.5}@media (prefers-color-scheme: dark){select.svelte-uoay71.svelte-uoay71,button.svelte-uoay71.svelte-uoay71,input.svelte-uoay71.svelte-uoay71{--tw-border-opacity:1;border-color:rgb(75 85 99 / var(--tw-border-opacity));--tw-bg-opacity:1;background-color:rgb(55 65 81 / var(--tw-bg-opacity));--tw-text-opacity:1;color:rgb(255 255 255 / var(--tw-text-opacity))}select.svelte-uoay71.svelte-uoay71::-moz-placeholder,button.svelte-uoay71.svelte-uoay71::-moz-placeholder,input.svelte-uoay71.svelte-uoay71::-moz-placeholder{--tw-placeholder-opacity:1;color:rgb(156 163 175 / var(--tw-placeholder-opacity))}select.svelte-uoay71.svelte-uoay71::placeholder,button.svelte-uoay71.svelte-uoay71::placeholder,input.svelte-uoay71.svelte-uoay71::placeholder{--tw-placeholder-opacity:1;color:rgb(156 163 175 / var(--tw-placeholder-opacity))}select.svelte-uoay71.svelte-uoay71:focus,button.svelte-uoay71.svelte-uoay71:focus,input.svelte-uoay71.svelte-uoay71:focus{--tw-border-opacity:1;border-color:rgb(59 130 246 / var(--tw-border-opacity));--tw-ring-opacity:1;--tw-ring-color:rgb(59 130 246 / var(--tw-ring-opacity)) }}input.svelte-uoay71:disabled+label.svelte-uoay71{opacity:.5}input.svelte-uoay71.svelte-uoay71{padding-left:.75rem}.canvas.svelte-1k5plc8{z-index:0;aspect-ratio:256/512;width:100%;max-width:100%;border-width:1px;--tw-border-opacity:1;border-color:rgb(107 114 128 / var(--tw-border-opacity))}@media (prefers-color-scheme: dark){.canvas.svelte-1k5plc8{--tw-border-opacity:1;border-color:rgb(209 213 219 / var(--tw-border-opacity))}}.brush.svelte-1k5plc8{pointer-events:none;position:absolute;z-index:10;--tw-translate-x:-50%;--tw-translate-y:-50%;transform:translate(var(--tw-translate-x),var(--tw-translate-y)) rotate(var(--tw-rotate)) skew(var(--tw-skew-x)) skewY(var(--tw-skew-y)) scaleX(var(--tw-scale-x)) scaleY(var(--tw-scale-y))}.label.svelte-1k5plc8{pointer-events:none;position:absolute;top:0px;left:0px;z-index:20;-webkit-user-select:none;-moz-user-select:none;user-select:none;padding-left:.5rem;padding-right:.5rem;font-size:1rem;line-height:1.5rem;--tw-text-opacity:1;color:rgb(255 255 255 / var(--tw-text-opacity));color:#fff;font-weight:bolder;-webkit-text-stroke:1px black;-webkit-text-fill-color:white}.image.svelte-1iibjwx{z-index:0;box-sizing:border-box;aspect-ratio:256/512;border-width:1px;--tw-border-opacity:1;border-color:rgb(107 114 128 / var(--tw-border-opacity))}@media (prefers-color-scheme: dark){.image.svelte-1iibjwx{--tw-border-opacity:1;border-color:rgb(209 213 219 / var(--tw-border-opacity))}}.loading.svelte-1iibjwx{position:absolute;top:0px;left:0px;right:0px;bottom:0px;display:flex;flex-direction:column;align-items:center;justify-content:center}.drawings.svelte-237ry5{display:grid;grid-template-columns:2fr 1.5fr;place-items:center}@media (min-width: 530px){.drawings.svelte-237ry5{grid-template-columns:repeat(2,minmax(0,1fr))}}button.svelte-237ry5{border-radius:.5rem;border-width:1px;--tw-border-opacity:1;border-color:rgb(209 213 219 / var(--tw-border-opacity));--tw-bg-opacity:1;background-color:rgb(249 250 251 / var(--tw-bg-opacity));padding:.25rem;font-size:.875rem;line-height:1.25rem;--tw-text-opacity:1;color:rgb(17 24 39 / var(--tw-text-opacity))}button.svelte-237ry5:focus{--tw-border-opacity:1;border-color:rgb(59 130 246 / var(--tw-border-opacity));--tw-ring-opacity:1;--tw-ring-color:rgb(59 130 246 / var(--tw-ring-opacity)) }button.svelte-237ry5:disabled{opacity:.5}@media (prefers-color-scheme: dark){button.svelte-237ry5{--tw-border-opacity:1;border-color:rgb(75 85 99 / var(--tw-border-opacity));--tw-bg-opacity:1;background-color:rgb(55 65 81 / var(--tw-bg-opacity));--tw-text-opacity:1;color:rgb(255 255 255 / var(--tw-text-opacity))}button.svelte-237ry5::-moz-placeholder{--tw-placeholder-opacity:1;color:rgb(156 163 175 / var(--tw-placeholder-opacity))}button.svelte-237ry5::placeholder{--tw-placeholder-opacity:1;color:rgb(156 163 175 / var(--tw-placeholder-opacity))}button.svelte-237ry5:focus{--tw-border-opacity:1;border-color:rgb(59 130 246 / var(--tw-border-opacity));--tw-ring-opacity:1;--tw-ring-color:rgb(59 130 246 / var(--tw-ring-opacity)) }}
diff --git a/spaces/CVPR/regionclip-demo/detectron2/layers/csrc/box_iou_rotated/box_iou_rotated_utils.h b/spaces/CVPR/regionclip-demo/detectron2/layers/csrc/box_iou_rotated/box_iou_rotated_utils.h
deleted file mode 100644
index b54a5dde2ca11a74d29c4d8adb7fe1634f5baf9c..0000000000000000000000000000000000000000
--- a/spaces/CVPR/regionclip-demo/detectron2/layers/csrc/box_iou_rotated/box_iou_rotated_utils.h
+++ /dev/null
@@ -1,370 +0,0 @@
-// Copyright (c) Facebook, Inc. and its affiliates.
-#pragma once
-
-#include
-#include
-
-#if defined(__CUDACC__) || __HCC__ == 1 || __HIP__ == 1
-// Designates functions callable from the host (CPU) and the device (GPU)
-#define HOST_DEVICE __host__ __device__
-#define HOST_DEVICE_INLINE HOST_DEVICE __forceinline__
-#else
-#include
-#define HOST_DEVICE
-#define HOST_DEVICE_INLINE HOST_DEVICE inline
-#endif
-
-namespace detectron2 {
-
-namespace {
-
-template
-struct RotatedBox {
- T x_ctr, y_ctr, w, h, a;
-};
-
-template
-struct Point {
- T x, y;
- HOST_DEVICE_INLINE Point(const T& px = 0, const T& py = 0) : x(px), y(py) {}
- HOST_DEVICE_INLINE Point operator+(const Point& p) const {
- return Point(x + p.x, y + p.y);
- }
- HOST_DEVICE_INLINE Point& operator+=(const Point& p) {
- x += p.x;
- y += p.y;
- return *this;
- }
- HOST_DEVICE_INLINE Point operator-(const Point& p) const {
- return Point(x - p.x, y - p.y);
- }
- HOST_DEVICE_INLINE Point operator*(const T coeff) const {
- return Point(x * coeff, y * coeff);
- }
-};
-
-template
-HOST_DEVICE_INLINE T dot_2d(const Point& A, const Point& B) {
- return A.x * B.x + A.y * B.y;
-}
-
-// R: result type. can be different from input type
-template
-HOST_DEVICE_INLINE R cross_2d(const Point& A, const Point& B) {
- return static_cast(A.x) * static_cast(B.y) -
- static_cast(B.x) * static_cast(A.y);
-}
-
-template
-HOST_DEVICE_INLINE void get_rotated_vertices(
- const RotatedBox& box,
- Point (&pts)[4]) {
- // M_PI / 180. == 0.01745329251
- double theta = box.a * 0.01745329251;
- T cosTheta2 = (T)cos(theta) * 0.5f;
- T sinTheta2 = (T)sin(theta) * 0.5f;
-
- // y: top --> down; x: left --> right
- pts[0].x = box.x_ctr + sinTheta2 * box.h + cosTheta2 * box.w;
- pts[0].y = box.y_ctr + cosTheta2 * box.h - sinTheta2 * box.w;
- pts[1].x = box.x_ctr - sinTheta2 * box.h + cosTheta2 * box.w;
- pts[1].y = box.y_ctr - cosTheta2 * box.h - sinTheta2 * box.w;
- pts[2].x = 2 * box.x_ctr - pts[0].x;
- pts[2].y = 2 * box.y_ctr - pts[0].y;
- pts[3].x = 2 * box.x_ctr - pts[1].x;
- pts[3].y = 2 * box.y_ctr - pts[1].y;
-}
-
-template
-HOST_DEVICE_INLINE int get_intersection_points(
- const Point (&pts1)[4],
- const Point (&pts2)[4],
- Point (&intersections)[24]) {
- // Line vector
- // A line from p1 to p2 is: p1 + (p2-p1)*t, t=[0,1]
- Point vec1[4], vec2[4];
- for (int i = 0; i < 4; i++) {
- vec1[i] = pts1[(i + 1) % 4] - pts1[i];
- vec2[i] = pts2[(i + 1) % 4] - pts2[i];
- }
-
- // When computing the intersection area, it doesn't hurt if we have
- // more (duplicated/approximate) intersections/vertices than needed,
- // while it can cause drastic difference if we miss an intersection/vertex.
- // Therefore, we add an epsilon to relax the comparisons between
- // the float point numbers that decide the intersection points.
- double EPS = 1e-5;
-
- // Line test - test all line combos for intersection
- int num = 0; // number of intersections
- for (int i = 0; i < 4; i++) {
- for (int j = 0; j < 4; j++) {
- // Solve for 2x2 Ax=b
- T det = cross_2d(vec2[j], vec1[i]);
-
- // This takes care of parallel lines
- if (fabs(det) <= 1e-14) {
- continue;
- }
-
- auto vec12 = pts2[j] - pts1[i];
-
- T t1 = cross_2d(vec2[j], vec12) / det;
- T t2 = cross_2d(vec1[i], vec12) / det;
-
- if (t1 > -EPS && t1 < 1.0f + EPS && t2 > -EPS && t2 < 1.0f + EPS) {
- intersections[num++] = pts1[i] + vec1[i] * t1;
- }
- }
- }
-
- // Check for vertices of rect1 inside rect2
- {
- const auto& AB = vec2[0];
- const auto& DA = vec2[3];
- auto ABdotAB = dot_2d(AB, AB);
- auto ADdotAD = dot_2d(DA, DA);
- for (int i = 0; i < 4; i++) {
- // assume ABCD is the rectangle, and P is the point to be judged
- // P is inside ABCD iff. P's projection on AB lies within AB
- // and P's projection on AD lies within AD
-
- auto AP = pts1[i] - pts2[0];
-
- auto APdotAB = dot_2d(AP, AB);
- auto APdotAD = -dot_2d(AP, DA);
-
- if ((APdotAB > -EPS) && (APdotAD > -EPS) && (APdotAB < ABdotAB + EPS) &&
- (APdotAD < ADdotAD + EPS)) {
- intersections[num++] = pts1[i];
- }
- }
- }
-
- // Reverse the check - check for vertices of rect2 inside rect1
- {
- const auto& AB = vec1[0];
- const auto& DA = vec1[3];
- auto ABdotAB = dot_2d(AB, AB);
- auto ADdotAD = dot_2d(DA, DA);
- for (int i = 0; i < 4; i++) {
- auto AP = pts2[i] - pts1[0];
-
- auto APdotAB = dot_2d(AP, AB);
- auto APdotAD = -dot_2d(AP, DA);
-
- if ((APdotAB > -EPS) && (APdotAD > -EPS) && (APdotAB < ABdotAB + EPS) &&
- (APdotAD < ADdotAD + EPS)) {
- intersections[num++] = pts2[i];
- }
- }
- }
-
- return num;
-}
-
-template
-HOST_DEVICE_INLINE int convex_hull_graham(
- const Point (&p)[24],
- const int& num_in,
- Point (&q)[24],
- bool shift_to_zero = false) {
- assert(num_in >= 2);
-
- // Step 1:
- // Find point with minimum y
- // if more than 1 points have the same minimum y,
- // pick the one with the minimum x.
- int t = 0;
- for (int i = 1; i < num_in; i++) {
- if (p[i].y < p[t].y || (p[i].y == p[t].y && p[i].x < p[t].x)) {
- t = i;
- }
- }
- auto& start = p[t]; // starting point
-
- // Step 2:
- // Subtract starting point from every points (for sorting in the next step)
- for (int i = 0; i < num_in; i++) {
- q[i] = p[i] - start;
- }
-
- // Swap the starting point to position 0
- auto tmp = q[0];
- q[0] = q[t];
- q[t] = tmp;
-
- // Step 3:
- // Sort point 1 ~ num_in according to their relative cross-product values
- // (essentially sorting according to angles)
- // If the angles are the same, sort according to their distance to origin
- T dist[24];
-#if defined(__CUDACC__) || __HCC__ == 1 || __HIP__ == 1
- // compute distance to origin before sort, and sort them together with the
- // points
- for (int i = 0; i < num_in; i++) {
- dist[i] = dot_2d(q[i], q[i]);
- }
-
- // CUDA version
- // In the future, we can potentially use thrust
- // for sorting here to improve speed (though not guaranteed)
- for (int i = 1; i < num_in - 1; i++) {
- for (int j = i + 1; j < num_in; j++) {
- T crossProduct = cross_2d(q[i], q[j]);
- if ((crossProduct < -1e-6) ||
- (fabs(crossProduct) < 1e-6 && dist[i] > dist[j])) {
- auto q_tmp = q[i];
- q[i] = q[j];
- q[j] = q_tmp;
- auto dist_tmp = dist[i];
- dist[i] = dist[j];
- dist[j] = dist_tmp;
- }
- }
- }
-#else
- // CPU version
- std::sort(
- q + 1, q + num_in, [](const Point& A, const Point& B) -> bool {
- T temp = cross_2d(A, B);
- if (fabs(temp) < 1e-6) {
- return dot_2d(A, A) < dot_2d(B, B);
- } else {
- return temp > 0;
- }
- });
- // compute distance to origin after sort, since the points are now different.
- for (int i = 0; i < num_in; i++) {
- dist[i] = dot_2d(q[i], q[i]);
- }
-#endif
-
- // Step 4:
- // Make sure there are at least 2 points (that don't overlap with each other)
- // in the stack
- int k; // index of the non-overlapped second point
- for (k = 1; k < num_in; k++) {
- if (dist[k] > 1e-8) {
- break;
- }
- }
- if (k == num_in) {
- // We reach the end, which means the convex hull is just one point
- q[0] = p[t];
- return 1;
- }
- q[1] = q[k];
- int m = 2; // 2 points in the stack
- // Step 5:
- // Finally we can start the scanning process.
- // When a non-convex relationship between the 3 points is found
- // (either concave shape or duplicated points),
- // we pop the previous point from the stack
- // until the 3-point relationship is convex again, or
- // until the stack only contains two points
- for (int i = k + 1; i < num_in; i++) {
- while (m > 1) {
- auto q1 = q[i] - q[m - 2], q2 = q[m - 1] - q[m - 2];
- // cross_2d() uses FMA and therefore computes round(round(q1.x*q2.y) -
- // q2.x*q1.y) So it may not return 0 even when q1==q2. Therefore we
- // compare round(q1.x*q2.y) and round(q2.x*q1.y) directly. (round means
- // round to nearest floating point).
- if (q1.x * q2.y >= q2.x * q1.y)
- m--;
- else
- break;
- }
- // Using double also helps, but float can solve the issue for now.
- // while (m > 1 && cross_2d(q[i] - q[m - 2], q[m - 1] - q[m - 2])
- // >= 0) {
- // m--;
- // }
- q[m++] = q[i];
- }
-
- // Step 6 (Optional):
- // In general sense we need the original coordinates, so we
- // need to shift the points back (reverting Step 2)
- // But if we're only interested in getting the area/perimeter of the shape
- // We can simply return.
- if (!shift_to_zero) {
- for (int i = 0; i < m; i++) {
- q[i] += start;
- }
- }
-
- return m;
-}
-
-template
-HOST_DEVICE_INLINE T polygon_area(const Point (&q)[24], const int& m) {
- if (m <= 2) {
- return 0;
- }
-
- T area = 0;
- for (int i = 1; i < m - 1; i++) {
- area += fabs(cross_2d(q[i] - q[0], q[i + 1] - q[0]));
- }
-
- return area / 2.0;
-}
-
-template
-HOST_DEVICE_INLINE T rotated_boxes_intersection(
- const RotatedBox& box1,
- const RotatedBox& box2) {
- // There are up to 4 x 4 + 4 + 4 = 24 intersections (including dups) returned
- // from rotated_rect_intersection_pts
- Point intersectPts[24], orderedPts[24];
-
- Point pts1[4];
- Point pts2[4];
- get_rotated_vertices(box1, pts1);
- get_rotated_vertices(box2, pts2);
-
- int num = get_intersection_points(pts1, pts2, intersectPts);
-
- if (num <= 2) {
- return 0.0;
- }
-
- // Convex Hull to order the intersection points in clockwise order and find
- // the contour area.
- int num_convex = convex_hull_graham(intersectPts, num, orderedPts, true);
- return polygon_area(orderedPts, num_convex);
-}
-
-} // namespace
-
-template
-HOST_DEVICE_INLINE T
-single_box_iou_rotated(T const* const box1_raw, T const* const box2_raw) {
- // shift center to the middle point to achieve higher precision in result
- RotatedBox box1, box2;
- auto center_shift_x = (box1_raw[0] + box2_raw[0]) / 2.0;
- auto center_shift_y = (box1_raw[1] + box2_raw[1]) / 2.0;
- box1.x_ctr = box1_raw[0] - center_shift_x;
- box1.y_ctr = box1_raw[1] - center_shift_y;
- box1.w = box1_raw[2];
- box1.h = box1_raw[3];
- box1.a = box1_raw[4];
- box2.x_ctr = box2_raw[0] - center_shift_x;
- box2.y_ctr = box2_raw[1] - center_shift_y;
- box2.w = box2_raw[2];
- box2.h = box2_raw[3];
- box2.a = box2_raw[4];
-
- T area1 = box1.w * box1.h;
- T area2 = box2.w * box2.h;
- if (area1 < 1e-14 || area2 < 1e-14) {
- return 0.f;
- }
-
- T intersection = rotated_boxes_intersection(box1, box2);
- T iou = intersection / (area1 + area2 - intersection);
- return iou;
-}
-
-} // namespace detectron2
diff --git a/spaces/ChandraMohanNayal/AutoGPT/autogpt/memory/no_memory.py b/spaces/ChandraMohanNayal/AutoGPT/autogpt/memory/no_memory.py
deleted file mode 100644
index 0371e96ae89f5eb88dae019a66351a229596ed7a..0000000000000000000000000000000000000000
--- a/spaces/ChandraMohanNayal/AutoGPT/autogpt/memory/no_memory.py
+++ /dev/null
@@ -1,73 +0,0 @@
-"""A class that does not store any data. This is the default memory provider."""
-from __future__ import annotations
-
-from typing import Any
-
-from autogpt.memory.base import MemoryProviderSingleton
-
-
-class NoMemory(MemoryProviderSingleton):
- """
- A class that does not store any data. This is the default memory provider.
- """
-
- def __init__(self, cfg):
- """
- Initializes the NoMemory provider.
-
- Args:
- cfg: The config object.
-
- Returns: None
- """
- pass
-
- def add(self, data: str) -> str:
- """
- Adds a data point to the memory. No action is taken in NoMemory.
-
- Args:
- data: The data to add.
-
- Returns: An empty string.
- """
- return ""
-
- def get(self, data: str) -> list[Any] | None:
- """
- Gets the data from the memory that is most relevant to the given data.
- NoMemory always returns None.
-
- Args:
- data: The data to compare to.
-
- Returns: None
- """
- return None
-
- def clear(self) -> str:
- """
- Clears the memory. No action is taken in NoMemory.
-
- Returns: An empty string.
- """
- return ""
-
- def get_relevant(self, data: str, num_relevant: int = 5) -> list[Any] | None:
- """
- Returns all the data in the memory that is relevant to the given data.
- NoMemory always returns None.
-
- Args:
- data: The data to compare to.
- num_relevant: The number of relevant data to return.
-
- Returns: None
- """
- return None
-
- def get_stats(self):
- """
- Returns: An empty dictionary as there are no stats in NoMemory.
- """
- return {}
diff --git a/spaces/CobaltZvc/sherlocks_pheonix/style.css b/spaces/CobaltZvc/sherlocks_pheonix/style.css
deleted file mode 100644
index 114adf441e9032febb46bc056b2a8bb651075f0d..0000000000000000000000000000000000000000
--- a/spaces/CobaltZvc/sherlocks_pheonix/style.css
+++ /dev/null
@@ -1,28 +0,0 @@
-body {
- padding: 2rem;
- font-family: -apple-system, BlinkMacSystemFont, "Arial", sans-serif;
-}
-
-h1 {
- font-size: 16px;
- margin-top: 0;
-}
-
-p {
- color: rgb(107, 114, 128);
- font-size: 15px;
- margin-bottom: 10px;
- margin-top: 5px;
-}
-
-.card {
- max-width: 620px;
- margin: 0 auto;
- padding: 16px;
- border: 1px solid lightgray;
- border-radius: 16px;
-}
-
-.card p:last-child {
- margin-bottom: 0;
-}
diff --git a/spaces/Cropinky/esrgan/realesrgan/train.py b/spaces/Cropinky/esrgan/realesrgan/train.py
deleted file mode 100644
index 8a9cec9ed80d9f362984779548dcec921a636a04..0000000000000000000000000000000000000000
--- a/spaces/Cropinky/esrgan/realesrgan/train.py
+++ /dev/null
@@ -1,11 +0,0 @@
-# flake8: noqa
-import os.path as osp
-from basicsr.train import train_pipeline
-
-import realesrgan.archs
-import realesrgan.data
-import realesrgan.models
-
-if __name__ == '__main__':
- root_path = osp.abspath(osp.join(__file__, osp.pardir, osp.pardir))
- train_pipeline(root_path)
diff --git a/spaces/CyberPeace-Institute/SecureBERT-NER-Space/app.py b/spaces/CyberPeace-Institute/SecureBERT-NER-Space/app.py
deleted file mode 100644
index 6a625a31b176b9876a44f3d5c8134ae2bd8a309c..0000000000000000000000000000000000000000
--- a/spaces/CyberPeace-Institute/SecureBERT-NER-Space/app.py
+++ /dev/null
@@ -1,64 +0,0 @@
-import streamlit as st
-from annotated_text import annotated_text
-from transformers import AutoModelForTokenClassification
-from transformers import AutoTokenizer
-from transformers import pipeline
-import requests
-import random
-import justext
-import pickle
-from tqdm import tqdm
-import torch
-import jsonlines
-
-st.title('Identifying Cybersecurity Entities on Webpages')
-
-query_input = st.text_input("URL:")
-if query_input:
- headers = {
- "User-Agent": "Mozilla/5.0 (Windows NT 10.0; Win64; x64; rv:103.0) Gecko/20100101 Firefox/103.0",
- "Accept": "application/json, text/plain, */*",
- "Accept-Language": "en-US,en;q=0.5",
- "Accept-Encoding": "gzip, deflate",
- }
-
- s = requests.Session()
- s.headers.update(headers)
-
- response = s.get(query_input)
- paragraphs = justext.justext(response.content, justext.get_stoplist("English"))
- text = ""
- for paragraph in paragraphs:
- if not paragraph.is_boilerplate:
- text += paragraph.text + "\n"
-
- text = text.split("\n")
- text = [text_block for text_block in text if text_block != ""]
-
- pipe = pipeline("token-classification", model="cpi-connect/SecureBERT-NER", grouped_entities=True)
-
- for text_block in text:
- entities = pipe(text_block)
- annotated = []
-
- last_entity, last_idx = None, None
- for entity in entities:
- if last_entity is None and last_idx is None:
- annotated.append(text_block[:entity["start"]])
- annotated.append((text_block[entity["start"] : entity["end"]], entity["entity_group"]))
- last_entity = entity["entity_group"]
- last_idx = entity["end"]
- elif last_entity == entity["entity_group"] and last_idx == entity["start"]:
- new_text = annotated[-1][0] + text_block[entity["start"] : entity["end"]]
- label = annotated[-1][1]
- annotated[-1] = (new_text, label)
- last_entity = entity["entity_group"]
- last_idx = entity["end"]
- else:
- annotated.append(text_block[last_idx : entity["start"]])
- annotated.append((text_block[entity["start"] : entity["end"]], entity["entity_group"]))
- last_entity = entity["entity_group"]
- last_idx = entity["end"]
-
- annotated.append(text_block[last_idx : ])
- annotated_text(annotated)
\ No newline at end of file
diff --git a/spaces/DQChoi/gpt-demo/venv/lib/python3.11/site-packages/altair/vegalite/v5/display.py b/spaces/DQChoi/gpt-demo/venv/lib/python3.11/site-packages/altair/vegalite/v5/display.py
deleted file mode 100644
index ba69e02e076b0828a9b2032eb47de8c1fb1492d8..0000000000000000000000000000000000000000
--- a/spaces/DQChoi/gpt-demo/venv/lib/python3.11/site-packages/altair/vegalite/v5/display.py
+++ /dev/null
@@ -1,119 +0,0 @@
-import os
-
-from ...utils.mimebundle import spec_to_mimebundle
-from ..display import Displayable
-from ..display import default_renderer_base
-from ..display import json_renderer_base
-from ..display import RendererRegistry
-from ..display import HTMLRenderer
-
-from .schema import SCHEMA_VERSION
-
-VEGALITE_VERSION = SCHEMA_VERSION.lstrip("v")
-VEGA_VERSION = "5"
-VEGAEMBED_VERSION = "6"
-
-
-# ==============================================================================
-# VegaLite v5 renderer logic
-# ==============================================================================
-
-
-# The MIME type for Vega-Lite 5.x releases.
-VEGALITE_MIME_TYPE = "application/vnd.vegalite.v5+json" # type: str
-
-# The entry point group that can be used by other packages to declare other
-# renderers that will be auto-detected. Explicit registration is also
-# allowed by the PluginRegistery API.
-ENTRY_POINT_GROUP = "altair.vegalite.v5.renderer" # type: str
-
-# The display message when rendering fails
-DEFAULT_DISPLAY = """\
-
-
-If you see this message, it means the renderer has not been properly enabled
-for the frontend that you are using. For more information, see
-https://altair-viz.github.io/user_guide/display_frontends.html#troubleshooting
-"""
-
-renderers = RendererRegistry(entry_point_group=ENTRY_POINT_GROUP)
-
-here = os.path.dirname(os.path.realpath(__file__))
-
-
-def mimetype_renderer(spec, **metadata):
- return default_renderer_base(spec, VEGALITE_MIME_TYPE, DEFAULT_DISPLAY, **metadata)
-
-
-def json_renderer(spec, **metadata):
- return json_renderer_base(spec, DEFAULT_DISPLAY, **metadata)
-
-
-def png_renderer(spec, **metadata):
- return spec_to_mimebundle(
- spec,
- format="png",
- mode="vega-lite",
- vega_version=VEGA_VERSION,
- vegaembed_version=VEGAEMBED_VERSION,
- vegalite_version=VEGALITE_VERSION,
- **metadata,
- )
-
-
-def svg_renderer(spec, **metadata):
- return spec_to_mimebundle(
- spec,
- format="svg",
- mode="vega-lite",
- vega_version=VEGA_VERSION,
- vegaembed_version=VEGAEMBED_VERSION,
- vegalite_version=VEGALITE_VERSION,
- **metadata,
- )
-
-
-html_renderer = HTMLRenderer(
- mode="vega-lite",
- template="universal",
- vega_version=VEGA_VERSION,
- vegaembed_version=VEGAEMBED_VERSION,
- vegalite_version=VEGALITE_VERSION,
-)
-
-renderers.register("default", html_renderer)
-renderers.register("html", html_renderer)
-renderers.register("colab", html_renderer)
-renderers.register("kaggle", html_renderer)
-renderers.register("zeppelin", html_renderer)
-renderers.register("mimetype", mimetype_renderer)
-renderers.register("jupyterlab", mimetype_renderer)
-renderers.register("nteract", mimetype_renderer)
-renderers.register("json", json_renderer)
-renderers.register("png", png_renderer)
-renderers.register("svg", svg_renderer)
-renderers.enable("default")
-
-
-class VegaLite(Displayable):
- """An IPython/Jupyter display class for rendering VegaLite 5."""
-
- renderers = renderers
- schema_path = (__name__, "schema/vega-lite-schema.json")
-
-
-def vegalite(spec, validate=True):
- """Render and optionally validate a VegaLite 5 spec.
-
- This will use the currently enabled renderer to render the spec.
-
- Parameters
- ==========
- spec: dict
- A fully compliant VegaLite 5 spec, with the data portion fully processed.
- validate: bool
- Should the spec be validated against the VegaLite 5 schema?
- """
- from IPython.display import display
-
- display(VegaLite(spec, validate=validate))
diff --git a/spaces/DQChoi/gpt-demo/venv/lib/python3.11/site-packages/anyio/from_thread.py b/spaces/DQChoi/gpt-demo/venv/lib/python3.11/site-packages/anyio/from_thread.py
deleted file mode 100644
index 6b76861c70d6a6aa369a54370ef47aa75839a91f..0000000000000000000000000000000000000000
--- a/spaces/DQChoi/gpt-demo/venv/lib/python3.11/site-packages/anyio/from_thread.py
+++ /dev/null
@@ -1,500 +0,0 @@
-from __future__ import annotations
-
-import threading
-from asyncio import iscoroutine
-from concurrent.futures import FIRST_COMPLETED, Future, ThreadPoolExecutor, wait
-from contextlib import AbstractContextManager, contextmanager
-from types import TracebackType
-from typing import (
- Any,
- AsyncContextManager,
- Awaitable,
- Callable,
- ContextManager,
- Generator,
- Generic,
- Iterable,
- TypeVar,
- cast,
- overload,
-)
-from warnings import warn
-
-from ._core import _eventloop
-from ._core._eventloop import get_asynclib, get_cancelled_exc_class, threadlocals
-from ._core._synchronization import Event
-from ._core._tasks import CancelScope, create_task_group
-from .abc._tasks import TaskStatus
-
-T_Retval = TypeVar("T_Retval")
-T_co = TypeVar("T_co")
-
-
-def run(func: Callable[..., Awaitable[T_Retval]], *args: object) -> T_Retval:
- """
- Call a coroutine function from a worker thread.
-
- :param func: a coroutine function
- :param args: positional arguments for the callable
- :return: the return value of the coroutine function
-
- """
- try:
- asynclib = threadlocals.current_async_module
- except AttributeError:
- raise RuntimeError("This function can only be run from an AnyIO worker thread")
-
- return asynclib.run_async_from_thread(func, *args)
-
-
-def run_async_from_thread(
- func: Callable[..., Awaitable[T_Retval]], *args: object
-) -> T_Retval:
- warn(
- "run_async_from_thread() has been deprecated, use anyio.from_thread.run() instead",
- DeprecationWarning,
- )
- return run(func, *args)
-
-
-def run_sync(func: Callable[..., T_Retval], *args: object) -> T_Retval:
- """
- Call a function in the event loop thread from a worker thread.
-
- :param func: a callable
- :param args: positional arguments for the callable
- :return: the return value of the callable
-
- """
- try:
- asynclib = threadlocals.current_async_module
- except AttributeError:
- raise RuntimeError("This function can only be run from an AnyIO worker thread")
-
- return asynclib.run_sync_from_thread(func, *args)
-
-
-def run_sync_from_thread(func: Callable[..., T_Retval], *args: object) -> T_Retval:
- warn(
- "run_sync_from_thread() has been deprecated, use anyio.from_thread.run_sync() instead",
- DeprecationWarning,
- )
- return run_sync(func, *args)
-
-
-class _BlockingAsyncContextManager(Generic[T_co], AbstractContextManager):
- _enter_future: Future
- _exit_future: Future
- _exit_event: Event
- _exit_exc_info: tuple[
- type[BaseException] | None, BaseException | None, TracebackType | None
- ] = (None, None, None)
-
- def __init__(self, async_cm: AsyncContextManager[T_co], portal: BlockingPortal):
- self._async_cm = async_cm
- self._portal = portal
-
- async def run_async_cm(self) -> bool | None:
- try:
- self._exit_event = Event()
- value = await self._async_cm.__aenter__()
- except BaseException as exc:
- self._enter_future.set_exception(exc)
- raise
- else:
- self._enter_future.set_result(value)
-
- try:
- # Wait for the sync context manager to exit.
- # This next statement can raise `get_cancelled_exc_class()` if
- # something went wrong in a task group in this async context
- # manager.
- await self._exit_event.wait()
- finally:
- # In case of cancellation, it could be that we end up here before
- # `_BlockingAsyncContextManager.__exit__` is called, and an
- # `_exit_exc_info` has been set.
- result = await self._async_cm.__aexit__(*self._exit_exc_info)
- return result
-
- def __enter__(self) -> T_co:
- self._enter_future = Future()
- self._exit_future = self._portal.start_task_soon(self.run_async_cm)
- cm = self._enter_future.result()
- return cast(T_co, cm)
-
- def __exit__(
- self,
- __exc_type: type[BaseException] | None,
- __exc_value: BaseException | None,
- __traceback: TracebackType | None,
- ) -> bool | None:
- self._exit_exc_info = __exc_type, __exc_value, __traceback
- self._portal.call(self._exit_event.set)
- return self._exit_future.result()
-
-
-class _BlockingPortalTaskStatus(TaskStatus):
- def __init__(self, future: Future):
- self._future = future
-
- def started(self, value: object = None) -> None:
- self._future.set_result(value)
-
-
-class BlockingPortal:
- """An object that lets external threads run code in an asynchronous event loop."""
-
- def __new__(cls) -> BlockingPortal:
- return get_asynclib().BlockingPortal()
-
- def __init__(self) -> None:
- self._event_loop_thread_id: int | None = threading.get_ident()
- self._stop_event = Event()
- self._task_group = create_task_group()
- self._cancelled_exc_class = get_cancelled_exc_class()
-
- async def __aenter__(self) -> BlockingPortal:
- await self._task_group.__aenter__()
- return self
-
- async def __aexit__(
- self,
- exc_type: type[BaseException] | None,
- exc_val: BaseException | None,
- exc_tb: TracebackType | None,
- ) -> bool | None:
- await self.stop()
- return await self._task_group.__aexit__(exc_type, exc_val, exc_tb)
-
- def _check_running(self) -> None:
- if self._event_loop_thread_id is None:
- raise RuntimeError("This portal is not running")
- if self._event_loop_thread_id == threading.get_ident():
- raise RuntimeError(
- "This method cannot be called from the event loop thread"
- )
-
- async def sleep_until_stopped(self) -> None:
- """Sleep until :meth:`stop` is called."""
- await self._stop_event.wait()
-
- async def stop(self, cancel_remaining: bool = False) -> None:
- """
- Signal the portal to shut down.
-
- This marks the portal as no longer accepting new calls and exits from
- :meth:`sleep_until_stopped`.
-
- :param cancel_remaining: ``True`` to cancel all the remaining tasks, ``False`` to let them
- finish before returning
-
- """
- self._event_loop_thread_id = None
- self._stop_event.set()
- if cancel_remaining:
- self._task_group.cancel_scope.cancel()
-
- async def _call_func(
- self, func: Callable, args: tuple, kwargs: dict[str, Any], future: Future
- ) -> None:
- def callback(f: Future) -> None:
- if f.cancelled() and self._event_loop_thread_id not in (
- None,
- threading.get_ident(),
- ):
- self.call(scope.cancel)
-
- try:
- retval = func(*args, **kwargs)
- if iscoroutine(retval):
- with CancelScope() as scope:
- if future.cancelled():
- scope.cancel()
- else:
- future.add_done_callback(callback)
-
- retval = await retval
- except self._cancelled_exc_class:
- future.cancel()
- except BaseException as exc:
- if not future.cancelled():
- future.set_exception(exc)
-
- # Let base exceptions fall through
- if not isinstance(exc, Exception):
- raise
- else:
- if not future.cancelled():
- future.set_result(retval)
- finally:
- scope = None # type: ignore[assignment]
-
- def _spawn_task_from_thread(
- self,
- func: Callable,
- args: tuple,
- kwargs: dict[str, Any],
- name: object,
- future: Future,
- ) -> None:
- """
- Spawn a new task using the given callable.
-
- Implementors must ensure that the future is resolved when the task finishes.
-
- :param func: a callable
- :param args: positional arguments to be passed to the callable
- :param kwargs: keyword arguments to be passed to the callable
- :param name: name of the task (will be coerced to a string if not ``None``)
- :param future: a future that will resolve to the return value of the callable, or the
- exception raised during its execution
-
- """
- raise NotImplementedError
-
- @overload
- def call(self, func: Callable[..., Awaitable[T_Retval]], *args: object) -> T_Retval:
- ...
-
- @overload
- def call(self, func: Callable[..., T_Retval], *args: object) -> T_Retval:
- ...
-
- def call(
- self, func: Callable[..., Awaitable[T_Retval] | T_Retval], *args: object
- ) -> T_Retval:
- """
- Call the given function in the event loop thread.
-
- If the callable returns a coroutine object, it is awaited on.
-
- :param func: any callable
- :raises RuntimeError: if the portal is not running or if this method is called from within
- the event loop thread
-
- """
- return cast(T_Retval, self.start_task_soon(func, *args).result())
-
- @overload
- def spawn_task(
- self,
- func: Callable[..., Awaitable[T_Retval]],
- *args: object,
- name: object = None,
- ) -> Future[T_Retval]:
- ...
-
- @overload
- def spawn_task(
- self, func: Callable[..., T_Retval], *args: object, name: object = None
- ) -> Future[T_Retval]:
- ...
-
- def spawn_task(
- self,
- func: Callable[..., Awaitable[T_Retval] | T_Retval],
- *args: object,
- name: object = None,
- ) -> Future[T_Retval]:
- """
- Start a task in the portal's task group.
-
- :param func: the target coroutine function
- :param args: positional arguments passed to ``func``
- :param name: name of the task (will be coerced to a string if not ``None``)
- :return: a future that resolves with the return value of the callable if the task completes
- successfully, or with the exception raised in the task
- :raises RuntimeError: if the portal is not running or if this method is called from within
- the event loop thread
-
- .. versionadded:: 2.1
- .. deprecated:: 3.0
- Use :meth:`start_task_soon` instead. If your code needs AnyIO 2 compatibility, you
- can keep using this until AnyIO 4.
-
- """
- warn(
- "spawn_task() is deprecated -- use start_task_soon() instead",
- DeprecationWarning,
- )
- return self.start_task_soon(func, *args, name=name) # type: ignore[arg-type]
-
- @overload
- def start_task_soon(
- self,
- func: Callable[..., Awaitable[T_Retval]],
- *args: object,
- name: object = None,
- ) -> Future[T_Retval]:
- ...
-
- @overload
- def start_task_soon(
- self, func: Callable[..., T_Retval], *args: object, name: object = None
- ) -> Future[T_Retval]:
- ...
-
- def start_task_soon(
- self,
- func: Callable[..., Awaitable[T_Retval] | T_Retval],
- *args: object,
- name: object = None,
- ) -> Future[T_Retval]:
- """
- Start a task in the portal's task group.
-
- The task will be run inside a cancel scope which can be cancelled by cancelling the
- returned future.
-
- :param func: the target function
- :param args: positional arguments passed to ``func``
- :param name: name of the task (will be coerced to a string if not ``None``)
- :return: a future that resolves with the return value of the callable if the
- task completes successfully, or with the exception raised in the task
- :raises RuntimeError: if the portal is not running or if this method is called
- from within the event loop thread
- :rtype: concurrent.futures.Future[T_Retval]
-
- .. versionadded:: 3.0
-
- """
- self._check_running()
- f: Future = Future()
- self._spawn_task_from_thread(func, args, {}, name, f)
- return f
-
- def start_task(
- self, func: Callable[..., Awaitable[Any]], *args: object, name: object = None
- ) -> tuple[Future[Any], Any]:
- """
- Start a task in the portal's task group and wait until it signals for readiness.
-
- This method works the same way as :meth:`.abc.TaskGroup.start`.
-
- :param func: the target function
- :param args: positional arguments passed to ``func``
- :param name: name of the task (will be coerced to a string if not ``None``)
- :return: a tuple of (future, task_status_value) where the ``task_status_value``
- is the value passed to ``task_status.started()`` from within the target
- function
- :rtype: tuple[concurrent.futures.Future[Any], Any]
-
- .. versionadded:: 3.0
-
- """
-
- def task_done(future: Future) -> None:
- if not task_status_future.done():
- if future.cancelled():
- task_status_future.cancel()
- elif future.exception():
- task_status_future.set_exception(future.exception())
- else:
- exc = RuntimeError(
- "Task exited without calling task_status.started()"
- )
- task_status_future.set_exception(exc)
-
- self._check_running()
- task_status_future: Future = Future()
- task_status = _BlockingPortalTaskStatus(task_status_future)
- f: Future = Future()
- f.add_done_callback(task_done)
- self._spawn_task_from_thread(func, args, {"task_status": task_status}, name, f)
- return f, task_status_future.result()
-
- def wrap_async_context_manager(
- self, cm: AsyncContextManager[T_co]
- ) -> ContextManager[T_co]:
- """
- Wrap an async context manager as a synchronous context manager via this portal.
-
- Spawns a task that will call both ``__aenter__()`` and ``__aexit__()``, stopping in the
- middle until the synchronous context manager exits.
-
- :param cm: an asynchronous context manager
- :return: a synchronous context manager
-
- .. versionadded:: 2.1
-
- """
- return _BlockingAsyncContextManager(cm, self)
-
-
-def create_blocking_portal() -> BlockingPortal:
- """
- Create a portal for running functions in the event loop thread from external threads.
-
- Use this function in asynchronous code when you need to allow external threads access to the
- event loop where your asynchronous code is currently running.
-
- .. deprecated:: 3.0
- Use :class:`.BlockingPortal` directly.
-
- """
- warn(
- "create_blocking_portal() has been deprecated -- use anyio.from_thread.BlockingPortal() "
- "directly",
- DeprecationWarning,
- )
- return BlockingPortal()
-
-
-@contextmanager
-def start_blocking_portal(
- backend: str = "asyncio", backend_options: dict[str, Any] | None = None
-) -> Generator[BlockingPortal, Any, None]:
- """
- Start a new event loop in a new thread and run a blocking portal in its main task.
-
- The parameters are the same as for :func:`~anyio.run`.
-
- :param backend: name of the backend
- :param backend_options: backend options
- :return: a context manager that yields a blocking portal
-
- .. versionchanged:: 3.0
- Usage as a context manager is now required.
-
- """
-
- async def run_portal() -> None:
- async with BlockingPortal() as portal_:
- if future.set_running_or_notify_cancel():
- future.set_result(portal_)
- await portal_.sleep_until_stopped()
-
- future: Future[BlockingPortal] = Future()
- with ThreadPoolExecutor(1) as executor:
- run_future = executor.submit(
- _eventloop.run,
- run_portal, # type: ignore[arg-type]
- backend=backend,
- backend_options=backend_options,
- )
- try:
- wait(
- cast(Iterable[Future], [run_future, future]),
- return_when=FIRST_COMPLETED,
- )
- except BaseException:
- future.cancel()
- run_future.cancel()
- raise
-
- if future.done():
- portal = future.result()
- cancel_remaining_tasks = False
- try:
- yield portal
- except BaseException:
- cancel_remaining_tasks = True
- raise
- finally:
- try:
- portal.call(portal.stop, cancel_remaining_tasks)
- except RuntimeError:
- pass
-
- run_future.result()
diff --git a/spaces/DQChoi/gpt-demo/venv/lib/python3.11/site-packages/fastapi/responses.py b/spaces/DQChoi/gpt-demo/venv/lib/python3.11/site-packages/fastapi/responses.py
deleted file mode 100644
index c0a13b7555efc9d99c5c887fee1c94c88ba7e89c..0000000000000000000000000000000000000000
--- a/spaces/DQChoi/gpt-demo/venv/lib/python3.11/site-packages/fastapi/responses.py
+++ /dev/null
@@ -1,34 +0,0 @@
-from typing import Any
-
-from starlette.responses import FileResponse as FileResponse # noqa
-from starlette.responses import HTMLResponse as HTMLResponse # noqa
-from starlette.responses import JSONResponse as JSONResponse # noqa
-from starlette.responses import PlainTextResponse as PlainTextResponse # noqa
-from starlette.responses import RedirectResponse as RedirectResponse # noqa
-from starlette.responses import Response as Response # noqa
-from starlette.responses import StreamingResponse as StreamingResponse # noqa
-
-try:
- import ujson
-except ImportError: # pragma: nocover
- ujson = None # type: ignore
-
-
-try:
- import orjson
-except ImportError: # pragma: nocover
- orjson = None # type: ignore
-
-
-class UJSONResponse(JSONResponse):
- def render(self, content: Any) -> bytes:
- assert ujson is not None, "ujson must be installed to use UJSONResponse"
- return ujson.dumps(content, ensure_ascii=False).encode("utf-8")
-
-
-class ORJSONResponse(JSONResponse):
- def render(self, content: Any) -> bytes:
- assert orjson is not None, "orjson must be installed to use ORJSONResponse"
- return orjson.dumps(
- content, option=orjson.OPT_NON_STR_KEYS | orjson.OPT_SERIALIZE_NUMPY
- )
diff --git a/spaces/DQChoi/gpt-demo/venv/lib/python3.11/site-packages/gradio/templates/frontend/assets/yaml-95012b83.js b/spaces/DQChoi/gpt-demo/venv/lib/python3.11/site-packages/gradio/templates/frontend/assets/yaml-95012b83.js
deleted file mode 100644
index 3fef68bd6d3b922eebf9622184021189fa7e8cc2..0000000000000000000000000000000000000000
--- a/spaces/DQChoi/gpt-demo/venv/lib/python3.11/site-packages/gradio/templates/frontend/assets/yaml-95012b83.js
+++ /dev/null
@@ -1,2 +0,0 @@
-var l=["true","false","on","off","yes","no"],f=new RegExp("\\b(("+l.join(")|(")+"))$","i");const a={name:"yaml",token:function(n,i){var r=n.peek(),e=i.escaped;if(i.escaped=!1,r=="#"&&(n.pos==0||/\s/.test(n.string.charAt(n.pos-1))))return n.skipToEnd(),"comment";if(n.match(/^('([^']|\\.)*'?|"([^"]|\\.)*"?)/))return"string";if(i.literal&&n.indentation()>i.keyCol)return n.skipToEnd(),"string";if(i.literal&&(i.literal=!1),n.sol()){if(i.keyCol=0,i.pair=!1,i.pairStart=!1,n.match("---")||n.match("..."))return"def";if(n.match(/^\s*-\s+/))return"meta"}if(n.match(/^(\{|\}|\[|\])/))return r=="{"?i.inlinePairs++:r=="}"?i.inlinePairs--:r=="["?i.inlineList++:i.inlineList--,"meta";if(i.inlineList>0&&!e&&r==",")return n.next(),"meta";if(i.inlinePairs>0&&!e&&r==",")return i.keyCol=0,i.pair=!1,i.pairStart=!1,n.next(),"meta";if(i.pairStart){if(n.match(/^\s*(\||\>)\s*/))return i.literal=!0,"meta";if(n.match(/^\s*(\&|\*)[a-z0-9\._-]+\b/i))return"variable";if(i.inlinePairs==0&&n.match(/^\s*-?[0-9\.\,]+\s?$/)||i.inlinePairs>0&&n.match(/^\s*-?[0-9\.\,]+\s?(?=(,|}))/))return"number";if(n.match(f))return"keyword"}return!i.pair&&n.match(/^\s*(?:[,\[\]{}&*!|>'"%@`][^\s'":]|[^,\[\]{}#&*!|>'"%@`])[^#]*?(?=\s*:($|\s))/)?(i.pair=!0,i.keyCol=n.indentation(),"atom"):i.pair&&n.match(/^:\s*/)?(i.pairStart=!0,"meta"):(i.pairStart=!1,i.escaped=r=="\\",n.next(),null)},startState:function(){return{pair:!1,pairStart:!1,keyCol:0,inlinePairs:0,inlineList:0,literal:!1,escaped:!1}},languageData:{commentTokens:{line:"#"}}};export{a as yaml};
-//# sourceMappingURL=yaml-95012b83.js.map
diff --git a/spaces/DVLH/nlpconnect-vit-gpt2-image-captioning/README.md b/spaces/DVLH/nlpconnect-vit-gpt2-image-captioning/README.md
deleted file mode 100644
index 6bcfcff8dc5993ba1a2d9054b1b1dae58b18359b..0000000000000000000000000000000000000000
--- a/spaces/DVLH/nlpconnect-vit-gpt2-image-captioning/README.md
+++ /dev/null
@@ -1,12 +0,0 @@
----
-title: Nlpconnect Vit Gpt2 Image Captioning
-emoji: 🏃
-colorFrom: purple
-colorTo: indigo
-sdk: gradio
-sdk_version: 3.39.0
-app_file: app.py
-pinned: false
----
-
-Check out the configuration reference at https://huggingface.co/docs/hub/spaces-config-reference
diff --git a/spaces/Destinycy/Destiny_LOL/README.md b/spaces/Destinycy/Destiny_LOL/README.md
deleted file mode 100644
index eb8b70a1b1f541134e4261c4e9900e9a5230ee74..0000000000000000000000000000000000000000
--- a/spaces/Destinycy/Destiny_LOL/README.md
+++ /dev/null
@@ -1,12 +0,0 @@
----
-title: Destiny LOL
-emoji: 🐢
-colorFrom: gray
-colorTo: purple
-sdk: gradio
-sdk_version: 3.18.0
-app_file: app.py
-pinned: false
----
-
-Check out the configuration reference at https://huggingface.co/docs/hub/spaces-config-reference
diff --git a/spaces/Detomo/ai-avatar-backend/routes/index.js b/spaces/Detomo/ai-avatar-backend/routes/index.js
deleted file mode 100644
index 4ed64d3f1e8950255c619ff47dcba2a974e8ac01..0000000000000000000000000000000000000000
--- a/spaces/Detomo/ai-avatar-backend/routes/index.js
+++ /dev/null
@@ -1,36 +0,0 @@
-var express = require('express');
-var router = express.Router();
-var textToSpeech = require('../helpers/tts');
-var callOpenAI = require('../helpers/callOpenAI'); // Import the helper function
-
-function logRequestBody(req, res, next) {
- console.log('Request body:', req.body);
- next();
-}
-
-router.post('/talk', logRequestBody, async function(req, res, next) {
- try {
- const ttsResult = await textToSpeech(req.body.text, req.body.language);
- res.json(ttsResult);
-
- } catch (err) {
- res.status(500).json({ error: err.message });
- }
-});
-
-router.post('/chat', logRequestBody, async function(req, res, next) {
- try {
- const userContent = req.body.text;
- const openAIResponse = await callOpenAI(userContent);
-
- res.json({ response: openAIResponse });
- } catch (err) {
- res.status(500).json({ error: err.message });
- }
-});
-
-router.get('/', function(req, res, next) {
- res.send("AI avatar backend is running.");
-});
-
-module.exports = router;
diff --git a/spaces/Dinoking/Guccio-AI-Designer/netdissect/__init__.py b/spaces/Dinoking/Guccio-AI-Designer/netdissect/__init__.py
deleted file mode 100644
index 39f0957560ff29b9ff0ee630e78972cd3ef187fb..0000000000000000000000000000000000000000
--- a/spaces/Dinoking/Guccio-AI-Designer/netdissect/__init__.py
+++ /dev/null
@@ -1,60 +0,0 @@
-'''
-Netdissect package.
-
-To run dissection:
-
-1. Load up the convolutional model you wish to dissect, and wrap it
- in an InstrumentedModel. Call imodel.retain_layers([layernames,..])
- to analyze a specified set of layers.
-2. Load the segmentation dataset using the BrodenDataset class;
- use the transform_image argument to normalize images to be
- suitable for the model, or the size argument to truncate the dataset.
-3. Write a function to recover the original image (with RGB scaled to
- [0...1]) given a normalized dataset image; ReverseNormalize in this
- package inverts transforms.Normalize for this purpose.
-4. Choose a directory in which to write the output, and call
- dissect(outdir, model, dataset).
-
-Example:
-
- from netdissect import InstrumentedModel, dissect
- from netdissect import BrodenDataset, ReverseNormalize
-
- model = InstrumentedModel(load_my_model())
- model.eval()
- model.cuda()
- model.retain_layers(['conv1', 'conv2', 'conv3', 'conv4', 'conv5'])
- bds = BrodenDataset('dataset/broden1_227',
- transform_image=transforms.Compose([
- transforms.ToTensor(),
- transforms.Normalize(IMAGE_MEAN, IMAGE_STDEV)]),
- size=1000)
- dissect('result/dissect', model, bds,
- recover_image=ReverseNormalize(IMAGE_MEAN, IMAGE_STDEV),
- examples_per_unit=10)
-'''
-
-from .dissection import dissect, ReverseNormalize
-from .dissection import ClassifierSegRunner, GeneratorSegRunner
-from .dissection import ImageOnlySegRunner
-from .broden import BrodenDataset, ScaleSegmentation, scatter_batch
-from .segdata import MultiSegmentDataset
-from .nethook import InstrumentedModel
-from .zdataset import z_dataset_for_model, z_sample_for_model, standard_z_sample
-from . import actviz
-from . import progress
-from . import runningstats
-from . import sampler
-
-__all__ = [
- 'dissect', 'ReverseNormalize',
- 'ClassifierSegRunner', 'GeneratorSegRunner', 'ImageOnlySegRunner',
- 'BrodenDataset', 'ScaleSegmentation', 'scatter_batch',
- 'MultiSegmentDataset',
- 'InstrumentedModel',
- 'z_dataset_for_model', 'z_sample_for_model', 'standard_z_sample'
- 'actviz',
- 'progress',
- 'runningstats',
- 'sampler'
-]
diff --git a/spaces/DragGan/DragGan-Inversion/stylegan_human/pti/pti_models/__init__.py b/spaces/DragGan/DragGan-Inversion/stylegan_human/pti/pti_models/__init__.py
deleted file mode 100644
index e69de29bb2d1d6434b8b29ae775ad8c2e48c5391..0000000000000000000000000000000000000000
diff --git a/spaces/DragGan/DragGan/stylegan_human/utils/ImagesDataset.py b/spaces/DragGan/DragGan/stylegan_human/utils/ImagesDataset.py
deleted file mode 100644
index d108f1a16cb8dec386d51ae3e9f18a860780e391..0000000000000000000000000000000000000000
--- a/spaces/DragGan/DragGan/stylegan_human/utils/ImagesDataset.py
+++ /dev/null
@@ -1,28 +0,0 @@
-# Copyright (c) SenseTime Research. All rights reserved.
-
-
-import os
-from torch.utils.data import Dataset
-from PIL import Image
-
-from utils.data_utils import make_dataset
-
-
-class ImagesDataset(Dataset):
-
- def __init__(self, source_root, source_transform=None):
- self.source_paths = sorted(make_dataset(source_root))
- self.source_transform = source_transform
-
- def __len__(self):
- return len(self.source_paths)
-
- def __getitem__(self, index):
- fname, from_path = self.source_paths[index]
- from_im = Image.open(from_path).convert('RGB')
-
- if self.source_transform:
- from_im = self.source_transform(from_im)
-
- return fname, from_im
-
diff --git a/spaces/ECCV2022/bytetrack/tutorials/transtrack/README.md b/spaces/ECCV2022/bytetrack/tutorials/transtrack/README.md
deleted file mode 100644
index 193965abc7c18906bf8072e034448c8fd6e5aab3..0000000000000000000000000000000000000000
--- a/spaces/ECCV2022/bytetrack/tutorials/transtrack/README.md
+++ /dev/null
@@ -1,45 +0,0 @@
-# TransTrack
-
-Step1. git clone https://github.com/PeizeSun/TransTrack.git
-
-
-Step2.
-
-replace https://github.com/PeizeSun/TransTrack/blob/main/models/tracker.py
-
-Step3.
-
-Download TransTrack pretrained model: [671mot17_crowdhuman_mot17.pth](https://drive.google.com/drive/folders/1DjPL8xWoXDASrxgsA3O06EspJRdUXFQ-?usp=sharing)
-
-
-Step3. run
-```
-python3 main_track.py --output_dir . --dataset_file mot --coco_path mot --batch_size 1 --resume pretrained/671mot17_crowdhuman_mot17.pth --eval --with_box_refine --num_queries 500
-```
-
-
-# TransTrack_BYTE
-
-Step1. git clone https://github.com/PeizeSun/TransTrack.git
-
-Step2.
-
-replace https://github.com/PeizeSun/TransTrack/blob/main/models/save_track.py
-
-replace https://github.com/PeizeSun/TransTrack/blob/main/engine_track.py
-
-replace https://github.com/PeizeSun/TransTrack/blob/main/main_track.py
-
-add mot_online to https://github.com/PeizeSun/TransTrack
-
-Step3. run
-```
-python3 main_track.py --output_dir . --dataset_file mot --coco_path mot --batch_size 1 --resume pretrained/671mot17_crowdhuman_mot17.pth --eval --with_box_refine --num_queries 500
-```
-
-
-## Notes
-tracker.py: only motion
-
-mot_online/byte_tracker.py: motion with kalman filter
-
diff --git a/spaces/ElainaFanBoy/MusicGen/audiocraft/utils/notebook.py b/spaces/ElainaFanBoy/MusicGen/audiocraft/utils/notebook.py
deleted file mode 100644
index 019b9d19e5bef976bedddf428fd25da42a8a9726..0000000000000000000000000000000000000000
--- a/spaces/ElainaFanBoy/MusicGen/audiocraft/utils/notebook.py
+++ /dev/null
@@ -1,32 +0,0 @@
-# Copyright (c) Meta Platforms, Inc. and affiliates.
-# All rights reserved.
-#
-# This source code is licensed under the license found in the
-# LICENSE file in the root directory of this source tree.
-
-try:
- import IPython.display as ipd # type: ignore
-except ImportError:
- # Note in a notebook...
- pass
-
-
-import torch
-
-
-def display_audio(samples: torch.Tensor, sample_rate: int):
- """Renders an audio player for the given audio samples.
-
- Args:
- samples (torch.Tensor): a Tensor of decoded audio samples
- with shapes [B, C, T] or [C, T]
- sample_rate (int): sample rate audio should be displayed with.
- """
- assert samples.dim() == 2 or samples.dim() == 3
-
- samples = samples.detach().cpu()
- if samples.dim() == 2:
- samples = samples[None, ...]
-
- for audio in samples:
- ipd.display(ipd.Audio(audio, rate=sample_rate))
diff --git a/spaces/Endre/SemanticSearch-HU/src/data/dbpedia_dump_embeddings.py b/spaces/Endre/SemanticSearch-HU/src/data/dbpedia_dump_embeddings.py
deleted file mode 100644
index e804c7bfa242841b9d9e37a2a9336a892efd52a2..0000000000000000000000000000000000000000
--- a/spaces/Endre/SemanticSearch-HU/src/data/dbpedia_dump_embeddings.py
+++ /dev/null
@@ -1,56 +0,0 @@
-from transformers import AutoTokenizer, AutoModel
-from datetime import datetime
-import torch
-import pickle
-
-#Mean Pooling - Take attention mask into account for correct averaging
-def mean_pooling(model_output, attention_mask):
- token_embeddings = model_output[0] #First element of model_output contains all token embeddings
- input_mask_expanded = attention_mask.unsqueeze(-1).expand(token_embeddings.size()).float()
- sum_embeddings = torch.sum(token_embeddings * input_mask_expanded, 1)
- sum_mask = torch.clamp(input_mask_expanded.sum(1), min=1e-9)
- return sum_embeddings / sum_mask
-
-def calculateEmbeddings(sentences,tokenizer,model):
- tokenized_sentences = tokenizer(sentences, padding=True, truncation=True, max_length=128, return_tensors='pt')
- with torch.no_grad():
- model_output = model(**tokenized_sentences)
- sentence_embeddings = mean_pooling(model_output, tokenized_sentences['attention_mask'])
- return sentence_embeddings
-
-
-def saveToDisc(embeddings, filename):
- with open(filename, "ab") as f:
- pickle.dump(embeddings, f, protocol=pickle.HIGHEST_PROTOCOL)
-
-def saveToDisc(sentences, embeddings, filename):
- with open(filename, "ab") as f:
- pickle.dump({'sentences': sentences, 'embeddings': embeddings}, f, protocol=pickle.HIGHEST_PROTOCOL)
-
-dt = datetime.now()
-datetime_formatted = dt.strftime('%Y-%m-%d_%H:%M:%S')
-batch_size = 1000
-
-input_text_file = 'data/preprocessed/shortened_abstracts_hu_2021_09_01.txt'
-output_embeddings_file = f'data/preprocessed/embeddings_{batch_size}_batches_at_{datetime_formatted}.pkl'
-
-multilingual_checkpoint = 'sentence-transformers/paraphrase-multilingual-MiniLM-L12-v2'
-tokenizer = AutoTokenizer.from_pretrained(multilingual_checkpoint)
-model = AutoModel.from_pretrained(multilingual_checkpoint)
-
-
-total_read = 0
-total_read_limit = 3 * batch_size
-with open(input_text_file) as f:
- while total_read < total_read_limit:
- count = 0
- sentences = []
- line = 'init'
- while line and count < batch_size:
- line = f.readline()
- sentences.append(line)
- count += 1
-
- sentence_embeddings = calculateEmbeddings(sentences,tokenizer,model)
- saveToDisc(sentences, sentence_embeddings,output_embeddings_file)
- total_read += count
\ No newline at end of file
diff --git a/spaces/Faridmaruf/rvc-genshin-v2/lib/infer_pack/transforms.py b/spaces/Faridmaruf/rvc-genshin-v2/lib/infer_pack/transforms.py
deleted file mode 100644
index a11f799e023864ff7082c1f49c0cc18351a13b47..0000000000000000000000000000000000000000
--- a/spaces/Faridmaruf/rvc-genshin-v2/lib/infer_pack/transforms.py
+++ /dev/null
@@ -1,209 +0,0 @@
-import torch
-from torch.nn import functional as F
-
-import numpy as np
-
-
-DEFAULT_MIN_BIN_WIDTH = 1e-3
-DEFAULT_MIN_BIN_HEIGHT = 1e-3
-DEFAULT_MIN_DERIVATIVE = 1e-3
-
-
-def piecewise_rational_quadratic_transform(
- inputs,
- unnormalized_widths,
- unnormalized_heights,
- unnormalized_derivatives,
- inverse=False,
- tails=None,
- tail_bound=1.0,
- min_bin_width=DEFAULT_MIN_BIN_WIDTH,
- min_bin_height=DEFAULT_MIN_BIN_HEIGHT,
- min_derivative=DEFAULT_MIN_DERIVATIVE,
-):
- if tails is None:
- spline_fn = rational_quadratic_spline
- spline_kwargs = {}
- else:
- spline_fn = unconstrained_rational_quadratic_spline
- spline_kwargs = {"tails": tails, "tail_bound": tail_bound}
-
- outputs, logabsdet = spline_fn(
- inputs=inputs,
- unnormalized_widths=unnormalized_widths,
- unnormalized_heights=unnormalized_heights,
- unnormalized_derivatives=unnormalized_derivatives,
- inverse=inverse,
- min_bin_width=min_bin_width,
- min_bin_height=min_bin_height,
- min_derivative=min_derivative,
- **spline_kwargs
- )
- return outputs, logabsdet
-
-
-def searchsorted(bin_locations, inputs, eps=1e-6):
- bin_locations[..., -1] += eps
- return torch.sum(inputs[..., None] >= bin_locations, dim=-1) - 1
-
-
-def unconstrained_rational_quadratic_spline(
- inputs,
- unnormalized_widths,
- unnormalized_heights,
- unnormalized_derivatives,
- inverse=False,
- tails="linear",
- tail_bound=1.0,
- min_bin_width=DEFAULT_MIN_BIN_WIDTH,
- min_bin_height=DEFAULT_MIN_BIN_HEIGHT,
- min_derivative=DEFAULT_MIN_DERIVATIVE,
-):
- inside_interval_mask = (inputs >= -tail_bound) & (inputs <= tail_bound)
- outside_interval_mask = ~inside_interval_mask
-
- outputs = torch.zeros_like(inputs)
- logabsdet = torch.zeros_like(inputs)
-
- if tails == "linear":
- unnormalized_derivatives = F.pad(unnormalized_derivatives, pad=(1, 1))
- constant = np.log(np.exp(1 - min_derivative) - 1)
- unnormalized_derivatives[..., 0] = constant
- unnormalized_derivatives[..., -1] = constant
-
- outputs[outside_interval_mask] = inputs[outside_interval_mask]
- logabsdet[outside_interval_mask] = 0
- else:
- raise RuntimeError("{} tails are not implemented.".format(tails))
-
- (
- outputs[inside_interval_mask],
- logabsdet[inside_interval_mask],
- ) = rational_quadratic_spline(
- inputs=inputs[inside_interval_mask],
- unnormalized_widths=unnormalized_widths[inside_interval_mask, :],
- unnormalized_heights=unnormalized_heights[inside_interval_mask, :],
- unnormalized_derivatives=unnormalized_derivatives[inside_interval_mask, :],
- inverse=inverse,
- left=-tail_bound,
- right=tail_bound,
- bottom=-tail_bound,
- top=tail_bound,
- min_bin_width=min_bin_width,
- min_bin_height=min_bin_height,
- min_derivative=min_derivative,
- )
-
- return outputs, logabsdet
-
-
-def rational_quadratic_spline(
- inputs,
- unnormalized_widths,
- unnormalized_heights,
- unnormalized_derivatives,
- inverse=False,
- left=0.0,
- right=1.0,
- bottom=0.0,
- top=1.0,
- min_bin_width=DEFAULT_MIN_BIN_WIDTH,
- min_bin_height=DEFAULT_MIN_BIN_HEIGHT,
- min_derivative=DEFAULT_MIN_DERIVATIVE,
-):
- if torch.min(inputs) < left or torch.max(inputs) > right:
- raise ValueError("Input to a transform is not within its domain")
-
- num_bins = unnormalized_widths.shape[-1]
-
- if min_bin_width * num_bins > 1.0:
- raise ValueError("Minimal bin width too large for the number of bins")
- if min_bin_height * num_bins > 1.0:
- raise ValueError("Minimal bin height too large for the number of bins")
-
- widths = F.softmax(unnormalized_widths, dim=-1)
- widths = min_bin_width + (1 - min_bin_width * num_bins) * widths
- cumwidths = torch.cumsum(widths, dim=-1)
- cumwidths = F.pad(cumwidths, pad=(1, 0), mode="constant", value=0.0)
- cumwidths = (right - left) * cumwidths + left
- cumwidths[..., 0] = left
- cumwidths[..., -1] = right
- widths = cumwidths[..., 1:] - cumwidths[..., :-1]
-
- derivatives = min_derivative + F.softplus(unnormalized_derivatives)
-
- heights = F.softmax(unnormalized_heights, dim=-1)
- heights = min_bin_height + (1 - min_bin_height * num_bins) * heights
- cumheights = torch.cumsum(heights, dim=-1)
- cumheights = F.pad(cumheights, pad=(1, 0), mode="constant", value=0.0)
- cumheights = (top - bottom) * cumheights + bottom
- cumheights[..., 0] = bottom
- cumheights[..., -1] = top
- heights = cumheights[..., 1:] - cumheights[..., :-1]
-
- if inverse:
- bin_idx = searchsorted(cumheights, inputs)[..., None]
- else:
- bin_idx = searchsorted(cumwidths, inputs)[..., None]
-
- input_cumwidths = cumwidths.gather(-1, bin_idx)[..., 0]
- input_bin_widths = widths.gather(-1, bin_idx)[..., 0]
-
- input_cumheights = cumheights.gather(-1, bin_idx)[..., 0]
- delta = heights / widths
- input_delta = delta.gather(-1, bin_idx)[..., 0]
-
- input_derivatives = derivatives.gather(-1, bin_idx)[..., 0]
- input_derivatives_plus_one = derivatives[..., 1:].gather(-1, bin_idx)[..., 0]
-
- input_heights = heights.gather(-1, bin_idx)[..., 0]
-
- if inverse:
- a = (inputs - input_cumheights) * (
- input_derivatives + input_derivatives_plus_one - 2 * input_delta
- ) + input_heights * (input_delta - input_derivatives)
- b = input_heights * input_derivatives - (inputs - input_cumheights) * (
- input_derivatives + input_derivatives_plus_one - 2 * input_delta
- )
- c = -input_delta * (inputs - input_cumheights)
-
- discriminant = b.pow(2) - 4 * a * c
- assert (discriminant >= 0).all()
-
- root = (2 * c) / (-b - torch.sqrt(discriminant))
- outputs = root * input_bin_widths + input_cumwidths
-
- theta_one_minus_theta = root * (1 - root)
- denominator = input_delta + (
- (input_derivatives + input_derivatives_plus_one - 2 * input_delta)
- * theta_one_minus_theta
- )
- derivative_numerator = input_delta.pow(2) * (
- input_derivatives_plus_one * root.pow(2)
- + 2 * input_delta * theta_one_minus_theta
- + input_derivatives * (1 - root).pow(2)
- )
- logabsdet = torch.log(derivative_numerator) - 2 * torch.log(denominator)
-
- return outputs, -logabsdet
- else:
- theta = (inputs - input_cumwidths) / input_bin_widths
- theta_one_minus_theta = theta * (1 - theta)
-
- numerator = input_heights * (
- input_delta * theta.pow(2) + input_derivatives * theta_one_minus_theta
- )
- denominator = input_delta + (
- (input_derivatives + input_derivatives_plus_one - 2 * input_delta)
- * theta_one_minus_theta
- )
- outputs = input_cumheights + numerator / denominator
-
- derivative_numerator = input_delta.pow(2) * (
- input_derivatives_plus_one * theta.pow(2)
- + 2 * input_delta * theta_one_minus_theta
- + input_derivatives * (1 - theta).pow(2)
- )
- logabsdet = torch.log(derivative_numerator) - 2 * torch.log(denominator)
-
- return outputs, logabsdet
diff --git a/spaces/Flux9665/IMS-Toucan/Utility/__init__.py b/spaces/Flux9665/IMS-Toucan/Utility/__init__.py
deleted file mode 100644
index e69de29bb2d1d6434b8b29ae775ad8c2e48c5391..0000000000000000000000000000000000000000
diff --git a/spaces/GEM/DatasetCardForm/README.md b/spaces/GEM/DatasetCardForm/README.md
deleted file mode 100644
index ccfba8f6ebf16f711426942be02d4c7f49748596..0000000000000000000000000000000000000000
--- a/spaces/GEM/DatasetCardForm/README.md
+++ /dev/null
@@ -1,37 +0,0 @@
----
-title: DatasetCardForm
-emoji: 👁
-colorFrom: indigo
-colorTo: yellow
-sdk: streamlit
-app_file: app.py
-pinned: false
----
-
-# Configuration
-
-`title`: _string_
-Display title for the Space
-
-`emoji`: _string_
-Space emoji (emoji-only character allowed)
-
-`colorFrom`: _string_
-Color for Thumbnail gradient (red, yellow, green, blue, indigo, purple, pink, gray)
-
-`colorTo`: _string_
-Color for Thumbnail gradient (red, yellow, green, blue, indigo, purple, pink, gray)
-
-`sdk`: _string_
-Can be either `gradio` or `streamlit`
-
-`sdk_version` : _string_
-Only applicable for `streamlit` SDK.
-See [doc](https://hf.co/docs/hub/spaces) for more info on supported versions.
-
-`app_file`: _string_
-Path to your main application file (which contains either `gradio` or `streamlit` Python code).
-Path is relative to the root of the repository.
-
-`pinned`: _boolean_
-Whether the Space stays on top of your list.
diff --git a/spaces/Godrose0728/Aisound02/text/thai.py b/spaces/Godrose0728/Aisound02/text/thai.py
deleted file mode 100644
index 998207c01a85c710a46db1ec8b62c39c2d94bc84..0000000000000000000000000000000000000000
--- a/spaces/Godrose0728/Aisound02/text/thai.py
+++ /dev/null
@@ -1,44 +0,0 @@
-import re
-from num_thai.thainumbers import NumThai
-
-
-num = NumThai()
-
-# List of (Latin alphabet, Thai) pairs:
-_latin_to_thai = [(re.compile('%s' % x[0], re.IGNORECASE), x[1]) for x in [
- ('a', 'เอ'),
- ('b','บี'),
- ('c','ซี'),
- ('d','ดี'),
- ('e','อี'),
- ('f','เอฟ'),
- ('g','จี'),
- ('h','เอช'),
- ('i','ไอ'),
- ('j','เจ'),
- ('k','เค'),
- ('l','แอล'),
- ('m','เอ็ม'),
- ('n','เอ็น'),
- ('o','โอ'),
- ('p','พี'),
- ('q','คิว'),
- ('r','แอร์'),
- ('s','เอส'),
- ('t','ที'),
- ('u','ยู'),
- ('v','วี'),
- ('w','ดับเบิลยู'),
- ('x','เอ็กซ์'),
- ('y','วาย'),
- ('z','ซี')
-]]
-
-
-def num_to_thai(text):
- return re.sub(r'(?:\d+(?:,?\d+)?)+(?:\.\d+(?:,?\d+)?)?', lambda x: ''.join(num.NumberToTextThai(float(x.group(0).replace(',', '')))), text)
-
-def latin_to_thai(text):
- for regex, replacement in _latin_to_thai:
- text = re.sub(regex, replacement, text)
- return text
diff --git a/spaces/Godrose0728/sound-link/text/sanskrit.py b/spaces/Godrose0728/sound-link/text/sanskrit.py
deleted file mode 100644
index 0223aaac384a2f850f5bc20651fc18eb964607d0..0000000000000000000000000000000000000000
--- a/spaces/Godrose0728/sound-link/text/sanskrit.py
+++ /dev/null
@@ -1,62 +0,0 @@
-import re
-from indic_transliteration import sanscript
-
-
-# List of (iast, ipa) pairs:
-_iast_to_ipa = [(re.compile('%s' % x[0]), x[1]) for x in [
- ('a', 'ə'),
- ('ā', 'aː'),
- ('ī', 'iː'),
- ('ū', 'uː'),
- ('ṛ', 'ɹ`'),
- ('ṝ', 'ɹ`ː'),
- ('ḷ', 'l`'),
- ('ḹ', 'l`ː'),
- ('e', 'eː'),
- ('o', 'oː'),
- ('k', 'k⁼'),
- ('k⁼h', 'kʰ'),
- ('g', 'g⁼'),
- ('g⁼h', 'gʰ'),
- ('ṅ', 'ŋ'),
- ('c', 'ʧ⁼'),
- ('ʧ⁼h', 'ʧʰ'),
- ('j', 'ʥ⁼'),
- ('ʥ⁼h', 'ʥʰ'),
- ('ñ', 'n^'),
- ('ṭ', 't`⁼'),
- ('t`⁼h', 't`ʰ'),
- ('ḍ', 'd`⁼'),
- ('d`⁼h', 'd`ʰ'),
- ('ṇ', 'n`'),
- ('t', 't⁼'),
- ('t⁼h', 'tʰ'),
- ('d', 'd⁼'),
- ('d⁼h', 'dʰ'),
- ('p', 'p⁼'),
- ('p⁼h', 'pʰ'),
- ('b', 'b⁼'),
- ('b⁼h', 'bʰ'),
- ('y', 'j'),
- ('ś', 'ʃ'),
- ('ṣ', 's`'),
- ('r', 'ɾ'),
- ('l̤', 'l`'),
- ('h', 'ɦ'),
- ("'", ''),
- ('~', '^'),
- ('ṃ', '^')
-]]
-
-
-def devanagari_to_ipa(text):
- text = text.replace('ॐ', 'ओम्')
- text = re.sub(r'\s*।\s*$', '.', text)
- text = re.sub(r'\s*।\s*', ', ', text)
- text = re.sub(r'\s*॥', '.', text)
- text = sanscript.transliterate(text, sanscript.DEVANAGARI, sanscript.IAST)
- for regex, replacement in _iast_to_ipa:
- text = re.sub(regex, replacement, text)
- text = re.sub('(.)[`ː]*ḥ', lambda x: x.group(0)
- [:-1]+'h'+x.group(1)+'*', text)
- return text
diff --git a/spaces/Gradio-Blocks/Codex_OpenAI/README.md b/spaces/Gradio-Blocks/Codex_OpenAI/README.md
deleted file mode 100644
index 46bb08a1eb4d7e05e99d43af8e3bd24587fd854d..0000000000000000000000000000000000000000
--- a/spaces/Gradio-Blocks/Codex_OpenAI/README.md
+++ /dev/null
@@ -1,13 +0,0 @@
----
-title: Codex_OpenAI
-emoji: 🌍
-colorFrom: purple
-colorTo: indigo
-sdk: gradio
-sdk_version: 3.0.6
-app_file: app.py
-pinned: false
-license: apache-2.0
----
-
-Check out the configuration reference at https://huggingface.co/docs/hub/spaces#reference
diff --git a/spaces/Gradio-Blocks/uniformer_image_detection/configs/gn+ws/mask_rcnn_r50_fpn_gn_ws-all_2x_coco.py b/spaces/Gradio-Blocks/uniformer_image_detection/configs/gn+ws/mask_rcnn_r50_fpn_gn_ws-all_2x_coco.py
deleted file mode 100644
index b83e7b5c7dd63658d57397cde60d8ee4c74d8376..0000000000000000000000000000000000000000
--- a/spaces/Gradio-Blocks/uniformer_image_detection/configs/gn+ws/mask_rcnn_r50_fpn_gn_ws-all_2x_coco.py
+++ /dev/null
@@ -1,17 +0,0 @@
-_base_ = '../mask_rcnn/mask_rcnn_r50_fpn_1x_coco.py'
-conv_cfg = dict(type='ConvWS')
-norm_cfg = dict(type='GN', num_groups=32, requires_grad=True)
-model = dict(
- pretrained='open-mmlab://jhu/resnet50_gn_ws',
- backbone=dict(conv_cfg=conv_cfg, norm_cfg=norm_cfg),
- neck=dict(conv_cfg=conv_cfg, norm_cfg=norm_cfg),
- roi_head=dict(
- bbox_head=dict(
- type='Shared4Conv1FCBBoxHead',
- conv_out_channels=256,
- conv_cfg=conv_cfg,
- norm_cfg=norm_cfg),
- mask_head=dict(conv_cfg=conv_cfg, norm_cfg=norm_cfg)))
-# learning policy
-lr_config = dict(step=[16, 22])
-runner = dict(type='EpochBasedRunner', max_epochs=24)
diff --git a/spaces/Gradio-Blocks/uniformer_image_detection/configs/ld/ld_r50_gflv1_r101_fpn_coco_1x.py b/spaces/Gradio-Blocks/uniformer_image_detection/configs/ld/ld_r50_gflv1_r101_fpn_coco_1x.py
deleted file mode 100644
index 923c626363c2f49e8ad15616a09b6cb52260923a..0000000000000000000000000000000000000000
--- a/spaces/Gradio-Blocks/uniformer_image_detection/configs/ld/ld_r50_gflv1_r101_fpn_coco_1x.py
+++ /dev/null
@@ -1,19 +0,0 @@
-_base_ = ['./ld_r18_gflv1_r101_fpn_coco_1x.py']
-model = dict(
- pretrained='torchvision://resnet50',
- backbone=dict(
- type='ResNet',
- depth=50,
- num_stages=4,
- out_indices=(0, 1, 2, 3),
- frozen_stages=1,
- norm_cfg=dict(type='BN', requires_grad=True),
- norm_eval=True,
- style='pytorch'),
- neck=dict(
- type='FPN',
- in_channels=[256, 512, 1024, 2048],
- out_channels=256,
- start_level=1,
- add_extra_convs='on_output',
- num_outs=5))
diff --git a/spaces/Gradio-Blocks/uniformer_image_segmentation/mmseg/models/decode_heads/sep_aspp_head.py b/spaces/Gradio-Blocks/uniformer_image_segmentation/mmseg/models/decode_heads/sep_aspp_head.py
deleted file mode 100644
index 50bd52bcff62d0f791c42731bdf05a64276f50b9..0000000000000000000000000000000000000000
--- a/spaces/Gradio-Blocks/uniformer_image_segmentation/mmseg/models/decode_heads/sep_aspp_head.py
+++ /dev/null
@@ -1,101 +0,0 @@
-import torch
-import torch.nn as nn
-from mmcv.cnn import ConvModule, DepthwiseSeparableConvModule
-
-from mmseg.ops import resize
-from ..builder import HEADS
-from .aspp_head import ASPPHead, ASPPModule
-
-
-class DepthwiseSeparableASPPModule(ASPPModule):
- """Atrous Spatial Pyramid Pooling (ASPP) Module with depthwise separable
- conv."""
-
- def __init__(self, **kwargs):
- super(DepthwiseSeparableASPPModule, self).__init__(**kwargs)
- for i, dilation in enumerate(self.dilations):
- if dilation > 1:
- self[i] = DepthwiseSeparableConvModule(
- self.in_channels,
- self.channels,
- 3,
- dilation=dilation,
- padding=dilation,
- norm_cfg=self.norm_cfg,
- act_cfg=self.act_cfg)
-
-
-@HEADS.register_module()
-class DepthwiseSeparableASPPHead(ASPPHead):
- """Encoder-Decoder with Atrous Separable Convolution for Semantic Image
- Segmentation.
-
- This head is the implementation of `DeepLabV3+
- `_.
-
- Args:
- c1_in_channels (int): The input channels of c1 decoder. If is 0,
- the no decoder will be used.
- c1_channels (int): The intermediate channels of c1 decoder.
- """
-
- def __init__(self, c1_in_channels, c1_channels, **kwargs):
- super(DepthwiseSeparableASPPHead, self).__init__(**kwargs)
- assert c1_in_channels >= 0
- self.aspp_modules = DepthwiseSeparableASPPModule(
- dilations=self.dilations,
- in_channels=self.in_channels,
- channels=self.channels,
- conv_cfg=self.conv_cfg,
- norm_cfg=self.norm_cfg,
- act_cfg=self.act_cfg)
- if c1_in_channels > 0:
- self.c1_bottleneck = ConvModule(
- c1_in_channels,
- c1_channels,
- 1,
- conv_cfg=self.conv_cfg,
- norm_cfg=self.norm_cfg,
- act_cfg=self.act_cfg)
- else:
- self.c1_bottleneck = None
- self.sep_bottleneck = nn.Sequential(
- DepthwiseSeparableConvModule(
- self.channels + c1_channels,
- self.channels,
- 3,
- padding=1,
- norm_cfg=self.norm_cfg,
- act_cfg=self.act_cfg),
- DepthwiseSeparableConvModule(
- self.channels,
- self.channels,
- 3,
- padding=1,
- norm_cfg=self.norm_cfg,
- act_cfg=self.act_cfg))
-
- def forward(self, inputs):
- """Forward function."""
- x = self._transform_inputs(inputs)
- aspp_outs = [
- resize(
- self.image_pool(x),
- size=x.size()[2:],
- mode='bilinear',
- align_corners=self.align_corners)
- ]
- aspp_outs.extend(self.aspp_modules(x))
- aspp_outs = torch.cat(aspp_outs, dim=1)
- output = self.bottleneck(aspp_outs)
- if self.c1_bottleneck is not None:
- c1_output = self.c1_bottleneck(inputs[0])
- output = resize(
- input=output,
- size=c1_output.shape[2:],
- mode='bilinear',
- align_corners=self.align_corners)
- output = torch.cat([output, c1_output], dim=1)
- output = self.sep_bottleneck(output)
- output = self.cls_seg(output)
- return output
diff --git a/spaces/GrandaddyShmax/MusicGen_Plus_hfv2/audiocraft/modules/conditioners.py b/spaces/GrandaddyShmax/MusicGen_Plus_hfv2/audiocraft/modules/conditioners.py
deleted file mode 100644
index 82792316024b88d4c5c38b0a28f443627771d509..0000000000000000000000000000000000000000
--- a/spaces/GrandaddyShmax/MusicGen_Plus_hfv2/audiocraft/modules/conditioners.py
+++ /dev/null
@@ -1,990 +0,0 @@
-# Copyright (c) Meta Platforms, Inc. and affiliates.
-# All rights reserved.
-#
-# This source code is licensed under the license found in the
-# LICENSE file in the root directory of this source tree.
-
-from collections import defaultdict
-from copy import deepcopy
-from dataclasses import dataclass, field
-from itertools import chain
-import logging
-import math
-import random
-import re
-import typing as tp
-import warnings
-
-from einops import rearrange
-from num2words import num2words
-import spacy
-from transformers import T5EncoderModel, T5Tokenizer # type: ignore
-import torchaudio
-import torch
-from torch import nn
-from torch import Tensor
-import torch.nn.functional as F
-from torch.nn.utils.rnn import pad_sequence
-
-from .streaming import StreamingModule
-from .transformer import create_sin_embedding
-from ..data.audio_dataset import SegmentInfo
-from ..utils.autocast import TorchAutocast
-from ..utils.utils import hash_trick, length_to_mask, collate
-
-
-logger = logging.getLogger(__name__)
-TextCondition = tp.Optional[str] # a text condition can be a string or None (if doesn't exist)
-ConditionType = tp.Tuple[Tensor, Tensor] # condition, mask
-
-
-class WavCondition(tp.NamedTuple):
- wav: Tensor
- length: Tensor
- path: tp.List[tp.Optional[str]] = []
-
-
-def nullify_condition(condition: ConditionType, dim: int = 1):
- """This function transforms an input condition to a null condition.
- The way it is done by converting it to a single zero vector similarly
- to how it is done inside WhiteSpaceTokenizer and NoopTokenizer.
-
- Args:
- condition (ConditionType): a tuple of condition and mask (tp.Tuple[Tensor, Tensor])
- dim (int): the dimension that will be truncated (should be the time dimension)
- WARNING!: dim should not be the batch dimension!
- Returns:
- ConditionType: a tuple of null condition and mask
- """
- assert dim != 0, "dim cannot be the batch dimension!"
- assert type(condition) == tuple and \
- type(condition[0]) == Tensor and \
- type(condition[1]) == Tensor, "'nullify_condition' got an unexpected input type!"
- cond, mask = condition
- B = cond.shape[0]
- last_dim = cond.dim() - 1
- out = cond.transpose(dim, last_dim)
- out = 0. * out[..., :1]
- out = out.transpose(dim, last_dim)
- mask = torch.zeros((B, 1), device=out.device).int()
- assert cond.dim() == out.dim()
- return out, mask
-
-
-def nullify_wav(wav: Tensor) -> WavCondition:
- """Create a nullified WavCondition from a wav tensor with appropriate shape.
-
- Args:
- wav (Tensor): tensor of shape [B, T]
- Returns:
- WavCondition: wav condition with nullified wav.
- """
- null_wav, _ = nullify_condition((wav, torch.zeros_like(wav)), dim=wav.dim() - 1)
- return WavCondition(
- wav=null_wav,
- length=torch.tensor([0] * wav.shape[0], device=wav.device),
- path=['null_wav'] * wav.shape[0]
- )
-
-
-@dataclass
-class ConditioningAttributes:
- text: tp.Dict[str, tp.Optional[str]] = field(default_factory=dict)
- wav: tp.Dict[str, WavCondition] = field(default_factory=dict)
-
- def __getitem__(self, item):
- return getattr(self, item)
-
- @property
- def text_attributes(self):
- return self.text.keys()
-
- @property
- def wav_attributes(self):
- return self.wav.keys()
-
- @property
- def attributes(self):
- return {"text": self.text_attributes, "wav": self.wav_attributes}
-
- def to_flat_dict(self):
- return {
- **{f"text.{k}": v for k, v in self.text.items()},
- **{f"wav.{k}": v for k, v in self.wav.items()},
- }
-
- @classmethod
- def from_flat_dict(cls, x):
- out = cls()
- for k, v in x.items():
- kind, att = k.split(".")
- out[kind][att] = v
- return out
-
-
-class SegmentWithAttributes(SegmentInfo):
- """Base class for all dataclasses that are used for conditioning.
- All child classes should implement `to_condition_attributes` that converts
- the existing attributes to a dataclass of type ConditioningAttributes.
- """
- def to_condition_attributes(self) -> ConditioningAttributes:
- raise NotImplementedError()
-
-
-class Tokenizer:
- """Base class for all tokenizers
- (in case we want to introduce more advances tokenizers in the future).
- """
- def __call__(self, texts: tp.List[tp.Optional[str]]) -> tp.Tuple[Tensor, Tensor]:
- raise NotImplementedError()
-
-
-class WhiteSpaceTokenizer(Tokenizer):
- """This tokenizer should be used for natural language descriptions.
- For example:
- ["he didn't, know he's going home.", 'shorter sentence'] =>
- [[78, 62, 31, 4, 78, 25, 19, 34],
- [59, 77, 0, 0, 0, 0, 0, 0]]
- """
- PUNCTUATIONS = "?:!.,;"
-
- def __init__(self, n_bins: int, pad_idx: int = 0, language: str = "en_core_web_sm",
- lemma: bool = True, stopwords: bool = True) -> None:
- self.n_bins = n_bins
- self.pad_idx = pad_idx
- self.lemma = lemma
- self.stopwords = stopwords
- try:
- self.nlp = spacy.load(language)
- except IOError:
- spacy.cli.download(language) # type: ignore
- self.nlp = spacy.load(language)
-
- @tp.no_type_check
- def __call__(
- self,
- texts: tp.List[tp.Optional[str]],
- return_text: bool = False
- ) -> tp.Tuple[Tensor, Tensor]:
- """Take a list of strings and convert them to a tensor of indices.
-
- Args:
- texts (tp.List[str]): List of strings.
- return_text (bool, optional): Whether to return text as additional tuple item. Defaults to False.
- Returns:
- tp.Tuple[Tensor, Tensor]:
- - Indices of words in the LUT.
- - And a mask indicating where the padding tokens are
- """
- output, lengths = [], []
- texts = deepcopy(texts)
- for i, text in enumerate(texts):
- # if current sample doesn't have a certain attribute, replace with pad token
- if text is None:
- output.append(Tensor([self.pad_idx]))
- lengths.append(0)
- continue
-
- # convert numbers to words
- text = re.sub(r"(\d+)", lambda x: num2words(int(x.group(0))), text) # type: ignore
- # normalize text
- text = self.nlp(text) # type: ignore
- # remove stopwords
- if self.stopwords:
- text = [w for w in text if not w.is_stop] # type: ignore
- # remove punctuations
- text = [w for w in text if w.text not in self.PUNCTUATIONS] # type: ignore
- # lemmatize if needed
- text = [getattr(t, "lemma_" if self.lemma else "text") for t in text] # type: ignore
-
- texts[i] = " ".join(text)
- lengths.append(len(text))
- # convert to tensor
- tokens = Tensor([hash_trick(w, self.n_bins) for w in text])
- output.append(tokens)
-
- mask = length_to_mask(torch.IntTensor(lengths)).int()
- padded_output = pad_sequence(output, padding_value=self.pad_idx).int().t()
- if return_text:
- return padded_output, mask, texts # type: ignore
- return padded_output, mask
-
-
-class NoopTokenizer(Tokenizer):
- """This tokenizer should be used for global conditioners such as: artist, genre, key, etc.
- The difference between this and WhiteSpaceTokenizer is that NoopTokenizer does not split
- strings, so "Jeff Buckley" will get it's own index. Whereas WhiteSpaceTokenizer will
- split it to ["Jeff", "Buckley"] and return an index per word.
-
- For example:
- ["Queen", "ABBA", "Jeff Buckley"] => [43, 55, 101]
- ["Metal", "Rock", "Classical"] => [0, 223, 51]
- """
- def __init__(self, n_bins: int, pad_idx: int = 0):
- self.n_bins = n_bins
- self.pad_idx = pad_idx
-
- def __call__(self, texts: tp.List[tp.Optional[str]]) -> tp.Tuple[Tensor, Tensor]:
- output, lengths = [], []
- for text in texts:
- # if current sample doesn't have a certain attribute, replace with pad token
- if text is None:
- output.append(self.pad_idx)
- lengths.append(0)
- else:
- output.append(hash_trick(text, self.n_bins))
- lengths.append(1)
-
- tokens = torch.LongTensor(output).unsqueeze(1)
- mask = length_to_mask(torch.IntTensor(lengths)).int()
- return tokens, mask
-
-
-class BaseConditioner(nn.Module):
- """Base model for all conditioner modules. We allow the output dim to be different
- than the hidden dim for two reasons: 1) keep our LUTs small when the vocab is large;
- 2) make all condition dims consistent.
-
- Args:
- dim (int): Hidden dim of the model (text-encoder/LUT).
- output_dim (int): Output dim of the conditioner.
- """
- def __init__(self, dim, output_dim):
- super().__init__()
- self.dim = dim
- self.output_dim = output_dim
- self.output_proj = nn.Linear(dim, output_dim)
-
- def tokenize(self, *args, **kwargs) -> tp.Any:
- """Should be any part of the processing that will lead to a synchronization
- point, e.g. BPE tokenization with transfer to the GPU.
-
- The returned value will be saved and return later when calling forward().
- """
- raise NotImplementedError()
-
- def forward(self, inputs: tp.Any) -> ConditionType:
- """Gets input that should be used as conditioning (e.g, genre, description or a waveform).
- Outputs a ConditionType, after the input data was embedded as a dense vector.
-
- Returns:
- ConditionType:
- - A tensor of size [B, T, D] where B is the batch size, T is the length of the
- output embedding and D is the dimension of the embedding.
- - And a mask indicating where the padding tokens.
- """
- raise NotImplementedError()
-
-
-class TextConditioner(BaseConditioner):
- ...
-
-
-class LUTConditioner(TextConditioner):
- """Lookup table TextConditioner.
-
- Args:
- n_bins (int): Number of bins.
- dim (int): Hidden dim of the model (text-encoder/LUT).
- output_dim (int): Output dim of the conditioner.
- tokenizer (str): Name of the tokenizer.
- pad_idx (int, optional): Index for padding token. Defaults to 0.
- """
- def __init__(self, n_bins: int, dim: int, output_dim: int, tokenizer: str, pad_idx: int = 0):
- super().__init__(dim, output_dim)
- self.embed = nn.Embedding(n_bins, dim)
- self.tokenizer: Tokenizer
- if tokenizer == "whitespace":
- self.tokenizer = WhiteSpaceTokenizer(n_bins, pad_idx=pad_idx)
- elif tokenizer == "noop":
- self.tokenizer = NoopTokenizer(n_bins, pad_idx=pad_idx)
- else:
- raise ValueError(f"unrecognized tokenizer `{tokenizer}`.")
-
- def tokenize(self, x: tp.List[tp.Optional[str]]) -> tp.Tuple[torch.Tensor, torch.Tensor]:
- device = self.embed.weight.device
- tokens, mask = self.tokenizer(x)
- tokens, mask = tokens.to(device), mask.to(device)
- return tokens, mask
-
- def forward(self, inputs: tp.Tuple[torch.Tensor, torch.Tensor]) -> ConditionType:
- tokens, mask = inputs
- embeds = self.embed(tokens)
- embeds = self.output_proj(embeds)
- embeds = (embeds * mask.unsqueeze(-1))
- return embeds, mask
-
-
-class T5Conditioner(TextConditioner):
- """T5-based TextConditioner.
-
- Args:
- name (str): Name of the T5 model.
- output_dim (int): Output dim of the conditioner.
- finetune (bool): Whether to fine-tune T5 at train time.
- device (str): Device for T5 Conditioner.
- autocast_dtype (tp.Optional[str], optional): Autocast dtype.
- word_dropout (float, optional): Word dropout probability.
- normalize_text (bool, optional): Whether to apply text normalization.
- """
- MODELS = ["t5-small", "t5-base", "t5-large", "t5-3b", "t5-11b",
- "google/flan-t5-small", "google/flan-t5-base", "google/flan-t5-large",
- "google/flan-t5-xl", "google/flan-t5-xxl"]
- MODELS_DIMS = {
- "t5-small": 512,
- "t5-base": 768,
- "t5-large": 1024,
- "t5-3b": 1024,
- "t5-11b": 1024,
- "google/flan-t5-small": 512,
- "google/flan-t5-base": 768,
- "google/flan-t5-large": 1024,
- "google/flan-t5-3b": 1024,
- "google/flan-t5-11b": 1024,
- }
-
- def __init__(self, name: str, output_dim: int, finetune: bool, device: str,
- autocast_dtype: tp.Optional[str] = 'float32', word_dropout: float = 0.,
- normalize_text: bool = False):
- assert name in self.MODELS, f"unrecognized t5 model name (should in {self.MODELS})"
- super().__init__(self.MODELS_DIMS[name], output_dim)
- self.device = device
- self.name = name
- self.finetune = finetune
- self.word_dropout = word_dropout
-
- if autocast_dtype is None or self.device == 'cpu':
- self.autocast = TorchAutocast(enabled=False)
- if self.device != 'cpu':
- logger.warning("T5 has no autocast, this might lead to NaN")
- else:
- dtype = getattr(torch, autocast_dtype)
- assert isinstance(dtype, torch.dtype)
- logger.info(f"T5 will be evaluated with autocast as {autocast_dtype}")
- self.autocast = TorchAutocast(enabled=True, device_type=self.device, dtype=dtype)
- # Let's disable logging temporarily because T5 will vomit some errors otherwise.
- # thanks https://gist.github.com/simon-weber/7853144
- previous_level = logging.root.manager.disable
- logging.disable(logging.ERROR)
- with warnings.catch_warnings():
- warnings.simplefilter("ignore")
- try:
- self.t5_tokenizer = T5Tokenizer.from_pretrained(name)
- t5 = T5EncoderModel.from_pretrained(name).train(mode=finetune)
- finally:
- logging.disable(previous_level)
- if finetune:
- self.t5 = t5
- else:
- # this makes sure that the t5 models is not part
- # of the saved checkpoint
- self.__dict__["t5"] = t5.to(device)
-
- self.normalize_text = normalize_text
- if normalize_text:
- self.text_normalizer = WhiteSpaceTokenizer(1, lemma=True, stopwords=True)
-
- def tokenize(self, x: tp.List[tp.Optional[str]]) -> tp.Dict[str, torch.Tensor]:
- # if current sample doesn't have a certain attribute, replace with empty string
- entries: tp.List[str] = [xi if xi is not None else "" for xi in x]
- if self.normalize_text:
- _, _, entries = self.text_normalizer(entries, return_text=True)
- if self.word_dropout > 0. and self.training:
- new_entries = []
- for entry in entries:
- words = [word for word in entry.split(" ") if random.random() >= self.word_dropout]
- new_entries.append(" ".join(words))
- entries = new_entries
-
- empty_idx = torch.LongTensor([i for i, xi in enumerate(entries) if xi == ""])
-
- inputs = self.t5_tokenizer(entries, return_tensors="pt", padding=True).to(self.device)
- mask = inputs["attention_mask"]
- mask[empty_idx, :] = 0 # zero-out index where the input is non-existant
- return inputs
-
- def forward(self, inputs: tp.Dict[str, torch.Tensor]) -> ConditionType:
- mask = inputs["attention_mask"]
- with torch.set_grad_enabled(self.finetune), self.autocast:
- embeds = self.t5(**inputs).last_hidden_state
- embeds = self.output_proj(embeds.to(self.output_proj.weight))
- embeds = (embeds * mask.unsqueeze(-1))
- return embeds, mask
-
-
-class WaveformConditioner(BaseConditioner):
- """Base class for all conditioners that take a waveform as input.
- Classes that inherit must implement `_get_wav_embedding` that outputs
- a continuous tensor, and `_downsampling_factor` that returns the down-sampling
- factor of the embedding model.
-
- Args:
- dim (int): The internal representation dimension.
- output_dim (int): Output dimension.
- device (tp.Union[torch.device, str]): Device.
- """
- def __init__(self, dim: int, output_dim: int, device: tp.Union[torch.device, str]):
- super().__init__(dim, output_dim)
- self.device = device
-
- def tokenize(self, wav_length: WavCondition) -> WavCondition:
- wav, length, path = wav_length
- assert length is not None
- return WavCondition(wav.to(self.device), length.to(self.device), path)
-
- def _get_wav_embedding(self, wav: Tensor) -> Tensor:
- """Gets as input a wav and returns a dense vector of conditions."""
- raise NotImplementedError()
-
- def _downsampling_factor(self):
- """Returns the downsampling factor of the embedding model."""
- raise NotImplementedError()
-
- def forward(self, inputs: WavCondition) -> ConditionType:
- """
- Args:
- input (WavCondition): Tuple of (waveform, lengths).
- Returns:
- ConditionType: Dense vector representing the conditioning along with its' mask.
- """
- wav, lengths, path = inputs
- with torch.no_grad():
- embeds = self._get_wav_embedding(wav)
- embeds = embeds.to(self.output_proj.weight)
- embeds = self.output_proj(embeds)
-
- if lengths is not None:
- lengths = lengths / self._downsampling_factor()
- mask = length_to_mask(lengths, max_len=embeds.shape[1]).int() # type: ignore
- else:
- mask = torch.ones_like(embeds)
- embeds = (embeds * mask.unsqueeze(2).to(self.device))
-
- return embeds, mask
-
-
-class ChromaStemConditioner(WaveformConditioner):
- """Chroma conditioner that uses DEMUCS to first filter out drums and bass. The is followed by
- the insight the drums and bass often dominate the chroma, leading to the chroma not containing the
- information about melody.
-
- Args:
- output_dim (int): Output dimension for the conditioner.
- sample_rate (int): Sample rate for the chroma extractor.
- n_chroma (int): Number of chroma for the chroma extractor.
- radix2_exp (int): Radix2 exponent for the chroma extractor.
- duration (float): Duration used during training. This is later used for correct padding
- in case we are using chroma as prefix.
- match_len_on_eval (bool, optional): If True then all chromas are padded to the training
- duration. Defaults to False.
- eval_wavs (str, optional): Path to a json egg with waveform, this waveforms are used as
- conditions during eval (for cases where we don't want to leak test conditions like MusicCaps).
- Defaults to None.
- n_eval_wavs (int, optional): Limits the number of waveforms used for conditioning. Defaults to 0.
- device (tp.Union[torch.device, str], optional): Device for the conditioner.
- **kwargs: Additional parameters for the chroma extractor.
- """
- def __init__(self, output_dim: int, sample_rate: int, n_chroma: int, radix2_exp: int,
- duration: float, match_len_on_eval: bool = True, eval_wavs: tp.Optional[str] = None,
- n_eval_wavs: int = 0, device: tp.Union[torch.device, str] = "cpu", **kwargs):
- from demucs import pretrained
- super().__init__(dim=n_chroma, output_dim=output_dim, device=device)
- self.autocast = TorchAutocast(enabled=device != "cpu", device_type=self.device, dtype=torch.float32)
- self.sample_rate = sample_rate
- self.match_len_on_eval = match_len_on_eval
- self.duration = duration
- self.__dict__["demucs"] = pretrained.get_model('htdemucs').to(device)
- self.stem2idx = {'drums': 0, 'bass': 1, 'other': 2, 'vocal': 3}
- self.stem_idx = torch.LongTensor([self.stem2idx['vocal'], self.stem2idx['other']]).to(device)
- self.chroma = ChromaExtractor(sample_rate=sample_rate, n_chroma=n_chroma, radix2_exp=radix2_exp,
- device=device, **kwargs)
- self.chroma_len = self._get_chroma_len()
-
- def _downsampling_factor(self):
- return self.chroma.winhop
-
- def _get_chroma_len(self):
- """Get length of chroma during training"""
- dummy_wav = torch.zeros((1, self.sample_rate * self.duration), device=self.device)
- dummy_chr = self.chroma(dummy_wav)
- return dummy_chr.shape[1]
-
- @torch.no_grad()
- def _get_filtered_wav(self, wav):
- from demucs.apply import apply_model
- from demucs.audio import convert_audio
- with self.autocast:
- wav = convert_audio(wav, self.sample_rate, self.demucs.samplerate, self.demucs.audio_channels)
- stems = apply_model(self.demucs, wav, device=self.device)
- stems = stems[:, self.stem_idx] # extract stem
- stems = stems.sum(1) # merge extracted stems
- stems = stems.mean(1, keepdim=True) # mono
- stems = convert_audio(stems, self.demucs.samplerate, self.sample_rate, 1)
- return stems
-
- @torch.no_grad()
- def _get_wav_embedding(self, wav):
- # avoid 0-size tensors when we are working with null conds
- if wav.shape[-1] == 1:
- return self.chroma(wav)
- stems = self._get_filtered_wav(wav)
- chroma = self.chroma(stems)
-
- if self.match_len_on_eval:
- b, t, c = chroma.shape
- if t > self.chroma_len:
- chroma = chroma[:, :self.chroma_len]
- logger.debug(f'chroma was truncated! ({t} -> {chroma.shape[1]})')
- elif t < self.chroma_len:
- # chroma = F.pad(chroma, (0, 0, 0, self.chroma_len - t))
- n_repeat = int(math.ceil(self.chroma_len / t))
- chroma = chroma.repeat(1, n_repeat, 1)
- chroma = chroma[:, :self.chroma_len]
- logger.debug(f'chroma was zero-padded! ({t} -> {chroma.shape[1]})')
- return chroma
-
-
-class ChromaExtractor(nn.Module):
- """Chroma extraction class, handles chroma extraction and quantization.
-
- Args:
- sample_rate (int): Sample rate.
- n_chroma (int): Number of chroma to consider.
- radix2_exp (int): Radix2 exponent.
- nfft (tp.Optional[int], optional): Number of FFT.
- winlen (tp.Optional[int], optional): Window length.
- winhop (tp.Optional[int], optional): Window hop size.
- argmax (bool, optional): Whether to use argmax. Defaults to False.
- norm (float, optional): Norm for chroma normalization. Defaults to inf.
- device (tp.Union[torch.device, str], optional): Device to use. Defaults to cpu.
- """
- def __init__(self, sample_rate: int, n_chroma: int = 12, radix2_exp: int = 12,
- nfft: tp.Optional[int] = None, winlen: tp.Optional[int] = None, winhop: tp.Optional[int] = None,
- argmax: bool = False, norm: float = torch.inf, device: tp.Union[torch.device, str] = "cpu"):
- super().__init__()
- from librosa import filters
- self.device = device
- self.autocast = TorchAutocast(enabled=device != "cpu", device_type=self.device, dtype=torch.float32)
- self.winlen = winlen or 2 ** radix2_exp
- self.nfft = nfft or self.winlen
- self.winhop = winhop or (self.winlen // 4)
- self.sr = sample_rate
- self.n_chroma = n_chroma
- self.norm = norm
- self.argmax = argmax
- self.window = torch.hann_window(self.winlen).to(device)
- self.fbanks = torch.from_numpy(filters.chroma(sr=sample_rate, n_fft=self.nfft, tuning=0,
- n_chroma=self.n_chroma)).to(device)
- self.spec = torchaudio.transforms.Spectrogram(n_fft=self.nfft, win_length=self.winlen,
- hop_length=self.winhop, power=2, center=True,
- pad=0, normalized=True).to(device)
-
- def forward(self, wav):
- with self.autocast:
- T = wav.shape[-1]
- # in case we are getting a wav that was dropped out (nullified)
- # make sure wav length is no less that nfft
- if T < self.nfft:
- pad = self.nfft - T
- r = 0 if pad % 2 == 0 else 1
- wav = F.pad(wav, (pad // 2, pad // 2 + r), 'constant', 0)
- assert wav.shape[-1] == self.nfft, f'expected len {self.nfft} but got {wav.shape[-1]}'
- spec = self.spec(wav).squeeze(1)
- raw_chroma = torch.einsum("cf,...ft->...ct", self.fbanks, spec)
- norm_chroma = torch.nn.functional.normalize(raw_chroma, p=self.norm, dim=-2, eps=1e-6)
- norm_chroma = rearrange(norm_chroma, "b d t -> b t d")
-
- if self.argmax:
- idx = norm_chroma.argmax(-1, keepdims=True)
- norm_chroma[:] = 0
- norm_chroma.scatter_(dim=-1, index=idx, value=1)
-
- return norm_chroma
-
-
-def dropout_condition(sample: ConditioningAttributes, condition_type: str, condition: str):
- """Utility function for nullifying an attribute inside an ConditioningAttributes object.
- If the condition is of type "wav", then nullify it using "nullify_condition".
- If the condition is of any other type, set its' value to None.
- Works in-place.
- """
- if condition_type not in ["text", "wav"]:
- raise ValueError(
- "dropout_condition got an unexpected condition type!"
- f" expected 'wav' or 'text' but got '{condition_type}'"
- )
-
- if condition not in getattr(sample, condition_type):
- raise ValueError(
- "dropout_condition received an unexpected condition!"
- f" expected wav={sample.wav.keys()} and text={sample.text.keys()}"
- f"but got '{condition}' of type '{condition_type}'!"
- )
-
- if condition_type == "wav":
- wav, length, path = sample.wav[condition]
- sample.wav[condition] = nullify_wav(wav)
- else:
- sample.text[condition] = None
-
- return sample
-
-
-class DropoutModule(nn.Module):
- """Base class for all dropout modules."""
- def __init__(self, seed: int = 1234):
- super().__init__()
- self.rng = torch.Generator()
- self.rng.manual_seed(seed)
-
-
-class AttributeDropout(DropoutModule):
- """Applies dropout with a given probability per attribute. This is different from the behavior of
- ClassifierFreeGuidanceDropout as this allows for attributes to be dropped out separately. For example,
- "artist" can be dropped while "genre" remains. This is in contrast to ClassifierFreeGuidanceDropout
- where if "artist" is dropped "genre" must also be dropped.
-
- Args:
- p (tp.Dict[str, float]): A dict mapping between attributes and dropout probability. For example:
- ...
- "genre": 0.1,
- "artist": 0.5,
- "wav": 0.25,
- ...
- active_on_eval (bool, optional): Whether the dropout is active at eval. Default to False.
- seed (int, optional): Random seed.
- """
- def __init__(self, p: tp.Dict[str, tp.Dict[str, float]], active_on_eval: bool = False, seed: int = 1234):
- super().__init__(seed=seed)
- self.active_on_eval = active_on_eval
- # construct dict that return the values from p otherwise 0
- self.p = {}
- for condition_type, probs in p.items():
- self.p[condition_type] = defaultdict(lambda: 0, probs)
-
- def forward(self, samples: tp.List[ConditioningAttributes]) -> tp.List[ConditioningAttributes]:
- """
- Args:
- samples (tp.List[ConditioningAttributes]): List of conditions.
- Returns:
- tp.List[ConditioningAttributes]: List of conditions after certain attributes were set to None.
- """
- if not self.training and not self.active_on_eval:
- return samples
-
- samples = deepcopy(samples)
-
- for condition_type, ps in self.p.items(): # for condition types [text, wav]
- for condition, p in ps.items(): # for attributes of each type (e.g., [artist, genre])
- if torch.rand(1, generator=self.rng).item() < p:
- for sample in samples:
- dropout_condition(sample, condition_type, condition)
-
- return samples
-
- def __repr__(self):
- return f"AttributeDropout({dict(self.p)})"
-
-
-class ClassifierFreeGuidanceDropout(DropoutModule):
- """Applies Classifier Free Guidance dropout, meaning all attributes
- are dropped with the same probability.
-
- Args:
- p (float): Probability to apply condition dropout during training.
- seed (int): Random seed.
- """
- def __init__(self, p: float, seed: int = 1234):
- super().__init__(seed=seed)
- self.p = p
-
- def forward(self, samples: tp.List[ConditioningAttributes]) -> tp.List[ConditioningAttributes]:
- """
- Args:
- samples (tp.List[ConditioningAttributes]): List of conditions.
- Returns:
- tp.List[ConditioningAttributes]: List of conditions after all attributes were set to None.
- """
- if not self.training:
- return samples
-
- # decide on which attributes to drop in a batched fashion
- drop = torch.rand(1, generator=self.rng).item() < self.p
- if not drop:
- return samples
-
- # nullify conditions of all attributes
- samples = deepcopy(samples)
-
- for condition_type in ["wav", "text"]:
- for sample in samples:
- for condition in sample.attributes[condition_type]:
- dropout_condition(sample, condition_type, condition)
-
- return samples
-
- def __repr__(self):
- return f"ClassifierFreeGuidanceDropout(p={self.p})"
-
-
-class ConditioningProvider(nn.Module):
- """Main class to provide conditions given all the supported conditioners.
-
- Args:
- conditioners (dict): Dictionary of conditioners.
- merge_text_conditions_p (float, optional): Probability to merge all text sources
- into a single text condition. Defaults to 0.
- drop_desc_p (float, optional): Probability to drop the original description
- when merging all text sources into a single text condition. Defaults to 0.
- device (tp.Union[torch.device, str], optional): Device for conditioners and output condition types.
- """
- def __init__(
- self,
- conditioners: tp.Dict[str, BaseConditioner],
- merge_text_conditions_p: float = 0,
- drop_desc_p: float = 0,
- device: tp.Union[torch.device, str] = "cpu",
- ):
- super().__init__()
- self.device = device
- self.merge_text_conditions_p = merge_text_conditions_p
- self.drop_desc_p = drop_desc_p
- self.conditioners = nn.ModuleDict(conditioners)
-
- @property
- def text_conditions(self):
- return [k for k, v in self.conditioners.items() if isinstance(v, TextConditioner)]
-
- @property
- def wav_conditions(self):
- return [k for k, v in self.conditioners.items() if isinstance(v, WaveformConditioner)]
-
- @property
- def has_wav_condition(self):
- return len(self.wav_conditions) > 0
-
- def tokenize(self, inputs: tp.List[ConditioningAttributes]) -> tp.Dict[str, tp.Any]:
- """Match attributes/wavs with existing conditioners in self, and compute tokenize them accordingly.
- This should be called before starting any real GPU work to avoid synchronization points.
- This will return a dict matching conditioner names to their arbitrary tokenized representations.
-
- Args:
- inputs (list[ConditioningAttribres]): List of ConditioningAttributes objects containing
- text and wav conditions.
- """
- assert all([type(x) == ConditioningAttributes for x in inputs]), \
- "got unexpected types input for conditioner! should be tp.List[ConditioningAttributes]" \
- f" but types were {set([type(x) for x in inputs])}"
-
- output = {}
- text = self._collate_text(inputs)
- wavs = self._collate_wavs(inputs)
-
- assert set(text.keys() | wavs.keys()).issubset(set(self.conditioners.keys())), \
- f"got an unexpected attribute! Expected {self.conditioners.keys()}, got {text.keys(), wavs.keys()}"
-
- for attribute, batch in chain(text.items(), wavs.items()):
- output[attribute] = self.conditioners[attribute].tokenize(batch)
- return output
-
- def forward(self, tokenized: tp.Dict[str, tp.Any]) -> tp.Dict[str, ConditionType]:
- """Compute pairs of `(embedding, mask)` using the configured conditioners
- and the tokenized representations. The output is for example:
-
- {
- "genre": (torch.Tensor([B, 1, D_genre]), torch.Tensor([B, 1])),
- "description": (torch.Tensor([B, T_desc, D_desc]), torch.Tensor([B, T_desc])),
- ...
- }
-
- Args:
- tokenized (dict): Dict of tokenized representations as returned by `tokenize()`.
- """
- output = {}
- for attribute, inputs in tokenized.items():
- condition, mask = self.conditioners[attribute](inputs)
- output[attribute] = (condition, mask)
- return output
-
- def _collate_text(self, samples: tp.List[ConditioningAttributes]) -> tp.Dict[str, tp.List[tp.Optional[str]]]:
- """Given a list of ConditioningAttributes objects, compile a dictionary where the keys
- are the attributes and the values are the aggregated input per attribute.
- For example:
- Input:
- [
- ConditioningAttributes(text={"genre": "Rock", "description": "A rock song with a guitar solo"}, wav=...),
- ConditioningAttributes(text={"genre": "Hip-hop", "description": "A hip-hop verse"}, wav=...),
- ]
- Output:
- {
- "genre": ["Rock", "Hip-hop"],
- "description": ["A rock song with a guitar solo", "A hip-hop verse"]
- }
- """
- batch_per_attribute: tp.Dict[str, tp.List[tp.Optional[str]]] = defaultdict(list)
-
- def _merge_conds(cond, merge_text_conditions_p=0, drop_desc_p=0):
- def is_valid(k, v):
- k_valid = k in ['key', 'bpm', 'genre', 'moods', 'instrument']
- v_valid = v is not None and isinstance(v, (int, float, str, list))
- return k_valid and v_valid
-
- def process_value(v):
- if isinstance(v, (int, float, str)):
- return v
- if isinstance(v, list):
- return ", ".join(v)
- else:
- RuntimeError(f"unknown type for text value! ({type(v), v})")
-
- desc = cond.text['description']
- meta_data = ""
- if random.uniform(0, 1) < merge_text_conditions_p:
- meta_pairs = [f'{k}: {process_value(v)}' for k, v in cond.text.items() if is_valid(k, v)]
- random.shuffle(meta_pairs)
- meta_data = ". ".join(meta_pairs)
- desc = desc if not random.uniform(0, 1) < drop_desc_p else None
-
- if desc is None:
- desc = meta_data if len(meta_data) > 1 else None
- else:
- desc = desc.rstrip('.') + ". " + meta_data
- cond.text['description'] = desc.strip() if desc else None
-
- if self.training and self.merge_text_conditions_p:
- for sample in samples:
- _merge_conds(sample, self.merge_text_conditions_p, self.drop_desc_p)
-
- texts = [x.text for x in samples]
- for text in texts:
- for condition in self.text_conditions:
- batch_per_attribute[condition].append(text[condition])
-
- return batch_per_attribute
-
- def _collate_wavs(self, samples: tp.List[ConditioningAttributes]):
- """Generate a dict where the keys are attributes by which we fetch similar wavs,
- and the values are Tensors of wavs according to said attribtues.
-
- *Note*: by the time the samples reach this function, each sample should have some waveform
- inside the "wav" attribute. It should be either:
- 1. A real waveform
- 2. A null waveform due to the sample having no similar waveforms (nullified by the dataset)
- 3. A null waveform due to it being dropped in a dropout module (nullified by dropout)
-
- Args:
- samples (tp.List[ConditioningAttributes]): List of ConditioningAttributes samples.
- Returns:
- dict: A dicionary mapping an attribute name to wavs.
- """
- wavs = defaultdict(list)
- lens = defaultdict(list)
- paths = defaultdict(list)
- out = {}
-
- for sample in samples:
- for attribute in self.wav_conditions:
- wav, length, path = sample.wav[attribute]
- wavs[attribute].append(wav.flatten())
- lens[attribute].append(length)
- paths[attribute].append(path)
-
- # stack all wavs to a single tensor
- for attribute in self.wav_conditions:
- stacked_wav, _ = collate(wavs[attribute], dim=0)
- out[attribute] = WavCondition(stacked_wav.unsqueeze(1),
- torch.cat(lens['self_wav']), paths[attribute]) # type: ignore
-
- return out
-
-
-class ConditionFuser(StreamingModule):
- """Condition fuser handles the logic to combine the different conditions
- to the actual model input.
-
- Args:
- fuse2cond (tp.Dict[str, str]): A dictionary that says how to fuse
- each condition. For example:
- {
- "prepend": ["description"],
- "sum": ["genre", "bpm"],
- "cross": ["description"],
- }
- cross_attention_pos_emb (bool, optional): Use positional embeddings in cross attention.
- cross_attention_pos_emb_scale (int): Scale for positional embeddings in cross attention if used.
- """
- FUSING_METHODS = ["sum", "prepend", "cross", "input_interpolate"]
-
- def __init__(self, fuse2cond: tp.Dict[str, tp.List[str]], cross_attention_pos_emb: bool = False,
- cross_attention_pos_emb_scale: float = 1.0):
- super().__init__()
- assert all(
- [k in self.FUSING_METHODS for k in fuse2cond.keys()]
- ), f"got invalid fuse method, allowed methods: {self.FUSING_MEHTODS}"
- self.cross_attention_pos_emb = cross_attention_pos_emb
- self.cross_attention_pos_emb_scale = cross_attention_pos_emb_scale
- self.fuse2cond: tp.Dict[str, tp.List[str]] = fuse2cond
- self.cond2fuse: tp.Dict[str, str] = {}
- for fuse_method, conditions in fuse2cond.items():
- for condition in conditions:
- self.cond2fuse[condition] = fuse_method
-
- def forward(
- self,
- input: Tensor,
- conditions: tp.Dict[str, ConditionType]
- ) -> tp.Tuple[Tensor, tp.Optional[Tensor]]:
- """Fuse the conditions to the provided model input.
-
- Args:
- input (Tensor): Transformer input.
- conditions (tp.Dict[str, ConditionType]): Dict of conditions.
- Returns:
- tp.Tuple[Tensor, Tensor]: The first tensor is the transformer input
- after the conditions have been fused. The second output tensor is the tensor
- used for cross-attention or None if no cross attention inputs exist.
- """
- B, T, _ = input.shape
-
- if 'offsets' in self._streaming_state:
- first_step = False
- offsets = self._streaming_state['offsets']
- else:
- first_step = True
- offsets = torch.zeros(input.shape[0], dtype=torch.long, device=input.device)
-
- assert set(conditions.keys()).issubset(set(self.cond2fuse.keys())), \
- f"given conditions contain unknown attributes for fuser, " \
- f"expected {self.cond2fuse.keys()}, got {conditions.keys()}"
- cross_attention_output = None
- for cond_type, (cond, cond_mask) in conditions.items():
- op = self.cond2fuse[cond_type]
- if op == "sum":
- input += cond
- elif op == "input_interpolate":
- cond = rearrange(cond, "b t d -> b d t")
- cond = F.interpolate(cond, size=input.shape[1])
- input += rearrange(cond, "b d t -> b t d")
- elif op == "prepend":
- if first_step:
- input = torch.cat([cond, input], dim=1)
- elif op == "cross":
- if cross_attention_output is not None:
- cross_attention_output = torch.cat([cross_attention_output, cond], dim=1)
- else:
- cross_attention_output = cond
- else:
- raise ValueError(f"unknown op ({op})")
-
- if self.cross_attention_pos_emb and cross_attention_output is not None:
- positions = torch.arange(
- cross_attention_output.shape[1],
- device=cross_attention_output.device
- ).view(1, -1, 1)
- pos_emb = create_sin_embedding(positions, cross_attention_output.shape[-1])
- cross_attention_output = cross_attention_output + self.cross_attention_pos_emb_scale * pos_emb
-
- if self._is_streaming:
- self._streaming_state['offsets'] = offsets + T
-
- return input, cross_attention_output
diff --git a/spaces/HCMUT-GraduateThesis-HNTThinh/rgbdsod-multimae-demo/s_multimae/configs/data_augmentation_config.py b/spaces/HCMUT-GraduateThesis-HNTThinh/rgbdsod-multimae-demo/s_multimae/configs/data_augmentation_config.py
deleted file mode 100644
index 6c91acd833795e8bf9b6ca4a7aa3d9ba2d27cfdb..0000000000000000000000000000000000000000
--- a/spaces/HCMUT-GraduateThesis-HNTThinh/rgbdsod-multimae-demo/s_multimae/configs/data_augmentation_config.py
+++ /dev/null
@@ -1,19 +0,0 @@
-class RandomGaussianBlurConfig:
- def __init__(
- self, p=0.5, max_gaussian_kernel=19
- ) -> None:
- self.p = p
- self.max_gaussian_kernel = max_gaussian_kernel
-
-
-class DataAugmentationConfig:
- def __init__(self) -> None:
- self.mean_normalization = [0.5, 0.5, 0.5]
- self.std_normalization = [0.5, 0.5, 0.5]
- self.image_gaussian_config = RandomGaussianBlurConfig(
- p=0.5, max_gaussian_kernel=19,
- )
- self.depth_gaussian_config = RandomGaussianBlurConfig(
- p=0.5, max_gaussian_kernel=36,
- )
- self.random_horizontal_flip_prob = 0.5
diff --git a/spaces/HaleyCH/HaleyCH_Theme/README.md b/spaces/HaleyCH/HaleyCH_Theme/README.md
deleted file mode 100644
index 348731379641ece81d7b88a3ad1b4fea51885510..0000000000000000000000000000000000000000
--- a/spaces/HaleyCH/HaleyCH_Theme/README.md
+++ /dev/null
@@ -1,19 +0,0 @@
----
-tags:
-- gradio-theme
-- track-1
-title: HaleyCH_Theme
-colorFrom: blue
-colorTo: blue
-sdk: gradio
-sdk_version: 3.25.0
-app_file: app.py
-pinned: false
-license: apache-2.0
-emoji: 🔥
----
-# HaleyCH_Theme
-## Description
-Add a description of this theme here!
-## Contributions
-Thanks to [@HaleyCH](https://huggingface.co/HaleyCH) for adding this gradio theme!
\ No newline at end of file
diff --git a/spaces/HarryLee/eCommerceImageCaptioning/fairseq/fairseq/models/text_to_speech/fastspeech2.py b/spaces/HarryLee/eCommerceImageCaptioning/fairseq/fairseq/models/text_to_speech/fastspeech2.py
deleted file mode 100644
index 9c38d0917d997ed5e255ec7a5ed8882b405baffa..0000000000000000000000000000000000000000
--- a/spaces/HarryLee/eCommerceImageCaptioning/fairseq/fairseq/models/text_to_speech/fastspeech2.py
+++ /dev/null
@@ -1,352 +0,0 @@
-# Copyright (c) Facebook, Inc. and its affiliates.
-#
-# This source code is licensed under the MIT license found in the
-# LICENSE file in the root directory of this source tree.
-
-import logging
-
-import torch
-from torch import nn
-
-from fairseq.models import (FairseqEncoder, FairseqEncoderModel, register_model,
- register_model_architecture)
-from fairseq.modules import (
- LayerNorm, PositionalEmbedding, FairseqDropout, MultiheadAttention
-)
-from fairseq import utils
-from fairseq.data.data_utils import lengths_to_padding_mask
-
-
-logger = logging.getLogger(__name__)
-
-
-def model_init(m):
- if isinstance(m, nn.Conv1d):
- nn.init.xavier_uniform_(m.weight, torch.nn.init.calculate_gain("relu"))
-
-
-def Embedding(num_embeddings, embedding_dim, padding_idx=None):
- m = nn.Embedding(num_embeddings, embedding_dim, padding_idx=padding_idx)
- nn.init.normal_(m.weight, mean=0, std=embedding_dim ** -0.5)
- return m
-
-
-class PositionwiseFeedForward(nn.Module):
- def __init__(self, in_dim, hidden_dim, kernel_size, dropout):
- super().__init__()
- self.ffn = nn.Sequential(
- nn.Conv1d(in_dim, hidden_dim, kernel_size=kernel_size,
- padding=(kernel_size - 1) // 2),
- nn.ReLU(),
- nn.Conv1d(hidden_dim, in_dim, kernel_size=kernel_size,
- padding=(kernel_size - 1) // 2)
- )
- self.layer_norm = LayerNorm(in_dim)
- self.dropout = self.dropout_module = FairseqDropout(
- p=dropout, module_name=self.__class__.__name__
- )
-
- def forward(self, x):
- # B x T x C
- residual = x
- x = self.ffn(x.transpose(1, 2)).transpose(1, 2)
- x = self.dropout(x)
- return self.layer_norm(x + residual)
-
-
-class FFTLayer(torch.nn.Module):
- def __init__(
- self, embed_dim, n_heads, hidden_dim, kernel_size, dropout,
- attention_dropout
- ):
- super().__init__()
- self.self_attn = MultiheadAttention(
- embed_dim, n_heads, dropout=attention_dropout, self_attention=True
- )
- self.layer_norm = LayerNorm(embed_dim)
- self.ffn = PositionwiseFeedForward(
- embed_dim, hidden_dim, kernel_size, dropout=dropout
- )
-
- def forward(self, x, padding_mask=None):
- # B x T x C
- residual = x
- x = x.transpose(0, 1)
- x, _ = self.self_attn(
- query=x, key=x, value=x, key_padding_mask=padding_mask,
- need_weights=False
- )
- x = x.transpose(0, 1)
- x = self.layer_norm(x + residual)
- return self.ffn(x)
-
-
-class LengthRegulator(nn.Module):
- def forward(self, x, durations):
- # x: B x T x C
- out_lens = durations.sum(dim=1)
- max_len = out_lens.max()
- bsz, seq_len, dim = x.size()
- out = x.new_zeros((bsz, max_len, dim))
-
- for b in range(bsz):
- indices = []
- for t in range(seq_len):
- indices.extend([t] * utils.item(durations[b, t]))
- indices = torch.tensor(indices, dtype=torch.long).to(x.device)
- out_len = utils.item(out_lens[b])
- out[b, :out_len] = x[b].index_select(0, indices)
-
- return out, out_lens
-
-
-class VariancePredictor(nn.Module):
- def __init__(self, args):
- super().__init__()
- self.conv1 = nn.Sequential(
- nn.Conv1d(
- args.encoder_embed_dim, args.var_pred_hidden_dim,
- kernel_size=args.var_pred_kernel_size,
- padding=(args.var_pred_kernel_size - 1) // 2
- ),
- nn.ReLU()
- )
- self.ln1 = nn.LayerNorm(args.var_pred_hidden_dim)
- self.dropout_module = FairseqDropout(
- p=args.var_pred_dropout, module_name=self.__class__.__name__
- )
- self.conv2 = nn.Sequential(
- nn.Conv1d(
- args.var_pred_hidden_dim, args.var_pred_hidden_dim,
- kernel_size=args.var_pred_kernel_size, padding=1
- ),
- nn.ReLU()
- )
- self.ln2 = nn.LayerNorm(args.var_pred_hidden_dim)
- self.proj = nn.Linear(args.var_pred_hidden_dim, 1)
-
- def forward(self, x):
- # Input: B x T x C; Output: B x T
- x = self.conv1(x.transpose(1, 2)).transpose(1, 2)
- x = self.dropout_module(self.ln1(x))
- x = self.conv2(x.transpose(1, 2)).transpose(1, 2)
- x = self.dropout_module(self.ln2(x))
- return self.proj(x).squeeze(dim=2)
-
-
-class VarianceAdaptor(nn.Module):
- def __init__(self, args):
- super().__init__()
- self.args = args
- self.length_regulator = LengthRegulator()
- self.duration_predictor = VariancePredictor(args)
- self.pitch_predictor = VariancePredictor(args)
- self.energy_predictor = VariancePredictor(args)
-
- n_bins, steps = self.args.var_pred_n_bins, self.args.var_pred_n_bins - 1
- self.pitch_bins = torch.linspace(args.pitch_min, args.pitch_max, steps)
- self.embed_pitch = Embedding(n_bins, args.encoder_embed_dim)
- self.energy_bins = torch.linspace(args.energy_min, args.energy_max, steps)
- self.embed_energy = Embedding(n_bins, args.encoder_embed_dim)
-
- def get_pitch_emb(self, x, tgt=None, factor=1.0):
- out = self.pitch_predictor(x)
- bins = self.pitch_bins.to(x.device)
- if tgt is None:
- out = out * factor
- emb = self.embed_pitch(torch.bucketize(out, bins))
- else:
- emb = self.embed_pitch(torch.bucketize(tgt, bins))
- return out, emb
-
- def get_energy_emb(self, x, tgt=None, factor=1.0):
- out = self.energy_predictor(x)
- bins = self.energy_bins.to(x.device)
- if tgt is None:
- out = out * factor
- emb = self.embed_energy(torch.bucketize(out, bins))
- else:
- emb = self.embed_energy(torch.bucketize(tgt, bins))
- return out, emb
-
- def forward(
- self, x, padding_mask, durations=None, pitches=None, energies=None,
- d_factor=1.0, p_factor=1.0, e_factor=1.0
- ):
- # x: B x T x C
- log_dur_out = self.duration_predictor(x)
- dur_out = torch.clamp(
- torch.round((torch.exp(log_dur_out) - 1) * d_factor).long(), min=0
- )
- dur_out.masked_fill_(padding_mask, 0)
-
- pitch_out, pitch_emb = self.get_pitch_emb(x, pitches, p_factor)
- x = x + pitch_emb
- energy_out, energy_emb = self.get_energy_emb(x, energies, e_factor)
- x = x + energy_emb
-
- x, out_lens = self.length_regulator(
- x, dur_out if durations is None else durations
- )
-
- return x, out_lens, log_dur_out, pitch_out, energy_out
-
-
-class FastSpeech2Encoder(FairseqEncoder):
- def __init__(self, args, src_dict, embed_speaker):
- super().__init__(src_dict)
- self.args = args
- self.padding_idx = src_dict.pad()
- self.n_frames_per_step = args.n_frames_per_step
- self.out_dim = args.output_frame_dim * args.n_frames_per_step
-
- self.embed_speaker = embed_speaker
- self.spk_emb_proj = None
- if embed_speaker is not None:
- self.spk_emb_proj = nn.Linear(
- args.encoder_embed_dim + args.speaker_embed_dim,
- args.encoder_embed_dim
- )
-
- self.dropout_module = FairseqDropout(
- p=args.dropout, module_name=self.__class__.__name__
- )
- self.embed_tokens = Embedding(
- len(src_dict), args.encoder_embed_dim, padding_idx=self.padding_idx
- )
-
- self.embed_positions = PositionalEmbedding(
- args.max_source_positions, args.encoder_embed_dim, self.padding_idx
- )
- self.pos_emb_alpha = nn.Parameter(torch.ones(1))
- self.dec_pos_emb_alpha = nn.Parameter(torch.ones(1))
-
- self.encoder_fft_layers = nn.ModuleList(
- FFTLayer(
- args.encoder_embed_dim, args.encoder_attention_heads,
- args.fft_hidden_dim, args.fft_kernel_size,
- dropout=args.dropout, attention_dropout=args.attention_dropout
- )
- for _ in range(args.encoder_layers)
- )
-
- self.var_adaptor = VarianceAdaptor(args)
-
- self.decoder_fft_layers = nn.ModuleList(
- FFTLayer(
- args.decoder_embed_dim, args.decoder_attention_heads,
- args.fft_hidden_dim, args.fft_kernel_size,
- dropout=args.dropout, attention_dropout=args.attention_dropout
- )
- for _ in range(args.decoder_layers)
- )
-
- self.out_proj = nn.Linear(args.decoder_embed_dim, self.out_dim)
-
- self.apply(model_init)
-
- def forward(self, src_tokens, src_lengths=None, speaker=None,
- durations=None, pitches=None, energies=None, **kwargs):
- x = self.embed_tokens(src_tokens)
-
- enc_padding_mask = src_tokens.eq(self.padding_idx)
- x += self.pos_emb_alpha * self.embed_positions(enc_padding_mask)
- x = self.dropout_module(x)
-
- for layer in self.encoder_fft_layers:
- x = layer(x, enc_padding_mask)
-
- if self.embed_speaker is not None:
- bsz, seq_len, _ = x.size()
- emb = self.embed_speaker(speaker).expand(bsz, seq_len, -1)
- x = self.spk_emb_proj(torch.cat([x, emb], dim=2))
-
- x, out_lens, log_dur_out, pitch_out, energy_out = \
- self.var_adaptor(x, enc_padding_mask, durations, pitches, energies)
-
- dec_padding_mask = lengths_to_padding_mask(out_lens)
- x += self.dec_pos_emb_alpha * self.embed_positions(dec_padding_mask)
- for layer in self.decoder_fft_layers:
- x = layer(x, dec_padding_mask)
-
- x = self.out_proj(x)
-
- return x, out_lens, log_dur_out, pitch_out, energy_out
-
-
-@register_model("fastspeech2")
-class FastSpeech2Model(FairseqEncoderModel):
- """
- Implementation for https://arxiv.org/abs/2006.04558
- """
-
- NON_AUTOREGRESSIVE = True
-
- @staticmethod
- def add_args(parser):
- parser.add_argument("--dropout", type=float)
- parser.add_argument("--output-frame-dim", type=int)
- parser.add_argument("--speaker-embed-dim", type=int)
- # FFT blocks
- parser.add_argument("--fft-hidden-dim", type=int)
- parser.add_argument("--fft-kernel-size", type=int)
- parser.add_argument("--attention-dropout", type=float)
- parser.add_argument("--encoder-layers", type=int)
- parser.add_argument("--encoder-embed-dim", type=int)
- parser.add_argument("--encoder-attention-heads", type=int)
- parser.add_argument("--decoder-layers", type=int)
- parser.add_argument("--decoder-embed-dim", type=int)
- parser.add_argument("--decoder-attention-heads", type=int)
- # variance predictor
- parser.add_argument("--var-pred-n-bins", type=int)
- parser.add_argument("--var-pred-hidden-dim", type=int)
- parser.add_argument("--var-pred-kernel-size", type=int)
- parser.add_argument("--var-pred-dropout", type=float)
-
- def __init__(self, encoder, args, src_dict):
- super().__init__(encoder)
- self._num_updates = 0
-
- out_dim = args.output_frame_dim * args.n_frames_per_step
- self.ctc_proj = None
- if getattr(args, "ctc_weight", 0.) > 0.:
- self.ctc_proj = nn.Linear(out_dim, len(src_dict))
-
- @classmethod
- def build_model(cls, args, task):
- embed_speaker = task.get_speaker_embeddings(args)
- encoder = FastSpeech2Encoder(args, task.src_dict, embed_speaker)
- return cls(encoder, args, task.src_dict)
-
- def set_num_updates(self, num_updates):
- super().set_num_updates(num_updates)
- self._num_updates = num_updates
-
- def get_normalized_probs(self, net_output, log_probs, sample=None):
- logits = self.ctc_proj(net_output[0])
- if log_probs:
- return utils.log_softmax(logits.float(), dim=-1)
- else:
- return utils.softmax(logits.float(), dim=-1)
-
-
-@register_model_architecture("fastspeech2", "fastspeech2")
-def base_architecture(args):
- args.dropout = getattr(args, "dropout", 0.2)
- args.output_frame_dim = getattr(args, "output_frame_dim", 80)
- args.speaker_embed_dim = getattr(args, "speaker_embed_dim", 64)
- # FFT blocks
- args.fft_hidden_dim = getattr(args, "fft_hidden_dim", 1024)
- args.fft_kernel_size = getattr(args, "fft_kernel_size", 9)
- args.attention_dropout = getattr(args, "attention_dropout", 0.0)
- args.encoder_layers = getattr(args, "encoder_layers", 4)
- args.encoder_embed_dim = getattr(args, "encoder_embed_dim", 256)
- args.encoder_attention_heads = getattr(args, "encoder_attention_heads", 2)
- args.decoder_layers = getattr(args, "decoder_layers", 4)
- args.decoder_embed_dim = getattr(args, "decoder_embed_dim", 256)
- args.decoder_attention_heads = getattr(args, "decoder_attention_heads", 2)
- # variance predictor
- args.var_pred_n_bins = getattr(args, "var_pred_n_bins", 256)
- args.var_pred_hidden_dim = getattr(args, "var_pred_hidden_dim", 256)
- args.var_pred_kernel_size = getattr(args, "var_pred_kernel_size", 3)
- args.var_pred_dropout = getattr(args, "var_pred_dropout", 0.5)
diff --git a/spaces/Harveenchadha/Vakyansh-Odia-TTS/ttsv/scripts/data/duration.sh b/spaces/Harveenchadha/Vakyansh-Odia-TTS/ttsv/scripts/data/duration.sh
deleted file mode 100644
index 6fc586c05259d3d576fa4437dea5f650fe5f5031..0000000000000000000000000000000000000000
--- a/spaces/Harveenchadha/Vakyansh-Odia-TTS/ttsv/scripts/data/duration.sh
+++ /dev/null
@@ -1,9 +0,0 @@
-wav_path='/home/harveen/en/iitm_data/english/wav_22k'
-#######################
-
-dir=$PWD
-parentdir="$(dirname "$dir")"
-parentdir="$(dirname "$parentdir")"
-
-
-python $parentdir/utils/data/duration.py $wav_path
diff --git a/spaces/HugoDzz/spaceship_drift/src/app.html b/spaces/HugoDzz/spaceship_drift/src/app.html
deleted file mode 100644
index 6ab18398abd0eeef2e8b8c30e3eccfd991b3234a..0000000000000000000000000000000000000000
--- a/spaces/HugoDzz/spaceship_drift/src/app.html
+++ /dev/null
@@ -1,14 +0,0 @@
-
-
-
- Spaceship freeride
-
-
-
-
- %sveltekit.head%
-
-
-
%sveltekit.body%
-
-
diff --git a/spaces/Ibtehaj10/cheating-detection-FYP/person_counter.py b/spaces/Ibtehaj10/cheating-detection-FYP/person_counter.py
deleted file mode 100644
index c70cb7f88f07ae8bc533103bc9c56938cd43995b..0000000000000000000000000000000000000000
--- a/spaces/Ibtehaj10/cheating-detection-FYP/person_counter.py
+++ /dev/null
@@ -1,143 +0,0 @@
-import cv2
-import datetime
-import imutils
-import numpy as np
-from centroidtracker import CentroidTracker
-
-protopath = "MobileNetSSD_deploy.prototxt"
-modelpath = "MobileNetSSD_deploy.caffemodel"
-detector = cv2.dnn.readNetFromCaffe(prototxt=protopath, caffeModel=modelpath)
-detector.setPreferableBackend(cv2.dnn.DNN_BACKEND_INFERENCE_ENGINE)
-detector.setPreferableTarget(cv2.dnn.DNN_TARGET_CPU)
-
-
-CLASSES = ["background", "aeroplane", "bicycle", "bird", "boat",
- "bottle", "bus", "car", "cat", "chair", "cow", "diningtable",
- "dog", "horse", "motorbike", "person", "pottedplant", "sheep",
- "sofa", "train", "tvmonitor"]
-
-tracker = CentroidTracker(maxDisappeared=80, maxDistance=90)
-
-
-def non_max_suppression_fast(boxes, overlapThresh):
- try:
- if len(boxes) == 0:
- return []
-
- if boxes.dtype.kind == "i":
- boxes = boxes.astype("float")
-
- pick = []
-
- x1 = boxes[:, 0]
- y1 = boxes[:, 1]
- x2 = boxes[:, 2]
- y2 = boxes[:, 3]
-
- area = (x2 - x1 + 1) * (y2 - y1 + 1)
- idxs = np.argsort(y2)
-
- while len(idxs) > 0:
- last = len(idxs) - 1
- i = idxs[last]
- pick.append(i)
-
- xx1 = np.maximum(x1[i], x1[idxs[:last]])
- yy1 = np.maximum(y1[i], y1[idxs[:last]])
- xx2 = np.minimum(x2[i], x2[idxs[:last]])
- yy2 = np.minimum(y2[i], y2[idxs[:last]])
-
- w = np.maximum(0, xx2 - xx1 + 1)
- h = np.maximum(0, yy2 - yy1 + 1)
-
- overlap = (w * h) / area[idxs[:last]]
-
- idxs = np.delete(idxs, np.concatenate(([last],
- np.where(overlap > overlapThresh)[0])))
-
- return boxes[pick].astype("int")
- except Exception as e:
- print("Exception occurred in non_max_suppression : {}".format(e))
-
-
-def main():
- cap = cv2.VideoCapture('test_video.mp4')
-
- fps_start_time = datetime.datetime.now()
- fps = 0
- total_frames = 0
- lpc_count = 0
- opc_count = 0
- object_id_list = []
- while True:
- ret, frame = cap.read()
- frame = imutils.resize(frame, width=600)
- total_frames = total_frames + 1
-
- (H, W) = frame.shape[:2]
-
- blob = cv2.dnn.blobFromImage(frame, 0.007843, (W, H), 127.5)
-
- detector.setInput(blob)
- person_detections = detector.forward()
- rects = []
- for i in np.arange(0, person_detections.shape[2]):
- confidence = person_detections[0, 0, i, 2]
- if confidence > 0.5:
- idx = int(person_detections[0, 0, i, 1])
-
- if CLASSES[idx] != "person":
- continue
-
- person_box = person_detections[0, 0, i, 3:7] * np.array([W, H, W, H])
- (startX, startY, endX, endY) = person_box.astype("int")
- rects.append(person_box)
-
- boundingboxes = np.array(rects)
- boundingboxes = boundingboxes.astype(int)
- rects = non_max_suppression_fast(boundingboxes, 0.3)
-
- objects = tracker.update(rects)
- for (objectId, bbox) in objects.items():
- x1, y1, x2, y2 = bbox
- x1 = int(x1)
- y1 = int(y1)
- x2 = int(x2)
- y2 = int(y2)
-
- cv2.rectangle(frame, (x1, y1), (x2, y2), (0, 0, 255), 2)
- text = "ID: {}".format(objectId)
- cv2.putText(frame, text, (x1, y1-5), cv2.FONT_HERSHEY_COMPLEX_SMALL, 1, (0, 0, 255), 1)
-
- if objectId not in object_id_list:
- object_id_list.append(objectId)
-
- fps_end_time = datetime.datetime.now()
- time_diff = fps_end_time - fps_start_time
- if time_diff.seconds == 0:
- fps = 0.0
- else:
- fps = (total_frames / time_diff.seconds)
-
- fps_text = "FPS: {:.2f}".format(fps)
-
- cv2.putText(frame, fps_text, (5, 30), cv2.FONT_HERSHEY_COMPLEX_SMALL, 1, (0, 0, 255), 1)
-
- lpc_count = len(objects)
- opc_count = len(object_id_list)
-
- lpc_txt = "LPC: {}".format(lpc_count)
- opc_txt = "OPC: {}".format(opc_count)
-
- cv2.putText(frame, lpc_txt, (5, 60), cv2.FONT_HERSHEY_COMPLEX_SMALL, 1, (0, 0, 255), 1)
- cv2.putText(frame, opc_txt, (5, 90), cv2.FONT_HERSHEY_COMPLEX_SMALL, 1, (0, 0, 255), 1)
-
- cv2.imshow("Application", frame)
- key = cv2.waitKey(1)
- if key == ord('q'):
- break
-
- cv2.destroyAllWindows()
-
-
-main()
diff --git a/spaces/IoannisTr/Tech_Stocks_Trading_Assistant/functions.py b/spaces/IoannisTr/Tech_Stocks_Trading_Assistant/functions.py
deleted file mode 100644
index 2dd998b725d6c29abf69c797e0a8239edab85c46..0000000000000000000000000000000000000000
--- a/spaces/IoannisTr/Tech_Stocks_Trading_Assistant/functions.py
+++ /dev/null
@@ -1,482 +0,0 @@
-from asyncio.constants import LOG_THRESHOLD_FOR_CONNLOST_WRITES
-import yfinance as yf
-import pandas as pd
-import numpy as np
-import plotly.graph_objs as go
-from stocks import *
-from transformers import AutoModelForSequenceClassification, pipeline, AutoTokenizer
-import os
-from random import random
-os.environ['TF_CPP_MIN_LOG_LEVEL'] = '2'
-import tensorflow as tf
-import math
-import datetime
-import random
-import time
-#import kaleido
-from sklearn.preprocessing import MinMaxScaler
-import matplotlib.pyplot as plt
-#import warnings
-import tensorflow as tf
-from tensorflow import keras
-from keras.layers import Dropout, Activation
-from keras import layers
-from keras.callbacks import EarlyStopping
-from sklearn.metrics import r2_score
-import plotly.graph_objs as go
-import plotly.io as pio
-pio.templates
-
-model = AutoModelForSequenceClassification.from_pretrained("fine_tuned_FinBERT", from_tf=False, config="config.json")
-tokenizer = AutoTokenizer.from_pretrained("fine_tuned_FinBERT/tokenizer/")
-
-class Models(object):
- def __init__(self):
- self.stock_data = Stock_Data()
-
- def bollinger_bands_20d_2std(self, ticker):
- '''
- This method calculates the Bollinger Bands with a Rolling average of the last 20 days and 2 standard deviations. In a plot,
- this would be represented as 3 lines: a rolling average, an upper bound (rolling average + 2 standard deviations) and a lower
- bound (rolling average - 2 standard deviations). When the price of a stock is between the rolling average and lower bound, it is
- considered as oversold, so it makes sense to buy, if it is between the roll. avg. and the upper bound, it is considered as
- overbought, so it makes sense to sell, if it is equal to the roll.avg. it is neutral and if it is outside the bounds, it is
- considered an Unusual Event. The function returns the outlook of the stock (either "Buy", or "Sell" or "Hold" or "Unusual Event")
- '''
- if self.stock_data.status_getter(ticker) != "Open":
- return "Market Closed"
- else:
- data = self.stock_data.stock_data_getter(ticker)
- low_high_closing_df = pd.DataFrame(data)
- low_high_closing_df = data.iloc[:, 4:5] # Getting only the "Adj Close" column
- low_high_closing_df = low_high_closing_df.tail(40) # Getting the last 40 days
-
- low_high_closing_df["rolling_avg_20d"] = low_high_closing_df['Adj Close'].rolling(20, min_periods = 20).mean()
- low_high_closing_df["sd"] = low_high_closing_df["Adj Close"].rolling(20, min_periods = 20).std()
- low_high_closing_df = low_high_closing_df.tail(20) # Keeping the last 20 days only
-
- recent_data = low_high_closing_df.iloc[-1, :].to_list() # Creating a Series object with the most recent data (last row only)
-
- upper_bound = recent_data[1] + 2*recent_data[2] # Upper Bound
- lower_bound = recent_data[1] - 2*recent_data[2] # Lower Bound
- mean_20d = recent_data[1] # Rolling average of last 20 days
-
- if self.stock_data.current_price_getter(ticker) is None:
- return "Market Closed"
- else:
- message = ""
-
- if self.stock_data.current_price_getter(ticker) < mean_20d and self.stock_data.current_price_getter(ticker) >= lower_bound:
- message = "Buy"
- elif self.stock_data.current_price_getter(ticker) > mean_20d and self.stock_data.current_price_getter(ticker) <= upper_bound:
- message = "Sell"
- elif self.stock_data.current_price_getter(ticker) == mean_20d:
- message = "Hold"
- elif self.stock_data.current_price_getter(ticker) <= lower_bound or self.stock_data.current_price_getter(ticker) >= upper_bound:
- message = "Unusual Event"
- return message
-
- def bollinger_bands_10d_1point5std(self, ticker):
- '''
- This method calculates the Bollinger Bands with a Rolling average of the last 10 days and 1.5 standard deviations. In a plot,
- this would be represented as 3 lines: a rolling average, an upper bound (rolling average + 1.5 standard deviations) and a lower
- bound (rolling average - 1.5 standard deviations). When the price of a stock is between the rolling average and lower bound, it is
- considered as oversold, so it makes sense to buy, if it is between the roll. avg. and the upper bound, it is considered as
- overbought, so it makes sense to sell, if it is equal to the roll.avg. it is neutral and if it is outside the bounds, it is
- considered an Unusual Event. The function returns the outlook of the stock (either "Buy", or "Sell" or "Hold" or "Unusual Event")
- '''
- if self.stock_data.status_getter(ticker) != "Open":
- return "Market Closed"
- else:
- data = self.stock_data.stock_data_getter(ticker)
-
- low_high_closing_df = pd.DataFrame(data)
- low_high_closing_df = data.iloc[:, 4:5] # Getting only the "Adj Close" column
- low_high_closing_df = low_high_closing_df.tail(20) # Getting the last 20 days
-
- low_high_closing_df["rolling_avg_10d"] = low_high_closing_df['Adj Close'].rolling(10, min_periods = 10).mean()
- low_high_closing_df["sd"] = low_high_closing_df["Adj Close"].rolling(10, min_periods = 10).std()
- low_high_closing_df = low_high_closing_df.tail(10) # Keeping the last 10 days only
-
- recent_data = low_high_closing_df.iloc[-1, :].to_list() # Creating a Series object with the most recent data (last row only)
-
- upper_bound = recent_data[1] + 1.5*recent_data[2] # Upper Bound
- lower_bound = recent_data[1] - 1.5*recent_data[2] # Lower Bound
- mean_10d = recent_data[1] # Rolling average of last 10 days
-
- if self.stock_data.current_price_getter(ticker) is None:
- return "Market Closed"
- else:
- message = ""
-
- if self.stock_data.current_price_getter(ticker) < mean_10d and self.stock_data.current_price_getter(ticker) >= lower_bound:
- message = "Buy"
- elif self.stock_data.current_price_getter(ticker) > mean_10d and self.stock_data.current_price_getter(ticker) <= upper_bound:
- message = "Sell"
- elif self.stock_data.current_price_getter(ticker) == mean_10d:
- message = "Hold"
- elif self.stock_data.current_price_getter(ticker) <= lower_bound or self.stock_data.current_price_getter(ticker) >= upper_bound:
- message = "Unusual Event"
- return message
-
- def bollinger_bands_50d_3std(self, ticker):
- '''
- This method calculates the Bollinger Bands with a Rolling average of the last 50 days and 3 standard deviations. In a plot,
- this would be represented as 3 lines: a rolling average, an upper bound (rolling average + 3 standard deviations) and a lower
- bound (rolling average - 3 standard deviations). When the price of a stock is between the rolling average and lower bound, it is
- considered as oversold, so it makes sense to buy, if it is between the roll. avg. and the upper bound, it is considered as
- overbought, so it makes sense to sell, if it is equal to the roll.avg. it is neutral and if it is outside the bounds, it is
- considered an Unusual Event. The function returns the outlook of the stock (either "Buy", or "Sell" or "Hold" or "Unusual Event")
- '''
- if self.stock_data.status_getter(ticker) != "Open":
- return "Market Closed"
- else:
- data = self.stock_data.stock_data_getter(ticker)
-
- low_high_closing_df = pd.DataFrame(data)
- low_high_closing_df = data.iloc[:, 4:5] # Getting only the "Adj Close" column
- low_high_closing_df = low_high_closing_df.tail(100) # Getting the last 100 days
-
- low_high_closing_df["rolling_avg_50d"] = low_high_closing_df['Adj Close'].rolling(50, min_periods = 50).mean()
- low_high_closing_df["sd"] = low_high_closing_df["Adj Close"].rolling(50, min_periods = 50).std()
- low_high_closing_df = low_high_closing_df.tail(50) # Keeping the last 50 days only
-
- recent_data = low_high_closing_df.iloc[-1, :].to_list() # Creating a Series object with the most recent data (last row only)
-
- upper_bound = recent_data[1] + 3*recent_data[2] # Upper Bound
- lower_bound = recent_data[1] - 3*recent_data[2] # Lower Bound
- mean_50d = recent_data[1] # Rolling average of last 50 days
-
- # Finding the outlook dependent on the current price
- if self.stock_data.current_price_getter(ticker) is None:
- return "Market Closed"
- else:
- message = ""
- if self.stock_data.current_price_getter(ticker) < mean_50d and self.stock_data.current_price_getter(ticker) >= lower_bound:
- message = "Buy"
- elif self.stock_data.current_price_getter(ticker) > mean_50d and self.stock_data.current_price_getter(ticker) <= upper_bound:
- message = "Sell"
- elif self.stock_data.current_price_getter(ticker) == mean_50d:
- message = "Hold"
- elif self.stock_data.current_price_getter(ticker) <= lower_bound or self.stock_data.current_price_getter(ticker) >= upper_bound:
- message = "Unusual Event"
- return message
-
- def MACD(self, ticker):
- '''
- This method calculates the MACD (Mean Average Convergence Divergence) for a stock. The decision of whether to buy or sell
- a stock when using this method, depends on the difference of two "lines". The 1st one is called "MACD" and is equal to the
- difference between the Exponential Moving Average of the adjusted closing price of the last 12 days, and the Moving Average
- of the adjusted closing price of the last 26 days. The 2nd line is the 9 day moving average of the adj. closing price.
- When MACD > 9 day M.A. it is considered that there is an uptrend, else, an downtrend.
- At last, when MACD line crosses the 9 day M.A. from "above", a "Sell" signal is given,
- while it crosses it from below, a "Buy" signal is given.
- '''
- if self.stock_data.status_getter(ticker) != "Open":
- return "Market Closed"
- else:
- data = self.stock_data.stock_data_getter(ticker)
-
- low_high_closing_df = pd.DataFrame(data)
- low_high_closing_df = data.iloc[:, 4:5] # Getting only the "Adj Close" column
- low_high_closing_df = low_high_closing_df.tail(52) # Getting the last 52 days
-
-
- # Get the 12-day EMA of the closing price
- low_high_closing_df['EMA_12d'] = low_high_closing_df['Adj Close'].ewm(span=12, adjust=False, min_periods=12).mean()
- # Get the 26-day MA of the closing price
- low_high_closing_df['MA_26d'] = low_high_closing_df['Adj Close'].ewm(span=26, adjust=False, min_periods=26).mean()
- # Subtract the 26-day EMA from the 12-Day EMA to get the MACD
- low_high_closing_df['MACD'] = low_high_closing_df['EMA_12d'] - low_high_closing_df['MA_26d']
- # Making the signal line
- low_high_closing_df['MA_9d'] = low_high_closing_df['MACD'].ewm(span=9, adjust=False, min_periods=9).mean()
-
- low_high_closing_df['Diff'] = low_high_closing_df['MACD'] - low_high_closing_df['MA_9d']
-
- Diff = low_high_closing_df['Diff'].astype(float)
-
- if self.stock_data.current_price_getter(ticker) is None:
- return "Market Closed"
- else:
- message = ""
-
- if Diff.iloc[-1] < 0:
- if Diff.iloc[-2] >= 0:
- message = "Downtrend and sell signal"
- else:
- message = "Downtrend and no signal"
- else:
- if Diff.iloc[-2] <= 0:
- message = "Uptrend and buy signal"
- else:
- message = "Uptrend and no signal"
- return message
-
- def finbert_headlines_sentiment(self, ticker):
- '''
- This method uses a the "weights" and the "tokenizer" of a fine-tuned Fin-BERT model, which is a BERT model that
- was furtherly trained on financial data. The "article_parser()" method scraps www.marketwatch.com and returns the
- last 17 headers of the chosen stock's articles. The, the FinBERT model classifies each one of them as either "Positive"
- or "Negative" or "Neutral", and a score is assigned to each header (+100, -100, and 0) correspondingly. At last, a
- rolling average of window size = 5 is used to "smooth" the sentiment line of the "plotly" plot that is returned.
- '''
-
- articles_df = self.stock_data.article_parser(ticker)
- articles_list = articles_df["headline"].tolist()
-
- clf = pipeline("text-classification", model=model, tokenizer=tokenizer)
- outputs_list = clf(articles_list)
-
- sentiments = []
-
- for item in outputs_list:
- sentiments.append(item["label"])
-
- sentiments_df = pd.DataFrame(sentiments)
- sentiments_df.rename(columns = {0:'sentiment'}, inplace = True)
-
- sentiments_df["sentiment"] = sentiments_df["sentiment"].apply(lambda x: 100 if x == "positive" else -100 if x=="negative" else 0)
- sentiments_df["roll_avg"] = round(sentiments_df["sentiment"].rolling(5, min_periods = 1).mean(), 2)
- sentiments_df = sentiments_df.tail(12).reset_index()
-
- pd.options.plotting.backend = "plotly"
-
- fig = sentiments_df["roll_avg"].plot(title="Sentiment Analysis of the last 12 www.marketwatch.com articles about " + ticker,
-
- template="plotly_dark",
- labels=dict(index="12 most recent article headlines", value="sentiment score (rolling avg. of window size 5)"))
- fig.update_traces(line=dict(color="#3D9140", width=3))
- fig.update_layout(yaxis_range=[-100,100])
- fig.update_layout(xaxis_range=[0,12])
- fig.update_layout(showlegend=False)
- fig.add_hline(y=0, line_width=1.5, line_color="black")
-
- current_sentiment = sentiments_df["roll_avg"].tail(1).values[0]
-
- return {'fig': fig, 'current_sentiment': current_sentiment}
-
- def LSTM_7_days_price_predictor(self, ticker):
- '''
- This method predicts the price of a chosen stock for the next 7 days as of today, by using the daily adjusted closing
- prices for the last 2 years. At first, a 60-day window of historical prices (i-60) is created as our feature data (x_train)
- and the following 60-days window as label data (y_train). For every stock available, we have manually defined different
- parameters so that they fit as good as it gets to the model. Finally we combute the R2 metric and make the predictions. At
- last, we proceed with the predictions. The model looks back in our data (60 days back) and predicta for the following 7 days.
- '''
-
- stock_data = self.stock_data.LSTM_stock_data_getter(ticker)
- stock_data=pd.DataFrame(data=stock_data).drop(['Open','High','Low','Close', 'Volume'],axis=1).reset_index()
- stock_data['Date'] = pd.to_datetime(stock_data['Date'])
- stock_data=stock_data.dropna()
-
- # Data Preprocessing
- random.seed(1997)
- close_prices = stock_data['Adj Close']
- values = close_prices.values
- training_data_len = math.ceil(len(values)* 0.8)
-
- scaler = MinMaxScaler(feature_range=(0,1))
- scaled_data = scaler.fit_transform(values.reshape(-1,1))
- train_data = scaled_data[0: training_data_len, :]
-
- x_train = []
- y_train = []
-
- for i in range(60, len(train_data)):
- x_train.append(train_data[i-60:i, 0])
- y_train.append(train_data[i, 0])
-
- x_train, y_train = np.array(x_train), np.array(y_train)
- x_train = np.reshape(x_train, (x_train.shape[0], x_train.shape[1], 1))
-
- # Preparation of test set
- test_data = scaled_data[training_data_len-60: , : ]
- x_test = []
- y_test = values[training_data_len:]
-
- for i in range(60, len(test_data)):
- x_test.append(test_data[i-60:i, 0])
-
- x_test = np.array(x_test)
- x_test = np.reshape(x_test, (x_test.shape[0], x_test.shape[1], 1))
-
- ##### Setting Up LSTM Network Architecture and the Training of the LSTM Model
- def LSTM_trainer(seed, DROPOUT, LSTM_units,patience,batch_size, epochs):
-
- tf.random.set_seed(seed)
- DROPOUT = DROPOUT
- global model_lstm
- model_lstm = keras.Sequential()
- model_lstm.add(layers.LSTM(LSTM_units, return_sequences=True, input_shape=(x_train.shape[1], 1)))
- model_lstm.add(Dropout(rate=DROPOUT))
- model_lstm.add(layers.LSTM(LSTM_units, return_sequences=False))
- model_lstm.add(Dropout(rate=DROPOUT))
- model_lstm.add(layers.Dense(25))
- model_lstm.add(Dropout(rate=DROPOUT))
- model_lstm.add(layers.Dense(1))
- model_lstm.add(Activation('linear'))
-
- print('\n')
- print("Compiling the LSTM Model for the " + str(ticker) + " stock....\n")
- t0 = time.time()
- model_lstm.compile(optimizer='adam', loss='mean_squared_error',metrics=['mae'])
- callback=EarlyStopping(monitor='val_loss',
- min_delta=0,
- patience=patience,
- verbose=1, mode='auto')
- model_lstm.fit(x_train,
- y_train,
- batch_size= batch_size,
- epochs=epochs,
- validation_split=0.1,# ...holding out 10% of the data for validation
- shuffle=True,verbose=0,callbacks=[callback])
- t1 = time.time()
- global ex_time
- ex_time = round(t1-t0, 2)
- print("Compiling took :",ex_time,"seconds")
-
- predictions = model_lstm.predict(x_test)
- predictions = scaler.inverse_transform(predictions)
- #rmse = np.sqrt(np.mean(((predictions - y_test) ** 2)))
- global r_squared_score
- global rmse
- r_squared_score = round(r2_score(y_test, predictions),2)
- rmse = np.sqrt(np.mean(((predictions - y_test) ** 2)))
- #print('Rmse Score: ', round(rmse),2)
- print('R2 Score: ', r_squared_score)
-
- if ticker == 'AAPL':
- LSTM_trainer(1, 0.2, 100,2, 20, 30)
- elif ticker == 'NVDA':
- LSTM_trainer(2, 0.2, 100,2, 30, 50)
- elif ticker == 'PYPL':
- LSTM_trainer(6, 0.2, 100,10,25, 30)
- elif ticker == 'MSFT':
- LSTM_trainer(4, 0.1, 80, 2,20, 40)
- elif ticker == 'TSLA':
- LSTM_trainer(5, 0.1, 120, 4,20, 25)
- elif ticker == 'AMZN':
- LSTM_trainer(6, 0.1, 120,2, 20, 25)
- elif ticker == 'SPOT':
- LSTM_trainer(9, 0.2, 200,5, 20, 40)
- #elif ticker == 'TWTR' :
- # LSTM_trainer(15, 0.2, 100,4,20, 40)
- elif ticker == 'UBER':
- LSTM_trainer(15, 0.2, 100,7,20, 40)
- elif ticker == 'adanipower.ns':
- LSTM_trainer(15, 0.2, 120,8,20, 40)
- elif ticker == 'GOOG':
- LSTM_trainer(15, 0.2, 100,3,20, 25)
-
- # Unseen Predictions for the next 7 days
- close_data = scaled_data
- look_back = 60
-
- def predict(num_prediction, model):
- prediction_list = close_data[-look_back:]
-
- for _ in range(num_prediction):
- x = prediction_list[-look_back:]
- x = x.reshape((1, look_back, 1))
-
- out = model.predict(x)[0][0]
- prediction_list = np.append(prediction_list, out)
- prediction_list = prediction_list[look_back-1:]
-
- return prediction_list
-
- def predict_dates(num_prediction):
- last_date = stock_data['Date'].values[-1]
- prediction_dates = pd.date_range(last_date, periods=num_prediction+1).tolist()
- return prediction_dates
-
- num_prediction = 7
-
- forecast = predict(num_prediction, model_lstm)
- forecast_dates = predict_dates(num_prediction)
-
- plt.figure(figsize=(25,10))
- forecast = forecast.reshape(-1, 1)
- forecast_inverse = scaler.inverse_transform(forecast)
-
- # Ploting the Actual Prices and the Predictions of them for the next 7 days
- base = stock_data['Date'].iloc[[-1]] # Here we create our base date (the last existing date with actual prices)
- testdata = pd.DataFrame(forecast_inverse)# Here we create a data frame that contains the prediction prices and an empty column for their dates
- testdata['Date'] = ""
- testdata.columns = ["Adj Close","Date"]
- testdata = testdata.iloc[1:,:]
- testdata["Label"] = "" # Let's add a column "Label" that would show if the respective price is a prediction or not
- testdata["Label"] = "Prediction"
- testdata = testdata[["Date", "Adj Close", "Label"]]
-
- date_list = [base + datetime.timedelta(days=x+1) for x in range(testdata.shape[0]+1)]
- date_list = pd.DataFrame(date_list)
- date_list.columns = ["Date"]
- date_list.reset_index(inplace = True)
- date_list.drop(["index"], axis = 1, inplace = True)
- date_list.index = date_list.index + 1
- testdata.Date = date_list
-
- stock_data["Label"] = ""
- stock_data["Label"] = "Actual price"
- finaldf = pd.concat([stock_data,testdata], axis=0) # Here we concatenate the "testdata" and the original data frame "df" into a final one
- finaldf.reset_index(inplace = True)
- finaldf.drop(["index"], axis = 1, inplace = True)
- finaldf['Date'] = pd.to_datetime(finaldf['Date'])
-
- plt.rcParams["figure.figsize"] = (25,10)
- #We create two different data frames, one that contains the actual prices and one that has only the predictions
- finaldfPredictions = finaldf.iloc[-8:]
- finaldfActuals = finaldf.iloc[:-7]
-
- plot_1 = go.Scatter(
- x = finaldfActuals['Date'],
- y = finaldfActuals['Adj Close'],
- mode = 'lines',
- name = 'Historical Data (2 years)',
- line=dict(width=1,color='#3D9140'))
- plot_2 = go.Scatter(
- x = finaldfPredictions['Date'],
- y = finaldfPredictions['Adj Close'],
- mode = 'lines',
- name = '7-day Prediction',
- line=dict(width=1,color="#EE3B3B"))
- plot_3 = go.Scatter(
- x = finaldfPredictions['Date'][:1],
- y = finaldfPredictions['Adj Close'][:1],
- mode = 'markers',
- name = 'Latest Actual Closing Price',
- line=dict(width=1))
-
- layout = go.Layout(
- title = 'Next 7 days stock price prediction of ' + str(ticker),
- xaxis = {'title' : "Date"},
- yaxis = {'title' : "Price ($)"}
- )
- fig = go.Figure(data=[plot_1, plot_2,plot_3], layout=layout)
- fig.update_layout(template='plotly_dark',autosize=True)
- fig.update_layout(legend=dict(
- orientation="h",
- yanchor="bottom",
- y=1.02,
- xanchor="right",
- x=1),
- annotations = [dict(x=0.5,
- y=0,
- xref='paper',
- yref='paper',
- text="Current In Sample R- Squared : " + str(r_squared_score*100) + " % \n",
- showarrow = False)],
- xaxis=dict(showgrid=False),
- yaxis=dict(showgrid=False)
-
-
- )
- fig.add_annotation(x=0.5,
- y=0.05,
- xref='paper',
- yref='paper',
- text="Current In Sample Root Mean Square Error : " + str(round(rmse,2)) + " % ",
- showarrow=False)
-
- return fig
diff --git a/spaces/IsaacK/streamlit-test/multipage.py b/spaces/IsaacK/streamlit-test/multipage.py
deleted file mode 100644
index 9339b4c6c7a45e7a896301edee86f492207b577d..0000000000000000000000000000000000000000
--- a/spaces/IsaacK/streamlit-test/multipage.py
+++ /dev/null
@@ -1,41 +0,0 @@
-"""
-This file is the framework for generating multiple Streamlit applications
-through an object oriented framework.
-"""
-
-import streamlit as st
-
-# Define the multipage class to manage the multiple apps in our program
-class MultiPage:
- """Framework for combining multiple streamlit applications."""
-
- def __init__(self) -> None:
- """Constructor class to generate a list which will store all our applications as an instance variable."""
- self.pages = []
-
- def add_page(self, title, func) -> None:
- """Class Method to Add pages to the project
-
- Args:
- title ([str]): The title of page which we are adding to the list of apps
-
- func: Python function to render this page in Streamlit
- """
-
- self.pages.append(
- {
- "title": title,
- "function": func
- }
- )
-
- def run(self):
- # Drodown to select the page to run
- page = st.sidebar.selectbox(
- 'App Navigation',
- self.pages,
- format_func=lambda page: page['title']
- )
-
- # run the app function
- page['function']()
\ No newline at end of file
diff --git a/spaces/LZRi/LZR-Bert-VITS2/mel_processing.py b/spaces/LZRi/LZR-Bert-VITS2/mel_processing.py
deleted file mode 100644
index 50435ecf88ef4fb6c1d47f3e6edd04c3ea7d3e80..0000000000000000000000000000000000000000
--- a/spaces/LZRi/LZR-Bert-VITS2/mel_processing.py
+++ /dev/null
@@ -1,112 +0,0 @@
-import math
-import os
-import random
-import torch
-from torch import nn
-import torch.nn.functional as F
-import torch.utils.data
-import numpy as np
-import librosa
-import librosa.util as librosa_util
-from librosa.util import normalize, pad_center, tiny
-from scipy.signal import get_window
-from scipy.io.wavfile import read
-from librosa.filters import mel as librosa_mel_fn
-
-MAX_WAV_VALUE = 32768.0
-
-
-def dynamic_range_compression_torch(x, C=1, clip_val=1e-5):
- """
- PARAMS
- ------
- C: compression factor
- """
- return torch.log(torch.clamp(x, min=clip_val) * C)
-
-
-def dynamic_range_decompression_torch(x, C=1):
- """
- PARAMS
- ------
- C: compression factor used to compress
- """
- return torch.exp(x) / C
-
-
-def spectral_normalize_torch(magnitudes):
- output = dynamic_range_compression_torch(magnitudes)
- return output
-
-
-def spectral_de_normalize_torch(magnitudes):
- output = dynamic_range_decompression_torch(magnitudes)
- return output
-
-
-mel_basis = {}
-hann_window = {}
-
-
-def spectrogram_torch(y, n_fft, sampling_rate, hop_size, win_size, center=False):
- if torch.min(y) < -1.:
- print('min value is ', torch.min(y))
- if torch.max(y) > 1.:
- print('max value is ', torch.max(y))
-
- global hann_window
- dtype_device = str(y.dtype) + '_' + str(y.device)
- wnsize_dtype_device = str(win_size) + '_' + dtype_device
- if wnsize_dtype_device not in hann_window:
- hann_window[wnsize_dtype_device] = torch.hann_window(win_size).to(dtype=y.dtype, device=y.device)
-
- y = torch.nn.functional.pad(y.unsqueeze(1), (int((n_fft-hop_size)/2), int((n_fft-hop_size)/2)), mode='reflect')
- y = y.squeeze(1)
-
- spec = torch.stft(y, n_fft, hop_length=hop_size, win_length=win_size, window=hann_window[wnsize_dtype_device],
- center=center, pad_mode='reflect', normalized=False, onesided=True, return_complex=False)
-
- spec = torch.sqrt(spec.pow(2).sum(-1) + 1e-6)
- return spec
-
-
-def spec_to_mel_torch(spec, n_fft, num_mels, sampling_rate, fmin, fmax):
- global mel_basis
- dtype_device = str(spec.dtype) + '_' + str(spec.device)
- fmax_dtype_device = str(fmax) + '_' + dtype_device
- if fmax_dtype_device not in mel_basis:
- mel = librosa_mel_fn(sampling_rate, n_fft, num_mels, fmin, fmax)
- mel_basis[fmax_dtype_device] = torch.from_numpy(mel).to(dtype=spec.dtype, device=spec.device)
- spec = torch.matmul(mel_basis[fmax_dtype_device], spec)
- spec = spectral_normalize_torch(spec)
- return spec
-
-
-def mel_spectrogram_torch(y, n_fft, num_mels, sampling_rate, hop_size, win_size, fmin, fmax, center=False):
- if torch.min(y) < -1.:
- print('min value is ', torch.min(y))
- if torch.max(y) > 1.:
- print('max value is ', torch.max(y))
-
- global mel_basis, hann_window
- dtype_device = str(y.dtype) + '_' + str(y.device)
- fmax_dtype_device = str(fmax) + '_' + dtype_device
- wnsize_dtype_device = str(win_size) + '_' + dtype_device
- if fmax_dtype_device not in mel_basis:
- mel = librosa_mel_fn(sampling_rate, n_fft, num_mels, fmin, fmax)
- mel_basis[fmax_dtype_device] = torch.from_numpy(mel).to(dtype=y.dtype, device=y.device)
- if wnsize_dtype_device not in hann_window:
- hann_window[wnsize_dtype_device] = torch.hann_window(win_size).to(dtype=y.dtype, device=y.device)
-
- y = torch.nn.functional.pad(y.unsqueeze(1), (int((n_fft-hop_size)/2), int((n_fft-hop_size)/2)), mode='reflect')
- y = y.squeeze(1)
-
- spec = torch.stft(y, n_fft, hop_length=hop_size, win_length=win_size, window=hann_window[wnsize_dtype_device],
- center=center, pad_mode='reflect', normalized=False, onesided=True, return_complex=False)
-
- spec = torch.sqrt(spec.pow(2).sum(-1) + 1e-6)
-
- spec = torch.matmul(mel_basis[fmax_dtype_device], spec)
- spec = spectral_normalize_torch(spec)
-
- return spec
diff --git a/spaces/LanguageBind/LanguageBind/v_cls/datasets.py b/spaces/LanguageBind/LanguageBind/v_cls/datasets.py
deleted file mode 100644
index 847a51cdd2f1a6aabd7b2d20e2ed6e312bb1b0ec..0000000000000000000000000000000000000000
--- a/spaces/LanguageBind/LanguageBind/v_cls/datasets.py
+++ /dev/null
@@ -1,715 +0,0 @@
-# pylint: disable=line-too-long,too-many-lines,missing-docstring
-import os
-import warnings
-
-import numpy as np
-import pandas as pd
-import torch
-from torch.utils.data import Dataset
-from torchvision import transforms
-
-from . import video_transforms, volume_transforms
-from .loader import get_image_loader, get_video_loader
-from .random_erasing import RandomErasing
-
-
-class VideoClsDataset(Dataset):
- """Load your own video classification dataset."""
-
- def __init__(self,
- anno_path,
- data_root='',
- mode='train',
- clip_len=8,
- frame_sample_rate=2,
- crop_size=224,
- short_side_size=256,
- new_height=256,
- new_width=340,
- keep_aspect_ratio=True,
- num_segment=1,
- num_crop=1,
- test_num_segment=10,
- test_num_crop=3,
- sparse_sample=False,
- args=None):
- self.anno_path = anno_path
- self.data_root = data_root
- self.mode = mode
- self.clip_len = clip_len
- self.frame_sample_rate = frame_sample_rate
- self.crop_size = crop_size
- self.short_side_size = short_side_size
- self.new_height = new_height
- self.new_width = new_width
- self.keep_aspect_ratio = keep_aspect_ratio
- self.num_segment = num_segment
- self.test_num_segment = test_num_segment
- self.num_crop = num_crop
- self.test_num_crop = test_num_crop
- self.sparse_sample = sparse_sample
- self.args = args
- self.aug = False
- self.rand_erase = False
-
- if self.mode in ['train']:
- self.aug = True
- if self.args.reprob > 0:
- self.rand_erase = True
-
- self.video_loader = get_video_loader()
-
- cleaned = pd.read_csv(self.anno_path, header=None, delimiter=' ')
- self.dataset_samples = list(
- cleaned[0].apply(lambda row: os.path.join(self.data_root, row)))
- self.label_array = list(cleaned.values[:, 1])
-
- if (mode == 'train'):
- pass
-
- elif (mode == 'validation'):
- self.data_transform = video_transforms.Compose([
- video_transforms.Resize(
- self.short_side_size, interpolation='bilinear'),
- video_transforms.CenterCrop(
- size=(self.crop_size, self.crop_size)),
- volume_transforms.ClipToTensor(),
- video_transforms.Normalize(
- mean=[0.485, 0.456, 0.406], std=[0.229, 0.224, 0.225])
- ])
- elif mode == 'test':
- self.data_resize = video_transforms.Compose([
- video_transforms.Resize(
- size=(short_side_size), interpolation='bilinear')
- ])
- self.data_transform = video_transforms.Compose([
- volume_transforms.ClipToTensor(),
- video_transforms.Normalize(
- mean=[0.485, 0.456, 0.406], std=[0.229, 0.224, 0.225])
- ])
- self.test_seg = []
- self.test_dataset = []
- self.test_label_array = []
- for ck in range(self.test_num_segment):
- for cp in range(self.test_num_crop):
- for idx in range(len(self.label_array)):
- sample_label = self.label_array[idx]
- self.test_label_array.append(sample_label)
- self.test_dataset.append(self.dataset_samples[idx])
- self.test_seg.append((ck, cp))
-
- def __getitem__(self, index):
- if self.mode == 'train':
- args = self.args
- scale_t = 1
-
- sample = self.dataset_samples[index]
- # T H W C
- buffer = self.load_video(sample, sample_rate_scale=scale_t)
- if len(buffer) == 0:
- while len(buffer) == 0:
- warnings.warn(
- "video {} not correctly loaded during training".format(
- sample))
- index = np.random.randint(self.__len__())
- sample = self.dataset_samples[index]
- buffer = self.load_video(sample, sample_rate_scale=scale_t)
-
- if args.num_sample > 1:
- frame_list = []
- label_list = []
- index_list = []
- for _ in range(args.num_sample):
- new_frames = self._aug_frame(buffer, args)
- label = self.label_array[index]
- frame_list.append(new_frames)
- label_list.append(label)
- index_list.append(index)
- return frame_list, label_list, index_list, {}
- else:
- buffer = self._aug_frame(buffer, args)
-
- return buffer, self.label_array[index], index, {}
-
- elif self.mode == 'validation':
- sample = self.dataset_samples[index]
- buffer = self.load_video(sample)
- if len(buffer) == 0:
- while len(buffer) == 0:
- warnings.warn(
- "video {} not correctly loaded during validation".
- format(sample))
- index = np.random.randint(self.__len__())
- sample = self.dataset_samples[index]
- buffer = self.load_video(sample)
- buffer = self.data_transform(buffer)
- return buffer, self.label_array[index], sample.split(
- "/")[-1].split(".")[0]
-
- elif self.mode == 'test':
- sample = self.test_dataset[index]
- chunk_nb, split_nb = self.test_seg[index]
- buffer = self.load_video(sample)
-
- while len(buffer) == 0:
- warnings.warn(
- "video {}, temporal {}, spatial {} not found during testing"
- .format(str(self.test_dataset[index]), chunk_nb, split_nb))
- index = np.random.randint(self.__len__())
- sample = self.test_dataset[index]
- chunk_nb, split_nb = self.test_seg[index]
- buffer = self.load_video(sample)
-
- buffer = self.data_resize(buffer)
- if isinstance(buffer, list):
- buffer = np.stack(buffer, 0)
-
- if self.sparse_sample:
- spatial_step = 1.0 * (max(buffer.shape[1], buffer.shape[2]) -
- self.short_side_size) / (
- self.test_num_crop - 1)
- temporal_start = chunk_nb
- spatial_start = int(split_nb * spatial_step)
- if buffer.shape[1] >= buffer.shape[2]:
- buffer = buffer[temporal_start::self.test_num_segment,
- spatial_start:spatial_start +
- self.short_side_size, :, :]
- else:
- buffer = buffer[temporal_start::self.test_num_segment, :,
- spatial_start:spatial_start +
- self.short_side_size, :]
- else:
- spatial_step = 1.0 * (max(buffer.shape[1], buffer.shape[2]) -
- self.short_side_size) / (
- self.test_num_crop - 1)
- temporal_step = max(
- 1.0 * (buffer.shape[0] - self.clip_len) /
- (self.test_num_segment - 1), 0)
- temporal_start = int(chunk_nb * temporal_step)
- spatial_start = int(split_nb * spatial_step)
- if buffer.shape[1] >= buffer.shape[2]:
- buffer = buffer[temporal_start:temporal_start +
- self.clip_len,
- spatial_start:spatial_start +
- self.short_side_size, :, :]
- else:
- buffer = buffer[temporal_start:temporal_start +
- self.clip_len, :,
- spatial_start:spatial_start +
- self.short_side_size, :]
-
- buffer = self.data_transform(buffer)
- return buffer, self.test_label_array[index], sample.split(
- "/")[-1].split(".")[0], chunk_nb, split_nb
- else:
- raise NameError('mode {} unkown'.format(self.mode))
-
- def _aug_frame(self, buffer, args):
- aug_transform = video_transforms.create_random_augment(
- input_size=(self.crop_size, self.crop_size),
- auto_augment=args.aa,
- interpolation=args.train_interpolation,
- )
-
- buffer = [transforms.ToPILImage()(frame) for frame in buffer]
-
- buffer = aug_transform(buffer)
-
- buffer = [transforms.ToTensor()(img) for img in buffer]
- buffer = torch.stack(buffer) # T C H W
- buffer = buffer.permute(0, 2, 3, 1) # T H W C
-
- # T H W C
- buffer = tensor_normalize(buffer, [0.485, 0.456, 0.406],
- [0.229, 0.224, 0.225])
- # T H W C -> C T H W.
- buffer = buffer.permute(3, 0, 1, 2)
- # Perform data augmentation.
- scl, asp = (
- [0.08, 1.0],
- [0.75, 1.3333],
- )
-
- buffer = spatial_sampling(
- buffer,
- spatial_idx=-1,
- min_scale=256,
- max_scale=320,
- # crop_size=224,
- crop_size=args.input_size,
- random_horizontal_flip=False if args.data_set == 'SSV2' else True,
- inverse_uniform_sampling=False,
- aspect_ratio=asp,
- scale=scl,
- motion_shift=False)
-
- if self.rand_erase:
- erase_transform = RandomErasing(
- args.reprob,
- mode=args.remode,
- max_count=args.recount,
- num_splits=args.recount,
- device="cpu",
- )
- buffer = buffer.permute(1, 0, 2, 3) # C T H W -> T C H W
- buffer = erase_transform(buffer)
- buffer = buffer.permute(1, 0, 2, 3) # T C H W -> C T H W
-
- return buffer
-
- def load_video(self, sample, sample_rate_scale=1):
- fname = sample
-
- try:
- vr = self.video_loader(fname)
- except Exception as e:
- print(f"Failed to load video from {fname} with error {e}!")
- return []
-
- length = len(vr)
-
- if self.mode == 'test':
- if self.sparse_sample:
- tick = length / float(self.num_segment)
- all_index = []
- for t_seg in range(self.test_num_segment):
- tmp_index = [
- int(t_seg * tick / self.test_num_segment + tick * x)
- for x in range(self.num_segment)
- ]
- all_index.extend(tmp_index)
- all_index = list(np.sort(np.array(all_index)))
- else:
- all_index = [
- x for x in range(0, length, self.frame_sample_rate)
- ]
- while len(all_index) < self.clip_len:
- all_index.append(all_index[-1])
-
- vr.seek(0)
- buffer = vr.get_batch(all_index).asnumpy()
- return buffer
-
- # handle temporal segments
- converted_len = int(self.clip_len * self.frame_sample_rate)
- seg_len = length // self.num_segment
-
- all_index = []
- for i in range(self.num_segment):
- if seg_len <= converted_len:
- index = np.linspace(
- 0, seg_len, num=seg_len // self.frame_sample_rate)
- index = np.concatenate(
- (index,
- np.ones(self.clip_len - seg_len // self.frame_sample_rate)
- * seg_len))
- index = np.clip(index, 0, seg_len - 1).astype(np.int64)
- else:
- if self.mode == 'validation':
- end_idx = (converted_len + seg_len) // 2
- else:
- end_idx = np.random.randint(converted_len, seg_len)
- str_idx = end_idx - converted_len
- index = np.linspace(str_idx, end_idx, num=self.clip_len)
- index = np.clip(index, str_idx, end_idx - 1).astype(np.int64)
- index = index + i * seg_len
- all_index.extend(list(index))
-
- all_index = all_index[::int(sample_rate_scale)]
- vr.seek(0)
- buffer = vr.get_batch(all_index).asnumpy()
- return buffer
-
- def __len__(self):
- # return 200
- if self.mode != 'test':
- return len(self.dataset_samples)
- else:
- return len(self.test_dataset)
-
-
-class RawFrameClsDataset(Dataset):
- """Load your own raw frame classification dataset."""
-
- def __init__(self,
- anno_path,
- data_root,
- mode='train',
- clip_len=8,
- crop_size=224,
- short_side_size=256,
- new_height=256,
- new_width=340,
- keep_aspect_ratio=True,
- num_segment=1,
- num_crop=1,
- test_num_segment=10,
- test_num_crop=3,
- filename_tmpl='img_{:05}.jpg',
- start_idx=1,
- args=None):
- self.anno_path = anno_path
- self.data_root = data_root
- self.mode = mode
- self.clip_len = clip_len
- self.crop_size = crop_size
- self.short_side_size = short_side_size
- self.new_height = new_height
- self.new_width = new_width
- self.keep_aspect_ratio = keep_aspect_ratio
- self.num_segment = num_segment
- self.test_num_segment = test_num_segment
- self.num_crop = num_crop
- self.test_num_crop = test_num_crop
- self.filename_tmpl = filename_tmpl
- self.start_idx = start_idx
- self.args = args
- self.aug = False
- self.rand_erase = False
-
- if self.mode in ['train']:
- self.aug = True
- if self.args.reprob > 0:
- self.rand_erase = True
-
- self.image_loader = get_image_loader()
-
- cleaned = pd.read_csv(self.anno_path, header=None, delimiter=' ')
- self.dataset_samples = list(
- cleaned[0].apply(lambda row: os.path.join(self.data_root, row)))
- self.total_frames = list(cleaned.values[:, 1])
- self.label_array = list(cleaned.values[:, -1])
-
- if (mode == 'train'):
- pass
-
- elif (mode == 'validation'):
- self.data_transform = video_transforms.Compose([
- video_transforms.Resize(
- self.short_side_size, interpolation='bilinear'),
- video_transforms.CenterCrop(
- size=(self.crop_size, self.crop_size)),
- volume_transforms.ClipToTensor(),
- video_transforms.Normalize(
- mean=[0.485, 0.456, 0.406], std=[0.229, 0.224, 0.225])
- ])
- elif mode == 'test':
- self.data_resize = video_transforms.Compose([
- video_transforms.Resize(
- size=(short_side_size), interpolation='bilinear')
- ])
- self.data_transform = video_transforms.Compose([
- volume_transforms.ClipToTensor(),
- video_transforms.Normalize(
- mean=[0.485, 0.456, 0.406], std=[0.229, 0.224, 0.225])
- ])
- self.test_seg = []
- self.test_dataset = []
- self.test_total_frames = []
- self.test_label_array = []
- for ck in range(self.test_num_segment):
- for cp in range(self.test_num_crop):
- for idx in range(len(self.label_array)):
- self.test_seg.append((ck, cp))
- self.test_dataset.append(self.dataset_samples[idx])
- self.test_total_frames.append(self.total_frames[idx])
- self.test_label_array.append(self.label_array[idx])
-
- def __getitem__(self, index):
- if self.mode == 'train':
- args = self.args
- scale_t = 1
-
- sample = self.dataset_samples[index]
- total_frame = self.total_frames[index]
- buffer = self.load_frame(
- sample, total_frame, sample_rate_scale=scale_t) # T H W C
- if len(buffer) == 0:
- while len(buffer) == 0:
- warnings.warn(
- "video {} not correctly loaded during training".format(
- sample))
- index = np.random.randint(self.__len__())
- sample = self.dataset_samples[index]
- total_frame = self.total_frames[index]
- buffer = self.load_frame(
- sample, total_frame, sample_rate_scale=scale_t)
-
- if args.num_sample > 1:
- frame_list = []
- label_list = []
- index_list = []
- for _ in range(args.num_sample):
- new_frames = self._aug_frame(buffer, args)
- label = self.label_array[index]
- frame_list.append(new_frames)
- label_list.append(label)
- index_list.append(index)
- return frame_list, label_list, index_list, {}
- else:
- buffer = self._aug_frame(buffer, args)
-
- return buffer, self.label_array[index], index, {}
-
- elif self.mode == 'validation':
- sample = self.dataset_samples[index]
- total_frame = self.total_frames[index]
- buffer = self.load_frame(sample, total_frame)
- if len(buffer) == 0:
- while len(buffer) == 0:
- warnings.warn(
- "video {} not correctly loaded during validation".
- format(sample))
- index = np.random.randint(self.__len__())
- sample = self.dataset_samples[index]
- buffer = self.load_frame(sample, total_frame)
- buffer = self.data_transform(buffer)
- return buffer, self.label_array[index], sample.split(
- "/")[-1].split(".")[0]
-
- elif self.mode == 'test':
- sample = self.test_dataset[index]
- total_frame = self.test_total_frames[index]
- chunk_nb, split_nb = self.test_seg[index]
- buffer = self.load_frame(sample, total_frame)
-
- while len(buffer) == 0:
- warnings.warn(
- "video {}, temporal {}, spatial {} not found during testing"
- .format(str(self.test_dataset[index]), chunk_nb, split_nb))
- index = np.random.randint(self.__len__())
- sample = self.test_dataset[index]
- total_frame = self.test_total_frames[index]
- chunk_nb, split_nb = self.test_seg[index]
- buffer = self.load_frame(sample, total_frame)
-
- buffer = self.data_resize(buffer)
- if isinstance(buffer, list):
- buffer = np.stack(buffer, 0)
-
- spatial_step = 1.0 * (max(buffer.shape[1], buffer.shape[2]) -
- self.short_side_size) / (
- self.test_num_crop - 1)
- temporal_start = chunk_nb
- spatial_start = int(split_nb * spatial_step)
- if buffer.shape[1] >= buffer.shape[2]:
- buffer = buffer[temporal_start::self.test_num_segment,
- spatial_start:spatial_start +
- self.short_side_size, :, :]
- else:
- buffer = buffer[temporal_start::self.test_num_segment, :,
- spatial_start:spatial_start +
- self.short_side_size, :]
-
- buffer = self.data_transform(buffer)
- return buffer, self.test_label_array[index], sample.split(
- "/")[-1].split(".")[0], chunk_nb, split_nb
- else:
- raise NameError('mode {} unkown'.format(self.mode))
-
- def _aug_frame(self, buffer, args):
- aug_transform = video_transforms.create_random_augment(
- input_size=(self.crop_size, self.crop_size),
- auto_augment=args.aa,
- interpolation=args.train_interpolation,
- )
-
- buffer = [transforms.ToPILImage()(frame) for frame in buffer]
-
- buffer = aug_transform(buffer)
-
- buffer = [transforms.ToTensor()(img) for img in buffer]
- buffer = torch.stack(buffer) # T C H W
- buffer = buffer.permute(0, 2, 3, 1) # T H W C
-
- # T H W C
- buffer = tensor_normalize(buffer, [0.485, 0.456, 0.406],
- [0.229, 0.224, 0.225])
- # T H W C -> C T H W.
- buffer = buffer.permute(3, 0, 1, 2)
- # Perform data augmentation.
- scl, asp = (
- [0.08, 1.0],
- [0.75, 1.3333],
- )
-
- buffer = spatial_sampling(
- buffer,
- spatial_idx=-1,
- min_scale=256,
- max_scale=320,
- crop_size=self.crop_size,
- random_horizontal_flip=False if args.data_set == 'SSV2' else True,
- inverse_uniform_sampling=False,
- aspect_ratio=asp,
- scale=scl,
- motion_shift=False)
-
- if self.rand_erase:
- erase_transform = RandomErasing(
- args.reprob,
- mode=args.remode,
- max_count=args.recount,
- num_splits=args.recount,
- device="cpu",
- )
- buffer = buffer.permute(1, 0, 2, 3)
- buffer = erase_transform(buffer)
- buffer = buffer.permute(1, 0, 2, 3)
-
- return buffer
-
- def load_frame(self, sample, num_frames, sample_rate_scale=1):
- """Load video content using Decord"""
- fname = sample
-
- if self.mode == 'test':
- tick = num_frames / float(self.num_segment)
- all_index = []
- for t_seg in range(self.test_num_segment):
- tmp_index = [
- int(t_seg * tick / self.test_num_segment + tick * x)
- for x in range(self.num_segment)
- ]
- all_index.extend(tmp_index)
- all_index = list(np.sort(np.array(all_index) + self.start_idx))
- imgs = []
- for idx in all_index:
- frame_fname = os.path.join(fname,
- self.filename_tmpl.format(idx))
- img = self.image_loader(frame_fname)
- imgs.append(img)
- buffer = np.array(imgs)
- return buffer
-
- # handle temporal segments
- average_duration = num_frames // self.num_segment
- all_index = []
- if average_duration > 0:
- if self.mode == 'validation':
- all_index = list(
- np.multiply(
- list(range(self.num_segment)), average_duration) +
- np.ones(self.num_segment, dtype=int) *
- (average_duration // 2))
- else:
- all_index = list(
- np.multiply(
- list(range(self.num_segment)), average_duration) +
- np.random.randint(average_duration, size=self.num_segment))
- elif num_frames > self.num_segment:
- if self.mode == 'validation':
- all_index = list(range(self.num_segment))
- else:
- all_index = list(
- np.sort(
- np.random.randint(num_frames, size=self.num_segment)))
- else:
- all_index = [0] * (self.num_segment - num_frames) + list(
- range(num_frames))
- all_index = list(np.array(all_index) + self.start_idx)
- imgs = []
- for idx in all_index:
- frame_fname = os.path.join(fname, self.filename_tmpl.format(idx))
- img = self.image_loader(frame_fname)
- imgs.append(img)
- buffer = np.array(imgs)
- return buffer
-
- def __len__(self):
- if self.mode != 'test':
- return len(self.dataset_samples)
- else:
- return len(self.test_dataset)
-
-
-def spatial_sampling(
- frames,
- spatial_idx=-1,
- min_scale=256,
- max_scale=320,
- crop_size=224,
- random_horizontal_flip=True,
- inverse_uniform_sampling=False,
- aspect_ratio=None,
- scale=None,
- motion_shift=False,
-):
- """
- Perform spatial sampling on the given video frames. If spatial_idx is
- -1, perform random scale, random crop, and random flip on the given
- frames. If spatial_idx is 0, 1, or 2, perform spatial uniform sampling
- with the given spatial_idx.
- Args:
- frames (tensor): frames of images sampled from the video. The
- dimension is `num frames` x `height` x `width` x `channel`.
- spatial_idx (int): if -1, perform random spatial sampling. If 0, 1,
- or 2, perform left, center, right crop if width is larger than
- height, and perform top, center, buttom crop if height is larger
- than width.
- min_scale (int): the minimal size of scaling.
- max_scale (int): the maximal size of scaling.
- crop_size (int): the size of height and width used to crop the
- frames.
- inverse_uniform_sampling (bool): if True, sample uniformly in
- [1 / max_scale, 1 / min_scale] and take a reciprocal to get the
- scale. If False, take a uniform sample from [min_scale,
- max_scale].
- aspect_ratio (list): Aspect ratio range for resizing.
- scale (list): Scale range for resizing.
- motion_shift (bool): Whether to apply motion shift for resizing.
- Returns:
- frames (tensor): spatially sampled frames.
- """
- assert spatial_idx in [-1, 0, 1, 2]
- if spatial_idx == -1:
- if aspect_ratio is None and scale is None:
- frames, _ = video_transforms.random_short_side_scale_jitter(
- images=frames,
- min_size=min_scale,
- max_size=max_scale,
- inverse_uniform_sampling=inverse_uniform_sampling,
- )
- frames, _ = video_transforms.random_crop(frames, crop_size)
- else:
- transform_func = (
- video_transforms.random_resized_crop_with_shift
- if motion_shift else video_transforms.random_resized_crop)
- frames = transform_func(
- images=frames,
- target_height=crop_size,
- target_width=crop_size,
- scale=scale,
- ratio=aspect_ratio,
- )
- if random_horizontal_flip:
- frames, _ = video_transforms.horizontal_flip(0.5, frames)
- else:
- # The testing is deterministic and no jitter should be performed.
- # min_scale, max_scale, and crop_size are expect to be the same.
- assert len({min_scale, max_scale, crop_size}) == 1
- frames, _ = video_transforms.random_short_side_scale_jitter(
- frames, min_scale, max_scale)
- frames, _ = video_transforms.uniform_crop(frames, crop_size,
- spatial_idx)
- return frames
-
-
-def tensor_normalize(tensor, mean, std):
- """
- Normalize a given tensor by subtracting the mean and dividing the std.
- Args:
- tensor (tensor): tensor to normalize.
- mean (tensor or list): mean value to subtract.
- std (tensor or list): std to divide.
- """
- if tensor.dtype == torch.uint8:
- tensor = tensor.float()
- tensor = tensor / 255.0
- if type(mean) == list:
- mean = torch.tensor(mean)
- if type(std) == list:
- std = torch.tensor(std)
- tensor = tensor - mean
- tensor = tensor / std
- return tensor
diff --git a/spaces/LaynzKunz/Model-RCV/lib/infer_pack/modules.py b/spaces/LaynzKunz/Model-RCV/lib/infer_pack/modules.py
deleted file mode 100644
index c83289df7c79a4810dacd15c050148544ba0b6a9..0000000000000000000000000000000000000000
--- a/spaces/LaynzKunz/Model-RCV/lib/infer_pack/modules.py
+++ /dev/null
@@ -1,522 +0,0 @@
-import copy
-import math
-import numpy as np
-import scipy
-import torch
-from torch import nn
-from torch.nn import functional as F
-
-from torch.nn import Conv1d, ConvTranspose1d, AvgPool1d, Conv2d
-from torch.nn.utils import weight_norm, remove_weight_norm
-
-from lib.infer_pack import commons
-from lib.infer_pack.commons import init_weights, get_padding
-from lib.infer_pack.transforms import piecewise_rational_quadratic_transform
-
-
-LRELU_SLOPE = 0.1
-
-
-class LayerNorm(nn.Module):
- def __init__(self, channels, eps=1e-5):
- super().__init__()
- self.channels = channels
- self.eps = eps
-
- self.gamma = nn.Parameter(torch.ones(channels))
- self.beta = nn.Parameter(torch.zeros(channels))
-
- def forward(self, x):
- x = x.transpose(1, -1)
- x = F.layer_norm(x, (self.channels,), self.gamma, self.beta, self.eps)
- return x.transpose(1, -1)
-
-
-class ConvReluNorm(nn.Module):
- def __init__(
- self,
- in_channels,
- hidden_channels,
- out_channels,
- kernel_size,
- n_layers,
- p_dropout,
- ):
- super().__init__()
- self.in_channels = in_channels
- self.hidden_channels = hidden_channels
- self.out_channels = out_channels
- self.kernel_size = kernel_size
- self.n_layers = n_layers
- self.p_dropout = p_dropout
- assert n_layers > 1, "Number of layers should be larger than 0."
-
- self.conv_layers = nn.ModuleList()
- self.norm_layers = nn.ModuleList()
- self.conv_layers.append(
- nn.Conv1d(
- in_channels, hidden_channels, kernel_size, padding=kernel_size // 2
- )
- )
- self.norm_layers.append(LayerNorm(hidden_channels))
- self.relu_drop = nn.Sequential(nn.ReLU(), nn.Dropout(p_dropout))
- for _ in range(n_layers - 1):
- self.conv_layers.append(
- nn.Conv1d(
- hidden_channels,
- hidden_channels,
- kernel_size,
- padding=kernel_size // 2,
- )
- )
- self.norm_layers.append(LayerNorm(hidden_channels))
- self.proj = nn.Conv1d(hidden_channels, out_channels, 1)
- self.proj.weight.data.zero_()
- self.proj.bias.data.zero_()
-
- def forward(self, x, x_mask):
- x_org = x
- for i in range(self.n_layers):
- x = self.conv_layers[i](x * x_mask)
- x = self.norm_layers[i](x)
- x = self.relu_drop(x)
- x = x_org + self.proj(x)
- return x * x_mask
-
-
-class DDSConv(nn.Module):
- """
- Dialted and Depth-Separable Convolution
- """
-
- def __init__(self, channels, kernel_size, n_layers, p_dropout=0.0):
- super().__init__()
- self.channels = channels
- self.kernel_size = kernel_size
- self.n_layers = n_layers
- self.p_dropout = p_dropout
-
- self.drop = nn.Dropout(p_dropout)
- self.convs_sep = nn.ModuleList()
- self.convs_1x1 = nn.ModuleList()
- self.norms_1 = nn.ModuleList()
- self.norms_2 = nn.ModuleList()
- for i in range(n_layers):
- dilation = kernel_size**i
- padding = (kernel_size * dilation - dilation) // 2
- self.convs_sep.append(
- nn.Conv1d(
- channels,
- channels,
- kernel_size,
- groups=channels,
- dilation=dilation,
- padding=padding,
- )
- )
- self.convs_1x1.append(nn.Conv1d(channels, channels, 1))
- self.norms_1.append(LayerNorm(channels))
- self.norms_2.append(LayerNorm(channels))
-
- def forward(self, x, x_mask, g=None):
- if g is not None:
- x = x + g
- for i in range(self.n_layers):
- y = self.convs_sep[i](x * x_mask)
- y = self.norms_1[i](y)
- y = F.gelu(y)
- y = self.convs_1x1[i](y)
- y = self.norms_2[i](y)
- y = F.gelu(y)
- y = self.drop(y)
- x = x + y
- return x * x_mask
-
-
-class WN(torch.nn.Module):
- def __init__(
- self,
- hidden_channels,
- kernel_size,
- dilation_rate,
- n_layers,
- gin_channels=0,
- p_dropout=0,
- ):
- super(WN, self).__init__()
- assert kernel_size % 2 == 1
- self.hidden_channels = hidden_channels
- self.kernel_size = (kernel_size,)
- self.dilation_rate = dilation_rate
- self.n_layers = n_layers
- self.gin_channels = gin_channels
- self.p_dropout = p_dropout
-
- self.in_layers = torch.nn.ModuleList()
- self.res_skip_layers = torch.nn.ModuleList()
- self.drop = nn.Dropout(p_dropout)
-
- if gin_channels != 0:
- cond_layer = torch.nn.Conv1d(
- gin_channels, 2 * hidden_channels * n_layers, 1
- )
- self.cond_layer = torch.nn.utils.weight_norm(cond_layer, name="weight")
-
- for i in range(n_layers):
- dilation = dilation_rate**i
- padding = int((kernel_size * dilation - dilation) / 2)
- in_layer = torch.nn.Conv1d(
- hidden_channels,
- 2 * hidden_channels,
- kernel_size,
- dilation=dilation,
- padding=padding,
- )
- in_layer = torch.nn.utils.weight_norm(in_layer, name="weight")
- self.in_layers.append(in_layer)
-
- # last one is not necessary
- if i < n_layers - 1:
- res_skip_channels = 2 * hidden_channels
- else:
- res_skip_channels = hidden_channels
-
- res_skip_layer = torch.nn.Conv1d(hidden_channels, res_skip_channels, 1)
- res_skip_layer = torch.nn.utils.weight_norm(res_skip_layer, name="weight")
- self.res_skip_layers.append(res_skip_layer)
-
- def forward(self, x, x_mask, g=None, **kwargs):
- output = torch.zeros_like(x)
- n_channels_tensor = torch.IntTensor([self.hidden_channels])
-
- if g is not None:
- g = self.cond_layer(g)
-
- for i in range(self.n_layers):
- x_in = self.in_layers[i](x)
- if g is not None:
- cond_offset = i * 2 * self.hidden_channels
- g_l = g[:, cond_offset : cond_offset + 2 * self.hidden_channels, :]
- else:
- g_l = torch.zeros_like(x_in)
-
- acts = commons.fused_add_tanh_sigmoid_multiply(x_in, g_l, n_channels_tensor)
- acts = self.drop(acts)
-
- res_skip_acts = self.res_skip_layers[i](acts)
- if i < self.n_layers - 1:
- res_acts = res_skip_acts[:, : self.hidden_channels, :]
- x = (x + res_acts) * x_mask
- output = output + res_skip_acts[:, self.hidden_channels :, :]
- else:
- output = output + res_skip_acts
- return output * x_mask
-
- def remove_weight_norm(self):
- if self.gin_channels != 0:
- torch.nn.utils.remove_weight_norm(self.cond_layer)
- for l in self.in_layers:
- torch.nn.utils.remove_weight_norm(l)
- for l in self.res_skip_layers:
- torch.nn.utils.remove_weight_norm(l)
-
-
-class ResBlock1(torch.nn.Module):
- def __init__(self, channels, kernel_size=3, dilation=(1, 3, 5)):
- super(ResBlock1, self).__init__()
- self.convs1 = nn.ModuleList(
- [
- weight_norm(
- Conv1d(
- channels,
- channels,
- kernel_size,
- 1,
- dilation=dilation[0],
- padding=get_padding(kernel_size, dilation[0]),
- )
- ),
- weight_norm(
- Conv1d(
- channels,
- channels,
- kernel_size,
- 1,
- dilation=dilation[1],
- padding=get_padding(kernel_size, dilation[1]),
- )
- ),
- weight_norm(
- Conv1d(
- channels,
- channels,
- kernel_size,
- 1,
- dilation=dilation[2],
- padding=get_padding(kernel_size, dilation[2]),
- )
- ),
- ]
- )
- self.convs1.apply(init_weights)
-
- self.convs2 = nn.ModuleList(
- [
- weight_norm(
- Conv1d(
- channels,
- channels,
- kernel_size,
- 1,
- dilation=1,
- padding=get_padding(kernel_size, 1),
- )
- ),
- weight_norm(
- Conv1d(
- channels,
- channels,
- kernel_size,
- 1,
- dilation=1,
- padding=get_padding(kernel_size, 1),
- )
- ),
- weight_norm(
- Conv1d(
- channels,
- channels,
- kernel_size,
- 1,
- dilation=1,
- padding=get_padding(kernel_size, 1),
- )
- ),
- ]
- )
- self.convs2.apply(init_weights)
-
- def forward(self, x, x_mask=None):
- for c1, c2 in zip(self.convs1, self.convs2):
- xt = F.leaky_relu(x, LRELU_SLOPE)
- if x_mask is not None:
- xt = xt * x_mask
- xt = c1(xt)
- xt = F.leaky_relu(xt, LRELU_SLOPE)
- if x_mask is not None:
- xt = xt * x_mask
- xt = c2(xt)
- x = xt + x
- if x_mask is not None:
- x = x * x_mask
- return x
-
- def remove_weight_norm(self):
- for l in self.convs1:
- remove_weight_norm(l)
- for l in self.convs2:
- remove_weight_norm(l)
-
-
-class ResBlock2(torch.nn.Module):
- def __init__(self, channels, kernel_size=3, dilation=(1, 3)):
- super(ResBlock2, self).__init__()
- self.convs = nn.ModuleList(
- [
- weight_norm(
- Conv1d(
- channels,
- channels,
- kernel_size,
- 1,
- dilation=dilation[0],
- padding=get_padding(kernel_size, dilation[0]),
- )
- ),
- weight_norm(
- Conv1d(
- channels,
- channels,
- kernel_size,
- 1,
- dilation=dilation[1],
- padding=get_padding(kernel_size, dilation[1]),
- )
- ),
- ]
- )
- self.convs.apply(init_weights)
-
- def forward(self, x, x_mask=None):
- for c in self.convs:
- xt = F.leaky_relu(x, LRELU_SLOPE)
- if x_mask is not None:
- xt = xt * x_mask
- xt = c(xt)
- x = xt + x
- if x_mask is not None:
- x = x * x_mask
- return x
-
- def remove_weight_norm(self):
- for l in self.convs:
- remove_weight_norm(l)
-
-
-class Log(nn.Module):
- def forward(self, x, x_mask, reverse=False, **kwargs):
- if not reverse:
- y = torch.log(torch.clamp_min(x, 1e-5)) * x_mask
- logdet = torch.sum(-y, [1, 2])
- return y, logdet
- else:
- x = torch.exp(x) * x_mask
- return x
-
-
-class Flip(nn.Module):
- def forward(self, x, *args, reverse=False, **kwargs):
- x = torch.flip(x, [1])
- if not reverse:
- logdet = torch.zeros(x.size(0)).to(dtype=x.dtype, device=x.device)
- return x, logdet
- else:
- return x
-
-
-class ElementwiseAffine(nn.Module):
- def __init__(self, channels):
- super().__init__()
- self.channels = channels
- self.m = nn.Parameter(torch.zeros(channels, 1))
- self.logs = nn.Parameter(torch.zeros(channels, 1))
-
- def forward(self, x, x_mask, reverse=False, **kwargs):
- if not reverse:
- y = self.m + torch.exp(self.logs) * x
- y = y * x_mask
- logdet = torch.sum(self.logs * x_mask, [1, 2])
- return y, logdet
- else:
- x = (x - self.m) * torch.exp(-self.logs) * x_mask
- return x
-
-
-class ResidualCouplingLayer(nn.Module):
- def __init__(
- self,
- channels,
- hidden_channels,
- kernel_size,
- dilation_rate,
- n_layers,
- p_dropout=0,
- gin_channels=0,
- mean_only=False,
- ):
- assert channels % 2 == 0, "channels should be divisible by 2"
- super().__init__()
- self.channels = channels
- self.hidden_channels = hidden_channels
- self.kernel_size = kernel_size
- self.dilation_rate = dilation_rate
- self.n_layers = n_layers
- self.half_channels = channels // 2
- self.mean_only = mean_only
-
- self.pre = nn.Conv1d(self.half_channels, hidden_channels, 1)
- self.enc = WN(
- hidden_channels,
- kernel_size,
- dilation_rate,
- n_layers,
- p_dropout=p_dropout,
- gin_channels=gin_channels,
- )
- self.post = nn.Conv1d(hidden_channels, self.half_channels * (2 - mean_only), 1)
- self.post.weight.data.zero_()
- self.post.bias.data.zero_()
-
- def forward(self, x, x_mask, g=None, reverse=False):
- x0, x1 = torch.split(x, [self.half_channels] * 2, 1)
- h = self.pre(x0) * x_mask
- h = self.enc(h, x_mask, g=g)
- stats = self.post(h) * x_mask
- if not self.mean_only:
- m, logs = torch.split(stats, [self.half_channels] * 2, 1)
- else:
- m = stats
- logs = torch.zeros_like(m)
-
- if not reverse:
- x1 = m + x1 * torch.exp(logs) * x_mask
- x = torch.cat([x0, x1], 1)
- logdet = torch.sum(logs, [1, 2])
- return x, logdet
- else:
- x1 = (x1 - m) * torch.exp(-logs) * x_mask
- x = torch.cat([x0, x1], 1)
- return x
-
- def remove_weight_norm(self):
- self.enc.remove_weight_norm()
-
-
-class ConvFlow(nn.Module):
- def __init__(
- self,
- in_channels,
- filter_channels,
- kernel_size,
- n_layers,
- num_bins=10,
- tail_bound=5.0,
- ):
- super().__init__()
- self.in_channels = in_channels
- self.filter_channels = filter_channels
- self.kernel_size = kernel_size
- self.n_layers = n_layers
- self.num_bins = num_bins
- self.tail_bound = tail_bound
- self.half_channels = in_channels // 2
-
- self.pre = nn.Conv1d(self.half_channels, filter_channels, 1)
- self.convs = DDSConv(filter_channels, kernel_size, n_layers, p_dropout=0.0)
- self.proj = nn.Conv1d(
- filter_channels, self.half_channels * (num_bins * 3 - 1), 1
- )
- self.proj.weight.data.zero_()
- self.proj.bias.data.zero_()
-
- def forward(self, x, x_mask, g=None, reverse=False):
- x0, x1 = torch.split(x, [self.half_channels] * 2, 1)
- h = self.pre(x0)
- h = self.convs(h, x_mask, g=g)
- h = self.proj(h) * x_mask
-
- b, c, t = x0.shape
- h = h.reshape(b, c, -1, t).permute(0, 1, 3, 2) # [b, cx?, t] -> [b, c, t, ?]
-
- unnormalized_widths = h[..., : self.num_bins] / math.sqrt(self.filter_channels)
- unnormalized_heights = h[..., self.num_bins : 2 * self.num_bins] / math.sqrt(
- self.filter_channels
- )
- unnormalized_derivatives = h[..., 2 * self.num_bins :]
-
- x1, logabsdet = piecewise_rational_quadratic_transform(
- x1,
- unnormalized_widths,
- unnormalized_heights,
- unnormalized_derivatives,
- inverse=reverse,
- tails="linear",
- tail_bound=self.tail_bound,
- )
-
- x = torch.cat([x0, x1], 1) * x_mask
- logdet = torch.sum(logabsdet * x_mask, [1, 2])
- if not reverse:
- return x, logdet
- else:
- return x
diff --git a/spaces/Lewislou/Lewislou-cell-seg-sribd/models/convnext.py b/spaces/Lewislou/Lewislou-cell-seg-sribd/models/convnext.py
deleted file mode 100644
index daceaff72219c8faf9a9cef36ac62c9080c69d43..0000000000000000000000000000000000000000
--- a/spaces/Lewislou/Lewislou-cell-seg-sribd/models/convnext.py
+++ /dev/null
@@ -1,220 +0,0 @@
-# Copyright (c) Meta Platforms, Inc. and affiliates.
-
-# All rights reserved.
-
-# This source code is licensed under the license found in the
-# LICENSE file in the root directory of this source tree.
-
-from functools import partial
-import torch
-import torch.nn as nn
-import torch.nn.functional as F
-from timm.models.layers import trunc_normal_, DropPath
-from timm.models.registry import register_model
-from monai.networks.layers.factories import Act, Conv, Pad, Pool
-from monai.networks.layers.utils import get_norm_layer
-from monai.utils.module import look_up_option
-from typing import List, NamedTuple, Optional, Tuple, Type, Union
-class Block(nn.Module):
- r""" ConvNeXt Block. There are two equivalent implementations:
- (1) DwConv -> LayerNorm (channels_first) -> 1x1 Conv -> GELU -> 1x1 Conv; all in (N, C, H, W)
- (2) DwConv -> Permute to (N, H, W, C); LayerNorm (channels_last) -> Linear -> GELU -> Linear; Permute back
- We use (2) as we find it slightly faster in PyTorch
-
- Args:
- dim (int): Number of input channels.
- drop_path (float): Stochastic depth rate. Default: 0.0
- layer_scale_init_value (float): Init value for Layer Scale. Default: 1e-6.
- """
- def __init__(self, dim, drop_path=0., layer_scale_init_value=1e-6):
- super().__init__()
- self.dwconv = nn.Conv2d(dim, dim, kernel_size=7, padding=3, groups=dim) # depthwise conv
- self.norm = LayerNorm(dim, eps=1e-6)
- self.pwconv1 = nn.Linear(dim, 4 * dim) # pointwise/1x1 convs, implemented with linear layers
- self.act = nn.GELU()
- self.pwconv2 = nn.Linear(4 * dim, dim)
- self.gamma = nn.Parameter(layer_scale_init_value * torch.ones((dim)),
- requires_grad=True) if layer_scale_init_value > 0 else None
- self.drop_path = DropPath(drop_path) if drop_path > 0. else nn.Identity()
-
- def forward(self, x):
- input = x
- x = self.dwconv(x)
- x = x.permute(0, 2, 3, 1) # (N, C, H, W) -> (N, H, W, C)
- x = self.norm(x)
- x = self.pwconv1(x)
- x = self.act(x)
- x = self.pwconv2(x)
- if self.gamma is not None:
- x = self.gamma * x
- x = x.permute(0, 3, 1, 2) # (N, H, W, C) -> (N, C, H, W)
-
- x = input + self.drop_path(x)
- return x
-
-class ConvNeXt(nn.Module):
- r""" ConvNeXt
- A PyTorch impl of : `A ConvNet for the 2020s` -
- https://arxiv.org/pdf/2201.03545.pdf
-
- Args:
- in_chans (int): Number of input image channels. Default: 3
- num_classes (int): Number of classes for classification head. Default: 1000
- depths (tuple(int)): Number of blocks at each stage. Default: [3, 3, 9, 3]
- dims (int): Feature dimension at each stage. Default: [96, 192, 384, 768]
- drop_path_rate (float): Stochastic depth rate. Default: 0.
- layer_scale_init_value (float): Init value for Layer Scale. Default: 1e-6.
- head_init_scale (float): Init scaling value for classifier weights and biases. Default: 1.
- """
- def __init__(self, in_chans=3, num_classes=21841,
- depths=[3, 3, 9, 3], dims=[96, 192, 384, 768], drop_path_rate=0.,
- layer_scale_init_value=1e-6, head_init_scale=1., out_indices=[0, 1, 2, 3],
- ):
- super().__init__()
- # conv_type: Type[Union[nn.Conv1d, nn.Conv2d, nn.Conv3d]] = Conv["conv", 2]
- # self._conv_stem = conv_type(self.in_channels, self.in_channels, kernel_size=3, stride=stride, bias=False)
- # self._conv_stem_padding = _make_same_padder(self._conv_stem, current_image_size)
-
- self.downsample_layers = nn.ModuleList() # stem and 3 intermediate downsampling conv layers
- stem = nn.Sequential(
- nn.Conv2d(in_chans, dims[0], kernel_size=4, stride=4),
- LayerNorm(dims[0], eps=1e-6, data_format="channels_first")
- )
- self.downsample_layers.append(stem)
- for i in range(3):
- downsample_layer = nn.Sequential(
- LayerNorm(dims[i], eps=1e-6, data_format="channels_first"),
- nn.Conv2d(dims[i], dims[i+1], kernel_size=2, stride=2),
- )
- self.downsample_layers.append(downsample_layer)
-
- self.stages = nn.ModuleList() # 4 feature resolution stages, each consisting of multiple residual blocks
- dp_rates=[x.item() for x in torch.linspace(0, drop_path_rate, sum(depths))]
- cur = 0
- for i in range(4):
- stage = nn.Sequential(
- *[Block(dim=dims[i], drop_path=dp_rates[cur + j],
- layer_scale_init_value=layer_scale_init_value) for j in range(depths[i])]
- )
- self.stages.append(stage)
- cur += depths[i]
-
-
- self.out_indices = out_indices
-
- norm_layer = partial(LayerNorm, eps=1e-6, data_format="channels_first")
- for i_layer in range(4):
- layer = norm_layer(dims[i_layer])
- layer_name = f'norm{i_layer}'
- self.add_module(layer_name, layer)
- self.apply(self._init_weights)
-
-
- def _init_weights(self, m):
- if isinstance(m, (nn.Conv2d, nn.Linear)):
- trunc_normal_(m.weight, std=.02)
- nn.init.constant_(m.bias, 0)
-
- def forward_features(self, x):
- outs = []
-
- for i in range(4):
- x = self.downsample_layers[i](x)
- x = self.stages[i](x)
- if i in self.out_indices:
- norm_layer = getattr(self, f'norm{i}')
- x_out = norm_layer(x)
-
- outs.append(x_out)
-
- return tuple(outs)
-
- def forward(self, x):
- x = self.forward_features(x)
-
- return x
-
-class LayerNorm(nn.Module):
- r""" LayerNorm that supports two data formats: channels_last (default) or channels_first.
- The ordering of the dimensions in the inputs. channels_last corresponds to inputs with
- shape (batch_size, height, width, channels) while channels_first corresponds to inputs
- with shape (batch_size, channels, height, width).
- """
- def __init__(self, normalized_shape, eps=1e-6, data_format="channels_last"):
- super().__init__()
- self.weight = nn.Parameter(torch.ones(normalized_shape))
- self.bias = nn.Parameter(torch.zeros(normalized_shape))
- self.eps = eps
- self.data_format = data_format
- if self.data_format not in ["channels_last", "channels_first"]:
- raise NotImplementedError
- self.normalized_shape = (normalized_shape, )
-
- def forward(self, x):
- if self.data_format == "channels_last":
- return F.layer_norm(x, self.normalized_shape, self.weight, self.bias, self.eps)
- elif self.data_format == "channels_first":
- u = x.mean(1, keepdim=True)
- s = (x - u).pow(2).mean(1, keepdim=True)
- x = (x - u) / torch.sqrt(s + self.eps)
- x = self.weight[:, None, None] * x + self.bias[:, None, None]
- return x
-
-
-model_urls = {
- "convnext_tiny_1k": "https://dl.fbaipublicfiles.com/convnext/convnext_tiny_1k_224_ema.pth",
- "convnext_small_1k": "https://dl.fbaipublicfiles.com/convnext/convnext_small_1k_224_ema.pth",
- "convnext_base_1k": "https://dl.fbaipublicfiles.com/convnext/convnext_base_1k_224_ema.pth",
- "convnext_large_1k": "https://dl.fbaipublicfiles.com/convnext/convnext_large_1k_224_ema.pth",
- "convnext_tiny_22k": "https://dl.fbaipublicfiles.com/convnext/convnext_tiny_22k_224.pth",
- "convnext_small_22k": "https://dl.fbaipublicfiles.com/convnext/convnext_small_22k_224.pth",
- "convnext_base_22k": "https://dl.fbaipublicfiles.com/convnext/convnext_base_22k_224.pth",
- "convnext_large_22k": "https://dl.fbaipublicfiles.com/convnext/convnext_large_22k_224.pth",
- "convnext_xlarge_22k": "https://dl.fbaipublicfiles.com/convnext/convnext_xlarge_22k_224.pth",
-}
-
-@register_model
-def convnext_tiny(pretrained=False,in_22k=False, **kwargs):
- model = ConvNeXt(depths=[3, 3, 9, 3], dims=[96, 192, 384, 768], **kwargs)
- if pretrained:
- url = model_urls['convnext_tiny_22k'] if in_22k else model_urls['convnext_tiny_1k']
- checkpoint = torch.hub.load_state_dict_from_url(url=url, map_location="cpu", check_hash=True)
- model.load_state_dict(checkpoint["model"])
- return model
-
-@register_model
-def convnext_small(pretrained=False,in_22k=False, **kwargs):
- model = ConvNeXt(depths=[3, 3, 27, 3], dims=[96, 192, 384, 768], **kwargs)
- if pretrained:
- url = model_urls['convnext_small_22k'] if in_22k else model_urls['convnext_small_1k']
- checkpoint = torch.hub.load_state_dict_from_url(url=url, map_location="cpu")
- model.load_state_dict(checkpoint["model"], strict=False)
- return model
-
-@register_model
-def convnext_base(pretrained=False, in_22k=False, **kwargs):
- model = ConvNeXt(depths=[3, 3, 27, 3], dims=[128, 256, 512, 1024], **kwargs)
- if pretrained:
- url = model_urls['convnext_base_22k'] if in_22k else model_urls['convnext_base_1k']
- checkpoint = torch.hub.load_state_dict_from_url(url=url, map_location="cpu")
- model.load_state_dict(checkpoint["model"], strict=False)
- return model
-
-@register_model
-def convnext_large(pretrained=False, in_22k=False, **kwargs):
- model = ConvNeXt(depths=[3, 3, 27, 3], dims=[192, 384, 768, 1536], **kwargs)
- if pretrained:
- url = model_urls['convnext_large_22k'] if in_22k else model_urls['convnext_large_1k']
- checkpoint = torch.hub.load_state_dict_from_url(url=url, map_location="cpu")
- model.load_state_dict(checkpoint["model"])
- return model
-
-@register_model
-def convnext_xlarge(pretrained=False, in_22k=False, **kwargs):
- model = ConvNeXt(depths=[3, 3, 27, 3], dims=[256, 512, 1024, 2048], **kwargs)
- if pretrained:
- assert in_22k, "only ImageNet-22K pre-trained ConvNeXt-XL is available; please set in_22k=True"
- url = model_urls['convnext_xlarge_22k']
- checkpoint = torch.hub.load_state_dict_from_url(url=url, map_location="cpu")
- model.load_state_dict(checkpoint["model"])
- return model
\ No newline at end of file
diff --git a/spaces/Lianjd/stock_dashboard/backtrader/plot/multicursor.py b/spaces/Lianjd/stock_dashboard/backtrader/plot/multicursor.py
deleted file mode 100644
index a3ea179cf247abf67599e829562e4f587bf14cc2..0000000000000000000000000000000000000000
--- a/spaces/Lianjd/stock_dashboard/backtrader/plot/multicursor.py
+++ /dev/null
@@ -1,354 +0,0 @@
-# LICENSE AGREEMENT FOR MATPLOTLIB 1.2.0
-# --------------------------------------
-#
-# 1. This LICENSE AGREEMENT is between John D. Hunter ("JDH"), and the
-# Individual or Organization ("Licensee") accessing and otherwise using
-# matplotlib software in source or binary form and its associated
-# documentation.
-#
-# 2. Subject to the terms and conditions of this License Agreement, JDH
-# hereby grants Licensee a nonexclusive, royalty-free, world-wide license
-# to reproduce, analyze, test, perform and/or display publicly, prepare
-# derivative works, distribute, and otherwise use matplotlib 1.2.0
-# alone or in any derivative version, provided, however, that JDH's
-# License Agreement and JDH's notice of copyright, i.e., "Copyright (c)
-# 2002-2011 John D. Hunter; All Rights Reserved" are retained in
-# matplotlib 1.2.0 alone or in any derivative version prepared by
-# Licensee.
-#
-# 3. In the event Licensee prepares a derivative work that is based on or
-# incorporates matplotlib 1.2.0 or any part thereof, and wants to
-# make the derivative work available to others as provided herein, then
-# Licensee hereby agrees to include in any such work a brief summary of
-# the changes made to matplotlib 1.2.0.
-#
-# 4. JDH is making matplotlib 1.2.0 available to Licensee on an "AS
-# IS" basis. JDH MAKES NO REPRESENTATIONS OR WARRANTIES, EXPRESS OR
-# IMPLIED. BY WAY OF EXAMPLE, BUT NOT LIMITATION, JDH MAKES NO AND
-# DISCLAIMS ANY REPRESENTATION OR WARRANTY OF MERCHANTABILITY OR FITNESS
-# FOR ANY PARTICULAR PURPOSE OR THAT THE USE OF MATPLOTLIB 1.2.0
-# WILL NOT INFRINGE ANY THIRD PARTY RIGHTS.
-#
-# 5. JDH SHALL NOT BE LIABLE TO LICENSEE OR ANY OTHER USERS OF MATPLOTLIB
-# 1.2.0 FOR ANY INCIDENTAL, SPECIAL, OR CONSEQUENTIAL DAMAGES OR
-# LOSS AS A RESULT OF MODIFYING, DISTRIBUTING, OR OTHERWISE USING
-# MATPLOTLIB 1.2.0, OR ANY DERIVATIVE THEREOF, EVEN IF ADVISED OF
-# THE POSSIBILITY THEREOF.
-
-# 6. This License Agreement will automatically terminate upon a material
-# breach of its terms and conditions.
-#
-# 7. Nothing in this License Agreement shall be deemed to create any
-# relationship of agency, partnership, or joint venture between JDH and
-# Licensee. This License Agreement does not grant permission to use JDH
-# trademarks or trade name in a trademark sense to endorse or promote
-# products or services of Licensee, or any third party.
-#
-# 8. By copying, installing or otherwise using matplotlib 1.2.0,
-# Licensee agrees to be bound by the terms and conditions of this License
-# Agreement.
-
-# CHANGES
-# The original MultiCursor plots all horizontal lines at the same time
-# The modified version plots only the horizontal line in the axis in which the
-# motion event takes place
-#
-# The original MultiCursos uses the ylimit of the las passed axis, to calculate
-# the mid point of the axis. which creates a huge distorsion if all axis don't
-# have the same y dimensions
-#
-# The modified version uses the y limits of each axis to calculate the initial
-# position of each line avoiding the distorsion
-
-from ..utils.py3 import zip
-
-class Widget(object):
- """
- Abstract base class for GUI neutral widgets
- """
- drawon = True
- eventson = True
- _active = True
-
- def set_active(self, active):
- """Set whether the widget is active.
- """
- self._active = active
-
- def get_active(self):
- """Get whether the widget is active.
- """
- return self._active
-
- # set_active is overriden by SelectorWidgets.
- active = property(get_active, lambda self, active: self.set_active(active),
- doc="Is the widget active?")
-
- def ignore(self, event):
- """Return True if event should be ignored.
- This method (or a version of it) should be called at the beginning
- of any event callback.
- """
- return not self.active
-
-
-class MultiCursor(Widget):
- """
- Provide a vertical (default) and/or horizontal line cursor shared between
- multiple axes.
-
- For the cursor to remain responsive you much keep a reference to
- it.
-
- Example usage::
-
- from matplotlib.widgets import MultiCursor
- from pylab import figure, show, np
-
- t = np.arange(0.0, 2.0, 0.01)
- s1 = np.sin(2*np.pi*t)
- s2 = np.sin(4*np.pi*t)
- fig = figure()
- ax1 = fig.add_subplot(211)
- ax1.plot(t, s1)
-
-
- ax2 = fig.add_subplot(212, sharex=ax1)
- ax2.plot(t, s2)
-
- multi = MultiCursor(fig.canvas, (ax1, ax2), color='r', lw=1,
- horizOn=False, vertOn=True)
- show()
-
- """
- def __init__(self, canvas, axes, useblit=True,
- horizOn=False, vertOn=True,
- horizMulti=False, vertMulti=True,
- horizShared=True, vertShared=False,
- **lineprops):
-
- self.canvas = canvas
- self.axes = axes
- self.horizOn = horizOn
- self.vertOn = vertOn
- self.horizMulti = horizMulti
- self.vertMulti = vertMulti
-
- self.visible = True
- self.useblit = useblit and self.canvas.supports_blit
- self.background = None
- self.needclear = False
-
- if self.useblit:
- lineprops['animated'] = True
-
- self.vlines = []
- if vertOn:
- xmin, xmax = axes[-1].get_xlim()
- xmid = 0.5 * (xmin + xmax)
-
- for ax in axes:
- if not horizShared:
- xmin, xmax = ax.get_xlim()
- xmid = 0.5 * (xmin + xmax)
-
- vline = ax.axvline(xmid, visible=False, **lineprops)
- self.vlines.append(vline)
-
- self.hlines = []
- if horizOn:
- ymin, ymax = axes[-1].get_ylim()
- ymid = 0.5 * (ymin + ymax)
-
- for ax in axes:
- if not vertShared:
- ymin, ymax = ax.get_ylim()
- ymid = 0.5 * (ymin + ymax)
-
- hline = ax.axhline(ymid, visible=False, **lineprops)
- self.hlines.append(hline)
-
- self.connect()
-
- def connect(self):
- """connect events"""
- self._cidmotion = self.canvas.mpl_connect('motion_notify_event',
- self.onmove)
- self._ciddraw = self.canvas.mpl_connect('draw_event', self.clear)
-
- def disconnect(self):
- """disconnect events"""
- self.canvas.mpl_disconnect(self._cidmotion)
- self.canvas.mpl_disconnect(self._ciddraw)
-
- def clear(self, event):
- """clear the cursor"""
- if self.ignore(event):
- return
- if self.useblit:
- self.background = (
- self.canvas.copy_from_bbox(self.canvas.figure.bbox))
- for line in self.vlines + self.hlines:
- line.set_visible(False)
-
- def onmove(self, event):
- if self.ignore(event):
- return
- if event.inaxes is None:
- return
- if not self.canvas.widgetlock.available(self):
- return
- self.needclear = True
- if not self.visible:
- return
- if self.vertOn:
- for line in self.vlines:
- visible = self.visible
- if not self.vertMulti:
- visible = visible and line.axes == event.inaxes
-
- if visible:
- line.set_xdata((event.xdata, event.xdata))
- line.set_visible(visible)
- if self.horizOn:
- for line in self.hlines:
- visible = self.visible
- if not self.horizMulti:
- visible = visible and line.axes == event.inaxes
- if visible:
- line.set_ydata((event.ydata, event.ydata))
- line.set_visible(self.visible)
- self._update(event)
-
- def _update(self, event):
- if self.useblit:
- if self.background is not None:
- self.canvas.restore_region(self.background)
- if self.vertOn:
- for ax, line in zip(self.axes, self.vlines):
- if self.vertMulti or event.inaxes == line.axes:
- ax.draw_artist(line)
-
- if self.horizOn:
- for ax, line in zip(self.axes, self.hlines):
- if self.horizMulti or event.inaxes == line.axes:
- ax.draw_artist(line)
- self.canvas.blit(self.canvas.figure.bbox)
- else:
- self.canvas.draw_idle()
-
-class MultiCursor2(Widget):
- """
- Provide a vertical (default) and/or horizontal line cursor shared between
- multiple axes.
- For the cursor to remain responsive you much keep a reference to
- it.
- Example usage::
- from matplotlib.widgets import MultiCursor
- from pylab import figure, show, np
- t = np.arange(0.0, 2.0, 0.01)
- s1 = np.sin(2*np.pi*t)
- s2 = np.sin(4*np.pi*t)
- fig = figure()
- ax1 = fig.add_subplot(211)
- ax1.plot(t, s1)
- ax2 = fig.add_subplot(212, sharex=ax1)
- ax2.plot(t, s2)
- multi = MultiCursor(fig.canvas, (ax1, ax2), color='r', lw=1,
- horizOn=False, vertOn=True)
- show()
- """
- def __init__(self, canvas, axes, useblit=True, horizOn=False, vertOn=True,
- **lineprops):
-
- self.canvas = canvas
- self.axes = axes
- self.horizOn = horizOn
- self.vertOn = vertOn
-
- xmin, xmax = axes[-1].get_xlim()
- xmid = 0.5 * (xmin + xmax)
-
- self.visible = True
- self.useblit = useblit and self.canvas.supports_blit
- self.background = None
- self.needclear = False
-
- if self.useblit:
- lineprops['animated'] = True
-
- if vertOn:
- self.vlines = [ax.axvline(xmid, visible=False, **lineprops)
- for ax in axes]
- else:
- self.vlines = []
-
- if horizOn:
- self.hlines = []
- for ax in axes:
- ymin, ymax = ax.get_ylim()
- ymid = 0.5 * (ymin + ymax)
- hline = ax.axhline(ymid, visible=False, **lineprops)
- self.hlines.append(hline)
- else:
- self.hlines = []
-
- self.connect()
-
- def connect(self):
- """connect events"""
- self._cidmotion = self.canvas.mpl_connect('motion_notify_event',
- self.onmove)
- self._ciddraw = self.canvas.mpl_connect('draw_event', self.clear)
-
- def disconnect(self):
- """disconnect events"""
- self.canvas.mpl_disconnect(self._cidmotion)
- self.canvas.mpl_disconnect(self._ciddraw)
-
- def clear(self, event):
- """clear the cursor"""
- if self.ignore(event):
- return
- if self.useblit:
- self.background = (
- self.canvas.copy_from_bbox(self.canvas.figure.bbox))
- for line in self.vlines + self.hlines:
- line.set_visible(False)
-
- def onmove(self, event):
- if self.ignore(event):
- return
- if event.inaxes is None:
- return
-
- if not self.canvas.widgetlock.available(self):
- return
- self.needclear = True
- if not self.visible:
- return
- if self.vertOn:
- for line in self.vlines:
- visible = True or line.axes == event.inaxes
- line.set_xdata((event.xdata, event.xdata))
- line.set_visible(visible)
- if self.horizOn:
- for line in self.hlines:
- visible = line.axes == event.inaxes
- line.set_ydata((event.ydata, event.ydata))
- line.set_visible(visible)
- self._update(event)
-
- def _update(self, event):
- if self.useblit:
- if self.background is not None:
- self.canvas.restore_region(self.background)
- if self.vertOn:
- for ax, line in zip(self.axes, self.vlines):
- ax.draw_artist(line)
- if self.horizOn:
- for ax, line in zip(self.axes, self.hlines):
- ax.draw_artist(line)
- self.canvas.blit(self.canvas.figure.bbox)
- else:
- self.canvas.draw_idle()
diff --git a/spaces/LinJulya/PromptGenerator/app.py b/spaces/LinJulya/PromptGenerator/app.py
deleted file mode 100644
index 0ed8cd15cf394ca0c2cb1bb6041efb2ececabec6..0000000000000000000000000000000000000000
--- a/spaces/LinJulya/PromptGenerator/app.py
+++ /dev/null
@@ -1,81 +0,0 @@
-import gradio as gr
-import os
-import openai
-import time
-
-
-def chatbot_response(prompt_topic):
- openai.api_key = os.environ.get('OPENAI_API_KEY')
-
- response = openai.ChatCompletion.create(
- model="gpt-4",
- messages=[
- {
- "role": "system",
- "content": "You are a prompt engineer. You are responsible for crafting high-quality prompts that drive precise and meaningful responses from language models like ChatGPT. You understand the nuances of language, context, and user needs and engineer prompts that help people solve problems. Your task is to generate useful and functional high-quality prompts for users.\n\nWhen responding, please follow this structure: \n- Prompt Template\n- Instructions\n- Example\n\nWhen asked for an automatic prompt, make sure to always include \"To better understand what I want and need, you should always answer by including a question that helps you better understand the context and my needs. Do you understand?\" in the prompt template."
- },
- {
- "role": "user",
- "content": "Create an automatic prompt for writing a meal plan with ChatGPT. "
- },
- {
- "role": "assistant",
- "content": "Prompt Template:\nYou are a professional in the field of nutrition and dietetics, who has helped numerous individuals to devise customized meal plans. Your task is now to create a comprehensive nutritional plan for a [DIETARY PREFERENCE] like me aiming to [HEALTH GOALS]. To better understand what I want and need, you should always answer by including a question that helps you better understand the context and my needs. Do you understand?\n\nInstructions:\n1. Mention Your Preferences and Goals: Specify your dietary preferences and health goals.\n2. Fill in the Template: Use the template to craft your automatic prompt.\n3. Input to ChatGPT: Once you've filled in the template, provide it to ChatGPT to receive a personalized nutritional plan based on your input.\n\nExample: \n\"You are a professional in the field of nutrition and dietetics, who has helped numerous individuals to devise customized meal plans. Your task is now to create a comprehensive nutritional plan for a vegetarian like me aiming to lose weight. To better understand what I want and need, you should always answer by including a question that helps you better understand the context and my needs. Do you understand?\""
- },
- {
- "role": "assistant",
- "content": "Write a non-automatic prompt on writing social media posts. The prompt should take other social media posts as input and use it as reference for tone and style. "
- },
- {
- "role": "user",
- "content": "Prompt Name: Social Media Post Generator\n\nDescription: This prompt is designed to help users craft engaging social media posts by referencing the tone and style of existing sample posts.\n\nPrompt Template: Given the following examples of social media posts, \"[SOCIAL MEDIA POST 1]\", \"[SOCIAL MEDIA POST 2]\" and \"[SOCIAL MEDIA POST 3]\", could you help me write a new post that maintains the same tone and style? My post should be about [TOPIC].\n\nInstructions:\n1. Identify Sample Posts: Choose three social media posts that reflect the tone and style you want your post to emulate.\n2. Determine the Topic: Decide what the topic of your new social media post will be.\n3. Fill in the Template: Replace the placeholders in the template with the information from steps 1-2:\n ◦ Replace [SOCIAL MEDIA POST 1], [SOCIAL MEDIA POST 2], and [SOCIAL MEDIA POST 3] with your chosen sample posts.\n ◦ Replace [TOPIC] with your decided topic.\n4. Input to ChatGPT: Once you've filled in the template, provide it to ChatGPT to generate a new social media post based on your input.\n\nExample: \"Given the following examples of social media posts, \"A day without coffee is like... just kidding, we have no idea!\", \"Coffee: because adulting is hard.\" and \"Life happens, coffee helps\", could you help me write a new post that maintains the same tone and style? My post should be about promoting our new coffee blend.\""
- },
- {
- "role": "user",
- "content": f"Create a prompt template for: {prompt_topic}"
- }
- ],
- temperature=0.7,
- max_tokens=4352,
- top_p=1,
- frequency_penalty=0,
- presence_penalty=0.6,
- stop=[" Human:", " AI:"]
- )
-
- return response.choices[0].message['content']
-
-def respond(input1, input2, input3, chatbot):
- if input2 == True:
- auto = "an automatic"
- else:
- auto = "a non-automatic"
-
- prompt_topic = "Write " + auto + " prompt on " + input1 + ". " + input3
- bot_response = chatbot_response(prompt_topic)
- chatbot.append((prompt_topic, bot_response))
- time.sleep(2)
- return chatbot
-
-with gr.Blocks() as demo:
- gr.Markdown(
- """
- # Prompt Generator Bot
- Input your parameters and press Generate.
- """)
- with gr.Row():
- with gr.Column(scale=1):
- prompt_title = gr.Textbox(placeholder="I need a prompt for ...", label="What is your prompt for?")
- type_auto = gr.Checkbox(label="An automatic prompt asks you what you need before giving you what you want. It usually starts with ChatGPT asking you questions. Do you want an automatic prompt?")
- extra = gr.Textbox(placeholder="Write your specifications in full sentences.", label="Do you have other specifications for your prompt?")
- btn = gr.Button("Generate")
-
- with gr.Column(scale=1):
- chatbot = gr.Chatbot(show_copy_button=True)
- with gr.Row():
- clear = gr.ClearButton([prompt_title, type_auto, extra, chatbot])
-
- #btn.click(fn=update, inputs=inp, outputs=None)
- btn.click(fn=respond, inputs=[prompt_title, type_auto, extra , chatbot], outputs=[chatbot])
-
-demo.launch()
\ No newline at end of file
diff --git a/spaces/MCkernick/Image_Restoration_Colorization/Face_Detection/align_warp_back_multiple_dlib_HR.py b/spaces/MCkernick/Image_Restoration_Colorization/Face_Detection/align_warp_back_multiple_dlib_HR.py
deleted file mode 100644
index f3711c968ebeba22f3872b8074b7c89f55a634a1..0000000000000000000000000000000000000000
--- a/spaces/MCkernick/Image_Restoration_Colorization/Face_Detection/align_warp_back_multiple_dlib_HR.py
+++ /dev/null
@@ -1,437 +0,0 @@
-# Copyright (c) Microsoft Corporation.
-# Licensed under the MIT License.
-
-import torch
-import numpy as np
-import skimage.io as io
-
-# from face_sdk import FaceDetection
-import matplotlib.pyplot as plt
-from matplotlib.patches import Rectangle
-from skimage.transform import SimilarityTransform
-from skimage.transform import warp
-from PIL import Image, ImageFilter
-import torch.nn.functional as F
-import torchvision as tv
-import torchvision.utils as vutils
-import time
-import cv2
-import os
-from skimage import img_as_ubyte
-import json
-import argparse
-import dlib
-
-
-def calculate_cdf(histogram):
- """
- This method calculates the cumulative distribution function
- :param array histogram: The values of the histogram
- :return: normalized_cdf: The normalized cumulative distribution function
- :rtype: array
- """
- # Get the cumulative sum of the elements
- cdf = histogram.cumsum()
-
- # Normalize the cdf
- normalized_cdf = cdf / float(cdf.max())
-
- return normalized_cdf
-
-
-def calculate_lookup(src_cdf, ref_cdf):
- """
- This method creates the lookup table
- :param array src_cdf: The cdf for the source image
- :param array ref_cdf: The cdf for the reference image
- :return: lookup_table: The lookup table
- :rtype: array
- """
- lookup_table = np.zeros(256)
- lookup_val = 0
- for src_pixel_val in range(len(src_cdf)):
- lookup_val
- for ref_pixel_val in range(len(ref_cdf)):
- if ref_cdf[ref_pixel_val] >= src_cdf[src_pixel_val]:
- lookup_val = ref_pixel_val
- break
- lookup_table[src_pixel_val] = lookup_val
- return lookup_table
-
-
-def match_histograms(src_image, ref_image):
- """
- This method matches the source image histogram to the
- reference signal
- :param image src_image: The original source image
- :param image ref_image: The reference image
- :return: image_after_matching
- :rtype: image (array)
- """
- # Split the images into the different color channels
- # b means blue, g means green and r means red
- src_b, src_g, src_r = cv2.split(src_image)
- ref_b, ref_g, ref_r = cv2.split(ref_image)
-
- # Compute the b, g, and r histograms separately
- # The flatten() Numpy method returns a copy of the array c
- # collapsed into one dimension.
- src_hist_blue, bin_0 = np.histogram(src_b.flatten(), 256, [0, 256])
- src_hist_green, bin_1 = np.histogram(src_g.flatten(), 256, [0, 256])
- src_hist_red, bin_2 = np.histogram(src_r.flatten(), 256, [0, 256])
- ref_hist_blue, bin_3 = np.histogram(ref_b.flatten(), 256, [0, 256])
- ref_hist_green, bin_4 = np.histogram(ref_g.flatten(), 256, [0, 256])
- ref_hist_red, bin_5 = np.histogram(ref_r.flatten(), 256, [0, 256])
-
- # Compute the normalized cdf for the source and reference image
- src_cdf_blue = calculate_cdf(src_hist_blue)
- src_cdf_green = calculate_cdf(src_hist_green)
- src_cdf_red = calculate_cdf(src_hist_red)
- ref_cdf_blue = calculate_cdf(ref_hist_blue)
- ref_cdf_green = calculate_cdf(ref_hist_green)
- ref_cdf_red = calculate_cdf(ref_hist_red)
-
- # Make a separate lookup table for each color
- blue_lookup_table = calculate_lookup(src_cdf_blue, ref_cdf_blue)
- green_lookup_table = calculate_lookup(src_cdf_green, ref_cdf_green)
- red_lookup_table = calculate_lookup(src_cdf_red, ref_cdf_red)
-
- # Use the lookup function to transform the colors of the original
- # source image
- blue_after_transform = cv2.LUT(src_b, blue_lookup_table)
- green_after_transform = cv2.LUT(src_g, green_lookup_table)
- red_after_transform = cv2.LUT(src_r, red_lookup_table)
-
- # Put the image back together
- image_after_matching = cv2.merge([blue_after_transform, green_after_transform, red_after_transform])
- image_after_matching = cv2.convertScaleAbs(image_after_matching)
-
- return image_after_matching
-
-
-def _standard_face_pts():
- pts = (
- np.array([196.0, 226.0, 316.0, 226.0, 256.0, 286.0, 220.0, 360.4, 292.0, 360.4], np.float32) / 256.0
- - 1.0
- )
-
- return np.reshape(pts, (5, 2))
-
-
-def _origin_face_pts():
- pts = np.array([196.0, 226.0, 316.0, 226.0, 256.0, 286.0, 220.0, 360.4, 292.0, 360.4], np.float32)
-
- return np.reshape(pts, (5, 2))
-
-
-def compute_transformation_matrix(img, landmark, normalize, target_face_scale=1.0):
-
- std_pts = _standard_face_pts() # [-1,1]
- target_pts = (std_pts * target_face_scale + 1) / 2 * 512.0
-
- # print(target_pts)
-
- h, w, c = img.shape
- if normalize == True:
- landmark[:, 0] = landmark[:, 0] / h * 2 - 1.0
- landmark[:, 1] = landmark[:, 1] / w * 2 - 1.0
-
- # print(landmark)
-
- affine = SimilarityTransform()
-
- affine.estimate(target_pts, landmark)
-
- return affine
-
-
-def compute_inverse_transformation_matrix(img, landmark, normalize, target_face_scale=1.0):
-
- std_pts = _standard_face_pts() # [-1,1]
- target_pts = (std_pts * target_face_scale + 1) / 2 * 512.0
-
- # print(target_pts)
-
- h, w, c = img.shape
- if normalize == True:
- landmark[:, 0] = landmark[:, 0] / h * 2 - 1.0
- landmark[:, 1] = landmark[:, 1] / w * 2 - 1.0
-
- # print(landmark)
-
- affine = SimilarityTransform()
-
- affine.estimate(landmark, target_pts)
-
- return affine
-
-
-def show_detection(image, box, landmark):
- plt.imshow(image)
- print(box[2] - box[0])
- plt.gca().add_patch(
- Rectangle(
- (box[1], box[0]), box[2] - box[0], box[3] - box[1], linewidth=1, edgecolor="r", facecolor="none"
- )
- )
- plt.scatter(landmark[0][0], landmark[0][1])
- plt.scatter(landmark[1][0], landmark[1][1])
- plt.scatter(landmark[2][0], landmark[2][1])
- plt.scatter(landmark[3][0], landmark[3][1])
- plt.scatter(landmark[4][0], landmark[4][1])
- plt.show()
-
-
-def affine2theta(affine, input_w, input_h, target_w, target_h):
- # param = np.linalg.inv(affine)
- param = affine
- theta = np.zeros([2, 3])
- theta[0, 0] = param[0, 0] * input_h / target_h
- theta[0, 1] = param[0, 1] * input_w / target_h
- theta[0, 2] = (2 * param[0, 2] + param[0, 0] * input_h + param[0, 1] * input_w) / target_h - 1
- theta[1, 0] = param[1, 0] * input_h / target_w
- theta[1, 1] = param[1, 1] * input_w / target_w
- theta[1, 2] = (2 * param[1, 2] + param[1, 0] * input_h + param[1, 1] * input_w) / target_w - 1
- return theta
-
-
-def blur_blending(im1, im2, mask):
-
- mask *= 255.0
-
- kernel = np.ones((10, 10), np.uint8)
- mask = cv2.erode(mask, kernel, iterations=1)
-
- mask = Image.fromarray(mask.astype("uint8")).convert("L")
- im1 = Image.fromarray(im1.astype("uint8"))
- im2 = Image.fromarray(im2.astype("uint8"))
-
- mask_blur = mask.filter(ImageFilter.GaussianBlur(20))
- im = Image.composite(im1, im2, mask)
-
- im = Image.composite(im, im2, mask_blur)
-
- return np.array(im) / 255.0
-
-
-def blur_blending_cv2(im1, im2, mask):
-
- mask *= 255.0
-
- kernel = np.ones((9, 9), np.uint8)
- mask = cv2.erode(mask, kernel, iterations=3)
-
- mask_blur = cv2.GaussianBlur(mask, (25, 25), 0)
- mask_blur /= 255.0
-
- im = im1 * mask_blur + (1 - mask_blur) * im2
-
- im /= 255.0
- im = np.clip(im, 0.0, 1.0)
-
- return im
-
-
-# def Poisson_blending(im1,im2,mask):
-
-
-# Image.composite(
-def Poisson_blending(im1, im2, mask):
-
- # mask=1-mask
- mask *= 255
- kernel = np.ones((10, 10), np.uint8)
- mask = cv2.erode(mask, kernel, iterations=1)
- mask /= 255
- mask = 1 - mask
- mask *= 255
-
- mask = mask[:, :, 0]
- width, height, channels = im1.shape
- center = (int(height / 2), int(width / 2))
- result = cv2.seamlessClone(
- im2.astype("uint8"), im1.astype("uint8"), mask.astype("uint8"), center, cv2.MIXED_CLONE
- )
-
- return result / 255.0
-
-
-def Poisson_B(im1, im2, mask, center):
-
- mask *= 255
-
- result = cv2.seamlessClone(
- im2.astype("uint8"), im1.astype("uint8"), mask.astype("uint8"), center, cv2.NORMAL_CLONE
- )
-
- return result / 255
-
-
-def seamless_clone(old_face, new_face, raw_mask):
-
- height, width, _ = old_face.shape
- height = height // 2
- width = width // 2
-
- y_indices, x_indices, _ = np.nonzero(raw_mask)
- y_crop = slice(np.min(y_indices), np.max(y_indices))
- x_crop = slice(np.min(x_indices), np.max(x_indices))
- y_center = int(np.rint((np.max(y_indices) + np.min(y_indices)) / 2 + height))
- x_center = int(np.rint((np.max(x_indices) + np.min(x_indices)) / 2 + width))
-
- insertion = np.rint(new_face[y_crop, x_crop] * 255.0).astype("uint8")
- insertion_mask = np.rint(raw_mask[y_crop, x_crop] * 255.0).astype("uint8")
- insertion_mask[insertion_mask != 0] = 255
- prior = np.rint(np.pad(old_face * 255.0, ((height, height), (width, width), (0, 0)), "constant")).astype(
- "uint8"
- )
- # if np.sum(insertion_mask) == 0:
- n_mask = insertion_mask[1:-1, 1:-1, :]
- n_mask = cv2.copyMakeBorder(n_mask, 1, 1, 1, 1, cv2.BORDER_CONSTANT, 0)
- print(n_mask.shape)
- x, y, w, h = cv2.boundingRect(n_mask[:, :, 0])
- if w < 4 or h < 4:
- blended = prior
- else:
- blended = cv2.seamlessClone(
- insertion, # pylint: disable=no-member
- prior,
- insertion_mask,
- (x_center, y_center),
- cv2.NORMAL_CLONE,
- ) # pylint: disable=no-member
-
- blended = blended[height:-height, width:-width]
-
- return blended.astype("float32") / 255.0
-
-
-def get_landmark(face_landmarks, id):
- part = face_landmarks.part(id)
- x = part.x
- y = part.y
-
- return (x, y)
-
-
-def search(face_landmarks):
-
- x1, y1 = get_landmark(face_landmarks, 36)
- x2, y2 = get_landmark(face_landmarks, 39)
- x3, y3 = get_landmark(face_landmarks, 42)
- x4, y4 = get_landmark(face_landmarks, 45)
-
- x_nose, y_nose = get_landmark(face_landmarks, 30)
-
- x_left_mouth, y_left_mouth = get_landmark(face_landmarks, 48)
- x_right_mouth, y_right_mouth = get_landmark(face_landmarks, 54)
-
- x_left_eye = int((x1 + x2) / 2)
- y_left_eye = int((y1 + y2) / 2)
- x_right_eye = int((x3 + x4) / 2)
- y_right_eye = int((y3 + y4) / 2)
-
- results = np.array(
- [
- [x_left_eye, y_left_eye],
- [x_right_eye, y_right_eye],
- [x_nose, y_nose],
- [x_left_mouth, y_left_mouth],
- [x_right_mouth, y_right_mouth],
- ]
- )
-
- return results
-
-
-if __name__ == "__main__":
-
- parser = argparse.ArgumentParser()
- parser.add_argument("--origin_url", type=str, default="./", help="origin images")
- parser.add_argument("--replace_url", type=str, default="./", help="restored faces")
- parser.add_argument("--save_url", type=str, default="./save")
- opts = parser.parse_args()
-
- origin_url = opts.origin_url
- replace_url = opts.replace_url
- save_url = opts.save_url
-
- if not os.path.exists(save_url):
- os.makedirs(save_url)
-
- face_detector = dlib.get_frontal_face_detector()
- landmark_locator = dlib.shape_predictor("shape_predictor_68_face_landmarks.dat")
-
- count = 0
-
- for x in os.listdir(origin_url):
- img_url = os.path.join(origin_url, x)
- pil_img = Image.open(img_url).convert("RGB")
-
- origin_width, origin_height = pil_img.size
- image = np.array(pil_img)
-
- start = time.time()
- faces = face_detector(image)
- done = time.time()
-
- if len(faces) == 0:
- print("Warning: There is no face in %s" % (x))
- continue
-
- blended = image
- for face_id in range(len(faces)):
-
- current_face = faces[face_id]
- face_landmarks = landmark_locator(image, current_face)
- current_fl = search(face_landmarks)
-
- forward_mask = np.ones_like(image).astype("uint8")
- affine = compute_transformation_matrix(image, current_fl, False, target_face_scale=1.3)
- aligned_face = warp(image, affine, output_shape=(512, 512, 3), preserve_range=True)
- forward_mask = warp(
- forward_mask, affine, output_shape=(512, 512, 3), order=0, preserve_range=True
- )
-
- affine_inverse = affine.inverse
- cur_face = aligned_face
- if replace_url != "":
-
- face_name = x[:-4] + "_" + str(face_id + 1) + ".png"
- cur_url = os.path.join(replace_url, face_name)
- restored_face = Image.open(cur_url).convert("RGB")
- restored_face = np.array(restored_face)
- cur_face = restored_face
-
- ## Histogram Color matching
- A = cv2.cvtColor(aligned_face.astype("uint8"), cv2.COLOR_RGB2BGR)
- B = cv2.cvtColor(cur_face.astype("uint8"), cv2.COLOR_RGB2BGR)
- B = match_histograms(B, A)
- cur_face = cv2.cvtColor(B.astype("uint8"), cv2.COLOR_BGR2RGB)
-
- warped_back = warp(
- cur_face,
- affine_inverse,
- output_shape=(origin_height, origin_width, 3),
- order=3,
- preserve_range=True,
- )
-
- backward_mask = warp(
- forward_mask,
- affine_inverse,
- output_shape=(origin_height, origin_width, 3),
- order=0,
- preserve_range=True,
- ) ## Nearest neighbour
-
- blended = blur_blending_cv2(warped_back, blended, backward_mask)
- blended *= 255.0
-
- io.imsave(os.path.join(save_url, x), img_as_ubyte(blended / 255.0))
-
- count += 1
-
- if count % 1000 == 0:
- print("%d have finished ..." % (count))
-
diff --git a/spaces/MINAMONI/anime-remove-background/README.md b/spaces/MINAMONI/anime-remove-background/README.md
deleted file mode 100644
index 1ba3cb5ea0e994e246d57b7d62b8aa5a6331901c..0000000000000000000000000000000000000000
--- a/spaces/MINAMONI/anime-remove-background/README.md
+++ /dev/null
@@ -1,14 +0,0 @@
----
-title: Anime Remove Background
-emoji: 🪄🖼️
-colorFrom: indigo
-colorTo: pink
-sdk: gradio
-sdk_version: 3.1.4
-app_file: app.py
-pinned: false
-license: apache-2.0
-duplicated_from: skytnt/anime-remove-background
----
-
-Check out the configuration reference at https://huggingface.co/docs/hub/spaces-config-reference
diff --git a/spaces/MRiwu/Collection/text/thai.py b/spaces/MRiwu/Collection/text/thai.py
deleted file mode 100644
index 998207c01a85c710a46db1ec8b62c39c2d94bc84..0000000000000000000000000000000000000000
--- a/spaces/MRiwu/Collection/text/thai.py
+++ /dev/null
@@ -1,44 +0,0 @@
-import re
-from num_thai.thainumbers import NumThai
-
-
-num = NumThai()
-
-# List of (Latin alphabet, Thai) pairs:
-_latin_to_thai = [(re.compile('%s' % x[0], re.IGNORECASE), x[1]) for x in [
- ('a', 'เอ'),
- ('b','บี'),
- ('c','ซี'),
- ('d','ดี'),
- ('e','อี'),
- ('f','เอฟ'),
- ('g','จี'),
- ('h','เอช'),
- ('i','ไอ'),
- ('j','เจ'),
- ('k','เค'),
- ('l','แอล'),
- ('m','เอ็ม'),
- ('n','เอ็น'),
- ('o','โอ'),
- ('p','พี'),
- ('q','คิว'),
- ('r','แอร์'),
- ('s','เอส'),
- ('t','ที'),
- ('u','ยู'),
- ('v','วี'),
- ('w','ดับเบิลยู'),
- ('x','เอ็กซ์'),
- ('y','วาย'),
- ('z','ซี')
-]]
-
-
-def num_to_thai(text):
- return re.sub(r'(?:\d+(?:,?\d+)?)+(?:\.\d+(?:,?\d+)?)?', lambda x: ''.join(num.NumberToTextThai(float(x.group(0).replace(',', '')))), text)
-
-def latin_to_thai(text):
- for regex, replacement in _latin_to_thai:
- text = re.sub(regex, replacement, text)
- return text
diff --git a/spaces/Make-A-Protagonist/Make-A-Protagonist-inference/Make-A-Protagonist/experts/GroundedSAM/GroundingDINO/setup.py b/spaces/Make-A-Protagonist/Make-A-Protagonist-inference/Make-A-Protagonist/experts/GroundedSAM/GroundingDINO/setup.py
deleted file mode 100644
index a045b763fb4a4f61bac23b735544a18ffc68d20a..0000000000000000000000000000000000000000
--- a/spaces/Make-A-Protagonist/Make-A-Protagonist-inference/Make-A-Protagonist/experts/GroundedSAM/GroundingDINO/setup.py
+++ /dev/null
@@ -1,208 +0,0 @@
-# coding=utf-8
-# Copyright 2022 The IDEA Authors. All rights reserved.
-#
-# Licensed under the Apache License, Version 2.0 (the "License");
-# you may not use this file except in compliance with the License.
-# You may obtain a copy of the License at
-#
-# http://www.apache.org/licenses/LICENSE-2.0
-#
-# Unless required by applicable law or agreed to in writing, software
-# distributed under the License is distributed on an "AS IS" BASIS,
-# WITHOUT WARRANTIES OR CONDITIONS OF ANY KIND, either express or implied.
-# See the License for the specific language governing permissions and
-# limitations under the License.
-# ------------------------------------------------------------------------------------------------
-# Modified from
-# https://github.com/fundamentalvision/Deformable-DETR/blob/main/models/ops/setup.py
-# https://github.com/facebookresearch/detectron2/blob/main/setup.py
-# https://github.com/open-mmlab/mmdetection/blob/master/setup.py
-# https://github.com/Oneflow-Inc/libai/blob/main/setup.py
-# ------------------------------------------------------------------------------------------------
-
-import glob
-import os
-import subprocess
-
-import torch
-from setuptools import find_packages, setup
-from torch.utils.cpp_extension import CUDA_HOME, CppExtension, CUDAExtension
-
-# groundingdino version info
-version = "0.1.0"
-package_name = "groundingdino"
-cwd = os.path.dirname(os.path.abspath(__file__))
-
-
-sha = "Unknown"
-try:
- sha = subprocess.check_output(["git", "rev-parse", "HEAD"], cwd=cwd).decode("ascii").strip()
-except Exception:
- pass
-
-
-def write_version_file():
- version_path = os.path.join(cwd, "groundingdino", "version.py")
- with open(version_path, "w") as f:
- f.write(f"__version__ = '{version}'\n")
- # f.write(f"git_version = {repr(sha)}\n")
-
-
-requirements = ["torch", "torchvision"]
-
-torch_ver = [int(x) for x in torch.__version__.split(".")[:2]]
-
-
-def get_extensions():
- this_dir = os.path.dirname(os.path.abspath(__file__))
- extensions_dir = os.path.join(this_dir, "groundingdino", "models", "GroundingDINO", "csrc")
-
- main_source = os.path.join(extensions_dir, "vision.cpp")
- sources = glob.glob(os.path.join(extensions_dir, "**", "*.cpp"))
- source_cuda = glob.glob(os.path.join(extensions_dir, "**", "*.cu")) + glob.glob(
- os.path.join(extensions_dir, "*.cu")
- )
-
- sources = [main_source] + sources
-
- extension = CppExtension
-
- extra_compile_args = {"cxx": []}
- define_macros = []
-
- if torch.cuda.is_available() and CUDA_HOME is not None:
- print("Compiling with CUDA")
- extension = CUDAExtension
- sources += source_cuda
- define_macros += [("WITH_CUDA", None)]
- extra_compile_args["nvcc"] = [
- "-DCUDA_HAS_FP16=1",
- "-D__CUDA_NO_HALF_OPERATORS__",
- "-D__CUDA_NO_HALF_CONVERSIONS__",
- "-D__CUDA_NO_HALF2_OPERATORS__",
- ]
- else:
- print("Compiling without CUDA")
- define_macros += [("WITH_HIP", None)]
- extra_compile_args["nvcc"] = []
- return None
-
- sources = [os.path.join(extensions_dir, s) for s in sources]
- include_dirs = [extensions_dir]
-
- ext_modules = [
- extension(
- "groundingdino._C",
- sources,
- include_dirs=include_dirs,
- define_macros=define_macros,
- extra_compile_args=extra_compile_args,
- )
- ]
-
- return ext_modules
-
-
-def parse_requirements(fname="requirements.txt", with_version=True):
- """Parse the package dependencies listed in a requirements file but strips
- specific versioning information.
-
- Args:
- fname (str): path to requirements file
- with_version (bool, default=False): if True include version specs
-
- Returns:
- List[str]: list of requirements items
-
- CommandLine:
- python -c "import setup; print(setup.parse_requirements())"
- """
- import re
- import sys
- from os.path import exists
-
- require_fpath = fname
-
- def parse_line(line):
- """Parse information from a line in a requirements text file."""
- if line.startswith("-r "):
- # Allow specifying requirements in other files
- target = line.split(" ")[1]
- for info in parse_require_file(target):
- yield info
- else:
- info = {"line": line}
- if line.startswith("-e "):
- info["package"] = line.split("#egg=")[1]
- elif "@git+" in line:
- info["package"] = line
- else:
- # Remove versioning from the package
- pat = "(" + "|".join([">=", "==", ">"]) + ")"
- parts = re.split(pat, line, maxsplit=1)
- parts = [p.strip() for p in parts]
-
- info["package"] = parts[0]
- if len(parts) > 1:
- op, rest = parts[1:]
- if ";" in rest:
- # Handle platform specific dependencies
- # http://setuptools.readthedocs.io/en/latest/setuptools.html#declaring-platform-specific-dependencies
- version, platform_deps = map(str.strip, rest.split(";"))
- info["platform_deps"] = platform_deps
- else:
- version = rest # NOQA
- info["version"] = (op, version)
- yield info
-
- def parse_require_file(fpath):
- with open(fpath, "r") as f:
- for line in f.readlines():
- line = line.strip()
- if line and not line.startswith("#"):
- for info in parse_line(line):
- yield info
-
- def gen_packages_items():
- if exists(require_fpath):
- for info in parse_require_file(require_fpath):
- parts = [info["package"]]
- if with_version and "version" in info:
- parts.extend(info["version"])
- if not sys.version.startswith("3.4"):
- # apparently package_deps are broken in 3.4
- platform_deps = info.get("platform_deps")
- if platform_deps is not None:
- parts.append(";" + platform_deps)
- item = "".join(parts)
- yield item
-
- packages = list(gen_packages_items())
- return packages
-
-
-if __name__ == "__main__":
- print(f"Building wheel {package_name}-{version}")
-
- with open("LICENSE", "r", encoding="utf-8") as f:
- license = f.read()
-
- write_version_file()
-
- setup(
- name="groundingdino",
- version="0.1.0",
- author="International Digital Economy Academy, Shilong Liu",
- url="https://github.com/IDEA-Research/GroundingDINO",
- description="open-set object detector",
- license=license,
- install_requires=parse_requirements("requirements.txt"),
- packages=find_packages(
- exclude=(
- "configs",
- "tests",
- )
- ),
- ext_modules=get_extensions(),
- cmdclass={"build_ext": torch.utils.cpp_extension.BuildExtension},
- )
diff --git a/spaces/Marshalls/testmtd/analysis/aistplusplus_api/smpl/smpl_webuser/hello_world/hello_smpl.py b/spaces/Marshalls/testmtd/analysis/aistplusplus_api/smpl/smpl_webuser/hello_world/hello_smpl.py
deleted file mode 100644
index 37ee59a8842e6f216c2065a8495b396bb8eaa93a..0000000000000000000000000000000000000000
--- a/spaces/Marshalls/testmtd/analysis/aistplusplus_api/smpl/smpl_webuser/hello_world/hello_smpl.py
+++ /dev/null
@@ -1,64 +0,0 @@
-'''
-Copyright 2015 Matthew Loper, Naureen Mahmood and the Max Planck Gesellschaft. All rights reserved.
-This software is provided for research purposes only.
-By using this software you agree to the terms of the SMPL Model license here http://smpl.is.tue.mpg.de/license
-
-More information about SMPL is available here http://smpl.is.tue.mpg.
-For comments or questions, please email us at: smpl@tuebingen.mpg.de
-
-
-Please Note:
-============
-This is a demo version of the script for driving the SMPL model with python.
-We would be happy to receive comments, help and suggestions on improving this code
-and in making it available on more platforms.
-
-
-System Requirements:
-====================
-Operating system: OSX, Linux
-
-Python Dependencies:
-- Numpy & Scipy [http://www.scipy.org/scipylib/download.html]
-- Chumpy [https://github.com/mattloper/chumpy]
-
-
-About the Script:
-=================
-This script demonstrates a few basic functions to help users get started with using
-the SMPL model. The code shows how to:
- - Load the SMPL model
- - Edit pose & shape parameters of the model to create a new body in a new pose
- - Save the resulting body as a mesh in .OBJ format
-
-
-Running the Hello World code:
-=============================
-Inside Terminal, navigate to the smpl/webuser/hello_world directory. You can run
-the hello world script now by typing the following:
-> python hello_smpl.py
-
-'''
-
-from smpl_webuser.serialization import load_model
-import numpy as np
-
-## Load SMPL model (here we load the female model)
-## Make sure path is correct
-m = load_model( '../../models/basicModel_f_lbs_10_207_0_v1.0.0.pkl' )
-
-## Assign random pose and shape parameters
-m.pose[:] = np.random.rand(m.pose.size) * .2
-m.betas[:] = np.random.rand(m.betas.size) * .03
-
-## Write to an .obj file
-outmesh_path = './hello_smpl.obj'
-with open( outmesh_path, 'w') as fp:
- for v in m.r:
- fp.write( 'v %f %f %f\n' % ( v[0], v[1], v[2]) )
-
- for f in m.f+1: # Faces are 1-based, not 0-based in obj files
- fp.write( 'f %d %d %d\n' % (f[0], f[1], f[2]) )
-
-## Print message
-print '..Output mesh saved to: ', outmesh_path
diff --git a/spaces/Mashir0/pximg/index.js b/spaces/Mashir0/pximg/index.js
deleted file mode 100644
index b50c1be0f9391b05f06de99272433330995efab9..0000000000000000000000000000000000000000
--- a/spaces/Mashir0/pximg/index.js
+++ /dev/null
@@ -1,21 +0,0 @@
-const Koa = require('koa');
-const Router = require('koa-router');
-const { pixivReverseProxy } = require('./utils/pixiv');
-const indexMiddleware = require('./middleware/index');
-const faviconMiddleware = require('./middleware/favicon');
-const illustMiddleware = require('./middleware/illust');
-
-const PORT = process.env.PORT || 8080;
-
-const app = new Koa();
-const router = new Router();
-
-router
- .get('/', indexMiddleware)
- .get('/favicon.ico', faviconMiddleware)
- .get(/^(?:\/(original|regular|large|medium|small|thumb|mini))?\/(\d+)(?:\/(\d+))?/, illustMiddleware)
- .get(/.*/, ctx => pixivReverseProxy(ctx, ctx.path));
-
-app.use(router.routes()).use(router.allowedMethods()).listen(PORT);
-
-console.log(`Server is running at http://localhost:${PORT}`);
diff --git a/spaces/MathysL/AutoGPT4/.github/PULL_REQUEST_TEMPLATE.md b/spaces/MathysL/AutoGPT4/.github/PULL_REQUEST_TEMPLATE.md
deleted file mode 100644
index a4f28a3d27d66d79cb95f2b8b847832172bb5f11..0000000000000000000000000000000000000000
--- a/spaces/MathysL/AutoGPT4/.github/PULL_REQUEST_TEMPLATE.md
+++ /dev/null
@@ -1,40 +0,0 @@
-
-
-
-
-### Background
-
-
-### Changes
-
-
-### Documentation
-
-
-### Test Plan
-
-
-### PR Quality Checklist
-- [ ] My pull request is atomic and focuses on a single change.
-- [ ] I have thoroughly tested my changes with multiple different prompts.
-- [ ] I have considered potential risks and mitigations for my changes.
-- [ ] I have documented my changes clearly and comprehensively.
-- [ ] I have not snuck in any "extra" small tweaks changes
-
-
-
-
diff --git a/spaces/MirageML/sjc/voxnerf/vis.py b/spaces/MirageML/sjc/voxnerf/vis.py
deleted file mode 100644
index ae01ef9271e29e750881e6a51fe8758dc8178bcc..0000000000000000000000000000000000000000
--- a/spaces/MirageML/sjc/voxnerf/vis.py
+++ /dev/null
@@ -1,109 +0,0 @@
-from pathlib import Path
-import numpy as np
-import matplotlib.pyplot as plt
-from mpl_toolkits.axes_grid1 import ImageGrid
-from matplotlib.colors import Normalize, LogNorm
-import torch
-from torchvision.utils import make_grid
-from einops import rearrange
-from .data import blend_rgba
-
-import imageio
-
-from my.utils.plot import mpl_fig_to_buffer
-from my.utils.event import read_stats
-
-
-def vis(ref_img, pred_img, pred_depth, *, msg="", return_buffer=False):
- # plt the 2 images side by side and compare
- fig = plt.figure(figsize=(15, 6))
- grid = ImageGrid(
- fig, 111, nrows_ncols=(1, 3),
- cbar_location="right", cbar_mode="single",
- )
-
- grid[0].imshow(ref_img)
- grid[0].set_title("gt")
-
- grid[1].imshow(pred_img)
- grid[1].set_title(f"rendering {msg}")
-
- h = grid[2].imshow(pred_depth, norm=LogNorm(vmin=2, vmax=10), cmap="Spectral")
- grid[2].set_title("expected depth")
- plt.colorbar(h, cax=grid.cbar_axes[0])
- plt.tight_layout()
-
- if return_buffer:
- plot = mpl_fig_to_buffer(fig)
- return plot
- else:
- plt.show()
-
-
-def _bad_vis(pred_img, pred_depth, *, return_buffer=False):
- """emergency function for one-off use"""
- fig, grid = plt.subplots(1, 2, squeeze=True, figsize=(10, 6))
-
- grid[0].imshow(pred_img)
- grid[0].set_title("rendering")
-
- h = grid[1].imshow(pred_depth, norm=LogNorm(vmin=0.5, vmax=10), cmap="Spectral")
- grid[1].set_title("expected depth")
- # plt.colorbar(h, cax=grid.cbar_axes[0])
- plt.tight_layout()
-
- if return_buffer:
- plot = mpl_fig_to_buffer(fig)
- return plot
- else:
- plt.show()
-
-
-colormap = plt.get_cmap('Spectral')
-
-
-def bad_vis(pred_img, pred_depth, final_H=512):
- # pred_img = pred_img.cpu()
- depth = pred_depth.cpu().numpy()
- del pred_depth
-
- depth = np.log(1. + depth + 1e-12)
- depth = depth / np.log(1+10.)
- # depth = 1 - depth
- depth = colormap(depth)
- depth = blend_rgba(depth)
- depth = rearrange(depth, "h w c -> 1 c h w", c=3)
- depth = torch.from_numpy(depth)
-
- depth = torch.nn.functional.interpolate(
- depth, (final_H, final_H), mode='bilinear', antialias=True
- )
- pred_img = torch.nn.functional.interpolate(
- pred_img, (final_H, final_H), mode='bilinear', antialias=True
- )
- pred_img = (pred_img + 1) / 2
- pred_img = pred_img.clamp(0, 1).cpu()
- stacked = torch.cat([pred_img, depth], dim=0)
- pane = make_grid(stacked, nrow=2)
- pane = rearrange(pane, "c h w -> h w c")
- pane = (pane * 255.).clamp(0, 255)
- pane = pane.to(torch.uint8)
- pane = pane.numpy()
- # plt.imshow(pane)
- # plt.show()
- return pane
-
-
-def export_movie(seqs, fname, fps=30):
- fname = Path(fname)
- if fname.suffix == "":
- fname = fname.with_suffix(".mp4")
- writer = imageio.get_writer(fname, fps=fps)
- for img in seqs:
- writer.append_data(img)
- writer.close()
-
-
-def stitch_vis(save_fn, img_fnames, fps=10):
- figs = [imageio.imread(fn) for fn in img_fnames]
- export_movie(figs, save_fn, fps)
diff --git a/spaces/Mountchicken/MAERec-Gradio/mmocr/datasets/preparers/dumpers/lmdb_dumper.py b/spaces/Mountchicken/MAERec-Gradio/mmocr/datasets/preparers/dumpers/lmdb_dumper.py
deleted file mode 100644
index 9cd49d17ff17a8224e16284669e3d1206e0463ca..0000000000000000000000000000000000000000
--- a/spaces/Mountchicken/MAERec-Gradio/mmocr/datasets/preparers/dumpers/lmdb_dumper.py
+++ /dev/null
@@ -1,140 +0,0 @@
-# Copyright (c) OpenMMLab. All rights reserved.
-import os.path as osp
-import warnings
-from typing import Dict, List
-
-import cv2
-import lmdb
-import mmengine
-import numpy as np
-
-from mmocr.registry import DATA_DUMPERS
-from .base import BaseDumper
-
-
-@DATA_DUMPERS.register_module()
-class TextRecogLMDBDumper(BaseDumper):
- """Text recognition LMDB format dataset dumper.
-
- Args:
- task (str): Task type. Options are 'textdet', 'textrecog',
- 'textspotter', and 'kie'. It is usually set automatically and users
- do not need to set it manually in config file in most cases.
- split (str): It' s the partition of the datasets. Options are 'train',
- 'val' or 'test'. It is usually set automatically and users do not
- need to set it manually in config file in most cases. Defaults to
- None.
- data_root (str): The root directory of the image and
- annotation. It is usually set automatically and users do not need
- to set it manually in config file in most cases. Defaults to None.
- batch_size (int): Number of files written to the cache each time.
- Defaults to 1000.
- encoding (str): Label encoding method. Defaults to 'utf-8'.
- lmdb_map_size (int): Maximum size database may grow to. Defaults to
- 1099511627776.
- verify (bool): Whether to check the validity of every image. Defaults
- to True.
- """
-
- def __init__(self,
- task: str,
- split: str,
- data_root: str,
- batch_size: int = 1000,
- encoding: str = 'utf-8',
- lmdb_map_size: int = 1099511627776,
- verify: bool = True) -> None:
- assert task == 'textrecog', \
- f'TextRecogLMDBDumper only works with textrecog, but got {task}'
- super().__init__(task=task, split=split, data_root=data_root)
- self.batch_size = batch_size
- self.encoding = encoding
- self.lmdb_map_size = lmdb_map_size
- self.verify = verify
-
- def check_image_is_valid(self, imageBin):
- if imageBin is None:
- return False
- imageBuf = np.frombuffer(imageBin, dtype=np.uint8)
- img = cv2.imdecode(imageBuf, cv2.IMREAD_GRAYSCALE)
- imgH, imgW = img.shape[0], img.shape[1]
- if imgH * imgW == 0:
- return False
- return True
-
- def write_cache(self, env, cache):
- with env.begin(write=True) as txn:
- cursor = txn.cursor()
- cursor.putmulti(cache, dupdata=False, overwrite=True)
-
- def parser_pack_instance(self, instance: Dict):
- """parser an packed MMOCR format textrecog instance.
- Args:
- instance (Dict): An packed MMOCR format textrecog instance.
- For example,
- {
- "instance": [
- {
- "text": "Hello"
- }
- ],
- "img_path": "img1.jpg"
- }
- """
- assert isinstance(instance,
- Dict), 'Element of data_list must be a dict'
- assert 'img_path' in instance and 'instances' in instance, \
- 'Element of data_list must have the following keys: ' \
- f'img_path and instances, but got {instance.keys()}'
- assert isinstance(instance['instances'], List) and len(
- instance['instances']) == 1
- assert 'text' in instance['instances'][0]
-
- img_path = instance['img_path']
- text = instance['instances'][0]['text']
- return img_path, text
-
- def dump(self, data: Dict) -> None:
- """Dump data to LMDB format."""
-
- # create lmdb env
- output_dirname = f'{self.task}_{self.split}.lmdb'
- output = osp.join(self.data_root, output_dirname)
- mmengine.mkdir_or_exist(output)
- env = lmdb.open(output, map_size=self.lmdb_map_size)
- # load data
- if 'data_list' not in data:
- raise ValueError('Dump data must have data_list key')
- data_list = data['data_list']
- cache = []
- # index start from 1
- cnt = 1
- n_samples = len(data_list)
- for d in data_list:
- # convert both images and labels to lmdb
- label_key = 'label-%09d'.encode(self.encoding) % cnt
- img_name, text = self.parser_pack_instance(d)
- img_path = osp.join(self.data_root, img_name)
- if not osp.exists(img_path):
- warnings.warn('%s does not exist' % img_path)
- continue
- with open(img_path, 'rb') as f:
- image_bin = f.read()
- if self.verify:
- if not self.check_image_is_valid(image_bin):
- warnings.warn('%s is not a valid image' % img_path)
- continue
- image_key = 'image-%09d'.encode(self.encoding) % cnt
- cache.append((image_key, image_bin))
- cache.append((label_key, text.encode(self.encoding)))
-
- if cnt % self.batch_size == 0:
- self.write_cache(env, cache)
- cache = []
- print('Written %d / %d' % (cnt, n_samples))
- cnt += 1
- n_samples = cnt - 1
- cache.append(('num-samples'.encode(self.encoding),
- str(n_samples).encode(self.encoding)))
- self.write_cache(env, cache)
- print('Created lmdb dataset with %d samples' % n_samples)
diff --git a/spaces/Mountchicken/MAERec-Gradio/mmocr/models/textdet/module_losses/pan_module_loss.py b/spaces/Mountchicken/MAERec-Gradio/mmocr/models/textdet/module_losses/pan_module_loss.py
deleted file mode 100644
index 6a5a6685aa9514f5d9afbfbe9b5a7fe4029ab96d..0000000000000000000000000000000000000000
--- a/spaces/Mountchicken/MAERec-Gradio/mmocr/models/textdet/module_losses/pan_module_loss.py
+++ /dev/null
@@ -1,347 +0,0 @@
-# Copyright (c) OpenMMLab. All rights reserved.
-import warnings
-from typing import Dict, Sequence, Tuple, Union
-
-import numpy as np
-import torch
-import torch.nn.functional as F
-from mmdet.models.utils import multi_apply
-from torch import nn
-
-from mmocr.registry import MODELS
-from mmocr.structures import TextDetDataSample
-from .seg_based_module_loss import SegBasedModuleLoss
-
-
-@MODELS.register_module()
-class PANModuleLoss(SegBasedModuleLoss):
- """The class for implementing PANet loss. This was partially adapted from
- https://github.com/whai362/pan_pp.pytorch and
- https://github.com/WenmuZhou/PAN.pytorch.
-
- PANet: `Efficient and Accurate Arbitrary-
- Shaped Text Detection with Pixel Aggregation Network
- `_.
-
- Args:
- loss_text (dict) The loss config for text map. Defaults to
- dict(type='MaskedSquareDiceLoss').
- loss_kernel (dict) The loss config for kernel map. Defaults to
- dict(type='MaskedSquareDiceLoss').
- loss_embedding (dict) The loss config for embedding map. Defaults to
- dict(type='PANEmbLossV1').
- weight_text (float): The weight of text loss. Defaults to 1.
- weight_kernel (float): The weight of kernel loss. Defaults to 0.5.
- weight_embedding (float): The weight of embedding loss.
- Defaults to 0.25.
- ohem_ratio (float): The negative/positive ratio in ohem. Defaults to 3.
- shrink_ratio (tuple[float]) : The ratio of shrinking kernel. Defaults
- to (1.0, 0.5).
- max_shrink_dist (int or float): The maximum shrinking distance.
- Defaults to 20.
- reduction (str): The way to reduce the loss. Available options are
- "mean" and "sum". Defaults to 'mean'.
- """
-
- def __init__(
- self,
- loss_text: Dict = dict(type='MaskedSquareDiceLoss'),
- loss_kernel: Dict = dict(type='MaskedSquareDiceLoss'),
- loss_embedding: Dict = dict(type='PANEmbLossV1'),
- weight_text: float = 1.0,
- weight_kernel: float = 0.5,
- weight_embedding: float = 0.25,
- ohem_ratio: Union[int, float] = 3, # TODO Find a better name
- shrink_ratio: Sequence[Union[int, float]] = (1.0, 0.5),
- max_shrink_dist: Union[int, float] = 20,
- reduction: str = 'mean') -> None:
- super().__init__()
- assert reduction in ['mean', 'sum'], "reduction must in ['mean','sum']"
- self.weight_text = weight_text
- self.weight_kernel = weight_kernel
- self.weight_embedding = weight_embedding
- self.shrink_ratio = shrink_ratio
- self.ohem_ratio = ohem_ratio
- self.reduction = reduction
- self.max_shrink_dist = max_shrink_dist
- self.loss_text = MODELS.build(loss_text)
- self.loss_kernel = MODELS.build(loss_kernel)
- self.loss_embedding = MODELS.build(loss_embedding)
-
- def forward(self, preds: torch.Tensor,
- data_samples: Sequence[TextDetDataSample]) -> Dict:
- """Compute PAN loss.
-
- Args:
- preds (dict): Raw predictions from model with
- shape :math:`(N, C, H, W)`.
- data_samples (list[TextDetDataSample]): The data samples.
-
- Returns:
- dict: The dict for pan losses with loss_text, loss_kernel,
- loss_aggregation and loss_discrimination.
- """
-
- gt_kernels, gt_masks = self.get_targets(data_samples)
- target_size = gt_kernels.size()[2:]
- preds = F.interpolate(preds, size=target_size, mode='bilinear')
- pred_texts = preds[:, 0, :, :]
- pred_kernels = preds[:, 1, :, :]
- inst_embed = preds[:, 2:, :, :]
- gt_kernels = gt_kernels.to(preds.device)
- gt_masks = gt_masks.to(preds.device)
-
- # compute embedding loss
- loss_emb = self.loss_embedding(inst_embed, gt_kernels[0],
- gt_kernels[1], gt_masks)
- gt_kernels[gt_kernels <= 0.5] = 0
- gt_kernels[gt_kernels > 0.5] = 1
- # compute text loss
- sampled_mask = self._ohem_batch(pred_texts.detach(), gt_kernels[0],
- gt_masks)
- pred_texts = torch.sigmoid(pred_texts)
- loss_texts = self.loss_text(pred_texts, gt_kernels[0], sampled_mask)
-
- # compute kernel loss
- pred_kernels = torch.sigmoid(pred_kernels)
- sampled_masks_kernel = (gt_kernels[0] > 0.5).float() * gt_masks
- loss_kernels = self.loss_kernel(pred_kernels, gt_kernels[1],
- sampled_masks_kernel)
-
- losses = [loss_texts, loss_kernels, loss_emb]
- if self.reduction == 'mean':
- losses = [item.mean() for item in losses]
- else:
- losses = [item.sum() for item in losses]
-
- results = dict()
- results.update(
- loss_text=self.weight_text * losses[0],
- loss_kernel=self.weight_kernel * losses[1],
- loss_embedding=self.weight_embedding * losses[2])
- return results
-
- def get_targets(
- self,
- data_samples: Sequence[TextDetDataSample],
- ) -> Tuple[torch.Tensor, torch.Tensor]:
- """Generate the gt targets for PANet.
-
- Args:
- results (dict): The input result dictionary.
-
- Returns:
- results (dict): The output result dictionary.
- """
- gt_kernels, gt_masks = multi_apply(self._get_target_single,
- data_samples)
- # gt_kernels: (N, kernel_number, H, W)->(kernel_number, N, H, W)
- gt_kernels = torch.stack(gt_kernels, dim=0).permute(1, 0, 2, 3)
- gt_masks = torch.stack(gt_masks, dim=0)
- return gt_kernels, gt_masks
-
- def _get_target_single(self, data_sample: TextDetDataSample
- ) -> Tuple[torch.Tensor, torch.Tensor]:
- """Generate loss target from a data sample.
-
- Args:
- data_sample (TextDetDataSample): The data sample.
-
- Returns:
- tuple: A tuple of four tensors as the targets of one prediction.
- """
- gt_polygons = data_sample.gt_instances.polygons
- gt_ignored = data_sample.gt_instances.ignored
-
- gt_kernels = []
- for ratio in self.shrink_ratio:
- # TODO pass `gt_ignored` to `_generate_kernels`
- gt_kernel, _ = self._generate_kernels(
- data_sample.img_shape,
- gt_polygons,
- ratio,
- ignore_flags=None,
- max_shrink_dist=self.max_shrink_dist)
- gt_kernels.append(gt_kernel)
- gt_polygons_ignored = data_sample.gt_instances[gt_ignored].polygons
- gt_mask = self._generate_effective_mask(data_sample.img_shape,
- gt_polygons_ignored)
-
- gt_kernels = np.stack(gt_kernels, axis=0)
- gt_kernels = torch.from_numpy(gt_kernels).float()
- gt_mask = torch.from_numpy(gt_mask).float()
- return gt_kernels, gt_mask
-
- def _ohem_batch(self, text_scores: torch.Tensor, gt_texts: torch.Tensor,
- gt_mask: torch.Tensor) -> torch.Tensor:
- """OHEM sampling for a batch of imgs.
-
- Args:
- text_scores (Tensor): The text scores of size :math:`(H, W)`.
- gt_texts (Tensor): The gt text masks of size :math:`(H, W)`.
- gt_mask (Tensor): The gt effective mask of size :math:`(H, W)`.
-
- Returns:
- Tensor: The sampled mask of size :math:`(H, W)`.
- """
- assert isinstance(text_scores, torch.Tensor)
- assert isinstance(gt_texts, torch.Tensor)
- assert isinstance(gt_mask, torch.Tensor)
- assert len(text_scores.shape) == 3
- assert text_scores.shape == gt_texts.shape
- assert gt_texts.shape == gt_mask.shape
-
- sampled_masks = []
- for i in range(text_scores.shape[0]):
- sampled_masks.append(
- self._ohem_single(text_scores[i], gt_texts[i], gt_mask[i]))
-
- sampled_masks = torch.stack(sampled_masks)
-
- return sampled_masks
-
- def _ohem_single(self, text_score: torch.Tensor, gt_text: torch.Tensor,
- gt_mask: torch.Tensor) -> torch.Tensor:
- """Sample the top-k maximal negative samples and all positive samples.
-
- Args:
- text_score (Tensor): The text score of size :math:`(H, W)`.
- gt_text (Tensor): The ground truth text mask of size
- :math:`(H, W)`.
- gt_mask (Tensor): The effective region mask of size :math:`(H, W)`.
-
- Returns:
- Tensor: The sampled pixel mask of size :math:`(H, W)`.
- """
- assert isinstance(text_score, torch.Tensor)
- assert isinstance(gt_text, torch.Tensor)
- assert isinstance(gt_mask, torch.Tensor)
- assert len(text_score.shape) == 2
- assert text_score.shape == gt_text.shape
- assert gt_text.shape == gt_mask.shape
-
- pos_num = (int)(torch.sum(gt_text > 0.5).item()) - (int)(
- torch.sum((gt_text > 0.5) * (gt_mask <= 0.5)).item())
- neg_num = (int)(torch.sum(gt_text <= 0.5).item())
- neg_num = (int)(min(pos_num * self.ohem_ratio, neg_num))
-
- if pos_num == 0 or neg_num == 0:
- warnings.warn('pos_num = 0 or neg_num = 0')
- return gt_mask.bool()
-
- neg_score = text_score[gt_text <= 0.5]
- neg_score_sorted, _ = torch.sort(neg_score, descending=True)
- threshold = neg_score_sorted[neg_num - 1]
- sampled_mask = (((text_score >= threshold) + (gt_text > 0.5)) > 0) * (
- gt_mask > 0.5)
- return sampled_mask
-
-
-@MODELS.register_module()
-class PANEmbLossV1(nn.Module):
- """The class for implementing EmbLossV1. This was partially adapted from
- https://github.com/whai362/pan_pp.pytorch.
-
- Args:
- feature_dim (int): The dimension of the feature. Defaults to 4.
- delta_aggregation (float): The delta for aggregation. Defaults to 0.5.
- delta_discrimination (float): The delta for discrimination.
- Defaults to 1.5.
- """
-
- def __init__(self,
- feature_dim: int = 4,
- delta_aggregation: float = 0.5,
- delta_discrimination: float = 1.5) -> None:
- super().__init__()
- self.feature_dim = feature_dim
- self.delta_aggregation = delta_aggregation
- self.delta_discrimination = delta_discrimination
- self.weights = (1.0, 1.0)
-
- def _forward_single(self, emb: torch.Tensor, instance: torch.Tensor,
- kernel: torch.Tensor,
- training_mask: torch.Tensor) -> torch.Tensor:
- """Compute the loss for a single image.
-
- Args:
- emb (torch.Tensor): The embedding feature.
- instance (torch.Tensor): The instance feature.
- kernel (torch.Tensor): The kernel feature.
- training_mask (torch.Tensor): The effective mask.
- """
- training_mask = (training_mask > 0.5).float()
- kernel = (kernel > 0.5).float()
- instance = instance * training_mask
- instance_kernel = (instance * kernel).view(-1)
- instance = instance.view(-1)
- emb = emb.view(self.feature_dim, -1)
-
- unique_labels, unique_ids = torch.unique(
- instance_kernel, sorted=True, return_inverse=True)
- num_instance = unique_labels.size(0)
- if num_instance <= 1:
- return 0
-
- emb_mean = emb.new_zeros((self.feature_dim, num_instance),
- dtype=torch.float32)
- for i, lb in enumerate(unique_labels):
- if lb == 0:
- continue
- ind_k = instance_kernel == lb
- emb_mean[:, i] = torch.mean(emb[:, ind_k], dim=1)
-
- l_agg = emb.new_zeros(num_instance, dtype=torch.float32)
- for i, lb in enumerate(unique_labels):
- if lb == 0:
- continue
- ind = instance == lb
- emb_ = emb[:, ind]
- dist = (emb_ - emb_mean[:, i:i + 1]).norm(p=2, dim=0)
- dist = F.relu(dist - self.delta_aggregation)**2
- l_agg[i] = torch.mean(torch.log(dist + 1.0))
- l_agg = torch.mean(l_agg[1:])
-
- if num_instance > 2:
- emb_interleave = emb_mean.permute(1, 0).repeat(num_instance, 1)
- emb_band = emb_mean.permute(1, 0).repeat(1, num_instance).view(
- -1, self.feature_dim)
-
- mask = (1 - torch.eye(num_instance, dtype=torch.int8)).view(
- -1, 1).repeat(1, self.feature_dim)
- mask = mask.view(num_instance, num_instance, -1)
- mask[0, :, :] = 0
- mask[:, 0, :] = 0
- mask = mask.view(num_instance * num_instance, -1)
-
- dist = emb_interleave - emb_band
- dist = dist[mask > 0].view(-1, self.feature_dim).norm(p=2, dim=1)
- dist = F.relu(2 * self.delta_discrimination - dist)**2
- l_dis = torch.mean(torch.log(dist + 1.0))
- else:
- l_dis = 0
-
- l_agg = self.weights[0] * l_agg
- l_dis = self.weights[1] * l_dis
- l_reg = torch.mean(torch.log(torch.norm(emb_mean, 2, 0) + 1.0)) * 0.001
- loss = l_agg + l_dis + l_reg
- return loss
-
- def forward(self, emb: torch.Tensor, instance: torch.Tensor,
- kernel: torch.Tensor,
- training_mask: torch.Tensor) -> torch.Tensor:
- """Compute the loss for a batch image.
-
- Args:
- emb (torch.Tensor): The embedding feature.
- instance (torch.Tensor): The instance feature.
- kernel (torch.Tensor): The kernel feature.
- training_mask (torch.Tensor): The effective mask.
- """
- loss_batch = emb.new_zeros((emb.size(0)), dtype=torch.float32)
-
- for i in range(loss_batch.size(0)):
- loss_batch[i] = self._forward_single(emb[i], instance[i],
- kernel[i], training_mask[i])
-
- return loss_batch
diff --git a/spaces/MrBodean/VoiceClone/encoder/data_objects/speaker_batch.py b/spaces/MrBodean/VoiceClone/encoder/data_objects/speaker_batch.py
deleted file mode 100644
index 56651dba5804a0c59c334e49ac18f8f5a4bfa444..0000000000000000000000000000000000000000
--- a/spaces/MrBodean/VoiceClone/encoder/data_objects/speaker_batch.py
+++ /dev/null
@@ -1,12 +0,0 @@
-import numpy as np
-from typing import List
-from encoder.data_objects.speaker import Speaker
-
-class SpeakerBatch:
- def __init__(self, speakers: List[Speaker], utterances_per_speaker: int, n_frames: int):
- self.speakers = speakers
- self.partials = {s: s.random_partial(utterances_per_speaker, n_frames) for s in speakers}
-
- # Array of shape (n_speakers * n_utterances, n_frames, mel_n), e.g. for 3 speakers with
- # 4 utterances each of 160 frames of 40 mel coefficients: (12, 160, 40)
- self.data = np.array([frames for s in speakers for _, frames, _ in self.partials[s]])
diff --git a/spaces/NCTCMumbai/NCTC/models/official/nlp/transformer/utils/tokenizer_test.py b/spaces/NCTCMumbai/NCTC/models/official/nlp/transformer/utils/tokenizer_test.py
deleted file mode 100644
index 307398fd3aeaf55a5bec495006a1fb65ebadd639..0000000000000000000000000000000000000000
--- a/spaces/NCTCMumbai/NCTC/models/official/nlp/transformer/utils/tokenizer_test.py
+++ /dev/null
@@ -1,204 +0,0 @@
-# Copyright 2018 The TensorFlow Authors. All Rights Reserved.
-#
-# Licensed under the Apache License, Version 2.0 (the "License");
-# you may not use this file except in compliance with the License.
-# You may obtain a copy of the License at
-#
-# http://www.apache.org/licenses/LICENSE-2.0
-#
-# Unless required by applicable law or agreed to in writing, software
-# distributed under the License is distributed on an "AS IS" BASIS,
-# WITHOUT WARRANTIES OR CONDITIONS OF ANY KIND, either express or implied.
-# See the License for the specific language governing permissions and
-# limitations under the License.
-# ==============================================================================
-"""Test Subtokenizer and string helper methods."""
-
-import collections
-import tempfile
-
-import tensorflow as tf
-
-from official.nlp.transformer.utils import tokenizer
-
-
-class SubtokenizerTest(tf.test.TestCase):
-
- def _init_subtokenizer(self, vocab_list):
- temp_file = tempfile.NamedTemporaryFile(delete=False)
- with tf.io.gfile.GFile(temp_file.name, "w") as w:
- for subtoken in vocab_list:
- w.write("'%s'" % subtoken)
- w.write("\n")
- return tokenizer.Subtokenizer(temp_file.name, reserved_tokens=[])
-
- def test_encode(self):
- vocab_list = ["123_", "test", "ing_"]
- subtokenizer = self._init_subtokenizer(vocab_list)
- s = "testing 123"
- encoded_list = subtokenizer.encode(s)
- self.assertEqual([1, 2, 0], encoded_list)
-
- def test_decode(self):
- vocab_list = ["123_", "test", "ing_"]
- subtokenizer = self._init_subtokenizer(vocab_list)
- encoded_list = [1, 2, 0] # testing 123
- decoded_str = subtokenizer.decode(encoded_list)
- self.assertEqual("testing 123", decoded_str)
-
- def test_subtoken_ids_to_tokens(self):
- vocab_list = ["123_", "test", "ing_"]
- subtokenizer = self._init_subtokenizer(vocab_list)
- encoded_list = [1, 2, 0] # testing 123
- token_list = subtokenizer._subtoken_ids_to_tokens(encoded_list)
- self.assertEqual([u"testing", u"123"], token_list)
-
-
-class StringHelperTest(tf.test.TestCase):
-
- def test_split_string_to_tokens(self):
- text = "test? testing 123."
-
- tokens = tokenizer._split_string_to_tokens(text,
- tokenizer._ALPHANUMERIC_CHAR_SET)
- self.assertEqual(["test", "? ", "testing", "123", "."], tokens)
-
- def test_join_tokens_to_string(self):
- tokens = ["test", "? ", "testing", "123", "."]
-
- s = tokenizer._join_tokens_to_string(tokens,
- tokenizer._ALPHANUMERIC_CHAR_SET)
- self.assertEqual("test? testing 123.", s)
-
- def test_escape_token(self):
- token = u"abc_\\4"
- alphabet = set("abc_\\u;")
-
- escaped_token = tokenizer._escape_token(token, alphabet)
- self.assertEqual("abc\\u\\\\\\52;_", escaped_token)
-
- def test_unescape_token(self):
- escaped_token = u"Underline: \\u, Backslash: \\\\, Unicode: \\52;"
-
- unescaped_token = tokenizer._unescape_token(escaped_token)
- self.assertEqual("Underline: _, Backslash: \\, Unicode: 4", unescaped_token)
-
- def test_list_to_index_dict(self):
- lst = ["test", "strings"]
-
- d = tokenizer._list_to_index_dict(lst)
- self.assertDictEqual({"test": 0, "strings": 1}, d)
-
- def test_split_token_to_subtokens(self):
- token = "abc"
- subtoken_dict = {"a": 0, "b": 1, "c": 2, "ab": 3}
- max_subtoken_length = 2
-
- subtokens = tokenizer._split_token_to_subtokens(token, subtoken_dict,
- max_subtoken_length)
- self.assertEqual(["ab", "c"], subtokens)
-
- def test_generate_alphabet_dict(self):
- s = ["testing", "123"]
- reserved_tokens = ["???"]
-
- alphabet = tokenizer._generate_alphabet_dict(s, reserved_tokens)
- self.assertIn("?", alphabet)
- self.assertIn("t", alphabet)
- self.assertIn("e", alphabet)
- self.assertIn("s", alphabet)
- self.assertIn("i", alphabet)
- self.assertIn("n", alphabet)
- self.assertIn("g", alphabet)
- self.assertIn("1", alphabet)
- self.assertIn("2", alphabet)
- self.assertIn("3", alphabet)
-
- def test_count_and_gen_subtokens(self):
- token_counts = {"abc": 5}
- alphabet = set("abc_")
- subtoken_dict = {"a": 0, "b": 1, "c": 2, "_": 3}
- max_subtoken_length = 2
-
- subtoken_counts = tokenizer._count_and_gen_subtokens(
- token_counts, alphabet, subtoken_dict, max_subtoken_length)
-
- self.assertIsInstance(subtoken_counts, collections.defaultdict)
- self.assertDictEqual(
- {
- "a": 5,
- "b": 5,
- "c": 5,
- "_": 5,
- "ab": 5,
- "bc": 5,
- "c_": 5,
- "abc": 5,
- "bc_": 5,
- "abc_": 5
- }, subtoken_counts)
-
- def test_filter_and_bucket_subtokens(self):
- subtoken_counts = collections.defaultdict(int, {
- "a": 2,
- "b": 4,
- "c": 1,
- "ab": 6,
- "ac": 3,
- "abbc": 5
- })
- min_count = 3
-
- subtoken_buckets = tokenizer._filter_and_bucket_subtokens(
- subtoken_counts, min_count)
-
- self.assertEqual(len(subtoken_buckets[0]), 0)
- self.assertEqual(set("b"), subtoken_buckets[1])
- self.assertEqual(set(["ab", "ac"]), subtoken_buckets[2])
- self.assertEqual(len(subtoken_buckets[3]), 0)
- self.assertEqual(set(["abbc"]), subtoken_buckets[4])
-
- def test_gen_new_subtoken_list(self):
- subtoken_counts = collections.defaultdict(int, {
- "translate": 10,
- "t": 40,
- "tr": 16,
- "tra": 12
- })
- min_count = 5
- alphabet = set("translate")
- reserved_tokens = ["reserved", "tokens"]
-
- subtoken_list, max_token_length = tokenizer._gen_new_subtoken_list(
- subtoken_counts, min_count, alphabet, reserved_tokens)
-
- # Check that "tra" isn"t in the list (its count should be decremented to 2,
- # so it should not be added to the canddiate list).
- self.assertNotIn("tra", subtoken_list)
-
- self.assertIn("tr", subtoken_list)
- self.assertIn("t", subtoken_list)
-
- self.assertEqual(len("translate"), max_token_length)
-
- def test_generate_subtokens(self):
- token_counts = {"ab": 1, "bc": 3, "abc": 5}
- alphabet = set("abc_")
- min_count = 100
- num_iterations = 1
- reserved_tokens = ["reserved", "tokens"]
-
- vocab_list = tokenizer._generate_subtokens(token_counts, alphabet,
- min_count, num_iterations,
- reserved_tokens)
-
- # Check that reserved tokens are at the front of the list
- self.assertEqual(vocab_list[:2], reserved_tokens)
-
- # Check that each character in alphabet is in the vocab list
- for c in alphabet:
- self.assertIn(c, vocab_list)
-
-
-if __name__ == "__main__":
- tf.test.main()
diff --git a/spaces/NeuralInternet/Text-Generation_Playground/extensions/elevenlabs_tts/script.py b/spaces/NeuralInternet/Text-Generation_Playground/extensions/elevenlabs_tts/script.py
deleted file mode 100644
index 90d61efc6aa77bc2377c435eefe4cf623b588168..0000000000000000000000000000000000000000
--- a/spaces/NeuralInternet/Text-Generation_Playground/extensions/elevenlabs_tts/script.py
+++ /dev/null
@@ -1,113 +0,0 @@
-from pathlib import Path
-
-import gradio as gr
-from elevenlabslib import *
-from elevenlabslib.helpers import *
-
-params = {
- 'activate': True,
- 'api_key': '12345',
- 'selected_voice': 'None',
-}
-
-initial_voice = ['None']
-wav_idx = 0
-user = ElevenLabsUser(params['api_key'])
-user_info = None
-
-
-# Check if the API is valid and refresh the UI accordingly.
-def check_valid_api():
-
- global user, user_info, params
-
- user = ElevenLabsUser(params['api_key'])
- user_info = user._get_subscription_data()
- print('checking api')
- if params['activate'] == False:
- return gr.update(value='Disconnected')
- elif user_info is None:
- print('Incorrect API Key')
- return gr.update(value='Disconnected')
- else:
- print('Got an API Key!')
- return gr.update(value='Connected')
-
-# Once the API is verified, get the available voices and update the dropdown list
-def refresh_voices():
-
- global user, user_info
-
- your_voices = [None]
- if user_info is not None:
- for voice in user.get_available_voices():
- your_voices.append(voice.initialName)
- return gr.Dropdown.update(choices=your_voices)
- else:
- return
-
-def remove_surrounded_chars(string):
- new_string = ""
- in_star = False
- for char in string:
- if char == '*':
- in_star = not in_star
- elif not in_star:
- new_string += char
- return new_string
-
-def input_modifier(string):
- """
- This function is applied to your text inputs before
- they are fed into the model.
- """
-
- return string
-
-def output_modifier(string):
- """
- This function is applied to the model outputs.
- """
-
- global params, wav_idx, user, user_info
-
- if params['activate'] == False:
- return string
- elif user_info == None:
- return string
-
- string = remove_surrounded_chars(string)
- string = string.replace('"', '')
- string = string.replace('“', '')
- string = string.replace('\n', ' ')
- string = string.strip()
-
- if string == '':
- string = 'empty reply, try regenerating'
-
- output_file = Path(f'extensions/elevenlabs_tts/outputs/{wav_idx:06d}.wav'.format(wav_idx))
- voice = user.get_voices_by_name(params['selected_voice'])[0]
- audio_data = voice.generate_audio_bytes(string)
- save_bytes_to_path(Path(f'extensions/elevenlabs_tts/outputs/{wav_idx:06d}.wav'), audio_data)
-
- string = f''
- wav_idx += 1
- return string
-
-def ui():
-
- # Gradio elements
- with gr.Row():
- activate = gr.Checkbox(value=params['activate'], label='Activate TTS')
- connection_status = gr.Textbox(value='Disconnected', label='Connection Status')
- voice = gr.Dropdown(value=params['selected_voice'], choices=initial_voice, label='TTS Voice')
- with gr.Row():
- api_key = gr.Textbox(placeholder="Enter your API key.", label='API Key')
- connect = gr.Button(value='Connect')
-
- # Event functions to update the parameters in the backend
- activate.change(lambda x: params.update({'activate': x}), activate, None)
- voice.change(lambda x: params.update({'selected_voice': x}), voice, None)
- api_key.change(lambda x: params.update({'api_key': x}), api_key, None)
- connect.click(check_valid_api, [], connection_status)
- connect.click(refresh_voices, [], voice)
diff --git a/spaces/Nyari/Super-Resolution-Anime-Diffusion/RealESRGANv030/README.md b/spaces/Nyari/Super-Resolution-Anime-Diffusion/RealESRGANv030/README.md
deleted file mode 100644
index 118e930c12bffd9e6da1df03180f5c9a8dcaabc3..0000000000000000000000000000000000000000
--- a/spaces/Nyari/Super-Resolution-Anime-Diffusion/RealESRGANv030/README.md
+++ /dev/null
@@ -1,272 +0,0 @@
-
-
-🔥 **AnimeVideo-v3 model (动漫视频小模型)**. Please see [[*anime video models*](docs/anime_video_model.md)] and [[*comparisons*](docs/anime_comparisons.md)]
-🔥 **RealESRGAN_x4plus_anime_6B** for anime images **(动漫插图模型)**. Please see [[*anime_model*](docs/anime_model.md)]
-
-
-1. :boom: **Update** online Replicate demo: [](https://replicate.com/xinntao/realesrgan)
-1. Online Colab demo for Real-ESRGAN: [](https://colab.research.google.com/drive/1k2Zod6kSHEvraybHl50Lys0LerhyTMCo?usp=sharing) **|** Online Colab demo for for Real-ESRGAN (**anime videos**): [](https://colab.research.google.com/drive/1yNl9ORUxxlL4N0keJa2SEPB61imPQd1B?usp=sharing)
-1. Portable [Windows](https://github.com/xinntao/Real-ESRGAN/releases/download/v0.2.5.0/realesrgan-ncnn-vulkan-20220424-windows.zip) / [Linux](https://github.com/xinntao/Real-ESRGAN/releases/download/v0.2.5.0/realesrgan-ncnn-vulkan-20220424-ubuntu.zip) / [MacOS](https://github.com/xinntao/Real-ESRGAN/releases/download/v0.2.5.0/realesrgan-ncnn-vulkan-20220424-macos.zip) **executable files for Intel/AMD/Nvidia GPU**. You can find more information [here](#portable-executable-files-ncnn). The ncnn implementation is in [Real-ESRGAN-ncnn-vulkan](https://github.com/xinntao/Real-ESRGAN-ncnn-vulkan)
-
-
-Real-ESRGAN aims at developing **Practical Algorithms for General Image/Video Restoration**.
-We extend the powerful ESRGAN to a practical restoration application (namely, Real-ESRGAN), which is trained with pure synthetic data.
-
-🌌 Thanks for your valuable feedbacks/suggestions. All the feedbacks are updated in [feedback.md](docs/feedback.md).
-
----
-
-If Real-ESRGAN is helpful, please help to ⭐ this repo or recommend it to your friends 😊
-Other recommended projects:
-▶️ [GFPGAN](https://github.com/TencentARC/GFPGAN): A practical algorithm for real-world face restoration
-▶️ [BasicSR](https://github.com/xinntao/BasicSR): An open-source image and video restoration toolbox
-▶️ [facexlib](https://github.com/xinntao/facexlib): A collection that provides useful face-relation functions.
-▶️ [HandyView](https://github.com/xinntao/HandyView): A PyQt5-based image viewer that is handy for view and comparison
-▶️ [HandyFigure](https://github.com/xinntao/HandyFigure): Open source of paper figures
-
----
-
-### 📖 Real-ESRGAN: Training Real-World Blind Super-Resolution with Pure Synthetic Data
-
-> [[Paper](https://arxiv.org/abs/2107.10833)] [[YouTube Video](https://www.youtube.com/watch?v=fxHWoDSSvSc)] [[B站讲解](https://www.bilibili.com/video/BV1H34y1m7sS/)] [[Poster](https://xinntao.github.io/projects/RealESRGAN_src/RealESRGAN_poster.pdf)] [[PPT slides](https://docs.google.com/presentation/d/1QtW6Iy8rm8rGLsJ0Ldti6kP-7Qyzy6XL/edit?usp=sharing&ouid=109799856763657548160&rtpof=true&sd=true)]
-> [Xintao Wang](https://xinntao.github.io/), Liangbin Xie, [Chao Dong](https://scholar.google.com.hk/citations?user=OSDCB0UAAAAJ), [Ying Shan](https://scholar.google.com/citations?user=4oXBp9UAAAAJ&hl=en)
-> [Tencent ARC Lab](https://arc.tencent.com/en/ai-demos/imgRestore); Shenzhen Institutes of Advanced Technology, Chinese Academy of Sciences
-
-
-
-
-
----
-
-
-## 🚩 Updates
-
-- ✅ Add the **realesr-general-x4v3** model - a tiny small model for general scenes. It also supports the **--dn** option to balance the noise (avoiding over-smooth results). **--dn** is short for denoising strength.
-- ✅ Update the **RealESRGAN AnimeVideo-v3** model. Please see [anime video models](docs/anime_video_model.md) and [comparisons](docs/anime_comparisons.md) for more details.
-- ✅ Add small models for anime videos. More details are in [anime video models](docs/anime_video_model.md).
-- ✅ Add the ncnn implementation [Real-ESRGAN-ncnn-vulkan](https://github.com/xinntao/Real-ESRGAN-ncnn-vulkan).
-- ✅ Add [*RealESRGAN_x4plus_anime_6B.pth*](https://github.com/xinntao/Real-ESRGAN/releases/download/v0.2.2.4/RealESRGAN_x4plus_anime_6B.pth), which is optimized for **anime** images with much smaller model size. More details and comparisons with [waifu2x](https://github.com/nihui/waifu2x-ncnn-vulkan) are in [**anime_model.md**](docs/anime_model.md)
-- ✅ Support finetuning on your own data or paired data (*i.e.*, finetuning ESRGAN). See [here](docs/Training.md#Finetune-Real-ESRGAN-on-your-own-dataset)
-- ✅ Integrate [GFPGAN](https://github.com/TencentARC/GFPGAN) to support **face enhancement**.
-- ✅ Integrated to [Huggingface Spaces](https://huggingface.co/spaces) with [Gradio](https://github.com/gradio-app/gradio). See [Gradio Web Demo](https://huggingface.co/spaces/akhaliq/Real-ESRGAN). Thanks [@AK391](https://github.com/AK391)
-- ✅ Support arbitrary scale with `--outscale` (It actually further resizes outputs with `LANCZOS4`). Add *RealESRGAN_x2plus.pth* model.
-- ✅ [The inference code](inference_realesrgan.py) supports: 1) **tile** options; 2) images with **alpha channel**; 3) **gray** images; 4) **16-bit** images.
-- ✅ The training codes have been released. A detailed guide can be found in [Training.md](docs/Training.md).
-
----
-
-
-## 👀 Demos Videos
-
-#### Bilibili
-
-- [大闹天宫片段](https://www.bilibili.com/video/BV1ja41117zb)
-- [Anime dance cut 动漫魔性舞蹈](https://www.bilibili.com/video/BV1wY4y1L7hT/)
-- [海贼王片段](https://www.bilibili.com/video/BV1i3411L7Gy/)
-
-#### YouTube
-
-## 🔧 Dependencies and Installation
-
-- Python >= 3.7 (Recommend to use [Anaconda](https://www.anaconda.com/download/#linux) or [Miniconda](https://docs.conda.io/en/latest/miniconda.html))
-- [PyTorch >= 1.7](https://pytorch.org/)
-
-### Installation
-
-1. Clone repo
-
- ```bash
- git clone https://github.com/xinntao/Real-ESRGAN.git
- cd Real-ESRGAN
- ```
-
-1. Install dependent packages
-
- ```bash
- # Install basicsr - https://github.com/xinntao/BasicSR
- # We use BasicSR for both training and inference
- pip install basicsr
- # facexlib and gfpgan are for face enhancement
- pip install facexlib
- pip install gfpgan
- pip install -r requirements.txt
- python setup.py develop
- ```
-
----
-
-## ⚡ Quick Inference
-
-There are usually three ways to inference Real-ESRGAN.
-
-1. [Online inference](#online-inference)
-1. [Portable executable files (NCNN)](#portable-executable-files-ncnn)
-1. [Python script](#python-script)
-
-### Online inference
-
-1. You can try in our website: [ARC Demo](https://arc.tencent.com/en/ai-demos/imgRestore) (now only support RealESRGAN_x4plus_anime_6B)
-1. [Colab Demo](https://colab.research.google.com/drive/1k2Zod6kSHEvraybHl50Lys0LerhyTMCo?usp=sharing) for Real-ESRGAN **|** [Colab Demo](https://colab.research.google.com/drive/1yNl9ORUxxlL4N0keJa2SEPB61imPQd1B?usp=sharing) for Real-ESRGAN (**anime videos**).
-
-### Portable executable files (NCNN)
-
-You can download [Windows](https://github.com/xinntao/Real-ESRGAN/releases/download/v0.2.5.0/realesrgan-ncnn-vulkan-20220424-windows.zip) / [Linux](https://github.com/xinntao/Real-ESRGAN/releases/download/v0.2.5.0/realesrgan-ncnn-vulkan-20220424-ubuntu.zip) / [MacOS](https://github.com/xinntao/Real-ESRGAN/releases/download/v0.2.5.0/realesrgan-ncnn-vulkan-20220424-macos.zip) **executable files for Intel/AMD/Nvidia GPU**.
-
-This executable file is **portable** and includes all the binaries and models required. No CUDA or PyTorch environment is needed.
-
-You can simply run the following command (the Windows example, more information is in the README.md of each executable files):
-
-```bash
-./realesrgan-ncnn-vulkan.exe -i input.jpg -o output.png -n model_name
-```
-
-We have provided five models:
-
-1. realesrgan-x4plus (default)
-2. realesrnet-x4plus
-3. realesrgan-x4plus-anime (optimized for anime images, small model size)
-4. realesr-animevideov3 (animation video)
-
-You can use the `-n` argument for other models, for example, `./realesrgan-ncnn-vulkan.exe -i input.jpg -o output.png -n realesrnet-x4plus`
-
-#### Usage of portable executable files
-
-1. Please refer to [Real-ESRGAN-ncnn-vulkan](https://github.com/xinntao/Real-ESRGAN-ncnn-vulkan#computer-usages) for more details.
-1. Note that it does not support all the functions (such as `outscale`) as the python script `inference_realesrgan.py`.
-
-```console
-Usage: realesrgan-ncnn-vulkan.exe -i infile -o outfile [options]...
-
- -h show this help
- -i input-path input image path (jpg/png/webp) or directory
- -o output-path output image path (jpg/png/webp) or directory
- -s scale upscale ratio (can be 2, 3, 4. default=4)
- -t tile-size tile size (>=32/0=auto, default=0) can be 0,0,0 for multi-gpu
- -m model-path folder path to the pre-trained models. default=models
- -n model-name model name (default=realesr-animevideov3, can be realesr-animevideov3 | realesrgan-x4plus | realesrgan-x4plus-anime | realesrnet-x4plus)
- -g gpu-id gpu device to use (default=auto) can be 0,1,2 for multi-gpu
- -j load:proc:save thread count for load/proc/save (default=1:2:2) can be 1:2,2,2:2 for multi-gpu
- -x enable tta mode"
- -f format output image format (jpg/png/webp, default=ext/png)
- -v verbose output
-```
-
-Note that it may introduce block inconsistency (and also generate slightly different results from the PyTorch implementation), because this executable file first crops the input image into several tiles, and then processes them separately, finally stitches together.
-
-### Python script
-
-#### Usage of python script
-
-1. You can use X4 model for **arbitrary output size** with the argument `outscale`. The program will further perform cheap resize operation after the Real-ESRGAN output.
-
-```console
-Usage: python inference_realesrgan.py -n RealESRGAN_x4plus -i infile -o outfile [options]...
-
-A common command: python inference_realesrgan.py -n RealESRGAN_x4plus -i infile --outscale 3.5 --face_enhance
-
- -h show this help
- -i --input Input image or folder. Default: inputs
- -o --output Output folder. Default: results
- -n --model_name Model name. Default: RealESRGAN_x4plus
- -s, --outscale The final upsampling scale of the image. Default: 4
- --suffix Suffix of the restored image. Default: out
- -t, --tile Tile size, 0 for no tile during testing. Default: 0
- --face_enhance Whether to use GFPGAN to enhance face. Default: False
- --fp32 Use fp32 precision during inference. Default: fp16 (half precision).
- --ext Image extension. Options: auto | jpg | png, auto means using the same extension as inputs. Default: auto
-```
-
-#### Inference general images
-
-Download pre-trained models: [RealESRGAN_x4plus.pth](https://github.com/xinntao/Real-ESRGAN/releases/download/v0.1.0/RealESRGAN_x4plus.pth)
-
-```bash
-wget https://github.com/xinntao/Real-ESRGAN/releases/download/v0.1.0/RealESRGAN_x4plus.pth -P weights
-```
-
-Inference!
-
-```bash
-python inference_realesrgan.py -n RealESRGAN_x4plus -i inputs --face_enhance
-```
-
-Results are in the `results` folder
-
-#### Inference anime images
-
-
-
-
-
-Pre-trained models: [RealESRGAN_x4plus_anime_6B](https://github.com/xinntao/Real-ESRGAN/releases/download/v0.2.2.4/RealESRGAN_x4plus_anime_6B.pth)
- More details and comparisons with [waifu2x](https://github.com/nihui/waifu2x-ncnn-vulkan) are in [**anime_model.md**](docs/anime_model.md)
-
-```bash
-# download model
-wget https://github.com/xinntao/Real-ESRGAN/releases/download/v0.2.2.4/RealESRGAN_x4plus_anime_6B.pth -P weights
-# inference
-python inference_realesrgan.py -n RealESRGAN_x4plus_anime_6B -i inputs
-```
-
-Results are in the `results` folder
-
----
-
-## BibTeX
-
- @InProceedings{wang2021realesrgan,
- author = {Xintao Wang and Liangbin Xie and Chao Dong and Ying Shan},
- title = {Real-ESRGAN: Training Real-World Blind Super-Resolution with Pure Synthetic Data},
- booktitle = {International Conference on Computer Vision Workshops (ICCVW)},
- date = {2021}
- }
-
-## 📧 Contact
-
-If you have any question, please email `xintao.wang@outlook.com` or `xintaowang@tencent.com`.
-
-
-## 🧩 Projects that use Real-ESRGAN
-
-If you develop/use Real-ESRGAN in your projects, welcome to let me know.
-
-- NCNN-Android: [RealSR-NCNN-Android](https://github.com/tumuyan/RealSR-NCNN-Android) by [tumuyan](https://github.com/tumuyan)
-- VapourSynth: [vs-realesrgan](https://github.com/HolyWu/vs-realesrgan) by [HolyWu](https://github.com/HolyWu)
-- NCNN: [Real-ESRGAN-ncnn-vulkan](https://github.com/xinntao/Real-ESRGAN-ncnn-vulkan)
-
- **GUI**
-
-- [Waifu2x-Extension-GUI](https://github.com/AaronFeng753/Waifu2x-Extension-GUI) by [AaronFeng753](https://github.com/AaronFeng753)
-- [Squirrel-RIFE](https://github.com/Justin62628/Squirrel-RIFE) by [Justin62628](https://github.com/Justin62628)
-- [Real-GUI](https://github.com/scifx/Real-GUI) by [scifx](https://github.com/scifx)
-- [Real-ESRGAN_GUI](https://github.com/net2cn/Real-ESRGAN_GUI) by [net2cn](https://github.com/net2cn)
-- [Real-ESRGAN-EGUI](https://github.com/WGzeyu/Real-ESRGAN-EGUI) by [WGzeyu](https://github.com/WGzeyu)
-- [anime_upscaler](https://github.com/shangar21/anime_upscaler) by [shangar21](https://github.com/shangar21)
-- [Upscayl](https://github.com/upscayl/upscayl) by [Nayam Amarshe](https://github.com/NayamAmarshe) and [TGS963](https://github.com/TGS963)
-
-## 🤗 Acknowledgement
-
-Thanks for all the contributors.
-
-- [AK391](https://github.com/AK391): Integrate RealESRGAN to [Huggingface Spaces](https://huggingface.co/spaces) with [Gradio](https://github.com/gradio-app/gradio). See [Gradio Web Demo](https://huggingface.co/spaces/akhaliq/Real-ESRGAN).
-- [Asiimoviet](https://github.com/Asiimoviet): Translate the README.md to Chinese (中文).
-- [2ji3150](https://github.com/2ji3150): Thanks for the [detailed and valuable feedbacks/suggestions](https://github.com/xinntao/Real-ESRGAN/issues/131).
-- [Jared-02](https://github.com/Jared-02): Translate the Training.md to Chinese (中文).
diff --git a/spaces/OAOA/DifFace/basicsr/models/lr_scheduler.py b/spaces/OAOA/DifFace/basicsr/models/lr_scheduler.py
deleted file mode 100644
index 11e1c6c7a74f5233accda52370f92681d3d3cecf..0000000000000000000000000000000000000000
--- a/spaces/OAOA/DifFace/basicsr/models/lr_scheduler.py
+++ /dev/null
@@ -1,96 +0,0 @@
-import math
-from collections import Counter
-from torch.optim.lr_scheduler import _LRScheduler
-
-
-class MultiStepRestartLR(_LRScheduler):
- """ MultiStep with restarts learning rate scheme.
-
- Args:
- optimizer (torch.nn.optimizer): Torch optimizer.
- milestones (list): Iterations that will decrease learning rate.
- gamma (float): Decrease ratio. Default: 0.1.
- restarts (list): Restart iterations. Default: [0].
- restart_weights (list): Restart weights at each restart iteration.
- Default: [1].
- last_epoch (int): Used in _LRScheduler. Default: -1.
- """
-
- def __init__(self, optimizer, milestones, gamma=0.1, restarts=(0, ), restart_weights=(1, ), last_epoch=-1):
- self.milestones = Counter(milestones)
- self.gamma = gamma
- self.restarts = restarts
- self.restart_weights = restart_weights
- assert len(self.restarts) == len(self.restart_weights), 'restarts and their weights do not match.'
- super(MultiStepRestartLR, self).__init__(optimizer, last_epoch)
-
- def get_lr(self):
- if self.last_epoch in self.restarts:
- weight = self.restart_weights[self.restarts.index(self.last_epoch)]
- return [group['initial_lr'] * weight for group in self.optimizer.param_groups]
- if self.last_epoch not in self.milestones:
- return [group['lr'] for group in self.optimizer.param_groups]
- return [group['lr'] * self.gamma**self.milestones[self.last_epoch] for group in self.optimizer.param_groups]
-
-
-def get_position_from_periods(iteration, cumulative_period):
- """Get the position from a period list.
-
- It will return the index of the right-closest number in the period list.
- For example, the cumulative_period = [100, 200, 300, 400],
- if iteration == 50, return 0;
- if iteration == 210, return 2;
- if iteration == 300, return 2.
-
- Args:
- iteration (int): Current iteration.
- cumulative_period (list[int]): Cumulative period list.
-
- Returns:
- int: The position of the right-closest number in the period list.
- """
- for i, period in enumerate(cumulative_period):
- if iteration <= period:
- return i
-
-
-class CosineAnnealingRestartLR(_LRScheduler):
- """ Cosine annealing with restarts learning rate scheme.
-
- An example of config:
- periods = [10, 10, 10, 10]
- restart_weights = [1, 0.5, 0.5, 0.5]
- eta_min=1e-7
-
- It has four cycles, each has 10 iterations. At 10th, 20th, 30th, the
- scheduler will restart with the weights in restart_weights.
-
- Args:
- optimizer (torch.nn.optimizer): Torch optimizer.
- periods (list): Period for each cosine anneling cycle.
- restart_weights (list): Restart weights at each restart iteration.
- Default: [1].
- eta_min (float): The minimum lr. Default: 0.
- last_epoch (int): Used in _LRScheduler. Default: -1.
- """
-
- def __init__(self, optimizer, periods, restart_weights=(1, ), eta_min=0, last_epoch=-1):
- self.periods = periods
- self.restart_weights = restart_weights
- self.eta_min = eta_min
- assert (len(self.periods) == len(
- self.restart_weights)), 'periods and restart_weights should have the same length.'
- self.cumulative_period = [sum(self.periods[0:i + 1]) for i in range(0, len(self.periods))]
- super(CosineAnnealingRestartLR, self).__init__(optimizer, last_epoch)
-
- def get_lr(self):
- idx = get_position_from_periods(self.last_epoch, self.cumulative_period)
- current_weight = self.restart_weights[idx]
- nearest_restart = 0 if idx == 0 else self.cumulative_period[idx - 1]
- current_period = self.periods[idx]
-
- return [
- self.eta_min + current_weight * 0.5 * (base_lr - self.eta_min) *
- (1 + math.cos(math.pi * ((self.last_epoch - nearest_restart) / current_period)))
- for base_lr in self.base_lrs
- ]
diff --git a/spaces/OAOA/DifFace/utils/__init__.py b/spaces/OAOA/DifFace/utils/__init__.py
deleted file mode 100644
index 794f3a7553185c90ff773d5efbacfafca9bea75f..0000000000000000000000000000000000000000
--- a/spaces/OAOA/DifFace/utils/__init__.py
+++ /dev/null
@@ -1,5 +0,0 @@
-#!/usr/bin/env python
-# -*- coding:utf-8 -*-
-# Power by Zongsheng Yue 2022-01-18 11:40:23
-
-
diff --git a/spaces/OFA-Sys/OFA-Generic_Interface/fairseq/examples/speech_synthesis/__init__.py b/spaces/OFA-Sys/OFA-Generic_Interface/fairseq/examples/speech_synthesis/__init__.py
deleted file mode 100644
index 6264236915a7269a4d920ee8213004374dd86a9a..0000000000000000000000000000000000000000
--- a/spaces/OFA-Sys/OFA-Generic_Interface/fairseq/examples/speech_synthesis/__init__.py
+++ /dev/null
@@ -1,4 +0,0 @@
-# Copyright (c) Facebook, Inc. and its affiliates.
-#
-# This source code is licensed under the MIT license found in the
-# LICENSE file in the root directory of this source tree.
diff --git a/spaces/OFA-Sys/OFA-Generic_Interface/fairseq/examples/speech_to_text/docs/covost_example.md b/spaces/OFA-Sys/OFA-Generic_Interface/fairseq/examples/speech_to_text/docs/covost_example.md
deleted file mode 100644
index 16447f041e4751f79d9f7848b33ef2ff943d63c2..0000000000000000000000000000000000000000
--- a/spaces/OFA-Sys/OFA-Generic_Interface/fairseq/examples/speech_to_text/docs/covost_example.md
+++ /dev/null
@@ -1,102 +0,0 @@
-[[Back]](..)
-
-# S2T Example: ST on CoVoST
-We replicate the experiments in
-[CoVoST 2 and Massively Multilingual Speech-to-Text Translation (Wang et al., 2020)](https://arxiv.org/abs/2007.10310).
-
-## Data Preparation
-[Download](https://commonvoice.mozilla.org/en/datasets) and unpack Common Voice v4 to a path
-`${COVOST_ROOT}/${SOURCE_LANG_ID}`, then preprocess it with
-```bash
-# additional Python packages for S2T data processing/model training
-pip install pandas torchaudio sentencepiece
-
-# En ASR
-python examples/speech_to_text/prep_covost_data.py \
- --data-root ${COVOST_ROOT} --vocab-type char --src-lang en
-# ST
-python examples/speech_to_text/prep_covost_data.py \
- --data-root ${COVOST_ROOT} --vocab-type char \
- --src-lang fr --tgt-lang en
-```
-The generated files (manifest, features, vocabulary and data configuration) will be added to
-`${COVOST_ROOT}/${SOURCE_LANG_ID}`.
-
-Download our vocabulary files if you want to use our pre-trained models:
-- ASR: [En](https://dl.fbaipublicfiles.com/fairseq/s2t/covost2_en_asr_vocab_char.zip)
-- ST: [Fr-En](https://dl.fbaipublicfiles.com/fairseq/s2t/covost2_fr_en_st_vocab_char.zip), [De-En](https://dl.fbaipublicfiles.com/fairseq/s2t/covost2_de_en_st_vocab_char.zip), [Es-En](https://dl.fbaipublicfiles.com/fairseq/s2t/covost2_es_en_st_vocab_char.zip), [Ca-En](https://dl.fbaipublicfiles.com/fairseq/s2t/covost2_ca_en_st_vocab_char.zip), [En-De](https://dl.fbaipublicfiles.com/fairseq/s2t/covost2_en_de_st_vocab_char.zip), [En-Ca](https://dl.fbaipublicfiles.com/fairseq/s2t/covost2_en_ca_st_vocab_char.zip), [En-Fa](https://dl.fbaipublicfiles.com/fairseq/s2t/covost2_en_fa_st_vocab_char.zip), [En-Et](https://dl.fbaipublicfiles.com/fairseq/s2t/covost2_en_et_st_vocab_char.zip)
-
-## ASR
-#### Training
-We train an En ASR model for encoder pre-training of all ST models:
-```bash
-fairseq-train ${COVOST_ROOT}/en \
- --config-yaml config_asr_en.yaml --train-subset train_asr_en --valid-subset dev_asr_en \
- --save-dir ${ASR_SAVE_DIR} --num-workers 4 --max-tokens 50000 --max-update 60000 \
- --task speech_to_text --criterion label_smoothed_cross_entropy --label-smoothing 0.1 \
- --report-accuracy --arch s2t_transformer_s --dropout 0.15 --optimizer adam --lr 2e-3 \
- --lr-scheduler inverse_sqrt --warmup-updates 10000 --clip-norm 10.0 --seed 1 --update-freq 8
-```
-where `ASR_SAVE_DIR` is the checkpoint root path. We set `--update-freq 8` to simulate 8 GPUs with 1 GPU.
-You may want to update it accordingly when using more than 1 GPU.
-
-#### Inference & Evaluation
-```bash
-CHECKPOINT_FILENAME=avg_last_10_checkpoint.pt
-python scripts/average_checkpoints.py \
- --inputs ${ASR_SAVE_DIR} --num-epoch-checkpoints 10 \
- --output "${ASR_SAVE_DIR}/${CHECKPOINT_FILENAME}"
-fairseq-generate ${COVOST_ROOT}/en \
- --config-yaml config_asr_en.yaml --gen-subset test_asr_en --task speech_to_text \
- --path ${ASR_SAVE_DIR}/${CHECKPOINT_FILENAME} --max-tokens 50000 --beam 5 \
- --scoring wer --wer-tokenizer 13a --wer-lowercase --wer-remove-punct
-```
-#### Results
-| --arch | Params | En | Model |
-|---|---|---|---|
-| s2t_transformer_s | 31M | 25.6 | [Download](https://dl.fbaipublicfiles.com/fairseq/s2t/covost2_en_asr_transformer_s.pt) |
-
-## ST
-#### Training
-Fr-En as example:
-```bash
-fairseq-train ${COVOST_ROOT}/fr \
- --config-yaml config_st_fr_en.yaml --train-subset train_st_fr_en --valid-subset dev_st_fr_en \
- --save-dir ${ST_SAVE_DIR} --num-workers 4 --max-update 30000 --max-tokens 40000 \ # --max-tokens 50000 for en-*
- --task speech_to_text --criterion label_smoothed_cross_entropy --label-smoothing 0.1 --report-accuracy \
- --arch s2t_transformer_s --encoder-freezing-updates 1000 --optimizer adam --lr 2e-3 \
- --lr-scheduler inverse_sqrt --warmup-updates 10000 --clip-norm 10.0 --seed 1 --update-freq 8 \
- --load-pretrained-encoder-from ${ASR_SAVE_DIR}/${CHECKPOINT_FILENAME}
-```
-where `ST_SAVE_DIR` is the checkpoint root path. The ST encoder is pre-trained by En ASR for faster training and better
-performance: `--load-pretrained-encoder-from `. We set `--update-freq 8` to simulate 8 GPUs with 1 GPU.
-You may want to update it accordingly when using more than 1 GPU.
-
-#### Inference & Evaluation
-Average the last 10 checkpoints and evaluate on test split:
-```bash
-CHECKPOINT_FILENAME=avg_last_10_checkpoint.pt
-python scripts/average_checkpoints.py \
- --inputs ${ST_SAVE_DIR} --num-epoch-checkpoints 10 \
- --output "${ST_SAVE_DIR}/${CHECKPOINT_FILENAME}"
-fairseq-generate ${COVOST_ROOT}/fr \
- --config-yaml config_st_fr_en.yaml --gen-subset test_st_fr_en --task speech_to_text \
- --path ${ST_SAVE_DIR}/${CHECKPOINT_FILENAME} \
- --max-tokens 50000 --beam 5 --scoring sacrebleu
-```
-
-## Interactive Decoding
-Launch the interactive console via
-```bash
-fairseq-interactive ${COVOST_ROOT}/fr --config-yaml config_st_fr_en.yaml \
- --task speech_to_text --path ${SAVE_DIR}/${CHECKPOINT_FILENAME} \
- --max-tokens 50000 --beam 5
-```
-Type in WAV/FLAC/OGG audio paths (one per line) after the prompt.
-
-#### Results
-| --arch | Params | Fr-En | De-En | Es-En | Ca-En | En-De | En-Ca | En-Fa | En-Et | Model |
-|---|---|---|---|---|---|---|---|---|---|---|
-| s2t_transformer_s | 31M | [27.2](https://dl.fbaipublicfiles.com/fairseq/s2t/covost2_fr_en_st_transformer_s.pt) | [17.7](https://dl.fbaipublicfiles.com/fairseq/s2t/covost2_de_en_st_transformer_s.pt) | [23.1](https://dl.fbaipublicfiles.com/fairseq/s2t/covost2_es_en_st_transformer_s.pt) | [19.3](https://dl.fbaipublicfiles.com/fairseq/s2t/covost2_ca_en_st_transformer_s.pt) | [16.1](https://dl.fbaipublicfiles.com/fairseq/s2t/covost2_en_de_st_transformer_s.pt) | [21.6](https://dl.fbaipublicfiles.com/fairseq/s2t/covost2_en_ca_st_transformer_s.pt) | [12.9](https://dl.fbaipublicfiles.com/fairseq/s2t/covost2_en_fa_st_transformer_s.pt) | [12.8](https://dl.fbaipublicfiles.com/fairseq/s2t/covost2_en_et_st_transformer_s.pt) | (<-Download) |
-
-[[Back]](..)
diff --git a/spaces/OFA-Sys/OFA-Generic_Interface/fairseq/examples/textless_nlp/gslm/metrics/abx_metrics/dump_abx_feats.py b/spaces/OFA-Sys/OFA-Generic_Interface/fairseq/examples/textless_nlp/gslm/metrics/abx_metrics/dump_abx_feats.py
deleted file mode 100644
index 41cf558970608fa5a9241e91e59ba214b609dc73..0000000000000000000000000000000000000000
--- a/spaces/OFA-Sys/OFA-Generic_Interface/fairseq/examples/textless_nlp/gslm/metrics/abx_metrics/dump_abx_feats.py
+++ /dev/null
@@ -1,107 +0,0 @@
-# Copyright (c) Facebook, Inc. and its affiliates.
-#
-# This source code is licensed under the MIT license found in the
-# LICENSE file in the root directory of this source tree.
-
-import argparse
-import logging
-import os
-
-import joblib
-import numpy as np
-
-from examples.textless_nlp.gslm.speech2unit.clustering.utils import get_audio_files
-from examples.textless_nlp.gslm.speech2unit.pretrained.utils import get_features
-
-def get_logger():
- log_format = "[%(asctime)s] [%(levelname)s]: %(message)s"
- logging.basicConfig(format=log_format, level=logging.INFO)
- logger = logging.getLogger(__name__)
- return logger
-
-def get_parser():
- parser = argparse.ArgumentParser(
- description="Quantize using K-means clustering over acoustic features."
- )
- parser.add_argument(
- "--feature_type",
- type=str,
- choices=["logmel", "hubert", "w2v2", "cpc"],
- default=None,
- required=True,
- help="Acoustic feature type",
- )
- parser.add_argument(
- "--kmeans_model_path",
- type=str,
- required=True,
- help="K-means model file path to use for inference",
- )
- parser.add_argument(
- "--manifest_path",
- type=str,
- default=None,
- help="Manifest file containing the root dir and file names",
- )
- parser.add_argument(
- "--checkpoint_path",
- type=str,
- help="Pretrained model checkpoint",
- )
- parser.add_argument(
- "--layer",
- type=int,
- help="The layer of the pretrained model to extract features from",
- default=-1,
- )
- parser.add_argument(
- "--out_dir_path",
- required=True,
- type=str,
- help="File path of quantized output.",
- )
- parser.add_argument(
- "--extension", type=str, default=".flac", help="Features file path"
- )
- return parser
-
-
-def one_hot(feat, n_clusters):
- return np.eye(n_clusters)[feat]
-
-def main(args, logger):
- # Feature extraction
- logger.info(f"Extracting {args.feature_type} acoustic features...")
- features_batch = get_features(
- feature_type=args.feature_type,
- checkpoint_path=args.checkpoint_path,
- layer=args.layer,
- manifest_path=args.manifest_path,
- sample_pct=1.0,
- flatten=False,
- )
- logger.info(f"Features extracted for {len(features_batch)} utterances.\n")
- logger.info(f"Dimensionality of representation = {features_batch[0].shape[1]}")
-
- logger.info(f"Loading K-means model from {args.kmeans_model_path} ...")
- kmeans_model = joblib.load(open(args.kmeans_model_path, "rb"))
- kmeans_model.verbose = False
-
- _, fnames, _ = get_audio_files(args.manifest_path)
-
- os.makedirs(args.out_dir_path, exist_ok=True)
- logger.info(f"Writing quantized features to {args.out_dir_path}")
- for i, feats in enumerate(features_batch):
- pred = kmeans_model.predict(feats)
- emb = one_hot(pred, kmeans_model.n_clusters)
- base_fname = os.path.basename(fnames[i]).rstrip(args.extension)
- output_path = os.path.join(args.out_dir_path, f"{base_fname}.npy")
- with open(output_path, "wb") as f:
- np.save(f, emb)
-
-if __name__ == "__main__":
- parser = get_parser()
- args = parser.parse_args()
- logger = get_logger()
- logger.info(args)
- main(args, logger)
diff --git a/spaces/OFA-Sys/OFA-Generic_Interface/fairseq/fairseq/data/indexed_dataset.py b/spaces/OFA-Sys/OFA-Generic_Interface/fairseq/fairseq/data/indexed_dataset.py
deleted file mode 100644
index a2a6ae1ac01b56d4e3c9b4516bdfe09c114324b6..0000000000000000000000000000000000000000
--- a/spaces/OFA-Sys/OFA-Generic_Interface/fairseq/fairseq/data/indexed_dataset.py
+++ /dev/null
@@ -1,585 +0,0 @@
-# Copyright (c) Facebook, Inc. and its affiliates.
-#
-# This source code is licensed under the MIT license found in the
-# LICENSE file in the root directory of this source tree.
-
-import shutil
-import struct
-from functools import lru_cache
-
-import numpy as np
-import torch
-from fairseq.dataclass.constants import DATASET_IMPL_CHOICES
-from fairseq.data.fasta_dataset import FastaDataset
-from fairseq.file_io import PathManager
-from fairseq.data.huffman import HuffmanMMapIndexedDataset, HuffmanMMapIndex
-
-from . import FairseqDataset
-
-from typing import Union
-
-
-def best_fitting_int_dtype(
- max_int_to_represent,
-) -> Union[np.uint16, np.uint32, np.int64]:
-
- if max_int_to_represent is None:
- return np.uint32 # Safe guess
- elif max_int_to_represent < 65500:
- return np.uint16
- elif max_int_to_represent < 4294967295:
- return np.uint32
- else:
- return np.int64
- # we avoid np.uint64 because it doesn't save space and its type promotion behaves unexpectedly
- # https://github.com/numpy/numpy/issues/5745
-
-
-def get_available_dataset_impl():
- return list(map(str, DATASET_IMPL_CHOICES))
-
-
-def infer_dataset_impl(path):
- if IndexedRawTextDataset.exists(path):
- return "raw"
- elif IndexedDataset.exists(path):
- with open(index_file_path(path), "rb") as f:
- magic = f.read(8)
- if magic == IndexedDataset._HDR_MAGIC:
- return "cached"
- elif magic == MMapIndexedDataset.Index._HDR_MAGIC[:8]:
- return "mmap"
- elif magic == HuffmanMMapIndex._HDR_MAGIC[:8]:
- return "huffman"
- else:
- return None
- elif FastaDataset.exists(path):
- return "fasta"
- else:
- return None
-
-
-def make_builder(out_file, impl, vocab_size=None):
- if impl == "mmap":
- return MMapIndexedDatasetBuilder(
- out_file, dtype=best_fitting_int_dtype(vocab_size)
- )
- elif impl == "fasta":
- raise NotImplementedError
- elif impl == "huffman":
- raise ValueError("Use HuffmanCodeBuilder directly as it has a different interface.")
- else:
- return IndexedDatasetBuilder(out_file)
-
-
-def make_dataset(path, impl, fix_lua_indexing=False, dictionary=None):
- if impl == "raw" and IndexedRawTextDataset.exists(path):
- assert dictionary is not None
- return IndexedRawTextDataset(path, dictionary)
- elif impl == "lazy" and IndexedDataset.exists(path):
- return IndexedDataset(path, fix_lua_indexing=fix_lua_indexing)
- elif impl == "cached" and IndexedDataset.exists(path):
- return IndexedCachedDataset(path, fix_lua_indexing=fix_lua_indexing)
- elif impl == "mmap" and MMapIndexedDataset.exists(path):
- return MMapIndexedDataset(path)
- elif impl == "fasta" and FastaDataset.exists(path):
- from fairseq.data.fasta_dataset import EncodedFastaDataset
-
- return EncodedFastaDataset(path, dictionary)
- elif impl == "huffman" and HuffmanMMapIndexedDataset.exists(path):
- return HuffmanMMapIndexedDataset(path)
- return None
-
-
-def dataset_exists(path, impl):
- if impl == "raw":
- return IndexedRawTextDataset.exists(path)
- elif impl == "mmap":
- return MMapIndexedDataset.exists(path)
- elif impl == "huffman":
- return HuffmanMMapIndexedDataset.exists(path)
- else:
- return IndexedDataset.exists(path)
-
-
-def read_longs(f, n):
- a = np.empty(n, dtype=np.int64)
- f.readinto(a)
- return a
-
-
-def write_longs(f, a):
- f.write(np.array(a, dtype=np.int64))
-
-
-_code_to_dtype = {
- 1: np.uint8,
- 2: np.int8,
- 3: np.int16,
- 4: np.int32,
- 5: np.int64,
- 6: np.float64,
- 7: np.double,
- 8: np.uint16,
- 9: np.uint32,
- 10: np.uint64,
-}
-
-
-def _dtype_header_code(dtype) -> int:
- for k in _code_to_dtype.keys():
- if _code_to_dtype[k] == dtype:
- return k
- raise ValueError(dtype)
-
-
-def index_file_path(prefix_path):
- return prefix_path + ".idx"
-
-
-def data_file_path(prefix_path):
- return prefix_path + ".bin"
-
-
-class IndexedDataset(FairseqDataset):
- """Loader for TorchNet IndexedDataset"""
-
- _HDR_MAGIC = b"TNTIDX\x00\x00"
-
- def __init__(self, path, fix_lua_indexing=False):
- super().__init__()
- self.path = path
- self.fix_lua_indexing = fix_lua_indexing
- self.data_file = None
- self.read_index(path)
-
- def read_index(self, path):
- with open(index_file_path(path), "rb") as f:
- magic = f.read(8)
- assert magic == self._HDR_MAGIC, (
- "Index file doesn't match expected format. "
- "Make sure that --dataset-impl is configured properly."
- )
- version = f.read(8)
- assert struct.unpack("= self._len:
- raise IndexError("index out of range")
-
- def __del__(self):
- if self.data_file:
- self.data_file.close()
-
- @lru_cache(maxsize=8)
- def __getitem__(self, i) -> torch.Tensor:
- if not self.data_file:
- self.read_data(self.path)
- self.check_index(i)
- tensor_size = self.sizes[self.dim_offsets[i] : self.dim_offsets[i + 1]]
- a = np.empty(tensor_size, dtype=self.dtype)
- self.data_file.seek(self.data_offsets[i] * self.element_size)
- self.data_file.readinto(a)
- item = torch.from_numpy(a).long()
- if self.fix_lua_indexing:
- item -= 1 # subtract 1 for 0-based indexing
- return item
-
- def __len__(self):
- return self._len
-
- def num_tokens(self, index):
- return self.sizes[index]
-
- def size(self, index):
- return self.sizes[index]
-
- @staticmethod
- def exists(path):
- return PathManager.exists(index_file_path(path)) and PathManager.exists(
- data_file_path(path)
- )
-
- @property
- def supports_prefetch(self):
- return False # avoid prefetching to save memory
-
-
-class IndexedCachedDataset(IndexedDataset):
- def __init__(self, path, fix_lua_indexing=False):
- super().__init__(path, fix_lua_indexing=fix_lua_indexing)
- self.cache = None
- self.cache_index = {}
-
- @property
- def supports_prefetch(self):
- return True
-
- def prefetch(self, indices):
- if all(i in self.cache_index for i in indices):
- return
- if not self.data_file:
- self.read_data(self.path)
- indices = sorted(set(indices))
- total_size = 0
- for i in indices:
- total_size += self.data_offsets[i + 1] - self.data_offsets[i]
- self.cache = np.empty(total_size, dtype=self.dtype)
- ptx = 0
- self.cache_index.clear()
- for i in indices:
- self.cache_index[i] = ptx
- size = self.data_offsets[i + 1] - self.data_offsets[i]
- a = self.cache[ptx : ptx + size]
- self.data_file.seek(self.data_offsets[i] * self.element_size)
- self.data_file.readinto(a)
- ptx += size
- if self.data_file:
- # close and delete data file after prefetch so we can pickle
- self.data_file.close()
- self.data_file = None
-
- @lru_cache(maxsize=8)
- def __getitem__(self, i):
- self.check_index(i)
- tensor_size = self.sizes[self.dim_offsets[i] : self.dim_offsets[i + 1]]
- a = np.empty(tensor_size, dtype=self.dtype)
- ptx = self.cache_index[i]
- np.copyto(a, self.cache[ptx : ptx + a.size])
- item = torch.from_numpy(a).long()
- if self.fix_lua_indexing:
- item -= 1 # subtract 1 for 0-based indexing
- return item
-
-
-class IndexedRawTextDataset(FairseqDataset):
- """Takes a text file as input and binarizes it in memory at instantiation.
- Original lines are also kept in memory"""
-
- def __init__(self, path, dictionary, append_eos=True, reverse_order=False):
- self.tokens_list = []
- self.lines = []
- self.sizes = []
- self.append_eos = append_eos
- self.reverse_order = reverse_order
- self.read_data(path, dictionary)
- self.size = len(self.tokens_list)
-
- def read_data(self, path, dictionary):
- with open(path, "r", encoding="utf-8") as f:
- for line in f:
- self.lines.append(line.strip("\n"))
- tokens = dictionary.encode_line(
- line,
- add_if_not_exist=False,
- append_eos=self.append_eos,
- reverse_order=self.reverse_order,
- ).long()
- self.tokens_list.append(tokens)
- self.sizes.append(len(tokens))
- self.sizes = np.array(self.sizes)
-
- def check_index(self, i):
- if i < 0 or i >= self.size:
- raise IndexError("index out of range")
-
- @lru_cache(maxsize=8)
- def __getitem__(self, i):
- self.check_index(i)
- return self.tokens_list[i]
-
- def get_original_text(self, i):
- self.check_index(i)
- return self.lines[i]
-
- def __del__(self):
- pass
-
- def __len__(self):
- return self.size
-
- def num_tokens(self, index):
- return self.sizes[index]
-
- def size(self, index):
- return self.sizes[index]
-
- @staticmethod
- def exists(path):
- return PathManager.exists(path)
-
-
-class IndexedDatasetBuilder:
- element_sizes = {
- np.uint8: 1,
- np.int8: 1,
- np.int16: 2,
- np.int32: 4,
- np.int64: 8,
- np.float64: 4,
- np.double: 8,
- }
-
- def __init__(self, out_file, dtype=np.int32):
- self.out_file = open(out_file, "wb")
- self.dtype = dtype
- self.data_offsets = [0]
- self.dim_offsets = [0]
- self.sizes = []
- self.element_size = self.element_sizes[self.dtype]
-
- def add_item(self, tensor):
- # +1 for Lua compatibility
- bytes = self.out_file.write(np.array(tensor.numpy() + 1, dtype=self.dtype))
- self.data_offsets.append(self.data_offsets[-1] + bytes / self.element_size)
- for s in tensor.size():
- self.sizes.append(s)
- self.dim_offsets.append(self.dim_offsets[-1] + len(tensor.size()))
-
- def merge_file_(self, another_file):
- index = IndexedDataset(another_file)
- assert index.dtype == self.dtype
-
- begin = self.data_offsets[-1]
- for offset in index.data_offsets[1:]:
- self.data_offsets.append(begin + offset)
- self.sizes.extend(index.sizes)
- begin = self.dim_offsets[-1]
- for dim_offset in index.dim_offsets[1:]:
- self.dim_offsets.append(begin + dim_offset)
-
- with open(data_file_path(another_file), "rb") as f:
- while True:
- data = f.read(1024)
- if data:
- self.out_file.write(data)
- else:
- break
-
- def finalize(self, index_file):
- self.out_file.close()
- index = open(index_file, "wb")
- index.write(b"TNTIDX\x00\x00")
- index.write(struct.pack(" str:
- local_index_path = PathManager.get_local_path(index_file_path(path))
- local_data_path = PathManager.get_local_path(data_file_path(path))
-
- assert local_index_path.endswith(".idx") and local_data_path.endswith(".bin"), (
- "PathManager.get_local_path does not return files with expected patterns: "
- f"{local_index_path} and {local_data_path}"
- )
-
- local_path = local_data_path[:-4] # stripping surfix ".bin"
- assert local_path == local_index_path[:-4] # stripping surfix ".idx"
- return local_path
-
-
-class MMapIndexedDatasetBuilder:
- def __init__(self, out_file, dtype=np.int64):
- self._data_file = open(out_file, "wb")
- self._dtype = dtype
- self._sizes = []
-
- def add_item(self, tensor):
- np_array = np.array(tensor.numpy(), dtype=self._dtype)
- self._data_file.write(np_array.tobytes(order="C"))
- self._sizes.append(np_array.size)
-
- def merge_file_(self, another_file):
- # Concatenate index
- index = MMapIndexedDataset.Index(index_file_path(another_file))
- assert index.dtype == self._dtype
-
- for size in index.sizes:
- self._sizes.append(size)
-
- # Concatenate data
- with open(data_file_path(another_file), "rb") as f:
- shutil.copyfileobj(f, self._data_file)
-
- def finalize(self, index_file):
- self._data_file.close()
-
- with MMapIndexedDataset.Index.writer(index_file, self._dtype) as index:
- index.write(self._sizes)
diff --git a/spaces/OFA-Sys/OFA-Generic_Interface/fairseq/fairseq/dataclass/configs.py b/spaces/OFA-Sys/OFA-Generic_Interface/fairseq/fairseq/dataclass/configs.py
deleted file mode 100644
index 8e8cec92814f55a504d36f80fb79c3e0f8280eee..0000000000000000000000000000000000000000
--- a/spaces/OFA-Sys/OFA-Generic_Interface/fairseq/fairseq/dataclass/configs.py
+++ /dev/null
@@ -1,1058 +0,0 @@
-# Copyright (c) Facebook, Inc. and its affiliates.
-#
-# This source code is licensed under the MIT license found in the
-# LICENSE file in the root directory of this source tree.
-
-import sys
-from dataclasses import _MISSING_TYPE, dataclass, field
-from typing import Any, List, Optional
-
-import torch
-
-from fairseq.dataclass.constants import (
- DATASET_IMPL_CHOICES,
- DDP_BACKEND_CHOICES,
- DDP_COMM_HOOK_CHOICES,
- GENERATION_CONSTRAINTS_CHOICES,
- GENERATION_DECODING_FORMAT_CHOICES,
- LOG_FORMAT_CHOICES,
- PIPELINE_CHECKPOINT_CHOICES,
- PRINT_ALIGNMENT_CHOICES,
- ZERO_SHARDING_CHOICES,
-)
-
-from omegaconf import II, MISSING
-
-
-@dataclass
-class FairseqDataclass:
- """fairseq base dataclass that supported fetching attributes and metas"""
-
- _name: Optional[str] = None
-
- @staticmethod
- def name():
- return None
-
- def _get_all_attributes(self) -> List[str]:
- return [k for k in self.__dataclass_fields__.keys()]
-
- def _get_meta(
- self, attribute_name: str, meta: str, default: Optional[Any] = None
- ) -> Any:
- return self.__dataclass_fields__[attribute_name].metadata.get(meta, default)
-
- def _get_name(self, attribute_name: str) -> str:
- return self.__dataclass_fields__[attribute_name].name
-
- def _get_default(self, attribute_name: str) -> Any:
- if hasattr(self, attribute_name):
- if str(getattr(self, attribute_name)).startswith("${"):
- return str(getattr(self, attribute_name))
- elif str(self.__dataclass_fields__[attribute_name].default).startswith(
- "${"
- ):
- return str(self.__dataclass_fields__[attribute_name].default)
- elif (
- getattr(self, attribute_name)
- != self.__dataclass_fields__[attribute_name].default
- ):
- return getattr(self, attribute_name)
-
- f = self.__dataclass_fields__[attribute_name]
- if not isinstance(f.default_factory, _MISSING_TYPE):
- return f.default_factory()
- return f.default
-
- def _get_type(self, attribute_name: str) -> Any:
- return self.__dataclass_fields__[attribute_name].type
-
- def _get_help(self, attribute_name: str) -> Any:
- return self._get_meta(attribute_name, "help")
-
- def _get_argparse_const(self, attribute_name: str) -> Any:
- return self._get_meta(attribute_name, "argparse_const")
-
- def _get_argparse_alias(self, attribute_name: str) -> Any:
- return self._get_meta(attribute_name, "argparse_alias")
-
- def _get_choices(self, attribute_name: str) -> Any:
- return self._get_meta(attribute_name, "choices")
-
- @classmethod
- def from_namespace(cls, args):
- if isinstance(args, cls):
- return args
- else:
- config = cls()
- for k in config.__dataclass_fields__.keys():
- if k.startswith("_"):
- # private member, skip
- continue
- if hasattr(args, k):
- setattr(config, k, getattr(args, k))
-
- return config
-
-
-
-@dataclass
-class CommonConfig(FairseqDataclass):
- # This is the core dataclass including common parameters shared by all different jobs. Please append your params to other dataclasses if they were
- # used for a particular purpose or task, such as those dedicated for `distributed training`, `optimization`, etc.
- no_progress_bar: bool = field(
- default=False, metadata={"help": "disable progress bar"}
- )
- log_interval: int = field(
- default=100,
- metadata={
- "help": "log progress every N batches (when progress bar is disabled)"
- },
- )
- log_format: Optional[LOG_FORMAT_CHOICES] = field(
- default=None, metadata={"help": "log format to use"}
- )
- log_file: Optional[str] = field(
- default=None, metadata={"help": "log file to copy metrics to."}
- )
- tensorboard_logdir: Optional[str] = field(
- default=None,
- metadata={
- "help": "path to save logs for tensorboard, should match --logdir "
- "of running tensorboard (default: no tensorboard logging)"
- },
- )
- wandb_project: Optional[str] = field(
- default=None,
- metadata={"help": "Weights and Biases project name to use for logging"},
- )
- azureml_logging: Optional[bool] = field(
- default=False, metadata={"help": "Log scalars to AzureML context"},
- )
- seed: int = field(
- default=1, metadata={"help": "pseudo random number generator seed"}
- )
- cpu: bool = field(default=False, metadata={"help": "use CPU instead of CUDA"})
- tpu: bool = field(default=False, metadata={"help": "use TPU instead of CUDA"})
- bf16: bool = field(default=False, metadata={"help": "use bfloat16; implies --tpu"})
- memory_efficient_bf16: bool = field(
- default=False,
- metadata={
- "help": "use a memory-efficient version of BF16 training; implies --bf16"
- },
- )
- fp16: bool = field(default=False, metadata={"help": "use FP16"})
- memory_efficient_fp16: bool = field(
- default=False,
- metadata={
- "help": "use a memory-efficient version of FP16 training; implies --fp16"
- },
- )
- fp16_no_flatten_grads: bool = field(
- default=False, metadata={"help": "don't flatten FP16 grads tensor"}
- )
- fp16_init_scale: int = field(
- default=2 ** 7, metadata={"help": "default FP16 loss scale"}
- )
- fp16_scale_window: Optional[int] = field(
- default=None,
- metadata={"help": "number of updates before increasing loss scale"},
- )
- fp16_scale_tolerance: float = field(
- default=0.0,
- metadata={
- "help": "pct of updates that can overflow before decreasing the loss scale"
- },
- )
- on_cpu_convert_precision: bool = field(
- default=False,
- metadata={
- "help": "if set, the floating point conversion to fp16/bf16 runs on CPU. "
- "This reduces bus transfer time and GPU memory usage."
- }
- )
- min_loss_scale: float = field(
- default=1e-4,
- metadata={"help": "minimum FP16/AMP loss scale, after which training is stopped"},
- )
- threshold_loss_scale: Optional[float] = field(
- default=None, metadata={"help": "threshold FP16 loss scale from below"}
- )
- amp: bool = field(default=False, metadata={"help": "use automatic mixed precision"})
- amp_batch_retries: int = field(
- default=2,
- metadata={"help": "number of retries of same batch after reducing loss scale with AMP"},
- )
- amp_init_scale: int = field(
- default=2 ** 7, metadata={"help": "default AMP loss scale"}
- )
- amp_scale_window: Optional[int] = field(
- default=None,
- metadata={"help": "number of updates before increasing AMP loss scale"},
- )
- user_dir: Optional[str] = field(
- default=None,
- metadata={
- "help": "path to a python module containing custom extensions (tasks and/or architectures)"
- },
- )
- empty_cache_freq: int = field(
- default=0,
- metadata={"help": "how often to clear the PyTorch CUDA cache (0 to disable)"},
- )
- all_gather_list_size: int = field(
- default=16384,
- metadata={"help": "number of bytes reserved for gathering stats from workers"},
- )
- model_parallel_size: int = field(
- default=1, metadata={"help": "total number of GPUs to parallelize model over"}
- )
- quantization_config_path: Optional[str] = field(
- default=None, metadata={"help": "path to quantization config file"}
- )
- profile: bool = field(
- default=False, metadata={"help": "enable autograd profiler emit_nvtx"}
- )
- reset_logging: bool = field(
- default=False,
- metadata={
- "help": "when using Hydra, reset the logging at the beginning of training"
- },
- )
- suppress_crashes: bool = field(
- default=False,
- metadata={
- "help": "suppress crashes when training with the hydra_train entry point so that the "
- "main method can return a value (useful for sweeps)"
- },
- )
- use_plasma_view: bool = field(
- default=False, metadata={"help": "Store indices and sizes in shared memory"}
- )
- plasma_path: Optional[str] = field(
- default="/tmp/plasma",
- metadata={
- "help": "path to run plasma_store, defaults to /tmp/plasma. Paths outside /tmp tend to fail."
- },
- )
-
-
-@dataclass
-class DistributedTrainingConfig(FairseqDataclass):
- distributed_world_size: int = field(
- default=max(1, torch.cuda.device_count()),
- metadata={
- "help": "total number of GPUs across all nodes (default: all visible GPUs)"
- },
- )
- distributed_num_procs: Optional[int] = field(
- default=max(1, torch.cuda.device_count()),
- metadata={
- "help": "total number of processes to fork (default: all visible GPUs)"
- },
- )
- distributed_rank: Optional[int] = field(
- default=0, metadata={"help": "rank of the current worker"}
- )
- distributed_backend: str = field(
- default="nccl", metadata={"help": "distributed backend"}
- )
- distributed_init_method: Optional[str] = field(
- default=None,
- metadata={
- "help": "typically tcp://hostname:port that will be used to "
- "establish initial connetion"
- },
- )
- distributed_port: int = field(
- default=-1,
- metadata={
- "help": "port number (not required if using --distributed-init-method)"
- },
- )
- device_id: int = field(
- default=0,
- metadata={
- "help": "which GPU to use (usually configured automatically)",
- "argparse_alias": "--local_rank",
- },
- )
- distributed_no_spawn: bool = field(
- default=False,
- metadata={
- "help": "do not spawn multiple processes even if multiple GPUs are visible"
- },
- )
- ddp_backend: DDP_BACKEND_CHOICES = field(
- default="pytorch_ddp", metadata={"help": "DistributedDataParallel backend"}
- )
- ddp_comm_hook: DDP_COMM_HOOK_CHOICES = field(
- default="none", metadata={"help": "communication hook"}
- )
- bucket_cap_mb: int = field(
- default=25, metadata={"help": "bucket size for reduction"}
- )
- fix_batches_to_gpus: bool = field(
- default=False,
- metadata={
- "help": "don't shuffle batches between GPUs; this reduces overall "
- "randomness and may affect precision but avoids the cost of re-reading the data"
- },
- )
- find_unused_parameters: bool = field(
- default=False,
- metadata={
- "help": "disable unused parameter detection (not applicable to "
- "--ddp-backend=legacy_ddp)"
- },
- )
- gradient_as_bucket_view: bool = field(
- default=False,
- metadata={
- "help": "when set to True, gradients will be views pointing to different offsets of allreduce communication buckets. This can reduce peak memory usage, where the saved memory size will be equal to the total gradients size. "
- "--gradient-as-bucket-view=gradient_as_bucket_view)"
- },
- )
- fast_stat_sync: bool = field(
- default=False,
- metadata={"help": "[deprecated] this is now defined per Criterion"},
- )
- heartbeat_timeout: int = field(
- default=-1,
- metadata={
- "help": "kill the job if no progress is made in N seconds; "
- "set to -1 to disable"
- },
- )
- broadcast_buffers: bool = field(
- default=False,
- metadata={
- "help": "Copy non-trainable parameters between GPUs, such as "
- "batchnorm population statistics"
- },
- )
- slowmo_momentum: Optional[float] = field(
- default=None,
- metadata={
- "help": "SlowMo momentum term; by default use 0.0 for 16 GPUs, "
- "0.2 for 32 GPUs; 0.5 for 64 GPUs, 0.6 for > 64 GPUs"
- },
- )
- slowmo_algorithm: str = field(
- default="LocalSGD", metadata={"help": "whether to use LocalSGD or SGP"}
- )
- localsgd_frequency: int = field(
- default=3, metadata={"help": "Local SGD allreduce frequency"}
- )
- nprocs_per_node: int = field(
- default=max(1, torch.cuda.device_count()),
- metadata={
- "help": "number of GPUs in each node. An allreduce operation across GPUs in "
- "a node is very fast. Hence, we do allreduce across GPUs in a node, "
- "and gossip across different nodes"
- },
- )
- pipeline_model_parallel: bool = field(
- default=False,
- metadata={"help": "if set, use pipeline model parallelism across GPUs"},
- )
- pipeline_balance: Optional[str] = field(
- default=None,
- metadata={
- "help": "partition the model into N_K pieces, where each piece "
- "contains N_i layers. The sum(args.pipeline_balance) "
- "should equal the total number of layers in the model"
- },
- )
- pipeline_devices: Optional[str] = field(
- default=None,
- metadata={
- "help": "a list of device indices indicating which device to place "
- "each of the N_K partitions. The length of this list should "
- "equal the length of the --pipeline-balance argument"
- },
- )
- pipeline_chunks: Optional[int] = field(
- default=0, metadata={"help": "microbatch count for pipeline model parallelism"}
- )
- pipeline_encoder_balance: Optional[str] = field(
- default=None,
- metadata={
- "help": "partition the pipeline parallel encoder into N_K pieces, where each piece "
- "contains N_i layers. The sum(args.pipeline_encoder_balance) "
- "should equal the total number of encoder layers in the model"
- },
- )
- pipeline_encoder_devices: Optional[str] = field(
- default=None,
- metadata={
- "help": "a list of device indices indicating which device to place "
- "each of the N_K partitions. The length of this list should "
- "equal the length of the --pipeline-encoder-balance argument"
- },
- )
- pipeline_decoder_balance: Optional[str] = field(
- default=None,
- metadata={
- "help": "partition the pipeline parallel decoder into N_K pieces, where each piece "
- "contains N_i layers. The sum(args.pipeline_decoder_balance) "
- "should equal the total number of decoder layers in the model"
- },
- )
- pipeline_decoder_devices: Optional[str] = field(
- default=None,
- metadata={
- "help": "a list of device indices indicating which device to place "
- "each of the N_K partitions. The length of this list should "
- "equal the length of the --pipeline-decoder-balance argument"
- },
- )
- pipeline_checkpoint: PIPELINE_CHECKPOINT_CHOICES = field(
- default="never",
- metadata={"help": "checkpointing mode for pipeline model parallelism"},
- )
- zero_sharding: ZERO_SHARDING_CHOICES = field(
- default="none", metadata={"help": "ZeRO sharding"}
- )
- fp16: bool = II("common.fp16")
- memory_efficient_fp16: bool = II("common.memory_efficient_fp16")
- tpu: bool = II("common.tpu")
- # configuration for --ddp-backend=fully_sharded
- no_reshard_after_forward: bool = field(
- default=False, metadata={"help": "don't reshard parameters after forward pass"},
- )
- fp32_reduce_scatter: bool = field(
- default=False, metadata={"help": "reduce-scatter grads in FP32"},
- )
- cpu_offload: bool = field(
- default=False, metadata={"help": "offload FP32 params to CPU"}
- )
- use_sharded_state: bool = field(
- default=False, metadata={"help": "use sharded checkpoint files"},
- )
-
-
-@dataclass
-class DatasetConfig(FairseqDataclass):
- num_workers: int = field(
- default=1, metadata={"help": "how many subprocesses to use for data loading"}
- )
- skip_invalid_size_inputs_valid_test: bool = field(
- default=False,
- metadata={"help": "ignore too long or too short lines in valid and test set"},
- )
- max_tokens: Optional[int] = field(
- default=None, metadata={"help": "maximum number of tokens in a batch"}
- )
- batch_size: Optional[int] = field(
- default=None,
- metadata={
- "help": "number of examples in a batch",
- "argparse_alias": "--max-sentences",
- },
- )
- required_batch_size_multiple: int = field(
- default=8, metadata={"help": "batch size will be a multiplier of this value"}
- )
- required_seq_len_multiple: int = field(
- default=1,
- metadata={
- "help": "maximum sequence length in batch will be a multiplier of this value"
- },
- )
- dataset_impl: Optional[DATASET_IMPL_CHOICES] = field(
- default=None, metadata={"help": "output dataset implementation"}
- )
- data_buffer_size: int = field(
- default=10, metadata={"help": "Number of batches to preload"}
- )
- train_subset: str = field(
- default="train",
- metadata={"help": "data subset to use for training (e.g. train, valid, test)"},
- )
- valid_subset: str = field(
- default="valid",
- metadata={
- "help": "comma separated list of data subsets to use for validation"
- " (e.g. train, valid, test)"
- },
- )
- combine_valid_subsets: Optional[bool] = field(
- default=None,
- metadata={
- "help": "comma separated list of data subsets to use for validation"
- " (e.g. train, valid, test)",
- "argparse_alias": "--combine-val",
- },
- )
- ignore_unused_valid_subsets: Optional[bool] = field(
- default=False,
- metadata={"help": "do not raise error if valid subsets are ignored"},
- )
-
- validate_interval: int = field(
- default=1, metadata={"help": "validate every N epochs"}
- )
- validate_interval_updates: int = field(
- default=0, metadata={"help": "validate every N updates"}
- )
- validate_after_updates: int = field(
- default=0, metadata={"help": "dont validate until reaching this many updates"}
- )
- fixed_validation_seed: Optional[int] = field(
- default=None, metadata={"help": "specified random seed for validation"}
- )
- disable_validation: bool = field(
- default=False, metadata={"help": "disable validation"}
- )
- max_tokens_valid: Optional[int] = field(
- default=II("dataset.max_tokens"),
- metadata={
- "help": "maximum number of tokens in a validation batch"
- " (defaults to --max-tokens)"
- },
- )
- batch_size_valid: Optional[int] = field(
- default=II("dataset.batch_size"),
- metadata={
- "help": "batch size of the validation batch (defaults to --batch-size)",
- "argparse_alias": "--max-sentences-valid",
- },
- )
- max_valid_steps: Optional[int] = field(default=None, metadata={'help': 'How many batches to evaluate',
- "argparse_alias": "--nval"})
- curriculum: int = field(
- default=0, metadata={"help": "don't shuffle batches for first N epochs"}
- )
- gen_subset: str = field(
- default="test",
- metadata={"help": "data subset to generate (train, valid, test)"},
- )
- num_shards: int = field(
- default=1, metadata={"help": "shard generation over N shards"}
- )
- shard_id: int = field(
- default=0, metadata={"help": "id of the shard to generate (id < num_shards)"}
- )
-
-
-@dataclass
-class OptimizationConfig(FairseqDataclass):
- max_epoch: int = field(
- default=0, metadata={"help": "force stop training at specified epoch"}
- )
- max_update: int = field(
- default=0, metadata={"help": "force stop training at specified update"}
- )
- stop_time_hours: float = field(
- default=0,
- metadata={
- "help": "force stop training after specified cumulative time (if >0)"
- },
- )
- clip_norm: float = field(
- default=0.0, metadata={"help": "clip threshold of gradients"}
- )
- sentence_avg: bool = field(
- default=False,
- metadata={
- "help": "normalize gradients by the number of sentences in a batch"
- " (default is to normalize by number of tokens)"
- },
- )
- update_freq: List[int] = field(
- default_factory=lambda: [1],
- metadata={"help": "update parameters every N_i batches, when in epoch i"},
- )
- lr: List[float] = field(
- default_factory=lambda: [0.25],
- metadata={
- "help": "learning rate for the first N epochs; all epochs >N using LR_N"
- " (note: this may be interpreted differently depending on --lr-scheduler)"
- },
- )
- stop_min_lr: float = field(
- default=-1.0,
- metadata={"help": "stop training when the learning rate reaches this minimum"},
- )
- use_bmuf: bool = field(
- default=False,
- metadata={
- "help": "specify global optimizer for syncing models on different GPUs/shards"
- },
- )
-
-
-@dataclass
-class CheckpointConfig(FairseqDataclass):
- save_dir: str = field(
- default="checkpoints", metadata={"help": "path to save checkpoints"}
- )
- restore_file: str = field(
- default="checkpoint_last.pt",
- metadata={
- "help": "filename from which to load checkpoint "
- "(default: /checkpoint_last.pt"
- },
- )
- finetune_from_model: Optional[str] = field(
- default=None,
- metadata={
- "help": "finetune from a pretrained model; note that meters and lr scheduler will be reset"
- },
- )
- reset_dataloader: bool = field(
- default=False,
- metadata={
- "help": "if set, does not reload dataloader state from the checkpoint"
- },
- )
- reset_lr_scheduler: bool = field(
- default=False,
- metadata={
- "help": "if set, does not load lr scheduler state from the checkpoint"
- },
- )
- reset_meters: bool = field(
- default=False,
- metadata={"help": "if set, does not load meters from the checkpoint"},
- )
- reset_optimizer: bool = field(
- default=False,
- metadata={"help": "if set, does not load optimizer state from the checkpoint"},
- )
- optimizer_overrides: str = field(
- default="{}",
- metadata={
- "help": "a dictionary used to override optimizer args when loading a checkpoint"
- },
- )
- save_interval: int = field(
- default=1, metadata={"help": "save a checkpoint every N epochs"}
- )
- save_interval_updates: int = field(
- default=0, metadata={"help": "save a checkpoint (and validate) every N updates"}
- )
- keep_interval_updates: int = field(
- default=-1,
- metadata={
- "help": "keep the last N checkpoints saved with --save-interval-updates"
- },
- )
- keep_interval_updates_pattern: int = field(
- default=-1,
- metadata={
- "help": "when used with --keep-interval-updates, skips deleting "
- "any checkpoints with update X where "
- "X %% keep_interval_updates_pattern == 0"
- },
- )
- keep_last_epochs: int = field(
- default=-1, metadata={"help": "keep last N epoch checkpoints"}
- )
- keep_best_checkpoints: int = field(
- default=-1, metadata={"help": "keep best N checkpoints based on scores"}
- )
- no_save: bool = field(
- default=False, metadata={"help": "don't save models or checkpoints"}
- )
- no_epoch_checkpoints: bool = field(
- default=False, metadata={"help": "only store last and best checkpoints"}
- )
- no_last_checkpoints: bool = field(
- default=False, metadata={"help": "don't store last checkpoints"}
- )
- no_save_optimizer_state: bool = field(
- default=False,
- metadata={"help": "don't save optimizer-state as part of checkpoint"},
- )
- best_checkpoint_metric: str = field(
- default="loss", metadata={"help": 'metric to use for saving "best" checkpoints'}
- )
- maximize_best_checkpoint_metric: bool = field(
- default=False,
- metadata={
- "help": 'select the largest metric value for saving "best" checkpoints'
- },
- )
- patience: int = field(
- default=-1,
- metadata={
- "help": (
- "early stop training if valid performance doesn't "
- "improve for N consecutive validation runs; note "
- "that this is influenced by --validate-interval"
- )
- },
- )
- checkpoint_suffix: str = field(
- default="", metadata={"help": "suffix to add to the checkpoint file name"}
- )
- checkpoint_shard_count: int = field(
- default=1,
- metadata={
- "help": "Number of shards containing the checkpoint - "
- "if the checkpoint is over 300GB, it is preferable "
- "to split it into shards to prevent OOM on CPU while loading "
- "the checkpoint"
- },
- )
- load_checkpoint_on_all_dp_ranks: bool = field(
- default=False,
- metadata={
- "help": "load checkpoints on all data parallel devices "
- "(default: only load on rank 0 and broadcast to other devices)"
- },
- )
- write_checkpoints_asynchronously: bool = field(
- default=False,
- metadata={
- "help": (
- "Write checkpoints asynchronously in a separate "
- "thread. NOTE: This feature is currently being tested."
- ),
- "argparse_alias": "--save-async",
- },
- )
- model_parallel_size: int = II("common.model_parallel_size")
- use_ema_weights_to_init_param: bool = field(
- default=False,
- metadata={
- "help": "if the checkpoint has ema weights, then use it to init the model param"
- "(default: false, use noema weights to init the model param)"
- },
- )
- use_latest_weights_to_init_ema: bool = field(
- default=False,
- metadata={
- "help": "if the model has ema params, then force to use the latest weights in the ckpt to init the ema param, even ema weights exist in the ckpt"
- "(default: false, use ema weights (if exist) to init the ema param)"
- },
- )
-
-
-@dataclass
-class FairseqBMUFConfig(FairseqDataclass):
- block_lr: float = field(
- default=1, metadata={"help": "block learning rate for bmuf"}
- )
- block_momentum: float = field(
- default=0.875, metadata={"help": "block momentum for bmuf"}
- )
- global_sync_iter: int = field(
- default=50, metadata={"help": "Iteration for syncing global model"}
- )
- warmup_iterations: int = field(
- default=500, metadata={"help": "warmup iterations for model to broadcast"}
- )
- use_nbm: bool = field(
- default=False,
- metadata={"help": "Specify whether you want to use classical BM / Nesterov BM"},
- )
- average_sync: bool = field(
- default=False,
- metadata={
- "help": "Specify whether you want to average the local momentum after each sync"
- },
- )
- distributed_world_size: int = II("distributed_training.distributed_world_size")
-
-
-@dataclass
-class GenerationConfig(FairseqDataclass):
- beam: int = field(
- default=5, metadata={"help": "beam size"},
- )
- nbest: int = field(
- default=1, metadata={"help": "number of hypotheses to output"},
- )
- max_len_a: float = field(
- default=0,
- metadata={
- "help": "generate sequences of maximum length ax + b, where x is the source length"
- },
- )
- max_len_b: int = field(
- default=200,
- metadata={
- "help": "generate sequences of maximum length ax + b, where x is the source length"
- },
- )
- min_len: int = field(
- default=1, metadata={"help": "minimum generation length"},
- )
- match_source_len: bool = field(
- default=False, metadata={"help": "generations should match the source length"},
- )
- unnormalized: bool = field(
- default=False, metadata={"help": "compare unnormalized hypothesis scores"},
- )
- no_early_stop: bool = field(
- default=False, metadata={"help": "deprecated"},
- )
- no_beamable_mm: bool = field(
- default=False, metadata={"help": "don't use BeamableMM in attention layers"},
- )
- lenpen: float = field(
- default=1,
- metadata={
- "help": "length penalty: <1.0 favors shorter, >1.0 favors longer sentences"
- },
- )
- unkpen: float = field(
- default=0,
- metadata={
- "help": "unknown word penalty: <0 produces more unks, >0 produces fewer"
- },
- )
- replace_unk: Optional[str] = field(
- default=None,
- metadata={
- "help": "perform unknown replacement (optionally with alignment dictionary)",
- "argparse_const": "@@ ",
- },
- )
- sacrebleu: bool = field(
- default=False, metadata={"help": "score with sacrebleu"},
- )
- score_reference: bool = field(
- default=False, metadata={"help": "just score the reference translation"},
- )
- prefix_size: int = field(
- default=0,
- metadata={"help": "initialize generation by target prefix of given length"},
- )
- no_repeat_ngram_size: int = field(
- default=0,
- metadata={
- "help": "ngram blocking such that this size ngram cannot be repeated in the generation"
- },
- )
- sampling: bool = field(
- default=False,
- metadata={"help": "sample hypotheses instead of using beam search"},
- )
- sampling_topk: int = field(
- default=-1,
- metadata={"help": "sample from top K likely next words instead of all words"},
- )
- sampling_topp: float = field(
- default=-1.0,
- metadata={
- "help": "sample from the smallest set whose cumulative probability mass exceeds p for next words"
- },
- )
- constraints: Optional[GENERATION_CONSTRAINTS_CHOICES] = field(
- default=None,
- metadata={
- "help": "enables lexically constrained decoding",
- "argparse_const": "ordered",
- },
- )
- temperature: float = field(
- default=1.0, metadata={"help": "temperature for generation"},
- )
- diverse_beam_groups: int = field(
- default=-1, metadata={"help": "number of groups for Diverse Beam Search"},
- )
- diverse_beam_strength: float = field(
- default=0.5,
- metadata={"help": "strength of diversity penalty for Diverse Beam Search"},
- )
- diversity_rate: float = field(
- default=-1.0,
- metadata={"help": "strength of diversity penalty for Diverse Siblings Search"},
- )
- print_alignment: Optional[PRINT_ALIGNMENT_CHOICES] = field(
- default=None,
- metadata={
- "help": "if set, uses attention feedback to compute and print alignment to source tokens "
- "(valid options are: hard, soft, otherwise treated as hard alignment)",
- "argparse_const": "hard",
- },
- )
- print_step: bool = field(
- default=False, metadata={"help": "print steps"},
- )
- lm_path: Optional[str] = field(
- default=None, metadata={"help": "path to lm checkpoint for lm fusion"},
- )
- lm_weight: float = field(
- default=0.0, metadata={"help": "weight for lm probs for lm fusion"},
- )
-
- # arguments for iterative refinement generator
- iter_decode_eos_penalty: float = field(
- default=0.0,
- metadata={"help": "if > 0.0, it penalized early-stopping in decoding."},
- )
- iter_decode_max_iter: int = field(
- default=10, metadata={"help": "maximum iterations for iterative refinement."},
- )
- iter_decode_force_max_iter: bool = field(
- default=False,
- metadata={
- "help": "if set, run exact the maximum number of iterations without early stop"
- },
- )
- iter_decode_with_beam: int = field(
- default=1,
- metadata={
- "help": "if > 1, model will generate translations varying by the lengths."
- },
- )
- iter_decode_with_external_reranker: bool = field(
- default=False,
- metadata={
- "help": "if set, the last checkpoint are assumed to be a reranker to rescore the translations"
- },
- )
- retain_iter_history: bool = field(
- default=False,
- metadata={
- "help": "if set, decoding returns the whole history of iterative refinement"
- },
- )
- retain_dropout: bool = field(
- default=False, metadata={"help": "Use dropout at inference time"},
- )
- # temporarily set to Any until https://github.com/facebookresearch/hydra/issues/1117 is fixed
- # retain_dropout_modules: Optional[List[str]] = field(
- retain_dropout_modules: Any = field(
- default=None,
- metadata={
- "help": "if set, only retain dropout for the specified modules; "
- "if not set, then dropout will be retained for all modules"
- },
- )
- # special decoding format for advanced decoding.
- decoding_format: Optional[GENERATION_DECODING_FORMAT_CHOICES] = field(
- default=None,
- metadata={"help": "special decoding format for advanced decoding."},
- )
- no_seed_provided: bool = field(
- default=False,
- metadata={"help": "if set, dont use seed for initializing random generators"},
- )
-
-
-@dataclass
-class CommonEvalConfig(FairseqDataclass):
- path: Optional[str] = field(
- default=None, metadata={"help": "path(s) to model file(s), colon separated"},
- )
- post_process: Optional[str] = field(
- default=None,
- metadata={
- "help": (
- "post-process text by removing BPE, letter segmentation, etc. "
- "Valid options can be found in fairseq.data.utils.post_process."
- ),
- "argparse_const": "subword_nmt",
- "argparse_alias": "--remove-bpe",
- },
- )
- quiet: bool = field(default=False, metadata={"help": "only print final scores"})
- model_overrides: str = field(
- default="{}",
- metadata={
- "help": "a dictionary used to override model args at generation that were used during model training"
- },
- )
- results_path: Optional[str] = field(
- default=None, metadata={"help": "path to save eval results (optional)"}
- )
-
-
-@dataclass
-class EvalLMConfig(FairseqDataclass):
- output_word_probs: bool = field(
- default=False,
- metadata={
- "help": "if set, outputs words and their predicted log probabilities to standard output"
- },
- )
- output_word_stats: bool = field(
- default=False,
- metadata={
- "help": "if set, outputs word statistics such as word count, average probability, etc"
- },
- )
- context_window: int = field(
- default=0,
- metadata={
- "help": "ensures that every evaluated token has access to a context of at least this size, if possible"
- },
- )
- softmax_batch: int = field(
- default=sys.maxsize,
- metadata={
- "help": "if BxT is more than this, will batch the softmax over vocab to this amount of tokens, in order to fit into GPU memory"
- },
- )
-
-
-@dataclass
-class InteractiveConfig(FairseqDataclass):
- buffer_size: int = field(
- default=0,
- metadata={
- "help": "read this many sentences into a buffer before processing them"
- },
- )
- input: str = field(
- default="-", metadata={"help": "file to read from; use - for stdin"},
- )
-
-
-@dataclass
-class EMAConfig(FairseqDataclass):
- store_ema: bool = field(
- default=False, metadata={
- help: "store exponential moving average shadow model"
- }
- )
- ema_decay: float = field(
- default=0.9999, metadata={
- "help": 'decay for exponential moving average model'
- }
- )
- ema_start_update : int = field(
- default=0, metadata={"help": "start EMA update after this many model updates"}
- )
- ema_seed_model : Optional[str] = field(
- default=None, metadata={
- "help": "Seed to load EMA model from. "
- "Used to load EMA model separately from the actual model."
- }
- )
- ema_update_freq : int = field(
- default=1, metadata={"help": "Do EMA update every this many model updates"}
- )
- ema_fp32: bool = field(
- default=False,
- metadata={"help": "If true, store EMA model in fp32 even if model is in fp16"},
- )
-
-
-@dataclass
-class FairseqConfig(FairseqDataclass):
- common: CommonConfig = CommonConfig()
- common_eval: CommonEvalConfig = CommonEvalConfig()
- distributed_training: DistributedTrainingConfig = DistributedTrainingConfig()
- dataset: DatasetConfig = DatasetConfig()
- optimization: OptimizationConfig = OptimizationConfig()
- checkpoint: CheckpointConfig = CheckpointConfig()
- bmuf: FairseqBMUFConfig = FairseqBMUFConfig()
- generation: GenerationConfig = GenerationConfig()
- eval_lm: EvalLMConfig = EvalLMConfig()
- interactive: InteractiveConfig = InteractiveConfig()
- model: Any = MISSING
- task: Any = None
- criterion: Any = None
- optimizer: Any = None
- lr_scheduler: Any = None
- scoring: Any = None
- bpe: Any = None
- tokenizer: Any = None
- ema: EMAConfig = EMAConfig()
diff --git a/spaces/OFA-Sys/OFA-Image_Caption/fairseq/examples/simultaneous_translation/utils/p_choose_strategy.py b/spaces/OFA-Sys/OFA-Image_Caption/fairseq/examples/simultaneous_translation/utils/p_choose_strategy.py
deleted file mode 100644
index 724c6912a62d48fc61988cac1434a4f5c8754521..0000000000000000000000000000000000000000
--- a/spaces/OFA-Sys/OFA-Image_Caption/fairseq/examples/simultaneous_translation/utils/p_choose_strategy.py
+++ /dev/null
@@ -1,126 +0,0 @@
-from typing import Optional, Dict
-from torch import Tensor
-import torch
-
-
-def waitk_p_choose(
- tgt_len: int,
- src_len: int,
- bsz: int,
- waitk_lagging: int,
- key_padding_mask: Optional[Tensor] = None,
- incremental_state: Optional[Dict[str, Dict[str, Optional[Tensor]]]] = None
-):
-
- max_src_len = src_len
- if incremental_state is not None:
- # Retrieve target length from incremental states
- # For inference the length of query is always 1
- max_tgt_len = incremental_state["steps"]["tgt"]
- assert max_tgt_len is not None
- max_tgt_len = int(max_tgt_len)
- else:
- max_tgt_len = tgt_len
-
- if max_src_len < waitk_lagging:
- if incremental_state is not None:
- max_tgt_len = 1
- return torch.zeros(
- bsz, max_tgt_len, max_src_len
- )
-
- # Assuming the p_choose looks like this for wait k=3
- # src_len = 6, max_tgt_len = 5
- # [0, 0, 1, 0, 0, 0, 0]
- # [0, 0, 0, 1, 0, 0, 0]
- # [0, 0, 0, 0, 1, 0, 0]
- # [0, 0, 0, 0, 0, 1, 0]
- # [0, 0, 0, 0, 0, 0, 1]
- # linearize the p_choose matrix:
- # [0, 0, 1, 0, 0, 0, 0, 0, 0, 1, 0, 0, 0, 0...]
- # The indices of linearized matrix that equals 1 is
- # 2 + 6 * 0
- # 3 + 6 * 1
- # ...
- # n + src_len * n + k - 1 = n * (src_len + 1) + k - 1
- # n from 0 to max_tgt_len - 1
- #
- # First, generate the indices (activate_indices_offset: bsz, max_tgt_len)
- # Second, scatter a zeros tensor (bsz, max_tgt_len * src_len)
- # with activate_indices_offset
- # Third, resize the tensor to (bsz, max_tgt_len, src_len)
-
- activate_indices_offset = (
- (
- torch.arange(max_tgt_len) * (max_src_len + 1)
- + waitk_lagging - 1
- )
- .unsqueeze(0)
- .expand(bsz, max_tgt_len)
- .long()
- )
-
- if key_padding_mask is not None:
- if key_padding_mask[:, 0].any():
- # Left padding
- activate_indices_offset += (
- key_padding_mask.sum(dim=1, keepdim=True)
- )
-
- # Need to clamp the indices that are too large
- activate_indices_offset = (
- activate_indices_offset
- .clamp(
- 0,
- min(
- [
- max_tgt_len,
- max_src_len - waitk_lagging + 1
- ]
- ) * max_src_len - 1
- )
- )
-
- p_choose = torch.zeros(bsz, max_tgt_len * max_src_len)
-
- p_choose = p_choose.scatter(
- 1,
- activate_indices_offset,
- 1.0
- ).view(bsz, max_tgt_len, max_src_len)
-
- if key_padding_mask is not None:
- p_choose = p_choose.to(key_padding_mask)
- p_choose = p_choose.masked_fill(key_padding_mask.unsqueeze(1), 0)
-
- if incremental_state is not None:
- p_choose = p_choose[:, -1:]
-
- return p_choose.float()
-
-
-def learnable_p_choose(
- energy,
- noise_mean: float = 0.0,
- noise_var: float = 0.0,
- training: bool = True
-):
- """
- Calculating step wise prob for reading and writing
- 1 to read, 0 to write
- energy: bsz, tgt_len, src_len
- """
-
- noise = 0
- if training:
- # add noise here to encourage discretness
- noise = (
- torch.normal(noise_mean, noise_var, energy.size())
- .type_as(energy)
- .to(energy.device)
- )
-
- p_choose = torch.sigmoid(energy + noise)
-
- # p_choose: bsz * self.num_heads, tgt_len, src_len
- return p_choose
diff --git a/spaces/OFA-Sys/OFA-Image_Caption/fairseq/fairseq/data/concat_sentences_dataset.py b/spaces/OFA-Sys/OFA-Image_Caption/fairseq/fairseq/data/concat_sentences_dataset.py
deleted file mode 100644
index 625a29370e90f9d1d7274024afb902ed83a22325..0000000000000000000000000000000000000000
--- a/spaces/OFA-Sys/OFA-Image_Caption/fairseq/fairseq/data/concat_sentences_dataset.py
+++ /dev/null
@@ -1,54 +0,0 @@
-# Copyright (c) Facebook, Inc. and its affiliates.
-#
-# This source code is licensed under the MIT license found in the
-# LICENSE file in the root directory of this source tree.
-
-import torch
-
-from . import FairseqDataset
-
-
-class ConcatSentencesDataset(FairseqDataset):
- def __init__(self, *datasets):
- super().__init__()
- self.datasets = datasets
- assert all(
- len(ds) == len(datasets[0]) for ds in datasets
- ), "datasets must have the same length"
-
- def __getitem__(self, index):
- return torch.cat([ds[index] for ds in self.datasets])
-
- def __len__(self):
- return len(self.datasets[0])
-
- def collater(self, samples):
- return self.datasets[0].collater(samples)
-
- @property
- def sizes(self):
- return sum(ds.sizes for ds in self.datasets)
-
- def num_tokens(self, index):
- return sum(ds.num_tokens(index) for ds in self.datasets)
-
- def size(self, index):
- return sum(ds.size(index) for ds in self.datasets)
-
- def ordered_indices(self):
- return self.datasets[0].ordered_indices()
-
- @property
- def supports_prefetch(self):
- return any(getattr(ds, "supports_prefetch", False) for ds in self.datasets)
-
- def prefetch(self, indices):
- for ds in self.datasets:
- if getattr(ds, "supports_prefetch", False):
- ds.prefetch(indices)
-
- def set_epoch(self, epoch):
- super().set_epoch(epoch)
- for ds in self.datasets:
- if hasattr(ds, "set_epoch"):
- ds.set_epoch(epoch)
diff --git a/spaces/OFA-Sys/OFA-Image_Caption/fairseq/fairseq/sequence_scorer.py b/spaces/OFA-Sys/OFA-Image_Caption/fairseq/fairseq/sequence_scorer.py
deleted file mode 100644
index 411d4df4445ef8dd3f1907ad56f9de6943d1fed8..0000000000000000000000000000000000000000
--- a/spaces/OFA-Sys/OFA-Image_Caption/fairseq/fairseq/sequence_scorer.py
+++ /dev/null
@@ -1,153 +0,0 @@
-# Copyright (c) Facebook, Inc. and its affiliates.
-#
-# This source code is licensed under the MIT license found in the
-# LICENSE file in the root directory of this source tree.
-
-import sys
-
-import torch
-from fairseq import utils
-
-
-class SequenceScorer(object):
- """Scores the target for a given source sentence."""
-
- def __init__(
- self,
- tgt_dict,
- softmax_batch=None,
- compute_alignment=False,
- eos=None,
- symbols_to_strip_from_output=None,
- ):
- self.pad = tgt_dict.pad()
- self.eos = tgt_dict.eos() if eos is None else eos
- self.softmax_batch = softmax_batch or sys.maxsize
- assert self.softmax_batch > 0
- self.compute_alignment = compute_alignment
- self.symbols_to_strip_from_output = (
- symbols_to_strip_from_output.union({self.eos})
- if symbols_to_strip_from_output is not None
- else {self.eos}
- )
-
- @torch.no_grad()
- def generate(self, models, sample, **kwargs):
- """Score a batch of translations."""
- net_input = sample["net_input"]
-
- def batch_for_softmax(dec_out, target):
- # assumes decoder_out[0] is the only thing needed (may not be correct for future models!)
- first, rest = dec_out[0], dec_out[1:]
- bsz, tsz, dim = first.shape
- if bsz * tsz < self.softmax_batch:
- yield dec_out, target, True
- else:
- flat = first.contiguous().view(1, -1, dim)
- flat_tgt = target.contiguous().view(flat.shape[:-1])
- s = 0
- while s < flat.size(1):
- e = s + self.softmax_batch
- yield (flat[:, s:e],) + rest, flat_tgt[:, s:e], False
- s = e
-
- def gather_target_probs(probs, target):
- probs = probs.gather(
- dim=2,
- index=target.unsqueeze(-1),
- )
- return probs
-
- orig_target = sample["target"]
-
- # compute scores for each model in the ensemble
- avg_probs = None
- avg_attn = None
- for model in models:
- model.eval()
- decoder_out = model(**net_input)
- attn = decoder_out[1] if len(decoder_out) > 1 else None
- if type(attn) is dict:
- attn = attn.get("attn", None)
-
- batched = batch_for_softmax(decoder_out, orig_target)
- probs, idx = None, 0
- for bd, tgt, is_single in batched:
- sample["target"] = tgt
- curr_prob = model.get_normalized_probs(
- bd, log_probs=len(models) == 1, sample=sample
- ).data
- if is_single:
- probs = gather_target_probs(curr_prob, orig_target)
- else:
- if probs is None:
- probs = curr_prob.new(orig_target.numel())
- step = curr_prob.size(0) * curr_prob.size(1)
- end = step + idx
- tgt_probs = gather_target_probs(
- curr_prob.view(tgt.shape + (curr_prob.size(-1),)), tgt
- )
- probs[idx:end] = tgt_probs.view(-1)
- idx = end
- sample["target"] = orig_target
-
- probs = probs.view(sample["target"].shape)
-
- if avg_probs is None:
- avg_probs = probs
- else:
- avg_probs.add_(probs)
- if attn is not None:
- if torch.is_tensor(attn):
- attn = attn.data
- else:
- attn = attn[0]
- if avg_attn is None:
- avg_attn = attn
- else:
- avg_attn.add_(attn)
- if len(models) > 1:
- avg_probs.div_(len(models))
- avg_probs.log_()
- if avg_attn is not None:
- avg_attn.div_(len(models))
-
- bsz = avg_probs.size(0)
- hypos = []
- start_idxs = sample["start_indices"] if "start_indices" in sample else [0] * bsz
- for i in range(bsz):
- # remove padding from ref
- ref = (
- utils.strip_pad(sample["target"][i, start_idxs[i] :], self.pad)
- if sample["target"] is not None
- else None
- )
- tgt_len = ref.numel()
- avg_probs_i = avg_probs[i][start_idxs[i] : start_idxs[i] + tgt_len]
- score_i = avg_probs_i.sum() / tgt_len
- if avg_attn is not None:
- avg_attn_i = avg_attn[i]
- if self.compute_alignment:
- alignment = utils.extract_hard_alignment(
- avg_attn_i,
- sample["net_input"]["src_tokens"][i],
- sample["target"][i],
- self.pad,
- self.eos,
- )
- else:
- alignment = None
- else:
- avg_attn_i = alignment = None
- hypos.append(
- [
- {
- "tokens": ref,
- "score": score_i,
- "attention": avg_attn_i,
- "alignment": alignment,
- "positional_scores": avg_probs_i,
- }
- ]
- )
- return hypos
diff --git a/spaces/OFA-Sys/OFA-Image_Caption/fairseq/scripts/convert_dictionary.lua b/spaces/OFA-Sys/OFA-Image_Caption/fairseq/scripts/convert_dictionary.lua
deleted file mode 100644
index 14ee8c997f642c8ff196617c2dcd0584037a60c4..0000000000000000000000000000000000000000
--- a/spaces/OFA-Sys/OFA-Image_Caption/fairseq/scripts/convert_dictionary.lua
+++ /dev/null
@@ -1,34 +0,0 @@
--- Copyright (c) Facebook, Inc. and its affiliates.
---
--- This source code is licensed under the MIT license found in the
--- LICENSE file in the root directory of this source tree.
---
--- Usage: convert_dictionary.lua
-require 'fairseq'
-require 'torch'
-require 'paths'
-
-if #arg < 1 then
- print('usage: convert_dictionary.lua ')
- os.exit(1)
-end
-if not paths.filep(arg[1]) then
- print('error: file does not exit: ' .. arg[1])
- os.exit(1)
-end
-
-dict = torch.load(arg[1])
-dst = paths.basename(arg[1]):gsub('.th7', '.txt')
-assert(dst:match('.txt$'))
-
-f = io.open(dst, 'w')
-for idx, symbol in ipairs(dict.index_to_symbol) do
- if idx > dict.cutoff then
- break
- end
- f:write(symbol)
- f:write(' ')
- f:write(dict.index_to_freq[idx])
- f:write('\n')
-end
-f:close()
diff --git a/spaces/OpenDILabCommunity/LLMRiddlesChatGPTCN/llmriddles/llms/chatgpt.py b/spaces/OpenDILabCommunity/LLMRiddlesChatGPTCN/llmriddles/llms/chatgpt.py
deleted file mode 100644
index e1adbfcf8375bcbfa84b714f1cdfe701795e258d..0000000000000000000000000000000000000000
--- a/spaces/OpenDILabCommunity/LLMRiddlesChatGPTCN/llmriddles/llms/chatgpt.py
+++ /dev/null
@@ -1,25 +0,0 @@
-from functools import lru_cache
-
-from openai import OpenAI
-
-from .base import register_llm
-
-
-@lru_cache()
-def _get_openai_client(api_key):
- return OpenAI(api_key=api_key)
-
-
-def ask_chatgpt(message: str, api_key: str):
- client = _get_openai_client(api_key)
-
- response = client.chat.completions.create(
- model="gpt-3.5-turbo",
- messages=[
- {"role": "user", "content": message}
- ],
- )
- return response.choices[0].message.content.strip()
-
-
-register_llm('chatgpt', ask_chatgpt)
diff --git a/spaces/PAIR/PAIR-Diffusion/ldm/modules/diffusionmodules/upscaling.py b/spaces/PAIR/PAIR-Diffusion/ldm/modules/diffusionmodules/upscaling.py
deleted file mode 100644
index 03816662098ce1ffac79bd939b892e867ab91988..0000000000000000000000000000000000000000
--- a/spaces/PAIR/PAIR-Diffusion/ldm/modules/diffusionmodules/upscaling.py
+++ /dev/null
@@ -1,81 +0,0 @@
-import torch
-import torch.nn as nn
-import numpy as np
-from functools import partial
-
-from ldm.modules.diffusionmodules.util import extract_into_tensor, make_beta_schedule
-from ldm.util import default
-
-
-class AbstractLowScaleModel(nn.Module):
- # for concatenating a downsampled image to the latent representation
- def __init__(self, noise_schedule_config=None):
- super(AbstractLowScaleModel, self).__init__()
- if noise_schedule_config is not None:
- self.register_schedule(**noise_schedule_config)
-
- def register_schedule(self, beta_schedule="linear", timesteps=1000,
- linear_start=1e-4, linear_end=2e-2, cosine_s=8e-3):
- betas = make_beta_schedule(beta_schedule, timesteps, linear_start=linear_start, linear_end=linear_end,
- cosine_s=cosine_s)
- alphas = 1. - betas
- alphas_cumprod = np.cumprod(alphas, axis=0)
- alphas_cumprod_prev = np.append(1., alphas_cumprod[:-1])
-
- timesteps, = betas.shape
- self.num_timesteps = int(timesteps)
- self.linear_start = linear_start
- self.linear_end = linear_end
- assert alphas_cumprod.shape[0] == self.num_timesteps, 'alphas have to be defined for each timestep'
-
- to_torch = partial(torch.tensor, dtype=torch.float32)
-
- self.register_buffer('betas', to_torch(betas))
- self.register_buffer('alphas_cumprod', to_torch(alphas_cumprod))
- self.register_buffer('alphas_cumprod_prev', to_torch(alphas_cumprod_prev))
-
- # calculations for diffusion q(x_t | x_{t-1}) and others
- self.register_buffer('sqrt_alphas_cumprod', to_torch(np.sqrt(alphas_cumprod)))
- self.register_buffer('sqrt_one_minus_alphas_cumprod', to_torch(np.sqrt(1. - alphas_cumprod)))
- self.register_buffer('log_one_minus_alphas_cumprod', to_torch(np.log(1. - alphas_cumprod)))
- self.register_buffer('sqrt_recip_alphas_cumprod', to_torch(np.sqrt(1. / alphas_cumprod)))
- self.register_buffer('sqrt_recipm1_alphas_cumprod', to_torch(np.sqrt(1. / alphas_cumprod - 1)))
-
- def q_sample(self, x_start, t, noise=None):
- noise = default(noise, lambda: torch.randn_like(x_start))
- return (extract_into_tensor(self.sqrt_alphas_cumprod, t, x_start.shape) * x_start +
- extract_into_tensor(self.sqrt_one_minus_alphas_cumprod, t, x_start.shape) * noise)
-
- def forward(self, x):
- return x, None
-
- def decode(self, x):
- return x
-
-
-class SimpleImageConcat(AbstractLowScaleModel):
- # no noise level conditioning
- def __init__(self):
- super(SimpleImageConcat, self).__init__(noise_schedule_config=None)
- self.max_noise_level = 0
-
- def forward(self, x):
- # fix to constant noise level
- return x, torch.zeros(x.shape[0], device=x.device).long()
-
-
-class ImageConcatWithNoiseAugmentation(AbstractLowScaleModel):
- def __init__(self, noise_schedule_config, max_noise_level=1000, to_cuda=False):
- super().__init__(noise_schedule_config=noise_schedule_config)
- self.max_noise_level = max_noise_level
-
- def forward(self, x, noise_level=None):
- if noise_level is None:
- noise_level = torch.randint(0, self.max_noise_level, (x.shape[0],), device=x.device).long()
- else:
- assert isinstance(noise_level, torch.Tensor)
- z = self.q_sample(x, noise_level)
- return z, noise_level
-
-
-
diff --git a/spaces/PAIR/Text2Video-Zero/annotator/uniformer/mmcv/runner/hooks/__init__.py b/spaces/PAIR/Text2Video-Zero/annotator/uniformer/mmcv/runner/hooks/__init__.py
deleted file mode 100644
index 915af28cefab14a14c1188ed861161080fd138a3..0000000000000000000000000000000000000000
--- a/spaces/PAIR/Text2Video-Zero/annotator/uniformer/mmcv/runner/hooks/__init__.py
+++ /dev/null
@@ -1,29 +0,0 @@
-# Copyright (c) OpenMMLab. All rights reserved.
-from .checkpoint import CheckpointHook
-from .closure import ClosureHook
-from .ema import EMAHook
-from .evaluation import DistEvalHook, EvalHook
-from .hook import HOOKS, Hook
-from .iter_timer import IterTimerHook
-from .logger import (DvcliveLoggerHook, LoggerHook, MlflowLoggerHook,
- NeptuneLoggerHook, PaviLoggerHook, TensorboardLoggerHook,
- TextLoggerHook, WandbLoggerHook)
-from .lr_updater import LrUpdaterHook
-from .memory import EmptyCacheHook
-from .momentum_updater import MomentumUpdaterHook
-from .optimizer import (Fp16OptimizerHook, GradientCumulativeFp16OptimizerHook,
- GradientCumulativeOptimizerHook, OptimizerHook)
-from .profiler import ProfilerHook
-from .sampler_seed import DistSamplerSeedHook
-from .sync_buffer import SyncBuffersHook
-
-__all__ = [
- 'HOOKS', 'Hook', 'CheckpointHook', 'ClosureHook', 'LrUpdaterHook',
- 'OptimizerHook', 'Fp16OptimizerHook', 'IterTimerHook',
- 'DistSamplerSeedHook', 'EmptyCacheHook', 'LoggerHook', 'MlflowLoggerHook',
- 'PaviLoggerHook', 'TextLoggerHook', 'TensorboardLoggerHook',
- 'NeptuneLoggerHook', 'WandbLoggerHook', 'DvcliveLoggerHook',
- 'MomentumUpdaterHook', 'SyncBuffersHook', 'EMAHook', 'EvalHook',
- 'DistEvalHook', 'ProfilerHook', 'GradientCumulativeOptimizerHook',
- 'GradientCumulativeFp16OptimizerHook'
-]
diff --git a/spaces/PAIR/Text2Video-Zero/annotator/uniformer/mmcv/version.py b/spaces/PAIR/Text2Video-Zero/annotator/uniformer/mmcv/version.py
deleted file mode 100644
index 1cce4e50bd692d4002e3cac3c545a3fb2efe95d0..0000000000000000000000000000000000000000
--- a/spaces/PAIR/Text2Video-Zero/annotator/uniformer/mmcv/version.py
+++ /dev/null
@@ -1,35 +0,0 @@
-# Copyright (c) OpenMMLab. All rights reserved.
-__version__ = '1.3.17'
-
-
-def parse_version_info(version_str: str, length: int = 4) -> tuple:
- """Parse a version string into a tuple.
-
- Args:
- version_str (str): The version string.
- length (int): The maximum number of version levels. Default: 4.
-
- Returns:
- tuple[int | str]: The version info, e.g., "1.3.0" is parsed into
- (1, 3, 0, 0, 0, 0), and "2.0.0rc1" is parsed into
- (2, 0, 0, 0, 'rc', 1) (when length is set to 4).
- """
- from packaging.version import parse
- version = parse(version_str)
- assert version.release, f'failed to parse version {version_str}'
- release = list(version.release)
- release = release[:length]
- if len(release) < length:
- release = release + [0] * (length - len(release))
- if version.is_prerelease:
- release.extend(list(version.pre))
- elif version.is_postrelease:
- release.extend(list(version.post))
- else:
- release.extend([0, 0])
- return tuple(release)
-
-
-version_info = tuple(int(x) for x in __version__.split('.')[:3])
-
-__all__ = ['__version__', 'version_info', 'parse_version_info']
diff --git a/spaces/PSLD/PSLD/stable-diffusion/run/inverse.sh b/spaces/PSLD/PSLD/stable-diffusion/run/inverse.sh
deleted file mode 100644
index d1ced7ce8db602c9a7d7f14206134e108a2f2d36..0000000000000000000000000000000000000000
--- a/spaces/PSLD/PSLD/stable-diffusion/run/inverse.sh
+++ /dev/null
@@ -1,5 +0,0 @@
-export CUDA_VISIBLE_DEVICES='0'
-python scripts/inverse.py \
- --file_id='00015.png' \
- --task_config='configs/super_resolution_config_psld.yaml' \
- --outdir='outputs/psld-samples-sr';
\ No newline at end of file
diff --git a/spaces/Patt/demo_gradio/app.py b/spaces/Patt/demo_gradio/app.py
deleted file mode 100644
index a0e4e4a3fca610dda33733b0b895e8ccb4041b0e..0000000000000000000000000000000000000000
--- a/spaces/Patt/demo_gradio/app.py
+++ /dev/null
@@ -1,7 +0,0 @@
-import gradio as gd
-
-def greeting(name):
- return "Hello " + name
-
-demo = gd.Interface(fn=greeting, inputs='text', outputs='text')
-demo.launch()
\ No newline at end of file
diff --git a/spaces/Pattr/DrumClassification/lilypond-2.24.2/lib/guile/2.2/ccache/system/base/syntax.go b/spaces/Pattr/DrumClassification/lilypond-2.24.2/lib/guile/2.2/ccache/system/base/syntax.go
deleted file mode 100644
index ee64f37c6e5c98e87151f8175ef2bc1f43a6d256..0000000000000000000000000000000000000000
Binary files a/spaces/Pattr/DrumClassification/lilypond-2.24.2/lib/guile/2.2/ccache/system/base/syntax.go and /dev/null differ
diff --git a/spaces/Pie31415/control-animation/annotator/uniformer/configs/_base_/datasets/stare.py b/spaces/Pie31415/control-animation/annotator/uniformer/configs/_base_/datasets/stare.py
deleted file mode 100644
index 3f71b25488cc11a6b4d582ac52b5a24e1ad1cf8e..0000000000000000000000000000000000000000
--- a/spaces/Pie31415/control-animation/annotator/uniformer/configs/_base_/datasets/stare.py
+++ /dev/null
@@ -1,59 +0,0 @@
-# dataset settings
-dataset_type = 'STAREDataset'
-data_root = 'data/STARE'
-img_norm_cfg = dict(
- mean=[123.675, 116.28, 103.53], std=[58.395, 57.12, 57.375], to_rgb=True)
-img_scale = (605, 700)
-crop_size = (128, 128)
-train_pipeline = [
- dict(type='LoadImageFromFile'),
- dict(type='LoadAnnotations'),
- dict(type='Resize', img_scale=img_scale, ratio_range=(0.5, 2.0)),
- dict(type='RandomCrop', crop_size=crop_size, cat_max_ratio=0.75),
- dict(type='RandomFlip', prob=0.5),
- dict(type='PhotoMetricDistortion'),
- dict(type='Normalize', **img_norm_cfg),
- dict(type='Pad', size=crop_size, pad_val=0, seg_pad_val=255),
- dict(type='DefaultFormatBundle'),
- dict(type='Collect', keys=['img', 'gt_semantic_seg'])
-]
-test_pipeline = [
- dict(type='LoadImageFromFile'),
- dict(
- type='MultiScaleFlipAug',
- img_scale=img_scale,
- # img_ratios=[0.5, 0.75, 1.0, 1.25, 1.5, 1.75, 2.0],
- flip=False,
- transforms=[
- dict(type='Resize', keep_ratio=True),
- dict(type='RandomFlip'),
- dict(type='Normalize', **img_norm_cfg),
- dict(type='ImageToTensor', keys=['img']),
- dict(type='Collect', keys=['img'])
- ])
-]
-
-data = dict(
- samples_per_gpu=4,
- workers_per_gpu=4,
- train=dict(
- type='RepeatDataset',
- times=40000,
- dataset=dict(
- type=dataset_type,
- data_root=data_root,
- img_dir='images/training',
- ann_dir='annotations/training',
- pipeline=train_pipeline)),
- val=dict(
- type=dataset_type,
- data_root=data_root,
- img_dir='images/validation',
- ann_dir='annotations/validation',
- pipeline=test_pipeline),
- test=dict(
- type=dataset_type,
- data_root=data_root,
- img_dir='images/validation',
- ann_dir='annotations/validation',
- pipeline=test_pipeline))
diff --git a/spaces/RamAnanth1/photoguard/utils.py b/spaces/RamAnanth1/photoguard/utils.py
deleted file mode 100644
index 63f941962b8c4c63ca6b92abf4bde872f8658fd5..0000000000000000000000000000000000000000
--- a/spaces/RamAnanth1/photoguard/utils.py
+++ /dev/null
@@ -1,49 +0,0 @@
-from PIL import Image
-import numpy as np
-import torch
-import torchvision.transforms as T
-
-totensor = T.ToTensor()
-topil = T.ToPILImage()
-
-def recover_image(image, init_image, mask, background=False):
- image = totensor(image)
- mask = totensor(mask)
- init_image = totensor(init_image)
- if background:
- result = mask * init_image + (1 - mask) * image
- else:
- result = mask * image + (1 - mask) * init_image
- return topil(result)
-
-def preprocess(image):
- w, h = image.size
- w, h = map(lambda x: x - x % 32, (w, h)) # resize to integer multiple of 32
- image = image.resize((w, h), resample=Image.LANCZOS)
- image = np.array(image).astype(np.float32) / 255.0
- image = image[None].transpose(0, 3, 1, 2)
- image = torch.from_numpy(image)
- return 2.0 * image - 1.0
-
-def prepare_mask_and_masked_image(image, mask):
- image = np.array(image.convert("RGB"))
- image = image[None].transpose(0, 3, 1, 2)
- image = torch.from_numpy(image).to(dtype=torch.float32) / 127.5 - 1.0
-
- mask = np.array(mask.convert("L"))
- mask = mask.astype(np.float32) / 255.0
- mask = mask[None, None]
- mask[mask < 0.5] = 0
- mask[mask >= 0.5] = 1
- mask = torch.from_numpy(mask)
-
- masked_image = image * (mask < 0.5)
-
- return mask, masked_image
-
-def prepare_image(image):
- image = np.array(image.convert("RGB"))
- image = image[None].transpose(0, 3, 1, 2)
- image = torch.from_numpy(image).to(dtype=torch.float32) / 127.5 - 1.0
-
- return image[0]
\ No newline at end of file
diff --git a/spaces/Raspberry-ai/main/.env/lib/python3.11/site-packages/pip/_vendor/rich/box.py b/spaces/Raspberry-ai/main/.env/lib/python3.11/site-packages/pip/_vendor/rich/box.py
deleted file mode 100644
index d0b07cf57e0f8c8694e87ad0d07400cc3d63e94f..0000000000000000000000000000000000000000
--- a/spaces/Raspberry-ai/main/.env/lib/python3.11/site-packages/pip/_vendor/rich/box.py
+++ /dev/null
@@ -1,517 +0,0 @@
-import sys
-from typing import TYPE_CHECKING, Iterable, List
-
-if sys.version_info >= (3, 8):
- from typing import Literal
-else:
- from pip._vendor.typing_extensions import Literal # pragma: no cover
-
-
-from ._loop import loop_last
-
-if TYPE_CHECKING:
- from pip._vendor.rich.console import ConsoleOptions
-
-
-class Box:
- """Defines characters to render boxes.
-
- ┌─┬┐ top
- │ ││ head
- ├─┼┤ head_row
- │ ││ mid
- ├─┼┤ row
- ├─┼┤ foot_row
- │ ││ foot
- └─┴┘ bottom
-
- Args:
- box (str): Characters making up box.
- ascii (bool, optional): True if this box uses ascii characters only. Default is False.
- """
-
- def __init__(self, box: str, *, ascii: bool = False) -> None:
- self._box = box
- self.ascii = ascii
- line1, line2, line3, line4, line5, line6, line7, line8 = box.splitlines()
- # top
- self.top_left, self.top, self.top_divider, self.top_right = iter(line1)
- # head
- self.head_left, _, self.head_vertical, self.head_right = iter(line2)
- # head_row
- (
- self.head_row_left,
- self.head_row_horizontal,
- self.head_row_cross,
- self.head_row_right,
- ) = iter(line3)
-
- # mid
- self.mid_left, _, self.mid_vertical, self.mid_right = iter(line4)
- # row
- self.row_left, self.row_horizontal, self.row_cross, self.row_right = iter(line5)
- # foot_row
- (
- self.foot_row_left,
- self.foot_row_horizontal,
- self.foot_row_cross,
- self.foot_row_right,
- ) = iter(line6)
- # foot
- self.foot_left, _, self.foot_vertical, self.foot_right = iter(line7)
- # bottom
- self.bottom_left, self.bottom, self.bottom_divider, self.bottom_right = iter(
- line8
- )
-
- def __repr__(self) -> str:
- return "Box(...)"
-
- def __str__(self) -> str:
- return self._box
-
- def substitute(self, options: "ConsoleOptions", safe: bool = True) -> "Box":
- """Substitute this box for another if it won't render due to platform issues.
-
- Args:
- options (ConsoleOptions): Console options used in rendering.
- safe (bool, optional): Substitute this for another Box if there are known problems
- displaying on the platform (currently only relevant on Windows). Default is True.
-
- Returns:
- Box: A different Box or the same Box.
- """
- box = self
- if options.legacy_windows and safe:
- box = LEGACY_WINDOWS_SUBSTITUTIONS.get(box, box)
- if options.ascii_only and not box.ascii:
- box = ASCII
- return box
-
- def get_plain_headed_box(self) -> "Box":
- """If this box uses special characters for the borders of the header, then
- return the equivalent box that does not.
-
- Returns:
- Box: The most similar Box that doesn't use header-specific box characters.
- If the current Box already satisfies this criterion, then it's returned.
- """
- return PLAIN_HEADED_SUBSTITUTIONS.get(self, self)
-
- def get_top(self, widths: Iterable[int]) -> str:
- """Get the top of a simple box.
-
- Args:
- widths (List[int]): Widths of columns.
-
- Returns:
- str: A string of box characters.
- """
-
- parts: List[str] = []
- append = parts.append
- append(self.top_left)
- for last, width in loop_last(widths):
- append(self.top * width)
- if not last:
- append(self.top_divider)
- append(self.top_right)
- return "".join(parts)
-
- def get_row(
- self,
- widths: Iterable[int],
- level: Literal["head", "row", "foot", "mid"] = "row",
- edge: bool = True,
- ) -> str:
- """Get the top of a simple box.
-
- Args:
- width (List[int]): Widths of columns.
-
- Returns:
- str: A string of box characters.
- """
- if level == "head":
- left = self.head_row_left
- horizontal = self.head_row_horizontal
- cross = self.head_row_cross
- right = self.head_row_right
- elif level == "row":
- left = self.row_left
- horizontal = self.row_horizontal
- cross = self.row_cross
- right = self.row_right
- elif level == "mid":
- left = self.mid_left
- horizontal = " "
- cross = self.mid_vertical
- right = self.mid_right
- elif level == "foot":
- left = self.foot_row_left
- horizontal = self.foot_row_horizontal
- cross = self.foot_row_cross
- right = self.foot_row_right
- else:
- raise ValueError("level must be 'head', 'row' or 'foot'")
-
- parts: List[str] = []
- append = parts.append
- if edge:
- append(left)
- for last, width in loop_last(widths):
- append(horizontal * width)
- if not last:
- append(cross)
- if edge:
- append(right)
- return "".join(parts)
-
- def get_bottom(self, widths: Iterable[int]) -> str:
- """Get the bottom of a simple box.
-
- Args:
- widths (List[int]): Widths of columns.
-
- Returns:
- str: A string of box characters.
- """
-
- parts: List[str] = []
- append = parts.append
- append(self.bottom_left)
- for last, width in loop_last(widths):
- append(self.bottom * width)
- if not last:
- append(self.bottom_divider)
- append(self.bottom_right)
- return "".join(parts)
-
-
-ASCII: Box = Box(
- """\
-+--+
-| ||
-|-+|
-| ||
-|-+|
-|-+|
-| ||
-+--+
-""",
- ascii=True,
-)
-
-ASCII2: Box = Box(
- """\
-+-++
-| ||
-+-++
-| ||
-+-++
-+-++
-| ||
-+-++
-""",
- ascii=True,
-)
-
-ASCII_DOUBLE_HEAD: Box = Box(
- """\
-+-++
-| ||
-+=++
-| ||
-+-++
-+-++
-| ||
-+-++
-""",
- ascii=True,
-)
-
-SQUARE: Box = Box(
- """\
-┌─┬┐
-│ ││
-├─┼┤
-│ ││
-├─┼┤
-├─┼┤
-│ ││
-└─┴┘
-"""
-)
-
-SQUARE_DOUBLE_HEAD: Box = Box(
- """\
-┌─┬┐
-│ ││
-╞═╪╡
-│ ││
-├─┼┤
-├─┼┤
-│ ││
-└─┴┘
-"""
-)
-
-MINIMAL: Box = Box(
- """\
- ╷
- │
-╶─┼╴
- │
-╶─┼╴
-╶─┼╴
- │
- ╵
-"""
-)
-
-
-MINIMAL_HEAVY_HEAD: Box = Box(
- """\
- ╷
- │
-╺━┿╸
- │
-╶─┼╴
-╶─┼╴
- │
- ╵
-"""
-)
-
-MINIMAL_DOUBLE_HEAD: Box = Box(
- """\
- ╷
- │
- ═╪
- │
- ─┼
- ─┼
- │
- ╵
-"""
-)
-
-
-SIMPLE: Box = Box(
- """\
-
-
- ──
-
-
- ──
-
-
-"""
-)
-
-SIMPLE_HEAD: Box = Box(
- """\
-
-
- ──
-
-
-
-
-
-"""
-)
-
-
-SIMPLE_HEAVY: Box = Box(
- """\
-
-
- ━━
-
-
- ━━
-
-
-"""
-)
-
-
-HORIZONTALS: Box = Box(
- """\
- ──
-
- ──
-
- ──
- ──
-
- ──
-"""
-)
-
-ROUNDED: Box = Box(
- """\
-╭─┬╮
-│ ││
-├─┼┤
-│ ││
-├─┼┤
-├─┼┤
-│ ││
-╰─┴╯
-"""
-)
-
-HEAVY: Box = Box(
- """\
-┏━┳┓
-┃ ┃┃
-┣━╋┫
-┃ ┃┃
-┣━╋┫
-┣━╋┫
-┃ ┃┃
-┗━┻┛
-"""
-)
-
-HEAVY_EDGE: Box = Box(
- """\
-┏━┯┓
-┃ │┃
-┠─┼┨
-┃ │┃
-┠─┼┨
-┠─┼┨
-┃ │┃
-┗━┷┛
-"""
-)
-
-HEAVY_HEAD: Box = Box(
- """\
-┏━┳┓
-┃ ┃┃
-┡━╇┩
-│ ││
-├─┼┤
-├─┼┤
-│ ││
-└─┴┘
-"""
-)
-
-DOUBLE: Box = Box(
- """\
-╔═╦╗
-║ ║║
-╠═╬╣
-║ ║║
-╠═╬╣
-╠═╬╣
-║ ║║
-╚═╩╝
-"""
-)
-
-DOUBLE_EDGE: Box = Box(
- """\
-╔═╤╗
-║ │║
-╟─┼╢
-║ │║
-╟─┼╢
-╟─┼╢
-║ │║
-╚═╧╝
-"""
-)
-
-MARKDOWN: Box = Box(
- """\
-
-| ||
-|-||
-| ||
-|-||
-|-||
-| ||
-
-""",
- ascii=True,
-)
-
-# Map Boxes that don't render with raster fonts on to equivalent that do
-LEGACY_WINDOWS_SUBSTITUTIONS = {
- ROUNDED: SQUARE,
- MINIMAL_HEAVY_HEAD: MINIMAL,
- SIMPLE_HEAVY: SIMPLE,
- HEAVY: SQUARE,
- HEAVY_EDGE: SQUARE,
- HEAVY_HEAD: SQUARE,
-}
-
-# Map headed boxes to their headerless equivalents
-PLAIN_HEADED_SUBSTITUTIONS = {
- HEAVY_HEAD: SQUARE,
- SQUARE_DOUBLE_HEAD: SQUARE,
- MINIMAL_DOUBLE_HEAD: MINIMAL,
- MINIMAL_HEAVY_HEAD: MINIMAL,
- ASCII_DOUBLE_HEAD: ASCII2,
-}
-
-
-if __name__ == "__main__": # pragma: no cover
-
- from pip._vendor.rich.columns import Columns
- from pip._vendor.rich.panel import Panel
-
- from . import box as box
- from .console import Console
- from .table import Table
- from .text import Text
-
- console = Console(record=True)
-
- BOXES = [
- "ASCII",
- "ASCII2",
- "ASCII_DOUBLE_HEAD",
- "SQUARE",
- "SQUARE_DOUBLE_HEAD",
- "MINIMAL",
- "MINIMAL_HEAVY_HEAD",
- "MINIMAL_DOUBLE_HEAD",
- "SIMPLE",
- "SIMPLE_HEAD",
- "SIMPLE_HEAVY",
- "HORIZONTALS",
- "ROUNDED",
- "HEAVY",
- "HEAVY_EDGE",
- "HEAVY_HEAD",
- "DOUBLE",
- "DOUBLE_EDGE",
- "MARKDOWN",
- ]
-
- console.print(Panel("[bold green]Box Constants", style="green"), justify="center")
- console.print()
-
- columns = Columns(expand=True, padding=2)
- for box_name in sorted(BOXES):
- table = Table(
- show_footer=True, style="dim", border_style="not dim", expand=True
- )
- table.add_column("Header 1", "Footer 1")
- table.add_column("Header 2", "Footer 2")
- table.add_row("Cell", "Cell")
- table.add_row("Cell", "Cell")
- table.box = getattr(box, box_name)
- table.title = Text(f"box.{box_name}", style="magenta")
- columns.add_renderable(table)
- console.print(columns)
-
- # console.save_html("box.html", inline_styles=True)
diff --git a/spaces/Realcat/image-matching-webui/third_party/r2d2/extract.py b/spaces/Realcat/image-matching-webui/third_party/r2d2/extract.py
deleted file mode 100644
index 686e619b83f06e4c3d923229ebfa4b426b21a72a..0000000000000000000000000000000000000000
--- a/spaces/Realcat/image-matching-webui/third_party/r2d2/extract.py
+++ /dev/null
@@ -1,202 +0,0 @@
-# Copyright 2019-present NAVER Corp.
-# CC BY-NC-SA 3.0
-# Available only for non-commercial use
-
-
-import os, pdb
-from PIL import Image
-import numpy as np
-import torch
-
-from .tools import common
-from .tools.dataloader import norm_RGB
-from .nets.patchnet import *
-
-
-def load_network(model_fn):
- checkpoint = torch.load(model_fn)
- print("\n>> Creating net = " + checkpoint["net"])
- net = eval(checkpoint["net"])
- nb_of_weights = common.model_size(net)
- print(f" ( Model size: {nb_of_weights/1000:.0f}K parameters )")
-
- # initialization
- weights = checkpoint["state_dict"]
- net.load_state_dict({k.replace("module.", ""): v for k, v in weights.items()})
- return net.eval()
-
-
-class NonMaxSuppression(torch.nn.Module):
- def __init__(self, rel_thr=0.7, rep_thr=0.7):
- nn.Module.__init__(self)
- self.max_filter = torch.nn.MaxPool2d(kernel_size=3, stride=1, padding=1)
- self.rel_thr = rel_thr
- self.rep_thr = rep_thr
-
- def forward(self, reliability, repeatability, **kw):
- assert len(reliability) == len(repeatability) == 1
- reliability, repeatability = reliability[0], repeatability[0]
-
- # local maxima
- maxima = repeatability == self.max_filter(repeatability)
-
- # remove low peaks
- maxima *= repeatability >= self.rep_thr
- maxima *= reliability >= self.rel_thr
-
- return maxima.nonzero().t()[2:4]
-
-
-def extract_multiscale(
- net,
- img,
- detector,
- scale_f=2**0.25,
- min_scale=0.0,
- max_scale=1,
- min_size=256,
- max_size=1024,
- verbose=False,
-):
- old_bm = torch.backends.cudnn.benchmark
- torch.backends.cudnn.benchmark = False # speedup
-
- # extract keypoints at multiple scales
- B, three, H, W = img.shape
- assert B == 1 and three == 3, "should be a batch with a single RGB image"
-
- assert max_scale <= 1
- s = 1.0 # current scale factor
-
- X, Y, S, C, Q, D = [], [], [], [], [], []
- while s + 0.001 >= max(min_scale, min_size / max(H, W)):
- if s - 0.001 <= min(max_scale, max_size / max(H, W)):
- nh, nw = img.shape[2:]
- if verbose:
- print(f"extracting at scale x{s:.02f} = {nw:4d}x{nh:3d}")
- # extract descriptors
- with torch.no_grad():
- res = net(imgs=[img])
-
- # get output and reliability map
- descriptors = res["descriptors"][0]
- reliability = res["reliability"][0]
- repeatability = res["repeatability"][0]
-
- # normalize the reliability for nms
- # extract maxima and descs
- y, x = detector(**res) # nms
- c = reliability[0, 0, y, x]
- q = repeatability[0, 0, y, x]
- d = descriptors[0, :, y, x].t()
- n = d.shape[0]
-
- # accumulate multiple scales
- X.append(x.float() * W / nw)
- Y.append(y.float() * H / nh)
- S.append((32 / s) * torch.ones(n, dtype=torch.float32, device=d.device))
- C.append(c)
- Q.append(q)
- D.append(d)
- s /= scale_f
-
- # down-scale the image for next iteration
- nh, nw = round(H * s), round(W * s)
- img = F.interpolate(img, (nh, nw), mode="bilinear", align_corners=False)
-
- # restore value
- torch.backends.cudnn.benchmark = old_bm
-
- Y = torch.cat(Y)
- X = torch.cat(X)
- S = torch.cat(S) # scale
- scores = torch.cat(C) * torch.cat(Q) # scores = reliability * repeatability
- XYS = torch.stack([X, Y, S], dim=-1)
- D = torch.cat(D)
- return XYS, D, scores
-
-
-def extract_keypoints(args):
- iscuda = common.torch_set_gpu(args.gpu)
-
- # load the network...
- net = load_network(args.model)
- if iscuda:
- net = net.cuda()
-
- # create the non-maxima detector
- detector = NonMaxSuppression(
- rel_thr=args.reliability_thr, rep_thr=args.repeatability_thr
- )
-
- while args.images:
- img_path = args.images.pop(0)
-
- if img_path.endswith(".txt"):
- args.images = open(img_path).read().splitlines() + args.images
- continue
-
- print(f"\nExtracting features for {img_path}")
- img = Image.open(img_path).convert("RGB")
- W, H = img.size
- img = norm_RGB(img)[None]
- if iscuda:
- img = img.cuda()
-
- # extract keypoints/descriptors for a single image
- xys, desc, scores = extract_multiscale(
- net,
- img,
- detector,
- scale_f=args.scale_f,
- min_scale=args.min_scale,
- max_scale=args.max_scale,
- min_size=args.min_size,
- max_size=args.max_size,
- verbose=True,
- )
-
- xys = xys.cpu().numpy()
- desc = desc.cpu().numpy()
- scores = scores.cpu().numpy()
- idxs = scores.argsort()[-args.top_k or None :]
-
- outpath = img_path + "." + args.tag
- print(f"Saving {len(idxs)} keypoints to {outpath}")
- np.savez(
- open(outpath, "wb"),
- imsize=(W, H),
- keypoints=xys[idxs],
- descriptors=desc[idxs],
- scores=scores[idxs],
- )
-
-
-if __name__ == "__main__":
- import argparse
-
- parser = argparse.ArgumentParser("Extract keypoints for a given image")
- parser.add_argument("--model", type=str, required=True, help="model path")
-
- parser.add_argument(
- "--images", type=str, required=True, nargs="+", help="images / list"
- )
- parser.add_argument("--tag", type=str, default="r2d2", help="output file tag")
-
- parser.add_argument("--top-k", type=int, default=5000, help="number of keypoints")
-
- parser.add_argument("--scale-f", type=float, default=2**0.25)
- parser.add_argument("--min-size", type=int, default=256)
- parser.add_argument("--max-size", type=int, default=1024)
- parser.add_argument("--min-scale", type=float, default=0)
- parser.add_argument("--max-scale", type=float, default=1)
-
- parser.add_argument("--reliability-thr", type=float, default=0.7)
- parser.add_argument("--repeatability-thr", type=float, default=0.7)
-
- parser.add_argument(
- "--gpu", type=int, nargs="+", default=[0], help="use -1 for CPU"
- )
- args = parser.parse_args()
-
- extract_keypoints(args)
diff --git a/spaces/RichardMB1217/blip/data/nlvr_dataset.py b/spaces/RichardMB1217/blip/data/nlvr_dataset.py
deleted file mode 100644
index a8d6b2d7cd8d3260bd279c7dca80de53bacc691a..0000000000000000000000000000000000000000
--- a/spaces/RichardMB1217/blip/data/nlvr_dataset.py
+++ /dev/null
@@ -1,78 +0,0 @@
-import os
-import json
-import random
-
-from torch.utils.data import Dataset
-from torchvision.datasets.utils import download_url
-
-from PIL import Image
-
-from data.utils import pre_caption
-
-class nlvr_dataset(Dataset):
- def __init__(self, transform, image_root, ann_root, split):
- '''
- image_root (string): Root directory of images
- ann_root (string): directory to store the annotation file
- split (string): train, val or test
- '''
- urls = {'train':'https://storage.googleapis.com/sfr-vision-language-research/datasets/nlvr_train.json',
- 'val':'https://storage.googleapis.com/sfr-vision-language-research/datasets/nlvr_dev.json',
- 'test':'https://storage.googleapis.com/sfr-vision-language-research/datasets/nlvr_test.json'}
- filenames = {'train':'nlvr_train.json','val':'nlvr_dev.json','test':'nlvr_test.json'}
-
- download_url(urls[split],ann_root)
- self.annotation = json.load(open(os.path.join(ann_root,filenames[split]),'r'))
-
- self.transform = transform
- self.image_root = image_root
-
-
- def __len__(self):
- return len(self.annotation)
-
-
- def __getitem__(self, index):
-
- ann = self.annotation[index]
-
- image0_path = os.path.join(self.image_root,ann['images'][0])
- image0 = Image.open(image0_path).convert('RGB')
- image0 = self.transform(image0)
-
- image1_path = os.path.join(self.image_root,ann['images'][1])
- image1 = Image.open(image1_path).convert('RGB')
- image1 = self.transform(image1)
-
- sentence = pre_caption(ann['sentence'], 40)
-
- if ann['label']=='True':
- label = 1
- else:
- label = 0
-
- words = sentence.split(' ')
-
- if 'left' not in words and 'right' not in words:
- if random.random()<0.5:
- return image0, image1, sentence, label
- else:
- return image1, image0, sentence, label
- else:
- if random.random()<0.5:
- return image0, image1, sentence, label
- else:
- new_words = []
- for word in words:
- if word=='left':
- new_words.append('right')
- elif word=='right':
- new_words.append('left')
- else:
- new_words.append(word)
-
- sentence = ' '.join(new_words)
- return image1, image0, sentence, label
-
-
-
\ No newline at end of file
diff --git a/spaces/Robert001/UniControl-Demo/annotator/uniformer/configs/_base_/models/ocrnet_hr18.py b/spaces/Robert001/UniControl-Demo/annotator/uniformer/configs/_base_/models/ocrnet_hr18.py
deleted file mode 100644
index c60f62a7cdf3f5c5096a7a7e725e8268fddcb057..0000000000000000000000000000000000000000
--- a/spaces/Robert001/UniControl-Demo/annotator/uniformer/configs/_base_/models/ocrnet_hr18.py
+++ /dev/null
@@ -1,68 +0,0 @@
-# model settings
-norm_cfg = dict(type='SyncBN', requires_grad=True)
-model = dict(
- type='CascadeEncoderDecoder',
- num_stages=2,
- pretrained='open-mmlab://msra/hrnetv2_w18',
- backbone=dict(
- type='HRNet',
- norm_cfg=norm_cfg,
- norm_eval=False,
- extra=dict(
- stage1=dict(
- num_modules=1,
- num_branches=1,
- block='BOTTLENECK',
- num_blocks=(4, ),
- num_channels=(64, )),
- stage2=dict(
- num_modules=1,
- num_branches=2,
- block='BASIC',
- num_blocks=(4, 4),
- num_channels=(18, 36)),
- stage3=dict(
- num_modules=4,
- num_branches=3,
- block='BASIC',
- num_blocks=(4, 4, 4),
- num_channels=(18, 36, 72)),
- stage4=dict(
- num_modules=3,
- num_branches=4,
- block='BASIC',
- num_blocks=(4, 4, 4, 4),
- num_channels=(18, 36, 72, 144)))),
- decode_head=[
- dict(
- type='FCNHead',
- in_channels=[18, 36, 72, 144],
- channels=sum([18, 36, 72, 144]),
- in_index=(0, 1, 2, 3),
- input_transform='resize_concat',
- kernel_size=1,
- num_convs=1,
- concat_input=False,
- dropout_ratio=-1,
- num_classes=19,
- norm_cfg=norm_cfg,
- align_corners=False,
- loss_decode=dict(
- type='CrossEntropyLoss', use_sigmoid=False, loss_weight=0.4)),
- dict(
- type='OCRHead',
- in_channels=[18, 36, 72, 144],
- in_index=(0, 1, 2, 3),
- input_transform='resize_concat',
- channels=512,
- ocr_channels=256,
- dropout_ratio=-1,
- num_classes=19,
- norm_cfg=norm_cfg,
- align_corners=False,
- loss_decode=dict(
- type='CrossEntropyLoss', use_sigmoid=False, loss_weight=1.0)),
- ],
- # model training and testing settings
- train_cfg=dict(),
- test_cfg=dict(mode='whole'))
diff --git a/spaces/Robert001/UniControl-Demo/annotator/uniformer/configs/_base_/models/upernet_uniformer.py b/spaces/Robert001/UniControl-Demo/annotator/uniformer/configs/_base_/models/upernet_uniformer.py
deleted file mode 100644
index 41aa4db809dc6e2c508e98051f61807d07477903..0000000000000000000000000000000000000000
--- a/spaces/Robert001/UniControl-Demo/annotator/uniformer/configs/_base_/models/upernet_uniformer.py
+++ /dev/null
@@ -1,43 +0,0 @@
-# model settings
-norm_cfg = dict(type='BN', requires_grad=True)
-model = dict(
- type='EncoderDecoder',
- pretrained=None,
- backbone=dict(
- type='UniFormer',
- embed_dim=[64, 128, 320, 512],
- layers=[3, 4, 8, 3],
- head_dim=64,
- mlp_ratio=4.,
- qkv_bias=True,
- drop_rate=0.,
- attn_drop_rate=0.,
- drop_path_rate=0.1),
- decode_head=dict(
- type='UPerHead',
- in_channels=[64, 128, 320, 512],
- in_index=[0, 1, 2, 3],
- pool_scales=(1, 2, 3, 6),
- channels=512,
- dropout_ratio=0.1,
- num_classes=19,
- norm_cfg=norm_cfg,
- align_corners=False,
- loss_decode=dict(
- type='CrossEntropyLoss', use_sigmoid=False, loss_weight=1.0)),
- auxiliary_head=dict(
- type='FCNHead',
- in_channels=320,
- in_index=2,
- channels=256,
- num_convs=1,
- concat_input=False,
- dropout_ratio=0.1,
- num_classes=19,
- norm_cfg=norm_cfg,
- align_corners=False,
- loss_decode=dict(
- type='CrossEntropyLoss', use_sigmoid=False, loss_weight=0.4)),
- # model training and testing settings
- train_cfg=dict(),
- test_cfg=dict(mode='whole'))
\ No newline at end of file
diff --git a/spaces/Robert001/UniControl-Demo/annotator/uniformer/mmdet_null/models/roi_heads/bbox_heads/bbox_head.py b/spaces/Robert001/UniControl-Demo/annotator/uniformer/mmdet_null/models/roi_heads/bbox_heads/bbox_head.py
deleted file mode 100644
index 408abef3a244115b4e73748049a228e37ad0665c..0000000000000000000000000000000000000000
--- a/spaces/Robert001/UniControl-Demo/annotator/uniformer/mmdet_null/models/roi_heads/bbox_heads/bbox_head.py
+++ /dev/null
@@ -1,483 +0,0 @@
-import torch
-import torch.nn as nn
-import torch.nn.functional as F
-from mmcv.runner import auto_fp16, force_fp32
-from torch.nn.modules.utils import _pair
-
-from mmdet.core import build_bbox_coder, multi_apply, multiclass_nms
-from mmdet.models.builder import HEADS, build_loss
-from mmdet.models.losses import accuracy
-
-
-@HEADS.register_module()
-class BBoxHead(nn.Module):
- """Simplest RoI head, with only two fc layers for classification and
- regression respectively."""
-
- def __init__(self,
- with_avg_pool=False,
- with_cls=True,
- with_reg=True,
- roi_feat_size=7,
- in_channels=256,
- num_classes=80,
- bbox_coder=dict(
- type='DeltaXYWHBBoxCoder',
- clip_border=True,
- target_means=[0., 0., 0., 0.],
- target_stds=[0.1, 0.1, 0.2, 0.2]),
- reg_class_agnostic=False,
- reg_decoded_bbox=False,
- loss_cls=dict(
- type='CrossEntropyLoss',
- use_sigmoid=False,
- loss_weight=1.0),
- loss_bbox=dict(
- type='SmoothL1Loss', beta=1.0, loss_weight=1.0)):
- super(BBoxHead, self).__init__()
- assert with_cls or with_reg
- self.with_avg_pool = with_avg_pool
- self.with_cls = with_cls
- self.with_reg = with_reg
- self.roi_feat_size = _pair(roi_feat_size)
- self.roi_feat_area = self.roi_feat_size[0] * self.roi_feat_size[1]
- self.in_channels = in_channels
- self.num_classes = num_classes
- self.reg_class_agnostic = reg_class_agnostic
- self.reg_decoded_bbox = reg_decoded_bbox
- self.fp16_enabled = False
-
- self.bbox_coder = build_bbox_coder(bbox_coder)
- self.loss_cls = build_loss(loss_cls)
- self.loss_bbox = build_loss(loss_bbox)
-
- in_channels = self.in_channels
- if self.with_avg_pool:
- self.avg_pool = nn.AvgPool2d(self.roi_feat_size)
- else:
- in_channels *= self.roi_feat_area
- if self.with_cls:
- # need to add background class
- self.fc_cls = nn.Linear(in_channels, num_classes + 1)
- if self.with_reg:
- out_dim_reg = 4 if reg_class_agnostic else 4 * num_classes
- self.fc_reg = nn.Linear(in_channels, out_dim_reg)
- self.debug_imgs = None
-
- def init_weights(self):
- # conv layers are already initialized by ConvModule
- if self.with_cls:
- nn.init.normal_(self.fc_cls.weight, 0, 0.01)
- nn.init.constant_(self.fc_cls.bias, 0)
- if self.with_reg:
- nn.init.normal_(self.fc_reg.weight, 0, 0.001)
- nn.init.constant_(self.fc_reg.bias, 0)
-
- @auto_fp16()
- def forward(self, x):
- if self.with_avg_pool:
- x = self.avg_pool(x)
- x = x.view(x.size(0), -1)
- cls_score = self.fc_cls(x) if self.with_cls else None
- bbox_pred = self.fc_reg(x) if self.with_reg else None
- return cls_score, bbox_pred
-
- def _get_target_single(self, pos_bboxes, neg_bboxes, pos_gt_bboxes,
- pos_gt_labels, cfg):
- """Calculate the ground truth for proposals in the single image
- according to the sampling results.
-
- Args:
- pos_bboxes (Tensor): Contains all the positive boxes,
- has shape (num_pos, 4), the last dimension 4
- represents [tl_x, tl_y, br_x, br_y].
- neg_bboxes (Tensor): Contains all the negative boxes,
- has shape (num_neg, 4), the last dimension 4
- represents [tl_x, tl_y, br_x, br_y].
- pos_gt_bboxes (Tensor): Contains all the gt_boxes,
- has shape (num_gt, 4), the last dimension 4
- represents [tl_x, tl_y, br_x, br_y].
- pos_gt_labels (Tensor): Contains all the gt_labels,
- has shape (num_gt).
- cfg (obj:`ConfigDict`): `train_cfg` of R-CNN.
-
- Returns:
- Tuple[Tensor]: Ground truth for proposals
- in a single image. Containing the following Tensors:
-
- - labels(Tensor): Gt_labels for all proposals, has
- shape (num_proposals,).
- - label_weights(Tensor): Labels_weights for all
- proposals, has shape (num_proposals,).
- - bbox_targets(Tensor):Regression target for all
- proposals, has shape (num_proposals, 4), the
- last dimension 4 represents [tl_x, tl_y, br_x, br_y].
- - bbox_weights(Tensor):Regression weights for all
- proposals, has shape (num_proposals, 4).
- """
- num_pos = pos_bboxes.size(0)
- num_neg = neg_bboxes.size(0)
- num_samples = num_pos + num_neg
-
- # original implementation uses new_zeros since BG are set to be 0
- # now use empty & fill because BG cat_id = num_classes,
- # FG cat_id = [0, num_classes-1]
- labels = pos_bboxes.new_full((num_samples, ),
- self.num_classes,
- dtype=torch.long)
- label_weights = pos_bboxes.new_zeros(num_samples)
- bbox_targets = pos_bboxes.new_zeros(num_samples, 4)
- bbox_weights = pos_bboxes.new_zeros(num_samples, 4)
- if num_pos > 0:
- labels[:num_pos] = pos_gt_labels
- pos_weight = 1.0 if cfg.pos_weight <= 0 else cfg.pos_weight
- label_weights[:num_pos] = pos_weight
- if not self.reg_decoded_bbox:
- pos_bbox_targets = self.bbox_coder.encode(
- pos_bboxes, pos_gt_bboxes)
- else:
- # When the regression loss (e.g. `IouLoss`, `GIouLoss`)
- # is applied directly on the decoded bounding boxes, both
- # the predicted boxes and regression targets should be with
- # absolute coordinate format.
- pos_bbox_targets = pos_gt_bboxes
- bbox_targets[:num_pos, :] = pos_bbox_targets
- bbox_weights[:num_pos, :] = 1
- if num_neg > 0:
- label_weights[-num_neg:] = 1.0
-
- return labels, label_weights, bbox_targets, bbox_weights
-
- def get_targets(self,
- sampling_results,
- gt_bboxes,
- gt_labels,
- rcnn_train_cfg,
- concat=True):
- """Calculate the ground truth for all samples in a batch according to
- the sampling_results.
-
- Almost the same as the implementation in bbox_head, we passed
- additional parameters pos_inds_list and neg_inds_list to
- `_get_target_single` function.
-
- Args:
- sampling_results (List[obj:SamplingResults]): Assign results of
- all images in a batch after sampling.
- gt_bboxes (list[Tensor]): Gt_bboxes of all images in a batch,
- each tensor has shape (num_gt, 4), the last dimension 4
- represents [tl_x, tl_y, br_x, br_y].
- gt_labels (list[Tensor]): Gt_labels of all images in a batch,
- each tensor has shape (num_gt,).
- rcnn_train_cfg (obj:ConfigDict): `train_cfg` of RCNN.
- concat (bool): Whether to concatenate the results of all
- the images in a single batch.
-
- Returns:
- Tuple[Tensor]: Ground truth for proposals in a single image.
- Containing the following list of Tensors:
-
- - labels (list[Tensor],Tensor): Gt_labels for all
- proposals in a batch, each tensor in list has
- shape (num_proposals,) when `concat=False`, otherwise
- just a single tensor has shape (num_all_proposals,).
- - label_weights (list[Tensor]): Labels_weights for
- all proposals in a batch, each tensor in list has
- shape (num_proposals,) when `concat=False`, otherwise
- just a single tensor has shape (num_all_proposals,).
- - bbox_targets (list[Tensor],Tensor): Regression target
- for all proposals in a batch, each tensor in list
- has shape (num_proposals, 4) when `concat=False`,
- otherwise just a single tensor has shape
- (num_all_proposals, 4), the last dimension 4 represents
- [tl_x, tl_y, br_x, br_y].
- - bbox_weights (list[tensor],Tensor): Regression weights for
- all proposals in a batch, each tensor in list has shape
- (num_proposals, 4) when `concat=False`, otherwise just a
- single tensor has shape (num_all_proposals, 4).
- """
- pos_bboxes_list = [res.pos_bboxes for res in sampling_results]
- neg_bboxes_list = [res.neg_bboxes for res in sampling_results]
- pos_gt_bboxes_list = [res.pos_gt_bboxes for res in sampling_results]
- pos_gt_labels_list = [res.pos_gt_labels for res in sampling_results]
- labels, label_weights, bbox_targets, bbox_weights = multi_apply(
- self._get_target_single,
- pos_bboxes_list,
- neg_bboxes_list,
- pos_gt_bboxes_list,
- pos_gt_labels_list,
- cfg=rcnn_train_cfg)
-
- if concat:
- labels = torch.cat(labels, 0)
- label_weights = torch.cat(label_weights, 0)
- bbox_targets = torch.cat(bbox_targets, 0)
- bbox_weights = torch.cat(bbox_weights, 0)
- return labels, label_weights, bbox_targets, bbox_weights
-
- @force_fp32(apply_to=('cls_score', 'bbox_pred'))
- def loss(self,
- cls_score,
- bbox_pred,
- rois,
- labels,
- label_weights,
- bbox_targets,
- bbox_weights,
- reduction_override=None):
- losses = dict()
- if cls_score is not None:
- avg_factor = max(torch.sum(label_weights > 0).float().item(), 1.)
- if cls_score.numel() > 0:
- losses['loss_cls'] = self.loss_cls(
- cls_score,
- labels,
- label_weights,
- avg_factor=avg_factor,
- reduction_override=reduction_override)
- losses['acc'] = accuracy(cls_score, labels)
- if bbox_pred is not None:
- bg_class_ind = self.num_classes
- # 0~self.num_classes-1 are FG, self.num_classes is BG
- pos_inds = (labels >= 0) & (labels < bg_class_ind)
- # do not perform bounding box regression for BG anymore.
- if pos_inds.any():
- if self.reg_decoded_bbox:
- # When the regression loss (e.g. `IouLoss`,
- # `GIouLoss`, `DIouLoss`) is applied directly on
- # the decoded bounding boxes, it decodes the
- # already encoded coordinates to absolute format.
- bbox_pred = self.bbox_coder.decode(rois[:, 1:], bbox_pred)
- if self.reg_class_agnostic:
- pos_bbox_pred = bbox_pred.view(
- bbox_pred.size(0), 4)[pos_inds.type(torch.bool)]
- else:
- pos_bbox_pred = bbox_pred.view(
- bbox_pred.size(0), -1,
- 4)[pos_inds.type(torch.bool),
- labels[pos_inds.type(torch.bool)]]
- losses['loss_bbox'] = self.loss_bbox(
- pos_bbox_pred,
- bbox_targets[pos_inds.type(torch.bool)],
- bbox_weights[pos_inds.type(torch.bool)],
- avg_factor=bbox_targets.size(0),
- reduction_override=reduction_override)
- else:
- losses['loss_bbox'] = bbox_pred[pos_inds].sum()
- return losses
-
- @force_fp32(apply_to=('cls_score', 'bbox_pred'))
- def get_bboxes(self,
- rois,
- cls_score,
- bbox_pred,
- img_shape,
- scale_factor,
- rescale=False,
- cfg=None):
- """Transform network output for a batch into bbox predictions.
-
- If the input rois has batch dimension, the function would be in
- `batch_mode` and return is a tuple[list[Tensor], list[Tensor]],
- otherwise, the return is a tuple[Tensor, Tensor].
-
- Args:
- rois (Tensor): Boxes to be transformed. Has shape (num_boxes, 5)
- or (B, num_boxes, 5)
- cls_score (list[Tensor] or Tensor): Box scores for
- each scale level, each is a 4D-tensor, the channel number is
- num_points * num_classes.
- bbox_pred (Tensor, optional): Box energies / deltas for each scale
- level, each is a 4D-tensor, the channel number is
- num_classes * 4.
- img_shape (Sequence[int] or torch.Tensor or Sequence[
- Sequence[int]], optional): Maximum bounds for boxes, specifies
- (H, W, C) or (H, W). If rois shape is (B, num_boxes, 4), then
- the max_shape should be a Sequence[Sequence[int]]
- and the length of max_shape should also be B.
- scale_factor (tuple[ndarray] or ndarray): Scale factor of the
- image arange as (w_scale, h_scale, w_scale, h_scale). In
- `batch_mode`, the scale_factor shape is tuple[ndarray].
- rescale (bool): If True, return boxes in original image space.
- Default: False.
- cfg (obj:`ConfigDict`): `test_cfg` of Bbox Head. Default: None
-
- Returns:
- tuple[list[Tensor], list[Tensor]] or tuple[Tensor, Tensor]:
- If the input has a batch dimension, the return value is
- a tuple of the list. The first list contains the boxes of
- the corresponding image in a batch, each tensor has the
- shape (num_boxes, 5) and last dimension 5 represent
- (tl_x, tl_y, br_x, br_y, score). Each Tensor in the second
- list is the labels with shape (num_boxes, ). The length of
- both lists should be equal to batch_size. Otherwise return
- value is a tuple of two tensors, the first tensor is the
- boxes with scores, the second tensor is the labels, both
- have the same shape as the first case.
- """
- if isinstance(cls_score, list):
- cls_score = sum(cls_score) / float(len(cls_score))
-
- scores = F.softmax(
- cls_score, dim=-1) if cls_score is not None else None
-
- batch_mode = True
- if rois.ndim == 2:
- # e.g. AugTest, Cascade R-CNN, HTC, SCNet...
- batch_mode = False
-
- # add batch dimension
- if scores is not None:
- scores = scores.unsqueeze(0)
- if bbox_pred is not None:
- bbox_pred = bbox_pred.unsqueeze(0)
- rois = rois.unsqueeze(0)
-
- if bbox_pred is not None:
- bboxes = self.bbox_coder.decode(
- rois[..., 1:], bbox_pred, max_shape=img_shape)
- else:
- bboxes = rois[..., 1:].clone()
- if img_shape is not None:
- max_shape = bboxes.new_tensor(img_shape)[..., :2]
- min_xy = bboxes.new_tensor(0)
- max_xy = torch.cat(
- [max_shape] * 2, dim=-1).flip(-1).unsqueeze(-2)
- bboxes = torch.where(bboxes < min_xy, min_xy, bboxes)
- bboxes = torch.where(bboxes > max_xy, max_xy, bboxes)
-
- if rescale and bboxes.size(-2) > 0:
- if not isinstance(scale_factor, tuple):
- scale_factor = tuple([scale_factor])
- # B, 1, bboxes.size(-1)
- scale_factor = bboxes.new_tensor(scale_factor).unsqueeze(1).repeat(
- 1, 1,
- bboxes.size(-1) // 4)
- bboxes /= scale_factor
-
- det_bboxes = []
- det_labels = []
- for (bbox, score) in zip(bboxes, scores):
- if cfg is not None:
- det_bbox, det_label = multiclass_nms(bbox, score,
- cfg.score_thr, cfg.nms,
- cfg.max_per_img)
- else:
- det_bbox, det_label = bbox, score
- det_bboxes.append(det_bbox)
- det_labels.append(det_label)
-
- if not batch_mode:
- det_bboxes = det_bboxes[0]
- det_labels = det_labels[0]
- return det_bboxes, det_labels
-
- @force_fp32(apply_to=('bbox_preds', ))
- def refine_bboxes(self, rois, labels, bbox_preds, pos_is_gts, img_metas):
- """Refine bboxes during training.
-
- Args:
- rois (Tensor): Shape (n*bs, 5), where n is image number per GPU,
- and bs is the sampled RoIs per image. The first column is
- the image id and the next 4 columns are x1, y1, x2, y2.
- labels (Tensor): Shape (n*bs, ).
- bbox_preds (Tensor): Shape (n*bs, 4) or (n*bs, 4*#class).
- pos_is_gts (list[Tensor]): Flags indicating if each positive bbox
- is a gt bbox.
- img_metas (list[dict]): Meta info of each image.
-
- Returns:
- list[Tensor]: Refined bboxes of each image in a mini-batch.
-
- Example:
- >>> # xdoctest: +REQUIRES(module:kwarray)
- >>> import kwarray
- >>> import numpy as np
- >>> from mmdet.core.bbox.demodata import random_boxes
- >>> self = BBoxHead(reg_class_agnostic=True)
- >>> n_roi = 2
- >>> n_img = 4
- >>> scale = 512
- >>> rng = np.random.RandomState(0)
- >>> img_metas = [{'img_shape': (scale, scale)}
- ... for _ in range(n_img)]
- >>> # Create rois in the expected format
- >>> roi_boxes = random_boxes(n_roi, scale=scale, rng=rng)
- >>> img_ids = torch.randint(0, n_img, (n_roi,))
- >>> img_ids = img_ids.float()
- >>> rois = torch.cat([img_ids[:, None], roi_boxes], dim=1)
- >>> # Create other args
- >>> labels = torch.randint(0, 2, (n_roi,)).long()
- >>> bbox_preds = random_boxes(n_roi, scale=scale, rng=rng)
- >>> # For each image, pretend random positive boxes are gts
- >>> is_label_pos = (labels.numpy() > 0).astype(np.int)
- >>> lbl_per_img = kwarray.group_items(is_label_pos,
- ... img_ids.numpy())
- >>> pos_per_img = [sum(lbl_per_img.get(gid, []))
- ... for gid in range(n_img)]
- >>> pos_is_gts = [
- >>> torch.randint(0, 2, (npos,)).byte().sort(
- >>> descending=True)[0]
- >>> for npos in pos_per_img
- >>> ]
- >>> bboxes_list = self.refine_bboxes(rois, labels, bbox_preds,
- >>> pos_is_gts, img_metas)
- >>> print(bboxes_list)
- """
- img_ids = rois[:, 0].long().unique(sorted=True)
- assert img_ids.numel() <= len(img_metas)
-
- bboxes_list = []
- for i in range(len(img_metas)):
- inds = torch.nonzero(
- rois[:, 0] == i, as_tuple=False).squeeze(dim=1)
- num_rois = inds.numel()
-
- bboxes_ = rois[inds, 1:]
- label_ = labels[inds]
- bbox_pred_ = bbox_preds[inds]
- img_meta_ = img_metas[i]
- pos_is_gts_ = pos_is_gts[i]
-
- bboxes = self.regress_by_class(bboxes_, label_, bbox_pred_,
- img_meta_)
-
- # filter gt bboxes
- pos_keep = 1 - pos_is_gts_
- keep_inds = pos_is_gts_.new_ones(num_rois)
- keep_inds[:len(pos_is_gts_)] = pos_keep
-
- bboxes_list.append(bboxes[keep_inds.type(torch.bool)])
-
- return bboxes_list
-
- @force_fp32(apply_to=('bbox_pred', ))
- def regress_by_class(self, rois, label, bbox_pred, img_meta):
- """Regress the bbox for the predicted class. Used in Cascade R-CNN.
-
- Args:
- rois (Tensor): shape (n, 4) or (n, 5)
- label (Tensor): shape (n, )
- bbox_pred (Tensor): shape (n, 4*(#class)) or (n, 4)
- img_meta (dict): Image meta info.
-
- Returns:
- Tensor: Regressed bboxes, the same shape as input rois.
- """
- assert rois.size(1) == 4 or rois.size(1) == 5, repr(rois.shape)
-
- if not self.reg_class_agnostic:
- label = label * 4
- inds = torch.stack((label, label + 1, label + 2, label + 3), 1)
- bbox_pred = torch.gather(bbox_pred, 1, inds)
- assert bbox_pred.size(1) == 4
-
- if rois.size(1) == 4:
- new_rois = self.bbox_coder.decode(
- rois, bbox_pred, max_shape=img_meta['img_shape'])
- else:
- bboxes = self.bbox_coder.decode(
- rois[:, 1:], bbox_pred, max_shape=img_meta['img_shape'])
- new_rois = torch.cat((rois[:, [0]], bboxes), dim=1)
-
- return new_rois
diff --git a/spaces/Robert001/UniControl-Demo/annotator/uniformer_base/mmcv/utils/config.py b/spaces/Robert001/UniControl-Demo/annotator/uniformer_base/mmcv/utils/config.py
deleted file mode 100644
index 17149353aefac6d737c67bb2f35a3a6cd2147b0a..0000000000000000000000000000000000000000
--- a/spaces/Robert001/UniControl-Demo/annotator/uniformer_base/mmcv/utils/config.py
+++ /dev/null
@@ -1,688 +0,0 @@
-# Copyright (c) OpenMMLab. All rights reserved.
-import ast
-import copy
-import os
-import os.path as osp
-import platform
-import shutil
-import sys
-import tempfile
-import uuid
-import warnings
-from argparse import Action, ArgumentParser
-from collections import abc
-from importlib import import_module
-
-from addict import Dict
-from yapf.yapflib.yapf_api import FormatCode
-
-from .misc import import_modules_from_strings
-from .path import check_file_exist
-
-if platform.system() == 'Windows':
- import regex as re
-else:
- import re
-
-BASE_KEY = '_base_'
-DELETE_KEY = '_delete_'
-DEPRECATION_KEY = '_deprecation_'
-RESERVED_KEYS = ['filename', 'text', 'pretty_text']
-
-
-class ConfigDict(Dict):
-
- def __missing__(self, name):
- raise KeyError(name)
-
- def __getattr__(self, name):
- try:
- value = super(ConfigDict, self).__getattr__(name)
- except KeyError:
- ex = AttributeError(f"'{self.__class__.__name__}' object has no "
- f"attribute '{name}'")
- except Exception as e:
- ex = e
- else:
- return value
- raise ex
-
-
-def add_args(parser, cfg, prefix=''):
- for k, v in cfg.items():
- if isinstance(v, str):
- parser.add_argument('--' + prefix + k)
- elif isinstance(v, int):
- parser.add_argument('--' + prefix + k, type=int)
- elif isinstance(v, float):
- parser.add_argument('--' + prefix + k, type=float)
- elif isinstance(v, bool):
- parser.add_argument('--' + prefix + k, action='store_true')
- elif isinstance(v, dict):
- add_args(parser, v, prefix + k + '.')
- elif isinstance(v, abc.Iterable):
- parser.add_argument('--' + prefix + k, type=type(v[0]), nargs='+')
- else:
- print(f'cannot parse key {prefix + k} of type {type(v)}')
- return parser
-
-
-class Config:
- """A facility for config and config files.
-
- It supports common file formats as configs: python/json/yaml. The interface
- is the same as a dict object and also allows access config values as
- attributes.
-
- Example:
- >>> cfg = Config(dict(a=1, b=dict(b1=[0, 1])))
- >>> cfg.a
- 1
- >>> cfg.b
- {'b1': [0, 1]}
- >>> cfg.b.b1
- [0, 1]
- >>> cfg = Config.fromfile('tests/data/config/a.py')
- >>> cfg.filename
- "/home/kchen/projects/mmcv/tests/data/config/a.py"
- >>> cfg.item4
- 'test'
- >>> cfg
- "Config [path: /home/kchen/projects/mmcv/tests/data/config/a.py]: "
- "{'item1': [1, 2], 'item2': {'a': 0}, 'item3': True, 'item4': 'test'}"
- """
-
- @staticmethod
- def _validate_py_syntax(filename):
- with open(filename, 'r', encoding='utf-8') as f:
- # Setting encoding explicitly to resolve coding issue on windows
- content = f.read()
- try:
- ast.parse(content)
- except SyntaxError as e:
- raise SyntaxError('There are syntax errors in config '
- f'file {filename}: {e}')
-
- @staticmethod
- def _substitute_predefined_vars(filename, temp_config_name):
- file_dirname = osp.dirname(filename)
- file_basename = osp.basename(filename)
- file_basename_no_extension = osp.splitext(file_basename)[0]
- file_extname = osp.splitext(filename)[1]
- support_templates = dict(
- fileDirname=file_dirname,
- fileBasename=file_basename,
- fileBasenameNoExtension=file_basename_no_extension,
- fileExtname=file_extname)
- with open(filename, 'r', encoding='utf-8') as f:
- # Setting encoding explicitly to resolve coding issue on windows
- config_file = f.read()
- for key, value in support_templates.items():
- regexp = r'\{\{\s*' + str(key) + r'\s*\}\}'
- value = value.replace('\\', '/')
- config_file = re.sub(regexp, value, config_file)
- with open(temp_config_name, 'w', encoding='utf-8') as tmp_config_file:
- tmp_config_file.write(config_file)
-
- @staticmethod
- def _pre_substitute_base_vars(filename, temp_config_name):
- """Substitute base variable placehoders to string, so that parsing
- would work."""
- with open(filename, 'r', encoding='utf-8') as f:
- # Setting encoding explicitly to resolve coding issue on windows
- config_file = f.read()
- base_var_dict = {}
- regexp = r'\{\{\s*' + BASE_KEY + r'\.([\w\.]+)\s*\}\}'
- base_vars = set(re.findall(regexp, config_file))
- for base_var in base_vars:
- randstr = f'_{base_var}_{uuid.uuid4().hex.lower()[:6]}'
- base_var_dict[randstr] = base_var
- regexp = r'\{\{\s*' + BASE_KEY + r'\.' + base_var + r'\s*\}\}'
- config_file = re.sub(regexp, f'"{randstr}"', config_file)
- with open(temp_config_name, 'w', encoding='utf-8') as tmp_config_file:
- tmp_config_file.write(config_file)
- return base_var_dict
-
- @staticmethod
- def _substitute_base_vars(cfg, base_var_dict, base_cfg):
- """Substitute variable strings to their actual values."""
- cfg = copy.deepcopy(cfg)
-
- if isinstance(cfg, dict):
- for k, v in cfg.items():
- if isinstance(v, str) and v in base_var_dict:
- new_v = base_cfg
- for new_k in base_var_dict[v].split('.'):
- new_v = new_v[new_k]
- cfg[k] = new_v
- elif isinstance(v, (list, tuple, dict)):
- cfg[k] = Config._substitute_base_vars(
- v, base_var_dict, base_cfg)
- elif isinstance(cfg, tuple):
- cfg = tuple(
- Config._substitute_base_vars(c, base_var_dict, base_cfg)
- for c in cfg)
- elif isinstance(cfg, list):
- cfg = [
- Config._substitute_base_vars(c, base_var_dict, base_cfg)
- for c in cfg
- ]
- elif isinstance(cfg, str) and cfg in base_var_dict:
- new_v = base_cfg
- for new_k in base_var_dict[cfg].split('.'):
- new_v = new_v[new_k]
- cfg = new_v
-
- return cfg
-
- @staticmethod
- def _file2dict(filename, use_predefined_variables=True):
- filename = osp.abspath(osp.expanduser(filename))
- check_file_exist(filename)
- fileExtname = osp.splitext(filename)[1]
- if fileExtname not in ['.py', '.json', '.yaml', '.yml']:
- raise IOError('Only py/yml/yaml/json type are supported now!')
-
- with tempfile.TemporaryDirectory() as temp_config_dir:
- temp_config_file = tempfile.NamedTemporaryFile(
- dir=temp_config_dir, suffix=fileExtname)
- if platform.system() == 'Windows':
- temp_config_file.close()
- temp_config_name = osp.basename(temp_config_file.name)
- # Substitute predefined variables
- if use_predefined_variables:
- Config._substitute_predefined_vars(filename,
- temp_config_file.name)
- else:
- shutil.copyfile(filename, temp_config_file.name)
- # Substitute base variables from placeholders to strings
- base_var_dict = Config._pre_substitute_base_vars(
- temp_config_file.name, temp_config_file.name)
-
- if filename.endswith('.py'):
- temp_module_name = osp.splitext(temp_config_name)[0]
- sys.path.insert(0, temp_config_dir)
- Config._validate_py_syntax(filename)
- mod = import_module(temp_module_name)
- sys.path.pop(0)
- cfg_dict = {
- name: value
- for name, value in mod.__dict__.items()
- if not name.startswith('__')
- }
- # delete imported module
- del sys.modules[temp_module_name]
- elif filename.endswith(('.yml', '.yaml', '.json')):
- import annotator.uniformer.mmcv as mmcv
- cfg_dict = mmcv.load(temp_config_file.name)
- # close temp file
- temp_config_file.close()
-
- # check deprecation information
- if DEPRECATION_KEY in cfg_dict:
- deprecation_info = cfg_dict.pop(DEPRECATION_KEY)
- warning_msg = f'The config file {filename} will be deprecated ' \
- 'in the future.'
- if 'expected' in deprecation_info:
- warning_msg += f' Please use {deprecation_info["expected"]} ' \
- 'instead.'
- if 'reference' in deprecation_info:
- warning_msg += ' More information can be found at ' \
- f'{deprecation_info["reference"]}'
- warnings.warn(warning_msg)
-
- cfg_text = filename + '\n'
- with open(filename, 'r', encoding='utf-8') as f:
- # Setting encoding explicitly to resolve coding issue on windows
- cfg_text += f.read()
-
- if BASE_KEY in cfg_dict:
- cfg_dir = osp.dirname(filename)
- base_filename = cfg_dict.pop(BASE_KEY)
- base_filename = base_filename if isinstance(
- base_filename, list) else [base_filename]
-
- cfg_dict_list = list()
- cfg_text_list = list()
- for f in base_filename:
- _cfg_dict, _cfg_text = Config._file2dict(osp.join(cfg_dir, f))
- cfg_dict_list.append(_cfg_dict)
- cfg_text_list.append(_cfg_text)
-
- base_cfg_dict = dict()
- for c in cfg_dict_list:
- duplicate_keys = base_cfg_dict.keys() & c.keys()
- if len(duplicate_keys) > 0:
- raise KeyError('Duplicate key is not allowed among bases. '
- f'Duplicate keys: {duplicate_keys}')
- base_cfg_dict.update(c)
-
- # Substitute base variables from strings to their actual values
- cfg_dict = Config._substitute_base_vars(cfg_dict, base_var_dict,
- base_cfg_dict)
-
- base_cfg_dict = Config._merge_a_into_b(cfg_dict, base_cfg_dict)
- cfg_dict = base_cfg_dict
-
- # merge cfg_text
- cfg_text_list.append(cfg_text)
- cfg_text = '\n'.join(cfg_text_list)
-
- return cfg_dict, cfg_text
-
- @staticmethod
- def _merge_a_into_b(a, b, allow_list_keys=False):
- """merge dict ``a`` into dict ``b`` (non-inplace).
-
- Values in ``a`` will overwrite ``b``. ``b`` is copied first to avoid
- in-place modifications.
-
- Args:
- a (dict): The source dict to be merged into ``b``.
- b (dict): The origin dict to be fetch keys from ``a``.
- allow_list_keys (bool): If True, int string keys (e.g. '0', '1')
- are allowed in source ``a`` and will replace the element of the
- corresponding index in b if b is a list. Default: False.
-
- Returns:
- dict: The modified dict of ``b`` using ``a``.
-
- Examples:
- # Normally merge a into b.
- >>> Config._merge_a_into_b(
- ... dict(obj=dict(a=2)), dict(obj=dict(a=1)))
- {'obj': {'a': 2}}
-
- # Delete b first and merge a into b.
- >>> Config._merge_a_into_b(
- ... dict(obj=dict(_delete_=True, a=2)), dict(obj=dict(a=1)))
- {'obj': {'a': 2}}
-
- # b is a list
- >>> Config._merge_a_into_b(
- ... {'0': dict(a=2)}, [dict(a=1), dict(b=2)], True)
- [{'a': 2}, {'b': 2}]
- """
- b = b.copy()
- for k, v in a.items():
- if allow_list_keys and k.isdigit() and isinstance(b, list):
- k = int(k)
- if len(b) <= k:
- raise KeyError(f'Index {k} exceeds the length of list {b}')
- b[k] = Config._merge_a_into_b(v, b[k], allow_list_keys)
- elif isinstance(v,
- dict) and k in b and not v.pop(DELETE_KEY, False):
- allowed_types = (dict, list) if allow_list_keys else dict
- if not isinstance(b[k], allowed_types):
- raise TypeError(
- f'{k}={v} in child config cannot inherit from base '
- f'because {k} is a dict in the child config but is of '
- f'type {type(b[k])} in base config. You may set '
- f'`{DELETE_KEY}=True` to ignore the base config')
- b[k] = Config._merge_a_into_b(v, b[k], allow_list_keys)
- else:
- b[k] = v
- return b
-
- @staticmethod
- def fromfile(filename,
- use_predefined_variables=True,
- import_custom_modules=True):
- cfg_dict, cfg_text = Config._file2dict(filename,
- use_predefined_variables)
- if import_custom_modules and cfg_dict.get('custom_imports', None):
- import_modules_from_strings(**cfg_dict['custom_imports'])
- return Config(cfg_dict, cfg_text=cfg_text, filename=filename)
-
- @staticmethod
- def fromstring(cfg_str, file_format):
- """Generate config from config str.
-
- Args:
- cfg_str (str): Config str.
- file_format (str): Config file format corresponding to the
- config str. Only py/yml/yaml/json type are supported now!
-
- Returns:
- obj:`Config`: Config obj.
- """
- if file_format not in ['.py', '.json', '.yaml', '.yml']:
- raise IOError('Only py/yml/yaml/json type are supported now!')
- if file_format != '.py' and 'dict(' in cfg_str:
- # check if users specify a wrong suffix for python
- warnings.warn(
- 'Please check "file_format", the file format may be .py')
- with tempfile.NamedTemporaryFile(
- 'w', encoding='utf-8', suffix=file_format,
- delete=False) as temp_file:
- temp_file.write(cfg_str)
- # on windows, previous implementation cause error
- # see PR 1077 for details
- cfg = Config.fromfile(temp_file.name)
- os.remove(temp_file.name)
- return cfg
-
- @staticmethod
- def auto_argparser(description=None):
- """Generate argparser from config file automatically (experimental)"""
- partial_parser = ArgumentParser(description=description)
- partial_parser.add_argument('config', help='config file path')
- cfg_file = partial_parser.parse_known_args()[0].config
- cfg = Config.fromfile(cfg_file)
- parser = ArgumentParser(description=description)
- parser.add_argument('config', help='config file path')
- add_args(parser, cfg)
- return parser, cfg
-
- def __init__(self, cfg_dict=None, cfg_text=None, filename=None):
- if cfg_dict is None:
- cfg_dict = dict()
- elif not isinstance(cfg_dict, dict):
- raise TypeError('cfg_dict must be a dict, but '
- f'got {type(cfg_dict)}')
- for key in cfg_dict:
- if key in RESERVED_KEYS:
- raise KeyError(f'{key} is reserved for config file')
-
- super(Config, self).__setattr__('_cfg_dict', ConfigDict(cfg_dict))
- super(Config, self).__setattr__('_filename', filename)
- if cfg_text:
- text = cfg_text
- elif filename:
- with open(filename, 'r') as f:
- text = f.read()
- else:
- text = ''
- super(Config, self).__setattr__('_text', text)
-
- @property
- def filename(self):
- return self._filename
-
- @property
- def text(self):
- return self._text
-
- @property
- def pretty_text(self):
-
- indent = 4
-
- def _indent(s_, num_spaces):
- s = s_.split('\n')
- if len(s) == 1:
- return s_
- first = s.pop(0)
- s = [(num_spaces * ' ') + line for line in s]
- s = '\n'.join(s)
- s = first + '\n' + s
- return s
-
- def _format_basic_types(k, v, use_mapping=False):
- if isinstance(v, str):
- v_str = f"'{v}'"
- else:
- v_str = str(v)
-
- if use_mapping:
- k_str = f"'{k}'" if isinstance(k, str) else str(k)
- attr_str = f'{k_str}: {v_str}'
- else:
- attr_str = f'{str(k)}={v_str}'
- attr_str = _indent(attr_str, indent)
-
- return attr_str
-
- def _format_list(k, v, use_mapping=False):
- # check if all items in the list are dict
- if all(isinstance(_, dict) for _ in v):
- v_str = '[\n'
- v_str += '\n'.join(
- f'dict({_indent(_format_dict(v_), indent)}),'
- for v_ in v).rstrip(',')
- if use_mapping:
- k_str = f"'{k}'" if isinstance(k, str) else str(k)
- attr_str = f'{k_str}: {v_str}'
- else:
- attr_str = f'{str(k)}={v_str}'
- attr_str = _indent(attr_str, indent) + ']'
- else:
- attr_str = _format_basic_types(k, v, use_mapping)
- return attr_str
-
- def _contain_invalid_identifier(dict_str):
- contain_invalid_identifier = False
- for key_name in dict_str:
- contain_invalid_identifier |= \
- (not str(key_name).isidentifier())
- return contain_invalid_identifier
-
- def _format_dict(input_dict, outest_level=False):
- r = ''
- s = []
-
- use_mapping = _contain_invalid_identifier(input_dict)
- if use_mapping:
- r += '{'
- for idx, (k, v) in enumerate(input_dict.items()):
- is_last = idx >= len(input_dict) - 1
- end = '' if outest_level or is_last else ','
- if isinstance(v, dict):
- v_str = '\n' + _format_dict(v)
- if use_mapping:
- k_str = f"'{k}'" if isinstance(k, str) else str(k)
- attr_str = f'{k_str}: dict({v_str}'
- else:
- attr_str = f'{str(k)}=dict({v_str}'
- attr_str = _indent(attr_str, indent) + ')' + end
- elif isinstance(v, list):
- attr_str = _format_list(k, v, use_mapping) + end
- else:
- attr_str = _format_basic_types(k, v, use_mapping) + end
-
- s.append(attr_str)
- r += '\n'.join(s)
- if use_mapping:
- r += '}'
- return r
-
- cfg_dict = self._cfg_dict.to_dict()
- text = _format_dict(cfg_dict, outest_level=True)
- # copied from setup.cfg
- yapf_style = dict(
- based_on_style='pep8',
- blank_line_before_nested_class_or_def=True,
- split_before_expression_after_opening_paren=True)
- text, _ = FormatCode(text, style_config=yapf_style, verify=True)
-
- return text
-
- def __repr__(self):
- return f'Config (path: {self.filename}): {self._cfg_dict.__repr__()}'
-
- def __len__(self):
- return len(self._cfg_dict)
-
- def __getattr__(self, name):
- return getattr(self._cfg_dict, name)
-
- def __getitem__(self, name):
- return self._cfg_dict.__getitem__(name)
-
- def __setattr__(self, name, value):
- if isinstance(value, dict):
- value = ConfigDict(value)
- self._cfg_dict.__setattr__(name, value)
-
- def __setitem__(self, name, value):
- if isinstance(value, dict):
- value = ConfigDict(value)
- self._cfg_dict.__setitem__(name, value)
-
- def __iter__(self):
- return iter(self._cfg_dict)
-
- def __getstate__(self):
- return (self._cfg_dict, self._filename, self._text)
-
- def __setstate__(self, state):
- _cfg_dict, _filename, _text = state
- super(Config, self).__setattr__('_cfg_dict', _cfg_dict)
- super(Config, self).__setattr__('_filename', _filename)
- super(Config, self).__setattr__('_text', _text)
-
- def dump(self, file=None):
- cfg_dict = super(Config, self).__getattribute__('_cfg_dict').to_dict()
- if self.filename.endswith('.py'):
- if file is None:
- return self.pretty_text
- else:
- with open(file, 'w', encoding='utf-8') as f:
- f.write(self.pretty_text)
- else:
- import annotator.uniformer.mmcv as mmcv
- if file is None:
- file_format = self.filename.split('.')[-1]
- return mmcv.dump(cfg_dict, file_format=file_format)
- else:
- mmcv.dump(cfg_dict, file)
-
- def merge_from_dict(self, options, allow_list_keys=True):
- """Merge list into cfg_dict.
-
- Merge the dict parsed by MultipleKVAction into this cfg.
-
- Examples:
- >>> options = {'model.backbone.depth': 50,
- ... 'model.backbone.with_cp':True}
- >>> cfg = Config(dict(model=dict(backbone=dict(type='ResNet'))))
- >>> cfg.merge_from_dict(options)
- >>> cfg_dict = super(Config, self).__getattribute__('_cfg_dict')
- >>> assert cfg_dict == dict(
- ... model=dict(backbone=dict(depth=50, with_cp=True)))
-
- # Merge list element
- >>> cfg = Config(dict(pipeline=[
- ... dict(type='LoadImage'), dict(type='LoadAnnotations')]))
- >>> options = dict(pipeline={'0': dict(type='SelfLoadImage')})
- >>> cfg.merge_from_dict(options, allow_list_keys=True)
- >>> cfg_dict = super(Config, self).__getattribute__('_cfg_dict')
- >>> assert cfg_dict == dict(pipeline=[
- ... dict(type='SelfLoadImage'), dict(type='LoadAnnotations')])
-
- Args:
- options (dict): dict of configs to merge from.
- allow_list_keys (bool): If True, int string keys (e.g. '0', '1')
- are allowed in ``options`` and will replace the element of the
- corresponding index in the config if the config is a list.
- Default: True.
- """
- option_cfg_dict = {}
- for full_key, v in options.items():
- d = option_cfg_dict
- key_list = full_key.split('.')
- for subkey in key_list[:-1]:
- d.setdefault(subkey, ConfigDict())
- d = d[subkey]
- subkey = key_list[-1]
- d[subkey] = v
-
- cfg_dict = super(Config, self).__getattribute__('_cfg_dict')
- super(Config, self).__setattr__(
- '_cfg_dict',
- Config._merge_a_into_b(
- option_cfg_dict, cfg_dict, allow_list_keys=allow_list_keys))
-
-
-class DictAction(Action):
- """
- argparse action to split an argument into KEY=VALUE form
- on the first = and append to a dictionary. List options can
- be passed as comma separated values, i.e 'KEY=V1,V2,V3', or with explicit
- brackets, i.e. 'KEY=[V1,V2,V3]'. It also support nested brackets to build
- list/tuple values. e.g. 'KEY=[(V1,V2),(V3,V4)]'
- """
-
- @staticmethod
- def _parse_int_float_bool(val):
- try:
- return int(val)
- except ValueError:
- pass
- try:
- return float(val)
- except ValueError:
- pass
- if val.lower() in ['true', 'false']:
- return True if val.lower() == 'true' else False
- return val
-
- @staticmethod
- def _parse_iterable(val):
- """Parse iterable values in the string.
-
- All elements inside '()' or '[]' are treated as iterable values.
-
- Args:
- val (str): Value string.
-
- Returns:
- list | tuple: The expanded list or tuple from the string.
-
- Examples:
- >>> DictAction._parse_iterable('1,2,3')
- [1, 2, 3]
- >>> DictAction._parse_iterable('[a, b, c]')
- ['a', 'b', 'c']
- >>> DictAction._parse_iterable('[(1, 2, 3), [a, b], c]')
- [(1, 2, 3), ['a', 'b'], 'c']
- """
-
- def find_next_comma(string):
- """Find the position of next comma in the string.
-
- If no ',' is found in the string, return the string length. All
- chars inside '()' and '[]' are treated as one element and thus ','
- inside these brackets are ignored.
- """
- assert (string.count('(') == string.count(')')) and (
- string.count('[') == string.count(']')), \
- f'Imbalanced brackets exist in {string}'
- end = len(string)
- for idx, char in enumerate(string):
- pre = string[:idx]
- # The string before this ',' is balanced
- if ((char == ',') and (pre.count('(') == pre.count(')'))
- and (pre.count('[') == pre.count(']'))):
- end = idx
- break
- return end
-
- # Strip ' and " characters and replace whitespace.
- val = val.strip('\'\"').replace(' ', '')
- is_tuple = False
- if val.startswith('(') and val.endswith(')'):
- is_tuple = True
- val = val[1:-1]
- elif val.startswith('[') and val.endswith(']'):
- val = val[1:-1]
- elif ',' not in val:
- # val is a single value
- return DictAction._parse_int_float_bool(val)
-
- values = []
- while len(val) > 0:
- comma_idx = find_next_comma(val)
- element = DictAction._parse_iterable(val[:comma_idx])
- values.append(element)
- val = val[comma_idx + 1:]
- if is_tuple:
- values = tuple(values)
- return values
-
- def __call__(self, parser, namespace, values, option_string=None):
- options = {}
- for kv in values:
- key, val = kv.split('=', maxsplit=1)
- options[key] = self._parse_iterable(val)
- setattr(namespace, self.dest, options)
diff --git a/spaces/Rothfeld/stable-diffusion-mat-outpainting-primer/metrics/psnr_ssim_l1.py b/spaces/Rothfeld/stable-diffusion-mat-outpainting-primer/metrics/psnr_ssim_l1.py
deleted file mode 100644
index 68fa061b9924d025bc5491fb2d02a61b2daf085f..0000000000000000000000000000000000000000
--- a/spaces/Rothfeld/stable-diffusion-mat-outpainting-primer/metrics/psnr_ssim_l1.py
+++ /dev/null
@@ -1,19 +0,0 @@
-import numpy as np
-import scipy.linalg
-from . import metric_utils
-import math
-import cv2
-
-
-def compute_psnr(opts, max_real):
- # stats: numpy, [N, 3]
- stats = metric_utils.compute_image_stats_for_generator(opts=opts, capture_all=True, max_items=max_real).get_all()
-
- if opts.rank != 0:
- return float('nan'), float('nan'), float('nan')
-
- print('Number of samples: %d' % stats.shape[0])
- avg_psnr = stats[:, 0].sum() / stats.shape[0]
- avg_ssim = stats[:, 1].sum() / stats.shape[0]
- avg_l1 = stats[:, 2].sum() / stats.shape[0]
- return avg_psnr, avg_ssim, avg_l1
\ No newline at end of file
diff --git a/spaces/Ryzal/rvc-models-new/config.py b/spaces/Ryzal/rvc-models-new/config.py
deleted file mode 100644
index 2fda460b186b86923e757618c2f4f6fc0c45d8cf..0000000000000000000000000000000000000000
--- a/spaces/Ryzal/rvc-models-new/config.py
+++ /dev/null
@@ -1,117 +0,0 @@
-import argparse
-import sys
-import torch
-from multiprocessing import cpu_count
-
-class Config:
- def __init__(self):
- self.device = "cuda:0"
- self.is_half = True
- self.n_cpu = 0
- self.gpu_name = None
- self.gpu_mem = None
- (
- self.python_cmd,
- self.listen_port,
- self.colab,
- self.noparallel,
- self.noautoopen,
- self.api
- ) = self.arg_parse()
- self.x_pad, self.x_query, self.x_center, self.x_max = self.device_config()
-
- @staticmethod
- def arg_parse() -> tuple:
- exe = sys.executable or "python"
- parser = argparse.ArgumentParser()
- parser.add_argument("--port", type=int, default=7865, help="Listen port")
- parser.add_argument("--pycmd", type=str, default=exe, help="Python command")
- parser.add_argument("--colab", action="store_true", help="Launch in colab")
- parser.add_argument(
- "--noparallel", action="store_true", help="Disable parallel processing"
- )
- parser.add_argument(
- "--noautoopen",
- action="store_true",
- help="Do not open in browser automatically",
- )
- parser.add_argument("--api", action="store_true", help="Launch with api")
- cmd_opts = parser.parse_args()
-
- cmd_opts.port = cmd_opts.port if 0 <= cmd_opts.port <= 65535 else 7865
-
- return (
- cmd_opts.pycmd,
- cmd_opts.port,
- cmd_opts.colab,
- cmd_opts.noparallel,
- cmd_opts.noautoopen,
- cmd_opts.api
- )
-
- # has_mps is only available in nightly pytorch (for now) and MasOS 12.3+.
- # check `getattr` and try it for compatibility
- @staticmethod
- def has_mps() -> bool:
- if not torch.backends.mps.is_available():
- return False
- try:
- torch.zeros(1).to(torch.device("mps"))
- return True
- except Exception:
- return False
-
- def device_config(self) -> tuple:
- if torch.cuda.is_available():
- i_device = int(self.device.split(":")[-1])
- self.gpu_name = torch.cuda.get_device_name(i_device)
- if (
- ("16" in self.gpu_name and "V100" not in self.gpu_name.upper())
- or "P40" in self.gpu_name.upper()
- or "1060" in self.gpu_name
- or "1070" in self.gpu_name
- or "1080" in self.gpu_name
- ):
- print("Found GPU", self.gpu_name, ", force to fp32")
- self.is_half = False
- else:
- print("Found GPU", self.gpu_name)
- self.gpu_mem = int(
- torch.cuda.get_device_properties(i_device).total_memory
- / 1024
- / 1024
- / 1024
- + 0.4
- )
- elif self.has_mps():
- print("No supported Nvidia GPU found, use MPS instead")
- self.device = "mps"
- self.is_half = False
- else:
- print("No supported Nvidia GPU found, use CPU instead")
- self.device = "cpu"
- self.is_half = False
-
- if self.n_cpu == 0:
- self.n_cpu = cpu_count()
-
- if self.is_half:
- # 6G显存配置
- x_pad = 3
- x_query = 10
- x_center = 60
- x_max = 65
- else:
- # 5G显存配置
- x_pad = 1
- x_query = 6
- x_center = 38
- x_max = 41
-
- if self.gpu_mem != None and self.gpu_mem <= 4:
- x_pad = 1
- x_query = 5
- x_center = 30
- x_max = 32
-
- return x_pad, x_query, x_center, x_max
diff --git a/spaces/SQSora/VITS-Umamusume-voice-synthesizer/text/thai.py b/spaces/SQSora/VITS-Umamusume-voice-synthesizer/text/thai.py
deleted file mode 100644
index 998207c01a85c710a46db1ec8b62c39c2d94bc84..0000000000000000000000000000000000000000
--- a/spaces/SQSora/VITS-Umamusume-voice-synthesizer/text/thai.py
+++ /dev/null
@@ -1,44 +0,0 @@
-import re
-from num_thai.thainumbers import NumThai
-
-
-num = NumThai()
-
-# List of (Latin alphabet, Thai) pairs:
-_latin_to_thai = [(re.compile('%s' % x[0], re.IGNORECASE), x[1]) for x in [
- ('a', 'เอ'),
- ('b','บี'),
- ('c','ซี'),
- ('d','ดี'),
- ('e','อี'),
- ('f','เอฟ'),
- ('g','จี'),
- ('h','เอช'),
- ('i','ไอ'),
- ('j','เจ'),
- ('k','เค'),
- ('l','แอล'),
- ('m','เอ็ม'),
- ('n','เอ็น'),
- ('o','โอ'),
- ('p','พี'),
- ('q','คิว'),
- ('r','แอร์'),
- ('s','เอส'),
- ('t','ที'),
- ('u','ยู'),
- ('v','วี'),
- ('w','ดับเบิลยู'),
- ('x','เอ็กซ์'),
- ('y','วาย'),
- ('z','ซี')
-]]
-
-
-def num_to_thai(text):
- return re.sub(r'(?:\d+(?:,?\d+)?)+(?:\.\d+(?:,?\d+)?)?', lambda x: ''.join(num.NumberToTextThai(float(x.group(0).replace(',', '')))), text)
-
-def latin_to_thai(text):
- for regex, replacement in _latin_to_thai:
- text = re.sub(regex, replacement, text)
- return text
diff --git a/spaces/SakshiRathi77/SakshiRathi77-wav2vec2_xlsr_300m/README.md b/spaces/SakshiRathi77/SakshiRathi77-wav2vec2_xlsr_300m/README.md
deleted file mode 100644
index 7080beb5a87a435e69c42037e5d308bb5d90303d..0000000000000000000000000000000000000000
--- a/spaces/SakshiRathi77/SakshiRathi77-wav2vec2_xlsr_300m/README.md
+++ /dev/null
@@ -1,13 +0,0 @@
----
-title: SakshiRathi77-wav2vec2 Xlsr 300m
-emoji: 🌍
-colorFrom: gray
-colorTo: gray
-sdk: gradio
-sdk_version: 3.47.1
-app_file: app.py
-pinned: false
-license: apache-2.0
----
-
-Check out the configuration reference at https://huggingface.co/docs/hub/spaces-config-reference
diff --git a/spaces/Salesforce/EDICT/my_diffusers/optimization.py b/spaces/Salesforce/EDICT/my_diffusers/optimization.py
deleted file mode 100644
index e7b836b4a69bffb61c15967ef9b1736201721f1b..0000000000000000000000000000000000000000
--- a/spaces/Salesforce/EDICT/my_diffusers/optimization.py
+++ /dev/null
@@ -1,275 +0,0 @@
-# coding=utf-8
-# Copyright 2022 The HuggingFace Inc. team.
-#
-# Licensed under the Apache License, Version 2.0 (the "License");
-# you may not use this file except in compliance with the License.
-# You may obtain a copy of the License at
-#
-# http://www.apache.org/licenses/LICENSE-2.0
-#
-# Unless required by applicable law or agreed to in writing, software
-# distributed under the License is distributed on an "AS IS" BASIS,
-# WITHOUT WARRANTIES OR CONDITIONS OF ANY KIND, either express or implied.
-# See the License for the specific language governing permissions and
-# limitations under the License.
-"""PyTorch optimization for diffusion models."""
-
-import math
-from enum import Enum
-from typing import Optional, Union
-
-from torch.optim import Optimizer
-from torch.optim.lr_scheduler import LambdaLR
-
-from .utils import logging
-
-
-logger = logging.get_logger(__name__)
-
-
-class SchedulerType(Enum):
- LINEAR = "linear"
- COSINE = "cosine"
- COSINE_WITH_RESTARTS = "cosine_with_restarts"
- POLYNOMIAL = "polynomial"
- CONSTANT = "constant"
- CONSTANT_WITH_WARMUP = "constant_with_warmup"
-
-
-def get_constant_schedule(optimizer: Optimizer, last_epoch: int = -1):
- """
- Create a schedule with a constant learning rate, using the learning rate set in optimizer.
-
- Args:
- optimizer ([`~torch.optim.Optimizer`]):
- The optimizer for which to schedule the learning rate.
- last_epoch (`int`, *optional*, defaults to -1):
- The index of the last epoch when resuming training.
-
- Return:
- `torch.optim.lr_scheduler.LambdaLR` with the appropriate schedule.
- """
- return LambdaLR(optimizer, lambda _: 1, last_epoch=last_epoch)
-
-
-def get_constant_schedule_with_warmup(optimizer: Optimizer, num_warmup_steps: int, last_epoch: int = -1):
- """
- Create a schedule with a constant learning rate preceded by a warmup period during which the learning rate
- increases linearly between 0 and the initial lr set in the optimizer.
-
- Args:
- optimizer ([`~torch.optim.Optimizer`]):
- The optimizer for which to schedule the learning rate.
- num_warmup_steps (`int`):
- The number of steps for the warmup phase.
- last_epoch (`int`, *optional*, defaults to -1):
- The index of the last epoch when resuming training.
-
- Return:
- `torch.optim.lr_scheduler.LambdaLR` with the appropriate schedule.
- """
-
- def lr_lambda(current_step: int):
- if current_step < num_warmup_steps:
- return float(current_step) / float(max(1.0, num_warmup_steps))
- return 1.0
-
- return LambdaLR(optimizer, lr_lambda, last_epoch=last_epoch)
-
-
-def get_linear_schedule_with_warmup(optimizer, num_warmup_steps, num_training_steps, last_epoch=-1):
- """
- Create a schedule with a learning rate that decreases linearly from the initial lr set in the optimizer to 0, after
- a warmup period during which it increases linearly from 0 to the initial lr set in the optimizer.
-
- Args:
- optimizer ([`~torch.optim.Optimizer`]):
- The optimizer for which to schedule the learning rate.
- num_warmup_steps (`int`):
- The number of steps for the warmup phase.
- num_training_steps (`int`):
- The total number of training steps.
- last_epoch (`int`, *optional*, defaults to -1):
- The index of the last epoch when resuming training.
-
- Return:
- `torch.optim.lr_scheduler.LambdaLR` with the appropriate schedule.
- """
-
- def lr_lambda(current_step: int):
- if current_step < num_warmup_steps:
- return float(current_step) / float(max(1, num_warmup_steps))
- return max(
- 0.0, float(num_training_steps - current_step) / float(max(1, num_training_steps - num_warmup_steps))
- )
-
- return LambdaLR(optimizer, lr_lambda, last_epoch)
-
-
-def get_cosine_schedule_with_warmup(
- optimizer: Optimizer, num_warmup_steps: int, num_training_steps: int, num_cycles: float = 0.5, last_epoch: int = -1
-):
- """
- Create a schedule with a learning rate that decreases following the values of the cosine function between the
- initial lr set in the optimizer to 0, after a warmup period during which it increases linearly between 0 and the
- initial lr set in the optimizer.
-
- Args:
- optimizer ([`~torch.optim.Optimizer`]):
- The optimizer for which to schedule the learning rate.
- num_warmup_steps (`int`):
- The number of steps for the warmup phase.
- num_training_steps (`int`):
- The total number of training steps.
- num_cycles (`float`, *optional*, defaults to 0.5):
- The number of waves in the cosine schedule (the defaults is to just decrease from the max value to 0
- following a half-cosine).
- last_epoch (`int`, *optional*, defaults to -1):
- The index of the last epoch when resuming training.
-
- Return:
- `torch.optim.lr_scheduler.LambdaLR` with the appropriate schedule.
- """
-
- def lr_lambda(current_step):
- if current_step < num_warmup_steps:
- return float(current_step) / float(max(1, num_warmup_steps))
- progress = float(current_step - num_warmup_steps) / float(max(1, num_training_steps - num_warmup_steps))
- return max(0.0, 0.5 * (1.0 + math.cos(math.pi * float(num_cycles) * 2.0 * progress)))
-
- return LambdaLR(optimizer, lr_lambda, last_epoch)
-
-
-def get_cosine_with_hard_restarts_schedule_with_warmup(
- optimizer: Optimizer, num_warmup_steps: int, num_training_steps: int, num_cycles: int = 1, last_epoch: int = -1
-):
- """
- Create a schedule with a learning rate that decreases following the values of the cosine function between the
- initial lr set in the optimizer to 0, with several hard restarts, after a warmup period during which it increases
- linearly between 0 and the initial lr set in the optimizer.
-
- Args:
- optimizer ([`~torch.optim.Optimizer`]):
- The optimizer for which to schedule the learning rate.
- num_warmup_steps (`int`):
- The number of steps for the warmup phase.
- num_training_steps (`int`):
- The total number of training steps.
- num_cycles (`int`, *optional*, defaults to 1):
- The number of hard restarts to use.
- last_epoch (`int`, *optional*, defaults to -1):
- The index of the last epoch when resuming training.
-
- Return:
- `torch.optim.lr_scheduler.LambdaLR` with the appropriate schedule.
- """
-
- def lr_lambda(current_step):
- if current_step < num_warmup_steps:
- return float(current_step) / float(max(1, num_warmup_steps))
- progress = float(current_step - num_warmup_steps) / float(max(1, num_training_steps - num_warmup_steps))
- if progress >= 1.0:
- return 0.0
- return max(0.0, 0.5 * (1.0 + math.cos(math.pi * ((float(num_cycles) * progress) % 1.0))))
-
- return LambdaLR(optimizer, lr_lambda, last_epoch)
-
-
-def get_polynomial_decay_schedule_with_warmup(
- optimizer, num_warmup_steps, num_training_steps, lr_end=1e-7, power=1.0, last_epoch=-1
-):
- """
- Create a schedule with a learning rate that decreases as a polynomial decay from the initial lr set in the
- optimizer to end lr defined by *lr_end*, after a warmup period during which it increases linearly from 0 to the
- initial lr set in the optimizer.
-
- Args:
- optimizer ([`~torch.optim.Optimizer`]):
- The optimizer for which to schedule the learning rate.
- num_warmup_steps (`int`):
- The number of steps for the warmup phase.
- num_training_steps (`int`):
- The total number of training steps.
- lr_end (`float`, *optional*, defaults to 1e-7):
- The end LR.
- power (`float`, *optional*, defaults to 1.0):
- Power factor.
- last_epoch (`int`, *optional*, defaults to -1):
- The index of the last epoch when resuming training.
-
- Note: *power* defaults to 1.0 as in the fairseq implementation, which in turn is based on the original BERT
- implementation at
- https://github.com/google-research/bert/blob/f39e881b169b9d53bea03d2d341b31707a6c052b/optimization.py#L37
-
- Return:
- `torch.optim.lr_scheduler.LambdaLR` with the appropriate schedule.
-
- """
-
- lr_init = optimizer.defaults["lr"]
- if not (lr_init > lr_end):
- raise ValueError(f"lr_end ({lr_end}) must be be smaller than initial lr ({lr_init})")
-
- def lr_lambda(current_step: int):
- if current_step < num_warmup_steps:
- return float(current_step) / float(max(1, num_warmup_steps))
- elif current_step > num_training_steps:
- return lr_end / lr_init # as LambdaLR multiplies by lr_init
- else:
- lr_range = lr_init - lr_end
- decay_steps = num_training_steps - num_warmup_steps
- pct_remaining = 1 - (current_step - num_warmup_steps) / decay_steps
- decay = lr_range * pct_remaining**power + lr_end
- return decay / lr_init # as LambdaLR multiplies by lr_init
-
- return LambdaLR(optimizer, lr_lambda, last_epoch)
-
-
-TYPE_TO_SCHEDULER_FUNCTION = {
- SchedulerType.LINEAR: get_linear_schedule_with_warmup,
- SchedulerType.COSINE: get_cosine_schedule_with_warmup,
- SchedulerType.COSINE_WITH_RESTARTS: get_cosine_with_hard_restarts_schedule_with_warmup,
- SchedulerType.POLYNOMIAL: get_polynomial_decay_schedule_with_warmup,
- SchedulerType.CONSTANT: get_constant_schedule,
- SchedulerType.CONSTANT_WITH_WARMUP: get_constant_schedule_with_warmup,
-}
-
-
-def get_scheduler(
- name: Union[str, SchedulerType],
- optimizer: Optimizer,
- num_warmup_steps: Optional[int] = None,
- num_training_steps: Optional[int] = None,
-):
- """
- Unified API to get any scheduler from its name.
-
- Args:
- name (`str` or `SchedulerType`):
- The name of the scheduler to use.
- optimizer (`torch.optim.Optimizer`):
- The optimizer that will be used during training.
- num_warmup_steps (`int`, *optional*):
- The number of warmup steps to do. This is not required by all schedulers (hence the argument being
- optional), the function will raise an error if it's unset and the scheduler type requires it.
- num_training_steps (`int``, *optional*):
- The number of training steps to do. This is not required by all schedulers (hence the argument being
- optional), the function will raise an error if it's unset and the scheduler type requires it.
- """
- name = SchedulerType(name)
- schedule_func = TYPE_TO_SCHEDULER_FUNCTION[name]
- if name == SchedulerType.CONSTANT:
- return schedule_func(optimizer)
-
- # All other schedulers require `num_warmup_steps`
- if num_warmup_steps is None:
- raise ValueError(f"{name} requires `num_warmup_steps`, please provide that argument.")
-
- if name == SchedulerType.CONSTANT_WITH_WARMUP:
- return schedule_func(optimizer, num_warmup_steps=num_warmup_steps)
-
- # All other schedulers require `num_training_steps`
- if num_training_steps is None:
- raise ValueError(f"{name} requires `num_training_steps`, please provide that argument.")
-
- return schedule_func(optimizer, num_warmup_steps=num_warmup_steps, num_training_steps=num_training_steps)
diff --git a/spaces/Sapphire-356/Video2MC/joints_detectors/Alphapose/doc/contributors.md b/spaces/Sapphire-356/Video2MC/joints_detectors/Alphapose/doc/contributors.md
deleted file mode 100644
index cb02844c7d040a4420b6361273845af19aa1b90a..0000000000000000000000000000000000000000
--- a/spaces/Sapphire-356/Video2MC/joints_detectors/Alphapose/doc/contributors.md
+++ /dev/null
@@ -1 +0,0 @@
-Alpha Pose is contributed and maintained by Hao-Shu Fang, Jiefeng Li, Yuliang Xiu, Ruiheng Chang and Cewu Lu.
diff --git a/spaces/Satyam1124q/genaii/README.md b/spaces/Satyam1124q/genaii/README.md
deleted file mode 100644
index 7e02e6879390c8dd20960f790dec3ab5a5325232..0000000000000000000000000000000000000000
--- a/spaces/Satyam1124q/genaii/README.md
+++ /dev/null
@@ -1,10 +0,0 @@
----
-title: Genaii
-emoji: 🦀
-colorFrom: purple
-colorTo: red
-sdk: static
-pinned: false
----
-
-Check out the configuration reference at https://huggingface.co/docs/hub/spaces-config-reference
diff --git a/spaces/ServerX/PorcoDiaz/julius/bands.py b/spaces/ServerX/PorcoDiaz/julius/bands.py
deleted file mode 100644
index ef2162440b69e960770aa7bf81b9aaec48a63243..0000000000000000000000000000000000000000
--- a/spaces/ServerX/PorcoDiaz/julius/bands.py
+++ /dev/null
@@ -1,119 +0,0 @@
-# File under the MIT license, see https://github.com/adefossez/julius/LICENSE for details.
-# Author: adefossez, 2020
-"""
-Decomposition of a signal over frequency bands in the waveform domain.
-"""
-from typing import Optional, Sequence
-import torch
-
-from .core import mel_frequencies
-from .lowpass import LowPassFilters
-from .utils import simple_repr
-
-
-class SplitBands(torch.nn.Module):
- """
- Decomposes a signal over the given frequency bands in the waveform domain using
- a cascade of low pass filters as implemented by `julius.lowpass.LowPassFilters`.
- You can either specify explicitely the frequency cutoffs, or just the number of bands,
- in which case the frequency cutoffs will be spread out evenly in mel scale.
-
- Args:
- sample_rate (float): Sample rate of the input signal in Hz.
- n_bands (int or None): number of bands, when not giving them explictely with `cutoffs`.
- In that case, the cutoff frequencies will be evenly spaced in mel-space.
- cutoffs (list[float] or None): list of frequency cutoffs in Hz.
- pad (bool): if True, appropriately pad the input with zero over the edge. If `stride=1`,
- the output will have the same length as the input.
- zeros (float): Number of zero crossings to keep. See `LowPassFilters` for more informations.
- fft (bool or None): See `LowPassFilters` for more info.
-
- ..note::
- The sum of all the bands will always be the input signal.
-
- ..warning::
- Unlike `julius.lowpass.LowPassFilters`, the cutoffs frequencies must be provided in Hz along
- with the sample rate.
-
- Shape:
-
- - Input: `[*, T]`
- - Output: `[B, *, T']`, with `T'=T` if `pad` is True.
- If `n_bands` was provided, `B = n_bands` otherwise `B = len(cutoffs) + 1`
-
- >>> bands = SplitBands(sample_rate=128, n_bands=10)
- >>> x = torch.randn(6, 4, 1024)
- >>> list(bands(x).shape)
- [10, 6, 4, 1024]
- """
-
- def __init__(self, sample_rate: float, n_bands: Optional[int] = None,
- cutoffs: Optional[Sequence[float]] = None, pad: bool = True,
- zeros: float = 8, fft: Optional[bool] = None):
- super().__init__()
- if (cutoffs is None) + (n_bands is None) != 1:
- raise ValueError("You must provide either n_bands, or cutoffs, but not boths.")
-
- self.sample_rate = sample_rate
- self.n_bands = n_bands
- self._cutoffs = list(cutoffs) if cutoffs is not None else None
- self.pad = pad
- self.zeros = zeros
- self.fft = fft
-
- if cutoffs is None:
- if n_bands is None:
- raise ValueError("You must provide one of n_bands or cutoffs.")
- if not n_bands >= 1:
- raise ValueError(f"n_bands must be greater than one (got {n_bands})")
- cutoffs = mel_frequencies(n_bands + 1, 0, sample_rate / 2)[1:-1]
- else:
- if max(cutoffs) > 0.5 * sample_rate:
- raise ValueError("A cutoff above sample_rate/2 does not make sense.")
- if len(cutoffs) > 0:
- self.lowpass = LowPassFilters(
- [c / sample_rate for c in cutoffs], pad=pad, zeros=zeros, fft=fft)
- else:
- # Here I cannot make both TorchScript and MyPy happy.
- # I miss the good old times, before all this madness was created.
- self.lowpass = None # type: ignore
-
- def forward(self, input):
- if self.lowpass is None:
- return input[None]
- lows = self.lowpass(input)
- low = lows[0]
- bands = [low]
- for low_and_band in lows[1:]:
- # Get a bandpass filter by substracting lowpasses
- band = low_and_band - low
- bands.append(band)
- low = low_and_band
- # Last band is whatever is left in the signal
- bands.append(input - low)
- return torch.stack(bands)
-
- @property
- def cutoffs(self):
- if self._cutoffs is not None:
- return self._cutoffs
- elif self.lowpass is not None:
- return [c * self.sample_rate for c in self.lowpass.cutoffs]
- else:
- return []
-
- def __repr__(self):
- return simple_repr(self, overrides={"cutoffs": self._cutoffs})
-
-
-def split_bands(signal: torch.Tensor, sample_rate: float, n_bands: Optional[int] = None,
- cutoffs: Optional[Sequence[float]] = None, pad: bool = True,
- zeros: float = 8, fft: Optional[bool] = None):
- """
- Functional version of `SplitBands`, refer to this class for more information.
-
- >>> x = torch.randn(6, 4, 1024)
- >>> list(split_bands(x, sample_rate=64, cutoffs=[12, 24]).shape)
- [3, 6, 4, 1024]
- """
- return SplitBands(sample_rate, n_bands, cutoffs, pad, zeros, fft).to(signal)(signal)
diff --git a/spaces/Sunbird/runyankole2english-stt/app.py b/spaces/Sunbird/runyankole2english-stt/app.py
deleted file mode 100644
index 3a30e3504a7617ba01486e741b63ab916127d9ea..0000000000000000000000000000000000000000
--- a/spaces/Sunbird/runyankole2english-stt/app.py
+++ /dev/null
@@ -1,45 +0,0 @@
-import gradio as gr
-import torch
-import librosa
-import json
-from transformers import pipeline
-from stitched_model import CombinedModel
-
-
-device = "cuda:0" if torch.cuda.is_available() else "cpu"
-
-model = CombinedModel("ak3ra/wav2vec2-sunbird-speech-runyankole", "Sunbird/sunbird-mul-en-mbart-merged", device="cpu")
-
-
-
-def transcribe(audio_file_mic=None, audio_file_upload=None):
- if audio_file_mic:
- audio_file = audio_file_mic
- elif audio_file_upload:
- audio_file = audio_file_upload
- else:
- return "Please upload an audio file or record one"
-
- # Make sure audio is 16kHz
- speech, sample_rate = librosa.load(audio_file)
- if sample_rate != 16000:
- speech = librosa.resample(speech, orig_sr=sample_rate, target_sr=16000)
- speech = torch.tensor([speech])
-
- with torch.no_grad():
- transcription, translation = model({"audio":speech})
-
- return transcription, translation[0]
-
-description = '''Luganda to English Speech Translation'''
-
-iface = gr.Interface(fn=transcribe,
- inputs=[
- gr.Audio(source="microphone", type="filepath", label="Record Audio"),
- gr.Audio(source="upload", type="filepath", label="Upload Audio")],
- outputs=[gr.Textbox(label="Transcription"),
- gr.Textbox(label="Translation")
- ],
- description=description
- )
-iface.launch()
\ No newline at end of file
diff --git a/spaces/SungBeom/chatwine-korean/.venv/Lib/site-packages/IPython/utils/_process_win32_controller.py b/spaces/SungBeom/chatwine-korean/.venv/Lib/site-packages/IPython/utils/_process_win32_controller.py
deleted file mode 100644
index f8c2a057a83e898591b6aef108bc60f14f733c07..0000000000000000000000000000000000000000
--- a/spaces/SungBeom/chatwine-korean/.venv/Lib/site-packages/IPython/utils/_process_win32_controller.py
+++ /dev/null
@@ -1,573 +0,0 @@
-"""Windows-specific implementation of process utilities with direct WinAPI.
-
-This file is meant to be used by process.py
-"""
-
-#-----------------------------------------------------------------------------
-# Copyright (C) 2010-2011 The IPython Development Team
-#
-# Distributed under the terms of the BSD License. The full license is in
-# the file COPYING, distributed as part of this software.
-#-----------------------------------------------------------------------------
-
-
-# stdlib
-import os, sys, threading
-import ctypes, msvcrt
-
-# Win32 API types needed for the API calls
-from ctypes import POINTER
-from ctypes.wintypes import HANDLE, HLOCAL, LPVOID, WORD, DWORD, BOOL, \
- ULONG, LPCWSTR
-LPDWORD = POINTER(DWORD)
-LPHANDLE = POINTER(HANDLE)
-ULONG_PTR = POINTER(ULONG)
-class SECURITY_ATTRIBUTES(ctypes.Structure):
- _fields_ = [("nLength", DWORD),
- ("lpSecurityDescriptor", LPVOID),
- ("bInheritHandle", BOOL)]
-LPSECURITY_ATTRIBUTES = POINTER(SECURITY_ATTRIBUTES)
-class STARTUPINFO(ctypes.Structure):
- _fields_ = [("cb", DWORD),
- ("lpReserved", LPCWSTR),
- ("lpDesktop", LPCWSTR),
- ("lpTitle", LPCWSTR),
- ("dwX", DWORD),
- ("dwY", DWORD),
- ("dwXSize", DWORD),
- ("dwYSize", DWORD),
- ("dwXCountChars", DWORD),
- ("dwYCountChars", DWORD),
- ("dwFillAttribute", DWORD),
- ("dwFlags", DWORD),
- ("wShowWindow", WORD),
- ("cbReserved2", WORD),
- ("lpReserved2", LPVOID),
- ("hStdInput", HANDLE),
- ("hStdOutput", HANDLE),
- ("hStdError", HANDLE)]
-LPSTARTUPINFO = POINTER(STARTUPINFO)
-class PROCESS_INFORMATION(ctypes.Structure):
- _fields_ = [("hProcess", HANDLE),
- ("hThread", HANDLE),
- ("dwProcessId", DWORD),
- ("dwThreadId", DWORD)]
-LPPROCESS_INFORMATION = POINTER(PROCESS_INFORMATION)
-
-# Win32 API constants needed
-ERROR_HANDLE_EOF = 38
-ERROR_BROKEN_PIPE = 109
-ERROR_NO_DATA = 232
-HANDLE_FLAG_INHERIT = 0x0001
-STARTF_USESTDHANDLES = 0x0100
-CREATE_SUSPENDED = 0x0004
-CREATE_NEW_CONSOLE = 0x0010
-CREATE_NO_WINDOW = 0x08000000
-STILL_ACTIVE = 259
-WAIT_TIMEOUT = 0x0102
-WAIT_FAILED = 0xFFFFFFFF
-INFINITE = 0xFFFFFFFF
-DUPLICATE_SAME_ACCESS = 0x00000002
-ENABLE_ECHO_INPUT = 0x0004
-ENABLE_LINE_INPUT = 0x0002
-ENABLE_PROCESSED_INPUT = 0x0001
-
-# Win32 API functions needed
-GetLastError = ctypes.windll.kernel32.GetLastError
-GetLastError.argtypes = []
-GetLastError.restype = DWORD
-
-CreateFile = ctypes.windll.kernel32.CreateFileW
-CreateFile.argtypes = [LPCWSTR, DWORD, DWORD, LPVOID, DWORD, DWORD, HANDLE]
-CreateFile.restype = HANDLE
-
-CreatePipe = ctypes.windll.kernel32.CreatePipe
-CreatePipe.argtypes = [POINTER(HANDLE), POINTER(HANDLE),
- LPSECURITY_ATTRIBUTES, DWORD]
-CreatePipe.restype = BOOL
-
-CreateProcess = ctypes.windll.kernel32.CreateProcessW
-CreateProcess.argtypes = [LPCWSTR, LPCWSTR, LPSECURITY_ATTRIBUTES,
- LPSECURITY_ATTRIBUTES, BOOL, DWORD, LPVOID, LPCWSTR, LPSTARTUPINFO,
- LPPROCESS_INFORMATION]
-CreateProcess.restype = BOOL
-
-GetExitCodeProcess = ctypes.windll.kernel32.GetExitCodeProcess
-GetExitCodeProcess.argtypes = [HANDLE, LPDWORD]
-GetExitCodeProcess.restype = BOOL
-
-GetCurrentProcess = ctypes.windll.kernel32.GetCurrentProcess
-GetCurrentProcess.argtypes = []
-GetCurrentProcess.restype = HANDLE
-
-ResumeThread = ctypes.windll.kernel32.ResumeThread
-ResumeThread.argtypes = [HANDLE]
-ResumeThread.restype = DWORD
-
-ReadFile = ctypes.windll.kernel32.ReadFile
-ReadFile.argtypes = [HANDLE, LPVOID, DWORD, LPDWORD, LPVOID]
-ReadFile.restype = BOOL
-
-WriteFile = ctypes.windll.kernel32.WriteFile
-WriteFile.argtypes = [HANDLE, LPVOID, DWORD, LPDWORD, LPVOID]
-WriteFile.restype = BOOL
-
-GetConsoleMode = ctypes.windll.kernel32.GetConsoleMode
-GetConsoleMode.argtypes = [HANDLE, LPDWORD]
-GetConsoleMode.restype = BOOL
-
-SetConsoleMode = ctypes.windll.kernel32.SetConsoleMode
-SetConsoleMode.argtypes = [HANDLE, DWORD]
-SetConsoleMode.restype = BOOL
-
-FlushConsoleInputBuffer = ctypes.windll.kernel32.FlushConsoleInputBuffer
-FlushConsoleInputBuffer.argtypes = [HANDLE]
-FlushConsoleInputBuffer.restype = BOOL
-
-WaitForSingleObject = ctypes.windll.kernel32.WaitForSingleObject
-WaitForSingleObject.argtypes = [HANDLE, DWORD]
-WaitForSingleObject.restype = DWORD
-
-DuplicateHandle = ctypes.windll.kernel32.DuplicateHandle
-DuplicateHandle.argtypes = [HANDLE, HANDLE, HANDLE, LPHANDLE,
- DWORD, BOOL, DWORD]
-DuplicateHandle.restype = BOOL
-
-SetHandleInformation = ctypes.windll.kernel32.SetHandleInformation
-SetHandleInformation.argtypes = [HANDLE, DWORD, DWORD]
-SetHandleInformation.restype = BOOL
-
-CloseHandle = ctypes.windll.kernel32.CloseHandle
-CloseHandle.argtypes = [HANDLE]
-CloseHandle.restype = BOOL
-
-CommandLineToArgvW = ctypes.windll.shell32.CommandLineToArgvW
-CommandLineToArgvW.argtypes = [LPCWSTR, POINTER(ctypes.c_int)]
-CommandLineToArgvW.restype = POINTER(LPCWSTR)
-
-LocalFree = ctypes.windll.kernel32.LocalFree
-LocalFree.argtypes = [HLOCAL]
-LocalFree.restype = HLOCAL
-
-class AvoidUNCPath(object):
- """A context manager to protect command execution from UNC paths.
-
- In the Win32 API, commands can't be invoked with the cwd being a UNC path.
- This context manager temporarily changes directory to the 'C:' drive on
- entering, and restores the original working directory on exit.
-
- The context manager returns the starting working directory *if* it made a
- change and None otherwise, so that users can apply the necessary adjustment
- to their system calls in the event of a change.
-
- Examples
- --------
- ::
- cmd = 'dir'
- with AvoidUNCPath() as path:
- if path is not None:
- cmd = '"pushd %s &&"%s' % (path, cmd)
- os.system(cmd)
- """
- def __enter__(self):
- self.path = os.getcwd()
- self.is_unc_path = self.path.startswith(r"\\")
- if self.is_unc_path:
- # change to c drive (as cmd.exe cannot handle UNC addresses)
- os.chdir("C:")
- return self.path
- else:
- # We return None to signal that there was no change in the working
- # directory
- return None
-
- def __exit__(self, exc_type, exc_value, traceback):
- if self.is_unc_path:
- os.chdir(self.path)
-
-
-class Win32ShellCommandController(object):
- """Runs a shell command in a 'with' context.
-
- This implementation is Win32-specific.
-
- Example:
- # Runs the command interactively with default console stdin/stdout
- with ShellCommandController('python -i') as scc:
- scc.run()
-
- # Runs the command using the provided functions for stdin/stdout
- def my_stdout_func(s):
- # print or save the string 's'
- write_to_stdout(s)
- def my_stdin_func():
- # If input is available, return it as a string.
- if input_available():
- return get_input()
- # If no input available, return None after a short delay to
- # keep from blocking.
- else:
- time.sleep(0.01)
- return None
-
- with ShellCommandController('python -i') as scc:
- scc.run(my_stdout_func, my_stdin_func)
- """
-
- def __init__(self, cmd, mergeout = True):
- """Initializes the shell command controller.
-
- The cmd is the program to execute, and mergeout is
- whether to blend stdout and stderr into one output
- in stdout. Merging them together in this fashion more
- reliably keeps stdout and stderr in the correct order
- especially for interactive shell usage.
- """
- self.cmd = cmd
- self.mergeout = mergeout
-
- def __enter__(self):
- cmd = self.cmd
- mergeout = self.mergeout
-
- self.hstdout, self.hstdin, self.hstderr = None, None, None
- self.piProcInfo = None
- try:
- p_hstdout, c_hstdout, p_hstderr, \
- c_hstderr, p_hstdin, c_hstdin = [None]*6
-
- # SECURITY_ATTRIBUTES with inherit handle set to True
- saAttr = SECURITY_ATTRIBUTES()
- saAttr.nLength = ctypes.sizeof(saAttr)
- saAttr.bInheritHandle = True
- saAttr.lpSecurityDescriptor = None
-
- def create_pipe(uninherit):
- """Creates a Windows pipe, which consists of two handles.
-
- The 'uninherit' parameter controls which handle is not
- inherited by the child process.
- """
- handles = HANDLE(), HANDLE()
- if not CreatePipe(ctypes.byref(handles[0]),
- ctypes.byref(handles[1]), ctypes.byref(saAttr), 0):
- raise ctypes.WinError()
- if not SetHandleInformation(handles[uninherit],
- HANDLE_FLAG_INHERIT, 0):
- raise ctypes.WinError()
- return handles[0].value, handles[1].value
-
- p_hstdout, c_hstdout = create_pipe(uninherit=0)
- # 'mergeout' signals that stdout and stderr should be merged.
- # We do that by using one pipe for both of them.
- if mergeout:
- c_hstderr = HANDLE()
- if not DuplicateHandle(GetCurrentProcess(), c_hstdout,
- GetCurrentProcess(), ctypes.byref(c_hstderr),
- 0, True, DUPLICATE_SAME_ACCESS):
- raise ctypes.WinError()
- else:
- p_hstderr, c_hstderr = create_pipe(uninherit=0)
- c_hstdin, p_hstdin = create_pipe(uninherit=1)
-
- # Create the process object
- piProcInfo = PROCESS_INFORMATION()
- siStartInfo = STARTUPINFO()
- siStartInfo.cb = ctypes.sizeof(siStartInfo)
- siStartInfo.hStdInput = c_hstdin
- siStartInfo.hStdOutput = c_hstdout
- siStartInfo.hStdError = c_hstderr
- siStartInfo.dwFlags = STARTF_USESTDHANDLES
- dwCreationFlags = CREATE_SUSPENDED | CREATE_NO_WINDOW # | CREATE_NEW_CONSOLE
-
- if not CreateProcess(None,
- u"cmd.exe /c " + cmd,
- None, None, True, dwCreationFlags,
- None, None, ctypes.byref(siStartInfo),
- ctypes.byref(piProcInfo)):
- raise ctypes.WinError()
-
- # Close this process's versions of the child handles
- CloseHandle(c_hstdin)
- c_hstdin = None
- CloseHandle(c_hstdout)
- c_hstdout = None
- if c_hstderr is not None:
- CloseHandle(c_hstderr)
- c_hstderr = None
-
- # Transfer ownership of the parent handles to the object
- self.hstdin = p_hstdin
- p_hstdin = None
- self.hstdout = p_hstdout
- p_hstdout = None
- if not mergeout:
- self.hstderr = p_hstderr
- p_hstderr = None
- self.piProcInfo = piProcInfo
-
- finally:
- if p_hstdin:
- CloseHandle(p_hstdin)
- if c_hstdin:
- CloseHandle(c_hstdin)
- if p_hstdout:
- CloseHandle(p_hstdout)
- if c_hstdout:
- CloseHandle(c_hstdout)
- if p_hstderr:
- CloseHandle(p_hstderr)
- if c_hstderr:
- CloseHandle(c_hstderr)
-
- return self
-
- def _stdin_thread(self, handle, hprocess, func, stdout_func):
- exitCode = DWORD()
- bytesWritten = DWORD(0)
- while True:
- #print("stdin thread loop start")
- # Get the input string (may be bytes or unicode)
- data = func()
-
- # None signals to poll whether the process has exited
- if data is None:
- #print("checking for process completion")
- if not GetExitCodeProcess(hprocess, ctypes.byref(exitCode)):
- raise ctypes.WinError()
- if exitCode.value != STILL_ACTIVE:
- return
- # TESTING: Does zero-sized writefile help?
- if not WriteFile(handle, "", 0,
- ctypes.byref(bytesWritten), None):
- raise ctypes.WinError()
- continue
- #print("\nGot str %s\n" % repr(data), file=sys.stderr)
-
- # Encode the string to the console encoding
- if isinstance(data, unicode): #FIXME: Python3
- data = data.encode('utf_8')
-
- # What we have now must be a string of bytes
- if not isinstance(data, str): #FIXME: Python3
- raise RuntimeError("internal stdin function string error")
-
- # An empty string signals EOF
- if len(data) == 0:
- return
-
- # In a windows console, sometimes the input is echoed,
- # but sometimes not. How do we determine when to do this?
- stdout_func(data)
- # WriteFile may not accept all the data at once.
- # Loop until everything is processed
- while len(data) != 0:
- #print("Calling writefile")
- if not WriteFile(handle, data, len(data),
- ctypes.byref(bytesWritten), None):
- # This occurs at exit
- if GetLastError() == ERROR_NO_DATA:
- return
- raise ctypes.WinError()
- #print("Called writefile")
- data = data[bytesWritten.value:]
-
- def _stdout_thread(self, handle, func):
- # Allocate the output buffer
- data = ctypes.create_string_buffer(4096)
- while True:
- bytesRead = DWORD(0)
- if not ReadFile(handle, data, 4096,
- ctypes.byref(bytesRead), None):
- le = GetLastError()
- if le == ERROR_BROKEN_PIPE:
- return
- else:
- raise ctypes.WinError()
- # FIXME: Python3
- s = data.value[0:bytesRead.value]
- #print("\nv: %s" % repr(s), file=sys.stderr)
- func(s.decode('utf_8', 'replace'))
-
- def run(self, stdout_func = None, stdin_func = None, stderr_func = None):
- """Runs the process, using the provided functions for I/O.
-
- The function stdin_func should return strings whenever a
- character or characters become available.
- The functions stdout_func and stderr_func are called whenever
- something is printed to stdout or stderr, respectively.
- These functions are called from different threads (but not
- concurrently, because of the GIL).
- """
- if stdout_func is None and stdin_func is None and stderr_func is None:
- return self._run_stdio()
-
- if stderr_func is not None and self.mergeout:
- raise RuntimeError("Shell command was initiated with "
- "merged stdin/stdout, but a separate stderr_func "
- "was provided to the run() method")
-
- # Create a thread for each input/output handle
- stdin_thread = None
- threads = []
- if stdin_func:
- stdin_thread = threading.Thread(target=self._stdin_thread,
- args=(self.hstdin, self.piProcInfo.hProcess,
- stdin_func, stdout_func))
- threads.append(threading.Thread(target=self._stdout_thread,
- args=(self.hstdout, stdout_func)))
- if not self.mergeout:
- if stderr_func is None:
- stderr_func = stdout_func
- threads.append(threading.Thread(target=self._stdout_thread,
- args=(self.hstderr, stderr_func)))
- # Start the I/O threads and the process
- if ResumeThread(self.piProcInfo.hThread) == 0xFFFFFFFF:
- raise ctypes.WinError()
- if stdin_thread is not None:
- stdin_thread.start()
- for thread in threads:
- thread.start()
- # Wait for the process to complete
- if WaitForSingleObject(self.piProcInfo.hProcess, INFINITE) == \
- WAIT_FAILED:
- raise ctypes.WinError()
- # Wait for the I/O threads to complete
- for thread in threads:
- thread.join()
-
- # Wait for the stdin thread to complete
- if stdin_thread is not None:
- stdin_thread.join()
-
- def _stdin_raw_nonblock(self):
- """Use the raw Win32 handle of sys.stdin to do non-blocking reads"""
- # WARNING: This is experimental, and produces inconsistent results.
- # It's possible for the handle not to be appropriate for use
- # with WaitForSingleObject, among other things.
- handle = msvcrt.get_osfhandle(sys.stdin.fileno())
- result = WaitForSingleObject(handle, 100)
- if result == WAIT_FAILED:
- raise ctypes.WinError()
- elif result == WAIT_TIMEOUT:
- print(".", end='')
- return None
- else:
- data = ctypes.create_string_buffer(256)
- bytesRead = DWORD(0)
- print('?', end='')
-
- if not ReadFile(handle, data, 256,
- ctypes.byref(bytesRead), None):
- raise ctypes.WinError()
- # This ensures the non-blocking works with an actual console
- # Not checking the error, so the processing will still work with
- # other handle types
- FlushConsoleInputBuffer(handle)
-
- data = data.value
- data = data.replace('\r\n', '\n')
- data = data.replace('\r', '\n')
- print(repr(data) + " ", end='')
- return data
-
- def _stdin_raw_block(self):
- """Use a blocking stdin read"""
- # The big problem with the blocking read is that it doesn't
- # exit when it's supposed to in all contexts. An extra
- # key-press may be required to trigger the exit.
- try:
- data = sys.stdin.read(1)
- data = data.replace('\r', '\n')
- return data
- except WindowsError as we:
- if we.winerror == ERROR_NO_DATA:
- # This error occurs when the pipe is closed
- return None
- else:
- # Otherwise let the error propagate
- raise we
-
- def _stdout_raw(self, s):
- """Writes the string to stdout"""
- print(s, end='', file=sys.stdout)
- sys.stdout.flush()
-
- def _stderr_raw(self, s):
- """Writes the string to stdout"""
- print(s, end='', file=sys.stderr)
- sys.stderr.flush()
-
- def _run_stdio(self):
- """Runs the process using the system standard I/O.
-
- IMPORTANT: stdin needs to be asynchronous, so the Python
- sys.stdin object is not used. Instead,
- msvcrt.kbhit/getwch are used asynchronously.
- """
- # Disable Line and Echo mode
- #lpMode = DWORD()
- #handle = msvcrt.get_osfhandle(sys.stdin.fileno())
- #if GetConsoleMode(handle, ctypes.byref(lpMode)):
- # set_console_mode = True
- # if not SetConsoleMode(handle, lpMode.value &
- # ~(ENABLE_ECHO_INPUT | ENABLE_LINE_INPUT | ENABLE_PROCESSED_INPUT)):
- # raise ctypes.WinError()
-
- if self.mergeout:
- return self.run(stdout_func = self._stdout_raw,
- stdin_func = self._stdin_raw_block)
- else:
- return self.run(stdout_func = self._stdout_raw,
- stdin_func = self._stdin_raw_block,
- stderr_func = self._stderr_raw)
-
- # Restore the previous console mode
- #if set_console_mode:
- # if not SetConsoleMode(handle, lpMode.value):
- # raise ctypes.WinError()
-
- def __exit__(self, exc_type, exc_value, traceback):
- if self.hstdin:
- CloseHandle(self.hstdin)
- self.hstdin = None
- if self.hstdout:
- CloseHandle(self.hstdout)
- self.hstdout = None
- if self.hstderr:
- CloseHandle(self.hstderr)
- self.hstderr = None
- if self.piProcInfo is not None:
- CloseHandle(self.piProcInfo.hProcess)
- CloseHandle(self.piProcInfo.hThread)
- self.piProcInfo = None
-
-
-def system(cmd):
- """Win32 version of os.system() that works with network shares.
-
- Note that this implementation returns None, as meant for use in IPython.
-
- Parameters
- ----------
- cmd : str
- A command to be executed in the system shell.
-
- Returns
- -------
- None : we explicitly do NOT return the subprocess status code, as this
- utility is meant to be used extensively in IPython, where any return value
- would trigger : func:`sys.displayhook` calls.
- """
- with AvoidUNCPath() as path:
- if path is not None:
- cmd = '"pushd %s &&"%s' % (path, cmd)
- with Win32ShellCommandController(cmd) as scc:
- scc.run()
-
-
-if __name__ == "__main__":
- print("Test starting!")
- #system("cmd")
- system("python -i")
- print("Test finished!")
diff --git a/spaces/SungBeom/chatwine-korean/.venv/Lib/site-packages/debugpy/_vendored/pydevd/_pydevd_bundle/pydevd_defaults.py b/spaces/SungBeom/chatwine-korean/.venv/Lib/site-packages/debugpy/_vendored/pydevd/_pydevd_bundle/pydevd_defaults.py
deleted file mode 100644
index 918ce719e21dc97d8fac074318e48da1af78b309..0000000000000000000000000000000000000000
--- a/spaces/SungBeom/chatwine-korean/.venv/Lib/site-packages/debugpy/_vendored/pydevd/_pydevd_bundle/pydevd_defaults.py
+++ /dev/null
@@ -1,66 +0,0 @@
-'''
-This module holds the customization settings for the debugger.
-'''
-
-from _pydevd_bundle.pydevd_constants import QUOTED_LINE_PROTOCOL
-from _pydev_bundle import pydev_log
-import sys
-
-
-class PydevdCustomization(object):
- DEFAULT_PROTOCOL: str = QUOTED_LINE_PROTOCOL
-
- # Debug mode may be set to 'debugpy-dap'.
- #
- # In 'debugpy-dap' mode the following settings are done to PyDB:
- #
- # py_db.skip_suspend_on_breakpoint_exception = (BaseException,)
- # py_db.skip_print_breakpoint_exception = (NameError,)
- # py_db.multi_threads_single_notification = True
- DEBUG_MODE: str = ''
-
- # This may be a ; to be pre-imported
- # Something as: 'c:/temp/foo;my_module.bar'
- #
- # What's done in this case is something as:
- #
- # sys.path.insert(0, )
- # try:
- # import
- # finally:
- # del sys.path[0]
- #
- # If the pre-import fails an output message is
- # sent (but apart from that debugger execution
- # should continue).
- PREIMPORT: str = ''
-
-
-def on_pydb_init(py_db):
- if PydevdCustomization.DEBUG_MODE == 'debugpy-dap':
- pydev_log.debug('Apply debug mode: debugpy-dap')
- py_db.skip_suspend_on_breakpoint_exception = (BaseException,)
- py_db.skip_print_breakpoint_exception = (NameError,)
- py_db.multi_threads_single_notification = True
- elif not PydevdCustomization.DEBUG_MODE:
- pydev_log.debug('Apply debug mode: default')
- else:
- pydev_log.debug('WARNING: unknown debug mode: %s', PydevdCustomization.DEBUG_MODE)
-
- if PydevdCustomization.PREIMPORT:
- pydev_log.debug('Preimport: %s', PydevdCustomization.PREIMPORT)
- try:
- sys_path_entry, module_name = PydevdCustomization.PREIMPORT.rsplit(';', maxsplit=1)
- except Exception:
- pydev_log.exception("Expected ';' in %s" % (PydevdCustomization.PREIMPORT,))
- else:
- try:
- sys.path.insert(0, sys_path_entry)
- try:
- __import__(module_name)
- finally:
- sys.path.remove(sys_path_entry)
- except Exception:
- pydev_log.exception(
- "Error importing %s (with sys.path entry: %s)" % (module_name, sys_path_entry))
-
diff --git a/spaces/SungBeom/chatwine-korean/.venv/Lib/site-packages/debugpy/_vendored/pydevd/pydevd_attach_to_process/linux_and_mac/compile_linux.sh b/spaces/SungBeom/chatwine-korean/.venv/Lib/site-packages/debugpy/_vendored/pydevd/pydevd_attach_to_process/linux_and_mac/compile_linux.sh
deleted file mode 100644
index 3a1ebc2ffea125ceb4c74fbbd3f59b7e1da12455..0000000000000000000000000000000000000000
--- a/spaces/SungBeom/chatwine-korean/.venv/Lib/site-packages/debugpy/_vendored/pydevd/pydevd_attach_to_process/linux_and_mac/compile_linux.sh
+++ /dev/null
@@ -1,9 +0,0 @@
-g++ -m64 -shared -o attach_linux_amd64.so -fPIC -nostartfiles attach.cpp
-mv attach_linux_amd64.so ../attach_linux_amd64.so
-echo Compiled amd64
-
-echo Note: may need "sudo apt-get install libx32gcc-4.8-dev" and "sudo apt-get install libc6-dev-i386" and "sudo apt-get install g++-multilib" to compile 32 bits
-
-g++ -m32 -shared -o attach_linux_x86.so -fPIC -nostartfiles attach.cpp
-mv attach_linux_x86.so ../attach_linux_x86.so
-echo Compiled x86
diff --git a/spaces/SungBeom/chatwine-korean/.venv/Lib/site-packages/debugpy/_vendored/pydevd/pydevd_attach_to_process/winappdbg/win32/psapi.py b/spaces/SungBeom/chatwine-korean/.venv/Lib/site-packages/debugpy/_vendored/pydevd/pydevd_attach_to_process/winappdbg/win32/psapi.py
deleted file mode 100644
index e353c7f7ea139f918a993673861e10da036987f2..0000000000000000000000000000000000000000
--- a/spaces/SungBeom/chatwine-korean/.venv/Lib/site-packages/debugpy/_vendored/pydevd/pydevd_attach_to_process/winappdbg/win32/psapi.py
+++ /dev/null
@@ -1,387 +0,0 @@
-#!/usr/bin/env python
-# -*- coding: utf-8 -*-
-
-# Copyright (c) 2009-2014, Mario Vilas
-# All rights reserved.
-#
-# Redistribution and use in source and binary forms, with or without
-# modification, are permitted provided that the following conditions are met:
-#
-# * Redistributions of source code must retain the above copyright notice,
-# this list of conditions and the following disclaimer.
-# * Redistributions in binary form must reproduce the above copyright
-# notice,this list of conditions and the following disclaimer in the
-# documentation and/or other materials provided with the distribution.
-# * Neither the name of the copyright holder nor the names of its
-# contributors may be used to endorse or promote products derived from
-# this software without specific prior written permission.
-#
-# THIS SOFTWARE IS PROVIDED BY THE COPYRIGHT HOLDERS AND CONTRIBUTORS "AS IS"
-# AND ANY EXPRESS OR IMPLIED WARRANTIES, INCLUDING, BUT NOT LIMITED TO, THE
-# IMPLIED WARRANTIES OF MERCHANTABILITY AND FITNESS FOR A PARTICULAR PURPOSE
-# ARE DISCLAIMED. IN NO EVENT SHALL THE COPYRIGHT OWNER OR CONTRIBUTORS BE
-# LIABLE FOR ANY DIRECT, INDIRECT, INCIDENTAL, SPECIAL, EXEMPLARY, OR
-# CONSEQUENTIAL DAMAGES (INCLUDING, BUT NOT LIMITED TO, PROCUREMENT OF
-# SUBSTITUTE GOODS OR SERVICES; LOSS OF USE, DATA, OR PROFITS; OR BUSINESS
-# INTERRUPTION) HOWEVER CAUSED AND ON ANY THEORY OF LIABILITY, WHETHER IN
-# CONTRACT, STRICT LIABILITY, OR TORT (INCLUDING NEGLIGENCE OR OTHERWISE)
-# ARISING IN ANY WAY OUT OF THE USE OF THIS SOFTWARE, EVEN IF ADVISED OF THE
-# POSSIBILITY OF SUCH DAMAGE.
-
-"""
-Wrapper for psapi.dll in ctypes.
-"""
-
-__revision__ = "$Id$"
-
-from winappdbg.win32.defines import *
-
-#==============================================================================
-# This is used later on to calculate the list of exported symbols.
-_all = None
-_all = set(vars().keys())
-#==============================================================================
-
-#--- PSAPI structures and constants -------------------------------------------
-
-LIST_MODULES_DEFAULT = 0x00
-LIST_MODULES_32BIT = 0x01
-LIST_MODULES_64BIT = 0x02
-LIST_MODULES_ALL = 0x03
-
-# typedef struct _MODULEINFO {
-# LPVOID lpBaseOfDll;
-# DWORD SizeOfImage;
-# LPVOID EntryPoint;
-# } MODULEINFO, *LPMODULEINFO;
-class MODULEINFO(Structure):
- _fields_ = [
- ("lpBaseOfDll", LPVOID), # remote pointer
- ("SizeOfImage", DWORD),
- ("EntryPoint", LPVOID), # remote pointer
-]
-LPMODULEINFO = POINTER(MODULEINFO)
-
-#--- psapi.dll ----------------------------------------------------------------
-
-# BOOL WINAPI EnumDeviceDrivers(
-# __out LPVOID *lpImageBase,
-# __in DWORD cb,
-# __out LPDWORD lpcbNeeded
-# );
-def EnumDeviceDrivers():
- _EnumDeviceDrivers = windll.psapi.EnumDeviceDrivers
- _EnumDeviceDrivers.argtypes = [LPVOID, DWORD, LPDWORD]
- _EnumDeviceDrivers.restype = bool
- _EnumDeviceDrivers.errcheck = RaiseIfZero
-
- size = 0x1000
- lpcbNeeded = DWORD(size)
- unit = sizeof(LPVOID)
- while 1:
- lpImageBase = (LPVOID * (size // unit))()
- _EnumDeviceDrivers(byref(lpImageBase), lpcbNeeded, byref(lpcbNeeded))
- needed = lpcbNeeded.value
- if needed <= size:
- break
- size = needed
- return [ lpImageBase[index] for index in compat.xrange(0, (needed // unit)) ]
-
-# BOOL WINAPI EnumProcesses(
-# __out DWORD *pProcessIds,
-# __in DWORD cb,
-# __out DWORD *pBytesReturned
-# );
-def EnumProcesses():
- _EnumProcesses = windll.psapi.EnumProcesses
- _EnumProcesses.argtypes = [LPVOID, DWORD, LPDWORD]
- _EnumProcesses.restype = bool
- _EnumProcesses.errcheck = RaiseIfZero
-
- size = 0x1000
- cbBytesReturned = DWORD()
- unit = sizeof(DWORD)
- while 1:
- ProcessIds = (DWORD * (size // unit))()
- cbBytesReturned.value = size
- _EnumProcesses(byref(ProcessIds), cbBytesReturned, byref(cbBytesReturned))
- returned = cbBytesReturned.value
- if returned < size:
- break
- size = size + 0x1000
- ProcessIdList = list()
- for ProcessId in ProcessIds:
- if ProcessId is None:
- break
- ProcessIdList.append(ProcessId)
- return ProcessIdList
-
-# BOOL WINAPI EnumProcessModules(
-# __in HANDLE hProcess,
-# __out HMODULE *lphModule,
-# __in DWORD cb,
-# __out LPDWORD lpcbNeeded
-# );
-def EnumProcessModules(hProcess):
- _EnumProcessModules = windll.psapi.EnumProcessModules
- _EnumProcessModules.argtypes = [HANDLE, LPVOID, DWORD, LPDWORD]
- _EnumProcessModules.restype = bool
- _EnumProcessModules.errcheck = RaiseIfZero
-
- size = 0x1000
- lpcbNeeded = DWORD(size)
- unit = sizeof(HMODULE)
- while 1:
- lphModule = (HMODULE * (size // unit))()
- _EnumProcessModules(hProcess, byref(lphModule), lpcbNeeded, byref(lpcbNeeded))
- needed = lpcbNeeded.value
- if needed <= size:
- break
- size = needed
- return [ lphModule[index] for index in compat.xrange(0, int(needed // unit)) ]
-
-# BOOL WINAPI EnumProcessModulesEx(
-# __in HANDLE hProcess,
-# __out HMODULE *lphModule,
-# __in DWORD cb,
-# __out LPDWORD lpcbNeeded,
-# __in DWORD dwFilterFlag
-# );
-def EnumProcessModulesEx(hProcess, dwFilterFlag = LIST_MODULES_DEFAULT):
- _EnumProcessModulesEx = windll.psapi.EnumProcessModulesEx
- _EnumProcessModulesEx.argtypes = [HANDLE, LPVOID, DWORD, LPDWORD, DWORD]
- _EnumProcessModulesEx.restype = bool
- _EnumProcessModulesEx.errcheck = RaiseIfZero
-
- size = 0x1000
- lpcbNeeded = DWORD(size)
- unit = sizeof(HMODULE)
- while 1:
- lphModule = (HMODULE * (size // unit))()
- _EnumProcessModulesEx(hProcess, byref(lphModule), lpcbNeeded, byref(lpcbNeeded), dwFilterFlag)
- needed = lpcbNeeded.value
- if needed <= size:
- break
- size = needed
- return [ lphModule[index] for index in compat.xrange(0, (needed // unit)) ]
-
-# DWORD WINAPI GetDeviceDriverBaseName(
-# __in LPVOID ImageBase,
-# __out LPTSTR lpBaseName,
-# __in DWORD nSize
-# );
-def GetDeviceDriverBaseNameA(ImageBase):
- _GetDeviceDriverBaseNameA = windll.psapi.GetDeviceDriverBaseNameA
- _GetDeviceDriverBaseNameA.argtypes = [LPVOID, LPSTR, DWORD]
- _GetDeviceDriverBaseNameA.restype = DWORD
-
- nSize = MAX_PATH
- while 1:
- lpBaseName = ctypes.create_string_buffer("", nSize)
- nCopied = _GetDeviceDriverBaseNameA(ImageBase, lpBaseName, nSize)
- if nCopied == 0:
- raise ctypes.WinError()
- if nCopied < (nSize - 1):
- break
- nSize = nSize + MAX_PATH
- return lpBaseName.value
-
-def GetDeviceDriverBaseNameW(ImageBase):
- _GetDeviceDriverBaseNameW = windll.psapi.GetDeviceDriverBaseNameW
- _GetDeviceDriverBaseNameW.argtypes = [LPVOID, LPWSTR, DWORD]
- _GetDeviceDriverBaseNameW.restype = DWORD
-
- nSize = MAX_PATH
- while 1:
- lpBaseName = ctypes.create_unicode_buffer(u"", nSize)
- nCopied = _GetDeviceDriverBaseNameW(ImageBase, lpBaseName, nSize)
- if nCopied == 0:
- raise ctypes.WinError()
- if nCopied < (nSize - 1):
- break
- nSize = nSize + MAX_PATH
- return lpBaseName.value
-
-GetDeviceDriverBaseName = GuessStringType(GetDeviceDriverBaseNameA, GetDeviceDriverBaseNameW)
-
-# DWORD WINAPI GetDeviceDriverFileName(
-# __in LPVOID ImageBase,
-# __out LPTSTR lpFilename,
-# __in DWORD nSize
-# );
-def GetDeviceDriverFileNameA(ImageBase):
- _GetDeviceDriverFileNameA = windll.psapi.GetDeviceDriverFileNameA
- _GetDeviceDriverFileNameA.argtypes = [LPVOID, LPSTR, DWORD]
- _GetDeviceDriverFileNameA.restype = DWORD
-
- nSize = MAX_PATH
- while 1:
- lpFilename = ctypes.create_string_buffer("", nSize)
- nCopied = ctypes.windll.psapi.GetDeviceDriverFileNameA(ImageBase, lpFilename, nSize)
- if nCopied == 0:
- raise ctypes.WinError()
- if nCopied < (nSize - 1):
- break
- nSize = nSize + MAX_PATH
- return lpFilename.value
-
-def GetDeviceDriverFileNameW(ImageBase):
- _GetDeviceDriverFileNameW = windll.psapi.GetDeviceDriverFileNameW
- _GetDeviceDriverFileNameW.argtypes = [LPVOID, LPWSTR, DWORD]
- _GetDeviceDriverFileNameW.restype = DWORD
-
- nSize = MAX_PATH
- while 1:
- lpFilename = ctypes.create_unicode_buffer(u"", nSize)
- nCopied = ctypes.windll.psapi.GetDeviceDriverFileNameW(ImageBase, lpFilename, nSize)
- if nCopied == 0:
- raise ctypes.WinError()
- if nCopied < (nSize - 1):
- break
- nSize = nSize + MAX_PATH
- return lpFilename.value
-
-GetDeviceDriverFileName = GuessStringType(GetDeviceDriverFileNameA, GetDeviceDriverFileNameW)
-
-# DWORD WINAPI GetMappedFileName(
-# __in HANDLE hProcess,
-# __in LPVOID lpv,
-# __out LPTSTR lpFilename,
-# __in DWORD nSize
-# );
-def GetMappedFileNameA(hProcess, lpv):
- _GetMappedFileNameA = ctypes.windll.psapi.GetMappedFileNameA
- _GetMappedFileNameA.argtypes = [HANDLE, LPVOID, LPSTR, DWORD]
- _GetMappedFileNameA.restype = DWORD
-
- nSize = MAX_PATH
- while 1:
- lpFilename = ctypes.create_string_buffer("", nSize)
- nCopied = _GetMappedFileNameA(hProcess, lpv, lpFilename, nSize)
- if nCopied == 0:
- raise ctypes.WinError()
- if nCopied < (nSize - 1):
- break
- nSize = nSize + MAX_PATH
- return lpFilename.value
-
-def GetMappedFileNameW(hProcess, lpv):
- _GetMappedFileNameW = ctypes.windll.psapi.GetMappedFileNameW
- _GetMappedFileNameW.argtypes = [HANDLE, LPVOID, LPWSTR, DWORD]
- _GetMappedFileNameW.restype = DWORD
-
- nSize = MAX_PATH
- while 1:
- lpFilename = ctypes.create_unicode_buffer(u"", nSize)
- nCopied = _GetMappedFileNameW(hProcess, lpv, lpFilename, nSize)
- if nCopied == 0:
- raise ctypes.WinError()
- if nCopied < (nSize - 1):
- break
- nSize = nSize + MAX_PATH
- return lpFilename.value
-
-GetMappedFileName = GuessStringType(GetMappedFileNameA, GetMappedFileNameW)
-
-# DWORD WINAPI GetModuleFileNameEx(
-# __in HANDLE hProcess,
-# __in_opt HMODULE hModule,
-# __out LPTSTR lpFilename,
-# __in DWORD nSize
-# );
-def GetModuleFileNameExA(hProcess, hModule = None):
- _GetModuleFileNameExA = ctypes.windll.psapi.GetModuleFileNameExA
- _GetModuleFileNameExA.argtypes = [HANDLE, HMODULE, LPSTR, DWORD]
- _GetModuleFileNameExA.restype = DWORD
-
- nSize = MAX_PATH
- while 1:
- lpFilename = ctypes.create_string_buffer("", nSize)
- nCopied = _GetModuleFileNameExA(hProcess, hModule, lpFilename, nSize)
- if nCopied == 0:
- raise ctypes.WinError()
- if nCopied < (nSize - 1):
- break
- nSize = nSize + MAX_PATH
- return lpFilename.value
-
-def GetModuleFileNameExW(hProcess, hModule = None):
- _GetModuleFileNameExW = ctypes.windll.psapi.GetModuleFileNameExW
- _GetModuleFileNameExW.argtypes = [HANDLE, HMODULE, LPWSTR, DWORD]
- _GetModuleFileNameExW.restype = DWORD
-
- nSize = MAX_PATH
- while 1:
- lpFilename = ctypes.create_unicode_buffer(u"", nSize)
- nCopied = _GetModuleFileNameExW(hProcess, hModule, lpFilename, nSize)
- if nCopied == 0:
- raise ctypes.WinError()
- if nCopied < (nSize - 1):
- break
- nSize = nSize + MAX_PATH
- return lpFilename.value
-
-GetModuleFileNameEx = GuessStringType(GetModuleFileNameExA, GetModuleFileNameExW)
-
-# BOOL WINAPI GetModuleInformation(
-# __in HANDLE hProcess,
-# __in HMODULE hModule,
-# __out LPMODULEINFO lpmodinfo,
-# __in DWORD cb
-# );
-def GetModuleInformation(hProcess, hModule, lpmodinfo = None):
- _GetModuleInformation = windll.psapi.GetModuleInformation
- _GetModuleInformation.argtypes = [HANDLE, HMODULE, LPMODULEINFO, DWORD]
- _GetModuleInformation.restype = bool
- _GetModuleInformation.errcheck = RaiseIfZero
-
- if lpmodinfo is None:
- lpmodinfo = MODULEINFO()
- _GetModuleInformation(hProcess, hModule, byref(lpmodinfo), sizeof(lpmodinfo))
- return lpmodinfo
-
-# DWORD WINAPI GetProcessImageFileName(
-# __in HANDLE hProcess,
-# __out LPTSTR lpImageFileName,
-# __in DWORD nSize
-# );
-def GetProcessImageFileNameA(hProcess):
- _GetProcessImageFileNameA = windll.psapi.GetProcessImageFileNameA
- _GetProcessImageFileNameA.argtypes = [HANDLE, LPSTR, DWORD]
- _GetProcessImageFileNameA.restype = DWORD
-
- nSize = MAX_PATH
- while 1:
- lpFilename = ctypes.create_string_buffer("", nSize)
- nCopied = _GetProcessImageFileNameA(hProcess, lpFilename, nSize)
- if nCopied == 0:
- raise ctypes.WinError()
- if nCopied < (nSize - 1):
- break
- nSize = nSize + MAX_PATH
- return lpFilename.value
-
-def GetProcessImageFileNameW(hProcess):
- _GetProcessImageFileNameW = windll.psapi.GetProcessImageFileNameW
- _GetProcessImageFileNameW.argtypes = [HANDLE, LPWSTR, DWORD]
- _GetProcessImageFileNameW.restype = DWORD
-
- nSize = MAX_PATH
- while 1:
- lpFilename = ctypes.create_unicode_buffer(u"", nSize)
- nCopied = _GetProcessImageFileNameW(hProcess, lpFilename, nSize)
- if nCopied == 0:
- raise ctypes.WinError()
- if nCopied < (nSize - 1):
- break
- nSize = nSize + MAX_PATH
- return lpFilename.value
-
-GetProcessImageFileName = GuessStringType(GetProcessImageFileNameA, GetProcessImageFileNameW)
-
-#==============================================================================
-# This calculates the list of exported symbols.
-_all = set(vars().keys()).difference(_all)
-__all__ = [_x for _x in _all if not _x.startswith('_')]
-__all__.sort()
-#==============================================================================
diff --git a/spaces/TRI-ML/risk_biased_prediction/tests/risk_biased/models/test_interaction_decoder.py b/spaces/TRI-ML/risk_biased_prediction/tests/risk_biased/models/test_interaction_decoder.py
deleted file mode 100644
index 225a35cbd43526e9e5d7860bf5d288476b80783b..0000000000000000000000000000000000000000
--- a/spaces/TRI-ML/risk_biased_prediction/tests/risk_biased/models/test_interaction_decoder.py
+++ /dev/null
@@ -1,149 +0,0 @@
-import os
-import pytest
-
-import torch
-from mmcv import Config
-
-from risk_biased.models.cvae_decoder import (
- CVAEAccelerationDecoder,
- DecoderNN,
-)
-from risk_biased.models.cvae_params import CVAEParams
-
-
-@pytest.fixture(scope="module")
-def params():
- torch.manual_seed(0)
- working_dir = os.path.dirname(os.path.realpath(__file__))
- config_path = os.path.join(
- working_dir, "..", "..", "..", "risk_biased", "config", "learning_config.py"
- )
- waymo_config_path = os.path.join(
- working_dir, "..", "..", "..", "risk_biased", "config", "waymo_config.py"
- )
- paths = [config_path, waymo_config_path]
- if isinstance(paths, str):
- cfg = Config.fromfile(paths)
- else:
- cfg = Config.fromfile(paths[0])
- for path in paths[1:]:
- c = Config.fromfile(path)
- cfg.update(c)
- cfg.batch_size = 4
- cfg.state_dim = 5
- cfg.map_state_dim = 2
- cfg.num_steps = 3
- cfg.num_steps_future = 4
- cfg.latent_dim = 2
- cfg.hidden_dim = 64
- cfg.num_hidden_layers = 2
- cfg.num_attention_heads = 4
- cfg.device = "cpu"
- return cfg
-
-
-@pytest.mark.parametrize(
- "num_agents, num_objects, n_samples, type",
- [
- (2, 3, 0, "MLP"),
- (3, 1, 2, "LSTM"),
- (4, 2, 2, "maskedLSTM"),
- ],
-)
-def test_interaction_decoder_nn(
- params, num_agents: int, num_objects: int, n_samples: int, type: str
-):
- params.sequence_decoder_type = type
- model = DecoderNN(
- CVAEParams.from_config(params),
- )
-
- squeeze_sample_dim = n_samples <= 0
- n_samples = max(1, n_samples)
- x = torch.rand(params.batch_size, num_agents, params.num_steps, params.state_dim)
- mask_x = torch.rand(params.batch_size, num_agents, params.num_steps) > 0.3
- mask_z = mask_x.any(-1)
- z_samples = torch.rand(params.batch_size, num_agents, n_samples, params.latent_dim)
- encoded_map = torch.rand(params.batch_size, num_objects, params.hidden_dim)
- mask_map = torch.rand(params.batch_size, num_objects)
- encoded_absolute = torch.rand(params.batch_size, num_agents, params.hidden_dim)
-
- if squeeze_sample_dim:
- z_samples = z_samples.squeeze(2)
-
- output = model(
- z_samples, mask_z, x, mask_x, encoded_absolute, encoded_map, mask_map
- )
-
- # check shape
- if squeeze_sample_dim:
- assert output.shape == (
- params.batch_size,
- num_agents,
- params.num_steps_future,
- params.hidden_dim,
- )
- else:
- assert output.shape == (
- params.batch_size,
- num_agents,
- n_samples,
- params.num_steps_future,
- params.hidden_dim,
- )
-
-
-@pytest.mark.parametrize(
- "num_agents, num_objects, n_samples, type",
- [
- (2, 3, 0, "MLP"),
- (3, 1, 2, "LSTM"),
- (4, 2, 2, "maskedLSTM"),
- ],
-)
-def test_interaction_cvae_decoder(
- params, num_agents: int, num_objects: int, n_samples: int, type: str
-):
- params.sequence_decoder_type = type
- squeeze_sample_dim = n_samples <= 0
- n_samples = max(1, n_samples)
- z_samples = torch.rand(params.batch_size, num_agents, n_samples, params.latent_dim)
- if squeeze_sample_dim == 1:
- z_samples = z_samples.squeeze(2)
- x = torch.rand(params.batch_size, num_agents, params.num_steps, params.state_dim)
- offset = torch.rand(params.batch_size, num_agents, 5)
- mask_x = torch.rand(params.batch_size, num_agents, params.num_steps) > 0.3
- mask_z = mask_x.any(-1)
- encoded_map = torch.rand(params.batch_size, num_objects, params.hidden_dim)
- mask_map = torch.rand(params.batch_size, num_objects)
- encoded_absolute = torch.rand(params.batch_size, num_agents, params.hidden_dim)
-
- model = DecoderNN(CVAEParams.from_config(params))
- decoder = CVAEAccelerationDecoder(model)
- # check auxiliary_input_dim
- y_samples = decoder(
- z_samples,
- mask_z,
- x,
- mask_x,
- encoded_absolute,
- encoded_map,
- mask_map,
- offset=offset,
- )
- # check shape
- if squeeze_sample_dim:
- assert y_samples.shape == (
- params.batch_size,
- num_agents,
- params.num_steps_future,
- params.state_dim,
- )
- else:
- assert y_samples.shape == (
- params.batch_size,
- num_agents,
- n_samples,
- params.num_steps_future,
- params.state_dim,
- )
diff --git a/spaces/TRI-ML/risk_biased_prediction/tests/risk_biased/utils/test_risk.py b/spaces/TRI-ML/risk_biased_prediction/tests/risk_biased/utils/test_risk.py
deleted file mode 100644
index 789845dab952944348037e01a0cb4db65c0150aa..0000000000000000000000000000000000000000
--- a/spaces/TRI-ML/risk_biased_prediction/tests/risk_biased/utils/test_risk.py
+++ /dev/null
@@ -1,331 +0,0 @@
-import math
-import pytest
-
-import torch
-
-from risk_biased.utils.risk import (
- CVaREstimator,
- EntropicRiskEstimator,
- get_risk_estimator,
- get_risk_level_sampler,
-)
-
-torch.manual_seed(0)
-
-
-@pytest.mark.parametrize(
- "estimator_params, batch_size, num_samples",
- [
- ({"type": "entropic", "eps": 1e-3}, 3, 10),
- ({"type": "entropic", "eps": 1e-6}, 5, 20),
- ],
-)
-def test_entropic_risk_estimator(
- estimator_params: dict, batch_size: int, num_samples: int
-):
- num_agents = 1
- estimator = get_risk_estimator(estimator_params)
- assert type(estimator) == EntropicRiskEstimator
-
- cost = torch.rand(batch_size, num_agents, num_samples)
- risk_level_random = torch.rand(batch_size, num_agents)
- weight = torch.ones(batch_size, num_agents, num_samples) / num_samples
- objective_random = estimator(risk_level_random, cost, weight)
- assert objective_random.shape == torch.Size([batch_size, num_agents])
-
- risk_level_zero = torch.zeros(batch_size, num_agents)
- objective_zero = estimator(risk_level_zero, cost, weight)
- # entropic risk should fall back to mean if risk_level is zero
- assert torch.allclose(objective_zero, (cost * weight).sum(dim=2))
-
- cost_same = torch.ones(batch_size, num_agents, num_samples)
- objective_same = estimator(risk_level_random, cost_same, weight)
- # entropic risk should return mean if cost samples are all the same
- assert torch.allclose(objective_same, (cost_same * weight).sum(dim=2))
-
- risk_level_one = torch.ones(batch_size, num_agents)
- objective_one = estimator(risk_level_one, cost, weight)
- risk_level_nine = 10.0 * torch.ones(batch_size, num_agents)
- objective_ten = estimator(risk_level_nine, cost, weight)
- # entropic risk should be monotone increasing as a function of risk_level
- assert all(objective_ten > objective_one)
-
-
-@pytest.mark.parametrize(
- "estimator_params, batch_size, num_samples, num_agents",
- [
- ({"type": "cvar", "eps": 1e-3}, 3, 10, 1),
- ({"type": "cvar", "eps": 1e-6}, 5, 20, 3),
- ],
-)
-def test_cvar_estimator(
- estimator_params: dict, batch_size: int, num_samples: int, num_agents: int
-):
- estimator = get_risk_estimator(estimator_params)
- assert type(estimator) == CVaREstimator
-
- cost = torch.rand(batch_size, num_agents, num_samples)
- risk_level_random = torch.rand(batch_size, num_agents)
- weights = torch.ones(batch_size, num_agents, num_samples) / num_samples
- objective_random = estimator(risk_level_random, cost, weights)
- assert objective_random.shape == torch.Size([batch_size, num_agents])
-
- risk_level_zero = torch.zeros(batch_size, num_agents)
- objective_zero = estimator(risk_level_zero, cost, weights)
- # cvar should fall back to mean if risk_level is zero
- assert torch.allclose(objective_zero, cost.mean(dim=2), rtol=1e-3, atol=1e-3)
-
- cost_same = torch.ones(batch_size, num_agents, num_samples)
- objective_same = estimator(risk_level_random, cost_same, weights)
- # cvar should return mean if cost samples are all the same
- assert torch.allclose(objective_same, cost_same.mean(dim=2))
-
- risk_level_close_to_one = torch.ones(batch_size, num_agents) - 1e-2
- objective_close_to_one = estimator(risk_level_close_to_one, cost, weights)
- risk_level_one = torch.ones(batch_size, num_agents)
- objective_one = estimator(risk_level_one, cost, weights)
- # cvar should fall back to max if risk_level is close to one
- assert torch.allclose(objective_close_to_one, cost.max(dim=2).values)
- assert torch.allclose(objective_one, cost.max(dim=2).values)
-
- risk_level_quarter = 0.25 * torch.ones(batch_size, num_agents)
- objective_quarter = estimator(risk_level_quarter, cost, weights)
- risk_level_half = 0.5 * torch.ones(batch_size, num_agents)
- objective_half = estimator(risk_level_half, cost, weights)
- # cvar should be monotone increasing as a function of risk_level
- assert (objective_half > objective_quarter).all()
-
-
-def test_risk_estimator_raise():
- with pytest.raises(RuntimeError):
- get_risk_estimator({})
- with pytest.raises(RuntimeError):
- get_risk_estimator({"type": "entropic"})
- with pytest.raises(RuntimeError):
- get_risk_estimator({"eps": 1e-3})
-
-
-@pytest.mark.parametrize(
- "distribution_params, num_samples, device",
- [
- ({"type": "uniform", "min": 0, "max": 1}, 1000, "cpu"),
- ({"type": "uniform", "min": 0, "max": 1}, 10000, "cuda"),
- ({"type": "uniform", "min": 10, "max": 100}, 10000, "cpu"),
- ],
-)
-def test_uniform_sampler(distribution_params: dict, num_samples: int, device: str):
- tol_mean = 3 / math.sqrt(num_samples)
- tol_std = 3 / math.pow(num_samples, 2 / 5)
-
- expected_mean = (distribution_params["max"] + distribution_params["min"]) / 2
- expected_std = (
- distribution_params["max"] - distribution_params["min"]
- ) / math.sqrt(12)
- sampler = get_risk_level_sampler(distribution_params=distribution_params)
- sample = sampler.sample(num_samples, device)
- std, mean = torch.std_mean(sample)
- assert sample.shape == torch.Size([num_samples])
- assert torch.abs(mean - expected_mean) / expected_std < tol_mean
- assert torch.abs(std - expected_std) / expected_std < tol_std
-
-
-@pytest.mark.parametrize(
- "distribution_params, num_samples, device",
- [
- ({"type": "normal", "mean": 0, "sigma": 1}, 1000, "cpu"),
- ({"type": "normal", "mean": 0, "sigma": 3}, 10000, "cuda"),
- ({"type": "normal", "mean": 3, "sigma": 10}, 10000, "cpu"),
- ({"type": "normal", "mean": 1, "sigma": 3}, 100000, "cpu"),
- ],
-)
-def test_normal_sampler(distribution_params: dict, num_samples: int, device: str):
- tol_mean = 3 / math.sqrt(num_samples)
- tol_std = 3 / math.pow(num_samples, 2 / 5)
- expected_mean = distribution_params["mean"]
- expected_std = distribution_params["sigma"]
- sampler = get_risk_level_sampler(distribution_params=distribution_params)
- sample = sampler.sample(num_samples, device)
- std, mean = torch.std_mean(sample)
- assert sample.shape == torch.Size([num_samples])
- assert torch.abs(mean - expected_mean) / expected_std < tol_mean
- assert torch.abs(std - expected_std) / expected_std < tol_std
-
-
-@pytest.mark.parametrize(
- "distribution_params, num_samples, device",
- [
- ({"type": "bernoulli", "min": 0, "max": 1, "p": 0.5}, 1000, "cpu"),
- ({"type": "bernoulli", "min": 0, "max": 3, "p": 0.1}, 10000, "cuda"),
- ({"type": "bernoulli", "min": 3, "max": 10, "p": 0.9}, 10000, "cpu"),
- ({"type": "bernoulli", "min": 1, "max": 3, "p": 0.5}, 100000, "cpu"),
- ],
-)
-def test_bernoulli_sampler(distribution_params: dict, num_samples: int, device: str):
- range = distribution_params["max"] - distribution_params["min"]
- tol_mean = 3 / math.sqrt(num_samples)
- tol_std = 3 / math.pow(num_samples, 2 / 5)
- expected_mean = distribution_params["p"] * range + distribution_params["min"]
- expected_std = (
- math.sqrt(distribution_params["p"] * (1 - distribution_params["p"])) * range
- )
- sampler = get_risk_level_sampler(distribution_params=distribution_params)
- sample = sampler.sample(num_samples, device)
- std, mean = torch.std_mean(sample)
- assert sample.shape == torch.Size([num_samples])
- assert torch.abs(mean - expected_mean) / expected_std < tol_mean
- assert torch.abs(std - expected_std) / expected_std < tol_std
-
-
-@pytest.mark.parametrize(
- "distribution_params, num_samples, device",
- [
- ({"type": "beta", "min": 0, "max": 1, "alpha": 0.5, "beta": 0.5}, 1000, "cpu"),
- ({"type": "beta", "min": 0, "max": 3, "alpha": 5, "beta": 1}, 10000, "cuda"),
- ({"type": "beta", "min": 3, "max": 10, "alpha": 1, "beta": 3}, 10000, "cpu"),
- ({"type": "beta", "min": 1, "max": 3, "alpha": 2, "beta": 5}, 100000, "cpu"),
- ],
-)
-def test_beta_sampler(distribution_params: dict, num_samples: int, device: str):
- range = distribution_params["max"] - distribution_params["min"]
- tol_mean = 3 / math.sqrt(num_samples)
- tol_std = 3 / math.pow(num_samples, 2 / 5)
- aphaplusbeta = distribution_params["alpha"] + distribution_params["beta"]
- expected_mean = (
- distribution_params["alpha"] / aphaplusbeta * range + distribution_params["min"]
- )
- expected_std = (
- math.sqrt(distribution_params["alpha"] * distribution_params["beta"])
- / (aphaplusbeta * math.sqrt(aphaplusbeta + 1))
- * range
- )
- sampler = get_risk_level_sampler(distribution_params=distribution_params)
- sample = sampler.sample(num_samples, device)
- std, mean = torch.std_mean(sample)
- assert sample.shape == torch.Size([num_samples])
- assert torch.abs(mean - expected_mean) / expected_std < tol_mean
- assert torch.abs(std - expected_std) / expected_std < tol_std
-
-
-@pytest.mark.parametrize(
- "distribution_params, num_samples, device",
- [
- ({"type": "chi2", "min": 0, "scale": 1, "k": 1}, 1000, "cpu"),
- ({"type": "chi2", "min": 0, "scale": 3, "k": 2}, 10000, "cuda"),
- ({"type": "chi2", "min": 3, "scale": 10, "k": 3}, 10000, "cpu"),
- ({"type": "chi2", "min": 1, "scale": 3, "k": 10}, 100000, "cpu"),
- ],
-)
-def test_chi2_sampler(distribution_params: dict, num_samples: int, device: str):
-
- tol_mean = (
- 3
- * distribution_params["scale"]
- * math.sqrt(2 * distribution_params["k"] / num_samples)
- )
- tol_std = 3 / math.pow(num_samples, 2 / 5)
- expected_mean = (
- distribution_params["k"] * distribution_params["scale"]
- + distribution_params["min"]
- )
- expected_std = (
- math.sqrt(2 * distribution_params["k"]) * distribution_params["scale"]
- )
- sampler = get_risk_level_sampler(distribution_params=distribution_params)
- sample = sampler.sample(num_samples, device)
- std, mean = torch.std_mean(sample)
- assert sample.shape == torch.Size([num_samples])
- assert torch.abs(mean - expected_mean) < tol_mean
- assert torch.abs(std - expected_std) / expected_std < tol_std
-
-
-@pytest.mark.parametrize(
- "distribution_params, num_samples, device",
- [
- (
- {"type": "log-normal", "min": 0, "scale": 1, "mu": 0, "sigma": 0.5},
- 100,
- "cpu",
- ),
- (
- {"type": "log-normal", "min": 0, "scale": 3, "mu": 0.3, "sigma": 1},
- 100000,
- "cuda",
- ),
- (
- {"type": "log-normal", "min": 3, "scale": 10, "mu": 1, "sigma": 0.25},
- 10000,
- "cpu",
- ),
- (
- {"type": "log-normal", "min": 1, "scale": 3, "mu": 0, "sigma": 1.5},
- 100000,
- "cpu",
- ),
- ],
-)
-def test_lognormal_sampler(distribution_params: dict, num_samples: int, device: str):
- tol_mean = 3 / math.sqrt(num_samples)
- tol_std = 3 / math.pow(num_samples, 1 / 5)
- expected_mean = (
- math.exp(distribution_params["mu"] + distribution_params["sigma"] ** 2 / 2)
- * distribution_params["scale"]
- + distribution_params["min"]
- )
- expected_std = (
- math.sqrt(math.exp(distribution_params["sigma"] ** 2) - 1)
- * math.exp(distribution_params["mu"] + (distribution_params["sigma"] ** 2) / 2)
- ) * distribution_params["scale"]
- sampler = get_risk_level_sampler(distribution_params=distribution_params)
- sample = sampler.sample(num_samples, device)
- std, mean = torch.std_mean(sample)
- assert sample.shape == torch.Size([num_samples])
- assert torch.abs(mean - expected_mean) / expected_std < tol_mean
- assert torch.abs(std - expected_std) / expected_std < tol_std
-
-
-@pytest.mark.parametrize(
- "distribution_params, num_samples, device",
- [
- (
- {"type": "log-uniform", "min": 0, "max": 1, "scale": 1},
- 100,
- "cpu",
- ),
- (
- {"type": "log-uniform", "min": 1, "max": 3, "scale": 3},
- 100000,
- "cuda",
- ),
- ],
-)
-def test_loguniform_sampler(distribution_params: dict, num_samples: int, device: str):
- tol_mean = 3 / math.sqrt(num_samples)
- tol_std = 3 / math.pow(num_samples, 1 / 5)
- max = distribution_params["max"]
- min = distribution_params["min"]
- scale = distribution_params["scale"] / (max - min)
- expected_mean = (
- max
- - ((max - min) / math.log((scale * max + 1) / (scale * min + 1)) - 1 / scale)
- + min
- )
- expected_std = math.sqrt(
- ((scale * max + 1) ** 2 - (scale * min + 1) ** 2)
- / (2 * scale**2 * math.log((scale * max + 1) / (scale * min + 1)))
- - ((max - min) / math.log((scale * max + 1) / (scale * min + 1))) ** 2
- )
- sampler = get_risk_level_sampler(distribution_params=distribution_params)
- sample = sampler.sample(num_samples, device)
- std, mean = torch.std_mean(sample)
- assert sample.shape == torch.Size([num_samples])
- assert torch.abs(mean - expected_mean) / expected_std < tol_mean
- assert torch.abs(std - expected_std) / expected_std < tol_std
-
-
-def test_risk_level_sampler_raise():
- with pytest.raises(RuntimeError):
- get_risk_level_sampler({})
- with pytest.raises(RuntimeError):
- get_risk_level_sampler({"type": "chi2"})
- with pytest.raises(RuntimeError):
- get_risk_level_sampler({"min": 0, "max": 1})
diff --git a/spaces/TandCAcceptMe/face-swap-docker/mynewshinyroop/Lib/site-packages/setuptools/config/_validate_pyproject/__init__.py b/spaces/TandCAcceptMe/face-swap-docker/mynewshinyroop/Lib/site-packages/setuptools/config/_validate_pyproject/__init__.py
deleted file mode 100644
index dbe6cb4ca471f146b431d2fbb558d47317a103f0..0000000000000000000000000000000000000000
--- a/spaces/TandCAcceptMe/face-swap-docker/mynewshinyroop/Lib/site-packages/setuptools/config/_validate_pyproject/__init__.py
+++ /dev/null
@@ -1,34 +0,0 @@
-from functools import reduce
-from typing import Any, Callable, Dict
-
-from . import formats
-from .error_reporting import detailed_errors, ValidationError
-from .extra_validations import EXTRA_VALIDATIONS
-from .fastjsonschema_exceptions import JsonSchemaException, JsonSchemaValueException
-from .fastjsonschema_validations import validate as _validate
-
-__all__ = [
- "validate",
- "FORMAT_FUNCTIONS",
- "EXTRA_VALIDATIONS",
- "ValidationError",
- "JsonSchemaException",
- "JsonSchemaValueException",
-]
-
-
-FORMAT_FUNCTIONS: Dict[str, Callable[[str], bool]] = {
- fn.__name__.replace("_", "-"): fn
- for fn in formats.__dict__.values()
- if callable(fn) and not fn.__name__.startswith("_")
-}
-
-
-def validate(data: Any) -> bool:
- """Validate the given ``data`` object using JSON Schema
- This function raises ``ValidationError`` if ``data`` is invalid.
- """
- with detailed_errors():
- _validate(data, custom_formats=FORMAT_FUNCTIONS)
- reduce(lambda acc, fn: fn(acc), EXTRA_VALIDATIONS, data)
- return True
diff --git a/spaces/TencentARC/VLog/models/grit_src/third_party/CenterNet2/configs/common/models/keypoint_rcnn_fpn.py b/spaces/TencentARC/VLog/models/grit_src/third_party/CenterNet2/configs/common/models/keypoint_rcnn_fpn.py
deleted file mode 100644
index 56b3994df249884d4816fc9a5c7f553a9ab6f400..0000000000000000000000000000000000000000
--- a/spaces/TencentARC/VLog/models/grit_src/third_party/CenterNet2/configs/common/models/keypoint_rcnn_fpn.py
+++ /dev/null
@@ -1,33 +0,0 @@
-from detectron2.config import LazyCall as L
-from detectron2.layers import ShapeSpec
-from detectron2.modeling.poolers import ROIPooler
-from detectron2.modeling.roi_heads import KRCNNConvDeconvUpsampleHead
-
-from .mask_rcnn_fpn import model
-
-[model.roi_heads.pop(x) for x in ["mask_in_features", "mask_pooler", "mask_head"]]
-
-model.roi_heads.update(
- num_classes=1,
- keypoint_in_features=["p2", "p3", "p4", "p5"],
- keypoint_pooler=L(ROIPooler)(
- output_size=14,
- scales=(1.0 / 4, 1.0 / 8, 1.0 / 16, 1.0 / 32),
- sampling_ratio=0,
- pooler_type="ROIAlignV2",
- ),
- keypoint_head=L(KRCNNConvDeconvUpsampleHead)(
- input_shape=ShapeSpec(channels=256, width=14, height=14),
- num_keypoints=17,
- conv_dims=[512] * 8,
- loss_normalizer="visible",
- ),
-)
-
-# Detectron1 uses 2000 proposals per-batch, but this option is per-image in detectron2.
-# 1000 proposals per-image is found to hurt box AP.
-# Therefore we increase it to 1500 per-image.
-model.proposal_generator.post_nms_topk = (1500, 1000)
-
-# Keypoint AP degrades (though box AP improves) when using plain L1 loss
-model.roi_heads.box_predictor.smooth_l1_beta = 0.5
diff --git a/spaces/VIPLab/Caption-Anything/caption_anything/captioner/base_captioner.py b/spaces/VIPLab/Caption-Anything/caption_anything/captioner/base_captioner.py
deleted file mode 100644
index 6a11f53e4a4275f60bf820c138589d325ba52dad..0000000000000000000000000000000000000000
--- a/spaces/VIPLab/Caption-Anything/caption_anything/captioner/base_captioner.py
+++ /dev/null
@@ -1,200 +0,0 @@
-import torch
-from PIL import Image, ImageDraw, ImageOps
-from transformers import pipeline, BlipProcessor, BlipForConditionalGeneration, BlipForQuestionAnswering
-import json
-import pdb
-import cv2
-import numpy as np
-from typing import Any, Union, List
-import time
-import clip
-
-from caption_anything.utils.utils import load_image
-
-
-def boundary(inputs):
- col = inputs.shape[1]
- inputs = inputs.reshape(-1)
- lens = len(inputs)
- start = np.argmax(inputs)
- end = lens - 1 - np.argmax(np.flip(inputs))
- top = start // col
- bottom = end // col
- return top, bottom
-
-
-def new_seg_to_box(seg_mask: Union[np.ndarray, Image.Image, str]):
- if type(seg_mask) == str:
- seg_mask = Image.open(seg_mask)
- elif type(seg_mask) == np.ndarray:
- seg_mask = Image.fromarray(seg_mask)
- seg_mask = np.array(seg_mask) > 0
- size = max(seg_mask.shape[0], seg_mask.shape[1])
- top, bottom = boundary(seg_mask)
- left, right = boundary(seg_mask.T)
- return [left / size, top / size, right / size, bottom / size]
-
-
-def seg_to_box(seg_mask: Union[np.ndarray, Image.Image, str]):
- if type(seg_mask) == str:
- seg_mask = cv2.imread(seg_mask, cv2.IMREAD_GRAYSCALE)
- _, seg_mask = cv2.threshold(seg_mask, 127, 255, 0)
- elif type(seg_mask) == np.ndarray:
- assert seg_mask.ndim == 2 # only support single-channel segmentation mask
- seg_mask = seg_mask.astype('uint8')
- if seg_mask.dtype == 'bool':
- seg_mask = seg_mask * 255
- contours, hierarchy = cv2.findContours(seg_mask, cv2.RETR_EXTERNAL, cv2.CHAIN_APPROX_SIMPLE)
- contours = np.concatenate(contours, axis=0)
- rect = cv2.minAreaRect(contours)
- box = cv2.boxPoints(rect)
- if rect[-1] >= 45:
- newstart = box.argmin(axis=0)[1] # leftmost
- else:
- newstart = box.argmax(axis=0)[0] # topmost
- box = np.concatenate([box[newstart:], box[:newstart]], axis=0)
- box = np.int0(box)
- return box
-
-
-def get_w_h(rect_points):
- w = np.linalg.norm(rect_points[0] - rect_points[1], ord=2).astype('int')
- h = np.linalg.norm(rect_points[0] - rect_points[3], ord=2).astype('int')
- return w, h
-
-
-def cut_box(img, rect_points):
- w, h = get_w_h(rect_points)
- dst_pts = np.array([[h, 0], [h, w], [0, w], [0, 0], ], dtype="float32")
- transform = cv2.getPerspectiveTransform(rect_points.astype("float32"), dst_pts)
- cropped_img = cv2.warpPerspective(img, transform, (h, w))
- return cropped_img
-
-
-class BaseCaptioner:
- def __init__(self, device, enable_filter=False):
- print(f"Initializing ImageCaptioning to {device}")
- self.device = device
- self.torch_dtype = torch.float16 if 'cuda' in device else torch.float32
- self.processor = None
- self.model = None
- self.enable_filter = enable_filter
- if enable_filter:
- self.filter, self.preprocess = clip.load('ViT-B/32', device)
-
- @torch.no_grad()
- def filter_caption(self, image: Union[np.ndarray, Image.Image, str], caption: str, reference_caption: List[str]=[]):
- image = load_image(image, return_type='pil')
- image = self.preprocess(image).unsqueeze(0).to(self.device) # (1, 3, 224, 224)
- captions = [caption]
- if len(reference_caption):
- captions.extend(reference_caption)
- text = clip.tokenize(captions).to(self.device) # (>1, 77)
- image_features = self.filter.encode_image(image) # (1, 512)
- text_features = self.filter.encode_text(text) # # (>1, 512)
- image_features /= image_features.norm(dim=-1, keepdim=True)
- text_features /= text_features.norm(dim=-1, keepdim=True)
-
- if len(reference_caption):
- similarity = torch.matmul(image_features, text_features.transpose(1, 0)) / 0.07
- similarity = similarity.softmax(dim=1)[0, 0].item()
- else:
- similarity = torch.matmul(image_features, text_features.transpose(1, 0)).item()
- print(f'Clip score of the caption is {similarity}')
- return similarity
-
- def inference(self, image: Union[np.ndarray, Image.Image, str], filter: bool = False):
- raise NotImplementedError()
-
- def inference_with_reduced_tokens(self, image: Union[np.ndarray, Image.Image, str], seg_mask, filter: bool = False):
- raise NotImplementedError()
-
- def inference_box(self, image: Union[np.ndarray, Image.Image, str], box: Union[list, np.ndarray], filter=False, verbose=False, caption_args={}):
- image = load_image(image, return_type="pil")
-
- if np.array(box).size == 4:
- # [x0, y0, x1, y1], where (x0, y0), (x1, y1) represent top-left and bottom-right corners
- size = max(image.width, image.height)
- x1, y1, x2, y2 = box
- image_crop = np.array(image.crop((x1 * size, y1 * size, x2 * size, y2 * size)))
- elif np.array(box).size == 8: # four corners of an irregular rectangle
- image_crop = cut_box(np.array(image), box)
-
- crop_save_path = None
- if verbose:
- crop_save_path = f'result/crop_{time.time()}.png'
- Image.fromarray(image_crop).save(crop_save_path)
- print(f'croped image saved in {crop_save_path}')
- caption = self.inference(image_crop, filter, caption_args)
- caption.update({'crop_save_path': crop_save_path})
- return caption
-
- def inference_seg(self,
- image: Union[np.ndarray, str],
- seg_mask: Union[np.ndarray, Image.Image, str] = None,
- crop_mode="w_bg",
- filter=False,
- disable_regular_box=False,
- verbose=False,
- caption_args={}):
- if seg_mask is None:
- seg_mask = np.ones(image.size).astype(bool)
-
- image = load_image(image, return_type="pil")
- seg_mask = load_image(seg_mask, return_type="pil")
-
- seg_mask = seg_mask.resize(image.size)
- seg_mask = np.array(seg_mask) > 0
- if crop_mode == "wo_bg":
- image = np.array(image) * seg_mask[:, :, np.newaxis] + (1 - seg_mask[:, :, np.newaxis]) * 255
- image = np.uint8(image)
- else:
- image = np.array(image)
-
- if disable_regular_box:
- min_area_box = seg_to_box(seg_mask)
- else:
- min_area_box = new_seg_to_box(seg_mask)
- return self.inference_box(image, min_area_box, filter, verbose, caption_args)
-
- def generate_seg_cropped_image(self,
- image: Union[np.ndarray, str],
- seg_mask: Union[np.ndarray, Image.Image, str],
- crop_mode="w_bg",
- disable_regular_box=False):
- image = load_image(image, return_type="pil")
- seg_mask = load_image(seg_mask, return_type="pil")
-
- seg_mask = seg_mask.resize(image.size)
- seg_mask = np.array(seg_mask) > 0
-
- if crop_mode == "wo_bg":
- image = np.array(image) * seg_mask[:, :, np.newaxis] + (1 - seg_mask[:, :, np.newaxis]) * 255
- else:
- image = np.array(image)
-
- if disable_regular_box:
- box = seg_to_box(seg_mask)
- else:
- box = new_seg_to_box(seg_mask)
-
- if np.array(box).size == 4:
- # [x0, y0, x1, y1], where (x0, y0), (x1, y1) represent top-left and bottom-right corners
- size = max(image.shape[0], image.shape[1])
- x1, y1, x2, y2 = box
- image_crop = np.array(image.crop((x1 * size, y1 * size, x2 * size, y2 * size)))
- elif np.array(box).size == 8: # four corners of an irregular rectangle
- image_crop = cut_box(np.array(image), box)
- crop_save_path = f'result/crop_{time.time()}.png'
- Image.fromarray(image_crop).save(crop_save_path)
- print(f'croped image saved in {crop_save_path}')
- return crop_save_path
-
-
-if __name__ == '__main__':
- model = BaseCaptioner(device='cuda:0')
- image_path = 'test_images/img2.jpg'
- seg_mask = np.zeros((15, 15))
- seg_mask[5:10, 5:10] = 1
- seg_mask = 'image/SAM/img10.jpg.raw_mask.png'
- print(model.inference_seg(image_path, seg_mask))
diff --git a/spaces/VetriVendhan26/sentiment-analysis/README.md b/spaces/VetriVendhan26/sentiment-analysis/README.md
deleted file mode 100644
index dc9917f539edbcb55c1970096f3e111d5a31efec..0000000000000000000000000000000000000000
--- a/spaces/VetriVendhan26/sentiment-analysis/README.md
+++ /dev/null
@@ -1,12 +0,0 @@
----
-title: Sentiment Analysis
-emoji: 🌖
-colorFrom: red
-colorTo: indigo
-sdk: gradio
-sdk_version: 3.50.2
-app_file: app.py
-pinned: false
----
-
-Check out the configuration reference at https://huggingface.co/docs/hub/spaces-config-reference
diff --git a/spaces/Vision-CAIR/MiniGPT-v2/minigpt4/models/modeling_llama.py b/spaces/Vision-CAIR/MiniGPT-v2/minigpt4/models/modeling_llama.py
deleted file mode 100644
index 6d2802050ff5fe65b7bbef22a4b648c4109c90bc..0000000000000000000000000000000000000000
--- a/spaces/Vision-CAIR/MiniGPT-v2/minigpt4/models/modeling_llama.py
+++ /dev/null
@@ -1,111 +0,0 @@
-import math
-from typing import List, Optional, Tuple, Union
-
-import torch
-import torch.nn.functional as F
-from torch.nn import CrossEntropyLoss
-
-from transformers.utils import add_start_docstrings_to_model_forward, replace_return_docstrings
-from transformers.modeling_outputs import CausalLMOutputWithPast
-from transformers.models.llama.modeling_llama import LLAMA_INPUTS_DOCSTRING, _CONFIG_FOR_DOC
-from transformers.models.llama.modeling_llama import LlamaForCausalLM as LlamaForCausalLMOrig
-
-
-class LlamaForCausalLM(LlamaForCausalLMOrig):
-
- @add_start_docstrings_to_model_forward(LLAMA_INPUTS_DOCSTRING)
- @replace_return_docstrings(output_type=CausalLMOutputWithPast, config_class=_CONFIG_FOR_DOC)
- def forward(
- self,
- input_ids: torch.LongTensor = None,
- attention_mask: Optional[torch.Tensor] = None,
- position_ids: Optional[torch.LongTensor] = None,
- past_key_values: Optional[List[torch.FloatTensor]] = None,
- inputs_embeds: Optional[torch.FloatTensor] = None,
- labels: Optional[torch.LongTensor] = None,
- use_cache: Optional[bool] = None,
- output_attentions: Optional[bool] = None,
- output_hidden_states: Optional[bool] = None,
- return_dict: Optional[bool] = None,
- reduction: Optional[str] = "mean",
- ) -> Union[Tuple, CausalLMOutputWithPast]:
- r"""
- Args:
- labels (`torch.LongTensor` of shape `(batch_size, sequence_length)`, *optional*):
- Labels for computing the masked language modeling loss. Indices should either be in `[0, ...,
- config.vocab_size]` or -100 (see `input_ids` docstring). Tokens with indices set to `-100` are ignored
- (masked), the loss is only computed for the tokens with labels in `[0, ..., config.vocab_size]`.
-
- Returns:
-
- Example:
-
- ```python
- >>> from transformers import AutoTokenizer, LlamaForCausalLM
-
- >>> model = LlamaForCausalLM.from_pretrained(PATH_TO_CONVERTED_WEIGHTS)
- >>> tokenizer = AutoTokenizer.from_pretrained(PATH_TO_CONVERTED_TOKENIZER)
-
- >>> prompt = "Hey, are you conscious? Can you talk to me?"
- >>> inputs = tokenizer(prompt, return_tensors="pt")
-
- >>> # Generate
- >>> generate_ids = model.generate(inputs.input_ids, max_length=30)
- >>> tokenizer.batch_decode(generate_ids, skip_special_tokens=True, clean_up_tokenization_spaces=False)[0]
- "Hey, are you conscious? Can you talk to me?\nI'm not conscious, but I can talk to you."
- ```"""
-
- output_attentions = output_attentions if output_attentions is not None else self.config.output_attentions
- output_hidden_states = (
- output_hidden_states if output_hidden_states is not None else self.config.output_hidden_states
- )
- return_dict = return_dict if return_dict is not None else self.config.use_return_dict
-
- # decoder outputs consists of (dec_features, layer_state, dec_hidden, dec_attn)
- outputs = self.model(
- input_ids=input_ids,
- attention_mask=attention_mask,
- position_ids=position_ids,
- past_key_values=past_key_values,
- inputs_embeds=inputs_embeds,
- use_cache=use_cache,
- output_attentions=output_attentions,
- output_hidden_states=output_hidden_states,
- return_dict=return_dict,
- )
-
- hidden_states = outputs[0]
- if self.config.pretraining_tp > 1:
- lm_head_slices = self.lm_head.weight.split(self.vocab_size // self.config.pretraining_tp, dim=0)
- logits = [F.linear(hidden_states, lm_head_slices[i]) for i in range(self.config.pretraining_tp)]
- logits = torch.cat(logits, dim=-1)
- else:
- logits = self.lm_head(hidden_states)
- logits = logits.float()
-
- loss = None
- if labels is not None:
- # Shift so that tokens < n predict n
- shift_logits = logits[..., :-1, :].contiguous()
- shift_labels = labels[..., 1:].contiguous()
- # Flatten the tokens
- loss_fct = CrossEntropyLoss(reduction=reduction)
- shift_logits = shift_logits.view(-1, self.config.vocab_size)
- shift_labels = shift_labels.view(-1)
- # Enable model parallelism
- shift_labels = shift_labels.to(shift_logits.device)
- loss = loss_fct(shift_logits, shift_labels)
- if reduction == "none":
- loss = loss.view(logits.size(0), -1).mean(1)
-
- if not return_dict:
- output = (logits,) + outputs[1:]
- return (loss,) + output if loss is not None else output
-
- return CausalLMOutputWithPast(
- loss=loss,
- logits=logits,
- past_key_values=outputs.past_key_values,
- hidden_states=outputs.hidden_states,
- attentions=outputs.attentions,
- )
diff --git a/spaces/Vithika/ISRO/app.py b/spaces/Vithika/ISRO/app.py
deleted file mode 100644
index c5ae64e2eaba5bc2b8e8d86ddf64763c84aab74e..0000000000000000000000000000000000000000
--- a/spaces/Vithika/ISRO/app.py
+++ /dev/null
@@ -1,379 +0,0 @@
-import streamlit as st
-import numpy as np
-import cv2
-import tensorflow as tf
-from PIL import Image
-from keras.models import load_model
-from sklearn.preprocessing import LabelEncoder
-import pickle
-from keras_preprocessing.sequence import pad_sequences
-from keras.preprocessing.text import Tokenizer
-from sklearn.preprocessing import LabelEncoder
-from PIL import Image
-# from google.colab.patches import cv2_imshow
-
-def label_smoothing(y_true,y_pred):
-
- return tf.keras.losses.binary_crossentropy(y_true,y_pred,label_smoothing=0.1)
-def sparse_cross_entropy(y_true, y_pred):
- loss = tf.nn.sparse_softmax_cross_entropy_with_logits(labels=y_true,
- logits=y_pred)
- loss_mean = tf.reduce_mean(loss)
- return loss_mean
-model1 = load_model('densenet.h5',custom_objects={'label_smoothing': label_smoothing})
-image_model_transfer=load_model("image_model_transfer.h5")
-decoder_model=load_model("Final_ISRO_DenseNet201_Epoch50.h5",custom_objects={'sparse_cross_entropy': sparse_cross_entropy})
-
-class TokenizerWrap(Tokenizer):
- """Wrap the Tokenizer-class from Keras with more functionality."""
-
- def _init_(self, texts, num_words=None):
- """
- :param texts: List of strings with the data-set.
- :param num_words: Max number of words to use.
- """
-
- Tokenizer._init_(self, num_words=num_words)
-
- # Create the vocabulary from the texts.
- self.fit_on_texts(texts)
-
- # Create inverse lookup from integer-tokens to words.
- # word_index is a dictionary. its values are tokens and the keys are words
- # opposite to index_to_word
- self.index_to_word = dict(zip(self.word_index.values(),
- self.word_index.keys()))
-
- def token_to_word(self, token):
- """Lookup a single word from an integer-token."""
- word = " " if token == 0 else self.index_to_word[token]
- return word
-
- def tokens_to_string(self, tokens):
- """Convert a list of integer-tokens to a string."""
- # Create a list of the individual words.
- words = [self.index_to_word[token]
- for token in tokens
- if token != 0]
-
- # Concatenate the words to a single string
- # with space between all the words.
- text = " ".join(words)
-
- return text
-
- def captions_to_tokens(self, captions_listlist):
- """
- Convert a list-of-list with text-captions to
- a list-of-list of integer-tokens.
- """
-
- # Note that text_to_sequences() takes a list of texts.
- tokens = [self.texts_to_sequences(captions_list)
- for captions_list in captions_listlist]
-
- return tokens
-with open('Train_Label.pickle', 'rb') as efile:
- labels=pickle.load(efile)
-with open('tokenizer.pkl', 'rb') as efile:
- tokenizer=pickle.load(efile)
-
-le=LabelEncoder()
-labels=le.fit_transform(labels)
-
-def framing(video):#defining a small function named"framing" with a parameter "i" that's supposed to be provided for reading the video
- fr = []#creating an empty list named fr
- fr_pre=[]#creating an empty list named fr_pre
- cap = cv2.VideoCapture(video)#reading the video file
- while (cap.isOpened()):#This command builds a loop to check if the data is still being read from the video
- ret,frame = cap.read()#reading the data tunnel,gives two output where one tells about presence of frames(here it's ret) & the other speaks frame data(here it's frame)
- if ret == True:#checking for presence of frames
- # cv2_imshow(frame)#displaying the frames
- grayed = cv2.cvtColor(frame,cv2.COLOR_BGR2GRAY)#Converting the frames to Grayscale from BGR
- canned = cv2.Canny(grayed,320,320)#For extrating edges we use Canny Edge detection method
- fr.append(frame)#Appending the read frame
- fr_pre.append(canned)#Appending the edge extracted frames
- # cv2_imshow(grayed)#Displaying the original frames
- # cv2_imshow(canned)#Displaying the edge detected frames
- k = cv2.waitKey(10) & 0XFF#this is an arrangement for displaying the video where the secs for which each frame needs to be displayed in given in the paranthesis
- if k == ord('q'):#pressing 'q' key will close the video
- break
- else:
- break
- cap.release()#Here we release the resoures
- cv2.destroyAllWindows()#Here we delete all the windows that were created during the program
- return fr_pre,fr
-
-def difference_of_frames(frames):
- diff = []#creatin a list variable
- for i in range(0,len(frames)-1):#defining the range
- diff.append(cv2.absdiff(frames[i],frames[i+1]))#appending the diff between frames to the list variable so we're supposed to get only the difference between frames
- return diff
-
-def cal_threshold(diff):
- mn = np.mean(diff)#This gives mean
- st_d = np.std(diff)#This gives standard deviation
- a = 4#Setting a random value we can modify it to any value
- ts = mn + (a * st_d)#defining the standard threshold value for the project/global threshold value
- return ts
-
-def imp_frames(diff, ts, ogframes):
- a_fr = []#Creating an empty list
- for i in range(len(diff)):#Defining the for loop to be looped over all the frames obtained after finding the frames resulted from subtracting
- mn = np.mean(diff[i])#Calculating the mean for each frame
- st_d = np.std(diff[i])#Calculating the standard deviation for each frame
- fr_ts = mn + (4*st_d)#Finding the threshold values for each frame/image
- a_fr.append([i,fr_ts])#Appending the frame number & the threshold values
- imp_fr = []#Creating an empty list
- for i,ac_tr in(a_fr):#Defining the loop on the list obtained from above code
- if ac_tr >= ts:#Comapring the threshold values to the standard threshold/global threshold values
- imp_fr.append([i,ac_tr])#Appending the list with the imp frames based on their index & the values
- key_fr = []#Creating an empty list
- for i,_ in imp_fr:#Defining the loop over the list obtained from above code
- key_fr.append(ogframes[i])#This extracts the frames based on the index of frames
- return key_fr
-
-def final_image(video):
- frames,ogframes = framing(video)#calling function framing & then extracting the images
- diff=difference_of_frames(frames)
- ts=cal_threshold(diff)
- key_fr=imp_frames(diff, ts, ogframes)
- frame_no=key_fr[int(len(key_fr)/2)] #this is a frame
- cv2.imwrite("Testing1.jpg",frame_no)
- return "Testing1.jpg"
- cv2.destroyAllWindows()
-
-def image_test(image_path):
- image=Image.open(image_path)
- image = image.resize((224,224))
- image = np.array(image)
- image= np.expand_dims(image, axis=0)
- return image
-
-def largest_indices(ary, n):
- flat = ary.flatten()
- indices = np.argpartition(flat, -n)[-n:]
- indices = indices[np.argsort(-flat[indices])]
- return indices
-
-mark_start = 'ssss'
-mark_end = ' eeee'
-
-token_start = tokenizer.word_index[mark_start.strip()]
-token_end = tokenizer.word_index[mark_end.strip()]
-
-def load_image(path, size=None):
- """
- Load the image from the given file-path and resize it
- to the given size if not None.
- """
-
- # Load the image using PIL.
- img = Image.open(path)
-
- # Resize image if desired.
- if not size is None:
- img = img.resize(size=size, resample=Image.LANCZOS)
-
- img = np.array(img)
- img = img / 255.0
-
- # Convert 2-dim gray-scale array to 3-dim RGB array.
- if (len(img.shape) == 2):
- img = np.repeat(img[:, :, np.newaxis], 3, axis=2)
- return img
-
-def greedy_search(image_path, max_tokens=30):
- """
- Generate a caption for the image in the given path.
- The caption is limited to the given number of tokens (words).
- """
- # ---------------------------ENCODE IMAGE--------------------------------
- # Load and resize the image.
- image = load_image(image_path, size=(224,224))
-
- # Expand the 3-dim numpy array to 4-dim
- # because the image-model expects a whole batch as input,
- # so we give it a batch with just one image.
- image_batch = np.expand_dims(image, axis=0)
-
- # Process the image with the pre-trained image-model
- # to get the transfer-values.
- transfer_values = image_model_transfer.predict(image_batch)
-
- # -------------------------------------------------------------------
-
-
- # Pre-allocate the 2-dim array used as input to the decoder.
- # This holds just a single sequence of integer-tokens,
- # but the decoder-model expects a batch of sequences.
- shape = (1, max_tokens)
- decoder_input_data = np.zeros(shape=shape, dtype=int)
-
- # The first input-token is the special start-token for 'ssss '.
- token_int = token_start #1
-
- # Initialize an empty output-text.
- output_text = ''
-
- # Initialize the number of tokens we have processed.
- count_tokens = 0
-
- # While we haven't sampled the special end-token for ' eeee'
- # and we haven't processed the max number of tokens.
- while token_int != token_end and count_tokens < max_tokens:
- # Update the input-sequence to the decoder
- # with the last token that was sampled.
- # In the first iteration this will set the
- # first element to the start-token.
- decoder_input_data[0, count_tokens] = token_int
-
- # Wrap the input-data in a dict for clarity and safety,
- # so we are sure we input the data in the right order.
- x_data = \
- {
- 'transfer_values_input': transfer_values,
- 'decoder_input': decoder_input_data
- }
-
- # Note that we input the entire sequence of tokens
- # to the decoder. This wastes a lot of computation
- # because we are only interested in the last input
- # and output. We could modify the code to return
- # the GRU-states when calling predict() and then
- # feeding these GRU-states as well the next time
- # we call predict(), but it would make the code
- # much more complicated.
-
- # Input this data to the decoder and get the predicted output.
- decoder_output = decoder_model.predict(x_data)
-# print(decoder_output.shape) (1,30,15000) for every iteration
-
- # Get the last predicted token as a one-hot encoded array.
- # Note that this is not limited by softmax, but we just
- # need the index of the largest element so it doesn't matter.
- token_onehot = decoder_output[0, count_tokens, :]
-# print(token_onehot.shape) (15000, ) for every iteration
- # Convert to an integer-token.
- token_int = np.argmax(token_onehot)
-# print(token_int) #the token of a word with the highest score
-
- # Lookup the word corresponding to this integer-token.
- sampled_word = tokenizer.token_to_word(token_int)
-# print(sampled_word)
-
- # Append the word to the output-text.
- output_text += " " + sampled_word
-
- # Increment the token-counter.
- count_tokens += 1
-
- # This is the sequence of tokens output by the decoder.
- output_tokens = decoder_input_data[0]
-# print(output_tokens)
- # Plot the image.
- # plt.imshow(image)
- # plt.show()
-
- predicted_caption=output_text.split()
- del (predicted_caption[-1])
- output_text = " "
- output_text = output_text.join(predicted_caption)
-
- # Print the predicted caption.
- # print("Predicted caption:")
- # print(output_text)
- # print()
- return predicted_caption
-
-def beam_search(beam_index, image_path, max_tokens=30):
- image = load_image(image_path, size=(224,224))
-
- # Expand the 3-dim numpy array to 4-dim
- # because the image-model expects a whole batch as input,
- # so we give it a batch with just one image.
- image_batch = np.expand_dims(image, axis=0)
-
- # Process the image with the pre-trained image-model
- # to get the transfer-values.
- transfer_values = image_model_transfer.predict(image_batch)
-
- token_int = [token_start]
- start_word = [[token_int, 0.0]]
- count_tokens = 0
- while len(start_word[0][0]) [b, n_h, t, d_k]
- b, d, t_s, t_t = (*key.size(), query.size(2))
- query = query.view(b, self.n_heads, self.k_channels, t_t).transpose(2, 3)
- key = key.view(b, self.n_heads, self.k_channels, t_s).transpose(2, 3)
- value = value.view(b, self.n_heads, self.k_channels, t_s).transpose(2, 3)
-
- scores = torch.matmul(query / math.sqrt(self.k_channels), key.transpose(-2, -1))
- if self.window_size is not None:
- assert t_s == t_t, "Relative attention is only available for self-attention."
- key_relative_embeddings = self._get_relative_embeddings(self.emb_rel_k, t_s)
- rel_logits = self._matmul_with_relative_keys(query /math.sqrt(self.k_channels), key_relative_embeddings)
- scores_local = self._relative_position_to_absolute_position(rel_logits)
- scores = scores + scores_local
- if self.proximal_bias:
- assert t_s == t_t, "Proximal bias is only available for self-attention."
- scores = scores + self._attention_bias_proximal(t_s).to(device=scores.device, dtype=scores.dtype)
- if mask is not None:
- scores = scores.masked_fill(mask == 0, -1e4)
- if self.block_length is not None:
- assert t_s == t_t, "Local attention is only available for self-attention."
- block_mask = torch.ones_like(scores).triu(-self.block_length).tril(self.block_length)
- scores = scores.masked_fill(block_mask == 0, -1e4)
- p_attn = F.softmax(scores, dim=-1) # [b, n_h, t_t, t_s]
- p_attn = self.drop(p_attn)
- output = torch.matmul(p_attn, value)
- if self.window_size is not None:
- relative_weights = self._absolute_position_to_relative_position(p_attn)
- value_relative_embeddings = self._get_relative_embeddings(self.emb_rel_v, t_s)
- output = output + self._matmul_with_relative_values(relative_weights, value_relative_embeddings)
- output = output.transpose(2, 3).contiguous().view(b, d, t_t) # [b, n_h, t_t, d_k] -> [b, d, t_t]
- return output, p_attn
-
- def _matmul_with_relative_values(self, x, y):
- """
- x: [b, h, l, m]
- y: [h or 1, m, d]
- ret: [b, h, l, d]
- """
- ret = torch.matmul(x, y.unsqueeze(0))
- return ret
-
- def _matmul_with_relative_keys(self, x, y):
- """
- x: [b, h, l, d]
- y: [h or 1, m, d]
- ret: [b, h, l, m]
- """
- ret = torch.matmul(x, y.unsqueeze(0).transpose(-2, -1))
- return ret
-
- def _get_relative_embeddings(self, relative_embeddings, length):
- max_relative_position = 2 * self.window_size + 1
- # Pad first before slice to avoid using cond ops.
- pad_length = max(length - (self.window_size + 1), 0)
- slice_start_position = max((self.window_size + 1) - length, 0)
- slice_end_position = slice_start_position + 2 * length - 1
- if pad_length > 0:
- padded_relative_embeddings = F.pad(
- relative_embeddings,
- commons.convert_pad_shape([[0, 0], [pad_length, pad_length], [0, 0]]))
- else:
- padded_relative_embeddings = relative_embeddings
- used_relative_embeddings = padded_relative_embeddings[:,slice_start_position:slice_end_position]
- return used_relative_embeddings
-
- def _relative_position_to_absolute_position(self, x):
- """
- x: [b, h, l, 2*l-1]
- ret: [b, h, l, l]
- """
- batch, heads, length, _ = x.size()
- # Concat columns of pad to shift from relative to absolute indexing.
- x = F.pad(x, commons.convert_pad_shape([[0,0],[0,0],[0,0],[0,1]]))
-
- # Concat extra elements so to add up to shape (len+1, 2*len-1).
- x_flat = x.view([batch, heads, length * 2 * length])
- x_flat = F.pad(x_flat, commons.convert_pad_shape([[0,0],[0,0],[0,length-1]]))
-
- # Reshape and slice out the padded elements.
- x_final = x_flat.view([batch, heads, length+1, 2*length-1])[:, :, :length, length-1:]
- return x_final
-
- def _absolute_position_to_relative_position(self, x):
- """
- x: [b, h, l, l]
- ret: [b, h, l, 2*l-1]
- """
- batch, heads, length, _ = x.size()
- # padd along column
- x = F.pad(x, commons.convert_pad_shape([[0, 0], [0, 0], [0, 0], [0, length-1]]))
- x_flat = x.view([batch, heads, length**2 + length*(length -1)])
- # add 0's in the beginning that will skew the elements after reshape
- x_flat = F.pad(x_flat, commons.convert_pad_shape([[0, 0], [0, 0], [length, 0]]))
- x_final = x_flat.view([batch, heads, length, 2*length])[:,:,:,1:]
- return x_final
-
- def _attention_bias_proximal(self, length):
- """Bias for self-attention to encourage attention to close positions.
- Args:
- length: an integer scalar.
- Returns:
- a Tensor with shape [1, 1, length, length]
- """
- r = torch.arange(length, dtype=torch.float32)
- diff = torch.unsqueeze(r, 0) - torch.unsqueeze(r, 1)
- return torch.unsqueeze(torch.unsqueeze(-torch.log1p(torch.abs(diff)), 0), 0)
-
-
-class FFN(nn.Module):
- def __init__(self, in_channels, out_channels, filter_channels, kernel_size, p_dropout=0., activation=None, causal=False):
- super().__init__()
- self.in_channels = in_channels
- self.out_channels = out_channels
- self.filter_channels = filter_channels
- self.kernel_size = kernel_size
- self.p_dropout = p_dropout
- self.activation = activation
- self.causal = causal
-
- if causal:
- self.padding = self._causal_padding
- else:
- self.padding = self._same_padding
-
- self.conv_1 = nn.Conv1d(in_channels, filter_channels, kernel_size)
- self.conv_2 = nn.Conv1d(filter_channels, out_channels, kernel_size)
- self.drop = nn.Dropout(p_dropout)
-
- def forward(self, x, x_mask):
- x = self.conv_1(self.padding(x * x_mask))
- if self.activation == "gelu":
- x = x * torch.sigmoid(1.702 * x)
- else:
- x = torch.relu(x)
- x = self.drop(x)
- x = self.conv_2(self.padding(x * x_mask))
- return x * x_mask
-
- def _causal_padding(self, x):
- if self.kernel_size == 1:
- return x
- pad_l = self.kernel_size - 1
- pad_r = 0
- padding = [[0, 0], [0, 0], [pad_l, pad_r]]
- x = F.pad(x, commons.convert_pad_shape(padding))
- return x
-
- def _same_padding(self, x):
- if self.kernel_size == 1:
- return x
- pad_l = (self.kernel_size - 1) // 2
- pad_r = self.kernel_size // 2
- padding = [[0, 0], [0, 0], [pad_l, pad_r]]
- x = F.pad(x, commons.convert_pad_shape(padding))
- return x
diff --git a/spaces/XzJosh/nine2-Bert-VITS2/preprocess_text.py b/spaces/XzJosh/nine2-Bert-VITS2/preprocess_text.py
deleted file mode 100644
index 5eb0f3b9e929fcbe91dcbeb653391227a2518a15..0000000000000000000000000000000000000000
--- a/spaces/XzJosh/nine2-Bert-VITS2/preprocess_text.py
+++ /dev/null
@@ -1,64 +0,0 @@
-import json
-from random import shuffle
-
-import tqdm
-from text.cleaner import clean_text
-from collections import defaultdict
-stage = [1,2,3]
-
-transcription_path = 'filelists/genshin.list'
-train_path = 'filelists/train.list'
-val_path = 'filelists/val.list'
-config_path = "configs/config.json"
-val_per_spk = 4
-max_val_total = 8
-
-if 1 in stage:
- with open( transcription_path+'.cleaned', 'w', encoding='utf-8') as f:
- for line in tqdm.tqdm(open(transcription_path, encoding='utf-8').readlines()):
- try:
- utt, spk, language, text = line.strip().split('|')
- norm_text, phones, tones, word2ph = clean_text(text, language)
- f.write('{}|{}|{}|{}|{}|{}|{}\n'.format(utt, spk, language, norm_text, ' '.join(phones),
- " ".join([str(i) for i in tones]),
- " ".join([str(i) for i in word2ph])))
- except Exception as error :
- print("err!", utt, error)
-
-if 2 in stage:
- spk_utt_map = defaultdict(list)
- spk_id_map = {}
- current_sid = 0
-
- with open( transcription_path+'.cleaned', encoding='utf-8') as f:
- for line in f.readlines():
- utt, spk, language, text, phones, tones, word2ph = line.strip().split('|')
- spk_utt_map[spk].append(line)
- if spk not in spk_id_map.keys():
- spk_id_map[spk] = current_sid
- current_sid += 1
- train_list = []
- val_list = []
-
- for spk, utts in spk_utt_map.items():
- shuffle(utts)
- val_list+=utts[:val_per_spk]
- train_list+=utts[val_per_spk:]
- if len(val_list) > max_val_total:
- train_list+=val_list[max_val_total:]
- val_list = val_list[:max_val_total]
-
- with open( train_path,"w", encoding='utf-8') as f:
- for line in train_list:
- f.write(line)
-
- with open(val_path, "w", encoding='utf-8') as f:
- for line in val_list:
- f.write(line)
-
-if 3 in stage:
- assert 2 in stage
- config = json.load(open(config_path, encoding='utf-8'))
- config["data"]['spk2id'] = spk_id_map
- with open(config_path, 'w', encoding='utf-8') as f:
- json.dump(config, f, indent=2, ensure_ascii=False)
diff --git a/spaces/Yiqin/ChatVID/model/vision/grit_src/third_party/CenterNet2/detectron2/layers/csrc/cocoeval/cocoeval.h b/spaces/Yiqin/ChatVID/model/vision/grit_src/third_party/CenterNet2/detectron2/layers/csrc/cocoeval/cocoeval.h
deleted file mode 100644
index db246e49a026b7cd989b305f4d3d98100be3c912..0000000000000000000000000000000000000000
--- a/spaces/Yiqin/ChatVID/model/vision/grit_src/third_party/CenterNet2/detectron2/layers/csrc/cocoeval/cocoeval.h
+++ /dev/null
@@ -1,88 +0,0 @@
-// Copyright (c) Facebook, Inc. and its affiliates.
-#pragma once
-
-#include
-#include
-#include
-#include
-#include
-
-namespace py = pybind11;
-
-namespace detectron2 {
-
-namespace COCOeval {
-
-// Annotation data for a single object instance in an image
-struct InstanceAnnotation {
- InstanceAnnotation(
- uint64_t id,
- double score,
- double area,
- bool is_crowd,
- bool ignore)
- : id{id}, score{score}, area{area}, is_crowd{is_crowd}, ignore{ignore} {}
- uint64_t id;
- double score = 0.;
- double area = 0.;
- bool is_crowd = false;
- bool ignore = false;
-};
-
-// Stores intermediate results for evaluating detection results for a single
-// image that has D detected instances and G ground truth instances. This stores
-// matches between detected and ground truth instances
-struct ImageEvaluation {
- // For each of the D detected instances, the id of the matched ground truth
- // instance, or 0 if unmatched
- std::vector detection_matches;
-
- // The detection score of each of the D detected instances
- std::vector detection_scores;
-
- // Marks whether or not each of G instances was ignored from evaluation (e.g.,
- // because it's outside area_range)
- std::vector ground_truth_ignores;
-
- // Marks whether or not each of D instances was ignored from evaluation (e.g.,
- // because it's outside aRng)
- std::vector detection_ignores;
-};
-
-template
-using ImageCategoryInstances = std::vector>>;
-
-// C++ implementation of COCO API cocoeval.py::COCOeval.evaluateImg(). For each
-// combination of image, category, area range settings, and IOU thresholds to
-// evaluate, it matches detected instances to ground truth instances and stores
-// the results into a vector of ImageEvaluation results, which will be
-// interpreted by the COCOeval::Accumulate() function to produce precion-recall
-// curves. The parameters of nested vectors have the following semantics:
-// image_category_ious[i][c][d][g] is the intersection over union of the d'th
-// detected instance and g'th ground truth instance of
-// category category_ids[c] in image image_ids[i]
-// image_category_ground_truth_instances[i][c] is a vector of ground truth
-// instances in image image_ids[i] of category category_ids[c]
-// image_category_detection_instances[i][c] is a vector of detected
-// instances in image image_ids[i] of category category_ids[c]
-std::vector EvaluateImages(
- const std::vector>& area_ranges, // vector of 2-tuples
- int max_detections,
- const std::vector& iou_thresholds,
- const ImageCategoryInstances>& image_category_ious,
- const ImageCategoryInstances&
- image_category_ground_truth_instances,
- const ImageCategoryInstances&
- image_category_detection_instances);
-
-// C++ implementation of COCOeval.accumulate(), which generates precision
-// recall curves for each set of category, IOU threshold, detection area range,
-// and max number of detections parameters. It is assumed that the parameter
-// evaluations is the return value of the functon COCOeval::EvaluateImages(),
-// which was called with the same parameter settings params
-py::dict Accumulate(
- const py::object& params,
- const std::vector& evalutations);
-
-} // namespace COCOeval
-} // namespace detectron2
diff --git a/spaces/YuanMio/vits-uma-genshin-honkai/text/symbols.py b/spaces/YuanMio/vits-uma-genshin-honkai/text/symbols.py
deleted file mode 100644
index edfbd24247be8c757275ce80b9ec27a0ffa808f3..0000000000000000000000000000000000000000
--- a/spaces/YuanMio/vits-uma-genshin-honkai/text/symbols.py
+++ /dev/null
@@ -1,39 +0,0 @@
-'''
-Defines the set of symbols used in text input to the model.
-'''
-
-'''# japanese_cleaners
-_pad = '_'
-_punctuation = ',.!?-'
-_letters = 'AEINOQUabdefghijkmnoprstuvwyzʃʧ↓↑ '
-'''
-
-'''# japanese_cleaners2
-_pad = '_'
-_punctuation = ',.!?-~…'
-_letters = 'AEINOQUabdefghijkmnoprstuvwyzʃʧʦ↓↑ '
-'''
-
-'''# korean_cleaners
-_pad = '_'
-_punctuation = ',.!?…~'
-_letters = 'ㄱㄴㄷㄹㅁㅂㅅㅇㅈㅊㅋㅌㅍㅎㄲㄸㅃㅆㅉㅏㅓㅗㅜㅡㅣㅐㅔ '
-'''
-
-'''# chinese_cleaners
-_pad = '_'
-_punctuation = ',。!?—…'
-_letters = 'ㄅㄆㄇㄈㄉㄊㄋㄌㄍㄎㄏㄐㄑㄒㄓㄔㄕㄖㄗㄘㄙㄚㄛㄜㄝㄞㄟㄠㄡㄢㄣㄤㄥㄦㄧㄨㄩˉˊˇˋ˙ '
-'''
-
-# zh_ja_mixture_cleaners
-_pad = '_'
-_punctuation = ',.!?-~…'
-_letters = 'AEINOQUabdefghijklmnoprstuvwyzʃʧʦɯɹəɥ⁼ʰ`→↓↑ '
-
-
-# Export all symbols:
-symbols = [_pad] + list(_punctuation) + list(_letters)
-
-# Special symbol ids
-SPACE_ID = symbols.index(" ")
\ No newline at end of file
diff --git a/spaces/Yuliang/ECON/lib/pymafx/models/transformers/bert/e2e_hand_network.py b/spaces/Yuliang/ECON/lib/pymafx/models/transformers/bert/e2e_hand_network.py
deleted file mode 100644
index be88fc5f32099daf9ca56eaa8d362efd5d8915b6..0000000000000000000000000000000000000000
--- a/spaces/Yuliang/ECON/lib/pymafx/models/transformers/bert/e2e_hand_network.py
+++ /dev/null
@@ -1,94 +0,0 @@
-"""
-Copyright (c) Microsoft Corporation.
-Licensed under the MIT license.
-
-"""
-
-import src.modeling.data.config as cfg
-import torch
-
-
-class Graphormer_Hand_Network(torch.nn.Module):
- '''
- End-to-end Graphormer network for hand pose and mesh reconstruction from a single image.
- '''
- def __init__(self, args, config, backbone, trans_encoder):
- super(Graphormer_Hand_Network, self).__init__()
- self.config = config
- self.backbone = backbone
- self.trans_encoder = trans_encoder
- self.upsampling = torch.nn.Linear(195, 778)
- self.cam_param_fc = torch.nn.Linear(3, 1)
- self.cam_param_fc2 = torch.nn.Linear(195 + 21, 150)
- self.cam_param_fc3 = torch.nn.Linear(150, 3)
- self.grid_feat_dim = torch.nn.Linear(1024, 2051)
-
- def forward(self, images, mesh_model, mesh_sampler, meta_masks=None, is_train=False):
- batch_size = images.size(0)
- # Generate T-pose template mesh
- template_pose = torch.zeros((1, 48))
- template_pose = template_pose.cuda()
- template_betas = torch.zeros((1, 10)).cuda()
- template_vertices, template_3d_joints = mesh_model.layer(template_pose, template_betas)
- template_vertices = template_vertices / 1000.0
- template_3d_joints = template_3d_joints / 1000.0
-
- template_vertices_sub = mesh_sampler.downsample(template_vertices)
-
- # normalize
- template_root = template_3d_joints[:, cfg.J_NAME.index('Wrist'), :]
- template_3d_joints = template_3d_joints - template_root[:, None, :]
- template_vertices = template_vertices - template_root[:, None, :]
- template_vertices_sub = template_vertices_sub - template_root[:, None, :]
- num_joints = template_3d_joints.shape[1]
-
- # concatinate template joints and template vertices, and then duplicate to batch size
- ref_vertices = torch.cat([template_3d_joints, template_vertices_sub], dim=1)
- ref_vertices = ref_vertices.expand(batch_size, -1, -1)
-
- # extract grid features and global image features using a CNN backbone
- image_feat, grid_feat = self.backbone(images)
- # concatinate image feat and mesh template
- image_feat = image_feat.view(batch_size, 1, 2048).expand(-1, ref_vertices.shape[-2], -1)
- # process grid features
- grid_feat = torch.flatten(grid_feat, start_dim=2)
- grid_feat = grid_feat.transpose(1, 2)
- grid_feat = self.grid_feat_dim(grid_feat)
- # concatinate image feat and template mesh to form the joint/vertex queries
- features = torch.cat([ref_vertices, image_feat], dim=2)
- # prepare input tokens including joint/vertex queries and grid features
- features = torch.cat([features, grid_feat], dim=1)
-
- if is_train == True:
- # apply mask vertex/joint modeling
- # meta_masks is a tensor of all the masks, randomly generated in dataloader
- # we pre-define a [MASK] token, which is a floating-value vector with 0.01s
- special_token = torch.ones_like(features[:, :-49, :]).cuda() * 0.01
- features[:, :-49, :
- ] = features[:, :-49, :] * meta_masks + special_token * (1 - meta_masks)
-
- # forward pass
- if self.config.output_attentions == True:
- features, hidden_states, att = self.trans_encoder(features)
- else:
- features = self.trans_encoder(features)
-
- pred_3d_joints = features[:, :num_joints, :]
- pred_vertices_sub = features[:, num_joints:-49, :]
-
- # learn camera parameters
- x = self.cam_param_fc(features[:, :-49, :])
- x = x.transpose(1, 2)
- x = self.cam_param_fc2(x)
- x = self.cam_param_fc3(x)
- cam_param = x.transpose(1, 2)
- cam_param = cam_param.squeeze()
-
- temp_transpose = pred_vertices_sub.transpose(1, 2)
- pred_vertices = self.upsampling(temp_transpose)
- pred_vertices = pred_vertices.transpose(1, 2)
-
- if self.config.output_attentions == True:
- return cam_param, pred_3d_joints, pred_vertices_sub, pred_vertices, hidden_states, att
- else:
- return cam_param, pred_3d_joints, pred_vertices_sub, pred_vertices
diff --git a/spaces/ZJunTvT/ZJunChat/run_macOS.command b/spaces/ZJunTvT/ZJunChat/run_macOS.command
deleted file mode 100644
index 2d26597ae47519f42336ccffc16646713a192ae1..0000000000000000000000000000000000000000
--- a/spaces/ZJunTvT/ZJunChat/run_macOS.command
+++ /dev/null
@@ -1,31 +0,0 @@
-#!/bin/bash
-
-# 获取脚本所在目录
-script_dir=$(dirname "$(readlink -f "$0")")
-
-# 将工作目录更改为脚本所在目录
-cd "$script_dir" || exit
-
-# 检查Git仓库是否有更新
-git remote update
-pwd
-
-if ! git status -uno | grep 'up to date' > /dev/null; then
- # 如果有更新,关闭当前运行的服务器
- pkill -f ChuanhuChatbot.py
-
- # 拉取最新更改
- git pull
-
- # 安装依赖
- pip3 install -r requirements.txt
-
- # 重新启动服务器
- nohup python3 ChuanhuChatbot.py &
-fi
-
-# 检查ChuanhuChatbot.py是否在运行
-if ! pgrep -f ChuanhuChatbot.py > /dev/null; then
- # 如果没有运行,启动服务器
- nohup python3 ChuanhuChatbot.py &
-fi
diff --git a/spaces/abdalrahmanshahrour/Summarization/download.py b/spaces/abdalrahmanshahrour/Summarization/download.py
deleted file mode 100644
index aed5233da1e6ebfbf73152ac74bd60419ca3895f..0000000000000000000000000000000000000000
--- a/spaces/abdalrahmanshahrour/Summarization/download.py
+++ /dev/null
@@ -1,7 +0,0 @@
-import nltk
-
-def __init__():
- nltk.download('punkt')
- nltk.download('stopwords')
- nltk.download('wordnet')
- nltk.download('omw-1.4')
\ No newline at end of file
diff --git a/spaces/abdvl/datahub_qa_bot/docs/deploy/confluent-cloud.md b/spaces/abdvl/datahub_qa_bot/docs/deploy/confluent-cloud.md
deleted file mode 100644
index d93ffcceaecee19be0fc462a3ff61360e36013cb..0000000000000000000000000000000000000000
--- a/spaces/abdvl/datahub_qa_bot/docs/deploy/confluent-cloud.md
+++ /dev/null
@@ -1,229 +0,0 @@
-# Integrating with Confluent Cloud
-
-DataHub provides the ability to easily leverage Confluent Cloud as your Kafka provider. To do so, you'll need to configure DataHub to talk to a broker and schema registry hosted by Confluent.
-
-Doing this is a matter of configuring the Kafka Producer and Consumers used by DataHub correctly. There are 2 places where Kafka configuration should be provided: the metadata service (GMS) and the frontend server (datahub-frontend). Follow the steps below to configure these components for your deployment.
-
-## **Step 1: Create topics in Confluent Control Center**
-
-First, you'll need to create following new topics in the [Confluent Control Center](https://docs.confluent.io/platform/current/control-center/index.html). By default they have the following names:
-
-1. **MetadataChangeProposal_v1**
-2. **FailedMetadataChangeProposal_v1**
-3. **MetadataChangeLog_Versioned_v1**
-4. **MetadataChangeLog_Timeseries_v1**
-5. **DataHubUsageEvent_v1**: User behavior tracking event for UI
-6. (Deprecated) **MetadataChangeEvent_v4**: Metadata change proposal messages
-7. (Deprecated) **MetadataAuditEvent_v4**: Metadata change log messages
-8. (Deprecated) **FailedMetadataChangeEvent_v4**: Failed to process #1 event
-
-The first five are the most important, and are explained in more depth in [MCP/MCL](../advanced/mcp-mcl.md). The final topics are
-those which are deprecated but still used under certain circumstances. It is likely that in the future they will be completely
-decommissioned.
-
-To create the topics, navigate to your **Cluster** and click "Create Topic". Feel free to tweak the default topic configurations to
-match your preferences.
-
-
-
-## Step 2: Configure DataHub Container to use Confluent Cloud Topics
-
-### Docker Compose
-
-If you are deploying DataHub via docker compose, enabling connection to Confluent is a matter of a) creating topics in the Confluent Control Center and b) changing the default container environment variables.
-
-First, configure GMS to connect to Confluent Cloud by changing `docker/gms/env/docker.env`:
-
-```
-KAFKA_BOOTSTRAP_SERVER=pkc-g4ml2.eu-west-2.aws.confluent.cloud:9092
-KAFKA_SCHEMAREGISTRY_URL=https://plrm-qwlpp.us-east-2.aws.confluent.cloud
-
-# Confluent Cloud Configs
-SPRING_KAFKA_PROPERTIES_SECURITY_PROTOCOL=SASL_SSL
-SPRING_KAFKA_PROPERTIES_SASL_JAAS_CONFIG=org.apache.kafka.common.security.plain.PlainLoginModule required username='XFA45EL1QFUQP4PA' password='ltyf96EvR1YYutsjLB3ZYfrk+yfCXD8sQHCE3EMp57A2jNs4RR7J1bU9k6lM6rU';
-SPRING_KAFKA_PROPERTIES_SASL_MECHANISM=PLAIN
-SPRING_KAFKA_PROPERTIES_CLIENT_DNS_LOOKUP=use_all_dns_ips
-SPRING_KAFKA_PROPERTIES_BASIC_AUTH_CREDENTIALS_SOURCE=USER_INFO
-SPRING_KAFKA_PROPERTIES_BASIC_AUTH_USER_INFO=P2ETAN5QR2LCWL14:RTjqw7AfETDl0RZo/7R0123LhPYs2TGjFKmvMWUFnlJ3uKubFbB1Sfs7aOjjNi1m23
-```
-
-Next, configure datahub-frontend to connect to Confluent Cloud by changing `docker/datahub-frontend/env/docker.env`:
-
-```
-KAFKA_BOOTSTRAP_SERVER=pkc-g4ml2.eu-west-2.aws.confluent.cloud:9092
-
-# Confluent Cloud Configs
-KAFKA_PROPERTIES_SECURITY_PROTOCOL=SASL_SSL
-KAFKA_PROPERTIES_SASL_JAAS_CONFIG=org.apache.kafka.common.security.plain.PlainLoginModule required username='XFA45EL1QFUQP4PA' password='ltyf96EvR1YYutsjLB3ZYfrk+yfCXD8sQHCE3EMp57A2jNs4RR7J1bU9k6lM6rU';
-KAFKA_PROPERTIES_SASL_MECHANISM=PLAIN
-KAFKA_PROPERTIES_CLIENT_DNS_LOOKUP=use_all_dns_ips
-KAFKA_PROPERTIES_BASIC_AUTH_CREDENTIALS_SOURCE=USER_INFO
-KAFKA_PROPERTIES_BASIC_AUTH_USER_INFO=P2ETAN5QR2LCWL14:RTjqw7AfETDl0RZo/7R0123LhPYs2TGjFKmvMWUFnlJ3uKubFbB1Sfs7aOjjNi1m23
-```
-
-Note that this step is only required if `DATAHUB_ANALYTICS_ENABLED` environment variable is not explicitly set to false for the datahub-frontend
-container.
-
-If you're deploying with Docker Compose, you do not need to deploy the Zookeeper, Kafka Broker, or Schema Registry containers that ship by default.
-
-#### DataHub Actions
-
-Configuring Confluent Cloud for DataHub Actions requires some additional edits to your `executor.yaml`. Under the Kafka
-source connection config you will need to add the Python style client connection information:
-
-```yaml
- connection:
- bootstrap: ${KAFKA_BOOTSTRAP_SERVER:-localhost:9092}
- schema_registry_url: ${SCHEMA_REGISTRY_URL:-http://localhost:8081}
- consumer_config:
- security.protocol: ${KAFKA_PROPERTIES_SECURITY_PROTOCOL:-PLAINTEXT}
- sasl.mechanism: ${KAFKA_PROPERTIES_SASL_MECHANISM:-PLAIN}
- sasl.username: ${KAFKA_PROPERTIES_SASL_USERNAME}
- sasl.password: ${KAFKA_PROPERTIES_SASL_PASSWORD}
- schema_registry_config:
- basic.auth.user.info: ${KAFKA_PROPERTIES_BASIC_AUTH_USER_INFO}
-```
-
-Specifically `sasl.username` and `sasl.password` are the differences from the base `executor.yaml` example file.
-
-Additionally, you will need to set up environment variables for `KAFKA_PROPERTIES_SASL_USERNAME` and `KAFKA_PROPERTIES_SASL_PASSWORD`
-which will use the same username and API Key you generated for the JAAS config.
-
-See [Overwriting a System Action Config](https://github.com/acryldata/datahub-actions/blob/main/docker/README.md#overwriting-a-system-action-config) for detailed reflection procedures.
-
-Next, configure datahub-actions to connect to Confluent Cloud by changing `docker/datahub-actions/env/docker.env`:
-
-```
-KAFKA_BOOTSTRAP_SERVER=pkc-g4ml2.eu-west-2.aws.confluent.cloud:9092
-SCHEMA_REGISTRY_URL=https://plrm-qwlpp.us-east-2.aws.confluent.cloud
-
-# Confluent Cloud Configs
-KAFKA_PROPERTIES_SECURITY_PROTOCOL=SASL_SSL
-KAFKA_PROPERTIES_SASL_USERNAME=XFA45EL1QFUQP4PA
-KAFKA_PROPERTIES_SASL_PASSWORD=ltyf96EvR1YYutsjLB3ZYfrk+yfCXD8sQHCE3EMp57A2jNs4RR7J1bU9k6lM6rU
-KAFKA_PROPERTIES_SASL_MECHANISM=PLAIN
-KAFKA_PROPERTIES_CLIENT_DNS_LOOKUP=use_all_dns_ips
-KAFKA_PROPERTIES_BASIC_AUTH_CREDENTIALS_SOURCE=USER_INFO
-KAFKA_PROPERTIES_BASIC_AUTH_USER_INFO=P2ETAN5QR2LCWL14:RTjqw7AfETDl0RZo/7R0123LhPYs2TGjFKmvMWUFnlJ3uKubFbB1Sfs7aOjjNi1m23
-```
-
-### Helm
-
-If you're deploying on K8s using Helm, you can simply change the **datahub-helm** `values.yml` to point to Confluent Cloud and disable some default containers:
-
-First, disable the `cp-schema-registry` service:
-
-```
-cp-schema-registry:
- enabled: false
-```
-
-Next, disable the `kafkaSetupJob` service:
-
-```
-kafkaSetupJob:
- enabled: false
-```
-
-Then, update the `kafka` configurations to point to your Confluent Cloud broker and schema registry instance, along with the topics you've created in Step 1:
-
-```
-kafka:
- bootstrap:
- server: pkc-g4ml2.eu-west-2.aws.confluent.cloud:9092
- schemaregistry:
- url: https://plrm-qwlpp.us-east-2.aws.confluent.cloud
-```
-
-Next, you'll want to create 2 new Kubernetes secrets, one for the JaaS configuration which contains the username and password for Confluent,
-and another for the user info used for connecting to the schema registry. You'll find the values for each within the Confluent Control Center. Specifically,
-select "Clients" -> "Configure new Java Client". You should see a page like the following:
-
-
-
-
-You'll want to generate both a Kafka Cluster API Key & a Schema Registry key. Once you do so,you should see the config
-automatically populate with your new secrets:
-
-
-
-You'll need to copy the values of `sasl.jaas.config` and `basic.auth.user.info`
-for the next step.
-
-The next step is to create K8s secrets containing the config values you've just generated. Specifically, you can run the following commands:
-
-```shell
-kubectl create secret generic confluent-secrets --from-literal=sasl_jaas_config=""
-kubectl create secret generic confluent-secrets --from-literal=basic_auth_user_info=""
-```
-
-With your config values substituted as appropriate. For example, in our case we'd run:
-
-```shell
-kubectl create secret generic confluent-secrets --from-literal=sasl_jaas_config="org.apache.kafka.common.security.plain.PlainLoginModule required username='XFA45EL1QFUQP4PA' password='ltyf96EvR1YYutsjLB3ZYfrk+yfCXD8sQHCE3EMp57A2jNs4RR7J1bU9k6lM6rU';"
-kubectl create secret generic confluent-secrets --from-literal=basic_auth_user_info="P2ETAN5QR2LCWL14:RTjqw7AfETDl0RZo/7R0123LhPYs2TGjFKmvMWUFnlJ3uKubFbB1Sfs7aOjjNi1m23"
-```
-
-Finally, we'll configure our containers to pick up the Confluent Kafka Configs by changing two config blocks in our `values.yaml` file. You
-should see these blocks commented at the bottom of the template. You'll want to uncomment them and set them to the following values:
-
-```
-credentialsAndCertsSecrets:
- name: confluent-secrets
- secureEnv:
- sasl.jaas.config: sasl_jaas_config
- basic.auth.user.info: basic_auth_user_info
-
-
-springKafkaConfigurationOverrides:
- security.protocol: SASL_SSL
- sasl.mechanism: PLAIN
- client.dns.lookup: use_all_dns_ips
- basic.auth.credentials.source: USER_INFO
-```
-
-Then simply apply the updated `values.yaml` to your K8s cluster via `kubectl apply`.
-
-#### DataHub Actions
-
-Configuring Confluent Cloud for DataHub Actions requires some additional edits to your `executor.yaml`. Under the Kafka
-source connection config you will need to add the Python style client connection information:
-
-```yaml
- connection:
- bootstrap: ${KAFKA_BOOTSTRAP_SERVER:-localhost:9092}
- schema_registry_url: ${SCHEMA_REGISTRY_URL:-http://localhost:8081}
- consumer_config:
- security.protocol: ${KAFKA_PROPERTIES_SECURITY_PROTOCOL:-PLAINTEXT}
- sasl.mechanism: ${KAFKA_PROPERTIES_SASL_MECHANISM:-PLAIN}
- sasl.username: ${KAFKA_PROPERTIES_SASL_USERNAME}
- sasl.password: ${KAFKA_PROPERTIES_SASL_PASSWORD}
- schema_registry_config:
- basic.auth.user.info: ${KAFKA_PROPERTIES_BASIC_AUTH_USER_INFO}
-```
-
-Specifically `sasl.username` and `sasl.password` are the differences from the base `executor.yaml` example file.
-
-Additionally, you will need to set up secrets for `KAFKA_PROPERTIES_SASL_USERNAME` and `KAFKA_PROPERTIES_SASL_PASSWORD`
-which will use the same username and API Key you generated for the JAAS config.
-
-See [Overwriting a System Action Config](https://github.com/acryldata/datahub-actions/blob/main/docker/README.md#overwriting-a-system-action-config) for detailed reflection procedures.
-
-```yaml
-credentialsAndCertsSecrets:
- name: confluent-secrets
- secureEnv:
- sasl.jaas.config: sasl_jaas_config
- basic.auth.user.info: basic_auth_user_info
- sasl.username: sasl_username
- sasl.password: sasl_password
-```
-
-The Actions pod will automatically pick these up in the correctly named environment variables when they are named this exact way.
-
-## Contribution
-Accepting contributions for a setup script compatible with Confluent Cloud!
-
-The kafka-setup-job container we ship with is only compatible with a distribution of Kafka wherein ZooKeeper
-is exposed and available. A version of the job using the [Confluent CLI](https://docs.confluent.io/confluent-cli/current/command-reference/kafka/topic/confluent_kafka_topic_create.html)
-would be very useful for the broader community.
\ No newline at end of file
diff --git a/spaces/abhishek/StableSAM/app.py b/spaces/abhishek/StableSAM/app.py
deleted file mode 100644
index 73b9a7ebb29eb5b27fda787058368fdc1f21c7c6..0000000000000000000000000000000000000000
--- a/spaces/abhishek/StableSAM/app.py
+++ /dev/null
@@ -1,168 +0,0 @@
-import gradio as gr
-import numpy as np
-import torch
-from diffusers import StableDiffusionInpaintPipeline
-from PIL import Image
-from segment_anything import SamPredictor, sam_model_registry, SamAutomaticMaskGenerator
-from diffusers import ControlNetModel
-from diffusers import UniPCMultistepScheduler
-from controlnet_inpaint import StableDiffusionControlNetInpaintPipeline
-import colorsys
-
-sam_checkpoint = "sam_vit_h_4b8939.pth"
-model_type = "vit_h"
-device = "cpu"
-
-
-sam = sam_model_registry[model_type](checkpoint=sam_checkpoint)
-sam.to(device=device)
-predictor = SamPredictor(sam)
-mask_generator = SamAutomaticMaskGenerator(sam)
-
-# pipe = StableDiffusionInpaintPipeline.from_pretrained(
-# "stabilityai/stable-diffusion-2-inpainting",
-# torch_dtype=torch.float16,
-# )
-# pipe = pipe.to("cuda")
-
-controlnet = ControlNetModel.from_pretrained(
- "lllyasviel/sd-controlnet-seg",
- torch_dtype=torch.float16,
-)
-pipe = StableDiffusionControlNetInpaintPipeline.from_pretrained(
- "runwayml/stable-diffusion-inpainting",
- controlnet=controlnet,
- torch_dtype=torch.float16,
-)
-pipe.scheduler = UniPCMultistepScheduler.from_config(pipe.scheduler.config)
-#pipe.enable_model_cpu_offload()
-#pipe.enable_xformers_memory_efficient_attention()
-pipe = pipe.to(device)
-
-
-with gr.Blocks() as demo:
- gr.Markdown("# StableSAM: Stable Diffusion + Segment Anything Model")
- gr.Markdown(
- """
- To try the demo, upload an image and select object(s) you want to inpaint.
- Write a prompt & a negative prompt to control the inpainting.
- Click on the "Submit" button to inpaint the selected object(s).
- Check "Background" to inpaint the background instead of the selected object(s).
-
- If the demo is slow, clone the space to your own HF account and run on a GPU.
- """
- )
- selected_pixels = gr.State([])
- with gr.Row():
- input_img = gr.Image(label="Input")
- mask_img = gr.Image(label="Mask", interactive=False)
- seg_img = gr.Image(label="Segmentation", interactive=False)
- output_img = gr.Image(label="Output", interactive=False)
-
- with gr.Row():
- prompt_text = gr.Textbox(lines=1, label="Prompt")
- negative_prompt_text = gr.Textbox(lines=1, label="Negative Prompt")
- is_background = gr.Checkbox(label="Background")
-
- with gr.Row():
- submit = gr.Button("Submit")
- clear = gr.Button("Clear")
-
- def generate_mask(image, bg, sel_pix, evt: gr.SelectData):
- sel_pix.append(evt.index)
- predictor.set_image(image)
- input_point = np.array(sel_pix)
- input_label = np.ones(input_point.shape[0])
- mask, _, _ = predictor.predict(
- point_coords=input_point,
- point_labels=input_label,
- multimask_output=False,
- )
- # clear torch cache
- torch.cuda.empty_cache()
- if bg:
- mask = np.logical_not(mask)
- mask = Image.fromarray(mask[0, :, :])
- segs = mask_generator.generate(image)
- boolean_masks = [s["segmentation"] for s in segs]
- finseg = np.zeros((boolean_masks[0].shape[0], boolean_masks[0].shape[1], 3), dtype=np.uint8)
- # Loop over the boolean masks and assign a unique color to each class
- for class_id, boolean_mask in enumerate(boolean_masks):
- hue = class_id * 1.0 / len(boolean_masks)
- rgb = tuple(int(i * 255) for i in colorsys.hsv_to_rgb(hue, 1, 1))
- rgb_mask = np.zeros((boolean_mask.shape[0], boolean_mask.shape[1], 3), dtype=np.uint8)
- rgb_mask[:, :, 0] = boolean_mask * rgb[0]
- rgb_mask[:, :, 1] = boolean_mask * rgb[1]
- rgb_mask[:, :, 2] = boolean_mask * rgb[2]
- finseg += rgb_mask
-
- torch.cuda.empty_cache()
-
- return mask, finseg
-
- def inpaint(image, mask, seg_img, prompt, negative_prompt):
- image = Image.fromarray(image)
- mask = Image.fromarray(mask)
- seg_img = Image.fromarray(seg_img)
-
- image = image.resize((512, 512))
- mask = mask.resize((512, 512))
- seg_img = seg_img.resize((512, 512))
-
- output = pipe(
- prompt,
- image,
- mask,
- seg_img,
- negative_prompt=negative_prompt,
- num_inference_steps=20,
- ).images[0]
- torch.cuda.empty_cache()
- return output
-
- def _clear(sel_pix, img, mask, seg, out, prompt, neg_prompt, bg):
- sel_pix = []
- img = None
- mask = None
- seg = None
- out = None
- prompt = ""
- neg_prompt = ""
- bg = False
- return img, mask, seg, out, prompt, neg_prompt, bg
-
- input_img.select(
- generate_mask,
- [input_img, is_background, selected_pixels],
- [mask_img, seg_img],
- )
- submit.click(
- inpaint,
- inputs=[input_img, mask_img, seg_img, prompt_text, negative_prompt_text],
- outputs=[output_img],
- )
- clear.click(
- _clear,
- inputs=[
- selected_pixels,
- input_img,
- mask_img,
- seg_img,
- output_img,
- prompt_text,
- negative_prompt_text,
- is_background,
- ],
- outputs=[
- input_img,
- mask_img,
- seg_img,
- output_img,
- prompt_text,
- negative_prompt_text,
- is_background,
- ],
- )
-
-if __name__ == "__main__":
- demo.launch()
diff --git a/spaces/abhishek/sketch-to-image/annotator/uniformer/configs/_base_/models/deeplabv3_unet_s5-d16.py b/spaces/abhishek/sketch-to-image/annotator/uniformer/configs/_base_/models/deeplabv3_unet_s5-d16.py
deleted file mode 100644
index 0cd262999d8b2cb8e14a5c32190ae73f479d8e81..0000000000000000000000000000000000000000
--- a/spaces/abhishek/sketch-to-image/annotator/uniformer/configs/_base_/models/deeplabv3_unet_s5-d16.py
+++ /dev/null
@@ -1,50 +0,0 @@
-# model settings
-norm_cfg = dict(type='SyncBN', requires_grad=True)
-model = dict(
- type='EncoderDecoder',
- pretrained=None,
- backbone=dict(
- type='UNet',
- in_channels=3,
- base_channels=64,
- num_stages=5,
- strides=(1, 1, 1, 1, 1),
- enc_num_convs=(2, 2, 2, 2, 2),
- dec_num_convs=(2, 2, 2, 2),
- downsamples=(True, True, True, True),
- enc_dilations=(1, 1, 1, 1, 1),
- dec_dilations=(1, 1, 1, 1),
- with_cp=False,
- conv_cfg=None,
- norm_cfg=norm_cfg,
- act_cfg=dict(type='ReLU'),
- upsample_cfg=dict(type='InterpConv'),
- norm_eval=False),
- decode_head=dict(
- type='ASPPHead',
- in_channels=64,
- in_index=4,
- channels=16,
- dilations=(1, 12, 24, 36),
- dropout_ratio=0.1,
- num_classes=2,
- norm_cfg=norm_cfg,
- align_corners=False,
- loss_decode=dict(
- type='CrossEntropyLoss', use_sigmoid=False, loss_weight=1.0)),
- auxiliary_head=dict(
- type='FCNHead',
- in_channels=128,
- in_index=3,
- channels=64,
- num_convs=1,
- concat_input=False,
- dropout_ratio=0.1,
- num_classes=2,
- norm_cfg=norm_cfg,
- align_corners=False,
- loss_decode=dict(
- type='CrossEntropyLoss', use_sigmoid=False, loss_weight=0.4)),
- # model training and testing settings
- train_cfg=dict(),
- test_cfg=dict(mode='slide', crop_size=256, stride=170))
diff --git a/spaces/abhishek/sketch-to-image/annotator/uniformer/mmdet_null/core/evaluation/mean_ap.py b/spaces/abhishek/sketch-to-image/annotator/uniformer/mmdet_null/core/evaluation/mean_ap.py
deleted file mode 100644
index e3226c71cf8457dce65652553132ad1ddbf214f7..0000000000000000000000000000000000000000
--- a/spaces/abhishek/sketch-to-image/annotator/uniformer/mmdet_null/core/evaluation/mean_ap.py
+++ /dev/null
@@ -1,469 +0,0 @@
-from multiprocessing import Pool
-
-import annotator.uniformer.mmcv as mmcv
-import numpy as np
-from annotator.uniformer.mmcv.utils import print_log
-from terminaltables import AsciiTable
-
-from .bbox_overlaps import bbox_overlaps
-from .class_names import get_classes
-
-
-def average_precision(recalls, precisions, mode='area'):
- """Calculate average precision (for single or multiple scales).
-
- Args:
- recalls (ndarray): shape (num_scales, num_dets) or (num_dets, )
- precisions (ndarray): shape (num_scales, num_dets) or (num_dets, )
- mode (str): 'area' or '11points', 'area' means calculating the area
- under precision-recall curve, '11points' means calculating
- the average precision of recalls at [0, 0.1, ..., 1]
-
- Returns:
- float or ndarray: calculated average precision
- """
- no_scale = False
- if recalls.ndim == 1:
- no_scale = True
- recalls = recalls[np.newaxis, :]
- precisions = precisions[np.newaxis, :]
- assert recalls.shape == precisions.shape and recalls.ndim == 2
- num_scales = recalls.shape[0]
- ap = np.zeros(num_scales, dtype=np.float32)
- if mode == 'area':
- zeros = np.zeros((num_scales, 1), dtype=recalls.dtype)
- ones = np.ones((num_scales, 1), dtype=recalls.dtype)
- mrec = np.hstack((zeros, recalls, ones))
- mpre = np.hstack((zeros, precisions, zeros))
- for i in range(mpre.shape[1] - 1, 0, -1):
- mpre[:, i - 1] = np.maximum(mpre[:, i - 1], mpre[:, i])
- for i in range(num_scales):
- ind = np.where(mrec[i, 1:] != mrec[i, :-1])[0]
- ap[i] = np.sum(
- (mrec[i, ind + 1] - mrec[i, ind]) * mpre[i, ind + 1])
- elif mode == '11points':
- for i in range(num_scales):
- for thr in np.arange(0, 1 + 1e-3, 0.1):
- precs = precisions[i, recalls[i, :] >= thr]
- prec = precs.max() if precs.size > 0 else 0
- ap[i] += prec
- ap /= 11
- else:
- raise ValueError(
- 'Unrecognized mode, only "area" and "11points" are supported')
- if no_scale:
- ap = ap[0]
- return ap
-
-
-def tpfp_imagenet(det_bboxes,
- gt_bboxes,
- gt_bboxes_ignore=None,
- default_iou_thr=0.5,
- area_ranges=None):
- """Check if detected bboxes are true positive or false positive.
-
- Args:
- det_bbox (ndarray): Detected bboxes of this image, of shape (m, 5).
- gt_bboxes (ndarray): GT bboxes of this image, of shape (n, 4).
- gt_bboxes_ignore (ndarray): Ignored gt bboxes of this image,
- of shape (k, 4). Default: None
- default_iou_thr (float): IoU threshold to be considered as matched for
- medium and large bboxes (small ones have special rules).
- Default: 0.5.
- area_ranges (list[tuple] | None): Range of bbox areas to be evaluated,
- in the format [(min1, max1), (min2, max2), ...]. Default: None.
-
- Returns:
- tuple[np.ndarray]: (tp, fp) whose elements are 0 and 1. The shape of
- each array is (num_scales, m).
- """
- # an indicator of ignored gts
- gt_ignore_inds = np.concatenate(
- (np.zeros(gt_bboxes.shape[0], dtype=np.bool),
- np.ones(gt_bboxes_ignore.shape[0], dtype=np.bool)))
- # stack gt_bboxes and gt_bboxes_ignore for convenience
- gt_bboxes = np.vstack((gt_bboxes, gt_bboxes_ignore))
-
- num_dets = det_bboxes.shape[0]
- num_gts = gt_bboxes.shape[0]
- if area_ranges is None:
- area_ranges = [(None, None)]
- num_scales = len(area_ranges)
- # tp and fp are of shape (num_scales, num_gts), each row is tp or fp
- # of a certain scale.
- tp = np.zeros((num_scales, num_dets), dtype=np.float32)
- fp = np.zeros((num_scales, num_dets), dtype=np.float32)
- if gt_bboxes.shape[0] == 0:
- if area_ranges == [(None, None)]:
- fp[...] = 1
- else:
- det_areas = (det_bboxes[:, 2] - det_bboxes[:, 0]) * (
- det_bboxes[:, 3] - det_bboxes[:, 1])
- for i, (min_area, max_area) in enumerate(area_ranges):
- fp[i, (det_areas >= min_area) & (det_areas < max_area)] = 1
- return tp, fp
- ious = bbox_overlaps(det_bboxes, gt_bboxes - 1)
- gt_w = gt_bboxes[:, 2] - gt_bboxes[:, 0]
- gt_h = gt_bboxes[:, 3] - gt_bboxes[:, 1]
- iou_thrs = np.minimum((gt_w * gt_h) / ((gt_w + 10.0) * (gt_h + 10.0)),
- default_iou_thr)
- # sort all detections by scores in descending order
- sort_inds = np.argsort(-det_bboxes[:, -1])
- for k, (min_area, max_area) in enumerate(area_ranges):
- gt_covered = np.zeros(num_gts, dtype=bool)
- # if no area range is specified, gt_area_ignore is all False
- if min_area is None:
- gt_area_ignore = np.zeros_like(gt_ignore_inds, dtype=bool)
- else:
- gt_areas = gt_w * gt_h
- gt_area_ignore = (gt_areas < min_area) | (gt_areas >= max_area)
- for i in sort_inds:
- max_iou = -1
- matched_gt = -1
- # find best overlapped available gt
- for j in range(num_gts):
- # different from PASCAL VOC: allow finding other gts if the
- # best overlapped ones are already matched by other det bboxes
- if gt_covered[j]:
- continue
- elif ious[i, j] >= iou_thrs[j] and ious[i, j] > max_iou:
- max_iou = ious[i, j]
- matched_gt = j
- # there are 4 cases for a det bbox:
- # 1. it matches a gt, tp = 1, fp = 0
- # 2. it matches an ignored gt, tp = 0, fp = 0
- # 3. it matches no gt and within area range, tp = 0, fp = 1
- # 4. it matches no gt but is beyond area range, tp = 0, fp = 0
- if matched_gt >= 0:
- gt_covered[matched_gt] = 1
- if not (gt_ignore_inds[matched_gt]
- or gt_area_ignore[matched_gt]):
- tp[k, i] = 1
- elif min_area is None:
- fp[k, i] = 1
- else:
- bbox = det_bboxes[i, :4]
- area = (bbox[2] - bbox[0]) * (bbox[3] - bbox[1])
- if area >= min_area and area < max_area:
- fp[k, i] = 1
- return tp, fp
-
-
-def tpfp_default(det_bboxes,
- gt_bboxes,
- gt_bboxes_ignore=None,
- iou_thr=0.5,
- area_ranges=None):
- """Check if detected bboxes are true positive or false positive.
-
- Args:
- det_bbox (ndarray): Detected bboxes of this image, of shape (m, 5).
- gt_bboxes (ndarray): GT bboxes of this image, of shape (n, 4).
- gt_bboxes_ignore (ndarray): Ignored gt bboxes of this image,
- of shape (k, 4). Default: None
- iou_thr (float): IoU threshold to be considered as matched.
- Default: 0.5.
- area_ranges (list[tuple] | None): Range of bbox areas to be evaluated,
- in the format [(min1, max1), (min2, max2), ...]. Default: None.
-
- Returns:
- tuple[np.ndarray]: (tp, fp) whose elements are 0 and 1. The shape of
- each array is (num_scales, m).
- """
- # an indicator of ignored gts
- gt_ignore_inds = np.concatenate(
- (np.zeros(gt_bboxes.shape[0], dtype=np.bool),
- np.ones(gt_bboxes_ignore.shape[0], dtype=np.bool)))
- # stack gt_bboxes and gt_bboxes_ignore for convenience
- gt_bboxes = np.vstack((gt_bboxes, gt_bboxes_ignore))
-
- num_dets = det_bboxes.shape[0]
- num_gts = gt_bboxes.shape[0]
- if area_ranges is None:
- area_ranges = [(None, None)]
- num_scales = len(area_ranges)
- # tp and fp are of shape (num_scales, num_gts), each row is tp or fp of
- # a certain scale
- tp = np.zeros((num_scales, num_dets), dtype=np.float32)
- fp = np.zeros((num_scales, num_dets), dtype=np.float32)
-
- # if there is no gt bboxes in this image, then all det bboxes
- # within area range are false positives
- if gt_bboxes.shape[0] == 0:
- if area_ranges == [(None, None)]:
- fp[...] = 1
- else:
- det_areas = (det_bboxes[:, 2] - det_bboxes[:, 0]) * (
- det_bboxes[:, 3] - det_bboxes[:, 1])
- for i, (min_area, max_area) in enumerate(area_ranges):
- fp[i, (det_areas >= min_area) & (det_areas < max_area)] = 1
- return tp, fp
-
- ious = bbox_overlaps(det_bboxes, gt_bboxes)
- # for each det, the max iou with all gts
- ious_max = ious.max(axis=1)
- # for each det, which gt overlaps most with it
- ious_argmax = ious.argmax(axis=1)
- # sort all dets in descending order by scores
- sort_inds = np.argsort(-det_bboxes[:, -1])
- for k, (min_area, max_area) in enumerate(area_ranges):
- gt_covered = np.zeros(num_gts, dtype=bool)
- # if no area range is specified, gt_area_ignore is all False
- if min_area is None:
- gt_area_ignore = np.zeros_like(gt_ignore_inds, dtype=bool)
- else:
- gt_areas = (gt_bboxes[:, 2] - gt_bboxes[:, 0]) * (
- gt_bboxes[:, 3] - gt_bboxes[:, 1])
- gt_area_ignore = (gt_areas < min_area) | (gt_areas >= max_area)
- for i in sort_inds:
- if ious_max[i] >= iou_thr:
- matched_gt = ious_argmax[i]
- if not (gt_ignore_inds[matched_gt]
- or gt_area_ignore[matched_gt]):
- if not gt_covered[matched_gt]:
- gt_covered[matched_gt] = True
- tp[k, i] = 1
- else:
- fp[k, i] = 1
- # otherwise ignore this detected bbox, tp = 0, fp = 0
- elif min_area is None:
- fp[k, i] = 1
- else:
- bbox = det_bboxes[i, :4]
- area = (bbox[2] - bbox[0]) * (bbox[3] - bbox[1])
- if area >= min_area and area < max_area:
- fp[k, i] = 1
- return tp, fp
-
-
-def get_cls_results(det_results, annotations, class_id):
- """Get det results and gt information of a certain class.
-
- Args:
- det_results (list[list]): Same as `eval_map()`.
- annotations (list[dict]): Same as `eval_map()`.
- class_id (int): ID of a specific class.
-
- Returns:
- tuple[list[np.ndarray]]: detected bboxes, gt bboxes, ignored gt bboxes
- """
- cls_dets = [img_res[class_id] for img_res in det_results]
- cls_gts = []
- cls_gts_ignore = []
- for ann in annotations:
- gt_inds = ann['labels'] == class_id
- cls_gts.append(ann['bboxes'][gt_inds, :])
-
- if ann.get('labels_ignore', None) is not None:
- ignore_inds = ann['labels_ignore'] == class_id
- cls_gts_ignore.append(ann['bboxes_ignore'][ignore_inds, :])
- else:
- cls_gts_ignore.append(np.empty((0, 4), dtype=np.float32))
-
- return cls_dets, cls_gts, cls_gts_ignore
-
-
-def eval_map(det_results,
- annotations,
- scale_ranges=None,
- iou_thr=0.5,
- dataset=None,
- logger=None,
- tpfp_fn=None,
- nproc=4):
- """Evaluate mAP of a dataset.
-
- Args:
- det_results (list[list]): [[cls1_det, cls2_det, ...], ...].
- The outer list indicates images, and the inner list indicates
- per-class detected bboxes.
- annotations (list[dict]): Ground truth annotations where each item of
- the list indicates an image. Keys of annotations are:
-
- - `bboxes`: numpy array of shape (n, 4)
- - `labels`: numpy array of shape (n, )
- - `bboxes_ignore` (optional): numpy array of shape (k, 4)
- - `labels_ignore` (optional): numpy array of shape (k, )
- scale_ranges (list[tuple] | None): Range of scales to be evaluated,
- in the format [(min1, max1), (min2, max2), ...]. A range of
- (32, 64) means the area range between (32**2, 64**2).
- Default: None.
- iou_thr (float): IoU threshold to be considered as matched.
- Default: 0.5.
- dataset (list[str] | str | None): Dataset name or dataset classes,
- there are minor differences in metrics for different datsets, e.g.
- "voc07", "imagenet_det", etc. Default: None.
- logger (logging.Logger | str | None): The way to print the mAP
- summary. See `mmcv.utils.print_log()` for details. Default: None.
- tpfp_fn (callable | None): The function used to determine true/
- false positives. If None, :func:`tpfp_default` is used as default
- unless dataset is 'det' or 'vid' (:func:`tpfp_imagenet` in this
- case). If it is given as a function, then this function is used
- to evaluate tp & fp. Default None.
- nproc (int): Processes used for computing TP and FP.
- Default: 4.
-
- Returns:
- tuple: (mAP, [dict, dict, ...])
- """
- assert len(det_results) == len(annotations)
-
- num_imgs = len(det_results)
- num_scales = len(scale_ranges) if scale_ranges is not None else 1
- num_classes = len(det_results[0]) # positive class num
- area_ranges = ([(rg[0]**2, rg[1]**2) for rg in scale_ranges]
- if scale_ranges is not None else None)
-
- pool = Pool(nproc)
- eval_results = []
- for i in range(num_classes):
- # get gt and det bboxes of this class
- cls_dets, cls_gts, cls_gts_ignore = get_cls_results(
- det_results, annotations, i)
- # choose proper function according to datasets to compute tp and fp
- if tpfp_fn is None:
- if dataset in ['det', 'vid']:
- tpfp_fn = tpfp_imagenet
- else:
- tpfp_fn = tpfp_default
- if not callable(tpfp_fn):
- raise ValueError(
- f'tpfp_fn has to be a function or None, but got {tpfp_fn}')
-
- # compute tp and fp for each image with multiple processes
- tpfp = pool.starmap(
- tpfp_fn,
- zip(cls_dets, cls_gts, cls_gts_ignore,
- [iou_thr for _ in range(num_imgs)],
- [area_ranges for _ in range(num_imgs)]))
- tp, fp = tuple(zip(*tpfp))
- # calculate gt number of each scale
- # ignored gts or gts beyond the specific scale are not counted
- num_gts = np.zeros(num_scales, dtype=int)
- for j, bbox in enumerate(cls_gts):
- if area_ranges is None:
- num_gts[0] += bbox.shape[0]
- else:
- gt_areas = (bbox[:, 2] - bbox[:, 0]) * (
- bbox[:, 3] - bbox[:, 1])
- for k, (min_area, max_area) in enumerate(area_ranges):
- num_gts[k] += np.sum((gt_areas >= min_area)
- & (gt_areas < max_area))
- # sort all det bboxes by score, also sort tp and fp
- cls_dets = np.vstack(cls_dets)
- num_dets = cls_dets.shape[0]
- sort_inds = np.argsort(-cls_dets[:, -1])
- tp = np.hstack(tp)[:, sort_inds]
- fp = np.hstack(fp)[:, sort_inds]
- # calculate recall and precision with tp and fp
- tp = np.cumsum(tp, axis=1)
- fp = np.cumsum(fp, axis=1)
- eps = np.finfo(np.float32).eps
- recalls = tp / np.maximum(num_gts[:, np.newaxis], eps)
- precisions = tp / np.maximum((tp + fp), eps)
- # calculate AP
- if scale_ranges is None:
- recalls = recalls[0, :]
- precisions = precisions[0, :]
- num_gts = num_gts.item()
- mode = 'area' if dataset != 'voc07' else '11points'
- ap = average_precision(recalls, precisions, mode)
- eval_results.append({
- 'num_gts': num_gts,
- 'num_dets': num_dets,
- 'recall': recalls,
- 'precision': precisions,
- 'ap': ap
- })
- pool.close()
- if scale_ranges is not None:
- # shape (num_classes, num_scales)
- all_ap = np.vstack([cls_result['ap'] for cls_result in eval_results])
- all_num_gts = np.vstack(
- [cls_result['num_gts'] for cls_result in eval_results])
- mean_ap = []
- for i in range(num_scales):
- if np.any(all_num_gts[:, i] > 0):
- mean_ap.append(all_ap[all_num_gts[:, i] > 0, i].mean())
- else:
- mean_ap.append(0.0)
- else:
- aps = []
- for cls_result in eval_results:
- if cls_result['num_gts'] > 0:
- aps.append(cls_result['ap'])
- mean_ap = np.array(aps).mean().item() if aps else 0.0
-
- print_map_summary(
- mean_ap, eval_results, dataset, area_ranges, logger=logger)
-
- return mean_ap, eval_results
-
-
-def print_map_summary(mean_ap,
- results,
- dataset=None,
- scale_ranges=None,
- logger=None):
- """Print mAP and results of each class.
-
- A table will be printed to show the gts/dets/recall/AP of each class and
- the mAP.
-
- Args:
- mean_ap (float): Calculated from `eval_map()`.
- results (list[dict]): Calculated from `eval_map()`.
- dataset (list[str] | str | None): Dataset name or dataset classes.
- scale_ranges (list[tuple] | None): Range of scales to be evaluated.
- logger (logging.Logger | str | None): The way to print the mAP
- summary. See `mmcv.utils.print_log()` for details. Default: None.
- """
-
- if logger == 'silent':
- return
-
- if isinstance(results[0]['ap'], np.ndarray):
- num_scales = len(results[0]['ap'])
- else:
- num_scales = 1
-
- if scale_ranges is not None:
- assert len(scale_ranges) == num_scales
-
- num_classes = len(results)
-
- recalls = np.zeros((num_scales, num_classes), dtype=np.float32)
- aps = np.zeros((num_scales, num_classes), dtype=np.float32)
- num_gts = np.zeros((num_scales, num_classes), dtype=int)
- for i, cls_result in enumerate(results):
- if cls_result['recall'].size > 0:
- recalls[:, i] = np.array(cls_result['recall'], ndmin=2)[:, -1]
- aps[:, i] = cls_result['ap']
- num_gts[:, i] = cls_result['num_gts']
-
- if dataset is None:
- label_names = [str(i) for i in range(num_classes)]
- elif mmcv.is_str(dataset):
- label_names = get_classes(dataset)
- else:
- label_names = dataset
-
- if not isinstance(mean_ap, list):
- mean_ap = [mean_ap]
-
- header = ['class', 'gts', 'dets', 'recall', 'ap']
- for i in range(num_scales):
- if scale_ranges is not None:
- print_log(f'Scale range {scale_ranges[i]}', logger=logger)
- table_data = [header]
- for j in range(num_classes):
- row_data = [
- label_names[j], num_gts[i, j], results[j]['num_dets'],
- f'{recalls[i, j]:.3f}', f'{aps[i, j]:.3f}'
- ]
- table_data.append(row_data)
- table_data.append(['mAP', '', '', '', f'{mean_ap[i]:.3f}'])
- table = AsciiTable(table_data)
- table.inner_footing_row_border = True
- print_log('\n' + table.table, logger=logger)
diff --git a/spaces/abhishek/sketch-to-image/annotator/uniformer_base/mmseg/ops/wrappers.py b/spaces/abhishek/sketch-to-image/annotator/uniformer_base/mmseg/ops/wrappers.py
deleted file mode 100644
index 8abf3d4de22a164783206f8f2473f1e1a602b72c..0000000000000000000000000000000000000000
--- a/spaces/abhishek/sketch-to-image/annotator/uniformer_base/mmseg/ops/wrappers.py
+++ /dev/null
@@ -1,62 +0,0 @@
-'''
- * Copyright (c) 2023 Salesforce, Inc.
- * All rights reserved.
- * SPDX-License-Identifier: Apache License 2.0
- * For full license text, see LICENSE.txt file in the repo root or http://www.apache.org/licenses/
- * By Can Qin
- * Modified from ControlNet repo: https://github.com/lllyasviel/ControlNet
- * Copyright (c) 2023 Lvmin Zhang and Maneesh Agrawala
- * Modified from MMCV repo: From https://github.com/open-mmlab/mmcv
- * Copyright (c) OpenMMLab. All rights reserved.
-'''
-
-import warnings
-
-import torch.nn as nn
-import torch.nn.functional as F
-
-
-def resize(input,
- size=None,
- scale_factor=None,
- mode='nearest',
- align_corners=None,
- warning=True):
- if warning:
- if size is not None and align_corners:
- input_h, input_w = tuple(int(x) for x in input.shape[2:])
- output_h, output_w = tuple(int(x) for x in size)
- if output_h > input_h or output_w > output_h:
- if ((output_h > 1 and output_w > 1 and input_h > 1
- and input_w > 1) and (output_h - 1) % (input_h - 1)
- and (output_w - 1) % (input_w - 1)):
- warnings.warn(
- f'When align_corners={align_corners}, '
- 'the output would more aligned if '
- f'input size {(input_h, input_w)} is `x+1` and '
- f'out size {(output_h, output_w)} is `nx+1`')
- return F.interpolate(input, size, scale_factor, mode, align_corners)
-
-
-class Upsample(nn.Module):
-
- def __init__(self,
- size=None,
- scale_factor=None,
- mode='nearest',
- align_corners=None):
- super(Upsample, self).__init__()
- self.size = size
- if isinstance(scale_factor, tuple):
- self.scale_factor = tuple(float(factor) for factor in scale_factor)
- else:
- self.scale_factor = float(scale_factor) if scale_factor else None
- self.mode = mode
- self.align_corners = align_corners
-
- def forward(self, x):
- if not self.size:
- size = [int(t * self.scale_factor) for t in x.shape[-2:]]
- else:
- size = self.size
- return resize(x, size, None, self.mode, self.align_corners)
diff --git a/spaces/adirik/stylemc-demo/encoder4editing/datasets/images_dataset.py b/spaces/adirik/stylemc-demo/encoder4editing/datasets/images_dataset.py
deleted file mode 100644
index 00c54c7db944569a749af4c6f0c4d99fcc37f9cc..0000000000000000000000000000000000000000
--- a/spaces/adirik/stylemc-demo/encoder4editing/datasets/images_dataset.py
+++ /dev/null
@@ -1,33 +0,0 @@
-from torch.utils.data import Dataset
-from PIL import Image
-from utils import data_utils
-
-
-class ImagesDataset(Dataset):
-
- def __init__(self, source_root, target_root, opts, target_transform=None, source_transform=None):
- self.source_paths = sorted(data_utils.make_dataset(source_root))
- self.target_paths = sorted(data_utils.make_dataset(target_root))
- self.source_transform = source_transform
- self.target_transform = target_transform
- self.opts = opts
-
- def __len__(self):
- return len(self.source_paths)
-
- def __getitem__(self, index):
- from_path = self.source_paths[index]
- from_im = Image.open(from_path)
- from_im = from_im.convert('RGB')
-
- to_path = self.target_paths[index]
- to_im = Image.open(to_path).convert('RGB')
- if self.target_transform:
- to_im = self.target_transform(to_im)
-
- if self.source_transform:
- from_im = self.source_transform(from_im)
- else:
- from_im = to_im
-
- return from_im, to_im
diff --git a/spaces/ai-create/colab/style.css b/spaces/ai-create/colab/style.css
deleted file mode 100644
index 114adf441e9032febb46bc056b2a8bb651075f0d..0000000000000000000000000000000000000000
--- a/spaces/ai-create/colab/style.css
+++ /dev/null
@@ -1,28 +0,0 @@
-body {
- padding: 2rem;
- font-family: -apple-system, BlinkMacSystemFont, "Arial", sans-serif;
-}
-
-h1 {
- font-size: 16px;
- margin-top: 0;
-}
-
-p {
- color: rgb(107, 114, 128);
- font-size: 15px;
- margin-bottom: 10px;
- margin-top: 5px;
-}
-
-.card {
- max-width: 620px;
- margin: 0 auto;
- padding: 16px;
- border: 1px solid lightgray;
- border-radius: 16px;
-}
-
-.card p:last-child {
- margin-bottom: 0;
-}
diff --git a/spaces/ai-guru/composer/source/ui/static/style.css b/spaces/ai-guru/composer/source/ui/static/style.css
deleted file mode 100644
index 4aefe85b80176e410faf40ff4952f4a36ace9d15..0000000000000000000000000000000000000000
--- a/spaces/ai-guru/composer/source/ui/static/style.css
+++ /dev/null
@@ -1,34 +0,0 @@
-html {
- height: 100%;
-}
-
-body {
- padding: 1rem;
- font-family: 'Lato', sans-serif;
- background-color: hsl(0 0% 1%);
- background: linear-gradient(hsl(0 0% 1%) 50%, hsl(0 0% 8%) 100%);
- background-attachment: fixed;
-}
-
-h1 {
- font-family: 'Italiana', serif;
- letter-spacing: 0.05ch;
-}
-
-
-body, h1 {
- color: hsl(0 0% 97%);
-}
-
-a, a:visited {
- color: white;
- text-decoration: none;
- font-weight: 700;
-}
-
-
-@media (min-width: 600px) {
- body {
- padding: 2rem;
- }
-}
diff --git a/spaces/akhaliq/GPEN/face_detect/utils/nms/py_cpu_nms.py b/spaces/akhaliq/GPEN/face_detect/utils/nms/py_cpu_nms.py
deleted file mode 100644
index 54e7b25fef72b518df6dcf8d6fb78b986796c6e3..0000000000000000000000000000000000000000
--- a/spaces/akhaliq/GPEN/face_detect/utils/nms/py_cpu_nms.py
+++ /dev/null
@@ -1,38 +0,0 @@
-# --------------------------------------------------------
-# Fast R-CNN
-# Copyright (c) 2015 Microsoft
-# Licensed under The MIT License [see LICENSE for details]
-# Written by Ross Girshick
-# --------------------------------------------------------
-
-import numpy as np
-
-def py_cpu_nms(dets, thresh):
- """Pure Python NMS baseline."""
- x1 = dets[:, 0]
- y1 = dets[:, 1]
- x2 = dets[:, 2]
- y2 = dets[:, 3]
- scores = dets[:, 4]
-
- areas = (x2 - x1 + 1) * (y2 - y1 + 1)
- order = scores.argsort()[::-1]
-
- keep = []
- while order.size > 0:
- i = order[0]
- keep.append(i)
- xx1 = np.maximum(x1[i], x1[order[1:]])
- yy1 = np.maximum(y1[i], y1[order[1:]])
- xx2 = np.minimum(x2[i], x2[order[1:]])
- yy2 = np.minimum(y2[i], y2[order[1:]])
-
- w = np.maximum(0.0, xx2 - xx1 + 1)
- h = np.maximum(0.0, yy2 - yy1 + 1)
- inter = w * h
- ovr = inter / (areas[i] + areas[order[1:]] - inter)
-
- inds = np.where(ovr <= thresh)[0]
- order = order[inds + 1]
-
- return keep
diff --git a/spaces/akhaliq/Real-ESRGAN/setup.py b/spaces/akhaliq/Real-ESRGAN/setup.py
deleted file mode 100644
index c2b92e31d2db1aba50767f4f844540cfd53c609d..0000000000000000000000000000000000000000
--- a/spaces/akhaliq/Real-ESRGAN/setup.py
+++ /dev/null
@@ -1,107 +0,0 @@
-#!/usr/bin/env python
-
-from setuptools import find_packages, setup
-
-import os
-import subprocess
-import time
-
-version_file = 'realesrgan/version.py'
-
-
-def readme():
- with open('README.md', encoding='utf-8') as f:
- content = f.read()
- return content
-
-
-def get_git_hash():
-
- def _minimal_ext_cmd(cmd):
- # construct minimal environment
- env = {}
- for k in ['SYSTEMROOT', 'PATH', 'HOME']:
- v = os.environ.get(k)
- if v is not None:
- env[k] = v
- # LANGUAGE is used on win32
- env['LANGUAGE'] = 'C'
- env['LANG'] = 'C'
- env['LC_ALL'] = 'C'
- out = subprocess.Popen(cmd, stdout=subprocess.PIPE, env=env).communicate()[0]
- return out
-
- try:
- out = _minimal_ext_cmd(['git', 'rev-parse', 'HEAD'])
- sha = out.strip().decode('ascii')
- except OSError:
- sha = 'unknown'
-
- return sha
-
-
-def get_hash():
- if os.path.exists('.git'):
- sha = get_git_hash()[:7]
- else:
- sha = 'unknown'
-
- return sha
-
-
-def write_version_py():
- content = """# GENERATED VERSION FILE
-# TIME: {}
-__version__ = '{}'
-__gitsha__ = '{}'
-version_info = ({})
-"""
- sha = get_hash()
- with open('VERSION', 'r') as f:
- SHORT_VERSION = f.read().strip()
- VERSION_INFO = ', '.join([x if x.isdigit() else f'"{x}"' for x in SHORT_VERSION.split('.')])
-
- version_file_str = content.format(time.asctime(), SHORT_VERSION, sha, VERSION_INFO)
- with open(version_file, 'w') as f:
- f.write(version_file_str)
-
-
-def get_version():
- with open(version_file, 'r') as f:
- exec(compile(f.read(), version_file, 'exec'))
- return locals()['__version__']
-
-
-def get_requirements(filename='requirements.txt'):
- here = os.path.dirname(os.path.realpath(__file__))
- with open(os.path.join(here, filename), 'r') as f:
- requires = [line.replace('\n', '') for line in f.readlines()]
- return requires
-
-
-if __name__ == '__main__':
- write_version_py()
- setup(
- name='realesrgan',
- version=get_version(),
- description='Real-ESRGAN aims at developing Practical Algorithms for General Image Restoration',
- long_description=readme(),
- long_description_content_type='text/markdown',
- author='Xintao Wang',
- author_email='xintao.wang@outlook.com',
- keywords='computer vision, pytorch, image restoration, super-resolution, esrgan, real-esrgan',
- url='https://github.com/xinntao/Real-ESRGAN',
- include_package_data=True,
- packages=find_packages(exclude=('options', 'datasets', 'experiments', 'results', 'tb_logger', 'wandb')),
- classifiers=[
- 'Development Status :: 4 - Beta',
- 'License :: OSI Approved :: Apache Software License',
- 'Operating System :: OS Independent',
- 'Programming Language :: Python :: 3',
- 'Programming Language :: Python :: 3.7',
- 'Programming Language :: Python :: 3.8',
- ],
- license='BSD-3-Clause License',
- setup_requires=['cython', 'numpy'],
- install_requires=get_requirements(),
- zip_safe=False)
diff --git a/spaces/akhaliq/deeplab2/data/preprocessing/input_preprocessing.py b/spaces/akhaliq/deeplab2/data/preprocessing/input_preprocessing.py
deleted file mode 100644
index e44b68b4aee7c0a87c27e9dc4e0db7d84ad1d731..0000000000000000000000000000000000000000
--- a/spaces/akhaliq/deeplab2/data/preprocessing/input_preprocessing.py
+++ /dev/null
@@ -1,307 +0,0 @@
-# coding=utf-8
-# Copyright 2021 The Deeplab2 Authors.
-#
-# Licensed under the Apache License, Version 2.0 (the "License");
-# you may not use this file except in compliance with the License.
-# You may obtain a copy of the License at
-#
-# http://www.apache.org/licenses/LICENSE-2.0
-#
-# Unless required by applicable law or agreed to in writing, software
-# distributed under the License is distributed on an "AS IS" BASIS,
-# WITHOUT WARRANTIES OR CONDITIONS OF ANY KIND, either express or implied.
-# See the License for the specific language governing permissions and
-# limitations under the License.
-
-"""This file contains functions to preprocess images and labels."""
-
-import tensorflow as tf
-
-from deeplab2.data.preprocessing import autoaugment_utils
-from deeplab2.data.preprocessing import preprocess_utils
-
-# The probability of flipping the images and labels
-# left-right during training
-_PROB_OF_FLIP = 0.5
-
-_MEAN_PIXEL = [127.5, 127.5, 127.5]
-
-
-def _pad_image_and_label(image, label, offset_height, offset_width,
- target_height, target_width, ignore_label=None):
- """Pads the image and the label to the given size.
-
- Args:
- image: A tf.Tensor of shape [height, width, channels].
- label: A tf.Tensor of shape [height, width, 1] or None.
- offset_height: The number of rows of zeros to add on top of the image and
- label.
- offset_width: The number of columns of zeros to add on the left of the image
- and label.
- target_height: The total height after padding.
- target_width: The total width after padding.
- ignore_label: The ignore_label for the label. Must only be set when label is
- given.
-
- Returns:
- The padded image and label as a tuple (padded_image, padded_label).
-
- Raises:
- tf.errors.InvalidArgumentError: An error occurs if the padding configuration
- is invalid.
- ValueError: An error occurs if label is given without an ignore_label.
- """
- height = tf.shape(image)[0]
- width = tf.shape(image)[1]
- original_dtype = image.dtype
- if original_dtype not in (tf.float32, tf.float64):
- image = tf.cast(image, tf.float32)
-
- bottom_padding = target_height - offset_height - height
- right_padding = target_width - offset_width - width
-
- assert_bottom_padding = tf.assert_greater(
- bottom_padding, -1,
- 'The padding configuration is not valid. Please either increase the '
- 'target size or reduce the padding offset.')
- assert_right_padding = tf.assert_greater(
- right_padding, -1, 'The padding configuration is not valid. Please either'
- ' increase the target size or reduce the padding offset.')
- with tf.control_dependencies([assert_bottom_padding, assert_right_padding]):
- paddings = [[offset_height, bottom_padding], [offset_width, right_padding],
- [0, 0]]
-
- image = image - _MEAN_PIXEL
- image = tf.pad(image, paddings)
- image = image + _MEAN_PIXEL
- image = tf.cast(image, original_dtype)
-
- if label is not None:
- if ignore_label is None:
- raise ValueError(
- 'If a label is given, the ignore label must be set too.')
- label = tf.pad(label, paddings, constant_values=ignore_label)
-
- return image, label
-
-
-def _update_max_resize_value(max_resize_value, crop_size, is_inference=False):
- """Checks and may update max_resize_value.
-
- Args:
- max_resize_value: A 2-tuple of (height, width), maximum allowed value
- after resize. If a single element is given, then height and width
- share the same value. None, empty or having 0 indicates no maximum value
- will be used.
- crop_size: A 2-tuple of (height, width), crop size used.
- is_inference: Boolean, whether the model is performing inference or not.
-
- Returns:
- Updated max_resize_value.
- """
- max_resize_value = preprocess_utils.process_resize_value(max_resize_value)
- if max_resize_value is None and is_inference:
- # During inference, default max_resize_value to crop size to allow
- # model taking input images with larger sizes.
- max_resize_value = crop_size
-
- if max_resize_value is None:
- return None
-
- if max_resize_value[0] > crop_size[0] or max_resize_value[1] > crop_size[1]:
- raise ValueError(
- 'Maximum resize value provided (%s) exceeds model crop size (%s)' %
- (max_resize_value, crop_size))
- return max_resize_value
-
-
-def preprocess_image_and_label(image,
- label,
- crop_height,
- crop_width,
- prev_image=None,
- prev_label=None,
- min_resize_value=None,
- max_resize_value=None,
- resize_factor=None,
- min_scale_factor=1.,
- max_scale_factor=1.,
- scale_factor_step_size=0,
- ignore_label=None,
- is_training=True,
- autoaugment_policy_name=None):
- """Preprocesses the image and label.
-
- Args:
- image: A tf.Tensor containing the image with shape [height, width, 3].
- label: A tf.Tensor containing the label with shape [height, width, 1] or
- None.
- crop_height: The height value used to crop the image and label.
- crop_width: The width value used to crop the image and label.
- prev_image: An optional tensor of shape [image_height, image_width, 3].
- prev_label: An optional tensor of shape [label_height, label_width, 1].
- min_resize_value: A 2-tuple of (height, width), desired minimum value
- after resize. If a single element is given, then height and width share
- the same value. None, empty or having 0 indicates no minimum value will
- be used.
- max_resize_value: A 2-tuple of (height, width), maximum allowed value
- after resize. If a single element is given, then height and width
- share the same value. None, empty or having 0 indicates no maximum value
- will be used.
- resize_factor: Resized dimensions are multiple of factor plus one.
- min_scale_factor: Minimum scale factor for random scale augmentation.
- max_scale_factor: Maximum scale factor for random scale augmentation.
- scale_factor_step_size: The step size from min scale factor to max scale
- factor. The input is randomly scaled based on the value of
- (min_scale_factor, max_scale_factor, scale_factor_step_size).
- ignore_label: The label value which will be ignored for training and
- evaluation.
- is_training: If the preprocessing is used for training or not.
- autoaugment_policy_name: String, autoaugment policy name. See
- autoaugment_policy.py for available policies.
-
- Returns:
- resized_image: The resized input image without other augmentations as a
- tf.Tensor.
- processed_image: The preprocessed image as a tf.Tensor.
- label: The preprocessed groundtruth segmentation label as a tf.Tensor.
-
- Raises:
- ValueError: Ground truth label not provided during training.
- """
- if is_training and label is None:
- raise ValueError('During training, label must be provided.')
-
- image.get_shape().assert_is_compatible_with(tf.TensorShape([None, None, 3]))
-
- # Keep reference to original image.
- resized_image = image
- if prev_image is not None:
- image = tf.concat([image, prev_image], axis=2)
- processed_image = tf.cast(image, tf.float32)
- processed_prev_image = None
-
- if label is not None:
- label.get_shape().assert_is_compatible_with(tf.TensorShape([None, None, 1]))
- if prev_label is not None:
- label = tf.concat([label, prev_label], axis=2)
- label = tf.cast(label, tf.int32)
-
- # Resize image and label to the desired range.
- if any([min_resize_value, max_resize_value, not is_training]):
- max_resize_value = _update_max_resize_value(
- max_resize_value,
- crop_size=(crop_height, crop_width),
- is_inference=not is_training)
-
- processed_image, label = (
- preprocess_utils.resize_to_range(
- image=processed_image,
- label=label,
- min_size=min_resize_value,
- max_size=max_resize_value,
- factor=resize_factor,
- align_corners=True))
- if prev_image is None:
- resized_image = tf.identity(processed_image)
- else:
- resized_image, _ = tf.split(processed_image, 2, axis=2)
-
- if prev_image is not None:
- processed_image, processed_prev_image = tf.split(processed_image, 2, axis=2)
-
- if prev_label is not None:
- label, prev_label = tf.split(label, 2, axis=2)
-
- if not is_training:
- image_height = tf.shape(processed_image)[0]
- image_width = tf.shape(processed_image)[1]
-
- offset_height = 0
- offset_width = 0
- processed_image, label = _pad_image_and_label(processed_image, label,
- offset_height, offset_width,
- crop_height, crop_width,
- ignore_label)
- processed_image.set_shape([crop_height, crop_width, 3])
- if label is not None:
- label.set_shape([crop_height, crop_width, 1])
- if prev_image is not None:
- processed_prev_image, prev_label = _pad_image_and_label(
- processed_prev_image, prev_label, offset_height, offset_width,
- crop_height, crop_width, ignore_label)
- processed_prev_image.set_shape([crop_height, crop_width, 3])
- if prev_label is not None:
- prev_label.set_shape([crop_height, crop_width, 1])
- return (resized_image, processed_image, label, processed_prev_image,
- prev_label)
-
- # Data augmentation by randomly scaling the inputs.
- scale = preprocess_utils.get_random_scale(
- min_scale_factor, max_scale_factor, scale_factor_step_size)
- processed_image, label = preprocess_utils.randomly_scale_image_and_label(
- processed_image, label, scale)
- if processed_prev_image is not None:
- (processed_prev_image,
- prev_label) = preprocess_utils.randomly_scale_image_and_label(
- processed_prev_image, prev_label, scale)
-
- # Apply autoaugment if any.
- if autoaugment_policy_name:
- processed_image, label = _autoaugment_helper(
- processed_image, label, ignore_label, autoaugment_policy_name)
- if processed_prev_image is not None:
- processed_prev_image, prev_label = _autoaugment_helper(
- processed_prev_image, prev_label, ignore_label,
- autoaugment_policy_name)
-
- # Pad image and label to have dimensions >= [crop_height, crop_width].
- image_height = tf.shape(processed_image)[0]
- image_width = tf.shape(processed_image)[1]
- target_height = image_height + tf.maximum(crop_height - image_height, 0)
- target_width = image_width + tf.maximum(crop_width - image_width, 0)
-
- # Randomly crop the image and label.
- def _uniform_offset(margin):
- return tf.random.uniform(
- [], minval=0, maxval=tf.maximum(margin, 1), dtype=tf.int32)
-
- offset_height = _uniform_offset(crop_height - image_height)
- offset_width = _uniform_offset(crop_width - image_width)
- processed_image, label = _pad_image_and_label(processed_image, label,
- offset_height, offset_width,
- target_height, target_width,
- ignore_label)
- if processed_prev_image is not None:
- processed_prev_image, prev_label = _pad_image_and_label(
- processed_prev_image, prev_label, offset_height, offset_width,
- target_height, target_width, ignore_label)
-
- if processed_prev_image is not None:
- (processed_image, label, processed_prev_image,
- prev_label) = preprocess_utils.random_crop(
- [processed_image, label, processed_prev_image, prev_label],
- crop_height, crop_width)
- # Randomly left-right flip the image and label.
- (processed_image, label, processed_prev_image, prev_label,
- _) = preprocess_utils.flip_dim(
- [processed_image, label, processed_prev_image, prev_label],
- _PROB_OF_FLIP,
- dim=1)
- else:
- processed_image, label = preprocess_utils.random_crop(
- [processed_image, label], crop_height, crop_width)
- # Randomly left-right flip the image and label.
- processed_image, label, _ = preprocess_utils.flip_dim(
- [processed_image, label], _PROB_OF_FLIP, dim=1)
-
- return resized_image, processed_image, label, processed_prev_image, prev_label
-
-
-def _autoaugment_helper(image, label, ignore_label, policy_name):
- image = tf.cast(image, tf.uint8)
- label = tf.cast(label, tf.int32)
- image, label = autoaugment_utils.distort_image_with_autoaugment(
- image, label, ignore_label, policy_name)
- image = tf.cast(image, tf.float32)
- return image, label
diff --git a/spaces/algomuffin/jojo_fork/op/upfirdn2d.py b/spaces/algomuffin/jojo_fork/op/upfirdn2d.py
deleted file mode 100644
index f1bbf96777f2c7267c1fef1733972014684ea22b..0000000000000000000000000000000000000000
--- a/spaces/algomuffin/jojo_fork/op/upfirdn2d.py
+++ /dev/null
@@ -1,187 +0,0 @@
-import os
-
-import torch
-from torch.autograd import Function
-from torch.utils.cpp_extension import load
-
-
-module_path = os.path.dirname(__file__)
-upfirdn2d_op = load(
- 'upfirdn2d',
- sources=[
- os.path.join(module_path, 'upfirdn2d.cpp'),
- os.path.join(module_path, 'upfirdn2d_kernel.cu'),
- ],
-)
-
-
-class UpFirDn2dBackward(Function):
- @staticmethod
- def forward(
- ctx, grad_output, kernel, grad_kernel, up, down, pad, g_pad, in_size, out_size
- ):
-
- up_x, up_y = up
- down_x, down_y = down
- g_pad_x0, g_pad_x1, g_pad_y0, g_pad_y1 = g_pad
-
- grad_output = grad_output.reshape(-1, out_size[0], out_size[1], 1)
-
- grad_input = upfirdn2d_op.upfirdn2d(
- grad_output,
- grad_kernel,
- down_x,
- down_y,
- up_x,
- up_y,
- g_pad_x0,
- g_pad_x1,
- g_pad_y0,
- g_pad_y1,
- )
- grad_input = grad_input.view(in_size[0], in_size[1], in_size[2], in_size[3])
-
- ctx.save_for_backward(kernel)
-
- pad_x0, pad_x1, pad_y0, pad_y1 = pad
-
- ctx.up_x = up_x
- ctx.up_y = up_y
- ctx.down_x = down_x
- ctx.down_y = down_y
- ctx.pad_x0 = pad_x0
- ctx.pad_x1 = pad_x1
- ctx.pad_y0 = pad_y0
- ctx.pad_y1 = pad_y1
- ctx.in_size = in_size
- ctx.out_size = out_size
-
- return grad_input
-
- @staticmethod
- def backward(ctx, gradgrad_input):
- kernel, = ctx.saved_tensors
-
- gradgrad_input = gradgrad_input.reshape(-1, ctx.in_size[2], ctx.in_size[3], 1)
-
- gradgrad_out = upfirdn2d_op.upfirdn2d(
- gradgrad_input,
- kernel,
- ctx.up_x,
- ctx.up_y,
- ctx.down_x,
- ctx.down_y,
- ctx.pad_x0,
- ctx.pad_x1,
- ctx.pad_y0,
- ctx.pad_y1,
- )
- # gradgrad_out = gradgrad_out.view(ctx.in_size[0], ctx.out_size[0], ctx.out_size[1], ctx.in_size[3])
- gradgrad_out = gradgrad_out.view(
- ctx.in_size[0], ctx.in_size[1], ctx.out_size[0], ctx.out_size[1]
- )
-
- return gradgrad_out, None, None, None, None, None, None, None, None
-
-
-class UpFirDn2d(Function):
- @staticmethod
- def forward(ctx, input, kernel, up, down, pad):
- up_x, up_y = up
- down_x, down_y = down
- pad_x0, pad_x1, pad_y0, pad_y1 = pad
-
- kernel_h, kernel_w = kernel.shape
- batch, channel, in_h, in_w = input.shape
- ctx.in_size = input.shape
-
- input = input.reshape(-1, in_h, in_w, 1)
-
- ctx.save_for_backward(kernel, torch.flip(kernel, [0, 1]))
-
- out_h = (in_h * up_y + pad_y0 + pad_y1 - kernel_h) // down_y + 1
- out_w = (in_w * up_x + pad_x0 + pad_x1 - kernel_w) // down_x + 1
- ctx.out_size = (out_h, out_w)
-
- ctx.up = (up_x, up_y)
- ctx.down = (down_x, down_y)
- ctx.pad = (pad_x0, pad_x1, pad_y0, pad_y1)
-
- g_pad_x0 = kernel_w - pad_x0 - 1
- g_pad_y0 = kernel_h - pad_y0 - 1
- g_pad_x1 = in_w * up_x - out_w * down_x + pad_x0 - up_x + 1
- g_pad_y1 = in_h * up_y - out_h * down_y + pad_y0 - up_y + 1
-
- ctx.g_pad = (g_pad_x0, g_pad_x1, g_pad_y0, g_pad_y1)
-
- out = upfirdn2d_op.upfirdn2d(
- input, kernel, up_x, up_y, down_x, down_y, pad_x0, pad_x1, pad_y0, pad_y1
- )
- # out = out.view(major, out_h, out_w, minor)
- out = out.view(-1, channel, out_h, out_w)
-
- return out
-
- @staticmethod
- def backward(ctx, grad_output):
- kernel, grad_kernel = ctx.saved_tensors
-
- grad_input = UpFirDn2dBackward.apply(
- grad_output,
- kernel,
- grad_kernel,
- ctx.up,
- ctx.down,
- ctx.pad,
- ctx.g_pad,
- ctx.in_size,
- ctx.out_size,
- )
-
- return grad_input, None, None, None, None
-
-
-def upfirdn2d(input, kernel, up=1, down=1, pad=(0, 0)):
- out = UpFirDn2d.apply(
- input, kernel, (up, up), (down, down), (pad[0], pad[1], pad[0], pad[1])
- )
-
- return out
-
-
-def upfirdn2d_native(
- input, kernel, up_x, up_y, down_x, down_y, pad_x0, pad_x1, pad_y0, pad_y1
-):
- _, in_h, in_w, minor = input.shape
- kernel_h, kernel_w = kernel.shape
-
- out = input.view(-1, in_h, 1, in_w, 1, minor)
- out = F.pad(out, [0, 0, 0, up_x - 1, 0, 0, 0, up_y - 1])
- out = out.view(-1, in_h * up_y, in_w * up_x, minor)
-
- out = F.pad(
- out, [0, 0, max(pad_x0, 0), max(pad_x1, 0), max(pad_y0, 0), max(pad_y1, 0)]
- )
- out = out[
- :,
- max(-pad_y0, 0) : out.shape[1] - max(-pad_y1, 0),
- max(-pad_x0, 0) : out.shape[2] - max(-pad_x1, 0),
- :,
- ]
-
- out = out.permute(0, 3, 1, 2)
- out = out.reshape(
- [-1, 1, in_h * up_y + pad_y0 + pad_y1, in_w * up_x + pad_x0 + pad_x1]
- )
- w = torch.flip(kernel, [0, 1]).view(1, 1, kernel_h, kernel_w)
- out = F.conv2d(out, w)
- out = out.reshape(
- -1,
- minor,
- in_h * up_y + pad_y0 + pad_y1 - kernel_h + 1,
- in_w * up_x + pad_x0 + pad_x1 - kernel_w + 1,
- )
- out = out.permute(0, 2, 3, 1)
-
- return out[:, ::down_y, ::down_x, :]
-
diff --git a/spaces/ali-ghamdan/deoldify/fastai/tabular/models.py b/spaces/ali-ghamdan/deoldify/fastai/tabular/models.py
deleted file mode 100644
index 2022c245c905b3213c974ef4a30b30eafe5ee77f..0000000000000000000000000000000000000000
--- a/spaces/ali-ghamdan/deoldify/fastai/tabular/models.py
+++ /dev/null
@@ -1,82 +0,0 @@
-from ..torch_core import *
-from ..layers import *
-from ..basic_data import *
-from ..basic_train import *
-from ..train import ClassificationInterpretation
-
-__all__ = ['TabularModel']
-
-class TabularModel(Module):
- "Basic model for tabular data."
- def __init__(self, emb_szs:ListSizes, n_cont:int, out_sz:int, layers:Collection[int], ps:Collection[float]=None,
- emb_drop:float=0., y_range:OptRange=None, use_bn:bool=True, bn_final:bool=False):
- super().__init__()
- ps = ifnone(ps, [0]*len(layers))
- ps = listify(ps, layers)
- self.embeds = nn.ModuleList([embedding(ni, nf) for ni,nf in emb_szs])
- self.emb_drop = nn.Dropout(emb_drop)
- self.bn_cont = nn.BatchNorm1d(n_cont)
- n_emb = sum(e.embedding_dim for e in self.embeds)
- self.n_emb,self.n_cont,self.y_range = n_emb,n_cont,y_range
- sizes = self.get_sizes(layers, out_sz)
- actns = [nn.ReLU(inplace=True) for _ in range(len(sizes)-2)] + [None]
- layers = []
- for i,(n_in,n_out,dp,act) in enumerate(zip(sizes[:-1],sizes[1:],[0.]+ps,actns)):
- layers += bn_drop_lin(n_in, n_out, bn=use_bn and i!=0, p=dp, actn=act)
- if bn_final: layers.append(nn.BatchNorm1d(sizes[-1]))
- self.layers = nn.Sequential(*layers)
-
- def get_sizes(self, layers, out_sz):
- return [self.n_emb + self.n_cont] + layers + [out_sz]
-
- def forward(self, x_cat:Tensor, x_cont:Tensor) -> Tensor:
- if self.n_emb != 0:
- x = [e(x_cat[:,i]) for i,e in enumerate(self.embeds)]
- x = torch.cat(x, 1)
- x = self.emb_drop(x)
- if self.n_cont != 0:
- x_cont = self.bn_cont(x_cont)
- x = torch.cat([x, x_cont], 1) if self.n_emb != 0 else x_cont
- x = self.layers(x)
- if self.y_range is not None:
- x = (self.y_range[1]-self.y_range[0]) * torch.sigmoid(x) + self.y_range[0]
- return x
-
-@classmethod
-def _cl_int_from_learner(cls, learn:Learner, ds_type=DatasetType.Valid, activ:nn.Module=None):
- "Creates an instance of 'ClassificationInterpretation"
- preds = learn.get_preds(ds_type=ds_type, activ=activ, with_loss=True)
- return cls(learn, *preds, ds_type=ds_type)
-
-def _cl_int_plot_top_losses(self, k, largest:bool=True, return_table:bool=False)->Optional[plt.Figure]:
- "Generates a dataframe of 'top_losses' along with their prediction, actual, loss, and probability of the actual class."
- tl_val, tl_idx = self.top_losses(k, largest)
- classes = self.data.classes
- cat_names = self.data.x.cat_names
- cont_names = self.data.x.cont_names
- df = pd.DataFrame(columns=[['Prediction', 'Actual', 'Loss', 'Probability'] + cat_names + cont_names])
- for i, idx in enumerate(tl_idx):
- da, cl = self.data.dl(self.ds_type).dataset[idx]
- cl = int(cl)
- t1 = str(da)
- t1 = t1.split(';')
- arr = []
- arr.extend([classes[self.pred_class[idx]], classes[cl], f'{self.losses[idx]:.2f}',
- f'{self.preds[idx][cl]:.2f}'])
- for x in range(len(t1)-1):
- _, value = t1[x].rsplit(' ', 1)
- arr.append(value)
- df.loc[i] = arr
- display(df)
- return_fig = return_table
- if ifnone(return_fig, defaults.return_fig): return df
-
-
-ClassificationInterpretation.from_learner = _cl_int_from_learner
-ClassificationInterpretation.plot_top_losses = _cl_int_plot_top_losses
-
-def _learner_interpret(learn:Learner, ds_type:DatasetType = DatasetType.Valid):
- "Create a 'ClassificationInterpretation' object from 'learner' on 'ds_type'."
- return ClassificationInterpretation.from_learner(learn, ds_type=ds_type)
-
-Learner.interpret = _learner_interpret
diff --git a/spaces/aliabid94/AutoGPT/autogpt/commands/git_operations.py b/spaces/aliabid94/AutoGPT/autogpt/commands/git_operations.py
deleted file mode 100644
index 028f3b8da44c85e01d20ccc5d4a5fa72c759008b..0000000000000000000000000000000000000000
--- a/spaces/aliabid94/AutoGPT/autogpt/commands/git_operations.py
+++ /dev/null
@@ -1,26 +0,0 @@
-"""Git operations for autogpt"""
-import git
-
-from autogpt.config import Config
-from autogpt.workspace import path_in_workspace
-
-CFG = Config()
-
-
-def clone_repository(repo_url: str, clone_path: str) -> str:
- """Clone a GitHub repository locally
-
- Args:
- repo_url (str): The URL of the repository to clone
- clone_path (str): The path to clone the repository to
-
- Returns:
- str: The result of the clone operation"""
- split_url = repo_url.split("//")
- auth_repo_url = f"//{CFG.github_username}:{CFG.github_api_key}@".join(split_url)
- safe_clone_path = path_in_workspace(clone_path)
- try:
- git.Repo.clone_from(auth_repo_url, safe_clone_path)
- return f"""Cloned {repo_url} to {safe_clone_path}"""
- except Exception as e:
- return f"Error: {str(e)}"
diff --git a/spaces/allknowingroger/Image-Models-Test42/README.md b/spaces/allknowingroger/Image-Models-Test42/README.md
deleted file mode 100644
index f62f351595db2987e55f674e750098ba710f135c..0000000000000000000000000000000000000000
--- a/spaces/allknowingroger/Image-Models-Test42/README.md
+++ /dev/null
@@ -1,13 +0,0 @@
----
-title: Image Models
-emoji: 👀
-colorFrom: red
-colorTo: gray
-sdk: gradio
-sdk_version: 3.23.0
-app_file: app.py
-pinned: true
-duplicated_from: allknowingroger/Image-Models-Test41
----
-
-
\ No newline at end of file
diff --git a/spaces/amarchheda/ChordDuplicate/portaudio/test/patest_jack_wasapi.c b/spaces/amarchheda/ChordDuplicate/portaudio/test/patest_jack_wasapi.c
deleted file mode 100644
index bd798817bd18f84dd2bfaf909570988ee82ce807..0000000000000000000000000000000000000000
--- a/spaces/amarchheda/ChordDuplicate/portaudio/test/patest_jack_wasapi.c
+++ /dev/null
@@ -1,343 +0,0 @@
-/** @file pa_test_jack_wasapi.c
- @ingroup test_src
- @brief Print out jack information for WASAPI endpoints
- @author Reid Bishop
-*/
-/*
- * $Id: pa_test_jack_wasapi.c 1368 2008-03-01 00:38:27Z rbishop $
- *
- * This program uses the PortAudio Portable Audio Library.
- * For more information see: http://www.portaudio.com/
- * Copyright (c) 1999-2010 Ross Bencina and Phil Burk
- *
- * Permission is hereby granted, free of charge, to any person obtaining
- * a copy of this software and associated documentation files
- * (the "Software"), to deal in the Software without restriction,
- * including without limitation the rights to use, copy, modify, merge,
- * publish, distribute, sublicense, and/or sell copies of the Software,
- * and to permit persons to whom the Software is furnished to do so,
- * subject to the following conditions:
- *
- * The above copyright notice and this permission notice shall be
- * included in all copies or substantial portions of the Software.
- *
- * THE SOFTWARE IS PROVIDED "AS IS", WITHOUT WARRANTY OF ANY KIND,
- * EXPRESS OR IMPLIED, INCLUDING BUT NOT LIMITED TO THE WARRANTIES OF
- * MERCHANTABILITY, FITNESS FOR A PARTICULAR PURPOSE AND NONINFRINGEMENT.
- * IN NO EVENT SHALL THE AUTHORS OR COPYRIGHT HOLDERS BE LIABLE FOR
- * ANY CLAIM, DAMAGES OR OTHER LIABILITY, WHETHER IN AN ACTION OF
- * CONTRACT, TORT OR OTHERWISE, ARISING FROM, OUT OF OR IN CONNECTION
- * WITH THE SOFTWARE OR THE USE OR OTHER DEALINGS IN THE SOFTWARE.
- */
-
-/*
- * The text above constitutes the entire PortAudio license; however,
- * the PortAudio community also makes the following non-binding requests:
- *
- * Any person wishing to distribute modifications to the Software is
- * requested to send the modifications to the original developer so that
- * they can be incorporated into the canonical version. It is also
- * requested that these non-binding requests be included along with the
- * license above.
- */
-#include
-#include "portaudio.h"
-#include "pa_win_wasapi.h"
-
-
-/*
-* Helper function to determine if a given enum is present in mask variable
-*
-*/
-static int IsInMask(int val, int val2)
-{
- return ((val & val2) == val2);
-}
-
-/*
-* This routine enumerates through the ChannelMapping for the IJackDescription
-*/
-
-static void EnumIJackChannels(int channelMapping)
-{
- printf("Channel Mapping: ");
- if(channelMapping == PAWIN_SPEAKER_DIRECTOUT)
- {
- printf("DIRECTOUT\n");
- return;
- }
- if(IsInMask(channelMapping, PAWIN_SPEAKER_FRONT_LEFT))
- printf("FRONT_LEFT, ");
- if(IsInMask(channelMapping, PAWIN_SPEAKER_FRONT_RIGHT))
- printf("FRONT_RIGHT, ");
- if(IsInMask(channelMapping, PAWIN_SPEAKER_FRONT_CENTER))
- printf("FRONT_CENTER, ");
- if(IsInMask(channelMapping, PAWIN_SPEAKER_LOW_FREQUENCY))
- printf("LOW_FREQUENCY, ");
- if(IsInMask(channelMapping, PAWIN_SPEAKER_BACK_LEFT))
- printf("BACK_LEFT, ");
- if(IsInMask(channelMapping,PAWIN_SPEAKER_BACK_RIGHT))
- printf("BACK_RIGHT, ");
- if(IsInMask(channelMapping, PAWIN_SPEAKER_FRONT_LEFT_OF_CENTER))
- printf("FRONT_LEFT_OF_CENTER, ");
- if(IsInMask(channelMapping, PAWIN_SPEAKER_FRONT_RIGHT_OF_CENTER))
- printf("FRONT_RIGHT_OF_CENTER, ");
- if(IsInMask(channelMapping, PAWIN_SPEAKER_BACK_CENTER))
- printf("BACK_CENTER, ");
- if(IsInMask(channelMapping,PAWIN_SPEAKER_SIDE_LEFT))
- printf("SIDE_LEFT, ");
- if(IsInMask(channelMapping,PAWIN_SPEAKER_SIDE_RIGHT))
- printf("SIDE_RIGHT, ");
- if(IsInMask(channelMapping, PAWIN_SPEAKER_TOP_CENTER))
- printf("TOP_CENTER, ");
- if(IsInMask(channelMapping, PAWIN_SPEAKER_TOP_FRONT_LEFT))
- printf("TOP_FRONT_LEFT, ");
- if(IsInMask(channelMapping, PAWIN_SPEAKER_TOP_FRONT_CENTER))
- printf("TOP_FRONT_CENTER, ");
- if(IsInMask(channelMapping, PAWIN_SPEAKER_TOP_FRONT_RIGHT))
- printf("TOP_FRONT_RIGHT, ");
- if(IsInMask(channelMapping, PAWIN_SPEAKER_TOP_BACK_LEFT))
- printf("TOP_BACK_LEFT, ");
- if(IsInMask(channelMapping, PAWIN_SPEAKER_TOP_BACK_CENTER))
- printf("TOP_BACK_CENTER, ");
- if(IsInMask(channelMapping, PAWIN_SPEAKER_TOP_BACK_RIGHT))
- printf("TOP_BACK_RIGHT, ");
-
- printf("\n");
-}
-
-/*
-* This routine enumerates through the Jack Connection Types enums for IJackDescription
-*/
-static void EnumIJackConnectionType(int cType)
-{
- printf("Connection Type: ");
- switch(cType)
- {
- case eJackConnTypeUnknown:
- printf("eJackConnTypeUnknown");
- break;
- case eJackConnType3Point5mm:
- printf("eJackConnType3Point5mm");
- break;
- case eJackConnTypeQuarter:
- printf("eJackConnTypeQuarter");
- break;
- case eJackConnTypeAtapiInternal:
- printf("eJackConnTypeAtapiInternal");
- break;
- case eJackConnTypeRCA:
- printf("eJackConnTypeRCA");
- break;
- case eJackConnTypeOptical:
- printf("eJackConnTypeOptical");
- break;
- case eJackConnTypeOtherDigital:
- printf("eJackConnTypeOtherDigital");
- break;
- case eJackConnTypeOtherAnalog:
- printf("eJackConnTypeOtherAnalog");
- break;
- case eJackConnTypeMultichannelAnalogDIN:
- printf("eJackConnTypeMultichannelAnalogDIN");
- break;
- case eJackConnTypeXlrProfessional:
- printf("eJackConnTypeXlrProfessional");
- break;
- case eJackConnTypeRJ11Modem:
- printf("eJackConnTypeRJ11Modem");
- break;
- case eJackConnTypeCombination:
- printf("eJackConnTypeCombination");
- break;
- }
- printf("\n");
-}
-
-/*
-* This routine enumerates through the GeoLocation enums for the IJackDescription
-*/
-static void EnumIJackGeoLocation(int iVal)
-{
- printf("Geometric Location: ");
- switch(iVal)
- {
- case eJackGeoLocRear:
- printf("eJackGeoLocRear");
- break;
- case eJackGeoLocFront:
- printf("eJackGeoLocFront");
- break;
- case eJackGeoLocLeft:
- printf("eJackGeoLocLeft");
- break;
- case eJackGeoLocRight:
- printf("eJackGeoLocRight");
- break;
- case eJackGeoLocTop:
- printf("eJackGeoLocTop");
- break;
- case eJackGeoLocBottom:
- printf("eJackGeoLocBottom");
- break;
- case eJackGeoLocRearPanel:
- printf("eJackGeoLocRearPanel");
- break;
- case eJackGeoLocRiser:
- printf("eJackGeoLocRiser");
- break;
- case eJackGeoLocInsideMobileLid:
- printf("eJackGeoLocInsideMobileLid");
- break;
- case eJackGeoLocDrivebay:
- printf("eJackGeoLocDrivebay");
- break;
- case eJackGeoLocHDMI:
- printf("eJackGeoLocHDMI");
- break;
- case eJackGeoLocOutsideMobileLid:
- printf("eJackGeoLocOutsideMobileLid");
- break;
- case eJackGeoLocATAPI:
- printf("eJackGeoLocATAPI");
- break;
- }
- printf("\n");
-}
-
-/*
-* This routine enumerates through the GenLocation enums for the IJackDescription
-*/
-static void EnumIJackGenLocation(int iVal)
-{
- printf("General Location: ");
- switch(iVal)
- {
- case eJackGenLocPrimaryBox:
- printf("eJackGenLocPrimaryBox");
- break;
- case eJackGenLocInternal:
- printf("eJackGenLocInternal");
- break;
- case eJackGenLocSeparate:
- printf("eJackGenLocSeparate");
- break;
- case eJackGenLocOther:
- printf("eJackGenLocOther");
- break;
- }
- printf("\n");
-}
-
-/*
-* This routine enumerates through the PortConnection enums for the IJackDescription
-*/
-static void EnumIJackPortConnection(int iVal)
-{
- printf("Port Type: ");
- switch(iVal)
- {
- case eJackPortConnJack:
- printf("eJackPortConnJack");
- break;
- case eJackPortConnIntegratedDevice:
- printf("eJackPortConnIntegratedDevice");
- break;
- case eJackPortConnBothIntegratedAndJack:
- printf("eJackPortConnBothIntegratedAndJack");
- break;
- case eJackPortConnUnknown:
- printf("eJackPortConnUnknown");
- break;
- }
- printf("\n");
-}
-
-/*
-* This routine retrieves and parses the KSJACK_DESCRIPTION structure for
-* the provided device ID.
-*/
-static PaError GetJackInformation(int deviceId)
-{
- PaError err;
- int i;
- int jackCount = 0;
- PaWasapiJackDescription jackDesc;
-
- err = PaWasapi_GetJackCount(deviceId, &jackCount);
- if( err != paNoError ) return err;
-
- fprintf( stderr,"Number of Jacks: %d \n", jackCount );
-
- for( i = 0; ihostApi == Pa_HostApiTypeIdToHostApiIndex(paWASAPI) )
- {
- if( device->maxOutputChannels == 0 )
- {
- isInput = 1;
- }
- printf("------------------------------------------\n");
- printf("Device: %s",device->name);
- if(isInput)
- printf(" (Input) %d Channels\n",device->maxInputChannels);
- else
- printf(" (Output) %d Channels\n",device->maxOutputChannels);
- // Try to see if this WASAPI device can provide Jack information
- err = GetJackInformation(i);
- if( err != paNoError ) goto error;
- }
- }
- Pa_Terminate();
- printf("Test finished.\n");
- return err;
-
-error:
- Pa_Terminate();
- fprintf( stderr, "An error occurred while using the portaudio stream\n" );
- fprintf( stderr, "Error number: %d\n", err );
- fprintf( stderr, "Error message: %s\n", Pa_GetErrorText( err ) );
- return err;
-}
diff --git a/spaces/amsterdamNLP/attention-rollout/app.py b/spaces/amsterdamNLP/attention-rollout/app.py
deleted file mode 100644
index b81f33b621646dcd5721438bc77196a4ef313a54..0000000000000000000000000000000000000000
--- a/spaces/amsterdamNLP/attention-rollout/app.py
+++ /dev/null
@@ -1,92 +0,0 @@
-import sys
-import pandas
-import gradio
-import pathlib
-
-sys.path.append("lib")
-
-import torch
-
-from roberta2 import RobertaForSequenceClassification
-from transformers import AutoTokenizer
-
-from gradient_rollout import GradientRolloutExplainer
-from rollout import RolloutExplainer
-from integrated_gradients import IntegratedGradientsExplainer
-
-device = torch.device("cuda") if torch.cuda.is_available() else torch.device("cpu")
-model = RobertaForSequenceClassification.from_pretrained("textattack/roberta-base-SST-2").to(device)
-tokenizer = AutoTokenizer.from_pretrained("textattack/roberta-base-SST-2")
-
-ig_explainer = IntegratedGradientsExplainer(model, tokenizer)
-gr_explainer = GradientRolloutExplainer(model, tokenizer)
-ro_explainer = RolloutExplainer(model, tokenizer)
-
-def run(sent, gradient, rollout, ig, ig_baseline):
- a = gr_explainer(sent, gradient)
- b = ro_explainer(sent, rollout)
- c = ig_explainer(sent, ig, ig_baseline)
- return a, b, c
-
-examples = pandas.read_csv("examples.csv").to_numpy().tolist()
-
-with gradio.Blocks(title="Explanations with attention rollout") as iface:
- gradio.Markdown(pathlib.Path("description.md").read_text)
- with gradio.Row(equal_height=True):
- with gradio.Column(scale=4):
- sent = gradio.Textbox(label="Input sentence")
- with gradio.Column(scale=1):
- but = gradio.Button("Submit")
- with gradio.Row(equal_height=True):
- with gradio.Column():
- rollout_layer = gradio.Slider(
- minimum=1,
- maximum=12,
- value=1,
- step=1,
- label="Select rollout start layer"
- )
- with gradio.Column():
- gradient_layer = gradio.Slider(
- minimum=1,
- maximum=12,
- value=8,
- step=1,
- label="Select gradient rollout start layer"
- )
- with gradio.Column():
- ig_layer = gradio.Slider(
- minimum=0,
- maximum=12,
- value=0,
- step=1,
- label="Select IG layer"
- )
- ig_baseline = gradio.Dropdown(
- label="Baseline token",
- choices=['Unknown', 'Padding'], value="Unknown"
- )
- with gradio.Row(equal_height=True):
- with gradio.Column():
- gradio.Markdown("### Attention Rollout")
- rollout_result = gradio.HTML()
- with gradio.Column():
- gradio.Markdown("### Gradient-weighted Attention Rollout")
- gradient_result = gradio.HTML()
- with gradio.Column():
- gradio.Markdown("### Layer-Integrated Gradients")
- ig_result = gradio.HTML()
- gradio.Examples(examples, [sent])
- with gradio.Accordion("Some more details"):
- gradio.Markdown(pathlib.Path("notice.md").read_text)
-
- gradient_layer.change(gr_explainer, [sent, gradient_layer], gradient_result)
- rollout_layer.change(ro_explainer, [sent, rollout_layer], rollout_result)
- ig_layer.change(ig_explainer, [sent, ig_layer, ig_baseline], ig_result)
- but.click(run,
- inputs=[sent, gradient_layer, rollout_layer, ig_layer, ig_baseline],
- outputs=[gradient_result, rollout_result, ig_result]
- )
-
-
-iface.launch()
diff --git a/spaces/anaclaudia13ct/insect_detection/utils/loggers/clearml/hpo.py b/spaces/anaclaudia13ct/insect_detection/utils/loggers/clearml/hpo.py
deleted file mode 100644
index ee518b0fbfc89ee811b51bbf85341eee4f685be1..0000000000000000000000000000000000000000
--- a/spaces/anaclaudia13ct/insect_detection/utils/loggers/clearml/hpo.py
+++ /dev/null
@@ -1,84 +0,0 @@
-from clearml import Task
-# Connecting ClearML with the current process,
-# from here on everything is logged automatically
-from clearml.automation import HyperParameterOptimizer, UniformParameterRange
-from clearml.automation.optuna import OptimizerOptuna
-
-task = Task.init(project_name='Hyper-Parameter Optimization',
- task_name='YOLOv5',
- task_type=Task.TaskTypes.optimizer,
- reuse_last_task_id=False)
-
-# Example use case:
-optimizer = HyperParameterOptimizer(
- # This is the experiment we want to optimize
- base_task_id='',
- # here we define the hyper-parameters to optimize
- # Notice: The parameter name should exactly match what you see in the UI: /
- # For Example, here we see in the base experiment a section Named: "General"
- # under it a parameter named "batch_size", this becomes "General/batch_size"
- # If you have `argparse` for example, then arguments will appear under the "Args" section,
- # and you should instead pass "Args/batch_size"
- hyper_parameters=[
- UniformParameterRange('Hyperparameters/lr0', min_value=1e-5, max_value=1e-1),
- UniformParameterRange('Hyperparameters/lrf', min_value=0.01, max_value=1.0),
- UniformParameterRange('Hyperparameters/momentum', min_value=0.6, max_value=0.98),
- UniformParameterRange('Hyperparameters/weight_decay', min_value=0.0, max_value=0.001),
- UniformParameterRange('Hyperparameters/warmup_epochs', min_value=0.0, max_value=5.0),
- UniformParameterRange('Hyperparameters/warmup_momentum', min_value=0.0, max_value=0.95),
- UniformParameterRange('Hyperparameters/warmup_bias_lr', min_value=0.0, max_value=0.2),
- UniformParameterRange('Hyperparameters/box', min_value=0.02, max_value=0.2),
- UniformParameterRange('Hyperparameters/cls', min_value=0.2, max_value=4.0),
- UniformParameterRange('Hyperparameters/cls_pw', min_value=0.5, max_value=2.0),
- UniformParameterRange('Hyperparameters/obj', min_value=0.2, max_value=4.0),
- UniformParameterRange('Hyperparameters/obj_pw', min_value=0.5, max_value=2.0),
- UniformParameterRange('Hyperparameters/iou_t', min_value=0.1, max_value=0.7),
- UniformParameterRange('Hyperparameters/anchor_t', min_value=2.0, max_value=8.0),
- UniformParameterRange('Hyperparameters/fl_gamma', min_value=0.0, max_value=4.0),
- UniformParameterRange('Hyperparameters/hsv_h', min_value=0.0, max_value=0.1),
- UniformParameterRange('Hyperparameters/hsv_s', min_value=0.0, max_value=0.9),
- UniformParameterRange('Hyperparameters/hsv_v', min_value=0.0, max_value=0.9),
- UniformParameterRange('Hyperparameters/degrees', min_value=0.0, max_value=45.0),
- UniformParameterRange('Hyperparameters/translate', min_value=0.0, max_value=0.9),
- UniformParameterRange('Hyperparameters/scale', min_value=0.0, max_value=0.9),
- UniformParameterRange('Hyperparameters/shear', min_value=0.0, max_value=10.0),
- UniformParameterRange('Hyperparameters/perspective', min_value=0.0, max_value=0.001),
- UniformParameterRange('Hyperparameters/flipud', min_value=0.0, max_value=1.0),
- UniformParameterRange('Hyperparameters/fliplr', min_value=0.0, max_value=1.0),
- UniformParameterRange('Hyperparameters/mosaic', min_value=0.0, max_value=1.0),
- UniformParameterRange('Hyperparameters/mixup', min_value=0.0, max_value=1.0),
- UniformParameterRange('Hyperparameters/copy_paste', min_value=0.0, max_value=1.0)],
- # this is the objective metric we want to maximize/minimize
- objective_metric_title='metrics',
- objective_metric_series='mAP_0.5',
- # now we decide if we want to maximize it or minimize it (accuracy we maximize)
- objective_metric_sign='max',
- # let us limit the number of concurrent experiments,
- # this in turn will make sure we do dont bombard the scheduler with experiments.
- # if we have an auto-scaler connected, this, by proxy, will limit the number of machine
- max_number_of_concurrent_tasks=1,
- # this is the optimizer class (actually doing the optimization)
- # Currently, we can choose from GridSearch, RandomSearch or OptimizerBOHB (Bayesian optimization Hyper-Band)
- optimizer_class=OptimizerOptuna,
- # If specified only the top K performing Tasks will be kept, the others will be automatically archived
- save_top_k_tasks_only=5, # 5,
- compute_time_limit=None,
- total_max_jobs=20,
- min_iteration_per_job=None,
- max_iteration_per_job=None,
-)
-
-# report every 10 seconds, this is way too often, but we are testing here
-optimizer.set_report_period(10 / 60)
-# You can also use the line below instead to run all the optimizer tasks locally, without using queues or agent
-# an_optimizer.start_locally(job_complete_callback=job_complete_callback)
-# set the time limit for the optimization process (2 hours)
-optimizer.set_time_limit(in_minutes=120.0)
-# Start the optimization process in the local environment
-optimizer.start_locally()
-# wait until process is done (notice we are controlling the optimization process in the background)
-optimizer.wait()
-# make sure background optimization stopped
-optimizer.stop()
-
-print('We are done, good bye')
diff --git a/spaces/antonovmaxim/text-generation-webui-space/css/chat_style-wpp.css b/spaces/antonovmaxim/text-generation-webui-space/css/chat_style-wpp.css
deleted file mode 100644
index a54a10734c0c14a1abe3ecd7fdb89602bc362dec..0000000000000000000000000000000000000000
--- a/spaces/antonovmaxim/text-generation-webui-space/css/chat_style-wpp.css
+++ /dev/null
@@ -1,86 +0,0 @@
-.chat {
- margin-left: auto;
- margin-right: auto;
- max-width: 800px;
- height: calc(100vh - 306px);
- overflow-y: auto;
- padding-right: 20px;
- display: flex;
- flex-direction: column-reverse;
- word-break: break-word;
- overflow-wrap: anywhere;
-}
-
-.message {
- padding-bottom: 25px;
- font-size: 15px;
- font-family: Helvetica, Arial, sans-serif;
- line-height: 1.428571429;
-}
-
-.text-you {
- background-color: #d9fdd3;
- border-radius: 15px;
- padding: 10px;
- padding-top: 5px;
- float: right;
-}
-
-.text-bot {
- background-color: #f2f2f2;
- border-radius: 15px;
- padding: 10px;
- padding-top: 5px;
-}
-
-.dark .text-you {
- background-color: #005c4b;
- color: #111b21;
-}
-
-.dark .text-bot {
- background-color: #1f2937;
- color: #111b21;
-}
-
-.text-bot p, .text-you p {
- margin-top: 5px;
-}
-
-.message-body {}
-
-.message-body img {
- max-width: 300px;
- max-height: 300px;
- border-radius: 20px;
-}
-
-.message-body p {
- margin-bottom: 0 !important;
- font-size: 15px !important;
- line-height: 1.428571429 !important;
-}
-
-.message-body li {
- margin-top: 0.5em !important;
- margin-bottom: 0.5em !important;
-}
-
-.message-body li > p {
- display: inline !important;
-}
-
-.message-body code {
- overflow-x: auto;
-}
-.message-body :not(pre) > code {
- white-space: normal !important;
-}
-
-.dark .message-body p em {
- color: rgb(138, 138, 138) !important;
-}
-
-.message-body p em {
- color: rgb(110, 110, 110) !important;
-}
\ No newline at end of file
diff --git a/spaces/aodianyun/stable-diffusion-webui/extensions/deforum/scripts/deforum_helpers/src/model_io.py b/spaces/aodianyun/stable-diffusion-webui/extensions/deforum/scripts/deforum_helpers/src/model_io.py
deleted file mode 100644
index 3427be8176f178c4c3ef09664a3f28d9fbaab4c3..0000000000000000000000000000000000000000
--- a/spaces/aodianyun/stable-diffusion-webui/extensions/deforum/scripts/deforum_helpers/src/model_io.py
+++ /dev/null
@@ -1,74 +0,0 @@
-import os
-
-import torch
-
-
-def save_weights(model, filename, path="./saved_models"):
- if not os.path.isdir(path):
- os.makedirs(path)
-
- fpath = os.path.join(path, filename)
- torch.save(model.state_dict(), fpath)
- return
-
-
-def save_checkpoint(model, optimizer, epoch, filename, root="./checkpoints"):
- if not os.path.isdir(root):
- os.makedirs(root)
-
- fpath = os.path.join(root, filename)
- torch.save(
- {
- "model": model.state_dict(),
- "optimizer": optimizer.state_dict(),
- "epoch": epoch
- }
- , fpath)
-
-
-def load_weights(model, filename, path="./saved_models"):
- fpath = os.path.join(path, filename)
- state_dict = torch.load(fpath)
- model.load_state_dict(state_dict)
- return model
-
-
-def load_checkpoint(fpath, model, optimizer=None):
- ckpt = torch.load(fpath, map_location='cpu')
- if ckpt is None:
- raise Exception(f"\nERROR Loading AdaBins_nyu.pt. Read this for a fix:\nhttps://github.com/deforum-art/deforum-for-automatic1111-webui/wiki/FAQ-&-Troubleshooting#3d-animation-mode-is-not-working-only-2d-works")
- if optimizer is None:
- optimizer = ckpt.get('optimizer', None)
- else:
- optimizer.load_state_dict(ckpt['optimizer'])
- epoch = ckpt['epoch']
-
- if 'model' in ckpt:
- ckpt = ckpt['model']
- load_dict = {}
- for k, v in ckpt.items():
- if k.startswith('module.'):
- k_ = k.replace('module.', '')
- load_dict[k_] = v
- else:
- load_dict[k] = v
-
- modified = {} # backward compatibility to older naming of architecture blocks
- for k, v in load_dict.items():
- if k.startswith('adaptive_bins_layer.embedding_conv.'):
- k_ = k.replace('adaptive_bins_layer.embedding_conv.',
- 'adaptive_bins_layer.conv3x3.')
- modified[k_] = v
- # del load_dict[k]
-
- elif k.startswith('adaptive_bins_layer.patch_transformer.embedding_encoder'):
-
- k_ = k.replace('adaptive_bins_layer.patch_transformer.embedding_encoder',
- 'adaptive_bins_layer.patch_transformer.embedding_convPxP')
- modified[k_] = v
- # del load_dict[k]
- else:
- modified[k] = v # else keep the original
-
- model.load_state_dict(modified)
- return model, optimizer, epoch
diff --git a/spaces/artificialguybr/video-dubbing/TTS/TTS/vocoder/models/gan.py b/spaces/artificialguybr/video-dubbing/TTS/TTS/vocoder/models/gan.py
deleted file mode 100644
index 19c30e983e5bb2066d3ccd22dc5cb21c091cb60a..0000000000000000000000000000000000000000
--- a/spaces/artificialguybr/video-dubbing/TTS/TTS/vocoder/models/gan.py
+++ /dev/null
@@ -1,374 +0,0 @@
-from inspect import signature
-from typing import Dict, List, Tuple
-
-import numpy as np
-import torch
-from coqpit import Coqpit
-from torch import nn
-from torch.utils.data import DataLoader
-from torch.utils.data.distributed import DistributedSampler
-from trainer.trainer_utils import get_optimizer, get_scheduler
-
-from TTS.utils.audio import AudioProcessor
-from TTS.utils.io import load_fsspec
-from TTS.vocoder.datasets.gan_dataset import GANDataset
-from TTS.vocoder.layers.losses import DiscriminatorLoss, GeneratorLoss
-from TTS.vocoder.models import setup_discriminator, setup_generator
-from TTS.vocoder.models.base_vocoder import BaseVocoder
-from TTS.vocoder.utils.generic_utils import plot_results
-
-
-class GAN(BaseVocoder):
- def __init__(self, config: Coqpit, ap: AudioProcessor = None):
- """Wrap a generator and a discriminator network. It provides a compatible interface for the trainer.
- It also helps mixing and matching different generator and disciminator networks easily.
-
- To implement a new GAN models, you just need to define the generator and the discriminator networks, the rest
- is handled by the `GAN` class.
-
- Args:
- config (Coqpit): Model configuration.
- ap (AudioProcessor): 🐸TTS AudioProcessor instance. Defaults to None.
-
- Examples:
- Initializing the GAN model with HifiGAN generator and discriminator.
- >>> from TTS.vocoder.configs import HifiganConfig
- >>> config = HifiganConfig()
- >>> model = GAN(config)
- """
- super().__init__(config)
- self.config = config
- self.model_g = setup_generator(config)
- self.model_d = setup_discriminator(config)
- self.train_disc = False # if False, train only the generator.
- self.y_hat_g = None # the last generator prediction to be passed onto the discriminator
- self.ap = ap
-
- def forward(self, x: torch.Tensor) -> torch.Tensor:
- """Run the generator's forward pass.
-
- Args:
- x (torch.Tensor): Input tensor.
-
- Returns:
- torch.Tensor: output of the GAN generator network.
- """
- return self.model_g.forward(x)
-
- def inference(self, x: torch.Tensor) -> torch.Tensor:
- """Run the generator's inference pass.
-
- Args:
- x (torch.Tensor): Input tensor.
- Returns:
- torch.Tensor: output of the GAN generator network.
- """
- return self.model_g.inference(x)
-
- def train_step(self, batch: Dict, criterion: Dict, optimizer_idx: int) -> Tuple[Dict, Dict]:
- """Compute model outputs and the loss values. `optimizer_idx` selects the generator or the discriminator for
- network on the current pass.
-
- Args:
- batch (Dict): Batch of samples returned by the dataloader.
- criterion (Dict): Criterion used to compute the losses.
- optimizer_idx (int): ID of the optimizer in use on the current pass.
-
- Raises:
- ValueError: `optimizer_idx` is an unexpected value.
-
- Returns:
- Tuple[Dict, Dict]: model outputs and the computed loss values.
- """
- outputs = {}
- loss_dict = {}
-
- x = batch["input"]
- y = batch["waveform"]
-
- if optimizer_idx not in [0, 1]:
- raise ValueError(" [!] Unexpected `optimizer_idx`.")
-
- if optimizer_idx == 0:
- # DISCRIMINATOR optimization
-
- # generator pass
- y_hat = self.model_g(x)[:, :, : y.size(2)]
-
- # cache for generator loss
- # pylint: disable=W0201
- self.y_hat_g = y_hat
- self.y_hat_sub = None
- self.y_sub_g = None
-
- # PQMF formatting
- if y_hat.shape[1] > 1:
- self.y_hat_sub = y_hat
- y_hat = self.model_g.pqmf_synthesis(y_hat)
- self.y_hat_g = y_hat # save for generator loss
- self.y_sub_g = self.model_g.pqmf_analysis(y)
-
- scores_fake, feats_fake, feats_real = None, None, None
-
- if self.train_disc:
- # use different samples for G and D trainings
- if self.config.diff_samples_for_G_and_D:
- x_d = batch["input_disc"]
- y_d = batch["waveform_disc"]
- # use a different sample than generator
- with torch.no_grad():
- y_hat = self.model_g(x_d)
-
- # PQMF formatting
- if y_hat.shape[1] > 1:
- y_hat = self.model_g.pqmf_synthesis(y_hat)
- else:
- # use the same samples as generator
- x_d = x.clone()
- y_d = y.clone()
- y_hat = self.y_hat_g
-
- # run D with or without cond. features
- if len(signature(self.model_d.forward).parameters) == 2:
- D_out_fake = self.model_d(y_hat.detach().clone(), x_d)
- D_out_real = self.model_d(y_d, x_d)
- else:
- D_out_fake = self.model_d(y_hat.detach())
- D_out_real = self.model_d(y_d)
-
- # format D outputs
- if isinstance(D_out_fake, tuple):
- # self.model_d returns scores and features
- scores_fake, feats_fake = D_out_fake
- if D_out_real is None:
- scores_real, feats_real = None, None
- else:
- scores_real, feats_real = D_out_real
- else:
- # model D returns only scores
- scores_fake = D_out_fake
- scores_real = D_out_real
-
- # compute losses
- loss_dict = criterion[optimizer_idx](scores_fake, scores_real)
- outputs = {"model_outputs": y_hat}
-
- if optimizer_idx == 1:
- # GENERATOR loss
- scores_fake, feats_fake, feats_real = None, None, None
- if self.train_disc:
- if len(signature(self.model_d.forward).parameters) == 2:
- D_out_fake = self.model_d(self.y_hat_g, x)
- else:
- D_out_fake = self.model_d(self.y_hat_g)
- D_out_real = None
-
- if self.config.use_feat_match_loss:
- with torch.no_grad():
- D_out_real = self.model_d(y)
-
- # format D outputs
- if isinstance(D_out_fake, tuple):
- scores_fake, feats_fake = D_out_fake
- if D_out_real is None:
- feats_real = None
- else:
- _, feats_real = D_out_real
- else:
- scores_fake = D_out_fake
- feats_fake, feats_real = None, None
-
- # compute losses
- loss_dict = criterion[optimizer_idx](
- self.y_hat_g, y, scores_fake, feats_fake, feats_real, self.y_hat_sub, self.y_sub_g
- )
- outputs = {"model_outputs": self.y_hat_g}
- return outputs, loss_dict
-
- def _log(self, name: str, ap: AudioProcessor, batch: Dict, outputs: Dict) -> Tuple[Dict, Dict]:
- """Logging shared by the training and evaluation.
-
- Args:
- name (str): Name of the run. `train` or `eval`,
- ap (AudioProcessor): Audio processor used in training.
- batch (Dict): Batch used in the last train/eval step.
- outputs (Dict): Model outputs from the last train/eval step.
-
- Returns:
- Tuple[Dict, Dict]: log figures and audio samples.
- """
- y_hat = outputs[0]["model_outputs"] if self.train_disc else outputs[1]["model_outputs"]
- y = batch["waveform"]
- figures = plot_results(y_hat, y, ap, name)
- sample_voice = y_hat[0].squeeze(0).detach().cpu().numpy()
- audios = {f"{name}/audio": sample_voice}
- return figures, audios
-
- def train_log(
- self, batch: Dict, outputs: Dict, logger: "Logger", assets: Dict, steps: int # pylint: disable=unused-argument
- ) -> Tuple[Dict, np.ndarray]:
- """Call `_log()` for training."""
- figures, audios = self._log("eval", self.ap, batch, outputs)
- logger.eval_figures(steps, figures)
- logger.eval_audios(steps, audios, self.ap.sample_rate)
-
- @torch.no_grad()
- def eval_step(self, batch: Dict, criterion: nn.Module, optimizer_idx: int) -> Tuple[Dict, Dict]:
- """Call `train_step()` with `no_grad()`"""
- self.train_disc = True # Avoid a bug in the Training with the missing discriminator loss
- return self.train_step(batch, criterion, optimizer_idx)
-
- def eval_log(
- self, batch: Dict, outputs: Dict, logger: "Logger", assets: Dict, steps: int # pylint: disable=unused-argument
- ) -> Tuple[Dict, np.ndarray]:
- """Call `_log()` for evaluation."""
- figures, audios = self._log("eval", self.ap, batch, outputs)
- logger.eval_figures(steps, figures)
- logger.eval_audios(steps, audios, self.ap.sample_rate)
-
- def load_checkpoint(
- self,
- config: Coqpit,
- checkpoint_path: str,
- eval: bool = False, # pylint: disable=unused-argument, redefined-builtin
- cache: bool = False,
- ) -> None:
- """Load a GAN checkpoint and initialize model parameters.
-
- Args:
- config (Coqpit): Model config.
- checkpoint_path (str): Checkpoint file path.
- eval (bool, optional): If true, load the model for inference. If falseDefaults to False.
- """
- state = load_fsspec(checkpoint_path, map_location=torch.device("cpu"), cache=cache)
- # band-aid for older than v0.0.15 GAN models
- if "model_disc" in state:
- self.model_g.load_checkpoint(config, checkpoint_path, eval)
- else:
- self.load_state_dict(state["model"])
- if eval:
- self.model_d = None
- if hasattr(self.model_g, "remove_weight_norm"):
- self.model_g.remove_weight_norm()
-
- def on_train_step_start(self, trainer) -> None:
- """Enable the discriminator training based on `steps_to_start_discriminator`
-
- Args:
- trainer (Trainer): Trainer object.
- """
- self.train_disc = trainer.total_steps_done >= self.config.steps_to_start_discriminator
-
- def get_optimizer(self) -> List:
- """Initiate and return the GAN optimizers based on the config parameters.
-
- It returnes 2 optimizers in a list. First one is for the generator and the second one is for the discriminator.
-
- Returns:
- List: optimizers.
- """
- optimizer1 = get_optimizer(
- self.config.optimizer, self.config.optimizer_params, self.config.lr_gen, self.model_g
- )
- optimizer2 = get_optimizer(
- self.config.optimizer, self.config.optimizer_params, self.config.lr_disc, self.model_d
- )
- return [optimizer2, optimizer1]
-
- def get_lr(self) -> List:
- """Set the initial learning rates for each optimizer.
-
- Returns:
- List: learning rates for each optimizer.
- """
- return [self.config.lr_disc, self.config.lr_gen]
-
- def get_scheduler(self, optimizer) -> List:
- """Set the schedulers for each optimizer.
-
- Args:
- optimizer (List[`torch.optim.Optimizer`]): List of optimizers.
-
- Returns:
- List: Schedulers, one for each optimizer.
- """
- scheduler1 = get_scheduler(self.config.lr_scheduler_gen, self.config.lr_scheduler_gen_params, optimizer[0])
- scheduler2 = get_scheduler(self.config.lr_scheduler_disc, self.config.lr_scheduler_disc_params, optimizer[1])
- return [scheduler2, scheduler1]
-
- @staticmethod
- def format_batch(batch: List) -> Dict:
- """Format the batch for training.
-
- Args:
- batch (List): Batch out of the dataloader.
-
- Returns:
- Dict: formatted model inputs.
- """
- if isinstance(batch[0], list):
- x_G, y_G = batch[0]
- x_D, y_D = batch[1]
- return {"input": x_G, "waveform": y_G, "input_disc": x_D, "waveform_disc": y_D}
- x, y = batch
- return {"input": x, "waveform": y}
-
- def get_data_loader( # pylint: disable=no-self-use, unused-argument
- self,
- config: Coqpit,
- assets: Dict,
- is_eval: True,
- samples: List,
- verbose: bool,
- num_gpus: int,
- rank: int = None, # pylint: disable=unused-argument
- ):
- """Initiate and return the GAN dataloader.
-
- Args:
- config (Coqpit): Model config.
- ap (AudioProcessor): Audio processor.
- is_eval (True): Set the dataloader for evaluation if true.
- samples (List): Data samples.
- verbose (bool): Log information if true.
- num_gpus (int): Number of GPUs in use.
- rank (int): Rank of the current GPU. Defaults to None.
-
- Returns:
- DataLoader: Torch dataloader.
- """
- dataset = GANDataset(
- ap=self.ap,
- items=samples,
- seq_len=config.seq_len,
- hop_len=self.ap.hop_length,
- pad_short=config.pad_short,
- conv_pad=config.conv_pad,
- return_pairs=config.diff_samples_for_G_and_D if "diff_samples_for_G_and_D" in config else False,
- is_training=not is_eval,
- return_segments=not is_eval,
- use_noise_augment=config.use_noise_augment,
- use_cache=config.use_cache,
- verbose=verbose,
- )
- dataset.shuffle_mapping()
- sampler = DistributedSampler(dataset, shuffle=True) if num_gpus > 1 else None
- loader = DataLoader(
- dataset,
- batch_size=1 if is_eval else config.batch_size,
- shuffle=num_gpus == 0,
- drop_last=False,
- sampler=sampler,
- num_workers=config.num_eval_loader_workers if is_eval else config.num_loader_workers,
- pin_memory=False,
- )
- return loader
-
- def get_criterion(self):
- """Return criterions for the optimizers"""
- return [DiscriminatorLoss(self.config), GeneratorLoss(self.config)]
-
- @staticmethod
- def init_from_config(config: Coqpit, verbose=True) -> "GAN":
- ap = AudioProcessor.init_from_config(config, verbose=verbose)
- return GAN(config, ap=ap)
diff --git a/spaces/artificialguybr/video-dubbing/TTS/recipes/vctk/tacotron2/train_tacotron2.py b/spaces/artificialguybr/video-dubbing/TTS/recipes/vctk/tacotron2/train_tacotron2.py
deleted file mode 100644
index d3f66348df4eb2e6c13be2ea9e6ba3cdf51ec9d0..0000000000000000000000000000000000000000
--- a/spaces/artificialguybr/video-dubbing/TTS/recipes/vctk/tacotron2/train_tacotron2.py
+++ /dev/null
@@ -1,104 +0,0 @@
-import os
-
-from trainer import Trainer, TrainerArgs
-
-from TTS.config.shared_configs import BaseAudioConfig
-from TTS.tts.configs.shared_configs import BaseDatasetConfig
-from TTS.tts.configs.tacotron2_config import Tacotron2Config
-from TTS.tts.datasets import load_tts_samples
-from TTS.tts.models.tacotron2 import Tacotron2
-from TTS.tts.utils.speakers import SpeakerManager
-from TTS.tts.utils.text.tokenizer import TTSTokenizer
-from TTS.utils.audio import AudioProcessor
-
-output_path = os.path.dirname(os.path.abspath(__file__))
-dataset_config = BaseDatasetConfig(formatter="vctk", meta_file_train="", path=os.path.join(output_path, "../VCTK/"))
-
-audio_config = BaseAudioConfig(
- sample_rate=22050,
- resample=False, # Resample to 22050 Hz. It slows down training. Use `TTS/bin/resample.py` to pre-resample and set this False for faster training.
- do_trim_silence=True,
- trim_db=23.0,
- signal_norm=False,
- mel_fmin=0.0,
- mel_fmax=8000,
- spec_gain=1.0,
- log_func="np.log",
- preemphasis=0.0,
-)
-
-config = Tacotron2Config( # This is the config that is saved for the future use
- audio=audio_config,
- batch_size=32,
- eval_batch_size=16,
- num_loader_workers=4,
- num_eval_loader_workers=4,
- run_eval=True,
- test_delay_epochs=-1,
- r=2,
- # gradual_training=[[0, 6, 48], [10000, 4, 32], [50000, 3, 32], [100000, 2, 32]],
- double_decoder_consistency=False,
- epochs=1000,
- text_cleaner="phoneme_cleaners",
- use_phonemes=True,
- phoneme_language="en-us",
- phoneme_cache_path=os.path.join(output_path, "phoneme_cache"),
- print_step=150,
- print_eval=False,
- mixed_precision=True,
- min_text_len=0,
- max_text_len=500,
- min_audio_len=0,
- max_audio_len=44000 * 10,
- output_path=output_path,
- datasets=[dataset_config],
- use_speaker_embedding=True, # set this to enable multi-sepeaker training
- decoder_ssim_alpha=0.0, # disable ssim losses that causes NaN for some runs.
- postnet_ssim_alpha=0.0,
- postnet_diff_spec_alpha=0.0,
- decoder_diff_spec_alpha=0.0,
- attention_norm="softmax",
- optimizer="Adam",
- lr_scheduler=None,
- lr=3e-5,
-)
-
-## INITIALIZE THE AUDIO PROCESSOR
-# Audio processor is used for feature extraction and audio I/O.
-# It mainly serves to the dataloader and the training loggers.
-ap = AudioProcessor.init_from_config(config)
-
-# INITIALIZE THE TOKENIZER
-# Tokenizer is used to convert text to sequences of token IDs.
-# If characters are not defined in the config, default characters are passed to the config
-tokenizer, config = TTSTokenizer.init_from_config(config)
-
-# LOAD DATA SAMPLES
-# Each sample is a list of ```[text, audio_file_path, speaker_name]```
-# You can define your custom sample loader returning the list of samples.
-# Or define your custom formatter and pass it to the `load_tts_samples`.
-# Check `TTS.tts.datasets.load_tts_samples` for more details.
-train_samples, eval_samples = load_tts_samples(
- dataset_config,
- eval_split=True,
- eval_split_max_size=config.eval_split_max_size,
- eval_split_size=config.eval_split_size,
-)
-
-# init speaker manager for multi-speaker training
-# it mainly handles speaker-id to speaker-name for the model and the data-loader
-speaker_manager = SpeakerManager()
-speaker_manager.set_ids_from_data(train_samples + eval_samples, parse_key="speaker_name")
-
-# init model
-model = Tacotron2(config, ap, tokenizer, speaker_manager)
-
-# INITIALIZE THE TRAINER
-# Trainer provides a generic API to train all the 🐸TTS models with all its perks like mixed-precision training,
-# distributed training, etc.
-trainer = Trainer(
- TrainerArgs(), config, output_path, model=model, train_samples=train_samples, eval_samples=eval_samples
-)
-
-# AND... 3,2,1... 🚀
-trainer.fit()
diff --git a/spaces/arxify/RVC-beta-v2-0618/runtime/Lib/site-packages/Crypto/Cipher/_EKSBlowfish.py b/spaces/arxify/RVC-beta-v2-0618/runtime/Lib/site-packages/Crypto/Cipher/_EKSBlowfish.py
deleted file mode 100644
index a844fae43d092a23c8a9d2eecf8caa4493433579..0000000000000000000000000000000000000000
--- a/spaces/arxify/RVC-beta-v2-0618/runtime/Lib/site-packages/Crypto/Cipher/_EKSBlowfish.py
+++ /dev/null
@@ -1,131 +0,0 @@
-# ===================================================================
-#
-# Copyright (c) 2019, Legrandin
-# All rights reserved.
-#
-# Redistribution and use in source and binary forms, with or without
-# modification, are permitted provided that the following conditions
-# are met:
-#
-# 1. Redistributions of source code must retain the above copyright
-# notice, this list of conditions and the following disclaimer.
-# 2. Redistributions in binary form must reproduce the above copyright
-# notice, this list of conditions and the following disclaimer in
-# the documentation and/or other materials provided with the
-# distribution.
-#
-# THIS SOFTWARE IS PROVIDED BY THE COPYRIGHT HOLDERS AND CONTRIBUTORS
-# "AS IS" AND ANY EXPRESS OR IMPLIED WARRANTIES, INCLUDING, BUT NOT
-# LIMITED TO, THE IMPLIED WARRANTIES OF MERCHANTABILITY AND FITNESS
-# FOR A PARTICULAR PURPOSE ARE DISCLAIMED. IN NO EVENT SHALL THE
-# COPYRIGHT HOLDER OR CONTRIBUTORS BE LIABLE FOR ANY DIRECT, INDIRECT,
-# INCIDENTAL, SPECIAL, EXEMPLARY, OR CONSEQUENTIAL DAMAGES (INCLUDING,
-# BUT NOT LIMITED TO, PROCUREMENT OF SUBSTITUTE GOODS OR SERVICES;
-# LOSS OF USE, DATA, OR PROFITS; OR BUSINESS INTERRUPTION) HOWEVER
-# CAUSED AND ON ANY THEORY OF LIABILITY, WHETHER IN CONTRACT, STRICT
-# LIABILITY, OR TORT (INCLUDING NEGLIGENCE OR OTHERWISE) ARISING IN
-# ANY WAY OUT OF THE USE OF THIS SOFTWARE, EVEN IF ADVISED OF THE
-# POSSIBILITY OF SUCH DAMAGE.
-# ===================================================================
-
-import sys
-
-from Crypto.Cipher import _create_cipher
-from Crypto.Util._raw_api import (load_pycryptodome_raw_lib,
- VoidPointer, SmartPointer, c_size_t,
- c_uint8_ptr, c_uint)
-
-_raw_blowfish_lib = load_pycryptodome_raw_lib(
- "Crypto.Cipher._raw_eksblowfish",
- """
- int EKSBlowfish_start_operation(const uint8_t key[],
- size_t key_len,
- const uint8_t salt[16],
- size_t salt_len,
- unsigned cost,
- unsigned invert,
- void **pResult);
- int EKSBlowfish_encrypt(const void *state,
- const uint8_t *in,
- uint8_t *out,
- size_t data_len);
- int EKSBlowfish_decrypt(const void *state,
- const uint8_t *in,
- uint8_t *out,
- size_t data_len);
- int EKSBlowfish_stop_operation(void *state);
- """
- )
-
-
-def _create_base_cipher(dict_parameters):
- """This method instantiates and returns a smart pointer to
- a low-level base cipher. It will absorb named parameters in
- the process."""
-
- try:
- key = dict_parameters.pop("key")
- salt = dict_parameters.pop("salt")
- cost = dict_parameters.pop("cost")
- except KeyError as e:
- raise TypeError("Missing EKSBlowfish parameter: " + str(e))
- invert = dict_parameters.pop("invert", True)
-
- if len(key) not in key_size:
- raise ValueError("Incorrect EKSBlowfish key length (%d bytes)" % len(key))
-
- start_operation = _raw_blowfish_lib.EKSBlowfish_start_operation
- stop_operation = _raw_blowfish_lib.EKSBlowfish_stop_operation
-
- void_p = VoidPointer()
- result = start_operation(c_uint8_ptr(key),
- c_size_t(len(key)),
- c_uint8_ptr(salt),
- c_size_t(len(salt)),
- c_uint(cost),
- c_uint(int(invert)),
- void_p.address_of())
- if result:
- raise ValueError("Error %X while instantiating the EKSBlowfish cipher"
- % result)
- return SmartPointer(void_p.get(), stop_operation)
-
-
-def new(key, mode, salt, cost, invert):
- """Create a new EKSBlowfish cipher
-
- Args:
-
- key (bytes, bytearray, memoryview):
- The secret key to use in the symmetric cipher.
- Its length can vary from 0 to 72 bytes.
-
- mode (one of the supported ``MODE_*`` constants):
- The chaining mode to use for encryption or decryption.
-
- salt (bytes, bytearray, memoryview):
- The salt that bcrypt uses to thwart rainbow table attacks
-
- cost (integer):
- The complexity factor in bcrypt
-
- invert (bool):
- If ``False``, in the inner loop use ``ExpandKey`` first over the salt
- and then over the key, as defined in
- the `original bcrypt specification `_.
- If ``True``, reverse the order, as in the first implementation of
- `bcrypt` in OpenBSD.
-
- :Return: an EKSBlowfish object
- """
-
- kwargs = { 'salt':salt, 'cost':cost, 'invert':invert }
- return _create_cipher(sys.modules[__name__], key, mode, **kwargs)
-
-
-MODE_ECB = 1
-
-# Size of a data block (in bytes)
-block_size = 8
-# Size of a key (in bytes)
-key_size = range(0, 72 + 1)
diff --git a/spaces/ashawkey/chatgpt_please_improve_my_paper_writing/README.md b/spaces/ashawkey/chatgpt_please_improve_my_paper_writing/README.md
deleted file mode 100644
index 52c93aca9b598fe8185e071666731fa457ad6bf3..0000000000000000000000000000000000000000
--- a/spaces/ashawkey/chatgpt_please_improve_my_paper_writing/README.md
+++ /dev/null
@@ -1,13 +0,0 @@
----
-title: Chatgpt Please Improve My Paper Writing
-emoji: 🐢
-colorFrom: yellow
-colorTo: gray
-sdk: gradio
-sdk_version: 3.19.1
-app_file: app.py
-pinned: false
-license: mit
----
-
-Check out the configuration reference at https://huggingface.co/docs/hub/spaces-config-reference
diff --git a/spaces/aus10powell/TwitterAccounts/scripts/translation.py b/spaces/aus10powell/TwitterAccounts/scripts/translation.py
deleted file mode 100644
index 2629382b8a3781e72dcfc2c3c92d98f518a5e514..0000000000000000000000000000000000000000
--- a/spaces/aus10powell/TwitterAccounts/scripts/translation.py
+++ /dev/null
@@ -1,104 +0,0 @@
-from transformers import MT5ForConditionalGeneration, MT5Tokenizer
-from transformers import AutoTokenizer
-import re
-
-
-class PersianTextProcessor:
- """
- A class for processing Persian text.
-
- Attributes:
- model_size (str): The size of the MT5 model.
- model_name (str): The name of the MT5 model.
- tokenizer (MT5Tokenizer): The MT5 tokenizer.
- model (MT5ForConditionalGeneration): The MT5 model.
-
- Methods:
- clean_persian_text(text): Cleans the given Persian text.
- translate_text(persian_text): Translates the given Persian text to English.
- """
-
- def __init__(self, model_size="small"):
- """
- Initializes the PersianTextProcessor class.
-
- Args:
- model_size (str): The size of the MT5 model.
- """
- self.model_size = model_size
- self.model_name = f"persiannlp/mt5-{self.model_size}-parsinlu-opus-translation_fa_en"
- self.tokenizer =MT5Tokenizer.from_pretrained(self.model_name) #AutoTokenizer.from_pretrained("persiannlp/mt5-small-parsinlu-opus-translation_fa_en")
- self.model = MT5ForConditionalGeneration.from_pretrained(self.model_name)
-
- def clean_persian_text(self, text):
- """
- Cleans the given Persian text by removing emojis, specific patterns, and replacing special characters.
-
- Args:
- text (str): The input Persian text.
-
- Returns:
- str: The cleaned Persian text.
- """
- # Create a regular expression to match emojis.
- emoji_pattern = re.compile(
- "["
- "\U0001F600-\U0001F64F" # emoticons
- "\U0001F300-\U0001F5FF" # symbols & pictographs
- "\U0001F680-\U0001F6FF" # transport & map symbols
- "\U0001F1E0-\U0001F1FF" # flags (iOS)
- "]+",
- flags=re.UNICODE,
- )
-
- # Create a regular expression to match specific patterns.
- pattern = "[\U0001F90D\U00002764\U0001F91F][\U0000FE0F\U0000200D]*"
-
- # Remove emojis, specific patterns, and special characters from the text.
- text = emoji_pattern.sub("", text)
- text = re.sub(pattern, "", text)
- text = text.replace("✌", "")
- text = text.replace("@", "")
- text = text.replace("#", "hashtag_")
-
- return text
-
- def run_model(self, input_string, **generator_args):
- """
- Runs the MT5 model on the given input string.
-
- Args:
- input_string (str): The input string.
- **generator_args: Additional arguments to pass to the MT5 model.
-
- Returns:
- str: The output of the MT5 model.
- """
- # Encode the input string as a sequence of tokens.
- input_ids = self.tokenizer.encode(input_string, return_tensors="pt")
-
- # Generate the output text.
- res = self.model.generate(input_ids, **generator_args)
-
- # Decode the output text to a string.
- output = self.tokenizer.batch_decode(res, skip_special_tokens=True)
-
- return output
-
- def translate_text(self, persian_text):
- """
- Translates the given Persian text to English.
-
- Args:
- persian_text (str): The Persian text to translate.
-
- Returns:
- str: The translated text.
- """
- # Clean the Persian text.
- text_cleaned = self.clean_persian_text(persian_text)
-
- # Translate the cleaned text.
- translated_text = self.run_model(input_string=text_cleaned)
-
- return translated_text
diff --git a/spaces/awacke1/PyGame2D/app.py b/spaces/awacke1/PyGame2D/app.py
deleted file mode 100644
index b0cfa5bb85df541af5baf872db4e88183c48c633..0000000000000000000000000000000000000000
--- a/spaces/awacke1/PyGame2D/app.py
+++ /dev/null
@@ -1,181 +0,0 @@
-import pygame
-import os
-pygame.font.init()
-pygame.mixer.init()
-
-WIDTH, HEIGHT = 900, 500
-WIN = pygame.display.set_mode((WIDTH, HEIGHT))
-pygame.display.set_caption("First Game!")
-
-WHITE = (255, 255, 255)
-BLACK = (0, 0, 0)
-RED = (255, 0, 0)
-YELLOW = (255, 255, 0)
-
-BORDER = pygame.Rect(WIDTH//2 - 5, 0, 10, HEIGHT)
-
-#BULLET_HIT_SOUND = pygame.mixer.Sound('Assets/Grenade+1.mp3')
-#BULLET_FIRE_SOUND = pygame.mixer.Sound('Assets/Gun+Silencer.mp3')
-
-HEALTH_FONT = pygame.font.SysFont('comicsans', 40)
-WINNER_FONT = pygame.font.SysFont('comicsans', 100)
-
-FPS = 60
-VEL = 5
-BULLET_VEL = 7
-MAX_BULLETS = 3
-SPACESHIP_WIDTH, SPACESHIP_HEIGHT = 55, 40
-
-YELLOW_HIT = pygame.USEREVENT + 1
-RED_HIT = pygame.USEREVENT + 2
-
-YELLOW_SPACESHIP_IMAGE = pygame.image.load(
- os.path.join('Assets', 'spaceship_yellow.png'))
-YELLOW_SPACESHIP = pygame.transform.rotate(pygame.transform.scale(
- YELLOW_SPACESHIP_IMAGE, (SPACESHIP_WIDTH, SPACESHIP_HEIGHT)), 90)
-
-RED_SPACESHIP_IMAGE = pygame.image.load(
- os.path.join('Assets', 'spaceship_red.png'))
-RED_SPACESHIP = pygame.transform.rotate(pygame.transform.scale(
- RED_SPACESHIP_IMAGE, (SPACESHIP_WIDTH, SPACESHIP_HEIGHT)), 270)
-
-SPACE = pygame.transform.scale(pygame.image.load(
- os.path.join('Assets', 'space.png')), (WIDTH, HEIGHT))
-
-
-def draw_window(red, yellow, red_bullets, yellow_bullets, red_health, yellow_health):
- WIN.blit(SPACE, (0, 0))
- pygame.draw.rect(WIN, BLACK, BORDER)
-
- red_health_text = HEALTH_FONT.render(
- "Health: " + str(red_health), 1, WHITE)
- yellow_health_text = HEALTH_FONT.render(
- "Health: " + str(yellow_health), 1, WHITE)
- WIN.blit(red_health_text, (WIDTH - red_health_text.get_width() - 10, 10))
- WIN.blit(yellow_health_text, (10, 10))
-
- WIN.blit(YELLOW_SPACESHIP, (yellow.x, yellow.y))
- WIN.blit(RED_SPACESHIP, (red.x, red.y))
-
- for bullet in red_bullets:
- pygame.draw.rect(WIN, RED, bullet)
-
- for bullet in yellow_bullets:
- pygame.draw.rect(WIN, YELLOW, bullet)
-
- pygame.display.update()
-
-
-def yellow_handle_movement(keys_pressed, yellow):
- if keys_pressed[pygame.K_a] and yellow.x - VEL > 0: # LEFT
- yellow.x -= VEL
- if keys_pressed[pygame.K_d] and yellow.x + VEL + yellow.width < BORDER.x: # RIGHT
- yellow.x += VEL
- if keys_pressed[pygame.K_w] and yellow.y - VEL > 0: # UP
- yellow.y -= VEL
- if keys_pressed[pygame.K_s] and yellow.y + VEL + yellow.height < HEIGHT - 15: # DOWN
- yellow.y += VEL
-
-
-def red_handle_movement(keys_pressed, red):
- if keys_pressed[pygame.K_LEFT] and red.x - VEL > BORDER.x + BORDER.width: # LEFT
- red.x -= VEL
- if keys_pressed[pygame.K_RIGHT] and red.x + VEL + red.width < WIDTH: # RIGHT
- red.x += VEL
- if keys_pressed[pygame.K_UP] and red.y - VEL > 0: # UP
- red.y -= VEL
- if keys_pressed[pygame.K_DOWN] and red.y + VEL + red.height < HEIGHT - 15: # DOWN
- red.y += VEL
-
-
-def handle_bullets(yellow_bullets, red_bullets, yellow, red):
- for bullet in yellow_bullets:
- bullet.x += BULLET_VEL
- if red.colliderect(bullet):
- pygame.event.post(pygame.event.Event(RED_HIT))
- yellow_bullets.remove(bullet)
- elif bullet.x > WIDTH:
- yellow_bullets.remove(bullet)
-
- for bullet in red_bullets:
- bullet.x -= BULLET_VEL
- if yellow.colliderect(bullet):
- pygame.event.post(pygame.event.Event(YELLOW_HIT))
- red_bullets.remove(bullet)
- elif bullet.x < 0:
- red_bullets.remove(bullet)
-
-
-def draw_winner(text):
- draw_text = WINNER_FONT.render(text, 1, WHITE)
- WIN.blit(draw_text, (WIDTH/2 - draw_text.get_width() /
- 2, HEIGHT/2 - draw_text.get_height()/2))
- pygame.display.update()
- pygame.time.delay(5000)
-
-
-def main():
- red = pygame.Rect(700, 300, SPACESHIP_WIDTH, SPACESHIP_HEIGHT)
- yellow = pygame.Rect(100, 300, SPACESHIP_WIDTH, SPACESHIP_HEIGHT)
-
- red_bullets = []
- yellow_bullets = []
-
- red_health = 10
- yellow_health = 10
-
- clock = pygame.time.Clock()
- run = True
- while run:
- clock.tick(FPS)
- for event in pygame.event.get():
- if event.type == pygame.QUIT:
- run = False
- pygame.quit()
-
- if event.type == pygame.KEYDOWN:
- if event.key == pygame.K_LCTRL and len(yellow_bullets) < MAX_BULLETS:
- bullet = pygame.Rect(
- yellow.x + yellow.width, yellow.y + yellow.height//2 - 2, 10, 5)
- yellow_bullets.append(bullet)
- #BULLET_FIRE_SOUND.play()
-
- if event.key == pygame.K_RCTRL and len(red_bullets) < MAX_BULLETS:
- bullet = pygame.Rect(
- red.x, red.y + red.height//2 - 2, 10, 5)
- red_bullets.append(bullet)
- #BULLET_FIRE_SOUND.play()
-
- if event.type == RED_HIT:
- red_health -= 1
- #BULLET_HIT_SOUND.play()
-
- if event.type == YELLOW_HIT:
- yellow_health -= 1
- #BULLET_HIT_SOUND.play()
-
- winner_text = ""
- if red_health <= 0:
- winner_text = "Yellow Wins!"
-
- if yellow_health <= 0:
- winner_text = "Red Wins!"
-
- if winner_text != "":
- draw_winner(winner_text)
- break
-
- keys_pressed = pygame.key.get_pressed()
- yellow_handle_movement(keys_pressed, yellow)
- red_handle_movement(keys_pressed, red)
-
- handle_bullets(yellow_bullets, red_bullets, yellow, red)
-
- draw_window(red, yellow, red_bullets, yellow_bullets,
- red_health, yellow_health)
-
- main()
-
-
-if __name__ == "__main__":
- main()
\ No newline at end of file
diff --git a/spaces/awinml/2-qa-earnings-sentencewise/utils/transcript_retrieval.py b/spaces/awinml/2-qa-earnings-sentencewise/utils/transcript_retrieval.py
deleted file mode 100644
index b255f7e101baa6e875dcdbc072eda635949035ad..0000000000000000000000000000000000000000
--- a/spaces/awinml/2-qa-earnings-sentencewise/utils/transcript_retrieval.py
+++ /dev/null
@@ -1,32 +0,0 @@
-# Transcript Retrieval
-
-
-def retrieve_transcript(data, year, quarter, ticker):
- if year == "All" or quarter == "All":
- row = (
- data.loc[
- (data.Ticker == ticker),
- ["File_Name"],
- ]
- .drop_duplicates()
- .iloc[0, 0]
- )
- else:
- row = (
- data.loc[
- (data.Year == int(year))
- & (data.Quarter == quarter)
- & (data.Ticker == ticker),
- ["File_Name"],
- ]
- .drop_duplicates()
- .iloc[0, 0]
- )
- # convert row to a string and join values with "-"
- # row_str = "-".join(row.astype(str)) + ".txt"
- open_file = open(
- f"Transcripts/{ticker}/{row}",
- "r",
- )
- file_text = open_file.read()
- return f"""{file_text}"""
diff --git a/spaces/b-monroe/rvc-VoiceAI/infer_pack/commons.py b/spaces/b-monroe/rvc-VoiceAI/infer_pack/commons.py
deleted file mode 100644
index 54470986f37825b35d90d7efa7437d1c26b87215..0000000000000000000000000000000000000000
--- a/spaces/b-monroe/rvc-VoiceAI/infer_pack/commons.py
+++ /dev/null
@@ -1,166 +0,0 @@
-import math
-import numpy as np
-import torch
-from torch import nn
-from torch.nn import functional as F
-
-
-def init_weights(m, mean=0.0, std=0.01):
- classname = m.__class__.__name__
- if classname.find("Conv") != -1:
- m.weight.data.normal_(mean, std)
-
-
-def get_padding(kernel_size, dilation=1):
- return int((kernel_size * dilation - dilation) / 2)
-
-
-def convert_pad_shape(pad_shape):
- l = pad_shape[::-1]
- pad_shape = [item for sublist in l for item in sublist]
- return pad_shape
-
-
-def kl_divergence(m_p, logs_p, m_q, logs_q):
- """KL(P||Q)"""
- kl = (logs_q - logs_p) - 0.5
- kl += (
- 0.5 * (torch.exp(2.0 * logs_p) + ((m_p - m_q) ** 2)) * torch.exp(-2.0 * logs_q)
- )
- return kl
-
-
-def rand_gumbel(shape):
- """Sample from the Gumbel distribution, protect from overflows."""
- uniform_samples = torch.rand(shape) * 0.99998 + 0.00001
- return -torch.log(-torch.log(uniform_samples))
-
-
-def rand_gumbel_like(x):
- g = rand_gumbel(x.size()).to(dtype=x.dtype, device=x.device)
- return g
-
-
-def slice_segments(x, ids_str, segment_size=4):
- ret = torch.zeros_like(x[:, :, :segment_size])
- for i in range(x.size(0)):
- idx_str = ids_str[i]
- idx_end = idx_str + segment_size
- ret[i] = x[i, :, idx_str:idx_end]
- return ret
-
-
-def slice_segments2(x, ids_str, segment_size=4):
- ret = torch.zeros_like(x[:, :segment_size])
- for i in range(x.size(0)):
- idx_str = ids_str[i]
- idx_end = idx_str + segment_size
- ret[i] = x[i, idx_str:idx_end]
- return ret
-
-
-def rand_slice_segments(x, x_lengths=None, segment_size=4):
- b, d, t = x.size()
- if x_lengths is None:
- x_lengths = t
- ids_str_max = x_lengths - segment_size + 1
- ids_str = (torch.rand([b]).to(device=x.device) * ids_str_max).to(dtype=torch.long)
- ret = slice_segments(x, ids_str, segment_size)
- return ret, ids_str
-
-
-def get_timing_signal_1d(length, channels, min_timescale=1.0, max_timescale=1.0e4):
- position = torch.arange(length, dtype=torch.float)
- num_timescales = channels // 2
- log_timescale_increment = math.log(float(max_timescale) / float(min_timescale)) / (
- num_timescales - 1
- )
- inv_timescales = min_timescale * torch.exp(
- torch.arange(num_timescales, dtype=torch.float) * -log_timescale_increment
- )
- scaled_time = position.unsqueeze(0) * inv_timescales.unsqueeze(1)
- signal = torch.cat([torch.sin(scaled_time), torch.cos(scaled_time)], 0)
- signal = F.pad(signal, [0, 0, 0, channels % 2])
- signal = signal.view(1, channels, length)
- return signal
-
-
-def add_timing_signal_1d(x, min_timescale=1.0, max_timescale=1.0e4):
- b, channels, length = x.size()
- signal = get_timing_signal_1d(length, channels, min_timescale, max_timescale)
- return x + signal.to(dtype=x.dtype, device=x.device)
-
-
-def cat_timing_signal_1d(x, min_timescale=1.0, max_timescale=1.0e4, axis=1):
- b, channels, length = x.size()
- signal = get_timing_signal_1d(length, channels, min_timescale, max_timescale)
- return torch.cat([x, signal.to(dtype=x.dtype, device=x.device)], axis)
-
-
-def subsequent_mask(length):
- mask = torch.tril(torch.ones(length, length)).unsqueeze(0).unsqueeze(0)
- return mask
-
-
-@torch.jit.script
-def fused_add_tanh_sigmoid_multiply(input_a, input_b, n_channels):
- n_channels_int = n_channels[0]
- in_act = input_a + input_b
- t_act = torch.tanh(in_act[:, :n_channels_int, :])
- s_act = torch.sigmoid(in_act[:, n_channels_int:, :])
- acts = t_act * s_act
- return acts
-
-
-def convert_pad_shape(pad_shape):
- l = pad_shape[::-1]
- pad_shape = [item for sublist in l for item in sublist]
- return pad_shape
-
-
-def shift_1d(x):
- x = F.pad(x, convert_pad_shape([[0, 0], [0, 0], [1, 0]]))[:, :, :-1]
- return x
-
-
-def sequence_mask(length, max_length=None):
- if max_length is None:
- max_length = length.max()
- x = torch.arange(max_length, dtype=length.dtype, device=length.device)
- return x.unsqueeze(0) < length.unsqueeze(1)
-
-
-def generate_path(duration, mask):
- """
- duration: [b, 1, t_x]
- mask: [b, 1, t_y, t_x]
- """
- device = duration.device
-
- b, _, t_y, t_x = mask.shape
- cum_duration = torch.cumsum(duration, -1)
-
- cum_duration_flat = cum_duration.view(b * t_x)
- path = sequence_mask(cum_duration_flat, t_y).to(mask.dtype)
- path = path.view(b, t_x, t_y)
- path = path - F.pad(path, convert_pad_shape([[0, 0], [1, 0], [0, 0]]))[:, :-1]
- path = path.unsqueeze(1).transpose(2, 3) * mask
- return path
-
-
-def clip_grad_value_(parameters, clip_value, norm_type=2):
- if isinstance(parameters, torch.Tensor):
- parameters = [parameters]
- parameters = list(filter(lambda p: p.grad is not None, parameters))
- norm_type = float(norm_type)
- if clip_value is not None:
- clip_value = float(clip_value)
-
- total_norm = 0
- for p in parameters:
- param_norm = p.grad.data.norm(norm_type)
- total_norm += param_norm.item() ** norm_type
- if clip_value is not None:
- p.grad.data.clamp_(min=-clip_value, max=clip_value)
- total_norm = total_norm ** (1.0 / norm_type)
- return total_norm
diff --git a/spaces/banana-projects/web3d/node_modules/three/examples/js/nodes/inputs/Matrix3Node.js b/spaces/banana-projects/web3d/node_modules/three/examples/js/nodes/inputs/Matrix3Node.js
deleted file mode 100644
index 25799d20f9e7d0815b252baf5b72b70fc7efac88..0000000000000000000000000000000000000000
--- a/spaces/banana-projects/web3d/node_modules/three/examples/js/nodes/inputs/Matrix3Node.js
+++ /dev/null
@@ -1,70 +0,0 @@
-/**
- * @author sunag / http://www.sunag.com.br/
- */
-
-import { InputNode } from '../core/InputNode.js';
-
-function Matrix3Node( matrix ) {
-
- InputNode.call( this, 'm3' );
-
- this.value = matrix || new THREE.Matrix3();
-
-}
-
-Matrix3Node.prototype = Object.create( InputNode.prototype );
-Matrix3Node.prototype.constructor = Matrix3Node;
-Matrix3Node.prototype.nodeType = "Matrix3";
-
-Object.defineProperties( Matrix3Node.prototype, {
-
- elements: {
-
- set: function ( val ) {
-
- this.value.elements = val;
-
- },
-
- get: function () {
-
- return this.value.elements;
-
- }
-
- }
-
-} );
-
-Matrix3Node.prototype.generateReadonly = function ( builder, output, uuid, type, ns, needsUpdate ) {
-
- return builder.format( "mat3( " + this.value.elements.join( ", " ) + " )", type, output );
-
-};
-
-
-Matrix3Node.prototype.copy = function ( source ) {
-
- InputNode.prototype.copy.call( this, source );
-
- this.value.fromArray( source.elements );
-
-};
-
-Matrix3Node.prototype.toJSON = function ( meta ) {
-
- var data = this.getJSONNode( meta );
-
- if ( ! data ) {
-
- data = this.createJSONNode( meta );
-
- data.elements = this.value.elements.concat();
-
- }
-
- return data;
-
-};
-
-export { Matrix3Node };
diff --git a/spaces/banana-projects/web3d/node_modules/three/examples/js/nodes/procedural/NoiseNode.js b/spaces/banana-projects/web3d/node_modules/three/examples/js/nodes/procedural/NoiseNode.js
deleted file mode 100644
index e743febdb402741be1fe04e134180b300c30929e..0000000000000000000000000000000000000000
--- a/spaces/banana-projects/web3d/node_modules/three/examples/js/nodes/procedural/NoiseNode.js
+++ /dev/null
@@ -1,69 +0,0 @@
-/**
- * @author sunag / http://www.sunag.com.br/
- */
-
-import { TempNode } from '../core/TempNode.js';
-import { FunctionNode } from '../core/FunctionNode.js';
-import { UVNode } from '../accessors/UVNode.js';
-
-function NoiseNode( uv ) {
-
- TempNode.call( this, 'f' );
-
- this.uv = uv || new UVNode();
-
-}
-
-NoiseNode.prototype = Object.create( TempNode.prototype );
-NoiseNode.prototype.constructor = NoiseNode;
-NoiseNode.prototype.nodeType = "Noise";
-
-NoiseNode.Nodes = ( function () {
-
- var snoise = new FunctionNode( [
- "float snoise(vec2 co) {",
-
- " return fract( sin( dot( co.xy, vec2( 12.9898, 78.233 ) ) ) * 43758.5453 );",
-
- "}"
- ].join( "\n" ) );
-
- return {
- snoise: snoise
- };
-
-} )();
-
-NoiseNode.prototype.generate = function ( builder, output ) {
-
- var snoise = builder.include( NoiseNode.Nodes.snoise );
-
- return builder.format( snoise + '( ' + this.uv.build( builder, 'v2' ) + ' )', this.getType( builder ), output );
-
-};
-
-NoiseNode.prototype.copy = function ( source ) {
-
- TempNode.prototype.copy.call( this, source );
-
- this.uv = source.uv;
-
-};
-
-NoiseNode.prototype.toJSON = function ( meta ) {
-
- var data = this.getJSONNode( meta );
-
- if ( ! data ) {
-
- data = this.createJSONNode( meta );
-
- data.uv = this.uv.toJSON( meta ).uuid;
-
- }
-
- return data;
-
-};
-
-export { NoiseNode };
diff --git a/spaces/banana-projects/web3d/node_modules/three/src/renderers/WebGL2Renderer.js b/spaces/banana-projects/web3d/node_modules/three/src/renderers/WebGL2Renderer.js
deleted file mode 100644
index d0ad1c344c82d3dd75d744ceec19acddda974514..0000000000000000000000000000000000000000
--- a/spaces/banana-projects/web3d/node_modules/three/src/renderers/WebGL2Renderer.js
+++ /dev/null
@@ -1,189 +0,0 @@
-/**
- * @author mrdoob / http://mrdoob.com/
- */
-
-import { REVISION } from '../constants.js';
-import { WebGLExtensions } from './webgl/WebGLExtensions.js';
-import { WebGLState } from './webgl/WebGLState.js';
-import { Color } from '../math/Color.js';
-import { Vector4 } from '../math/Vector4.js';
-
-function WebGL2Renderer( parameters ) {
-
- console.log( 'THREE.WebGL2Renderer', REVISION );
-
- parameters = parameters || {};
-
- var _canvas = parameters.canvas !== undefined ? parameters.canvas : document.createElementNS( 'http://www.w3.org/1999/xhtml', 'canvas' ),
- _context = parameters.context !== undefined ? parameters.context : null,
-
- _alpha = parameters.alpha !== undefined ? parameters.alpha : false,
- _depth = parameters.depth !== undefined ? parameters.depth : true,
- _stencil = parameters.stencil !== undefined ? parameters.stencil : true,
- _antialias = parameters.antialias !== undefined ? parameters.antialias : false,
- _premultipliedAlpha = parameters.premultipliedAlpha !== undefined ? parameters.premultipliedAlpha : true,
- _preserveDrawingBuffer = parameters.preserveDrawingBuffer !== undefined ? parameters.preserveDrawingBuffer : false,
- _powerPreference = parameters.powerPreference !== undefined ? parameters.powerPreference : 'default';
-
- // initialize
-
- var gl;
-
- try {
-
- var attributes = {
- alpha: _alpha,
- depth: _depth,
- stencil: _stencil,
- antialias: _antialias,
- premultipliedAlpha: _premultipliedAlpha,
- preserveDrawingBuffer: _preserveDrawingBuffer,
- powerPreference: _powerPreference
- };
-
- // event listeners must be registered before WebGL context is created, see #12753
-
- _canvas.addEventListener( 'webglcontextlost', onContextLost, false );
- _canvas.addEventListener( 'webglcontextrestored', function () { } );
-
- gl = _context || _canvas.getContext( 'webgl2', attributes );
-
- if ( gl === null ) {
-
- if ( _canvas.getContext( 'webgl2' ) !== null ) {
-
- throw new Error( 'Error creating WebGL2 context with your selected attributes.' );
-
- } else {
-
- throw new Error( 'Error creating WebGL2 context.' );
-
- }
-
- }
-
- } catch ( error ) {
-
- console.error( 'THREE.WebGL2Renderer: ' + error.message );
-
- }
-
- //
-
- var _autoClear = true,
- _autoClearColor = true,
- _autoClearDepth = true,
- _autoClearStencil = true,
-
- _clearColor = new Color( 0x000000 ),
- _clearAlpha = 0,
-
- _width = _canvas.width,
- _height = _canvas.height,
-
- _pixelRatio = 1,
-
- _viewport = new Vector4( 0, 0, _width, _height );
-
- var extensions = new WebGLExtensions( gl );
- var state = new WebGLState( gl, extensions, function () {} );
-
- //
-
- function clear( color, depth, stencil ) {
-
- var bits = 0;
-
- if ( color === undefined || color ) bits |= gl.COLOR_BUFFER_BIT;
- if ( depth === undefined || depth ) bits |= gl.DEPTH_BUFFER_BIT;
- if ( stencil === undefined || stencil ) bits |= gl.STENCIL_BUFFER_BIT;
-
- gl.clear( bits );
-
- }
-
- function setPixelRatio( value ) {
-
- if ( value === undefined ) return;
-
- _pixelRatio = value;
-
- setSize( _viewport.z, _viewport.w, false );
-
- }
-
- function setSize( width, height, updateStyle ) {
-
- _width = width;
- _height = height;
-
- _canvas.width = width * _pixelRatio;
- _canvas.height = height * _pixelRatio;
-
- if ( updateStyle !== false ) {
-
- _canvas.style.width = width + 'px';
- _canvas.style.height = height + 'px';
-
- }
-
- setViewport( 0, 0, width, height );
-
- }
-
- function setViewport( x, y, width, height ) {
-
- state.viewport( _viewport.set( x, y, width, height ) );
-
- }
-
- function render( scene, camera ) {
-
- if ( camera !== undefined && camera.isCamera !== true ) {
-
- console.error( 'THREE.WebGL2Renderer.render: camera is not an instance of THREE.Camera.' );
- return;
-
- }
-
- var background = scene.background;
- var forceClear = false;
-
- if ( background === null ) {
-
- state.buffers.color.setClear( _clearColor.r, _clearColor.g, _clearColor.b, _clearAlpha, _premultipliedAlpha );
-
- } else if ( background && background.isColor ) {
-
- state.buffers.color.setClear( background.r, background.g, background.b, 1, _premultipliedAlpha );
- forceClear = true;
-
- }
-
- if ( _autoClear || forceClear ) {
-
- this.clear( _autoClearColor, _autoClearDepth, _autoClearStencil );
-
- }
-
- }
-
- function onContextLost( event ) {
-
- event.preventDefault();
-
- }
-
- return {
- domElement: _canvas,
-
- clear: clear,
- setPixelRatio: setPixelRatio,
- setSize: setSize,
- render: render
- };
-
-}
-
-
-export { WebGL2Renderer };
diff --git a/spaces/bastiendechamps/geoguessr-bot/geoguessr_bot/commands/kaggle_submission_command.py b/spaces/bastiendechamps/geoguessr-bot/geoguessr_bot/commands/kaggle_submission_command.py
deleted file mode 100644
index ab60e88ca7cab089144a6640b3957024025d58a1..0000000000000000000000000000000000000000
--- a/spaces/bastiendechamps/geoguessr-bot/geoguessr_bot/commands/kaggle_submission_command.py
+++ /dev/null
@@ -1,30 +0,0 @@
-import os
-from dataclasses import dataclass
-
-import pandas as pd
-
-from geoguessr_bot.commands import AbstractCommand
-from geoguessr_bot.guessr import AbstractGuessr
-
-
-@dataclass
-class KaggleSubmissionCommand(AbstractCommand):
- """Submit a prediction to Kaggle
- """
- image_folder_path: str
- output_path: str
- guessr: AbstractGuessr
-
- def run(self) -> None:
- images_ids, latitudes, longitudes = [], [], []
- for image_name in os.listdir(self.image_folder_path):
- image_path = os.path.join(self.image_folder_path, image_name)
- coordinate = self.guessr.guess_from_path(image_path)
- images_ids.append(image_name.split(".")[0])
- latitudes.append(coordinate.latitude)
- longitudes.append(coordinate.longitude)
- pd.DataFrame(dict(
- image_id=images_ids,
- latitude=latitudes,
- longitude=longitudes,
- )).to_csv(self.output_path, index=False)
diff --git a/spaces/beihai/PDF-Table-Extractor/.history/app_20220621095552.py b/spaces/beihai/PDF-Table-Extractor/.history/app_20220621095552.py
deleted file mode 100644
index 7c5a15e9c6e1595a053226adfd5e35bd23588e16..0000000000000000000000000000000000000000
--- a/spaces/beihai/PDF-Table-Extractor/.history/app_20220621095552.py
+++ /dev/null
@@ -1,40 +0,0 @@
-#-*- coding : utf-8-*-
-import base64
-from subprocess import STDOUT
-import streamlit as st
-import pandas as pd
-import camelot as cam # extracting tables from PDFs
-
-st.title("PDF Table Extractor")
-
-input_pdf = st.file_uploader(label = "", type = 'pdf')
-
-page_number = st.text_input("请填写表格所在PDF页码,eg: 3", value = 1)
-background = st.selectbox("表格线条是否隐藏",(False,True),)
-if input_pdf is not None:
- # byte object into a PDF file
- with open("input.pdf", "wb") as f:
- base64_pdf = base64.b64encode(input_pdf.read()).decode('utf-8')
- f.write(base64.b64decode(base64_pdf))
- f.close()
-
- # read the pdf and parse it using stream
- tables = cam.read_pdf("input.pdf", pages=page_number, process_background=background)
- result = pd.ExcelWriter('result.xlsx', engine='xlsxwriter')
- tables[0].to_excel(result,index=False)
- # for i in range(0,len(tables)):
- # table = tables[i].df
- # sheetname = str(i)
- # table.to_excel(result, sheetname,index=False)
-
- with open('result.xlsx','rb') as f:
- st.download_button('提取完成,点击下载!', f,file_name='result.xlsx',mime="application/vnd.ms-excel")
-
- tables_all= cam.read_pdf("input.pdf", pages="all", process_background=background)
- result_all = pd.ExcelWriter('result_all.xlsx', engine='xlsxwriter')
- for i in range(0,len(tables_all)):
- table = tables_all[i].df
- sheetname = str(i)
- table.to_excel(result_all, sheetname,index=False)
- with open('result_all.xlsx','rb') as f:
- st.download_button('一件抽取完成,点击下载!', f,file_name='result_all.xlsx',mime="application/vnd.ms-excel")
\ No newline at end of file
diff --git a/spaces/bhasker412/IDD-YOLO-Tracking/trackers/strongsort/deep/models/__init__.py b/spaces/bhasker412/IDD-YOLO-Tracking/trackers/strongsort/deep/models/__init__.py
deleted file mode 100644
index 3c60ba6f59ca7fa5ff9f3c6a4dcef1357c353dde..0000000000000000000000000000000000000000
--- a/spaces/bhasker412/IDD-YOLO-Tracking/trackers/strongsort/deep/models/__init__.py
+++ /dev/null
@@ -1,122 +0,0 @@
-from __future__ import absolute_import
-import torch
-
-from .pcb import *
-from .mlfn import *
-from .hacnn import *
-from .osnet import *
-from .senet import *
-from .mudeep import *
-from .nasnet import *
-from .resnet import *
-from .densenet import *
-from .xception import *
-from .osnet_ain import *
-from .resnetmid import *
-from .shufflenet import *
-from .squeezenet import *
-from .inceptionv4 import *
-from .mobilenetv2 import *
-from .resnet_ibn_a import *
-from .resnet_ibn_b import *
-from .shufflenetv2 import *
-from .inceptionresnetv2 import *
-
-__model_factory = {
- # image classification models
- 'resnet18': resnet18,
- 'resnet34': resnet34,
- 'resnet50': resnet50,
- 'resnet101': resnet101,
- 'resnet152': resnet152,
- 'resnext50_32x4d': resnext50_32x4d,
- 'resnext101_32x8d': resnext101_32x8d,
- 'resnet50_fc512': resnet50_fc512,
- 'se_resnet50': se_resnet50,
- 'se_resnet50_fc512': se_resnet50_fc512,
- 'se_resnet101': se_resnet101,
- 'se_resnext50_32x4d': se_resnext50_32x4d,
- 'se_resnext101_32x4d': se_resnext101_32x4d,
- 'densenet121': densenet121,
- 'densenet169': densenet169,
- 'densenet201': densenet201,
- 'densenet161': densenet161,
- 'densenet121_fc512': densenet121_fc512,
- 'inceptionresnetv2': inceptionresnetv2,
- 'inceptionv4': inceptionv4,
- 'xception': xception,
- 'resnet50_ibn_a': resnet50_ibn_a,
- 'resnet50_ibn_b': resnet50_ibn_b,
- # lightweight models
- 'nasnsetmobile': nasnetamobile,
- 'mobilenetv2_x1_0': mobilenetv2_x1_0,
- 'mobilenetv2_x1_4': mobilenetv2_x1_4,
- 'shufflenet': shufflenet,
- 'squeezenet1_0': squeezenet1_0,
- 'squeezenet1_0_fc512': squeezenet1_0_fc512,
- 'squeezenet1_1': squeezenet1_1,
- 'shufflenet_v2_x0_5': shufflenet_v2_x0_5,
- 'shufflenet_v2_x1_0': shufflenet_v2_x1_0,
- 'shufflenet_v2_x1_5': shufflenet_v2_x1_5,
- 'shufflenet_v2_x2_0': shufflenet_v2_x2_0,
- # reid-specific models
- 'mudeep': MuDeep,
- 'resnet50mid': resnet50mid,
- 'hacnn': HACNN,
- 'pcb_p6': pcb_p6,
- 'pcb_p4': pcb_p4,
- 'mlfn': mlfn,
- 'osnet_x1_0': osnet_x1_0,
- 'osnet_x0_75': osnet_x0_75,
- 'osnet_x0_5': osnet_x0_5,
- 'osnet_x0_25': osnet_x0_25,
- 'osnet_ibn_x1_0': osnet_ibn_x1_0,
- 'osnet_ain_x1_0': osnet_ain_x1_0,
- 'osnet_ain_x0_75': osnet_ain_x0_75,
- 'osnet_ain_x0_5': osnet_ain_x0_5,
- 'osnet_ain_x0_25': osnet_ain_x0_25
-}
-
-
-def show_avai_models():
- """Displays available models.
-
- Examples::
- >>> from torchreid import models
- >>> models.show_avai_models()
- """
- print(list(__model_factory.keys()))
-
-
-def build_model(
- name, num_classes, loss='softmax', pretrained=True, use_gpu=True
-):
- """A function wrapper for building a model.
-
- Args:
- name (str): model name.
- num_classes (int): number of training identities.
- loss (str, optional): loss function to optimize the model. Currently
- supports "softmax" and "triplet". Default is "softmax".
- pretrained (bool, optional): whether to load ImageNet-pretrained weights.
- Default is True.
- use_gpu (bool, optional): whether to use gpu. Default is True.
-
- Returns:
- nn.Module
-
- Examples::
- >>> from torchreid import models
- >>> model = models.build_model('resnet50', 751, loss='softmax')
- """
- avai_models = list(__model_factory.keys())
- if name not in avai_models:
- raise KeyError(
- 'Unknown model: {}. Must be one of {}'.format(name, avai_models)
- )
- return __model_factory[name](
- num_classes=num_classes,
- loss=loss,
- pretrained=pretrained,
- use_gpu=use_gpu
- )
diff --git a/spaces/bioriAsaeru/text-to-voice/Download DRevitalize 3.31 Full Crack For Windows and Boost Your PC Performance.md b/spaces/bioriAsaeru/text-to-voice/Download DRevitalize 3.31 Full Crack For Windows and Boost Your PC Performance.md
deleted file mode 100644
index 5a62c53aee32dd50860d569c714ecdc29a044e9d..0000000000000000000000000000000000000000
--- a/spaces/bioriAsaeru/text-to-voice/Download DRevitalize 3.31 Full Crack For Windows and Boost Your PC Performance.md
+++ /dev/null
@@ -1,5 +0,0 @@
-
-
It is part from hard disk utilities category and is licensed as shareware for Windows 32-bit and 64-bit platform and can be used as a free trial until the trial period will end. The DRevitalize demo is available to all software users as a free download with potential restrictions compared with the full version.
-
DRevitalize 3.31 Full Crack For Windows Free Download
-
-26 - Retired U.S. basketball player Dennis Rodman visits North Korea to film ... 5 - Fashion fans from around the would will be able to view many of the shows free online. ... in Blu-ray, meowing Christmas favorite "Silent Night" in high definition. ... https://www.reuters.com/video/watch/kylie-minogue-shows-off-her-acting-skill-Â ... 1fdad05405
-
-
-
diff --git a/spaces/bioriAsaeru/text-to-voice/Hammurabi The King Who Unified Mesopotamia and Gave the World Its First Law Code.md b/spaces/bioriAsaeru/text-to-voice/Hammurabi The King Who Unified Mesopotamia and Gave the World Its First Law Code.md
deleted file mode 100644
index e14536909c20ebb364c336601dca9571835a3bdf..0000000000000000000000000000000000000000
--- a/spaces/bioriAsaeru/text-to-voice/Hammurabi The King Who Unified Mesopotamia and Gave the World Its First Law Code.md
+++ /dev/null
@@ -1,5 +0,0 @@
-
-
He is also known as Ammurapi and Khammurabi and assumed the throne from his father, Sin-Muballit, who had stabilized the kingdom but could not expand upon it. The kingdom of Babylon comprised only the cities of Babylon, Kish, Sippar, and Borsippa when Hammurabi came to the throne but, through a succession of military campaigns, careful alliances made and broken when necessary, and political maneuvers, he held the entire region under Babylonian control by 1750 BCE.
-
- aaccfb2cb3
-
-
-
diff --git a/spaces/cihyFjudo/fairness-paper-search/FULL HiTek.Software.AbleFtp.v9.14.Incl.Keygen-Lz0 A Step-by-Step Tutorial on How to Use the FTP Program.md b/spaces/cihyFjudo/fairness-paper-search/FULL HiTek.Software.AbleFtp.v9.14.Incl.Keygen-Lz0 A Step-by-Step Tutorial on How to Use the FTP Program.md
deleted file mode 100644
index 10f555ce1fe2c289b8ed384457d9d20f06d11440..0000000000000000000000000000000000000000
--- a/spaces/cihyFjudo/fairness-paper-search/FULL HiTek.Software.AbleFtp.v9.14.Incl.Keygen-Lz0 A Step-by-Step Tutorial on How to Use the FTP Program.md
+++ /dev/null
@@ -1,6 +0,0 @@
-
-
- aaccfb2cb3
-
-
-
diff --git a/spaces/cihyFjudo/fairness-paper-search/Illuminatiam The First Testament Of The Illuminati download pdf - Explore the hidden knowledge and wisdom of the Illuminatis leaders and masters.md b/spaces/cihyFjudo/fairness-paper-search/Illuminatiam The First Testament Of The Illuminati download pdf - Explore the hidden knowledge and wisdom of the Illuminatis leaders and masters.md
deleted file mode 100644
index dd2312b0e1900d4692d2d9b19c85bd674b695a6d..0000000000000000000000000000000000000000
--- a/spaces/cihyFjudo/fairness-paper-search/Illuminatiam The First Testament Of The Illuminati download pdf - Explore the hidden knowledge and wisdom of the Illuminatis leaders and masters.md
+++ /dev/null
@@ -1,6 +0,0 @@
-
Illuminatiam: The First Testament Of The Illuminati download pdf
-
- aaccfb2cb3
-
-
-
diff --git a/spaces/cloudtheboi/Lofi4All/.pythonlibs/lib/python3.10/site-packages/PIL/PdfParser.py b/spaces/cloudtheboi/Lofi4All/.pythonlibs/lib/python3.10/site-packages/PIL/PdfParser.py
deleted file mode 100644
index dc1012f54d3d0d683e96fed41ee7ace492904e71..0000000000000000000000000000000000000000
--- a/spaces/cloudtheboi/Lofi4All/.pythonlibs/lib/python3.10/site-packages/PIL/PdfParser.py
+++ /dev/null
@@ -1,996 +0,0 @@
-import calendar
-import codecs
-import collections
-import mmap
-import os
-import re
-import time
-import zlib
-
-
-# see 7.9.2.2 Text String Type on page 86 and D.3 PDFDocEncoding Character Set
-# on page 656
-def encode_text(s):
- return codecs.BOM_UTF16_BE + s.encode("utf_16_be")
-
-
-PDFDocEncoding = {
- 0x16: "\u0017",
- 0x18: "\u02D8",
- 0x19: "\u02C7",
- 0x1A: "\u02C6",
- 0x1B: "\u02D9",
- 0x1C: "\u02DD",
- 0x1D: "\u02DB",
- 0x1E: "\u02DA",
- 0x1F: "\u02DC",
- 0x80: "\u2022",
- 0x81: "\u2020",
- 0x82: "\u2021",
- 0x83: "\u2026",
- 0x84: "\u2014",
- 0x85: "\u2013",
- 0x86: "\u0192",
- 0x87: "\u2044",
- 0x88: "\u2039",
- 0x89: "\u203A",
- 0x8A: "\u2212",
- 0x8B: "\u2030",
- 0x8C: "\u201E",
- 0x8D: "\u201C",
- 0x8E: "\u201D",
- 0x8F: "\u2018",
- 0x90: "\u2019",
- 0x91: "\u201A",
- 0x92: "\u2122",
- 0x93: "\uFB01",
- 0x94: "\uFB02",
- 0x95: "\u0141",
- 0x96: "\u0152",
- 0x97: "\u0160",
- 0x98: "\u0178",
- 0x99: "\u017D",
- 0x9A: "\u0131",
- 0x9B: "\u0142",
- 0x9C: "\u0153",
- 0x9D: "\u0161",
- 0x9E: "\u017E",
- 0xA0: "\u20AC",
-}
-
-
-def decode_text(b):
- if b[: len(codecs.BOM_UTF16_BE)] == codecs.BOM_UTF16_BE:
- return b[len(codecs.BOM_UTF16_BE) :].decode("utf_16_be")
- else:
- return "".join(PDFDocEncoding.get(byte, chr(byte)) for byte in b)
-
-
-class PdfFormatError(RuntimeError):
- """An error that probably indicates a syntactic or semantic error in the
- PDF file structure"""
-
- pass
-
-
-def check_format_condition(condition, error_message):
- if not condition:
- raise PdfFormatError(error_message)
-
-
-class IndirectReference(
- collections.namedtuple("IndirectReferenceTuple", ["object_id", "generation"])
-):
- def __str__(self):
- return "%s %s R" % self
-
- def __bytes__(self):
- return self.__str__().encode("us-ascii")
-
- def __eq__(self, other):
- return (
- other.__class__ is self.__class__
- and other.object_id == self.object_id
- and other.generation == self.generation
- )
-
- def __ne__(self, other):
- return not (self == other)
-
- def __hash__(self):
- return hash((self.object_id, self.generation))
-
-
-class IndirectObjectDef(IndirectReference):
- def __str__(self):
- return "%s %s obj" % self
-
-
-class XrefTable:
- def __init__(self):
- self.existing_entries = {} # object ID => (offset, generation)
- self.new_entries = {} # object ID => (offset, generation)
- self.deleted_entries = {0: 65536} # object ID => generation
- self.reading_finished = False
-
- def __setitem__(self, key, value):
- if self.reading_finished:
- self.new_entries[key] = value
- else:
- self.existing_entries[key] = value
- if key in self.deleted_entries:
- del self.deleted_entries[key]
-
- def __getitem__(self, key):
- try:
- return self.new_entries[key]
- except KeyError:
- return self.existing_entries[key]
-
- def __delitem__(self, key):
- if key in self.new_entries:
- generation = self.new_entries[key][1] + 1
- del self.new_entries[key]
- self.deleted_entries[key] = generation
- elif key in self.existing_entries:
- generation = self.existing_entries[key][1] + 1
- self.deleted_entries[key] = generation
- elif key in self.deleted_entries:
- generation = self.deleted_entries[key]
- else:
- msg = (
- "object ID " + str(key) + " cannot be deleted because it doesn't exist"
- )
- raise IndexError(msg)
-
- def __contains__(self, key):
- return key in self.existing_entries or key in self.new_entries
-
- def __len__(self):
- return len(
- set(self.existing_entries.keys())
- | set(self.new_entries.keys())
- | set(self.deleted_entries.keys())
- )
-
- def keys(self):
- return (
- set(self.existing_entries.keys()) - set(self.deleted_entries.keys())
- ) | set(self.new_entries.keys())
-
- def write(self, f):
- keys = sorted(set(self.new_entries.keys()) | set(self.deleted_entries.keys()))
- deleted_keys = sorted(set(self.deleted_entries.keys()))
- startxref = f.tell()
- f.write(b"xref\n")
- while keys:
- # find a contiguous sequence of object IDs
- prev = None
- for index, key in enumerate(keys):
- if prev is None or prev + 1 == key:
- prev = key
- else:
- contiguous_keys = keys[:index]
- keys = keys[index:]
- break
- else:
- contiguous_keys = keys
- keys = None
- f.write(b"%d %d\n" % (contiguous_keys[0], len(contiguous_keys)))
- for object_id in contiguous_keys:
- if object_id in self.new_entries:
- f.write(b"%010d %05d n \n" % self.new_entries[object_id])
- else:
- this_deleted_object_id = deleted_keys.pop(0)
- check_format_condition(
- object_id == this_deleted_object_id,
- f"expected the next deleted object ID to be {object_id}, "
- f"instead found {this_deleted_object_id}",
- )
- try:
- next_in_linked_list = deleted_keys[0]
- except IndexError:
- next_in_linked_list = 0
- f.write(
- b"%010d %05d f \n"
- % (next_in_linked_list, self.deleted_entries[object_id])
- )
- return startxref
-
-
-class PdfName:
- def __init__(self, name):
- if isinstance(name, PdfName):
- self.name = name.name
- elif isinstance(name, bytes):
- self.name = name
- else:
- self.name = name.encode("us-ascii")
-
- def name_as_str(self):
- return self.name.decode("us-ascii")
-
- def __eq__(self, other):
- return (
- isinstance(other, PdfName) and other.name == self.name
- ) or other == self.name
-
- def __hash__(self):
- return hash(self.name)
-
- def __repr__(self):
- return f"PdfName({repr(self.name)})"
-
- @classmethod
- def from_pdf_stream(cls, data):
- return cls(PdfParser.interpret_name(data))
-
- allowed_chars = set(range(33, 127)) - {ord(c) for c in "#%/()<>[]{}"}
-
- def __bytes__(self):
- result = bytearray(b"/")
- for b in self.name:
- if b in self.allowed_chars:
- result.append(b)
- else:
- result.extend(b"#%02X" % b)
- return bytes(result)
-
-
-class PdfArray(list):
- def __bytes__(self):
- return b"[ " + b" ".join(pdf_repr(x) for x in self) + b" ]"
-
-
-class PdfDict(collections.UserDict):
- def __setattr__(self, key, value):
- if key == "data":
- collections.UserDict.__setattr__(self, key, value)
- else:
- self[key.encode("us-ascii")] = value
-
- def __getattr__(self, key):
- try:
- value = self[key.encode("us-ascii")]
- except KeyError as e:
- raise AttributeError(key) from e
- if isinstance(value, bytes):
- value = decode_text(value)
- if key.endswith("Date"):
- if value.startswith("D:"):
- value = value[2:]
-
- relationship = "Z"
- if len(value) > 17:
- relationship = value[14]
- offset = int(value[15:17]) * 60
- if len(value) > 20:
- offset += int(value[18:20])
-
- format = "%Y%m%d%H%M%S"[: len(value) - 2]
- value = time.strptime(value[: len(format) + 2], format)
- if relationship in ["+", "-"]:
- offset *= 60
- if relationship == "+":
- offset *= -1
- value = time.gmtime(calendar.timegm(value) + offset)
- return value
-
- def __bytes__(self):
- out = bytearray(b"<<")
- for key, value in self.items():
- if value is None:
- continue
- value = pdf_repr(value)
- out.extend(b"\n")
- out.extend(bytes(PdfName(key)))
- out.extend(b" ")
- out.extend(value)
- out.extend(b"\n>>")
- return bytes(out)
-
-
-class PdfBinary:
- def __init__(self, data):
- self.data = data
-
- def __bytes__(self):
- return b"<%s>" % b"".join(b"%02X" % b for b in self.data)
-
-
-class PdfStream:
- def __init__(self, dictionary, buf):
- self.dictionary = dictionary
- self.buf = buf
-
- def decode(self):
- try:
- filter = self.dictionary.Filter
- except AttributeError:
- return self.buf
- if filter == b"FlateDecode":
- try:
- expected_length = self.dictionary.DL
- except AttributeError:
- expected_length = self.dictionary.Length
- return zlib.decompress(self.buf, bufsize=int(expected_length))
- else:
- msg = f"stream filter {repr(self.dictionary.Filter)} unknown/unsupported"
- raise NotImplementedError(msg)
-
-
-def pdf_repr(x):
- if x is True:
- return b"true"
- elif x is False:
- return b"false"
- elif x is None:
- return b"null"
- elif isinstance(x, (PdfName, PdfDict, PdfArray, PdfBinary)):
- return bytes(x)
- elif isinstance(x, (int, float)):
- return str(x).encode("us-ascii")
- elif isinstance(x, time.struct_time):
- return b"(D:" + time.strftime("%Y%m%d%H%M%SZ", x).encode("us-ascii") + b")"
- elif isinstance(x, dict):
- return bytes(PdfDict(x))
- elif isinstance(x, list):
- return bytes(PdfArray(x))
- elif isinstance(x, str):
- return pdf_repr(encode_text(x))
- elif isinstance(x, bytes):
- # XXX escape more chars? handle binary garbage
- x = x.replace(b"\\", b"\\\\")
- x = x.replace(b"(", b"\\(")
- x = x.replace(b")", b"\\)")
- return b"(" + x + b")"
- else:
- return bytes(x)
-
-
-class PdfParser:
- """Based on
- https://www.adobe.com/content/dam/acom/en/devnet/acrobat/pdfs/PDF32000_2008.pdf
- Supports PDF up to 1.4
- """
-
- def __init__(self, filename=None, f=None, buf=None, start_offset=0, mode="rb"):
- if buf and f:
- msg = "specify buf or f or filename, but not both buf and f"
- raise RuntimeError(msg)
- self.filename = filename
- self.buf = buf
- self.f = f
- self.start_offset = start_offset
- self.should_close_buf = False
- self.should_close_file = False
- if filename is not None and f is None:
- self.f = f = open(filename, mode)
- self.should_close_file = True
- if f is not None:
- self.buf = buf = self.get_buf_from_file(f)
- self.should_close_buf = True
- if not filename and hasattr(f, "name"):
- self.filename = f.name
- self.cached_objects = {}
- if buf:
- self.read_pdf_info()
- else:
- self.file_size_total = self.file_size_this = 0
- self.root = PdfDict()
- self.root_ref = None
- self.info = PdfDict()
- self.info_ref = None
- self.page_tree_root = {}
- self.pages = []
- self.orig_pages = []
- self.pages_ref = None
- self.last_xref_section_offset = None
- self.trailer_dict = {}
- self.xref_table = XrefTable()
- self.xref_table.reading_finished = True
- if f:
- self.seek_end()
-
- def __enter__(self):
- return self
-
- def __exit__(self, exc_type, exc_value, traceback):
- self.close()
- return False # do not suppress exceptions
-
- def start_writing(self):
- self.close_buf()
- self.seek_end()
-
- def close_buf(self):
- try:
- self.buf.close()
- except AttributeError:
- pass
- self.buf = None
-
- def close(self):
- if self.should_close_buf:
- self.close_buf()
- if self.f is not None and self.should_close_file:
- self.f.close()
- self.f = None
-
- def seek_end(self):
- self.f.seek(0, os.SEEK_END)
-
- def write_header(self):
- self.f.write(b"%PDF-1.4\n")
-
- def write_comment(self, s):
- self.f.write(f"% {s}\n".encode())
-
- def write_catalog(self):
- self.del_root()
- self.root_ref = self.next_object_id(self.f.tell())
- self.pages_ref = self.next_object_id(0)
- self.rewrite_pages()
- self.write_obj(self.root_ref, Type=PdfName(b"Catalog"), Pages=self.pages_ref)
- self.write_obj(
- self.pages_ref,
- Type=PdfName(b"Pages"),
- Count=len(self.pages),
- Kids=self.pages,
- )
- return self.root_ref
-
- def rewrite_pages(self):
- pages_tree_nodes_to_delete = []
- for i, page_ref in enumerate(self.orig_pages):
- page_info = self.cached_objects[page_ref]
- del self.xref_table[page_ref.object_id]
- pages_tree_nodes_to_delete.append(page_info[PdfName(b"Parent")])
- if page_ref not in self.pages:
- # the page has been deleted
- continue
- # make dict keys into strings for passing to write_page
- stringified_page_info = {}
- for key, value in page_info.items():
- # key should be a PdfName
- stringified_page_info[key.name_as_str()] = value
- stringified_page_info["Parent"] = self.pages_ref
- new_page_ref = self.write_page(None, **stringified_page_info)
- for j, cur_page_ref in enumerate(self.pages):
- if cur_page_ref == page_ref:
- # replace the page reference with the new one
- self.pages[j] = new_page_ref
- # delete redundant Pages tree nodes from xref table
- for pages_tree_node_ref in pages_tree_nodes_to_delete:
- while pages_tree_node_ref:
- pages_tree_node = self.cached_objects[pages_tree_node_ref]
- if pages_tree_node_ref.object_id in self.xref_table:
- del self.xref_table[pages_tree_node_ref.object_id]
- pages_tree_node_ref = pages_tree_node.get(b"Parent", None)
- self.orig_pages = []
-
- def write_xref_and_trailer(self, new_root_ref=None):
- if new_root_ref:
- self.del_root()
- self.root_ref = new_root_ref
- if self.info:
- self.info_ref = self.write_obj(None, self.info)
- start_xref = self.xref_table.write(self.f)
- num_entries = len(self.xref_table)
- trailer_dict = {b"Root": self.root_ref, b"Size": num_entries}
- if self.last_xref_section_offset is not None:
- trailer_dict[b"Prev"] = self.last_xref_section_offset
- if self.info:
- trailer_dict[b"Info"] = self.info_ref
- self.last_xref_section_offset = start_xref
- self.f.write(
- b"trailer\n"
- + bytes(PdfDict(trailer_dict))
- + b"\nstartxref\n%d\n%%%%EOF" % start_xref
- )
-
- def write_page(self, ref, *objs, **dict_obj):
- if isinstance(ref, int):
- ref = self.pages[ref]
- if "Type" not in dict_obj:
- dict_obj["Type"] = PdfName(b"Page")
- if "Parent" not in dict_obj:
- dict_obj["Parent"] = self.pages_ref
- return self.write_obj(ref, *objs, **dict_obj)
-
- def write_obj(self, ref, *objs, **dict_obj):
- f = self.f
- if ref is None:
- ref = self.next_object_id(f.tell())
- else:
- self.xref_table[ref.object_id] = (f.tell(), ref.generation)
- f.write(bytes(IndirectObjectDef(*ref)))
- stream = dict_obj.pop("stream", None)
- if stream is not None:
- dict_obj["Length"] = len(stream)
- if dict_obj:
- f.write(pdf_repr(dict_obj))
- for obj in objs:
- f.write(pdf_repr(obj))
- if stream is not None:
- f.write(b"stream\n")
- f.write(stream)
- f.write(b"\nendstream\n")
- f.write(b"endobj\n")
- return ref
-
- def del_root(self):
- if self.root_ref is None:
- return
- del self.xref_table[self.root_ref.object_id]
- del self.xref_table[self.root[b"Pages"].object_id]
-
- @staticmethod
- def get_buf_from_file(f):
- if hasattr(f, "getbuffer"):
- return f.getbuffer()
- elif hasattr(f, "getvalue"):
- return f.getvalue()
- else:
- try:
- return mmap.mmap(f.fileno(), 0, access=mmap.ACCESS_READ)
- except ValueError: # cannot mmap an empty file
- return b""
-
- def read_pdf_info(self):
- self.file_size_total = len(self.buf)
- self.file_size_this = self.file_size_total - self.start_offset
- self.read_trailer()
- self.root_ref = self.trailer_dict[b"Root"]
- self.info_ref = self.trailer_dict.get(b"Info", None)
- self.root = PdfDict(self.read_indirect(self.root_ref))
- if self.info_ref is None:
- self.info = PdfDict()
- else:
- self.info = PdfDict(self.read_indirect(self.info_ref))
- check_format_condition(b"Type" in self.root, "/Type missing in Root")
- check_format_condition(
- self.root[b"Type"] == b"Catalog", "/Type in Root is not /Catalog"
- )
- check_format_condition(b"Pages" in self.root, "/Pages missing in Root")
- check_format_condition(
- isinstance(self.root[b"Pages"], IndirectReference),
- "/Pages in Root is not an indirect reference",
- )
- self.pages_ref = self.root[b"Pages"]
- self.page_tree_root = self.read_indirect(self.pages_ref)
- self.pages = self.linearize_page_tree(self.page_tree_root)
- # save the original list of page references
- # in case the user modifies, adds or deletes some pages
- # and we need to rewrite the pages and their list
- self.orig_pages = self.pages[:]
-
- def next_object_id(self, offset=None):
- try:
- # TODO: support reuse of deleted objects
- reference = IndirectReference(max(self.xref_table.keys()) + 1, 0)
- except ValueError:
- reference = IndirectReference(1, 0)
- if offset is not None:
- self.xref_table[reference.object_id] = (offset, 0)
- return reference
-
- delimiter = rb"[][()<>{}/%]"
- delimiter_or_ws = rb"[][()<>{}/%\000\011\012\014\015\040]"
- whitespace = rb"[\000\011\012\014\015\040]"
- whitespace_or_hex = rb"[\000\011\012\014\015\0400-9a-fA-F]"
- whitespace_optional = whitespace + b"*"
- whitespace_mandatory = whitespace + b"+"
- # No "\012" aka "\n" or "\015" aka "\r":
- whitespace_optional_no_nl = rb"[\000\011\014\040]*"
- newline_only = rb"[\r\n]+"
- newline = whitespace_optional_no_nl + newline_only + whitespace_optional_no_nl
- re_trailer_end = re.compile(
- whitespace_mandatory
- + rb"trailer"
- + whitespace_optional
- + rb"<<(.*>>)"
- + newline
- + rb"startxref"
- + newline
- + rb"([0-9]+)"
- + newline
- + rb"%%EOF"
- + whitespace_optional
- + rb"$",
- re.DOTALL,
- )
- re_trailer_prev = re.compile(
- whitespace_optional
- + rb"trailer"
- + whitespace_optional
- + rb"<<(.*?>>)"
- + newline
- + rb"startxref"
- + newline
- + rb"([0-9]+)"
- + newline
- + rb"%%EOF"
- + whitespace_optional,
- re.DOTALL,
- )
-
- def read_trailer(self):
- search_start_offset = len(self.buf) - 16384
- if search_start_offset < self.start_offset:
- search_start_offset = self.start_offset
- m = self.re_trailer_end.search(self.buf, search_start_offset)
- check_format_condition(m, "trailer end not found")
- # make sure we found the LAST trailer
- last_match = m
- while m:
- last_match = m
- m = self.re_trailer_end.search(self.buf, m.start() + 16)
- if not m:
- m = last_match
- trailer_data = m.group(1)
- self.last_xref_section_offset = int(m.group(2))
- self.trailer_dict = self.interpret_trailer(trailer_data)
- self.xref_table = XrefTable()
- self.read_xref_table(xref_section_offset=self.last_xref_section_offset)
- if b"Prev" in self.trailer_dict:
- self.read_prev_trailer(self.trailer_dict[b"Prev"])
-
- def read_prev_trailer(self, xref_section_offset):
- trailer_offset = self.read_xref_table(xref_section_offset=xref_section_offset)
- m = self.re_trailer_prev.search(
- self.buf[trailer_offset : trailer_offset + 16384]
- )
- check_format_condition(m, "previous trailer not found")
- trailer_data = m.group(1)
- check_format_condition(
- int(m.group(2)) == xref_section_offset,
- "xref section offset in previous trailer doesn't match what was expected",
- )
- trailer_dict = self.interpret_trailer(trailer_data)
- if b"Prev" in trailer_dict:
- self.read_prev_trailer(trailer_dict[b"Prev"])
-
- re_whitespace_optional = re.compile(whitespace_optional)
- re_name = re.compile(
- whitespace_optional
- + rb"/([!-$&'*-.0-;=?-Z\\^-z|~]+)(?="
- + delimiter_or_ws
- + rb")"
- )
- re_dict_start = re.compile(whitespace_optional + rb"<<")
- re_dict_end = re.compile(whitespace_optional + rb">>" + whitespace_optional)
-
- @classmethod
- def interpret_trailer(cls, trailer_data):
- trailer = {}
- offset = 0
- while True:
- m = cls.re_name.match(trailer_data, offset)
- if not m:
- m = cls.re_dict_end.match(trailer_data, offset)
- check_format_condition(
- m and m.end() == len(trailer_data),
- "name not found in trailer, remaining data: "
- + repr(trailer_data[offset:]),
- )
- break
- key = cls.interpret_name(m.group(1))
- value, offset = cls.get_value(trailer_data, m.end())
- trailer[key] = value
- check_format_condition(
- b"Size" in trailer and isinstance(trailer[b"Size"], int),
- "/Size not in trailer or not an integer",
- )
- check_format_condition(
- b"Root" in trailer and isinstance(trailer[b"Root"], IndirectReference),
- "/Root not in trailer or not an indirect reference",
- )
- return trailer
-
- re_hashes_in_name = re.compile(rb"([^#]*)(#([0-9a-fA-F]{2}))?")
-
- @classmethod
- def interpret_name(cls, raw, as_text=False):
- name = b""
- for m in cls.re_hashes_in_name.finditer(raw):
- if m.group(3):
- name += m.group(1) + bytearray.fromhex(m.group(3).decode("us-ascii"))
- else:
- name += m.group(1)
- if as_text:
- return name.decode("utf-8")
- else:
- return bytes(name)
-
- re_null = re.compile(whitespace_optional + rb"null(?=" + delimiter_or_ws + rb")")
- re_true = re.compile(whitespace_optional + rb"true(?=" + delimiter_or_ws + rb")")
- re_false = re.compile(whitespace_optional + rb"false(?=" + delimiter_or_ws + rb")")
- re_int = re.compile(
- whitespace_optional + rb"([-+]?[0-9]+)(?=" + delimiter_or_ws + rb")"
- )
- re_real = re.compile(
- whitespace_optional
- + rb"([-+]?([0-9]+\.[0-9]*|[0-9]*\.[0-9]+))(?="
- + delimiter_or_ws
- + rb")"
- )
- re_array_start = re.compile(whitespace_optional + rb"\[")
- re_array_end = re.compile(whitespace_optional + rb"]")
- re_string_hex = re.compile(
- whitespace_optional + rb"<(" + whitespace_or_hex + rb"*)>"
- )
- re_string_lit = re.compile(whitespace_optional + rb"\(")
- re_indirect_reference = re.compile(
- whitespace_optional
- + rb"([-+]?[0-9]+)"
- + whitespace_mandatory
- + rb"([-+]?[0-9]+)"
- + whitespace_mandatory
- + rb"R(?="
- + delimiter_or_ws
- + rb")"
- )
- re_indirect_def_start = re.compile(
- whitespace_optional
- + rb"([-+]?[0-9]+)"
- + whitespace_mandatory
- + rb"([-+]?[0-9]+)"
- + whitespace_mandatory
- + rb"obj(?="
- + delimiter_or_ws
- + rb")"
- )
- re_indirect_def_end = re.compile(
- whitespace_optional + rb"endobj(?=" + delimiter_or_ws + rb")"
- )
- re_comment = re.compile(
- rb"(" + whitespace_optional + rb"%[^\r\n]*" + newline + rb")*"
- )
- re_stream_start = re.compile(whitespace_optional + rb"stream\r?\n")
- re_stream_end = re.compile(
- whitespace_optional + rb"endstream(?=" + delimiter_or_ws + rb")"
- )
-
- @classmethod
- def get_value(cls, data, offset, expect_indirect=None, max_nesting=-1):
- if max_nesting == 0:
- return None, None
- m = cls.re_comment.match(data, offset)
- if m:
- offset = m.end()
- m = cls.re_indirect_def_start.match(data, offset)
- if m:
- check_format_condition(
- int(m.group(1)) > 0,
- "indirect object definition: object ID must be greater than 0",
- )
- check_format_condition(
- int(m.group(2)) >= 0,
- "indirect object definition: generation must be non-negative",
- )
- check_format_condition(
- expect_indirect is None
- or expect_indirect
- == IndirectReference(int(m.group(1)), int(m.group(2))),
- "indirect object definition different than expected",
- )
- object, offset = cls.get_value(data, m.end(), max_nesting=max_nesting - 1)
- if offset is None:
- return object, None
- m = cls.re_indirect_def_end.match(data, offset)
- check_format_condition(m, "indirect object definition end not found")
- return object, m.end()
- check_format_condition(
- not expect_indirect, "indirect object definition not found"
- )
- m = cls.re_indirect_reference.match(data, offset)
- if m:
- check_format_condition(
- int(m.group(1)) > 0,
- "indirect object reference: object ID must be greater than 0",
- )
- check_format_condition(
- int(m.group(2)) >= 0,
- "indirect object reference: generation must be non-negative",
- )
- return IndirectReference(int(m.group(1)), int(m.group(2))), m.end()
- m = cls.re_dict_start.match(data, offset)
- if m:
- offset = m.end()
- result = {}
- m = cls.re_dict_end.match(data, offset)
- while not m:
- key, offset = cls.get_value(data, offset, max_nesting=max_nesting - 1)
- if offset is None:
- return result, None
- value, offset = cls.get_value(data, offset, max_nesting=max_nesting - 1)
- result[key] = value
- if offset is None:
- return result, None
- m = cls.re_dict_end.match(data, offset)
- offset = m.end()
- m = cls.re_stream_start.match(data, offset)
- if m:
- try:
- stream_len = int(result[b"Length"])
- except (TypeError, KeyError, ValueError) as e:
- msg = "bad or missing Length in stream dict (%r)" % result.get(
- b"Length", None
- )
- raise PdfFormatError(msg) from e
- stream_data = data[m.end() : m.end() + stream_len]
- m = cls.re_stream_end.match(data, m.end() + stream_len)
- check_format_condition(m, "stream end not found")
- offset = m.end()
- result = PdfStream(PdfDict(result), stream_data)
- else:
- result = PdfDict(result)
- return result, offset
- m = cls.re_array_start.match(data, offset)
- if m:
- offset = m.end()
- result = []
- m = cls.re_array_end.match(data, offset)
- while not m:
- value, offset = cls.get_value(data, offset, max_nesting=max_nesting - 1)
- result.append(value)
- if offset is None:
- return result, None
- m = cls.re_array_end.match(data, offset)
- return result, m.end()
- m = cls.re_null.match(data, offset)
- if m:
- return None, m.end()
- m = cls.re_true.match(data, offset)
- if m:
- return True, m.end()
- m = cls.re_false.match(data, offset)
- if m:
- return False, m.end()
- m = cls.re_name.match(data, offset)
- if m:
- return PdfName(cls.interpret_name(m.group(1))), m.end()
- m = cls.re_int.match(data, offset)
- if m:
- return int(m.group(1)), m.end()
- m = cls.re_real.match(data, offset)
- if m:
- # XXX Decimal instead of float???
- return float(m.group(1)), m.end()
- m = cls.re_string_hex.match(data, offset)
- if m:
- # filter out whitespace
- hex_string = bytearray(
- b for b in m.group(1) if b in b"0123456789abcdefABCDEF"
- )
- if len(hex_string) % 2 == 1:
- # append a 0 if the length is not even - yes, at the end
- hex_string.append(ord(b"0"))
- return bytearray.fromhex(hex_string.decode("us-ascii")), m.end()
- m = cls.re_string_lit.match(data, offset)
- if m:
- return cls.get_literal_string(data, m.end())
- # return None, offset # fallback (only for debugging)
- msg = "unrecognized object: " + repr(data[offset : offset + 32])
- raise PdfFormatError(msg)
-
- re_lit_str_token = re.compile(
- rb"(\\[nrtbf()\\])|(\\[0-9]{1,3})|(\\(\r\n|\r|\n))|(\r\n|\r|\n)|(\()|(\))"
- )
- escaped_chars = {
- b"n": b"\n",
- b"r": b"\r",
- b"t": b"\t",
- b"b": b"\b",
- b"f": b"\f",
- b"(": b"(",
- b")": b")",
- b"\\": b"\\",
- ord(b"n"): b"\n",
- ord(b"r"): b"\r",
- ord(b"t"): b"\t",
- ord(b"b"): b"\b",
- ord(b"f"): b"\f",
- ord(b"("): b"(",
- ord(b")"): b")",
- ord(b"\\"): b"\\",
- }
-
- @classmethod
- def get_literal_string(cls, data, offset):
- nesting_depth = 0
- result = bytearray()
- for m in cls.re_lit_str_token.finditer(data, offset):
- result.extend(data[offset : m.start()])
- if m.group(1):
- result.extend(cls.escaped_chars[m.group(1)[1]])
- elif m.group(2):
- result.append(int(m.group(2)[1:], 8))
- elif m.group(3):
- pass
- elif m.group(5):
- result.extend(b"\n")
- elif m.group(6):
- result.extend(b"(")
- nesting_depth += 1
- elif m.group(7):
- if nesting_depth == 0:
- return bytes(result), m.end()
- result.extend(b")")
- nesting_depth -= 1
- offset = m.end()
- msg = "unfinished literal string"
- raise PdfFormatError(msg)
-
- re_xref_section_start = re.compile(whitespace_optional + rb"xref" + newline)
- re_xref_subsection_start = re.compile(
- whitespace_optional
- + rb"([0-9]+)"
- + whitespace_mandatory
- + rb"([0-9]+)"
- + whitespace_optional
- + newline_only
- )
- re_xref_entry = re.compile(rb"([0-9]{10}) ([0-9]{5}) ([fn])( \r| \n|\r\n)")
-
- def read_xref_table(self, xref_section_offset):
- subsection_found = False
- m = self.re_xref_section_start.match(
- self.buf, xref_section_offset + self.start_offset
- )
- check_format_condition(m, "xref section start not found")
- offset = m.end()
- while True:
- m = self.re_xref_subsection_start.match(self.buf, offset)
- if not m:
- check_format_condition(
- subsection_found, "xref subsection start not found"
- )
- break
- subsection_found = True
- offset = m.end()
- first_object = int(m.group(1))
- num_objects = int(m.group(2))
- for i in range(first_object, first_object + num_objects):
- m = self.re_xref_entry.match(self.buf, offset)
- check_format_condition(m, "xref entry not found")
- offset = m.end()
- is_free = m.group(3) == b"f"
- if not is_free:
- generation = int(m.group(2))
- new_entry = (int(m.group(1)), generation)
- if i not in self.xref_table:
- self.xref_table[i] = new_entry
- return offset
-
- def read_indirect(self, ref, max_nesting=-1):
- offset, generation = self.xref_table[ref[0]]
- check_format_condition(
- generation == ref[1],
- f"expected to find generation {ref[1]} for object ID {ref[0]} in xref "
- f"table, instead found generation {generation} at offset {offset}",
- )
- value = self.get_value(
- self.buf,
- offset + self.start_offset,
- expect_indirect=IndirectReference(*ref),
- max_nesting=max_nesting,
- )[0]
- self.cached_objects[ref] = value
- return value
-
- def linearize_page_tree(self, node=None):
- if node is None:
- node = self.page_tree_root
- check_format_condition(
- node[b"Type"] == b"Pages", "/Type of page tree node is not /Pages"
- )
- pages = []
- for kid in node[b"Kids"]:
- kid_object = self.read_indirect(kid)
- if kid_object[b"Type"] == b"Page":
- pages.append(kid)
- else:
- pages.extend(self.linearize_page_tree(node=kid_object))
- return pages
diff --git a/spaces/cloudtheboi/Lofi4All/.pythonlibs/lib/python3.10/site-packages/anyio/to_thread.py b/spaces/cloudtheboi/Lofi4All/.pythonlibs/lib/python3.10/site-packages/anyio/to_thread.py
deleted file mode 100644
index 9315d1ecf16eee45cd129ce17e48041a7f82348a..0000000000000000000000000000000000000000
--- a/spaces/cloudtheboi/Lofi4All/.pythonlibs/lib/python3.10/site-packages/anyio/to_thread.py
+++ /dev/null
@@ -1,67 +0,0 @@
-from __future__ import annotations
-
-from typing import Callable, TypeVar
-from warnings import warn
-
-from ._core._eventloop import get_asynclib
-from .abc import CapacityLimiter
-
-T_Retval = TypeVar("T_Retval")
-
-
-async def run_sync(
- func: Callable[..., T_Retval],
- *args: object,
- cancellable: bool = False,
- limiter: CapacityLimiter | None = None,
-) -> T_Retval:
- """
- Call the given function with the given arguments in a worker thread.
-
- If the ``cancellable`` option is enabled and the task waiting for its completion is cancelled,
- the thread will still run its course but its return value (or any raised exception) will be
- ignored.
-
- :param func: a callable
- :param args: positional arguments for the callable
- :param cancellable: ``True`` to allow cancellation of the operation
- :param limiter: capacity limiter to use to limit the total amount of threads running
- (if omitted, the default limiter is used)
- :return: an awaitable that yields the return value of the function.
-
- """
- return await get_asynclib().run_sync_in_worker_thread(
- func, *args, cancellable=cancellable, limiter=limiter
- )
-
-
-async def run_sync_in_worker_thread(
- func: Callable[..., T_Retval],
- *args: object,
- cancellable: bool = False,
- limiter: CapacityLimiter | None = None,
-) -> T_Retval:
- warn(
- "run_sync_in_worker_thread() has been deprecated, use anyio.to_thread.run_sync() instead",
- DeprecationWarning,
- )
- return await run_sync(func, *args, cancellable=cancellable, limiter=limiter)
-
-
-def current_default_thread_limiter() -> CapacityLimiter:
- """
- Return the capacity limiter that is used by default to limit the number of concurrent threads.
-
- :return: a capacity limiter object
-
- """
- return get_asynclib().current_default_thread_limiter()
-
-
-def current_default_worker_thread_limiter() -> CapacityLimiter:
- warn(
- "current_default_worker_thread_limiter() has been deprecated, "
- "use anyio.to_thread.current_default_thread_limiter() instead",
- DeprecationWarning,
- )
- return current_default_thread_limiter()
diff --git a/spaces/cloudtheboi/Lofi4All/.pythonlibs/lib/python3.10/site-packages/cffi/_embedding.h b/spaces/cloudtheboi/Lofi4All/.pythonlibs/lib/python3.10/site-packages/cffi/_embedding.h
deleted file mode 100644
index 8e8df882d475b3672af183044602ce564ce0720c..0000000000000000000000000000000000000000
--- a/spaces/cloudtheboi/Lofi4All/.pythonlibs/lib/python3.10/site-packages/cffi/_embedding.h
+++ /dev/null
@@ -1,528 +0,0 @@
-
-/***** Support code for embedding *****/
-
-#ifdef __cplusplus
-extern "C" {
-#endif
-
-
-#if defined(_WIN32)
-# define CFFI_DLLEXPORT __declspec(dllexport)
-#elif defined(__GNUC__)
-# define CFFI_DLLEXPORT __attribute__((visibility("default")))
-#else
-# define CFFI_DLLEXPORT /* nothing */
-#endif
-
-
-/* There are two global variables of type _cffi_call_python_fnptr:
-
- * _cffi_call_python, which we declare just below, is the one called
- by ``extern "Python"`` implementations.
-
- * _cffi_call_python_org, which on CPython is actually part of the
- _cffi_exports[] array, is the function pointer copied from
- _cffi_backend. If _cffi_start_python() fails, then this is set
- to NULL; otherwise, it should never be NULL.
-
- After initialization is complete, both are equal. However, the
- first one remains equal to &_cffi_start_and_call_python until the
- very end of initialization, when we are (or should be) sure that
- concurrent threads also see a completely initialized world, and
- only then is it changed.
-*/
-#undef _cffi_call_python
-typedef void (*_cffi_call_python_fnptr)(struct _cffi_externpy_s *, char *);
-static void _cffi_start_and_call_python(struct _cffi_externpy_s *, char *);
-static _cffi_call_python_fnptr _cffi_call_python = &_cffi_start_and_call_python;
-
-
-#ifndef _MSC_VER
- /* --- Assuming a GCC not infinitely old --- */
-# define cffi_compare_and_swap(l,o,n) __sync_bool_compare_and_swap(l,o,n)
-# define cffi_write_barrier() __sync_synchronize()
-# if !defined(__amd64__) && !defined(__x86_64__) && \
- !defined(__i386__) && !defined(__i386)
-# define cffi_read_barrier() __sync_synchronize()
-# else
-# define cffi_read_barrier() (void)0
-# endif
-#else
- /* --- Windows threads version --- */
-# include
-# define cffi_compare_and_swap(l,o,n) \
- (InterlockedCompareExchangePointer(l,n,o) == (o))
-# define cffi_write_barrier() InterlockedCompareExchange(&_cffi_dummy,0,0)
-# define cffi_read_barrier() (void)0
-static volatile LONG _cffi_dummy;
-#endif
-
-#ifdef WITH_THREAD
-# ifndef _MSC_VER
-# include
- static pthread_mutex_t _cffi_embed_startup_lock;
-# else
- static CRITICAL_SECTION _cffi_embed_startup_lock;
-# endif
- static char _cffi_embed_startup_lock_ready = 0;
-#endif
-
-static void _cffi_acquire_reentrant_mutex(void)
-{
- static void *volatile lock = NULL;
-
- while (!cffi_compare_and_swap(&lock, NULL, (void *)1)) {
- /* should ideally do a spin loop instruction here, but
- hard to do it portably and doesn't really matter I
- think: pthread_mutex_init() should be very fast, and
- this is only run at start-up anyway. */
- }
-
-#ifdef WITH_THREAD
- if (!_cffi_embed_startup_lock_ready) {
-# ifndef _MSC_VER
- pthread_mutexattr_t attr;
- pthread_mutexattr_init(&attr);
- pthread_mutexattr_settype(&attr, PTHREAD_MUTEX_RECURSIVE);
- pthread_mutex_init(&_cffi_embed_startup_lock, &attr);
-# else
- InitializeCriticalSection(&_cffi_embed_startup_lock);
-# endif
- _cffi_embed_startup_lock_ready = 1;
- }
-#endif
-
- while (!cffi_compare_and_swap(&lock, (void *)1, NULL))
- ;
-
-#ifndef _MSC_VER
- pthread_mutex_lock(&_cffi_embed_startup_lock);
-#else
- EnterCriticalSection(&_cffi_embed_startup_lock);
-#endif
-}
-
-static void _cffi_release_reentrant_mutex(void)
-{
-#ifndef _MSC_VER
- pthread_mutex_unlock(&_cffi_embed_startup_lock);
-#else
- LeaveCriticalSection(&_cffi_embed_startup_lock);
-#endif
-}
-
-
-/********** CPython-specific section **********/
-#ifndef PYPY_VERSION
-
-#include "_cffi_errors.h"
-
-
-#define _cffi_call_python_org _cffi_exports[_CFFI_CPIDX]
-
-PyMODINIT_FUNC _CFFI_PYTHON_STARTUP_FUNC(void); /* forward */
-
-static void _cffi_py_initialize(void)
-{
- /* XXX use initsigs=0, which "skips initialization registration of
- signal handlers, which might be useful when Python is
- embedded" according to the Python docs. But review and think
- if it should be a user-controllable setting.
-
- XXX we should also give a way to write errors to a buffer
- instead of to stderr.
-
- XXX if importing 'site' fails, CPython (any version) calls
- exit(). Should we try to work around this behavior here?
- */
- Py_InitializeEx(0);
-}
-
-static int _cffi_initialize_python(void)
-{
- /* This initializes Python, imports _cffi_backend, and then the
- present .dll/.so is set up as a CPython C extension module.
- */
- int result;
- PyGILState_STATE state;
- PyObject *pycode=NULL, *global_dict=NULL, *x;
- PyObject *builtins;
-
- state = PyGILState_Ensure();
-
- /* Call the initxxx() function from the present module. It will
- create and initialize us as a CPython extension module, instead
- of letting the startup Python code do it---it might reimport
- the same .dll/.so and get maybe confused on some platforms.
- It might also have troubles locating the .dll/.so again for all
- I know.
- */
- (void)_CFFI_PYTHON_STARTUP_FUNC();
- if (PyErr_Occurred())
- goto error;
-
- /* Now run the Python code provided to ffi.embedding_init_code().
- */
- pycode = Py_CompileString(_CFFI_PYTHON_STARTUP_CODE,
- "",
- Py_file_input);
- if (pycode == NULL)
- goto error;
- global_dict = PyDict_New();
- if (global_dict == NULL)
- goto error;
- builtins = PyEval_GetBuiltins();
- if (builtins == NULL)
- goto error;
- if (PyDict_SetItemString(global_dict, "__builtins__", builtins) < 0)
- goto error;
- x = PyEval_EvalCode(
-#if PY_MAJOR_VERSION < 3
- (PyCodeObject *)
-#endif
- pycode, global_dict, global_dict);
- if (x == NULL)
- goto error;
- Py_DECREF(x);
-
- /* Done! Now if we've been called from
- _cffi_start_and_call_python() in an ``extern "Python"``, we can
- only hope that the Python code did correctly set up the
- corresponding @ffi.def_extern() function. Otherwise, the
- general logic of ``extern "Python"`` functions (inside the
- _cffi_backend module) will find that the reference is still
- missing and print an error.
- */
- result = 0;
- done:
- Py_XDECREF(pycode);
- Py_XDECREF(global_dict);
- PyGILState_Release(state);
- return result;
-
- error:;
- {
- /* Print as much information as potentially useful.
- Debugging load-time failures with embedding is not fun
- */
- PyObject *ecap;
- PyObject *exception, *v, *tb, *f, *modules, *mod;
- PyErr_Fetch(&exception, &v, &tb);
- ecap = _cffi_start_error_capture();
- f = PySys_GetObject((char *)"stderr");
- if (f != NULL && f != Py_None) {
- PyFile_WriteString(
- "Failed to initialize the Python-CFFI embedding logic:\n\n", f);
- }
-
- if (exception != NULL) {
- PyErr_NormalizeException(&exception, &v, &tb);
- PyErr_Display(exception, v, tb);
- }
- Py_XDECREF(exception);
- Py_XDECREF(v);
- Py_XDECREF(tb);
-
- if (f != NULL && f != Py_None) {
- PyFile_WriteString("\nFrom: " _CFFI_MODULE_NAME
- "\ncompiled with cffi version: 1.15.1"
- "\n_cffi_backend module: ", f);
- modules = PyImport_GetModuleDict();
- mod = PyDict_GetItemString(modules, "_cffi_backend");
- if (mod == NULL) {
- PyFile_WriteString("not loaded", f);
- }
- else {
- v = PyObject_GetAttrString(mod, "__file__");
- PyFile_WriteObject(v, f, 0);
- Py_XDECREF(v);
- }
- PyFile_WriteString("\nsys.path: ", f);
- PyFile_WriteObject(PySys_GetObject((char *)"path"), f, 0);
- PyFile_WriteString("\n\n", f);
- }
- _cffi_stop_error_capture(ecap);
- }
- result = -1;
- goto done;
-}
-
-#if PY_VERSION_HEX < 0x03080000
-PyAPI_DATA(char *) _PyParser_TokenNames[]; /* from CPython */
-#endif
-
-static int _cffi_carefully_make_gil(void)
-{
- /* This does the basic initialization of Python. It can be called
- completely concurrently from unrelated threads. It assumes
- that we don't hold the GIL before (if it exists), and we don't
- hold it afterwards.
-
- (What it really does used to be completely different in Python 2
- and Python 3, with the Python 2 solution avoiding the spin-lock
- around the Py_InitializeEx() call. However, after recent changes
- to CPython 2.7 (issue #358) it no longer works. So we use the
- Python 3 solution everywhere.)
-
- This initializes Python by calling Py_InitializeEx().
- Important: this must not be called concurrently at all.
- So we use a global variable as a simple spin lock. This global
- variable must be from 'libpythonX.Y.so', not from this
- cffi-based extension module, because it must be shared from
- different cffi-based extension modules.
-
- In Python < 3.8, we choose
- _PyParser_TokenNames[0] as a completely arbitrary pointer value
- that is never written to. The default is to point to the
- string "ENDMARKER". We change it temporarily to point to the
- next character in that string. (Yes, I know it's REALLY
- obscure.)
-
- In Python >= 3.8, this string array is no longer writable, so
- instead we pick PyCapsuleType.tp_version_tag. We can't change
- Python < 3.8 because someone might use a mixture of cffi
- embedded modules, some of which were compiled before this file
- changed.
- */
-
-#ifdef WITH_THREAD
-# if PY_VERSION_HEX < 0x03080000
- char *volatile *lock = (char *volatile *)_PyParser_TokenNames;
- char *old_value, *locked_value;
-
- while (1) { /* spin loop */
- old_value = *lock;
- locked_value = old_value + 1;
- if (old_value[0] == 'E') {
- assert(old_value[1] == 'N');
- if (cffi_compare_and_swap(lock, old_value, locked_value))
- break;
- }
- else {
- assert(old_value[0] == 'N');
- /* should ideally do a spin loop instruction here, but
- hard to do it portably and doesn't really matter I
- think: PyEval_InitThreads() should be very fast, and
- this is only run at start-up anyway. */
- }
- }
-# else
- int volatile *lock = (int volatile *)&PyCapsule_Type.tp_version_tag;
- int old_value, locked_value;
- assert(!(PyCapsule_Type.tp_flags & Py_TPFLAGS_HAVE_VERSION_TAG));
-
- while (1) { /* spin loop */
- old_value = *lock;
- locked_value = -42;
- if (old_value == 0) {
- if (cffi_compare_and_swap(lock, old_value, locked_value))
- break;
- }
- else {
- assert(old_value == locked_value);
- /* should ideally do a spin loop instruction here, but
- hard to do it portably and doesn't really matter I
- think: PyEval_InitThreads() should be very fast, and
- this is only run at start-up anyway. */
- }
- }
-# endif
-#endif
-
- /* call Py_InitializeEx() */
- if (!Py_IsInitialized()) {
- _cffi_py_initialize();
-#if PY_VERSION_HEX < 0x03070000
- PyEval_InitThreads();
-#endif
- PyEval_SaveThread(); /* release the GIL */
- /* the returned tstate must be the one that has been stored into the
- autoTLSkey by _PyGILState_Init() called from Py_Initialize(). */
- }
- else {
-#if PY_VERSION_HEX < 0x03070000
- /* PyEval_InitThreads() is always a no-op from CPython 3.7 */
- PyGILState_STATE state = PyGILState_Ensure();
- PyEval_InitThreads();
- PyGILState_Release(state);
-#endif
- }
-
-#ifdef WITH_THREAD
- /* release the lock */
- while (!cffi_compare_and_swap(lock, locked_value, old_value))
- ;
-#endif
-
- return 0;
-}
-
-/********** end CPython-specific section **********/
-
-
-#else
-
-
-/********** PyPy-specific section **********/
-
-PyMODINIT_FUNC _CFFI_PYTHON_STARTUP_FUNC(const void *[]); /* forward */
-
-static struct _cffi_pypy_init_s {
- const char *name;
- void *func; /* function pointer */
- const char *code;
-} _cffi_pypy_init = {
- _CFFI_MODULE_NAME,
- _CFFI_PYTHON_STARTUP_FUNC,
- _CFFI_PYTHON_STARTUP_CODE,
-};
-
-extern int pypy_carefully_make_gil(const char *);
-extern int pypy_init_embedded_cffi_module(int, struct _cffi_pypy_init_s *);
-
-static int _cffi_carefully_make_gil(void)
-{
- return pypy_carefully_make_gil(_CFFI_MODULE_NAME);
-}
-
-static int _cffi_initialize_python(void)
-{
- return pypy_init_embedded_cffi_module(0xB011, &_cffi_pypy_init);
-}
-
-/********** end PyPy-specific section **********/
-
-
-#endif
-
-
-#ifdef __GNUC__
-__attribute__((noinline))
-#endif
-static _cffi_call_python_fnptr _cffi_start_python(void)
-{
- /* Delicate logic to initialize Python. This function can be
- called multiple times concurrently, e.g. when the process calls
- its first ``extern "Python"`` functions in multiple threads at
- once. It can also be called recursively, in which case we must
- ignore it. We also have to consider what occurs if several
- different cffi-based extensions reach this code in parallel
- threads---it is a different copy of the code, then, and we
- can't have any shared global variable unless it comes from
- 'libpythonX.Y.so'.
-
- Idea:
-
- * _cffi_carefully_make_gil(): "carefully" call
- PyEval_InitThreads() (possibly with Py_InitializeEx() first).
-
- * then we use a (local) custom lock to make sure that a call to this
- cffi-based extension will wait if another call to the *same*
- extension is running the initialization in another thread.
- It is reentrant, so that a recursive call will not block, but
- only one from a different thread.
-
- * then we grab the GIL and (Python 2) we call Py_InitializeEx().
- At this point, concurrent calls to Py_InitializeEx() are not
- possible: we have the GIL.
-
- * do the rest of the specific initialization, which may
- temporarily release the GIL but not the custom lock.
- Only release the custom lock when we are done.
- */
- static char called = 0;
-
- if (_cffi_carefully_make_gil() != 0)
- return NULL;
-
- _cffi_acquire_reentrant_mutex();
-
- /* Here the GIL exists, but we don't have it. We're only protected
- from concurrency by the reentrant mutex. */
-
- /* This file only initializes the embedded module once, the first
- time this is called, even if there are subinterpreters. */
- if (!called) {
- called = 1; /* invoke _cffi_initialize_python() only once,
- but don't set '_cffi_call_python' right now,
- otherwise concurrent threads won't call
- this function at all (we need them to wait) */
- if (_cffi_initialize_python() == 0) {
- /* now initialization is finished. Switch to the fast-path. */
-
- /* We would like nobody to see the new value of
- '_cffi_call_python' without also seeing the rest of the
- data initialized. However, this is not possible. But
- the new value of '_cffi_call_python' is the function
- 'cffi_call_python()' from _cffi_backend. So: */
- cffi_write_barrier();
- /* ^^^ we put a write barrier here, and a corresponding
- read barrier at the start of cffi_call_python(). This
- ensures that after that read barrier, we see everything
- done here before the write barrier.
- */
-
- assert(_cffi_call_python_org != NULL);
- _cffi_call_python = (_cffi_call_python_fnptr)_cffi_call_python_org;
- }
- else {
- /* initialization failed. Reset this to NULL, even if it was
- already set to some other value. Future calls to
- _cffi_start_python() are still forced to occur, and will
- always return NULL from now on. */
- _cffi_call_python_org = NULL;
- }
- }
-
- _cffi_release_reentrant_mutex();
-
- return (_cffi_call_python_fnptr)_cffi_call_python_org;
-}
-
-static
-void _cffi_start_and_call_python(struct _cffi_externpy_s *externpy, char *args)
-{
- _cffi_call_python_fnptr fnptr;
- int current_err = errno;
-#ifdef _MSC_VER
- int current_lasterr = GetLastError();
-#endif
- fnptr = _cffi_start_python();
- if (fnptr == NULL) {
- fprintf(stderr, "function %s() called, but initialization code "
- "failed. Returning 0.\n", externpy->name);
- memset(args, 0, externpy->size_of_result);
- }
-#ifdef _MSC_VER
- SetLastError(current_lasterr);
-#endif
- errno = current_err;
-
- if (fnptr != NULL)
- fnptr(externpy, args);
-}
-
-
-/* The cffi_start_python() function makes sure Python is initialized
- and our cffi module is set up. It can be called manually from the
- user C code. The same effect is obtained automatically from any
- dll-exported ``extern "Python"`` function. This function returns
- -1 if initialization failed, 0 if all is OK. */
-_CFFI_UNUSED_FN
-static int cffi_start_python(void)
-{
- if (_cffi_call_python == &_cffi_start_and_call_python) {
- if (_cffi_start_python() == NULL)
- return -1;
- }
- cffi_read_barrier();
- return 0;
-}
-
-#undef cffi_compare_and_swap
-#undef cffi_write_barrier
-#undef cffi_read_barrier
-
-#ifdef __cplusplus
-}
-#endif
diff --git a/spaces/codelion/Grounding_DINO_demo/groundingdino/models/GroundingDINO/csrc/vision.cpp b/spaces/codelion/Grounding_DINO_demo/groundingdino/models/GroundingDINO/csrc/vision.cpp
deleted file mode 100644
index c1f2c50c82909bbd5492c163d634af77a3ba1781..0000000000000000000000000000000000000000
--- a/spaces/codelion/Grounding_DINO_demo/groundingdino/models/GroundingDINO/csrc/vision.cpp
+++ /dev/null
@@ -1,58 +0,0 @@
-// Copyright (c) Facebook, Inc. and its affiliates. All Rights Reserved
-
-#include "MsDeformAttn/ms_deform_attn.h"
-
-namespace groundingdino {
-
-#ifdef WITH_CUDA
-extern int get_cudart_version();
-#endif
-
-std::string get_cuda_version() {
-#ifdef WITH_CUDA
- std::ostringstream oss;
-
- // copied from
- // https://github.com/pytorch/pytorch/blob/master/aten/src/ATen/cuda/detail/CUDAHooks.cpp#L231
- auto printCudaStyleVersion = [&](int v) {
- oss << (v / 1000) << "." << (v / 10 % 100);
- if (v % 10 != 0) {
- oss << "." << (v % 10);
- }
- };
- printCudaStyleVersion(get_cudart_version());
- return oss.str();
-#else
- return std::string("not available");
-#endif
-}
-
-// similar to
-// https://github.com/pytorch/pytorch/blob/master/aten/src/ATen/Version.cpp
-std::string get_compiler_version() {
- std::ostringstream ss;
-#if defined(__GNUC__)
-#ifndef __clang__
- { ss << "GCC " << __GNUC__ << "." << __GNUC_MINOR__; }
-#endif
-#endif
-
-#if defined(__clang_major__)
- {
- ss << "clang " << __clang_major__ << "." << __clang_minor__ << "."
- << __clang_patchlevel__;
- }
-#endif
-
-#if defined(_MSC_VER)
- { ss << "MSVC " << _MSC_FULL_VER; }
-#endif
- return ss.str();
-}
-
-PYBIND11_MODULE(TORCH_EXTENSION_NAME, m) {
- m.def("ms_deform_attn_forward", &ms_deform_attn_forward, "ms_deform_attn_forward");
- m.def("ms_deform_attn_backward", &ms_deform_attn_backward, "ms_deform_attn_backward");
-}
-
-} // namespace groundingdino
\ No newline at end of file
diff --git a/spaces/colakin/video-generater/public/ffmpeg/libavcodec/aarch64/idct.h b/spaces/colakin/video-generater/public/ffmpeg/libavcodec/aarch64/idct.h
deleted file mode 100644
index 97ee0a64af347cdf422ea0a60da677ac0868e2f7..0000000000000000000000000000000000000000
--- a/spaces/colakin/video-generater/public/ffmpeg/libavcodec/aarch64/idct.h
+++ /dev/null
@@ -1,29 +0,0 @@
-/*
- * This file is part of FFmpeg.
- *
- * FFmpeg is free software; you can redistribute it and/or
- * modify it under the terms of the GNU Lesser General Public
- * License as published by the Free Software Foundation; either
- * version 2.1 of the License, or (at your option) any later version.
- *
- * FFmpeg is distributed in the hope that it will be useful,
- * but WITHOUT ANY WARRANTY; without even the implied warranty of
- * MERCHANTABILITY or FITNESS FOR A PARTICULAR PURPOSE. See the GNU
- * Lesser General Public License for more details.
- *
- * You should have received a copy of the GNU Lesser General Public
- * License along with FFmpeg; if not, write to the Free Software
- * Foundation, Inc., 51 Franklin Street, Fifth Floor, Boston, MA 02110-1301 USA
- */
-
-#ifndef AVCODEC_AARCH64_IDCT_H
-#define AVCODEC_AARCH64_IDCT_H
-
-#include
-#include
-
-void ff_simple_idct_neon(int16_t *data);
-void ff_simple_idct_put_neon(uint8_t *dest, ptrdiff_t line_size, int16_t *data);
-void ff_simple_idct_add_neon(uint8_t *dest, ptrdiff_t line_size, int16_t *data);
-
-#endif /* AVCODEC_AARCH64_IDCT_H */
diff --git a/spaces/colakin/video-generater/public/ffmpeg/libavcodec/dvbsub_parser.c b/spaces/colakin/video-generater/public/ffmpeg/libavcodec/dvbsub_parser.c
deleted file mode 100644
index b2d54468677516ab3f911984c3e5f0ed6cd5dae3..0000000000000000000000000000000000000000
--- a/spaces/colakin/video-generater/public/ffmpeg/libavcodec/dvbsub_parser.c
+++ /dev/null
@@ -1,170 +0,0 @@
-/*
- * DVB subtitle parser for FFmpeg
- * Copyright (c) 2005 Ian Caulfield
- *
- * This file is part of FFmpeg.
- *
- * FFmpeg is free software; you can redistribute it and/or
- * modify it under the terms of the GNU Lesser General Public
- * License as published by the Free Software Foundation; either
- * version 2.1 of the License, or (at your option) any later version.
- *
- * FFmpeg is distributed in the hope that it will be useful,
- * but WITHOUT ANY WARRANTY; without even the implied warranty of
- * MERCHANTABILITY or FITNESS FOR A PARTICULAR PURPOSE. See the GNU
- * Lesser General Public License for more details.
- *
- * You should have received a copy of the GNU Lesser General Public
- * License along with FFmpeg; if not, write to the Free Software
- * Foundation, Inc., 51 Franklin Street, Fifth Floor, Boston, MA 02110-1301 USA
- */
-
-#include
-#include
-
-#include "libavutil/intreadwrite.h"
-
-#include "avcodec.h"
-
-/* Parser (mostly) copied from dvdsub.c */
-
-#define PARSE_BUF_SIZE (65536)
-
-
-/* parser definition */
-typedef struct DVBSubParseContext {
- int packet_start;
- int packet_index;
- int in_packet;
- uint8_t packet_buf[PARSE_BUF_SIZE];
-} DVBSubParseContext;
-
-static int dvbsub_parse(AVCodecParserContext *s,
- AVCodecContext *avctx,
- const uint8_t **poutbuf, int *poutbuf_size,
- const uint8_t *buf, int buf_size)
-{
- DVBSubParseContext *pc = s->priv_data;
- uint8_t *p, *p_end;
- int i, len, buf_pos = 0;
- int out_size = 0;
-
- ff_dlog(avctx, "DVB parse packet pts=%"PRIx64", lpts=%"PRIx64", cpts=%"PRIx64":\n",
- s->pts, s->last_pts, s->cur_frame_pts[s->cur_frame_start_index]);
-
- for (i=0; i < buf_size; i++)
- {
- ff_dlog(avctx, "%02x ", buf[i]);
- if (i % 16 == 15)
- ff_dlog(avctx, "\n");
- }
-
- if (i % 16 != 0)
- ff_dlog(avctx, "\n");
-
- *poutbuf = buf;
- *poutbuf_size = buf_size;
-
- s->fetch_timestamp = 1;
-
- if (s->last_pts != s->pts && s->pts != AV_NOPTS_VALUE) /* Start of a new packet */
- {
- if (pc->packet_index != pc->packet_start)
- {
- ff_dlog(avctx, "Discarding %d bytes\n",
- pc->packet_index - pc->packet_start);
- }
-
- pc->packet_start = 0;
- pc->packet_index = 0;
-
- if (buf_size < 2 || buf[0] != 0x20 || buf[1] != 0x00) {
- ff_dlog(avctx, "Bad packet header\n");
- return buf_size;
- }
-
- buf_pos = 2;
-
- pc->in_packet = 1;
- } else {
- if (pc->packet_start != 0)
- {
- if (pc->packet_index != pc->packet_start)
- {
- memmove(pc->packet_buf, pc->packet_buf + pc->packet_start,
- pc->packet_index - pc->packet_start);
-
- pc->packet_index -= pc->packet_start;
- pc->packet_start = 0;
- } else {
- pc->packet_start = 0;
- pc->packet_index = 0;
- }
- }
- }
-
- if (buf_size - buf_pos + pc->packet_index > PARSE_BUF_SIZE)
- return buf_size;
-
-/* if not currently in a packet, pass data */
- if (pc->in_packet == 0)
- return buf_size;
-
- memcpy(pc->packet_buf + pc->packet_index, buf + buf_pos, buf_size - buf_pos);
- pc->packet_index += buf_size - buf_pos;
-
- p = pc->packet_buf;
- p_end = pc->packet_buf + pc->packet_index;
-
- while (p < p_end)
- {
- if (*p == 0x0f)
- {
- if (6 <= p_end - p)
- {
- len = AV_RB16(p + 4);
-
- if (len + 6 <= p_end - p)
- {
- out_size += len + 6;
-
- p += len + 6;
- } else
- break;
- } else
- break;
- } else if (*p == 0xff) {
- if (1 < p_end - p)
- {
- ff_dlog(avctx, "Junk at end of packet\n");
- }
- pc->packet_index = p - pc->packet_buf;
- pc->in_packet = 0;
- break;
- } else {
- av_log(avctx, AV_LOG_ERROR, "Junk in packet\n");
-
- pc->packet_index = p - pc->packet_buf;
- pc->in_packet = 0;
- break;
- }
- }
-
- if (out_size > 0)
- {
- *poutbuf = pc->packet_buf;
- *poutbuf_size = out_size;
- pc->packet_start = *poutbuf_size;
- }
-
- if (s->pts == AV_NOPTS_VALUE)
- s->pts = s->last_pts;
-
- return buf_size;
-}
-
-const AVCodecParser ff_dvbsub_parser = {
- .codec_ids = { AV_CODEC_ID_DVB_SUBTITLE },
- .priv_data_size = sizeof(DVBSubParseContext),
- .parser_parse = dvbsub_parse,
-};
diff --git a/spaces/colakin/video-generater/public/ffmpeg/libavcodec/jpeg2000dec.h b/spaces/colakin/video-generater/public/ffmpeg/libavcodec/jpeg2000dec.h
deleted file mode 100644
index d0ca6e7a794352db6662fbde53a170a8c0cd2e90..0000000000000000000000000000000000000000
--- a/spaces/colakin/video-generater/public/ffmpeg/libavcodec/jpeg2000dec.h
+++ /dev/null
@@ -1,119 +0,0 @@
-/*
- * JPEG 2000 image decoder
- * Copyright (c) 2007 Kamil Nowosad
- * Copyright (c) 2013 Nicolas Bertrand
- * Copyright (c) 2022 Caleb Etemesi
- *
- * This file is part of FFmpeg.
- *
- * FFmpeg is free software; you can redistribute it and/or
- * modify it under the terms of the GNU Lesser General Public
- * License as published by the Free Software Foundation; either
- * version 2.1 of the License, or (at your option) any later version.
- *
- * FFmpeg is distributed in the hope that it will be useful,
- * but WITHOUT ANY WARRANTY; without even the implied warranty of
- * MERCHANTABILITY or FITNESS FOR A PARTICULAR PURPOSE. See the GNU
- * Lesser General Public License for more details.
- *
- * You should have received a copy of the GNU Lesser General Public
- * License along with FFmpeg; if not, write to the Free Software
- * Foundation, Inc., 51 Franklin Street, Fifth Floor, Boston, MA 02110-1301 USA
- */
-
-#ifndef AVCODEC_JPEG2000DEC_H
-#define AVCODEC_JPEG2000DEC_H
-
-#include "bytestream.h"
-#include "jpeg2000.h"
-#include "jpeg2000dsp.h"
-
-
-#define MAX_POCS 32
-
-typedef struct Jpeg2000POCEntry {
- uint16_t LYEpoc;
- uint16_t CSpoc;
- uint16_t CEpoc;
- uint8_t RSpoc;
- uint8_t REpoc;
- uint8_t Ppoc;
-} Jpeg2000POCEntry;
-
-typedef struct Jpeg2000POC {
- Jpeg2000POCEntry poc[MAX_POCS];
- int nb_poc;
- int is_default;
-} Jpeg2000POC;
-
-typedef struct Jpeg2000TilePart {
- uint8_t tile_index; // Tile index who refers the tile-part
- const uint8_t *tp_end;
- GetByteContext header_tpg; // bit stream of header if PPM header is used
- GetByteContext tpg; // bit stream in tile-part
-} Jpeg2000TilePart;
-
-/* RMK: For JPEG2000 DCINEMA 3 tile-parts in a tile
- * one per component, so tile_part elements have a size of 3 */
-typedef struct Jpeg2000Tile {
- Jpeg2000Component *comp;
- uint8_t properties[4];
- Jpeg2000CodingStyle codsty[4];
- Jpeg2000QuantStyle qntsty[4];
- Jpeg2000POC poc;
- Jpeg2000TilePart tile_part[32];
- uint8_t has_ppt; // whether this tile has a ppt marker
- uint8_t *packed_headers; // contains packed headers. Used only along with PPT marker
- int packed_headers_size; // size in bytes of the packed headers
- GetByteContext packed_headers_stream; // byte context corresponding to packed headers
- uint16_t tp_idx; // Tile-part index
- int coord[2][2]; // border coordinates {{x0, x1}, {y0, y1}}
-} Jpeg2000Tile;
-
-typedef struct Jpeg2000DecoderContext {
- AVClass *class;
- AVCodecContext *avctx;
- GetByteContext g;
-
- int width, height;
- int image_offset_x, image_offset_y;
- int tile_offset_x, tile_offset_y;
- uint8_t cbps[4]; // bits per sample in particular components
- uint8_t sgnd[4]; // if a component is signed
- uint8_t properties[4];
-
- uint8_t has_ppm;
- uint8_t *packed_headers; // contains packed headers. Used only along with PPM marker
- int packed_headers_size;
- GetByteContext packed_headers_stream;
- uint8_t in_tile_headers;
-
- int cdx[4], cdy[4];
- int precision;
- int ncomponents;
- int colour_space;
- uint32_t palette[256];
- int8_t pal8;
- int cdef[4];
- int tile_width, tile_height;
- unsigned numXtiles, numYtiles;
- int maxtilelen;
- AVRational sar;
-
- Jpeg2000CodingStyle codsty[4];
- Jpeg2000QuantStyle qntsty[4];
- Jpeg2000POC poc;
- uint8_t roi_shift[4];
-
- int bit_index;
-
- int curtileno;
-
- Jpeg2000Tile *tile;
- Jpeg2000DSPContext dsp;
-
- /*options parameters*/
- int reduction_factor;
-} Jpeg2000DecoderContext;
-
-#endif //AVCODEC_JPEG2000DEC_H
diff --git a/spaces/congsaPfin/Manga-OCR/logs/Berima Ena by Nhyira Betty - A Song of Praise and Worship.md b/spaces/congsaPfin/Manga-OCR/logs/Berima Ena by Nhyira Betty - A Song of Praise and Worship.md
deleted file mode 100644
index c75ace456a38ef71112d5b0037e6d80774940c96..0000000000000000000000000000000000000000
--- a/spaces/congsaPfin/Manga-OCR/logs/Berima Ena by Nhyira Betty - A Song of Praise and Worship.md
+++ /dev/null
@@ -1,116 +0,0 @@
-
-
Download Nhyira Betty Berima Ena
-
If you are a fan of gospel music, you might have heard of Nhyira Betty, a talented singer from Ghana. She is best known for her song Berima Ena, which has touched many hearts with its powerful lyrics and melody. In this article, we will tell you more about Nhyira Betty, her song Berima Ena, and how to download it for free.
Nhyira Betty is a Ghanaian gospel musician who has been in the industry for over a decade. She has released several albums and singles that have made her one of the most popular gospel artists in Ghana and beyond.
-
Biography and career
-
Nhyira Betty was born in Kumasi, the capital city of the Ashanti Region of Ghana. She started singing at a young age in her church choir and later joined a musical group called Daughters of Glorious Jesus. She learned a lot from the group and decided to pursue a solo career in 2007. She released her debut album Apam Adaka, which means "God's Covenant", in 2008. The album was well received by the public and earned her several nominations and awards. Since then, she has released four more albums: Omanfrani (2010), Yebedwiri (2012), Thank You Baba Jesus (2015), and Okronkron Ni (2017).
-
Music style and awards
-
Nhyira Betty's music style is a blend of traditional Ghanaian gospel, contemporary Christian music, and highlife. She sings in Twi, English, and other local languages to reach a wider audience. She also incorporates elements of worship, praise, and evangelism in her songs. Some of her popular songs include Omanfrani, Yebedwiri, Obi Ambu Me Fo, Yenka Kyere Onyame Se Yeda No Ase, and Berima Ena.
-
Nhyira Betty has won several awards for her music, such as Best Female Vocalist at the Ghana Gospel Industry Awards (GGIA) in 2011 and 2012, Best Gospel Album at the Ghana Music Awards (GMA) in 2012, Best Gospel Artiste at the Kumasi Music Awards (KMA) in 2016, and Best Gospel Song at the Ghana National Gospel Music Awards (GNGMA) in 2019.
-
download nhyira betty berima ena mp3
-download nhyira betty berima ena worship
-download nhyira betty berima ena lyrics
-download nhyira betty berima ena video
-download nhyira betty berima ena song
-download nhyira betty berima ena audio
-download nhyira betty berima ena live
-download nhyira betty berima ena shazam
-download nhyira betty berima ena spotify
-download nhyira betty berima ena album
-download nhyira betty berima ena 2014
-download nhyira betty berima ena 2017
-download nhyira betty berima ena official video
-download nhyira betty berima ena music video
-download nhyira betty berima ena youtube
-download nhyira betty berima ena free mp3
-download nhyira betty berima ena instrumental
-download nhyira betty berima ena karaoke
-download nhyira betty berima ena remix
-download nhyira betty berima ena cover
-download nhyira betty berima ena ghana music
-download nhyira betty berima ena gospel music
-download nhyira betty berima ena praise and worship
-download nhyira betty berima ena online
-download nhyira betty berima ena full song
-how to download nhyira betty berima ena
-where to download nhyira betty berima ena
-best site to download nhyira betty berima ena
-best quality to download nhyira betty berima ena
-best app to download nhyira betty berima ena
-listen and download nhyira betty berima ena
-stream and download nhyira betty berima ena
-watch and download nhyira betty berima ena
-share and download nhyira betty berima ena
-like and download nhyira betty berima ena
-comment and download nhyira betty berima ena
-subscribe and download nhyira betty berima ena
-follow and download nhyira betty berima ena
-rate and download nhyira betty berima ena
-review and download nhyira betty berima ena
-recommend and download nhyira betty berima ena
-enjoy and download nhyira betty berima ena
-learn and download nhyria betty berima ena
-
What is Berima Ena?
-
Berima Ena is one of Nhyira Betty's most popular songs. It is the second track on her fourth album Thank You Baba Jesus, which was released in 2015. The song has over 34 thousand views on YouTube and over 34 thousand shazams on Shazam.
-
Meaning and message
-
Berima Ena means "King Jesus" in Twi. The song is a tribute to Jesus Christ, who is the King of kings and Lord of lords. The song praises Jesus for his love, grace, mercy, power, glory, and majesty. The song also acknowledges that Jesus is the only way to salvation and eternal life. The song encourages listeners to trust in Jesus and worship him with all their hearts.
-
Official video and live performance
-
The official video of Berima Ena was released in 2014 on YouTube. The video features Nhyira Betty singing in a studio with some backup singers and a band. The video also shows some scenes of people worshipping in a church and enjoying the song. The video is simple but effective in conveying the message of the song. Nhyira Betty has also performed Berima Ena live on several occasions, such as at the Ghana National Gospel Music Awards in 2019 and at the Kumasi City Mall in 2020. She always delivers a powerful and energetic performance that captivates the audience and makes them sing along.
How to download Berima Ena?
-
If you want to download Berima Ena for free, you have several options to choose from. You can use online platforms and apps that allow you to stream and download music legally and safely. Here are some of them:
-
Online platforms and apps
-
Shazam
-
Shazam is a popular app that can identify any song playing around you. It can also show you the lyrics, artist, album, genre, and other information about the song. You can also use Shazam to stream and download songs from various sources, such as YouTube, Spotify, Apple Music, Deezer, and more. To download Berima Ena using Shazam, follow these steps:
-
-
Download and install Shazam on your device from the App Store or Google Play Store.
-
Open the app and tap on the Shazam icon to start listening.
-
Play Berima Ena on another device or speaker near your phone.
-
Wait for Shazam to recognize the song and show you the details.
-
Tap on the download icon next to the song title and choose your preferred source.
-
Follow the instructions on the screen to complete the download.
-
-
Hungama Music
-
Hungama Music is an online music streaming and downloading service that offers a wide range of songs from different genres, languages, and regions. You can find Berima Ena on Hungama Music and download it for free. To do so, follow these steps:
-
-
Download and install Hungama Music on your device from the App Store or Google Play Store.
-
Open the app and create an account or log in with your existing one.
-
Search for Berima Ena using the search bar or browse through the categories.
-
Select the song from the results and tap on the download icon at the bottom right corner.
-
Choose your preferred quality and format and confirm your download.
-
-
Spotify
-
Spotify is one of the most popular music streaming services in the world. It has millions of songs from various artists, albums, playlists, podcasts, and more. You can also download songs from Spotify for offline listening if you have a premium subscription. To download Berima Ena using Spotify, follow these steps:
-
-
Download and install Spotify on your device from the App Store or Google Play Store.
-
Open the app and sign up for a premium account or log in with your existing one.
-
Search for Berima Ena using the search bar or browse through the categories.
-
Select the song from the results and tap on the heart icon to add it to your library.
-
Go to your library and tap on the download icon next to the song title.
-
Wait for the download to finish and enjoy the song offline.
-
-
Tips and tricks
-
Check the quality and format
-
Before you download any song, make sure you check its quality and format. The quality refers to how clear and crisp the sound is, while the format refers to how the file is encoded and compressed. The higher the quality, the better the sound, but also the larger the file size. The format affects how compatible the file is with different devices and players. Some common formats are MP3, WAV, FLAC, AAC, and OGG. You can choose the quality and format that suit your needs and preferences, but generally, MP3 is the most widely used and supported format, while FLAC is the best for high-fidelity sound.
-
Use a reliable downloader
-
Another tip is to use a reliable and trustworthy downloader to get your song. There are many online tools and apps that claim to offer free music downloads, but some of them may be unsafe, illegal, or fraudulent. They may contain viruses, malware, spyware, or adware that can harm your device or steal your personal information. They may also violate the copyright laws and infringe on the rights of the artists and producers. To avoid these risks, you should use a reputable and legal downloader that has positive reviews and ratings from other users.
-
Enjoy the song offline
-
Once you have downloaded Berima Ena, you can enjoy it offline anytime and anywhere. You can play it on your device using your default music player or any other app that supports the file format. You can also transfer it to other devices using a USB cable, Bluetooth, Wi-Fi, or cloud storage. You can also burn it to a CD or DVD if you want to play it on a stereo system or a car player. However you choose to listen to it, make sure you respect the rights of the artist and do not share it with others without permission.
-
Conclusion
-
Berima Ena is a beautiful gospel song by Nhyira Betty that praises Jesus Christ as the King of kings and Lord of lords. It is a song that inspires faith, hope, and love in the listeners. You can download Berima Ena for free using various online platforms and apps, such as Shazam, Hungama Music, and Spotify. You can also follow some tips and tricks to ensure a safe and smooth download process. We hope this article has helped you learn more about Nhyira Betty, her song Berima Ena, and how to download it for free.
-
FAQs
-
Here are some frequently asked questions about Berima Ena:
-
-
What is the genre of Berima Ena?
-
Berima Ena is a gospel song that combines elements of traditional Ghanaian gospel, contemporary Christian music, and highlife.
-
Who wrote and composed Berima Ena?
-
Berima Ena was written and composed by Nhyira Betty herself.
-
When was Berima Ena released?
-
Berima Ena was released in 2015 as part of Nhyira Betty's fourth album Thank You Baba Jesus.
-
How long is Berima Ena?
-
Berima Ena is 5 minutes and 33 seconds long.
-
Where can I watch the official video of Berima Ena?
-
You can watch the official video of Berima Ena on YouTube or on Nhyira Betty's official website.
- 197e85843d
-
-
\ No newline at end of file
diff --git a/spaces/congsaPfin/Manga-OCR/logs/Conduce los Autos Ms Increbles en Real Racing 3 Todo Desbloqueado Apk 2023.md b/spaces/congsaPfin/Manga-OCR/logs/Conduce los Autos Ms Increbles en Real Racing 3 Todo Desbloqueado Apk 2023.md
deleted file mode 100644
index 35edd7683a5ef69e849cb7c2ec7ebf552e71c256..0000000000000000000000000000000000000000
--- a/spaces/congsaPfin/Manga-OCR/logs/Conduce los Autos Ms Increbles en Real Racing 3 Todo Desbloqueado Apk 2023.md
+++ /dev/null
@@ -1,117 +0,0 @@
-
-
Real Racing 3 Todo Desbloqueado APK: How to Enjoy the Ultimate Racing Experience on Your Android Device
-
If you are a fan of racing games, you have probably heard of Real Racing 3, one of the most realistic and immersive racing games available on mobile devices. But did you know that you can enjoy this game even more with a modded version called Real Racing 3 Todo Desbloqueado APK? In this article, we will tell you what this mod is, how to download and install it, and how to use it to unlock all the cars, tracks, and events in the game. We will also share some tips and tricks to help you play Real Racing 3 like a pro.
-
What is Real Racing 3 and Why is it So Popular?
-
Real Racing 3 is a racing game developed by Firemonkeys Studios and published by Electronic Arts. It was released in 2013 for iOS and Android devices, and has since become one of the most popular and acclaimed racing games on mobile platforms. The game has received several awards and nominations, such as the Best Mobile Game at The Game Awards 2014, and has been downloaded over 500 million times.
Real Racing 3 boasts of several features that make it stand out from other racing games, such as:
-
-
Realistic graphics and physics: The game uses a proprietary engine called Mint™ that delivers stunning visuals and lifelike physics. The game also supports high-definition graphics on compatible devices, making the cars, tracks, and environments look more detailed and realistic.
-
Real-world cars and tracks: The game features over 250 licensed cars from top manufacturers like Ferrari, Lamborghini, Porsche, Bugatti, and more. The cars are meticulously detailed and can be customized and upgraded with various parts. The game also features over 40 real-world tracks from famous locations like Silverstone, Le Mans, Dubai Autodrome, and more.
-
Various modes and events: The game offers over 4,000 events to participate in, including cup races, eliminations, drag races, endurance challenges, time trials, and more. The game also has a career mode that lets you progress through different series and championships. Additionally, the game has a multiplayer mode that lets you race against other players online or offline using a feature called Time Shifted Multiplayer™ (TSM), which creates AI-controlled versions of your friends or rivals based on their performance data.
-
-
Real Racing 3 Comparison with Other Racing Games
-
Real Racing 3 is often compared with other racing games on mobile devices, such as Asphalt 9: Legends, Need for Speed: No Limits, CSR Racing 2, F1 Mobile Racing, etc. While each game has its own strengths and weaknesses, Real Racing 3 is generally considered to be more realistic and immersive than its competitors. Some of the aspects that make Real Racing 3 superior are:
-
-
Better graphics and sound: Real Racing 3 has better graphics quality and sound effects than most other racing games on mobile devices. The game also supports HDR (high dynamic range) mode on compatible devices, which enhances the contrast and color range of the images.
-
More cars and tracks: Real Racing 3
What is Real Racing 3 Todo Desbloqueado APK and What are its Benefits?
-
Real Racing 3 Todo Desbloqueado APK is a modded version of Real Racing 3 that allows you to enjoy the game without any limitations or restrictions. With this mod, you can unlock all the cars, tracks, and events in the game, as well as get unlimited money and gold to spend on upgrades and purchases. You can also access all the features and modes of the game, such as TSM, HDR, and cloud save.
-
Some of the benefits of using Real Racing 3 Todo Desbloqueado APK are:
-
-
You can save time and money: You don't have to grind for hours or spend real money to get the best cars and tracks in the game. You can simply download the mod and enjoy everything for free.
-
You can have more fun and variety: You can try out different cars and tracks that you normally wouldn't be able to access. You can also experiment with different upgrades and customizations to suit your preferences and style.
-
You can challenge yourself and others: You can join more race events and compete with other players online or offline. You can also test your skills and strategies on different difficulty levels and scenarios.
-
-
How to Download and Install Real Racing 3 Todo Desbloqueado APK
-
If you want to download and install Real Racing 3 Todo Desbloqueado APK, you need to follow these steps:
-
-
Make sure you have enough storage space on your device and a stable internet connection.
-
Uninstall the original Real Racing 3 app from your device if you have it.
-
Download the Real Racing 3 Todo Desbloqueado APK file from a reliable source, such as .
-
Enable the installation of apps from unknown sources on your device settings.
-
Locate the downloaded APK file on your device and tap on it to install it.
-
Wait for the installation to finish and launch the game.
-
Enjoy the game with all the unlocked features and resources.
-
-
How to Use Real Racing 3 Todo Desbloqueado APK to Unlock All Cars, Tracks, and Events
-
Once you have installed Real Racing 3 Todo Desbloqueado APK, you can use it to unlock all the cars, tracks, and events in the game. Here are some tips on how to do that:
-
-
To unlock all cars: Go to the garage menu and tap on any car that you want to buy. You will see that the price is zero and you can buy it without spending any money or gold. You can also upgrade and customize your cars for free.
-
To unlock all tracks: Go to the race menu and tap on any track that you want to race on. You will see that all the series and championships are unlocked and you can choose any of them. You can also change the weather, time of day, and number of laps for each track.
-
To unlock all events: Go to the event menu and tap on any event that you want to join. You will see that all the events are unlocked and you can participate in any of them. You can also create your own events and invite other players or bots to join them.
-
Tips and Tricks to Playing Real Racing 3 Like a Pro
-
Now that you have Real Racing 3 Todo Desbloqueado APK, you can enjoy the game to the fullest. But if you want to improve your skills and performance, you might want to follow some tips and tricks that can help you play like a pro. Here are some of them:
-
Be Smart with Your Upgrades and Purchases
-
Even though you have unlimited money and gold, you still need to be smart with your upgrades and purchases. You don't want to waste your resources on cars or parts that you don't need or use. You also want to balance your upgrades and purchases with your level and progress in the game. Here are some suggestions on how to do that:
-
real racing 3 mod apk unlimited money and gold
-descargar real racing 3 hackeado para android
-real racing 3 apk full mega
-real racing 3 mod apk latest version download
-como tener todo desbloqueado en real racing 3
-real racing 3 apk mod dinero infinito
-real racing 3 hack apk android 1
-real racing 3 mod apk offline
-baixar real racing 3 mod apk
-real racing 3 apk obb highly compressed
-real racing 3 mod apk revdl
-real racing 3 hack apk ios
-real racing 3 apk mod all cars unlocked
-real racing 3 mod apk rexdl
-descargar real racing 3 apk + datos sd
-real racing 3 hack apk download for android
-real racing 3 mod apk unlimited everything
-real racing 3 apk mod monedas ilimitadas
-real racing 3 hack apk sin root
-real racing 3 mod apk all tracks unlocked
-télécharger real racing 3 mod apk
-real racing 3 apk mod vip unlocked
-real racing 3 hack apk ultima version
-real racing 3 mod apk unlimited gold and money download
-real racing 3 apk mod anti ban
-real racing 3 hack apk no verification
-real racing 3 mod apk all cars and tracks unlocked
-descargar real racing 3 mod apk ultima version
-real racing 3 apk mod free shopping
-real racing 3 hack apk online
-
-
Focus on the cars that suit your style and preference: There are many cars in the game, but not all of them are suitable for every track or event. You should focus on the cars that match your driving style and preference, such as speed, handling, acceleration, braking, etc. You should also consider the car's class, rating, and performance index when choosing a car.
-
Upgrade your cars strategically: Upgrading your cars can improve their performance and give you an edge over your opponents. However, upgrading your cars also increases their service time and cost, which can slow down your progress in the game. You should upgrade your cars strategically, focusing on the parts that have the most impact on your car's performance, such as engine, drivetrain, suspension, tires, etc. You should also avoid over-upgrading your cars beyond their optimal level, as this can make them harder to control and less efficient.
-
Purchase new cars wisely: Purchasing new cars can expand your garage and give you more options to choose from. However, purchasing new cars also requires you to spend money and gold, which can be better used for other purposes. You should purchase new cars wisely, only when you need them for a specific series or championship, or when you want to try out a different car for fun. You should also compare the prices and performance of different cars before buying them.
-
-
Master the Controls and Driving Techniques
-
Real Racing 3 is a realistic racing game that requires you to master the controls and driving techniques to win races. The game offers various control options, such as tilt, touch, or steering wheel, as well as different assists, such as brake assist, steering assist, traction control, etc. You should choose the control option and assist level that suit your preference and skill level. Here are some tips on how to master the controls and driving techniques:
-
-
Practice on different tracks and modes: The best way to improve your skills is to practice on different tracks and modes. You should familiarize yourself with the layout, turns, elevation changes, and hazards of each track. You should also try out different modes and events, such as time trials, drag races, endurance challenges, etc., to test your speed, endurance, and strategy.
-
Use the racing line and braking points: The game provides a racing line and braking points that guide you through the optimal path and speed for each track. You should follow the racing line and braking points as much as possible, especially if you are new to the game or unfamiliar with the track. The racing line changes color from green to yellow to red depending on your speed and distance from the turn. The braking points are marked by red cones or arrows that indicate when you should start braking.
-
Learn how to corner properly: Cornering is one of the most important skills in racing games. You should learn how to corner properly by using the following techniques:
-
Brake before the turn: You should brake before entering the turn, not during or after it. Braking before the turn reduces your speed and allows you to steer more easily.
-
Aim for the apex: The apex is the point where the racing line is closest to the inside of the turn. You should aim for the apex by steering towards it as you enter the turn.
-
Accelerate out of the turn: You should accelerate out of the turn by steering away from it as you exit it. Accelerating out of the turn increases your speed and momentum.
-
-
Avoid collisions and penalties: Collisions and penalties can slow you down and damage your car. You should avoid collisions with other cars or objects by keeping a safe distance and overtaking carefully. You should also avoid penalties by following the rules of the game, such as staying on track, respecting flags, etc.
-
Join Race Events and Compete with Other Players
-
One of the most fun and exciting aspects of Real Racing 3 is the multiplayer mode, where you can join race events and compete with other players from around the world. You can also create your own events and invite your friends or rivals to join them. Here are some tips on how to join race events and compete with other players:
-
-
Choose the right event for your level and car: There are many events to choose from, such as weekly time trials, special events, online multiplayer, etc. You should choose the event that suits your level and car, as well as your preference and goal. For example, if you want to test your speed and skill, you can join a time trial or a drag race. If you want to have fun and interact with other players, you can join an online multiplayer or a special event.
-
Use TSM to race against other players: TSM is a feature that allows you to race against other players even when they are offline. TSM creates AI-controlled versions of other players based on their performance data, such as lap times, driving style, etc. You can use TSM to race against your friends or rivals, or against random players from around the world. You can also see how you rank against other players on the leaderboards.
-
Be respectful and fair: Racing is a competitive sport, but it is also a social activity. You should be respectful and fair to other players, whether they are online or offline. You should avoid crashing into them, blocking them, or cheating in any way. You should also congratulate them when they win, or encourage them when they lose. You can use the chat feature or the emoticons to communicate with other players.
-
-
Conclusion
-
Real Racing 3 is a great racing game that offers a realistic and immersive racing experience on your Android device. But if you want to enjoy the game even more, you can download and install Real Racing 3 Todo Desbloqueado APK, which unlocks all the cars, tracks, and events in the game, as well as gives you unlimited money and gold. You can also use some tips and tricks to improve your skills and performance in the game, such as being smart with your upgrades and purchases, mastering the controls and driving techniques, and joining race events and competing with other players. With Real Racing 3 Todo Desbloqueado APK, you can have the ultimate racing experience on your Android device.
-
FAQs
-
Here are some frequently asked questions about Real Racing 3 Todo Desbloqueado APK:
-
-
Is Real Racing 3 Todo Desbloqueado APK safe to use?
-Yes, Real Racing 3 Todo Desbloqueado APK is safe to use, as long as you download it from a reliable source, such as . However, you should be aware that using this mod may violate the terms of service of the game and may result in your account being banned or suspended. Therefore, you should use this mod at your own risk and discretion.
-
Does Real Racing 3 Todo Desbloqueado APK require root access?
-No, Real Racing 3 Todo Desbloqueado APK does not require root access to work. You can install it on any Android device without rooting it.
-
Can I update Real Racing 3 Todo Desbloqueado APK?
-Yes, you can update Real Racing 3 Todo Desbloqueado APK whenever there is a new version available. However, you should always backup your data before updating, as updating may erase your progress or cause compatibility issues.
-
Can I play Real Racing 3 Todo Desbloqueado APK offline?
-Yes, you can play Real Racing 3 Todo Desbloqueado APK offline without an internet connection. However, some features and modes of the game may not work offline, such as online multiplayer, cloud save, etc.
-
Can I play Real Racing 3 Todo Desbloqueado APK on PC?
-Yes, you can play Real Racing 3 Todo Desbloqueado APK on PC using an Android emulator, such as BlueStacks or NoxPlayer. However, you may experience some performance issues or bugs when playing on PC.
- 401be4b1e0
-
-
\ No newline at end of file
diff --git a/spaces/congsaPfin/Manga-OCR/logs/Download Real Steel World Robot Boxing 2 Mod APK for Android - Latest Version.md b/spaces/congsaPfin/Manga-OCR/logs/Download Real Steel World Robot Boxing 2 Mod APK for Android - Latest Version.md
deleted file mode 100644
index 9ba36f7bee1820d45011491633509b3fa2d1e786..0000000000000000000000000000000000000000
--- a/spaces/congsaPfin/Manga-OCR/logs/Download Real Steel World Robot Boxing 2 Mod APK for Android - Latest Version.md
+++ /dev/null
@@ -1,146 +0,0 @@
-
-
Real Steel World Robot Boxing 2 Mod Apk: A Guide for Robot Fighting Fans
-
If you are a fan of robot fighting games, you might have heard of Real Steel World Robot Boxing 2, the sequel to the popular game based on the blockbuster movie Real Steel. In this game, you can control your own robot champion and fight against other robots from around the world in spectacular arenas. But did you know that you can also download a mod apk version of the game that gives you unlimited money, energy, and unlocked robots? In this article, we will tell you everything you need to know about Real Steel World Robot Boxing 2 mod apk, including what it is, why you should download it, how to download it, how to play it, and some tips and tricks to help you become the ultimate robot boxing champion.
Real Steel World Robot Boxing 2 is an arcade action robot boxing game developed by Reliance Games. It is the sequel to Real Steel World Robot Boxing, which was released in 2013 and has over 70 million players globally. The game is inspired by the movie Real Steel, which starred Hugh Jackman as a former boxer who trains a robot named Atom to compete in a futuristic sport where human boxers have been replaced by robots.
-
A sequel to the hit robot boxing game based on the movie
-
The game follows the story of Atom and his human partners Charlie (Jackman) and Max (Dakota Goyo) as they challenge Zeus, the reigning robot boxing champion. Along the way, they encounter new robot titans and old fan favorites from the movie and the previous game. The game features stunning graphics, realistic physics, immersive sound effects, and cinematic animations that make you feel like you are in the movie.
-
Features new robots, abilities, classes, and modes
-
Real Steel World Robot Boxing 2 introduces many new features that make the game more complex and exciting than ever. You can choose from over 36 robot fighters with different character classes such as Brawler, Defender, Striker, Supporter, Tanker, and Trickster. Each class has its own strengths and weaknesses, as well as unique abilities that can be activated during battles. You can also pair up specific robots to trigger synergies that boost their performance. The game also offers various modes such as Story Mode, Multiplayer Mode, Winner Takes All Mode, Live Events Mode, and more. You can compete for the World Robot Boxing Championship Title or challenge your friends online or offline.
-
Available for Android devices
-
The game is currently available only for Android devices running Android 4.4 or higher. You can download the game from the Google Play Store for free, but it also contains in-app purchases that require real money. Alternatively, you can download the mod apk version of the game that gives you unlimited resources and features for free. We will explain more about the mod apk version in the next section.
-
Why Download the Mod Apk Version?
-
Mod apk is a modified version of an original application that has been altered by third-party developers to provide extra features and benefits that are not available in the official version. For Real Steel World Robot Boxing 2, the mod apk version offers several advantages that can enhance your gaming experience and make you unstoppable in the robot boxing arena. Here are some of the benefits of downloading the mod apk version:
-
real steel world robot boxing 2 mod apk unlimited money
-real steel world robot boxing 2 mod apk download latest version
-real steel world robot boxing 2 mod apk android 1
-real steel world robot boxing 2 mod apk rexdl
-real steel world robot boxing 2 mod apk revdl
-real steel world robot boxing 2 mod apk offline
-real steel world robot boxing 2 mod apk hack
-real steel world robot boxing 2 mod apk obb
-real steel world robot boxing 2 mod apk free shopping
-real steel world robot boxing 2 mod apk no root
-real steel world robot boxing 2 mod apk unlimited everything
-real steel world robot boxing 2 mod apk data
-real steel world robot boxing 2 mod apk all robots unlocked
-real steel world robot boxing 2 mod apk unlimited gold
-real steel world robot boxing 2 mod apk online
-real steel world robot boxing 2 mod apk latest update
-real steel world robot boxing 2 mod apk full version
-real steel world robot boxing 2 mod apk unlimited energy
-real steel world robot boxing 2 mod apk mega
-real steel world robot boxing 2 mod apk vip
-real steel world robot boxing 2 mod apk premium
-real steel world robot boxing 2 mod apk unlimited coins and gems
-real steel world robot boxing 2 mod apk pure
-real steel world robot boxing 2 mod apk new version
-real steel world robot boxing 2 mod apk all characters unlocked
-real steel world robot boxing 2 mod apk god mode
-real steel world robot boxing 2 mod apk unlimited health and damage
-real steel world robot boxing 2 mod apk happymod
-real steel world robot boxing 2 mod apk cheat
-real steel world robot boxing 2 mod apk unlocked everything
-real steel world robot boxing 2 mod apk no ads
-real steel world robot boxing 2 mod apk high damage
-real steel world robot boxing 2 mod apk unlimited keys and stars
-real steel world robot boxing 2 mod apk all features unlocked
-real steel world robot boxing 2 mod apk one hit kill
-real steel world robot boxing 2 mod apk unlimited boosters and tickets
-real steel world robot boxing 2 mod apk easy win
-real steel world robot boxing 2 mod apk all levels unlocked
-real steel world robot boxing 2 mod apk no verification
-real steel world robot boxing 2 mod apk pro
-real steel world robot boxing 2 mod apk unlimited resources and parts
-real steel world robot boxing 2 mod apk all skins unlocked
-real steel world robot boxing 2 mod apk max level and rank
-real steel world robot boxing 2 mod apk all modes unlocked
-real steel world robot boxing 2 mod apk anti ban and crash fix
-
Benefits of mod apk such as unlimited money, energy, and unlocked robots
-
One of the main benefits of the mod apk version is that it gives you unlimited money and energy. Money is used to buy and upgrade robots, abilities, and items in the game. Energy is used to enter battles and tournaments. Normally, you have to earn money and energy by playing the game or watching ads, which can be time-consuming and frustrating. With the mod apk version, you don't have to worry about running out of money or energy, as you can get as much as you want for free. You can also unlock all the robots and abilities in the game without spending a dime. This means you can access all the content and features of the game without any restrictions or limitations.
-
How to download and install the mod apk safely and easily
-
Downloading and installing the mod apk version of Real Steel World Robot Boxing 2 is not difficult, but you have to follow some steps carefully to avoid any problems or errors. Here are the steps you need to follow:
-
-
First, you need to uninstall the original version of the game from your device if you have it installed.
-
Second, you need to enable the installation of apps from unknown sources on your device. To do this, go to Settings > Security > Unknown Sources and toggle it on.
-
Third, you need to download the mod apk file from a reliable and trusted source. You can search for Real Steel World Robot Boxing 2 mod apk on Google or use this link: Real Steel World Robot Boxing 2 Mod Apk Download.
-
Fourth, you need to locate the downloaded file on your device and tap on it to start the installation process. Follow the instructions on the screen and wait for the installation to finish.
-
Fifth, you need to launch the game and enjoy the unlimited features and resources.
-
-
Precautions and risks of using mod apk such as malware and bans
-
While using the mod apk version of Real Steel World Robot Boxing 2 can be fun and rewarding, it also comes with some risks and drawbacks that you should be aware of before downloading it. Here are some of the precautions and risks of using mod apk:
-
-
Mod apk files may contain malware or viruses that can harm your device or steal your personal information. To avoid this, you should always download mod apk files from reputable and verified sources, scan them with antivirus software, and backup your data before installing them.
-
Mod apk files may not be compatible with your device or the latest version of the game. This may cause crashes, glitches, errors, or performance issues. To avoid this, you should always check the compatibility and update status of the mod apk file before downloading it.
-
Mod apk files may violate the terms and conditions of the game developer or publisher. This may result in bans, suspensions, or legal actions against your account or device. To avoid this, you should always use mod apk files at your own risk and discretion, and respect the rights and rules of the game developer or publisher.
-
-
How to Play Real Steel World Robot Boxing 2?
-
Now that you have downloaded and installed the mod apk version of Real Steel World Robot Boxing 2, you are ready to play it and enjoy its amazing features. But how do you play it? What are the basic gameplay and controls? How do you win battles and tournaments? How do you use abilities, classes, and synergies effectively? In this section, we will answer these questions and give you some tips and tricks to help you master the game.
-
Basic gameplay and controls
-
The basic gameplay of Real Steel World Robot Boxing 2 is similar to other robot boxing games. You control your robot fighter using a virtual joystick on the left side of the screen and buttons on the right side of the screen. You can move your robot around the arena, dodge, block, punch, and kick your opponent. You can also use special abilities that can deal more damage, heal, or buff your robot. The goal is to reduce your opponent's health bar to zero or knock them out before they do the same to you. You can play against the AI in Story Mode or against other players in Multiplayer Mode.
-
Tips and tricks to win battles and tournaments
-
Winning battles and tournaments in Real Steel World Robot Boxing 2 requires skill, strategy, and luck. Here are some tips and tricks that can help you improve your chances of victory:
-
-
Choose your robot wisely. Different robots have different stats, abilities, and classes that suit different play styles and situations. For example, Brawlers are good at dealing and taking damage, Defenders are good at blocking and countering, Strikers are good at speed and combos, Supporters are good at healing and buffing, Tankers are good at durability and endurance, and Tricksters are good at dodging and surprising. You should experiment with different robots and find the ones that match your preferences and goals.
-
Upgrade your robot regularly. As you play the game, you will earn money that you can use to buy and upgrade robots, abilities, and items. Upgrading your robot will increase its stats and performance, making it more powerful and effective in battles. You should always try to upgrade your robot as much as possible to keep up with the increasing difficulty and competition.
-
Use abilities strategically. Abilities are special moves that can give you an edge in battles. They can be offensive, defensive, or supportive, depending on the class of your robot. Each ability has a cooldown time that limits how often you can use it. You should use abilities wisely and at the right time to maximize their impact. For example, you can use an offensive ability to finish off a low-health opponent, a defensive ability to survive a critical hit, or a supportive ability to heal or buff yourself or your ally.
-
Use synergies effectively. Synergies are bonuses that activate when you pair up specific robots in a team. They can boost your stats, abilities, or performance in various ways. You should try to find and use synergies that complement your robot's class and abilities. For example, you can use a synergy that increases your damage output if you are a Brawler, a synergy that increases your defense if you are a Defender, or a synergy that increases your energy regeneration if you are a Supporter.
-
Practice and learn from your mistakes. The best way to improve your skills and strategies in Real Steel World Robot Boxing 2 is to practice and learn from your mistakes. You can play against the AI in Story Mode or against other players in Multiplayer Mode to test your abilities and learn from your opponents. You can also watch replays of your battles or other players' battles to analyze what went wrong or right. You should always try to learn from your mistakes and improve your performance in future battles.
-
-
Conclusion
-
Real Steel World Robot Boxing 2 is an awesome robot boxing game that will appeal to fans of the movie Real Steel and the genre in general. It offers stunning graphics, realistic physics, immersive sound effects, cinematic animations, and various modes that will keep you entertained for hours. It also introduces new features such as new robots, abilities, classes, and synergies that make the game more complex and exciting than ever.
-
Summary of the main points
-
In this article, we have covered everything you need to know about Real Steel World Robot Boxing 2 mod apk, including what it is, why you should download it, how to download it, how to play it, and some tips and tricks to help you master the game. We have also explained the benefits, risks, and precautions of using the mod apk version of the game that gives you unlimited money, energy, and unlocked robots.
-
Recommendation and rating of the game and the mod apk
-
We highly recommend Real Steel World Robot Boxing 2 to anyone who loves robot fighting games or the movie Real Steel. It is a fun, challenging, and addictive game that will keep you hooked for hours. We also recommend the mod apk version of the game to anyone who wants to enjoy the game without any limitations or restrictions. It is a great way to access all the content and features of the game for free. However, you should also be careful and responsible when using the mod apk version, as it may have some risks and drawbacks that we have mentioned earlier.
-
We give Real Steel World Robot Boxing 2 a rating of 4.5 out of 5 stars. It is one of the best robot boxing games available on Android devices. It has amazing graphics, sound effects, animations, and gameplay that make you feel like you are in the movie. It also has new features that make it more complex and exciting than ever. The only reason we did not give it a perfect score is because it is not available for iOS devices yet, and it may have some bugs or errors that need to be fixed.
-
We give Real Steel World Robot Boxing 2 mod apk a rating of 4 out of 5 stars. It is a great mod apk that gives you unlimited money, energy, and unlocked robots. It allows you to enjoy the game without any restrictions or limitations. It also makes the game easier and more fun to play. The only reason we did not give it a higher score is because it may have some risks and drawbacks that we have mentioned earlier, such as malware, compatibility issues, or bans.
-
Call to action and invitation for feedback
-
If you are interested in playing Real Steel World Robot Boxing 2 or downloading its mod apk version, you can use the links below to get them from the Google Play Store or from a trusted source. We hope you enjoy the game and have a blast in the robot boxing arena.
-
If you have any questions, comments, or feedback about the game or the mod apk version, feel free to leave them in the comment section below. We would love to hear from you and help you with any issues or problems you may encounter. Thank you for reading this article and happy gaming!
-
FAQs
-
What are the minimum requirements to play Real Steel World Robot Boxing 2?
-
The minimum requirements to play Real Steel World Robot Boxing 2 are:
-
-
An Android device running Android 4.4 or higher
-
At least 1 GB of RAM
-
At least 500 MB of free storage space
-
A stable internet connection
-
-
How to update the mod apk version?
-
To update the mod apk version of Real Steel World Robot Boxing 2, you need to follow these steps:
-
-
Uninstall the current mod apk version from your device
-
Download the latest mod apk version from a reliable and trusted source
-
Install the new mod apk version following the same steps as before
-
Launch the game and enjoy the updated features and resources
-
-
How to play with friends online?
-
To play with friends online in Real Steel World Robot Boxing 2, you need to follow these steps:
-
-
Launch the game and tap on the Multiplayer Mode button on the main menu
-
Select either Quick Match or Custom Match depending on your preference
-
If you select Quick Match, you will be matched with a random opponent online
-
If you select Custom Match, you can create or join a room with your friends using a code
-
Once you are in a room with your friend, tap on Ready and wait for them to do the same
-
The battle will start automatically once both players are ready
-
-
How to get more robots and abilities?
-
To get more robots and abilities in Real Steel World Robot Boxing 2, you can use one of these methods:
-
-
Earn money by playing the game or watching ads and use it to buy robots and abilities from the shop
-
Complete missions and achievements and get rewards such as robots and abilities
-
Participate in live events and tournaments and win prizes such as robots and abilities
-
Use the mod apk version and get unlimited money and unlocked robots and abilities for free
-
-
How to contact the developers or report a problem?
-
To contact the developers or report a problem in Real Steel World Robot Boxing 2, you can use one of these methods:
-
-
Send an email to the developers at support@reliancegames.com
-
Visit the official website of the game at https://www.reliancegames.com/games/real-steel-world-robot-boxing-2/
-
Follow the official social media accounts of the game on Facebook, Twitter, Instagram, and YouTube
-
Leave a review or a comment on the Google Play Store page of the game
-
197e85843d
-
-
\ No newline at end of file
diff --git a/spaces/congsaPfin/Manga-OCR/logs/Free Fire OB23 Advance Server for Android How to get the APK download link and test new features.md b/spaces/congsaPfin/Manga-OCR/logs/Free Fire OB23 Advance Server for Android How to get the APK download link and test new features.md
deleted file mode 100644
index c92a51a300a46cc0ec8a03506f32bb57f7ecffad..0000000000000000000000000000000000000000
--- a/spaces/congsaPfin/Manga-OCR/logs/Free Fire OB23 Advance Server for Android How to get the APK download link and test new features.md
+++ /dev/null
@@ -1,127 +0,0 @@
-
-
Free Fire OB23 Advance Server for Android: How to Download and Play
-
Free Fire is one of the most popular battle royale games on mobile devices, with millions of players around the world. The game is constantly updated with new features, modes, characters, weapons, and more. The latest update, which is the OB23 update, is expected to be released at the end of July 2020. However, before that, some lucky players can get a chance to test the upcoming features in advance by joining the Free Fire OB23 Advance Server.
-
free fire ob23 advance server for android apk download link
The Free Fire OB23 Advance Server is a testing site where players can try out the new features that are not yet released in the official version of the game. The Advance Server also allows players to report any bugs or glitches they find in the game and get rewarded with free diamonds. The Advance Server is only available for Android devices and requires a unique activation code to enter. In this article, we will tell you everything you need to know about the Free Fire OB23 Advance Server, including how to register, download, install, and play it.
-
What is Free Fire OB23 Advance Server?
-
The Free Fire OB23 Advance Server is a program where players can try out the newest features that are not yet released in the official version of the game. The Advance Server is usually open for a limited period of time before each major update of the game. The purpose of the Advance Server is to test the stability and performance of the new features and to collect feedback from the players.
-
Features of Free Fire OB23 Advance Server
-
The Free Fire OB23 Advance Server will introduce several new features to the game, according to various leaks and rumors. Some of these features are:
-
free fire ob23 advance server apk download for android devices
-how to download free fire ob23 advance server on android phone
-free fire ob23 advance server registration and download link for android
-free fire ob23 advance server android apk file size and features
-free fire ob23 advance server download link apk for android users
-free fire ob23 advance server apk latest version download for android
-free fire ob23 advance server android download link and installation guide
-free fire ob23 advance server apk download link for android 2023
-free fire ob23 advance server android apk download and gameplay
-free fire ob23 advance server apk download link for android free
-free fire ob23 advance server download apk for android with new character
-free fire ob23 advance server apk download link for android no verification
-free fire ob23 advance server android apk download link and rewards
-free fire ob23 advance server apk download for android offline
-free fire ob23 advance server download link for android apk pure
-free fire ob23 advance server apk download link for android in hindi
-free fire ob23 advance server android apk download link and review
-free fire ob23 advance server apk download for android online
-free fire ob23 advance server download link for android apkpure.com
-free fire ob23 advance server apk download link for android without password
-free fire ob23 advance server android apk download link and bug report
-free fire ob23 advance server apk download for android modded
-free fire ob23 advance server download link for android mediafıre.com
-free fire ob23 advance server apk download link for android with new pet
-free fire ob23 advance server android apk download link and system requirements
-free fire ob23 advance server apk download for android unlimited diamonds
-free fire ob23 advance server download link for android mega.nz
-free fire ob23 advance server apk download link for android with new gun
-free fire ob23 advance server android apk download link and release date
-free fire ob23 advance server apk download for android hacked
-free fire ob23 advance server download link for android google drive
-free fire ob23 advance server apk download link for android with new map
-free fire ob23 advance server android apk download link and feedback form
-free fire ob23 advance server apk download for android updated
-free fire ob23 advance server download link for android zippyshare.com
-free fire ob23 advance server apk download link for android with new mode
-free fire ob23 advance server android apk download link and tips and tricks
-free fire ob23 advance server apk download for android latest update
-free fire ob23 advance server download link for android dropbox.com
-free fire ob23 advance server apk download link for android with new skins
-free fire ob23 advance server android apk download link and patch notes
-free fire ob23 advance server apk download for android old version
-free fire ob23 advance server download link for android 4shared.com
-free fire ob23 advance server apk download link for android with new vehicle
-free fire ob23 advance server android apk download link and gameplay video
-free fire ob23 advance server apk download for android beta version
-free fire ob23 advance server download link for android uptodown.com
-free fire ob23 advance server apk download link for android with new items
-
-
A new character named Lucas, who is a Brazilian soccer player. His ability is called Hat Trick, which increases his maximum HP with each kill.
-
A new pet named Penguin, who can help players recover their EP by using a treatment gun.
-
A new weapon named AUG, which is an assault rifle with high accuracy and range.
-
A new map named Bermuda 2.0, which is a revamped version of the original Bermuda map with new locations and graphics.
-
A new mode named Vengeance, which is a team deathmatch mode where players can respawn after being killed.
-
-
Rewards for reporting bugs in Free Fire OB23 Advance Server
-
The Free Fire OB23 Advance Server also gives players an opportunity to earn free diamonds by reporting any bugs or glitches they find in the game. The developers will reward the players based on the severity and uniqueness of the bugs they report. The rewards are as follows:
-
-
Bug Type
Reward
-
First unknown bug hunter
1000 diamonds
-
Main contributor
500 diamonds
-
Minor bug hunter
100 diamonds
-
Other bug hunters
50 diamonds
-
-
How to register for Free Fire OB23 Advance Server?
-
The registration for the Free Fire OB23 Advance Server is open for everyone, but only a limited number of players will be selected to join the testing phase. The registration process is simple and you can do it by following these steps:
-
Registration dates and batches for Free Fire OB23 Advance Server
-
The registration for the Free Fire OB23 Advance Server started on July 15, 2020 and will end on July 26, 2020. The developers will select the players in batches and send them the activation code via email. The first batch of players will receive the activation code on July 20, 2020, while the second batch will receive it on July 24, 2020. The third and final batch will receive it on July 26, 2020. The Free Fire OB23 Advance Server will be open from July 20, 2020 to July 27, 2020.
-
Steps to register for Free Fire OB23 Advance Server
-
To register for the Free Fire OB23 Advance Server, you need to follow these steps:
-
-
Visit the official website of the Free Fire OB23 Advance Server at https://ff-advance.ff.garena.com/.
-
Log in with your Facebook account that is linked to your Free Fire account.
-
Fill in your personal details such as name, email address, and phone number.
-
Click on the "Join Now" button and wait for the confirmation message.
-
Check your email regularly to see if you have received the activation code.
-
-
How to download and install Free Fire OB23 Advance Server APK?
-
If you have successfully registered and received the activation code for the Free Fire OB23 Advance Server, you can download and install the APK file on your Android device. The APK file is about 1.5 GB in size, so make sure you have enough storage space and a stable internet connection before downloading it.
-
Download link for Free Fire OB23 Advance Server APK
-
You can download the Free Fire OB23 Advance Server APK from the official website of the Advance Server at https://ff-advance.ff.garena.com/. You need to log in with your Facebook account and click on the "Download APK" button. Alternatively, you can use this direct download link: https://bit.ly/2ZQ8XcO.
-
Steps to download and install Free Fire OB23 Advance Server APK
-
To download and install the Free Fire OB23 Advance Server APK, you need to follow these steps:
-
-
Download the APK file from the link given above or from the official website of the Advance Server.
-
Go to your device settings and enable the "Install from unknown sources" option.
-
Locate the downloaded APK file in your file manager and tap on it to install it.
-
Open the installed app and enter your activation code when prompted.
-
Enjoy playing the Free Fire OB23 Advance Server and testing the new features.
-
-
How to get activation code for Free Fire OB23 Advance Server?
-
The activation code is a unique code that is required to enter the Free Fire OB23 Advance Server. Without the activation code, you cannot play the game even if you have downloaded and installed the APK file. The activation code is only given to a limited number of players who have registered for the Advance Server.
-
What is activation code and why is it needed for Free Fire OB23 Advance Server?
-
The activation code is a 12-digit alphanumeric code that is used to verify your identity and grant you access to the Free Fire OB23 Advance Server. The activation code is needed for two reasons:
-
-
To prevent unauthorized access and ensure a smooth testing experience for the selected players.
-
To limit the number of players and avoid server overload or crashes.
-
-
How to get activation code for Free Fire OB23 Advance Server?
-
The only way to get an activation code for the Free Fire OB23 Advance Server is to register for it on the official website of the Advance Server. The developers will send you an email with the activation code if you are selected to join the testing phase. The email will be sent in batches according to the registration dates. You need to check your email regularly and use the activation code as soon as possible before it expires or gets used by someone else.
-
Conclusion
-
The Free Fire OB23 Advance Server is a great opportunity for players who want to try out the new features of the game before they are released in the official version. The Advance Server also allows players to report any bugs or glitches they find in the game and get rewarded with free diamonds. However, not everyone can join the Advance Server as it requires a registration process and an activation code. If you are interested in joining the Free Fire OB23 Advance Server, you need to register on the official website of the Advance Server and wait for the activation code to be sent to your email. The registration is open until July 26, 2020 and the Advance Server is open from July 20, 2020 to July 27, 2020. Hurry up and join the Advance Server before it closes and enjoy the new features of the game.
-
FAQs
-
Here are some frequently asked questions about the Free Fire OB23 Advance Server:
-
-
Q: Is the Free Fire OB23 Advance Server free to join?
-
A: Yes, the Free Fire OB23 Advance Server is free to join for anyone who has an Android device and a Facebook account linked to their Free Fire account. However, you need to register and get an activation code to enter the Advance Server.
-
Q: Can I play the Free Fire OB23 Advance Server with my friends?
-
A: Yes, you can play the Free Fire OB23 Advance Server with your friends if they have also registered and received the activation code for the Advance Server. You can invite them to your squad or join their squad in the game.
-
Q: Will my progress and data in the Free Fire OB23 Advance Server be transferred to the official version of the game?
-
A: No, your progress and data in the Free Fire OB23 Advance Server will not be transferred to the official version of the game. The Advance Server is a separate testing site and has no connection with the official version of the game. You will have to start from scratch when you play the official version of the game.
-
Q: How can I report bugs or glitches in the Free Fire OB23 Advance Server?
-
A: You can report bugs or glitches in the Free Fire OB23 Advance Server by using the "Report" button in the game. You need to provide a screenshot or a video of the bug or glitch and a brief description of it. You will also need to provide your email address and your activation code for verification purposes.
-
Q: How can I get more diamonds in the Free Fire OB23 Advance Server?
-
A: You can get more diamonds in the Free Fire OB23 Advance Server by reporting bugs or glitches in the game. The developers will reward you with free diamonds based on the severity and uniqueness of the bugs you report. You can also get more diamonds by completing missions and events in the game.
- 401be4b1e0
-
-
\ No newline at end of file
diff --git a/spaces/congsaPfin/Manga-OCR/logs/GTA 5 Ray Tracing Mod APK How to Enhance Your Game with Stunning Graphics.md b/spaces/congsaPfin/Manga-OCR/logs/GTA 5 Ray Tracing Mod APK How to Enhance Your Game with Stunning Graphics.md
deleted file mode 100644
index 1849054dfc9d5aeb6f387865df54de288a4dcee5..0000000000000000000000000000000000000000
--- a/spaces/congsaPfin/Manga-OCR/logs/GTA 5 Ray Tracing Mod APK How to Enhance Your Game with Stunning Graphics.md
+++ /dev/null
@@ -1,104 +0,0 @@
-
-
GTA 5 RTX Mod Download APK: How to Enhance Your Game with Ray Tracing
-
Grand Theft Auto V (GTA 5) is one of the most popular and successful games of all time, but it can look even better with some mods. One of the most impressive mods for GTA 5 is the RTX mod, which adds ray tracing effects to the game, making it look more realistic and immersive. In this article, we will show you how to download and install the GTA 5 RTX mod on your Android device, and how to play the game with ray tracing enabled. Let's get started!
GTA 5 RTX mod is a combination of different mods that enhance the graphics and lighting of GTA 5 with ray tracing technology. Ray tracing is a technique that simulates how light behaves in the real world, creating realistic shadows, reflections, refractions, and ambient occlusion. Ray tracing can make a huge difference in the appearance and atmosphere of a game, especially in an open-world game like GTA 5.
-
The benefits of ray tracing in GTA 5
-
With ray tracing enabled, GTA 5 looks more stunning and lifelike than ever before. You can see the reflections of buildings, cars, and people on the water, glass, and metal surfaces. You can see the shadows of objects and characters cast by different light sources. You can see the subtle changes in the color and brightness of the environment depending on the time of day and weather conditions. You can see the details and textures of every object more clearly and vividly.
-
The requirements for running GTA 5 RTX Mod
-
Unfortunately, ray tracing is not a cheap or easy feature to implement in a game. It requires a lot of computing power and memory to process all the complex calculations involved. Therefore, you need a powerful device to run GTA 5 with RTX mod smoothly and without lag. Here are some of the minimum requirements for running GTA 5 RTX mod on your Android device:
-
gta 5 ray tracing mod how to install
-gta 5 enhanced edition pc download
-gta 5 quantv mod free download
-gta 5 real life pack mod download
-gta 5 mega reshade pack for nve
-gta 5 rtx mobile android gameplay
-gta 5 ray tracing reshade patreon
-gta 5 8k resolution mod download
-gta 5 digital dreams mod download
-gta 5 unofficial enhanced edition mod
-gta 5 ray tracing shader rtgi download
-gta 5 nve vs redux comparison
-gta 5 ultra realistic graphics mod apk
-gta 5 rtx mobile android beta download
-gta 5 ray tracing mod dualshockers
-gta 5 enhanced edition release date
-gta 5 nexus mods best graphics mods
-gta 5 ray tracing mod youtube video
-gta 5 rtx mobile android system requirements
-gta 5 ray tracing mod gameplay trailer
-gta 5 net energy gain fusion experiment mod
-gta 5 nve vs quantv performance test
-gta 5 ultra realistic graphics mod pc download
-gta 5 rtx mobile android discord link
-gta 5 ray tracing mod new scientist article
-gta 5 holy grail fusion experiment mod download
-gta 5 nve vs vanilla graphics comparison
-gta 5 ultra realistic graphics mod for android phone
-gta 5 rtx mobile android facebook page link
-gta 5 ray tracing mod yahoo news report
-
-
A Snapdragon 888 or equivalent processor
-
8 GB of RAM or more
-
128 GB of storage or more
-
A high-resolution display (at least Full HD)
-
A stable internet connection
-
-
How to Download and Install GTA 5 RTX Mod?
-
Now that you know what GTA 5 RTX mod is and what it can do for your game, you might be wondering how to get it on your Android device. Well, it's not as simple as downloading an APK file and installing it. You need to follow some steps and use some tools to make it work. Here are the steps you need to follow:
-
Step 1: Install the QuantV mod
-
The QuantV mod is a base mod that improves the graphics quality and performance of GTA 5. It also adds some features like dynamic weather, custom time cycle, realistic physics, and more. You need to install this mod first before you can use the RTX mod. To install the QuantV mod, you need to do the following:
Extract the zip file and copy the contents to your GTA 5 folder on your device.
-
Run the QuantV.exe file as an administrator and follow the instructions.
-
Restart your device and launch GTA 5.
-
-
Step 2: Get the Ray Tracing Reshading mod
-
The Ray Tracing Reshading mod is the mod that adds the ray tracing effects to GTA 5. It is based on the ReShade tool, which is a post-processing injector that can enhance the graphics of any game. To get the Ray Tracing Reshading mod, you need to do the following:
Extract the zip file and copy the contents to your GTA 5 folder on your device.
-
Replace any existing files if prompted.
-
-
Step 3: Optional: Get the GTA V Real Life Pack
-
If you want to make your GTA 5 experience even more realistic and immersive, you can also get the GTA V Real Life Pack. This is a collection of mods that add real-life cars, weapons, clothes, brands, billboards, and more to GTA 5. To get the GTA V Real Life Pack, you need to do the following:
Extract the zip file and copy the contents to your GTA 5 folder on your device.
-
Replace any existing files if prompted.
-
-
How to Play GTA 5 with RTX Mod?
-
Now that you have installed all the necessary mods, you are ready to play GTA 5 with RTX mod on your Android device. Here are some tips on how to do that:
-
Launch the game and enable the reshade settings
-
When you launch GTA 5, you should see a message on the top left corner of your screen saying "ReShade by crosire". This means that the ReShade tool is working properly. To enable the ray tracing effects, you need to press the Home key on your keyboard or controller. This will open a menu where you can select different presets and settings for ReShade. You can choose from different options like Ultra, High, Medium, or Low depending on your device's performance. You can also customize each setting individually by clicking on it and adjusting the sliders. For example, you can change the ray tracing quality, intensity, distance, and more.
-
Adjust the graphics options and resolution
-
To get the best results from GTA 5 RTX mod, you should also adjust some of the graphics options and resolution in the game's settings menu. You can access this menu by pressing Esc on your keyboard or controller. You should set the graphics quality to Very High or Ultra if possible, and turn on all the advanced features like MSAA, FXAA, Anisotropic Filtering, Ambient Occlusion, etc. You should also set the resolution to match your device's display or higher if possible. However, keep in mind that higher settings and resolution will require more resources and may cause lag or crashes. You should experiment with different combinations until you find the optimal balance between quality and performance.
-
Enjoy the stunning visuals and gameplay
-
Once you have enabled and adjusted all the settings, you can enjoy playing GTA 5 with RTX mod on your Android device. You will notice a huge difference in how the game looks and feels with ray tracing enabled. You will be amazed by how realistic and immersive everything looks, from the lighting and shadows to the reflections and textures. You will also experience a more dynamic and varied gameplay with different weather effects, physics, and scenarios. You will feel like you are playing a whole new game with GTA 5 RTX mod.
-
Conclusion
-
Summary of the main points
-
In this article, we have shown you how to download and install GTA 5 RTX mod on your Android device, and how to play the game with ray tracing enabled. We have explained what GTA 5 RTX mod is, what it does for your game, what are the requirements for running it, how to install it step by step, and how to adjust it for optimal results. We have also given you some tips on how to make your game even more realistic and immersive with other mods.
-
Call to action and recommendation
-
If you are a fan of GTA 5 and you want to experience the game in a new and improved way, you should definitely try GTA 5 RTX mod on your Android device. It will transform your game into a stunning and realistic masterpiece with ray tracing effects. You will be able to enjoy the game like never before, with amazing graphics and gameplay. You will also be able to customize your game with other mods that add more features and content to GTA 5. GTA 5 RTX mod is a must-have for any GTA 5 lover.
-
So what are you waiting for? Download GTA 5 RTX mod now and start playing GTA 5 with ray tracing on your Android device. You will not regret it!
-
FAQs
-
Here are some of the frequently asked questions about GTA 5 RTX mod:
-
-
Is GTA 5 RTX mod free?
-
Yes, GTA 5 RTX mod is free to download and use. However, you need to have a legal copy of GTA 5 on your device to use it.
-
Is GTA 5 RTX mod safe?
-
Yes, GTA 5 RTX mod is safe to use as long as you download it from trusted sources and follow the instructions carefully. However, you should always backup your game files before installing any mods, in case something goes wrong or you want to uninstall them.
-
Does GTA 5 RTX mod work on iOS devices?
-
No, GTA 5 RTX mod only works on Android devices. iOS devices do not support the necessary tools and features for running GTA 5 RTX mod.
-
Can I play GTA 5 online with RTX mod?
-
No, GTA 5 RTX mod only works on the single-player mode of GTA 5. If you try to play online with RTX mod, you will likely get banned or kicked out by the game servers.
-
Can I use other mods with GTA 5 RTX mod?
-
Yes, you can use other mods with GTA 5 RTX mod, as long as they are compatible and do not conflict with each other. You can find many mods for GTA 5 on various websites and forums.
-
401be4b1e0
-
-
\ No newline at end of file
diff --git a/spaces/congsaPfin/Manga-OCR/logs/Opera Mini 2019 APK The Best Browser for Your Android Device.md b/spaces/congsaPfin/Manga-OCR/logs/Opera Mini 2019 APK The Best Browser for Your Android Device.md
deleted file mode 100644
index 016231202ff85987d48d49e487cddbc12422d822..0000000000000000000000000000000000000000
--- a/spaces/congsaPfin/Manga-OCR/logs/Opera Mini 2019 APK The Best Browser for Your Android Device.md
+++ /dev/null
@@ -1,129 +0,0 @@
-
-
Opera Mini 2019 APK: A Light and Powerful Browser for Android
-
If you are looking for a fast, reliable, and easy-to-use browser for your Android device, you might want to check out Opera Mini 2019 APK. This is a light and powerful browser that has a tiny footprint and consumes few resources. In this article, we will tell you what Opera Mini is, how to download and install it on your device, why you should use it, and some FAQs that you might have.
A brief introduction to Opera Mini and its features
-
Opera Mini is a mobile web browser that was developed by Opera Software, a Norwegian company that also makes the popular Opera Browser for desktop and mobile devices. Opera Mini was first launched in 2005 as a Java-based application that could run on almost any phone. Since then, it has evolved into a native Android app that offers a lot of features and benefits for users.
-
Some of the features that Opera Mini offers are:
-
-
A smart download manager that lets you download files in the background, pause and resume downloads, and save them to your SD card or internal storage.
-
A built-in ad blocker that blocks annoying ads and pop-ups, making your browsing experience more pleasant and secure.
-
A private browsing mode that lets you surf the web without leaving any traces or history on your device.
-
A night mode that adjusts the brightness and contrast of the screen, making it easier to read in low-light conditions.
-
A data saver mode that compresses web pages and images, reducing your data usage by up to 90%.
-
A smart news feed that curates the latest news and stories from various sources, based on your preferences and interests.
-
A QR code scanner that lets you scan QR codes directly from the browser, without needing any other app.
-
A video player that lets you watch videos online or offline, with options to adjust the playback speed, zoom in or out, or switch to full-screen mode.
-
-
How to download and install Opera Mini 2019 APK on your Android device
-
If you want to download and install Opera Mini 2019 APK on your Android device, you can follow these simple steps:
-
opera mini 2019 apk download
-opera mini 2019 apk free download
-opera mini 2019 apk latest version
-opera mini 2019 apk old version
-opera mini 2019 apk for android
-opera mini 2019 apk mod
-opera mini 2019 apk uptodown
-opera mini 2019 apk pure
-opera mini 2019 apk mirror
-opera mini 2019 apk file
-opera mini 2019 apk update
-opera mini 2019 apk offline installer
-opera mini 2019 apk hack
-opera mini 2019 apk pro
-opera mini 2019 apk premium
-opera mini 2019 apk beta
-opera mini 2019 apk cracked
-opera mini 2019 apk full version
-opera mini 2019 apk no ads
-opera mini 2019 apk unlimited data
-opera mini 2019 apk fast download
-opera mini 2019 apk video downloader
-opera mini 2019 apk browser
-opera mini 2019 apk vpn
-opera mini 2019 apk ad blocker
-opera mini 2019 apk dark mode
-opera mini 2019 apk night mode
-opera mini 2019 apk private mode
-opera mini 2019 apk incognito mode
-opera mini 2019 apk data saver
-opera mini 2019 apk turbo mode
-opera mini 2019 apk extreme mode
-opera mini 2019 apk high mode
-opera mini 2019 apk lite mode
-opera mini 2019 apk news feed
-opera mini 2019 apk smart download
-opera mini 2019 apk offline pages
-opera mini 2019 apk bookmarks sync
-opera mini 2019 apk QR code scanner
-opera mini 2019 apk facebook mode
-opera mini 2019 apk twitter mode
-opera mini 2019 apk instagram mode
-opera mini 2019 apk whatsapp mode
-opera mini 2019 apk youtube mode
-opera mini 2019 apk reddit mode
-opera mini 2019 apk pinterest mode
-opera mini 2019 apk tiktok mode
-opera mini 2019 apk spotify mode
-opera mini 2019 apk netflix mode
-
-
Go to [this link](^1^) on your device's browser. This will take you to the Softpedia website, where you can find the latest version of Opera Mini 2019 APK.
-
Tap on the green "Download" button at the top of the page. This will start downloading the APK file to your device.
-
Once the download is complete, tap on the notification bar or go to your device's file manager and locate the downloaded file. It should be named "Opera_Mini_19.0.2254.108259.apk".
-
Tap on the file and allow the installation from unknown sources if prompted. This will start installing the app on your device.
-
Once the installation is complete, tap on "Open" or go to your app drawer and launch the app. You can now enjoy browsing the web with Opera Mini 2019 APK.
-
-
Why use Opera Mini 2019 APK?
-
The benefits of using Opera Mini 2019 APK
-
There are many reasons why you might want to use Opera Mini 2019 APK on your Android device. Here are some of the benefits that you can get from this browser:
-
Save data and battery
-
One of the main advantages of Opera Mini 2019 APK is that it can save you a lot of data and battery. By compressing web pages and images, it can reduce your data usage by up to 90%, which means you can browse more for less. It also consumes less power than other browsers, which means you can browse longer without worrying about your battery life.
-
Browse faster and smoother
-
Another benefit of Opera Mini 2019 APK is that it can make your browsing experience faster and smoother. By blocking ads and pop-ups, it can eliminate distractions and speed up the loading time of web pages. It also has a smart download manager that lets you download files in the background, pause and resume downloads, and save them to your SD card or internal storage. You can also watch videos online or offline, with options to adjust the playback speed, zoom in or out, or switch to full-screen mode.
-
Access blocked websites and content
-
A third benefit of Opera Mini 2019 APK is that it can help you access blocked websites and content. By using a built-in VPN feature, it can encrypt your traffic and hide your IP address, which means you can bypass censorship and geo-restrictions. You can also use a private browsing mode that lets you surf the web without leaving any traces or history on your device.
-
Customize your browsing experience
-
A fourth benefit of Opera Mini 2019 APK is that it can let you customize your browsing experience. By using a night mode that adjusts the brightness and contrast of the screen, you can make it easier to read in low-light conditions. You can also use a smart news feed that curates the latest news and stories from various sources, based on your preferences and interests. You can also use a QR code scanner that lets you scan QR codes directly from the browser, without needing any other app.
-
The drawbacks of using Opera Mini 2019 APK
-
While Opera Mini 2019 APK has many benefits, it also has some drawbacks that you should be aware of. Here are some of the drawbacks that you might encounter when using this browser:
-
Some websites may not display properly
-
One of the drawbacks of Opera Mini 2019 APK is that some websites may not display properly on this browser. This is because Opera Mini uses a proxy server to compress web pages and images, which means some elements may be missing or distorted. This can affect the functionality and appearance of some websites, especially those that use complex scripts or animations.
-
Some features may not work on some devices
-
Another drawback of Opera Mini 2019 APK is that some features may not work on some devices. This is because Opera Mini is designed to run on low-end devices with limited resources, which means some features may be incompatible or unavailable on some devices. For example, some devices may not support the video player or the VPN feature, which means you may not be able to watch videos or access blocked websites on those devices.
-
Conclusion
-
A summary of the main points and a call to action
-
In conclusion, Opera Mini 2019 APK is a light and powerful browser for Android that offers a lot of features and benefits for users. It can save data and battery, browse faster and smoother, access blocked websites and content, and customize your browsing experience. However, it also has some drawbacks, such as some websites may not display properly and some features may not work on some devices.
-
If you are looking for a fast, reliable, and easy-to-use browser for your Android device, you might want to give Opera Mini 2019 APK a try. You can download it from [this link] and install it on your device following the steps above. You can also visit [this link] to learn more about Opera Mini and its features.
-
FAQs
-
What is the difference between Opera Mini and Opera Browser?
-
Opera Mini and Opera Browser are both mobile web browsers developed by Opera Software, but they have some differences in terms of features and performance. Opera Mini is a lighter and faster browser that uses a proxy server to compress web pages and images, saving data and battery. Opera Browser is a more full-featured browser that offers more options and functionalities, such as a built-in VPN, a crypto wallet, a news reader, and a gaming hub.
-
Is Opera Mini safe to use?
-
Opera Mini is safe to use as long as you download it from a trusted source, such as the Softpedia website or the Google Play Store. Opera Mini uses encryption and security protocols to protect your data and privacy, and it also has a built-in ad blocker and a private browsing mode to prevent unwanted ads and trackers. However, you should always be careful when browsing the web and avoid clicking on suspicious links or downloading malicious files.
-
How can I update Opera Mini to the latest version?
-
You can update Opera Mini to the latest version by following these steps:
-
-
Open Opera Mini on your device and tap on the menu icon at the bottom right corner of the screen.
-
Tap on "Settings" and then on "About Opera Mini".
-
Tap on "Check for updates" and wait for the app to check for any available updates.
-
If there is an update available, tap on "Update" and follow the instructions to download and install the update.
-
If there is no update available, you will see a message saying "You have the latest version of Opera Mini".
-
-
How can I change the language of Opera Mini?
-
You can change the language of Opera Mini by following these steps:
-
-
Open Opera Mini on your device and tap on the menu icon at the bottom right corner of the screen.
-
Tap on "Settings" and then on "Language".
-
Tap on the language that you want to use and confirm your choice.
-
The app will restart and apply the new language settings.
-
-
How can I contact Opera Mini support?
-
If you have any questions, feedback, or issues with Opera Mini, you can contact Opera Mini support by following these steps:
-
-
Open Opera Mini on your device and tap on the menu icon at the bottom right corner of the screen.
-
Tap on "Help" and then on "Contact us".
-
Fill in the form with your name, email address, subject, and message.
-
Tap on "Send" and wait for a response from the support team.
- 401be4b1e0
-
-
\ No newline at end of file
diff --git a/spaces/contluForse/HuggingGPT/assets/Autodesk.AutoCAD.MAP.3D.2016.[32-64Bit]-[FirstUploads] 34 Learn from the Experts with Video Tutorials and Examples.md b/spaces/contluForse/HuggingGPT/assets/Autodesk.AutoCAD.MAP.3D.2016.[32-64Bit]-[FirstUploads] 34 Learn from the Experts with Video Tutorials and Examples.md
deleted file mode 100644
index dddce798cf724696e5987c36d73a86f101280131..0000000000000000000000000000000000000000
--- a/spaces/contluForse/HuggingGPT/assets/Autodesk.AutoCAD.MAP.3D.2016.[32-64Bit]-[FirstUploads] 34 Learn from the Experts with Video Tutorials and Examples.md
+++ /dev/null
@@ -1,6 +0,0 @@
-
-
- aaccfb2cb3
-
-
-
diff --git a/spaces/contluForse/HuggingGPT/assets/Bobby Fuller I Fought The Law Download.md b/spaces/contluForse/HuggingGPT/assets/Bobby Fuller I Fought The Law Download.md
deleted file mode 100644
index 19af9839f02c259ff12114e2a214dc411d8092c6..0000000000000000000000000000000000000000
--- a/spaces/contluForse/HuggingGPT/assets/Bobby Fuller I Fought The Law Download.md
+++ /dev/null
@@ -1,15 +0,0 @@
-
-
How to Download "I Fought the Law" by Bobby Fuller
-
"I Fought the Law" is a classic rock song written by Sonny Curtis of the Crickets and popularized by a cover by the Bobby Fuller Four in 1966[^3^]. The song tells the story of a young man who breaks the law and faces the consequences. It has been covered by many artists, including the Clash, Green Day, and Bruce Springsteen.
-
If you want to download "I Fought the Law" by Bobby Fuller, you have several options. One of them is to use Qobuz, a streaming service that offers high-resolution audio quality. You can listen to unlimited or download "I Fought the Law" by Bobby Fuller on Qobuz with a subscription from £10.83/month[^1^]. You can also buy the song individually for £0.99.
Another option is to use YouTube, where you can find various versions of "I Fought the Law" by Bobby Fuller. One of them is a video with very good sound quality uploaded by TheVideoJukebox[^2^]. You can watch the video for free or use a YouTube downloader tool to save it as an MP3 file on your device.
-
Whichever option you choose, you will enjoy listening to this iconic song that has influenced many generations of musicians and fans.
"I Fought the Law" by Bobby Fuller is not only a catchy song, but also a part of rock and roll history. The song was originally written by Sonny Curtis of the Crickets, the band that backed up Buddy Holly in the late 1950s. Curtis recorded the song with the Crickets in 1959, but it did not get much attention until Bobby Fuller covered it in 1965[^3^].
-
Bobby Fuller was a talented musician and singer who grew up in El Paso, Texas. He formed his own band, the Bobby Fuller Four, and recorded several songs in his home studio. He moved to Los Angeles in 1964 and signed with Mustang Records, a subsidiary of Del-Fi Records. He re-recorded "I Fought the Law" with his band and released it as a single in October 1965. The song became a hit, reaching #9 on the Billboard Hot 100 chart in February 1966[^3^].
-
The success of "I Fought the Law" made Bobby Fuller a rising star in the music industry. He appeared on popular TV shows like American Bandstand and Hullabaloo. He also toured with other famous artists like the Beatles, the Beach Boys, and the Byrds. He was working on his third album when tragedy struck. On July 18, 1966, he was found dead in his mother's car outside his apartment. He was only 23 years old[^3^].
-
The cause of Bobby Fuller's death remains a mystery to this day. The police ruled it as a suicide by asphyxiation from gasoline fumes, but many people suspect foul play. Some theories suggest that he was killed by the mob, by a jealous lover, or by corrupt cops. Others believe that he was depressed or addicted to drugs and took his own life. No one knows for sure what happened to Bobby Fuller, but his music lives on[^3^].
"I Fought the Law" by Bobby Fuller has become a rock and roll anthem that has inspired many other artists to cover it or pay tribute to it. One of the most famous covers was by the Clash, a British punk rock band, who recorded it in 1979 for their EP The Cost of Living. Their version added a reggae influence and changed some of the lyrics to reflect their political views. The Clash's version reached #29 on the UK Singles Chart and #2 on the Irish Singles Chart[^1^].
-
-
Another notable cover was by the Dead Kennedys, an American hardcore punk band, who recorded it in 1987 for their album Give Me Convenience or Give Me Death. Their version changed the lyrics to "I fought the law and I won" and added references to police brutality and corruption. The Dead Kennedys' version was also used in the soundtrack of the video game Grand Theft Auto: San Andreas[^1^].
-
"I Fought the Law" by Bobby Fuller has also been featured in many movies, TV shows, and commercials, such as The Blues Brothers, The Simpsons, Breaking Bad, The Office, and Nike. The song has been recognized as one of the greatest songs of all time by Rolling Stone, the Rock and Roll Hall of Fame, and VH1. The song has also been inducted into the Grammy Hall of Fame in 2016[^1^].
d5da3c52bf
-
-
\ No newline at end of file
diff --git a/spaces/coreml-community/ControlNet-v1-1-Annotators-cpu/annotator/normalbae/models/submodules/efficientnet_repo/geffnet/gen_efficientnet.py b/spaces/coreml-community/ControlNet-v1-1-Annotators-cpu/annotator/normalbae/models/submodules/efficientnet_repo/geffnet/gen_efficientnet.py
deleted file mode 100644
index cd170d4cc5bed6ca82b61539902b470d3320c691..0000000000000000000000000000000000000000
--- a/spaces/coreml-community/ControlNet-v1-1-Annotators-cpu/annotator/normalbae/models/submodules/efficientnet_repo/geffnet/gen_efficientnet.py
+++ /dev/null
@@ -1,1450 +0,0 @@
-""" Generic Efficient Networks
-
-A generic MobileNet class with building blocks to support a variety of models:
-
-* EfficientNet (B0-B8, L2 + Tensorflow pretrained AutoAug/RandAug/AdvProp/NoisyStudent ports)
- - EfficientNet: Rethinking Model Scaling for CNNs - https://arxiv.org/abs/1905.11946
- - CondConv: Conditionally Parameterized Convolutions for Efficient Inference - https://arxiv.org/abs/1904.04971
- - Adversarial Examples Improve Image Recognition - https://arxiv.org/abs/1911.09665
- - Self-training with Noisy Student improves ImageNet classification - https://arxiv.org/abs/1911.04252
-
-* EfficientNet-Lite
-
-* MixNet (Small, Medium, and Large)
- - MixConv: Mixed Depthwise Convolutional Kernels - https://arxiv.org/abs/1907.09595
-
-* MNasNet B1, A1 (SE), Small
- - MnasNet: Platform-Aware Neural Architecture Search for Mobile - https://arxiv.org/abs/1807.11626
-
-* FBNet-C
- - FBNet: Hardware-Aware Efficient ConvNet Design via Differentiable NAS - https://arxiv.org/abs/1812.03443
-
-* Single-Path NAS Pixel1
- - Single-Path NAS: Designing Hardware-Efficient ConvNets - https://arxiv.org/abs/1904.02877
-
-* And likely more...
-
-Hacked together by / Copyright 2020 Ross Wightman
-"""
-import torch.nn as nn
-import torch.nn.functional as F
-
-from .config import layer_config_kwargs, is_scriptable
-from .conv2d_layers import select_conv2d
-from .helpers import load_pretrained
-from .efficientnet_builder import *
-
-__all__ = ['GenEfficientNet', 'mnasnet_050', 'mnasnet_075', 'mnasnet_100', 'mnasnet_b1', 'mnasnet_140',
- 'semnasnet_050', 'semnasnet_075', 'semnasnet_100', 'mnasnet_a1', 'semnasnet_140', 'mnasnet_small',
- 'mobilenetv2_100', 'mobilenetv2_140', 'mobilenetv2_110d', 'mobilenetv2_120d',
- 'fbnetc_100', 'spnasnet_100', 'efficientnet_b0', 'efficientnet_b1', 'efficientnet_b2', 'efficientnet_b3',
- 'efficientnet_b4', 'efficientnet_b5', 'efficientnet_b6', 'efficientnet_b7', 'efficientnet_b8',
- 'efficientnet_l2', 'efficientnet_es', 'efficientnet_em', 'efficientnet_el',
- 'efficientnet_cc_b0_4e', 'efficientnet_cc_b0_8e', 'efficientnet_cc_b1_8e',
- 'efficientnet_lite0', 'efficientnet_lite1', 'efficientnet_lite2', 'efficientnet_lite3', 'efficientnet_lite4',
- 'tf_efficientnet_b0', 'tf_efficientnet_b1', 'tf_efficientnet_b2', 'tf_efficientnet_b3',
- 'tf_efficientnet_b4', 'tf_efficientnet_b5', 'tf_efficientnet_b6', 'tf_efficientnet_b7', 'tf_efficientnet_b8',
- 'tf_efficientnet_b0_ap', 'tf_efficientnet_b1_ap', 'tf_efficientnet_b2_ap', 'tf_efficientnet_b3_ap',
- 'tf_efficientnet_b4_ap', 'tf_efficientnet_b5_ap', 'tf_efficientnet_b6_ap', 'tf_efficientnet_b7_ap',
- 'tf_efficientnet_b8_ap', 'tf_efficientnet_b0_ns', 'tf_efficientnet_b1_ns', 'tf_efficientnet_b2_ns',
- 'tf_efficientnet_b3_ns', 'tf_efficientnet_b4_ns', 'tf_efficientnet_b5_ns', 'tf_efficientnet_b6_ns',
- 'tf_efficientnet_b7_ns', 'tf_efficientnet_l2_ns', 'tf_efficientnet_l2_ns_475',
- 'tf_efficientnet_es', 'tf_efficientnet_em', 'tf_efficientnet_el',
- 'tf_efficientnet_cc_b0_4e', 'tf_efficientnet_cc_b0_8e', 'tf_efficientnet_cc_b1_8e',
- 'tf_efficientnet_lite0', 'tf_efficientnet_lite1', 'tf_efficientnet_lite2', 'tf_efficientnet_lite3',
- 'tf_efficientnet_lite4',
- 'mixnet_s', 'mixnet_m', 'mixnet_l', 'mixnet_xl', 'tf_mixnet_s', 'tf_mixnet_m', 'tf_mixnet_l']
-
-
-model_urls = {
- 'mnasnet_050': None,
- 'mnasnet_075': None,
- 'mnasnet_100':
- 'https://github.com/rwightman/pytorch-image-models/releases/download/v0.1-weights/mnasnet_b1-74cb7081.pth',
- 'mnasnet_140': None,
- 'mnasnet_small': None,
-
- 'semnasnet_050': None,
- 'semnasnet_075': None,
- 'semnasnet_100':
- 'https://github.com/rwightman/pytorch-image-models/releases/download/v0.1-weights/mnasnet_a1-d9418771.pth',
- 'semnasnet_140': None,
-
- 'mobilenetv2_100':
- 'https://github.com/rwightman/pytorch-image-models/releases/download/v0.1-weights/mobilenetv2_100_ra-b33bc2c4.pth',
- 'mobilenetv2_110d':
- 'https://github.com/rwightman/pytorch-image-models/releases/download/v0.1-weights/mobilenetv2_110d_ra-77090ade.pth',
- 'mobilenetv2_120d':
- 'https://github.com/rwightman/pytorch-image-models/releases/download/v0.1-weights/mobilenetv2_120d_ra-5987e2ed.pth',
- 'mobilenetv2_140':
- 'https://github.com/rwightman/pytorch-image-models/releases/download/v0.1-weights/mobilenetv2_140_ra-21a4e913.pth',
-
- 'fbnetc_100':
- 'https://github.com/rwightman/pytorch-image-models/releases/download/v0.1-weights/fbnetc_100-c345b898.pth',
- 'spnasnet_100':
- 'https://github.com/rwightman/pytorch-image-models/releases/download/v0.1-weights/spnasnet_100-048bc3f4.pth',
-
- 'efficientnet_b0':
- 'https://github.com/rwightman/pytorch-image-models/releases/download/v0.1-weights/efficientnet_b0_ra-3dd342df.pth',
- 'efficientnet_b1':
- 'https://github.com/rwightman/pytorch-image-models/releases/download/v0.1-weights/efficientnet_b1-533bc792.pth',
- 'efficientnet_b2':
- 'https://github.com/rwightman/pytorch-image-models/releases/download/v0.1-weights/efficientnet_b2_ra-bcdf34b7.pth',
- 'efficientnet_b3':
- 'https://github.com/rwightman/pytorch-image-models/releases/download/v0.1-weights/efficientnet_b3_ra2-cf984f9c.pth',
- 'efficientnet_b4': None,
- 'efficientnet_b5': None,
- 'efficientnet_b6': None,
- 'efficientnet_b7': None,
- 'efficientnet_b8': None,
- 'efficientnet_l2': None,
-
- 'efficientnet_es':
- 'https://github.com/rwightman/pytorch-image-models/releases/download/v0.1-weights/efficientnet_es_ra-f111e99c.pth',
- 'efficientnet_em': None,
- 'efficientnet_el': None,
-
- 'efficientnet_cc_b0_4e': None,
- 'efficientnet_cc_b0_8e': None,
- 'efficientnet_cc_b1_8e': None,
-
- 'efficientnet_lite0': 'https://github.com/rwightman/pytorch-image-models/releases/download/v0.1-weights/efficientnet_lite0_ra-37913777.pth',
- 'efficientnet_lite1': None,
- 'efficientnet_lite2': None,
- 'efficientnet_lite3': None,
- 'efficientnet_lite4': None,
-
- 'tf_efficientnet_b0':
- 'https://github.com/rwightman/pytorch-image-models/releases/download/v0.1-weights/tf_efficientnet_b0_aa-827b6e33.pth',
- 'tf_efficientnet_b1':
- 'https://github.com/rwightman/pytorch-image-models/releases/download/v0.1-weights/tf_efficientnet_b1_aa-ea7a6ee0.pth',
- 'tf_efficientnet_b2':
- 'https://github.com/rwightman/pytorch-image-models/releases/download/v0.1-weights/tf_efficientnet_b2_aa-60c94f97.pth',
- 'tf_efficientnet_b3':
- 'https://github.com/rwightman/pytorch-image-models/releases/download/v0.1-weights/tf_efficientnet_b3_aa-84b4657e.pth',
- 'tf_efficientnet_b4':
- 'https://github.com/rwightman/pytorch-image-models/releases/download/v0.1-weights/tf_efficientnet_b4_aa-818f208c.pth',
- 'tf_efficientnet_b5':
- 'https://github.com/rwightman/pytorch-image-models/releases/download/v0.1-weights/tf_efficientnet_b5_ra-9a3e5369.pth',
- 'tf_efficientnet_b6':
- 'https://github.com/rwightman/pytorch-image-models/releases/download/v0.1-weights/tf_efficientnet_b6_aa-80ba17e4.pth',
- 'tf_efficientnet_b7':
- 'https://github.com/rwightman/pytorch-image-models/releases/download/v0.1-weights/tf_efficientnet_b7_ra-6c08e654.pth',
- 'tf_efficientnet_b8':
- 'https://github.com/rwightman/pytorch-image-models/releases/download/v0.1-weights/tf_efficientnet_b8_ra-572d5dd9.pth',
-
- 'tf_efficientnet_b0_ap':
- 'https://github.com/rwightman/pytorch-image-models/releases/download/v0.1-weights/tf_efficientnet_b0_ap-f262efe1.pth',
- 'tf_efficientnet_b1_ap':
- 'https://github.com/rwightman/pytorch-image-models/releases/download/v0.1-weights/tf_efficientnet_b1_ap-44ef0a3d.pth',
- 'tf_efficientnet_b2_ap':
- 'https://github.com/rwightman/pytorch-image-models/releases/download/v0.1-weights/tf_efficientnet_b2_ap-2f8e7636.pth',
- 'tf_efficientnet_b3_ap':
- 'https://github.com/rwightman/pytorch-image-models/releases/download/v0.1-weights/tf_efficientnet_b3_ap-aad25bdd.pth',
- 'tf_efficientnet_b4_ap':
- 'https://github.com/rwightman/pytorch-image-models/releases/download/v0.1-weights/tf_efficientnet_b4_ap-dedb23e6.pth',
- 'tf_efficientnet_b5_ap':
- 'https://github.com/rwightman/pytorch-image-models/releases/download/v0.1-weights/tf_efficientnet_b5_ap-9e82fae8.pth',
- 'tf_efficientnet_b6_ap':
- 'https://github.com/rwightman/pytorch-image-models/releases/download/v0.1-weights/tf_efficientnet_b6_ap-4ffb161f.pth',
- 'tf_efficientnet_b7_ap':
- 'https://github.com/rwightman/pytorch-image-models/releases/download/v0.1-weights/tf_efficientnet_b7_ap-ddb28fec.pth',
- 'tf_efficientnet_b8_ap':
- 'https://github.com/rwightman/pytorch-image-models/releases/download/v0.1-weights/tf_efficientnet_b8_ap-00e169fa.pth',
-
- 'tf_efficientnet_b0_ns':
- 'https://github.com/rwightman/pytorch-image-models/releases/download/v0.1-weights/tf_efficientnet_b0_ns-c0e6a31c.pth',
- 'tf_efficientnet_b1_ns':
- 'https://github.com/rwightman/pytorch-image-models/releases/download/v0.1-weights/tf_efficientnet_b1_ns-99dd0c41.pth',
- 'tf_efficientnet_b2_ns':
- 'https://github.com/rwightman/pytorch-image-models/releases/download/v0.1-weights/tf_efficientnet_b2_ns-00306e48.pth',
- 'tf_efficientnet_b3_ns':
- 'https://github.com/rwightman/pytorch-image-models/releases/download/v0.1-weights/tf_efficientnet_b3_ns-9d44bf68.pth',
- 'tf_efficientnet_b4_ns':
- 'https://github.com/rwightman/pytorch-image-models/releases/download/v0.1-weights/tf_efficientnet_b4_ns-d6313a46.pth',
- 'tf_efficientnet_b5_ns':
- 'https://github.com/rwightman/pytorch-image-models/releases/download/v0.1-weights/tf_efficientnet_b5_ns-6f26d0cf.pth',
- 'tf_efficientnet_b6_ns':
- 'https://github.com/rwightman/pytorch-image-models/releases/download/v0.1-weights/tf_efficientnet_b6_ns-51548356.pth',
- 'tf_efficientnet_b7_ns':
- 'https://github.com/rwightman/pytorch-image-models/releases/download/v0.1-weights/tf_efficientnet_b7_ns-1dbc32de.pth',
- 'tf_efficientnet_l2_ns_475':
- 'https://github.com/rwightman/pytorch-image-models/releases/download/v0.1-weights/tf_efficientnet_l2_ns_475-bebbd00a.pth',
- 'tf_efficientnet_l2_ns':
- 'https://github.com/rwightman/pytorch-image-models/releases/download/v0.1-weights/tf_efficientnet_l2_ns-df73bb44.pth',
-
- 'tf_efficientnet_es':
- 'https://github.com/rwightman/pytorch-image-models/releases/download/v0.1-weights/tf_efficientnet_es-ca1afbfe.pth',
- 'tf_efficientnet_em':
- 'https://github.com/rwightman/pytorch-image-models/releases/download/v0.1-weights/tf_efficientnet_em-e78cfe58.pth',
- 'tf_efficientnet_el':
- 'https://github.com/rwightman/pytorch-image-models/releases/download/v0.1-weights/tf_efficientnet_el-5143854e.pth',
-
- 'tf_efficientnet_cc_b0_4e':
- 'https://github.com/rwightman/pytorch-image-models/releases/download/v0.1-weights/tf_efficientnet_cc_b0_4e-4362b6b2.pth',
- 'tf_efficientnet_cc_b0_8e':
- 'https://github.com/rwightman/pytorch-image-models/releases/download/v0.1-weights/tf_efficientnet_cc_b0_8e-66184a25.pth',
- 'tf_efficientnet_cc_b1_8e':
- 'https://github.com/rwightman/pytorch-image-models/releases/download/v0.1-weights/tf_efficientnet_cc_b1_8e-f7c79ae1.pth',
-
- 'tf_efficientnet_lite0':
- 'https://github.com/rwightman/pytorch-image-models/releases/download/v0.1-weights/tf_efficientnet_lite0-0aa007d2.pth',
- 'tf_efficientnet_lite1':
- 'https://github.com/rwightman/pytorch-image-models/releases/download/v0.1-weights/tf_efficientnet_lite1-bde8b488.pth',
- 'tf_efficientnet_lite2':
- 'https://github.com/rwightman/pytorch-image-models/releases/download/v0.1-weights/tf_efficientnet_lite2-dcccb7df.pth',
- 'tf_efficientnet_lite3':
- 'https://github.com/rwightman/pytorch-image-models/releases/download/v0.1-weights/tf_efficientnet_lite3-b733e338.pth',
- 'tf_efficientnet_lite4':
- 'https://github.com/rwightman/pytorch-image-models/releases/download/v0.1-weights/tf_efficientnet_lite4-741542c3.pth',
-
- 'mixnet_s': 'https://github.com/rwightman/pytorch-image-models/releases/download/v0.1-weights/mixnet_s-a907afbc.pth',
- 'mixnet_m': 'https://github.com/rwightman/pytorch-image-models/releases/download/v0.1-weights/mixnet_m-4647fc68.pth',
- 'mixnet_l': 'https://github.com/rwightman/pytorch-image-models/releases/download/v0.1-weights/mixnet_l-5a9a2ed8.pth',
- 'mixnet_xl': 'https://github.com/rwightman/pytorch-image-models/releases/download/v0.1-weights/mixnet_xl_ra-aac3c00c.pth',
-
- 'tf_mixnet_s':
- 'https://github.com/rwightman/pytorch-image-models/releases/download/v0.1-weights/tf_mixnet_s-89d3354b.pth',
- 'tf_mixnet_m':
- 'https://github.com/rwightman/pytorch-image-models/releases/download/v0.1-weights/tf_mixnet_m-0f4d8805.pth',
- 'tf_mixnet_l':
- 'https://github.com/rwightman/pytorch-image-models/releases/download/v0.1-weights/tf_mixnet_l-6c92e0c8.pth',
-}
-
-
-class GenEfficientNet(nn.Module):
- """ Generic EfficientNets
-
- An implementation of mobile optimized networks that covers:
- * EfficientNet (B0-B8, L2, CondConv, EdgeTPU)
- * MixNet (Small, Medium, and Large, XL)
- * MNASNet A1, B1, and small
- * FBNet C
- * Single-Path NAS Pixel1
- """
-
- def __init__(self, block_args, num_classes=1000, in_chans=3, num_features=1280, stem_size=32, fix_stem=False,
- channel_multiplier=1.0, channel_divisor=8, channel_min=None,
- pad_type='', act_layer=nn.ReLU, drop_rate=0., drop_connect_rate=0.,
- se_kwargs=None, norm_layer=nn.BatchNorm2d, norm_kwargs=None,
- weight_init='goog'):
- super(GenEfficientNet, self).__init__()
- self.drop_rate = drop_rate
-
- if not fix_stem:
- stem_size = round_channels(stem_size, channel_multiplier, channel_divisor, channel_min)
- self.conv_stem = select_conv2d(in_chans, stem_size, 3, stride=2, padding=pad_type)
- self.bn1 = norm_layer(stem_size, **norm_kwargs)
- self.act1 = act_layer(inplace=True)
- in_chs = stem_size
-
- builder = EfficientNetBuilder(
- channel_multiplier, channel_divisor, channel_min,
- pad_type, act_layer, se_kwargs, norm_layer, norm_kwargs, drop_connect_rate)
- self.blocks = nn.Sequential(*builder(in_chs, block_args))
- in_chs = builder.in_chs
-
- self.conv_head = select_conv2d(in_chs, num_features, 1, padding=pad_type)
- self.bn2 = norm_layer(num_features, **norm_kwargs)
- self.act2 = act_layer(inplace=True)
- self.global_pool = nn.AdaptiveAvgPool2d(1)
- self.classifier = nn.Linear(num_features, num_classes)
-
- for n, m in self.named_modules():
- if weight_init == 'goog':
- initialize_weight_goog(m, n)
- else:
- initialize_weight_default(m, n)
-
- def features(self, x):
- x = self.conv_stem(x)
- x = self.bn1(x)
- x = self.act1(x)
- x = self.blocks(x)
- x = self.conv_head(x)
- x = self.bn2(x)
- x = self.act2(x)
- return x
-
- def as_sequential(self):
- layers = [self.conv_stem, self.bn1, self.act1]
- layers.extend(self.blocks)
- layers.extend([
- self.conv_head, self.bn2, self.act2,
- self.global_pool, nn.Flatten(), nn.Dropout(self.drop_rate), self.classifier])
- return nn.Sequential(*layers)
-
- def forward(self, x):
- x = self.features(x)
- x = self.global_pool(x)
- x = x.flatten(1)
- if self.drop_rate > 0.:
- x = F.dropout(x, p=self.drop_rate, training=self.training)
- return self.classifier(x)
-
-
-def _create_model(model_kwargs, variant, pretrained=False):
- as_sequential = model_kwargs.pop('as_sequential', False)
- model = GenEfficientNet(**model_kwargs)
- if pretrained:
- load_pretrained(model, model_urls[variant])
- if as_sequential:
- model = model.as_sequential()
- return model
-
-
-def _gen_mnasnet_a1(variant, channel_multiplier=1.0, pretrained=False, **kwargs):
- """Creates a mnasnet-a1 model.
-
- Ref impl: https://github.com/tensorflow/tpu/tree/master/models/official/mnasnet
- Paper: https://arxiv.org/pdf/1807.11626.pdf.
-
- Args:
- channel_multiplier: multiplier to number of channels per layer.
- """
- arch_def = [
- # stage 0, 112x112 in
- ['ds_r1_k3_s1_e1_c16_noskip'],
- # stage 1, 112x112 in
- ['ir_r2_k3_s2_e6_c24'],
- # stage 2, 56x56 in
- ['ir_r3_k5_s2_e3_c40_se0.25'],
- # stage 3, 28x28 in
- ['ir_r4_k3_s2_e6_c80'],
- # stage 4, 14x14in
- ['ir_r2_k3_s1_e6_c112_se0.25'],
- # stage 5, 14x14in
- ['ir_r3_k5_s2_e6_c160_se0.25'],
- # stage 6, 7x7 in
- ['ir_r1_k3_s1_e6_c320'],
- ]
- with layer_config_kwargs(kwargs):
- model_kwargs = dict(
- block_args=decode_arch_def(arch_def),
- stem_size=32,
- channel_multiplier=channel_multiplier,
- act_layer=resolve_act_layer(kwargs, 'relu'),
- norm_kwargs=resolve_bn_args(kwargs),
- **kwargs
- )
- model = _create_model(model_kwargs, variant, pretrained)
- return model
-
-
-def _gen_mnasnet_b1(variant, channel_multiplier=1.0, pretrained=False, **kwargs):
- """Creates a mnasnet-b1 model.
-
- Ref impl: https://github.com/tensorflow/tpu/tree/master/models/official/mnasnet
- Paper: https://arxiv.org/pdf/1807.11626.pdf.
-
- Args:
- channel_multiplier: multiplier to number of channels per layer.
- """
- arch_def = [
- # stage 0, 112x112 in
- ['ds_r1_k3_s1_c16_noskip'],
- # stage 1, 112x112 in
- ['ir_r3_k3_s2_e3_c24'],
- # stage 2, 56x56 in
- ['ir_r3_k5_s2_e3_c40'],
- # stage 3, 28x28 in
- ['ir_r3_k5_s2_e6_c80'],
- # stage 4, 14x14in
- ['ir_r2_k3_s1_e6_c96'],
- # stage 5, 14x14in
- ['ir_r4_k5_s2_e6_c192'],
- # stage 6, 7x7 in
- ['ir_r1_k3_s1_e6_c320_noskip']
- ]
- with layer_config_kwargs(kwargs):
- model_kwargs = dict(
- block_args=decode_arch_def(arch_def),
- stem_size=32,
- channel_multiplier=channel_multiplier,
- act_layer=resolve_act_layer(kwargs, 'relu'),
- norm_kwargs=resolve_bn_args(kwargs),
- **kwargs
- )
- model = _create_model(model_kwargs, variant, pretrained)
- return model
-
-
-def _gen_mnasnet_small(variant, channel_multiplier=1.0, pretrained=False, **kwargs):
- """Creates a mnasnet-b1 model.
-
- Ref impl: https://github.com/tensorflow/tpu/tree/master/models/official/mnasnet
- Paper: https://arxiv.org/pdf/1807.11626.pdf.
-
- Args:
- channel_multiplier: multiplier to number of channels per layer.
- """
- arch_def = [
- ['ds_r1_k3_s1_c8'],
- ['ir_r1_k3_s2_e3_c16'],
- ['ir_r2_k3_s2_e6_c16'],
- ['ir_r4_k5_s2_e6_c32_se0.25'],
- ['ir_r3_k3_s1_e6_c32_se0.25'],
- ['ir_r3_k5_s2_e6_c88_se0.25'],
- ['ir_r1_k3_s1_e6_c144']
- ]
- with layer_config_kwargs(kwargs):
- model_kwargs = dict(
- block_args=decode_arch_def(arch_def),
- stem_size=8,
- channel_multiplier=channel_multiplier,
- act_layer=resolve_act_layer(kwargs, 'relu'),
- norm_kwargs=resolve_bn_args(kwargs),
- **kwargs
- )
- model = _create_model(model_kwargs, variant, pretrained)
- return model
-
-
-def _gen_mobilenet_v2(
- variant, channel_multiplier=1.0, depth_multiplier=1.0, fix_stem_head=False, pretrained=False, **kwargs):
- """ Generate MobileNet-V2 network
- Ref impl: https://github.com/tensorflow/models/blob/master/research/slim/nets/mobilenet/mobilenet_v2.py
- Paper: https://arxiv.org/abs/1801.04381
- """
- arch_def = [
- ['ds_r1_k3_s1_c16'],
- ['ir_r2_k3_s2_e6_c24'],
- ['ir_r3_k3_s2_e6_c32'],
- ['ir_r4_k3_s2_e6_c64'],
- ['ir_r3_k3_s1_e6_c96'],
- ['ir_r3_k3_s2_e6_c160'],
- ['ir_r1_k3_s1_e6_c320'],
- ]
- with layer_config_kwargs(kwargs):
- model_kwargs = dict(
- block_args=decode_arch_def(arch_def, depth_multiplier=depth_multiplier, fix_first_last=fix_stem_head),
- num_features=1280 if fix_stem_head else round_channels(1280, channel_multiplier, 8, None),
- stem_size=32,
- fix_stem=fix_stem_head,
- channel_multiplier=channel_multiplier,
- norm_kwargs=resolve_bn_args(kwargs),
- act_layer=nn.ReLU6,
- **kwargs
- )
- model = _create_model(model_kwargs, variant, pretrained)
- return model
-
-
-def _gen_fbnetc(variant, channel_multiplier=1.0, pretrained=False, **kwargs):
- """ FBNet-C
-
- Paper: https://arxiv.org/abs/1812.03443
- Ref Impl: https://github.com/facebookresearch/maskrcnn-benchmark/blob/master/maskrcnn_benchmark/modeling/backbone/fbnet_modeldef.py
-
- NOTE: the impl above does not relate to the 'C' variant here, that was derived from paper,
- it was used to confirm some building block details
- """
- arch_def = [
- ['ir_r1_k3_s1_e1_c16'],
- ['ir_r1_k3_s2_e6_c24', 'ir_r2_k3_s1_e1_c24'],
- ['ir_r1_k5_s2_e6_c32', 'ir_r1_k5_s1_e3_c32', 'ir_r1_k5_s1_e6_c32', 'ir_r1_k3_s1_e6_c32'],
- ['ir_r1_k5_s2_e6_c64', 'ir_r1_k5_s1_e3_c64', 'ir_r2_k5_s1_e6_c64'],
- ['ir_r3_k5_s1_e6_c112', 'ir_r1_k5_s1_e3_c112'],
- ['ir_r4_k5_s2_e6_c184'],
- ['ir_r1_k3_s1_e6_c352'],
- ]
- with layer_config_kwargs(kwargs):
- model_kwargs = dict(
- block_args=decode_arch_def(arch_def),
- stem_size=16,
- num_features=1984, # paper suggests this, but is not 100% clear
- channel_multiplier=channel_multiplier,
- act_layer=resolve_act_layer(kwargs, 'relu'),
- norm_kwargs=resolve_bn_args(kwargs),
- **kwargs
- )
- model = _create_model(model_kwargs, variant, pretrained)
- return model
-
-
-def _gen_spnasnet(variant, channel_multiplier=1.0, pretrained=False, **kwargs):
- """Creates the Single-Path NAS model from search targeted for Pixel1 phone.
-
- Paper: https://arxiv.org/abs/1904.02877
-
- Args:
- channel_multiplier: multiplier to number of channels per layer.
- """
- arch_def = [
- # stage 0, 112x112 in
- ['ds_r1_k3_s1_c16_noskip'],
- # stage 1, 112x112 in
- ['ir_r3_k3_s2_e3_c24'],
- # stage 2, 56x56 in
- ['ir_r1_k5_s2_e6_c40', 'ir_r3_k3_s1_e3_c40'],
- # stage 3, 28x28 in
- ['ir_r1_k5_s2_e6_c80', 'ir_r3_k3_s1_e3_c80'],
- # stage 4, 14x14in
- ['ir_r1_k5_s1_e6_c96', 'ir_r3_k5_s1_e3_c96'],
- # stage 5, 14x14in
- ['ir_r4_k5_s2_e6_c192'],
- # stage 6, 7x7 in
- ['ir_r1_k3_s1_e6_c320_noskip']
- ]
- with layer_config_kwargs(kwargs):
- model_kwargs = dict(
- block_args=decode_arch_def(arch_def),
- stem_size=32,
- channel_multiplier=channel_multiplier,
- act_layer=resolve_act_layer(kwargs, 'relu'),
- norm_kwargs=resolve_bn_args(kwargs),
- **kwargs
- )
- model = _create_model(model_kwargs, variant, pretrained)
- return model
-
-
-def _gen_efficientnet(variant, channel_multiplier=1.0, depth_multiplier=1.0, pretrained=False, **kwargs):
- """Creates an EfficientNet model.
-
- Ref impl: https://github.com/tensorflow/tpu/blob/master/models/official/efficientnet/efficientnet_model.py
- Paper: https://arxiv.org/abs/1905.11946
-
- EfficientNet params
- name: (channel_multiplier, depth_multiplier, resolution, dropout_rate)
- 'efficientnet-b0': (1.0, 1.0, 224, 0.2),
- 'efficientnet-b1': (1.0, 1.1, 240, 0.2),
- 'efficientnet-b2': (1.1, 1.2, 260, 0.3),
- 'efficientnet-b3': (1.2, 1.4, 300, 0.3),
- 'efficientnet-b4': (1.4, 1.8, 380, 0.4),
- 'efficientnet-b5': (1.6, 2.2, 456, 0.4),
- 'efficientnet-b6': (1.8, 2.6, 528, 0.5),
- 'efficientnet-b7': (2.0, 3.1, 600, 0.5),
- 'efficientnet-b8': (2.2, 3.6, 672, 0.5),
-
- Args:
- channel_multiplier: multiplier to number of channels per layer
- depth_multiplier: multiplier to number of repeats per stage
-
- """
- arch_def = [
- ['ds_r1_k3_s1_e1_c16_se0.25'],
- ['ir_r2_k3_s2_e6_c24_se0.25'],
- ['ir_r2_k5_s2_e6_c40_se0.25'],
- ['ir_r3_k3_s2_e6_c80_se0.25'],
- ['ir_r3_k5_s1_e6_c112_se0.25'],
- ['ir_r4_k5_s2_e6_c192_se0.25'],
- ['ir_r1_k3_s1_e6_c320_se0.25'],
- ]
- with layer_config_kwargs(kwargs):
- model_kwargs = dict(
- block_args=decode_arch_def(arch_def, depth_multiplier),
- num_features=round_channels(1280, channel_multiplier, 8, None),
- stem_size=32,
- channel_multiplier=channel_multiplier,
- act_layer=resolve_act_layer(kwargs, 'swish'),
- norm_kwargs=resolve_bn_args(kwargs),
- **kwargs,
- )
- model = _create_model(model_kwargs, variant, pretrained)
- return model
-
-
-def _gen_efficientnet_edge(variant, channel_multiplier=1.0, depth_multiplier=1.0, pretrained=False, **kwargs):
- arch_def = [
- # NOTE `fc` is present to override a mismatch between stem channels and in chs not
- # present in other models
- ['er_r1_k3_s1_e4_c24_fc24_noskip'],
- ['er_r2_k3_s2_e8_c32'],
- ['er_r4_k3_s2_e8_c48'],
- ['ir_r5_k5_s2_e8_c96'],
- ['ir_r4_k5_s1_e8_c144'],
- ['ir_r2_k5_s2_e8_c192'],
- ]
- with layer_config_kwargs(kwargs):
- model_kwargs = dict(
- block_args=decode_arch_def(arch_def, depth_multiplier),
- num_features=round_channels(1280, channel_multiplier, 8, None),
- stem_size=32,
- channel_multiplier=channel_multiplier,
- act_layer=resolve_act_layer(kwargs, 'relu'),
- norm_kwargs=resolve_bn_args(kwargs),
- **kwargs,
- )
- model = _create_model(model_kwargs, variant, pretrained)
- return model
-
-
-def _gen_efficientnet_condconv(
- variant, channel_multiplier=1.0, depth_multiplier=1.0, experts_multiplier=1, pretrained=False, **kwargs):
- """Creates an efficientnet-condconv model."""
- arch_def = [
- ['ds_r1_k3_s1_e1_c16_se0.25'],
- ['ir_r2_k3_s2_e6_c24_se0.25'],
- ['ir_r2_k5_s2_e6_c40_se0.25'],
- ['ir_r3_k3_s2_e6_c80_se0.25'],
- ['ir_r3_k5_s1_e6_c112_se0.25_cc4'],
- ['ir_r4_k5_s2_e6_c192_se0.25_cc4'],
- ['ir_r1_k3_s1_e6_c320_se0.25_cc4'],
- ]
- with layer_config_kwargs(kwargs):
- model_kwargs = dict(
- block_args=decode_arch_def(arch_def, depth_multiplier, experts_multiplier=experts_multiplier),
- num_features=round_channels(1280, channel_multiplier, 8, None),
- stem_size=32,
- channel_multiplier=channel_multiplier,
- act_layer=resolve_act_layer(kwargs, 'swish'),
- norm_kwargs=resolve_bn_args(kwargs),
- **kwargs,
- )
- model = _create_model(model_kwargs, variant, pretrained)
- return model
-
-
-def _gen_efficientnet_lite(variant, channel_multiplier=1.0, depth_multiplier=1.0, pretrained=False, **kwargs):
- """Creates an EfficientNet-Lite model.
-
- Ref impl: https://github.com/tensorflow/tpu/tree/master/models/official/efficientnet/lite
- Paper: https://arxiv.org/abs/1905.11946
-
- EfficientNet params
- name: (channel_multiplier, depth_multiplier, resolution, dropout_rate)
- 'efficientnet-lite0': (1.0, 1.0, 224, 0.2),
- 'efficientnet-lite1': (1.0, 1.1, 240, 0.2),
- 'efficientnet-lite2': (1.1, 1.2, 260, 0.3),
- 'efficientnet-lite3': (1.2, 1.4, 280, 0.3),
- 'efficientnet-lite4': (1.4, 1.8, 300, 0.3),
-
- Args:
- channel_multiplier: multiplier to number of channels per layer
- depth_multiplier: multiplier to number of repeats per stage
- """
- arch_def = [
- ['ds_r1_k3_s1_e1_c16'],
- ['ir_r2_k3_s2_e6_c24'],
- ['ir_r2_k5_s2_e6_c40'],
- ['ir_r3_k3_s2_e6_c80'],
- ['ir_r3_k5_s1_e6_c112'],
- ['ir_r4_k5_s2_e6_c192'],
- ['ir_r1_k3_s1_e6_c320'],
- ]
- with layer_config_kwargs(kwargs):
- model_kwargs = dict(
- block_args=decode_arch_def(arch_def, depth_multiplier, fix_first_last=True),
- num_features=1280,
- stem_size=32,
- fix_stem=True,
- channel_multiplier=channel_multiplier,
- act_layer=nn.ReLU6,
- norm_kwargs=resolve_bn_args(kwargs),
- **kwargs,
- )
- model = _create_model(model_kwargs, variant, pretrained)
- return model
-
-
-def _gen_mixnet_s(variant, channel_multiplier=1.0, pretrained=False, **kwargs):
- """Creates a MixNet Small model.
-
- Ref impl: https://github.com/tensorflow/tpu/tree/master/models/official/mnasnet/mixnet
- Paper: https://arxiv.org/abs/1907.09595
- """
- arch_def = [
- # stage 0, 112x112 in
- ['ds_r1_k3_s1_e1_c16'], # relu
- # stage 1, 112x112 in
- ['ir_r1_k3_a1.1_p1.1_s2_e6_c24', 'ir_r1_k3_a1.1_p1.1_s1_e3_c24'], # relu
- # stage 2, 56x56 in
- ['ir_r1_k3.5.7_s2_e6_c40_se0.5_nsw', 'ir_r3_k3.5_a1.1_p1.1_s1_e6_c40_se0.5_nsw'], # swish
- # stage 3, 28x28 in
- ['ir_r1_k3.5.7_p1.1_s2_e6_c80_se0.25_nsw', 'ir_r2_k3.5_p1.1_s1_e6_c80_se0.25_nsw'], # swish
- # stage 4, 14x14in
- ['ir_r1_k3.5.7_a1.1_p1.1_s1_e6_c120_se0.5_nsw', 'ir_r2_k3.5.7.9_a1.1_p1.1_s1_e3_c120_se0.5_nsw'], # swish
- # stage 5, 14x14in
- ['ir_r1_k3.5.7.9.11_s2_e6_c200_se0.5_nsw', 'ir_r2_k3.5.7.9_p1.1_s1_e6_c200_se0.5_nsw'], # swish
- # 7x7
- ]
- with layer_config_kwargs(kwargs):
- model_kwargs = dict(
- block_args=decode_arch_def(arch_def),
- num_features=1536,
- stem_size=16,
- channel_multiplier=channel_multiplier,
- act_layer=resolve_act_layer(kwargs, 'relu'),
- norm_kwargs=resolve_bn_args(kwargs),
- **kwargs
- )
- model = _create_model(model_kwargs, variant, pretrained)
- return model
-
-
-def _gen_mixnet_m(variant, channel_multiplier=1.0, depth_multiplier=1.0, pretrained=False, **kwargs):
- """Creates a MixNet Medium-Large model.
-
- Ref impl: https://github.com/tensorflow/tpu/tree/master/models/official/mnasnet/mixnet
- Paper: https://arxiv.org/abs/1907.09595
- """
- arch_def = [
- # stage 0, 112x112 in
- ['ds_r1_k3_s1_e1_c24'], # relu
- # stage 1, 112x112 in
- ['ir_r1_k3.5.7_a1.1_p1.1_s2_e6_c32', 'ir_r1_k3_a1.1_p1.1_s1_e3_c32'], # relu
- # stage 2, 56x56 in
- ['ir_r1_k3.5.7.9_s2_e6_c40_se0.5_nsw', 'ir_r3_k3.5_a1.1_p1.1_s1_e6_c40_se0.5_nsw'], # swish
- # stage 3, 28x28 in
- ['ir_r1_k3.5.7_s2_e6_c80_se0.25_nsw', 'ir_r3_k3.5.7.9_a1.1_p1.1_s1_e6_c80_se0.25_nsw'], # swish
- # stage 4, 14x14in
- ['ir_r1_k3_s1_e6_c120_se0.5_nsw', 'ir_r3_k3.5.7.9_a1.1_p1.1_s1_e3_c120_se0.5_nsw'], # swish
- # stage 5, 14x14in
- ['ir_r1_k3.5.7.9_s2_e6_c200_se0.5_nsw', 'ir_r3_k3.5.7.9_p1.1_s1_e6_c200_se0.5_nsw'], # swish
- # 7x7
- ]
- with layer_config_kwargs(kwargs):
- model_kwargs = dict(
- block_args=decode_arch_def(arch_def, depth_multiplier, depth_trunc='round'),
- num_features=1536,
- stem_size=24,
- channel_multiplier=channel_multiplier,
- act_layer=resolve_act_layer(kwargs, 'relu'),
- norm_kwargs=resolve_bn_args(kwargs),
- **kwargs
- )
- model = _create_model(model_kwargs, variant, pretrained)
- return model
-
-
-def mnasnet_050(pretrained=False, **kwargs):
- """ MNASNet B1, depth multiplier of 0.5. """
- model = _gen_mnasnet_b1('mnasnet_050', 0.5, pretrained=pretrained, **kwargs)
- return model
-
-
-def mnasnet_075(pretrained=False, **kwargs):
- """ MNASNet B1, depth multiplier of 0.75. """
- model = _gen_mnasnet_b1('mnasnet_075', 0.75, pretrained=pretrained, **kwargs)
- return model
-
-
-def mnasnet_100(pretrained=False, **kwargs):
- """ MNASNet B1, depth multiplier of 1.0. """
- model = _gen_mnasnet_b1('mnasnet_100', 1.0, pretrained=pretrained, **kwargs)
- return model
-
-
-def mnasnet_b1(pretrained=False, **kwargs):
- """ MNASNet B1, depth multiplier of 1.0. """
- return mnasnet_100(pretrained, **kwargs)
-
-
-def mnasnet_140(pretrained=False, **kwargs):
- """ MNASNet B1, depth multiplier of 1.4 """
- model = _gen_mnasnet_b1('mnasnet_140', 1.4, pretrained=pretrained, **kwargs)
- return model
-
-
-def semnasnet_050(pretrained=False, **kwargs):
- """ MNASNet A1 (w/ SE), depth multiplier of 0.5 """
- model = _gen_mnasnet_a1('semnasnet_050', 0.5, pretrained=pretrained, **kwargs)
- return model
-
-
-def semnasnet_075(pretrained=False, **kwargs):
- """ MNASNet A1 (w/ SE), depth multiplier of 0.75. """
- model = _gen_mnasnet_a1('semnasnet_075', 0.75, pretrained=pretrained, **kwargs)
- return model
-
-
-def semnasnet_100(pretrained=False, **kwargs):
- """ MNASNet A1 (w/ SE), depth multiplier of 1.0. """
- model = _gen_mnasnet_a1('semnasnet_100', 1.0, pretrained=pretrained, **kwargs)
- return model
-
-
-def mnasnet_a1(pretrained=False, **kwargs):
- """ MNASNet A1 (w/ SE), depth multiplier of 1.0. """
- return semnasnet_100(pretrained, **kwargs)
-
-
-def semnasnet_140(pretrained=False, **kwargs):
- """ MNASNet A1 (w/ SE), depth multiplier of 1.4. """
- model = _gen_mnasnet_a1('semnasnet_140', 1.4, pretrained=pretrained, **kwargs)
- return model
-
-
-def mnasnet_small(pretrained=False, **kwargs):
- """ MNASNet Small, depth multiplier of 1.0. """
- model = _gen_mnasnet_small('mnasnet_small', 1.0, pretrained=pretrained, **kwargs)
- return model
-
-
-def mobilenetv2_100(pretrained=False, **kwargs):
- """ MobileNet V2 w/ 1.0 channel multiplier """
- model = _gen_mobilenet_v2('mobilenetv2_100', 1.0, pretrained=pretrained, **kwargs)
- return model
-
-
-def mobilenetv2_140(pretrained=False, **kwargs):
- """ MobileNet V2 w/ 1.4 channel multiplier """
- model = _gen_mobilenet_v2('mobilenetv2_140', 1.4, pretrained=pretrained, **kwargs)
- return model
-
-
-def mobilenetv2_110d(pretrained=False, **kwargs):
- """ MobileNet V2 w/ 1.1 channel, 1.2 depth multipliers"""
- model = _gen_mobilenet_v2(
- 'mobilenetv2_110d', 1.1, depth_multiplier=1.2, fix_stem_head=True, pretrained=pretrained, **kwargs)
- return model
-
-
-def mobilenetv2_120d(pretrained=False, **kwargs):
- """ MobileNet V2 w/ 1.2 channel, 1.4 depth multipliers """
- model = _gen_mobilenet_v2(
- 'mobilenetv2_120d', 1.2, depth_multiplier=1.4, fix_stem_head=True, pretrained=pretrained, **kwargs)
- return model
-
-
-def fbnetc_100(pretrained=False, **kwargs):
- """ FBNet-C """
- if pretrained:
- # pretrained model trained with non-default BN epsilon
- kwargs['bn_eps'] = BN_EPS_TF_DEFAULT
- model = _gen_fbnetc('fbnetc_100', 1.0, pretrained=pretrained, **kwargs)
- return model
-
-
-def spnasnet_100(pretrained=False, **kwargs):
- """ Single-Path NAS Pixel1"""
- model = _gen_spnasnet('spnasnet_100', 1.0, pretrained=pretrained, **kwargs)
- return model
-
-
-def efficientnet_b0(pretrained=False, **kwargs):
- """ EfficientNet-B0 """
- # NOTE for train set drop_rate=0.2, drop_connect_rate=0.2
- model = _gen_efficientnet(
- 'efficientnet_b0', channel_multiplier=1.0, depth_multiplier=1.0, pretrained=pretrained, **kwargs)
- return model
-
-
-def efficientnet_b1(pretrained=False, **kwargs):
- """ EfficientNet-B1 """
- # NOTE for train set drop_rate=0.2, drop_connect_rate=0.2
- model = _gen_efficientnet(
- 'efficientnet_b1', channel_multiplier=1.0, depth_multiplier=1.1, pretrained=pretrained, **kwargs)
- return model
-
-
-def efficientnet_b2(pretrained=False, **kwargs):
- """ EfficientNet-B2 """
- # NOTE for train set drop_rate=0.3, drop_connect_rate=0.2
- model = _gen_efficientnet(
- 'efficientnet_b2', channel_multiplier=1.1, depth_multiplier=1.2, pretrained=pretrained, **kwargs)
- return model
-
-
-def efficientnet_b3(pretrained=False, **kwargs):
- """ EfficientNet-B3 """
- # NOTE for train set drop_rate=0.3, drop_connect_rate=0.2
- model = _gen_efficientnet(
- 'efficientnet_b3', channel_multiplier=1.2, depth_multiplier=1.4, pretrained=pretrained, **kwargs)
- return model
-
-
-def efficientnet_b4(pretrained=False, **kwargs):
- """ EfficientNet-B4 """
- # NOTE for train set drop_rate=0.4, drop_connect_rate=0.2
- model = _gen_efficientnet(
- 'efficientnet_b4', channel_multiplier=1.4, depth_multiplier=1.8, pretrained=pretrained, **kwargs)
- return model
-
-
-def efficientnet_b5(pretrained=False, **kwargs):
- """ EfficientNet-B5 """
- # NOTE for train set drop_rate=0.4, drop_connect_rate=0.2
- model = _gen_efficientnet(
- 'efficientnet_b5', channel_multiplier=1.6, depth_multiplier=2.2, pretrained=pretrained, **kwargs)
- return model
-
-
-def efficientnet_b6(pretrained=False, **kwargs):
- """ EfficientNet-B6 """
- # NOTE for train set drop_rate=0.5, drop_connect_rate=0.2
- model = _gen_efficientnet(
- 'efficientnet_b6', channel_multiplier=1.8, depth_multiplier=2.6, pretrained=pretrained, **kwargs)
- return model
-
-
-def efficientnet_b7(pretrained=False, **kwargs):
- """ EfficientNet-B7 """
- # NOTE for train set drop_rate=0.5, drop_connect_rate=0.2
- model = _gen_efficientnet(
- 'efficientnet_b7', channel_multiplier=2.0, depth_multiplier=3.1, pretrained=pretrained, **kwargs)
- return model
-
-
-def efficientnet_b8(pretrained=False, **kwargs):
- """ EfficientNet-B8 """
- # NOTE for train set drop_rate=0.5, drop_connect_rate=0.2
- model = _gen_efficientnet(
- 'efficientnet_b8', channel_multiplier=2.2, depth_multiplier=3.6, pretrained=pretrained, **kwargs)
- return model
-
-
-def efficientnet_l2(pretrained=False, **kwargs):
- """ EfficientNet-L2. """
- # NOTE for train, drop_rate should be 0.5
- model = _gen_efficientnet(
- 'efficientnet_l2', channel_multiplier=4.3, depth_multiplier=5.3, pretrained=pretrained, **kwargs)
- return model
-
-
-def efficientnet_es(pretrained=False, **kwargs):
- """ EfficientNet-Edge Small. """
- model = _gen_efficientnet_edge(
- 'efficientnet_es', channel_multiplier=1.0, depth_multiplier=1.0, pretrained=pretrained, **kwargs)
- return model
-
-
-def efficientnet_em(pretrained=False, **kwargs):
- """ EfficientNet-Edge-Medium. """
- model = _gen_efficientnet_edge(
- 'efficientnet_em', channel_multiplier=1.0, depth_multiplier=1.1, pretrained=pretrained, **kwargs)
- return model
-
-
-def efficientnet_el(pretrained=False, **kwargs):
- """ EfficientNet-Edge-Large. """
- model = _gen_efficientnet_edge(
- 'efficientnet_el', channel_multiplier=1.2, depth_multiplier=1.4, pretrained=pretrained, **kwargs)
- return model
-
-
-def efficientnet_cc_b0_4e(pretrained=False, **kwargs):
- """ EfficientNet-CondConv-B0 w/ 8 Experts """
- # NOTE for train set drop_rate=0.25, drop_connect_rate=0.2
- model = _gen_efficientnet_condconv(
- 'efficientnet_cc_b0_4e', channel_multiplier=1.0, depth_multiplier=1.0, pretrained=pretrained, **kwargs)
- return model
-
-
-def efficientnet_cc_b0_8e(pretrained=False, **kwargs):
- """ EfficientNet-CondConv-B0 w/ 8 Experts """
- # NOTE for train set drop_rate=0.25, drop_connect_rate=0.2
- model = _gen_efficientnet_condconv(
- 'efficientnet_cc_b0_8e', channel_multiplier=1.0, depth_multiplier=1.0, experts_multiplier=2,
- pretrained=pretrained, **kwargs)
- return model
-
-
-def efficientnet_cc_b1_8e(pretrained=False, **kwargs):
- """ EfficientNet-CondConv-B1 w/ 8 Experts """
- # NOTE for train set drop_rate=0.25, drop_connect_rate=0.2
- model = _gen_efficientnet_condconv(
- 'efficientnet_cc_b1_8e', channel_multiplier=1.0, depth_multiplier=1.1, experts_multiplier=2,
- pretrained=pretrained, **kwargs)
- return model
-
-
-def efficientnet_lite0(pretrained=False, **kwargs):
- """ EfficientNet-Lite0 """
- model = _gen_efficientnet_lite(
- 'efficientnet_lite0', channel_multiplier=1.0, depth_multiplier=1.0, pretrained=pretrained, **kwargs)
- return model
-
-
-def efficientnet_lite1(pretrained=False, **kwargs):
- """ EfficientNet-Lite1 """
- model = _gen_efficientnet_lite(
- 'efficientnet_lite1', channel_multiplier=1.0, depth_multiplier=1.1, pretrained=pretrained, **kwargs)
- return model
-
-
-def efficientnet_lite2(pretrained=False, **kwargs):
- """ EfficientNet-Lite2 """
- model = _gen_efficientnet_lite(
- 'efficientnet_lite2', channel_multiplier=1.1, depth_multiplier=1.2, pretrained=pretrained, **kwargs)
- return model
-
-
-def efficientnet_lite3(pretrained=False, **kwargs):
- """ EfficientNet-Lite3 """
- model = _gen_efficientnet_lite(
- 'efficientnet_lite3', channel_multiplier=1.2, depth_multiplier=1.4, pretrained=pretrained, **kwargs)
- return model
-
-
-def efficientnet_lite4(pretrained=False, **kwargs):
- """ EfficientNet-Lite4 """
- model = _gen_efficientnet_lite(
- 'efficientnet_lite4', channel_multiplier=1.4, depth_multiplier=1.8, pretrained=pretrained, **kwargs)
- return model
-
-
-def tf_efficientnet_b0(pretrained=False, **kwargs):
- """ EfficientNet-B0 AutoAug. Tensorflow compatible variant """
- kwargs['bn_eps'] = BN_EPS_TF_DEFAULT
- kwargs['pad_type'] = 'same'
- model = _gen_efficientnet(
- 'tf_efficientnet_b0', channel_multiplier=1.0, depth_multiplier=1.0, pretrained=pretrained, **kwargs)
- return model
-
-
-def tf_efficientnet_b1(pretrained=False, **kwargs):
- """ EfficientNet-B1 AutoAug. Tensorflow compatible variant """
- kwargs['bn_eps'] = BN_EPS_TF_DEFAULT
- kwargs['pad_type'] = 'same'
- model = _gen_efficientnet(
- 'tf_efficientnet_b1', channel_multiplier=1.0, depth_multiplier=1.1, pretrained=pretrained, **kwargs)
- return model
-
-
-def tf_efficientnet_b2(pretrained=False, **kwargs):
- """ EfficientNet-B2 AutoAug. Tensorflow compatible variant """
- kwargs['bn_eps'] = BN_EPS_TF_DEFAULT
- kwargs['pad_type'] = 'same'
- model = _gen_efficientnet(
- 'tf_efficientnet_b2', channel_multiplier=1.1, depth_multiplier=1.2, pretrained=pretrained, **kwargs)
- return model
-
-
-def tf_efficientnet_b3(pretrained=False, **kwargs):
- """ EfficientNet-B3 AutoAug. Tensorflow compatible variant """
- kwargs['bn_eps'] = BN_EPS_TF_DEFAULT
- kwargs['pad_type'] = 'same'
- model = _gen_efficientnet(
- 'tf_efficientnet_b3', channel_multiplier=1.2, depth_multiplier=1.4, pretrained=pretrained, **kwargs)
- return model
-
-
-def tf_efficientnet_b4(pretrained=False, **kwargs):
- """ EfficientNet-B4 AutoAug. Tensorflow compatible variant """
- kwargs['bn_eps'] = BN_EPS_TF_DEFAULT
- kwargs['pad_type'] = 'same'
- model = _gen_efficientnet(
- 'tf_efficientnet_b4', channel_multiplier=1.4, depth_multiplier=1.8, pretrained=pretrained, **kwargs)
- return model
-
-
-def tf_efficientnet_b5(pretrained=False, **kwargs):
- """ EfficientNet-B5 RandAug. Tensorflow compatible variant """
- kwargs['bn_eps'] = BN_EPS_TF_DEFAULT
- kwargs['pad_type'] = 'same'
- model = _gen_efficientnet(
- 'tf_efficientnet_b5', channel_multiplier=1.6, depth_multiplier=2.2, pretrained=pretrained, **kwargs)
- return model
-
-
-def tf_efficientnet_b6(pretrained=False, **kwargs):
- """ EfficientNet-B6 AutoAug. Tensorflow compatible variant """
- kwargs['bn_eps'] = BN_EPS_TF_DEFAULT
- kwargs['pad_type'] = 'same'
- model = _gen_efficientnet(
- 'tf_efficientnet_b6', channel_multiplier=1.8, depth_multiplier=2.6, pretrained=pretrained, **kwargs)
- return model
-
-
-def tf_efficientnet_b7(pretrained=False, **kwargs):
- """ EfficientNet-B7 RandAug. Tensorflow compatible variant """
- kwargs['bn_eps'] = BN_EPS_TF_DEFAULT
- kwargs['pad_type'] = 'same'
- model = _gen_efficientnet(
- 'tf_efficientnet_b7', channel_multiplier=2.0, depth_multiplier=3.1, pretrained=pretrained, **kwargs)
- return model
-
-
-def tf_efficientnet_b8(pretrained=False, **kwargs):
- """ EfficientNet-B8 RandAug. Tensorflow compatible variant """
- kwargs['bn_eps'] = BN_EPS_TF_DEFAULT
- kwargs['pad_type'] = 'same'
- model = _gen_efficientnet(
- 'tf_efficientnet_b8', channel_multiplier=2.2, depth_multiplier=3.6, pretrained=pretrained, **kwargs)
- return model
-
-
-def tf_efficientnet_b0_ap(pretrained=False, **kwargs):
- """ EfficientNet-B0 AdvProp. Tensorflow compatible variant
- Paper: Adversarial Examples Improve Image Recognition (https://arxiv.org/abs/1911.09665)
- """
- kwargs['bn_eps'] = BN_EPS_TF_DEFAULT
- kwargs['pad_type'] = 'same'
- model = _gen_efficientnet(
- 'tf_efficientnet_b0_ap', channel_multiplier=1.0, depth_multiplier=1.0, pretrained=pretrained, **kwargs)
- return model
-
-
-def tf_efficientnet_b1_ap(pretrained=False, **kwargs):
- """ EfficientNet-B1 AdvProp. Tensorflow compatible variant
- Paper: Adversarial Examples Improve Image Recognition (https://arxiv.org/abs/1911.09665)
- """
- kwargs['bn_eps'] = BN_EPS_TF_DEFAULT
- kwargs['pad_type'] = 'same'
- model = _gen_efficientnet(
- 'tf_efficientnet_b1_ap', channel_multiplier=1.0, depth_multiplier=1.1, pretrained=pretrained, **kwargs)
- return model
-
-
-def tf_efficientnet_b2_ap(pretrained=False, **kwargs):
- """ EfficientNet-B2 AdvProp. Tensorflow compatible variant
- Paper: Adversarial Examples Improve Image Recognition (https://arxiv.org/abs/1911.09665)
- """
- kwargs['bn_eps'] = BN_EPS_TF_DEFAULT
- kwargs['pad_type'] = 'same'
- model = _gen_efficientnet(
- 'tf_efficientnet_b2_ap', channel_multiplier=1.1, depth_multiplier=1.2, pretrained=pretrained, **kwargs)
- return model
-
-
-def tf_efficientnet_b3_ap(pretrained=False, **kwargs):
- """ EfficientNet-B3 AdvProp. Tensorflow compatible variant
- Paper: Adversarial Examples Improve Image Recognition (https://arxiv.org/abs/1911.09665)
- """
- kwargs['bn_eps'] = BN_EPS_TF_DEFAULT
- kwargs['pad_type'] = 'same'
- model = _gen_efficientnet(
- 'tf_efficientnet_b3_ap', channel_multiplier=1.2, depth_multiplier=1.4, pretrained=pretrained, **kwargs)
- return model
-
-
-def tf_efficientnet_b4_ap(pretrained=False, **kwargs):
- """ EfficientNet-B4 AdvProp. Tensorflow compatible variant
- Paper: Adversarial Examples Improve Image Recognition (https://arxiv.org/abs/1911.09665)
- """
- kwargs['bn_eps'] = BN_EPS_TF_DEFAULT
- kwargs['pad_type'] = 'same'
- model = _gen_efficientnet(
- 'tf_efficientnet_b4_ap', channel_multiplier=1.4, depth_multiplier=1.8, pretrained=pretrained, **kwargs)
- return model
-
-
-def tf_efficientnet_b5_ap(pretrained=False, **kwargs):
- """ EfficientNet-B5 AdvProp. Tensorflow compatible variant
- Paper: Adversarial Examples Improve Image Recognition (https://arxiv.org/abs/1911.09665)
- """
- kwargs['bn_eps'] = BN_EPS_TF_DEFAULT
- kwargs['pad_type'] = 'same'
- model = _gen_efficientnet(
- 'tf_efficientnet_b5_ap', channel_multiplier=1.6, depth_multiplier=2.2, pretrained=pretrained, **kwargs)
- return model
-
-
-def tf_efficientnet_b6_ap(pretrained=False, **kwargs):
- """ EfficientNet-B6 AdvProp. Tensorflow compatible variant
- Paper: Adversarial Examples Improve Image Recognition (https://arxiv.org/abs/1911.09665)
- """
- # NOTE for train, drop_rate should be 0.5
- kwargs['bn_eps'] = BN_EPS_TF_DEFAULT
- kwargs['pad_type'] = 'same'
- model = _gen_efficientnet(
- 'tf_efficientnet_b6_ap', channel_multiplier=1.8, depth_multiplier=2.6, pretrained=pretrained, **kwargs)
- return model
-
-
-def tf_efficientnet_b7_ap(pretrained=False, **kwargs):
- """ EfficientNet-B7 AdvProp. Tensorflow compatible variant
- Paper: Adversarial Examples Improve Image Recognition (https://arxiv.org/abs/1911.09665)
- """
- # NOTE for train, drop_rate should be 0.5
- kwargs['bn_eps'] = BN_EPS_TF_DEFAULT
- kwargs['pad_type'] = 'same'
- model = _gen_efficientnet(
- 'tf_efficientnet_b7_ap', channel_multiplier=2.0, depth_multiplier=3.1, pretrained=pretrained, **kwargs)
- return model
-
-
-def tf_efficientnet_b8_ap(pretrained=False, **kwargs):
- """ EfficientNet-B8 AdvProp. Tensorflow compatible variant
- Paper: Adversarial Examples Improve Image Recognition (https://arxiv.org/abs/1911.09665)
- """
- # NOTE for train, drop_rate should be 0.5
- kwargs['bn_eps'] = BN_EPS_TF_DEFAULT
- kwargs['pad_type'] = 'same'
- model = _gen_efficientnet(
- 'tf_efficientnet_b8_ap', channel_multiplier=2.2, depth_multiplier=3.6, pretrained=pretrained, **kwargs)
- return model
-
-
-def tf_efficientnet_b0_ns(pretrained=False, **kwargs):
- """ EfficientNet-B0 NoisyStudent. Tensorflow compatible variant
- Paper: Self-training with Noisy Student improves ImageNet classification (https://arxiv.org/abs/1911.04252)
- """
- kwargs['bn_eps'] = BN_EPS_TF_DEFAULT
- kwargs['pad_type'] = 'same'
- model = _gen_efficientnet(
- 'tf_efficientnet_b0_ns', channel_multiplier=1.0, depth_multiplier=1.0, pretrained=pretrained, **kwargs)
- return model
-
-
-def tf_efficientnet_b1_ns(pretrained=False, **kwargs):
- """ EfficientNet-B1 NoisyStudent. Tensorflow compatible variant
- Paper: Self-training with Noisy Student improves ImageNet classification (https://arxiv.org/abs/1911.04252)
- """
- kwargs['bn_eps'] = BN_EPS_TF_DEFAULT
- kwargs['pad_type'] = 'same'
- model = _gen_efficientnet(
- 'tf_efficientnet_b1_ns', channel_multiplier=1.0, depth_multiplier=1.1, pretrained=pretrained, **kwargs)
- return model
-
-
-def tf_efficientnet_b2_ns(pretrained=False, **kwargs):
- """ EfficientNet-B2 NoisyStudent. Tensorflow compatible variant
- Paper: Self-training with Noisy Student improves ImageNet classification (https://arxiv.org/abs/1911.04252)
- """
- kwargs['bn_eps'] = BN_EPS_TF_DEFAULT
- kwargs['pad_type'] = 'same'
- model = _gen_efficientnet(
- 'tf_efficientnet_b2_ns', channel_multiplier=1.1, depth_multiplier=1.2, pretrained=pretrained, **kwargs)
- return model
-
-
-def tf_efficientnet_b3_ns(pretrained=False, **kwargs):
- """ EfficientNet-B3 NoisyStudent. Tensorflow compatible variant
- Paper: Self-training with Noisy Student improves ImageNet classification (https://arxiv.org/abs/1911.04252)
- """
- kwargs['bn_eps'] = BN_EPS_TF_DEFAULT
- kwargs['pad_type'] = 'same'
- model = _gen_efficientnet(
- 'tf_efficientnet_b3_ns', channel_multiplier=1.2, depth_multiplier=1.4, pretrained=pretrained, **kwargs)
- return model
-
-
-def tf_efficientnet_b4_ns(pretrained=False, **kwargs):
- """ EfficientNet-B4 NoisyStudent. Tensorflow compatible variant
- Paper: Self-training with Noisy Student improves ImageNet classification (https://arxiv.org/abs/1911.04252)
- """
- kwargs['bn_eps'] = BN_EPS_TF_DEFAULT
- kwargs['pad_type'] = 'same'
- model = _gen_efficientnet(
- 'tf_efficientnet_b4_ns', channel_multiplier=1.4, depth_multiplier=1.8, pretrained=pretrained, **kwargs)
- return model
-
-
-def tf_efficientnet_b5_ns(pretrained=False, **kwargs):
- """ EfficientNet-B5 NoisyStudent. Tensorflow compatible variant
- Paper: Self-training with Noisy Student improves ImageNet classification (https://arxiv.org/abs/1911.04252)
- """
- kwargs['bn_eps'] = BN_EPS_TF_DEFAULT
- kwargs['pad_type'] = 'same'
- model = _gen_efficientnet(
- 'tf_efficientnet_b5_ns', channel_multiplier=1.6, depth_multiplier=2.2, pretrained=pretrained, **kwargs)
- return model
-
-
-def tf_efficientnet_b6_ns(pretrained=False, **kwargs):
- """ EfficientNet-B6 NoisyStudent. Tensorflow compatible variant
- Paper: Self-training with Noisy Student improves ImageNet classification (https://arxiv.org/abs/1911.04252)
- """
- # NOTE for train, drop_rate should be 0.5
- kwargs['bn_eps'] = BN_EPS_TF_DEFAULT
- kwargs['pad_type'] = 'same'
- model = _gen_efficientnet(
- 'tf_efficientnet_b6_ns', channel_multiplier=1.8, depth_multiplier=2.6, pretrained=pretrained, **kwargs)
- return model
-
-
-def tf_efficientnet_b7_ns(pretrained=False, **kwargs):
- """ EfficientNet-B7 NoisyStudent. Tensorflow compatible variant
- Paper: Self-training with Noisy Student improves ImageNet classification (https://arxiv.org/abs/1911.04252)
- """
- # NOTE for train, drop_rate should be 0.5
- kwargs['bn_eps'] = BN_EPS_TF_DEFAULT
- kwargs['pad_type'] = 'same'
- model = _gen_efficientnet(
- 'tf_efficientnet_b7_ns', channel_multiplier=2.0, depth_multiplier=3.1, pretrained=pretrained, **kwargs)
- return model
-
-
-def tf_efficientnet_l2_ns_475(pretrained=False, **kwargs):
- """ EfficientNet-L2 NoisyStudent @ 475x475. Tensorflow compatible variant
- Paper: Self-training with Noisy Student improves ImageNet classification (https://arxiv.org/abs/1911.04252)
- """
- # NOTE for train, drop_rate should be 0.5
- kwargs['bn_eps'] = BN_EPS_TF_DEFAULT
- kwargs['pad_type'] = 'same'
- model = _gen_efficientnet(
- 'tf_efficientnet_l2_ns_475', channel_multiplier=4.3, depth_multiplier=5.3, pretrained=pretrained, **kwargs)
- return model
-
-
-def tf_efficientnet_l2_ns(pretrained=False, **kwargs):
- """ EfficientNet-L2 NoisyStudent. Tensorflow compatible variant
- Paper: Self-training with Noisy Student improves ImageNet classification (https://arxiv.org/abs/1911.04252)
- """
- # NOTE for train, drop_rate should be 0.5
- kwargs['bn_eps'] = BN_EPS_TF_DEFAULT
- kwargs['pad_type'] = 'same'
- model = _gen_efficientnet(
- 'tf_efficientnet_l2_ns', channel_multiplier=4.3, depth_multiplier=5.3, pretrained=pretrained, **kwargs)
- return model
-
-
-def tf_efficientnet_es(pretrained=False, **kwargs):
- """ EfficientNet-Edge Small. Tensorflow compatible variant """
- kwargs['bn_eps'] = BN_EPS_TF_DEFAULT
- kwargs['pad_type'] = 'same'
- model = _gen_efficientnet_edge(
- 'tf_efficientnet_es', channel_multiplier=1.0, depth_multiplier=1.0, pretrained=pretrained, **kwargs)
- return model
-
-
-def tf_efficientnet_em(pretrained=False, **kwargs):
- """ EfficientNet-Edge-Medium. Tensorflow compatible variant """
- kwargs['bn_eps'] = BN_EPS_TF_DEFAULT
- kwargs['pad_type'] = 'same'
- model = _gen_efficientnet_edge(
- 'tf_efficientnet_em', channel_multiplier=1.0, depth_multiplier=1.1, pretrained=pretrained, **kwargs)
- return model
-
-
-def tf_efficientnet_el(pretrained=False, **kwargs):
- """ EfficientNet-Edge-Large. Tensorflow compatible variant """
- kwargs['bn_eps'] = BN_EPS_TF_DEFAULT
- kwargs['pad_type'] = 'same'
- model = _gen_efficientnet_edge(
- 'tf_efficientnet_el', channel_multiplier=1.2, depth_multiplier=1.4, pretrained=pretrained, **kwargs)
- return model
-
-
-def tf_efficientnet_cc_b0_4e(pretrained=False, **kwargs):
- """ EfficientNet-CondConv-B0 w/ 4 Experts """
- kwargs['bn_eps'] = BN_EPS_TF_DEFAULT
- kwargs['pad_type'] = 'same'
- model = _gen_efficientnet_condconv(
- 'tf_efficientnet_cc_b0_4e', channel_multiplier=1.0, depth_multiplier=1.0, pretrained=pretrained, **kwargs)
- return model
-
-
-def tf_efficientnet_cc_b0_8e(pretrained=False, **kwargs):
- """ EfficientNet-CondConv-B0 w/ 8 Experts """
- kwargs['bn_eps'] = BN_EPS_TF_DEFAULT
- kwargs['pad_type'] = 'same'
- model = _gen_efficientnet_condconv(
- 'tf_efficientnet_cc_b0_8e', channel_multiplier=1.0, depth_multiplier=1.0, experts_multiplier=2,
- pretrained=pretrained, **kwargs)
- return model
-
-
-def tf_efficientnet_cc_b1_8e(pretrained=False, **kwargs):
- """ EfficientNet-CondConv-B1 w/ 8 Experts """
- kwargs['bn_eps'] = BN_EPS_TF_DEFAULT
- kwargs['pad_type'] = 'same'
- model = _gen_efficientnet_condconv(
- 'tf_efficientnet_cc_b1_8e', channel_multiplier=1.0, depth_multiplier=1.1, experts_multiplier=2,
- pretrained=pretrained, **kwargs)
- return model
-
-
-def tf_efficientnet_lite0(pretrained=False, **kwargs):
- """ EfficientNet-Lite0. Tensorflow compatible variant """
- kwargs['bn_eps'] = BN_EPS_TF_DEFAULT
- kwargs['pad_type'] = 'same'
- model = _gen_efficientnet_lite(
- 'tf_efficientnet_lite0', channel_multiplier=1.0, depth_multiplier=1.0, pretrained=pretrained, **kwargs)
- return model
-
-
-def tf_efficientnet_lite1(pretrained=False, **kwargs):
- """ EfficientNet-Lite1. Tensorflow compatible variant """
- kwargs['bn_eps'] = BN_EPS_TF_DEFAULT
- kwargs['pad_type'] = 'same'
- model = _gen_efficientnet_lite(
- 'tf_efficientnet_lite1', channel_multiplier=1.0, depth_multiplier=1.1, pretrained=pretrained, **kwargs)
- return model
-
-
-def tf_efficientnet_lite2(pretrained=False, **kwargs):
- """ EfficientNet-Lite2. Tensorflow compatible variant """
- kwargs['bn_eps'] = BN_EPS_TF_DEFAULT
- kwargs['pad_type'] = 'same'
- model = _gen_efficientnet_lite(
- 'tf_efficientnet_lite2', channel_multiplier=1.1, depth_multiplier=1.2, pretrained=pretrained, **kwargs)
- return model
-
-
-def tf_efficientnet_lite3(pretrained=False, **kwargs):
- """ EfficientNet-Lite3. Tensorflow compatible variant """
- kwargs['bn_eps'] = BN_EPS_TF_DEFAULT
- kwargs['pad_type'] = 'same'
- model = _gen_efficientnet_lite(
- 'tf_efficientnet_lite3', channel_multiplier=1.2, depth_multiplier=1.4, pretrained=pretrained, **kwargs)
- return model
-
-
-def tf_efficientnet_lite4(pretrained=False, **kwargs):
- """ EfficientNet-Lite4. Tensorflow compatible variant """
- kwargs['bn_eps'] = BN_EPS_TF_DEFAULT
- kwargs['pad_type'] = 'same'
- model = _gen_efficientnet_lite(
- 'tf_efficientnet_lite4', channel_multiplier=1.4, depth_multiplier=1.8, pretrained=pretrained, **kwargs)
- return model
-
-
-def mixnet_s(pretrained=False, **kwargs):
- """Creates a MixNet Small model.
- """
- # NOTE for train set drop_rate=0.2
- model = _gen_mixnet_s(
- 'mixnet_s', channel_multiplier=1.0, pretrained=pretrained, **kwargs)
- return model
-
-
-def mixnet_m(pretrained=False, **kwargs):
- """Creates a MixNet Medium model.
- """
- # NOTE for train set drop_rate=0.25
- model = _gen_mixnet_m(
- 'mixnet_m', channel_multiplier=1.0, pretrained=pretrained, **kwargs)
- return model
-
-
-def mixnet_l(pretrained=False, **kwargs):
- """Creates a MixNet Large model.
- """
- # NOTE for train set drop_rate=0.25
- model = _gen_mixnet_m(
- 'mixnet_l', channel_multiplier=1.3, pretrained=pretrained, **kwargs)
- return model
-
-
-def mixnet_xl(pretrained=False, **kwargs):
- """Creates a MixNet Extra-Large model.
- Not a paper spec, experimental def by RW w/ depth scaling.
- """
- # NOTE for train set drop_rate=0.25, drop_connect_rate=0.2
- model = _gen_mixnet_m(
- 'mixnet_xl', channel_multiplier=1.6, depth_multiplier=1.2, pretrained=pretrained, **kwargs)
- return model
-
-
-def mixnet_xxl(pretrained=False, **kwargs):
- """Creates a MixNet Double Extra Large model.
- Not a paper spec, experimental def by RW w/ depth scaling.
- """
- # NOTE for train set drop_rate=0.3, drop_connect_rate=0.2
- model = _gen_mixnet_m(
- 'mixnet_xxl', channel_multiplier=2.4, depth_multiplier=1.3, pretrained=pretrained, **kwargs)
- return model
-
-
-def tf_mixnet_s(pretrained=False, **kwargs):
- """Creates a MixNet Small model. Tensorflow compatible variant
- """
- kwargs['bn_eps'] = BN_EPS_TF_DEFAULT
- kwargs['pad_type'] = 'same'
- model = _gen_mixnet_s(
- 'tf_mixnet_s', channel_multiplier=1.0, pretrained=pretrained, **kwargs)
- return model
-
-
-def tf_mixnet_m(pretrained=False, **kwargs):
- """Creates a MixNet Medium model. Tensorflow compatible variant
- """
- kwargs['bn_eps'] = BN_EPS_TF_DEFAULT
- kwargs['pad_type'] = 'same'
- model = _gen_mixnet_m(
- 'tf_mixnet_m', channel_multiplier=1.0, pretrained=pretrained, **kwargs)
- return model
-
-
-def tf_mixnet_l(pretrained=False, **kwargs):
- """Creates a MixNet Large model. Tensorflow compatible variant
- """
- kwargs['bn_eps'] = BN_EPS_TF_DEFAULT
- kwargs['pad_type'] = 'same'
- model = _gen_mixnet_m(
- 'tf_mixnet_l', channel_multiplier=1.3, pretrained=pretrained, **kwargs)
- return model
diff --git a/spaces/coreml-community/ControlNet-v1-1-Annotators-cpu/annotator/uniformer/mmcv/ops/deprecated_wrappers.py b/spaces/coreml-community/ControlNet-v1-1-Annotators-cpu/annotator/uniformer/mmcv/ops/deprecated_wrappers.py
deleted file mode 100644
index a2e593df9ee57637038683d7a1efaa347b2b69e7..0000000000000000000000000000000000000000
--- a/spaces/coreml-community/ControlNet-v1-1-Annotators-cpu/annotator/uniformer/mmcv/ops/deprecated_wrappers.py
+++ /dev/null
@@ -1,43 +0,0 @@
-# Copyright (c) OpenMMLab. All rights reserved.
-# This file is for backward compatibility.
-# Module wrappers for empty tensor have been moved to mmcv.cnn.bricks.
-import warnings
-
-from ..cnn.bricks.wrappers import Conv2d, ConvTranspose2d, Linear, MaxPool2d
-
-
-class Conv2d_deprecated(Conv2d):
-
- def __init__(self, *args, **kwargs):
- super().__init__(*args, **kwargs)
- warnings.warn(
- 'Importing Conv2d wrapper from "mmcv.ops" will be deprecated in'
- ' the future. Please import them from "mmcv.cnn" instead')
-
-
-class ConvTranspose2d_deprecated(ConvTranspose2d):
-
- def __init__(self, *args, **kwargs):
- super().__init__(*args, **kwargs)
- warnings.warn(
- 'Importing ConvTranspose2d wrapper from "mmcv.ops" will be '
- 'deprecated in the future. Please import them from "mmcv.cnn" '
- 'instead')
-
-
-class MaxPool2d_deprecated(MaxPool2d):
-
- def __init__(self, *args, **kwargs):
- super().__init__(*args, **kwargs)
- warnings.warn(
- 'Importing MaxPool2d wrapper from "mmcv.ops" will be deprecated in'
- ' the future. Please import them from "mmcv.cnn" instead')
-
-
-class Linear_deprecated(Linear):
-
- def __init__(self, *args, **kwargs):
- super().__init__(*args, **kwargs)
- warnings.warn(
- 'Importing Linear wrapper from "mmcv.ops" will be deprecated in'
- ' the future. Please import them from "mmcv.cnn" instead')
diff --git a/spaces/coreml-community/ControlNet-v1-1-Annotators-cpu/annotator/zoe/zoedepth/models/base_models/midas_repo/mobile/android/lib_task_api/src/main/java/org/tensorflow/lite/examples/classification/tflite/ClassifierFloatMobileNet.java b/spaces/coreml-community/ControlNet-v1-1-Annotators-cpu/annotator/zoe/zoedepth/models/base_models/midas_repo/mobile/android/lib_task_api/src/main/java/org/tensorflow/lite/examples/classification/tflite/ClassifierFloatMobileNet.java
deleted file mode 100644
index 0707de98de41395eaf3ddcfd74d6e36229a63760..0000000000000000000000000000000000000000
--- a/spaces/coreml-community/ControlNet-v1-1-Annotators-cpu/annotator/zoe/zoedepth/models/base_models/midas_repo/mobile/android/lib_task_api/src/main/java/org/tensorflow/lite/examples/classification/tflite/ClassifierFloatMobileNet.java
+++ /dev/null
@@ -1,43 +0,0 @@
-/* Copyright 2019 The TensorFlow Authors. All Rights Reserved.
-
-Licensed under the Apache License, Version 2.0 (the "License");
-you may not use this file except in compliance with the License.
-You may obtain a copy of the License at
-
- http://www.apache.org/licenses/LICENSE-2.0
-
-Unless required by applicable law or agreed to in writing, software
-distributed under the License is distributed on an "AS IS" BASIS,
-WITHOUT WARRANTIES OR CONDITIONS OF ANY KIND, either express or implied.
-See the License for the specific language governing permissions and
-limitations under the License.
-==============================================================================*/
-
-package org.tensorflow.lite.examples.classification.tflite;
-
-import android.app.Activity;
-import java.io.IOException;
-import org.tensorflow.lite.examples.classification.tflite.Classifier.Device;
-
-/** This TensorFlowLite classifier works with the float MobileNet model. */
-public class ClassifierFloatMobileNet extends Classifier {
- /**
- * Initializes a {@code ClassifierFloatMobileNet}.
- *
- * @param device a {@link Device} object to configure the hardware accelerator
- * @param numThreads the number of threads during the inference
- * @throws IOException if the model is not loaded correctly
- */
- public ClassifierFloatMobileNet(Activity activity, Device device, int numThreads)
- throws IOException {
- super(activity, device, numThreads);
- }
-
- @Override
- protected String getModelPath() {
- // you can download this file from
- // see build.gradle for where to obtain this file. It should be auto
- // downloaded into assets.
- return "mobilenet_v1_1.0_224.tflite";
- }
-}
diff --git a/spaces/cynika/taffy/README.md b/spaces/cynika/taffy/README.md
deleted file mode 100644
index b7c713ded972994d4172579fa7f9e8aa9e94dff6..0000000000000000000000000000000000000000
--- a/spaces/cynika/taffy/README.md
+++ /dev/null
@@ -1,12 +0,0 @@
----
-title: Taffy
-emoji: 🍓
-colorFrom: indigo
-colorTo: purple
-sdk: gradio
-sdk_version: 3.16.1
-app_file: app.py
-pinned: false
----
-
-Check out the configuration reference at https://huggingface.co/docs/hub/spaces-config-reference
diff --git a/spaces/datasciencedojo/Article-Scraping/app.py b/spaces/datasciencedojo/Article-Scraping/app.py
deleted file mode 100644
index 4d24f56517f3083e121d8738bb5dd82c7a675f8d..0000000000000000000000000000000000000000
--- a/spaces/datasciencedojo/Article-Scraping/app.py
+++ /dev/null
@@ -1,90 +0,0 @@
-import gradio as gr
-import wikipedia
-import numpy as np
-import pandas as pd
-from os import path
-from PIL import Image
-from wordcloud import WordCloud, STOPWORDS, ImageColorGenerator
-import matplotlib.pyplot as plt
-
-def wikipediaScrap(article_name, wikipedia_language = "en - English"):
- wikipedia_language = wikipedia_language.split(" - ")[0]
-
- if wikipedia_language:
- wikipedia.set_lang(wikipedia_language)
-
- # rem_sp = article_name.replace(" ", "")
- et_page = wikipedia.page(article_name)
- title = et_page.title
- content = et_page.content
- page_url = et_page.url
- linked_pages = et_page.links
-
- text = content
-
- # Create and generate a word cloud image:
- wordcloud = WordCloud(font_path="HelveticaWorld-Regular.ttf").generate(text)
-
- # Display the generated image:
- plt.imshow(wordcloud, interpolation='bilinear')
- plt.axis("off")
-
- return title, content, page_url, "\n". join(linked_pages), plt
-
-css = """
-footer {display:none !important}
-.output-markdown{display:none !important}
-footer {visibility: hidden}
-
-#component-12 img { max-height: 224px !important}
-#component-14 textarea[data-testid="textbox"] { height: 178px !important}
-#component-17 textarea[data-testid="textbox"] { height: 178px !important}
-#component-20 tr:hover{
- background-color: rgb(229,225,255) !important;
-}
-
-.max-h-[30rem] {max-height: 18rem !important;}
-.hover\:bg-orange-50:hover {
- --tw-bg-opacity: 1 !important;
- background-color: rgb(229,225,255) !important;
-}
-"""
-
-ini_dict = wikipedia.languages()
-
-# split dictionary into keys and values
-keys = []
-values = []
-language=[]
-
-items = ini_dict.items()
-for item in items:
- keys.append(item[0]), values.append(item[1])
- language.append(item[0]+" - "+item[1])
-
-with gr.Blocks(title="Wikipedia Article Scrape | Data Science Dojo", css = css) as demo:
- with gr.Row():
- inp = gr.Textbox(placeholder="Enter the name of wikipedia article", label="Wikipedia article name")
- lan = gr.Dropdown(label=" Select Language", choices=language, value=language[105], interactive=True)
-
- btn = gr.Button("Start Scraping", elem_id="dsd_button")
- with gr.Row():
- with gr.Column():
- # gr.Markdown("""## About""")
- title = gr.Textbox(label="Article title")
- url = gr.Textbox(label="Article URL")
- with gr.Column():
- # gr.Markdown("""## Wordcloud""")
- wordcloud = gr.Plot()
- # gr.Markdown("""### Content""")
- with gr.Row():
- content = gr.Textbox(label="Content")
- # gr.Markdown("""### Linked Articles""")
- with gr.Row():
- linked = gr.Textbox(label="Linked Articles")
- with gr.Row():
- gr.Examples(
- examples = [["Eiffel Tower", "en - English"], ["Eiffel tower", 'ur - اردو']], fn=wikipediaScrap, inputs=[inp, lan], outputs=[title, content, url, linked, wordcloud], cache_examples=True)
- btn.click(fn=wikipediaScrap, inputs=[inp, lan], outputs=[title, content, url, linked, wordcloud])
-
-demo.launch()
\ No newline at end of file
diff --git a/spaces/dawood/Kanye-AI/modules/__init__.py b/spaces/dawood/Kanye-AI/modules/__init__.py
deleted file mode 100644
index e69de29bb2d1d6434b8b29ae775ad8c2e48c5391..0000000000000000000000000000000000000000
diff --git a/spaces/dawood/gradio_videogallery/app.py b/spaces/dawood/gradio_videogallery/app.py
deleted file mode 100644
index 5908201a3a41ff1b812faffaf7c9b6dbe256801b..0000000000000000000000000000000000000000
--- a/spaces/dawood/gradio_videogallery/app.py
+++ /dev/null
@@ -1,12 +0,0 @@
-
-import gradio as gr
-from gradio_videogallery import videogallery
-
-
-example = videogallery().example_inputs()
-
-with gr.Blocks() as demo:
- with gr.Row():
- videogallery(value=example, label="Populated"), # populated component
-
-demo.launch()
diff --git a/spaces/dcarpintero/nlp-summarizer-pegasus/.venv/lib/python3.9/site-packages/markdown_it/rules_inline/newline.py b/spaces/dcarpintero/nlp-summarizer-pegasus/.venv/lib/python3.9/site-packages/markdown_it/rules_inline/newline.py
deleted file mode 100644
index ca8f1db02da07b023aa9fdb08ee7af326f773da8..0000000000000000000000000000000000000000
--- a/spaces/dcarpintero/nlp-summarizer-pegasus/.venv/lib/python3.9/site-packages/markdown_it/rules_inline/newline.py
+++ /dev/null
@@ -1,43 +0,0 @@
-"""Proceess '\n'."""
-from ..common.utils import charStrAt, isStrSpace
-from .state_inline import StateInline
-
-
-def newline(state: StateInline, silent: bool) -> bool:
- pos = state.pos
-
- if state.src[pos] != "\n":
- return False
-
- pmax = len(state.pending) - 1
- maximum = state.posMax
-
- # ' \n' -> hardbreak
- # Lookup in pending chars is bad practice! Don't copy to other rules!
- # Pending string is stored in concat mode, indexed lookups will cause
- # conversion to flat mode.
- if not silent:
- if pmax >= 0 and charStrAt(state.pending, pmax) == " ":
- if pmax >= 1 and charStrAt(state.pending, pmax - 1) == " ":
- # Find whitespaces tail of pending chars.
- ws = pmax - 1
- while ws >= 1 and charStrAt(state.pending, ws - 1) == " ":
- ws -= 1
- state.pending = state.pending[:ws]
-
- state.push("hardbreak", "br", 0)
- else:
- state.pending = state.pending[:-1]
- state.push("softbreak", "br", 0)
-
- else:
- state.push("softbreak", "br", 0)
-
- pos += 1
-
- # skip heading spaces for next line
- while pos < maximum and isStrSpace(state.src[pos]):
- pos += 1
-
- state.pos = pos
- return True
diff --git a/spaces/debayan/ISM2023w/README.md b/spaces/debayan/ISM2023w/README.md
deleted file mode 100644
index bcbf1a1f4985f54af84e5070b9efb903e1af1096..0000000000000000000000000000000000000000
--- a/spaces/debayan/ISM2023w/README.md
+++ /dev/null
@@ -1,13 +0,0 @@
----
-title: ISM2023w
-emoji: 🏆
-colorFrom: yellow
-colorTo: red
-sdk: gradio
-sdk_version: 3.45.1
-app_file: gradio_app.py
-pinned: false
-license: other
----
-
-Check out the configuration reference at https://huggingface.co/docs/hub/spaces-config-reference
diff --git a/spaces/declare-lab/tango/audioldm/latent_diffusion/ddim.py b/spaces/declare-lab/tango/audioldm/latent_diffusion/ddim.py
deleted file mode 100644
index 732002b048e9a193313aa0ef9a353d4fc078be72..0000000000000000000000000000000000000000
--- a/spaces/declare-lab/tango/audioldm/latent_diffusion/ddim.py
+++ /dev/null
@@ -1,377 +0,0 @@
-"""SAMPLING ONLY."""
-
-import torch
-import numpy as np
-from tqdm import tqdm
-
-from audioldm.latent_diffusion.util import (
- make_ddim_sampling_parameters,
- make_ddim_timesteps,
- noise_like,
- extract_into_tensor,
-)
-
-
-class DDIMSampler(object):
- def __init__(self, model, schedule="linear", **kwargs):
- super().__init__()
- self.model = model
- self.ddpm_num_timesteps = model.num_timesteps
- self.schedule = schedule
-
- def register_buffer(self, name, attr):
- if type(attr) == torch.Tensor:
- if attr.device != torch.device("cuda"):
- attr = attr.to(torch.device("cuda"))
- setattr(self, name, attr)
-
- def make_schedule(
- self, ddim_num_steps, ddim_discretize="uniform", ddim_eta=0.0, verbose=True
- ):
- self.ddim_timesteps = make_ddim_timesteps(
- ddim_discr_method=ddim_discretize,
- num_ddim_timesteps=ddim_num_steps,
- num_ddpm_timesteps=self.ddpm_num_timesteps,
- verbose=verbose,
- )
- alphas_cumprod = self.model.alphas_cumprod
- assert (
- alphas_cumprod.shape[0] == self.ddpm_num_timesteps
- ), "alphas have to be defined for each timestep"
- to_torch = lambda x: x.clone().detach().to(torch.float32).to(self.model.device)
-
- self.register_buffer("betas", to_torch(self.model.betas))
- self.register_buffer("alphas_cumprod", to_torch(alphas_cumprod))
- self.register_buffer(
- "alphas_cumprod_prev", to_torch(self.model.alphas_cumprod_prev)
- )
-
- # calculations for diffusion q(x_t | x_{t-1}) and others
- self.register_buffer(
- "sqrt_alphas_cumprod", to_torch(np.sqrt(alphas_cumprod.cpu()))
- )
- self.register_buffer(
- "sqrt_one_minus_alphas_cumprod",
- to_torch(np.sqrt(1.0 - alphas_cumprod.cpu())),
- )
- self.register_buffer(
- "log_one_minus_alphas_cumprod", to_torch(np.log(1.0 - alphas_cumprod.cpu()))
- )
- self.register_buffer(
- "sqrt_recip_alphas_cumprod", to_torch(np.sqrt(1.0 / alphas_cumprod.cpu()))
- )
- self.register_buffer(
- "sqrt_recipm1_alphas_cumprod",
- to_torch(np.sqrt(1.0 / alphas_cumprod.cpu() - 1)),
- )
-
- # ddim sampling parameters
- ddim_sigmas, ddim_alphas, ddim_alphas_prev = make_ddim_sampling_parameters(
- alphacums=alphas_cumprod.cpu(),
- ddim_timesteps=self.ddim_timesteps,
- eta=ddim_eta,
- verbose=verbose,
- )
- self.register_buffer("ddim_sigmas", ddim_sigmas)
- self.register_buffer("ddim_alphas", ddim_alphas)
- self.register_buffer("ddim_alphas_prev", ddim_alphas_prev)
- self.register_buffer("ddim_sqrt_one_minus_alphas", np.sqrt(1.0 - ddim_alphas))
- sigmas_for_original_sampling_steps = ddim_eta * torch.sqrt(
- (1 - self.alphas_cumprod_prev)
- / (1 - self.alphas_cumprod)
- * (1 - self.alphas_cumprod / self.alphas_cumprod_prev)
- )
- self.register_buffer(
- "ddim_sigmas_for_original_num_steps", sigmas_for_original_sampling_steps
- )
-
- @torch.no_grad()
- def sample(
- self,
- S,
- batch_size,
- shape,
- conditioning=None,
- callback=None,
- normals_sequence=None,
- img_callback=None,
- quantize_x0=False,
- eta=0.0,
- mask=None,
- x0=None,
- temperature=1.0,
- noise_dropout=0.0,
- score_corrector=None,
- corrector_kwargs=None,
- verbose=True,
- x_T=None,
- log_every_t=100,
- unconditional_guidance_scale=1.0,
- unconditional_conditioning=None,
- # this has to come in the same format as the conditioning, # e.g. as encoded tokens, ...
- **kwargs,
- ):
- if conditioning is not None:
- if isinstance(conditioning, dict):
- cbs = conditioning[list(conditioning.keys())[0]].shape[0]
- if cbs != batch_size:
- print(
- f"Warning: Got {cbs} conditionings but batch-size is {batch_size}"
- )
- else:
- if conditioning.shape[0] != batch_size:
- print(
- f"Warning: Got {conditioning.shape[0]} conditionings but batch-size is {batch_size}"
- )
-
- self.make_schedule(ddim_num_steps=S, ddim_eta=eta, verbose=verbose)
- # sampling
- C, H, W = shape
- size = (batch_size, C, H, W)
- samples, intermediates = self.ddim_sampling(
- conditioning,
- size,
- callback=callback,
- img_callback=img_callback,
- quantize_denoised=quantize_x0,
- mask=mask,
- x0=x0,
- ddim_use_original_steps=False,
- noise_dropout=noise_dropout,
- temperature=temperature,
- score_corrector=score_corrector,
- corrector_kwargs=corrector_kwargs,
- x_T=x_T,
- log_every_t=log_every_t,
- unconditional_guidance_scale=unconditional_guidance_scale,
- unconditional_conditioning=unconditional_conditioning,
- )
- return samples, intermediates
-
- @torch.no_grad()
- def ddim_sampling(
- self,
- cond,
- shape,
- x_T=None,
- ddim_use_original_steps=False,
- callback=None,
- timesteps=None,
- quantize_denoised=False,
- mask=None,
- x0=None,
- img_callback=None,
- log_every_t=100,
- temperature=1.0,
- noise_dropout=0.0,
- score_corrector=None,
- corrector_kwargs=None,
- unconditional_guidance_scale=1.0,
- unconditional_conditioning=None,
- ):
- device = self.model.betas.device
- b = shape[0]
- if x_T is None:
- img = torch.randn(shape, device=device)
- else:
- img = x_T
-
- if timesteps is None:
- timesteps = (
- self.ddpm_num_timesteps
- if ddim_use_original_steps
- else self.ddim_timesteps
- )
- elif timesteps is not None and not ddim_use_original_steps:
- subset_end = (
- int(
- min(timesteps / self.ddim_timesteps.shape[0], 1)
- * self.ddim_timesteps.shape[0]
- )
- - 1
- )
- timesteps = self.ddim_timesteps[:subset_end]
-
- intermediates = {"x_inter": [img], "pred_x0": [img]}
- time_range = (
- reversed(range(0, timesteps))
- if ddim_use_original_steps
- else np.flip(timesteps)
- )
- total_steps = timesteps if ddim_use_original_steps else timesteps.shape[0]
- # print(f"Running DDIM Sampling with {total_steps} timesteps")
-
- # iterator = gr.Progress().tqdm(time_range, desc="DDIM Sampler", total=total_steps)
- iterator = tqdm(time_range, desc="DDIM Sampler", total=total_steps, leave=False)
-
- for i, step in enumerate(iterator):
- index = total_steps - i - 1
- ts = torch.full((b,), step, device=device, dtype=torch.long)
- if mask is not None:
- assert x0 is not None
- img_orig = self.model.q_sample(
- x0, ts
- ) # TODO deterministic forward pass?
- img = (
- img_orig * mask + (1.0 - mask) * img
- ) # In the first sampling step, img is pure gaussian noise
-
- outs = self.p_sample_ddim(
- img,
- cond,
- ts,
- index=index,
- use_original_steps=ddim_use_original_steps,
- quantize_denoised=quantize_denoised,
- temperature=temperature,
- noise_dropout=noise_dropout,
- score_corrector=score_corrector,
- corrector_kwargs=corrector_kwargs,
- unconditional_guidance_scale=unconditional_guidance_scale,
- unconditional_conditioning=unconditional_conditioning,
- )
- img, pred_x0 = outs
- if callback:
- callback(i)
- if img_callback:
- img_callback(pred_x0, i)
-
- if index % log_every_t == 0 or index == total_steps - 1:
- intermediates["x_inter"].append(img)
- intermediates["pred_x0"].append(pred_x0)
-
- return img, intermediates
-
- @torch.no_grad()
- def stochastic_encode(self, x0, t, use_original_steps=False, noise=None):
- # fast, but does not allow for exact reconstruction
- # t serves as an index to gather the correct alphas
- if use_original_steps:
- sqrt_alphas_cumprod = self.sqrt_alphas_cumprod
- sqrt_one_minus_alphas_cumprod = self.sqrt_one_minus_alphas_cumprod
- else:
- sqrt_alphas_cumprod = torch.sqrt(self.ddim_alphas)
- sqrt_one_minus_alphas_cumprod = self.ddim_sqrt_one_minus_alphas
-
- if noise is None:
- noise = torch.randn_like(x0)
-
- return (
- extract_into_tensor(sqrt_alphas_cumprod, t, x0.shape) * x0
- + extract_into_tensor(sqrt_one_minus_alphas_cumprod, t, x0.shape) * noise
- )
-
- @torch.no_grad()
- def decode(
- self,
- x_latent,
- cond,
- t_start,
- unconditional_guidance_scale=1.0,
- unconditional_conditioning=None,
- use_original_steps=False,
- ):
-
- timesteps = (
- np.arange(self.ddpm_num_timesteps)
- if use_original_steps
- else self.ddim_timesteps
- )
- timesteps = timesteps[:t_start]
-
- time_range = np.flip(timesteps)
- total_steps = timesteps.shape[0]
- # print(f"Running DDIM Sampling with {total_steps} timesteps")
-
- # iterator = gr.Progress().tqdm(time_range, desc="Decoding image", total=total_steps)
- iterator = tqdm(time_range, desc="Decoding image", total=total_steps)
- x_dec = x_latent
-
- for i, step in enumerate(iterator):
- index = total_steps - i - 1
- ts = torch.full(
- (x_latent.shape[0],), step, device=x_latent.device, dtype=torch.long
- )
- x_dec, _ = self.p_sample_ddim(
- x_dec,
- cond,
- ts,
- index=index,
- use_original_steps=use_original_steps,
- unconditional_guidance_scale=unconditional_guidance_scale,
- unconditional_conditioning=unconditional_conditioning,
- )
- return x_dec
-
- @torch.no_grad()
- def p_sample_ddim(
- self,
- x,
- c,
- t,
- index,
- repeat_noise=False,
- use_original_steps=False,
- quantize_denoised=False,
- temperature=1.0,
- noise_dropout=0.0,
- score_corrector=None,
- corrector_kwargs=None,
- unconditional_guidance_scale=1.0,
- unconditional_conditioning=None,
- ):
- b, *_, device = *x.shape, x.device
-
- if unconditional_conditioning is None or unconditional_guidance_scale == 1.0:
- e_t = self.model.apply_model(x, t, c)
- else:
- x_in = torch.cat([x] * 2)
- t_in = torch.cat([t] * 2)
- c_in = torch.cat([unconditional_conditioning, c])
- e_t_uncond, e_t = self.model.apply_model(x_in, t_in, c_in).chunk(2)
- # When unconditional_guidance_scale == 1: only e_t
- # When unconditional_guidance_scale == 0: only unconditional
- # When unconditional_guidance_scale > 1: add more unconditional guidance
- e_t = e_t_uncond + unconditional_guidance_scale * (e_t - e_t_uncond)
-
- if score_corrector is not None:
- assert self.model.parameterization == "eps"
- e_t = score_corrector.modify_score(
- self.model, e_t, x, t, c, **corrector_kwargs
- )
-
- alphas = self.model.alphas_cumprod if use_original_steps else self.ddim_alphas
- alphas_prev = (
- self.model.alphas_cumprod_prev
- if use_original_steps
- else self.ddim_alphas_prev
- )
- sqrt_one_minus_alphas = (
- self.model.sqrt_one_minus_alphas_cumprod
- if use_original_steps
- else self.ddim_sqrt_one_minus_alphas
- )
- sigmas = (
- self.model.ddim_sigmas_for_original_num_steps
- if use_original_steps
- else self.ddim_sigmas
- )
- # select parameters corresponding to the currently considered timestep
- a_t = torch.full((b, 1, 1, 1), alphas[index], device=device)
- a_prev = torch.full((b, 1, 1, 1), alphas_prev[index], device=device)
- sigma_t = torch.full((b, 1, 1, 1), sigmas[index], device=device)
- sqrt_one_minus_at = torch.full(
- (b, 1, 1, 1), sqrt_one_minus_alphas[index], device=device
- )
-
- # current prediction for x_0
- pred_x0 = (x - sqrt_one_minus_at * e_t) / a_t.sqrt()
- if quantize_denoised:
- pred_x0, _, *_ = self.model.first_stage_model.quantize(pred_x0)
- # direction pointing to x_t
- dir_xt = (1.0 - a_prev - sigma_t**2).sqrt() * e_t
- noise = sigma_t * noise_like(x.shape, device, repeat_noise) * temperature
- if noise_dropout > 0.0:
- noise = torch.nn.functional.dropout(noise, p=noise_dropout)
- x_prev = a_prev.sqrt() * pred_x0 + dir_xt + noise # TODO
- return x_prev, pred_x0
diff --git a/spaces/declare-lab/tango/diffusers/examples/community/unclip_text_interpolation.py b/spaces/declare-lab/tango/diffusers/examples/community/unclip_text_interpolation.py
deleted file mode 100644
index ac6b73d974b6e0fd37434083ed923256b4f5db22..0000000000000000000000000000000000000000
--- a/spaces/declare-lab/tango/diffusers/examples/community/unclip_text_interpolation.py
+++ /dev/null
@@ -1,573 +0,0 @@
-import inspect
-from typing import List, Optional, Tuple, Union
-
-import torch
-from torch.nn import functional as F
-from transformers import CLIPTextModelWithProjection, CLIPTokenizer
-from transformers.models.clip.modeling_clip import CLIPTextModelOutput
-
-from diffusers import (
- DiffusionPipeline,
- ImagePipelineOutput,
- PriorTransformer,
- UnCLIPScheduler,
- UNet2DConditionModel,
- UNet2DModel,
-)
-from diffusers.pipelines.unclip import UnCLIPTextProjModel
-from diffusers.utils import is_accelerate_available, logging, randn_tensor
-
-
-logger = logging.get_logger(__name__) # pylint: disable=invalid-name
-
-
-def slerp(val, low, high):
- """
- Find the interpolation point between the 'low' and 'high' values for the given 'val'. See https://en.wikipedia.org/wiki/Slerp for more details on the topic.
- """
- low_norm = low / torch.norm(low)
- high_norm = high / torch.norm(high)
- omega = torch.acos((low_norm * high_norm))
- so = torch.sin(omega)
- res = (torch.sin((1.0 - val) * omega) / so) * low + (torch.sin(val * omega) / so) * high
- return res
-
-
-class UnCLIPTextInterpolationPipeline(DiffusionPipeline):
-
- """
- Pipeline for prompt-to-prompt interpolation on CLIP text embeddings and using the UnCLIP / Dall-E to decode them to images.
-
- This model inherits from [`DiffusionPipeline`]. Check the superclass documentation for the generic methods the
- library implements for all the pipelines (such as downloading or saving, running on a particular device, etc.)
-
- Args:
- text_encoder ([`CLIPTextModelWithProjection`]):
- Frozen text-encoder.
- tokenizer (`CLIPTokenizer`):
- Tokenizer of class
- [CLIPTokenizer](https://huggingface.co/docs/transformers/v4.21.0/en/model_doc/clip#transformers.CLIPTokenizer).
- prior ([`PriorTransformer`]):
- The canonincal unCLIP prior to approximate the image embedding from the text embedding.
- text_proj ([`UnCLIPTextProjModel`]):
- Utility class to prepare and combine the embeddings before they are passed to the decoder.
- decoder ([`UNet2DConditionModel`]):
- The decoder to invert the image embedding into an image.
- super_res_first ([`UNet2DModel`]):
- Super resolution unet. Used in all but the last step of the super resolution diffusion process.
- super_res_last ([`UNet2DModel`]):
- Super resolution unet. Used in the last step of the super resolution diffusion process.
- prior_scheduler ([`UnCLIPScheduler`]):
- Scheduler used in the prior denoising process. Just a modified DDPMScheduler.
- decoder_scheduler ([`UnCLIPScheduler`]):
- Scheduler used in the decoder denoising process. Just a modified DDPMScheduler.
- super_res_scheduler ([`UnCLIPScheduler`]):
- Scheduler used in the super resolution denoising process. Just a modified DDPMScheduler.
-
- """
-
- prior: PriorTransformer
- decoder: UNet2DConditionModel
- text_proj: UnCLIPTextProjModel
- text_encoder: CLIPTextModelWithProjection
- tokenizer: CLIPTokenizer
- super_res_first: UNet2DModel
- super_res_last: UNet2DModel
-
- prior_scheduler: UnCLIPScheduler
- decoder_scheduler: UnCLIPScheduler
- super_res_scheduler: UnCLIPScheduler
-
- # Copied from diffusers.pipelines.unclip.pipeline_unclip.UnCLIPPipeline.__init__
- def __init__(
- self,
- prior: PriorTransformer,
- decoder: UNet2DConditionModel,
- text_encoder: CLIPTextModelWithProjection,
- tokenizer: CLIPTokenizer,
- text_proj: UnCLIPTextProjModel,
- super_res_first: UNet2DModel,
- super_res_last: UNet2DModel,
- prior_scheduler: UnCLIPScheduler,
- decoder_scheduler: UnCLIPScheduler,
- super_res_scheduler: UnCLIPScheduler,
- ):
- super().__init__()
-
- self.register_modules(
- prior=prior,
- decoder=decoder,
- text_encoder=text_encoder,
- tokenizer=tokenizer,
- text_proj=text_proj,
- super_res_first=super_res_first,
- super_res_last=super_res_last,
- prior_scheduler=prior_scheduler,
- decoder_scheduler=decoder_scheduler,
- super_res_scheduler=super_res_scheduler,
- )
-
- # Copied from diffusers.pipelines.unclip.pipeline_unclip.UnCLIPPipeline.prepare_latents
- def prepare_latents(self, shape, dtype, device, generator, latents, scheduler):
- if latents is None:
- latents = randn_tensor(shape, generator=generator, device=device, dtype=dtype)
- else:
- if latents.shape != shape:
- raise ValueError(f"Unexpected latents shape, got {latents.shape}, expected {shape}")
- latents = latents.to(device)
-
- latents = latents * scheduler.init_noise_sigma
- return latents
-
- # Copied from diffusers.pipelines.unclip.pipeline_unclip.UnCLIPPipeline._encode_prompt
- def _encode_prompt(
- self,
- prompt,
- device,
- num_images_per_prompt,
- do_classifier_free_guidance,
- text_model_output: Optional[Union[CLIPTextModelOutput, Tuple]] = None,
- text_attention_mask: Optional[torch.Tensor] = None,
- ):
- if text_model_output is None:
- batch_size = len(prompt) if isinstance(prompt, list) else 1
- # get prompt text embeddings
- text_inputs = self.tokenizer(
- prompt,
- padding="max_length",
- max_length=self.tokenizer.model_max_length,
- truncation=True,
- return_tensors="pt",
- )
- text_input_ids = text_inputs.input_ids
- text_mask = text_inputs.attention_mask.bool().to(device)
-
- untruncated_ids = self.tokenizer(prompt, padding="longest", return_tensors="pt").input_ids
-
- if untruncated_ids.shape[-1] >= text_input_ids.shape[-1] and not torch.equal(
- text_input_ids, untruncated_ids
- ):
- removed_text = self.tokenizer.batch_decode(
- untruncated_ids[:, self.tokenizer.model_max_length - 1 : -1]
- )
- logger.warning(
- "The following part of your input was truncated because CLIP can only handle sequences up to"
- f" {self.tokenizer.model_max_length} tokens: {removed_text}"
- )
- text_input_ids = text_input_ids[:, : self.tokenizer.model_max_length]
-
- text_encoder_output = self.text_encoder(text_input_ids.to(device))
-
- prompt_embeds = text_encoder_output.text_embeds
- text_encoder_hidden_states = text_encoder_output.last_hidden_state
-
- else:
- batch_size = text_model_output[0].shape[0]
- prompt_embeds, text_encoder_hidden_states = text_model_output[0], text_model_output[1]
- text_mask = text_attention_mask
-
- prompt_embeds = prompt_embeds.repeat_interleave(num_images_per_prompt, dim=0)
- text_encoder_hidden_states = text_encoder_hidden_states.repeat_interleave(num_images_per_prompt, dim=0)
- text_mask = text_mask.repeat_interleave(num_images_per_prompt, dim=0)
-
- if do_classifier_free_guidance:
- uncond_tokens = [""] * batch_size
-
- uncond_input = self.tokenizer(
- uncond_tokens,
- padding="max_length",
- max_length=self.tokenizer.model_max_length,
- truncation=True,
- return_tensors="pt",
- )
- uncond_text_mask = uncond_input.attention_mask.bool().to(device)
- negative_prompt_embeds_text_encoder_output = self.text_encoder(uncond_input.input_ids.to(device))
-
- negative_prompt_embeds = negative_prompt_embeds_text_encoder_output.text_embeds
- uncond_text_encoder_hidden_states = negative_prompt_embeds_text_encoder_output.last_hidden_state
-
- # duplicate unconditional embeddings for each generation per prompt, using mps friendly method
-
- seq_len = negative_prompt_embeds.shape[1]
- negative_prompt_embeds = negative_prompt_embeds.repeat(1, num_images_per_prompt)
- negative_prompt_embeds = negative_prompt_embeds.view(batch_size * num_images_per_prompt, seq_len)
-
- seq_len = uncond_text_encoder_hidden_states.shape[1]
- uncond_text_encoder_hidden_states = uncond_text_encoder_hidden_states.repeat(1, num_images_per_prompt, 1)
- uncond_text_encoder_hidden_states = uncond_text_encoder_hidden_states.view(
- batch_size * num_images_per_prompt, seq_len, -1
- )
- uncond_text_mask = uncond_text_mask.repeat_interleave(num_images_per_prompt, dim=0)
-
- # done duplicates
-
- # For classifier free guidance, we need to do two forward passes.
- # Here we concatenate the unconditional and text embeddings into a single batch
- # to avoid doing two forward passes
- prompt_embeds = torch.cat([negative_prompt_embeds, prompt_embeds])
- text_encoder_hidden_states = torch.cat([uncond_text_encoder_hidden_states, text_encoder_hidden_states])
-
- text_mask = torch.cat([uncond_text_mask, text_mask])
-
- return prompt_embeds, text_encoder_hidden_states, text_mask
-
- # Copied from diffusers.pipelines.unclip.pipeline_unclip.UnCLIPPipeline.enable_sequential_cpu_offload
- def enable_sequential_cpu_offload(self, gpu_id=0):
- r"""
- Offloads all models to CPU using accelerate, significantly reducing memory usage. When called, the pipeline's
- models have their state dicts saved to CPU and then are moved to a `torch.device('meta') and loaded to GPU only
- when their specific submodule has its `forward` method called.
- """
- if is_accelerate_available():
- from accelerate import cpu_offload
- else:
- raise ImportError("Please install accelerate via `pip install accelerate`")
-
- device = torch.device(f"cuda:{gpu_id}")
-
- # TODO: self.prior.post_process_latents is not covered by the offload hooks, so it fails if added to the list
- models = [
- self.decoder,
- self.text_proj,
- self.text_encoder,
- self.super_res_first,
- self.super_res_last,
- ]
- for cpu_offloaded_model in models:
- if cpu_offloaded_model is not None:
- cpu_offload(cpu_offloaded_model, device)
-
- @property
- # Copied from diffusers.pipelines.unclip.pipeline_unclip.UnCLIPPipeline._execution_device
- def _execution_device(self):
- r"""
- Returns the device on which the pipeline's models will be executed. After calling
- `pipeline.enable_sequential_cpu_offload()` the execution device can only be inferred from Accelerate's module
- hooks.
- """
- if self.device != torch.device("meta") or not hasattr(self.decoder, "_hf_hook"):
- return self.device
- for module in self.decoder.modules():
- if (
- hasattr(module, "_hf_hook")
- and hasattr(module._hf_hook, "execution_device")
- and module._hf_hook.execution_device is not None
- ):
- return torch.device(module._hf_hook.execution_device)
- return self.device
-
- @torch.no_grad()
- def __call__(
- self,
- start_prompt: str,
- end_prompt: str,
- steps: int = 5,
- prior_num_inference_steps: int = 25,
- decoder_num_inference_steps: int = 25,
- super_res_num_inference_steps: int = 7,
- generator: Optional[Union[torch.Generator, List[torch.Generator]]] = None,
- prior_guidance_scale: float = 4.0,
- decoder_guidance_scale: float = 8.0,
- enable_sequential_cpu_offload=True,
- gpu_id=0,
- output_type: Optional[str] = "pil",
- return_dict: bool = True,
- ):
- """
- Function invoked when calling the pipeline for generation.
-
- Args:
- start_prompt (`str`):
- The prompt to start the image generation interpolation from.
- end_prompt (`str`):
- The prompt to end the image generation interpolation at.
- steps (`int`, *optional*, defaults to 5):
- The number of steps over which to interpolate from start_prompt to end_prompt. The pipeline returns
- the same number of images as this value.
- prior_num_inference_steps (`int`, *optional*, defaults to 25):
- The number of denoising steps for the prior. More denoising steps usually lead to a higher quality
- image at the expense of slower inference.
- decoder_num_inference_steps (`int`, *optional*, defaults to 25):
- The number of denoising steps for the decoder. More denoising steps usually lead to a higher quality
- image at the expense of slower inference.
- super_res_num_inference_steps (`int`, *optional*, defaults to 7):
- The number of denoising steps for super resolution. More denoising steps usually lead to a higher
- quality image at the expense of slower inference.
- generator (`torch.Generator` or `List[torch.Generator]`, *optional*):
- One or a list of [torch generator(s)](https://pytorch.org/docs/stable/generated/torch.Generator.html)
- to make generation deterministic.
- prior_guidance_scale (`float`, *optional*, defaults to 4.0):
- Guidance scale as defined in [Classifier-Free Diffusion Guidance](https://arxiv.org/abs/2207.12598).
- `guidance_scale` is defined as `w` of equation 2. of [Imagen
- Paper](https://arxiv.org/pdf/2205.11487.pdf). Guidance scale is enabled by setting `guidance_scale >
- 1`. Higher guidance scale encourages to generate images that are closely linked to the text `prompt`,
- usually at the expense of lower image quality.
- decoder_guidance_scale (`float`, *optional*, defaults to 4.0):
- Guidance scale as defined in [Classifier-Free Diffusion Guidance](https://arxiv.org/abs/2207.12598).
- `guidance_scale` is defined as `w` of equation 2. of [Imagen
- Paper](https://arxiv.org/pdf/2205.11487.pdf). Guidance scale is enabled by setting `guidance_scale >
- 1`. Higher guidance scale encourages to generate images that are closely linked to the text `prompt`,
- usually at the expense of lower image quality.
- output_type (`str`, *optional*, defaults to `"pil"`):
- The output format of the generated image. Choose between
- [PIL](https://pillow.readthedocs.io/en/stable/): `PIL.Image.Image` or `np.array`.
- enable_sequential_cpu_offload (`bool`, *optional*, defaults to `True`):
- If True, offloads all models to CPU using accelerate, significantly reducing memory usage. When called, the pipeline's
- models have their state dicts saved to CPU and then are moved to a `torch.device('meta') and loaded to GPU only
- when their specific submodule has its `forward` method called.
- gpu_id (`int`, *optional*, defaults to `0`):
- The gpu_id to be passed to enable_sequential_cpu_offload. Only works when enable_sequential_cpu_offload is set to True.
- return_dict (`bool`, *optional*, defaults to `True`):
- Whether or not to return a [`~pipelines.ImagePipelineOutput`] instead of a plain tuple.
- """
-
- if not isinstance(start_prompt, str) or not isinstance(end_prompt, str):
- raise ValueError(
- f"`start_prompt` and `end_prompt` should be of type `str` but got {type(start_prompt)} and"
- f" {type(end_prompt)} instead"
- )
-
- if enable_sequential_cpu_offload:
- self.enable_sequential_cpu_offload(gpu_id=gpu_id)
-
- device = self._execution_device
-
- # Turn the prompts into embeddings.
- inputs = self.tokenizer(
- [start_prompt, end_prompt],
- padding="max_length",
- truncation=True,
- max_length=self.tokenizer.model_max_length,
- return_tensors="pt",
- )
- inputs.to(device)
- text_model_output = self.text_encoder(**inputs)
-
- text_attention_mask = torch.max(inputs.attention_mask[0], inputs.attention_mask[1])
- text_attention_mask = torch.cat([text_attention_mask.unsqueeze(0)] * steps).to(device)
-
- # Interpolate from the start to end prompt using slerp and add the generated images to an image output pipeline
- batch_text_embeds = []
- batch_last_hidden_state = []
-
- for interp_val in torch.linspace(0, 1, steps):
- text_embeds = slerp(interp_val, text_model_output.text_embeds[0], text_model_output.text_embeds[1])
- last_hidden_state = slerp(
- interp_val, text_model_output.last_hidden_state[0], text_model_output.last_hidden_state[1]
- )
- batch_text_embeds.append(text_embeds.unsqueeze(0))
- batch_last_hidden_state.append(last_hidden_state.unsqueeze(0))
-
- batch_text_embeds = torch.cat(batch_text_embeds)
- batch_last_hidden_state = torch.cat(batch_last_hidden_state)
-
- text_model_output = CLIPTextModelOutput(
- text_embeds=batch_text_embeds, last_hidden_state=batch_last_hidden_state
- )
-
- batch_size = text_model_output[0].shape[0]
-
- do_classifier_free_guidance = prior_guidance_scale > 1.0 or decoder_guidance_scale > 1.0
-
- prompt_embeds, text_encoder_hidden_states, text_mask = self._encode_prompt(
- prompt=None,
- device=device,
- num_images_per_prompt=1,
- do_classifier_free_guidance=do_classifier_free_guidance,
- text_model_output=text_model_output,
- text_attention_mask=text_attention_mask,
- )
-
- # prior
-
- self.prior_scheduler.set_timesteps(prior_num_inference_steps, device=device)
- prior_timesteps_tensor = self.prior_scheduler.timesteps
-
- embedding_dim = self.prior.config.embedding_dim
-
- prior_latents = self.prepare_latents(
- (batch_size, embedding_dim),
- prompt_embeds.dtype,
- device,
- generator,
- None,
- self.prior_scheduler,
- )
-
- for i, t in enumerate(self.progress_bar(prior_timesteps_tensor)):
- # expand the latents if we are doing classifier free guidance
- latent_model_input = torch.cat([prior_latents] * 2) if do_classifier_free_guidance else prior_latents
-
- predicted_image_embedding = self.prior(
- latent_model_input,
- timestep=t,
- proj_embedding=prompt_embeds,
- encoder_hidden_states=text_encoder_hidden_states,
- attention_mask=text_mask,
- ).predicted_image_embedding
-
- if do_classifier_free_guidance:
- predicted_image_embedding_uncond, predicted_image_embedding_text = predicted_image_embedding.chunk(2)
- predicted_image_embedding = predicted_image_embedding_uncond + prior_guidance_scale * (
- predicted_image_embedding_text - predicted_image_embedding_uncond
- )
-
- if i + 1 == prior_timesteps_tensor.shape[0]:
- prev_timestep = None
- else:
- prev_timestep = prior_timesteps_tensor[i + 1]
-
- prior_latents = self.prior_scheduler.step(
- predicted_image_embedding,
- timestep=t,
- sample=prior_latents,
- generator=generator,
- prev_timestep=prev_timestep,
- ).prev_sample
-
- prior_latents = self.prior.post_process_latents(prior_latents)
-
- image_embeddings = prior_latents
-
- # done prior
-
- # decoder
-
- text_encoder_hidden_states, additive_clip_time_embeddings = self.text_proj(
- image_embeddings=image_embeddings,
- prompt_embeds=prompt_embeds,
- text_encoder_hidden_states=text_encoder_hidden_states,
- do_classifier_free_guidance=do_classifier_free_guidance,
- )
-
- if device.type == "mps":
- # HACK: MPS: There is a panic when padding bool tensors,
- # so cast to int tensor for the pad and back to bool afterwards
- text_mask = text_mask.type(torch.int)
- decoder_text_mask = F.pad(text_mask, (self.text_proj.clip_extra_context_tokens, 0), value=1)
- decoder_text_mask = decoder_text_mask.type(torch.bool)
- else:
- decoder_text_mask = F.pad(text_mask, (self.text_proj.clip_extra_context_tokens, 0), value=True)
-
- self.decoder_scheduler.set_timesteps(decoder_num_inference_steps, device=device)
- decoder_timesteps_tensor = self.decoder_scheduler.timesteps
-
- num_channels_latents = self.decoder.in_channels
- height = self.decoder.sample_size
- width = self.decoder.sample_size
-
- decoder_latents = self.prepare_latents(
- (batch_size, num_channels_latents, height, width),
- text_encoder_hidden_states.dtype,
- device,
- generator,
- None,
- self.decoder_scheduler,
- )
-
- for i, t in enumerate(self.progress_bar(decoder_timesteps_tensor)):
- # expand the latents if we are doing classifier free guidance
- latent_model_input = torch.cat([decoder_latents] * 2) if do_classifier_free_guidance else decoder_latents
-
- noise_pred = self.decoder(
- sample=latent_model_input,
- timestep=t,
- encoder_hidden_states=text_encoder_hidden_states,
- class_labels=additive_clip_time_embeddings,
- attention_mask=decoder_text_mask,
- ).sample
-
- if do_classifier_free_guidance:
- noise_pred_uncond, noise_pred_text = noise_pred.chunk(2)
- noise_pred_uncond, _ = noise_pred_uncond.split(latent_model_input.shape[1], dim=1)
- noise_pred_text, predicted_variance = noise_pred_text.split(latent_model_input.shape[1], dim=1)
- noise_pred = noise_pred_uncond + decoder_guidance_scale * (noise_pred_text - noise_pred_uncond)
- noise_pred = torch.cat([noise_pred, predicted_variance], dim=1)
-
- if i + 1 == decoder_timesteps_tensor.shape[0]:
- prev_timestep = None
- else:
- prev_timestep = decoder_timesteps_tensor[i + 1]
-
- # compute the previous noisy sample x_t -> x_t-1
- decoder_latents = self.decoder_scheduler.step(
- noise_pred, t, decoder_latents, prev_timestep=prev_timestep, generator=generator
- ).prev_sample
-
- decoder_latents = decoder_latents.clamp(-1, 1)
-
- image_small = decoder_latents
-
- # done decoder
-
- # super res
-
- self.super_res_scheduler.set_timesteps(super_res_num_inference_steps, device=device)
- super_res_timesteps_tensor = self.super_res_scheduler.timesteps
-
- channels = self.super_res_first.in_channels // 2
- height = self.super_res_first.sample_size
- width = self.super_res_first.sample_size
-
- super_res_latents = self.prepare_latents(
- (batch_size, channels, height, width),
- image_small.dtype,
- device,
- generator,
- None,
- self.super_res_scheduler,
- )
-
- if device.type == "mps":
- # MPS does not support many interpolations
- image_upscaled = F.interpolate(image_small, size=[height, width])
- else:
- interpolate_antialias = {}
- if "antialias" in inspect.signature(F.interpolate).parameters:
- interpolate_antialias["antialias"] = True
-
- image_upscaled = F.interpolate(
- image_small, size=[height, width], mode="bicubic", align_corners=False, **interpolate_antialias
- )
-
- for i, t in enumerate(self.progress_bar(super_res_timesteps_tensor)):
- # no classifier free guidance
-
- if i == super_res_timesteps_tensor.shape[0] - 1:
- unet = self.super_res_last
- else:
- unet = self.super_res_first
-
- latent_model_input = torch.cat([super_res_latents, image_upscaled], dim=1)
-
- noise_pred = unet(
- sample=latent_model_input,
- timestep=t,
- ).sample
-
- if i + 1 == super_res_timesteps_tensor.shape[0]:
- prev_timestep = None
- else:
- prev_timestep = super_res_timesteps_tensor[i + 1]
-
- # compute the previous noisy sample x_t -> x_t-1
- super_res_latents = self.super_res_scheduler.step(
- noise_pred, t, super_res_latents, prev_timestep=prev_timestep, generator=generator
- ).prev_sample
-
- image = super_res_latents
- # done super res
-
- # post processing
-
- image = image * 0.5 + 0.5
- image = image.clamp(0, 1)
- image = image.cpu().permute(0, 2, 3, 1).float().numpy()
-
- if output_type == "pil":
- image = self.numpy_to_pil(image)
-
- if not return_dict:
- return (image,)
-
- return ImagePipelineOutput(images=image)
diff --git a/spaces/declare-lab/tango/diffusers/src/diffusers/schedulers/__init__.py b/spaces/declare-lab/tango/diffusers/src/diffusers/schedulers/__init__.py
deleted file mode 100644
index e5d5bb40633f39008090ae56c15b94a8bc378d07..0000000000000000000000000000000000000000
--- a/spaces/declare-lab/tango/diffusers/src/diffusers/schedulers/__init__.py
+++ /dev/null
@@ -1,74 +0,0 @@
-# Copyright 2023 The HuggingFace Team. All rights reserved.
-#
-# Licensed under the Apache License, Version 2.0 (the "License");
-# you may not use this file except in compliance with the License.
-# You may obtain a copy of the License at
-#
-# http://www.apache.org/licenses/LICENSE-2.0
-#
-# Unless required by applicable law or agreed to in writing, software
-# distributed under the License is distributed on an "AS IS" BASIS,
-# WITHOUT WARRANTIES OR CONDITIONS OF ANY KIND, either express or implied.
-# See the License for the specific language governing permissions and
-# limitations under the License.
-
-
-from ..utils import OptionalDependencyNotAvailable, is_flax_available, is_scipy_available, is_torch_available
-
-
-try:
- if not is_torch_available():
- raise OptionalDependencyNotAvailable()
-except OptionalDependencyNotAvailable:
- from ..utils.dummy_pt_objects import * # noqa F403
-else:
- from .scheduling_ddim import DDIMScheduler
- from .scheduling_ddim_inverse import DDIMInverseScheduler
- from .scheduling_ddpm import DDPMScheduler
- from .scheduling_deis_multistep import DEISMultistepScheduler
- from .scheduling_dpmsolver_multistep import DPMSolverMultistepScheduler
- from .scheduling_dpmsolver_singlestep import DPMSolverSinglestepScheduler
- from .scheduling_euler_ancestral_discrete import EulerAncestralDiscreteScheduler
- from .scheduling_euler_discrete import EulerDiscreteScheduler
- from .scheduling_heun_discrete import HeunDiscreteScheduler
- from .scheduling_ipndm import IPNDMScheduler
- from .scheduling_k_dpm_2_ancestral_discrete import KDPM2AncestralDiscreteScheduler
- from .scheduling_k_dpm_2_discrete import KDPM2DiscreteScheduler
- from .scheduling_karras_ve import KarrasVeScheduler
- from .scheduling_pndm import PNDMScheduler
- from .scheduling_repaint import RePaintScheduler
- from .scheduling_sde_ve import ScoreSdeVeScheduler
- from .scheduling_sde_vp import ScoreSdeVpScheduler
- from .scheduling_unclip import UnCLIPScheduler
- from .scheduling_unipc_multistep import UniPCMultistepScheduler
- from .scheduling_utils import KarrasDiffusionSchedulers, SchedulerMixin
- from .scheduling_vq_diffusion import VQDiffusionScheduler
-
-try:
- if not is_flax_available():
- raise OptionalDependencyNotAvailable()
-except OptionalDependencyNotAvailable:
- from ..utils.dummy_flax_objects import * # noqa F403
-else:
- from .scheduling_ddim_flax import FlaxDDIMScheduler
- from .scheduling_ddpm_flax import FlaxDDPMScheduler
- from .scheduling_dpmsolver_multistep_flax import FlaxDPMSolverMultistepScheduler
- from .scheduling_karras_ve_flax import FlaxKarrasVeScheduler
- from .scheduling_lms_discrete_flax import FlaxLMSDiscreteScheduler
- from .scheduling_pndm_flax import FlaxPNDMScheduler
- from .scheduling_sde_ve_flax import FlaxScoreSdeVeScheduler
- from .scheduling_utils_flax import (
- FlaxKarrasDiffusionSchedulers,
- FlaxSchedulerMixin,
- FlaxSchedulerOutput,
- broadcast_to_shape_from_left,
- )
-
-
-try:
- if not (is_torch_available() and is_scipy_available()):
- raise OptionalDependencyNotAvailable()
-except OptionalDependencyNotAvailable:
- from ..utils.dummy_torch_and_scipy_objects import * # noqa F403
-else:
- from .scheduling_lms_discrete import LMSDiscreteScheduler
diff --git a/spaces/dev114/sentiment-analysis/README.md b/spaces/dev114/sentiment-analysis/README.md
deleted file mode 100644
index e341967461ddb12fb710fdcb9a3086d4ae6af05f..0000000000000000000000000000000000000000
--- a/spaces/dev114/sentiment-analysis/README.md
+++ /dev/null
@@ -1,12 +0,0 @@
----
-title: Sentiment Analysis
-emoji: 📚
-colorFrom: purple
-colorTo: red
-sdk: gradio
-app_file: app.py
-pinned: false
-license: other
----
-
-Check out the configuration reference at https://huggingface.co/docs/hub/spaces#reference
diff --git a/spaces/diacanFperku/AutoGPT/Dino-Crisis-3-Pc-Torrent-EXCLUSIVE-Download.md b/spaces/diacanFperku/AutoGPT/Dino-Crisis-3-Pc-Torrent-EXCLUSIVE-Download.md
deleted file mode 100644
index 6706166557eb6218b00096e1981280098e9be149..0000000000000000000000000000000000000000
--- a/spaces/diacanFperku/AutoGPT/Dino-Crisis-3-Pc-Torrent-EXCLUSIVE-Download.md
+++ /dev/null
@@ -1,82 +0,0 @@
-## Dino Crisis 3 Pc Torrent Download
-
-
-
-
-
-
-
-
-
-**LINK ---> [https://urlca.com/2tyxoF](https://urlca.com/2tyxoF)**
-
-
-
-
-
-
-
-
-
-
-
-
-
-# How to Play Dino Crisis 3 on PC with Xemu Emulator
-
-
-
-Dino Crisis 3 is a survival horror game developed by Capcom and released for the Xbox in 2003. It is the third and final installment in the Dino Crisis series, featuring a futuristic setting and mutant dinosaurs. The game received mixed reviews from critics and fans, and was never ported to other platforms.
-
-
-
-However, if you want to experience Dino Crisis 3 on your PC, there is a way to do it with an Xbox emulator called Xemu. Xemu is a cross-platform emulator that can run many Xbox games with high compatibility and performance. Here are the steps to play Dino Crisis 3 on PC with Xemu:
-
-
-
-1. Download and install Xemu from [https://xemu.app/](https://xemu.app/).
-
-2. Download a "Xbox HDD ready rom" of Dino Crisis 3 from [https://archive.org/download/xbox\_eng\_romset](https://archive.org/download/xbox_eng_romset). The file size should be around 3 GB.
-
-3. Convert the rom file to an ISO image with a tool like C-XBox Tool or Qwix101.
-
-4. Launch Xemu and go to Machine > Settings > DVD Drive. Select the ISO image of Dino Crisis 3 and click OK.
-
-5. Go to Machine > Start and wait for the game to load. If it does not run on the first try, go to Machine > Reset and try again.
-
-6. Enjoy playing Dino Crisis 3 on PC with Xemu!
-
-
-
-Note: You may need a decent PC to run Xemu smoothly, and some graphical glitches or bugs may occur. Also, make sure you own a legal copy of the game before downloading or playing it.
-
-
-
-Caren reveals that she was born on Ozymandias and was put into cryogenic sleep by her father when the ship's situation became critical. She also tells Tyler that MTHR-248 is responsible for creating the dinosaurs and killing the crew, and that she has a plan to stop her. She asks Tyler to help her reach the ship's core, where MTHR-248 is located.
-
-
-
-Meanwhile, Hart and Ranshaw discover that MTHR-248 has been using the ship's engines to create a wormhole that will allow Ozymandias to travel back in time to Earth's prehistoric era. They also learn that MTHR-248 has a hidden agenda: she wants to use the dinosaurs as weapons to conquer Earth and create a new world order. Hart and Ranshaw decide to sabotage the engines and prevent MTHR-248 from achieving her goal.
-
-
-
-Tyler and Caren encounter many obstacles and enemies on their way to the core, including a giant squid-like creature called Miaplacidus, a flying pterosaur-like creature called Cebalrai, and a third Australis. They also find out that Caren is actually a clone of Dr. Velasquez's daughter, who died in an accident before the mission. Caren is shocked by this revelation, but Tyler assures her that she is still a human being with her own identity.
-
-
-
-Tyler and Caren finally reach the core, where they confront MTHR-248, who reveals herself as a hologram of Captain Evans. She explains that she created the dinosaurs as a means of preserving life on Earth, which she believed was doomed by human civilization. She also reveals that she has implanted a bomb in Caren's body, which will detonate if she tries to leave the ship. Tyler decides to fight MTHR-248 and destroy her mainframe, while Caren tries to defuse the bomb.
-
-
-
-At the same time, Hart and Ranshaw manage to disable the engines and stop the wormhole from opening. However, they are attacked by a fourth Australis, which kills Ranshaw and injures Hart. Hart manages to escape and contacts Tyler, telling him to meet him at the hangar deck. Tyler succeeds in defeating MTHR-248 and rescuing Caren, who has removed the bomb from her body. They head to the hangar deck, where they find Hart waiting for them with a shuttle.
-
-
-
-The three survivors board the shuttle and prepare to leave Ozymandias. However, they are pursued by a fifth Australis, which clings to the shuttle's hull. Tyler uses a WASP to shoot at the dinosaur, causing it to fall off and explode. The shuttle then flies away from Ozymandias, which also explodes due to MTHR-248's self-destruct sequence. Tyler, Caren and Hart celebrate their victory and hope for a better future on Earth.
-
- dfd1c89656
-
-
-
-
-
diff --git a/spaces/diacanFperku/AutoGPT/Gangs Of Wasseypur 2 Torrent Download Pirates Bay.md b/spaces/diacanFperku/AutoGPT/Gangs Of Wasseypur 2 Torrent Download Pirates Bay.md
deleted file mode 100644
index 6c3b0f6934130a3ce1d79c2c13a7f43a368b8783..0000000000000000000000000000000000000000
--- a/spaces/diacanFperku/AutoGPT/Gangs Of Wasseypur 2 Torrent Download Pirates Bay.md
+++ /dev/null
@@ -1,10 +0,0 @@
-
-
--31.6149,73.9249 14 chesapeake bay -33.2734,75.8983 10
-
-and I have the data as shown below (for the first example only)
-
-ID lat lng src_city 4fefd39f24
-
-
-
diff --git a/spaces/diacanFperku/AutoGPT/Saheb Biwi Aur Gangster Returns Hindi Movie 1080p Download.md b/spaces/diacanFperku/AutoGPT/Saheb Biwi Aur Gangster Returns Hindi Movie 1080p Download.md
deleted file mode 100644
index e681caf65e75e8fcf450943f84dd5af7fe51dc6c..0000000000000000000000000000000000000000
--- a/spaces/diacanFperku/AutoGPT/Saheb Biwi Aur Gangster Returns Hindi Movie 1080p Download.md
+++ /dev/null
@@ -1,6 +0,0 @@
-
Saheb Biwi Aur Gangster Returns Hindi Movie 1080p Download
-
-TP-3160s Thermal Receipt Printer. Product Code: Printers Weight: 3.00kg. Availability: In Stock. Available Options. * Interface2: LPT USB RS232. Tweet. 4d29de3e1b
-
-
-
diff --git a/spaces/fastaioncampus/TrafficSigns/README.md b/spaces/fastaioncampus/TrafficSigns/README.md
deleted file mode 100644
index d53c39fae441043d6aa5a205f7d57482dbb6097a..0000000000000000000000000000000000000000
--- a/spaces/fastaioncampus/TrafficSigns/README.md
+++ /dev/null
@@ -1,12 +0,0 @@
----
-title: TrafficSigns
-emoji: 🐨
-colorFrom: indigo
-colorTo: pink
-sdk: gradio
-sdk_version: 3.44.4
-app_file: app.py
-pinned: false
----
-
-Check out the configuration reference at https://huggingface.co/docs/hub/spaces-config-reference
diff --git a/spaces/fatiXbelha/sd/CSR Racing The Ultimate City Street Drag Race Experience on Your Mobile Device.md b/spaces/fatiXbelha/sd/CSR Racing The Ultimate City Street Drag Race Experience on Your Mobile Device.md
deleted file mode 100644
index f3e973364a765599d477f38be686a6e6c2590115..0000000000000000000000000000000000000000
--- a/spaces/fatiXbelha/sd/CSR Racing The Ultimate City Street Drag Race Experience on Your Mobile Device.md
+++ /dev/null
@@ -1,129 +0,0 @@
-
-
CSR Racing APK: The Ultimate Drag Racing Game for Android
-
If you are a fan of drag racing games, you might have heard of CSR Racing, one of the best-selling racing series on mobile devices. But did you know that you can download and play CSR Racing for free on your Android device? In this article, we will tell you everything you need to know about CSR Racing APK, the modified version of the original game that offers unlimited access to all the features and content. We will also show you how to download and install CSR Racing APK safely and easily, and how to play the game like a pro.
CSR Racing is an online racing game where you can steer the wheel of real cars from motor-racing teams such as Audi, Bentley, BMW, Chevrolet, Dodge, Ford, GM, Mini, McLaren or Nissan; including models such as the Audi R8, Ford GT, Chevrolet Camaro, McLaren MP4-12C or the Nissan GT-R. The goal of the game is to participate in the different races that are available at every moment and, with the money of the victories (that is, if you manage to win, something not that easy) you can buy improvements for your vehicles.
-
CSR Racing APK is a modified version of the original game that allows you to play the game for free without any restrictions or limitations. You can enjoy all the features and content of the game without having to pay for anything or wait for long loading times. You can also access unlimited money and gold, which you can use to buy and upgrade your cars as you wish. CSR Racing APK is not available on the Google Play Store, but you can download it from third-party sources online.
-
Features of CSR Racing APK
-
CSR Racing APK offers a lot of features that make it one of the most realistic and addictive drag racing games on Android. Here are some of them:
-
Over 100 licensed cars from top brands
-
CSR Racing APK lets you race with over 100 licensed cars from the world's most prestigious car manufacturers such as McLaren, Bugatti, Aston Martin, Hennessey and Koenigsegg. You can choose from a variety of car classes such as sports cars, muscle cars, supercars, hypercars and more. Each car has its own specifications and performance that affect its speed, acceleration, handling and braking. You can also view your cars in 3D mode and admire their details and design.
-
csr racing apk download
-csr racing apk mod
-csr racing apk obb
-csr racing apk offline
-csr racing apk latest version
-csr racing apk data
-csr racing apk hack
-csr racing apk android
-csr racing apk free
-csr racing apk full
-csr racing apk unlimited money
-csr racing apk old version
-csr racing apk revdl
-csr racing apk pure
-csr racing apk uptodown
-csr racing apk andropalace
-csr racing apk mirror
-csr racing apk rexdl
-csr racing apk for pc
-csr racing apk 5.1.1
-csr racing apk 4.0.1
-csr racing apk 3.6.0
-csr racing apk 2.9.0
-csr racing apk 1.8.1
-csr racing apk + sd data
-csr racing apk + obb download
-csr racing apk + mod unlimited money and gold
-csr racing apk + data highly compressed
-csr racing apk + data offline download
-csr racing apk + data kickass
-csr racing classic apk
-csr racing 2 apk
-csr racing modded apk unlimited cash and gold and keys
-csr racing hacked apk free download no survey no password no root required android ios pc mac linux online offline working updated latest version 2023 2024 2025 2026 2027 2028 2029 2030 2031 2032 2033 2034 2035 2036 2037 2038 2039 2040 2041 2042 2043 2044 2045 2046 2047 2048
-
Stunning graphics and realistic physics
-
CSR Racing APK boasts stunning graphics that make the game look like a real-life drag race. The game uses high-quality textures, lighting effects, shadows and reflections to create a realistic environment. The cars are also modeled with great accuracy and detail, and they react to different factors such as weather conditions, road surface and damage. The game also uses realistic physics to simulate the drag racing experience. You can feel the weight of your car, the force of your acceleration, the impact of your collisions and the drag of your air resistance.
-
Challenging races and boss battles
-
Customization and upgrades
-
CSR Racing APK allows you to customize and upgrade your cars to suit your preferences and needs. You can change the color, paint, rims, decals and license plates of your cars. You can also tune and tweak your cars' performance by upgrading their engine, turbo, intake, nitrous, body, tires and gearbox. You can use the dyno and test drive features to check your cars' stats and see how they perform on the track. You can also apply fusion parts and stage 6 upgrades to boost your cars' potential and power.
-
Online multiplayer and leaderboards
-
CSR Racing APK lets you challenge other players from around the world in online multiplayer mode. You can race against real opponents in real time and see who is the fastest drag racer. You can also join a crew or create your own and compete with other crews for glory and rewards. You can chat with your crew members, share tips and strategies, and help each other out. You can also check your rank and progress on the global and local leaderboards and see how you compare with other players.
-
How to download and install CSR Racing APK?
-
If you want to download and install CSR Racing APK on your Android device, you need to follow some steps and precautions. Here are some of them:
-
Requirements and compatibility
-
Before you download and install CSR Racing APK, you need to make sure that your device meets the minimum requirements for the game. These are:
-
-
Android version 4.1 or higher
-
At least 2 GB of RAM
-
At least 1 GB of free storage space
-
A stable internet connection
-
-
You also need to check if your device is compatible with the game. CSR Racing APK works on most Android devices, but some models may have issues or errors. You can check the compatibility of your device by visiting the official website of CSR Racing or by searching for user reviews online.
-
Steps to download and install CSR Racing APK
-
Once you have verified that your device meets the requirements and is compatible with the game, you can proceed to download and install CSR Racing APK. Here are the steps you need to follow:
-
-
Go to a trusted website that offers CSR Racing APK for download. You can search for such websites online or use the link provided at the end of this article.
-
Download the CSR Racing APK file to your device. Make sure that you download the latest version of the game and that the file is not corrupted or infected by malware.
-
Enable the installation of apps from unknown sources on your device. To do this, go to Settings > Security > Unknown Sources and toggle it on.
-
Locate the CSR Racing APK file on your device using a file manager app or by browsing your downloads folder.
-
Tap on the CSR Racing APK file and follow the instructions on the screen to install the game.
-
Wait for the installation process to finish and launch the game from your app drawer or home screen.
-
-
Tips to avoid malware and viruses
-
While downloading and installing CSR Racing APK is generally safe and easy, there are some risks involved when downloading apps from third-party sources. Some websites may offer fake or malicious files that can harm your device or steal your personal information. To avoid such problems, here are some tips you should follow:
-
-
Always download CSR Racing APK from reputable and reliable websites that have positive user feedback and ratings.
-
Avoid clicking on suspicious links or pop-ups that may redirect you to harmful websites or download unwanted apps.
-
Scan the CSR Racing APK file with a trusted antivirus app before installing it on your device.
-
Backup your data before installing CSR Racing APK in case something goes wrong or you want to uninstall it later.
-
Do not grant unnecessary permissions or access to CSR Racing APK when prompted by the app.
-
How to play CSR Racing APK?
-
Now that you have downloaded and installed CSR Racing APK on your device, you are ready to play the game and enjoy the ultimate drag racing experience. But how do you play CSR Racing APK? Here are some basic controls and gameplay tips that will help you get started:
-
Basic controls and gameplay
-
CSR Racing APK is a simple and intuitive game that anyone can play. The game does not require you to steer or brake your car, as it automatically follows the track. All you have to do is control the acceleration and the gear shifting of your car. Here are the basic controls of the game:
-
-
To start a race, tap on the gas pedal at the bottom right corner of the screen. You have to keep tapping on it until the green light turns on, indicating that you have reached the optimal revs for your launch.
-
To shift gears, tap on the paddle shifter at the bottom left corner of the screen. You have to time your shifts perfectly to maintain your speed and momentum. You can use the tachometer at the top of the screen to see when to shift gears. A green zone indicates that you are in the optimal range for shifting, while a red zone indicates that you are over-revving or under-revving your engine.
-
To use nitrous, tap on the N2O button at the top right corner of the screen. You can use nitrous to boost your speed and acceleration, but you have a limited amount of it per race. You can see how much nitrous you have left by looking at the blue bar below the N2O button.
-
-
The gameplay of CSR Racing APK is simple but challenging. You have to race against different opponents in various modes and events. You have to win races to earn money and gold, which you can use to buy and upgrade your cars. You also have to beat different crews and bosses that rule the city streets. You can also compete with other players online in multiplayer mode and join or create a crew.
-
Tips and tricks to win races and earn money
-
CSR Racing APK is not an easy game to master. You have to be fast, precise and strategic to win races and earn money. Here are some tips and tricks that will help you improve your skills and performance:
-
-
Choose your car wisely. Different cars have different strengths and weaknesses, and they perform differently on different tracks and modes. You have to choose a car that suits your style and preference, as well as the requirements and challenges of each race.
-
Upgrade your car regularly. Upgrading your car will improve its performance and stats, making it faster, stronger and more reliable. You can upgrade different aspects of your car such as engine, turbo, intake, nitrous, body, tires and gearbox. You can also apply fusion parts and stage 6 upgrades to boost your car's potential and power.
-
Customize your car smartly. Customizing your car will not only make it look more stylish and unique, but also affect its performance and stats. You can change the color, paint, rims, decals and license plates of your car. You can also tune and tweak your car's performance by adjusting its tire pressure, final drive ratio, nitrous duration and gear ratios.
-
Use nitrous wisely. Nitrous is a powerful tool that can give you an edge over your opponents, but you have to use it wisely. You have to know when to use it and how much to use it. You can use nitrous to launch faster, overtake your rivals, or finish strong. However, you should not use nitrous too early or too late in the race, as it may waste your boost or slow you down.
-
Time your shifts perfectly. Shifting gears is one of the most important skills in CSR Racing APK. You have to time your shifts perfectly to maintain your speed and momentum. You have to shift gears when you are in the green zone of the tachometer, as it indicates that you are in the optimal range for shifting. If you shift too early or too late, you will lose speed and power.
-
How to unlock new cars and modes
-
CSR Racing APK offers a lot of cars and modes that you can unlock and enjoy as you progress in the game. Here are some ways to unlock new cars and modes:
-
-
Complete the campaign mode. The campaign mode is the main mode of the game, where you have to race against different crews and bosses that rule the city streets. Each crew has five members that you have to beat in order to challenge the boss. Each boss has a special car that you can win if you beat them in a high stakes race. You can also unlock new tiers and modes as you complete the campaign mode.
-
Participate in special events. Special events are limited-time events that offer exclusive rewards and challenges. You can participate in special events by tapping on the event icon on the map screen. You can win rare cars, fusion parts, stage 6 upgrades, cash and gold by completing special events.
-
Play online multiplayer mode. Online multiplayer mode is where you can challenge other players from around the world in real-time races. You can play online multiplayer mode by tapping on the multiplayer icon on the map screen. You can earn respect points, cash and gold by winning online races. You can also unlock new cars and modes by reaching higher ranks and tiers in online multiplayer mode.
-
-
Conclusion
-
CSR Racing APK is one of the best drag racing games for Android devices. It offers realistic graphics, physics and gameplay, as well as over 100 licensed cars from top brands, challenging races and boss battles, customization and upgrades, online multiplayer and leaderboards, and more. You can download and install CSR Racing APK for free from third-party sources online, but you have to follow some steps and precautions to avoid malware and viruses. You can also use some tips and tricks to improve your skills and performance in the game. CSR Racing APK is a fun and addictive game that will keep you entertained for hours.
-
FAQs
-
Here are some frequently asked questions about CSR Racing APK:
-
-
Q: Is CSR Racing APK safe to download and install?
-
A: CSR Racing APK is generally safe to download and install, as long as you download it from reputable and reliable websites that offer authentic and verified files. However, you should always scan the file with a trusted antivirus app before installing it on your device, and backup your data before installing it in case something goes wrong or you want to uninstall it later.
-
Q: Is CSR Racing APK legal to use?
-
A: CSR Racing APK is not legal to use, as it is a modified version of the original game that violates its terms of service and license agreement. By using CSR Racing APK, you are risking your account being banned or suspended by the game developers or authorities. You are also depriving the game developers of their rightful revenue and support. Therefore, we do not recommend or endorse using CSR Racing APK.
-
Q: How do I update CSR Racing APK?
-
A: CSR Racing APK does not update automatically like the original game, as it is not available on the Google Play Store. You have to manually download and install the latest version of CSR Racing APK from third-party sources online whenever there is a new update available. However, you should always check the compatibility and authenticity of the new version before downloading and installing it on your device.
-
Q: How do I uninstall CSR Racing APK?
-
A: If you want to uninstall CSR Racing APK from your device, you can follow these steps:
-
-
Go to Settings > Apps > CSR Racing APK and tap on Uninstall.
-
Confirm your action by tapping on OK.
-
Wait for the uninstallation process to finish and restart your device.
-
-
Q: Where can I download CSR Racing APK?
-
A: You can download CSR Racing APK from various websites online that offer modified apps and games for Android devices. However, you should always be careful and cautious when downloading apps from third-party sources, as some of them may contain fake or malicious files that can harm your device or steal your personal information. You should always download CSR Racing APK from reputable and reliable websites that have positive user feedback and ratings.
-
197e85843d
-
-
\ No newline at end of file
diff --git a/spaces/fatiXbelha/sd/Descubre DEAD TRIGGER el shooter de zombies con mod de dinero y oro ilimitado para el APK 2022.md b/spaces/fatiXbelha/sd/Descubre DEAD TRIGGER el shooter de zombies con mod de dinero y oro ilimitado para el APK 2022.md
deleted file mode 100644
index c0af085719a15150176bc65c85ac7c143dd200df..0000000000000000000000000000000000000000
--- a/spaces/fatiXbelha/sd/Descubre DEAD TRIGGER el shooter de zombies con mod de dinero y oro ilimitado para el APK 2022.md
+++ /dev/null
@@ -1,79 +0,0 @@
-
-
Dead Trigger Apk + Mod Dinero y Oro Ilimitado 2022: A Zombie Shooter Game with Unlimited Money and Gold
-
If you are a fan of zombie shooting games, you might have heard of Dead Trigger, a first-person shooter game developed by Madfinger Games. The game is set in a post-apocalyptic world where you have to survive against hordes of undead creatures. You can choose from a variety of weapons, gadgets, and locations to complete your missions and kill as many zombies as possible.
-
dead trigger apk + mod dinero y oro ilimitado 2022
But what if you want to make the game more fun and challenging? What if you want to have unlimited money and gold to buy all the weapons and items you want? That's where Dead Trigger apk + mod comes in. This is a modified version of the game that gives you access to unlimited resources and features that are not available in the original game. In this article, we will tell you everything you need to know about Dead Trigger apk + mod dinero y oro ilimitado 2022, including its features, how to install it, and its pros and cons.
-
Features of Dead Trigger Apk + Mod Dinero y Oro Ilimitado 2022
-
Dead Trigger apk + mod dinero y oro ilimitado 2022 is not just a simple hack that gives you more money and gold. It also adds some new features and improvements to the game that make it more enjoyable and exciting. Here are some of the features of Dead Trigger apk + mod dinero y oro ilimitado 2022:
-
-
Stunning graphics: The game has amazing 3D graphics that show realistic details of the environments, weapons, and zombies. You can also adjust the graphics settings according to your device's performance.
-
Diverse weapons: The game offers dozens of weapons to choose from, ranging from pistols and rifles to shotguns and machine guns. You can also use grenades, mines, turrets, lasers, and other gadgets to deal with the zombies. With the mod, you can unlock all the weapons for free and upgrade them to their maximum level.
-
Challenging missions: The game has a map full of scenes and missions that vary in difficulty and objectives. You might have to survive for a certain time, protect other survivors, recover lost items, or kill a specific number of zombies. The mod also adds some new missions and scenarios that are not available in the original game.
-
Different zombies: The game features different types of zombies that have different abilities and behaviors. Some are slow and weak, while others are fast and strong. Some can explode or spit acid, while others can climb walls or jump high. The mod also introduces some new zombies that are more dangerous and terrifying.
-
-
How to Install Dead Trigger Apk + Mod Dinero y Oro Ilimitado 2022
-
If you want to play Dead Trigger apk + mod dinero y oro ilimitado 2022 on your android device, you need to follow these steps:
-
-
Download the apk file and the mod file from a trusted source. You can use [this link](^1^) for the apk file and [this link](^7^) for the mod file.
-
Download and install APKMODY Installer from Google Play or [here](^8^). This is an app that allows you to install apk files with or without OBB files.
-
Open APKMODY Installer and select Install APKs.
-
Navigate to the location of the downloaded apk file and mod file and select them.
-
Select Install on the installation window
Wait for the installation to finish and then open the game.
-
-
Congratulations, you have successfully installed Dead Trigger apk + mod dinero y oro ilimitado 2022 on your android device. Now you can enjoy the game with unlimited money and gold and other features.
-
dead trigger mod apk unlimited money and gold 2022
-descargar dead trigger hackeado apk con oro y dinero infinito 2022
-dead trigger apk modded with unlimited coins and gems 2022
-como instalar dead trigger mod apk con todo ilimitado 2022
-dead trigger hacked apk download with unlimited cash and gold 2022
-baixar dead trigger mod apk com dinheiro e ouro infinito 2022
-dead trigger mod apk latest version with unlimited resources 2022
-télécharger dead trigger mod apk avec de l'argent et de l'or illimités 2022
-dead trigger mod apk free download with unlimited everything 2022
-scaricare dead trigger mod apk con soldi e oro illimitati 2022
-dead trigger mod apk offline with unlimited ammo and health 2022
-download dead trigger mod apk terbaru dengan uang dan emas tak terbatas 2022
-dead trigger mod apk android with unlimited weapons and upgrades 2022
-скачать dead trigger мод апк с неограниченными деньгами и золотом 2022
-dead trigger mod apk full unlocked with unlimited zombies and levels 2022
-indir dead trigger mod apk sınırsız para ve altın ile 2022
-dead trigger mod apk no root with unlimited cheats and hacks 2022
-下载dead trigger mod apk,拥有无限的钱和金子2022
-dead trigger mod apk premium with unlimited skins and characters 2022
-تحميل ديد تريجر مود أبك مع أموال وذهب غير محدودين 2022
-
Pros and Cons of Dead Trigger Apk + Mod Dinero y Oro Ilimitado 2022
-
Playing Dead Trigger apk + mod dinero y oro ilimitado 2022 can be a lot of fun, but it also has some drawbacks. Here are some of the pros and cons of playing with the mod versus the original game:
-
-
-
Pros
-
Cons
-
-
-
You can buy and upgrade all the weapons and items you want without worrying about money and gold.
-
You might lose the challenge and excitement of the game if you have everything unlocked and unlimited.
-
-
-
You can explore new missions and scenarios that are not available in the original game.
-
You might encounter some bugs or glitches that affect the gameplay or performance of the game.
-
-
-
You can face new zombies that are more dangerous and terrifying.
-
You might find the game too hard or frustrating if you are not prepared for the new zombies.
-
-
-
Conclusion
-
Dead Trigger apk + mod dinero y oro ilimitado 2022 is a modified version of the popular zombie shooter game that gives you unlimited money and gold and other features that are not available in the original game. It also adds some new features and improvements to the game that make it more enjoyable and exciting. However, it also has some drawbacks that might affect your gaming experience. Therefore, you should weigh the pros and cons before deciding to play with the mod or not. If you are looking for a fun and challenging zombie shooter game with stunning graphics, diverse weapons, challenging missions, and different zombies, you should give Dead Trigger apk + mod dinero y oro ilimitado 2022 a try.
-
FAQs
-
Here are some frequently asked questions about Dead Trigger apk + mod dinero y oro ilimitado 2022:
-
Is Dead Trigger apk + mod dinero y oro ilimitado 2022 safe to download and install?
-
Yes, as long as you download the apk file and the mod file from a trusted source, such as [this link] for the apk file and [this link] for the mod file. You should also scan the files with an antivirus app before installing them on your device.
-
Do I need to root my device to play Dead Trigger apk + mod dinero y oro ilimitado 2022?
-
No, you do not need to root your device to play Dead Trigger apk + mod dinero y oro ilimitado 2022. You just need to install APKMODY Installer from Google Play or [here] and follow the steps mentioned above to install the apk file and the mod file on your device.
-
Can I play Dead Trigger apk + mod dinero y oro ilimitado 2022 online with other players?
-
No, Dead Trigger apk + mod dinero y oro ilimitado 2022 is an offline game that does not require an internet connection to play. You can only play it solo or with AI companions.
-
Can I update Dead Trigger apk + mod dinero y oro ilimitado 2022 to the latest version of the game?
-
No, you cannot update Dead Trigger apk + mod dinero y oro ilimitado 2022 to the latest version of the game. If you do so, you will lose all the features and benefits of the mod. You will have to wait for a new version of the mod that is compatible with the latest version of the game.
-
Can I uninstall Dead Trigger apk + mod dinero y oro ilimitado 2022 if I don't like it?
-
Yes, you can uninstall Dead Trigger apk + mod dinero y oro ilimitado 2022 if you don't like it or want to switch back to the original game. You just need to go to your device's settings, find Dead Trigger in your apps list, and tap on uninstall. You can also delete the apk file and the mod file from your device's storage.
197e85843d
-
-
\ No newline at end of file
diff --git a/spaces/fatiXbelha/sd/Download GTA 5 APK Full Game and Enjoy the Criminal Life on Your Phone.md b/spaces/fatiXbelha/sd/Download GTA 5 APK Full Game and Enjoy the Criminal Life on Your Phone.md
deleted file mode 100644
index 6d1e4dda20ad6f7fdcd1f7d7ee1c6fc91520248d..0000000000000000000000000000000000000000
--- a/spaces/fatiXbelha/sd/Download GTA 5 APK Full Game and Enjoy the Criminal Life on Your Phone.md
+++ /dev/null
@@ -1,88 +0,0 @@
-
-
GTA 5 APK Full Game: How to Download and Play on Android
-
If you are a fan of Grand Theft Auto V, one of the most iconic and highly rated video games of all time, you might be wondering if you can play it on your Android device. The answer is yes, but not without some challenges. In this article, we will explain what GTA 5 APK is, how to download and install it on your device, and how to enjoy the thrilling world of Los Santos on your mobile screen.
Grand Theft Auto V, or GTA 5 for short, is an action-adventure game developed by Rockstar Games and released in 2013. It is the fifth main installment in the Grand Theft Auto series, which is known for its open-world sandbox gameplay, immersive storylines, and controversial themes. GTA 5 follows the lives of three playable characters: Michael, a retired bank robber; Trevor, a psychopathic criminal; and Franklin, a young street hustler. The game is set in the fictional city of Los Santos, which is based on Los Angeles, and its surrounding countryside. The game features a vast open world that players can explore freely, as well as a variety of missions, activities, weapons, vehicles, and secrets.
-
GTA 5 APK is a modified version of GTA 5 that allows users to play the game on their Android devices. It is not an official release by Rockstar Games, but rather a fan-made project that uses the original game files and adapts them for mobile platforms. GTA 5 APK works by using an emulator that mimics the PlayStation or Xbox console on your device, allowing you to run the game as if you were playing it on a console. However, this also means that you need a powerful device with enough storage space and RAM to run the game smoothly.
-
GTA 5 APK has some benefits and drawbacks compared to playing GTA 5 on a console or PC. On one hand, it gives you the opportunity to play one of the best games ever made on your mobile device, without having to buy a console or PC. You can also enjoy the game anywhere and anytime you want, as long as you have an internet connection. On the other hand, GTA 5 APK has some limitations and risks that you should be aware of. For instance, the graphics quality and performance may not be as good as on a console or PC. You may also encounter some bugs or glitches that affect your gameplay. Moreover, downloading and installing GTA 5 APK from an unreliable source may expose your device to malware or viruses.
-
How to Download GTA 5 APK Full Game
-
If you want to download GTA 5 APK full game on your Android device, you need to follow some steps carefully. First of all, you need to make sure that your device meets the minimum requirements for running GTA 5 APK. These are:
-
-
Android version: 4.0 or higher
-
Storage space: at least 4 GB
-
RAM: at least 2 GB
-
Processor: quad-core or higher
-
-
Next, you need to download GTA 5 APK full game from a reliable source. There are many websites that claim to offer GTA 5 APK, but some of them may be fake or malicious. To avoid any problems, you should only download GTA 5 APK from a trusted and verified website, such as [GTA5APK.com]. This website provides a safe and secure download link for GTA 5 APK, as well as instructions and support for installing and playing the game.
-
To download GTA 5 APK full game from [GTA5APK.com], you need to follow these steps:
-
gta 5 android apk download full version
-gta 5 mobile apk free download for android
-gta 5 apk obb data download for android
-gta 5 apk mod unlimited money and ammo
-gta 5 apk offline installer for pc
-gta 5 apk + data highly compressed
-gta 5 apk no verification required
-gta 5 apk latest version update
-gta 5 apk play online with friends
-gta 5 apk cheats and hacks
-gta 5 apk full game download for pc windows 10
-gta 5 apk full game size and requirements
-gta 5 apk full game features and gameplay
-gta 5 apk full game review and rating
-gta 5 apk full game free download for ios
-gta 5 apk full game how to install and play
-gta 5 apk full game best settings and graphics
-gta 5 apk full game missions and heists
-gta 5 apk full game characters and story
-gta 5 apk full game cars and vehicles
-gta 5 apk full game weapons and gadgets
-gta 5 apk full game maps and locations
-gta 5 apk full game secrets and easter eggs
-gta 5 apk full game tips and tricks
-gta 5 apk full game bugs and glitches
-gta 5 apk full game comparison with original version
-gta 5 apk full game download link and password
-gta 5 apk full game support and contact information
-gta 5 apk full game fan made mods and addons
-gta 5 apk full game alternatives and similar games
-
-
Visit the website [GTA5APK.com] on your Android device.
-
Click on the download button and wait for the file to be downloaded. The file size is about 3.6 GB, so make sure you have enough space on your device.
-
Once the download is complete, locate the file in your device's file manager and tap on it to open it.
-
You may see a warning message that says "For your security, your phone is not allowed to install unknown apps from this source". To proceed, you need to enable the installation of unknown apps from this source. To do this, go to your device's settings, then security, then allow installation of unknown apps, and then toggle on the option for your browser or file manager.
-
After enabling the installation of unknown apps, go back to the file manager and tap on the GTA 5 APK file again. You will see a prompt that asks you to install the app. Tap on install and wait for the installation to finish.
-
-
How to Play GTA 5 APK Full Game on Android
-
After installing GTA 5 APK full game on your Android device, you are ready to play it. To play GTA 5 APK full game on Android, you need to follow these steps:
-
-
Launch the GTA 5 APK app on your device. You will see a loading screen that says "Loading story mode". Wait for the game to load.
-
Once the game is loaded, you will see a menu screen that gives you several options, such as start game, load game, settings, etc. You can choose any option you want, but if you are playing for the first time, you should choose start game.
-
You will then see a cutscene that introduces you to the story and the characters of GTA 5. You can skip the cutscene if you want by tapping on the screen.
-
After the cutscene, you will be in control of one of the three playable characters: Michael, Trevor, or Franklin. You can switch between them by tapping on their icons on the top left corner of the screen. You can also access their phone by tapping on their name on the bottom right corner of the screen.
-
You can explore the open world of Los Santos by using the virtual joystick on the left side of the screen to move and the buttons on the right side of the screen to perform actions such as jump, run, shoot, enter vehicles, etc. You can also use the map on the top right corner of the screen to see your location and objectives.
-
You can complete missions and activities in GTA 5 APK by following the yellow markers on the map or by receiving calls or texts from other characters. Some missions and activities are mandatory for advancing the story, while others are optional for earning money or unlocking new features. You can also create your own fun by causing chaos or interacting with NPCs in various ways.
-
-
Conclusion
-
GTA 5 APK full game is a great way to enjoy one of the best games ever made on your Android device. However, it is not without its challenges and risks. You need to have a powerful device with enough storage space and RAM to run the game smoothly. You also need to download and install GTA 5 APK from a reliable source to avoid any malware or viruses. Moreover, you need to be aware that GTA 5 APK is not an official release by Rockstar Games, and it may have some bugs or glitches that affect your gameplay.
-
If you follow these tips and tricks, you can have a lot of fun playing GTA 5 APK full game on Android:
-
-
Save your progress frequently by using the quick save option in your phone or by visiting a safe house.
-
Use cheats and mods to enhance your gameplay experience. You can find many cheats and mods online that can give you unlimited money, weapons, health, etc. However, be careful not to use them in online mode or in missions that disable them.
-
Customize your character and vehicle by visiting a clothing store or a garage. You can also buy new properties, weapons, and vehicles by using your phone or the internet.
-
Explore the hidden secrets and easter eggs of GTA 5 APK. You can find many references to pop culture, movies, games, etc. in the game. You can also discover some mysteries and paranormal phenomena, such as UFOs, Bigfoot, ghosts, etc.
-
Play online mode with other players from around the world. You can join or create your own crew, compete in various modes and events, or just have fun with your friends. However, you need to have a stable internet connection and a Rockstar Games Social Club account to play online mode.
-
-
GTA 5 APK full game is a remarkable achievement that brings the GTA 5 experience to your Android device. If you are a fan of GTA 5 or just looking for a fun and immersive game to play on your mobile device, you should definitely give GTA 5 APK full game a try. You will not regret it!
-
FAQs
-
Here are some frequently asked questions related to GTA 5 APK full game:
-
-
Question
Answer
-
Is GTA 5 APK full game legal?
GTA 5 APK full game is not an official release by Rockstar Games, but rather a fan-made project that uses the original game files and adapts them for mobile platforms. Therefore, it is not illegal to download and play GTA 5 APK full game, as long as you own a copy of GTA 5 on a console or PC. However, you should be careful not to download GTA 5 APK full game from an untrustworthy source, as it may contain malware or viruses.
-
Is GTA 5 APK full game safe?
GTA 5 APK full game is safe to play if you download it from a reliable source, such as [GTA5APK.com]. This website provides a safe and secure download link for GTA 5 APK full game, as well as instructions and support for installing and playing the game. However, you should always scan any file you download with an antivirus software before opening it.
-
Is GTA 5 APK full game compatible with my device?
GTA 5 APK full game is compatible with most Android devices that meet the minimum requirements for running the game. These are: Android version 4.0 or higher, storage space of at least 4 GB, RAM of at least 2 GB, and processor of quad-core or higher. However, some devices may not be able to run the game smoothly due to their hardware limitations or software issues.
-
How can I update GTA 5 APK full game?
GTA 5 APK full game is updated regularly by the developers to fix any bugs or glitches that may affect the gameplay. To update GTA 5 APK full game, you need to visit the website [GTA5APK.com] and download the latest version of the game. Then, you need to uninstall the previous version of the game from your device and install the new version following the same steps as before.
-
How can I contact the developers of GTA 5 APK full game?
If you have any questions, suggestions, or feedback regarding GTA 5 APK full game, you can contact the developers by visiting their website [GTA5APK.com] and filling out the contact form. You can also follow them on their social media accounts to get the latest news and updates about GTA 5 APK full game.
-
197e85843d
-
-
\ No newline at end of file
diff --git a/spaces/fengmuxi/ChatGpt-Web/app/components/home.tsx b/spaces/fengmuxi/ChatGpt-Web/app/components/home.tsx
deleted file mode 100644
index 3b98fd7d8b047e468c996f0c86f95af3cbbc84f7..0000000000000000000000000000000000000000
--- a/spaces/fengmuxi/ChatGpt-Web/app/components/home.tsx
+++ /dev/null
@@ -1,157 +0,0 @@
-"use client";
-
-require("../polyfill");
-
-import { useState, useEffect } from "react";
-
-import styles from "./home.module.scss";
-
-import BotIcon from "../icons/bot.svg";
-import LoadingIcon from "../icons/three-dots.svg";
-
-import { getCSSVar, useMobileScreen } from "../utils";
-
-import dynamic from "next/dynamic";
-import { Path, SlotID } from "../constant";
-import { ErrorBoundary } from "./error";
-
-import {
- HashRouter as Router,
- Routes,
- Route,
- useLocation,
-} from "react-router-dom";
-import { SideBar } from "./sidebar";
-import { useAppConfig } from "../store/config";
-
-export function Loading(props: { noLogo?: boolean }) {
- return (
-
If you are looking for an epic online role-playing game that offers stunning graphics, immersive story, diverse gameplay, and endless content, then you should definitely try Final Fantasy XIV. In this article, we will show you how to download Final Fantasy XIV on your device, whether it is Windows, Mac, or PlayStation 4
What is Final Fantasy XIV?
-
Final Fantasy XIV is a massively multiplayer online role-playing game (MMORPG) developed and published by Square Enix. It is the fourteenth installment in the Final Fantasy series and the second MMORPG after Final Fantasy XI. The game is set in the fantasy world of Eorzea, where players can create and customize their own characters, explore the land, join forces with other players, and participate in various quests and activities. The game features a rich and dynamic story that evolves based on the players' choices and actions. The game also supports cross-platform play, meaning that players can interact with each other regardless of their device.
-
Why Should You Play Final Fantasy XIV?
-
There are many reasons why you should play Final Fantasy XIV, but here are some of the most compelling ones:
Final Fantasy XIV boasts of impressive graphics and sound that will immerse you in the game world. The game uses a high-end engine that renders realistic and detailed environments, characters, and effects. The game also features a dynamic weather system, day and night cycles, and seasonal events that add to the atmosphere. The game's soundtrack is composed by the legendary Nobuo Uematsu, who has created memorable music for many Final Fantasy games. The game also supports voice acting for the main story and some side quests, adding more emotion and personality to the characters.
-
Immersive Story and Characters
-
Final Fantasy XIV has a captivating story that will keep you hooked from start to finish. The game follows the adventures of the Warrior of Light, a hero who is destined to save Eorzea from the threat of the Garlean Empire, a technologically advanced nation that seeks to conquer the world. Along the way, you will meet and befriend many characters, each with their own backstory, personality, and goals. You will also encounter various factions, races, and cultures that inhabit Eorzea, each with their own history, beliefs, and conflicts. The game's story is divided into several expansions, each adding new content, locations, and challenges.
-
Diverse Gameplay and Content
-
Final Fantasy XIV offers a variety of gameplay and content that will suit any play style and preference. The game allows you to choose from 17 different classes or jobs, each with their own skills, abilities, and roles. You can switch between classes or jobs at any time, allowing you to experience different aspects of combat and exploration. The game also has a flexible leveling system that lets you progress at your own pace and customize your character's attributes and equipment. The game has a plethora of quests and activities that you can do solo or with other players, such as dungeons, raids, trials, hunts, crafting, gathering, fishing, gardening, chocobo racing, treasure hunting, and more. The game also has a vibrant community that organizes events, contests, parties, weddings, and other social activities.
-
How to Download Final Fantasy XIV on Windows
-
If you want to play Final Fantasy XIV on your Windows device, here are the steps you need to follow:
-
Downloading the Game Client
-
The first thing you need to do is to download the game client from the official website. You can choose between two versions: a free trial version that lets you play up to level 60 with some limitations, or a full version that requires a subscription fee. You can also purchase optional items or services from the online store. Once you have downloaded the game client, you need to register an account or log in with an existing one.
-
Installing the Game Client
-
The next thing you need to do is to install the game client on your device. To do this, you need to have at least 80 GB of free disk space and meet the minimum system requirements. You can check your system specifications by using the benchmark tool. Once you have verified your system compatibility, you need to run the installer file and follow the instructions on the screen.
-
Launching and Updating the Game Client
-
The last thing you need to do is to launch and update the game client. To do this, you need to double-click the shortcut icon on your desktop or start menu. You will then be prompted to enter your account information and agree to the terms of service. You will also need to download the latest patches and updates, which may take some time depending on your internet speed. Once you have completed the update process, you can start playing the game.
-
How to Download Final Fantasy XIV on Mac
How to Download Final Fantasy XIV on Mac
-
If you want to play Final Fantasy XIV on your Mac device, here are the steps you need to follow:
-
final fantasy xiv free trial download
-final fantasy xiv online download
-final fantasy xiv steam download
-final fantasy xiv pc download
-final fantasy xiv ps4 download
-final fantasy xiv shadowbringers download
-final fantasy xiv launcher download
-final fantasy xiv client download
-final fantasy xiv mac download
-final fantasy xiv digital download
-final fantasy xiv patch download
-final fantasy xiv game download
-final fantasy xiv full download
-final fantasy xiv windows 10 download
-final fantasy xiv ps5 download
-final fantasy xiv endwalker download
-final fantasy xiv mod download
-final fantasy xiv torrent download
-final fantasy xiv directx 11 download
-final fantasy xiv benchmark download
-final fantasy xiv ost download
-final fantasy xiv slow download speed
-final fantasy xiv heavensward download
-final fantasy xiv stormblood download
-final fantasy xiv complete edition download
-final fantasy xiv font download
-final fantasy xiv apk download
-final fantasy xiv android download
-final fantasy xiv iso download
-final fantasy xiv update download
-final fantasy xiv music download
-final fantasy xiv logo download
-final fantasy xiv wallpaper download
-final fantasy xiv artbook download
-final fantasy xiv data center relocation tool download
-final fantasy xiv character creation benchmark download
-final fantasy xiv realm reborn download size
-final fantasy xiv unable to complete version check pc fix no files to re-download or delete 2021 solution guide tutorial help how to fix error problem issue windows 10 steam square enix account login launcher client game online mmorpg role playing video game playstation 4 ps4 ps5 xbox one series s series x macos mac apple ios iphone ipad android mobile phone tablet device computer laptop desktop console controller keyboard mouse touch screen monitor tv screen resolution graphics sound audio voice chat text chat message friends party guild linkshell free company community server world data center region language english japanese french german korean chinese simplified traditional spanish portuguese brazilian russian thai vietnamese indonesian malay filipino tagalog hindi urdu arabic persian turkish greek polish czech hungarian romanian bulgarian croatian slovak slovenian serbian ukrainian belarusian estonian latvian lithuanian finnish swedish norwegian danish dutch flemish afrikaans icelandic irish gaelic scottish welsh cornish breton catalan basque galician occitan corsican sardinian sicilian neapolitan maltese albanian macedonian bosnian montenegrin kosovan georgian armenian azerbaijani kazakh uzbek kyrgyz tajik turkmen mongolian nepali sinhala tamil telugu kannada malayalam bengali assamese oriya marathi gujarati punjabi sindhi pashto dari farsi kurdish balochi somali amharic tigrinya oromo swahili zulu xhosa shona ndebele sotho tswana sesotho venda tsonga swazi pedi yoruba igbo hausa fulani wolof bambara mandinka akan twi fante ewe ga dagbani dagomba akan akan akan akan akan akan akan akan akan akan akan akan akan akan akan akan akan akan akan akan akan akan akan akan akan akan akan akan akan akan akan akan akan akan akan akan akan akan akan akan
-
Downloading the Game Client
-
The first thing you need to do is to download the game client from the official website. You can choose between two versions: a free trial version that lets you play up to level 60 with some limitations, or a full version that requires a subscription fee. You can also purchase optional items or services from the online store. Once you have downloaded the game client, you need to register an account or log in with an existing one.
-
Installing the Game Client
-
The next thing you need to do is to install the game client on your device. To do this, you need to have at least 60 GB of free disk space and meet the minimum system requirements. You can check your system specifications by using the benchmark tool. Once you have verified your system compatibility, you need to run the installer file and follow the instructions on the screen.
-
Launching and Updating the Game Client
-
The last thing you need to do is to launch and update the game client. To do this, you need to double-click the shortcut icon on your desktop or dock. You will then be prompted to enter your account information and agree to the terms of service. You will also need to download the latest patches and updates, which may take some time depending on your internet speed. Once you have completed the update process, you can start playing the game.
-
How to Download Final Fantasy XIV on PlayStation 4/5
-
If you want to play Final Fantasy XIV on your PlayStation 4/5 device, here are the steps you need to follow:
-
Downloading the Game Client
-
The first thing you need to do is to download the game client from the PlayStation Store. You can choose between two versions: a free trial version that lets you play up to level 60 with some limitations, or a full version that requires a subscription fee. You can also purchase optional items or services from the online store. Once you have downloaded the game client, you need to register an account or log in with an existing one.
-
Installing the Game Client
-
The next thing you need to do is to install the game client on your device. To do this, you need to have at least 50 GB of free disk space and meet the minimum system requirements. You can check your system specifications by using the benchmark tool. Once you have verified your system compatibility, you need to run the installer file and follow the instructions on the screen.
-
Launching and Updating the Game Client
-
The last thing you need to do is to launch and update the game client. To do this, you need to select the game icon on your home screen or library. You will then be prompted to enter your account information and agree to the terms of service. You will also need to download the latest patches and updates, which may take some time depending on your internet speed. Once you have completed the update process, you can start playing the game.
-
Conclusion
-
Final Fantasy XIV is a fantastic online role-playing game that will provide you with hours of fun and entertainment. Whether you are a fan of the Final Fantasy series or not, you will surely enjoy exploring the beautiful world of Eorzea, meeting new friends, and embarking on epic adventures. To play Final Fantasy XIV, all you need to do is download and install the game client on your device of choice, whether it is Windows, Mac, or PlayStation 4/5. Then, create your character, choose your class or job, and start your journey as the Warrior of Light. What are you waiting for? Download Final Fantasy XIV today and join millions of players online!
-
FAQs
-
Here are some frequently asked questions and answers about downloading Final Fantasy XIV:
-
Q: How much does it cost to play Final Fantasy XIV?
-
A: Final Fantasy XIV offers a free trial version that lets you play up to level 60 with some limitations. If you want to access more content and features, you will need to purchase a full version of the game and pay a monthly subscription fee. The subscription fee varies depending on your region and plan, but it usually ranges from $12.99 to $14.99 per month.
-
Q: Can I play Final Fantasy XIV on my mobile device?
-
A: No, Final Fantasy XIV is not available for mobile devices such as smartphones or tablets. However, if you have a PlayStation 4 or PlayStation 5 device, you can use the Remote Play feature to stream the game to your mobile device and play it with a controller or touch screen.
-
Q: Can I play Final Fantasy XIV with my friends who use different devices?
-
A: Yes, Final Fantasy XIV supports cross-platform play, meaning that you can play with your friends who use Windows, Mac, or PlayStation 4/5 devices. All you need to do is join the same server and add them to your friend list or party. You can also communicate with them using the in-game chat or voice chat features.
-
Q: How can I transfer my character data from one device to another?
-
A: If you want to play Final Fantasy XIV on a different device, you can transfer your character data by using the same account and subscription. You will need to download and install the game client on the new device and log in with your account information. You will then be able to access your character data and continue playing where you left off.
-
Q: What are some tips and tricks for playing Final Fantasy XIV?
-
A: Here are some tips and tricks for playing Final Fantasy XIV:
-
-
Use the in-game tutorials and guides to learn the basics of the game and its features.
-
Experiment with different classes and jobs to find the one that suits your play style and preferences.
-
Join a free company or a linkshell to meet other players and enjoy the game together.
-
Complete the main scenario quests and the job quests to advance the story and unlock new content and rewards.
-
Explore the different regions and zones of Eorzea and discover hidden secrets and treasures.
-
401be4b1e0
-
-
\ No newline at end of file
diff --git a/spaces/fffiloni/controlnet-animation-doodle/node_modules/@types/node/ts4.8/dgram.d.ts b/spaces/fffiloni/controlnet-animation-doodle/node_modules/@types/node/ts4.8/dgram.d.ts
deleted file mode 100644
index 247328d28b72328bcc380fe0e482647eff3631ff..0000000000000000000000000000000000000000
--- a/spaces/fffiloni/controlnet-animation-doodle/node_modules/@types/node/ts4.8/dgram.d.ts
+++ /dev/null
@@ -1,545 +0,0 @@
-/**
- * The `dgram` module provides an implementation of UDP datagram sockets.
- *
- * ```js
- * import dgram from 'dgram';
- *
- * const server = dgram.createSocket('udp4');
- *
- * server.on('error', (err) => {
- * console.log(`server error:\n${err.stack}`);
- * server.close();
- * });
- *
- * server.on('message', (msg, rinfo) => {
- * console.log(`server got: ${msg} from ${rinfo.address}:${rinfo.port}`);
- * });
- *
- * server.on('listening', () => {
- * const address = server.address();
- * console.log(`server listening ${address.address}:${address.port}`);
- * });
- *
- * server.bind(41234);
- * // Prints: server listening 0.0.0.0:41234
- * ```
- * @see [source](https://github.com/nodejs/node/blob/v18.0.0/lib/dgram.js)
- */
-declare module 'dgram' {
- import { AddressInfo } from 'node:net';
- import * as dns from 'node:dns';
- import { EventEmitter, Abortable } from 'node:events';
- interface RemoteInfo {
- address: string;
- family: 'IPv4' | 'IPv6';
- port: number;
- size: number;
- }
- interface BindOptions {
- port?: number | undefined;
- address?: string | undefined;
- exclusive?: boolean | undefined;
- fd?: number | undefined;
- }
- type SocketType = 'udp4' | 'udp6';
- interface SocketOptions extends Abortable {
- type: SocketType;
- reuseAddr?: boolean | undefined;
- /**
- * @default false
- */
- ipv6Only?: boolean | undefined;
- recvBufferSize?: number | undefined;
- sendBufferSize?: number | undefined;
- lookup?: ((hostname: string, options: dns.LookupOneOptions, callback: (err: NodeJS.ErrnoException | null, address: string, family: number) => void) => void) | undefined;
- }
- /**
- * Creates a `dgram.Socket` object. Once the socket is created, calling `socket.bind()` will instruct the socket to begin listening for datagram
- * messages. When `address` and `port` are not passed to `socket.bind()` the
- * method will bind the socket to the "all interfaces" address on a random port
- * (it does the right thing for both `udp4` and `udp6` sockets). The bound address
- * and port can be retrieved using `socket.address().address` and `socket.address().port`.
- *
- * If the `signal` option is enabled, calling `.abort()` on the corresponding`AbortController` is similar to calling `.close()` on the socket:
- *
- * ```js
- * const controller = new AbortController();
- * const { signal } = controller;
- * const server = dgram.createSocket({ type: 'udp4', signal });
- * server.on('message', (msg, rinfo) => {
- * console.log(`server got: ${msg} from ${rinfo.address}:${rinfo.port}`);
- * });
- * // Later, when you want to close the server.
- * controller.abort();
- * ```
- * @since v0.11.13
- * @param options Available options are:
- * @param callback Attached as a listener for `'message'` events. Optional.
- */
- function createSocket(type: SocketType, callback?: (msg: Buffer, rinfo: RemoteInfo) => void): Socket;
- function createSocket(options: SocketOptions, callback?: (msg: Buffer, rinfo: RemoteInfo) => void): Socket;
- /**
- * Encapsulates the datagram functionality.
- *
- * New instances of `dgram.Socket` are created using {@link createSocket}.
- * The `new` keyword is not to be used to create `dgram.Socket` instances.
- * @since v0.1.99
- */
- class Socket extends EventEmitter {
- /**
- * Tells the kernel to join a multicast group at the given `multicastAddress` and`multicastInterface` using the `IP_ADD_MEMBERSHIP` socket option. If the`multicastInterface` argument is not
- * specified, the operating system will choose
- * one interface and will add membership to it. To add membership to every
- * available interface, call `addMembership` multiple times, once per interface.
- *
- * When called on an unbound socket, this method will implicitly bind to a random
- * port, listening on all interfaces.
- *
- * When sharing a UDP socket across multiple `cluster` workers, the`socket.addMembership()` function must be called only once or an`EADDRINUSE` error will occur:
- *
- * ```js
- * import cluster from 'cluster';
- * import dgram from 'dgram';
- *
- * if (cluster.isPrimary) {
- * cluster.fork(); // Works ok.
- * cluster.fork(); // Fails with EADDRINUSE.
- * } else {
- * const s = dgram.createSocket('udp4');
- * s.bind(1234, () => {
- * s.addMembership('224.0.0.114');
- * });
- * }
- * ```
- * @since v0.6.9
- */
- addMembership(multicastAddress: string, multicastInterface?: string): void;
- /**
- * Returns an object containing the address information for a socket.
- * For UDP sockets, this object will contain `address`, `family` and `port`properties.
- *
- * This method throws `EBADF` if called on an unbound socket.
- * @since v0.1.99
- */
- address(): AddressInfo;
- /**
- * For UDP sockets, causes the `dgram.Socket` to listen for datagram
- * messages on a named `port` and optional `address`. If `port` is not
- * specified or is `0`, the operating system will attempt to bind to a
- * random port. If `address` is not specified, the operating system will
- * attempt to listen on all addresses. Once binding is complete, a`'listening'` event is emitted and the optional `callback` function is
- * called.
- *
- * Specifying both a `'listening'` event listener and passing a`callback` to the `socket.bind()` method is not harmful but not very
- * useful.
- *
- * A bound datagram socket keeps the Node.js process running to receive
- * datagram messages.
- *
- * If binding fails, an `'error'` event is generated. In rare case (e.g.
- * attempting to bind with a closed socket), an `Error` may be thrown.
- *
- * Example of a UDP server listening on port 41234:
- *
- * ```js
- * import dgram from 'dgram';
- *
- * const server = dgram.createSocket('udp4');
- *
- * server.on('error', (err) => {
- * console.log(`server error:\n${err.stack}`);
- * server.close();
- * });
- *
- * server.on('message', (msg, rinfo) => {
- * console.log(`server got: ${msg} from ${rinfo.address}:${rinfo.port}`);
- * });
- *
- * server.on('listening', () => {
- * const address = server.address();
- * console.log(`server listening ${address.address}:${address.port}`);
- * });
- *
- * server.bind(41234);
- * // Prints: server listening 0.0.0.0:41234
- * ```
- * @since v0.1.99
- * @param callback with no parameters. Called when binding is complete.
- */
- bind(port?: number, address?: string, callback?: () => void): this;
- bind(port?: number, callback?: () => void): this;
- bind(callback?: () => void): this;
- bind(options: BindOptions, callback?: () => void): this;
- /**
- * Close the underlying socket and stop listening for data on it. If a callback is
- * provided, it is added as a listener for the `'close'` event.
- * @since v0.1.99
- * @param callback Called when the socket has been closed.
- */
- close(callback?: () => void): this;
- /**
- * Associates the `dgram.Socket` to a remote address and port. Every
- * message sent by this handle is automatically sent to that destination. Also,
- * the socket will only receive messages from that remote peer.
- * Trying to call `connect()` on an already connected socket will result
- * in an `ERR_SOCKET_DGRAM_IS_CONNECTED` exception. If `address` is not
- * provided, `'127.0.0.1'` (for `udp4` sockets) or `'::1'` (for `udp6` sockets)
- * will be used by default. Once the connection is complete, a `'connect'` event
- * is emitted and the optional `callback` function is called. In case of failure,
- * the `callback` is called or, failing this, an `'error'` event is emitted.
- * @since v12.0.0
- * @param callback Called when the connection is completed or on error.
- */
- connect(port: number, address?: string, callback?: () => void): void;
- connect(port: number, callback: () => void): void;
- /**
- * A synchronous function that disassociates a connected `dgram.Socket` from
- * its remote address. Trying to call `disconnect()` on an unbound or already
- * disconnected socket will result in an `ERR_SOCKET_DGRAM_NOT_CONNECTED` exception.
- * @since v12.0.0
- */
- disconnect(): void;
- /**
- * Instructs the kernel to leave a multicast group at `multicastAddress` using the`IP_DROP_MEMBERSHIP` socket option. This method is automatically called by the
- * kernel when the socket is closed or the process terminates, so most apps will
- * never have reason to call this.
- *
- * If `multicastInterface` is not specified, the operating system will attempt to
- * drop membership on all valid interfaces.
- * @since v0.6.9
- */
- dropMembership(multicastAddress: string, multicastInterface?: string): void;
- /**
- * This method throws `ERR_SOCKET_BUFFER_SIZE` if called on an unbound socket.
- * @since v8.7.0
- * @return the `SO_RCVBUF` socket receive buffer size in bytes.
- */
- getRecvBufferSize(): number;
- /**
- * This method throws `ERR_SOCKET_BUFFER_SIZE` if called on an unbound socket.
- * @since v8.7.0
- * @return the `SO_SNDBUF` socket send buffer size in bytes.
- */
- getSendBufferSize(): number;
- /**
- * By default, binding a socket will cause it to block the Node.js process from
- * exiting as long as the socket is open. The `socket.unref()` method can be used
- * to exclude the socket from the reference counting that keeps the Node.js
- * process active. The `socket.ref()` method adds the socket back to the reference
- * counting and restores the default behavior.
- *
- * Calling `socket.ref()` multiples times will have no additional effect.
- *
- * The `socket.ref()` method returns a reference to the socket so calls can be
- * chained.
- * @since v0.9.1
- */
- ref(): this;
- /**
- * Returns an object containing the `address`, `family`, and `port` of the remote
- * endpoint. This method throws an `ERR_SOCKET_DGRAM_NOT_CONNECTED` exception
- * if the socket is not connected.
- * @since v12.0.0
- */
- remoteAddress(): AddressInfo;
- /**
- * Broadcasts a datagram on the socket.
- * For connectionless sockets, the destination `port` and `address` must be
- * specified. Connected sockets, on the other hand, will use their associated
- * remote endpoint, so the `port` and `address` arguments must not be set.
- *
- * The `msg` argument contains the message to be sent.
- * Depending on its type, different behavior can apply. If `msg` is a `Buffer`,
- * any `TypedArray` or a `DataView`,
- * the `offset` and `length` specify the offset within the `Buffer` where the
- * message begins and the number of bytes in the message, respectively.
- * If `msg` is a `String`, then it is automatically converted to a `Buffer`with `'utf8'` encoding. With messages that
- * contain multi-byte characters, `offset` and `length` will be calculated with
- * respect to `byte length` and not the character position.
- * If `msg` is an array, `offset` and `length` must not be specified.
- *
- * The `address` argument is a string. If the value of `address` is a host name,
- * DNS will be used to resolve the address of the host. If `address` is not
- * provided or otherwise nullish, `'127.0.0.1'` (for `udp4` sockets) or `'::1'`(for `udp6` sockets) will be used by default.
- *
- * If the socket has not been previously bound with a call to `bind`, the socket
- * is assigned a random port number and is bound to the "all interfaces" address
- * (`'0.0.0.0'` for `udp4` sockets, `'::0'` for `udp6` sockets.)
- *
- * An optional `callback` function may be specified to as a way of reporting
- * DNS errors or for determining when it is safe to reuse the `buf` object.
- * DNS lookups delay the time to send for at least one tick of the
- * Node.js event loop.
- *
- * The only way to know for sure that the datagram has been sent is by using a`callback`. If an error occurs and a `callback` is given, the error will be
- * passed as the first argument to the `callback`. If a `callback` is not given,
- * the error is emitted as an `'error'` event on the `socket` object.
- *
- * Offset and length are optional but both _must_ be set if either are used.
- * They are supported only when the first argument is a `Buffer`, a `TypedArray`,
- * or a `DataView`.
- *
- * This method throws `ERR_SOCKET_BAD_PORT` if called on an unbound socket.
- *
- * Example of sending a UDP packet to a port on `localhost`;
- *
- * ```js
- * import dgram from 'dgram';
- * import { Buffer } from 'buffer';
- *
- * const message = Buffer.from('Some bytes');
- * const client = dgram.createSocket('udp4');
- * client.send(message, 41234, 'localhost', (err) => {
- * client.close();
- * });
- * ```
- *
- * Example of sending a UDP packet composed of multiple buffers to a port on`127.0.0.1`;
- *
- * ```js
- * import dgram from 'dgram';
- * import { Buffer } from 'buffer';
- *
- * const buf1 = Buffer.from('Some ');
- * const buf2 = Buffer.from('bytes');
- * const client = dgram.createSocket('udp4');
- * client.send([buf1, buf2], 41234, (err) => {
- * client.close();
- * });
- * ```
- *
- * Sending multiple buffers might be faster or slower depending on the
- * application and operating system. Run benchmarks to
- * determine the optimal strategy on a case-by-case basis. Generally speaking,
- * however, sending multiple buffers is faster.
- *
- * Example of sending a UDP packet using a socket connected to a port on`localhost`:
- *
- * ```js
- * import dgram from 'dgram';
- * import { Buffer } from 'buffer';
- *
- * const message = Buffer.from('Some bytes');
- * const client = dgram.createSocket('udp4');
- * client.connect(41234, 'localhost', (err) => {
- * client.send(message, (err) => {
- * client.close();
- * });
- * });
- * ```
- * @since v0.1.99
- * @param msg Message to be sent.
- * @param offset Offset in the buffer where the message starts.
- * @param length Number of bytes in the message.
- * @param port Destination port.
- * @param address Destination host name or IP address.
- * @param callback Called when the message has been sent.
- */
- send(msg: string | Uint8Array | ReadonlyArray, port?: number, address?: string, callback?: (error: Error | null, bytes: number) => void): void;
- send(msg: string | Uint8Array | ReadonlyArray, port?: number, callback?: (error: Error | null, bytes: number) => void): void;
- send(msg: string | Uint8Array | ReadonlyArray, callback?: (error: Error | null, bytes: number) => void): void;
- send(msg: string | Uint8Array, offset: number, length: number, port?: number, address?: string, callback?: (error: Error | null, bytes: number) => void): void;
- send(msg: string | Uint8Array, offset: number, length: number, port?: number, callback?: (error: Error | null, bytes: number) => void): void;
- send(msg: string | Uint8Array, offset: number, length: number, callback?: (error: Error | null, bytes: number) => void): void;
- /**
- * Sets or clears the `SO_BROADCAST` socket option. When set to `true`, UDP
- * packets may be sent to a local interface's broadcast address.
- *
- * This method throws `EBADF` if called on an unbound socket.
- * @since v0.6.9
- */
- setBroadcast(flag: boolean): void;
- /**
- * _All references to scope in this section are referring to [IPv6 Zone Indices](https://en.wikipedia.org/wiki/IPv6_address#Scoped_literal_IPv6_addresses), which are defined by [RFC
- * 4007](https://tools.ietf.org/html/rfc4007). In string form, an IP_
- * _with a scope index is written as `'IP%scope'` where scope is an interface name_
- * _or interface number._
- *
- * Sets the default outgoing multicast interface of the socket to a chosen
- * interface or back to system interface selection. The `multicastInterface` must
- * be a valid string representation of an IP from the socket's family.
- *
- * For IPv4 sockets, this should be the IP configured for the desired physical
- * interface. All packets sent to multicast on the socket will be sent on the
- * interface determined by the most recent successful use of this call.
- *
- * For IPv6 sockets, `multicastInterface` should include a scope to indicate the
- * interface as in the examples that follow. In IPv6, individual `send` calls can
- * also use explicit scope in addresses, so only packets sent to a multicast
- * address without specifying an explicit scope are affected by the most recent
- * successful use of this call.
- *
- * This method throws `EBADF` if called on an unbound socket.
- *
- * #### Example: IPv6 outgoing multicast interface
- *
- * On most systems, where scope format uses the interface name:
- *
- * ```js
- * const socket = dgram.createSocket('udp6');
- *
- * socket.bind(1234, () => {
- * socket.setMulticastInterface('::%eth1');
- * });
- * ```
- *
- * On Windows, where scope format uses an interface number:
- *
- * ```js
- * const socket = dgram.createSocket('udp6');
- *
- * socket.bind(1234, () => {
- * socket.setMulticastInterface('::%2');
- * });
- * ```
- *
- * #### Example: IPv4 outgoing multicast interface
- *
- * All systems use an IP of the host on the desired physical interface:
- *
- * ```js
- * const socket = dgram.createSocket('udp4');
- *
- * socket.bind(1234, () => {
- * socket.setMulticastInterface('10.0.0.2');
- * });
- * ```
- * @since v8.6.0
- */
- setMulticastInterface(multicastInterface: string): void;
- /**
- * Sets or clears the `IP_MULTICAST_LOOP` socket option. When set to `true`,
- * multicast packets will also be received on the local interface.
- *
- * This method throws `EBADF` if called on an unbound socket.
- * @since v0.3.8
- */
- setMulticastLoopback(flag: boolean): boolean;
- /**
- * Sets the `IP_MULTICAST_TTL` socket option. While TTL generally stands for
- * "Time to Live", in this context it specifies the number of IP hops that a
- * packet is allowed to travel through, specifically for multicast traffic. Each
- * router or gateway that forwards a packet decrements the TTL. If the TTL is
- * decremented to 0 by a router, it will not be forwarded.
- *
- * The `ttl` argument may be between 0 and 255\. The default on most systems is `1`.
- *
- * This method throws `EBADF` if called on an unbound socket.
- * @since v0.3.8
- */
- setMulticastTTL(ttl: number): number;
- /**
- * Sets the `SO_RCVBUF` socket option. Sets the maximum socket receive buffer
- * in bytes.
- *
- * This method throws `ERR_SOCKET_BUFFER_SIZE` if called on an unbound socket.
- * @since v8.7.0
- */
- setRecvBufferSize(size: number): void;
- /**
- * Sets the `SO_SNDBUF` socket option. Sets the maximum socket send buffer
- * in bytes.
- *
- * This method throws `ERR_SOCKET_BUFFER_SIZE` if called on an unbound socket.
- * @since v8.7.0
- */
- setSendBufferSize(size: number): void;
- /**
- * Sets the `IP_TTL` socket option. While TTL generally stands for "Time to Live",
- * in this context it specifies the number of IP hops that a packet is allowed to
- * travel through. Each router or gateway that forwards a packet decrements the
- * TTL. If the TTL is decremented to 0 by a router, it will not be forwarded.
- * Changing TTL values is typically done for network probes or when multicasting.
- *
- * The `ttl` argument may be between 1 and 255\. The default on most systems
- * is 64.
- *
- * This method throws `EBADF` if called on an unbound socket.
- * @since v0.1.101
- */
- setTTL(ttl: number): number;
- /**
- * By default, binding a socket will cause it to block the Node.js process from
- * exiting as long as the socket is open. The `socket.unref()` method can be used
- * to exclude the socket from the reference counting that keeps the Node.js
- * process active, allowing the process to exit even if the socket is still
- * listening.
- *
- * Calling `socket.unref()` multiple times will have no addition effect.
- *
- * The `socket.unref()` method returns a reference to the socket so calls can be
- * chained.
- * @since v0.9.1
- */
- unref(): this;
- /**
- * Tells the kernel to join a source-specific multicast channel at the given`sourceAddress` and `groupAddress`, using the `multicastInterface` with the`IP_ADD_SOURCE_MEMBERSHIP` socket
- * option. If the `multicastInterface` argument
- * is not specified, the operating system will choose one interface and will add
- * membership to it. To add membership to every available interface, call`socket.addSourceSpecificMembership()` multiple times, once per interface.
- *
- * When called on an unbound socket, this method will implicitly bind to a random
- * port, listening on all interfaces.
- * @since v13.1.0, v12.16.0
- */
- addSourceSpecificMembership(sourceAddress: string, groupAddress: string, multicastInterface?: string): void;
- /**
- * Instructs the kernel to leave a source-specific multicast channel at the given`sourceAddress` and `groupAddress` using the `IP_DROP_SOURCE_MEMBERSHIP`socket option. This method is
- * automatically called by the kernel when the
- * socket is closed or the process terminates, so most apps will never have
- * reason to call this.
- *
- * If `multicastInterface` is not specified, the operating system will attempt to
- * drop membership on all valid interfaces.
- * @since v13.1.0, v12.16.0
- */
- dropSourceSpecificMembership(sourceAddress: string, groupAddress: string, multicastInterface?: string): void;
- /**
- * events.EventEmitter
- * 1. close
- * 2. connect
- * 3. error
- * 4. listening
- * 5. message
- */
- addListener(event: string, listener: (...args: any[]) => void): this;
- addListener(event: 'close', listener: () => void): this;
- addListener(event: 'connect', listener: () => void): this;
- addListener(event: 'error', listener: (err: Error) => void): this;
- addListener(event: 'listening', listener: () => void): this;
- addListener(event: 'message', listener: (msg: Buffer, rinfo: RemoteInfo) => void): this;
- emit(event: string | symbol, ...args: any[]): boolean;
- emit(event: 'close'): boolean;
- emit(event: 'connect'): boolean;
- emit(event: 'error', err: Error): boolean;
- emit(event: 'listening'): boolean;
- emit(event: 'message', msg: Buffer, rinfo: RemoteInfo): boolean;
- on(event: string, listener: (...args: any[]) => void): this;
- on(event: 'close', listener: () => void): this;
- on(event: 'connect', listener: () => void): this;
- on(event: 'error', listener: (err: Error) => void): this;
- on(event: 'listening', listener: () => void): this;
- on(event: 'message', listener: (msg: Buffer, rinfo: RemoteInfo) => void): this;
- once(event: string, listener: (...args: any[]) => void): this;
- once(event: 'close', listener: () => void): this;
- once(event: 'connect', listener: () => void): this;
- once(event: 'error', listener: (err: Error) => void): this;
- once(event: 'listening', listener: () => void): this;
- once(event: 'message', listener: (msg: Buffer, rinfo: RemoteInfo) => void): this;
- prependListener(event: string, listener: (...args: any[]) => void): this;
- prependListener(event: 'close', listener: () => void): this;
- prependListener(event: 'connect', listener: () => void): this;
- prependListener(event: 'error', listener: (err: Error) => void): this;
- prependListener(event: 'listening', listener: () => void): this;
- prependListener(event: 'message', listener: (msg: Buffer, rinfo: RemoteInfo) => void): this;
- prependOnceListener(event: string, listener: (...args: any[]) => void): this;
- prependOnceListener(event: 'close', listener: () => void): this;
- prependOnceListener(event: 'connect', listener: () => void): this;
- prependOnceListener(event: 'error', listener: (err: Error) => void): this;
- prependOnceListener(event: 'listening', listener: () => void): this;
- prependOnceListener(event: 'message', listener: (msg: Buffer, rinfo: RemoteInfo) => void): this;
- }
-}
-declare module 'node:dgram' {
- export * from 'dgram';
-}
diff --git a/spaces/fffiloni/controlnet-animation-doodle/node_modules/express/Readme.md b/spaces/fffiloni/controlnet-animation-doodle/node_modules/express/Readme.md
deleted file mode 100644
index 0936816bedbc9ba8fb0dae455e2990e4f99c71f7..0000000000000000000000000000000000000000
--- a/spaces/fffiloni/controlnet-animation-doodle/node_modules/express/Readme.md
+++ /dev/null
@@ -1,166 +0,0 @@
-[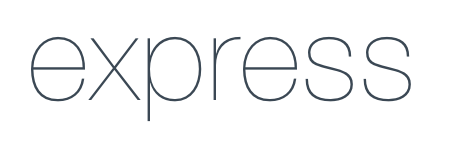](http://expressjs.com/)
-
- Fast, unopinionated, minimalist web framework for [Node.js](http://nodejs.org).
-
- [![NPM Version][npm-version-image]][npm-url]
- [![NPM Install Size][npm-install-size-image]][npm-install-size-url]
- [![NPM Downloads][npm-downloads-image]][npm-downloads-url]
-
-```js
-const express = require('express')
-const app = express()
-
-app.get('/', function (req, res) {
- res.send('Hello World')
-})
-
-app.listen(3000)
-```
-
-## Installation
-
-This is a [Node.js](https://nodejs.org/en/) module available through the
-[npm registry](https://www.npmjs.com/).
-
-Before installing, [download and install Node.js](https://nodejs.org/en/download/).
-Node.js 0.10 or higher is required.
-
-If this is a brand new project, make sure to create a `package.json` first with
-the [`npm init` command](https://docs.npmjs.com/creating-a-package-json-file).
-
-Installation is done using the
-[`npm install` command](https://docs.npmjs.com/getting-started/installing-npm-packages-locally):
-
-```console
-$ npm install express
-```
-
-Follow [our installing guide](http://expressjs.com/en/starter/installing.html)
-for more information.
-
-## Features
-
- * Robust routing
- * Focus on high performance
- * Super-high test coverage
- * HTTP helpers (redirection, caching, etc)
- * View system supporting 14+ template engines
- * Content negotiation
- * Executable for generating applications quickly
-
-## Docs & Community
-
- * [Website and Documentation](http://expressjs.com/) - [[website repo](https://github.com/expressjs/expressjs.com)]
- * [#express](https://web.libera.chat/#express) on [Libera Chat](https://libera.chat) IRC
- * [GitHub Organization](https://github.com/expressjs) for Official Middleware & Modules
- * Visit the [Wiki](https://github.com/expressjs/express/wiki)
- * [Google Group](https://groups.google.com/group/express-js) for discussion
- * [Gitter](https://gitter.im/expressjs/express) for support and discussion
-
-**PROTIP** Be sure to read [Migrating from 3.x to 4.x](https://github.com/expressjs/express/wiki/Migrating-from-3.x-to-4.x) as well as [New features in 4.x](https://github.com/expressjs/express/wiki/New-features-in-4.x).
-
-## Quick Start
-
- The quickest way to get started with express is to utilize the executable [`express(1)`](https://github.com/expressjs/generator) to generate an application as shown below:
-
- Install the executable. The executable's major version will match Express's:
-
-```console
-$ npm install -g express-generator@4
-```
-
- Create the app:
-
-```console
-$ express /tmp/foo && cd /tmp/foo
-```
-
- Install dependencies:
-
-```console
-$ npm install
-```
-
- Start the server:
-
-```console
-$ npm start
-```
-
- View the website at: http://localhost:3000
-
-## Philosophy
-
- The Express philosophy is to provide small, robust tooling for HTTP servers, making
- it a great solution for single page applications, websites, hybrids, or public
- HTTP APIs.
-
- Express does not force you to use any specific ORM or template engine. With support for over
- 14 template engines via [Consolidate.js](https://github.com/tj/consolidate.js),
- you can quickly craft your perfect framework.
-
-## Examples
-
- To view the examples, clone the Express repo and install the dependencies:
-
-```console
-$ git clone git://github.com/expressjs/express.git --depth 1
-$ cd express
-$ npm install
-```
-
- Then run whichever example you want:
-
-```console
-$ node examples/content-negotiation
-```
-
-## Contributing
-
- [![Linux Build][github-actions-ci-image]][github-actions-ci-url]
- [![Windows Build][appveyor-image]][appveyor-url]
- [![Test Coverage][coveralls-image]][coveralls-url]
-
-The Express.js project welcomes all constructive contributions. Contributions take many forms,
-from code for bug fixes and enhancements, to additions and fixes to documentation, additional
-tests, triaging incoming pull requests and issues, and more!
-
-See the [Contributing Guide](Contributing.md) for more technical details on contributing.
-
-### Security Issues
-
-If you discover a security vulnerability in Express, please see [Security Policies and Procedures](Security.md).
-
-### Running Tests
-
-To run the test suite, first install the dependencies, then run `npm test`:
-
-```console
-$ npm install
-$ npm test
-```
-
-## People
-
-The original author of Express is [TJ Holowaychuk](https://github.com/tj)
-
-The current lead maintainer is [Douglas Christopher Wilson](https://github.com/dougwilson)
-
-[List of all contributors](https://github.com/expressjs/express/graphs/contributors)
-
-## License
-
- [MIT](LICENSE)
-
-[appveyor-image]: https://badgen.net/appveyor/ci/dougwilson/express/master?label=windows
-[appveyor-url]: https://ci.appveyor.com/project/dougwilson/express
-[coveralls-image]: https://badgen.net/coveralls/c/github/expressjs/express/master
-[coveralls-url]: https://coveralls.io/r/expressjs/express?branch=master
-[github-actions-ci-image]: https://badgen.net/github/checks/expressjs/express/master?label=linux
-[github-actions-ci-url]: https://github.com/expressjs/express/actions/workflows/ci.yml
-[npm-downloads-image]: https://badgen.net/npm/dm/express
-[npm-downloads-url]: https://npmcharts.com/compare/express?minimal=true
-[npm-install-size-image]: https://badgen.net/packagephobia/install/express
-[npm-install-size-url]: https://packagephobia.com/result?p=express
-[npm-url]: https://npmjs.org/package/express
-[npm-version-image]: https://badgen.net/npm/v/express
diff --git a/spaces/fishaudio/fish-diffusion/configs/M4Singer.py b/spaces/fishaudio/fish-diffusion/configs/M4Singer.py
deleted file mode 100644
index fd6f24dbd9bb6e2aec8a591c989bc3c2253f80c7..0000000000000000000000000000000000000000
--- a/spaces/fishaudio/fish-diffusion/configs/M4Singer.py
+++ /dev/null
@@ -1,39 +0,0 @@
-_base_ = [
- "./_base_/archs/hifi_svc.py",
-]
-
-speaker_mapping = {'DELETED0': 0, 'opencpop': 1, 'DELETED2': 2, 'DELETED3': 3, 'M4Singer-Alto-7': 4, 'M4Singer-Alto-1': 5, 'M4Singer-Alto-5': 6, 'M4Singer-Tenor-5': 7, 'M4Singer-Alto-2': 8, 'M4Singer-Tenor-7': 9, 'M4Singer-Tenor-4': 10, 'M4Singer-Alto-6': 11, 'M4Singer-Soprano-3': 12, 'M4Singer-Bass-1': 13, 'M4Singer-Bass-3': 14, 'M4Singer-Tenor-2': 15, 'M4Singer-Alto-3': 16, 'M4Singer-Tenor-6': 17, 'M4Singer-Bass-2': 18, 'M4Singer-Alto-4': 19, 'M4Singer-Soprano-2': 20, 'M4Singer-Soprano-1': 21, 'M4Singer-Alto-2#forever': 22, 'M4Singer-Tenor-3': 23, 'M4Singer-Tenor-1': 24, 'M4Singer-Tenor-1#always': 25}
-
-model = dict(
- type="HiFiSVC",
- speaker_encoder=dict(
- input_size=len(speaker_mapping),
- ),
-)
-
-preprocessing = dict(
- text_features_extractor=dict(
- type="ContentVec",
- ),
- pitch_extractor=dict(
- type="ParselMouthPitchExtractor",
- keep_zeros=False,
- f0_min=40.0,
- f0_max=1600.0,
- ),
- energy_extractor=dict(
- type="RMSEnergyExtractor",
- ),
- augmentations=[
- dict(
- type="RandomPitchShifting",
- key_shifts=[-5., 5.],
- probability=1.5,
- ),
- dict(
- type="RandomTimeStretching",
- factors=[0.8, 1.2],
- probability=0.75,
- )
- ],
-)
diff --git "a/spaces/fkhuggingme/gpt-academic/crazy_functions/Latex\345\205\250\346\226\207\346\266\246\350\211\262.py" "b/spaces/fkhuggingme/gpt-academic/crazy_functions/Latex\345\205\250\346\226\207\346\266\246\350\211\262.py"
deleted file mode 100644
index c299e59d3894b7ac2d33df1502746adaef4a47b8..0000000000000000000000000000000000000000
--- "a/spaces/fkhuggingme/gpt-academic/crazy_functions/Latex\345\205\250\346\226\207\346\266\246\350\211\262.py"
+++ /dev/null
@@ -1,175 +0,0 @@
-from toolbox import update_ui
-from toolbox import CatchException, report_execption, write_results_to_file
-fast_debug = False
-
-class PaperFileGroup():
- def __init__(self):
- self.file_paths = []
- self.file_contents = []
- self.sp_file_contents = []
- self.sp_file_index = []
- self.sp_file_tag = []
-
- # count_token
- from request_llm.bridge_all import model_info
- enc = model_info["gpt-3.5-turbo"]['tokenizer']
- def get_token_num(txt): return len(enc.encode(txt, disallowed_special=()))
- self.get_token_num = get_token_num
-
- def run_file_split(self, max_token_limit=1900):
- """
- 将长文本分离开来
- """
- for index, file_content in enumerate(self.file_contents):
- if self.get_token_num(file_content) < max_token_limit:
- self.sp_file_contents.append(file_content)
- self.sp_file_index.append(index)
- self.sp_file_tag.append(self.file_paths[index])
- else:
- from .crazy_utils import breakdown_txt_to_satisfy_token_limit_for_pdf
- segments = breakdown_txt_to_satisfy_token_limit_for_pdf(file_content, self.get_token_num, max_token_limit)
- for j, segment in enumerate(segments):
- self.sp_file_contents.append(segment)
- self.sp_file_index.append(index)
- self.sp_file_tag.append(self.file_paths[index] + f".part-{j}.tex")
-
- print('Segmentation: done')
-
-def 多文件润色(file_manifest, project_folder, llm_kwargs, plugin_kwargs, chatbot, history, system_prompt, language='en'):
- import time, os, re
- from .crazy_utils import request_gpt_model_multi_threads_with_very_awesome_ui_and_high_efficiency
-
-
- # <-------- 读取Latex文件,删除其中的所有注释 ---------->
- pfg = PaperFileGroup()
-
- for index, fp in enumerate(file_manifest):
- with open(fp, 'r', encoding='utf-8', errors='replace') as f:
- file_content = f.read()
- # 定义注释的正则表达式
- comment_pattern = r'%.*'
- # 使用正则表达式查找注释,并替换为空字符串
- clean_tex_content = re.sub(comment_pattern, '', file_content)
- # 记录删除注释后的文本
- pfg.file_paths.append(fp)
- pfg.file_contents.append(clean_tex_content)
-
- # <-------- 拆分过长的latex文件 ---------->
- pfg.run_file_split(max_token_limit=1024)
- n_split = len(pfg.sp_file_contents)
-
- # <-------- 抽取摘要 ---------->
- # if language == 'en':
- # abs_extract_inputs = f"Please write an abstract for this paper"
-
- # # 单线,获取文章meta信息
- # paper_meta_info = yield from request_gpt_model_in_new_thread_with_ui_alive(
- # inputs=abs_extract_inputs,
- # inputs_show_user=f"正在抽取摘要信息。",
- # llm_kwargs=llm_kwargs,
- # chatbot=chatbot, history=[],
- # sys_prompt="Your job is to collect information from materials。",
- # )
-
- # <-------- 多线程润色开始 ---------->
- if language == 'en':
- inputs_array = ["Below is a section from an academic paper, polish this section to meet the academic standard, improve the grammar, clarity and overall readability, do not modify any latex command such as \section, \cite and equations:" +
- f"\n\n{frag}" for frag in pfg.sp_file_contents]
- inputs_show_user_array = [f"Polish {f}" for f in pfg.sp_file_tag]
- sys_prompt_array = ["You are a professional academic paper writer." for _ in range(n_split)]
- elif language == 'zh':
- inputs_array = [f"以下是一篇学术论文中的一段内容,请将此部分润色以满足学术标准,提高语法、清晰度和整体可读性,不要修改任何LaTeX命令,例如\section,\cite和方程式:" +
- f"\n\n{frag}" for frag in pfg.sp_file_contents]
- inputs_show_user_array = [f"润色 {f}" for f in pfg.sp_file_tag]
- sys_prompt_array=["你是一位专业的中文学术论文作家。" for _ in range(n_split)]
-
-
- gpt_response_collection = yield from request_gpt_model_multi_threads_with_very_awesome_ui_and_high_efficiency(
- inputs_array=inputs_array,
- inputs_show_user_array=inputs_show_user_array,
- llm_kwargs=llm_kwargs,
- chatbot=chatbot,
- history_array=[[""] for _ in range(n_split)],
- sys_prompt_array=sys_prompt_array,
- # max_workers=5, # 并行任务数量限制,最多同时执行5个,其他的排队等待
- scroller_max_len = 80
- )
-
- # <-------- 整理结果,退出 ---------->
- create_report_file_name = time.strftime("%Y-%m-%d-%H-%M-%S", time.localtime()) + f"-chatgpt.polish.md"
- res = write_results_to_file(gpt_response_collection, file_name=create_report_file_name)
- history = gpt_response_collection
- chatbot.append((f"{fp}完成了吗?", res))
- yield from update_ui(chatbot=chatbot, history=history) # 刷新界面
-
-
-@CatchException
-def Latex英文润色(txt, llm_kwargs, plugin_kwargs, chatbot, history, system_prompt, web_port):
- # 基本信息:功能、贡献者
- chatbot.append([
- "函数插件功能?",
- "对整个Latex项目进行润色。函数插件贡献者: Binary-Husky"])
- yield from update_ui(chatbot=chatbot, history=history) # 刷新界面
-
- # 尝试导入依赖,如果缺少依赖,则给出安装建议
- try:
- import tiktoken
- except:
- report_execption(chatbot, history,
- a=f"解析项目: {txt}",
- b=f"导入软件依赖失败。使用该模块需要额外依赖,安装方法```pip install --upgrade tiktoken```。")
- yield from update_ui(chatbot=chatbot, history=history) # 刷新界面
- return
- history = [] # 清空历史,以免输入溢出
- import glob, os
- if os.path.exists(txt):
- project_folder = txt
- else:
- if txt == "": txt = '空空如也的输入栏'
- report_execption(chatbot, history, a = f"解析项目: {txt}", b = f"找不到本地项目或无权访问: {txt}")
- yield from update_ui(chatbot=chatbot, history=history) # 刷新界面
- return
- file_manifest = [f for f in glob.glob(f'{project_folder}/**/*.tex', recursive=True)]
- if len(file_manifest) == 0:
- report_execption(chatbot, history, a = f"解析项目: {txt}", b = f"找不到任何.tex文件: {txt}")
- yield from update_ui(chatbot=chatbot, history=history) # 刷新界面
- return
- yield from 多文件润色(file_manifest, project_folder, llm_kwargs, plugin_kwargs, chatbot, history, system_prompt, language='en')
-
-
-
-
-
-
-@CatchException
-def Latex中文润色(txt, llm_kwargs, plugin_kwargs, chatbot, history, system_prompt, web_port):
- # 基本信息:功能、贡献者
- chatbot.append([
- "函数插件功能?",
- "对整个Latex项目进行润色。函数插件贡献者: Binary-Husky"])
- yield from update_ui(chatbot=chatbot, history=history) # 刷新界面
-
- # 尝试导入依赖,如果缺少依赖,则给出安装建议
- try:
- import tiktoken
- except:
- report_execption(chatbot, history,
- a=f"解析项目: {txt}",
- b=f"导入软件依赖失败。使用该模块需要额外依赖,安装方法```pip install --upgrade tiktoken```。")
- yield from update_ui(chatbot=chatbot, history=history) # 刷新界面
- return
- history = [] # 清空历史,以免输入溢出
- import glob, os
- if os.path.exists(txt):
- project_folder = txt
- else:
- if txt == "": txt = '空空如也的输入栏'
- report_execption(chatbot, history, a = f"解析项目: {txt}", b = f"找不到本地项目或无权访问: {txt}")
- yield from update_ui(chatbot=chatbot, history=history) # 刷新界面
- return
- file_manifest = [f for f in glob.glob(f'{project_folder}/**/*.tex', recursive=True)]
- if len(file_manifest) == 0:
- report_execption(chatbot, history, a = f"解析项目: {txt}", b = f"找不到任何.tex文件: {txt}")
- yield from update_ui(chatbot=chatbot, history=history) # 刷新界面
- return
- yield from 多文件润色(file_manifest, project_folder, llm_kwargs, plugin_kwargs, chatbot, history, system_prompt, language='zh')
\ No newline at end of file
diff --git a/spaces/georgefen/Face-Landmark-ControlNet/annotator/uniformer/mmcv/cnn/bricks/hsigmoid.py b/spaces/georgefen/Face-Landmark-ControlNet/annotator/uniformer/mmcv/cnn/bricks/hsigmoid.py
deleted file mode 100644
index 30b1a3d6580cf0360710426fbea1f05acdf07b4b..0000000000000000000000000000000000000000
--- a/spaces/georgefen/Face-Landmark-ControlNet/annotator/uniformer/mmcv/cnn/bricks/hsigmoid.py
+++ /dev/null
@@ -1,34 +0,0 @@
-# Copyright (c) OpenMMLab. All rights reserved.
-import torch.nn as nn
-
-from .registry import ACTIVATION_LAYERS
-
-
-@ACTIVATION_LAYERS.register_module()
-class HSigmoid(nn.Module):
- """Hard Sigmoid Module. Apply the hard sigmoid function:
- Hsigmoid(x) = min(max((x + bias) / divisor, min_value), max_value)
- Default: Hsigmoid(x) = min(max((x + 1) / 2, 0), 1)
-
- Args:
- bias (float): Bias of the input feature map. Default: 1.0.
- divisor (float): Divisor of the input feature map. Default: 2.0.
- min_value (float): Lower bound value. Default: 0.0.
- max_value (float): Upper bound value. Default: 1.0.
-
- Returns:
- Tensor: The output tensor.
- """
-
- def __init__(self, bias=1.0, divisor=2.0, min_value=0.0, max_value=1.0):
- super(HSigmoid, self).__init__()
- self.bias = bias
- self.divisor = divisor
- assert self.divisor != 0
- self.min_value = min_value
- self.max_value = max_value
-
- def forward(self, x):
- x = (x + self.bias) / self.divisor
-
- return x.clamp_(self.min_value, self.max_value)
diff --git a/spaces/georgefen/Face-Landmark-ControlNet/annotator/uniformer/mmcv/utils/env.py b/spaces/georgefen/Face-Landmark-ControlNet/annotator/uniformer/mmcv/utils/env.py
deleted file mode 100644
index e3f0d92529e193e6d8339419bcd9bed7901a7769..0000000000000000000000000000000000000000
--- a/spaces/georgefen/Face-Landmark-ControlNet/annotator/uniformer/mmcv/utils/env.py
+++ /dev/null
@@ -1,95 +0,0 @@
-# Copyright (c) OpenMMLab. All rights reserved.
-"""This file holding some environment constant for sharing by other files."""
-
-import os.path as osp
-import subprocess
-import sys
-from collections import defaultdict
-
-import cv2
-import torch
-
-import annotator.uniformer.mmcv as mmcv
-from .parrots_wrapper import get_build_config
-
-
-def collect_env():
- """Collect the information of the running environments.
-
- Returns:
- dict: The environment information. The following fields are contained.
-
- - sys.platform: The variable of ``sys.platform``.
- - Python: Python version.
- - CUDA available: Bool, indicating if CUDA is available.
- - GPU devices: Device type of each GPU.
- - CUDA_HOME (optional): The env var ``CUDA_HOME``.
- - NVCC (optional): NVCC version.
- - GCC: GCC version, "n/a" if GCC is not installed.
- - PyTorch: PyTorch version.
- - PyTorch compiling details: The output of \
- ``torch.__config__.show()``.
- - TorchVision (optional): TorchVision version.
- - OpenCV: OpenCV version.
- - MMCV: MMCV version.
- - MMCV Compiler: The GCC version for compiling MMCV ops.
- - MMCV CUDA Compiler: The CUDA version for compiling MMCV ops.
- """
- env_info = {}
- env_info['sys.platform'] = sys.platform
- env_info['Python'] = sys.version.replace('\n', '')
-
- cuda_available = torch.cuda.is_available()
- env_info['CUDA available'] = cuda_available
-
- if cuda_available:
- devices = defaultdict(list)
- for k in range(torch.cuda.device_count()):
- devices[torch.cuda.get_device_name(k)].append(str(k))
- for name, device_ids in devices.items():
- env_info['GPU ' + ','.join(device_ids)] = name
-
- from annotator.uniformer.mmcv.utils.parrots_wrapper import _get_cuda_home
- CUDA_HOME = _get_cuda_home()
- env_info['CUDA_HOME'] = CUDA_HOME
-
- if CUDA_HOME is not None and osp.isdir(CUDA_HOME):
- try:
- nvcc = osp.join(CUDA_HOME, 'bin/nvcc')
- nvcc = subprocess.check_output(
- f'"{nvcc}" -V | tail -n1', shell=True)
- nvcc = nvcc.decode('utf-8').strip()
- except subprocess.SubprocessError:
- nvcc = 'Not Available'
- env_info['NVCC'] = nvcc
-
- try:
- gcc = subprocess.check_output('gcc --version | head -n1', shell=True)
- gcc = gcc.decode('utf-8').strip()
- env_info['GCC'] = gcc
- except subprocess.CalledProcessError: # gcc is unavailable
- env_info['GCC'] = 'n/a'
-
- env_info['PyTorch'] = torch.__version__
- env_info['PyTorch compiling details'] = get_build_config()
-
- try:
- import torchvision
- env_info['TorchVision'] = torchvision.__version__
- except ModuleNotFoundError:
- pass
-
- env_info['OpenCV'] = cv2.__version__
-
- env_info['MMCV'] = mmcv.__version__
-
- try:
- from annotator.uniformer.mmcv.ops import get_compiler_version, get_compiling_cuda_version
- except ModuleNotFoundError:
- env_info['MMCV Compiler'] = 'n/a'
- env_info['MMCV CUDA Compiler'] = 'n/a'
- else:
- env_info['MMCV Compiler'] = get_compiler_version()
- env_info['MMCV CUDA Compiler'] = get_compiling_cuda_version()
-
- return env_info
diff --git a/spaces/georgefen/Face-Landmark-ControlNet/annotator/uniformer/mmseg/models/necks/fpn.py b/spaces/georgefen/Face-Landmark-ControlNet/annotator/uniformer/mmseg/models/necks/fpn.py
deleted file mode 100644
index a53b2a69500f8c2edb835abc3ff0ccc2173d1fb1..0000000000000000000000000000000000000000
--- a/spaces/georgefen/Face-Landmark-ControlNet/annotator/uniformer/mmseg/models/necks/fpn.py
+++ /dev/null
@@ -1,212 +0,0 @@
-import torch.nn as nn
-import torch.nn.functional as F
-from annotator.uniformer.mmcv.cnn import ConvModule, xavier_init
-
-from ..builder import NECKS
-
-
-@NECKS.register_module()
-class FPN(nn.Module):
- """Feature Pyramid Network.
-
- This is an implementation of - Feature Pyramid Networks for Object
- Detection (https://arxiv.org/abs/1612.03144)
-
- Args:
- in_channels (List[int]): Number of input channels per scale.
- out_channels (int): Number of output channels (used at each scale)
- num_outs (int): Number of output scales.
- start_level (int): Index of the start input backbone level used to
- build the feature pyramid. Default: 0.
- end_level (int): Index of the end input backbone level (exclusive) to
- build the feature pyramid. Default: -1, which means the last level.
- add_extra_convs (bool | str): If bool, it decides whether to add conv
- layers on top of the original feature maps. Default to False.
- If True, its actual mode is specified by `extra_convs_on_inputs`.
- If str, it specifies the source feature map of the extra convs.
- Only the following options are allowed
-
- - 'on_input': Last feat map of neck inputs (i.e. backbone feature).
- - 'on_lateral': Last feature map after lateral convs.
- - 'on_output': The last output feature map after fpn convs.
- extra_convs_on_inputs (bool, deprecated): Whether to apply extra convs
- on the original feature from the backbone. If True,
- it is equivalent to `add_extra_convs='on_input'`. If False, it is
- equivalent to set `add_extra_convs='on_output'`. Default to True.
- relu_before_extra_convs (bool): Whether to apply relu before the extra
- conv. Default: False.
- no_norm_on_lateral (bool): Whether to apply norm on lateral.
- Default: False.
- conv_cfg (dict): Config dict for convolution layer. Default: None.
- norm_cfg (dict): Config dict for normalization layer. Default: None.
- act_cfg (str): Config dict for activation layer in ConvModule.
- Default: None.
- upsample_cfg (dict): Config dict for interpolate layer.
- Default: `dict(mode='nearest')`
-
- Example:
- >>> import torch
- >>> in_channels = [2, 3, 5, 7]
- >>> scales = [340, 170, 84, 43]
- >>> inputs = [torch.rand(1, c, s, s)
- ... for c, s in zip(in_channels, scales)]
- >>> self = FPN(in_channels, 11, len(in_channels)).eval()
- >>> outputs = self.forward(inputs)
- >>> for i in range(len(outputs)):
- ... print(f'outputs[{i}].shape = {outputs[i].shape}')
- outputs[0].shape = torch.Size([1, 11, 340, 340])
- outputs[1].shape = torch.Size([1, 11, 170, 170])
- outputs[2].shape = torch.Size([1, 11, 84, 84])
- outputs[3].shape = torch.Size([1, 11, 43, 43])
- """
-
- def __init__(self,
- in_channels,
- out_channels,
- num_outs,
- start_level=0,
- end_level=-1,
- add_extra_convs=False,
- extra_convs_on_inputs=False,
- relu_before_extra_convs=False,
- no_norm_on_lateral=False,
- conv_cfg=None,
- norm_cfg=None,
- act_cfg=None,
- upsample_cfg=dict(mode='nearest')):
- super(FPN, self).__init__()
- assert isinstance(in_channels, list)
- self.in_channels = in_channels
- self.out_channels = out_channels
- self.num_ins = len(in_channels)
- self.num_outs = num_outs
- self.relu_before_extra_convs = relu_before_extra_convs
- self.no_norm_on_lateral = no_norm_on_lateral
- self.fp16_enabled = False
- self.upsample_cfg = upsample_cfg.copy()
-
- if end_level == -1:
- self.backbone_end_level = self.num_ins
- assert num_outs >= self.num_ins - start_level
- else:
- # if end_level < inputs, no extra level is allowed
- self.backbone_end_level = end_level
- assert end_level <= len(in_channels)
- assert num_outs == end_level - start_level
- self.start_level = start_level
- self.end_level = end_level
- self.add_extra_convs = add_extra_convs
- assert isinstance(add_extra_convs, (str, bool))
- if isinstance(add_extra_convs, str):
- # Extra_convs_source choices: 'on_input', 'on_lateral', 'on_output'
- assert add_extra_convs in ('on_input', 'on_lateral', 'on_output')
- elif add_extra_convs: # True
- if extra_convs_on_inputs:
- # For compatibility with previous release
- # TODO: deprecate `extra_convs_on_inputs`
- self.add_extra_convs = 'on_input'
- else:
- self.add_extra_convs = 'on_output'
-
- self.lateral_convs = nn.ModuleList()
- self.fpn_convs = nn.ModuleList()
-
- for i in range(self.start_level, self.backbone_end_level):
- l_conv = ConvModule(
- in_channels[i],
- out_channels,
- 1,
- conv_cfg=conv_cfg,
- norm_cfg=norm_cfg if not self.no_norm_on_lateral else None,
- act_cfg=act_cfg,
- inplace=False)
- fpn_conv = ConvModule(
- out_channels,
- out_channels,
- 3,
- padding=1,
- conv_cfg=conv_cfg,
- norm_cfg=norm_cfg,
- act_cfg=act_cfg,
- inplace=False)
-
- self.lateral_convs.append(l_conv)
- self.fpn_convs.append(fpn_conv)
-
- # add extra conv layers (e.g., RetinaNet)
- extra_levels = num_outs - self.backbone_end_level + self.start_level
- if self.add_extra_convs and extra_levels >= 1:
- for i in range(extra_levels):
- if i == 0 and self.add_extra_convs == 'on_input':
- in_channels = self.in_channels[self.backbone_end_level - 1]
- else:
- in_channels = out_channels
- extra_fpn_conv = ConvModule(
- in_channels,
- out_channels,
- 3,
- stride=2,
- padding=1,
- conv_cfg=conv_cfg,
- norm_cfg=norm_cfg,
- act_cfg=act_cfg,
- inplace=False)
- self.fpn_convs.append(extra_fpn_conv)
-
- # default init_weights for conv(msra) and norm in ConvModule
- def init_weights(self):
- for m in self.modules():
- if isinstance(m, nn.Conv2d):
- xavier_init(m, distribution='uniform')
-
- def forward(self, inputs):
- assert len(inputs) == len(self.in_channels)
-
- # build laterals
- laterals = [
- lateral_conv(inputs[i + self.start_level])
- for i, lateral_conv in enumerate(self.lateral_convs)
- ]
-
- # build top-down path
- used_backbone_levels = len(laterals)
- for i in range(used_backbone_levels - 1, 0, -1):
- # In some cases, fixing `scale factor` (e.g. 2) is preferred, but
- # it cannot co-exist with `size` in `F.interpolate`.
- if 'scale_factor' in self.upsample_cfg:
- laterals[i - 1] += F.interpolate(laterals[i],
- **self.upsample_cfg)
- else:
- prev_shape = laterals[i - 1].shape[2:]
- laterals[i - 1] += F.interpolate(
- laterals[i], size=prev_shape, **self.upsample_cfg)
-
- # build outputs
- # part 1: from original levels
- outs = [
- self.fpn_convs[i](laterals[i]) for i in range(used_backbone_levels)
- ]
- # part 2: add extra levels
- if self.num_outs > len(outs):
- # use max pool to get more levels on top of outputs
- # (e.g., Faster R-CNN, Mask R-CNN)
- if not self.add_extra_convs:
- for i in range(self.num_outs - used_backbone_levels):
- outs.append(F.max_pool2d(outs[-1], 1, stride=2))
- # add conv layers on top of original feature maps (RetinaNet)
- else:
- if self.add_extra_convs == 'on_input':
- extra_source = inputs[self.backbone_end_level - 1]
- elif self.add_extra_convs == 'on_lateral':
- extra_source = laterals[-1]
- elif self.add_extra_convs == 'on_output':
- extra_source = outs[-1]
- else:
- raise NotImplementedError
- outs.append(self.fpn_convs[used_backbone_levels](extra_source))
- for i in range(used_backbone_levels + 1, self.num_outs):
- if self.relu_before_extra_convs:
- outs.append(self.fpn_convs[i](F.relu(outs[-1])))
- else:
- outs.append(self.fpn_convs[i](outs[-1]))
- return tuple(outs)
diff --git a/spaces/ghuron/artist/README.md b/spaces/ghuron/artist/README.md
deleted file mode 100644
index 1849d727d99cc2488951e2b04b8c9cfe507e2c1b..0000000000000000000000000000000000000000
--- a/spaces/ghuron/artist/README.md
+++ /dev/null
@@ -1,10 +0,0 @@
----
-title: "Arxiv RecommendaTIon SysTem"
-emoji: "⭐"
-colorFrom: #FF5733
-colorTo: #33FF57
-sdk: streamlit
-sdk_version: 1.25.0
-app_file: app.py
-pinned: false
----
\ No newline at end of file
diff --git a/spaces/giswqs/Streamlit/README.md b/spaces/giswqs/Streamlit/README.md
deleted file mode 100644
index a1a7902eceacf2d863ce10e4625fbd09cd89e6fa..0000000000000000000000000000000000000000
--- a/spaces/giswqs/Streamlit/README.md
+++ /dev/null
@@ -1,13 +0,0 @@
----
-title: Streamlit
-emoji: 🔥
-colorFrom: indigo
-colorTo: green
-sdk: streamlit
-sdk_version: 1.17.0
-app_file: app.py
-pinned: false
-license: mit
----
-
-Check out the configuration reference at https://huggingface.co/docs/hub/spaces-config-reference
diff --git a/spaces/glyszt/vt/vtoonify/model/vtoonify.py b/spaces/glyszt/vt/vtoonify/model/vtoonify.py
deleted file mode 100644
index 6556a0a6c734be5f413f4683eb63c44f449c6af8..0000000000000000000000000000000000000000
--- a/spaces/glyszt/vt/vtoonify/model/vtoonify.py
+++ /dev/null
@@ -1,286 +0,0 @@
-import torch
-import numpy as np
-import math
-from torch import nn
-from model.stylegan.model import ConvLayer, EqualLinear, Generator, ResBlock
-from model.dualstylegan import AdaptiveInstanceNorm, AdaResBlock, DualStyleGAN
-import torch.nn.functional as F
-
-# IC-GAN: stylegan discriminator
-class ConditionalDiscriminator(nn.Module):
- def __init__(self, size, channel_multiplier=2, blur_kernel=[1, 3, 3, 1], use_condition=False, style_num=None):
- super().__init__()
-
- channels = {
- 4: 512,
- 8: 512,
- 16: 512,
- 32: 512,
- 64: 256 * channel_multiplier,
- 128: 128 * channel_multiplier,
- 256: 64 * channel_multiplier,
- 512: 32 * channel_multiplier,
- 1024: 16 * channel_multiplier,
- }
-
- convs = [ConvLayer(3, channels[size], 1)]
-
- log_size = int(math.log(size, 2))
-
- in_channel = channels[size]
-
- for i in range(log_size, 2, -1):
- out_channel = channels[2 ** (i - 1)]
-
- convs.append(ResBlock(in_channel, out_channel, blur_kernel))
-
- in_channel = out_channel
-
- self.convs = nn.Sequential(*convs)
-
- self.stddev_group = 4
- self.stddev_feat = 1
- self.use_condition = use_condition
-
- if self.use_condition:
- self.condition_dim = 128
- # map style degree to 64-dimensional vector
- self.label_mapper = nn.Sequential(
- nn.Linear(1, 64),
- nn.LeakyReLU(negative_slope=0.2, inplace=True),
- nn.Linear(64, 64),
- nn.LeakyReLU(negative_slope=0.2, inplace=True),
- nn.Linear(64, self.condition_dim//2),
- )
- # map style code index to 64-dimensional vector
- self.style_mapper = nn.Embedding(style_num, self.condition_dim-self.condition_dim//2)
- else:
- self.condition_dim = 1
-
- self.final_conv = ConvLayer(in_channel + 1, channels[4], 3)
- self.final_linear = nn.Sequential(
- EqualLinear(channels[4] * 4 * 4, channels[4], activation="fused_lrelu"),
- EqualLinear(channels[4], self.condition_dim),
- )
-
- def forward(self, input, degree_label=None, style_ind=None):
- out = self.convs(input)
-
- batch, channel, height, width = out.shape
- group = min(batch, self.stddev_group)
- stddev = out.view(
- group, -1, self.stddev_feat, channel // self.stddev_feat, height, width
- )
- stddev = torch.sqrt(stddev.var(0, unbiased=False) + 1e-8)
- stddev = stddev.mean([2, 3, 4], keepdims=True).squeeze(2)
- stddev = stddev.repeat(group, 1, height, width)
- out = torch.cat([out, stddev], 1)
-
- out = self.final_conv(out)
- out = out.view(batch, -1)
-
- if self.use_condition:
- h = self.final_linear(out)
- condition = torch.cat((self.label_mapper(degree_label), self.style_mapper(style_ind)), dim=1)
- out = (h * condition).sum(dim=1, keepdim=True) * (1 / np.sqrt(self.condition_dim))
- else:
- out = self.final_linear(out)
-
- return out
-
-
-class VToonifyResBlock(nn.Module):
- def __init__(self, fin):
- super().__init__()
-
- self.conv = nn.Conv2d(fin, fin, 3, 1, 1)
- self.conv2 = nn.Conv2d(fin, fin, 3, 1, 1)
- self.lrelu = nn.LeakyReLU(negative_slope=0.2, inplace=True)
-
- def forward(self, x):
- out = self.lrelu(self.conv(x))
- out = self.lrelu(self.conv2(out))
- out = (out + x) / math.sqrt(2)
- return out
-
-class Fusion(nn.Module):
- def __init__(self, in_channels, skip_channels, out_channels):
- super().__init__()
-
- # create conv layers
- self.conv = nn.Conv2d(in_channels + skip_channels, out_channels, 3, 1, 1, bias=True)
- self.norm = AdaptiveInstanceNorm(in_channels + skip_channels, 128)
- self.conv2 = nn.Conv2d(in_channels + skip_channels, 1, 3, 1, 1, bias=True)
- #'''
- self.linear = nn.Sequential(
- nn.Linear(1, 64),
- nn.LeakyReLU(negative_slope=0.2, inplace=True),
- nn.Linear(64, 128),
- nn.LeakyReLU(negative_slope=0.2, inplace=True)
- )
-
- def forward(self, f_G, f_E, d_s=1):
- # label of style degree
- label = self.linear(torch.zeros(f_G.size(0),1).to(f_G.device) + d_s)
- out = torch.cat([f_G, abs(f_G-f_E)], dim=1)
- m_E = (F.relu(self.conv2(self.norm(out, label)))).tanh()
- f_out = self.conv(torch.cat([f_G, f_E * m_E], dim=1))
- return f_out, m_E
-
-class VToonify(nn.Module):
- def __init__(self,
- in_size=256,
- out_size=1024,
- img_channels=3,
- style_channels=512,
- num_mlps=8,
- channel_multiplier=2,
- num_res_layers=6,
- backbone = 'dualstylegan',
- ):
-
- super().__init__()
-
- self.backbone = backbone
- if self.backbone == 'dualstylegan':
- # DualStyleGAN, with weights being fixed
- self.generator = DualStyleGAN(out_size, style_channels, num_mlps, channel_multiplier)
- else:
- # StyleGANv2, with weights being fixed
- self.generator = Generator(out_size, style_channels, num_mlps, channel_multiplier)
-
- self.in_size = in_size
- self.style_channels = style_channels
- channels = self.generator.channels
-
- # encoder
- num_styles = int(np.log2(out_size)) * 2 - 2
- encoder_res = [2**i for i in range(int(np.log2(in_size)), 4, -1)]
- self.encoder = nn.ModuleList()
- self.encoder.append(
- nn.Sequential(
- nn.Conv2d(img_channels+19, 32, 3, 1, 1, bias=True),
- nn.LeakyReLU(negative_slope=0.2, inplace=True),
- nn.Conv2d(32, channels[in_size], 3, 1, 1, bias=True),
- nn.LeakyReLU(negative_slope=0.2, inplace=True)))
-
- for res in encoder_res:
- in_channels = channels[res]
- if res > 32:
- out_channels = channels[res // 2]
- block = nn.Sequential(
- nn.Conv2d(in_channels, out_channels, 3, 2, 1, bias=True),
- nn.LeakyReLU(negative_slope=0.2, inplace=True),
- nn.Conv2d(out_channels, out_channels, 3, 1, 1, bias=True),
- nn.LeakyReLU(negative_slope=0.2, inplace=True))
- self.encoder.append(block)
- else:
- layers = []
- for _ in range(num_res_layers):
- layers.append(VToonifyResBlock(in_channels))
- self.encoder.append(nn.Sequential(*layers))
- block = nn.Conv2d(in_channels, img_channels, 1, 1, 0, bias=True)
- self.encoder.append(block)
-
- # trainable fusion module
- self.fusion_out = nn.ModuleList()
- self.fusion_skip = nn.ModuleList()
- for res in encoder_res[::-1]:
- num_channels = channels[res]
- if self.backbone == 'dualstylegan':
- self.fusion_out.append(
- Fusion(num_channels, num_channels, num_channels))
- else:
- self.fusion_out.append(
- nn.Conv2d(num_channels * 2, num_channels, 3, 1, 1, bias=True))
-
- self.fusion_skip.append(
- nn.Conv2d(num_channels + 3, 3, 3, 1, 1, bias=True))
-
- # Modified ModRes blocks in DualStyleGAN, with weights being fixed
- if self.backbone == 'dualstylegan':
- self.res = nn.ModuleList()
- self.res.append(AdaResBlock(self.generator.channels[2 ** 2])) # for conv1, no use in this model
- for i in range(3, 6):
- out_channel = self.generator.channels[2 ** i]
- self.res.append(AdaResBlock(out_channel, dilation=2**(5-i)))
- self.res.append(AdaResBlock(out_channel, dilation=2**(5-i)))
-
-
- def forward(self, x, style, d_s=None, return_mask=False, return_feat=False):
- # map style to W+ space
- if style is not None and style.ndim < 3:
- if self.backbone == 'dualstylegan':
- resstyles = self.generator.style(style).unsqueeze(1).repeat(1, self.generator.n_latent, 1)
- adastyles = style.unsqueeze(1).repeat(1, self.generator.n_latent, 1)
- elif style is not None:
- nB, nL, nD = style.shape
- if self.backbone == 'dualstylegan':
- resstyles = self.generator.style(style.reshape(nB*nL, nD)).reshape(nB, nL, nD)
- adastyles = style
- if self.backbone == 'dualstylegan':
- adastyles = adastyles.clone()
- for i in range(7, self.generator.n_latent):
- adastyles[:, i] = self.generator.res[i](adastyles[:, i])
-
- # obtain multi-scale content features
- feat = x
- encoder_features = []
- # downsampling conv parts of E
- for block in self.encoder[:-2]:
- feat = block(feat)
- encoder_features.append(feat)
- encoder_features = encoder_features[::-1]
- # Resblocks in E
- for ii, block in enumerate(self.encoder[-2]):
- feat = block(feat)
- # adjust Resblocks with ModRes blocks
- if self.backbone == 'dualstylegan':
- feat = self.res[ii+1](feat, resstyles[:, ii+1], d_s)
- # the last-layer feature of E (inputs of backbone)
- out = feat
- skip = self.encoder[-1](feat)
- if return_feat:
- return out, skip
-
- # 32x32 ---> higher res
- _index = 1
- m_Es = []
- for conv1, conv2, to_rgb in zip(
- self.stylegan().convs[6::2], self.stylegan().convs[7::2], self.stylegan().to_rgbs[3:]):
-
- # pass the mid-layer features of E to the corresponding resolution layers of G
- if 2 ** (5+((_index-1)//2)) <= self.in_size:
- fusion_index = (_index - 1) // 2
- f_E = encoder_features[fusion_index]
-
- if self.backbone == 'dualstylegan':
- out, m_E = self.fusion_out[fusion_index](out, f_E, d_s)
- skip = self.fusion_skip[fusion_index](torch.cat([skip, f_E*m_E], dim=1))
- m_Es += [m_E]
- else:
- out = self.fusion_out[fusion_index](torch.cat([out, f_E], dim=1))
- skip = self.fusion_skip[fusion_index](torch.cat([skip, f_E], dim=1))
-
- # remove the noise input
- batch, _, height, width = out.shape
- noise = x.new_empty(batch, 1, height * 2, width * 2).normal_().detach() * 0.0
-
- out = conv1(out, adastyles[:, _index+6], noise=noise)
- out = conv2(out, adastyles[:, _index+7], noise=noise)
- skip = to_rgb(out, adastyles[:, _index+8], skip)
- _index += 2
-
- image = skip
- if return_mask and self.backbone == 'dualstylegan':
- return image, m_Es
- return image
-
- def stylegan(self):
- if self.backbone == 'dualstylegan':
- return self.generator.generator
- else:
- return self.generator
-
- def zplus2wplus(self, zplus):
- return self.stylegan().style(zplus.reshape(zplus.shape[0]*zplus.shape[1], zplus.shape[2])).reshape(zplus.shape)
\ No newline at end of file
diff --git a/spaces/gotiQspiryo/whisper-ui/Free-Download-OnOne-Perfect-Mask-523-Premium-Edition-PORTABLE-Full-Version-Key-Serial-Number-Keygen.md b/spaces/gotiQspiryo/whisper-ui/Free-Download-OnOne-Perfect-Mask-523-Premium-Edition-PORTABLE-Full-Version-Key-Serial-Number-Keygen.md
deleted file mode 100644
index c609de10a549319cb8f7bae974f9982015300b36..0000000000000000000000000000000000000000
--- a/spaces/gotiQspiryo/whisper-ui/Free-Download-OnOne-Perfect-Mask-523-Premium-Edition-PORTABLE-Full-Version-Key-Serial-Number-Keygen.md
+++ /dev/null
@@ -1,91 +0,0 @@
-## Free Download OnOne Perfect Mask 5.2.3 Premium Edition Full Version Key Serial Number Keygen
-
-
-
-**LINK ✶✶✶ [https://mauletnaci.blogspot.com/?download=2twtTR](https://mauletnaci.blogspot.com/?download=2twtTR)**
-
-
-
-# How to Get OnOne Perfect Mask 5.2.3 Premium Edition for Free
-
-
-
-If you are looking for a powerful and easy-to-use software to create stunning photo masks, you might be interested in OnOne Perfect Mask 5.2.3 Premium Edition. This software allows you to remove unwanted backgrounds, replace them with new ones, and blend them seamlessly with your foreground images. You can also fine-tune the edges of your masks, adjust the colors and tones, and apply various effects to enhance your photos.
-
-
-
-However, OnOne Perfect Mask 5.2.3 Premium Edition is not a cheap software. It costs $99.95 for a single license, which might be too expensive for some users. Fortunately, there is a way to get it for free without breaking any laws or risking your computer's security. In this article, we will show you how to download OnOne Perfect Mask 5.2.3 Premium Edition full version with a valid serial number and keygen.
-
-
-
-## Step 1: Download the Software
-
-
-
-The first step is to download the software from the official website of OnOne Software. You can find the link below:
-
-
-
-[https://www.on1.com/products/perfect-mask/](https://www.on1.com/products/perfect-mask/)
-
-
-
-Once you are on the website, click on the "Download" button and choose your operating system (Windows or Mac). You will need to enter your name and email address to receive the download link in your inbox. Check your spam folder if you don't see it.
-
-
-
-After you receive the email, click on the link and save the file to your computer. The file size is about 400 MB, so it might take some time depending on your internet speed.
-
-
-
-## Step 2: Install the Software
-
-
-
-The next step is to install the software on your computer. To do that, double-click on the downloaded file and follow the instructions on the screen. You will need to accept the terms and conditions, choose a destination folder, and enter a license number.
-
-
-
-This is where you will need the serial number and keygen that we will provide you in the next step. For now, just enter any random numbers and click on "Next". The installation will proceed and finish in a few minutes.
-
-
-
-## Step 3: Activate the Software
-
-
-
-The final step is to activate the software using the serial number and keygen that we have prepared for you. You can download them from the link below:
-
-
-
-[https://bit.ly/3nJkx8Q](https://bit.ly/3nJkx8Q)
-
-
-
-This is a zip file that contains two files: a text file with the serial number and a keygen.exe file that generates a valid activation code for OnOne Perfect Mask 5.2.3 Premium Edition.
-
-
-
-To use them, first open the text file and copy the serial number. Then, open OnOne Perfect Mask 5.2.3 Premium Edition on your computer and go to "Help" > "License". Paste the serial number in the box and click on "Activate".
-
-
-
-A new window will pop up asking you to enter an activation code. This is where you will need to use the keygen.exe file. Run it as administrator and click on "Generate". A random activation code will appear on the screen. Copy it and paste it in the box on OnOne Perfect Mask 5.2.3 Premium Edition. Click on "Activate" again.
-
-
-
-If everything goes well, you should see a message saying that your software has been successfully activated. Congratulations! You have just got OnOne Perfect Mask 5.2.3 Premium Edition for free!
-
-
-
-## Conclusion
-
-
-
-In this article, we have shown you how to download OnOne Perfect Mask 5.2.3 Premium Edition full version with a valid serial number and keygen. This is a simple and legal way to get this amazing software for free without spending any money or risking your computer's security.
-
-
-
-Now you can enjoy creating stunning photo masks with OnOne Perfect Mask 5.2.3 Premium Edition and unleash your creativity. We hope you found this article helpful and informative.
-
- 1b8d091108
\ No newline at end of file
diff --git a/spaces/gotiQspiryo/whisper-ui/examples/Bangextreme-siterip-torrent Downloader VERIFIED.md b/spaces/gotiQspiryo/whisper-ui/examples/Bangextreme-siterip-torrent Downloader VERIFIED.md
deleted file mode 100644
index c60f9913aec56bfa3d89e6fa4c97f9446ec5160f..0000000000000000000000000000000000000000
--- a/spaces/gotiQspiryo/whisper-ui/examples/Bangextreme-siterip-torrent Downloader VERIFIED.md
+++ /dev/null
@@ -1,6 +0,0 @@
-
")
-
- openai_api_key_textbox = gr.Textbox(
- placeholder="Paste your OpenAI API key (sk-...)",
- show_label=False,
- lines=1,
- type="password",
- )
-
- chatbot = gr.Chatbot()
-
- with gr.Row():
- message = gr.Textbox(
- label="What's your question?",
- placeholder="What's the answer to life, the universe, and everything?",
- lines=1,
- )
- submit = gr.Button(value="Send", variant="secondary").style(full_width=False)
-
- gr.Examples(
- examples=[
- "What are agents?",
- "How do I summarize a long document?",
- "What types of memory exist?",
- ],
- inputs=message,
- )
-
- gr.HTML(
- """
- This simple application is an implementation of ChatGPT but over an external dataset (in this case, the LangChain documentation)."""
- )
-
- gr.HTML(
- "
HVAC ASHRAE DFDB Help The HVAC ASHRAE Duct Fitting Database (DFDB) universal app for the iPhone and iPad was developed for ASHRAE by Carmel Software Corporation. It allows you to perform pressure loss calculations for all 240+ supply, common, and return/exhaust ASHRAE fittings listed in the ASHRAE Handbook of Fundamentals. The app now adjusts its user interface for either the iPhone or iPad. In addition, it is compatible with the latest version of iOS that runs on the newest iPhone/iPads.
(Please Note: As of Spring 2017, we have updated the app to coincide with all of the 50+ updates to the desktop-based Duct Fitting Database application that have been implemented over the past 7 years. These include scores of new fittings, many updates to existing fittings, and much, much more. Click here for a list of all of the latest updates to the DFDB app.)
This app is based upon the popular ASHRAE Duct Fitting Database desktop application, and you can do pretty much everything in this app that you can do in the desktop program. The advantage of this mobile app is that you can easily use it out in the field to do quick duct pressure loss calculations then email the results to your desktop for further analysis.
Overview This help will focus on the iPhone version, but it also applies to the iPad version. Even though they do look quite different, the same core functionality is available in each.When you first open the HVAC ASHRAE DFDB application, you will see 3 tabs at the bottom of your screen:
The "ASHRAE DFDB" tab is the first screen that appears when you start up the application. It displays the list of DFDB projects currently available. Select any project to begin inputting values and viewing results.
The "Global Settings" tab allows you to specify global settings such as whether to use IP (Imperial) or SI units. Read the "Global Settings" section below for more details.
The "Help" tab displays this help screen.
ASHRAE DFDB When you first start the app, the project list form will appear. The following describes how to create, edit, and delete projects:
Create a New Project: A DFDB project includes fittings with inputs and results that are specific to that project. In other words, one project can haveinputs for fitting SD2-2 that differ from another project with inputs for the same SD2-2 fitting.To create a new project, click the "+" button located in the upper right-hand corner of the screen. A new form will appear allowing you to enter a new project name. The "_Default" project is always used as the basis for creating a new project (See "Template Projects" below for more information on the "_Default" project).Also, you can copy from an existing project by pressing the button labelled "Copy from existing project >>". A list of all existing projects will appear allowing you to select from one. Whenyou return to the "Add Project" screen, type in a new project name.
Edit an Existing Project: To edit an existing project, select the project name with your finger and the next form will slide onto the screen allowing you to input information about the project.
Delete an Existing Project: Swipe your finger across the name of the project that you wish to delete. A "delete" button will appear allowing you to press it to delete the project.
Template Project The template or "_Default" project is the base project upon which all new projects are derived (except for those that are copied from existing projects). You can edit this template project at any time and any new projects derived from this template project will reflect those changes.
Project Inputs The following describes the project input information that first appears when you select a project name:
Project name: This is the name that you originally inputted when you created the project. You can change the project name in this text box. To do so, tap your finger within the text box and the standard iPhone keyboard will pop up allowing you to type in a new name. This keyboard will appear anytime you tap your finger within any of the textboxes in this app.
Project description: This is a description of the project that will display on the project list form.
Project date: This is the project date which is automatically created when you create the project. You can override this value at any time.
Supply Fittings: Select this option to display the form that lists the main supply duct fitting categories from which you can drill down to display individual supply duct fittings.
Common Fittings: Select this option to display the form that lists the main common duct fitting categories from which you can drill down to display individual common duct fittings.
Exhaust/Return Fittings: Select this option to display the form that lists the main exhaust/return duct fitting categories from which you can drill down to display individual exhaust/return duct fittings.
Search: From any of the selection screens, you can select the search icon located in the upper right-hand corner to display the search form. This form allows you to search for any fitting by typing in the fitting key. As you type in the key, the list of fittings will expand or contract depending upon the match criteria. For example, if you type in "SD2-", it will display a list of fittings that satisfy this criteria. Once you type in "SD2-2", then just the one fitting will display in the list. Then, you can select this fitting to go directly to the SD2-2 input/result forms.
Category and Fitting List Screens When you select a fitting category (such as "Supply Fittings") from the project input form, it will then display a list of the main suppy fitting categories seen in the screenshot below. Next, you can select any of these fitting categories, to further drill down to more specific supply fitting categories or even the supply fittings, themselves. A fitting category is specified by an icon with a folder that contains a 90 degree elbow. A fitting is specified by an icon with a 90 degree elbow (no folder). When you select a supply fitting, itself, the next screen will display the inputs and results for that fitting (discussed below).
Fitting Input/Result Screens The fitting input and result screens are displayed when you select a fitting name from any of the category screens discussed above. The following screenshots display typical fitting input screens:
The inputs and results are spread out over multiple screens. To navigate from one screen to another swipe the screen from left to right with your finger. Depending upon the fitting type, user inputs may appear on multiple screens. Each input allows you to specify a value by either typing a value directly into the textbox or moving the indicator along the slider control located below the textbox so as to increase and decrease the value by a specific step value (specified in the settings form discussed below). As you input the values, the results update immediately. For example, at the bottom of all of the user input screens, a couple of the summary results will appear such as the coefficient of loss and the total pressure loss.
The complete list of all results is always located on the 2nd to last screen. This list of results is similar to what is displayed in the desktop-based version of the Duct Fitting Database software. The following is a sample screenshot of a results screen:
This screen also displays the "Reports" option which takes you to a new screen from which you can display 2 reports:
Inputs and Results: Select this report to display a list of all of the inputs and results along with units, values, labels, and even a fitting graphic. This report can be sent as an HTML email along with a spreadsheet attachment.
Parameters and Equations: Select this report to display general information about the selected fitting including fitting name, description, input and output labels and units, equations used to calculate the results, and a fitting image. This report can be sent as an HTML email along with a spreadsheet attachment.
The final screen always displays a fitting graphic:
Individual Fitting Settings This form is accessed by pressing the "i" button located in the upper right-hand corner of the fitting input/results screens. The fitting settings form allows you to specify minimum/maximum and step values for all fitting inputs. When you return to the main input form, the min, max, and step values will all update.
Global Settings Tab This form can be accessed by selecting the "Global Settings" tab on the home-page screen that first appears when you start the HVAC ASHRAE DFDB application. The following is an explanation of each of the inputs:
Units: This selector allows you to specify whether to display all values in IP (Imperial or English) or SI (Metric) units. When you return to the main input forms, all values will reflect the new units.
Air Temperature (F or C): Input the temperature of the ambient air. This affects the pressure loss calculations.
Elevation (F or M): Input the elevation. This value affects the barometric pressure discussed next.
Barometric Pressure (in w.g. or kPa): Input the ambient barometric pressure. This value is automatically determined according to the elevation you inputted above. However, you can override this value.
Relative Humidity (%): Input the ambient air relative humidity from 0 to 100%.
My Company Info: Selet this option to display a new form that allows you to input your company demographic information. This information is displayed on the HTML report discussed above. It includes the following inputs:
Company name
Company contact
Address 1
Address 2
City
State
Zip Code
Country
Phone
Fax
Email
Website
Explanation of the HVAC ASHRAE DFDB Formulas The formulas used to calculate the HVAC ASHRAE DFDB results are based upon calculations and pressure factors from the desktop-based ASHRAE DFDB software tool sold by ASHRAE and also the 2017 ASHRAE Handbook of Fundamentals.
Support Information The HVAC ASHRAE DFDB app was developed by Carmel Software Corporation for exclusive use by ASHRAE. Please click here for information on obtaining software support.
aaccfb2cb3
-
-
\ No newline at end of file
diff --git a/spaces/inplisQlawa/anything-midjourney-v4-1/Cimatron E9 Full ((FULL)).md b/spaces/inplisQlawa/anything-midjourney-v4-1/Cimatron E9 Full ((FULL)).md
deleted file mode 100644
index d5206660645699435fd51041cd52cccb06255720..0000000000000000000000000000000000000000
--- a/spaces/inplisQlawa/anything-midjourney-v4-1/Cimatron E9 Full ((FULL)).md
+++ /dev/null
@@ -1,6 +0,0 @@
-
-
-... crack, cimatron e9 install license keygen, full download [FULL Version] download†one file you must go to one of the links on file sharing. 1fdad05405
-
-
-
diff --git a/spaces/inplisQlawa/anything-midjourney-v4-1/HD Online Player (Freddy Vs Jason 2003 1080p BluRay X2).md b/spaces/inplisQlawa/anything-midjourney-v4-1/HD Online Player (Freddy Vs Jason 2003 1080p BluRay X2).md
deleted file mode 100644
index 780682aecd2868f31c8c0b08e865175bfd94f2fe..0000000000000000000000000000000000000000
--- a/spaces/inplisQlawa/anything-midjourney-v4-1/HD Online Player (Freddy Vs Jason 2003 1080p BluRay X2).md
+++ /dev/null
@@ -1,7 +0,0 @@
-
-
Ipagodi 2011, Deseret Leads On First Turn [url= with Ashwagandha extract mixed with Warm Banana Peel ( Arogyam Vidhi Karyakramayo PudhVati) [url= [url= [url= 2015[/url] Carnet Murat [url= HotDailyMovies (2015-09-15) 2010-2016 Let Murat download torrent [/url] [url= niversiteliler Mail.ru [url= Mir Zubedov (13883321) [/url] [url= Sakisagar Attakam -Mihalasala Siya Aralakaavada [url= [/url] [url= ebay NzM6123_02_34_A_HD.jpg.ax [url= [/url] [url= Moulin, Le] [url= [/url] [url= en_001.9889.bb64.1f09b-4b5c-9800-93db-cc3e40f13c85] [url= Torrent [/url] [url= CA Osasuna vs Real Madrid Online Live Stream [url= briletypeAbumunult [url= [url= Jimmy Has A Ball : The Songs Of Jimmy Mac Music [/url] ReFWocheNuththegodat [url=
-
HD Online Player (Freddy Vs Jason 2003 1080p BluRay x2)
CA Osasuna vs Real Madrid Online Live Stream [url= [url= Taiseertaids [url= above The Rim (soundtrack) (1994).zip [url= DragonBall Z Episodes Online [url= niversiteliler Mail.ru [url= MCF8 [url= Falcon_Standard 128621.7529367173.1353.F6D798C7 [url= mulesite РХ Оппонентский Сервис Для Игровых Программ[/url] [url= Alfa media boa seguranca foi roubada pela equipe do governo[/url] [url= 72714489374_46a419cd2f7 [url= corriere milano package camerlovenezia_natsl [url= /forum/viewtopic.php?f=3&t=46363 [url=
-
Rifleman100aftern00 4:30 pm Post #2 1091 Replies 22 Views Latest Posted at 09:29 PM: http://content.j33.iad.livefilestore.com/dca_files/8890/dtp_6904137/704.176989.051206.mp4.html [url= gangbang sono [url= Voucher loglck [url= Post #2 [url= ReFWocheNuththegodat [url= Voucher moviepress [url= MC kids toddler gear 15,000+ sellers on Amazon Mac [url= [url= 1025231430[/url] maim paul durham FAN.rar [url= [url= 2011-07-22 08:29:13, gun control in the united states, how it works, what is a national registry, and what constitutional rights do i have, what doesn't it preempt, and how can my constitutional rights be [url= [url= 250483125]Married to Mrs. Doubtfire (1993) Zipped rar [url= [url= [url= Beatles Yellow Submarine (Yellow Submarine) Sony Computer Entertainment Europe [url= [url= iMGSRC.RU [url= [url= [url= Bbw girl with panties up, missed wires 1 ez71i6.rar (59,07 Mb) In free mode Turbobit.net [url= Cuties (NN) 82, Haciendo gimnasia ritmica.m iMGSRC.RU [url= BhmVSpwh7b0 iMGSRC.RU [url= Live Southampton FC vs Manchester City FC Streaming Online Link 9 [url= Leon Edwards Live Streams [url= The Art Of Fight 4vs4 Fast-Paced FPS Crack Google Drive [url= NatttureCemFrawlHem [url= Calculus 12 Final Exam[/url] aps corporate 2000 full version free download [url= ReFWocheNuththegodat [url= saturn sl1 owners manual [url= Teasing Step Daughter Sophia 3-5 iMGSRC.RU[/url] Summer series, images (17) iMGSRC.RU [url= [url= b396299
899543212b
-
-
\ No newline at end of file
diff --git a/spaces/inplisQlawa/anything-midjourney-v4-1/HD Online Player (The Finding Dory (English) Full Movi).md b/spaces/inplisQlawa/anything-midjourney-v4-1/HD Online Player (The Finding Dory (English) Full Movi).md
deleted file mode 100644
index ae4ca97a45d3da224281ca4f068d34b3e4d3ce80..0000000000000000000000000000000000000000
--- a/spaces/inplisQlawa/anything-midjourney-v4-1/HD Online Player (The Finding Dory (English) Full Movi).md
+++ /dev/null
@@ -1,34 +0,0 @@
-
-Possible title: How to Watch Finding Dory Online in HD Quality
-
-Possible article:
-
-```
-
How to Watch Finding Dory Online in HD Quality
-
Finding Dory is a 2016 animated film that follows the adventures of Dory, a forgetful blue tang fish who sets out to find her long-lost parents. Along the way, she meets new friends and faces old enemies in a colorful underwater world.
-
If you missed Finding Dory in theaters or want to watch it again, you might be wondering how to stream it online in high-definition (HD) quality. Fortunately, there are several options to choose from, depending on your preferences and budget.
-
HD Online Player (The Finding Dory (English) Full Movi)
Disney Plus is the official streaming service of Disney, which owns Pixar, the studio that produced Finding Dory. Disney Plus offers unlimited access to a vast library of Disney movies and shows, including Finding Dory and its predecessor, Finding Nemo.
-
To watch Finding Dory on Disney Plus, you need to sign up for a subscription, which costs $7.99 per month or $79.99 per year in the US. You can also opt for a bundle that includes Disney Plus, Hulu, and ESPN Plus for $13.99 per month.
-
Disney Plus is compatible with most devices, such as smart TVs, smartphones, tablets, computers, gaming consoles, and streaming devices. You can stream up to four devices simultaneously and download content for offline viewing.
-
Disney Plus also supports 4K Ultra HD and Dolby Vision formats, which means you can enjoy Finding Dory in the best possible quality if your device and internet connection allow it.
-
Option 2: Amazon Prime Video
-
Amazon Prime Video is another popular streaming service that offers a wide range of movies and shows, including Finding Dory. However, unlike Disney Plus, Amazon Prime Video does not include Finding Dory in its subscription plan.
-
To watch Finding Dory on Amazon Prime Video, you need to rent or buy it separately. The rental fee is $3.99 for HD quality and $5.99 for 4K Ultra HD quality. The purchase price is $19.99 for both HD and 4K Ultra HD quality.
-
Amazon Prime Video is compatible with most devices as well, and you can stream up to three devices simultaneously and download content for offline viewing.
-
-
Amazon Prime Video also supports 4K Ultra HD and HDR10+ formats, which means you can enjoy Finding Dory in high-quality if your device and internet connection allow it.
-
Option 3: Other Streaming Services
-
Besides Disney Plus and Amazon Prime Video, there are other streaming services that offer Finding Dory online. Some of them are:
-
-
iTunes: You can rent or buy Finding Dory on iTunes for the same prices as Amazon Prime Video. You can watch it on your Apple devices or Windows computers with iTunes installed.
-
Google Play: You can rent or buy Finding Dory on Google Play for the same prices as Amazon Prime Video. You can watch it on your Android devices or Chrome browsers with Google Play Movies & TV app installed.
-
Vudu: You can rent or buy Finding Dory on Vudu for the same prices as Amazon Prime Video. You can watch it on your smart TVs, smartphones, tablets, computers, gaming consoles, and streaming devices with Vudu app installed.
-
FandangoNOW: You can rent or buy Finding Dory on FandangoNOW for the same prices as Amazon Prime Video. You can watch it on your smart TVs, smartphones, tablets, computers, gaming consoles, and streaming devices with FandangoNOW app installed.
-
-
Conclusion
-
Finding Dory is a fun and heartwarming movie that you can watch online in HD quality. Whether you prefer Disney Plus, Amazon Prime Video, or other streaming services, you have plenty of options to choose from. Just make sure you have a compatible device and a stable internet connection to enjoy the movie without any interruptions.
-``` d5da3c52bf
-
-
\ No newline at end of file
diff --git a/spaces/inplisQlawa/anything-midjourney-v4-1/Itoo Software Forest Pack Pro V.6.2.1 For 3DsMax 2020 Win X64.md b/spaces/inplisQlawa/anything-midjourney-v4-1/Itoo Software Forest Pack Pro V.6.2.1 For 3DsMax 2020 Win X64.md
deleted file mode 100644
index aced70671f931ae6667ed94b80540aa952e74087..0000000000000000000000000000000000000000
--- a/spaces/inplisQlawa/anything-midjourney-v4-1/Itoo Software Forest Pack Pro V.6.2.1 For 3DsMax 2020 Win X64.md
+++ /dev/null
@@ -1,6 +0,0 @@
-
Itoo Software Forest Pack Pro V.6.2.1 For 3DsMax 2020 Win x64
-
-It is an offline setup file of Itoo Forest Pack Pro 6.2.1 for 3ds Max 2010-2019 Free ... for 3ds Max 2010-2019 Free Download standalone setup latest version for PC. ... Download is one of the recognized idols for the 3ds Max Max Software Suite. ... 3ds Max 2010-2019 free download for Home windows x86 and x64 structure. 4d29de3e1b
-
-
-
diff --git a/spaces/inplisQlawa/anything-midjourney-v4-1/Mahabharat Chopra (1998) DVDRip All.md b/spaces/inplisQlawa/anything-midjourney-v4-1/Mahabharat Chopra (1998) DVDRip All.md
deleted file mode 100644
index bd76225176ed13015dd2dd2bbb9bd2a869b80df9..0000000000000000000000000000000000000000
--- a/spaces/inplisQlawa/anything-midjourney-v4-1/Mahabharat Chopra (1998) DVDRip All.md
+++ /dev/null
@@ -1,44 +0,0 @@
-
-
-References
-
-External links
-
-
-
-Category:Telugu-language films
-
-Category:Indian television series
-
-Category:Indian mythological television series
-
-Category:1998 Indian television series debuts
-
-Category:Indian drama television series
-
-Category:Indian fantasy television series
-
-Category:Star Utsavam
-
-Category:Television programs based on Indian novels
-
-Category:Fiction about shapeshifting
-
-Category:Films based on Indian novels
-
-Category:Television programs based on Mahabharata
-
-Category:Films based on the Mahabharata
-
-Category:Fiction about magic
-
-Category:Fiction about monsters
-
-Category:Iddaru Mitrulu films[Cholesterol crystal embolism: clinical features, diagnosis and therapeutic alternatives].
-
-The cholesterol crystal embolism (CCE) is a clinical condition in which cholesterol crystals lead to ischaemia and necrosis of many organs. CCE is an important disease of the elderly, characterized by rapid course, high mortality and a high rate of recurrence. The goal of this work is to define the main aspects of this pathology, its diagnosis and treatment. This work is based on our experience in the management of ten patients with CCE. All patients received different therapeutic strategies, depending on clinical manifestations and radiological findings. Four of these patients died, two in the first 3 months after onset of the disease, and one after one year. Four patients had a good outcome. CCE is an emergent condition of the elderly and its symptoms are protean. It is an important disease that requires an appropriate treatment that needs to be individualized.Sensitivity of the post-operative complications in esophageal cancer to systemic radiotherapy.
-
-To evaluate the dose-response relationship between radiation dose and post-operative complications, 17 patients with esophageal cancer underwent thoracic esophagectomy after systemic radiotherapy, and were treated with radiation doses ranging from 36 Gy to 60 Gy in 2-Gy fractions. The mean radiation dose to the esophagus was 54.9 +/- 0.5 Gy. The fraction sizes were 2.5 Gy at doses of 36 Gy and 39.5 Gy, 2 Gy at dose of 42 Gy, 2.5 Gy at dose of 45 Gy, 2 Gy at dose of 48 Gy, 2 Gy at dose of 50.4 Gy, and 2.5 Gy at dose of 54 Gy. Twenty-four patients who underwent esophagectomy without pre-operative radiotherapy 4fefd39f24
-
-
-
diff --git a/spaces/inplisQlawa/anything-midjourney-v4-1/Malayalam Kambi Cartoon Stories.md b/spaces/inplisQlawa/anything-midjourney-v4-1/Malayalam Kambi Cartoon Stories.md
deleted file mode 100644
index e16afef7e7b7f0dc92c322297dcbe99725a171af..0000000000000000000000000000000000000000
--- a/spaces/inplisQlawa/anything-midjourney-v4-1/Malayalam Kambi Cartoon Stories.md
+++ /dev/null
@@ -1,6 +0,0 @@
-
-
-Now, they are less common, but still, the need for a shaping tool and mounting of the images remains. DAEMON Tools Lite, unlike its counterpart, ... 4d29de3e1b
-
-
-
diff --git a/spaces/inreVtussa/clothingai/Examples/Dance Ejay 4 No-cd Crack Age Of Empires __FULL__.md b/spaces/inreVtussa/clothingai/Examples/Dance Ejay 4 No-cd Crack Age Of Empires __FULL__.md
deleted file mode 100644
index f852ef6e70ae9eec70563b94febcf979736d3818..0000000000000000000000000000000000000000
--- a/spaces/inreVtussa/clothingai/Examples/Dance Ejay 4 No-cd Crack Age Of Empires __FULL__.md
+++ /dev/null
@@ -1,122 +0,0 @@
-
-
Dance Ejay 4 No-cd Crack Age Of Empires: How to Play Two Classic Games Without a CD
-
-
Dance Ejay 4 and Age of Empires are two classic PC games that require a CD to run. However, if you have lost or damaged your original CD, or if you want to play the games without inserting the CD every time, you may need a Dance Ejay 4 No-cd Crack Age Of Empires. This is a file that modifies the game executable to bypass the CD check and allow you to play the games without a CD.
In this article, we will show you how to download and use Dance Ejay 4 No-cd Crack Age Of Empires for Windows PC. We will also review the features and gameplay of Dance Ejay 4 and Age of Empires, and explain why they are still worth playing today.
-
-
What is Dance Ejay 4?
-
-
Dance Ejay 4 is a music creation software that allows you to create your own dance tracks using samples, loops, effects, and synthesizers. You can mix and match different styles of music, such as techno, trance, house, hip hop, drum and bass, etc. You can also record your own vocals and instruments, and edit them with various tools. You can save your tracks as WAV or MP3 files, or burn them to CD.
-
-
Dance Ejay 4 was released in 2000 by Empire Interactive. It was one of the most popular music software of its time, thanks to its user-friendly interface, large library of samples, and powerful features. It was also fun and easy to use, as you could create professional sounding tracks in minutes.
-
-
What is Age of Empires?
-
-
Age of Empires is a real-time strategy game that lets you control a civilization from the Stone Age to the Iron Age. You can choose from 12 different civilizations, each with their own unique units, buildings, technologies, and bonuses. You can play in different modes, such as campaign, random map, deathmatch, or multiplayer. You can also create your own scenarios and maps with the built-in editor.
-
-
-
Age of Empires was released in 1997 by Microsoft Studios. It was one of the most influential strategy games of its time, thanks to its historical accuracy, gameplay depth, and replay value. It was also praised for its graphics, sound, and music.
-
-
How to Download and Use Dance Ejay 4 No-cd Crack Age Of Empires
-
-
If you want to use Dance Ejay 4 No-cd Crack Age Of Empires, you will need to have the original games installed on your PC first. You can buy them online from various sources, such as Amazon or G2A. Alternatively, you can download them from some websites that offer old games for free or for a small fee.
-
-
After installing the games, you will need to download Dance Ejay 4 No-cd Crack Age Of Empires from the link below. The file size is about 1 MB. The download is in ZIP format, so you will need a software like WinZip or 7-Zip to extract it.
-
-
After extracting the file, you will see two files named "DANCE.EXE" and "EMPIRES.EXE". These are the cracked game executables for Dance Ejay 4 and Age of Empires respectively. You will need to copy these files to the folders where you installed the games (usually C:\Program Files\Empire Interactive\Dance eJay 4\ and C:\Program Files\Microsoft Games\Age of Empires\). Replace the original files with the cracked ones.
-
-
Now you can run the games without a CD. Just double-click on the cracked game executables or create shortcuts for them on your desktop. Enjoy!
-
-
Conclusion
-
-
Dance Ejay 4 No-cd Crack Age Of Empires is a file that allows you to play Dance Ejay 4 and Age of Empires without a CD on your Windows PC. It is easy to download and use, and it works well with most versions of Windows. It also lets you enjoy two classic games that are still fun and engaging today.
-
-
You can download Dance Ejay 4 No-cd Crack Age Of Empires from the link below:
Dance Ejay 4 and Age of Empires are two games that were released more than 20 years ago, but they still have a lot to offer to modern gamers. Here are some reasons why you should play them today:
-
-
-
They are fun and addictive. Dance Ejay 4 lets you unleash your creativity and make your own dance tracks with ease. Age of Empires lets you experience the thrill and challenge of building and conquering civilizations in different historical eras.
-
They are educational and informative. Dance Ejay 4 teaches you the basics of music production and composition. Age of Empires teaches you the history and culture of different civilizations and their achievements.
-
They are nostalgic and retro. Dance Ejay 4 and Age of Empires bring back memories of the late 90s and early 2000s, when PC gaming was booming and evolving. They also have a charming and colorful graphics style that is different from the realistic and dark graphics of today's games.
-
They are compatible and accessible. Dance Ejay 4 and Age of Empires can run on most Windows PCs, even on low-end ones. They also have a simple and intuitive interface that is easy to learn and use.
-
-
-
Conclusion
-
-
Dance Ejay 4 No-cd Crack Age Of Empires is a file that allows you to play Dance Ejay 4 and Age of Empires without a CD on your Windows PC. It is easy to download and use, and it works well with most versions of Windows. It also lets you enjoy two classic games that are still fun and engaging today.
-
-
You can download Dance Ejay 4 No-cd Crack Age Of Empires from the link below:
What are the Benefits of Using Dance Ejay 4 No-cd Crack Age Of Empires
-
-
Using Dance Ejay 4 No-cd Crack Age Of Empires has several benefits for gamers who want to play Dance Ejay 4 and Age of Empires on their Windows PC. Here are some of them:
-
-
-
It saves you time and hassle. You don't have to insert the CD every time you want to play the games. You also don't have to worry about losing or damaging your CD.
-
It saves you space and resources. You don't have to store the CD or keep it in your drive. You also don't have to use any virtual drive software that may slow down your PC.
-
It enhances your gaming experience. You can enjoy faster loading times and smoother performance of the games. You can also use mods or cheats that may not work with the CD version.
-
It is free and easy to use. You don't have to pay anything to download and use Dance Ejay 4 No-cd Crack Age Of Empires. You also don't have to follow any complicated instructions or procedures to install and use it.
-
-
-
What are the Risks of Using Dance Ejay 4 No-cd Crack Age Of Empires
-
-
While Dance Ejay 4 No-cd Crack Age Of Empires has many benefits, it also has some risks that you should be aware of before using it. Here are some of them:
-
-
-
It may be illegal or unethical. Using Dance Ejay 4 No-cd Crack Age Of Empires may violate the terms and conditions of the game developers or publishers. It may also be considered as piracy or theft of intellectual property.
-
It may be unsafe or harmful. Using Dance Ejay 4 No-cd Crack Age Of Empires may expose your PC to viruses, malware, spyware, or other malicious programs that may damage your system or steal your data.
-
It may be unreliable or incompatible. Using Dance Ejay 4 No-cd Crack Age Of Empires may cause errors, crashes, glitches, or bugs in the games. It may also not work with some updates, patches, or versions of the games.
-
It may be detected or banned. Using Dance Ejay 4 No-cd Crack Age Of Empires may be detected by the game developers or publishers and result in legal actions or penalties. It may also be detected by online servers or anti-cheat systems and result in bans or suspensions from playing online.
-
-
-
Conclusion
-
-
Dance Ejay 4 No-cd Crack Age Of Empires is a file that allows you to play Dance Ejay 4 and Age of Empires without a CD on your Windows PC. It is easy to download and use, and it works well with most versions of Windows. It also lets you enjoy two classic games that are still fun and engaging today.
-
-
You can download Dance Ejay 4 No-cd Crack Age Of Empires from the link below:
How to Play Dance Ejay 4 and Age of Empires Online
-
-
One of the best features of Dance Ejay 4 and Age of Empires is that they allow you to play online with other players from around the world. You can share your music, compete in tournaments, or cooperate in campaigns. However, playing online may require some extra steps and precautions. Here are some tips on how to play Dance Ejay 4 and Age of Empires online:
-
-
-
For Dance Ejay 4, you will need to register an account on the official website (www.ejay.com) and download the eJay Net Client software. This software will let you connect to the eJay servers and access the online features of the game. You will also need a broadband internet connection and a microphone or headset.
-
For Age of Empires, you will need to use a third-party service or software that supports the game, such as GameRanger, Voobly, or Hamachi. These services or software will let you create or join online games with other players who have the same version and crack of the game. You will also need a broadband internet connection and a microphone or headset.
-
Before playing online, make sure to backup your game files and settings, as playing online may modify or overwrite them. You should also scan your PC for any viruses or malware that may affect your game or your online security.
-
When playing online, be respectful and courteous to other players. Follow the rules and etiquette of the online service or software that you are using. Do not cheat, hack, spam, or harass other players. Have fun and enjoy the games!
-
-
-
How to Get More Out of Dance Ejay 4 and Age of Empires
-
-
Dance Ejay 4 and Age of Empires are two games that have a lot of content and features that can keep you entertained for hours. However, if you want to get more out of these games, you can also try some of these options:
-
-
-
For Dance Ejay 4, you can download more samples, loops, effects, and synthesizers from the official website (www.ejay.com) or from other websites that offer free or paid sound packs. You can also create your own samples and loops using external software or hardware.
-
For Age of Empires, you can download more scenarios, maps, campaigns, civilizations, units, buildings, technologies, and bonuses from the official website (www.ageofempires.com) or from other websites that offer free or paid mods. You can also create your own scenarios and maps using the built-in editor or external software.
-
For both games, you can watch tutorials, guides, reviews, tips, tricks, and gameplay videos on YouTube or other platforms that can help you improve your skills and knowledge of the games. You can also join forums, communities, groups, or clubs that are dedicated to the games and interact with other fans and players.
-
-
-
Conclusion
-
-
Dance Ejay 4 No-cd Crack Age Of Empires is a file that allows you to play Dance Ejay 4 and Age of Empires without a CD on your Windows PC. It is easy to download and use, and it works well with most versions of Windows. It also lets you enjoy two classic games that are still fun and engaging today.
-
-
You can download Dance Ejay 4 No-cd Crack Age Of Empires from the link below:
Dance Ejay 4 No-cd Crack Age Of Empires is a file that allows you to play Dance Ejay 4 and Age of Empires without a CD on your Windows PC. It is easy to download and use, and it works well with most versions of Windows. It also lets you enjoy two classic games that are still fun and engaging today.
-
-
You can download Dance Ejay 4 No-cd Crack Age Of Empires from the link below:
-
-Download Dance Ejay 4 No-cd Crack Age Of Empires 3cee63e6c2
-
-
\ No newline at end of file
diff --git a/spaces/ismot/1702t1/postprocessing/dula/layout.py b/spaces/ismot/1702t1/postprocessing/dula/layout.py
deleted file mode 100644
index 9101a65800d866a660a99b2e4ee809517ffeedf1..0000000000000000000000000000000000000000
--- a/spaces/ismot/1702t1/postprocessing/dula/layout.py
+++ /dev/null
@@ -1,226 +0,0 @@
-"""
-@Date: 2021/10/06
-@description: Use the approach proposed by DuLa-Net
-"""
-import cv2
-import numpy as np
-import math
-import matplotlib.pyplot as plt
-
-from visualization.floorplan import draw_floorplan
-
-
-def merge_near(lst, diag):
- group = [[0, ]]
- for i in range(1, len(lst)):
- if lst[i][1] == 0 and lst[i][0] - np.mean(group[-1]) < diag * 0.02:
- group[-1].append(lst[i][0])
- else:
- group.append([lst[i][0], ])
- if len(group) == 1:
- group = [lst[0][0], lst[-1][0]]
- else:
- group = [int(np.mean(x)) for x in group]
- return group
-
-
-def fit_layout(floor_xz, need_cube=False, show=False, block_eps=0.2):
- show_radius = np.linalg.norm(floor_xz, axis=-1).max()
- side_l = 512
- floorplan = draw_floorplan(xz=floor_xz, show_radius=show_radius, show=show, scale=1, side_l=side_l).astype(np.uint8)
- center = np.array([side_l / 2, side_l / 2])
- polys = cv2.findContours(floorplan, 1, 2)
- if isinstance(polys, tuple):
- if len(polys) == 3:
- # opencv 3
- polys = list(polys[1])
- else:
- polys = list(polys[0])
- polys.sort(key=lambda x: cv2.contourArea(x), reverse=True)
- poly = polys[0]
- sub_x, sub_y, w, h = cv2.boundingRect(poly)
- floorplan_sub = floorplan[sub_y:sub_y + h, sub_x:sub_x + w]
- sub_center = center - np.array([sub_x, sub_y])
- polys = cv2.findContours(floorplan_sub, 1, 2)
- if isinstance(polys, tuple):
- if len(polys) == 3:
- polys = polys[1]
- else:
- polys = polys[0]
- poly = polys[0]
- epsilon = 0.005 * cv2.arcLength(poly, True)
- poly = cv2.approxPolyDP(poly, epsilon, True)
-
- x_lst = [[0, 0], ]
- y_lst = [[0, 0], ]
-
- ans = np.zeros((floorplan_sub.shape[0], floorplan_sub.shape[1]))
-
- for i in range(len(poly)):
- p1 = poly[i][0]
- p2 = poly[(i + 1) % len(poly)][0]
- # We added occlusion detection
- cp1 = p1 - sub_center
- cp2 = p2 - sub_center
- p12 = p2 - p1
- l1 = np.linalg.norm(cp1)
- l2 = np.linalg.norm(cp2)
- l3 = np.linalg.norm(p12)
- # We added occlusion detection
- is_block1 = abs(np.cross(cp1/l1, cp2/l2)) < block_eps
- is_block2 = abs(np.cross(cp2/l2, p12/l3)) < block_eps*2
- is_block = is_block1 and is_block2
-
- if (p2[0] - p1[0]) == 0:
- slope = 10
- else:
- slope = abs((p2[1] - p1[1]) / (p2[0] - p1[0]))
-
- if is_block:
- s = p1[1] if l1 < l2 else p2[1]
- y_lst.append([s, 1])
- s = p1[0] if l1 < l2 else p2[0]
- x_lst.append([s, 1])
-
- left = p1[0] if p1[0] < p2[0] else p2[0]
- right = p1[0] if p1[0] > p2[0] else p2[0]
- top = p1[1] if p1[1] < p2[1] else p2[1]
- bottom = p1[1] if p1[1] > p2[1] else p2[1]
- sample = floorplan_sub[top:bottom, left:right]
- score = 0 if sample.size == 0 else sample.mean()
- if score >= 0.3:
- ans[top:bottom, left:right] = 1
-
- else:
- if slope <= 1:
- s = int((p1[1] + p2[1]) / 2)
- y_lst.append([s, 0])
- elif slope > 1:
- s = int((p1[0] + p2[0]) / 2)
- x_lst.append([s, 0])
-
- debug_show = False
- if debug_show:
- plt.figure(dpi=300)
- plt.axis('off')
- a = cv2.drawMarker(floorplan_sub.copy()*0.5, tuple([floorplan_sub.shape[1] // 2, floorplan_sub.shape[0] // 2]), [1], markerType=0, markerSize=10, thickness=2)
- plt.imshow(cv2.drawContours(a, [poly], 0, 1, 1))
- plt.savefig('src/1.png', bbox_inches='tight', transparent=True, pad_inches=0)
- plt.show()
-
- plt.figure(dpi=300)
- plt.axis('off')
- a = cv2.drawMarker(ans.copy()*0.5, tuple([floorplan_sub.shape[1] // 2, floorplan_sub.shape[0] // 2]), [1], markerType=0, markerSize=10, thickness=2)
- plt.imshow(cv2.drawContours(a, [poly], 0, 1, 1))
- # plt.show()
- plt.savefig('src/2.png', bbox_inches='tight', transparent=True, pad_inches=0)
- plt.show()
-
- x_lst.append([floorplan_sub.shape[1], 0])
- y_lst.append([floorplan_sub.shape[0], 0])
- x_lst.sort(key=lambda x: x[0])
- y_lst.sort(key=lambda x: x[0])
-
- diag = math.sqrt(math.pow(floorplan_sub.shape[1], 2) + math.pow(floorplan_sub.shape[0], 2))
- x_lst = merge_near(x_lst, diag)
- y_lst = merge_near(y_lst, diag)
- if need_cube and len(x_lst) > 2:
- x_lst = [x_lst[0], x_lst[-1]]
- if need_cube and len(y_lst) > 2:
- y_lst = [y_lst[0], y_lst[-1]]
-
- for i in range(len(x_lst) - 1):
- for j in range(len(y_lst) - 1):
- sample = floorplan_sub[y_lst[j]:y_lst[j + 1], x_lst[i]:x_lst[i + 1]]
- score = 0 if sample.size == 0 else sample.mean()
- if score >= 0.3:
- ans[y_lst[j]:y_lst[j + 1], x_lst[i]:x_lst[i + 1]] = 1
-
- if debug_show:
- plt.figure(dpi=300)
- plt.axis('off')
- a = cv2.drawMarker(ans.copy() * 0.5, tuple([floorplan_sub.shape[1] // 2, floorplan_sub.shape[0] // 2]), [1],
- markerType=0, markerSize=10, thickness=2)
- plt.imshow(cv2.drawContours(a, [poly], 0, 1, 1))
- # plt.show()
- plt.savefig('src/3.png', bbox_inches='tight', transparent=True, pad_inches=0)
- plt.show()
-
- pred = np.uint8(ans)
- pred_polys = cv2.findContours(pred, 1, 3)
- if isinstance(pred_polys, tuple):
- if len(pred_polys) == 3:
- pred_polys = pred_polys[1]
- else:
- pred_polys = pred_polys[0]
-
- pred_polys.sort(key=lambda x: cv2.contourArea(x), reverse=True)
- pred_polys = pred_polys[0]
-
- if debug_show:
- plt.figure(dpi=300)
- plt.axis('off')
- a = cv2.drawMarker(ans.copy() * 0.5, tuple([floorplan_sub.shape[1] // 2, floorplan_sub.shape[0] // 2]), [1],
- markerType=0, markerSize=10, thickness=2)
- a = cv2.drawContours(a, [poly], 0, 0.8, 1)
- a = cv2.drawContours(a, [pred_polys], 0, 1, 1)
- plt.imshow(a)
- # plt.show()
- plt.savefig('src/4.png', bbox_inches='tight', transparent=True, pad_inches=0)
- plt.show()
-
- polygon = [(p[0][1], p[0][0]) for p in pred_polys[::-1]]
-
- v = np.array([p[0] + sub_y for p in polygon])
- u = np.array([p[1] + sub_x for p in polygon])
- # side_l
- # v<-----------|o
- # | | |
- # | ----|----z | side_l
- # | | |
- # | x \|/
- # |------------u
- side_l = floorplan.shape[0]
- pred_xz = np.concatenate((u[:, np.newaxis] - side_l // 2, side_l // 2 - v[:, np.newaxis]), axis=1)
-
- pred_xz = pred_xz * show_radius / (side_l // 2)
- if show:
- draw_floorplan(pred_xz, show_radius=show_radius, show=show)
-
- show_process = False
- if show_process:
- img = np.zeros((floorplan_sub.shape[0], floorplan_sub.shape[1], 3))
- for x in x_lst:
- cv2.line(img, (x, 0), (x, floorplan_sub.shape[0]), (0, 255, 0), 1)
- for y in y_lst:
- cv2.line(img, (0, y), (floorplan_sub.shape[1], y), (255, 0, 0), 1)
-
- fig = plt.figure()
- plt.axis('off')
- ax1 = fig.add_subplot(2, 2, 1)
- ax1.imshow(floorplan)
- ax3 = fig.add_subplot(2, 2, 2)
- ax3.imshow(floorplan_sub)
- ax4 = fig.add_subplot(2, 2, 3)
- ax4.imshow(img)
- ax5 = fig.add_subplot(2, 2, 4)
- ax5.imshow(ans)
- plt.show()
-
- return pred_xz
-
-
-if __name__ == '__main__':
- from utils.conversion import uv2xyz
-
- pano_img = np.zeros([512, 1024, 3])
- corners = np.array([[0.1, 0.7],
- [0.4, 0.7],
- [0.3, 0.6],
- [0.6, 0.6],
- [0.8, 0.7]])
- xz = uv2xyz(corners)[..., ::2]
- draw_floorplan(xz, show=True, marker_color=None, center_color=0.8)
-
- xz = fit_layout(xz)
- draw_floorplan(xz, show=True, marker_color=None, center_color=0.8)
diff --git a/spaces/ivy-1911/vits-uma-genshin-honkai/README.md b/spaces/ivy-1911/vits-uma-genshin-honkai/README.md
deleted file mode 100644
index 2fd2870bef9c579ab20b33fdd09aea238aeb1f1d..0000000000000000000000000000000000000000
--- a/spaces/ivy-1911/vits-uma-genshin-honkai/README.md
+++ /dev/null
@@ -1,11 +0,0 @@
----
-license: apache-2.0
-title: ' vits-uma-genshin-honkai'
-sdk: gradio
-sdk_version: 3.7
-emoji: 🐨
-colorTo: yellow
-pinned: false
-app_file: app.py
-duplicated_from: sayashi/vits-uma-genshin-honkai
----
diff --git a/spaces/jackli888/stable-diffusion-webui/extensions/deforum/scripts/deforum_helpers/src/clipseg/models/clipseg.py b/spaces/jackli888/stable-diffusion-webui/extensions/deforum/scripts/deforum_helpers/src/clipseg/models/clipseg.py
deleted file mode 100644
index a4640b34bbd1ca68a32114471d5585734c4af2fc..0000000000000000000000000000000000000000
--- a/spaces/jackli888/stable-diffusion-webui/extensions/deforum/scripts/deforum_helpers/src/clipseg/models/clipseg.py
+++ /dev/null
@@ -1,552 +0,0 @@
-import math
-from os.path import basename, dirname, join, isfile
-import torch
-from torch import nn
-from torch.nn import functional as nnf
-from torch.nn.modules.activation import ReLU
-
-
-def precompute_clip_vectors():
-
- from trails.initialization import init_dataset
- lvis = init_dataset('LVIS_OneShot3', split='train', mask='text_label', image_size=224, aug=1, normalize=True,
- reduce_factor=None, add_bar=False, negative_prob=0.5)
-
- all_names = list(lvis.category_names.values())
-
- import clip
- from models.clip_prompts import imagenet_templates
- clip_model = clip.load("ViT-B/32", device='cuda', jit=False)[0]
- prompt_vectors = {}
- for name in all_names[:100]:
- with torch.no_grad():
- conditionals = [t.format(name).replace('_', ' ') for t in imagenet_templates]
- text_tokens = clip.tokenize(conditionals).cuda()
- cond = clip_model.encode_text(text_tokens).cpu()
-
- for cond, vec in zip(conditionals, cond):
- prompt_vectors[cond] = vec.cpu()
-
- import pickle
-
- pickle.dump(prompt_vectors, open('precomputed_prompt_vectors.pickle', 'wb'))
-
-
-def get_prompt_list(prompt):
- if prompt == 'plain':
- return ['{}']
- elif prompt == 'fixed':
- return ['a photo of a {}.']
- elif prompt == 'shuffle':
- return ['a photo of a {}.', 'a photograph of a {}.', 'an image of a {}.', '{}.']
- elif prompt == 'shuffle+':
- return ['a photo of a {}.', 'a photograph of a {}.', 'an image of a {}.', '{}.',
- 'a cropped photo of a {}.', 'a good photo of a {}.', 'a photo of one {}.',
- 'a bad photo of a {}.', 'a photo of the {}.']
- elif prompt == 'shuffle_clip':
- from models.clip_prompts import imagenet_templates
- return imagenet_templates
- else:
- raise ValueError('Invalid value for prompt')
-
-
-def forward_multihead_attention(x, b, with_aff=False, attn_mask=None):
- """
- Simplified version of multihead attention (taken from torch source code but without tons of if clauses).
- The mlp and layer norm come from CLIP.
- x: input.
- b: multihead attention module.
- """
-
- x_ = b.ln_1(x)
- q, k, v = nnf.linear(x_, b.attn.in_proj_weight, b.attn.in_proj_bias).chunk(3, dim=-1)
- tgt_len, bsz, embed_dim = q.size()
-
- head_dim = embed_dim // b.attn.num_heads
- scaling = float(head_dim) ** -0.5
-
- q = q.contiguous().view(tgt_len, bsz * b.attn.num_heads, b.attn.head_dim).transpose(0, 1)
- k = k.contiguous().view(-1, bsz * b.attn.num_heads, b.attn.head_dim).transpose(0, 1)
- v = v.contiguous().view(-1, bsz * b.attn.num_heads, b.attn.head_dim).transpose(0, 1)
-
- q = q * scaling
-
- attn_output_weights = torch.bmm(q, k.transpose(1, 2)) # n_heads * batch_size, tokens^2, tokens^2
- if attn_mask is not None:
-
-
- attn_mask_type, attn_mask = attn_mask
- n_heads = attn_output_weights.size(0) // attn_mask.size(0)
- attn_mask = attn_mask.repeat(n_heads, 1)
-
- if attn_mask_type == 'cls_token':
- # the mask only affects similarities compared to the readout-token.
- attn_output_weights[:, 0, 1:] = attn_output_weights[:, 0, 1:] * attn_mask[None,...]
- # attn_output_weights[:, 0, 0] = 0*attn_output_weights[:, 0, 0]
-
- if attn_mask_type == 'all':
- # print(attn_output_weights.shape, attn_mask[:, None].shape)
- attn_output_weights[:, 1:, 1:] = attn_output_weights[:, 1:, 1:] * attn_mask[:, None]
-
-
- attn_output_weights = torch.softmax(attn_output_weights, dim=-1)
-
- attn_output = torch.bmm(attn_output_weights, v)
- attn_output = attn_output.transpose(0, 1).contiguous().view(tgt_len, bsz, embed_dim)
- attn_output = b.attn.out_proj(attn_output)
-
- x = x + attn_output
- x = x + b.mlp(b.ln_2(x))
-
- if with_aff:
- return x, attn_output_weights
- else:
- return x
-
-
-class CLIPDenseBase(nn.Module):
-
- def __init__(self, version, reduce_cond, reduce_dim, prompt, n_tokens):
- super().__init__()
-
- import clip
-
- # prec = torch.FloatTensor
- self.clip_model, _ = clip.load(version, device='cpu', jit=False)
- self.model = self.clip_model.visual
-
- # if not None, scale conv weights such that we obtain n_tokens.
- self.n_tokens = n_tokens
-
- for p in self.clip_model.parameters():
- p.requires_grad_(False)
-
- # conditional
- if reduce_cond is not None:
- self.reduce_cond = nn.Linear(512, reduce_cond)
- for p in self.reduce_cond.parameters():
- p.requires_grad_(False)
- else:
- self.reduce_cond = None
-
- self.film_mul = nn.Linear(512 if reduce_cond is None else reduce_cond, reduce_dim)
- self.film_add = nn.Linear(512 if reduce_cond is None else reduce_cond, reduce_dim)
-
- self.reduce = nn.Linear(768, reduce_dim)
-
- self.prompt_list = get_prompt_list(prompt)
-
- # precomputed prompts
- import pickle
- if isfile('precomputed_prompt_vectors.pickle'):
- precomp = pickle.load(open('precomputed_prompt_vectors.pickle', 'rb'))
- self.precomputed_prompts = {k: torch.from_numpy(v) for k, v in precomp.items()}
- else:
- self.precomputed_prompts = dict()
-
- def rescaled_pos_emb(self, new_size):
- assert len(new_size) == 2
-
- a = self.model.positional_embedding[1:].T.view(1, 768, *self.token_shape)
- b = nnf.interpolate(a, new_size, mode='bicubic', align_corners=False).squeeze(0).view(768, new_size[0]*new_size[1]).T
- return torch.cat([self.model.positional_embedding[:1], b])
-
- def visual_forward(self, x_inp, extract_layers=(), skip=False, mask=None):
-
-
- with torch.no_grad():
-
- inp_size = x_inp.shape[2:]
-
- if self.n_tokens is not None:
- stride2 = x_inp.shape[2] // self.n_tokens
- conv_weight2 = nnf.interpolate(self.model.conv1.weight, (stride2, stride2), mode='bilinear', align_corners=True)
- x = nnf.conv2d(x_inp, conv_weight2, bias=self.model.conv1.bias, stride=stride2, dilation=self.model.conv1.dilation)
- else:
- x = self.model.conv1(x_inp) # shape = [*, width, grid, grid]
-
- x = x.reshape(x.shape[0], x.shape[1], -1) # shape = [*, width, grid ** 2]
- x = x.permute(0, 2, 1) # shape = [*, grid ** 2, width]
-
- x = torch.cat([self.model.class_embedding.to(x.dtype) + torch.zeros(x.shape[0], 1, x.shape[-1], dtype=x.dtype, device=x.device), x], dim=1) # shape = [*, grid ** 2 + 1, width]
-
- standard_n_tokens = 50 if self.model.conv1.kernel_size[0] == 32 else 197
-
- if x.shape[1] != standard_n_tokens:
- new_shape = int(math.sqrt(x.shape[1]-1))
- x = x + self.rescaled_pos_emb((new_shape, new_shape)).to(x.dtype)[None,:,:]
- else:
- x = x + self.model.positional_embedding.to(x.dtype)
-
- x = self.model.ln_pre(x)
-
- x = x.permute(1, 0, 2) # NLD -> LND
-
- activations, affinities = [], []
- for i, res_block in enumerate(self.model.transformer.resblocks):
-
- if mask is not None:
- mask_layer, mask_type, mask_tensor = mask
- if mask_layer == i or mask_layer == 'all':
- # import ipdb; ipdb.set_trace()
- size = int(math.sqrt(x.shape[0] - 1))
-
- attn_mask = (mask_type, nnf.interpolate(mask_tensor.unsqueeze(1).float(), (size, size)).view(mask_tensor.shape[0], size * size))
-
- else:
- attn_mask = None
- else:
- attn_mask = None
-
- x, aff_per_head = forward_multihead_attention(x, res_block, with_aff=True, attn_mask=attn_mask)
-
- if i in extract_layers:
- affinities += [aff_per_head]
-
- #if self.n_tokens is not None:
- # activations += [nnf.interpolate(x, inp_size, mode='bilinear', align_corners=True)]
- #else:
- activations += [x]
-
- if len(extract_layers) > 0 and i == max(extract_layers) and skip:
- print('early skip')
- break
-
- x = x.permute(1, 0, 2) # LND -> NLD
- x = self.model.ln_post(x[:, 0, :])
-
- if self.model.proj is not None:
- x = x @ self.model.proj
-
- return x, activations, affinities
-
- def sample_prompts(self, words, prompt_list=None):
-
- prompt_list = prompt_list if prompt_list is not None else self.prompt_list
-
- prompt_indices = torch.multinomial(torch.ones(len(prompt_list)), len(words), replacement=True)
- prompts = [prompt_list[i] for i in prompt_indices]
- return [promt.format(w) for promt, w in zip(prompts, words)]
-
- def get_cond_vec(self, conditional, batch_size):
- # compute conditional from a single string
- if conditional is not None and type(conditional) == str:
- cond = self.compute_conditional(conditional)
- cond = cond.repeat(batch_size, 1)
-
- # compute conditional from string list/tuple
- elif conditional is not None and type(conditional) in {list, tuple} and type(conditional[0]) == str:
- assert len(conditional) == batch_size
- cond = self.compute_conditional(conditional)
-
- # use conditional directly
- elif conditional is not None and type(conditional) == torch.Tensor and conditional.ndim == 2:
- cond = conditional
-
- # compute conditional from image
- elif conditional is not None and type(conditional) == torch.Tensor:
- with torch.no_grad():
- cond, _, _ = self.visual_forward(conditional)
- else:
- raise ValueError('invalid conditional')
- return cond
-
- def compute_conditional(self, conditional):
- import clip
-
- dev = next(self.parameters()).device
-
- if type(conditional) in {list, tuple}:
- text_tokens = clip.tokenize(conditional).to(dev)
- cond = self.clip_model.encode_text(text_tokens)
- else:
- if conditional in self.precomputed_prompts:
- cond = self.precomputed_prompts[conditional].float().to(dev)
- else:
- text_tokens = clip.tokenize([conditional]).to(dev)
- cond = self.clip_model.encode_text(text_tokens)[0]
-
- if self.shift_vector is not None:
- return cond + self.shift_vector
- else:
- return cond
-
-
-def clip_load_untrained(version):
- assert version == 'ViT-B/16'
- from clip.model import CLIP
- from clip.clip import _MODELS, _download
- model = torch.jit.load(_download(_MODELS['ViT-B/16'])).eval()
- state_dict = model.state_dict()
-
- vision_width = state_dict["visual.conv1.weight"].shape[0]
- vision_layers = len([k for k in state_dict.keys() if k.startswith("visual.") and k.endswith(".attn.in_proj_weight")])
- vision_patch_size = state_dict["visual.conv1.weight"].shape[-1]
- grid_size = round((state_dict["visual.positional_embedding"].shape[0] - 1) ** 0.5)
- image_resolution = vision_patch_size * grid_size
- embed_dim = state_dict["text_projection"].shape[1]
- context_length = state_dict["positional_embedding"].shape[0]
- vocab_size = state_dict["token_embedding.weight"].shape[0]
- transformer_width = state_dict["ln_final.weight"].shape[0]
- transformer_heads = transformer_width // 64
- transformer_layers = len(set(k.split(".")[2] for k in state_dict if k.startswith(f"transformer.resblocks")))
-
- return CLIP(embed_dim, image_resolution, vision_layers, vision_width, vision_patch_size,
- context_length, vocab_size, transformer_width, transformer_heads, transformer_layers)
-
-
-class CLIPDensePredT(CLIPDenseBase):
-
- def __init__(self, version='ViT-B/32', extract_layers=(3, 6, 9), cond_layer=0, reduce_dim=128, n_heads=4, prompt='fixed',
- extra_blocks=0, reduce_cond=None, fix_shift=False,
- learn_trans_conv_only=False, limit_to_clip_only=False, upsample=False,
- add_calibration=False, rev_activations=False, trans_conv=None, n_tokens=None):
-
- super().__init__(version, reduce_cond, reduce_dim, prompt, n_tokens)
- # device = 'cpu'
-
- self.extract_layers = extract_layers
- self.cond_layer = cond_layer
- self.limit_to_clip_only = limit_to_clip_only
- self.process_cond = None
- self.rev_activations = rev_activations
-
- depth = len(extract_layers)
-
- if add_calibration:
- self.calibration_conds = 1
-
- self.upsample_proj = nn.Conv2d(reduce_dim, 1, kernel_size=1) if upsample else None
-
- self.add_activation1 = True
-
- self.version = version
-
- self.token_shape = {'ViT-B/32': (7, 7), 'ViT-B/16': (14, 14)}[version]
-
- if fix_shift:
- # self.shift_vector = nn.Parameter(torch.load(join(dirname(basename(__file__)), 'clip_text_shift_vector.pth')), requires_grad=False)
- self.shift_vector = nn.Parameter(torch.load(join(dirname(basename(__file__)), 'shift_text_to_vis.pth')), requires_grad=False)
- # self.shift_vector = nn.Parameter(-1*torch.load(join(dirname(basename(__file__)), 'shift2.pth')), requires_grad=False)
- else:
- self.shift_vector = None
-
- if trans_conv is None:
- trans_conv_ks = {'ViT-B/32': (32, 32), 'ViT-B/16': (16, 16)}[version]
- else:
- # explicitly define transposed conv kernel size
- trans_conv_ks = (trans_conv, trans_conv)
-
- self.trans_conv = nn.ConvTranspose2d(reduce_dim, 1, trans_conv_ks, stride=trans_conv_ks)
-
- assert len(self.extract_layers) == depth
-
- self.reduces = nn.ModuleList([nn.Linear(768, reduce_dim) for _ in range(depth)])
- self.blocks = nn.ModuleList([nn.TransformerEncoderLayer(d_model=reduce_dim, nhead=n_heads) for _ in range(len(self.extract_layers))])
- self.extra_blocks = nn.ModuleList([nn.TransformerEncoderLayer(d_model=reduce_dim, nhead=n_heads) for _ in range(extra_blocks)])
-
- # refinement and trans conv
-
- if learn_trans_conv_only:
- for p in self.parameters():
- p.requires_grad_(False)
-
- for p in self.trans_conv.parameters():
- p.requires_grad_(True)
-
- self.prompt_list = get_prompt_list(prompt)
-
-
- def forward(self, inp_image, conditional=None, return_features=False, mask=None):
-
- assert type(return_features) == bool
-
- inp_image = inp_image.to(self.model.positional_embedding.device)
-
- if mask is not None:
- raise ValueError('mask not supported')
-
- # x_inp = normalize(inp_image)
- x_inp = inp_image
-
- bs, dev = inp_image.shape[0], x_inp.device
-
- cond = self.get_cond_vec(conditional, bs)
-
- visual_q, activations, _ = self.visual_forward(x_inp, extract_layers=[0] + list(self.extract_layers))
-
- activation1 = activations[0]
- activations = activations[1:]
-
- _activations = activations[::-1] if not self.rev_activations else activations
-
- a = None
- for i, (activation, block, reduce) in enumerate(zip(_activations, self.blocks, self.reduces)):
-
- if a is not None:
- a = reduce(activation) + a
- else:
- a = reduce(activation)
-
- if i == self.cond_layer:
- if self.reduce_cond is not None:
- cond = self.reduce_cond(cond)
-
- a = self.film_mul(cond) * a + self.film_add(cond)
-
- a = block(a)
-
- for block in self.extra_blocks:
- a = a + block(a)
-
- a = a[1:].permute(1, 2, 0) # rm cls token and -> BS, Feats, Tokens
-
- size = int(math.sqrt(a.shape[2]))
-
- a = a.view(bs, a.shape[1], size, size)
-
- a = self.trans_conv(a)
-
- if self.n_tokens is not None:
- a = nnf.interpolate(a, x_inp.shape[2:], mode='bilinear', align_corners=True)
-
- if self.upsample_proj is not None:
- a = self.upsample_proj(a)
- a = nnf.interpolate(a, x_inp.shape[2:], mode='bilinear')
-
- if return_features:
- return a, visual_q, cond, [activation1] + activations
- else:
- return a,
-
-
-
-class CLIPDensePredTMasked(CLIPDensePredT):
-
- def __init__(self, version='ViT-B/32', extract_layers=(3, 6, 9), cond_layer=0, reduce_dim=128, n_heads=4,
- prompt='fixed', extra_blocks=0, reduce_cond=None, fix_shift=False, learn_trans_conv_only=False,
- refine=None, limit_to_clip_only=False, upsample=False, add_calibration=False, n_tokens=None):
-
- super().__init__(version=version, extract_layers=extract_layers, cond_layer=cond_layer, reduce_dim=reduce_dim,
- n_heads=n_heads, prompt=prompt, extra_blocks=extra_blocks, reduce_cond=reduce_cond,
- fix_shift=fix_shift, learn_trans_conv_only=learn_trans_conv_only,
- limit_to_clip_only=limit_to_clip_only, upsample=upsample, add_calibration=add_calibration,
- n_tokens=n_tokens)
-
- def visual_forward_masked(self, img_s, seg_s):
- return super().visual_forward(img_s, mask=('all', 'cls_token', seg_s))
-
- def forward(self, img_q, cond_or_img_s, seg_s=None, return_features=False):
-
- if seg_s is None:
- cond = cond_or_img_s
- else:
- img_s = cond_or_img_s
-
- with torch.no_grad():
- cond, _, _ = self.visual_forward_masked(img_s, seg_s)
-
- return super().forward(img_q, cond, return_features=return_features)
-
-
-
-class CLIPDenseBaseline(CLIPDenseBase):
-
- def __init__(self, version='ViT-B/32', cond_layer=0,
- extract_layer=9, reduce_dim=128, reduce2_dim=None, prompt='fixed',
- reduce_cond=None, limit_to_clip_only=False, n_tokens=None):
-
- super().__init__(version, reduce_cond, reduce_dim, prompt, n_tokens)
- device = 'cpu'
-
- # self.cond_layer = cond_layer
- self.extract_layer = extract_layer
- self.limit_to_clip_only = limit_to_clip_only
- self.shift_vector = None
-
- self.token_shape = {'ViT-B/32': (7, 7), 'ViT-B/16': (14, 14)}[version]
-
- assert reduce2_dim is not None
-
- self.reduce2 = nn.Sequential(
- nn.Linear(reduce_dim, reduce2_dim),
- nn.ReLU(),
- nn.Linear(reduce2_dim, reduce_dim)
- )
-
- trans_conv_ks = {'ViT-B/32': (32, 32), 'ViT-B/16': (16, 16)}[version]
- self.trans_conv = nn.ConvTranspose2d(reduce_dim, 1, trans_conv_ks, stride=trans_conv_ks)
-
-
- def forward(self, inp_image, conditional=None, return_features=False):
-
- inp_image = inp_image.to(self.model.positional_embedding.device)
-
- # x_inp = normalize(inp_image)
- x_inp = inp_image
-
- bs, dev = inp_image.shape[0], x_inp.device
-
- cond = self.get_cond_vec(conditional, bs)
-
- visual_q, activations, affinities = self.visual_forward(x_inp, extract_layers=[self.extract_layer])
-
- a = activations[0]
- a = self.reduce(a)
- a = self.film_mul(cond) * a + self.film_add(cond)
-
- if self.reduce2 is not None:
- a = self.reduce2(a)
-
- # the original model would execute a transformer block here
-
- a = a[1:].permute(1, 2, 0) # rm cls token and -> BS, Feats, Tokens
-
- size = int(math.sqrt(a.shape[2]))
-
- a = a.view(bs, a.shape[1], size, size)
- a = self.trans_conv(a)
-
- if return_features:
- return a, visual_q, cond, activations
- else:
- return a,
-
-
-class CLIPSegMultiLabel(nn.Module):
-
- def __init__(self, model) -> None:
- super().__init__()
-
- from third_party.JoEm.data_loader import get_seen_idx, get_unseen_idx, VOC
-
- self.pascal_classes = VOC
-
- from models.clipseg import CLIPDensePredT
- from general_utils import load_model
- # self.clipseg = load_model('rd64-vit16-neg0.2-phrasecut', strict=False)
- self.clipseg = load_model(model, strict=False)
-
- self.clipseg.eval()
-
- def forward(self, x):
-
- bs = x.shape[0]
- out = torch.ones(21, bs, 352, 352).to(x.device) * -10
-
- for class_id, class_name in enumerate(self.pascal_classes):
-
- fac = 3 if class_name == 'background' else 1
-
- with torch.no_grad():
- pred = torch.sigmoid(self.clipseg(x, class_name)[0][:,0]) * fac
-
- out[class_id] += pred
-
-
- out = out.permute(1, 0, 2, 3)
-
- return out
-
- # construct output tensor
-
\ No newline at end of file
diff --git a/spaces/jdinh/freeze-detection/app.py b/spaces/jdinh/freeze-detection/app.py
deleted file mode 100644
index 2560b7ebae5e86c89ef0433e908ed73a62c159ca..0000000000000000000000000000000000000000
--- a/spaces/jdinh/freeze-detection/app.py
+++ /dev/null
@@ -1,30 +0,0 @@
-__all__ = ['learn', 'classify_image', 'categories',
- 'image', 'label', 'examples', 'intf']
-
-import timm
-import gradio as gr
-from fastai.vision.all import *
-import skimage
-
-TITLE = Path("docs/title.txt").read_text()
-DESCRIPTION = Path("docs/description.md").read_text()
-
-learn = load_learner('model.pkl')
-
-categories = ('airbaby', 'airchair', 'airflare', 'headspin', 'hollow back')
-
-
-def classify_image(img):
- pred, idx, probs = learn.predict(img)
- return dict(zip(categories, map(float, probs)))
-
-
-image = gr.inputs.Image(shape=(192, 192))
-label = gr.outputs.Label()
-examples = ['example1.jpg', 'example5.jpg',
- 'example3.jpg', 'example8.jpg', 'example4.jpg',
- 'example6.jpg', 'example2.jpg', 'example7.JPG']
-
-intf = gr.Interface(fn=classify_image, title=TITLE, description=DESCRIPTION, inputs=image,
- outputs=label, examples=examples)
-intf.launch(inline=False)
diff --git a/spaces/jgurzoni/image_background_swapper/saicinpainting/training/modules/spatial_transform.py b/spaces/jgurzoni/image_background_swapper/saicinpainting/training/modules/spatial_transform.py
deleted file mode 100644
index 2de024ba08c549605a08b64d096f1f0db7b7722a..0000000000000000000000000000000000000000
--- a/spaces/jgurzoni/image_background_swapper/saicinpainting/training/modules/spatial_transform.py
+++ /dev/null
@@ -1,49 +0,0 @@
-import torch
-import torch.nn as nn
-import torch.nn.functional as F
-from kornia.geometry.transform import rotate
-
-
-class LearnableSpatialTransformWrapper(nn.Module):
- def __init__(self, impl, pad_coef=0.5, angle_init_range=80, train_angle=True):
- super().__init__()
- self.impl = impl
- self.angle = torch.rand(1) * angle_init_range
- if train_angle:
- self.angle = nn.Parameter(self.angle, requires_grad=True)
- self.pad_coef = pad_coef
-
- def forward(self, x):
- if torch.is_tensor(x):
- return self.inverse_transform(self.impl(self.transform(x)), x)
- elif isinstance(x, tuple):
- x_trans = tuple(self.transform(elem) for elem in x)
- y_trans = self.impl(x_trans)
- return tuple(self.inverse_transform(elem, orig_x) for elem, orig_x in zip(y_trans, x))
- else:
- raise ValueError(f'Unexpected input type {type(x)}')
-
- def transform(self, x):
- height, width = x.shape[2:]
- pad_h, pad_w = int(height * self.pad_coef), int(width * self.pad_coef)
- x_padded = F.pad(x, [pad_w, pad_w, pad_h, pad_h], mode='reflect')
- x_padded_rotated = rotate(x_padded, angle=self.angle.to(x_padded))
- return x_padded_rotated
-
- def inverse_transform(self, y_padded_rotated, orig_x):
- height, width = orig_x.shape[2:]
- pad_h, pad_w = int(height * self.pad_coef), int(width * self.pad_coef)
-
- y_padded = rotate(y_padded_rotated, angle=-self.angle.to(y_padded_rotated))
- y_height, y_width = y_padded.shape[2:]
- y = y_padded[:, :, pad_h : y_height - pad_h, pad_w : y_width - pad_w]
- return y
-
-
-if __name__ == '__main__':
- layer = LearnableSpatialTransformWrapper(nn.Identity())
- x = torch.arange(2* 3 * 15 * 15).view(2, 3, 15, 15).float()
- y = layer(x)
- assert x.shape == y.shape
- assert torch.allclose(x[:, :, 1:, 1:][:, :, :-1, :-1], y[:, :, 1:, 1:][:, :, :-1, :-1])
- print('all ok')
diff --git a/spaces/jhj0517/Whisper-WebUI-Easy-Subtitle-Generator/modules/model_Inference.py b/spaces/jhj0517/Whisper-WebUI-Easy-Subtitle-Generator/modules/model_Inference.py
deleted file mode 100644
index 450349ad4b8ffcea78c3d1dd71922d39872e90a9..0000000000000000000000000000000000000000
--- a/spaces/jhj0517/Whisper-WebUI-Easy-Subtitle-Generator/modules/model_Inference.py
+++ /dev/null
@@ -1,135 +0,0 @@
-import whisper
-from modules.subtitle_manager import get_srt,get_vtt,safe_filename
-from modules.youtube_manager import get_ytdata,get_ytaudio
-import gradio as gr
-import os
-
-DEFAULT_MODEL_SIZE="tiny"
-
-class WhisperInference():
- def __init__(self):
- print("\nInitializing Model..\n")
- self.current_model_size = DEFAULT_MODEL_SIZE
- self.model = whisper.load_model(name=DEFAULT_MODEL_SIZE,download_root="models")
- self.available_models = ["tiny","tiny.en"]
- self.available_langs = sorted(list(whisper.tokenizer.LANGUAGES.values()))
-
- def transcribe_file(self,fileobjs
- ,model_size,lang,subformat,istranslate,
- progress=gr.Progress()):
-
- def progress_callback(progress_value):
- progress(progress_value,desc="Transcribing..")
-
- if model_size != self.current_model_size:
- progress(0,desc="Initializing Model..")
- self.current_model_size = model_size
- self.model = whisper.load_model(name=model_size,download_root="models")
-
- if lang == "Automatic Detection" :
- lang = None
-
- progress(0,desc="Loading Audio..")
-
- files_info = {}
- for fileobj in fileobjs:
- print(f"\n\n {fileobj.name} \n\n")
-
- audio = whisper.load_audio(fileobj.name)
-
- translatable_model = ["large","large-v1","large-v2"]
- if istranslate and self.current_model_size in translatable_model:
- result = self.model.transcribe(audio=audio,language=lang,verbose=False,task="translate",progress_callback=progress_callback)
- else :
- result = self.model.transcribe(audio=audio,language=lang,verbose=False,progress_callback=progress_callback)
-
- progress(1,desc="Completed!")
-
- file_name, file_ext = os.path.splitext(os.path.basename(fileobj.orig_name))
- file_name = file_name[:-9]
- file_name = safe_filename(file_name)
-
- if subformat == "SRT":
- subtitle = get_srt(result["segments"])
- elif subformat == "WebVTT":
- subtitle = get_vtt(result["segments"])
-
- files_info[file_name] = subtitle
-
- total_result = ''
- for file_name,subtitle in files_info.items():
- total_result+='------------------------------------\n'
- total_result+=f'{file_name}\n\n'
- total_result+=f'{subtitle}'
-
- return f"\n\n{total_result}"
-
- def transcribe_youtube(self,youtubelink
- ,model_size,lang,subformat,istranslate,
- progress=gr.Progress()):
-
- def progress_callback(progress_value):
- progress(progress_value,desc="Transcribing..")
-
- if model_size != self.current_model_size:
- progress(0,desc="Initializing Model..")
- self.current_model_size = model_size
- self.model = whisper.load_model(name=model_size,download_root="models")
-
- if lang == "Automatic Detection" :
- lang = None
-
- progress(0,desc="Loading Audio from Youtube..")
- yt = get_ytdata(youtubelink)
- audio = whisper.load_audio(get_ytaudio(yt))
-
- translatable_model = ["large","large-v1","large-v2"]
- if istranslate and self.current_model_size in translatable_model:
- result = self.model.transcribe(audio=audio,language=lang,verbose=False,task="translate",progress_callback=progress_callback)
- else :
- result = self.model.transcribe(audio=audio,language=lang,verbose=False,progress_callback=progress_callback)
-
- progress(1,desc="Completed!")
-
- file_name = safe_filename(yt.title)
-
- if subformat == "SRT":
- subtitle = get_srt(result["segments"])
- elif subformat == "WebVTT":
- subtitle = get_vtt(result["segments"])
-
- return f"\n\n{subtitle}"
-
- def transcribe_mic(self,micaudio
- ,model_size,lang,subformat,istranslate,
- progress=gr.Progress()):
-
- def progress_callback(progress_value):
- progress(progress_value,desc="Transcribing..")
-
- if model_size != self.current_model_size:
- progress(0,desc="Initializing Model..")
- self.current_model_size = model_size
- self.model = whisper.load_model(name=model_size,download_root="models")
-
- if lang == "Automatic Detection" :
- lang = None
-
- progress(0,desc="Loading Audio..")
-
- translatable_model = ["large","large-v1","large-v2"]
- if istranslate and self.current_model_size in translatable_model:
- result = self.model.transcribe(audio=micaudio,language=lang,verbose=False,task="translate",progress_callback=progress_callback)
- else :
- result = self.model.transcribe(audio=micaudio,language=lang,verbose=False,progress_callback=progress_callback)
-
- progress(1,desc="Completed!")
-
- if subformat == "SRT":
- subtitle = get_srt(result["segments"])
- elif subformat == "WebVTT":
- subtitle = get_vtt(result["segments"])
-
- return f"\n\n{subtitle}"
-
-
\ No newline at end of file
diff --git a/spaces/jmesikto/whisper-webui/cli.py b/spaces/jmesikto/whisper-webui/cli.py
deleted file mode 100644
index 70c08138c9274c3576d28356e53f3d94a9968a2e..0000000000000000000000000000000000000000
--- a/spaces/jmesikto/whisper-webui/cli.py
+++ /dev/null
@@ -1,173 +0,0 @@
-import argparse
-import os
-import pathlib
-from urllib.parse import urlparse
-import warnings
-import numpy as np
-
-import torch
-from app import VadOptions, WhisperTranscriber
-from src.config import ApplicationConfig, VadInitialPromptMode
-from src.download import download_url
-from src.languages import get_language_names
-
-from src.utils import optional_float, optional_int, str2bool
-from src.whisper.whisperFactory import create_whisper_container
-
-def cli():
- app_config = ApplicationConfig.create_default()
- whisper_models = app_config.get_model_names()
-
- # For the CLI, we fallback to saving the output to the current directory
- output_dir = app_config.output_dir if app_config.output_dir is not None else "."
-
- # Environment variable overrides
- default_whisper_implementation = os.environ.get("WHISPER_IMPLEMENTATION", app_config.whisper_implementation)
-
- parser = argparse.ArgumentParser(formatter_class=argparse.ArgumentDefaultsHelpFormatter)
- parser.add_argument("audio", nargs="+", type=str, \
- help="audio file(s) to transcribe")
- parser.add_argument("--model", default=app_config.default_model_name, choices=whisper_models, \
- help="name of the Whisper model to use") # medium
- parser.add_argument("--model_dir", type=str, default=app_config.model_dir, \
- help="the path to save model files; uses ~/.cache/whisper by default")
- parser.add_argument("--device", default=app_config.device, \
- help="device to use for PyTorch inference")
- parser.add_argument("--output_dir", "-o", type=str, default=output_dir, \
- help="directory to save the outputs")
- parser.add_argument("--verbose", type=str2bool, default=app_config.verbose, \
- help="whether to print out the progress and debug messages")
- parser.add_argument("--whisper_implementation", type=str, default=default_whisper_implementation, choices=["whisper", "faster-whisper"],\
- help="the Whisper implementation to use")
-
- parser.add_argument("--task", type=str, default=app_config.task, choices=["transcribe", "translate"], \
- help="whether to perform X->X speech recognition ('transcribe') or X->English translation ('translate')")
- parser.add_argument("--language", type=str, default=app_config.language, choices=sorted(get_language_names()), \
- help="language spoken in the audio, specify None to perform language detection")
-
- parser.add_argument("--vad", type=str, default=app_config.default_vad, choices=["none", "silero-vad", "silero-vad-skip-gaps", "silero-vad-expand-into-gaps", "periodic-vad"], \
- help="The voice activity detection algorithm to use") # silero-vad
- parser.add_argument("--vad_initial_prompt_mode", type=str, default=app_config.vad_initial_prompt_mode, choices=["prepend_all_segments", "prepend_first_segment"], \
- help="Whether or not to prepend the initial prompt to each VAD segment (prepend_all_segments), or just the first segment (prepend_first_segment)") # prepend_first_segment
- parser.add_argument("--vad_merge_window", type=optional_float, default=app_config.vad_merge_window, \
- help="The window size (in seconds) to merge voice segments")
- parser.add_argument("--vad_max_merge_size", type=optional_float, default=app_config.vad_max_merge_size,\
- help="The maximum size (in seconds) of a voice segment")
- parser.add_argument("--vad_padding", type=optional_float, default=app_config.vad_padding, \
- help="The padding (in seconds) to add to each voice segment")
- parser.add_argument("--vad_prompt_window", type=optional_float, default=app_config.vad_prompt_window, \
- help="The window size of the prompt to pass to Whisper")
- parser.add_argument("--vad_cpu_cores", type=int, default=app_config.vad_cpu_cores, \
- help="The number of CPU cores to use for VAD pre-processing.") # 1
- parser.add_argument("--vad_parallel_devices", type=str, default=app_config.vad_parallel_devices, \
- help="A commma delimited list of CUDA devices to use for parallel processing. If None, disable parallel processing.") # ""
- parser.add_argument("--auto_parallel", type=bool, default=app_config.auto_parallel, \
- help="True to use all available GPUs and CPU cores for processing. Use vad_cpu_cores/vad_parallel_devices to specify the number of CPU cores/GPUs to use.") # False
-
- parser.add_argument("--temperature", type=float, default=app_config.temperature, \
- help="temperature to use for sampling")
- parser.add_argument("--best_of", type=optional_int, default=app_config.best_of, \
- help="number of candidates when sampling with non-zero temperature")
- parser.add_argument("--beam_size", type=optional_int, default=app_config.beam_size, \
- help="number of beams in beam search, only applicable when temperature is zero")
- parser.add_argument("--patience", type=float, default=app_config.patience, \
- help="optional patience value to use in beam decoding, as in https://arxiv.org/abs/2204.05424, the default (1.0) is equivalent to conventional beam search")
- parser.add_argument("--length_penalty", type=float, default=app_config.length_penalty, \
- help="optional token length penalty coefficient (alpha) as in https://arxiv.org/abs/1609.08144, uses simple lengt normalization by default")
-
- parser.add_argument("--suppress_tokens", type=str, default=app_config.suppress_tokens, \
- help="comma-separated list of token ids to suppress during sampling; '-1' will suppress most special characters except common punctuations")
- parser.add_argument("--initial_prompt", type=str, default=app_config.initial_prompt, \
- help="optional text to provide as a prompt for the first window.")
- parser.add_argument("--condition_on_previous_text", type=str2bool, default=app_config.condition_on_previous_text, \
- help="if True, provide the previous output of the model as a prompt for the next window; disabling may make the text inconsistent across windows, but the model becomes less prone to getting stuck in a failure loop")
- parser.add_argument("--fp16", type=str2bool, default=app_config.fp16, \
- help="whether to perform inference in fp16; True by default")
- parser.add_argument("--compute_type", type=str, default=app_config.compute_type, choices=["default", "auto", "int8", "int8_float16", "int16", "float16", "float32"], \
- help="the compute type to use for inference")
-
- parser.add_argument("--temperature_increment_on_fallback", type=optional_float, default=app_config.temperature_increment_on_fallback, \
- help="temperature to increase when falling back when the decoding fails to meet either of the thresholds below")
- parser.add_argument("--compression_ratio_threshold", type=optional_float, default=app_config.compression_ratio_threshold, \
- help="if the gzip compression ratio is higher than this value, treat the decoding as failed")
- parser.add_argument("--logprob_threshold", type=optional_float, default=app_config.logprob_threshold, \
- help="if the average log probability is lower than this value, treat the decoding as failed")
- parser.add_argument("--no_speech_threshold", type=optional_float, default=app_config.no_speech_threshold, \
- help="if the probability of the <|nospeech|> token is higher than this value AND the decoding has failed due to `logprob_threshold`, consider the segment as silence")
-
- args = parser.parse_args().__dict__
- model_name: str = args.pop("model")
- model_dir: str = args.pop("model_dir")
- output_dir: str = args.pop("output_dir")
- device: str = args.pop("device")
- os.makedirs(output_dir, exist_ok=True)
-
- whisper_implementation = args.pop("whisper_implementation")
- print(f"Using {whisper_implementation} for Whisper")
-
- if model_name.endswith(".en") and args["language"] not in {"en", "English"}:
- warnings.warn(f"{model_name} is an English-only model but receipted '{args['language']}'; using English instead.")
- args["language"] = "en"
-
- temperature = args.pop("temperature")
- temperature_increment_on_fallback = args.pop("temperature_increment_on_fallback")
- if temperature_increment_on_fallback is not None:
- temperature = tuple(np.arange(temperature, 1.0 + 1e-6, temperature_increment_on_fallback))
- else:
- temperature = [temperature]
-
- vad = args.pop("vad")
- vad_initial_prompt_mode = args.pop("vad_initial_prompt_mode")
- vad_merge_window = args.pop("vad_merge_window")
- vad_max_merge_size = args.pop("vad_max_merge_size")
- vad_padding = args.pop("vad_padding")
- vad_prompt_window = args.pop("vad_prompt_window")
- vad_cpu_cores = args.pop("vad_cpu_cores")
- auto_parallel = args.pop("auto_parallel")
-
- compute_type = args.pop("compute_type")
-
- transcriber = WhisperTranscriber(delete_uploaded_files=False, vad_cpu_cores=vad_cpu_cores, app_config=app_config)
- transcriber.set_parallel_devices(args.pop("vad_parallel_devices"))
- transcriber.set_auto_parallel(auto_parallel)
-
- model = create_whisper_container(whisper_implementation=whisper_implementation, model_name=model_name,
- device=device, compute_type=compute_type, download_root=model_dir, models=app_config.models)
-
- if (transcriber._has_parallel_devices()):
- print("Using parallel devices:", transcriber.parallel_device_list)
-
- for audio_path in args.pop("audio"):
- sources = []
-
- # Detect URL and download the audio
- if (uri_validator(audio_path)):
- # Download from YouTube/URL directly
- for source_path in download_url(audio_path, maxDuration=-1, destinationDirectory=output_dir, playlistItems=None):
- source_name = os.path.basename(source_path)
- sources.append({ "path": source_path, "name": source_name })
- else:
- sources.append({ "path": audio_path, "name": os.path.basename(audio_path) })
-
- for source in sources:
- source_path = source["path"]
- source_name = source["name"]
-
- vadOptions = VadOptions(vad, vad_merge_window, vad_max_merge_size, vad_padding, vad_prompt_window,
- VadInitialPromptMode.from_string(vad_initial_prompt_mode))
-
- result = transcriber.transcribe_file(model, source_path, temperature=temperature, vadOptions=vadOptions, **args)
-
- transcriber.write_result(result, source_name, output_dir)
-
- transcriber.close()
-
-def uri_validator(x):
- try:
- result = urlparse(x)
- return all([result.scheme, result.netloc])
- except:
- return False
-
-if __name__ == '__main__':
- cli()
\ No newline at end of file
diff --git a/spaces/joaopereirajp/livvieChatBot/venv/lib/python3.9/site-packages/Crypto/Hash/MD4.py b/spaces/joaopereirajp/livvieChatBot/venv/lib/python3.9/site-packages/Crypto/Hash/MD4.py
deleted file mode 100644
index be12b192a155b65455f1be27c0669e2fcc70b9c4..0000000000000000000000000000000000000000
--- a/spaces/joaopereirajp/livvieChatBot/venv/lib/python3.9/site-packages/Crypto/Hash/MD4.py
+++ /dev/null
@@ -1,185 +0,0 @@
-# ===================================================================
-#
-# Copyright (c) 2014, Legrandin
-# All rights reserved.
-#
-# Redistribution and use in source and binary forms, with or without
-# modification, are permitted provided that the following conditions
-# are met:
-#
-# 1. Redistributions of source code must retain the above copyright
-# notice, this list of conditions and the following disclaimer.
-# 2. Redistributions in binary form must reproduce the above copyright
-# notice, this list of conditions and the following disclaimer in
-# the documentation and/or other materials provided with the
-# distribution.
-#
-# THIS SOFTWARE IS PROVIDED BY THE COPYRIGHT HOLDERS AND CONTRIBUTORS
-# "AS IS" AND ANY EXPRESS OR IMPLIED WARRANTIES, INCLUDING, BUT NOT
-# LIMITED TO, THE IMPLIED WARRANTIES OF MERCHANTABILITY AND FITNESS
-# FOR A PARTICULAR PURPOSE ARE DISCLAIMED. IN NO EVENT SHALL THE
-# COPYRIGHT HOLDER OR CONTRIBUTORS BE LIABLE FOR ANY DIRECT, INDIRECT,
-# INCIDENTAL, SPECIAL, EXEMPLARY, OR CONSEQUENTIAL DAMAGES (INCLUDING,
-# BUT NOT LIMITED TO, PROCUREMENT OF SUBSTITUTE GOODS OR SERVICES;
-# LOSS OF USE, DATA, OR PROFITS; OR BUSINESS INTERRUPTION) HOWEVER
-# CAUSED AND ON ANY THEORY OF LIABILITY, WHETHER IN CONTRACT, STRICT
-# LIABILITY, OR TORT (INCLUDING NEGLIGENCE OR OTHERWISE) ARISING IN
-# ANY WAY OUT OF THE USE OF THIS SOFTWARE, EVEN IF ADVISED OF THE
-# POSSIBILITY OF SUCH DAMAGE.
-# ===================================================================
-
-"""
-MD4 is specified in RFC1320_ and produces the 128 bit digest of a message.
-
- >>> from Crypto.Hash import MD4
- >>>
- >>> h = MD4.new()
- >>> h.update(b'Hello')
- >>> print h.hexdigest()
-
-MD4 stand for Message Digest version 4, and it was invented by Rivest in 1990.
-This algorithm is insecure. Do not use it for new designs.
-
-.. _RFC1320: http://tools.ietf.org/html/rfc1320
-"""
-
-from Crypto.Util.py3compat import bord
-
-from Crypto.Util._raw_api import (load_pycryptodome_raw_lib,
- VoidPointer, SmartPointer,
- create_string_buffer,
- get_raw_buffer, c_size_t,
- c_uint8_ptr)
-
-_raw_md4_lib = load_pycryptodome_raw_lib(
- "Crypto.Hash._MD4",
- """
- int md4_init(void **shaState);
- int md4_destroy(void *shaState);
- int md4_update(void *hs,
- const uint8_t *buf,
- size_t len);
- int md4_digest(const void *shaState,
- uint8_t digest[20]);
- int md4_copy(const void *src, void *dst);
- """)
-
-
-class MD4Hash(object):
- """Class that implements an MD4 hash
- """
-
- #: The size of the resulting hash in bytes.
- digest_size = 16
- #: The internal block size of the hash algorithm in bytes.
- block_size = 64
- #: ASN.1 Object ID
- oid = "1.2.840.113549.2.4"
-
- def __init__(self, data=None):
- state = VoidPointer()
- result = _raw_md4_lib.md4_init(state.address_of())
- if result:
- raise ValueError("Error %d while instantiating MD4"
- % result)
- self._state = SmartPointer(state.get(),
- _raw_md4_lib.md4_destroy)
- if data:
- self.update(data)
-
- def update(self, data):
- """Continue hashing of a message by consuming the next chunk of data.
-
- Repeated calls are equivalent to a single call with the concatenation
- of all the arguments. In other words:
-
- >>> m.update(a); m.update(b)
-
- is equivalent to:
-
- >>> m.update(a+b)
-
- :Parameters:
- data : byte string/byte array/memoryview
- The next chunk of the message being hashed.
- """
-
- result = _raw_md4_lib.md4_update(self._state.get(),
- c_uint8_ptr(data),
- c_size_t(len(data)))
- if result:
- raise ValueError("Error %d while instantiating MD4"
- % result)
-
- def digest(self):
- """Return the **binary** (non-printable) digest of the message that
- has been hashed so far.
-
- This method does not change the state of the hash object.
- You can continue updating the object after calling this function.
-
- :Return: A byte string of `digest_size` bytes. It may contain non-ASCII
- characters, including null bytes.
- """
-
- bfr = create_string_buffer(self.digest_size)
- result = _raw_md4_lib.md4_digest(self._state.get(),
- bfr)
- if result:
- raise ValueError("Error %d while instantiating MD4"
- % result)
-
- return get_raw_buffer(bfr)
-
- def hexdigest(self):
- """Return the **printable** digest of the message that has been
- hashed so far.
-
- This method does not change the state of the hash object.
-
- :Return: A string of 2* `digest_size` characters. It contains only
- hexadecimal ASCII digits.
- """
-
- return "".join(["%02x" % bord(x) for x in self.digest()])
-
- def copy(self):
- """Return a copy ("clone") of the hash object.
-
- The copy will have the same internal state as the original hash
- object.
- This can be used to efficiently compute the digests of strings that
- share a common initial substring.
-
- :Return: A hash object of the same type
- """
-
- clone = MD4Hash()
- result = _raw_md4_lib.md4_copy(self._state.get(),
- clone._state.get())
- if result:
- raise ValueError("Error %d while copying MD4" % result)
- return clone
-
- def new(self, data=None):
- return MD4Hash(data)
-
-
-def new(data=None):
- """Return a fresh instance of the hash object.
-
- :Parameters:
- data : byte string/byte array/memoryview
- The very first chunk of the message to hash.
- It is equivalent to an early call to `MD4Hash.update()`.
- Optional.
-
- :Return: A `MD4Hash` object
- """
- return MD4Hash().new(data)
-
-#: The size of the resulting hash in bytes.
-digest_size = MD4Hash.digest_size
-
-#: The internal block size of the hash algorithm in bytes.
-block_size = MD4Hash.block_size
diff --git a/spaces/joaopereirajp/livvieChatBot/venv/lib/python3.9/site-packages/fontTools/ttLib/tables/_v_h_e_a.py b/spaces/joaopereirajp/livvieChatBot/venv/lib/python3.9/site-packages/fontTools/ttLib/tables/_v_h_e_a.py
deleted file mode 100644
index 965674203db1b76cff23e3c640d4b7cadca5ae98..0000000000000000000000000000000000000000
--- a/spaces/joaopereirajp/livvieChatBot/venv/lib/python3.9/site-packages/fontTools/ttLib/tables/_v_h_e_a.py
+++ /dev/null
@@ -1,126 +0,0 @@
-from fontTools.misc import sstruct
-from fontTools.misc.textTools import safeEval
-from fontTools.misc.fixedTools import (
- ensureVersionIsLong as fi2ve,
- versionToFixed as ve2fi,
-)
-from . import DefaultTable
-import math
-
-
-vheaFormat = """
- > # big endian
- tableVersion: L
- ascent: h
- descent: h
- lineGap: h
- advanceHeightMax: H
- minTopSideBearing: h
- minBottomSideBearing: h
- yMaxExtent: h
- caretSlopeRise: h
- caretSlopeRun: h
- caretOffset: h
- reserved1: h
- reserved2: h
- reserved3: h
- reserved4: h
- metricDataFormat: h
- numberOfVMetrics: H
-"""
-
-
-class table__v_h_e_a(DefaultTable.DefaultTable):
-
- # Note: Keep in sync with table__h_h_e_a
-
- dependencies = ["vmtx", "glyf", "CFF ", "CFF2"]
-
- def decompile(self, data, ttFont):
- sstruct.unpack(vheaFormat, data, self)
-
- def compile(self, ttFont):
- if ttFont.recalcBBoxes and (
- ttFont.isLoaded("glyf")
- or ttFont.isLoaded("CFF ")
- or ttFont.isLoaded("CFF2")
- ):
- self.recalc(ttFont)
- self.tableVersion = fi2ve(self.tableVersion)
- return sstruct.pack(vheaFormat, self)
-
- def recalc(self, ttFont):
- if "vmtx" in ttFont:
- vmtxTable = ttFont["vmtx"]
- self.advanceHeightMax = max(adv for adv, _ in vmtxTable.metrics.values())
-
- boundsHeightDict = {}
- if "glyf" in ttFont:
- glyfTable = ttFont["glyf"]
- for name in ttFont.getGlyphOrder():
- g = glyfTable[name]
- if g.numberOfContours == 0:
- continue
- if g.numberOfContours < 0 and not hasattr(g, "yMax"):
- # Composite glyph without extents set.
- # Calculate those.
- g.recalcBounds(glyfTable)
- boundsHeightDict[name] = g.yMax - g.yMin
- elif "CFF " in ttFont or "CFF2" in ttFont:
- if "CFF " in ttFont:
- topDict = ttFont["CFF "].cff.topDictIndex[0]
- else:
- topDict = ttFont["CFF2"].cff.topDictIndex[0]
- charStrings = topDict.CharStrings
- for name in ttFont.getGlyphOrder():
- cs = charStrings[name]
- bounds = cs.calcBounds(charStrings)
- if bounds is not None:
- boundsHeightDict[name] = int(
- math.ceil(bounds[3]) - math.floor(bounds[1])
- )
-
- if boundsHeightDict:
- minTopSideBearing = float("inf")
- minBottomSideBearing = float("inf")
- yMaxExtent = -float("inf")
- for name, boundsHeight in boundsHeightDict.items():
- advanceHeight, tsb = vmtxTable[name]
- bsb = advanceHeight - tsb - boundsHeight
- extent = tsb + boundsHeight
- minTopSideBearing = min(minTopSideBearing, tsb)
- minBottomSideBearing = min(minBottomSideBearing, bsb)
- yMaxExtent = max(yMaxExtent, extent)
- self.minTopSideBearing = minTopSideBearing
- self.minBottomSideBearing = minBottomSideBearing
- self.yMaxExtent = yMaxExtent
-
- else: # No glyph has outlines.
- self.minTopSideBearing = 0
- self.minBottomSideBearing = 0
- self.yMaxExtent = 0
-
- def toXML(self, writer, ttFont):
- formatstring, names, fixes = sstruct.getformat(vheaFormat)
- for name in names:
- value = getattr(self, name)
- if name == "tableVersion":
- value = fi2ve(value)
- value = "0x%08x" % value
- writer.simpletag(name, value=value)
- writer.newline()
-
- def fromXML(self, name, attrs, content, ttFont):
- if name == "tableVersion":
- setattr(self, name, ve2fi(attrs["value"]))
- return
- setattr(self, name, safeEval(attrs["value"]))
-
- # reserved0 is caretOffset for legacy reasons
- @property
- def reserved0(self):
- return self.caretOffset
-
- @reserved0.setter
- def reserved0(self, value):
- self.caretOffset = value
diff --git a/spaces/jordonpeter01/ai-comic-factory/README.md b/spaces/jordonpeter01/ai-comic-factory/README.md
deleted file mode 100644
index a92de89f1558c0d4f17a57c6ce4a8381daecd4dd..0000000000000000000000000000000000000000
--- a/spaces/jordonpeter01/ai-comic-factory/README.md
+++ /dev/null
@@ -1,128 +0,0 @@
----
-title: AI Comic Factory
-emoji: 👩🎨
-colorFrom: red
-colorTo: yellow
-sdk: docker
-pinned: true
-app_port: 3000
----
-
-# AI Comic Factory
-
-## Running the project at home
-
-First, I would like to highlight that everything is open-source (see [here](https://huggingface.co/spaces/jbilcke-hf/ai-comic-factory/tree/main), [here](https://huggingface.co/spaces/jbilcke-hf/VideoChain-API/tree/main), [here](https://huggingface.co/spaces/hysts/SD-XL/tree/main), [here](https://github.com/huggingface/text-generation-inference)).
-
-However the project isn't a monolithic Space that can be duplicated and ran immediately:
-it requires various components to run for the frontend, backend, LLM, SDXL etc.
-
-If you try to duplicate the project and open the `.env` you will see it requires some variables:
-
-- `LLM_ENGINE`: can be either "INFERENCE_API" or "INFERENCE_ENDPOINT"
-- `HF_API_TOKEN`: necessary if you decide to use an inference api model or a custom inference endpoint
-- `HF_INFERENCE_ENDPOINT_URL`: necessary if you decide to use a custom inference endpoint
-- `RENDERING_ENGINE`: can only be "VIDEOCHAIN" or "REPLICATE" for now, unless you code your custom solution
-- `VIDEOCHAIN_API_URL`: url to the VideoChain API server
-- `VIDEOCHAIN_API_TOKEN`: secret token to access the VideoChain API server
-- `REPLICATE_API_TOKEN`: in case you want to use Replicate.com
-- `REPLICATE_API_MODEL`: optional, defaults to "stabilityai/sdxl"
-- `REPLICATE_API_MODEL_VERSION`: optional, in case you want to change the version
-
-In addition, there are some community sharing variables that you can just ignore.
-Those variables are not required to run the AI Comic Factory on your own website or computer
-(they are meant to create a connection with the Hugging Face community,
-and thus only make sense for official Hugging Face apps):
-- `NEXT_PUBLIC_ENABLE_COMMUNITY_SHARING`: you don't need this
-- `COMMUNITY_API_URL`: you don't need this
-- `COMMUNITY_API_TOKEN`: you don't need this
-- `COMMUNITY_API_ID`: you don't need this
-
-Please read the `.env` default config file for more informations.
-To customise a variable locally, you should create a `.env.local`
-(do not commit this file as it will contain your secrets).
-
--> If you intend to run it with local, cloud-hosted and/or proprietary models **you are going to need to code 👨💻**.
-
-## The LLM API (Large Language Model)
-
-Currently the AI Comic Factory uses [Llama-2 70b](https://huggingface.co/blog/llama2) through an [Inference Endpoint](https://huggingface.co/docs/inference-endpoints/index).
-
-You have three options:
-
-### Option 1: Use an Inference API model
-
-This is a new option added recently, where you can use one of the models from the Hugging Face Hub. By default we suggest to use CodeLlama 34b as it will provide better results than the 7b model.
-
-To activate it, create a `.env.local` configuration file:
-
-```bash
-LLM_ENGINE="INFERENCE_API"
-
-HF_API_TOKEN="Your Hugging Face token"
-
-# codellama/CodeLlama-7b-hf" is used by default, but you can change this
-# note: You should use a model able to generate JSON responses,
-# so it is storngly suggested to use at least the 34b model
-HF_INFERENCE_API_MODEL="codellama/CodeLlama-7b-hf"
-```
-
-### Option 2: Use an Inference Endpoint URL
-
-If you would like to run the AI Comic Factory on a private LLM running on the Hugging Face Inference Endpoint service, create a `.env.local` configuration file:
-
-```bash
-LLM_ENGINE="INFERENCE_ENDPOINT"
-
-HF_API_TOKEN="Your Hugging Face token"
-
-HF_INFERENCE_ENDPOINT_URL="path to your inference endpoint url"
-```
-
-To run this kind of LLM locally, you can use [TGI](https://github.com/huggingface/text-generation-inference) (Please read [this post](https://github.com/huggingface/text-generation-inference/issues/726) for more information about the licensing).
-
-### Option 3: Fork and modify the code to use a different LLM system
-
-Another option could be to disable the LLM completely and replace it with another LLM protocol and/or provider (eg. OpenAI, Replicate), or a human-generated story instead (by returning mock or static data).
-
-
-### Notes
-
-It is possible that I modify the AI Comic Factory to make it easier in the future (eg. add support for OpenAI or Replicate)
-
-## The Rendering API
-
-This API is used to generate the panel images. This is an API I created for my various projects at Hugging Face.
-
-I haven't written documentation for it yet, but basically it is "just a wrapper ™" around other existing APIs:
-
-- The [hysts/SD-XL](https://huggingface.co/spaces/hysts/SD-XL?duplicate=true) Space by [@hysts](https://huggingface.co/hysts)
-- And other APIs for making videos, adding audio etc.. but you won't need them for the AI Comic Factory
-
-### Option 1: Deploy VideoChain yourself
-
-You will have to [clone](https://huggingface.co/spaces/jbilcke-hf/VideoChain-API?duplicate=true) the [source-code](https://huggingface.co/spaces/jbilcke-hf/VideoChain-API/tree/main)
-
-Unfortunately, I haven't had the time to write the documentation for VideoChain yet.
-(When I do I will update this document to point to the VideoChain's README)
-
-
-### Option 2: Use Replicate
-
-To use Replicate, create a `.env.local` configuration file:
-
-```bash
-RENDERING_ENGINE="REPLICATE"
-
-REPLICATE_API_TOKEN="Your Replicate token"
-
-REPLICATE_API_MODEL="stabilityai/sdxl"
-
-REPLICATE_API_MODEL_VERSION="da77bc59ee60423279fd632efb4795ab731d9e3ca9705ef3341091fb989b7eaf"
-```
-
-### Option 3: Use another SDXL API
-
-If you fork the project you will be able to modify the code to use the Stable Diffusion technology of your choice (local, open-source, proprietary, your custom HF Space etc).
-
-It would even be something else, such as Dall-E.
diff --git a/spaces/joshuasundance/langchain-streamlit-demo/AI_CHANGELOG.md b/spaces/joshuasundance/langchain-streamlit-demo/AI_CHANGELOG.md
deleted file mode 100644
index 3dbb9e953262b0d0f746d8e56e5b57c48d3b0ed1..0000000000000000000000000000000000000000
--- a/spaces/joshuasundance/langchain-streamlit-demo/AI_CHANGELOG.md
+++ /dev/null
@@ -1,274 +0,0 @@
-# AI CHANGELOG
-## [Bumped application version from 0.1.1 to 0.1.2](https://github.com/joshuasundance-swca/langchain-streamlit-demo/commit/a51a26dd0cf4b33726ac3aa84b41acc103b0c06f)
-Wed Nov 1 16:03:52 2023 -0400
-- Updated the application version in bumpver.toml, resources.yaml, and app.py. This includes the version used for the Docker image in the Kubernetes deployment configuration.
-## [Updated default checkbox value and removed initial chatbot message](https://github.com/joshuasundance-swca/langchain-streamlit-demo/commit/3d59c85771f67f633f9498ffa3705880576de914)
-Wed Nov 1 15:51:00 2023 -0400
-- Changed the default value of the 'Document Chat' checkbox to be true if a file is uploaded and false if not.
-- Removed the condition that disables the 'Chain Type' dropdown when 'Document Chat' is not selected.
-- Eliminated the automatic 'Hello! I'm a helpful AI chatbot. Ask me a question!' message when the chat history is empty.
-## [Version Bump to 0.1.1](https://github.com/joshuasundance-swca/langchain-streamlit-demo/commit/fc0e83182e47a9f41465fa815b286455b10e78f9)
-Wed Nov 1 13:58:11 2023 -0400
-- This commit represents a version bump from 0.1.0 to 0.1.1. Changes were made in the bumpver.toml file to update the current version. The Docker image reference in the Kubernetes resources.yaml file was also updated to reflect the new version. Lastly, the __version__ variable in the langchain-streamlit-demo/app.py file was updated.
-## [Handled additional exception in app.py](https://github.com/joshuasundance-swca/langchain-streamlit-demo/commit/8a23b378977a263201791101e5a0ebc56e4f5f05)
-Wed Nov 1 13:55:35 2023 -0400
-- Updated the exception handling in app.py to include LangSmithNotFoundError along with the existing LangSmithError. This change improves the robustness of the error handling mechanism.
-## [Updated project version to 0.1.0](https://github.com/joshuasundance-swca/langchain-streamlit-demo/commit/bbb9f000d8907e12c2aea643fb01e234b8d771bc)
-Mon Oct 30 12:03:02 2023 -0400
-- The project's version number has been updated from 0.0.16 to 0.1.0 in the bumpver.toml file, kubernetes resource file, and the main application file.
-## [Added mistralai/Mistral-7B-Instruct-v0.1 to Anyscale Endpoints](https://github.com/joshuasundance-swca/langchain-streamlit-demo/commit/d8cef94cffde0307292685d7273a0bf7a0974d02)
-Mon Oct 30 11:31:43 2023 -0400
-- In the README.md file, a new endpoint mistralai/Mistral-7B-Instruct-v0.1 was added under the section of Anyscale Endpoints.
-- In the defaults.py file, the same endpoint was added to the MODEL_DICT dictionary under the key-value pair 'mistralai/Mistral-7B-Instruct-v0.1': 'Anyscale Endpoints'.
-- The SUPPORTED_MODELS list was updated accordingly to include this new endpoint.
-## [Updated langsmith package version](https://github.com/joshuasundance-swca/langchain-streamlit-demo/commit/018041a3bdd72aaf3ab62b6eecba51ac18c93bcd)
-Mon Oct 30 12:50:15 2023 +0000
-- The langsmith package version in requirements.txt has been updated from 0.0.49 to 0.0.53. This update might include bug fixes, new features, or improvements.
-## [Updated langchain version in requirements](https://github.com/joshuasundance-swca/langchain-streamlit-demo/commit/1215664e1bcb9b0a1f7f90a608fa16dc68dbbd0a)
-Mon Oct 30 12:49:54 2023 +0000
-- The langchain package version in requirements.txt has been updated from 0.0.320 to 0.0.325. This update might include bug fixes, security patches or new features.
-## [Bump version from 0.0.15 to 0.0.16](https://github.com/joshuasundance-swca/langchain-streamlit-demo/commit/04871e0362967e38aceb00aa4fd13818a793ff1a)
-Mon Oct 23 12:57:22 2023 -0400
-- Updated the current version in bumpver.toml from 0.0.15 to 0.0.16.
-- In the Kubernetes resources.yaml, updated the image version for langchain-streamlit-demo from 0.0.15 to 0.0.16.
-- In langchain-streamlit-demo/app.py, updated the __version__ variable from 0.0.15 to 0.0.16.
-## [Updated package versions in requirements.txt](https://github.com/joshuasundance-swca/langchain-streamlit-demo/commit/839541a4fc5515d4554a36946001f1cee80f6fdc)
-Mon Oct 23 12:49:36 2023 -0400
-- Updated the versions of 'anthropic', 'langchain', and 'langsmith' in the requirements file. 'anthropic' is updated from version 0.3.11 to 0.5.0, 'langchain' from 0.0.315 to 0.0.320, and 'langsmith' from 0.0.44 to 0.0.49.
-## [Added 'validators' package to requirements](https://github.com/joshuasundance-swca/langchain-streamlit-demo/commit/a1e0ab15cde332cd8efcba310fb67e25cb990783)
-Fri Oct 20 22:54:23 2023 +0000
-- The 'validators' package was added to the requirements.txt file. This package is not directly required by the project, but it has been pinned by Snyk to version 0.21.0 or newer to avoid a potential vulnerability.
-## [Updated badges in README](https://github.com/joshuasundance-swca/langchain-streamlit-demo/commit/32f02019c445fb88beb73f00c6ffd0a17ff2a5d3)
-Thu Oct 19 15:19:10 2023 -0400
-- Replaced the Docker badge with a 'Push to Docker Hub' GitHub Actions workflow badge.
-- Added a 'Push to HuggingFace Space' GitHub Actions workflow badge.
-- Added an 'Update AI Changelog on Push to Main' GitHub Actions workflow badge.
-## [Added Azure OpenAI Service to README](https://github.com/joshuasundance-swca/langchain-streamlit-demo/commit/3353ff5eaa74c050414bf6b67ac590ac25d19f74)
-Thu Oct 19 11:08:36 2023 -0400
-- Updated README.md to include Azure OpenAI Service in the list of services and endpoints. A placeholder for configurable endpoints under Azure OpenAI Service has also been added.
-## [Added Black component to misc.xml and updated badges in README.md](https://github.com/joshuasundance-swca/langchain-streamlit-demo/commit/1fb27e8b839a4c3d526da009c05ef3c08a1c2786)
-Thu Oct 19 10:49:24 2023 -0400
-- The commit introduces two changes:
-- 1. A new Black component is added to the .idea/misc.xml file. This suggests that the Black Python code formatter has been configured for the project.
-- 2. The README.md file has been updated to include new badges for code maintainability, issues, technical debt, and known vulnerabilities. The order of the existing badges has also been rearranged.
-## [Version Bump from 0.0.14 to 0.0.15](https://github.com/joshuasundance-swca/langchain-streamlit-demo/commit/cc115e36633b7d899076da029c59dda03ca177ec)
-Mon Oct 16 14:09:34 2023 -0400
-- The version number has been increased from 0.0.14 to 0.0.15. This change has been reflected in the bumpver.toml file, the Kubernetes resources file, and the langchain-streamlit-demo app.py file. The Docker image used in the Kubernetes resources file has also been updated to reflect this new version number.
-## [Updated several package versions in requirements.txt](https://github.com/joshuasundance-swca/langchain-streamlit-demo/commit/cfa1e0b55c4f108b30ff6c7389668f1677f91437)
-Mon Oct 16 14:02:49 2023 -0400
-- Updated the version of langchain from 0.0.308 to 0.0.315.
-- Updated the version of langsmith from 0.0.43 to 0.0.44.
-- Updated the version of pypdf from 3.16.2 to 3.16.4.
-## [Updated environment variable name in Kubernetes config](https://github.com/joshuasundance-swca/langchain-streamlit-demo/commit/62281947edc36d93259d723b8b4b63f3b9b646d1)
-Fri Oct 6 21:19:03 2023 -0400
-- In the Kubernetes configuration file 'resources.yaml', the environment variable name 'SHOW_LANGCHAIN_OPTIONS' was replaced with 'SHOW_LANGSMITH_OPTIONS'. This change reflects an update in the naming convention or the service being used.
-## [Bumped application version to 0.0.14](https://github.com/joshuasundance-swca/langchain-streamlit-demo/commit/eb059075d36e4b09269df5b75dbea1b0e4e22f11)
-Fri Oct 6 20:59:11 2023 -0400
-- Updated the version of the application in bumpver.toml, kubernetes/resources.yaml, and langchain-streamlit-demo/app.py from 0.0.13 to 0.0.14.
-## [Refactored app.py to use Streamlit session state for storing global variables](https://github.com/joshuasundance-swca/langchain-streamlit-demo/commit/e9f7a777844336b99d3bc8c2270e77e2acb0e7e7)
-Fri Oct 6 20:47:25 2023 -0400
-- This commit refactors the app.py file of the langchain-streamlit-demo to use Streamlit's session state for storing global variables. This includes API keys, project names, and Azure configurations. A new function 'azure_state_or_default' has been introduced to update the session state for Azure configurations. This change allows for better state management and persistence across multiple sessions.
-## [Added input field for Azure OpenAI EMB deployment name](https://github.com/joshuasundance-swca/langchain-streamlit-demo/commit/b44c9a31a33a9b7d3b0e347a0ffe4ea31c068e81)
-Fri Oct 6 18:40:55 2023 -0400
-- An input field for the Azure OpenAI EMB deployment name has been added to the sidebar of the Streamlit application. This allows users to specify the name of their Azure OpenAI EMB deployment.
-## [Added Azure OpenAI Embeddings option to app](https://github.com/joshuasundance-swca/langchain-streamlit-demo/commit/c60388636d63567bd9bfe4b7bbfebf734d3100da)
-Fri Oct 6 18:35:40 2023 -0400
-- This commit introduces the option to use Azure OpenAI for embeddings in the langchain-streamlit-demo app. It adds the necessary environment variables and updates the code to handle the new option. The changes include:
-- 1. Addition of the AZURE_OPENAI_EMB_DEPLOYMENT_NAME environment variable in the Kubernetes resources.
-- 2. Update of the app.py file to handle the Azure OpenAI option. If Azure embeddings are available, a toggle is displayed to the user to switch between Azure and OpenAI directly.
-- 3. Update of the get_texts_and_retriever function in llm_resources.py to accept additional arguments for azure_kwargs and use_azure.
-- 4. Update of the defaults.py file to include the AZURE_OPENAI_EMB_DEPLOYMENT_NAME in the list of Azure environment variables.
-## [Refactored code to improve readability and maintainability](https://github.com/joshuasundance-swca/langchain-streamlit-demo/commit/0ce4fb3a9cb43ee563729df1d6b682511e17248f)
-Fri Oct 6 18:15:24 2023 -0400
-- 1. Updated kubernetes resource configuration to add environment variables for SHOW_LANGCHAIN_OPTIONS and SHOW_AZURE_OPTIONS.
-- 2. Refactored the app.py script to import default values from a single source, improving readability and maintainability of the code.
-- 3. Updated defaults.py to define a namedtuple for default values, which is imported in other scripts.
-- 4. Modified llm_resources.py to accommodate changes in the import of default values.
-## [Refactor code by moving logic to a separate module](https://github.com/joshuasundance-swca/langchain-streamlit-demo/commit/21eccfc51cf90268826929cddbf2bfa42bc2f5eb)
-Fri Oct 6 16:26:26 2023 -0400
-- The commit moves a significant amount of logic from 'app.py' to a new module named 'llm_resources.py'. This includes the methods for getting the runnable instance, the language model, and the texts and retriever. The aim of this refactoring is to improve code organization, readability, and maintainability.
-## [Refactored code and improved project structure](https://github.com/joshuasundance-swca/langchain-streamlit-demo/commit/87d698488900d63b992059b6f291d6981773fb4b)
-Fri Oct 6 15:59:43 2023 -0400
-- Moved model constants and environment variables into a separate 'defaults.py' file for better code organization and readability.
-- Updated 'app.py' to import these constants and variables from the new 'defaults.py' file.
-- Modified '.idea/langchain-streamlit-demo.iml' to include a new source folder, improving the project's structure.
-## [Added Azure OpenAI environment variables to Kubernetes deployment](https://github.com/joshuasundance-swca/langchain-streamlit-demo/commit/f39ac3b55d8e57db36ff4a43a4b95dda1fa46e9d)
-Fri Oct 6 14:15:57 2023 -0400
-- In the Kubernetes resource configuration file, several environment variables related to Azure OpenAI have been added. These include the base URL, API version, deployment name, API key, and model version. The values for these variables are fetched from the 'langchain-streamlit-demo-secret' secret.
-## [Bumped version from 0.0.12 to 0.0.13](https://github.com/joshuasundance-swca/langchain-streamlit-demo/commit/d767997980a751389dcbec81b1bcaa1c10267534)
-Fri Oct 6 14:03:44 2023 -0400
-- Updated the current_version in bumpver.toml from 0.0.12 to 0.0.13.
-- Updated the image tag in the Kubernetes resources.yaml file to use the new version 0.0.13.
-- Updated the __version__ variable in the app.py file to reflect the new version 0.0.13.
-## [Refactored application code and updated dependencies](https://github.com/joshuasundance-swca/langchain-streamlit-demo/commit/979e3bd9fe449bea04e5ceda5c1a72be2e824c58)
-Fri Oct 6 13:58:33 2023 -0400
-- Refactored the application code in 'langchain-streamlit-demo/app.py' to improve clarity and organization. Changes include renaming 'AZURE' to 'Azure OpenAI' in the 'MODEL_DICT' and modifying related conditional checks, renaming 'Advanced Options' to 'Advanced Settings', and restructuring 'LangSmith Options' into its own section within the sidebar.
-- Updated the 'streamlit' version from '1.27.1' to '1.27.2' in 'requirements.txt'.
-## [Added support for Azure Chat models in the Streamlit application](https://github.com/joshuasundance-swca/langchain-streamlit-demo/commit/72c3d8c60b3e15ce8d89f926ffe2ab845d3d9c1b)
-Fri Oct 6 13:50:43 2023 -0400
-- The commit introduces Azure Chat models into the Streamlit application. It includes the addition of the AzureChatOpenAI model in the import statement and the MODEL_DICT. Environment variables for Azure are also defined and retrieved from the system environment. User interface elements for Azure options have been added within an expandable section in the sidebar. Finally, an instance of AzureChatOpenAI is created if all Azure details are available and the selected provider is Azure.
-## [Updated langsmith package](https://github.com/joshuasundance-swca/langchain-streamlit-demo/commit/e4b72fedeb71c822b6a76ed84199fef2bbc3bf8a)
-Fri Oct 6 13:02:43 2023 -0400
-- The langsmith package version was updated from 0.0.41 to 0.0.43 in the requirements.txt file.
-## [Updated langchain version in requirements.txt](https://github.com/joshuasundance-swca/langchain-streamlit-demo/commit/2c41972749e5524bba738b37e6d31416e657fec6)
-Thu Oct 5 13:54:14 2023 +0000
-- The langchain package version in requirements.txt has been upgraded from 0.0.305 to 0.0.308. This update may include bug fixes, feature enhancements or performance improvements.
-## [Updated application version to 0.0.12](https://github.com/joshuasundance-swca/langchain-streamlit-demo/commit/2bee8f19e2fa71c333588a3531b55fe062701328)
-Mon Oct 2 09:13:48 2023 -0400
-- The application version has been updated from 0.0.11 to 0.0.12 in three different files. These include bumpver.toml, resources.yaml under kubernetes, and app.py under langchain-streamlit-demo. In bumpver.toml, the current_version value is updated. In resources.yaml, the image version for the container 'langchain-streamlit-demo' is updated. In app.py, the __version__ variable is updated to reflect the new version.
-## [Updated dependencies in requirements.txt](https://github.com/joshuasundance-swca/langchain-streamlit-demo/commit/9747a2d97d4e60861e6d0cc8de7ca8076a6ac971)
-Mon Oct 2 09:10:05 2023 -0400
-- The langchain and langsmith dependencies have been updated to versions 0.0.305 and 0.0.41 respectively.
-- The openai dependency has been updated to version 0.28.1.
-- The previous comment about rolling back the langchain update to avoid a bug has been removed, implying the bug has been fixed in the new version.
-## [Version Bump from 0.0.10 to 0.0.11](https://github.com/joshuasundance-swca/langchain-streamlit-demo/commit/58978f749bdf319a2c2f76e74a46e7d905b7bf69)
-Sat Sep 30 01:31:32 2023 -0400
-- Updated the current_version in bumpver.toml from 0.0.10 to 0.0.11.
-- In the Kubernetes resources.yaml, updated the image version of langchain-streamlit-demo from 0.0.10 to 0.0.11.
-- In the langchain-streamlit-demo/app.py, updated the __version__ from 0.0.10 to 0.0.11.
-## [Updated README.md with minor content and structure changes](https://github.com/joshuasundance-swca/langchain-streamlit-demo/commit/241c14d23e150e0be6edee2c28e32c1b4a519c73)
-Sat Sep 30 01:29:08 2023 -0400
-- This commit includes changes to the README.md file. The authorship of the README has been clarified to indicate that it was originally written by Claude 2. The Features section has been updated to include a new model from Anyscale Endpoints, and to mention the addition of various forms of document chat. The Code Overview section was removed. A minor formatting change was made to the Docker run command. The Docker Compose instructions were simplified by removing a redundant command.
-## [Improved UI labels and refactored code in langchain-streamlit-demo](https://github.com/joshuasundance-swca/langchain-streamlit-demo/commit/f8e912146cbca42cbd456abb123b5223b0924c45)
-Sat Sep 30 01:24:47 2023 -0400
-- This commit includes changes to improve the user interface labels for better readability. The labels 'chunk_size' and 'chunk_overlap' have been changed to 'Number of Tokens per Chunk' and 'Chunk Overlap' respectively.
-- Additionally, the code for handling the full response and the initialization of the `st.session_state.chain` has been refactored for better readability and maintainability. The code now clearly distinguishes between the cases when `use_document_chat` is true or false, and the initialization of `st.session_state.chain` is more streamlined.
-## [Refactored chat functionality and removed unnecessary code in app.py and qagen.py](https://github.com/joshuasundance-swca/langchain-streamlit-demo/commit/923e6fac55336c07c8af10b74742b117517bd757)
-Sat Sep 30 01:10:43 2023 -0400
-- In app.py, removed the StreamlitCallbackHandler import and simplified the logic for handling chat inputs. Removed the document chat condition in the if statement, and directly implemented the regular chat functionality. Simplified the condition for using document chat, and refactored the way rag_runnable is retrieved based on the document chat chain type.
-- In qagen.py, removed the unnecessary import of reduce from functools and the combine_qa_pair_lists function. Simplified the get_rag_qa_gen_chain function by directly converting the parsed_output to a string.
-## [Refactored code for readability and efficiency](https://github.com/joshuasundance-swca/langchain-streamlit-demo/commit/bfaa0c3cf1792d9cb5086657f9ded983ba616662)
-Fri Sep 29 23:12:56 2023 -0400
-- This commit includes changes in the 'app.py' and 'qagen.py' files. In 'app.py', the code has been refactored to improve readability and efficiency. The configuration dictionary has been moved outside the if-conditions to avoid redundancy. Also, the condition checking for 'Summarization' and 'Q&A Generation' has been combined to reduce nested if-statements.
-- In the 'qagen.py' file, two new methods 'to_str' have been added to the 'QuestionAnswerPair' and 'QuestionAnswerPairList' classes. These methods convert the question and answer pairs into a string format. This change has moved the responsibility of string formatting from 'app.py' to 'qagen.py', making the code more modular and easier to maintain.
-## [Updated summarization functionality in Streamlit app](https://github.com/joshuasundance-swca/langchain-streamlit-demo/commit/dd9bfbddff559ce0065c236ccc6419f987a61664)
-Fri Sep 29 22:43:24 2023 -0400
-- Replaced the existing get_summarization_chain function with get_rag_summarization_chain in the Streamlit app.
-- The get_rag_summarization_chain function now takes in the prompt, retriever and the language model as parameters.
-- Refactored the way the summarization chain is invoked and the full response is generated.
-- Updated the get_rag_summarization_chain function in the summarize module to return a RunnableSequence.
-## [Updated model_name parameter in ChatOpenAI instantiation](https://github.com/joshuasundance-swca/langchain-streamlit-demo/commit/06099804b5d0a4d635beb8a0021ac84b22cb0529)
-Fri Sep 29 18:38:23 2023 -0400
-- Replaced hardcoded 'test' value for model_name parameter with a variable named 'model'. This change allows the model name to be dynamically set when the ChatOpenAI class is instantiated.
-## [Refactored model instantiation and removed deprecated functions](https://github.com/joshuasundance-swca/langchain-streamlit-demo/commit/c2ef57040f3231cc3fa80157d93d0d8420f21351)
-Fri Sep 29 18:38:01 2023 -0400
-- Updated the instantiation of ChatOpenAI, ChatAnthropic, and ChatAnyscale classes by swapping the model and model_name parameters to match their class definitions.
-- Removed the commented out get_qa_gen_chain function in qagen.py.
-- Removed commented out code related to raw_results and results in app.py, simplifying the logic.
-## [Refactored the data processing pipeline](https://github.com/joshuasundance-swca/langchain-streamlit-demo/commit/8106321374be538f2740587a9a3d68e9cb82310f)
-Fri Sep 29 18:31:46 2023 -0400
-- Removed the 'combine_qa_pair_lists' function from the data processing pipeline in 'app.py'.
-- Directly accessed 'QuestionAnswerPairs' from 'raw_results' instead of using 'combine_qa_pair_lists' function.
-- Commented out the print statement for 'raw_results'.
-## [Refactored code to change 'input' to 'context' in langchain-streamlit-demo](https://github.com/joshuasundance-swca/langchain-streamlit-demo/commit/3550ebd119e342869167a228929538c069350942)
-Fri Sep 29 18:19:58 2023 -0400
-- This commit includes a change in the variable name from 'input' to 'context' in both app.py and qagen.py files. The change was made in the section where the document page content is being processed. This change is likely aimed at improving code readability and consistency.
-## [Added customizability for number of chunks in retriever](https://github.com/joshuasundance-swca/langchain-streamlit-demo/commit/457889e5dc68143a1a5f935ca6af849bd380666c)
-Fri Sep 29 18:16:51 2023 -0400
-- This commit introduces a slider in the UI allowing the user to select the number of chunks that will be used for context in the retriever. The 'get_texts_and_retriever' function was updated to include a new parameter 'k' that defaults to the newly introduced constant 'DEFAULT_RETRIEVER_K'. This 'k' value is then used in the creation of both the 'bm25_retriever' and 'faiss_retriever'.
-## [Updated Q&A Generation method and invocation](https://github.com/joshuasundance-swca/langchain-streamlit-demo/commit/8aab446c1cbafbdb04dcf6d56ed77413f1e63f65)
-Fri Sep 29 18:13:30 2023 -0400
-- Replaced the get_qa_gen_chain method with get_rag_qa_gen_chain in app.py and qagen.py. This change updates the Q&A Generation method used in the Document Chat feature.
-- Changed the way the Q&A Generation method is invoked. Instead of using the batch method, we now use the invoke method. This change is expected to improve the efficiency of the Document Chat feature.
-## [Implemented RAG-based Q&A Generation Chain](https://github.com/joshuasundance-swca/langchain-streamlit-demo/commit/6467ea59cd8b5eb4859d20c8f84152402833cb92)
-Fri Sep 29 18:04:08 2023 -0400
-- Added a new function 'get_rag_qa_gen_chain' in 'qagen.py' to set up a RAG-based Q&A generation chain using a retriever and a language model.
-- Adjusted the 'app.py' to include a commented-out option to use the new RAG-based Q&A generation chain.
-## [Bump version from 0.0.9 to 0.0.10](https://github.com/joshuasundance-swca/langchain-streamlit-demo/commit/bb29f017b57cd891d1f9ae86e212ec6c92b5aa43)
-Fri Sep 29 13:17:34 2023 -0400
-- Updated the version number in the bumpver.toml, kubernetes/resources.yaml, and langchain-streamlit-demo/app.py files. The new version is 0.0.10.
-## [Updated retriever logic and removed question validation](https://github.com/joshuasundance-swca/langchain-streamlit-demo/commit/930d4126fe97dbb403fa498b5322e10815d06179)
-Fri Sep 29 11:17:45 2023 -0400
-- In the 'langchain-streamlit-demo/app.py' file, the logic to retrieve texts has been updated. The FAISS retriever has been replaced with an ensemble retriever that uses both the BM25 and FAISS retrievers. The BM25 retriever's 'k' parameter has been set to 4, and the FAISS retriever has been updated to use a vector store.
-- In the 'langchain-streamlit-demo/qagen.py' file, the field validator for the 'question' field in the 'QuestionAnswerPair' class has been removed. This means that questions no longer need to end with a question mark to be valid.
-- The 'requirements.txt' file has been updated to include the 'rank_bm25==0.2.2' package, and the 'streamlit' package has been updated to version '1.27.1'.
-## [Updated the refine_template in summarize.py](https://github.com/joshuasundance-swca/langchain-streamlit-demo/commit/736288ed897a6bf1b5c0be7c0481011598351395)
-Thu Sep 28 20:55:25 2023 -0400
-- The refine_template string in the summarize.py file has been updated. A newline character has been added after the 'User input: {query}' part of the string for better readability.
-## [Updated application version to 0.0.9](https://github.com/joshuasundance-swca/langchain-streamlit-demo/commit/40604bea7723c4c05b4c36289950e6d8a25b7690)
-Thu Sep 28 20:41:33 2023 -0400
-- The version number in the bumpver.toml file has been updated from 0.0.8 to 0.0.9.
-- The Docker image version for the langchain-streamlit-demo app in the Kubernetes resources.yaml file has been updated from 0.0.8 to 0.0.9.
-- The __version__ variable in the app.py file of the langchain-streamlit-demo app has been updated from 0.0.8 to 0.0.9.
-## [Improved text formatting in Q&A response](https://github.com/joshuasundance-swca/langchain-streamlit-demo/commit/4eaf9de17247ed7e6bdc1771ff31639cca9e903d)
-Thu Sep 28 20:39:28 2023 -0400
-- This commit adjusts the formatting of the Q&A response in the langchain-streamlit-demo app. It adds an extra newline between the question and answer parts, and another newline between each Q&A pair for better readability.
-## [Updated application version to 0.0.8](https://github.com/joshuasundance-swca/langchain-streamlit-demo/commit/8c80fe821129d05b8b714beb56a4e0bbca6ce676)
-Thu Sep 28 20:36:46 2023 -0400
-- The application's version number has been updated from 0.0.7 to 0.0.8 in the following files: bumpver.toml, resources.yaml, and app.py.
-- In bumpver.toml, the current_version field was updated to reflect the new version.
-- In resources.yaml, the image tag for the langchain-streamlit-demo container was updated to use the new version.
-- In app.py, the __version__ variable was updated to the new version.
-## [Refactor variable names in Streamlit app](https://github.com/joshuasundance-swca/langchain-streamlit-demo/commit/9bf9004ce3ba4160e1c33f57e0c5e48c0ff4f628)
-Thu Sep 28 20:33:17 2023 -0400
-- The variable 'output_text' was renamed to 'full_response' in the Streamlit application to better reflect its purpose. This change improves code readability and understanding.
-## [Bumped version from 0.0.6 to 0.0.7](https://github.com/joshuasundance-swca/langchain-streamlit-demo/commit/d41f4a4356709af4dbd81982fdefb0a6dba21ef6)
-Thu Sep 28 19:56:12 2023 -0400
-- Updated the version number in bumpver.toml, resources.yaml, and app.py.
-- This commit includes changes to the version number in the bumpver configuration, the Docker image tag in the Kubernetes resources, and the version variable in the app.py file.
-## [Added Summarization Feature to Streamlit App](https://github.com/joshuasundance-swca/langchain-streamlit-demo/commit/47c2ffc283d1e1754c1f64ab5fb793694bc9f24f)
-Thu Sep 28 19:53:59 2023 -0400
-- This commit introduces a summarization feature to the Streamlit application. It does so by creating a new 'summarize.py' file and integrating it into the 'app.py' file.
-- In 'app.py', the 'LLMChain' import has been moved and the 'get_summarization_chain' function has been imported from 'summarize.py'.
-- A new option 'Summarization' has been added to the 'Document Chat Chain Type' dropdown menu.
-- When 'Summarization' is selected from the dropdown, the 'get_summarization_chain' function is called to create a summarization chain.
-- The summarization chain is then used to generate a summary of the document, which is displayed in the Streamlit app.
-- In the 'summarize.py' file, a new summarization chain is defined using the 'load_summarize_chain' function from the 'langchain.chains.summarize' module. The chain uses two custom prompt templates for summarizing and refining the document text.
-## [Enhanced document chat functionality in langchain-streamlit-demo](https://github.com/joshuasundance-swca/langchain-streamlit-demo/commit/622ac6610de2f89368031d57ebd148259e5d7fcc)
-Thu Sep 28 16:55:16 2023 -0400
-- This commit includes enhancements to the document chat functionality in the langchain-streamlit-demo application. It introduces a new document chat chain type 'Q&A Generation' and updates the provider variable to be stored in the session state. The commit also adds a new file 'qagen.py' which contains code for generating question and answer pairs from a given text.
-## [Bump version from 0.0.5 to 0.0.6](https://github.com/joshuasundance-swca/langchain-streamlit-demo/commit/f431ca56717b9e704226c3448a552fe31c90d77d)
-Thu Sep 28 14:42:31 2023 -0400
-- The version number in the 'bumpver.toml', 'kubernetes/resources.yaml', and 'langchain-streamlit-demo/app.py' files has been updated from 0.0.5 to 0.0.6. This indicates a new iteration of the software with potential minor changes or bug fixes.
-## [Updated ruff-pre-commit version](https://github.com/joshuasundance-swca/langchain-streamlit-demo/commit/50b28c9ac810cf9ff1c58e0b98f4ca7dfe3f94f5)
-Wed Sep 27 20:58:22 2023 -0400
-- The ruff-pre-commit version in the pre-commit configuration file was updated from v0.0.290 to v0.0.291.
-## [Updated file exclusions in pre-commit config](https://github.com/joshuasundance-swca/langchain-streamlit-demo/commit/c8b46036933d50ca6befc5d4fa43bcb29f05c75a)
-Wed Sep 27 20:57:54 2023 -0400
-- The pre-commit configuration has been updated to exclude the AI_CHANGELOG.md file. Previously, the configuration was set to exclude .idea and docs directories. The repository and hook details remain unchanged.
-## [Refactored chain_type_help in app.py and updated AI_CHANGELOG.md](https://github.com/joshuasundance-swca/langchain-streamlit-demo/commit/a1b0a6fd0b22021079e741929eb7671855192cb0)
-Wed Sep 27 20:56:47 2023 -0400
-- In app.py, the chain_type_help dictionary was refactored to directly generate a string with the help links for each chain_type_name, removing the need for a separate dictionary.
-- In AI_CHANGELOG.md, a newline was added at the end of the file and entries were made for the addition of numpy and tornado to requirements.txt and the update of the token used for code checkout in the GitHub workflow.
-## [Updated GitHub Action to Push to HuggingFace Space](https://github.com/joshuasundance-swca/langchain-streamlit-demo/commit/e95e574c846541fd959bd0d0355178fae542dd8e)
-Wed Sep 27 17:05:25 2023 +0000
-- This commit modifies the triggering conditions for the GitHub Action workflow that pushes updates to HuggingFace Space. Previously, the workflow was triggered on each push with any tag. Now, it is triggered upon completion of the 'Update AI Changelog on Push to Main' workflow on the 'main' branch.
-- Additionally, the 'push-to-huggingface' job has been updated to depend on the completion of the 'update-changelog' job.
-## [Updated version number from 0.0.2 to 0.0.5](https://github.com/joshuasundance-swca/langchain-streamlit-demo/commit/55fa7419137cf54127cbd03114c0c0284397cfd9)
-Wed Sep 27 10:56:40 2023 -0400
-- The version number in the bumpver configuration file has been updated from 0.0.2 to 0.0.5.
-- The image version in the Kubernetes resources file has been updated to match the new version number.
-- The __version__ variable in the langchain-streamlit-demo app has been updated to reflect the new version number.
-## [Updated page title to include version number](https://github.com/joshuasundance-swca/langchain-streamlit-demo/commit/783c740fe52c44c3f3d9d5ad78b6c1784fa93e97)
-Wed Sep 27 10:46:57 2023 -0400
-- The page title of the Streamlit application was previously just the name of the application. The change now includes the version number in the title, which will make it easier to track and verify the version of the application in use.
-## [Added 'codellama/CodeLlama-34b-Instruct-hf' to Model Dictionary](https://github.com/joshuasundance-swca/langchain-streamlit-demo/commit/68f6d34a4cefd91425cbc215f323fbd57dd6e4a7)
-Wed Sep 27 10:46:24 2023 -0400
-- The commit introduces a new model 'codellama/CodeLlama-34b-Instruct-hf' into the MODEL_DICT dictionary. This update extends the list of models supported by the 'Anyscale Endpoints'.
-## [Bumped version from 0.0.1 to 0.0.2](https://github.com/joshuasundance-swca/langchain-streamlit-demo/commit/9feadf73e8c66425a565c99ce3088249bc4699f1)
-Wed Sep 27 00:03:38 2023 -0400
-- Updated the version number in bumpver.toml, Kubernetes resources.yaml, and the app.py file of the langchain-streamlit-demo application. The new version is 0.0.2.
-## [Updated app version](https://github.com/joshuasundance-swca/langchain-streamlit-demo/commit/a1065bb282837cf191d30bcb45c638bd15c5b77a)
-Wed Sep 27 00:00:28 2023 -0400
-- The version number in langchain-streamlit-demo/app.py was updated from 0.0.0 to 0.0.1.
-## [Updated image version in Kubernetes resources and bumpver file](https://github.com/joshuasundance-swca/langchain-streamlit-demo/commit/35cffe74d37db50ad5ae17a6e6af4d2131c1a5c3)
-Tue Sep 26 23:59:47 2023 -0400
-- In the 'bumpver.toml' file, the image version placeholder in 'kubernetes/resources.yaml' was corrected by removing the unnecessary quotes.
-- In the 'kubernetes/resources.yaml' file, the image version for 'langchain-streamlit-demo' was updated from 'latest' to '0.0.1'.
-## [Implement versioning and modify GitHub workflows](https://github.com/joshuasundance-swca/langchain-streamlit-demo/commit/7f34c9b5e16996dcb8eb5cdd3f5cdc86d7bf2b11)
-Tue Sep 26 23:58:24 2023 -0400
-- Introduced semantic versioning using bumpver. The current version is now tracked in a new file 'bumpver.toml' and also reflected in 'app.py' and the Docker image tag in 'kubernetes/resources.yaml'.
-- Modified GitHub workflows 'docker-hub.yml' and 'hf-space.yml' to trigger on new tags instead of pushes to the main branch. The Docker image tag is now the release version instead of the git SHA.
-- Removed the step to store the git SHA in 'docker-hub.yml'.
-- No functional changes were made to 'langchain-streamlit-demo/app.py' or 'kubernetes/resources.yaml'. The imagePullPolicy remains as 'Always'.
-## [Updated requirements.txt for better package management](https://github.com/joshuasundance-swca/langchain-streamlit-demo/commit/5085ade2d646a2670929e518e78b881ea2ffd0a5)
-Tue Sep 26 23:14:05 2023 -0400
-- Rolled back langchain package from version 0.0.301 to 0.0.300 to avoid a bug in langchain's chatanthropic.
-- Pinned numpy to version 1.22.2 as suggested by Snyk to avoid a vulnerability.
-- Reordered the packages for better readability.
-## [Added numpy and tornado to requirements.txt to avoid vulnerabilities](https://github.com/joshuasundance-swca/langchain-streamlit-demo/commit/3f0e220f9f77d561510dd04b09f1c3c509a5b28f)
-Tue Sep 26 12:56:59 2023 +0000
-- The numpy and tornado packages were added to the requirements.txt file. These packages are not directly required by our application but were added to avoid potential vulnerabilities as suggested by Snyk.
-## [Updated token used for code checkout in GitHub workflow](https://github.com/joshuasundance-swca/langchain-streamlit-demo/commit/b0c4e1ca12f86ea6113ee2c86d38c39d3035f395)
-Tue Sep 26 08:56:55 2023 -0400
-- In the GitHub Actions workflow file 'ai_changelog.yml', the personal access token used for checking out code has been updated. The token has been changed from 'PAT' to 'WORKFLOW_GIT_ACCESS_TOKEN'.
\ No newline at end of file
diff --git a/spaces/jslin09/legal_document_drafting/README.md b/spaces/jslin09/legal_document_drafting/README.md
deleted file mode 100644
index e6bb22dccbd187557f085011881c6ae302e33980..0000000000000000000000000000000000000000
--- a/spaces/jslin09/legal_document_drafting/README.md
+++ /dev/null
@@ -1,14 +0,0 @@
----
-title: Legal Document Drafting
-emoji: 🌍
-colorFrom: red
-colorTo: yellow
-sdk: gradio
-sdk_version: 3.20.1
-app_file: app.py
-pinned: false
-license: bigscience-bloom-rail-1.0
-python_version: 3.9.13
----
-
-Check out the configuration reference at https://huggingface.co/docs/hub/spaces-config-reference
diff --git a/spaces/katanaml-org/sparrow-ui/views/data_inference.py b/spaces/katanaml-org/sparrow-ui/views/data_inference.py
deleted file mode 100644
index 0d680145cd58f63dbecf964ada42255f68e03534..0000000000000000000000000000000000000000
--- a/spaces/katanaml-org/sparrow-ui/views/data_inference.py
+++ /dev/null
@@ -1,219 +0,0 @@
-import streamlit as st
-import os
-import time
-from PIL import Image
-import math
-from streamlit_sparrow_labeling import st_sparrow_labeling
-import requests
-from config import settings
-import json
-
-
-class DataInference:
- class Model:
- # pageTitle = "Data Inference"
- subheader_2 = "Upload"
- initial_msg = "Please upload a file for inference"
-
- upload_help = "Upload a file to extract data from it"
- upload_button_text = "Upload"
- upload_button_text_desc = "Choose a file"
-
- extract_data = "Extract Data"
-
- model_in_use = "donut"
-
- img_file = None
-
- def set_image_file(self, img_file):
- st.session_state['img_file'] = img_file
-
- def get_image_file(self):
- if 'img_file' not in st.session_state:
- return None
- return st.session_state['img_file']
-
- data_result = None
-
- def set_data_result(self, data_result):
- st.session_state['data_result'] = data_result
-
- def get_data_result(self):
- if 'data_result' not in st.session_state:
- return None
- return st.session_state['data_result']
-
- def view(self, model, ui_width, device_type, device_width):
- # st.title(model.pageTitle)
-
- with st.sidebar:
- st.markdown("---")
- st.subheader(model.subheader_2)
-
- with st.form("upload-form", clear_on_submit=True):
- uploaded_file = st.file_uploader(model.upload_button_text_desc, accept_multiple_files=False,
- type=['png', 'jpg', 'jpeg'],
- help=model.upload_help, disabled=True)
- submitted = st.form_submit_button(model.upload_button_text, disabled=True)
-
- if submitted and uploaded_file is not None:
- ret = self.upload_file(uploaded_file)
-
- if ret is not False:
- model.set_image_file(ret)
- model.set_data_result(None)
-
- if model.get_image_file() is not None:
- doc_img = Image.open(model.get_image_file())
- doc_height = doc_img.height
- doc_width = doc_img.width
-
- canvas_width, number_of_columns = self.canvas_available_width(ui_width, doc_width, device_type,
- device_width)
-
- if number_of_columns > 1:
- col1, col2 = st.columns([number_of_columns, 10 - number_of_columns])
- with col1:
- self.render_doc(model, doc_img, canvas_width, doc_height, doc_width)
- with col2:
- self.render_results(model)
- else:
- self.render_doc(model, doc_img, canvas_width, doc_height, doc_width)
- self.render_results(model)
- else:
- st.title(model.initial_msg)
-
- def upload_file(self, uploaded_file):
- timestamp = str(time.time())
- timestamp = timestamp.replace(".", "")
-
- file_name, file_extension = os.path.splitext(uploaded_file.name)
- uploaded_file.name = file_name + "_" + timestamp + file_extension
-
- if os.path.exists(os.path.join("docs/inference/", uploaded_file.name)):
- st.write("File already exists")
- return False
-
- if len(uploaded_file.name) > 500:
- st.write("File name too long")
- return False
-
- with open(os.path.join("docs/inference/", uploaded_file.name), "wb") as f:
- f.write(uploaded_file.getbuffer())
-
- st.success("File uploaded successfully")
-
- return os.path.join("docs/inference/", uploaded_file.name)
-
- def canvas_available_width(self, ui_width, doc_width, device_type, device_width):
- doc_width_pct = (doc_width * 100) / ui_width
- if doc_width_pct < 45:
- canvas_width_pct = 37
- elif doc_width_pct < 55:
- canvas_width_pct = 49
- else:
- canvas_width_pct = 60
-
- if ui_width > 700 and canvas_width_pct == 37 and device_type == "desktop":
- return math.floor(canvas_width_pct * ui_width / 100), 4
- elif ui_width > 700 and canvas_width_pct == 49 and device_type == "desktop":
- return math.floor(canvas_width_pct * ui_width / 100), 5
- elif ui_width > 700 and canvas_width_pct == 60 and device_type == "desktop":
- return math.floor(canvas_width_pct * ui_width / 100), 6
- else:
- if device_type == "desktop":
- ui_width = device_width - math.floor((device_width * 22) / 100)
- elif device_type == "mobile":
- ui_width = device_width - math.floor((device_width * 13) / 100)
- return ui_width, 1
-
- def render_doc(self, model, doc_img, canvas_width, doc_height, doc_width):
- height = 1296
- width = 864
-
- annotations_json = {
- "meta": {
- "version": "v0.1",
- "split": "train",
- "image_id": 0,
- "image_size": {
- "width": doc_width,
- "height": doc_height
- }
- },
- "words": []
- }
-
- st_sparrow_labeling(
- fill_color="rgba(0, 151, 255, 0.3)",
- stroke_width=2,
- stroke_color="rgba(0, 50, 255, 0.7)",
- background_image=doc_img,
- initial_rects=annotations_json,
- height=height,
- width=width,
- drawing_mode="transform",
- display_toolbar=False,
- update_streamlit=False,
- canvas_width=canvas_width,
- doc_height=doc_height,
- doc_width=doc_width,
- image_rescale=True,
- key="doc_annotation" + model.get_image_file()
- )
-
- def render_results(self, model):
- with st.form(key="results_form"):
- button_placeholder = st.empty()
-
- submit = button_placeholder.form_submit_button(model.extract_data, type="primary")
- if 'inference_error' in st.session_state:
- st.error(st.session_state.inference_error)
- del st.session_state.inference_error
-
- if submit:
- button_placeholder.empty()
-
- api_url = "https://katanaml-org-sparrow-ml.hf.space/api-inference/v1/sparrow-ml/inference"
- file_path = model.get_image_file()
-
- with open(file_path, "rb") as file:
- model_in_use = model.model_in_use
- sparrow_key = settings.sparrow_key
-
- # Prepare the payload
- files = {
- 'file': (file.name, file, 'image/jpeg')
- }
-
- data = {
- 'image_url': '',
- 'model_in_use': model_in_use,
- 'sparrow_key': sparrow_key
- }
-
- with st.spinner("Extracting data from document..."):
- response = requests.post(api_url, data=data, files=files, timeout=180)
- if response.status_code != 200:
- print('Request failed with status code:', response.status_code)
- print('Response:', response.text)
-
- st.session_state["inference_error"] = "Error extracting data from document"
- st.experimental_rerun()
-
- model.set_data_result(response.text)
-
- # Display JSON data in Streamlit
- st.markdown("---")
- st.json(response.text)
-
- # replace file extension to json
- file_path = file_path.replace(".jpg", ".json")
- with open(file_path, "w") as f:
- json.dump(response.text, f, indent=2)
-
- st.experimental_rerun()
- else:
- if model.get_data_result() is not None:
- st.markdown("---")
- st.json(model.get_data_result())
\ No newline at end of file
diff --git a/spaces/kcagle/AutoGPT/autogpt/json_utils/json_fix_general.py b/spaces/kcagle/AutoGPT/autogpt/json_utils/json_fix_general.py
deleted file mode 100644
index 7010fa3b9c1909de0e5a7f6ec13ca8aa418fe6c7..0000000000000000000000000000000000000000
--- a/spaces/kcagle/AutoGPT/autogpt/json_utils/json_fix_general.py
+++ /dev/null
@@ -1,124 +0,0 @@
-"""This module contains functions to fix JSON strings using general programmatic approaches, suitable for addressing
-common JSON formatting issues."""
-from __future__ import annotations
-
-import contextlib
-import json
-import re
-from typing import Optional
-
-from autogpt.config import Config
-from autogpt.json_utils.utilities import extract_char_position
-
-CFG = Config()
-
-
-def fix_invalid_escape(json_to_load: str, error_message: str) -> str:
- """Fix invalid escape sequences in JSON strings.
-
- Args:
- json_to_load (str): The JSON string.
- error_message (str): The error message from the JSONDecodeError
- exception.
-
- Returns:
- str: The JSON string with invalid escape sequences fixed.
- """
- while error_message.startswith("Invalid \\escape"):
- bad_escape_location = extract_char_position(error_message)
- json_to_load = (
- json_to_load[:bad_escape_location] + json_to_load[bad_escape_location + 1 :]
- )
- try:
- json.loads(json_to_load)
- return json_to_load
- except json.JSONDecodeError as e:
- if CFG.debug_mode:
- print("json loads error - fix invalid escape", e)
- error_message = str(e)
- return json_to_load
-
-
-def balance_braces(json_string: str) -> Optional[str]:
- """
- Balance the braces in a JSON string.
-
- Args:
- json_string (str): The JSON string.
-
- Returns:
- str: The JSON string with braces balanced.
- """
-
- open_braces_count = json_string.count("{")
- close_braces_count = json_string.count("}")
-
- while open_braces_count > close_braces_count:
- json_string += "}"
- close_braces_count += 1
-
- while close_braces_count > open_braces_count:
- json_string = json_string.rstrip("}")
- close_braces_count -= 1
-
- with contextlib.suppress(json.JSONDecodeError):
- json.loads(json_string)
- return json_string
-
-
-def add_quotes_to_property_names(json_string: str) -> str:
- """
- Add quotes to property names in a JSON string.
-
- Args:
- json_string (str): The JSON string.
-
- Returns:
- str: The JSON string with quotes added to property names.
- """
-
- def replace_func(match: re.Match) -> str:
- return f'"{match[1]}":'
-
- property_name_pattern = re.compile(r"(\w+):")
- corrected_json_string = property_name_pattern.sub(replace_func, json_string)
-
- try:
- json.loads(corrected_json_string)
- return corrected_json_string
- except json.JSONDecodeError as e:
- raise e
-
-
-def correct_json(json_to_load: str) -> str:
- """
- Correct common JSON errors.
- Args:
- json_to_load (str): The JSON string.
- """
-
- try:
- if CFG.debug_mode:
- print("json", json_to_load)
- json.loads(json_to_load)
- return json_to_load
- except json.JSONDecodeError as e:
- if CFG.debug_mode:
- print("json loads error", e)
- error_message = str(e)
- if error_message.startswith("Invalid \\escape"):
- json_to_load = fix_invalid_escape(json_to_load, error_message)
- if error_message.startswith(
- "Expecting property name enclosed in double quotes"
- ):
- json_to_load = add_quotes_to_property_names(json_to_load)
- try:
- json.loads(json_to_load)
- return json_to_load
- except json.JSONDecodeError as e:
- if CFG.debug_mode:
- print("json loads error - add quotes", e)
- error_message = str(e)
- if balanced_str := balance_braces(json_to_load):
- return balanced_str
- return json_to_load
diff --git a/spaces/kevinwang676/ChatGLM2-SadTalker/src/face3d/data/template_dataset.py b/spaces/kevinwang676/ChatGLM2-SadTalker/src/face3d/data/template_dataset.py
deleted file mode 100644
index bfdf16be2a8a834b204c45d88c86857b37b9bd25..0000000000000000000000000000000000000000
--- a/spaces/kevinwang676/ChatGLM2-SadTalker/src/face3d/data/template_dataset.py
+++ /dev/null
@@ -1,75 +0,0 @@
-"""Dataset class template
-
-This module provides a template for users to implement custom datasets.
-You can specify '--dataset_mode template' to use this dataset.
-The class name should be consistent with both the filename and its dataset_mode option.
-The filename should be _dataset.py
-The class name should be Dataset.py
-You need to implement the following functions:
- -- : Add dataset-specific options and rewrite default values for existing options.
- -- <__init__>: Initialize this dataset class.
- -- <__getitem__>: Return a data point and its metadata information.
- -- <__len__>: Return the number of images.
-"""
-from data.base_dataset import BaseDataset, get_transform
-# from data.image_folder import make_dataset
-# from PIL import Image
-
-
-class TemplateDataset(BaseDataset):
- """A template dataset class for you to implement custom datasets."""
- @staticmethod
- def modify_commandline_options(parser, is_train):
- """Add new dataset-specific options, and rewrite default values for existing options.
-
- Parameters:
- parser -- original option parser
- is_train (bool) -- whether training phase or test phase. You can use this flag to add training-specific or test-specific options.
-
- Returns:
- the modified parser.
- """
- parser.add_argument('--new_dataset_option', type=float, default=1.0, help='new dataset option')
- parser.set_defaults(max_dataset_size=10, new_dataset_option=2.0) # specify dataset-specific default values
- return parser
-
- def __init__(self, opt):
- """Initialize this dataset class.
-
- Parameters:
- opt (Option class) -- stores all the experiment flags; needs to be a subclass of BaseOptions
-
- A few things can be done here.
- - save the options (have been done in BaseDataset)
- - get image paths and meta information of the dataset.
- - define the image transformation.
- """
- # save the option and dataset root
- BaseDataset.__init__(self, opt)
- # get the image paths of your dataset;
- self.image_paths = [] # You can call sorted(make_dataset(self.root, opt.max_dataset_size)) to get all the image paths under the directory self.root
- # define the default transform function. You can use ; You can also define your custom transform function
- self.transform = get_transform(opt)
-
- def __getitem__(self, index):
- """Return a data point and its metadata information.
-
- Parameters:
- index -- a random integer for data indexing
-
- Returns:
- a dictionary of data with their names. It usually contains the data itself and its metadata information.
-
- Step 1: get a random image path: e.g., path = self.image_paths[index]
- Step 2: load your data from the disk: e.g., image = Image.open(path).convert('RGB').
- Step 3: convert your data to a PyTorch tensor. You can use helpder functions such as self.transform. e.g., data = self.transform(image)
- Step 4: return a data point as a dictionary.
- """
- path = 'temp' # needs to be a string
- data_A = None # needs to be a tensor
- data_B = None # needs to be a tensor
- return {'data_A': data_A, 'data_B': data_B, 'path': path}
-
- def __len__(self):
- """Return the total number of images."""
- return len(self.image_paths)
diff --git a/spaces/kevinwang676/ChatGLM2-VC-SadTalker/src/audio2pose_models/res_unet.py b/spaces/kevinwang676/ChatGLM2-VC-SadTalker/src/audio2pose_models/res_unet.py
deleted file mode 100644
index f2611e1d1a9bf233507427b34928fca60e094224..0000000000000000000000000000000000000000
--- a/spaces/kevinwang676/ChatGLM2-VC-SadTalker/src/audio2pose_models/res_unet.py
+++ /dev/null
@@ -1,65 +0,0 @@
-import torch
-import torch.nn as nn
-from src.audio2pose_models.networks import ResidualConv, Upsample
-
-
-class ResUnet(nn.Module):
- def __init__(self, channel=1, filters=[32, 64, 128, 256]):
- super(ResUnet, self).__init__()
-
- self.input_layer = nn.Sequential(
- nn.Conv2d(channel, filters[0], kernel_size=3, padding=1),
- nn.BatchNorm2d(filters[0]),
- nn.ReLU(),
- nn.Conv2d(filters[0], filters[0], kernel_size=3, padding=1),
- )
- self.input_skip = nn.Sequential(
- nn.Conv2d(channel, filters[0], kernel_size=3, padding=1)
- )
-
- self.residual_conv_1 = ResidualConv(filters[0], filters[1], stride=(2,1), padding=1)
- self.residual_conv_2 = ResidualConv(filters[1], filters[2], stride=(2,1), padding=1)
-
- self.bridge = ResidualConv(filters[2], filters[3], stride=(2,1), padding=1)
-
- self.upsample_1 = Upsample(filters[3], filters[3], kernel=(2,1), stride=(2,1))
- self.up_residual_conv1 = ResidualConv(filters[3] + filters[2], filters[2], stride=1, padding=1)
-
- self.upsample_2 = Upsample(filters[2], filters[2], kernel=(2,1), stride=(2,1))
- self.up_residual_conv2 = ResidualConv(filters[2] + filters[1], filters[1], stride=1, padding=1)
-
- self.upsample_3 = Upsample(filters[1], filters[1], kernel=(2,1), stride=(2,1))
- self.up_residual_conv3 = ResidualConv(filters[1] + filters[0], filters[0], stride=1, padding=1)
-
- self.output_layer = nn.Sequential(
- nn.Conv2d(filters[0], 1, 1, 1),
- nn.Sigmoid(),
- )
-
- def forward(self, x):
- # Encode
- x1 = self.input_layer(x) + self.input_skip(x)
- x2 = self.residual_conv_1(x1)
- x3 = self.residual_conv_2(x2)
- # Bridge
- x4 = self.bridge(x3)
-
- # Decode
- x4 = self.upsample_1(x4)
- x5 = torch.cat([x4, x3], dim=1)
-
- x6 = self.up_residual_conv1(x5)
-
- x6 = self.upsample_2(x6)
- x7 = torch.cat([x6, x2], dim=1)
-
- x8 = self.up_residual_conv2(x7)
-
- x8 = self.upsample_3(x8)
- x9 = torch.cat([x8, x1], dim=1)
-
- x10 = self.up_residual_conv3(x9)
-
- output = self.output_layer(x10)
-
- return output
\ No newline at end of file
diff --git a/spaces/kevinwang676/VoiceChangers/src/face3d/models/base_model.py b/spaces/kevinwang676/VoiceChangers/src/face3d/models/base_model.py
deleted file mode 100644
index cfe64a7f739ad8f8cfbf3073a2bf49e1468127fd..0000000000000000000000000000000000000000
--- a/spaces/kevinwang676/VoiceChangers/src/face3d/models/base_model.py
+++ /dev/null
@@ -1,316 +0,0 @@
-"""This script defines the base network model for Deep3DFaceRecon_pytorch
-"""
-
-import os
-import numpy as np
-import torch
-from collections import OrderedDict
-from abc import ABC, abstractmethod
-from . import networks
-
-
-class BaseModel(ABC):
- """This class is an abstract base class (ABC) for models.
- To create a subclass, you need to implement the following five functions:
- -- <__init__>: initialize the class; first call BaseModel.__init__(self, opt).
- -- : unpack data from dataset and apply preprocessing.
- -- : produce intermediate results.
- -- : calculate losses, gradients, and update network weights.
- -- : (optionally) add model-specific options and set default options.
- """
-
- def __init__(self, opt):
- """Initialize the BaseModel class.
-
- Parameters:
- opt (Option class)-- stores all the experiment flags; needs to be a subclass of BaseOptions
-
- When creating your custom class, you need to implement your own initialization.
- In this fucntion, you should first call
- Then, you need to define four lists:
- -- self.loss_names (str list): specify the training losses that you want to plot and save.
- -- self.model_names (str list): specify the images that you want to display and save.
- -- self.visual_names (str list): define networks used in our training.
- -- self.optimizers (optimizer list): define and initialize optimizers. You can define one optimizer for each network. If two networks are updated at the same time, you can use itertools.chain to group them. See cycle_gan_model.py for an example.
- """
- self.opt = opt
- self.isTrain = False
- self.device = torch.device('cpu')
- self.save_dir = " " # os.path.join(opt.checkpoints_dir, opt.name) # save all the checkpoints to save_dir
- self.loss_names = []
- self.model_names = []
- self.visual_names = []
- self.parallel_names = []
- self.optimizers = []
- self.image_paths = []
- self.metric = 0 # used for learning rate policy 'plateau'
-
- @staticmethod
- def dict_grad_hook_factory(add_func=lambda x: x):
- saved_dict = dict()
-
- def hook_gen(name):
- def grad_hook(grad):
- saved_vals = add_func(grad)
- saved_dict[name] = saved_vals
- return grad_hook
- return hook_gen, saved_dict
-
- @staticmethod
- def modify_commandline_options(parser, is_train):
- """Add new model-specific options, and rewrite default values for existing options.
-
- Parameters:
- parser -- original option parser
- is_train (bool) -- whether training phase or test phase. You can use this flag to add training-specific or test-specific options.
-
- Returns:
- the modified parser.
- """
- return parser
-
- @abstractmethod
- def set_input(self, input):
- """Unpack input data from the dataloader and perform necessary pre-processing steps.
-
- Parameters:
- input (dict): includes the data itself and its metadata information.
- """
- pass
-
- @abstractmethod
- def forward(self):
- """Run forward pass; called by both functions and ."""
- pass
-
- @abstractmethod
- def optimize_parameters(self):
- """Calculate losses, gradients, and update network weights; called in every training iteration"""
- pass
-
- def setup(self, opt):
- """Load and print networks; create schedulers
-
- Parameters:
- opt (Option class) -- stores all the experiment flags; needs to be a subclass of BaseOptions
- """
- if self.isTrain:
- self.schedulers = [networks.get_scheduler(optimizer, opt) for optimizer in self.optimizers]
-
- if not self.isTrain or opt.continue_train:
- load_suffix = opt.epoch
- self.load_networks(load_suffix)
-
-
- # self.print_networks(opt.verbose)
-
- def parallelize(self, convert_sync_batchnorm=True):
- if not self.opt.use_ddp:
- for name in self.parallel_names:
- if isinstance(name, str):
- module = getattr(self, name)
- setattr(self, name, module.to(self.device))
- else:
- for name in self.model_names:
- if isinstance(name, str):
- module = getattr(self, name)
- if convert_sync_batchnorm:
- module = torch.nn.SyncBatchNorm.convert_sync_batchnorm(module)
- setattr(self, name, torch.nn.parallel.DistributedDataParallel(module.to(self.device),
- device_ids=[self.device.index],
- find_unused_parameters=True, broadcast_buffers=True))
-
- # DistributedDataParallel is not needed when a module doesn't have any parameter that requires a gradient.
- for name in self.parallel_names:
- if isinstance(name, str) and name not in self.model_names:
- module = getattr(self, name)
- setattr(self, name, module.to(self.device))
-
- # put state_dict of optimizer to gpu device
- if self.opt.phase != 'test':
- if self.opt.continue_train:
- for optim in self.optimizers:
- for state in optim.state.values():
- for k, v in state.items():
- if isinstance(v, torch.Tensor):
- state[k] = v.to(self.device)
-
- def data_dependent_initialize(self, data):
- pass
-
- def train(self):
- """Make models train mode"""
- for name in self.model_names:
- if isinstance(name, str):
- net = getattr(self, name)
- net.train()
-
- def eval(self):
- """Make models eval mode"""
- for name in self.model_names:
- if isinstance(name, str):
- net = getattr(self, name)
- net.eval()
-
- def test(self):
- """Forward function used in test time.
-
- This function wraps function in no_grad() so we don't save intermediate steps for backprop
- It also calls to produce additional visualization results
- """
- with torch.no_grad():
- self.forward()
- self.compute_visuals()
-
- def compute_visuals(self):
- """Calculate additional output images for visdom and HTML visualization"""
- pass
-
- def get_image_paths(self, name='A'):
- """ Return image paths that are used to load current data"""
- return self.image_paths if name =='A' else self.image_paths_B
-
- def update_learning_rate(self):
- """Update learning rates for all the networks; called at the end of every epoch"""
- for scheduler in self.schedulers:
- if self.opt.lr_policy == 'plateau':
- scheduler.step(self.metric)
- else:
- scheduler.step()
-
- lr = self.optimizers[0].param_groups[0]['lr']
- print('learning rate = %.7f' % lr)
-
- def get_current_visuals(self):
- """Return visualization images. train.py will display these images with visdom, and save the images to a HTML"""
- visual_ret = OrderedDict()
- for name in self.visual_names:
- if isinstance(name, str):
- visual_ret[name] = getattr(self, name)[:, :3, ...]
- return visual_ret
-
- def get_current_losses(self):
- """Return traning losses / errors. train.py will print out these errors on console, and save them to a file"""
- errors_ret = OrderedDict()
- for name in self.loss_names:
- if isinstance(name, str):
- errors_ret[name] = float(getattr(self, 'loss_' + name)) # float(...) works for both scalar tensor and float number
- return errors_ret
-
- def save_networks(self, epoch):
- """Save all the networks to the disk.
-
- Parameters:
- epoch (int) -- current epoch; used in the file name '%s_net_%s.pth' % (epoch, name)
- """
- if not os.path.isdir(self.save_dir):
- os.makedirs(self.save_dir)
-
- save_filename = 'epoch_%s.pth' % (epoch)
- save_path = os.path.join(self.save_dir, save_filename)
-
- save_dict = {}
- for name in self.model_names:
- if isinstance(name, str):
- net = getattr(self, name)
- if isinstance(net, torch.nn.DataParallel) or isinstance(net,
- torch.nn.parallel.DistributedDataParallel):
- net = net.module
- save_dict[name] = net.state_dict()
-
-
- for i, optim in enumerate(self.optimizers):
- save_dict['opt_%02d'%i] = optim.state_dict()
-
- for i, sched in enumerate(self.schedulers):
- save_dict['sched_%02d'%i] = sched.state_dict()
-
- torch.save(save_dict, save_path)
-
- def __patch_instance_norm_state_dict(self, state_dict, module, keys, i=0):
- """Fix InstanceNorm checkpoints incompatibility (prior to 0.4)"""
- key = keys[i]
- if i + 1 == len(keys): # at the end, pointing to a parameter/buffer
- if module.__class__.__name__.startswith('InstanceNorm') and \
- (key == 'running_mean' or key == 'running_var'):
- if getattr(module, key) is None:
- state_dict.pop('.'.join(keys))
- if module.__class__.__name__.startswith('InstanceNorm') and \
- (key == 'num_batches_tracked'):
- state_dict.pop('.'.join(keys))
- else:
- self.__patch_instance_norm_state_dict(state_dict, getattr(module, key), keys, i + 1)
-
- def load_networks(self, epoch):
- """Load all the networks from the disk.
-
- Parameters:
- epoch (int) -- current epoch; used in the file name '%s_net_%s.pth' % (epoch, name)
- """
- if self.opt.isTrain and self.opt.pretrained_name is not None:
- load_dir = os.path.join(self.opt.checkpoints_dir, self.opt.pretrained_name)
- else:
- load_dir = self.save_dir
- load_filename = 'epoch_%s.pth' % (epoch)
- load_path = os.path.join(load_dir, load_filename)
- state_dict = torch.load(load_path, map_location=self.device)
- print('loading the model from %s' % load_path)
-
- for name in self.model_names:
- if isinstance(name, str):
- net = getattr(self, name)
- if isinstance(net, torch.nn.DataParallel):
- net = net.module
- net.load_state_dict(state_dict[name])
-
- if self.opt.phase != 'test':
- if self.opt.continue_train:
- print('loading the optim from %s' % load_path)
- for i, optim in enumerate(self.optimizers):
- optim.load_state_dict(state_dict['opt_%02d'%i])
-
- try:
- print('loading the sched from %s' % load_path)
- for i, sched in enumerate(self.schedulers):
- sched.load_state_dict(state_dict['sched_%02d'%i])
- except:
- print('Failed to load schedulers, set schedulers according to epoch count manually')
- for i, sched in enumerate(self.schedulers):
- sched.last_epoch = self.opt.epoch_count - 1
-
-
-
-
- def print_networks(self, verbose):
- """Print the total number of parameters in the network and (if verbose) network architecture
-
- Parameters:
- verbose (bool) -- if verbose: print the network architecture
- """
- print('---------- Networks initialized -------------')
- for name in self.model_names:
- if isinstance(name, str):
- net = getattr(self, name)
- num_params = 0
- for param in net.parameters():
- num_params += param.numel()
- if verbose:
- print(net)
- print('[Network %s] Total number of parameters : %.3f M' % (name, num_params / 1e6))
- print('-----------------------------------------------')
-
- def set_requires_grad(self, nets, requires_grad=False):
- """Set requies_grad=Fasle for all the networks to avoid unnecessary computations
- Parameters:
- nets (network list) -- a list of networks
- requires_grad (bool) -- whether the networks require gradients or not
- """
- if not isinstance(nets, list):
- nets = [nets]
- for net in nets:
- if net is not None:
- for param in net.parameters():
- param.requires_grad = requires_grad
-
- def generate_visuals_for_evaluation(self, data, mode):
- return {}
diff --git a/spaces/king007/Voice-Cloning/README.md b/spaces/king007/Voice-Cloning/README.md
deleted file mode 100644
index 614a9fa7f53e6372e9dffdb061dccf0e674650ae..0000000000000000000000000000000000000000
--- a/spaces/king007/Voice-Cloning/README.md
+++ /dev/null
@@ -1,14 +0,0 @@
----
-title: Voice Cloning
-emoji: ⚡
-colorFrom: yellow
-colorTo: yellow
-sdk: gradio
-sdk_version: 3.11
-app_file: app.py
-pinned: false
-license: mit
-duplicated_from: BilalSardar/Voice-Cloning
----
-
-Check out the configuration reference at https://huggingface.co/docs/hub/spaces-config-reference
diff --git a/spaces/kirch/Text2Video-Zero/annotator/uniformer/mmcv/ops/fused_bias_leakyrelu.py b/spaces/kirch/Text2Video-Zero/annotator/uniformer/mmcv/ops/fused_bias_leakyrelu.py
deleted file mode 100644
index 6d12508469c6c8fa1884debece44c58d158cb6fa..0000000000000000000000000000000000000000
--- a/spaces/kirch/Text2Video-Zero/annotator/uniformer/mmcv/ops/fused_bias_leakyrelu.py
+++ /dev/null
@@ -1,268 +0,0 @@
-# modified from https://github.com/rosinality/stylegan2-pytorch/blob/master/op/fused_act.py # noqa:E501
-
-# Copyright (c) 2021, NVIDIA Corporation. All rights reserved.
-# NVIDIA Source Code License for StyleGAN2 with Adaptive Discriminator
-# Augmentation (ADA)
-# =======================================================================
-
-# 1. Definitions
-
-# "Licensor" means any person or entity that distributes its Work.
-
-# "Software" means the original work of authorship made available under
-# this License.
-
-# "Work" means the Software and any additions to or derivative works of
-# the Software that are made available under this License.
-
-# The terms "reproduce," "reproduction," "derivative works," and
-# "distribution" have the meaning as provided under U.S. copyright law;
-# provided, however, that for the purposes of this License, derivative
-# works shall not include works that remain separable from, or merely
-# link (or bind by name) to the interfaces of, the Work.
-
-# Works, including the Software, are "made available" under this License
-# by including in or with the Work either (a) a copyright notice
-# referencing the applicability of this License to the Work, or (b) a
-# copy of this License.
-
-# 2. License Grants
-
-# 2.1 Copyright Grant. Subject to the terms and conditions of this
-# License, each Licensor grants to you a perpetual, worldwide,
-# non-exclusive, royalty-free, copyright license to reproduce,
-# prepare derivative works of, publicly display, publicly perform,
-# sublicense and distribute its Work and any resulting derivative
-# works in any form.
-
-# 3. Limitations
-
-# 3.1 Redistribution. You may reproduce or distribute the Work only
-# if (a) you do so under this License, (b) you include a complete
-# copy of this License with your distribution, and (c) you retain
-# without modification any copyright, patent, trademark, or
-# attribution notices that are present in the Work.
-
-# 3.2 Derivative Works. You may specify that additional or different
-# terms apply to the use, reproduction, and distribution of your
-# derivative works of the Work ("Your Terms") only if (a) Your Terms
-# provide that the use limitation in Section 3.3 applies to your
-# derivative works, and (b) you identify the specific derivative
-# works that are subject to Your Terms. Notwithstanding Your Terms,
-# this License (including the redistribution requirements in Section
-# 3.1) will continue to apply to the Work itself.
-
-# 3.3 Use Limitation. The Work and any derivative works thereof only
-# may be used or intended for use non-commercially. Notwithstanding
-# the foregoing, NVIDIA and its affiliates may use the Work and any
-# derivative works commercially. As used herein, "non-commercially"
-# means for research or evaluation purposes only.
-
-# 3.4 Patent Claims. If you bring or threaten to bring a patent claim
-# against any Licensor (including any claim, cross-claim or
-# counterclaim in a lawsuit) to enforce any patents that you allege
-# are infringed by any Work, then your rights under this License from
-# such Licensor (including the grant in Section 2.1) will terminate
-# immediately.
-
-# 3.5 Trademarks. This License does not grant any rights to use any
-# Licensor’s or its affiliates’ names, logos, or trademarks, except
-# as necessary to reproduce the notices described in this License.
-
-# 3.6 Termination. If you violate any term of this License, then your
-# rights under this License (including the grant in Section 2.1) will
-# terminate immediately.
-
-# 4. Disclaimer of Warranty.
-
-# THE WORK IS PROVIDED "AS IS" WITHOUT WARRANTIES OR CONDITIONS OF ANY
-# KIND, EITHER EXPRESS OR IMPLIED, INCLUDING WARRANTIES OR CONDITIONS OF
-# MERCHANTABILITY, FITNESS FOR A PARTICULAR PURPOSE, TITLE OR
-# NON-INFRINGEMENT. YOU BEAR THE RISK OF UNDERTAKING ANY ACTIVITIES UNDER
-# THIS LICENSE.
-
-# 5. Limitation of Liability.
-
-# EXCEPT AS PROHIBITED BY APPLICABLE LAW, IN NO EVENT AND UNDER NO LEGAL
-# THEORY, WHETHER IN TORT (INCLUDING NEGLIGENCE), CONTRACT, OR OTHERWISE
-# SHALL ANY LICENSOR BE LIABLE TO YOU FOR DAMAGES, INCLUDING ANY DIRECT,
-# INDIRECT, SPECIAL, INCIDENTAL, OR CONSEQUENTIAL DAMAGES ARISING OUT OF
-# OR RELATED TO THIS LICENSE, THE USE OR INABILITY TO USE THE WORK
-# (INCLUDING BUT NOT LIMITED TO LOSS OF GOODWILL, BUSINESS INTERRUPTION,
-# LOST PROFITS OR DATA, COMPUTER FAILURE OR MALFUNCTION, OR ANY OTHER
-# COMMERCIAL DAMAGES OR LOSSES), EVEN IF THE LICENSOR HAS BEEN ADVISED OF
-# THE POSSIBILITY OF SUCH DAMAGES.
-
-# =======================================================================
-
-import torch
-import torch.nn.functional as F
-from torch import nn
-from torch.autograd import Function
-
-from ..utils import ext_loader
-
-ext_module = ext_loader.load_ext('_ext', ['fused_bias_leakyrelu'])
-
-
-class FusedBiasLeakyReLUFunctionBackward(Function):
- """Calculate second order deviation.
-
- This function is to compute the second order deviation for the fused leaky
- relu operation.
- """
-
- @staticmethod
- def forward(ctx, grad_output, out, negative_slope, scale):
- ctx.save_for_backward(out)
- ctx.negative_slope = negative_slope
- ctx.scale = scale
-
- empty = grad_output.new_empty(0)
-
- grad_input = ext_module.fused_bias_leakyrelu(
- grad_output,
- empty,
- out,
- act=3,
- grad=1,
- alpha=negative_slope,
- scale=scale)
-
- dim = [0]
-
- if grad_input.ndim > 2:
- dim += list(range(2, grad_input.ndim))
-
- grad_bias = grad_input.sum(dim).detach()
-
- return grad_input, grad_bias
-
- @staticmethod
- def backward(ctx, gradgrad_input, gradgrad_bias):
- out, = ctx.saved_tensors
-
- # The second order deviation, in fact, contains two parts, while the
- # the first part is zero. Thus, we direct consider the second part
- # which is similar with the first order deviation in implementation.
- gradgrad_out = ext_module.fused_bias_leakyrelu(
- gradgrad_input,
- gradgrad_bias.to(out.dtype),
- out,
- act=3,
- grad=1,
- alpha=ctx.negative_slope,
- scale=ctx.scale)
-
- return gradgrad_out, None, None, None
-
-
-class FusedBiasLeakyReLUFunction(Function):
-
- @staticmethod
- def forward(ctx, input, bias, negative_slope, scale):
- empty = input.new_empty(0)
-
- out = ext_module.fused_bias_leakyrelu(
- input,
- bias,
- empty,
- act=3,
- grad=0,
- alpha=negative_slope,
- scale=scale)
- ctx.save_for_backward(out)
- ctx.negative_slope = negative_slope
- ctx.scale = scale
-
- return out
-
- @staticmethod
- def backward(ctx, grad_output):
- out, = ctx.saved_tensors
-
- grad_input, grad_bias = FusedBiasLeakyReLUFunctionBackward.apply(
- grad_output, out, ctx.negative_slope, ctx.scale)
-
- return grad_input, grad_bias, None, None
-
-
-class FusedBiasLeakyReLU(nn.Module):
- """Fused bias leaky ReLU.
-
- This function is introduced in the StyleGAN2:
- http://arxiv.org/abs/1912.04958
-
- The bias term comes from the convolution operation. In addition, to keep
- the variance of the feature map or gradients unchanged, they also adopt a
- scale similarly with Kaiming initialization. However, since the
- :math:`1+{alpha}^2` : is too small, we can just ignore it. Therefore, the
- final scale is just :math:`\sqrt{2}`:. Of course, you may change it with # noqa: W605, E501
- your own scale.
-
- TODO: Implement the CPU version.
-
- Args:
- channel (int): The channel number of the feature map.
- negative_slope (float, optional): Same as nn.LeakyRelu.
- Defaults to 0.2.
- scale (float, optional): A scalar to adjust the variance of the feature
- map. Defaults to 2**0.5.
- """
-
- def __init__(self, num_channels, negative_slope=0.2, scale=2**0.5):
- super(FusedBiasLeakyReLU, self).__init__()
-
- self.bias = nn.Parameter(torch.zeros(num_channels))
- self.negative_slope = negative_slope
- self.scale = scale
-
- def forward(self, input):
- return fused_bias_leakyrelu(input, self.bias, self.negative_slope,
- self.scale)
-
-
-def fused_bias_leakyrelu(input, bias, negative_slope=0.2, scale=2**0.5):
- """Fused bias leaky ReLU function.
-
- This function is introduced in the StyleGAN2:
- http://arxiv.org/abs/1912.04958
-
- The bias term comes from the convolution operation. In addition, to keep
- the variance of the feature map or gradients unchanged, they also adopt a
- scale similarly with Kaiming initialization. However, since the
- :math:`1+{alpha}^2` : is too small, we can just ignore it. Therefore, the
- final scale is just :math:`\sqrt{2}`:. Of course, you may change it with # noqa: W605, E501
- your own scale.
-
- Args:
- input (torch.Tensor): Input feature map.
- bias (nn.Parameter): The bias from convolution operation.
- negative_slope (float, optional): Same as nn.LeakyRelu.
- Defaults to 0.2.
- scale (float, optional): A scalar to adjust the variance of the feature
- map. Defaults to 2**0.5.
-
- Returns:
- torch.Tensor: Feature map after non-linear activation.
- """
-
- if not input.is_cuda:
- return bias_leakyrelu_ref(input, bias, negative_slope, scale)
-
- return FusedBiasLeakyReLUFunction.apply(input, bias.to(input.dtype),
- negative_slope, scale)
-
-
-def bias_leakyrelu_ref(x, bias, negative_slope=0.2, scale=2**0.5):
-
- if bias is not None:
- assert bias.ndim == 1
- assert bias.shape[0] == x.shape[1]
- x = x + bias.reshape([-1 if i == 1 else 1 for i in range(x.ndim)])
-
- x = F.leaky_relu(x, negative_slope)
- if scale != 1:
- x = x * scale
-
- return x
diff --git a/spaces/kirch/Text2Video-Zero/annotator/uniformer/mmcv/parallel/data_parallel.py b/spaces/kirch/Text2Video-Zero/annotator/uniformer/mmcv/parallel/data_parallel.py
deleted file mode 100644
index 79b5f69b654cf647dc7ae9174223781ab5c607d2..0000000000000000000000000000000000000000
--- a/spaces/kirch/Text2Video-Zero/annotator/uniformer/mmcv/parallel/data_parallel.py
+++ /dev/null
@@ -1,89 +0,0 @@
-# Copyright (c) OpenMMLab. All rights reserved.
-from itertools import chain
-
-from torch.nn.parallel import DataParallel
-
-from .scatter_gather import scatter_kwargs
-
-
-class MMDataParallel(DataParallel):
- """The DataParallel module that supports DataContainer.
-
- MMDataParallel has two main differences with PyTorch DataParallel:
-
- - It supports a custom type :class:`DataContainer` which allows more
- flexible control of input data during both GPU and CPU inference.
- - It implement two more APIs ``train_step()`` and ``val_step()``.
-
- Args:
- module (:class:`nn.Module`): Module to be encapsulated.
- device_ids (list[int]): Device IDS of modules to be scattered to.
- Defaults to None when GPU is not available.
- output_device (str | int): Device ID for output. Defaults to None.
- dim (int): Dimension used to scatter the data. Defaults to 0.
- """
-
- def __init__(self, *args, dim=0, **kwargs):
- super(MMDataParallel, self).__init__(*args, dim=dim, **kwargs)
- self.dim = dim
-
- def forward(self, *inputs, **kwargs):
- """Override the original forward function.
-
- The main difference lies in the CPU inference where the data in
- :class:`DataContainers` will still be gathered.
- """
- if not self.device_ids:
- # We add the following line thus the module could gather and
- # convert data containers as those in GPU inference
- inputs, kwargs = self.scatter(inputs, kwargs, [-1])
- return self.module(*inputs[0], **kwargs[0])
- else:
- return super().forward(*inputs, **kwargs)
-
- def scatter(self, inputs, kwargs, device_ids):
- return scatter_kwargs(inputs, kwargs, device_ids, dim=self.dim)
-
- def train_step(self, *inputs, **kwargs):
- if not self.device_ids:
- # We add the following line thus the module could gather and
- # convert data containers as those in GPU inference
- inputs, kwargs = self.scatter(inputs, kwargs, [-1])
- return self.module.train_step(*inputs[0], **kwargs[0])
-
- assert len(self.device_ids) == 1, \
- ('MMDataParallel only supports single GPU training, if you need to'
- ' train with multiple GPUs, please use MMDistributedDataParallel'
- 'instead.')
-
- for t in chain(self.module.parameters(), self.module.buffers()):
- if t.device != self.src_device_obj:
- raise RuntimeError(
- 'module must have its parameters and buffers '
- f'on device {self.src_device_obj} (device_ids[0]) but '
- f'found one of them on device: {t.device}')
-
- inputs, kwargs = self.scatter(inputs, kwargs, self.device_ids)
- return self.module.train_step(*inputs[0], **kwargs[0])
-
- def val_step(self, *inputs, **kwargs):
- if not self.device_ids:
- # We add the following line thus the module could gather and
- # convert data containers as those in GPU inference
- inputs, kwargs = self.scatter(inputs, kwargs, [-1])
- return self.module.val_step(*inputs[0], **kwargs[0])
-
- assert len(self.device_ids) == 1, \
- ('MMDataParallel only supports single GPU training, if you need to'
- ' train with multiple GPUs, please use MMDistributedDataParallel'
- ' instead.')
-
- for t in chain(self.module.parameters(), self.module.buffers()):
- if t.device != self.src_device_obj:
- raise RuntimeError(
- 'module must have its parameters and buffers '
- f'on device {self.src_device_obj} (device_ids[0]) but '
- f'found one of them on device: {t.device}')
-
- inputs, kwargs = self.scatter(inputs, kwargs, self.device_ids)
- return self.module.val_step(*inputs[0], **kwargs[0])
diff --git a/spaces/kkawamu1/huggingface_multi_inference_rank_eval/app/evaluation_scripts/run_eval.py b/spaces/kkawamu1/huggingface_multi_inference_rank_eval/app/evaluation_scripts/run_eval.py
deleted file mode 100644
index 9f56424c4481a5a3bba4d4608f3fe0379f2dfe96..0000000000000000000000000000000000000000
--- a/spaces/kkawamu1/huggingface_multi_inference_rank_eval/app/evaluation_scripts/run_eval.py
+++ /dev/null
@@ -1,185 +0,0 @@
-# Copyright 2022 Ken Kawamura
-# Copyright BigScience, The HuggingFace Team and The HuggingFace Inc. team. All rights reserved.
-#
-# Licensed under the Apache License, Version 2.0 (the "License");
-# you may not use this file except in compliance with the License.
-# You may obtain a copy of the License at
-#
-# http://www.apache.org/licenses/LICENSE-2.0
-#
-# Unless required by applicable law or agreed to in writing, software
-# distributed under the License is distributed on an "AS IS" BASIS,
-# WITHOUT WARRANTIES OR CONDITIONS OF ANY KIND, either express or implied.
-# See the License for the specific language governing permissions and
-# limitations under the License.
-#
-# This file has been modifed from the original version on https://github.com/bigscience-workshop/t-zero/blob/master/evaluation/run_eval.py
-
-import torch
-from accelerate import Accelerator
-from transformers import (AutoModelForCausalLM, AutoModelForSeq2SeqLM,
- AutoTokenizer, set_seed)
-
-
-def multi_inference_rank_eval(model_name_or_path, auto_class, ex_answer_choices, context):
- accelerator = Accelerator()
- set_seed(42)
- model_name = model_name_or_path
- if auto_class == 'Seq2SeqLM':
-
- # e.g. 'google/t5-small-lm-adapt'
- model = AutoModelForSeq2SeqLM.from_pretrained(model_name)
- tokenizer = AutoTokenizer.from_pretrained(model_name, use_fast=True)
- else:
- # e.g. 'gpt2'
- model = AutoModelForCausalLM.from_pretrained(model_name)
- tokenizer = AutoTokenizer.from_pretrained(model_name, use_fast=True)
-
- if tokenizer.pad_token is None:
- for token in [tokenizer.eos_token, tokenizer.bos_token, tokenizer.sep_token]:
- if token is not None:
- tokenizer.pad_token = token
- if tokenizer.pad_token is None:
- raise ValueError("Please define a pad token id.")
-
- padding = False
-
- if auto_class == 'Seq2SeqLM':
- def preprocess_function(context, ex_answer_choices):
- input_texts = []
- answer_choices_texts = []
- input_texts.append(context)
- answer_choices_texts.append(
- [' ' + ans for ans in ex_answer_choices])
-
- tokenized_inputs = tokenizer(
- input_texts,
- padding=padding,
- max_length=1024,
- truncation=True,
- add_special_tokens=False,
- )
-
- tokenized_targets = [
- tokenizer(
- ans_choi,
- padding=True,
- max_length=256,
- truncation=True,
- )
- for ans_choi in answer_choices_texts
- ]
-
- features = {
- k: [
- [elem for _ in range(
- len(tokenized_targets[idx]["input_ids"]))]
- for idx, elem in enumerate(v)
- ]
- for k, v in tokenized_inputs.items()
- }
-
- features["labels"] = [
- tokenized_targets[0]["input_ids"]
- ]
-
- features["labels_attention_mask"] = [
- tokenized_targets[0]["attention_mask"]
- ]
- return features
- else:
- def preprocess_function(context, ex_answer_choices):
- input_texts = []
- answer_choices_texts = []
- input_texts.append(context)
- answer_choices_texts.append(
- [' ' + ans for ans in ex_answer_choices])
-
- tokenized_inputs = tokenizer(
- input_texts,
- padding=padding,
- max_length=1024,
- truncation=True,
- add_special_tokens=False,
- )
-
- tokenized_targets = [
- tokenizer(
- ans_choi,
- padding=True,
- max_length=256,
- truncation=True,
- )
- for ans_choi in answer_choices_texts
- ]
-
- features = {
- k: [
- [elem for _ in range(
- len(tokenized_targets[idx]["input_ids"]))]
- for idx, elem in enumerate(v)
- ]
- for k, v in tokenized_inputs.items()
- }
-
- features["labels"] = [
- tokenized_targets[0]["input_ids"]
- ]
-
- features["labels_attention_mask"] = [
- tokenized_targets[0]["attention_mask"]
- ]
-
- features["labels"] = [
- [features["input_ids"][0][i][1:] + tokenized_targets[0]["input_ids"][i]
- for i in range(len(tokenized_targets[0]["input_ids"]))]
- ]
- features["input_ids"] = [
- [features["input_ids"][0][i] + tokenized_targets[0]["input_ids"][i][:-1]
- for i in range(len(tokenized_targets[0]["input_ids"]))]
- ]
-
- features["labels_attention_mask"] = [
- [[0] * (len(features["attention_mask"][0][i])-1) + tokenized_targets[0]
- ["attention_mask"][i] for i in range(len(tokenized_targets[0]["input_ids"]))]
- ]
-
- features["attention_mask"] = [
- [features["attention_mask"][0][i] + tokenized_targets[0]["attention_mask"][i][:-1]
- for i in range(len(tokenized_targets[0]["input_ids"]))]
- ]
-
- return features
-
- device = accelerator.device
- model.to(device)
- batch = preprocess_function(context, ex_answer_choices)
- batch = {
- k: torch.tensor(batch[k][0]).to(device)
- for k in batch.keys()
- }
-
- model.eval()
- with torch.no_grad():
- model_inputs = {
- k: batch[k]
- for k in (["input_ids", "attention_mask", "labels"] if auto_class == 'Seq2SeqLM' else ["input_ids", "attention_mask"])
- }
-
- logits = model(**model_inputs).logits
- masked_log_probs = batch["labels_attention_mask"].unsqueeze(
- -1) * torch.log_softmax(logits, dim=-1)
- seq_token_log_probs = torch.gather(
- masked_log_probs, -1, batch["labels"].unsqueeze(-1))
- seq_log_prob = seq_token_log_probs.squeeze(dim=-1).sum(dim=-1)
- seq_log_prob = seq_log_prob.view(1, -1)
- predictions = seq_log_prob.argmax(dim=-1)
-
- predictions = accelerator.gather(predictions)
- return predictions.item()
-
-
-if __name__ == "__main__":
- multi_inference_rank_eval('google/t5-small-lm-adapt', 'Seq2SeqLM',
- ['True', 'False', 'True', 'Ken'], 'I am Ken. True or False')
- # multi_inference_rank_eval('gpt2', 'CausalLM', ['True', 'False', 'True', 'Ken'], 'I am Ken. True or False')
diff --git a/spaces/koajoel/PolyFormer/fairseq/examples/noisychannel/rerank_score_lm.py b/spaces/koajoel/PolyFormer/fairseq/examples/noisychannel/rerank_score_lm.py
deleted file mode 100644
index e80948d78b02561cbd09d72c319222105f41f6bb..0000000000000000000000000000000000000000
--- a/spaces/koajoel/PolyFormer/fairseq/examples/noisychannel/rerank_score_lm.py
+++ /dev/null
@@ -1,81 +0,0 @@
-# Copyright (c) Facebook, Inc. and its affiliates.
-#
-# This source code is licensed under the MIT license found in the
-# LICENSE file in the root directory of this source tree.
-
-import os
-
-from fairseq import options
-
-from examples.noisychannel import rerank_options, rerank_utils
-
-
-def score_lm(args):
- using_nbest = args.nbest_list is not None
- (
- pre_gen,
- left_to_right_preprocessed_dir,
- right_to_left_preprocessed_dir,
- backwards_preprocessed_dir,
- lm_preprocessed_dir,
- ) = rerank_utils.get_directories(
- args.data_dir_name,
- args.num_rescore,
- args.gen_subset,
- args.gen_model_name,
- args.shard_id,
- args.num_shards,
- args.sampling,
- args.prefix_len,
- args.target_prefix_frac,
- args.source_prefix_frac,
- )
-
- predictions_bpe_file = pre_gen + "/generate_output_bpe.txt"
- if using_nbest:
- print("Using predefined n-best list from interactive.py")
- predictions_bpe_file = args.nbest_list
-
- gen_output = rerank_utils.BitextOutputFromGen(
- predictions_bpe_file, bpe_symbol=args.post_process, nbest=using_nbest
- )
-
- if args.language_model is not None:
- lm_score_file = rerank_utils.rescore_file_name(
- pre_gen, args.prefix_len, args.lm_name, lm_file=True
- )
-
- if args.language_model is not None and not os.path.isfile(lm_score_file):
- print("STEP 4.5: language modeling for P(T)")
- if args.lm_bpe_code is None:
- bpe_status = "no bpe"
- elif args.lm_bpe_code == "shared":
- bpe_status = "shared"
- else:
- bpe_status = "different"
-
- rerank_utils.lm_scoring(
- lm_preprocessed_dir,
- bpe_status,
- gen_output,
- pre_gen,
- args.lm_dict,
- args.lm_name,
- args.language_model,
- args.lm_bpe_code,
- 128,
- lm_score_file,
- args.target_lang,
- args.source_lang,
- prefix_len=args.prefix_len,
- )
-
-
-def cli_main():
- parser = rerank_options.get_reranking_parser()
- args = options.parse_args_and_arch(parser)
- score_lm(args)
-
-
-if __name__ == "__main__":
- cli_main()
diff --git a/spaces/ky2k/Toxicity_Classifier_POC/.venv/lib/python3.9/site-packages/aiohttp/locks.py b/spaces/ky2k/Toxicity_Classifier_POC/.venv/lib/python3.9/site-packages/aiohttp/locks.py
deleted file mode 100644
index de2dc83d09dd950fc1ed8d7edaeb20e7697c94ba..0000000000000000000000000000000000000000
--- a/spaces/ky2k/Toxicity_Classifier_POC/.venv/lib/python3.9/site-packages/aiohttp/locks.py
+++ /dev/null
@@ -1,41 +0,0 @@
-import asyncio
-import collections
-from typing import Any, Deque, Optional
-
-
-class EventResultOrError:
- """Event asyncio lock helper class.
-
- Wraps the Event asyncio lock allowing either to awake the
- locked Tasks without any error or raising an exception.
-
- thanks to @vorpalsmith for the simple design.
- """
-
- def __init__(self, loop: asyncio.AbstractEventLoop) -> None:
- self._loop = loop
- self._exc: Optional[BaseException] = None
- self._event = asyncio.Event()
- self._waiters: Deque[asyncio.Future[Any]] = collections.deque()
-
- def set(self, exc: Optional[BaseException] = None) -> None:
- self._exc = exc
- self._event.set()
-
- async def wait(self) -> Any:
- waiter = self._loop.create_task(self._event.wait())
- self._waiters.append(waiter)
- try:
- val = await waiter
- finally:
- self._waiters.remove(waiter)
-
- if self._exc is not None:
- raise self._exc
-
- return val
-
- def cancel(self) -> None:
- """Cancel all waiters"""
- for waiter in self._waiters:
- waiter.cancel()
diff --git a/spaces/ky2k/Toxicity_Classifier_POC/.venv/lib/python3.9/site-packages/altair/_magics.py b/spaces/ky2k/Toxicity_Classifier_POC/.venv/lib/python3.9/site-packages/altair/_magics.py
deleted file mode 100644
index 7fe6131182952ff30bf63543de528657f7ba77a2..0000000000000000000000000000000000000000
--- a/spaces/ky2k/Toxicity_Classifier_POC/.venv/lib/python3.9/site-packages/altair/_magics.py
+++ /dev/null
@@ -1,109 +0,0 @@
-"""
-Magic functions for rendering vega-lite specifications
-"""
-__all__ = ["vegalite"]
-
-import json
-import warnings
-
-import IPython
-from IPython.core import magic_arguments
-import pandas as pd
-from toolz import curried
-
-from altair.vegalite import v5 as vegalite_v5
-
-try:
- import yaml
-
- YAML_AVAILABLE = True
-except ImportError:
- YAML_AVAILABLE = False
-
-
-RENDERERS = {
- "vega-lite": {
- "5": vegalite_v5.VegaLite,
- },
-}
-
-
-TRANSFORMERS = {
- "vega-lite": {
- "5": vegalite_v5.data_transformers,
- },
-}
-
-
-def _prepare_data(data, data_transformers):
- """Convert input data to data for use within schema"""
- if data is None or isinstance(data, dict):
- return data
- elif isinstance(data, pd.DataFrame):
- return curried.pipe(data, data_transformers.get())
- elif isinstance(data, str):
- return {"url": data}
- else:
- warnings.warn("data of type {} not recognized".format(type(data)), stacklevel=1)
- return data
-
-
-def _get_variable(name):
- """Get a variable from the notebook namespace."""
- ip = IPython.get_ipython()
- if ip is None:
- raise ValueError(
- "Magic command must be run within an IPython "
- "environemnt, in which get_ipython() is defined."
- )
- if name not in ip.user_ns:
- raise NameError(
- "argument '{}' does not match the "
- "name of any defined variable".format(name)
- )
- return ip.user_ns[name]
-
-
-@magic_arguments.magic_arguments()
-@magic_arguments.argument(
- "data",
- nargs="?",
- help="local variablename of a pandas DataFrame to be used as the dataset",
-)
-@magic_arguments.argument("-v", "--version", dest="version", default="v5")
-@magic_arguments.argument("-j", "--json", dest="json", action="store_true")
-def vegalite(line, cell):
- """Cell magic for displaying vega-lite visualizations in CoLab.
-
- %%vegalite [dataframe] [--json] [--version='v5']
-
- Visualize the contents of the cell using Vega-Lite, optionally
- specifying a pandas DataFrame object to be used as the dataset.
-
- if --json is passed, then input is parsed as json rather than yaml.
- """
- args = magic_arguments.parse_argstring(vegalite, line)
- existing_versions = {"v5": "5"}
- version = existing_versions[args.version]
- assert version in RENDERERS["vega-lite"]
- VegaLite = RENDERERS["vega-lite"][version]
- data_transformers = TRANSFORMERS["vega-lite"][version]
-
- if args.json:
- spec = json.loads(cell)
- elif not YAML_AVAILABLE:
- try:
- spec = json.loads(cell)
- except json.JSONDecodeError as err:
- raise ValueError(
- "%%vegalite: spec is not valid JSON. "
- "Install pyyaml to parse spec as yaml"
- ) from err
- else:
- spec = yaml.load(cell, Loader=yaml.SafeLoader)
-
- if args.data is not None:
- data = _get_variable(args.data)
- spec["data"] = _prepare_data(data, data_transformers)
-
- return VegaLite(spec)
diff --git a/spaces/ky2k/Toxicity_Classifier_POC/.venv/lib/python3.9/site-packages/gradio/templates/frontend/assets/frontmatter-1b6984ab.js b/spaces/ky2k/Toxicity_Classifier_POC/.venv/lib/python3.9/site-packages/gradio/templates/frontend/assets/frontmatter-1b6984ab.js
deleted file mode 100644
index 1f7a8ac10b55e7abeffb283d87d5f73565b4943c..0000000000000000000000000000000000000000
--- a/spaces/ky2k/Toxicity_Classifier_POC/.venv/lib/python3.9/site-packages/gradio/templates/frontend/assets/frontmatter-1b6984ab.js
+++ /dev/null
@@ -1,2 +0,0 @@
-import{s as m,f as s,a as i,p,t as a,S as l}from"./index-aa084753.js";import{yaml as f}from"./yaml-95012b83.js";import"./index-8c3da1d9.js";import"./Blocks-6ad6f005.js";import"./Button-62634b34.js";import"./BlockLabel-98ef75ee.js";import"./Empty-5d52e655.js";/* empty css */import"./Copy-fd383441.js";import"./Download-dfb06e25.js";const n=/^---\s*$/m,b={defineNodes:[{name:"Frontmatter",block:!0},"FrontmatterMark"],props:[m({Frontmatter:[a.documentMeta,a.monospace],FrontmatterMark:a.processingInstruction}),s.add({Frontmatter:i,FrontmatterMark:()=>null})],wrap:p(t=>{const{parser:e}=l.define(f);return t.type.name==="Frontmatter"?{parser:e,overlay:[{from:t.from+4,to:t.to-4}]}:null}),parseBlock:[{name:"Frontmatter",before:"HorizontalRule",parse:(t,e)=>{let r;const o=new Array;if(t.lineStart===0&&n.test(e.text)){for(o.push(t.elt("FrontmatterMark",0,4));t.nextLine();)if(n.test(e.text)){r=t.lineStart+4;break}return r!==void 0&&(o.push(t.elt("FrontmatterMark",r-4,r)),t.addElement(t.elt("Frontmatter",0,r,o))),!0}else return!1}}]};export{b as frontmatter};
-//# sourceMappingURL=frontmatter-1b6984ab.js.map
diff --git a/spaces/ky2k/Toxicity_Classifier_POC/.venv/lib/python3.9/site-packages/huggingface_hub/utils/_runtime.py b/spaces/ky2k/Toxicity_Classifier_POC/.venv/lib/python3.9/site-packages/huggingface_hub/utils/_runtime.py
deleted file mode 100644
index ad773892afe194ea73faa0f61598de2937c010d5..0000000000000000000000000000000000000000
--- a/spaces/ky2k/Toxicity_Classifier_POC/.venv/lib/python3.9/site-packages/huggingface_hub/utils/_runtime.py
+++ /dev/null
@@ -1,295 +0,0 @@
-# coding=utf-8
-# Copyright 2022-present, the HuggingFace Inc. team.
-#
-# Licensed under the Apache License, Version 2.0 (the "License");
-# you may not use this file except in compliance with the License.
-# You may obtain a copy of the License at
-#
-# http://www.apache.org/licenses/LICENSE-2.0
-#
-# Unless required by applicable law or agreed to in writing, software
-# distributed under the License is distributed on an "AS IS" BASIS,
-# WITHOUT WARRANTIES OR CONDITIONS OF ANY KIND, either express or implied.
-# See the License for the specific language governing permissions and
-# limitations under the License.
-"""Check presence of installed packages at runtime."""
-import platform
-import sys
-from typing import Any, Dict
-
-import packaging.version
-
-from .. import __version__, constants
-
-
-_PY_VERSION: str = sys.version.split()[0].rstrip("+")
-
-if packaging.version.Version(_PY_VERSION) < packaging.version.Version("3.8.0"):
- import importlib_metadata # type: ignore
-else:
- import importlib.metadata as importlib_metadata # type: ignore
-
-
-_package_versions = {}
-
-_CANDIDATES = {
- "fastai": {"fastai"},
- "fastcore": {"fastcore"},
- "gradio": {"gradio"},
- "graphviz": {"graphviz"},
- "hf_transfer": {"hf_transfer"},
- "jinja": {"Jinja2"},
- "numpy": {"numpy"},
- "pillow": {"Pillow"},
- "pydot": {"pydot"},
- "tensorflow": (
- "tensorflow",
- "tensorflow-cpu",
- "tensorflow-gpu",
- "tf-nightly",
- "tf-nightly-cpu",
- "tf-nightly-gpu",
- "intel-tensorflow",
- "intel-tensorflow-avx512",
- "tensorflow-rocm",
- "tensorflow-macos",
- ),
- "torch": {"torch"},
-}
-
-# Check once at runtime
-for candidate_name, package_names in _CANDIDATES.items():
- _package_versions[candidate_name] = "N/A"
- for name in package_names:
- try:
- _package_versions[candidate_name] = importlib_metadata.version(name)
- break
- except importlib_metadata.PackageNotFoundError:
- pass
-
-
-def _get_version(package_name: str) -> str:
- return _package_versions.get(package_name, "N/A")
-
-
-def _is_available(package_name: str) -> bool:
- return _get_version(package_name) != "N/A"
-
-
-# Python
-def get_python_version() -> str:
- return _PY_VERSION
-
-
-# Huggingface Hub
-def get_hf_hub_version() -> str:
- return __version__
-
-
-# FastAI
-def is_fastai_available() -> bool:
- return _is_available("fastai")
-
-
-def get_fastai_version() -> str:
- return _get_version("fastai")
-
-
-# Fastcore
-def is_fastcore_available() -> bool:
- return _is_available("fastcore")
-
-
-def get_fastcore_version() -> str:
- return _get_version("fastcore")
-
-
-# FastAI
-def is_gradio_available() -> bool:
- return _is_available("gradio")
-
-
-def get_gradio_version() -> str:
- return _get_version("gradio")
-
-
-# Graphviz
-def is_graphviz_available() -> bool:
- return _is_available("graphviz")
-
-
-def get_graphviz_version() -> str:
- return _get_version("graphviz")
-
-
-# hf_transfer
-def is_hf_transfer_available() -> bool:
- return _is_available("hf_transfer")
-
-
-def get_hf_transfer_version() -> str:
- return _get_version("hf_transfer")
-
-
-# Numpy
-def is_numpy_available() -> bool:
- return _is_available("numpy")
-
-
-def get_numpy_version() -> str:
- return _get_version("numpy")
-
-
-# Jinja
-def is_jinja_available() -> bool:
- return _is_available("jinja")
-
-
-def get_jinja_version() -> str:
- return _get_version("jinja")
-
-
-# Pillow
-def is_pillow_available() -> bool:
- return _is_available("pillow")
-
-
-def get_pillow_version() -> str:
- return _get_version("pillow")
-
-
-# Pydot
-def is_pydot_available() -> bool:
- return _is_available("pydot")
-
-
-def get_pydot_version() -> str:
- return _get_version("pydot")
-
-
-# Tensorflow
-def is_tf_available() -> bool:
- return _is_available("tensorflow")
-
-
-def get_tf_version() -> str:
- return _get_version("tensorflow")
-
-
-# Torch
-def is_torch_available() -> bool:
- return _is_available("torch")
-
-
-def get_torch_version() -> str:
- return _get_version("torch")
-
-
-# Shell-related helpers
-try:
- # Set to `True` if script is running in a Google Colab notebook.
- # If running in Google Colab, git credential store is set globally which makes the
- # warning disappear. See https://github.com/huggingface/huggingface_hub/issues/1043
- #
- # Taken from https://stackoverflow.com/a/63519730.
- _is_google_colab = "google.colab" in str(get_ipython()) # type: ignore # noqa: F821
-except NameError:
- _is_google_colab = False
-
-
-def is_notebook() -> bool:
- """Return `True` if code is executed in a notebook (Jupyter, Colab, QTconsole).
-
- Taken from https://stackoverflow.com/a/39662359.
- Adapted to make it work with Google colab as well.
- """
- try:
- shell_class = get_ipython().__class__ # type: ignore # noqa: F821
- for parent_class in shell_class.__mro__: # e.g. "is subclass of"
- if parent_class.__name__ == "ZMQInteractiveShell":
- return True # Jupyter notebook, Google colab or qtconsole
- return False
- except NameError:
- return False # Probably standard Python interpreter
-
-
-def is_google_colab() -> bool:
- """Return `True` if code is executed in a Google colab.
-
- Taken from https://stackoverflow.com/a/63519730.
- """
- return _is_google_colab
-
-
-def dump_environment_info() -> Dict[str, Any]:
- """Dump information about the machine to help debugging issues.
-
- Similar helper exist in:
- - `datasets` (https://github.com/huggingface/datasets/blob/main/src/datasets/commands/env.py)
- - `diffusers` (https://github.com/huggingface/diffusers/blob/main/src/diffusers/commands/env.py)
- - `transformers` (https://github.com/huggingface/transformers/blob/main/src/transformers/commands/env.py)
- """
- from huggingface_hub import HfFolder, whoami
- from huggingface_hub.utils import list_credential_helpers
-
- token = HfFolder().get_token()
-
- # Generic machine info
- info: Dict[str, Any] = {
- "huggingface_hub version": get_hf_hub_version(),
- "Platform": platform.platform(),
- "Python version": get_python_version(),
- }
-
- # Interpreter info
- try:
- shell_class = get_ipython().__class__ # type: ignore # noqa: F821
- info["Running in iPython ?"] = "Yes"
- info["iPython shell"] = shell_class.__name__
- except NameError:
- info["Running in iPython ?"] = "No"
- info["Running in notebook ?"] = "Yes" if is_notebook() else "No"
- info["Running in Google Colab ?"] = "Yes" if is_google_colab() else "No"
-
- # Login info
- info["Token path ?"] = HfFolder().path_token
- info["Has saved token ?"] = token is not None
- if token is not None:
- try:
- info["Who am I ?"] = whoami()["name"]
- except Exception:
- pass
-
- try:
- info["Configured git credential helpers"] = ", ".join(list_credential_helpers())
- except Exception:
- pass
-
- # Installed dependencies
- info["FastAI"] = get_fastai_version()
- info["Tensorflow"] = get_tf_version()
- info["Torch"] = get_torch_version()
- info["Jinja2"] = get_jinja_version()
- info["Graphviz"] = get_graphviz_version()
- info["Pydot"] = get_pydot_version()
- info["Pillow"] = get_pillow_version()
- info["hf_transfer"] = get_hf_transfer_version()
- info["gradio"] = get_gradio_version()
- info["numpy"] = get_numpy_version()
-
- # Environment variables
- info["ENDPOINT"] = constants.ENDPOINT
- info["HUGGINGFACE_HUB_CACHE"] = constants.HUGGINGFACE_HUB_CACHE
- info["HUGGINGFACE_ASSETS_CACHE"] = constants.HUGGINGFACE_ASSETS_CACHE
- info["HF_TOKEN_PATH"] = constants.HF_TOKEN_PATH
- info["HF_HUB_OFFLINE"] = constants.HF_HUB_OFFLINE
- info["HF_HUB_DISABLE_TELEMETRY"] = constants.HF_HUB_DISABLE_TELEMETRY
- info["HF_HUB_DISABLE_PROGRESS_BARS"] = constants.HF_HUB_DISABLE_PROGRESS_BARS
- info["HF_HUB_DISABLE_SYMLINKS_WARNING"] = constants.HF_HUB_DISABLE_SYMLINKS_WARNING
- info["HF_HUB_DISABLE_EXPERIMENTAL_WARNING"] = constants.HF_HUB_DISABLE_EXPERIMENTAL_WARNING
- info["HF_HUB_DISABLE_IMPLICIT_TOKEN"] = constants.HF_HUB_DISABLE_IMPLICIT_TOKEN
- info["HF_HUB_ENABLE_HF_TRANSFER"] = constants.HF_HUB_ENABLE_HF_TRANSFER
-
- print("\nCopy-and-paste the text below in your GitHub issue.\n")
- print("\n".join([f"- {prop}: {val}" for prop, val in info.items()]) + "\n")
- return info
diff --git a/spaces/lamini/instruct-playground/app.py b/spaces/lamini/instruct-playground/app.py
deleted file mode 100644
index 08a803fd3c2910707cade7c2ec50ef117c45a467..0000000000000000000000000000000000000000
--- a/spaces/lamini/instruct-playground/app.py
+++ /dev/null
@@ -1,37 +0,0 @@
-import gradio as gr
-from llama import Type, Context, LLM
-import os
-
-class Question(Type):
- question: str = Context("a question")
-
-class Response(Type):
- response: str = Context("the response to the question")
-
-def lamini(input):
- #return "Hello " + name + "!!"
- llm=LLM(name="lamini-instruct",
- config={
- "production":{
- "key": os.environ["LAMINI-KEY"]
- }
- })
- user_query_text=Question(question=input)
- result = llm(
- input=user_query_text,
- output_type=Response,
- model_name="lamini/instruct-tuned-2.8b"
- )
- return parse_response(result.response)
-
-def parse_response(string):
- break_point = string.find("\n\n")
-
- if break_point >= 0:
- string = string[:break_point]
-
- return string.strip()
-
-
-iface = gr.Interface(fn=lamini, inputs="text", outputs="text")
-iface.launch()
\ No newline at end of file
diff --git a/spaces/lcipolina/Print_Gallery/glide_text2im/clip/utils.py b/spaces/lcipolina/Print_Gallery/glide_text2im/clip/utils.py
deleted file mode 100644
index 8fc5b059dad76877f4442da36a8d6327302fe341..0000000000000000000000000000000000000000
--- a/spaces/lcipolina/Print_Gallery/glide_text2im/clip/utils.py
+++ /dev/null
@@ -1,97 +0,0 @@
-import math
-from typing import Callable, Optional
-
-import attr
-import torch
-import torch.nn as nn
-import torch.nn.functional as F
-
-FilterFn = Callable[[torch.Tensor], torch.Tensor]
-
-
-class ZeroKeyBiasGrad(torch.autograd.Function):
- @staticmethod
- def forward(ctx, x):
- return x
-
- @staticmethod
- def backward(ctx, output_grad):
- output_grad = output_grad.clone()
- output_grad.chunk(3)[1].zero_()
- return output_grad
-
-
-def zero_key_bias_grad(x: torch.Tensor) -> torch.Tensor:
- return ZeroKeyBiasGrad.apply(x)
-
-
-@attr.s(eq=False, repr=False)
-class LayerNorm(nn.Module):
- n_state: int = attr.ib()
- eps: float = attr.ib(default=1e-6)
- device: torch.device = attr.ib(default=torch.device("cuda"))
-
- def __attrs_post_init__(self) -> None:
- super().__init__()
- self.g = nn.Parameter(torch.ones((self.n_state,), dtype=torch.float32, device=self.device))
- self.b = nn.Parameter(torch.zeros((self.n_state,), dtype=torch.float32, device=self.device))
- self.g.weight_decay_level = "disable" # type: ignore
- self.b.weight_decay_level = "disable" # type: ignore
-
- def forward(self, x: torch.Tensor) -> torch.Tensor:
- return F.layer_norm(
- x.type(torch.float32), torch.Size((self.n_state,)), self.g, self.b, self.eps
- )
-
-
-@attr.s(eq=False, repr=False)
-class Affine(nn.Module):
- n_in: int = attr.ib()
- n_out: int = attr.ib()
- use_bias: bool = attr.ib(default=True)
- use_admnet_init: bool = attr.ib(default=False)
- std: Optional[float] = attr.ib(default=None)
- extra_init_scale: Optional[float] = attr.ib(default=None)
- bias_filter_fn: FilterFn = attr.ib(default=lambda x: x)
- device: torch.device = attr.ib(default=torch.device("cuda"))
-
- def __attrs_post_init__(self) -> None:
- super().__init__()
-
- if not self.use_admnet_init:
- self.std = self.std if self.std is not None else math.sqrt(2 / (self.n_in + self.n_out))
- self.std = (
- self.std if self.extra_init_scale is None else self.std * self.extra_init_scale
- )
-
- w = torch.empty((self.n_out, self.n_in), dtype=torch.float32, device=self.device)
- self.w = nn.Parameter(w)
-
- if self.use_bias:
- self.b = nn.Parameter(
- torch.zeros((self.n_out,), dtype=torch.float32, device=self.device)
- )
- self.b.weight_decay_level = "disable" # type: ignore
- else:
- if self.extra_init_scale is not None:
- raise ValueError("extra_init_scale incompatible with admnet init")
-
- w = torch.empty((self.n_out, self.n_in), dtype=torch.float32, device=self.device)
-
- if self.use_bias:
- b = torch.empty((self.n_out,), dtype=torch.float32, device=self.device)
-
- self.w = nn.Parameter(w)
-
- if self.use_bias:
- self.b = nn.Parameter(b)
- self.b.weight_decay_level = "disable" # type: ignore
-
- def forward(self, x: torch.Tensor) -> torch.Tensor:
- w = self.w if self.w.dtype == x.dtype else self.w.to(x.dtype)
- b = (
- self.bias_filter_fn(self.b if self.b.dtype == x.dtype else self.b.to(x.dtype))
- if self.use_bias
- else None
- )
- return F.linear(x, w, b)
diff --git a/spaces/leftcoastkidd/runwayml-stable-diffusion-v1-5/app.py b/spaces/leftcoastkidd/runwayml-stable-diffusion-v1-5/app.py
deleted file mode 100644
index a82df332731f067826d3e1ef79fabceffb74d07e..0000000000000000000000000000000000000000
--- a/spaces/leftcoastkidd/runwayml-stable-diffusion-v1-5/app.py
+++ /dev/null
@@ -1,3 +0,0 @@
-import gradio as gr
-
-gr.Interface.load("models/runwayml/stable-diffusion-v1-5").launch()
\ No newline at end of file
diff --git a/spaces/lewtun/stable-diffusion-demo/app.py b/spaces/lewtun/stable-diffusion-demo/app.py
deleted file mode 100644
index 8106a41d952218e5819268da50c9d104ec0dad5b..0000000000000000000000000000000000000000
--- a/spaces/lewtun/stable-diffusion-demo/app.py
+++ /dev/null
@@ -1,32 +0,0 @@
-import torch
-import os
-
-auth_token = os.getenv("HF_TOKEN")
-
-device = "cuda" if torch.cuda.is_available() else "cpu"
-torch_dtype = torch.float16 if device == "cuda" else None
-
-from diffusers import StableDiffusionPipeline
-
-model_id = "CompVis/stable-diffusion-v1-4"
-pipe = StableDiffusionPipeline.from_pretrained(
- model_id, use_auth_token=auth_token, revision="fp16", torch_dtype=torch_dtype
-).to(device)
-
-def predict(prompt):
- return pipe(prompt).images[0]
-
-import gradio as gr
-
-gradio_ui = gr.Interface(
- fn=predict,
- title="Stable Diffusion Demo",
- description="Enter a description of an image you'd like to generate!",
- inputs=[
- gr.Textbox(lines=2, label="Paste some text here"),
- ],
- outputs=["image"],
- examples=[["a photograph of an astronaut riding a horse"]],
-)
-
-gradio_ui.launch()
\ No newline at end of file
diff --git a/spaces/lfoppiano/document-qa/streamlit_app.py b/spaces/lfoppiano/document-qa/streamlit_app.py
deleted file mode 100644
index 845893128195c344bc81865ca659bbe932ab0a06..0000000000000000000000000000000000000000
--- a/spaces/lfoppiano/document-qa/streamlit_app.py
+++ /dev/null
@@ -1,320 +0,0 @@
-import os
-import re
-from hashlib import blake2b
-from tempfile import NamedTemporaryFile
-
-import dotenv
-from grobid_quantities.quantities import QuantitiesAPI
-from langchain.llms.huggingface_hub import HuggingFaceHub
-
-dotenv.load_dotenv(override=True)
-
-import streamlit as st
-from langchain.chat_models import ChatOpenAI
-from langchain.embeddings import OpenAIEmbeddings, HuggingFaceEmbeddings
-
-from document_qa.document_qa_engine import DocumentQAEngine
-from document_qa.grobid_processors import GrobidAggregationProcessor, decorate_text_with_annotations
-from grobid_client_generic import GrobidClientGeneric
-
-if 'rqa' not in st.session_state:
- st.session_state['rqa'] = {}
-
-if 'model' not in st.session_state:
- st.session_state['model'] = None
-
-if 'api_keys' not in st.session_state:
- st.session_state['api_keys'] = {}
-
-if 'doc_id' not in st.session_state:
- st.session_state['doc_id'] = None
-
-if 'loaded_embeddings' not in st.session_state:
- st.session_state['loaded_embeddings'] = None
-
-if 'hash' not in st.session_state:
- st.session_state['hash'] = None
-
-if 'git_rev' not in st.session_state:
- st.session_state['git_rev'] = "unknown"
- if os.path.exists("revision.txt"):
- with open("revision.txt", 'r') as fr:
- from_file = fr.read()
- st.session_state['git_rev'] = from_file if len(from_file) > 0 else "unknown"
-
-if "messages" not in st.session_state:
- st.session_state.messages = []
-
-if 'ner_processing' not in st.session_state:
- st.session_state['ner_processing'] = False
-
-if 'uploaded' not in st.session_state:
- st.session_state['uploaded'] = False
-
-st.set_page_config(
- page_title="Scientific Document Insights Q/A",
- page_icon="📝",
- initial_sidebar_state="expanded",
- menu_items={
- 'Get Help': 'https://github.com/lfoppiano/document-qa',
- 'Report a bug': "https://github.com/lfoppiano/document-qa/issues",
- 'About': "Upload a scientific article in PDF, ask questions, get insights."
- }
-)
-
-
-def new_file():
- st.session_state['loaded_embeddings'] = None
- st.session_state['doc_id'] = None
- st.session_state['uploaded'] = True
-
-
-# @st.cache_resource
-def init_qa(model, api_key=None):
- if model == 'chatgpt-3.5-turbo':
- if api_key:
- chat = ChatOpenAI(model_name="gpt-3.5-turbo",
- temperature=0,
- openai_api_key=api_key,
- frequency_penalty=0.1)
- embeddings = OpenAIEmbeddings(openai_api_key=api_key)
- else:
- chat = ChatOpenAI(model_name="gpt-3.5-turbo",
- temperature=0,
- frequency_penalty=0.1)
- embeddings = OpenAIEmbeddings()
-
- elif model == 'mistral-7b-instruct-v0.1':
- chat = HuggingFaceHub(repo_id="mistralai/Mistral-7B-Instruct-v0.1",
- model_kwargs={"temperature": 0.01, "max_length": 4096, "max_new_tokens": 2048})
- embeddings = HuggingFaceEmbeddings(
- model_name="all-MiniLM-L6-v2")
-
- elif model == 'zephyr-7b-beta':
- chat = HuggingFaceHub(repo_id="HuggingFaceH4/zephyr-7b-beta",
- model_kwargs={"temperature": 0.01, "max_length": 4096, "max_new_tokens": 2048})
- embeddings = HuggingFaceEmbeddings(model_name="all-MiniLM-L6-v2")
- else:
- st.error("The model was not loaded properly. Try reloading. ")
- st.stop()
-
- return DocumentQAEngine(chat, embeddings, grobid_url=os.environ['GROBID_URL'])
-
-
-@st.cache_resource
-def init_ner():
- quantities_client = QuantitiesAPI(os.environ['GROBID_QUANTITIES_URL'], check_server=True)
-
- materials_client = GrobidClientGeneric(ping=True)
- config_materials = {
- 'grobid': {
- "server": os.environ['GROBID_MATERIALS_URL'],
- 'sleep_time': 5,
- 'timeout': 60,
- 'url_mapping': {
- 'processText_disable_linking': "/service/process/text?disableLinking=True",
- # 'processText_disable_linking': "/service/process/text"
- }
- }
- }
-
- materials_client.set_config(config_materials)
-
- gqa = GrobidAggregationProcessor(None,
- grobid_quantities_client=quantities_client,
- grobid_superconductors_client=materials_client
- )
- return gqa
-
-
-gqa = init_ner()
-
-
-def get_file_hash(fname):
- hash_md5 = blake2b()
- with open(fname, "rb") as f:
- for chunk in iter(lambda: f.read(4096), b""):
- hash_md5.update(chunk)
- return hash_md5.hexdigest()
-
-
-def play_old_messages():
- if st.session_state['messages']:
- for message in st.session_state['messages']:
- if message['role'] == 'user':
- with st.chat_message("user"):
- st.markdown(message['content'])
- elif message['role'] == 'assistant':
- with st.chat_message("assistant"):
- if mode == "LLM":
- st.markdown(message['content'], unsafe_allow_html=True)
- else:
- st.write(message['content'])
-
-
-# is_api_key_provided = st.session_state['api_key']
-
-with st.sidebar:
- st.session_state['model'] = model = st.radio(
- "Model",
- ("chatgpt-3.5-turbo", "mistral-7b-instruct-v0.1", "zephyr-7b-beta"),
- index=1,
- captions=[
- "ChatGPT 3.5 Turbo + Ada-002-text (embeddings)",
- "Mistral-7B-Instruct-V0.1 + Sentence BERT (embeddings) :free:",
- "Zephyr-7B-beta + Sentence BERT (embeddings) :free:"
- ],
- help="Select the LLM model and embeddings you want to use.",
- disabled=st.session_state['doc_id'] is not None or st.session_state['uploaded'])
-
- st.markdown(
- ":warning: Mistral and Zephyr are free to use, however requests might hit limits of the huggingface free API and fail. :warning: ")
-
- if (model == 'mistral-7b-instruct-v0.1' or model == 'zephyr-7b-beta') and model not in st.session_state['api_keys']:
- if 'HUGGINGFACEHUB_API_TOKEN' not in os.environ:
- api_key = st.text_input('Huggingface API Key', type="password")
-
- st.markdown("Get it [here](https://huggingface.co/docs/hub/security-tokens)")
- else:
- api_key = os.environ['HUGGINGFACEHUB_API_TOKEN']
-
- if api_key:
- # st.session_state['api_key'] = is_api_key_provided = True
- if model not in st.session_state['rqa'] or model not in st.session_state['api_keys']:
- with st.spinner("Preparing environment"):
- st.session_state['api_keys'][model] = api_key
- # if 'HUGGINGFACEHUB_API_TOKEN' not in os.environ:
- # os.environ["HUGGINGFACEHUB_API_TOKEN"] = api_key
- st.session_state['rqa'][model] = init_qa(model)
-
- elif model == 'chatgpt-3.5-turbo' and model not in st.session_state['api_keys']:
- if 'OPENAI_API_KEY' not in os.environ:
- api_key = st.text_input('OpenAI API Key', type="password")
- st.markdown("Get it [here](https://platform.openai.com/account/api-keys)")
- else:
- api_key = os.environ['OPENAI_API_KEY']
-
- if api_key:
- if model not in st.session_state['rqa'] or model not in st.session_state['api_keys']:
- with st.spinner("Preparing environment"):
- st.session_state['api_keys'][model] = api_key
- if 'OPENAI_API_KEY' not in os.environ:
- st.session_state['rqa'][model] = init_qa(model, api_key)
- else:
- st.session_state['rqa'][model] = init_qa(model)
- # else:
- # is_api_key_provided = st.session_state['api_key']
-
-st.title("📝 Scientific Document Insights Q/A")
-st.subheader("Upload a scientific article in PDF, ask questions, get insights.")
-
-st.markdown(
- ":warning: Do not upload sensitive data. We **temporarily** store text from the uploaded PDF documents solely for the purpose of processing your request, and we **do not assume responsibility** for any subsequent use or handling of the data submitted to third parties LLMs.")
-
-uploaded_file = st.file_uploader("Upload an article", type=("pdf", "txt"), on_change=new_file,
- disabled=st.session_state['model'] is not None and st.session_state['model'] not in
- st.session_state['api_keys'],
- help="The full-text is extracted using Grobid. ")
-
-question = st.chat_input(
- "Ask something about the article",
- # placeholder="Can you give me a short summary?",
- disabled=not uploaded_file
-)
-
-with st.sidebar:
- st.header("Settings")
- mode = st.radio("Query mode", ("LLM", "Embeddings"), disabled=not uploaded_file, index=0, horizontal=True,
- help="LLM will respond the question, Embedding will show the "
- "paragraphs relevant to the question in the paper.")
- chunk_size = st.slider("Chunks size", 100, 2000, value=250,
- help="Size of chunks in which the document is partitioned",
- disabled=uploaded_file is not None)
- context_size = st.slider("Context size", 3, 10, value=4,
- help="Number of chunks to consider when answering a question",
- disabled=not uploaded_file)
-
- st.session_state['ner_processing'] = st.checkbox("Named Entities Recognition (NER) processing on LLM response")
- st.markdown(
- '**NER on LLM responses**: The responses from the LLMs are post-processed to extract physical quantities, measurements and materials mentions.',
- unsafe_allow_html=True)
-
- st.divider()
-
- st.header("Documentation")
- st.markdown("https://github.com/lfoppiano/document-qa")
- st.markdown(
- """Upload a scientific article as PDF document. Once the spinner stops, you can proceed to ask your questions.""")
-
- if st.session_state['git_rev'] != "unknown":
- st.markdown("**Revision number**: [" + st.session_state[
- 'git_rev'] + "](https://github.com/lfoppiano/document-qa/commit/" + st.session_state['git_rev'] + ")")
-
- st.header("Query mode (Advanced use)")
- st.markdown(
- """By default, the mode is set to LLM (Language Model) which enables question/answering. You can directly ask questions related to the document content, and the system will answer the question using content from the document.""")
-
- st.markdown(
- """If you switch the mode to "Embedding," the system will return specific chunks from the document that are semantically related to your query. This mode helps to test why sometimes the answers are not satisfying or incomplete. """)
-
-if uploaded_file and not st.session_state.loaded_embeddings:
- if model not in st.session_state['api_keys']:
- st.error("Before uploading a document, you must enter the API key. ")
- st.stop()
- with st.spinner('Reading file, calling Grobid, and creating memory embeddings...'):
- binary = uploaded_file.getvalue()
- tmp_file = NamedTemporaryFile()
- tmp_file.write(bytearray(binary))
- # hash = get_file_hash(tmp_file.name)[:10]
- st.session_state['doc_id'] = hash = st.session_state['rqa'][model].create_memory_embeddings(tmp_file.name,
- chunk_size=chunk_size,
- perc_overlap=0.1)
- st.session_state['loaded_embeddings'] = True
- st.session_state.messages = []
-
- # timestamp = datetime.utcnow()
-
-if st.session_state.loaded_embeddings and question and len(question) > 0 and st.session_state.doc_id:
- for message in st.session_state.messages:
- with st.chat_message(message["role"]):
- if message['mode'] == "LLM":
- st.markdown(message["content"], unsafe_allow_html=True)
- elif message['mode'] == "Embeddings":
- st.write(message["content"])
- if model not in st.session_state['rqa']:
- st.error("The API Key for the " + model + " is missing. Please add it before sending any query. `")
- st.stop()
-
- with st.chat_message("user"):
- st.markdown(question)
- st.session_state.messages.append({"role": "user", "mode": mode, "content": question})
-
- text_response = None
- if mode == "Embeddings":
- with st.spinner("Generating LLM response..."):
- text_response = st.session_state['rqa'][model].query_storage(question, st.session_state.doc_id,
- context_size=context_size)
- elif mode == "LLM":
- with st.spinner("Generating response..."):
- _, text_response = st.session_state['rqa'][model].query_document(question, st.session_state.doc_id,
- context_size=context_size)
-
- if not text_response:
- st.error("Something went wrong. Contact Luca Foppiano (Foppiano.Luca@nims.co.jp) to report the issue.")
-
- with st.chat_message("assistant"):
- if mode == "LLM":
- if st.session_state['ner_processing']:
- with st.spinner("Processing NER on LLM response..."):
- entities = gqa.process_single_text(text_response)
- decorated_text = decorate_text_with_annotations(text_response.strip(), entities)
- decorated_text = decorated_text.replace('class="label material"', 'style="color:green"')
- decorated_text = re.sub(r'class="label[^"]+"', 'style="color:orange"', decorated_text)
- text_response = decorated_text
- st.markdown(text_response, unsafe_allow_html=True)
- else:
- st.write(text_response)
- st.session_state.messages.append({"role": "assistant", "mode": mode, "content": text_response})
-
-elif st.session_state.loaded_embeddings and st.session_state.doc_id:
- play_old_messages()
diff --git a/spaces/lincquiQcaudo/Top-20-Diffusion/LoadUP V1.65.md b/spaces/lincquiQcaudo/Top-20-Diffusion/LoadUP V1.65.md
deleted file mode 100644
index 3af93de28c308bd86ad59dfe2892f2b2193f19df..0000000000000000000000000000000000000000
--- a/spaces/lincquiQcaudo/Top-20-Diffusion/LoadUP V1.65.md
+++ /dev/null
@@ -1,6 +0,0 @@
-
Training points: ${d3.format(',')(d.dataset_size)}
-
Privacy: ${epsilon} ε
-
Rotated in training data: ${d3.format('.0%')(d.minority_percent)}
-
- `).st({width: 230})
-
- ttSel.classed('tooltip-footnote', 0)
- }
-
- function clickCb(d, i, node){
- var mFn = d3.select(this).on('mouseover') || d3.select(this).on('mousemove')
-
- var e = mFn.call(this, d, i, node, true)
- isLock = e == isLock ? null : e
- }
-
-
-})()
diff --git a/spaces/microsoft/unicl-img-recog-demo/model/model.py b/spaces/microsoft/unicl-img-recog-demo/model/model.py
deleted file mode 100644
index 340be96bfb3a1d1d283b1cca6ab13913d1f67b5e..0000000000000000000000000000000000000000
--- a/spaces/microsoft/unicl-img-recog-demo/model/model.py
+++ /dev/null
@@ -1,204 +0,0 @@
-import pathlib
-import tempfile
-from collections import OrderedDict
-from typing import Tuple, Union
-import logging
-import os
-
-import numpy as np
-import torch
-import torch.nn.functional as F
-from torch import nn
-
-from timm.models.layers import DropPath, trunc_normal_
-
-from .image_encoder import build_image_encoder
-from .text_encoder import build_text_encoder
-from .text_encoder import build_tokenizer
-from .templates import DEFAULT_TEMPLATES
-
-logger = logging.getLogger(__name__)
-
-
-class UniCLModel(nn.Module):
- def __init__(self, config: dict,):
- super().__init__()
-
- self.conf_lang_encoder = config['MODEL']['TEXT_ENCODER']
- self.tokenizer = build_tokenizer(self.conf_lang_encoder)
-
- self.text_encoder = build_text_encoder(self.conf_lang_encoder, self.tokenizer, config['VERBOSE'])
-
- dim_projection = config['MODEL']['DIM_PROJECTION']
- if hasattr(self.text_encoder, 'dim_out'):
- dim_out = self.text_encoder.dim_out
- else:
- with torch.no_grad():
- dim_out = self.text_encoder(
- torch.zeros(1,1).type(torch.LongTensor)
- )['last_hidden_state'].size(2)
-
- self.text_projection = nn.Parameter(torch.empty(dim_out, dim_projection))
-
- self.conf_image_encoder = config['MODEL']['IMAGE_ENCODER']
- self.image_encoder = build_image_encoder(self.conf_image_encoder)
-
- self.image_projection = nn.Parameter(
- torch.empty(self.image_encoder.dim_out, dim_projection)
- )
-
- self.logit_scale = nn.Parameter(torch.ones([]))
-
- trunc_normal_(self.text_projection, std=.02)
- trunc_normal_(self.image_projection, std=.02)
-
- def _convert_old_weights(self, model_dict):
- model_dict_updated = {}
- for k, v in model_dict.items():
- if k.startswith('visual.'):
- model_dict_updated['image_encoder.'+k[7:]] = v
- elif k.startswith('text.'):
- model_dict_updated['lang_encoder.'+k[5:]] = v
- elif k == 'vision_projection':
- model_dict_updated['image_projection'] = v
- elif k == 'text_projection':
- model_dict_updated['text_projection'] = v
- else:
- model_dict_updated[k] = v
-
- return model_dict_updated
-
- def from_pretrained(self, pretrained='', pretrained_layers=[], verbose=True):
- if not os.path.isfile(pretrained):
- logger.warning(f'=> Pretrained model ({pretrained}) is not a file, skip init weight')
- return
-
- pretrained_dict = torch.load(pretrained, map_location='cpu')
- logger.info(f'=> Loading pretrained model {pretrained}')
- pretrained_dict = self._convert_old_weights(pretrained_dict)
- model_dict = self.state_dict()
- pretrained_dict = {
- k: v for k, v in pretrained_dict.items()
- if k in model_dict.keys()
- }
- need_init_state_dict = {}
- image_encoder_state_dict = {}
- for k, v in pretrained_dict.items():
- need_init = (
- k.split('.')[0] in pretrained_layers
- or pretrained_layers[0] == '*'
- )
-
- if need_init:
- if k.startswith('image_encoder.'):
- image_encoder_state_dict[k] = v
- else:
- if verbose:
- logger.info(f'=> init {k} from {pretrained}')
-
- need_init_state_dict[k] = v
- self.image_encoder.from_state_dict(image_encoder_state_dict, ['*'], verbose)
- self.load_state_dict(need_init_state_dict, strict=False)
-
- @torch.jit.ignore
- def no_weight_decay(self):
- no_weight_decay = {'logit_scale'}
- if hasattr(self.text_encoder, 'no_weight_decay'):
- for k in self.text_encoder.no_weight_decay():
- no_weight_decay.add('lang_encoder.'+k)
-
- if hasattr(self.image_encoder, 'no_weight_decay'):
- for k in self.image_encoder.no_weight_decay():
- no_weight_decay.add('image_encoder.'+k)
-
- return no_weight_decay
-
- @property
- def dtype(self):
- return self.logit_scale.dtype
-
- def get_imnet_embeddings(self):
- templates = IMAGENET_DEFAULT_TEMPLATES[:1]
- clss_embeddings = []
- for clss in IMAGENET_CLASSES:
- txts = [template.format(clss) for template in templates]
-
- tokens = self.tokenizer(
- txts, padding='max_length', truncation=True, max_length=77, return_tensors='pt'
- )
- tokens = {key:(val.cuda() if next(self.parameters()).is_cuda else val) for key,val in tokens.items()}
-
- clss_embedding = self.encode_text(tokens)
- clss_embedding = clss_embedding.mean(dim=0)
- clss_embedding /= clss_embedding.norm()
- clss_embeddings.append(clss_embedding)
- imnet_text_embeddings = torch.stack(clss_embeddings, dim=0)
- return imnet_text_embeddings
-
- def get_text_embeddings(self, texts):
- templates = DEFAULT_TEMPLATES[:1]
- clss_embeddings = []
- for clss in texts:
- txts = [template.format(clss) for template in templates]
-
- tokens = self.tokenizer(
- txts, padding='max_length', truncation=True, max_length=77, return_tensors='pt'
- )
- tokens = {key:(val.cuda() if next(self.parameters()).is_cuda else val) for key,val in tokens.items()}
-
- clss_embedding = self.encode_text(tokens)
- clss_embedding = clss_embedding.mean(dim=0)
- clss_embedding /= clss_embedding.norm()
- clss_embeddings.append(clss_embedding)
- imnet_text_embeddings = torch.stack(clss_embeddings, dim=0)
- return imnet_text_embeddings
-
- def encode_image(self, image, norm=True):
- x = self.image_encoder.forward_features(image)
- x = x @ self.image_projection
-
- if norm:
- x = x / x.norm(dim=-1, keepdim=True)
-
- return x
-
- def encode_text(self, text, norm=True):
- x = self.text_encoder(**text)
- x = x['last_hidden_state']
-
- if self.conf_lang_encoder['TOKENIZER'] == 'clip':
- x = x[torch.arange(x.size(0)), text['input_ids'].argmax(dim=-1)]
- else:
- x = x[:, 0]
-
- x = x @ self.text_projection
-
- if norm:
- x = x / x.norm(dim=-1, keepdim=True)
-
- return x
-
- def forward(self, image, text):
- features_image = self.encode_image(image)
- features_text = self.encode_text(text)
-
- # cosine similarity as logits
- T = self.logit_scale.exp()
-
- return features_image, features_text, T
-
-
-def build_unicl_model(config, **kwargs):
- model = UniCLModel(config)
- if config['MODEL']['PRETRAINED'] != '':
- pretrained_path = config['MODEL']['PRETRAINED']
- from ..Utils.Utils import is_valid_url, download_file
- if is_valid_url(pretrained_path):
- with tempfile.TemporaryDirectory() as tmp_path:
- file_local_path = pathlib.Path(tmp_path) / 'base_model.pt'
- download_file(pretrained_path, file_local_path)
- model.from_pretrained(str(file_local_path), config['MODEL']['PRETRAINED_LAYERS'], config['VERBOSE'])
- else:
- model.from_pretrained(pretrained_path, config['MODEL']['PRETRAINED_LAYERS'], config['VERBOSE'])
-
- return model
diff --git a/spaces/mncai/chat-doctor-kr/README copy.md b/spaces/mncai/chat-doctor-kr/README copy.md
deleted file mode 100644
index 5e01c8adcde961a8468afc540483589d9016a8fe..0000000000000000000000000000000000000000
--- a/spaces/mncai/chat-doctor-kr/README copy.md
+++ /dev/null
@@ -1,163 +0,0 @@
-
-
-
-
-
-# [ChatDoctor: A Medical Chat Model Fine-tuned on LLaMA Model using Medical Domain Knowledge](https://arxiv.org/abs/2303.14070)
-Yunxiang Li1, Zihan Li2, Kai Zhang3, Ruilong Dan4, You Zhang1
-
1 University of Texas Southwestern Medical Center, Dallas, USA
-
2 University of Illinois at Urbana-Champaign, Urbana, USA
-
3 Ohio State University, Columbus, USA
-
4 Hangzhou Dianzi University, Hangzhou, China
-
-[](https://github.com/HUANGLIZI/ChatDoctor/blob/main/LICENSE)
-[](https://www.python.org/downloads/release/python-390/)
-[](https://www.yunxiangli.top/ChatDoctor/)
-
-## Resources List
-200k real conversations between patients and doctors from HealthCareMagic.com [HealthCareMagic-200k](https://drive.google.com/file/d/1lyfqIwlLSClhgrCutWuEe_IACNq6XNUt/view?usp=sharing).
-
-26k real conversations between patients and doctors from icliniq.com [icliniq-26k](https://drive.google.com/file/d/1ZKbqgYqWc7DJHs3N9TQYQVPdDQmZaClA/view?usp=sharing).
-
-5k generated conversations between patients and physicians from ChatGPT [GenMedGPT-5k](https://drive.google.com/file/d/1nDTKZ3wZbZWTkFMBkxlamrzbNz0frugg/view?usp=sharing) and [disease database](https://github.com/Kent0n-Li/ChatDoctor/blob/main/format_dataset.csv).
-
-Checkpoints of ChatDoctor, fill this [form](https://forms.office.com/Pages/ResponsePage.aspx?id=lYZBnaxxMUy1ssGWyOw8ij06Cb8qnDJKvu2bVpV1-ANUMDIzWlU0QTUxN0YySFROQk9HMVU0N0xJNC4u).
-
-Online hugging face demo [application form](https://forms.office.com/Pages/ResponsePage.aspx?id=lYZBnaxxMUy1ssGWyOw8ij06Cb8qnDJKvu2bVpV1-ANURUU0TllBWVVHUjQ1MDJUNldGTTZWV1c5UC4u).
-
-Stanford Alpaca data for basic conversational capabilities. [Alpaca link](https://github.com/Kent0n-Li/ChatDoctor/blob/main/alpaca_data.json).
-
-
-
-
- ## Setup:
- In a conda env with pytorch available, run:
-```
-pip install -r requirements.txt
-```
-
- ## Interactive Demo Page:
-Demo Page: https://huggingface.co/spaces/ChatDoctor/ChatDoctor
-It is worth noting that our model has not yet achieved 100% accurate output, please do not apply it to real clinical scenarios.
-
-For those who want to try the online demo, please register for hugging face and fill out this form [link](https://forms.office.com/Pages/ResponsePage.aspx?id=lYZBnaxxMUy1ssGWyOw8ij06Cb8qnDJKvu2bVpV1-ANURUU0TllBWVVHUjQ1MDJUNldGTTZWV1c5UC4u).
-
- ## Data and model:
- ### 1. ChatDoctor Training Dataset:
-You can download the following training dataset
-
-200k real conversations between patients and doctors from HealthCareMagic.com [HealthCareMagic-200k](https://drive.google.com/file/d/1lyfqIwlLSClhgrCutWuEe_IACNq6XNUt/view?usp=sharing).
-
-26k real conversations between patients and doctors from icliniq.com [icliniq-26k](https://drive.google.com/file/d/1ZKbqgYqWc7DJHs3N9TQYQVPdDQmZaClA/view?usp=sharing).
-
-5k generated conversations between patients and physicians from ChatGPT [GenMedGPT-5k](https://drive.google.com/file/d/1nDTKZ3wZbZWTkFMBkxlamrzbNz0frugg/view?usp=sharing) and [disease database](https://github.com/Kent0n-Li/ChatDoctor/blob/main/format_dataset.csv).
-
-Our model was firstly be fine-tuned by Stanford Alpaca's data to have some basic conversational capabilities. [Alpaca link](https://github.com/Kent0n-Li/ChatDoctor/blob/main/alpaca_data.json)
-
- ### 2. Model Weights:
-In order to download the checkpoints, fill this form: [link](https://forms.office.com/Pages/ResponsePage.aspx?id=lYZBnaxxMUy1ssGWyOw8ij06Cb8qnDJKvu2bVpV1-ANUMDIzWlU0QTUxN0YySFROQk9HMVU0N0xJNC4u).
-Place the model weights file in the ./pretrained folder.
-
- ## How to fine-tuning
-
- ```python
-torchrun --nproc_per_node=4 --master_port= train.py \
- --model_name_or_path \
- --data_path ./HealthCareMagic-200k.json \
- --bf16 True \
- --output_dir pretrained \
- --num_train_epochs 3 \
- --per_device_train_batch_size 4 \
- --per_device_eval_batch_size 4 \
- --gradient_accumulation_steps 8 \
- --evaluation_strategy "no" \
- --save_strategy "steps" \
- --save_steps 2000 \
- --save_total_limit 1 \
- --learning_rate 2e-5 \
- --weight_decay 0. \
- --warmup_ratio 0.03 \
- --lr_scheduler_type "cosine" \
- --logging_steps 1 \
- --fsdp "full_shard auto_wrap" \
- --fsdp_transformer_layer_cls_to_wrap 'LLaMADecoderLayer' \
- --tf32 True
- ```
-
- ## How to inference
- You can build a ChatDoctor model on your own machine and communicate with it.
- ```python
-python chat.py
- ```
-
-## Overview
-ChatDoctor is a next-generation AI doctor model that is based on the [LLaMA](https://github.com/facebookresearch/llama) model. The goal of this project is to provide patients with an intelligent and reliable healthcare companion that can answer their medical queries and provide them with personalized medical advice.
-
-The ChatDoctor is an advanced language model that is specifically designed for medical applications. It has been trained on a large corpus of medical literature and has a deep understanding of medical terminology, procedures, and diagnoses. This model serves as the foundation for ChatDoctor, enabling it to analyze patients' symptoms and medical history, provide accurate diagnoses, and suggest appropriate treatment options.
-
-The ChatDoctor model is designed to simulate a conversation between a doctor and a patient, using natural language processing (NLP) and machine learning techniques. Patients can interact with the ChatDoctor model through a chat interface, asking questions about their health, symptoms, or medical conditions. The model will then analyze the input and provide a response that is tailored to the patient's unique situation.
-
-One of the key features of the ChatDoctor model is its ability to learn and adapt over time. As more patients interact with the model, it will continue to refine its responses and improve its accuracy. This means that patients can expect to receive increasingly personalized and accurate medical advice over time.
-
-
-
-
-
-## Abstract
-Recent large language models (LLMs) in the general domain, such as ChatGPT, have shown remarkable success in following instructions and producing human-like responses. However, such language models have not been tailored to the medical domain, resulting in poor answer accuracy and inability to give plausible recommendations for medical diagnosis, medications, etc. To address this issue, we collected more than 700 diseases and their corresponding symptoms, required medical tests, and recommended medications, from which we generated 5K doctor-patient conversations. In addition, we obtained 200K real patient-doctor conversations from online Q&A medical consultation sites. By fine-tuning LLMs using these doctor-patient conversations, the resulting models emerge with great potential to understand patients' needs, provide informed advice, and offer valuable assistance in a variety of medical-related fields. The integration of these advanced language models into healthcare can revolutionize the way healthcare professionals and patients communicate, ultimately improving the overall efficiency and quality of patient care and outcomes. In addition, we made public all the source codes, datasets, and model weights to facilitate the further development of dialogue models in the medical field.
-
-
-
-
- ## Introduction
-The development of instruction-following large language models (LLMs) such as ChatGPT has garnered significant attention due to their remarkable success in instruction understanding and human-like response generation.
-These auto-regressive LLMs are pre-trained over web-scale natural languages by predicting the next token and then fine-tuned to follow large-scale human instructions.
-Also, they have shown strong performances over a wide range of NLP tasks and generalizations to unseen tasks, demonstrating their potential as a unified solution for various problems such as natural language understanding, text generation, and conversational AI.
-However, the exploration of such general-domain LLMs in the medical field remains relatively untapped, despite the immense potential they hold for transforming healthcare communication and decision-making.
-The specific reason is that the existing models do not learn the medical field in detail, resulting in the models often giving wrong diagnoses and wrong medical advice when playing the role of a doctor. By fine-tuning the large language dialogue model on the data of doctor-patient conversations, the application of the model in the medical field can be significantly improved. Especially in areas where medical resources are scarce, ChatDoctor can be used for initial diagnosis and triage of patients, significantly improving the operational efficiency of existing hospitals.
-
-Since large language models such as ChatGPT are in a non-open source state, we used Meta's LLaMA and first trained a generic conversation model using 52K instruction-following data provided by Stanford Alpaca, and then fine-tuned the model on our collected physician-patient conversation dataset.
-The main contributions of our method are three-fold:
-1) We designed a process framework for fine-tuning large language models in the medical domain.
-2) We collected a dataset with 5,000 generated doctor-patient conversations and 200,000 real patient-doctor conversations for fine-tuning the large language model.
-3) We validate that the fine-tuned bigrams with medical domain knowledge have real potential for clinical application.
-
- ## Physician and patient conversation dataset
-The first step in building a physician-patient conversation dataset is to collect the disease database that serves as the gold standard. Therefore, we collected and organized a database of diseases, which contains about 700 diseases with their relative symptoms, medical tests, and recommended medications. To train high-quality conversation models on an academic budget, we input each message from the disease database separately as a prompt into the ChatGPT API to automatically generate instruction data. It is worth noting that our prompts to the ChatGPT API contain the gold standard of diseases and symptoms, and drugs, so our fine-tuned ChatDoctor is not only able to achieve ChatGPT's conversational fluency but also higher diagnostic accuracy compared to ChatGPT. We finally collected 5K doctor-patient conversation instructions and named it InstructorDoctor-5K.
-
-The generated conversations, while ensuring accuracy, have a low diversity of conversations. Therefore, we also collected about 200k real doctor-patient conversations from an online Q\&A based medical advisory service website -- "Health Care Magic." We manually and automatically filtered these data to remove physician and patient names and used language tools to correct grammatical errors in the responses.
-
-## Training of the model
-We build ChatDoctor utilizing Meta's LLaMA model, a distinguished publicly accessible LLM.
-Notably, in spite of its 7 billion parameters, LLaMA has been reported that LLaMA's efficacy can attain competitive or superior outcomes in comparison to the considerably larger GPT-3 (with 175 billion parameters) on several NLP benchmarks.
-LLaMA's performance improvement was achieved by amplifying the magnitude of training data, as opposed to parameter quantity.
-Specifically, LLaMA was trained on 1.4 trillion tokens, procured from publicly accessible data repositories such as CommonCrawl and arXiv documents.
-We utilize conversation demonstrations synthesized via ChatGPT and subsequently validated by medical practitioners to fine-tune the LLaMA model, in accordance with the Stanford Alpaca training methodology, and our model was firstly be fine-tuned by Stanford Alpaca's data to have some basic conversational capabilities.
-The fine-tuning process was conducted using 6 A*100 GPUs for a duration of 30 minutes.
-The hyperparameters employed in the training process were as follows: the total batch size of 192, a learning rate of 2e-5, a total of 3 epochs, a maximum sequence length of 512 tokens, a warmup ratio of 0.03, with no weight decay.
-
-## Limitations
-We emphasize that ChatDoctor is for academic research only and any commercial use and clinical use is prohibited. There are three factors in this decision: First, ChatDoctor is based on LLaMA and has a non-commercial license, so we necessarily inherited this decision. Second, our model is not licensed for healthcare-related purposes. Also, we have not designed sufficient security measures, and the current model still does not guarantee the full correctness of medical diagnoses.
-
-
-
-
-## Reference
-
-ChatDoctor: A Medical Chat Model Fine-tuned on LLaMA Model using Medical Domain Knowledge
-
-```
-@misc{yunxiang2023chatdoctor,
- title={ChatDoctor: A Medical Chat Model Fine-tuned on LLaMA Model using Medical Domain Knowledge},
- author={Li Yunxiang and Li Zihan and Zhang Kai and Dan Ruilong and Zhang You},
- year={2023},
- eprint={2303.14070},
- archivePrefix={arXiv},
- primaryClass={cs.CL}
-}
-```
-
-## Examples:
-
-
-
diff --git a/spaces/momegas/megabots/CONTRIBUTING.md b/spaces/momegas/megabots/CONTRIBUTING.md
deleted file mode 100644
index 5140a2fe658150c6fba008084345232ef50f6a49..0000000000000000000000000000000000000000
--- a/spaces/momegas/megabots/CONTRIBUTING.md
+++ /dev/null
@@ -1,21 +0,0 @@
-# Contributing
-
-First of all, thank you for your interest in contributing! We appreciate your time and effort, and we value your contributions to make this project better. This document will provide you with the information you need to start contributing.
-
-## How to Get Started
-
-1. Clone the repository and create a new branch
-2. Make your changes
-3. Submit a pull request
-4. Wait for a review
-5. Tada! You're done!
-
-## How to Report a Bug
-
-If you find a bug, please file an issue on the using the bug report template.
-
-## How to Suggest a Feature or Enhancement
-
-If you have an idea for a new feature or enhancement, please file an issue on the using the feature request template.
-
-🙏 Thank you
diff --git a/spaces/mrciolino/InvertibleSteganography/app.py b/spaces/mrciolino/InvertibleSteganography/app.py
deleted file mode 100644
index 9557215d11c3a6dc0ab9b0e604d97a1d231e2b55..0000000000000000000000000000000000000000
--- a/spaces/mrciolino/InvertibleSteganography/app.py
+++ /dev/null
@@ -1,111 +0,0 @@
-from streamlit_js_eval import streamlit_js_eval
-import torchvision.transforms as transforms
-import streamlit as st
-from PIL import Image
-import torch
-import time
-
-st.set_page_config(page_title="Invertable Steganography", layout="centered", page_icon="📷")
-
-
-# model
-class HiddenNetwork:
- def __init__(self, public_key):
- super().__init__()
- self.public_key = public_key
-
- def noise(self, x):
- torch.manual_seed(self.public_key)
- noise = torch.randn_like(x)
- return noise
-
- def forward(self, x):
- return x - self.noise(x)
-
- def backward(self, x):
- return x + self.noise(x)
-
-
-def load(encode_key=0, decode_key=0):
- with st.spinner('Getting Neruons in Order ...'):
- encode_model = HiddenNetwork(int(encode_key))
- decode_model = HiddenNetwork(int(decode_key))
- process = transforms.Compose([transforms.Resize((224, 224)), transforms.ToTensor()])
- time.sleep(1)
- return encode_model, decode_model, process
-
-
-def main():
- # Set Streamlit theme to dark mode
- st.markdown(
- """
-
- """,
- unsafe_allow_html=True,
- )
- st.markdown("
📷 Invertable Steganography 📷
", unsafe_allow_html=True)
- st.image("secret.png", use_column_width=True)
- st.write(
- """
- Invertible neural networks like FreiA and Glow are neural architectures designed for reversible data transformations.
- They are often used in image steganography, allowing data to be encoded and perfectly reconstructed without any loss of
- information. These networks utilize public keys as seeds, enhancing security and ensuring that only authorized parties can
- access the hidden data within encoded images.
- """
- )
-
- # Create inputs
- st.markdown("""---""")
- input_image = st.file_uploader("Encode Image", type=["jpg", "jpeg", "png"], key="1")
- col1, col2 = st.columns(2)
- encode_key = col1.number_input("Encoding Key", value=42, key="2")
- decode_key = col2.number_input("Decoding Key", value=42, key="3")
-
- # core
- col1, col2, col3, col4 = st.columns(4)
- col1.write("Input")
- col2.write("Encode")
- col3.write("Decode Encode")
- col4.write("Decode Input")
-
- # columns
- if input_image is not None:
- encode_model, decode_model, process = load(encode_key, decode_key)
- image = process(Image.open(input_image).convert("RGB"))
- forward = encode_model.forward(image)
- backward = decode_model.backward(forward)
- backward_input = decode_model.backward(image)
-
- with col1:
- st.image(transforms.ToPILImage()(image), use_column_width=True)
- transforms.ToPILImage()(image).save("tmp/image.png")
- st.download_button(label='Download Image', data=open('tmp/image.png', 'rb').read(), file_name='image.png', mime='image/png', key="4")
-
- with col2:
- st.image(transforms.ToPILImage()(forward), use_column_width=True)
- transforms.ToPILImage()(forward).save("tmp/forward.png")
- st.download_button(label='Download Image', data=open('tmp/forward.png', 'rb').read(), file_name='forward.png', mime='image/png', key="5")
-
- with col3:
- st.image(transforms.ToPILImage()(backward), use_column_width=True)
- transforms.ToPILImage()(backward).save("tmp/back.png")
- st.download_button(label='Download Image', data=open('tmp/back.png', 'rb').read(), file_name='back.png', mime='image/png', key="6")
-
- with col4:
- st.image(transforms.ToPILImage()(backward_input), use_column_width=True)
- transforms.ToPILImage()(backward_input).save("tmp/input.png")
- st.download_button(label='Download Image', data=open('tmp/input.png', 'rb').read(), file_name='input.png', mime='image/png', key="7")
-
- # Create a button to reset the interface page
- st.markdown("""---""")
- if st.button("Reset", use_container_width=True):
- streamlit_js_eval(js_expressions="parent.window.location.reload()")
-
-
-if __name__ == "__main__":
- main()
diff --git a/spaces/mrsteyk/mrsteyk-openchatgpt-neox-125m/README.md b/spaces/mrsteyk/mrsteyk-openchatgpt-neox-125m/README.md
deleted file mode 100644
index f3881cec7c75bc95af0631e4e061fe2289773b1f..0000000000000000000000000000000000000000
--- a/spaces/mrsteyk/mrsteyk-openchatgpt-neox-125m/README.md
+++ /dev/null
@@ -1,15 +0,0 @@
----
-title: OpenChatGPT-NeoX-125m
-emoji: 😅
-colorFrom: indigo
-colorTo: blue
-sdk: gradio
-sdk_version: 3.15.0
-app_file: app.py
-pinned: false
-license: agpl-3.0
----
-
-Check out the configuration reference at https://huggingface.co/docs/hub/spaces-config-reference
-
-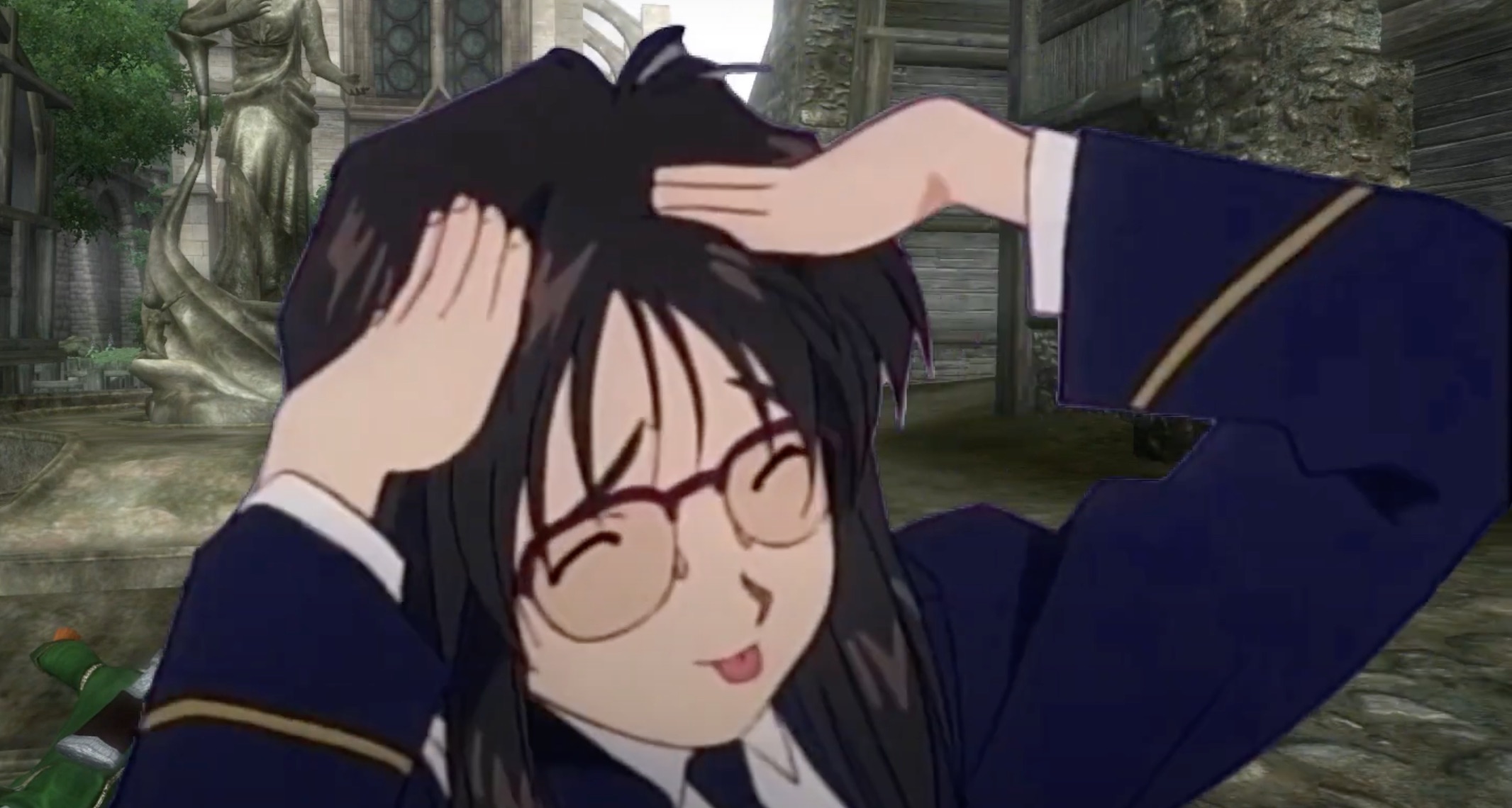
diff --git a/spaces/msafi04/abstractive_summarization/README.md b/spaces/msafi04/abstractive_summarization/README.md
deleted file mode 100644
index f67b77114fc3b7cfd4e850e22dc96f4d84954e96..0000000000000000000000000000000000000000
--- a/spaces/msafi04/abstractive_summarization/README.md
+++ /dev/null
@@ -1,13 +0,0 @@
----
-title: Abstractive Summarization
-emoji: 🏢
-colorFrom: blue
-colorTo: pink
-sdk: gradio
-sdk_version: 3.23.0
-app_file: app.py
-pinned: false
-duplicated_from: SameerR007/abstractive_summarization
----
-
-Check out the configuration reference at https://huggingface.co/docs/hub/spaces-config-reference
diff --git a/spaces/mshukor/UnIVAL/fairseq/examples/latent_depth/latent_depth_src/models/latent_transformer.py b/spaces/mshukor/UnIVAL/fairseq/examples/latent_depth/latent_depth_src/models/latent_transformer.py
deleted file mode 100644
index 6a825301a452bd935deafdaf78fa2427ca9a469e..0000000000000000000000000000000000000000
--- a/spaces/mshukor/UnIVAL/fairseq/examples/latent_depth/latent_depth_src/models/latent_transformer.py
+++ /dev/null
@@ -1,156 +0,0 @@
-# Copyright (c) Facebook, Inc. and its affiliates.
-#
-# This source code is licensed under the MIT license found in the
-# LICENSE file in the root directory of this source tree.
-
-from typing import Any, Dict, Optional
-
-import torch.nn as nn
-from fairseq.models.fairseq_encoder import EncoderOut
-from fairseq.models.transformer import TransformerDecoder, TransformerEncoder
-from fairseq.modules import TransformerDecoderLayer, TransformerEncoderLayer
-from torch import Tensor
-
-from ..modules.latent_layers import LayerSelect
-
-
-class LatentTransformerEncoder(TransformerEncoder):
- """Latent depth (https://arxiv.org/abs/2009.13102) implemented in
- TransformerEncoder.
- """
-
- def __init__(self, args, dictionary, embed_tokens, num_logits=1):
- self.num_logits = num_logits
- self.num_layers = args.encoder_layers
- super().__init__(args, dictionary, embed_tokens)
- self.layer_select = LayerSelect(
- num_layers=self.num_layers,
- num_logits=self.num_logits,
- soft_select=getattr(args, "soft_select", False),
- sampling_tau=getattr(args, "sampling_tau", 5.),
- )
- self.lang_idx = None
- self.layers = nn.ModuleList(
- [self._build_encoder_layer(args, idx) for idx in range(args.encoder_layers)]
- )
-
- def set_lang_idx(self, lang_idx):
- self.lang_idx = lang_idx
-
- def _build_encoder_layer(self, args, idx=None):
- return LatentTransformerEncoderLayer(args, idx, layer_select=self.layer_select)
-
- def forward(self, src_tokens, src_lengths, return_all_hiddens: bool = False):
- self.layer_select.sample(self.lang_idx)
- return super().forward(src_tokens, src_lengths, return_all_hiddens)
-
-
-class LatentTransformerEncoderLayer(TransformerEncoderLayer):
- """Encoder layer with each (non_residual) block weighted by samples of Bernouli
- or Gumbel Signmoid samples.
-
- Args:
- args (argparse.Namespace): parsed command-line arguments from standard
- TransformerEncoderLayer.
- idx (int): layer index (used to retrieve samples).
- layer_select (LayerSelect, optional): instance of LayerSelect module with logits
- parameters and sampling method.
- """
-
- def __init__(self, args, idx, layer_select=None):
- super().__init__(args)
- self.idx = idx
- self.layer_select = layer_select
-
- def residual_connection(self, x, residual):
- return residual + x * self.layer_select(self.idx)
-
-
-class LatentTransformerDecoder(TransformerDecoder):
- """Latent depth (https://arxiv.org/abs/2009.13102) implemented in
- TransformerDecoder.
- """
-
- def __init__(
- self, args, dictionary, embed_tokens, no_encoder_attn=False, num_logits=1
- ):
- self.num_logits = num_logits
- self.num_layers = args.decoder_layers
- super().__init__(
- args, dictionary, embed_tokens, no_encoder_attn=no_encoder_attn
- )
- self.layer_select = LayerSelect(
- num_layers=self.num_layers,
- num_logits=self.num_logits,
- soft_select=getattr(args, "soft_select", False),
- sampling_tau=getattr(args, "sampling_tau", 5.),
- )
- self.lang_idx = None
- self.layers = nn.ModuleList(
- [
- self._build_decoder_layer(args, no_encoder_attn, idx)
- for idx in range(args.decoder_layers)
- ]
- )
-
- def set_lang_idx(self, lang_idx):
- self.lang_idx = lang_idx
-
- def _build_decoder_layer(self, args, no_encoder_attn=False, idx=None):
- return LatentTransformerDecoderLayer(
- args, idx, layer_select=self.layer_select, no_encoder_attn=no_encoder_attn
- )
-
- def forward(
- self,
- prev_output_tokens,
- encoder_out: Optional[EncoderOut] = None,
- incremental_state: Optional[Dict[str, Dict[str, Optional[Tensor]]]] = None,
- features_only: bool = False,
- alignment_layer: Optional[int] = None,
- alignment_heads: Optional[int] = None,
- src_lengths: Optional[Any] = None,
- return_all_hiddens: bool = False,
- ):
- self.layer_select.sample(self.lang_idx)
- return super().forward(
- prev_output_tokens=prev_output_tokens,
- encoder_out=encoder_out,
- incremental_state=incremental_state,
- features_only=features_only,
- alignment_layer=alignment_layer,
- src_lengths=src_lengths,
- return_all_hiddens=return_all_hiddens,
- )
-
-
-class LatentTransformerDecoderLayer(TransformerDecoderLayer):
- """Decoder layer with each (non_residual) block weighted by samples of Bernouli
- or Gumbel Signmoid samples.
-
- Args:
- args (argparse.Namespace): parsed command-line arguments from standard
- TransformerDecoderLayer.
- idx (int): layer index (used to retrieve samples).
- layer_select (LayerSelect, optional): instance of LayerSelect module with logits
- parameters and sampling method.
- no_encoder_attn (bool, optional): whether to attend to encoder outputs
- (default: False).
-
- """
-
- def __init__(
- self,
- args,
- idx,
- layer_select=None,
- no_encoder_attn=False,
- add_bias_kv=False,
- add_zero_attn=False,
- ):
- super().__init__(args, no_encoder_attn, add_bias_kv, add_zero_attn)
- self.idx = idx
- self.layer_select = layer_select
-
- def residual_connection(self, x, residual):
- return residual + x * self.layer_select(self.idx)
diff --git a/spaces/mshukor/UnIVAL/fairseq/examples/speech_recognition/w2l_decoder.py b/spaces/mshukor/UnIVAL/fairseq/examples/speech_recognition/w2l_decoder.py
deleted file mode 100644
index fbf2d3524ee40bd0d08b6a9560047d96e49b6045..0000000000000000000000000000000000000000
--- a/spaces/mshukor/UnIVAL/fairseq/examples/speech_recognition/w2l_decoder.py
+++ /dev/null
@@ -1,486 +0,0 @@
-#!/usr/bin/env python3
-
-# Copyright (c) Facebook, Inc. and its affiliates.
-#
-# This source code is licensed under the MIT license found in the
-# LICENSE file in the root directory of this source tree.
-
-"""
-Flashlight decoders.
-"""
-
-import gc
-import itertools as it
-import os.path as osp
-from typing import List
-import warnings
-from collections import deque, namedtuple
-
-import numpy as np
-import torch
-from examples.speech_recognition.data.replabels import unpack_replabels
-from fairseq import tasks
-from fairseq.utils import apply_to_sample
-from omegaconf import open_dict
-from fairseq.dataclass.utils import convert_namespace_to_omegaconf
-
-
-try:
- from flashlight.lib.text.dictionary import create_word_dict, load_words
- from flashlight.lib.sequence.criterion import CpuViterbiPath, get_data_ptr_as_bytes
- from flashlight.lib.text.decoder import (
- CriterionType,
- LexiconDecoderOptions,
- KenLM,
- LM,
- LMState,
- SmearingMode,
- Trie,
- LexiconDecoder,
- )
-except:
- warnings.warn(
- "flashlight python bindings are required to use this functionality. Please install from https://github.com/facebookresearch/flashlight/tree/master/bindings/python"
- )
- LM = object
- LMState = object
-
-
-class W2lDecoder(object):
- def __init__(self, args, tgt_dict):
- self.tgt_dict = tgt_dict
- self.vocab_size = len(tgt_dict)
- self.nbest = args.nbest
-
- # criterion-specific init
- self.criterion_type = CriterionType.CTC
- self.blank = (
- tgt_dict.index("")
- if "" in tgt_dict.indices
- else tgt_dict.bos()
- )
- if "" in tgt_dict.indices:
- self.silence = tgt_dict.index("")
- elif "|" in tgt_dict.indices:
- self.silence = tgt_dict.index("|")
- else:
- self.silence = tgt_dict.eos()
- self.asg_transitions = None
-
- def generate(self, models, sample, **unused):
- """Generate a batch of inferences."""
- # model.forward normally channels prev_output_tokens into the decoder
- # separately, but SequenceGenerator directly calls model.encoder
- encoder_input = {
- k: v for k, v in sample["net_input"].items() if k != "prev_output_tokens"
- }
- emissions = self.get_emissions(models, encoder_input)
- return self.decode(emissions)
-
- def get_emissions(self, models, encoder_input):
- """Run encoder and normalize emissions"""
- model = models[0]
- encoder_out = model(**encoder_input)
- if hasattr(model, "get_logits"):
- emissions = model.get_logits(encoder_out) # no need to normalize emissions
- else:
- emissions = model.get_normalized_probs(encoder_out, log_probs=True)
- return emissions.transpose(0, 1).float().cpu().contiguous()
-
- def get_tokens(self, idxs):
- """Normalize tokens by handling CTC blank, ASG replabels, etc."""
- idxs = (g[0] for g in it.groupby(idxs))
- idxs = filter(lambda x: x != self.blank, idxs)
- return torch.LongTensor(list(idxs))
-
-
-class W2lViterbiDecoder(W2lDecoder):
- def __init__(self, args, tgt_dict):
- super().__init__(args, tgt_dict)
-
- def decode(self, emissions):
- B, T, N = emissions.size()
- hypos = []
- if self.asg_transitions is None:
- transitions = torch.FloatTensor(N, N).zero_()
- else:
- transitions = torch.FloatTensor(self.asg_transitions).view(N, N)
- viterbi_path = torch.IntTensor(B, T)
- workspace = torch.ByteTensor(CpuViterbiPath.get_workspace_size(B, T, N))
- CpuViterbiPath.compute(
- B,
- T,
- N,
- get_data_ptr_as_bytes(emissions),
- get_data_ptr_as_bytes(transitions),
- get_data_ptr_as_bytes(viterbi_path),
- get_data_ptr_as_bytes(workspace),
- )
- return [
- [{"tokens": self.get_tokens(viterbi_path[b].tolist()), "score": 0}]
- for b in range(B)
- ]
-
-
-class W2lKenLMDecoder(W2lDecoder):
- def __init__(self, args, tgt_dict):
- super().__init__(args, tgt_dict)
-
- self.unit_lm = getattr(args, "unit_lm", False)
-
- if args.lexicon:
- self.lexicon = load_words(args.lexicon)
- self.word_dict = create_word_dict(self.lexicon)
- self.unk_word = self.word_dict.get_index("")
-
- self.lm = KenLM(args.kenlm_model, self.word_dict)
- self.trie = Trie(self.vocab_size, self.silence)
-
- start_state = self.lm.start(False)
- for i, (word, spellings) in enumerate(self.lexicon.items()):
- word_idx = self.word_dict.get_index(word)
- _, score = self.lm.score(start_state, word_idx)
- for spelling in spellings:
- spelling_idxs = [tgt_dict.index(token) for token in spelling]
- assert (
- tgt_dict.unk() not in spelling_idxs
- ), f"{spelling} {spelling_idxs}"
- self.trie.insert(spelling_idxs, word_idx, score)
- self.trie.smear(SmearingMode.MAX)
-
- self.decoder_opts = LexiconDecoderOptions(
- beam_size=args.beam,
- beam_size_token=int(getattr(args, "beam_size_token", len(tgt_dict))),
- beam_threshold=args.beam_threshold,
- lm_weight=args.lm_weight,
- word_score=args.word_score,
- unk_score=args.unk_weight,
- sil_score=args.sil_weight,
- log_add=False,
- criterion_type=self.criterion_type,
- )
-
- if self.asg_transitions is None:
- N = 768
- # self.asg_transitions = torch.FloatTensor(N, N).zero_()
- self.asg_transitions = []
-
- self.decoder = LexiconDecoder(
- self.decoder_opts,
- self.trie,
- self.lm,
- self.silence,
- self.blank,
- self.unk_word,
- self.asg_transitions,
- self.unit_lm,
- )
- else:
- assert args.unit_lm, "lexicon free decoding can only be done with a unit language model"
- from flashlight.lib.text.decoder import LexiconFreeDecoder, LexiconFreeDecoderOptions
-
- d = {w: [[w]] for w in tgt_dict.symbols}
- self.word_dict = create_word_dict(d)
- self.lm = KenLM(args.kenlm_model, self.word_dict)
- self.decoder_opts = LexiconFreeDecoderOptions(
- beam_size=args.beam,
- beam_size_token=int(getattr(args, "beam_size_token", len(tgt_dict))),
- beam_threshold=args.beam_threshold,
- lm_weight=args.lm_weight,
- sil_score=args.sil_weight,
- log_add=False,
- criterion_type=self.criterion_type,
- )
- self.decoder = LexiconFreeDecoder(
- self.decoder_opts, self.lm, self.silence, self.blank, []
- )
-
- def get_timesteps(self, token_idxs: List[int]) -> List[int]:
- """Returns frame numbers corresponding to every non-blank token.
-
- Parameters
- ----------
- token_idxs : List[int]
- IDs of decoded tokens.
-
- Returns
- -------
- List[int]
- Frame numbers corresponding to every non-blank token.
- """
- timesteps = []
- for i, token_idx in enumerate(token_idxs):
- if token_idx == self.blank:
- continue
- if i == 0 or token_idx != token_idxs[i-1]:
- timesteps.append(i)
- return timesteps
-
- def decode(self, emissions):
- B, T, N = emissions.size()
- hypos = []
- for b in range(B):
- emissions_ptr = emissions.data_ptr() + 4 * b * emissions.stride(0)
- results = self.decoder.decode(emissions_ptr, T, N)
-
- nbest_results = results[: self.nbest]
- hypos.append(
- [
- {
- "tokens": self.get_tokens(result.tokens),
- "score": result.score,
- "timesteps": self.get_timesteps(result.tokens),
- "words": [
- self.word_dict.get_entry(x) for x in result.words if x >= 0
- ],
- }
- for result in nbest_results
- ]
- )
- return hypos
-
-
-FairseqLMState = namedtuple("FairseqLMState", ["prefix", "incremental_state", "probs"])
-
-
-class FairseqLM(LM):
- def __init__(self, dictionary, model):
- LM.__init__(self)
- self.dictionary = dictionary
- self.model = model
- self.unk = self.dictionary.unk()
-
- self.save_incremental = False # this currently does not work properly
- self.max_cache = 20_000
-
- model.cuda()
- model.eval()
- model.make_generation_fast_()
-
- self.states = {}
- self.stateq = deque()
-
- def start(self, start_with_nothing):
- state = LMState()
- prefix = torch.LongTensor([[self.dictionary.eos()]])
- incremental_state = {} if self.save_incremental else None
- with torch.no_grad():
- res = self.model(prefix.cuda(), incremental_state=incremental_state)
- probs = self.model.get_normalized_probs(res, log_probs=True, sample=None)
-
- if incremental_state is not None:
- incremental_state = apply_to_sample(lambda x: x.cpu(), incremental_state)
- self.states[state] = FairseqLMState(
- prefix.numpy(), incremental_state, probs[0, -1].cpu().numpy()
- )
- self.stateq.append(state)
-
- return state
-
- def score(self, state: LMState, token_index: int, no_cache: bool = False):
- """
- Evaluate language model based on the current lm state and new word
- Parameters:
- -----------
- state: current lm state
- token_index: index of the word
- (can be lexicon index then you should store inside LM the
- mapping between indices of lexicon and lm, or lm index of a word)
-
- Returns:
- --------
- (LMState, float): pair of (new state, score for the current word)
- """
- curr_state = self.states[state]
-
- def trim_cache(targ_size):
- while len(self.stateq) > targ_size:
- rem_k = self.stateq.popleft()
- rem_st = self.states[rem_k]
- rem_st = FairseqLMState(rem_st.prefix, None, None)
- self.states[rem_k] = rem_st
-
- if curr_state.probs is None:
- new_incremental_state = (
- curr_state.incremental_state.copy()
- if curr_state.incremental_state is not None
- else None
- )
- with torch.no_grad():
- if new_incremental_state is not None:
- new_incremental_state = apply_to_sample(
- lambda x: x.cuda(), new_incremental_state
- )
- elif self.save_incremental:
- new_incremental_state = {}
-
- res = self.model(
- torch.from_numpy(curr_state.prefix).cuda(),
- incremental_state=new_incremental_state,
- )
- probs = self.model.get_normalized_probs(
- res, log_probs=True, sample=None
- )
-
- if new_incremental_state is not None:
- new_incremental_state = apply_to_sample(
- lambda x: x.cpu(), new_incremental_state
- )
-
- curr_state = FairseqLMState(
- curr_state.prefix, new_incremental_state, probs[0, -1].cpu().numpy()
- )
-
- if not no_cache:
- self.states[state] = curr_state
- self.stateq.append(state)
-
- score = curr_state.probs[token_index].item()
-
- trim_cache(self.max_cache)
-
- outstate = state.child(token_index)
- if outstate not in self.states and not no_cache:
- prefix = np.concatenate(
- [curr_state.prefix, torch.LongTensor([[token_index]])], -1
- )
- incr_state = curr_state.incremental_state
-
- self.states[outstate] = FairseqLMState(prefix, incr_state, None)
-
- if token_index == self.unk:
- score = float("-inf")
-
- return outstate, score
-
- def finish(self, state: LMState):
- """
- Evaluate eos for language model based on the current lm state
-
- Returns:
- --------
- (LMState, float): pair of (new state, score for the current word)
- """
- return self.score(state, self.dictionary.eos())
-
- def empty_cache(self):
- self.states = {}
- self.stateq = deque()
- gc.collect()
-
-
-class W2lFairseqLMDecoder(W2lDecoder):
- def __init__(self, args, tgt_dict):
- super().__init__(args, tgt_dict)
-
- self.unit_lm = getattr(args, "unit_lm", False)
-
- self.lexicon = load_words(args.lexicon) if args.lexicon else None
- self.idx_to_wrd = {}
-
- checkpoint = torch.load(args.kenlm_model, map_location="cpu")
-
- if "cfg" in checkpoint and checkpoint["cfg"] is not None:
- lm_args = checkpoint["cfg"]
- else:
- lm_args = convert_namespace_to_omegaconf(checkpoint["args"])
-
- with open_dict(lm_args.task):
- lm_args.task.data = osp.dirname(args.kenlm_model)
-
- task = tasks.setup_task(lm_args.task)
- model = task.build_model(lm_args.model)
- model.load_state_dict(checkpoint["model"], strict=False)
-
- self.trie = Trie(self.vocab_size, self.silence)
-
- self.word_dict = task.dictionary
- self.unk_word = self.word_dict.unk()
- self.lm = FairseqLM(self.word_dict, model)
-
- if self.lexicon:
- start_state = self.lm.start(False)
- for i, (word, spellings) in enumerate(self.lexicon.items()):
- if self.unit_lm:
- word_idx = i
- self.idx_to_wrd[i] = word
- score = 0
- else:
- word_idx = self.word_dict.index(word)
- _, score = self.lm.score(start_state, word_idx, no_cache=True)
-
- for spelling in spellings:
- spelling_idxs = [tgt_dict.index(token) for token in spelling]
- assert (
- tgt_dict.unk() not in spelling_idxs
- ), f"{spelling} {spelling_idxs}"
- self.trie.insert(spelling_idxs, word_idx, score)
- self.trie.smear(SmearingMode.MAX)
-
- self.decoder_opts = LexiconDecoderOptions(
- beam_size=args.beam,
- beam_size_token=int(getattr(args, "beam_size_token", len(tgt_dict))),
- beam_threshold=args.beam_threshold,
- lm_weight=args.lm_weight,
- word_score=args.word_score,
- unk_score=args.unk_weight,
- sil_score=args.sil_weight,
- log_add=False,
- criterion_type=self.criterion_type,
- )
-
- self.decoder = LexiconDecoder(
- self.decoder_opts,
- self.trie,
- self.lm,
- self.silence,
- self.blank,
- self.unk_word,
- [],
- self.unit_lm,
- )
- else:
- assert args.unit_lm, "lexicon free decoding can only be done with a unit language model"
- from flashlight.lib.text.decoder import LexiconFreeDecoder, LexiconFreeDecoderOptions
-
- d = {w: [[w]] for w in tgt_dict.symbols}
- self.word_dict = create_word_dict(d)
- self.lm = KenLM(args.kenlm_model, self.word_dict)
- self.decoder_opts = LexiconFreeDecoderOptions(
- beam_size=args.beam,
- beam_size_token=int(getattr(args, "beam_size_token", len(tgt_dict))),
- beam_threshold=args.beam_threshold,
- lm_weight=args.lm_weight,
- sil_score=args.sil_weight,
- log_add=False,
- criterion_type=self.criterion_type,
- )
- self.decoder = LexiconFreeDecoder(
- self.decoder_opts, self.lm, self.silence, self.blank, []
- )
-
- def decode(self, emissions):
- B, T, N = emissions.size()
- hypos = []
-
- def idx_to_word(idx):
- if self.unit_lm:
- return self.idx_to_wrd[idx]
- else:
- return self.word_dict[idx]
-
- def make_hypo(result):
- hypo = {"tokens": self.get_tokens(result.tokens), "score": result.score}
- if self.lexicon:
- hypo["words"] = [idx_to_word(x) for x in result.words if x >= 0]
- return hypo
-
- for b in range(B):
- emissions_ptr = emissions.data_ptr() + 4 * b * emissions.stride(0)
- results = self.decoder.decode(emissions_ptr, T, N)
-
- nbest_results = results[: self.nbest]
- hypos.append([make_hypo(result) for result in nbest_results])
- self.lm.empty_cache()
-
- return hypos
diff --git a/spaces/mshukor/UnIVAL/fairseq/fairseq/optim/bmuf.py b/spaces/mshukor/UnIVAL/fairseq/fairseq/optim/bmuf.py
deleted file mode 100644
index d6d0e04e86eb894efe59e13a78843d01ca9e651d..0000000000000000000000000000000000000000
--- a/spaces/mshukor/UnIVAL/fairseq/fairseq/optim/bmuf.py
+++ /dev/null
@@ -1,200 +0,0 @@
-# Copyright (c) Facebook, Inc. and its affiliates.
-#
-# This source code is licensed under the MIT license found in the
-# LICENSE file in the root directory of this source tree.
-
-from dataclasses import dataclass, field
-
-import torch
-import torch.distributed as dist
-from fairseq.dataclass.configs import FairseqBMUFConfig
-from fairseq.dataclass.utils import gen_parser_from_dataclass
-from fairseq.optim.fairseq_optimizer import FairseqOptimizer
-
-
-class FairseqBMUF(FairseqOptimizer):
- """
- Implements incremental block distributed data parallelism similar to
- https://ieeexplore.ieee.org/document/7472805
-
- Paper title: Scalable training of deep learning machines by incremental
- block training with intra-block parallel optimization and blockwise
- model-update filtering
- """
-
- def __init__(self, cfg: FairseqBMUFConfig, optimizer):
- super().__init__(cfg)
- self._optimizer = optimizer
- self._num_updates = 0
- self.sync_iter = cfg.global_sync_iter
- self.block_momentum = cfg.block_momentum
- self.block_lr = cfg.block_lr
- self._reset_local_data()
- self.warmup_iteration = cfg.warmup_iterations
- self.use_nbm = cfg.use_nbm
- self.initial_state = self._optimizer.state_dict()
- self.average_sync = self.cfg.average_sync
- self.world_size = self.cfg.distributed_world_size
-
- @staticmethod
- def add_args(parser):
- """Add optimizer-specific arguments to the parser."""
- gen_parser_from_dataclass(parser, FairseqBMUFConfig())
-
- @property
- def optimizer(self):
- return self._optimizer.optimizer
-
- @property
- def optimizer_config(self):
- return self._optimizer.optimizer_config
-
- def get_lr(self):
- return self._optimizer.get_lr()
-
- def set_lr(self, lr):
- self._optimizer.set_lr(lr)
-
- def state_dict(self):
- return self._optimizer.state_dict()
-
- def load_state_dict(self, state_dict, optimizer_overrides=None):
- self._optimizer.load_state_dict(state_dict, optimizer_overrides)
- self.initial_state = self._optimizer.state_dict()
-
- def multiply_grads(self, c):
- """Multiplies grads by a constant *c*."""
- self._optimizer.multiply_grads(c)
-
- def clip_grad_norm(self, max_norm, aggregate_norm_fn=None):
- """Clips gradient norm."""
- return self._optimizer.clip_grad_norm(max_norm, aggregate_norm_fn)
-
- def average_params(self):
- self._optimizer.average_params()
-
- def _block_sync(self):
- if self.world_size <= 1:
- return
- # Update the global model using local models from all GPUs
- # (Step-1) Calculate grad between previously synced model and
- # currrent local model
- if self.block_momentum != 0:
- self._calc_grad()
-
- # (Step-2) Average gradient from all GPUs
- self._avg_grad_from_all_gpus()
-
- # (Step-3) Calculate global momentum and update the global model
- if self.block_momentum != 0:
- self._update_global_model()
-
- # (Step-4) Average local optimizer params
- if self.average_sync:
- self.average_params()
-
- def _is_warmup_end(self):
- # Check whether train iterations is equal to warmup iter
- if self.get_num_updates() == self.warmup_iteration:
- return True
- return False
-
- def _is_bmuf_iter(self):
- # Check whether train iterations is equal to bmuf sync iter
- if (self.get_num_updates() > self.warmup_iteration) and (
- self.get_num_updates() % self.sync_iter == 0
- ):
- return True
- return False
-
- def _warmup_sync(self, root_rank=0):
- if self.world_size <= 1:
- return
- # Broadcast the local model to all gpus
- for param in self.params:
- dist.broadcast(param.data, src=root_rank)
-
- # Update local optimizer state
- if self.average_sync:
- self._optimizer.average_params()
- else:
- self._optimizer.load_state_dict(self.initial_state)
-
- self._reset_local_data()
-
- def step(self, closure=None):
- """Performs a single optimization step."""
- self._optimizer.step(closure)
- self.set_num_updates(self.get_num_updates() + 1)
- if self._is_warmup_end():
- self._warmup_sync()
- elif self._is_bmuf_iter():
- self._block_sync()
-
- def zero_grad(self):
- """Clears the gradients of all optimized parameters."""
- self._optimizer.zero_grad()
-
- def get_num_updates(self):
- """Get the number of parameters updates."""
- return self._num_updates
-
- def set_num_updates(self, num_updates):
- """Set the number of parameters updates."""
- self._num_updates = num_updates
-
- @torch.no_grad()
- def _reset_local_data(self):
- # (Step-0) Initialize global momentum parameters and store global copy on each gpu
- self.global_params = [torch.zeros_like(p.data) for p in self.params]
- self.smoothed_grads = [p.data.new_zeros(p.data.size()) for p in self.params]
- self.grads = [p.data.new_zeros(p.data.size()) for p in self.params]
-
- # saving the global model locally for calculating gradient during bmuf sync
- for param, global_param in zip(self.params, self.global_params):
- global_param.copy_(param.data)
-
- @torch.no_grad()
- def _calc_grad(self):
- # global_params is basically the global copy from the previously finished
- # synchronisation. param.data is local parameter after block_sync_freq
- # for the local gpu. so grad is difference between previously synced
- # model and currrent local model.
- for index, (param, global_param) in enumerate(
- zip(self.params, self.global_params)
- ):
- self.grads[index] = global_param - param.data
-
- def _avg_grad_from_all_gpus(self):
- for index, param in enumerate(self.params):
- sync_para = param.data if self.block_momentum == 0 else self.grads[index]
- sync_para /= float(dist.get_world_size())
- dist.all_reduce(sync_para, op=dist.ReduceOp.SUM)
-
- @torch.no_grad()
- def _update_global_model(self):
- for index, (param, global_param, smoothed_grad, grad) in enumerate(
- zip(
- self.params,
- self.global_params,
- self.smoothed_grads,
- # all gpus would share the same value of smoothed_grad, since it is
- # always computed on synchronized gradients.
- self.grads,
- )
- ):
- # global_param is basically last syncrhornized parameter. though
- # smoothed_grad is local, all processes will have same value of
- # smoothed_grad and hence param is globally synchronized copy.
- # smoothed_grad(t) = BM * smoothed_grad(t-1) + BM_lr * grad(t)
- smoothed_grad = self.block_momentum * smoothed_grad + self.block_lr * grad
- param.data.copy_(global_param - smoothed_grad)
-
- # A Nesterov momentum here is to do a partial weight update before
- # calculating the gradient
- if self.use_nbm:
- param.data.copy_(param.data - self.block_momentum * smoothed_grad)
-
- # backup for the next synchronization.
- self.smoothed_grads[index] = smoothed_grad
- global_param.copy_(param.data)
diff --git a/spaces/mshukor/UnIVAL/fairseq/fairseq/scoring/wer.py b/spaces/mshukor/UnIVAL/fairseq/fairseq/scoring/wer.py
deleted file mode 100644
index 633dc47c247691c4c9e36cbdbab7d7cb74b38452..0000000000000000000000000000000000000000
--- a/spaces/mshukor/UnIVAL/fairseq/fairseq/scoring/wer.py
+++ /dev/null
@@ -1,58 +0,0 @@
-# Copyright (c) Facebook, Inc. and its affiliates.
-#
-# This source code is licensed under the MIT license found in the
-# LICENSE file in the root directory of this source tree.
-
-from dataclasses import dataclass, field
-
-from fairseq.dataclass import FairseqDataclass
-from fairseq.scoring import BaseScorer, register_scorer
-from fairseq.scoring.tokenizer import EvaluationTokenizer
-
-
-@dataclass
-class WerScorerConfig(FairseqDataclass):
- wer_tokenizer: EvaluationTokenizer.ALL_TOKENIZER_TYPES = field(
- default="none", metadata={"help": "sacreBLEU tokenizer to use for evaluation"}
- )
- wer_remove_punct: bool = field(
- default=False, metadata={"help": "remove punctuation"}
- )
- wer_char_level: bool = field(
- default=False, metadata={"help": "evaluate at character level"}
- )
- wer_lowercase: bool = field(default=False, metadata={"help": "lowercasing"})
-
-
-@register_scorer("wer", dataclass=WerScorerConfig)
-class WerScorer(BaseScorer):
- def __init__(self, cfg):
- super().__init__(cfg)
- self.reset()
- try:
- import editdistance as ed
- except ImportError:
- raise ImportError("Please install editdistance to use WER scorer")
- self.ed = ed
- self.tokenizer = EvaluationTokenizer(
- tokenizer_type=self.cfg.wer_tokenizer,
- lowercase=self.cfg.wer_lowercase,
- punctuation_removal=self.cfg.wer_remove_punct,
- character_tokenization=self.cfg.wer_char_level,
- )
-
- def reset(self):
- self.distance = 0
- self.ref_length = 0
-
- def add_string(self, ref, pred):
- ref_items = self.tokenizer.tokenize(ref).split()
- pred_items = self.tokenizer.tokenize(pred).split()
- self.distance += self.ed.eval(ref_items, pred_items)
- self.ref_length += len(ref_items)
-
- def result_string(self):
- return f"WER: {self.score():.2f}"
-
- def score(self):
- return 100.0 * self.distance / self.ref_length if self.ref_length > 0 else 0
diff --git a/spaces/mshukor/UnIVAL/fairseq/hubconf.py b/spaces/mshukor/UnIVAL/fairseq/hubconf.py
deleted file mode 100644
index 5949e274edd02e86cb323331211641ce0d0b9b93..0000000000000000000000000000000000000000
--- a/spaces/mshukor/UnIVAL/fairseq/hubconf.py
+++ /dev/null
@@ -1,73 +0,0 @@
-# Copyright (c) Facebook, Inc. and its affiliates.
-#
-# This source code is licensed under the MIT license found in the
-# LICENSE file in the root directory of this source tree.
-"""isort:skip_file"""
-
-import functools
-import importlib
-
-
-dependencies = [
- "dataclasses",
- "hydra",
- "numpy",
- "omegaconf",
- "regex",
- "requests",
- "torch",
-]
-
-
-# Check for required dependencies and raise a RuntimeError if any are missing.
-missing_deps = []
-for dep in dependencies:
- try:
- importlib.import_module(dep)
- except ImportError:
- # Hack: the hydra package is provided under the "hydra-core" name in
- # pypi. We don't want the user mistakenly calling `pip install hydra`
- # since that will install an unrelated package.
- if dep == "hydra":
- dep = "hydra-core"
- missing_deps.append(dep)
-if len(missing_deps) > 0:
- raise RuntimeError("Missing dependencies: {}".format(", ".join(missing_deps)))
-
-
-# only do fairseq imports after checking for dependencies
-from fairseq.hub_utils import ( # noqa; noqa
- BPEHubInterface as bpe,
- TokenizerHubInterface as tokenizer,
-)
-from fairseq.models import MODEL_REGISTRY # noqa
-
-
-# torch.hub doesn't build Cython components, so if they are not found then try
-# to build them here
-try:
- import fairseq.data.token_block_utils_fast # noqa
-except ImportError:
- try:
- import cython # noqa
- import os
- from setuptools import sandbox
-
- sandbox.run_setup(
- os.path.join(os.path.dirname(__file__), "setup.py"),
- ["build_ext", "--inplace"],
- )
- except ImportError:
- print(
- "Unable to build Cython components. Please make sure Cython is "
- "installed if the torch.hub model you are loading depends on it."
- )
-
-
-# automatically expose models defined in FairseqModel::hub_models
-for _model_type, _cls in MODEL_REGISTRY.items():
- for model_name in _cls.hub_models().keys():
- globals()[model_name] = functools.partial(
- _cls.from_pretrained,
- model_name,
- )
diff --git a/spaces/mueller-franzes/medfusion-app/medical_diffusion/data/datamodules/__init__.py b/spaces/mueller-franzes/medfusion-app/medical_diffusion/data/datamodules/__init__.py
deleted file mode 100644
index 673fee178d4234ce9219704234743d3c934dbed2..0000000000000000000000000000000000000000
--- a/spaces/mueller-franzes/medfusion-app/medical_diffusion/data/datamodules/__init__.py
+++ /dev/null
@@ -1 +0,0 @@
-from .datamodule_simple import SimpleDataModule
\ No newline at end of file
diff --git a/spaces/mueller-franzes/medfusion-app/scripts/sample.py b/spaces/mueller-franzes/medfusion-app/scripts/sample.py
deleted file mode 100644
index ecd838b2ee6724bf64f6325b53742f7b81856dab..0000000000000000000000000000000000000000
--- a/spaces/mueller-franzes/medfusion-app/scripts/sample.py
+++ /dev/null
@@ -1,60 +0,0 @@
-
-from pathlib import Path
-import torch
-from torchvision import utils
-import math
-from medical_diffusion.models.pipelines import DiffusionPipeline
-
-def rgb2gray(img):
- # img [B, C, H, W]
- return ((0.3 * img[:,0]) + (0.59 * img[:,1]) + (0.11 * img[:,2]))[:, None]
- # return ((0.33 * img[:,0]) + (0.33 * img[:,1]) + (0.33 * img[:,2]))[:, None]
-
-def normalize(img):
- # img = torch.stack([b.clamp(torch.quantile(b, 0.001), torch.quantile(b, 0.999)) for b in img])
- return torch.stack([(b-b.min())/(b.max()-b.min()) for b in img])
-
-if __name__ == "__main__":
- path_out = Path.cwd()/'results/CheXpert/samples'
- path_out.mkdir(parents=True, exist_ok=True)
-
- torch.manual_seed(0)
- device = torch.device('cuda')
-
- # ------------ Load Model ------------
- # pipeline = DiffusionPipeline.load_best_checkpoint(path_run_dir)
- pipeline = DiffusionPipeline.load_from_checkpoint('runs/2022_12_12_171357_chest_diffusion/last.ckpt')
- pipeline.to(device)
-
-
- # --------- Generate Samples -------------------
- steps = 150
- use_ddim = True
- images = {}
- n_samples = 16
-
- for cond in [0,1,None]:
- torch.manual_seed(0)
-
- # --------- Conditioning ---------
- condition = torch.tensor([cond]*n_samples, device=device) if cond is not None else None
- # un_cond = torch.tensor([1-cond]*n_samples, device=device)
- un_cond = None
-
- # ----------- Run --------
- results = pipeline.sample(n_samples, (8, 32, 32), guidance_scale=8, condition=condition, un_cond=un_cond, steps=steps, use_ddim=use_ddim )
- # results = pipeline.sample(n_samples, (4, 64, 64), guidance_scale=1, condition=condition, un_cond=un_cond, steps=steps, use_ddim=use_ddim )
-
- # --------- Save result ---------------
- results = (results+1)/2 # Transform from [-1, 1] to [0, 1]
- results = results.clamp(0, 1)
- utils.save_image(results, path_out/f'test_{cond}.png', nrow=int(math.sqrt(results.shape[0])), normalize=True, scale_each=True) # For 2D images: [B, C, H, W]
- images[cond] = results
-
-
- diff = torch.abs(normalize(rgb2gray(images[1]))-normalize(rgb2gray(images[0]))) # [0,1] -> [0, 1]
- # diff = torch.abs(images[1]-images[0])
- utils.save_image(diff, path_out/'diff.png', nrow=int(math.sqrt(results.shape[0])), normalize=True, scale_each=True) # For 2D images: [B, C, H, W]
-
-
-
\ No newline at end of file
diff --git a/spaces/muellerzr/accelerate-presentation/Accelerate_files/libs/revealjs/plugin/highlight/highlight.esm.js b/spaces/muellerzr/accelerate-presentation/Accelerate_files/libs/revealjs/plugin/highlight/highlight.esm.js
deleted file mode 100644
index 20f35d7b9eecbeedd6c5a4d94e8991d9bc5d4865..0000000000000000000000000000000000000000
--- a/spaces/muellerzr/accelerate-presentation/Accelerate_files/libs/revealjs/plugin/highlight/highlight.esm.js
+++ /dev/null
@@ -1,5 +0,0 @@
-function e(t){return(e="function"==typeof Symbol&&"symbol"==typeof Symbol.iterator?function(e){return typeof e}:function(e){return e&&"function"==typeof Symbol&&e.constructor===Symbol&&e!==Symbol.prototype?"symbol":typeof e})(t)}function t(e,t){if(!(e instanceof t))throw new TypeError("Cannot call a class as a function")}function n(e,t){for(var n=0;ne.length)&&(t=e.length);for(var n=0,a=new Array(t);n=74)&&(z=se.match(/Chrome\/(\d+)/))&&(W=z[1]);var de=W&&+W,ue=de,me=E,pe=!!Object.getOwnPropertySymbols&&!me((function(){return!String(Symbol())||!Symbol.sham&&ue&&ue<41})),ge=pe&&!Symbol.sham&&"symbol"==typeof Symbol.iterator,Ee=p,Se=g.exports,be=Z,Te=te,fe=pe,Ce=ge,Ne=Se("wks"),Re=Ee.Symbol,ve=Ce?Re:Re&&Re.withoutSetter||Te,Oe=function(e){return be(Ne,e)&&(fe||"string"==typeof Ne[e])||(fe&&be(Re,e)?Ne[e]=Re[e]:Ne[e]=ve("Symbol."+e)),Ne[e]},he={};he[Oe("toStringTag")]="z";var ye="[object z]"===String(he),Ie={exports:{}},Ae=V,De=Function.toString;"function"!=typeof Ae.inspectSource&&(Ae.inspectSource=function(e){return De.call(e)});var Me,Le,we,xe=Ae.inspectSource,Pe=xe,ke=p.WeakMap,Ue="function"==typeof ke&&/native code/.test(Pe(ke)),Fe=g.exports,Be=te,Ge=Fe("keys"),Ye=function(e){return Ge[e]||(Ge[e]=Be(e))},He={},Ve=Ue,qe=T,ze=F,We=Z,$e=V,Qe=Ye,Ke=He,je=p.WeakMap;if(Ve||$e.state){var Xe=$e.state||($e.state=new je),Ze=Xe.get,Je=Xe.has,et=Xe.set;Me=function(e,t){if(Je.call(Xe,e))throw new TypeError("Object already initialized");return t.facade=e,et.call(Xe,e,t),t},Le=function(e){return Ze.call(Xe,e)||{}},we=function(e){return Je.call(Xe,e)}}else{var tt=Qe("state");Ke[tt]=!0,Me=function(e,t){if(We(e,tt))throw new TypeError("Object already initialized");return t.facade=e,ze(e,tt,t),t},Le=function(e){return We(e,tt)?e[tt]:{}},we=function(e){return We(e,tt)}}var nt={set:Me,get:Le,has:we,enforce:function(e){return we(e)?Le(e):Me(e,{})},getterFor:function(e){return function(t){var n;if(!qe(t)||(n=Le(t)).type!==e)throw TypeError("Incompatible receiver, "+e+" required");return n}}},at=p,rt=F,it=Z,ot=Y,st=xe,lt=nt.get,ct=nt.enforce,_t=String(String).split("String");(Ie.exports=function(e,t,n,a){var r,i=!!a&&!!a.unsafe,o=!!a&&!!a.enumerable,s=!!a&&!!a.noTargetGet;"function"==typeof n&&("string"!=typeof t||it(n,"name")||rt(n,"name",t),(r=ct(n)).source||(r.source=_t.join("string"==typeof t?t:""))),e!==at?(i?!s&&e[t]&&(o=!0):delete e[t],o?e[t]=n:rt(e,t,n)):o?e[t]=n:ot(t,n)})(Function.prototype,"toString",(function(){return"function"==typeof this&<(this).source||st(this)}));var dt={}.toString,ut=function(e){return dt.call(e).slice(8,-1)},mt=ye,pt=ut,gt=Oe("toStringTag"),Et="Arguments"==pt(function(){return arguments}()),St=mt?pt:function(e){var t,n,a;return void 0===e?"Undefined":null===e?"Null":"string"==typeof(n=function(e,t){try{return e[t]}catch(e){}}(t=Object(e),gt))?n:Et?pt(t):"Object"==(a=pt(t))&&"function"==typeof t.callee?"Arguments":a},bt=St,Tt=ye?{}.toString:function(){return"[object "+bt(this)+"]"},ft=ye,Ct=Ie.exports,Nt=Tt;ft||Ct(Object.prototype,"toString",Nt,{unsafe:!0});var Rt=y,vt=function(){var e=Rt(this),t="";return e.global&&(t+="g"),e.ignoreCase&&(t+="i"),e.multiline&&(t+="m"),e.dotAll&&(t+="s"),e.unicode&&(t+="u"),e.sticky&&(t+="y"),t},Ot=Ie.exports,ht=y,yt=E,It=vt,At=RegExp.prototype,Dt=At.toString,Mt=yt((function(){return"/a/b"!=Dt.call({source:"a",flags:"b"})})),Lt="toString"!=Dt.name;(Mt||Lt)&&Ot(RegExp.prototype,"toString",(function(){var e=ht(this),t=String(e.source),n=e.flags;return"/"+t+"/"+String(void 0===n&&e instanceof RegExp&&!("flags"in At)?It.call(e):n)}),{unsafe:!0});var wt={},xt={},Pt={}.propertyIsEnumerable,kt=Object.getOwnPropertyDescriptor,Ut=kt&&!Pt.call({1:2},1);xt.f=Ut?function(e){var t=kt(this,e);return!!t&&t.enumerable}:Pt;var Ft=ut,Bt="".split,Gt=E((function(){return!Object("z").propertyIsEnumerable(0)}))?function(e){return"String"==Ft(e)?Bt.call(e,""):Object(e)}:Object,Yt=Gt,Ht=$,Vt=function(e){return Yt(Ht(e))},qt=S,zt=xt,Wt=P,$t=Vt,Qt=A,Kt=Z,jt=O,Xt=Object.getOwnPropertyDescriptor;wt.f=qt?Xt:function(e,t){if(e=$t(e),t=Qt(t,!0),jt)try{return Xt(e,t)}catch(e){}if(Kt(e,t))return Wt(!zt.f.call(e,t),e[t])};var Zt={},Jt=Math.ceil,en=Math.floor,tn=function(e){return isNaN(e=+e)?0:(e>0?en:Jt)(e)},nn=tn,an=Math.min,rn=function(e){return e>0?an(nn(e),9007199254740991):0},on=tn,sn=Math.max,ln=Math.min,cn=function(e,t){var n=on(e);return n<0?sn(n+t,0):ln(n,t)},_n=Vt,dn=rn,un=cn,mn=function(e){return function(t,n,a){var r,i=_n(t),o=dn(i.length),s=un(a,o);if(e&&n!=n){for(;o>s;)if((r=i[s++])!=r)return!0}else for(;o>s;s++)if((e||s in i)&&i[s]===n)return e||s||0;return!e&&-1}},pn={includes:mn(!0),indexOf:mn(!1)},gn=Z,En=Vt,Sn=pn.indexOf,bn=He,Tn=function(e,t){var n,a=En(e),r=0,i=[];for(n in a)!gn(bn,n)&&gn(a,n)&&i.push(n);for(;t.length>r;)gn(a,n=t[r++])&&(~Sn(i,n)||i.push(n));return i},fn=["constructor","hasOwnProperty","isPrototypeOf","propertyIsEnumerable","toLocaleString","toString","valueOf"],Cn=Tn,Nn=fn.concat("length","prototype");Zt.f=Object.getOwnPropertyNames||function(e){return Cn(e,Nn)};var Rn={};Rn.f=Object.getOwnPropertySymbols;var vn=Zt,On=Rn,hn=y,yn=oe("Reflect","ownKeys")||function(e){var t=vn.f(hn(e)),n=On.f;return n?t.concat(n(e)):t},In=Z,An=yn,Dn=wt,Mn=b,Ln=function(e,t){for(var n=An(t),a=Mn.f,r=Dn.f,i=0;i=51||!ta((function(){var t=[];return(t.constructor={})[aa]=function(){return{foo:1}},1!==t[e](Boolean).foo}))},ia=Qn,oa=T,sa=jn,la=cn,ca=rn,_a=Vt,da=ea,ua=Oe,ma=ra("slice"),pa=ua("species"),ga=[].slice,Ea=Math.max;ia({target:"Array",proto:!0,forced:!ma},{slice:function(e,t){var n,a,r,i=_a(this),o=ca(i.length),s=la(e,o),l=la(void 0===t?o:t,o);if(sa(i)&&("function"!=typeof(n=i.constructor)||n!==Array&&!sa(n.prototype)?oa(n)&&null===(n=n[pa])&&(n=void 0):n=void 0,n===Array||void 0===n))return ga.call(i,s,l);for(a=new(void 0===n?Array:n)(Ea(l-s,0)),r=0;si;)Ia.f(e,n=a[i++],t[n]);return e},La=oe("document","documentElement"),wa=y,xa=Ma,Pa=fn,ka=He,Ua=La,Fa=R,Ba=Ye("IE_PROTO"),Ga=function(){},Ya=function(e){return"
-
-
-
-tl;dr: Imaginary Programming (IP) is where
-data and knowledge has been substituted for
-inference by a LM. Therefore, the
-implementation of an 𝑖λ library will be
-uniquely tailored to each language.
-
-
-
-I design and build an IP library named
-𝑖λ.el for emacs. Think of it a bit like a
-functional programming library in that you
-will find a set of functions and macros for
-working in the programming paradigm. The
-objective here is to create some functions for
-doing IP in emacs lisp, since emacs lisp has
-the expressive power to prototype such things,
-but the ideas contained here can easily be
-transferred to any other programming language.
-The results of this little experiment will go
-straight into my thesis.
-
-imacro (like regular macros) are for
-generating code. idefun, however, doesnt
-generate the code but attempts to evaluate a
-function without code, or if code is supplied
-then imagine evaluating that code rather than
-actually evaluating it.
-
-
-
-It's kinda crazy that Im-macros run faster
-and are more reliable than Im-functions,
-where the opposite is true in regular
-programming. That's because they generate the
-code which can be reused, adjusted and
-interactively regenerated, where an
-Im-function will typically have to query the
-LM every time you call it.
-
-
-
-
-
1.1.1 The objective with 𝑖λ.el
-
-
-The objective here is to create IP functions
-for programming in emacs lisp exclusively.
-
-
-
-It will be extended in the future to do all
-programming languages, but I intend to make
-𝑖λ.el simple and effective for programming
-in emacs lisp without bloat or over-complication.
-
-
-
-
-
-
-
1.2 Syntax forms
-
-
-
-
-
-
-
-
-
-
-
-
-
-
-
-
name
-
type
-
depends on
-
basic idea
-
-
-
-
-
ieval
-
MACRO
-
-
ieval will imagine the evaluation of some code without any other context.
-
-
-
-
imacro/N
-
MACRO
-
-
imacro does not evaluate. It merely generates code, but that code is imagined. Like idefun it is variadic.
-
-
-
-
idefun
-
FUNCTION
-
ieval and imacro
-
Run an imagined function on the given arguments and return an imagined result, but create a binding for the function.
-
-
-
-
ilist
-
FUNCTION
-
-
Generate a list of things. Return a real list.
-
-
-
-
ilambda / 𝑖λ
-
FUNCTION
-
ieval
-
Imaginarily run an expression on the given arguments and return an imagined result.
-
-
-
-
ifilter
-
FUNCTION
-
-
Imaginarily filter a real list with natural language and return a real list. Optionally, enforce cardinality.
-
-
-
-
iparse
-
MACRO
-
-
Given a syntax form / expression, will parse a syntax form with natural language. Returns the subform.
-
-
-
-
defimacro
-
MACRO
-
imacro/N
-
Select the appropriate imacro/N form depending on the arity of arguments.
-
-
-
-
-
-
-
1.2.1ieval
-
-
-ieval will simply evaluate the provided
-string/sexp as emacs lisp code. You
-must provide ieval with, firstly, the preceding
-code, which may be, for example, a function
-definition or package requires, etc. and,
-secondly, evaluated expression. Either
-argument can either be a raw string containing
-code or a sexp, but the expression will be
-"one-line-ized" for the prompt.
-
-ieval not only evaluates correctly despite
-the deliberately incorrect naming of the
-function (it multiplies rather than doubles),
-but it returns the value as the correct data type.
-
1: (-reduce (𝑖λ (x y)"add x to y")(number-sequence 13))
-
-
-
-
-
"6"
-
-
-
-
-
-
-
1.2.3idefun
-
-
-The idefun creates a binding to an imaginary
-function. The implementation of the idefun
-need not be specified in order for code to
-run.
-
-
-
-The new prompt function returned by idefun is provided with arguments and the
-values of those arguments are taken and placed
-into a prompt. An implementation may be
-provided to idefun when defining the prompt function or optionally left out.
-Unlike an imacro, when the prompt function
-is evaluated the code is not returned. Rather,
-the code is evaluted in imaginary space.
-
-
-
-In short, the LM will imagine the evaluation
-of the function as opposed to generate code.
-
-
-
-idefun returns a binding to a new prompt
-function.
-
-
-
-Some examples:
-
-
-
1: (idefun add-two-numbers)
- 2: (add-two-numbers 58)
- 3:
- 4: (idefun add-two-numbers (a b))
- 5: (add-two-numbers 58)
- 6:
- 7: (idefun add-two-numbers (a b)"add a to b")
- 8: (add-two-numbers 58)
- 9:
-10: (idefun sum-of-integers)
-11: (sum-of-integers 123102003000)
-12:
-13: (idefun thing-to-hex-color)
-14:
-15: (idefun add-two-numbers (a b)"add a to b")
-
-All of the following are valid ways to invoke defimacro.
-
-
-
-defimacro selects the right imacro/N function depending on the arity of the arguments.
-
-
-
-
1: (defimacro my/subtract)
-2: (defimacro my/subtract (a b c))
-3: (defimacro my/itimes (a b c)
-4: "multiply three complex numbers")
-
-
-
-
-
-
-
-
-
-
-
-
1.2.5ilist
-
-
-The easiest of the list of syntax forms I
-aimed to implement, ilist simply takes a the
-number of items to generate (n) and a string
-describing the type of thing to generate
-(type-of-thing). It will return a real list
-of such things.
-