Commit
·
0fec13f
1
Parent(s):
de68c1d
Update parquet files (step 40 of 249)
Browse filesThis view is limited to 50 files because it contains too many changes.
See raw diff
- spaces/1acneusushi/gradio-2dmoleculeeditor/Damodarastakam-In-Malayalam-Pdf-31.md +0 -70
- spaces/1acneusushi/gradio-2dmoleculeeditor/data/Agbot Silkroad.md +0 -25
- spaces/1acneusushi/gradio-2dmoleculeeditor/data/Bhaag Johnny Movie Download In Hindi 720p Download BEST.md +0 -16
- spaces/1acneusushi/gradio-2dmoleculeeditor/data/Fifa 08 Xbox.md +0 -28
- spaces/1gistliPinn/ChatGPT4/Examples/Age Of Empires 3 NO CD Crack [2021].dmg Hack Torrent.md +0 -10
- spaces/1phancelerku/anime-remove-background/Download Cars Fast as Lightning APK for Android and Race with Lightning McQueen.md +0 -135
- spaces/1phancelerku/anime-remove-background/Download Euro Truck Simulator 2 and Customize Your Truck with Tons of Tuning Options.md +0 -133
- spaces/1phancelerku/anime-remove-background/Download Nebulous io Mod Apk Now and Unlock Unlimited Plasma and All Features.md +0 -82
- spaces/1phancelerku/anime-remove-background/EA SPORTS FIFA 23 Companion Build Manage and Compete in FUT.md +0 -88
- spaces/52Hz/HWMNet_lowlight_enhancement/WT/transform.py +0 -53
- spaces/7hao/bingo/src/components/chat.tsx +0 -93
- spaces/7hao/bingo/src/components/ui/dialog.tsx +0 -128
- spaces/ADUPA/README/README.md +0 -10
- spaces/AIGC-Audio/AudioGPT/text_to_audio/Make_An_Audio/ldm/modules/diffusionmodules/__init__.py +0 -0
- spaces/AIGC-Audio/AudioGPT/text_to_speech/data_gen/tts/txt_processors/zh_aishell_no_tone_sing.py +0 -126
- spaces/AIGC-Audio/AudioGPT/text_to_speech/modules/vocoder/parallel_wavegan/layers/tf_layers.py +0 -129
- spaces/AIGC-Audio/Make_An_Audio_inpaint/ldm/data/extract_mel_spectrogram.py +0 -151
- spaces/AILab-CVC/SEED-LLaMA/scripts/start_frontend_8b.sh +0 -1
- spaces/AIWaves/SOP_Generation-single/gradio_backend.py +0 -123
- spaces/AIZeroToHero/02-Transformers-Sentence2Paragraph/README.md +0 -13
- spaces/ANILYADAV/mygenaichatbot/README.md +0 -12
- spaces/ATang0729/Forecast4Muses/Model/Model6/Model6_1_ClothesKeyPoint/mmpose_1_x/configs/__init__.py +0 -0
- spaces/After-the-Dark/paragraph-similarity/app.py +0 -56
- spaces/AgentVerse/agentVerse/ui/src/phaser3-rex-plugins/templates/ui/basesizer/LayoutBackgrounds.js +0 -41
- spaces/AgentVerse/agentVerse/ui/src/phaser3-rex-plugins/templates/ui/knob/input/IsLocalPointInKnob.js +0 -8
- spaces/AgentVerse/agentVerse/ui/src/phaser3-rex-plugins/templates/ui/namevaluelabel/NameValueLabel.d.ts +0 -94
- spaces/Ajay07pandey/Netfilx_Movie_Recommendation_System/app.py +0 -58
- spaces/Akmyradov/TurkmenTTSweSTT/uroman/bin/string-distance.pl +0 -99
- spaces/AlawnCN/webui-docker/README.md +0 -19
- spaces/Alpaca233/SadTalker/src/facerender/modules/util.py +0 -564
- spaces/Androidonnxfork/CivitAi-to-Diffusers/diffusers/src/diffusers/models/controlnet_flax.py +0 -394
- spaces/Androidonnxfork/CivitAi-to-Diffusers/diffusers/tests/pipelines/stable_diffusion/test_onnx_stable_diffusion_inpaint_legacy.py +0 -97
- spaces/Androidonnxfork/CivitAi-to-Diffusers/diffusers/utils/check_table.py +0 -185
- spaces/Andy1621/uniformer_image_detection/mmdet/models/detectors/cascade_rcnn.py +0 -46
- spaces/Anew1007/extras/README.md +0 -11
- spaces/Anonymous-sub/Rerender/ControlNet/share.py +0 -8
- spaces/Ataturk-Chatbot/HuggingFaceChat/venv/lib/python3.11/site-packages/pip/_vendor/colorama/ansitowin32.py +0 -277
- spaces/Awiny/Image2Paragraph/models/grit_src/third_party/CenterNet2/detectron2/checkpoint/c2_model_loading.py +0 -407
- spaces/BAAI/AltDiffusion-m9/style.css +0 -81
- spaces/BAAI/vid2vid-zero/vid2vid_zero/models/resnet_2d.py +0 -209
- spaces/Badaleeloveashley/badaleeloveashley/README.md +0 -12
- spaces/Banbri/zcvzcv/postcss.config.js +0 -6
- spaces/Banbri/zcvzcv/src/app/interface/grid/index.tsx +0 -26
- spaces/BartPoint/VoiceChange_Beta/infer_pack/models.py +0 -1124
- spaces/BartPoint/VoiceChange_Beta/infer_pack/models_onnx.py +0 -818
- spaces/BeeMon/dreambooth-training/README.md +0 -14
- spaces/Benson/text-generation/Examples/Descargar Gratis Brawlhalla Steamunlocked.md +0 -64
- spaces/Betacuckgpt/togethercomputer-GPT-JT-Moderation-6B/README.md +0 -12
- spaces/BetterAPI/BetterChat/src/lib/types/Conversation.ts +0 -17
- spaces/BetterAPI/BetterChat_new/src/lib/types/UrlDependency.ts +0 -5
spaces/1acneusushi/gradio-2dmoleculeeditor/Damodarastakam-In-Malayalam-Pdf-31.md
DELETED
@@ -1,70 +0,0 @@
|
|
1 |
-
## Damodarastakam In Malayalam Pdf 31
|
2 |
-
|
3 |
-
|
4 |
-
|
5 |
-
|
6 |
-
|
7 |
-
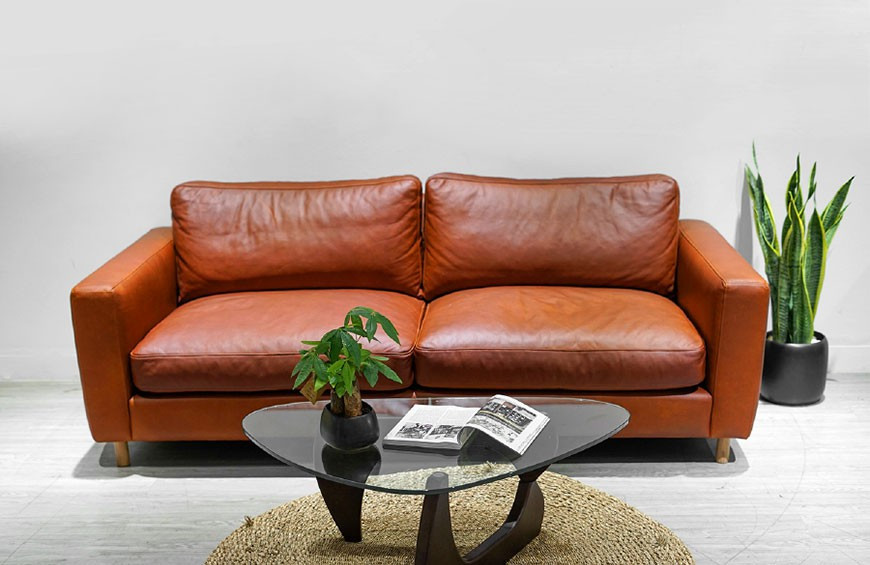
|
8 |
-
|
9 |
-
|
10 |
-
|
11 |
-
|
12 |
-
|
13 |
-
**LINK 🆓 [https://eromdesre.blogspot.com/?d=2txKRe](https://eromdesre.blogspot.com/?d=2txKRe)**
|
14 |
-
|
15 |
-
|
16 |
-
|
17 |
-
|
18 |
-
|
19 |
-
|
20 |
-
|
21 |
-
|
22 |
-
|
23 |
-
|
24 |
-
|
25 |
-
```
|
26 |
-
|
27 |
-
# Damodarastakam In Malayalam Pdf 31: A Devotional Song for Kartik Maas
|
28 |
-
|
29 |
-
|
30 |
-
|
31 |
-
Damodarastakam is a Sanskrit hymn composed by Satyavrata Muni, a great devotee of Lord Krishna. It describes the pastime of Krishna being bound by a rope (damodara) by His mother Yashoda as a punishment for stealing butter. This pastime is celebrated during the month of Kartik (October-November), also known as Damodara Maas, when devotees offer lamps to Krishna and sing Damodarastakam every day.
|
32 |
-
|
33 |
-
|
34 |
-
|
35 |
-
Damodarastakam consists of eight verses, each ending with the refrain "namo namah tulasi krishna-preyasi", which means "I offer my respectful obeisances to Tulasi Devi, who is very dear to Lord Krishna". The hymn expresses the mood of surrender, humility, and love for Krishna, and also reveals the glories of Tulasi Devi, the sacred plant that is worshiped by Vaishnavas.
|
36 |
-
|
37 |
-
|
38 |
-
|
39 |
-
Damodarastakam has been translated into many languages, including Malayalam, the official language of Kerala state in India. A PDF file of Damodarastakam in Malayalam with transliteration and meaning can be downloaded from [this link](https://sway.office.com/wk1QiLzrQegRtCRb). The PDF file contains 31 pages, with one verse per page. The file also has an introduction and a conclusion that explain the significance and benefits of Damodarastakam.
|
40 |
-
|
41 |
-
|
42 |
-
|
43 |
-
Damodarastakam in Malayalam can also be listened to on YouTube, where several videos have been uploaded by devotees. One such video is [this one](https://www.youtube.com/watch?v=oVon63hGYtQ), which features the voice of Nimais Media, a channel dedicated to spreading Krishna consciousness. The video has over 42,000 views and 55 comments as of April 2023.
|
44 |
-
|
45 |
-
|
46 |
-
|
47 |
-
Damodarastakam in Malayalam is a beautiful way to connect with Krishna and His devotees during Kartik Maas. By singing or hearing this hymn, one can attain the mercy of Krishna and Tulasi Devi, and become free from all material bondage.
|
48 |
-
|
49 |
-
``` ```
|
50 |
-
|
51 |
-
If you are wondering why Damodarastakam is so important and beneficial, here are some reasons. First of all, Damodarastakam is a hymn that was composed by Satyavrata Muni, a great sage and devotee of Lord Krishna. He sang this hymn in a conversation with Narada Muni and Saunaka Rishi, who were also great authorities on spiritual knowledge. Therefore, Damodarastakam has the potency to bestow the highest realization of Krishna's sweetness and mercy.
|
52 |
-
|
53 |
-
|
54 |
-
|
55 |
-
Secondly, Damodarastakam is recommended to be recited during the month of Kartik, which is also known as Damodara Maas. This month is very dear to Lord Krishna, as it commemorates His pastime of being bound by His mother's love. During this month, devotees offer lamps to Krishna and sing Damodarastakam every day. By doing so, they please Krishna and attract His special blessings. It is said that any devotional service performed in this month is multiplied a thousand times.
|
56 |
-
|
57 |
-
|
58 |
-
|
59 |
-
Thirdly, Damodarastakam reveals the essence of pure devotion to Krishna. It shows how Krishna is conquered by the love of His devotees, especially His mother Yashoda. It also shows how Krishna reciprocates with His devotees by manifesting His most charming and playful form as a child. It also shows how Krishna grants His devotees the highest benediction of prema-bhakti, or pure love for Him.
|
60 |
-
|
61 |
-
|
62 |
-
|
63 |
-
Therefore, Damodarastakam is a treasure for all devotees of Krishna. By reading, hearing, or singing this hymn, one can experience the bliss of Krishna's pastimes and develop a deep attachment to Him.
|
64 |
-
|
65 |
-
``` 1b8d091108
|
66 |
-
|
67 |
-
|
68 |
-
|
69 |
-
|
70 |
-
|
|
|
|
|
|
|
|
|
|
|
|
|
|
|
|
|
|
|
|
|
|
|
|
|
|
|
|
|
|
|
|
|
|
|
|
|
|
|
|
|
|
|
|
|
|
|
|
|
|
|
|
|
|
|
|
|
|
|
|
|
|
|
|
|
|
|
|
|
|
|
|
|
|
|
|
|
|
|
|
|
|
|
|
|
|
|
|
|
|
|
|
|
|
|
|
|
|
|
|
|
|
|
|
|
|
|
|
|
|
|
|
|
|
|
|
|
|
|
|
|
|
|
|
|
|
|
|
|
|
|
|
|
|
|
|
|
|
|
|
|
|
spaces/1acneusushi/gradio-2dmoleculeeditor/data/Agbot Silkroad.md
DELETED
@@ -1,25 +0,0 @@
|
|
1 |
-
|
2 |
-
<h1>Agbot: A Popular Bot for Silkroad Online</h1>
|
3 |
-
<p>Silkroad Online is a massively multiplayer online role-playing game that takes place in a historical fantasy world inspired by the ancient Silk Road trade route. Players can choose from three races: Chinese, European, and Arabian, and explore a vast open world full of quests, dungeons, and PvP battles.</p>
|
4 |
-
<p>However, some players may find the game too grindy or repetitive, and may want to use a bot to automate some of the tasks. A bot is a software program that can perform certain actions in the game without human input, such as fighting monsters, collecting loot, selling items, or using skills.</p>
|
5 |
-
<h2>agbot silkroad</h2><br /><p><b><b>Download File</b> --->>> <a href="https://byltly.com/2uKwDt">https://byltly.com/2uKwDt</a></b></p><br /><br />
|
6 |
-
<p>One of the most popular bots for Silkroad Online is Agbot, which works for version 1.227 of the game. Agbot is a free bot that can be downloaded from various websites, such as <a href="https://www.gamefront.com/games/silkroad-online/file/agbot">GameFront</a> or <a href="https://www.elitepvpers.com/forum/sro-hacks-bots-cheats-exploits/1611445-sro-r-agbot-release.html">Elitepvpers</a>. Agbot has many features and options that allow players to customize their botting experience, such as:</p>
|
7 |
-
<ul>
|
8 |
-
<li>Choosing different hunting areas and scripts</li>
|
9 |
-
<li>Setting up skills and buffs for combat</li>
|
10 |
-
<li>Managing inventory and storage space</li>
|
11 |
-
<li>Using potions and scrolls when needed</li>
|
12 |
-
<li>Returning to town when low on health or arrows</li>
|
13 |
-
<li>Switching weapons and shields</li>
|
14 |
-
<li>Buying and selling items from NPCs</li>
|
15 |
-
<li>Using media patcher and nuconnector to bypass security checks</li>
|
16 |
-
</ul>
|
17 |
-
<p>To use Agbot, players need to follow some steps to set it up correctly. First, they need to use the media patcher in the Silkroad Online folder to patch the port and redirect the DNS using the hosts file. Then, they need to delete the data folder in Agbot and rename the datar folder to data. Next, they need to open Agbot.exe and configure the nuconnector.ini file with the IP address 31.193.168.140 or 31.193.168.141. Finally, they need to open nuconnector1.3 in Agbot folder and then open Silkroad Online, which will connect to nuconnector and load the modified nuconnector.ini file.</p>
|
18 |
-
<p>Once in the game, players can use Agbot to start botting by selecting their character name, choosing a hunting script, setting up their skills and options, and clicking on start. Agbot will then take over and perform the actions specified by the player.</p>
|
19 |
-
<p>Agbot is a useful tool for players who want to level up faster, earn more gold, or enjoy other aspects of Silkroad Online without spending too much time on grinding. However, players should also be aware of the risks involved in using a bot, such as getting banned by the game developers or losing their account information to hackers. Therefore, players should always use Agbot at their own discretion and responsibility.</p>
|
20 |
-
|
21 |
-
<p>Using bots in online games can have both benefits and drawbacks for players and publishers. On one hand, bots can provide a convenient and fun way to practice skills, learn strategies, or enjoy the game without the pressure of competing with other human players. Bots can also help fill up servers, create diversity, and enhance the social aspects of online gaming by simulating different personalities and behaviors.</p>
|
22 |
-
<p>On the other hand, bots can also ruin the online gaming experience for many players and publishers by creating unfair advantages, disrupting the game balance, and violating the game rules. Bots can be used to cheat, spam, farm, or grief other players, which can lower their satisfaction and engagement with the game. Bots can also harm the game economy by inflating or deflating the value of in-game items and currencies, which can affect the revenue and reputation of the game publishers.</p>
|
23 |
-
<p>Therefore, it is important for game developers and designers to consider the impact of bots on their online games and to implement appropriate measures to prevent or mitigate their negative effects. Some possible solutions include detecting and banning bots, designing bot-proof game mechanics, educating and rewarding players for fair play, and creating official or authorized bots that can enhance the game experience without harming it.</p> 7b8c122e87<br />
|
24 |
-
<br />
|
25 |
-
<br />
|
|
|
|
|
|
|
|
|
|
|
|
|
|
|
|
|
|
|
|
|
|
|
|
|
|
|
|
|
|
|
|
|
|
|
|
|
|
|
|
|
|
|
|
|
|
|
|
|
|
|
|
spaces/1acneusushi/gradio-2dmoleculeeditor/data/Bhaag Johnny Movie Download In Hindi 720p Download BEST.md
DELETED
@@ -1,16 +0,0 @@
|
|
1 |
-
<br />
|
2 |
-
<h1>Bhaag Johnny Movie Download in Hindi 720p: How to Watch Online for Free</h1>
|
3 |
-
<p>Bhaag Johnny is a 2015 Bollywood thriller movie starring Kunal Khemu, Zoa Morani and Mandana Karimi. The movie revolves around Johnny, a casanova who is blackmailed by his boss to kill a girl or lose his job. However, he gets a chance to live two lives: one where he commits the crime and another where he refuses and goes on the run. The movie explores the consequences of his choices and how they affect his love life and destiny.</p>
|
4 |
-
<h2>Bhaag Johnny movie download in hindi 720p download</h2><br /><p><b><b>Download</b> ✦✦✦ <a href="https://byltly.com/2uKxtj">https://byltly.com/2uKxtj</a></b></p><br /><br />
|
5 |
-
<p>If you are looking for Bhaag Johnny movie download in Hindi 720p, you might be disappointed to know that the movie is not available on any legal streaming platforms. The movie was released on Disney+ Hotstar, but it has been removed from the service due to some issues. Therefore, you cannot watch Bhaag Johnny online for free legally.</p>
|
6 |
-
<p>However, there are some illegal websites that claim to offer Bhaag Johnny movie download in Hindi 720p. These websites are not authorized by the makers or distributors of the movie and may contain viruses or malware that can harm your device. Moreover, downloading or streaming movies from such websites is a violation of the Indian Copyright Act and can land you in legal trouble.</p>
|
7 |
-
<p>Therefore, we advise you to stay away from such websites and watch Bhaag Johnny movie online only on Disney+ Hotstar when it becomes available again. You can subscribe to Disney+ Hotstar for a nominal fee and enjoy unlimited access to a vast library of movies, shows, sports and more. You can also watch Bhaag Johnny movie online on your smartphone, laptop, tablet or smart TV with a high-speed internet connection.</p>
|
8 |
-
<p>Bhaag Johnny is a thrilling and entertaining movie that will keep you hooked till the end. The movie has some amazing action sequences, suspenseful twists and turns, and a romantic angle that will make you root for Johnny. The movie also has some catchy songs composed by Mithoon, Yo Yo Honey Singh and Devi Sri Prasad. The movie is directed by Shivam Nair and produced by Bhushan Kumar, Krishan Kumar and Vikram Bhatt.</p>
|
9 |
-
<p>So, what are you waiting for? Watch Bhaag Johnny movie online on Disney+ Hotstar as soon as it becomes available and enjoy this thrilling ride with Johnny.</p>
|
10 |
-
|
11 |
-
<p>Bhaag Johnny movie has an interesting plot that explores the concept of parallel lives and alternate realities. The movie is inspired by the German film Run Lola Run (1998), which also had a similar theme of a woman running to save her boyfriend's life in three different scenarios. Bhaag Johnny movie adds a twist to this idea by introducing a genie who gives Johnny the choice to live two lives simultaneously and see the outcomes of his actions.</p>
|
12 |
-
<p></p>
|
13 |
-
<p>Bhaag Johnny movie has a talented cast and crew who have worked hard to make this movie a success. The movie features Kunal Khemu as Johnny, who is known for his comic timing and versatile acting skills. He has previously worked in movies like Golmaal 3, Go Goa Gone and Lootcase. Zoa Morani plays Tanya, Johnny's love interest, who is an aspiring singer. She made her debut with Always Kabhi Kabhi (2011) and also appeared in Zindagi Na Milegi Dobara (2011). Mandana Karimi plays Rachel, the girl who Johnny is supposed to kill. She is an Iranian model and actress who was seen in Roy (2015) and Kyaa Kool Hain Hum 3 (2016). She also participated in Bigg Boss 9.</p>
|
14 |
-
<p>Bhaag Johnny movie is directed by Shivam Nair, who has helmed movies like Maharathi (2008), Ahista Ahista (2006) and Naam Shabana (2017). He has also directed several TV shows like Sea Hawks, CID and Special Squad. The movie is written by Vikram Bhatt, who is a renowned filmmaker and producer of movies like Raaz, 1920, Haunted and more. He also plays the role of the genie in the movie. The movie is produced by Bhushan Kumar, Krishan Kumar and Vikram Bhatt under the banners of T-Series and BVG Films.</p> 7b8c122e87<br />
|
15 |
-
<br />
|
16 |
-
<br />
|
|
|
|
|
|
|
|
|
|
|
|
|
|
|
|
|
|
|
|
|
|
|
|
|
|
|
|
|
|
|
|
|
|
spaces/1acneusushi/gradio-2dmoleculeeditor/data/Fifa 08 Xbox.md
DELETED
@@ -1,28 +0,0 @@
|
|
1 |
-
|
2 |
-
<h1>FIFA 08 Xbox: A Review of the Classic Soccer Game</h1>
|
3 |
-
<p>If you are a fan of soccer games, you may have played or heard of FIFA 08, a football simulation video game developed by EA Canada and published by Electronic Arts under the EA Sports label. FIFA 08 was released on all popular gaming formats in September 2007 in Europe, Australia and Asia, and in October 2007 in North America. The PlayStation 3 and Xbox 360 versions of the game feature an improved game engine with superior graphics and different commentators and are dubbed "next-generation" by EA. In this article, we will focus on the Xbox 360 version of FIFA 08 and review its features, gameplay, and pros and cons.</p>
|
4 |
-
<h2>Features of FIFA 08 Xbox</h2>
|
5 |
-
<p>FIFA 08 Xbox has many features that make it a realistic and enjoyable soccer game. Some of the main features are:</p>
|
6 |
-
<h2>fifa 08 xbox</h2><br /><p><b><b>DOWNLOAD</b> ✑ <a href="https://byltly.com/2uKwtZ">https://byltly.com/2uKwtZ</a></b></p><br /><br />
|
7 |
-
<ul>
|
8 |
-
<li><strong>Be a Pro Mode</strong>: This mode allows you to play as only one player (the player can be changed) throughout the entire match. You can control your player's movements, passes, shots, tackles, and positioning. You can also customize your player's appearance, attributes, and skills.</li>
|
9 |
-
<li><strong>Cooperative Online Play</strong>: This feature enables you to play online with up to three friends on the same team against another team of human players. You can communicate with your teammates using voice chat and coordinate your strategies.</li>
|
10 |
-
<li><strong>Interactive Leagues</strong>: This feature lets you choose a league, a team, and play against real-life opponents online. You can compete in the official fixtures of the league and try to win the title. You can also track your progress and stats on the online leaderboard.</li>
|
11 |
-
<li><strong>Trick Moves</strong>: FIFA 08 Xbox includes new trick moves that can be used by using the right analog stick. You can combine tricks and skill moves together to recreate signature superstar moves or invent your own. Trick moves can help you beat defenders, create space, and score spectacular goals.</li>
|
12 |
-
<li><strong>Goalkeeper AI</strong>: FIFA 08 Xbox has improved the artificial intelligence of the goalkeeper, making him more responsive and realistic. You can also control the goalkeeper manually by pushing the right analog stick and have complete control of his movements and actions.</li>
|
13 |
-
</ul>
|
14 |
-
<h2>Gameplay of FIFA 08 Xbox</h2>
|
15 |
-
<p>FIFA 08 Xbox has a smooth and realistic gameplay that simulates the unpredictability and excitement of soccer. The game has various modes that cater to different preferences and skill levels. Some of the main modes are:</p>
|
16 |
-
<ul>
|
17 |
-
<li><strong>Kick Off</strong>: This mode allows you to play a quick match against the computer or a friend. You can choose from 620 licensed teams, 30 official leagues, and more than 15,000 players. You can also customize the match settings, such as difficulty, time, weather, stadium, etc.</li>
|
18 |
-
<li><strong>Career</strong>: This mode allows you to create your own player or manager and guide him through a 15-year career. You can start from the lower leagues or join a top club. You can also transfer players, negotiate contracts, scout talents, train your team, etc.</li>
|
19 |
-
<li><strong>Tournament</strong>: This mode allows you to create or join a tournament with up to 32 teams. You can choose from various tournament formats, such as knockout, league, group stage, etc. You can also set the rules, prizes, fixtures, etc.</li>
|
20 |
-
<li><strong>Lounge</strong>: This mode allows you to play with up to eight friends on the same console. You can create your own custom teams and players and compete in various mini-games and challenges.</li>
|
21 |
-
<li><strong>Online</strong>: This mode allows you to play online with other players around the world. You can join or create an interactive league, a cooperative team, or a ranked match. You can also chat with other players, view your stats and achievements, upload your highlights, etc.</li>
|
22 |
-
</ul>
|
23 |
-
<h2>Pros and Cons of FIFA 08 Xbox</h2>
|
24 |
-
<p>FIFA 08 Xbox is a great soccer game that offers many features and modes for different tastes and preferences. However, it also has some drawbacks that may affect your enjoyment of the game. Here are some of the pros and cons of FIFA 08 Xbox:</p>
|
25 |
-
<table border</p>
|
26 |
-
<p></p> ddb901b051<br />
|
27 |
-
<br />
|
28 |
-
<br />
|
|
|
|
|
|
|
|
|
|
|
|
|
|
|
|
|
|
|
|
|
|
|
|
|
|
|
|
|
|
|
|
|
|
|
|
|
|
|
|
|
|
|
|
|
|
|
|
|
|
|
|
|
|
|
|
|
|
spaces/1gistliPinn/ChatGPT4/Examples/Age Of Empires 3 NO CD Crack [2021].dmg Hack Torrent.md
DELETED
@@ -1,10 +0,0 @@
|
|
1 |
-
<br />
|
2 |
-
<p>the age of empires 3 crack free cheat code is super easy to use. in fact, we have built this site to be as easy to use as possible. our intuitive interface and easy to follow prompts should make this the first choice for anyone looking to make the jump from the demo to the full version of the game. you can play the game immediately after downloading.</p>
|
3 |
-
<p>this is the best program to get free age of empires 3 cd key. with this tool, you can download your age of empires 3 cd key for free. we are sure that you will enjoy playing age of empires 3 free. <strong>it's time to let our free games download engine work for you.</strong></p>
|
4 |
-
<h2>Age of Empires 3 NO CD Crack.dmg hack torrent</h2><br /><p><b><b>DOWNLOAD</b> » <a href="https://imgfil.com/2uxXUN">https://imgfil.com/2uxXUN</a></b></p><br /><br />
|
5 |
-
<p>anyone can play age of empires 3 cd key for free. if you have the cd key, all you have to do is run the program, choose your cd key and click the generate code button. once you have the cd key, you can download the game from the website and play it for free.</p>
|
6 |
-
<p>age of empires 3 key is an online crack program which allows you to download the game free of cost. with the help of this program, you can get the product key of age of empires 3 for free. the age of empires 3 key is an online crack which allows you to download the game free of cost.</p>
|
7 |
-
<p>this is a powerful tool that will allow you to download free age of empires 3 cd key without having to go through all the hassle of searching for the code online. this tool is completely safe and it will not mess up your system. it is compatible with both 32bit and 64bit versions of windows xp and windows vista.</p>
|
8 |
-
<p> <aside role=region class=portable-infobox pi-background pi-border-color pi-theme-wikia pi-layout-default > <i>age of empires iii: the warchiefs</i> <figure class=pi-item pi-image data-source=image> </figure> main series - expansion pack base game <i> age of empires iii </i> general information developers ensemble studios publishers microsoft game studios genre real-time strategy platform windows<br/>mac osx release date windows <b>north america</b>: october 17, 2006<br/><b>europe:</b> october 20, 2006 mac osx <b>north america:</b> june 13, 2007<br/> <b>europe:</b> june 25, 2007 compilations <i>age of empires iii: gold edition</i> <br/> <i>age of empires iii: complete collection</i> </aside> <i><b>age of empires iii: the warchiefs</b></i> is the first official expansion pack for the real-time strategy game <i> age of empires iii </i>. it was announced by ensemble studios and microsoft game studios on march 7, 2006. </p> 899543212b<br />
|
9 |
-
<br />
|
10 |
-
<br />
|
|
|
|
|
|
|
|
|
|
|
|
|
|
|
|
|
|
|
|
|
|
spaces/1phancelerku/anime-remove-background/Download Cars Fast as Lightning APK for Android and Race with Lightning McQueen.md
DELETED
@@ -1,135 +0,0 @@
|
|
1 |
-
<br />
|
2 |
-
<h1>How to Download Cars Fast as Lightning on Android</h1>
|
3 |
-
<p>Do you love the Cars movie franchise and want to experience the thrill of racing with your favorite characters? If so, you might want to check out Cars Fast as Lightning, a fun and exciting racing game that lets you play as 20 different characters from the movies, customize your cars and tracks, build your own Radiator Springs, and enjoy animated cutscenes and voice acting. In this article, we will show you how to download and install this game on your android device, as well as some tips and tricks for playing it.</p>
|
4 |
-
<h2>What is Cars Fast as Lightning?</h2>
|
5 |
-
<p>Cars Fast as Lightning is a racing game based on the Cars movie franchise, developed by Gameloft. The game features a variety of characters from the movies, such as Lightning McQueen, Mater, Francesco Bernoulli, Sally, Doc Hudson, and more. You can choose your favorite character and race against other characters in different locations, such as Radiator Springs, Tokyo, Porto Corsa, London, Paris, and more. You can also customize your cars and tracks with different paint jobs, stickers, accessories, jumps, loops, and props.</p>
|
6 |
-
<h2>how to download cars fast as lightning on android</h2><br /><p><b><b>Download</b> >> <a href="https://jinyurl.com/2uNPoV">https://jinyurl.com/2uNPoV</a></b></p><br /><br />
|
7 |
-
<h3>Features of the game</h3>
|
8 |
-
<p>The game has many features that make it fun and engaging for players of all ages. Some of these features are:</p>
|
9 |
-
<h4>Play as 20 different characters</h4>
|
10 |
-
<p>You can play as 20 different characters from the Cars movies, each with their own personality, voice, and style. You can also unlock new characters by winning races and cups. Some of the characters you can play as are:</p>
|
11 |
-
<ul>
|
12 |
-
<li>Lightning McQueen</li>
|
13 |
-
<li>Mater</li>
|
14 |
-
<li>Francesco Bernoulli</li>
|
15 |
-
<li>Sally</li>
|
16 |
-
<li>Doc Hudson</li>
|
17 |
-
<li>Ramone</li>
|
18 |
-
<li>Flo</li>
|
19 |
-
<li>Sheriff</li>
|
20 |
-
<li>Finn McMissile</li>
|
21 |
-
<li>Holley Shiftwell</li>
|
22 |
-
<li>And more!</li>
|
23 |
-
</ul>
|
24 |
-
<h4>Customize your cars and tracks</h4>
|
25 |
-
<p>You can customize your cars and tracks with different paint jobs, stickers, accessories, jumps, loops, and props. You can make your cars look cool and unique with different colors, patterns, decals, spoilers, wheels, exhausts, lights, horns, and more. You can also make your tracks more fun and challenging with different ramps, bridges, tunnels, cacti, dinosaurs, rockets, fireworks, and more.</p>
|
26 |
-
<h4>Build your own Radiator Springs</h4 <p>You can build your own Radiator Springs by placing different buildings, landmarks, and decorations. You can create your own version of the town from the movies, or make your own original design. You can also visit other players' towns and see how they have built their own Radiator Springs.</p>
|
27 |
-
<h4>Enjoy animated cutscenes and voice acting</h4>
|
28 |
-
<p>The game has animated cutscenes and voice acting that make it feel like you are watching a Cars movie. You can see your favorite characters interact with each other, crack jokes, and show their emotions. You can also hear their original voices from the movies, such as Owen Wilson as Lightning McQueen, Larry the Cable Guy as Mater, John Turturro as Francesco Bernoulli, and more.</p>
|
29 |
-
<h2>How to download and install the game on your android device</h2>
|
30 |
-
<p>If you are interested in playing Cars Fast as Lightning on your android device, you will need to download and install the game from the Google Play Store. Here are the requirements and steps for doing so.</p>
|
31 |
-
<h3>Requirements for the game</h3>
|
32 |
-
<p>Before you download and install the game, you will need to make sure that your device meets the following requirements:</p>
|
33 |
-
<h4>Android version 4.0 or higher</h4>
|
34 |
-
<p>The game requires Android version 4.0 or higher to run smoothly. You can check your device's Android version by going to Settings > About phone > Software information.</p>
|
35 |
-
<p>How to install cars fast as lightning on android<br />
|
36 |
-
How to get cars fast as lightning for android<br />
|
37 |
-
How to play cars fast as lightning on android<br />
|
38 |
-
How to download cars fast as lightning apk for android<br />
|
39 |
-
How to download cars fast as lightning game on android<br />
|
40 |
-
How to download and install cars fast as lightning on android<br />
|
41 |
-
How to download cars fast as lightning mod apk for android<br />
|
42 |
-
How to download cars fast as lightning hack for android<br />
|
43 |
-
How to download cars fast as lightning latest version for android<br />
|
44 |
-
How to download cars fast as lightning offline for android<br />
|
45 |
-
How to download cars fast as lightning from play store on android<br />
|
46 |
-
How to download cars fast as lightning without internet on android<br />
|
47 |
-
How to download cars fast as lightning unlimited money for android<br />
|
48 |
-
How to download cars fast as lightning cheats for android<br />
|
49 |
-
How to download cars fast as lightning free for android<br />
|
50 |
-
How to download cars fast as lightning full version for android<br />
|
51 |
-
How to download cars fast as lightning in pc for android<br />
|
52 |
-
How to download cars fast as lightning in bluestacks for android<br />
|
53 |
-
How to download cars fast as lightning in laptop for android<br />
|
54 |
-
How to download cars fast as lightning in windows 10 for android<br />
|
55 |
-
How to download cars fast as lightning disney pixar game for android<br />
|
56 |
-
How to download cars fast as lightning gameloft game for android<br />
|
57 |
-
How to download cars fast as lightning racing game for android<br />
|
58 |
-
How to download cars fast as lightning city building game for android<br />
|
59 |
-
How to download cars fast as lightning 3d game for android<br />
|
60 |
-
How to download cars fast as lightning with nitro boost for android<br />
|
61 |
-
How to download cars fast as lightning with stunts for android<br />
|
62 |
-
How to download cars fast as lightning with Owen Wilson voice for android<br />
|
63 |
-
How to download cars fast as lightning with Lightning McQueen character for android<br />
|
64 |
-
How to download cars fast as lightning with Mater character for android<br />
|
65 |
-
How to download cars fast as lightning with Francesco character for android<br />
|
66 |
-
How to download cars fast as lightning with Radiator Springs theme for android<br />
|
67 |
-
How to download cars fast as lightning with Rocky Loops theme for android<br />
|
68 |
-
How to download cars fast as lightning with Roller Coasters theme for android<br />
|
69 |
-
How to download cars fast as lightning with Luigi's Casa Della Tires theme for android<br />
|
70 |
-
How to download cars fast as lightning with Fillmore's Taste-In theme for android<br />
|
71 |
-
How to download cars fast as lightning with 20 Cars characters for android<br />
|
72 |
-
How to download cars fast as lightning with 30 town buildings for android<br />
|
73 |
-
How to download cars fast as lightning with animated cutscenes for android<br />
|
74 |
-
How to download cars fast as lightning with voice acting for android<br />
|
75 |
-
How to download cars fast as lightning with easy controls for android<br />
|
76 |
-
How to download cars fast as lightning with high-quality graphics for android<br />
|
77 |
-
How to download cars fast as lightning with fun animations for android<br />
|
78 |
-
How to download cars fast as lightning with kids-friendly gameplay for android<br />
|
79 |
-
How to download cars fast as lightning with fans-favorite gameplay for android <br />
|
80 |
-
How to download cars fast as lightning with free arcade gameplay for android <br />
|
81 |
-
How to download cars fast as lightning with customizable gameplay for android <br />
|
82 |
-
How to download cars fast as lightning with virtual currency gameplay for android <br />
|
83 |
-
How to download cars fast as lightning with in-app purchases gameplay for android</p>
|
84 |
-
<h4>At least 1 GB of free storage space</h4>
|
85 |
-
<p>The game takes up about 1 GB of storage space on your device. You will need to have at least that much free space available to download and install the game. You can check your device's storage space by going to Settings > Storage.</p>
|
86 |
-
<h4>A stable internet connection</h4>
|
87 |
-
<p>The game requires a stable internet connection to download and play. You will need to have a Wi-Fi or mobile data connection that is fast and reliable. You can check your device's internet connection by going to Settings > Network & internet.</p>
|
88 |
-
<h3>Steps to download and install the game</h3>
|
89 |
-
<p>Once you have made sure that your device meets the requirements, you can follow these steps to download and install the game:</p>
|
90 |
-
<h4>Go to the Google Play Store app on your device</h4>
|
91 |
-
<p>The Google Play Store app is where you can find and download apps and games for your android device. You can access it by tapping on its icon on your home screen or app drawer.</p>
|
92 |
-
<h4>Search for Cars Fast as Lightning or use this link</h4>
|
93 |
-
<p>You can search for Cars Fast as Lightning by typing its name in the search bar at the top of the app. Alternatively, you can use this link to go directly to the game's page on the Google Play Store.</p>
|
94 |
-
<h4>Tap on the Install button and wait for the download to finish</h4 <p>Tap on the Install button and wait for the download to finish</p>
|
95 |
-
<p>Once you have found the game on the Google Play Store, you can tap on the Install button to start the download process. You will see a progress bar that shows how much of the game has been downloaded. You will need to wait for the download to finish before you can install and play the game.</p>
|
96 |
-
<h4>Tap on the Open button and enjoy the game</h4>
|
97 |
-
<p>After the download is complete, you will see an Open button that lets you launch the game. You can tap on it to start the game and enjoy racing with your favorite Cars characters. You can also find the game's icon on your home screen or app drawer and tap on it to open the game anytime.</p>
|
98 |
-
<h2>Tips and tricks for playing the game</h2>
|
99 |
-
<p>Now that you have downloaded and installed Cars Fast as Lightning on your android device, you might want to know some tips and tricks for playing the game. Here are some of them:</p>
|
100 |
-
<h3>How to win races and earn coins</h3>
|
101 |
-
<p>Races are the main mode of gameplay in Cars Fast as Lightning. You can race against other characters in different locations and try to beat them to the finish line. You can also earn coins by winning races, which you can use to customize your cars and tracks. Here are some tips for winning races and earning coins:</p>
|
102 |
-
<h4>Tap on the screen to accelerate and release to drift</h4>
|
103 |
-
<p>The game has a simple control scheme that lets you control your car's speed and direction. You can tap on the screen to accelerate and release to drift. Drifting helps you turn corners faster and avoid obstacles. You can also use drifting to perform stunts and tricks, which we will discuss later.</p>
|
104 |
-
<h4>Collect lightning bolts and use them to boost your speed</h4 <h4>Collect lightning bolts and use them to boost your speed</h4>
|
105 |
-
<p>As you race, you will see lightning bolts on the track. These are power-ups that can help you boost your speed and gain an advantage over your opponents. You can collect them by driving over them or by performing stunts and tricks. You can use them by tapping on the lightning icon on the bottom right corner of the screen. You can also save them for later by tapping on the pause icon next to the lightning icon.</p>
|
106 |
-
<h4>Perform stunts and tricks to fill up your turbo meter</h4>
|
107 |
-
<p>Another way to boost your speed is by filling up your turbo meter. You can do this by performing stunts and tricks on the track, such as jumping, flipping, spinning, and drifting. You will see a blue bar on the top left corner of the screen that shows how much turbo you have. When it is full, you can tap on it to activate turbo mode, which makes your car go faster and glow with sparks. You can also use turbo mode to smash through obstacles and opponents.</p>
|
108 |
-
<h4>Upgrade your cars and tracks to improve your performance</h4>
|
109 |
-
<p>You can use the coins you earn from winning races to upgrade your cars and tracks. You can improve your car's speed, acceleration, handling, and nitro by buying new parts and accessories. You can also improve your track's difficulty, length, and fun factor by buying new props and decorations. Upgrading your cars and tracks can help you win more races and earn more coins.</p>
|
110 |
-
<h3>How to unlock new characters and locations</h3>
|
111 |
-
<p>The game has a lot of characters and locations to unlock and explore. You can unlock new characters by winning races and cups, and new locations by completing missions and challenges. Here are some tips for unlocking new characters and locations:</p>
|
112 |
-
<h4>Complete missions and challenges to earn stars</h4 <h4>Complete missions and challenges to earn stars</h4>
|
113 |
-
<p>Missions and challenges are tasks that you can complete to earn stars. Stars are used to unlock new cups and tournaments, which in turn unlock new characters and tracks. You can see your missions and challenges by tapping on the map icon on the bottom left corner of the screen. You can also see how many stars you have and how many you need to unlock the next cup or tournament by tapping on the trophy icon on the top right corner of the screen.</p>
|
114 |
-
<h4>Use stars to unlock new cups and tournaments</h4>
|
115 |
-
<p>Cups and tournaments are series of races that you can compete in to win prizes and unlock new characters and tracks. You can access them by tapping on the cup icon on the bottom right corner of the screen. You will see different cups and tournaments with different themes, such as Radiator Springs Cup, Tokyo Cup, World Grand Prix, and more. You will need a certain number of stars to unlock each cup or tournament. You will also need to have a specific character to enter each cup or tournament.</p>
|
116 |
-
<h4>Win races and cups to unlock new characters and tracks</h4>
|
117 |
-
<p>Winning races and cups is the main way to unlock new characters and tracks. You will see a lock icon on the characters and tracks that you have not unlocked yet. You will need to win a specific race or cup to unlock them. For example, you will need to win the Radiator Springs Cup to unlock Mater, or the Tokyo Cup to unlock Shu Todoroki. You will also see a star icon on the characters and tracks that you have unlocked but not played yet. You can tap on them to play as them or race on them.</p>
|
118 |
-
<h4>Visit other players' towns and race against them</h4>
|
119 |
-
<p>You can also unlock new characters and tracks by visiting other players' towns and racing against them. You can access this feature by tapping on the social icon on the top left corner of the screen. You will see a list of your friends who play the game, as well as random players from around the world. You can tap on their names to visit their towns and see how they have built their own Radiator Springs. You can also tap on the race icon next to their names to challenge them to a race. You can win coins, stars, and sometimes new characters and tracks by racing against other players.</p>
|
120 |
-
<h2>Conclusion and FAQs</h2>
|
121 |
-
<p>Cars Fast as Lightning is a fun and exciting racing game that lets you play as 20 different characters from the Cars movie franchise, customize your cars and tracks, build your own Radiator Springs, and enjoy animated cutscenes and voice acting. You can download and install this game on your android device by following the steps we have shown you in this article. You can also improve your skills and experience by following the tips and tricks we have shared with you. We hope you enjoy playing this game as much as we do.</p>
|
122 |
-
<p>If you have any questions or doubts about this game, you might find the answers in these FAQs:</p>
|
123 |
-
<table>
|
124 |
-
<tr><td><b>Q: How do I save my progress in the game?</b></td></tr>
|
125 |
-
<tr><td>A: The game automatically saves your progress every time you finish a race or make a change in your town. You can also manually save your progress by tapping on the settings icon on the top right corner of the screen and then tapping on the save icon.</td></tr>
|
126 |
-
<tr><td><b>Q: How do I restore my progress if I lose it or change my device?</b></td></tr>
|
127 |
-
<tr><td>A: You can restore your progress by connecting your game to your Facebook account. You can do this by tapping on the settings icon on the top right corner of the screen and then tapping on the connect icon. This will allow you to sync your progress across different devices and recover it if you lose it.</td></tr>
|
128 |
-
<tr><td><b>Q: How do I get more coins without spending real money?</b></td></tr <tr><td>A: You can get more coins by winning races, completing missions and challenges, visiting other players' towns, and watching ads. You can also get free coins by tapping on the gift icon on the top right corner of the screen and claiming your daily reward.</td></tr>
|
129 |
-
<tr><td><b>Q: How do I change the language of the game?</b></td></tr>
|
130 |
-
<tr><td>A: You can change the language of the game by tapping on the settings icon on the top right corner of the screen and then tapping on the language icon. You will see a list of available languages that you can choose from, such as English, Spanish, French, German, Italian, Portuguese, Russian, Turkish, Arabic, Chinese, Japanese, Korean, and more.</td></tr>
|
131 |
-
<tr><td><b>Q: How do I contact the support team if I have a problem with the game?</b></td></tr>
|
132 |
-
<tr><td>A: You can contact the support team by tapping on the settings icon on the top right corner of the screen and then tapping on the help icon. You will see a list of frequently asked questions and answers that might solve your problem. If you still need help, you can tap on the contact us icon and fill out a form with your name, email, device model, game version, and description of your problem. You can also attach a screenshot or a video of your problem if you have one. The support team will get back to you as soon as possible.</td></tr>
|
133 |
-
</table></p> 197e85843d<br />
|
134 |
-
<br />
|
135 |
-
<br />
|
|
|
|
|
|
|
|
|
|
|
|
|
|
|
|
|
|
|
|
|
|
|
|
|
|
|
|
|
|
|
|
|
|
|
|
|
|
|
|
|
|
|
|
|
|
|
|
|
|
|
|
|
|
|
|
|
|
|
|
|
|
|
|
|
|
|
|
|
|
|
|
|
|
|
|
|
|
|
|
|
|
|
|
|
|
|
|
|
|
|
|
|
|
|
|
|
|
|
|
|
|
|
|
|
|
|
|
|
|
|
|
|
|
|
|
|
|
|
|
|
|
|
|
|
|
|
|
|
|
|
|
|
|
|
|
|
|
|
|
|
|
|
|
|
|
|
|
|
|
|
|
|
|
|
|
|
|
|
|
|
|
|
|
|
|
|
|
|
|
|
|
|
|
|
|
|
|
|
|
|
|
|
|
|
|
|
|
|
|
|
|
|
|
|
|
|
|
|
|
|
|
|
|
|
|
|
|
|
|
|
|
|
|
|
|
|
|
|
|
|
|
|
|
|
|
|
|
|
|
|
|
|
|
|
|
|
|
|
|
|
|
|
|
|
|
|
|
|
|
|
|
|
|
|
|
|
|
|
|
|
|
|
|
|
|
|
|
|
|
|
|
spaces/1phancelerku/anime-remove-background/Download Euro Truck Simulator 2 and Customize Your Truck with Tons of Tuning Options.md
DELETED
@@ -1,133 +0,0 @@
|
|
1 |
-
<br />
|
2 |
-
<h1>How to Download Euro Truck Simulator 2</h1>
|
3 |
-
<p>Have you ever dreamed of becoming a truck driver and traveling across Europe? If so, you might want to check out Euro Truck Simulator 2, a popular simulation game that lets you do just that. Euro Truck Simulator 2 is a game that gives you the chance to become a real truck driver from the comfort of your home. Featuring licensed trucks with countless customization options and advanced driving physics, the game delivers an unparalleled driving experience which has put it in the spot of the most popular truck driving simulator on the market. In this article, we will show you how to download Euro Truck Simulator 2 and enjoy its amazing features.</p>
|
4 |
-
<h2>Why You Should Play Euro Truck Simulator 2</h2>
|
5 |
-
<p>Euro Truck Simulator 2 is not only about driving - it's also about exploring, managing, and customizing. Here are some of the reasons why you should play this game:</p>
|
6 |
-
<h2>download euro truck simulator 2</h2><br /><p><b><b>Download</b> ✅ <a href="https://jinyurl.com/2uNJNs">https://jinyurl.com/2uNJNs</a></b></p><br /><br />
|
7 |
-
<ul>
|
8 |
-
<li>You can transport a vast variety of cargo across more than 60 European cities, from London to Lisbon, from Berlin to Bucharest.</li>
|
9 |
-
<li>You can run your own business which continues to grow even as you complete your freight deliveries. You can build your own fleet of trucks, buy garages, hire drivers, and manage your company for maximum profits.</li>
|
10 |
-
<li>You can customize every truck in a countless number of ways, from performance to cosmetic changes. You can choose from different chassis configurations, cabs, colors, accessories, and more.</li>
|
11 |
-
<li>You can experience realistic driving physics and dynamic weather conditions that affect your driving. You can also adjust the difficulty level and settings to suit your preferences.</li>
|
12 |
-
<li>You can discover numerous landmarks and precisely recreated territories that make you feel as if you were driving in real life. You can also enjoy beautiful scenery and diverse landscapes, from mountains to coastlines.</li>
|
13 |
-
</ul>
|
14 |
-
<h3>The Benefits of Driving Across Europe</h3>
|
15 |
-
<p>One of the best things about Euro Truck Simulator 2 is that it allows you to explore different countries and cultures in Europe. You can learn about their history, geography, architecture, cuisine, and more. You can also admire their unique attractions such as the Eiffel Tower, the Colosseum, the Big Ben, and more. You can also experience different driving rules and regulations, such as speed limits, traffic signs, tolls, and fines. Driving across Europe is a great way to broaden your horizons and have fun at the same time.</p>
|
16 |
-
<h3>The Challenges of Running Your Own Trucking Business</h3>
|
17 |
-
<p>Another aspect of Euro Truck Simulator 2 is that it lets you run your own trucking business. You can start from scratch and work your way up to become a successful entrepreneur. You can buy new trucks, upgrade them, hire drivers, assign them routes, and monitor their performance. You can also manage your finances, loans, expenses, and income. You can compete with other companies and try to get the best contracts and reputation. Running your own trucking business is a rewarding and challenging experience that will test your skills and strategy.</p>
|
18 |
-
<h3>The Customization Options for Your Trucks</h3>
|
19 |
-
<p>One of the most enjoyable features of Euro Truck Simulator 2 is that it allows you to customize your trucks in a variety of ways. You can choose from over 40 licensed truck models from famous brands such as Volvo, Scania, MAN, DAF, Renault, and more. You can also tune your trucks to improve their performance, such as engine power, fuel efficiency, braking, suspension, and more. You can also paint your trucks with different colors and patterns, and add accessories such as lights, horns, exhausts, bumpers, and more. You can even create your own custom decals and logos to make your trucks stand out. Customizing your trucks is a fun and creative way to express yourself and show off your style.</p>
|
20 |
-
<h2>Where to Download Euro Truck Simulator 2</h2>
|
21 |
-
<p>Now that you know why you should play Euro Truck Simulator 2, you might be wondering where to download it. There are several platforms and sources where you can get the game, each with its own advantages and disadvantages. Here are some of the most popular ones:</p>
|
22 |
-
<h3>Steam</h3>
|
23 |
-
<p>Steam is one of the most popular and reliable platforms where you can download Euro Truck Simulator 2. Steam is a digital distribution service that offers a large library of games, including Euro Truck Simulator 2 and its expansions. Steam also provides automatic updates, cloud saving, achievements, multiplayer support, community features, and more. Steam is easy to use and secure, and you can access your games from any device with your Steam account.</p>
|
24 |
-
<h4>How to Install Euro Truck Simulator 2 from Steam</h4>
|
25 |
-
<p>To install Euro Truck Simulator 2 from Steam, you need to follow these steps:</p>
|
26 |
-
<p>download euro truck simulator 2 free<br />
|
27 |
-
download euro truck simulator 2 full version<br />
|
28 |
-
download euro truck simulator 2 mods<br />
|
29 |
-
download euro truck simulator 2 for pc<br />
|
30 |
-
download euro truck simulator 2 apk<br />
|
31 |
-
download euro truck simulator 2 demo<br />
|
32 |
-
download euro truck simulator 2 multiplayer<br />
|
33 |
-
download euro truck simulator 2 crack<br />
|
34 |
-
download euro truck simulator 2 steam<br />
|
35 |
-
download euro truck simulator 2 android<br />
|
36 |
-
download euro truck simulator 2 mac<br />
|
37 |
-
download euro truck simulator 2 dlc<br />
|
38 |
-
download euro truck simulator 2 highly compressed<br />
|
39 |
-
download euro truck simulator 2 latest version<br />
|
40 |
-
download euro truck simulator 2 online<br />
|
41 |
-
download euro truck simulator 2 torrent<br />
|
42 |
-
download euro truck simulator 2 update<br />
|
43 |
-
download euro truck simulator 2 windows 10<br />
|
44 |
-
download euro truck simulator 2 bus mod<br />
|
45 |
-
download euro truck simulator 2 map editor<br />
|
46 |
-
download euro truck simulator 2 save game<br />
|
47 |
-
download euro truck simulator 2 product key<br />
|
48 |
-
download euro truck simulator 2 activation code<br />
|
49 |
-
download euro truck simulator 2 going east<br />
|
50 |
-
download euro truck simulator 2 scandinavia<br />
|
51 |
-
download euro truck simulator 2 vive la france<br />
|
52 |
-
download euro truck simulator 2 italia<br />
|
53 |
-
download euro truck simulator 2 road to the black sea<br />
|
54 |
-
download euro truck simulator 2 beyond the baltic sea<br />
|
55 |
-
download euro truck simulator 2 promods<br />
|
56 |
-
download euro truck simulator 2 trainer<br />
|
57 |
-
download euro truck simulator 2 cheat engine<br />
|
58 |
-
download euro truck simulator 2 money hack<br />
|
59 |
-
download euro truck simulator 2 profile editor<br />
|
60 |
-
download euro truck simulator 2 realistic physics mod<br />
|
61 |
-
download euro truck simulator 2 graphics mod<br />
|
62 |
-
download euro truck simulator 2 sound mod<br />
|
63 |
-
download euro truck simulator 2 traffic mod<br />
|
64 |
-
download euro truck simulator 2 weather mod<br />
|
65 |
-
download euro truck simulator 2 skin pack<br />
|
66 |
-
download euro truck simulator 2 car mod<br />
|
67 |
-
download euro truck simulator 2 volvo mod<br />
|
68 |
-
download euro truck simulator 2 scania mod<br />
|
69 |
-
download euro truck simulator 2 mercedes mod<br />
|
70 |
-
download euro truck simulator 2 renault mod<br />
|
71 |
-
download euro truck simulator 2 man mod<br />
|
72 |
-
download euro truck simulator 2 daf mod<br />
|
73 |
-
download euro truck simulator 2 iveco mod<br />
|
74 |
-
download euro truck simulator 2 trailer mod</p>
|
75 |
-
<ol>
|
76 |
-
<li>Create a Steam account if you don't have one already.</li>
|
77 |
-
<li>Download and install the Steam client from the official website.</li>
|
78 |
-
<li>Launch the Steam client and log in with your account.</li>
|
79 |
-
<li>Go to the Store tab and search for Euro Truck Simulator 2.</li>
|
80 |
-
<li>Select the game and click on Add to Cart.</li>
|
81 |
-
<li>Proceed to checkout and choose your payment method.</li>
|
82 |
-
<li>After the payment is confirmed, the game will be added to your Library.</li>
|
83 |
-
<li>Go to your Library and select Euro Truck Simulator 2.</li>
|
84 |
-
<li>Click on Install and choose the destination folder for the game.</li>
|
85 |
-
<li>Wait for the download and installation to finish.</li>
|
86 |
-
<li>Click on Play and enjoy the game!</li>
|
87 |
-
</ol>
|
88 |
-
<h3>Official Website</h3>
|
89 |
-
<p>Another option where you can download Euro Truck Simulator 2 is the official website of the game. The official website offers direct downloads of the game and its expansions, as well as news, updates, support, merchandise, and more. The official website also has a blog where you can read about the development of the game and its future plans. The official website is a great source of information and resources for Euro Truck Simulator 2 fans.</p>
|
90 |
-
<h4>How to Install Euro Truck Simulator 2 from the Official Website</h4>
|
91 |
-
<p>To install Euro Truck Simulator 2 from the official website, you need to follow these steps:</p>
|
92 |
-
<ol>
|
93 |
-
<li>Go to the official website of Euro Truck Simulator 2.</li>
|
94 |
-
<li>Click on Buy Now and choose your edition of the game.</li>
|
95 |
-
<li>Select your payment method and complete the purchase.</li>
|
96 |
-
<li>You will receive an email with a link to download the game installer.</li>
|
97 |
-
<li>Download the game installer and run it on your computer.</li>
|
98 |
-
<li>Follow the instructions on the screen to install the game.</li>
|
99 |
-
<li>Activate the game with the product key that you received in your email.</li>
|
100 |
-
<li>Launch the game and enjoy!</li>
|
101 |
-
</ol>
|
102 |
-
<h3>Other , or just have fun with them. You can also join online forums and communities where you can discuss the game, share your experiences, ask for help, give feedback, and more. You can find multiplayer servers and online forums on websites such as TruckersMP, ETS2MP, SCS Software Forum, and more. Connecting with other players is a great way to make new friends and enjoy the game together.</p>
|
103 |
-
<h1>Conclusion</h1>
|
104 |
-
<p>Euro Truck Simulator 2 is a game that offers you a unique and immersive experience of driving a truck across Europe. You can explore different countries and cultures, run your own business, customize your trucks, and connect with other players. You can download the game from various platforms and sources, such as Steam, the official website, or other sources. However, you should be careful when choosing your source, as some of them may be unsafe or illegal. You can also use mods and community content to enhance your game in many ways. You can find them on websites such as Steam Workshop, ETS2 Mods, ETS2 World, and more. However, you should also backup your game files before using them, as they may not be compatible with your game version or other mods. Euro Truck Simulator 2 is a game that will keep you entertained for hours and hours. If you are looking for a realistic and fun simulation game, you should definitely give it a try.</p>
|
105 |
-
<h2>FAQs</h2>
|
106 |
-
<p>Here are some of the frequently asked questions about Euro Truck Simulator 2:</p>
|
107 |
-
<ol>
|
108 |
-
<li>Q: How much does Euro Truck Simulator 2 cost?<br>
|
109 |
-
A: The base game costs $19.99 on Steam and the official website. However, you can also buy bundles that include the game and its expansions for a discounted price. You can also wait for sales and promotions that offer the game for a lower price.</li>
|
110 |
-
<li>Q: What are the system requirements for Euro Truck Simulator 2?<br>
|
111 |
-
A: The minimum system requirements for Euro Truck Simulator 2 are: <ul>
|
112 |
-
<li>OS: Windows 7 or higher</li>
|
113 |
-
<li>Processor: Dual core CPU 2.4 GHz</li>
|
114 |
-
<li>Memory: 4 GB RAM</li>
|
115 |
-
<li>Graphics: GeForce GTS 450-class (Intel HD 4000)</li>
|
116 |
-
<li>Storage: 5 GB available space</li>
|
117 |
-
</ul>
|
118 |
-
The recommended system requirements for Euro Truck Simulator 2 are: <ul>
|
119 |
-
<li>OS: Windows 7 or higher</li>
|
120 |
-
<li>Processor: Quad core CPU 3.0 GHz</li>
|
121 |
-
<li>Memory: 6 GB RAM</li>
|
122 |
-
<li>Graphics: GeForce GTX 760-class (2 GB)</li>
|
123 |
-
<li>Storage: 5 GB available space</li>
|
124 |
-
</ul></li>
|
125 |
-
<li>Q: How many trucks are there in Euro Truck Simulator 2?<br>
|
126 |
-
A: There are over 40 licensed truck models from famous brands such as Volvo, Scania, MAN, DAF, Renault, and more. You can also download mods that add more trucks to the game.</li>
|
127 |
-
<li>Q: How many countries are there in Euro Truck Simulator 2?<br>
|
128 |
-
A: The base game includes 13 countries in Europe: Austria, Belgium, Czech Republic, France, Germany, Italy, Luxembourg, Netherlands, Poland, Slovakia, Switzerland, Hungary, and United Kingdom. You can also buy expansions that add more countries to the game, such as Scandinavia, Going East!, Vive la France!, Italia, Beyond the Baltic Sea, Road to the Black Sea, and Iberia.</li>
|
129 |
-
<li>Q: How do I update Euro Truck Simulator 2?<br>
|
130 |
-
A: If you have downloaded the game from Steam or the official website, you will receive automatic updates whenever there is a new version of the game available. However, if you have downloaded the game from other sources , you may need to manually check for updates on the source's website or download the latest version of the game. However, you should be careful when updating the game from other sources, as they may not be compatible with your game version or other mods.</li>
|
131 |
-
</ol></p> 401be4b1e0<br />
|
132 |
-
<br />
|
133 |
-
<br />
|
|
|
|
|
|
|
|
|
|
|
|
|
|
|
|
|
|
|
|
|
|
|
|
|
|
|
|
|
|
|
|
|
|
|
|
|
|
|
|
|
|
|
|
|
|
|
|
|
|
|
|
|
|
|
|
|
|
|
|
|
|
|
|
|
|
|
|
|
|
|
|
|
|
|
|
|
|
|
|
|
|
|
|
|
|
|
|
|
|
|
|
|
|
|
|
|
|
|
|
|
|
|
|
|
|
|
|
|
|
|
|
|
|
|
|
|
|
|
|
|
|
|
|
|
|
|
|
|
|
|
|
|
|
|
|
|
|
|
|
|
|
|
|
|
|
|
|
|
|
|
|
|
|
|
|
|
|
|
|
|
|
|
|
|
|
|
|
|
|
|
|
|
|
|
|
|
|
|
|
|
|
|
|
|
|
|
|
|
|
|
|
|
|
|
|
|
|
|
|
|
|
|
|
|
|
|
|
|
|
|
|
|
|
|
|
|
|
|
|
|
|
|
|
|
|
|
|
|
|
|
|
|
|
|
|
|
|
|
|
|
|
|
|
|
|
|
|
|
|
|
|
|
|
|
|
|
|
|
|
|
|
|
|
|
|
|
|
spaces/1phancelerku/anime-remove-background/Download Nebulous io Mod Apk Now and Unlock Unlimited Plasma and All Features.md
DELETED
@@ -1,82 +0,0 @@
|
|
1 |
-
<br />
|
2 |
-
<h1>Download Nebulous IO Mod APK Unlimited Plasma - A Fun and Addictive Mobile Game</h1>
|
3 |
-
<p>If you are looking for a fun and addictive mobile game that will keep you entertained for hours, then you should try Nebulous IO. Nebulous IO is a multiplayer online game where you control a blob and try to grow bigger by eating other blobs. Sounds simple, right? Well, not so fast. There are also other players who want to eat you, as well as viruses, black holes, and other obstacles that can make your life difficult. In this article, we will tell you everything you need to know about Nebulous IO, and how you can download Nebulous IO Mod APK Unlimited Plasma to enjoy the game with more features and advantages.</p>
|
4 |
-
<h2>What is Nebulous IO?</h2>
|
5 |
-
<p>Nebulous IO is a mobile game that was inspired by the popular web game Agar.io. The game was developed by Simplicial Software and released in 2015. The game has over 10 million downloads on Google Play Store and has a rating of 4.4 out of 5 stars. The game is compatible with Android 4.1 and up devices.</p>
|
6 |
-
<h2>download nebulous io mod apk unlimited plasma</h2><br /><p><b><b>Download</b> ⚹⚹⚹ <a href="https://jinyurl.com/2uNUin">https://jinyurl.com/2uNUin</a></b></p><br /><br />
|
7 |
-
<h3>Features of Nebulous IO</h3>
|
8 |
-
<p>Nebulous IO has many features that make it an enjoyable and challenging game. Some of these features are:</p>
|
9 |
-
<ul>
|
10 |
-
<li>Over 500 skins to customize your blob</li>
|
11 |
-
<li>Over 20 game modes to choose from, such as free-for-all, teams, capture the flag, soccer, and more</li>
|
12 |
-
<li>Online multiplayer with up to 27 players per server</li>
|
13 |
-
<li>Offline single-player with bots</li>
|
14 |
-
<li>Chat and voice chat with other players</li>
|
15 |
-
<li>Create your own clan and compete with others</li>
|
16 |
-
<li>Rank up and earn achievements</li>
|
17 |
-
<li>Use special items such as bombs, speed boosts, portals, and more</li>
|
18 |
-
<li>Create your own custom maps and share them with others</li>
|
19 |
-
</ul>
|
20 |
-
<h3>How to play Nebulous IO</h3>
|
21 |
-
<p>The gameplay of Nebulous IO is simple but addictive. You start as a small blob and you have to move around the map and eat smaller blobs to grow bigger. You can also split your blob into smaller pieces to move faster or to escape from bigger blobs. However, be careful not to get eaten by bigger blobs or get trapped by viruses or black holes. The goal is to become the biggest blob on the server and dominate the leaderboard.</p>
|
22 |
-
<h2>Why download Nebulous IO Mod APK Unlimited Plasma?</h2>
|
23 |
-
<p>Nebulous IO is a free game that you can download from Google Play Store or App Store. However, if you want to enjoy the game with more features and advantages, you should download Nebulous IO Mod APK Unlimited Plasma. This is a modified version of the game that gives you unlimited plasma, which is the in-game currency that you can use to buy skins, items, clan tokens, and more. With unlimited plasma, you can unlock all the skins and items that you want and customize your blob however you like. You can also create your own clan and invite your friends to join you.</p>
|
24 |
-
<h3>Benefits of Nebulous IO Mod APK Unlimited Plasma</h3>
|
25 |
-
<p>Some of the benefits of downloading Nebulous IO Mod APK Unlimited Plasma are:</p>
|
26 |
-
<ul>
|
27 |
-
<li>You can get unlimited plasma without spending real money</li>
|
28 |
-
<li>You can unlock all the skins and items that you want</li>
|
29 |
-
<li>You can create your own clan and invite your friends</li>
|
30 |
-
<li>You can access all the game modes and maps without restrictions</li>
|
31 |
-
<li>You can enjoy the game without ads or pop-ups</li>
|
32 |
-
</ul>
|
33 |
-
<h3>How to download and install Nebulous IO Mod APK Unlimited Plasma</h3>
|
34 |
-
<p>If you want to download and install Nebulous IO Mod APK Unlimited Plasma on your Android device, you need to follow these steps:</p>
|
35 |
-
<ol>
|
36 |
-
<li>Click on this link to download the mod <li>Allow your device to install apps from unknown sources by going to Settings > Security > Unknown Sources and enable it</li>
|
37 |
-
<li>Locate the downloaded file in your file manager and tap on it to install it</li>
|
38 |
-
<li>Launch the game and enjoy unlimited plasma and other features</li>
|
39 |
-
</ol>
|
40 |
-
<h2>Conclusion</h2>
|
41 |
-
<p>Nebulous IO is a fun and addictive mobile game that you can play online or offline with millions of players around the world. You can customize your blob with hundreds of skins and items, and compete in various game modes and maps. If you want to have more fun and advantages, you should download Nebulous IO Mod APK Unlimited Plasma, which gives you unlimited plasma and access to all the features of the game. Download it now and have a blast!</p>
|
42 |
-
<h3>FAQs</h3>
|
43 |
-
<p>Here are some frequently asked questions about Nebulous IO Mod APK Unlimited Plasma:</p>
|
44 |
-
<ul>
|
45 |
-
<li>Q: Is Nebulous IO Mod APK Unlimited Plasma safe to download and install?</li>
|
46 |
-
<li>A: Yes, it is safe and virus-free. However, you should always download it from a trusted source and scan it before installing it.</li>
|
47 |
-
<li>Q: Do I need to root my device to use Nebulous IO Mod APK Unlimited Plasma?</li>
|
48 |
-
<li>A: No, you do not need to root your device to use this mod. It works on both rooted and non-rooted devices.</li>
|
49 |
-
<li>Q: Will I get banned from the game if I use Nebulous IO Mod APK Unlimited Plasma?</li>
|
50 |
-
<li>A: No, you will not get banned from the game if you use this mod. However, you should not abuse it or use it to cheat or harass other players.</li>
|
51 |
-
<li>Q: Can I play online with other players who do not have Nebulous IO Mod APK Unlimited Plasma?</li>
|
52 |
-
<li>A: Yes, you can play online with other players who do not have this mod. However, they will not have the same features and advantages as you.</li>
|
53 |
-
<li>Q: Can I update Nebulous IO Mod APK Unlimited Plasma when a new version of the game is released?</li>
|
54 |
-
<li>A: Yes, you can update this mod when a new version of the game is released. However, you may need to wait for a few days until the mod is updated as well.</li>
|
55 |
-
</ul></p>
|
56 |
-
<p>How to get nebulous io mod apk with unlimited plasma and skins<br />
|
57 |
-
Nebulous io mod apk latest version free download for android<br />
|
58 |
-
Nebulous io hack mod apk unlimited plasma and coins<br />
|
59 |
-
Download nebulous io mod apk unlocked everything 2023<br />
|
60 |
-
Nebulous io mod apk online multiplayer game with unlimited plasma<br />
|
61 |
-
Nebulous io mod apk no root required for unlimited plasma<br />
|
62 |
-
Nebulous io mod apk offline mode with unlimited plasma and levels<br />
|
63 |
-
Nebulous io mod apk premium features unlocked for free<br />
|
64 |
-
Nebulous io mod apk unlimited plasma generator tool<br />
|
65 |
-
Nebulous io mod apk cheats and tips for unlimited plasma<br />
|
66 |
-
Nebulous io mod apk download link and installation guide<br />
|
67 |
-
Nebulous io mod apk review and rating by users<br />
|
68 |
-
Nebulous io mod apk gameplay and features overview<br />
|
69 |
-
Nebulous io mod apk comparison with original nebulous io game<br />
|
70 |
-
Nebulous io mod apk best settings and options for unlimited plasma<br />
|
71 |
-
Nebulous io mod apk unlimited plasma and custom skins creator<br />
|
72 |
-
Nebulous io mod apk challenges and achievements with unlimited plasma<br />
|
73 |
-
Nebulous io mod apk support and feedback from developers<br />
|
74 |
-
Nebulous io mod apk update and new features with unlimited plasma<br />
|
75 |
-
Nebulous io mod apk download for PC and Mac with unlimited plasma<br />
|
76 |
-
Nebulous io mod apk alternative apps and games with unlimited plasma<br />
|
77 |
-
Nebulous io mod apk benefits and advantages of unlimited plasma<br />
|
78 |
-
Nebulous io mod apk disadvantages and risks of unlimited plasma<br />
|
79 |
-
Nebulous io mod apk legal and ethical issues of unlimited plasma<br />
|
80 |
-
Nebulous io mod apk safety and security measures for unlimited plasma</p> 197e85843d<br />
|
81 |
-
<br />
|
82 |
-
<br />
|
|
|
|
|
|
|
|
|
|
|
|
|
|
|
|
|
|
|
|
|
|
|
|
|
|
|
|
|
|
|
|
|
|
|
|
|
|
|
|
|
|
|
|
|
|
|
|
|
|
|
|
|
|
|
|
|
|
|
|
|
|
|
|
|
|
|
|
|
|
|
|
|
|
|
|
|
|
|
|
|
|
|
|
|
|
|
|
|
|
|
|
|
|
|
|
|
|
|
|
|
|
|
|
|
|
|
|
|
|
|
|
|
|
|
|
|
|
|
|
|
|
|
|
|
|
|
|
|
|
|
|
|
|
|
|
|
|
|
|
|
|
|
|
|
|
|
|
|
|
|
|
|
|
|
|
|
|
|
|
|
|
|
|
|
|
spaces/1phancelerku/anime-remove-background/EA SPORTS FIFA 23 Companion Build Manage and Compete in FUT.md
DELETED
@@ -1,88 +0,0 @@
|
|
1 |
-
<br />
|
2 |
-
<h1>EA SPORTS™ FIFA 23 Companion Download: How to Manage Your FUT Club on the Go</h1>
|
3 |
-
<p>If you are a fan of FIFA Ultimate Team (FUT), you might want to download the official EA SPORTS™ FIFA 23 Companion App on your mobile device. This app allows you to access and manage your FUT Club from anywhere, anytime, without having to log into your console or PC. In this article, we will explain what the FIFA 23 Companion App is, why you should use it, and how to download and use it.</p>
|
4 |
-
<h2>What is the FIFA 23 Companion App?</h2>
|
5 |
-
<p>The FIFA 23 Companion App is a mobile extension of FIFA Ultimate Team, the most popular mode in FIFA 23. It lets you build your dream squad from thousands of players past and present, customize your FUT Stadium, participate in FUT Events, trade on the Transfer Market, complete Squad Building Challenges, claim rewards, and more.</p>
|
6 |
-
<h2>ea sportstm fifa 23 companion download</h2><br /><p><b><b>Download</b> »»» <a href="https://jinyurl.com/2uNLDl">https://jinyurl.com/2uNLDl</a></b></p><br /><br />
|
7 |
-
<h3>A mobile extension of FIFA Ultimate Team</h3>
|
8 |
-
<p>The FIFA 23 Companion App gives you access to all the features and functions of FIFA Ultimate Team on your mobile device. You can create and edit your squads, buy and sell players, open packs, check your progress, and much more. You can also sync your app with your console or PC version of FIFA 23, so you can switch between devices seamlessly.</p>
|
9 |
-
<h3>Compatible with Android and iOS devices</h3>
|
10 |
-
<p>The FIFA 23 Companion App is available for both Android and iOS mobile devices and tablets. You can download it for free from Google Play or the App Store. The app requires an Internet connection (network fees may apply) and a compatible device. You can check the minimum requirements on the app's page before downloading it.</p>
|
11 |
-
<h3>Requires FIFA 23 and an EA account to use</h3>
|
12 |
-
<p>To use the FIFA 23 Companion App, you need to have FIFA 23 for PlayStation 4, PlayStation 5, Xbox One, Xbox Series X|S, PC, or Stadia (sold separately) and an EA account. You also need to create a FUT Club and a FUT Security Question and Answer on your console or PC. Then, you can log in to your EA account from the app and connect to your FUT Club.</p>
|
13 |
-
<h2>Why should you use the FIFA 23 Companion App?</h2>
|
14 |
-
<p>The FIFA 23 Companion App offers many benefits for FUT enthusiasts. Here are some of the main reasons why you should use it:</p>
|
15 |
-
<h3>FUT Stadium Customisation</h3>
|
16 |
-
<p>The FIFA 23 Companion App allows you to customize every aspect of your FUT Stadium on the go. You can change your walkout music, goal celebrations, pyrotechnics, Tifos, banners, pitch patterns, seat colors, net shapes, and more. You can also flaunt your achievements and show off your style to your opponents.</p>
|
17 |
-
<h3>FUT Events</h3>
|
18 |
-
<p>The FIFA 23 Companion App lets you compete or collaborate in all new FUT Events to unlock rewards for your Club and the wider FUT Community. You can choose a side in Team Events and compete against other players in various challenges. Or you can join forces with other players in Community Events and track the collective progress towards a common goal.</p>
|
19 |
-
<p>ea sportstm fifa 23 companion app android<br />
|
20 |
-
ea sportstm fifa 23 companion app ios<br />
|
21 |
-
ea sportstm fifa 23 companion web app<br />
|
22 |
-
ea sportstm fifa 23 companion apk<br />
|
23 |
-
ea sportstm fifa 23 companion app pc<br />
|
24 |
-
ea sportstm fifa 23 companion app login<br />
|
25 |
-
ea sportstm fifa 23 companion app not working<br />
|
26 |
-
ea sportstm fifa 23 companion app release date<br />
|
27 |
-
ea sportstm fifa 23 companion app features<br />
|
28 |
-
ea sportstm fifa 23 companion app fut stadium customisation<br />
|
29 |
-
ea sportstm fifa 23 companion app events<br />
|
30 |
-
ea sportstm fifa 23 companion app rewards<br />
|
31 |
-
ea sportstm fifa 23 companion app transfer market<br />
|
32 |
-
ea sportstm fifa 23 companion app squad building challenges<br />
|
33 |
-
ea sportstm fifa 23 companion app help<br />
|
34 |
-
ea sportstm fifa 23 companion app review<br />
|
35 |
-
ea sportstm fifa 23 companion app tips<br />
|
36 |
-
ea sportstm fifa 23 companion app guide<br />
|
37 |
-
ea sportstm fifa 23 companion app tutorial<br />
|
38 |
-
ea sportstm fifa 23 companion app hack<br />
|
39 |
-
ea sportstm fifa 23 companion app mod<br />
|
40 |
-
ea sportstm fifa 23 companion app cheats<br />
|
41 |
-
ea sportstm fifa 23 companion app free coins<br />
|
42 |
-
ea sportstm fifa 23 companion app free packs<br />
|
43 |
-
ea sportstm fifa 23 companion app free download<br />
|
44 |
-
download ea sportstm fifa 23 companion for android<br />
|
45 |
-
download ea sportstm fifa 23 companion for ios<br />
|
46 |
-
download ea sportstm fifa 23 companion for pc<br />
|
47 |
-
download ea sportstm fifa 23 companion for windows<br />
|
48 |
-
download ea sportstm fifa 23 companion for mac<br />
|
49 |
-
download ea sportstm fifa 23 companion latest version<br />
|
50 |
-
download ea sportstm fifa 23 companion update<br />
|
51 |
-
download ea sportstm fifa 23 companion offline<br />
|
52 |
-
download ea sportstm fifa 23 companion online<br />
|
53 |
-
download ea sportstm fifa 23 companion without verification<br />
|
54 |
-
how to download ea sportstm fifa 23 companion app<br />
|
55 |
-
how to download ea sportstm fifa 23 companion on pc<br />
|
56 |
-
how to download ea sportstm fifa 23 companion on laptop<br />
|
57 |
-
how to download ea sportstm fifa 23 companion on macbook<br />
|
58 |
-
how to download ea sportstm fifa 23 companion on iphone<br />
|
59 |
-
how to download ea sportstm fifa 23 companion on ipad<br />
|
60 |
-
how to download ea sportstm fifa 23 companion on android phone<br />
|
61 |
-
how to download ea sportstm fifa 23 companion on android tablet<br />
|
62 |
-
how to download ea sportstm fifa 23 companion on ps4<br />
|
63 |
-
how to download ea sportstm fifa 23 companion on ps5<br />
|
64 |
-
how to download ea sportstm fifa 23 companion on xbox one<br />
|
65 |
-
how to download ea sportstm fifa 23 companion on xbox series x|s</p>
|
66 |
-
<h3>Transfer Market</h3>
|
67 |
-
<p>The FIFA 23 Companion App enables you to buy and sell players with the global FUT Community in the Transfer Market. You can search for players by name, rating, position, league, club, nationality, chemistry style, or price range. You can also bid on auctions, list your own players, and monitor your transactions. The Transfer Market is the best way to improve your squad and make some coins.</p>
|
68 |
-
<h3>Squad Building Challenges</h3>
|
69 |
-
<p>The FIFA 23 Companion App allows you to complete Squad Building Challenges (SBCs) on your mobile device. SBCs are puzzles that require you to build a squad that meets certain criteria, such as chemistry, rating, or nationality. You can exchange your squad for rewards, such as packs, coins, or special players. You can also browse and track the progress of all the available SBCs, including the ones that are exclusive to the app.</p>
|
70 |
-
<h3>Rewards and Objectives</h3>
|
71 |
-
<p>The FIFA 23 Companion App lets you claim your rewards and track your objectives on the go. You can collect your rewards from Division Rivals, FUT Champions, FUT Events, SBCs, and more. You can also view your active and completed objectives, such as Season Objectives, Milestones, Foundations, and Daily Objectives. You can earn XP, coins, packs, players, and other items by completing objectives.</p>
|
72 |
-
<h2>How to download and use the FIFA 23 Companion App?</h2>
|
73 |
-
<p>Downloading and using the FIFA 23 Companion App is easy and convenient. Here are the steps you need to follow:</p>
|
74 |
-
<h3>Download from Google Play or App Store</h3>
|
75 |
-
<p>The first step is to download the FIFA 23 Companion App from Google Play or the App Store on your mobile device. The app is free to download and use, but it may require some storage space and data usage. You can check the app's page for the minimum requirements and ratings before downloading it.</p>
|
76 |
-
<h3>Log in with your EA account</h3>
|
77 |
-
<p>The next step is to log in with your EA account on the app. If you don't have an EA account, you can create one for free on the app or on the EA website. You need to use the same EA account that you use for FIFA 23 on your console or PC. You also need to accept the User Agreement and Privacy Policy of EA.</p>
|
78 |
-
<h3>Connect to your FUT Club</h3>
|
79 |
-
<p>The final step is to connect to your FUT Club on the app. You need to have FIFA 23 for PlayStation 4, PlayStation 5, Xbox One, Xbox Series X|S, PC, or Stadia (sold separately) and a FUT Club created on your console or PC. You also need to set up a FUT Security Question and Answer on your console or PC. Then, you can select your platform and FUT Club on the app and start managing it.</p>
|
80 |
-
<h3>Enjoy the features and benefits</h3>
|
81 |
-
<p>Once you have connected to your FUT Club on the app, you can enjoy all the features and benefits that we have mentioned above. You can build your squad, customize your stadium, participate in events, trade on the market, complete challenges, claim rewards, and more. You can also sync your app with your console or PC version of FIFA 23, so you can switch between devices without losing any progress.</p>
|
82 |
-
<h2>Conclusion</h2>
|
83 |
-
<p>The FIFA 23 Companion App is a must-have for any FUT fan who wants to manage their FUT Club on the go. It offers many features and benefits that enhance your FUT experience and help you achieve your goals. You can download it for free from Google Play or the App Store and connect it to your EA account and FUT Club. Then, you can enjoy all the aspects of FIFA Ultimate Team on your mobile device.</p>
|
84 |
-
<h2>FAQs</h2>
|
85 |
-
<p>Here are some of the frequently asked questions about the FIFA 23 Companion App:</p>
|
86 |
-
- Q: Is the FIFA 23 Companion App safe to use? - A: Yes, the FIFA 23 Companion App is safe to use as long as you download it from official sources (Google Play or App Store) and log in with a secure EA account. The app uses encryption and authentication methods to protect your data and transactions. - Q: Can I use the FIFA 23 Companion App without FIFA 23? - A: No, you cannot use the FIFA 23 Companion App without FIFA 23. You need to have FIFA 23 for PlayStation 4, PlayStation 5, Xbox One, Xbox Series X|S, PC, or Stadia (sold separately) and a FUT Club created on your console or PC to use the app. You also need to log in with the same EA account that you use for FIFA 23. - Q: Can I play matches on the FIFA 23 Companion App? - A: No, you cannot play matches on the FIFA 23 Companion App. The app is designed to help you manage your FUT Club, not to play the game itself. You can only play matches on your console or PC version of FIFA 23. - Q: How can I contact EA support if I have any issues with the FIFA 23 Companion App? - A: If you have any issues with the FIFA 23 Companion App, you can contact EA support through the app itself or through the EA website. You can also check the EA Help Center for FAQs, guides, and troubleshooting tips. - Q: How can I update the FIFA 23 Companion App? - A: The FIFA 23 Companion App will automatically update itself when there is a new version available. You can also check for updates manually on Google Play or the App Store. You should always keep your app updated to enjoy the latest features and improvements.</p> 197e85843d<br />
|
87 |
-
<br />
|
88 |
-
<br />
|
|
|
|
|
|
|
|
|
|
|
|
|
|
|
|
|
|
|
|
|
|
|
|
|
|
|
|
|
|
|
|
|
|
|
|
|
|
|
|
|
|
|
|
|
|
|
|
|
|
|
|
|
|
|
|
|
|
|
|
|
|
|
|
|
|
|
|
|
|
|
|
|
|
|
|
|
|
|
|
|
|
|
|
|
|
|
|
|
|
|
|
|
|
|
|
|
|
|
|
|
|
|
|
|
|
|
|
|
|
|
|
|
|
|
|
|
|
|
|
|
|
|
|
|
|
|
|
|
|
|
|
|
|
|
|
|
|
|
|
|
|
|
|
|
|
|
|
|
|
|
|
|
|
|
|
|
|
|
|
|
|
|
|
|
|
|
|
|
|
|
|
|
|
|
|
|
|
spaces/52Hz/HWMNet_lowlight_enhancement/WT/transform.py
DELETED
@@ -1,53 +0,0 @@
|
|
1 |
-
import torch
|
2 |
-
import torch.nn as nn
|
3 |
-
|
4 |
-
def dwt_init(x):
|
5 |
-
x01 = x[:, :, 0::2, :] / 2
|
6 |
-
x02 = x[:, :, 1::2, :] / 2
|
7 |
-
x1 = x01[:, :, :, 0::2]
|
8 |
-
x2 = x02[:, :, :, 0::2]
|
9 |
-
x3 = x01[:, :, :, 1::2]
|
10 |
-
x4 = x02[:, :, :, 1::2]
|
11 |
-
x_LL = x1 + x2 + x3 + x4
|
12 |
-
x_HL = -x1 - x2 + x3 + x4
|
13 |
-
x_LH = -x1 + x2 - x3 + x4
|
14 |
-
x_HH = x1 - x2 - x3 + x4
|
15 |
-
# print(x_HH[:, 0, :, :])
|
16 |
-
return torch.cat((x_LL, x_HL, x_LH, x_HH), 1)
|
17 |
-
|
18 |
-
def iwt_init(x):
|
19 |
-
r = 2
|
20 |
-
in_batch, in_channel, in_height, in_width = x.size()
|
21 |
-
out_batch, out_channel, out_height, out_width = in_batch, int(in_channel / (r ** 2)), r * in_height, r * in_width
|
22 |
-
x1 = x[:, 0:out_channel, :, :] / 2
|
23 |
-
x2 = x[:, out_channel:out_channel * 2, :, :] / 2
|
24 |
-
x3 = x[:, out_channel * 2:out_channel * 3, :, :] / 2
|
25 |
-
x4 = x[:, out_channel * 3:out_channel * 4, :, :] / 2
|
26 |
-
h = torch.zeros([out_batch, out_channel, out_height, out_width])#.cuda() #
|
27 |
-
|
28 |
-
h[:, :, 0::2, 0::2] = x1 - x2 - x3 + x4
|
29 |
-
h[:, :, 1::2, 0::2] = x1 - x2 + x3 - x4
|
30 |
-
h[:, :, 0::2, 1::2] = x1 + x2 - x3 - x4
|
31 |
-
h[:, :, 1::2, 1::2] = x1 + x2 + x3 + x4
|
32 |
-
|
33 |
-
return h
|
34 |
-
|
35 |
-
|
36 |
-
class DWT(nn.Module):
|
37 |
-
def __init__(self):
|
38 |
-
super(DWT, self).__init__()
|
39 |
-
self.requires_grad = True
|
40 |
-
|
41 |
-
def forward(self, x):
|
42 |
-
return dwt_init(x)
|
43 |
-
|
44 |
-
|
45 |
-
class IWT(nn.Module):
|
46 |
-
def __init__(self):
|
47 |
-
super(IWT, self).__init__()
|
48 |
-
self.requires_grad = True
|
49 |
-
|
50 |
-
def forward(self, x):
|
51 |
-
return iwt_init(x)
|
52 |
-
|
53 |
-
|
|
|
|
|
|
|
|
|
|
|
|
|
|
|
|
|
|
|
|
|
|
|
|
|
|
|
|
|
|
|
|
|
|
|
|
|
|
|
|
|
|
|
|
|
|
|
|
|
|
|
|
|
|
|
|
|
|
|
|
|
|
|
|
|
|
|
|
|
|
|
|
|
|
|
|
|
|
|
|
|
|
|
|
|
|
|
|
|
|
|
|
|
|
|
|
|
|
|
|
|
|
|
|
|
|
|
|
spaces/7hao/bingo/src/components/chat.tsx
DELETED
@@ -1,93 +0,0 @@
|
|
1 |
-
'use client'
|
2 |
-
|
3 |
-
import { useCallback, useEffect, useMemo, useState } from 'react'
|
4 |
-
import { useAtom } from 'jotai'
|
5 |
-
import Image from 'next/image'
|
6 |
-
import { cn } from '@/lib/utils'
|
7 |
-
import { ChatList } from '@/components/chat-list'
|
8 |
-
import { ChatPanel } from '@/components/chat-panel'
|
9 |
-
import { WelcomeScreen } from '@/components/welcome-screen'
|
10 |
-
import { ChatScrollAnchor } from '@/components/chat-scroll-anchor'
|
11 |
-
import { ToneSelector } from './tone-selector'
|
12 |
-
import { ChatHeader } from './chat-header'
|
13 |
-
import { ChatSuggestions } from './chat-suggestions'
|
14 |
-
import { bingConversationStyleAtom } from '@/state'
|
15 |
-
import { ButtonScrollToBottom } from '@/components/button-scroll-to-bottom'
|
16 |
-
import StopIcon from '@/assets/images/stop.svg'
|
17 |
-
import { useBing } from '@/lib/hooks/use-bing'
|
18 |
-
import { ChatMessageModel } from '@/lib/bots/bing/types'
|
19 |
-
import { ChatNotification } from './chat-notification'
|
20 |
-
import { Settings } from './settings'
|
21 |
-
import { ChatHistory } from './chat-history'
|
22 |
-
|
23 |
-
export type ChatProps = React.ComponentProps<'div'> & { initialMessages?: ChatMessageModel[] }
|
24 |
-
|
25 |
-
export default function Chat({ className }: ChatProps) {
|
26 |
-
|
27 |
-
const [bingStyle, setBingStyle] = useAtom(bingConversationStyleAtom)
|
28 |
-
const {
|
29 |
-
messages,
|
30 |
-
sendMessage,
|
31 |
-
resetConversation,
|
32 |
-
stopGenerating,
|
33 |
-
setInput,
|
34 |
-
bot,
|
35 |
-
input,
|
36 |
-
generating,
|
37 |
-
isSpeaking,
|
38 |
-
uploadImage,
|
39 |
-
attachmentList,
|
40 |
-
setAttachmentList,
|
41 |
-
} = useBing()
|
42 |
-
|
43 |
-
useEffect(() => {
|
44 |
-
window.scrollTo({
|
45 |
-
top: document.body.offsetHeight,
|
46 |
-
behavior: 'smooth'
|
47 |
-
})
|
48 |
-
}, [])
|
49 |
-
|
50 |
-
return (
|
51 |
-
<div className="flex flex-1 flex-col">
|
52 |
-
<Settings />
|
53 |
-
<div className={cn('flex-1 pb-16', className)}>
|
54 |
-
<ChatHeader />
|
55 |
-
<WelcomeScreen setInput={setInput} />
|
56 |
-
<ToneSelector type={bingStyle} onChange={setBingStyle} />
|
57 |
-
{messages.length ? (
|
58 |
-
<>
|
59 |
-
<ChatList messages={messages} />
|
60 |
-
<ChatScrollAnchor trackVisibility={generating} />
|
61 |
-
<ChatNotification message={messages.at(-1)} bot={bot} />
|
62 |
-
<ChatSuggestions setInput={setInput} suggestions={messages.at(-1)?.suggestedResponses} />
|
63 |
-
|
64 |
-
{generating ? (
|
65 |
-
<div className="flex h-10 items-center justify-center my-4">
|
66 |
-
<button
|
67 |
-
onClick={stopGenerating}
|
68 |
-
className="typing-control-item stop"
|
69 |
-
>
|
70 |
-
<Image alt="stop" src={StopIcon} width={24} className="mr-1" />
|
71 |
-
<span>停止响应</span>
|
72 |
-
</button>
|
73 |
-
</div>
|
74 |
-
) : null}
|
75 |
-
</>
|
76 |
-
) : null}
|
77 |
-
</div>
|
78 |
-
<ChatPanel
|
79 |
-
className="pt-24 z-10"
|
80 |
-
isSpeaking={isSpeaking}
|
81 |
-
generating={generating}
|
82 |
-
sendMessage={sendMessage}
|
83 |
-
input={input}
|
84 |
-
setInput={setInput}
|
85 |
-
resetConversation={resetConversation}
|
86 |
-
uploadImage={uploadImage}
|
87 |
-
attachmentList={attachmentList}
|
88 |
-
setAttachmentList={setAttachmentList}
|
89 |
-
/>
|
90 |
-
<ButtonScrollToBottom />
|
91 |
-
</div>
|
92 |
-
)
|
93 |
-
}
|
|
|
|
|
|
|
|
|
|
|
|
|
|
|
|
|
|
|
|
|
|
|
|
|
|
|
|
|
|
|
|
|
|
|
|
|
|
|
|
|
|
|
|
|
|
|
|
|
|
|
|
|
|
|
|
|
|
|
|
|
|
|
|
|
|
|
|
|
|
|
|
|
|
|
|
|
|
|
|
|
|
|
|
|
|
|
|
|
|
|
|
|
|
|
|
|
|
|
|
|
|
|
|
|
|
|
|
|
|
|
|
|
|
|
|
|
|
|
|
|
|
|
|
|
|
|
|
|
|
|
|
|
|
|
|
|
|
|
|
|
|
|
|
|
|
|
|
|
|
|
|
|
|
|
|
|
|
|
|
|
|
|
|
|
|
|
|
|
|
|
|
|
|
|
|
|
|
|
|
|
|
|
|
|
|
|
|
spaces/7hao/bingo/src/components/ui/dialog.tsx
DELETED
@@ -1,128 +0,0 @@
|
|
1 |
-
'use client'
|
2 |
-
|
3 |
-
import * as React from 'react'
|
4 |
-
import * as DialogPrimitive from '@radix-ui/react-dialog'
|
5 |
-
|
6 |
-
import { cn } from '@/lib/utils'
|
7 |
-
import { IconClose } from '@/components/ui/icons'
|
8 |
-
|
9 |
-
const Dialog = DialogPrimitive.Root
|
10 |
-
|
11 |
-
const DialogTrigger = DialogPrimitive.Trigger
|
12 |
-
|
13 |
-
const DialogPortal = ({
|
14 |
-
className,
|
15 |
-
children,
|
16 |
-
...props
|
17 |
-
}: DialogPrimitive.DialogPortalProps) => (
|
18 |
-
<DialogPrimitive.Portal className={cn(className)} {...props}>
|
19 |
-
<div className="fixed inset-0 z-50 flex items-start justify-center sm:items-center">
|
20 |
-
{children}
|
21 |
-
</div>
|
22 |
-
</DialogPrimitive.Portal>
|
23 |
-
)
|
24 |
-
DialogPortal.displayName = DialogPrimitive.Portal.displayName
|
25 |
-
|
26 |
-
const DialogOverlay = React.forwardRef<
|
27 |
-
React.ElementRef<typeof DialogPrimitive.Overlay>,
|
28 |
-
React.ComponentPropsWithoutRef<typeof DialogPrimitive.Overlay>
|
29 |
-
>(({ className, ...props }, ref) => (
|
30 |
-
<DialogPrimitive.Overlay
|
31 |
-
ref={ref}
|
32 |
-
className={cn(
|
33 |
-
'bg-white fixed inset-0 z-50 bg-background/80 backdrop-blur-sm transition-all duration-100 data-[state=closed]:animate-out data-[state=closed]:fade-out data-[state=open]:fade-in',
|
34 |
-
className
|
35 |
-
)}
|
36 |
-
{...props}
|
37 |
-
/>
|
38 |
-
))
|
39 |
-
DialogOverlay.displayName = DialogPrimitive.Overlay.displayName
|
40 |
-
|
41 |
-
const DialogContent = React.forwardRef<
|
42 |
-
React.ElementRef<typeof DialogPrimitive.Content>,
|
43 |
-
React.ComponentPropsWithoutRef<typeof DialogPrimitive.Content>
|
44 |
-
>(({ className, children, ...props }, ref) => (
|
45 |
-
<DialogPortal>
|
46 |
-
<DialogOverlay />
|
47 |
-
<DialogPrimitive.Content
|
48 |
-
ref={ref}
|
49 |
-
className={cn(
|
50 |
-
'fixed z-50 grid w-full gap-4 rounded-b-lg border bg-background p-6 shadow-sm animate-in data-[state=open]:fade-in-90 data-[state=open]:slide-in-from-bottom-10 sm:max-w-lg sm:rounded-lg sm:zoom-in-90 data-[state=open]:sm:slide-in-from-bottom-0',
|
51 |
-
className
|
52 |
-
)}
|
53 |
-
{...props}
|
54 |
-
>
|
55 |
-
{children}
|
56 |
-
<DialogPrimitive.Close className="absolute right-4 top-4 rounded-sm opacity-70 ring-offset-background transition-opacity hover:opacity-100 focus:outline-none focus:ring-2 focus:ring-ring focus:ring-offset-2 disabled:pointer-events-none data-[state=open]:bg-accent data-[state=open]:text-muted-foreground">
|
57 |
-
<IconClose />
|
58 |
-
<span className="sr-only">Close</span>
|
59 |
-
</DialogPrimitive.Close>
|
60 |
-
</DialogPrimitive.Content>
|
61 |
-
</DialogPortal>
|
62 |
-
))
|
63 |
-
DialogContent.displayName = DialogPrimitive.Content.displayName
|
64 |
-
|
65 |
-
const DialogHeader = ({
|
66 |
-
className,
|
67 |
-
...props
|
68 |
-
}: React.HTMLAttributes<HTMLDivElement>) => (
|
69 |
-
<div
|
70 |
-
className={cn(
|
71 |
-
'flex flex-col space-y-1.5 text-center sm:text-left',
|
72 |
-
className
|
73 |
-
)}
|
74 |
-
{...props}
|
75 |
-
/>
|
76 |
-
)
|
77 |
-
DialogHeader.displayName = 'DialogHeader'
|
78 |
-
|
79 |
-
const DialogFooter = ({
|
80 |
-
className,
|
81 |
-
...props
|
82 |
-
}: React.HTMLAttributes<HTMLDivElement>) => (
|
83 |
-
<div
|
84 |
-
className={cn(
|
85 |
-
'flex flex-col-reverse sm:flex-row sm:justify-end sm:space-x-2',
|
86 |
-
className
|
87 |
-
)}
|
88 |
-
{...props}
|
89 |
-
/>
|
90 |
-
)
|
91 |
-
DialogFooter.displayName = 'DialogFooter'
|
92 |
-
|
93 |
-
const DialogTitle = React.forwardRef<
|
94 |
-
React.ElementRef<typeof DialogPrimitive.Title>,
|
95 |
-
React.ComponentPropsWithoutRef<typeof DialogPrimitive.Title>
|
96 |
-
>(({ className, ...props }, ref) => (
|
97 |
-
<DialogPrimitive.Title
|
98 |
-
ref={ref}
|
99 |
-
className={cn(
|
100 |
-
'text-lg font-semibold leading-none tracking-tight',
|
101 |
-
className
|
102 |
-
)}
|
103 |
-
{...props}
|
104 |
-
/>
|
105 |
-
))
|
106 |
-
DialogTitle.displayName = DialogPrimitive.Title.displayName
|
107 |
-
|
108 |
-
const DialogDescription = React.forwardRef<
|
109 |
-
React.ElementRef<typeof DialogPrimitive.Description>,
|
110 |
-
React.ComponentPropsWithoutRef<typeof DialogPrimitive.Description>
|
111 |
-
>(({ className, ...props }, ref) => (
|
112 |
-
<DialogPrimitive.Description
|
113 |
-
ref={ref}
|
114 |
-
className={cn('text-sm text-muted-foreground', className)}
|
115 |
-
{...props}
|
116 |
-
/>
|
117 |
-
))
|
118 |
-
DialogDescription.displayName = DialogPrimitive.Description.displayName
|
119 |
-
|
120 |
-
export {
|
121 |
-
Dialog,
|
122 |
-
DialogTrigger,
|
123 |
-
DialogContent,
|
124 |
-
DialogHeader,
|
125 |
-
DialogFooter,
|
126 |
-
DialogTitle,
|
127 |
-
DialogDescription
|
128 |
-
}
|
|
|
|
|
|
|
|
|
|
|
|
|
|
|
|
|
|
|
|
|
|
|
|
|
|
|
|
|
|
|
|
|
|
|
|
|
|
|
|
|
|
|
|
|
|
|
|
|
|
|
|
|
|
|
|
|
|
|
|
|
|
|
|
|
|
|
|
|
|
|
|
|
|
|
|
|
|
|
|
|
|
|
|
|
|
|
|
|
|
|
|
|
|
|
|
|
|
|
|
|
|
|
|
|
|
|
|
|
|
|
|
|
|
|
|
|
|
|
|
|
|
|
|
|
|
|
|
|
|
|
|
|
|
|
|
|
|
|
|
|
|
|
|
|
|
|
|
|
|
|
|
|
|
|
|
|
|
|
|
|
|
|
|
|
|
|
|
|
|
|
|
|
|
|
|
|
|
|
|
|
|
|
|
|
|
|
|
|
|
|
|
|
|
|
|
|
|
|
|
|
|
|
|
|
|
|
|
|
|
|
|
|
|
|
|
|
|
|
|
|
|
|
|
|
|
|
|
|
|
|
|
|
|
|
|
|
|
|
|
|
|
|
|
|
|
|
|
|
|
|
|
|
|
|
|
|
|
spaces/ADUPA/README/README.md
DELETED
@@ -1,10 +0,0 @@
|
|
1 |
-
---
|
2 |
-
title: README
|
3 |
-
emoji: 🔥
|
4 |
-
colorFrom: green
|
5 |
-
colorTo: green
|
6 |
-
sdk: static
|
7 |
-
pinned: false
|
8 |
-
---
|
9 |
-
|
10 |
-
Edit this `README.md` markdown file to author your organization card 🔥
|
|
|
|
|
|
|
|
|
|
|
|
|
|
|
|
|
|
|
|
|
|
spaces/AIGC-Audio/AudioGPT/text_to_audio/Make_An_Audio/ldm/modules/diffusionmodules/__init__.py
DELETED
File without changes
|
spaces/AIGC-Audio/AudioGPT/text_to_speech/data_gen/tts/txt_processors/zh_aishell_no_tone_sing.py
DELETED
@@ -1,126 +0,0 @@
|
|
1 |
-
import re
|
2 |
-
import jieba
|
3 |
-
from pypinyin import pinyin, Style
|
4 |
-
from text_to_speech.utils.text.text_norm import NSWNormalizer
|
5 |
-
from text_to_speech.data_gen.tts.txt_processors.base_text_processor import BaseTxtProcessor, register_txt_processors
|
6 |
-
from text_to_speech.utils.text.text_encoder import PUNCS, is_sil_phoneme
|
7 |
-
|
8 |
-
ALL_SHENMU = ['zh', 'ch', 'sh', 'b', 'p', 'm', 'f', 'd', 't', 'n', 'l', 'g', 'k', 'h', 'j',
|
9 |
-
'q', 'x', 'r', 'z', 'c', 's', 'y', 'w']
|
10 |
-
|
11 |
-
|
12 |
-
@register_txt_processors('zh')
|
13 |
-
class TxtProcessor(BaseTxtProcessor):
|
14 |
-
table = {ord(f): ord(t) for f, t in zip(
|
15 |
-
u':,。!?【】()%#@&1234567890',
|
16 |
-
u':,.!?[]()%#@&1234567890')}
|
17 |
-
|
18 |
-
@staticmethod
|
19 |
-
def sp_phonemes():
|
20 |
-
return ['|', '#']
|
21 |
-
|
22 |
-
@staticmethod
|
23 |
-
def preprocess_text(text):
|
24 |
-
text = text.translate(TxtProcessor.table)
|
25 |
-
text = NSWNormalizer(text).normalize(remove_punc=False).lower()
|
26 |
-
text = re.sub("[\'\"()]+", "", text)
|
27 |
-
text = re.sub("[-]+", " ", text)
|
28 |
-
text = re.sub(f"[^ A-Za-z\u4e00-\u9fff{PUNCS}]", "", text)
|
29 |
-
text = re.sub(f"([{PUNCS}])+", r"\1", text) # !! -> !
|
30 |
-
text = re.sub(f"([{PUNCS}])", r" \1 ", text)
|
31 |
-
text = re.sub(rf"\s+", r"", text)
|
32 |
-
text = re.sub(rf"[A-Za-z]+", r"$", text)
|
33 |
-
return text
|
34 |
-
|
35 |
-
@classmethod
|
36 |
-
def pinyin_with_en(cls, txt, style):
|
37 |
-
x = pinyin(txt, style)
|
38 |
-
x = [t[0] for t in x]
|
39 |
-
x_ = []
|
40 |
-
for t in x:
|
41 |
-
if '$' not in t:
|
42 |
-
x_.append(t)
|
43 |
-
else:
|
44 |
-
x_ += list(t)
|
45 |
-
x_ = [t if t != '$' else 'ENG' for t in x_]
|
46 |
-
return x_
|
47 |
-
|
48 |
-
@classmethod
|
49 |
-
def process(cls, txt, pre_align_args):
|
50 |
-
txt = cls.preprocess_text(txt)
|
51 |
-
txt = txt.replace("嗯", "蒽") # pypin会把嗯的声母韵母识别为'',导致ph2word出现错位。
|
52 |
-
# https://blog.csdn.net/zhoulei124/article/details/89055403
|
53 |
-
|
54 |
-
pre_align_args['use_tone'] = False
|
55 |
-
|
56 |
-
shengmu = cls.pinyin_with_en(txt, style=Style.INITIALS)
|
57 |
-
yunmu = cls.pinyin_with_en(txt, style=
|
58 |
-
Style.FINALS_TONE3 if pre_align_args['use_tone'] else Style.FINALS)
|
59 |
-
assert len(shengmu) == len(yunmu)
|
60 |
-
for i in range(len(shengmu)):
|
61 |
-
if shengmu[i] == '' and yunmu[i] == '':
|
62 |
-
print(f"发现了一个声母韵母都是空的文字:{txt[i]}")
|
63 |
-
ph_list = []
|
64 |
-
for a, b in zip(shengmu, yunmu):
|
65 |
-
|
66 |
-
if b == 'ueng': # 发现sing数据集里没有后鼻音
|
67 |
-
b = 'uen'
|
68 |
-
|
69 |
-
if a == b:
|
70 |
-
ph_list += [a]
|
71 |
-
else:
|
72 |
-
ph_list += [a + "%" + b]
|
73 |
-
seg_list = '#'.join(jieba.cut(txt))
|
74 |
-
assert len(ph_list) == len([s for s in seg_list if s != '#']), (ph_list, seg_list)
|
75 |
-
|
76 |
-
# 加入词边界'#'
|
77 |
-
ph_list_ = []
|
78 |
-
seg_idx = 0
|
79 |
-
for p in ph_list:
|
80 |
-
if seg_list[seg_idx] == '#':
|
81 |
-
ph_list_.append('#')
|
82 |
-
seg_idx += 1
|
83 |
-
elif len(ph_list_) > 0:
|
84 |
-
ph_list_.append("|")
|
85 |
-
seg_idx += 1
|
86 |
-
finished = False
|
87 |
-
if not finished:
|
88 |
-
ph_list_ += [x for x in p.split("%") if x != '']
|
89 |
-
|
90 |
-
ph_list = ph_list_
|
91 |
-
|
92 |
-
# 去除静音符号周围的词边界标记 [..., '#', ',', '#', ...]
|
93 |
-
sil_phonemes = list(PUNCS) + TxtProcessor.sp_phonemes()
|
94 |
-
ph_list_ = []
|
95 |
-
for i in range(0, len(ph_list), 1):
|
96 |
-
if ph_list[i] != '#' or (ph_list[i - 1] not in sil_phonemes and ph_list[i + 1] not in sil_phonemes):
|
97 |
-
ph_list_.append(ph_list[i])
|
98 |
-
ph_list = ph_list_
|
99 |
-
|
100 |
-
txt_struct = [[w, []] for w in txt]
|
101 |
-
i = 0
|
102 |
-
for ph in ph_list:
|
103 |
-
if ph == '|' or ph == '#':
|
104 |
-
i += 1
|
105 |
-
continue
|
106 |
-
# elif ph in [',', '.']:
|
107 |
-
elif ph in [',', '.', '?', '!', ':']:
|
108 |
-
i += 1
|
109 |
-
txt_struct[i][1].append(ph)
|
110 |
-
i += 1
|
111 |
-
continue
|
112 |
-
txt_struct[i][1].append(ph)
|
113 |
-
# return ph_list, txt
|
114 |
-
txt_struct.insert(0, ['_NONE', ['_NONE']])
|
115 |
-
txt_struct.append(['breathe', ['breathe']])
|
116 |
-
|
117 |
-
# txt_struct.insert(0, ['<BOS>', ['<BOS>']])
|
118 |
-
# txt_struct.append(['<EOS>', ['<EOS>']])
|
119 |
-
return txt_struct, txt
|
120 |
-
|
121 |
-
|
122 |
-
if __name__ == '__main__':
|
123 |
-
# t = 'simon演唱过后,simon还进行了simon精彩的文艺演出simon.'
|
124 |
-
t = '你当我傻啊?脑子那么大怎么塞进去???'
|
125 |
-
phs, txt = TxtProcessor.process(t, {'use_tone': True})
|
126 |
-
print(phs, txt)
|
|
|
|
|
|
|
|
|
|
|
|
|
|
|
|
|
|
|
|
|
|
|
|
|
|
|
|
|
|
|
|
|
|
|
|
|
|
|
|
|
|
|
|
|
|
|
|
|
|
|
|
|
|
|
|
|
|
|
|
|
|
|
|
|
|
|
|
|
|
|
|
|
|
|
|
|
|
|
|
|
|
|
|
|
|
|
|
|
|
|
|
|
|
|
|
|
|
|
|
|
|
|
|
|
|
|
|
|
|
|
|
|
|
|
|
|
|
|
|
|
|
|
|
|
|
|
|
|
|
|
|
|
|
|
|
|
|
|
|
|
|
|
|
|
|
|
|
|
|
|
|
|
|
|
|
|
|
|
|
|
|
|
|
|
|
|
|
|
|
|
|
|
|
|
|
|
|
|
|
|
|
|
|
|
|
|
|
|
|
|
|
|
|
|
|
|
|
|
|
|
|
|
|
|
|
|
|
|
|
|
|
|
|
|
|
|
|
|
|
|
|
|
|
|
|
|
|
|
|
|
|
|
|
|
|
|
|
|
|
|
|
|
|
|
|
|
|
|
|
|
|
|
|
spaces/AIGC-Audio/AudioGPT/text_to_speech/modules/vocoder/parallel_wavegan/layers/tf_layers.py
DELETED
@@ -1,129 +0,0 @@
|
|
1 |
-
# -*- coding: utf-8 -*-
|
2 |
-
|
3 |
-
# Copyright 2020 MINH ANH (@dathudeptrai)
|
4 |
-
# MIT License (https://opensource.org/licenses/MIT)
|
5 |
-
|
6 |
-
"""Tensorflow Layer modules complatible with pytorch."""
|
7 |
-
|
8 |
-
import tensorflow as tf
|
9 |
-
|
10 |
-
|
11 |
-
class TFReflectionPad1d(tf.keras.layers.Layer):
|
12 |
-
"""Tensorflow ReflectionPad1d module."""
|
13 |
-
|
14 |
-
def __init__(self, padding_size):
|
15 |
-
"""Initialize TFReflectionPad1d module.
|
16 |
-
|
17 |
-
Args:
|
18 |
-
padding_size (int): Padding size.
|
19 |
-
|
20 |
-
"""
|
21 |
-
super(TFReflectionPad1d, self).__init__()
|
22 |
-
self.padding_size = padding_size
|
23 |
-
|
24 |
-
@tf.function
|
25 |
-
def call(self, x):
|
26 |
-
"""Calculate forward propagation.
|
27 |
-
|
28 |
-
Args:
|
29 |
-
x (Tensor): Input tensor (B, T, 1, C).
|
30 |
-
|
31 |
-
Returns:
|
32 |
-
Tensor: Padded tensor (B, T + 2 * padding_size, 1, C).
|
33 |
-
|
34 |
-
"""
|
35 |
-
return tf.pad(x, [[0, 0], [self.padding_size, self.padding_size], [0, 0], [0, 0]], "REFLECT")
|
36 |
-
|
37 |
-
|
38 |
-
class TFConvTranspose1d(tf.keras.layers.Layer):
|
39 |
-
"""Tensorflow ConvTranspose1d module."""
|
40 |
-
|
41 |
-
def __init__(self, channels, kernel_size, stride, padding):
|
42 |
-
"""Initialize TFConvTranspose1d( module.
|
43 |
-
|
44 |
-
Args:
|
45 |
-
channels (int): Number of channels.
|
46 |
-
kernel_size (int): kernel size.
|
47 |
-
strides (int): Stride width.
|
48 |
-
padding (str): Padding type ("same" or "valid").
|
49 |
-
|
50 |
-
"""
|
51 |
-
super(TFConvTranspose1d, self).__init__()
|
52 |
-
self.conv1d_transpose = tf.keras.layers.Conv2DTranspose(
|
53 |
-
filters=channels,
|
54 |
-
kernel_size=(kernel_size, 1),
|
55 |
-
strides=(stride, 1),
|
56 |
-
padding=padding,
|
57 |
-
)
|
58 |
-
|
59 |
-
@tf.function
|
60 |
-
def call(self, x):
|
61 |
-
"""Calculate forward propagation.
|
62 |
-
|
63 |
-
Args:
|
64 |
-
x (Tensor): Input tensor (B, T, 1, C).
|
65 |
-
|
66 |
-
Returns:
|
67 |
-
Tensors: Output tensor (B, T', 1, C').
|
68 |
-
|
69 |
-
"""
|
70 |
-
x = self.conv1d_transpose(x)
|
71 |
-
return x
|
72 |
-
|
73 |
-
|
74 |
-
class TFResidualStack(tf.keras.layers.Layer):
|
75 |
-
"""Tensorflow ResidualStack module."""
|
76 |
-
|
77 |
-
def __init__(self,
|
78 |
-
kernel_size,
|
79 |
-
channels,
|
80 |
-
dilation,
|
81 |
-
bias,
|
82 |
-
nonlinear_activation,
|
83 |
-
nonlinear_activation_params,
|
84 |
-
padding,
|
85 |
-
):
|
86 |
-
"""Initialize TFResidualStack module.
|
87 |
-
|
88 |
-
Args:
|
89 |
-
kernel_size (int): Kernel size.
|
90 |
-
channles (int): Number of channels.
|
91 |
-
dilation (int): Dilation ine.
|
92 |
-
bias (bool): Whether to add bias parameter in convolution layers.
|
93 |
-
nonlinear_activation (str): Activation function module name.
|
94 |
-
nonlinear_activation_params (dict): Hyperparameters for activation function.
|
95 |
-
padding (str): Padding type ("same" or "valid").
|
96 |
-
|
97 |
-
"""
|
98 |
-
super(TFResidualStack, self).__init__()
|
99 |
-
self.block = [
|
100 |
-
getattr(tf.keras.layers, nonlinear_activation)(**nonlinear_activation_params),
|
101 |
-
TFReflectionPad1d(dilation),
|
102 |
-
tf.keras.layers.Conv2D(
|
103 |
-
filters=channels,
|
104 |
-
kernel_size=(kernel_size, 1),
|
105 |
-
dilation_rate=(dilation, 1),
|
106 |
-
use_bias=bias,
|
107 |
-
padding="valid",
|
108 |
-
),
|
109 |
-
getattr(tf.keras.layers, nonlinear_activation)(**nonlinear_activation_params),
|
110 |
-
tf.keras.layers.Conv2D(filters=channels, kernel_size=1, use_bias=bias)
|
111 |
-
]
|
112 |
-
self.shortcut = tf.keras.layers.Conv2D(filters=channels, kernel_size=1, use_bias=bias)
|
113 |
-
|
114 |
-
@tf.function
|
115 |
-
def call(self, x):
|
116 |
-
"""Calculate forward propagation.
|
117 |
-
|
118 |
-
Args:
|
119 |
-
x (Tensor): Input tensor (B, T, 1, C).
|
120 |
-
|
121 |
-
Returns:
|
122 |
-
Tensor: Output tensor (B, T, 1, C).
|
123 |
-
|
124 |
-
"""
|
125 |
-
_x = tf.identity(x)
|
126 |
-
for i, layer in enumerate(self.block):
|
127 |
-
_x = layer(_x)
|
128 |
-
shortcut = self.shortcut(x)
|
129 |
-
return shortcut + _x
|
|
|
|
|
|
|
|
|
|
|
|
|
|
|
|
|
|
|
|
|
|
|
|
|
|
|
|
|
|
|
|
|
|
|
|
|
|
|
|
|
|
|
|
|
|
|
|
|
|
|
|
|
|
|
|
|
|
|
|
|
|
|
|
|
|
|
|
|
|
|
|
|
|
|
|
|
|
|
|
|
|
|
|
|
|
|
|
|
|
|
|
|
|
|
|
|
|
|
|
|
|
|
|
|
|
|
|
|
|
|
|
|
|
|
|
|
|
|
|
|
|
|
|
|
|
|
|
|
|
|
|
|
|
|
|
|
|
|
|
|
|
|
|
|
|
|
|
|
|
|
|
|
|
|
|
|
|
|
|
|
|
|
|
|
|
|
|
|
|
|
|
|
|
|
|
|
|
|
|
|
|
|
|
|
|
|
|
|
|
|
|
|
|
|
|
|
|
|
|
|
|
|
|
|
|
|
|
|
|
|
|
|
|
|
|
|
|
|
|
|
|
|
|
|
|
|
|
|
|
|
|
|
|
|
|
|
|
|
|
|
|
|
|
|
|
|
|
|
|
|
|
|
|
|
|
|
|
|
|
spaces/AIGC-Audio/Make_An_Audio_inpaint/ldm/data/extract_mel_spectrogram.py
DELETED
@@ -1,151 +0,0 @@
|
|
1 |
-
import argparse
|
2 |
-
import os
|
3 |
-
import os.path as P
|
4 |
-
from copy import deepcopy
|
5 |
-
from functools import partial
|
6 |
-
from glob import glob
|
7 |
-
from multiprocessing import Pool
|
8 |
-
from pathlib import Path
|
9 |
-
|
10 |
-
import librosa
|
11 |
-
import numpy as np
|
12 |
-
import torchvision
|
13 |
-
|
14 |
-
|
15 |
-
class MelSpectrogram(object):
|
16 |
-
def __init__(self, sr, nfft, fmin, fmax, nmels, hoplen, spec_power, inverse=False):
|
17 |
-
self.sr = sr
|
18 |
-
self.nfft = nfft
|
19 |
-
self.fmin = fmin
|
20 |
-
self.fmax = fmax
|
21 |
-
self.nmels = nmels
|
22 |
-
self.hoplen = hoplen
|
23 |
-
self.spec_power = spec_power
|
24 |
-
self.inverse = inverse
|
25 |
-
|
26 |
-
self.mel_basis = librosa.filters.mel(sr=sr, n_fft=nfft, fmin=fmin, fmax=fmax, n_mels=nmels)
|
27 |
-
|
28 |
-
def __call__(self, x):
|
29 |
-
if self.inverse:
|
30 |
-
spec = librosa.feature.inverse.mel_to_stft(
|
31 |
-
x, sr=self.sr, n_fft=self.nfft, fmin=self.fmin, fmax=self.fmax, power=self.spec_power
|
32 |
-
)
|
33 |
-
wav = librosa.griffinlim(spec, hop_length=self.hoplen)
|
34 |
-
return wav
|
35 |
-
else:
|
36 |
-
spec = np.abs(librosa.stft(x, n_fft=self.nfft, hop_length=self.hoplen)) ** self.spec_power
|
37 |
-
mel_spec = np.dot(self.mel_basis, spec)
|
38 |
-
return mel_spec
|
39 |
-
|
40 |
-
class LowerThresh(object):
|
41 |
-
def __init__(self, min_val, inverse=False):
|
42 |
-
self.min_val = min_val
|
43 |
-
self.inverse = inverse
|
44 |
-
|
45 |
-
def __call__(self, x):
|
46 |
-
if self.inverse:
|
47 |
-
return x
|
48 |
-
else:
|
49 |
-
return np.maximum(self.min_val, x)
|
50 |
-
|
51 |
-
class Add(object):
|
52 |
-
def __init__(self, val, inverse=False):
|
53 |
-
self.inverse = inverse
|
54 |
-
self.val = val
|
55 |
-
|
56 |
-
def __call__(self, x):
|
57 |
-
if self.inverse:
|
58 |
-
return x - self.val
|
59 |
-
else:
|
60 |
-
return x + self.val
|
61 |
-
|
62 |
-
class Subtract(Add):
|
63 |
-
def __init__(self, val, inverse=False):
|
64 |
-
self.inverse = inverse
|
65 |
-
self.val = val
|
66 |
-
|
67 |
-
def __call__(self, x):
|
68 |
-
if self.inverse:
|
69 |
-
return x + self.val
|
70 |
-
else:
|
71 |
-
return x - self.val
|
72 |
-
|
73 |
-
class Multiply(object):
|
74 |
-
def __init__(self, val, inverse=False) -> None:
|
75 |
-
self.val = val
|
76 |
-
self.inverse = inverse
|
77 |
-
|
78 |
-
def __call__(self, x):
|
79 |
-
if self.inverse:
|
80 |
-
return x / self.val
|
81 |
-
else:
|
82 |
-
return x * self.val
|
83 |
-
|
84 |
-
class Divide(Multiply):
|
85 |
-
def __init__(self, val, inverse=False):
|
86 |
-
self.inverse = inverse
|
87 |
-
self.val = val
|
88 |
-
|
89 |
-
def __call__(self, x):
|
90 |
-
if self.inverse:
|
91 |
-
return x * self.val
|
92 |
-
else:
|
93 |
-
return x / self.val
|
94 |
-
|
95 |
-
class Log10(object):
|
96 |
-
def __init__(self, inverse=False):
|
97 |
-
self.inverse = inverse
|
98 |
-
|
99 |
-
def __call__(self, x):
|
100 |
-
if self.inverse:
|
101 |
-
return 10 ** x
|
102 |
-
else:
|
103 |
-
return np.log10(x)
|
104 |
-
|
105 |
-
class Clip(object):
|
106 |
-
def __init__(self, min_val, max_val, inverse=False):
|
107 |
-
self.min_val = min_val
|
108 |
-
self.max_val = max_val
|
109 |
-
self.inverse = inverse
|
110 |
-
|
111 |
-
def __call__(self, x):
|
112 |
-
if self.inverse:
|
113 |
-
return x
|
114 |
-
else:
|
115 |
-
return np.clip(x, self.min_val, self.max_val)
|
116 |
-
|
117 |
-
class TrimSpec(object):
|
118 |
-
def __init__(self, max_len, inverse=False):
|
119 |
-
self.max_len = max_len
|
120 |
-
self.inverse = inverse
|
121 |
-
|
122 |
-
def __call__(self, x):
|
123 |
-
if self.inverse:
|
124 |
-
return x
|
125 |
-
else:
|
126 |
-
return x[:, :self.max_len]
|
127 |
-
|
128 |
-
class MaxNorm(object):
|
129 |
-
def __init__(self, inverse=False):
|
130 |
-
self.inverse = inverse
|
131 |
-
self.eps = 1e-10
|
132 |
-
|
133 |
-
def __call__(self, x):
|
134 |
-
if self.inverse:
|
135 |
-
return x
|
136 |
-
else:
|
137 |
-
return x / (x.max() + self.eps)
|
138 |
-
|
139 |
-
|
140 |
-
TRANSFORMS_16000 = torchvision.transforms.Compose([
|
141 |
-
MelSpectrogram(sr=16000, nfft=1024, fmin=125, fmax=7600, nmels=80, hoplen=1024//4, spec_power=1),
|
142 |
-
LowerThresh(1e-5),
|
143 |
-
Log10(),
|
144 |
-
Multiply(20),
|
145 |
-
Subtract(20),
|
146 |
-
Add(100),
|
147 |
-
Divide(100),
|
148 |
-
Clip(0, 1.0)
|
149 |
-
# TrimSpec(860)
|
150 |
-
])
|
151 |
-
|
|
|
|
|
|
|
|
|
|
|
|
|
|
|
|
|
|
|
|
|
|
|
|
|
|
|
|
|
|
|
|
|
|
|
|
|
|
|
|
|
|
|
|
|
|
|
|
|
|
|
|
|
|
|
|
|
|
|
|
|
|
|
|
|
|
|
|
|
|
|
|
|
|
|
|
|
|
|
|
|
|
|
|
|
|
|
|
|
|
|
|
|
|
|
|
|
|
|
|
|
|
|
|
|
|
|
|
|
|
|
|
|
|
|
|
|
|
|
|
|
|
|
|
|
|
|
|
|
|
|
|
|
|
|
|
|
|
|
|
|
|
|
|
|
|
|
|
|
|
|
|
|
|
|
|
|
|
|
|
|
|
|
|
|
|
|
|
|
|
|
|
|
|
|
|
|
|
|
|
|
|
|
|
|
|
|
|
|
|
|
|
|
|
|
|
|
|
|
|
|
|
|
|
|
|
|
|
|
|
|
|
|
|
|
|
|
|
|
|
|
|
|
|
|
|
|
|
|
|
|
|
|
|
|
|
|
|
|
|
|
|
|
|
|
|
|
|
|
|
|
|
|
|
|
|
|
|
|
|
|
|
|
|
|
|
|
|
|
|
|
|
|
|
|
|
|
|
|
|
|
|
|
|
|
|
|
|
|
|
|
|
|
|
|
|
|
|
|
|
|
|
|
|
spaces/AILab-CVC/SEED-LLaMA/scripts/start_frontend_8b.sh
DELETED
@@ -1 +0,0 @@
|
|
1 |
-
python3 gradio_demo/seed_llama_gradio.py --server_port 80 --request_address http://127.0.0.1:7890/generate --model_type seed-llama-8b
|
|
|
|
spaces/AIWaves/SOP_Generation-single/gradio_backend.py
DELETED
@@ -1,123 +0,0 @@
|
|
1 |
-
import json
|
2 |
-
import os
|
3 |
-
import argparse
|
4 |
-
import sys
|
5 |
-
sys.path.append("Gradio_Config")
|
6 |
-
from SOP import SOP
|
7 |
-
from Agent import Agent
|
8 |
-
from Environment import Environment
|
9 |
-
from Memory import Memory
|
10 |
-
from gradio_base import Client, convert2list4agentname
|
11 |
-
|
12 |
-
# add ===================
|
13 |
-
def process(action):
|
14 |
-
response = action.response
|
15 |
-
send_name = action.name
|
16 |
-
send_role = action.role
|
17 |
-
if not action.is_user:
|
18 |
-
print(f"{send_name}({send_role}):{response}")
|
19 |
-
memory = Memory(send_role, send_name, response)
|
20 |
-
return memory
|
21 |
-
|
22 |
-
def gradio_process(action,current_state):
|
23 |
-
response = action.response
|
24 |
-
all = ""
|
25 |
-
for i,res in enumerate(response):
|
26 |
-
all+=res
|
27 |
-
state = 10
|
28 |
-
if action.is_user:
|
29 |
-
state = 30
|
30 |
-
elif action.state_begin:
|
31 |
-
state = 12
|
32 |
-
action.state_begin = False
|
33 |
-
elif i>0:
|
34 |
-
state = 11
|
35 |
-
send_name = f"{action.name}({action.role})"
|
36 |
-
Client.send_server(str([state, send_name, res, current_state.name]))
|
37 |
-
if state == 30:
|
38 |
-
# print("client: waiting for server")
|
39 |
-
data: list = next(Client.receive_server)
|
40 |
-
content = ""
|
41 |
-
for item in data:
|
42 |
-
if item.startswith("<USER>"):
|
43 |
-
content = item.split("<USER>")[1]
|
44 |
-
break
|
45 |
-
# print(f"client: received `{content}` from server.")
|
46 |
-
action.response = content
|
47 |
-
break
|
48 |
-
else:
|
49 |
-
action.response = all
|
50 |
-
|
51 |
-
def prepare(agents, sop, environment):
|
52 |
-
client = Client()
|
53 |
-
Client.send_server = client.send_message
|
54 |
-
|
55 |
-
client.send_message(
|
56 |
-
{
|
57 |
-
"agents_name": convert2list4agentname(sop)[0],
|
58 |
-
"api_key": os.environ["API_KEY"]
|
59 |
-
}
|
60 |
-
)
|
61 |
-
print(f"client: {list(agents.keys())}")
|
62 |
-
client.listening_for_start_()
|
63 |
-
client.mode = Client.mode = client.cache["mode"]
|
64 |
-
os.environ["API_KEY"] = client.cache["api_key"]
|
65 |
-
uploaded_sop = Client.cache['uploaded_sop']
|
66 |
-
agents,sop,environment = init(uploaded_sop)
|
67 |
-
run(agents,sop,environment)
|
68 |
-
|
69 |
-
def block_when_next(current_agent, current_state):
|
70 |
-
if Client.LAST_USER:
|
71 |
-
assert not current_agent.is_user
|
72 |
-
Client.LAST_USER = False
|
73 |
-
return
|
74 |
-
if current_agent.is_user:
|
75 |
-
# if next turn is user, we don't handle it here
|
76 |
-
Client.LAST_USER = True
|
77 |
-
return
|
78 |
-
if Client.FIRST_RUN:
|
79 |
-
Client.FIRST_RUN = False
|
80 |
-
else:
|
81 |
-
# block current process
|
82 |
-
if Client.mode == Client.SINGLE_MODE:
|
83 |
-
Client.send_server(str([98, f"{current_agent.name}({current_agent.state_roles[current_state.name]})", " ", current_state.name]))
|
84 |
-
data: list = next(Client.receive_server)
|
85 |
-
|
86 |
-
# =======================
|
87 |
-
|
88 |
-
def init(config):
|
89 |
-
if not os.path.exists("logs"):
|
90 |
-
os.mkdir("logs")
|
91 |
-
sop = SOP.from_config(config)
|
92 |
-
agents,roles_to_names,names_to_roles = Agent.from_config(config)
|
93 |
-
environment = Environment.from_config(config)
|
94 |
-
environment.agents = agents
|
95 |
-
environment.roles_to_names,environment.names_to_roles = roles_to_names,names_to_roles
|
96 |
-
sop.roles_to_names,sop.names_to_roles = roles_to_names,names_to_roles
|
97 |
-
for name,agent in agents.items():
|
98 |
-
agent.environment = environment
|
99 |
-
return agents,sop,environment
|
100 |
-
|
101 |
-
def run(agents,sop,environment):
|
102 |
-
while True:
|
103 |
-
current_state,current_agent= sop.next(environment,agents)
|
104 |
-
if sop.finished:
|
105 |
-
print("finished!")
|
106 |
-
Client.send_server(str([99, " ", " ", "done"]))
|
107 |
-
os.environ.clear()
|
108 |
-
break
|
109 |
-
block_when_next(current_agent, current_state)
|
110 |
-
action = current_agent.step(current_state) #component_dict = current_state[self.role[current_node.name]] current_agent.compile(component_dict)
|
111 |
-
gradio_process(action,current_state)
|
112 |
-
memory = process(action)
|
113 |
-
environment.update_memory(memory,current_state)
|
114 |
-
|
115 |
-
|
116 |
-
if __name__ == '__main__':
|
117 |
-
parser = argparse.ArgumentParser(description='A demo of chatbot')
|
118 |
-
parser.add_argument('--agent', type=str, help='path to SOP json',default="config.json")
|
119 |
-
args = parser.parse_args()
|
120 |
-
|
121 |
-
agents,sop,environment = init(args.agent)
|
122 |
-
prepare(agents, sop, environment)
|
123 |
-
# run(agents,sop,environment)
|
|
|
|
|
|
|
|
|
|
|
|
|
|
|
|
|
|
|
|
|
|
|
|
|
|
|
|
|
|
|
|
|
|
|
|
|
|
|
|
|
|
|
|
|
|
|
|
|
|
|
|
|
|
|
|
|
|
|
|
|
|
|
|
|
|
|
|
|
|
|
|
|
|
|
|
|
|
|
|
|
|
|
|
|
|
|
|
|
|
|
|
|
|
|
|
|
|
|
|
|
|
|
|
|
|
|
|
|
|
|
|
|
|
|
|
|
|
|
|
|
|
|
|
|
|
|
|
|
|
|
|
|
|
|
|
|
|
|
|
|
|
|
|
|
|
|
|
|
|
|
|
|
|
|
|
|
|
|
|
|
|
|
|
|
|
|
|
|
|
|
|
|
|
|
|
|
|
|
|
|
|
|
|
|
|
|
|
|
|
|
|
|
|
|
|
|
|
|
|
|
|
|
|
|
|
|
|
|
|
|
|
|
|
|
|
|
|
|
|
|
|
|
|
|
|
|
|
|
|
|
|
|
|
|
|
|
|
|
|
|
|
|
|
|
|
|
|
spaces/AIZeroToHero/02-Transformers-Sentence2Paragraph/README.md
DELETED
@@ -1,13 +0,0 @@
|
|
1 |
-
---
|
2 |
-
title: 02 Transformers Sentence2Paragraph
|
3 |
-
emoji: 🐨
|
4 |
-
colorFrom: blue
|
5 |
-
colorTo: purple
|
6 |
-
sdk: gradio
|
7 |
-
sdk_version: 3.1.5
|
8 |
-
app_file: app.py
|
9 |
-
pinned: false
|
10 |
-
license: mit
|
11 |
-
---
|
12 |
-
|
13 |
-
Check out the configuration reference at https://huggingface.co/docs/hub/spaces-config-reference
|
|
|
|
|
|
|
|
|
|
|
|
|
|
|
|
|
|
|
|
|
|
|
|
|
|
|
|
spaces/ANILYADAV/mygenaichatbot/README.md
DELETED
@@ -1,12 +0,0 @@
|
|
1 |
-
---
|
2 |
-
title: Mygenaichatbot
|
3 |
-
emoji: 📚
|
4 |
-
colorFrom: purple
|
5 |
-
colorTo: yellow
|
6 |
-
sdk: gradio
|
7 |
-
sdk_version: 3.39.0
|
8 |
-
app_file: app.py
|
9 |
-
pinned: false
|
10 |
-
---
|
11 |
-
|
12 |
-
Check out the configuration reference at https://huggingface.co/docs/hub/spaces-config-reference
|
|
|
|
|
|
|
|
|
|
|
|
|
|
|
|
|
|
|
|
|
|
|
|
|
|
spaces/ATang0729/Forecast4Muses/Model/Model6/Model6_1_ClothesKeyPoint/mmpose_1_x/configs/__init__.py
DELETED
File without changes
|
spaces/After-the-Dark/paragraph-similarity/app.py
DELETED
@@ -1,56 +0,0 @@
|
|
1 |
-
import gradio as gr
|
2 |
-
from sentence_transformers import SentenceTransformer, util
|
3 |
-
model_sentence = SentenceTransformer('all-MiniLM-L6-v2')
|
4 |
-
|
5 |
-
para_1 ="""
|
6 |
-
Natural language processing (NLP) is a field of computer science that studies how computers can understand and process human language. NLP is a subfield of artificial intelligence (AI) that deals with the interaction between computers and human (natural) languages.
|
7 |
-
|
8 |
-
NLP has a wide range of applications, including:
|
9 |
-
|
10 |
-
Machine translation: translating text from one language to another
|
11 |
-
Text summarization: extracting the main points of a text
|
12 |
-
Question answering: answering questions posed in natural language
|
13 |
-
Text classification: classifying text into categories, such as spam or ham
|
14 |
-
Sentiment analysis: determining the sentiment of a text, such as positive, negative, or neutral
|
15 |
-
Natural language generation: generating text that is similar to human-written text
|
16 |
-
NLP is a challenging field, as human language is complex and nuanced. However, NLP has made significant progress in recent years, and it is now a powerful tool that can be used to solve a wide range of problems.
|
17 |
-
|
18 |
-
|
19 |
-
"""
|
20 |
-
para_2 ="""
|
21 |
-
Generative adversarial networks (GANs) are a type of machine learning model that can be used to generate realistic and creative content. GANs were first introduced in 2014 by Ian Goodfellow, and they have since been used to generate a wide range of content, including images, text, and music.
|
22 |
-
|
23 |
-
GANs work by pitting two neural networks against each other in a game-like setting. One network, the generator, is responsible for creating new content. The other network, the discriminator, is responsible for determining whether the content created by the generator is real or fake.
|
24 |
-
|
25 |
-
The generator is trained to create content that is as realistic as possible, while the discriminator is trained to distinguish between real and fake content. As the two networks compete against each other, they both become better at their respective tasks.
|
26 |
-
|
27 |
-
GANs have been used to generate a wide range of content, including:
|
28 |
-
|
29 |
-
Images: GANs have been used to generate realistic images of people, animals, and objects.
|
30 |
-
Text: GANs have been used to generate realistic text, such as news articles, blog posts, and even poetry.
|
31 |
-
Music: GANs have been used to generate realistic music, such as songs, symphonies, and even jazz improvisations.
|
32 |
-
GANs are a powerful tool that can be used to generate realistic and creative content. As GANs continue to develop, they are likely to be used to create even more amazing and impressive content in the future.
|
33 |
-
|
34 |
-
|
35 |
-
"""
|
36 |
-
def paragraph_similar(text1, text2):
|
37 |
-
sentences = []
|
38 |
-
sentences.append(text1)
|
39 |
-
sentences.append(text2)
|
40 |
-
paraphrases = util.paraphrase_mining(model_sentence, sentences, corpus_chunk_size=len(sentences))
|
41 |
-
return {"Similarity": [round(paraphrases[0][0], 2)]}
|
42 |
-
|
43 |
-
|
44 |
-
with gr.Blocks(title="Paragraph",css="footer {visibility: hidden}") as demo:
|
45 |
-
with gr.Row():
|
46 |
-
with gr.Column():
|
47 |
-
gr.Markdown("## Paragraph Compare")
|
48 |
-
with gr.Row():
|
49 |
-
with gr.Column():
|
50 |
-
inputs_1 = gr.TextArea(label="Paragraph 1",value=para_1,interactive=True)
|
51 |
-
inputs_2 = gr.TextArea(label="Paragraph 2",value=para_2,interactive=True)
|
52 |
-
with gr.Column():
|
53 |
-
btn = gr.Button(value="RUN")
|
54 |
-
output = gr.Label(label="output")
|
55 |
-
btn.click(fn=paragraph_similar,inputs=[inputs_1,inputs_2],outputs=[output])
|
56 |
-
demo.launch()
|
|
|
|
|
|
|
|
|
|
|
|
|
|
|
|
|
|
|
|
|
|
|
|
|
|
|
|
|
|
|
|
|
|
|
|
|
|
|
|
|
|
|
|
|
|
|
|
|
|
|
|
|
|
|
|
|
|
|
|
|
|
|
|
|
|
|
|
|
|
|
|
|
|
|
|
|
|
|
|
|
|
|
|
|
|
|
|
|
|
|
|
|
|
|
|
|
|
|
|
|
|
|
|
|
|
|
|
|
|
|
|
|
|
spaces/AgentVerse/agentVerse/ui/src/phaser3-rex-plugins/templates/ui/basesizer/LayoutBackgrounds.js
DELETED
@@ -1,41 +0,0 @@
|
|
1 |
-
import ResizeGameObject from '../../../plugins/utils/size/ResizeGameObject.js';
|
2 |
-
import PreLayoutChild from './utils/PreLayoutChild.js';
|
3 |
-
import LayoutChild from './utils/LayoutChild.js';
|
4 |
-
|
5 |
-
const ALIGN_CENTER = Phaser.Display.Align.CENTER;
|
6 |
-
|
7 |
-
var LayoutBackgrounds = function () {
|
8 |
-
if (this.backgroundChildren === undefined) {
|
9 |
-
return;
|
10 |
-
}
|
11 |
-
var backgrounds = this.backgroundChildren;
|
12 |
-
|
13 |
-
var startX = this.left,
|
14 |
-
startY = this.top;
|
15 |
-
var parentWidth = this.width,
|
16 |
-
parentHeight = this.height;
|
17 |
-
var child, childConfig, padding,
|
18 |
-
x, y, width, height;
|
19 |
-
for (var i = 0, cnt = backgrounds.length; i < cnt; i++) {
|
20 |
-
child = backgrounds[i];
|
21 |
-
childConfig = child.rexSizer;
|
22 |
-
if (childConfig.hidden) {
|
23 |
-
continue;
|
24 |
-
}
|
25 |
-
|
26 |
-
padding = childConfig.padding;
|
27 |
-
|
28 |
-
PreLayoutChild.call(this, child);
|
29 |
-
|
30 |
-
x = startX + padding.left;
|
31 |
-
y = startY + padding.top;
|
32 |
-
width = parentWidth - padding.left - padding.right;
|
33 |
-
height = parentHeight - padding.top - padding.bottom;
|
34 |
-
|
35 |
-
ResizeGameObject(child, width, height);
|
36 |
-
|
37 |
-
LayoutChild.call(this, child, x, y, width, height, ALIGN_CENTER);
|
38 |
-
}
|
39 |
-
}
|
40 |
-
|
41 |
-
export default LayoutBackgrounds;
|
|
|
|
|
|
|
|
|
|
|
|
|
|
|
|
|
|
|
|
|
|
|
|
|
|
|
|
|
|
|
|
|
|
|
|
|
|
|
|
|
|
|
|
|
|
|
|
|
|
|
|
|
|
|
|
|
|
|
|
|
|
|
|
|
|
|
|
|
|
|
|
|
|
|
|
|
|
|
|
|
|
|
|
spaces/AgentVerse/agentVerse/ui/src/phaser3-rex-plugins/templates/ui/knob/input/IsLocalPointInKnob.js
DELETED
@@ -1,8 +0,0 @@
|
|
1 |
-
var GetDistance = Phaser.Math.Distance.Between;
|
2 |
-
|
3 |
-
var IsLocalPointInKnob = function (knob, localX, localY) {
|
4 |
-
var centerX = knob.width / 2;
|
5 |
-
return GetDistance(centerX, centerX, localX, localY) <= centerX;
|
6 |
-
}
|
7 |
-
|
8 |
-
export default IsLocalPointInKnob;
|
|
|
|
|
|
|
|
|
|
|
|
|
|
|
|
|
|
spaces/AgentVerse/agentVerse/ui/src/phaser3-rex-plugins/templates/ui/namevaluelabel/NameValueLabel.d.ts
DELETED
@@ -1,94 +0,0 @@
|
|
1 |
-
import LineProgressCanvas from '../lineprogresscanvas/LineProgressCanvas';
|
2 |
-
|
3 |
-
// import * as Phaser from 'phaser';
|
4 |
-
import Sizer from '../sizer/Sizer';
|
5 |
-
|
6 |
-
export default NameValueLabel;
|
7 |
-
|
8 |
-
declare namespace NameValueLabel {
|
9 |
-
|
10 |
-
interface IConfig extends Sizer.IConfig {
|
11 |
-
space?: {
|
12 |
-
left?: number, right?: number, top?: number, bottom?: number,
|
13 |
-
|
14 |
-
icon?: number, iconTop?: number, iconBottom?: number, iconLeft?: number, iconRight?: number,
|
15 |
-
|
16 |
-
name?: number,
|
17 |
-
value?: number,
|
18 |
-
|
19 |
-
bar?: number, barBottom?: number, barLeft?: number, barRight?: number,
|
20 |
-
|
21 |
-
action?: number, actionTop?: number, actionBottom?: number, actionLeft?: number, actionRight?: number,
|
22 |
-
},
|
23 |
-
|
24 |
-
background?: Phaser.GameObjects.GameObject,
|
25 |
-
|
26 |
-
icon?: Phaser.GameObjects.GameObject,
|
27 |
-
iconMask?: boolean,
|
28 |
-
|
29 |
-
nameText?: Phaser.GameObjects.GameObject,
|
30 |
-
valueText?: Phaser.GameObjects.GameObject,
|
31 |
-
bar?: Phaser.GameObjects.GameObject | LineProgressCanvas.IConfig,
|
32 |
-
|
33 |
-
action?: Phaser.GameObjects.GameObject,
|
34 |
-
actionMask?: boolean,
|
35 |
-
|
36 |
-
valueTextFormatCallback?: (
|
37 |
-
value: number,
|
38 |
-
min: number,
|
39 |
-
max: number
|
40 |
-
) => string,
|
41 |
-
|
42 |
-
align?: {
|
43 |
-
text?: 'left' | 'right' | 'center' | number,
|
44 |
-
title?: 'left' | 'right' | 'center' | number,
|
45 |
-
},
|
46 |
-
|
47 |
-
proportion?: {
|
48 |
-
title?: number,
|
49 |
-
separator?: number,
|
50 |
-
text?: number,
|
51 |
-
}
|
52 |
-
}
|
53 |
-
}
|
54 |
-
|
55 |
-
declare class NameValueLabel extends Sizer {
|
56 |
-
constructor(
|
57 |
-
scene: Phaser.Scene,
|
58 |
-
config?: NameValueLabel.IConfig
|
59 |
-
);
|
60 |
-
|
61 |
-
nameText: string;
|
62 |
-
setNameText(value?:string):this;
|
63 |
-
|
64 |
-
valueText: string;
|
65 |
-
setValueText(value?:string):this;
|
66 |
-
|
67 |
-
barValue: number;
|
68 |
-
setBarValue(
|
69 |
-
value: number,
|
70 |
-
min?: number,
|
71 |
-
max?: number
|
72 |
-
): this;
|
73 |
-
easeBarValueTo(
|
74 |
-
value: number,
|
75 |
-
min?: number,
|
76 |
-
max?: number
|
77 |
-
): this;
|
78 |
-
|
79 |
-
setTexture(
|
80 |
-
key: string | Phaser.Textures.Texture,
|
81 |
-
frame?: string | number
|
82 |
-
): this;
|
83 |
-
readonly texture: Phaser.Textures.Texture | Phaser.Textures.CanvasTexture;
|
84 |
-
readonly frame: Phaser.Textures.Frame;
|
85 |
-
|
86 |
-
setValue(
|
87 |
-
value: number,
|
88 |
-
min: number,
|
89 |
-
max: number
|
90 |
-
): this;
|
91 |
-
value: number;
|
92 |
-
minValue: number;
|
93 |
-
maxValue: number;
|
94 |
-
}
|
|
|
|
|
|
|
|
|
|
|
|
|
|
|
|
|
|
|
|
|
|
|
|
|
|
|
|
|
|
|
|
|
|
|
|
|
|
|
|
|
|
|
|
|
|
|
|
|
|
|
|
|
|
|
|
|
|
|
|
|
|
|
|
|
|
|
|
|
|
|
|
|
|
|
|
|
|
|
|
|
|
|
|
|
|
|
|
|
|
|
|
|
|
|
|
|
|
|
|
|
|
|
|
|
|
|
|
|
|
|
|
|
|
|
|
|
|
|
|
|
|
|
|
|
|
|
|
|
|
|
|
|
|
|
|
|
|
|
|
|
|
|
|
|
|
|
|
|
|
|
|
|
|
|
|
|
|
|
|
|
|
|
|
|
|
|
|
|
|
|
|
|
|
|
|
|
|
|
|
|
|
|
|
|
|
|
|
|
|
spaces/Ajay07pandey/Netfilx_Movie_Recommendation_System/app.py
DELETED
@@ -1,58 +0,0 @@
|
|
1 |
-
import streamlit as st
|
2 |
-
import pickle
|
3 |
-
import pandas as pd
|
4 |
-
|
5 |
-
|
6 |
-
st.image("Netflix.png")
|
7 |
-
|
8 |
-
movies_list = pickle.load(open("content_dict.pkl",'br'))
|
9 |
-
movies = pd.DataFrame(movies_list)
|
10 |
-
|
11 |
-
similarity= pickle.load(open('cosine_similarity.pkl','rb'))
|
12 |
-
|
13 |
-
def recommend(title, cosine_sim=similarity, data=movies):
|
14 |
-
recommended_content=[]
|
15 |
-
# Get the index of the input title in the programme_list
|
16 |
-
programme_list = data['title'].to_list()
|
17 |
-
index = programme_list.index(title)
|
18 |
-
|
19 |
-
|
20 |
-
# Create a list of tuples containing the similarity score and index
|
21 |
-
# between the input title and all other programs in the dataset
|
22 |
-
sim_scores = list(enumerate(cosine_sim[index]))
|
23 |
-
|
24 |
-
|
25 |
-
# Sort the list of tuples by similarity score in descending order
|
26 |
-
sim_scores = sorted(sim_scores, key=lambda x: x[1], reverse=True)[1:11]
|
27 |
-
|
28 |
-
|
29 |
-
# Get the recommended movie titles and their similarity scores
|
30 |
-
recommend_index = [i[0] for i in sim_scores]
|
31 |
-
rec_movie = data['title'].iloc[recommend_index]
|
32 |
-
rec_score = [round(i[1], 4) for i in sim_scores]
|
33 |
-
|
34 |
-
|
35 |
-
# Create a pandas DataFrame to display the recommendations
|
36 |
-
rec_table = pd.DataFrame(list(zip(rec_movie, rec_score)), columns=['Recommendation', 'Similarity_score(0-1)'])
|
37 |
-
# recommended_content.append(rec_table['Recommendation'].values)
|
38 |
-
|
39 |
-
|
40 |
-
return rec_table['Recommendation'].values
|
41 |
-
|
42 |
-
|
43 |
-
# Displaying title
|
44 |
-
st.title(" Movie Recommender System ")
|
45 |
-
|
46 |
-
|
47 |
-
movie_list = movies['title'].values
|
48 |
-
selected_movie = st.selectbox(
|
49 |
-
"Type or select a movie from the dropdown",
|
50 |
-
movie_list
|
51 |
-
)
|
52 |
-
|
53 |
-
# Setting a button
|
54 |
-
if st.button('Show Recommendation'):
|
55 |
-
recommended_movie_names = recommend(selected_movie)
|
56 |
-
st.balloons()
|
57 |
-
for j in recommended_movie_names:
|
58 |
-
st.write(j)
|
|
|
|
|
|
|
|
|
|
|
|
|
|
|
|
|
|
|
|
|
|
|
|
|
|
|
|
|
|
|
|
|
|
|
|
|
|
|
|
|
|
|
|
|
|
|
|
|
|
|
|
|
|
|
|
|
|
|
|
|
|
|
|
|
|
|
|
|
|
|
|
|
|
|
|
|
|
|
|
|
|
|
|
|
|
|
|
|
|
|
|
|
|
|
|
|
|
|
|
|
|
|
|
|
|
|
|
|
|
|
|
|
|
|
|
|
|
spaces/Akmyradov/TurkmenTTSweSTT/uroman/bin/string-distance.pl
DELETED
@@ -1,99 +0,0 @@
|
|
1 |
-
#!/usr/bin/perl -w
|
2 |
-
|
3 |
-
# Author: Ulf Hermjakob
|
4 |
-
# Release date: October 13, 2019
|
5 |
-
|
6 |
-
# Usage: string-distance.pl {-lc1 <language-code>} {-lc2 <language-code>} < STDIN > STDOUT
|
7 |
-
# Example: string-distance.pl -lc1 rus -lc2 ukr < STDIN > STDOUT
|
8 |
-
# Example: string-distance.pl < ../test/string-similarity-test-input.txt
|
9 |
-
# Input format: two strings per line (tab-separated, in Latin script)
|
10 |
-
# Strings in non-Latin scripts should first be romanized. (Recommended script: uroman.pl)
|
11 |
-
# Output format: repetition of the two input strings, plus the string distance between them (tab-separated).
|
12 |
-
# Additional output meta info lines at the top are marked with an initial #.
|
13 |
-
#
|
14 |
-
# The script uses data from a string-distance-cost-rules file that lists costs,
|
15 |
-
# where the default cost is "1" with lower costs for differences in vowels,
|
16 |
-
# duplicate consonants, "f" vs. "ph" etc.
|
17 |
-
# Language cost rules can be language-specific and context-sensitive.
|
18 |
-
|
19 |
-
$|=1;
|
20 |
-
|
21 |
-
use FindBin;
|
22 |
-
use Cwd "abs_path";
|
23 |
-
use File::Basename qw(dirname);
|
24 |
-
use File::Spec;
|
25 |
-
|
26 |
-
my $bin_dir = abs_path(dirname($0));
|
27 |
-
my $root_dir = File::Spec->catfile($bin_dir, File::Spec->updir());
|
28 |
-
my $data_dir = File::Spec->catfile($root_dir, "data");
|
29 |
-
my $lib_dir = File::Spec->catfile($root_dir, "lib");
|
30 |
-
|
31 |
-
use lib "$FindBin::Bin/../lib";
|
32 |
-
use List::Util qw(min max);
|
33 |
-
use NLP::utilities;
|
34 |
-
use NLP::stringDistance;
|
35 |
-
$util = NLP::utilities;
|
36 |
-
$sd = NLP::stringDistance;
|
37 |
-
$verbose = 0;
|
38 |
-
$separator = "\t";
|
39 |
-
|
40 |
-
$cost_rule_filename = File::Spec->catfile($data_dir, "string-distance-cost-rules.txt");
|
41 |
-
|
42 |
-
$lang_code1 = "eng";
|
43 |
-
$lang_code2 = "eng";
|
44 |
-
%ht = ();
|
45 |
-
|
46 |
-
while (@ARGV) {
|
47 |
-
$arg = shift @ARGV;
|
48 |
-
if ($arg =~ /^-+lc1$/) {
|
49 |
-
$lang_code_candidate = shift @ARGV;
|
50 |
-
$lang_code1 = $lang_code_candidate if $lang_code_candidate =~ /^[a-z]{3,3}$/;
|
51 |
-
} elsif ($arg =~ /^-+lc2$/) {
|
52 |
-
$lang_code_candidate = shift @ARGV;
|
53 |
-
$lang_code2 = $lang_code_candidate if $lang_code_candidate =~ /^[a-z]{3,3}$/;
|
54 |
-
} elsif ($arg =~ /^-+(v|verbose)$/) {
|
55 |
-
$verbose = shift @ARGV;
|
56 |
-
} else {
|
57 |
-
print STDERR "Ignoring unrecognized arg $arg\n";
|
58 |
-
}
|
59 |
-
}
|
60 |
-
|
61 |
-
$sd->load_string_distance_data($cost_rule_filename, *ht, $verbose);
|
62 |
-
print STDERR "Loaded resources.\n" if $verbose;
|
63 |
-
|
64 |
-
my $chart_id = 0;
|
65 |
-
my $line_number = 0;
|
66 |
-
print "# Lang-code-1: $lang_code1 Lang-code-2: $lang_code2\n";
|
67 |
-
while (<>) {
|
68 |
-
$line_number++;
|
69 |
-
if ($verbose) {
|
70 |
-
if ($line_number =~ /000$/) {
|
71 |
-
if ($line_number =~ /0000$/) {
|
72 |
-
print STDERR $line_number;
|
73 |
-
} else {
|
74 |
-
print STDERR ".";
|
75 |
-
}
|
76 |
-
}
|
77 |
-
}
|
78 |
-
my $line = $_;
|
79 |
-
$line =~ s/^\xEF\xBB\xBF//;
|
80 |
-
next if $line =~ /^\s*(\#.*)?$/;
|
81 |
-
my $s1;
|
82 |
-
my $s2;
|
83 |
-
if (($s1, $s2) = ($line =~ /^("(?:\\"|[^"])*"|\S+)$separator("(?:\\"|[^"])*"|\S+)\s*$/)) {
|
84 |
-
$s1 = $util->dequote_string($s1);
|
85 |
-
$s2 = $util->dequote_string($s2);
|
86 |
-
} elsif ($line =~ /^\s*(#.*)$/) {
|
87 |
-
} else {
|
88 |
-
print STDERR "Could not process line $line_number: $line" if $verbose;
|
89 |
-
print "\n";
|
90 |
-
next;
|
91 |
-
}
|
92 |
-
|
93 |
-
$cost = $sd->quick_romanized_string_distance_by_chart($s1, $s2, *ht, "", $lang_code1, $lang_code2);
|
94 |
-
print "$s1\t$s2\t$cost\n";
|
95 |
-
}
|
96 |
-
print STDERR "\n" if $verbose;
|
97 |
-
|
98 |
-
exit 0;
|
99 |
-
|
|
|
|
|
|
|
|
|
|
|
|
|
|
|
|
|
|
|
|
|
|
|
|
|
|
|
|
|
|
|
|
|
|
|
|
|
|
|
|
|
|
|
|
|
|
|
|
|
|
|
|
|
|
|
|
|
|
|
|
|
|
|
|
|
|
|
|
|
|
|
|
|
|
|
|
|
|
|
|
|
|
|
|
|
|
|
|
|
|
|
|
|
|
|
|
|
|
|
|
|
|
|
|
|
|
|
|
|
|
|
|
|
|
|
|
|
|
|
|
|
|
|
|
|
|
|
|
|
|
|
|
|
|
|
|
|
|
|
|
|
|
|
|
|
|
|
|
|
|
|
|
|
|
|
|
|
|
|
|
|
|
|
|
|
|
|
|
|
|
|
|
|
|
|
|
|
|
|
|
|
|
|
|
|
|
|
|
|
|
|
|
|
|
|
|
|
|
|
|
spaces/AlawnCN/webui-docker/README.md
DELETED
@@ -1,19 +0,0 @@
|
|
1 |
-
---
|
2 |
-
title: Stable Diffusion Web UI Docker
|
3 |
-
emoji: 🐳
|
4 |
-
colorFrom: blue
|
5 |
-
colorTo: blue
|
6 |
-
sdk: docker
|
7 |
-
sdk_version: 3.9
|
8 |
-
app_file: oh-no.py
|
9 |
-
pinned: false
|
10 |
-
---
|
11 |
-
|
12 |
-
## Stable Diffusion Web UI
|
13 |
-
https://github.com/AUTOMATIC1111/stable-diffusion-webui
|
14 |
-
|
15 |
-
## Documentation
|
16 |
-
https://github.com/AUTOMATIC1111/stable-diffusion-webui/wiki
|
17 |
-
|
18 |
-
## Models License
|
19 |
-
https://huggingface.co/spaces/CompVis/stable-diffusion-license
|
|
|
|
|
|
|
|
|
|
|
|
|
|
|
|
|
|
|
|
|
|
|
|
|
|
|
|
|
|
|
|
|
|
|
|
|
|
|
|
spaces/Alpaca233/SadTalker/src/facerender/modules/util.py
DELETED
@@ -1,564 +0,0 @@
|
|
1 |
-
from torch import nn
|
2 |
-
|
3 |
-
import torch.nn.functional as F
|
4 |
-
import torch
|
5 |
-
|
6 |
-
from src.facerender.sync_batchnorm import SynchronizedBatchNorm2d as BatchNorm2d
|
7 |
-
from src.facerender.sync_batchnorm import SynchronizedBatchNorm3d as BatchNorm3d
|
8 |
-
|
9 |
-
import torch.nn.utils.spectral_norm as spectral_norm
|
10 |
-
|
11 |
-
|
12 |
-
def kp2gaussian(kp, spatial_size, kp_variance):
|
13 |
-
"""
|
14 |
-
Transform a keypoint into gaussian like representation
|
15 |
-
"""
|
16 |
-
mean = kp['value']
|
17 |
-
|
18 |
-
coordinate_grid = make_coordinate_grid(spatial_size, mean.type())
|
19 |
-
number_of_leading_dimensions = len(mean.shape) - 1
|
20 |
-
shape = (1,) * number_of_leading_dimensions + coordinate_grid.shape
|
21 |
-
coordinate_grid = coordinate_grid.view(*shape)
|
22 |
-
repeats = mean.shape[:number_of_leading_dimensions] + (1, 1, 1, 1)
|
23 |
-
coordinate_grid = coordinate_grid.repeat(*repeats)
|
24 |
-
|
25 |
-
# Preprocess kp shape
|
26 |
-
shape = mean.shape[:number_of_leading_dimensions] + (1, 1, 1, 3)
|
27 |
-
mean = mean.view(*shape)
|
28 |
-
|
29 |
-
mean_sub = (coordinate_grid - mean)
|
30 |
-
|
31 |
-
out = torch.exp(-0.5 * (mean_sub ** 2).sum(-1) / kp_variance)
|
32 |
-
|
33 |
-
return out
|
34 |
-
|
35 |
-
def make_coordinate_grid_2d(spatial_size, type):
|
36 |
-
"""
|
37 |
-
Create a meshgrid [-1,1] x [-1,1] of given spatial_size.
|
38 |
-
"""
|
39 |
-
h, w = spatial_size
|
40 |
-
x = torch.arange(w).type(type)
|
41 |
-
y = torch.arange(h).type(type)
|
42 |
-
|
43 |
-
x = (2 * (x / (w - 1)) - 1)
|
44 |
-
y = (2 * (y / (h - 1)) - 1)
|
45 |
-
|
46 |
-
yy = y.view(-1, 1).repeat(1, w)
|
47 |
-
xx = x.view(1, -1).repeat(h, 1)
|
48 |
-
|
49 |
-
meshed = torch.cat([xx.unsqueeze_(2), yy.unsqueeze_(2)], 2)
|
50 |
-
|
51 |
-
return meshed
|
52 |
-
|
53 |
-
|
54 |
-
def make_coordinate_grid(spatial_size, type):
|
55 |
-
d, h, w = spatial_size
|
56 |
-
x = torch.arange(w).type(type)
|
57 |
-
y = torch.arange(h).type(type)
|
58 |
-
z = torch.arange(d).type(type)
|
59 |
-
|
60 |
-
x = (2 * (x / (w - 1)) - 1)
|
61 |
-
y = (2 * (y / (h - 1)) - 1)
|
62 |
-
z = (2 * (z / (d - 1)) - 1)
|
63 |
-
|
64 |
-
yy = y.view(1, -1, 1).repeat(d, 1, w)
|
65 |
-
xx = x.view(1, 1, -1).repeat(d, h, 1)
|
66 |
-
zz = z.view(-1, 1, 1).repeat(1, h, w)
|
67 |
-
|
68 |
-
meshed = torch.cat([xx.unsqueeze_(3), yy.unsqueeze_(3), zz.unsqueeze_(3)], 3)
|
69 |
-
|
70 |
-
return meshed
|
71 |
-
|
72 |
-
|
73 |
-
class ResBottleneck(nn.Module):
|
74 |
-
def __init__(self, in_features, stride):
|
75 |
-
super(ResBottleneck, self).__init__()
|
76 |
-
self.conv1 = nn.Conv2d(in_channels=in_features, out_channels=in_features//4, kernel_size=1)
|
77 |
-
self.conv2 = nn.Conv2d(in_channels=in_features//4, out_channels=in_features//4, kernel_size=3, padding=1, stride=stride)
|
78 |
-
self.conv3 = nn.Conv2d(in_channels=in_features//4, out_channels=in_features, kernel_size=1)
|
79 |
-
self.norm1 = BatchNorm2d(in_features//4, affine=True)
|
80 |
-
self.norm2 = BatchNorm2d(in_features//4, affine=True)
|
81 |
-
self.norm3 = BatchNorm2d(in_features, affine=True)
|
82 |
-
|
83 |
-
self.stride = stride
|
84 |
-
if self.stride != 1:
|
85 |
-
self.skip = nn.Conv2d(in_channels=in_features, out_channels=in_features, kernel_size=1, stride=stride)
|
86 |
-
self.norm4 = BatchNorm2d(in_features, affine=True)
|
87 |
-
|
88 |
-
def forward(self, x):
|
89 |
-
out = self.conv1(x)
|
90 |
-
out = self.norm1(out)
|
91 |
-
out = F.relu(out)
|
92 |
-
out = self.conv2(out)
|
93 |
-
out = self.norm2(out)
|
94 |
-
out = F.relu(out)
|
95 |
-
out = self.conv3(out)
|
96 |
-
out = self.norm3(out)
|
97 |
-
if self.stride != 1:
|
98 |
-
x = self.skip(x)
|
99 |
-
x = self.norm4(x)
|
100 |
-
out += x
|
101 |
-
out = F.relu(out)
|
102 |
-
return out
|
103 |
-
|
104 |
-
|
105 |
-
class ResBlock2d(nn.Module):
|
106 |
-
"""
|
107 |
-
Res block, preserve spatial resolution.
|
108 |
-
"""
|
109 |
-
|
110 |
-
def __init__(self, in_features, kernel_size, padding):
|
111 |
-
super(ResBlock2d, self).__init__()
|
112 |
-
self.conv1 = nn.Conv2d(in_channels=in_features, out_channels=in_features, kernel_size=kernel_size,
|
113 |
-
padding=padding)
|
114 |
-
self.conv2 = nn.Conv2d(in_channels=in_features, out_channels=in_features, kernel_size=kernel_size,
|
115 |
-
padding=padding)
|
116 |
-
self.norm1 = BatchNorm2d(in_features, affine=True)
|
117 |
-
self.norm2 = BatchNorm2d(in_features, affine=True)
|
118 |
-
|
119 |
-
def forward(self, x):
|
120 |
-
out = self.norm1(x)
|
121 |
-
out = F.relu(out)
|
122 |
-
out = self.conv1(out)
|
123 |
-
out = self.norm2(out)
|
124 |
-
out = F.relu(out)
|
125 |
-
out = self.conv2(out)
|
126 |
-
out += x
|
127 |
-
return out
|
128 |
-
|
129 |
-
|
130 |
-
class ResBlock3d(nn.Module):
|
131 |
-
"""
|
132 |
-
Res block, preserve spatial resolution.
|
133 |
-
"""
|
134 |
-
|
135 |
-
def __init__(self, in_features, kernel_size, padding):
|
136 |
-
super(ResBlock3d, self).__init__()
|
137 |
-
self.conv1 = nn.Conv3d(in_channels=in_features, out_channels=in_features, kernel_size=kernel_size,
|
138 |
-
padding=padding)
|
139 |
-
self.conv2 = nn.Conv3d(in_channels=in_features, out_channels=in_features, kernel_size=kernel_size,
|
140 |
-
padding=padding)
|
141 |
-
self.norm1 = BatchNorm3d(in_features, affine=True)
|
142 |
-
self.norm2 = BatchNorm3d(in_features, affine=True)
|
143 |
-
|
144 |
-
def forward(self, x):
|
145 |
-
out = self.norm1(x)
|
146 |
-
out = F.relu(out)
|
147 |
-
out = self.conv1(out)
|
148 |
-
out = self.norm2(out)
|
149 |
-
out = F.relu(out)
|
150 |
-
out = self.conv2(out)
|
151 |
-
out += x
|
152 |
-
return out
|
153 |
-
|
154 |
-
|
155 |
-
class UpBlock2d(nn.Module):
|
156 |
-
"""
|
157 |
-
Upsampling block for use in decoder.
|
158 |
-
"""
|
159 |
-
|
160 |
-
def __init__(self, in_features, out_features, kernel_size=3, padding=1, groups=1):
|
161 |
-
super(UpBlock2d, self).__init__()
|
162 |
-
|
163 |
-
self.conv = nn.Conv2d(in_channels=in_features, out_channels=out_features, kernel_size=kernel_size,
|
164 |
-
padding=padding, groups=groups)
|
165 |
-
self.norm = BatchNorm2d(out_features, affine=True)
|
166 |
-
|
167 |
-
def forward(self, x):
|
168 |
-
out = F.interpolate(x, scale_factor=2)
|
169 |
-
out = self.conv(out)
|
170 |
-
out = self.norm(out)
|
171 |
-
out = F.relu(out)
|
172 |
-
return out
|
173 |
-
|
174 |
-
class UpBlock3d(nn.Module):
|
175 |
-
"""
|
176 |
-
Upsampling block for use in decoder.
|
177 |
-
"""
|
178 |
-
|
179 |
-
def __init__(self, in_features, out_features, kernel_size=3, padding=1, groups=1):
|
180 |
-
super(UpBlock3d, self).__init__()
|
181 |
-
|
182 |
-
self.conv = nn.Conv3d(in_channels=in_features, out_channels=out_features, kernel_size=kernel_size,
|
183 |
-
padding=padding, groups=groups)
|
184 |
-
self.norm = BatchNorm3d(out_features, affine=True)
|
185 |
-
|
186 |
-
def forward(self, x):
|
187 |
-
# out = F.interpolate(x, scale_factor=(1, 2, 2), mode='trilinear')
|
188 |
-
out = F.interpolate(x, scale_factor=(1, 2, 2))
|
189 |
-
out = self.conv(out)
|
190 |
-
out = self.norm(out)
|
191 |
-
out = F.relu(out)
|
192 |
-
return out
|
193 |
-
|
194 |
-
|
195 |
-
class DownBlock2d(nn.Module):
|
196 |
-
"""
|
197 |
-
Downsampling block for use in encoder.
|
198 |
-
"""
|
199 |
-
|
200 |
-
def __init__(self, in_features, out_features, kernel_size=3, padding=1, groups=1):
|
201 |
-
super(DownBlock2d, self).__init__()
|
202 |
-
self.conv = nn.Conv2d(in_channels=in_features, out_channels=out_features, kernel_size=kernel_size,
|
203 |
-
padding=padding, groups=groups)
|
204 |
-
self.norm = BatchNorm2d(out_features, affine=True)
|
205 |
-
self.pool = nn.AvgPool2d(kernel_size=(2, 2))
|
206 |
-
|
207 |
-
def forward(self, x):
|
208 |
-
out = self.conv(x)
|
209 |
-
out = self.norm(out)
|
210 |
-
out = F.relu(out)
|
211 |
-
out = self.pool(out)
|
212 |
-
return out
|
213 |
-
|
214 |
-
|
215 |
-
class DownBlock3d(nn.Module):
|
216 |
-
"""
|
217 |
-
Downsampling block for use in encoder.
|
218 |
-
"""
|
219 |
-
|
220 |
-
def __init__(self, in_features, out_features, kernel_size=3, padding=1, groups=1):
|
221 |
-
super(DownBlock3d, self).__init__()
|
222 |
-
'''
|
223 |
-
self.conv = nn.Conv3d(in_channels=in_features, out_channels=out_features, kernel_size=kernel_size,
|
224 |
-
padding=padding, groups=groups, stride=(1, 2, 2))
|
225 |
-
'''
|
226 |
-
self.conv = nn.Conv3d(in_channels=in_features, out_channels=out_features, kernel_size=kernel_size,
|
227 |
-
padding=padding, groups=groups)
|
228 |
-
self.norm = BatchNorm3d(out_features, affine=True)
|
229 |
-
self.pool = nn.AvgPool3d(kernel_size=(1, 2, 2))
|
230 |
-
|
231 |
-
def forward(self, x):
|
232 |
-
out = self.conv(x)
|
233 |
-
out = self.norm(out)
|
234 |
-
out = F.relu(out)
|
235 |
-
out = self.pool(out)
|
236 |
-
return out
|
237 |
-
|
238 |
-
|
239 |
-
class SameBlock2d(nn.Module):
|
240 |
-
"""
|
241 |
-
Simple block, preserve spatial resolution.
|
242 |
-
"""
|
243 |
-
|
244 |
-
def __init__(self, in_features, out_features, groups=1, kernel_size=3, padding=1, lrelu=False):
|
245 |
-
super(SameBlock2d, self).__init__()
|
246 |
-
self.conv = nn.Conv2d(in_channels=in_features, out_channels=out_features,
|
247 |
-
kernel_size=kernel_size, padding=padding, groups=groups)
|
248 |
-
self.norm = BatchNorm2d(out_features, affine=True)
|
249 |
-
if lrelu:
|
250 |
-
self.ac = nn.LeakyReLU()
|
251 |
-
else:
|
252 |
-
self.ac = nn.ReLU()
|
253 |
-
|
254 |
-
def forward(self, x):
|
255 |
-
out = self.conv(x)
|
256 |
-
out = self.norm(out)
|
257 |
-
out = self.ac(out)
|
258 |
-
return out
|
259 |
-
|
260 |
-
|
261 |
-
class Encoder(nn.Module):
|
262 |
-
"""
|
263 |
-
Hourglass Encoder
|
264 |
-
"""
|
265 |
-
|
266 |
-
def __init__(self, block_expansion, in_features, num_blocks=3, max_features=256):
|
267 |
-
super(Encoder, self).__init__()
|
268 |
-
|
269 |
-
down_blocks = []
|
270 |
-
for i in range(num_blocks):
|
271 |
-
down_blocks.append(DownBlock3d(in_features if i == 0 else min(max_features, block_expansion * (2 ** i)),
|
272 |
-
min(max_features, block_expansion * (2 ** (i + 1))),
|
273 |
-
kernel_size=3, padding=1))
|
274 |
-
self.down_blocks = nn.ModuleList(down_blocks)
|
275 |
-
|
276 |
-
def forward(self, x):
|
277 |
-
outs = [x]
|
278 |
-
for down_block in self.down_blocks:
|
279 |
-
outs.append(down_block(outs[-1]))
|
280 |
-
return outs
|
281 |
-
|
282 |
-
|
283 |
-
class Decoder(nn.Module):
|
284 |
-
"""
|
285 |
-
Hourglass Decoder
|
286 |
-
"""
|
287 |
-
|
288 |
-
def __init__(self, block_expansion, in_features, num_blocks=3, max_features=256):
|
289 |
-
super(Decoder, self).__init__()
|
290 |
-
|
291 |
-
up_blocks = []
|
292 |
-
|
293 |
-
for i in range(num_blocks)[::-1]:
|
294 |
-
in_filters = (1 if i == num_blocks - 1 else 2) * min(max_features, block_expansion * (2 ** (i + 1)))
|
295 |
-
out_filters = min(max_features, block_expansion * (2 ** i))
|
296 |
-
up_blocks.append(UpBlock3d(in_filters, out_filters, kernel_size=3, padding=1))
|
297 |
-
|
298 |
-
self.up_blocks = nn.ModuleList(up_blocks)
|
299 |
-
# self.out_filters = block_expansion
|
300 |
-
self.out_filters = block_expansion + in_features
|
301 |
-
|
302 |
-
self.conv = nn.Conv3d(in_channels=self.out_filters, out_channels=self.out_filters, kernel_size=3, padding=1)
|
303 |
-
self.norm = BatchNorm3d(self.out_filters, affine=True)
|
304 |
-
|
305 |
-
def forward(self, x):
|
306 |
-
out = x.pop()
|
307 |
-
# for up_block in self.up_blocks[:-1]:
|
308 |
-
for up_block in self.up_blocks:
|
309 |
-
out = up_block(out)
|
310 |
-
skip = x.pop()
|
311 |
-
out = torch.cat([out, skip], dim=1)
|
312 |
-
# out = self.up_blocks[-1](out)
|
313 |
-
out = self.conv(out)
|
314 |
-
out = self.norm(out)
|
315 |
-
out = F.relu(out)
|
316 |
-
return out
|
317 |
-
|
318 |
-
|
319 |
-
class Hourglass(nn.Module):
|
320 |
-
"""
|
321 |
-
Hourglass architecture.
|
322 |
-
"""
|
323 |
-
|
324 |
-
def __init__(self, block_expansion, in_features, num_blocks=3, max_features=256):
|
325 |
-
super(Hourglass, self).__init__()
|
326 |
-
self.encoder = Encoder(block_expansion, in_features, num_blocks, max_features)
|
327 |
-
self.decoder = Decoder(block_expansion, in_features, num_blocks, max_features)
|
328 |
-
self.out_filters = self.decoder.out_filters
|
329 |
-
|
330 |
-
def forward(self, x):
|
331 |
-
return self.decoder(self.encoder(x))
|
332 |
-
|
333 |
-
|
334 |
-
class KPHourglass(nn.Module):
|
335 |
-
"""
|
336 |
-
Hourglass architecture.
|
337 |
-
"""
|
338 |
-
|
339 |
-
def __init__(self, block_expansion, in_features, reshape_features, reshape_depth, num_blocks=3, max_features=256):
|
340 |
-
super(KPHourglass, self).__init__()
|
341 |
-
|
342 |
-
self.down_blocks = nn.Sequential()
|
343 |
-
for i in range(num_blocks):
|
344 |
-
self.down_blocks.add_module('down'+ str(i), DownBlock2d(in_features if i == 0 else min(max_features, block_expansion * (2 ** i)),
|
345 |
-
min(max_features, block_expansion * (2 ** (i + 1))),
|
346 |
-
kernel_size=3, padding=1))
|
347 |
-
|
348 |
-
in_filters = min(max_features, block_expansion * (2 ** num_blocks))
|
349 |
-
self.conv = nn.Conv2d(in_channels=in_filters, out_channels=reshape_features, kernel_size=1)
|
350 |
-
|
351 |
-
self.up_blocks = nn.Sequential()
|
352 |
-
for i in range(num_blocks):
|
353 |
-
in_filters = min(max_features, block_expansion * (2 ** (num_blocks - i)))
|
354 |
-
out_filters = min(max_features, block_expansion * (2 ** (num_blocks - i - 1)))
|
355 |
-
self.up_blocks.add_module('up'+ str(i), UpBlock3d(in_filters, out_filters, kernel_size=3, padding=1))
|
356 |
-
|
357 |
-
self.reshape_depth = reshape_depth
|
358 |
-
self.out_filters = out_filters
|
359 |
-
|
360 |
-
def forward(self, x):
|
361 |
-
out = self.down_blocks(x)
|
362 |
-
out = self.conv(out)
|
363 |
-
bs, c, h, w = out.shape
|
364 |
-
out = out.view(bs, c//self.reshape_depth, self.reshape_depth, h, w)
|
365 |
-
out = self.up_blocks(out)
|
366 |
-
|
367 |
-
return out
|
368 |
-
|
369 |
-
|
370 |
-
|
371 |
-
class AntiAliasInterpolation2d(nn.Module):
|
372 |
-
"""
|
373 |
-
Band-limited downsampling, for better preservation of the input signal.
|
374 |
-
"""
|
375 |
-
def __init__(self, channels, scale):
|
376 |
-
super(AntiAliasInterpolation2d, self).__init__()
|
377 |
-
sigma = (1 / scale - 1) / 2
|
378 |
-
kernel_size = 2 * round(sigma * 4) + 1
|
379 |
-
self.ka = kernel_size // 2
|
380 |
-
self.kb = self.ka - 1 if kernel_size % 2 == 0 else self.ka
|
381 |
-
|
382 |
-
kernel_size = [kernel_size, kernel_size]
|
383 |
-
sigma = [sigma, sigma]
|
384 |
-
# The gaussian kernel is the product of the
|
385 |
-
# gaussian function of each dimension.
|
386 |
-
kernel = 1
|
387 |
-
meshgrids = torch.meshgrid(
|
388 |
-
[
|
389 |
-
torch.arange(size, dtype=torch.float32)
|
390 |
-
for size in kernel_size
|
391 |
-
]
|
392 |
-
)
|
393 |
-
for size, std, mgrid in zip(kernel_size, sigma, meshgrids):
|
394 |
-
mean = (size - 1) / 2
|
395 |
-
kernel *= torch.exp(-(mgrid - mean) ** 2 / (2 * std ** 2))
|
396 |
-
|
397 |
-
# Make sure sum of values in gaussian kernel equals 1.
|
398 |
-
kernel = kernel / torch.sum(kernel)
|
399 |
-
# Reshape to depthwise convolutional weight
|
400 |
-
kernel = kernel.view(1, 1, *kernel.size())
|
401 |
-
kernel = kernel.repeat(channels, *[1] * (kernel.dim() - 1))
|
402 |
-
|
403 |
-
self.register_buffer('weight', kernel)
|
404 |
-
self.groups = channels
|
405 |
-
self.scale = scale
|
406 |
-
inv_scale = 1 / scale
|
407 |
-
self.int_inv_scale = int(inv_scale)
|
408 |
-
|
409 |
-
def forward(self, input):
|
410 |
-
if self.scale == 1.0:
|
411 |
-
return input
|
412 |
-
|
413 |
-
out = F.pad(input, (self.ka, self.kb, self.ka, self.kb))
|
414 |
-
out = F.conv2d(out, weight=self.weight, groups=self.groups)
|
415 |
-
out = out[:, :, ::self.int_inv_scale, ::self.int_inv_scale]
|
416 |
-
|
417 |
-
return out
|
418 |
-
|
419 |
-
|
420 |
-
class SPADE(nn.Module):
|
421 |
-
def __init__(self, norm_nc, label_nc):
|
422 |
-
super().__init__()
|
423 |
-
|
424 |
-
self.param_free_norm = nn.InstanceNorm2d(norm_nc, affine=False)
|
425 |
-
nhidden = 128
|
426 |
-
|
427 |
-
self.mlp_shared = nn.Sequential(
|
428 |
-
nn.Conv2d(label_nc, nhidden, kernel_size=3, padding=1),
|
429 |
-
nn.ReLU())
|
430 |
-
self.mlp_gamma = nn.Conv2d(nhidden, norm_nc, kernel_size=3, padding=1)
|
431 |
-
self.mlp_beta = nn.Conv2d(nhidden, norm_nc, kernel_size=3, padding=1)
|
432 |
-
|
433 |
-
def forward(self, x, segmap):
|
434 |
-
normalized = self.param_free_norm(x)
|
435 |
-
segmap = F.interpolate(segmap, size=x.size()[2:], mode='nearest')
|
436 |
-
actv = self.mlp_shared(segmap)
|
437 |
-
gamma = self.mlp_gamma(actv)
|
438 |
-
beta = self.mlp_beta(actv)
|
439 |
-
out = normalized * (1 + gamma) + beta
|
440 |
-
return out
|
441 |
-
|
442 |
-
|
443 |
-
class SPADEResnetBlock(nn.Module):
|
444 |
-
def __init__(self, fin, fout, norm_G, label_nc, use_se=False, dilation=1):
|
445 |
-
super().__init__()
|
446 |
-
# Attributes
|
447 |
-
self.learned_shortcut = (fin != fout)
|
448 |
-
fmiddle = min(fin, fout)
|
449 |
-
self.use_se = use_se
|
450 |
-
# create conv layers
|
451 |
-
self.conv_0 = nn.Conv2d(fin, fmiddle, kernel_size=3, padding=dilation, dilation=dilation)
|
452 |
-
self.conv_1 = nn.Conv2d(fmiddle, fout, kernel_size=3, padding=dilation, dilation=dilation)
|
453 |
-
if self.learned_shortcut:
|
454 |
-
self.conv_s = nn.Conv2d(fin, fout, kernel_size=1, bias=False)
|
455 |
-
# apply spectral norm if specified
|
456 |
-
if 'spectral' in norm_G:
|
457 |
-
self.conv_0 = spectral_norm(self.conv_0)
|
458 |
-
self.conv_1 = spectral_norm(self.conv_1)
|
459 |
-
if self.learned_shortcut:
|
460 |
-
self.conv_s = spectral_norm(self.conv_s)
|
461 |
-
# define normalization layers
|
462 |
-
self.norm_0 = SPADE(fin, label_nc)
|
463 |
-
self.norm_1 = SPADE(fmiddle, label_nc)
|
464 |
-
if self.learned_shortcut:
|
465 |
-
self.norm_s = SPADE(fin, label_nc)
|
466 |
-
|
467 |
-
def forward(self, x, seg1):
|
468 |
-
x_s = self.shortcut(x, seg1)
|
469 |
-
dx = self.conv_0(self.actvn(self.norm_0(x, seg1)))
|
470 |
-
dx = self.conv_1(self.actvn(self.norm_1(dx, seg1)))
|
471 |
-
out = x_s + dx
|
472 |
-
return out
|
473 |
-
|
474 |
-
def shortcut(self, x, seg1):
|
475 |
-
if self.learned_shortcut:
|
476 |
-
x_s = self.conv_s(self.norm_s(x, seg1))
|
477 |
-
else:
|
478 |
-
x_s = x
|
479 |
-
return x_s
|
480 |
-
|
481 |
-
def actvn(self, x):
|
482 |
-
return F.leaky_relu(x, 2e-1)
|
483 |
-
|
484 |
-
class audio2image(nn.Module):
|
485 |
-
def __init__(self, generator, kp_extractor, he_estimator_video, he_estimator_audio, train_params):
|
486 |
-
super().__init__()
|
487 |
-
# Attributes
|
488 |
-
self.generator = generator
|
489 |
-
self.kp_extractor = kp_extractor
|
490 |
-
self.he_estimator_video = he_estimator_video
|
491 |
-
self.he_estimator_audio = he_estimator_audio
|
492 |
-
self.train_params = train_params
|
493 |
-
|
494 |
-
def headpose_pred_to_degree(self, pred):
|
495 |
-
device = pred.device
|
496 |
-
idx_tensor = [idx for idx in range(66)]
|
497 |
-
idx_tensor = torch.FloatTensor(idx_tensor).to(device)
|
498 |
-
pred = F.softmax(pred)
|
499 |
-
degree = torch.sum(pred*idx_tensor, 1) * 3 - 99
|
500 |
-
|
501 |
-
return degree
|
502 |
-
|
503 |
-
def get_rotation_matrix(self, yaw, pitch, roll):
|
504 |
-
yaw = yaw / 180 * 3.14
|
505 |
-
pitch = pitch / 180 * 3.14
|
506 |
-
roll = roll / 180 * 3.14
|
507 |
-
|
508 |
-
roll = roll.unsqueeze(1)
|
509 |
-
pitch = pitch.unsqueeze(1)
|
510 |
-
yaw = yaw.unsqueeze(1)
|
511 |
-
|
512 |
-
roll_mat = torch.cat([torch.ones_like(roll), torch.zeros_like(roll), torch.zeros_like(roll),
|
513 |
-
torch.zeros_like(roll), torch.cos(roll), -torch.sin(roll),
|
514 |
-
torch.zeros_like(roll), torch.sin(roll), torch.cos(roll)], dim=1)
|
515 |
-
roll_mat = roll_mat.view(roll_mat.shape[0], 3, 3)
|
516 |
-
|
517 |
-
pitch_mat = torch.cat([torch.cos(pitch), torch.zeros_like(pitch), torch.sin(pitch),
|
518 |
-
torch.zeros_like(pitch), torch.ones_like(pitch), torch.zeros_like(pitch),
|
519 |
-
-torch.sin(pitch), torch.zeros_like(pitch), torch.cos(pitch)], dim=1)
|
520 |
-
pitch_mat = pitch_mat.view(pitch_mat.shape[0], 3, 3)
|
521 |
-
|
522 |
-
yaw_mat = torch.cat([torch.cos(yaw), -torch.sin(yaw), torch.zeros_like(yaw),
|
523 |
-
torch.sin(yaw), torch.cos(yaw), torch.zeros_like(yaw),
|
524 |
-
torch.zeros_like(yaw), torch.zeros_like(yaw), torch.ones_like(yaw)], dim=1)
|
525 |
-
yaw_mat = yaw_mat.view(yaw_mat.shape[0], 3, 3)
|
526 |
-
|
527 |
-
rot_mat = torch.einsum('bij,bjk,bkm->bim', roll_mat, pitch_mat, yaw_mat)
|
528 |
-
|
529 |
-
return rot_mat
|
530 |
-
|
531 |
-
def keypoint_transformation(self, kp_canonical, he):
|
532 |
-
kp = kp_canonical['value'] # (bs, k, 3)
|
533 |
-
yaw, pitch, roll = he['yaw'], he['pitch'], he['roll']
|
534 |
-
t, exp = he['t'], he['exp']
|
535 |
-
|
536 |
-
yaw = self.headpose_pred_to_degree(yaw)
|
537 |
-
pitch = self.headpose_pred_to_degree(pitch)
|
538 |
-
roll = self.headpose_pred_to_degree(roll)
|
539 |
-
|
540 |
-
rot_mat = self.get_rotation_matrix(yaw, pitch, roll) # (bs, 3, 3)
|
541 |
-
|
542 |
-
# keypoint rotation
|
543 |
-
kp_rotated = torch.einsum('bmp,bkp->bkm', rot_mat, kp)
|
544 |
-
|
545 |
-
|
546 |
-
|
547 |
-
# keypoint translation
|
548 |
-
t = t.unsqueeze_(1).repeat(1, kp.shape[1], 1)
|
549 |
-
kp_t = kp_rotated + t
|
550 |
-
|
551 |
-
# add expression deviation
|
552 |
-
exp = exp.view(exp.shape[0], -1, 3)
|
553 |
-
kp_transformed = kp_t + exp
|
554 |
-
|
555 |
-
return {'value': kp_transformed}
|
556 |
-
|
557 |
-
def forward(self, source_image, target_audio):
|
558 |
-
pose_source = self.he_estimator_video(source_image)
|
559 |
-
pose_generated = self.he_estimator_audio(target_audio)
|
560 |
-
kp_canonical = self.kp_extractor(source_image)
|
561 |
-
kp_source = self.keypoint_transformation(kp_canonical, pose_source)
|
562 |
-
kp_transformed_generated = self.keypoint_transformation(kp_canonical, pose_generated)
|
563 |
-
generated = self.generator(source_image, kp_source=kp_source, kp_driving=kp_transformed_generated)
|
564 |
-
return generated
|
|
|
|
|
|
|
|
|
|
|
|
|
|
|
|
|
|
|
|
|
|
|
|
|
|
|
|
|
|
|
|
|
|
|
|
|
|
|
|
|
|
|
|
|
|
|
|
|
|
|
|
|
|
|
|
|
|
|
|
|
|
|
|
|
|
|
|
|
|
|
|
|
|
|
|
|
|
|
|
|
|
|
|
|
|
|
|
|
|
|
|
|
|
|
|
|
|
|
|
|
|
|
|
|
|
|
|
|
|
|
|
|
|
|
|
|
|
|
|
|
|
|
|
|
|
|
|
|
|
|
|
|
|
|
|
|
|
|
|
|
|
|
|
|
|
|
|
|
|
|
|
|
|
|
|
|
|
|
|
|
|
|
|
|
|
|
|
|
|
|
|
|
|
|
|
|
|
|
|
|
|
|
|
|
|
|
|
|
|
|
|
|
|
|
|
|
|
|
|
|
|
|
|
|
|
|
|
|
|
|
|
|
|
|
|
|
|
|
|
|
|
|
|
|
|
|
|
|
|
|
|
|
|
|
|
|
|
|
|
|
|
|
|
|
|
|
|
|
|
|
|
|
|
|
|
|
|
|
|
|
|
|
|
|
|
|
|
|
|
|
|
|
|
|
|
|
|
|
|
|
|
|
|
|
|
|
|
|
|
|
|
|
|
|
|
|
|
|
|
|
|
|
|
|
|
|
|
|
|
|
|
|
|
|
|
|
|
|
|
|
|
|
|
|
|
|
|
|
|
|
|
|
|
|
|
|
|
|
|
|
|
|
|
|
|
|
|
|
|
|
|
|
|
|
|
|
|
|
|
|
|
|
|
|
|
|
|
|
|
|
|
|
|
|
|
|
|
|
|
|
|
|
|
|
|
|
|
|
|
|
|
|
|
|
|
|
|
|
|
|
|
|
|
|
|
|
|
|
|
|
|
|
|
|
|
|
|
|
|
|
|
|
|
|
|
|
|
|
|
|
|
|
|
|
|
|
|
|
|
|
|
|
|
|
|
|
|
|
|
|
|
|
|
|
|
|
|
|
|
|
|
|
|
|
|
|
|
|
|
|
|
|
|
|
|
|
|
|
|
|
|
|
|
|
|
|
|
|
|
|
|
|
|
|
|
|
|
|
|
|
|
|
|
|
|
|
|
|
|
|
|
|
|
|
|
|
|
|
|
|
|
|
|
|
|
|
|
|
|
|
|
|
|
|
|
|
|
|
|
|
|
|
|
|
|
|
|
|
|
|
|
|
|
|
|
|
|
|
|
|
|
|
|
|
|
|
|
|
|
|
|
|
|
|
|
|
|
|
|
|
|
|
|
|
|
|
|
|
|
|
|
|
|
|
|
|
|
|
|
|
|
|
|
|
|
|
|
|
|
|
|
|
|
|
|
|
|
|
|
|
|
|
|
|
|
|
|
|
|
|
|
|
|
|
|
|
|
|
|
|
|
|
|
|
|
|
|
|
|
|
|
|
|
|
|
|
|
|
|
|
|
|
|
|
|
|
|
|
|
|
|
|
|
|
|
|
|
|
|
|
|
|
|
|
|
|
|
|
|
|
|
|
|
|
|
|
|
|
|
|
|
|
|
|
|
|
|
|
|
|
|
|
|
|
|
|
|
|
|
|
|
|
|
|
|
|
|
|
|
|
|
|
|
|
|
|
|
|
|
|
|
|
|
|
|
|
|
|
|
|
|
|
|
|
|
|
|
|
|
|
|
|
|
|
|
|
|
|
|
|
|
|
|
|
|
|
|
|
|
|
|
|
|
|
|
|
|
|
|
|
|
|
|
|
|
|
|
|
|
|
|
|
|
|
|
|
|
|
|
|
|
|
|
|
|
|
|
|
|
|
|
|
|
|
|
|
|
|
|
|
|
|
|
|
|
|
|
|
|
|
|
|
|
|
|
|
|
|
|
|
|
|
|
|
|
|
|
|
|
|
|
|
|
|
|
|
|
|
|
|
|
|
|
|
|
|
|
|
|
|
|
|
|
|
|
|
|
|
|
|
|
|
|
|
|
|
|
|
|
|
|
|
|
|
|
|
|
|
|
|
|
|
|
|
|
|
|
|
|
|
|
|
|
|
|
|
|
|
|
|
|
|
|
|
|
|
|
|
|
|
|
|
|
|
|
|
|
|
|
|
|
|
|
|
|
|
|
|
|
|
|
|
|
|
|
|
|
|
|
|
|
|
|
|
|
|
|
|
|
|
|
|
|
|
|
|
|
|
|
|
|
|
|
|
|
|
|
|
|
|
|
|
|
|
|
|
|
|
|
|
|
|
|
|
|
|
|
|
|
|
|
|
|
|
|
|
|
|
|
|
|
|
|
|
|
|
|
|
|
|
|
|
|
|
|
|
|
|
|
|
|
|
|
|
|
|
|
|
|
|
|
|
|
|
|
|
|
|
|
|
|
|
|
|
|
|
|
|
|
|
|
|
|
|
|
|
|
|
|
|
|
|
|
|
|
|
|
|
|
|
|
|
|
|
|
|
|
|
|
|
|
|
|
|
|
|
|
|
|
|
|
|
|
|
|
|
|
|
|
spaces/Androidonnxfork/CivitAi-to-Diffusers/diffusers/src/diffusers/models/controlnet_flax.py
DELETED
@@ -1,394 +0,0 @@
|
|
1 |
-
# Copyright 2023 The HuggingFace Team. All rights reserved.
|
2 |
-
#
|
3 |
-
# Licensed under the Apache License, Version 2.0 (the "License");
|
4 |
-
# you may not use this file except in compliance with the License.
|
5 |
-
# You may obtain a copy of the License at
|
6 |
-
#
|
7 |
-
# http://www.apache.org/licenses/LICENSE-2.0
|
8 |
-
#
|
9 |
-
# Unless required by applicable law or agreed to in writing, software
|
10 |
-
# distributed under the License is distributed on an "AS IS" BASIS,
|
11 |
-
# WITHOUT WARRANTIES OR CONDITIONS OF ANY KIND, either express or implied.
|
12 |
-
# See the License for the specific language governing permissions and
|
13 |
-
# limitations under the License.
|
14 |
-
from typing import Optional, Tuple, Union
|
15 |
-
|
16 |
-
import flax
|
17 |
-
import flax.linen as nn
|
18 |
-
import jax
|
19 |
-
import jax.numpy as jnp
|
20 |
-
from flax.core.frozen_dict import FrozenDict
|
21 |
-
|
22 |
-
from ..configuration_utils import ConfigMixin, flax_register_to_config
|
23 |
-
from ..utils import BaseOutput
|
24 |
-
from .embeddings_flax import FlaxTimestepEmbedding, FlaxTimesteps
|
25 |
-
from .modeling_flax_utils import FlaxModelMixin
|
26 |
-
from .unet_2d_blocks_flax import (
|
27 |
-
FlaxCrossAttnDownBlock2D,
|
28 |
-
FlaxDownBlock2D,
|
29 |
-
FlaxUNetMidBlock2DCrossAttn,
|
30 |
-
)
|
31 |
-
|
32 |
-
|
33 |
-
@flax.struct.dataclass
|
34 |
-
class FlaxControlNetOutput(BaseOutput):
|
35 |
-
"""
|
36 |
-
The output of [`FlaxControlNetModel`].
|
37 |
-
|
38 |
-
Args:
|
39 |
-
down_block_res_samples (`jnp.ndarray`):
|
40 |
-
mid_block_res_sample (`jnp.ndarray`):
|
41 |
-
"""
|
42 |
-
|
43 |
-
down_block_res_samples: jnp.ndarray
|
44 |
-
mid_block_res_sample: jnp.ndarray
|
45 |
-
|
46 |
-
|
47 |
-
class FlaxControlNetConditioningEmbedding(nn.Module):
|
48 |
-
conditioning_embedding_channels: int
|
49 |
-
block_out_channels: Tuple[int] = (16, 32, 96, 256)
|
50 |
-
dtype: jnp.dtype = jnp.float32
|
51 |
-
|
52 |
-
def setup(self):
|
53 |
-
self.conv_in = nn.Conv(
|
54 |
-
self.block_out_channels[0],
|
55 |
-
kernel_size=(3, 3),
|
56 |
-
padding=((1, 1), (1, 1)),
|
57 |
-
dtype=self.dtype,
|
58 |
-
)
|
59 |
-
|
60 |
-
blocks = []
|
61 |
-
for i in range(len(self.block_out_channels) - 1):
|
62 |
-
channel_in = self.block_out_channels[i]
|
63 |
-
channel_out = self.block_out_channels[i + 1]
|
64 |
-
conv1 = nn.Conv(
|
65 |
-
channel_in,
|
66 |
-
kernel_size=(3, 3),
|
67 |
-
padding=((1, 1), (1, 1)),
|
68 |
-
dtype=self.dtype,
|
69 |
-
)
|
70 |
-
blocks.append(conv1)
|
71 |
-
conv2 = nn.Conv(
|
72 |
-
channel_out,
|
73 |
-
kernel_size=(3, 3),
|
74 |
-
strides=(2, 2),
|
75 |
-
padding=((1, 1), (1, 1)),
|
76 |
-
dtype=self.dtype,
|
77 |
-
)
|
78 |
-
blocks.append(conv2)
|
79 |
-
self.blocks = blocks
|
80 |
-
|
81 |
-
self.conv_out = nn.Conv(
|
82 |
-
self.conditioning_embedding_channels,
|
83 |
-
kernel_size=(3, 3),
|
84 |
-
padding=((1, 1), (1, 1)),
|
85 |
-
kernel_init=nn.initializers.zeros_init(),
|
86 |
-
bias_init=nn.initializers.zeros_init(),
|
87 |
-
dtype=self.dtype,
|
88 |
-
)
|
89 |
-
|
90 |
-
def __call__(self, conditioning):
|
91 |
-
embedding = self.conv_in(conditioning)
|
92 |
-
embedding = nn.silu(embedding)
|
93 |
-
|
94 |
-
for block in self.blocks:
|
95 |
-
embedding = block(embedding)
|
96 |
-
embedding = nn.silu(embedding)
|
97 |
-
|
98 |
-
embedding = self.conv_out(embedding)
|
99 |
-
|
100 |
-
return embedding
|
101 |
-
|
102 |
-
|
103 |
-
@flax_register_to_config
|
104 |
-
class FlaxControlNetModel(nn.Module, FlaxModelMixin, ConfigMixin):
|
105 |
-
r"""
|
106 |
-
A ControlNet model.
|
107 |
-
|
108 |
-
This model inherits from [`FlaxModelMixin`]. Check the superclass documentation for it’s generic methods
|
109 |
-
implemented for all models (such as downloading or saving).
|
110 |
-
|
111 |
-
This model is also a Flax Linen [`flax.linen.Module`](https://flax.readthedocs.io/en/latest/flax.linen.html#module)
|
112 |
-
subclass. Use it as a regular Flax Linen module and refer to the Flax documentation for all matters related to its
|
113 |
-
general usage and behavior.
|
114 |
-
|
115 |
-
Inherent JAX features such as the following are supported:
|
116 |
-
|
117 |
-
- [Just-In-Time (JIT) compilation](https://jax.readthedocs.io/en/latest/jax.html#just-in-time-compilation-jit)
|
118 |
-
- [Automatic Differentiation](https://jax.readthedocs.io/en/latest/jax.html#automatic-differentiation)
|
119 |
-
- [Vectorization](https://jax.readthedocs.io/en/latest/jax.html#vectorization-vmap)
|
120 |
-
- [Parallelization](https://jax.readthedocs.io/en/latest/jax.html#parallelization-pmap)
|
121 |
-
|
122 |
-
Parameters:
|
123 |
-
sample_size (`int`, *optional*):
|
124 |
-
The size of the input sample.
|
125 |
-
in_channels (`int`, *optional*, defaults to 4):
|
126 |
-
The number of channels in the input sample.
|
127 |
-
down_block_types (`Tuple[str]`, *optional*, defaults to `("FlaxCrossAttnDownBlock2D", "FlaxCrossAttnDownBlock2D", "FlaxCrossAttnDownBlock2D", "FlaxDownBlock2D")`):
|
128 |
-
The tuple of downsample blocks to use.
|
129 |
-
block_out_channels (`Tuple[int]`, *optional*, defaults to `(320, 640, 1280, 1280)`):
|
130 |
-
The tuple of output channels for each block.
|
131 |
-
layers_per_block (`int`, *optional*, defaults to 2):
|
132 |
-
The number of layers per block.
|
133 |
-
attention_head_dim (`int` or `Tuple[int]`, *optional*, defaults to 8):
|
134 |
-
The dimension of the attention heads.
|
135 |
-
num_attention_heads (`int` or `Tuple[int]`, *optional*):
|
136 |
-
The number of attention heads.
|
137 |
-
cross_attention_dim (`int`, *optional*, defaults to 768):
|
138 |
-
The dimension of the cross attention features.
|
139 |
-
dropout (`float`, *optional*, defaults to 0):
|
140 |
-
Dropout probability for down, up and bottleneck blocks.
|
141 |
-
flip_sin_to_cos (`bool`, *optional*, defaults to `True`):
|
142 |
-
Whether to flip the sin to cos in the time embedding.
|
143 |
-
freq_shift (`int`, *optional*, defaults to 0): The frequency shift to apply to the time embedding.
|
144 |
-
controlnet_conditioning_channel_order (`str`, *optional*, defaults to `rgb`):
|
145 |
-
The channel order of conditional image. Will convert to `rgb` if it's `bgr`.
|
146 |
-
conditioning_embedding_out_channels (`tuple`, *optional*, defaults to `(16, 32, 96, 256)`):
|
147 |
-
The tuple of output channel for each block in the `conditioning_embedding` layer.
|
148 |
-
"""
|
149 |
-
sample_size: int = 32
|
150 |
-
in_channels: int = 4
|
151 |
-
down_block_types: Tuple[str] = (
|
152 |
-
"CrossAttnDownBlock2D",
|
153 |
-
"CrossAttnDownBlock2D",
|
154 |
-
"CrossAttnDownBlock2D",
|
155 |
-
"DownBlock2D",
|
156 |
-
)
|
157 |
-
only_cross_attention: Union[bool, Tuple[bool]] = False
|
158 |
-
block_out_channels: Tuple[int] = (320, 640, 1280, 1280)
|
159 |
-
layers_per_block: int = 2
|
160 |
-
attention_head_dim: Union[int, Tuple[int]] = 8
|
161 |
-
num_attention_heads: Optional[Union[int, Tuple[int]]] = None
|
162 |
-
cross_attention_dim: int = 1280
|
163 |
-
dropout: float = 0.0
|
164 |
-
use_linear_projection: bool = False
|
165 |
-
dtype: jnp.dtype = jnp.float32
|
166 |
-
flip_sin_to_cos: bool = True
|
167 |
-
freq_shift: int = 0
|
168 |
-
controlnet_conditioning_channel_order: str = "rgb"
|
169 |
-
conditioning_embedding_out_channels: Tuple[int] = (16, 32, 96, 256)
|
170 |
-
|
171 |
-
def init_weights(self, rng: jax.random.KeyArray) -> FrozenDict:
|
172 |
-
# init input tensors
|
173 |
-
sample_shape = (1, self.in_channels, self.sample_size, self.sample_size)
|
174 |
-
sample = jnp.zeros(sample_shape, dtype=jnp.float32)
|
175 |
-
timesteps = jnp.ones((1,), dtype=jnp.int32)
|
176 |
-
encoder_hidden_states = jnp.zeros((1, 1, self.cross_attention_dim), dtype=jnp.float32)
|
177 |
-
controlnet_cond_shape = (1, 3, self.sample_size * 8, self.sample_size * 8)
|
178 |
-
controlnet_cond = jnp.zeros(controlnet_cond_shape, dtype=jnp.float32)
|
179 |
-
|
180 |
-
params_rng, dropout_rng = jax.random.split(rng)
|
181 |
-
rngs = {"params": params_rng, "dropout": dropout_rng}
|
182 |
-
|
183 |
-
return self.init(rngs, sample, timesteps, encoder_hidden_states, controlnet_cond)["params"]
|
184 |
-
|
185 |
-
def setup(self):
|
186 |
-
block_out_channels = self.block_out_channels
|
187 |
-
time_embed_dim = block_out_channels[0] * 4
|
188 |
-
|
189 |
-
# If `num_attention_heads` is not defined (which is the case for most models)
|
190 |
-
# it will default to `attention_head_dim`. This looks weird upon first reading it and it is.
|
191 |
-
# The reason for this behavior is to correct for incorrectly named variables that were introduced
|
192 |
-
# when this library was created. The incorrect naming was only discovered much later in https://github.com/huggingface/diffusers/issues/2011#issuecomment-1547958131
|
193 |
-
# Changing `attention_head_dim` to `num_attention_heads` for 40,000+ configurations is too backwards breaking
|
194 |
-
# which is why we correct for the naming here.
|
195 |
-
num_attention_heads = self.num_attention_heads or self.attention_head_dim
|
196 |
-
|
197 |
-
# input
|
198 |
-
self.conv_in = nn.Conv(
|
199 |
-
block_out_channels[0],
|
200 |
-
kernel_size=(3, 3),
|
201 |
-
strides=(1, 1),
|
202 |
-
padding=((1, 1), (1, 1)),
|
203 |
-
dtype=self.dtype,
|
204 |
-
)
|
205 |
-
|
206 |
-
# time
|
207 |
-
self.time_proj = FlaxTimesteps(
|
208 |
-
block_out_channels[0], flip_sin_to_cos=self.flip_sin_to_cos, freq_shift=self.config.freq_shift
|
209 |
-
)
|
210 |
-
self.time_embedding = FlaxTimestepEmbedding(time_embed_dim, dtype=self.dtype)
|
211 |
-
|
212 |
-
self.controlnet_cond_embedding = FlaxControlNetConditioningEmbedding(
|
213 |
-
conditioning_embedding_channels=block_out_channels[0],
|
214 |
-
block_out_channels=self.conditioning_embedding_out_channels,
|
215 |
-
)
|
216 |
-
|
217 |
-
only_cross_attention = self.only_cross_attention
|
218 |
-
if isinstance(only_cross_attention, bool):
|
219 |
-
only_cross_attention = (only_cross_attention,) * len(self.down_block_types)
|
220 |
-
|
221 |
-
if isinstance(num_attention_heads, int):
|
222 |
-
num_attention_heads = (num_attention_heads,) * len(self.down_block_types)
|
223 |
-
|
224 |
-
# down
|
225 |
-
down_blocks = []
|
226 |
-
controlnet_down_blocks = []
|
227 |
-
|
228 |
-
output_channel = block_out_channels[0]
|
229 |
-
|
230 |
-
controlnet_block = nn.Conv(
|
231 |
-
output_channel,
|
232 |
-
kernel_size=(1, 1),
|
233 |
-
padding="VALID",
|
234 |
-
kernel_init=nn.initializers.zeros_init(),
|
235 |
-
bias_init=nn.initializers.zeros_init(),
|
236 |
-
dtype=self.dtype,
|
237 |
-
)
|
238 |
-
controlnet_down_blocks.append(controlnet_block)
|
239 |
-
|
240 |
-
for i, down_block_type in enumerate(self.down_block_types):
|
241 |
-
input_channel = output_channel
|
242 |
-
output_channel = block_out_channels[i]
|
243 |
-
is_final_block = i == len(block_out_channels) - 1
|
244 |
-
|
245 |
-
if down_block_type == "CrossAttnDownBlock2D":
|
246 |
-
down_block = FlaxCrossAttnDownBlock2D(
|
247 |
-
in_channels=input_channel,
|
248 |
-
out_channels=output_channel,
|
249 |
-
dropout=self.dropout,
|
250 |
-
num_layers=self.layers_per_block,
|
251 |
-
num_attention_heads=num_attention_heads[i],
|
252 |
-
add_downsample=not is_final_block,
|
253 |
-
use_linear_projection=self.use_linear_projection,
|
254 |
-
only_cross_attention=only_cross_attention[i],
|
255 |
-
dtype=self.dtype,
|
256 |
-
)
|
257 |
-
else:
|
258 |
-
down_block = FlaxDownBlock2D(
|
259 |
-
in_channels=input_channel,
|
260 |
-
out_channels=output_channel,
|
261 |
-
dropout=self.dropout,
|
262 |
-
num_layers=self.layers_per_block,
|
263 |
-
add_downsample=not is_final_block,
|
264 |
-
dtype=self.dtype,
|
265 |
-
)
|
266 |
-
|
267 |
-
down_blocks.append(down_block)
|
268 |
-
|
269 |
-
for _ in range(self.layers_per_block):
|
270 |
-
controlnet_block = nn.Conv(
|
271 |
-
output_channel,
|
272 |
-
kernel_size=(1, 1),
|
273 |
-
padding="VALID",
|
274 |
-
kernel_init=nn.initializers.zeros_init(),
|
275 |
-
bias_init=nn.initializers.zeros_init(),
|
276 |
-
dtype=self.dtype,
|
277 |
-
)
|
278 |
-
controlnet_down_blocks.append(controlnet_block)
|
279 |
-
|
280 |
-
if not is_final_block:
|
281 |
-
controlnet_block = nn.Conv(
|
282 |
-
output_channel,
|
283 |
-
kernel_size=(1, 1),
|
284 |
-
padding="VALID",
|
285 |
-
kernel_init=nn.initializers.zeros_init(),
|
286 |
-
bias_init=nn.initializers.zeros_init(),
|
287 |
-
dtype=self.dtype,
|
288 |
-
)
|
289 |
-
controlnet_down_blocks.append(controlnet_block)
|
290 |
-
|
291 |
-
self.down_blocks = down_blocks
|
292 |
-
self.controlnet_down_blocks = controlnet_down_blocks
|
293 |
-
|
294 |
-
# mid
|
295 |
-
mid_block_channel = block_out_channels[-1]
|
296 |
-
self.mid_block = FlaxUNetMidBlock2DCrossAttn(
|
297 |
-
in_channels=mid_block_channel,
|
298 |
-
dropout=self.dropout,
|
299 |
-
num_attention_heads=num_attention_heads[-1],
|
300 |
-
use_linear_projection=self.use_linear_projection,
|
301 |
-
dtype=self.dtype,
|
302 |
-
)
|
303 |
-
|
304 |
-
self.controlnet_mid_block = nn.Conv(
|
305 |
-
mid_block_channel,
|
306 |
-
kernel_size=(1, 1),
|
307 |
-
padding="VALID",
|
308 |
-
kernel_init=nn.initializers.zeros_init(),
|
309 |
-
bias_init=nn.initializers.zeros_init(),
|
310 |
-
dtype=self.dtype,
|
311 |
-
)
|
312 |
-
|
313 |
-
def __call__(
|
314 |
-
self,
|
315 |
-
sample,
|
316 |
-
timesteps,
|
317 |
-
encoder_hidden_states,
|
318 |
-
controlnet_cond,
|
319 |
-
conditioning_scale: float = 1.0,
|
320 |
-
return_dict: bool = True,
|
321 |
-
train: bool = False,
|
322 |
-
) -> Union[FlaxControlNetOutput, Tuple]:
|
323 |
-
r"""
|
324 |
-
Args:
|
325 |
-
sample (`jnp.ndarray`): (batch, channel, height, width) noisy inputs tensor
|
326 |
-
timestep (`jnp.ndarray` or `float` or `int`): timesteps
|
327 |
-
encoder_hidden_states (`jnp.ndarray`): (batch_size, sequence_length, hidden_size) encoder hidden states
|
328 |
-
controlnet_cond (`jnp.ndarray`): (batch, channel, height, width) the conditional input tensor
|
329 |
-
conditioning_scale: (`float`) the scale factor for controlnet outputs
|
330 |
-
return_dict (`bool`, *optional*, defaults to `True`):
|
331 |
-
Whether or not to return a [`models.unet_2d_condition_flax.FlaxUNet2DConditionOutput`] instead of a
|
332 |
-
plain tuple.
|
333 |
-
train (`bool`, *optional*, defaults to `False`):
|
334 |
-
Use deterministic functions and disable dropout when not training.
|
335 |
-
|
336 |
-
Returns:
|
337 |
-
[`~models.unet_2d_condition_flax.FlaxUNet2DConditionOutput`] or `tuple`:
|
338 |
-
[`~models.unet_2d_condition_flax.FlaxUNet2DConditionOutput`] if `return_dict` is True, otherwise a `tuple`.
|
339 |
-
When returning a tuple, the first element is the sample tensor.
|
340 |
-
"""
|
341 |
-
channel_order = self.controlnet_conditioning_channel_order
|
342 |
-
if channel_order == "bgr":
|
343 |
-
controlnet_cond = jnp.flip(controlnet_cond, axis=1)
|
344 |
-
|
345 |
-
# 1. time
|
346 |
-
if not isinstance(timesteps, jnp.ndarray):
|
347 |
-
timesteps = jnp.array([timesteps], dtype=jnp.int32)
|
348 |
-
elif isinstance(timesteps, jnp.ndarray) and len(timesteps.shape) == 0:
|
349 |
-
timesteps = timesteps.astype(dtype=jnp.float32)
|
350 |
-
timesteps = jnp.expand_dims(timesteps, 0)
|
351 |
-
|
352 |
-
t_emb = self.time_proj(timesteps)
|
353 |
-
t_emb = self.time_embedding(t_emb)
|
354 |
-
|
355 |
-
# 2. pre-process
|
356 |
-
sample = jnp.transpose(sample, (0, 2, 3, 1))
|
357 |
-
sample = self.conv_in(sample)
|
358 |
-
|
359 |
-
controlnet_cond = jnp.transpose(controlnet_cond, (0, 2, 3, 1))
|
360 |
-
controlnet_cond = self.controlnet_cond_embedding(controlnet_cond)
|
361 |
-
sample += controlnet_cond
|
362 |
-
|
363 |
-
# 3. down
|
364 |
-
down_block_res_samples = (sample,)
|
365 |
-
for down_block in self.down_blocks:
|
366 |
-
if isinstance(down_block, FlaxCrossAttnDownBlock2D):
|
367 |
-
sample, res_samples = down_block(sample, t_emb, encoder_hidden_states, deterministic=not train)
|
368 |
-
else:
|
369 |
-
sample, res_samples = down_block(sample, t_emb, deterministic=not train)
|
370 |
-
down_block_res_samples += res_samples
|
371 |
-
|
372 |
-
# 4. mid
|
373 |
-
sample = self.mid_block(sample, t_emb, encoder_hidden_states, deterministic=not train)
|
374 |
-
|
375 |
-
# 5. contronet blocks
|
376 |
-
controlnet_down_block_res_samples = ()
|
377 |
-
for down_block_res_sample, controlnet_block in zip(down_block_res_samples, self.controlnet_down_blocks):
|
378 |
-
down_block_res_sample = controlnet_block(down_block_res_sample)
|
379 |
-
controlnet_down_block_res_samples += (down_block_res_sample,)
|
380 |
-
|
381 |
-
down_block_res_samples = controlnet_down_block_res_samples
|
382 |
-
|
383 |
-
mid_block_res_sample = self.controlnet_mid_block(sample)
|
384 |
-
|
385 |
-
# 6. scaling
|
386 |
-
down_block_res_samples = [sample * conditioning_scale for sample in down_block_res_samples]
|
387 |
-
mid_block_res_sample *= conditioning_scale
|
388 |
-
|
389 |
-
if not return_dict:
|
390 |
-
return (down_block_res_samples, mid_block_res_sample)
|
391 |
-
|
392 |
-
return FlaxControlNetOutput(
|
393 |
-
down_block_res_samples=down_block_res_samples, mid_block_res_sample=mid_block_res_sample
|
394 |
-
)
|
|
|
|
|
|
|
|
|
|
|
|
|
|
|
|
|
|
|
|
|
|
|
|
|
|
|
|
|
|
|
|
|
|
|
|
|
|
|
|
|
|
|
|
|
|
|
|
|
|
|
|
|
|
|
|
|
|
|
|
|
|
|
|
|
|
|
|
|
|
|
|
|
|
|
|
|
|
|
|
|
|
|
|
|
|
|
|
|
|
|
|
|
|
|
|
|
|
|
|
|
|
|
|
|
|
|
|
|
|
|
|
|
|
|
|
|
|
|
|
|
|
|
|
|
|
|
|
|
|
|
|
|
|
|
|
|
|
|
|
|
|
|
|
|
|
|
|
|
|
|
|
|
|
|
|
|
|
|
|
|
|
|
|
|
|
|
|
|
|
|
|
|
|
|
|
|
|
|
|
|
|
|
|
|
|
|
|
|
|
|
|
|
|
|
|
|
|
|
|
|
|
|
|
|
|
|
|
|
|
|
|
|
|
|
|
|
|
|
|
|
|
|
|
|
|
|
|
|
|
|
|
|
|
|
|
|
|
|
|
|
|
|
|
|
|
|
|
|
|
|
|
|
|
|
|
|
|
|
|
|
|
|
|
|
|
|
|
|
|
|
|
|
|
|
|
|
|
|
|
|
|
|
|
|
|
|
|
|
|
|
|
|
|
|
|
|
|
|
|
|
|
|
|
|
|
|
|
|
|
|
|
|
|
|
|
|
|
|
|
|
|
|
|
|
|
|
|
|
|
|
|
|
|
|
|
|
|
|
|
|
|
|
|
|
|
|
|
|
|
|
|
|
|
|
|
|
|
|
|
|
|
|
|
|
|
|
|
|
|
|
|
|
|
|
|
|
|
|
|
|
|
|
|
|
|
|
|
|
|
|
|
|
|
|
|
|
|
|
|
|
|
|
|
|
|
|
|
|
|
|
|
|
|
|
|
|
|
|
|
|
|
|
|
|
|
|
|
|
|
|
|
|
|
|
|
|
|
|
|
|
|
|
|
|
|
|
|
|
|
|
|
|
|
|
|
|
|
|
|
|
|
|
|
|
|
|
|
|
|
|
|
|
|
|
|
|
|
|
|
|
|
|
|
|
|
|
|
|
|
|
|
|
|
|
|
|
|
|
|
|
|
|
|
|
|
|
|
|
|
|
|
|
|
|
|
|
|
|
|
|
|
|
|
|
|
|
|
|
|
|
|
|
|
|
|
|
|
|
|
|
|
|
|
|
|
|
|
|
|
|
|
|
|
|
|
|
|
|
|
|
|
|
|
|
|
|
|
|
|
|
|
|
|
|
|
|
|
|
|
|
|
|
|
|
|
|
|
|
|
|
|
|
|
|
|
|
|
|
|
|
|
|
|
|
|
|
|
|
|
|
|
|
|
|
|
|
|
|
|
|
|
|
|
|
|
|
|
|
|
|
|
|
|
|
|
|
|
|
|
|
|
|
|
|
|
|
|
|
|
|
|
|
|
|
|
|
|
|
|
|
|
|
|
|
|
|
|
|
|
|
|
|
|
|
|
|
|
|
|
|
|
|
|
|
|
|
|
|
|
|
|
|
|
|
|
|
|
|
|
|
|
|
|
|
|
|
|
|
|
|
|
|
|
|
|
|
|
|
|
|
|
|
|
|
|
|
|
|
|
|
|
|
|
|
|
|
|
|
|
|
|
|
|
|
|
|
|
|
|
|
|
|
|
|
|
|
|
|
|
|
|
|
|
|
|
|
|
|
|
|
|
|
|
|
|
|
|
|
|
|
|
|
|
|
|
|
|
|
|
spaces/Androidonnxfork/CivitAi-to-Diffusers/diffusers/tests/pipelines/stable_diffusion/test_onnx_stable_diffusion_inpaint_legacy.py
DELETED
@@ -1,97 +0,0 @@
|
|
1 |
-
# coding=utf-8
|
2 |
-
# Copyright 2023 HuggingFace Inc.
|
3 |
-
#
|
4 |
-
# Licensed under the Apache License, Version 2.0 (the "License");
|
5 |
-
# you may not use this file except in compliance with the License.
|
6 |
-
# You may obtain a copy of the License at
|
7 |
-
#
|
8 |
-
# http://www.apache.org/licenses/LICENSE-2.0
|
9 |
-
#
|
10 |
-
# Unless required by applicable law or agreed to in writing, software
|
11 |
-
# distributed under the License is distributed on an "AS IS" BASIS,
|
12 |
-
# WITHOUT WARRANTIES OR CONDITIONS OF ANY KIND, either express or implied.
|
13 |
-
# See the License for the specific language governing permissions and
|
14 |
-
# limitations under the License.
|
15 |
-
|
16 |
-
import unittest
|
17 |
-
|
18 |
-
import numpy as np
|
19 |
-
|
20 |
-
from diffusers import OnnxStableDiffusionInpaintPipelineLegacy
|
21 |
-
from diffusers.utils.testing_utils import (
|
22 |
-
is_onnx_available,
|
23 |
-
load_image,
|
24 |
-
load_numpy,
|
25 |
-
nightly,
|
26 |
-
require_onnxruntime,
|
27 |
-
require_torch_gpu,
|
28 |
-
)
|
29 |
-
|
30 |
-
|
31 |
-
if is_onnx_available():
|
32 |
-
import onnxruntime as ort
|
33 |
-
|
34 |
-
|
35 |
-
@nightly
|
36 |
-
@require_onnxruntime
|
37 |
-
@require_torch_gpu
|
38 |
-
class StableDiffusionOnnxInpaintLegacyPipelineIntegrationTests(unittest.TestCase):
|
39 |
-
@property
|
40 |
-
def gpu_provider(self):
|
41 |
-
return (
|
42 |
-
"CUDAExecutionProvider",
|
43 |
-
{
|
44 |
-
"gpu_mem_limit": "15000000000", # 15GB
|
45 |
-
"arena_extend_strategy": "kSameAsRequested",
|
46 |
-
},
|
47 |
-
)
|
48 |
-
|
49 |
-
@property
|
50 |
-
def gpu_options(self):
|
51 |
-
options = ort.SessionOptions()
|
52 |
-
options.enable_mem_pattern = False
|
53 |
-
return options
|
54 |
-
|
55 |
-
def test_inference(self):
|
56 |
-
init_image = load_image(
|
57 |
-
"https://huggingface.co/datasets/hf-internal-testing/diffusers-images/resolve/main"
|
58 |
-
"/in_paint/overture-creations-5sI6fQgYIuo.png"
|
59 |
-
)
|
60 |
-
mask_image = load_image(
|
61 |
-
"https://huggingface.co/datasets/hf-internal-testing/diffusers-images/resolve/main"
|
62 |
-
"/in_paint/overture-creations-5sI6fQgYIuo_mask.png"
|
63 |
-
)
|
64 |
-
expected_image = load_numpy(
|
65 |
-
"https://huggingface.co/datasets/hf-internal-testing/diffusers-images/resolve/main"
|
66 |
-
"/in_paint/red_cat_sitting_on_a_park_bench_onnx.npy"
|
67 |
-
)
|
68 |
-
|
69 |
-
# using the PNDM scheduler by default
|
70 |
-
pipe = OnnxStableDiffusionInpaintPipelineLegacy.from_pretrained(
|
71 |
-
"CompVis/stable-diffusion-v1-4",
|
72 |
-
revision="onnx",
|
73 |
-
safety_checker=None,
|
74 |
-
feature_extractor=None,
|
75 |
-
provider=self.gpu_provider,
|
76 |
-
sess_options=self.gpu_options,
|
77 |
-
)
|
78 |
-
pipe.set_progress_bar_config(disable=None)
|
79 |
-
|
80 |
-
prompt = "A red cat sitting on a park bench"
|
81 |
-
|
82 |
-
generator = np.random.RandomState(0)
|
83 |
-
output = pipe(
|
84 |
-
prompt=prompt,
|
85 |
-
image=init_image,
|
86 |
-
mask_image=mask_image,
|
87 |
-
strength=0.75,
|
88 |
-
guidance_scale=7.5,
|
89 |
-
num_inference_steps=15,
|
90 |
-
generator=generator,
|
91 |
-
output_type="np",
|
92 |
-
)
|
93 |
-
|
94 |
-
image = output.images[0]
|
95 |
-
|
96 |
-
assert image.shape == (512, 512, 3)
|
97 |
-
assert np.abs(expected_image - image).max() < 1e-2
|
|
|
|
|
|
|
|
|
|
|
|
|
|
|
|
|
|
|
|
|
|
|
|
|
|
|
|
|
|
|
|
|
|
|
|
|
|
|
|
|
|
|
|
|
|
|
|
|
|
|
|
|
|
|
|
|
|
|
|
|
|
|
|
|
|
|
|
|
|
|
|
|
|
|
|
|
|
|
|
|
|
|
|
|
|
|
|
|
|
|
|
|
|
|
|
|
|
|
|
|
|
|
|
|
|
|
|
|
|
|
|
|
|
|
|
|
|
|
|
|
|
|
|
|
|
|
|
|
|
|
|
|
|
|
|
|
|
|
|
|
|
|
|
|
|
|
|
|
|
|
|
|
|
|
|
|
|
|
|
|
|
|
|
|
|
|
|
|
|
|
|
|
|
|
|
|
|
|
|
|
|
|
|
|
|
|
|
|
|
|
|
|
|
|
|
spaces/Androidonnxfork/CivitAi-to-Diffusers/diffusers/utils/check_table.py
DELETED
@@ -1,185 +0,0 @@
|
|
1 |
-
# coding=utf-8
|
2 |
-
# Copyright 2023 The HuggingFace Inc. team.
|
3 |
-
#
|
4 |
-
# Licensed under the Apache License, Version 2.0 (the "License");
|
5 |
-
# you may not use this file except in compliance with the License.
|
6 |
-
# You may obtain a copy of the License at
|
7 |
-
#
|
8 |
-
# http://www.apache.org/licenses/LICENSE-2.0
|
9 |
-
#
|
10 |
-
# Unless required by applicable law or agreed to in writing, software
|
11 |
-
# distributed under the License is distributed on an "AS IS" BASIS,
|
12 |
-
# WITHOUT WARRANTIES OR CONDITIONS OF ANY KIND, either express or implied.
|
13 |
-
# See the License for the specific language governing permissions and
|
14 |
-
# limitations under the License.
|
15 |
-
|
16 |
-
import argparse
|
17 |
-
import collections
|
18 |
-
import importlib.util
|
19 |
-
import os
|
20 |
-
import re
|
21 |
-
|
22 |
-
|
23 |
-
# All paths are set with the intent you should run this script from the root of the repo with the command
|
24 |
-
# python utils/check_table.py
|
25 |
-
TRANSFORMERS_PATH = "src/diffusers"
|
26 |
-
PATH_TO_DOCS = "docs/source/en"
|
27 |
-
REPO_PATH = "."
|
28 |
-
|
29 |
-
|
30 |
-
def _find_text_in_file(filename, start_prompt, end_prompt):
|
31 |
-
"""
|
32 |
-
Find the text in `filename` between a line beginning with `start_prompt` and before `end_prompt`, removing empty
|
33 |
-
lines.
|
34 |
-
"""
|
35 |
-
with open(filename, "r", encoding="utf-8", newline="\n") as f:
|
36 |
-
lines = f.readlines()
|
37 |
-
# Find the start prompt.
|
38 |
-
start_index = 0
|
39 |
-
while not lines[start_index].startswith(start_prompt):
|
40 |
-
start_index += 1
|
41 |
-
start_index += 1
|
42 |
-
|
43 |
-
end_index = start_index
|
44 |
-
while not lines[end_index].startswith(end_prompt):
|
45 |
-
end_index += 1
|
46 |
-
end_index -= 1
|
47 |
-
|
48 |
-
while len(lines[start_index]) <= 1:
|
49 |
-
start_index += 1
|
50 |
-
while len(lines[end_index]) <= 1:
|
51 |
-
end_index -= 1
|
52 |
-
end_index += 1
|
53 |
-
return "".join(lines[start_index:end_index]), start_index, end_index, lines
|
54 |
-
|
55 |
-
|
56 |
-
# Add here suffixes that are used to identify models, separated by |
|
57 |
-
ALLOWED_MODEL_SUFFIXES = "Model|Encoder|Decoder|ForConditionalGeneration"
|
58 |
-
# Regexes that match TF/Flax/PT model names.
|
59 |
-
_re_tf_models = re.compile(r"TF(.*)(?:Model|Encoder|Decoder|ForConditionalGeneration)")
|
60 |
-
_re_flax_models = re.compile(r"Flax(.*)(?:Model|Encoder|Decoder|ForConditionalGeneration)")
|
61 |
-
# Will match any TF or Flax model too so need to be in an else branch afterthe two previous regexes.
|
62 |
-
_re_pt_models = re.compile(r"(.*)(?:Model|Encoder|Decoder|ForConditionalGeneration)")
|
63 |
-
|
64 |
-
|
65 |
-
# This is to make sure the diffusers module imported is the one in the repo.
|
66 |
-
spec = importlib.util.spec_from_file_location(
|
67 |
-
"diffusers",
|
68 |
-
os.path.join(TRANSFORMERS_PATH, "__init__.py"),
|
69 |
-
submodule_search_locations=[TRANSFORMERS_PATH],
|
70 |
-
)
|
71 |
-
diffusers_module = spec.loader.load_module()
|
72 |
-
|
73 |
-
|
74 |
-
# Thanks to https://stackoverflow.com/questions/29916065/how-to-do-camelcase-split-in-python
|
75 |
-
def camel_case_split(identifier):
|
76 |
-
"Split a camelcased `identifier` into words."
|
77 |
-
matches = re.finditer(".+?(?:(?<=[a-z])(?=[A-Z])|(?<=[A-Z])(?=[A-Z][a-z])|$)", identifier)
|
78 |
-
return [m.group(0) for m in matches]
|
79 |
-
|
80 |
-
|
81 |
-
def _center_text(text, width):
|
82 |
-
text_length = 2 if text == "✅" or text == "❌" else len(text)
|
83 |
-
left_indent = (width - text_length) // 2
|
84 |
-
right_indent = width - text_length - left_indent
|
85 |
-
return " " * left_indent + text + " " * right_indent
|
86 |
-
|
87 |
-
|
88 |
-
def get_model_table_from_auto_modules():
|
89 |
-
"""Generates an up-to-date model table from the content of the auto modules."""
|
90 |
-
# Dictionary model names to config.
|
91 |
-
config_mapping_names = diffusers_module.models.auto.configuration_auto.CONFIG_MAPPING_NAMES
|
92 |
-
model_name_to_config = {
|
93 |
-
name: config_mapping_names[code]
|
94 |
-
for code, name in diffusers_module.MODEL_NAMES_MAPPING.items()
|
95 |
-
if code in config_mapping_names
|
96 |
-
}
|
97 |
-
model_name_to_prefix = {name: config.replace("ConfigMixin", "") for name, config in model_name_to_config.items()}
|
98 |
-
|
99 |
-
# Dictionaries flagging if each model prefix has a slow/fast tokenizer, backend in PT/TF/Flax.
|
100 |
-
slow_tokenizers = collections.defaultdict(bool)
|
101 |
-
fast_tokenizers = collections.defaultdict(bool)
|
102 |
-
pt_models = collections.defaultdict(bool)
|
103 |
-
tf_models = collections.defaultdict(bool)
|
104 |
-
flax_models = collections.defaultdict(bool)
|
105 |
-
|
106 |
-
# Let's lookup through all diffusers object (once).
|
107 |
-
for attr_name in dir(diffusers_module):
|
108 |
-
lookup_dict = None
|
109 |
-
if attr_name.endswith("Tokenizer"):
|
110 |
-
lookup_dict = slow_tokenizers
|
111 |
-
attr_name = attr_name[:-9]
|
112 |
-
elif attr_name.endswith("TokenizerFast"):
|
113 |
-
lookup_dict = fast_tokenizers
|
114 |
-
attr_name = attr_name[:-13]
|
115 |
-
elif _re_tf_models.match(attr_name) is not None:
|
116 |
-
lookup_dict = tf_models
|
117 |
-
attr_name = _re_tf_models.match(attr_name).groups()[0]
|
118 |
-
elif _re_flax_models.match(attr_name) is not None:
|
119 |
-
lookup_dict = flax_models
|
120 |
-
attr_name = _re_flax_models.match(attr_name).groups()[0]
|
121 |
-
elif _re_pt_models.match(attr_name) is not None:
|
122 |
-
lookup_dict = pt_models
|
123 |
-
attr_name = _re_pt_models.match(attr_name).groups()[0]
|
124 |
-
|
125 |
-
if lookup_dict is not None:
|
126 |
-
while len(attr_name) > 0:
|
127 |
-
if attr_name in model_name_to_prefix.values():
|
128 |
-
lookup_dict[attr_name] = True
|
129 |
-
break
|
130 |
-
# Try again after removing the last word in the name
|
131 |
-
attr_name = "".join(camel_case_split(attr_name)[:-1])
|
132 |
-
|
133 |
-
# Let's build that table!
|
134 |
-
model_names = list(model_name_to_config.keys())
|
135 |
-
model_names.sort(key=str.lower)
|
136 |
-
columns = ["Model", "Tokenizer slow", "Tokenizer fast", "PyTorch support", "TensorFlow support", "Flax Support"]
|
137 |
-
# We'll need widths to properly display everything in the center (+2 is to leave one extra space on each side).
|
138 |
-
widths = [len(c) + 2 for c in columns]
|
139 |
-
widths[0] = max([len(name) for name in model_names]) + 2
|
140 |
-
|
141 |
-
# Build the table per se
|
142 |
-
table = "|" + "|".join([_center_text(c, w) for c, w in zip(columns, widths)]) + "|\n"
|
143 |
-
# Use ":-----:" format to center-aligned table cell texts
|
144 |
-
table += "|" + "|".join([":" + "-" * (w - 2) + ":" for w in widths]) + "|\n"
|
145 |
-
|
146 |
-
check = {True: "✅", False: "❌"}
|
147 |
-
for name in model_names:
|
148 |
-
prefix = model_name_to_prefix[name]
|
149 |
-
line = [
|
150 |
-
name,
|
151 |
-
check[slow_tokenizers[prefix]],
|
152 |
-
check[fast_tokenizers[prefix]],
|
153 |
-
check[pt_models[prefix]],
|
154 |
-
check[tf_models[prefix]],
|
155 |
-
check[flax_models[prefix]],
|
156 |
-
]
|
157 |
-
table += "|" + "|".join([_center_text(l, w) for l, w in zip(line, widths)]) + "|\n"
|
158 |
-
return table
|
159 |
-
|
160 |
-
|
161 |
-
def check_model_table(overwrite=False):
|
162 |
-
"""Check the model table in the index.rst is consistent with the state of the lib and maybe `overwrite`."""
|
163 |
-
current_table, start_index, end_index, lines = _find_text_in_file(
|
164 |
-
filename=os.path.join(PATH_TO_DOCS, "index.md"),
|
165 |
-
start_prompt="<!--This table is updated automatically from the auto modules",
|
166 |
-
end_prompt="<!-- End table-->",
|
167 |
-
)
|
168 |
-
new_table = get_model_table_from_auto_modules()
|
169 |
-
|
170 |
-
if current_table != new_table:
|
171 |
-
if overwrite:
|
172 |
-
with open(os.path.join(PATH_TO_DOCS, "index.md"), "w", encoding="utf-8", newline="\n") as f:
|
173 |
-
f.writelines(lines[:start_index] + [new_table] + lines[end_index:])
|
174 |
-
else:
|
175 |
-
raise ValueError(
|
176 |
-
"The model table in the `index.md` has not been updated. Run `make fix-copies` to fix this."
|
177 |
-
)
|
178 |
-
|
179 |
-
|
180 |
-
if __name__ == "__main__":
|
181 |
-
parser = argparse.ArgumentParser()
|
182 |
-
parser.add_argument("--fix_and_overwrite", action="store_true", help="Whether to fix inconsistencies.")
|
183 |
-
args = parser.parse_args()
|
184 |
-
|
185 |
-
check_model_table(args.fix_and_overwrite)
|
|
|
|
|
|
|
|
|
|
|
|
|
|
|
|
|
|
|
|
|
|
|
|
|
|
|
|
|
|
|
|
|
|
|
|
|
|
|
|
|
|
|
|
|
|
|
|
|
|
|
|
|
|
|
|
|
|
|
|
|
|
|
|
|
|
|
|
|
|
|
|
|
|
|
|
|
|
|
|
|
|
|
|
|
|
|
|
|
|
|
|
|
|
|
|
|
|
|
|
|
|
|
|
|
|
|
|
|
|
|
|
|
|
|
|
|
|
|
|
|
|
|
|
|
|
|
|
|
|
|
|
|
|
|
|
|
|
|
|
|
|
|
|
|
|
|
|
|
|
|
|
|
|
|
|
|
|
|
|
|
|
|
|
|
|
|
|
|
|
|
|
|
|
|
|
|
|
|
|
|
|
|
|
|
|
|
|
|
|
|
|
|
|
|
|
|
|
|
|
|
|
|
|
|
|
|
|
|
|
|
|
|
|
|
|
|
|
|
|
|
|
|
|
|
|
|
|
|
|
|
|
|
|
|
|
|
|
|
|
|
|
|
|
|
|
|
|
|
|
|
|
|
|
|
|
|
|
|
|
|
|
|
|
|
|
|
|
|
|
|
|
|
|
|
|
|
|
|
|
|
|
|
|
|
|
|
|
|
|
|
|
|
|
|
|
|
|
|
|
|
|
|
|
|
|
|
|
|
|
|
|
|
|
|
|
|
|
|
|
|
|
|
|
|
|
|
|
|
|
|
|
|
|
|
|
|
|
|
|
|
|
|
|
|
|
|
|
|
|
|
|
|
|
|
|
|
|
|
|
|
|
|
|
|
|
|
|
|
|
|
|
spaces/Andy1621/uniformer_image_detection/mmdet/models/detectors/cascade_rcnn.py
DELETED
@@ -1,46 +0,0 @@
|
|
1 |
-
from ..builder import DETECTORS
|
2 |
-
from .two_stage import TwoStageDetector
|
3 |
-
|
4 |
-
|
5 |
-
@DETECTORS.register_module()
|
6 |
-
class CascadeRCNN(TwoStageDetector):
|
7 |
-
r"""Implementation of `Cascade R-CNN: Delving into High Quality Object
|
8 |
-
Detection <https://arxiv.org/abs/1906.09756>`_"""
|
9 |
-
|
10 |
-
def __init__(self,
|
11 |
-
backbone,
|
12 |
-
neck=None,
|
13 |
-
rpn_head=None,
|
14 |
-
roi_head=None,
|
15 |
-
train_cfg=None,
|
16 |
-
test_cfg=None,
|
17 |
-
pretrained=None):
|
18 |
-
super(CascadeRCNN, self).__init__(
|
19 |
-
backbone=backbone,
|
20 |
-
neck=neck,
|
21 |
-
rpn_head=rpn_head,
|
22 |
-
roi_head=roi_head,
|
23 |
-
train_cfg=train_cfg,
|
24 |
-
test_cfg=test_cfg,
|
25 |
-
pretrained=pretrained)
|
26 |
-
|
27 |
-
def show_result(self, data, result, **kwargs):
|
28 |
-
"""Show prediction results of the detector.
|
29 |
-
|
30 |
-
Args:
|
31 |
-
data (str or np.ndarray): Image filename or loaded image.
|
32 |
-
result (Tensor or tuple): The results to draw over `img`
|
33 |
-
bbox_result or (bbox_result, segm_result).
|
34 |
-
|
35 |
-
Returns:
|
36 |
-
np.ndarray: The image with bboxes drawn on it.
|
37 |
-
"""
|
38 |
-
if self.with_mask:
|
39 |
-
ms_bbox_result, ms_segm_result = result
|
40 |
-
if isinstance(ms_bbox_result, dict):
|
41 |
-
result = (ms_bbox_result['ensemble'],
|
42 |
-
ms_segm_result['ensemble'])
|
43 |
-
else:
|
44 |
-
if isinstance(result, dict):
|
45 |
-
result = result['ensemble']
|
46 |
-
return super(CascadeRCNN, self).show_result(data, result, **kwargs)
|
|
|
|
|
|
|
|
|
|
|
|
|
|
|
|
|
|
|
|
|
|
|
|
|
|
|
|
|
|
|
|
|
|
|
|
|
|
|
|
|
|
|
|
|
|
|
|
|
|
|
|
|
|
|
|
|
|
|
|
|
|
|
|
|
|
|
|
|
|
|
|
|
|
|
|
|
|
|
|
|
|
|
|
|
|
|
|
|
|
|
|
|
|
spaces/Anew1007/extras/README.md
DELETED
@@ -1,11 +0,0 @@
|
|
1 |
-
---
|
2 |
-
title: extras
|
3 |
-
emoji: 🧊
|
4 |
-
colorFrom: blue
|
5 |
-
colorTo: green
|
6 |
-
sdk: docker
|
7 |
-
pinned: false
|
8 |
-
license: mit
|
9 |
-
duplicated_from: doctord98/extras
|
10 |
-
---
|
11 |
-
Fixed Server.JS Latest 2023/08/16
|
|
|
|
|
|
|
|
|
|
|
|
|
|
|
|
|
|
|
|
|
|
|
|
spaces/Anonymous-sub/Rerender/ControlNet/share.py
DELETED
@@ -1,8 +0,0 @@
|
|
1 |
-
import config
|
2 |
-
from cldm.hack import disable_verbosity, enable_sliced_attention
|
3 |
-
|
4 |
-
|
5 |
-
disable_verbosity()
|
6 |
-
|
7 |
-
if config.save_memory:
|
8 |
-
enable_sliced_attention()
|
|
|
|
|
|
|
|
|
|
|
|
|
|
|
|
|
|
spaces/Ataturk-Chatbot/HuggingFaceChat/venv/lib/python3.11/site-packages/pip/_vendor/colorama/ansitowin32.py
DELETED
@@ -1,277 +0,0 @@
|
|
1 |
-
# Copyright Jonathan Hartley 2013. BSD 3-Clause license, see LICENSE file.
|
2 |
-
import re
|
3 |
-
import sys
|
4 |
-
import os
|
5 |
-
|
6 |
-
from .ansi import AnsiFore, AnsiBack, AnsiStyle, Style, BEL
|
7 |
-
from .winterm import enable_vt_processing, WinTerm, WinColor, WinStyle
|
8 |
-
from .win32 import windll, winapi_test
|
9 |
-
|
10 |
-
|
11 |
-
winterm = None
|
12 |
-
if windll is not None:
|
13 |
-
winterm = WinTerm()
|
14 |
-
|
15 |
-
|
16 |
-
class StreamWrapper(object):
|
17 |
-
'''
|
18 |
-
Wraps a stream (such as stdout), acting as a transparent proxy for all
|
19 |
-
attribute access apart from method 'write()', which is delegated to our
|
20 |
-
Converter instance.
|
21 |
-
'''
|
22 |
-
def __init__(self, wrapped, converter):
|
23 |
-
# double-underscore everything to prevent clashes with names of
|
24 |
-
# attributes on the wrapped stream object.
|
25 |
-
self.__wrapped = wrapped
|
26 |
-
self.__convertor = converter
|
27 |
-
|
28 |
-
def __getattr__(self, name):
|
29 |
-
return getattr(self.__wrapped, name)
|
30 |
-
|
31 |
-
def __enter__(self, *args, **kwargs):
|
32 |
-
# special method lookup bypasses __getattr__/__getattribute__, see
|
33 |
-
# https://stackoverflow.com/questions/12632894/why-doesnt-getattr-work-with-exit
|
34 |
-
# thus, contextlib magic methods are not proxied via __getattr__
|
35 |
-
return self.__wrapped.__enter__(*args, **kwargs)
|
36 |
-
|
37 |
-
def __exit__(self, *args, **kwargs):
|
38 |
-
return self.__wrapped.__exit__(*args, **kwargs)
|
39 |
-
|
40 |
-
def __setstate__(self, state):
|
41 |
-
self.__dict__ = state
|
42 |
-
|
43 |
-
def __getstate__(self):
|
44 |
-
return self.__dict__
|
45 |
-
|
46 |
-
def write(self, text):
|
47 |
-
self.__convertor.write(text)
|
48 |
-
|
49 |
-
def isatty(self):
|
50 |
-
stream = self.__wrapped
|
51 |
-
if 'PYCHARM_HOSTED' in os.environ:
|
52 |
-
if stream is not None and (stream is sys.__stdout__ or stream is sys.__stderr__):
|
53 |
-
return True
|
54 |
-
try:
|
55 |
-
stream_isatty = stream.isatty
|
56 |
-
except AttributeError:
|
57 |
-
return False
|
58 |
-
else:
|
59 |
-
return stream_isatty()
|
60 |
-
|
61 |
-
@property
|
62 |
-
def closed(self):
|
63 |
-
stream = self.__wrapped
|
64 |
-
try:
|
65 |
-
return stream.closed
|
66 |
-
# AttributeError in the case that the stream doesn't support being closed
|
67 |
-
# ValueError for the case that the stream has already been detached when atexit runs
|
68 |
-
except (AttributeError, ValueError):
|
69 |
-
return True
|
70 |
-
|
71 |
-
|
72 |
-
class AnsiToWin32(object):
|
73 |
-
'''
|
74 |
-
Implements a 'write()' method which, on Windows, will strip ANSI character
|
75 |
-
sequences from the text, and if outputting to a tty, will convert them into
|
76 |
-
win32 function calls.
|
77 |
-
'''
|
78 |
-
ANSI_CSI_RE = re.compile('\001?\033\\[((?:\\d|;)*)([a-zA-Z])\002?') # Control Sequence Introducer
|
79 |
-
ANSI_OSC_RE = re.compile('\001?\033\\]([^\a]*)(\a)\002?') # Operating System Command
|
80 |
-
|
81 |
-
def __init__(self, wrapped, convert=None, strip=None, autoreset=False):
|
82 |
-
# The wrapped stream (normally sys.stdout or sys.stderr)
|
83 |
-
self.wrapped = wrapped
|
84 |
-
|
85 |
-
# should we reset colors to defaults after every .write()
|
86 |
-
self.autoreset = autoreset
|
87 |
-
|
88 |
-
# create the proxy wrapping our output stream
|
89 |
-
self.stream = StreamWrapper(wrapped, self)
|
90 |
-
|
91 |
-
on_windows = os.name == 'nt'
|
92 |
-
# We test if the WinAPI works, because even if we are on Windows
|
93 |
-
# we may be using a terminal that doesn't support the WinAPI
|
94 |
-
# (e.g. Cygwin Terminal). In this case it's up to the terminal
|
95 |
-
# to support the ANSI codes.
|
96 |
-
conversion_supported = on_windows and winapi_test()
|
97 |
-
try:
|
98 |
-
fd = wrapped.fileno()
|
99 |
-
except Exception:
|
100 |
-
fd = -1
|
101 |
-
system_has_native_ansi = not on_windows or enable_vt_processing(fd)
|
102 |
-
have_tty = not self.stream.closed and self.stream.isatty()
|
103 |
-
need_conversion = conversion_supported and not system_has_native_ansi
|
104 |
-
|
105 |
-
# should we strip ANSI sequences from our output?
|
106 |
-
if strip is None:
|
107 |
-
strip = need_conversion or not have_tty
|
108 |
-
self.strip = strip
|
109 |
-
|
110 |
-
# should we should convert ANSI sequences into win32 calls?
|
111 |
-
if convert is None:
|
112 |
-
convert = need_conversion and have_tty
|
113 |
-
self.convert = convert
|
114 |
-
|
115 |
-
# dict of ansi codes to win32 functions and parameters
|
116 |
-
self.win32_calls = self.get_win32_calls()
|
117 |
-
|
118 |
-
# are we wrapping stderr?
|
119 |
-
self.on_stderr = self.wrapped is sys.stderr
|
120 |
-
|
121 |
-
def should_wrap(self):
|
122 |
-
'''
|
123 |
-
True if this class is actually needed. If false, then the output
|
124 |
-
stream will not be affected, nor will win32 calls be issued, so
|
125 |
-
wrapping stdout is not actually required. This will generally be
|
126 |
-
False on non-Windows platforms, unless optional functionality like
|
127 |
-
autoreset has been requested using kwargs to init()
|
128 |
-
'''
|
129 |
-
return self.convert or self.strip or self.autoreset
|
130 |
-
|
131 |
-
def get_win32_calls(self):
|
132 |
-
if self.convert and winterm:
|
133 |
-
return {
|
134 |
-
AnsiStyle.RESET_ALL: (winterm.reset_all, ),
|
135 |
-
AnsiStyle.BRIGHT: (winterm.style, WinStyle.BRIGHT),
|
136 |
-
AnsiStyle.DIM: (winterm.style, WinStyle.NORMAL),
|
137 |
-
AnsiStyle.NORMAL: (winterm.style, WinStyle.NORMAL),
|
138 |
-
AnsiFore.BLACK: (winterm.fore, WinColor.BLACK),
|
139 |
-
AnsiFore.RED: (winterm.fore, WinColor.RED),
|
140 |
-
AnsiFore.GREEN: (winterm.fore, WinColor.GREEN),
|
141 |
-
AnsiFore.YELLOW: (winterm.fore, WinColor.YELLOW),
|
142 |
-
AnsiFore.BLUE: (winterm.fore, WinColor.BLUE),
|
143 |
-
AnsiFore.MAGENTA: (winterm.fore, WinColor.MAGENTA),
|
144 |
-
AnsiFore.CYAN: (winterm.fore, WinColor.CYAN),
|
145 |
-
AnsiFore.WHITE: (winterm.fore, WinColor.GREY),
|
146 |
-
AnsiFore.RESET: (winterm.fore, ),
|
147 |
-
AnsiFore.LIGHTBLACK_EX: (winterm.fore, WinColor.BLACK, True),
|
148 |
-
AnsiFore.LIGHTRED_EX: (winterm.fore, WinColor.RED, True),
|
149 |
-
AnsiFore.LIGHTGREEN_EX: (winterm.fore, WinColor.GREEN, True),
|
150 |
-
AnsiFore.LIGHTYELLOW_EX: (winterm.fore, WinColor.YELLOW, True),
|
151 |
-
AnsiFore.LIGHTBLUE_EX: (winterm.fore, WinColor.BLUE, True),
|
152 |
-
AnsiFore.LIGHTMAGENTA_EX: (winterm.fore, WinColor.MAGENTA, True),
|
153 |
-
AnsiFore.LIGHTCYAN_EX: (winterm.fore, WinColor.CYAN, True),
|
154 |
-
AnsiFore.LIGHTWHITE_EX: (winterm.fore, WinColor.GREY, True),
|
155 |
-
AnsiBack.BLACK: (winterm.back, WinColor.BLACK),
|
156 |
-
AnsiBack.RED: (winterm.back, WinColor.RED),
|
157 |
-
AnsiBack.GREEN: (winterm.back, WinColor.GREEN),
|
158 |
-
AnsiBack.YELLOW: (winterm.back, WinColor.YELLOW),
|
159 |
-
AnsiBack.BLUE: (winterm.back, WinColor.BLUE),
|
160 |
-
AnsiBack.MAGENTA: (winterm.back, WinColor.MAGENTA),
|
161 |
-
AnsiBack.CYAN: (winterm.back, WinColor.CYAN),
|
162 |
-
AnsiBack.WHITE: (winterm.back, WinColor.GREY),
|
163 |
-
AnsiBack.RESET: (winterm.back, ),
|
164 |
-
AnsiBack.LIGHTBLACK_EX: (winterm.back, WinColor.BLACK, True),
|
165 |
-
AnsiBack.LIGHTRED_EX: (winterm.back, WinColor.RED, True),
|
166 |
-
AnsiBack.LIGHTGREEN_EX: (winterm.back, WinColor.GREEN, True),
|
167 |
-
AnsiBack.LIGHTYELLOW_EX: (winterm.back, WinColor.YELLOW, True),
|
168 |
-
AnsiBack.LIGHTBLUE_EX: (winterm.back, WinColor.BLUE, True),
|
169 |
-
AnsiBack.LIGHTMAGENTA_EX: (winterm.back, WinColor.MAGENTA, True),
|
170 |
-
AnsiBack.LIGHTCYAN_EX: (winterm.back, WinColor.CYAN, True),
|
171 |
-
AnsiBack.LIGHTWHITE_EX: (winterm.back, WinColor.GREY, True),
|
172 |
-
}
|
173 |
-
return dict()
|
174 |
-
|
175 |
-
def write(self, text):
|
176 |
-
if self.strip or self.convert:
|
177 |
-
self.write_and_convert(text)
|
178 |
-
else:
|
179 |
-
self.wrapped.write(text)
|
180 |
-
self.wrapped.flush()
|
181 |
-
if self.autoreset:
|
182 |
-
self.reset_all()
|
183 |
-
|
184 |
-
|
185 |
-
def reset_all(self):
|
186 |
-
if self.convert:
|
187 |
-
self.call_win32('m', (0,))
|
188 |
-
elif not self.strip and not self.stream.closed:
|
189 |
-
self.wrapped.write(Style.RESET_ALL)
|
190 |
-
|
191 |
-
|
192 |
-
def write_and_convert(self, text):
|
193 |
-
'''
|
194 |
-
Write the given text to our wrapped stream, stripping any ANSI
|
195 |
-
sequences from the text, and optionally converting them into win32
|
196 |
-
calls.
|
197 |
-
'''
|
198 |
-
cursor = 0
|
199 |
-
text = self.convert_osc(text)
|
200 |
-
for match in self.ANSI_CSI_RE.finditer(text):
|
201 |
-
start, end = match.span()
|
202 |
-
self.write_plain_text(text, cursor, start)
|
203 |
-
self.convert_ansi(*match.groups())
|
204 |
-
cursor = end
|
205 |
-
self.write_plain_text(text, cursor, len(text))
|
206 |
-
|
207 |
-
|
208 |
-
def write_plain_text(self, text, start, end):
|
209 |
-
if start < end:
|
210 |
-
self.wrapped.write(text[start:end])
|
211 |
-
self.wrapped.flush()
|
212 |
-
|
213 |
-
|
214 |
-
def convert_ansi(self, paramstring, command):
|
215 |
-
if self.convert:
|
216 |
-
params = self.extract_params(command, paramstring)
|
217 |
-
self.call_win32(command, params)
|
218 |
-
|
219 |
-
|
220 |
-
def extract_params(self, command, paramstring):
|
221 |
-
if command in 'Hf':
|
222 |
-
params = tuple(int(p) if len(p) != 0 else 1 for p in paramstring.split(';'))
|
223 |
-
while len(params) < 2:
|
224 |
-
# defaults:
|
225 |
-
params = params + (1,)
|
226 |
-
else:
|
227 |
-
params = tuple(int(p) for p in paramstring.split(';') if len(p) != 0)
|
228 |
-
if len(params) == 0:
|
229 |
-
# defaults:
|
230 |
-
if command in 'JKm':
|
231 |
-
params = (0,)
|
232 |
-
elif command in 'ABCD':
|
233 |
-
params = (1,)
|
234 |
-
|
235 |
-
return params
|
236 |
-
|
237 |
-
|
238 |
-
def call_win32(self, command, params):
|
239 |
-
if command == 'm':
|
240 |
-
for param in params:
|
241 |
-
if param in self.win32_calls:
|
242 |
-
func_args = self.win32_calls[param]
|
243 |
-
func = func_args[0]
|
244 |
-
args = func_args[1:]
|
245 |
-
kwargs = dict(on_stderr=self.on_stderr)
|
246 |
-
func(*args, **kwargs)
|
247 |
-
elif command in 'J':
|
248 |
-
winterm.erase_screen(params[0], on_stderr=self.on_stderr)
|
249 |
-
elif command in 'K':
|
250 |
-
winterm.erase_line(params[0], on_stderr=self.on_stderr)
|
251 |
-
elif command in 'Hf': # cursor position - absolute
|
252 |
-
winterm.set_cursor_position(params, on_stderr=self.on_stderr)
|
253 |
-
elif command in 'ABCD': # cursor position - relative
|
254 |
-
n = params[0]
|
255 |
-
# A - up, B - down, C - forward, D - back
|
256 |
-
x, y = {'A': (0, -n), 'B': (0, n), 'C': (n, 0), 'D': (-n, 0)}[command]
|
257 |
-
winterm.cursor_adjust(x, y, on_stderr=self.on_stderr)
|
258 |
-
|
259 |
-
|
260 |
-
def convert_osc(self, text):
|
261 |
-
for match in self.ANSI_OSC_RE.finditer(text):
|
262 |
-
start, end = match.span()
|
263 |
-
text = text[:start] + text[end:]
|
264 |
-
paramstring, command = match.groups()
|
265 |
-
if command == BEL:
|
266 |
-
if paramstring.count(";") == 1:
|
267 |
-
params = paramstring.split(";")
|
268 |
-
# 0 - change title and icon (we will only change title)
|
269 |
-
# 1 - change icon (we don't support this)
|
270 |
-
# 2 - change title
|
271 |
-
if params[0] in '02':
|
272 |
-
winterm.set_title(params[1])
|
273 |
-
return text
|
274 |
-
|
275 |
-
|
276 |
-
def flush(self):
|
277 |
-
self.wrapped.flush()
|
|
|
|
|
|
|
|
|
|
|
|
|
|
|
|
|
|
|
|
|
|
|
|
|
|
|
|
|
|
|
|
|
|
|
|
|
|
|
|
|
|
|
|
|
|
|
|
|
|
|
|
|
|
|
|
|
|
|
|
|
|
|
|
|
|
|
|
|
|
|
|
|
|
|
|
|
|
|
|
|
|
|
|
|
|
|
|
|
|
|
|
|
|
|
|
|
|
|
|
|
|
|
|
|
|
|
|
|
|
|
|
|
|
|
|
|
|
|
|
|
|
|
|
|
|
|
|
|
|
|
|
|
|
|
|
|
|
|
|
|
|
|
|
|
|
|
|
|
|
|
|
|
|
|
|
|
|
|
|
|
|
|
|
|
|
|
|
|
|
|
|
|
|
|
|
|
|
|
|
|
|
|
|
|
|
|
|
|
|
|
|
|
|
|
|
|
|
|
|
|
|
|
|
|
|
|
|
|
|
|
|
|
|
|
|
|
|
|
|
|
|
|
|
|
|
|
|
|
|
|
|
|
|
|
|
|
|
|
|
|
|
|
|
|
|
|
|
|
|
|
|
|
|
|
|
|
|
|
|
|
|
|
|
|
|
|
|
|
|
|
|
|
|
|
|
|
|
|
|
|
|
|
|
|
|
|
|
|
|
|
|
|
|
|
|
|
|
|
|
|
|
|
|
|
|
|
|
|
|
|
|
|
|
|
|
|
|
|
|
|
|
|
|
|
|
|
|
|
|
|
|
|
|
|
|
|
|
|
|
|
|
|
|
|
|
|
|
|
|
|
|
|
|
|
|
|
|
|
|
|
|
|
|
|
|
|
|
|
|
|
|
|
|
|
|
|
|
|
|
|
|
|
|
|
|
|
|
|
|
|
|
|
|
|
|
|
|
|
|
|
|
|
|
|
|
|
|
|
|
|
|
|
|
|
|
|
|
|
|
|
|
|
|
|
|
|
|
|
|
|
|
|
|
|
|
|
|
|
|
|
|
|
|
|
|
|
|
|
|
|
|
|
|
|
|
|
|
|
|
|
|
|
|
|
|
|
|
|
|
|
|
|
|
|
|
|
|
|
|
|
|
|
|
|
|
|
|
|
|
|
|
|
|
|
|
|
|
|
|
|
|
|
|
|
|
|
|
|
|
|
|
|
|
|
|
|
|
|
|
|
|
|
|
|
|
|
|
|
|
|
|
|
|
|
|
|
|
|
|
|
|
|
|
|
|
|
|
|
|
|
|
|
|
|
|
spaces/Awiny/Image2Paragraph/models/grit_src/third_party/CenterNet2/detectron2/checkpoint/c2_model_loading.py
DELETED
@@ -1,407 +0,0 @@
|
|
1 |
-
# Copyright (c) Facebook, Inc. and its affiliates.
|
2 |
-
import copy
|
3 |
-
import logging
|
4 |
-
import re
|
5 |
-
from typing import Dict, List
|
6 |
-
import torch
|
7 |
-
from tabulate import tabulate
|
8 |
-
|
9 |
-
|
10 |
-
def convert_basic_c2_names(original_keys):
|
11 |
-
"""
|
12 |
-
Apply some basic name conversion to names in C2 weights.
|
13 |
-
It only deals with typical backbone models.
|
14 |
-
|
15 |
-
Args:
|
16 |
-
original_keys (list[str]):
|
17 |
-
Returns:
|
18 |
-
list[str]: The same number of strings matching those in original_keys.
|
19 |
-
"""
|
20 |
-
layer_keys = copy.deepcopy(original_keys)
|
21 |
-
layer_keys = [
|
22 |
-
{"pred_b": "linear_b", "pred_w": "linear_w"}.get(k, k) for k in layer_keys
|
23 |
-
] # some hard-coded mappings
|
24 |
-
|
25 |
-
layer_keys = [k.replace("_", ".") for k in layer_keys]
|
26 |
-
layer_keys = [re.sub("\\.b$", ".bias", k) for k in layer_keys]
|
27 |
-
layer_keys = [re.sub("\\.w$", ".weight", k) for k in layer_keys]
|
28 |
-
# Uniform both bn and gn names to "norm"
|
29 |
-
layer_keys = [re.sub("bn\\.s$", "norm.weight", k) for k in layer_keys]
|
30 |
-
layer_keys = [re.sub("bn\\.bias$", "norm.bias", k) for k in layer_keys]
|
31 |
-
layer_keys = [re.sub("bn\\.rm", "norm.running_mean", k) for k in layer_keys]
|
32 |
-
layer_keys = [re.sub("bn\\.running.mean$", "norm.running_mean", k) for k in layer_keys]
|
33 |
-
layer_keys = [re.sub("bn\\.riv$", "norm.running_var", k) for k in layer_keys]
|
34 |
-
layer_keys = [re.sub("bn\\.running.var$", "norm.running_var", k) for k in layer_keys]
|
35 |
-
layer_keys = [re.sub("bn\\.gamma$", "norm.weight", k) for k in layer_keys]
|
36 |
-
layer_keys = [re.sub("bn\\.beta$", "norm.bias", k) for k in layer_keys]
|
37 |
-
layer_keys = [re.sub("gn\\.s$", "norm.weight", k) for k in layer_keys]
|
38 |
-
layer_keys = [re.sub("gn\\.bias$", "norm.bias", k) for k in layer_keys]
|
39 |
-
|
40 |
-
# stem
|
41 |
-
layer_keys = [re.sub("^res\\.conv1\\.norm\\.", "conv1.norm.", k) for k in layer_keys]
|
42 |
-
# to avoid mis-matching with "conv1" in other components (e.g. detection head)
|
43 |
-
layer_keys = [re.sub("^conv1\\.", "stem.conv1.", k) for k in layer_keys]
|
44 |
-
|
45 |
-
# layer1-4 is used by torchvision, however we follow the C2 naming strategy (res2-5)
|
46 |
-
# layer_keys = [re.sub("^res2.", "layer1.", k) for k in layer_keys]
|
47 |
-
# layer_keys = [re.sub("^res3.", "layer2.", k) for k in layer_keys]
|
48 |
-
# layer_keys = [re.sub("^res4.", "layer3.", k) for k in layer_keys]
|
49 |
-
# layer_keys = [re.sub("^res5.", "layer4.", k) for k in layer_keys]
|
50 |
-
|
51 |
-
# blocks
|
52 |
-
layer_keys = [k.replace(".branch1.", ".shortcut.") for k in layer_keys]
|
53 |
-
layer_keys = [k.replace(".branch2a.", ".conv1.") for k in layer_keys]
|
54 |
-
layer_keys = [k.replace(".branch2b.", ".conv2.") for k in layer_keys]
|
55 |
-
layer_keys = [k.replace(".branch2c.", ".conv3.") for k in layer_keys]
|
56 |
-
|
57 |
-
# DensePose substitutions
|
58 |
-
layer_keys = [re.sub("^body.conv.fcn", "body_conv_fcn", k) for k in layer_keys]
|
59 |
-
layer_keys = [k.replace("AnnIndex.lowres", "ann_index_lowres") for k in layer_keys]
|
60 |
-
layer_keys = [k.replace("Index.UV.lowres", "index_uv_lowres") for k in layer_keys]
|
61 |
-
layer_keys = [k.replace("U.lowres", "u_lowres") for k in layer_keys]
|
62 |
-
layer_keys = [k.replace("V.lowres", "v_lowres") for k in layer_keys]
|
63 |
-
return layer_keys
|
64 |
-
|
65 |
-
|
66 |
-
def convert_c2_detectron_names(weights):
|
67 |
-
"""
|
68 |
-
Map Caffe2 Detectron weight names to Detectron2 names.
|
69 |
-
|
70 |
-
Args:
|
71 |
-
weights (dict): name -> tensor
|
72 |
-
|
73 |
-
Returns:
|
74 |
-
dict: detectron2 names -> tensor
|
75 |
-
dict: detectron2 names -> C2 names
|
76 |
-
"""
|
77 |
-
logger = logging.getLogger(__name__)
|
78 |
-
logger.info("Renaming Caffe2 weights ......")
|
79 |
-
original_keys = sorted(weights.keys())
|
80 |
-
layer_keys = copy.deepcopy(original_keys)
|
81 |
-
|
82 |
-
layer_keys = convert_basic_c2_names(layer_keys)
|
83 |
-
|
84 |
-
# --------------------------------------------------------------------------
|
85 |
-
# RPN hidden representation conv
|
86 |
-
# --------------------------------------------------------------------------
|
87 |
-
# FPN case
|
88 |
-
# In the C2 model, the RPN hidden layer conv is defined for FPN level 2 and then
|
89 |
-
# shared for all other levels, hence the appearance of "fpn2"
|
90 |
-
layer_keys = [
|
91 |
-
k.replace("conv.rpn.fpn2", "proposal_generator.rpn_head.conv") for k in layer_keys
|
92 |
-
]
|
93 |
-
# Non-FPN case
|
94 |
-
layer_keys = [k.replace("conv.rpn", "proposal_generator.rpn_head.conv") for k in layer_keys]
|
95 |
-
|
96 |
-
# --------------------------------------------------------------------------
|
97 |
-
# RPN box transformation conv
|
98 |
-
# --------------------------------------------------------------------------
|
99 |
-
# FPN case (see note above about "fpn2")
|
100 |
-
layer_keys = [
|
101 |
-
k.replace("rpn.bbox.pred.fpn2", "proposal_generator.rpn_head.anchor_deltas")
|
102 |
-
for k in layer_keys
|
103 |
-
]
|
104 |
-
layer_keys = [
|
105 |
-
k.replace("rpn.cls.logits.fpn2", "proposal_generator.rpn_head.objectness_logits")
|
106 |
-
for k in layer_keys
|
107 |
-
]
|
108 |
-
# Non-FPN case
|
109 |
-
layer_keys = [
|
110 |
-
k.replace("rpn.bbox.pred", "proposal_generator.rpn_head.anchor_deltas") for k in layer_keys
|
111 |
-
]
|
112 |
-
layer_keys = [
|
113 |
-
k.replace("rpn.cls.logits", "proposal_generator.rpn_head.objectness_logits")
|
114 |
-
for k in layer_keys
|
115 |
-
]
|
116 |
-
|
117 |
-
# --------------------------------------------------------------------------
|
118 |
-
# Fast R-CNN box head
|
119 |
-
# --------------------------------------------------------------------------
|
120 |
-
layer_keys = [re.sub("^bbox\\.pred", "bbox_pred", k) for k in layer_keys]
|
121 |
-
layer_keys = [re.sub("^cls\\.score", "cls_score", k) for k in layer_keys]
|
122 |
-
layer_keys = [re.sub("^fc6\\.", "box_head.fc1.", k) for k in layer_keys]
|
123 |
-
layer_keys = [re.sub("^fc7\\.", "box_head.fc2.", k) for k in layer_keys]
|
124 |
-
# 4conv1fc head tensor names: head_conv1_w, head_conv1_gn_s
|
125 |
-
layer_keys = [re.sub("^head\\.conv", "box_head.conv", k) for k in layer_keys]
|
126 |
-
|
127 |
-
# --------------------------------------------------------------------------
|
128 |
-
# FPN lateral and output convolutions
|
129 |
-
# --------------------------------------------------------------------------
|
130 |
-
def fpn_map(name):
|
131 |
-
"""
|
132 |
-
Look for keys with the following patterns:
|
133 |
-
1) Starts with "fpn.inner."
|
134 |
-
Example: "fpn.inner.res2.2.sum.lateral.weight"
|
135 |
-
Meaning: These are lateral pathway convolutions
|
136 |
-
2) Starts with "fpn.res"
|
137 |
-
Example: "fpn.res2.2.sum.weight"
|
138 |
-
Meaning: These are FPN output convolutions
|
139 |
-
"""
|
140 |
-
splits = name.split(".")
|
141 |
-
norm = ".norm" if "norm" in splits else ""
|
142 |
-
if name.startswith("fpn.inner."):
|
143 |
-
# splits example: ['fpn', 'inner', 'res2', '2', 'sum', 'lateral', 'weight']
|
144 |
-
stage = int(splits[2][len("res") :])
|
145 |
-
return "fpn_lateral{}{}.{}".format(stage, norm, splits[-1])
|
146 |
-
elif name.startswith("fpn.res"):
|
147 |
-
# splits example: ['fpn', 'res2', '2', 'sum', 'weight']
|
148 |
-
stage = int(splits[1][len("res") :])
|
149 |
-
return "fpn_output{}{}.{}".format(stage, norm, splits[-1])
|
150 |
-
return name
|
151 |
-
|
152 |
-
layer_keys = [fpn_map(k) for k in layer_keys]
|
153 |
-
|
154 |
-
# --------------------------------------------------------------------------
|
155 |
-
# Mask R-CNN mask head
|
156 |
-
# --------------------------------------------------------------------------
|
157 |
-
# roi_heads.StandardROIHeads case
|
158 |
-
layer_keys = [k.replace(".[mask].fcn", "mask_head.mask_fcn") for k in layer_keys]
|
159 |
-
layer_keys = [re.sub("^\\.mask\\.fcn", "mask_head.mask_fcn", k) for k in layer_keys]
|
160 |
-
layer_keys = [k.replace("mask.fcn.logits", "mask_head.predictor") for k in layer_keys]
|
161 |
-
# roi_heads.Res5ROIHeads case
|
162 |
-
layer_keys = [k.replace("conv5.mask", "mask_head.deconv") for k in layer_keys]
|
163 |
-
|
164 |
-
# --------------------------------------------------------------------------
|
165 |
-
# Keypoint R-CNN head
|
166 |
-
# --------------------------------------------------------------------------
|
167 |
-
# interestingly, the keypoint head convs have blob names that are simply "conv_fcnX"
|
168 |
-
layer_keys = [k.replace("conv.fcn", "roi_heads.keypoint_head.conv_fcn") for k in layer_keys]
|
169 |
-
layer_keys = [
|
170 |
-
k.replace("kps.score.lowres", "roi_heads.keypoint_head.score_lowres") for k in layer_keys
|
171 |
-
]
|
172 |
-
layer_keys = [k.replace("kps.score.", "roi_heads.keypoint_head.score.") for k in layer_keys]
|
173 |
-
|
174 |
-
# --------------------------------------------------------------------------
|
175 |
-
# Done with replacements
|
176 |
-
# --------------------------------------------------------------------------
|
177 |
-
assert len(set(layer_keys)) == len(layer_keys)
|
178 |
-
assert len(original_keys) == len(layer_keys)
|
179 |
-
|
180 |
-
new_weights = {}
|
181 |
-
new_keys_to_original_keys = {}
|
182 |
-
for orig, renamed in zip(original_keys, layer_keys):
|
183 |
-
new_keys_to_original_keys[renamed] = orig
|
184 |
-
if renamed.startswith("bbox_pred.") or renamed.startswith("mask_head.predictor."):
|
185 |
-
# remove the meaningless prediction weight for background class
|
186 |
-
new_start_idx = 4 if renamed.startswith("bbox_pred.") else 1
|
187 |
-
new_weights[renamed] = weights[orig][new_start_idx:]
|
188 |
-
logger.info(
|
189 |
-
"Remove prediction weight for background class in {}. The shape changes from "
|
190 |
-
"{} to {}.".format(
|
191 |
-
renamed, tuple(weights[orig].shape), tuple(new_weights[renamed].shape)
|
192 |
-
)
|
193 |
-
)
|
194 |
-
elif renamed.startswith("cls_score."):
|
195 |
-
# move weights of bg class from original index 0 to last index
|
196 |
-
logger.info(
|
197 |
-
"Move classification weights for background class in {} from index 0 to "
|
198 |
-
"index {}.".format(renamed, weights[orig].shape[0] - 1)
|
199 |
-
)
|
200 |
-
new_weights[renamed] = torch.cat([weights[orig][1:], weights[orig][:1]])
|
201 |
-
else:
|
202 |
-
new_weights[renamed] = weights[orig]
|
203 |
-
|
204 |
-
return new_weights, new_keys_to_original_keys
|
205 |
-
|
206 |
-
|
207 |
-
# Note the current matching is not symmetric.
|
208 |
-
# it assumes model_state_dict will have longer names.
|
209 |
-
def align_and_update_state_dicts(model_state_dict, ckpt_state_dict, c2_conversion=True):
|
210 |
-
"""
|
211 |
-
Match names between the two state-dict, and returns a new chkpt_state_dict with names
|
212 |
-
converted to match model_state_dict with heuristics. The returned dict can be later
|
213 |
-
loaded with fvcore checkpointer.
|
214 |
-
If `c2_conversion==True`, `ckpt_state_dict` is assumed to be a Caffe2
|
215 |
-
model and will be renamed at first.
|
216 |
-
|
217 |
-
Strategy: suppose that the models that we will create will have prefixes appended
|
218 |
-
to each of its keys, for example due to an extra level of nesting that the original
|
219 |
-
pre-trained weights from ImageNet won't contain. For example, model.state_dict()
|
220 |
-
might return backbone[0].body.res2.conv1.weight, while the pre-trained model contains
|
221 |
-
res2.conv1.weight. We thus want to match both parameters together.
|
222 |
-
For that, we look for each model weight, look among all loaded keys if there is one
|
223 |
-
that is a suffix of the current weight name, and use it if that's the case.
|
224 |
-
If multiple matches exist, take the one with longest size
|
225 |
-
of the corresponding name. For example, for the same model as before, the pretrained
|
226 |
-
weight file can contain both res2.conv1.weight, as well as conv1.weight. In this case,
|
227 |
-
we want to match backbone[0].body.conv1.weight to conv1.weight, and
|
228 |
-
backbone[0].body.res2.conv1.weight to res2.conv1.weight.
|
229 |
-
"""
|
230 |
-
model_keys = sorted(model_state_dict.keys())
|
231 |
-
if c2_conversion:
|
232 |
-
ckpt_state_dict, original_keys = convert_c2_detectron_names(ckpt_state_dict)
|
233 |
-
# original_keys: the name in the original dict (before renaming)
|
234 |
-
else:
|
235 |
-
original_keys = {x: x for x in ckpt_state_dict.keys()}
|
236 |
-
ckpt_keys = sorted(ckpt_state_dict.keys())
|
237 |
-
|
238 |
-
def match(a, b):
|
239 |
-
# Matched ckpt_key should be a complete (starts with '.') suffix.
|
240 |
-
# For example, roi_heads.mesh_head.whatever_conv1 does not match conv1,
|
241 |
-
# but matches whatever_conv1 or mesh_head.whatever_conv1.
|
242 |
-
return a == b or a.endswith("." + b)
|
243 |
-
|
244 |
-
# get a matrix of string matches, where each (i, j) entry correspond to the size of the
|
245 |
-
# ckpt_key string, if it matches
|
246 |
-
match_matrix = [len(j) if match(i, j) else 0 for i in model_keys for j in ckpt_keys]
|
247 |
-
match_matrix = torch.as_tensor(match_matrix).view(len(model_keys), len(ckpt_keys))
|
248 |
-
# use the matched one with longest size in case of multiple matches
|
249 |
-
max_match_size, idxs = match_matrix.max(1)
|
250 |
-
# remove indices that correspond to no-match
|
251 |
-
idxs[max_match_size == 0] = -1
|
252 |
-
|
253 |
-
logger = logging.getLogger(__name__)
|
254 |
-
# matched_pairs (matched checkpoint key --> matched model key)
|
255 |
-
matched_keys = {}
|
256 |
-
result_state_dict = {}
|
257 |
-
for idx_model, idx_ckpt in enumerate(idxs.tolist()):
|
258 |
-
if idx_ckpt == -1:
|
259 |
-
continue
|
260 |
-
key_model = model_keys[idx_model]
|
261 |
-
key_ckpt = ckpt_keys[idx_ckpt]
|
262 |
-
value_ckpt = ckpt_state_dict[key_ckpt]
|
263 |
-
shape_in_model = model_state_dict[key_model].shape
|
264 |
-
|
265 |
-
if shape_in_model != value_ckpt.shape:
|
266 |
-
logger.warning(
|
267 |
-
"Shape of {} in checkpoint is {}, while shape of {} in model is {}.".format(
|
268 |
-
key_ckpt, value_ckpt.shape, key_model, shape_in_model
|
269 |
-
)
|
270 |
-
)
|
271 |
-
logger.warning(
|
272 |
-
"{} will not be loaded. Please double check and see if this is desired.".format(
|
273 |
-
key_ckpt
|
274 |
-
)
|
275 |
-
)
|
276 |
-
continue
|
277 |
-
|
278 |
-
assert key_model not in result_state_dict
|
279 |
-
result_state_dict[key_model] = value_ckpt
|
280 |
-
if key_ckpt in matched_keys: # already added to matched_keys
|
281 |
-
logger.error(
|
282 |
-
"Ambiguity found for {} in checkpoint!"
|
283 |
-
"It matches at least two keys in the model ({} and {}).".format(
|
284 |
-
key_ckpt, key_model, matched_keys[key_ckpt]
|
285 |
-
)
|
286 |
-
)
|
287 |
-
raise ValueError("Cannot match one checkpoint key to multiple keys in the model.")
|
288 |
-
|
289 |
-
matched_keys[key_ckpt] = key_model
|
290 |
-
|
291 |
-
# logging:
|
292 |
-
matched_model_keys = sorted(matched_keys.values())
|
293 |
-
if len(matched_model_keys) == 0:
|
294 |
-
logger.warning("No weights in checkpoint matched with model.")
|
295 |
-
return ckpt_state_dict
|
296 |
-
common_prefix = _longest_common_prefix(matched_model_keys)
|
297 |
-
rev_matched_keys = {v: k for k, v in matched_keys.items()}
|
298 |
-
original_keys = {k: original_keys[rev_matched_keys[k]] for k in matched_model_keys}
|
299 |
-
|
300 |
-
model_key_groups = _group_keys_by_module(matched_model_keys, original_keys)
|
301 |
-
table = []
|
302 |
-
memo = set()
|
303 |
-
for key_model in matched_model_keys:
|
304 |
-
if key_model in memo:
|
305 |
-
continue
|
306 |
-
if key_model in model_key_groups:
|
307 |
-
group = model_key_groups[key_model]
|
308 |
-
memo |= set(group)
|
309 |
-
shapes = [tuple(model_state_dict[k].shape) for k in group]
|
310 |
-
table.append(
|
311 |
-
(
|
312 |
-
_longest_common_prefix([k[len(common_prefix) :] for k in group]) + "*",
|
313 |
-
_group_str([original_keys[k] for k in group]),
|
314 |
-
" ".join([str(x).replace(" ", "") for x in shapes]),
|
315 |
-
)
|
316 |
-
)
|
317 |
-
else:
|
318 |
-
key_checkpoint = original_keys[key_model]
|
319 |
-
shape = str(tuple(model_state_dict[key_model].shape))
|
320 |
-
table.append((key_model[len(common_prefix) :], key_checkpoint, shape))
|
321 |
-
table_str = tabulate(
|
322 |
-
table, tablefmt="pipe", headers=["Names in Model", "Names in Checkpoint", "Shapes"]
|
323 |
-
)
|
324 |
-
logger.info(
|
325 |
-
"Following weights matched with "
|
326 |
-
+ (f"submodule {common_prefix[:-1]}" if common_prefix else "model")
|
327 |
-
+ ":\n"
|
328 |
-
+ table_str
|
329 |
-
)
|
330 |
-
|
331 |
-
unmatched_ckpt_keys = [k for k in ckpt_keys if k not in set(matched_keys.keys())]
|
332 |
-
for k in unmatched_ckpt_keys:
|
333 |
-
result_state_dict[k] = ckpt_state_dict[k]
|
334 |
-
return result_state_dict
|
335 |
-
|
336 |
-
|
337 |
-
def _group_keys_by_module(keys: List[str], original_names: Dict[str, str]):
|
338 |
-
"""
|
339 |
-
Params in the same submodule are grouped together.
|
340 |
-
|
341 |
-
Args:
|
342 |
-
keys: names of all parameters
|
343 |
-
original_names: mapping from parameter name to their name in the checkpoint
|
344 |
-
|
345 |
-
Returns:
|
346 |
-
dict[name -> all other names in the same group]
|
347 |
-
"""
|
348 |
-
|
349 |
-
def _submodule_name(key):
|
350 |
-
pos = key.rfind(".")
|
351 |
-
if pos < 0:
|
352 |
-
return None
|
353 |
-
prefix = key[: pos + 1]
|
354 |
-
return prefix
|
355 |
-
|
356 |
-
all_submodules = [_submodule_name(k) for k in keys]
|
357 |
-
all_submodules = [x for x in all_submodules if x]
|
358 |
-
all_submodules = sorted(all_submodules, key=len)
|
359 |
-
|
360 |
-
ret = {}
|
361 |
-
for prefix in all_submodules:
|
362 |
-
group = [k for k in keys if k.startswith(prefix)]
|
363 |
-
if len(group) <= 1:
|
364 |
-
continue
|
365 |
-
original_name_lcp = _longest_common_prefix_str([original_names[k] for k in group])
|
366 |
-
if len(original_name_lcp) == 0:
|
367 |
-
# don't group weights if original names don't share prefix
|
368 |
-
continue
|
369 |
-
|
370 |
-
for k in group:
|
371 |
-
if k in ret:
|
372 |
-
continue
|
373 |
-
ret[k] = group
|
374 |
-
return ret
|
375 |
-
|
376 |
-
|
377 |
-
def _longest_common_prefix(names: List[str]) -> str:
|
378 |
-
"""
|
379 |
-
["abc.zfg", "abc.zef"] -> "abc."
|
380 |
-
"""
|
381 |
-
names = [n.split(".") for n in names]
|
382 |
-
m1, m2 = min(names), max(names)
|
383 |
-
ret = [a for a, b in zip(m1, m2) if a == b]
|
384 |
-
ret = ".".join(ret) + "." if len(ret) else ""
|
385 |
-
return ret
|
386 |
-
|
387 |
-
|
388 |
-
def _longest_common_prefix_str(names: List[str]) -> str:
|
389 |
-
m1, m2 = min(names), max(names)
|
390 |
-
lcp = [a for a, b in zip(m1, m2) if a == b]
|
391 |
-
lcp = "".join(lcp)
|
392 |
-
return lcp
|
393 |
-
|
394 |
-
|
395 |
-
def _group_str(names: List[str]) -> str:
|
396 |
-
"""
|
397 |
-
Turn "common1", "common2", "common3" into "common{1,2,3}"
|
398 |
-
"""
|
399 |
-
lcp = _longest_common_prefix_str(names)
|
400 |
-
rest = [x[len(lcp) :] for x in names]
|
401 |
-
rest = "{" + ",".join(rest) + "}"
|
402 |
-
ret = lcp + rest
|
403 |
-
|
404 |
-
# add some simplification for BN specifically
|
405 |
-
ret = ret.replace("bn_{beta,running_mean,running_var,gamma}", "bn_*")
|
406 |
-
ret = ret.replace("bn_beta,bn_running_mean,bn_running_var,bn_gamma", "bn_*")
|
407 |
-
return ret
|
|
|
|
|
|
|
|
|
|
|
|
|
|
|
|
|
|
|
|
|
|
|
|
|
|
|
|
|
|
|
|
|
|
|
|
|
|
|
|
|
|
|
|
|
|
|
|
|
|
|
|
|
|
|
|
|
|
|
|
|
|
|
|
|
|
|
|
|
|
|
|
|
|
|
|
|
|
|
|
|
|
|
|
|
|
|
|
|
|
|
|
|
|
|
|
|
|
|
|
|
|
|
|
|
|
|
|
|
|
|
|
|
|
|
|
|
|
|
|
|
|
|
|
|
|
|
|
|
|
|
|
|
|
|
|
|
|
|
|
|
|
|
|
|
|
|
|
|
|
|
|
|
|
|
|
|
|
|
|
|
|
|
|
|
|
|
|
|
|
|
|
|
|
|
|
|
|
|
|
|
|
|
|
|
|
|
|
|
|
|
|
|
|
|
|
|
|
|
|
|
|
|
|
|
|
|
|
|
|
|
|
|
|
|
|
|
|
|
|
|
|
|
|
|
|
|
|
|
|
|
|
|
|
|
|
|
|
|
|
|
|
|
|
|
|
|
|
|
|
|
|
|
|
|
|
|
|
|
|
|
|
|
|
|
|
|
|
|
|
|
|
|
|
|
|
|
|
|
|
|
|
|
|
|
|
|
|
|
|
|
|
|
|
|
|
|
|
|
|
|
|
|
|
|
|
|
|
|
|
|
|
|
|
|
|
|
|
|
|
|
|
|
|
|
|
|
|
|
|
|
|
|
|
|
|
|
|
|
|
|
|
|
|
|
|
|
|
|
|
|
|
|
|
|
|
|
|
|
|
|
|
|
|
|
|
|
|
|
|
|
|
|
|
|
|
|
|
|
|
|
|
|
|
|
|
|
|
|
|
|
|
|
|
|
|
|
|
|
|
|
|
|
|
|
|
|
|
|
|
|
|
|
|
|
|
|
|
|
|
|
|
|
|
|
|
|
|
|
|
|
|
|
|
|
|
|
|
|
|
|
|
|
|
|
|
|
|
|
|
|
|
|
|
|
|
|
|
|
|
|
|
|
|
|
|
|
|
|
|
|
|
|
|
|
|
|
|
|
|
|
|
|
|
|
|
|
|
|
|
|
|
|
|
|
|
|
|
|
|
|
|
|
|
|
|
|
|
|
|
|
|
|
|
|
|
|
|
|
|
|
|
|
|
|
|
|
|
|
|
|
|
|
|
|
|
|
|
|
|
|
|
|
|
|
|
|
|
|
|
|
|
|
|
|
|
|
|
|
|
|
|
|
|
|
|
|
|
|
|
|
|
|
|
|
|
|
|
|
|
|
|
|
|
|
|
|
|
|
|
|
|
|
|
|
|
|
|
|
|
|
|
|
|
|
|
|
|
|
|
|
|
|
|
|
|
|
|
|
|
|
|
|
|
|
|
|
|
|
|
|
|
|
|
|
|
|
|
|
|
|
|
|
|
|
|
|
|
|
|
|
|
|
|
|
|
|
|
|
|
|
|
|
|
|
|
|
|
|
|
|
|
|
|
|
|
|
|
|
|
|
|
|
|
|
|
|
|
|
|
|
|
|
|
|
|
|
|
|
|
|
|
|
|
|
|
|
|
|
|
|
|
|
|
|
|
|
|
|
|
|
|
|
|
|
|
|
|
|
|
|
|
|
|
|
|
|
|
|
|
|
|
|
|
|
|
|
|
|
|
|
|
|
|
|
|
|
|
|
|
|
|
|
|
|
|
|
|
|
|
|
|
|
|
|
|
|
|
|
|
|
|
|
|
|
|
|
|
|
|
|
|
|
|
|
|
|
|
|
|
|
|
|
|
|
|
|
|
|
|
|
|
|
|
|
|
spaces/BAAI/AltDiffusion-m9/style.css
DELETED
@@ -1,81 +0,0 @@
|
|
1 |
-
.gradio-container {
|
2 |
-
font-family: 'IBM Plex Sans', sans-serif;
|
3 |
-
}
|
4 |
-
.gr-button {
|
5 |
-
color: white;
|
6 |
-
/* border-color: black; */
|
7 |
-
/* background: black; */
|
8 |
-
background: rgb(60, 145, 238);
|
9 |
-
}
|
10 |
-
/* input[type='range'] {
|
11 |
-
accent-color: rgb(60, 145, 238);
|
12 |
-
}
|
13 |
-
.dark input[type='range'] {
|
14 |
-
accent-color: #dfdfdf;
|
15 |
-
} */
|
16 |
-
.container {
|
17 |
-
max-width: 900px;
|
18 |
-
margin: auto;
|
19 |
-
padding-top: 1.5rem;
|
20 |
-
}
|
21 |
-
#gallery {
|
22 |
-
min-height: 22rem;
|
23 |
-
margin-bottom: 15px;
|
24 |
-
margin-left: auto;
|
25 |
-
margin-right: auto;
|
26 |
-
border-bottom-right-radius: .5rem !important;
|
27 |
-
border-bottom-left-radius: .5rem !important;
|
28 |
-
}
|
29 |
-
#gallery>div>.h-full {
|
30 |
-
min-height: 20rem;
|
31 |
-
}
|
32 |
-
.details:hover {
|
33 |
-
text-decoration: underline;
|
34 |
-
}
|
35 |
-
.gr-button {
|
36 |
-
white-space: nowrap;
|
37 |
-
}
|
38 |
-
/* .gr-button:focus {
|
39 |
-
border-color: rgb(147 197 253 / var(--tw-border-opacity));
|
40 |
-
outline: none;
|
41 |
-
box-shadow: var(--tw-ring-offset-shadow), var(--tw-ring-shadow), var(--tw-shadow, 0 0 #0000);
|
42 |
-
--tw-border-opacity: 1;
|
43 |
-
--tw-ring-offset-shadow: var(--tw-ring-inset) 0 0 0 var(--tw-ring-offset-width) var(--tw-ring-offset-color);
|
44 |
-
--tw-ring-shadow: var(--tw-ring-inset) 0 0 0 calc(3px var(--tw-ring-offset-width)) var(--tw-ring-color);
|
45 |
-
--tw-ring-color: rgb(191 219 254 / var(--tw-ring-opacity));
|
46 |
-
--tw-ring-opacity: .5;
|
47 |
-
} */
|
48 |
-
.footer {
|
49 |
-
margin-bottom: 45px;
|
50 |
-
margin-top: 20px;
|
51 |
-
/* text-align: center; */
|
52 |
-
border-bottom: 1px solid #e5e5e5;
|
53 |
-
}
|
54 |
-
.footer>p {
|
55 |
-
font-size: .8rem;
|
56 |
-
display: inline-block;
|
57 |
-
padding: 0 10px;
|
58 |
-
transform: translateY(10px);
|
59 |
-
background: white;
|
60 |
-
}
|
61 |
-
.footer>p>h4 {
|
62 |
-
font-size: .20rem;
|
63 |
-
display: inline-block;
|
64 |
-
padding: 0 10px;
|
65 |
-
transform: translateY(10px);
|
66 |
-
background: white;
|
67 |
-
font-weight: bold;
|
68 |
-
}
|
69 |
-
.dark .footer {
|
70 |
-
/* border-color: #303030; */
|
71 |
-
border-color: rgb(60, 145, 238);
|
72 |
-
}
|
73 |
-
.dark .footer>p {
|
74 |
-
/* background: #0b0f19; */
|
75 |
-
background: rgb(60, 145, 238);
|
76 |
-
}
|
77 |
-
.prompt h4{
|
78 |
-
margin: 1.25em 0 .25em 0;
|
79 |
-
font-weight: bold;
|
80 |
-
font-size: 115%;
|
81 |
-
}
|
|
|
|
|
|
|
|
|
|
|
|
|
|
|
|
|
|
|
|
|
|
|
|
|
|
|
|
|
|
|
|
|
|
|
|
|
|
|
|
|
|
|
|
|
|
|
|
|
|
|
|
|
|
|
|
|
|
|
|
|
|
|
|
|
|
|
|
|
|
|
|
|
|
|
|
|
|
|
|
|
|
|
|
|
|
|
|
|
|
|
|
|
|
|
|
|
|
|
|
|
|
|
|
|
|
|
|
|
|
|
|
|
|
|
|
|
|
|
|
|
|
|
|
|
|
|
|
|
|
|
|
|
|
|
|
|
|
|
|
|
|
|
|
|
|
|
|
|
|
|
|
|
|
|
|
|
|
|
|
|
|
|
|
spaces/BAAI/vid2vid-zero/vid2vid_zero/models/resnet_2d.py
DELETED
@@ -1,209 +0,0 @@
|
|
1 |
-
# Adapted from https://github.com/huggingface/diffusers/blob/main/src/diffusers/models/resnet.py
|
2 |
-
|
3 |
-
import torch
|
4 |
-
import torch.nn as nn
|
5 |
-
import torch.nn.functional as F
|
6 |
-
|
7 |
-
from einops import rearrange
|
8 |
-
|
9 |
-
|
10 |
-
class InflatedConv3d(nn.Conv2d):
|
11 |
-
def forward(self, x):
|
12 |
-
video_length = x.shape[2]
|
13 |
-
|
14 |
-
x = rearrange(x, "b c f h w -> (b f) c h w")
|
15 |
-
x = super().forward(x)
|
16 |
-
x = rearrange(x, "(b f) c h w -> b c f h w", f=video_length)
|
17 |
-
|
18 |
-
return x
|
19 |
-
|
20 |
-
|
21 |
-
class Upsample2D(nn.Module):
|
22 |
-
def __init__(self, channels, use_conv=False, use_conv_transpose=False, out_channels=None, name="conv"):
|
23 |
-
super().__init__()
|
24 |
-
self.channels = channels
|
25 |
-
self.out_channels = out_channels or channels
|
26 |
-
self.use_conv = use_conv
|
27 |
-
self.use_conv_transpose = use_conv_transpose
|
28 |
-
self.name = name
|
29 |
-
|
30 |
-
conv = None
|
31 |
-
if use_conv_transpose:
|
32 |
-
raise NotImplementedError
|
33 |
-
elif use_conv:
|
34 |
-
conv = InflatedConv3d(self.channels, self.out_channels, 3, padding=1)
|
35 |
-
|
36 |
-
if name == "conv":
|
37 |
-
self.conv = conv
|
38 |
-
else:
|
39 |
-
self.Conv2d_0 = conv
|
40 |
-
|
41 |
-
def forward(self, hidden_states, output_size=None):
|
42 |
-
assert hidden_states.shape[1] == self.channels
|
43 |
-
|
44 |
-
if self.use_conv_transpose:
|
45 |
-
raise NotImplementedError
|
46 |
-
|
47 |
-
# Cast to float32 to as 'upsample_nearest2d_out_frame' op does not support bfloat16
|
48 |
-
dtype = hidden_states.dtype
|
49 |
-
if dtype == torch.bfloat16:
|
50 |
-
hidden_states = hidden_states.to(torch.float32)
|
51 |
-
|
52 |
-
# upsample_nearest_nhwc fails with large batch sizes. see https://github.com/huggingface/diffusers/issues/984
|
53 |
-
if hidden_states.shape[0] >= 64:
|
54 |
-
hidden_states = hidden_states.contiguous()
|
55 |
-
|
56 |
-
# if `output_size` is passed we force the interpolation output
|
57 |
-
# size and do not make use of `scale_factor=2`
|
58 |
-
if output_size is None:
|
59 |
-
hidden_states = F.interpolate(hidden_states, scale_factor=[1.0, 2.0, 2.0], mode="nearest")
|
60 |
-
else:
|
61 |
-
hidden_states = F.interpolate(hidden_states, size=output_size, mode="nearest")
|
62 |
-
|
63 |
-
# If the input is bfloat16, we cast back to bfloat16
|
64 |
-
if dtype == torch.bfloat16:
|
65 |
-
hidden_states = hidden_states.to(dtype)
|
66 |
-
|
67 |
-
if self.use_conv:
|
68 |
-
if self.name == "conv":
|
69 |
-
hidden_states = self.conv(hidden_states)
|
70 |
-
else:
|
71 |
-
hidden_states = self.Conv2d_0(hidden_states)
|
72 |
-
|
73 |
-
return hidden_states
|
74 |
-
|
75 |
-
|
76 |
-
class Downsample2D(nn.Module):
|
77 |
-
def __init__(self, channels, use_conv=False, out_channels=None, padding=1, name="conv"):
|
78 |
-
super().__init__()
|
79 |
-
self.channels = channels
|
80 |
-
self.out_channels = out_channels or channels
|
81 |
-
self.use_conv = use_conv
|
82 |
-
self.padding = padding
|
83 |
-
stride = 2
|
84 |
-
self.name = name
|
85 |
-
|
86 |
-
if use_conv:
|
87 |
-
conv = InflatedConv3d(self.channels, self.out_channels, 3, stride=stride, padding=padding)
|
88 |
-
else:
|
89 |
-
raise NotImplementedError
|
90 |
-
|
91 |
-
if name == "conv":
|
92 |
-
self.Conv2d_0 = conv
|
93 |
-
self.conv = conv
|
94 |
-
elif name == "Conv2d_0":
|
95 |
-
self.conv = conv
|
96 |
-
else:
|
97 |
-
self.conv = conv
|
98 |
-
|
99 |
-
def forward(self, hidden_states):
|
100 |
-
assert hidden_states.shape[1] == self.channels
|
101 |
-
if self.use_conv and self.padding == 0:
|
102 |
-
raise NotImplementedError
|
103 |
-
|
104 |
-
assert hidden_states.shape[1] == self.channels
|
105 |
-
hidden_states = self.conv(hidden_states)
|
106 |
-
|
107 |
-
return hidden_states
|
108 |
-
|
109 |
-
|
110 |
-
class ResnetBlock2D(nn.Module):
|
111 |
-
def __init__(
|
112 |
-
self,
|
113 |
-
*,
|
114 |
-
in_channels,
|
115 |
-
out_channels=None,
|
116 |
-
conv_shortcut=False,
|
117 |
-
dropout=0.0,
|
118 |
-
temb_channels=512,
|
119 |
-
groups=32,
|
120 |
-
groups_out=None,
|
121 |
-
pre_norm=True,
|
122 |
-
eps=1e-6,
|
123 |
-
non_linearity="swish",
|
124 |
-
time_embedding_norm="default",
|
125 |
-
output_scale_factor=1.0,
|
126 |
-
use_in_shortcut=None,
|
127 |
-
):
|
128 |
-
super().__init__()
|
129 |
-
self.pre_norm = pre_norm
|
130 |
-
self.pre_norm = True
|
131 |
-
self.in_channels = in_channels
|
132 |
-
out_channels = in_channels if out_channels is None else out_channels
|
133 |
-
self.out_channels = out_channels
|
134 |
-
self.use_conv_shortcut = conv_shortcut
|
135 |
-
self.time_embedding_norm = time_embedding_norm
|
136 |
-
self.output_scale_factor = output_scale_factor
|
137 |
-
|
138 |
-
if groups_out is None:
|
139 |
-
groups_out = groups
|
140 |
-
|
141 |
-
self.norm1 = torch.nn.GroupNorm(num_groups=groups, num_channels=in_channels, eps=eps, affine=True)
|
142 |
-
|
143 |
-
self.conv1 = InflatedConv3d(in_channels, out_channels, kernel_size=3, stride=1, padding=1)
|
144 |
-
|
145 |
-
if temb_channels is not None:
|
146 |
-
if self.time_embedding_norm == "default":
|
147 |
-
time_emb_proj_out_channels = out_channels
|
148 |
-
elif self.time_embedding_norm == "scale_shift":
|
149 |
-
time_emb_proj_out_channels = out_channels * 2
|
150 |
-
else:
|
151 |
-
raise ValueError(f"unknown time_embedding_norm : {self.time_embedding_norm} ")
|
152 |
-
|
153 |
-
self.time_emb_proj = torch.nn.Linear(temb_channels, time_emb_proj_out_channels)
|
154 |
-
else:
|
155 |
-
self.time_emb_proj = None
|
156 |
-
|
157 |
-
self.norm2 = torch.nn.GroupNorm(num_groups=groups_out, num_channels=out_channels, eps=eps, affine=True)
|
158 |
-
self.dropout = torch.nn.Dropout(dropout)
|
159 |
-
self.conv2 = InflatedConv3d(out_channels, out_channels, kernel_size=3, stride=1, padding=1)
|
160 |
-
|
161 |
-
if non_linearity == "swish":
|
162 |
-
self.nonlinearity = lambda x: F.silu(x)
|
163 |
-
elif non_linearity == "mish":
|
164 |
-
self.nonlinearity = Mish()
|
165 |
-
elif non_linearity == "silu":
|
166 |
-
self.nonlinearity = nn.SiLU()
|
167 |
-
|
168 |
-
self.use_in_shortcut = self.in_channels != self.out_channels if use_in_shortcut is None else use_in_shortcut
|
169 |
-
|
170 |
-
self.conv_shortcut = None
|
171 |
-
if self.use_in_shortcut:
|
172 |
-
self.conv_shortcut = InflatedConv3d(in_channels, out_channels, kernel_size=1, stride=1, padding=0)
|
173 |
-
|
174 |
-
def forward(self, input_tensor, temb):
|
175 |
-
hidden_states = input_tensor
|
176 |
-
|
177 |
-
hidden_states = self.norm1(hidden_states)
|
178 |
-
hidden_states = self.nonlinearity(hidden_states)
|
179 |
-
|
180 |
-
hidden_states = self.conv1(hidden_states)
|
181 |
-
|
182 |
-
if temb is not None:
|
183 |
-
temb = self.time_emb_proj(self.nonlinearity(temb))[:, :, None, None, None]
|
184 |
-
|
185 |
-
if temb is not None and self.time_embedding_norm == "default":
|
186 |
-
hidden_states = hidden_states + temb
|
187 |
-
|
188 |
-
hidden_states = self.norm2(hidden_states)
|
189 |
-
|
190 |
-
if temb is not None and self.time_embedding_norm == "scale_shift":
|
191 |
-
scale, shift = torch.chunk(temb, 2, dim=1)
|
192 |
-
hidden_states = hidden_states * (1 + scale) + shift
|
193 |
-
|
194 |
-
hidden_states = self.nonlinearity(hidden_states)
|
195 |
-
|
196 |
-
hidden_states = self.dropout(hidden_states)
|
197 |
-
hidden_states = self.conv2(hidden_states)
|
198 |
-
|
199 |
-
if self.conv_shortcut is not None:
|
200 |
-
input_tensor = self.conv_shortcut(input_tensor)
|
201 |
-
|
202 |
-
output_tensor = (input_tensor + hidden_states) / self.output_scale_factor
|
203 |
-
|
204 |
-
return output_tensor
|
205 |
-
|
206 |
-
|
207 |
-
class Mish(torch.nn.Module):
|
208 |
-
def forward(self, hidden_states):
|
209 |
-
return hidden_states * torch.tanh(torch.nn.functional.softplus(hidden_states))
|
|
|
|
|
|
|
|
|
|
|
|
|
|
|
|
|
|
|
|
|
|
|
|
|
|
|
|
|
|
|
|
|
|
|
|
|
|
|
|
|
|
|
|
|
|
|
|
|
|
|
|
|
|
|
|
|
|
|
|
|
|
|
|
|
|
|
|
|
|
|
|
|
|
|
|
|
|
|
|
|
|
|
|
|
|
|
|
|
|
|
|
|
|
|
|
|
|
|
|
|
|
|
|
|
|
|
|
|
|
|
|
|
|
|
|
|
|
|
|
|
|
|
|
|
|
|
|
|
|
|
|
|
|
|
|
|
|
|
|
|
|
|
|
|
|
|
|
|
|
|
|
|
|
|
|
|
|
|
|
|
|
|
|
|
|
|
|
|
|
|
|
|
|
|
|
|
|
|
|
|
|
|
|
|
|
|
|
|
|
|
|
|
|
|
|
|
|
|
|
|
|
|
|
|
|
|
|
|
|
|
|
|
|
|
|
|
|
|
|
|
|
|
|
|
|
|
|
|
|
|
|
|
|
|
|
|
|
|
|
|
|
|
|
|
|
|
|
|
|
|
|
|
|
|
|
|
|
|
|
|
|
|
|
|
|
|
|
|
|
|
|
|
|
|
|
|
|
|
|
|
|
|
|
|
|
|
|
|
|
|
|
|
|
|
|
|
|
|
|
|
|
|
|
|
|
|
|
|
|
|
|
|
|
|
|
|
|
|
|
|
|
|
|
|
|
|
|
|
|
|
|
|
|
|
|
|
|
|
|
|
|
|
|
|
|
|
|
|
|
|
|
|
|
|
|
|
|
|
|
|
|
|
|
|
|
|
|
|
|
|
|
|
|
|
|
|
|
|
|
|
|
|
|
|
|
|
|
|
|
|
|
|
|
|
|
|
|
|
|
|
|
|
|
|
|
|
|
|
|
|
|
|
|
|
|
|
|
|
|
spaces/Badaleeloveashley/badaleeloveashley/README.md
DELETED
@@ -1,12 +0,0 @@
|
|
1 |
-
---
|
2 |
-
title: Badaleeloveashley
|
3 |
-
emoji: 🚀
|
4 |
-
colorFrom: yellow
|
5 |
-
colorTo: purple
|
6 |
-
sdk: gradio
|
7 |
-
sdk_version: 3.12.0
|
8 |
-
app_file: app.py
|
9 |
-
pinned: false
|
10 |
-
---
|
11 |
-
|
12 |
-
Check out the configuration reference at https://huggingface.co/docs/hub/spaces-config-reference
|
|
|
|
|
|
|
|
|
|
|
|
|
|
|
|
|
|
|
|
|
|
|
|
|
|
spaces/Banbri/zcvzcv/postcss.config.js
DELETED
@@ -1,6 +0,0 @@
|
|
1 |
-
module.exports = {
|
2 |
-
plugins: {
|
3 |
-
tailwindcss: {},
|
4 |
-
autoprefixer: {},
|
5 |
-
},
|
6 |
-
}
|
|
|
|
|
|
|
|
|
|
|
|
|
|
spaces/Banbri/zcvzcv/src/app/interface/grid/index.tsx
DELETED
@@ -1,26 +0,0 @@
|
|
1 |
-
"use client"
|
2 |
-
|
3 |
-
import { ReactNode } from "react"
|
4 |
-
|
5 |
-
import { cn } from "@/lib/utils"
|
6 |
-
import { useStore } from "@/app/store"
|
7 |
-
|
8 |
-
export function Grid({ children, className }: { children: ReactNode; className: string }) {
|
9 |
-
const zoomLevel = useStore(state => state.zoomLevel)
|
10 |
-
|
11 |
-
return (
|
12 |
-
<div
|
13 |
-
// the "fixed" width ensure our comic keeps a consistent ratio
|
14 |
-
className={cn(
|
15 |
-
`w-full h-full grid`,
|
16 |
-
className
|
17 |
-
)}
|
18 |
-
style={{
|
19 |
-
gap: `${(zoomLevel / 100) * 0.7}vw`
|
20 |
-
}}
|
21 |
-
>
|
22 |
-
{children}
|
23 |
-
</div>
|
24 |
-
)
|
25 |
-
}
|
26 |
-
|
|
|
|
|
|
|
|
|
|
|
|
|
|
|
|
|
|
|
|
|
|
|
|
|
|
|
|
|
|
|
|
|
|
|
|
|
|
|
|
|
|
|
|
|
|
|
|
|
|
|
|
|
|
spaces/BartPoint/VoiceChange_Beta/infer_pack/models.py
DELETED
@@ -1,1124 +0,0 @@
|
|
1 |
-
import math, pdb, os
|
2 |
-
from time import time as ttime
|
3 |
-
import torch
|
4 |
-
from torch import nn
|
5 |
-
from torch.nn import functional as F
|
6 |
-
from infer_pack import modules
|
7 |
-
from infer_pack import attentions
|
8 |
-
from infer_pack import commons
|
9 |
-
from infer_pack.commons import init_weights, get_padding
|
10 |
-
from torch.nn import Conv1d, ConvTranspose1d, AvgPool1d, Conv2d
|
11 |
-
from torch.nn.utils import weight_norm, remove_weight_norm, spectral_norm
|
12 |
-
from infer_pack.commons import init_weights
|
13 |
-
import numpy as np
|
14 |
-
from infer_pack import commons
|
15 |
-
|
16 |
-
|
17 |
-
class TextEncoder256(nn.Module):
|
18 |
-
def __init__(
|
19 |
-
self,
|
20 |
-
out_channels,
|
21 |
-
hidden_channels,
|
22 |
-
filter_channels,
|
23 |
-
n_heads,
|
24 |
-
n_layers,
|
25 |
-
kernel_size,
|
26 |
-
p_dropout,
|
27 |
-
f0=True,
|
28 |
-
):
|
29 |
-
super().__init__()
|
30 |
-
self.out_channels = out_channels
|
31 |
-
self.hidden_channels = hidden_channels
|
32 |
-
self.filter_channels = filter_channels
|
33 |
-
self.n_heads = n_heads
|
34 |
-
self.n_layers = n_layers
|
35 |
-
self.kernel_size = kernel_size
|
36 |
-
self.p_dropout = p_dropout
|
37 |
-
self.emb_phone = nn.Linear(256, hidden_channels)
|
38 |
-
self.lrelu = nn.LeakyReLU(0.1, inplace=True)
|
39 |
-
if f0 == True:
|
40 |
-
self.emb_pitch = nn.Embedding(256, hidden_channels) # pitch 256
|
41 |
-
self.encoder = attentions.Encoder(
|
42 |
-
hidden_channels, filter_channels, n_heads, n_layers, kernel_size, p_dropout
|
43 |
-
)
|
44 |
-
self.proj = nn.Conv1d(hidden_channels, out_channels * 2, 1)
|
45 |
-
|
46 |
-
def forward(self, phone, pitch, lengths):
|
47 |
-
if pitch == None:
|
48 |
-
x = self.emb_phone(phone)
|
49 |
-
else:
|
50 |
-
x = self.emb_phone(phone) + self.emb_pitch(pitch)
|
51 |
-
x = x * math.sqrt(self.hidden_channels) # [b, t, h]
|
52 |
-
x = self.lrelu(x)
|
53 |
-
x = torch.transpose(x, 1, -1) # [b, h, t]
|
54 |
-
x_mask = torch.unsqueeze(commons.sequence_mask(lengths, x.size(2)), 1).to(
|
55 |
-
x.dtype
|
56 |
-
)
|
57 |
-
x = self.encoder(x * x_mask, x_mask)
|
58 |
-
stats = self.proj(x) * x_mask
|
59 |
-
|
60 |
-
m, logs = torch.split(stats, self.out_channels, dim=1)
|
61 |
-
return m, logs, x_mask
|
62 |
-
|
63 |
-
|
64 |
-
class TextEncoder768(nn.Module):
|
65 |
-
def __init__(
|
66 |
-
self,
|
67 |
-
out_channels,
|
68 |
-
hidden_channels,
|
69 |
-
filter_channels,
|
70 |
-
n_heads,
|
71 |
-
n_layers,
|
72 |
-
kernel_size,
|
73 |
-
p_dropout,
|
74 |
-
f0=True,
|
75 |
-
):
|
76 |
-
super().__init__()
|
77 |
-
self.out_channels = out_channels
|
78 |
-
self.hidden_channels = hidden_channels
|
79 |
-
self.filter_channels = filter_channels
|
80 |
-
self.n_heads = n_heads
|
81 |
-
self.n_layers = n_layers
|
82 |
-
self.kernel_size = kernel_size
|
83 |
-
self.p_dropout = p_dropout
|
84 |
-
self.emb_phone = nn.Linear(768, hidden_channels)
|
85 |
-
self.lrelu = nn.LeakyReLU(0.1, inplace=True)
|
86 |
-
if f0 == True:
|
87 |
-
self.emb_pitch = nn.Embedding(256, hidden_channels) # pitch 256
|
88 |
-
self.encoder = attentions.Encoder(
|
89 |
-
hidden_channels, filter_channels, n_heads, n_layers, kernel_size, p_dropout
|
90 |
-
)
|
91 |
-
self.proj = nn.Conv1d(hidden_channels, out_channels * 2, 1)
|
92 |
-
|
93 |
-
def forward(self, phone, pitch, lengths):
|
94 |
-
if pitch == None:
|
95 |
-
x = self.emb_phone(phone)
|
96 |
-
else:
|
97 |
-
x = self.emb_phone(phone) + self.emb_pitch(pitch)
|
98 |
-
x = x * math.sqrt(self.hidden_channels) # [b, t, h]
|
99 |
-
x = self.lrelu(x)
|
100 |
-
x = torch.transpose(x, 1, -1) # [b, h, t]
|
101 |
-
x_mask = torch.unsqueeze(commons.sequence_mask(lengths, x.size(2)), 1).to(
|
102 |
-
x.dtype
|
103 |
-
)
|
104 |
-
x = self.encoder(x * x_mask, x_mask)
|
105 |
-
stats = self.proj(x) * x_mask
|
106 |
-
|
107 |
-
m, logs = torch.split(stats, self.out_channels, dim=1)
|
108 |
-
return m, logs, x_mask
|
109 |
-
|
110 |
-
|
111 |
-
class ResidualCouplingBlock(nn.Module):
|
112 |
-
def __init__(
|
113 |
-
self,
|
114 |
-
channels,
|
115 |
-
hidden_channels,
|
116 |
-
kernel_size,
|
117 |
-
dilation_rate,
|
118 |
-
n_layers,
|
119 |
-
n_flows=4,
|
120 |
-
gin_channels=0,
|
121 |
-
):
|
122 |
-
super().__init__()
|
123 |
-
self.channels = channels
|
124 |
-
self.hidden_channels = hidden_channels
|
125 |
-
self.kernel_size = kernel_size
|
126 |
-
self.dilation_rate = dilation_rate
|
127 |
-
self.n_layers = n_layers
|
128 |
-
self.n_flows = n_flows
|
129 |
-
self.gin_channels = gin_channels
|
130 |
-
|
131 |
-
self.flows = nn.ModuleList()
|
132 |
-
for i in range(n_flows):
|
133 |
-
self.flows.append(
|
134 |
-
modules.ResidualCouplingLayer(
|
135 |
-
channels,
|
136 |
-
hidden_channels,
|
137 |
-
kernel_size,
|
138 |
-
dilation_rate,
|
139 |
-
n_layers,
|
140 |
-
gin_channels=gin_channels,
|
141 |
-
mean_only=True,
|
142 |
-
)
|
143 |
-
)
|
144 |
-
self.flows.append(modules.Flip())
|
145 |
-
|
146 |
-
def forward(self, x, x_mask, g=None, reverse=False):
|
147 |
-
if not reverse:
|
148 |
-
for flow in self.flows:
|
149 |
-
x, _ = flow(x, x_mask, g=g, reverse=reverse)
|
150 |
-
else:
|
151 |
-
for flow in reversed(self.flows):
|
152 |
-
x = flow(x, x_mask, g=g, reverse=reverse)
|
153 |
-
return x
|
154 |
-
|
155 |
-
def remove_weight_norm(self):
|
156 |
-
for i in range(self.n_flows):
|
157 |
-
self.flows[i * 2].remove_weight_norm()
|
158 |
-
|
159 |
-
|
160 |
-
class PosteriorEncoder(nn.Module):
|
161 |
-
def __init__(
|
162 |
-
self,
|
163 |
-
in_channels,
|
164 |
-
out_channels,
|
165 |
-
hidden_channels,
|
166 |
-
kernel_size,
|
167 |
-
dilation_rate,
|
168 |
-
n_layers,
|
169 |
-
gin_channels=0,
|
170 |
-
):
|
171 |
-
super().__init__()
|
172 |
-
self.in_channels = in_channels
|
173 |
-
self.out_channels = out_channels
|
174 |
-
self.hidden_channels = hidden_channels
|
175 |
-
self.kernel_size = kernel_size
|
176 |
-
self.dilation_rate = dilation_rate
|
177 |
-
self.n_layers = n_layers
|
178 |
-
self.gin_channels = gin_channels
|
179 |
-
|
180 |
-
self.pre = nn.Conv1d(in_channels, hidden_channels, 1)
|
181 |
-
self.enc = modules.WN(
|
182 |
-
hidden_channels,
|
183 |
-
kernel_size,
|
184 |
-
dilation_rate,
|
185 |
-
n_layers,
|
186 |
-
gin_channels=gin_channels,
|
187 |
-
)
|
188 |
-
self.proj = nn.Conv1d(hidden_channels, out_channels * 2, 1)
|
189 |
-
|
190 |
-
def forward(self, x, x_lengths, g=None):
|
191 |
-
x_mask = torch.unsqueeze(commons.sequence_mask(x_lengths, x.size(2)), 1).to(
|
192 |
-
x.dtype
|
193 |
-
)
|
194 |
-
x = self.pre(x) * x_mask
|
195 |
-
x = self.enc(x, x_mask, g=g)
|
196 |
-
stats = self.proj(x) * x_mask
|
197 |
-
m, logs = torch.split(stats, self.out_channels, dim=1)
|
198 |
-
z = (m + torch.randn_like(m) * torch.exp(logs)) * x_mask
|
199 |
-
return z, m, logs, x_mask
|
200 |
-
|
201 |
-
def remove_weight_norm(self):
|
202 |
-
self.enc.remove_weight_norm()
|
203 |
-
|
204 |
-
|
205 |
-
class Generator(torch.nn.Module):
|
206 |
-
def __init__(
|
207 |
-
self,
|
208 |
-
initial_channel,
|
209 |
-
resblock,
|
210 |
-
resblock_kernel_sizes,
|
211 |
-
resblock_dilation_sizes,
|
212 |
-
upsample_rates,
|
213 |
-
upsample_initial_channel,
|
214 |
-
upsample_kernel_sizes,
|
215 |
-
gin_channels=0,
|
216 |
-
):
|
217 |
-
super(Generator, self).__init__()
|
218 |
-
self.num_kernels = len(resblock_kernel_sizes)
|
219 |
-
self.num_upsamples = len(upsample_rates)
|
220 |
-
self.conv_pre = Conv1d(
|
221 |
-
initial_channel, upsample_initial_channel, 7, 1, padding=3
|
222 |
-
)
|
223 |
-
resblock = modules.ResBlock1 if resblock == "1" else modules.ResBlock2
|
224 |
-
|
225 |
-
self.ups = nn.ModuleList()
|
226 |
-
for i, (u, k) in enumerate(zip(upsample_rates, upsample_kernel_sizes)):
|
227 |
-
self.ups.append(
|
228 |
-
weight_norm(
|
229 |
-
ConvTranspose1d(
|
230 |
-
upsample_initial_channel // (2**i),
|
231 |
-
upsample_initial_channel // (2 ** (i + 1)),
|
232 |
-
k,
|
233 |
-
u,
|
234 |
-
padding=(k - u) // 2,
|
235 |
-
)
|
236 |
-
)
|
237 |
-
)
|
238 |
-
|
239 |
-
self.resblocks = nn.ModuleList()
|
240 |
-
for i in range(len(self.ups)):
|
241 |
-
ch = upsample_initial_channel // (2 ** (i + 1))
|
242 |
-
for j, (k, d) in enumerate(
|
243 |
-
zip(resblock_kernel_sizes, resblock_dilation_sizes)
|
244 |
-
):
|
245 |
-
self.resblocks.append(resblock(ch, k, d))
|
246 |
-
|
247 |
-
self.conv_post = Conv1d(ch, 1, 7, 1, padding=3, bias=False)
|
248 |
-
self.ups.apply(init_weights)
|
249 |
-
|
250 |
-
if gin_channels != 0:
|
251 |
-
self.cond = nn.Conv1d(gin_channels, upsample_initial_channel, 1)
|
252 |
-
|
253 |
-
def forward(self, x, g=None):
|
254 |
-
x = self.conv_pre(x)
|
255 |
-
if g is not None:
|
256 |
-
x = x + self.cond(g)
|
257 |
-
|
258 |
-
for i in range(self.num_upsamples):
|
259 |
-
x = F.leaky_relu(x, modules.LRELU_SLOPE)
|
260 |
-
x = self.ups[i](x)
|
261 |
-
xs = None
|
262 |
-
for j in range(self.num_kernels):
|
263 |
-
if xs is None:
|
264 |
-
xs = self.resblocks[i * self.num_kernels + j](x)
|
265 |
-
else:
|
266 |
-
xs += self.resblocks[i * self.num_kernels + j](x)
|
267 |
-
x = xs / self.num_kernels
|
268 |
-
x = F.leaky_relu(x)
|
269 |
-
x = self.conv_post(x)
|
270 |
-
x = torch.tanh(x)
|
271 |
-
|
272 |
-
return x
|
273 |
-
|
274 |
-
def remove_weight_norm(self):
|
275 |
-
for l in self.ups:
|
276 |
-
remove_weight_norm(l)
|
277 |
-
for l in self.resblocks:
|
278 |
-
l.remove_weight_norm()
|
279 |
-
|
280 |
-
|
281 |
-
class SineGen(torch.nn.Module):
|
282 |
-
"""Definition of sine generator
|
283 |
-
SineGen(samp_rate, harmonic_num = 0,
|
284 |
-
sine_amp = 0.1, noise_std = 0.003,
|
285 |
-
voiced_threshold = 0,
|
286 |
-
flag_for_pulse=False)
|
287 |
-
samp_rate: sampling rate in Hz
|
288 |
-
harmonic_num: number of harmonic overtones (default 0)
|
289 |
-
sine_amp: amplitude of sine-wavefrom (default 0.1)
|
290 |
-
noise_std: std of Gaussian noise (default 0.003)
|
291 |
-
voiced_thoreshold: F0 threshold for U/V classification (default 0)
|
292 |
-
flag_for_pulse: this SinGen is used inside PulseGen (default False)
|
293 |
-
Note: when flag_for_pulse is True, the first time step of a voiced
|
294 |
-
segment is always sin(np.pi) or cos(0)
|
295 |
-
"""
|
296 |
-
|
297 |
-
def __init__(
|
298 |
-
self,
|
299 |
-
samp_rate,
|
300 |
-
harmonic_num=0,
|
301 |
-
sine_amp=0.1,
|
302 |
-
noise_std=0.003,
|
303 |
-
voiced_threshold=0,
|
304 |
-
flag_for_pulse=False,
|
305 |
-
):
|
306 |
-
super(SineGen, self).__init__()
|
307 |
-
self.sine_amp = sine_amp
|
308 |
-
self.noise_std = noise_std
|
309 |
-
self.harmonic_num = harmonic_num
|
310 |
-
self.dim = self.harmonic_num + 1
|
311 |
-
self.sampling_rate = samp_rate
|
312 |
-
self.voiced_threshold = voiced_threshold
|
313 |
-
|
314 |
-
def _f02uv(self, f0):
|
315 |
-
# generate uv signal
|
316 |
-
uv = torch.ones_like(f0)
|
317 |
-
uv = uv * (f0 > self.voiced_threshold)
|
318 |
-
return uv
|
319 |
-
|
320 |
-
def forward(self, f0, upp):
|
321 |
-
"""sine_tensor, uv = forward(f0)
|
322 |
-
input F0: tensor(batchsize=1, length, dim=1)
|
323 |
-
f0 for unvoiced steps should be 0
|
324 |
-
output sine_tensor: tensor(batchsize=1, length, dim)
|
325 |
-
output uv: tensor(batchsize=1, length, 1)
|
326 |
-
"""
|
327 |
-
with torch.no_grad():
|
328 |
-
f0 = f0[:, None].transpose(1, 2)
|
329 |
-
f0_buf = torch.zeros(f0.shape[0], f0.shape[1], self.dim, device=f0.device)
|
330 |
-
# fundamental component
|
331 |
-
f0_buf[:, :, 0] = f0[:, :, 0]
|
332 |
-
for idx in np.arange(self.harmonic_num):
|
333 |
-
f0_buf[:, :, idx + 1] = f0_buf[:, :, 0] * (
|
334 |
-
idx + 2
|
335 |
-
) # idx + 2: the (idx+1)-th overtone, (idx+2)-th harmonic
|
336 |
-
rad_values = (f0_buf / self.sampling_rate) % 1 ###%1意味着n_har的乘积无法后处理优化
|
337 |
-
rand_ini = torch.rand(
|
338 |
-
f0_buf.shape[0], f0_buf.shape[2], device=f0_buf.device
|
339 |
-
)
|
340 |
-
rand_ini[:, 0] = 0
|
341 |
-
rad_values[:, 0, :] = rad_values[:, 0, :] + rand_ini
|
342 |
-
tmp_over_one = torch.cumsum(rad_values, 1) # % 1 #####%1意味着后面的cumsum无法再优化
|
343 |
-
tmp_over_one *= upp
|
344 |
-
tmp_over_one = F.interpolate(
|
345 |
-
tmp_over_one.transpose(2, 1),
|
346 |
-
scale_factor=upp,
|
347 |
-
mode="linear",
|
348 |
-
align_corners=True,
|
349 |
-
).transpose(2, 1)
|
350 |
-
rad_values = F.interpolate(
|
351 |
-
rad_values.transpose(2, 1), scale_factor=upp, mode="nearest"
|
352 |
-
).transpose(
|
353 |
-
2, 1
|
354 |
-
) #######
|
355 |
-
tmp_over_one %= 1
|
356 |
-
tmp_over_one_idx = (tmp_over_one[:, 1:, :] - tmp_over_one[:, :-1, :]) < 0
|
357 |
-
cumsum_shift = torch.zeros_like(rad_values)
|
358 |
-
cumsum_shift[:, 1:, :] = tmp_over_one_idx * -1.0
|
359 |
-
sine_waves = torch.sin(
|
360 |
-
torch.cumsum(rad_values + cumsum_shift, dim=1) * 2 * np.pi
|
361 |
-
)
|
362 |
-
sine_waves = sine_waves * self.sine_amp
|
363 |
-
uv = self._f02uv(f0)
|
364 |
-
uv = F.interpolate(
|
365 |
-
uv.transpose(2, 1), scale_factor=upp, mode="nearest"
|
366 |
-
).transpose(2, 1)
|
367 |
-
noise_amp = uv * self.noise_std + (1 - uv) * self.sine_amp / 3
|
368 |
-
noise = noise_amp * torch.randn_like(sine_waves)
|
369 |
-
sine_waves = sine_waves * uv + noise
|
370 |
-
return sine_waves, uv, noise
|
371 |
-
|
372 |
-
|
373 |
-
class SourceModuleHnNSF(torch.nn.Module):
|
374 |
-
"""SourceModule for hn-nsf
|
375 |
-
SourceModule(sampling_rate, harmonic_num=0, sine_amp=0.1,
|
376 |
-
add_noise_std=0.003, voiced_threshod=0)
|
377 |
-
sampling_rate: sampling_rate in Hz
|
378 |
-
harmonic_num: number of harmonic above F0 (default: 0)
|
379 |
-
sine_amp: amplitude of sine source signal (default: 0.1)
|
380 |
-
add_noise_std: std of additive Gaussian noise (default: 0.003)
|
381 |
-
note that amplitude of noise in unvoiced is decided
|
382 |
-
by sine_amp
|
383 |
-
voiced_threshold: threhold to set U/V given F0 (default: 0)
|
384 |
-
Sine_source, noise_source = SourceModuleHnNSF(F0_sampled)
|
385 |
-
F0_sampled (batchsize, length, 1)
|
386 |
-
Sine_source (batchsize, length, 1)
|
387 |
-
noise_source (batchsize, length 1)
|
388 |
-
uv (batchsize, length, 1)
|
389 |
-
"""
|
390 |
-
|
391 |
-
def __init__(
|
392 |
-
self,
|
393 |
-
sampling_rate,
|
394 |
-
harmonic_num=0,
|
395 |
-
sine_amp=0.1,
|
396 |
-
add_noise_std=0.003,
|
397 |
-
voiced_threshod=0,
|
398 |
-
is_half=True,
|
399 |
-
):
|
400 |
-
super(SourceModuleHnNSF, self).__init__()
|
401 |
-
|
402 |
-
self.sine_amp = sine_amp
|
403 |
-
self.noise_std = add_noise_std
|
404 |
-
self.is_half = is_half
|
405 |
-
# to produce sine waveforms
|
406 |
-
self.l_sin_gen = SineGen(
|
407 |
-
sampling_rate, harmonic_num, sine_amp, add_noise_std, voiced_threshod
|
408 |
-
)
|
409 |
-
|
410 |
-
# to merge source harmonics into a single excitation
|
411 |
-
self.l_linear = torch.nn.Linear(harmonic_num + 1, 1)
|
412 |
-
self.l_tanh = torch.nn.Tanh()
|
413 |
-
|
414 |
-
def forward(self, x, upp=None):
|
415 |
-
sine_wavs, uv, _ = self.l_sin_gen(x, upp)
|
416 |
-
if self.is_half:
|
417 |
-
sine_wavs = sine_wavs.half()
|
418 |
-
sine_merge = self.l_tanh(self.l_linear(sine_wavs))
|
419 |
-
return sine_merge, None, None # noise, uv
|
420 |
-
|
421 |
-
|
422 |
-
class GeneratorNSF(torch.nn.Module):
|
423 |
-
def __init__(
|
424 |
-
self,
|
425 |
-
initial_channel,
|
426 |
-
resblock,
|
427 |
-
resblock_kernel_sizes,
|
428 |
-
resblock_dilation_sizes,
|
429 |
-
upsample_rates,
|
430 |
-
upsample_initial_channel,
|
431 |
-
upsample_kernel_sizes,
|
432 |
-
gin_channels,
|
433 |
-
sr,
|
434 |
-
is_half=False,
|
435 |
-
):
|
436 |
-
super(GeneratorNSF, self).__init__()
|
437 |
-
self.num_kernels = len(resblock_kernel_sizes)
|
438 |
-
self.num_upsamples = len(upsample_rates)
|
439 |
-
|
440 |
-
self.f0_upsamp = torch.nn.Upsample(scale_factor=np.prod(upsample_rates))
|
441 |
-
self.m_source = SourceModuleHnNSF(
|
442 |
-
sampling_rate=sr, harmonic_num=0, is_half=is_half
|
443 |
-
)
|
444 |
-
self.noise_convs = nn.ModuleList()
|
445 |
-
self.conv_pre = Conv1d(
|
446 |
-
initial_channel, upsample_initial_channel, 7, 1, padding=3
|
447 |
-
)
|
448 |
-
resblock = modules.ResBlock1 if resblock == "1" else modules.ResBlock2
|
449 |
-
|
450 |
-
self.ups = nn.ModuleList()
|
451 |
-
for i, (u, k) in enumerate(zip(upsample_rates, upsample_kernel_sizes)):
|
452 |
-
c_cur = upsample_initial_channel // (2 ** (i + 1))
|
453 |
-
self.ups.append(
|
454 |
-
weight_norm(
|
455 |
-
ConvTranspose1d(
|
456 |
-
upsample_initial_channel // (2**i),
|
457 |
-
upsample_initial_channel // (2 ** (i + 1)),
|
458 |
-
k,
|
459 |
-
u,
|
460 |
-
padding=(k - u) // 2,
|
461 |
-
)
|
462 |
-
)
|
463 |
-
)
|
464 |
-
if i + 1 < len(upsample_rates):
|
465 |
-
stride_f0 = np.prod(upsample_rates[i + 1 :])
|
466 |
-
self.noise_convs.append(
|
467 |
-
Conv1d(
|
468 |
-
1,
|
469 |
-
c_cur,
|
470 |
-
kernel_size=stride_f0 * 2,
|
471 |
-
stride=stride_f0,
|
472 |
-
padding=stride_f0 // 2,
|
473 |
-
)
|
474 |
-
)
|
475 |
-
else:
|
476 |
-
self.noise_convs.append(Conv1d(1, c_cur, kernel_size=1))
|
477 |
-
|
478 |
-
self.resblocks = nn.ModuleList()
|
479 |
-
for i in range(len(self.ups)):
|
480 |
-
ch = upsample_initial_channel // (2 ** (i + 1))
|
481 |
-
for j, (k, d) in enumerate(
|
482 |
-
zip(resblock_kernel_sizes, resblock_dilation_sizes)
|
483 |
-
):
|
484 |
-
self.resblocks.append(resblock(ch, k, d))
|
485 |
-
|
486 |
-
self.conv_post = Conv1d(ch, 1, 7, 1, padding=3, bias=False)
|
487 |
-
self.ups.apply(init_weights)
|
488 |
-
|
489 |
-
if gin_channels != 0:
|
490 |
-
self.cond = nn.Conv1d(gin_channels, upsample_initial_channel, 1)
|
491 |
-
|
492 |
-
self.upp = np.prod(upsample_rates)
|
493 |
-
|
494 |
-
def forward(self, x, f0, g=None):
|
495 |
-
har_source, noi_source, uv = self.m_source(f0, self.upp)
|
496 |
-
har_source = har_source.transpose(1, 2)
|
497 |
-
x = self.conv_pre(x)
|
498 |
-
if g is not None:
|
499 |
-
x = x + self.cond(g)
|
500 |
-
|
501 |
-
for i in range(self.num_upsamples):
|
502 |
-
x = F.leaky_relu(x, modules.LRELU_SLOPE)
|
503 |
-
x = self.ups[i](x)
|
504 |
-
x_source = self.noise_convs[i](har_source)
|
505 |
-
x = x + x_source
|
506 |
-
xs = None
|
507 |
-
for j in range(self.num_kernels):
|
508 |
-
if xs is None:
|
509 |
-
xs = self.resblocks[i * self.num_kernels + j](x)
|
510 |
-
else:
|
511 |
-
xs += self.resblocks[i * self.num_kernels + j](x)
|
512 |
-
x = xs / self.num_kernels
|
513 |
-
x = F.leaky_relu(x)
|
514 |
-
x = self.conv_post(x)
|
515 |
-
x = torch.tanh(x)
|
516 |
-
return x
|
517 |
-
|
518 |
-
def remove_weight_norm(self):
|
519 |
-
for l in self.ups:
|
520 |
-
remove_weight_norm(l)
|
521 |
-
for l in self.resblocks:
|
522 |
-
l.remove_weight_norm()
|
523 |
-
|
524 |
-
|
525 |
-
sr2sr = {
|
526 |
-
"32k": 32000,
|
527 |
-
"40k": 40000,
|
528 |
-
"48k": 48000,
|
529 |
-
}
|
530 |
-
|
531 |
-
|
532 |
-
class SynthesizerTrnMs256NSFsid(nn.Module):
|
533 |
-
def __init__(
|
534 |
-
self,
|
535 |
-
spec_channels,
|
536 |
-
segment_size,
|
537 |
-
inter_channels,
|
538 |
-
hidden_channels,
|
539 |
-
filter_channels,
|
540 |
-
n_heads,
|
541 |
-
n_layers,
|
542 |
-
kernel_size,
|
543 |
-
p_dropout,
|
544 |
-
resblock,
|
545 |
-
resblock_kernel_sizes,
|
546 |
-
resblock_dilation_sizes,
|
547 |
-
upsample_rates,
|
548 |
-
upsample_initial_channel,
|
549 |
-
upsample_kernel_sizes,
|
550 |
-
spk_embed_dim,
|
551 |
-
gin_channels,
|
552 |
-
sr,
|
553 |
-
**kwargs
|
554 |
-
):
|
555 |
-
super().__init__()
|
556 |
-
if type(sr) == type("strr"):
|
557 |
-
sr = sr2sr[sr]
|
558 |
-
self.spec_channels = spec_channels
|
559 |
-
self.inter_channels = inter_channels
|
560 |
-
self.hidden_channels = hidden_channels
|
561 |
-
self.filter_channels = filter_channels
|
562 |
-
self.n_heads = n_heads
|
563 |
-
self.n_layers = n_layers
|
564 |
-
self.kernel_size = kernel_size
|
565 |
-
self.p_dropout = p_dropout
|
566 |
-
self.resblock = resblock
|
567 |
-
self.resblock_kernel_sizes = resblock_kernel_sizes
|
568 |
-
self.resblock_dilation_sizes = resblock_dilation_sizes
|
569 |
-
self.upsample_rates = upsample_rates
|
570 |
-
self.upsample_initial_channel = upsample_initial_channel
|
571 |
-
self.upsample_kernel_sizes = upsample_kernel_sizes
|
572 |
-
self.segment_size = segment_size
|
573 |
-
self.gin_channels = gin_channels
|
574 |
-
# self.hop_length = hop_length#
|
575 |
-
self.spk_embed_dim = spk_embed_dim
|
576 |
-
self.enc_p = TextEncoder256(
|
577 |
-
inter_channels,
|
578 |
-
hidden_channels,
|
579 |
-
filter_channels,
|
580 |
-
n_heads,
|
581 |
-
n_layers,
|
582 |
-
kernel_size,
|
583 |
-
p_dropout,
|
584 |
-
)
|
585 |
-
self.dec = GeneratorNSF(
|
586 |
-
inter_channels,
|
587 |
-
resblock,
|
588 |
-
resblock_kernel_sizes,
|
589 |
-
resblock_dilation_sizes,
|
590 |
-
upsample_rates,
|
591 |
-
upsample_initial_channel,
|
592 |
-
upsample_kernel_sizes,
|
593 |
-
gin_channels=gin_channels,
|
594 |
-
sr=sr,
|
595 |
-
is_half=kwargs["is_half"],
|
596 |
-
)
|
597 |
-
self.enc_q = PosteriorEncoder(
|
598 |
-
spec_channels,
|
599 |
-
inter_channels,
|
600 |
-
hidden_channels,
|
601 |
-
5,
|
602 |
-
1,
|
603 |
-
16,
|
604 |
-
gin_channels=gin_channels,
|
605 |
-
)
|
606 |
-
self.flow = ResidualCouplingBlock(
|
607 |
-
inter_channels, hidden_channels, 5, 1, 3, gin_channels=gin_channels
|
608 |
-
)
|
609 |
-
self.emb_g = nn.Embedding(self.spk_embed_dim, gin_channels)
|
610 |
-
print("gin_channels:", gin_channels, "self.spk_embed_dim:", self.spk_embed_dim)
|
611 |
-
|
612 |
-
def remove_weight_norm(self):
|
613 |
-
self.dec.remove_weight_norm()
|
614 |
-
self.flow.remove_weight_norm()
|
615 |
-
self.enc_q.remove_weight_norm()
|
616 |
-
|
617 |
-
def forward(
|
618 |
-
self, phone, phone_lengths, pitch, pitchf, y, y_lengths, ds
|
619 |
-
): # 这里ds是id,[bs,1]
|
620 |
-
# print(1,pitch.shape)#[bs,t]
|
621 |
-
g = self.emb_g(ds).unsqueeze(-1) # [b, 256, 1]##1是t,广播的
|
622 |
-
m_p, logs_p, x_mask = self.enc_p(phone, pitch, phone_lengths)
|
623 |
-
z, m_q, logs_q, y_mask = self.enc_q(y, y_lengths, g=g)
|
624 |
-
z_p = self.flow(z, y_mask, g=g)
|
625 |
-
z_slice, ids_slice = commons.rand_slice_segments(
|
626 |
-
z, y_lengths, self.segment_size
|
627 |
-
)
|
628 |
-
# print(-1,pitchf.shape,ids_slice,self.segment_size,self.hop_length,self.segment_size//self.hop_length)
|
629 |
-
pitchf = commons.slice_segments2(pitchf, ids_slice, self.segment_size)
|
630 |
-
# print(-2,pitchf.shape,z_slice.shape)
|
631 |
-
o = self.dec(z_slice, pitchf, g=g)
|
632 |
-
return o, ids_slice, x_mask, y_mask, (z, z_p, m_p, logs_p, m_q, logs_q)
|
633 |
-
|
634 |
-
def infer(self, phone, phone_lengths, pitch, nsff0, sid, max_len=None):
|
635 |
-
g = self.emb_g(sid).unsqueeze(-1)
|
636 |
-
m_p, logs_p, x_mask = self.enc_p(phone, pitch, phone_lengths)
|
637 |
-
z_p = (m_p + torch.exp(logs_p) * torch.randn_like(m_p) * 0.66666) * x_mask
|
638 |
-
z = self.flow(z_p, x_mask, g=g, reverse=True)
|
639 |
-
o = self.dec((z * x_mask)[:, :, :max_len], nsff0, g=g)
|
640 |
-
return o, x_mask, (z, z_p, m_p, logs_p)
|
641 |
-
|
642 |
-
|
643 |
-
class SynthesizerTrnMs768NSFsid(nn.Module):
|
644 |
-
def __init__(
|
645 |
-
self,
|
646 |
-
spec_channels,
|
647 |
-
segment_size,
|
648 |
-
inter_channels,
|
649 |
-
hidden_channels,
|
650 |
-
filter_channels,
|
651 |
-
n_heads,
|
652 |
-
n_layers,
|
653 |
-
kernel_size,
|
654 |
-
p_dropout,
|
655 |
-
resblock,
|
656 |
-
resblock_kernel_sizes,
|
657 |
-
resblock_dilation_sizes,
|
658 |
-
upsample_rates,
|
659 |
-
upsample_initial_channel,
|
660 |
-
upsample_kernel_sizes,
|
661 |
-
spk_embed_dim,
|
662 |
-
gin_channels,
|
663 |
-
sr,
|
664 |
-
**kwargs
|
665 |
-
):
|
666 |
-
super().__init__()
|
667 |
-
if type(sr) == type("strr"):
|
668 |
-
sr = sr2sr[sr]
|
669 |
-
self.spec_channels = spec_channels
|
670 |
-
self.inter_channels = inter_channels
|
671 |
-
self.hidden_channels = hidden_channels
|
672 |
-
self.filter_channels = filter_channels
|
673 |
-
self.n_heads = n_heads
|
674 |
-
self.n_layers = n_layers
|
675 |
-
self.kernel_size = kernel_size
|
676 |
-
self.p_dropout = p_dropout
|
677 |
-
self.resblock = resblock
|
678 |
-
self.resblock_kernel_sizes = resblock_kernel_sizes
|
679 |
-
self.resblock_dilation_sizes = resblock_dilation_sizes
|
680 |
-
self.upsample_rates = upsample_rates
|
681 |
-
self.upsample_initial_channel = upsample_initial_channel
|
682 |
-
self.upsample_kernel_sizes = upsample_kernel_sizes
|
683 |
-
self.segment_size = segment_size
|
684 |
-
self.gin_channels = gin_channels
|
685 |
-
# self.hop_length = hop_length#
|
686 |
-
self.spk_embed_dim = spk_embed_dim
|
687 |
-
self.enc_p = TextEncoder768(
|
688 |
-
inter_channels,
|
689 |
-
hidden_channels,
|
690 |
-
filter_channels,
|
691 |
-
n_heads,
|
692 |
-
n_layers,
|
693 |
-
kernel_size,
|
694 |
-
p_dropout,
|
695 |
-
)
|
696 |
-
self.dec = GeneratorNSF(
|
697 |
-
inter_channels,
|
698 |
-
resblock,
|
699 |
-
resblock_kernel_sizes,
|
700 |
-
resblock_dilation_sizes,
|
701 |
-
upsample_rates,
|
702 |
-
upsample_initial_channel,
|
703 |
-
upsample_kernel_sizes,
|
704 |
-
gin_channels=gin_channels,
|
705 |
-
sr=sr,
|
706 |
-
is_half=kwargs["is_half"],
|
707 |
-
)
|
708 |
-
self.enc_q = PosteriorEncoder(
|
709 |
-
spec_channels,
|
710 |
-
inter_channels,
|
711 |
-
hidden_channels,
|
712 |
-
5,
|
713 |
-
1,
|
714 |
-
16,
|
715 |
-
gin_channels=gin_channels,
|
716 |
-
)
|
717 |
-
self.flow = ResidualCouplingBlock(
|
718 |
-
inter_channels, hidden_channels, 5, 1, 3, gin_channels=gin_channels
|
719 |
-
)
|
720 |
-
self.emb_g = nn.Embedding(self.spk_embed_dim, gin_channels)
|
721 |
-
print("gin_channels:", gin_channels, "self.spk_embed_dim:", self.spk_embed_dim)
|
722 |
-
|
723 |
-
def remove_weight_norm(self):
|
724 |
-
self.dec.remove_weight_norm()
|
725 |
-
self.flow.remove_weight_norm()
|
726 |
-
self.enc_q.remove_weight_norm()
|
727 |
-
|
728 |
-
def forward(
|
729 |
-
self, phone, phone_lengths, pitch, pitchf, y, y_lengths, ds
|
730 |
-
): # 这里ds是id,[bs,1]
|
731 |
-
# print(1,pitch.shape)#[bs,t]
|
732 |
-
g = self.emb_g(ds).unsqueeze(-1) # [b, 256, 1]##1是t,广播的
|
733 |
-
m_p, logs_p, x_mask = self.enc_p(phone, pitch, phone_lengths)
|
734 |
-
z, m_q, logs_q, y_mask = self.enc_q(y, y_lengths, g=g)
|
735 |
-
z_p = self.flow(z, y_mask, g=g)
|
736 |
-
z_slice, ids_slice = commons.rand_slice_segments(
|
737 |
-
z, y_lengths, self.segment_size
|
738 |
-
)
|
739 |
-
# print(-1,pitchf.shape,ids_slice,self.segment_size,self.hop_length,self.segment_size//self.hop_length)
|
740 |
-
pitchf = commons.slice_segments2(pitchf, ids_slice, self.segment_size)
|
741 |
-
# print(-2,pitchf.shape,z_slice.shape)
|
742 |
-
o = self.dec(z_slice, pitchf, g=g)
|
743 |
-
return o, ids_slice, x_mask, y_mask, (z, z_p, m_p, logs_p, m_q, logs_q)
|
744 |
-
|
745 |
-
def infer(self, phone, phone_lengths, pitch, nsff0, sid, max_len=None):
|
746 |
-
g = self.emb_g(sid).unsqueeze(-1)
|
747 |
-
m_p, logs_p, x_mask = self.enc_p(phone, pitch, phone_lengths)
|
748 |
-
z_p = (m_p + torch.exp(logs_p) * torch.randn_like(m_p) * 0.66666) * x_mask
|
749 |
-
z = self.flow(z_p, x_mask, g=g, reverse=True)
|
750 |
-
o = self.dec((z * x_mask)[:, :, :max_len], nsff0, g=g)
|
751 |
-
return o, x_mask, (z, z_p, m_p, logs_p)
|
752 |
-
|
753 |
-
|
754 |
-
class SynthesizerTrnMs256NSFsid_nono(nn.Module):
|
755 |
-
def __init__(
|
756 |
-
self,
|
757 |
-
spec_channels,
|
758 |
-
segment_size,
|
759 |
-
inter_channels,
|
760 |
-
hidden_channels,
|
761 |
-
filter_channels,
|
762 |
-
n_heads,
|
763 |
-
n_layers,
|
764 |
-
kernel_size,
|
765 |
-
p_dropout,
|
766 |
-
resblock,
|
767 |
-
resblock_kernel_sizes,
|
768 |
-
resblock_dilation_sizes,
|
769 |
-
upsample_rates,
|
770 |
-
upsample_initial_channel,
|
771 |
-
upsample_kernel_sizes,
|
772 |
-
spk_embed_dim,
|
773 |
-
gin_channels,
|
774 |
-
sr=None,
|
775 |
-
**kwargs
|
776 |
-
):
|
777 |
-
super().__init__()
|
778 |
-
self.spec_channels = spec_channels
|
779 |
-
self.inter_channels = inter_channels
|
780 |
-
self.hidden_channels = hidden_channels
|
781 |
-
self.filter_channels = filter_channels
|
782 |
-
self.n_heads = n_heads
|
783 |
-
self.n_layers = n_layers
|
784 |
-
self.kernel_size = kernel_size
|
785 |
-
self.p_dropout = p_dropout
|
786 |
-
self.resblock = resblock
|
787 |
-
self.resblock_kernel_sizes = resblock_kernel_sizes
|
788 |
-
self.resblock_dilation_sizes = resblock_dilation_sizes
|
789 |
-
self.upsample_rates = upsample_rates
|
790 |
-
self.upsample_initial_channel = upsample_initial_channel
|
791 |
-
self.upsample_kernel_sizes = upsample_kernel_sizes
|
792 |
-
self.segment_size = segment_size
|
793 |
-
self.gin_channels = gin_channels
|
794 |
-
# self.hop_length = hop_length#
|
795 |
-
self.spk_embed_dim = spk_embed_dim
|
796 |
-
self.enc_p = TextEncoder256(
|
797 |
-
inter_channels,
|
798 |
-
hidden_channels,
|
799 |
-
filter_channels,
|
800 |
-
n_heads,
|
801 |
-
n_layers,
|
802 |
-
kernel_size,
|
803 |
-
p_dropout,
|
804 |
-
f0=False,
|
805 |
-
)
|
806 |
-
self.dec = Generator(
|
807 |
-
inter_channels,
|
808 |
-
resblock,
|
809 |
-
resblock_kernel_sizes,
|
810 |
-
resblock_dilation_sizes,
|
811 |
-
upsample_rates,
|
812 |
-
upsample_initial_channel,
|
813 |
-
upsample_kernel_sizes,
|
814 |
-
gin_channels=gin_channels,
|
815 |
-
)
|
816 |
-
self.enc_q = PosteriorEncoder(
|
817 |
-
spec_channels,
|
818 |
-
inter_channels,
|
819 |
-
hidden_channels,
|
820 |
-
5,
|
821 |
-
1,
|
822 |
-
16,
|
823 |
-
gin_channels=gin_channels,
|
824 |
-
)
|
825 |
-
self.flow = ResidualCouplingBlock(
|
826 |
-
inter_channels, hidden_channels, 5, 1, 3, gin_channels=gin_channels
|
827 |
-
)
|
828 |
-
self.emb_g = nn.Embedding(self.spk_embed_dim, gin_channels)
|
829 |
-
print("gin_channels:", gin_channels, "self.spk_embed_dim:", self.spk_embed_dim)
|
830 |
-
|
831 |
-
def remove_weight_norm(self):
|
832 |
-
self.dec.remove_weight_norm()
|
833 |
-
self.flow.remove_weight_norm()
|
834 |
-
self.enc_q.remove_weight_norm()
|
835 |
-
|
836 |
-
def forward(self, phone, phone_lengths, y, y_lengths, ds): # 这里ds是id,[bs,1]
|
837 |
-
g = self.emb_g(ds).unsqueeze(-1) # [b, 256, 1]##1是t,广播的
|
838 |
-
m_p, logs_p, x_mask = self.enc_p(phone, None, phone_lengths)
|
839 |
-
z, m_q, logs_q, y_mask = self.enc_q(y, y_lengths, g=g)
|
840 |
-
z_p = self.flow(z, y_mask, g=g)
|
841 |
-
z_slice, ids_slice = commons.rand_slice_segments(
|
842 |
-
z, y_lengths, self.segment_size
|
843 |
-
)
|
844 |
-
o = self.dec(z_slice, g=g)
|
845 |
-
return o, ids_slice, x_mask, y_mask, (z, z_p, m_p, logs_p, m_q, logs_q)
|
846 |
-
|
847 |
-
def infer(self, phone, phone_lengths, sid, max_len=None):
|
848 |
-
g = self.emb_g(sid).unsqueeze(-1)
|
849 |
-
m_p, logs_p, x_mask = self.enc_p(phone, None, phone_lengths)
|
850 |
-
z_p = (m_p + torch.exp(logs_p) * torch.randn_like(m_p) * 0.66666) * x_mask
|
851 |
-
z = self.flow(z_p, x_mask, g=g, reverse=True)
|
852 |
-
o = self.dec((z * x_mask)[:, :, :max_len], g=g)
|
853 |
-
return o, x_mask, (z, z_p, m_p, logs_p)
|
854 |
-
|
855 |
-
|
856 |
-
class SynthesizerTrnMs768NSFsid_nono(nn.Module):
|
857 |
-
def __init__(
|
858 |
-
self,
|
859 |
-
spec_channels,
|
860 |
-
segment_size,
|
861 |
-
inter_channels,
|
862 |
-
hidden_channels,
|
863 |
-
filter_channels,
|
864 |
-
n_heads,
|
865 |
-
n_layers,
|
866 |
-
kernel_size,
|
867 |
-
p_dropout,
|
868 |
-
resblock,
|
869 |
-
resblock_kernel_sizes,
|
870 |
-
resblock_dilation_sizes,
|
871 |
-
upsample_rates,
|
872 |
-
upsample_initial_channel,
|
873 |
-
upsample_kernel_sizes,
|
874 |
-
spk_embed_dim,
|
875 |
-
gin_channels,
|
876 |
-
sr=None,
|
877 |
-
**kwargs
|
878 |
-
):
|
879 |
-
super().__init__()
|
880 |
-
self.spec_channels = spec_channels
|
881 |
-
self.inter_channels = inter_channels
|
882 |
-
self.hidden_channels = hidden_channels
|
883 |
-
self.filter_channels = filter_channels
|
884 |
-
self.n_heads = n_heads
|
885 |
-
self.n_layers = n_layers
|
886 |
-
self.kernel_size = kernel_size
|
887 |
-
self.p_dropout = p_dropout
|
888 |
-
self.resblock = resblock
|
889 |
-
self.resblock_kernel_sizes = resblock_kernel_sizes
|
890 |
-
self.resblock_dilation_sizes = resblock_dilation_sizes
|
891 |
-
self.upsample_rates = upsample_rates
|
892 |
-
self.upsample_initial_channel = upsample_initial_channel
|
893 |
-
self.upsample_kernel_sizes = upsample_kernel_sizes
|
894 |
-
self.segment_size = segment_size
|
895 |
-
self.gin_channels = gin_channels
|
896 |
-
# self.hop_length = hop_length#
|
897 |
-
self.spk_embed_dim = spk_embed_dim
|
898 |
-
self.enc_p = TextEncoder768(
|
899 |
-
inter_channels,
|
900 |
-
hidden_channels,
|
901 |
-
filter_channels,
|
902 |
-
n_heads,
|
903 |
-
n_layers,
|
904 |
-
kernel_size,
|
905 |
-
p_dropout,
|
906 |
-
f0=False,
|
907 |
-
)
|
908 |
-
self.dec = Generator(
|
909 |
-
inter_channels,
|
910 |
-
resblock,
|
911 |
-
resblock_kernel_sizes,
|
912 |
-
resblock_dilation_sizes,
|
913 |
-
upsample_rates,
|
914 |
-
upsample_initial_channel,
|
915 |
-
upsample_kernel_sizes,
|
916 |
-
gin_channels=gin_channels,
|
917 |
-
)
|
918 |
-
self.enc_q = PosteriorEncoder(
|
919 |
-
spec_channels,
|
920 |
-
inter_channels,
|
921 |
-
hidden_channels,
|
922 |
-
5,
|
923 |
-
1,
|
924 |
-
16,
|
925 |
-
gin_channels=gin_channels,
|
926 |
-
)
|
927 |
-
self.flow = ResidualCouplingBlock(
|
928 |
-
inter_channels, hidden_channels, 5, 1, 3, gin_channels=gin_channels
|
929 |
-
)
|
930 |
-
self.emb_g = nn.Embedding(self.spk_embed_dim, gin_channels)
|
931 |
-
print("gin_channels:", gin_channels, "self.spk_embed_dim:", self.spk_embed_dim)
|
932 |
-
|
933 |
-
def remove_weight_norm(self):
|
934 |
-
self.dec.remove_weight_norm()
|
935 |
-
self.flow.remove_weight_norm()
|
936 |
-
self.enc_q.remove_weight_norm()
|
937 |
-
|
938 |
-
def forward(self, phone, phone_lengths, y, y_lengths, ds): # 这里ds是id,[bs,1]
|
939 |
-
g = self.emb_g(ds).unsqueeze(-1) # [b, 256, 1]##1是t,广播的
|
940 |
-
m_p, logs_p, x_mask = self.enc_p(phone, None, phone_lengths)
|
941 |
-
z, m_q, logs_q, y_mask = self.enc_q(y, y_lengths, g=g)
|
942 |
-
z_p = self.flow(z, y_mask, g=g)
|
943 |
-
z_slice, ids_slice = commons.rand_slice_segments(
|
944 |
-
z, y_lengths, self.segment_size
|
945 |
-
)
|
946 |
-
o = self.dec(z_slice, g=g)
|
947 |
-
return o, ids_slice, x_mask, y_mask, (z, z_p, m_p, logs_p, m_q, logs_q)
|
948 |
-
|
949 |
-
def infer(self, phone, phone_lengths, sid, max_len=None):
|
950 |
-
g = self.emb_g(sid).unsqueeze(-1)
|
951 |
-
m_p, logs_p, x_mask = self.enc_p(phone, None, phone_lengths)
|
952 |
-
z_p = (m_p + torch.exp(logs_p) * torch.randn_like(m_p) * 0.66666) * x_mask
|
953 |
-
z = self.flow(z_p, x_mask, g=g, reverse=True)
|
954 |
-
o = self.dec((z * x_mask)[:, :, :max_len], g=g)
|
955 |
-
return o, x_mask, (z, z_p, m_p, logs_p)
|
956 |
-
|
957 |
-
|
958 |
-
class MultiPeriodDiscriminator(torch.nn.Module):
|
959 |
-
def __init__(self, use_spectral_norm=False):
|
960 |
-
super(MultiPeriodDiscriminator, self).__init__()
|
961 |
-
periods = [2, 3, 5, 7, 11, 17]
|
962 |
-
# periods = [3, 5, 7, 11, 17, 23, 37]
|
963 |
-
|
964 |
-
discs = [DiscriminatorS(use_spectral_norm=use_spectral_norm)]
|
965 |
-
discs = discs + [
|
966 |
-
DiscriminatorP(i, use_spectral_norm=use_spectral_norm) for i in periods
|
967 |
-
]
|
968 |
-
self.discriminators = nn.ModuleList(discs)
|
969 |
-
|
970 |
-
def forward(self, y, y_hat):
|
971 |
-
y_d_rs = [] #
|
972 |
-
y_d_gs = []
|
973 |
-
fmap_rs = []
|
974 |
-
fmap_gs = []
|
975 |
-
for i, d in enumerate(self.discriminators):
|
976 |
-
y_d_r, fmap_r = d(y)
|
977 |
-
y_d_g, fmap_g = d(y_hat)
|
978 |
-
# for j in range(len(fmap_r)):
|
979 |
-
# print(i,j,y.shape,y_hat.shape,fmap_r[j].shape,fmap_g[j].shape)
|
980 |
-
y_d_rs.append(y_d_r)
|
981 |
-
y_d_gs.append(y_d_g)
|
982 |
-
fmap_rs.append(fmap_r)
|
983 |
-
fmap_gs.append(fmap_g)
|
984 |
-
|
985 |
-
return y_d_rs, y_d_gs, fmap_rs, fmap_gs
|
986 |
-
|
987 |
-
|
988 |
-
class MultiPeriodDiscriminatorV2(torch.nn.Module):
|
989 |
-
def __init__(self, use_spectral_norm=False):
|
990 |
-
super(MultiPeriodDiscriminatorV2, self).__init__()
|
991 |
-
# periods = [2, 3, 5, 7, 11, 17]
|
992 |
-
periods = [2, 3, 5, 7, 11, 17, 23, 37]
|
993 |
-
|
994 |
-
discs = [DiscriminatorS(use_spectral_norm=use_spectral_norm)]
|
995 |
-
discs = discs + [
|
996 |
-
DiscriminatorP(i, use_spectral_norm=use_spectral_norm) for i in periods
|
997 |
-
]
|
998 |
-
self.discriminators = nn.ModuleList(discs)
|
999 |
-
|
1000 |
-
def forward(self, y, y_hat):
|
1001 |
-
y_d_rs = [] #
|
1002 |
-
y_d_gs = []
|
1003 |
-
fmap_rs = []
|
1004 |
-
fmap_gs = []
|
1005 |
-
for i, d in enumerate(self.discriminators):
|
1006 |
-
y_d_r, fmap_r = d(y)
|
1007 |
-
y_d_g, fmap_g = d(y_hat)
|
1008 |
-
# for j in range(len(fmap_r)):
|
1009 |
-
# print(i,j,y.shape,y_hat.shape,fmap_r[j].shape,fmap_g[j].shape)
|
1010 |
-
y_d_rs.append(y_d_r)
|
1011 |
-
y_d_gs.append(y_d_g)
|
1012 |
-
fmap_rs.append(fmap_r)
|
1013 |
-
fmap_gs.append(fmap_g)
|
1014 |
-
|
1015 |
-
return y_d_rs, y_d_gs, fmap_rs, fmap_gs
|
1016 |
-
|
1017 |
-
|
1018 |
-
class DiscriminatorS(torch.nn.Module):
|
1019 |
-
def __init__(self, use_spectral_norm=False):
|
1020 |
-
super(DiscriminatorS, self).__init__()
|
1021 |
-
norm_f = weight_norm if use_spectral_norm == False else spectral_norm
|
1022 |
-
self.convs = nn.ModuleList(
|
1023 |
-
[
|
1024 |
-
norm_f(Conv1d(1, 16, 15, 1, padding=7)),
|
1025 |
-
norm_f(Conv1d(16, 64, 41, 4, groups=4, padding=20)),
|
1026 |
-
norm_f(Conv1d(64, 256, 41, 4, groups=16, padding=20)),
|
1027 |
-
norm_f(Conv1d(256, 1024, 41, 4, groups=64, padding=20)),
|
1028 |
-
norm_f(Conv1d(1024, 1024, 41, 4, groups=256, padding=20)),
|
1029 |
-
norm_f(Conv1d(1024, 1024, 5, 1, padding=2)),
|
1030 |
-
]
|
1031 |
-
)
|
1032 |
-
self.conv_post = norm_f(Conv1d(1024, 1, 3, 1, padding=1))
|
1033 |
-
|
1034 |
-
def forward(self, x):
|
1035 |
-
fmap = []
|
1036 |
-
|
1037 |
-
for l in self.convs:
|
1038 |
-
x = l(x)
|
1039 |
-
x = F.leaky_relu(x, modules.LRELU_SLOPE)
|
1040 |
-
fmap.append(x)
|
1041 |
-
x = self.conv_post(x)
|
1042 |
-
fmap.append(x)
|
1043 |
-
x = torch.flatten(x, 1, -1)
|
1044 |
-
|
1045 |
-
return x, fmap
|
1046 |
-
|
1047 |
-
|
1048 |
-
class DiscriminatorP(torch.nn.Module):
|
1049 |
-
def __init__(self, period, kernel_size=5, stride=3, use_spectral_norm=False):
|
1050 |
-
super(DiscriminatorP, self).__init__()
|
1051 |
-
self.period = period
|
1052 |
-
self.use_spectral_norm = use_spectral_norm
|
1053 |
-
norm_f = weight_norm if use_spectral_norm == False else spectral_norm
|
1054 |
-
self.convs = nn.ModuleList(
|
1055 |
-
[
|
1056 |
-
norm_f(
|
1057 |
-
Conv2d(
|
1058 |
-
1,
|
1059 |
-
32,
|
1060 |
-
(kernel_size, 1),
|
1061 |
-
(stride, 1),
|
1062 |
-
padding=(get_padding(kernel_size, 1), 0),
|
1063 |
-
)
|
1064 |
-
),
|
1065 |
-
norm_f(
|
1066 |
-
Conv2d(
|
1067 |
-
32,
|
1068 |
-
128,
|
1069 |
-
(kernel_size, 1),
|
1070 |
-
(stride, 1),
|
1071 |
-
padding=(get_padding(kernel_size, 1), 0),
|
1072 |
-
)
|
1073 |
-
),
|
1074 |
-
norm_f(
|
1075 |
-
Conv2d(
|
1076 |
-
128,
|
1077 |
-
512,
|
1078 |
-
(kernel_size, 1),
|
1079 |
-
(stride, 1),
|
1080 |
-
padding=(get_padding(kernel_size, 1), 0),
|
1081 |
-
)
|
1082 |
-
),
|
1083 |
-
norm_f(
|
1084 |
-
Conv2d(
|
1085 |
-
512,
|
1086 |
-
1024,
|
1087 |
-
(kernel_size, 1),
|
1088 |
-
(stride, 1),
|
1089 |
-
padding=(get_padding(kernel_size, 1), 0),
|
1090 |
-
)
|
1091 |
-
),
|
1092 |
-
norm_f(
|
1093 |
-
Conv2d(
|
1094 |
-
1024,
|
1095 |
-
1024,
|
1096 |
-
(kernel_size, 1),
|
1097 |
-
1,
|
1098 |
-
padding=(get_padding(kernel_size, 1), 0),
|
1099 |
-
)
|
1100 |
-
),
|
1101 |
-
]
|
1102 |
-
)
|
1103 |
-
self.conv_post = norm_f(Conv2d(1024, 1, (3, 1), 1, padding=(1, 0)))
|
1104 |
-
|
1105 |
-
def forward(self, x):
|
1106 |
-
fmap = []
|
1107 |
-
|
1108 |
-
# 1d to 2d
|
1109 |
-
b, c, t = x.shape
|
1110 |
-
if t % self.period != 0: # pad first
|
1111 |
-
n_pad = self.period - (t % self.period)
|
1112 |
-
x = F.pad(x, (0, n_pad), "reflect")
|
1113 |
-
t = t + n_pad
|
1114 |
-
x = x.view(b, c, t // self.period, self.period)
|
1115 |
-
|
1116 |
-
for l in self.convs:
|
1117 |
-
x = l(x)
|
1118 |
-
x = F.leaky_relu(x, modules.LRELU_SLOPE)
|
1119 |
-
fmap.append(x)
|
1120 |
-
x = self.conv_post(x)
|
1121 |
-
fmap.append(x)
|
1122 |
-
x = torch.flatten(x, 1, -1)
|
1123 |
-
|
1124 |
-
return x, fmap
|
|
|
|
|
|
|
|
|
|
|
|
|
|
|
|
|
|
|
|
|
|
|
|
|
|
|
|
|
|
|
|
|
|
|
|
|
|
|
|
|
|
|
|
|
|
|
|
|
|
|
|
|
|
|
|
|
|
|
|
|
|
|
|
|
|
|
|
|
|
|
|
|
|
|
|
|
|
|
|
|
|
|
|
|
|
|
|
|
|
|
|
|
|
|
|
|
|
|
|
|
|
|
|
|
|
|
|
|
|
|
|
|
|
|
|
|
|
|
|
|
|
|
|
|
|
|
|
|
|
|
|
|
|
|
|
|
|
|
|
|
|
|
|
|
|
|
|
|
|
|
|
|
|
|
|
|
|
|
|
|
|
|
|
|
|
|
|
|
|
|
|
|
|
|
|
|
|
|
|
|
|
|
|
|
|
|
|
|
|
|
|
|
|
|
|
|
|
|
|
|
|
|
|
|
|
|
|
|
|
|
|
|
|
|
|
|
|
|
|
|
|
|
|
|
|
|
|
|
|
|
|
|
|
|
|
|
|
|
|
|
|
|
|
|
|
|
|
|
|
|
|
|
|
|
|
|
|
|
|
|
|
|
|
|
|
|
|
|
|
|
|
|
|
|
|
|
|
|
|
|
|
|
|
|
|
|
|
|
|
|
|
|
|
|
|
|
|
|
|
|
|
|
|
|
|
|
|
|
|
|
|
|
|
|
|
|
|
|
|
|
|
|
|
|
|
|
|
|
|
|
|
|
|
|
|
|
|
|
|
|
|
|
|
|
|
|
|
|
|
|
|
|
|
|
|
|
|
|
|
|
|
|
|
|
|
|
|
|
|
|
|
|
|
|
|
|
|
|
|
|
|
|
|
|
|
|
|
|
|
|
|
|
|
|
|
|
|
|
|
|
|
|
|
|
|
|
|
|
|
|
|
|
|
|
|
|
|
|
|
|
|
|
|
|
|
|
|
|
|
|
|
|
|
|
|
|
|
|
|
|
|
|
|
|
|
|
|
|
|
|
|
|
|
|
|
|
|
|
|
|
|
|
|
|
|
|
|
|
|
|
|
|
|
|
|
|
|
|
|
|
|
|
|
|
|
|
|
|
|
|
|
|
|
|
|
|
|
|
|
|
|
|
|
|
|
|
|
|
|
|
|
|
|
|
|
|
|
|
|
|
|
|
|
|
|
|
|
|
|
|
|
|
|
|
|
|
|
|
|
|
|
|
|
|
|
|
|
|
|
|
|
|
|
|
|
|
|
|
|
|
|
|
|
|
|
|
|
|
|
|
|
|
|
|
|
|
|
|
|
|
|
|
|
|
|
|
|
|
|
|
|
|
|
|
|
|
|
|
|
|
|
|
|
|
|
|
|
|
|
|
|
|
|
|
|
|
|
|
|
|
|
|
|
|
|
|
|
|
|
|
|
|
|
|
|
|
|
|
|
|
|
|
|
|
|
|
|
|
|
|
|
|
|
|
|
|
|
|
|
|
|
|
|
|
|
|
|
|
|
|
|
|
|
|
|
|
|
|
|
|
|
|
|
|
|
|
|
|
|
|
|
|
|
|
|
|
|
|
|
|
|
|
|
|
|
|
|
|
|
|
|
|
|
|
|
|
|
|
|
|
|
|
|
|
|
|
|
|
|
|
|
|
|
|
|
|
|
|
|
|
|
|
|
|
|
|
|
|
|
|
|
|
|
|
|
|
|
|
|
|
|
|
|
|
|
|
|
|
|
|
|
|
|
|
|
|
|
|
|
|
|
|
|
|
|
|
|
|
|
|
|
|
|
|
|
|
|
|
|
|
|
|
|
|
|
|
|
|
|
|
|
|
|
|
|
|
|
|
|
|
|
|
|
|
|
|
|
|
|
|
|
|
|
|
|
|
|
|
|
|
|
|
|
|
|
|
|
|
|
|
|
|
|
|
|
|
|
|
|
|
|
|
|
|
|
|
|
|
|
|
|
|
|
|
|
|
|
|
|
|
|
|
|
|
|
|
|
|
|
|
|
|
|
|
|
|
|
|
|
|
|
|
|
|
|
|
|
|
|
|
|
|
|
|
|
|
|
|
|
|
|
|
|
|
|
|
|
|
|
|
|
|
|
|
|
|
|
|
|
|
|
|
|
|
|
|
|
|
|
|
|
|
|
|
|
|
|
|
|
|
|
|
|
|
|
|
|
|
|
|
|
|
|
|
|
|
|
|
|
|
|
|
|
|
|
|
|
|
|
|
|
|
|
|
|
|
|
|
|
|
|
|
|
|
|
|
|
|
|
|
|
|
|
|
|
|
|
|
|
|
|
|
|
|
|
|
|
|
|
|
|
|
|
|
|
|
|
|
|
|
|
|
|
|
|
|
|
|
|
|
|
|
|
|
|
|
|
|
|
|
|
|
|
|
|
|
|
|
|
|
|
|
|
|
|
|
|
|
|
|
|
|
|
|
|
|
|
|
|
|
|
|
|
|
|
|
|
|
|
|
|
|
|
|
|
|
|
|
|
|
|
|
|
|
|
|
|
|
|
|
|
|
|
|
|
|
|
|
|
|
|
|
|
|
|
|
|
|
|
|
|
|
|
|
|
|
|
|
|
|
|
|
|
|
|
|
|
|
|
|
|
|
|
|
|
|
|
|
|
|
|
|
|
|
|
|
|
|
|
|
|
|
|
|
|
|
|
|
|
|
|
|
|
|
|
|
|
|
|
|
|
|
|
|
|
|
|
|
|
|
|
|
|
|
|
|
|
|
|
|
|
|
|
|
|
|
|
|
|
|
|
|
|
|
|
|
|
|
|
|
|
|
|
|
|
|
|
|
|
|
|
|
|
|
|
|
|
|
|
|
|
|
|
|
|
|
|
|
|
|
|
|
|
|
|
|
|
|
|
|
|
|
|
|
|
|
|
|
|
|
|
|
|
|
|
|
|
|
|
|
|
|
|
|
|
|
|
|
|
|
|
|
|
|
|
|
|
|
|
|
|
|
|
|
|
|
|
|
|
|
|
|
|
|
|
|
|
|
|
|
|
|
|
|
|
|
|
|
|
|
|
|
|
|
|
|
|
|
|
|
|
|
|
|
|
|
|
|
|
|
|
|
|
|
|
|
|
|
|
|
|
|
|
|
|
|
|
|
|
|
|
|
|
|
|
|
|
|
|
|
|
|
|
|
|
|
|
|
|
|
|
|
|
|
|
|
|
|
|
|
|
|
|
|
|
|
|
|
|
|
|
|
|
|
|
|
|
|
|
|
|
|
|
|
|
|
|
|
|
|
|
|
|
|
|
|
|
|
|
|
|
|
|
|
|
|
|
|
|
|
|
|
|
|
|
|
|
|
|
|
|
|
|
|
|
|
|
|
|
|
|
|
|
|
|
|
|
|
|
|
|
|
|
|
|
|
|
|
|
|
|
|
|
|
|
|
|
|
|
|
|
|
|
|
|
|
|
|
|
|
|
|
|
|
|
|
|
|
|
|
|
|
|
|
|
|
|
|
|
|
|
|
|
|
|
|
|
|
|
|
|
|
|
|
|
|
|
|
|
|
|
|
|
|
|
|
|
|
|
|
|
|
|
|
|
|
|
|
|
|
|
|
|
|
|
|
|
|
|
|
|
|
|
|
|
|
|
|
|
|
|
|
|
|
|
|
|
|
|
|
|
|
|
|
|
|
|
|
|
|
|
|
|
|
|
|
|
|
|
|
|
|
|
|
|
|
|
|
|
|
|
|
|
|
|
|
|
|
|
|
|
|
|
|
|
|
|
|
|
|
|
|
|
|
|
|
|
|
|
|
|
|
|
|
|
|
|
|
|
|
|
|
|
|
|
|
|
|
|
|
|
|
|
|
|
|
|
|
|
|
|
|
|
|
|
|
|
|
|
|
|
|
|
|
|
|
|
|
|
|
|
|
|
|
|
|
|
|
|
|
|
|
|
|
|
|
|
|
|
|
|
|
|
|
|
|
|
|
|
|
|
|
|
|
|
|
|
|
|
|
|
|
|
|
|
|
|
|
|
|
|
|
|
|
|
|
|
|
|
|
|
|
|
|
|
|
|
|
|
|
|
|
|
|
|
|
|
|
|
|
|
|
|
|
|
|
|
|
|
|
|
|
|
|
|
|
|
|
|
|
|
|
|
|
|
|
|
|
|
|
|
|
|
|
|
|
|
|
|
|
|
|
|
|
|
|
|
|
|
|
|
|
|
|
|
|
|
|
|
|
|
|
|
|
|
|
|
|
|
|
|
|
|
|
|
|
|
|
|
|
|
|
|
|
|
|
|
|
|
|
|
|
|
|
|
|
|
|
|
|
|
|
|
|
|
|
|
|
|
|
|
|
|
|
|
|
|
|
|
|
|
|
|
|
|
|
|
|
|
|
|
|
|
|
|
|
|
|
|
|
|
|
|
|
|
|
|
|
|
|
|
|
|
|
|
|
|
|
|
|
|
|
|
|
|
|
|
|
|
|
|
|
|
|
|
|
|
|
|
|
|
|
|
|
|
|
|
|
|
|
|
|
|
|
|
|
|
|
|
|
|
|
|
|
|
|
|
|
|
|
|
|
|
|
|
|
|
|
|
|
|
|
|
|
|
|
|
|
|
|
|
|
|
|
|
|
|
|
|
|
|
|
|
|
|
|
|
|
|
|
|
|
|
|
|
|
|
|
|
|
|
|
|
|
|
|
|
|
|
|
|
|
|
|
|
|
|
|
|
|
|
|
|
|
|
|
|
|
|
|
|
|
|
|
|
|
|
|
|
|
|
|
|
|
|
|
|
|
|
|
|
|
|
|
|
|
|
|
|
|
|
|
|
|
|
|
|
|
|
|
|
|
|
|
|
|
|
|
|
|
|
|
|
|
|
|
|
|
|
|
|
|
|
|
|
|
|
|
|
|
|
|
|
|
|
|
|
|
|
|
|
|
|
|
|
|
|
|
|
|
|
|
|
|
|
|
|
|
|
|
|
|
|
|
|
|
|
|
|
|
|
|
|
|
|
|
|
|
|
|
|
|
|
|
|
|
|
|
|
|
|
|
|
|
|
|
|
|
|
|
|
|
|
|
|
|
|
|
|
spaces/BartPoint/VoiceChange_Beta/infer_pack/models_onnx.py
DELETED
@@ -1,818 +0,0 @@
|
|
1 |
-
import math, pdb, os
|
2 |
-
from time import time as ttime
|
3 |
-
import torch
|
4 |
-
from torch import nn
|
5 |
-
from torch.nn import functional as F
|
6 |
-
from infer_pack import modules
|
7 |
-
from infer_pack import attentions
|
8 |
-
from infer_pack import commons
|
9 |
-
from infer_pack.commons import init_weights, get_padding
|
10 |
-
from torch.nn import Conv1d, ConvTranspose1d, AvgPool1d, Conv2d
|
11 |
-
from torch.nn.utils import weight_norm, remove_weight_norm, spectral_norm
|
12 |
-
from infer_pack.commons import init_weights
|
13 |
-
import numpy as np
|
14 |
-
from infer_pack import commons
|
15 |
-
|
16 |
-
|
17 |
-
class TextEncoder256(nn.Module):
|
18 |
-
def __init__(
|
19 |
-
self,
|
20 |
-
out_channels,
|
21 |
-
hidden_channels,
|
22 |
-
filter_channels,
|
23 |
-
n_heads,
|
24 |
-
n_layers,
|
25 |
-
kernel_size,
|
26 |
-
p_dropout,
|
27 |
-
f0=True,
|
28 |
-
):
|
29 |
-
super().__init__()
|
30 |
-
self.out_channels = out_channels
|
31 |
-
self.hidden_channels = hidden_channels
|
32 |
-
self.filter_channels = filter_channels
|
33 |
-
self.n_heads = n_heads
|
34 |
-
self.n_layers = n_layers
|
35 |
-
self.kernel_size = kernel_size
|
36 |
-
self.p_dropout = p_dropout
|
37 |
-
self.emb_phone = nn.Linear(256, hidden_channels)
|
38 |
-
self.lrelu = nn.LeakyReLU(0.1, inplace=True)
|
39 |
-
if f0 == True:
|
40 |
-
self.emb_pitch = nn.Embedding(256, hidden_channels) # pitch 256
|
41 |
-
self.encoder = attentions.Encoder(
|
42 |
-
hidden_channels, filter_channels, n_heads, n_layers, kernel_size, p_dropout
|
43 |
-
)
|
44 |
-
self.proj = nn.Conv1d(hidden_channels, out_channels * 2, 1)
|
45 |
-
|
46 |
-
def forward(self, phone, pitch, lengths):
|
47 |
-
if pitch == None:
|
48 |
-
x = self.emb_phone(phone)
|
49 |
-
else:
|
50 |
-
x = self.emb_phone(phone) + self.emb_pitch(pitch)
|
51 |
-
x = x * math.sqrt(self.hidden_channels) # [b, t, h]
|
52 |
-
x = self.lrelu(x)
|
53 |
-
x = torch.transpose(x, 1, -1) # [b, h, t]
|
54 |
-
x_mask = torch.unsqueeze(commons.sequence_mask(lengths, x.size(2)), 1).to(
|
55 |
-
x.dtype
|
56 |
-
)
|
57 |
-
x = self.encoder(x * x_mask, x_mask)
|
58 |
-
stats = self.proj(x) * x_mask
|
59 |
-
|
60 |
-
m, logs = torch.split(stats, self.out_channels, dim=1)
|
61 |
-
return m, logs, x_mask
|
62 |
-
|
63 |
-
|
64 |
-
class TextEncoder768(nn.Module):
|
65 |
-
def __init__(
|
66 |
-
self,
|
67 |
-
out_channels,
|
68 |
-
hidden_channels,
|
69 |
-
filter_channels,
|
70 |
-
n_heads,
|
71 |
-
n_layers,
|
72 |
-
kernel_size,
|
73 |
-
p_dropout,
|
74 |
-
f0=True,
|
75 |
-
):
|
76 |
-
super().__init__()
|
77 |
-
self.out_channels = out_channels
|
78 |
-
self.hidden_channels = hidden_channels
|
79 |
-
self.filter_channels = filter_channels
|
80 |
-
self.n_heads = n_heads
|
81 |
-
self.n_layers = n_layers
|
82 |
-
self.kernel_size = kernel_size
|
83 |
-
self.p_dropout = p_dropout
|
84 |
-
self.emb_phone = nn.Linear(768, hidden_channels)
|
85 |
-
self.lrelu = nn.LeakyReLU(0.1, inplace=True)
|
86 |
-
if f0 == True:
|
87 |
-
self.emb_pitch = nn.Embedding(256, hidden_channels) # pitch 256
|
88 |
-
self.encoder = attentions.Encoder(
|
89 |
-
hidden_channels, filter_channels, n_heads, n_layers, kernel_size, p_dropout
|
90 |
-
)
|
91 |
-
self.proj = nn.Conv1d(hidden_channels, out_channels * 2, 1)
|
92 |
-
|
93 |
-
def forward(self, phone, pitch, lengths):
|
94 |
-
if pitch == None:
|
95 |
-
x = self.emb_phone(phone)
|
96 |
-
else:
|
97 |
-
x = self.emb_phone(phone) + self.emb_pitch(pitch)
|
98 |
-
x = x * math.sqrt(self.hidden_channels) # [b, t, h]
|
99 |
-
x = self.lrelu(x)
|
100 |
-
x = torch.transpose(x, 1, -1) # [b, h, t]
|
101 |
-
x_mask = torch.unsqueeze(commons.sequence_mask(lengths, x.size(2)), 1).to(
|
102 |
-
x.dtype
|
103 |
-
)
|
104 |
-
x = self.encoder(x * x_mask, x_mask)
|
105 |
-
stats = self.proj(x) * x_mask
|
106 |
-
|
107 |
-
m, logs = torch.split(stats, self.out_channels, dim=1)
|
108 |
-
return m, logs, x_mask
|
109 |
-
|
110 |
-
|
111 |
-
class ResidualCouplingBlock(nn.Module):
|
112 |
-
def __init__(
|
113 |
-
self,
|
114 |
-
channels,
|
115 |
-
hidden_channels,
|
116 |
-
kernel_size,
|
117 |
-
dilation_rate,
|
118 |
-
n_layers,
|
119 |
-
n_flows=4,
|
120 |
-
gin_channels=0,
|
121 |
-
):
|
122 |
-
super().__init__()
|
123 |
-
self.channels = channels
|
124 |
-
self.hidden_channels = hidden_channels
|
125 |
-
self.kernel_size = kernel_size
|
126 |
-
self.dilation_rate = dilation_rate
|
127 |
-
self.n_layers = n_layers
|
128 |
-
self.n_flows = n_flows
|
129 |
-
self.gin_channels = gin_channels
|
130 |
-
|
131 |
-
self.flows = nn.ModuleList()
|
132 |
-
for i in range(n_flows):
|
133 |
-
self.flows.append(
|
134 |
-
modules.ResidualCouplingLayer(
|
135 |
-
channels,
|
136 |
-
hidden_channels,
|
137 |
-
kernel_size,
|
138 |
-
dilation_rate,
|
139 |
-
n_layers,
|
140 |
-
gin_channels=gin_channels,
|
141 |
-
mean_only=True,
|
142 |
-
)
|
143 |
-
)
|
144 |
-
self.flows.append(modules.Flip())
|
145 |
-
|
146 |
-
def forward(self, x, x_mask, g=None, reverse=False):
|
147 |
-
if not reverse:
|
148 |
-
for flow in self.flows:
|
149 |
-
x, _ = flow(x, x_mask, g=g, reverse=reverse)
|
150 |
-
else:
|
151 |
-
for flow in reversed(self.flows):
|
152 |
-
x = flow(x, x_mask, g=g, reverse=reverse)
|
153 |
-
return x
|
154 |
-
|
155 |
-
def remove_weight_norm(self):
|
156 |
-
for i in range(self.n_flows):
|
157 |
-
self.flows[i * 2].remove_weight_norm()
|
158 |
-
|
159 |
-
|
160 |
-
class PosteriorEncoder(nn.Module):
|
161 |
-
def __init__(
|
162 |
-
self,
|
163 |
-
in_channels,
|
164 |
-
out_channels,
|
165 |
-
hidden_channels,
|
166 |
-
kernel_size,
|
167 |
-
dilation_rate,
|
168 |
-
n_layers,
|
169 |
-
gin_channels=0,
|
170 |
-
):
|
171 |
-
super().__init__()
|
172 |
-
self.in_channels = in_channels
|
173 |
-
self.out_channels = out_channels
|
174 |
-
self.hidden_channels = hidden_channels
|
175 |
-
self.kernel_size = kernel_size
|
176 |
-
self.dilation_rate = dilation_rate
|
177 |
-
self.n_layers = n_layers
|
178 |
-
self.gin_channels = gin_channels
|
179 |
-
|
180 |
-
self.pre = nn.Conv1d(in_channels, hidden_channels, 1)
|
181 |
-
self.enc = modules.WN(
|
182 |
-
hidden_channels,
|
183 |
-
kernel_size,
|
184 |
-
dilation_rate,
|
185 |
-
n_layers,
|
186 |
-
gin_channels=gin_channels,
|
187 |
-
)
|
188 |
-
self.proj = nn.Conv1d(hidden_channels, out_channels * 2, 1)
|
189 |
-
|
190 |
-
def forward(self, x, x_lengths, g=None):
|
191 |
-
x_mask = torch.unsqueeze(commons.sequence_mask(x_lengths, x.size(2)), 1).to(
|
192 |
-
x.dtype
|
193 |
-
)
|
194 |
-
x = self.pre(x) * x_mask
|
195 |
-
x = self.enc(x, x_mask, g=g)
|
196 |
-
stats = self.proj(x) * x_mask
|
197 |
-
m, logs = torch.split(stats, self.out_channels, dim=1)
|
198 |
-
z = (m + torch.randn_like(m) * torch.exp(logs)) * x_mask
|
199 |
-
return z, m, logs, x_mask
|
200 |
-
|
201 |
-
def remove_weight_norm(self):
|
202 |
-
self.enc.remove_weight_norm()
|
203 |
-
|
204 |
-
|
205 |
-
class Generator(torch.nn.Module):
|
206 |
-
def __init__(
|
207 |
-
self,
|
208 |
-
initial_channel,
|
209 |
-
resblock,
|
210 |
-
resblock_kernel_sizes,
|
211 |
-
resblock_dilation_sizes,
|
212 |
-
upsample_rates,
|
213 |
-
upsample_initial_channel,
|
214 |
-
upsample_kernel_sizes,
|
215 |
-
gin_channels=0,
|
216 |
-
):
|
217 |
-
super(Generator, self).__init__()
|
218 |
-
self.num_kernels = len(resblock_kernel_sizes)
|
219 |
-
self.num_upsamples = len(upsample_rates)
|
220 |
-
self.conv_pre = Conv1d(
|
221 |
-
initial_channel, upsample_initial_channel, 7, 1, padding=3
|
222 |
-
)
|
223 |
-
resblock = modules.ResBlock1 if resblock == "1" else modules.ResBlock2
|
224 |
-
|
225 |
-
self.ups = nn.ModuleList()
|
226 |
-
for i, (u, k) in enumerate(zip(upsample_rates, upsample_kernel_sizes)):
|
227 |
-
self.ups.append(
|
228 |
-
weight_norm(
|
229 |
-
ConvTranspose1d(
|
230 |
-
upsample_initial_channel // (2**i),
|
231 |
-
upsample_initial_channel // (2 ** (i + 1)),
|
232 |
-
k,
|
233 |
-
u,
|
234 |
-
padding=(k - u) // 2,
|
235 |
-
)
|
236 |
-
)
|
237 |
-
)
|
238 |
-
|
239 |
-
self.resblocks = nn.ModuleList()
|
240 |
-
for i in range(len(self.ups)):
|
241 |
-
ch = upsample_initial_channel // (2 ** (i + 1))
|
242 |
-
for j, (k, d) in enumerate(
|
243 |
-
zip(resblock_kernel_sizes, resblock_dilation_sizes)
|
244 |
-
):
|
245 |
-
self.resblocks.append(resblock(ch, k, d))
|
246 |
-
|
247 |
-
self.conv_post = Conv1d(ch, 1, 7, 1, padding=3, bias=False)
|
248 |
-
self.ups.apply(init_weights)
|
249 |
-
|
250 |
-
if gin_channels != 0:
|
251 |
-
self.cond = nn.Conv1d(gin_channels, upsample_initial_channel, 1)
|
252 |
-
|
253 |
-
def forward(self, x, g=None):
|
254 |
-
x = self.conv_pre(x)
|
255 |
-
if g is not None:
|
256 |
-
x = x + self.cond(g)
|
257 |
-
|
258 |
-
for i in range(self.num_upsamples):
|
259 |
-
x = F.leaky_relu(x, modules.LRELU_SLOPE)
|
260 |
-
x = self.ups[i](x)
|
261 |
-
xs = None
|
262 |
-
for j in range(self.num_kernels):
|
263 |
-
if xs is None:
|
264 |
-
xs = self.resblocks[i * self.num_kernels + j](x)
|
265 |
-
else:
|
266 |
-
xs += self.resblocks[i * self.num_kernels + j](x)
|
267 |
-
x = xs / self.num_kernels
|
268 |
-
x = F.leaky_relu(x)
|
269 |
-
x = self.conv_post(x)
|
270 |
-
x = torch.tanh(x)
|
271 |
-
|
272 |
-
return x
|
273 |
-
|
274 |
-
def remove_weight_norm(self):
|
275 |
-
for l in self.ups:
|
276 |
-
remove_weight_norm(l)
|
277 |
-
for l in self.resblocks:
|
278 |
-
l.remove_weight_norm()
|
279 |
-
|
280 |
-
|
281 |
-
class SineGen(torch.nn.Module):
|
282 |
-
"""Definition of sine generator
|
283 |
-
SineGen(samp_rate, harmonic_num = 0,
|
284 |
-
sine_amp = 0.1, noise_std = 0.003,
|
285 |
-
voiced_threshold = 0,
|
286 |
-
flag_for_pulse=False)
|
287 |
-
samp_rate: sampling rate in Hz
|
288 |
-
harmonic_num: number of harmonic overtones (default 0)
|
289 |
-
sine_amp: amplitude of sine-wavefrom (default 0.1)
|
290 |
-
noise_std: std of Gaussian noise (default 0.003)
|
291 |
-
voiced_thoreshold: F0 threshold for U/V classification (default 0)
|
292 |
-
flag_for_pulse: this SinGen is used inside PulseGen (default False)
|
293 |
-
Note: when flag_for_pulse is True, the first time step of a voiced
|
294 |
-
segment is always sin(np.pi) or cos(0)
|
295 |
-
"""
|
296 |
-
|
297 |
-
def __init__(
|
298 |
-
self,
|
299 |
-
samp_rate,
|
300 |
-
harmonic_num=0,
|
301 |
-
sine_amp=0.1,
|
302 |
-
noise_std=0.003,
|
303 |
-
voiced_threshold=0,
|
304 |
-
flag_for_pulse=False,
|
305 |
-
):
|
306 |
-
super(SineGen, self).__init__()
|
307 |
-
self.sine_amp = sine_amp
|
308 |
-
self.noise_std = noise_std
|
309 |
-
self.harmonic_num = harmonic_num
|
310 |
-
self.dim = self.harmonic_num + 1
|
311 |
-
self.sampling_rate = samp_rate
|
312 |
-
self.voiced_threshold = voiced_threshold
|
313 |
-
|
314 |
-
def _f02uv(self, f0):
|
315 |
-
# generate uv signal
|
316 |
-
uv = torch.ones_like(f0)
|
317 |
-
uv = uv * (f0 > self.voiced_threshold)
|
318 |
-
return uv
|
319 |
-
|
320 |
-
def forward(self, f0, upp):
|
321 |
-
"""sine_tensor, uv = forward(f0)
|
322 |
-
input F0: tensor(batchsize=1, length, dim=1)
|
323 |
-
f0 for unvoiced steps should be 0
|
324 |
-
output sine_tensor: tensor(batchsize=1, length, dim)
|
325 |
-
output uv: tensor(batchsize=1, length, 1)
|
326 |
-
"""
|
327 |
-
with torch.no_grad():
|
328 |
-
f0 = f0[:, None].transpose(1, 2)
|
329 |
-
f0_buf = torch.zeros(f0.shape[0], f0.shape[1], self.dim, device=f0.device)
|
330 |
-
# fundamental component
|
331 |
-
f0_buf[:, :, 0] = f0[:, :, 0]
|
332 |
-
for idx in np.arange(self.harmonic_num):
|
333 |
-
f0_buf[:, :, idx + 1] = f0_buf[:, :, 0] * (
|
334 |
-
idx + 2
|
335 |
-
) # idx + 2: the (idx+1)-th overtone, (idx+2)-th harmonic
|
336 |
-
rad_values = (f0_buf / self.sampling_rate) % 1 ###%1意味着n_har的乘积无法后处理优化
|
337 |
-
rand_ini = torch.rand(
|
338 |
-
f0_buf.shape[0], f0_buf.shape[2], device=f0_buf.device
|
339 |
-
)
|
340 |
-
rand_ini[:, 0] = 0
|
341 |
-
rad_values[:, 0, :] = rad_values[:, 0, :] + rand_ini
|
342 |
-
tmp_over_one = torch.cumsum(rad_values, 1) # % 1 #####%1意味着后面的cumsum无法再优化
|
343 |
-
tmp_over_one *= upp
|
344 |
-
tmp_over_one = F.interpolate(
|
345 |
-
tmp_over_one.transpose(2, 1),
|
346 |
-
scale_factor=upp,
|
347 |
-
mode="linear",
|
348 |
-
align_corners=True,
|
349 |
-
).transpose(2, 1)
|
350 |
-
rad_values = F.interpolate(
|
351 |
-
rad_values.transpose(2, 1), scale_factor=upp, mode="nearest"
|
352 |
-
).transpose(
|
353 |
-
2, 1
|
354 |
-
) #######
|
355 |
-
tmp_over_one %= 1
|
356 |
-
tmp_over_one_idx = (tmp_over_one[:, 1:, :] - tmp_over_one[:, :-1, :]) < 0
|
357 |
-
cumsum_shift = torch.zeros_like(rad_values)
|
358 |
-
cumsum_shift[:, 1:, :] = tmp_over_one_idx * -1.0
|
359 |
-
sine_waves = torch.sin(
|
360 |
-
torch.cumsum(rad_values + cumsum_shift, dim=1) * 2 * np.pi
|
361 |
-
)
|
362 |
-
sine_waves = sine_waves * self.sine_amp
|
363 |
-
uv = self._f02uv(f0)
|
364 |
-
uv = F.interpolate(
|
365 |
-
uv.transpose(2, 1), scale_factor=upp, mode="nearest"
|
366 |
-
).transpose(2, 1)
|
367 |
-
noise_amp = uv * self.noise_std + (1 - uv) * self.sine_amp / 3
|
368 |
-
noise = noise_amp * torch.randn_like(sine_waves)
|
369 |
-
sine_waves = sine_waves * uv + noise
|
370 |
-
return sine_waves, uv, noise
|
371 |
-
|
372 |
-
|
373 |
-
class SourceModuleHnNSF(torch.nn.Module):
|
374 |
-
"""SourceModule for hn-nsf
|
375 |
-
SourceModule(sampling_rate, harmonic_num=0, sine_amp=0.1,
|
376 |
-
add_noise_std=0.003, voiced_threshod=0)
|
377 |
-
sampling_rate: sampling_rate in Hz
|
378 |
-
harmonic_num: number of harmonic above F0 (default: 0)
|
379 |
-
sine_amp: amplitude of sine source signal (default: 0.1)
|
380 |
-
add_noise_std: std of additive Gaussian noise (default: 0.003)
|
381 |
-
note that amplitude of noise in unvoiced is decided
|
382 |
-
by sine_amp
|
383 |
-
voiced_threshold: threhold to set U/V given F0 (default: 0)
|
384 |
-
Sine_source, noise_source = SourceModuleHnNSF(F0_sampled)
|
385 |
-
F0_sampled (batchsize, length, 1)
|
386 |
-
Sine_source (batchsize, length, 1)
|
387 |
-
noise_source (batchsize, length 1)
|
388 |
-
uv (batchsize, length, 1)
|
389 |
-
"""
|
390 |
-
|
391 |
-
def __init__(
|
392 |
-
self,
|
393 |
-
sampling_rate,
|
394 |
-
harmonic_num=0,
|
395 |
-
sine_amp=0.1,
|
396 |
-
add_noise_std=0.003,
|
397 |
-
voiced_threshod=0,
|
398 |
-
is_half=True,
|
399 |
-
):
|
400 |
-
super(SourceModuleHnNSF, self).__init__()
|
401 |
-
|
402 |
-
self.sine_amp = sine_amp
|
403 |
-
self.noise_std = add_noise_std
|
404 |
-
self.is_half = is_half
|
405 |
-
# to produce sine waveforms
|
406 |
-
self.l_sin_gen = SineGen(
|
407 |
-
sampling_rate, harmonic_num, sine_amp, add_noise_std, voiced_threshod
|
408 |
-
)
|
409 |
-
|
410 |
-
# to merge source harmonics into a single excitation
|
411 |
-
self.l_linear = torch.nn.Linear(harmonic_num + 1, 1)
|
412 |
-
self.l_tanh = torch.nn.Tanh()
|
413 |
-
|
414 |
-
def forward(self, x, upp=None):
|
415 |
-
sine_wavs, uv, _ = self.l_sin_gen(x, upp)
|
416 |
-
if self.is_half:
|
417 |
-
sine_wavs = sine_wavs.half()
|
418 |
-
sine_merge = self.l_tanh(self.l_linear(sine_wavs))
|
419 |
-
return sine_merge, None, None # noise, uv
|
420 |
-
|
421 |
-
|
422 |
-
class GeneratorNSF(torch.nn.Module):
|
423 |
-
def __init__(
|
424 |
-
self,
|
425 |
-
initial_channel,
|
426 |
-
resblock,
|
427 |
-
resblock_kernel_sizes,
|
428 |
-
resblock_dilation_sizes,
|
429 |
-
upsample_rates,
|
430 |
-
upsample_initial_channel,
|
431 |
-
upsample_kernel_sizes,
|
432 |
-
gin_channels,
|
433 |
-
sr,
|
434 |
-
is_half=False,
|
435 |
-
):
|
436 |
-
super(GeneratorNSF, self).__init__()
|
437 |
-
self.num_kernels = len(resblock_kernel_sizes)
|
438 |
-
self.num_upsamples = len(upsample_rates)
|
439 |
-
|
440 |
-
self.f0_upsamp = torch.nn.Upsample(scale_factor=np.prod(upsample_rates))
|
441 |
-
self.m_source = SourceModuleHnNSF(
|
442 |
-
sampling_rate=sr, harmonic_num=0, is_half=is_half
|
443 |
-
)
|
444 |
-
self.noise_convs = nn.ModuleList()
|
445 |
-
self.conv_pre = Conv1d(
|
446 |
-
initial_channel, upsample_initial_channel, 7, 1, padding=3
|
447 |
-
)
|
448 |
-
resblock = modules.ResBlock1 if resblock == "1" else modules.ResBlock2
|
449 |
-
|
450 |
-
self.ups = nn.ModuleList()
|
451 |
-
for i, (u, k) in enumerate(zip(upsample_rates, upsample_kernel_sizes)):
|
452 |
-
c_cur = upsample_initial_channel // (2 ** (i + 1))
|
453 |
-
self.ups.append(
|
454 |
-
weight_norm(
|
455 |
-
ConvTranspose1d(
|
456 |
-
upsample_initial_channel // (2**i),
|
457 |
-
upsample_initial_channel // (2 ** (i + 1)),
|
458 |
-
k,
|
459 |
-
u,
|
460 |
-
padding=(k - u) // 2,
|
461 |
-
)
|
462 |
-
)
|
463 |
-
)
|
464 |
-
if i + 1 < len(upsample_rates):
|
465 |
-
stride_f0 = np.prod(upsample_rates[i + 1 :])
|
466 |
-
self.noise_convs.append(
|
467 |
-
Conv1d(
|
468 |
-
1,
|
469 |
-
c_cur,
|
470 |
-
kernel_size=stride_f0 * 2,
|
471 |
-
stride=stride_f0,
|
472 |
-
padding=stride_f0 // 2,
|
473 |
-
)
|
474 |
-
)
|
475 |
-
else:
|
476 |
-
self.noise_convs.append(Conv1d(1, c_cur, kernel_size=1))
|
477 |
-
|
478 |
-
self.resblocks = nn.ModuleList()
|
479 |
-
for i in range(len(self.ups)):
|
480 |
-
ch = upsample_initial_channel // (2 ** (i + 1))
|
481 |
-
for j, (k, d) in enumerate(
|
482 |
-
zip(resblock_kernel_sizes, resblock_dilation_sizes)
|
483 |
-
):
|
484 |
-
self.resblocks.append(resblock(ch, k, d))
|
485 |
-
|
486 |
-
self.conv_post = Conv1d(ch, 1, 7, 1, padding=3, bias=False)
|
487 |
-
self.ups.apply(init_weights)
|
488 |
-
|
489 |
-
if gin_channels != 0:
|
490 |
-
self.cond = nn.Conv1d(gin_channels, upsample_initial_channel, 1)
|
491 |
-
|
492 |
-
self.upp = np.prod(upsample_rates)
|
493 |
-
|
494 |
-
def forward(self, x, f0, g=None):
|
495 |
-
har_source, noi_source, uv = self.m_source(f0, self.upp)
|
496 |
-
har_source = har_source.transpose(1, 2)
|
497 |
-
x = self.conv_pre(x)
|
498 |
-
if g is not None:
|
499 |
-
x = x + self.cond(g)
|
500 |
-
|
501 |
-
for i in range(self.num_upsamples):
|
502 |
-
x = F.leaky_relu(x, modules.LRELU_SLOPE)
|
503 |
-
x = self.ups[i](x)
|
504 |
-
x_source = self.noise_convs[i](har_source)
|
505 |
-
x = x + x_source
|
506 |
-
xs = None
|
507 |
-
for j in range(self.num_kernels):
|
508 |
-
if xs is None:
|
509 |
-
xs = self.resblocks[i * self.num_kernels + j](x)
|
510 |
-
else:
|
511 |
-
xs += self.resblocks[i * self.num_kernels + j](x)
|
512 |
-
x = xs / self.num_kernels
|
513 |
-
x = F.leaky_relu(x)
|
514 |
-
x = self.conv_post(x)
|
515 |
-
x = torch.tanh(x)
|
516 |
-
return x
|
517 |
-
|
518 |
-
def remove_weight_norm(self):
|
519 |
-
for l in self.ups:
|
520 |
-
remove_weight_norm(l)
|
521 |
-
for l in self.resblocks:
|
522 |
-
l.remove_weight_norm()
|
523 |
-
|
524 |
-
|
525 |
-
sr2sr = {
|
526 |
-
"32k": 32000,
|
527 |
-
"40k": 40000,
|
528 |
-
"48k": 48000,
|
529 |
-
}
|
530 |
-
|
531 |
-
|
532 |
-
class SynthesizerTrnMsNSFsidM(nn.Module):
|
533 |
-
def __init__(
|
534 |
-
self,
|
535 |
-
spec_channels,
|
536 |
-
segment_size,
|
537 |
-
inter_channels,
|
538 |
-
hidden_channels,
|
539 |
-
filter_channels,
|
540 |
-
n_heads,
|
541 |
-
n_layers,
|
542 |
-
kernel_size,
|
543 |
-
p_dropout,
|
544 |
-
resblock,
|
545 |
-
resblock_kernel_sizes,
|
546 |
-
resblock_dilation_sizes,
|
547 |
-
upsample_rates,
|
548 |
-
upsample_initial_channel,
|
549 |
-
upsample_kernel_sizes,
|
550 |
-
spk_embed_dim,
|
551 |
-
gin_channels,
|
552 |
-
sr,
|
553 |
-
**kwargs
|
554 |
-
):
|
555 |
-
super().__init__()
|
556 |
-
if type(sr) == type("strr"):
|
557 |
-
sr = sr2sr[sr]
|
558 |
-
self.spec_channels = spec_channels
|
559 |
-
self.inter_channels = inter_channels
|
560 |
-
self.hidden_channels = hidden_channels
|
561 |
-
self.filter_channels = filter_channels
|
562 |
-
self.n_heads = n_heads
|
563 |
-
self.n_layers = n_layers
|
564 |
-
self.kernel_size = kernel_size
|
565 |
-
self.p_dropout = p_dropout
|
566 |
-
self.resblock = resblock
|
567 |
-
self.resblock_kernel_sizes = resblock_kernel_sizes
|
568 |
-
self.resblock_dilation_sizes = resblock_dilation_sizes
|
569 |
-
self.upsample_rates = upsample_rates
|
570 |
-
self.upsample_initial_channel = upsample_initial_channel
|
571 |
-
self.upsample_kernel_sizes = upsample_kernel_sizes
|
572 |
-
self.segment_size = segment_size
|
573 |
-
self.gin_channels = gin_channels
|
574 |
-
# self.hop_length = hop_length#
|
575 |
-
self.spk_embed_dim = spk_embed_dim
|
576 |
-
if self.gin_channels == 256:
|
577 |
-
self.enc_p = TextEncoder256(
|
578 |
-
inter_channels,
|
579 |
-
hidden_channels,
|
580 |
-
filter_channels,
|
581 |
-
n_heads,
|
582 |
-
n_layers,
|
583 |
-
kernel_size,
|
584 |
-
p_dropout,
|
585 |
-
)
|
586 |
-
else:
|
587 |
-
self.enc_p = TextEncoder768(
|
588 |
-
inter_channels,
|
589 |
-
hidden_channels,
|
590 |
-
filter_channels,
|
591 |
-
n_heads,
|
592 |
-
n_layers,
|
593 |
-
kernel_size,
|
594 |
-
p_dropout,
|
595 |
-
)
|
596 |
-
self.dec = GeneratorNSF(
|
597 |
-
inter_channels,
|
598 |
-
resblock,
|
599 |
-
resblock_kernel_sizes,
|
600 |
-
resblock_dilation_sizes,
|
601 |
-
upsample_rates,
|
602 |
-
upsample_initial_channel,
|
603 |
-
upsample_kernel_sizes,
|
604 |
-
gin_channels=gin_channels,
|
605 |
-
sr=sr,
|
606 |
-
is_half=kwargs["is_half"],
|
607 |
-
)
|
608 |
-
self.enc_q = PosteriorEncoder(
|
609 |
-
spec_channels,
|
610 |
-
inter_channels,
|
611 |
-
hidden_channels,
|
612 |
-
5,
|
613 |
-
1,
|
614 |
-
16,
|
615 |
-
gin_channels=gin_channels,
|
616 |
-
)
|
617 |
-
self.flow = ResidualCouplingBlock(
|
618 |
-
inter_channels, hidden_channels, 5, 1, 3, gin_channels=gin_channels
|
619 |
-
)
|
620 |
-
self.emb_g = nn.Embedding(self.spk_embed_dim, gin_channels)
|
621 |
-
self.speaker_map = None
|
622 |
-
print("gin_channels:", gin_channels, "self.spk_embed_dim:", self.spk_embed_dim)
|
623 |
-
|
624 |
-
def remove_weight_norm(self):
|
625 |
-
self.dec.remove_weight_norm()
|
626 |
-
self.flow.remove_weight_norm()
|
627 |
-
self.enc_q.remove_weight_norm()
|
628 |
-
|
629 |
-
def construct_spkmixmap(self, n_speaker):
|
630 |
-
self.speaker_map = torch.zeros((n_speaker, 1, 1, self.gin_channels))
|
631 |
-
for i in range(n_speaker):
|
632 |
-
self.speaker_map[i] = self.emb_g(torch.LongTensor([[i]]))
|
633 |
-
self.speaker_map = self.speaker_map.unsqueeze(0)
|
634 |
-
|
635 |
-
def forward(self, phone, phone_lengths, pitch, nsff0, g, rnd, max_len=None):
|
636 |
-
if self.speaker_map is not None: # [N, S] * [S, B, 1, H]
|
637 |
-
g = g.reshape((g.shape[0], g.shape[1], 1, 1, 1)) # [N, S, B, 1, 1]
|
638 |
-
g = g * self.speaker_map # [N, S, B, 1, H]
|
639 |
-
g = torch.sum(g, dim=1) # [N, 1, B, 1, H]
|
640 |
-
g = g.transpose(0, -1).transpose(0, -2).squeeze(0) # [B, H, N]
|
641 |
-
else:
|
642 |
-
g = g.unsqueeze(0)
|
643 |
-
g = self.emb_g(g).transpose(1, 2)
|
644 |
-
|
645 |
-
m_p, logs_p, x_mask = self.enc_p(phone, pitch, phone_lengths)
|
646 |
-
z_p = (m_p + torch.exp(logs_p) * rnd) * x_mask
|
647 |
-
z = self.flow(z_p, x_mask, g=g, reverse=True)
|
648 |
-
o = self.dec((z * x_mask)[:, :, :max_len], nsff0, g=g)
|
649 |
-
return o
|
650 |
-
|
651 |
-
|
652 |
-
class MultiPeriodDiscriminator(torch.nn.Module):
|
653 |
-
def __init__(self, use_spectral_norm=False):
|
654 |
-
super(MultiPeriodDiscriminator, self).__init__()
|
655 |
-
periods = [2, 3, 5, 7, 11, 17]
|
656 |
-
# periods = [3, 5, 7, 11, 17, 23, 37]
|
657 |
-
|
658 |
-
discs = [DiscriminatorS(use_spectral_norm=use_spectral_norm)]
|
659 |
-
discs = discs + [
|
660 |
-
DiscriminatorP(i, use_spectral_norm=use_spectral_norm) for i in periods
|
661 |
-
]
|
662 |
-
self.discriminators = nn.ModuleList(discs)
|
663 |
-
|
664 |
-
def forward(self, y, y_hat):
|
665 |
-
y_d_rs = [] #
|
666 |
-
y_d_gs = []
|
667 |
-
fmap_rs = []
|
668 |
-
fmap_gs = []
|
669 |
-
for i, d in enumerate(self.discriminators):
|
670 |
-
y_d_r, fmap_r = d(y)
|
671 |
-
y_d_g, fmap_g = d(y_hat)
|
672 |
-
# for j in range(len(fmap_r)):
|
673 |
-
# print(i,j,y.shape,y_hat.shape,fmap_r[j].shape,fmap_g[j].shape)
|
674 |
-
y_d_rs.append(y_d_r)
|
675 |
-
y_d_gs.append(y_d_g)
|
676 |
-
fmap_rs.append(fmap_r)
|
677 |
-
fmap_gs.append(fmap_g)
|
678 |
-
|
679 |
-
return y_d_rs, y_d_gs, fmap_rs, fmap_gs
|
680 |
-
|
681 |
-
|
682 |
-
class MultiPeriodDiscriminatorV2(torch.nn.Module):
|
683 |
-
def __init__(self, use_spectral_norm=False):
|
684 |
-
super(MultiPeriodDiscriminatorV2, self).__init__()
|
685 |
-
# periods = [2, 3, 5, 7, 11, 17]
|
686 |
-
periods = [2, 3, 5, 7, 11, 17, 23, 37]
|
687 |
-
|
688 |
-
discs = [DiscriminatorS(use_spectral_norm=use_spectral_norm)]
|
689 |
-
discs = discs + [
|
690 |
-
DiscriminatorP(i, use_spectral_norm=use_spectral_norm) for i in periods
|
691 |
-
]
|
692 |
-
self.discriminators = nn.ModuleList(discs)
|
693 |
-
|
694 |
-
def forward(self, y, y_hat):
|
695 |
-
y_d_rs = [] #
|
696 |
-
y_d_gs = []
|
697 |
-
fmap_rs = []
|
698 |
-
fmap_gs = []
|
699 |
-
for i, d in enumerate(self.discriminators):
|
700 |
-
y_d_r, fmap_r = d(y)
|
701 |
-
y_d_g, fmap_g = d(y_hat)
|
702 |
-
# for j in range(len(fmap_r)):
|
703 |
-
# print(i,j,y.shape,y_hat.shape,fmap_r[j].shape,fmap_g[j].shape)
|
704 |
-
y_d_rs.append(y_d_r)
|
705 |
-
y_d_gs.append(y_d_g)
|
706 |
-
fmap_rs.append(fmap_r)
|
707 |
-
fmap_gs.append(fmap_g)
|
708 |
-
|
709 |
-
return y_d_rs, y_d_gs, fmap_rs, fmap_gs
|
710 |
-
|
711 |
-
|
712 |
-
class DiscriminatorS(torch.nn.Module):
|
713 |
-
def __init__(self, use_spectral_norm=False):
|
714 |
-
super(DiscriminatorS, self).__init__()
|
715 |
-
norm_f = weight_norm if use_spectral_norm == False else spectral_norm
|
716 |
-
self.convs = nn.ModuleList(
|
717 |
-
[
|
718 |
-
norm_f(Conv1d(1, 16, 15, 1, padding=7)),
|
719 |
-
norm_f(Conv1d(16, 64, 41, 4, groups=4, padding=20)),
|
720 |
-
norm_f(Conv1d(64, 256, 41, 4, groups=16, padding=20)),
|
721 |
-
norm_f(Conv1d(256, 1024, 41, 4, groups=64, padding=20)),
|
722 |
-
norm_f(Conv1d(1024, 1024, 41, 4, groups=256, padding=20)),
|
723 |
-
norm_f(Conv1d(1024, 1024, 5, 1, padding=2)),
|
724 |
-
]
|
725 |
-
)
|
726 |
-
self.conv_post = norm_f(Conv1d(1024, 1, 3, 1, padding=1))
|
727 |
-
|
728 |
-
def forward(self, x):
|
729 |
-
fmap = []
|
730 |
-
|
731 |
-
for l in self.convs:
|
732 |
-
x = l(x)
|
733 |
-
x = F.leaky_relu(x, modules.LRELU_SLOPE)
|
734 |
-
fmap.append(x)
|
735 |
-
x = self.conv_post(x)
|
736 |
-
fmap.append(x)
|
737 |
-
x = torch.flatten(x, 1, -1)
|
738 |
-
|
739 |
-
return x, fmap
|
740 |
-
|
741 |
-
|
742 |
-
class DiscriminatorP(torch.nn.Module):
|
743 |
-
def __init__(self, period, kernel_size=5, stride=3, use_spectral_norm=False):
|
744 |
-
super(DiscriminatorP, self).__init__()
|
745 |
-
self.period = period
|
746 |
-
self.use_spectral_norm = use_spectral_norm
|
747 |
-
norm_f = weight_norm if use_spectral_norm == False else spectral_norm
|
748 |
-
self.convs = nn.ModuleList(
|
749 |
-
[
|
750 |
-
norm_f(
|
751 |
-
Conv2d(
|
752 |
-
1,
|
753 |
-
32,
|
754 |
-
(kernel_size, 1),
|
755 |
-
(stride, 1),
|
756 |
-
padding=(get_padding(kernel_size, 1), 0),
|
757 |
-
)
|
758 |
-
),
|
759 |
-
norm_f(
|
760 |
-
Conv2d(
|
761 |
-
32,
|
762 |
-
128,
|
763 |
-
(kernel_size, 1),
|
764 |
-
(stride, 1),
|
765 |
-
padding=(get_padding(kernel_size, 1), 0),
|
766 |
-
)
|
767 |
-
),
|
768 |
-
norm_f(
|
769 |
-
Conv2d(
|
770 |
-
128,
|
771 |
-
512,
|
772 |
-
(kernel_size, 1),
|
773 |
-
(stride, 1),
|
774 |
-
padding=(get_padding(kernel_size, 1), 0),
|
775 |
-
)
|
776 |
-
),
|
777 |
-
norm_f(
|
778 |
-
Conv2d(
|
779 |
-
512,
|
780 |
-
1024,
|
781 |
-
(kernel_size, 1),
|
782 |
-
(stride, 1),
|
783 |
-
padding=(get_padding(kernel_size, 1), 0),
|
784 |
-
)
|
785 |
-
),
|
786 |
-
norm_f(
|
787 |
-
Conv2d(
|
788 |
-
1024,
|
789 |
-
1024,
|
790 |
-
(kernel_size, 1),
|
791 |
-
1,
|
792 |
-
padding=(get_padding(kernel_size, 1), 0),
|
793 |
-
)
|
794 |
-
),
|
795 |
-
]
|
796 |
-
)
|
797 |
-
self.conv_post = norm_f(Conv2d(1024, 1, (3, 1), 1, padding=(1, 0)))
|
798 |
-
|
799 |
-
def forward(self, x):
|
800 |
-
fmap = []
|
801 |
-
|
802 |
-
# 1d to 2d
|
803 |
-
b, c, t = x.shape
|
804 |
-
if t % self.period != 0: # pad first
|
805 |
-
n_pad = self.period - (t % self.period)
|
806 |
-
x = F.pad(x, (0, n_pad), "reflect")
|
807 |
-
t = t + n_pad
|
808 |
-
x = x.view(b, c, t // self.period, self.period)
|
809 |
-
|
810 |
-
for l in self.convs:
|
811 |
-
x = l(x)
|
812 |
-
x = F.leaky_relu(x, modules.LRELU_SLOPE)
|
813 |
-
fmap.append(x)
|
814 |
-
x = self.conv_post(x)
|
815 |
-
fmap.append(x)
|
816 |
-
x = torch.flatten(x, 1, -1)
|
817 |
-
|
818 |
-
return x, fmap
|
|
|
|
|
|
|
|
|
|
|
|
|
|
|
|
|
|
|
|
|
|
|
|
|
|
|
|
|
|
|
|
|
|
|
|
|
|
|
|
|
|
|
|
|
|
|
|
|
|
|
|
|
|
|
|
|
|
|
|
|
|
|
|
|
|
|
|
|
|
|
|
|
|
|
|
|
|
|
|
|
|
|
|
|
|
|
|
|
|
|
|
|
|
|
|
|
|
|
|
|
|
|
|
|
|
|
|
|
|
|
|
|
|
|
|
|
|
|
|
|
|
|
|
|
|
|
|
|
|
|
|
|
|
|
|
|
|
|
|
|
|
|
|
|
|
|
|
|
|
|
|
|
|
|
|
|
|
|
|
|
|
|
|
|
|
|
|
|
|
|
|
|
|
|
|
|
|
|
|
|
|
|
|
|
|
|
|
|
|
|
|
|
|
|
|
|
|
|
|
|
|
|
|
|
|
|
|
|
|
|
|
|
|
|
|
|
|
|
|
|
|
|
|
|
|
|
|
|
|
|
|
|
|
|
|
|
|
|
|
|
|
|
|
|
|
|
|
|
|
|
|
|
|
|
|
|
|
|
|
|
|
|
|
|
|
|
|
|
|
|
|
|
|
|
|
|
|
|
|
|
|
|
|
|
|
|
|
|
|
|
|
|
|
|
|
|
|
|
|
|
|
|
|
|
|
|
|
|
|
|
|
|
|
|
|
|
|
|
|
|
|
|
|
|
|
|
|
|
|
|
|
|
|
|
|
|
|
|
|
|
|
|
|
|
|
|
|
|
|
|
|
|
|
|
|
|
|
|
|
|
|
|
|
|
|
|
|
|
|
|
|
|
|
|
|
|
|
|
|
|
|
|
|
|
|
|
|
|
|
|
|
|
|
|
|
|
|
|
|
|
|
|
|
|
|
|
|
|
|
|
|
|
|
|
|
|
|
|
|
|
|
|
|
|
|
|
|
|
|
|
|
|
|
|
|
|
|
|
|
|
|
|
|
|
|
|
|
|
|
|
|
|
|
|
|
|
|
|
|
|
|
|
|
|
|
|
|
|
|
|
|
|
|
|
|
|
|
|
|
|
|
|
|
|
|
|
|
|
|
|
|
|
|
|
|
|
|
|
|
|
|
|
|
|
|
|
|
|
|
|
|
|
|
|
|
|
|
|
|
|
|
|
|
|
|
|
|
|
|
|
|
|
|
|
|
|
|
|
|
|
|
|
|
|
|
|
|
|
|
|
|
|
|
|
|
|
|
|
|
|
|
|
|
|
|
|
|
|
|
|
|
|
|
|
|
|
|
|
|
|
|
|
|
|
|
|
|
|
|
|
|
|
|
|
|
|
|
|
|
|
|
|
|
|
|
|
|
|
|
|
|
|
|
|
|
|
|
|
|
|
|
|
|
|
|
|
|
|
|
|
|
|
|
|
|
|
|
|
|
|
|
|
|
|
|
|
|
|
|
|
|
|
|
|
|
|
|
|
|
|
|
|
|
|
|
|
|
|
|
|
|
|
|
|
|
|
|
|
|
|
|
|
|
|
|
|
|
|
|
|
|
|
|
|
|
|
|
|
|
|
|
|
|
|
|
|
|
|
|
|
|
|
|
|
|
|
|
|
|
|
|
|
|
|
|
|
|
|
|
|
|
|
|
|
|
|
|
|
|
|
|
|
|
|
|
|
|
|
|
|
|
|
|
|
|
|
|
|
|
|
|
|
|
|
|
|
|
|
|
|
|
|
|
|
|
|
|
|
|
|
|
|
|
|
|
|
|
|
|
|
|
|
|
|
|
|
|
|
|
|
|
|
|
|
|
|
|
|
|
|
|
|
|
|
|
|
|
|
|
|
|
|
|
|
|
|
|
|
|
|
|
|
|
|
|
|
|
|
|
|
|
|
|
|
|
|
|
|
|
|
|
|
|
|
|
|
|
|
|
|
|
|
|
|
|
|
|
|
|
|
|
|
|
|
|
|
|
|
|
|
|
|
|
|
|
|
|
|
|
|
|
|
|
|
|
|
|
|
|
|
|
|
|
|
|
|
|
|
|
|
|
|
|
|
|
|
|
|
|
|
|
|
|
|
|
|
|
|
|
|
|
|
|
|
|
|
|
|
|
|
|
|
|
|
|
|
|
|
|
|
|
|
|
|
|
|
|
|
|
|
|
|
|
|
|
|
|
|
|
|
|
|
|
|
|
|
|
|
|
|
|
|
|
|
|
|
|
|
|
|
|
|
|
|
|
|
|
|
|
|
|
|
|
|
|
|
|
|
|
|
|
|
|
|
|
|
|
|
|
|
|
|
|
|
|
|
|
|
|
|
|
|
|
|
|
|
|
|
|
|
|
|
|
|
|
|
|
|
|
|
|
|
|
|
|
|
|
|
|
|
|
|
|
|
|
|
|
|
|
|
|
|
|
|
|
|
|
|
|
|
|
|
|
|
|
|
|
|
|
|
|
|
|
|
|
|
|
|
|
|
|
|
|
|
|
|
|
|
|
|
|
|
|
|
|
|
|
|
|
|
|
|
|
|
|
|
|
|
|
|
|
|
|
|
|
|
|
|
|
|
|
|
|
|
|
|
|
|
|
|
|
|
|
|
|
|
|
|
|
|
|
|
|
|
|
|
|
|
|
|
|
|
|
|
|
|
|
|
|
|
|
|
|
|
|
|
|
|
|
|
|
|
|
|
|
|
|
|
|
|
|
|
|
|
|
|
|
|
|
|
|
|
|
|
|
|
|
|
|
|
|
|
|
|
|
|
|
|
|
|
|
|
|
|
|
|
|
|
|
|
|
|
|
|
|
|
|
|
|
|
|
|
|
|
|
|
|
|
|
|
|
|
|
|
|
|
|
|
|
|
|
|
|
|
|
|
|
|
|
|
|
|
|
|
|
|
|
|
|
|
|
|
|
|
|
|
|
|
|
|
|
|
|
|
|
|
|
|
|
|
|
|
|
|
|
|
|
|
|
|
|
|
|
|
|
|
|
|
|
|
|
|
|
|
|
|
|
|
|
|
|
|
|
|
|
|
|
|
|
|
|
|
|
|
|
|
|
|
|
|
|
|
|
|
|
|
|
|
|
|
|
|
|
|
|
|
|
|
|
|
|
|
|
|
|
|
|
|
|
|
|
|
|
|
|
|
|
|
|
|
|
|
|
|
|
|
|
|
|
|
|
|
|
|
|
|
|
|
|
|
|
|
|
|
|
|
|
|
|
|
|
|
|
|
|
|
|
|
|
|
|
|
|
|
|
|
|
|
|
|
|
|
|
|
|
|
|
|
|
|
|
|
|
|
|
|
|
|
|
|
|
|
|
|
|
|
|
|
|
|
|
|
|
|
|
|
|
|
|
|
|
|
|
|
|
|
|
|
|
|
|
|
|
|
|
|
|
|
|
|
|
|
|
|
|
|
|
|
|
|
|
|
|
|
|
|
|
|
|
|
|
|
|
|
|
|
|
|
|
|
|
|
|
|
|
|
|
|
|
|
|
|
|
|
|
|
|
|
|
|
|
|
|
|
|
|
|
|
|
|
|
|
|
|
|
|
|
|
|
|
|
|
|
|
|
|
|
|
|
|
|
|
|
|
|
|
|
|
|
|
|
|
|
|
|
|
|
|
|
|
|
|
|
|
|
|
|
|
|
|
|
|
|
|
|
|
|
|
|
|
|
|
|
|
|
|
|
spaces/BeeMon/dreambooth-training/README.md
DELETED
@@ -1,14 +0,0 @@
|
|
1 |
-
---
|
2 |
-
title: Dreambooth Training
|
3 |
-
emoji: ☁️
|
4 |
-
colorFrom: pink
|
5 |
-
colorTo: red
|
6 |
-
sdk: gradio
|
7 |
-
sdk_version: 3.16.2
|
8 |
-
app_file: app.py
|
9 |
-
pinned: false
|
10 |
-
license: mit
|
11 |
-
duplicated_from: multimodalart/dreambooth-training
|
12 |
-
---
|
13 |
-
|
14 |
-
Check out the configuration reference at https://huggingface.co/docs/hub/spaces-config-reference
|
|
|
|
|
|
|
|
|
|
|
|
|
|
|
|
|
|
|
|
|
|
|
|
|
|
|
|
|
|
spaces/Benson/text-generation/Examples/Descargar Gratis Brawlhalla Steamunlocked.md
DELETED
@@ -1,64 +0,0 @@
|
|
1 |
-
|
2 |
-
<h1>Descarga gratuita de Brawlhalla Steamunlocked: Una guía para los fans de Platform Fighter</h1>
|
3 |
-
<p>Si usted está buscando un juego de lucha divertido y emocionante que se puede jugar de forma gratuita en su PC, es posible que desee echa un vistazo a <strong>Brawlhalla</strong>. Este juego es un luchador de plataformas que soporta el juego cruzado en varios dispositivos, incluyendo PC, PlayStation, Xbox, Nintendo Switch, iOS y Android. En este artículo, te diremos qué es Brawlhalla, cómo descargarlo gratis en Steam, cómo desbloquear más contenido en el juego y cómo mejorar tus habilidades como luchador. </p>
|
4 |
-
<h2>descargar gratis brawlhalla steamunlocked</h2><br /><p><b><b>Download Zip</b> ––– <a href="https://bltlly.com/2v6K1g">https://bltlly.com/2v6K1g</a></b></p><br /><br />
|
5 |
-
<h2>¿Qué es Brawlhalla? </h2>
|
6 |
-
<p>Brawlhalla es un juego de lucha de plataformas 2D que fue desarrollado por Blue Mammoth Games y publicado por Ubisoft. Fue lanzado en 2017 después de un período beta que comenzó en 2015. El juego ha sido elogiado por su mecánica de juego simple pero profunda, su colorido y diverso elenco de personajes, sus frecuentes actualizaciones y eventos, y su falta de ventajas de pago para ganar. </p>
|
7 |
-
<h3>Un luchador de plataforma de juego libre con soporte de juego cruzado</h3>
|
8 |
-
<p>Uno de los principales puntos de venta de Brawlhalla es que es libre de jugar. Usted no necesita pagar nada para descargar y jugar el juego en cualquier plataforma. Tampoco necesitas un servicio de suscripción online como PlayStation Plus o Nintendo Switch Online para jugar online con otros jugadores. El juego también soporta cross-play en todas las plataformas, lo que significa que puedes jugar con o contra cualquier persona que tenga el juego en cualquier dispositivo. </p>
|
9 |
-
<h3>Una lista de más de 50 leyendas y actualizaciones frecuentes</h3>
|
10 |
-
|
11 |
-
<h3>Una variedad de modos de juego y características</h3>
|
12 |
-
<p>Brawlhalla también ofrece una variedad de modos de juego y características para mantenerte entretenido y desafiado. Puedes jugar online o localmente con hasta 8 jugadores en varios modos como Free-for-All, 1v1, 2v2, Brawlball, Kung Foot, Capture the Flag, Horde y más. También puedes personalizar tus partidos con diferentes configuraciones, mapas y modificadores. También puede unirse a los partidos clasificados y subir las tablas de clasificación, o participar en torneos y eventos para recompensas y gloria. El juego también tiene un modo para un solo jugador donde puedes luchar contra bots, completar misiones o jugar el modo historia. </p>
|
13 |
-
<h2>Cómo descargar Brawlhalla gratis en Steam? </h2>
|
14 |
-
<p>Si quieres jugar Brawlhalla en tu PC, puedes descargarlo gratis en Steam. Estos son los pasos para hacerlo:</p>
|
15 |
-
<h3>Visita la página de la tienda de Steam y haz clic en "Jugar"</h3>
|
16 |
-
<p>Primero, necesitas tener una cuenta de Steam y el cliente de Steam instalado en tu PC. Si aún no los tiene, puede crear una cuenta y descargar el cliente desde <a href="">https://store.steampowered.com/</a>. Una vez que los tengas, abre el cliente de Steam y busca Brawlhalla en la tienda. Alternativamente, puedes visitar la página de la tienda del juego directamente desde este enlace: <a href="">https://store.steampowered.com/app/291550/Brawlhalla/</a>. En la página de la tienda, verá un botón que dice "Jugar". Haga clic en él para comenzar a descargar el juego. </p>
|
17 |
-
<h3>Instalar el juego y lanzarlo desde su biblioteca</h3>
|
18 |
-
<p>Después de hacer clic en el botón "Jugar juego", verá una ventana emergente que le pide que confirme la instalación. Haga clic en "Siguiente" y siga las instrucciones para elegir la carpeta de instalación y aceptar los términos del servicio. El juego comenzará a descargarse e instalarse en su PC. El proceso puede tardar unos minutos dependiendo de su velocidad de Internet y espacio en disco. Una vez completada la instalación, puedes iniciar el juego desde tu biblioteca o desde el acceso directo del escritorio. </p>
|
19 |
-
<p></p>
|
20 |
-
|
21 |
-
<p>Cuando inicie el juego por primera vez, se le pedirá que cree una cuenta o enlace a la existente. Puedes usar tu dirección de correo electrónico o tus cuentas de redes sociales como Facebook, Twitter, Google o Apple para registrarte o iniciar sesión. Crear una cuenta te permitirá guardar tu progreso, personalizar tu perfil, acceder a funciones en línea y sincronizar tus datos entre dispositivos. Si ya tienes una cuenta en otra plataforma, puedes vincularla a tu cuenta de Steam y mantener tu progreso y tus compras. </p>
|
22 |
-
<h2>¿Cómo desbloquear más contenido en Brawlhalla? </h2>
|
23 |
-
<p>Brawlhalla es gratis para jugar, pero también tiene mucho contenido que puedes desbloquear jugando o gastando dinero real. Aquí hay algunas maneras de desbloquear más contenido en Brawlhalla:</p>
|
24 |
-
<h3>Gana monedas de oro y mamut jugando partidos y completando misiones</h3>
|
25 |
-
<p>La moneda principal en Brawlhalla es <strong>gold</strong>, que puedes ganar jugando partidas y completando misiones. Puedes usar oro para comprar leyendas, colores y avatares en la tienda del juego. También puede ganar monedas <strong>mammoth</strong>, que son moneda premium que puede comprar con dinero real o obtener de eventos especiales. Puedes usar monedas gigantescas para comprar pieles, burlas, compañeros y pases de batalla de la tienda. </p>
|
26 |
-
<h3>Usa oro para comprar leyendas, colores y avatares</h3>
|
27 |
-
<p>Una de las cosas más importantes para desbloquear en Brawlhalla es <strong>Legends</strong>, que son los personajes que puedes jugar. Hay más de 50 leyendas en el juego, cada una con sus propias habilidades, armas, estadísticas y personalidades. Puedes comprar Leyendas con monedas de oro o de mamut en la tienda. Cada Leyenda cuesta 5400 monedas de oro o 100 monedas de mamut. También puede probar cualquier leyenda de forma gratuita en el modo de entrenamiento o durante rotaciones semanales. </p>
|
28 |
-
|
29 |
-
<h3>Usa monedas gigantescas para comprar pieles, burlas, compañeros y pases de batalla</h3>
|
30 |
-
<p>Si quieres personalizar tus leyendas aún más, puedes usar monedas gigantescas para comprar <strong>skins</strong>, <strong>burlas</strong>, <strong>, <strong>compañeros</strong>, y <strong>pases de batalla</strong> desde la tienda. Las pieles son opciones cosméticas que cambian la apariencia del atuendo, las armas y los efectos de tu leyenda. Puedes comprar pieles con monedas gigantescas en la tienda o desbloquearlas comprando pases de batalla o durante eventos especiales. Las burlas son emotes que puedes usar para expresarte en el juego. Puedes comprar burlas con monedas gigantescas en la tienda o desbloquearlas comprando pases de batalla o durante eventos especiales. Los compañeros son mascotas que te acompañan en el juego. Puedes comprar compañeros con monedas de mamut en la tienda o desbloquearlos comprando pases de batalla o durante eventos especiales. Los pases de batalla son pases de temporada que te dan acceso a recompensas exclusivas como pieles, burlas, compañeros, colores, avatares y más. Puedes comprar pases de batalla con monedas gigantescas en la tienda o conseguirlos gratis jugando el juego. </p>
|
31 |
-
<h2>¿Cómo mejorar tus habilidades en Brawlhalla? </h2>
|
32 |
-
<p>Brawlhalla es un juego que es fácil de aprender pero difícil de dominar. Si quieres mejorar tus habilidades como luchador, aquí hay algunos consejos y trucos que puedes seguir:</p>
|
33 |
-
<h3>Aprende los conceptos básicos de movimiento, recuperación, esquivar y atacar</h3>
|
34 |
-
|
35 |
-
tecla de ataque pesado o el botón X mientras se mantiene pulsada la tecla de flecha hacia abajo o el botón B, que realiza un ataque especial y potente que es único para cada leyenda y arma. </p>
|
36 |
-
<h3>Experimenta con diferentes leyendas y armas</h3>
|
37 |
-
<p>Otra cosa que necesitas aprender en Brawlhalla es cómo usar diferentes leyendas y armas. Cada leyenda tiene sus propias habilidades, armas, estadísticas y personalidades. Puedes elegir una leyenda que se adapte a tu estilo de juego, preferencias y objetivos. También puedes cambiar entre leyendas para adaptarte a diferentes situaciones, oponentes y modos. Cada leyenda tiene dos armas que pueden usar en batalla. Puede recoger un arma pulsando la tecla de recogida o el botón Z cuando vea un arma en el escenario. También puedes lanzar tu arma presionando la tecla de recogida o el botón Z mientras sostienes un arma, lo que puede ser útil para golpear a tu oponente desde la distancia o desarmarlo. Cada arma tiene su propio movimiento, rango, velocidad y daño. Puedes usar diferentes armas para lidiar con diferentes escenarios, enemigos y estrategias. También puedes combinar tus armas con tus ataques desarmados, tu esquivar y tus ataques característicos para crear combos y cadenas. </p>
|
38 |
-
<h3>Practica en modo entrenamiento y mira tutoriales</h3>
|
39 |
-
<p>Lo último que tienes que hacer para mejorar tus habilidades en Brawlhalla es practicar y aprender de los demás. Puedes practicar en el modo de entrenamiento, que es un modo donde puedes probar tus habilidades contra un maniquí o un bot. Puedes personalizar los ajustes del modo de entrenamiento, como la leyenda, el arma, el mapa, el daño, la velocidad, los hitboxes y más. Puedes usar el modo de entrenamiento para aprender nuevos movimientos, practicar combos, probar daños y experimentar con diferentes opciones. También puedes ver tutoriales de otros jugadores o de los desarrolladores, que pueden enseñarte consejos, trucos, técnicas y estrategias para jugar a Brawlhalla. Puedes encontrar tutoriales en YouTube, Twitch, Reddit, Discord, o el sitio web oficial de Brawlhalla.</p>
|
40 |
-
|
41 |
-
<p>Brawlhalla es un divertido y accesible luchador de plataformas que puedes jugar gratis en Steam. Tiene una gran y diversa lista de leyendas, una variedad de modos de juego y características, y un sistema de juego simple pero profundo. Puede descargarlo ahora y unirse a millones de jugadores en línea o localmente. También puedes desbloquear más contenido en el juego jugando partidos y completando misiones, o gastando dinero real si quieres. También puedes mejorar tus habilidades en Brawlhalla aprendiendo los conceptos básicos de movimiento, recuperación, esquivar y atacar, experimentando con diferentes leyendas y armas, y practicando en modo entrenamiento y viendo tutoriales. Brawlhalla es un juego que te mantendrá entretenido y retado durante horas. ¡Disfruta del juego y conviértete en el mejor luchador del Valhalla! </p>
|
42 |
-
<h2>Preguntas frecuentes</h2>
|
43 |
-
<h3>¿Es Brawlhalla pago a ganar? </h3>
|
44 |
-
<p>No, Brawlhalla no es pagar para ganar. Todo el contenido que afecta al juego, como leyendas y armas, se puede desbloquear jugando el juego o gastando oro, que se gana jugando el juego. El único contenido que requiere dinero real para comprar es cosmético, como pieles, burlas, compañeros y pases de batalla. Estos elementos no dan ninguna ventaja de juego y son solo para personalización y expresión. </p>
|
45 |
-
<h3>¿Brawlhalla es multiplataforma? </h3>
|
46 |
-
<p>Sí, Brawlhalla es multiplataforma. Puedes jugar con o contra cualquier persona que tenga el juego en cualquier dispositivo, incluyendo PC, PlayStation, Xbox, Nintendo Switch, iOS y Android. También puedes sincronizar tu progreso y tus compras entre dispositivos mediante la vinculación de tu cuenta. Para habilitar el cross-play, necesita tener una conexión en línea y activar la opción de cross-play en la configuración. </p>
|
47 |
-
<h3>¿Cuántos jugadores pueden jugar Brawlhalla online o localmente? </h3>
|
48 |
-
|
49 |
-
<h3>¿Cuáles son los requisitos del sistema para Brawlhalla en PC? </h3>
|
50 |
-
<p>Brawlhalla es un juego que no requiere muchos recursos para ejecutarse en PC. Aquí están los requisitos mínimos y recomendados del sistema para Brawlhalla en PC:</p>
|
51 |
-
<tabla>
|
52 |
-
<tr><th>Mínimo</th><th>Recomendado</th></tr>
|
53 |
-
<tr><td>OS: Windows XP/Vista/7/8/10</td><td>OS: Windows 7/8/10</td></tr>
|
54 |
-
<tr><td>Procesador: 2.4 GHz Dual Core</td><td>Procesador: 2.8 GHz Quad Core</td></tr>
|
55 |
-
<tr><td>Memoria: 1 GB de RAM</td><td>Memoria: 4 GB de RAM</td></tr>
|
56 |
-
<tr><td>Gráficos: 512 MB VRAM</td><td>Gráficos: 1 GB VRAM</td></tr>
|
57 |
-
<tr><td>DirectX: Versión 9.0c</td><td>DirectX: Versión 9.0c</td></tr>
|
58 |
-
<tr><td>Red: Conexión a Internet de banda ancha</td><td>Red: Conexión a Internet de banda ancha</td></tr>
|
59 |
-
<tr><td>Almacenamiento: 350 MB de espacio disponible</td><td>Almacenamiento: 350 MB de espacio disponible</td></tr>
|
60 |
-
</tabla>
|
61 |
-
<h3>¿Dónde puedo encontrar más información sobre Brawlhalla? </h3>
|
62 |
-
<p>Si quieres saber más sobre Brawlhalla, puedes visitar el sitio web oficial del juego en <a href="">https://www.brawlhalla.com/</a>. Allí puedes encontrar noticias, actualizaciones, eventos, torneos, guías, videos y más. También puedes seguir el juego en plataformas de redes sociales como Facebook, Twitter, Instagram, YouTube, Twitch, Reddit, Discord y Steam. También puede ponerse en contacto con los desarrolladores o los administradores de la comunidad si tiene alguna pregunta, comentario o sugerencia. </p> 64aa2da5cf<br />
|
63 |
-
<br />
|
64 |
-
<br />
|
|
|
|
|
|
|
|
|
|
|
|
|
|
|
|
|
|
|
|
|
|
|
|
|
|
|
|
|
|
|
|
|
|
|
|
|
|
|
|
|
|
|
|
|
|
|
|
|
|
|
|
|
|
|
|
|
|
|
|
|
|
|
|
|
|
|
|
|
|
|
|
|
|
|
|
|
|
|
|
|
|
|
|
|
|
|
|
|
|
|
|
|
|
|
|
|
|
|
|
|
|
|
|
|
|
|
|
|
|
|
|
|
|
|
|
|
|
|
|
|
|
|
|
|
|
|
|
|
|
spaces/Betacuckgpt/togethercomputer-GPT-JT-Moderation-6B/README.md
DELETED
@@ -1,12 +0,0 @@
|
|
1 |
-
---
|
2 |
-
title: Togethercomputer GPT JT Moderation 6B
|
3 |
-
emoji: 🐨
|
4 |
-
colorFrom: pink
|
5 |
-
colorTo: pink
|
6 |
-
sdk: gradio
|
7 |
-
sdk_version: 3.21.0
|
8 |
-
app_file: app.py
|
9 |
-
pinned: false
|
10 |
-
---
|
11 |
-
|
12 |
-
Check out the configuration reference at https://huggingface.co/docs/hub/spaces-config-reference
|
|
|
|
|
|
|
|
|
|
|
|
|
|
|
|
|
|
|
|
|
|
|
|
|
|
spaces/BetterAPI/BetterChat/src/lib/types/Conversation.ts
DELETED
@@ -1,17 +0,0 @@
|
|
1 |
-
import type { ObjectId } from "mongodb";
|
2 |
-
import type { Message } from "./Message";
|
3 |
-
import type { Timestamps } from "./Timestamps";
|
4 |
-
|
5 |
-
export interface Conversation extends Timestamps {
|
6 |
-
_id: ObjectId;
|
7 |
-
|
8 |
-
// Can be undefined for shared convo then deleted
|
9 |
-
sessionId: string;
|
10 |
-
|
11 |
-
title: string;
|
12 |
-
messages: Message[];
|
13 |
-
|
14 |
-
meta?: {
|
15 |
-
fromShareId?: string;
|
16 |
-
};
|
17 |
-
}
|
|
|
|
|
|
|
|
|
|
|
|
|
|
|
|
|
|
|
|
|
|
|
|
|
|
|
|
|
|
|
|
|
|
|
|
spaces/BetterAPI/BetterChat_new/src/lib/types/UrlDependency.ts
DELETED
@@ -1,5 +0,0 @@
|
|
1 |
-
/* eslint-disable no-shadow */
|
2 |
-
export enum UrlDependency {
|
3 |
-
ConversationList = "conversation:list",
|
4 |
-
Settings = "settings:list",
|
5 |
-
}
|
|
|
|
|
|
|
|
|
|
|
|