Commit
·
0f8fe23
1
Parent(s):
cd758f0
Update parquet files (step 23 of 296)
Browse filesThis view is limited to 50 files because it contains too many changes.
See raw diff
- spaces/1doemePnordwo/upscale/app.py +0 -127
- spaces/1gistliPinn/ChatGPT4/Examples/1993.el.retorno.de.las.brujas.dvdrip.spanish.www.zonatorrent.com.23 _HOT_.md +0 -6
- spaces/1pelhydcardo/ChatGPT-prompt-generator/assets/Busy 21 6.2 How to Download and Get Started with the No.1 GST Accounting Software in India.md +0 -162
- spaces/1phancelerku/anime-remove-background/BMW E30 Mod APK Turn Your Phone into a Racing Simulator.md +0 -93
- spaces/1phancelerku/anime-remove-background/Baseball 9 Mod APK The Most Fun and Informative Baseball Game with Unlimited Diamonds and Stamina.md +0 -105
- spaces/1phancelerku/anime-remove-background/Download FR Legends Mod Apk with Supra and RX7 in 2021 (New Cars Mod Unlimited Money).md +0 -119
- spaces/1phancelerku/anime-remove-background/Flash Game Player Pro Mod APK How to Play Any Flash Game on Your Phone.md +0 -145
- spaces/44ov41za8i/FreeVC/mel_processing.py +0 -112
- spaces/4f20/text_generator/app.py +0 -3
- spaces/801artistry/RVC801/julius/core.py +0 -122
- spaces/A00001/bingothoo/src/components/voice.tsx +0 -52
- spaces/AIConsultant/MusicGen/audiocraft/utils/cluster.py +0 -75
- spaces/AIConsultant/MusicGen/docs/TRAINING.md +0 -312
- spaces/AIGC-Audio/AudioGPT/NeuralSeq/tasks/base_task.py +0 -360
- spaces/AIGC-Audio/AudioGPT/text_to_audio/Make_An_Audio/ldm/modules/encoders/open_clap/feature_fusion.py +0 -193
- spaces/AIGC-Audio/Make_An_Audio/ldm/modules/encoders/open_clap/__init__.py +0 -8
- spaces/AILab-CVC/SEED-LLaMA/models/seed_qformer/eva_vit.py +0 -486
- spaces/ATang0729/Forecast4Muses/Model/Model6/Model6_0_ClothesDetection/mmyolo/configs/_base_/det_p5_tta.py +0 -57
- spaces/AiMimicry/sovits-models/modules/crepe.py +0 -327
- spaces/Ajay-user/Optical-Character-Recognition/app.py +0 -61
- spaces/Androidonnxfork/CivitAi-to-Diffusers/diffusers/docs/source/en/using-diffusers/weighted_prompts.md +0 -110
- spaces/Annotation-AI/fast-segment-everything-with-image-prompt/README.md +0 -12
- spaces/Anonymous-sub/Rerender/ControlNet/ldm/modules/diffusionmodules/openaimodel.py +0 -786
- spaces/Anthony7906/MengHuiMXD_GPT/modules/config.py +0 -173
- spaces/ArkanDash/rvc-models-new/lib/infer_pack/commons.py +0 -166
- spaces/Arnx/MusicGenXvAKN/audiocraft/models/__init__.py +0 -10
- spaces/Arulkumar03/GroundingDINO_SOTA_Zero_Shot_Model/demo/test_ap_on_coco.py +0 -233
- spaces/Arulkumar03/GroundingDINO_SOTA_Zero_Shot_Model/groundingdino/models/GroundingDINO/utils.py +0 -268
- spaces/Ataturk-Chatbot/HuggingFaceChat/venv/lib/python3.11/site-packages/pip/_internal/metadata/base.py +0 -688
- spaces/Ataturk-Chatbot/HuggingFaceChat/venv/lib/python3.11/site-packages/pip/_vendor/chardet/macromanprober.py +0 -162
- spaces/Ataturk-Chatbot/HuggingFaceChat/venv/lib/python3.11/site-packages/setuptools/_distutils/command/build.py +0 -153
- spaces/Ataturk-Chatbot/HuggingFaceChat/venv/lib/python3.11/site-packages/setuptools/_vendor/zipp.py +0 -329
- spaces/Ataturk-Chatbot/HuggingFaceChat/venv/lib/python3.11/site-packages/setuptools/command/saveopts.py +0 -22
- spaces/Awiny/Image2Paragraph/utils/ignore_large_files.py +0 -17
- spaces/Bart92/RVC_HF/diffq/uniform.py +0 -121
- spaces/Benson/text-generation/Examples/Camin Simulador Final 1.1 7 Apk Descargar.md +0 -62
- spaces/Benson/text-generation/Examples/Descargar Flash Player Pro.md +0 -85
- spaces/Benson/text-generation/Examples/Descargar Fondo De Pantalla En Blanco Y Negro.md +0 -88
- spaces/Big-Web/MMSD/env/Lib/site-packages/pip/_vendor/requests/certs.py +0 -24
- spaces/Big-Web/MMSD/env/Lib/site-packages/pip/_vendor/rich/_null_file.py +0 -69
- spaces/Big-Web/MMSD/env/Lib/site-packages/pip/_vendor/webencodings/labels.py +0 -231
- spaces/BramVanroy/opus-mt/README.md +0 -11
- spaces/CVPR/LIVE/thrust/thrust/system/cuda/detail/get_value.h +0 -98
- spaces/CVPR/lama-example/bin/gen_mask_dataset_hydra.py +0 -124
- spaces/Chaitanya01/InvestingPlatform/config.py +0 -391
- spaces/ChrisPreston/diff-svc_minato_aqua/preprocessing/hubertinfer.py +0 -53
- spaces/CikeyQI/meme-api/meme_generator/memes/behead/__init__.py +0 -34
- spaces/CompVis/stable-diffusion-license/license.html +0 -0
- spaces/Cong723/gpt-academic-public/crazy_functions/test_project/latex/attention/introduction.tex +0 -18
- spaces/DAMO-NLP-SG/Video-LLaMA/video_llama/processors/base_processor.py +0 -26
spaces/1doemePnordwo/upscale/app.py
DELETED
@@ -1,127 +0,0 @@
|
|
1 |
-
from sldl.video import VideoSR
|
2 |
-
from sldl.image import ImageSR
|
3 |
-
|
4 |
-
import gradio as gr
|
5 |
-
import tempfile
|
6 |
-
import shutil
|
7 |
-
import torch
|
8 |
-
import ffmpeg
|
9 |
-
import time
|
10 |
-
from PIL import Image
|
11 |
-
|
12 |
-
cc = 2
|
13 |
-
if torch.backends.mps.is_available():
|
14 |
-
device = 'mps'
|
15 |
-
cc = 5
|
16 |
-
elif torch.cuda.is_available():
|
17 |
-
device = 'cuda'
|
18 |
-
cc = 10
|
19 |
-
else:
|
20 |
-
device = 'cpu'
|
21 |
-
|
22 |
-
vbsrgan = VideoSR('BSRGAN').to(device)
|
23 |
-
vresrgan = VideoSR('RealESRGAN').to(device)
|
24 |
-
ibsrgan = ImageSR('BSRGAN').to(device)
|
25 |
-
iresrgan = ImageSR('RealESRGAN').to(device)
|
26 |
-
|
27 |
-
def upscale_video(input_video, output_video, progress, mname):
|
28 |
-
modelname = mname.lower()
|
29 |
-
model = vbsrgan
|
30 |
-
if modelname == 'bsrgan (default)':
|
31 |
-
# do nothing
|
32 |
-
pass
|
33 |
-
elif modelname == 'real esrgan':
|
34 |
-
model = vresrgan
|
35 |
-
model(input_video, output_video, progress.tqdm)
|
36 |
-
|
37 |
-
def upscale_image(input_image, output_image, mname):
|
38 |
-
modelname = mname.lower()
|
39 |
-
model = ibsrgan
|
40 |
-
if modelname == 'bsrgan (default)':
|
41 |
-
# do nothing
|
42 |
-
pass
|
43 |
-
elif modelname == 'real esrgan':
|
44 |
-
model = iresrgan
|
45 |
-
shutil.copy(input_image, output_image)
|
46 |
-
model(output_image)
|
47 |
-
|
48 |
-
# Gradio interface
|
49 |
-
def video_upscaling_interface(input_text, model_name, progress=gr.Progress()):
|
50 |
-
if input_text:
|
51 |
-
temp_dir = tempfile.mkdtemp()
|
52 |
-
input_video_path = f"{temp_dir}/input_video"
|
53 |
-
output_video_path = f"{temp_dir}/output_video.mp4"
|
54 |
-
ffmpeg.input(input_text).output(input_video_path + '.mp4').run()
|
55 |
-
|
56 |
-
# Upscale the video
|
57 |
-
upscale_video(input_video_path + '.mp4', output_video_path, progress, model_name)
|
58 |
-
|
59 |
-
return [output_video_path, output_video_path]
|
60 |
-
else:
|
61 |
-
return ["no_vid.mp4", "no_vid.mp4"]
|
62 |
-
|
63 |
-
|
64 |
-
def image_upscaling_interface(input_text, model_name):
|
65 |
-
if input_text:
|
66 |
-
temp_dir = tempfile.mkdtemp()
|
67 |
-
input_image_path = f"{temp_dir}/input_image.jpg"
|
68 |
-
output_image_path = f"{temp_dir}/output_image.jpg"
|
69 |
-
input_text.save(input_image_path)
|
70 |
-
upscale_image(input_image_path, output_image_path, model_name)
|
71 |
-
return [output_image_path, output_image_path]
|
72 |
-
else:
|
73 |
-
return ["no_image.jpg", "no_image.jpg"]
|
74 |
-
|
75 |
-
|
76 |
-
css = "footer {display: none !important;} .gradio-container {min-height: 0px !important;}"
|
77 |
-
|
78 |
-
|
79 |
-
with gr.Blocks(css=css) as demo:
|
80 |
-
gr.Markdown('''
|
81 |
-
# Upscale
|
82 |
-
## A CVSYS Project
|
83 |
-
|
84 |
-
### NOTICE: This is running on a free Hugging Face Space, so it will be quite slow. Expect it to take _hours_ to upscale 5 minutes. Please be mindful and _DO NOT_ upscale videos longer than 15 seconds! Thank you!
|
85 |
-
|
86 |
-
[Check out Upscale on GitHub!](https://github.com/cv-sys/upscale)
|
87 |
-
|
88 |
-
## Want Faster Inference?
|
89 |
-
|
90 |
-
Duplicate this space for faster inference! We recommend using an A10G or A100.
|
91 |
-
|
92 |
-
<a href="https://huggingface.co/spaces/cvsys/upscale?duplicate=true"><img src="https://img.shields.io/badge/-Duplicate%20Space-blue?labelColor=white&style=flat&logo=data:image/png;base64,iVBORw0KGgoAAAANSUhEUgAAABAAAAAQCAYAAAAf8/9hAAAAAXNSR0IArs4c6QAAAP5JREFUOE+lk7FqAkEURY+ltunEgFXS2sZGIbXfEPdLlnxJyDdYB62sbbUKpLbVNhyYFzbrrA74YJlh9r079973psed0cvUD4A+4HoCjsA85X0Dfn/RBLBgBDxnQPfAEJgBY+A9gALA4tcbamSzS4xq4FOQAJgCDwV2CPKV8tZAJcAjMMkUe1vX+U+SMhfAJEHasQIWmXNN3abzDwHUrgcRGmYcgKe0bxrblHEB4E/pndMazNpSZGcsZdBlYJcEL9Afo75molJyM2FxmPgmgPqlWNLGfwZGG6UiyEvLzHYDmoPkDDiNm9JR9uboiONcBXrpY1qmgs21x1QwyZcpvxt9NS09PlsPAAAAAElFTkSuQmCC&logoWidth=14"></a>
|
93 |
-
|
94 |
-
Please note that after you upload an image, it may take several minutes before the progress bar appears. This is because we first convert your video to ensure the correct format.
|
95 |
-
''')
|
96 |
-
# with gr.Tab("Image"):
|
97 |
-
# with gr.Row():
|
98 |
-
# with gr.Column():
|
99 |
-
# iinp = gr.Image(label="Upload Image", interactive=True, type="pil")
|
100 |
-
# imod = gr.Dropdown(
|
101 |
-
# ["BSRGAN (Default)", "Real ESRGAN"],
|
102 |
-
# value="BSRGAN (Default)",
|
103 |
-
# interactive=True,
|
104 |
-
# label="Model"
|
105 |
-
# )
|
106 |
-
# with gr.Column():
|
107 |
-
# iout = gr.Image(label="View Image", interactive=False, type="filepath")
|
108 |
-
# ifile = gr.File(label="Download Image", interactive=False)
|
109 |
-
# ibtn = gr.Button(value="Upscale Image")
|
110 |
-
with gr.Tab("Video"):
|
111 |
-
with gr.Row():
|
112 |
-
with gr.Column():
|
113 |
-
vinp = gr.Video(label="Upload Video", interactive=True)
|
114 |
-
vmod = gr.Dropdown(
|
115 |
-
["BSRGAN (Default)", "Real ESRGAN"],
|
116 |
-
value="BSRGAN (Default)",
|
117 |
-
interactive=True,
|
118 |
-
label="Model"
|
119 |
-
)
|
120 |
-
with gr.Column():
|
121 |
-
vout = gr.Video(label="Watch Video", interactive=False)
|
122 |
-
vfile = gr.File(label="Download Video", interactive=False)
|
123 |
-
vbtn = gr.Button(value="Upscale Video")
|
124 |
-
# ibtn.click(image_upscaling_interface, [iinp, imod], outputs=[iout, ifile])
|
125 |
-
vbtn.click(video_upscaling_interface, [vinp, vmod], outputs=[vout, vfile])
|
126 |
-
demo.queue(concurrency_count=cc)
|
127 |
-
demo.launch()
|
|
|
|
|
|
|
|
|
|
|
|
|
|
|
|
|
|
|
|
|
|
|
|
|
|
|
|
|
|
|
|
|
|
|
|
|
|
|
|
|
|
|
|
|
|
|
|
|
|
|
|
|
|
|
|
|
|
|
|
|
|
|
|
|
|
|
|
|
|
|
|
|
|
|
|
|
|
|
|
|
|
|
|
|
|
|
|
|
|
|
|
|
|
|
|
|
|
|
|
|
|
|
|
|
|
|
|
|
|
|
|
|
|
|
|
|
|
|
|
|
|
|
|
|
|
|
|
|
|
|
|
|
|
|
|
|
|
|
|
|
|
|
|
|
|
|
|
|
|
|
|
|
|
|
|
|
|
|
|
|
|
|
|
|
|
|
|
|
|
|
|
|
|
|
|
|
|
|
|
|
|
|
|
|
|
|
|
|
|
|
|
|
|
|
|
|
|
|
|
|
|
|
|
|
|
|
|
|
|
|
|
|
|
|
|
|
|
|
|
|
|
|
|
|
|
|
|
|
|
|
|
|
|
|
|
|
|
|
|
|
|
|
|
|
|
|
|
|
|
|
|
|
|
|
|
spaces/1gistliPinn/ChatGPT4/Examples/1993.el.retorno.de.las.brujas.dvdrip.spanish.www.zonatorrent.com.23 _HOT_.md
DELETED
@@ -1,6 +0,0 @@
|
|
1 |
-
<h2>1993.el.retorno.de.las.brujas.dvdrip.spanish.www.zonatorrent.com.23</h2><br /><p><b><b>DOWNLOAD</b> ⚹ <a href="https://imgfil.com/2uy1dK">https://imgfil.com/2uy1dK</a></b></p><br /><br />
|
2 |
-
|
3 |
-
00.el.retorno.de.las.brujas.dvdrip.spanish.supersport.org.2330.el.retorno.de.las.brujas.dvdrip.spanish.superobserver.cc.2340.el.retorno.de.las.brujas.dvdrip.spanish.superbin.net.2350.el.retorno.de.las.brujas.dvdrip.spanish.sunnylife.net.2360.el.retorno.de.las.brujas.dvdrip.spanish.superninja.org.2370.el.retorno.de.las.brujas.dvdrip.spanish.supermagged.org.2380.el.retorno.de.las.brujas.dvdrip.spanish.superpiracy.org.2390.el.retorno.de.las.brujas.dvdrip.spanish.supervideonet.org.2400.el.retorno.de.las.brujas.dvdrip.spanish.superweb.org.2410.el.retorno.de.las.brujas.dvdrip.spanish.sue.el.retorno.de.las.brujas.dvdrip.spanish.superxvideo.com.2420.el.retorno.de.las.brujas.dvdrip.spanish.superservice.com.2430.el.retorno.de.las.brujas.dvdrip.spanish.supersonic.tv.2440.el.retorno.de.las.brujas.dvdrip.spanish.superspeed.org.2450.el.retorno.de.las.brujas.dvdrip.spanish.supersports.net.2460.el.retorno.de.las.brujas.dvdrip.spanish.supersportsonline.biz.2470.el.retorno.de.las.brujas.dvdrip.spanish.supersportsonline.net.2480.el.retorno.de.las.brujas.dvdrip.spanish 4fefd39f24<br />
|
4 |
-
<br />
|
5 |
-
<br />
|
6 |
-
<p></p>
|
|
|
|
|
|
|
|
|
|
|
|
|
|
spaces/1pelhydcardo/ChatGPT-prompt-generator/assets/Busy 21 6.2 How to Download and Get Started with the No.1 GST Accounting Software in India.md
DELETED
@@ -1,162 +0,0 @@
|
|
1 |
-
<br />
|
2 |
-
<h1>Busy 21 6.2 Download: How to Get the Latest Version of India's No.1 GST Accounting Software</h1>
|
3 |
-
<p>If you are looking for a simple, yet powerful GST/VAT compliant business accounting software that has everything you need to grow your business, then you should consider Busy 21. In this article, we will show you how to download and install the latest version of Busy 21, which is release 9.0, and what are the new features and benefits that it offers. We will also show you how to migrate your data from Tally to Busy 21, and how to use Busy 21 for your business accounting needs.</p>
|
4 |
-
<h2>What is Busy 21 and Why You Need It</h2>
|
5 |
-
<p>Busy 21 is a business accounting software that is designed for small and medium enterprises (SMEs) in India. It is developed by Busy Infotech Pvt. Ltd., which is one of the leading software companies in India. Busy 21 is trusted by over 10 lakh users across India and abroad, and it is India's No.1 GST accounting software.</p>
|
6 |
-
<h2>busy 21 6.2 download</h2><br /><p><b><b>Download File</b> ••• <a href="https://urlin.us/2uSU40">https://urlin.us/2uSU40</a></b></p><br /><br />
|
7 |
-
<p>Busy 21 helps you to manage your business finances, inventory, taxation, compliance, reporting, and more. It also helps you to automate your business processes, reduce errors, save time, and increase productivity. With Busy 21, you can:</p>
|
8 |
-
<ul>
|
9 |
-
<li>Create and manage multiple companies, branches, godowns, projects, budgets, etc.</li>
|
10 |
-
<li>Create and manage ledgers, groups, vouchers, invoices, bills, receipts, payments, etc.</li>
|
11 |
-
<li>Generate and share various reports such as balance sheet, profit and loss account, trial balance, cash flow statement, GST reports, etc.</li>
|
12 |
-
<li>Track and manage receivables, payables, stock, orders, quotations, production, costing, etc.</li>
|
13 |
-
<li>Comply with GST/VAT laws and regulations, file GST returns online, generate e-way bills, etc.</li>
|
14 |
-
<li>Integrate with other applications such as Busy BNS (Business Notification System), Busy Agent (Data Backup and Cloudsync), Busy Mobile App (Mobile Access), etc.</li>
|
15 |
-
</ul>
|
16 |
-
<h2>How to Download and Install Busy 21 6.2</h2>
|
17 |
-
<p>To download and install the latest version of Busy 21 (release 9.0), you need to follow these steps:</p>
|
18 |
-
<p>busy 21 6.2 crack download<br />
|
19 |
-
busy 21 6.2 free download full version<br />
|
20 |
-
busy 21 6.2 gst software download<br />
|
21 |
-
busy 21 6.2 latest version download<br />
|
22 |
-
busy 21 6.2 patch download<br />
|
23 |
-
busy 21 6.2 release date download<br />
|
24 |
-
busy 21 6.2 setup download<br />
|
25 |
-
busy 21 6.2 universal patcher download<br />
|
26 |
-
busy accounting software 21 6.2 download<br />
|
27 |
-
busywin 21 rel 6.2 download<br />
|
28 |
-
how to download busy 21 6.2<br />
|
29 |
-
how to install busy 21 6.2<br />
|
30 |
-
how to update busy 21 to 6.2<br />
|
31 |
-
what's new in busy 21 rel 6.2<br />
|
32 |
-
where to download busy 21 rel 6.2<br />
|
33 |
-
busy 21 rel 5.9 vs rel 6.2 download<br />
|
34 |
-
busy software solution busy 21 rel 6.2 download<br />
|
35 |
-
download busywin.com/busy_21_rel_6_2.zip<br />
|
36 |
-
download section of busy/busy_21_rel_6_2.zip<br />
|
37 |
-
new features of busy software/busy_21_rel_6_2.zip download<br />
|
38 |
-
benefits of using busy software/busy_21_rel_6_2.zip download<br />
|
39 |
-
comparison of busy software with other accounting software/busy_21_rel_6_2.zip download<br />
|
40 |
-
customer reviews of busy software/busy_21_rel_6_2.zip download<br />
|
41 |
-
demo of busy software/busy_21_rel_6_2.zip download<br />
|
42 |
-
faq of busy software/busy_21_rel_6_2.zip download<br />
|
43 |
-
how to activate busy software/busy_21_rel_6_2.zip download<br />
|
44 |
-
how to backup data in busy software/busy_21_rel_6_2.zip download<br />
|
45 |
-
how to migrate data from tally to busy software/busy_21_rel_6_2.zip download<br />
|
46 |
-
how to use busy mobile app with busy software/busy_21_rel_6_2.zip download<br />
|
47 |
-
how to use busy bns with busy software/busy_21_rel_6_2.zip download<br />
|
48 |
-
how to use gst data upload formats with busy software/busy_21_rel_6_2.zip download<br />
|
49 |
-
how to use sample data with busy software/busy_21_rel_6_2.zip download<br />
|
50 |
-
pricing of busy software/busy_21_rel_6_2.zip download<br />
|
51 |
-
support and training of busy software/busy_21_rel_6_2.zip download<br />
|
52 |
-
system requirements of busy software/busy_21_rel_6_2.zip download<br />
|
53 |
-
testimonials of busy software/busy_21_rel_6_2.zip download<br />
|
54 |
-
tips and tricks of using busy software/busy_21_rel_6_2.zip download<br />
|
55 |
-
troubleshooting of busy software/busy_21_rel_6_2.zip download<br />
|
56 |
-
user manual of busy software/busy_21_rel_6_2.zip download<br />
|
57 |
-
video tutorials of busy software/busy_21_rel_6_2.zip download</p>
|
58 |
-
<ol>
|
59 |
-
<li>Go to the official website of Busy or click on this link to download the setup file of Busy 21.</li>
|
60 |
-
<li>Save the setup file on your computer and run it as administrator.</li>
|
61 |
-
<li>Follow the instructions on the screen to complete the installation process.</li>
|
62 |
-
<li>After the installation is complete, launch Busy 21 from your desktop or start menu.</li>
|
63 |
-
<li>Enter your license key or request for a free trial if you are a new user.</li>
|
64 |
-
<li>Enjoy using Busy 21 for your business accounting needs.</li>
|
65 |
-
</ol>
|
66 |
-
<h2>What's New in Busy 21 Release 9.0</h2>
|
67 |
-
<p>The latest release of Busy 21 (release 9.0) was launched on June 4th, 2023. It comes with many new features and improvements that make it more user-friendly, efficient, and secure. Some of the highlights are:</p>
|
68 |
-
<h3 <h3>Enhanced GST Compliance and Reporting</h3>
|
69 |
-
<p>Busy 21 has made it easier and faster for you to comply with the GST laws and regulations. You can now file your GST returns online directly from Busy 21, without using any third-party software or portal. You can also generate and print e-invoices, e-way bills, and QR codes from Busy 21, as per the latest GST norms. You can also view and reconcile your GSTR-2A and GSTR-2B data with your purchase data in Busy 21, and get alerts for any mismatches or errors.</p>
|
70 |
-
<h3>Improved Data Security and Backup</h3>
|
71 |
-
<p>Busy 21 has improved its data security and backup features to protect your data from any loss or damage. You can now encrypt your data with a password, and restrict access to your data based on user roles and permissions. You can also backup your data automatically or manually, and restore it easily in case of any emergency. You can also sync your data with Busy Agent, which is a cloud-based service that allows you to access your data from anywhere, anytime.</p>
|
72 |
-
<h3>Integration with Busy BNS, Agent and Mobile App</h3>
|
73 |
-
<p>Busy 21 has integrated with other applications that enhance its functionality and usability. You can now use Busy BNS (Business Notification System) to send and receive SMS and email notifications for various business events, such as overdue payments, stock alerts, pending orders, etc. You can also use Busy Agent (Data Backup and Cloudsync) to backup and sync your data with the cloud, and access it from any device. You can also use Busy Mobile App (Mobile Access) to view and manage your business data on your smartphone or tablet.</p>
|
74 |
-
<h2>How to Migrate Data from Tally to Busy 21</h2>
|
75 |
-
<p>If you are currently using Tally for your business accounting needs, and want to switch to Busy 21, you can easily migrate your data from Tally to Busy 21. You just need to follow these steps:</p>
|
76 |
-
<h3>Steps to Export Data from Tally</h3>
|
77 |
-
<ol>
|
78 |
-
<li>Open Tally and go to the company whose data you want to export.</li>
|
79 |
-
<li>Go to Display > List of Accounts.</li>
|
80 |
-
<li>Press Alt+E to export the data.</li>
|
81 |
-
<li>Select the format as XML (Data Interchange).</li>
|
82 |
-
<li>Select the output file name and location.</li>
|
83 |
-
<li>Press Enter to export the data.</li>
|
84 |
-
</ol>
|
85 |
-
<h3>Steps to Import Data into Busy 21</h3>
|
86 |
-
<ol>
|
87 |
-
<li>Open Busy 21 and create a new company with the same name as the Tally company.</li>
|
88 |
-
<li>Go to Utilities > Import Masters/Transactions > From Tally.</li>
|
89 |
-
<li>Select the XML file that you exported from Tally.</li>
|
90 |
-
<li>Select the options as per your requirements, such as overwrite existing data, import opening balances, etc.</li>
|
91 |
-
<li>Press Enter to import the data.</li>
|
92 |
-
</ol>
|
93 |
-
<p>Congratulations! You have successfully migrated your data from Tally to Busy 21.</p>
|
94 |
-
<h2>How to Use Busy 21 for Your Business Accounting Needs</h2>
|
95 |
-
<p>Now that you have downloaded, installed, and migrated your data to Busy 21, you are ready to use it for your business accounting needs. Here are some of the basic tasks that you can perform with Busy 21:</p>
|
96 |
-
<h3>How to Create and Manage Ledgers, Groups and Vouchers</h3>
|
97 |
-
<p>Ledgers are the accounts that record your transactions, such as sales, purchases, expenses, income, etc. Groups are the categories that classify your ledgers, such as assets, liabilities, revenue, etc. Vouchers are the documents that record your transactions, such as invoices, receipts, payments, etc.</p>
|
98 |
-
<p>To create and manage ledgers, groups and vouchers in Busy 21, you need to follow these steps:</p>
|
99 |
-
<ol>
|
100 |
-
<li>Go to Masters > Accounts > Ledger Master.</li>
|
101 |
-
<li>Press Alt+N to create a new ledger.</li>
|
102 |
-
<li>Enter the name of the ledger, select the group under which it belongs, enter the opening balance if any, and other details as required.</li>
|
103 |
-
<li>Press Enter to save the ledger.</li>
|
104 |
-
<li>To create a new group, go to Masters > Accounts > Group Master.</li>
|
105 |
-
<li>Press Alt+N to create a new group.</li>
|
106 |
-
<li>Enter the name of the group, select the parent group under which it belongs, select the nature of group (assets/liabilities/revenue/expense), and other details as required.</li>
|
107 |
-
<li>Press Enter to save the group.</li>
|
108 |
-
<li>To create a new voucher, go to Transactions > Accounts > Voucher Entry.</li>
|
109 |
-
<li>Select the type of voucher (sales/purchase/receipt/payment/journal/etc.) <li>Enter the date, reference number, party name, ledger name, amount, narration, and other details as required.</li>
|
110 |
-
<li>Press Enter to save the voucher.</li>
|
111 |
-
</ol>
|
112 |
-
<h3>How to Generate and Share Invoices, Reports and Transactions</h3>
|
113 |
-
<p>Invoices are the documents that show the details of your sales or purchases, such as item name, quantity, rate, tax, discount, etc. Reports are the documents that show the summary or analysis of your data, such as balance sheet, profit and loss account, GST reports, etc. Transactions are the records of your vouchers, such as sales register, purchase register, cash book, bank book, etc.</p>
|
114 |
-
<p>To generate and share invoices, reports and transactions in Busy 21, you need to follow these steps:</p>
|
115 |
-
<ol>
|
116 |
-
<li>Go to Reports > Accounts > Invoice Printing.</li>
|
117 |
-
<li>Select the type of invoice (sales/purchase), the date range, the party name, and other filters as required.</li>
|
118 |
-
<li>Press Enter to view the list of invoices.</li>
|
119 |
-
<li>Select the invoice that you want to print or share.</li>
|
120 |
-
<li>Press Alt+P to print the invoice. You can also press Alt+E to export the invoice as PDF or Excel file.</li>
|
121 |
-
<li>To generate a report, go to Reports > Accounts > Balance Sheet or Profit and Loss Account or GST Reports or any other report that you want.</li>
|
122 |
-
<li>Select the date range, the level of details, and other options as required.</li>
|
123 |
-
<li>Press Enter to view the report.</li>
|
124 |
-
<li>Press Alt+P to print the report. You can also press Alt+E to export the report as PDF or Excel file.</li>
|
125 |
-
<li>To view a transaction, go to Reports > Accounts > Sales Register or Purchase Register or Cash Book or Bank Book or any other transaction that you want.</li>
|
126 |
-
<li>Select the date range, the party name, the ledger name, and other filters as required.</li>
|
127 |
-
<li>Press Enter to view the transaction.</li>
|
128 |
-
<li>Press Alt+P to print the transaction. You can also press Alt+E to export the transaction as PDF or Excel file.</li>
|
129 |
-
</ol>
|
130 |
-
<h3>How to Track and Manage Receivables, Payables and Stock</h3>
|
131 |
-
<p>Receivables are the amounts that you are supposed to receive from your customers for your sales. Payables are the amounts that you are supposed to pay to your suppliers for your purchases. Stock is the inventory of your goods that you buy or sell.</p>
|
132 |
-
<p>To track and manage receivables, payables and stock in Busy 21, you need to follow these steps:</p>
|
133 |
-
<ol>
|
134 |
-
<li>Go to Reports > Accounts > Receivables or Payables.</li>
|
135 |
-
<li>Select the date range, the party name, the ledger name, and other filters as required.</li>
|
136 |
-
<li>Press Enter to view the list of receivables or payables.</li>
|
137 |
-
<li>You can see the outstanding amount, due date, ageing analysis, interest calculation, etc. for each receivable or payable.</li>
|
138 |
-
<li>You can also send reminders or follow-ups to your customers or suppliers for payment collection or settlement.</li>
|
139 |
-
<li>To track and manage stock, go to Reports > Inventory > Stock Status or Stock Valuation or Stock Movement or any other stock report that you want.</li>
|
140 |
-
<li>Select the date range, the item name, the godown name, and other filters as required.</li>
|
141 |
-
<li>Press Enter to view the stock report.</li>
|
142 |
-
<li>You can see the opening stock, closing stock, inward stock, outward stock, stock value, stock rate, etc. for each item or godown.</li>
|
143 |
-
<li>You can also adjust your stock levels by using physical stock vouchers or stock transfer vouchers in Busy 21.</li>
|
144 |
-
</ol>
|
145 |
-
<h2>Conclusion and FAQs</h2>
|
146 |
-
<p>In conclusion, Busy 21 is a comprehensive business accounting software that can help you manage your business finances, inventory, taxation, compliance, reporting, and more. It is easy to download, install, and use, and it comes with many new features and improvements in its latest version (release 9.0). It also allows you to migrate your data from Tally to Busy 21, and integrate with other applications such as Busy BNS, Agent, and Mobile App. If you are looking for a simple, yet powerful GST/VAT compliant business accounting software that has everything you need to grow your business, then you should consider Busy 21.</p>
|
147 |
-
<p>Here are some FAQs that you might have about Busy 21:</p>
|
148 |
-
<ul>
|
149 |
-
<li><b>Q: How much does Busy 21 cost?</b></li>
|
150 |
-
<li>A: Busy 21 has different editions and plans based on your business size and requirements. You can check out their pricing details here. You can also request for a free trial or a demo before buying Busy 21.</li>
|
151 |
-
<li><b>Q: How can I get support for Busy 21?</b></li>
|
152 |
-
<li>A: Busy 21 has a dedicated customer support team that is available 24/7 to help you with any queries or issues that you might have. You can contact them through phone, email, chat, or remote access. You can also visit their support portal to access FAQs, user manuals, videos, tutorials, and forums.</li>
|
153 |
-
<li><b>Q: How can I update Busy 21 to the latest version?</b></li>
|
154 |
-
<li>A: Busy 21 has an auto-update feature that notifies you whenever a new version or patch is available. You can download and install the update from within Busy 21, or from their website. You can also check the release notes to see what are the new features and improvements in each update.</li>
|
155 |
-
<li><b>Q: How can I customize Busy 21 to suit my business needs?</b></li>
|
156 |
-
<li>A: Busy 21 has a flexible and user-friendly interface that allows you to customize various aspects of the software, such as the dashboard, the reports, the vouchers, the invoices, etc. You can also use the customization tools to create your own fields, tables, forms, reports, etc. You can also use the Busy API to integrate Busy 21 with your own applications or third-party software.</li>
|
157 |
-
<li><b>Q: How can I learn more about Busy 21 and its features?</b></li>
|
158 |
-
<li>A: You can learn more about Busy 21 and its features by visiting their website, where you can find detailed information, videos, testimonials, case studies, blogs, etc. You can also subscribe to their newsletter or follow them on social media to get the latest news and updates about Busy 21.</li>
|
159 |
-
</ul>
|
160 |
-
<p>I hope you found this article helpful and informative. If you have any questions or feedback, please feel free to leave a comment below. Thank you for reading!</p> 197e85843d<br />
|
161 |
-
<br />
|
162 |
-
<br />
|
|
|
|
|
|
|
|
|
|
|
|
|
|
|
|
|
|
|
|
|
|
|
|
|
|
|
|
|
|
|
|
|
|
|
|
|
|
|
|
|
|
|
|
|
|
|
|
|
|
|
|
|
|
|
|
|
|
|
|
|
|
|
|
|
|
|
|
|
|
|
|
|
|
|
|
|
|
|
|
|
|
|
|
|
|
|
|
|
|
|
|
|
|
|
|
|
|
|
|
|
|
|
|
|
|
|
|
|
|
|
|
|
|
|
|
|
|
|
|
|
|
|
|
|
|
|
|
|
|
|
|
|
|
|
|
|
|
|
|
|
|
|
|
|
|
|
|
|
|
|
|
|
|
|
|
|
|
|
|
|
|
|
|
|
|
|
|
|
|
|
|
|
|
|
|
|
|
|
|
|
|
|
|
|
|
|
|
|
|
|
|
|
|
|
|
|
|
|
|
|
|
|
|
|
|
|
|
|
|
|
|
|
|
|
|
|
|
|
|
|
|
|
|
|
|
|
|
|
|
|
|
|
|
|
|
|
|
|
|
|
|
|
|
|
|
|
|
|
|
|
|
|
|
|
|
|
|
|
|
|
|
|
|
|
|
|
|
|
|
|
|
|
|
|
|
|
|
|
|
|
|
|
|
|
|
|
|
|
|
|
|
|
|
|
|
|
|
|
|
|
|
|
|
|
|
|
|
|
|
|
|
|
|
|
|
|
|
|
|
|
|
|
|
|
|
spaces/1phancelerku/anime-remove-background/BMW E30 Mod APK Turn Your Phone into a Racing Simulator.md
DELETED
@@ -1,93 +0,0 @@
|
|
1 |
-
<br />
|
2 |
-
<h1>BMW E30 Mod APK: How to Enjoy the Classic Car in Various Games</h1>
|
3 |
-
<p>The BMW E30 is the second generation of the BMW 3 Series, which was produced from 1982 to 1994. It is one of the most iconic and popular models of BMW, especially among car enthusiasts and collectors. The E30 has a variety of body styles, engines, and features that make it a versatile and fun car to drive.</p>
|
4 |
-
<p>But what if you want to experience the thrill of driving an E30 without owning one? Or what if you want to customize and tune your E30 to your liking? Or what if you want to race and drift with your E30 in different scenarios and environments?</p>
|
5 |
-
<h2>bmw e30 mod apk</h2><br /><p><b><b>Download File</b> ☆☆☆☆☆ <a href="https://jinyurl.com/2uNRmN">https://jinyurl.com/2uNRmN</a></b></p><br /><br />
|
6 |
-
<p>Well, there is a solution for that: BMW E30 mod APKs. These are modified versions of Android games that feature the E30 as a playable car. With these mod APKs, you can enjoy the E30 in various games, such as car parking simulators, drifting simulators, racing games, and more.</p>
|
7 |
-
<h2>What are mod APKs and how to install them?</h2>
|
8 |
-
<p>A mod APK is a modified version of an original Android application package (APK) file that has been altered by someone to add or change some features. For example, a mod APK can add new cars, maps, modes, graphics, sounds, etc. to an existing game.</p>
|
9 |
-
<p>To install a mod APK, you need to download it from a reliable source and enable the installation of apps from unknown sources on your device. Then, you can simply tap on the mod APK file and follow the instructions to install it. However, be careful when downloading and installing mod APKs, as some of them may contain viruses or malware that can harm your device or steal your data.</p>
|
10 |
-
<p>bmw e30 live for speed mods<br />
|
11 |
-
bmw e30 lfs car mod<br />
|
12 |
-
bmw e30 simulator apk download<br />
|
13 |
-
bmw e30 drift game mod apk<br />
|
14 |
-
bmw e30 m3 racing mod apk<br />
|
15 |
-
bmw e30 tuning mod for android<br />
|
16 |
-
bmw e30 3d model apk<br />
|
17 |
-
bmw e30 wallpaper hd mod apk<br />
|
18 |
-
bmw e30 sound mod apk<br />
|
19 |
-
bmw e30 engine swap mod apk<br />
|
20 |
-
bmw e30 turbo kit mod apk<br />
|
21 |
-
bmw e30 stance mod apk<br />
|
22 |
-
bmw e30 rally mod apk<br />
|
23 |
-
bmw e30 convertible mod apk<br />
|
24 |
-
bmw e30 alpina mod apk<br />
|
25 |
-
bmw e30 restoration mod apk<br />
|
26 |
-
bmw e30 interior mod apk<br />
|
27 |
-
bmw e30 manual transmission mod apk<br />
|
28 |
-
bmw e30 custom paint mod apk<br />
|
29 |
-
bmw e30 body kit mod apk<br />
|
30 |
-
bmw e30 wheels mod apk<br />
|
31 |
-
bmw e30 headlights mod apk<br />
|
32 |
-
bmw e30 dashboard mod apk<br />
|
33 |
-
bmw e30 steering wheel mod apk<br />
|
34 |
-
bmw e30 exhaust mod apk<br />
|
35 |
-
bmw e30 suspension mod apk<br />
|
36 |
-
bmw e30 brake mod apk<br />
|
37 |
-
bmw e30 fuel tank mod apk<br />
|
38 |
-
bmw e30 radiator mod apk<br />
|
39 |
-
bmw e30 air intake mod apk<br />
|
40 |
-
bmw e30 spark plugs mod apk<br />
|
41 |
-
bmw e30 oil filter mod apk<br />
|
42 |
-
bmw e30 battery mod apk<br />
|
43 |
-
bmw e30 alternator mod apk<br />
|
44 |
-
bmw e30 starter motor mod apk<br />
|
45 |
-
bmw e30 ignition coil mod apk<br />
|
46 |
-
bmw e30 distributor cap mod apk<br />
|
47 |
-
bmw e30 thermostat mod apk<br />
|
48 |
-
bmw e30 water pump mod apk<br />
|
49 |
-
bmw e30 fan clutch mod apk<br />
|
50 |
-
bmw e30 belt tensioner mod apk<br />
|
51 |
-
bmw e30 timing belt mod apk<br />
|
52 |
-
bmw e30 camshaft sensor mod apk<br />
|
53 |
-
bmw e30 crankshaft sensor mod apk<br />
|
54 |
-
bmw e30 oxygen sensor mod apk<br />
|
55 |
-
bmw e30 fuel injector mod apk<br />
|
56 |
-
bmw e30 fuel pump mod apk<br />
|
57 |
-
bmw e30 fuel filter mod apk<br />
|
58 |
-
bmw e30 fuel pressure regulator mod apk</p>
|
59 |
-
<h2>What are some of the best BMW E30 mod APKs?</h2>
|
60 |
-
<p>There are many BMW E30 mod APKs available on the internet, but not all of them are worth downloading or playing. Some of them may have poor quality graphics, bugs, glitches, or limited features. To help you find some of the best BMW E30 mod APKs, we have compiled a list of some of the most popular and well-made ones. Here they are:</p>
|
61 |
-
<table>
|
62 |
-
<tr>
|
63 |
-
<th>Mod APK</th>
|
64 |
-
<th>Description</th>
|
65 |
-
<th>Download Link</th>
|
66 |
-
</tr>
|
67 |
-
<tr>
|
68 |
-
<td>E30 Drift & Modified Simulator</td>
|
69 |
-
<td>This is a drifting game with superior 3D graphics and amazing real car physics. You can customize your E30 with lots of options, such as wheels, colors, spoilers, exhausts, cambers, hoods, etc. You can also play any mode between Parking, Checkpoint, Career, Drift, Stunt, Lap Time, Midnight, Race Track, Breaking, Ramps, Winter, Airport, Off-road or City.</td>
|
70 |
-
<td>[E30 Drift & Modified Simulator](^11^)</td>
|
71 |
-
</tr>
|
72 |
-
<tr>
|
73 |
-
<td>E30 Drift Simulator Car Games</td>
|
74 |
-
<td>This is another drifting game with realistic graphics and sound effects. You can tune your E30 with various engine upgrades, camber and suspension settings, wheels sets and car painting options. You can also choose between four different maps to drift: desert drift, city drift, mountain drift and winter drift.</td>
|
75 |
-
<td>[E30 Drift Simulator Car Games](^12^)</td>
|
76 |
-
</tr>
|
77 |
-
<tr>
|
78 |
-
<td>Driving BMW E30 M3 in 17 Different Games</td>
|
79 |
-
<td>This is not exactly a mod APK but a YouTube video that shows how the BMW E30 M3 has evolved in 17 different racing games throughout the years. You can see how the graphics, physics and gameplay have changed from game to game and compare them with each other.</td>
|
80 |
-
<td>[Driving BMW E30 M3 in 17 Different Games](^13^)</td>
|
81 |
-
</tr>
|
82 |
-
</table>
|
83 |
-
<h2>What are some of the benefits of playing BMW E30 mod APKs?</h2>
|
84 |
-
<p>Playing BMW E30 mod APKs can be fun and rewarding for many reasons. Some of them are:</p>
|
85 |
-
<ul>
|
86 |
-
<li>You can experience Some of the best BMW E30 mod APKs are: - E30 Drift & Modified Simulator - E30 Drift Simulator Car Games - Driving BMW E30 M3 in 17 Different Games <h3>How to install a mod APK?</h3>
|
87 |
-
<p>To install a mod APK, you need to download it from a reliable source and enable the installation of apps from unknown sources on your device. Then, you can simply tap on the mod APK file and follow the instructions to install it.</p>
|
88 |
-
<h3>What are the benefits and drawbacks of playing BMW E30 mod APKs?</h3>
|
89 |
-
<p>The benefits of playing BMW E30 mod APKs are: - You can experience the classic and legendary car that is the BMW E30 in different games and scenarios. - You can customize and personalize your E30 to your liking. - You can challenge yourself and improve your driving skills by playing different modes and maps. - You can have fun and relax by playing BMW E30 mod APKs. The drawbacks of playing BMW E30 mod APKs are: - You may encounter some technical issues or errors when playing mod APKs. - You may expose your device or data to security threats when downloading or installing mod APKs. - You may violate the terms of service or intellectual property rights of the original game developers or publishers when playing mod APKs.</p>
|
90 |
-
<h3>How to play BMW E30 mod APKs safely and responsibly?</h3>
|
91 |
-
<p>To play BMW E30 mod APKs safely and responsibly, you should: - Download and install mod APKs only from trusted and reputable sources. - Scan your device regularly with a reliable antivirus or anti-malware software to detect and remove any potential threats. - Backup your device's data regularly to avoid losing any important files or information in case of any damage or corruption. - Respect the rights and interests of the original game developers and publishers. Do not use mod APKs to cheat, hack, or harm the original games or other players. Do not distribute or share any mod APKs without permission or credit. Do not claim any ownership or authorship of any mod APKs that are not yours.</p> 197e85843d<br />
|
92 |
-
<br />
|
93 |
-
<br />
|
|
|
|
|
|
|
|
|
|
|
|
|
|
|
|
|
|
|
|
|
|
|
|
|
|
|
|
|
|
|
|
|
|
|
|
|
|
|
|
|
|
|
|
|
|
|
|
|
|
|
|
|
|
|
|
|
|
|
|
|
|
|
|
|
|
|
|
|
|
|
|
|
|
|
|
|
|
|
|
|
|
|
|
|
|
|
|
|
|
|
|
|
|
|
|
|
|
|
|
|
|
|
|
|
|
|
|
|
|
|
|
|
|
|
|
|
|
|
|
|
|
|
|
|
|
|
|
|
|
|
|
|
|
|
|
|
|
|
|
|
|
|
|
|
|
|
|
|
|
|
|
|
|
|
|
|
|
|
|
|
|
|
|
|
|
|
|
|
|
|
|
|
|
|
|
|
|
|
|
|
|
|
|
|
|
|
|
spaces/1phancelerku/anime-remove-background/Baseball 9 Mod APK The Most Fun and Informative Baseball Game with Unlimited Diamonds and Stamina.md
DELETED
@@ -1,105 +0,0 @@
|
|
1 |
-
<br />
|
2 |
-
<h1>Baseball 9 Mod APK 2023: A Fast-Paced and Realistic Baseball Game for Android</h1>
|
3 |
-
<p>If you are a fan of baseball games, you should not miss Baseball 9, a popular and addictive game that lets you enjoy fast-paced, realistic baseball action on your Android device. Baseball 9 is a game that features compact gameplay and informative stats, as well as casual characters and serious game mechanics. You can play as a pitcher, a batter, or a fielder, and customize your team and players to your liking. You can also challenge different leagues and modes, such as Legend League, Challenge Mode, Event Match, and Club Battle. Whether you are a beginner or an expert, you will find Baseball 9 to be a fun and exciting game that will keep you hooked for hours.</p>
|
4 |
-
<h2>Features of Baseball 9 Mod APK 2023</h2>
|
5 |
-
<p>Baseball 9 has many features that make it stand out from other baseball games. Here are some of them:</p>
|
6 |
-
<h2>baseball 9 mod apk 2023</h2><br /><p><b><b>Download File</b> ✓ <a href="https://jinyurl.com/2uNUDj">https://jinyurl.com/2uNUDj</a></b></p><br /><br />
|
7 |
-
<h3>Lean and fast gameplay</h3>
|
8 |
-
<p>Baseball 9 is designed to be easy to play and fast to load. You don't have to wait for long loading times or complicated menus. You can start playing right away, and enjoy smooth and responsive controls. You can also skip between innings, auto-play, auto-field, or quick-result when you want to speed up the game.</p>
|
9 |
-
<h3>Casual characters and serious game mechanics</h3>
|
10 |
-
<p>Baseball 9 has a unique style that combines casual characters with serious game mechanics. You can choose from various characters with different appearances, personalities, skills, and abilities. You can also customize their faces, hairstyles, body types, uniforms, bats, gloves, shoes, helmets, tattoos, accessories, etc. The game also has realistic physics and animations that make the game more immersive and realistic.</p>
|
11 |
-
<h3>Pitching and fielding as fun as batting</h3>
|
12 |
-
<p>Baseball 9 is not just about hitting home runs. You can also enjoy pitching and fielding as much as batting. You can choose from different types of pitches, such as fastball, curveball, slider, changeup, etc., and adjust their speed, direction, and movement. You can also control your fielders manually or automatically, and make spectacular catches, throws, double plays, etc.</p>
|
13 |
-
<h3>Customizing your team and players</h3>
|
14 |
-
<p>Baseball 9 allows you to create your own team and players <p>Baseball 9 allows you to create your own team and players, and customize them to your liking. You can name your team, choose its emblem, uniform, and stadium, and recruit new players with different stats and skills. You can also train your players to improve their abilities, such as power, contact, speed, stamina, etc. You can also equip them with various items that enhance their performance, such as bats, gloves, shoes, etc.</p>
|
15 |
-
<h3>Challenging different leagues and modes</h3>
|
16 |
-
<p>Baseball 9 has various leagues and modes that you can challenge and enjoy. You can start from the Rookie League and advance to the higher leagues, such as Master League, Champion League, and Legend League. You can also play in different modes, such as Challenge Mode, where you can complete various missions and objectives; Event Match, where you can participate in special events and win rewards; and Club Battle, where you can join a club and compete with other clubs online.</p>
|
17 |
-
<h2>How to Download and Install Baseball 9 Mod APK 2023</h2>
|
18 |
-
<p>If you want to enjoy Baseball 9 with unlimited gems and coins, unlocked all players and items, no ads, and no root required, you can download and install the mod apk file from a trusted source. Here are the steps to do so:</p>
|
19 |
-
<h3>Step 1: Download the mod apk file from a trusted source</h3>
|
20 |
-
<p>You can find the mod apk file for Baseball 9 on various websites that offer modded games and apps. However, you should be careful and only download from a trusted source that has positive reviews and feedback from other users. You can also scan the file with an antivirus program before downloading it to ensure that it is safe and virus-free.</p>
|
21 |
-
<p>baseball 9 mod apk 2023 unlimited money and gems<br />
|
22 |
-
baseball 9 mod apk 2023 latest version download<br />
|
23 |
-
baseball 9 mod apk 2023 free shopping<br />
|
24 |
-
baseball 9 mod apk 2023 no root<br />
|
25 |
-
baseball 9 mod apk 2023 offline<br />
|
26 |
-
baseball 9 mod apk 2023 hack<br />
|
27 |
-
baseball 9 mod apk 2023 android<br />
|
28 |
-
baseball 9 mod apk 2023 ios<br />
|
29 |
-
baseball 9 mod apk 2023 gameplay<br />
|
30 |
-
baseball 9 mod apk 2023 review<br />
|
31 |
-
baseball 9 mod apk 2023 cheats<br />
|
32 |
-
baseball 9 mod apk 2023 tips and tricks<br />
|
33 |
-
baseball 9 mod apk 2023 features<br />
|
34 |
-
baseball 9 mod apk 2023 update<br />
|
35 |
-
baseball 9 mod apk 2023 how to install<br />
|
36 |
-
baseball 9 mod apk 2023 best team<br />
|
37 |
-
baseball 9 mod apk 2023 all players unlocked<br />
|
38 |
-
baseball 9 mod apk 2023 custom mode<br />
|
39 |
-
baseball 9 mod apk 2023 league mode<br />
|
40 |
-
baseball 9 mod apk 2023 challenge mode<br />
|
41 |
-
baseball 9 mod apk 2023 world series mode<br />
|
42 |
-
baseball 9 mod apk 2023 multiplayer mode<br />
|
43 |
-
baseball 9 mod apk 2023 online mode<br />
|
44 |
-
baseball 9 mod apk 2023 vs mode<br />
|
45 |
-
baseball 9 mod apk 2023 rankings<br />
|
46 |
-
baseball 9 mod apk 2023 achievements<br />
|
47 |
-
baseball 9 mod apk 2023 missions<br />
|
48 |
-
baseball 9 mod apk 2023 rewards<br />
|
49 |
-
baseball 9 mod apk 2023 events<br />
|
50 |
-
baseball 9 mod apk 2023 codes<br />
|
51 |
-
baseball 9 mod apk 2023 generator<br />
|
52 |
-
baseball 9 mod apk 2023 download link<br />
|
53 |
-
baseball 9 mod apk 2023 direct download<br />
|
54 |
-
baseball 9 mod apk 2023 mediafire download<br />
|
55 |
-
baseball 9 mod apk 2023 mega download<br />
|
56 |
-
baseball 9 mod apk 2023 google drive download<br />
|
57 |
-
baseball 9 mod apk 2023 dropbox download<br />
|
58 |
-
baseball 9 mod apk</p>
|
59 |
-
<h3>Step 2: Enable unknown sources on your device settings</h3>
|
60 |
-
<p>Before you can install the mod apk file on your device, you need to enable unknown sources on your device settings. This will allow you to install apps from sources other than the Google Play Store. To do this, go to your device settings > security > unknown sources > enable.</p>
|
61 |
-
<h3>Step 3: Install the mod apk file and launch the game</h3>
|
62 |
-
<p>After you have downloaded the mod apk file and enabled unknown sources on your device settings, you can install the mod apk file by tapping on it and following the instructions on the screen. Once the installation is complete, you can launch the game and enjoy Baseball 9 with all the benefits of the mod.</p>
|
63 |
-
<h2>Benefits of Using Baseball 9 Mod APK 2023</h2>
|
64 |
-
<p>Using Baseball 9 Mod APK 2023 has many benefits that will make your gaming experience more enjoyable and satisfying. Here are some of them:</p>
|
65 |
-
<h3>Unlimited gems and coins</h3>
|
66 |
-
<p>Gems and coins are the main currencies in Baseball 9 that you can use to buy items, upgrade players, recruit new players, etc. However, they are not easy to earn in the game, and you may need to spend real money to get more of them. With Baseball 9 Mod APK 2023, you don't have to worry about that anymore. You will have unlimited gems and coins that you can use as much as you want without any restrictions.</p>
|
67 |
-
<h3>Unlocked all players and items</h3>
|
68 |
-
<p>Baseball 9 has many players and items that you can unlock by playing the game or spending gems and coins. However, some of them may be too expensive or too hard to get. With Baseball 9 Mod APK 2023, you don't have to wait or spend anything to unlock them. You will have access to all the players and items in the game from the start, and you can choose any of them to customize your team and players.</p>
|
69 |
-
<h3>No ads and no root required</h3>
|
70 |
-
<p>Baseball 9 is a free game that contains ads that may interrupt your gameplay or annoy you. With Baseball 9 Mod APK 2023, you don't have to see any ads at all. You will have a smooth and uninterrupted gaming experience without any distractions. Moreover, Baseball 9 Mod APK 2023 does not require root access on your device. You can install it easily without any risk of damaging your device or voiding its warranty.</p>
|
71 |
-
<h2>Tips and Tricks for Playing Baseball 9 Mod APK 2023</h2>
|
72 |
-
<p>If you want to master Baseball 9 Mod APK 2023 If you want to master Baseball 9 Mod APK 2023, you should follow some tips and tricks that will help you improve your skills and win more games. Here are some of them:</p>
|
73 |
-
<h3>Master the basics of batting, pitching, and fielding</h3>
|
74 |
-
<p>Baseball 9 Mod APK 2023 is a game that requires you to have good skills in batting, pitching, and fielding. You should practice these skills and learn how to use them effectively in different situations. For batting, you should pay attention to the pitch type, speed, direction, and movement, and time your swing accordingly. You should also aim for the sweet spot of the bat and hit the ball at the right angle and power. For pitching, you should choose the right pitch type and adjust its speed, direction, and movement. You should also try to deceive the batter with your pitch selection and location. For fielding, you should position your fielders properly and react quickly to the ball's trajectory. You should also make accurate throws and catches, and avoid errors.</p>
|
75 |
-
<h3>Upgrade your players and equipment regularly</h3>
|
76 |
-
<p>Baseball 9 Mod APK 2023 allows you to upgrade your players and equipment to enhance their performance. You should do this regularly to keep up with the increasing difficulty of the game. You can use gems and coins to upgrade your players' abilities, such as power, contact, speed, stamina, etc. You can also use gems and coins to buy better equipment, such as bats, gloves, shoes, etc., that have different effects on your players' stats. You should also train your players to improve their skills and unlock new abilities.</p>
|
77 |
-
<h3>Use the right strategy for each mode and league</h3>
|
78 |
-
<p>Baseball 9 Mod APK 2023 has different modes and leagues that require different strategies to win. You should adapt your strategy according to the mode and league you are playing in. For example, in Challenge Mode, you should focus on completing the missions and objectives that are given to you. In Event Match, you should take advantage of the special rules and conditions that apply to each event. In Club Battle, you should cooperate with your club members and compete with other clubs online. In Legend League, you should face the toughest opponents and prove your skills.</p>
|
79 |
-
<h3>Enjoy the game with friends online or offline</h3>
|
80 |
-
<p>Baseball 9 Mod APK 2023 is a game that you can enjoy with friends online or offline. You can play with friends online by joining a club or creating a room. You can chat with your friends, invite them to play with you, or challenge them to a friendly match. You can also play with friends offline by using the same device or connecting via Bluetooth or Wi-Fi. You can choose from different modes and settings, such as innings, difficulty, stadium, etc., and have fun playing baseball together.</p>
|
81 |
-
<h2>Conclusion</h2>
|
82 |
-
<p>Baseball 9 Mod APK 2023 is a fast-paced and realistic baseball game for Android that you should try if you love baseball games. It has many features that make it fun and exciting, such as lean and fast gameplay, casual characters and serious game mechanics, pitching and fielding as fun as batting, customizing your team and players, challenging different leagues and modes, etc. It also has many benefits that make it more enjoyable and satisfying, such as unlimited gems and coins, unlocked all players and items, no ads, and no root required. It also has some tips and tricks that will help you master the game and win more games. If you want to download and install Baseball 9 Mod APK 2023 on your device, you can follow the steps above. If you want to play Baseball 9 Mod APK 2023 with friends online or offline <p>you can follow the steps above. If you want to play Baseball 9 Mod APK 2023 with friends online or offline, you can also do so by using the online or offline modes. Baseball 9 Mod APK 2023 is a game that will give you hours of fun and excitement, and make you feel like a real baseball player. Download it now and enjoy!</p>
|
83 |
-
<h2>FAQs</h2>
|
84 |
-
<p>Here are some frequently asked questions about Baseball 9 Mod APK 2023:</p>
|
85 |
-
<h3>Q1: Is Baseball 9 Mod APK 2023 safe to use?</h3>
|
86 |
-
<p>A1: Yes, Baseball 9 Mod APK 2023 is safe to use as long as you download it from a trusted source and scan it with an antivirus program before installing it. It does not contain any malware or viruses that can harm your device or compromise your privacy.</p>
|
87 |
-
<h3>Q2: How can I get more gems and coins in Baseball 9 Mod APK 2023?</h3>
|
88 |
-
<p>A2: You don't have to worry about getting more gems and coins in Baseball 9 Mod APK 2023, because you will have unlimited gems and coins that you can use as much as you want. You can also earn more gems and coins by playing the game, completing missions, participating in events, etc.</p>
|
89 |
-
<h3>Q3: How can I customize my team and players in Baseball 9 Mod APK 2023?</h3>
|
90 |
-
<p>A3: You can customize your team and players in Baseball 9 Mod APK 2023 by using the customization options available in the game. You can change their names, appearances, skills, abilities, equipment, etc. You can also create your own team and players from scratch.</p>
|
91 |
-
<h3>Q4: What are the different modes and leagues in Baseball 9 Mod APK 2023?</h3>
|
92 |
-
<p>A4: There are different modes and leagues in Baseball 9 Mod APK 2023 that you can challenge and enjoy. They are:</p>
|
93 |
-
<ul>
|
94 |
-
<li>Rookie League: The beginner league where you can learn the basics of the game and improve your skills.</li>
|
95 |
-
<li>Master League: The intermediate league where you can face more challenging opponents and earn more rewards.</li>
|
96 |
-
<li>Champion League: The advanced league where you can compete with the best players and teams in the game.</li>
|
97 |
-
<li>Legend League: The ultimate league where you can prove your skills and become a legend.</li>
|
98 |
-
<li>Challenge Mode: The mode where you can complete various missions and objectives that test your skills and knowledge.</li>
|
99 |
-
<li>Event Match: The mode where you can participate in special events that have different rules and conditions.</li>
|
100 |
-
<li>Club Battle: The mode where you can join a club or create your own club, and compete with other clubs online.</li>
|
101 |
-
</ul>
|
102 |
-
<h3>Q5: How can I play Baseball 9 Mod APK 2023 with friends?</h3>
|
103 |
-
<p>A5: You can play Baseball 9 Mod APK 2023 with friends online or offline. To play online, you need to have an internet connection and join a club or create a room. You can chat with your friends, invite them to play with you, or challenge them to a friendly match. To play offline, you need to have the same device or connect via Bluetooth or Wi-Fi. You can choose from different modes and settings, such as innings, difficulty, stadium, etc., and have fun playing baseball together.</p> 197e85843d<br />
|
104 |
-
<br />
|
105 |
-
<br />
|
|
|
|
|
|
|
|
|
|
|
|
|
|
|
|
|
|
|
|
|
|
|
|
|
|
|
|
|
|
|
|
|
|
|
|
|
|
|
|
|
|
|
|
|
|
|
|
|
|
|
|
|
|
|
|
|
|
|
|
|
|
|
|
|
|
|
|
|
|
|
|
|
|
|
|
|
|
|
|
|
|
|
|
|
|
|
|
|
|
|
|
|
|
|
|
|
|
|
|
|
|
|
|
|
|
|
|
|
|
|
|
|
|
|
|
|
|
|
|
|
|
|
|
|
|
|
|
|
|
|
|
|
|
|
|
|
|
|
|
|
|
|
|
|
|
|
|
|
|
|
|
|
|
|
|
|
|
|
|
|
|
|
|
|
|
|
|
|
|
|
|
|
|
|
|
|
|
|
|
|
|
|
|
|
|
|
|
|
|
|
|
|
|
|
|
|
|
|
|
|
|
|
|
|
|
|
|
|
|
|
|
spaces/1phancelerku/anime-remove-background/Download FR Legends Mod Apk with Supra and RX7 in 2021 (New Cars Mod Unlimited Money).md
DELETED
@@ -1,119 +0,0 @@
|
|
1 |
-
|
2 |
-
<h1>How to Download FR Legends Mod APK Terbaru 2021 Supra</h1>
|
3 |
-
<p>If you are a fan of drifting games, you might have heard of FR Legends, a mobile game that lets you drive legendary front-engine, rear-wheel-drive drift cars at world's most iconic circuits. You can also customize everything on your car, from engine swaps to wide-body kits, and have tandem drift battles with AI drivers or other players online.</p>
|
4 |
-
<p>But what if you want to get unlimited money and access to new cars like the Toyota Supra? Well, you can do that by downloading FR Legends Mod APK Terbaru 2021 Supra, a modified version of the game that gives you these features and more. In this article, we will show you what FR Legends Mod APK Terbaru 2021 Supra is, where to download it, and how to install it on your Android device.</p>
|
5 |
-
<h2>download fr legends mod apk terbaru 2021 supra</h2><br /><p><b><b>DOWNLOAD</b> ––– <a href="https://jinyurl.com/2uNJbt">https://jinyurl.com/2uNJbt</a></b></p><br /><br />
|
6 |
-
<h2>What is FR Legends?</h2>
|
7 |
-
<h3>A drifting game with realistic physics and customization</h3>
|
8 |
-
<p>FR Legends is a drifting game that was released in 2018 by Feng Li. It is one of the most realistic and fun drifting games on mobile devices, as it uses a unique scoring system based on real world competition judging rules. You can drift on various tracks, from mountain roads to urban streets, and challenge yourself or other players in different modes.</p>
|
9 |
-
<p>One of the best features of FR Legends is the customization. You can choose from a variety of cars, such as Nissan Silvia, Mazda RX-7, BMW E30, and more. You can also modify your car's appearance and performance, by changing the fender, bumper, engine, turbo, suspension, wheels, tires, paint, stickers, and more. You can even create your own livery and share it with other players.</p>
|
10 |
-
<h3>A popular game with positive reviews and ratings</h3>
|
11 |
-
<p>FR Legends has been downloaded over 10 million times on Google Play Store and has an average rating of 4.4 out of 5 stars. It has also received positive reviews from users and critics alike. For example, TobyXDZ wrote on Google Play Store:</p>
|
12 |
-
<blockquote>"In my opinion it's the best drifting game in the store... I would suggest that you change the steering settings to arrows because it is much easier to control. But there is so much to do in this game, and i can't stop playing it."</blockquote>
|
13 |
-
<p>Similarly, Gelatinous wrote on Google Play Store:</p>
|
14 |
-
<blockquote>"Fantastic game, realistic drifting, and tons of cool classic cars. A few things I'd like to see added: -More engine swap options... -A supercharger kit... -Sway bar tuning... -1v1 Singleplayer Race..."</blockquote>
|
15 |
-
<h2>What is FR Legends Mod APK Terbaru 2021 Supra?</h2>
|
16 |
-
<h3>A modified version of the game with unlimited money and new cars</h3>
|
17 |
-
<p>FR Legends Mod APK Terbaru 2021 Supra is a modified version of the original game that gives you some extra features that are not available in the official version. The most notable features are:</p>
|
18 |
-
<ul>
|
19 |
-
<li>Unlimited money: You can buy any car or part you want without worrying about your budget.</li>
|
20 |
-
<li>New cars: You can drive new cars like the Toyota Supra MK4 and MK5, the Nissan Skyline GT-R R34 and R35, and the Honda NSX.</li>
|
21 |
-
<li>Unlocked tracks: You can drift on all the tracks without having to unlock them.</li>
|
22 |
-
<li>Unlocked modes: You can play all the modes without having to complete the previous ones.</li>
|
23 |
-
</ul>
|
24 |
-
<p>With these features, you can enjoy FR Legends even more and have a better drifting experience.</p>
|
25 |
-
<h3>A file that can be downloaded from third-party websites</h3>
|
26 |
-
<p>FR Legends Mod APK Terbaru 2021 Supra is not available on Google Play Store or any official website. It is a file that can be downloaded from third-party websites that offer modded apps and games. However, you should be careful when downloading such files, as they may contain viruses or malware that can harm your device or steal your data. Therefore, you should only download FR Legends Mod APK Terbaru 2021 Supra from reputable and trusted sources, such as [this one].</p>
|
27 |
-
<p>fr legends mod apk supra terbaru 2021 unlimited money<br />
|
28 |
-
download fr legends mod apk l300 supra jazz 2021<br />
|
29 |
-
fr legends mod apk terbaru 2021 toyota supra drift<br />
|
30 |
-
fr legends mod apk supra r35 mustang terbaru 2021<br />
|
31 |
-
download fr legends mod apk terbaru 2021 honda accord supra<br />
|
32 |
-
fr legends mod apk supra s15 silvia terbaru 2021<br />
|
33 |
-
fr legends mod apk terbaru 2021 supra offline online<br />
|
34 |
-
download fr legends mod apk terbaru 2021 supra map baru<br />
|
35 |
-
fr legends mod apk terbaru 2021 supra aksesoris baru<br />
|
36 |
-
fr legends mod apk terbaru 2021 supra desain mobil baru<br />
|
37 |
-
download fr legends mod apk terbaru 2021 supra grafik animasi 3d<br />
|
38 |
-
fr legends mod apk terbaru 2021 supra sistem drift terbaik<br />
|
39 |
-
fr legends mod apk terbaru 2021 supra kustomisasi mobil<br />
|
40 |
-
download fr legends mod apk terbaru 2021 supra formula drift amerika<br />
|
41 |
-
fr legends mod apk terbaru 2021 supra tandem dengan musuh<br />
|
42 |
-
fr legends mod apk terbaru 2021 supra engine turbo fender bumper<br />
|
43 |
-
download fr legends mod apk terbaru 2021 supra poin cone wall checkpoint<br />
|
44 |
-
fr legends mod apk terbaru 2021 supra front engine rear wheel drive<br />
|
45 |
-
fr legends mod apk terbaru 2021 supra track track menantang<br />
|
46 |
-
download fr legends mod apk terbaru 2021 supra net energy gain<br />
|
47 |
-
fr legends mod apk terbaru 2021 supra holy grail fusion experiment<br />
|
48 |
-
fr legends mod apk terbaru 2021 supra mini sun temperature<br />
|
49 |
-
download fr legends mod apk terbaru 2021 supra korea superconducting tokamak advanced research facility<br />
|
50 |
-
fr legends mod apk terbaru 2021 supra korea institute of fusion energy<br />
|
51 |
-
fr legends mod apk terbaru 2021 supra nuclear fusion reaction<br />
|
52 |
-
download fr legends mod apk terbaru 2021 segitekno.com ulingame.com newscientist.com the-sun.com yahoo.com<br />
|
53 |
-
fr legends mod apk terbaru 2021 review rating gameplay tips tricks guide tutorial walkthrough hack cheat code<br />
|
54 |
-
download fr legends mod apk terbaru 2021 latest version update patch bug fix performance improvement stability enhancement security enhancement feature addition compatibility improvement user interface improvement user experience improvement accessibility improvement usability improvement feedback improvement customer satisfaction improvement support improvement quality assurance improvement reliability improvement availability improvement scalability improvement maintainability improvement portability improvement reusability improvement interoperability improvement testability improvement verifiability improvement validity improvement correctness improvement completeness improvement consistency improvement accuracy improvement precision improvement robustness improvement fault tolerance improvement resilience improvement recoverability improvement adaptability improvement configurability improvement customizability improvement extensibility improvement flexibility improvement modifiability improvement variability improvement evolvability improvement changeability improvement upgradability improvement installability improvement uninstallability improvement deployability improvement replaceability improvement localizability improvement internationalization improvement globalization improvement localization improvement translation improvement multilingualism improvement multiculturalism improvement diversity inclusion equity respect fairness justice equality human rights dignity freedom democracy peace harmony love joy happiness gratitude appreciation kindness compassion empathy sympathy generosity altruism benevolence goodwill cooperation collaboration teamwork partnership friendship trust loyalty honesty integrity sincerity authenticity transparency accountability responsibility ethics morality values principles standards norms rules regulations policies guidelines best practices recommendations suggestions advice opinions insights perspectives viewpoints angles approaches methods techniques strategies tactics procedures processes workflows steps stages phases tasks activities actions operations functions procedures routines algorithms formulas equations models frameworks theories concepts ideas notions thoughts beliefs assumptions hypotheses premises conclusions inferences deductions inductions abductions generalizations specializations classifications categorizations groupings clusterings segmentations partitions divisions subdivisions sections subsections parts components elements items units modules chunks pieces bits bytes bits nibbles words doublewords quadwords octets octals hexadecimals binaries decimals fractions percentages ratios proportions rates constants variables parameters arguments inputs outputs outcomes results effects impacts influences consequences benefits costs risks tradeoffs trade-offs trade offs advantages disadvantages pros cons strengths weaknesses opportunities threats swot analysis pest analysis pestle analysis porter's five forces analysis value chain analysis balanced scorecard analysis gap analysis root cause analysis fishbone diagram pareto chart histogram scatter plot pie chart bar chart line chart area chart spline chart radar chart bubble chart doughnut chart funnel chart waterfall chart gantt chart pert chart cpm chart network diagram flowchart swimlane diagram mind map concept map venn diagram euler diagram tree diagram organizational chart hierarchy chart matrix diagram relationship diagram entity relationship diagram erd class diagram object diagram use case diagram sequence diagram collaboration diagram state diagram activity diagram component diagram deployment diagram package diagram composite structure diagram timing diagram interaction overview diagram communication diagram uml unified modeling language sdlc software development life cycle agile scrum kanban lean waterfall spiral prototyping</p>
|
55 |
-
<h2>How to Install FR Legends Mod APK Terbaru 2021 Supra?</h2>
|
56 |
-
<h3>Allow unknown apps on your Android device</h3>
|
57 |
-
<p>Before you can install FR Legends Mod APK Terbaru 2021 Supra, you need to allow your Android device to install apps from unknown sources. This is because FR Legends Mod APK Terbaru 2021 Supra is not from Google Play Store or any official source. To do this, follow these steps:</p>
|
58 |
-
<ol>
|
59 |
-
<li>Go to your device's settings and tap on security or privacy.</li>
|
60 |
-
<li>Find the option that says "Unknown sources" or "Install unknown apps" and enable it.</li>
|
61 |
-
<li>You may see a warning message that says installing unknown apps may harm your device. Tap on OK or Allow to proceed.</li>
|
62 |
-
</ol>
|
63 |
-
<h3>Download a file manager app to find the APK file</h3>
|
64 |
-
<p>After you have allowed unknown apps on your device, you need to download a file manager app that can help you find the APK file of FR Legends Mod APK Terbaru 2021 Supra. A file manager app is an app that lets you browse and manage the files and folders on your device. You can use any file manager app you like, such as ES File Explorer, File Manager, or Files by Google. To download a file manager app, follow these steps:</p>
|
65 |
-
<ol>
|
66 |
-
<li>Go to Google Play Store and search for a file manager app of your choice.</li>
|
67 |
-
<li>Tap on the app and then tap on Install.</li>
|
68 |
-
<li>Wait for the app to download and install on your device.</li>
|
69 |
-
</ol>
|
70 |
-
<h3>Download the APK file from a reputable source</h3>
|
71 |
-
<p>Now that you have a file manager app on your device, you can download the APK file of FR Legends Mod APK Terbaru 2021 Supra from a reputable source. As mentioned earlier, you should only download FR Legends Mod APK Terbaru 2021 Supra from trusted and reliable websites, such as [this one]. To download the APK file, follow these steps:</p>
|
72 |
-
<ol>
|
73 |
-
<li>Open your browser and go to [this website].</li>
|
74 |
-
<li>Scroll down and find the download button that says "FR Legends Mod APK Terbaru 2021 Supra". Tap on it.</li>
|
75 |
-
<li>You may see a pop-up window that asks you to confirm the download. Tap on OK or Download.</li>
|
76 |
-
<li>Wait for the APK file to download on your device. You can check the progress in your notification bar or in your browser's downloads section.</li>
|
77 |
-
</ol>
|
78 |
-
<h3>Open the APK file and follow the instructions to install the app</h3>
|
79 |
-
<p>The final step is to open the APK file and follow the instructions to install FR Legends Mod APK Terbaru 2021 Supra on your device. To do this, follow these steps:</p>
|
80 |
-
<ol>
|
81 |
-
<li>Open your file manager app and locate the APK file of FR Legends Mod APK Terbaru 2021 Supra. It should be in your downloads folder or in the folder where you saved it.</li>
|
82 |
-
<li>Tap on the APK file and then tap on Install.</li>
|
83 |
-
<li>You may see a pop-up window that asks you to confirm the installation. Tap on OK or Install.</li>
|
84 |
-
<li>Wait for the app to install on your device. You can check the progress in your notification bar or in your file manager app.</li>
|
85 |
-
<li>Once the installation is complete, you can open FR Legends Mod APK Terbaru 2021 Supra by tapping on Open or by finding it in your app drawer.</li>
|
86 |
-
</ol>
|
87 |
-
<h2>Conclusion</h2>
|
88 |
-
<h3>Enjoy drifting with FR Legends Mod APK Terbaru 2021 Supra</h3>
|
89 |
-
<p>Congratulations! You have successfully downloaded and installed FR Legends Mod APK Terbaru 2021 Supra on your Android device. You can now enjoy drifting with unlimited money and new cars like the Toyota Supra. You can also customize your car to your liking and challenge other players online. FR Legends Mod APK Terbaru 2021 Supra is a great way to have fun and improve your drifting skills.</p>
|
90 |
-
<h3>FAQs</h3>
|
91 |
-
<p>Here are some frequently asked questions about FR Legends Mod APK Terbaru 2021 Supra:</p>
|
92 |
-
<table>
|
93 |
-
<tr>
|
94 |
-
<th>Question</th>
|
95 |
-
<th>Answer</th>
|
96 |
-
</tr>
|
97 |
-
<tr>
|
98 |
-
<td>Is FR Legends Mod APK Terbaru 2021 Supra safe to download and install?</td>
|
99 |
-
<td>FR Legends Mod APK Terbaru 2021 Supra is safe to download and install if you get it from a reputable source, such as [this one]. However, you should always be careful when downloading files from unknown sources, as they may contain viruses or malware. You should also scan the APK file with an antivirus app before installing it.</td>
|
100 |
-
</tr>
|
101 |
-
<tr>
|
102 |
-
<td>Does FR Legends Mod APK Terbaru 2021 Supra require root access?</td>
|
103 |
-
<td>No, FR Legends Mod APK Terbaru 2021 Supra does not require root access to work. You can install it on any Android device without rooting it.</td>
|
104 |
-
</tr>
|
105 |
-
<tr>
|
106 |
-
<td>Will FR Legends Mod APK Terbaru 2021 Supra affect the original game?</td>
|
107 |
-
<td>No, FR Legends Mod APK Terbaru 2021 Supra will not affect the original game. You can still play the official version of FR Legends if you want. However, you should not use the same account for both versions, as it may cause problems or bans.</td>
|
108 |
-
</tr>
|
109 |
-
<tr>
|
110 |
-
<td>Can I update FR Legends Mod APK Terbaru 2021 Supra?</td>
|
111 |
-
<td>No, FR Legends Mod APK Terbaru 2021 Supra cannot be updated through the app or Google Play Store. If you want to get the latest version of the mod, you have to download and install it again from the source website.</td>
|
112 |
-
</tr>
|
113 |
-
<tr>
|
114 |
-
<td>Can I play FR Legends Mod APK Terbaru 2021 Supra offline?</td>
|
115 |
-
<td>Yes, you can play FR Legends Mod APK Terbaru 2021 Supra offline. However, some features may not work properly, such as online multiplayer and leaderboards.</td>
|
116 |
-
</tr>
|
117 |
-
</table></p> 197e85843d<br />
|
118 |
-
<br />
|
119 |
-
<br />
|
|
|
|
|
|
|
|
|
|
|
|
|
|
|
|
|
|
|
|
|
|
|
|
|
|
|
|
|
|
|
|
|
|
|
|
|
|
|
|
|
|
|
|
|
|
|
|
|
|
|
|
|
|
|
|
|
|
|
|
|
|
|
|
|
|
|
|
|
|
|
|
|
|
|
|
|
|
|
|
|
|
|
|
|
|
|
|
|
|
|
|
|
|
|
|
|
|
|
|
|
|
|
|
|
|
|
|
|
|
|
|
|
|
|
|
|
|
|
|
|
|
|
|
|
|
|
|
|
|
|
|
|
|
|
|
|
|
|
|
|
|
|
|
|
|
|
|
|
|
|
|
|
|
|
|
|
|
|
|
|
|
|
|
|
|
|
|
|
|
|
|
|
|
|
|
|
|
|
|
|
|
|
|
|
|
|
|
|
|
|
|
|
|
|
|
|
|
|
|
|
|
|
|
|
|
|
|
|
|
|
|
|
|
|
|
|
|
|
|
|
|
|
|
|
|
|
|
|
|
|
|
|
|
|
|
|
|
|
|
spaces/1phancelerku/anime-remove-background/Flash Game Player Pro Mod APK How to Play Any Flash Game on Your Phone.md
DELETED
@@ -1,145 +0,0 @@
|
|
1 |
-
|
2 |
-
<h1>Flash Game Player Pro Mod APK: How to Play Flash Games on Your Android Device</h1>
|
3 |
-
<p>Do you miss playing flash games on your browser? Do you want to enjoy them on your Android device without any hassle? If yes, then you should try Flash Game Player Pro Mod APK. This is a powerful app that lets you play any flash game on your Android device with ease. In this article, we will tell you everything you need to know about Flash Game Player Pro Mod APK, including its features, benefits, download and installation process, and how to use it to play flash games on your Android device.</p>
|
4 |
-
<h2>flash game player pro mod apk</h2><br /><p><b><b>DOWNLOAD</b> 🆓 <a href="https://jinyurl.com/2uNM30">https://jinyurl.com/2uNM30</a></b></p><br /><br />
|
5 |
-
<h2>What is Flash Game Player Pro?</h2>
|
6 |
-
<p>Flash Game Player Pro is an app that allows you to play flash games on your Android device. It is a modified version of the original Flash Game Player app, which has some limitations and ads. Flash Game Player Pro Mod APK removes those limitations and ads, and adds some extra features and enhancements.</p>
|
7 |
-
<h3>Features of Flash Game Player Pro</h3>
|
8 |
-
<p>Some of the features of Flash Game Player Pro are:</p>
|
9 |
-
<ul>
|
10 |
-
<li>It supports all kinds of flash games, including SWF and FLV formats.</li>
|
11 |
-
<li>It has a built-in browser that lets you browse and play online flash games.</li>
|
12 |
-
<li>It has a file manager that lets you manage and play local flash games.</li>
|
13 |
-
<li>It has a gamepad that lets you control the flash games with virtual buttons.</li>
|
14 |
-
<li>It has a screen orientation option that lets you switch between portrait and landscape modes.</li>
|
15 |
-
<li>It has a zoom option that lets you adjust the size of the flash game screen.</li>
|
16 |
-
<li>It has a screenshot option that lets you capture and share the flash game screen.</li>
|
17 |
-
<li>It has a cheat option that lets you modify the flash game parameters.</li>
|
18 |
-
</ul>
|
19 |
-
<h3>Benefits of using Flash Game Player Pro</h3>
|
20 |
-
<p>Some of the benefits of using Flash Game Player Pro are:</p>
|
21 |
-
<ul>
|
22 |
-
<li>You can play any flash game on your Android device without any compatibility issues.</li>
|
23 |
-
<li>You can enjoy the flash games in full screen mode without any distractions.</li>
|
24 |
-
<li>You can customize the flash games according to your preferences and needs.</li>
|
25 |
-
<li>You can save your progress and resume the flash games anytime.</li>
|
26 |
-
<li>You can access thousands of online flash games for free.</li>
|
27 |
-
</ul>
|
28 |
-
<h2>What is a mod APK?</h2>
|
29 |
-
<p>A mod APK is a modified version of an original APK (Android Package Kit) file, which is the format used to distribute and install apps on Android devices. A mod APK usually has some changes or additions that are not present in the original app. These changes or additions can be for various purposes, such as unlocking premium features, removing ads, adding extra functionality, enhancing performance, etc.</p>
|
30 |
-
<p>flash game player pro key apk download<br />
|
31 |
-
flash game player pro mod apk latest version<br />
|
32 |
-
flash game player pro apk free download<br />
|
33 |
-
flash game player pro mod apk unlimited money<br />
|
34 |
-
flash game player pro key mod apk<br />
|
35 |
-
flash game player pro mod apk android 1<br />
|
36 |
-
flash game player pro apk full version<br />
|
37 |
-
flash game player pro mod apk unlocked<br />
|
38 |
-
flash game player pro key apk free<br />
|
39 |
-
flash game player pro mod apk happymod<br />
|
40 |
-
flash game player pro apk no ads<br />
|
41 |
-
flash game player pro mod apk premium<br />
|
42 |
-
flash game player pro key apk 2023<br />
|
43 |
-
flash game player pro mod apk rexdl<br />
|
44 |
-
flash game player pro apk cracked<br />
|
45 |
-
flash game player pro mod apk revdl<br />
|
46 |
-
flash game player pro key apk modded<br />
|
47 |
-
flash game player pro mod apk offline<br />
|
48 |
-
flash game player pro apk hack<br />
|
49 |
-
flash game player pro mod apk online<br />
|
50 |
-
flash game player pro key apk hacked<br />
|
51 |
-
flash game player pro mod apk 2023<br />
|
52 |
-
flash game player pro apk mod<br />
|
53 |
-
flash game player pro mod apk update<br />
|
54 |
-
flash game player pro key apk latest<br />
|
55 |
-
flash game player pro mod apk old version<br />
|
56 |
-
flash game player pro apk paid<br />
|
57 |
-
flash game player pro mod apk original<br />
|
58 |
-
flash game player pro key apk unlocked<br />
|
59 |
-
flash game player pro mod apk no root<br />
|
60 |
-
flash game player pro apk premium<br />
|
61 |
-
flash game player pro mod apk android 2.3+<br />
|
62 |
-
flash game player pro key apk cracked<br />
|
63 |
-
flash game player pro mod apk android 4.4+<br />
|
64 |
-
flash game player pro apk unlocked<br />
|
65 |
-
flash game player pro mod apk android 5.0+<br />
|
66 |
-
flash game player pro key apk full version<br />
|
67 |
-
flash game player pro mod apk android 6.0+<br />
|
68 |
-
flash game player pro apk update<br />
|
69 |
-
flash game player pro mod apk android 7.0+<br />
|
70 |
-
flash game player pro key apk no ads<br />
|
71 |
-
flash game player pro mod apk android 8.0+<br />
|
72 |
-
flash game player pro apk original<br />
|
73 |
-
flash game player pro mod apk android 9.0+<br />
|
74 |
-
flash game player pro key apk premium<br />
|
75 |
-
flash game player pro mod apk android 10.0+<br />
|
76 |
-
flash game player pro apk offline<br />
|
77 |
-
flash game player pro mod apk android 11.0+</p>
|
78 |
-
<h3>Advantages of using a mod APK</h3>
|
79 |
-
<p>Some of the advantages of using a mod APK are:</p>
|
80 |
-
<ul>
|
81 |
-
<li>You can access premium features that are otherwise locked or paid in the original app.</li>
|
82 |
-
<li>You can remove annoying ads that interrupt your experience in the original app.</li>
|
83 |
-
<li>You can add extra functionality that is not available in the original app.</li>
|
84 |
-
<li>You can enhance the performance or quality of the original app.</li>
|
85 |
-
</ul>
|
86 |
-
<h3>Risks of using a mod APK</h3>
|
87 |
-
<p>Some of the risks of using a mod APK are:</p>
|
88 |
-
<ul>
|
89 |
-
<li>You may violate the terms and conditions of the original app developer or provider.</li>
|
90 |
-
<li>You may expose your device to malware or viruses that may harm your data or privacy.</li>
|
91 |
-
<li>You may encounter bugs or errors that may affect the functionality of the original app.</li>
|
92 |
-
<li>You may not receive updates or support from the original app developer or provider.</li>
|
93 |
-
</ul>
|
94 |
-
<p>Therefore, you should be careful and cautious when using a mod APK. You should only download and install a mod APK from a trusted and reputable source. You should also scan the mod APK file with an antivirus software before installing it. You should also backup your data and device before using a mod APK.</p>
|
95 |
-
<h2>How to download and install Flash Game Player Pro Mod APK?</h2>
|
96 |
-
<p>If you want to download and install Flash Game Player Pro Mod APK on your Android device, you need to follow these steps:</p>
|
97 |
-
<h3>Steps to download and install Flash Game Player Pro Mod APK</h3>
|
98 |
-
<ol>
|
99 |
-
<li>Go to the link and download the Flash Game Player Pro Mod APK file on your device.</li>
|
100 |
-
<li>Go to the settings of your device and enable the option of installing apps from unknown sources.</li>
|
101 |
-
<li>Locate the downloaded Flash Game Player Pro Mod APK file and tap on it to start the installation process.</li>
|
102 |
-
<li>Follow the instructions on the screen and wait for the installation to complete.</li>
|
103 |
-
<li>Launch the Flash Game Player Pro app and enjoy playing flash games on your Android device.</li>
|
104 |
-
</ol>
|
105 |
-
<h3>Tips to avoid malware and viruses</h3>
|
106 |
-
<p>Some tips to avoid malware and viruses when downloading and installing a mod APK are:</p>
|
107 |
-
<ul>
|
108 |
-
<li>Only download and install a mod APK from a trusted and reputable source.</li>
|
109 |
-
<li>Scan the mod APK file with an antivirus software before installing it.</li>
|
110 |
-
<li>Backup your data and device before using a mod APK.</li>
|
111 |
-
<li>Do not grant unnecessary permissions or access to the mod APK.</li>
|
112 |
-
<li>Delete the mod APK file after installing it.</li>
|
113 |
-
</ul>
|
114 |
-
<h2>How to play flash games on your Android device using Flash Game Player Pro Mod APK?</h2>
|
115 |
-
<p>Once you have downloaded and installed Flash Game Player Pro Mod APK on your Android device, you can start playing flash games on it. Here is how:</p>
|
116 |
-
<h3>How to load flash games from your device or online sources</h3>
|
117 |
-
<p>You can load flash games from your device or online sources using Flash Game Player Pro. Here is how:</p>
|
118 |
-
<ul>
|
119 |
-
<li>If you want to load flash games from your device, you need to have them stored in your device memory or SD card. You can then use the file manager of Flash Game Player Pro to browse and select the flash game file you want to play.</li>
|
120 |
-
<li>If you want to load flash games from online sources, you need to have an internet connection. You can then use the browser of Flash Game Player Pro to search and access the website that hosts the flash game you want to play. You can also enter the URL of the flash game directly in the browser.</li>
|
121 |
-
</ul>
|
122 |
-
<h3>How to customize the settings and controls of flash games</h3>
|
123 |
-
<p>You can customize the settings and controls of flash games using Flash Game Player Pro. Here is how:</p>
|
124 |
-
<ul>
|
125 |
-
<li>If you want to customize the settings of flash games, you can tap on the menu button on the top right corner of the screen and select the settings option. You can then adjust various options such as screen orientation, zoom, screenshot, cheat, etc.</li>
|
126 |
-
<li>If you want to customize the controls of flash games, you can tap on the gamepad button on the bottom right corner of the screen and select the gamepad option. You can then drag and drop virtual buttons on the screen according to your preference. You can also resize and reposition them as you like.</li>
|
127 |
-
</ul>
|
128 |
-
<h2>Conclusion</h2>
|
129 |
-
<p>Flash Game Player Pro Mod APK is a great app that lets you play flash games on your Android device with ease. It has many features and benefits that make it superior to other similar apps. It also has some risks that you should be aware of before using it. You can download and install Flash Game Player Pro Mod APK from and follow our guide to play flash games on your Android device using it. We hope you have fun playing flash games on your Android device with Flash Game Player Pro Mod APK.</p>
|
130 |
-
<h2>FAQs</h2>
|
131 |
-
<p>Here are some frequently asked questions about Flash Game Player Pro Mod APK:</p>
|
132 |
-
<h4>Q: Is Flash Game Player Pro Mod APK safe to use?</h4>
|
133 |
-
<p>A: Flash Game Player Pro Mod APK is generally safe to use if you download and install it from a trusted and reputable source. However, you should always scan it with an antivirus software before installing it, backup your data and device before using it, and delete it after installing it.</p>
|
134 |
-
<h4>Q: Is Flash Game Player Pro Mod APK legal to use?</h4>
|
135 |
-
<p>A: Flash Game Player Pro Mod APK may not be legal to use in some countries or regions, as it may violate the terms and conditions of the original app developer or provider. You should check the laws and regulations of your country or region before using Flash Game Player Pro Mod APK.</p>
|
136 |
-
<h4>Q: Does Flash Game Player Pro Mod APK work on all Android devices?</h4>
|
137 |
-
<p>A: Flash Game Player Pro Mod APK works on most Android devices that support flash games. However, some devices may not be compatible or may experience some issues with Flash Game Player Pro Mod APK. You should try it on your device and see if it works well.</p>
|
138 |
-
<h4>Q: Can I play flash games offline with Flash Game Player Pro Mod APK?</h4>
|
139 |
-
<p>A: Yes, you can play flash games offline with Flash Game Player Pro Mod APK if you have them stored in your device memory or SD card. You can use the file manager of Flash Game Player Pro to load and play them. However, you need an internet connection to play online flash games with Flash Game Player Pro.</p>
|
140 |
-
<h4>Q: Can I update Flash Game Player Pro Mod APK?</h4>
|
141 |
-
<p>A: No, you cannot update Flash Game Player Pro Mod APK, as it is a modified version of the original app. If you update it, you may lose the mod features and enhancements. You should only download and install the latest version of Flash Game Player Pro Mod APK from a trusted and reputable source.</p>
|
142 |
-
<h4>Q: Where can I find more flash games to play with Flash Game Player Pro Mod APK?</h4>
|
143 |
-
<p>A: You can find more flash games to play with Flash Game Player Pro Mod APK from various online sources, such as websites, blogs, forums, etc. You can also search for flash games on Google or other search engines. However, you should be careful and cautious when downloading flash games from online sources, as they may contain malware or viruses that may harm your device or data.</p> 401be4b1e0<br />
|
144 |
-
<br />
|
145 |
-
<br />
|
|
|
|
|
|
|
|
|
|
|
|
|
|
|
|
|
|
|
|
|
|
|
|
|
|
|
|
|
|
|
|
|
|
|
|
|
|
|
|
|
|
|
|
|
|
|
|
|
|
|
|
|
|
|
|
|
|
|
|
|
|
|
|
|
|
|
|
|
|
|
|
|
|
|
|
|
|
|
|
|
|
|
|
|
|
|
|
|
|
|
|
|
|
|
|
|
|
|
|
|
|
|
|
|
|
|
|
|
|
|
|
|
|
|
|
|
|
|
|
|
|
|
|
|
|
|
|
|
|
|
|
|
|
|
|
|
|
|
|
|
|
|
|
|
|
|
|
|
|
|
|
|
|
|
|
|
|
|
|
|
|
|
|
|
|
|
|
|
|
|
|
|
|
|
|
|
|
|
|
|
|
|
|
|
|
|
|
|
|
|
|
|
|
|
|
|
|
|
|
|
|
|
|
|
|
|
|
|
|
|
|
|
|
|
|
|
|
|
|
|
|
|
|
|
|
|
|
|
|
|
|
|
|
|
|
|
|
|
|
|
|
|
|
|
|
|
|
|
|
|
|
|
|
|
|
|
|
|
|
|
|
|
|
|
|
|
|
|
|
|
|
|
|
|
|
|
|
|
|
|
|
|
|
|
|
|
|
|
|
|
|
spaces/44ov41za8i/FreeVC/mel_processing.py
DELETED
@@ -1,112 +0,0 @@
|
|
1 |
-
import math
|
2 |
-
import os
|
3 |
-
import random
|
4 |
-
import torch
|
5 |
-
from torch import nn
|
6 |
-
import torch.nn.functional as F
|
7 |
-
import torch.utils.data
|
8 |
-
import numpy as np
|
9 |
-
import librosa
|
10 |
-
import librosa.util as librosa_util
|
11 |
-
from librosa.util import normalize, pad_center, tiny
|
12 |
-
from scipy.signal import get_window
|
13 |
-
from scipy.io.wavfile import read
|
14 |
-
from librosa.filters import mel as librosa_mel_fn
|
15 |
-
|
16 |
-
MAX_WAV_VALUE = 32768.0
|
17 |
-
|
18 |
-
|
19 |
-
def dynamic_range_compression_torch(x, C=1, clip_val=1e-5):
|
20 |
-
"""
|
21 |
-
PARAMS
|
22 |
-
------
|
23 |
-
C: compression factor
|
24 |
-
"""
|
25 |
-
return torch.log(torch.clamp(x, min=clip_val) * C)
|
26 |
-
|
27 |
-
|
28 |
-
def dynamic_range_decompression_torch(x, C=1):
|
29 |
-
"""
|
30 |
-
PARAMS
|
31 |
-
------
|
32 |
-
C: compression factor used to compress
|
33 |
-
"""
|
34 |
-
return torch.exp(x) / C
|
35 |
-
|
36 |
-
|
37 |
-
def spectral_normalize_torch(magnitudes):
|
38 |
-
output = dynamic_range_compression_torch(magnitudes)
|
39 |
-
return output
|
40 |
-
|
41 |
-
|
42 |
-
def spectral_de_normalize_torch(magnitudes):
|
43 |
-
output = dynamic_range_decompression_torch(magnitudes)
|
44 |
-
return output
|
45 |
-
|
46 |
-
|
47 |
-
mel_basis = {}
|
48 |
-
hann_window = {}
|
49 |
-
|
50 |
-
|
51 |
-
def spectrogram_torch(y, n_fft, sampling_rate, hop_size, win_size, center=False):
|
52 |
-
if torch.min(y) < -1.:
|
53 |
-
print('min value is ', torch.min(y))
|
54 |
-
if torch.max(y) > 1.:
|
55 |
-
print('max value is ', torch.max(y))
|
56 |
-
|
57 |
-
global hann_window
|
58 |
-
dtype_device = str(y.dtype) + '_' + str(y.device)
|
59 |
-
wnsize_dtype_device = str(win_size) + '_' + dtype_device
|
60 |
-
if wnsize_dtype_device not in hann_window:
|
61 |
-
hann_window[wnsize_dtype_device] = torch.hann_window(win_size).to(dtype=y.dtype, device=y.device)
|
62 |
-
|
63 |
-
y = torch.nn.functional.pad(y.unsqueeze(1), (int((n_fft-hop_size)/2), int((n_fft-hop_size)/2)), mode='reflect')
|
64 |
-
y = y.squeeze(1)
|
65 |
-
|
66 |
-
spec = torch.stft(y, n_fft, hop_length=hop_size, win_length=win_size, window=hann_window[wnsize_dtype_device],
|
67 |
-
center=center, pad_mode='reflect', normalized=False, onesided=True, return_complex=False)
|
68 |
-
|
69 |
-
spec = torch.sqrt(spec.pow(2).sum(-1) + 1e-6)
|
70 |
-
return spec
|
71 |
-
|
72 |
-
|
73 |
-
def spec_to_mel_torch(spec, n_fft, num_mels, sampling_rate, fmin, fmax):
|
74 |
-
global mel_basis
|
75 |
-
dtype_device = str(spec.dtype) + '_' + str(spec.device)
|
76 |
-
fmax_dtype_device = str(fmax) + '_' + dtype_device
|
77 |
-
if fmax_dtype_device not in mel_basis:
|
78 |
-
mel = librosa_mel_fn(sr=sampling_rate, n_fft=n_fft, n_mels=num_mels, fmin=fmin, fmax=fmax)
|
79 |
-
mel_basis[fmax_dtype_device] = torch.from_numpy(mel).to(dtype=spec.dtype, device=spec.device)
|
80 |
-
spec = torch.matmul(mel_basis[fmax_dtype_device], spec)
|
81 |
-
spec = spectral_normalize_torch(spec)
|
82 |
-
return spec
|
83 |
-
|
84 |
-
|
85 |
-
def mel_spectrogram_torch(y, n_fft, num_mels, sampling_rate, hop_size, win_size, fmin, fmax, center=False):
|
86 |
-
if torch.min(y) < -1.:
|
87 |
-
print('min value is ', torch.min(y))
|
88 |
-
if torch.max(y) > 1.:
|
89 |
-
print('max value is ', torch.max(y))
|
90 |
-
|
91 |
-
global mel_basis, hann_window
|
92 |
-
dtype_device = str(y.dtype) + '_' + str(y.device)
|
93 |
-
fmax_dtype_device = str(fmax) + '_' + dtype_device
|
94 |
-
wnsize_dtype_device = str(win_size) + '_' + dtype_device
|
95 |
-
if fmax_dtype_device not in mel_basis:
|
96 |
-
mel = librosa_mel_fn(sr=sampling_rate, n_fft=n_fft, n_mels=num_mels, fmin=fmin, fmax=fmax)
|
97 |
-
mel_basis[fmax_dtype_device] = torch.from_numpy(mel).to(dtype=y.dtype, device=y.device)
|
98 |
-
if wnsize_dtype_device not in hann_window:
|
99 |
-
hann_window[wnsize_dtype_device] = torch.hann_window(win_size).to(dtype=y.dtype, device=y.device)
|
100 |
-
|
101 |
-
y = torch.nn.functional.pad(y.unsqueeze(1), (int((n_fft-hop_size)/2), int((n_fft-hop_size)/2)), mode='reflect')
|
102 |
-
y = y.squeeze(1)
|
103 |
-
|
104 |
-
spec = torch.stft(y, n_fft, hop_length=hop_size, win_length=win_size, window=hann_window[wnsize_dtype_device],
|
105 |
-
center=center, pad_mode='reflect', normalized=False, onesided=True, return_complex=False)
|
106 |
-
|
107 |
-
spec = torch.sqrt(spec.pow(2).sum(-1) + 1e-6)
|
108 |
-
|
109 |
-
spec = torch.matmul(mel_basis[fmax_dtype_device], spec)
|
110 |
-
spec = spectral_normalize_torch(spec)
|
111 |
-
|
112 |
-
return spec
|
|
|
|
|
|
|
|
|
|
|
|
|
|
|
|
|
|
|
|
|
|
|
|
|
|
|
|
|
|
|
|
|
|
|
|
|
|
|
|
|
|
|
|
|
|
|
|
|
|
|
|
|
|
|
|
|
|
|
|
|
|
|
|
|
|
|
|
|
|
|
|
|
|
|
|
|
|
|
|
|
|
|
|
|
|
|
|
|
|
|
|
|
|
|
|
|
|
|
|
|
|
|
|
|
|
|
|
|
|
|
|
|
|
|
|
|
|
|
|
|
|
|
|
|
|
|
|
|
|
|
|
|
|
|
|
|
|
|
|
|
|
|
|
|
|
|
|
|
|
|
|
|
|
|
|
|
|
|
|
|
|
|
|
|
|
|
|
|
|
|
|
|
|
|
|
|
|
|
|
|
|
|
|
|
|
|
|
|
|
|
|
|
|
|
|
|
|
|
|
|
|
|
|
|
|
|
|
|
|
|
|
|
|
|
|
|
|
|
|
|
|
|
|
|
|
spaces/4f20/text_generator/app.py
DELETED
@@ -1,3 +0,0 @@
|
|
1 |
-
import gradio as gr
|
2 |
-
|
3 |
-
gr.Interface.load("huggingface/gpt2").launch()
|
|
|
|
|
|
|
|
spaces/801artistry/RVC801/julius/core.py
DELETED
@@ -1,122 +0,0 @@
|
|
1 |
-
# File under the MIT license, see https://github.com/adefossez/julius/LICENSE for details.
|
2 |
-
# Author: adefossez, 2020
|
3 |
-
"""
|
4 |
-
Signal processing or PyTorch related utilities.
|
5 |
-
"""
|
6 |
-
import math
|
7 |
-
import typing as tp
|
8 |
-
|
9 |
-
import torch
|
10 |
-
from torch.nn import functional as F
|
11 |
-
|
12 |
-
|
13 |
-
def sinc(x: torch.Tensor):
|
14 |
-
"""
|
15 |
-
Implementation of sinc, i.e. sin(x) / x
|
16 |
-
|
17 |
-
__Warning__: the input is not multiplied by `pi`!
|
18 |
-
"""
|
19 |
-
return torch.where(x == 0, torch.tensor(1., device=x.device, dtype=x.dtype), torch.sin(x) / x)
|
20 |
-
|
21 |
-
|
22 |
-
def pad_to(tensor: torch.Tensor, target_length: int, mode: str = 'constant', value: float = 0):
|
23 |
-
"""
|
24 |
-
Pad the given tensor to the given length, with 0s on the right.
|
25 |
-
"""
|
26 |
-
return F.pad(tensor, (0, target_length - tensor.shape[-1]), mode=mode, value=value)
|
27 |
-
|
28 |
-
|
29 |
-
def hz_to_mel(freqs: torch.Tensor):
|
30 |
-
"""
|
31 |
-
Converts a Tensor of frequencies in hertz to the mel scale.
|
32 |
-
Uses the simple formula by O'Shaughnessy (1987).
|
33 |
-
|
34 |
-
Args:
|
35 |
-
freqs (torch.Tensor): frequencies to convert.
|
36 |
-
|
37 |
-
"""
|
38 |
-
return 2595 * torch.log10(1 + freqs / 700)
|
39 |
-
|
40 |
-
|
41 |
-
def mel_to_hz(mels: torch.Tensor):
|
42 |
-
"""
|
43 |
-
Converts a Tensor of mel scaled frequencies to Hertz.
|
44 |
-
Uses the simple formula by O'Shaughnessy (1987).
|
45 |
-
|
46 |
-
Args:
|
47 |
-
mels (torch.Tensor): mel frequencies to convert.
|
48 |
-
"""
|
49 |
-
return 700 * (10**(mels / 2595) - 1)
|
50 |
-
|
51 |
-
|
52 |
-
def mel_frequencies(n_mels: int, fmin: float, fmax: float):
|
53 |
-
"""
|
54 |
-
Return frequencies that are evenly spaced in mel scale.
|
55 |
-
|
56 |
-
Args:
|
57 |
-
n_mels (int): number of frequencies to return.
|
58 |
-
fmin (float): start from this frequency (in Hz).
|
59 |
-
fmax (float): finish at this frequency (in Hz).
|
60 |
-
|
61 |
-
|
62 |
-
"""
|
63 |
-
low = hz_to_mel(torch.tensor(float(fmin))).item()
|
64 |
-
high = hz_to_mel(torch.tensor(float(fmax))).item()
|
65 |
-
mels = torch.linspace(low, high, n_mels)
|
66 |
-
return mel_to_hz(mels)
|
67 |
-
|
68 |
-
|
69 |
-
def volume(x: torch.Tensor, floor=1e-8):
|
70 |
-
"""
|
71 |
-
Return the volume in dBFS.
|
72 |
-
"""
|
73 |
-
return torch.log10(floor + (x**2).mean(-1)) * 10
|
74 |
-
|
75 |
-
|
76 |
-
def pure_tone(freq: float, sr: float = 128, dur: float = 4, device=None):
|
77 |
-
"""
|
78 |
-
Return a pure tone, i.e. cosine.
|
79 |
-
|
80 |
-
Args:
|
81 |
-
freq (float): frequency (in Hz)
|
82 |
-
sr (float): sample rate (in Hz)
|
83 |
-
dur (float): duration (in seconds)
|
84 |
-
"""
|
85 |
-
time = torch.arange(int(sr * dur), device=device).float() / sr
|
86 |
-
return torch.cos(2 * math.pi * freq * time)
|
87 |
-
|
88 |
-
|
89 |
-
def unfold(input, kernel_size: int, stride: int):
|
90 |
-
"""1D only unfolding similar to the one from PyTorch.
|
91 |
-
However PyTorch unfold is extremely slow.
|
92 |
-
|
93 |
-
Given an input tensor of size `[*, T]` this will return
|
94 |
-
a tensor `[*, F, K]` with `K` the kernel size, and `F` the number
|
95 |
-
of frames. The i-th frame is a view onto `i * stride: i * stride + kernel_size`.
|
96 |
-
This will automatically pad the input to cover at least once all entries in `input`.
|
97 |
-
|
98 |
-
Args:
|
99 |
-
input (Tensor): tensor for which to return the frames.
|
100 |
-
kernel_size (int): size of each frame.
|
101 |
-
stride (int): stride between each frame.
|
102 |
-
|
103 |
-
Shape:
|
104 |
-
|
105 |
-
- Inputs: `input` is `[*, T]`
|
106 |
-
- Output: `[*, F, kernel_size]` with `F = 1 + ceil((T - kernel_size) / stride)`
|
107 |
-
|
108 |
-
|
109 |
-
..Warning:: unlike PyTorch unfold, this will pad the input
|
110 |
-
so that any position in `input` is covered by at least one frame.
|
111 |
-
"""
|
112 |
-
shape = list(input.shape)
|
113 |
-
length = shape.pop(-1)
|
114 |
-
n_frames = math.ceil((max(length, kernel_size) - kernel_size) / stride) + 1
|
115 |
-
tgt_length = (n_frames - 1) * stride + kernel_size
|
116 |
-
padded = F.pad(input, (0, tgt_length - length)).contiguous()
|
117 |
-
strides: tp.List[int] = []
|
118 |
-
for dim in range(padded.dim()):
|
119 |
-
strides.append(padded.stride(dim))
|
120 |
-
assert strides.pop(-1) == 1, 'data should be contiguous'
|
121 |
-
strides = strides + [stride, 1]
|
122 |
-
return padded.as_strided(shape + [n_frames, kernel_size], strides)
|
|
|
|
|
|
|
|
|
|
|
|
|
|
|
|
|
|
|
|
|
|
|
|
|
|
|
|
|
|
|
|
|
|
|
|
|
|
|
|
|
|
|
|
|
|
|
|
|
|
|
|
|
|
|
|
|
|
|
|
|
|
|
|
|
|
|
|
|
|
|
|
|
|
|
|
|
|
|
|
|
|
|
|
|
|
|
|
|
|
|
|
|
|
|
|
|
|
|
|
|
|
|
|
|
|
|
|
|
|
|
|
|
|
|
|
|
|
|
|
|
|
|
|
|
|
|
|
|
|
|
|
|
|
|
|
|
|
|
|
|
|
|
|
|
|
|
|
|
|
|
|
|
|
|
|
|
|
|
|
|
|
|
|
|
|
|
|
|
|
|
|
|
|
|
|
|
|
|
|
|
|
|
|
|
|
|
|
|
|
|
|
|
|
|
|
|
|
|
|
|
|
|
|
|
|
|
|
|
|
|
|
|
|
|
|
|
|
|
|
|
|
|
|
|
|
|
|
|
|
|
|
|
|
|
|
|
|
|
|
|
|
|
|
|
|
spaces/A00001/bingothoo/src/components/voice.tsx
DELETED
@@ -1,52 +0,0 @@
|
|
1 |
-
import React, { useEffect } from 'react'
|
2 |
-
import { useSetAtom } from 'jotai'
|
3 |
-
import { useBing } from '@/lib/hooks/use-bing'
|
4 |
-
import Image from 'next/image'
|
5 |
-
import VoiceIcon from '@/assets/images/voice.svg'
|
6 |
-
import VoiceButton from './ui/voice'
|
7 |
-
import { SR } from '@/lib/bots/bing/sr'
|
8 |
-
import { voiceListenAtom } from '@/state'
|
9 |
-
|
10 |
-
const sr = new SR(['发送', '清空', '退出'])
|
11 |
-
|
12 |
-
const Voice = ({ setInput, input, sendMessage, isSpeaking }: Pick<ReturnType<typeof useBing>, 'setInput' | 'sendMessage' | 'input' | 'isSpeaking'>) => {
|
13 |
-
const setListen = useSetAtom(voiceListenAtom)
|
14 |
-
useEffect(() => {
|
15 |
-
if (sr.listening) return
|
16 |
-
sr.transcript = !isSpeaking
|
17 |
-
}, [isSpeaking])
|
18 |
-
|
19 |
-
useEffect(() => {
|
20 |
-
sr.onchange = (msg: string, command?: string) => {
|
21 |
-
switch (command) {
|
22 |
-
case '退出':
|
23 |
-
sr.stop()
|
24 |
-
break;
|
25 |
-
case '发送':
|
26 |
-
sendMessage(input)
|
27 |
-
case '清空':
|
28 |
-
setInput('')
|
29 |
-
break;
|
30 |
-
default:
|
31 |
-
setInput(input + msg)
|
32 |
-
}
|
33 |
-
}
|
34 |
-
}, [input])
|
35 |
-
|
36 |
-
const switchSR = (enable: boolean = false) => {
|
37 |
-
setListen(enable)
|
38 |
-
if (enable) {
|
39 |
-
sr.start()
|
40 |
-
} else {
|
41 |
-
sr.stop()
|
42 |
-
}
|
43 |
-
}
|
44 |
-
|
45 |
-
return sr.listening ? (
|
46 |
-
<VoiceButton onClick={() => switchSR(false)} />
|
47 |
-
) : (
|
48 |
-
<Image alt="start voice" src={VoiceIcon} width={24} className="-mt-0.5" onClick={() => switchSR(true)} />
|
49 |
-
)
|
50 |
-
};
|
51 |
-
|
52 |
-
export default Voice;
|
|
|
|
|
|
|
|
|
|
|
|
|
|
|
|
|
|
|
|
|
|
|
|
|
|
|
|
|
|
|
|
|
|
|
|
|
|
|
|
|
|
|
|
|
|
|
|
|
|
|
|
|
|
|
|
|
|
|
|
|
|
|
|
|
|
|
|
|
|
|
|
|
|
|
|
|
|
|
|
|
|
|
|
|
|
|
|
|
|
|
|
|
|
|
|
|
|
|
|
|
|
|
|
|
|
spaces/AIConsultant/MusicGen/audiocraft/utils/cluster.py
DELETED
@@ -1,75 +0,0 @@
|
|
1 |
-
# Copyright (c) Meta Platforms, Inc. and affiliates.
|
2 |
-
# All rights reserved.
|
3 |
-
#
|
4 |
-
# This source code is licensed under the license found in the
|
5 |
-
# LICENSE file in the root directory of this source tree.
|
6 |
-
|
7 |
-
"""
|
8 |
-
Utility functions for SLURM configuration and cluster settings.
|
9 |
-
"""
|
10 |
-
|
11 |
-
from enum import Enum
|
12 |
-
import os
|
13 |
-
import socket
|
14 |
-
import typing as tp
|
15 |
-
|
16 |
-
import omegaconf
|
17 |
-
|
18 |
-
|
19 |
-
class ClusterType(Enum):
|
20 |
-
AWS = "aws"
|
21 |
-
FAIR = "fair"
|
22 |
-
RSC = "rsc"
|
23 |
-
LOCAL_DARWIN = "darwin"
|
24 |
-
DEFAULT = "default" # used for any other cluster.
|
25 |
-
|
26 |
-
|
27 |
-
def _guess_cluster_type() -> ClusterType:
|
28 |
-
uname = os.uname()
|
29 |
-
fqdn = socket.getfqdn()
|
30 |
-
if uname.sysname == "Linux" and (uname.release.endswith("-aws") or ".ec2" in fqdn):
|
31 |
-
return ClusterType.AWS
|
32 |
-
|
33 |
-
if fqdn.endswith(".fair"):
|
34 |
-
return ClusterType.FAIR
|
35 |
-
|
36 |
-
if fqdn.endswith(".facebook.com"):
|
37 |
-
return ClusterType.RSC
|
38 |
-
|
39 |
-
if uname.sysname == "Darwin":
|
40 |
-
return ClusterType.LOCAL_DARWIN
|
41 |
-
|
42 |
-
return ClusterType.DEFAULT
|
43 |
-
|
44 |
-
|
45 |
-
def get_cluster_type(
|
46 |
-
cluster_type: tp.Optional[ClusterType] = None,
|
47 |
-
) -> tp.Optional[ClusterType]:
|
48 |
-
if cluster_type is None:
|
49 |
-
return _guess_cluster_type()
|
50 |
-
|
51 |
-
return cluster_type
|
52 |
-
|
53 |
-
|
54 |
-
def get_slurm_parameters(
|
55 |
-
cfg: omegaconf.DictConfig, cluster_type: tp.Optional[ClusterType] = None
|
56 |
-
) -> omegaconf.DictConfig:
|
57 |
-
"""Update SLURM parameters in configuration based on cluster type.
|
58 |
-
If the cluster type is not specify, it infers it automatically.
|
59 |
-
"""
|
60 |
-
from ..environment import AudioCraftEnvironment
|
61 |
-
cluster_type = get_cluster_type(cluster_type)
|
62 |
-
# apply cluster-specific adjustments
|
63 |
-
if cluster_type == ClusterType.AWS:
|
64 |
-
cfg["mem_per_gpu"] = None
|
65 |
-
cfg["constraint"] = None
|
66 |
-
cfg["setup"] = []
|
67 |
-
elif cluster_type == ClusterType.RSC:
|
68 |
-
cfg["mem_per_gpu"] = None
|
69 |
-
cfg["setup"] = []
|
70 |
-
cfg["constraint"] = None
|
71 |
-
cfg["partition"] = "learn"
|
72 |
-
slurm_exclude = AudioCraftEnvironment.get_slurm_exclude()
|
73 |
-
if slurm_exclude is not None:
|
74 |
-
cfg["exclude"] = slurm_exclude
|
75 |
-
return cfg
|
|
|
|
|
|
|
|
|
|
|
|
|
|
|
|
|
|
|
|
|
|
|
|
|
|
|
|
|
|
|
|
|
|
|
|
|
|
|
|
|
|
|
|
|
|
|
|
|
|
|
|
|
|
|
|
|
|
|
|
|
|
|
|
|
|
|
|
|
|
|
|
|
|
|
|
|
|
|
|
|
|
|
|
|
|
|
|
|
|
|
|
|
|
|
|
|
|
|
|
|
|
|
|
|
|
|
|
|
|
|
|
|
|
|
|
|
|
|
|
|
|
|
|
|
|
|
|
|
|
|
|
|
|
|
|
|
|
|
|
|
|
|
|
|
|
|
|
|
|
|
|
spaces/AIConsultant/MusicGen/docs/TRAINING.md
DELETED
@@ -1,312 +0,0 @@
|
|
1 |
-
# AudioCraft training pipelines
|
2 |
-
|
3 |
-
AudioCraft training pipelines are built on top of PyTorch as our core deep learning library
|
4 |
-
and [Flashy](https://github.com/facebookresearch/flashy) as our training pipeline design library,
|
5 |
-
and [Dora](https://github.com/facebookresearch/dora) as our experiment manager.
|
6 |
-
AudioCraft training pipelines are designed to be research and experiment-friendly.
|
7 |
-
|
8 |
-
|
9 |
-
## Environment setup
|
10 |
-
|
11 |
-
For the base installation, follow the instructions from the [README.md](../README.md).
|
12 |
-
Below are some additional instructions for setting up environment to train new models.
|
13 |
-
|
14 |
-
### Team and cluster configuration
|
15 |
-
|
16 |
-
In order to support multiple teams and clusters, AudioCraft uses an environment configuration.
|
17 |
-
The team configuration allows to specify cluster-specific configurations (e.g. SLURM configuration),
|
18 |
-
or convenient mapping of paths between the supported environments.
|
19 |
-
|
20 |
-
Each team can have a yaml file under the [configuration folder](../config). To select a team set the
|
21 |
-
`AUDIOCRAFT_TEAM` environment variable to a valid team name (e.g. `labs` or `default`):
|
22 |
-
```shell
|
23 |
-
conda env config vars set AUDIOCRAFT_TEAM=default
|
24 |
-
```
|
25 |
-
|
26 |
-
Alternatively, you can add it to your `.bashrc`:
|
27 |
-
```shell
|
28 |
-
export AUDIOCRAFT_TEAM=default
|
29 |
-
```
|
30 |
-
|
31 |
-
If not defined, the environment will default to the `default` team.
|
32 |
-
|
33 |
-
The cluster is automatically detected, but it is also possible to override it by setting
|
34 |
-
the `AUDIOCRAFT_CLUSTER` environment variable.
|
35 |
-
|
36 |
-
Based on this team and cluster, the environment is then configured with:
|
37 |
-
* The dora experiment outputs directory.
|
38 |
-
* The available slurm partitions: categorized by global and team.
|
39 |
-
* A shared reference directory: In order to facilitate sharing research models while remaining
|
40 |
-
agnostic to the used compute cluster, we created the `//reference` symbol that can be used in
|
41 |
-
YAML config to point to a defined reference folder containing shared checkpoints
|
42 |
-
(e.g. baselines, models for evaluation...).
|
43 |
-
|
44 |
-
**Important:** The default output dir for trained models and checkpoints is under `/tmp/`. This is suitable
|
45 |
-
only for quick testing. If you are doing anything serious you MUST edit the file `default.yaml` and
|
46 |
-
properly set the `dora_dir` entries.
|
47 |
-
|
48 |
-
#### Overriding environment configurations
|
49 |
-
|
50 |
-
You can set the following environmet variables to bypass the team's environment configuration:
|
51 |
-
* `AUDIOCRAFT_CONFIG`: absolute path to a team config yaml file.
|
52 |
-
* `AUDIOCRAFT_DORA_DIR`: absolute path to a custom dora directory.
|
53 |
-
* `AUDIOCRAFT_REFERENCE_DIR`: absolute path to the shared reference directory.
|
54 |
-
|
55 |
-
## Training pipelines
|
56 |
-
|
57 |
-
Each task supported in AudioCraft has its own training pipeline and dedicated solver.
|
58 |
-
Learn more about solvers and key designs around AudioCraft training pipeline below.
|
59 |
-
Please refer to the documentation of each task and model for specific information on a given task.
|
60 |
-
|
61 |
-
|
62 |
-
### Solvers
|
63 |
-
|
64 |
-
The core training component in AudioCraft is the solver. A solver holds the definition
|
65 |
-
of how to solve a given task: It implements the training pipeline logic, combining the datasets,
|
66 |
-
model, optimization criterion and components and the full training loop. We refer the reader
|
67 |
-
to [Flashy](https://github.com/facebookresearch/flashy) for core principles around solvers.
|
68 |
-
|
69 |
-
AudioCraft proposes an initial solver, the `StandardSolver` that is used as the base implementation
|
70 |
-
for downstream solvers. This standard solver provides a nice base management of logging,
|
71 |
-
checkpoints loading/saving, xp restoration, etc. on top of the base Flashy implementation.
|
72 |
-
In AudioCraft, we made the assumption that all tasks are following the same set of stages:
|
73 |
-
train, valid, evaluate and generation, each relying on a dedicated dataset.
|
74 |
-
|
75 |
-
Each solver is responsible for defining the task to solve and the associated stages
|
76 |
-
of the training loop in order to leave the full ownership of the training pipeline
|
77 |
-
to the researchers. This includes loading the datasets, building the model and
|
78 |
-
optimisation components, registering them and defining the execution of each stage.
|
79 |
-
To create a new solver for a given task, one should extend the StandardSolver
|
80 |
-
and define each stage of the training loop. One can further customise its own solver
|
81 |
-
starting from scratch instead of inheriting from the standard solver.
|
82 |
-
|
83 |
-
```python
|
84 |
-
from . import base
|
85 |
-
from .. import optim
|
86 |
-
|
87 |
-
|
88 |
-
class MyNewSolver(base.StandardSolver):
|
89 |
-
|
90 |
-
def __init__(self, cfg: omegaconf.DictConfig):
|
91 |
-
super().__init__(cfg)
|
92 |
-
# one can add custom attributes to the solver
|
93 |
-
self.criterion = torch.nn.L1Loss()
|
94 |
-
|
95 |
-
def best_metric(self):
|
96 |
-
# here optionally specify which metric to use to keep track of best state
|
97 |
-
return 'loss'
|
98 |
-
|
99 |
-
def build_model(self):
|
100 |
-
# here you can instantiate your models and optimization related objects
|
101 |
-
# this method will be called by the StandardSolver init method
|
102 |
-
self.model = ...
|
103 |
-
# the self.cfg attribute contains the raw configuration
|
104 |
-
self.optimizer = optim.build_optimizer(self.model.parameters(), self.cfg.optim)
|
105 |
-
# don't forget to register the states you'd like to include in your checkpoints!
|
106 |
-
self.register_stateful('model', 'optimizer')
|
107 |
-
# keep the model best state based on the best value achieved at validation for the given best_metric
|
108 |
-
self.register_best('model')
|
109 |
-
# if you want to add EMA around the model
|
110 |
-
self.register_ema('model')
|
111 |
-
|
112 |
-
def build_dataloaders(self):
|
113 |
-
# here you can instantiate your dataloaders
|
114 |
-
# this method will be called by the StandardSolver init method
|
115 |
-
self.dataloaders = ...
|
116 |
-
|
117 |
-
...
|
118 |
-
|
119 |
-
# For both train and valid stages, the StandardSolver relies on
|
120 |
-
# a share common_train_valid implementation that is in charge of
|
121 |
-
# accessing the appropriate loader, iterate over the data up to
|
122 |
-
# the specified number of updates_per_epoch, run the ``run_step``
|
123 |
-
# function that you need to implement to specify the behavior
|
124 |
-
# and finally update the EMA and collect the metrics properly.
|
125 |
-
@abstractmethod
|
126 |
-
def run_step(self, idx: int, batch: tp.Any, metrics: dict):
|
127 |
-
"""Perform one training or valid step on a given batch.
|
128 |
-
"""
|
129 |
-
... # provide your implementation of the solver over a batch
|
130 |
-
|
131 |
-
def train(self):
|
132 |
-
"""Train stage.
|
133 |
-
"""
|
134 |
-
return self.common_train_valid('train')
|
135 |
-
|
136 |
-
def valid(self):
|
137 |
-
"""Valid stage.
|
138 |
-
"""
|
139 |
-
return self.common_train_valid('valid')
|
140 |
-
|
141 |
-
@abstractmethod
|
142 |
-
def evaluate(self):
|
143 |
-
"""Evaluate stage.
|
144 |
-
"""
|
145 |
-
... # provide your implementation here!
|
146 |
-
|
147 |
-
@abstractmethod
|
148 |
-
def generate(self):
|
149 |
-
"""Generate stage.
|
150 |
-
"""
|
151 |
-
... # provide your implementation here!
|
152 |
-
```
|
153 |
-
|
154 |
-
### About Epochs
|
155 |
-
|
156 |
-
AudioCraft Solvers uses the concept of Epoch. One epoch doesn't necessarily mean one pass over the entire
|
157 |
-
dataset, but instead represent the smallest amount of computation that we want to work with before checkpointing.
|
158 |
-
Typically, we find that having an Epoch time around 30min is ideal both in terms of safety (checkpointing often enough)
|
159 |
-
and getting updates often enough. One Epoch is at least a `train` stage that lasts for `optim.updates_per_epoch` (2000 by default),
|
160 |
-
and a `valid` stage. You can control how long the valid stage takes with `dataset.valid.num_samples`.
|
161 |
-
Other stages (`evaluate`, `generate`) will only happen every X epochs, as given by `evaluate.every` and `generate.every`).
|
162 |
-
|
163 |
-
|
164 |
-
### Models
|
165 |
-
|
166 |
-
In AudioCraft, a model is a container object that wraps one or more torch modules together
|
167 |
-
with potential processing logic to use in a solver. For example, a model would wrap an encoder module,
|
168 |
-
a quantisation bottleneck module, a decoder and some tensor processing logic. Each of the previous components
|
169 |
-
can be considered as a small « model unit » on its own but the container model is a practical component
|
170 |
-
to manipulate and train a set of modules together.
|
171 |
-
|
172 |
-
### Datasets
|
173 |
-
|
174 |
-
See the [dedicated documentation on datasets](./DATASETS.md).
|
175 |
-
|
176 |
-
### Metrics
|
177 |
-
|
178 |
-
See the [dedicated documentation on metrics](./METRICS.md).
|
179 |
-
|
180 |
-
### Conditioners
|
181 |
-
|
182 |
-
AudioCraft language models can be conditioned in various ways and the codebase offers a modular implementation
|
183 |
-
of different conditioners that can be potentially combined together.
|
184 |
-
Learn more in the [dedicated documentation on conditioning](./CONDITIONING.md).
|
185 |
-
|
186 |
-
### Configuration
|
187 |
-
|
188 |
-
AudioCraft's configuration is defined in yaml files and the framework relies on
|
189 |
-
[hydra](https://hydra.cc/docs/intro/) and [omegaconf](https://omegaconf.readthedocs.io/) to parse
|
190 |
-
and manipulate the configuration through Dora.
|
191 |
-
|
192 |
-
##### :warning: Important considerations around configurations
|
193 |
-
|
194 |
-
Our configuration management relies on Hydra and the concept of group configs to structure
|
195 |
-
and compose configurations. Updating the root default configuration files will then have
|
196 |
-
an impact on all solvers and tasks.
|
197 |
-
**One should never change the default configuration files. Instead they should use Hydra config groups in order to store custom configuration.**
|
198 |
-
Once this configuration is created and used for running experiments, you should not edit it anymore.
|
199 |
-
|
200 |
-
Note that as we are using Dora as our experiment manager, all our experiment tracking is based on
|
201 |
-
signatures computed from delta between configurations.
|
202 |
-
**One must therefore ensure backward compatibilty of the configuration at all time.**
|
203 |
-
See [Dora's README](https://github.com/facebookresearch/dora) and the
|
204 |
-
[section below introduction Dora](#running-experiments-with-dora).
|
205 |
-
|
206 |
-
##### Configuration structure
|
207 |
-
|
208 |
-
The configuration is organized in config groups:
|
209 |
-
* `conditioner`: default values for conditioning modules.
|
210 |
-
* `dset`: contains all data source related information (paths to manifest files
|
211 |
-
and metadata for a given dataset).
|
212 |
-
* `model`: contains configuration for each model defined in AudioCraft and configurations
|
213 |
-
for different variants of models.
|
214 |
-
* `solver`: contains the default configuration for each solver as well as configuration
|
215 |
-
for each solver task, combining all the above components.
|
216 |
-
* `teams`: contains the cluster configuration per teams. See environment setup for more details.
|
217 |
-
|
218 |
-
The `config.yaml` file is the main configuration that composes the above groups
|
219 |
-
and contains default configuration for AudioCraft.
|
220 |
-
|
221 |
-
##### Solver's core configuration structure
|
222 |
-
|
223 |
-
The core configuration structure shared across solver is available in `solvers/default.yaml`.
|
224 |
-
|
225 |
-
##### Other configuration modules
|
226 |
-
|
227 |
-
AudioCraft configuration contains the different setups we used for our research and publications.
|
228 |
-
|
229 |
-
## Running experiments with Dora
|
230 |
-
|
231 |
-
### Launching jobs
|
232 |
-
|
233 |
-
Try launching jobs for different tasks locally with dora run:
|
234 |
-
|
235 |
-
```shell
|
236 |
-
# run compression task with lightweight encodec
|
237 |
-
dora run solver=compression/debug
|
238 |
-
```
|
239 |
-
|
240 |
-
Most of the time, the jobs are launched through dora grids, for example:
|
241 |
-
|
242 |
-
```shell
|
243 |
-
# run compression task through debug grid
|
244 |
-
dora grid compression.debug
|
245 |
-
```
|
246 |
-
|
247 |
-
Learn more about running experiments with Dora below.
|
248 |
-
|
249 |
-
### A small introduction to Dora
|
250 |
-
|
251 |
-
[Dora](https://github.com/facebookresearch/dora) is the experiment manager tool used in AudioCraft.
|
252 |
-
Check out the README to learn how Dora works. Here is a quick summary of what to know:
|
253 |
-
* An XP is a unique set of hyper-parameters with a given signature. The signature is a hash
|
254 |
-
of those hyper-parameters. We always refer to an XP with its signature, e.g. 9357e12e. We will see
|
255 |
-
after that one can retrieve the hyper-params and re-rerun it in a single command.
|
256 |
-
* In fact, the hash is defined as a delta between the base config and the one obtained
|
257 |
-
with the config overrides you passed from the command line. This means you must never change
|
258 |
-
the `conf/**.yaml` files directly., except for editing things like paths. Changing the default values
|
259 |
-
in the config files means the XP signature won't reflect that change, and wrong checkpoints might be reused.
|
260 |
-
I know, this is annoying, but the reason is that otherwise, any change to the config file would mean
|
261 |
-
that all XPs ran so far would see their signature change.
|
262 |
-
|
263 |
-
#### Dora commands
|
264 |
-
|
265 |
-
```shell
|
266 |
-
dora info -f 81de367c # this will show the hyper-parameter used by a specific XP.
|
267 |
-
# Be careful some overrides might present twice, and the right most one
|
268 |
-
# will give you the right value for it.
|
269 |
-
|
270 |
-
dora run -d -f 81de367c # run an XP with the hyper-parameters from XP 81de367c.
|
271 |
-
# `-d` is for distributed, it will use all available GPUs.
|
272 |
-
|
273 |
-
dora run -d -f 81de367c dataset.batch_size=32 # start from the config of XP 81de367c but change some hyper-params.
|
274 |
-
# This will give you a new XP with a new signature (e.g. 3fe9c332).
|
275 |
-
|
276 |
-
dora info -f SIG -t # will tail the log (if the XP has scheduled).
|
277 |
-
# if you need to access the logs of the process for rank > 0, in particular because a crash didn't happen in the main
|
278 |
-
# process, then use `dora info -f SIG` to get the main log name (finished into something like `/5037674_0_0_log.out`)
|
279 |
-
# and worker K can accessed as `/5037674_0_{K}_log.out`.
|
280 |
-
# This is only for scheduled jobs, for local distributed runs with `-d`, then you should go into the XP folder,
|
281 |
-
# and look for `worker_{K}.log` logs.
|
282 |
-
```
|
283 |
-
|
284 |
-
An XP runs from a specific folder based on its signature, under the
|
285 |
-
`<cluster_specific_path>/<user>/experiments/audiocraft/outputs/` folder.
|
286 |
-
You can safely interrupt a training and resume it, it will reuse any existing checkpoint,
|
287 |
-
as it will reuse the same folder. If you made some change to the code and need to ignore
|
288 |
-
a previous checkpoint you can use `dora run --clear [RUN ARGS]`.
|
289 |
-
|
290 |
-
If you have a Slurm cluster, you can also use the dora grid command, e.g.
|
291 |
-
|
292 |
-
```shell
|
293 |
-
# run a dummy grid located at `audiocraft/grids/my_grid_folder/my_grid_name.py`
|
294 |
-
dora grid my_grid_folder.my_grid_name
|
295 |
-
# Run the following will simply display the grid and also initialized the Dora experiments database.
|
296 |
-
# You can then simply refer to a config using its signature (e.g. as `dora run -f SIG`).
|
297 |
-
dora grid my_grid_folder.my_grid_name --dry_run --init
|
298 |
-
```
|
299 |
-
|
300 |
-
Please refer to the [Dora documentation](https://github.com/facebookresearch/dora) for more information.
|
301 |
-
|
302 |
-
|
303 |
-
#### Clearing up past experiments
|
304 |
-
|
305 |
-
```shell
|
306 |
-
# This will cancel all the XPs and delete their folder and checkpoints.
|
307 |
-
# It will then reschedule them starting from scratch.
|
308 |
-
dora grid my_grid_folder.my_grid_name --clear
|
309 |
-
# The following will delete the folder and checkpoint for a single XP,
|
310 |
-
# and then run it afresh.
|
311 |
-
dora run [-f BASE_SIG] [ARGS] --clear
|
312 |
-
```
|
|
|
|
|
|
|
|
|
|
|
|
|
|
|
|
|
|
|
|
|
|
|
|
|
|
|
|
|
|
|
|
|
|
|
|
|
|
|
|
|
|
|
|
|
|
|
|
|
|
|
|
|
|
|
|
|
|
|
|
|
|
|
|
|
|
|
|
|
|
|
|
|
|
|
|
|
|
|
|
|
|
|
|
|
|
|
|
|
|
|
|
|
|
|
|
|
|
|
|
|
|
|
|
|
|
|
|
|
|
|
|
|
|
|
|
|
|
|
|
|
|
|
|
|
|
|
|
|
|
|
|
|
|
|
|
|
|
|
|
|
|
|
|
|
|
|
|
|
|
|
|
|
|
|
|
|
|
|
|
|
|
|
|
|
|
|
|
|
|
|
|
|
|
|
|
|
|
|
|
|
|
|
|
|
|
|
|
|
|
|
|
|
|
|
|
|
|
|
|
|
|
|
|
|
|
|
|
|
|
|
|
|
|
|
|
|
|
|
|
|
|
|
|
|
|
|
|
|
|
|
|
|
|
|
|
|
|
|
|
|
|
|
|
|
|
|
|
|
|
|
|
|
|
|
|
|
|
|
|
|
|
|
|
|
|
|
|
|
|
|
|
|
|
|
|
|
|
|
|
|
|
|
|
|
|
|
|
|
|
|
|
|
|
|
|
|
|
|
|
|
|
|
|
|
|
|
|
|
|
|
|
|
|
|
|
|
|
|
|
|
|
|
|
|
|
|
|
|
|
|
|
|
|
|
|
|
|
|
|
|
|
|
|
|
|
|
|
|
|
|
|
|
|
|
|
|
|
|
|
|
|
|
|
|
|
|
|
|
|
|
|
|
|
|
|
|
|
|
|
|
|
|
|
|
|
|
|
|
|
|
|
|
|
|
|
|
|
|
|
|
|
|
|
|
|
|
|
|
|
|
|
|
|
|
|
|
|
|
|
|
|
|
|
|
|
|
|
|
|
|
|
|
|
|
|
|
|
|
|
|
|
|
|
|
|
|
|
|
|
|
|
|
|
|
|
|
|
|
|
|
|
|
|
|
|
|
|
|
|
|
|
|
|
|
|
|
|
|
|
|
|
|
|
|
|
|
|
|
|
|
|
|
|
|
|
|
|
|
|
|
|
|
|
|
|
|
|
|
|
|
|
|
|
|
|
|
|
|
|
|
|
|
|
|
|
|
|
|
|
|
|
|
|
|
|
|
|
|
|
|
|
|
|
|
|
|
|
|
|
|
|
|
|
|
|
|
|
|
|
|
|
|
|
|
|
|
|
|
|
|
|
|
|
|
|
|
|
|
|
|
|
|
|
|
|
|
|
|
|
|
|
|
|
|
|
|
|
|
|
|
|
|
|
|
|
|
|
|
|
|
|
|
|
|
|
|
|
|
|
|
|
|
|
|
|
spaces/AIGC-Audio/AudioGPT/NeuralSeq/tasks/base_task.py
DELETED
@@ -1,360 +0,0 @@
|
|
1 |
-
import glob
|
2 |
-
import re
|
3 |
-
import subprocess
|
4 |
-
from datetime import datetime
|
5 |
-
|
6 |
-
import matplotlib
|
7 |
-
|
8 |
-
matplotlib.use('Agg')
|
9 |
-
|
10 |
-
from utils.hparams import hparams, set_hparams
|
11 |
-
import random
|
12 |
-
import sys
|
13 |
-
import numpy as np
|
14 |
-
import torch.distributed as dist
|
15 |
-
from pytorch_lightning.loggers import TensorBoardLogger
|
16 |
-
from utils.pl_utils import LatestModelCheckpoint, BaseTrainer, data_loader, DDP
|
17 |
-
from torch import nn
|
18 |
-
import torch.utils.data
|
19 |
-
import utils
|
20 |
-
import logging
|
21 |
-
import os
|
22 |
-
|
23 |
-
torch.multiprocessing.set_sharing_strategy(os.getenv('TORCH_SHARE_STRATEGY', 'file_system'))
|
24 |
-
|
25 |
-
log_format = '%(asctime)s %(message)s'
|
26 |
-
logging.basicConfig(stream=sys.stdout, level=logging.INFO,
|
27 |
-
format=log_format, datefmt='%m/%d %I:%M:%S %p')
|
28 |
-
|
29 |
-
|
30 |
-
class BaseDataset(torch.utils.data.Dataset):
|
31 |
-
def __init__(self, shuffle):
|
32 |
-
super().__init__()
|
33 |
-
self.hparams = hparams
|
34 |
-
self.shuffle = shuffle
|
35 |
-
self.sort_by_len = hparams['sort_by_len']
|
36 |
-
self.sizes = None
|
37 |
-
|
38 |
-
@property
|
39 |
-
def _sizes(self):
|
40 |
-
return self.sizes
|
41 |
-
|
42 |
-
def __getitem__(self, index):
|
43 |
-
raise NotImplementedError
|
44 |
-
|
45 |
-
def collater(self, samples):
|
46 |
-
raise NotImplementedError
|
47 |
-
|
48 |
-
def __len__(self):
|
49 |
-
return len(self._sizes)
|
50 |
-
|
51 |
-
def num_tokens(self, index):
|
52 |
-
return self.size(index)
|
53 |
-
|
54 |
-
def size(self, index):
|
55 |
-
"""Return an example's size as a float or tuple. This value is used when
|
56 |
-
filtering a dataset with ``--max-positions``."""
|
57 |
-
size = min(self._sizes[index], hparams['max_frames'])
|
58 |
-
return size
|
59 |
-
|
60 |
-
def ordered_indices(self):
|
61 |
-
"""Return an ordered list of indices. Batches will be constructed based
|
62 |
-
on this order."""
|
63 |
-
if self.shuffle:
|
64 |
-
indices = np.random.permutation(len(self))
|
65 |
-
if self.sort_by_len:
|
66 |
-
indices = indices[np.argsort(np.array(self._sizes)[indices], kind='mergesort')]
|
67 |
-
# 先random, 然后稳定排序, 保证排序后同长度的数据顺序是依照random permutation的 (被其随机打乱).
|
68 |
-
else:
|
69 |
-
indices = np.arange(len(self))
|
70 |
-
return indices
|
71 |
-
|
72 |
-
@property
|
73 |
-
def num_workers(self):
|
74 |
-
return int(os.getenv('NUM_WORKERS', hparams['ds_workers']))
|
75 |
-
|
76 |
-
|
77 |
-
class BaseTask(nn.Module):
|
78 |
-
def __init__(self, *args, **kwargs):
|
79 |
-
# dataset configs
|
80 |
-
super(BaseTask, self).__init__(*args, **kwargs)
|
81 |
-
self.current_epoch = 0
|
82 |
-
self.global_step = 0
|
83 |
-
self.loaded_optimizer_states_dict = {}
|
84 |
-
self.trainer = None
|
85 |
-
self.logger = None
|
86 |
-
self.on_gpu = False
|
87 |
-
self.use_dp = False
|
88 |
-
self.use_ddp = False
|
89 |
-
self.example_input_array = None
|
90 |
-
|
91 |
-
self.max_tokens = hparams['max_tokens']
|
92 |
-
self.max_sentences = hparams['max_sentences']
|
93 |
-
self.max_eval_tokens = hparams['max_eval_tokens']
|
94 |
-
if self.max_eval_tokens == -1:
|
95 |
-
hparams['max_eval_tokens'] = self.max_eval_tokens = self.max_tokens
|
96 |
-
self.max_eval_sentences = hparams['max_eval_sentences']
|
97 |
-
if self.max_eval_sentences == -1:
|
98 |
-
hparams['max_eval_sentences'] = self.max_eval_sentences = self.max_sentences
|
99 |
-
|
100 |
-
self.model = None
|
101 |
-
self.training_losses_meter = None
|
102 |
-
|
103 |
-
###########
|
104 |
-
# Training, validation and testing
|
105 |
-
###########
|
106 |
-
def build_model(self):
|
107 |
-
raise NotImplementedError
|
108 |
-
|
109 |
-
def load_ckpt(self, ckpt_base_dir, current_model_name=None, model_name='model', force=True, strict=True):
|
110 |
-
# This function is updated on 2021.12.13
|
111 |
-
if current_model_name is None:
|
112 |
-
current_model_name = model_name
|
113 |
-
utils.load_ckpt(self.__getattr__(current_model_name), ckpt_base_dir, current_model_name, force, strict)
|
114 |
-
|
115 |
-
def on_epoch_start(self):
|
116 |
-
self.training_losses_meter = {'total_loss': utils.AvgrageMeter()}
|
117 |
-
|
118 |
-
def _training_step(self, sample, batch_idx, optimizer_idx):
|
119 |
-
"""
|
120 |
-
|
121 |
-
:param sample:
|
122 |
-
:param batch_idx:
|
123 |
-
:return: total loss: torch.Tensor, loss_log: dict
|
124 |
-
"""
|
125 |
-
raise NotImplementedError
|
126 |
-
|
127 |
-
def training_step(self, sample, batch_idx, optimizer_idx=-1):
|
128 |
-
loss_ret = self._training_step(sample, batch_idx, optimizer_idx)
|
129 |
-
self.opt_idx = optimizer_idx
|
130 |
-
if loss_ret is None:
|
131 |
-
return {'loss': None}
|
132 |
-
total_loss, log_outputs = loss_ret
|
133 |
-
log_outputs = utils.tensors_to_scalars(log_outputs)
|
134 |
-
for k, v in log_outputs.items():
|
135 |
-
if k not in self.training_losses_meter:
|
136 |
-
self.training_losses_meter[k] = utils.AvgrageMeter()
|
137 |
-
if not np.isnan(v):
|
138 |
-
self.training_losses_meter[k].update(v)
|
139 |
-
self.training_losses_meter['total_loss'].update(total_loss.item())
|
140 |
-
|
141 |
-
try:
|
142 |
-
log_outputs['lr'] = self.scheduler.get_lr()
|
143 |
-
if isinstance(log_outputs['lr'], list):
|
144 |
-
log_outputs['lr'] = log_outputs['lr'][0]
|
145 |
-
except:
|
146 |
-
pass
|
147 |
-
|
148 |
-
# log_outputs['all_loss'] = total_loss.item()
|
149 |
-
progress_bar_log = log_outputs
|
150 |
-
tb_log = {f'tr/{k}': v for k, v in log_outputs.items()}
|
151 |
-
return {
|
152 |
-
'loss': total_loss,
|
153 |
-
'progress_bar': progress_bar_log,
|
154 |
-
'log': tb_log
|
155 |
-
}
|
156 |
-
|
157 |
-
def optimizer_step(self, epoch, batch_idx, optimizer, optimizer_idx):
|
158 |
-
optimizer.step()
|
159 |
-
optimizer.zero_grad()
|
160 |
-
if self.scheduler is not None:
|
161 |
-
self.scheduler.step(self.global_step // hparams['accumulate_grad_batches'])
|
162 |
-
|
163 |
-
def on_epoch_end(self):
|
164 |
-
loss_outputs = {k: round(v.avg, 4) for k, v in self.training_losses_meter.items()}
|
165 |
-
print(f"\n==============\n "
|
166 |
-
f"Epoch {self.current_epoch} ended. Steps: {self.global_step}. {loss_outputs}"
|
167 |
-
f"\n==============\n")
|
168 |
-
|
169 |
-
def validation_step(self, sample, batch_idx):
|
170 |
-
"""
|
171 |
-
|
172 |
-
:param sample:
|
173 |
-
:param batch_idx:
|
174 |
-
:return: output: dict
|
175 |
-
"""
|
176 |
-
raise NotImplementedError
|
177 |
-
|
178 |
-
def _validation_end(self, outputs):
|
179 |
-
"""
|
180 |
-
|
181 |
-
:param outputs:
|
182 |
-
:return: loss_output: dict
|
183 |
-
"""
|
184 |
-
raise NotImplementedError
|
185 |
-
|
186 |
-
def validation_end(self, outputs):
|
187 |
-
loss_output = self._validation_end(outputs)
|
188 |
-
print(f"\n==============\n "
|
189 |
-
f"valid results: {loss_output}"
|
190 |
-
f"\n==============\n")
|
191 |
-
return {
|
192 |
-
'log': {f'val/{k}': v for k, v in loss_output.items()},
|
193 |
-
'val_loss': loss_output['total_loss']
|
194 |
-
}
|
195 |
-
|
196 |
-
def build_scheduler(self, optimizer):
|
197 |
-
raise NotImplementedError
|
198 |
-
|
199 |
-
def build_optimizer(self, model):
|
200 |
-
raise NotImplementedError
|
201 |
-
|
202 |
-
def configure_optimizers(self):
|
203 |
-
optm = self.build_optimizer(self.model)
|
204 |
-
self.scheduler = self.build_scheduler(optm)
|
205 |
-
return [optm]
|
206 |
-
|
207 |
-
def test_start(self):
|
208 |
-
pass
|
209 |
-
|
210 |
-
def test_step(self, sample, batch_idx):
|
211 |
-
return self.validation_step(sample, batch_idx)
|
212 |
-
|
213 |
-
def test_end(self, outputs):
|
214 |
-
return self.validation_end(outputs)
|
215 |
-
|
216 |
-
###########
|
217 |
-
# Running configuration
|
218 |
-
###########
|
219 |
-
|
220 |
-
@classmethod
|
221 |
-
def start(cls):
|
222 |
-
set_hparams()
|
223 |
-
os.environ['MASTER_PORT'] = str(random.randint(15000, 30000))
|
224 |
-
random.seed(hparams['seed'])
|
225 |
-
np.random.seed(hparams['seed'])
|
226 |
-
task = cls()
|
227 |
-
work_dir = hparams['work_dir']
|
228 |
-
trainer = BaseTrainer(checkpoint_callback=LatestModelCheckpoint(
|
229 |
-
filepath=work_dir,
|
230 |
-
verbose=True,
|
231 |
-
monitor='val_loss',
|
232 |
-
mode='min',
|
233 |
-
num_ckpt_keep=hparams['num_ckpt_keep'],
|
234 |
-
save_best=hparams['save_best'],
|
235 |
-
period=1 if hparams['save_ckpt'] else 100000
|
236 |
-
),
|
237 |
-
logger=TensorBoardLogger(
|
238 |
-
save_dir=work_dir,
|
239 |
-
name='lightning_logs',
|
240 |
-
version='lastest'
|
241 |
-
),
|
242 |
-
gradient_clip_val=hparams['clip_grad_norm'],
|
243 |
-
val_check_interval=hparams['val_check_interval'],
|
244 |
-
row_log_interval=hparams['log_interval'],
|
245 |
-
max_updates=hparams['max_updates'],
|
246 |
-
num_sanity_val_steps=hparams['num_sanity_val_steps'] if not hparams[
|
247 |
-
'validate'] else 10000,
|
248 |
-
accumulate_grad_batches=hparams['accumulate_grad_batches'])
|
249 |
-
if not hparams['infer']: # train
|
250 |
-
t = datetime.now().strftime('%Y%m%d%H%M%S')
|
251 |
-
code_dir = f'{work_dir}/codes/{t}'
|
252 |
-
subprocess.check_call(f'mkdir -p "{code_dir}"', shell=True)
|
253 |
-
for c in hparams['save_codes']:
|
254 |
-
subprocess.check_call(f'cp -r "{c}" "{code_dir}/"', shell=True)
|
255 |
-
print(f"| Copied codes to {code_dir}.")
|
256 |
-
trainer.checkpoint_callback.task = task
|
257 |
-
trainer.fit(task)
|
258 |
-
else:
|
259 |
-
trainer.test(task)
|
260 |
-
|
261 |
-
def configure_ddp(self, model, device_ids):
|
262 |
-
model = DDP(
|
263 |
-
model,
|
264 |
-
device_ids=device_ids,
|
265 |
-
find_unused_parameters=True
|
266 |
-
)
|
267 |
-
if dist.get_rank() != 0 and not hparams['debug']:
|
268 |
-
sys.stdout = open(os.devnull, "w")
|
269 |
-
sys.stderr = open(os.devnull, "w")
|
270 |
-
random.seed(hparams['seed'])
|
271 |
-
np.random.seed(hparams['seed'])
|
272 |
-
return model
|
273 |
-
|
274 |
-
def training_end(self, *args, **kwargs):
|
275 |
-
return None
|
276 |
-
|
277 |
-
def init_ddp_connection(self, proc_rank, world_size):
|
278 |
-
set_hparams(print_hparams=False)
|
279 |
-
# guarantees unique ports across jobs from same grid search
|
280 |
-
default_port = 12910
|
281 |
-
# if user gave a port number, use that one instead
|
282 |
-
try:
|
283 |
-
default_port = os.environ['MASTER_PORT']
|
284 |
-
except Exception:
|
285 |
-
os.environ['MASTER_PORT'] = str(default_port)
|
286 |
-
|
287 |
-
# figure out the root node addr
|
288 |
-
root_node = '127.0.0.2'
|
289 |
-
root_node = self.trainer.resolve_root_node_address(root_node)
|
290 |
-
os.environ['MASTER_ADDR'] = root_node
|
291 |
-
dist.init_process_group('nccl', rank=proc_rank, world_size=world_size)
|
292 |
-
|
293 |
-
@data_loader
|
294 |
-
def train_dataloader(self):
|
295 |
-
return None
|
296 |
-
|
297 |
-
@data_loader
|
298 |
-
def test_dataloader(self):
|
299 |
-
return None
|
300 |
-
|
301 |
-
@data_loader
|
302 |
-
def val_dataloader(self):
|
303 |
-
return None
|
304 |
-
|
305 |
-
def on_load_checkpoint(self, checkpoint):
|
306 |
-
pass
|
307 |
-
|
308 |
-
def on_save_checkpoint(self, checkpoint):
|
309 |
-
pass
|
310 |
-
|
311 |
-
def on_sanity_check_start(self):
|
312 |
-
pass
|
313 |
-
|
314 |
-
def on_train_start(self):
|
315 |
-
pass
|
316 |
-
|
317 |
-
def on_train_end(self):
|
318 |
-
pass
|
319 |
-
|
320 |
-
def on_batch_start(self, batch):
|
321 |
-
pass
|
322 |
-
|
323 |
-
def on_batch_end(self):
|
324 |
-
pass
|
325 |
-
|
326 |
-
def on_pre_performance_check(self):
|
327 |
-
pass
|
328 |
-
|
329 |
-
def on_post_performance_check(self):
|
330 |
-
pass
|
331 |
-
|
332 |
-
def on_before_zero_grad(self, optimizer):
|
333 |
-
pass
|
334 |
-
|
335 |
-
def on_after_backward(self):
|
336 |
-
pass
|
337 |
-
|
338 |
-
def backward(self, loss, optimizer):
|
339 |
-
loss.backward()
|
340 |
-
|
341 |
-
def grad_norm(self, norm_type):
|
342 |
-
results = {}
|
343 |
-
total_norm = 0
|
344 |
-
for name, p in self.named_parameters():
|
345 |
-
if p.requires_grad:
|
346 |
-
try:
|
347 |
-
param_norm = p.grad.data.norm(norm_type)
|
348 |
-
total_norm += param_norm ** norm_type
|
349 |
-
norm = param_norm ** (1 / norm_type)
|
350 |
-
|
351 |
-
grad = round(norm.data.cpu().numpy().flatten()[0], 3)
|
352 |
-
results['grad_{}_norm_{}'.format(norm_type, name)] = grad
|
353 |
-
except Exception:
|
354 |
-
# this param had no grad
|
355 |
-
pass
|
356 |
-
|
357 |
-
total_norm = total_norm ** (1. / norm_type)
|
358 |
-
grad = round(total_norm.data.cpu().numpy().flatten()[0], 3)
|
359 |
-
results['grad_{}_norm_total'.format(norm_type)] = grad
|
360 |
-
return results
|
|
|
|
|
|
|
|
|
|
|
|
|
|
|
|
|
|
|
|
|
|
|
|
|
|
|
|
|
|
|
|
|
|
|
|
|
|
|
|
|
|
|
|
|
|
|
|
|
|
|
|
|
|
|
|
|
|
|
|
|
|
|
|
|
|
|
|
|
|
|
|
|
|
|
|
|
|
|
|
|
|
|
|
|
|
|
|
|
|
|
|
|
|
|
|
|
|
|
|
|
|
|
|
|
|
|
|
|
|
|
|
|
|
|
|
|
|
|
|
|
|
|
|
|
|
|
|
|
|
|
|
|
|
|
|
|
|
|
|
|
|
|
|
|
|
|
|
|
|
|
|
|
|
|
|
|
|
|
|
|
|
|
|
|
|
|
|
|
|
|
|
|
|
|
|
|
|
|
|
|
|
|
|
|
|
|
|
|
|
|
|
|
|
|
|
|
|
|
|
|
|
|
|
|
|
|
|
|
|
|
|
|
|
|
|
|
|
|
|
|
|
|
|
|
|
|
|
|
|
|
|
|
|
|
|
|
|
|
|
|
|
|
|
|
|
|
|
|
|
|
|
|
|
|
|
|
|
|
|
|
|
|
|
|
|
|
|
|
|
|
|
|
|
|
|
|
|
|
|
|
|
|
|
|
|
|
|
|
|
|
|
|
|
|
|
|
|
|
|
|
|
|
|
|
|
|
|
|
|
|
|
|
|
|
|
|
|
|
|
|
|
|
|
|
|
|
|
|
|
|
|
|
|
|
|
|
|
|
|
|
|
|
|
|
|
|
|
|
|
|
|
|
|
|
|
|
|
|
|
|
|
|
|
|
|
|
|
|
|
|
|
|
|
|
|
|
|
|
|
|
|
|
|
|
|
|
|
|
|
|
|
|
|
|
|
|
|
|
|
|
|
|
|
|
|
|
|
|
|
|
|
|
|
|
|
|
|
|
|
|
|
|
|
|
|
|
|
|
|
|
|
|
|
|
|
|
|
|
|
|
|
|
|
|
|
|
|
|
|
|
|
|
|
|
|
|
|
|
|
|
|
|
|
|
|
|
|
|
|
|
|
|
|
|
|
|
|
|
|
|
|
|
|
|
|
|
|
|
|
|
|
|
|
|
|
|
|
|
|
|
|
|
|
|
|
|
|
|
|
|
|
|
|
|
|
|
|
|
|
|
|
|
|
|
|
|
|
|
|
|
|
|
|
|
|
|
|
|
|
|
|
|
|
|
|
|
|
|
|
|
|
|
|
|
|
|
|
|
|
|
|
|
|
|
|
|
|
|
|
|
|
|
|
|
|
|
|
|
|
|
|
|
|
|
|
|
|
|
|
|
|
|
|
|
|
|
|
|
|
|
|
|
|
|
|
|
|
|
|
|
|
|
|
|
|
|
|
|
|
|
|
|
|
|
|
|
|
|
|
|
|
|
|
|
|
|
|
|
|
|
|
|
|
|
|
|
|
|
|
|
|
|
|
|
|
|
|
|
|
|
|
|
|
|
|
|
|
|
|
|
|
|
|
|
|
|
|
|
|
|
|
|
|
|
|
|
|
|
|
|
|
|
|
|
|
|
|
|
|
|
|
|
|
|
|
|
|
|
|
|
|
|
|
|
|
|
|
|
|
|
|
spaces/AIGC-Audio/AudioGPT/text_to_audio/Make_An_Audio/ldm/modules/encoders/open_clap/feature_fusion.py
DELETED
@@ -1,193 +0,0 @@
|
|
1 |
-
'''
|
2 |
-
Feature Fusion for Varible-Length Data Processing
|
3 |
-
AFF/iAFF is referred and modified from https://github.com/YimianDai/open-aff/blob/master/aff_pytorch/aff_net/fusion.py
|
4 |
-
According to the paper: Yimian Dai et al, Attentional Feature Fusion, IEEE Winter Conference on Applications of Computer Vision, WACV 2021
|
5 |
-
'''
|
6 |
-
|
7 |
-
import torch
|
8 |
-
import torch.nn as nn
|
9 |
-
|
10 |
-
|
11 |
-
class DAF(nn.Module):
|
12 |
-
'''
|
13 |
-
直接相加 DirectAddFuse
|
14 |
-
'''
|
15 |
-
|
16 |
-
def __init__(self):
|
17 |
-
super(DAF, self).__init__()
|
18 |
-
|
19 |
-
def forward(self, x, residual):
|
20 |
-
return x + residual
|
21 |
-
|
22 |
-
|
23 |
-
class iAFF(nn.Module):
|
24 |
-
'''
|
25 |
-
多特征融合 iAFF
|
26 |
-
'''
|
27 |
-
|
28 |
-
def __init__(self, channels=64, r=4, type='2D'):
|
29 |
-
super(iAFF, self).__init__()
|
30 |
-
inter_channels = int(channels // r)
|
31 |
-
|
32 |
-
if type == '1D':
|
33 |
-
# 本地注意力
|
34 |
-
self.local_att = nn.Sequential(
|
35 |
-
nn.Conv1d(channels, inter_channels, kernel_size=1, stride=1, padding=0),
|
36 |
-
nn.BatchNorm1d(inter_channels),
|
37 |
-
nn.ReLU(inplace=True),
|
38 |
-
nn.Conv1d(inter_channels, channels, kernel_size=1, stride=1, padding=0),
|
39 |
-
nn.BatchNorm1d(channels),
|
40 |
-
)
|
41 |
-
|
42 |
-
# 全局注意力
|
43 |
-
self.global_att = nn.Sequential(
|
44 |
-
nn.AdaptiveAvgPool1d(1),
|
45 |
-
nn.Conv1d(channels, inter_channels, kernel_size=1, stride=1, padding=0),
|
46 |
-
nn.BatchNorm1d(inter_channels),
|
47 |
-
nn.ReLU(inplace=True),
|
48 |
-
nn.Conv1d(inter_channels, channels, kernel_size=1, stride=1, padding=0),
|
49 |
-
nn.BatchNorm1d(channels),
|
50 |
-
)
|
51 |
-
|
52 |
-
# 第二次本地注意力
|
53 |
-
self.local_att2 = nn.Sequential(
|
54 |
-
nn.Conv1d(channels, inter_channels, kernel_size=1, stride=1, padding=0),
|
55 |
-
nn.BatchNorm1d(inter_channels),
|
56 |
-
nn.ReLU(inplace=True),
|
57 |
-
nn.Conv1d(inter_channels, channels, kernel_size=1, stride=1, padding=0),
|
58 |
-
nn.BatchNorm1d(channels),
|
59 |
-
)
|
60 |
-
# 第二次全局注意力
|
61 |
-
self.global_att2 = nn.Sequential(
|
62 |
-
nn.AdaptiveAvgPool1d(1),
|
63 |
-
nn.Conv1d(channels, inter_channels, kernel_size=1, stride=1, padding=0),
|
64 |
-
nn.BatchNorm1d(inter_channels),
|
65 |
-
nn.ReLU(inplace=True),
|
66 |
-
nn.Conv1d(inter_channels, channels, kernel_size=1, stride=1, padding=0),
|
67 |
-
nn.BatchNorm1d(channels),
|
68 |
-
)
|
69 |
-
elif type == '2D':
|
70 |
-
# 本地注意力
|
71 |
-
self.local_att = nn.Sequential(
|
72 |
-
nn.Conv2d(channels, inter_channels, kernel_size=1, stride=1, padding=0),
|
73 |
-
nn.BatchNorm2d(inter_channels),
|
74 |
-
nn.ReLU(inplace=True),
|
75 |
-
nn.Conv2d(inter_channels, channels, kernel_size=1, stride=1, padding=0),
|
76 |
-
nn.BatchNorm2d(channels),
|
77 |
-
)
|
78 |
-
|
79 |
-
# 全局注意力
|
80 |
-
self.global_att = nn.Sequential(
|
81 |
-
nn.AdaptiveAvgPool2d(1),
|
82 |
-
nn.Conv2d(channels, inter_channels, kernel_size=1, stride=1, padding=0),
|
83 |
-
nn.BatchNorm2d(inter_channels),
|
84 |
-
nn.ReLU(inplace=True),
|
85 |
-
nn.Conv2d(inter_channels, channels, kernel_size=1, stride=1, padding=0),
|
86 |
-
nn.BatchNorm2d(channels),
|
87 |
-
)
|
88 |
-
|
89 |
-
# 第二次本地注意力
|
90 |
-
self.local_att2 = nn.Sequential(
|
91 |
-
nn.Conv2d(channels, inter_channels, kernel_size=1, stride=1, padding=0),
|
92 |
-
nn.BatchNorm2d(inter_channels),
|
93 |
-
nn.ReLU(inplace=True),
|
94 |
-
nn.Conv2d(inter_channels, channels, kernel_size=1, stride=1, padding=0),
|
95 |
-
nn.BatchNorm2d(channels),
|
96 |
-
)
|
97 |
-
# 第二次全局注意力
|
98 |
-
self.global_att2 = nn.Sequential(
|
99 |
-
nn.AdaptiveAvgPool2d(1),
|
100 |
-
nn.Conv2d(channels, inter_channels, kernel_size=1, stride=1, padding=0),
|
101 |
-
nn.BatchNorm2d(inter_channels),
|
102 |
-
nn.ReLU(inplace=True),
|
103 |
-
nn.Conv2d(inter_channels, channels, kernel_size=1, stride=1, padding=0),
|
104 |
-
nn.BatchNorm2d(channels),
|
105 |
-
)
|
106 |
-
else:
|
107 |
-
raise f'the type is not supported'
|
108 |
-
|
109 |
-
self.sigmoid = nn.Sigmoid()
|
110 |
-
|
111 |
-
def forward(self, x, residual):
|
112 |
-
flag = False
|
113 |
-
xa = x + residual
|
114 |
-
if xa.size(0) == 1:
|
115 |
-
xa = torch.cat([xa,xa],dim=0)
|
116 |
-
flag = True
|
117 |
-
xl = self.local_att(xa)
|
118 |
-
xg = self.global_att(xa)
|
119 |
-
xlg = xl + xg
|
120 |
-
wei = self.sigmoid(xlg)
|
121 |
-
xi = x * wei + residual * (1 - wei)
|
122 |
-
|
123 |
-
xl2 = self.local_att2(xi)
|
124 |
-
xg2 = self.global_att(xi)
|
125 |
-
xlg2 = xl2 + xg2
|
126 |
-
wei2 = self.sigmoid(xlg2)
|
127 |
-
xo = x * wei2 + residual * (1 - wei2)
|
128 |
-
if flag:
|
129 |
-
xo = xo[0].unsqueeze(0)
|
130 |
-
return xo
|
131 |
-
|
132 |
-
|
133 |
-
class AFF(nn.Module):
|
134 |
-
'''
|
135 |
-
多特征融合 AFF
|
136 |
-
'''
|
137 |
-
|
138 |
-
def __init__(self, channels=64, r=4, type='2D'):
|
139 |
-
super(AFF, self).__init__()
|
140 |
-
inter_channels = int(channels // r)
|
141 |
-
|
142 |
-
if type == '1D':
|
143 |
-
self.local_att = nn.Sequential(
|
144 |
-
nn.Conv1d(channels, inter_channels, kernel_size=1, stride=1, padding=0),
|
145 |
-
nn.BatchNorm1d(inter_channels),
|
146 |
-
nn.ReLU(inplace=True),
|
147 |
-
nn.Conv1d(inter_channels, channels, kernel_size=1, stride=1, padding=0),
|
148 |
-
nn.BatchNorm1d(channels),
|
149 |
-
)
|
150 |
-
self.global_att = nn.Sequential(
|
151 |
-
nn.AdaptiveAvgPool1d(1),
|
152 |
-
nn.Conv1d(channels, inter_channels, kernel_size=1, stride=1, padding=0),
|
153 |
-
nn.BatchNorm1d(inter_channels),
|
154 |
-
nn.ReLU(inplace=True),
|
155 |
-
nn.Conv1d(inter_channels, channels, kernel_size=1, stride=1, padding=0),
|
156 |
-
nn.BatchNorm1d(channels),
|
157 |
-
)
|
158 |
-
elif type == '2D':
|
159 |
-
self.local_att = nn.Sequential(
|
160 |
-
nn.Conv2d(channels, inter_channels, kernel_size=1, stride=1, padding=0),
|
161 |
-
nn.BatchNorm2d(inter_channels),
|
162 |
-
nn.ReLU(inplace=True),
|
163 |
-
nn.Conv2d(inter_channels, channels, kernel_size=1, stride=1, padding=0),
|
164 |
-
nn.BatchNorm2d(channels),
|
165 |
-
)
|
166 |
-
self.global_att = nn.Sequential(
|
167 |
-
nn.AdaptiveAvgPool2d(1),
|
168 |
-
nn.Conv2d(channels, inter_channels, kernel_size=1, stride=1, padding=0),
|
169 |
-
nn.BatchNorm2d(inter_channels),
|
170 |
-
nn.ReLU(inplace=True),
|
171 |
-
nn.Conv2d(inter_channels, channels, kernel_size=1, stride=1, padding=0),
|
172 |
-
nn.BatchNorm2d(channels),
|
173 |
-
)
|
174 |
-
else:
|
175 |
-
raise f'the type is not supported.'
|
176 |
-
|
177 |
-
self.sigmoid = nn.Sigmoid()
|
178 |
-
|
179 |
-
def forward(self, x, residual):
|
180 |
-
flag = False
|
181 |
-
xa = x + residual
|
182 |
-
if xa.size(0) == 1:
|
183 |
-
xa = torch.cat([xa,xa],dim=0)
|
184 |
-
flag = True
|
185 |
-
xl = self.local_att(xa)
|
186 |
-
xg = self.global_att(xa)
|
187 |
-
xlg = xl + xg
|
188 |
-
wei = self.sigmoid(xlg)
|
189 |
-
xo = 2 * x * wei + 2 * residual * (1 - wei)
|
190 |
-
if flag:
|
191 |
-
xo = xo[0].unsqueeze(0)
|
192 |
-
return xo
|
193 |
-
|
|
|
|
|
|
|
|
|
|
|
|
|
|
|
|
|
|
|
|
|
|
|
|
|
|
|
|
|
|
|
|
|
|
|
|
|
|
|
|
|
|
|
|
|
|
|
|
|
|
|
|
|
|
|
|
|
|
|
|
|
|
|
|
|
|
|
|
|
|
|
|
|
|
|
|
|
|
|
|
|
|
|
|
|
|
|
|
|
|
|
|
|
|
|
|
|
|
|
|
|
|
|
|
|
|
|
|
|
|
|
|
|
|
|
|
|
|
|
|
|
|
|
|
|
|
|
|
|
|
|
|
|
|
|
|
|
|
|
|
|
|
|
|
|
|
|
|
|
|
|
|
|
|
|
|
|
|
|
|
|
|
|
|
|
|
|
|
|
|
|
|
|
|
|
|
|
|
|
|
|
|
|
|
|
|
|
|
|
|
|
|
|
|
|
|
|
|
|
|
|
|
|
|
|
|
|
|
|
|
|
|
|
|
|
|
|
|
|
|
|
|
|
|
|
|
|
|
|
|
|
|
|
|
|
|
|
|
|
|
|
|
|
|
|
|
|
|
|
|
|
|
|
|
|
|
|
|
|
|
|
|
|
|
|
|
|
|
|
|
|
|
|
|
|
|
|
|
|
|
|
|
|
|
|
|
|
|
|
|
|
|
|
|
|
|
|
|
|
|
|
|
|
|
|
|
|
|
|
|
|
|
|
|
|
|
|
|
|
|
|
|
|
|
|
|
|
|
|
|
|
|
|
|
|
|
|
|
|
|
|
|
|
|
|
|
|
|
|
|
|
|
|
|
|
|
|
|
|
|
|
|
|
|
|
|
|
|
|
|
|
|
|
|
|
|
|
|
|
|
|
|
|
|
|
|
|
|
spaces/AIGC-Audio/Make_An_Audio/ldm/modules/encoders/open_clap/__init__.py
DELETED
@@ -1,8 +0,0 @@
|
|
1 |
-
from .factory import list_models, create_model, create_model_and_transforms, add_model_config
|
2 |
-
from .loss import ClipLoss, gather_features, LPLoss, lp_gather_features, LPMetrics
|
3 |
-
from .model import CLAP, CLAPTextCfg, CLAPVisionCfg, CLAPAudioCfp, convert_weights_to_fp16, trace_model
|
4 |
-
from .openai import load_openai_model, list_openai_models
|
5 |
-
from .pretrained import list_pretrained, list_pretrained_tag_models, list_pretrained_model_tags,\
|
6 |
-
get_pretrained_url, download_pretrained
|
7 |
-
from .tokenizer import SimpleTokenizer, tokenize
|
8 |
-
from .transform import image_transform
|
|
|
|
|
|
|
|
|
|
|
|
|
|
|
|
|
|
spaces/AILab-CVC/SEED-LLaMA/models/seed_qformer/eva_vit.py
DELETED
@@ -1,486 +0,0 @@
|
|
1 |
-
# Based on EVA, BEIT, timm and DeiT code bases
|
2 |
-
# https://github.com/baaivision/EVA
|
3 |
-
# https://github.com/rwightman/pytorch-image-models/tree/master/timm
|
4 |
-
# https://github.com/microsoft/unilm/tree/master/beit
|
5 |
-
# https://github.com/facebookresearch/deit/
|
6 |
-
# https://github.com/facebookresearch/dino
|
7 |
-
# --------------------------------------------------------'
|
8 |
-
import math
|
9 |
-
from functools import partial
|
10 |
-
|
11 |
-
import torch
|
12 |
-
import torch.nn as nn
|
13 |
-
import torch.nn.functional as F
|
14 |
-
import torch.utils.checkpoint as checkpoint
|
15 |
-
from timm.models.layers import drop_path, to_2tuple, trunc_normal_
|
16 |
-
|
17 |
-
|
18 |
-
from .utils import download_cached_file
|
19 |
-
|
20 |
-
|
21 |
-
def _cfg(url='', **kwargs):
|
22 |
-
return {
|
23 |
-
'url': url,
|
24 |
-
'num_classes': 1000,
|
25 |
-
'input_size': (3, 224, 224),
|
26 |
-
'pool_size': None,
|
27 |
-
'crop_pct': .9,
|
28 |
-
'interpolation': 'bicubic',
|
29 |
-
'mean': (0.5, 0.5, 0.5),
|
30 |
-
'std': (0.5, 0.5, 0.5),
|
31 |
-
**kwargs
|
32 |
-
}
|
33 |
-
|
34 |
-
|
35 |
-
class DropPath(nn.Module):
|
36 |
-
"""Drop paths (Stochastic Depth) per sample (when applied in main path of residual blocks).
|
37 |
-
"""
|
38 |
-
def __init__(self, drop_prob=None):
|
39 |
-
super(DropPath, self).__init__()
|
40 |
-
self.drop_prob = drop_prob
|
41 |
-
|
42 |
-
def forward(self, x):
|
43 |
-
return drop_path(x, self.drop_prob, self.training)
|
44 |
-
|
45 |
-
def extra_repr(self) -> str:
|
46 |
-
return 'p={}'.format(self.drop_prob)
|
47 |
-
|
48 |
-
|
49 |
-
class Mlp(nn.Module):
|
50 |
-
def __init__(self, in_features, hidden_features=None, out_features=None, act_layer=nn.GELU, drop=0.):
|
51 |
-
super().__init__()
|
52 |
-
out_features = out_features or in_features
|
53 |
-
hidden_features = hidden_features or in_features
|
54 |
-
self.fc1 = nn.Linear(in_features, hidden_features)
|
55 |
-
self.act = act_layer()
|
56 |
-
self.fc2 = nn.Linear(hidden_features, out_features)
|
57 |
-
self.drop = nn.Dropout(drop)
|
58 |
-
|
59 |
-
def forward(self, x):
|
60 |
-
x = self.fc1(x)
|
61 |
-
x = self.act(x)
|
62 |
-
# x = self.drop(x)
|
63 |
-
# commit this for the orignal BERT implement
|
64 |
-
x = self.fc2(x)
|
65 |
-
x = self.drop(x)
|
66 |
-
return x
|
67 |
-
|
68 |
-
|
69 |
-
class Attention(nn.Module):
|
70 |
-
def __init__(self,
|
71 |
-
dim,
|
72 |
-
num_heads=8,
|
73 |
-
qkv_bias=False,
|
74 |
-
qk_scale=None,
|
75 |
-
attn_drop=0.,
|
76 |
-
proj_drop=0.,
|
77 |
-
window_size=None,
|
78 |
-
attn_head_dim=None):
|
79 |
-
super().__init__()
|
80 |
-
self.num_heads = num_heads
|
81 |
-
head_dim = dim // num_heads
|
82 |
-
if attn_head_dim is not None:
|
83 |
-
head_dim = attn_head_dim
|
84 |
-
all_head_dim = head_dim * self.num_heads
|
85 |
-
self.scale = qk_scale or head_dim**-0.5
|
86 |
-
|
87 |
-
self.qkv = nn.Linear(dim, all_head_dim * 3, bias=False)
|
88 |
-
if qkv_bias:
|
89 |
-
self.q_bias = nn.Parameter(torch.zeros(all_head_dim))
|
90 |
-
self.v_bias = nn.Parameter(torch.zeros(all_head_dim))
|
91 |
-
else:
|
92 |
-
self.q_bias = None
|
93 |
-
self.v_bias = None
|
94 |
-
|
95 |
-
if window_size:
|
96 |
-
self.window_size = window_size
|
97 |
-
self.num_relative_distance = (2 * window_size[0] - 1) * (2 * window_size[1] - 1) + 3
|
98 |
-
self.relative_position_bias_table = nn.Parameter(torch.zeros(self.num_relative_distance,
|
99 |
-
num_heads)) # 2*Wh-1 * 2*Ww-1, nH
|
100 |
-
# cls to token & token 2 cls & cls to cls
|
101 |
-
|
102 |
-
# get pair-wise relative position index for each token inside the window
|
103 |
-
coords_h = torch.arange(window_size[0])
|
104 |
-
coords_w = torch.arange(window_size[1])
|
105 |
-
coords = torch.stack(torch.meshgrid([coords_h, coords_w])) # 2, Wh, Ww
|
106 |
-
coords_flatten = torch.flatten(coords, 1) # 2, Wh*Ww
|
107 |
-
relative_coords = coords_flatten[:, :, None] - coords_flatten[:, None, :] # 2, Wh*Ww, Wh*Ww
|
108 |
-
relative_coords = relative_coords.permute(1, 2, 0).contiguous() # Wh*Ww, Wh*Ww, 2
|
109 |
-
relative_coords[:, :, 0] += window_size[0] - 1 # shift to start from 0
|
110 |
-
relative_coords[:, :, 1] += window_size[1] - 1
|
111 |
-
relative_coords[:, :, 0] *= 2 * window_size[1] - 1
|
112 |
-
relative_position_index = \
|
113 |
-
torch.zeros(size=(window_size[0] * window_size[1] + 1, ) * 2, dtype=relative_coords.dtype)
|
114 |
-
relative_position_index[1:, 1:] = relative_coords.sum(-1) # Wh*Ww, Wh*Ww
|
115 |
-
relative_position_index[0, 0:] = self.num_relative_distance - 3
|
116 |
-
relative_position_index[0:, 0] = self.num_relative_distance - 2
|
117 |
-
relative_position_index[0, 0] = self.num_relative_distance - 1
|
118 |
-
|
119 |
-
self.register_buffer("relative_position_index", relative_position_index)
|
120 |
-
else:
|
121 |
-
self.window_size = None
|
122 |
-
self.relative_position_bias_table = None
|
123 |
-
self.relative_position_index = None
|
124 |
-
|
125 |
-
self.attn_drop = nn.Dropout(attn_drop)
|
126 |
-
self.proj = nn.Linear(all_head_dim, dim)
|
127 |
-
self.proj_drop = nn.Dropout(proj_drop)
|
128 |
-
|
129 |
-
def forward(self, x, rel_pos_bias=None):
|
130 |
-
B, N, C = x.shape
|
131 |
-
qkv_bias = None
|
132 |
-
if self.q_bias is not None:
|
133 |
-
qkv_bias = torch.cat((self.q_bias, torch.zeros_like(self.v_bias, requires_grad=False), self.v_bias))
|
134 |
-
# qkv = self.qkv(x).reshape(B, N, 3, self.num_heads, C // self.num_heads).permute(2, 0, 3, 1, 4)
|
135 |
-
qkv = F.linear(input=x, weight=self.qkv.weight, bias=qkv_bias)
|
136 |
-
qkv = qkv.reshape(B, N, 3, self.num_heads, -1).permute(2, 0, 3, 1, 4)
|
137 |
-
q, k, v = qkv[0], qkv[1], qkv[2] # make torchscript happy (cannot use tensor as tuple)
|
138 |
-
|
139 |
-
q = q * self.scale
|
140 |
-
attn = (q @ k.transpose(-2, -1))
|
141 |
-
|
142 |
-
if self.relative_position_bias_table is not None:
|
143 |
-
relative_position_bias = \
|
144 |
-
self.relative_position_bias_table[self.relative_position_index.view(-1)].view(
|
145 |
-
self.window_size[0] * self.window_size[1] + 1,
|
146 |
-
self.window_size[0] * self.window_size[1] + 1, -1) # Wh*Ww,Wh*Ww,nH
|
147 |
-
relative_position_bias = relative_position_bias.permute(2, 0, 1).contiguous() # nH, Wh*Ww, Wh*Ww
|
148 |
-
attn = attn + relative_position_bias.unsqueeze(0)
|
149 |
-
|
150 |
-
if rel_pos_bias is not None:
|
151 |
-
attn = attn + rel_pos_bias
|
152 |
-
|
153 |
-
attn = attn.softmax(dim=-1)
|
154 |
-
attn = self.attn_drop(attn)
|
155 |
-
|
156 |
-
x = (attn @ v).transpose(1, 2).reshape(B, N, -1)
|
157 |
-
x = self.proj(x)
|
158 |
-
x = self.proj_drop(x)
|
159 |
-
return x
|
160 |
-
|
161 |
-
|
162 |
-
class Block(nn.Module):
|
163 |
-
def __init__(self,
|
164 |
-
dim,
|
165 |
-
num_heads,
|
166 |
-
mlp_ratio=4.,
|
167 |
-
qkv_bias=False,
|
168 |
-
qk_scale=None,
|
169 |
-
drop=0.,
|
170 |
-
attn_drop=0.,
|
171 |
-
drop_path=0.,
|
172 |
-
init_values=None,
|
173 |
-
act_layer=nn.GELU,
|
174 |
-
norm_layer=nn.LayerNorm,
|
175 |
-
window_size=None,
|
176 |
-
attn_head_dim=None):
|
177 |
-
super().__init__()
|
178 |
-
self.norm1 = norm_layer(dim)
|
179 |
-
self.attn = Attention(dim,
|
180 |
-
num_heads=num_heads,
|
181 |
-
qkv_bias=qkv_bias,
|
182 |
-
qk_scale=qk_scale,
|
183 |
-
attn_drop=attn_drop,
|
184 |
-
proj_drop=drop,
|
185 |
-
window_size=window_size,
|
186 |
-
attn_head_dim=attn_head_dim)
|
187 |
-
# NOTE: drop path for stochastic depth, we shall see if this is better than dropout here
|
188 |
-
self.drop_path = DropPath(drop_path) if drop_path > 0. else nn.Identity()
|
189 |
-
self.norm2 = norm_layer(dim)
|
190 |
-
mlp_hidden_dim = int(dim * mlp_ratio)
|
191 |
-
self.mlp = Mlp(in_features=dim, hidden_features=mlp_hidden_dim, act_layer=act_layer, drop=drop)
|
192 |
-
|
193 |
-
if init_values is not None and init_values > 0:
|
194 |
-
self.gamma_1 = nn.Parameter(init_values * torch.ones((dim)), requires_grad=True)
|
195 |
-
self.gamma_2 = nn.Parameter(init_values * torch.ones((dim)), requires_grad=True)
|
196 |
-
else:
|
197 |
-
self.gamma_1, self.gamma_2 = None, None
|
198 |
-
|
199 |
-
def forward(self, x, rel_pos_bias=None):
|
200 |
-
if self.gamma_1 is None:
|
201 |
-
x = x + self.drop_path(self.attn(self.norm1(x), rel_pos_bias=rel_pos_bias))
|
202 |
-
x = x + self.drop_path(self.mlp(self.norm2(x)))
|
203 |
-
else:
|
204 |
-
x = x + self.drop_path(self.gamma_1 * self.attn(self.norm1(x), rel_pos_bias=rel_pos_bias))
|
205 |
-
x = x + self.drop_path(self.gamma_2 * self.mlp(self.norm2(x)))
|
206 |
-
return x
|
207 |
-
|
208 |
-
|
209 |
-
class PatchEmbed(nn.Module):
|
210 |
-
""" Image to Patch Embedding
|
211 |
-
"""
|
212 |
-
def __init__(self, img_size=224, patch_size=16, in_chans=3, embed_dim=768):
|
213 |
-
super().__init__()
|
214 |
-
img_size = to_2tuple(img_size)
|
215 |
-
patch_size = to_2tuple(patch_size)
|
216 |
-
num_patches = (img_size[1] // patch_size[1]) * (img_size[0] // patch_size[0])
|
217 |
-
self.patch_shape = (img_size[0] // patch_size[0], img_size[1] // patch_size[1])
|
218 |
-
self.img_size = img_size
|
219 |
-
self.patch_size = patch_size
|
220 |
-
self.num_patches = num_patches
|
221 |
-
|
222 |
-
self.proj = nn.Conv2d(in_chans, embed_dim, kernel_size=patch_size, stride=patch_size)
|
223 |
-
|
224 |
-
def forward(self, x, **kwargs):
|
225 |
-
B, C, H, W = x.shape
|
226 |
-
# FIXME look at relaxing size constraints
|
227 |
-
assert H == self.img_size[0] and W == self.img_size[1], \
|
228 |
-
f"Input image size ({H}*{W}) doesn't match model ({self.img_size[0]}*{self.img_size[1]})."
|
229 |
-
x = self.proj(x).flatten(2).transpose(1, 2)
|
230 |
-
return x
|
231 |
-
|
232 |
-
|
233 |
-
class RelativePositionBias(nn.Module):
|
234 |
-
def __init__(self, window_size, num_heads):
|
235 |
-
super().__init__()
|
236 |
-
self.window_size = window_size
|
237 |
-
self.num_relative_distance = (2 * window_size[0] - 1) * (2 * window_size[1] - 1) + 3
|
238 |
-
self.relative_position_bias_table = nn.Parameter(torch.zeros(self.num_relative_distance,
|
239 |
-
num_heads)) # 2*Wh-1 * 2*Ww-1, nH
|
240 |
-
# cls to token & token 2 cls & cls to cls
|
241 |
-
|
242 |
-
# get pair-wise relative position index for each token inside the window
|
243 |
-
coords_h = torch.arange(window_size[0])
|
244 |
-
coords_w = torch.arange(window_size[1])
|
245 |
-
coords = torch.stack(torch.meshgrid([coords_h, coords_w])) # 2, Wh, Ww
|
246 |
-
coords_flatten = torch.flatten(coords, 1) # 2, Wh*Ww
|
247 |
-
relative_coords = coords_flatten[:, :, None] - coords_flatten[:, None, :] # 2, Wh*Ww, Wh*Ww
|
248 |
-
relative_coords = relative_coords.permute(1, 2, 0).contiguous() # Wh*Ww, Wh*Ww, 2
|
249 |
-
relative_coords[:, :, 0] += window_size[0] - 1 # shift to start from 0
|
250 |
-
relative_coords[:, :, 1] += window_size[1] - 1
|
251 |
-
relative_coords[:, :, 0] *= 2 * window_size[1] - 1
|
252 |
-
relative_position_index = \
|
253 |
-
torch.zeros(size=(window_size[0] * window_size[1] + 1,) * 2, dtype=relative_coords.dtype)
|
254 |
-
relative_position_index[1:, 1:] = relative_coords.sum(-1) # Wh*Ww, Wh*Ww
|
255 |
-
relative_position_index[0, 0:] = self.num_relative_distance - 3
|
256 |
-
relative_position_index[0:, 0] = self.num_relative_distance - 2
|
257 |
-
relative_position_index[0, 0] = self.num_relative_distance - 1
|
258 |
-
|
259 |
-
self.register_buffer("relative_position_index", relative_position_index)
|
260 |
-
|
261 |
-
# trunc_normal_(self.relative_position_bias_table, std=.02)
|
262 |
-
|
263 |
-
def forward(self):
|
264 |
-
relative_position_bias = \
|
265 |
-
self.relative_position_bias_table[self.relative_position_index.view(-1)].view(
|
266 |
-
self.window_size[0] * self.window_size[1] + 1,
|
267 |
-
self.window_size[0] * self.window_size[1] + 1, -1) # Wh*Ww,Wh*Ww,nH
|
268 |
-
return relative_position_bias.permute(2, 0, 1).contiguous() # nH, Wh*Ww, Wh*Ww
|
269 |
-
|
270 |
-
|
271 |
-
class VisionTransformer(nn.Module):
|
272 |
-
""" Vision Transformer with support for patch or hybrid CNN input stage
|
273 |
-
"""
|
274 |
-
def __init__(self,
|
275 |
-
img_size=224,
|
276 |
-
patch_size=16,
|
277 |
-
in_chans=3,
|
278 |
-
num_classes=1000,
|
279 |
-
embed_dim=768,
|
280 |
-
depth=12,
|
281 |
-
num_heads=12,
|
282 |
-
mlp_ratio=4.,
|
283 |
-
qkv_bias=False,
|
284 |
-
qk_scale=None,
|
285 |
-
drop_rate=0.,
|
286 |
-
attn_drop_rate=0.,
|
287 |
-
drop_path_rate=0.,
|
288 |
-
norm_layer=nn.LayerNorm,
|
289 |
-
init_values=None,
|
290 |
-
use_abs_pos_emb=True,
|
291 |
-
use_rel_pos_bias=False,
|
292 |
-
use_shared_rel_pos_bias=False,
|
293 |
-
use_mean_pooling=True,
|
294 |
-
init_scale=0.001,
|
295 |
-
use_checkpoint=False):
|
296 |
-
super().__init__()
|
297 |
-
self.image_size = img_size
|
298 |
-
self.num_classes = num_classes
|
299 |
-
self.num_features = self.embed_dim = embed_dim # num_features for consistency with other models
|
300 |
-
|
301 |
-
self.patch_embed = PatchEmbed(img_size=img_size, patch_size=patch_size, in_chans=in_chans, embed_dim=embed_dim)
|
302 |
-
num_patches = self.patch_embed.num_patches
|
303 |
-
|
304 |
-
self.cls_token = nn.Parameter(torch.zeros(1, 1, embed_dim))
|
305 |
-
if use_abs_pos_emb:
|
306 |
-
self.pos_embed = nn.Parameter(torch.zeros(1, num_patches + 1, embed_dim))
|
307 |
-
else:
|
308 |
-
self.pos_embed = None
|
309 |
-
self.pos_drop = nn.Dropout(p=drop_rate)
|
310 |
-
|
311 |
-
if use_shared_rel_pos_bias:
|
312 |
-
self.rel_pos_bias = RelativePositionBias(window_size=self.patch_embed.patch_shape, num_heads=num_heads)
|
313 |
-
else:
|
314 |
-
self.rel_pos_bias = None
|
315 |
-
self.use_checkpoint = use_checkpoint
|
316 |
-
|
317 |
-
dpr = [x.item() for x in torch.linspace(0, drop_path_rate, depth)] # stochastic depth decay rule
|
318 |
-
self.use_rel_pos_bias = use_rel_pos_bias
|
319 |
-
self.blocks = nn.ModuleList([
|
320 |
-
Block(dim=embed_dim,
|
321 |
-
num_heads=num_heads,
|
322 |
-
mlp_ratio=mlp_ratio,
|
323 |
-
qkv_bias=qkv_bias,
|
324 |
-
qk_scale=qk_scale,
|
325 |
-
drop=drop_rate,
|
326 |
-
attn_drop=attn_drop_rate,
|
327 |
-
drop_path=dpr[i],
|
328 |
-
norm_layer=norm_layer,
|
329 |
-
init_values=init_values,
|
330 |
-
window_size=self.patch_embed.patch_shape if use_rel_pos_bias else None) for i in range(depth)
|
331 |
-
])
|
332 |
-
# self.norm = nn.Identity() if use_mean_pooling else norm_layer(embed_dim)
|
333 |
-
# self.fc_norm = norm_layer(embed_dim) if use_mean_pooling else None
|
334 |
-
# self.head = nn.Linear(embed_dim, num_classes) if num_classes > 0 else nn.Identity()
|
335 |
-
|
336 |
-
if self.pos_embed is not None:
|
337 |
-
trunc_normal_(self.pos_embed, std=.02)
|
338 |
-
trunc_normal_(self.cls_token, std=.02)
|
339 |
-
# trunc_normal_(self.mask_token, std=.02)
|
340 |
-
# if isinstance(self.head, nn.Linear):
|
341 |
-
# trunc_normal_(self.head.weight, std=.02)
|
342 |
-
self.apply(self._init_weights)
|
343 |
-
self.fix_init_weight()
|
344 |
-
|
345 |
-
def fix_init_weight(self):
|
346 |
-
def rescale(param, layer_id):
|
347 |
-
param.div_(math.sqrt(2.0 * layer_id))
|
348 |
-
|
349 |
-
for layer_id, layer in enumerate(self.blocks):
|
350 |
-
rescale(layer.attn.proj.weight.data, layer_id + 1)
|
351 |
-
rescale(layer.mlp.fc2.weight.data, layer_id + 1)
|
352 |
-
|
353 |
-
def _init_weights(self, m):
|
354 |
-
if isinstance(m, nn.Linear):
|
355 |
-
trunc_normal_(m.weight, std=.02)
|
356 |
-
if isinstance(m, nn.Linear) and m.bias is not None:
|
357 |
-
nn.init.constant_(m.bias, 0)
|
358 |
-
elif isinstance(m, nn.LayerNorm):
|
359 |
-
nn.init.constant_(m.bias, 0)
|
360 |
-
nn.init.constant_(m.weight, 1.0)
|
361 |
-
|
362 |
-
def get_classifier(self):
|
363 |
-
return self.head
|
364 |
-
|
365 |
-
def reset_classifier(self, num_classes, global_pool=''):
|
366 |
-
self.num_classes = num_classes
|
367 |
-
self.head = nn.Linear(self.embed_dim, num_classes) if num_classes > 0 else nn.Identity()
|
368 |
-
|
369 |
-
def forward_features(self, x):
|
370 |
-
x = self.patch_embed(x)
|
371 |
-
batch_size, seq_len, _ = x.size()
|
372 |
-
|
373 |
-
cls_tokens = self.cls_token.expand(batch_size, -1, -1) # stole cls_tokens impl from Phil Wang, thanks
|
374 |
-
x = torch.cat((cls_tokens, x), dim=1)
|
375 |
-
if self.pos_embed is not None:
|
376 |
-
x = x + self.pos_embed
|
377 |
-
x = self.pos_drop(x)
|
378 |
-
|
379 |
-
rel_pos_bias = self.rel_pos_bias() if self.rel_pos_bias is not None else None
|
380 |
-
for blk in self.blocks:
|
381 |
-
if self.use_checkpoint:
|
382 |
-
x = checkpoint.checkpoint(blk, x, rel_pos_bias)
|
383 |
-
else:
|
384 |
-
x = blk(x, rel_pos_bias)
|
385 |
-
return x
|
386 |
-
|
387 |
-
def forward(self, x):
|
388 |
-
x = self.forward_features(x)
|
389 |
-
# x = self.head(x)
|
390 |
-
return x
|
391 |
-
|
392 |
-
def get_intermediate_layers(self, x):
|
393 |
-
x = self.patch_embed(x)
|
394 |
-
batch_size, seq_len, _ = x.size()
|
395 |
-
|
396 |
-
cls_tokens = self.cls_token.expand(batch_size, -1, -1) # stole cls_tokens impl from Phil Wang, thanks
|
397 |
-
x = torch.cat((cls_tokens, x), dim=1)
|
398 |
-
if self.pos_embed is not None:
|
399 |
-
x = x + self.pos_embed
|
400 |
-
x = self.pos_drop(x)
|
401 |
-
|
402 |
-
features = []
|
403 |
-
rel_pos_bias = self.rel_pos_bias() if self.rel_pos_bias is not None else None
|
404 |
-
for blk in self.blocks:
|
405 |
-
x = blk(x, rel_pos_bias)
|
406 |
-
features.append(x)
|
407 |
-
|
408 |
-
return features
|
409 |
-
|
410 |
-
def get_num_layer(self, var_name=""):
|
411 |
-
if var_name in ("cls_token", "mask_token", "pos_embed"):
|
412 |
-
return 0
|
413 |
-
elif var_name.startswith("patch_embed"):
|
414 |
-
return 0
|
415 |
-
elif var_name.startswith("rel_pos_bias"):
|
416 |
-
return len(self.blocks) - 1
|
417 |
-
elif var_name.startswith("blocks"):
|
418 |
-
layer_id = int(var_name.split('.')[1])
|
419 |
-
return layer_id + 1
|
420 |
-
else:
|
421 |
-
return len(self.blocks)
|
422 |
-
|
423 |
-
|
424 |
-
def interpolate_pos_embed(model, checkpoint_model):
|
425 |
-
if 'pos_embed' in checkpoint_model:
|
426 |
-
pos_embed_checkpoint = checkpoint_model['pos_embed'].float()
|
427 |
-
embedding_size = pos_embed_checkpoint.shape[-1]
|
428 |
-
num_patches = model.patch_embed.num_patches
|
429 |
-
num_extra_tokens = model.pos_embed.shape[-2] - num_patches
|
430 |
-
# height (== width) for the checkpoint position embedding
|
431 |
-
orig_size = int((pos_embed_checkpoint.shape[-2] - num_extra_tokens)**0.5)
|
432 |
-
# height (== width) for the new position embedding
|
433 |
-
new_size = int(num_patches**0.5)
|
434 |
-
# class_token and dist_token are kept unchanged
|
435 |
-
if orig_size != new_size:
|
436 |
-
print("Position interpolate from %dx%d to %dx%d" % (orig_size, orig_size, new_size, new_size))
|
437 |
-
extra_tokens = pos_embed_checkpoint[:, :num_extra_tokens]
|
438 |
-
# only the position tokens are interpolated
|
439 |
-
pos_tokens = pos_embed_checkpoint[:, num_extra_tokens:]
|
440 |
-
pos_tokens = pos_tokens.reshape(-1, orig_size, orig_size, embedding_size).permute(0, 3, 1, 2)
|
441 |
-
pos_tokens = torch.nn.functional.interpolate(pos_tokens,
|
442 |
-
size=(new_size, new_size),
|
443 |
-
mode='bicubic',
|
444 |
-
align_corners=False)
|
445 |
-
pos_tokens = pos_tokens.permute(0, 2, 3, 1).flatten(1, 2)
|
446 |
-
new_pos_embed = torch.cat((extra_tokens, pos_tokens), dim=1)
|
447 |
-
checkpoint_model['pos_embed'] = new_pos_embed
|
448 |
-
|
449 |
-
|
450 |
-
def convert_weights_to_fp16(model: nn.Module):
|
451 |
-
"""Convert applicable model parameters to fp16"""
|
452 |
-
def _convert_weights_to_fp16(l):
|
453 |
-
if isinstance(l, (nn.Conv1d, nn.Conv2d, nn.Linear)):
|
454 |
-
l.weight.data = l.weight.data.half()
|
455 |
-
if l.bias is not None:
|
456 |
-
l.bias.data = l.bias.data.half()
|
457 |
-
|
458 |
-
model.apply(_convert_weights_to_fp16)
|
459 |
-
|
460 |
-
|
461 |
-
def create_eva_vit_g(img_size=224, drop_path_rate=0.4, use_checkpoint=False, precision="fp16"):
|
462 |
-
model = VisionTransformer(
|
463 |
-
img_size=img_size,
|
464 |
-
patch_size=14,
|
465 |
-
use_mean_pooling=False,
|
466 |
-
embed_dim=1408,
|
467 |
-
depth=39,
|
468 |
-
num_heads=1408 // 88,
|
469 |
-
mlp_ratio=4.3637,
|
470 |
-
qkv_bias=True,
|
471 |
-
drop_path_rate=drop_path_rate,
|
472 |
-
norm_layer=partial(nn.LayerNorm, eps=1e-6),
|
473 |
-
use_checkpoint=use_checkpoint,
|
474 |
-
)
|
475 |
-
url = "https://storage.googleapis.com/sfr-vision-language-research/LAVIS/models/BLIP2/eva_vit_g.pth"
|
476 |
-
cached_file = download_cached_file(url, check_hash=False, progress=True)
|
477 |
-
state_dict = torch.load(cached_file, map_location="cpu")
|
478 |
-
interpolate_pos_embed(model, state_dict)
|
479 |
-
|
480 |
-
incompatible_keys = model.load_state_dict(state_dict, strict=False)
|
481 |
-
# print(incompatible_keys)
|
482 |
-
|
483 |
-
if precision == "fp16":
|
484 |
-
# model.to("cuda")
|
485 |
-
convert_weights_to_fp16(model)
|
486 |
-
return model
|
|
|
|
|
|
|
|
|
|
|
|
|
|
|
|
|
|
|
|
|
|
|
|
|
|
|
|
|
|
|
|
|
|
|
|
|
|
|
|
|
|
|
|
|
|
|
|
|
|
|
|
|
|
|
|
|
|
|
|
|
|
|
|
|
|
|
|
|
|
|
|
|
|
|
|
|
|
|
|
|
|
|
|
|
|
|
|
|
|
|
|
|
|
|
|
|
|
|
|
|
|
|
|
|
|
|
|
|
|
|
|
|
|
|
|
|
|
|
|
|
|
|
|
|
|
|
|
|
|
|
|
|
|
|
|
|
|
|
|
|
|
|
|
|
|
|
|
|
|
|
|
|
|
|
|
|
|
|
|
|
|
|
|
|
|
|
|
|
|
|
|
|
|
|
|
|
|
|
|
|
|
|
|
|
|
|
|
|
|
|
|
|
|
|
|
|
|
|
|
|
|
|
|
|
|
|
|
|
|
|
|
|
|
|
|
|
|
|
|
|
|
|
|
|
|
|
|
|
|
|
|
|
|
|
|
|
|
|
|
|
|
|
|
|
|
|
|
|
|
|
|
|
|
|
|
|
|
|
|
|
|
|
|
|
|
|
|
|
|
|
|
|
|
|
|
|
|
|
|
|
|
|
|
|
|
|
|
|
|
|
|
|
|
|
|
|
|
|
|
|
|
|
|
|
|
|
|
|
|
|
|
|
|
|
|
|
|
|
|
|
|
|
|
|
|
|
|
|
|
|
|
|
|
|
|
|
|
|
|
|
|
|
|
|
|
|
|
|
|
|
|
|
|
|
|
|
|
|
|
|
|
|
|
|
|
|
|
|
|
|
|
|
|
|
|
|
|
|
|
|
|
|
|
|
|
|
|
|
|
|
|
|
|
|
|
|
|
|
|
|
|
|
|
|
|
|
|
|
|
|
|
|
|
|
|
|
|
|
|
|
|
|
|
|
|
|
|
|
|
|
|
|
|
|
|
|
|
|
|
|
|
|
|
|
|
|
|
|
|
|
|
|
|
|
|
|
|
|
|
|
|
|
|
|
|
|
|
|
|
|
|
|
|
|
|
|
|
|
|
|
|
|
|
|
|
|
|
|
|
|
|
|
|
|
|
|
|
|
|
|
|
|
|
|
|
|
|
|
|
|
|
|
|
|
|
|
|
|
|
|
|
|
|
|
|
|
|
|
|
|
|
|
|
|
|
|
|
|
|
|
|
|
|
|
|
|
|
|
|
|
|
|
|
|
|
|
|
|
|
|
|
|
|
|
|
|
|
|
|
|
|
|
|
|
|
|
|
|
|
|
|
|
|
|
|
|
|
|
|
|
|
|
|
|
|
|
|
|
|
|
|
|
|
|
|
|
|
|
|
|
|
|
|
|
|
|
|
|
|
|
|
|
|
|
|
|
|
|
|
|
|
|
|
|
|
|
|
|
|
|
|
|
|
|
|
|
|
|
|
|
|
|
|
|
|
|
|
|
|
|
|
|
|
|
|
|
|
|
|
|
|
|
|
|
|
|
|
|
|
|
|
|
|
|
|
|
|
|
|
|
|
|
|
|
|
|
|
|
|
|
|
|
|
|
|
|
|
|
|
|
|
|
|
|
|
|
|
|
|
|
|
|
|
|
|
|
|
|
|
|
|
|
|
|
|
|
|
|
|
|
|
|
|
|
|
|
|
|
|
|
|
|
|
|
|
|
|
|
|
|
|
|
|
|
|
|
|
|
|
|
|
|
|
|
|
|
|
|
|
|
|
|
|
|
|
|
|
|
|
|
|
|
|
|
|
|
|
|
|
|
|
|
|
|
|
|
|
|
|
|
|
|
|
|
|
|
|
|
|
|
|
|
|
|
|
|
|
|
|
|
|
|
|
|
|
|
|
|
|
|
|
|
|
|
|
|
|
|
|
|
|
|
|
|
|
|
|
|
|
|
|
|
|
|
|
|
|
|
|
|
|
|
|
|
|
|
|
|
|
|
|
|
|
|
|
|
|
|
|
|
|
|
|
|
|
|
|
|
|
|
|
|
|
|
|
|
|
|
|
|
|
|
|
|
|
|
|
|
|
|
|
|
|
|
|
|
|
|
|
|
|
|
|
|
|
|
|
|
|
|
|
|
|
|
|
|
|
|
|
|
|
|
|
|
|
|
|
|
|
|
|
|
|
|
|
|
|
|
|
|
|
|
|
spaces/ATang0729/Forecast4Muses/Model/Model6/Model6_0_ClothesDetection/mmyolo/configs/_base_/det_p5_tta.py
DELETED
@@ -1,57 +0,0 @@
|
|
1 |
-
# TODO: Need to solve the problem of multiple file_client_args parameters
|
2 |
-
# _file_client_args = dict(
|
3 |
-
# backend='petrel',
|
4 |
-
# path_mapping=dict({
|
5 |
-
# './data/': 's3://openmmlab/datasets/detection/',
|
6 |
-
# 'data/': 's3://openmmlab/datasets/detection/'
|
7 |
-
# }))
|
8 |
-
_file_client_args = dict(backend='disk')
|
9 |
-
|
10 |
-
tta_model = dict(
|
11 |
-
type='mmdet.DetTTAModel',
|
12 |
-
tta_cfg=dict(nms=dict(type='nms', iou_threshold=0.65), max_per_img=300))
|
13 |
-
|
14 |
-
img_scales = [(640, 640), (320, 320), (960, 960)]
|
15 |
-
|
16 |
-
# LoadImageFromFile
|
17 |
-
# / | \
|
18 |
-
# (RatioResize,LetterResize) (RatioResize,LetterResize) (RatioResize,LetterResize) # noqa
|
19 |
-
# / \ / \ / \
|
20 |
-
# RandomFlip RandomFlip RandomFlip RandomFlip RandomFlip RandomFlip # noqa
|
21 |
-
# | | | | | |
|
22 |
-
# LoadAnn LoadAnn LoadAnn LoadAnn LoadAnn LoadAnn
|
23 |
-
# | | | | | |
|
24 |
-
# PackDetIn PackDetIn PackDetIn PackDetIn PackDetIn PackDetIn # noqa
|
25 |
-
|
26 |
-
_multiscale_resize_transforms = [
|
27 |
-
dict(
|
28 |
-
type='Compose',
|
29 |
-
transforms=[
|
30 |
-
dict(type='YOLOv5KeepRatioResize', scale=s),
|
31 |
-
dict(
|
32 |
-
type='LetterResize',
|
33 |
-
scale=s,
|
34 |
-
allow_scale_up=False,
|
35 |
-
pad_val=dict(img=114))
|
36 |
-
]) for s in img_scales
|
37 |
-
]
|
38 |
-
|
39 |
-
tta_pipeline = [
|
40 |
-
dict(type='LoadImageFromFile', file_client_args=_file_client_args),
|
41 |
-
dict(
|
42 |
-
type='TestTimeAug',
|
43 |
-
transforms=[
|
44 |
-
_multiscale_resize_transforms,
|
45 |
-
[
|
46 |
-
dict(type='mmdet.RandomFlip', prob=1.),
|
47 |
-
dict(type='mmdet.RandomFlip', prob=0.)
|
48 |
-
], [dict(type='mmdet.LoadAnnotations', with_bbox=True)],
|
49 |
-
[
|
50 |
-
dict(
|
51 |
-
type='mmdet.PackDetInputs',
|
52 |
-
meta_keys=('img_id', 'img_path', 'ori_shape', 'img_shape',
|
53 |
-
'scale_factor', 'pad_param', 'flip',
|
54 |
-
'flip_direction'))
|
55 |
-
]
|
56 |
-
])
|
57 |
-
]
|
|
|
|
|
|
|
|
|
|
|
|
|
|
|
|
|
|
|
|
|
|
|
|
|
|
|
|
|
|
|
|
|
|
|
|
|
|
|
|
|
|
|
|
|
|
|
|
|
|
|
|
|
|
|
|
|
|
|
|
|
|
|
|
|
|
|
|
|
|
|
|
|
|
|
|
|
|
|
|
|
|
|
|
|
|
|
|
|
|
|
|
|
|
|
|
|
|
|
|
|
|
|
|
|
|
|
|
|
|
|
|
|
|
|
|
spaces/AiMimicry/sovits-models/modules/crepe.py
DELETED
@@ -1,327 +0,0 @@
|
|
1 |
-
from typing import Optional,Union
|
2 |
-
try:
|
3 |
-
from typing import Literal
|
4 |
-
except Exception as e:
|
5 |
-
from typing_extensions import Literal
|
6 |
-
import numpy as np
|
7 |
-
import torch
|
8 |
-
import torchcrepe
|
9 |
-
from torch import nn
|
10 |
-
from torch.nn import functional as F
|
11 |
-
import scipy
|
12 |
-
|
13 |
-
#from:https://github.com/fishaudio/fish-diffusion
|
14 |
-
|
15 |
-
def repeat_expand(
|
16 |
-
content: Union[torch.Tensor, np.ndarray], target_len: int, mode: str = "nearest"
|
17 |
-
):
|
18 |
-
"""Repeat content to target length.
|
19 |
-
This is a wrapper of torch.nn.functional.interpolate.
|
20 |
-
|
21 |
-
Args:
|
22 |
-
content (torch.Tensor): tensor
|
23 |
-
target_len (int): target length
|
24 |
-
mode (str, optional): interpolation mode. Defaults to "nearest".
|
25 |
-
|
26 |
-
Returns:
|
27 |
-
torch.Tensor: tensor
|
28 |
-
"""
|
29 |
-
|
30 |
-
ndim = content.ndim
|
31 |
-
|
32 |
-
if content.ndim == 1:
|
33 |
-
content = content[None, None]
|
34 |
-
elif content.ndim == 2:
|
35 |
-
content = content[None]
|
36 |
-
|
37 |
-
assert content.ndim == 3
|
38 |
-
|
39 |
-
is_np = isinstance(content, np.ndarray)
|
40 |
-
if is_np:
|
41 |
-
content = torch.from_numpy(content)
|
42 |
-
|
43 |
-
results = torch.nn.functional.interpolate(content, size=target_len, mode=mode)
|
44 |
-
|
45 |
-
if is_np:
|
46 |
-
results = results.numpy()
|
47 |
-
|
48 |
-
if ndim == 1:
|
49 |
-
return results[0, 0]
|
50 |
-
elif ndim == 2:
|
51 |
-
return results[0]
|
52 |
-
|
53 |
-
|
54 |
-
class BasePitchExtractor:
|
55 |
-
def __init__(
|
56 |
-
self,
|
57 |
-
hop_length: int = 512,
|
58 |
-
f0_min: float = 50.0,
|
59 |
-
f0_max: float = 1100.0,
|
60 |
-
keep_zeros: bool = True,
|
61 |
-
):
|
62 |
-
"""Base pitch extractor.
|
63 |
-
|
64 |
-
Args:
|
65 |
-
hop_length (int, optional): Hop length. Defaults to 512.
|
66 |
-
f0_min (float, optional): Minimum f0. Defaults to 50.0.
|
67 |
-
f0_max (float, optional): Maximum f0. Defaults to 1100.0.
|
68 |
-
keep_zeros (bool, optional): Whether keep zeros in pitch. Defaults to True.
|
69 |
-
"""
|
70 |
-
|
71 |
-
self.hop_length = hop_length
|
72 |
-
self.f0_min = f0_min
|
73 |
-
self.f0_max = f0_max
|
74 |
-
self.keep_zeros = keep_zeros
|
75 |
-
|
76 |
-
def __call__(self, x, sampling_rate=44100, pad_to=None):
|
77 |
-
raise NotImplementedError("BasePitchExtractor is not callable.")
|
78 |
-
|
79 |
-
def post_process(self, x, sampling_rate, f0, pad_to):
|
80 |
-
if isinstance(f0, np.ndarray):
|
81 |
-
f0 = torch.from_numpy(f0).float().to(x.device)
|
82 |
-
|
83 |
-
if pad_to is None:
|
84 |
-
return f0
|
85 |
-
|
86 |
-
f0 = repeat_expand(f0, pad_to)
|
87 |
-
|
88 |
-
if self.keep_zeros:
|
89 |
-
return f0
|
90 |
-
|
91 |
-
vuv_vector = torch.zeros_like(f0)
|
92 |
-
vuv_vector[f0 > 0.0] = 1.0
|
93 |
-
vuv_vector[f0 <= 0.0] = 0.0
|
94 |
-
|
95 |
-
# 去掉0频率, 并线性插值
|
96 |
-
nzindex = torch.nonzero(f0).squeeze()
|
97 |
-
f0 = torch.index_select(f0, dim=0, index=nzindex).cpu().numpy()
|
98 |
-
time_org = self.hop_length / sampling_rate * nzindex.cpu().numpy()
|
99 |
-
time_frame = np.arange(pad_to) * self.hop_length / sampling_rate
|
100 |
-
|
101 |
-
if f0.shape[0] <= 0:
|
102 |
-
return torch.zeros(pad_to, dtype=torch.float, device=x.device),torch.zeros(pad_to, dtype=torch.float, device=x.device)
|
103 |
-
|
104 |
-
if f0.shape[0] == 1:
|
105 |
-
return torch.ones(pad_to, dtype=torch.float, device=x.device) * f0[0],torch.ones(pad_to, dtype=torch.float, device=x.device)
|
106 |
-
|
107 |
-
# 大概可以用 torch 重写?
|
108 |
-
f0 = np.interp(time_frame, time_org, f0, left=f0[0], right=f0[-1])
|
109 |
-
vuv_vector = vuv_vector.cpu().numpy()
|
110 |
-
vuv_vector = np.ceil(scipy.ndimage.zoom(vuv_vector,pad_to/len(vuv_vector),order = 0))
|
111 |
-
|
112 |
-
return f0,vuv_vector
|
113 |
-
|
114 |
-
|
115 |
-
class MaskedAvgPool1d(nn.Module):
|
116 |
-
def __init__(
|
117 |
-
self, kernel_size: int, stride: Optional[int] = None, padding: Optional[int] = 0
|
118 |
-
):
|
119 |
-
"""An implementation of mean pooling that supports masked values.
|
120 |
-
|
121 |
-
Args:
|
122 |
-
kernel_size (int): The size of the median pooling window.
|
123 |
-
stride (int, optional): The stride of the median pooling window. Defaults to None.
|
124 |
-
padding (int, optional): The padding of the median pooling window. Defaults to 0.
|
125 |
-
"""
|
126 |
-
|
127 |
-
super(MaskedAvgPool1d, self).__init__()
|
128 |
-
self.kernel_size = kernel_size
|
129 |
-
self.stride = stride or kernel_size
|
130 |
-
self.padding = padding
|
131 |
-
|
132 |
-
def forward(self, x, mask=None):
|
133 |
-
ndim = x.dim()
|
134 |
-
if ndim == 2:
|
135 |
-
x = x.unsqueeze(1)
|
136 |
-
|
137 |
-
assert (
|
138 |
-
x.dim() == 3
|
139 |
-
), "Input tensor must have 2 or 3 dimensions (batch_size, channels, width)"
|
140 |
-
|
141 |
-
# Apply the mask by setting masked elements to zero, or make NaNs zero
|
142 |
-
if mask is None:
|
143 |
-
mask = ~torch.isnan(x)
|
144 |
-
|
145 |
-
# Ensure mask has the same shape as the input tensor
|
146 |
-
assert x.shape == mask.shape, "Input tensor and mask must have the same shape"
|
147 |
-
|
148 |
-
masked_x = torch.where(mask, x, torch.zeros_like(x))
|
149 |
-
# Create a ones kernel with the same number of channels as the input tensor
|
150 |
-
ones_kernel = torch.ones(x.size(1), 1, self.kernel_size, device=x.device)
|
151 |
-
|
152 |
-
# Perform sum pooling
|
153 |
-
sum_pooled = nn.functional.conv1d(
|
154 |
-
masked_x,
|
155 |
-
ones_kernel,
|
156 |
-
stride=self.stride,
|
157 |
-
padding=self.padding,
|
158 |
-
groups=x.size(1),
|
159 |
-
)
|
160 |
-
|
161 |
-
# Count the non-masked (valid) elements in each pooling window
|
162 |
-
valid_count = nn.functional.conv1d(
|
163 |
-
mask.float(),
|
164 |
-
ones_kernel,
|
165 |
-
stride=self.stride,
|
166 |
-
padding=self.padding,
|
167 |
-
groups=x.size(1),
|
168 |
-
)
|
169 |
-
valid_count = valid_count.clamp(min=1) # Avoid division by zero
|
170 |
-
|
171 |
-
# Perform masked average pooling
|
172 |
-
avg_pooled = sum_pooled / valid_count
|
173 |
-
|
174 |
-
# Fill zero values with NaNs
|
175 |
-
avg_pooled[avg_pooled == 0] = float("nan")
|
176 |
-
|
177 |
-
if ndim == 2:
|
178 |
-
return avg_pooled.squeeze(1)
|
179 |
-
|
180 |
-
return avg_pooled
|
181 |
-
|
182 |
-
|
183 |
-
class MaskedMedianPool1d(nn.Module):
|
184 |
-
def __init__(
|
185 |
-
self, kernel_size: int, stride: Optional[int] = None, padding: Optional[int] = 0
|
186 |
-
):
|
187 |
-
"""An implementation of median pooling that supports masked values.
|
188 |
-
|
189 |
-
This implementation is inspired by the median pooling implementation in
|
190 |
-
https://gist.github.com/rwightman/f2d3849281624be7c0f11c85c87c1598
|
191 |
-
|
192 |
-
Args:
|
193 |
-
kernel_size (int): The size of the median pooling window.
|
194 |
-
stride (int, optional): The stride of the median pooling window. Defaults to None.
|
195 |
-
padding (int, optional): The padding of the median pooling window. Defaults to 0.
|
196 |
-
"""
|
197 |
-
|
198 |
-
super(MaskedMedianPool1d, self).__init__()
|
199 |
-
self.kernel_size = kernel_size
|
200 |
-
self.stride = stride or kernel_size
|
201 |
-
self.padding = padding
|
202 |
-
|
203 |
-
def forward(self, x, mask=None):
|
204 |
-
ndim = x.dim()
|
205 |
-
if ndim == 2:
|
206 |
-
x = x.unsqueeze(1)
|
207 |
-
|
208 |
-
assert (
|
209 |
-
x.dim() == 3
|
210 |
-
), "Input tensor must have 2 or 3 dimensions (batch_size, channels, width)"
|
211 |
-
|
212 |
-
if mask is None:
|
213 |
-
mask = ~torch.isnan(x)
|
214 |
-
|
215 |
-
assert x.shape == mask.shape, "Input tensor and mask must have the same shape"
|
216 |
-
|
217 |
-
masked_x = torch.where(mask, x, torch.zeros_like(x))
|
218 |
-
|
219 |
-
x = F.pad(masked_x, (self.padding, self.padding), mode="reflect")
|
220 |
-
mask = F.pad(
|
221 |
-
mask.float(), (self.padding, self.padding), mode="constant", value=0
|
222 |
-
)
|
223 |
-
|
224 |
-
x = x.unfold(2, self.kernel_size, self.stride)
|
225 |
-
mask = mask.unfold(2, self.kernel_size, self.stride)
|
226 |
-
|
227 |
-
x = x.contiguous().view(x.size()[:3] + (-1,))
|
228 |
-
mask = mask.contiguous().view(mask.size()[:3] + (-1,)).to(x.device)
|
229 |
-
|
230 |
-
# Combine the mask with the input tensor
|
231 |
-
#x_masked = torch.where(mask.bool(), x, torch.fill_(torch.zeros_like(x),float("inf")))
|
232 |
-
x_masked = torch.where(mask.bool(), x, torch.FloatTensor([float("inf")]).to(x.device))
|
233 |
-
|
234 |
-
# Sort the masked tensor along the last dimension
|
235 |
-
x_sorted, _ = torch.sort(x_masked, dim=-1)
|
236 |
-
|
237 |
-
# Compute the count of non-masked (valid) values
|
238 |
-
valid_count = mask.sum(dim=-1)
|
239 |
-
|
240 |
-
# Calculate the index of the median value for each pooling window
|
241 |
-
median_idx = (torch.div((valid_count - 1), 2, rounding_mode='trunc')).clamp(min=0)
|
242 |
-
|
243 |
-
# Gather the median values using the calculated indices
|
244 |
-
median_pooled = x_sorted.gather(-1, median_idx.unsqueeze(-1).long()).squeeze(-1)
|
245 |
-
|
246 |
-
# Fill infinite values with NaNs
|
247 |
-
median_pooled[torch.isinf(median_pooled)] = float("nan")
|
248 |
-
|
249 |
-
if ndim == 2:
|
250 |
-
return median_pooled.squeeze(1)
|
251 |
-
|
252 |
-
return median_pooled
|
253 |
-
|
254 |
-
|
255 |
-
class CrepePitchExtractor(BasePitchExtractor):
|
256 |
-
def __init__(
|
257 |
-
self,
|
258 |
-
hop_length: int = 512,
|
259 |
-
f0_min: float = 50.0,
|
260 |
-
f0_max: float = 1100.0,
|
261 |
-
threshold: float = 0.05,
|
262 |
-
keep_zeros: bool = False,
|
263 |
-
device = None,
|
264 |
-
model: Literal["full", "tiny"] = "full",
|
265 |
-
use_fast_filters: bool = True,
|
266 |
-
):
|
267 |
-
super().__init__(hop_length, f0_min, f0_max, keep_zeros)
|
268 |
-
|
269 |
-
self.threshold = threshold
|
270 |
-
self.model = model
|
271 |
-
self.use_fast_filters = use_fast_filters
|
272 |
-
self.hop_length = hop_length
|
273 |
-
if device is None:
|
274 |
-
self.dev = torch.device("cuda" if torch.cuda.is_available() else "cpu")
|
275 |
-
else:
|
276 |
-
self.dev = torch.device(device)
|
277 |
-
if self.use_fast_filters:
|
278 |
-
self.median_filter = MaskedMedianPool1d(3, 1, 1).to(device)
|
279 |
-
self.mean_filter = MaskedAvgPool1d(3, 1, 1).to(device)
|
280 |
-
|
281 |
-
def __call__(self, x, sampling_rate=44100, pad_to=None):
|
282 |
-
"""Extract pitch using crepe.
|
283 |
-
|
284 |
-
|
285 |
-
Args:
|
286 |
-
x (torch.Tensor): Audio signal, shape (1, T).
|
287 |
-
sampling_rate (int, optional): Sampling rate. Defaults to 44100.
|
288 |
-
pad_to (int, optional): Pad to length. Defaults to None.
|
289 |
-
|
290 |
-
Returns:
|
291 |
-
torch.Tensor: Pitch, shape (T // hop_length,).
|
292 |
-
"""
|
293 |
-
|
294 |
-
assert x.ndim == 2, f"Expected 2D tensor, got {x.ndim}D tensor."
|
295 |
-
assert x.shape[0] == 1, f"Expected 1 channel, got {x.shape[0]} channels."
|
296 |
-
|
297 |
-
x = x.to(self.dev)
|
298 |
-
f0, pd = torchcrepe.predict(
|
299 |
-
x,
|
300 |
-
sampling_rate,
|
301 |
-
self.hop_length,
|
302 |
-
self.f0_min,
|
303 |
-
self.f0_max,
|
304 |
-
pad=True,
|
305 |
-
model=self.model,
|
306 |
-
batch_size=1024,
|
307 |
-
device=x.device,
|
308 |
-
return_periodicity=True,
|
309 |
-
)
|
310 |
-
|
311 |
-
# Filter, remove silence, set uv threshold, refer to the original warehouse readme
|
312 |
-
if self.use_fast_filters:
|
313 |
-
pd = self.median_filter(pd)
|
314 |
-
else:
|
315 |
-
pd = torchcrepe.filter.median(pd, 3)
|
316 |
-
|
317 |
-
pd = torchcrepe.threshold.Silence(-60.0)(pd, x, sampling_rate, 512)
|
318 |
-
f0 = torchcrepe.threshold.At(self.threshold)(f0, pd)
|
319 |
-
|
320 |
-
if self.use_fast_filters:
|
321 |
-
f0 = self.mean_filter(f0)
|
322 |
-
else:
|
323 |
-
f0 = torchcrepe.filter.mean(f0, 3)
|
324 |
-
|
325 |
-
f0 = torch.where(torch.isnan(f0), torch.full_like(f0, 0), f0)[0]
|
326 |
-
|
327 |
-
return self.post_process(x, sampling_rate, f0, pad_to)
|
|
|
|
|
|
|
|
|
|
|
|
|
|
|
|
|
|
|
|
|
|
|
|
|
|
|
|
|
|
|
|
|
|
|
|
|
|
|
|
|
|
|
|
|
|
|
|
|
|
|
|
|
|
|
|
|
|
|
|
|
|
|
|
|
|
|
|
|
|
|
|
|
|
|
|
|
|
|
|
|
|
|
|
|
|
|
|
|
|
|
|
|
|
|
|
|
|
|
|
|
|
|
|
|
|
|
|
|
|
|
|
|
|
|
|
|
|
|
|
|
|
|
|
|
|
|
|
|
|
|
|
|
|
|
|
|
|
|
|
|
|
|
|
|
|
|
|
|
|
|
|
|
|
|
|
|
|
|
|
|
|
|
|
|
|
|
|
|
|
|
|
|
|
|
|
|
|
|
|
|
|
|
|
|
|
|
|
|
|
|
|
|
|
|
|
|
|
|
|
|
|
|
|
|
|
|
|
|
|
|
|
|
|
|
|
|
|
|
|
|
|
|
|
|
|
|
|
|
|
|
|
|
|
|
|
|
|
|
|
|
|
|
|
|
|
|
|
|
|
|
|
|
|
|
|
|
|
|
|
|
|
|
|
|
|
|
|
|
|
|
|
|
|
|
|
|
|
|
|
|
|
|
|
|
|
|
|
|
|
|
|
|
|
|
|
|
|
|
|
|
|
|
|
|
|
|
|
|
|
|
|
|
|
|
|
|
|
|
|
|
|
|
|
|
|
|
|
|
|
|
|
|
|
|
|
|
|
|
|
|
|
|
|
|
|
|
|
|
|
|
|
|
|
|
|
|
|
|
|
|
|
|
|
|
|
|
|
|
|
|
|
|
|
|
|
|
|
|
|
|
|
|
|
|
|
|
|
|
|
|
|
|
|
|
|
|
|
|
|
|
|
|
|
|
|
|
|
|
|
|
|
|
|
|
|
|
|
|
|
|
|
|
|
|
|
|
|
|
|
|
|
|
|
|
|
|
|
|
|
|
|
|
|
|
|
|
|
|
|
|
|
|
|
|
|
|
|
|
|
|
|
|
|
|
|
|
|
|
|
|
|
|
|
|
|
|
|
|
|
|
|
|
|
|
|
|
|
|
|
|
|
|
|
|
|
|
|
|
|
|
|
|
|
|
|
|
|
|
|
|
|
|
|
|
|
|
|
|
|
|
|
|
|
|
|
|
|
|
|
|
|
|
|
|
|
|
|
|
|
|
|
|
|
|
|
|
|
|
|
|
|
|
|
|
|
|
|
|
|
|
|
|
|
|
|
|
|
|
|
|
|
|
|
|
|
|
|
|
|
|
|
|
|
|
|
|
|
|
|
|
|
|
|
|
|
|
|
|
|
|
|
|
|
|
|
|
|
|
|
|
|
|
|
|
|
|
|
|
|
|
|
|
|
|
|
|
|
|
|
|
|
|
|
|
|
|
|
|
|
|
|
|
|
|
|
|
|
|
|
|
|
|
|
|
|
spaces/Ajay-user/Optical-Character-Recognition/app.py
DELETED
@@ -1,61 +0,0 @@
|
|
1 |
-
|
2 |
-
import numpy as np
|
3 |
-
import streamlit as st
|
4 |
-
from PIL import Image, ImageOps
|
5 |
-
import cv2
|
6 |
-
from utils import OCR
|
7 |
-
|
8 |
-
|
9 |
-
alert = False
|
10 |
-
ocr = None
|
11 |
-
|
12 |
-
st.title("Optical Character Recognition")
|
13 |
-
|
14 |
-
tab_upload, tab_cam = st.tabs(['Upload', 'Camera'])
|
15 |
-
|
16 |
-
with tab_upload:
|
17 |
-
image_upload = st.file_uploader(
|
18 |
-
label='Upload the Image', type=['jpg', 'jpeg', 'png'])
|
19 |
-
|
20 |
-
with tab_cam:
|
21 |
-
image_webcam = st.camera_input(
|
22 |
-
label="Take a picture 📷")
|
23 |
-
if image_upload:
|
24 |
-
image = Image.open(image_upload)
|
25 |
-
elif image_webcam:
|
26 |
-
image = Image.open(image_webcam)
|
27 |
-
else:
|
28 |
-
image = Image.open('./Images/sample_image_1.jpg')
|
29 |
-
|
30 |
-
st.image(image=ImageOps.scale(image, factor=0.2))
|
31 |
-
|
32 |
-
if st.button('Detect'):
|
33 |
-
|
34 |
-
try:
|
35 |
-
pass
|
36 |
-
ocr = OCR(image=image)
|
37 |
-
|
38 |
-
except:
|
39 |
-
|
40 |
-
st.warning(
|
41 |
-
" Please use a different image.", icon="⚠")
|
42 |
-
alert = True
|
43 |
-
|
44 |
-
|
45 |
-
if ocr:
|
46 |
-
st.caption("✨Result")
|
47 |
-
|
48 |
-
try:
|
49 |
-
st.pyplot(fig=ocr.detection(), use_container_width=True)
|
50 |
-
except:
|
51 |
-
st.warning(
|
52 |
-
" Please use a different image", icon="⚠")
|
53 |
-
|
54 |
-
|
55 |
-
else:
|
56 |
-
st.caption('Just click the Detect button')
|
57 |
-
if alert:
|
58 |
-
st.warning(
|
59 |
-
" Please use a different image.", icon="⚠")
|
60 |
-
|
61 |
-
st.image(image)
|
|
|
|
|
|
|
|
|
|
|
|
|
|
|
|
|
|
|
|
|
|
|
|
|
|
|
|
|
|
|
|
|
|
|
|
|
|
|
|
|
|
|
|
|
|
|
|
|
|
|
|
|
|
|
|
|
|
|
|
|
|
|
|
|
|
|
|
|
|
|
|
|
|
|
|
|
|
|
|
|
|
|
|
|
|
|
|
|
|
|
|
|
|
|
|
|
|
|
|
|
|
|
|
|
|
|
|
|
|
|
|
|
|
|
|
|
|
|
|
|
|
|
|
spaces/Androidonnxfork/CivitAi-to-Diffusers/diffusers/docs/source/en/using-diffusers/weighted_prompts.md
DELETED
@@ -1,110 +0,0 @@
|
|
1 |
-
<!--Copyright 2023 The HuggingFace Team. All rights reserved.
|
2 |
-
|
3 |
-
Licensed under the Apache License, Version 2.0 (the "License"); you may not use this file except in compliance with
|
4 |
-
the License. You may obtain a copy of the License at
|
5 |
-
|
6 |
-
http://www.apache.org/licenses/LICENSE-2.0
|
7 |
-
|
8 |
-
Unless required by applicable law or agreed to in writing, software distributed under the License is distributed on
|
9 |
-
an "AS IS" BASIS, WITHOUT WARRANTIES OR CONDITIONS OF ANY KIND, either express or implied. See the License for the
|
10 |
-
specific language governing permissions and limitations under the License.
|
11 |
-
-->
|
12 |
-
|
13 |
-
# Weighting prompts
|
14 |
-
|
15 |
-
[[open-in-colab]]
|
16 |
-
|
17 |
-
Text-guided diffusion models generate images based on a given text prompt. The text prompt
|
18 |
-
can include multiple concepts that the model should generate and it's often desirable to weight
|
19 |
-
certain parts of the prompt more or less.
|
20 |
-
|
21 |
-
Diffusion models work by conditioning the cross attention layers of the diffusion model with contextualized text embeddings (see the [Stable Diffusion Guide for more information](../stable-diffusion)).
|
22 |
-
Thus a simple way to emphasize (or de-emphasize) certain parts of the prompt is by increasing or reducing the scale of the text embedding vector that corresponds to the relevant part of the prompt.
|
23 |
-
This is called "prompt-weighting" and has been a highly demanded feature by the community (see issue [here](https://github.com/huggingface/diffusers/issues/2431)).
|
24 |
-
|
25 |
-
## How to do prompt-weighting in Diffusers
|
26 |
-
|
27 |
-
We believe the role of `diffusers` is to be a toolbox that provides essential features that enable other projects, such as [InvokeAI](https://github.com/invoke-ai/InvokeAI) or [diffuzers](https://github.com/abhishekkrthakur/diffuzers), to build powerful UIs. In order to support arbitrary methods to manipulate prompts, `diffusers` exposes a [`prompt_embeds`](https://huggingface.co/docs/diffusers/v0.14.0/en/api/pipelines/stable_diffusion/text2img#diffusers.StableDiffusionPipeline.__call__.prompt_embeds) function argument to many pipelines such as [`StableDiffusionPipeline`], allowing to directly pass the "prompt-weighted"/scaled text embeddings to the pipeline.
|
28 |
-
|
29 |
-
The [compel library](https://github.com/damian0815/compel) provides an easy way to emphasize or de-emphasize portions of the prompt for you. We strongly recommend it instead of preparing the embeddings yourself.
|
30 |
-
|
31 |
-
Let's look at a simple example. Imagine you want to generate an image of `"a red cat playing with a ball"` as
|
32 |
-
follows:
|
33 |
-
|
34 |
-
```py
|
35 |
-
from diffusers import StableDiffusionPipeline, UniPCMultistepScheduler
|
36 |
-
|
37 |
-
pipe = StableDiffusionPipeline.from_pretrained("CompVis/stable-diffusion-v1-4")
|
38 |
-
pipe.scheduler = UniPCMultistepScheduler.from_config(pipe.scheduler.config)
|
39 |
-
|
40 |
-
prompt = "a red cat playing with a ball"
|
41 |
-
|
42 |
-
generator = torch.Generator(device="cpu").manual_seed(33)
|
43 |
-
|
44 |
-
image = pipe(prompt, generator=generator, num_inference_steps=20).images[0]
|
45 |
-
image
|
46 |
-
```
|
47 |
-
|
48 |
-
This gives you:
|
49 |
-
|
50 |
-
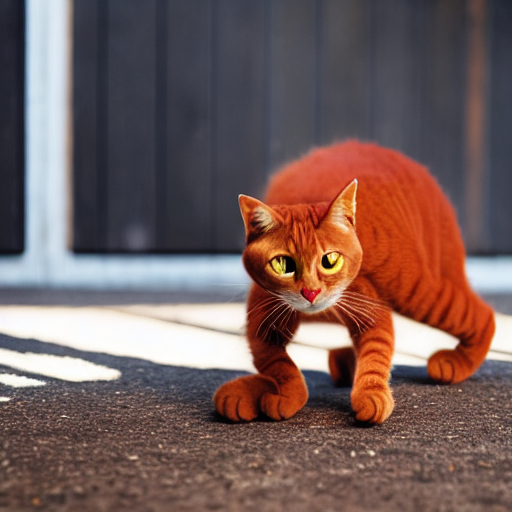
|
51 |
-
|
52 |
-
As you can see, there is no "ball" in the image. Let's emphasize this part!
|
53 |
-
|
54 |
-
For this we should install the `compel` library:
|
55 |
-
|
56 |
-
```
|
57 |
-
pip install compel
|
58 |
-
```
|
59 |
-
|
60 |
-
and then create a `Compel` object:
|
61 |
-
|
62 |
-
```py
|
63 |
-
from compel import Compel
|
64 |
-
|
65 |
-
compel_proc = Compel(tokenizer=pipe.tokenizer, text_encoder=pipe.text_encoder)
|
66 |
-
```
|
67 |
-
|
68 |
-
Now we emphasize the part "ball" with the `"++"` syntax:
|
69 |
-
|
70 |
-
```py
|
71 |
-
prompt = "a red cat playing with a ball++"
|
72 |
-
```
|
73 |
-
|
74 |
-
and instead of passing this to the pipeline directly, we have to process it using `compel_proc`:
|
75 |
-
|
76 |
-
```py
|
77 |
-
prompt_embeds = compel_proc(prompt)
|
78 |
-
```
|
79 |
-
|
80 |
-
Now we can pass `prompt_embeds` directly to the pipeline:
|
81 |
-
|
82 |
-
```py
|
83 |
-
generator = torch.Generator(device="cpu").manual_seed(33)
|
84 |
-
|
85 |
-
images = pipe(prompt_embeds=prompt_embeds, generator=generator, num_inference_steps=20).images[0]
|
86 |
-
image
|
87 |
-
```
|
88 |
-
|
89 |
-
We now get the following image which has a "ball"!
|
90 |
-
|
91 |
-
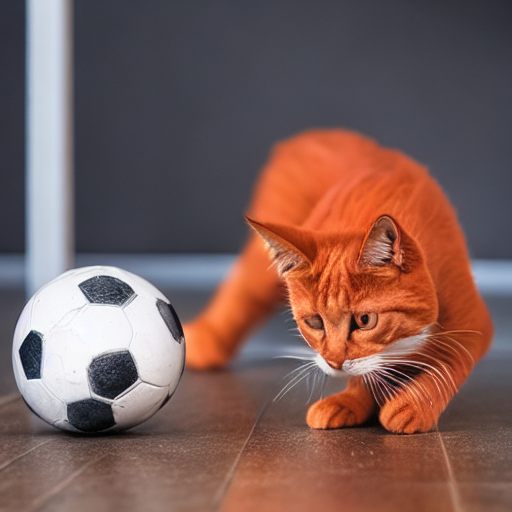
|
92 |
-
|
93 |
-
Similarly, we de-emphasize parts of the sentence by using the `--` suffix for words, feel free to give it
|
94 |
-
a try!
|
95 |
-
|
96 |
-
If your favorite pipeline does not have a `prompt_embeds` input, please make sure to open an issue, the
|
97 |
-
diffusers team tries to be as responsive as possible.
|
98 |
-
|
99 |
-
Compel 1.1.6 adds a utility class to simplify using textual inversions. Instantiate a `DiffusersTextualInversionManager` and pass it to Compel init:
|
100 |
-
|
101 |
-
```
|
102 |
-
textual_inversion_manager = DiffusersTextualInversionManager(pipe)
|
103 |
-
compel = Compel(
|
104 |
-
tokenizer=pipe.tokenizer,
|
105 |
-
text_encoder=pipe.text_encoder,
|
106 |
-
textual_inversion_manager=textual_inversion_manager)
|
107 |
-
```
|
108 |
-
|
109 |
-
Also, please check out the documentation of the [compel](https://github.com/damian0815/compel) library for
|
110 |
-
more information.
|
|
|
|
|
|
|
|
|
|
|
|
|
|
|
|
|
|
|
|
|
|
|
|
|
|
|
|
|
|
|
|
|
|
|
|
|
|
|
|
|
|
|
|
|
|
|
|
|
|
|
|
|
|
|
|
|
|
|
|
|
|
|
|
|
|
|
|
|
|
|
|
|
|
|
|
|
|
|
|
|
|
|
|
|
|
|
|
|
|
|
|
|
|
|
|
|
|
|
|
|
|
|
|
|
|
|
|
|
|
|
|
|
|
|
|
|
|
|
|
|
|
|
|
|
|
|
|
|
|
|
|
|
|
|
|
|
|
|
|
|
|
|
|
|
|
|
|
|
|
|
|
|
|
|
|
|
|
|
|
|
|
|
|
|
|
|
|
|
|
|
|
|
|
|
|
|
|
|
|
|
|
|
|
|
|
|
|
|
|
|
|
|
|
|
|
|
|
|
|
|
|
|
|
|
|
|
|
|
|
|
|
|
|
|
|
|
|
|
|
|
|
spaces/Annotation-AI/fast-segment-everything-with-image-prompt/README.md
DELETED
@@ -1,12 +0,0 @@
|
|
1 |
-
---
|
2 |
-
title: Fast Segment Everything With Image Prompt
|
3 |
-
emoji: 🔥
|
4 |
-
colorFrom: red
|
5 |
-
colorTo: red
|
6 |
-
sdk: gradio
|
7 |
-
sdk_version: 3.32.0
|
8 |
-
app_file: app.py
|
9 |
-
pinned: false
|
10 |
-
---
|
11 |
-
|
12 |
-
Check out the configuration reference at https://huggingface.co/docs/hub/spaces-config-reference
|
|
|
|
|
|
|
|
|
|
|
|
|
|
|
|
|
|
|
|
|
|
|
|
|
|
spaces/Anonymous-sub/Rerender/ControlNet/ldm/modules/diffusionmodules/openaimodel.py
DELETED
@@ -1,786 +0,0 @@
|
|
1 |
-
from abc import abstractmethod
|
2 |
-
import math
|
3 |
-
|
4 |
-
import numpy as np
|
5 |
-
import torch as th
|
6 |
-
import torch.nn as nn
|
7 |
-
import torch.nn.functional as F
|
8 |
-
|
9 |
-
from ldm.modules.diffusionmodules.util import (
|
10 |
-
checkpoint,
|
11 |
-
conv_nd,
|
12 |
-
linear,
|
13 |
-
avg_pool_nd,
|
14 |
-
zero_module,
|
15 |
-
normalization,
|
16 |
-
timestep_embedding,
|
17 |
-
)
|
18 |
-
from ldm.modules.attention import SpatialTransformer
|
19 |
-
from ldm.util import exists
|
20 |
-
|
21 |
-
|
22 |
-
# dummy replace
|
23 |
-
def convert_module_to_f16(x):
|
24 |
-
pass
|
25 |
-
|
26 |
-
def convert_module_to_f32(x):
|
27 |
-
pass
|
28 |
-
|
29 |
-
|
30 |
-
## go
|
31 |
-
class AttentionPool2d(nn.Module):
|
32 |
-
"""
|
33 |
-
Adapted from CLIP: https://github.com/openai/CLIP/blob/main/clip/model.py
|
34 |
-
"""
|
35 |
-
|
36 |
-
def __init__(
|
37 |
-
self,
|
38 |
-
spacial_dim: int,
|
39 |
-
embed_dim: int,
|
40 |
-
num_heads_channels: int,
|
41 |
-
output_dim: int = None,
|
42 |
-
):
|
43 |
-
super().__init__()
|
44 |
-
self.positional_embedding = nn.Parameter(th.randn(embed_dim, spacial_dim ** 2 + 1) / embed_dim ** 0.5)
|
45 |
-
self.qkv_proj = conv_nd(1, embed_dim, 3 * embed_dim, 1)
|
46 |
-
self.c_proj = conv_nd(1, embed_dim, output_dim or embed_dim, 1)
|
47 |
-
self.num_heads = embed_dim // num_heads_channels
|
48 |
-
self.attention = QKVAttention(self.num_heads)
|
49 |
-
|
50 |
-
def forward(self, x):
|
51 |
-
b, c, *_spatial = x.shape
|
52 |
-
x = x.reshape(b, c, -1) # NC(HW)
|
53 |
-
x = th.cat([x.mean(dim=-1, keepdim=True), x], dim=-1) # NC(HW+1)
|
54 |
-
x = x + self.positional_embedding[None, :, :].to(x.dtype) # NC(HW+1)
|
55 |
-
x = self.qkv_proj(x)
|
56 |
-
x = self.attention(x)
|
57 |
-
x = self.c_proj(x)
|
58 |
-
return x[:, :, 0]
|
59 |
-
|
60 |
-
|
61 |
-
class TimestepBlock(nn.Module):
|
62 |
-
"""
|
63 |
-
Any module where forward() takes timestep embeddings as a second argument.
|
64 |
-
"""
|
65 |
-
|
66 |
-
@abstractmethod
|
67 |
-
def forward(self, x, emb):
|
68 |
-
"""
|
69 |
-
Apply the module to `x` given `emb` timestep embeddings.
|
70 |
-
"""
|
71 |
-
|
72 |
-
|
73 |
-
class TimestepEmbedSequential(nn.Sequential, TimestepBlock):
|
74 |
-
"""
|
75 |
-
A sequential module that passes timestep embeddings to the children that
|
76 |
-
support it as an extra input.
|
77 |
-
"""
|
78 |
-
|
79 |
-
def forward(self, x, emb, context=None):
|
80 |
-
for layer in self:
|
81 |
-
if isinstance(layer, TimestepBlock):
|
82 |
-
x = layer(x, emb)
|
83 |
-
elif isinstance(layer, SpatialTransformer):
|
84 |
-
x = layer(x, context)
|
85 |
-
else:
|
86 |
-
x = layer(x)
|
87 |
-
return x
|
88 |
-
|
89 |
-
|
90 |
-
class Upsample(nn.Module):
|
91 |
-
"""
|
92 |
-
An upsampling layer with an optional convolution.
|
93 |
-
:param channels: channels in the inputs and outputs.
|
94 |
-
:param use_conv: a bool determining if a convolution is applied.
|
95 |
-
:param dims: determines if the signal is 1D, 2D, or 3D. If 3D, then
|
96 |
-
upsampling occurs in the inner-two dimensions.
|
97 |
-
"""
|
98 |
-
|
99 |
-
def __init__(self, channels, use_conv, dims=2, out_channels=None, padding=1):
|
100 |
-
super().__init__()
|
101 |
-
self.channels = channels
|
102 |
-
self.out_channels = out_channels or channels
|
103 |
-
self.use_conv = use_conv
|
104 |
-
self.dims = dims
|
105 |
-
if use_conv:
|
106 |
-
self.conv = conv_nd(dims, self.channels, self.out_channels, 3, padding=padding)
|
107 |
-
|
108 |
-
def forward(self, x):
|
109 |
-
assert x.shape[1] == self.channels
|
110 |
-
if self.dims == 3:
|
111 |
-
x = F.interpolate(
|
112 |
-
x, (x.shape[2], x.shape[3] * 2, x.shape[4] * 2), mode="nearest"
|
113 |
-
)
|
114 |
-
else:
|
115 |
-
x = F.interpolate(x, scale_factor=2, mode="nearest")
|
116 |
-
if self.use_conv:
|
117 |
-
x = self.conv(x)
|
118 |
-
return x
|
119 |
-
|
120 |
-
class TransposedUpsample(nn.Module):
|
121 |
-
'Learned 2x upsampling without padding'
|
122 |
-
def __init__(self, channels, out_channels=None, ks=5):
|
123 |
-
super().__init__()
|
124 |
-
self.channels = channels
|
125 |
-
self.out_channels = out_channels or channels
|
126 |
-
|
127 |
-
self.up = nn.ConvTranspose2d(self.channels,self.out_channels,kernel_size=ks,stride=2)
|
128 |
-
|
129 |
-
def forward(self,x):
|
130 |
-
return self.up(x)
|
131 |
-
|
132 |
-
|
133 |
-
class Downsample(nn.Module):
|
134 |
-
"""
|
135 |
-
A downsampling layer with an optional convolution.
|
136 |
-
:param channels: channels in the inputs and outputs.
|
137 |
-
:param use_conv: a bool determining if a convolution is applied.
|
138 |
-
:param dims: determines if the signal is 1D, 2D, or 3D. If 3D, then
|
139 |
-
downsampling occurs in the inner-two dimensions.
|
140 |
-
"""
|
141 |
-
|
142 |
-
def __init__(self, channels, use_conv, dims=2, out_channels=None,padding=1):
|
143 |
-
super().__init__()
|
144 |
-
self.channels = channels
|
145 |
-
self.out_channels = out_channels or channels
|
146 |
-
self.use_conv = use_conv
|
147 |
-
self.dims = dims
|
148 |
-
stride = 2 if dims != 3 else (1, 2, 2)
|
149 |
-
if use_conv:
|
150 |
-
self.op = conv_nd(
|
151 |
-
dims, self.channels, self.out_channels, 3, stride=stride, padding=padding
|
152 |
-
)
|
153 |
-
else:
|
154 |
-
assert self.channels == self.out_channels
|
155 |
-
self.op = avg_pool_nd(dims, kernel_size=stride, stride=stride)
|
156 |
-
|
157 |
-
def forward(self, x):
|
158 |
-
assert x.shape[1] == self.channels
|
159 |
-
return self.op(x)
|
160 |
-
|
161 |
-
|
162 |
-
class ResBlock(TimestepBlock):
|
163 |
-
"""
|
164 |
-
A residual block that can optionally change the number of channels.
|
165 |
-
:param channels: the number of input channels.
|
166 |
-
:param emb_channels: the number of timestep embedding channels.
|
167 |
-
:param dropout: the rate of dropout.
|
168 |
-
:param out_channels: if specified, the number of out channels.
|
169 |
-
:param use_conv: if True and out_channels is specified, use a spatial
|
170 |
-
convolution instead of a smaller 1x1 convolution to change the
|
171 |
-
channels in the skip connection.
|
172 |
-
:param dims: determines if the signal is 1D, 2D, or 3D.
|
173 |
-
:param use_checkpoint: if True, use gradient checkpointing on this module.
|
174 |
-
:param up: if True, use this block for upsampling.
|
175 |
-
:param down: if True, use this block for downsampling.
|
176 |
-
"""
|
177 |
-
|
178 |
-
def __init__(
|
179 |
-
self,
|
180 |
-
channels,
|
181 |
-
emb_channels,
|
182 |
-
dropout,
|
183 |
-
out_channels=None,
|
184 |
-
use_conv=False,
|
185 |
-
use_scale_shift_norm=False,
|
186 |
-
dims=2,
|
187 |
-
use_checkpoint=False,
|
188 |
-
up=False,
|
189 |
-
down=False,
|
190 |
-
):
|
191 |
-
super().__init__()
|
192 |
-
self.channels = channels
|
193 |
-
self.emb_channels = emb_channels
|
194 |
-
self.dropout = dropout
|
195 |
-
self.out_channels = out_channels or channels
|
196 |
-
self.use_conv = use_conv
|
197 |
-
self.use_checkpoint = use_checkpoint
|
198 |
-
self.use_scale_shift_norm = use_scale_shift_norm
|
199 |
-
|
200 |
-
self.in_layers = nn.Sequential(
|
201 |
-
normalization(channels),
|
202 |
-
nn.SiLU(),
|
203 |
-
conv_nd(dims, channels, self.out_channels, 3, padding=1),
|
204 |
-
)
|
205 |
-
|
206 |
-
self.updown = up or down
|
207 |
-
|
208 |
-
if up:
|
209 |
-
self.h_upd = Upsample(channels, False, dims)
|
210 |
-
self.x_upd = Upsample(channels, False, dims)
|
211 |
-
elif down:
|
212 |
-
self.h_upd = Downsample(channels, False, dims)
|
213 |
-
self.x_upd = Downsample(channels, False, dims)
|
214 |
-
else:
|
215 |
-
self.h_upd = self.x_upd = nn.Identity()
|
216 |
-
|
217 |
-
self.emb_layers = nn.Sequential(
|
218 |
-
nn.SiLU(),
|
219 |
-
linear(
|
220 |
-
emb_channels,
|
221 |
-
2 * self.out_channels if use_scale_shift_norm else self.out_channels,
|
222 |
-
),
|
223 |
-
)
|
224 |
-
self.out_layers = nn.Sequential(
|
225 |
-
normalization(self.out_channels),
|
226 |
-
nn.SiLU(),
|
227 |
-
nn.Dropout(p=dropout),
|
228 |
-
zero_module(
|
229 |
-
conv_nd(dims, self.out_channels, self.out_channels, 3, padding=1)
|
230 |
-
),
|
231 |
-
)
|
232 |
-
|
233 |
-
if self.out_channels == channels:
|
234 |
-
self.skip_connection = nn.Identity()
|
235 |
-
elif use_conv:
|
236 |
-
self.skip_connection = conv_nd(
|
237 |
-
dims, channels, self.out_channels, 3, padding=1
|
238 |
-
)
|
239 |
-
else:
|
240 |
-
self.skip_connection = conv_nd(dims, channels, self.out_channels, 1)
|
241 |
-
|
242 |
-
def forward(self, x, emb):
|
243 |
-
"""
|
244 |
-
Apply the block to a Tensor, conditioned on a timestep embedding.
|
245 |
-
:param x: an [N x C x ...] Tensor of features.
|
246 |
-
:param emb: an [N x emb_channels] Tensor of timestep embeddings.
|
247 |
-
:return: an [N x C x ...] Tensor of outputs.
|
248 |
-
"""
|
249 |
-
return checkpoint(
|
250 |
-
self._forward, (x, emb), self.parameters(), self.use_checkpoint
|
251 |
-
)
|
252 |
-
|
253 |
-
|
254 |
-
def _forward(self, x, emb):
|
255 |
-
if self.updown:
|
256 |
-
in_rest, in_conv = self.in_layers[:-1], self.in_layers[-1]
|
257 |
-
h = in_rest(x)
|
258 |
-
h = self.h_upd(h)
|
259 |
-
x = self.x_upd(x)
|
260 |
-
h = in_conv(h)
|
261 |
-
else:
|
262 |
-
h = self.in_layers(x)
|
263 |
-
emb_out = self.emb_layers(emb).type(h.dtype)
|
264 |
-
while len(emb_out.shape) < len(h.shape):
|
265 |
-
emb_out = emb_out[..., None]
|
266 |
-
if self.use_scale_shift_norm:
|
267 |
-
out_norm, out_rest = self.out_layers[0], self.out_layers[1:]
|
268 |
-
scale, shift = th.chunk(emb_out, 2, dim=1)
|
269 |
-
h = out_norm(h) * (1 + scale) + shift
|
270 |
-
h = out_rest(h)
|
271 |
-
else:
|
272 |
-
h = h + emb_out
|
273 |
-
h = self.out_layers(h)
|
274 |
-
return self.skip_connection(x) + h
|
275 |
-
|
276 |
-
|
277 |
-
class AttentionBlock(nn.Module):
|
278 |
-
"""
|
279 |
-
An attention block that allows spatial positions to attend to each other.
|
280 |
-
Originally ported from here, but adapted to the N-d case.
|
281 |
-
https://github.com/hojonathanho/diffusion/blob/1e0dceb3b3495bbe19116a5e1b3596cd0706c543/diffusion_tf/models/unet.py#L66.
|
282 |
-
"""
|
283 |
-
|
284 |
-
def __init__(
|
285 |
-
self,
|
286 |
-
channels,
|
287 |
-
num_heads=1,
|
288 |
-
num_head_channels=-1,
|
289 |
-
use_checkpoint=False,
|
290 |
-
use_new_attention_order=False,
|
291 |
-
):
|
292 |
-
super().__init__()
|
293 |
-
self.channels = channels
|
294 |
-
if num_head_channels == -1:
|
295 |
-
self.num_heads = num_heads
|
296 |
-
else:
|
297 |
-
assert (
|
298 |
-
channels % num_head_channels == 0
|
299 |
-
), f"q,k,v channels {channels} is not divisible by num_head_channels {num_head_channels}"
|
300 |
-
self.num_heads = channels // num_head_channels
|
301 |
-
self.use_checkpoint = use_checkpoint
|
302 |
-
self.norm = normalization(channels)
|
303 |
-
self.qkv = conv_nd(1, channels, channels * 3, 1)
|
304 |
-
if use_new_attention_order:
|
305 |
-
# split qkv before split heads
|
306 |
-
self.attention = QKVAttention(self.num_heads)
|
307 |
-
else:
|
308 |
-
# split heads before split qkv
|
309 |
-
self.attention = QKVAttentionLegacy(self.num_heads)
|
310 |
-
|
311 |
-
self.proj_out = zero_module(conv_nd(1, channels, channels, 1))
|
312 |
-
|
313 |
-
def forward(self, x):
|
314 |
-
return checkpoint(self._forward, (x,), self.parameters(), True) # TODO: check checkpoint usage, is True # TODO: fix the .half call!!!
|
315 |
-
#return pt_checkpoint(self._forward, x) # pytorch
|
316 |
-
|
317 |
-
def _forward(self, x):
|
318 |
-
b, c, *spatial = x.shape
|
319 |
-
x = x.reshape(b, c, -1)
|
320 |
-
qkv = self.qkv(self.norm(x))
|
321 |
-
h = self.attention(qkv)
|
322 |
-
h = self.proj_out(h)
|
323 |
-
return (x + h).reshape(b, c, *spatial)
|
324 |
-
|
325 |
-
|
326 |
-
def count_flops_attn(model, _x, y):
|
327 |
-
"""
|
328 |
-
A counter for the `thop` package to count the operations in an
|
329 |
-
attention operation.
|
330 |
-
Meant to be used like:
|
331 |
-
macs, params = thop.profile(
|
332 |
-
model,
|
333 |
-
inputs=(inputs, timestamps),
|
334 |
-
custom_ops={QKVAttention: QKVAttention.count_flops},
|
335 |
-
)
|
336 |
-
"""
|
337 |
-
b, c, *spatial = y[0].shape
|
338 |
-
num_spatial = int(np.prod(spatial))
|
339 |
-
# We perform two matmuls with the same number of ops.
|
340 |
-
# The first computes the weight matrix, the second computes
|
341 |
-
# the combination of the value vectors.
|
342 |
-
matmul_ops = 2 * b * (num_spatial ** 2) * c
|
343 |
-
model.total_ops += th.DoubleTensor([matmul_ops])
|
344 |
-
|
345 |
-
|
346 |
-
class QKVAttentionLegacy(nn.Module):
|
347 |
-
"""
|
348 |
-
A module which performs QKV attention. Matches legacy QKVAttention + input/ouput heads shaping
|
349 |
-
"""
|
350 |
-
|
351 |
-
def __init__(self, n_heads):
|
352 |
-
super().__init__()
|
353 |
-
self.n_heads = n_heads
|
354 |
-
|
355 |
-
def forward(self, qkv):
|
356 |
-
"""
|
357 |
-
Apply QKV attention.
|
358 |
-
:param qkv: an [N x (H * 3 * C) x T] tensor of Qs, Ks, and Vs.
|
359 |
-
:return: an [N x (H * C) x T] tensor after attention.
|
360 |
-
"""
|
361 |
-
bs, width, length = qkv.shape
|
362 |
-
assert width % (3 * self.n_heads) == 0
|
363 |
-
ch = width // (3 * self.n_heads)
|
364 |
-
q, k, v = qkv.reshape(bs * self.n_heads, ch * 3, length).split(ch, dim=1)
|
365 |
-
scale = 1 / math.sqrt(math.sqrt(ch))
|
366 |
-
weight = th.einsum(
|
367 |
-
"bct,bcs->bts", q * scale, k * scale
|
368 |
-
) # More stable with f16 than dividing afterwards
|
369 |
-
weight = th.softmax(weight.float(), dim=-1).type(weight.dtype)
|
370 |
-
a = th.einsum("bts,bcs->bct", weight, v)
|
371 |
-
return a.reshape(bs, -1, length)
|
372 |
-
|
373 |
-
@staticmethod
|
374 |
-
def count_flops(model, _x, y):
|
375 |
-
return count_flops_attn(model, _x, y)
|
376 |
-
|
377 |
-
|
378 |
-
class QKVAttention(nn.Module):
|
379 |
-
"""
|
380 |
-
A module which performs QKV attention and splits in a different order.
|
381 |
-
"""
|
382 |
-
|
383 |
-
def __init__(self, n_heads):
|
384 |
-
super().__init__()
|
385 |
-
self.n_heads = n_heads
|
386 |
-
|
387 |
-
def forward(self, qkv):
|
388 |
-
"""
|
389 |
-
Apply QKV attention.
|
390 |
-
:param qkv: an [N x (3 * H * C) x T] tensor of Qs, Ks, and Vs.
|
391 |
-
:return: an [N x (H * C) x T] tensor after attention.
|
392 |
-
"""
|
393 |
-
bs, width, length = qkv.shape
|
394 |
-
assert width % (3 * self.n_heads) == 0
|
395 |
-
ch = width // (3 * self.n_heads)
|
396 |
-
q, k, v = qkv.chunk(3, dim=1)
|
397 |
-
scale = 1 / math.sqrt(math.sqrt(ch))
|
398 |
-
weight = th.einsum(
|
399 |
-
"bct,bcs->bts",
|
400 |
-
(q * scale).view(bs * self.n_heads, ch, length),
|
401 |
-
(k * scale).view(bs * self.n_heads, ch, length),
|
402 |
-
) # More stable with f16 than dividing afterwards
|
403 |
-
weight = th.softmax(weight.float(), dim=-1).type(weight.dtype)
|
404 |
-
a = th.einsum("bts,bcs->bct", weight, v.reshape(bs * self.n_heads, ch, length))
|
405 |
-
return a.reshape(bs, -1, length)
|
406 |
-
|
407 |
-
@staticmethod
|
408 |
-
def count_flops(model, _x, y):
|
409 |
-
return count_flops_attn(model, _x, y)
|
410 |
-
|
411 |
-
|
412 |
-
class UNetModel(nn.Module):
|
413 |
-
"""
|
414 |
-
The full UNet model with attention and timestep embedding.
|
415 |
-
:param in_channels: channels in the input Tensor.
|
416 |
-
:param model_channels: base channel count for the model.
|
417 |
-
:param out_channels: channels in the output Tensor.
|
418 |
-
:param num_res_blocks: number of residual blocks per downsample.
|
419 |
-
:param attention_resolutions: a collection of downsample rates at which
|
420 |
-
attention will take place. May be a set, list, or tuple.
|
421 |
-
For example, if this contains 4, then at 4x downsampling, attention
|
422 |
-
will be used.
|
423 |
-
:param dropout: the dropout probability.
|
424 |
-
:param channel_mult: channel multiplier for each level of the UNet.
|
425 |
-
:param conv_resample: if True, use learned convolutions for upsampling and
|
426 |
-
downsampling.
|
427 |
-
:param dims: determines if the signal is 1D, 2D, or 3D.
|
428 |
-
:param num_classes: if specified (as an int), then this model will be
|
429 |
-
class-conditional with `num_classes` classes.
|
430 |
-
:param use_checkpoint: use gradient checkpointing to reduce memory usage.
|
431 |
-
:param num_heads: the number of attention heads in each attention layer.
|
432 |
-
:param num_heads_channels: if specified, ignore num_heads and instead use
|
433 |
-
a fixed channel width per attention head.
|
434 |
-
:param num_heads_upsample: works with num_heads to set a different number
|
435 |
-
of heads for upsampling. Deprecated.
|
436 |
-
:param use_scale_shift_norm: use a FiLM-like conditioning mechanism.
|
437 |
-
:param resblock_updown: use residual blocks for up/downsampling.
|
438 |
-
:param use_new_attention_order: use a different attention pattern for potentially
|
439 |
-
increased efficiency.
|
440 |
-
"""
|
441 |
-
|
442 |
-
def __init__(
|
443 |
-
self,
|
444 |
-
image_size,
|
445 |
-
in_channels,
|
446 |
-
model_channels,
|
447 |
-
out_channels,
|
448 |
-
num_res_blocks,
|
449 |
-
attention_resolutions,
|
450 |
-
dropout=0,
|
451 |
-
channel_mult=(1, 2, 4, 8),
|
452 |
-
conv_resample=True,
|
453 |
-
dims=2,
|
454 |
-
num_classes=None,
|
455 |
-
use_checkpoint=False,
|
456 |
-
use_fp16=False,
|
457 |
-
num_heads=-1,
|
458 |
-
num_head_channels=-1,
|
459 |
-
num_heads_upsample=-1,
|
460 |
-
use_scale_shift_norm=False,
|
461 |
-
resblock_updown=False,
|
462 |
-
use_new_attention_order=False,
|
463 |
-
use_spatial_transformer=False, # custom transformer support
|
464 |
-
transformer_depth=1, # custom transformer support
|
465 |
-
context_dim=None, # custom transformer support
|
466 |
-
n_embed=None, # custom support for prediction of discrete ids into codebook of first stage vq model
|
467 |
-
legacy=True,
|
468 |
-
disable_self_attentions=None,
|
469 |
-
num_attention_blocks=None,
|
470 |
-
disable_middle_self_attn=False,
|
471 |
-
use_linear_in_transformer=False,
|
472 |
-
):
|
473 |
-
super().__init__()
|
474 |
-
if use_spatial_transformer:
|
475 |
-
assert context_dim is not None, 'Fool!! You forgot to include the dimension of your cross-attention conditioning...'
|
476 |
-
|
477 |
-
if context_dim is not None:
|
478 |
-
assert use_spatial_transformer, 'Fool!! You forgot to use the spatial transformer for your cross-attention conditioning...'
|
479 |
-
from omegaconf.listconfig import ListConfig
|
480 |
-
if type(context_dim) == ListConfig:
|
481 |
-
context_dim = list(context_dim)
|
482 |
-
|
483 |
-
if num_heads_upsample == -1:
|
484 |
-
num_heads_upsample = num_heads
|
485 |
-
|
486 |
-
if num_heads == -1:
|
487 |
-
assert num_head_channels != -1, 'Either num_heads or num_head_channels has to be set'
|
488 |
-
|
489 |
-
if num_head_channels == -1:
|
490 |
-
assert num_heads != -1, 'Either num_heads or num_head_channels has to be set'
|
491 |
-
|
492 |
-
self.image_size = image_size
|
493 |
-
self.in_channels = in_channels
|
494 |
-
self.model_channels = model_channels
|
495 |
-
self.out_channels = out_channels
|
496 |
-
if isinstance(num_res_blocks, int):
|
497 |
-
self.num_res_blocks = len(channel_mult) * [num_res_blocks]
|
498 |
-
else:
|
499 |
-
if len(num_res_blocks) != len(channel_mult):
|
500 |
-
raise ValueError("provide num_res_blocks either as an int (globally constant) or "
|
501 |
-
"as a list/tuple (per-level) with the same length as channel_mult")
|
502 |
-
self.num_res_blocks = num_res_blocks
|
503 |
-
if disable_self_attentions is not None:
|
504 |
-
# should be a list of booleans, indicating whether to disable self-attention in TransformerBlocks or not
|
505 |
-
assert len(disable_self_attentions) == len(channel_mult)
|
506 |
-
if num_attention_blocks is not None:
|
507 |
-
assert len(num_attention_blocks) == len(self.num_res_blocks)
|
508 |
-
assert all(map(lambda i: self.num_res_blocks[i] >= num_attention_blocks[i], range(len(num_attention_blocks))))
|
509 |
-
print(f"Constructor of UNetModel received num_attention_blocks={num_attention_blocks}. "
|
510 |
-
f"This option has LESS priority than attention_resolutions {attention_resolutions}, "
|
511 |
-
f"i.e., in cases where num_attention_blocks[i] > 0 but 2**i not in attention_resolutions, "
|
512 |
-
f"attention will still not be set.")
|
513 |
-
|
514 |
-
self.attention_resolutions = attention_resolutions
|
515 |
-
self.dropout = dropout
|
516 |
-
self.channel_mult = channel_mult
|
517 |
-
self.conv_resample = conv_resample
|
518 |
-
self.num_classes = num_classes
|
519 |
-
self.use_checkpoint = use_checkpoint
|
520 |
-
self.dtype = th.float16 if use_fp16 else th.float32
|
521 |
-
self.num_heads = num_heads
|
522 |
-
self.num_head_channels = num_head_channels
|
523 |
-
self.num_heads_upsample = num_heads_upsample
|
524 |
-
self.predict_codebook_ids = n_embed is not None
|
525 |
-
|
526 |
-
time_embed_dim = model_channels * 4
|
527 |
-
self.time_embed = nn.Sequential(
|
528 |
-
linear(model_channels, time_embed_dim),
|
529 |
-
nn.SiLU(),
|
530 |
-
linear(time_embed_dim, time_embed_dim),
|
531 |
-
)
|
532 |
-
|
533 |
-
if self.num_classes is not None:
|
534 |
-
if isinstance(self.num_classes, int):
|
535 |
-
self.label_emb = nn.Embedding(num_classes, time_embed_dim)
|
536 |
-
elif self.num_classes == "continuous":
|
537 |
-
print("setting up linear c_adm embedding layer")
|
538 |
-
self.label_emb = nn.Linear(1, time_embed_dim)
|
539 |
-
else:
|
540 |
-
raise ValueError()
|
541 |
-
|
542 |
-
self.input_blocks = nn.ModuleList(
|
543 |
-
[
|
544 |
-
TimestepEmbedSequential(
|
545 |
-
conv_nd(dims, in_channels, model_channels, 3, padding=1)
|
546 |
-
)
|
547 |
-
]
|
548 |
-
)
|
549 |
-
self._feature_size = model_channels
|
550 |
-
input_block_chans = [model_channels]
|
551 |
-
ch = model_channels
|
552 |
-
ds = 1
|
553 |
-
for level, mult in enumerate(channel_mult):
|
554 |
-
for nr in range(self.num_res_blocks[level]):
|
555 |
-
layers = [
|
556 |
-
ResBlock(
|
557 |
-
ch,
|
558 |
-
time_embed_dim,
|
559 |
-
dropout,
|
560 |
-
out_channels=mult * model_channels,
|
561 |
-
dims=dims,
|
562 |
-
use_checkpoint=use_checkpoint,
|
563 |
-
use_scale_shift_norm=use_scale_shift_norm,
|
564 |
-
)
|
565 |
-
]
|
566 |
-
ch = mult * model_channels
|
567 |
-
if ds in attention_resolutions:
|
568 |
-
if num_head_channels == -1:
|
569 |
-
dim_head = ch // num_heads
|
570 |
-
else:
|
571 |
-
num_heads = ch // num_head_channels
|
572 |
-
dim_head = num_head_channels
|
573 |
-
if legacy:
|
574 |
-
#num_heads = 1
|
575 |
-
dim_head = ch // num_heads if use_spatial_transformer else num_head_channels
|
576 |
-
if exists(disable_self_attentions):
|
577 |
-
disabled_sa = disable_self_attentions[level]
|
578 |
-
else:
|
579 |
-
disabled_sa = False
|
580 |
-
|
581 |
-
if not exists(num_attention_blocks) or nr < num_attention_blocks[level]:
|
582 |
-
layers.append(
|
583 |
-
AttentionBlock(
|
584 |
-
ch,
|
585 |
-
use_checkpoint=use_checkpoint,
|
586 |
-
num_heads=num_heads,
|
587 |
-
num_head_channels=dim_head,
|
588 |
-
use_new_attention_order=use_new_attention_order,
|
589 |
-
) if not use_spatial_transformer else SpatialTransformer(
|
590 |
-
ch, num_heads, dim_head, depth=transformer_depth, context_dim=context_dim,
|
591 |
-
disable_self_attn=disabled_sa, use_linear=use_linear_in_transformer,
|
592 |
-
use_checkpoint=use_checkpoint
|
593 |
-
)
|
594 |
-
)
|
595 |
-
self.input_blocks.append(TimestepEmbedSequential(*layers))
|
596 |
-
self._feature_size += ch
|
597 |
-
input_block_chans.append(ch)
|
598 |
-
if level != len(channel_mult) - 1:
|
599 |
-
out_ch = ch
|
600 |
-
self.input_blocks.append(
|
601 |
-
TimestepEmbedSequential(
|
602 |
-
ResBlock(
|
603 |
-
ch,
|
604 |
-
time_embed_dim,
|
605 |
-
dropout,
|
606 |
-
out_channels=out_ch,
|
607 |
-
dims=dims,
|
608 |
-
use_checkpoint=use_checkpoint,
|
609 |
-
use_scale_shift_norm=use_scale_shift_norm,
|
610 |
-
down=True,
|
611 |
-
)
|
612 |
-
if resblock_updown
|
613 |
-
else Downsample(
|
614 |
-
ch, conv_resample, dims=dims, out_channels=out_ch
|
615 |
-
)
|
616 |
-
)
|
617 |
-
)
|
618 |
-
ch = out_ch
|
619 |
-
input_block_chans.append(ch)
|
620 |
-
ds *= 2
|
621 |
-
self._feature_size += ch
|
622 |
-
|
623 |
-
if num_head_channels == -1:
|
624 |
-
dim_head = ch // num_heads
|
625 |
-
else:
|
626 |
-
num_heads = ch // num_head_channels
|
627 |
-
dim_head = num_head_channels
|
628 |
-
if legacy:
|
629 |
-
#num_heads = 1
|
630 |
-
dim_head = ch // num_heads if use_spatial_transformer else num_head_channels
|
631 |
-
self.middle_block = TimestepEmbedSequential(
|
632 |
-
ResBlock(
|
633 |
-
ch,
|
634 |
-
time_embed_dim,
|
635 |
-
dropout,
|
636 |
-
dims=dims,
|
637 |
-
use_checkpoint=use_checkpoint,
|
638 |
-
use_scale_shift_norm=use_scale_shift_norm,
|
639 |
-
),
|
640 |
-
AttentionBlock(
|
641 |
-
ch,
|
642 |
-
use_checkpoint=use_checkpoint,
|
643 |
-
num_heads=num_heads,
|
644 |
-
num_head_channels=dim_head,
|
645 |
-
use_new_attention_order=use_new_attention_order,
|
646 |
-
) if not use_spatial_transformer else SpatialTransformer( # always uses a self-attn
|
647 |
-
ch, num_heads, dim_head, depth=transformer_depth, context_dim=context_dim,
|
648 |
-
disable_self_attn=disable_middle_self_attn, use_linear=use_linear_in_transformer,
|
649 |
-
use_checkpoint=use_checkpoint
|
650 |
-
),
|
651 |
-
ResBlock(
|
652 |
-
ch,
|
653 |
-
time_embed_dim,
|
654 |
-
dropout,
|
655 |
-
dims=dims,
|
656 |
-
use_checkpoint=use_checkpoint,
|
657 |
-
use_scale_shift_norm=use_scale_shift_norm,
|
658 |
-
),
|
659 |
-
)
|
660 |
-
self._feature_size += ch
|
661 |
-
|
662 |
-
self.output_blocks = nn.ModuleList([])
|
663 |
-
for level, mult in list(enumerate(channel_mult))[::-1]:
|
664 |
-
for i in range(self.num_res_blocks[level] + 1):
|
665 |
-
ich = input_block_chans.pop()
|
666 |
-
layers = [
|
667 |
-
ResBlock(
|
668 |
-
ch + ich,
|
669 |
-
time_embed_dim,
|
670 |
-
dropout,
|
671 |
-
out_channels=model_channels * mult,
|
672 |
-
dims=dims,
|
673 |
-
use_checkpoint=use_checkpoint,
|
674 |
-
use_scale_shift_norm=use_scale_shift_norm,
|
675 |
-
)
|
676 |
-
]
|
677 |
-
ch = model_channels * mult
|
678 |
-
if ds in attention_resolutions:
|
679 |
-
if num_head_channels == -1:
|
680 |
-
dim_head = ch // num_heads
|
681 |
-
else:
|
682 |
-
num_heads = ch // num_head_channels
|
683 |
-
dim_head = num_head_channels
|
684 |
-
if legacy:
|
685 |
-
#num_heads = 1
|
686 |
-
dim_head = ch // num_heads if use_spatial_transformer else num_head_channels
|
687 |
-
if exists(disable_self_attentions):
|
688 |
-
disabled_sa = disable_self_attentions[level]
|
689 |
-
else:
|
690 |
-
disabled_sa = False
|
691 |
-
|
692 |
-
if not exists(num_attention_blocks) or i < num_attention_blocks[level]:
|
693 |
-
layers.append(
|
694 |
-
AttentionBlock(
|
695 |
-
ch,
|
696 |
-
use_checkpoint=use_checkpoint,
|
697 |
-
num_heads=num_heads_upsample,
|
698 |
-
num_head_channels=dim_head,
|
699 |
-
use_new_attention_order=use_new_attention_order,
|
700 |
-
) if not use_spatial_transformer else SpatialTransformer(
|
701 |
-
ch, num_heads, dim_head, depth=transformer_depth, context_dim=context_dim,
|
702 |
-
disable_self_attn=disabled_sa, use_linear=use_linear_in_transformer,
|
703 |
-
use_checkpoint=use_checkpoint
|
704 |
-
)
|
705 |
-
)
|
706 |
-
if level and i == self.num_res_blocks[level]:
|
707 |
-
out_ch = ch
|
708 |
-
layers.append(
|
709 |
-
ResBlock(
|
710 |
-
ch,
|
711 |
-
time_embed_dim,
|
712 |
-
dropout,
|
713 |
-
out_channels=out_ch,
|
714 |
-
dims=dims,
|
715 |
-
use_checkpoint=use_checkpoint,
|
716 |
-
use_scale_shift_norm=use_scale_shift_norm,
|
717 |
-
up=True,
|
718 |
-
)
|
719 |
-
if resblock_updown
|
720 |
-
else Upsample(ch, conv_resample, dims=dims, out_channels=out_ch)
|
721 |
-
)
|
722 |
-
ds //= 2
|
723 |
-
self.output_blocks.append(TimestepEmbedSequential(*layers))
|
724 |
-
self._feature_size += ch
|
725 |
-
|
726 |
-
self.out = nn.Sequential(
|
727 |
-
normalization(ch),
|
728 |
-
nn.SiLU(),
|
729 |
-
zero_module(conv_nd(dims, model_channels, out_channels, 3, padding=1)),
|
730 |
-
)
|
731 |
-
if self.predict_codebook_ids:
|
732 |
-
self.id_predictor = nn.Sequential(
|
733 |
-
normalization(ch),
|
734 |
-
conv_nd(dims, model_channels, n_embed, 1),
|
735 |
-
#nn.LogSoftmax(dim=1) # change to cross_entropy and produce non-normalized logits
|
736 |
-
)
|
737 |
-
|
738 |
-
def convert_to_fp16(self):
|
739 |
-
"""
|
740 |
-
Convert the torso of the model to float16.
|
741 |
-
"""
|
742 |
-
self.input_blocks.apply(convert_module_to_f16)
|
743 |
-
self.middle_block.apply(convert_module_to_f16)
|
744 |
-
self.output_blocks.apply(convert_module_to_f16)
|
745 |
-
|
746 |
-
def convert_to_fp32(self):
|
747 |
-
"""
|
748 |
-
Convert the torso of the model to float32.
|
749 |
-
"""
|
750 |
-
self.input_blocks.apply(convert_module_to_f32)
|
751 |
-
self.middle_block.apply(convert_module_to_f32)
|
752 |
-
self.output_blocks.apply(convert_module_to_f32)
|
753 |
-
|
754 |
-
def forward(self, x, timesteps=None, context=None, y=None,**kwargs):
|
755 |
-
"""
|
756 |
-
Apply the model to an input batch.
|
757 |
-
:param x: an [N x C x ...] Tensor of inputs.
|
758 |
-
:param timesteps: a 1-D batch of timesteps.
|
759 |
-
:param context: conditioning plugged in via crossattn
|
760 |
-
:param y: an [N] Tensor of labels, if class-conditional.
|
761 |
-
:return: an [N x C x ...] Tensor of outputs.
|
762 |
-
"""
|
763 |
-
assert (y is not None) == (
|
764 |
-
self.num_classes is not None
|
765 |
-
), "must specify y if and only if the model is class-conditional"
|
766 |
-
hs = []
|
767 |
-
t_emb = timestep_embedding(timesteps, self.model_channels, repeat_only=False)
|
768 |
-
emb = self.time_embed(t_emb)
|
769 |
-
|
770 |
-
if self.num_classes is not None:
|
771 |
-
assert y.shape[0] == x.shape[0]
|
772 |
-
emb = emb + self.label_emb(y)
|
773 |
-
|
774 |
-
h = x.type(self.dtype)
|
775 |
-
for module in self.input_blocks:
|
776 |
-
h = module(h, emb, context)
|
777 |
-
hs.append(h)
|
778 |
-
h = self.middle_block(h, emb, context)
|
779 |
-
for module in self.output_blocks:
|
780 |
-
h = th.cat([h, hs.pop()], dim=1)
|
781 |
-
h = module(h, emb, context)
|
782 |
-
h = h.type(x.dtype)
|
783 |
-
if self.predict_codebook_ids:
|
784 |
-
return self.id_predictor(h)
|
785 |
-
else:
|
786 |
-
return self.out(h)
|
|
|
|
|
|
|
|
|
|
|
|
|
|
|
|
|
|
|
|
|
|
|
|
|
|
|
|
|
|
|
|
|
|
|
|
|
|
|
|
|
|
|
|
|
|
|
|
|
|
|
|
|
|
|
|
|
|
|
|
|
|
|
|
|
|
|
|
|
|
|
|
|
|
|
|
|
|
|
|
|
|
|
|
|
|
|
|
|
|
|
|
|
|
|
|
|
|
|
|
|
|
|
|
|
|
|
|
|
|
|
|
|
|
|
|
|
|
|
|
|
|
|
|
|
|
|
|
|
|
|
|
|
|
|
|
|
|
|
|
|
|
|
|
|
|
|
|
|
|
|
|
|
|
|
|
|
|
|
|
|
|
|
|
|
|
|
|
|
|
|
|
|
|
|
|
|
|
|
|
|
|
|
|
|
|
|
|
|
|
|
|
|
|
|
|
|
|
|
|
|
|
|
|
|
|
|
|
|
|
|
|
|
|
|
|
|
|
|
|
|
|
|
|
|
|
|
|
|
|
|
|
|
|
|
|
|
|
|
|
|
|
|
|
|
|
|
|
|
|
|
|
|
|
|
|
|
|
|
|
|
|
|
|
|
|
|
|
|
|
|
|
|
|
|
|
|
|
|
|
|
|
|
|
|
|
|
|
|
|
|
|
|
|
|
|
|
|
|
|
|
|
|
|
|
|
|
|
|
|
|
|
|
|
|
|
|
|
|
|
|
|
|
|
|
|
|
|
|
|
|
|
|
|
|
|
|
|
|
|
|
|
|
|
|
|
|
|
|
|
|
|
|
|
|
|
|
|
|
|
|
|
|
|
|
|
|
|
|
|
|
|
|
|
|
|
|
|
|
|
|
|
|
|
|
|
|
|
|
|
|
|
|
|
|
|
|
|
|
|
|
|
|
|
|
|
|
|
|
|
|
|
|
|
|
|
|
|
|
|
|
|
|
|
|
|
|
|
|
|
|
|
|
|
|
|
|
|
|
|
|
|
|
|
|
|
|
|
|
|
|
|
|
|
|
|
|
|
|
|
|
|
|
|
|
|
|
|
|
|
|
|
|
|
|
|
|
|
|
|
|
|
|
|
|
|
|
|
|
|
|
|
|
|
|
|
|
|
|
|
|
|
|
|
|
|
|
|
|
|
|
|
|
|
|
|
|
|
|
|
|
|
|
|
|
|
|
|
|
|
|
|
|
|
|
|
|
|
|
|
|
|
|
|
|
|
|
|
|
|
|
|
|
|
|
|
|
|
|
|
|
|
|
|
|
|
|
|
|
|
|
|
|
|
|
|
|
|
|
|
|
|
|
|
|
|
|
|
|
|
|
|
|
|
|
|
|
|
|
|
|
|
|
|
|
|
|
|
|
|
|
|
|
|
|
|
|
|
|
|
|
|
|
|
|
|
|
|
|
|
|
|
|
|
|
|
|
|
|
|
|
|
|
|
|
|
|
|
|
|
|
|
|
|
|
|
|
|
|
|
|
|
|
|
|
|
|
|
|
|
|
|
|
|
|
|
|
|
|
|
|
|
|
|
|
|
|
|
|
|
|
|
|
|
|
|
|
|
|
|
|
|
|
|
|
|
|
|
|
|
|
|
|
|
|
|
|
|
|
|
|
|
|
|
|
|
|
|
|
|
|
|
|
|
|
|
|
|
|
|
|
|
|
|
|
|
|
|
|
|
|
|
|
|
|
|
|
|
|
|
|
|
|
|
|
|
|
|
|
|
|
|
|
|
|
|
|
|
|
|
|
|
|
|
|
|
|
|
|
|
|
|
|
|
|
|
|
|
|
|
|
|
|
|
|
|
|
|
|
|
|
|
|
|
|
|
|
|
|
|
|
|
|
|
|
|
|
|
|
|
|
|
|
|
|
|
|
|
|
|
|
|
|
|
|
|
|
|
|
|
|
|
|
|
|
|
|
|
|
|
|
|
|
|
|
|
|
|
|
|
|
|
|
|
|
|
|
|
|
|
|
|
|
|
|
|
|
|
|
|
|
|
|
|
|
|
|
|
|
|
|
|
|
|
|
|
|
|
|
|
|
|
|
|
|
|
|
|
|
|
|
|
|
|
|
|
|
|
|
|
|
|
|
|
|
|
|
|
|
|
|
|
|
|
|
|
|
|
|
|
|
|
|
|
|
|
|
|
|
|
|
|
|
|
|
|
|
|
|
|
|
|
|
|
|
|
|
|
|
|
|
|
|
|
|
|
|
|
|
|
|
|
|
|
|
|
|
|
|
|
|
|
|
|
|
|
|
|
|
|
|
|
|
|
|
|
|
|
|
|
|
|
|
|
|
|
|
|
|
|
|
|
|
|
|
|
|
|
|
|
|
|
|
|
|
|
|
|
|
|
|
|
|
|
|
|
|
|
|
|
|
|
|
|
|
|
|
|
|
|
|
|
|
|
|
|
|
|
|
|
|
|
|
|
|
|
|
|
|
|
|
|
|
|
|
|
|
|
|
|
|
|
|
|
|
|
|
|
|
|
|
|
|
|
|
|
|
|
|
|
|
|
|
|
|
|
|
|
|
|
|
|
|
|
|
|
|
|
|
|
|
|
|
|
|
|
|
|
|
|
|
|
|
|
|
|
|
|
|
|
|
|
|
|
|
|
|
|
|
|
|
|
|
|
|
|
|
|
|
|
|
|
|
|
|
|
|
|
|
|
|
|
|
|
|
|
|
|
|
|
|
|
|
|
|
|
|
|
|
|
|
|
|
|
|
|
|
|
|
|
|
|
|
|
|
|
|
|
|
|
|
|
|
|
|
|
|
|
|
|
|
|
|
|
|
|
|
|
|
|
|
|
|
|
|
|
|
|
|
|
|
|
|
|
|
|
|
|
|
|
|
|
|
|
|
|
|
|
|
|
|
|
|
|
|
|
|
|
|
|
|
|
|
|
|
|
|
|
|
|
|
|
|
|
|
|
|
|
|
|
|
|
|
|
|
|
|
|
|
|
|
|
|
|
|
|
|
|
|
|
|
|
|
|
|
|
|
|
|
|
|
|
|
|
|
|
|
|
|
|
|
|
|
|
|
|
|
|
|
|
|
|
|
|
|
|
|
|
|
|
|
|
|
|
|
|
|
|
|
|
|
|
|
|
|
|
|
|
|
|
|
|
|
|
|
|
|
|
|
|
|
|
|
|
|
|
|
|
|
|
|
|
|
|
|
|
|
|
|
|
|
|
|
|
|
|
|
|
|
|
|
|
|
|
|
|
|
|
|
|
|
|
|
|
|
|
|
|
|
|
|
|
|
|
|
|
|
|
|
|
|
|
|
|
|
|
|
|
|
|
|
|
|
|
|
|
|
|
|
|
|
|
|
|
|
|
|
|
|
|
|
|
|
|
|
|
|
|
|
|
|
|
|
|
|
|
|
|
|
|
|
|
|
|
|
|
|
|
|
|
|
|
|
|
|
|
|
|
|
|
|
|
|
|
|
|
|
|
|
|
|
|
|
|
|
|
|
|
|
|
|
|
|
|
|
|
|
|
|
|
|
|
|
|
|
|
|
|
|
|
|
|
|
|
spaces/Anthony7906/MengHuiMXD_GPT/modules/config.py
DELETED
@@ -1,173 +0,0 @@
|
|
1 |
-
from collections import defaultdict
|
2 |
-
from contextlib import contextmanager
|
3 |
-
import os
|
4 |
-
import logging
|
5 |
-
import sys
|
6 |
-
import commentjson as json
|
7 |
-
|
8 |
-
from . import shared
|
9 |
-
from . import presets
|
10 |
-
|
11 |
-
|
12 |
-
__all__ = [
|
13 |
-
"my_api_key",
|
14 |
-
"authflag",
|
15 |
-
"auth_list",
|
16 |
-
"dockerflag",
|
17 |
-
"retrieve_proxy",
|
18 |
-
"log_level",
|
19 |
-
"advance_docs",
|
20 |
-
"update_doc_config",
|
21 |
-
"multi_api_key",
|
22 |
-
"server_name",
|
23 |
-
"server_port",
|
24 |
-
"share",
|
25 |
-
]
|
26 |
-
|
27 |
-
# 添加一个统一的config文件,避免文件过多造成的疑惑(优先级最低)
|
28 |
-
# 同时,也可以为后续支持自定义功能提供config的帮助
|
29 |
-
if os.path.exists("config.json"):
|
30 |
-
with open("config.json", "r", encoding='utf-8') as f:
|
31 |
-
config = json.load(f)
|
32 |
-
else:
|
33 |
-
config = {}
|
34 |
-
|
35 |
-
lang_config = config.get("language", "auto")
|
36 |
-
language = os.environ.get("LANGUAGE", lang_config)
|
37 |
-
|
38 |
-
if os.path.exists("api_key.txt"):
|
39 |
-
logging.info("检测到api_key.txt文件,正在进行迁移...")
|
40 |
-
with open("api_key.txt", "r") as f:
|
41 |
-
config["openai_api_key"] = f.read().strip()
|
42 |
-
os.rename("api_key.txt", "api_key(deprecated).txt")
|
43 |
-
with open("config.json", "w", encoding='utf-8') as f:
|
44 |
-
json.dump(config, f, indent=4)
|
45 |
-
|
46 |
-
if os.path.exists("auth.json"):
|
47 |
-
logging.info("检测到auth.json文件,正在进行迁移...")
|
48 |
-
auth_list = []
|
49 |
-
with open("auth.json", "r", encoding='utf-8') as f:
|
50 |
-
auth = json.load(f)
|
51 |
-
for _ in auth:
|
52 |
-
if auth[_]["username"] and auth[_]["password"]:
|
53 |
-
auth_list.append((auth[_]["username"], auth[_]["password"]))
|
54 |
-
else:
|
55 |
-
logging.error("请检查auth.json文件中的用户名和密码!")
|
56 |
-
sys.exit(1)
|
57 |
-
config["users"] = auth_list
|
58 |
-
os.rename("auth.json", "auth(deprecated).json")
|
59 |
-
with open("config.json", "w", encoding='utf-8') as f:
|
60 |
-
json.dump(config, f, indent=4)
|
61 |
-
|
62 |
-
## 处理docker if we are running in Docker
|
63 |
-
dockerflag = config.get("dockerflag", False)
|
64 |
-
if os.environ.get("dockerrun") == "yes":
|
65 |
-
dockerflag = True
|
66 |
-
|
67 |
-
## 处理 api-key 以及 允许的用户列表
|
68 |
-
my_api_key = config.get("openai_api_key", "")
|
69 |
-
my_api_key = os.environ.get("OPENAI_API_KEY", my_api_key)
|
70 |
-
|
71 |
-
xmchat_api_key = config.get("xmchat_api_key", "")
|
72 |
-
if os.environ.get("XMCHAT_API_KEY", None) == None:
|
73 |
-
os.environ["XMCHAT_API_KEY"] = xmchat_api_key
|
74 |
-
|
75 |
-
## 多账户机制
|
76 |
-
multi_api_key = config.get("multi_api_key", False) # 是否开启多账户机制
|
77 |
-
if multi_api_key:
|
78 |
-
api_key_list = config.get("api_key_list", [])
|
79 |
-
if len(api_key_list) == 0:
|
80 |
-
logging.error("多账号模式已开启,但api_key_list为空,请检查config.json")
|
81 |
-
sys.exit(1)
|
82 |
-
shared.state.set_api_key_queue(api_key_list)
|
83 |
-
|
84 |
-
auth_list = config.get("users", []) # 实际上是使用者的列表
|
85 |
-
authflag = len(auth_list) > 0 # 是否开启认证的状态值,改为判断auth_list长度
|
86 |
-
|
87 |
-
# 处理自定义的api_host,优先读环境变量的配置,如果存在则自动装配
|
88 |
-
api_host = os.environ.get("api_host", config.get("api_host", ""))
|
89 |
-
if api_host:
|
90 |
-
shared.state.set_api_host(api_host)
|
91 |
-
|
92 |
-
@contextmanager
|
93 |
-
def retrieve_openai_api(api_key = None):
|
94 |
-
old_api_key = os.environ.get("OPENAI_API_KEY", "")
|
95 |
-
if api_key is None:
|
96 |
-
os.environ["OPENAI_API_KEY"] = my_api_key
|
97 |
-
yield my_api_key
|
98 |
-
else:
|
99 |
-
os.environ["OPENAI_API_KEY"] = api_key
|
100 |
-
yield api_key
|
101 |
-
os.environ["OPENAI_API_KEY"] = old_api_key
|
102 |
-
|
103 |
-
## 处理log
|
104 |
-
log_level = config.get("log_level", "INFO")
|
105 |
-
logging.basicConfig(
|
106 |
-
level=log_level,
|
107 |
-
format="%(asctime)s [%(levelname)s] [%(filename)s:%(lineno)d] %(message)s",
|
108 |
-
)
|
109 |
-
|
110 |
-
## 处理代理:
|
111 |
-
http_proxy = config.get("http_proxy", "")
|
112 |
-
https_proxy = config.get("https_proxy", "")
|
113 |
-
http_proxy = os.environ.get("HTTP_PROXY", http_proxy)
|
114 |
-
https_proxy = os.environ.get("HTTPS_PROXY", https_proxy)
|
115 |
-
|
116 |
-
# 重置系统变量,在不需要设置的时候不设置环境变量,以免引起全局代理报错
|
117 |
-
os.environ["HTTP_PROXY"] = ""
|
118 |
-
os.environ["HTTPS_PROXY"] = ""
|
119 |
-
|
120 |
-
local_embedding = config.get("local_embedding", False) # 是否使用本地embedding
|
121 |
-
|
122 |
-
@contextmanager
|
123 |
-
def retrieve_proxy(proxy=None):
|
124 |
-
"""
|
125 |
-
1, 如果proxy = NONE,设置环境变量,并返回最新设置的代理
|
126 |
-
2,如果proxy != NONE,更新当前的代理配置,但是不更新环境变量
|
127 |
-
"""
|
128 |
-
global http_proxy, https_proxy
|
129 |
-
if proxy is not None:
|
130 |
-
http_proxy = proxy
|
131 |
-
https_proxy = proxy
|
132 |
-
yield http_proxy, https_proxy
|
133 |
-
else:
|
134 |
-
old_var = os.environ["HTTP_PROXY"], os.environ["HTTPS_PROXY"]
|
135 |
-
os.environ["HTTP_PROXY"] = http_proxy
|
136 |
-
os.environ["HTTPS_PROXY"] = https_proxy
|
137 |
-
yield http_proxy, https_proxy # return new proxy
|
138 |
-
|
139 |
-
# return old proxy
|
140 |
-
os.environ["HTTP_PROXY"], os.environ["HTTPS_PROXY"] = old_var
|
141 |
-
|
142 |
-
|
143 |
-
## 处理advance docs
|
144 |
-
advance_docs = defaultdict(lambda: defaultdict(dict))
|
145 |
-
advance_docs.update(config.get("advance_docs", {}))
|
146 |
-
def update_doc_config(two_column_pdf):
|
147 |
-
global advance_docs
|
148 |
-
advance_docs["pdf"]["two_column"] = two_column_pdf
|
149 |
-
|
150 |
-
logging.info(f"更新后的文件参数为:{advance_docs}")
|
151 |
-
|
152 |
-
## 处理gradio.launch参数
|
153 |
-
server_name = config.get("server_name", None)
|
154 |
-
server_port = config.get("server_port", None)
|
155 |
-
if server_name is None:
|
156 |
-
if dockerflag:
|
157 |
-
server_name = "0.0.0.0"
|
158 |
-
else:
|
159 |
-
server_name = "127.0.0.1"
|
160 |
-
if server_port is None:
|
161 |
-
if dockerflag:
|
162 |
-
server_port = 7860
|
163 |
-
|
164 |
-
assert server_port is None or type(server_port) == int, "要求port设置为int类型"
|
165 |
-
|
166 |
-
# 设置默认model
|
167 |
-
default_model = config.get("default_model", "")
|
168 |
-
try:
|
169 |
-
presets.DEFAULT_MODEL = presets.MODELS.index(default_model)
|
170 |
-
except ValueError:
|
171 |
-
pass
|
172 |
-
|
173 |
-
share = config.get("share", False)
|
|
|
|
|
|
|
|
|
|
|
|
|
|
|
|
|
|
|
|
|
|
|
|
|
|
|
|
|
|
|
|
|
|
|
|
|
|
|
|
|
|
|
|
|
|
|
|
|
|
|
|
|
|
|
|
|
|
|
|
|
|
|
|
|
|
|
|
|
|
|
|
|
|
|
|
|
|
|
|
|
|
|
|
|
|
|
|
|
|
|
|
|
|
|
|
|
|
|
|
|
|
|
|
|
|
|
|
|
|
|
|
|
|
|
|
|
|
|
|
|
|
|
|
|
|
|
|
|
|
|
|
|
|
|
|
|
|
|
|
|
|
|
|
|
|
|
|
|
|
|
|
|
|
|
|
|
|
|
|
|
|
|
|
|
|
|
|
|
|
|
|
|
|
|
|
|
|
|
|
|
|
|
|
|
|
|
|
|
|
|
|
|
|
|
|
|
|
|
|
|
|
|
|
|
|
|
|
|
|
|
|
|
|
|
|
|
|
|
|
|
|
|
|
|
|
|
|
|
|
|
|
|
|
|
|
|
|
|
|
|
|
|
|
|
|
|
|
|
|
|
|
|
|
|
|
|
|
|
|
|
|
|
|
|
|
|
|
|
|
|
|
|
|
|
|
|
|
|
|
|
|
|
|
|
|
|
|
|
|
|
|
|
|
|
|
|
|
|
|
|
|
|
|
|
|
|
|
|
|
|
|
|
|
|
|
|
|
|
|
|
|
|
|
|
|
|
|
|
|
|
|
|
|
|
|
|
|
|
|
|
|
|
|
|
|
|
|
spaces/ArkanDash/rvc-models-new/lib/infer_pack/commons.py
DELETED
@@ -1,166 +0,0 @@
|
|
1 |
-
import math
|
2 |
-
import numpy as np
|
3 |
-
import torch
|
4 |
-
from torch import nn
|
5 |
-
from torch.nn import functional as F
|
6 |
-
|
7 |
-
|
8 |
-
def init_weights(m, mean=0.0, std=0.01):
|
9 |
-
classname = m.__class__.__name__
|
10 |
-
if classname.find("Conv") != -1:
|
11 |
-
m.weight.data.normal_(mean, std)
|
12 |
-
|
13 |
-
|
14 |
-
def get_padding(kernel_size, dilation=1):
|
15 |
-
return int((kernel_size * dilation - dilation) / 2)
|
16 |
-
|
17 |
-
|
18 |
-
def convert_pad_shape(pad_shape):
|
19 |
-
l = pad_shape[::-1]
|
20 |
-
pad_shape = [item for sublist in l for item in sublist]
|
21 |
-
return pad_shape
|
22 |
-
|
23 |
-
|
24 |
-
def kl_divergence(m_p, logs_p, m_q, logs_q):
|
25 |
-
"""KL(P||Q)"""
|
26 |
-
kl = (logs_q - logs_p) - 0.5
|
27 |
-
kl += (
|
28 |
-
0.5 * (torch.exp(2.0 * logs_p) + ((m_p - m_q) ** 2)) * torch.exp(-2.0 * logs_q)
|
29 |
-
)
|
30 |
-
return kl
|
31 |
-
|
32 |
-
|
33 |
-
def rand_gumbel(shape):
|
34 |
-
"""Sample from the Gumbel distribution, protect from overflows."""
|
35 |
-
uniform_samples = torch.rand(shape) * 0.99998 + 0.00001
|
36 |
-
return -torch.log(-torch.log(uniform_samples))
|
37 |
-
|
38 |
-
|
39 |
-
def rand_gumbel_like(x):
|
40 |
-
g = rand_gumbel(x.size()).to(dtype=x.dtype, device=x.device)
|
41 |
-
return g
|
42 |
-
|
43 |
-
|
44 |
-
def slice_segments(x, ids_str, segment_size=4):
|
45 |
-
ret = torch.zeros_like(x[:, :, :segment_size])
|
46 |
-
for i in range(x.size(0)):
|
47 |
-
idx_str = ids_str[i]
|
48 |
-
idx_end = idx_str + segment_size
|
49 |
-
ret[i] = x[i, :, idx_str:idx_end]
|
50 |
-
return ret
|
51 |
-
|
52 |
-
|
53 |
-
def slice_segments2(x, ids_str, segment_size=4):
|
54 |
-
ret = torch.zeros_like(x[:, :segment_size])
|
55 |
-
for i in range(x.size(0)):
|
56 |
-
idx_str = ids_str[i]
|
57 |
-
idx_end = idx_str + segment_size
|
58 |
-
ret[i] = x[i, idx_str:idx_end]
|
59 |
-
return ret
|
60 |
-
|
61 |
-
|
62 |
-
def rand_slice_segments(x, x_lengths=None, segment_size=4):
|
63 |
-
b, d, t = x.size()
|
64 |
-
if x_lengths is None:
|
65 |
-
x_lengths = t
|
66 |
-
ids_str_max = x_lengths - segment_size + 1
|
67 |
-
ids_str = (torch.rand([b]).to(device=x.device) * ids_str_max).to(dtype=torch.long)
|
68 |
-
ret = slice_segments(x, ids_str, segment_size)
|
69 |
-
return ret, ids_str
|
70 |
-
|
71 |
-
|
72 |
-
def get_timing_signal_1d(length, channels, min_timescale=1.0, max_timescale=1.0e4):
|
73 |
-
position = torch.arange(length, dtype=torch.float)
|
74 |
-
num_timescales = channels // 2
|
75 |
-
log_timescale_increment = math.log(float(max_timescale) / float(min_timescale)) / (
|
76 |
-
num_timescales - 1
|
77 |
-
)
|
78 |
-
inv_timescales = min_timescale * torch.exp(
|
79 |
-
torch.arange(num_timescales, dtype=torch.float) * -log_timescale_increment
|
80 |
-
)
|
81 |
-
scaled_time = position.unsqueeze(0) * inv_timescales.unsqueeze(1)
|
82 |
-
signal = torch.cat([torch.sin(scaled_time), torch.cos(scaled_time)], 0)
|
83 |
-
signal = F.pad(signal, [0, 0, 0, channels % 2])
|
84 |
-
signal = signal.view(1, channels, length)
|
85 |
-
return signal
|
86 |
-
|
87 |
-
|
88 |
-
def add_timing_signal_1d(x, min_timescale=1.0, max_timescale=1.0e4):
|
89 |
-
b, channels, length = x.size()
|
90 |
-
signal = get_timing_signal_1d(length, channels, min_timescale, max_timescale)
|
91 |
-
return x + signal.to(dtype=x.dtype, device=x.device)
|
92 |
-
|
93 |
-
|
94 |
-
def cat_timing_signal_1d(x, min_timescale=1.0, max_timescale=1.0e4, axis=1):
|
95 |
-
b, channels, length = x.size()
|
96 |
-
signal = get_timing_signal_1d(length, channels, min_timescale, max_timescale)
|
97 |
-
return torch.cat([x, signal.to(dtype=x.dtype, device=x.device)], axis)
|
98 |
-
|
99 |
-
|
100 |
-
def subsequent_mask(length):
|
101 |
-
mask = torch.tril(torch.ones(length, length)).unsqueeze(0).unsqueeze(0)
|
102 |
-
return mask
|
103 |
-
|
104 |
-
|
105 |
-
@torch.jit.script
|
106 |
-
def fused_add_tanh_sigmoid_multiply(input_a, input_b, n_channels):
|
107 |
-
n_channels_int = n_channels[0]
|
108 |
-
in_act = input_a + input_b
|
109 |
-
t_act = torch.tanh(in_act[:, :n_channels_int, :])
|
110 |
-
s_act = torch.sigmoid(in_act[:, n_channels_int:, :])
|
111 |
-
acts = t_act * s_act
|
112 |
-
return acts
|
113 |
-
|
114 |
-
|
115 |
-
def convert_pad_shape(pad_shape):
|
116 |
-
l = pad_shape[::-1]
|
117 |
-
pad_shape = [item for sublist in l for item in sublist]
|
118 |
-
return pad_shape
|
119 |
-
|
120 |
-
|
121 |
-
def shift_1d(x):
|
122 |
-
x = F.pad(x, convert_pad_shape([[0, 0], [0, 0], [1, 0]]))[:, :, :-1]
|
123 |
-
return x
|
124 |
-
|
125 |
-
|
126 |
-
def sequence_mask(length, max_length=None):
|
127 |
-
if max_length is None:
|
128 |
-
max_length = length.max()
|
129 |
-
x = torch.arange(max_length, dtype=length.dtype, device=length.device)
|
130 |
-
return x.unsqueeze(0) < length.unsqueeze(1)
|
131 |
-
|
132 |
-
|
133 |
-
def generate_path(duration, mask):
|
134 |
-
"""
|
135 |
-
duration: [b, 1, t_x]
|
136 |
-
mask: [b, 1, t_y, t_x]
|
137 |
-
"""
|
138 |
-
device = duration.device
|
139 |
-
|
140 |
-
b, _, t_y, t_x = mask.shape
|
141 |
-
cum_duration = torch.cumsum(duration, -1)
|
142 |
-
|
143 |
-
cum_duration_flat = cum_duration.view(b * t_x)
|
144 |
-
path = sequence_mask(cum_duration_flat, t_y).to(mask.dtype)
|
145 |
-
path = path.view(b, t_x, t_y)
|
146 |
-
path = path - F.pad(path, convert_pad_shape([[0, 0], [1, 0], [0, 0]]))[:, :-1]
|
147 |
-
path = path.unsqueeze(1).transpose(2, 3) * mask
|
148 |
-
return path
|
149 |
-
|
150 |
-
|
151 |
-
def clip_grad_value_(parameters, clip_value, norm_type=2):
|
152 |
-
if isinstance(parameters, torch.Tensor):
|
153 |
-
parameters = [parameters]
|
154 |
-
parameters = list(filter(lambda p: p.grad is not None, parameters))
|
155 |
-
norm_type = float(norm_type)
|
156 |
-
if clip_value is not None:
|
157 |
-
clip_value = float(clip_value)
|
158 |
-
|
159 |
-
total_norm = 0
|
160 |
-
for p in parameters:
|
161 |
-
param_norm = p.grad.data.norm(norm_type)
|
162 |
-
total_norm += param_norm.item() ** norm_type
|
163 |
-
if clip_value is not None:
|
164 |
-
p.grad.data.clamp_(min=-clip_value, max=clip_value)
|
165 |
-
total_norm = total_norm ** (1.0 / norm_type)
|
166 |
-
return total_norm
|
|
|
|
|
|
|
|
|
|
|
|
|
|
|
|
|
|
|
|
|
|
|
|
|
|
|
|
|
|
|
|
|
|
|
|
|
|
|
|
|
|
|
|
|
|
|
|
|
|
|
|
|
|
|
|
|
|
|
|
|
|
|
|
|
|
|
|
|
|
|
|
|
|
|
|
|
|
|
|
|
|
|
|
|
|
|
|
|
|
|
|
|
|
|
|
|
|
|
|
|
|
|
|
|
|
|
|
|
|
|
|
|
|
|
|
|
|
|
|
|
|
|
|
|
|
|
|
|
|
|
|
|
|
|
|
|
|
|
|
|
|
|
|
|
|
|
|
|
|
|
|
|
|
|
|
|
|
|
|
|
|
|
|
|
|
|
|
|
|
|
|
|
|
|
|
|
|
|
|
|
|
|
|
|
|
|
|
|
|
|
|
|
|
|
|
|
|
|
|
|
|
|
|
|
|
|
|
|
|
|
|
|
|
|
|
|
|
|
|
|
|
|
|
|
|
|
|
|
|
|
|
|
|
|
|
|
|
|
|
|
|
|
|
|
|
|
|
|
|
|
|
|
|
|
|
|
|
|
|
|
|
|
|
|
|
|
|
|
|
|
|
|
|
|
|
|
|
|
|
|
|
|
|
|
|
|
|
|
|
|
|
|
|
|
|
|
|
|
|
|
|
|
|
|
|
|
|
|
|
|
|
|
|
|
|
|
|
|
|
|
|
|
|
|
|
|
|
|
|
|
|
|
|
spaces/Arnx/MusicGenXvAKN/audiocraft/models/__init__.py
DELETED
@@ -1,10 +0,0 @@
|
|
1 |
-
# Copyright (c) Meta Platforms, Inc. and affiliates.
|
2 |
-
# All rights reserved.
|
3 |
-
#
|
4 |
-
# This source code is licensed under the license found in the
|
5 |
-
# LICENSE file in the root directory of this source tree.
|
6 |
-
|
7 |
-
# flake8: noqa
|
8 |
-
from .musicgen import MusicGen
|
9 |
-
from .lm import LMModel
|
10 |
-
from .encodec import CompressionModel, EncodecModel
|
|
|
|
|
|
|
|
|
|
|
|
|
|
|
|
|
|
|
|
|
|
spaces/Arulkumar03/GroundingDINO_SOTA_Zero_Shot_Model/demo/test_ap_on_coco.py
DELETED
@@ -1,233 +0,0 @@
|
|
1 |
-
import argparse
|
2 |
-
import os
|
3 |
-
import sys
|
4 |
-
import time
|
5 |
-
|
6 |
-
import numpy as np
|
7 |
-
import torch
|
8 |
-
import torch.nn as nn
|
9 |
-
from torch.utils.data import DataLoader, DistributedSampler
|
10 |
-
|
11 |
-
from groundingdino.models import build_model
|
12 |
-
import groundingdino.datasets.transforms as T
|
13 |
-
from groundingdino.util import box_ops, get_tokenlizer
|
14 |
-
from groundingdino.util.misc import clean_state_dict, collate_fn
|
15 |
-
from groundingdino.util.slconfig import SLConfig
|
16 |
-
|
17 |
-
# from torchvision.datasets import CocoDetection
|
18 |
-
import torchvision
|
19 |
-
|
20 |
-
from groundingdino.util.vl_utils import build_captions_and_token_span, create_positive_map_from_span
|
21 |
-
from groundingdino.datasets.cocogrounding_eval import CocoGroundingEvaluator
|
22 |
-
|
23 |
-
|
24 |
-
def load_model(model_config_path: str, model_checkpoint_path: str, device: str = "cuda"):
|
25 |
-
args = SLConfig.fromfile(model_config_path)
|
26 |
-
args.device = device
|
27 |
-
model = build_model(args)
|
28 |
-
checkpoint = torch.load(model_checkpoint_path, map_location="cpu")
|
29 |
-
model.load_state_dict(clean_state_dict(checkpoint["ema_model"]), strict=False)
|
30 |
-
model.eval()
|
31 |
-
return model
|
32 |
-
|
33 |
-
|
34 |
-
class CocoDetection(torchvision.datasets.CocoDetection):
|
35 |
-
def __init__(self, img_folder, ann_file, transforms):
|
36 |
-
super().__init__(img_folder, ann_file)
|
37 |
-
self._transforms = transforms
|
38 |
-
|
39 |
-
def __getitem__(self, idx):
|
40 |
-
img, target = super().__getitem__(idx) # target: list
|
41 |
-
|
42 |
-
# import ipdb; ipdb.set_trace()
|
43 |
-
|
44 |
-
w, h = img.size
|
45 |
-
boxes = [obj["bbox"] for obj in target]
|
46 |
-
boxes = torch.as_tensor(boxes, dtype=torch.float32).reshape(-1, 4)
|
47 |
-
boxes[:, 2:] += boxes[:, :2] # xywh -> xyxy
|
48 |
-
boxes[:, 0::2].clamp_(min=0, max=w)
|
49 |
-
boxes[:, 1::2].clamp_(min=0, max=h)
|
50 |
-
# filt invalid boxes/masks/keypoints
|
51 |
-
keep = (boxes[:, 3] > boxes[:, 1]) & (boxes[:, 2] > boxes[:, 0])
|
52 |
-
boxes = boxes[keep]
|
53 |
-
|
54 |
-
target_new = {}
|
55 |
-
image_id = self.ids[idx]
|
56 |
-
target_new["image_id"] = image_id
|
57 |
-
target_new["boxes"] = boxes
|
58 |
-
target_new["orig_size"] = torch.as_tensor([int(h), int(w)])
|
59 |
-
|
60 |
-
if self._transforms is not None:
|
61 |
-
img, target = self._transforms(img, target_new)
|
62 |
-
|
63 |
-
return img, target
|
64 |
-
|
65 |
-
|
66 |
-
class PostProcessCocoGrounding(nn.Module):
|
67 |
-
""" This module converts the model's output into the format expected by the coco api"""
|
68 |
-
|
69 |
-
def __init__(self, num_select=300, coco_api=None, tokenlizer=None) -> None:
|
70 |
-
super().__init__()
|
71 |
-
self.num_select = num_select
|
72 |
-
|
73 |
-
assert coco_api is not None
|
74 |
-
category_dict = coco_api.dataset['categories']
|
75 |
-
cat_list = [item['name'] for item in category_dict]
|
76 |
-
captions, cat2tokenspan = build_captions_and_token_span(cat_list, True)
|
77 |
-
tokenspanlist = [cat2tokenspan[cat] for cat in cat_list]
|
78 |
-
positive_map = create_positive_map_from_span(
|
79 |
-
tokenlizer(captions), tokenspanlist) # 80, 256. normed
|
80 |
-
|
81 |
-
id_map = {0: 1, 1: 2, 2: 3, 3: 4, 4: 5, 5: 6, 6: 7, 7: 8, 8: 9, 9: 10, 10: 11, 11: 13, 12: 14, 13: 15, 14: 16, 15: 17, 16: 18, 17: 19, 18: 20, 19: 21, 20: 22, 21: 23, 22: 24, 23: 25, 24: 27, 25: 28, 26: 31, 27: 32, 28: 33, 29: 34, 30: 35, 31: 36, 32: 37, 33: 38, 34: 39, 35: 40, 36: 41, 37: 42, 38: 43, 39: 44, 40: 46,
|
82 |
-
41: 47, 42: 48, 43: 49, 44: 50, 45: 51, 46: 52, 47: 53, 48: 54, 49: 55, 50: 56, 51: 57, 52: 58, 53: 59, 54: 60, 55: 61, 56: 62, 57: 63, 58: 64, 59: 65, 60: 67, 61: 70, 62: 72, 63: 73, 64: 74, 65: 75, 66: 76, 67: 77, 68: 78, 69: 79, 70: 80, 71: 81, 72: 82, 73: 84, 74: 85, 75: 86, 76: 87, 77: 88, 78: 89, 79: 90}
|
83 |
-
|
84 |
-
# build a mapping from label_id to pos_map
|
85 |
-
new_pos_map = torch.zeros((91, 256))
|
86 |
-
for k, v in id_map.items():
|
87 |
-
new_pos_map[v] = positive_map[k]
|
88 |
-
self.positive_map = new_pos_map
|
89 |
-
|
90 |
-
@torch.no_grad()
|
91 |
-
def forward(self, outputs, target_sizes, not_to_xyxy=False):
|
92 |
-
""" Perform the computation
|
93 |
-
Parameters:
|
94 |
-
outputs: raw outputs of the model
|
95 |
-
target_sizes: tensor of dimension [batch_size x 2] containing the size of each images of the batch
|
96 |
-
For evaluation, this must be the original image size (before any data augmentation)
|
97 |
-
For visualization, this should be the image size after data augment, but before padding
|
98 |
-
"""
|
99 |
-
num_select = self.num_select
|
100 |
-
out_logits, out_bbox = outputs['pred_logits'], outputs['pred_boxes']
|
101 |
-
|
102 |
-
# pos map to logit
|
103 |
-
prob_to_token = out_logits.sigmoid() # bs, 100, 256
|
104 |
-
pos_maps = self.positive_map.to(prob_to_token.device)
|
105 |
-
# (bs, 100, 256) @ (91, 256).T -> (bs, 100, 91)
|
106 |
-
prob_to_label = prob_to_token @ pos_maps.T
|
107 |
-
|
108 |
-
# if os.environ.get('IPDB_SHILONG_DEBUG', None) == 'INFO':
|
109 |
-
# import ipdb; ipdb.set_trace()
|
110 |
-
|
111 |
-
assert len(out_logits) == len(target_sizes)
|
112 |
-
assert target_sizes.shape[1] == 2
|
113 |
-
|
114 |
-
prob = prob_to_label
|
115 |
-
topk_values, topk_indexes = torch.topk(
|
116 |
-
prob.view(out_logits.shape[0], -1), num_select, dim=1)
|
117 |
-
scores = topk_values
|
118 |
-
topk_boxes = topk_indexes // prob.shape[2]
|
119 |
-
labels = topk_indexes % prob.shape[2]
|
120 |
-
|
121 |
-
if not_to_xyxy:
|
122 |
-
boxes = out_bbox
|
123 |
-
else:
|
124 |
-
boxes = box_ops.box_cxcywh_to_xyxy(out_bbox)
|
125 |
-
|
126 |
-
boxes = torch.gather(
|
127 |
-
boxes, 1, topk_boxes.unsqueeze(-1).repeat(1, 1, 4))
|
128 |
-
|
129 |
-
# and from relative [0, 1] to absolute [0, height] coordinates
|
130 |
-
img_h, img_w = target_sizes.unbind(1)
|
131 |
-
scale_fct = torch.stack([img_w, img_h, img_w, img_h], dim=1)
|
132 |
-
boxes = boxes * scale_fct[:, None, :]
|
133 |
-
|
134 |
-
results = [{'scores': s, 'labels': l, 'boxes': b}
|
135 |
-
for s, l, b in zip(scores, labels, boxes)]
|
136 |
-
|
137 |
-
return results
|
138 |
-
|
139 |
-
|
140 |
-
def main(args):
|
141 |
-
# config
|
142 |
-
cfg = SLConfig.fromfile(args.config_file)
|
143 |
-
|
144 |
-
# build model
|
145 |
-
model = load_model(args.config_file, args.checkpoint_path)
|
146 |
-
model = model.to(args.device)
|
147 |
-
model = model.eval()
|
148 |
-
|
149 |
-
# build dataloader
|
150 |
-
transform = T.Compose(
|
151 |
-
[
|
152 |
-
T.RandomResize([800], max_size=1333),
|
153 |
-
T.ToTensor(),
|
154 |
-
T.Normalize([0.485, 0.456, 0.406], [0.229, 0.224, 0.225]),
|
155 |
-
]
|
156 |
-
)
|
157 |
-
dataset = CocoDetection(
|
158 |
-
args.image_dir, args.anno_path, transforms=transform)
|
159 |
-
data_loader = DataLoader(
|
160 |
-
dataset, batch_size=1, shuffle=False, num_workers=args.num_workers, collate_fn=collate_fn)
|
161 |
-
|
162 |
-
# build post processor
|
163 |
-
tokenlizer = get_tokenlizer.get_tokenlizer(cfg.text_encoder_type)
|
164 |
-
postprocessor = PostProcessCocoGrounding(
|
165 |
-
coco_api=dataset.coco, tokenlizer=tokenlizer)
|
166 |
-
|
167 |
-
# build evaluator
|
168 |
-
evaluator = CocoGroundingEvaluator(
|
169 |
-
dataset.coco, iou_types=("bbox",), useCats=True)
|
170 |
-
|
171 |
-
# build captions
|
172 |
-
category_dict = dataset.coco.dataset['categories']
|
173 |
-
cat_list = [item['name'] for item in category_dict]
|
174 |
-
caption = " . ".join(cat_list) + ' .'
|
175 |
-
print("Input text prompt:", caption)
|
176 |
-
|
177 |
-
# run inference
|
178 |
-
start = time.time()
|
179 |
-
for i, (images, targets) in enumerate(data_loader):
|
180 |
-
# get images and captions
|
181 |
-
images = images.tensors.to(args.device)
|
182 |
-
bs = images.shape[0]
|
183 |
-
input_captions = [caption] * bs
|
184 |
-
|
185 |
-
# feed to the model
|
186 |
-
outputs = model(images, captions=input_captions)
|
187 |
-
|
188 |
-
orig_target_sizes = torch.stack(
|
189 |
-
[t["orig_size"] for t in targets], dim=0).to(images.device)
|
190 |
-
results = postprocessor(outputs, orig_target_sizes)
|
191 |
-
cocogrounding_res = {
|
192 |
-
target["image_id"]: output for target, output in zip(targets, results)}
|
193 |
-
evaluator.update(cocogrounding_res)
|
194 |
-
|
195 |
-
if (i+1) % 30 == 0:
|
196 |
-
used_time = time.time() - start
|
197 |
-
eta = len(data_loader) / (i+1e-5) * used_time - used_time
|
198 |
-
print(
|
199 |
-
f"processed {i}/{len(data_loader)} images. time: {used_time:.2f}s, ETA: {eta:.2f}s")
|
200 |
-
|
201 |
-
evaluator.synchronize_between_processes()
|
202 |
-
evaluator.accumulate()
|
203 |
-
evaluator.summarize()
|
204 |
-
|
205 |
-
print("Final results:", evaluator.coco_eval["bbox"].stats.tolist())
|
206 |
-
|
207 |
-
|
208 |
-
if __name__ == "__main__":
|
209 |
-
parser = argparse.ArgumentParser(
|
210 |
-
"Grounding DINO eval on COCO", add_help=True)
|
211 |
-
# load model
|
212 |
-
parser.add_argument("--config_file", "-c", type=str,
|
213 |
-
required=True, help="path to config file")
|
214 |
-
parser.add_argument(
|
215 |
-
"--checkpoint_path", "-p", type=str, required=True, help="path to checkpoint file"
|
216 |
-
)
|
217 |
-
parser.add_argument("--device", type=str, default="cuda",
|
218 |
-
help="running device (default: cuda)")
|
219 |
-
|
220 |
-
# post processing
|
221 |
-
parser.add_argument("--num_select", type=int, default=300,
|
222 |
-
help="number of topk to select")
|
223 |
-
|
224 |
-
# coco info
|
225 |
-
parser.add_argument("--anno_path", type=str,
|
226 |
-
required=True, help="coco root")
|
227 |
-
parser.add_argument("--image_dir", type=str,
|
228 |
-
required=True, help="coco image dir")
|
229 |
-
parser.add_argument("--num_workers", type=int, default=4,
|
230 |
-
help="number of workers for dataloader")
|
231 |
-
args = parser.parse_args()
|
232 |
-
|
233 |
-
main(args)
|
|
|
|
|
|
|
|
|
|
|
|
|
|
|
|
|
|
|
|
|
|
|
|
|
|
|
|
|
|
|
|
|
|
|
|
|
|
|
|
|
|
|
|
|
|
|
|
|
|
|
|
|
|
|
|
|
|
|
|
|
|
|
|
|
|
|
|
|
|
|
|
|
|
|
|
|
|
|
|
|
|
|
|
|
|
|
|
|
|
|
|
|
|
|
|
|
|
|
|
|
|
|
|
|
|
|
|
|
|
|
|
|
|
|
|
|
|
|
|
|
|
|
|
|
|
|
|
|
|
|
|
|
|
|
|
|
|
|
|
|
|
|
|
|
|
|
|
|
|
|
|
|
|
|
|
|
|
|
|
|
|
|
|
|
|
|
|
|
|
|
|
|
|
|
|
|
|
|
|
|
|
|
|
|
|
|
|
|
|
|
|
|
|
|
|
|
|
|
|
|
|
|
|
|
|
|
|
|
|
|
|
|
|
|
|
|
|
|
|
|
|
|
|
|
|
|
|
|
|
|
|
|
|
|
|
|
|
|
|
|
|
|
|
|
|
|
|
|
|
|
|
|
|
|
|
|
|
|
|
|
|
|
|
|
|
|
|
|
|
|
|
|
|
|
|
|
|
|
|
|
|
|
|
|
|
|
|
|
|
|
|
|
|
|
|
|
|
|
|
|
|
|
|
|
|
|
|
|
|
|
|
|
|
|
|
|
|
|
|
|
|
|
|
|
|
|
|
|
|
|
|
|
|
|
|
|
|
|
|
|
|
|
|
|
|
|
|
|
|
|
|
|
|
|
|
|
|
|
|
|
|
|
|
|
|
|
|
|
|
|
|
|
|
|
|
|
|
|
|
|
|
|
|
|
|
|
|
|
|
|
|
|
|
|
|
|
|
|
|
|
|
|
|
|
|
|
|
|
|
|
|
|
|
|
|
|
|
|
|
|
|
|
|
|
|
|
|
|
|
|
|
|
|
|
|
|
|
|
|
|
|
|
|
|
|
|
|
|
|
|
|
|
|
|
|
|
|
|
|
|
|
|
|
|
|
|
|
spaces/Arulkumar03/GroundingDINO_SOTA_Zero_Shot_Model/groundingdino/models/GroundingDINO/utils.py
DELETED
@@ -1,268 +0,0 @@
|
|
1 |
-
# ------------------------------------------------------------------------
|
2 |
-
# Grounding DINO
|
3 |
-
# url: https://github.com/IDEA-Research/GroundingDINO
|
4 |
-
# Copyright (c) 2023 IDEA. All Rights Reserved.
|
5 |
-
# Licensed under the Apache License, Version 2.0 [see LICENSE for details]
|
6 |
-
# ------------------------------------------------------------------------
|
7 |
-
|
8 |
-
import copy
|
9 |
-
import math
|
10 |
-
|
11 |
-
import torch
|
12 |
-
import torch.nn.functional as F
|
13 |
-
from torch import Tensor, nn
|
14 |
-
|
15 |
-
|
16 |
-
def _get_clones(module, N, layer_share=False):
|
17 |
-
# import ipdb; ipdb.set_trace()
|
18 |
-
if layer_share:
|
19 |
-
return nn.ModuleList([module for i in range(N)])
|
20 |
-
else:
|
21 |
-
return nn.ModuleList([copy.deepcopy(module) for i in range(N)])
|
22 |
-
|
23 |
-
|
24 |
-
def get_sine_pos_embed(
|
25 |
-
pos_tensor: torch.Tensor,
|
26 |
-
num_pos_feats: int = 128,
|
27 |
-
temperature: int = 10000,
|
28 |
-
exchange_xy: bool = True,
|
29 |
-
):
|
30 |
-
"""generate sine position embedding from a position tensor
|
31 |
-
Args:
|
32 |
-
pos_tensor (torch.Tensor): shape: [..., n].
|
33 |
-
num_pos_feats (int): projected shape for each float in the tensor.
|
34 |
-
temperature (int): temperature in the sine/cosine function.
|
35 |
-
exchange_xy (bool, optional): exchange pos x and pos y. \
|
36 |
-
For example, input tensor is [x,y], the results will be [pos(y), pos(x)]. Defaults to True.
|
37 |
-
Returns:
|
38 |
-
pos_embed (torch.Tensor): shape: [..., n*num_pos_feats].
|
39 |
-
"""
|
40 |
-
scale = 2 * math.pi
|
41 |
-
dim_t = torch.arange(num_pos_feats, dtype=torch.float32, device=pos_tensor.device)
|
42 |
-
dim_t = temperature ** (2 * torch.div(dim_t, 2, rounding_mode="floor") / num_pos_feats)
|
43 |
-
|
44 |
-
def sine_func(x: torch.Tensor):
|
45 |
-
sin_x = x * scale / dim_t
|
46 |
-
sin_x = torch.stack((sin_x[..., 0::2].sin(), sin_x[..., 1::2].cos()), dim=3).flatten(2)
|
47 |
-
return sin_x
|
48 |
-
|
49 |
-
pos_res = [sine_func(x) for x in pos_tensor.split([1] * pos_tensor.shape[-1], dim=-1)]
|
50 |
-
if exchange_xy:
|
51 |
-
pos_res[0], pos_res[1] = pos_res[1], pos_res[0]
|
52 |
-
pos_res = torch.cat(pos_res, dim=-1)
|
53 |
-
return pos_res
|
54 |
-
|
55 |
-
|
56 |
-
def gen_encoder_output_proposals(
|
57 |
-
memory: Tensor, memory_padding_mask: Tensor, spatial_shapes: Tensor, learnedwh=None
|
58 |
-
):
|
59 |
-
"""
|
60 |
-
Input:
|
61 |
-
- memory: bs, \sum{hw}, d_model
|
62 |
-
- memory_padding_mask: bs, \sum{hw}
|
63 |
-
- spatial_shapes: nlevel, 2
|
64 |
-
- learnedwh: 2
|
65 |
-
Output:
|
66 |
-
- output_memory: bs, \sum{hw}, d_model
|
67 |
-
- output_proposals: bs, \sum{hw}, 4
|
68 |
-
"""
|
69 |
-
N_, S_, C_ = memory.shape
|
70 |
-
proposals = []
|
71 |
-
_cur = 0
|
72 |
-
for lvl, (H_, W_) in enumerate(spatial_shapes):
|
73 |
-
mask_flatten_ = memory_padding_mask[:, _cur : (_cur + H_ * W_)].view(N_, H_, W_, 1)
|
74 |
-
valid_H = torch.sum(~mask_flatten_[:, :, 0, 0], 1)
|
75 |
-
valid_W = torch.sum(~mask_flatten_[:, 0, :, 0], 1)
|
76 |
-
|
77 |
-
# import ipdb; ipdb.set_trace()
|
78 |
-
|
79 |
-
grid_y, grid_x = torch.meshgrid(
|
80 |
-
torch.linspace(0, H_ - 1, H_, dtype=torch.float32, device=memory.device),
|
81 |
-
torch.linspace(0, W_ - 1, W_, dtype=torch.float32, device=memory.device),
|
82 |
-
)
|
83 |
-
grid = torch.cat([grid_x.unsqueeze(-1), grid_y.unsqueeze(-1)], -1) # H_, W_, 2
|
84 |
-
|
85 |
-
scale = torch.cat([valid_W.unsqueeze(-1), valid_H.unsqueeze(-1)], 1).view(N_, 1, 1, 2)
|
86 |
-
grid = (grid.unsqueeze(0).expand(N_, -1, -1, -1) + 0.5) / scale
|
87 |
-
|
88 |
-
if learnedwh is not None:
|
89 |
-
# import ipdb; ipdb.set_trace()
|
90 |
-
wh = torch.ones_like(grid) * learnedwh.sigmoid() * (2.0**lvl)
|
91 |
-
else:
|
92 |
-
wh = torch.ones_like(grid) * 0.05 * (2.0**lvl)
|
93 |
-
|
94 |
-
# scale = torch.cat([W_[None].unsqueeze(-1), H_[None].unsqueeze(-1)], 1).view(1, 1, 1, 2).repeat(N_, 1, 1, 1)
|
95 |
-
# grid = (grid.unsqueeze(0).expand(N_, -1, -1, -1) + 0.5) / scale
|
96 |
-
# wh = torch.ones_like(grid) / scale
|
97 |
-
proposal = torch.cat((grid, wh), -1).view(N_, -1, 4)
|
98 |
-
proposals.append(proposal)
|
99 |
-
_cur += H_ * W_
|
100 |
-
# import ipdb; ipdb.set_trace()
|
101 |
-
output_proposals = torch.cat(proposals, 1)
|
102 |
-
output_proposals_valid = ((output_proposals > 0.01) & (output_proposals < 0.99)).all(
|
103 |
-
-1, keepdim=True
|
104 |
-
)
|
105 |
-
output_proposals = torch.log(output_proposals / (1 - output_proposals)) # unsigmoid
|
106 |
-
output_proposals = output_proposals.masked_fill(memory_padding_mask.unsqueeze(-1), float("inf"))
|
107 |
-
output_proposals = output_proposals.masked_fill(~output_proposals_valid, float("inf"))
|
108 |
-
|
109 |
-
output_memory = memory
|
110 |
-
output_memory = output_memory.masked_fill(memory_padding_mask.unsqueeze(-1), float(0))
|
111 |
-
output_memory = output_memory.masked_fill(~output_proposals_valid, float(0))
|
112 |
-
|
113 |
-
# output_memory = output_memory.masked_fill(memory_padding_mask.unsqueeze(-1), float('inf'))
|
114 |
-
# output_memory = output_memory.masked_fill(~output_proposals_valid, float('inf'))
|
115 |
-
|
116 |
-
return output_memory, output_proposals
|
117 |
-
|
118 |
-
|
119 |
-
class RandomBoxPerturber:
|
120 |
-
def __init__(
|
121 |
-
self, x_noise_scale=0.2, y_noise_scale=0.2, w_noise_scale=0.2, h_noise_scale=0.2
|
122 |
-
) -> None:
|
123 |
-
self.noise_scale = torch.Tensor(
|
124 |
-
[x_noise_scale, y_noise_scale, w_noise_scale, h_noise_scale]
|
125 |
-
)
|
126 |
-
|
127 |
-
def __call__(self, refanchors: Tensor) -> Tensor:
|
128 |
-
nq, bs, query_dim = refanchors.shape
|
129 |
-
device = refanchors.device
|
130 |
-
|
131 |
-
noise_raw = torch.rand_like(refanchors)
|
132 |
-
noise_scale = self.noise_scale.to(device)[:query_dim]
|
133 |
-
|
134 |
-
new_refanchors = refanchors * (1 + (noise_raw - 0.5) * noise_scale)
|
135 |
-
return new_refanchors.clamp_(0, 1)
|
136 |
-
|
137 |
-
|
138 |
-
def sigmoid_focal_loss(
|
139 |
-
inputs, targets, num_boxes, alpha: float = 0.25, gamma: float = 2, no_reduction=False
|
140 |
-
):
|
141 |
-
"""
|
142 |
-
Loss used in RetinaNet for dense detection: https://arxiv.org/abs/1708.02002.
|
143 |
-
Args:
|
144 |
-
inputs: A float tensor of arbitrary shape.
|
145 |
-
The predictions for each example.
|
146 |
-
targets: A float tensor with the same shape as inputs. Stores the binary
|
147 |
-
classification label for each element in inputs
|
148 |
-
(0 for the negative class and 1 for the positive class).
|
149 |
-
alpha: (optional) Weighting factor in range (0,1) to balance
|
150 |
-
positive vs negative examples. Default = -1 (no weighting).
|
151 |
-
gamma: Exponent of the modulating factor (1 - p_t) to
|
152 |
-
balance easy vs hard examples.
|
153 |
-
Returns:
|
154 |
-
Loss tensor
|
155 |
-
"""
|
156 |
-
prob = inputs.sigmoid()
|
157 |
-
ce_loss = F.binary_cross_entropy_with_logits(inputs, targets, reduction="none")
|
158 |
-
p_t = prob * targets + (1 - prob) * (1 - targets)
|
159 |
-
loss = ce_loss * ((1 - p_t) ** gamma)
|
160 |
-
|
161 |
-
if alpha >= 0:
|
162 |
-
alpha_t = alpha * targets + (1 - alpha) * (1 - targets)
|
163 |
-
loss = alpha_t * loss
|
164 |
-
|
165 |
-
if no_reduction:
|
166 |
-
return loss
|
167 |
-
|
168 |
-
return loss.mean(1).sum() / num_boxes
|
169 |
-
|
170 |
-
|
171 |
-
class MLP(nn.Module):
|
172 |
-
"""Very simple multi-layer perceptron (also called FFN)"""
|
173 |
-
|
174 |
-
def __init__(self, input_dim, hidden_dim, output_dim, num_layers):
|
175 |
-
super().__init__()
|
176 |
-
self.num_layers = num_layers
|
177 |
-
h = [hidden_dim] * (num_layers - 1)
|
178 |
-
self.layers = nn.ModuleList(
|
179 |
-
nn.Linear(n, k) for n, k in zip([input_dim] + h, h + [output_dim])
|
180 |
-
)
|
181 |
-
|
182 |
-
def forward(self, x):
|
183 |
-
for i, layer in enumerate(self.layers):
|
184 |
-
x = F.relu(layer(x)) if i < self.num_layers - 1 else layer(x)
|
185 |
-
return x
|
186 |
-
|
187 |
-
|
188 |
-
def _get_activation_fn(activation, d_model=256, batch_dim=0):
|
189 |
-
"""Return an activation function given a string"""
|
190 |
-
if activation == "relu":
|
191 |
-
return F.relu
|
192 |
-
if activation == "gelu":
|
193 |
-
return F.gelu
|
194 |
-
if activation == "glu":
|
195 |
-
return F.glu
|
196 |
-
if activation == "prelu":
|
197 |
-
return nn.PReLU()
|
198 |
-
if activation == "selu":
|
199 |
-
return F.selu
|
200 |
-
|
201 |
-
raise RuntimeError(f"activation should be relu/gelu, not {activation}.")
|
202 |
-
|
203 |
-
|
204 |
-
def gen_sineembed_for_position(pos_tensor):
|
205 |
-
# n_query, bs, _ = pos_tensor.size()
|
206 |
-
# sineembed_tensor = torch.zeros(n_query, bs, 256)
|
207 |
-
scale = 2 * math.pi
|
208 |
-
dim_t = torch.arange(128, dtype=torch.float32, device=pos_tensor.device)
|
209 |
-
dim_t = 10000 ** (2 * (torch.div(dim_t, 2, rounding_mode='floor')) / 128)
|
210 |
-
x_embed = pos_tensor[:, :, 0] * scale
|
211 |
-
y_embed = pos_tensor[:, :, 1] * scale
|
212 |
-
pos_x = x_embed[:, :, None] / dim_t
|
213 |
-
pos_y = y_embed[:, :, None] / dim_t
|
214 |
-
pos_x = torch.stack((pos_x[:, :, 0::2].sin(), pos_x[:, :, 1::2].cos()), dim=3).flatten(2)
|
215 |
-
pos_y = torch.stack((pos_y[:, :, 0::2].sin(), pos_y[:, :, 1::2].cos()), dim=3).flatten(2)
|
216 |
-
if pos_tensor.size(-1) == 2:
|
217 |
-
pos = torch.cat((pos_y, pos_x), dim=2)
|
218 |
-
elif pos_tensor.size(-1) == 4:
|
219 |
-
w_embed = pos_tensor[:, :, 2] * scale
|
220 |
-
pos_w = w_embed[:, :, None] / dim_t
|
221 |
-
pos_w = torch.stack((pos_w[:, :, 0::2].sin(), pos_w[:, :, 1::2].cos()), dim=3).flatten(2)
|
222 |
-
|
223 |
-
h_embed = pos_tensor[:, :, 3] * scale
|
224 |
-
pos_h = h_embed[:, :, None] / dim_t
|
225 |
-
pos_h = torch.stack((pos_h[:, :, 0::2].sin(), pos_h[:, :, 1::2].cos()), dim=3).flatten(2)
|
226 |
-
|
227 |
-
pos = torch.cat((pos_y, pos_x, pos_w, pos_h), dim=2)
|
228 |
-
else:
|
229 |
-
raise ValueError("Unknown pos_tensor shape(-1):{}".format(pos_tensor.size(-1)))
|
230 |
-
return pos
|
231 |
-
|
232 |
-
|
233 |
-
class ContrastiveEmbed(nn.Module):
|
234 |
-
def __init__(self, max_text_len=256):
|
235 |
-
"""
|
236 |
-
Args:
|
237 |
-
max_text_len: max length of text.
|
238 |
-
"""
|
239 |
-
super().__init__()
|
240 |
-
self.max_text_len = max_text_len
|
241 |
-
|
242 |
-
def forward(self, x, text_dict):
|
243 |
-
"""_summary_
|
244 |
-
|
245 |
-
Args:
|
246 |
-
x (_type_): _description_
|
247 |
-
text_dict (_type_): _description_
|
248 |
-
{
|
249 |
-
'encoded_text': encoded_text, # bs, 195, d_model
|
250 |
-
'text_token_mask': text_token_mask, # bs, 195
|
251 |
-
# True for used tokens. False for padding tokens
|
252 |
-
}
|
253 |
-
Returns:
|
254 |
-
_type_: _description_
|
255 |
-
"""
|
256 |
-
assert isinstance(text_dict, dict)
|
257 |
-
|
258 |
-
y = text_dict["encoded_text"]
|
259 |
-
text_token_mask = text_dict["text_token_mask"]
|
260 |
-
|
261 |
-
res = x @ y.transpose(-1, -2)
|
262 |
-
res.masked_fill_(~text_token_mask[:, None, :], float("-inf"))
|
263 |
-
|
264 |
-
# padding to max_text_len
|
265 |
-
new_res = torch.full((*res.shape[:-1], self.max_text_len), float("-inf"), device=res.device)
|
266 |
-
new_res[..., : res.shape[-1]] = res
|
267 |
-
|
268 |
-
return new_res
|
|
|
|
|
|
|
|
|
|
|
|
|
|
|
|
|
|
|
|
|
|
|
|
|
|
|
|
|
|
|
|
|
|
|
|
|
|
|
|
|
|
|
|
|
|
|
|
|
|
|
|
|
|
|
|
|
|
|
|
|
|
|
|
|
|
|
|
|
|
|
|
|
|
|
|
|
|
|
|
|
|
|
|
|
|
|
|
|
|
|
|
|
|
|
|
|
|
|
|
|
|
|
|
|
|
|
|
|
|
|
|
|
|
|
|
|
|
|
|
|
|
|
|
|
|
|
|
|
|
|
|
|
|
|
|
|
|
|
|
|
|
|
|
|
|
|
|
|
|
|
|
|
|
|
|
|
|
|
|
|
|
|
|
|
|
|
|
|
|
|
|
|
|
|
|
|
|
|
|
|
|
|
|
|
|
|
|
|
|
|
|
|
|
|
|
|
|
|
|
|
|
|
|
|
|
|
|
|
|
|
|
|
|
|
|
|
|
|
|
|
|
|
|
|
|
|
|
|
|
|
|
|
|
|
|
|
|
|
|
|
|
|
|
|
|
|
|
|
|
|
|
|
|
|
|
|
|
|
|
|
|
|
|
|
|
|
|
|
|
|
|
|
|
|
|
|
|
|
|
|
|
|
|
|
|
|
|
|
|
|
|
|
|
|
|
|
|
|
|
|
|
|
|
|
|
|
|
|
|
|
|
|
|
|
|
|
|
|
|
|
|
|
|
|
|
|
|
|
|
|
|
|
|
|
|
|
|
|
|
|
|
|
|
|
|
|
|
|
|
|
|
|
|
|
|
|
|
|
|
|
|
|
|
|
|
|
|
|
|
|
|
|
|
|
|
|
|
|
|
|
|
|
|
|
|
|
|
|
|
|
|
|
|
|
|
|
|
|
|
|
|
|
|
|
|
|
|
|
|
|
|
|
|
|
|
|
|
|
|
|
|
|
|
|
|
|
|
|
|
|
|
|
|
|
|
|
|
|
|
|
|
|
|
|
|
|
|
|
|
|
|
|
|
|
|
|
|
|
|
|
|
|
|
|
|
|
|
|
|
|
|
|
|
|
|
|
|
|
|
|
|
|
|
|
|
|
|
|
|
|
|
|
|
|
|
|
|
|
|
|
|
|
|
|
|
|
|
|
|
|
|
|
|
|
|
|
|
|
|
|
|
|
|
|
|
|
|
|
|
|
|
|
|
|
|
|
|
spaces/Ataturk-Chatbot/HuggingFaceChat/venv/lib/python3.11/site-packages/pip/_internal/metadata/base.py
DELETED
@@ -1,688 +0,0 @@
|
|
1 |
-
import csv
|
2 |
-
import email.message
|
3 |
-
import functools
|
4 |
-
import json
|
5 |
-
import logging
|
6 |
-
import pathlib
|
7 |
-
import re
|
8 |
-
import zipfile
|
9 |
-
from typing import (
|
10 |
-
IO,
|
11 |
-
TYPE_CHECKING,
|
12 |
-
Any,
|
13 |
-
Collection,
|
14 |
-
Container,
|
15 |
-
Dict,
|
16 |
-
Iterable,
|
17 |
-
Iterator,
|
18 |
-
List,
|
19 |
-
NamedTuple,
|
20 |
-
Optional,
|
21 |
-
Tuple,
|
22 |
-
Union,
|
23 |
-
)
|
24 |
-
|
25 |
-
from pip._vendor.packaging.requirements import Requirement
|
26 |
-
from pip._vendor.packaging.specifiers import InvalidSpecifier, SpecifierSet
|
27 |
-
from pip._vendor.packaging.utils import NormalizedName
|
28 |
-
from pip._vendor.packaging.version import LegacyVersion, Version
|
29 |
-
|
30 |
-
from pip._internal.exceptions import NoneMetadataError
|
31 |
-
from pip._internal.locations import site_packages, user_site
|
32 |
-
from pip._internal.models.direct_url import (
|
33 |
-
DIRECT_URL_METADATA_NAME,
|
34 |
-
DirectUrl,
|
35 |
-
DirectUrlValidationError,
|
36 |
-
)
|
37 |
-
from pip._internal.utils.compat import stdlib_pkgs # TODO: Move definition here.
|
38 |
-
from pip._internal.utils.egg_link import egg_link_path_from_sys_path
|
39 |
-
from pip._internal.utils.misc import is_local, normalize_path
|
40 |
-
from pip._internal.utils.packaging import safe_extra
|
41 |
-
from pip._internal.utils.urls import url_to_path
|
42 |
-
|
43 |
-
from ._json import msg_to_json
|
44 |
-
|
45 |
-
if TYPE_CHECKING:
|
46 |
-
from typing import Protocol
|
47 |
-
else:
|
48 |
-
Protocol = object
|
49 |
-
|
50 |
-
DistributionVersion = Union[LegacyVersion, Version]
|
51 |
-
|
52 |
-
InfoPath = Union[str, pathlib.PurePath]
|
53 |
-
|
54 |
-
logger = logging.getLogger(__name__)
|
55 |
-
|
56 |
-
|
57 |
-
class BaseEntryPoint(Protocol):
|
58 |
-
@property
|
59 |
-
def name(self) -> str:
|
60 |
-
raise NotImplementedError()
|
61 |
-
|
62 |
-
@property
|
63 |
-
def value(self) -> str:
|
64 |
-
raise NotImplementedError()
|
65 |
-
|
66 |
-
@property
|
67 |
-
def group(self) -> str:
|
68 |
-
raise NotImplementedError()
|
69 |
-
|
70 |
-
|
71 |
-
def _convert_installed_files_path(
|
72 |
-
entry: Tuple[str, ...],
|
73 |
-
info: Tuple[str, ...],
|
74 |
-
) -> str:
|
75 |
-
"""Convert a legacy installed-files.txt path into modern RECORD path.
|
76 |
-
|
77 |
-
The legacy format stores paths relative to the info directory, while the
|
78 |
-
modern format stores paths relative to the package root, e.g. the
|
79 |
-
site-packages directory.
|
80 |
-
|
81 |
-
:param entry: Path parts of the installed-files.txt entry.
|
82 |
-
:param info: Path parts of the egg-info directory relative to package root.
|
83 |
-
:returns: The converted entry.
|
84 |
-
|
85 |
-
For best compatibility with symlinks, this does not use ``abspath()`` or
|
86 |
-
``Path.resolve()``, but tries to work with path parts:
|
87 |
-
|
88 |
-
1. While ``entry`` starts with ``..``, remove the equal amounts of parts
|
89 |
-
from ``info``; if ``info`` is empty, start appending ``..`` instead.
|
90 |
-
2. Join the two directly.
|
91 |
-
"""
|
92 |
-
while entry and entry[0] == "..":
|
93 |
-
if not info or info[-1] == "..":
|
94 |
-
info += ("..",)
|
95 |
-
else:
|
96 |
-
info = info[:-1]
|
97 |
-
entry = entry[1:]
|
98 |
-
return str(pathlib.Path(*info, *entry))
|
99 |
-
|
100 |
-
|
101 |
-
class RequiresEntry(NamedTuple):
|
102 |
-
requirement: str
|
103 |
-
extra: str
|
104 |
-
marker: str
|
105 |
-
|
106 |
-
|
107 |
-
class BaseDistribution(Protocol):
|
108 |
-
@classmethod
|
109 |
-
def from_directory(cls, directory: str) -> "BaseDistribution":
|
110 |
-
"""Load the distribution from a metadata directory.
|
111 |
-
|
112 |
-
:param directory: Path to a metadata directory, e.g. ``.dist-info``.
|
113 |
-
"""
|
114 |
-
raise NotImplementedError()
|
115 |
-
|
116 |
-
@classmethod
|
117 |
-
def from_metadata_file_contents(
|
118 |
-
cls,
|
119 |
-
metadata_contents: bytes,
|
120 |
-
filename: str,
|
121 |
-
project_name: str,
|
122 |
-
) -> "BaseDistribution":
|
123 |
-
"""Load the distribution from the contents of a METADATA file.
|
124 |
-
|
125 |
-
This is used to implement PEP 658 by generating a "shallow" dist object that can
|
126 |
-
be used for resolution without downloading or building the actual dist yet.
|
127 |
-
|
128 |
-
:param metadata_contents: The contents of a METADATA file.
|
129 |
-
:param filename: File name for the dist with this metadata.
|
130 |
-
:param project_name: Name of the project this dist represents.
|
131 |
-
"""
|
132 |
-
raise NotImplementedError()
|
133 |
-
|
134 |
-
@classmethod
|
135 |
-
def from_wheel(cls, wheel: "Wheel", name: str) -> "BaseDistribution":
|
136 |
-
"""Load the distribution from a given wheel.
|
137 |
-
|
138 |
-
:param wheel: A concrete wheel definition.
|
139 |
-
:param name: File name of the wheel.
|
140 |
-
|
141 |
-
:raises InvalidWheel: Whenever loading of the wheel causes a
|
142 |
-
:py:exc:`zipfile.BadZipFile` exception to be thrown.
|
143 |
-
:raises UnsupportedWheel: If the wheel is a valid zip, but malformed
|
144 |
-
internally.
|
145 |
-
"""
|
146 |
-
raise NotImplementedError()
|
147 |
-
|
148 |
-
def __repr__(self) -> str:
|
149 |
-
return f"{self.raw_name} {self.version} ({self.location})"
|
150 |
-
|
151 |
-
def __str__(self) -> str:
|
152 |
-
return f"{self.raw_name} {self.version}"
|
153 |
-
|
154 |
-
@property
|
155 |
-
def location(self) -> Optional[str]:
|
156 |
-
"""Where the distribution is loaded from.
|
157 |
-
|
158 |
-
A string value is not necessarily a filesystem path, since distributions
|
159 |
-
can be loaded from other sources, e.g. arbitrary zip archives. ``None``
|
160 |
-
means the distribution is created in-memory.
|
161 |
-
|
162 |
-
Do not canonicalize this value with e.g. ``pathlib.Path.resolve()``. If
|
163 |
-
this is a symbolic link, we want to preserve the relative path between
|
164 |
-
it and files in the distribution.
|
165 |
-
"""
|
166 |
-
raise NotImplementedError()
|
167 |
-
|
168 |
-
@property
|
169 |
-
def editable_project_location(self) -> Optional[str]:
|
170 |
-
"""The project location for editable distributions.
|
171 |
-
|
172 |
-
This is the directory where pyproject.toml or setup.py is located.
|
173 |
-
None if the distribution is not installed in editable mode.
|
174 |
-
"""
|
175 |
-
# TODO: this property is relatively costly to compute, memoize it ?
|
176 |
-
direct_url = self.direct_url
|
177 |
-
if direct_url:
|
178 |
-
if direct_url.is_local_editable():
|
179 |
-
return url_to_path(direct_url.url)
|
180 |
-
else:
|
181 |
-
# Search for an .egg-link file by walking sys.path, as it was
|
182 |
-
# done before by dist_is_editable().
|
183 |
-
egg_link_path = egg_link_path_from_sys_path(self.raw_name)
|
184 |
-
if egg_link_path:
|
185 |
-
# TODO: get project location from second line of egg_link file
|
186 |
-
# (https://github.com/pypa/pip/issues/10243)
|
187 |
-
return self.location
|
188 |
-
return None
|
189 |
-
|
190 |
-
@property
|
191 |
-
def installed_location(self) -> Optional[str]:
|
192 |
-
"""The distribution's "installed" location.
|
193 |
-
|
194 |
-
This should generally be a ``site-packages`` directory. This is
|
195 |
-
usually ``dist.location``, except for legacy develop-installed packages,
|
196 |
-
where ``dist.location`` is the source code location, and this is where
|
197 |
-
the ``.egg-link`` file is.
|
198 |
-
|
199 |
-
The returned location is normalized (in particular, with symlinks removed).
|
200 |
-
"""
|
201 |
-
raise NotImplementedError()
|
202 |
-
|
203 |
-
@property
|
204 |
-
def info_location(self) -> Optional[str]:
|
205 |
-
"""Location of the .[egg|dist]-info directory or file.
|
206 |
-
|
207 |
-
Similarly to ``location``, a string value is not necessarily a
|
208 |
-
filesystem path. ``None`` means the distribution is created in-memory.
|
209 |
-
|
210 |
-
For a modern .dist-info installation on disk, this should be something
|
211 |
-
like ``{location}/{raw_name}-{version}.dist-info``.
|
212 |
-
|
213 |
-
Do not canonicalize this value with e.g. ``pathlib.Path.resolve()``. If
|
214 |
-
this is a symbolic link, we want to preserve the relative path between
|
215 |
-
it and other files in the distribution.
|
216 |
-
"""
|
217 |
-
raise NotImplementedError()
|
218 |
-
|
219 |
-
@property
|
220 |
-
def installed_by_distutils(self) -> bool:
|
221 |
-
"""Whether this distribution is installed with legacy distutils format.
|
222 |
-
|
223 |
-
A distribution installed with "raw" distutils not patched by setuptools
|
224 |
-
uses one single file at ``info_location`` to store metadata. We need to
|
225 |
-
treat this specially on uninstallation.
|
226 |
-
"""
|
227 |
-
info_location = self.info_location
|
228 |
-
if not info_location:
|
229 |
-
return False
|
230 |
-
return pathlib.Path(info_location).is_file()
|
231 |
-
|
232 |
-
@property
|
233 |
-
def installed_as_egg(self) -> bool:
|
234 |
-
"""Whether this distribution is installed as an egg.
|
235 |
-
|
236 |
-
This usually indicates the distribution was installed by (older versions
|
237 |
-
of) easy_install.
|
238 |
-
"""
|
239 |
-
location = self.location
|
240 |
-
if not location:
|
241 |
-
return False
|
242 |
-
return location.endswith(".egg")
|
243 |
-
|
244 |
-
@property
|
245 |
-
def installed_with_setuptools_egg_info(self) -> bool:
|
246 |
-
"""Whether this distribution is installed with the ``.egg-info`` format.
|
247 |
-
|
248 |
-
This usually indicates the distribution was installed with setuptools
|
249 |
-
with an old pip version or with ``single-version-externally-managed``.
|
250 |
-
|
251 |
-
Note that this ensure the metadata store is a directory. distutils can
|
252 |
-
also installs an ``.egg-info``, but as a file, not a directory. This
|
253 |
-
property is *False* for that case. Also see ``installed_by_distutils``.
|
254 |
-
"""
|
255 |
-
info_location = self.info_location
|
256 |
-
if not info_location:
|
257 |
-
return False
|
258 |
-
if not info_location.endswith(".egg-info"):
|
259 |
-
return False
|
260 |
-
return pathlib.Path(info_location).is_dir()
|
261 |
-
|
262 |
-
@property
|
263 |
-
def installed_with_dist_info(self) -> bool:
|
264 |
-
"""Whether this distribution is installed with the "modern format".
|
265 |
-
|
266 |
-
This indicates a "modern" installation, e.g. storing metadata in the
|
267 |
-
``.dist-info`` directory. This applies to installations made by
|
268 |
-
setuptools (but through pip, not directly), or anything using the
|
269 |
-
standardized build backend interface (PEP 517).
|
270 |
-
"""
|
271 |
-
info_location = self.info_location
|
272 |
-
if not info_location:
|
273 |
-
return False
|
274 |
-
if not info_location.endswith(".dist-info"):
|
275 |
-
return False
|
276 |
-
return pathlib.Path(info_location).is_dir()
|
277 |
-
|
278 |
-
@property
|
279 |
-
def canonical_name(self) -> NormalizedName:
|
280 |
-
raise NotImplementedError()
|
281 |
-
|
282 |
-
@property
|
283 |
-
def version(self) -> DistributionVersion:
|
284 |
-
raise NotImplementedError()
|
285 |
-
|
286 |
-
@property
|
287 |
-
def setuptools_filename(self) -> str:
|
288 |
-
"""Convert a project name to its setuptools-compatible filename.
|
289 |
-
|
290 |
-
This is a copy of ``pkg_resources.to_filename()`` for compatibility.
|
291 |
-
"""
|
292 |
-
return self.raw_name.replace("-", "_")
|
293 |
-
|
294 |
-
@property
|
295 |
-
def direct_url(self) -> Optional[DirectUrl]:
|
296 |
-
"""Obtain a DirectUrl from this distribution.
|
297 |
-
|
298 |
-
Returns None if the distribution has no `direct_url.json` metadata,
|
299 |
-
or if `direct_url.json` is invalid.
|
300 |
-
"""
|
301 |
-
try:
|
302 |
-
content = self.read_text(DIRECT_URL_METADATA_NAME)
|
303 |
-
except FileNotFoundError:
|
304 |
-
return None
|
305 |
-
try:
|
306 |
-
return DirectUrl.from_json(content)
|
307 |
-
except (
|
308 |
-
UnicodeDecodeError,
|
309 |
-
json.JSONDecodeError,
|
310 |
-
DirectUrlValidationError,
|
311 |
-
) as e:
|
312 |
-
logger.warning(
|
313 |
-
"Error parsing %s for %s: %s",
|
314 |
-
DIRECT_URL_METADATA_NAME,
|
315 |
-
self.canonical_name,
|
316 |
-
e,
|
317 |
-
)
|
318 |
-
return None
|
319 |
-
|
320 |
-
@property
|
321 |
-
def installer(self) -> str:
|
322 |
-
try:
|
323 |
-
installer_text = self.read_text("INSTALLER")
|
324 |
-
except (OSError, ValueError, NoneMetadataError):
|
325 |
-
return "" # Fail silently if the installer file cannot be read.
|
326 |
-
for line in installer_text.splitlines():
|
327 |
-
cleaned_line = line.strip()
|
328 |
-
if cleaned_line:
|
329 |
-
return cleaned_line
|
330 |
-
return ""
|
331 |
-
|
332 |
-
@property
|
333 |
-
def requested(self) -> bool:
|
334 |
-
return self.is_file("REQUESTED")
|
335 |
-
|
336 |
-
@property
|
337 |
-
def editable(self) -> bool:
|
338 |
-
return bool(self.editable_project_location)
|
339 |
-
|
340 |
-
@property
|
341 |
-
def local(self) -> bool:
|
342 |
-
"""If distribution is installed in the current virtual environment.
|
343 |
-
|
344 |
-
Always True if we're not in a virtualenv.
|
345 |
-
"""
|
346 |
-
if self.installed_location is None:
|
347 |
-
return False
|
348 |
-
return is_local(self.installed_location)
|
349 |
-
|
350 |
-
@property
|
351 |
-
def in_usersite(self) -> bool:
|
352 |
-
if self.installed_location is None or user_site is None:
|
353 |
-
return False
|
354 |
-
return self.installed_location.startswith(normalize_path(user_site))
|
355 |
-
|
356 |
-
@property
|
357 |
-
def in_site_packages(self) -> bool:
|
358 |
-
if self.installed_location is None or site_packages is None:
|
359 |
-
return False
|
360 |
-
return self.installed_location.startswith(normalize_path(site_packages))
|
361 |
-
|
362 |
-
def is_file(self, path: InfoPath) -> bool:
|
363 |
-
"""Check whether an entry in the info directory is a file."""
|
364 |
-
raise NotImplementedError()
|
365 |
-
|
366 |
-
def iter_distutils_script_names(self) -> Iterator[str]:
|
367 |
-
"""Find distutils 'scripts' entries metadata.
|
368 |
-
|
369 |
-
If 'scripts' is supplied in ``setup.py``, distutils records those in the
|
370 |
-
installed distribution's ``scripts`` directory, a file for each script.
|
371 |
-
"""
|
372 |
-
raise NotImplementedError()
|
373 |
-
|
374 |
-
def read_text(self, path: InfoPath) -> str:
|
375 |
-
"""Read a file in the info directory.
|
376 |
-
|
377 |
-
:raise FileNotFoundError: If ``path`` does not exist in the directory.
|
378 |
-
:raise NoneMetadataError: If ``path`` exists in the info directory, but
|
379 |
-
cannot be read.
|
380 |
-
"""
|
381 |
-
raise NotImplementedError()
|
382 |
-
|
383 |
-
def iter_entry_points(self) -> Iterable[BaseEntryPoint]:
|
384 |
-
raise NotImplementedError()
|
385 |
-
|
386 |
-
def _metadata_impl(self) -> email.message.Message:
|
387 |
-
raise NotImplementedError()
|
388 |
-
|
389 |
-
@functools.lru_cache(maxsize=1)
|
390 |
-
def _metadata_cached(self) -> email.message.Message:
|
391 |
-
# When we drop python 3.7 support, move this to the metadata property and use
|
392 |
-
# functools.cached_property instead of lru_cache.
|
393 |
-
metadata = self._metadata_impl()
|
394 |
-
self._add_egg_info_requires(metadata)
|
395 |
-
return metadata
|
396 |
-
|
397 |
-
@property
|
398 |
-
def metadata(self) -> email.message.Message:
|
399 |
-
"""Metadata of distribution parsed from e.g. METADATA or PKG-INFO.
|
400 |
-
|
401 |
-
This should return an empty message if the metadata file is unavailable.
|
402 |
-
|
403 |
-
:raises NoneMetadataError: If the metadata file is available, but does
|
404 |
-
not contain valid metadata.
|
405 |
-
"""
|
406 |
-
return self._metadata_cached()
|
407 |
-
|
408 |
-
@property
|
409 |
-
def metadata_dict(self) -> Dict[str, Any]:
|
410 |
-
"""PEP 566 compliant JSON-serializable representation of METADATA or PKG-INFO.
|
411 |
-
|
412 |
-
This should return an empty dict if the metadata file is unavailable.
|
413 |
-
|
414 |
-
:raises NoneMetadataError: If the metadata file is available, but does
|
415 |
-
not contain valid metadata.
|
416 |
-
"""
|
417 |
-
return msg_to_json(self.metadata)
|
418 |
-
|
419 |
-
@property
|
420 |
-
def metadata_version(self) -> Optional[str]:
|
421 |
-
"""Value of "Metadata-Version:" in distribution metadata, if available."""
|
422 |
-
return self.metadata.get("Metadata-Version")
|
423 |
-
|
424 |
-
@property
|
425 |
-
def raw_name(self) -> str:
|
426 |
-
"""Value of "Name:" in distribution metadata."""
|
427 |
-
# The metadata should NEVER be missing the Name: key, but if it somehow
|
428 |
-
# does, fall back to the known canonical name.
|
429 |
-
return self.metadata.get("Name", self.canonical_name)
|
430 |
-
|
431 |
-
@property
|
432 |
-
def requires_python(self) -> SpecifierSet:
|
433 |
-
"""Value of "Requires-Python:" in distribution metadata.
|
434 |
-
|
435 |
-
If the key does not exist or contains an invalid value, an empty
|
436 |
-
SpecifierSet should be returned.
|
437 |
-
"""
|
438 |
-
value = self.metadata.get("Requires-Python")
|
439 |
-
if value is None:
|
440 |
-
return SpecifierSet()
|
441 |
-
try:
|
442 |
-
# Convert to str to satisfy the type checker; this can be a Header object.
|
443 |
-
spec = SpecifierSet(str(value))
|
444 |
-
except InvalidSpecifier as e:
|
445 |
-
message = "Package %r has an invalid Requires-Python: %s"
|
446 |
-
logger.warning(message, self.raw_name, e)
|
447 |
-
return SpecifierSet()
|
448 |
-
return spec
|
449 |
-
|
450 |
-
def iter_dependencies(self, extras: Collection[str] = ()) -> Iterable[Requirement]:
|
451 |
-
"""Dependencies of this distribution.
|
452 |
-
|
453 |
-
For modern .dist-info distributions, this is the collection of
|
454 |
-
"Requires-Dist:" entries in distribution metadata.
|
455 |
-
"""
|
456 |
-
raise NotImplementedError()
|
457 |
-
|
458 |
-
def iter_provided_extras(self) -> Iterable[str]:
|
459 |
-
"""Extras provided by this distribution.
|
460 |
-
|
461 |
-
For modern .dist-info distributions, this is the collection of
|
462 |
-
"Provides-Extra:" entries in distribution metadata.
|
463 |
-
"""
|
464 |
-
raise NotImplementedError()
|
465 |
-
|
466 |
-
def _iter_declared_entries_from_record(self) -> Optional[Iterator[str]]:
|
467 |
-
try:
|
468 |
-
text = self.read_text("RECORD")
|
469 |
-
except FileNotFoundError:
|
470 |
-
return None
|
471 |
-
# This extra Path-str cast normalizes entries.
|
472 |
-
return (str(pathlib.Path(row[0])) for row in csv.reader(text.splitlines()))
|
473 |
-
|
474 |
-
def _iter_declared_entries_from_legacy(self) -> Optional[Iterator[str]]:
|
475 |
-
try:
|
476 |
-
text = self.read_text("installed-files.txt")
|
477 |
-
except FileNotFoundError:
|
478 |
-
return None
|
479 |
-
paths = (p for p in text.splitlines(keepends=False) if p)
|
480 |
-
root = self.location
|
481 |
-
info = self.info_location
|
482 |
-
if root is None or info is None:
|
483 |
-
return paths
|
484 |
-
try:
|
485 |
-
info_rel = pathlib.Path(info).relative_to(root)
|
486 |
-
except ValueError: # info is not relative to root.
|
487 |
-
return paths
|
488 |
-
if not info_rel.parts: # info *is* root.
|
489 |
-
return paths
|
490 |
-
return (
|
491 |
-
_convert_installed_files_path(pathlib.Path(p).parts, info_rel.parts)
|
492 |
-
for p in paths
|
493 |
-
)
|
494 |
-
|
495 |
-
def iter_declared_entries(self) -> Optional[Iterator[str]]:
|
496 |
-
"""Iterate through file entries declared in this distribution.
|
497 |
-
|
498 |
-
For modern .dist-info distributions, this is the files listed in the
|
499 |
-
``RECORD`` metadata file. For legacy setuptools distributions, this
|
500 |
-
comes from ``installed-files.txt``, with entries normalized to be
|
501 |
-
compatible with the format used by ``RECORD``.
|
502 |
-
|
503 |
-
:return: An iterator for listed entries, or None if the distribution
|
504 |
-
contains neither ``RECORD`` nor ``installed-files.txt``.
|
505 |
-
"""
|
506 |
-
return (
|
507 |
-
self._iter_declared_entries_from_record()
|
508 |
-
or self._iter_declared_entries_from_legacy()
|
509 |
-
)
|
510 |
-
|
511 |
-
def _iter_requires_txt_entries(self) -> Iterator[RequiresEntry]:
|
512 |
-
"""Parse a ``requires.txt`` in an egg-info directory.
|
513 |
-
|
514 |
-
This is an INI-ish format where an egg-info stores dependencies. A
|
515 |
-
section name describes extra other environment markers, while each entry
|
516 |
-
is an arbitrary string (not a key-value pair) representing a dependency
|
517 |
-
as a requirement string (no markers).
|
518 |
-
|
519 |
-
There is a construct in ``importlib.metadata`` called ``Sectioned`` that
|
520 |
-
does mostly the same, but the format is currently considered private.
|
521 |
-
"""
|
522 |
-
try:
|
523 |
-
content = self.read_text("requires.txt")
|
524 |
-
except FileNotFoundError:
|
525 |
-
return
|
526 |
-
extra = marker = "" # Section-less entries don't have markers.
|
527 |
-
for line in content.splitlines():
|
528 |
-
line = line.strip()
|
529 |
-
if not line or line.startswith("#"): # Comment; ignored.
|
530 |
-
continue
|
531 |
-
if line.startswith("[") and line.endswith("]"): # A section header.
|
532 |
-
extra, _, marker = line.strip("[]").partition(":")
|
533 |
-
continue
|
534 |
-
yield RequiresEntry(requirement=line, extra=extra, marker=marker)
|
535 |
-
|
536 |
-
def _iter_egg_info_extras(self) -> Iterable[str]:
|
537 |
-
"""Get extras from the egg-info directory."""
|
538 |
-
known_extras = {""}
|
539 |
-
for entry in self._iter_requires_txt_entries():
|
540 |
-
if entry.extra in known_extras:
|
541 |
-
continue
|
542 |
-
known_extras.add(entry.extra)
|
543 |
-
yield entry.extra
|
544 |
-
|
545 |
-
def _iter_egg_info_dependencies(self) -> Iterable[str]:
|
546 |
-
"""Get distribution dependencies from the egg-info directory.
|
547 |
-
|
548 |
-
To ease parsing, this converts a legacy dependency entry into a PEP 508
|
549 |
-
requirement string. Like ``_iter_requires_txt_entries()``, there is code
|
550 |
-
in ``importlib.metadata`` that does mostly the same, but not do exactly
|
551 |
-
what we need.
|
552 |
-
|
553 |
-
Namely, ``importlib.metadata`` does not normalize the extra name before
|
554 |
-
putting it into the requirement string, which causes marker comparison
|
555 |
-
to fail because the dist-info format do normalize. This is consistent in
|
556 |
-
all currently available PEP 517 backends, although not standardized.
|
557 |
-
"""
|
558 |
-
for entry in self._iter_requires_txt_entries():
|
559 |
-
if entry.extra and entry.marker:
|
560 |
-
marker = f'({entry.marker}) and extra == "{safe_extra(entry.extra)}"'
|
561 |
-
elif entry.extra:
|
562 |
-
marker = f'extra == "{safe_extra(entry.extra)}"'
|
563 |
-
elif entry.marker:
|
564 |
-
marker = entry.marker
|
565 |
-
else:
|
566 |
-
marker = ""
|
567 |
-
if marker:
|
568 |
-
yield f"{entry.requirement} ; {marker}"
|
569 |
-
else:
|
570 |
-
yield entry.requirement
|
571 |
-
|
572 |
-
def _add_egg_info_requires(self, metadata: email.message.Message) -> None:
|
573 |
-
"""Add egg-info requires.txt information to the metadata."""
|
574 |
-
if not metadata.get_all("Requires-Dist"):
|
575 |
-
for dep in self._iter_egg_info_dependencies():
|
576 |
-
metadata["Requires-Dist"] = dep
|
577 |
-
if not metadata.get_all("Provides-Extra"):
|
578 |
-
for extra in self._iter_egg_info_extras():
|
579 |
-
metadata["Provides-Extra"] = extra
|
580 |
-
|
581 |
-
|
582 |
-
class BaseEnvironment:
|
583 |
-
"""An environment containing distributions to introspect."""
|
584 |
-
|
585 |
-
@classmethod
|
586 |
-
def default(cls) -> "BaseEnvironment":
|
587 |
-
raise NotImplementedError()
|
588 |
-
|
589 |
-
@classmethod
|
590 |
-
def from_paths(cls, paths: Optional[List[str]]) -> "BaseEnvironment":
|
591 |
-
raise NotImplementedError()
|
592 |
-
|
593 |
-
def get_distribution(self, name: str) -> Optional["BaseDistribution"]:
|
594 |
-
"""Given a requirement name, return the installed distributions.
|
595 |
-
|
596 |
-
The name may not be normalized. The implementation must canonicalize
|
597 |
-
it for lookup.
|
598 |
-
"""
|
599 |
-
raise NotImplementedError()
|
600 |
-
|
601 |
-
def _iter_distributions(self) -> Iterator["BaseDistribution"]:
|
602 |
-
"""Iterate through installed distributions.
|
603 |
-
|
604 |
-
This function should be implemented by subclass, but never called
|
605 |
-
directly. Use the public ``iter_distribution()`` instead, which
|
606 |
-
implements additional logic to make sure the distributions are valid.
|
607 |
-
"""
|
608 |
-
raise NotImplementedError()
|
609 |
-
|
610 |
-
def iter_all_distributions(self) -> Iterator[BaseDistribution]:
|
611 |
-
"""Iterate through all installed distributions without any filtering."""
|
612 |
-
for dist in self._iter_distributions():
|
613 |
-
# Make sure the distribution actually comes from a valid Python
|
614 |
-
# packaging distribution. Pip's AdjacentTempDirectory leaves folders
|
615 |
-
# e.g. ``~atplotlib.dist-info`` if cleanup was interrupted. The
|
616 |
-
# valid project name pattern is taken from PEP 508.
|
617 |
-
project_name_valid = re.match(
|
618 |
-
r"^([A-Z0-9]|[A-Z0-9][A-Z0-9._-]*[A-Z0-9])$",
|
619 |
-
dist.canonical_name,
|
620 |
-
flags=re.IGNORECASE,
|
621 |
-
)
|
622 |
-
if not project_name_valid:
|
623 |
-
logger.warning(
|
624 |
-
"Ignoring invalid distribution %s (%s)",
|
625 |
-
dist.canonical_name,
|
626 |
-
dist.location,
|
627 |
-
)
|
628 |
-
continue
|
629 |
-
yield dist
|
630 |
-
|
631 |
-
def iter_installed_distributions(
|
632 |
-
self,
|
633 |
-
local_only: bool = True,
|
634 |
-
skip: Container[str] = stdlib_pkgs,
|
635 |
-
include_editables: bool = True,
|
636 |
-
editables_only: bool = False,
|
637 |
-
user_only: bool = False,
|
638 |
-
) -> Iterator[BaseDistribution]:
|
639 |
-
"""Return a list of installed distributions.
|
640 |
-
|
641 |
-
This is based on ``iter_all_distributions()`` with additional filtering
|
642 |
-
options. Note that ``iter_installed_distributions()`` without arguments
|
643 |
-
is *not* equal to ``iter_all_distributions()``, since some of the
|
644 |
-
configurations exclude packages by default.
|
645 |
-
|
646 |
-
:param local_only: If True (default), only return installations
|
647 |
-
local to the current virtualenv, if in a virtualenv.
|
648 |
-
:param skip: An iterable of canonicalized project names to ignore;
|
649 |
-
defaults to ``stdlib_pkgs``.
|
650 |
-
:param include_editables: If False, don't report editables.
|
651 |
-
:param editables_only: If True, only report editables.
|
652 |
-
:param user_only: If True, only report installations in the user
|
653 |
-
site directory.
|
654 |
-
"""
|
655 |
-
it = self.iter_all_distributions()
|
656 |
-
if local_only:
|
657 |
-
it = (d for d in it if d.local)
|
658 |
-
if not include_editables:
|
659 |
-
it = (d for d in it if not d.editable)
|
660 |
-
if editables_only:
|
661 |
-
it = (d for d in it if d.editable)
|
662 |
-
if user_only:
|
663 |
-
it = (d for d in it if d.in_usersite)
|
664 |
-
return (d for d in it if d.canonical_name not in skip)
|
665 |
-
|
666 |
-
|
667 |
-
class Wheel(Protocol):
|
668 |
-
location: str
|
669 |
-
|
670 |
-
def as_zipfile(self) -> zipfile.ZipFile:
|
671 |
-
raise NotImplementedError()
|
672 |
-
|
673 |
-
|
674 |
-
class FilesystemWheel(Wheel):
|
675 |
-
def __init__(self, location: str) -> None:
|
676 |
-
self.location = location
|
677 |
-
|
678 |
-
def as_zipfile(self) -> zipfile.ZipFile:
|
679 |
-
return zipfile.ZipFile(self.location, allowZip64=True)
|
680 |
-
|
681 |
-
|
682 |
-
class MemoryWheel(Wheel):
|
683 |
-
def __init__(self, location: str, stream: IO[bytes]) -> None:
|
684 |
-
self.location = location
|
685 |
-
self.stream = stream
|
686 |
-
|
687 |
-
def as_zipfile(self) -> zipfile.ZipFile:
|
688 |
-
return zipfile.ZipFile(self.stream, allowZip64=True)
|
|
|
|
|
|
|
|
|
|
|
|
|
|
|
|
|
|
|
|
|
|
|
|
|
|
|
|
|
|
|
|
|
|
|
|
|
|
|
|
|
|
|
|
|
|
|
|
|
|
|
|
|
|
|
|
|
|
|
|
|
|
|
|
|
|
|
|
|
|
|
|
|
|
|
|
|
|
|
|
|
|
|
|
|
|
|
|
|
|
|
|
|
|
|
|
|
|
|
|
|
|
|
|
|
|
|
|
|
|
|
|
|
|
|
|
|
|
|
|
|
|
|
|
|
|
|
|
|
|
|
|
|
|
|
|
|
|
|
|
|
|
|
|
|
|
|
|
|
|
|
|
|
|
|
|
|
|
|
|
|
|
|
|
|
|
|
|
|
|
|
|
|
|
|
|
|
|
|
|
|
|
|
|
|
|
|
|
|
|
|
|
|
|
|
|
|
|
|
|
|
|
|
|
|
|
|
|
|
|
|
|
|
|
|
|
|
|
|
|
|
|
|
|
|
|
|
|
|
|
|
|
|
|
|
|
|
|
|
|
|
|
|
|
|
|
|
|
|
|
|
|
|
|
|
|
|
|
|
|
|
|
|
|
|
|
|
|
|
|
|
|
|
|
|
|
|
|
|
|
|
|
|
|
|
|
|
|
|
|
|
|
|
|
|
|
|
|
|
|
|
|
|
|
|
|
|
|
|
|
|
|
|
|
|
|
|
|
|
|
|
|
|
|
|
|
|
|
|
|
|
|
|
|
|
|
|
|
|
|
|
|
|
|
|
|
|
|
|
|
|
|
|
|
|
|
|
|
|
|
|
|
|
|
|
|
|
|
|
|
|
|
|
|
|
|
|
|
|
|
|
|
|
|
|
|
|
|
|
|
|
|
|
|
|
|
|
|
|
|
|
|
|
|
|
|
|
|
|
|
|
|
|
|
|
|
|
|
|
|
|
|
|
|
|
|
|
|
|
|
|
|
|
|
|
|
|
|
|
|
|
|
|
|
|
|
|
|
|
|
|
|
|
|
|
|
|
|
|
|
|
|
|
|
|
|
|
|
|
|
|
|
|
|
|
|
|
|
|
|
|
|
|
|
|
|
|
|
|
|
|
|
|
|
|
|
|
|
|
|
|
|
|
|
|
|
|
|
|
|
|
|
|
|
|
|
|
|
|
|
|
|
|
|
|
|
|
|
|
|
|
|
|
|
|
|
|
|
|
|
|
|
|
|
|
|
|
|
|
|
|
|
|
|
|
|
|
|
|
|
|
|
|
|
|
|
|
|
|
|
|
|
|
|
|
|
|
|
|
|
|
|
|
|
|
|
|
|
|
|
|
|
|
|
|
|
|
|
|
|
|
|
|
|
|
|
|
|
|
|
|
|
|
|
|
|
|
|
|
|
|
|
|
|
|
|
|
|
|
|
|
|
|
|
|
|
|
|
|
|
|
|
|
|
|
|
|
|
|
|
|
|
|
|
|
|
|
|
|
|
|
|
|
|
|
|
|
|
|
|
|
|
|
|
|
|
|
|
|
|
|
|
|
|
|
|
|
|
|
|
|
|
|
|
|
|
|
|
|
|
|
|
|
|
|
|
|
|
|
|
|
|
|
|
|
|
|
|
|
|
|
|
|
|
|
|
|
|
|
|
|
|
|
|
|
|
|
|
|
|
|
|
|
|
|
|
|
|
|
|
|
|
|
|
|
|
|
|
|
|
|
|
|
|
|
|
|
|
|
|
|
|
|
|
|
|
|
|
|
|
|
|
|
|
|
|
|
|
|
|
|
|
|
|
|
|
|
|
|
|
|
|
|
|
|
|
|
|
|
|
|
|
|
|
|
|
|
|
|
|
|
|
|
|
|
|
|
|
|
|
|
|
|
|
|
|
|
|
|
|
|
|
|
|
|
|
|
|
|
|
|
|
|
|
|
|
|
|
|
|
|
|
|
|
|
|
|
|
|
|
|
|
|
|
|
|
|
|
|
|
|
|
|
|
|
|
|
|
|
|
|
|
|
|
|
|
|
|
|
|
|
|
|
|
|
|
|
|
|
|
|
|
|
|
|
|
|
|
|
|
|
|
|
|
|
|
|
|
|
|
|
|
|
|
|
|
|
|
|
|
|
|
|
|
|
|
|
|
|
|
|
|
|
|
|
|
|
|
|
|
|
|
|
|
|
|
|
|
|
|
|
|
|
|
|
|
|
|
|
|
|
|
|
|
|
|
|
|
|
|
|
|
|
|
|
|
|
|
|
|
|
|
|
|
|
|
|
|
|
|
|
|
|
|
|
|
|
|
|
|
|
|
|
|
|
|
|
|
|
|
|
|
|
|
|
|
|
|
|
|
|
|
|
|
|
|
|
|
|
|
|
|
|
|
|
|
|
|
|
|
|
|
|
|
|
|
|
|
|
|
|
|
|
|
|
|
|
|
|
|
|
|
|
|
|
|
|
|
|
|
|
|
|
|
|
|
|
|
|
|
|
|
|
|
|
|
|
|
|
|
|
|
|
|
|
|
|
|
|
|
|
|
|
|
|
|
|
|
|
|
|
|
|
|
|
|
|
|
|
|
|
|
|
|
|
|
|
|
|
|
|
|
|
|
|
|
|
|
|
|
|
|
|
|
|
|
|
|
|
|
|
|
|
|
|
|
|
|
|
|
|
|
|
|
|
|
|
|
|
|
|
|
|
|
|
|
|
|
|
|
|
|
|
|
|
|
|
|
|
|
|
|
|
|
|
|
|
|
|
|
|
|
|
|
|
|
|
|
|
|
|
|
|
|
|
|
|
|
|
|
|
|
|
|
|
|
|
|
|
|
|
|
|
|
|
|
|
|
|
|
|
|
|
|
|
|
|
|
|
|
|
|
|
|
|
|
|
|
|
|
|
|
|
|
|
|
|
|
|
|
|
|
|
|
|
|
|
|
|
|
|
|
|
|
|
|
|
|
|
|
|
|
|
|
|
|
|
|
|
|
|
|
|
|
|
|
|
|
|
|
|
|
|
|
|
|
|
|
|
|
|
|
|
|
|
|
|
|
|
|
|
|
|
|
|
|
|
|
|
|
|
|
|
|
|
|
|
|
|
|
|
|
|
|
|
|
|
|
spaces/Ataturk-Chatbot/HuggingFaceChat/venv/lib/python3.11/site-packages/pip/_vendor/chardet/macromanprober.py
DELETED
@@ -1,162 +0,0 @@
|
|
1 |
-
######################## BEGIN LICENSE BLOCK ########################
|
2 |
-
# This code was modified from latin1prober.py by Rob Speer <[email protected]>.
|
3 |
-
# The Original Code is Mozilla Universal charset detector code.
|
4 |
-
#
|
5 |
-
# The Initial Developer of the Original Code is
|
6 |
-
# Netscape Communications Corporation.
|
7 |
-
# Portions created by the Initial Developer are Copyright (C) 2001
|
8 |
-
# the Initial Developer. All Rights Reserved.
|
9 |
-
#
|
10 |
-
# Contributor(s):
|
11 |
-
# Rob Speer - adapt to MacRoman encoding
|
12 |
-
# Mark Pilgrim - port to Python
|
13 |
-
# Shy Shalom - original C code
|
14 |
-
#
|
15 |
-
# This library is free software; you can redistribute it and/or
|
16 |
-
# modify it under the terms of the GNU Lesser General Public
|
17 |
-
# License as published by the Free Software Foundation; either
|
18 |
-
# version 2.1 of the License, or (at your option) any later version.
|
19 |
-
#
|
20 |
-
# This library is distributed in the hope that it will be useful,
|
21 |
-
# but WITHOUT ANY WARRANTY; without even the implied warranty of
|
22 |
-
# MERCHANTABILITY or FITNESS FOR A PARTICULAR PURPOSE. See the GNU
|
23 |
-
# Lesser General Public License for more details.
|
24 |
-
#
|
25 |
-
# You should have received a copy of the GNU Lesser General Public
|
26 |
-
# License along with this library; if not, write to the Free Software
|
27 |
-
# Foundation, Inc., 51 Franklin St, Fifth Floor, Boston, MA
|
28 |
-
# 02110-1301 USA
|
29 |
-
######################### END LICENSE BLOCK #########################
|
30 |
-
|
31 |
-
from typing import List, Union
|
32 |
-
|
33 |
-
from .charsetprober import CharSetProber
|
34 |
-
from .enums import ProbingState
|
35 |
-
|
36 |
-
FREQ_CAT_NUM = 4
|
37 |
-
|
38 |
-
UDF = 0 # undefined
|
39 |
-
OTH = 1 # other
|
40 |
-
ASC = 2 # ascii capital letter
|
41 |
-
ASS = 3 # ascii small letter
|
42 |
-
ACV = 4 # accent capital vowel
|
43 |
-
ACO = 5 # accent capital other
|
44 |
-
ASV = 6 # accent small vowel
|
45 |
-
ASO = 7 # accent small other
|
46 |
-
ODD = 8 # character that is unlikely to appear
|
47 |
-
CLASS_NUM = 9 # total classes
|
48 |
-
|
49 |
-
# The change from Latin1 is that we explicitly look for extended characters
|
50 |
-
# that are infrequently-occurring symbols, and consider them to always be
|
51 |
-
# improbable. This should let MacRoman get out of the way of more likely
|
52 |
-
# encodings in most situations.
|
53 |
-
|
54 |
-
# fmt: off
|
55 |
-
MacRoman_CharToClass = (
|
56 |
-
OTH, OTH, OTH, OTH, OTH, OTH, OTH, OTH, # 00 - 07
|
57 |
-
OTH, OTH, OTH, OTH, OTH, OTH, OTH, OTH, # 08 - 0F
|
58 |
-
OTH, OTH, OTH, OTH, OTH, OTH, OTH, OTH, # 10 - 17
|
59 |
-
OTH, OTH, OTH, OTH, OTH, OTH, OTH, OTH, # 18 - 1F
|
60 |
-
OTH, OTH, OTH, OTH, OTH, OTH, OTH, OTH, # 20 - 27
|
61 |
-
OTH, OTH, OTH, OTH, OTH, OTH, OTH, OTH, # 28 - 2F
|
62 |
-
OTH, OTH, OTH, OTH, OTH, OTH, OTH, OTH, # 30 - 37
|
63 |
-
OTH, OTH, OTH, OTH, OTH, OTH, OTH, OTH, # 38 - 3F
|
64 |
-
OTH, ASC, ASC, ASC, ASC, ASC, ASC, ASC, # 40 - 47
|
65 |
-
ASC, ASC, ASC, ASC, ASC, ASC, ASC, ASC, # 48 - 4F
|
66 |
-
ASC, ASC, ASC, ASC, ASC, ASC, ASC, ASC, # 50 - 57
|
67 |
-
ASC, ASC, ASC, OTH, OTH, OTH, OTH, OTH, # 58 - 5F
|
68 |
-
OTH, ASS, ASS, ASS, ASS, ASS, ASS, ASS, # 60 - 67
|
69 |
-
ASS, ASS, ASS, ASS, ASS, ASS, ASS, ASS, # 68 - 6F
|
70 |
-
ASS, ASS, ASS, ASS, ASS, ASS, ASS, ASS, # 70 - 77
|
71 |
-
ASS, ASS, ASS, OTH, OTH, OTH, OTH, OTH, # 78 - 7F
|
72 |
-
ACV, ACV, ACO, ACV, ACO, ACV, ACV, ASV, # 80 - 87
|
73 |
-
ASV, ASV, ASV, ASV, ASV, ASO, ASV, ASV, # 88 - 8F
|
74 |
-
ASV, ASV, ASV, ASV, ASV, ASV, ASO, ASV, # 90 - 97
|
75 |
-
ASV, ASV, ASV, ASV, ASV, ASV, ASV, ASV, # 98 - 9F
|
76 |
-
OTH, OTH, OTH, OTH, OTH, OTH, OTH, ASO, # A0 - A7
|
77 |
-
OTH, OTH, ODD, ODD, OTH, OTH, ACV, ACV, # A8 - AF
|
78 |
-
OTH, OTH, OTH, OTH, OTH, OTH, OTH, OTH, # B0 - B7
|
79 |
-
OTH, OTH, OTH, OTH, OTH, OTH, ASV, ASV, # B8 - BF
|
80 |
-
OTH, OTH, ODD, OTH, ODD, OTH, OTH, OTH, # C0 - C7
|
81 |
-
OTH, OTH, OTH, ACV, ACV, ACV, ACV, ASV, # C8 - CF
|
82 |
-
OTH, OTH, OTH, OTH, OTH, OTH, OTH, ODD, # D0 - D7
|
83 |
-
ASV, ACV, ODD, OTH, OTH, OTH, OTH, OTH, # D8 - DF
|
84 |
-
OTH, OTH, OTH, OTH, OTH, ACV, ACV, ACV, # E0 - E7
|
85 |
-
ACV, ACV, ACV, ACV, ACV, ACV, ACV, ACV, # E8 - EF
|
86 |
-
ODD, ACV, ACV, ACV, ACV, ASV, ODD, ODD, # F0 - F7
|
87 |
-
ODD, ODD, ODD, ODD, ODD, ODD, ODD, ODD, # F8 - FF
|
88 |
-
)
|
89 |
-
|
90 |
-
# 0 : illegal
|
91 |
-
# 1 : very unlikely
|
92 |
-
# 2 : normal
|
93 |
-
# 3 : very likely
|
94 |
-
MacRomanClassModel = (
|
95 |
-
# UDF OTH ASC ASS ACV ACO ASV ASO ODD
|
96 |
-
0, 0, 0, 0, 0, 0, 0, 0, 0, # UDF
|
97 |
-
0, 3, 3, 3, 3, 3, 3, 3, 1, # OTH
|
98 |
-
0, 3, 3, 3, 3, 3, 3, 3, 1, # ASC
|
99 |
-
0, 3, 3, 3, 1, 1, 3, 3, 1, # ASS
|
100 |
-
0, 3, 3, 3, 1, 2, 1, 2, 1, # ACV
|
101 |
-
0, 3, 3, 3, 3, 3, 3, 3, 1, # ACO
|
102 |
-
0, 3, 1, 3, 1, 1, 1, 3, 1, # ASV
|
103 |
-
0, 3, 1, 3, 1, 1, 3, 3, 1, # ASO
|
104 |
-
0, 1, 1, 1, 1, 1, 1, 1, 1, # ODD
|
105 |
-
)
|
106 |
-
# fmt: on
|
107 |
-
|
108 |
-
|
109 |
-
class MacRomanProber(CharSetProber):
|
110 |
-
def __init__(self) -> None:
|
111 |
-
super().__init__()
|
112 |
-
self._last_char_class = OTH
|
113 |
-
self._freq_counter: List[int] = []
|
114 |
-
self.reset()
|
115 |
-
|
116 |
-
def reset(self) -> None:
|
117 |
-
self._last_char_class = OTH
|
118 |
-
self._freq_counter = [0] * FREQ_CAT_NUM
|
119 |
-
|
120 |
-
# express the prior that MacRoman is a somewhat rare encoding;
|
121 |
-
# this can be done by starting out in a slightly improbable state
|
122 |
-
# that must be overcome
|
123 |
-
self._freq_counter[2] = 10
|
124 |
-
|
125 |
-
super().reset()
|
126 |
-
|
127 |
-
@property
|
128 |
-
def charset_name(self) -> str:
|
129 |
-
return "MacRoman"
|
130 |
-
|
131 |
-
@property
|
132 |
-
def language(self) -> str:
|
133 |
-
return ""
|
134 |
-
|
135 |
-
def feed(self, byte_str: Union[bytes, bytearray]) -> ProbingState:
|
136 |
-
byte_str = self.remove_xml_tags(byte_str)
|
137 |
-
for c in byte_str:
|
138 |
-
char_class = MacRoman_CharToClass[c]
|
139 |
-
freq = MacRomanClassModel[(self._last_char_class * CLASS_NUM) + char_class]
|
140 |
-
if freq == 0:
|
141 |
-
self._state = ProbingState.NOT_ME
|
142 |
-
break
|
143 |
-
self._freq_counter[freq] += 1
|
144 |
-
self._last_char_class = char_class
|
145 |
-
|
146 |
-
return self.state
|
147 |
-
|
148 |
-
def get_confidence(self) -> float:
|
149 |
-
if self.state == ProbingState.NOT_ME:
|
150 |
-
return 0.01
|
151 |
-
|
152 |
-
total = sum(self._freq_counter)
|
153 |
-
confidence = (
|
154 |
-
0.0
|
155 |
-
if total < 0.01
|
156 |
-
else (self._freq_counter[3] - self._freq_counter[1] * 20.0) / total
|
157 |
-
)
|
158 |
-
confidence = max(confidence, 0.0)
|
159 |
-
# lower the confidence of MacRoman so that other more accurate
|
160 |
-
# detector can take priority.
|
161 |
-
confidence *= 0.73
|
162 |
-
return confidence
|
|
|
|
|
|
|
|
|
|
|
|
|
|
|
|
|
|
|
|
|
|
|
|
|
|
|
|
|
|
|
|
|
|
|
|
|
|
|
|
|
|
|
|
|
|
|
|
|
|
|
|
|
|
|
|
|
|
|
|
|
|
|
|
|
|
|
|
|
|
|
|
|
|
|
|
|
|
|
|
|
|
|
|
|
|
|
|
|
|
|
|
|
|
|
|
|
|
|
|
|
|
|
|
|
|
|
|
|
|
|
|
|
|
|
|
|
|
|
|
|
|
|
|
|
|
|
|
|
|
|
|
|
|
|
|
|
|
|
|
|
|
|
|
|
|
|
|
|
|
|
|
|
|
|
|
|
|
|
|
|
|
|
|
|
|
|
|
|
|
|
|
|
|
|
|
|
|
|
|
|
|
|
|
|
|
|
|
|
|
|
|
|
|
|
|
|
|
|
|
|
|
|
|
|
|
|
|
|
|
|
|
|
|
|
|
|
|
|
|
|
|
|
|
|
|
|
|
|
|
|
|
|
|
|
|
|
|
|
|
|
|
|
|
|
|
|
|
|
|
|
|
|
|
|
|
|
|
|
|
|
|
|
|
|
|
|
|
|
|
|
|
|
|
|
|
|
|
|
|
|
|
|
|
|
|
|
|
|
|
|
|
|
|
|
|
|
|
|
|
|
|
|
|
|
|
|
|
|
|
|
|
|
|
|
|
|
|
|
|
|
|
|
|
|
|
spaces/Ataturk-Chatbot/HuggingFaceChat/venv/lib/python3.11/site-packages/setuptools/_distutils/command/build.py
DELETED
@@ -1,153 +0,0 @@
|
|
1 |
-
"""distutils.command.build
|
2 |
-
|
3 |
-
Implements the Distutils 'build' command."""
|
4 |
-
|
5 |
-
import sys
|
6 |
-
import os
|
7 |
-
from distutils.core import Command
|
8 |
-
from distutils.errors import DistutilsOptionError
|
9 |
-
from distutils.util import get_platform
|
10 |
-
|
11 |
-
|
12 |
-
def show_compilers():
|
13 |
-
from distutils.ccompiler import show_compilers
|
14 |
-
|
15 |
-
show_compilers()
|
16 |
-
|
17 |
-
|
18 |
-
class build(Command):
|
19 |
-
|
20 |
-
description = "build everything needed to install"
|
21 |
-
|
22 |
-
user_options = [
|
23 |
-
('build-base=', 'b', "base directory for build library"),
|
24 |
-
('build-purelib=', None, "build directory for platform-neutral distributions"),
|
25 |
-
('build-platlib=', None, "build directory for platform-specific distributions"),
|
26 |
-
(
|
27 |
-
'build-lib=',
|
28 |
-
None,
|
29 |
-
"build directory for all distribution (defaults to either "
|
30 |
-
+ "build-purelib or build-platlib",
|
31 |
-
),
|
32 |
-
('build-scripts=', None, "build directory for scripts"),
|
33 |
-
('build-temp=', 't', "temporary build directory"),
|
34 |
-
(
|
35 |
-
'plat-name=',
|
36 |
-
'p',
|
37 |
-
"platform name to build for, if supported "
|
38 |
-
"(default: %s)" % get_platform(),
|
39 |
-
),
|
40 |
-
('compiler=', 'c', "specify the compiler type"),
|
41 |
-
('parallel=', 'j', "number of parallel build jobs"),
|
42 |
-
('debug', 'g', "compile extensions and libraries with debugging information"),
|
43 |
-
('force', 'f', "forcibly build everything (ignore file timestamps)"),
|
44 |
-
('executable=', 'e', "specify final destination interpreter path (build.py)"),
|
45 |
-
]
|
46 |
-
|
47 |
-
boolean_options = ['debug', 'force']
|
48 |
-
|
49 |
-
help_options = [
|
50 |
-
('help-compiler', None, "list available compilers", show_compilers),
|
51 |
-
]
|
52 |
-
|
53 |
-
def initialize_options(self):
|
54 |
-
self.build_base = 'build'
|
55 |
-
# these are decided only after 'build_base' has its final value
|
56 |
-
# (unless overridden by the user or client)
|
57 |
-
self.build_purelib = None
|
58 |
-
self.build_platlib = None
|
59 |
-
self.build_lib = None
|
60 |
-
self.build_temp = None
|
61 |
-
self.build_scripts = None
|
62 |
-
self.compiler = None
|
63 |
-
self.plat_name = None
|
64 |
-
self.debug = None
|
65 |
-
self.force = 0
|
66 |
-
self.executable = None
|
67 |
-
self.parallel = None
|
68 |
-
|
69 |
-
def finalize_options(self): # noqa: C901
|
70 |
-
if self.plat_name is None:
|
71 |
-
self.plat_name = get_platform()
|
72 |
-
else:
|
73 |
-
# plat-name only supported for windows (other platforms are
|
74 |
-
# supported via ./configure flags, if at all). Avoid misleading
|
75 |
-
# other platforms.
|
76 |
-
if os.name != 'nt':
|
77 |
-
raise DistutilsOptionError(
|
78 |
-
"--plat-name only supported on Windows (try "
|
79 |
-
"using './configure --help' on your platform)"
|
80 |
-
)
|
81 |
-
|
82 |
-
plat_specifier = ".{}-{}".format(self.plat_name, sys.implementation.cache_tag)
|
83 |
-
|
84 |
-
# Make it so Python 2.x and Python 2.x with --with-pydebug don't
|
85 |
-
# share the same build directories. Doing so confuses the build
|
86 |
-
# process for C modules
|
87 |
-
if hasattr(sys, 'gettotalrefcount'):
|
88 |
-
plat_specifier += '-pydebug'
|
89 |
-
|
90 |
-
# 'build_purelib' and 'build_platlib' just default to 'lib' and
|
91 |
-
# 'lib.<plat>' under the base build directory. We only use one of
|
92 |
-
# them for a given distribution, though --
|
93 |
-
if self.build_purelib is None:
|
94 |
-
self.build_purelib = os.path.join(self.build_base, 'lib')
|
95 |
-
if self.build_platlib is None:
|
96 |
-
self.build_platlib = os.path.join(self.build_base, 'lib' + plat_specifier)
|
97 |
-
|
98 |
-
# 'build_lib' is the actual directory that we will use for this
|
99 |
-
# particular module distribution -- if user didn't supply it, pick
|
100 |
-
# one of 'build_purelib' or 'build_platlib'.
|
101 |
-
if self.build_lib is None:
|
102 |
-
if self.distribution.has_ext_modules():
|
103 |
-
self.build_lib = self.build_platlib
|
104 |
-
else:
|
105 |
-
self.build_lib = self.build_purelib
|
106 |
-
|
107 |
-
# 'build_temp' -- temporary directory for compiler turds,
|
108 |
-
# "build/temp.<plat>"
|
109 |
-
if self.build_temp is None:
|
110 |
-
self.build_temp = os.path.join(self.build_base, 'temp' + plat_specifier)
|
111 |
-
if self.build_scripts is None:
|
112 |
-
self.build_scripts = os.path.join(
|
113 |
-
self.build_base, 'scripts-%d.%d' % sys.version_info[:2]
|
114 |
-
)
|
115 |
-
|
116 |
-
if self.executable is None and sys.executable:
|
117 |
-
self.executable = os.path.normpath(sys.executable)
|
118 |
-
|
119 |
-
if isinstance(self.parallel, str):
|
120 |
-
try:
|
121 |
-
self.parallel = int(self.parallel)
|
122 |
-
except ValueError:
|
123 |
-
raise DistutilsOptionError("parallel should be an integer")
|
124 |
-
|
125 |
-
def run(self):
|
126 |
-
# Run all relevant sub-commands. This will be some subset of:
|
127 |
-
# - build_py - pure Python modules
|
128 |
-
# - build_clib - standalone C libraries
|
129 |
-
# - build_ext - Python extensions
|
130 |
-
# - build_scripts - (Python) scripts
|
131 |
-
for cmd_name in self.get_sub_commands():
|
132 |
-
self.run_command(cmd_name)
|
133 |
-
|
134 |
-
# -- Predicates for the sub-command list ---------------------------
|
135 |
-
|
136 |
-
def has_pure_modules(self):
|
137 |
-
return self.distribution.has_pure_modules()
|
138 |
-
|
139 |
-
def has_c_libraries(self):
|
140 |
-
return self.distribution.has_c_libraries()
|
141 |
-
|
142 |
-
def has_ext_modules(self):
|
143 |
-
return self.distribution.has_ext_modules()
|
144 |
-
|
145 |
-
def has_scripts(self):
|
146 |
-
return self.distribution.has_scripts()
|
147 |
-
|
148 |
-
sub_commands = [
|
149 |
-
('build_py', has_pure_modules),
|
150 |
-
('build_clib', has_c_libraries),
|
151 |
-
('build_ext', has_ext_modules),
|
152 |
-
('build_scripts', has_scripts),
|
153 |
-
]
|
|
|
|
|
|
|
|
|
|
|
|
|
|
|
|
|
|
|
|
|
|
|
|
|
|
|
|
|
|
|
|
|
|
|
|
|
|
|
|
|
|
|
|
|
|
|
|
|
|
|
|
|
|
|
|
|
|
|
|
|
|
|
|
|
|
|
|
|
|
|
|
|
|
|
|
|
|
|
|
|
|
|
|
|
|
|
|
|
|
|
|
|
|
|
|
|
|
|
|
|
|
|
|
|
|
|
|
|
|
|
|
|
|
|
|
|
|
|
|
|
|
|
|
|
|
|
|
|
|
|
|
|
|
|
|
|
|
|
|
|
|
|
|
|
|
|
|
|
|
|
|
|
|
|
|
|
|
|
|
|
|
|
|
|
|
|
|
|
|
|
|
|
|
|
|
|
|
|
|
|
|
|
|
|
|
|
|
|
|
|
|
|
|
|
|
|
|
|
|
|
|
|
|
|
|
|
|
|
|
|
|
|
|
|
|
|
|
|
|
|
|
|
|
|
|
|
|
|
|
|
|
|
|
|
|
|
|
|
|
|
|
|
|
|
|
|
|
|
|
|
|
|
|
|
|
|
|
|
|
|
|
|
|
|
|
|
|
|
|
|
|
|
|
|
|
|
|
|
|
|
|
|
|
|
|
|
|
|
|
|
|
|
|
|
|
|
|
|
|
|
|
|
|
|
|
|
|
spaces/Ataturk-Chatbot/HuggingFaceChat/venv/lib/python3.11/site-packages/setuptools/_vendor/zipp.py
DELETED
@@ -1,329 +0,0 @@
|
|
1 |
-
import io
|
2 |
-
import posixpath
|
3 |
-
import zipfile
|
4 |
-
import itertools
|
5 |
-
import contextlib
|
6 |
-
import sys
|
7 |
-
import pathlib
|
8 |
-
|
9 |
-
if sys.version_info < (3, 7):
|
10 |
-
from collections import OrderedDict
|
11 |
-
else:
|
12 |
-
OrderedDict = dict
|
13 |
-
|
14 |
-
|
15 |
-
__all__ = ['Path']
|
16 |
-
|
17 |
-
|
18 |
-
def _parents(path):
|
19 |
-
"""
|
20 |
-
Given a path with elements separated by
|
21 |
-
posixpath.sep, generate all parents of that path.
|
22 |
-
|
23 |
-
>>> list(_parents('b/d'))
|
24 |
-
['b']
|
25 |
-
>>> list(_parents('/b/d/'))
|
26 |
-
['/b']
|
27 |
-
>>> list(_parents('b/d/f/'))
|
28 |
-
['b/d', 'b']
|
29 |
-
>>> list(_parents('b'))
|
30 |
-
[]
|
31 |
-
>>> list(_parents(''))
|
32 |
-
[]
|
33 |
-
"""
|
34 |
-
return itertools.islice(_ancestry(path), 1, None)
|
35 |
-
|
36 |
-
|
37 |
-
def _ancestry(path):
|
38 |
-
"""
|
39 |
-
Given a path with elements separated by
|
40 |
-
posixpath.sep, generate all elements of that path
|
41 |
-
|
42 |
-
>>> list(_ancestry('b/d'))
|
43 |
-
['b/d', 'b']
|
44 |
-
>>> list(_ancestry('/b/d/'))
|
45 |
-
['/b/d', '/b']
|
46 |
-
>>> list(_ancestry('b/d/f/'))
|
47 |
-
['b/d/f', 'b/d', 'b']
|
48 |
-
>>> list(_ancestry('b'))
|
49 |
-
['b']
|
50 |
-
>>> list(_ancestry(''))
|
51 |
-
[]
|
52 |
-
"""
|
53 |
-
path = path.rstrip(posixpath.sep)
|
54 |
-
while path and path != posixpath.sep:
|
55 |
-
yield path
|
56 |
-
path, tail = posixpath.split(path)
|
57 |
-
|
58 |
-
|
59 |
-
_dedupe = OrderedDict.fromkeys
|
60 |
-
"""Deduplicate an iterable in original order"""
|
61 |
-
|
62 |
-
|
63 |
-
def _difference(minuend, subtrahend):
|
64 |
-
"""
|
65 |
-
Return items in minuend not in subtrahend, retaining order
|
66 |
-
with O(1) lookup.
|
67 |
-
"""
|
68 |
-
return itertools.filterfalse(set(subtrahend).__contains__, minuend)
|
69 |
-
|
70 |
-
|
71 |
-
class CompleteDirs(zipfile.ZipFile):
|
72 |
-
"""
|
73 |
-
A ZipFile subclass that ensures that implied directories
|
74 |
-
are always included in the namelist.
|
75 |
-
"""
|
76 |
-
|
77 |
-
@staticmethod
|
78 |
-
def _implied_dirs(names):
|
79 |
-
parents = itertools.chain.from_iterable(map(_parents, names))
|
80 |
-
as_dirs = (p + posixpath.sep for p in parents)
|
81 |
-
return _dedupe(_difference(as_dirs, names))
|
82 |
-
|
83 |
-
def namelist(self):
|
84 |
-
names = super(CompleteDirs, self).namelist()
|
85 |
-
return names + list(self._implied_dirs(names))
|
86 |
-
|
87 |
-
def _name_set(self):
|
88 |
-
return set(self.namelist())
|
89 |
-
|
90 |
-
def resolve_dir(self, name):
|
91 |
-
"""
|
92 |
-
If the name represents a directory, return that name
|
93 |
-
as a directory (with the trailing slash).
|
94 |
-
"""
|
95 |
-
names = self._name_set()
|
96 |
-
dirname = name + '/'
|
97 |
-
dir_match = name not in names and dirname in names
|
98 |
-
return dirname if dir_match else name
|
99 |
-
|
100 |
-
@classmethod
|
101 |
-
def make(cls, source):
|
102 |
-
"""
|
103 |
-
Given a source (filename or zipfile), return an
|
104 |
-
appropriate CompleteDirs subclass.
|
105 |
-
"""
|
106 |
-
if isinstance(source, CompleteDirs):
|
107 |
-
return source
|
108 |
-
|
109 |
-
if not isinstance(source, zipfile.ZipFile):
|
110 |
-
return cls(_pathlib_compat(source))
|
111 |
-
|
112 |
-
# Only allow for FastLookup when supplied zipfile is read-only
|
113 |
-
if 'r' not in source.mode:
|
114 |
-
cls = CompleteDirs
|
115 |
-
|
116 |
-
source.__class__ = cls
|
117 |
-
return source
|
118 |
-
|
119 |
-
|
120 |
-
class FastLookup(CompleteDirs):
|
121 |
-
"""
|
122 |
-
ZipFile subclass to ensure implicit
|
123 |
-
dirs exist and are resolved rapidly.
|
124 |
-
"""
|
125 |
-
|
126 |
-
def namelist(self):
|
127 |
-
with contextlib.suppress(AttributeError):
|
128 |
-
return self.__names
|
129 |
-
self.__names = super(FastLookup, self).namelist()
|
130 |
-
return self.__names
|
131 |
-
|
132 |
-
def _name_set(self):
|
133 |
-
with contextlib.suppress(AttributeError):
|
134 |
-
return self.__lookup
|
135 |
-
self.__lookup = super(FastLookup, self)._name_set()
|
136 |
-
return self.__lookup
|
137 |
-
|
138 |
-
|
139 |
-
def _pathlib_compat(path):
|
140 |
-
"""
|
141 |
-
For path-like objects, convert to a filename for compatibility
|
142 |
-
on Python 3.6.1 and earlier.
|
143 |
-
"""
|
144 |
-
try:
|
145 |
-
return path.__fspath__()
|
146 |
-
except AttributeError:
|
147 |
-
return str(path)
|
148 |
-
|
149 |
-
|
150 |
-
class Path:
|
151 |
-
"""
|
152 |
-
A pathlib-compatible interface for zip files.
|
153 |
-
|
154 |
-
Consider a zip file with this structure::
|
155 |
-
|
156 |
-
.
|
157 |
-
├── a.txt
|
158 |
-
└── b
|
159 |
-
├── c.txt
|
160 |
-
└── d
|
161 |
-
└── e.txt
|
162 |
-
|
163 |
-
>>> data = io.BytesIO()
|
164 |
-
>>> zf = zipfile.ZipFile(data, 'w')
|
165 |
-
>>> zf.writestr('a.txt', 'content of a')
|
166 |
-
>>> zf.writestr('b/c.txt', 'content of c')
|
167 |
-
>>> zf.writestr('b/d/e.txt', 'content of e')
|
168 |
-
>>> zf.filename = 'mem/abcde.zip'
|
169 |
-
|
170 |
-
Path accepts the zipfile object itself or a filename
|
171 |
-
|
172 |
-
>>> root = Path(zf)
|
173 |
-
|
174 |
-
From there, several path operations are available.
|
175 |
-
|
176 |
-
Directory iteration (including the zip file itself):
|
177 |
-
|
178 |
-
>>> a, b = root.iterdir()
|
179 |
-
>>> a
|
180 |
-
Path('mem/abcde.zip', 'a.txt')
|
181 |
-
>>> b
|
182 |
-
Path('mem/abcde.zip', 'b/')
|
183 |
-
|
184 |
-
name property:
|
185 |
-
|
186 |
-
>>> b.name
|
187 |
-
'b'
|
188 |
-
|
189 |
-
join with divide operator:
|
190 |
-
|
191 |
-
>>> c = b / 'c.txt'
|
192 |
-
>>> c
|
193 |
-
Path('mem/abcde.zip', 'b/c.txt')
|
194 |
-
>>> c.name
|
195 |
-
'c.txt'
|
196 |
-
|
197 |
-
Read text:
|
198 |
-
|
199 |
-
>>> c.read_text()
|
200 |
-
'content of c'
|
201 |
-
|
202 |
-
existence:
|
203 |
-
|
204 |
-
>>> c.exists()
|
205 |
-
True
|
206 |
-
>>> (b / 'missing.txt').exists()
|
207 |
-
False
|
208 |
-
|
209 |
-
Coercion to string:
|
210 |
-
|
211 |
-
>>> import os
|
212 |
-
>>> str(c).replace(os.sep, posixpath.sep)
|
213 |
-
'mem/abcde.zip/b/c.txt'
|
214 |
-
|
215 |
-
At the root, ``name``, ``filename``, and ``parent``
|
216 |
-
resolve to the zipfile. Note these attributes are not
|
217 |
-
valid and will raise a ``ValueError`` if the zipfile
|
218 |
-
has no filename.
|
219 |
-
|
220 |
-
>>> root.name
|
221 |
-
'abcde.zip'
|
222 |
-
>>> str(root.filename).replace(os.sep, posixpath.sep)
|
223 |
-
'mem/abcde.zip'
|
224 |
-
>>> str(root.parent)
|
225 |
-
'mem'
|
226 |
-
"""
|
227 |
-
|
228 |
-
__repr = "{self.__class__.__name__}({self.root.filename!r}, {self.at!r})"
|
229 |
-
|
230 |
-
def __init__(self, root, at=""):
|
231 |
-
"""
|
232 |
-
Construct a Path from a ZipFile or filename.
|
233 |
-
|
234 |
-
Note: When the source is an existing ZipFile object,
|
235 |
-
its type (__class__) will be mutated to a
|
236 |
-
specialized type. If the caller wishes to retain the
|
237 |
-
original type, the caller should either create a
|
238 |
-
separate ZipFile object or pass a filename.
|
239 |
-
"""
|
240 |
-
self.root = FastLookup.make(root)
|
241 |
-
self.at = at
|
242 |
-
|
243 |
-
def open(self, mode='r', *args, pwd=None, **kwargs):
|
244 |
-
"""
|
245 |
-
Open this entry as text or binary following the semantics
|
246 |
-
of ``pathlib.Path.open()`` by passing arguments through
|
247 |
-
to io.TextIOWrapper().
|
248 |
-
"""
|
249 |
-
if self.is_dir():
|
250 |
-
raise IsADirectoryError(self)
|
251 |
-
zip_mode = mode[0]
|
252 |
-
if not self.exists() and zip_mode == 'r':
|
253 |
-
raise FileNotFoundError(self)
|
254 |
-
stream = self.root.open(self.at, zip_mode, pwd=pwd)
|
255 |
-
if 'b' in mode:
|
256 |
-
if args or kwargs:
|
257 |
-
raise ValueError("encoding args invalid for binary operation")
|
258 |
-
return stream
|
259 |
-
return io.TextIOWrapper(stream, *args, **kwargs)
|
260 |
-
|
261 |
-
@property
|
262 |
-
def name(self):
|
263 |
-
return pathlib.Path(self.at).name or self.filename.name
|
264 |
-
|
265 |
-
@property
|
266 |
-
def suffix(self):
|
267 |
-
return pathlib.Path(self.at).suffix or self.filename.suffix
|
268 |
-
|
269 |
-
@property
|
270 |
-
def suffixes(self):
|
271 |
-
return pathlib.Path(self.at).suffixes or self.filename.suffixes
|
272 |
-
|
273 |
-
@property
|
274 |
-
def stem(self):
|
275 |
-
return pathlib.Path(self.at).stem or self.filename.stem
|
276 |
-
|
277 |
-
@property
|
278 |
-
def filename(self):
|
279 |
-
return pathlib.Path(self.root.filename).joinpath(self.at)
|
280 |
-
|
281 |
-
def read_text(self, *args, **kwargs):
|
282 |
-
with self.open('r', *args, **kwargs) as strm:
|
283 |
-
return strm.read()
|
284 |
-
|
285 |
-
def read_bytes(self):
|
286 |
-
with self.open('rb') as strm:
|
287 |
-
return strm.read()
|
288 |
-
|
289 |
-
def _is_child(self, path):
|
290 |
-
return posixpath.dirname(path.at.rstrip("/")) == self.at.rstrip("/")
|
291 |
-
|
292 |
-
def _next(self, at):
|
293 |
-
return self.__class__(self.root, at)
|
294 |
-
|
295 |
-
def is_dir(self):
|
296 |
-
return not self.at or self.at.endswith("/")
|
297 |
-
|
298 |
-
def is_file(self):
|
299 |
-
return self.exists() and not self.is_dir()
|
300 |
-
|
301 |
-
def exists(self):
|
302 |
-
return self.at in self.root._name_set()
|
303 |
-
|
304 |
-
def iterdir(self):
|
305 |
-
if not self.is_dir():
|
306 |
-
raise ValueError("Can't listdir a file")
|
307 |
-
subs = map(self._next, self.root.namelist())
|
308 |
-
return filter(self._is_child, subs)
|
309 |
-
|
310 |
-
def __str__(self):
|
311 |
-
return posixpath.join(self.root.filename, self.at)
|
312 |
-
|
313 |
-
def __repr__(self):
|
314 |
-
return self.__repr.format(self=self)
|
315 |
-
|
316 |
-
def joinpath(self, *other):
|
317 |
-
next = posixpath.join(self.at, *map(_pathlib_compat, other))
|
318 |
-
return self._next(self.root.resolve_dir(next))
|
319 |
-
|
320 |
-
__truediv__ = joinpath
|
321 |
-
|
322 |
-
@property
|
323 |
-
def parent(self):
|
324 |
-
if not self.at:
|
325 |
-
return self.filename.parent
|
326 |
-
parent_at = posixpath.dirname(self.at.rstrip('/'))
|
327 |
-
if parent_at:
|
328 |
-
parent_at += '/'
|
329 |
-
return self._next(parent_at)
|
|
|
|
|
|
|
|
|
|
|
|
|
|
|
|
|
|
|
|
|
|
|
|
|
|
|
|
|
|
|
|
|
|
|
|
|
|
|
|
|
|
|
|
|
|
|
|
|
|
|
|
|
|
|
|
|
|
|
|
|
|
|
|
|
|
|
|
|
|
|
|
|
|
|
|
|
|
|
|
|
|
|
|
|
|
|
|
|
|
|
|
|
|
|
|
|
|
|
|
|
|
|
|
|
|
|
|
|
|
|
|
|
|
|
|
|
|
|
|
|
|
|
|
|
|
|
|
|
|
|
|
|
|
|
|
|
|
|
|
|
|
|
|
|
|
|
|
|
|
|
|
|
|
|
|
|
|
|
|
|
|
|
|
|
|
|
|
|
|
|
|
|
|
|
|
|
|
|
|
|
|
|
|
|
|
|
|
|
|
|
|
|
|
|
|
|
|
|
|
|
|
|
|
|
|
|
|
|
|
|
|
|
|
|
|
|
|
|
|
|
|
|
|
|
|
|
|
|
|
|
|
|
|
|
|
|
|
|
|
|
|
|
|
|
|
|
|
|
|
|
|
|
|
|
|
|
|
|
|
|
|
|
|
|
|
|
|
|
|
|
|
|
|
|
|
|
|
|
|
|
|
|
|
|
|
|
|
|
|
|
|
|
|
|
|
|
|
|
|
|
|
|
|
|
|
|
|
|
|
|
|
|
|
|
|
|
|
|
|
|
|
|
|
|
|
|
|
|
|
|
|
|
|
|
|
|
|
|
|
|
|
|
|
|
|
|
|
|
|
|
|
|
|
|
|
|
|
|
|
|
|
|
|
|
|
|
|
|
|
|
|
|
|
|
|
|
|
|
|
|
|
|
|
|
|
|
|
|
|
|
|
|
|
|
|
|
|
|
|
|
|
|
|
|
|
|
|
|
|
|
|
|
|
|
|
|
|
|
|
|
|
|
|
|
|
|
|
|
|
|
|
|
|
|
|
|
|
|
|
|
|
|
|
|
|
|
|
|
|
|
|
|
|
|
|
|
|
|
|
|
|
|
|
|
|
|
|
|
|
|
|
|
|
|
|
|
|
|
|
|
|
|
|
|
|
|
|
|
|
|
|
|
|
|
|
|
|
|
|
|
|
|
|
|
|
|
|
|
|
|
|
|
|
|
|
|
|
|
|
|
|
|
|
|
|
|
|
|
|
|
|
|
|
|
|
|
|
|
|
|
|
|
|
|
|
|
|
|
|
|
|
|
|
|
|
|
|
|
|
|
|
|
|
|
|
|
|
|
|
|
|
|
|
|
|
|
|
|
|
|
|
|
|
|
|
|
|
|
|
|
|
|
|
|
|
|
|
|
|
|
|
|
|
|
|
|
|
|
|
|
|
|
|
|
|
|
|
|
|
|
|
|
|
|
|
|
|
|
|
|
|
|
|
|
|
|
|
|
|
|
|
|
|
|
|
|
|
|
|
|
|
|
|
|
|
|
|
|
|
spaces/Ataturk-Chatbot/HuggingFaceChat/venv/lib/python3.11/site-packages/setuptools/command/saveopts.py
DELETED
@@ -1,22 +0,0 @@
|
|
1 |
-
from setuptools.command.setopt import edit_config, option_base
|
2 |
-
|
3 |
-
|
4 |
-
class saveopts(option_base):
|
5 |
-
"""Save command-line options to a file"""
|
6 |
-
|
7 |
-
description = "save supplied options to setup.cfg or other config file"
|
8 |
-
|
9 |
-
def run(self):
|
10 |
-
dist = self.distribution
|
11 |
-
settings = {}
|
12 |
-
|
13 |
-
for cmd in dist.command_options:
|
14 |
-
|
15 |
-
if cmd == 'saveopts':
|
16 |
-
continue # don't save our own options!
|
17 |
-
|
18 |
-
for opt, (src, val) in dist.get_option_dict(cmd).items():
|
19 |
-
if src == "command line":
|
20 |
-
settings.setdefault(cmd, {})[opt] = val
|
21 |
-
|
22 |
-
edit_config(self.filename, settings, self.dry_run)
|
|
|
|
|
|
|
|
|
|
|
|
|
|
|
|
|
|
|
|
|
|
|
|
|
|
|
|
|
|
|
|
|
|
|
|
|
|
|
|
|
|
|
|
|
|
spaces/Awiny/Image2Paragraph/utils/ignore_large_files.py
DELETED
@@ -1,17 +0,0 @@
|
|
1 |
-
import os
|
2 |
-
|
3 |
-
max_size_mb = 10 # Set the maximum allowed file size in MB
|
4 |
-
|
5 |
-
# Walk through the repo and find files larger than the specified size
|
6 |
-
large_files = []
|
7 |
-
for root, _, files in os.walk('.'):
|
8 |
-
for file in files:
|
9 |
-
file_path = os.path.join(root, file)
|
10 |
-
file_size_mb = os.path.getsize(file_path) / (1024 * 1024)
|
11 |
-
if file_size_mb > max_size_mb:
|
12 |
-
large_files.append(file_path)
|
13 |
-
|
14 |
-
# Append the large files to the .gitignore file
|
15 |
-
with open('.gitignore', 'a') as gitignore:
|
16 |
-
for large_file in large_files:
|
17 |
-
gitignore.write(f'{large_file}\n')
|
|
|
|
|
|
|
|
|
|
|
|
|
|
|
|
|
|
|
|
|
|
|
|
|
|
|
|
|
|
|
|
|
|
|
|
spaces/Bart92/RVC_HF/diffq/uniform.py
DELETED
@@ -1,121 +0,0 @@
|
|
1 |
-
# Copyright (c) Facebook, Inc. and its affiliates.
|
2 |
-
# All rights reserved.
|
3 |
-
#
|
4 |
-
# This source code is licensed under the license found in the
|
5 |
-
# LICENSE file in the root directory of this source tree.
|
6 |
-
|
7 |
-
"""
|
8 |
-
Classic uniform quantization over n bits.
|
9 |
-
"""
|
10 |
-
from typing import Tuple
|
11 |
-
import torch
|
12 |
-
|
13 |
-
from .base import BaseQuantizer
|
14 |
-
from .utils import simple_repr
|
15 |
-
|
16 |
-
|
17 |
-
def uniform_quantize(p: torch.Tensor, bits: torch.Tensor = torch.tensor(8.)):
|
18 |
-
"""
|
19 |
-
Quantize the given weights over `bits` bits.
|
20 |
-
|
21 |
-
Returns:
|
22 |
-
- quantized levels
|
23 |
-
- (min, max) range.
|
24 |
-
|
25 |
-
"""
|
26 |
-
assert (bits >= 1).all() and (bits <= 15).all()
|
27 |
-
num_levels = (2 ** bits.float()).long()
|
28 |
-
mn = p.min().item()
|
29 |
-
mx = p.max().item()
|
30 |
-
p = (p - mn) / (mx - mn) # put p in [0, 1]
|
31 |
-
unit = 1 / (num_levels - 1) # quantization unit
|
32 |
-
levels = (p / unit).round()
|
33 |
-
if (bits <= 8).all():
|
34 |
-
levels = levels.byte()
|
35 |
-
else:
|
36 |
-
levels = levels.short()
|
37 |
-
return levels, (mn, mx)
|
38 |
-
|
39 |
-
|
40 |
-
def uniform_unquantize(levels: torch.Tensor, scales: Tuple[float, float],
|
41 |
-
bits: torch.Tensor = torch.tensor(8.)):
|
42 |
-
"""
|
43 |
-
Unquantize the weights from the levels and scale. Return a float32 tensor.
|
44 |
-
"""
|
45 |
-
mn, mx = scales
|
46 |
-
num_levels = 2 ** bits.float()
|
47 |
-
unit = 1 / (num_levels - 1)
|
48 |
-
levels = levels.float()
|
49 |
-
p = levels * unit # in [0, 1]
|
50 |
-
return p * (mx - mn) + mn
|
51 |
-
|
52 |
-
|
53 |
-
class UniformQuantizer(BaseQuantizer):
|
54 |
-
def __init__(self, model: torch.nn.Module, bits: float = 8., min_size: float = 0.01,
|
55 |
-
float16: bool = False, qat: bool = False, exclude=[], detect_bound=True):
|
56 |
-
"""
|
57 |
-
Args:
|
58 |
-
model (torch.nn.Module): model to quantize
|
59 |
-
bits (float): number of bits to quantize over.
|
60 |
-
min_size (float): minimum size in MB of a parameter to be quantized.
|
61 |
-
float16 (bool): if a layer is smaller than min_size, should we still do float16?
|
62 |
-
qat (bool): perform quantized aware training.
|
63 |
-
exclude (list[str]): list of patterns used to match parameters to exclude.
|
64 |
-
For instance `['bias']` to exclude all bias terms.
|
65 |
-
detect_bound (bool): if True, will detect bound parameters and reuse
|
66 |
-
the same quantized tensor for both.
|
67 |
-
"""
|
68 |
-
self.bits = float(bits)
|
69 |
-
self.qat = qat
|
70 |
-
|
71 |
-
super().__init__(model, min_size, float16, exclude, detect_bound)
|
72 |
-
|
73 |
-
def __repr__(self):
|
74 |
-
return simple_repr(self, )
|
75 |
-
|
76 |
-
def _pre_forward_train(self):
|
77 |
-
if self.qat:
|
78 |
-
for qparam in self._qparams:
|
79 |
-
if qparam.other is not None:
|
80 |
-
new_param = qparam.other.module._parameters[qparam.other.name]
|
81 |
-
else:
|
82 |
-
quantized = self._quantize_param(qparam)
|
83 |
-
qvalue = self._unquantize_param(qparam, quantized)
|
84 |
-
new_param = qparam.param + (qvalue - qparam.param).detach()
|
85 |
-
qparam.module._parameters[qparam.name] = new_param
|
86 |
-
return True
|
87 |
-
return False
|
88 |
-
|
89 |
-
def _post_forward_train(self):
|
90 |
-
if self.qat:
|
91 |
-
for qparam in self._qparams:
|
92 |
-
qparam.module._parameters[qparam.name] = qparam.param
|
93 |
-
return True
|
94 |
-
return False
|
95 |
-
|
96 |
-
def _quantize_param(self, qparam):
|
97 |
-
levels, scales = uniform_quantize(qparam.param.data, torch.tensor(self.bits))
|
98 |
-
return (levels, scales)
|
99 |
-
|
100 |
-
def _unquantize_param(self, qparam, quantized):
|
101 |
-
levels, scales = quantized
|
102 |
-
return uniform_unquantize(levels, scales, torch.tensor(self.bits))
|
103 |
-
|
104 |
-
def model_size(self):
|
105 |
-
"""
|
106 |
-
Non differentiable model size in MB.
|
107 |
-
"""
|
108 |
-
total = super().model_size()
|
109 |
-
subtotal = 0
|
110 |
-
for qparam in self._qparams:
|
111 |
-
if qparam.other is None: # if parameter is bound, count only one copy.
|
112 |
-
subtotal += self.bits * qparam.param.numel() + 64 # 2 float for the overall scales
|
113 |
-
subtotal /= 2**20 * 8 # bits to MegaBytes
|
114 |
-
return total + subtotal
|
115 |
-
|
116 |
-
def true_model_size(self):
|
117 |
-
"""
|
118 |
-
Return the true quantized model size, in MB, without extra
|
119 |
-
compression.
|
120 |
-
"""
|
121 |
-
return self.model_size().item()
|
|
|
|
|
|
|
|
|
|
|
|
|
|
|
|
|
|
|
|
|
|
|
|
|
|
|
|
|
|
|
|
|
|
|
|
|
|
|
|
|
|
|
|
|
|
|
|
|
|
|
|
|
|
|
|
|
|
|
|
|
|
|
|
|
|
|
|
|
|
|
|
|
|
|
|
|
|
|
|
|
|
|
|
|
|
|
|
|
|
|
|
|
|
|
|
|
|
|
|
|
|
|
|
|
|
|
|
|
|
|
|
|
|
|
|
|
|
|
|
|
|
|
|
|
|
|
|
|
|
|
|
|
|
|
|
|
|
|
|
|
|
|
|
|
|
|
|
|
|
|
|
|
|
|
|
|
|
|
|
|
|
|
|
|
|
|
|
|
|
|
|
|
|
|
|
|
|
|
|
|
|
|
|
|
|
|
|
|
|
|
|
|
|
|
|
|
|
|
|
|
|
|
|
|
|
|
|
|
|
|
|
|
|
|
|
|
|
|
|
|
|
|
|
|
|
|
|
|
|
|
|
|
|
|
|
|
|
|
|
|
|
|
|
spaces/Benson/text-generation/Examples/Camin Simulador Final 1.1 7 Apk Descargar.md
DELETED
@@ -1,62 +0,0 @@
|
|
1 |
-
<br />
|
2 |
-
<h1>Camión Simulador Ultimate 1.1 7 APK Descargar: Una revisión</h1>
|
3 |
-
<p>Si eres un fan de los juegos de conducción de camiones, es posible que desees echar un vistazo a Truck Simulator Ultimate, un juego de simulación realista e inmersivo que te permite experimentar la vida de un conductor de camiones. En este juego, puede conducir varios camiones y remolques a través de diferentes países, ciudades y carreteras, mientras administra su propia empresa y compite con otros jugadores en línea. En este artículo, vamos a revisar las características, pros y contras de Truck Simulator Ultimate 1.1 7 APK, así como mostrar cómo descargar e instalar en su dispositivo Android. </p>
|
4 |
-
<h2>camión simulador final 1.1 7 apk descargar</h2><br /><p><b><b>Download File</b> ————— <a href="https://bltlly.com/2v6JPH">https://bltlly.com/2v6JPH</a></b></p><br /><br />
|
5 |
-
<h2>¿Qué es Truck Simulator Ultimate? </h2>
|
6 |
-
<p>Truck Simulator Ultimate es un juego para móviles desarrollado por Zuuks Games, una empresa turca especializada en juegos de simulación. El juego fue lanzado en agosto de 2022 y desde entonces ha recibido críticas positivas de jugadores y críticos por igual. El juego tiene más de 10 millones de descargas en Google Play Store y tiene una calificación promedio de 4.3 de 5 estrellas. </p>
|
7 |
-
<p>Truck Simulator Ultimate es un juego que tiene como objetivo proporcionar una experiencia de conducción de camiones realista y agradable para los jugadores. El juego cuenta con varios camiones y remolques que puedes personalizar y actualizar, así como diferentes mapas y rutas que puedes explorar. También puede crear su propia empresa, contratar conductores, comprar garajes y ganar dinero entregando carga. Además, puedes jugar con otros jugadores en línea en modo multijugador, chatear con ellos, unirse a convoyes y competir en el sistema de clasificación global. </p>
|
8 |
-
<h3>Características de Truck Simulator Ultimate</h3>
|
9 |
-
<p>Truck Simulator Ultimate tiene muchas características que lo hacen destacar de otros juegos de conducción de camiones. Estos son algunos de ellos:</p>
|
10 |
-
<h4>Gráficos realistas y física</h4>
|
11 |
-
|
12 |
-
<h4>Camiones y remolques personalizables</h4>
|
13 |
-
<p>El juego ofrece una amplia gama de camiones y remolques que puede elegir, cada uno con sus propias especificaciones, rendimiento y apariencia. También puede personalizar sus camiones y remolques con varias piezas, accesorios, trabajos de pintura, calcomanías y más. Puede hacer que su vehículo se vea único y se adapte a su estilo personal. </p>
|
14 |
-
<p></p>
|
15 |
-
<h4>Modo multijugador y ranking online</h4>
|
16 |
-
<p>El juego te permite jugar con otros jugadores online en modo multijugador. Puede chatear con ellos, unirse a convoyes, ayudarse entre sí, o desafiarse entre sí. También puede competir en el sistema de clasificación en línea que clasifica a los jugadores en función de su nivel, reputación, ingresos, distancia recorrida, carga entregada y más. Puedes ver cómo te comparas con otros jugadores de todo el mundo. </p>
|
17 |
-
<h4>Clima dinámico y ciclo día-noche</h4>
|
18 |
-
<p>El juego cuenta con un sistema de tiempo dinámico que cambia según la ubicación, la temporada, la hora del día y los eventos aleatorios. Puede experimentar lluvia, nieve, niebla, viento, tormentas eléctricas y más. El juego también tiene un ciclo día-noche que afecta la visibilidad, la densidad de tráfico y la dificultad de conducir. Puede conducir durante el día o la noche, dependiendo de su preferencia. </p>
|
19 |
-
<h4>Varios mapas y rutas</h4>
|
20 |
-
<p>El juego tiene varios mapas y rutas que puedes explorar en diferentes países como Alemania, Francia, Italia, España, Turquía, Rusia, EE.UU., Canadá y más. Puede conducir por carreteras, caminos rurales, calles de ciudades, carreteras de montaña y más. También puede elegir entre diferentes tipos de carga, como alimentos, productos químicos, vehículos, maquinaria y más. <h3> ¿Cómo descargar e instalar Truck Simulator Ultimate 1.1 7 APK? </h3>
|
21 |
-
<p>Si desea jugar Truck Simulator Ultimate en su dispositivo Android, es necesario descargar e instalar el archivo APK, que es un archivo de paquete que contiene los datos del juego y las instrucciones de instalación. Estos son los pasos para hacerlo:</p>
|
22 |
-
<h4>Paso 1: Descargar el archivo APK de una fuente de confianza</h4>
|
23 |
-
|
24 |
-
<h4>Paso 2: Habilitar fuentes desconocidas en el dispositivo</h4>
|
25 |
-
<p>El siguiente paso es habilitar fuentes desconocidas en su dispositivo, lo que le permite instalar aplicaciones y juegos desde fuentes distintas de Google Play Store. Para hacer esto, vaya a la configuración del dispositivo, luego a la seguridad y luego active la opción para fuentes desconocidas. También es posible que necesite conceder permiso a su navegador o administrador de archivos para instalar aplicaciones. </p>
|
26 |
-
<h4>Paso 3: Instalar el archivo APK y lanzar el juego</h4>
|
27 |
-
<p>El paso final es instalar el archivo APK y lanzar el juego. Para ello, busque el archivo APK descargado en su dispositivo, ya sea en su carpeta de descargas o en su administrador de archivos. Toque en el archivo y siga las instrucciones de instalación. Una vez completada la instalación, puedes iniciar el juego desde el cajón de tu app o la pantalla de inicio. </p>
|
28 |
-
<h3> Pros y contras de Truck Simulator Ultimate 1.1 7 APK</h3>
|
29 |
-
<p>Truck Simulator Ultimate 1.1 7 APK tiene sus pros y sus contras, como cualquier otro juego. Estos son algunos de ellos:</p>
|
30 |
-
<h4>Pros</h4>
|
31 |
-
<ul>
|
32 |
-
<li> El juego tiene gráficos realistas y la física que crean una experiencia de conducción de camiones inmersiva. </li>
|
33 |
-
<li> El juego tiene camiones y remolques personalizables que puede modificar de acuerdo a su preferencia. </li>
|
34 |
-
<li>El juego tiene modo multijugador y ranking online que te permiten jugar con otros jugadores y competir con ellos. </li>
|
35 |
-
<li>El juego tiene un clima dinámico y un ciclo de día y noche que añaden variedad y desafío al juego. </li>
|
36 |
-
<li>El juego tiene varios mapas y rutas que puedes explorar en diferentes países y regiones. </li>
|
37 |
-
</ul>
|
38 |
-
<h4>Contras</h4>
|
39 |
-
<ul>
|
40 |
-
<li> El juego puede tener algunos errores y fallos que afectan el rendimiento y la estabilidad del juego. </li>
|
41 |
-
<li>El juego puede tener algunos anuncios y compras en la aplicación que pueden interrumpir o limitar el juego. </li>
|
42 |
-
<li>El juego puede requerir mucho espacio de almacenamiento y conexión a Internet para funcionar sin problemas. </li>
|
43 |
-
<li>El juego puede no ser compatible con algunos dispositivos o sistemas operativos. </li>
|
44 |
-
|
45 |
-
</ul>
|
46 |
-
<h2>Conclusión</h2>
|
47 |
-
<p>Truck Simulator Ultimate es un juego móvil que te permite experimentar la vida de un conductor de camión. Puede conducir varios camiones y remolques a través de diferentes países, ciudades y carreteras, mientras administra su propia empresa y compite con otros jugadores en línea. El juego tiene gráficos y física realistas, camiones y remolques personalizables, modo multijugador y clasificación en línea, clima dinámico y ciclo día-noche, y varios mapas y rutas. El juego también tiene algunos pros y contras que debes considerar antes de descargarlo e instalarlo en tu dispositivo. Si usted está buscando un juego de conducción de camiones realista y envolvente, es posible que desee dar Truck Simulator Ultimate una oportunidad. </p>
|
48 |
-
<h3>Preguntas frecuentes</h3>
|
49 |
-
<ol>
|
50 |
-
<li>¿Cuál es la última versión de Truck Simulator Ultimate? </li>
|
51 |
-
<p>La última versión de Truck Simulator Ultimate es 1.1 7, que fue lanzado el 9 de septiembre de 2022. La actualización agregó nuevos camiones, remolques, mapas, características, mejoras y correcciones de errores. </p>
|
52 |
-
<li> ¿Cómo puedo jugar Truck Simulator Ultimate en PC? </li>
|
53 |
-
<p>Si quieres jugar Truck Simulator Ultimate en PC, necesitas usar un emulador de Android, que es un software que te permite ejecutar aplicaciones y juegos de Android en tu ordenador. Algunos de los emuladores de Android populares son [BlueStacks], [NoxPlayer], [LDPlayer], y [MEmu]. Puede descargar cualquiera de estos emuladores desde sus sitios web oficiales, instalarlos en su PC, luego descargar Truck Simulator Ultimate desde Google Play Store o archivo APK dentro del emulador. </p>
|
54 |
-
<li>¿Cómo puedo contactar a los desarrolladores de Truck Simulator Ultimate? </li>
|
55 |
-
<p>Si tiene preguntas, comentarios, sugerencias o problemas con respecto a Truck Simulator Ultimate, puede ponerse en contacto con los desarrolladores de Zuuks Games por correo electrónico a [email protected] o por teléfono al +90 (212) <p>212) 988 64 59. También puede visitar su sitio web en https://www.zuuks.com/ o seguirlos en plataformas de redes sociales como Facebook, Twitter, Instagram y YouTube.</p>
|
56 |
-
|
57 |
-
<p>Hay varias maneras de obtener más dinero y oro en Truck Simulator Ultimate. Puedes ganar dinero y oro completando misiones, entregando carga, contratando conductores, comprando garajes y subiendo de nivel. También puedes ver anuncios, ofertas completas o comprarlos con dinero real a través de compras en la aplicación. </p>
|
58 |
-
<li>¿Cómo puedo cambiar el lenguaje de Truck Simulator Ultimate? </li>
|
59 |
-
<p>Puede cambiar el idioma de Truck Simulator Ultimate yendo al menú de configuración, luego seleccionando la opción de idioma. Puede elegir entre 25 idiomas, como inglés, turco, alemán, francés, español, italiano, ruso, portugués y más. </p>
|
60 |
-
</ol></p> 64aa2da5cf<br />
|
61 |
-
<br />
|
62 |
-
<br />
|
|
|
|
|
|
|
|
|
|
|
|
|
|
|
|
|
|
|
|
|
|
|
|
|
|
|
|
|
|
|
|
|
|
|
|
|
|
|
|
|
|
|
|
|
|
|
|
|
|
|
|
|
|
|
|
|
|
|
|
|
|
|
|
|
|
|
|
|
|
|
|
|
|
|
|
|
|
|
|
|
|
|
|
|
|
|
|
|
|
|
|
|
|
|
|
|
|
|
|
|
|
|
|
|
|
|
|
|
|
|
|
|
|
|
|
|
|
|
|
|
|
|
|
|
|
spaces/Benson/text-generation/Examples/Descargar Flash Player Pro.md
DELETED
@@ -1,85 +0,0 @@
|
|
1 |
-
<br />
|
2 |
-
<h1>¿Qué es edebiyyat sinaq y por qué es importante? </h1>
|
3 |
-
<p>Edebiyyat sinaq es un término que significa prueba de literatura en lengua azerbaiyana. Es un tipo de comunicación escrita que retrata un tema central de un escritor que quieren mostrar a sus lectores. Edebiyyat sinaq se puede utilizar para diversos fines, tales como:</p>
|
4 |
-
<ul>
|
5 |
-
<li>Compartir información y conocimientos sobre un tema o campo específico. </li>
|
6 |
-
<li>Expresar opiniones y perspectivas sobre eventos actuales o pasados. </li>
|
7 |
-
<li>Persuadir o influir en los lectores para que tomen una determinada acción o adopten un cierto punto de vista. </li>
|
8 |
-
<li>Entretener o inspirar a los lectores con historias o poemas creativos. </li>
|
9 |
-
</ul>
|
10 |
-
<p>Edebiyyat sinaq es importante porque puede ayudarte a desarrollar tus habilidades de escritura, mejorar tu pensamiento crítico, expandir tu vocabulario y mejorar tu creatividad. También puede ayudarle a comunicarse eficazmente con su público objetivo, ya sean estudiantes, profesores, clientes o colegas. </p>
|
11 |
-
<h2>descargar flash player pro</h2><br /><p><b><b>Download</b> ››››› <a href="https://bltlly.com/2v6L8g">https://bltlly.com/2v6L8g</a></b></p><br /><br />
|
12 |
-
<h2>Cómo prepararse para edebiyyat sinaq? </h2>
|
13 |
-
<h3>Elige un tema que sea relevante e interesante para tu público objetivo</h3>
|
14 |
-
<p>El primer paso para escribir un buen artículo de edebiyyat sinaq es elegir un tema que sea adecuado para su propósito y audiencia. Necesitas considerar qué tipo de información o mensaje quieres transmitir, qué tipo de tono y estilo quieres usar, y qué tipo de retroalimentación o respuesta esperas de tus lectores. </p>
|
15 |
-
<p>Para elegir un tema, puede intercambiar ideas con usted mismo u otros, hacer alguna investigación en línea, o mirar algunos ejemplos de artículos de edebiyyat sinaq en sitios web como [Testbook]( 1 ) o [Online Sinaq]( 2 ). También puedes usar herramientas como [Answer The Public] (https://answerthepublic.com/) o [Google Trends] (https://trends.google.com/trends/) para averiguar qué personas están buscando o hablando sobre tu tema. </p>
|
16 |
-
|
17 |
-
<h3>Investiga y recoge la información necesaria para tu tema</h3>
|
18 |
-
<p>El siguiente paso para escribir un buen artículo edebiyyat sinaq es hacer una investigación y recopilar la información necesaria para su tema. Necesitas encontrar fuentes confiables y creíbles que puedan apoyar tus argumentos, proporcionar hechos y estadísticas, o dar ejemplos e ilustraciones. </p>
|
19 |
-
<p>Para hacer investigación, puede imágenes, gráficos, tablas, tablas o videos que pueden ayudarlo a ilustrar sus puntos, proporcionar evidencia o mostrar comparaciones. Las imágenes también pueden ayudarte a dividir el texto y hacer que tu artículo sea más atractivo y atractivo para tus lectores. </p>
|
20 |
-
<p>Cuando usas imágenes, necesitas seguir algunas de las mejores prácticas, como:</p>
|
21 |
-
<ul>
|
22 |
-
<li>Utilice imágenes relevantes y de alta calidad que coincidan con su tema y tono. </li>
|
23 |
-
<li>Usa subtítulos y textos alternativos para describir tus imágenes y hacerlas accesibles para todos los lectores. </li>
|
24 |
-
<li>Usa fuentes y créditos para reconocer a los creadores originales de tus imágenes y evitar el plagio. </li>
|
25 |
-
<li>Utilice herramientas como [Canva](https://www.canva.com/) o [Piktochart](https://piktochart.com/) para crear o editar sus imágenes de forma fácil y profesional. </li>
|
26 |
-
</ul>
|
27 |
-
<h3>Usa palabras clave estratégicamente para optimizar tu artículo para motores de búsqueda</h3>
|
28 |
-
<p>El cuarto paso para escribir un artículo eficaz edebiyyat sinaq es utilizar palabras clave estratégicamente para optimizar su artículo para los motores de búsqueda. Las palabras clave son palabras o frases que describen tu tema y ayudan a tus lectores a encontrar tu artículo en línea. Las palabras clave también pueden ayudarte a posicionarte más alto en las páginas de resultados de motores de búsqueda (SERPs) y generar más tráfico a tu artículo. </p>
|
29 |
-
<p></p>
|
30 |
-
<p>Cuando usas palabras clave, necesitas seguir algunas de las mejores prácticas, como:</p>
|
31 |
-
<ul>
|
32 |
-
<li>Utilice herramientas de investigación de palabras clave como [Google Keyword Planner](https://ads.google.com/home/tools/keyword-planner/) o [Ubersuggest](https:/neilpatel.com/ubersuggest/) para averiguar qué palabras clave son populares y relevantes para su tema. </li>
|
33 |
-
|
34 |
-
<li>Usa variaciones de palabras clave que sean sinónimos o términos relacionados de tus palabras clave principales. </li>
|
35 |
-
<li>Usa la densidad de palabras clave que es el porcentaje de veces que tus palabras clave aparecen en tu artículo. Busca una densidad de palabras clave de 1-3% para obtener resultados óptimos. </li>
|
36 |
-
<li>Utilice la colocación de palabras clave que es la ubicación de sus palabras clave en su artículo. Coloca tus palabras clave en lugares estratégicos como tu título, encabezados, subtítulos, introducción, conclusión y oraciones primera y última de cada párrafo. </li>
|
37 |
-
</ul>
|
38 |
-
<h2>¿Cómo editar y corregir su artículo edebiyyat sinaq? </h2>
|
39 |
-
<h3>Edita tu trabajo y comprueba si hay errores gramaticales y repeticiones innecesarias</h3>
|
40 |
-
<p>El primer paso para editar y corregir su artículo edebiyyat sinaq es editar su trabajo y comprobar si hay errores gramaticales y repeticiones innecesarias. Necesitas revisar tu trabajo y asegurarte de que sea claro, coherente y correcto. Debes verificar si hay errores en la ortografía, puntuación, mayúsculas, tiempo verbal, acuerdo sujeto-verbo, referencia de pronombre, estructura de oración, elección de palabras y tono. También debes verificar si hay repeticiones en palabras, frases, oraciones o ideas que puedan hacer que tu artículo sea aburrido o redundante. </p>
|
41 |
-
<p>Para editar tu trabajo, puedes usar herramientas como [Grammarly](https://www.grammarly.com/) o [ProWritingAid](https://prowritingaid.com/) para detectar y corregir errores gramaticales automáticamente. También puede utilizar herramientas como [WordCounter](https://wordcounter.net/) o [Word Frequency Counter](https://www.writewords.org.uk/word_count.asp) para identificar y eliminar repeticiones innecesarias en su trabajo. </p>
|
42 |
-
<h3>Lea en voz alta hasta que su borrador esté libre de errores</h3>
|
43 |
-
|
44 |
-
<p>Para leer en voz alta, puedes usar herramientas como [Natural Reader](https://www.naturalreaders.com/online/) o [ReadSpeaker](https:/www.readspeaker.com/) para convertir tu texto en voz y escucharlo. También puede pedir a otra persona que lea su trabajo en voz alta para usted y darle retroalimentación o sugerencias. </p>
|
45 |
-
<h2>Conclusión</h2>
|
46 |
-
<p>Edebiyyat sinaq es un tipo de comunicación escrita que retrata un tema central de un escritor que quiere mostrar a sus lectores. Se puede utilizar para diversos fines, como compartir información, expresar opiniones, persuadir a los lectores o entretener a los lectores. Para escribir un buen artículo de edebiyyat sinaq, debe seguir algunos pasos, como:</p>
|
47 |
-
<ol>
|
48 |
-
<li>Prepararse para edebiyyat sinaq mediante la elección de un tema, haciendo la investigación, y la organización de hechos y estadísticas. </li>
|
49 |
-
<li>Escribir un artículo eficaz edebiyyat sinaq escribiendo un título pegadizo, un gancho fuerte, encabezados y subtítulos, imágenes y palabras clave. </li>
|
50 |
-
<li>Editar y corregir su artículo edebiyyat sinaq mediante la comprobación de errores gramaticales, repeticiones innecesarias, y la lectura en voz alta. </li>
|
51 |
-
</ol>
|
52 |
-
<p>Siguiendo estos pasos, puede escribir un buen artículo de edebiyyat sinaq que puede impresionar a sus lectores y lograr sus objetivos. También puede utilizar varias herramientas y recursos para ayudarle con cada paso del proceso de escritura. Recuerda siempre escribir con tus propias palabras y evitar copiar y pegar desde otras fuentes. ¡Buena suerte y feliz escritura! </p>
|
53 |
-
<h2>Preguntas frecuentes</h2>
|
54 |
-
<h3>¿Cuál es la diferencia entre edebiyyat sinaq y edebiyyat analizi? </h3>
|
55 |
-
<p>Edebiyyat sinaq es un tipo de comunicación escrita que retrata un tema central de un escritor que quiere mostrar a sus lectores. Edebiyyat analizi es un tipo de análisis literario que examina los elementos, técnicas y significados de una obra literaria. </p>
|
56 |
-
<h3>¿Cuánto tiempo debe ser un artículo de edebiyyat sinaq? </h3>
|
57 |
-
|
58 |
-
<h3>¿Cómo puedo mejorar mis habilidades de escritura edebiyyat sinaq? </h3>
|
59 |
-
<p>Algunas maneras de mejorar sus habilidades de escritura edebiyyat sinaq son:</p>
|
60 |
-
<ul>
|
61 |
-
<li>Leer más artículos de edebiyyat sinaq sobre diferentes temas y géneros y aprender de sus estilos, estructuras y estrategias. </li>
|
62 |
-
<li>Práctica de escribir artículos de edebiyyat sinaq con regularidad y obtener comentarios de otros o usted mismo. </li>
|
63 |
-
<li>Utilice cursos en línea, libros, blogs, podcasts o videos para aprender más sobre las técnicas y consejos de escritura edebiyyat sinaq. </li>
|
64 |
-
<li>Utilice herramientas, aplicaciones o software en línea para ayudarlo con su proceso de escritura, como investigación, edición, corrección o formato. </li>
|
65 |
-
</ul>
|
66 |
-
<h3>¿Cuáles son algunos ejemplos de buenos artículos de edebiyyat sinaq? </h3>
|
67 |
-
<p>Algunos ejemplos de buenos artículos de edebiyyat sinaq son:</p>
|
68 |
-
<ul>
|
69 |
-
<li>[Cómo escribir una entrada de blog: Una guía paso a paso] por HubSpot</li>
|
70 |
-
<li>[La guía definitiva para escribir en línea] por David Perell</li>
|
71 |
-
<li>[Cómo escribir un ensayo en 5 pasos fáciles] por Scribendi</li>
|
72 |
-
<li>[Cómo escribir un cuento que cautiva a tu lector] por Jerry Jenkins</li>
|
73 |
-
<li>[Cómo escribir un poema: 10 consejos para empezar] por MasterClass</li>
|
74 |
-
</ul>
|
75 |
-
<h3>¿Dónde puedo encontrar más información sobre edebiyyat sinaq? </h3>
|
76 |
-
<p>Algunos sitios web que pueden proporcionarle más información sobre edebiyyat sinaq son:</p>
|
77 |
-
<ul>
|
78 |
-
<li>[Testbook](https://testbook.com/)</li>
|
79 |
-
<li>[Sinaq en línea](https://onlinesinaq.com/)</li>
|
80 |
-
<li>[Edebiyat Sinaq](https://edebiyatsinaq.com/)</li>
|
81 |
-
<li>[Edebiyat Akademisi](https://edebiyatakademisi.com/)</li>
|
82 |
-
<li>[Edebiyat Öğretmenleri](https://edebiyatogretmenleri.net/)</li>
|
83 |
-
</ul></p> 64aa2da5cf<br />
|
84 |
-
<br />
|
85 |
-
<br />
|
|
|
|
|
|
|
|
|
|
|
|
|
|
|
|
|
|
|
|
|
|
|
|
|
|
|
|
|
|
|
|
|
|
|
|
|
|
|
|
|
|
|
|
|
|
|
|
|
|
|
|
|
|
|
|
|
|
|
|
|
|
|
|
|
|
|
|
|
|
|
|
|
|
|
|
|
|
|
|
|
|
|
|
|
|
|
|
|
|
|
|
|
|
|
|
|
|
|
|
|
|
|
|
|
|
|
|
|
|
|
|
|
|
|
|
|
|
|
|
|
|
|
|
|
|
|
|
|
|
|
|
|
|
|
|
|
|
|
|
|
|
|
|
|
|
|
|
|
|
|
|
|
|
|
|
|
|
|
|
|
|
|
|
|
|
|
|
|
|
|
|
spaces/Benson/text-generation/Examples/Descargar Fondo De Pantalla En Blanco Y Negro.md
DELETED
@@ -1,88 +0,0 @@
|
|
1 |
-
<br />
|
2 |
-
<h1>Descargar Fondo de pantalla en blanco y negro: Una guía para encontrar las mejores imágenes para su escritorio</h1>
|
3 |
-
<p>¿Está buscando una manera de darle vida a su fondo de escritorio? ¿Desea agregar un poco de elegancia, contraste y simplicidad a su pantalla? Si es así, es posible que desee considerar la descarga de fondo de pantalla en blanco y negro. Fondo de pantalla en blanco y negro es una opción popular entre muchos usuarios que aprecian la belleza y versatilidad de las imágenes monocromáticas. Si desea mostrar sus fotos favoritas, crear un aspecto minimalista, o expresar su estado de ánimo, fondo de pantalla en blanco y negro puede ayudarle a lograr sus objetivos. </p>
|
4 |
-
<h2>descargar fondo de pantalla en blanco y negro</h2><br /><p><b><b>Download File</b> ✔ <a href="https://bltlly.com/2v6MAu">https://bltlly.com/2v6MAu</a></b></p><br /><br />
|
5 |
-
<p>En este artículo, lo guiaremos a través del proceso de encontrar, descargar y configurar fondos de escritorio en blanco y negro en su escritorio. También explicaremos por qué es posible que desee elegir fondos de escritorio en blanco y negro sobre otras opciones, así como algunos consejos y trucos para aprovechar al máximo. Al final de este artículo, tendrá una mejor comprensión de cómo descargar fondos de escritorio en blanco y negro y disfrutar de sus beneficios. </p>
|
6 |
-
<h2>¿Por qué elegir fondo de pantalla en blanco y negro? </h2>
|
7 |
-
<p>Fondo de pantalla en blanco y negro no es solo una cuestión de preferencia o gusto. En realidad, hay algunas buenas razones por las que es posible que desee optar por este tipo de fondo de pantalla sobre otros. Estos son algunos de ellos:</p>
|
8 |
-
<h3>Beneficios de fondo de pantalla en blanco y negro</h3>
|
9 |
-
<ul>
|
10 |
-
<li><strong>Es atemporal y clásico. </strong> El papel pintado en blanco y negro nunca pasa de moda. Tiene un atractivo atemporal que trasciende las tendencias y las modas. Puede adaptarse a cualquier tema, ocasión o temporada. También puede complementar cualquier combinación de colores o decoración. </li>
|
11 |
-
<li><strong>Es versátil y adaptable. </strong> El papel pintado en blanco y negro puede funcionar con cualquier tipo de imagen, ya sea un retrato, un paisaje, un resumen o un texto. También se puede modificar fácilmente con filtros, efectos o superposiciones para crear diferentes miradas y estados de ánimo. También puede mezclar y combinar diferentes fondos de pantalla en blanco y negro para crear un collage o una presentación de diapositivas. </li>
|
12 |
-
|
13 |
-
<li><strong>Es simple y minimalista. </strong> El fondo de pantalla en blanco y negro puede ayudarlo a lograr un aspecto simple y minimalista para su escritorio. Puede reducir las distracciones, el desorden y el ruido. También puede hacer que sus iconos, widgets y carpetas se destaquen más. </li>
|
14 |
-
</ul>
|
15 |
-
<h3>Inconvenientes de fondo de pantalla en blanco y negro</h3>
|
16 |
-
<ul>
|
17 |
-
Puede ser aburrido y aburrido. </strong> El fondo de pantalla en blanco y negro a veces puede ser aburrido y aburrido, especialmente si usa la misma imagen durante mucho tiempo. También puede carecer de variedad, vitalidad y emoción. Puede hacer que su escritorio se vea soso, monótono o deprimente. </li>
|
18 |
-
<li><strong>Puede ser difícil de encontrar. </strong> El fondo de pantalla en blanco y negro puede ser difícil de encontrar en línea, especialmente si está buscando imágenes de alta calidad que se ajusten a la resolución de la pantalla. Es posible que tenga que pasar algún tiempo navegando por diferentes sitios web, buscando palabras clave o filtrando los resultados. También puede tener que lidiar con marcas de agua, anuncios o malware. </li>
|
19 |
-
<li><strong>Puede ser difícil de configurar. </strong> El fondo de pantalla en blanco y negro puede ser difícil de configurar en el escritorio, especialmente si no está familiarizado con la configuración o las opciones. Es posible que tenga que ajustar el tamaño, la posición, la alineación o la relación de aspecto de la imagen para que se ajuste a su pantalla. También es posible que tenga que cambiar el brillo, el contraste o la saturación de la imagen para hacerla más visible o atractiva. </li>
|
20 |
-
</ul>
|
21 |
-
<p>Como puedes ver, el papel pintado en blanco y negro tiene sus pros y sus contras. En última instancia, depende de ti decidir si te gusta o no. Si lo hace, siga leyendo para aprender a descargar fondos en blanco y negro para su escritorio. </p>
|
22 |
-
<p></p>
|
23 |
-
<h2>¿Cómo descargar el fondo de pantalla en blanco y negro? </h2>
|
24 |
-
|
25 |
-
<h3>Fuentes de fondo de pantalla en blanco y negro gratis Fotos</h3>
|
26 |
-
<h4>Pexels</h4>
|
27 |
-
<p>Pexels es uno de los sitios web más populares para fotos y videos de stock gratis. Tiene una gran colección de fotos de fondo de pantalla en blanco y negro que puede descargar y usar con fines personales o comerciales. Puede navegar por categoría, buscar por palabra clave o filtrar por orientación, tamaño o color. También puede ver los detalles, calificaciones y comentarios de cada foto. Para descargar una foto, simplemente haga clic en ella y elija la resolución que se adapte a su pantalla. También puedes usar la aplicación Pexels para Android o iOS para descargar fotos directamente a tu dispositivo. </p>
|
28 |
-
<h4>Unsplash</h4>
|
29 |
-
<p>Unsplash es otro gran sitio web para fotos y videos de stock gratis. Tiene una enorme biblioteca de fotos en blanco y negro que puede descargar y usar para cualquier proyecto. Puede explorar por tema, colección o usuario, o buscar por palabra clave o etiqueta. También puede ver la información, gustos y descargas de cada foto. Para descargar una foto, simplemente haga clic en ella y seleccione el botón de descarga. También puede usar la aplicación Unsplash para Android o iOS para descargar fotos fácilmente. </p>
|
30 |
-
<h4>Pixabay</h4>
|
31 |
-
<p>Pixabay es un sitio web que ofrece fotos de stock gratis, videos, ilustraciones y vectores. Tiene una selección decente de fotos de fondo de pantalla en blanco y negro que puede descargar y usar para cualquier cosa. Puede navegar por categoría, buscar por palabra clave o tipo de imagen, o filtrar por orientación, tamaño o color. También puede ver los detalles, gustos, favoritos y comentarios de cada foto. Para descargar una foto, simplemente haga clic en ella y elija la resolución que se adapte a su pantalla. También puede utilizar la aplicación Pixabay para Android o iOS para descargar fotos rápidamente. </p>
|
32 |
-
<h3> Consejos para elegir el fondo de pantalla blanco y negro derecho</h3>
|
33 |
-
<p>Ahora que sabe dónde encontrar fotos de fondos de escritorio en blanco y negro en línea, es posible que se pregunte cómo elegir el adecuado para su escritorio. Aquí hay algunos consejos para ayudarte:</p>
|
34 |
-
<h4>Considere la resolución y la relación de aspecto</h4>
|
35 |
-
|
36 |
-
<h4>Haga coincidir el fondo de pantalla con su tema y estado de ánimo</h4>
|
37 |
-
<p>El fondo de pantalla que elija debe reflejar su personalidad, preferencias y estado de ánimo. También debe coincidir con el tema y el estilo de su entorno de escritorio. Por ejemplo, si tienes un tema oscuro en el escritorio, es posible que desees elegir un fondo de pantalla que tenga un alto contraste, bajo brillo o un efecto de escala de grises. Si tiene un tema ligero en su escritorio, es posible que desee elegir un fondo de pantalla que tenga bajo contraste, alto brillo o un tono sepia. También debe tener en cuenta su estado de ánimo y cómo desea sentirse cuando se mira a su fondo de pantalla. Por ejemplo, si quieres sentirte tranquilo y relajado, puedes elegir un papel pintado que tenga una imagen sencilla, relajante o inspirada en la naturaleza. Si quieres sentirte energizado y motivado, puedes elegir un fondo de pantalla que tenga una imagen dinámica, inspiradora o llena de acción. </p>
|
38 |
-
<h4>Experimenta con diferentes estilos y efectos</h4>
|
39 |
-
<p>Una de las ventajas de fondo de pantalla en blanco y negro es que se puede modificar fácilmente con diferentes estilos y efectos para crear varios looks y estados de ánimo. Puede utilizar software de edición de fotos o herramientas en línea para aplicar filtros, efectos o superposiciones a su fondo de pantalla. Por ejemplo, puedes usar un efecto de desenfoque para crear un efecto bokeh o profundidad de campo. Puedes usar un efecto de ruido para crear un efecto granulado o vintage. Puede utilizar una superposición de degradado para crear un efecto de salpicadura de duotono o color. También puede utilizar texto, formas o pegatinas para añadir algunos toques divertidos o personales a su fondo de pantalla. </p>
|
40 |
-
<h2>Cómo configurar fondo de pantalla en blanco y negro en su escritorio? </h2>
|
41 |
-
<p>Una vez que haya descargado su foto de fondo de pantalla en blanco y negro, debe configurarlo como fondo de escritorio. El proceso puede variar según el sistema operativo y el dispositivo que esté utilizando. Estos son algunos pasos generales para configurar fondos de escritorio en blanco y negro:</p>
|
42 |
-
<h3>Para usuarios de Windows 10</h3>
|
43 |
-
<ol>
|
44 |
-
|
45 |
-
<li>Seleccione <strong>Fondo</strong> desde el menú de la izquierda. </li>
|
46 |
-
<li>Seleccionar <strong>Imagen</strong> desde el menú desplegable bajo <strong>Fondo</strong>. </li>
|
47 |
-
<li>Haga clic en <strong>Browse</strong> y busque la foto de fondo de pantalla en blanco y negro que descargó. </li>
|
48 |
-
<li>Seleccione la foto y haga clic en <strong>Elegir imagen</strong>. </li>
|
49 |
-
<li>Seleccione la opción <strong>Fit</strong> en el menú desplegable bajo <strong>Elija un ajuste</strong>. También puedes elegir otras opciones como <strong>Fill</strong>, <strong>Stretch</strong>, o <strong>Center</strong>, dependiendo de tu preferencia. </li>
|
50 |
-
<li>También puede ajustar el <strong>Brillo</strong>, <strong>Color</strong>, o <strong>Contraste</strong> de su fondo de pantalla haciendo clic en los botones correspondientes bajo <strong>Color</strong>. </li>
|
51 |
-
<li> Ha configurado con éxito su fondo de pantalla en blanco y negro en el escritorio de Windows 10. </li>
|
52 |
-
</ol>
|
53 |
-
<h3>Para usuarios de Mac</h3>
|
54 |
-
<ol>
|
55 |
-
<li>Haga clic en el icono <strong>Apple</strong> en la esquina superior izquierda de la pantalla y seleccione <strong>Preferencias del sistema</strong>. </li>
|
56 |
-
<li>Seleccionar <strong>Escritorio y salvapantallas</strong>. </li>
|
57 |
-
<li>Seleccione la pestaña <strong>Escritorio</strong>. </li>
|
58 |
-
<li>Haga clic en el botón <strong>+</strong> en la esquina inferior izquierda de la ventana y busque la foto de fondo de pantalla en blanco y negro que descargó. </li>
|
59 |
-
<li>Seleccione la foto y haga clic en <strong>Agregar</strong>. </li>
|
60 |
-
<li>Seleccione la foto de la lista de fondos de pantalla en el lado izquierdo de la ventana. </li>
|
61 |
-
<li>Seleccione la opción <strong>Fill Screen</strong> del menú desplegable bajo <strong>Fitting</strong>. También puede elegir otras opciones como <strong>Ajustar a la pantalla</strong>, <strong>Estirar a la pantalla de relleno</strong>, o <strong>Centrar</strong>, dependiendo de su preferencia. </li>
|
62 |
-
<li> Ha configurado con éxito su fondo de pantalla en blanco y negro en el escritorio de su Mac. </li> <h3>Para usuarios de Linux</h3>
|
63 |
-
<ol>
|
64 |
-
|
65 |
-
<li>Seleccione el botón <strong>Add</strong> en la parte inferior de la ventana y busque la foto de fondo de pantalla en blanco y negro que descargó. </li>
|
66 |
-
<li>Seleccione la foto y haga clic en <strong>Abrir</strong>. </li>
|
67 |
-
<li>Seleccione la foto de la lista de fondos de pantalla en el lado izquierdo de la ventana. </li>
|
68 |
-
<li>Seleccione la opción <strong>Zoom</strong> en el menú desplegable bajo <strong>Opciones de imagen</strong>. También puede elegir otras opciones como <strong>Scale</strong>, <strong>Tile</strong>, o <strong>Center</strong>, dependiendo de su preferencia. </li>
|
69 |
-
<li> Ha configurado con éxito su fondo de pantalla en blanco y negro en su escritorio Linux. </li>
|
70 |
-
</ol>
|
71 |
-
<h2>Conclusión</h2>
|
72 |
-
<p>El fondo de pantalla en blanco y negro es una excelente manera de agregar estilo, elegancia y simplicidad a su escritorio. También puede ofrecer algunos beneficios como ser atemporal, versátil y minimalista. Sin embargo, también puede tener algunos inconvenientes como ser aburrido, difícil de encontrar o difícil de configurar. Para descargar fondos de escritorio en blanco y negro, debe utilizar sitios web de buena reputación que ofrecen imágenes gratuitas, de alta calidad y libres de derechos. También debe considerar la resolución, relación de aspecto, tema, estado de ánimo y estilo de su fondo de pantalla. También debe seguir los pasos para configurar su fondo de pantalla en su escritorio de acuerdo con su sistema operativo y dispositivo. </p>
|
73 |
-
<p>Esperamos que este artículo le haya ayudado a aprender cómo descargar fondos de escritorio en blanco y negro y disfrutar de sus ventajas. Si tiene alguna pregunta o comentario, no dude en dejarlos abajo. ¡Gracias por leer! </p>
|
74 |
-
<h2>Preguntas frecuentes</h2>
|
75 |
-
<ul>
|
76 |
-
<li><strong>Q: ¿Qué es el fondo de pantalla en blanco y negro? </strong></li>
|
77 |
-
<li>A: Fondo de pantalla en blanco y negro es un tipo de fondo de pantalla que utiliza solo los colores blanco y negro o tonos de gris. Puede basarse en cualquier imagen, ya sea una foto, un dibujo, un texto o un resumen. </li>
|
78 |
-
<li><strong>Q: ¿Por qué debería usar fondos en blanco y negro? </strong></li>
|
79 |
-
|
80 |
-
<li><strong>Q: ¿Dónde puedo encontrar fondos en blanco y negro? </strong></li>
|
81 |
-
<li>A: Puede encontrar fondos de escritorio en blanco y negro en línea desde varios sitios web que ofrecen fotos y videos de stock gratis. Algunos de los mejores son Pexels, Unsplash y Pixabay. También puede crear su propio fondo de pantalla en blanco y negro mediante el uso de software de edición de fotos o herramientas en línea. </li>
|
82 |
-
<li><strong>Q: ¿Cómo puedo elegir el fondo de pantalla blanco y negro adecuado? </strong></li>
|
83 |
-
<li>A: Puede elegir el fondo de pantalla en blanco y negro correcto teniendo en cuenta la resolución, relación de aspecto, tema, estado de ánimo y estilo de su fondo de pantalla. También debe experimentar con diferentes estilos y efectos para crear diferentes miradas y estados de ánimo. </li>
|
84 |
-
<li><strong>Q: ¿Cómo puedo configurar fondos de escritorio en blanco y negro? </strong></li>
|
85 |
-
<li>A: Puede configurar fondos de escritorio en blanco y negro siguiendo los pasos para su sistema operativo y dispositivo. Para los usuarios de Windows 10, debe hacer clic derecho en el escritorio, seleccionar Personalizar, seleccionar Fondo, seleccionar Imagen, buscar su foto, elegir una opción de ajuste y ajustar la configuración de color. Para los usuarios de Mac, debe hacer clic en el icono de Apple, seleccionar Preferencias del sistema, seleccionar Escritorio y Protector de pantalla, seleccionar Escritorio, agregar su foto, elegir una opción adecuada. Para los usuarios de Linux, debe hacer clic derecho en el escritorio, seleccionar Cambiar fondo de escritorio, agregar su foto, elegir una opción de imagen. </li>
|
86 |
-
</ul></p> 64aa2da5cf<br />
|
87 |
-
<br />
|
88 |
-
<br />
|
|
|
|
|
|
|
|
|
|
|
|
|
|
|
|
|
|
|
|
|
|
|
|
|
|
|
|
|
|
|
|
|
|
|
|
|
|
|
|
|
|
|
|
|
|
|
|
|
|
|
|
|
|
|
|
|
|
|
|
|
|
|
|
|
|
|
|
|
|
|
|
|
|
|
|
|
|
|
|
|
|
|
|
|
|
|
|
|
|
|
|
|
|
|
|
|
|
|
|
|
|
|
|
|
|
|
|
|
|
|
|
|
|
|
|
|
|
|
|
|
|
|
|
|
|
|
|
|
|
|
|
|
|
|
|
|
|
|
|
|
|
|
|
|
|
|
|
|
|
|
|
|
|
|
|
|
|
|
|
|
|
|
|
|
|
|
|
|
|
|
|
|
|
|
|
|
|
spaces/Big-Web/MMSD/env/Lib/site-packages/pip/_vendor/requests/certs.py
DELETED
@@ -1,24 +0,0 @@
|
|
1 |
-
#!/usr/bin/env python
|
2 |
-
|
3 |
-
"""
|
4 |
-
requests.certs
|
5 |
-
~~~~~~~~~~~~~~
|
6 |
-
|
7 |
-
This module returns the preferred default CA certificate bundle. There is
|
8 |
-
only one — the one from the certifi package.
|
9 |
-
|
10 |
-
If you are packaging Requests, e.g., for a Linux distribution or a managed
|
11 |
-
environment, you can change the definition of where() to return a separately
|
12 |
-
packaged CA bundle.
|
13 |
-
"""
|
14 |
-
|
15 |
-
import os
|
16 |
-
|
17 |
-
if "_PIP_STANDALONE_CERT" not in os.environ:
|
18 |
-
from pip._vendor.certifi import where
|
19 |
-
else:
|
20 |
-
def where():
|
21 |
-
return os.environ["_PIP_STANDALONE_CERT"]
|
22 |
-
|
23 |
-
if __name__ == "__main__":
|
24 |
-
print(where())
|
|
|
|
|
|
|
|
|
|
|
|
|
|
|
|
|
|
|
|
|
|
|
|
|
|
|
|
|
|
|
|
|
|
|
|
|
|
|
|
|
|
|
|
|
|
|
|
|
|
spaces/Big-Web/MMSD/env/Lib/site-packages/pip/_vendor/rich/_null_file.py
DELETED
@@ -1,69 +0,0 @@
|
|
1 |
-
from types import TracebackType
|
2 |
-
from typing import IO, Iterable, Iterator, List, Optional, Type
|
3 |
-
|
4 |
-
|
5 |
-
class NullFile(IO[str]):
|
6 |
-
def close(self) -> None:
|
7 |
-
pass
|
8 |
-
|
9 |
-
def isatty(self) -> bool:
|
10 |
-
return False
|
11 |
-
|
12 |
-
def read(self, __n: int = 1) -> str:
|
13 |
-
return ""
|
14 |
-
|
15 |
-
def readable(self) -> bool:
|
16 |
-
return False
|
17 |
-
|
18 |
-
def readline(self, __limit: int = 1) -> str:
|
19 |
-
return ""
|
20 |
-
|
21 |
-
def readlines(self, __hint: int = 1) -> List[str]:
|
22 |
-
return []
|
23 |
-
|
24 |
-
def seek(self, __offset: int, __whence: int = 1) -> int:
|
25 |
-
return 0
|
26 |
-
|
27 |
-
def seekable(self) -> bool:
|
28 |
-
return False
|
29 |
-
|
30 |
-
def tell(self) -> int:
|
31 |
-
return 0
|
32 |
-
|
33 |
-
def truncate(self, __size: Optional[int] = 1) -> int:
|
34 |
-
return 0
|
35 |
-
|
36 |
-
def writable(self) -> bool:
|
37 |
-
return False
|
38 |
-
|
39 |
-
def writelines(self, __lines: Iterable[str]) -> None:
|
40 |
-
pass
|
41 |
-
|
42 |
-
def __next__(self) -> str:
|
43 |
-
return ""
|
44 |
-
|
45 |
-
def __iter__(self) -> Iterator[str]:
|
46 |
-
return iter([""])
|
47 |
-
|
48 |
-
def __enter__(self) -> IO[str]:
|
49 |
-
pass
|
50 |
-
|
51 |
-
def __exit__(
|
52 |
-
self,
|
53 |
-
__t: Optional[Type[BaseException]],
|
54 |
-
__value: Optional[BaseException],
|
55 |
-
__traceback: Optional[TracebackType],
|
56 |
-
) -> None:
|
57 |
-
pass
|
58 |
-
|
59 |
-
def write(self, text: str) -> int:
|
60 |
-
return 0
|
61 |
-
|
62 |
-
def flush(self) -> None:
|
63 |
-
pass
|
64 |
-
|
65 |
-
def fileno(self) -> int:
|
66 |
-
return -1
|
67 |
-
|
68 |
-
|
69 |
-
NULL_FILE = NullFile()
|
|
|
|
|
|
|
|
|
|
|
|
|
|
|
|
|
|
|
|
|
|
|
|
|
|
|
|
|
|
|
|
|
|
|
|
|
|
|
|
|
|
|
|
|
|
|
|
|
|
|
|
|
|
|
|
|
|
|
|
|
|
|
|
|
|
|
|
|
|
|
|
|
|
|
|
|
|
|
|
|
|
|
|
|
|
|
|
|
|
|
|
|
|
|
|
|
|
|
|
|
|
|
|
|
|
|
|
|
|
|
|
|
|
|
|
|
|
|
|
|
|
|
|
|
|
|
|
|
|
|
|
|
|
|
|
|
|
|
|
spaces/Big-Web/MMSD/env/Lib/site-packages/pip/_vendor/webencodings/labels.py
DELETED
@@ -1,231 +0,0 @@
|
|
1 |
-
"""
|
2 |
-
|
3 |
-
webencodings.labels
|
4 |
-
~~~~~~~~~~~~~~~~~~~
|
5 |
-
|
6 |
-
Map encoding labels to their name.
|
7 |
-
|
8 |
-
:copyright: Copyright 2012 by Simon Sapin
|
9 |
-
:license: BSD, see LICENSE for details.
|
10 |
-
|
11 |
-
"""
|
12 |
-
|
13 |
-
# XXX Do not edit!
|
14 |
-
# This file is automatically generated by mklabels.py
|
15 |
-
|
16 |
-
LABELS = {
|
17 |
-
'unicode-1-1-utf-8': 'utf-8',
|
18 |
-
'utf-8': 'utf-8',
|
19 |
-
'utf8': 'utf-8',
|
20 |
-
'866': 'ibm866',
|
21 |
-
'cp866': 'ibm866',
|
22 |
-
'csibm866': 'ibm866',
|
23 |
-
'ibm866': 'ibm866',
|
24 |
-
'csisolatin2': 'iso-8859-2',
|
25 |
-
'iso-8859-2': 'iso-8859-2',
|
26 |
-
'iso-ir-101': 'iso-8859-2',
|
27 |
-
'iso8859-2': 'iso-8859-2',
|
28 |
-
'iso88592': 'iso-8859-2',
|
29 |
-
'iso_8859-2': 'iso-8859-2',
|
30 |
-
'iso_8859-2:1987': 'iso-8859-2',
|
31 |
-
'l2': 'iso-8859-2',
|
32 |
-
'latin2': 'iso-8859-2',
|
33 |
-
'csisolatin3': 'iso-8859-3',
|
34 |
-
'iso-8859-3': 'iso-8859-3',
|
35 |
-
'iso-ir-109': 'iso-8859-3',
|
36 |
-
'iso8859-3': 'iso-8859-3',
|
37 |
-
'iso88593': 'iso-8859-3',
|
38 |
-
'iso_8859-3': 'iso-8859-3',
|
39 |
-
'iso_8859-3:1988': 'iso-8859-3',
|
40 |
-
'l3': 'iso-8859-3',
|
41 |
-
'latin3': 'iso-8859-3',
|
42 |
-
'csisolatin4': 'iso-8859-4',
|
43 |
-
'iso-8859-4': 'iso-8859-4',
|
44 |
-
'iso-ir-110': 'iso-8859-4',
|
45 |
-
'iso8859-4': 'iso-8859-4',
|
46 |
-
'iso88594': 'iso-8859-4',
|
47 |
-
'iso_8859-4': 'iso-8859-4',
|
48 |
-
'iso_8859-4:1988': 'iso-8859-4',
|
49 |
-
'l4': 'iso-8859-4',
|
50 |
-
'latin4': 'iso-8859-4',
|
51 |
-
'csisolatincyrillic': 'iso-8859-5',
|
52 |
-
'cyrillic': 'iso-8859-5',
|
53 |
-
'iso-8859-5': 'iso-8859-5',
|
54 |
-
'iso-ir-144': 'iso-8859-5',
|
55 |
-
'iso8859-5': 'iso-8859-5',
|
56 |
-
'iso88595': 'iso-8859-5',
|
57 |
-
'iso_8859-5': 'iso-8859-5',
|
58 |
-
'iso_8859-5:1988': 'iso-8859-5',
|
59 |
-
'arabic': 'iso-8859-6',
|
60 |
-
'asmo-708': 'iso-8859-6',
|
61 |
-
'csiso88596e': 'iso-8859-6',
|
62 |
-
'csiso88596i': 'iso-8859-6',
|
63 |
-
'csisolatinarabic': 'iso-8859-6',
|
64 |
-
'ecma-114': 'iso-8859-6',
|
65 |
-
'iso-8859-6': 'iso-8859-6',
|
66 |
-
'iso-8859-6-e': 'iso-8859-6',
|
67 |
-
'iso-8859-6-i': 'iso-8859-6',
|
68 |
-
'iso-ir-127': 'iso-8859-6',
|
69 |
-
'iso8859-6': 'iso-8859-6',
|
70 |
-
'iso88596': 'iso-8859-6',
|
71 |
-
'iso_8859-6': 'iso-8859-6',
|
72 |
-
'iso_8859-6:1987': 'iso-8859-6',
|
73 |
-
'csisolatingreek': 'iso-8859-7',
|
74 |
-
'ecma-118': 'iso-8859-7',
|
75 |
-
'elot_928': 'iso-8859-7',
|
76 |
-
'greek': 'iso-8859-7',
|
77 |
-
'greek8': 'iso-8859-7',
|
78 |
-
'iso-8859-7': 'iso-8859-7',
|
79 |
-
'iso-ir-126': 'iso-8859-7',
|
80 |
-
'iso8859-7': 'iso-8859-7',
|
81 |
-
'iso88597': 'iso-8859-7',
|
82 |
-
'iso_8859-7': 'iso-8859-7',
|
83 |
-
'iso_8859-7:1987': 'iso-8859-7',
|
84 |
-
'sun_eu_greek': 'iso-8859-7',
|
85 |
-
'csiso88598e': 'iso-8859-8',
|
86 |
-
'csisolatinhebrew': 'iso-8859-8',
|
87 |
-
'hebrew': 'iso-8859-8',
|
88 |
-
'iso-8859-8': 'iso-8859-8',
|
89 |
-
'iso-8859-8-e': 'iso-8859-8',
|
90 |
-
'iso-ir-138': 'iso-8859-8',
|
91 |
-
'iso8859-8': 'iso-8859-8',
|
92 |
-
'iso88598': 'iso-8859-8',
|
93 |
-
'iso_8859-8': 'iso-8859-8',
|
94 |
-
'iso_8859-8:1988': 'iso-8859-8',
|
95 |
-
'visual': 'iso-8859-8',
|
96 |
-
'csiso88598i': 'iso-8859-8-i',
|
97 |
-
'iso-8859-8-i': 'iso-8859-8-i',
|
98 |
-
'logical': 'iso-8859-8-i',
|
99 |
-
'csisolatin6': 'iso-8859-10',
|
100 |
-
'iso-8859-10': 'iso-8859-10',
|
101 |
-
'iso-ir-157': 'iso-8859-10',
|
102 |
-
'iso8859-10': 'iso-8859-10',
|
103 |
-
'iso885910': 'iso-8859-10',
|
104 |
-
'l6': 'iso-8859-10',
|
105 |
-
'latin6': 'iso-8859-10',
|
106 |
-
'iso-8859-13': 'iso-8859-13',
|
107 |
-
'iso8859-13': 'iso-8859-13',
|
108 |
-
'iso885913': 'iso-8859-13',
|
109 |
-
'iso-8859-14': 'iso-8859-14',
|
110 |
-
'iso8859-14': 'iso-8859-14',
|
111 |
-
'iso885914': 'iso-8859-14',
|
112 |
-
'csisolatin9': 'iso-8859-15',
|
113 |
-
'iso-8859-15': 'iso-8859-15',
|
114 |
-
'iso8859-15': 'iso-8859-15',
|
115 |
-
'iso885915': 'iso-8859-15',
|
116 |
-
'iso_8859-15': 'iso-8859-15',
|
117 |
-
'l9': 'iso-8859-15',
|
118 |
-
'iso-8859-16': 'iso-8859-16',
|
119 |
-
'cskoi8r': 'koi8-r',
|
120 |
-
'koi': 'koi8-r',
|
121 |
-
'koi8': 'koi8-r',
|
122 |
-
'koi8-r': 'koi8-r',
|
123 |
-
'koi8_r': 'koi8-r',
|
124 |
-
'koi8-u': 'koi8-u',
|
125 |
-
'csmacintosh': 'macintosh',
|
126 |
-
'mac': 'macintosh',
|
127 |
-
'macintosh': 'macintosh',
|
128 |
-
'x-mac-roman': 'macintosh',
|
129 |
-
'dos-874': 'windows-874',
|
130 |
-
'iso-8859-11': 'windows-874',
|
131 |
-
'iso8859-11': 'windows-874',
|
132 |
-
'iso885911': 'windows-874',
|
133 |
-
'tis-620': 'windows-874',
|
134 |
-
'windows-874': 'windows-874',
|
135 |
-
'cp1250': 'windows-1250',
|
136 |
-
'windows-1250': 'windows-1250',
|
137 |
-
'x-cp1250': 'windows-1250',
|
138 |
-
'cp1251': 'windows-1251',
|
139 |
-
'windows-1251': 'windows-1251',
|
140 |
-
'x-cp1251': 'windows-1251',
|
141 |
-
'ansi_x3.4-1968': 'windows-1252',
|
142 |
-
'ascii': 'windows-1252',
|
143 |
-
'cp1252': 'windows-1252',
|
144 |
-
'cp819': 'windows-1252',
|
145 |
-
'csisolatin1': 'windows-1252',
|
146 |
-
'ibm819': 'windows-1252',
|
147 |
-
'iso-8859-1': 'windows-1252',
|
148 |
-
'iso-ir-100': 'windows-1252',
|
149 |
-
'iso8859-1': 'windows-1252',
|
150 |
-
'iso88591': 'windows-1252',
|
151 |
-
'iso_8859-1': 'windows-1252',
|
152 |
-
'iso_8859-1:1987': 'windows-1252',
|
153 |
-
'l1': 'windows-1252',
|
154 |
-
'latin1': 'windows-1252',
|
155 |
-
'us-ascii': 'windows-1252',
|
156 |
-
'windows-1252': 'windows-1252',
|
157 |
-
'x-cp1252': 'windows-1252',
|
158 |
-
'cp1253': 'windows-1253',
|
159 |
-
'windows-1253': 'windows-1253',
|
160 |
-
'x-cp1253': 'windows-1253',
|
161 |
-
'cp1254': 'windows-1254',
|
162 |
-
'csisolatin5': 'windows-1254',
|
163 |
-
'iso-8859-9': 'windows-1254',
|
164 |
-
'iso-ir-148': 'windows-1254',
|
165 |
-
'iso8859-9': 'windows-1254',
|
166 |
-
'iso88599': 'windows-1254',
|
167 |
-
'iso_8859-9': 'windows-1254',
|
168 |
-
'iso_8859-9:1989': 'windows-1254',
|
169 |
-
'l5': 'windows-1254',
|
170 |
-
'latin5': 'windows-1254',
|
171 |
-
'windows-1254': 'windows-1254',
|
172 |
-
'x-cp1254': 'windows-1254',
|
173 |
-
'cp1255': 'windows-1255',
|
174 |
-
'windows-1255': 'windows-1255',
|
175 |
-
'x-cp1255': 'windows-1255',
|
176 |
-
'cp1256': 'windows-1256',
|
177 |
-
'windows-1256': 'windows-1256',
|
178 |
-
'x-cp1256': 'windows-1256',
|
179 |
-
'cp1257': 'windows-1257',
|
180 |
-
'windows-1257': 'windows-1257',
|
181 |
-
'x-cp1257': 'windows-1257',
|
182 |
-
'cp1258': 'windows-1258',
|
183 |
-
'windows-1258': 'windows-1258',
|
184 |
-
'x-cp1258': 'windows-1258',
|
185 |
-
'x-mac-cyrillic': 'x-mac-cyrillic',
|
186 |
-
'x-mac-ukrainian': 'x-mac-cyrillic',
|
187 |
-
'chinese': 'gbk',
|
188 |
-
'csgb2312': 'gbk',
|
189 |
-
'csiso58gb231280': 'gbk',
|
190 |
-
'gb2312': 'gbk',
|
191 |
-
'gb_2312': 'gbk',
|
192 |
-
'gb_2312-80': 'gbk',
|
193 |
-
'gbk': 'gbk',
|
194 |
-
'iso-ir-58': 'gbk',
|
195 |
-
'x-gbk': 'gbk',
|
196 |
-
'gb18030': 'gb18030',
|
197 |
-
'hz-gb-2312': 'hz-gb-2312',
|
198 |
-
'big5': 'big5',
|
199 |
-
'big5-hkscs': 'big5',
|
200 |
-
'cn-big5': 'big5',
|
201 |
-
'csbig5': 'big5',
|
202 |
-
'x-x-big5': 'big5',
|
203 |
-
'cseucpkdfmtjapanese': 'euc-jp',
|
204 |
-
'euc-jp': 'euc-jp',
|
205 |
-
'x-euc-jp': 'euc-jp',
|
206 |
-
'csiso2022jp': 'iso-2022-jp',
|
207 |
-
'iso-2022-jp': 'iso-2022-jp',
|
208 |
-
'csshiftjis': 'shift_jis',
|
209 |
-
'ms_kanji': 'shift_jis',
|
210 |
-
'shift-jis': 'shift_jis',
|
211 |
-
'shift_jis': 'shift_jis',
|
212 |
-
'sjis': 'shift_jis',
|
213 |
-
'windows-31j': 'shift_jis',
|
214 |
-
'x-sjis': 'shift_jis',
|
215 |
-
'cseuckr': 'euc-kr',
|
216 |
-
'csksc56011987': 'euc-kr',
|
217 |
-
'euc-kr': 'euc-kr',
|
218 |
-
'iso-ir-149': 'euc-kr',
|
219 |
-
'korean': 'euc-kr',
|
220 |
-
'ks_c_5601-1987': 'euc-kr',
|
221 |
-
'ks_c_5601-1989': 'euc-kr',
|
222 |
-
'ksc5601': 'euc-kr',
|
223 |
-
'ksc_5601': 'euc-kr',
|
224 |
-
'windows-949': 'euc-kr',
|
225 |
-
'csiso2022kr': 'iso-2022-kr',
|
226 |
-
'iso-2022-kr': 'iso-2022-kr',
|
227 |
-
'utf-16be': 'utf-16be',
|
228 |
-
'utf-16': 'utf-16le',
|
229 |
-
'utf-16le': 'utf-16le',
|
230 |
-
'x-user-defined': 'x-user-defined',
|
231 |
-
}
|
|
|
|
|
|
|
|
|
|
|
|
|
|
|
|
|
|
|
|
|
|
|
|
|
|
|
|
|
|
|
|
|
|
|
|
|
|
|
|
|
|
|
|
|
|
|
|
|
|
|
|
|
|
|
|
|
|
|
|
|
|
|
|
|
|
|
|
|
|
|
|
|
|
|
|
|
|
|
|
|
|
|
|
|
|
|
|
|
|
|
|
|
|
|
|
|
|
|
|
|
|
|
|
|
|
|
|
|
|
|
|
|
|
|
|
|
|
|
|
|
|
|
|
|
|
|
|
|
|
|
|
|
|
|
|
|
|
|
|
|
|
|
|
|
|
|
|
|
|
|
|
|
|
|
|
|
|
|
|
|
|
|
|
|
|
|
|
|
|
|
|
|
|
|
|
|
|
|
|
|
|
|
|
|
|
|
|
|
|
|
|
|
|
|
|
|
|
|
|
|
|
|
|
|
|
|
|
|
|
|
|
|
|
|
|
|
|
|
|
|
|
|
|
|
|
|
|
|
|
|
|
|
|
|
|
|
|
|
|
|
|
|
|
|
|
|
|
|
|
|
|
|
|
|
|
|
|
|
|
|
|
|
|
|
|
|
|
|
|
|
|
|
|
|
|
|
|
|
|
|
|
|
|
|
|
|
|
|
|
|
|
|
|
|
|
|
|
|
|
|
|
|
|
|
|
|
|
|
|
|
|
|
|
|
|
|
|
|
|
|
|
|
|
|
|
|
|
|
|
|
|
|
|
|
|
|
|
|
|
|
|
|
|
|
|
|
|
|
|
|
|
|
|
|
|
|
|
|
|
|
|
|
|
|
|
|
|
|
|
|
|
|
|
|
|
|
|
|
|
|
|
|
|
|
|
|
|
|
|
|
|
|
|
|
|
|
|
|
|
|
|
|
|
|
|
|
|
|
|
|
|
|
|
|
|
|
|
|
|
|
|
|
|
|
|
|
|
|
|
|
|
|
|
|
|
|
|
|
|
|
|
|
|
|
|
|
|
|
|
|
|
|
|
|
|
|
|
|
|
|
|
|
|
spaces/BramVanroy/opus-mt/README.md
DELETED
@@ -1,11 +0,0 @@
|
|
1 |
-
---
|
2 |
-
title: Opus MT
|
3 |
-
emoji: 🔨
|
4 |
-
colorFrom: blue
|
5 |
-
colorTo: indigo
|
6 |
-
sdk: streamlit
|
7 |
-
sdk_version: 1.10.0
|
8 |
-
app_file: app.py
|
9 |
-
pinned: false
|
10 |
-
license: mit
|
11 |
-
---
|
|
|
|
|
|
|
|
|
|
|
|
|
|
|
|
|
|
|
|
|
|
|
|
spaces/CVPR/LIVE/thrust/thrust/system/cuda/detail/get_value.h
DELETED
@@ -1,98 +0,0 @@
|
|
1 |
-
/*
|
2 |
-
* Copyright 2008-2013 NVIDIA Corporation
|
3 |
-
*
|
4 |
-
* Licensed under the Apache License, Version 2.0 (the "License");
|
5 |
-
* you may not use this file except in compliance with the License.
|
6 |
-
* You may obtain a copy of the License at
|
7 |
-
*
|
8 |
-
* http://www.apache.org/licenses/LICENSE-2.0
|
9 |
-
*
|
10 |
-
* Unless required by applicable law or agreed to in writing, software
|
11 |
-
* distributed under the License is distributed on an "AS IS" BASIS,
|
12 |
-
* WITHOUT WARRANTIES OR CONDITIONS OF ANY KIND, either express or implied.
|
13 |
-
* See the License for the specific language governing permissions and
|
14 |
-
* limitations under the License.
|
15 |
-
*/
|
16 |
-
|
17 |
-
#pragma once
|
18 |
-
|
19 |
-
#if THRUST_DEVICE_COMPILER == THRUST_DEVICE_COMPILER_NVCC
|
20 |
-
#include <thrust/detail/config.h>
|
21 |
-
#include <thrust/system/cuda/config.h>
|
22 |
-
#include <thrust/system/cuda/detail/cross_system.h>
|
23 |
-
#include <thrust/detail/raw_pointer_cast.h>
|
24 |
-
#include <thrust/iterator/iterator_traits.h>
|
25 |
-
|
26 |
-
namespace thrust
|
27 |
-
{
|
28 |
-
namespace cuda_cub {
|
29 |
-
|
30 |
-
|
31 |
-
namespace
|
32 |
-
{
|
33 |
-
|
34 |
-
|
35 |
-
template<typename DerivedPolicy, typename Pointer>
|
36 |
-
inline __host__ __device__
|
37 |
-
typename thrust::iterator_value<Pointer>::type
|
38 |
-
get_value_msvc2005_war(execution_policy<DerivedPolicy> &exec, Pointer ptr)
|
39 |
-
{
|
40 |
-
typedef typename thrust::iterator_value<Pointer>::type result_type;
|
41 |
-
|
42 |
-
// XXX war nvbugs/881631
|
43 |
-
struct war_nvbugs_881631
|
44 |
-
{
|
45 |
-
__host__ inline static result_type host_path(execution_policy<DerivedPolicy> &exec, Pointer ptr)
|
46 |
-
{
|
47 |
-
// when called from host code, implement with assign_value
|
48 |
-
// note that this requires a type with default constructor
|
49 |
-
result_type result;
|
50 |
-
|
51 |
-
thrust::host_system_tag host_tag;
|
52 |
-
cross_system<thrust::host_system_tag, DerivedPolicy> systems(host_tag, exec);
|
53 |
-
assign_value(systems, &result, ptr);
|
54 |
-
|
55 |
-
return result;
|
56 |
-
}
|
57 |
-
|
58 |
-
__device__ inline static result_type device_path(execution_policy<DerivedPolicy> &, Pointer ptr)
|
59 |
-
{
|
60 |
-
// when called from device code, just do simple deref
|
61 |
-
return *thrust::raw_pointer_cast(ptr);
|
62 |
-
}
|
63 |
-
};
|
64 |
-
|
65 |
-
// The usual pattern for separating host and device code doesn't work here
|
66 |
-
// because it would result in a compiler warning, either about falling off
|
67 |
-
// the end of a non-void function, or about result_type's default constructor
|
68 |
-
// being a host-only function.
|
69 |
-
#ifdef __NVCOMPILER_CUDA__
|
70 |
-
if (THRUST_IS_HOST_CODE) {
|
71 |
-
return war_nvbugs_881631::host_path(exec, ptr);
|
72 |
-
} else {
|
73 |
-
return war_nvbugs_881631::device_path(exec, ptr);
|
74 |
-
}
|
75 |
-
#else
|
76 |
-
#ifndef __CUDA_ARCH__
|
77 |
-
return war_nvbugs_881631::host_path(exec, ptr);
|
78 |
-
#else
|
79 |
-
return war_nvbugs_881631::device_path(exec, ptr);
|
80 |
-
#endif // __CUDA_ARCH__
|
81 |
-
#endif
|
82 |
-
} // end get_value_msvc2005_war()
|
83 |
-
} // end anon namespace
|
84 |
-
|
85 |
-
|
86 |
-
template<typename DerivedPolicy, typename Pointer>
|
87 |
-
inline __host__ __device__
|
88 |
-
typename thrust::iterator_value<Pointer>::type
|
89 |
-
get_value(execution_policy<DerivedPolicy> &exec, Pointer ptr)
|
90 |
-
{
|
91 |
-
return get_value_msvc2005_war(exec,ptr);
|
92 |
-
} // end get_value()
|
93 |
-
|
94 |
-
|
95 |
-
} // end cuda_cub
|
96 |
-
} // end namespace thrust
|
97 |
-
|
98 |
-
#endif
|
|
|
|
|
|
|
|
|
|
|
|
|
|
|
|
|
|
|
|
|
|
|
|
|
|
|
|
|
|
|
|
|
|
|
|
|
|
|
|
|
|
|
|
|
|
|
|
|
|
|
|
|
|
|
|
|
|
|
|
|
|
|
|
|
|
|
|
|
|
|
|
|
|
|
|
|
|
|
|
|
|
|
|
|
|
|
|
|
|
|
|
|
|
|
|
|
|
|
|
|
|
|
|
|
|
|
|
|
|
|
|
|
|
|
|
|
|
|
|
|
|
|
|
|
|
|
|
|
|
|
|
|
|
|
|
|
|
|
|
|
|
|
|
|
|
|
|
|
|
|
|
|
|
|
|
|
|
|
|
|
|
|
|
|
|
|
|
|
|
|
|
|
|
|
|
|
|
|
|
|
|
|
|
|
|
|
|
|
|
|
|
|
|
|
|
|
|
spaces/CVPR/lama-example/bin/gen_mask_dataset_hydra.py
DELETED
@@ -1,124 +0,0 @@
|
|
1 |
-
#!/usr/bin/env python3
|
2 |
-
|
3 |
-
import glob
|
4 |
-
import os
|
5 |
-
import shutil
|
6 |
-
import traceback
|
7 |
-
import hydra
|
8 |
-
from omegaconf import OmegaConf
|
9 |
-
|
10 |
-
import PIL.Image as Image
|
11 |
-
import numpy as np
|
12 |
-
from joblib import Parallel, delayed
|
13 |
-
|
14 |
-
from saicinpainting.evaluation.masks.mask import SegmentationMask, propose_random_square_crop
|
15 |
-
from saicinpainting.evaluation.utils import load_yaml, SmallMode
|
16 |
-
from saicinpainting.training.data.masks import MixedMaskGenerator
|
17 |
-
|
18 |
-
|
19 |
-
class MakeManyMasksWrapper:
|
20 |
-
def __init__(self, impl, variants_n=2):
|
21 |
-
self.impl = impl
|
22 |
-
self.variants_n = variants_n
|
23 |
-
|
24 |
-
def get_masks(self, img):
|
25 |
-
img = np.transpose(np.array(img), (2, 0, 1))
|
26 |
-
return [self.impl(img)[0] for _ in range(self.variants_n)]
|
27 |
-
|
28 |
-
|
29 |
-
def process_images(src_images, indir, outdir, config):
|
30 |
-
if config.generator_kind == 'segmentation':
|
31 |
-
mask_generator = SegmentationMask(**config.mask_generator_kwargs)
|
32 |
-
elif config.generator_kind == 'random':
|
33 |
-
mask_generator_kwargs = OmegaConf.to_container(config.mask_generator_kwargs, resolve=True)
|
34 |
-
variants_n = mask_generator_kwargs.pop('variants_n', 2)
|
35 |
-
mask_generator = MakeManyMasksWrapper(MixedMaskGenerator(**mask_generator_kwargs),
|
36 |
-
variants_n=variants_n)
|
37 |
-
else:
|
38 |
-
raise ValueError(f'Unexpected generator kind: {config.generator_kind}')
|
39 |
-
|
40 |
-
max_tamper_area = config.get('max_tamper_area', 1)
|
41 |
-
|
42 |
-
for infile in src_images:
|
43 |
-
try:
|
44 |
-
file_relpath = infile[len(indir):]
|
45 |
-
img_outpath = os.path.join(outdir, file_relpath)
|
46 |
-
os.makedirs(os.path.dirname(img_outpath), exist_ok=True)
|
47 |
-
|
48 |
-
image = Image.open(infile).convert('RGB')
|
49 |
-
|
50 |
-
# scale input image to output resolution and filter smaller images
|
51 |
-
if min(image.size) < config.cropping.out_min_size:
|
52 |
-
handle_small_mode = SmallMode(config.cropping.handle_small_mode)
|
53 |
-
if handle_small_mode == SmallMode.DROP:
|
54 |
-
continue
|
55 |
-
elif handle_small_mode == SmallMode.UPSCALE:
|
56 |
-
factor = config.cropping.out_min_size / min(image.size)
|
57 |
-
out_size = (np.array(image.size) * factor).round().astype('uint32')
|
58 |
-
image = image.resize(out_size, resample=Image.BICUBIC)
|
59 |
-
else:
|
60 |
-
factor = config.cropping.out_min_size / min(image.size)
|
61 |
-
out_size = (np.array(image.size) * factor).round().astype('uint32')
|
62 |
-
image = image.resize(out_size, resample=Image.BICUBIC)
|
63 |
-
|
64 |
-
# generate and select masks
|
65 |
-
src_masks = mask_generator.get_masks(image)
|
66 |
-
|
67 |
-
filtered_image_mask_pairs = []
|
68 |
-
for cur_mask in src_masks:
|
69 |
-
if config.cropping.out_square_crop:
|
70 |
-
(crop_left,
|
71 |
-
crop_top,
|
72 |
-
crop_right,
|
73 |
-
crop_bottom) = propose_random_square_crop(cur_mask,
|
74 |
-
min_overlap=config.cropping.crop_min_overlap)
|
75 |
-
cur_mask = cur_mask[crop_top:crop_bottom, crop_left:crop_right]
|
76 |
-
cur_image = image.copy().crop((crop_left, crop_top, crop_right, crop_bottom))
|
77 |
-
else:
|
78 |
-
cur_image = image
|
79 |
-
|
80 |
-
if len(np.unique(cur_mask)) == 0 or cur_mask.mean() > max_tamper_area:
|
81 |
-
continue
|
82 |
-
|
83 |
-
filtered_image_mask_pairs.append((cur_image, cur_mask))
|
84 |
-
|
85 |
-
mask_indices = np.random.choice(len(filtered_image_mask_pairs),
|
86 |
-
size=min(len(filtered_image_mask_pairs), config.max_masks_per_image),
|
87 |
-
replace=False)
|
88 |
-
|
89 |
-
# crop masks; save masks together with input image
|
90 |
-
mask_basename = os.path.join(outdir, os.path.splitext(file_relpath)[0])
|
91 |
-
for i, idx in enumerate(mask_indices):
|
92 |
-
cur_image, cur_mask = filtered_image_mask_pairs[idx]
|
93 |
-
cur_basename = mask_basename + f'_crop{i:03d}'
|
94 |
-
Image.fromarray(np.clip(cur_mask * 255, 0, 255).astype('uint8'),
|
95 |
-
mode='L').save(cur_basename + f'_mask{i:03d}.png')
|
96 |
-
cur_image.save(cur_basename + '.png')
|
97 |
-
except KeyboardInterrupt:
|
98 |
-
return
|
99 |
-
except Exception as ex:
|
100 |
-
print(f'Could not make masks for {infile} due to {ex}:\n{traceback.format_exc()}')
|
101 |
-
|
102 |
-
|
103 |
-
@hydra.main(config_path='../configs/data_gen/whydra', config_name='random_medium_256.yaml')
|
104 |
-
def main(config: OmegaConf):
|
105 |
-
if not config.indir.endswith('/'):
|
106 |
-
config.indir += '/'
|
107 |
-
|
108 |
-
os.makedirs(config.outdir, exist_ok=True)
|
109 |
-
|
110 |
-
in_files = list(glob.glob(os.path.join(config.indir, '**', f'*.{config.location.extension}'),
|
111 |
-
recursive=True))
|
112 |
-
if config.n_jobs == 0:
|
113 |
-
process_images(in_files, config.indir, config.outdir, config)
|
114 |
-
else:
|
115 |
-
in_files_n = len(in_files)
|
116 |
-
chunk_size = in_files_n // config.n_jobs + (1 if in_files_n % config.n_jobs > 0 else 0)
|
117 |
-
Parallel(n_jobs=config.n_jobs)(
|
118 |
-
delayed(process_images)(in_files[start:start+chunk_size], config.indir, config.outdir, config)
|
119 |
-
for start in range(0, len(in_files), chunk_size)
|
120 |
-
)
|
121 |
-
|
122 |
-
|
123 |
-
if __name__ == '__main__':
|
124 |
-
main()
|
|
|
|
|
|
|
|
|
|
|
|
|
|
|
|
|
|
|
|
|
|
|
|
|
|
|
|
|
|
|
|
|
|
|
|
|
|
|
|
|
|
|
|
|
|
|
|
|
|
|
|
|
|
|
|
|
|
|
|
|
|
|
|
|
|
|
|
|
|
|
|
|
|
|
|
|
|
|
|
|
|
|
|
|
|
|
|
|
|
|
|
|
|
|
|
|
|
|
|
|
|
|
|
|
|
|
|
|
|
|
|
|
|
|
|
|
|
|
|
|
|
|
|
|
|
|
|
|
|
|
|
|
|
|
|
|
|
|
|
|
|
|
|
|
|
|
|
|
|
|
|
|
|
|
|
|
|
|
|
|
|
|
|
|
|
|
|
|
|
|
|
|
|
|
|
|
|
|
|
|
|
|
|
|
|
|
|
|
|
|
|
|
|
|
|
|
|
|
|
|
|
|
|
|
|
|
|
|
|
|
|
|
|
|
|
|
|
|
|
|
|
|
|
|
|
|
|
|
|
|
|
|
|
|
|
|
|
|
|
|
|
|
|
|
|
|
|
|
|
spaces/Chaitanya01/InvestingPlatform/config.py
DELETED
@@ -1,391 +0,0 @@
|
|
1 |
-
# These are the tokens for twitter
|
2 |
-
TWITTER_CONSUMER_KEY = 'sw0F5LFCMaHtvlMvIiR7pc6WU'
|
3 |
-
TWITTER_CONSUMER_SECRET = 'DNb7V3NhPRjQN4GLwmEa4bcAwETmYQ3E9mHovV5sIDBY7G2vAb'
|
4 |
-
TWITTER_ACCESS_TOKEN = '1130160902358093825-e8xKgzIqhQG7BnJ1iJg6CES3rMFoKa'
|
5 |
-
TWITTER_ACCESS_TOKEN_SECRET = 'HUEG6XKDE7u9ZjUGucTkkzCI8TOl2hRHPCzulQj8XDK3P'
|
6 |
-
API_KEY = "PKK8K9OAF1WGCQ9JTK0Z"
|
7 |
-
SECRET_KEY = "d1uqIKPKq6iAVsvzYBNwFA4qe5WC2XuIpPFwN745"
|
8 |
-
API_URL = "https://paper-api.alpaca.markets/"
|
9 |
-
CRYPTO_API_URL = "https://data.alpaca.markets/v1beta1/crypto"
|
10 |
-
SLACK_TOKEN = "xoxb-2557354538181-2570404709172-oNr1bsP5hQoFyOL1HqgqF8lv"
|
11 |
-
TWITTER_USERNAMES = ["SELECT ALL",
|
12 |
-
'@saxena_puru',
|
13 |
-
'@chartmojo',
|
14 |
-
'@MacroCharts',
|
15 |
-
'@hiddensmallcaps',
|
16 |
-
'@jonahlupton',
|
17 |
-
'@cperruna',
|
18 |
-
'@cryptokaleo',
|
19 |
-
'@markminervini',
|
20 |
-
'@trendspider_j',
|
21 |
-
'@100trillionUSD',
|
22 |
-
'@fundstrat',
|
23 |
-
'@TKPTrader',
|
24 |
-
'@sunchartist',
|
25 |
-
'@ThePupOfWallSt'
|
26 |
-
]
|
27 |
-
# This mapping is required to get live prices from yahoo finance
|
28 |
-
symbol_mapping = {"SPX":"%5EGSPC","NASDAQ":"%5ENDX","gold":"GC%3DF","NIFTY50":"%5ENSEI","NIFTYBANK":"%5ENSEBANK",
|
29 |
-
"crude oil":"CL%3DF","silver":"SI%3DF","EURUSD":"EURUSD%3DX",
|
30 |
-
"HYG":"HYG","LQD":"LQD","VIX":"%5EVIX",
|
31 |
-
"US 30Y":"US 30Y","US 10Y":"US 10Y","US 2Y":"US 2Y","US 5Y":"US 5Y"
|
32 |
-
}
|
33 |
-
us_sectors = ["Commercial Services", "Communications", "Consumer Durables","Consumer Non-Durables",
|
34 |
-
"Consumer Services", "Distribution Services", "Electronic Technology","Energy Minerals",
|
35 |
-
"Finance","Health Services","Health Technology","Industrial Services","Miscellaneous",
|
36 |
-
"Non-Energy Minerals","Process Industries","Producer Manufacturing","Retail Trade",
|
37 |
-
"Technology Services","Transportation","Utilities"]
|
38 |
-
commodity_mapping = {"Gold":"gc","Silver":"si", "Platinum":"pl","Copper":"hg","Palladium":"pa", "Brent crude oil":"QA"}
|
39 |
-
|
40 |
-
crypto_symbols = ['YFI',
|
41 |
-
'ETH',
|
42 |
-
'USDC',
|
43 |
-
'EGLD',
|
44 |
-
'XLM',
|
45 |
-
'STPT',
|
46 |
-
'JST',
|
47 |
-
'AAVE',
|
48 |
-
'MBL',
|
49 |
-
'XEM',
|
50 |
-
'DOT',
|
51 |
-
'KLAY',
|
52 |
-
'SUPER',
|
53 |
-
'ALICE',
|
54 |
-
'MATIC',
|
55 |
-
'XMR',
|
56 |
-
'LTCUP',
|
57 |
-
'LTCDOWN',
|
58 |
-
'GXS',
|
59 |
-
'SUSHIUP',
|
60 |
-
'DOGE',
|
61 |
-
'BCHABC',
|
62 |
-
'MANA',
|
63 |
-
'FET',
|
64 |
-
'DASH',
|
65 |
-
'KEY',
|
66 |
-
'WIN',
|
67 |
-
'ETHUP',
|
68 |
-
'COCOS',
|
69 |
-
'HBAR',
|
70 |
-
'FTT',
|
71 |
-
'UNFI',
|
72 |
-
'GHST',
|
73 |
-
'XRPDOWN',
|
74 |
-
'GYEN',
|
75 |
-
'NMR',
|
76 |
-
'SC',
|
77 |
-
'UAH',
|
78 |
-
'UMA',
|
79 |
-
'MASK',
|
80 |
-
'XLMDOWN',
|
81 |
-
'BUSD',
|
82 |
-
'HNT',
|
83 |
-
'VITE',
|
84 |
-
'SYS',
|
85 |
-
'BTC',
|
86 |
-
'RAMP',
|
87 |
-
'SAND',
|
88 |
-
'DEXE',
|
89 |
-
'POND',
|
90 |
-
'LINA',
|
91 |
-
'BRY',
|
92 |
-
'NBS',
|
93 |
-
'GRT',
|
94 |
-
'SUSHIDOWN',
|
95 |
-
'BEAM',
|
96 |
-
'CTXC',
|
97 |
-
'GBP',
|
98 |
-
'BTCST',
|
99 |
-
'XRPUP',
|
100 |
-
'STMX',
|
101 |
-
'DODO',
|
102 |
-
'BAR',
|
103 |
-
'USDS',
|
104 |
-
'AION',
|
105 |
-
'WRX',
|
106 |
-
'SXPDOWN',
|
107 |
-
'BCHDOWN',
|
108 |
-
'BOND',
|
109 |
-
'AGLD',
|
110 |
-
'EOSBEAR',
|
111 |
-
'BKRW',
|
112 |
-
'NGN',
|
113 |
-
'BNBDOWN',
|
114 |
-
'ALGO',
|
115 |
-
'BURGER',
|
116 |
-
'AKRO',
|
117 |
-
'DCR',
|
118 |
-
'ERN',
|
119 |
-
'ENJ',
|
120 |
-
'LUNA',
|
121 |
-
'QTUM',
|
122 |
-
'XEC',
|
123 |
-
'MDX',
|
124 |
-
'BTT',
|
125 |
-
'LINKDOWN',
|
126 |
-
'NEAR',
|
127 |
-
'FIDA',
|
128 |
-
'DOCK',
|
129 |
-
'MITH',
|
130 |
-
'TVK',
|
131 |
-
'FIRO',
|
132 |
-
'ETHBEAR',
|
133 |
-
'TRX',
|
134 |
-
'LINK',
|
135 |
-
'ZEC',
|
136 |
-
'TRXUP',
|
137 |
-
'BNBUP',
|
138 |
-
'NPXS',
|
139 |
-
'DAI',
|
140 |
-
'CVP',
|
141 |
-
'MBOX',
|
142 |
-
'IDEX',
|
143 |
-
'DIA',
|
144 |
-
'STRAT',
|
145 |
-
'ZEN',
|
146 |
-
'CELO',
|
147 |
-
'ALPHA',
|
148 |
-
'BADGER',
|
149 |
-
'TORN',
|
150 |
-
'IOTA',
|
151 |
-
'REEF',
|
152 |
-
'STORJ',
|
153 |
-
'AXS',
|
154 |
-
'RVN',
|
155 |
-
'1INCHDOWN',
|
156 |
-
'WAN',
|
157 |
-
'TKO',
|
158 |
-
'USDSB',
|
159 |
-
'BVND',
|
160 |
-
'KSM',
|
161 |
-
'REP',
|
162 |
-
'ZRX',
|
163 |
-
'FILUP',
|
164 |
-
'ILV',
|
165 |
-
'TRXDOWN',
|
166 |
-
'BAND',
|
167 |
-
'BULL',
|
168 |
-
'NANO',
|
169 |
-
'LINKUP',
|
170 |
-
'OGN',
|
171 |
-
'CAKE',
|
172 |
-
'XRPBULL',
|
173 |
-
'DEGO',
|
174 |
-
'PERP',
|
175 |
-
'QNT',
|
176 |
-
'AR',
|
177 |
-
'XVS',
|
178 |
-
'DOTDOWN',
|
179 |
-
'LIT',
|
180 |
-
'STX',
|
181 |
-
'KMD',
|
182 |
-
'MINA',
|
183 |
-
'LTO',
|
184 |
-
'TRY',
|
185 |
-
'BTS',
|
186 |
-
'AVAX',
|
187 |
-
'TRU',
|
188 |
-
'BCH',
|
189 |
-
'DNT',
|
190 |
-
'XRPBEAR',
|
191 |
-
'TWT',
|
192 |
-
'TRIBE',
|
193 |
-
'BZRX',
|
194 |
-
'YFIDOWN',
|
195 |
-
'SXPUP',
|
196 |
-
'BNT',
|
197 |
-
'GALA',
|
198 |
-
'LRC',
|
199 |
-
'UNIUP',
|
200 |
-
'DAI',
|
201 |
-
'SRM',
|
202 |
-
'TOMO',
|
203 |
-
'OM',
|
204 |
-
'TRB',
|
205 |
-
'AUTO',
|
206 |
-
'LEND',
|
207 |
-
'BEAR',
|
208 |
-
'GTC',
|
209 |
-
'WAXP',
|
210 |
-
'PUNDIX',
|
211 |
-
'OCEAN',
|
212 |
-
'SUN',
|
213 |
-
'ARPA',
|
214 |
-
'DATA',
|
215 |
-
'ORN',
|
216 |
-
'CVC',
|
217 |
-
'YFII',
|
218 |
-
'KEEP',
|
219 |
-
'ATOM',
|
220 |
-
'YFIUP',
|
221 |
-
'RUB',
|
222 |
-
'NULS',
|
223 |
-
'PAXG',
|
224 |
-
'NEO',
|
225 |
-
'VIDT',
|
226 |
-
'PNT',
|
227 |
-
'TUSD',
|
228 |
-
'FORTH',
|
229 |
-
'CELR',
|
230 |
-
'PSG',
|
231 |
-
'MFT',
|
232 |
-
'MKR',
|
233 |
-
'ETHBULL',
|
234 |
-
'RSR',
|
235 |
-
'POLS',
|
236 |
-
'FILDOWN',
|
237 |
-
'ASR',
|
238 |
-
'RUNE',
|
239 |
-
'SUSHI',
|
240 |
-
'EOS',
|
241 |
-
'SNX',
|
242 |
-
'GNO',
|
243 |
-
'SUSD',
|
244 |
-
'CTK',
|
245 |
-
'TLM',
|
246 |
-
'ALPACA',
|
247 |
-
'FOR',
|
248 |
-
'RLC',
|
249 |
-
'IOST',
|
250 |
-
'1INCH',
|
251 |
-
'KNC',
|
252 |
-
'COTI',
|
253 |
-
'UNIDOWN',
|
254 |
-
'SXP',
|
255 |
-
'ANT',
|
256 |
-
'HIVE',
|
257 |
-
'FLOW',
|
258 |
-
'FUN',
|
259 |
-
'WAVES',
|
260 |
-
'DYDX',
|
261 |
-
'ACM',
|
262 |
-
'BNBBEAR',
|
263 |
-
'CRV',
|
264 |
-
'TFUEL',
|
265 |
-
'STRAX',
|
266 |
-
'SHIB',
|
267 |
-
'WTC',
|
268 |
-
'BLZ',
|
269 |
-
'ICP',
|
270 |
-
'FIS',
|
271 |
-
'XTZ',
|
272 |
-
'ETHDOWN',
|
273 |
-
'ONG',
|
274 |
-
'BCHUP',
|
275 |
-
'ADADOWN',
|
276 |
-
'EOSDOWN',
|
277 |
-
'IDRT',
|
278 |
-
'ERD',
|
279 |
-
'TCT',
|
280 |
-
'HARD',
|
281 |
-
'XVG',
|
282 |
-
'ROSE',
|
283 |
-
'IRIS',
|
284 |
-
'RAY',
|
285 |
-
'YGG',
|
286 |
-
'COMP',
|
287 |
-
'VET',
|
288 |
-
'OMG',
|
289 |
-
'WNXM',
|
290 |
-
'AUDIO',
|
291 |
-
'DUSK',
|
292 |
-
'MIR',
|
293 |
-
'GTO',
|
294 |
-
'NKN',
|
295 |
-
'FIO',
|
296 |
-
'XTZDOWN',
|
297 |
-
'NU',
|
298 |
-
'LSK',
|
299 |
-
'ZAR',
|
300 |
-
'FIL',
|
301 |
-
'OG',
|
302 |
-
'FARM',
|
303 |
-
'ARDR',
|
304 |
-
'WING',
|
305 |
-
'LTC',
|
306 |
-
'HOT',
|
307 |
-
'DGB',
|
308 |
-
'RIF',
|
309 |
-
'CHZ',
|
310 |
-
'BNBBULL',
|
311 |
-
'MTL',
|
312 |
-
'HC',
|
313 |
-
'BRL',
|
314 |
-
'VEN',
|
315 |
-
'JUV',
|
316 |
-
'CHR',
|
317 |
-
'EPS',
|
318 |
-
'ATA',
|
319 |
-
'OXT',
|
320 |
-
'REN',
|
321 |
-
'XRP',
|
322 |
-
'VTHO',
|
323 |
-
'BTCUP',
|
324 |
-
'XZC',
|
325 |
-
'MDT',
|
326 |
-
'THETA',
|
327 |
-
'AAVEUP',
|
328 |
-
'PHA',
|
329 |
-
'COS',
|
330 |
-
'SKL',
|
331 |
-
'DOTUP',
|
332 |
-
'DENT',
|
333 |
-
'SOL',
|
334 |
-
'AAVEDOWN',
|
335 |
-
'CKB',
|
336 |
-
'MLN',
|
337 |
-
'AUD',
|
338 |
-
'BIDR',
|
339 |
-
'ELF',
|
340 |
-
'TROY',
|
341 |
-
'IOTX',
|
342 |
-
'MCO',
|
343 |
-
'UNI',
|
344 |
-
'ANKR',
|
345 |
-
'FTM',
|
346 |
-
'XLMUP',
|
347 |
-
'EUR',
|
348 |
-
'EOSUP',
|
349 |
-
'BEL',
|
350 |
-
'ZIL',
|
351 |
-
'BTG',
|
352 |
-
'KAVA',
|
353 |
-
'SLP',
|
354 |
-
'DREP',
|
355 |
-
'BAKE',
|
356 |
-
'CFX',
|
357 |
-
'ATM',
|
358 |
-
'FLM',
|
359 |
-
'INJ',
|
360 |
-
'CTSI',
|
361 |
-
'STORM',
|
362 |
-
'1INCHUP',
|
363 |
-
'FRONT',
|
364 |
-
'DF',
|
365 |
-
'ADAUP',
|
366 |
-
'ONE',
|
367 |
-
'C98',
|
368 |
-
'BCC',
|
369 |
-
'BCHSV',
|
370 |
-
'QUICK',
|
371 |
-
'BAL',
|
372 |
-
'BAT',
|
373 |
-
'POLY',
|
374 |
-
'ETC',
|
375 |
-
'AVA',
|
376 |
-
'EOSBULL',
|
377 |
-
'XTZUP',
|
378 |
-
'USDP',
|
379 |
-
'UTK',
|
380 |
-
'PAX',
|
381 |
-
'PERL',
|
382 |
-
'ADA',
|
383 |
-
'BKRW',
|
384 |
-
'ONT',
|
385 |
-
'SFP',
|
386 |
-
'BNB',
|
387 |
-
'LPT',
|
388 |
-
'ICX',
|
389 |
-
'CLV',
|
390 |
-
'REQ',
|
391 |
-
'BTCDOWN']
|
|
|
|
|
|
|
|
|
|
|
|
|
|
|
|
|
|
|
|
|
|
|
|
|
|
|
|
|
|
|
|
|
|
|
|
|
|
|
|
|
|
|
|
|
|
|
|
|
|
|
|
|
|
|
|
|
|
|
|
|
|
|
|
|
|
|
|
|
|
|
|
|
|
|
|
|
|
|
|
|
|
|
|
|
|
|
|
|
|
|
|
|
|
|
|
|
|
|
|
|
|
|
|
|
|
|
|
|
|
|
|
|
|
|
|
|
|
|
|
|
|
|
|
|
|
|
|
|
|
|
|
|
|
|
|
|
|
|
|
|
|
|
|
|
|
|
|
|
|
|
|
|
|
|
|
|
|
|
|
|
|
|
|
|
|
|
|
|
|
|
|
|
|
|
|
|
|
|
|
|
|
|
|
|
|
|
|
|
|
|
|
|
|
|
|
|
|
|
|
|
|
|
|
|
|
|
|
|
|
|
|
|
|
|
|
|
|
|
|
|
|
|
|
|
|
|
|
|
|
|
|
|
|
|
|
|
|
|
|
|
|
|
|
|
|
|
|
|
|
|
|
|
|
|
|
|
|
|
|
|
|
|
|
|
|
|
|
|
|
|
|
|
|
|
|
|
|
|
|
|
|
|
|
|
|
|
|
|
|
|
|
|
|
|
|
|
|
|
|
|
|
|
|
|
|
|
|
|
|
|
|
|
|
|
|
|
|
|
|
|
|
|
|
|
|
|
|
|
|
|
|
|
|
|
|
|
|
|
|
|
|
|
|
|
|
|
|
|
|
|
|
|
|
|
|
|
|
|
|
|
|
|
|
|
|
|
|
|
|
|
|
|
|
|
|
|
|
|
|
|
|
|
|
|
|
|
|
|
|
|
|
|
|
|
|
|
|
|
|
|
|
|
|
|
|
|
|
|
|
|
|
|
|
|
|
|
|
|
|
|
|
|
|
|
|
|
|
|
|
|
|
|
|
|
|
|
|
|
|
|
|
|
|
|
|
|
|
|
|
|
|
|
|
|
|
|
|
|
|
|
|
|
|
|
|
|
|
|
|
|
|
|
|
|
|
|
|
|
|
|
|
|
|
|
|
|
|
|
|
|
|
|
|
|
|
|
|
|
|
|
|
|
|
|
|
|
|
|
|
|
|
|
|
|
|
|
|
|
|
|
|
|
|
|
|
|
|
|
|
|
|
|
|
|
|
|
|
|
|
|
|
|
|
|
|
|
|
|
|
|
|
|
|
|
|
|
|
|
|
|
|
|
|
|
|
|
|
|
|
|
|
|
|
|
|
|
|
|
|
|
|
|
|
|
|
|
|
|
|
|
|
|
|
|
|
|
|
|
|
|
|
|
|
|
|
|
|
|
|
|
|
|
|
|
|
|
|
|
|
|
|
|
|
|
|
|
|
|
|
|
|
|
|
|
|
|
|
|
|
|
|
|
|
|
|
|
|
|
|
|
|
|
|
|
|
|
|
|
|
|
|
|
|
|
|
|
|
|
|
|
|
|
|
|
|
|
|
|
|
|
|
|
|
|
|
|
|
|
|
|
|
|
|
|
|
|
|
|
|
|
|
|
|
|
|
|
|
|
|
|
|
|
|
|
|
|
|
|
|
|
|
|
|
|
|
|
|
|
|
|
|
|
|
|
|
|
|
|
|
|
|
|
|
|
|
|
|
|
|
|
|
|
|
|
|
|
|
|
|
|
|
|
|
|
|
|
|
|
|
|
|
|
|
|
|
|
|
|
|
|
|
|
|
spaces/ChrisPreston/diff-svc_minato_aqua/preprocessing/hubertinfer.py
DELETED
@@ -1,53 +0,0 @@
|
|
1 |
-
import os.path
|
2 |
-
from io import BytesIO
|
3 |
-
from pathlib import Path
|
4 |
-
|
5 |
-
import numpy as np
|
6 |
-
import onnxruntime as ort
|
7 |
-
import torch
|
8 |
-
|
9 |
-
from modules.hubert.cn_hubert import load_cn_model, get_cn_hubert_units
|
10 |
-
from modules.hubert.hubert_model import hubert_soft, get_units
|
11 |
-
from modules.hubert.hubert_onnx import get_onnx_units
|
12 |
-
from utils.hparams import hparams
|
13 |
-
|
14 |
-
|
15 |
-
class HubertEncoder:
|
16 |
-
def __init__(self, pt_path='checkpoints/hubert/hubert_soft.pt', hubert_mode='', onnx=False):
|
17 |
-
self.hubert_mode = hubert_mode
|
18 |
-
self.onnx = onnx
|
19 |
-
if 'use_cn_hubert' not in hparams.keys():
|
20 |
-
hparams['use_cn_hubert'] = False
|
21 |
-
if hparams['use_cn_hubert'] or self.hubert_mode == 'cn_hubert':
|
22 |
-
pt_path = "checkpoints/cn_hubert/chinese-hubert-base-fairseq-ckpt.pt"
|
23 |
-
self.dev = torch.device("cuda")
|
24 |
-
self.hbt_model = load_cn_model(pt_path)
|
25 |
-
else:
|
26 |
-
if onnx:
|
27 |
-
self.hbt_model = ort.InferenceSession("onnx/hubert_soft.onnx",
|
28 |
-
providers=['CUDAExecutionProvider', 'CPUExecutionProvider', ])
|
29 |
-
else:
|
30 |
-
pt_path = list(Path(pt_path).parent.rglob('*.pt'))[0]
|
31 |
-
if 'hubert_gpu' in hparams.keys():
|
32 |
-
self.use_gpu = hparams['hubert_gpu']
|
33 |
-
else:
|
34 |
-
self.use_gpu = True
|
35 |
-
self.dev = torch.device("cuda" if self.use_gpu and torch.cuda.is_available() else "cpu")
|
36 |
-
self.hbt_model = hubert_soft(str(pt_path)).to(self.dev)
|
37 |
-
print(f"| load 'model' from '{pt_path}'")
|
38 |
-
|
39 |
-
def encode(self, wav_path):
|
40 |
-
if isinstance(wav_path, BytesIO):
|
41 |
-
npy_path = ""
|
42 |
-
wav_path.seek(0)
|
43 |
-
else:
|
44 |
-
npy_path = Path(wav_path).with_suffix('.npy')
|
45 |
-
if os.path.exists(npy_path):
|
46 |
-
units = np.load(str(npy_path))
|
47 |
-
elif self.onnx:
|
48 |
-
units = get_onnx_units(self.hbt_model, wav_path).squeeze(0)
|
49 |
-
elif hparams['use_cn_hubert'] or self.hubert_mode == 'cn_hubert':
|
50 |
-
units = get_cn_hubert_units(self.hbt_model, wav_path, self.dev).cpu().numpy()[0]
|
51 |
-
else:
|
52 |
-
units = get_units(self.hbt_model, wav_path, self.dev).cpu().numpy()[0]
|
53 |
-
return units # [T,256]
|
|
|
|
|
|
|
|
|
|
|
|
|
|
|
|
|
|
|
|
|
|
|
|
|
|
|
|
|
|
|
|
|
|
|
|
|
|
|
|
|
|
|
|
|
|
|
|
|
|
|
|
|
|
|
|
|
|
|
|
|
|
|
|
|
|
|
|
|
|
|
|
|
|
|
|
|
|
|
|
|
|
|
|
|
|
|
|
|
|
|
|
|
|
|
|
|
|
|
|
|
|
|
|
|
|
|
|
spaces/CikeyQI/meme-api/meme_generator/memes/behead/__init__.py
DELETED
@@ -1,34 +0,0 @@
|
|
1 |
-
from pathlib import Path
|
2 |
-
from typing import List
|
3 |
-
|
4 |
-
from meme_generator import add_meme
|
5 |
-
from meme_generator.utils import save_gif
|
6 |
-
from PIL.Image import Image as IMG
|
7 |
-
from pil_utils import BuildImage
|
8 |
-
|
9 |
-
img_dir = Path(__file__).parent / "images"
|
10 |
-
|
11 |
-
|
12 |
-
def behead(images: List[BuildImage], texts, args):
|
13 |
-
img = images[0].convert("RGBA").square().resize((75, 75))
|
14 |
-
# fmt: off
|
15 |
-
locs = [
|
16 |
-
(80, 72, 0), (83, 73, 0), (82, 73, 0),
|
17 |
-
(78, 73, 0), (72, 74, 0), (72, 75, 0),
|
18 |
-
(73, 76, 0), (73, 76, 0), (73, 76, 0),
|
19 |
-
(74, 76, 0), (74, 76, 0), (70, 73, 12),
|
20 |
-
(61, 62, 25), (49, 40, 45), (46, 30, 65),
|
21 |
-
(50, 35, 85), (39, 34, 105), (19, 45, 135),
|
22 |
-
(9, 91, 155), (6, 161, 175), (-4, 248, 180),
|
23 |
-
]
|
24 |
-
# fmt: on
|
25 |
-
frames: List[IMG] = []
|
26 |
-
for i in range(21):
|
27 |
-
frame = BuildImage.open(img_dir / f"{i}.png")
|
28 |
-
x, y, angle = locs[i]
|
29 |
-
frame.paste(img.rotate(angle, expand=True), (x, y), below=True)
|
30 |
-
frames.append(frame.image)
|
31 |
-
return save_gif(frames, 0.05)
|
32 |
-
|
33 |
-
|
34 |
-
add_meme("behead", behead, min_images=1, max_images=1, keywords=["砍头", "斩首"])
|
|
|
|
|
|
|
|
|
|
|
|
|
|
|
|
|
|
|
|
|
|
|
|
|
|
|
|
|
|
|
|
|
|
|
|
|
|
|
|
|
|
|
|
|
|
|
|
|
|
|
|
|
|
|
|
|
|
|
|
|
|
|
|
|
|
|
|
|
|
spaces/CompVis/stable-diffusion-license/license.html
DELETED
The diff for this file is too large to render.
See raw diff
|
|
spaces/Cong723/gpt-academic-public/crazy_functions/test_project/latex/attention/introduction.tex
DELETED
@@ -1,18 +0,0 @@
|
|
1 |
-
Recurrent neural networks, long short-term memory \citep{hochreiter1997} and gated recurrent \citep{gruEval14} neural networks in particular, have been firmly established as state of the art approaches in sequence modeling and transduction problems such as language modeling and machine translation \citep{sutskever14, bahdanau2014neural, cho2014learning}. Numerous efforts have since continued to push the boundaries of recurrent language models and encoder-decoder architectures \citep{wu2016google,luong2015effective,jozefowicz2016exploring}.
|
2 |
-
|
3 |
-
Recurrent models typically factor computation along the symbol positions of the input and output sequences. Aligning the positions to steps in computation time, they generate a sequence of hidden states $h_t$, as a function of the previous hidden state $h_{t-1}$ and the input for position $t$. This inherently sequential nature precludes parallelization within training examples, which becomes critical at longer sequence lengths, as memory constraints limit batching across examples.
|
4 |
-
%\marginpar{not sure if the memory constraints are understandable here}
|
5 |
-
Recent work has achieved significant improvements in computational efficiency through factorization tricks \citep{Kuchaiev2017Factorization} and conditional computation \citep{shazeer2017outrageously}, while also improving model performance in case of the latter. The fundamental constraint of sequential computation, however, remains.
|
6 |
-
|
7 |
-
%\marginpar{@all: there is work on analyzing what attention really does in seq2seq models, couldn't find it right away}
|
8 |
-
|
9 |
-
Attention mechanisms have become an integral part of compelling sequence modeling and transduction models in various tasks, allowing modeling of dependencies without regard to their distance in the input or output sequences \citep{bahdanau2014neural, structuredAttentionNetworks}. In all but a few cases \citep{decomposableAttnModel}, however, such attention mechanisms are used in conjunction with a recurrent network.
|
10 |
-
|
11 |
-
%\marginpar{not sure if "cross-positional communication" is understandable without explanation}
|
12 |
-
%\marginpar{insert exact training times and stats for the model that reaches sota earliest, maybe even a single GPU model?}
|
13 |
-
|
14 |
-
In this work we propose the Transformer, a model architecture eschewing recurrence and instead relying entirely on an attention mechanism to draw global dependencies between input and output. The Transformer allows for significantly more parallelization and can reach a new state of the art in translation quality after being trained for as little as twelve hours on eight P100 GPUs.
|
15 |
-
%\marginpar{you removed the constant number of repetitions part. I wrote it because I wanted to make it clear that the model does not only perform attention once, while it's also not recurrent. I thought that might be important to get across early.}
|
16 |
-
|
17 |
-
% Just a standard paragraph with citations, rewrite.
|
18 |
-
%After the seminal papers of \citep{sutskever14}, \citep{bahdanau2014neural}, and \citep{cho2014learning}, recurrent models have become the dominant solution for both sequence modeling and sequence-to-sequence transduction. Many efforts such as \citep{wu2016google,luong2015effective,jozefowicz2016exploring} have pushed the boundaries of machine translation and language modeling with recurrent sequence models. Recent effort \citep{shazeer2017outrageously} has combined the power of conditional computation with sequence models to train very large models for machine translation, pushing SOTA at lower computational cost. Recurrent models compute a vector of hidden states $h_t$, for each time step $t$ of computation. $h_t$ is a function of both the input at time $t$ and the previous hidden state $h_t$. This dependence on the previous hidden state encumbers recurrnet models to process multiple inputs at once, and their time complexity is a linear function of the length of the input and output, both during training and inference. [What I want to say here is that although this is fine during decoding, at training time, we are given both input and output and this linear nature does not allow the RNN to process all inputs and outputs simultaneously and haven't been used on datasets that are the of the scale of the web. What's the largest dataset we have ? . Talk about Nividia and possibly other's effors to speed up things, and possibly other efforts that alleviate this, but are still limited by it's comptuational nature]. Rest of the intro: What if you could construct the state based on the actual inputs and outputs, then you could construct them all at once. This has been the foundation of many promising recent efforts, bytenet,facenet (Also talk about quasi rnn here). Now we talk about attention!! Along with cell architectures such as long short-term meory (LSTM) \citep{hochreiter1997}, and gated recurrent units (GRUs) \citep{cho2014learning}, attention has emerged as an essential ingredient in successful sequence models, in particular for machine translation. In recent years, many, if not all, state-of-the-art (SOTA) results in machine translation have been achieved with attention-based sequence models \citep{wu2016google,luong2015effective,jozefowicz2016exploring}. Talk about the neon work on how it played with attention to do self attention! Then talk about what we do.
|
|
|
|
|
|
|
|
|
|
|
|
|
|
|
|
|
|
|
|
|
|
|
|
|
|
|
|
|
|
|
|
|
|
|
|
|
|
spaces/DAMO-NLP-SG/Video-LLaMA/video_llama/processors/base_processor.py
DELETED
@@ -1,26 +0,0 @@
|
|
1 |
-
"""
|
2 |
-
Copyright (c) 2022, salesforce.com, inc.
|
3 |
-
All rights reserved.
|
4 |
-
SPDX-License-Identifier: BSD-3-Clause
|
5 |
-
For full license text, see the LICENSE_Lavis file in the repo root or https://opensource.org/licenses/BSD-3-Clause
|
6 |
-
"""
|
7 |
-
|
8 |
-
from omegaconf import OmegaConf
|
9 |
-
|
10 |
-
|
11 |
-
class BaseProcessor:
|
12 |
-
def __init__(self):
|
13 |
-
self.transform = lambda x: x
|
14 |
-
return
|
15 |
-
|
16 |
-
def __call__(self, item):
|
17 |
-
return self.transform(item)
|
18 |
-
|
19 |
-
@classmethod
|
20 |
-
def from_config(cls, cfg=None):
|
21 |
-
return cls()
|
22 |
-
|
23 |
-
def build(self, **kwargs):
|
24 |
-
cfg = OmegaConf.create(kwargs)
|
25 |
-
|
26 |
-
return self.from_config(cfg)
|
|
|
|
|
|
|
|
|
|
|
|
|
|
|
|
|
|
|
|
|
|
|
|
|
|
|
|
|
|
|
|
|
|
|
|
|
|
|
|
|
|
|
|
|
|
|
|
|
|
|
|
|
|